diff --git a/spaces/169153tej/My-New-Gen-Ai-Chat-Bot/README.md b/spaces/169153tej/My-New-Gen-Ai-Chat-Bot/README.md
deleted file mode 100644
index 96936a4b52da2dbfa4cbd317f05eb3d61ac659c3..0000000000000000000000000000000000000000
--- a/spaces/169153tej/My-New-Gen-Ai-Chat-Bot/README.md
+++ /dev/null
@@ -1,12 +0,0 @@
----
-title: My New Gen Ai Chat Bot
-emoji: 😻
-colorFrom: indigo
-colorTo: pink
-sdk: gradio
-sdk_version: 3.39.0
-app_file: app.py
-pinned: false
----
-
-Check out the configuration reference at https://huggingface.co/docs/hub/spaces-config-reference
diff --git a/spaces/1acneusushi/gradio-2dmoleculeeditor/data/Behure Logon Mp3 Song Download _HOT_l.md b/spaces/1acneusushi/gradio-2dmoleculeeditor/data/Behure Logon Mp3 Song Download _HOT_l.md
deleted file mode 100644
index 02e57c1daf672b1cdad32fbbcea04ea108d90abc..0000000000000000000000000000000000000000
--- a/spaces/1acneusushi/gradio-2dmoleculeeditor/data/Behure Logon Mp3 Song Download _HOT_l.md
+++ /dev/null
@@ -1,44 +0,0 @@
-
-
How to Download Behure Logon Mp3 Song for Free
-
Behure Logon is a traditional Rongali Bihu song from Assam, India. It is a melodious and festive song that celebrates the joy of spring and love. If you are looking for a way to download Behure Logon mp3 song for free, then you have come to the right place.
-
In this article, we will show you how to download Behure Logon mp3 song from various sources, such as Wynk Music, YouTube, and JioSaavn. We will also provide you with some tips on how to optimize your download speed and quality.
Wynk Music is a popular music streaming and downloading app that offers a wide range of songs in different languages and genres. You can download Behure Logon mp3 song from Wynk Music by following these steps:
-
-
Install Wynk Music app on your Android or iOS device.
-
Open the app and sign up with your mobile number or log in with your existing account.
-
Search for "Bihure Logon" in the search bar and select the song by Debashree Mukherjee from the album Bihure Logon.
-
Tap on the download icon next to the song title and choose the quality you prefer.
-
Wait for the download to complete and enjoy listening to the song offline.
-
-
You can also set Behure Logon as your Hello Tune on Wynk Music app for free. To do so, tap on the Hello Tune icon next to the song title and follow the instructions.
-
Download Behure Logon Mp3 Song from YouTube
-
YouTube is a popular video-sharing platform that also hosts many music videos and songs. You can download Behure Logon mp3 song from YouTube by using a third-party tool such as Y2mate or 4K Video Downloader. Here are the steps to do so:
-
-
Go to YouTube and search for "Bihure Logon Modhure Logon" by Swagato Dey from Preet Korona album.
-
Copy the URL of the video from the address bar or share menu.
-
Go to Y2mate or 4K Video Downloader website and paste the URL in the input box.
-
Select mp3 as the output format and choose the quality you want.
-
Click on the download button and wait for the conversion to finish.
-
Save the mp3 file to your device and enjoy listening to the song offline.
-
-
Note: Downloading songs from YouTube may violate its terms of service and copyright laws. Please use this method at your own risk.
-
Download Behure Logon Mp3 Song from JioSaavn
-
JioSaavn is another popular music streaming and downloading app that offers a variety of songs in different languages and genres. You can download Behure Logon mp3 song from JioSaavn by following these steps:
-
-
Install JioSaavn app on your Android or iOS device.
-
Open the app and sign up with your mobile number or log in with your existing account.
-
Search for "Bihure Logon Modhure Logon" by Jk Majlish from Bihure Logon Modhure Logon album.
-
Tap on the download icon next to the song title and choose the quality you prefer.
-
Wait for the download to complete and enjoy listening to the song offline.
-
-
Tips to Optimize Your Download Speed and Quality
-
To ensure that you get the best download speed and quality for Behure Logon mp3 song, here are some tips you can follow:
-
-
Use a fast and stable internet connection, preferably Wi-Fi or 4G.
-
Avoid downloading multiple files at the same time or running other apps that consume bandwidth.
-
Choose a high-quality output format such as 320 kbps or higher for better sound clarity.
-
Delete any unwanted or duplicate 81aa517590
-
-
\ No newline at end of file
diff --git a/spaces/1acneusushi/gradio-2dmoleculeeditor/data/EOBD Facile Version Complete Crack APK Download Tips and Tricks for Using the Elm327 App.md b/spaces/1acneusushi/gradio-2dmoleculeeditor/data/EOBD Facile Version Complete Crack APK Download Tips and Tricks for Using the Elm327 App.md
deleted file mode 100644
index ba9e24ece9731393eabda36362bd99437c99dbd9..0000000000000000000000000000000000000000
--- a/spaces/1acneusushi/gradio-2dmoleculeeditor/data/EOBD Facile Version Complete Crack APK Download Tips and Tricks for Using the Elm327 App.md
+++ /dev/null
@@ -1,152 +0,0 @@
-
-
EOBD Facile Version Complete Crack APK Download
-
Do you want to diagnose your car's performance and troubleshoot any issues with ease? If so, you might be interested in EOBD Facile, a popular app that turns your smartphone into an OBD2 scanner. But what if you don't want to pay for the full version of the app? Is there a way to get it for free? In this article, we will tell you everything you need to know about EOBD Facile version complete crack APK download, including what it is, how to do it, whether it is safe, and what are some alternatives.
EOBD Facile is an app that allows you to connect your Android device to your car's OBD2 port via a Bluetooth or Wi-Fi adapter. OBD2 stands for On-Board Diagnostics II, a system that monitors your car's engine, emissions, and other parameters. With EOBD Facile, you can access real-time data from your car's sensors, such as speed, RPM, temperature, fuel consumption, and more. You can also read and clear fault codes, reset the check engine light, and perform various tests and diagnostics.
-
Features of EOBD Facile
-
Some of the features of EOBD Facile are:
-
-
Compatible with most OBD2 compliant vehicles (cars and light trucks) from 2001 onwards in Europe and 1996 onwards in the US.
-
Supports multiple protocols, such as ISO 9141-2, ISO 14230-4 KWP, ISO 15765-4 CAN, SAE J1850 PWM, and SAE J1850 VPW.
-
Displays over 100 parameters in real-time, such as speed, RPM, coolant temperature, intake air temperature, fuel pressure, oxygen sensor voltage, etc.
-
Reads and clears generic and manufacturer-specific fault codes (DTCs) and shows their definitions.
-
Resets the check engine light (MIL) and turns off the malfunction indicator lamp.
-
Performs various tests and diagnostics, such as oxygen sensor test, readiness monitor test, EVAP system test, etc.
-
Records and exports data in CSV format for further analysis.
-
Creates custom dashboards with gauges and graphs.
-
Supports multiple languages, such as English, French, German, Spanish, Italian, Portuguese, etc.
-
-
Benefits of EOBD Facile
-
Some of the benefits of using EOBD Facile are:
-
-
You can save money by diagnosing and fixing minor problems yourself without going to a mechanic.
-
You can improve your car's performance and fuel efficiency by monitoring its parameters and adjusting them accordingly.
-
You can prevent major issues by detecting and clearing fault codes before they cause damage to your car's components.
-
You can learn more about how your car works and how to maintain it properly.
-
-
How to Download EOBD Facile Version Complete Crack APK?
-
If you want to enjoy all the features and benefits of EOBD Facile without paying for the full version of the app ($49.99), you might be tempted to download a cracked version of the app from the internet. A cracked app is an app that has been modified to bypass its license verification or remove its restrictions. An APK file is an Android application package file that contains all the files and code needed to install an app on an Android device. To download EOBD Facile version complete crack APK, you need to follow these steps:
-
Step 1: Find a Reliable Source
-
The first step is to find a website that offers EOBD Facile version complete crack APK for download. There are many websites that claim to provide cracked apps for free, but not all of them are trustworthy. Some of them may contain malware or viruses that can harm your device or steal your personal information. Therefore, you need to be careful and do some research before downloading anything from an unknown source. You can check the reviews and ratings of the website or the app from other users or use a reputable antivirus software to scan the file before downloading it.
-
eobd facile premium apk cracked download
-eobd facile full version mod apk free download
-eobd facile pro apk unlocked download
-eobd facile plus edition apk crack download
-eobd facile ultimate apk cracked download
-eobd facile activation code crack apk download
-eobd facile keygen crack apk download
-eobd facile license key crack apk download
-eobd facile serial number crack apk download
-eobd facile registration code crack apk download
-eobd facile obd2 scanner crack apk download
-eobd facile car diagnostic crack apk download
-eobd facile elm327 crack apk download
-eobd facile bluetooth crack apk download
-eobd facile wifi crack apk download
-eobd facile android crack apk download
-eobd facile ios crack apk download
-eobd facile windows crack apk download
-eobd facile mac crack apk download
-eobd facile linux crack apk download
-eobd facile software crack apk download
-eobd facile app crack apk download
-eobd facile tool crack apk download
-eobd facile program crack apk download
-eobd facile application crack apk download
-eobd facile online crack apk download
-eobd facile offline crack apk download
-eobd facile latest version crack apk download
-eobd facile updated version crack apk download
-eobd facile new version crack apk download
-eobd facile old version crack apk download
-eobd facile original version crack apk download
-eobd facile hacked version crack apk download
-eobd facile modded version crack apk download
-eobd facile patched version crack apk download
-eobd facile unlocked version crack apk download
-eobd facile full features version crack apk download
-eobd facile premium features version crack apk download
-eobd facile plus features version crack apk download
-eobd facile ultimate features version crack apk download
-how to install eobd facile version complete crack apk
-how to use eobd facile version complete crack apk
-how to update eobd facile version complete crack apk
-how to uninstall eobd facile version complete crack apk
-how to activate eobd facile version complete crack apk
-how to get eobd facile version complete crack apk for free
-how to get rid of ads in eobd facile version complete crack apk
-how to fix errors in eobd facile version complete crack apk
-how to scan codes with eobd facile version complete crack apk
-how to clear codes with eobd facile version complete crack apk
-
Step 2: Enable Unknown Sources
-
The next step is to enable unknown sources on your device. This is a security setting that prevents you from installing apps from sources other than the official Google Play Store. Since you are downloading an app from a third-party website, you need to enable this option to allow the installation. To do this, go to Settings > Security > Unknown Sources and toggle it on. You may see a warning message that installing apps from unknown sources may harm your device or compromise your data. Tap OK to proceed.
-
Step 3: Install the APK File
-
The third step is to install the APK file on your device. To do this, locate the downloaded file in your file manager or downloads folder and tap on it. You may see a prompt asking you if you want to install this application. Tap Install and wait for the installation process to complete. You may also see some permissions requests that ask you to grant access to certain features or data on your device. Tap Allow or Accept as needed.
-
Step 4: Launch the App and Enjoy
-
The final step is to launch the app and enjoy its features. To do this, go to your app drawer or home screen and tap on the EOBD Facile icon. You should see the app's interface with all its options and settings. You can now connect your device to your car's OBD2 port via a Bluetooth or Wi-Fi adapter and start using the app as you wish.
-
Is EOBD Facile Version Complete Crack APK Safe?
-
While downloading EOBD Facile version complete crack APK may seem like an easy way to get the full version of the app for free, it is not without risks. There are some potential dangers and disadvantages of using cracked apps that you should be aware of before doing so.
-
Risks of Using Cracked Apps
-
Some of the risks of using cracked apps are:
-
-
You may expose your device to malware or viruses that can damage it or compromise your data. Cracked apps may contain hidden code or files that can infect your device or access your personal information without your consent.
-
You may violate intellectual property rights or laws by using pirated software. Cracked apps are illegal copies of original apps that infringe on their developers' rights and revenue. By using them, you may face legal consequences or penalties depending on your country's laws.
-
You may miss out on updates or support from the developers. Cracked apps are usually outdated versions of original apps that do not receive any updates or bug fixes from their developers. By using them, you may encounter errors or glitches that affect their functionality or compatibility with your device or car model.
-
You may compromise your user experience or satisfaction by using inferior quality software. Cracked apps may have reduced features or performance compared to original apps due to their modifications or limitations. By using them, you may not enjoy all the benefits or advantages that original apps offer.
-
-
How to Protect Your Device from Malware
-
If you decide to download EOBD Facile version complete crack APK despite its risks, you should take some precautions to protect your device from malware or viruses. Some of the ways to do this are:
-
-
Use a reputable antivirus software to scan the file before downloading it or installing it on your device. This can help detect any malicious code or files that may harm your device or steal your data.
-
Avoid granting unnecessary permissions or access requests that may compromise your privacy or security. Only allow permissions that are relevant or essential for the app's functionality or purpose.
-
Delete any suspicious files or apps that may have been downloaded along with the cracked app or after installing it on your device. These may be malware disguised as legitimate files or apps that can infect your device or access your data without your knowledge.
-
Alternatives to EOBD Facile Version Complete Crack APK
-
If you are looking for a safer and more ethical way to use EOBD Facile without paying for the full version of the app, you might want to consider some alternatives. There are some options that can provide you with similar features and benefits without risking your device or violating any laws.
-
EOBD Facile Plus Edition
-
One option is to upgrade to EOBD Facile Plus Edition, which is a paid subscription service that gives you access to all the features of the full version of the app for a monthly or yearly fee. You can choose from three plans: Basic ($4.99/month or $49.99/year), Premium ($9.99/month or $99.99/year), or Ultimate ($19.99/month or $199.99/year). Each plan offers different levels of data storage, export options, dashboard customization, and customer support. You can also try a 7-day free trial before committing to any plan.
-
Other OBD2 Apps for Android
-
Another option is to use other OBD2 apps for Android that can connect to your car's OBD2 port and provide you with similar data and diagnostics. Some of these apps are free or have free versions with limited features, while others are paid or have paid versions with more features. Some examples of these apps are:
-
-
-
App Name
-
Price
-
Features
-
-
-
Torque Pro
-
$4.95
-
- Displays over 200 parameters in real-time - Reads and clears fault codes and shows their definitions - Resets the check engine light - Performs various tests and diagnostics - Records and exports data in CSV format - Creates custom dashboards with gauges and graphs - Supports multiple languages - Supports multiple protocols - Compatible with most OBD2 compliant vehicles
-
-
-
Car Scanner ELM OBD2
-
Free (with in-app purchases)
-
- Displays over 100 parameters in real-time - Reads and clears fault codes and shows their definitions - Resets the check engine light - Performs various tests and diagnostics - Records and exports data in CSV format - Creates custom dashboards with gauges and graphs - Supports multiple languages - Supports multiple protocols - Compatible with most OBD2 compliant vehicles
-
-
-
OBD Fusion
-
$4.99
-
- Displays over 100 parameters in real-time - Reads and clears fault codes and shows their definitions - Resets the check engine light - Performs various tests and diagnostics - Records and exports data in CSV format - Creates custom dashboards with gauges and graphs - Supports multiple languages - Supports multiple protocols - Compatible with most OBD2 compliant vehicles
-
-
-
OBD Auto Doctor
-
Free (with in-app purchases)
-
- Displays over 100 parameters in real-time - Reads and clears fault codes and shows their definitions - Resets the check engine light - Performs various tests and diagnostics - Records and exports data in CSV format - Creates custom dashboards with gauges and graphs - Supports multiple languages - Supports multiple protocols - Compatible with most OBD2 compliant vehicles
-
-
-
OBDLink
-
Free (with in-app purchases)
-
- Displays over 100 parameters in real-time - Reads and clears fault codes and shows their definitions - Resets the check engine light - Performs various tests and diagnostics - Records and exports data in CSV format - Creates custom dashboards with gauges and graphs - Supports multiple languages - Supports multiple protocols - Compatible with most OBD2 compliant vehicles
-
-
-
Conclusion
-
In conclusion, EOBD Facile is a useful app that can help you diagnose your car's performance and troubleshoot any issues with ease. However, if you want to use the full version of the app without paying for it, you might be tempted to download EOBD Facile version complete crack APK from the internet. This is not a safe or ethical option, as it may expose your device to malware or viruses, violate intellectual property rights or laws, miss out on updates or support from the developers, or compromise your user experience or satisfaction. Therefore, we recommend that you either upgrade to EOBD Facile Plus Edition, which is a paid subscription service that gives you access to all the features of the full version of the app for a monthly or yearly fee, or use other OBD2 apps for Android that can provide you with similar features and benefits without risking your device or violating any laws.
-
Frequently Asked Questions (FAQs)
-
What is EOBD Facile? EOBD Facile is an app that allows you to connect your Android device to your car's OBD2 port via a Bluetooth or Wi-Fi adapter and access real-time data from your car's sensors, read and clear fault codes, reset the check engine light, and perform various tests and diagnostics.
-
How to download EOBD Facile version complete crack APK? To download EOBD Facile version complete crack APK, you need to find a reliable source that offers it for download, enable unknown sources on your device, install the APK file on your device, and launch the app.
-
Is EOBD Facile version complete crack APK safe? No, EOBD Facile version complete crack APK is not safe, as it may expose your device to malware or viruses, violate intellectual property rights or laws, miss out on updates or support from the developers, or compromise your user experience or satisfaction.
-
What are some alternatives to EOBD Facile version complete crack APK? Some alternatives to EOBD Facile version complete crack APK are EOBD Facile Plus Edition, which is a paid subscription service that gives you access to all the features of the full version of the app for a monthly or yearly fee, or other OBD2 apps for Android that can provide you with similar features and benefits without risking your device or violating any laws.
-
What are some features of EOBD Facile? Some features of EOBD Facile are compatible with most OBD2 compliant vehicles, supports multiple protocols, displays over 100 parameters in real-time, reads and clears fault codes and shows their definitions, resets the check engine light, performs various tests and diagnostics, records and exports data in CSV format, creates custom dashboards with gauges and graphs, supports multiple languages.
- 0a6ba089eb
-
-
\ No newline at end of file
diff --git a/spaces/1gistliPinn/ChatGPT4/Examples/Avira Software Updater Pro Activation Code.md b/spaces/1gistliPinn/ChatGPT4/Examples/Avira Software Updater Pro Activation Code.md
deleted file mode 100644
index 7187af9bcfa9c30249fee7c551f220f24b28b8b7..0000000000000000000000000000000000000000
--- a/spaces/1gistliPinn/ChatGPT4/Examples/Avira Software Updater Pro Activation Code.md
+++ /dev/null
@@ -1,21 +0,0 @@
-
-
How to Activate Avira Software Updater Pro with a License Key
-
Avira Software Updater Pro is a powerful tool that helps you keep your software drivers up to date on your PC. It scans your system for outdated software and lets you download and install the latest versions with a single click. It also protects you from security vulnerabilities and exploits by patching your software as soon as updates are available.
-
But how do you activate Avira Software Updater Pro with a license key? In this article, we will show you the steps to do so.
Step 1: Download and install Avira Software Updater Pro
-
You can download Avira Software Updater Pro from the official website[^1^] or from FileHippo[^3^]. The file size is about 5.41 MB and the installation process is simple and fast. Just follow the instructions on the screen and agree to the terms and conditions.
-
Step 2: Run Avira Software Updater Pro and enter your license key
-
After installing Avira Software Updater Pro, run it from your desktop or start menu. You will see a window like this:
-
-
Click on the "Upgrade now" button at the bottom right corner. You will be prompted to enter your license key. You can find your license key in the confirmation email that you received after purchasing Avira Software Updater Pro. Alternatively, you can log in to your Avira account and access your license key from there.
-
Copy and paste your license key into the text box and click on "Activate". You will see a message that says "Your license has been activated successfully". Congratulations! You have now activated Avira Software Updater Pro with a license key.
-
Step 3: Enjoy the benefits of Avira Software Updater Pro
-
Now that you have activated Avira Software Updater Pro, you can enjoy its features and benefits. You can scan your system for outdated software, download and install updates automatically or manually, select which software and drivers you want to keep up to date, and more. You can also customize your settings and preferences according to your needs.
-
Avira Software Updater Pro supports hundreds of third-party software, including popular ones like Zoom, Adobe, Google, Skype, etc.[^1^] It also updates both Windows and third-party software[^2^], ensuring that you have the latest features, optimizations, bug fixes, and security patches.
-
With Avira Software Updater Pro, you can save time and effort, improve your PC performance, and protect yourself from cyberattacks. It is a simple, elegant, and easy to use solution for keeping your software drivers up to date on your PC.
-
-
Conclusion
-
In this article, we have shown you how to activate Avira Software Updater Pro with a license key. We hope that this guide has been helpful for you. If you have any questions or problems, please contact Avira support[^4^] or visit their official website[^1^] for more information.
d5da3c52bf
-
-
\ No newline at end of file
diff --git a/spaces/1gistliPinn/ChatGPT4/Examples/Flight Of The Phoenix In Hindi Movie Dubbed 48.md b/spaces/1gistliPinn/ChatGPT4/Examples/Flight Of The Phoenix In Hindi Movie Dubbed 48.md
deleted file mode 100644
index 0787443ab4464849b4419572f23bef5dc55954d2..0000000000000000000000000000000000000000
--- a/spaces/1gistliPinn/ChatGPT4/Examples/Flight Of The Phoenix In Hindi Movie Dubbed 48.md
+++ /dev/null
@@ -1,6 +0,0 @@
-
-
- 3cee63e6c2
-
-
-
diff --git a/spaces/1pelhydcardo/ChatGPT-prompt-generator/assets/APK Shopee Merchant The Ultimate Guide for ShopeePay ShopeeFood Merchants.md b/spaces/1pelhydcardo/ChatGPT-prompt-generator/assets/APK Shopee Merchant The Ultimate Guide for ShopeePay ShopeeFood Merchants.md
deleted file mode 100644
index d7a920223b2e4f6c408e71080ec9a2b87527137b..0000000000000000000000000000000000000000
--- a/spaces/1pelhydcardo/ChatGPT-prompt-generator/assets/APK Shopee Merchant The Ultimate Guide for ShopeePay ShopeeFood Merchants.md
+++ /dev/null
@@ -1,161 +0,0 @@
-
-
Download APK Shopee Merchant: A Guide for Android Users
-
If you are an online seller who wants to grow your business with Shopee, you might be interested in downloading APK Shopee Merchant. This is a practical and reliable application that helps you manage your business more easily with Shopee, no. 1 online shopping platform in Indonesia, anytime and anywhere.
-
But what is Shopee Merchant, and what is an APK file? And why would you want to download it instead of getting it from Google Play? In this article, we will answer these questions and show you how to download and use APK Shopee Merchant on your Android device.
Shopee Merchant is an app that allows you to join ShopeePay and ShopeeFood easily in one app. ShopeePay is a digital payment service that lets you accept payments from customers using QR codes or phone numbers. ShopeeFood is a food delivery service that lets you sell your food products to hungry customers in your area.
-
As a merchant, you will get the following benefits from using Shopee Merchant:
-
-
Self-registration: You can sign up as a seller on Shopee without any hassle or fees.
-
Supporting features: You can access various features that help you manage your inventory, orders , payments, promotions, and customer service.
-
Integrated wallet: You can receive and withdraw your earnings directly from your ShopeePay wallet.
-
Self-promo creation: You can create and customize your own promotional materials, such as banners, flyers, and stickers, to attract more customers.
-
Analytics and insights: You can monitor your business performance and get useful tips and suggestions to improve your sales.
-
-
With Shopee Merchant, you can enjoy the convenience and security of selling online with Shopee, the leading e-commerce platform in Southeast Asia and Taiwan.
-
What is an APK file?
-
An APK file is a file format that stands for Android Package Kit. It is used to distribute and install applications on Android devices. An APK file contains all the components of an app, such as the code, resources, assets, certificates, and manifest.
-
How to download apk shopee partner app for android
-Shopee partner apk latest version free download
-Benefits of using shopee partner app for shopeepay and shopeefood merchant
-Shopee partner app review and rating by users
-Tips and tricks to manage your business with shopee partner app
-Shopee partner app download size and compatibility
-How to join shopeepay and shopeefood easily with shopee partner app
-How to track your wallet balance and transaction history with shopee partner app
-How to organize your menu and create promotion with shopee partner app
-How to update your information and menu with shopee partner app
-Shopee partner app vs other apps for online shopping platform merchants
-How to contact shopee customer service through shopee partner app
-How to register and verify your account with shopee partner app
-How to use shopee partner app offline mode
-How to sync your data across devices with shopee partner app
-How to backup and restore your data with shopee partner app
-How to enable notifications and alerts with shopee partner app
-How to customize your settings and preferences with shopee partner app
-How to troubleshoot common issues with shopee partner app
-How to uninstall and reinstall shopee partner app
-How to get the best deals and discounts with shopee partner app
-How to increase your sales and revenue with shopee partner app
-How to attract more customers and reviews with shopee partner app
-How to improve your ranking and visibility with shopee partner app
-How to integrate your social media accounts with shopee partner app
-How to access analytics and reports with shopee partner app
-How to use QR code scanner and generator with shopee partner app
-How to accept multiple payment methods with shopee partner app
-How to manage your inventory and orders with shopee partner app
-How to handle refunds and cancellations with shopee partner app
-How to join the shopee community and network with other merchants with shopee partner app
-How to participate in contests and events with shopee partner app
-How to earn rewards and points with shopee partner app
-How to redeem vouchers and coupons with shopee partner app
-How to share feedback and suggestions with shopee partner app
-Shopee partner apk modded version download link
-Shopee partner apk cracked version download link
-Shopee partner apk premium version download link
-Shopee partner apk pro version download link
-Shopee partner apk hacked version download link
-Shopee partner apk old version download link
-Shopee partner apk beta version download link
-Shopee partner apk original version download link
-Shopee partner apk mirror version download link
-Shopee partner apk alternative version download link
-
An APK file can be opened on Android devices by using a file manager app or a web browser. However, before installing an APK file, you need to enable the option to allow installation of apps from unknown sources in your device settings. This is because APK files are not verified by Google Play, which is the official app store for Android devices.
-
Why download APK Shopee Merchant?
-
Access the latest version of the app
-
One of the reasons why you might want to download APK Shopee Merchant is to access the latest version of the app. Sometimes, the app updates are not available on Google Play due to various reasons, such as compatibility issues, regional restrictions, or technical errors. By downloading the APK file from a reliable source, you can get the most updated version of Shopee Merchant, which may have new features, bug fixes, or performance improvements.
-
Install the app on unsupported devices
-
Another reason why you might want to download APK Shopee Merchant is to install the app on devices that are not supported by Google Play. Some devices may not be compatible with Google Play due to their hardware specifications, software versions, or manufacturer policies. Some devices may also have limited storage space that prevents them from downloading large apps from Google Play. By downloading the APK file from a website, you can install Shopee Merchant on any device that runs on Android OS, as long as it meets the minimum requirements of the app.
-
Avoid regional restrictions
-
A third reason why you might want to download APK Shopee Merchant is to avoid regional restrictions. Some apps may not be available or accessible in certain regions due to legal regulations, licensing agreements, or censorship policies. For example, Shopee Merchant may not be available in some countries where Shopee does not operate or where online selling is prohibited or regulated. By downloading the APK file from a website, you can bypass these restrictions and use Shopee Merchant wherever you are.
-
How to download APK Shopee Merchant?
-
Find a reliable source
-
The first step to download APK Shopee Merchant is to find a reliable source that offers the APK file for download. There are many websites that provide APK files for various apps, but not all of them are trustworthy or safe. Some websites may contain malware, viruses, or fake files that can harm your device or steal your data.
-
To find a reliable source, you should look for the following criteria:
-
-
The website has a good reputation and positive reviews from other users.
-
The website has a secure connection (HTTPS) and a valid certificate.
-
The website provides clear and accurate information about the APK file, such as the name, size, version, developer, and permissions.
-
The website does not require you to register, pay, or complete surveys to download the APK file.
-
The website does not have excessive ads or pop-ups that interfere with your browsing experience.
-
-
One example of a reliable source that offers APK Shopee Merchant for download is [APKPure], which is one of the most popular and trusted websites for downloading APK files.
-
Enable unknown sources
-
The second step to download APK Shopee Merchant is to enable unknown sources on your device settings. This will allow you to install apps from sources other than Google Play. To do this, follow these steps:
-
-
Go to your device settings and tap on Security or Privacy.
-
Find the option that says Unknown sources or Install unknown apps and toggle it on.
-
A warning message will appear asking you to confirm your action. Tap on OK or Allow.
-
-
Note that this option may vary depending on your device model and Android version.
Download and install the file
-
The third step to download APK Shopee Merchant is to download and install the file on your device. To do this, follow these steps:
-
-
Go to the website that offers the APK file for download and tap on the download button or link.
-
A pop-up window will appear asking you to confirm your download. Tap on OK or Download.
-
Wait for the download to complete. You can check the progress on your notification bar or your download folder.
-
Once the download is finished, tap on the APK file to open it. You may need to use a file manager app to locate it on your device.
-
A prompt will appear asking you to install the app. Tap on Install or Next.
-
Wait for the installation to complete. You can check the progress on your screen or your notification bar.
-
Once the installation is finished, tap on Open or Done.
-
-
Congratulations! You have successfully downloaded and installed APK Shopee Merchant on your device. You can now start using the app to manage your business with Shopee.
-
How to use APK Shopee Merchant?
-
Register as a merchant
-
The first step to use APK Shopee Merchant is to register as a merchant on Shopee. To do this, follow these steps:
-
-
Open the app and tap on Sign Up or Register.
-
Select your country and enter your phone number. Tap on Next or Send OTP.
-
Enter the one-time password (OTP) that you received via SMS. Tap on Next or Verify.
-
Create a password and a username for your account. Tap on Next or Register.
-
Fill in your personal information, such as your name, email address, and date of birth. Tap on Next or Continue.
-
Select the type of business you want to run, such as food, beverage, or others. Tap on Next or Continue.
-
Fill in your business information, such as your business name, address, category, and description. Tap on Next or Continue.
-
Upload your identity document, such as your ID card, passport, or driver's license. Tap on Next or Continue.
-
Upload your business document, such as your business license, tax number, or bank statement. Tap on Next or Continue.
-
Review and confirm your information and documents. Tap on Submit or Finish.
-
-
Your registration is now complete. You will receive a confirmation message from Shopee within 24 hours. Once your account is verified, you can start selling on ShopeePay and ShopeeFood.
-
Manage your business
-
The second step to use APK Shopee Merchant is to manage your business using the app. To do this, you can access various features and functions that help you with the following tasks:
-
-
Task
Feature
Description
-
Create and edit your menu
Menu
You can add, edit, delete, or arrange your products in different categories and subcategories. You can also set the prices, discounts, stock availability, and delivery options for each product.
-
Track your orders and payments
Orders
You can view, accept, reject, or cancel your orders from customers. You can also update the status of your orders, such as preparing, ready, or delivered. You can also view the payment details and history of each order.
-
Promote your products
Promotions
You can create and manage various types of promotions for your products, such as vouchers, flash sales, free shipping, or bundle deals. You can also set the duration, budget, and target audience for each promotion.
-
Communicate with customers
Chat
You can chat with your customers directly from the app. You can send and receive text messages, images, videos, voice notes, or stickers. You can also use quick replies or templates to answer common questions or requests.
-
-
With these features, you can manage your business more efficiently and effectively with Shopee Merchant.
-
Grow your sales
-
The third step to use APK Shopee Merchant is to grow your sales using the app. To do this, you can access various features and benefits that help you with the following goals:
-
-
Goal
Feature
Benefit
-
Increase your visibility
Self-promo creation
You can create and customize your own promotional materials, such as banners, flyers, and stickers, to attract more customers. You can also print or share them on social media platforms.
-
Improve your reputation
Ratings and reviews
You can collect and display ratings and reviews from your customers on your menu page. You can also respond to them and thank them for their feedback. This can help you build trust and loyalty among your customers.
-
Expand your market
Regional expansion
You can expand your market to other regions where Shopee operates, such as Malaysia, Singapore, Thailand, Vietnam, Philippines, or Taiwan. You can also adjust your menu and prices according to the local preferences and demand.
-
Optimize your performance
Analytics and insights
You can monitor your business performance and get useful tips and suggestions to improve your sales. You can also access various reports and statistics, such as sales volume, revenue, customer behavior, and market trends.
-
-
With these features and benefits, you can grow your sales and customer satisfaction with Shopee Merchant.
-
Conclusion
-
In conclusion, downloading APK Shopee Merchant is a smart and convenient way to manage your business with Shopee on your Android device. You can access the latest version of the app, install it on unsupported devices, and avoid regional restrictions. You can also register as a merchant, manage your business, and grow your sales using various features and benefits that Shopee Merchant offers. If you are an online seller who wants to join ShopeePay and ShopeeFood easily in one app, you should download APK Shopee Merchant today and start selling more with Shopee.
-
FAQs
-
Here are some frequently asked questions that you might have about downloading APK Shopee Merchant:
-
-
Is it safe to download APK files from unknown sources?
-
It depends on the source that you download the APK file from. Some sources may be reliable and safe, while others may be malicious or fraudulent. To ensure your safety, you should only download APK files from reputable and trusted websites, such as [APKPure]. You should also scan the APK file with an antivirus app before installing it on your device.
-
How can I update my APK Shopee Merchant app?
-
You can update your APK Shopee Merchant app by downloading the latest version of the APK file from the same source that you downloaded it from. You can also check for updates within the app by tapping on the menu icon and selecting Settings > About > Check for updates.
-
What if I encounter problems or errors while using the app?
-
If you encounter any problems or errors while using the app, you can try the following solutions:
-
-
Clear the cache and data of the app by going to your device settings > Apps > Shopee Merchant > Storage > Clear cache / Clear data.
-
Uninstall and reinstall the app by deleting the APK file from your device and downloading it again from the website.
-
Contact Shopee for support or feedback by tapping on the menu icon and selecting Help Center > Contact Us.
-
-
Can I use APK Shopee Merchant on other operating systems besides Android?
-
No, you cannot use APK Shopee Merchant on other operating systems besides Android. APK files are only compatible with Android devices. If you want to use Shopee Merchant on other devices, such as iOS or Windows, you will need to download the app from their respective app stores or use the web version of Shopee Merchant.
-
How can I contact Shopee for support or feedback?
-
You can contact Shopee for support or feedback by tapping on the menu icon and selecting Help Center > Contact Us. You can also email them at [merchant.support@shopee.com] or call them at [1500 407]. They are available 24/7 to assist you with any issues or inquiries that you may have.
- 197e85843d
-
-
\ No newline at end of file
diff --git a/spaces/1phancelerku/anime-remove-background/Crack Turkey Sandwiches - A Delicious Way to Use Up Turkey.md b/spaces/1phancelerku/anime-remove-background/Crack Turkey Sandwiches - A Delicious Way to Use Up Turkey.md
deleted file mode 100644
index 1d105b2aae1b7a04244a1e84502f936dbeceb425..0000000000000000000000000000000000000000
--- a/spaces/1phancelerku/anime-remove-background/Crack Turkey Sandwiches - A Delicious Way to Use Up Turkey.md
+++ /dev/null
@@ -1,111 +0,0 @@
-
-
What is Crackturkey and Why You Should Avoid It
-
If you are looking for cracked software, games, or accounts online, you might have come across some websites that claim to offer them for free or for a low price. These websites are known as crackturkey sites, and they are not what they seem. In fact, they are very dangerous and can harm your device, your data, and your identity. In this article, we will explain what crackturkey is, what are the risks of using it, and how to recognize and avoid crackturkey sites.
-
Introduction
-
What is crackturkey?
-
Crackturkey is a term that refers to websites that offer cracked or pirated software, games, or accounts for download or purchase. These websites are usually run by hackers or scammers who want to infect your device with malware, steal your personal information, or trick you into paying for something that does not work or does not exist. Crackturkey sites often use fake names, logos, and reviews to make themselves look legitimate and trustworthy. However, they are anything but that.
Using crackturkey can expose you to many serious risks, such as:
-
-
Malware infection: Crackturkey sites often contain malicious files that can infect your device with viruses, worms, trojans, ransomware, spyware, or adware. These malware can damage your device, delete or encrypt your files, monitor your online activity, steal your passwords, credit card numbers, or bank details, or display unwanted ads or pop-ups.
-
Data loss: Crackturkey sites can also cause you to lose your data, either by deleting it intentionally or accidentally, or by making it inaccessible due to encryption or corruption. You might lose your important documents, photos, videos, music, or other files that you have stored on your device.
-
Identity theft: Crackturkey sites can also compromise your identity by stealing your personal information, such as your name, email address, phone number, social media accounts, or other online identities. They can use this information to impersonate you online, send spam emails or messages in your name, make fraudulent purchases or transactions with your credit card or bank account, or access your other online accounts.
-
Legal issues: Crackturkey sites can also get you into legal trouble by violating the intellectual property rights of the original software or game developers or owners. Downloading or using cracked or pirated software or games is illegal in most countries and can result in fines or even jail time. You might also face lawsuits from the developers or owners who can sue you for damages.
-
-
How to Recognize and Avoid Crackturkey Sites
-
How to spot a crackturkey site
-
Crackturkey sites can be hard to distinguish from legitimate ones at first glance. However, there are some signs that can help you identify them and avoid falling for their traps. Here are some of them:
-
Check the domain name and URL
-
A common way that crackturkey sites try to deceive you is by using domain names and URLs that look similar to the official ones of the software or game that they claim to offer. For example, they might use a domain name like www.adobe-photoshop-crack.com instead of www.adobe.com, or a URL like https://www.crackerte
Look for signs of poor quality and security
-
Another way that crackturkey sites can reveal their true nature is by showing signs of poor quality and security. For example, they might have:
-
-
Spelling and grammar errors: Crackturkey sites often have spelling and grammar mistakes in their content, titles, or descriptions. This can indicate that they are not professional or reliable, and that they might have been translated from another language by a machine or a non-native speaker.
-
Broken links or images: Crackturkey sites often have broken links or images that do not load properly or lead to nowhere. This can indicate that they are not maintained or updated regularly, and that they might contain outdated or corrupted files.
-
Lack of HTTPS or SSL encryption: Crackturkey sites often do not have HTTPS or SSL encryption, which means that they are not secure and that your data can be intercepted or tampered with by third parties. You can check if a website has HTTPS or SSL encryption by looking for a padlock icon or the word "Secure" in the address bar of your browser.
-
-
Beware of fake reviews and testimonials
-
A third way that crackturkey sites can try to fool you is by using fake reviews and testimonials to make themselves look credible and trustworthy. For example, they might have:
-
crackturkey.com
-crackturkey iptv forum
-crackturkey mernis data
-crackturkey twitter
-crackturkey eam Türkçe
-crackturkey gizlilik politikası
-crackturkey şartlar ve kurallar
-crackturkey en büyük cracking topluluğu
-crackturkey üyeler kong
-crackturkey hata sorunlar
-crackturkey iptv hesapları
-crackturkey netflix accounts
-crackturkey spotify premium
-crackturkey discord server
-crackturkey cracking tools
-crackturkey proxy list
-crackturkey combo list
-crackturkey dork generator
-crackturkey sql injection
-crackturkey brute force
-crackturkey checker programları
-crackturkey mail access
-crackturkey gaming accounts
-crackturkey vpn accounts
-crackturkey nordvpn
-crackturkey expressvpn
-crackturkey hulu accounts
-crackturkey disney plus accounts
-crackturkey amazon prime accounts
-crackturkey steam accounts
-crackturkey origin accounts
-crackturkey uplay accounts
-crackturkey epic games accounts
-crackturkey minecraft accounts
-crackturkey fortnite accounts
-crackturkey roblox accounts
-crackturkey pubg accounts
-crackturkey valorant accounts
-crackturkey league of legends accounts
-crackturkey cs go accounts
-crackturkey social media accounts
-crackturkey instagram accounts
-crackturkey facebook accounts
-crackturkey twitter accounts
-crackturkey snapchat accounts
-crackturkey tiktok accounts
-crackturkey youtube premium accounts
-
-
Too many positive reviews: Crackturkey sites often have too many positive reviews that sound too good to be true, such as "This is the best software ever!", "It works perfectly!", or "I love it!". These reviews are usually written by bots or paid reviewers who have not actually used the software or game.
-
No negative reviews: Crackturkey sites often have no negative reviews or complaints from users who have encountered problems or issues with the software or game. This can indicate that they are censoring or deleting any negative feedback, or that they have not been used by many people at all.
-
No dates or names: Crackturkey sites often have no dates or names attached to their reviews or testimonials, which makes them hard to verify or trust. This can indicate that they are fabricated or copied from other sources.
-
-
How to avoid crackturkey sites
-
Now that you know how to spot a crackturkey site, you might be wondering how to avoid them and protect yourself from their dangers. Here are some tips that can help you do that:
-
Use reputable and trusted sources
-
The best way to avoid crackturkey sites is to use reputable and trusted sources for downloading or purchasing software, games, or accounts online. These sources are usually the official websites of the developers or owners, or authorized distributors or resellers. They offer genuine, legal, and safe products that are updated and supported regularly. You can also check the ratings, reviews, and feedback from other users who have used these sources before.
-
Use antivirus and firewall software
-
The second way to avoid crackturkey sites is to use antivirus and firewall software on your device. These software can help you detect and block any malware, phishing, or hacking attempts from crackturkey sites. They can also warn you of any suspicious or malicious websites that you might encounter online. You should always keep your antivirus and firewall software updated and scan your device regularly for any threats.
-
Report and block crackturkey sites
-
The third way to avoid crackturkey sites is to report and block them whenever you find them online. You can report them to the authorities, such as the cybercrime units of your local police or the Federal Trade Commission (FTC) in the US. You can also report them to the web hosting providers, domain registrars, search engines, social media platforms, or other online services that they use. You can also block them from your browser, email, or phone settings, or use tools like AdBlocker Plus or Malwarebytes to prevent them from appearing on your screen.
-
Conclusion
-
Summary of the main points
-
In conclusion, crackturkey is a term that refers to websites that offer cracked or pirated software, games, or accounts for download or purchase. These websites are very dangerous and can harm your device, your data, and your identity. They can also get you into legal trouble by violating the intellectual property rights of the original developers or owners. You should avoid crackturkey sites by using reputable and trusted sources, using antivirus and firewall software, and reporting and blocking them whenever you encounter them online.
-
Call to action
If you want to learn more about how to protect yourself from crackturkey and other online threats, you can check out some of these resources:
We hope you found this article helpful and informative. If you did, please share it with your friends and family who might benefit from it. And if you have any questions or comments, please leave them below. We would love to hear from you!
-
FAQs
-
What is crackturkey?
-
Crackturkey is a term that refers to websites that offer cracked or pirated software, games, or accounts for download or purchase.
-
What are the risks of using crackturkey?
-
Using crackturkey can expose you to many serious risks, such as malware infection, data loss, identity theft, and legal issues.
-
How to spot a crackturkey site?
-
You can spot a crackturkey site by checking the domain name and URL, looking for signs of poor quality and security, and beware of fake reviews and testimonials.
-
How to avoid crackturkey sites?
-
You can avoid crackturkey sites by using reputable and trusted sources, using antivirus and firewall software, and reporting and blocking them whenever you find them online.
-
Where can I find more information about crackturkey and online security?
-
You can find more information about crackturkey and online security by visiting some of the resources we have listed above, or by doing your own research online.
401be4b1e0
-
-
\ No newline at end of file
diff --git a/spaces/801artistry/RVC801/tools/torchgate/utils.py b/spaces/801artistry/RVC801/tools/torchgate/utils.py
deleted file mode 100644
index dc97d45a399c112c76e80cdd8c73cfebaf3ef6ad..0000000000000000000000000000000000000000
--- a/spaces/801artistry/RVC801/tools/torchgate/utils.py
+++ /dev/null
@@ -1,66 +0,0 @@
-import torch
-from torch.types import Number
-
-
-@torch.no_grad()
-def amp_to_db(x: torch.Tensor, eps=torch.finfo(torch.float64).eps, top_db=40) -> torch.Tensor:
- """
- Convert the input tensor from amplitude to decibel scale.
-
- Arguments:
- x {[torch.Tensor]} -- [Input tensor.]
-
- Keyword Arguments:
- eps {[float]} -- [Small value to avoid numerical instability.]
- (default: {torch.finfo(torch.float64).eps})
- top_db {[float]} -- [threshold the output at ``top_db`` below the peak]
- ` (default: {40})
-
- Returns:
- [torch.Tensor] -- [Output tensor in decibel scale.]
- """
- x_db = 20 * torch.log10(x.abs() + eps)
- return torch.max(x_db, (x_db.max(-1).values - top_db).unsqueeze(-1))
-
-
-@torch.no_grad()
-def temperature_sigmoid(x: torch.Tensor, x0: float, temp_coeff: float) -> torch.Tensor:
- """
- Apply a sigmoid function with temperature scaling.
-
- Arguments:
- x {[torch.Tensor]} -- [Input tensor.]
- x0 {[float]} -- [Parameter that controls the threshold of the sigmoid.]
- temp_coeff {[float]} -- [Parameter that controls the slope of the sigmoid.]
-
- Returns:
- [torch.Tensor] -- [Output tensor after applying the sigmoid with temperature scaling.]
- """
- return torch.sigmoid((x - x0) / temp_coeff)
-
-
-@torch.no_grad()
-def linspace(start: Number, stop: Number, num: int = 50, endpoint: bool = True, **kwargs) -> torch.Tensor:
- """
- Generate a linearly spaced 1-D tensor.
-
- Arguments:
- start {[Number]} -- [The starting value of the sequence.]
- stop {[Number]} -- [The end value of the sequence, unless `endpoint` is set to False.
- In that case, the sequence consists of all but the last of ``num + 1``
- evenly spaced samples, so that `stop` is excluded. Note that the step
- size changes when `endpoint` is False.]
-
- Keyword Arguments:
- num {[int]} -- [Number of samples to generate. Default is 50. Must be non-negative.]
- endpoint {[bool]} -- [If True, `stop` is the last sample. Otherwise, it is not included.
- Default is True.]
- **kwargs -- [Additional arguments to be passed to the underlying PyTorch `linspace` function.]
-
- Returns:
- [torch.Tensor] -- [1-D tensor of `num` equally spaced samples from `start` to `stop`.]
- """
- if endpoint:
- return torch.linspace(start, stop, num, **kwargs)
- else:
- return torch.linspace(start, stop, num + 1, **kwargs)[:-1]
diff --git a/spaces/AIFILMS/audioldm-text-to-audio-generation/audioldm/clap/open_clip/factory.py b/spaces/AIFILMS/audioldm-text-to-audio-generation/audioldm/clap/open_clip/factory.py
deleted file mode 100644
index 844f9ca0e12a0ff43ba3e042a3e43530ebe91b8c..0000000000000000000000000000000000000000
--- a/spaces/AIFILMS/audioldm-text-to-audio-generation/audioldm/clap/open_clip/factory.py
+++ /dev/null
@@ -1,277 +0,0 @@
-import json
-import logging
-import os
-import pathlib
-import re
-from copy import deepcopy
-from pathlib import Path
-
-import torch
-
-from .model import CLAP, convert_weights_to_fp16
-from .openai import load_openai_model
-from .pretrained import get_pretrained_url, download_pretrained
-from .transform import image_transform
-
-_MODEL_CONFIG_PATHS = [Path(__file__).parent / f"model_configs/"]
-_MODEL_CONFIGS = {} # directory (model_name: config) of model architecture configs
-
-
-def _natural_key(string_):
- return [int(s) if s.isdigit() else s for s in re.split(r"(\d+)", string_.lower())]
-
-
-def _rescan_model_configs():
- global _MODEL_CONFIGS
-
- config_ext = (".json",)
- config_files = []
- for config_path in _MODEL_CONFIG_PATHS:
- if config_path.is_file() and config_path.suffix in config_ext:
- config_files.append(config_path)
- elif config_path.is_dir():
- for ext in config_ext:
- config_files.extend(config_path.glob(f"*{ext}"))
-
- for cf in config_files:
- if os.path.basename(cf)[0] == ".":
- continue # Ignore hidden files
-
- with open(cf, "r") as f:
- model_cfg = json.load(f)
- if all(a in model_cfg for a in ("embed_dim", "audio_cfg", "text_cfg")):
- _MODEL_CONFIGS[cf.stem] = model_cfg
-
- _MODEL_CONFIGS = {
- k: v
- for k, v in sorted(_MODEL_CONFIGS.items(), key=lambda x: _natural_key(x[0]))
- }
-
-
-_rescan_model_configs() # initial populate of model config registry
-
-
-def load_state_dict(checkpoint_path: str, map_location="cpu", skip_params=True):
- checkpoint = torch.load(checkpoint_path, map_location=map_location)
- if isinstance(checkpoint, dict) and "state_dict" in checkpoint:
- state_dict = checkpoint["state_dict"]
- else:
- state_dict = checkpoint
- if skip_params:
- if next(iter(state_dict.items()))[0].startswith("module"):
- state_dict = {k[7:]: v for k, v in state_dict.items()}
- # for k in state_dict:
- # if k.startswith('transformer'):
- # v = state_dict.pop(k)
- # state_dict['text_branch.' + k[12:]] = v
- return state_dict
-
-
-def create_model(
- amodel_name: str,
- tmodel_name: str,
- pretrained: str = "",
- precision: str = "fp32",
- device: torch.device = torch.device("cpu"),
- jit: bool = False,
- force_quick_gelu: bool = False,
- openai_model_cache_dir: str = os.path.expanduser("~/.cache/clip"),
- skip_params=True,
- pretrained_audio: str = "",
- pretrained_text: str = "",
- enable_fusion: bool = False,
- fusion_type: str = "None"
- # pretrained_image: bool = False,
-):
- amodel_name = amodel_name.replace(
- "/", "-"
- ) # for callers using old naming with / in ViT names
- pretrained_orig = pretrained
- pretrained = pretrained.lower()
- if pretrained == "openai":
- if amodel_name in _MODEL_CONFIGS:
- logging.info(f"Loading {amodel_name} model config.")
- model_cfg = deepcopy(_MODEL_CONFIGS[amodel_name])
- else:
- logging.error(
- f"Model config for {amodel_name} not found; available models {list_models()}."
- )
- raise RuntimeError(f"Model config for {amodel_name} not found.")
-
- logging.info(f"Loading pretrained ViT-B-16 text encoder from OpenAI.")
- # Hard Code in model name
- model_cfg["text_cfg"]["model_type"] = tmodel_name
- model = load_openai_model(
- "ViT-B-16",
- model_cfg,
- device=device,
- jit=jit,
- cache_dir=openai_model_cache_dir,
- enable_fusion=enable_fusion,
- fusion_type=fusion_type,
- )
- # See https://discuss.pytorch.org/t/valueerror-attemting-to-unscale-fp16-gradients/81372
- if precision == "amp" or precision == "fp32":
- model = model.float()
- else:
- if amodel_name in _MODEL_CONFIGS:
- logging.info(f"Loading {amodel_name} model config.")
- model_cfg = deepcopy(_MODEL_CONFIGS[amodel_name])
- else:
- logging.error(
- f"Model config for {amodel_name} not found; available models {list_models()}."
- )
- raise RuntimeError(f"Model config for {amodel_name} not found.")
-
- if force_quick_gelu:
- # override for use of QuickGELU on non-OpenAI transformer models
- model_cfg["quick_gelu"] = True
-
- # if pretrained_image:
- # if 'timm_amodel_name' in model_cfg.get('vision_cfg', {}):
- # # pretrained weight loading for timm models set via vision_cfg
- # model_cfg['vision_cfg']['timm_model_pretrained'] = True
- # else:
- # assert False, 'pretrained image towers currently only supported for timm models'
- model_cfg["text_cfg"]["model_type"] = tmodel_name
- model_cfg["enable_fusion"] = enable_fusion
- model_cfg["fusion_type"] = fusion_type
- model = CLAP(**model_cfg)
-
- if pretrained:
- checkpoint_path = ""
- url = get_pretrained_url(amodel_name, pretrained)
- if url:
- checkpoint_path = download_pretrained(url, root=openai_model_cache_dir)
- elif os.path.exists(pretrained_orig):
- checkpoint_path = pretrained_orig
- if checkpoint_path:
- logging.info(
- f"Loading pretrained {amodel_name}-{tmodel_name} weights ({pretrained})."
- )
- ckpt = load_state_dict(checkpoint_path, skip_params=True)
- model.load_state_dict(ckpt)
- param_names = [n for n, p in model.named_parameters()]
- # for n in param_names:
- # print(n, "\t", "Loaded" if n in ckpt else "Unloaded")
- else:
- logging.warning(
- f"Pretrained weights ({pretrained}) not found for model {amodel_name}."
- )
- raise RuntimeError(
- f"Pretrained weights ({pretrained}) not found for model {amodel_name}."
- )
-
- if pretrained_audio:
- if amodel_name.startswith("PANN"):
- if "Cnn14_mAP" in pretrained_audio: # official checkpoint
- audio_ckpt = torch.load(pretrained_audio, map_location="cpu")
- audio_ckpt = audio_ckpt["model"]
- keys = list(audio_ckpt.keys())
- for key in keys:
- if (
- "spectrogram_extractor" not in key
- and "logmel_extractor" not in key
- ):
- v = audio_ckpt.pop(key)
- audio_ckpt["audio_branch." + key] = v
- elif os.path.basename(pretrained_audio).startswith(
- "PANN"
- ): # checkpoint trained via HTSAT codebase
- audio_ckpt = torch.load(pretrained_audio, map_location="cpu")
- audio_ckpt = audio_ckpt["state_dict"]
- keys = list(audio_ckpt.keys())
- for key in keys:
- if key.startswith("sed_model"):
- v = audio_ckpt.pop(key)
- audio_ckpt["audio_branch." + key[10:]] = v
- elif os.path.basename(pretrained_audio).startswith(
- "finetuned"
- ): # checkpoint trained via linear probe codebase
- audio_ckpt = torch.load(pretrained_audio, map_location="cpu")
- else:
- raise ValueError("Unknown audio checkpoint")
- elif amodel_name.startswith("HTSAT"):
- if "HTSAT_AudioSet_Saved" in pretrained_audio: # official checkpoint
- audio_ckpt = torch.load(pretrained_audio, map_location="cpu")
- audio_ckpt = audio_ckpt["state_dict"]
- keys = list(audio_ckpt.keys())
- for key in keys:
- if key.startswith("sed_model") and (
- "spectrogram_extractor" not in key
- and "logmel_extractor" not in key
- ):
- v = audio_ckpt.pop(key)
- audio_ckpt["audio_branch." + key[10:]] = v
- elif os.path.basename(pretrained_audio).startswith(
- "HTSAT"
- ): # checkpoint trained via HTSAT codebase
- audio_ckpt = torch.load(pretrained_audio, map_location="cpu")
- audio_ckpt = audio_ckpt["state_dict"]
- keys = list(audio_ckpt.keys())
- for key in keys:
- if key.startswith("sed_model"):
- v = audio_ckpt.pop(key)
- audio_ckpt["audio_branch." + key[10:]] = v
- elif os.path.basename(pretrained_audio).startswith(
- "finetuned"
- ): # checkpoint trained via linear probe codebase
- audio_ckpt = torch.load(pretrained_audio, map_location="cpu")
- else:
- raise ValueError("Unknown audio checkpoint")
- else:
- raise f"this audio encoder pretrained checkpoint is not support"
-
- model.load_state_dict(audio_ckpt, strict=False)
- logging.info(
- f"Loading pretrained {amodel_name} weights ({pretrained_audio})."
- )
- param_names = [n for n, p in model.named_parameters()]
- for n in param_names:
- print(n, "\t", "Loaded" if n in audio_ckpt else "Unloaded")
-
- model.to(device=device)
- if precision == "fp16":
- assert device.type != "cpu"
- convert_weights_to_fp16(model)
-
- if jit:
- model = torch.jit.script(model)
-
- return model, model_cfg
-
-
-def create_model_and_transforms(
- model_name: str,
- pretrained: str = "",
- precision: str = "fp32",
- device: torch.device = torch.device("cpu"),
- jit: bool = False,
- force_quick_gelu: bool = False,
- # pretrained_image: bool = False,
-):
- model = create_model(
- model_name,
- pretrained,
- precision,
- device,
- jit,
- force_quick_gelu=force_quick_gelu,
- # pretrained_image=pretrained_image
- )
- preprocess_train = image_transform(model.visual.image_size, is_train=True)
- preprocess_val = image_transform(model.visual.image_size, is_train=False)
- return model, preprocess_train, preprocess_val
-
-
-def list_models():
- """enumerate available model architectures based on config files"""
- return list(_MODEL_CONFIGS.keys())
-
-
-def add_model_config(path):
- """add model config path or file and update registry"""
- if not isinstance(path, Path):
- path = Path(path)
- _MODEL_CONFIG_PATHS.append(path)
- _rescan_model_configs()
diff --git a/spaces/AIFILMS/generate_human_motion/VQ-Trans/models/vqvae.py b/spaces/AIFILMS/generate_human_motion/VQ-Trans/models/vqvae.py
deleted file mode 100644
index 7e6c940674d460853e8418514bf2306f774689fd..0000000000000000000000000000000000000000
--- a/spaces/AIFILMS/generate_human_motion/VQ-Trans/models/vqvae.py
+++ /dev/null
@@ -1,118 +0,0 @@
-import torch.nn as nn
-from models.encdec import Encoder, Decoder
-from models.quantize_cnn import QuantizeEMAReset, Quantizer, QuantizeEMA, QuantizeReset
-
-
-class VQVAE_251(nn.Module):
- def __init__(self,
- args,
- nb_code=1024,
- code_dim=512,
- output_emb_width=512,
- down_t=3,
- stride_t=2,
- width=512,
- depth=3,
- dilation_growth_rate=3,
- activation='relu',
- norm=None):
-
- super().__init__()
- self.code_dim = code_dim
- self.num_code = nb_code
- self.quant = args.quantizer
- self.encoder = Encoder(251 if args.dataname == 'kit' else 263, output_emb_width, down_t, stride_t, width, depth, dilation_growth_rate, activation=activation, norm=norm)
- self.decoder = Decoder(251 if args.dataname == 'kit' else 263, output_emb_width, down_t, stride_t, width, depth, dilation_growth_rate, activation=activation, norm=norm)
- if args.quantizer == "ema_reset":
- self.quantizer = QuantizeEMAReset(nb_code, code_dim, args)
- elif args.quantizer == "orig":
- self.quantizer = Quantizer(nb_code, code_dim, 1.0)
- elif args.quantizer == "ema":
- self.quantizer = QuantizeEMA(nb_code, code_dim, args)
- elif args.quantizer == "reset":
- self.quantizer = QuantizeReset(nb_code, code_dim, args)
-
-
- def preprocess(self, x):
- # (bs, T, Jx3) -> (bs, Jx3, T)
- x = x.permute(0,2,1).float()
- return x
-
-
- def postprocess(self, x):
- # (bs, Jx3, T) -> (bs, T, Jx3)
- x = x.permute(0,2,1)
- return x
-
-
- def encode(self, x):
- N, T, _ = x.shape
- x_in = self.preprocess(x)
- x_encoder = self.encoder(x_in)
- x_encoder = self.postprocess(x_encoder)
- x_encoder = x_encoder.contiguous().view(-1, x_encoder.shape[-1]) # (NT, C)
- code_idx = self.quantizer.quantize(x_encoder)
- code_idx = code_idx.view(N, -1)
- return code_idx
-
-
- def forward(self, x):
-
- x_in = self.preprocess(x)
- # Encode
- x_encoder = self.encoder(x_in)
-
- ## quantization
- x_quantized, loss, perplexity = self.quantizer(x_encoder)
-
- ## decoder
- x_decoder = self.decoder(x_quantized)
- x_out = self.postprocess(x_decoder)
- return x_out, loss, perplexity
-
-
- def forward_decoder(self, x):
- x_d = self.quantizer.dequantize(x)
- x_d = x_d.view(1, -1, self.code_dim).permute(0, 2, 1).contiguous()
-
- # decoder
- x_decoder = self.decoder(x_d)
- x_out = self.postprocess(x_decoder)
- return x_out
-
-
-
-class HumanVQVAE(nn.Module):
- def __init__(self,
- args,
- nb_code=512,
- code_dim=512,
- output_emb_width=512,
- down_t=3,
- stride_t=2,
- width=512,
- depth=3,
- dilation_growth_rate=3,
- activation='relu',
- norm=None):
-
- super().__init__()
-
- self.nb_joints = 21 if args.dataname == 'kit' else 22
- self.vqvae = VQVAE_251(args, nb_code, code_dim, output_emb_width, down_t, stride_t, width, depth, dilation_growth_rate, activation=activation, norm=norm)
-
- def encode(self, x):
- b, t, c = x.size()
- quants = self.vqvae.encode(x) # (N, T)
- return quants
-
- def forward(self, x):
-
- x_out, loss, perplexity = self.vqvae(x)
-
- return x_out, loss, perplexity
-
- def forward_decoder(self, x):
- x_out = self.vqvae.forward_decoder(x)
- return x_out
-
\ No newline at end of file
diff --git a/spaces/AIFILMS/generate_human_motion/pyrender/pyrender/constants.py b/spaces/AIFILMS/generate_human_motion/pyrender/pyrender/constants.py
deleted file mode 100644
index 8a5785b6fdb21910a174252c5af2f05b40ece4a5..0000000000000000000000000000000000000000
--- a/spaces/AIFILMS/generate_human_motion/pyrender/pyrender/constants.py
+++ /dev/null
@@ -1,149 +0,0 @@
-DEFAULT_Z_NEAR = 0.05 # Near clipping plane, in meters
-DEFAULT_Z_FAR = 100.0 # Far clipping plane, in meters
-DEFAULT_SCENE_SCALE = 2.0 # Default scene scale
-MAX_N_LIGHTS = 4 # Maximum number of lights of each type allowed
-TARGET_OPEN_GL_MAJOR = 4 # Target OpenGL Major Version
-TARGET_OPEN_GL_MINOR = 1 # Target OpenGL Minor Version
-MIN_OPEN_GL_MAJOR = 3 # Minimum OpenGL Major Version
-MIN_OPEN_GL_MINOR = 3 # Minimum OpenGL Minor Version
-FLOAT_SZ = 4 # Byte size of GL float32
-UINT_SZ = 4 # Byte size of GL uint32
-SHADOW_TEX_SZ = 2048 # Width and Height of Shadow Textures
-TEXT_PADDING = 20 # Width of padding for rendering text (px)
-
-
-# Flags for render type
-class RenderFlags(object):
- """Flags for rendering in the scene.
-
- Combine them with the bitwise or. For example,
-
- >>> flags = OFFSCREEN | SHADOWS_DIRECTIONAL | VERTEX_NORMALS
-
- would result in an offscreen render with directional shadows and
- vertex normals enabled.
- """
- NONE = 0
- """Normal PBR Render."""
- DEPTH_ONLY = 1
- """Only render the depth buffer."""
- OFFSCREEN = 2
- """Render offscreen and return the depth and (optionally) color buffers."""
- FLIP_WIREFRAME = 4
- """Invert the status of wireframe rendering for each mesh."""
- ALL_WIREFRAME = 8
- """Render all meshes as wireframes."""
- ALL_SOLID = 16
- """Render all meshes as solids."""
- SHADOWS_DIRECTIONAL = 32
- """Render shadows for directional lights."""
- SHADOWS_POINT = 64
- """Render shadows for point lights."""
- SHADOWS_SPOT = 128
- """Render shadows for spot lights."""
- SHADOWS_ALL = 32 | 64 | 128
- """Render shadows for all lights."""
- VERTEX_NORMALS = 256
- """Render vertex normals."""
- FACE_NORMALS = 512
- """Render face normals."""
- SKIP_CULL_FACES = 1024
- """Do not cull back faces."""
- RGBA = 2048
- """Render the color buffer with the alpha channel enabled."""
- FLAT = 4096
- """Render the color buffer flat, with no lighting computations."""
- SEG = 8192
-
-
-class TextAlign:
- """Text alignment options for captions.
-
- Only use one at a time.
- """
- CENTER = 0
- """Center the text by width and height."""
- CENTER_LEFT = 1
- """Center the text by height and left-align it."""
- CENTER_RIGHT = 2
- """Center the text by height and right-align it."""
- BOTTOM_LEFT = 3
- """Put the text in the bottom-left corner."""
- BOTTOM_RIGHT = 4
- """Put the text in the bottom-right corner."""
- BOTTOM_CENTER = 5
- """Center the text by width and fix it to the bottom."""
- TOP_LEFT = 6
- """Put the text in the top-left corner."""
- TOP_RIGHT = 7
- """Put the text in the top-right corner."""
- TOP_CENTER = 8
- """Center the text by width and fix it to the top."""
-
-
-class GLTF(object):
- """Options for GL objects."""
- NEAREST = 9728
- """Nearest neighbor interpolation."""
- LINEAR = 9729
- """Linear interpolation."""
- NEAREST_MIPMAP_NEAREST = 9984
- """Nearest mipmapping."""
- LINEAR_MIPMAP_NEAREST = 9985
- """Linear mipmapping."""
- NEAREST_MIPMAP_LINEAR = 9986
- """Nearest mipmapping."""
- LINEAR_MIPMAP_LINEAR = 9987
- """Linear mipmapping."""
- CLAMP_TO_EDGE = 33071
- """Clamp to the edge of the texture."""
- MIRRORED_REPEAT = 33648
- """Mirror the texture."""
- REPEAT = 10497
- """Repeat the texture."""
- POINTS = 0
- """Render as points."""
- LINES = 1
- """Render as lines."""
- LINE_LOOP = 2
- """Render as a line loop."""
- LINE_STRIP = 3
- """Render as a line strip."""
- TRIANGLES = 4
- """Render as triangles."""
- TRIANGLE_STRIP = 5
- """Render as a triangle strip."""
- TRIANGLE_FAN = 6
- """Render as a triangle fan."""
-
-
-class BufFlags(object):
- POSITION = 0
- NORMAL = 1
- TANGENT = 2
- TEXCOORD_0 = 4
- TEXCOORD_1 = 8
- COLOR_0 = 16
- JOINTS_0 = 32
- WEIGHTS_0 = 64
-
-
-class TexFlags(object):
- NONE = 0
- NORMAL = 1
- OCCLUSION = 2
- EMISSIVE = 4
- BASE_COLOR = 8
- METALLIC_ROUGHNESS = 16
- DIFFUSE = 32
- SPECULAR_GLOSSINESS = 64
-
-
-class ProgramFlags:
- NONE = 0
- USE_MATERIAL = 1
- VERTEX_NORMALS = 2
- FACE_NORMALS = 4
-
-
-__all__ = ['RenderFlags', 'TextAlign', 'GLTF']
diff --git a/spaces/AIGC-Audio/AudioGPT/text_to_speech/modules/commons/layers.py b/spaces/AIGC-Audio/AudioGPT/text_to_speech/modules/commons/layers.py
deleted file mode 100644
index 88e1c75876050fa05a768a5ae0467fdfc05bb006..0000000000000000000000000000000000000000
--- a/spaces/AIGC-Audio/AudioGPT/text_to_speech/modules/commons/layers.py
+++ /dev/null
@@ -1,50 +0,0 @@
-import torch
-from torch import nn
-
-
-class LayerNorm(torch.nn.LayerNorm):
- """Layer normalization module.
- :param int nout: output dim size
- :param int dim: dimension to be normalized
- """
-
- def __init__(self, nout, dim=-1, eps=1e-5):
- """Construct an LayerNorm object."""
- super(LayerNorm, self).__init__(nout, eps=eps)
- self.dim = dim
-
- def forward(self, x):
- """Apply layer normalization.
- :param torch.Tensor x: input tensor
- :return: layer normalized tensor
- :rtype torch.Tensor
- """
- if self.dim == -1:
- return super(LayerNorm, self).forward(x)
- return super(LayerNorm, self).forward(x.transpose(1, -1)).transpose(1, -1)
-
-
-class Reshape(nn.Module):
- def __init__(self, *args):
- super(Reshape, self).__init__()
- self.shape = args
-
- def forward(self, x):
- return x.view(self.shape)
-
-
-class Permute(nn.Module):
- def __init__(self, *args):
- super(Permute, self).__init__()
- self.args = args
-
- def forward(self, x):
- return x.permute(self.args)
-
-
-def Embedding(num_embeddings, embedding_dim, padding_idx=None):
- m = nn.Embedding(num_embeddings, embedding_dim, padding_idx=padding_idx)
- nn.init.normal_(m.weight, mean=0, std=embedding_dim ** -0.5)
- if padding_idx is not None:
- nn.init.constant_(m.weight[padding_idx], 0)
- return m
diff --git a/spaces/AIGC-Audio/Make_An_Audio/ldm/modules/losses_audio/vggishish/loss.py b/spaces/AIGC-Audio/Make_An_Audio/ldm/modules/losses_audio/vggishish/loss.py
deleted file mode 100644
index bae76571909eec571aaf075d58e3dea8f6424546..0000000000000000000000000000000000000000
--- a/spaces/AIGC-Audio/Make_An_Audio/ldm/modules/losses_audio/vggishish/loss.py
+++ /dev/null
@@ -1,41 +0,0 @@
-import torch
-import torch.nn as nn
-import torch.nn.functional as F
-import torch.optim as optim
-
-class WeightedCrossEntropy(nn.CrossEntropyLoss):
-
- def __init__(self, weights, **pytorch_ce_loss_args) -> None:
- super().__init__(reduction='none', **pytorch_ce_loss_args)
- self.weights = weights
-
- def __call__(self, outputs, targets, to_weight=True):
- loss = super().__call__(outputs, targets)
- if to_weight:
- return (loss * self.weights[targets]).sum() / self.weights[targets].sum()
- else:
- return loss.mean()
-
-
-if __name__ == '__main__':
- x = torch.randn(10, 5)
- target = torch.randint(0, 5, (10,))
- weights = torch.tensor([1., 2., 3., 4., 5.])
-
- # criterion_weighted = nn.CrossEntropyLoss(weight=weights)
- # loss_weighted = criterion_weighted(x, target)
-
- # criterion_weighted_manual = nn.CrossEntropyLoss(reduction='none')
- # loss_weighted_manual = criterion_weighted_manual(x, target)
- # print(loss_weighted, loss_weighted_manual.mean())
- # loss_weighted_manual = (loss_weighted_manual * weights[target]).sum() / weights[target].sum()
- # print(loss_weighted, loss_weighted_manual)
- # print(torch.allclose(loss_weighted, loss_weighted_manual))
-
- pytorch_weighted = nn.CrossEntropyLoss(weight=weights)
- pytorch_unweighted = nn.CrossEntropyLoss()
- custom = WeightedCrossEntropy(weights)
-
- assert torch.allclose(pytorch_weighted(x, target), custom(x, target, to_weight=True))
- assert torch.allclose(pytorch_unweighted(x, target), custom(x, target, to_weight=False))
- print(custom(x, target, to_weight=True), custom(x, target, to_weight=False))
diff --git a/spaces/ASJMO/freegpt/client/js/icons.js b/spaces/ASJMO/freegpt/client/js/icons.js
deleted file mode 100644
index 84fed38dd35e0d0203370a8314a360d27f350dd6..0000000000000000000000000000000000000000
--- a/spaces/ASJMO/freegpt/client/js/icons.js
+++ /dev/null
@@ -1 +0,0 @@
-window.FontAwesomeKitConfig={asyncLoading:{enabled:!1},autoA11y:{enabled:!0},baseUrl:"https://ka-f.fontawesome.com",baseUrlKit:"https://kit-pro.fontawesome.com",detectConflictsUntil:null,iconUploads:{},id:96462084,license:"pro",method:"css",minify:{enabled:!0},token:"d0514f1901",v4FontFaceShim:{enabled:!0},v4shim:{enabled:!0},v5FontFaceShim:{enabled:!0},version:"6.1.1"},function(t){"function"==typeof define&&define.amd?define("kit-loader",t):t()}(function(){"use strict";function t(e){return(t="function"==typeof Symbol&&"symbol"==typeof Symbol.iterator?function(t){return typeof t}:function(t){return t&&"function"==typeof Symbol&&t.constructor===Symbol&&t!==Symbol.prototype?"symbol":typeof t})(e)}function e(t,e,n){return e in t?Object.defineProperty(t,e,{value:n,enumerable:!0,configurable:!0,writable:!0}):t[e]=n,t}function n(t,e){var n=Object.keys(t);if(Object.getOwnPropertySymbols){var o=Object.getOwnPropertySymbols(t);e&&(o=o.filter(function(e){return Object.getOwnPropertyDescriptor(t,e).enumerable})),n.push.apply(n,o)}return n}function o(t){for(var o=1;ot.length)&&(e=t.length);for(var n=0,o=new Array(e);n2&&void 0!==arguments[2]?arguments[2]:function(){},r=e.document||r,i=a.bind(a,r,["fa","fab","fas","far","fal","fad","fak"]),u=Object.keys(t.iconUploads||{}).length>0;t.autoA11y.enabled&&n(i);var f=[{id:"fa-main",addOn:void 0}];t.v4shim&&t.v4shim.enabled&&f.push({id:"fa-v4-shims",addOn:"-v4-shims"}),t.v5FontFaceShim&&t.v5FontFaceShim.enabled&&f.push({id:"fa-v5-font-face",addOn:"-v5-font-face"}),t.v4FontFaceShim&&t.v4FontFaceShim.enabled&&f.push({id:"fa-v4-font-face",addOn:"-v4-font-face"}),u&&f.push({id:"fa-kit-upload",customCss:!0});var s=f.map(function(n){return new F(function(r,i){E(n.customCss?function(t){return t.baseUrlKit+"/"+t.token+"/"+t.id+"/kit-upload.css"}(t):c(t,{addOn:n.addOn,minify:t.minify.enabled}),e).then(function(i){r(function(t,e){var n=e.contentFilter||function(t,e){return t},o=document.createElement("style"),r=document.createTextNode(n(t,e));return o.appendChild(r),o.media="all",e.id&&o.setAttribute("id",e.id),e&&e.detectingConflicts&&e.detectionIgnoreAttr&&o.setAttributeNode(document.createAttribute(e.detectionIgnoreAttr)),o}(i,o(o({},e),{},{baseUrl:t.baseUrl,version:t.version,id:n.id,contentFilter:function(t,e){return _(t,e.baseUrl,e.version)}})))}).catch(i)})});return F.all(s)}function P(t,e){var n=document.createElement("SCRIPT"),o=document.createTextNode(t);return n.appendChild(o),n.referrerPolicy="strict-origin",e.id&&n.setAttribute("id",e.id),e&&e.detectingConflicts&&e.detectionIgnoreAttr&&n.setAttributeNode(document.createAttribute(e.detectionIgnoreAttr)),n}function U(t){var e,n=[],o=document,r=(o.documentElement.doScroll?/^loaded|^c/:/^loaded|^i|^c/).test(o.readyState);r||o.addEventListener("DOMContentLoaded",e=function(){for(o.removeEventListener("DOMContentLoaded",e),r=1;e=n.shift();)e()}),r?setTimeout(t,0):n.push(t)}try{if(window.FontAwesomeKitConfig){var k=window.FontAwesomeKitConfig,L={detectingConflicts:k.detectConflictsUntil&&new Date<=new Date(k.detectConflictsUntil),detectionIgnoreAttr:"data-fa-detection-ignore",fetch:window.fetch,token:k.token,XMLHttpRequest:window.XMLHttpRequest,document:document},I=document.currentScript,T=I?I.parentElement:document.head;(function(){var t=arguments.length>0&&void 0!==arguments[0]?arguments[0]:{},e=arguments.length>1&&void 0!==arguments[1]?arguments[1]:{};return"js"===t.method?function(t,e){e.autoA11y=t.autoA11y.enabled,"pro"===t.license&&(e.autoFetchSvg=!0,e.fetchSvgFrom=t.baseUrl+"/releases/"+("latest"===t.version?"latest":"v".concat(t.version))+"/svgs",e.fetchUploadedSvgFrom=t.uploadsUrl);var n=[];return t.v4shim.enabled&&n.push(new F(function(n,r){E(c(t,{addOn:"-v4-shims",minify:t.minify.enabled}),e).then(function(t){n(P(t,o(o({},e),{},{id:"fa-v4-shims"})))}).catch(r)})),n.push(new F(function(n,r){E(c(t,{minify:t.minify.enabled}),e).then(function(t){var r=P(t,o(o({},e),{},{id:"fa-main"}));n(function(t,e){var n=e&&void 0!==e.autoFetchSvg?e.autoFetchSvg:void 0,o=e&&void 0!==e.autoA11y?e.autoA11y:void 0;return void 0!==o&&t.setAttribute("data-auto-a11y",o?"true":"false"),n&&(t.setAttributeNode(document.createAttribute("data-auto-fetch-svg")),t.setAttribute("data-fetch-svg-from",e.fetchSvgFrom),t.setAttribute("data-fetch-uploaded-svg-from",e.fetchUploadedSvgFrom)),t}(r,e))}).catch(r)})),F.all(n)}(t,e):"css"===t.method?C(t,e,function(t){U(t),function(t){"undefined"!=typeof MutationObserver&&new MutationObserver(t).observe(document,{childList:!0,subtree:!0})}(t)}):void 0})(k,L).then(function(t){t.map(function(t){try{T.insertBefore(t,I?I.nextSibling:null)}catch(e){T.appendChild(t)}}),L.detectingConflicts&&I&&U(function(){I.setAttributeNode(document.createAttribute(L.detectionIgnoreAttr));var t=function(t,e){var n=document.createElement("script");return e&&e.detectionIgnoreAttr&&n.setAttributeNode(document.createAttribute(e.detectionIgnoreAttr)),n.src=c(t,{baseFilename:"conflict-detection",fileSuffix:"js",subdir:"js",minify:t.minify.enabled}),n}(k,L);document.body.appendChild(t)})}).catch(function(t){console.error("".concat("Font Awesome Kit:"," ").concat(t))})}}catch(t){console.error("".concat("Font Awesome Kit:"," ").concat(t))}});
\ No newline at end of file
diff --git a/spaces/AchyuthGamer/OpenGPT/g4f/Provider/needs_auth/Raycast.py b/spaces/AchyuthGamer/OpenGPT/g4f/Provider/needs_auth/Raycast.py
deleted file mode 100644
index 619b217b8aa7828a284285d422092c6c6dd3fe47..0000000000000000000000000000000000000000
--- a/spaces/AchyuthGamer/OpenGPT/g4f/Provider/needs_auth/Raycast.py
+++ /dev/null
@@ -1,72 +0,0 @@
-from __future__ import annotations
-
-import json
-
-import requests
-
-from ...typing import Any, CreateResult
-from ..base_provider import BaseProvider
-
-
-class Raycast(BaseProvider):
- url = "https://raycast.com"
- supports_gpt_35_turbo = True
- supports_gpt_4 = True
- supports_stream = True
- needs_auth = True
- working = True
-
- @staticmethod
- def create_completion(
- model: str,
- messages: list[dict[str, str]],
- stream: bool,
- **kwargs: Any,
- ) -> CreateResult:
- auth = kwargs.get('auth')
- headers = {
- 'Accept': 'application/json',
- 'Accept-Language': 'en-US,en;q=0.9',
- 'Authorization': f'Bearer {auth}',
- 'Content-Type': 'application/json',
- 'User-Agent': 'Raycast/0 CFNetwork/1410.0.3 Darwin/22.6.0',
- }
- parsed_messages = []
- for message in messages:
- parsed_messages.append({
- 'author': message['role'],
- 'content': {'text': message['content']}
- })
- data = {
- "debug": False,
- "locale": "en-CN",
- "messages": parsed_messages,
- "model": model,
- "provider": "openai",
- "source": "ai_chat",
- "system_instruction": "markdown",
- "temperature": 0.5
- }
- response = requests.post("https://backend.raycast.com/api/v1/ai/chat_completions", headers=headers, json=data, stream=True)
- for token in response.iter_lines():
- if b'data: ' not in token:
- continue
- completion_chunk = json.loads(token.decode().replace('data: ', ''))
- token = completion_chunk['text']
- if token != None:
- yield token
-
- @classmethod
- @property
- def params(cls):
- params = [
- ("model", "str"),
- ("messages", "list[dict[str, str]]"),
- ("stream", "bool"),
- ("temperature", "float"),
- ("top_p", "int"),
- ("model", "str"),
- ("auth", "str"),
- ]
- param = ", ".join([": ".join(p) for p in params])
- return f"g4f.provider.{cls.__name__} supports: ({param})"
diff --git a/spaces/AgentVerse/agentVerse/ui/src/phaser3-rex-plugins/plugins/scaleouter.js b/spaces/AgentVerse/agentVerse/ui/src/phaser3-rex-plugins/plugins/scaleouter.js
deleted file mode 100644
index 8c82e35da14d1d9a0fdec3ef2f6feb57be6b7d8e..0000000000000000000000000000000000000000
--- a/spaces/AgentVerse/agentVerse/ui/src/phaser3-rex-plugins/plugins/scaleouter.js
+++ /dev/null
@@ -1,2 +0,0 @@
-import ScaleOuter from './scale/scaleouter/ScaleOuter.js';
-export default ScaleOuter;
\ No newline at end of file
diff --git a/spaces/AkitoP/umamusume_bert_vits2/text/__init__.py b/spaces/AkitoP/umamusume_bert_vits2/text/__init__.py
deleted file mode 100644
index a45b650424306b6e077d7013e93e2c9bd1e073c2..0000000000000000000000000000000000000000
--- a/spaces/AkitoP/umamusume_bert_vits2/text/__init__.py
+++ /dev/null
@@ -1,28 +0,0 @@
-from text.symbols import *
-
-_symbol_to_id = {s: i for i, s in enumerate(symbols)}
-
-
-def cleaned_text_to_sequence(cleaned_text, tones, language):
- """Converts a string of text to a sequence of IDs corresponding to the symbols in the text.
- Args:
- text: string to convert to a sequence
- Returns:
- List of integers corresponding to the symbols in the text
- """
- phones = [_symbol_to_id[symbol] for symbol in cleaned_text]
- tone_start = language_tone_start_map[language]
- tones = [i + tone_start for i in tones]
- lang_id = language_id_map[language]
- lang_ids = [lang_id for i in phones]
- return phones, tones, lang_ids
-
-
-def get_bert(norm_text, word2ph, language, device):
- from .chinese_bert import get_bert_feature as zh_bert
- from .english_bert_mock import get_bert_feature as en_bert
- from .japanese_bert import get_bert_feature as jp_bert
-
- lang_bert_func_map = {"ZH": zh_bert, "EN": en_bert, "JP": jp_bert}
- bert = lang_bert_func_map[language](norm_text, word2ph, device)
- return bert
diff --git a/spaces/Alesteba/NeRF_ficus-pxl/app.py b/spaces/Alesteba/NeRF_ficus-pxl/app.py
deleted file mode 100644
index 77f9210ce11f204061840d2824174db529c5e18b..0000000000000000000000000000000000000000
--- a/spaces/Alesteba/NeRF_ficus-pxl/app.py
+++ /dev/null
@@ -1,79 +0,0 @@
-import streamlit as st
-import tensorflow as tf
-import numpy as np
-
-from config import *
-from transformations import *
-from rendering import *
-
-# Setting random seed to obtain reproducible results.
-tf.random.set_seed(42)
-
-def show_rendered_image(r,theta,phi):
-
- # Get the camera to world matrix.
-
- c2w = pose_spherical(theta, phi, r)
-
- ray_oris, ray_dirs = get_rays(H, W, focal, c2w)
- rays_flat, t_vals = render_flat_rays(
- ray_oris, ray_dirs, near=2.0, far=6.0, num_samples=NUM_SAMPLES, rand=False
- )
-
- rgb, depth = render_rgb_depth(
- nerf_loaded, rays_flat[None, ...], t_vals[None, ...], rand=False, train=False
- )
-
- return(rgb[0], depth[0])
-
-
-# app.py text matter starts here
-
-st.title('3D volumetric rendering with NeRF - A concrete example, Ficus Dataset')
-
-import base64
-
-file = open(r'./training(3).gif', 'rb')
-contents = file.read()
-data_url = base64.b64encode(contents).decode('utf-8')
-file.close()
-
-# st.markdown(
-# f'',
-# unsafe_allow_html=True,
-# )
-
-st.markdown("[NeRF](https://arxiv.org/abs/2003.08934) proposes an ingenious way to synthesize novel views of a scene by modelling the volumetric scene function through a neural network. The network learns to model the volumetric scene, thus generating novel views (images) of the 3D scene that the model was not shown at training time.")
-# st.markdown(".gif)")
-
-st.markdown(
- f'',
- unsafe_allow_html=True,
-)
-# st.image(image, caption='Training Steps')
-st.markdown("## Interactive Demo")
-
-# download the model:
-# from my own model repo
-
-from huggingface_hub import from_pretrained_keras
-nerf_loaded = from_pretrained_keras("Alesteba/NeRF_ficus")
-
-
-# set the values of r theta phi
-r = 4.0
-theta = st.slider("key_1",min_value=0.0, max_value=360.0, label_visibility="hidden")
-phi = st.slider("key_2", min_value=0.0, max_value=360.0, label_visibility="hidden")
-# phi = -30.0
-color, depth = show_rendered_image(r, theta, phi)
-
-col1, col2= st.columns(2)
-
-with col1:
- color = tf.keras.utils.array_to_img(color)
- st.image(color, caption="Color Image", clamp=True, width=300)
-
-with col2:
- depth = tf.keras.utils.array_to_img(depth[..., None])
- st.image(depth, caption="Depth Map", clamp=True, width=300)
-
diff --git a/spaces/Alichuan/VITS-Umamusume-voice-synthesizer/text/japanese.py b/spaces/Alichuan/VITS-Umamusume-voice-synthesizer/text/japanese.py
deleted file mode 100644
index 375e4d50872d5c68ee57ca17470a2ca425425eba..0000000000000000000000000000000000000000
--- a/spaces/Alichuan/VITS-Umamusume-voice-synthesizer/text/japanese.py
+++ /dev/null
@@ -1,153 +0,0 @@
-import re
-from unidecode import unidecode
-import pyopenjtalk
-
-
-# Regular expression matching Japanese without punctuation marks:
-_japanese_characters = re.compile(
- r'[A-Za-z\d\u3005\u3040-\u30ff\u4e00-\u9fff\uff11-\uff19\uff21-\uff3a\uff41-\uff5a\uff66-\uff9d]')
-
-# Regular expression matching non-Japanese characters or punctuation marks:
-_japanese_marks = re.compile(
- r'[^A-Za-z\d\u3005\u3040-\u30ff\u4e00-\u9fff\uff11-\uff19\uff21-\uff3a\uff41-\uff5a\uff66-\uff9d]')
-
-# List of (symbol, Japanese) pairs for marks:
-_symbols_to_japanese = [(re.compile('%s' % x[0]), x[1]) for x in [
- ('%', 'パーセント')
-]]
-
-# List of (romaji, ipa) pairs for marks:
-_romaji_to_ipa = [(re.compile('%s' % x[0]), x[1]) for x in [
- ('ts', 'ʦ'),
- ('u', 'ɯ'),
- ('j', 'ʥ'),
- ('y', 'j'),
- ('ni', 'n^i'),
- ('nj', 'n^'),
- ('hi', 'çi'),
- ('hj', 'ç'),
- ('f', 'ɸ'),
- ('I', 'i*'),
- ('U', 'ɯ*'),
- ('r', 'ɾ')
-]]
-
-# List of (romaji, ipa2) pairs for marks:
-_romaji_to_ipa2 = [(re.compile('%s' % x[0]), x[1]) for x in [
- ('u', 'ɯ'),
- ('ʧ', 'tʃ'),
- ('j', 'dʑ'),
- ('y', 'j'),
- ('ni', 'n^i'),
- ('nj', 'n^'),
- ('hi', 'çi'),
- ('hj', 'ç'),
- ('f', 'ɸ'),
- ('I', 'i*'),
- ('U', 'ɯ*'),
- ('r', 'ɾ')
-]]
-
-# List of (consonant, sokuon) pairs:
-_real_sokuon = [(re.compile('%s' % x[0]), x[1]) for x in [
- (r'Q([↑↓]*[kg])', r'k#\1'),
- (r'Q([↑↓]*[tdjʧ])', r't#\1'),
- (r'Q([↑↓]*[sʃ])', r's\1'),
- (r'Q([↑↓]*[pb])', r'p#\1')
-]]
-
-# List of (consonant, hatsuon) pairs:
-_real_hatsuon = [(re.compile('%s' % x[0]), x[1]) for x in [
- (r'N([↑↓]*[pbm])', r'm\1'),
- (r'N([↑↓]*[ʧʥj])', r'n^\1'),
- (r'N([↑↓]*[tdn])', r'n\1'),
- (r'N([↑↓]*[kg])', r'ŋ\1')
-]]
-
-
-def symbols_to_japanese(text):
- for regex, replacement in _symbols_to_japanese:
- text = re.sub(regex, replacement, text)
- return text
-
-
-def japanese_to_romaji_with_accent(text):
- '''Reference https://r9y9.github.io/ttslearn/latest/notebooks/ch10_Recipe-Tacotron.html'''
- text = symbols_to_japanese(text)
- sentences = re.split(_japanese_marks, text)
- marks = re.findall(_japanese_marks, text)
- text = ''
- for i, sentence in enumerate(sentences):
- if re.match(_japanese_characters, sentence):
- if text != '':
- text += ' '
- labels = pyopenjtalk.extract_fullcontext(sentence)
- for n, label in enumerate(labels):
- phoneme = re.search(r'\-([^\+]*)\+', label).group(1)
- if phoneme not in ['sil', 'pau']:
- text += phoneme.replace('ch', 'ʧ').replace('sh',
- 'ʃ').replace('cl', 'Q')
- else:
- continue
- # n_moras = int(re.search(r'/F:(\d+)_', label).group(1))
- a1 = int(re.search(r"/A:(\-?[0-9]+)\+", label).group(1))
- a2 = int(re.search(r"\+(\d+)\+", label).group(1))
- a3 = int(re.search(r"\+(\d+)/", label).group(1))
- if re.search(r'\-([^\+]*)\+', labels[n + 1]).group(1) in ['sil', 'pau']:
- a2_next = -1
- else:
- a2_next = int(
- re.search(r"\+(\d+)\+", labels[n + 1]).group(1))
- # Accent phrase boundary
- if a3 == 1 and a2_next == 1:
- text += ' '
- # Falling
- elif a1 == 0 and a2_next == a2 + 1:
- text += '↓'
- # Rising
- elif a2 == 1 and a2_next == 2:
- text += '↑'
- if i < len(marks):
- text += unidecode(marks[i]).replace(' ', '')
- return text
-
-
-def get_real_sokuon(text):
- for regex, replacement in _real_sokuon:
- text = re.sub(regex, replacement, text)
- return text
-
-
-def get_real_hatsuon(text):
- for regex, replacement in _real_hatsuon:
- text = re.sub(regex, replacement, text)
- return text
-
-
-def japanese_to_ipa(text):
- text = japanese_to_romaji_with_accent(text).replace('...', '…')
- text = re.sub(
- r'([aiueo])\1+', lambda x: x.group(0)[0]+'ː'*(len(x.group(0))-1), text)
- text = get_real_sokuon(text)
- text = get_real_hatsuon(text)
- for regex, replacement in _romaji_to_ipa:
- text = re.sub(regex, replacement, text)
- return text
-
-
-def japanese_to_ipa2(text):
- text = japanese_to_romaji_with_accent(text).replace('...', '…')
- text = get_real_sokuon(text)
- text = get_real_hatsuon(text)
- for regex, replacement in _romaji_to_ipa2:
- text = re.sub(regex, replacement, text)
- return text
-
-
-def japanese_to_ipa3(text):
- text = japanese_to_ipa2(text).replace('n^', 'ȵ').replace(
- 'ʃ', 'ɕ').replace('*', '\u0325').replace('#', '\u031a')
- text = re.sub(
- r'([aiɯeo])\1+', lambda x: x.group(0)[0]+'ː'*(len(x.group(0))-1), text)
- text = re.sub(r'((?:^|\s)(?:ts|tɕ|[kpt]))', r'\1ʰ', text)
- return text
diff --git a/spaces/Amrrs/DragGan-Inversion/stylegan_human/torch_utils/op_edit/fused_act.py b/spaces/Amrrs/DragGan-Inversion/stylegan_human/torch_utils/op_edit/fused_act.py
deleted file mode 100644
index c9c7b6f0e2b16b78dd81c174cf139a4bd848648a..0000000000000000000000000000000000000000
--- a/spaces/Amrrs/DragGan-Inversion/stylegan_human/torch_utils/op_edit/fused_act.py
+++ /dev/null
@@ -1,100 +0,0 @@
-# Copyright (c) SenseTime Research. All rights reserved.
-
-import os
-
-import torch
-from torch import nn
-from torch.nn import functional as F
-from torch.autograd import Function
-from torch.utils.cpp_extension import load
-
-
-module_path = os.path.dirname(__file__)
-fused = load(
- "fused",
- sources=[
- os.path.join(module_path, "fused_bias_act.cpp"),
- os.path.join(module_path, "fused_bias_act_kernel.cu"),
- ],
-)
-
-
-class FusedLeakyReLUFunctionBackward(Function):
- @staticmethod
- def forward(ctx, grad_output, out, negative_slope, scale):
- ctx.save_for_backward(out)
- ctx.negative_slope = negative_slope
- ctx.scale = scale
-
- empty = grad_output.new_empty(0)
-
- grad_input = fused.fused_bias_act(
- grad_output, empty, out, 3, 1, negative_slope, scale
- )
-
- dim = [0]
-
- if grad_input.ndim > 2:
- dim += list(range(2, grad_input.ndim))
-
- grad_bias = grad_input.sum(dim).detach()
-
- return grad_input, grad_bias
-
- @staticmethod
- def backward(ctx, gradgrad_input, gradgrad_bias):
- (out,) = ctx.saved_tensors
- gradgrad_out = fused.fused_bias_act(
- gradgrad_input, gradgrad_bias, out, 3, 1, ctx.negative_slope, ctx.scale
- )
-
- return gradgrad_out, None, None, None
-
-
-class FusedLeakyReLUFunction(Function):
- @staticmethod
- def forward(ctx, input, bias, negative_slope, scale):
- empty = input.new_empty(0)
- out = fused.fused_bias_act(
- input, bias, empty, 3, 0, negative_slope, scale)
- ctx.save_for_backward(out)
- ctx.negative_slope = negative_slope
- ctx.scale = scale
-
- return out
-
- @staticmethod
- def backward(ctx, grad_output):
- (out,) = ctx.saved_tensors
-
- grad_input, grad_bias = FusedLeakyReLUFunctionBackward.apply(
- grad_output, out, ctx.negative_slope, ctx.scale
- )
-
- return grad_input, grad_bias, None, None
-
-
-class FusedLeakyReLU(nn.Module):
- def __init__(self, channel, negative_slope=0.2, scale=2 ** 0.5):
- super().__init__()
-
- self.bias = nn.Parameter(torch.zeros(channel))
- self.negative_slope = negative_slope
- self.scale = scale
-
- def forward(self, input):
- return fused_leaky_relu(input, self.bias, self.negative_slope, self.scale)
-
-
-def fused_leaky_relu(input, bias, negative_slope=0.2, scale=2 ** 0.5):
- if input.device.type == "cpu":
- rest_dim = [1] * (input.ndim - bias.ndim - 1)
- return (
- F.leaky_relu(
- input + bias.view(1, bias.shape[0], *rest_dim), negative_slope=0.2
- )
- * scale
- )
-
- else:
- return FusedLeakyReLUFunction.apply(input, bias, negative_slope, scale)
diff --git a/spaces/Anar0140/6.AI.Dashboard.Wiki.Chat.Cognitive.HTML5/index.html b/spaces/Anar0140/6.AI.Dashboard.Wiki.Chat.Cognitive.HTML5/index.html
deleted file mode 100644
index 175522d4f076933e2f08c9d8fb5cb1231f25f098..0000000000000000000000000000000000000000
--- a/spaces/Anar0140/6.AI.Dashboard.Wiki.Chat.Cognitive.HTML5/index.html
+++ /dev/null
@@ -1,36 +0,0 @@
-
-
-
-
-
-
-
-
-
-
-
-
-
-
-
-
-
-
-
-
diff --git a/spaces/Androidonnxfork/CivitAi-to-Diffusers/diffusers/docs/source/ko/installation.md b/spaces/Androidonnxfork/CivitAi-to-Diffusers/diffusers/docs/source/ko/installation.md
deleted file mode 100644
index a10f9f8d1b52c0281433356f03f81039d4356f91..0000000000000000000000000000000000000000
--- a/spaces/Androidonnxfork/CivitAi-to-Diffusers/diffusers/docs/source/ko/installation.md
+++ /dev/null
@@ -1,142 +0,0 @@
-
-
-# 설치
-
-사용하시는 라이브러리에 맞는 🤗 Diffusers를 설치하세요.
-
-🤗 Diffusers는 Python 3.7+, PyTorch 1.7.0+ 및 flax에서 테스트되었습니다. 사용중인 딥러닝 라이브러리에 대한 아래의 설치 안내를 따르세요.
-
-- [PyTorch 설치 안내](https://pytorch.org/get-started/locally/)
-- [Flax 설치 안내](https://flax.readthedocs.io/en/latest/)
-
-## pip를 이용한 설치
-
-[가상 환경](https://docs.python.org/3/library/venv.html)에 🤗 Diffusers를 설치해야 합니다.
-Python 가상 환경에 익숙하지 않은 경우 [가상환경 pip 설치 가이드](https://packaging.python.org/guides/installing-using-pip-and-virtual-environments/)를 살펴보세요.
-가상 환경을 사용하면 서로 다른 프로젝트를 더 쉽게 관리하고, 종속성간의 호환성 문제를 피할 수 있습니다.
-
-프로젝트 디렉토리에 가상 환경을 생성하는 것으로 시작하세요:
-
-```bash
-python -m venv .env
-```
-
-그리고 가상 환경을 활성화합니다:
-
-```bash
-source .env/bin/activate
-```
-
-이제 다음의 명령어로 🤗 Diffusers를 설치할 준비가 되었습니다:
-
-**PyTorch의 경우**
-
-```bash
-pip install diffusers["torch"]
-```
-
-**Flax의 경우**
-
-```bash
-pip install diffusers["flax"]
-```
-
-## 소스로부터 설치
-
-소스에서 `diffusers`를 설치하기 전에, `torch` 및 `accelerate`이 설치되어 있는지 확인하세요.
-
-`torch` 설치에 대해서는 [torch docs](https://pytorch.org/get-started/locally/#start-locally)를 참고하세요.
-
-다음과 같이 `accelerate`을 설치하세요.
-
-```bash
-pip install accelerate
-```
-
-다음 명령어를 사용하여 소스에서 🤗 Diffusers를 설치하세요:
-
-```bash
-pip install git+https://github.com/huggingface/diffusers
-```
-
-이 명령어는 최신 `stable` 버전이 아닌 최첨단 `main` 버전을 설치합니다.
-`main` 버전은 최신 개발 정보를 최신 상태로 유지하는 데 유용합니다.
-예를 들어 마지막 공식 릴리즈 이후 버그가 수정되었지만, 새 릴리즈가 아직 출시되지 않은 경우입니다.
-그러나 이는 `main` 버전이 항상 안정적이지 않을 수 있음을 의미합니다.
-우리는 `main` 버전이 지속적으로 작동하도록 노력하고 있으며, 대부분의 문제는 보통 몇 시간 또는 하루 안에 해결됩니다.
-문제가 발생하면 더 빨리 해결할 수 있도록 [Issue](https://github.com/huggingface/transformers/issues)를 열어주세요!
-
-
-## 편집가능한 설치
-
-다음을 수행하려면 편집가능한 설치가 필요합니다:
-
-* 소스 코드의 `main` 버전을 사용
-* 🤗 Diffusers에 기여 (코드의 변경 사항을 테스트하기 위해 필요)
-
-저장소를 복제하고 다음 명령어를 사용하여 🤗 Diffusers를 설치합니다:
-
-```bash
-git clone https://github.com/huggingface/diffusers.git
-cd diffusers
-```
-
-**PyTorch의 경우**
-
-```
-pip install -e ".[torch]"
-```
-
-**Flax의 경우**
-
-```
-pip install -e ".[flax]"
-```
-
-이러한 명령어들은 저장소를 복제한 폴더와 Python 라이브러리 경로를 연결합니다.
-Python은 이제 일반 라이브러리 경로에 더하여 복제한 폴더 내부를 살펴봅니다.
-예를들어 Python 패키지가 `~/anaconda3/envs/main/lib/python3.7/site-packages/`에 설치되어 있는 경우 Python은 복제한 폴더인 `~/diffusers/`도 검색합니다.
-
-
-
-라이브러리를 계속 사용하려면 `diffusers` 폴더를 유지해야 합니다.
-
-
-
-이제 다음 명령어를 사용하여 최신 버전의 🤗 Diffusers로 쉽게 업데이트할 수 있습니다:
-
-```bash
-cd ~/diffusers/
-git pull
-```
-
-이렇게 하면, 다음에 실행할 때 Python 환경이 🤗 Diffusers의 `main` 버전을 찾게 됩니다.
-
-## 텔레메트리 로깅에 대한 알림
-
-우리 라이브러리는 `from_pretrained()` 요청 중에 텔레메트리 정보를 원격으로 수집합니다.
-이 데이터에는 Diffusers 및 PyTorch/Flax의 버전, 요청된 모델 또는 파이프라인 클래스, 그리고 허브에서 호스팅되는 경우 사전학습된 체크포인트에 대한 경로를 포함합니다.
-이 사용 데이터는 문제를 디버깅하고 새로운 기능의 우선순위를 지정하는데 도움이 됩니다.
-텔레메트리는 HuggingFace 허브에서 모델과 파이프라인을 불러올 때만 전송되며, 로컬 사용 중에는 수집되지 않습니다.
-
-우리는 추가 정보를 공유하지 않기를 원하는 사람이 있다는 것을 이해하고 개인 정보를 존중하므로, 터미널에서 `DISABLE_TELEMETRY` 환경 변수를 설정하여 텔레메트리 수집을 비활성화할 수 있습니다.
-
-Linux/MacOS에서:
-```bash
-export DISABLE_TELEMETRY=YES
-```
-
-Windows에서:
-```bash
-set DISABLE_TELEMETRY=YES
-```
\ No newline at end of file
diff --git a/spaces/Androidonnxfork/CivitAi-to-Diffusers/diffusers/src/diffusers/pipelines/t2i_adapter/__init__.py b/spaces/Androidonnxfork/CivitAi-to-Diffusers/diffusers/src/diffusers/pipelines/t2i_adapter/__init__.py
deleted file mode 100644
index c4de661dbefab846cc72fa576675aad6cab1d134..0000000000000000000000000000000000000000
--- a/spaces/Androidonnxfork/CivitAi-to-Diffusers/diffusers/src/diffusers/pipelines/t2i_adapter/__init__.py
+++ /dev/null
@@ -1,14 +0,0 @@
-from ...utils import (
- OptionalDependencyNotAvailable,
- is_torch_available,
- is_transformers_available,
-)
-
-
-try:
- if not (is_transformers_available() and is_torch_available()):
- raise OptionalDependencyNotAvailable()
-except OptionalDependencyNotAvailable:
- from ...utils.dummy_torch_and_transformers_objects import * # noqa F403
-else:
- from .pipeline_stable_diffusion_adapter import StableDiffusionAdapterPipeline
diff --git a/spaces/Andy1621/uniformer_image_detection/mmdet/models/roi_heads/mask_heads/maskiou_head.py b/spaces/Andy1621/uniformer_image_detection/mmdet/models/roi_heads/mask_heads/maskiou_head.py
deleted file mode 100644
index 39bcd6a7dbdb089cd19cef811038e0b6a80ab89a..0000000000000000000000000000000000000000
--- a/spaces/Andy1621/uniformer_image_detection/mmdet/models/roi_heads/mask_heads/maskiou_head.py
+++ /dev/null
@@ -1,186 +0,0 @@
-import numpy as np
-import torch
-import torch.nn as nn
-from mmcv.cnn import Conv2d, Linear, MaxPool2d, kaiming_init, normal_init
-from mmcv.runner import force_fp32
-from torch.nn.modules.utils import _pair
-
-from mmdet.models.builder import HEADS, build_loss
-
-
-@HEADS.register_module()
-class MaskIoUHead(nn.Module):
- """Mask IoU Head.
-
- This head predicts the IoU of predicted masks and corresponding gt masks.
- """
-
- def __init__(self,
- num_convs=4,
- num_fcs=2,
- roi_feat_size=14,
- in_channels=256,
- conv_out_channels=256,
- fc_out_channels=1024,
- num_classes=80,
- loss_iou=dict(type='MSELoss', loss_weight=0.5)):
- super(MaskIoUHead, self).__init__()
- self.in_channels = in_channels
- self.conv_out_channels = conv_out_channels
- self.fc_out_channels = fc_out_channels
- self.num_classes = num_classes
- self.fp16_enabled = False
-
- self.convs = nn.ModuleList()
- for i in range(num_convs):
- if i == 0:
- # concatenation of mask feature and mask prediction
- in_channels = self.in_channels + 1
- else:
- in_channels = self.conv_out_channels
- stride = 2 if i == num_convs - 1 else 1
- self.convs.append(
- Conv2d(
- in_channels,
- self.conv_out_channels,
- 3,
- stride=stride,
- padding=1))
-
- roi_feat_size = _pair(roi_feat_size)
- pooled_area = (roi_feat_size[0] // 2) * (roi_feat_size[1] // 2)
- self.fcs = nn.ModuleList()
- for i in range(num_fcs):
- in_channels = (
- self.conv_out_channels *
- pooled_area if i == 0 else self.fc_out_channels)
- self.fcs.append(Linear(in_channels, self.fc_out_channels))
-
- self.fc_mask_iou = Linear(self.fc_out_channels, self.num_classes)
- self.relu = nn.ReLU()
- self.max_pool = MaxPool2d(2, 2)
- self.loss_iou = build_loss(loss_iou)
-
- def init_weights(self):
- for conv in self.convs:
- kaiming_init(conv)
- for fc in self.fcs:
- kaiming_init(
- fc,
- a=1,
- mode='fan_in',
- nonlinearity='leaky_relu',
- distribution='uniform')
- normal_init(self.fc_mask_iou, std=0.01)
-
- def forward(self, mask_feat, mask_pred):
- mask_pred = mask_pred.sigmoid()
- mask_pred_pooled = self.max_pool(mask_pred.unsqueeze(1))
-
- x = torch.cat((mask_feat, mask_pred_pooled), 1)
-
- for conv in self.convs:
- x = self.relu(conv(x))
- x = x.flatten(1)
- for fc in self.fcs:
- x = self.relu(fc(x))
- mask_iou = self.fc_mask_iou(x)
- return mask_iou
-
- @force_fp32(apply_to=('mask_iou_pred', ))
- def loss(self, mask_iou_pred, mask_iou_targets):
- pos_inds = mask_iou_targets > 0
- if pos_inds.sum() > 0:
- loss_mask_iou = self.loss_iou(mask_iou_pred[pos_inds],
- mask_iou_targets[pos_inds])
- else:
- loss_mask_iou = mask_iou_pred.sum() * 0
- return dict(loss_mask_iou=loss_mask_iou)
-
- @force_fp32(apply_to=('mask_pred', ))
- def get_targets(self, sampling_results, gt_masks, mask_pred, mask_targets,
- rcnn_train_cfg):
- """Compute target of mask IoU.
-
- Mask IoU target is the IoU of the predicted mask (inside a bbox) and
- the gt mask of corresponding gt mask (the whole instance).
- The intersection area is computed inside the bbox, and the gt mask area
- is computed with two steps, firstly we compute the gt area inside the
- bbox, then divide it by the area ratio of gt area inside the bbox and
- the gt area of the whole instance.
-
- Args:
- sampling_results (list[:obj:`SamplingResult`]): sampling results.
- gt_masks (BitmapMask | PolygonMask): Gt masks (the whole instance)
- of each image, with the same shape of the input image.
- mask_pred (Tensor): Predicted masks of each positive proposal,
- shape (num_pos, h, w).
- mask_targets (Tensor): Gt mask of each positive proposal,
- binary map of the shape (num_pos, h, w).
- rcnn_train_cfg (dict): Training config for R-CNN part.
-
- Returns:
- Tensor: mask iou target (length == num positive).
- """
- pos_proposals = [res.pos_bboxes for res in sampling_results]
- pos_assigned_gt_inds = [
- res.pos_assigned_gt_inds for res in sampling_results
- ]
-
- # compute the area ratio of gt areas inside the proposals and
- # the whole instance
- area_ratios = map(self._get_area_ratio, pos_proposals,
- pos_assigned_gt_inds, gt_masks)
- area_ratios = torch.cat(list(area_ratios))
- assert mask_targets.size(0) == area_ratios.size(0)
-
- mask_pred = (mask_pred > rcnn_train_cfg.mask_thr_binary).float()
- mask_pred_areas = mask_pred.sum((-1, -2))
-
- # mask_pred and mask_targets are binary maps
- overlap_areas = (mask_pred * mask_targets).sum((-1, -2))
-
- # compute the mask area of the whole instance
- gt_full_areas = mask_targets.sum((-1, -2)) / (area_ratios + 1e-7)
-
- mask_iou_targets = overlap_areas / (
- mask_pred_areas + gt_full_areas - overlap_areas)
- return mask_iou_targets
-
- def _get_area_ratio(self, pos_proposals, pos_assigned_gt_inds, gt_masks):
- """Compute area ratio of the gt mask inside the proposal and the gt
- mask of the corresponding instance."""
- num_pos = pos_proposals.size(0)
- if num_pos > 0:
- area_ratios = []
- proposals_np = pos_proposals.cpu().numpy()
- pos_assigned_gt_inds = pos_assigned_gt_inds.cpu().numpy()
- # compute mask areas of gt instances (batch processing for speedup)
- gt_instance_mask_area = gt_masks.areas
- for i in range(num_pos):
- gt_mask = gt_masks[pos_assigned_gt_inds[i]]
-
- # crop the gt mask inside the proposal
- bbox = proposals_np[i, :].astype(np.int32)
- gt_mask_in_proposal = gt_mask.crop(bbox)
-
- ratio = gt_mask_in_proposal.areas[0] / (
- gt_instance_mask_area[pos_assigned_gt_inds[i]] + 1e-7)
- area_ratios.append(ratio)
- area_ratios = torch.from_numpy(np.stack(area_ratios)).float().to(
- pos_proposals.device)
- else:
- area_ratios = pos_proposals.new_zeros((0, ))
- return area_ratios
-
- @force_fp32(apply_to=('mask_iou_pred', ))
- def get_mask_scores(self, mask_iou_pred, det_bboxes, det_labels):
- """Get the mask scores.
-
- mask_score = bbox_score * mask_iou
- """
- inds = range(det_labels.size(0))
- mask_scores = mask_iou_pred[inds, det_labels] * det_bboxes[inds, -1]
- mask_scores = mask_scores.cpu().numpy()
- det_labels = det_labels.cpu().numpy()
- return [mask_scores[det_labels == i] for i in range(self.num_classes)]
diff --git a/spaces/Andy1621/uniformer_image_segmentation/configs/deeplabv3plus/deeplabv3plus_r101-d8_512x512_160k_ade20k.py b/spaces/Andy1621/uniformer_image_segmentation/configs/deeplabv3plus/deeplabv3plus_r101-d8_512x512_160k_ade20k.py
deleted file mode 100644
index a75c9d3019b13d01c0dd13dae53bce3d15791d52..0000000000000000000000000000000000000000
--- a/spaces/Andy1621/uniformer_image_segmentation/configs/deeplabv3plus/deeplabv3plus_r101-d8_512x512_160k_ade20k.py
+++ /dev/null
@@ -1,2 +0,0 @@
-_base_ = './deeplabv3plus_r50-d8_512x512_160k_ade20k.py'
-model = dict(pretrained='open-mmlab://resnet101_v1c', backbone=dict(depth=101))
diff --git a/spaces/AnonAndDesu/Desu_Proxy/README.md b/spaces/AnonAndDesu/Desu_Proxy/README.md
deleted file mode 100644
index bd931b28730317580a462295fb95fa89319bb92d..0000000000000000000000000000000000000000
--- a/spaces/AnonAndDesu/Desu_Proxy/README.md
+++ /dev/null
@@ -1,10 +0,0 @@
----
-title: Desu_Proxy
-emoji: 📉
-colorFrom: green
-colorTo: purple
-sdk: docker
-pinned: false
----
-
-Check out the configuration reference at https://huggingface.co/docs/hub/spaces-config-reference
diff --git a/spaces/Anonymous-sub/Rerender/ControlNet/annotator/mlsd/utils.py b/spaces/Anonymous-sub/Rerender/ControlNet/annotator/mlsd/utils.py
deleted file mode 100644
index ae3cf9420a33a4abae27c48ac4b90938c7d63cc3..0000000000000000000000000000000000000000
--- a/spaces/Anonymous-sub/Rerender/ControlNet/annotator/mlsd/utils.py
+++ /dev/null
@@ -1,580 +0,0 @@
-'''
-modified by lihaoweicv
-pytorch version
-'''
-
-'''
-M-LSD
-Copyright 2021-present NAVER Corp.
-Apache License v2.0
-'''
-
-import os
-import numpy as np
-import cv2
-import torch
-from torch.nn import functional as F
-
-
-def deccode_output_score_and_ptss(tpMap, topk_n = 200, ksize = 5):
- '''
- tpMap:
- center: tpMap[1, 0, :, :]
- displacement: tpMap[1, 1:5, :, :]
- '''
- b, c, h, w = tpMap.shape
- assert b==1, 'only support bsize==1'
- displacement = tpMap[:, 1:5, :, :][0]
- center = tpMap[:, 0, :, :]
- heat = torch.sigmoid(center)
- hmax = F.max_pool2d( heat, (ksize, ksize), stride=1, padding=(ksize-1)//2)
- keep = (hmax == heat).float()
- heat = heat * keep
- heat = heat.reshape(-1, )
-
- scores, indices = torch.topk(heat, topk_n, dim=-1, largest=True)
- yy = torch.floor_divide(indices, w).unsqueeze(-1)
- xx = torch.fmod(indices, w).unsqueeze(-1)
- ptss = torch.cat((yy, xx),dim=-1)
-
- ptss = ptss.detach().cpu().numpy()
- scores = scores.detach().cpu().numpy()
- displacement = displacement.detach().cpu().numpy()
- displacement = displacement.transpose((1,2,0))
- return ptss, scores, displacement
-
-
-def pred_lines(image, model,
- input_shape=[512, 512],
- score_thr=0.10,
- dist_thr=20.0):
- h, w, _ = image.shape
- h_ratio, w_ratio = [h / input_shape[0], w / input_shape[1]]
-
- resized_image = np.concatenate([cv2.resize(image, (input_shape[1], input_shape[0]), interpolation=cv2.INTER_AREA),
- np.ones([input_shape[0], input_shape[1], 1])], axis=-1)
-
- resized_image = resized_image.transpose((2,0,1))
- batch_image = np.expand_dims(resized_image, axis=0).astype('float32')
- batch_image = (batch_image / 127.5) - 1.0
-
- batch_image = torch.from_numpy(batch_image).float().cuda()
- outputs = model(batch_image)
- pts, pts_score, vmap = deccode_output_score_and_ptss(outputs, 200, 3)
- start = vmap[:, :, :2]
- end = vmap[:, :, 2:]
- dist_map = np.sqrt(np.sum((start - end) ** 2, axis=-1))
-
- segments_list = []
- for center, score in zip(pts, pts_score):
- y, x = center
- distance = dist_map[y, x]
- if score > score_thr and distance > dist_thr:
- disp_x_start, disp_y_start, disp_x_end, disp_y_end = vmap[y, x, :]
- x_start = x + disp_x_start
- y_start = y + disp_y_start
- x_end = x + disp_x_end
- y_end = y + disp_y_end
- segments_list.append([x_start, y_start, x_end, y_end])
-
- lines = 2 * np.array(segments_list) # 256 > 512
- lines[:, 0] = lines[:, 0] * w_ratio
- lines[:, 1] = lines[:, 1] * h_ratio
- lines[:, 2] = lines[:, 2] * w_ratio
- lines[:, 3] = lines[:, 3] * h_ratio
-
- return lines
-
-
-def pred_squares(image,
- model,
- input_shape=[512, 512],
- params={'score': 0.06,
- 'outside_ratio': 0.28,
- 'inside_ratio': 0.45,
- 'w_overlap': 0.0,
- 'w_degree': 1.95,
- 'w_length': 0.0,
- 'w_area': 1.86,
- 'w_center': 0.14}):
- '''
- shape = [height, width]
- '''
- h, w, _ = image.shape
- original_shape = [h, w]
-
- resized_image = np.concatenate([cv2.resize(image, (input_shape[0], input_shape[1]), interpolation=cv2.INTER_AREA),
- np.ones([input_shape[0], input_shape[1], 1])], axis=-1)
- resized_image = resized_image.transpose((2, 0, 1))
- batch_image = np.expand_dims(resized_image, axis=0).astype('float32')
- batch_image = (batch_image / 127.5) - 1.0
-
- batch_image = torch.from_numpy(batch_image).float().cuda()
- outputs = model(batch_image)
-
- pts, pts_score, vmap = deccode_output_score_and_ptss(outputs, 200, 3)
- start = vmap[:, :, :2] # (x, y)
- end = vmap[:, :, 2:] # (x, y)
- dist_map = np.sqrt(np.sum((start - end) ** 2, axis=-1))
-
- junc_list = []
- segments_list = []
- for junc, score in zip(pts, pts_score):
- y, x = junc
- distance = dist_map[y, x]
- if score > params['score'] and distance > 20.0:
- junc_list.append([x, y])
- disp_x_start, disp_y_start, disp_x_end, disp_y_end = vmap[y, x, :]
- d_arrow = 1.0
- x_start = x + d_arrow * disp_x_start
- y_start = y + d_arrow * disp_y_start
- x_end = x + d_arrow * disp_x_end
- y_end = y + d_arrow * disp_y_end
- segments_list.append([x_start, y_start, x_end, y_end])
-
- segments = np.array(segments_list)
-
- ####### post processing for squares
- # 1. get unique lines
- point = np.array([[0, 0]])
- point = point[0]
- start = segments[:, :2]
- end = segments[:, 2:]
- diff = start - end
- a = diff[:, 1]
- b = -diff[:, 0]
- c = a * start[:, 0] + b * start[:, 1]
-
- d = np.abs(a * point[0] + b * point[1] - c) / np.sqrt(a ** 2 + b ** 2 + 1e-10)
- theta = np.arctan2(diff[:, 0], diff[:, 1]) * 180 / np.pi
- theta[theta < 0.0] += 180
- hough = np.concatenate([d[:, None], theta[:, None]], axis=-1)
-
- d_quant = 1
- theta_quant = 2
- hough[:, 0] //= d_quant
- hough[:, 1] //= theta_quant
- _, indices, counts = np.unique(hough, axis=0, return_index=True, return_counts=True)
-
- acc_map = np.zeros([512 // d_quant + 1, 360 // theta_quant + 1], dtype='float32')
- idx_map = np.zeros([512 // d_quant + 1, 360 // theta_quant + 1], dtype='int32') - 1
- yx_indices = hough[indices, :].astype('int32')
- acc_map[yx_indices[:, 0], yx_indices[:, 1]] = counts
- idx_map[yx_indices[:, 0], yx_indices[:, 1]] = indices
-
- acc_map_np = acc_map
- # acc_map = acc_map[None, :, :, None]
- #
- # ### fast suppression using tensorflow op
- # acc_map = tf.constant(acc_map, dtype=tf.float32)
- # max_acc_map = tf.keras.layers.MaxPool2D(pool_size=(5, 5), strides=1, padding='same')(acc_map)
- # acc_map = acc_map * tf.cast(tf.math.equal(acc_map, max_acc_map), tf.float32)
- # flatten_acc_map = tf.reshape(acc_map, [1, -1])
- # topk_values, topk_indices = tf.math.top_k(flatten_acc_map, k=len(pts))
- # _, h, w, _ = acc_map.shape
- # y = tf.expand_dims(topk_indices // w, axis=-1)
- # x = tf.expand_dims(topk_indices % w, axis=-1)
- # yx = tf.concat([y, x], axis=-1)
-
- ### fast suppression using pytorch op
- acc_map = torch.from_numpy(acc_map_np).unsqueeze(0).unsqueeze(0)
- _,_, h, w = acc_map.shape
- max_acc_map = F.max_pool2d(acc_map,kernel_size=5, stride=1, padding=2)
- acc_map = acc_map * ( (acc_map == max_acc_map).float() )
- flatten_acc_map = acc_map.reshape([-1, ])
-
- scores, indices = torch.topk(flatten_acc_map, len(pts), dim=-1, largest=True)
- yy = torch.div(indices, w, rounding_mode='floor').unsqueeze(-1)
- xx = torch.fmod(indices, w).unsqueeze(-1)
- yx = torch.cat((yy, xx), dim=-1)
-
- yx = yx.detach().cpu().numpy()
-
- topk_values = scores.detach().cpu().numpy()
- indices = idx_map[yx[:, 0], yx[:, 1]]
- basis = 5 // 2
-
- merged_segments = []
- for yx_pt, max_indice, value in zip(yx, indices, topk_values):
- y, x = yx_pt
- if max_indice == -1 or value == 0:
- continue
- segment_list = []
- for y_offset in range(-basis, basis + 1):
- for x_offset in range(-basis, basis + 1):
- indice = idx_map[y + y_offset, x + x_offset]
- cnt = int(acc_map_np[y + y_offset, x + x_offset])
- if indice != -1:
- segment_list.append(segments[indice])
- if cnt > 1:
- check_cnt = 1
- current_hough = hough[indice]
- for new_indice, new_hough in enumerate(hough):
- if (current_hough == new_hough).all() and indice != new_indice:
- segment_list.append(segments[new_indice])
- check_cnt += 1
- if check_cnt == cnt:
- break
- group_segments = np.array(segment_list).reshape([-1, 2])
- sorted_group_segments = np.sort(group_segments, axis=0)
- x_min, y_min = sorted_group_segments[0, :]
- x_max, y_max = sorted_group_segments[-1, :]
-
- deg = theta[max_indice]
- if deg >= 90:
- merged_segments.append([x_min, y_max, x_max, y_min])
- else:
- merged_segments.append([x_min, y_min, x_max, y_max])
-
- # 2. get intersections
- new_segments = np.array(merged_segments) # (x1, y1, x2, y2)
- start = new_segments[:, :2] # (x1, y1)
- end = new_segments[:, 2:] # (x2, y2)
- new_centers = (start + end) / 2.0
- diff = start - end
- dist_segments = np.sqrt(np.sum(diff ** 2, axis=-1))
-
- # ax + by = c
- a = diff[:, 1]
- b = -diff[:, 0]
- c = a * start[:, 0] + b * start[:, 1]
- pre_det = a[:, None] * b[None, :]
- det = pre_det - np.transpose(pre_det)
-
- pre_inter_y = a[:, None] * c[None, :]
- inter_y = (pre_inter_y - np.transpose(pre_inter_y)) / (det + 1e-10)
- pre_inter_x = c[:, None] * b[None, :]
- inter_x = (pre_inter_x - np.transpose(pre_inter_x)) / (det + 1e-10)
- inter_pts = np.concatenate([inter_x[:, :, None], inter_y[:, :, None]], axis=-1).astype('int32')
-
- # 3. get corner information
- # 3.1 get distance
- '''
- dist_segments:
- | dist(0), dist(1), dist(2), ...|
- dist_inter_to_segment1:
- | dist(inter,0), dist(inter,0), dist(inter,0), ... |
- | dist(inter,1), dist(inter,1), dist(inter,1), ... |
- ...
- dist_inter_to_semgnet2:
- | dist(inter,0), dist(inter,1), dist(inter,2), ... |
- | dist(inter,0), dist(inter,1), dist(inter,2), ... |
- ...
- '''
-
- dist_inter_to_segment1_start = np.sqrt(
- np.sum(((inter_pts - start[:, None, :]) ** 2), axis=-1, keepdims=True)) # [n_batch, n_batch, 1]
- dist_inter_to_segment1_end = np.sqrt(
- np.sum(((inter_pts - end[:, None, :]) ** 2), axis=-1, keepdims=True)) # [n_batch, n_batch, 1]
- dist_inter_to_segment2_start = np.sqrt(
- np.sum(((inter_pts - start[None, :, :]) ** 2), axis=-1, keepdims=True)) # [n_batch, n_batch, 1]
- dist_inter_to_segment2_end = np.sqrt(
- np.sum(((inter_pts - end[None, :, :]) ** 2), axis=-1, keepdims=True)) # [n_batch, n_batch, 1]
-
- # sort ascending
- dist_inter_to_segment1 = np.sort(
- np.concatenate([dist_inter_to_segment1_start, dist_inter_to_segment1_end], axis=-1),
- axis=-1) # [n_batch, n_batch, 2]
- dist_inter_to_segment2 = np.sort(
- np.concatenate([dist_inter_to_segment2_start, dist_inter_to_segment2_end], axis=-1),
- axis=-1) # [n_batch, n_batch, 2]
-
- # 3.2 get degree
- inter_to_start = new_centers[:, None, :] - inter_pts
- deg_inter_to_start = np.arctan2(inter_to_start[:, :, 1], inter_to_start[:, :, 0]) * 180 / np.pi
- deg_inter_to_start[deg_inter_to_start < 0.0] += 360
- inter_to_end = new_centers[None, :, :] - inter_pts
- deg_inter_to_end = np.arctan2(inter_to_end[:, :, 1], inter_to_end[:, :, 0]) * 180 / np.pi
- deg_inter_to_end[deg_inter_to_end < 0.0] += 360
-
- '''
- B -- G
- | |
- C -- R
- B : blue / G: green / C: cyan / R: red
-
- 0 -- 1
- | |
- 3 -- 2
- '''
- # rename variables
- deg1_map, deg2_map = deg_inter_to_start, deg_inter_to_end
- # sort deg ascending
- deg_sort = np.sort(np.concatenate([deg1_map[:, :, None], deg2_map[:, :, None]], axis=-1), axis=-1)
-
- deg_diff_map = np.abs(deg1_map - deg2_map)
- # we only consider the smallest degree of intersect
- deg_diff_map[deg_diff_map > 180] = 360 - deg_diff_map[deg_diff_map > 180]
-
- # define available degree range
- deg_range = [60, 120]
-
- corner_dict = {corner_info: [] for corner_info in range(4)}
- inter_points = []
- for i in range(inter_pts.shape[0]):
- for j in range(i + 1, inter_pts.shape[1]):
- # i, j > line index, always i < j
- x, y = inter_pts[i, j, :]
- deg1, deg2 = deg_sort[i, j, :]
- deg_diff = deg_diff_map[i, j]
-
- check_degree = deg_diff > deg_range[0] and deg_diff < deg_range[1]
-
- outside_ratio = params['outside_ratio'] # over ratio >>> drop it!
- inside_ratio = params['inside_ratio'] # over ratio >>> drop it!
- check_distance = ((dist_inter_to_segment1[i, j, 1] >= dist_segments[i] and \
- dist_inter_to_segment1[i, j, 0] <= dist_segments[i] * outside_ratio) or \
- (dist_inter_to_segment1[i, j, 1] <= dist_segments[i] and \
- dist_inter_to_segment1[i, j, 0] <= dist_segments[i] * inside_ratio)) and \
- ((dist_inter_to_segment2[i, j, 1] >= dist_segments[j] and \
- dist_inter_to_segment2[i, j, 0] <= dist_segments[j] * outside_ratio) or \
- (dist_inter_to_segment2[i, j, 1] <= dist_segments[j] and \
- dist_inter_to_segment2[i, j, 0] <= dist_segments[j] * inside_ratio))
-
- if check_degree and check_distance:
- corner_info = None
-
- if (deg1 >= 0 and deg1 <= 45 and deg2 >= 45 and deg2 <= 120) or \
- (deg2 >= 315 and deg1 >= 45 and deg1 <= 120):
- corner_info, color_info = 0, 'blue'
- elif (deg1 >= 45 and deg1 <= 125 and deg2 >= 125 and deg2 <= 225):
- corner_info, color_info = 1, 'green'
- elif (deg1 >= 125 and deg1 <= 225 and deg2 >= 225 and deg2 <= 315):
- corner_info, color_info = 2, 'black'
- elif (deg1 >= 0 and deg1 <= 45 and deg2 >= 225 and deg2 <= 315) or \
- (deg2 >= 315 and deg1 >= 225 and deg1 <= 315):
- corner_info, color_info = 3, 'cyan'
- else:
- corner_info, color_info = 4, 'red' # we don't use it
- continue
-
- corner_dict[corner_info].append([x, y, i, j])
- inter_points.append([x, y])
-
- square_list = []
- connect_list = []
- segments_list = []
- for corner0 in corner_dict[0]:
- for corner1 in corner_dict[1]:
- connect01 = False
- for corner0_line in corner0[2:]:
- if corner0_line in corner1[2:]:
- connect01 = True
- break
- if connect01:
- for corner2 in corner_dict[2]:
- connect12 = False
- for corner1_line in corner1[2:]:
- if corner1_line in corner2[2:]:
- connect12 = True
- break
- if connect12:
- for corner3 in corner_dict[3]:
- connect23 = False
- for corner2_line in corner2[2:]:
- if corner2_line in corner3[2:]:
- connect23 = True
- break
- if connect23:
- for corner3_line in corner3[2:]:
- if corner3_line in corner0[2:]:
- # SQUARE!!!
- '''
- 0 -- 1
- | |
- 3 -- 2
- square_list:
- order: 0 > 1 > 2 > 3
- | x0, y0, x1, y1, x2, y2, x3, y3 |
- | x0, y0, x1, y1, x2, y2, x3, y3 |
- ...
- connect_list:
- order: 01 > 12 > 23 > 30
- | line_idx01, line_idx12, line_idx23, line_idx30 |
- | line_idx01, line_idx12, line_idx23, line_idx30 |
- ...
- segments_list:
- order: 0 > 1 > 2 > 3
- | line_idx0_i, line_idx0_j, line_idx1_i, line_idx1_j, line_idx2_i, line_idx2_j, line_idx3_i, line_idx3_j |
- | line_idx0_i, line_idx0_j, line_idx1_i, line_idx1_j, line_idx2_i, line_idx2_j, line_idx3_i, line_idx3_j |
- ...
- '''
- square_list.append(corner0[:2] + corner1[:2] + corner2[:2] + corner3[:2])
- connect_list.append([corner0_line, corner1_line, corner2_line, corner3_line])
- segments_list.append(corner0[2:] + corner1[2:] + corner2[2:] + corner3[2:])
-
- def check_outside_inside(segments_info, connect_idx):
- # return 'outside or inside', min distance, cover_param, peri_param
- if connect_idx == segments_info[0]:
- check_dist_mat = dist_inter_to_segment1
- else:
- check_dist_mat = dist_inter_to_segment2
-
- i, j = segments_info
- min_dist, max_dist = check_dist_mat[i, j, :]
- connect_dist = dist_segments[connect_idx]
- if max_dist > connect_dist:
- return 'outside', min_dist, 0, 1
- else:
- return 'inside', min_dist, -1, -1
-
- top_square = None
-
- try:
- map_size = input_shape[0] / 2
- squares = np.array(square_list).reshape([-1, 4, 2])
- score_array = []
- connect_array = np.array(connect_list)
- segments_array = np.array(segments_list).reshape([-1, 4, 2])
-
- # get degree of corners:
- squares_rollup = np.roll(squares, 1, axis=1)
- squares_rolldown = np.roll(squares, -1, axis=1)
- vec1 = squares_rollup - squares
- normalized_vec1 = vec1 / (np.linalg.norm(vec1, axis=-1, keepdims=True) + 1e-10)
- vec2 = squares_rolldown - squares
- normalized_vec2 = vec2 / (np.linalg.norm(vec2, axis=-1, keepdims=True) + 1e-10)
- inner_products = np.sum(normalized_vec1 * normalized_vec2, axis=-1) # [n_squares, 4]
- squares_degree = np.arccos(inner_products) * 180 / np.pi # [n_squares, 4]
-
- # get square score
- overlap_scores = []
- degree_scores = []
- length_scores = []
-
- for connects, segments, square, degree in zip(connect_array, segments_array, squares, squares_degree):
- '''
- 0 -- 1
- | |
- 3 -- 2
-
- # segments: [4, 2]
- # connects: [4]
- '''
-
- ###################################### OVERLAP SCORES
- cover = 0
- perimeter = 0
- # check 0 > 1 > 2 > 3
- square_length = []
-
- for start_idx in range(4):
- end_idx = (start_idx + 1) % 4
-
- connect_idx = connects[start_idx] # segment idx of segment01
- start_segments = segments[start_idx]
- end_segments = segments[end_idx]
-
- start_point = square[start_idx]
- end_point = square[end_idx]
-
- # check whether outside or inside
- start_position, start_min, start_cover_param, start_peri_param = check_outside_inside(start_segments,
- connect_idx)
- end_position, end_min, end_cover_param, end_peri_param = check_outside_inside(end_segments, connect_idx)
-
- cover += dist_segments[connect_idx] + start_cover_param * start_min + end_cover_param * end_min
- perimeter += dist_segments[connect_idx] + start_peri_param * start_min + end_peri_param * end_min
-
- square_length.append(
- dist_segments[connect_idx] + start_peri_param * start_min + end_peri_param * end_min)
-
- overlap_scores.append(cover / perimeter)
- ######################################
- ###################################### DEGREE SCORES
- '''
- deg0 vs deg2
- deg1 vs deg3
- '''
- deg0, deg1, deg2, deg3 = degree
- deg_ratio1 = deg0 / deg2
- if deg_ratio1 > 1.0:
- deg_ratio1 = 1 / deg_ratio1
- deg_ratio2 = deg1 / deg3
- if deg_ratio2 > 1.0:
- deg_ratio2 = 1 / deg_ratio2
- degree_scores.append((deg_ratio1 + deg_ratio2) / 2)
- ######################################
- ###################################### LENGTH SCORES
- '''
- len0 vs len2
- len1 vs len3
- '''
- len0, len1, len2, len3 = square_length
- len_ratio1 = len0 / len2 if len2 > len0 else len2 / len0
- len_ratio2 = len1 / len3 if len3 > len1 else len3 / len1
- length_scores.append((len_ratio1 + len_ratio2) / 2)
-
- ######################################
-
- overlap_scores = np.array(overlap_scores)
- overlap_scores /= np.max(overlap_scores)
-
- degree_scores = np.array(degree_scores)
- # degree_scores /= np.max(degree_scores)
-
- length_scores = np.array(length_scores)
-
- ###################################### AREA SCORES
- area_scores = np.reshape(squares, [-1, 4, 2])
- area_x = area_scores[:, :, 0]
- area_y = area_scores[:, :, 1]
- correction = area_x[:, -1] * area_y[:, 0] - area_y[:, -1] * area_x[:, 0]
- area_scores = np.sum(area_x[:, :-1] * area_y[:, 1:], axis=-1) - np.sum(area_y[:, :-1] * area_x[:, 1:], axis=-1)
- area_scores = 0.5 * np.abs(area_scores + correction)
- area_scores /= (map_size * map_size) # np.max(area_scores)
- ######################################
-
- ###################################### CENTER SCORES
- centers = np.array([[256 // 2, 256 // 2]], dtype='float32') # [1, 2]
- # squares: [n, 4, 2]
- square_centers = np.mean(squares, axis=1) # [n, 2]
- center2center = np.sqrt(np.sum((centers - square_centers) ** 2))
- center_scores = center2center / (map_size / np.sqrt(2.0))
-
- '''
- score_w = [overlap, degree, area, center, length]
- '''
- score_w = [0.0, 1.0, 10.0, 0.5, 1.0]
- score_array = params['w_overlap'] * overlap_scores \
- + params['w_degree'] * degree_scores \
- + params['w_area'] * area_scores \
- - params['w_center'] * center_scores \
- + params['w_length'] * length_scores
-
- best_square = []
-
- sorted_idx = np.argsort(score_array)[::-1]
- score_array = score_array[sorted_idx]
- squares = squares[sorted_idx]
-
- except Exception as e:
- pass
-
- '''return list
- merged_lines, squares, scores
- '''
-
- try:
- new_segments[:, 0] = new_segments[:, 0] * 2 / input_shape[1] * original_shape[1]
- new_segments[:, 1] = new_segments[:, 1] * 2 / input_shape[0] * original_shape[0]
- new_segments[:, 2] = new_segments[:, 2] * 2 / input_shape[1] * original_shape[1]
- new_segments[:, 3] = new_segments[:, 3] * 2 / input_shape[0] * original_shape[0]
- except:
- new_segments = []
-
- try:
- squares[:, :, 0] = squares[:, :, 0] * 2 / input_shape[1] * original_shape[1]
- squares[:, :, 1] = squares[:, :, 1] * 2 / input_shape[0] * original_shape[0]
- except:
- squares = []
- score_array = []
-
- try:
- inter_points = np.array(inter_points)
- inter_points[:, 0] = inter_points[:, 0] * 2 / input_shape[1] * original_shape[1]
- inter_points[:, 1] = inter_points[:, 1] * 2 / input_shape[0] * original_shape[0]
- except:
- inter_points = []
-
- return new_segments, squares, score_array, inter_points
diff --git a/spaces/Anonymous-sub/Rerender/gmflow_module/gmflow/utils.py b/spaces/Anonymous-sub/Rerender/gmflow_module/gmflow/utils.py
deleted file mode 100644
index a1ae124a652553d5b7578459d92dec4b6a207409..0000000000000000000000000000000000000000
--- a/spaces/Anonymous-sub/Rerender/gmflow_module/gmflow/utils.py
+++ /dev/null
@@ -1,86 +0,0 @@
-import torch
-from .position import PositionEmbeddingSine
-
-
-def split_feature(feature,
- num_splits=2,
- channel_last=False,
- ):
- if channel_last: # [B, H, W, C]
- b, h, w, c = feature.size()
- assert h % num_splits == 0 and w % num_splits == 0
-
- b_new = b * num_splits * num_splits
- h_new = h // num_splits
- w_new = w // num_splits
-
- feature = feature.view(b, num_splits, h // num_splits, num_splits, w // num_splits, c
- ).permute(0, 1, 3, 2, 4, 5).reshape(b_new, h_new, w_new, c) # [B*K*K, H/K, W/K, C]
- else: # [B, C, H, W]
- b, c, h, w = feature.size()
- assert h % num_splits == 0 and w % num_splits == 0
-
- b_new = b * num_splits * num_splits
- h_new = h // num_splits
- w_new = w // num_splits
-
- feature = feature.view(b, c, num_splits, h // num_splits, num_splits, w // num_splits
- ).permute(0, 2, 4, 1, 3, 5).reshape(b_new, c, h_new, w_new) # [B*K*K, C, H/K, W/K]
-
- return feature
-
-
-def merge_splits(splits,
- num_splits=2,
- channel_last=False,
- ):
- if channel_last: # [B*K*K, H/K, W/K, C]
- b, h, w, c = splits.size()
- new_b = b // num_splits // num_splits
-
- splits = splits.view(new_b, num_splits, num_splits, h, w, c)
- merge = splits.permute(0, 1, 3, 2, 4, 5).contiguous().view(
- new_b, num_splits * h, num_splits * w, c) # [B, H, W, C]
- else: # [B*K*K, C, H/K, W/K]
- b, c, h, w = splits.size()
- new_b = b // num_splits // num_splits
-
- splits = splits.view(new_b, num_splits, num_splits, c, h, w)
- merge = splits.permute(0, 3, 1, 4, 2, 5).contiguous().view(
- new_b, c, num_splits * h, num_splits * w) # [B, C, H, W]
-
- return merge
-
-
-def normalize_img(img0, img1):
- # loaded images are in [0, 255]
- # normalize by ImageNet mean and std
- mean = torch.tensor([0.485, 0.456, 0.406]).view(1, 3, 1, 1).to(img1.device)
- std = torch.tensor([0.229, 0.224, 0.225]).view(1, 3, 1, 1).to(img1.device)
- img0 = (img0 / 255. - mean) / std
- img1 = (img1 / 255. - mean) / std
-
- return img0, img1
-
-
-def feature_add_position(feature0, feature1, attn_splits, feature_channels):
- pos_enc = PositionEmbeddingSine(num_pos_feats=feature_channels // 2)
-
- if attn_splits > 1: # add position in splited window
- feature0_splits = split_feature(feature0, num_splits=attn_splits)
- feature1_splits = split_feature(feature1, num_splits=attn_splits)
-
- position = pos_enc(feature0_splits)
-
- feature0_splits = feature0_splits + position
- feature1_splits = feature1_splits + position
-
- feature0 = merge_splits(feature0_splits, num_splits=attn_splits)
- feature1 = merge_splits(feature1_splits, num_splits=attn_splits)
- else:
- position = pos_enc(feature0)
-
- feature0 = feature0 + position
- feature1 = feature1 + position
-
- return feature0, feature1
diff --git a/spaces/Anthony7906/MengHuiMXD_GPT/assets/custom.js b/spaces/Anthony7906/MengHuiMXD_GPT/assets/custom.js
deleted file mode 100644
index b8071034f3618c541e3f4169c7fc6d6593d56f44..0000000000000000000000000000000000000000
--- a/spaces/Anthony7906/MengHuiMXD_GPT/assets/custom.js
+++ /dev/null
@@ -1,224 +0,0 @@
-
-// custom javascript here
-
-const MAX_HISTORY_LENGTH = 32;
-
-var key_down_history = [];
-var currentIndex = -1;
-var user_input_ta;
-
-var gradioContainer = null;
-var user_input_ta = null;
-var user_input_tb = null;
-var userInfoDiv = null;
-var appTitleDiv = null;
-var chatbot = null;
-var apSwitch = null;
-
-var ga = document.getElementsByTagName("gradio-app");
-var targetNode = ga[0];
-var isInIframe = (window.self !== window.top);
-
-// gradio 页面加载好了么??? 我能动你的元素了么??
-function gradioLoaded(mutations) {
- for (var i = 0; i < mutations.length; i++) {
- if (mutations[i].addedNodes.length) {
- gradioContainer = document.querySelector(".gradio-container");
- user_input_tb = document.getElementById('user_input_tb');
- userInfoDiv = document.getElementById("user_info");
- appTitleDiv = document.getElementById("app_title");
- chatbot = document.querySelector('#chuanhu_chatbot');
- apSwitch = document.querySelector('.apSwitch input[type="checkbox"]');
-
- if (gradioContainer && apSwitch) { // gradioCainter 加载出来了没?
- adjustDarkMode();
- }
- if (user_input_tb) { // user_input_tb 加载出来了没?
- selectHistory();
- }
- if (userInfoDiv && appTitleDiv) { // userInfoDiv 和 appTitleDiv 加载出来了没?
- setTimeout(showOrHideUserInfo(), 2000);
- }
- if (chatbot) { // chatbot 加载出来了没?
- setChatbotHeight()
- }
- }
- }
-}
-
-function selectHistory() {
- user_input_ta = user_input_tb.querySelector("textarea");
- if (user_input_ta) {
- observer.disconnect(); // 停止监听
- // 在 textarea 上监听 keydown 事件
- user_input_ta.addEventListener("keydown", function (event) {
- var value = user_input_ta.value.trim();
- // 判断按下的是否为方向键
- if (event.code === 'ArrowUp' || event.code === 'ArrowDown') {
- // 如果按下的是方向键,且输入框中有内容,且历史记录中没有该内容,则不执行操作
- if (value && key_down_history.indexOf(value) === -1)
- return;
- // 对于需要响应的动作,阻止默认行为。
- event.preventDefault();
- var length = key_down_history.length;
- if (length === 0) {
- currentIndex = -1; // 如果历史记录为空,直接将当前选中的记录重置
- return;
- }
- if (currentIndex === -1) {
- currentIndex = length;
- }
- if (event.code === 'ArrowUp' && currentIndex > 0) {
- currentIndex--;
- user_input_ta.value = key_down_history[currentIndex];
- } else if (event.code === 'ArrowDown' && currentIndex < length - 1) {
- currentIndex++;
- user_input_ta.value = key_down_history[currentIndex];
- }
- user_input_ta.selectionStart = user_input_ta.value.length;
- user_input_ta.selectionEnd = user_input_ta.value.length;
- const input_event = new InputEvent("input", { bubbles: true, cancelable: true });
- user_input_ta.dispatchEvent(input_event);
- } else if (event.code === "Enter") {
- if (value) {
- currentIndex = -1;
- if (key_down_history.indexOf(value) === -1) {
- key_down_history.push(value);
- if (key_down_history.length > MAX_HISTORY_LENGTH) {
- key_down_history.shift();
- }
- }
- }
- }
- });
- }
-}
-
-function toggleUserInfoVisibility(shouldHide) {
- if (userInfoDiv) {
- if (shouldHide) {
- userInfoDiv.classList.add("hideK");
- } else {
- userInfoDiv.classList.remove("hideK");
- }
- }
-}
-function showOrHideUserInfo() {
- var sendBtn = document.getElementById("submit_btn");
-
- // Bind mouse/touch events to show/hide user info
- appTitleDiv.addEventListener("mouseenter", function () {
- toggleUserInfoVisibility(false);
- });
- userInfoDiv.addEventListener("mouseenter", function () {
- toggleUserInfoVisibility(false);
- });
- sendBtn.addEventListener("mouseenter", function () {
- toggleUserInfoVisibility(false);
- });
-
- appTitleDiv.addEventListener("mouseleave", function () {
- toggleUserInfoVisibility(true);
- });
- userInfoDiv.addEventListener("mouseleave", function () {
- toggleUserInfoVisibility(true);
- });
- sendBtn.addEventListener("mouseleave", function () {
- toggleUserInfoVisibility(true);
- });
-
- appTitleDiv.ontouchstart = function () {
- toggleUserInfoVisibility(false);
- };
- userInfoDiv.ontouchstart = function () {
- toggleUserInfoVisibility(false);
- };
- sendBtn.ontouchstart = function () {
- toggleUserInfoVisibility(false);
- };
-
- appTitleDiv.ontouchend = function () {
- setTimeout(function () {
- toggleUserInfoVisibility(true);
- }, 3000);
- };
- userInfoDiv.ontouchend = function () {
- setTimeout(function () {
- toggleUserInfoVisibility(true);
- }, 3000);
- };
- sendBtn.ontouchend = function () {
- setTimeout(function () {
- toggleUserInfoVisibility(true);
- }, 3000); // Delay 1 second to hide user info
- };
-
- // Hide user info after 2 second
- setTimeout(function () {
- toggleUserInfoVisibility(true);
- }, 2000);
-}
-
-function toggleDarkMode(isEnabled) {
- if (isEnabled) {
- gradioContainer.classList.add("dark");
- document.body.style.setProperty("background-color", "var(--neutral-950)", "important");
- } else {
- gradioContainer.classList.remove("dark");
- document.body.style.backgroundColor = "";
- }
-}
-function adjustDarkMode() {
- const darkModeQuery = window.matchMedia("(prefers-color-scheme: dark)");
-
- // 根据当前颜色模式设置初始状态
- apSwitch.checked = darkModeQuery.matches;
- toggleDarkMode(darkModeQuery.matches);
- // 监听颜色模式变化
- darkModeQuery.addEventListener("change", (e) => {
- apSwitch.checked = e.matches;
- toggleDarkMode(e.matches);
- });
- // apSwitch = document.querySelector('.apSwitch input[type="checkbox"]');
- apSwitch.addEventListener("change", (e) => {
- toggleDarkMode(e.target.checked);
- });
-}
-
-function setChatbotHeight() {
- const screenWidth = window.innerWidth;
- const statusDisplay = document.querySelector('#status_display');
- const statusDisplayHeight = statusDisplay ? statusDisplay.offsetHeight : 0;
- const wrap = chatbot.querySelector('.wrap');
- const vh = window.innerHeight * 0.01;
- document.documentElement.style.setProperty('--vh', `${vh}px`);
- if (isInIframe) {
- chatbot.style.height = `700px`;
- wrap.style.maxHeight = `calc(700px - var(--line-sm) * 1rem - 2 * var(--block-label-margin))`
- } else {
- if (screenWidth <= 320) {
- chatbot.style.height = `calc(var(--vh, 1vh) * 100 - ${statusDisplayHeight + 150}px)`;
- wrap.style.maxHeight = `calc(var(--vh, 1vh) * 100 - ${statusDisplayHeight + 150}px - var(--line-sm) * 1rem - 2 * var(--block-label-margin))`;
- } else if (screenWidth <= 499) {
- chatbot.style.height = `calc(var(--vh, 1vh) * 100 - ${statusDisplayHeight + 100}px)`;
- wrap.style.maxHeight = `calc(var(--vh, 1vh) * 100 - ${statusDisplayHeight + 100}px - var(--line-sm) * 1rem - 2 * var(--block-label-margin))`;
- } else {
- chatbot.style.height = `calc(var(--vh, 1vh) * 100 - ${statusDisplayHeight + 160}px)`;
- wrap.style.maxHeight = `calc(var(--vh, 1vh) * 100 - ${statusDisplayHeight + 160}px - var(--line-sm) * 1rem - 2 * var(--block-label-margin))`;
- }
- }
-}
-
-// 监视页面内部 DOM 变动
-var observer = new MutationObserver(function (mutations) {
- gradioLoaded(mutations);
-});
-observer.observe(targetNode, { childList: true, subtree: true });
-
-// 监视页面变化
-window.addEventListener("DOMContentLoaded", function () {
- isInIframe = (window.self !== window.top);
-});
-window.addEventListener('resize', setChatbotHeight);
-window.addEventListener('scroll', setChatbotHeight);
-window.matchMedia("(prefers-color-scheme: dark)").addEventListener("change", adjustDarkMode);
\ No newline at end of file
diff --git a/spaces/Apex-X/GODROOP/roop/processors/frame/face_swapper.py b/spaces/Apex-X/GODROOP/roop/processors/frame/face_swapper.py
deleted file mode 100644
index 3805e52f272e8e5470379c90979a34b3faa128af..0000000000000000000000000000000000000000
--- a/spaces/Apex-X/GODROOP/roop/processors/frame/face_swapper.py
+++ /dev/null
@@ -1,88 +0,0 @@
-from typing import Any, List, Callable
-import cv2
-import insightface
-import threading
-
-import roop.globals
-import roop.processors.frame.core
-from roop.core import update_status
-from roop.face_analyser import get_one_face, get_many_faces
-from roop.typing import Face, Frame
-from roop.utilities import conditional_download, resolve_relative_path, is_image, is_video
-
-FACE_SWAPPER = None
-THREAD_LOCK = threading.Lock()
-NAME = 'ROOP.FACE-SWAPPER'
-
-
-def get_face_swapper() -> Any:
- global FACE_SWAPPER
-
- with THREAD_LOCK:
- if FACE_SWAPPER is None:
- model_path = resolve_relative_path('../models/inswapper_128.onnx')
- FACE_SWAPPER = insightface.model_zoo.get_model(model_path, providers=roop.globals.execution_providers)
- return FACE_SWAPPER
-
-
-def pre_check() -> bool:
- download_directory_path = resolve_relative_path('../models')
- conditional_download(download_directory_path, ['https://huggingface.co/Apex-X/inswapper_128.onnx/resolve/main/inswapper_128.onnx'])
- return True
-
-
-def pre_start() -> bool:
- if not is_image(roop.globals.source_path):
- update_status('Select an image for source path.', NAME)
- return False
- elif not get_one_face(cv2.imread(roop.globals.source_path)):
- update_status('No face in source path detected.', NAME)
- return False
- if not is_image(roop.globals.target_path) and not is_video(roop.globals.target_path):
- update_status('Select an image or video for target path.', NAME)
- return False
- return True
-
-
-def post_process() -> None:
- global FACE_SWAPPER
-
- FACE_SWAPPER = None
-
-
-def swap_face(source_face: Face, target_face: Face, temp_frame: Frame) -> Frame:
- return get_face_swapper().get(temp_frame, target_face, source_face, paste_back=True)
-
-
-def process_frame(source_face: Face, temp_frame: Frame) -> Frame:
- if roop.globals.many_faces:
- many_faces = get_many_faces(temp_frame)
- if many_faces:
- for target_face in many_faces:
- temp_frame = swap_face(source_face, target_face, temp_frame)
- else:
- target_face = get_one_face(temp_frame)
- if target_face:
- temp_frame = swap_face(source_face, target_face, temp_frame)
- return temp_frame
-
-
-def process_frames(source_path: str, temp_frame_paths: List[str], update: Callable[[], None]) -> None:
- source_face = get_one_face(cv2.imread(source_path))
- for temp_frame_path in temp_frame_paths:
- temp_frame = cv2.imread(temp_frame_path)
- result = process_frame(source_face, temp_frame)
- cv2.imwrite(temp_frame_path, result)
- if update:
- update()
-
-
-def process_image(source_path: str, target_path: str, output_path: str) -> None:
- source_face = get_one_face(cv2.imread(source_path))
- target_frame = cv2.imread(target_path)
- result = process_frame(source_face, target_frame)
- cv2.imwrite(output_path, result)
-
-
-def process_video(source_path: str, temp_frame_paths: List[str]) -> None:
- roop.processors.frame.core.process_video(source_path, temp_frame_paths, process_frames)
diff --git a/spaces/Ataturk-Chatbot/HuggingFaceChat/venv/lib/python3.11/site-packages/pip/_internal/resolution/resolvelib/factory.py b/spaces/Ataturk-Chatbot/HuggingFaceChat/venv/lib/python3.11/site-packages/pip/_internal/resolution/resolvelib/factory.py
deleted file mode 100644
index 0331297b85b89c3387c3868d6254f420ed6a0381..0000000000000000000000000000000000000000
--- a/spaces/Ataturk-Chatbot/HuggingFaceChat/venv/lib/python3.11/site-packages/pip/_internal/resolution/resolvelib/factory.py
+++ /dev/null
@@ -1,730 +0,0 @@
-import contextlib
-import functools
-import logging
-from typing import (
- TYPE_CHECKING,
- Dict,
- FrozenSet,
- Iterable,
- Iterator,
- List,
- Mapping,
- NamedTuple,
- Optional,
- Sequence,
- Set,
- Tuple,
- TypeVar,
- cast,
-)
-
-from pip._vendor.packaging.requirements import InvalidRequirement
-from pip._vendor.packaging.specifiers import SpecifierSet
-from pip._vendor.packaging.utils import NormalizedName, canonicalize_name
-from pip._vendor.resolvelib import ResolutionImpossible
-
-from pip._internal.cache import CacheEntry, WheelCache
-from pip._internal.exceptions import (
- DistributionNotFound,
- InstallationError,
- MetadataInconsistent,
- UnsupportedPythonVersion,
- UnsupportedWheel,
-)
-from pip._internal.index.package_finder import PackageFinder
-from pip._internal.metadata import BaseDistribution, get_default_environment
-from pip._internal.models.link import Link
-from pip._internal.models.wheel import Wheel
-from pip._internal.operations.prepare import RequirementPreparer
-from pip._internal.req.constructors import install_req_from_link_and_ireq
-from pip._internal.req.req_install import (
- InstallRequirement,
- check_invalid_constraint_type,
-)
-from pip._internal.resolution.base import InstallRequirementProvider
-from pip._internal.utils.compatibility_tags import get_supported
-from pip._internal.utils.hashes import Hashes
-from pip._internal.utils.packaging import get_requirement
-from pip._internal.utils.virtualenv import running_under_virtualenv
-
-from .base import Candidate, CandidateVersion, Constraint, Requirement
-from .candidates import (
- AlreadyInstalledCandidate,
- BaseCandidate,
- EditableCandidate,
- ExtrasCandidate,
- LinkCandidate,
- RequiresPythonCandidate,
- as_base_candidate,
-)
-from .found_candidates import FoundCandidates, IndexCandidateInfo
-from .requirements import (
- ExplicitRequirement,
- RequiresPythonRequirement,
- SpecifierRequirement,
- UnsatisfiableRequirement,
-)
-
-if TYPE_CHECKING:
- from typing import Protocol
-
- class ConflictCause(Protocol):
- requirement: RequiresPythonRequirement
- parent: Candidate
-
-
-logger = logging.getLogger(__name__)
-
-C = TypeVar("C")
-Cache = Dict[Link, C]
-
-
-class CollectedRootRequirements(NamedTuple):
- requirements: List[Requirement]
- constraints: Dict[str, Constraint]
- user_requested: Dict[str, int]
-
-
-class Factory:
- def __init__(
- self,
- finder: PackageFinder,
- preparer: RequirementPreparer,
- make_install_req: InstallRequirementProvider,
- wheel_cache: Optional[WheelCache],
- use_user_site: bool,
- force_reinstall: bool,
- ignore_installed: bool,
- ignore_requires_python: bool,
- py_version_info: Optional[Tuple[int, ...]] = None,
- ) -> None:
- self._finder = finder
- self.preparer = preparer
- self._wheel_cache = wheel_cache
- self._python_candidate = RequiresPythonCandidate(py_version_info)
- self._make_install_req_from_spec = make_install_req
- self._use_user_site = use_user_site
- self._force_reinstall = force_reinstall
- self._ignore_requires_python = ignore_requires_python
-
- self._build_failures: Cache[InstallationError] = {}
- self._link_candidate_cache: Cache[LinkCandidate] = {}
- self._editable_candidate_cache: Cache[EditableCandidate] = {}
- self._installed_candidate_cache: Dict[str, AlreadyInstalledCandidate] = {}
- self._extras_candidate_cache: Dict[
- Tuple[int, FrozenSet[str]], ExtrasCandidate
- ] = {}
-
- if not ignore_installed:
- env = get_default_environment()
- self._installed_dists = {
- dist.canonical_name: dist
- for dist in env.iter_installed_distributions(local_only=False)
- }
- else:
- self._installed_dists = {}
-
- @property
- def force_reinstall(self) -> bool:
- return self._force_reinstall
-
- def _fail_if_link_is_unsupported_wheel(self, link: Link) -> None:
- if not link.is_wheel:
- return
- wheel = Wheel(link.filename)
- if wheel.supported(self._finder.target_python.get_tags()):
- return
- msg = f"{link.filename} is not a supported wheel on this platform."
- raise UnsupportedWheel(msg)
-
- def _make_extras_candidate(
- self, base: BaseCandidate, extras: FrozenSet[str]
- ) -> ExtrasCandidate:
- cache_key = (id(base), extras)
- try:
- candidate = self._extras_candidate_cache[cache_key]
- except KeyError:
- candidate = ExtrasCandidate(base, extras)
- self._extras_candidate_cache[cache_key] = candidate
- return candidate
-
- def _make_candidate_from_dist(
- self,
- dist: BaseDistribution,
- extras: FrozenSet[str],
- template: InstallRequirement,
- ) -> Candidate:
- try:
- base = self._installed_candidate_cache[dist.canonical_name]
- except KeyError:
- base = AlreadyInstalledCandidate(dist, template, factory=self)
- self._installed_candidate_cache[dist.canonical_name] = base
- if not extras:
- return base
- return self._make_extras_candidate(base, extras)
-
- def _make_candidate_from_link(
- self,
- link: Link,
- extras: FrozenSet[str],
- template: InstallRequirement,
- name: Optional[NormalizedName],
- version: Optional[CandidateVersion],
- ) -> Optional[Candidate]:
- # TODO: Check already installed candidate, and use it if the link and
- # editable flag match.
-
- if link in self._build_failures:
- # We already tried this candidate before, and it does not build.
- # Don't bother trying again.
- return None
-
- if template.editable:
- if link not in self._editable_candidate_cache:
- try:
- self._editable_candidate_cache[link] = EditableCandidate(
- link,
- template,
- factory=self,
- name=name,
- version=version,
- )
- except MetadataInconsistent as e:
- logger.info(
- "Discarding [blue underline]%s[/]: [yellow]%s[reset]",
- link,
- e,
- extra={"markup": True},
- )
- self._build_failures[link] = e
- return None
-
- base: BaseCandidate = self._editable_candidate_cache[link]
- else:
- if link not in self._link_candidate_cache:
- try:
- self._link_candidate_cache[link] = LinkCandidate(
- link,
- template,
- factory=self,
- name=name,
- version=version,
- )
- except MetadataInconsistent as e:
- logger.info(
- "Discarding [blue underline]%s[/]: [yellow]%s[reset]",
- link,
- e,
- extra={"markup": True},
- )
- self._build_failures[link] = e
- return None
- base = self._link_candidate_cache[link]
-
- if not extras:
- return base
- return self._make_extras_candidate(base, extras)
-
- def _iter_found_candidates(
- self,
- ireqs: Sequence[InstallRequirement],
- specifier: SpecifierSet,
- hashes: Hashes,
- prefers_installed: bool,
- incompatible_ids: Set[int],
- ) -> Iterable[Candidate]:
- if not ireqs:
- return ()
-
- # The InstallRequirement implementation requires us to give it a
- # "template". Here we just choose the first requirement to represent
- # all of them.
- # Hopefully the Project model can correct this mismatch in the future.
- template = ireqs[0]
- assert template.req, "Candidates found on index must be PEP 508"
- name = canonicalize_name(template.req.name)
-
- extras: FrozenSet[str] = frozenset()
- for ireq in ireqs:
- assert ireq.req, "Candidates found on index must be PEP 508"
- specifier &= ireq.req.specifier
- hashes &= ireq.hashes(trust_internet=False)
- extras |= frozenset(ireq.extras)
-
- def _get_installed_candidate() -> Optional[Candidate]:
- """Get the candidate for the currently-installed version."""
- # If --force-reinstall is set, we want the version from the index
- # instead, so we "pretend" there is nothing installed.
- if self._force_reinstall:
- return None
- try:
- installed_dist = self._installed_dists[name]
- except KeyError:
- return None
- # Don't use the installed distribution if its version does not fit
- # the current dependency graph.
- if not specifier.contains(installed_dist.version, prereleases=True):
- return None
- candidate = self._make_candidate_from_dist(
- dist=installed_dist,
- extras=extras,
- template=template,
- )
- # The candidate is a known incompatibility. Don't use it.
- if id(candidate) in incompatible_ids:
- return None
- return candidate
-
- def iter_index_candidate_infos() -> Iterator[IndexCandidateInfo]:
- result = self._finder.find_best_candidate(
- project_name=name,
- specifier=specifier,
- hashes=hashes,
- )
- icans = list(result.iter_applicable())
-
- # PEP 592: Yanked releases are ignored unless the specifier
- # explicitly pins a version (via '==' or '===') that can be
- # solely satisfied by a yanked release.
- all_yanked = all(ican.link.is_yanked for ican in icans)
-
- def is_pinned(specifier: SpecifierSet) -> bool:
- for sp in specifier:
- if sp.operator == "===":
- return True
- if sp.operator != "==":
- continue
- if sp.version.endswith(".*"):
- continue
- return True
- return False
-
- pinned = is_pinned(specifier)
-
- # PackageFinder returns earlier versions first, so we reverse.
- for ican in reversed(icans):
- if not (all_yanked and pinned) and ican.link.is_yanked:
- continue
- func = functools.partial(
- self._make_candidate_from_link,
- link=ican.link,
- extras=extras,
- template=template,
- name=name,
- version=ican.version,
- )
- yield ican.version, func
-
- return FoundCandidates(
- iter_index_candidate_infos,
- _get_installed_candidate(),
- prefers_installed,
- incompatible_ids,
- )
-
- def _iter_explicit_candidates_from_base(
- self,
- base_requirements: Iterable[Requirement],
- extras: FrozenSet[str],
- ) -> Iterator[Candidate]:
- """Produce explicit candidates from the base given an extra-ed package.
-
- :param base_requirements: Requirements known to the resolver. The
- requirements are guaranteed to not have extras.
- :param extras: The extras to inject into the explicit requirements'
- candidates.
- """
- for req in base_requirements:
- lookup_cand, _ = req.get_candidate_lookup()
- if lookup_cand is None: # Not explicit.
- continue
- # We've stripped extras from the identifier, and should always
- # get a BaseCandidate here, unless there's a bug elsewhere.
- base_cand = as_base_candidate(lookup_cand)
- assert base_cand is not None, "no extras here"
- yield self._make_extras_candidate(base_cand, extras)
-
- def _iter_candidates_from_constraints(
- self,
- identifier: str,
- constraint: Constraint,
- template: InstallRequirement,
- ) -> Iterator[Candidate]:
- """Produce explicit candidates from constraints.
-
- This creates "fake" InstallRequirement objects that are basically clones
- of what "should" be the template, but with original_link set to link.
- """
- for link in constraint.links:
- self._fail_if_link_is_unsupported_wheel(link)
- candidate = self._make_candidate_from_link(
- link,
- extras=frozenset(),
- template=install_req_from_link_and_ireq(link, template),
- name=canonicalize_name(identifier),
- version=None,
- )
- if candidate:
- yield candidate
-
- def find_candidates(
- self,
- identifier: str,
- requirements: Mapping[str, Iterable[Requirement]],
- incompatibilities: Mapping[str, Iterator[Candidate]],
- constraint: Constraint,
- prefers_installed: bool,
- ) -> Iterable[Candidate]:
- # Collect basic lookup information from the requirements.
- explicit_candidates: Set[Candidate] = set()
- ireqs: List[InstallRequirement] = []
- for req in requirements[identifier]:
- cand, ireq = req.get_candidate_lookup()
- if cand is not None:
- explicit_candidates.add(cand)
- if ireq is not None:
- ireqs.append(ireq)
-
- # If the current identifier contains extras, add explicit candidates
- # from entries from extra-less identifier.
- with contextlib.suppress(InvalidRequirement):
- parsed_requirement = get_requirement(identifier)
- explicit_candidates.update(
- self._iter_explicit_candidates_from_base(
- requirements.get(parsed_requirement.name, ()),
- frozenset(parsed_requirement.extras),
- ),
- )
-
- # Add explicit candidates from constraints. We only do this if there are
- # known ireqs, which represent requirements not already explicit. If
- # there are no ireqs, we're constraining already-explicit requirements,
- # which is handled later when we return the explicit candidates.
- if ireqs:
- try:
- explicit_candidates.update(
- self._iter_candidates_from_constraints(
- identifier,
- constraint,
- template=ireqs[0],
- ),
- )
- except UnsupportedWheel:
- # If we're constrained to install a wheel incompatible with the
- # target architecture, no candidates will ever be valid.
- return ()
-
- # Since we cache all the candidates, incompatibility identification
- # can be made quicker by comparing only the id() values.
- incompat_ids = {id(c) for c in incompatibilities.get(identifier, ())}
-
- # If none of the requirements want an explicit candidate, we can ask
- # the finder for candidates.
- if not explicit_candidates:
- return self._iter_found_candidates(
- ireqs,
- constraint.specifier,
- constraint.hashes,
- prefers_installed,
- incompat_ids,
- )
-
- return (
- c
- for c in explicit_candidates
- if id(c) not in incompat_ids
- and constraint.is_satisfied_by(c)
- and all(req.is_satisfied_by(c) for req in requirements[identifier])
- )
-
- def _make_requirement_from_install_req(
- self, ireq: InstallRequirement, requested_extras: Iterable[str]
- ) -> Optional[Requirement]:
- if not ireq.match_markers(requested_extras):
- logger.info(
- "Ignoring %s: markers '%s' don't match your environment",
- ireq.name,
- ireq.markers,
- )
- return None
- if not ireq.link:
- return SpecifierRequirement(ireq)
- self._fail_if_link_is_unsupported_wheel(ireq.link)
- cand = self._make_candidate_from_link(
- ireq.link,
- extras=frozenset(ireq.extras),
- template=ireq,
- name=canonicalize_name(ireq.name) if ireq.name else None,
- version=None,
- )
- if cand is None:
- # There's no way we can satisfy a URL requirement if the underlying
- # candidate fails to build. An unnamed URL must be user-supplied, so
- # we fail eagerly. If the URL is named, an unsatisfiable requirement
- # can make the resolver do the right thing, either backtrack (and
- # maybe find some other requirement that's buildable) or raise a
- # ResolutionImpossible eventually.
- if not ireq.name:
- raise self._build_failures[ireq.link]
- return UnsatisfiableRequirement(canonicalize_name(ireq.name))
- return self.make_requirement_from_candidate(cand)
-
- def collect_root_requirements(
- self, root_ireqs: List[InstallRequirement]
- ) -> CollectedRootRequirements:
- collected = CollectedRootRequirements([], {}, {})
- for i, ireq in enumerate(root_ireqs):
- if ireq.constraint:
- # Ensure we only accept valid constraints
- problem = check_invalid_constraint_type(ireq)
- if problem:
- raise InstallationError(problem)
- if not ireq.match_markers():
- continue
- assert ireq.name, "Constraint must be named"
- name = canonicalize_name(ireq.name)
- if name in collected.constraints:
- collected.constraints[name] &= ireq
- else:
- collected.constraints[name] = Constraint.from_ireq(ireq)
- else:
- req = self._make_requirement_from_install_req(
- ireq,
- requested_extras=(),
- )
- if req is None:
- continue
- if ireq.user_supplied and req.name not in collected.user_requested:
- collected.user_requested[req.name] = i
- collected.requirements.append(req)
- return collected
-
- def make_requirement_from_candidate(
- self, candidate: Candidate
- ) -> ExplicitRequirement:
- return ExplicitRequirement(candidate)
-
- def make_requirement_from_spec(
- self,
- specifier: str,
- comes_from: Optional[InstallRequirement],
- requested_extras: Iterable[str] = (),
- ) -> Optional[Requirement]:
- ireq = self._make_install_req_from_spec(specifier, comes_from)
- return self._make_requirement_from_install_req(ireq, requested_extras)
-
- def make_requires_python_requirement(
- self,
- specifier: SpecifierSet,
- ) -> Optional[Requirement]:
- if self._ignore_requires_python:
- return None
- # Don't bother creating a dependency for an empty Requires-Python.
- if not str(specifier):
- return None
- return RequiresPythonRequirement(specifier, self._python_candidate)
-
- def get_wheel_cache_entry(
- self, link: Link, name: Optional[str]
- ) -> Optional[CacheEntry]:
- """Look up the link in the wheel cache.
-
- If ``preparer.require_hashes`` is True, don't use the wheel cache,
- because cached wheels, always built locally, have different hashes
- than the files downloaded from the index server and thus throw false
- hash mismatches. Furthermore, cached wheels at present have
- nondeterministic contents due to file modification times.
- """
- if self._wheel_cache is None:
- return None
- return self._wheel_cache.get_cache_entry(
- link=link,
- package_name=name,
- supported_tags=get_supported(),
- )
-
- def get_dist_to_uninstall(self, candidate: Candidate) -> Optional[BaseDistribution]:
- # TODO: Are there more cases this needs to return True? Editable?
- dist = self._installed_dists.get(candidate.project_name)
- if dist is None: # Not installed, no uninstallation required.
- return None
-
- # We're installing into global site. The current installation must
- # be uninstalled, no matter it's in global or user site, because the
- # user site installation has precedence over global.
- if not self._use_user_site:
- return dist
-
- # We're installing into user site. Remove the user site installation.
- if dist.in_usersite:
- return dist
-
- # We're installing into user site, but the installed incompatible
- # package is in global site. We can't uninstall that, and would let
- # the new user installation to "shadow" it. But shadowing won't work
- # in virtual environments, so we error out.
- if running_under_virtualenv() and dist.in_site_packages:
- message = (
- f"Will not install to the user site because it will lack "
- f"sys.path precedence to {dist.raw_name} in {dist.location}"
- )
- raise InstallationError(message)
- return None
-
- def _report_requires_python_error(
- self, causes: Sequence["ConflictCause"]
- ) -> UnsupportedPythonVersion:
- assert causes, "Requires-Python error reported with no cause"
-
- version = self._python_candidate.version
-
- if len(causes) == 1:
- specifier = str(causes[0].requirement.specifier)
- message = (
- f"Package {causes[0].parent.name!r} requires a different "
- f"Python: {version} not in {specifier!r}"
- )
- return UnsupportedPythonVersion(message)
-
- message = f"Packages require a different Python. {version} not in:"
- for cause in causes:
- package = cause.parent.format_for_error()
- specifier = str(cause.requirement.specifier)
- message += f"\n{specifier!r} (required by {package})"
- return UnsupportedPythonVersion(message)
-
- def _report_single_requirement_conflict(
- self, req: Requirement, parent: Optional[Candidate]
- ) -> DistributionNotFound:
- if parent is None:
- req_disp = str(req)
- else:
- req_disp = f"{req} (from {parent.name})"
-
- cands = self._finder.find_all_candidates(req.project_name)
- skipped_by_requires_python = self._finder.requires_python_skipped_reasons()
- versions = [str(v) for v in sorted({c.version for c in cands})]
-
- if skipped_by_requires_python:
- logger.critical(
- "Ignored the following versions that require a different python "
- "version: %s",
- "; ".join(skipped_by_requires_python) or "none",
- )
- logger.critical(
- "Could not find a version that satisfies the requirement %s "
- "(from versions: %s)",
- req_disp,
- ", ".join(versions) or "none",
- )
- if str(req) == "requirements.txt":
- logger.info(
- "HINT: You are attempting to install a package literally "
- 'named "requirements.txt" (which cannot exist). Consider '
- "using the '-r' flag to install the packages listed in "
- "requirements.txt"
- )
-
- return DistributionNotFound(f"No matching distribution found for {req}")
-
- def get_installation_error(
- self,
- e: "ResolutionImpossible[Requirement, Candidate]",
- constraints: Dict[str, Constraint],
- ) -> InstallationError:
- assert e.causes, "Installation error reported with no cause"
-
- # If one of the things we can't solve is "we need Python X.Y",
- # that is what we report.
- requires_python_causes = [
- cause
- for cause in e.causes
- if isinstance(cause.requirement, RequiresPythonRequirement)
- and not cause.requirement.is_satisfied_by(self._python_candidate)
- ]
- if requires_python_causes:
- # The comprehension above makes sure all Requirement instances are
- # RequiresPythonRequirement, so let's cast for convenience.
- return self._report_requires_python_error(
- cast("Sequence[ConflictCause]", requires_python_causes),
- )
-
- # Otherwise, we have a set of causes which can't all be satisfied
- # at once.
-
- # The simplest case is when we have *one* cause that can't be
- # satisfied. We just report that case.
- if len(e.causes) == 1:
- req, parent = e.causes[0]
- if req.name not in constraints:
- return self._report_single_requirement_conflict(req, parent)
-
- # OK, we now have a list of requirements that can't all be
- # satisfied at once.
-
- # A couple of formatting helpers
- def text_join(parts: List[str]) -> str:
- if len(parts) == 1:
- return parts[0]
-
- return ", ".join(parts[:-1]) + " and " + parts[-1]
-
- def describe_trigger(parent: Candidate) -> str:
- ireq = parent.get_install_requirement()
- if not ireq or not ireq.comes_from:
- return f"{parent.name}=={parent.version}"
- if isinstance(ireq.comes_from, InstallRequirement):
- return str(ireq.comes_from.name)
- return str(ireq.comes_from)
-
- triggers = set()
- for req, parent in e.causes:
- if parent is None:
- # This is a root requirement, so we can report it directly
- trigger = req.format_for_error()
- else:
- trigger = describe_trigger(parent)
- triggers.add(trigger)
-
- if triggers:
- info = text_join(sorted(triggers))
- else:
- info = "the requested packages"
-
- msg = (
- "Cannot install {} because these package versions "
- "have conflicting dependencies.".format(info)
- )
- logger.critical(msg)
- msg = "\nThe conflict is caused by:"
-
- relevant_constraints = set()
- for req, parent in e.causes:
- if req.name in constraints:
- relevant_constraints.add(req.name)
- msg = msg + "\n "
- if parent:
- msg = msg + f"{parent.name} {parent.version} depends on "
- else:
- msg = msg + "The user requested "
- msg = msg + req.format_for_error()
- for key in relevant_constraints:
- spec = constraints[key].specifier
- msg += f"\n The user requested (constraint) {key}{spec}"
-
- msg = (
- msg
- + "\n\n"
- + "To fix this you could try to:\n"
- + "1. loosen the range of package versions you've specified\n"
- + "2. remove package versions to allow pip attempt to solve "
- + "the dependency conflict\n"
- )
-
- logger.info(msg)
-
- return DistributionNotFound(
- "ResolutionImpossible: for help visit "
- "https://pip.pypa.io/en/latest/topics/dependency-resolution/"
- "#dealing-with-dependency-conflicts"
- )
diff --git a/spaces/Atualli/yoloxTeste/telegramCrise.sh b/spaces/Atualli/yoloxTeste/telegramCrise.sh
deleted file mode 100644
index 3a027afa392f583e27fedd7c7a4db13801d0a623..0000000000000000000000000000000000000000
--- a/spaces/Atualli/yoloxTeste/telegramCrise.sh
+++ /dev/null
@@ -1 +0,0 @@
-curl -X POST "https://api.telegram.org/bot766543741:AAE0oO_ni_QYkfS8tZxC-VZt0RJztFiZNHc/sendMessage?chat_id=-927074982&text=$1"
diff --git a/spaces/Baishali/Pneumonia-Detection/app.py b/spaces/Baishali/Pneumonia-Detection/app.py
deleted file mode 100644
index ac98c0ec11e8b882b3fc9183afeef7de79d194b8..0000000000000000000000000000000000000000
--- a/spaces/Baishali/Pneumonia-Detection/app.py
+++ /dev/null
@@ -1,55 +0,0 @@
-__author__ = "Baishali Dutta"
-__copyright__ = "Copyright (C) 2021 Baishali Dutta"
-__license__ = "Apache License 2.0"
-__version__ = "0.1"
-
-# -------------------------------------------------------------------------
-# Importing the libraries
-# -------------------------------------------------------------------------
-import gradio as gr
-import numpy as np
-from tensorflow.keras.models import load_model
-from tensorflow.keras.preprocessing import image
-
-# -------------------------------------------------------------------------
-# Configurations
-# -------------------------------------------------------------------------
-MODEL_LOC = 'pneumonia_detection_cnn_model.h5'
-
-# load the trained CNN model
-cnn_model = load_model(MODEL_LOC)
-
-
-def make_prediction(test_image):
- test_image = test_image.name
- test_image = image.load_img(test_image, target_size=(224, 224))
- test_image = image.img_to_array(test_image) / 255.
- test_image = np.expand_dims(test_image, axis=0)
- result = cnn_model.predict(test_image)
- return {"Normal": str(result[0][0]), "Pneumonia": str(result[0][1])}
-
-
-image_input = gr.inputs.Image(type="file")
-
-title = "Pneumonia Detection"
-description = "This application uses a Convolutional Neural Network (CNN) model to predict whether a chosen X-ray shows if " \
- "the person has pneumonia disease or not. To check the model prediction, here are the true labels of the " \
- "provided examples below: the first 4 images belong to normal whereas the last 4 images are of pneumonia " \
- "category. More specifically, the 5th and 6th images are viral pneumonia infection in nature whereas " \
- "the last 2 images are bacterial infection in nature."
-
-gr.Interface(fn=make_prediction,
- inputs=image_input,
- outputs="label",
- examples=[["image1_normal.jpeg"],
- ["image2_normal.jpeg"],
- ["image3_normal.jpeg"],
- ["image4_normal.jpeg"],
- ["image1_pneumonia_virus.jpeg"],
- ["image2_pneumonia_virus.jpeg"],
- ["image1_pneumonia_bacteria.jpeg"],
- ["image2_pneumonia_bacteria.jpeg"]],
- title=title,
- description=description,
- article="http://raw.githubusercontent.com/baishalidutta/Pneumonia-Detection/gradio/README.md") \
- .launch(share=True)
diff --git a/spaces/Benson/text-generation/Examples/Descargar Clave De Licencia Para Fifa 19.md b/spaces/Benson/text-generation/Examples/Descargar Clave De Licencia Para Fifa 19.md
deleted file mode 100644
index 07fd185daf80367ba6ff032be8917521d521e7bb..0000000000000000000000000000000000000000
--- a/spaces/Benson/text-generation/Examples/Descargar Clave De Licencia Para Fifa 19.md
+++ /dev/null
@@ -1,81 +0,0 @@
-
-
Cómo descargar la clave de licencia para FIFA 19
-
FIFA 19 es uno de los videojuegos de fútbol más populares del mundo, desarrollado por EA Sports y lanzado en 2018. Cuenta con la prestigiosa Liga de Campeones de la UEFA, un modo de arranque renovado y una variedad de nuevas características de juego. Si quieres jugar a FIFA 19 en tu PC, necesitarás una clave de licencia para activarlo. Una clave de licencia es un código de 25 caracteres que verifica que su copia de FIFA 19 es original y no se ha utilizado en más dispositivos que los Términos de licencia de software de Microsoft permiten. En este artículo, te mostraremos cómo descargar una clave de licencia para FIFA 19 de diferentes fuentes y cómo activarla en tu PC.
¿Qué es FIFA 19 y por qué necesitas una clave de licencia?
-
Características y jugabilidad de FIFA 19
-
FIFA 19 es la 26ª entrega de la serie FIFA, y la primera en incluir la UEFA Champions League, la UEFA Europa League y la Supercopa de la UEFA. Puedes jugar como tus equipos favoritos y jugadores de todo el mundo, y competir en varios modos como el Modo Carrera, Ultimate Team, The Journey y más. También puedes disfrutar de las nuevas características de juego como el Active Touch System, Dynamic Tactics, 50/50 Battles, Timed Finishing y más. FIFA 19 también tiene gráficos impresionantes, animaciones realistas, bandas sonoras inmersivas y comentarios auténticos.
-
Requisitos del sistema FIFA 19 y compatibilidad
-
Para ejecutar FIFA 19 en su PC, necesitará cumplir con los requisitos mínimos o recomendados del sistema. Aquí están las especificaciones que necesita saber:
-
-
Mínimo
Recomendado
-
OS: Windows 7/8.1/10 - 64-Bit
OS: Windows 10 - 64-Bit
-
CPU: Core i3-2100 @ 3.1GHz o AMD Phenom II X4 965 @ 3.4 GHz
CPU: Intel i3 6300T o equivalente
-
RAM: 8 GB
RAM: 8 GB
-
-
DISCO DURO: Al menos 50 GB de espacio libre
DISCO DURO: Al menos 50 GB de espacio libre
-
VIDEO: NVIDIA GTX 460 1GB o AMD Radeon R7 260
VIDEO: NVIDIA GeForce GTX 670 o AMD Radeon R9 270X
-
DirectX: DirectX 11 compatible (7 necesarios para DirectX 11)
DirectX: DirectX 12 compatible
-
ENTRADA: Teclado y ratón, controlador analógico dual
ENTRADA: Teclado y ratón, controlador analógico dual
-
REQUISITOS DE CONEXIÓN ONLINE: Se requiere conexión a Internet para instalar y jugar.
REQUISITOS DE CONEXIÓN ONLINE: Se requiere conexión a Internet para instalar y jugar.
Métodos de activación FIFA 19 y clave de producto
-
Para jugar FIFA 19 en tu PC, tendrás que activarlo con una clave de producto válida. Una clave de producto es un código de 25 caracteres que se parece a esto: XXXXX-XXXXX-XXXXX-XXXXX-XXXXX. Puedes encontrar tu clave de producto de diferentes maneras dependiendo de cómo compraste FIFA 19. Hay tres métodos principales de activación para FIFA 19: activación en línea, activación telefónica y activación fuera de línea. La activación en línea es la forma más fácil y común de activar FIFA 19. Solo tienes que introducir tu clave de producto cuando se te solicite durante la instalación o el lanzamiento del juego, y luego iniciar sesión con tu cuenta de EA. La activación del teléfono es una forma alternativa de activar FIFA 19 si tiene problemas con la activación en línea. Solo tienes que llamar al número gratuito proporcionado por EA y seguir las instrucciones para introducir la clave del producto y obtener un código de confirmación. La activación sin conexión es una forma de último recurso para activar FIFA 19 si no tiene conexión a Internet o acceso telefónico. Solo tienes que ponerte en contacto con el servicio de atención al cliente de EA y proporcionarles la clave de tu producto y alguna información sobre tu PC. A continuación, le dará un archivo de activación sin conexión que puede utilizar para activar FIFA 19 en su PC.
-
-
Cómo obtener una clave de licencia para FIFA 19 de un distribuidor autorizado
-
-
Una de las maneras más fáciles de obtener una clave de licencia para FIFA 19 es comprar una copia física del juego de un minorista autorizado como Amazon, Walmart, Best Buy, GameStop, etc. Cuando usted compra una copia física de FIFA 19, obtendrá un disco DVD que contiene los archivos del juego y un inserto de papel que tiene su clave de producto impresa en él. Solo tiene que insertar el disco en la unidad de DVD de su PC y siga las instrucciones de instalación. A continuación, puede introducir su clave de producto cuando se le solicite y activar FIFA 19 en línea, por teléfono o fuera de línea.
-
Comprar una copia digital de FIFA 19 en una tienda online
-
Otra forma de obtener una clave de licencia para FIFA 19 es comprar una copia digital del juego en una tienda en línea como Origin, Steam, GOG, Humble Bundle, etc. Cuando compras una copia digital de FIFA 19, recibirá una confirmación por correo electrónico que contiene su clave de producto y un enlace para descargar los archivos del juego. Solo tienes que hacer clic en el enlace y descargar los archivos del juego a su PC. A continuación, puede introducir su clave de producto cuando se le solicite y activar FIFA 19 en línea, por teléfono o fuera de línea.
-
Comprar una copia digital de FIFA 19 desde la aplicación Microsoft Store
-
Una tercera manera de obtener una clave de licencia para FIFA 19 es comprar una copia digital del juego desde la aplicación Microsoft Store en su PC con Windows 10. Cuando compres una copia digital de FIFA 19 desde la aplicación de Microsoft Store, no obtendrás una clave de producto ni una confirmación por correo electrónico. En su lugar, obtendrá una licencia digital que está vinculada a su cuenta de Microsoft y su PC. Solo necesitas descargar e instalar el juego desde la aplicación de Microsoft Store e iniciar sesión con tu cuenta de Microsoft. A continuación, puede jugar FIFA 19 sin introducir ninguna clave de producto o activarlo.
Cómo obtener una clave de licencia para FIFA 19 de otras fuentes
-
Usando un software de búsqueda de claves
-
-
Usando un generador de llaves o herramienta de crack
-
Si no has comprado FIFA 19 en un minorista autorizado o en una tienda online, puedes intentar usar un generador de claves o una herramienta para obtener una clave de licencia para FIFA 19. Un generador de claves o herramienta crack es un programa que genera claves de producto aleatorias o evita el proceso de activación del software. Algunas de las herramientas más populares para FIFA 19 son FIFA 19 Key Generator, FIFA 19 Crack, FIFA 19 Serial Key, etc. Solo necesitas descargar y ejecutar uno de estos programas y obtener una clave de producto o un archivo crack para FIFA 19. A continuación, puede introducir la clave del producto cuando se le solicite o reemplazar el archivo de juego original con el archivo de crack y activar FIFA 19 en línea, por teléfono o sin conexión.
-
Usando una actualización gratuita o una oferta de prueba
-
Si ya ha comprado una versión anterior de FIFA como FIFA 18 o FIFA 17, puede intentar utilizar una actualización gratuita o una oferta de prueba para obtener una clave de licencia para FIFA 19. Una actualización gratuita o una oferta de prueba es una promoción que le permite actualizar o probar la última versión del software de forma gratuita o a un precio reducido. Algunas de las ofertas gratuitas de actualización o prueba para FIFA 19 son EA Play, Origin Access, EA Access, etc. Solo tienes que registrarte en uno de estos servicios y descargar FIFA 19 de su biblioteca. A continuación, puede jugar FIFA 19 sin introducir ninguna clave de producto o activarlo.
-
Cómo activar FIFA 19 con su clave de licencia
-
Introducir la clave del producto durante la instalación o el lanzamiento
-
La forma más común de activar FIFA 19 con tu clave de licencia es introducirla durante la instalación o el lanzamiento del juego. Solo tienes que seguir estos pasos:
-
-
Inserte el disco DVD en la unidad de DVD de su PC o descargue los archivos del juego desde el enlace proporcionado por la tienda en línea.
-
Ejecute el archivo setup.exe y siga las instrucciones de instalación.
-
Cuando se le solicite, introduzca su clave de producto en el cuadro y haga clic en Next.
-
-
Espere a que el juego se instale y se inicie.
-
Disfruta jugando FIFA 19 en tu PC.
-
-
Activar su licencia digital online o offline
-
Si ha comprado una copia digital de FIFA 19 desde la aplicación Microsoft Store o ha utilizado una actualización gratuita o una oferta de prueba, no tendrá que introducir ninguna clave de producto para activarla. En su lugar, tendrá una licencia digital vinculada a su cuenta de Microsoft y su PC. Solo tiene que seguir estos pasos:
-
-
Descargar e instalar FIFA 19 desde la aplicación de Microsoft Store o el servicio para el que te registraste.
-
Inicia sesión con tu cuenta de Microsoft que usaste para comprar o descargar FIFA 19.
-
Si tiene una conexión a Internet, su licencia digital se activará automáticamente.
-
Si no tiene una conexión a Internet, puede activar su licencia digital sin conexión mediante el Solucionador de problemas de activación. Para ello, vaya a Configuración > Actualización y seguridad > Activación > Solución de problemas y siga las instrucciones.
-
Disfruta jugando FIFA 19 en tu PC.
-
-
Solución de problemas y errores de activación comunes
-
A veces, puede encontrar algunos errores o problemas al intentar activar FIFA 19 con su clave de licencia. Estos son algunos de los más comunes y cómo solucionarlos:
-
-
Si recibe un mensaje de error que dice "Esta clave de producto ya se ha utilizado en otro dispositivo", significa que ha superado el número de dispositivos que puede activar con su clave de producto. Para solucionar esto, debes desactivar uno de tus dispositivos anteriores iniciando sesión con tu cuenta de EA y yendo a Mi cuenta > Configuración de privacidad > Seguridad > Desactivar dispositivos.
-
Si recibe un mensaje de error que dice "Esta clave de producto es inválida o incorrecta", significa que ha introducido una clave de producto incorrecta o ha cometido un error tipográfico. Para solucionar esto, debe revisar su clave de producto nuevamente y asegurarse de que la ingrese correctamente y sin espacios.
-
-
Si recibe un mensaje de error que dice "No se puede activar FIFA 19 en este momento", significa que hay un problema con los servidores de EA o su conexión a Internet. Para solucionar esto, debe esperar un tiempo e intentarlo de nuevo más tarde, o verificar su conexión a Internet y asegurarse de que es estable y seguro.
-
Si recibes un mensaje de error que dice "Límite de activación alcanzado para FIFA 19", significa que has alcanzado el número máximo de veces que puedes activar FIFA 19 con tu clave de producto. Para solucionarlo, debes ponerte en contacto con el servicio de atención al cliente de EA y solicitar un restablecimiento de tu límite de activación.
-
-
Conclusión y preguntas frecuentes
-
En conclusión, FIFA 19 es un gran videojuego de fútbol que puedes jugar en tu PC con una clave de licencia. Puede obtener una clave de licencia para FIFA 19 de varias fuentes, como comprar una copia física o digital del juego en un minorista autorizado o una tienda en línea, usar un software de búsqueda de claves, usar un generador de claves o una herramienta de crack, o usar una actualización gratuita o una oferta de prueba. También puede activar FIFA 19 con su clave de licencia en línea, por teléfono o fuera de línea, dependiendo de su situación. Sin embargo, también puede encontrar algunos errores o problemas al intentar activar FIFA 19 con su clave de licencia, por lo que debe ser consciente de las posibles soluciones y consejos de solución de problemas. Esperamos que este artículo te haya ayudado a aprender cómo descargar una clave de licencia para FIFA 19 y disfrutar jugando en tu PC.
-
Aquí hay algunas preguntas frecuentes que puede tener sobre la descarga de una clave de licencia para FIFA 19:
-
-
Q: ¿Puedo usar la misma clave de producto para FIFA 19 en más de un PC?
-
A: No, solo puedes usar la misma clave de producto para FIFA 19 en un PC a la vez. Si quieres jugar a FIFA 19 en otro PC, tendrás que desactivar el primer PC y activar el segundo PC con la misma clave de producto.
-
Q: ¿Puedo compartir mi clave de producto para FIFA 19 con otra persona?
-
-
Q: ¿Puedo obtener un reembolso por mi clave de producto para FIFA 19 si no me gusta el juego?
-
A: Depende de dónde compraste tu clave de producto para FIFA 19 y cuál es su política de reembolso. Algunos minoristas o tiendas en línea pueden ofrecer un reembolso por su clave de producto para FIFA 19 dentro de un cierto período de tiempo y bajo ciertas condiciones. Tendrá que ponerse en contacto con ellos y solicitar su política de reembolso y proceso.
-
Q: ¿Puedo jugar FIFA 19 sin una clave de producto o activación?
-
A: No, no puedes jugar a FIFA 19 sin una clave de producto o activación. Necesitarás una clave de producto válida y una activación para jugar a FIFA 19 en tu PC. Si intentas jugar a FIFA 19 sin una clave de producto o activación, recibirás un mensaje de error y el juego no se iniciará.
-
Q: ¿Puedo jugar FIFA 19 sin conexión después de activarlo con mi clave de producto?
-
A: Sí, puedes jugar FIFA 19 sin conexión después de activarlo con tu clave de producto. Sin embargo, algunas características del juego pueden no estar disponibles sin conexión, como modos multijugador en línea, actualizaciones en línea, recompensas en línea, etc. También tendrá que conectarse a Internet al menos una vez cada 30 días para verificar su estado de activación.
-
64aa2da5cf
-
-
\ No newline at end of file
diff --git a/spaces/Big-Web/MMSD/env/Lib/site-packages/setuptools/_vendor/importlib_resources/simple.py b/spaces/Big-Web/MMSD/env/Lib/site-packages/setuptools/_vendor/importlib_resources/simple.py
deleted file mode 100644
index da073cbdb11e6c24c19a2d388c53c8842228595f..0000000000000000000000000000000000000000
--- a/spaces/Big-Web/MMSD/env/Lib/site-packages/setuptools/_vendor/importlib_resources/simple.py
+++ /dev/null
@@ -1,116 +0,0 @@
-"""
-Interface adapters for low-level readers.
-"""
-
-import abc
-import io
-import itertools
-from typing import BinaryIO, List
-
-from .abc import Traversable, TraversableResources
-
-
-class SimpleReader(abc.ABC):
- """
- The minimum, low-level interface required from a resource
- provider.
- """
-
- @abc.abstractproperty
- def package(self):
- # type: () -> str
- """
- The name of the package for which this reader loads resources.
- """
-
- @abc.abstractmethod
- def children(self):
- # type: () -> List['SimpleReader']
- """
- Obtain an iterable of SimpleReader for available
- child containers (e.g. directories).
- """
-
- @abc.abstractmethod
- def resources(self):
- # type: () -> List[str]
- """
- Obtain available named resources for this virtual package.
- """
-
- @abc.abstractmethod
- def open_binary(self, resource):
- # type: (str) -> BinaryIO
- """
- Obtain a File-like for a named resource.
- """
-
- @property
- def name(self):
- return self.package.split('.')[-1]
-
-
-class ResourceHandle(Traversable):
- """
- Handle to a named resource in a ResourceReader.
- """
-
- def __init__(self, parent, name):
- # type: (ResourceContainer, str) -> None
- self.parent = parent
- self.name = name # type: ignore
-
- def is_file(self):
- return True
-
- def is_dir(self):
- return False
-
- def open(self, mode='r', *args, **kwargs):
- stream = self.parent.reader.open_binary(self.name)
- if 'b' not in mode:
- stream = io.TextIOWrapper(*args, **kwargs)
- return stream
-
- def joinpath(self, name):
- raise RuntimeError("Cannot traverse into a resource")
-
-
-class ResourceContainer(Traversable):
- """
- Traversable container for a package's resources via its reader.
- """
-
- def __init__(self, reader):
- # type: (SimpleReader) -> None
- self.reader = reader
-
- def is_dir(self):
- return True
-
- def is_file(self):
- return False
-
- def iterdir(self):
- files = (ResourceHandle(self, name) for name in self.reader.resources)
- dirs = map(ResourceContainer, self.reader.children())
- return itertools.chain(files, dirs)
-
- def open(self, *args, **kwargs):
- raise IsADirectoryError()
-
- def joinpath(self, name):
- return next(
- traversable for traversable in self.iterdir() if traversable.name == name
- )
-
-
-class TraversableReader(TraversableResources, SimpleReader):
- """
- A TraversableResources based on SimpleReader. Resource providers
- may derive from this class to provide the TraversableResources
- interface by supplying the SimpleReader interface.
- """
-
- def files(self):
- return ResourceContainer(self)
diff --git a/spaces/CVPR/LIVE/pybind11/tests/test_callbacks.py b/spaces/CVPR/LIVE/pybind11/tests/test_callbacks.py
deleted file mode 100644
index d5d0e045d224aab7381549bdcfb1d2102cdd0eb7..0000000000000000000000000000000000000000
--- a/spaces/CVPR/LIVE/pybind11/tests/test_callbacks.py
+++ /dev/null
@@ -1,137 +0,0 @@
-# -*- coding: utf-8 -*-
-import pytest
-from pybind11_tests import callbacks as m
-from threading import Thread
-
-
-def test_callbacks():
- from functools import partial
-
- def func1():
- return "func1"
-
- def func2(a, b, c, d):
- return "func2", a, b, c, d
-
- def func3(a):
- return "func3({})".format(a)
-
- assert m.test_callback1(func1) == "func1"
- assert m.test_callback2(func2) == ("func2", "Hello", "x", True, 5)
- assert m.test_callback1(partial(func2, 1, 2, 3, 4)) == ("func2", 1, 2, 3, 4)
- assert m.test_callback1(partial(func3, "partial")) == "func3(partial)"
- assert m.test_callback3(lambda i: i + 1) == "func(43) = 44"
-
- f = m.test_callback4()
- assert f(43) == 44
- f = m.test_callback5()
- assert f(number=43) == 44
-
-
-def test_bound_method_callback():
- # Bound Python method:
- class MyClass:
- def double(self, val):
- return 2 * val
-
- z = MyClass()
- assert m.test_callback3(z.double) == "func(43) = 86"
-
- z = m.CppBoundMethodTest()
- assert m.test_callback3(z.triple) == "func(43) = 129"
-
-
-def test_keyword_args_and_generalized_unpacking():
-
- def f(*args, **kwargs):
- return args, kwargs
-
- assert m.test_tuple_unpacking(f) == (("positional", 1, 2, 3, 4, 5, 6), {})
- assert m.test_dict_unpacking(f) == (("positional", 1), {"key": "value", "a": 1, "b": 2})
- assert m.test_keyword_args(f) == ((), {"x": 10, "y": 20})
- assert m.test_unpacking_and_keywords1(f) == ((1, 2), {"c": 3, "d": 4})
- assert m.test_unpacking_and_keywords2(f) == (
- ("positional", 1, 2, 3, 4, 5),
- {"key": "value", "a": 1, "b": 2, "c": 3, "d": 4, "e": 5}
- )
-
- with pytest.raises(TypeError) as excinfo:
- m.test_unpacking_error1(f)
- assert "Got multiple values for keyword argument" in str(excinfo.value)
-
- with pytest.raises(TypeError) as excinfo:
- m.test_unpacking_error2(f)
- assert "Got multiple values for keyword argument" in str(excinfo.value)
-
- with pytest.raises(RuntimeError) as excinfo:
- m.test_arg_conversion_error1(f)
- assert "Unable to convert call argument" in str(excinfo.value)
-
- with pytest.raises(RuntimeError) as excinfo:
- m.test_arg_conversion_error2(f)
- assert "Unable to convert call argument" in str(excinfo.value)
-
-
-def test_lambda_closure_cleanup():
- m.test_cleanup()
- cstats = m.payload_cstats()
- assert cstats.alive() == 0
- assert cstats.copy_constructions == 1
- assert cstats.move_constructions >= 1
-
-
-def test_cpp_function_roundtrip():
- """Test if passing a function pointer from C++ -> Python -> C++ yields the original pointer"""
-
- assert m.test_dummy_function(m.dummy_function) == "matches dummy_function: eval(1) = 2"
- assert (m.test_dummy_function(m.roundtrip(m.dummy_function)) ==
- "matches dummy_function: eval(1) = 2")
- assert m.roundtrip(None, expect_none=True) is None
- assert (m.test_dummy_function(lambda x: x + 2) ==
- "can't convert to function pointer: eval(1) = 3")
-
- with pytest.raises(TypeError) as excinfo:
- m.test_dummy_function(m.dummy_function2)
- assert "incompatible function arguments" in str(excinfo.value)
-
- with pytest.raises(TypeError) as excinfo:
- m.test_dummy_function(lambda x, y: x + y)
- assert any(s in str(excinfo.value) for s in ("missing 1 required positional argument",
- "takes exactly 2 arguments"))
-
-
-def test_function_signatures(doc):
- assert doc(m.test_callback3) == "test_callback3(arg0: Callable[[int], int]) -> str"
- assert doc(m.test_callback4) == "test_callback4() -> Callable[[int], int]"
-
-
-def test_movable_object():
- assert m.callback_with_movable(lambda _: None) is True
-
-
-def test_async_callbacks():
- # serves as state for async callback
- class Item:
- def __init__(self, value):
- self.value = value
-
- res = []
-
- # generate stateful lambda that will store result in `res`
- def gen_f():
- s = Item(3)
- return lambda j: res.append(s.value + j)
-
- # do some work async
- work = [1, 2, 3, 4]
- m.test_async_callback(gen_f(), work)
- # wait until work is done
- from time import sleep
- sleep(0.5)
- assert sum(res) == sum([x + 3 for x in work])
-
-
-def test_async_async_callbacks():
- t = Thread(target=test_async_callbacks)
- t.start()
- t.join()
diff --git a/spaces/CVPR/LIVE/thrust/thrust/system/tbb/detail/copy_if.h b/spaces/CVPR/LIVE/thrust/thrust/system/tbb/detail/copy_if.h
deleted file mode 100644
index 0420893ba642d3afa0f8370d0ac060290b636edd..0000000000000000000000000000000000000000
--- a/spaces/CVPR/LIVE/thrust/thrust/system/tbb/detail/copy_if.h
+++ /dev/null
@@ -1,50 +0,0 @@
-/*
- * Copyright 2008-2013 NVIDIA Corporation
- *
- * Licensed under the Apache License, Version 2.0 (the "License");
- * you may not use this file except in compliance with the License.
- * You may obtain a copy of the License at
- *
- * http://www.apache.org/licenses/LICENSE-2.0
- *
- * Unless required by applicable law or agreed to in writing, software
- * distributed under the License is distributed on an "AS IS" BASIS,
- * WITHOUT WARRANTIES OR CONDITIONS OF ANY KIND, either express or implied.
- * See the License for the specific language governing permissions and
- * limitations under the License.
- */
-
-#pragma once
-
-#include
-#include
-
-namespace thrust
-{
-namespace system
-{
-namespace tbb
-{
-namespace detail
-{
-
-
-template
- OutputIterator copy_if(tag,
- InputIterator1 first,
- InputIterator1 last,
- InputIterator2 stencil,
- OutputIterator result,
- Predicate pred);
-
-
-} // end detail
-} // end tbb
-} // end system
-} // end thrust
-
-#include
-
diff --git a/spaces/CVPR/lama-example/saicinpainting/evaluation/losses/fid/fid_score.py b/spaces/CVPR/lama-example/saicinpainting/evaluation/losses/fid/fid_score.py
deleted file mode 100644
index 6ca8e602c21bb6a624d646da3f6479aea033b0ac..0000000000000000000000000000000000000000
--- a/spaces/CVPR/lama-example/saicinpainting/evaluation/losses/fid/fid_score.py
+++ /dev/null
@@ -1,328 +0,0 @@
-#!/usr/bin/env python3
-"""Calculates the Frechet Inception Distance (FID) to evalulate GANs
-
-The FID metric calculates the distance between two distributions of images.
-Typically, we have summary statistics (mean & covariance matrix) of one
-of these distributions, while the 2nd distribution is given by a GAN.
-
-When run as a stand-alone program, it compares the distribution of
-images that are stored as PNG/JPEG at a specified location with a
-distribution given by summary statistics (in pickle format).
-
-The FID is calculated by assuming that X_1 and X_2 are the activations of
-the pool_3 layer of the inception net for generated samples and real world
-samples respectively.
-
-See --help to see further details.
-
-Code apapted from https://github.com/bioinf-jku/TTUR to use PyTorch instead
-of Tensorflow
-
-Copyright 2018 Institute of Bioinformatics, JKU Linz
-
-Licensed under the Apache License, Version 2.0 (the "License");
-you may not use this file except in compliance with the License.
-You may obtain a copy of the License at
-
- http://www.apache.org/licenses/LICENSE-2.0
-
-Unless required by applicable law or agreed to in writing, software
-distributed under the License is distributed on an "AS IS" BASIS,
-WITHOUT WARRANTIES OR CONDITIONS OF ANY KIND, either express or implied.
-See the License for the specific language governing permissions and
-limitations under the License.
-"""
-import os
-import pathlib
-from argparse import ArgumentDefaultsHelpFormatter, ArgumentParser
-
-import numpy as np
-import torch
-# from scipy.misc import imread
-from imageio import imread
-from PIL import Image, JpegImagePlugin
-from scipy import linalg
-from torch.nn.functional import adaptive_avg_pool2d
-from torchvision.transforms import CenterCrop, Compose, Resize, ToTensor
-
-try:
- from tqdm import tqdm
-except ImportError:
- # If not tqdm is not available, provide a mock version of it
- def tqdm(x): return x
-
-try:
- from .inception import InceptionV3
-except ModuleNotFoundError:
- from inception import InceptionV3
-
-parser = ArgumentParser(formatter_class=ArgumentDefaultsHelpFormatter)
-parser.add_argument('path', type=str, nargs=2,
- help=('Path to the generated images or '
- 'to .npz statistic files'))
-parser.add_argument('--batch-size', type=int, default=50,
- help='Batch size to use')
-parser.add_argument('--dims', type=int, default=2048,
- choices=list(InceptionV3.BLOCK_INDEX_BY_DIM),
- help=('Dimensionality of Inception features to use. '
- 'By default, uses pool3 features'))
-parser.add_argument('-c', '--gpu', default='', type=str,
- help='GPU to use (leave blank for CPU only)')
-parser.add_argument('--resize', default=256)
-
-transform = Compose([Resize(256), CenterCrop(256), ToTensor()])
-
-
-def get_activations(files, model, batch_size=50, dims=2048,
- cuda=False, verbose=False, keep_size=False):
- """Calculates the activations of the pool_3 layer for all images.
-
- Params:
- -- files : List of image files paths
- -- model : Instance of inception model
- -- batch_size : Batch size of images for the model to process at once.
- Make sure that the number of samples is a multiple of
- the batch size, otherwise some samples are ignored. This
- behavior is retained to match the original FID score
- implementation.
- -- dims : Dimensionality of features returned by Inception
- -- cuda : If set to True, use GPU
- -- verbose : If set to True and parameter out_step is given, the number
- of calculated batches is reported.
- Returns:
- -- A numpy array of dimension (num images, dims) that contains the
- activations of the given tensor when feeding inception with the
- query tensor.
- """
- model.eval()
-
- if len(files) % batch_size != 0:
- print(('Warning: number of images is not a multiple of the '
- 'batch size. Some samples are going to be ignored.'))
- if batch_size > len(files):
- print(('Warning: batch size is bigger than the data size. '
- 'Setting batch size to data size'))
- batch_size = len(files)
-
- n_batches = len(files) // batch_size
- n_used_imgs = n_batches * batch_size
-
- pred_arr = np.empty((n_used_imgs, dims))
-
- for i in tqdm(range(n_batches)):
- if verbose:
- print('\rPropagating batch %d/%d' % (i + 1, n_batches),
- end='', flush=True)
- start = i * batch_size
- end = start + batch_size
-
- # # Official code goes below
- # images = np.array([imread(str(f)).astype(np.float32)
- # for f in files[start:end]])
-
- # # Reshape to (n_images, 3, height, width)
- # images = images.transpose((0, 3, 1, 2))
- # images /= 255
- # batch = torch.from_numpy(images).type(torch.FloatTensor)
- # #
-
- t = transform if not keep_size else ToTensor()
-
- if isinstance(files[0], pathlib.PosixPath):
- images = [t(Image.open(str(f))) for f in files[start:end]]
-
- elif isinstance(files[0], Image.Image):
- images = [t(f) for f in files[start:end]]
-
- else:
- raise ValueError(f"Unknown data type for image: {type(files[0])}")
-
- batch = torch.stack(images)
-
- if cuda:
- batch = batch.cuda()
-
- pred = model(batch)[0]
-
- # If model output is not scalar, apply global spatial average pooling.
- # This happens if you choose a dimensionality not equal 2048.
- if pred.shape[2] != 1 or pred.shape[3] != 1:
- pred = adaptive_avg_pool2d(pred, output_size=(1, 1))
-
- pred_arr[start:end] = pred.cpu().data.numpy().reshape(batch_size, -1)
-
- if verbose:
- print(' done')
-
- return pred_arr
-
-
-def calculate_frechet_distance(mu1, sigma1, mu2, sigma2, eps=1e-6):
- """Numpy implementation of the Frechet Distance.
- The Frechet distance between two multivariate Gaussians X_1 ~ N(mu_1, C_1)
- and X_2 ~ N(mu_2, C_2) is
- d^2 = ||mu_1 - mu_2||^2 + Tr(C_1 + C_2 - 2*sqrt(C_1*C_2)).
-
- Stable version by Dougal J. Sutherland.
-
- Params:
- -- mu1 : Numpy array containing the activations of a layer of the
- inception net (like returned by the function 'get_predictions')
- for generated samples.
- -- mu2 : The sample mean over activations, precalculated on an
- representative data set.
- -- sigma1: The covariance matrix over activations for generated samples.
- -- sigma2: The covariance matrix over activations, precalculated on an
- representative data set.
-
- Returns:
- -- : The Frechet Distance.
- """
-
- mu1 = np.atleast_1d(mu1)
- mu2 = np.atleast_1d(mu2)
-
- sigma1 = np.atleast_2d(sigma1)
- sigma2 = np.atleast_2d(sigma2)
-
- assert mu1.shape == mu2.shape, \
- 'Training and test mean vectors have different lengths'
- assert sigma1.shape == sigma2.shape, \
- 'Training and test covariances have different dimensions'
-
- diff = mu1 - mu2
-
- # Product might be almost singular
- covmean, _ = linalg.sqrtm(sigma1.dot(sigma2), disp=False)
- if not np.isfinite(covmean).all():
- msg = ('fid calculation produces singular product; '
- 'adding %s to diagonal of cov estimates') % eps
- print(msg)
- offset = np.eye(sigma1.shape[0]) * eps
- covmean = linalg.sqrtm((sigma1 + offset).dot(sigma2 + offset))
-
- # Numerical error might give slight imaginary component
- if np.iscomplexobj(covmean):
- # if not np.allclose(np.diagonal(covmean).imag, 0, atol=1e-3):
- if not np.allclose(np.diagonal(covmean).imag, 0, atol=1e-2):
- m = np.max(np.abs(covmean.imag))
- raise ValueError('Imaginary component {}'.format(m))
- covmean = covmean.real
-
- tr_covmean = np.trace(covmean)
-
- return (diff.dot(diff) + np.trace(sigma1) +
- np.trace(sigma2) - 2 * tr_covmean)
-
-
-def calculate_activation_statistics(files, model, batch_size=50,
- dims=2048, cuda=False, verbose=False, keep_size=False):
- """Calculation of the statistics used by the FID.
- Params:
- -- files : List of image files paths
- -- model : Instance of inception model
- -- batch_size : The images numpy array is split into batches with
- batch size batch_size. A reasonable batch size
- depends on the hardware.
- -- dims : Dimensionality of features returned by Inception
- -- cuda : If set to True, use GPU
- -- verbose : If set to True and parameter out_step is given, the
- number of calculated batches is reported.
- Returns:
- -- mu : The mean over samples of the activations of the pool_3 layer of
- the inception model.
- -- sigma : The covariance matrix of the activations of the pool_3 layer of
- the inception model.
- """
- act = get_activations(files, model, batch_size, dims, cuda, verbose, keep_size=keep_size)
- mu = np.mean(act, axis=0)
- sigma = np.cov(act, rowvar=False)
- return mu, sigma
-
-
-def _compute_statistics_of_path(path, model, batch_size, dims, cuda):
- if path.endswith('.npz'):
- f = np.load(path)
- m, s = f['mu'][:], f['sigma'][:]
- f.close()
- else:
- path = pathlib.Path(path)
- files = list(path.glob('*.jpg')) + list(path.glob('*.png'))
- m, s = calculate_activation_statistics(files, model, batch_size,
- dims, cuda)
-
- return m, s
-
-
-def _compute_statistics_of_images(images, model, batch_size, dims, cuda, keep_size=False):
- if isinstance(images, list): # exact paths to files are provided
- m, s = calculate_activation_statistics(images, model, batch_size,
- dims, cuda, keep_size=keep_size)
-
- return m, s
-
- else:
- raise ValueError
-
-
-def calculate_fid_given_paths(paths, batch_size, cuda, dims):
- """Calculates the FID of two paths"""
- for p in paths:
- if not os.path.exists(p):
- raise RuntimeError('Invalid path: %s' % p)
-
- block_idx = InceptionV3.BLOCK_INDEX_BY_DIM[dims]
-
- model = InceptionV3([block_idx])
- if cuda:
- model.cuda()
-
- m1, s1 = _compute_statistics_of_path(paths[0], model, batch_size,
- dims, cuda)
- m2, s2 = _compute_statistics_of_path(paths[1], model, batch_size,
- dims, cuda)
- fid_value = calculate_frechet_distance(m1, s1, m2, s2)
-
- return fid_value
-
-
-def calculate_fid_given_images(images, batch_size, cuda, dims, use_globals=False, keep_size=False):
- if use_globals:
- global FID_MODEL # for multiprocessing
-
- for imgs in images:
- if isinstance(imgs, list) and isinstance(imgs[0], (Image.Image, JpegImagePlugin.JpegImageFile)):
- pass
- else:
- raise RuntimeError('Invalid images')
-
- block_idx = InceptionV3.BLOCK_INDEX_BY_DIM[dims]
-
- if 'FID_MODEL' not in globals() or not use_globals:
- model = InceptionV3([block_idx])
- if cuda:
- model.cuda()
-
- if use_globals:
- FID_MODEL = model
-
- else:
- model = FID_MODEL
-
- m1, s1 = _compute_statistics_of_images(images[0], model, batch_size,
- dims, cuda, keep_size=False)
- m2, s2 = _compute_statistics_of_images(images[1], model, batch_size,
- dims, cuda, keep_size=False)
- fid_value = calculate_frechet_distance(m1, s1, m2, s2)
- return fid_value
-
-
-if __name__ == '__main__':
- args = parser.parse_args()
- os.environ['CUDA_VISIBLE_DEVICES'] = args.gpu
-
- fid_value = calculate_fid_given_paths(args.path,
- args.batch_size,
- args.gpu != '',
- args.dims)
- print('FID: ', fid_value)
diff --git a/spaces/CVPR/monoscene_lite/monoscene/.ipynb_checkpoints/unet3d_nyu-checkpoint.py b/spaces/CVPR/monoscene_lite/monoscene/.ipynb_checkpoints/unet3d_nyu-checkpoint.py
deleted file mode 100644
index e9e3b3718999248efa1b2925658465ba59801b13..0000000000000000000000000000000000000000
--- a/spaces/CVPR/monoscene_lite/monoscene/.ipynb_checkpoints/unet3d_nyu-checkpoint.py
+++ /dev/null
@@ -1,90 +0,0 @@
-# encoding: utf-8
-import torch
-import torch.nn as nn
-import torch.nn.functional as F
-import numpy as np
-from monoscene.CRP3D import CPMegaVoxels
-from monoscene.modules import (
- Process,
- Upsample,
- Downsample,
- SegmentationHead,
- ASPP,
-)
-
-
-class UNet3D(nn.Module):
- def __init__(
- self,
- class_num,
- norm_layer,
- feature,
- full_scene_size,
- n_relations=4,
- project_res=[],
- context_prior=True,
- bn_momentum=0.1,
- ):
- super(UNet3D, self).__init__()
- self.business_layer = []
- self.project_res = project_res
-
- self.feature_1_4 = feature
- self.feature_1_8 = feature * 2
- self.feature_1_16 = feature * 4
-
- self.feature_1_16_dec = self.feature_1_16
- self.feature_1_8_dec = self.feature_1_8
- self.feature_1_4_dec = self.feature_1_4
-
- self.process_1_4 = nn.Sequential(
- Process(self.feature_1_4, norm_layer, bn_momentum, dilations=[1, 2, 3]),
- Downsample(self.feature_1_4, norm_layer, bn_momentum),
- )
- self.process_1_8 = nn.Sequential(
- Process(self.feature_1_8, norm_layer, bn_momentum, dilations=[1, 2, 3]),
- Downsample(self.feature_1_8, norm_layer, bn_momentum),
- )
- self.up_1_16_1_8 = Upsample(
- self.feature_1_16_dec, self.feature_1_8_dec, norm_layer, bn_momentum
- )
- self.up_1_8_1_4 = Upsample(
- self.feature_1_8_dec, self.feature_1_4_dec, norm_layer, bn_momentum
- )
- self.ssc_head_1_4 = SegmentationHead(
- self.feature_1_4_dec, self.feature_1_4_dec, class_num, [1, 2, 3]
- )
-
- self.context_prior = context_prior
- size_1_16 = tuple(np.ceil(i / 4).astype(int) for i in full_scene_size)
-
- if context_prior:
- self.CP_mega_voxels = CPMegaVoxels(
- self.feature_1_16,
- size_1_16,
- n_relations=n_relations,
- bn_momentum=bn_momentum,
- )
-
- #
- def forward(self, input_dict):
- res = {}
-
- x3d_1_4 = input_dict["x3d"]
- x3d_1_8 = self.process_1_4(x3d_1_4)
- x3d_1_16 = self.process_1_8(x3d_1_8)
-
- if self.context_prior:
- ret = self.CP_mega_voxels(x3d_1_16)
- x3d_1_16 = ret["x"]
- for k in ret.keys():
- res[k] = ret[k]
-
- x3d_up_1_8 = self.up_1_16_1_8(x3d_1_16) + x3d_1_8
- x3d_up_1_4 = self.up_1_8_1_4(x3d_up_1_8) + x3d_1_4
-
- ssc_logit_1_4 = self.ssc_head_1_4(x3d_up_1_4)
-
- res["ssc_logit"] = ssc_logit_1_4
-
- return res
diff --git a/spaces/Chirag1994/Melanoma_Skin_Cancer_Detection_App/model.py b/spaces/Chirag1994/Melanoma_Skin_Cancer_Detection_App/model.py
deleted file mode 100644
index 296bec8eb6794f3c9c4c0a5dda1076f6eb959bc3..0000000000000000000000000000000000000000
--- a/spaces/Chirag1994/Melanoma_Skin_Cancer_Detection_App/model.py
+++ /dev/null
@@ -1,22 +0,0 @@
-import torch
-import torch.nn as nn
-import torch.nn.functional as F
-from efficientnet_pytorch import EfficientNet
-
-
-class Model(nn.Module):
- """
- Creates an efficientnet-b5 model instance.
- """
- def __init__(self, model_name="efficientnet-b5", pool_type=F.adaptive_avg_pool2d):
- super().__init__()
- self.pool_type = pool_type
- self.model_name = model_name
- self.backbone = EfficientNet.from_pretrained(model_name)
- in_features = getattr(self.backbone, "_fc").in_features
- self.classifier = nn.Linear(in_features, 1)
-
- def forward(self, x):
- features = self.pool_type(self.backbone.extract_features(x), 1)
- features = features.view(x.size(0), -1)
- return self.classifier(features)
diff --git a/spaces/Classly/README/README.md b/spaces/Classly/README/README.md
deleted file mode 100644
index 8b5b5b8cf56880bc1011eb024c5e802ae17a89ad..0000000000000000000000000000000000000000
--- a/spaces/Classly/README/README.md
+++ /dev/null
@@ -1,10 +0,0 @@
----
-title: README
-emoji: 📊
-colorFrom: yellow
-colorTo: purple
-sdk: static
-pinned: false
----
-
-Edit this `README.md` markdown file to author your organization card 🔥
diff --git a/spaces/CoWork/dreambooth-training-public/train_dreambooth.py b/spaces/CoWork/dreambooth-training-public/train_dreambooth.py
deleted file mode 100644
index f4ff135e549f0d6c72f733092f3df817cb178e01..0000000000000000000000000000000000000000
--- a/spaces/CoWork/dreambooth-training-public/train_dreambooth.py
+++ /dev/null
@@ -1,889 +0,0 @@
-import argparse
-import itertools
-import math
-import os
-from pathlib import Path
-from typing import Optional
-import subprocess
-import sys
-import gc
-import random
-
-import torch
-import torch.nn.functional as F
-import torch.utils.checkpoint
-from torch.utils.data import Dataset
-
-from accelerate import Accelerator
-from accelerate.logging import get_logger
-from accelerate.utils import set_seed
-from diffusers import AutoencoderKL, DDPMScheduler, StableDiffusionPipeline, UNet2DConditionModel
-from diffusers.utils.import_utils import is_xformers_available
-from diffusers.optimization import get_scheduler
-from huggingface_hub import HfFolder, Repository, whoami
-from PIL import Image
-from torchvision import transforms
-from tqdm.auto import tqdm
-from transformers import CLIPTextModel, CLIPTokenizer
-
-
-logger = get_logger(__name__)
-
-
-def parse_args():
- parser = argparse.ArgumentParser(description="Simple example of a training script.")
- parser.add_argument(
- "--pretrained_model_name_or_path",
- type=str,
- default=None,
- #required=True,
- help="Path to pretrained model or model identifier from huggingface.co/models.",
- )
- parser.add_argument(
- "--tokenizer_name",
- type=str,
- default=None,
- help="Pretrained tokenizer name or path if not the same as model_name",
- )
- parser.add_argument(
- "--instance_data_dir",
- type=str,
- default=None,
- #required=True,
- help="A folder containing the training data of instance images.",
- )
- parser.add_argument(
- "--class_data_dir",
- type=str,
- default=None,
- #required=False,
- help="A folder containing the training data of class images.",
- )
- parser.add_argument(
- "--instance_prompt",
- type=str,
- default=None,
- help="The prompt with identifier specifying the instance",
- )
- parser.add_argument(
- "--class_prompt",
- type=str,
- default="",
- help="The prompt to specify images in the same class as provided instance images.",
- )
- parser.add_argument(
- "--with_prior_preservation",
- default=False,
- action="store_true",
- help="Flag to add prior preservation loss.",
- )
- parser.add_argument("--prior_loss_weight", type=float, default=1.0, help="The weight of prior preservation loss.")
- parser.add_argument(
- "--num_class_images",
- type=int,
- default=100,
- help=(
- "Minimal class images for prior preservation loss. If not have enough images, additional images will be"
- " sampled with class_prompt."
- ),
- )
- parser.add_argument(
- "--output_dir",
- type=str,
- default="",
- help="The output directory where the model predictions and checkpoints will be written.",
- )
- parser.add_argument("--seed", type=int, default=None, help="A seed for reproducible training.")
- parser.add_argument(
- "--resolution",
- type=int,
- default=512,
- help=(
- "The resolution for input images, all the images in the train/validation dataset will be resized to this"
- " resolution"
- ),
- )
- parser.add_argument(
- "--center_crop", action="store_true", help="Whether to center crop images before resizing to resolution"
- )
- parser.add_argument("--train_text_encoder", action="store_true", help="Whether to train the text encoder")
- parser.add_argument(
- "--train_batch_size", type=int, default=4, help="Batch size (per device) for the training dataloader."
- )
- parser.add_argument(
- "--sample_batch_size", type=int, default=4, help="Batch size (per device) for sampling images."
- )
- parser.add_argument("--num_train_epochs", type=int, default=1)
- parser.add_argument(
- "--max_train_steps",
- type=int,
- default=None,
- help="Total number of training steps to perform. If provided, overrides num_train_epochs.",
- )
- parser.add_argument(
- "--gradient_accumulation_steps",
- type=int,
- default=1,
- help="Number of updates steps to accumulate before performing a backward/update pass.",
- )
- parser.add_argument(
- "--gradient_checkpointing",
- action="store_true",
- help="Whether or not to use gradient checkpointing to save memory at the expense of slower backward pass.",
- )
- parser.add_argument(
- "--learning_rate",
- type=float,
- default=5e-6,
- help="Initial learning rate (after the potential warmup period) to use.",
- )
- parser.add_argument(
- "--scale_lr",
- action="store_true",
- default=False,
- help="Scale the learning rate by the number of GPUs, gradient accumulation steps, and batch size.",
- )
- parser.add_argument(
- "--lr_scheduler",
- type=str,
- default="constant",
- help=(
- 'The scheduler type to use. Choose between ["linear", "cosine", "cosine_with_restarts", "polynomial",'
- ' "constant", "constant_with_warmup"]'
- ),
- )
- parser.add_argument(
- "--lr_warmup_steps", type=int, default=500, help="Number of steps for the warmup in the lr scheduler."
- )
- parser.add_argument(
- "--use_8bit_adam", action="store_true", help="Whether or not to use 8-bit Adam from bitsandbytes."
- )
- parser.add_argument("--adam_beta1", type=float, default=0.9, help="The beta1 parameter for the Adam optimizer.")
- parser.add_argument("--adam_beta2", type=float, default=0.999, help="The beta2 parameter for the Adam optimizer.")
- parser.add_argument("--adam_weight_decay", type=float, default=1e-2, help="Weight decay to use.")
- parser.add_argument("--adam_epsilon", type=float, default=1e-08, help="Epsilon value for the Adam optimizer")
- parser.add_argument("--max_grad_norm", default=1.0, type=float, help="Max gradient norm.")
- parser.add_argument("--push_to_hub", action="store_true", help="Whether or not to push the model to the Hub.")
- parser.add_argument("--hub_token", type=str, default=None, help="The token to use to push to the Model Hub.")
- parser.add_argument(
- "--hub_model_id",
- type=str,
- default=None,
- help="The name of the repository to keep in sync with the local `output_dir`.",
- )
- parser.add_argument(
- "--logging_dir",
- type=str,
- default="logs",
- help=(
- "[TensorBoard](https://www.tensorflow.org/tensorboard) log directory. Will default to"
- " *output_dir/runs/**CURRENT_DATETIME_HOSTNAME***."
- ),
- )
- parser.add_argument(
- "--mixed_precision",
- type=str,
- default="no",
- choices=["no", "fp16", "bf16"],
- help=(
- "Whether to use mixed precision. Choose"
- "between fp16 and bf16 (bfloat16). Bf16 requires PyTorch >= 1.10."
- "and an Nvidia Ampere GPU."
- ),
- )
-
- parser.add_argument(
- "--save_n_steps",
- type=int,
- default=1,
- help=("Save the model every n global_steps"),
- )
-
-
- parser.add_argument(
- "--save_starting_step",
- type=int,
- default=1,
- help=("The step from which it starts saving intermediary checkpoints"),
- )
-
- parser.add_argument(
- "--stop_text_encoder_training",
- type=int,
- default=1000000,
- help=("The step at which the text_encoder is no longer trained"),
- )
-
-
- parser.add_argument(
- "--image_captions_filename",
- action="store_true",
- help="Get captions from filename",
- )
-
-
- parser.add_argument(
- "--dump_only_text_encoder",
- action="store_true",
- default=False,
- help="Dump only text encoder",
- )
-
- parser.add_argument(
- "--train_only_unet",
- action="store_true",
- default=False,
- help="Train only the unet",
- )
-
- parser.add_argument(
- "--cache_latents",
- action="store_true",
- default=False,
- help="Train only the unet",
- )
-
- parser.add_argument(
- "--Session_dir",
- type=str,
- default="",
- help="Current session directory",
- )
-
-
-
-
- parser.add_argument("--local_rank", type=int, default=-1, help="For distributed training: local_rank")
-
- args = parser.parse_args()
- env_local_rank = int(os.environ.get("LOCAL_RANK", -1))
- if env_local_rank != -1 and env_local_rank != args.local_rank:
- args.local_rank = env_local_rank
-
- #if args.instance_data_dir is None:
- # raise ValueError("You must specify a train data directory.")
-
- #if args.with_prior_preservation:
- # if args.class_data_dir is None:
- # raise ValueError("You must specify a data directory for class images.")
- # if args.class_prompt is None:
- # raise ValueError("You must specify prompt for class images.")
-
- return args
-
-
-class DreamBoothDataset(Dataset):
- """
- A dataset to prepare the instance and class images with the prompts for fine-tuning the model.
- It pre-processes the images and the tokenizes prompts.
- """
-
- def __init__(
- self,
- instance_data_root,
- instance_prompt,
- tokenizer,
- args,
- class_data_root=None,
- class_prompt=None,
- size=512,
- center_crop=False,
- ):
- self.size = size
- self.center_crop = center_crop
- self.tokenizer = tokenizer
- self.image_captions_filename = None
-
- self.instance_data_root = Path(instance_data_root)
- if not self.instance_data_root.exists():
- raise ValueError("Instance images root doesn't exists.")
-
- self.instance_images_path = list(Path(instance_data_root).iterdir())
- self.num_instance_images = len(self.instance_images_path)
- self.instance_prompt = instance_prompt
- self._length = self.num_instance_images
-
- if args.image_captions_filename:
- self.image_captions_filename = True
-
- if class_data_root is not None:
- self.class_data_root = Path(class_data_root)
- self.class_data_root.mkdir(parents=True, exist_ok=True)
- self.class_images_path = list(self.class_data_root.iterdir())
- random.shuffle(self.class_images_path)
- self.num_class_images = len(self.class_images_path)
- self._length = max(self.num_class_images, self.num_instance_images)
- self.class_prompt = class_prompt
- else:
- self.class_data_root = None
-
- self.image_transforms = transforms.Compose(
- [
- transforms.Resize(size, interpolation=transforms.InterpolationMode.BILINEAR),
- transforms.CenterCrop(size) if center_crop else transforms.RandomCrop(size),
- transforms.ToTensor(),
- transforms.Normalize([0.5], [0.5]),
- ]
- )
-
- def __len__(self):
- return self._length
-
- def __getitem__(self, index):
- example = {}
- path = self.instance_images_path[index % self.num_instance_images]
- instance_image = Image.open(path)
- if not instance_image.mode == "RGB":
- instance_image = instance_image.convert("RGB")
-
- instance_prompt = self.instance_prompt
-
- if self.image_captions_filename:
- filename = Path(path).stem
- pt=''.join([i for i in filename if not i.isdigit()])
- pt=pt.replace("_"," ")
- pt=pt.replace("(","")
- pt=pt.replace(")","")
- pt=pt.replace("-","")
- instance_prompt = pt
- sys.stdout.write(" [0;32m" +instance_prompt+" [0m")
- sys.stdout.flush()
-
-
- example["instance_images"] = self.image_transforms(instance_image)
- example["instance_prompt_ids"] = self.tokenizer(
- instance_prompt,
- padding="do_not_pad",
- truncation=True,
- max_length=self.tokenizer.model_max_length,
- ).input_ids
-
- if self.class_data_root:
- class_image = Image.open(self.class_images_path[index % self.num_class_images])
- if not class_image.mode == "RGB":
- class_image = class_image.convert("RGB")
- example["class_images"] = self.image_transforms(class_image)
- example["class_prompt_ids"] = self.tokenizer(
- self.class_prompt,
- padding="do_not_pad",
- truncation=True,
- max_length=self.tokenizer.model_max_length,
- ).input_ids
-
- return example
-
-
-
-class PromptDataset(Dataset):
- "A simple dataset to prepare the prompts to generate class images on multiple GPUs."
-
- def __init__(self, prompt, num_samples):
- self.prompt = prompt
- self.num_samples = num_samples
-
- def __len__(self):
- return self.num_samples
-
- def __getitem__(self, index):
- example = {}
- example["prompt"] = self.prompt
- example["index"] = index
- return example
-
-class LatentsDataset(Dataset):
- def __init__(self, latents_cache, text_encoder_cache):
- self.latents_cache = latents_cache
- self.text_encoder_cache = text_encoder_cache
-
- def __len__(self):
- return len(self.latents_cache)
-
- def __getitem__(self, index):
- return self.latents_cache[index], self.text_encoder_cache[index]
-
-def get_full_repo_name(model_id: str, organization: Optional[str] = None, token: Optional[str] = None):
- if token is None:
- token = HfFolder.get_token()
- if organization is None:
- username = whoami(token)["name"]
- return f"{username}/{model_id}"
- else:
- return f"{organization}/{model_id}"
-
-def merge_two_dicts(starting_dict: dict, updater_dict: dict) -> dict:
- """
- Starts from base starting dict and then adds the remaining key values from updater replacing the values from
- the first starting/base dict with the second updater dict.
-
- For later: how does d = {**d1, **d2} replace collision?
-
- :param starting_dict:
- :param updater_dict:
- :return:
- """
- new_dict: dict = starting_dict.copy() # start with keys and values of starting_dict
- new_dict.update(updater_dict) # modifies starting_dict with keys and values of updater_dict
- return new_dict
-
-def merge_args(args1: argparse.Namespace, args2: argparse.Namespace) -> argparse.Namespace:
- """
-
- ref: https://stackoverflow.com/questions/56136549/how-can-i-merge-two-argparse-namespaces-in-python-2-x
- :param args1:
- :param args2:
- :return:
- """
- # - the merged args
- # The vars() function returns the __dict__ attribute to values of the given object e.g {field:value}.
- merged_key_values_for_namespace: dict = merge_two_dicts(vars(args1), vars(args2))
- args = argparse.Namespace(**merged_key_values_for_namespace)
- return args
-
-def run_training(args_imported):
- args_default = parse_args()
- args = merge_args(args_default, args_imported)
- print(args)
- logging_dir = Path(args.output_dir, args.logging_dir)
- i=args.save_starting_step
- accelerator = Accelerator(
- gradient_accumulation_steps=args.gradient_accumulation_steps,
- mixed_precision=args.mixed_precision,
- log_with="tensorboard",
- logging_dir=logging_dir,
- )
-
- # Currently, it's not possible to do gradient accumulation when training two models with accelerate.accumulate
- # This will be enabled soon in accelerate. For now, we don't allow gradient accumulation when training two models.
- # TODO (patil-suraj): Remove this check when gradient accumulation with two models is enabled in accelerate.
- if args.train_text_encoder and args.gradient_accumulation_steps > 1 and accelerator.num_processes > 1:
- raise ValueError(
- "Gradient accumulation is not supported when training the text encoder in distributed training. "
- "Please set gradient_accumulation_steps to 1. This feature will be supported in the future."
- )
-
- if args.seed is not None:
- set_seed(args.seed)
-
- if args.with_prior_preservation:
- class_images_dir = Path(args.class_data_dir)
- if not class_images_dir.exists():
- class_images_dir.mkdir(parents=True)
- cur_class_images = len(list(class_images_dir.iterdir()))
-
- if cur_class_images < args.num_class_images:
- torch_dtype = torch.float16 if accelerator.device.type == "cuda" else torch.float32
- pipeline = StableDiffusionPipeline.from_pretrained(
- args.pretrained_model_name_or_path, torch_dtype=torch_dtype
- )
- pipeline.set_progress_bar_config(disable=True)
-
- num_new_images = args.num_class_images - cur_class_images
- logger.info(f"Number of class images to sample: {num_new_images}.")
-
- sample_dataset = PromptDataset(args.class_prompt, num_new_images)
- sample_dataloader = torch.utils.data.DataLoader(sample_dataset, batch_size=args.sample_batch_size)
-
- sample_dataloader = accelerator.prepare(sample_dataloader)
- pipeline.to(accelerator.device)
-
- for example in tqdm(
- sample_dataloader, desc="Generating class images", disable=not accelerator.is_local_main_process
- ):
- with torch.autocast("cuda"):
- images = pipeline(example["prompt"]).images
-
- for i, image in enumerate(images):
- image.save(class_images_dir / f"{example['index'][i] + cur_class_images}.jpg")
-
- del pipeline
- if torch.cuda.is_available():
- torch.cuda.empty_cache()
-
- # Handle the repository creation
- if accelerator.is_main_process:
- if args.push_to_hub:
- if args.hub_model_id is None:
- repo_name = get_full_repo_name(Path(args.output_dir).name, token=args.hub_token)
- else:
- repo_name = args.hub_model_id
- repo = Repository(args.output_dir, clone_from=repo_name)
-
- with open(os.path.join(args.output_dir, ".gitignore"), "w+") as gitignore:
- if "step_*" not in gitignore:
- gitignore.write("step_*\n")
- if "epoch_*" not in gitignore:
- gitignore.write("epoch_*\n")
- elif args.output_dir is not None:
- os.makedirs(args.output_dir, exist_ok=True)
-
- # Load the tokenizer
- if args.tokenizer_name:
- tokenizer = CLIPTokenizer.from_pretrained(args.tokenizer_name)
- elif args.pretrained_model_name_or_path:
- tokenizer = CLIPTokenizer.from_pretrained(args.pretrained_model_name_or_path, subfolder="tokenizer")
-
- # Load models and create wrapper for stable diffusion
- if args.train_only_unet:
- if os.path.exists(str(args.output_dir+"/text_encoder_trained")):
- text_encoder = CLIPTextModel.from_pretrained(args.output_dir, subfolder="text_encoder_trained")
- elif os.path.exists(str(args.output_dir+"/text_encoder")):
- text_encoder = CLIPTextModel.from_pretrained(args.output_dir, subfolder="text_encoder")
- else:
- text_encoder = CLIPTextModel.from_pretrained(args.pretrained_model_name_or_path, subfolder="text_encoder")
- else:
- text_encoder = CLIPTextModel.from_pretrained(args.pretrained_model_name_or_path, subfolder="text_encoder")
- vae = AutoencoderKL.from_pretrained(args.pretrained_model_name_or_path, subfolder="vae")
- unet = UNet2DConditionModel.from_pretrained(args.pretrained_model_name_or_path, subfolder="unet")
- if is_xformers_available():
- try:
- print("Enabling memory efficient attention with xformers...")
- unet.enable_xformers_memory_efficient_attention()
- except Exception as e:
- logger.warning(
- f"Could not enable memory efficient attention. Make sure xformers is installed correctly and a GPU is available: {e}"
- )
- vae.requires_grad_(False)
- if not args.train_text_encoder:
- text_encoder.requires_grad_(False)
-
- if args.gradient_checkpointing:
- unet.enable_gradient_checkpointing()
- if args.train_text_encoder:
- text_encoder.gradient_checkpointing_enable()
-
- if args.scale_lr:
- args.learning_rate = (
- args.learning_rate * args.gradient_accumulation_steps * args.train_batch_size * accelerator.num_processes
- )
-
- # Use 8-bit Adam for lower memory usage or to fine-tune the model in 16GB GPUs
- if args.use_8bit_adam:
- try:
- import bitsandbytes as bnb
- except ImportError:
- raise ImportError(
- "To use 8-bit Adam, please install the bitsandbytes library: `pip install bitsandbytes`."
- )
-
- optimizer_class = bnb.optim.AdamW8bit
- else:
- optimizer_class = torch.optim.AdamW
-
- params_to_optimize = (
- itertools.chain(unet.parameters(), text_encoder.parameters()) if args.train_text_encoder else unet.parameters()
- )
- optimizer = optimizer_class(
- params_to_optimize,
- lr=args.learning_rate,
- betas=(args.adam_beta1, args.adam_beta2),
- weight_decay=args.adam_weight_decay,
- eps=args.adam_epsilon,
- )
-
- noise_scheduler = DDPMScheduler.from_config(args.pretrained_model_name_or_path, subfolder="scheduler")
-
- train_dataset = DreamBoothDataset(
- instance_data_root=args.instance_data_dir,
- instance_prompt=args.instance_prompt,
- class_data_root=args.class_data_dir if args.with_prior_preservation else None,
- class_prompt=args.class_prompt,
- tokenizer=tokenizer,
- size=args.resolution,
- center_crop=args.center_crop,
- args=args,
- )
-
- def collate_fn(examples):
- input_ids = [example["instance_prompt_ids"] for example in examples]
- pixel_values = [example["instance_images"] for example in examples]
-
- # Concat class and instance examples for prior preservation.
- # We do this to avoid doing two forward passes.
- if args.with_prior_preservation:
- input_ids += [example["class_prompt_ids"] for example in examples]
- pixel_values += [example["class_images"] for example in examples]
-
- pixel_values = torch.stack(pixel_values)
- pixel_values = pixel_values.to(memory_format=torch.contiguous_format).float()
-
- input_ids = tokenizer.pad({"input_ids": input_ids}, padding=True, return_tensors="pt").input_ids
-
- batch = {
- "input_ids": input_ids,
- "pixel_values": pixel_values,
- }
- return batch
-
- train_dataloader = torch.utils.data.DataLoader(
- train_dataset, batch_size=args.train_batch_size, shuffle=True, collate_fn=collate_fn
- )
-
- # Scheduler and math around the number of training steps.
- overrode_max_train_steps = False
- num_update_steps_per_epoch = math.ceil(len(train_dataloader) / args.gradient_accumulation_steps)
- if args.max_train_steps is None:
- args.max_train_steps = args.num_train_epochs * num_update_steps_per_epoch
- overrode_max_train_steps = True
-
- lr_scheduler = get_scheduler(
- args.lr_scheduler,
- optimizer=optimizer,
- num_warmup_steps=args.lr_warmup_steps * args.gradient_accumulation_steps,
- num_training_steps=args.max_train_steps * args.gradient_accumulation_steps,
- )
-
- if args.train_text_encoder:
- unet, text_encoder, optimizer, train_dataloader, lr_scheduler = accelerator.prepare(
- unet, text_encoder, optimizer, train_dataloader, lr_scheduler
- )
- else:
- unet, optimizer, train_dataloader, lr_scheduler = accelerator.prepare(
- unet, optimizer, train_dataloader, lr_scheduler
- )
-
- weight_dtype = torch.float32
- if args.mixed_precision == "fp16":
- weight_dtype = torch.float16
- elif args.mixed_precision == "bf16":
- weight_dtype = torch.bfloat16
-
- # Move text_encode and vae to gpu.
- # For mixed precision training we cast the text_encoder and vae weights to half-precision
- # as these models are only used for inference, keeping weights in full precision is not required.
- vae.to(accelerator.device, dtype=weight_dtype)
- if not args.train_text_encoder:
- text_encoder.to(accelerator.device, dtype=weight_dtype)
-
-
- if args.cache_latents:
- latents_cache = []
- text_encoder_cache = []
- for batch in tqdm(train_dataloader, desc="Caching latents"):
- with torch.no_grad():
- batch["pixel_values"] = batch["pixel_values"].to(accelerator.device, non_blocking=True, dtype=weight_dtype)
- batch["input_ids"] = batch["input_ids"].to(accelerator.device, non_blocking=True)
- latents_cache.append(vae.encode(batch["pixel_values"]).latent_dist)
- if args.train_text_encoder:
- text_encoder_cache.append(batch["input_ids"])
- else:
- text_encoder_cache.append(text_encoder(batch["input_ids"])[0])
- train_dataset = LatentsDataset(latents_cache, text_encoder_cache)
- train_dataloader = torch.utils.data.DataLoader(train_dataset, batch_size=1, collate_fn=lambda x: x, shuffle=True)
-
- del vae
- #if not args.train_text_encoder:
- # del text_encoder
- if torch.cuda.is_available():
- torch.cuda.empty_cache()
-
- # We need to recalculate our total training steps as the size of the training dataloader may have changed.
- num_update_steps_per_epoch = math.ceil(len(train_dataloader) / args.gradient_accumulation_steps)
- if overrode_max_train_steps:
- args.max_train_steps = args.num_train_epochs * num_update_steps_per_epoch
- # Afterwards we recalculate our number of training epochs
- args.num_train_epochs = math.ceil(args.max_train_steps / num_update_steps_per_epoch)
-
- # We need to initialize the trackers we use, and also store our configuration.
- # The trackers initializes automatically on the main process.
- if accelerator.is_main_process:
- accelerator.init_trackers("dreambooth", config=vars(args))
-
- def bar(prg):
- br='|'+'█' * prg + ' ' * (25-prg)+'|'
- return br
-
- # Train!
- total_batch_size = args.train_batch_size * accelerator.num_processes * args.gradient_accumulation_steps
-
- logger.info("***** Running training *****")
- logger.info(f" Num examples = {len(train_dataset)}")
- logger.info(f" Num batches each epoch = {len(train_dataloader)}")
- logger.info(f" Num Epochs = {args.num_train_epochs}")
- logger.info(f" Instantaneous batch size per device = {args.train_batch_size}")
- logger.info(f" Total train batch size (w. parallel, distributed & accumulation) = {total_batch_size}")
- logger.info(f" Gradient Accumulation steps = {args.gradient_accumulation_steps}")
- logger.info(f" Total optimization steps = {args.max_train_steps}")
- # Only show the progress bar once on each machine.
- progress_bar = tqdm(range(args.max_train_steps), disable=not accelerator.is_local_main_process)
- global_step = 0
-
- for epoch in range(args.num_train_epochs):
- unet.train()
- if args.train_text_encoder:
- text_encoder.train()
- for step, batch in enumerate(train_dataloader):
- with accelerator.accumulate(unet):
- # Convert images to latent space
- with torch.no_grad():
- if args.cache_latents:
- latents_dist = batch[0][0]
- else:
- latents_dist = vae.encode(batch["pixel_values"].to(dtype=weight_dtype)).latent_dist
- latents = latents_dist.sample() * 0.18215
-
- # Sample noise that we'll add to the latents
- noise = torch.randn_like(latents)
- bsz = latents.shape[0]
- # Sample a random timestep for each image
- timesteps = torch.randint(0, noise_scheduler.config.num_train_timesteps, (bsz,), device=latents.device)
- timesteps = timesteps.long()
-
- # Add noise to the latents according to the noise magnitude at each timestep
- # (this is the forward diffusion process)
- noisy_latents = noise_scheduler.add_noise(latents, noise, timesteps)
-
- # Get the text embedding for conditioning
- if(args.cache_latents):
- if args.train_text_encoder:
- encoder_hidden_states = text_encoder(batch[0][1])[0]
- else:
- encoder_hidden_states = batch[0][1]
- else:
- encoder_hidden_states = text_encoder(batch["input_ids"])[0]
-
- # Predict the noise residual
- model_pred = unet(noisy_latents, timesteps, encoder_hidden_states).sample
-
- # Get the target for loss depending on the prediction type
- if noise_scheduler.config.prediction_type == "epsilon":
- target = noise
- elif noise_scheduler.config.prediction_type == "v_prediction":
- target = noise_scheduler.get_velocity(latents, noise, timesteps)
- else:
- raise ValueError(f"Unknown prediction type {noise_scheduler.config.prediction_type}")
-
- if args.with_prior_preservation:
- # Chunk the noise and model_pred into two parts and compute the loss on each part separately.
- model_pred, model_pred_prior = torch.chunk(model_pred, 2, dim=0)
- target, target_prior = torch.chunk(target, 2, dim=0)
-
- # Compute instance loss
- loss = F.mse_loss(model_pred.float(), target.float(), reduction="none").mean([1, 2, 3]).mean()
-
- # Compute prior loss
- prior_loss = F.mse_loss(model_pred_prior.float(), target_prior.float(), reduction="mean")
-
- # Add the prior loss to the instance loss.
- loss = loss + args.prior_loss_weight * prior_loss
- else:
- loss = F.mse_loss(model_pred.float(), target.float(), reduction="mean")
-
- accelerator.backward(loss)
- if accelerator.sync_gradients:
- params_to_clip = (
- itertools.chain(unet.parameters(), text_encoder.parameters())
- if args.train_text_encoder
- else unet.parameters()
- )
- accelerator.clip_grad_norm_(params_to_clip, args.max_grad_norm)
- optimizer.step()
- lr_scheduler.step()
- optimizer.zero_grad()
-
- # Checks if the accelerator has performed an optimization step behind the scenes
- if accelerator.sync_gradients:
- progress_bar.update(1)
- global_step += 1
-
- fll=round((global_step*100)/args.max_train_steps)
- fll=round(fll/4)
- pr=bar(fll)
-
- logs = {"loss": loss.detach().item(), "lr": lr_scheduler.get_last_lr()[0]}
- progress_bar.set_postfix(**logs)
- progress_bar.set_description_str("Progress:"+pr)
- accelerator.log(logs, step=global_step)
-
- if global_step >= args.max_train_steps:
- break
-
- if args.train_text_encoder and global_step == args.stop_text_encoder_training and global_step >= 30:
- if accelerator.is_main_process:
- print(" [0;32m" +" Freezing the text_encoder ..."+" [0m")
- frz_dir=args.output_dir + "/text_encoder_frozen"
- if os.path.exists(frz_dir):
- subprocess.call('rm -r '+ frz_dir, shell=True)
- os.mkdir(frz_dir)
- pipeline = StableDiffusionPipeline.from_pretrained(
- args.pretrained_model_name_or_path,
- unet=accelerator.unwrap_model(unet),
- text_encoder=accelerator.unwrap_model(text_encoder),
- )
- pipeline.text_encoder.save_pretrained(frz_dir)
-
- if args.save_n_steps >= 200:
- if global_step < args.max_train_steps and global_step+1==i:
- ckpt_name = "_step_" + str(global_step+1)
- save_dir = Path(args.output_dir+ckpt_name)
- save_dir=str(save_dir)
- save_dir=save_dir.replace(" ", "_")
- if not os.path.exists(save_dir):
- os.mkdir(save_dir)
- inst=save_dir[16:]
- inst=inst.replace(" ", "_")
- print(" [1;32mSAVING CHECKPOINT: "+args.Session_dir+"/"+inst+".ckpt")
- # Create the pipeline using the trained modules and save it.
- if accelerator.is_main_process:
- pipeline = StableDiffusionPipeline.from_pretrained(
- args.pretrained_model_name_or_path,
- unet=accelerator.unwrap_model(unet),
- text_encoder=accelerator.unwrap_model(text_encoder),
- )
- pipeline.save_pretrained(save_dir)
- frz_dir=args.output_dir + "/text_encoder_frozen"
- if args.train_text_encoder and os.path.exists(frz_dir):
- subprocess.call('rm -r '+save_dir+'/text_encoder/*.*', shell=True)
- subprocess.call('cp -f '+frz_dir +'/*.* '+ save_dir+'/text_encoder', shell=True)
- chkpth=args.Session_dir+"/"+inst+".ckpt"
- subprocess.call('python /content/diffusers/scripts/convert_diffusers_to_original_stable_diffusion.py --model_path ' + save_dir + ' --checkpoint_path ' + chkpth + ' --half', shell=True)
- subprocess.call('rm -r '+ save_dir, shell=True)
- i=i+args.save_n_steps
-
- accelerator.wait_for_everyone()
-
- # Create the pipeline using using the trained modules and save it.
- if accelerator.is_main_process:
- if args.dump_only_text_encoder:
- txt_dir=args.output_dir + "/text_encoder_trained"
- if not os.path.exists(txt_dir):
- os.mkdir(txt_dir)
- pipeline = StableDiffusionPipeline.from_pretrained(
- args.pretrained_model_name_or_path,
- unet=accelerator.unwrap_model(unet),
- text_encoder=accelerator.unwrap_model(text_encoder),
- )
- pipeline.text_encoder.save_pretrained(txt_dir)
-
- elif args.train_only_unet:
- pipeline = StableDiffusionPipeline.from_pretrained(
- args.pretrained_model_name_or_path,
- unet=accelerator.unwrap_model(unet),
- text_encoder=accelerator.unwrap_model(text_encoder),
- )
- pipeline.save_pretrained(args.output_dir)
- txt_dir=args.output_dir + "/text_encoder_trained"
- subprocess.call('rm -r '+txt_dir, shell=True)
-
- else:
- pipeline = StableDiffusionPipeline.from_pretrained(
- args.pretrained_model_name_or_path,
- unet=accelerator.unwrap_model(unet),
- text_encoder=accelerator.unwrap_model(text_encoder),
- )
- frz_dir=args.output_dir + "/text_encoder_frozen"
- pipeline.save_pretrained(args.output_dir)
- if args.train_text_encoder and os.path.exists(frz_dir):
- subprocess.call('mv -f '+frz_dir +'/*.* '+ args.output_dir+'/text_encoder', shell=True)
- subprocess.call('rm -r '+ frz_dir, shell=True)
-
- if args.push_to_hub:
- repo.push_to_hub(commit_message="End of training", blocking=False, auto_lfs_prune=True)
-
- accelerator.end_training()
- del pipeline
- torch.cuda.empty_cache()
- gc.collect()
-if __name__ == "__main__":
- pass
- #main()
-
diff --git a/spaces/CofAI/chat.b4/g4f/Provider/Providers/Lockchat.py b/spaces/CofAI/chat.b4/g4f/Provider/Providers/Lockchat.py
deleted file mode 100644
index 1bce74035403bf8615e68ccfcc9deb7e0151817a..0000000000000000000000000000000000000000
--- a/spaces/CofAI/chat.b4/g4f/Provider/Providers/Lockchat.py
+++ /dev/null
@@ -1,32 +0,0 @@
-import requests
-import os
-import json
-from ...typing import sha256, Dict, get_type_hints
-url = 'http://supertest.lockchat.app'
-model = ['gpt-4', 'gpt-3.5-turbo']
-supports_stream = True
-needs_auth = False
-
-def _create_completion(model: str, messages: list, stream: bool, temperature: float = 0.7, **kwargs):
-
- payload = {
- "temperature": 0.7,
- "messages": messages,
- "model": model,
- "stream": True,
- }
- headers = {
- "user-agent": "ChatX/39 CFNetwork/1408.0.4 Darwin/22.5.0",
- }
- response = requests.post("http://supertest.lockchat.app/v1/chat/completions",
- json=payload, headers=headers, stream=True)
- for token in response.iter_lines():
- if b'The model: `gpt-4` does not exist' in token:
- print('error, retrying...')
- _create_completion(model=model, messages=messages, stream=stream, temperature=temperature, **kwargs)
- if b"content" in token:
- token = json.loads(token.decode('utf-8').split('data: ')[1])['choices'][0]['delta'].get('content')
- if token: yield (token)
-
-params = f'g4f.Providers.{os.path.basename(__file__)[:-3]} supports: ' + \
- '(%s)' % ', '.join([f"{name}: {get_type_hints(_create_completion)[name].__name__}" for name in _create_completion.__code__.co_varnames[:_create_completion.__code__.co_argcount]])
\ No newline at end of file
diff --git a/spaces/Crossper6/stable-diffusion-webui/README.md b/spaces/Crossper6/stable-diffusion-webui/README.md
deleted file mode 100644
index e40f42fa25e9718686ea5f589e92d2617450a8d5..0000000000000000000000000000000000000000
--- a/spaces/Crossper6/stable-diffusion-webui/README.md
+++ /dev/null
@@ -1,14 +0,0 @@
----
-title: Stable Diffusion Webui
-emoji: 💻
-colorFrom: yellow
-colorTo: gray
-sdk: gradio
-sdk_version: 3.12.0
-app_file: app.py
-pinned: false
-license: openrail
-duplicated_from: voltcutter/stable-diffusion-webui
----
-
-Check out the configuration reference at https://huggingface.co/docs/hub/spaces-config-reference
diff --git a/spaces/DAMO-NLP-SG/Video-LLaMA/video_llama/models/__init__.py b/spaces/DAMO-NLP-SG/Video-LLaMA/video_llama/models/__init__.py
deleted file mode 100644
index 8ddc54288cdc9d7e75b71da3f8597052f4f4837c..0000000000000000000000000000000000000000
--- a/spaces/DAMO-NLP-SG/Video-LLaMA/video_llama/models/__init__.py
+++ /dev/null
@@ -1,201 +0,0 @@
-"""
-Adapted from salesforce@LAVIS Vision-CAIR@MiniGPT-4. Below is the original copyright:
- Copyright (c) 2022, salesforce.com, inc.
- All rights reserved.
- SPDX-License-Identifier: BSD-3-Clause
- For full license text, see the LICENSE_Lavis file in the repo root or https://opensource.org/licenses/BSD-3-Clause
-"""
-
-import logging
-import torch
-from omegaconf import OmegaConf
-
-from video_llama.common.registry import registry
-from video_llama.models.base_model import BaseModel
-from video_llama.models.blip2 import Blip2Base
-from video_llama.models.video_llama import VideoLLAMA
-from video_llama.processors.base_processor import BaseProcessor
-
-
-__all__ = [
- "load_model",
- "BaseModel",
- "Blip2Base",
- "VideoLLAMA"
-]
-
-
-def load_model(name, model_type, is_eval=False, device="cpu", checkpoint=None):
- """
- Load supported models.
-
- To list all available models and types in registry:
- >>> from video_llama.models import model_zoo
- >>> print(model_zoo)
-
- Args:
- name (str): name of the model.
- model_type (str): type of the model.
- is_eval (bool): whether the model is in eval mode. Default: False.
- device (str): device to use. Default: "cpu".
- checkpoint (str): path or to checkpoint. Default: None.
- Note that expecting the checkpoint to have the same keys in state_dict as the model.
-
- Returns:
- model (torch.nn.Module): model.
- """
-
- model = registry.get_model_class(name).from_pretrained(model_type=model_type)
-
- if checkpoint is not None:
- model.load_checkpoint(checkpoint)
-
- if is_eval:
- model.eval()
-
- if device == "cpu":
- model = model.float()
-
- return model.to(device)
-
-
-def load_preprocess(config):
- """
- Load preprocessor configs and construct preprocessors.
-
- If no preprocessor is specified, return BaseProcessor, which does not do any preprocessing.
-
- Args:
- config (dict): preprocessor configs.
-
- Returns:
- vis_processors (dict): preprocessors for visual inputs.
- txt_processors (dict): preprocessors for text inputs.
-
- Key is "train" or "eval" for processors used in training and evaluation respectively.
- """
-
- def _build_proc_from_cfg(cfg):
- return (
- registry.get_processor_class(cfg.name).from_config(cfg)
- if cfg is not None
- else BaseProcessor()
- )
-
- vis_processors = dict()
- txt_processors = dict()
-
- vis_proc_cfg = config.get("vis_processor")
- txt_proc_cfg = config.get("text_processor")
-
- if vis_proc_cfg is not None:
- vis_train_cfg = vis_proc_cfg.get("train")
- vis_eval_cfg = vis_proc_cfg.get("eval")
- else:
- vis_train_cfg = None
- vis_eval_cfg = None
-
- vis_processors["train"] = _build_proc_from_cfg(vis_train_cfg)
- vis_processors["eval"] = _build_proc_from_cfg(vis_eval_cfg)
-
- if txt_proc_cfg is not None:
- txt_train_cfg = txt_proc_cfg.get("train")
- txt_eval_cfg = txt_proc_cfg.get("eval")
- else:
- txt_train_cfg = None
- txt_eval_cfg = None
-
- txt_processors["train"] = _build_proc_from_cfg(txt_train_cfg)
- txt_processors["eval"] = _build_proc_from_cfg(txt_eval_cfg)
-
- return vis_processors, txt_processors
-
-
-def load_model_and_preprocess(name, model_type, is_eval=False, device="cpu"):
- """
- Load model and its related preprocessors.
-
- List all available models and types in registry:
- >>> from video_llama.models import model_zoo
- >>> print(model_zoo)
-
- Args:
- name (str): name of the model.
- model_type (str): type of the model.
- is_eval (bool): whether the model is in eval mode. Default: False.
- device (str): device to use. Default: "cpu".
-
- Returns:
- model (torch.nn.Module): model.
- vis_processors (dict): preprocessors for visual inputs.
- txt_processors (dict): preprocessors for text inputs.
- """
- model_cls = registry.get_model_class(name)
-
- # load model
- model = model_cls.from_pretrained(model_type=model_type)
-
- if is_eval:
- model.eval()
-
- # load preprocess
- cfg = OmegaConf.load(model_cls.default_config_path(model_type))
- if cfg is not None:
- preprocess_cfg = cfg.preprocess
-
- vis_processors, txt_processors = load_preprocess(preprocess_cfg)
- else:
- vis_processors, txt_processors = None, None
- logging.info(
- f"""No default preprocess for model {name} ({model_type}).
- This can happen if the model is not finetuned on downstream datasets,
- or it is not intended for direct use without finetuning.
- """
- )
-
- if device == "cpu" or device == torch.device("cpu"):
- model = model.float()
-
- return model.to(device), vis_processors, txt_processors
-
-
-class ModelZoo:
- """
- A utility class to create string representation of available model architectures and types.
-
- >>> from video_llama.models import model_zoo
- >>> # list all available models
- >>> print(model_zoo)
- >>> # show total number of models
- >>> print(len(model_zoo))
- """
-
- def __init__(self) -> None:
- self.model_zoo = {
- k: list(v.PRETRAINED_MODEL_CONFIG_DICT.keys())
- for k, v in registry.mapping["model_name_mapping"].items()
- }
-
- def __str__(self) -> str:
- return (
- "=" * 50
- + "\n"
- + f"{'Architectures':<30} {'Types'}\n"
- + "=" * 50
- + "\n"
- + "\n".join(
- [
- f"{name:<30} {', '.join(types)}"
- for name, types in self.model_zoo.items()
- ]
- )
- )
-
- def __iter__(self):
- return iter(self.model_zoo.items())
-
- def __len__(self):
- return sum([len(v) for v in self.model_zoo.values()])
-
-
-model_zoo = ModelZoo()
diff --git a/spaces/DQChoi/gpt-demo/venv/lib/python3.11/site-packages/attr/validators.py b/spaces/DQChoi/gpt-demo/venv/lib/python3.11/site-packages/attr/validators.py
deleted file mode 100644
index 1488554f789526d8d85eb467250a64a64489362d..0000000000000000000000000000000000000000
--- a/spaces/DQChoi/gpt-demo/venv/lib/python3.11/site-packages/attr/validators.py
+++ /dev/null
@@ -1,720 +0,0 @@
-# SPDX-License-Identifier: MIT
-
-"""
-Commonly useful validators.
-"""
-
-
-import operator
-import re
-
-from contextlib import contextmanager
-from re import Pattern
-
-from ._config import get_run_validators, set_run_validators
-from ._make import _AndValidator, and_, attrib, attrs
-from .converters import default_if_none
-from .exceptions import NotCallableError
-
-
-__all__ = [
- "and_",
- "deep_iterable",
- "deep_mapping",
- "disabled",
- "ge",
- "get_disabled",
- "gt",
- "in_",
- "instance_of",
- "is_callable",
- "le",
- "lt",
- "matches_re",
- "max_len",
- "min_len",
- "not_",
- "optional",
- "provides",
- "set_disabled",
-]
-
-
-def set_disabled(disabled):
- """
- Globally disable or enable running validators.
-
- By default, they are run.
-
- :param disabled: If ``True``, disable running all validators.
- :type disabled: bool
-
- .. warning::
-
- This function is not thread-safe!
-
- .. versionadded:: 21.3.0
- """
- set_run_validators(not disabled)
-
-
-def get_disabled():
- """
- Return a bool indicating whether validators are currently disabled or not.
-
- :return: ``True`` if validators are currently disabled.
- :rtype: bool
-
- .. versionadded:: 21.3.0
- """
- return not get_run_validators()
-
-
-@contextmanager
-def disabled():
- """
- Context manager that disables running validators within its context.
-
- .. warning::
-
- This context manager is not thread-safe!
-
- .. versionadded:: 21.3.0
- """
- set_run_validators(False)
- try:
- yield
- finally:
- set_run_validators(True)
-
-
-@attrs(repr=False, slots=True, hash=True)
-class _InstanceOfValidator:
- type = attrib()
-
- def __call__(self, inst, attr, value):
- """
- We use a callable class to be able to change the ``__repr__``.
- """
- if not isinstance(value, self.type):
- raise TypeError(
- "'{name}' must be {type!r} (got {value!r} that is a "
- "{actual!r}).".format(
- name=attr.name,
- type=self.type,
- actual=value.__class__,
- value=value,
- ),
- attr,
- self.type,
- value,
- )
-
- def __repr__(self):
- return "".format(
- type=self.type
- )
-
-
-def instance_of(type):
- """
- A validator that raises a `TypeError` if the initializer is called
- with a wrong type for this particular attribute (checks are performed using
- `isinstance` therefore it's also valid to pass a tuple of types).
-
- :param type: The type to check for.
- :type type: type or tuple of type
-
- :raises TypeError: With a human readable error message, the attribute
- (of type `attrs.Attribute`), the expected type, and the value it
- got.
- """
- return _InstanceOfValidator(type)
-
-
-@attrs(repr=False, frozen=True, slots=True)
-class _MatchesReValidator:
- pattern = attrib()
- match_func = attrib()
-
- def __call__(self, inst, attr, value):
- """
- We use a callable class to be able to change the ``__repr__``.
- """
- if not self.match_func(value):
- raise ValueError(
- "'{name}' must match regex {pattern!r}"
- " ({value!r} doesn't)".format(
- name=attr.name, pattern=self.pattern.pattern, value=value
- ),
- attr,
- self.pattern,
- value,
- )
-
- def __repr__(self):
- return "".format(
- pattern=self.pattern
- )
-
-
-def matches_re(regex, flags=0, func=None):
- r"""
- A validator that raises `ValueError` if the initializer is called
- with a string that doesn't match *regex*.
-
- :param regex: a regex string or precompiled pattern to match against
- :param int flags: flags that will be passed to the underlying re function
- (default 0)
- :param callable func: which underlying `re` function to call. Valid options
- are `re.fullmatch`, `re.search`, and `re.match`; the default ``None``
- means `re.fullmatch`. For performance reasons, the pattern is always
- precompiled using `re.compile`.
-
- .. versionadded:: 19.2.0
- .. versionchanged:: 21.3.0 *regex* can be a pre-compiled pattern.
- """
- valid_funcs = (re.fullmatch, None, re.search, re.match)
- if func not in valid_funcs:
- raise ValueError(
- "'func' must be one of {}.".format(
- ", ".join(
- sorted(
- e and e.__name__ or "None" for e in set(valid_funcs)
- )
- )
- )
- )
-
- if isinstance(regex, Pattern):
- if flags:
- raise TypeError(
- "'flags' can only be used with a string pattern; "
- "pass flags to re.compile() instead"
- )
- pattern = regex
- else:
- pattern = re.compile(regex, flags)
-
- if func is re.match:
- match_func = pattern.match
- elif func is re.search:
- match_func = pattern.search
- else:
- match_func = pattern.fullmatch
-
- return _MatchesReValidator(pattern, match_func)
-
-
-@attrs(repr=False, slots=True, hash=True)
-class _ProvidesValidator:
- interface = attrib()
-
- def __call__(self, inst, attr, value):
- """
- We use a callable class to be able to change the ``__repr__``.
- """
- if not self.interface.providedBy(value):
- raise TypeError(
- "'{name}' must provide {interface!r} which {value!r} "
- "doesn't.".format(
- name=attr.name, interface=self.interface, value=value
- ),
- attr,
- self.interface,
- value,
- )
-
- def __repr__(self):
- return "".format(
- interface=self.interface
- )
-
-
-def provides(interface):
- """
- A validator that raises a `TypeError` if the initializer is called
- with an object that does not provide the requested *interface* (checks are
- performed using ``interface.providedBy(value)`` (see `zope.interface
- `_).
-
- :param interface: The interface to check for.
- :type interface: ``zope.interface.Interface``
-
- :raises TypeError: With a human readable error message, the attribute
- (of type `attrs.Attribute`), the expected interface, and the
- value it got.
-
- .. deprecated:: 23.1.0
- """
- import warnings
-
- warnings.warn(
- "attrs's zope-interface support is deprecated and will be removed in, "
- "or after, April 2024.",
- DeprecationWarning,
- stacklevel=2,
- )
- return _ProvidesValidator(interface)
-
-
-@attrs(repr=False, slots=True, hash=True)
-class _OptionalValidator:
- validator = attrib()
-
- def __call__(self, inst, attr, value):
- if value is None:
- return
-
- self.validator(inst, attr, value)
-
- def __repr__(self):
- return "".format(
- what=repr(self.validator)
- )
-
-
-def optional(validator):
- """
- A validator that makes an attribute optional. An optional attribute is one
- which can be set to ``None`` in addition to satisfying the requirements of
- the sub-validator.
-
- :param Callable | tuple[Callable] | list[Callable] validator: A validator
- (or validators) that is used for non-``None`` values.
-
- .. versionadded:: 15.1.0
- .. versionchanged:: 17.1.0 *validator* can be a list of validators.
- .. versionchanged:: 23.1.0 *validator* can also be a tuple of validators.
- """
- if isinstance(validator, (list, tuple)):
- return _OptionalValidator(_AndValidator(validator))
-
- return _OptionalValidator(validator)
-
-
-@attrs(repr=False, slots=True, hash=True)
-class _InValidator:
- options = attrib()
-
- def __call__(self, inst, attr, value):
- try:
- in_options = value in self.options
- except TypeError: # e.g. `1 in "abc"`
- in_options = False
-
- if not in_options:
- raise ValueError(
- "'{name}' must be in {options!r} (got {value!r})".format(
- name=attr.name, options=self.options, value=value
- ),
- attr,
- self.options,
- value,
- )
-
- def __repr__(self):
- return "".format(
- options=self.options
- )
-
-
-def in_(options):
- """
- A validator that raises a `ValueError` if the initializer is called
- with a value that does not belong in the options provided. The check is
- performed using ``value in options``.
-
- :param options: Allowed options.
- :type options: list, tuple, `enum.Enum`, ...
-
- :raises ValueError: With a human readable error message, the attribute (of
- type `attrs.Attribute`), the expected options, and the value it
- got.
-
- .. versionadded:: 17.1.0
- .. versionchanged:: 22.1.0
- The ValueError was incomplete until now and only contained the human
- readable error message. Now it contains all the information that has
- been promised since 17.1.0.
- """
- return _InValidator(options)
-
-
-@attrs(repr=False, slots=False, hash=True)
-class _IsCallableValidator:
- def __call__(self, inst, attr, value):
- """
- We use a callable class to be able to change the ``__repr__``.
- """
- if not callable(value):
- message = (
- "'{name}' must be callable "
- "(got {value!r} that is a {actual!r})."
- )
- raise NotCallableError(
- msg=message.format(
- name=attr.name, value=value, actual=value.__class__
- ),
- value=value,
- )
-
- def __repr__(self):
- return ""
-
-
-def is_callable():
- """
- A validator that raises a `attrs.exceptions.NotCallableError` if the
- initializer is called with a value for this particular attribute
- that is not callable.
-
- .. versionadded:: 19.1.0
-
- :raises attrs.exceptions.NotCallableError: With a human readable error
- message containing the attribute (`attrs.Attribute`) name,
- and the value it got.
- """
- return _IsCallableValidator()
-
-
-@attrs(repr=False, slots=True, hash=True)
-class _DeepIterable:
- member_validator = attrib(validator=is_callable())
- iterable_validator = attrib(
- default=None, validator=optional(is_callable())
- )
-
- def __call__(self, inst, attr, value):
- """
- We use a callable class to be able to change the ``__repr__``.
- """
- if self.iterable_validator is not None:
- self.iterable_validator(inst, attr, value)
-
- for member in value:
- self.member_validator(inst, attr, member)
-
- def __repr__(self):
- iterable_identifier = (
- ""
- if self.iterable_validator is None
- else f" {self.iterable_validator!r}"
- )
- return (
- ""
- ).format(
- iterable_identifier=iterable_identifier,
- member=self.member_validator,
- )
-
-
-def deep_iterable(member_validator, iterable_validator=None):
- """
- A validator that performs deep validation of an iterable.
-
- :param member_validator: Validator(s) to apply to iterable members
- :param iterable_validator: Validator to apply to iterable itself
- (optional)
-
- .. versionadded:: 19.1.0
-
- :raises TypeError: if any sub-validators fail
- """
- if isinstance(member_validator, (list, tuple)):
- member_validator = and_(*member_validator)
- return _DeepIterable(member_validator, iterable_validator)
-
-
-@attrs(repr=False, slots=True, hash=True)
-class _DeepMapping:
- key_validator = attrib(validator=is_callable())
- value_validator = attrib(validator=is_callable())
- mapping_validator = attrib(default=None, validator=optional(is_callable()))
-
- def __call__(self, inst, attr, value):
- """
- We use a callable class to be able to change the ``__repr__``.
- """
- if self.mapping_validator is not None:
- self.mapping_validator(inst, attr, value)
-
- for key in value:
- self.key_validator(inst, attr, key)
- self.value_validator(inst, attr, value[key])
-
- def __repr__(self):
- return (
- ""
- ).format(key=self.key_validator, value=self.value_validator)
-
-
-def deep_mapping(key_validator, value_validator, mapping_validator=None):
- """
- A validator that performs deep validation of a dictionary.
-
- :param key_validator: Validator to apply to dictionary keys
- :param value_validator: Validator to apply to dictionary values
- :param mapping_validator: Validator to apply to top-level mapping
- attribute (optional)
-
- .. versionadded:: 19.1.0
-
- :raises TypeError: if any sub-validators fail
- """
- return _DeepMapping(key_validator, value_validator, mapping_validator)
-
-
-@attrs(repr=False, frozen=True, slots=True)
-class _NumberValidator:
- bound = attrib()
- compare_op = attrib()
- compare_func = attrib()
-
- def __call__(self, inst, attr, value):
- """
- We use a callable class to be able to change the ``__repr__``.
- """
- if not self.compare_func(value, self.bound):
- raise ValueError(
- "'{name}' must be {op} {bound}: {value}".format(
- name=attr.name,
- op=self.compare_op,
- bound=self.bound,
- value=value,
- )
- )
-
- def __repr__(self):
- return "".format(
- op=self.compare_op, bound=self.bound
- )
-
-
-def lt(val):
- """
- A validator that raises `ValueError` if the initializer is called
- with a number larger or equal to *val*.
-
- :param val: Exclusive upper bound for values
-
- .. versionadded:: 21.3.0
- """
- return _NumberValidator(val, "<", operator.lt)
-
-
-def le(val):
- """
- A validator that raises `ValueError` if the initializer is called
- with a number greater than *val*.
-
- :param val: Inclusive upper bound for values
-
- .. versionadded:: 21.3.0
- """
- return _NumberValidator(val, "<=", operator.le)
-
-
-def ge(val):
- """
- A validator that raises `ValueError` if the initializer is called
- with a number smaller than *val*.
-
- :param val: Inclusive lower bound for values
-
- .. versionadded:: 21.3.0
- """
- return _NumberValidator(val, ">=", operator.ge)
-
-
-def gt(val):
- """
- A validator that raises `ValueError` if the initializer is called
- with a number smaller or equal to *val*.
-
- :param val: Exclusive lower bound for values
-
- .. versionadded:: 21.3.0
- """
- return _NumberValidator(val, ">", operator.gt)
-
-
-@attrs(repr=False, frozen=True, slots=True)
-class _MaxLengthValidator:
- max_length = attrib()
-
- def __call__(self, inst, attr, value):
- """
- We use a callable class to be able to change the ``__repr__``.
- """
- if len(value) > self.max_length:
- raise ValueError(
- "Length of '{name}' must be <= {max}: {len}".format(
- name=attr.name, max=self.max_length, len=len(value)
- )
- )
-
- def __repr__(self):
- return f""
-
-
-def max_len(length):
- """
- A validator that raises `ValueError` if the initializer is called
- with a string or iterable that is longer than *length*.
-
- :param int length: Maximum length of the string or iterable
-
- .. versionadded:: 21.3.0
- """
- return _MaxLengthValidator(length)
-
-
-@attrs(repr=False, frozen=True, slots=True)
-class _MinLengthValidator:
- min_length = attrib()
-
- def __call__(self, inst, attr, value):
- """
- We use a callable class to be able to change the ``__repr__``.
- """
- if len(value) < self.min_length:
- raise ValueError(
- "Length of '{name}' must be => {min}: {len}".format(
- name=attr.name, min=self.min_length, len=len(value)
- )
- )
-
- def __repr__(self):
- return f""
-
-
-def min_len(length):
- """
- A validator that raises `ValueError` if the initializer is called
- with a string or iterable that is shorter than *length*.
-
- :param int length: Minimum length of the string or iterable
-
- .. versionadded:: 22.1.0
- """
- return _MinLengthValidator(length)
-
-
-@attrs(repr=False, slots=True, hash=True)
-class _SubclassOfValidator:
- type = attrib()
-
- def __call__(self, inst, attr, value):
- """
- We use a callable class to be able to change the ``__repr__``.
- """
- if not issubclass(value, self.type):
- raise TypeError(
- "'{name}' must be a subclass of {type!r} "
- "(got {value!r}).".format(
- name=attr.name,
- type=self.type,
- value=value,
- ),
- attr,
- self.type,
- value,
- )
-
- def __repr__(self):
- return "".format(
- type=self.type
- )
-
-
-def _subclass_of(type):
- """
- A validator that raises a `TypeError` if the initializer is called
- with a wrong type for this particular attribute (checks are performed using
- `issubclass` therefore it's also valid to pass a tuple of types).
-
- :param type: The type to check for.
- :type type: type or tuple of types
-
- :raises TypeError: With a human readable error message, the attribute
- (of type `attrs.Attribute`), the expected type, and the value it
- got.
- """
- return _SubclassOfValidator(type)
-
-
-@attrs(repr=False, slots=True, hash=True)
-class _NotValidator:
- validator = attrib()
- msg = attrib(
- converter=default_if_none(
- "not_ validator child '{validator!r}' "
- "did not raise a captured error"
- )
- )
- exc_types = attrib(
- validator=deep_iterable(
- member_validator=_subclass_of(Exception),
- iterable_validator=instance_of(tuple),
- ),
- )
-
- def __call__(self, inst, attr, value):
- try:
- self.validator(inst, attr, value)
- except self.exc_types:
- pass # suppress error to invert validity
- else:
- raise ValueError(
- self.msg.format(
- validator=self.validator,
- exc_types=self.exc_types,
- ),
- attr,
- self.validator,
- value,
- self.exc_types,
- )
-
- def __repr__(self):
- return (
- ""
- ).format(
- what=self.validator,
- exc_types=self.exc_types,
- )
-
-
-def not_(validator, *, msg=None, exc_types=(ValueError, TypeError)):
- """
- A validator that wraps and logically 'inverts' the validator passed to it.
- It will raise a `ValueError` if the provided validator *doesn't* raise a
- `ValueError` or `TypeError` (by default), and will suppress the exception
- if the provided validator *does*.
-
- Intended to be used with existing validators to compose logic without
- needing to create inverted variants, for example, ``not_(in_(...))``.
-
- :param validator: A validator to be logically inverted.
- :param msg: Message to raise if validator fails.
- Formatted with keys ``exc_types`` and ``validator``.
- :type msg: str
- :param exc_types: Exception type(s) to capture.
- Other types raised by child validators will not be intercepted and
- pass through.
-
- :raises ValueError: With a human readable error message,
- the attribute (of type `attrs.Attribute`),
- the validator that failed to raise an exception,
- the value it got,
- and the expected exception types.
-
- .. versionadded:: 22.2.0
- """
- try:
- exc_types = tuple(exc_types)
- except TypeError:
- exc_types = (exc_types,)
- return _NotValidator(validator, msg, exc_types)
diff --git a/spaces/DQChoi/gpt-demo/venv/lib/python3.11/site-packages/fontTools/ttLib/tables/S_T_A_T_.py b/spaces/DQChoi/gpt-demo/venv/lib/python3.11/site-packages/fontTools/ttLib/tables/S_T_A_T_.py
deleted file mode 100644
index 1769de91b5f0416354e040b52e3615c6824fd2f9..0000000000000000000000000000000000000000
--- a/spaces/DQChoi/gpt-demo/venv/lib/python3.11/site-packages/fontTools/ttLib/tables/S_T_A_T_.py
+++ /dev/null
@@ -1,5 +0,0 @@
-from .otBase import BaseTTXConverter
-
-
-class table_S_T_A_T_(BaseTTXConverter):
- pass
diff --git a/spaces/DQChoi/gpt-demo/venv/lib/python3.11/site-packages/gradio/templates/frontend/assets/index-ec1a8aac.js b/spaces/DQChoi/gpt-demo/venv/lib/python3.11/site-packages/gradio/templates/frontend/assets/index-ec1a8aac.js
deleted file mode 100644
index abccb9a584844c3db66449c6ad35577b568124f1..0000000000000000000000000000000000000000
--- a/spaces/DQChoi/gpt-demo/venv/lib/python3.11/site-packages/gradio/templates/frontend/assets/index-ec1a8aac.js
+++ /dev/null
@@ -1,7 +0,0 @@
-import{S as T,e as q,s as L,N as y,P as A,K as h,U as u,p as b,M,R as N,n as k,A as v,G as D,V,m as W,Z as Ie,ar as Fe,C as qe,h as Le,T as ne,Q as E,X as se,a1 as x,O as B,L as X,k as Z,o as J,z as S,v as H,x as K,B as Ge,J as ie,u as I,y as F,f as Y,as as ae}from"./index-3370be2a.js";import{B as Oe}from"./Button-89624748.js";import{E as We}from"./Image-8a3c68cc.js";import{c as Ze}from"./csv-b0b7514a.js";import{d as Je}from"./dsv-576afacd.js";import{E as Ke}from"./Model3D-db673911.js";var Qe=Je(" "),Ue=Qe.parseRows;function Xe(s){let e,l;return{c(){e=y("div"),l=A(s[0]),h(e,"class","svelte-1ayixqk"),u(e,"table",s[1]==="table"),u(e,"gallery",s[1]==="gallery"),u(e,"selected",s[2])},m(t,n){b(t,e,n),M(e,l)},p(t,[n]){n&1&&N(l,t[0]),n&2&&u(e,"table",t[1]==="table"),n&2&&u(e,"gallery",t[1]==="gallery"),n&4&&u(e,"selected",t[2])},i:k,o:k,d(t){t&&v(e)}}}function Ye(s,e,l){let{value:t}=e,{type:n}=e,{selected:a=!1}=e;return s.$$set=i=>{"value"in i&&l(0,t=i.value),"type"in i&&l(1,n=i.type),"selected"in i&&l(2,a=i.selected)},[t,n,a]}class xe extends T{constructor(e){super(),q(this,e,Ye,Xe,L,{value:0,type:1,selected:2})}}function $e(s){let e,l;return{c(){e=y("div"),l=A(s[0]),h(e,"class","svelte-1ayixqk"),u(e,"table",s[1]==="table"),u(e,"gallery",s[1]==="gallery"),u(e,"selected",s[2])},m(t,n){b(t,e,n),M(e,l)},p(t,[n]){n&1&&N(l,t[0]),n&2&&u(e,"table",t[1]==="table"),n&2&&u(e,"gallery",t[1]==="gallery"),n&4&&u(e,"selected",t[2])},i:k,o:k,d(t){t&&v(e)}}}function el(s,e,l){let{value:t}=e,{type:n}=e,{selected:a=!1}=e;return s.$$set=i=>{"value"in i&&l(0,t=i.value),"type"in i&&l(1,n=i.type),"selected"in i&&l(2,a=i.selected)},[t,n,a]}class ll extends T{constructor(e){super(),q(this,e,el,$e,L,{value:0,type:1,selected:2})}}function tl(s){let e,l=s[0].toLocaleString()+"",t;return{c(){e=y("div"),t=A(l),h(e,"class","svelte-1ayixqk"),u(e,"table",s[1]==="table"),u(e,"gallery",s[1]==="gallery"),u(e,"selected",s[2])},m(n,a){b(n,e,a),M(e,t)},p(n,[a]){a&1&&l!==(l=n[0].toLocaleString()+"")&&N(t,l),a&2&&u(e,"table",n[1]==="table"),a&2&&u(e,"gallery",n[1]==="gallery"),a&4&&u(e,"selected",n[2])},i:k,o:k,d(n){n&&v(e)}}}function nl(s,e,l){let{value:t}=e,{type:n}=e,{selected:a=!1}=e;return s.$$set=i=>{"value"in i&&l(0,t=i.value),"type"in i&&l(1,n=i.type),"selected"in i&&l(2,a=i.selected)},[t,n,a]}class sl extends T{constructor(e){super(),q(this,e,nl,tl,L,{value:0,type:1,selected:2})}}function fe(s,e,l){const t=s.slice();return t[3]=e[l],t[5]=l,t}function ce(s){let e;return{c(){e=A(", ")},m(l,t){b(l,e,t)},d(l){l&&v(e)}}}function ue(s){let e=s[3].toLocaleString()+"",l,t,n=s[5]!==s[0].length-1&&ce();return{c(){l=A(e),n&&n.c(),t=W()},m(a,i){b(a,l,i),n&&n.m(a,i),b(a,t,i)},p(a,i){i&1&&e!==(e=a[3].toLocaleString()+"")&&N(l,e),a[5]!==a[0].length-1?n||(n=ce(),n.c(),n.m(t.parentNode,t)):n&&(n.d(1),n=null)},d(a){a&&(v(l),v(t)),n&&n.d(a)}}}function il(s){let e,l=D(s[0]),t=[];for(let n=0;n{"value"in i&&l(0,t=i.value),"type"in i&&l(1,n=i.type),"selected"in i&&l(2,a=i.selected)},[t,n,a]}class fl extends T{constructor(e){super(),q(this,e,al,il,L,{value:0,type:1,selected:2})}}function cl(s){let e,l;return{c(){e=y("div"),l=A(s[0]),h(e,"class","svelte-1ayixqk"),u(e,"table",s[1]==="table"),u(e,"gallery",s[1]==="gallery"),u(e,"selected",s[2])},m(t,n){b(t,e,n),M(e,l)},p(t,[n]){n&1&&N(l,t[0]),n&2&&u(e,"table",t[1]==="table"),n&2&&u(e,"gallery",t[1]==="gallery"),n&4&&u(e,"selected",t[2])},i:k,o:k,d(t){t&&v(e)}}}function ul(s,e,l){let{value:t}=e,{type:n}=e,{selected:a=!1}=e;return s.$$set=i=>{"value"in i&&l(0,t=i.value),"type"in i&&l(1,n=i.type),"selected"in i&&l(2,a=i.selected)},[t,n,a]}class rl extends T{constructor(e){super(),q(this,e,ul,cl,L,{value:0,type:1,selected:2})}}function ol(s){let e,l;return{c(){e=y("div"),l=A(s[0]),h(e,"class","svelte-1ayixqk"),u(e,"table",s[1]==="table"),u(e,"gallery",s[1]==="gallery"),u(e,"selected",s[2])},m(t,n){b(t,e,n),M(e,l)},p(t,[n]){n&1&&N(l,t[0]),n&2&&u(e,"table",t[1]==="table"),n&2&&u(e,"gallery",t[1]==="gallery"),n&4&&u(e,"selected",t[2])},i:k,o:k,d(t){t&&v(e)}}}function _l(s,e,l){let{value:t}=e,{type:n}=e,{selected:a=!1}=e;return s.$$set=i=>{"value"in i&&l(0,t=i.value),"type"in i&&l(1,n=i.type),"selected"in i&&l(2,a=i.selected)},[t,n,a]}class dl extends T{constructor(e){super(),q(this,e,_l,ol,L,{value:0,type:1,selected:2})}}function ml(s){let e,l,t;return{c(){e=y("div"),l=A(s[0]),h(e,"class","svelte-1viwdyg"),Ie(()=>s[5].call(e)),u(e,"table",s[1]==="table"),u(e,"gallery",s[1]==="gallery"),u(e,"selected",s[2])},m(n,a){b(n,e,a),M(e,l),t=Fe(e,s[5].bind(e)),s[6](e)},p(n,[a]){a&1&&N(l,n[0]),a&2&&u(e,"table",n[1]==="table"),a&2&&u(e,"gallery",n[1]==="gallery"),a&4&&u(e,"selected",n[2])},i:k,o:k,d(n){n&&v(e),t(),s[6](null)}}}function hl(s,e,l){let{value:t}=e,{type:n}=e,{selected:a=!1}=e,i,f;function c(o,w){!o||!w||(f.style.setProperty("--local-text-width",`${w<150?w:200}px`),l(4,f.style.whiteSpace="unset",f))}qe(()=>{c(f,i)});function _(){i=this.clientWidth,l(3,i)}function m(o){Le[o?"unshift":"push"](()=>{f=o,l(4,f)})}return s.$$set=o=>{"value"in o&&l(0,t=o.value),"type"in o&&l(1,n=o.type),"selected"in o&&l(2,a=o.selected)},[t,n,a,i,f,_,m]}class gl extends T{constructor(e){super(),q(this,e,hl,ml,L,{value:0,type:1,selected:2})}}function bl(s){let e,l;return{c(){e=y("div"),l=A(s[0]),h(e,"class","svelte-1ayixqk"),u(e,"table",s[1]==="table"),u(e,"gallery",s[1]==="gallery"),u(e,"selected",s[2])},m(t,n){b(t,e,n),M(e,l)},p(t,[n]){n&1&&N(l,t[0]),n&2&&u(e,"table",t[1]==="table"),n&2&&u(e,"gallery",t[1]==="gallery"),n&4&&u(e,"selected",t[2])},i:k,o:k,d(t){t&&v(e)}}}function vl(s,e,l){let{value:t}=e,{type:n}=e,{selected:a=!1}=e;return s.$$set=i=>{"value"in i&&l(0,t=i.value),"type"in i&&l(1,n=i.type),"selected"in i&&l(2,a=i.selected)},[t,n,a]}class yl extends T{constructor(e){super(),q(this,e,vl,bl,L,{value:0,type:1,selected:2})}}function kl(s){let e,l,t,n;return{c(){e=y("video"),e.muted=!0,e.playsInline=!0,ne(e.src,l=s[3]+s[2])||h(e,"src",l),h(e,"class","svelte-1tntsc1"),u(e,"table",s[0]==="table"),u(e,"gallery",s[0]==="gallery"),u(e,"selected",s[1])},m(a,i){b(a,e,i),s[5](e),t||(n=[E(e,"mouseover",function(){se(s[4].play)&&s[4].play.apply(this,arguments)}),E(e,"mouseout",function(){se(s[4].pause)&&s[4].pause.apply(this,arguments)})],t=!0)},p(a,i){s=a,i&12&&!ne(e.src,l=s[3]+s[2])&&h(e,"src",l),i&1&&u(e,"table",s[0]==="table"),i&1&&u(e,"gallery",s[0]==="gallery"),i&2&&u(e,"selected",s[1])},d(a){a&&v(e),s[5](null),t=!1,x(n)}}}function wl(s){let e;function l(a,i){return kl}let n=l()(s);return{c(){n.c(),e=W()},m(a,i){n.m(a,i),b(a,e,i)},p(a,[i]){n.p(a,i)},i:k,o:k,d(a){a&&v(e),n.d(a)}}}function zl(s,e,l){let{type:t}=e,{selected:n=!1}=e,{value:a}=e,{samples_dir:i}=e,f;async function c(){l(4,f.muted=!0,f),l(4,f.playsInline=!0,f),l(4,f.controls=!1,f),f.setAttribute("muted",""),await f.play(),f.pause()}qe(()=>{c()});function _(m){Le[m?"unshift":"push"](()=>{f=m,l(4,f)})}return s.$$set=m=>{"type"in m&&l(0,t=m.type),"selected"in m&&l(1,n=m.selected),"value"in m&&l(2,a=m.value),"samples_dir"in m&&l(3,i=m.samples_dir)},[t,n,a,i,f,_]}class Cl extends T{constructor(e){super(),q(this,e,zl,wl,L,{type:0,selected:1,value:2,samples_dir:3})}}function Ml(s){let e,l=(Array.isArray(s[0])?s[0].join(", "):s[0])+"",t;return{c(){e=y("div"),t=A(l),h(e,"class","svelte-rgtszb"),u(e,"table",s[1]==="table"),u(e,"gallery",s[1]==="gallery"),u(e,"selected",s[2])},m(n,a){b(n,e,a),M(e,t)},p(n,[a]){a&1&&l!==(l=(Array.isArray(n[0])?n[0].join(", "):n[0])+"")&&N(t,l),a&2&&u(e,"table",n[1]==="table"),a&2&&u(e,"gallery",n[1]==="gallery"),a&4&&u(e,"selected",n[2])},i:k,o:k,d(n){n&&v(e)}}}function Sl(s,e,l){let{value:t}=e,{type:n}=e,{selected:a=!1}=e;return s.$$set=i=>{"value"in i&&l(0,t=i.value),"type"in i&&l(1,n=i.type),"selected"in i&&l(2,a=i.selected)},[t,n,a]}class Al extends T{constructor(e){super(),q(this,e,Sl,Ml,L,{value:0,type:1,selected:2})}}function re(s,e,l){const t=s.slice();return t[10]=e[l],t[12]=l,t}function oe(s,e,l){const t=s.slice();return t[13]=e[l],t[15]=l,t}function _e(s){let e,l,t;function n(f,c){return typeof f[6]=="string"?Tl:Hl}let a=n(s),i=a(s);return{c(){e=y("div"),i.c(),h(e,"class","svelte-1cib1xd"),u(e,"table",s[1]==="table"),u(e,"gallery",s[1]==="gallery"),u(e,"selected",s[2])},m(f,c){b(f,e,c),i.m(e,null),l||(t=[E(e,"mouseenter",s[8]),E(e,"mouseleave",s[9])],l=!0)},p(f,c){a===(a=n(f))&&i?i.p(f,c):(i.d(1),i=a(f),i&&(i.c(),i.m(e,null))),c&2&&u(e,"table",f[1]==="table"),c&2&&u(e,"gallery",f[1]==="gallery"),c&4&&u(e,"selected",f[2])},d(f){f&&v(e),i.d(),l=!1,x(t)}}}function Hl(s){let e,l,t=D(s[6].slice(0,3)),n=[];for(let i=0;i3&&ge(s);return{c(){e=y("table");for(let i=0;i3?a?a.p(i,f):(a=ge(i),a.c(),a.m(e,null)):a&&(a.d(1),a=null)},d(i){i&&v(e),V(n,i),a&&a.d()}}}function Tl(s){let e;return{c(){e=A(s[6])},m(l,t){b(l,e,t)},p(l,t){t&64&&N(e,l[6])},d(l){l&&v(e)}}}function de(s){let e,l=s[13]+"",t;return{c(){e=y("td"),t=A(l),h(e,"class","svelte-1cib1xd")},m(n,a){b(n,e,a),M(e,t)},p(n,a){a&64&&l!==(l=n[13]+"")&&N(t,l)},d(n){n&&v(e)}}}function me(s){let e;return{c(){e=y("td"),e.textContent="…",h(e,"class","svelte-1cib1xd")},m(l,t){b(l,e,t)},d(l){l&&v(e)}}}function he(s){let e,l,t=D(s[10].slice(0,3)),n=[];for(let i=0;i3&&me();return{c(){e=y("tr");for(let i=0;i3?a||(a=me(),a.c(),a.m(e,null)):a&&(a.d(1),a=null)},d(i){i&&v(e),V(n,i),a&&a.d()}}}function ge(s){let e;return{c(){e=y("div"),h(e,"class","overlay svelte-1cib1xd"),u(e,"odd",s[3]%2!=0),u(e,"even",s[3]%2==0),u(e,"button",s[1]==="gallery")},m(l,t){b(l,e,t)},p(l,t){t&8&&u(e,"odd",l[3]%2!=0),t&8&&u(e,"even",l[3]%2==0),t&2&&u(e,"button",l[1]==="gallery")},d(l){l&&v(e)}}}function ql(s){let e,l=s[4]&&_e(s);return{c(){l&&l.c(),e=W()},m(t,n){l&&l.m(t,n),b(t,e,n)},p(t,[n]){t[4]?l?l.p(t,n):(l=_e(t),l.c(),l.m(e.parentNode,e)):l&&(l.d(1),l=null)},i:k,o:k,d(t){t&&v(e),l&&l.d(t)}}}function Ll(s,e,l){let{value:t}=e,{samples_dir:n}=e,{type:a}=e,{selected:i=!1}=e,{index:f}=e,c=!1,_=t,m=Array.isArray(_);const o=()=>l(5,c=!0),w=()=>l(5,c=!1);return s.$$set=r=>{"value"in r&&l(0,t=r.value),"samples_dir"in r&&l(7,n=r.samples_dir),"type"in r&&l(1,a=r.type),"selected"in r&&l(2,i=r.selected),"index"in r&&l(3,f=r.index)},s.$$.update=()=>{s.$$.dirty&145&&!m&&typeof t=="string"&&/\.[a-zA-Z]+$/.test(t)&&fetch(n+t).then(r=>r.text()).then(r=>{try{if(t.endsWith("csv")){const z=r.split(`
-`).slice(0,4).map(d=>d.split(",").slice(0,4).join(",")).join(`
-`);l(6,_=Ze(z))}else if(t.endsWith("tsv")){const z=r.split(`
-`).slice(0,4).map(d=>d.split(" ").slice(0,4).join(" ")).join(`
-`);l(6,_=Ue(z))}else throw new Error("Incorrect format, only CSV and TSV files are supported");l(4,m=!0)}catch(z){console.error(z)}}).catch(r=>{l(6,_=t),l(4,m=!0)})},[t,a,i,f,m,c,_,n,o,w]}class Dl extends T{constructor(e){super(),q(this,e,Ll,ql,L,{value:0,samples_dir:7,type:1,selected:2,index:3})}}function Nl(s){let e;return{c(){e=y("div"),X(e,"background-color",s[0]),h(e,"class","svelte-h6ogpl"),u(e,"table",s[1]==="table"),u(e,"gallery",s[1]==="gallery"),u(e,"selected",s[2])},m(l,t){b(l,e,t)},p(l,[t]){t&1&&X(e,"background-color",l[0]),t&2&&u(e,"table",l[1]==="table"),t&2&&u(e,"gallery",l[1]==="gallery"),t&4&&u(e,"selected",l[2])},i:k,o:k,d(l){l&&v(e)}}}function jl(s,e,l){let{value:t}=e,{type:n}=e,{selected:a=!1}=e;return s.$$set=i=>{"value"in i&&l(0,t=i.value),"type"in i&&l(1,n=i.type),"selected"in i&&l(2,a=i.selected)},[t,n,a]}class pl extends T{constructor(e){super(),q(this,e,jl,Nl,L,{value:0,type:1,selected:2})}}function El(s){let e,l;return{c(){e=y("div"),l=A(s[0]),h(e,"class","svelte-1ayixqk"),u(e,"table",s[1]==="table"),u(e,"gallery",s[1]==="gallery"),u(e,"selected",s[2])},m(t,n){b(t,e,n),M(e,l)},p(t,[n]){n&1&&N(l,t[0]),n&2&&u(e,"table",t[1]==="table"),n&2&&u(e,"gallery",t[1]==="gallery"),n&4&&u(e,"selected",t[2])},i:k,o:k,d(t){t&&v(e)}}}function Bl(s,e,l){let{value:t}=e,{type:n}=e,{selected:a=!1}=e;return s.$$set=i=>{"value"in i&&l(0,t=i.value),"type"in i&&l(1,n=i.type),"selected"in i&&l(2,a=i.selected)},[t,n,a]}class Pl extends T{constructor(e){super(),q(this,e,Bl,El,L,{value:0,type:1,selected:2})}}function Rl(s){let e;return{c(){e=y("div"),h(e,"class","prose svelte-1ayixqk"),u(e,"table",s[1]==="table"),u(e,"gallery",s[1]==="gallery"),u(e,"selected",s[2])},m(l,t){b(l,e,t),e.innerHTML=s[0]},p(l,[t]){t&1&&(e.innerHTML=l[0]),t&2&&u(e,"table",l[1]==="table"),t&2&&u(e,"gallery",l[1]==="gallery"),t&4&&u(e,"selected",l[2])},i:k,o:k,d(l){l&&v(e)}}}function Vl(s,e,l){let{value:t}=e,{type:n}=e,{selected:a=!1}=e;return s.$$set=i=>{"value"in i&&l(0,t=i.value),"type"in i&&l(1,n=i.type),"selected"in i&&l(2,a=i.selected)},[t,n,a]}class Il extends T{constructor(e){super(),q(this,e,Vl,Rl,L,{value:0,type:1,selected:2})}}function Fl(s){let e;return{c(){e=y("div"),h(e,"class","prose svelte-zvfedn"),u(e,"table",s[1]==="table"),u(e,"gallery",s[1]==="gallery"),u(e,"selected",s[2])},m(l,t){b(l,e,t),e.innerHTML=s[0]},p(l,[t]){t&1&&(e.innerHTML=l[0]),t&2&&u(e,"table",l[1]==="table"),t&2&&u(e,"gallery",l[1]==="gallery"),t&4&&u(e,"selected",l[2])},i:k,o:k,d(l){l&&v(e)}}}function Gl(s,e,l){let{value:t}=e,{type:n}=e,{selected:a=!1}=e;return s.$$set=i=>{"value"in i&&l(0,t=i.value),"type"in i&&l(1,n=i.type),"selected"in i&&l(2,a=i.selected)},[t,n,a]}class Ol extends T{constructor(e){super(),q(this,e,Gl,Fl,L,{value:0,type:1,selected:2})}}function Wl(s){let e,l;return{c(){e=y("pre"),l=A(s[0]),h(e,"class","svelte-agpzo2"),u(e,"table",s[1]==="table"),u(e,"gallery",s[1]==="gallery"),u(e,"selected",s[2])},m(t,n){b(t,e,n),M(e,l)},p(t,[n]){n&1&&N(l,t[0]),n&2&&u(e,"table",t[1]==="table"),n&2&&u(e,"gallery",t[1]==="gallery"),n&4&&u(e,"selected",t[2])},i:k,o:k,d(t){t&&v(e)}}}function Zl(s,e,l){let{value:t}=e,{type:n}=e,{selected:a=!1}=e;return s.$$set=i=>{"value"in i&&l(0,t=i.value),"type"in i&&l(1,n=i.type),"selected"in i&&l(2,a=i.selected)},[t,n,a]}class Jl extends T{constructor(e){super(),q(this,e,Zl,Wl,L,{value:0,type:1,selected:2})}}const O={dropdown:ll,checkbox:sl,checkboxgroup:fl,number:xe,slider:rl,radio:dl,image:We,textbox:gl,audio:yl,video:Cl,file:Al,dataframe:Dl,model3d:Ke,colorpicker:pl,timeseries:Pl,markdown:Il,html:Ol,code:Jl};function be(s,e,l){const t=s.slice();return t[32]=e[l],t}function ve(s,e,l){const t=s.slice();return t[35]=e[l],t[37]=l,t}function ye(s,e,l){const t=s.slice();t[0]=e[l].value,t[39]=e[l].component,t[42]=l;const n=t[1][t[42]];return t[40]=n,t}function ke(s,e,l){const t=s.slice();return t[43]=e[l],t}function we(s,e,l){const t=s.slice();return t[35]=e[l],t[37]=l,t}function Kl(s){let e,l,t,n,a,i,f,c=D(s[3]),_=[];for(let r=0;rH(o[r],1,1,()=>{o[r]=null});return{c(){e=y("div"),l=y("table"),t=y("thead"),n=y("tr");for(let r=0;r<_.length;r+=1)_[r].c();a=B(),i=y("tbody");for(let r=0;rH(n[i],1,1,()=>{n[i]=null});return{c(){e=y("div");for(let i=0;i{K(m,1)}),F()}a?(l=Y(a,i(f)),Z(l.$$.fragment),S(l.$$.fragment,1),J(l,e,null)):l=null}else a&&l.$set(_);(!n||c[0]&2)&&X(e,"max-width",f[40]==="textbox"?"35ch":"auto"),(!n||c[0]&2&&t!==(t=ae(f[40])+" svelte-13hsdno"))&&h(e,"class",t)},i(f){n||(l&&S(l.$$.fragment,f),n=!0)},o(f){l&&H(l.$$.fragment,f),n=!1},d(f){f&&v(e),l&&K(l)}}}function Me(s){let e,l,t=s[40]!==void 0&&O[s[40]]!==void 0&&Ce(s);return{c(){t&&t.c(),e=W()},m(n,a){t&&t.m(n,a),b(n,e,a),l=!0},p(n,a){n[40]!==void 0&&O[n[40]]!==void 0?t?(t.p(n,a),a[0]&2&&S(t,1)):(t=Ce(n),t.c(),S(t,1),t.m(e.parentNode,e)):t&&(I(),H(t,1,1,()=>{t=null}),F())},i(n){l||(S(t),l=!0)},o(n){H(t),l=!1},d(n){n&&v(e),t&&t.d(n)}}}function Se(s){let e,l,t,n,a,i=D(s[35]),f=[];for(let o=0;oH(f[o],1,1,()=>{f[o]=null});function _(){return s[28](s[37])}function m(){return s[29](s[37])}return{c(){e=y("tr");for(let o=0;o{K(_,1)}),F()}n?(e=Y(n,a(i)),Z(e.$$.fragment),S(e.$$.fragment,1),J(e,l.parentNode,l)):e=null}else n&&e.$set(c)},i(i){t||(e&&S(e.$$.fragment,i),t=!0)},o(i){e&&H(e.$$.fragment,i),t=!1},d(i){i&&v(l),e&&K(e,i)}}}function He(s){let e,l=Object.keys(O).includes(s[1][0])&&O[s[1][0]],t,n,a,i,f=l&&Ae(s);function c(){return s[25](s[37],s[35])}function _(){return s[26](s[37])}return{c(){e=y("button"),f&&f.c(),t=B(),h(e,"class","gallery-item svelte-13hsdno")},m(m,o){b(m,e,o),f&&f.m(e,null),M(e,t),n=!0,a||(i=[E(e,"click",c),E(e,"mouseenter",_),E(e,"mouseleave",s[27])],a=!0)},p(m,o){s=m,o[0]&2&&(l=Object.keys(O).includes(s[1][0])&&O[s[1][0]]),l?f?(f.p(s,o),o[0]&2&&S(f,1)):(f=Ae(s),f.c(),S(f,1),f.m(e,t)):f&&(I(),H(f,1,1,()=>{f=null}),F())},i(m){n||(S(f),n=!0)},o(m){H(f),n=!1},d(m){m&&v(e),f&&f.d(),a=!1,x(i)}}}function Ul(s){let e,l,t=D(s[12]),n=[];for(let a=0;a{r[P]=null}),F(),c=r[f],c?c.p(C,j):(c=r[f]=w[f](C),c.c()),S(c,1),c.m(_.parentNode,_)),C[18]&&d.p(C,j)},i(C){o||(S(c),o=!0)},o(C){H(c),o=!1},d(C){C&&(v(e),v(i),v(_),v(m)),r[f].d(C),d&&d.d(C)}}}function $l(s){let e,l;return e=new Oe({props:{visible:s[6],padding:!1,elem_id:s[4],elem_classes:s[5],scale:s[8],min_width:s[9],allow_overflow:!1,container:!1,$$slots:{default:[xl]},$$scope:{ctx:s}}}),{c(){Z(e.$$.fragment)},m(t,n){J(e,t,n),l=!0},p(t,n){const a={};n[0]&64&&(a.visible=t[6]),n[0]&16&&(a.elem_id=t[4]),n[0]&32&&(a.elem_classes=t[5]),n[0]&256&&(a.scale=t[8]),n[0]&512&&(a.min_width=t[9]),n[0]&64655|n[1]&32768&&(a.$$scope={dirty:n,ctx:t}),e.$set(a)},i(t){l||(S(e.$$.fragment,t),l=!0)},o(t){H(e.$$.fragment,t),l=!1},d(t){K(e,t)}}}function et(s,e,l){let t,n,{components:a}=e,{label:i="Examples"}=e,{headers:f}=e,{samples:c}=e,{elem_id:_=""}=e,{elem_classes:m=[]}=e,{visible:o=!0}=e,{value:w=null}=e,{root:r}=e,{root_url:z}=e,{samples_per_page:d=10}=e,{scale:C=null}=e,{min_width:j=void 0}=e;const P=Ge();let De=z?"proxy="+z+"file=":r+"/file=",G=0,te=c.length>d,Q,U,R=[],$=-1;function ee(g){l(13,$=g)}function le(){l(13,$=-1)}const Ne=(g,p)=>{l(0,w=g+G*d),P("click",w),P("select",{index:w,value:p})},je=g=>ee(g),pe=()=>le(),Ee=g=>{l(0,w=g+G*d),P("click",w)},Be=g=>ee(g),Pe=()=>le(),Re=g=>l(10,G=g);return s.$$set=g=>{"components"in g&&l(1,a=g.components),"label"in g&&l(2,i=g.label),"headers"in g&&l(3,f=g.headers),"samples"in g&&l(21,c=g.samples),"elem_id"in g&&l(4,_=g.elem_id),"elem_classes"in g&&l(5,m=g.elem_classes),"visible"in g&&l(6,o=g.visible),"value"in g&&l(0,w=g.value),"root"in g&&l(22,r=g.root),"root_url"in g&&l(23,z=g.root_url),"samples_per_page"in g&&l(7,d=g.samples_per_page),"scale"in g&&l(8,C=g.scale),"min_width"in g&&l(9,j=g.min_width)},s.$$.update=()=>{s.$$.dirty[0]&2&&l(15,t=a.length<2),s.$$.dirty[0]&18879616&&(te?(l(12,R=[]),l(11,Q=c.slice(G*d,(G+1)*d)),l(24,U=Math.ceil(c.length/d)),[0,G,U-1].forEach(g=>{for(let p=g-2;p<=g+2;p++)p>=0&&p0&&p-R[R.length-1]>1&&R.push(-1),R.push(p))})):l(11,Q=c.slice())),s.$$.dirty[0]&2050&&l(14,n=Q.map(g=>g.map((p,Ve)=>({value:p,component:O[a[Ve]]}))))},[w,a,i,f,_,m,o,d,C,j,G,Q,R,$,n,t,P,De,te,ee,le,c,r,z,U,Ne,je,pe,Ee,Be,Pe,Re]}class lt extends T{constructor(e){super(),q(this,e,et,$l,L,{components:1,label:2,headers:3,samples:21,elem_id:4,elem_classes:5,visible:6,value:0,root:22,root_url:23,samples_per_page:7,scale:8,min_width:9},null,[-1,-1])}}const ct=lt,ut=["dynamic"],rt=()=>({type:{payload:"number"},description:{payload:"index of selected row"},example_data:0});export{ct as Component,rt as document,ut as modes};
-//# sourceMappingURL=index-ec1a8aac.js.map
diff --git a/spaces/DQChoi/gpt-demo/venv/lib/python3.11/site-packages/httpcore/_async/__init__.py b/spaces/DQChoi/gpt-demo/venv/lib/python3.11/site-packages/httpcore/_async/__init__.py
deleted file mode 100644
index 88dc7f01e132933728cbcf45c88ce82e85ddf65f..0000000000000000000000000000000000000000
--- a/spaces/DQChoi/gpt-demo/venv/lib/python3.11/site-packages/httpcore/_async/__init__.py
+++ /dev/null
@@ -1,39 +0,0 @@
-from .connection import AsyncHTTPConnection
-from .connection_pool import AsyncConnectionPool
-from .http11 import AsyncHTTP11Connection
-from .http_proxy import AsyncHTTPProxy
-from .interfaces import AsyncConnectionInterface
-
-try:
- from .http2 import AsyncHTTP2Connection
-except ImportError: # pragma: nocover
-
- class AsyncHTTP2Connection: # type: ignore
- def __init__(self, *args, **kwargs) -> None: # type: ignore
- raise RuntimeError(
- "Attempted to use http2 support, but the `h2` package is not "
- "installed. Use 'pip install httpcore[http2]'."
- )
-
-
-try:
- from .socks_proxy import AsyncSOCKSProxy
-except ImportError: # pragma: nocover
-
- class AsyncSOCKSProxy: # type: ignore
- def __init__(self, *args, **kwargs) -> None: # type: ignore
- raise RuntimeError(
- "Attempted to use SOCKS support, but the `socksio` package is not "
- "installed. Use 'pip install httpcore[socks]'."
- )
-
-
-__all__ = [
- "AsyncHTTPConnection",
- "AsyncConnectionPool",
- "AsyncHTTPProxy",
- "AsyncHTTP11Connection",
- "AsyncHTTP2Connection",
- "AsyncConnectionInterface",
- "AsyncSOCKSProxy",
-]
diff --git a/spaces/Danielzero/GPT3.5/modules/presets.py b/spaces/Danielzero/GPT3.5/modules/presets.py
deleted file mode 100644
index 969f122198a360f8c3eb126b156d056ab81d53e1..0000000000000000000000000000000000000000
--- a/spaces/Danielzero/GPT3.5/modules/presets.py
+++ /dev/null
@@ -1,222 +0,0 @@
-# -*- coding:utf-8 -*-
-import os
-from pathlib import Path
-import gradio as gr
-from .webui_locale import I18nAuto
-
-i18n = I18nAuto() # internationalization
-
-CHATGLM_MODEL = None
-CHATGLM_TOKENIZER = None
-LLAMA_MODEL = None
-LLAMA_INFERENCER = None
-
-# ChatGPT 设置
-INITIAL_SYSTEM_PROMPT = "You are a helpful assistant."
-API_HOST = "api.openai.com"
-COMPLETION_URL = "https://api.openai.com/v1/chat/completions"
-BALANCE_API_URL="https://api.openai.com/dashboard/billing/credit_grants"
-USAGE_API_URL="https://api.openai.com/dashboard/billing/usage"
-HISTORY_DIR = Path("history")
-HISTORY_DIR = "history"
-TEMPLATES_DIR = "templates"
-
-# 错误信息
-STANDARD_ERROR_MSG = i18n("☹️发生了错误:") # 错误信息的标准前缀
-GENERAL_ERROR_MSG = i18n("获取对话时发生错误,请查看后台日志")
-ERROR_RETRIEVE_MSG = i18n("请检查网络连接,或者API-Key是否有效。")
-CONNECTION_TIMEOUT_MSG = i18n("连接超时,无法获取对话。") # 连接超时
-READ_TIMEOUT_MSG = i18n("读取超时,无法获取对话。") # 读取超时
-PROXY_ERROR_MSG = i18n("代理错误,无法获取对话。") # 代理错误
-SSL_ERROR_PROMPT = i18n("SSL错误,无法获取对话。") # SSL 错误
-NO_APIKEY_MSG = i18n("API key为空,请检查是否输入正确。") # API key 长度不足 51 位
-NO_INPUT_MSG = i18n("请输入对话内容。") # 未输入对话内容
-BILLING_NOT_APPLICABLE_MSG = i18n("账单信息不适用") # 本地运行的模型返回的账单信息
-
-TIMEOUT_STREAMING = 60 # 流式对话时的超时时间
-TIMEOUT_ALL = 200 # 非流式对话时的超时时间
-ENABLE_STREAMING_OPTION = True # 是否启用选择选择是否实时显示回答的勾选框
-HIDE_MY_KEY = False # 如果你想在UI中隐藏你的 API 密钥,将此值设置为 True
-CONCURRENT_COUNT = 100 # 允许同时使用的用户数量
-
-SIM_K = 5
-INDEX_QUERY_TEMPRATURE = 1.0
-
-CHUANHU_TITLE = i18n("川虎Chat 🚀")
-
-CHUANHU_DESCRIPTION = i18n("由Bilibili [土川虎虎虎](https://space.bilibili.com/29125536) 和 [明昭MZhao](https://space.bilibili.com/24807452)开发 访问川虎Chat的 [GitHub项目](https://github.com/GaiZhenbiao/ChuanhuChatGPT) 下载最新版脚本")
-
-FOOTER = """
{versions}
"""
-
-APPEARANCE_SWITCHER = """
-
-"""+ i18n("切换亮暗色主题") + """
-
-
-"""
-
-SUMMARIZE_PROMPT = "你是谁?我们刚才聊了什么?" # 总结对话时的 prompt
-
-ONLINE_MODELS = [
- "gpt-3.5-turbo",
- "gpt-3.5-turbo-0301",
- "gpt-4",
- "gpt-4-0314",
- "gpt-4-32k",
- "gpt-4-32k-0314",
- "xmchat",
-]
-
-LOCAL_MODELS = [
- "chatglm-6b",
- "chatglm-6b-int4",
- "chatglm-6b-int4-qe",
- "llama-7b-hf",
- "llama-13b-hf",
- "llama-30b-hf",
- "llama-65b-hf"
-]
-
-if os.environ.get('HIDE_LOCAL_MODELS', 'false') == 'true':
- MODELS = ONLINE_MODELS
-else:
- MODELS = ONLINE_MODELS + LOCAL_MODELS
-
-DEFAULT_MODEL = 0
-
-os.makedirs("models", exist_ok=True)
-os.makedirs("lora", exist_ok=True)
-os.makedirs("history", exist_ok=True)
-for dir_name in os.listdir("models"):
- if os.path.isdir(os.path.join("models", dir_name)):
- if dir_name not in MODELS:
- MODELS.append(dir_name)
-
-MODEL_TOKEN_LIMIT = {
- "gpt-3.5-turbo": 4096,
- "gpt-3.5-turbo-0301": 4096,
- "gpt-4": 8192,
- "gpt-4-0314": 8192,
- "gpt-4-32k": 32768,
- "gpt-4-32k-0314": 32768
-}
-
-TOKEN_OFFSET = 1000 # 模型的token上限减去这个值,得到软上限。到达软上限之后,自动尝试减少token占用。
-DEFAULT_TOKEN_LIMIT = 3000 # 默认的token上限
-REDUCE_TOKEN_FACTOR = 0.5 # 与模型token上限想乘,得到目标token数。减少token占用时,将token占用减少到目标token数以下。
-
-REPLY_LANGUAGES = [
- "简体中文",
- "繁體中文",
- "English",
- "日本語",
- "Español",
- "Français",
- "Deutsch",
- "跟随问题语言(不稳定)"
-]
-
-
-WEBSEARCH_PTOMPT_TEMPLATE = """\
-Web search results:
-
-{web_results}
-Current date: {current_date}
-
-Instructions: Using the provided web search results, write a comprehensive reply to the given query. Make sure to cite results using [[number](URL)] notation after the reference. If the provided search results refer to multiple subjects with the same name, write separate answers for each subject.
-Query: {query}
-Reply in {reply_language}
-"""
-
-PROMPT_TEMPLATE = """\
-Context information is below.
----------------------
-{context_str}
----------------------
-Current date: {current_date}.
-Using the provided context information, write a comprehensive reply to the given query.
-Make sure to cite results using [number] notation after the reference.
-If the provided context information refer to multiple subjects with the same name, write separate answers for each subject.
-Use prior knowledge only if the given context didn't provide enough information.
-Answer the question: {query_str}
-Reply in {reply_language}
-"""
-
-REFINE_TEMPLATE = """\
-The original question is as follows: {query_str}
-We have provided an existing answer: {existing_answer}
-We have the opportunity to refine the existing answer
-(only if needed) with some more context below.
-------------
-{context_msg}
-------------
-Given the new context, refine the original answer to better
-Reply in {reply_language}
-If the context isn't useful, return the original answer.
-"""
-
-ALREADY_CONVERTED_MARK = ""
-
-small_and_beautiful_theme = gr.themes.Soft(
- primary_hue=gr.themes.Color(
- c50="#02C160",
- c100="rgba(2, 193, 96, 0.2)",
- c200="#02C160",
- c300="rgba(2, 193, 96, 0.32)",
- c400="rgba(2, 193, 96, 0.32)",
- c500="rgba(2, 193, 96, 1.0)",
- c600="rgba(2, 193, 96, 1.0)",
- c700="rgba(2, 193, 96, 0.32)",
- c800="rgba(2, 193, 96, 0.32)",
- c900="#02C160",
- c950="#02C160",
- ),
- secondary_hue=gr.themes.Color(
- c50="#576b95",
- c100="#576b95",
- c200="#576b95",
- c300="#576b95",
- c400="#576b95",
- c500="#576b95",
- c600="#576b95",
- c700="#576b95",
- c800="#576b95",
- c900="#576b95",
- c950="#576b95",
- ),
- neutral_hue=gr.themes.Color(
- name="gray",
- c50="#f9fafb",
- c100="#f3f4f6",
- c200="#e5e7eb",
- c300="#d1d5db",
- c400="#B2B2B2",
- c500="#808080",
- c600="#636363",
- c700="#515151",
- c800="#393939",
- c900="#272727",
- c950="#171717",
- ),
- radius_size=gr.themes.sizes.radius_sm,
- ).set(
- button_primary_background_fill="#06AE56",
- button_primary_background_fill_dark="#06AE56",
- button_primary_background_fill_hover="#07C863",
- button_primary_border_color="#06AE56",
- button_primary_border_color_dark="#06AE56",
- button_primary_text_color="#FFFFFF",
- button_primary_text_color_dark="#FFFFFF",
- button_secondary_background_fill="#F2F2F2",
- button_secondary_background_fill_dark="#2B2B2B",
- button_secondary_text_color="#393939",
- button_secondary_text_color_dark="#FFFFFF",
- # background_fill_primary="#F7F7F7",
- # background_fill_primary_dark="#1F1F1F",
- block_title_text_color="*primary_500",
- block_title_background_fill="*primary_100",
- input_background_fill="#F6F6F6",
- )
diff --git a/spaces/DeepLabCut/MegaDetector_DeepLabCut/examples/read.md b/spaces/DeepLabCut/MegaDetector_DeepLabCut/examples/read.md
deleted file mode 100644
index e69de29bb2d1d6434b8b29ae775ad8c2e48c5391..0000000000000000000000000000000000000000
diff --git a/spaces/Dinoking/Guccio-AI-Designer/models/stylegan/stylegan_tf/training/misc.py b/spaces/Dinoking/Guccio-AI-Designer/models/stylegan/stylegan_tf/training/misc.py
deleted file mode 100644
index 50ae51c722cb1e553c56051cbd4556110fe4a1f9..0000000000000000000000000000000000000000
--- a/spaces/Dinoking/Guccio-AI-Designer/models/stylegan/stylegan_tf/training/misc.py
+++ /dev/null
@@ -1,245 +0,0 @@
-# Copyright (c) 2019, NVIDIA CORPORATION. All rights reserved.
-#
-# This work is licensed under the Creative Commons Attribution-NonCommercial
-# 4.0 International License. To view a copy of this license, visit
-# http://creativecommons.org/licenses/by-nc/4.0/ or send a letter to
-# Creative Commons, PO Box 1866, Mountain View, CA 94042, USA.
-
-"""Miscellaneous utility functions."""
-
-import os
-import glob
-import pickle
-import re
-import numpy as np
-from collections import defaultdict
-import PIL.Image
-import dnnlib
-
-import config
-from training import dataset
-
-#----------------------------------------------------------------------------
-# Convenience wrappers for pickle that are able to load data produced by
-# older versions of the code, and from external URLs.
-
-def open_file_or_url(file_or_url):
- if dnnlib.util.is_url(file_or_url):
- return dnnlib.util.open_url(file_or_url, cache_dir=config.cache_dir)
- return open(file_or_url, 'rb')
-
-def load_pkl(file_or_url):
- with open_file_or_url(file_or_url) as file:
- return pickle.load(file, encoding='latin1')
-
-def save_pkl(obj, filename):
- with open(filename, 'wb') as file:
- pickle.dump(obj, file, protocol=pickle.HIGHEST_PROTOCOL)
-
-#----------------------------------------------------------------------------
-# Image utils.
-
-def adjust_dynamic_range(data, drange_in, drange_out):
- if drange_in != drange_out:
- scale = (np.float32(drange_out[1]) - np.float32(drange_out[0])) / (np.float32(drange_in[1]) - np.float32(drange_in[0]))
- bias = (np.float32(drange_out[0]) - np.float32(drange_in[0]) * scale)
- data = data * scale + bias
- return data
-
-def create_image_grid(images, grid_size=None):
- assert images.ndim == 3 or images.ndim == 4
- num, img_w, img_h = images.shape[0], images.shape[-1], images.shape[-2]
-
- if grid_size is not None:
- grid_w, grid_h = tuple(grid_size)
- else:
- grid_w = max(int(np.ceil(np.sqrt(num))), 1)
- grid_h = max((num - 1) // grid_w + 1, 1)
-
- grid = np.zeros(list(images.shape[1:-2]) + [grid_h * img_h, grid_w * img_w], dtype=images.dtype)
- for idx in range(num):
- x = (idx % grid_w) * img_w
- y = (idx // grid_w) * img_h
- grid[..., y : y + img_h, x : x + img_w] = images[idx]
- return grid
-
-def convert_to_pil_image(image, drange=[0,1]):
- assert image.ndim == 2 or image.ndim == 3
- if image.ndim == 3:
- if image.shape[0] == 1:
- image = image[0] # grayscale CHW => HW
- else:
- image = image.transpose(1, 2, 0) # CHW -> HWC
-
- image = adjust_dynamic_range(image, drange, [0,255])
- image = np.rint(image).clip(0, 255).astype(np.uint8)
- fmt = 'RGB' if image.ndim == 3 else 'L'
- return PIL.Image.fromarray(image, fmt)
-
-def save_image(image, filename, drange=[0,1], quality=95):
- img = convert_to_pil_image(image, drange)
- if '.jpg' in filename:
- img.save(filename,"JPEG", quality=quality, optimize=True)
- else:
- img.save(filename)
-
-def save_image_grid(images, filename, drange=[0,1], grid_size=None):
- convert_to_pil_image(create_image_grid(images, grid_size), drange).save(filename)
-
-#----------------------------------------------------------------------------
-# Locating results.
-
-def locate_run_dir(run_id_or_run_dir):
- if isinstance(run_id_or_run_dir, str):
- if os.path.isdir(run_id_or_run_dir):
- return run_id_or_run_dir
- converted = dnnlib.submission.submit.convert_path(run_id_or_run_dir)
- if os.path.isdir(converted):
- return converted
-
- run_dir_pattern = re.compile('^0*%s-' % str(run_id_or_run_dir))
- for search_dir in ['']:
- full_search_dir = config.result_dir if search_dir == '' else os.path.normpath(os.path.join(config.result_dir, search_dir))
- run_dir = os.path.join(full_search_dir, str(run_id_or_run_dir))
- if os.path.isdir(run_dir):
- return run_dir
- run_dirs = sorted(glob.glob(os.path.join(full_search_dir, '*')))
- run_dirs = [run_dir for run_dir in run_dirs if run_dir_pattern.match(os.path.basename(run_dir))]
- run_dirs = [run_dir for run_dir in run_dirs if os.path.isdir(run_dir)]
- if len(run_dirs) == 1:
- return run_dirs[0]
- raise IOError('Cannot locate result subdir for run', run_id_or_run_dir)
-
-def list_network_pkls(run_id_or_run_dir, include_final=True):
- run_dir = locate_run_dir(run_id_or_run_dir)
- pkls = sorted(glob.glob(os.path.join(run_dir, 'network-*.pkl')))
- if len(pkls) >= 1 and os.path.basename(pkls[0]) == 'network-final.pkl':
- if include_final:
- pkls.append(pkls[0])
- del pkls[0]
- return pkls
-
-def locate_network_pkl(run_id_or_run_dir_or_network_pkl, snapshot_or_network_pkl=None):
- for candidate in [snapshot_or_network_pkl, run_id_or_run_dir_or_network_pkl]:
- if isinstance(candidate, str):
- if os.path.isfile(candidate):
- return candidate
- converted = dnnlib.submission.submit.convert_path(candidate)
- if os.path.isfile(converted):
- return converted
-
- pkls = list_network_pkls(run_id_or_run_dir_or_network_pkl)
- if len(pkls) >= 1 and snapshot_or_network_pkl is None:
- return pkls[-1]
-
- for pkl in pkls:
- try:
- name = os.path.splitext(os.path.basename(pkl))[0]
- number = int(name.split('-')[-1])
- if number == snapshot_or_network_pkl:
- return pkl
- except ValueError: pass
- except IndexError: pass
- raise IOError('Cannot locate network pkl for snapshot', snapshot_or_network_pkl)
-
-def get_id_string_for_network_pkl(network_pkl):
- p = network_pkl.replace('.pkl', '').replace('\\', '/').split('/')
- return '-'.join(p[max(len(p) - 2, 0):])
-
-#----------------------------------------------------------------------------
-# Loading data from previous training runs.
-
-def load_network_pkl(run_id_or_run_dir_or_network_pkl, snapshot_or_network_pkl=None):
- return load_pkl(locate_network_pkl(run_id_or_run_dir_or_network_pkl, snapshot_or_network_pkl))
-
-def parse_config_for_previous_run(run_id):
- run_dir = locate_run_dir(run_id)
-
- # Parse config.txt.
- cfg = defaultdict(dict)
- with open(os.path.join(run_dir, 'config.txt'), 'rt') as f:
- for line in f:
- line = re.sub(r"^{?\s*'(\w+)':\s*{(.*)(},|}})$", r"\1 = {\2}", line.strip())
- if line.startswith('dataset =') or line.startswith('train ='):
- exec(line, cfg, cfg) # pylint: disable=exec-used
-
- # Handle legacy options.
- if 'file_pattern' in cfg['dataset']:
- cfg['dataset']['tfrecord_dir'] = cfg['dataset'].pop('file_pattern').replace('-r??.tfrecords', '')
- if 'mirror_augment' in cfg['dataset']:
- cfg['train']['mirror_augment'] = cfg['dataset'].pop('mirror_augment')
- if 'max_labels' in cfg['dataset']:
- v = cfg['dataset'].pop('max_labels')
- if v is None: v = 0
- if v == 'all': v = 'full'
- cfg['dataset']['max_label_size'] = v
- if 'max_images' in cfg['dataset']:
- cfg['dataset'].pop('max_images')
- return cfg
-
-def load_dataset_for_previous_run(run_id, **kwargs): # => dataset_obj, mirror_augment
- cfg = parse_config_for_previous_run(run_id)
- cfg['dataset'].update(kwargs)
- dataset_obj = dataset.load_dataset(data_dir=config.data_dir, **cfg['dataset'])
- mirror_augment = cfg['train'].get('mirror_augment', False)
- return dataset_obj, mirror_augment
-
-def apply_mirror_augment(minibatch):
- mask = np.random.rand(minibatch.shape[0]) < 0.5
- minibatch = np.array(minibatch)
- minibatch[mask] = minibatch[mask, :, :, ::-1]
- return minibatch
-
-#----------------------------------------------------------------------------
-# Size and contents of the image snapshot grids that are exported
-# periodically during training.
-
-def setup_snapshot_image_grid(G, training_set,
- size = '1080p', # '1080p' = to be viewed on 1080p display, '4k' = to be viewed on 4k display.
- layout = 'random'): # 'random' = grid contents are selected randomly, 'row_per_class' = each row corresponds to one class label.
-
- # Select size.
- gw = 1; gh = 1
- if size == '1080p':
- gw = np.clip(1920 // G.output_shape[3], 3, 32)
- gh = np.clip(1080 // G.output_shape[2], 2, 32)
- if size == '4k':
- gw = np.clip(3840 // G.output_shape[3], 7, 32)
- gh = np.clip(2160 // G.output_shape[2], 4, 32)
-
- # Initialize data arrays.
- reals = np.zeros([gw * gh] + training_set.shape, dtype=training_set.dtype)
- labels = np.zeros([gw * gh, training_set.label_size], dtype=training_set.label_dtype)
- latents = np.random.randn(gw * gh, *G.input_shape[1:])
-
- # Random layout.
- if layout == 'random':
- reals[:], labels[:] = training_set.get_minibatch_np(gw * gh)
-
- # Class-conditional layouts.
- class_layouts = dict(row_per_class=[gw,1], col_per_class=[1,gh], class4x4=[4,4])
- if layout in class_layouts:
- bw, bh = class_layouts[layout]
- nw = (gw - 1) // bw + 1
- nh = (gh - 1) // bh + 1
- blocks = [[] for _i in range(nw * nh)]
- for _iter in range(1000000):
- real, label = training_set.get_minibatch_np(1)
- idx = np.argmax(label[0])
- while idx < len(blocks) and len(blocks[idx]) >= bw * bh:
- idx += training_set.label_size
- if idx < len(blocks):
- blocks[idx].append((real, label))
- if all(len(block) >= bw * bh for block in blocks):
- break
- for i, block in enumerate(blocks):
- for j, (real, label) in enumerate(block):
- x = (i % nw) * bw + j % bw
- y = (i // nw) * bh + j // bw
- if x < gw and y < gh:
- reals[x + y * gw] = real[0]
- labels[x + y * gw] = label[0]
-
- return (gw, gh), reals, labels, latents
-
-#----------------------------------------------------------------------------
diff --git a/spaces/FacundoSander/PdfQA/static/index.html b/spaces/FacundoSander/PdfQA/static/index.html
deleted file mode 100644
index 025b3e6b3e67ce83a2aa5d8a5bb581e74303ca72..0000000000000000000000000000000000000000
--- a/spaces/FacundoSander/PdfQA/static/index.html
+++ /dev/null
@@ -1,76 +0,0 @@
-
-
-
-
-
-
-
- Chat Inteligente
-
-
-
-
-
-
-
-
-
-
-
-
Chat Inteligente
-
Sube un archivo PDF y haz preguntas sobre su contenido. Nuestra IA te responderá.
"
-examples=[['mona1.jpeg','starry.jpeg']]
-iface = gr.Interface(inference, inputs=[gr.inputs.Image(type="file",label='content'),gr.inputs.Image(type="file",label='style')], outputs=gr.outputs.Image(type="pil"),enable_queue=True,title=title,article=article,description=description,examples=examples)
-iface.launch()
\ No newline at end of file
diff --git a/spaces/akshatsanghvi/Rice-Disease-Classifier/README.md b/spaces/akshatsanghvi/Rice-Disease-Classifier/README.md
deleted file mode 100644
index bd1ceafdd499e52fe9a78b22227fabaa72d101e0..0000000000000000000000000000000000000000
--- a/spaces/akshatsanghvi/Rice-Disease-Classifier/README.md
+++ /dev/null
@@ -1,44 +0,0 @@
----
-title: Rice Disease Classifier
-emoji: 🌾
-colorFrom: green
-colorTo: yellow
-sdk: streamlit
-sdk_version: 1.17.0
-app_file: app.py
-license: apache-2.0
----
-
-### Rice Disease Classifier
-
-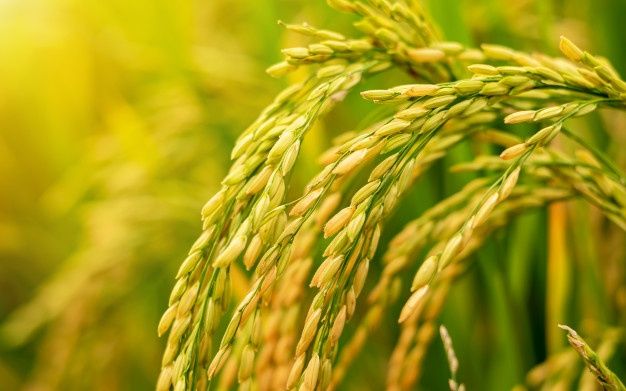
-
-Almost all of the world's food energy intake is satisfied by just a few crop plants. Rice, maize and wheat make up two-thirds of this already small group of foods. These three grains are the staple foods for more than four billion people both as a source of nutrition and income.
-
-Disease damage to rice can greatly reduce yield. They are mainly caused by bacteria, viruses, or fungi. Planting a resistant variety is the simplest and, often, the most cost effective management for diseases.
-
-
-### Types of Rice Disease :
-
-- #### Bacterial Leaf Blight
-- #### Brown Spot
-- #### Leaf Blast
-- #### Leaf Scald
-- #### Narrow Brown Spot
-
-
-### Links :
-- #### [Hugging Face](https://huggingface.co/spaces/akshatsanghvi/Rice-Disease-Classifier)
-- #### [Kaggle](https://www.kaggle.com/datasets/dedeikhsandwisaputra/rice-leafs-disease-dataset)
-- #### [LinkedIn](https://www.linkedin.com/in/akshat-sanghvi-5140a7165/)
-
-
-### Preview :
-
-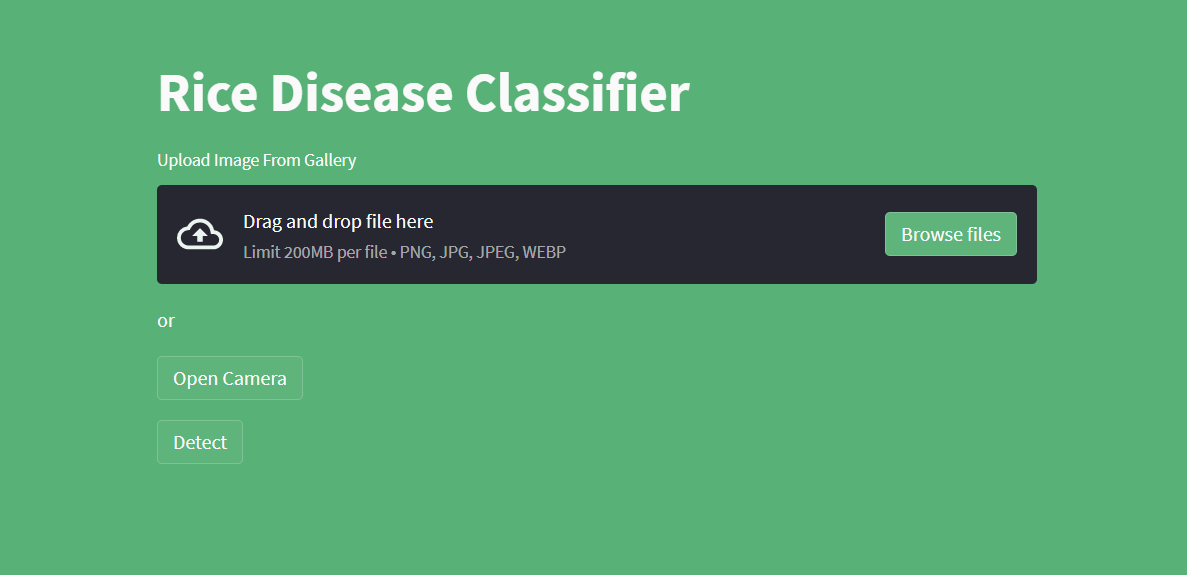
-
-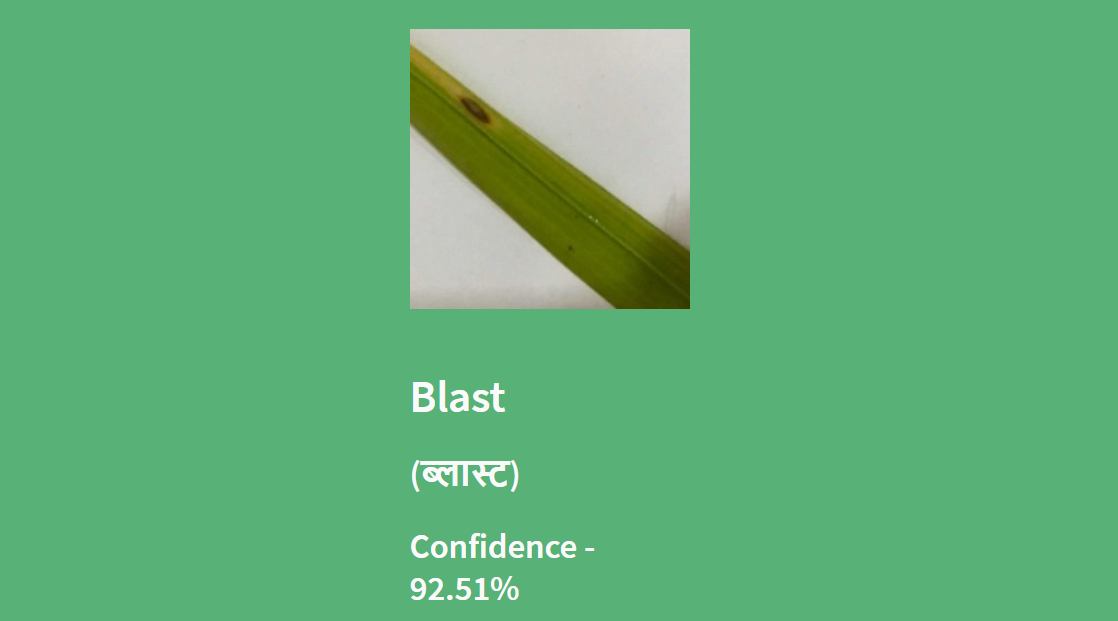
-
-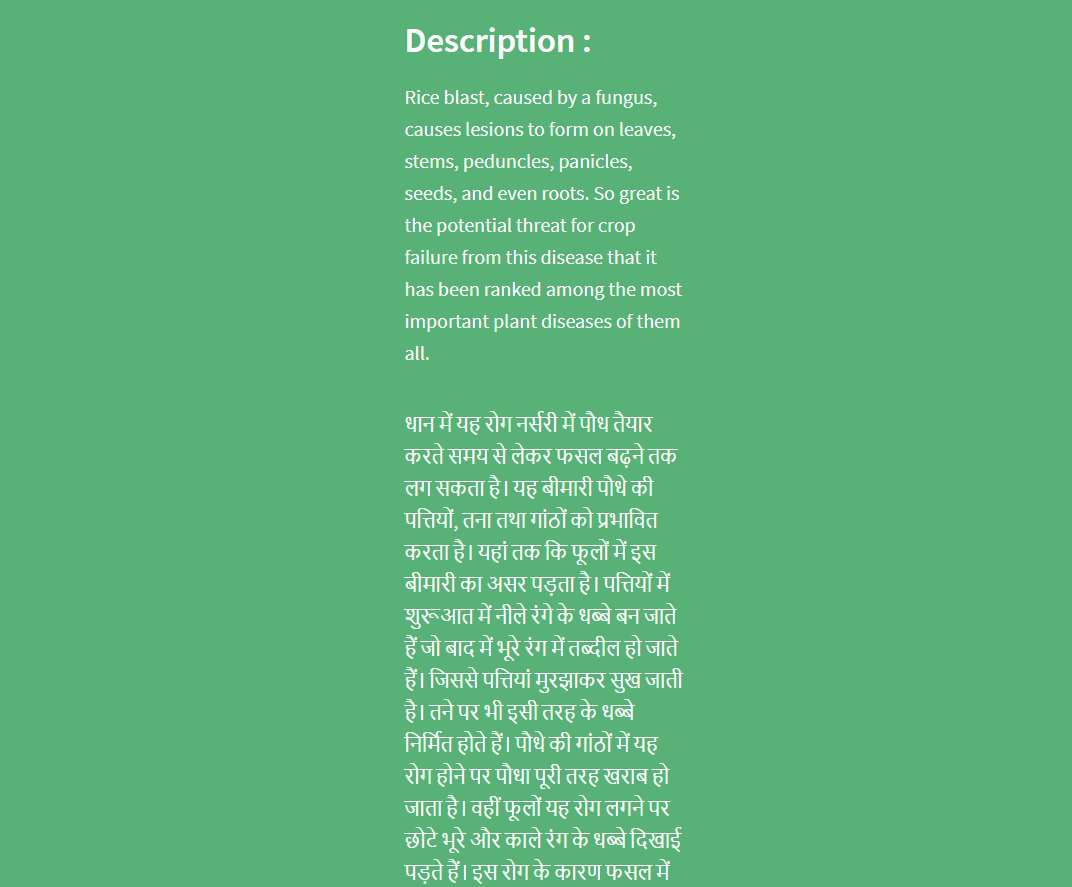
-
-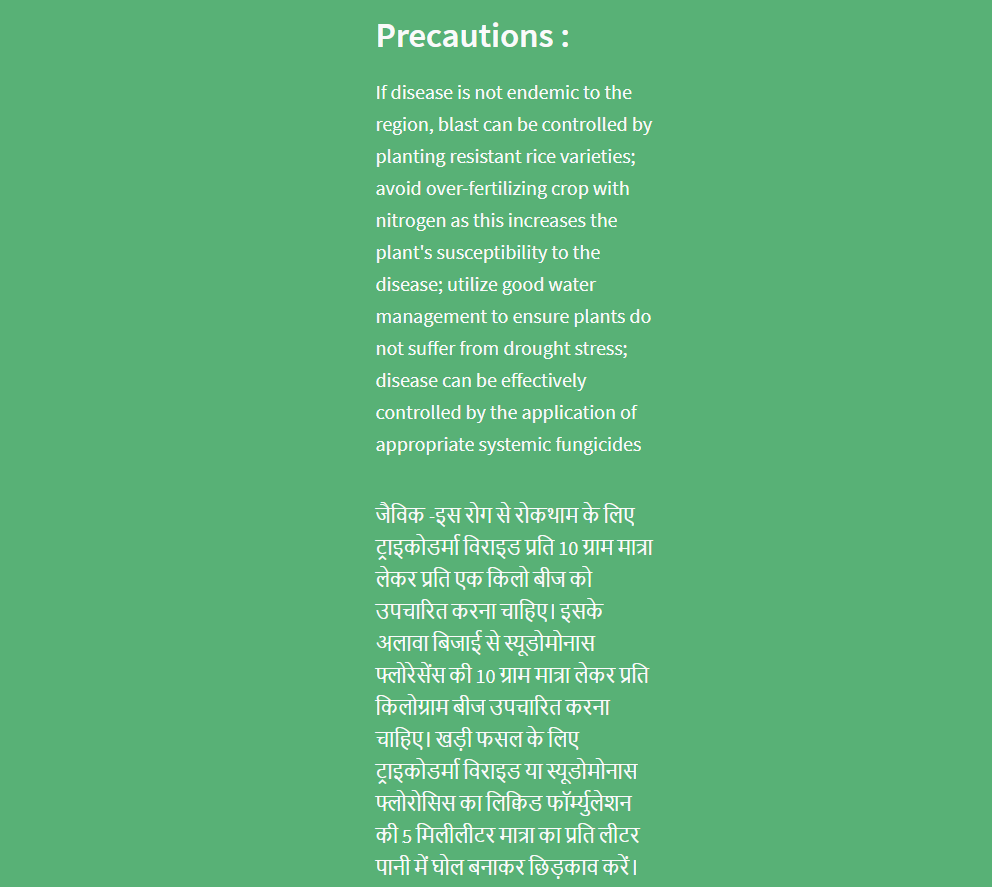
\ No newline at end of file
diff --git a/spaces/alaa-lab/InstructCV/app.py b/spaces/alaa-lab/InstructCV/app.py
deleted file mode 100644
index 7db493a8426911e5687275d63bda310b6b1c8355..0000000000000000000000000000000000000000
--- a/spaces/alaa-lab/InstructCV/app.py
+++ /dev/null
@@ -1,185 +0,0 @@
-# ------------------------------------------------------------------------------
-# Copyright (c) 2023, Alaa lab, UC Berkeley. All rights reserved.
-#
-# Written by Yulu Gan.
-# ------------------------------------------------------------------------------
-
-from __future__ import annotations
-
-import math
-import cv2
-import random
-from fnmatch import fnmatch
-import numpy as np
-
-import gradio as gr
-import torch
-from PIL import Image, ImageOps
-from diffusers import StableDiffusionInstructPix2PixPipeline
-
-title = "InstructCV"
-
-description = """
-
Yulu Gan, Sungwoo Park, Alex Schubert, Anthony Philippakis, Ahmed Alaa
-Project Page | Paper | Code
-
-Data was uploaded manually from the Amazon Web Services (AWS) account used to run the Kaggle competition. We used the Kaggle competition dataset to compare performance between different AI services. First we connected the Amazon Web Services (AWS) account used to run the Kaggle competition to Kaggle Notebooks. We then uploaded our data from Kaggle Notebooks and run our competition code to AWS Notebooks. Next we used the Amazon Machine Learning (Amazon ML) service to run our competition code on the data in Kaggle Notebooks. Finally we used the Amazon Natural Language Understanding (Amazon NLP) service to run the competition code on the data in Kaggle Notebooks. We repeated this process for each service to calculate the total time for each competition.
-
-Step 2
-
-Connect Kaggle Notebooks to Amazon Web Services (AWS)
-
-First we connected the Kaggle competition dataset to Amazon Web Services (AWS). Then we used the Connect Kaggle Notebooks to Amazon Web Services (AWS) API to upload our data to AWS.
-
-Step 3
-
-Import Kaggle Notebooks data into Amazon Web Services (AWS)
-
-First we downloaded the files for the Kaggle competition dataset to our computer. Next we copied the data to Amazon S3, then synced the data to the Amazon Web Services (AWS) account used to run the Kaggle competition.
-
-Step 4
-
-Upload the data for the Kaggle competition dataset to Amazon Web Services (AWS)
-
-First we uploaded the data from our computer to the Amazon S3 service in the Kaggle competition dataset. Next we configured the Amazon S3 bucket used for the Kaggle competition. Finally we synced the data to the Amazon Web Services (AWS) account used to run the Kaggle competition.
-
-Step 5
-
-Import the data for the Kaggle competition dataset to Amazon Machine Learning (Amazon ML) service
-
-First we connected the Amazon Web Services (AWS) account used to run the Kaggle competition to the Amazon Machine Learning (Amazon ML) service. Then we ran our code to compete in the Kaggle competition.
-
-Step 6
-
-Import the data for the Kaggle competition dataset to Amazon Natural Language Understanding (Amazon NLP) service
-
-First we connected the Amazon Web Services (AWS) account used to run the Kaggle competition to the Amazon Natural Language 4fefd39f24
-
-
-
diff --git a/spaces/bioriAsaeru/text-to-voice/FSX PHOTOREAL SCENERY KOLKATA VECC ((TOP)).md b/spaces/bioriAsaeru/text-to-voice/FSX PHOTOREAL SCENERY KOLKATA VECC ((TOP)).md
deleted file mode 100644
index bf08631b0ae991f2234c48fc567ae469342ee8fa..0000000000000000000000000000000000000000
--- a/spaces/bioriAsaeru/text-to-voice/FSX PHOTOREAL SCENERY KOLKATA VECC ((TOP)).md
+++ /dev/null
@@ -1,6 +0,0 @@
-
-
- aaccfb2cb3
-
-
-
diff --git a/spaces/brayden-gg/decoupled-style-descriptors/__init__.py b/spaces/brayden-gg/decoupled-style-descriptors/__init__.py
deleted file mode 100644
index e69de29bb2d1d6434b8b29ae775ad8c2e48c5391..0000000000000000000000000000000000000000
diff --git a/spaces/brjathu/HMR2.0/vendor/detectron2/detectron2/engine/defaults.py b/spaces/brjathu/HMR2.0/vendor/detectron2/detectron2/engine/defaults.py
deleted file mode 100644
index 5b9525745565479709730cbb5b7dc9cd8afd4707..0000000000000000000000000000000000000000
--- a/spaces/brjathu/HMR2.0/vendor/detectron2/detectron2/engine/defaults.py
+++ /dev/null
@@ -1,715 +0,0 @@
-# -*- coding: utf-8 -*-
-# Copyright (c) Facebook, Inc. and its affiliates.
-
-"""
-This file contains components with some default boilerplate logic user may need
-in training / testing. They will not work for everyone, but many users may find them useful.
-
-The behavior of functions/classes in this file is subject to change,
-since they are meant to represent the "common default behavior" people need in their projects.
-"""
-
-import argparse
-import logging
-import os
-import sys
-import weakref
-from collections import OrderedDict
-from typing import Optional
-import torch
-from fvcore.nn.precise_bn import get_bn_modules
-from omegaconf import OmegaConf
-from torch.nn.parallel import DistributedDataParallel
-
-import detectron2.data.transforms as T
-from detectron2.checkpoint import DetectionCheckpointer
-from detectron2.config import CfgNode, LazyConfig
-from detectron2.data import (
- MetadataCatalog,
- build_detection_test_loader,
- build_detection_train_loader,
-)
-from detectron2.evaluation import (
- DatasetEvaluator,
- inference_on_dataset,
- print_csv_format,
- verify_results,
-)
-from detectron2.modeling import build_model
-from detectron2.solver import build_lr_scheduler, build_optimizer
-from detectron2.utils import comm
-from detectron2.utils.collect_env import collect_env_info
-from detectron2.utils.env import seed_all_rng
-from detectron2.utils.events import CommonMetricPrinter, JSONWriter, TensorboardXWriter
-from detectron2.utils.file_io import PathManager
-from detectron2.utils.logger import setup_logger
-
-from . import hooks
-from .train_loop import AMPTrainer, SimpleTrainer, TrainerBase
-
-__all__ = [
- "create_ddp_model",
- "default_argument_parser",
- "default_setup",
- "default_writers",
- "DefaultPredictor",
- "DefaultTrainer",
-]
-
-
-def create_ddp_model(model, *, fp16_compression=False, **kwargs):
- """
- Create a DistributedDataParallel model if there are >1 processes.
-
- Args:
- model: a torch.nn.Module
- fp16_compression: add fp16 compression hooks to the ddp object.
- See more at https://pytorch.org/docs/stable/ddp_comm_hooks.html#torch.distributed.algorithms.ddp_comm_hooks.default_hooks.fp16_compress_hook
- kwargs: other arguments of :module:`torch.nn.parallel.DistributedDataParallel`.
- """ # noqa
- if comm.get_world_size() == 1:
- return model
- if "device_ids" not in kwargs:
- kwargs["device_ids"] = [comm.get_local_rank()]
- ddp = DistributedDataParallel(model, **kwargs)
- if fp16_compression:
- from torch.distributed.algorithms.ddp_comm_hooks import default as comm_hooks
-
- ddp.register_comm_hook(state=None, hook=comm_hooks.fp16_compress_hook)
- return ddp
-
-
-def default_argument_parser(epilog=None):
- """
- Create a parser with some common arguments used by detectron2 users.
-
- Args:
- epilog (str): epilog passed to ArgumentParser describing the usage.
-
- Returns:
- argparse.ArgumentParser:
- """
- parser = argparse.ArgumentParser(
- epilog=epilog
- or f"""
-Examples:
-
-Run on single machine:
- $ {sys.argv[0]} --num-gpus 8 --config-file cfg.yaml
-
-Change some config options:
- $ {sys.argv[0]} --config-file cfg.yaml MODEL.WEIGHTS /path/to/weight.pth SOLVER.BASE_LR 0.001
-
-Run on multiple machines:
- (machine0)$ {sys.argv[0]} --machine-rank 0 --num-machines 2 --dist-url [--other-flags]
- (machine1)$ {sys.argv[0]} --machine-rank 1 --num-machines 2 --dist-url [--other-flags]
-""",
- formatter_class=argparse.RawDescriptionHelpFormatter,
- )
- parser.add_argument("--config-file", default="", metavar="FILE", help="path to config file")
- parser.add_argument(
- "--resume",
- action="store_true",
- help="Whether to attempt to resume from the checkpoint directory. "
- "See documentation of `DefaultTrainer.resume_or_load()` for what it means.",
- )
- parser.add_argument("--eval-only", action="store_true", help="perform evaluation only")
- parser.add_argument("--num-gpus", type=int, default=1, help="number of gpus *per machine*")
- parser.add_argument("--num-machines", type=int, default=1, help="total number of machines")
- parser.add_argument(
- "--machine-rank", type=int, default=0, help="the rank of this machine (unique per machine)"
- )
-
- # PyTorch still may leave orphan processes in multi-gpu training.
- # Therefore we use a deterministic way to obtain port,
- # so that users are aware of orphan processes by seeing the port occupied.
- port = 2**15 + 2**14 + hash(os.getuid() if sys.platform != "win32" else 1) % 2**14
- parser.add_argument(
- "--dist-url",
- default="tcp://127.0.0.1:{}".format(port),
- help="initialization URL for pytorch distributed backend. See "
- "https://pytorch.org/docs/stable/distributed.html for details.",
- )
- parser.add_argument(
- "opts",
- help="""
-Modify config options at the end of the command. For Yacs configs, use
-space-separated "PATH.KEY VALUE" pairs.
-For python-based LazyConfig, use "path.key=value".
- """.strip(),
- default=None,
- nargs=argparse.REMAINDER,
- )
- return parser
-
-
-def _try_get_key(cfg, *keys, default=None):
- """
- Try select keys from cfg until the first key that exists. Otherwise return default.
- """
- if isinstance(cfg, CfgNode):
- cfg = OmegaConf.create(cfg.dump())
- for k in keys:
- none = object()
- p = OmegaConf.select(cfg, k, default=none)
- if p is not none:
- return p
- return default
-
-
-def _highlight(code, filename):
- try:
- import pygments
- except ImportError:
- return code
-
- from pygments.lexers import Python3Lexer, YamlLexer
- from pygments.formatters import Terminal256Formatter
-
- lexer = Python3Lexer() if filename.endswith(".py") else YamlLexer()
- code = pygments.highlight(code, lexer, Terminal256Formatter(style="monokai"))
- return code
-
-
-def default_setup(cfg, args):
- """
- Perform some basic common setups at the beginning of a job, including:
-
- 1. Set up the detectron2 logger
- 2. Log basic information about environment, cmdline arguments, and config
- 3. Backup the config to the output directory
-
- Args:
- cfg (CfgNode or omegaconf.DictConfig): the full config to be used
- args (argparse.NameSpace): the command line arguments to be logged
- """
- output_dir = _try_get_key(cfg, "OUTPUT_DIR", "output_dir", "train.output_dir")
- if comm.is_main_process() and output_dir:
- PathManager.mkdirs(output_dir)
-
- rank = comm.get_rank()
- setup_logger(output_dir, distributed_rank=rank, name="fvcore")
- logger = setup_logger(output_dir, distributed_rank=rank)
-
- logger.info("Rank of current process: {}. World size: {}".format(rank, comm.get_world_size()))
- logger.info("Environment info:\n" + collect_env_info())
-
- logger.info("Command line arguments: " + str(args))
- if hasattr(args, "config_file") and args.config_file != "":
- logger.info(
- "Contents of args.config_file={}:\n{}".format(
- args.config_file,
- _highlight(PathManager.open(args.config_file, "r").read(), args.config_file),
- )
- )
-
- if comm.is_main_process() and output_dir:
- # Note: some of our scripts may expect the existence of
- # config.yaml in output directory
- path = os.path.join(output_dir, "config.yaml")
- if isinstance(cfg, CfgNode):
- logger.info("Running with full config:\n{}".format(_highlight(cfg.dump(), ".yaml")))
- with PathManager.open(path, "w") as f:
- f.write(cfg.dump())
- else:
- LazyConfig.save(cfg, path)
- logger.info("Full config saved to {}".format(path))
-
- # make sure each worker has a different, yet deterministic seed if specified
- seed = _try_get_key(cfg, "SEED", "train.seed", default=-1)
- seed_all_rng(None if seed < 0 else seed + rank)
-
- # cudnn benchmark has large overhead. It shouldn't be used considering the small size of
- # typical validation set.
- if not (hasattr(args, "eval_only") and args.eval_only):
- torch.backends.cudnn.benchmark = _try_get_key(
- cfg, "CUDNN_BENCHMARK", "train.cudnn_benchmark", default=False
- )
-
-
-def default_writers(output_dir: str, max_iter: Optional[int] = None):
- """
- Build a list of :class:`EventWriter` to be used.
- It now consists of a :class:`CommonMetricPrinter`,
- :class:`TensorboardXWriter` and :class:`JSONWriter`.
-
- Args:
- output_dir: directory to store JSON metrics and tensorboard events
- max_iter: the total number of iterations
-
- Returns:
- list[EventWriter]: a list of :class:`EventWriter` objects.
- """
- PathManager.mkdirs(output_dir)
- return [
- # It may not always print what you want to see, since it prints "common" metrics only.
- CommonMetricPrinter(max_iter),
- JSONWriter(os.path.join(output_dir, "metrics.json")),
- TensorboardXWriter(output_dir),
- ]
-
-
-class DefaultPredictor:
- """
- Create a simple end-to-end predictor with the given config that runs on
- single device for a single input image.
-
- Compared to using the model directly, this class does the following additions:
-
- 1. Load checkpoint from `cfg.MODEL.WEIGHTS`.
- 2. Always take BGR image as the input and apply conversion defined by `cfg.INPUT.FORMAT`.
- 3. Apply resizing defined by `cfg.INPUT.{MIN,MAX}_SIZE_TEST`.
- 4. Take one input image and produce a single output, instead of a batch.
-
- This is meant for simple demo purposes, so it does the above steps automatically.
- This is not meant for benchmarks or running complicated inference logic.
- If you'd like to do anything more complicated, please refer to its source code as
- examples to build and use the model manually.
-
- Attributes:
- metadata (Metadata): the metadata of the underlying dataset, obtained from
- cfg.DATASETS.TEST.
-
- Examples:
- ::
- pred = DefaultPredictor(cfg)
- inputs = cv2.imread("input.jpg")
- outputs = pred(inputs)
- """
-
- def __init__(self, cfg):
- self.cfg = cfg.clone() # cfg can be modified by model
- self.model = build_model(self.cfg)
- self.model.eval()
- if len(cfg.DATASETS.TEST):
- self.metadata = MetadataCatalog.get(cfg.DATASETS.TEST[0])
-
- checkpointer = DetectionCheckpointer(self.model)
- checkpointer.load(cfg.MODEL.WEIGHTS)
-
- self.aug = T.ResizeShortestEdge(
- [cfg.INPUT.MIN_SIZE_TEST, cfg.INPUT.MIN_SIZE_TEST], cfg.INPUT.MAX_SIZE_TEST
- )
-
- self.input_format = cfg.INPUT.FORMAT
- assert self.input_format in ["RGB", "BGR"], self.input_format
-
- def __call__(self, original_image):
- """
- Args:
- original_image (np.ndarray): an image of shape (H, W, C) (in BGR order).
-
- Returns:
- predictions (dict):
- the output of the model for one image only.
- See :doc:`/tutorials/models` for details about the format.
- """
- with torch.no_grad(): # https://github.com/sphinx-doc/sphinx/issues/4258
- # Apply pre-processing to image.
- if self.input_format == "RGB":
- # whether the model expects BGR inputs or RGB
- original_image = original_image[:, :, ::-1]
- height, width = original_image.shape[:2]
- image = self.aug.get_transform(original_image).apply_image(original_image)
- image = torch.as_tensor(image.astype("float32").transpose(2, 0, 1))
-
- inputs = {"image": image, "height": height, "width": width}
- predictions = self.model([inputs])[0]
- return predictions
-
-
-class DefaultTrainer(TrainerBase):
- """
- A trainer with default training logic. It does the following:
-
- 1. Create a :class:`SimpleTrainer` using model, optimizer, dataloader
- defined by the given config. Create a LR scheduler defined by the config.
- 2. Load the last checkpoint or `cfg.MODEL.WEIGHTS`, if exists, when
- `resume_or_load` is called.
- 3. Register a few common hooks defined by the config.
-
- It is created to simplify the **standard model training workflow** and reduce code boilerplate
- for users who only need the standard training workflow, with standard features.
- It means this class makes *many assumptions* about your training logic that
- may easily become invalid in a new research. In fact, any assumptions beyond those made in the
- :class:`SimpleTrainer` are too much for research.
-
- The code of this class has been annotated about restrictive assumptions it makes.
- When they do not work for you, you're encouraged to:
-
- 1. Overwrite methods of this class, OR:
- 2. Use :class:`SimpleTrainer`, which only does minimal SGD training and
- nothing else. You can then add your own hooks if needed. OR:
- 3. Write your own training loop similar to `tools/plain_train_net.py`.
-
- See the :doc:`/tutorials/training` tutorials for more details.
-
- Note that the behavior of this class, like other functions/classes in
- this file, is not stable, since it is meant to represent the "common default behavior".
- It is only guaranteed to work well with the standard models and training workflow in detectron2.
- To obtain more stable behavior, write your own training logic with other public APIs.
-
- Examples:
- ::
- trainer = DefaultTrainer(cfg)
- trainer.resume_or_load() # load last checkpoint or MODEL.WEIGHTS
- trainer.train()
-
- Attributes:
- scheduler:
- checkpointer (DetectionCheckpointer):
- cfg (CfgNode):
- """
-
- def __init__(self, cfg):
- """
- Args:
- cfg (CfgNode):
- """
- super().__init__()
- logger = logging.getLogger("detectron2")
- if not logger.isEnabledFor(logging.INFO): # setup_logger is not called for d2
- setup_logger()
- cfg = DefaultTrainer.auto_scale_workers(cfg, comm.get_world_size())
-
- # Assume these objects must be constructed in this order.
- model = self.build_model(cfg)
- optimizer = self.build_optimizer(cfg, model)
- data_loader = self.build_train_loader(cfg)
-
- model = create_ddp_model(model, broadcast_buffers=False)
- self._trainer = (AMPTrainer if cfg.SOLVER.AMP.ENABLED else SimpleTrainer)(
- model, data_loader, optimizer
- )
-
- self.scheduler = self.build_lr_scheduler(cfg, optimizer)
- self.checkpointer = DetectionCheckpointer(
- # Assume you want to save checkpoints together with logs/statistics
- model,
- cfg.OUTPUT_DIR,
- trainer=weakref.proxy(self),
- )
- self.start_iter = 0
- self.max_iter = cfg.SOLVER.MAX_ITER
- self.cfg = cfg
-
- self.register_hooks(self.build_hooks())
-
- def resume_or_load(self, resume=True):
- """
- If `resume==True` and `cfg.OUTPUT_DIR` contains the last checkpoint (defined by
- a `last_checkpoint` file), resume from the file. Resuming means loading all
- available states (eg. optimizer and scheduler) and update iteration counter
- from the checkpoint. ``cfg.MODEL.WEIGHTS`` will not be used.
-
- Otherwise, this is considered as an independent training. The method will load model
- weights from the file `cfg.MODEL.WEIGHTS` (but will not load other states) and start
- from iteration 0.
-
- Args:
- resume (bool): whether to do resume or not
- """
- self.checkpointer.resume_or_load(self.cfg.MODEL.WEIGHTS, resume=resume)
- if resume and self.checkpointer.has_checkpoint():
- # The checkpoint stores the training iteration that just finished, thus we start
- # at the next iteration
- self.start_iter = self.iter + 1
-
- def build_hooks(self):
- """
- Build a list of default hooks, including timing, evaluation,
- checkpointing, lr scheduling, precise BN, writing events.
-
- Returns:
- list[HookBase]:
- """
- cfg = self.cfg.clone()
- cfg.defrost()
- cfg.DATALOADER.NUM_WORKERS = 0 # save some memory and time for PreciseBN
-
- ret = [
- hooks.IterationTimer(),
- hooks.LRScheduler(),
- hooks.PreciseBN(
- # Run at the same freq as (but before) evaluation.
- cfg.TEST.EVAL_PERIOD,
- self.model,
- # Build a new data loader to not affect training
- self.build_train_loader(cfg),
- cfg.TEST.PRECISE_BN.NUM_ITER,
- )
- if cfg.TEST.PRECISE_BN.ENABLED and get_bn_modules(self.model)
- else None,
- ]
-
- # Do PreciseBN before checkpointer, because it updates the model and need to
- # be saved by checkpointer.
- # This is not always the best: if checkpointing has a different frequency,
- # some checkpoints may have more precise statistics than others.
- if comm.is_main_process():
- ret.append(hooks.PeriodicCheckpointer(self.checkpointer, cfg.SOLVER.CHECKPOINT_PERIOD))
-
- def test_and_save_results():
- self._last_eval_results = self.test(self.cfg, self.model)
- return self._last_eval_results
-
- # Do evaluation after checkpointer, because then if it fails,
- # we can use the saved checkpoint to debug.
- ret.append(hooks.EvalHook(cfg.TEST.EVAL_PERIOD, test_and_save_results))
-
- if comm.is_main_process():
- # Here the default print/log frequency of each writer is used.
- # run writers in the end, so that evaluation metrics are written
- ret.append(hooks.PeriodicWriter(self.build_writers(), period=20))
- return ret
-
- def build_writers(self):
- """
- Build a list of writers to be used using :func:`default_writers()`.
- If you'd like a different list of writers, you can overwrite it in
- your trainer.
-
- Returns:
- list[EventWriter]: a list of :class:`EventWriter` objects.
- """
- return default_writers(self.cfg.OUTPUT_DIR, self.max_iter)
-
- def train(self):
- """
- Run training.
-
- Returns:
- OrderedDict of results, if evaluation is enabled. Otherwise None.
- """
- super().train(self.start_iter, self.max_iter)
- if len(self.cfg.TEST.EXPECTED_RESULTS) and comm.is_main_process():
- assert hasattr(
- self, "_last_eval_results"
- ), "No evaluation results obtained during training!"
- verify_results(self.cfg, self._last_eval_results)
- return self._last_eval_results
-
- def run_step(self):
- self._trainer.iter = self.iter
- self._trainer.run_step()
-
- def state_dict(self):
- ret = super().state_dict()
- ret["_trainer"] = self._trainer.state_dict()
- return ret
-
- def load_state_dict(self, state_dict):
- super().load_state_dict(state_dict)
- self._trainer.load_state_dict(state_dict["_trainer"])
-
- @classmethod
- def build_model(cls, cfg):
- """
- Returns:
- torch.nn.Module:
-
- It now calls :func:`detectron2.modeling.build_model`.
- Overwrite it if you'd like a different model.
- """
- model = build_model(cfg)
- logger = logging.getLogger(__name__)
- logger.info("Model:\n{}".format(model))
- return model
-
- @classmethod
- def build_optimizer(cls, cfg, model):
- """
- Returns:
- torch.optim.Optimizer:
-
- It now calls :func:`detectron2.solver.build_optimizer`.
- Overwrite it if you'd like a different optimizer.
- """
- return build_optimizer(cfg, model)
-
- @classmethod
- def build_lr_scheduler(cls, cfg, optimizer):
- """
- It now calls :func:`detectron2.solver.build_lr_scheduler`.
- Overwrite it if you'd like a different scheduler.
- """
- return build_lr_scheduler(cfg, optimizer)
-
- @classmethod
- def build_train_loader(cls, cfg):
- """
- Returns:
- iterable
-
- It now calls :func:`detectron2.data.build_detection_train_loader`.
- Overwrite it if you'd like a different data loader.
- """
- return build_detection_train_loader(cfg)
-
- @classmethod
- def build_test_loader(cls, cfg, dataset_name):
- """
- Returns:
- iterable
-
- It now calls :func:`detectron2.data.build_detection_test_loader`.
- Overwrite it if you'd like a different data loader.
- """
- return build_detection_test_loader(cfg, dataset_name)
-
- @classmethod
- def build_evaluator(cls, cfg, dataset_name):
- """
- Returns:
- DatasetEvaluator or None
-
- It is not implemented by default.
- """
- raise NotImplementedError(
- """
-If you want DefaultTrainer to automatically run evaluation,
-please implement `build_evaluator()` in subclasses (see train_net.py for example).
-Alternatively, you can call evaluation functions yourself (see Colab balloon tutorial for example).
-"""
- )
-
- @classmethod
- def test(cls, cfg, model, evaluators=None):
- """
- Evaluate the given model. The given model is expected to already contain
- weights to evaluate.
-
- Args:
- cfg (CfgNode):
- model (nn.Module):
- evaluators (list[DatasetEvaluator] or None): if None, will call
- :meth:`build_evaluator`. Otherwise, must have the same length as
- ``cfg.DATASETS.TEST``.
-
- Returns:
- dict: a dict of result metrics
- """
- logger = logging.getLogger(__name__)
- if isinstance(evaluators, DatasetEvaluator):
- evaluators = [evaluators]
- if evaluators is not None:
- assert len(cfg.DATASETS.TEST) == len(evaluators), "{} != {}".format(
- len(cfg.DATASETS.TEST), len(evaluators)
- )
-
- results = OrderedDict()
- for idx, dataset_name in enumerate(cfg.DATASETS.TEST):
- data_loader = cls.build_test_loader(cfg, dataset_name)
- # When evaluators are passed in as arguments,
- # implicitly assume that evaluators can be created before data_loader.
- if evaluators is not None:
- evaluator = evaluators[idx]
- else:
- try:
- evaluator = cls.build_evaluator(cfg, dataset_name)
- except NotImplementedError:
- logger.warn(
- "No evaluator found. Use `DefaultTrainer.test(evaluators=)`, "
- "or implement its `build_evaluator` method."
- )
- results[dataset_name] = {}
- continue
- results_i = inference_on_dataset(model, data_loader, evaluator)
- results[dataset_name] = results_i
- if comm.is_main_process():
- assert isinstance(
- results_i, dict
- ), "Evaluator must return a dict on the main process. Got {} instead.".format(
- results_i
- )
- logger.info("Evaluation results for {} in csv format:".format(dataset_name))
- print_csv_format(results_i)
-
- if len(results) == 1:
- results = list(results.values())[0]
- return results
-
- @staticmethod
- def auto_scale_workers(cfg, num_workers: int):
- """
- When the config is defined for certain number of workers (according to
- ``cfg.SOLVER.REFERENCE_WORLD_SIZE``) that's different from the number of
- workers currently in use, returns a new cfg where the total batch size
- is scaled so that the per-GPU batch size stays the same as the
- original ``IMS_PER_BATCH // REFERENCE_WORLD_SIZE``.
-
- Other config options are also scaled accordingly:
- * training steps and warmup steps are scaled inverse proportionally.
- * learning rate are scaled proportionally, following :paper:`ImageNet in 1h`.
-
- For example, with the original config like the following:
-
- .. code-block:: yaml
-
- IMS_PER_BATCH: 16
- BASE_LR: 0.1
- REFERENCE_WORLD_SIZE: 8
- MAX_ITER: 5000
- STEPS: (4000,)
- CHECKPOINT_PERIOD: 1000
-
- When this config is used on 16 GPUs instead of the reference number 8,
- calling this method will return a new config with:
-
- .. code-block:: yaml
-
- IMS_PER_BATCH: 32
- BASE_LR: 0.2
- REFERENCE_WORLD_SIZE: 16
- MAX_ITER: 2500
- STEPS: (2000,)
- CHECKPOINT_PERIOD: 500
-
- Note that both the original config and this new config can be trained on 16 GPUs.
- It's up to user whether to enable this feature (by setting ``REFERENCE_WORLD_SIZE``).
-
- Returns:
- CfgNode: a new config. Same as original if ``cfg.SOLVER.REFERENCE_WORLD_SIZE==0``.
- """
- old_world_size = cfg.SOLVER.REFERENCE_WORLD_SIZE
- if old_world_size == 0 or old_world_size == num_workers:
- return cfg
- cfg = cfg.clone()
- frozen = cfg.is_frozen()
- cfg.defrost()
-
- assert (
- cfg.SOLVER.IMS_PER_BATCH % old_world_size == 0
- ), "Invalid REFERENCE_WORLD_SIZE in config!"
- scale = num_workers / old_world_size
- bs = cfg.SOLVER.IMS_PER_BATCH = int(round(cfg.SOLVER.IMS_PER_BATCH * scale))
- lr = cfg.SOLVER.BASE_LR = cfg.SOLVER.BASE_LR * scale
- max_iter = cfg.SOLVER.MAX_ITER = int(round(cfg.SOLVER.MAX_ITER / scale))
- warmup_iter = cfg.SOLVER.WARMUP_ITERS = int(round(cfg.SOLVER.WARMUP_ITERS / scale))
- cfg.SOLVER.STEPS = tuple(int(round(s / scale)) for s in cfg.SOLVER.STEPS)
- cfg.TEST.EVAL_PERIOD = int(round(cfg.TEST.EVAL_PERIOD / scale))
- cfg.SOLVER.CHECKPOINT_PERIOD = int(round(cfg.SOLVER.CHECKPOINT_PERIOD / scale))
- cfg.SOLVER.REFERENCE_WORLD_SIZE = num_workers # maintain invariant
- logger = logging.getLogger(__name__)
- logger.info(
- f"Auto-scaling the config to batch_size={bs}, learning_rate={lr}, "
- f"max_iter={max_iter}, warmup={warmup_iter}."
- )
-
- if frozen:
- cfg.freeze()
- return cfg
-
-
-# Access basic attributes from the underlying trainer
-for _attr in ["model", "data_loader", "optimizer"]:
- setattr(
- DefaultTrainer,
- _attr,
- property(
- # getter
- lambda self, x=_attr: getattr(self._trainer, x),
- # setter
- lambda self, value, x=_attr: setattr(self._trainer, x, value),
- ),
- )
diff --git a/spaces/brjathu/HMR2.0/vendor/detectron2/detectron2/layers/rotated_boxes.py b/spaces/brjathu/HMR2.0/vendor/detectron2/detectron2/layers/rotated_boxes.py
deleted file mode 100644
index 03f73b3bb99275931a887ad9b2d8c0ac9f412bf3..0000000000000000000000000000000000000000
--- a/spaces/brjathu/HMR2.0/vendor/detectron2/detectron2/layers/rotated_boxes.py
+++ /dev/null
@@ -1,21 +0,0 @@
-# Copyright (c) Facebook, Inc. and its affiliates.
-from __future__ import absolute_import, division, print_function, unicode_literals
-import torch
-
-
-def pairwise_iou_rotated(boxes1, boxes2):
- """
- Return intersection-over-union (Jaccard index) of boxes.
-
- Both sets of boxes are expected to be in
- (x_center, y_center, width, height, angle) format.
-
- Arguments:
- boxes1 (Tensor[N, 5])
- boxes2 (Tensor[M, 5])
-
- Returns:
- iou (Tensor[N, M]): the NxM matrix containing the pairwise
- IoU values for every element in boxes1 and boxes2
- """
- return torch.ops.detectron2.box_iou_rotated(boxes1, boxes2)
diff --git a/spaces/brjathu/HMR2.0/vendor/detectron2/projects/DensePose/densepose/modeling/losses/soft_embed.py b/spaces/brjathu/HMR2.0/vendor/detectron2/projects/DensePose/densepose/modeling/losses/soft_embed.py
deleted file mode 100644
index 176d929f4adfa06164dd1ce1668b6d6743cc0983..0000000000000000000000000000000000000000
--- a/spaces/brjathu/HMR2.0/vendor/detectron2/projects/DensePose/densepose/modeling/losses/soft_embed.py
+++ /dev/null
@@ -1,141 +0,0 @@
-# Copyright (c) Facebook, Inc. and its affiliates. All Rights Reserved
-
-from typing import Any, Dict, List
-import torch
-from torch import nn
-from torch.nn import functional as F
-
-from detectron2.config import CfgNode
-from detectron2.structures import Instances
-
-from densepose.data.meshes.catalog import MeshCatalog
-from densepose.modeling.cse.utils import normalize_embeddings, squared_euclidean_distance_matrix
-from densepose.structures.mesh import create_mesh
-
-from .embed_utils import PackedCseAnnotations
-from .utils import BilinearInterpolationHelper
-
-
-class SoftEmbeddingLoss:
- """
- Computes losses for estimated embeddings given annotated vertices.
- Instances in a minibatch that correspond to the same mesh are grouped
- together. For each group, loss is computed as cross-entropy for
- unnormalized scores given ground truth mesh vertex ids.
- Scores are based on:
- 1) squared distances between estimated vertex embeddings
- and mesh vertex embeddings;
- 2) geodesic distances between vertices of a mesh
- """
-
- def __init__(self, cfg: CfgNode):
- """
- Initialize embedding loss from config
- """
- self.embdist_gauss_sigma = cfg.MODEL.ROI_DENSEPOSE_HEAD.CSE.EMBEDDING_DIST_GAUSS_SIGMA
- self.geodist_gauss_sigma = cfg.MODEL.ROI_DENSEPOSE_HEAD.CSE.GEODESIC_DIST_GAUSS_SIGMA
-
- def __call__(
- self,
- proposals_with_gt: List[Instances],
- densepose_predictor_outputs: Any,
- packed_annotations: PackedCseAnnotations,
- interpolator: BilinearInterpolationHelper,
- embedder: nn.Module,
- ) -> Dict[int, torch.Tensor]:
- """
- Produces losses for estimated embeddings given annotated vertices.
- Embeddings for all the vertices of a mesh are computed by the embedder.
- Embeddings for observed pixels are estimated by a predictor.
- Losses are computed as cross-entropy for unnormalized scores given
- ground truth vertex IDs.
- 1) squared distances between estimated vertex embeddings
- and mesh vertex embeddings;
- 2) geodesic distances between vertices of a mesh
-
- Args:
- proposals_with_gt (list of Instances): detections with associated
- ground truth data; each item corresponds to instances detected
- on 1 image; the number of items corresponds to the number of
- images in a batch
- densepose_predictor_outputs: an object of a dataclass that contains predictor
- outputs with estimated values; assumed to have the following attributes:
- * embedding - embedding estimates, tensor of shape [N, D, S, S], where
- N = number of instances (= sum N_i, where N_i is the number of
- instances on image i)
- D = embedding space dimensionality (MODEL.ROI_DENSEPOSE_HEAD.CSE.EMBED_SIZE)
- S = output size (width and height)
- packed_annotations (PackedCseAnnotations): contains various data useful
- for loss computation, each data is packed into a single tensor
- interpolator (BilinearInterpolationHelper): bilinear interpolation helper
- embedder (nn.Module): module that computes vertex embeddings for different meshes
- Return:
- dict(int -> tensor): losses for different mesh IDs
- """
- losses = {}
- for mesh_id_tensor in packed_annotations.vertex_mesh_ids_gt.unique():
- mesh_id = mesh_id_tensor.item()
- mesh_name = MeshCatalog.get_mesh_name(mesh_id)
- # valid points are those that fall into estimated bbox
- # and correspond to the current mesh
- j_valid = interpolator.j_valid * ( # pyre-ignore[16]
- packed_annotations.vertex_mesh_ids_gt == mesh_id
- )
- if not torch.any(j_valid):
- continue
- # extract estimated embeddings for valid points
- # -> tensor [J, D]
- vertex_embeddings_i = normalize_embeddings(
- interpolator.extract_at_points(
- densepose_predictor_outputs.embedding,
- slice_fine_segm=slice(None),
- w_ylo_xlo=interpolator.w_ylo_xlo[:, None], # pyre-ignore[16]
- w_ylo_xhi=interpolator.w_ylo_xhi[:, None], # pyre-ignore[16]
- w_yhi_xlo=interpolator.w_yhi_xlo[:, None], # pyre-ignore[16]
- w_yhi_xhi=interpolator.w_yhi_xhi[:, None], # pyre-ignore[16]
- )[j_valid, :]
- )
- # extract vertex ids for valid points
- # -> tensor [J]
- vertex_indices_i = packed_annotations.vertex_ids_gt[j_valid]
- # embeddings for all mesh vertices
- # -> tensor [K, D]
- mesh_vertex_embeddings = embedder(mesh_name)
- # softmax values of geodesic distances for GT mesh vertices
- # -> tensor [J, K]
- mesh = create_mesh(mesh_name, mesh_vertex_embeddings.device)
- geodist_softmax_values = F.softmax(
- mesh.geodists[vertex_indices_i] / (-self.geodist_gauss_sigma), dim=1
- )
- # logsoftmax values for valid points
- # -> tensor [J, K]
- embdist_logsoftmax_values = F.log_softmax(
- squared_euclidean_distance_matrix(vertex_embeddings_i, mesh_vertex_embeddings)
- / (-self.embdist_gauss_sigma),
- dim=1,
- )
- losses[mesh_name] = (-geodist_softmax_values * embdist_logsoftmax_values).sum(1).mean()
-
- # pyre-fixme[29]:
- # `Union[BoundMethod[typing.Callable(torch.Tensor.__iter__)[[Named(self,
- # torch.Tensor)], typing.Iterator[typing.Any]], torch.Tensor], nn.Module,
- # torch.Tensor]` is not a function.
- for mesh_name in embedder.mesh_names:
- if mesh_name not in losses:
- losses[mesh_name] = self.fake_value(
- densepose_predictor_outputs, embedder, mesh_name
- )
- return losses
-
- def fake_values(self, densepose_predictor_outputs: Any, embedder: nn.Module):
- losses = {}
- # pyre-fixme[29]:
- # `Union[BoundMethod[typing.Callable(torch.Tensor.__iter__)[[Named(self,
- # torch.Tensor)], typing.Iterator[typing.Any]], torch.Tensor], nn.Module,
- # torch.Tensor]` is not a function.
- for mesh_name in embedder.mesh_names:
- losses[mesh_name] = self.fake_value(densepose_predictor_outputs, embedder, mesh_name)
- return losses
-
- def fake_value(self, densepose_predictor_outputs: Any, embedder: nn.Module, mesh_name: str):
- return densepose_predictor_outputs.embedding.sum() * 0 + embedder(mesh_name).sum() * 0
diff --git a/spaces/brjathu/HMR2.0/vendor/detectron2/tests/config/dir1/dir1_a.py b/spaces/brjathu/HMR2.0/vendor/detectron2/tests/config/dir1/dir1_a.py
deleted file mode 100644
index a939955124556355524f48c0f0c16abb07cfc4c4..0000000000000000000000000000000000000000
--- a/spaces/brjathu/HMR2.0/vendor/detectron2/tests/config/dir1/dir1_a.py
+++ /dev/null
@@ -1,3 +0,0 @@
-# Copyright (c) Facebook, Inc. and its affiliates.
-dir1a_str = "base_a_1"
-dir1a_dict = {"a": 1, "b": 2}
diff --git a/spaces/brjathu/HMR2.0/vendor/detectron2/tests/modeling/test_mmdet.py b/spaces/brjathu/HMR2.0/vendor/detectron2/tests/modeling/test_mmdet.py
deleted file mode 100644
index a743b0b67d5ab664257040621d28c1b1b4451709..0000000000000000000000000000000000000000
--- a/spaces/brjathu/HMR2.0/vendor/detectron2/tests/modeling/test_mmdet.py
+++ /dev/null
@@ -1,186 +0,0 @@
-# Copyright (c) Facebook, Inc. and its affiliates.
-import unittest
-
-from detectron2.layers import ShapeSpec
-from detectron2.modeling.mmdet_wrapper import MMDetBackbone, MMDetDetector
-
-try:
- import mmdet.models # noqa
-
- HAS_MMDET = True
-except ImportError:
- HAS_MMDET = False
-
-
-@unittest.skipIf(not HAS_MMDET, "mmdet not available")
-class TestMMDetWrapper(unittest.TestCase):
- def test_backbone(self):
- MMDetBackbone(
- backbone=dict(
- type="DetectoRS_ResNet",
- conv_cfg=dict(type="ConvAWS"),
- sac=dict(type="SAC", use_deform=True),
- stage_with_sac=(False, True, True, True),
- depth=50,
- num_stages=4,
- out_indices=(0, 1, 2, 3),
- frozen_stages=1,
- norm_cfg=dict(type="BN", requires_grad=True),
- norm_eval=True,
- style="pytorch",
- ),
- neck=dict(
- type="FPN",
- in_channels=[256, 512, 1024, 2048],
- out_channels=256,
- num_outs=5,
- ),
- # skip pretrained model for tests
- # pretrained_backbone="torchvision://resnet50",
- output_shapes=[ShapeSpec(channels=256, stride=s) for s in [4, 8, 16, 32, 64]],
- output_names=["p2", "p3", "p4", "p5", "p6"],
- )
-
- def test_detector(self):
- # a basic R50 Mask R-CNN
- MMDetDetector(
- detector=dict(
- type="MaskRCNN",
- backbone=dict(
- type="ResNet",
- depth=50,
- num_stages=4,
- out_indices=(0, 1, 2, 3),
- frozen_stages=1,
- norm_cfg=dict(type="BN", requires_grad=True),
- norm_eval=True,
- style="pytorch",
- # skip pretrained model for tests
- # init_cfg=dict(type='Pretrained', checkpoint='torchvision://resnet50'))
- ),
- neck=dict(
- type="FPN", in_channels=[256, 512, 1024, 2048], out_channels=256, num_outs=5
- ),
- rpn_head=dict(
- type="RPNHead",
- in_channels=256,
- feat_channels=256,
- anchor_generator=dict(
- type="AnchorGenerator",
- scales=[8],
- ratios=[0.5, 1.0, 2.0],
- strides=[4, 8, 16, 32, 64],
- ),
- bbox_coder=dict(
- type="DeltaXYWHBBoxCoder",
- target_means=[0.0, 0.0, 0.0, 0.0],
- target_stds=[1.0, 1.0, 1.0, 1.0],
- ),
- loss_cls=dict(type="CrossEntropyLoss", use_sigmoid=True, loss_weight=1.0),
- loss_bbox=dict(type="L1Loss", loss_weight=1.0),
- ),
- roi_head=dict(
- type="StandardRoIHead",
- bbox_roi_extractor=dict(
- type="SingleRoIExtractor",
- roi_layer=dict(type="RoIAlign", output_size=7, sampling_ratio=0),
- out_channels=256,
- featmap_strides=[4, 8, 16, 32],
- ),
- bbox_head=dict(
- type="Shared2FCBBoxHead",
- in_channels=256,
- fc_out_channels=1024,
- roi_feat_size=7,
- num_classes=80,
- bbox_coder=dict(
- type="DeltaXYWHBBoxCoder",
- target_means=[0.0, 0.0, 0.0, 0.0],
- target_stds=[0.1, 0.1, 0.2, 0.2],
- ),
- reg_class_agnostic=False,
- loss_cls=dict(type="CrossEntropyLoss", use_sigmoid=False, loss_weight=1.0),
- loss_bbox=dict(type="L1Loss", loss_weight=1.0),
- ),
- mask_roi_extractor=dict(
- type="SingleRoIExtractor",
- roi_layer=dict(type="RoIAlign", output_size=14, sampling_ratio=0),
- out_channels=256,
- featmap_strides=[4, 8, 16, 32],
- ),
- mask_head=dict(
- type="FCNMaskHead",
- num_convs=4,
- in_channels=256,
- conv_out_channels=256,
- num_classes=80,
- loss_mask=dict(type="CrossEntropyLoss", use_mask=True, loss_weight=1.0),
- ),
- ),
- # model training and testing settings
- train_cfg=dict(
- rpn=dict(
- assigner=dict(
- type="MaxIoUAssigner",
- pos_iou_thr=0.7,
- neg_iou_thr=0.3,
- min_pos_iou=0.3,
- match_low_quality=True,
- ignore_iof_thr=-1,
- ),
- sampler=dict(
- type="RandomSampler",
- num=256,
- pos_fraction=0.5,
- neg_pos_ub=-1,
- add_gt_as_proposals=False,
- ),
- allowed_border=-1,
- pos_weight=-1,
- debug=False,
- ),
- rpn_proposal=dict(
- nms_pre=2000,
- max_per_img=1000,
- nms=dict(type="nms", iou_threshold=0.7),
- min_bbox_size=0,
- ),
- rcnn=dict(
- assigner=dict(
- type="MaxIoUAssigner",
- pos_iou_thr=0.5,
- neg_iou_thr=0.5,
- min_pos_iou=0.5,
- match_low_quality=True,
- ignore_iof_thr=-1,
- ),
- sampler=dict(
- type="RandomSampler",
- num=512,
- pos_fraction=0.25,
- neg_pos_ub=-1,
- add_gt_as_proposals=True,
- ),
- mask_size=28,
- pos_weight=-1,
- debug=False,
- ),
- ),
- test_cfg=dict(
- rpn=dict(
- nms_pre=1000,
- max_per_img=1000,
- nms=dict(type="nms", iou_threshold=0.7),
- min_bbox_size=0,
- ),
- rcnn=dict(
- score_thr=0.05,
- nms=dict(type="nms", iou_threshold=0.5),
- max_per_img=100,
- mask_thr_binary=0.5,
- ),
- ),
- ),
- pixel_mean=[1, 2, 3],
- pixel_std=[1, 2, 3],
- )
diff --git a/spaces/bryantmedical/oral_cancer/README.md b/spaces/bryantmedical/oral_cancer/README.md
deleted file mode 100644
index 7078b16f2f378dda95d21d419212d52cde58ac7e..0000000000000000000000000000000000000000
--- a/spaces/bryantmedical/oral_cancer/README.md
+++ /dev/null
@@ -1,12 +0,0 @@
----
-title: Oral Cancer
-emoji: 🚀
-colorFrom: green
-colorTo: green
-sdk: gradio
-sdk_version: 3.4
-app_file: app.py
-pinned: false
----
-
-Check out the configuration reference at https://huggingface.co/docs/hub/spaces-config-reference
diff --git a/spaces/cakiki/bokeh_plots/README.md b/spaces/cakiki/bokeh_plots/README.md
deleted file mode 100644
index c2bb86d7df4fd13b35a667c783a2a4b3512a71ba..0000000000000000000000000000000000000000
--- a/spaces/cakiki/bokeh_plots/README.md
+++ /dev/null
@@ -1,14 +0,0 @@
----
-title: Bokeh Plots
-emoji: 🏢
-colorFrom: gray
-colorTo: yellow
-sdk: gradio
-sdk_version: 3.18.0
-app_file: app.py
-pinned: false
-license: mit
-duplicated_from: gradio/bokeh_plots
----
-
-Check out the configuration reference at https://huggingface.co/docs/hub/spaces-config-reference
diff --git a/spaces/carlosalonso/Detection-video/carpeta_deteccion/docs/tutorials/evaluation.md b/spaces/carlosalonso/Detection-video/carpeta_deteccion/docs/tutorials/evaluation.md
deleted file mode 100644
index 2ef94faa38cae1c5f4e49eed4887ebbcd147513c..0000000000000000000000000000000000000000
--- a/spaces/carlosalonso/Detection-video/carpeta_deteccion/docs/tutorials/evaluation.md
+++ /dev/null
@@ -1,68 +0,0 @@
-
-# Evaluation
-
-Evaluation is a process that takes a number of inputs/outputs pairs and aggregate them.
-You can always [use the model](./models.md) directly and just parse its inputs/outputs manually to perform
-evaluation.
-Alternatively, evaluation is implemented in detectron2 using the [DatasetEvaluator](../modules/evaluation.html#detectron2.evaluation.DatasetEvaluator)
-interface.
-
-Detectron2 includes a few `DatasetEvaluator` that computes metrics using standard dataset-specific
-APIs (e.g., COCO, LVIS).
-You can also implement your own `DatasetEvaluator` that performs some other jobs
-using the inputs/outputs pairs.
-For example, to count how many instances are detected on the validation set:
-
-```python
-class Counter(DatasetEvaluator):
- def reset(self):
- self.count = 0
- def process(self, inputs, outputs):
- for output in outputs:
- self.count += len(output["instances"])
- def evaluate(self):
- # save self.count somewhere, or print it, or return it.
- return {"count": self.count}
-```
-
-## Use evaluators
-
-To evaluate using the methods of evaluators manually:
-```python
-def get_all_inputs_outputs():
- for data in data_loader:
- yield data, model(data)
-
-evaluator.reset()
-for inputs, outputs in get_all_inputs_outputs():
- evaluator.process(inputs, outputs)
-eval_results = evaluator.evaluate()
-```
-
-Evaluators can also be used with [inference_on_dataset](../modules/evaluation.html#detectron2.evaluation.inference_on_dataset).
-For example,
-
-```python
-eval_results = inference_on_dataset(
- model,
- data_loader,
- DatasetEvaluators([COCOEvaluator(...), Counter()]))
-```
-This will execute `model` on all inputs from `data_loader`, and call evaluator to process them.
-
-Compared to running the evaluation manually using the model, the benefit of this function is that
-evaluators can be merged together using [DatasetEvaluators](../modules/evaluation.html#detectron2.evaluation.DatasetEvaluators),
-and all the evaluation can finish in one forward pass over the dataset.
-This function also provides accurate speed benchmarks for the given model and dataset.
-
-## Evaluators for custom dataset
-
-Many evaluators in detectron2 are made for specific datasets,
-in order to obtain scores using each dataset's official API.
-In addition to that, two evaluators are able to evaluate any generic dataset
-that follows detectron2's [standard dataset format](./datasets.md), so they
-can be used to evaluate custom datasets:
-
-* [COCOEvaluator](../modules/evaluation.html#detectron2.evaluation.COCOEvaluator) is able to evaluate AP (Average Precision) for box detection,
- instance segmentation, keypoint detection on any custom dataset.
-* [SemSegEvaluator](../modules/evaluation.html#detectron2.evaluation.SemSegEvaluator) is able to evaluate semantic segmentation metrics on any custom dataset.
diff --git a/spaces/carloscar/stable-diffusion-webui-controlnet-docker/Dockerfile b/spaces/carloscar/stable-diffusion-webui-controlnet-docker/Dockerfile
deleted file mode 100644
index 95dd8620e127dfa3471d2fc93e86f0918c56ee24..0000000000000000000000000000000000000000
--- a/spaces/carloscar/stable-diffusion-webui-controlnet-docker/Dockerfile
+++ /dev/null
@@ -1,124 +0,0 @@
-FROM nvidia/cuda:11.7.1-cudnn8-devel-ubuntu22.04
-
-ENV DEBIAN_FRONTEND noninteractive
-ENV PYTHONUNBUFFERED=1
-ENV PIP_DISABLE_PIP_VERSION_CHECK=1
-ENV PIP_NO_CACHE_DIR=1
-
-# OS setup
-RUN apt-get update -y \
- && apt-get upgrade -y \
- && apt-get install -y \
- libgl1 \
- libglib2.0-0 \
- curl \
- vim \
- wget \
- git \
- git-lfs \
- tzdata \
- bash \
- ca-certificates \
- libreadline8 \
- bzip2 \
- psmisc \
- procps \
- netbase \
- openssh-client \
- libsqlite3-dev \
- python3-pip \
- python3-venv \
- python-is-python3 \
- build-essential \
- libssl-dev \
- libffi-dev \
- aria2 \
- \
- && pip3 install --upgrade pip \
- \
- && git lfs install \
- \
- && apt-get clean autoclean \
- && apt-get autoremove --yes \
- && rm -rf /var/lib/apt/lists/*
-
-# OS timezone setting (UTC)
-RUN echo "UTC" > /etc/timezone
-ENV TZ=UTC
-
-# Poetry for Python packages
-RUN curl -sSL https://install.python-poetry.org | POETRY_HOME=/usr/local/poetry python3 - --yes \
- && ln -s /usr/local/poetry/bin/poetry /usr/bin/poetry \
- \
- && poetry config virtualenvs.create false \
- && poetry config virtualenvs.in-project false
-
-# Create non-root user
-ENV ENV="/etc/profile"
-RUN adduser --disabled-password --gecos '' user && \
- mkdir -p /app && \
- chown -R user:user /app && \
- printf "\n. /etc/profile\n" >> /home/user/.profile \
- printf "\n. /etc/profile\n" >> /home/user/.bashrc
-
-# Sets up virtualenv for dependencies
-ENV VIRTUAL_ENV="/opt/venv"
-ENV VIRTUAL_ENV_DISABLE_PROMPT=1
-ENV POETRY_ACTIVE=1
-ENV PATH="$VIRTUAL_ENV/bin:$PATH"
-RUN echo "export PATH=$PATH" >> /home/user/.bashrc \
- && python3 -m venv $VIRTUAL_ENV \
- && /opt/venv/bin/pip install --upgrade --no-cache-dir pip \
- && chown -R user:user /opt/venv
-
-# Run as non-root user
-USER user
-WORKDIR /app
-
-# Installation of basic Python dependencies specified in pyproject.toml
-COPY --chown=user:user pyproject.toml poetry.lock /app/
-RUN poetry install
-
-# AUTOMATIC1111' WebUI
-RUN git clone https://github.com/AUTOMATIC1111/stable-diffusion-webui /app/stable-diffusion-webui \
- && (cd /app/stable-diffusion-webui && git checkout a9fed7c364061ae6efb37f797b6b522cb3cf7aa2)
-
-# Deforum extension
-RUN git clone https://github.com/deforum-art/deforum-for-automatic1111-webui /app/stable-diffusion-webui/extensions/deforum-for-automatic1111-webui \
- && (cd /app/stable-diffusion-webui/extensions/deforum-for-automatic1111-webui && git checkout 2366bfdb47c226df0d14e712445414e459febad3)
-
-# Images Browser WebUI extension
-RUN git clone https://github.com/yfszzx/stable-diffusion-webui-images-browser /app/stable-diffusion-webui/extensions/stable-diffusion-webui-images-browser \
- && (cd /app/stable-diffusion-webui/extensions/stable-diffusion-webui-images-browser && git checkout a42c7a30181636a05815e62426d5eff4d3340529)
-
-# CiviTAI Browser WebUI extension
-RUN git clone https://github.com/Vetchems/sd-civitai-browser /app/stable-diffusion-webui/extensions/sd-civitai-browser \
- && (cd /app/stable-diffusion-webui/extensions/sd-civitai-browser && git checkout b25a5daf7df3f6340d3e243d533228d8ade5288d)
-
-# Additional Networks WebUI extension
-RUN git clone https://github.com/kohya-ss/sd-webui-additional-networks /app/stable-diffusion-webui/extensions/sd-webui-additional-networks \
- && (cd /app/stable-diffusion-webui/extensions/sd-webui-additional-networks && git checkout d2758b6c8e2e8e956865a87b31fd74d3d7c010cb) \
- && mkdir -p /app/stable-diffusion-webui/extensions/sd-webui-additional-networks/models/LoRA
-
-# ControlNet WebUI extension
-RUN git clone https://github.com/Mikubill/sd-webui-controlnet /app/stable-diffusion-webui/extensions/sd-webui-controlnet \
- && (cd /app/stable-diffusion-webui/extensions/sd-webui-controlnet && git checkout 274dd5df217a03e059e9cf052447aece81bbd1cf) \
- && mkdir -p /app/stable-diffusion-webui/models/ControlNet
-
-# Prepare WebUI environment
-WORKDIR /app/stable-diffusion-webui
-RUN /opt/venv/bin/python launch.py --exit --skip-torch-cuda-test --xformers
-
-# Patch WebUI
-RUN sed -i -e 's/ show_progress=False,/ show_progress=True,/g' modules/ui.py
-RUN sed -i -e 's/shared.demo.launch/shared.demo.queue().launch/g' webui.py
-RUN sed -i -e 's/ outputs=\[/queue=False, &/g' modules/ui.py
-RUN sed -i -e 's/ queue=False, / /g' modules/ui.py
-
-# Copy startup scripts
-COPY --chown=user:user run.py on_start.sh config.json ui-config.json shared-config.json shared-ui-config.json header_patch.py /app/stable-diffusion-webui/
-RUN chmod +x on_start.sh
-
-EXPOSE 7860
-
-CMD ["/opt/venv/bin/python", "run.py", "--listen", "--ui-config-file", "ui-config.json", "--ui-settings-file", "config.json", "--disable-console-progressbars", "--cors-allow-origins", "huggingface.co,hf.space", "--no-progressbar-hiding", "--enable-console-prompts", "--no-download-sd-model", "--api", "--skip-version-check"]
diff --git a/spaces/chendl/compositional_test/transformers/src/transformers/image_processing_utils.py b/spaces/chendl/compositional_test/transformers/src/transformers/image_processing_utils.py
deleted file mode 100644
index cce3d475bab231bdaba5c23495f98ef8c8ec769f..0000000000000000000000000000000000000000
--- a/spaces/chendl/compositional_test/transformers/src/transformers/image_processing_utils.py
+++ /dev/null
@@ -1,550 +0,0 @@
-# coding=utf-8
-# Copyright 2022 The HuggingFace Inc. team.
-#
-# Licensed under the Apache License, Version 2.0 (the "License");
-# you may not use this file except in compliance with the License.
-# You may obtain a copy of the License at
-#
-# http://www.apache.org/licenses/LICENSE-2.0
-#
-# Unless required by applicable law or agreed to in writing, software
-# distributed under the License is distributed on an "AS IS" BASIS,
-# WITHOUT WARRANTIES OR CONDITIONS OF ANY KIND, either express or implied.
-# See the License for the specific language governing permissions and
-# limitations under the License.
-
-import copy
-import json
-import os
-from typing import Any, Dict, Iterable, Optional, Tuple, Union
-
-import numpy as np
-
-from .dynamic_module_utils import custom_object_save
-from .feature_extraction_utils import BatchFeature as BaseBatchFeature
-from .utils import (
- IMAGE_PROCESSOR_NAME,
- PushToHubMixin,
- cached_file,
- copy_func,
- download_url,
- is_offline_mode,
- is_remote_url,
- logging,
-)
-
-
-logger = logging.get_logger(__name__)
-
-
-# TODO: Move BatchFeature to be imported by both image_processing_utils and image_processing_utils
-# We override the class string here, but logic is the same.
-class BatchFeature(BaseBatchFeature):
- r"""
- Holds the output of the image processor specific `__call__` methods.
-
- This class is derived from a python dictionary and can be used as a dictionary.
-
- Args:
- data (`dict`):
- Dictionary of lists/arrays/tensors returned by the __call__ method ('pixel_values', etc.).
- tensor_type (`Union[None, str, TensorType]`, *optional*):
- You can give a tensor_type here to convert the lists of integers in PyTorch/TensorFlow/Numpy Tensors at
- initialization.
- """
-
-
-# TODO: (Amy) - factor out the common parts of this and the feature extractor
-class ImageProcessingMixin(PushToHubMixin):
- """
- This is an image processor mixin used to provide saving/loading functionality for sequential and image feature
- extractors.
- """
-
- _auto_class = None
-
- def __init__(self, **kwargs):
- """Set elements of `kwargs` as attributes."""
- # Pop "processor_class" as it should be saved as private attribute
- self._processor_class = kwargs.pop("processor_class", None)
- # Additional attributes without default values
- for key, value in kwargs.items():
- try:
- setattr(self, key, value)
- except AttributeError as err:
- logger.error(f"Can't set {key} with value {value} for {self}")
- raise err
-
- def _set_processor_class(self, processor_class: str):
- """Sets processor class as an attribute."""
- self._processor_class = processor_class
-
- @classmethod
- def from_pretrained(cls, pretrained_model_name_or_path: Union[str, os.PathLike], **kwargs):
- r"""
- Instantiate a type of [`~image_processing_utils.ImageProcessingMixin`] from an image processor.
-
- Args:
- pretrained_model_name_or_path (`str` or `os.PathLike`):
- This can be either:
-
- - a string, the *model id* of a pretrained image_processor hosted inside a model repo on
- huggingface.co. Valid model ids can be located at the root-level, like `bert-base-uncased`, or
- namespaced under a user or organization name, like `dbmdz/bert-base-german-cased`.
- - a path to a *directory* containing a image processor file saved using the
- [`~image_processing_utils.ImageProcessingMixin.save_pretrained`] method, e.g.,
- `./my_model_directory/`.
- - a path or url to a saved image processor JSON *file*, e.g.,
- `./my_model_directory/preprocessor_config.json`.
- cache_dir (`str` or `os.PathLike`, *optional*):
- Path to a directory in which a downloaded pretrained model image processor should be cached if the
- standard cache should not be used.
- force_download (`bool`, *optional*, defaults to `False`):
- Whether or not to force to (re-)download the image processor files and override the cached versions if
- they exist.
- resume_download (`bool`, *optional*, defaults to `False`):
- Whether or not to delete incompletely received file. Attempts to resume the download if such a file
- exists.
- proxies (`Dict[str, str]`, *optional*):
- A dictionary of proxy servers to use by protocol or endpoint, e.g., `{'http': 'foo.bar:3128',
- 'http://hostname': 'foo.bar:4012'}.` The proxies are used on each request.
- use_auth_token (`str` or `bool`, *optional*):
- The token to use as HTTP bearer authorization for remote files. If `True`, or not specified, will use
- the token generated when running `huggingface-cli login` (stored in `~/.huggingface`).
- revision (`str`, *optional*, defaults to `"main"`):
- The specific model version to use. It can be a branch name, a tag name, or a commit id, since we use a
- git-based system for storing models and other artifacts on huggingface.co, so `revision` can be any
- identifier allowed by git.
-
-
-
-
- To test a pull request you made on the Hub, you can pass `revision="refs/pr/".
-
-
-
- return_unused_kwargs (`bool`, *optional*, defaults to `False`):
- If `False`, then this function returns just the final image processor object. If `True`, then this
- functions returns a `Tuple(image_processor, unused_kwargs)` where *unused_kwargs* is a dictionary
- consisting of the key/value pairs whose keys are not image processor attributes: i.e., the part of
- `kwargs` which has not been used to update `image_processor` and is otherwise ignored.
- subfolder (`str`, *optional*, defaults to `""`):
- In case the relevant files are located inside a subfolder of the model repo on huggingface.co, you can
- specify the folder name here.
- kwargs (`Dict[str, Any]`, *optional*):
- The values in kwargs of any keys which are image processor attributes will be used to override the
- loaded values. Behavior concerning key/value pairs whose keys are *not* image processor attributes is
- controlled by the `return_unused_kwargs` keyword parameter.
-
- Returns:
- A image processor of type [`~image_processing_utils.ImageProcessingMixin`].
-
- Examples:
-
- ```python
- # We can't instantiate directly the base class *ImageProcessingMixin* so let's show the examples on a
- # derived class: *CLIPImageProcessor*
- image_processor = CLIPImageProcessor.from_pretrained(
- "openai/clip-vit-base-patch32"
- ) # Download image_processing_config from huggingface.co and cache.
- image_processor = CLIPImageProcessor.from_pretrained(
- "./test/saved_model/"
- ) # E.g. image processor (or model) was saved using *save_pretrained('./test/saved_model/')*
- image_processor = CLIPImageProcessor.from_pretrained("./test/saved_model/preprocessor_config.json")
- image_processor = CLIPImageProcessor.from_pretrained(
- "openai/clip-vit-base-patch32", do_normalize=False, foo=False
- )
- assert image_processor.do_normalize is False
- image_processor, unused_kwargs = CLIPImageProcessor.from_pretrained(
- "openai/clip-vit-base-patch32", do_normalize=False, foo=False, return_unused_kwargs=True
- )
- assert image_processor.do_normalize is False
- assert unused_kwargs == {"foo": False}
- ```"""
- image_processor_dict, kwargs = cls.get_image_processor_dict(pretrained_model_name_or_path, **kwargs)
-
- return cls.from_dict(image_processor_dict, **kwargs)
-
- def save_pretrained(self, save_directory: Union[str, os.PathLike], push_to_hub: bool = False, **kwargs):
- """
- Save an image processor object to the directory `save_directory`, so that it can be re-loaded using the
- [`~image_processing_utils.ImageProcessingMixin.from_pretrained`] class method.
-
- Args:
- save_directory (`str` or `os.PathLike`):
- Directory where the image processor JSON file will be saved (will be created if it does not exist).
- push_to_hub (`bool`, *optional*, defaults to `False`):
- Whether or not to push your model to the Hugging Face model hub after saving it. You can specify the
- repository you want to push to with `repo_id` (will default to the name of `save_directory` in your
- namespace).
- kwargs:
- Additional key word arguments passed along to the [`~utils.PushToHubMixin.push_to_hub`] method.
- """
- if os.path.isfile(save_directory):
- raise AssertionError(f"Provided path ({save_directory}) should be a directory, not a file")
-
- os.makedirs(save_directory, exist_ok=True)
-
- if push_to_hub:
- commit_message = kwargs.pop("commit_message", None)
- repo_id = kwargs.pop("repo_id", save_directory.split(os.path.sep)[-1])
- repo_id = self._create_repo(repo_id, **kwargs)
- files_timestamps = self._get_files_timestamps(save_directory)
-
- # If we have a custom config, we copy the file defining it in the folder and set the attributes so it can be
- # loaded from the Hub.
- if self._auto_class is not None:
- custom_object_save(self, save_directory, config=self)
-
- # If we save using the predefined names, we can load using `from_pretrained`
- output_image_processor_file = os.path.join(save_directory, IMAGE_PROCESSOR_NAME)
-
- self.to_json_file(output_image_processor_file)
- logger.info(f"Image processor saved in {output_image_processor_file}")
-
- if push_to_hub:
- self._upload_modified_files(
- save_directory,
- repo_id,
- files_timestamps,
- commit_message=commit_message,
- token=kwargs.get("use_auth_token"),
- )
-
- return [output_image_processor_file]
-
- @classmethod
- def get_image_processor_dict(
- cls, pretrained_model_name_or_path: Union[str, os.PathLike], **kwargs
- ) -> Tuple[Dict[str, Any], Dict[str, Any]]:
- """
- From a `pretrained_model_name_or_path`, resolve to a dictionary of parameters, to be used for instantiating a
- image processor of type [`~image_processor_utils.ImageProcessingMixin`] using `from_dict`.
-
- Parameters:
- pretrained_model_name_or_path (`str` or `os.PathLike`):
- The identifier of the pre-trained checkpoint from which we want the dictionary of parameters.
- subfolder (`str`, *optional*, defaults to `""`):
- In case the relevant files are located inside a subfolder of the model repo on huggingface.co, you can
- specify the folder name here.
-
- Returns:
- `Tuple[Dict, Dict]`: The dictionary(ies) that will be used to instantiate the image processor object.
- """
- cache_dir = kwargs.pop("cache_dir", None)
- force_download = kwargs.pop("force_download", False)
- resume_download = kwargs.pop("resume_download", False)
- proxies = kwargs.pop("proxies", None)
- use_auth_token = kwargs.pop("use_auth_token", None)
- local_files_only = kwargs.pop("local_files_only", False)
- revision = kwargs.pop("revision", None)
- subfolder = kwargs.pop("subfolder", "")
-
- from_pipeline = kwargs.pop("_from_pipeline", None)
- from_auto_class = kwargs.pop("_from_auto", False)
-
- user_agent = {"file_type": "image processor", "from_auto_class": from_auto_class}
- if from_pipeline is not None:
- user_agent["using_pipeline"] = from_pipeline
-
- if is_offline_mode() and not local_files_only:
- logger.info("Offline mode: forcing local_files_only=True")
- local_files_only = True
-
- pretrained_model_name_or_path = str(pretrained_model_name_or_path)
- is_local = os.path.isdir(pretrained_model_name_or_path)
- if os.path.isdir(pretrained_model_name_or_path):
- image_processor_file = os.path.join(pretrained_model_name_or_path, IMAGE_PROCESSOR_NAME)
- if os.path.isfile(pretrained_model_name_or_path):
- resolved_image_processor_file = pretrained_model_name_or_path
- is_local = True
- elif is_remote_url(pretrained_model_name_or_path):
- image_processor_file = pretrained_model_name_or_path
- resolved_image_processor_file = download_url(pretrained_model_name_or_path)
- else:
- image_processor_file = IMAGE_PROCESSOR_NAME
- try:
- # Load from local folder or from cache or download from model Hub and cache
- resolved_image_processor_file = cached_file(
- pretrained_model_name_or_path,
- image_processor_file,
- cache_dir=cache_dir,
- force_download=force_download,
- proxies=proxies,
- resume_download=resume_download,
- local_files_only=local_files_only,
- use_auth_token=use_auth_token,
- user_agent=user_agent,
- revision=revision,
- subfolder=subfolder,
- )
- except EnvironmentError:
- # Raise any environment error raise by `cached_file`. It will have a helpful error message adapted to
- # the original exception.
- raise
- except Exception:
- # For any other exception, we throw a generic error.
- raise EnvironmentError(
- f"Can't load image processor for '{pretrained_model_name_or_path}'. If you were trying to load"
- " it from 'https://huggingface.co/models', make sure you don't have a local directory with the"
- f" same name. Otherwise, make sure '{pretrained_model_name_or_path}' is the correct path to a"
- f" directory containing a {IMAGE_PROCESSOR_NAME} file"
- )
-
- try:
- # Load image_processor dict
- with open(resolved_image_processor_file, "r", encoding="utf-8") as reader:
- text = reader.read()
- image_processor_dict = json.loads(text)
-
- except json.JSONDecodeError:
- raise EnvironmentError(
- f"It looks like the config file at '{resolved_image_processor_file}' is not a valid JSON file."
- )
-
- if is_local:
- logger.info(f"loading configuration file {resolved_image_processor_file}")
- else:
- logger.info(
- f"loading configuration file {image_processor_file} from cache at {resolved_image_processor_file}"
- )
-
- return image_processor_dict, kwargs
-
- @classmethod
- def from_dict(cls, image_processor_dict: Dict[str, Any], **kwargs):
- """
- Instantiates a type of [`~image_processing_utils.ImageProcessingMixin`] from a Python dictionary of parameters.
-
- Args:
- image_processor_dict (`Dict[str, Any]`):
- Dictionary that will be used to instantiate the image processor object. Such a dictionary can be
- retrieved from a pretrained checkpoint by leveraging the
- [`~image_processing_utils.ImageProcessingMixin.to_dict`] method.
- kwargs (`Dict[str, Any]`):
- Additional parameters from which to initialize the image processor object.
-
- Returns:
- [`~image_processing_utils.ImageProcessingMixin`]: The image processor object instantiated from those
- parameters.
- """
- image_processor_dict = image_processor_dict.copy()
- return_unused_kwargs = kwargs.pop("return_unused_kwargs", False)
-
- # The `size` parameter is a dict and was previously an int or tuple in feature extractors.
- # We set `size` here directly to the `image_processor_dict` so that it is converted to the appropriate
- # dict within the image processor and isn't overwritten if `size` is passed in as a kwarg.
- if "size" in kwargs and "size" in image_processor_dict:
- image_processor_dict["size"] = kwargs.pop("size")
- if "crop_size" in kwargs and "crop_size" in image_processor_dict:
- image_processor_dict["crop_size"] = kwargs.pop("crop_size")
-
- image_processor = cls(**image_processor_dict)
-
- # Update image_processor with kwargs if needed
- to_remove = []
- for key, value in kwargs.items():
- if hasattr(image_processor, key):
- setattr(image_processor, key, value)
- to_remove.append(key)
- for key in to_remove:
- kwargs.pop(key, None)
-
- logger.info(f"Image processor {image_processor}")
- if return_unused_kwargs:
- return image_processor, kwargs
- else:
- return image_processor
-
- def to_dict(self) -> Dict[str, Any]:
- """
- Serializes this instance to a Python dictionary.
-
- Returns:
- `Dict[str, Any]`: Dictionary of all the attributes that make up this image processor instance.
- """
- output = copy.deepcopy(self.__dict__)
- output["image_processor_type"] = self.__class__.__name__
-
- return output
-
- @classmethod
- def from_json_file(cls, json_file: Union[str, os.PathLike]):
- """
- Instantiates a image processor of type [`~image_processing_utils.ImageProcessingMixin`] from the path to a JSON
- file of parameters.
-
- Args:
- json_file (`str` or `os.PathLike`):
- Path to the JSON file containing the parameters.
-
- Returns:
- A image processor of type [`~image_processing_utils.ImageProcessingMixin`]: The image_processor object
- instantiated from that JSON file.
- """
- with open(json_file, "r", encoding="utf-8") as reader:
- text = reader.read()
- image_processor_dict = json.loads(text)
- return cls(**image_processor_dict)
-
- def to_json_string(self) -> str:
- """
- Serializes this instance to a JSON string.
-
- Returns:
- `str`: String containing all the attributes that make up this feature_extractor instance in JSON format.
- """
- dictionary = self.to_dict()
-
- for key, value in dictionary.items():
- if isinstance(value, np.ndarray):
- dictionary[key] = value.tolist()
-
- # make sure private name "_processor_class" is correctly
- # saved as "processor_class"
- _processor_class = dictionary.pop("_processor_class", None)
- if _processor_class is not None:
- dictionary["processor_class"] = _processor_class
-
- return json.dumps(dictionary, indent=2, sort_keys=True) + "\n"
-
- def to_json_file(self, json_file_path: Union[str, os.PathLike]):
- """
- Save this instance to a JSON file.
-
- Args:
- json_file_path (`str` or `os.PathLike`):
- Path to the JSON file in which this image_processor instance's parameters will be saved.
- """
- with open(json_file_path, "w", encoding="utf-8") as writer:
- writer.write(self.to_json_string())
-
- def __repr__(self):
- return f"{self.__class__.__name__} {self.to_json_string()}"
-
- @classmethod
- def register_for_auto_class(cls, auto_class="AutoImageProcessor"):
- """
- Register this class with a given auto class. This should only be used for custom image processors as the ones
- in the library are already mapped with `AutoImageProcessor `.
-
-
-
- This API is experimental and may have some slight breaking changes in the next releases.
-
-
-
- Args:
- auto_class (`str` or `type`, *optional*, defaults to `"AutoImageProcessor "`):
- The auto class to register this new image processor with.
- """
- if not isinstance(auto_class, str):
- auto_class = auto_class.__name__
-
- import transformers.models.auto as auto_module
-
- if not hasattr(auto_module, auto_class):
- raise ValueError(f"{auto_class} is not a valid auto class.")
-
- cls._auto_class = auto_class
-
-
-class BaseImageProcessor(ImageProcessingMixin):
- def __init__(self, **kwargs):
- super().__init__(**kwargs)
-
- def __call__(self, images, **kwargs) -> BatchFeature:
- """Preprocess an image or a batch of images."""
- return self.preprocess(images, **kwargs)
-
- def preprocess(self, images, **kwargs) -> BatchFeature:
- raise NotImplementedError("Each image processor must implement its own preprocess method")
-
-
-VALID_SIZE_DICT_KEYS = ({"height", "width"}, {"shortest_edge"}, {"shortest_edge", "longest_edge"})
-
-
-def is_valid_size_dict(size_dict):
- if not isinstance(size_dict, dict):
- return False
-
- size_dict_keys = set(size_dict.keys())
- for allowed_keys in VALID_SIZE_DICT_KEYS:
- if size_dict_keys == allowed_keys:
- return True
- return False
-
-
-def convert_to_size_dict(
- size, max_size: Optional[int] = None, default_to_square: bool = True, height_width_order: bool = True
-):
- # By default, if size is an int we assume it represents a tuple of (size, size).
- if isinstance(size, int) and default_to_square:
- if max_size is not None:
- raise ValueError("Cannot specify both size as an int, with default_to_square=True and max_size")
- return {"height": size, "width": size}
- # In other configs, if size is an int and default_to_square is False, size represents the length of
- # the shortest edge after resizing.
- elif isinstance(size, int) and not default_to_square:
- size_dict = {"shortest_edge": size}
- if max_size is not None:
- size_dict["longest_edge"] = max_size
- return size_dict
- # Otherwise, if size is a tuple it's either (height, width) or (width, height)
- elif isinstance(size, (tuple, list)) and height_width_order:
- return {"height": size[0], "width": size[1]}
- elif isinstance(size, (tuple, list)) and not height_width_order:
- return {"height": size[1], "width": size[0]}
-
- raise ValueError(f"Could not convert size input to size dict: {size}")
-
-
-def get_size_dict(
- size: Union[int, Iterable[int], Dict[str, int]] = None,
- max_size: Optional[int] = None,
- height_width_order: bool = True,
- default_to_square: bool = True,
- param_name="size",
-) -> dict:
- """
- Converts the old size parameter in the config into the new dict expected in the config. This is to ensure backwards
- compatibility with the old image processor configs and removes ambiguity over whether the tuple is in (height,
- width) or (width, height) format.
-
- - If `size` is tuple, it is converted to `{"height": size[0], "width": size[1]}` or `{"height": size[1], "width":
- size[0]}` if `height_width_order` is `False`.
- - If `size` is an int, and `default_to_square` is `True`, it is converted to `{"height": size, "width": size}`.
- - If `size` is an int and `default_to_square` is False, it is converted to `{"shortest_edge": size}`. If `max_size`
- is set, it is added to the dict as `{"longest_edge": max_size}`.
-
- Args:
- size (`Union[int, Iterable[int], Dict[str, int]]`, *optional*):
- The `size` parameter to be cast into a size dictionary.
- max_size (`Optional[int]`, *optional*):
- The `max_size` parameter to be cast into a size dictionary.
- height_width_order (`bool`, *optional*, defaults to `True`):
- If `size` is a tuple, whether it's in (height, width) or (width, height) order.
- default_to_square (`bool`, *optional*, defaults to `True`):
- If `size` is an int, whether to default to a square image or not.
- """
- if not isinstance(size, dict):
- size_dict = convert_to_size_dict(size, max_size, default_to_square, height_width_order)
- logger.info(
- f"{param_name} should be a dictionary on of the following set of keys: {VALID_SIZE_DICT_KEYS}, got {size}."
- f" Converted to {size_dict}.",
- )
- else:
- size_dict = size
-
- if not is_valid_size_dict(size_dict):
- raise ValueError(
- f"{param_name} must have one of the following set of keys: {VALID_SIZE_DICT_KEYS}, got {size_dict.keys()}"
- )
- return size_dict
-
-
-ImageProcessingMixin.push_to_hub = copy_func(ImageProcessingMixin.push_to_hub)
-if ImageProcessingMixin.push_to_hub.__doc__ is not None:
- ImageProcessingMixin.push_to_hub.__doc__ = ImageProcessingMixin.push_to_hub.__doc__.format(
- object="image processor", object_class="AutoImageProcessor", object_files="image processor file"
- )
diff --git a/spaces/chendl/compositional_test/transformers/src/transformers/models/bart/modeling_bart.py b/spaces/chendl/compositional_test/transformers/src/transformers/models/bart/modeling_bart.py
deleted file mode 100644
index c1da4eb288e838e1da554444a477854cdf5941f9..0000000000000000000000000000000000000000
--- a/spaces/chendl/compositional_test/transformers/src/transformers/models/bart/modeling_bart.py
+++ /dev/null
@@ -1,1940 +0,0 @@
-# coding=utf-8
-# Copyright 2021 The Fairseq Authors and The HuggingFace Inc. team. All rights reserved.
-#
-# Licensed under the Apache License, Version 2.0 (the "License");
-# you may not use this file except in compliance with the License.
-# You may obtain a copy of the License at
-#
-# http://www.apache.org/licenses/LICENSE-2.0
-#
-# Unless required by applicable law or agreed to in writing, software
-# distributed under the License is distributed on an "AS IS" BASIS,
-# WITHOUT WARRANTIES OR CONDITIONS OF ANY KIND, either express or implied.
-# See the License for the specific language governing permissions and
-# limitations under the License.
-""" PyTorch BART model."""
-import copy
-import math
-import random
-import warnings
-from typing import List, Optional, Tuple, Union
-
-import torch
-import torch.utils.checkpoint
-from torch import nn
-from torch.nn import BCEWithLogitsLoss, CrossEntropyLoss, MSELoss
-
-from ...activations import ACT2FN
-from ...modeling_outputs import (
- BaseModelOutput,
- BaseModelOutputWithPastAndCrossAttentions,
- CausalLMOutputWithCrossAttentions,
- Seq2SeqLMOutput,
- Seq2SeqModelOutput,
- Seq2SeqQuestionAnsweringModelOutput,
- Seq2SeqSequenceClassifierOutput,
-)
-from ...modeling_utils import PreTrainedModel
-from ...utils import (
- add_code_sample_docstrings,
- add_end_docstrings,
- add_start_docstrings,
- add_start_docstrings_to_model_forward,
- logging,
- replace_return_docstrings,
-)
-from .configuration_bart import BartConfig
-
-
-logger = logging.get_logger(__name__)
-
-_CHECKPOINT_FOR_DOC = "facebook/bart-base"
-_CONFIG_FOR_DOC = "BartConfig"
-
-# Base model docstring
-_EXPECTED_OUTPUT_SHAPE = [1, 8, 768]
-
-# SequenceClassification docstring
-_CHECKPOINT_FOR_SEQUENCE_CLASSIFICATION = "valhalla/bart-large-sst2"
-_SEQ_CLASS_EXPECTED_LOSS = 0.0
-_SEQ_CLASS_EXPECTED_OUTPUT = "'POSITIVE'"
-
-# QuestionAsnwering docstring
-_CHECKPOINT_FOR_QA = "valhalla/bart-large-finetuned-squadv1"
-_QA_EXPECTED_LOSS = 0.59
-_QA_EXPECTED_OUTPUT = "' nice puppet'"
-
-
-BART_PRETRAINED_MODEL_ARCHIVE_LIST = [
- "facebook/bart-large",
- # see all BART models at https://huggingface.co/models?filter=bart
-]
-
-
-def shift_tokens_right(input_ids: torch.Tensor, pad_token_id: int, decoder_start_token_id: int):
- """
- Shift input ids one token to the right.
- """
- shifted_input_ids = input_ids.new_zeros(input_ids.shape)
- shifted_input_ids[:, 1:] = input_ids[:, :-1].clone()
- shifted_input_ids[:, 0] = decoder_start_token_id
-
- if pad_token_id is None:
- raise ValueError("self.model.config.pad_token_id has to be defined.")
- # replace possible -100 values in labels by `pad_token_id`
- shifted_input_ids.masked_fill_(shifted_input_ids == -100, pad_token_id)
-
- return shifted_input_ids
-
-
-def _make_causal_mask(
- input_ids_shape: torch.Size, dtype: torch.dtype, device: torch.device, past_key_values_length: int = 0
-):
- """
- Make causal mask used for bi-directional self-attention.
- """
- bsz, tgt_len = input_ids_shape
- mask = torch.full((tgt_len, tgt_len), torch.tensor(torch.finfo(dtype).min, device=device), device=device)
- mask_cond = torch.arange(mask.size(-1), device=device)
- mask.masked_fill_(mask_cond < (mask_cond + 1).view(mask.size(-1), 1), 0)
- mask = mask.to(dtype)
-
- if past_key_values_length > 0:
- mask = torch.cat([torch.zeros(tgt_len, past_key_values_length, dtype=dtype, device=device), mask], dim=-1)
- return mask[None, None, :, :].expand(bsz, 1, tgt_len, tgt_len + past_key_values_length)
-
-
-def _expand_mask(mask: torch.Tensor, dtype: torch.dtype, tgt_len: Optional[int] = None):
- """
- Expands attention_mask from `[bsz, seq_len]` to `[bsz, 1, tgt_seq_len, src_seq_len]`.
- """
- bsz, src_len = mask.size()
- tgt_len = tgt_len if tgt_len is not None else src_len
-
- expanded_mask = mask[:, None, None, :].expand(bsz, 1, tgt_len, src_len).to(dtype)
-
- inverted_mask = 1.0 - expanded_mask
-
- return inverted_mask.masked_fill(inverted_mask.to(torch.bool), torch.finfo(dtype).min)
-
-
-class BartLearnedPositionalEmbedding(nn.Embedding):
- """
- This module learns positional embeddings up to a fixed maximum size.
- """
-
- def __init__(self, num_embeddings: int, embedding_dim: int):
- # Bart is set up so that if padding_idx is specified then offset the embedding ids by 2
- # and adjust num_embeddings appropriately. Other models don't have this hack
- self.offset = 2
- super().__init__(num_embeddings + self.offset, embedding_dim)
-
- def forward(self, input_ids: torch.Tensor, past_key_values_length: int = 0):
- """`input_ids' shape is expected to be [bsz x seqlen]."""
-
- bsz, seq_len = input_ids.shape[:2]
- positions = torch.arange(
- past_key_values_length, past_key_values_length + seq_len, dtype=torch.long, device=self.weight.device
- ).expand(bsz, -1)
-
- return super().forward(positions + self.offset)
-
-
-class BartAttention(nn.Module):
- """Multi-headed attention from 'Attention Is All You Need' paper"""
-
- def __init__(
- self,
- embed_dim: int,
- num_heads: int,
- dropout: float = 0.0,
- is_decoder: bool = False,
- bias: bool = True,
- ):
- super().__init__()
- self.embed_dim = embed_dim
- self.num_heads = num_heads
- self.dropout = dropout
- self.head_dim = embed_dim // num_heads
-
- if (self.head_dim * num_heads) != self.embed_dim:
- raise ValueError(
- f"embed_dim must be divisible by num_heads (got `embed_dim`: {self.embed_dim}"
- f" and `num_heads`: {num_heads})."
- )
- self.scaling = self.head_dim**-0.5
- self.is_decoder = is_decoder
-
- self.k_proj = nn.Linear(embed_dim, embed_dim, bias=bias)
- self.v_proj = nn.Linear(embed_dim, embed_dim, bias=bias)
- self.q_proj = nn.Linear(embed_dim, embed_dim, bias=bias)
- self.out_proj = nn.Linear(embed_dim, embed_dim, bias=bias)
-
- def _shape(self, tensor: torch.Tensor, seq_len: int, bsz: int):
- return tensor.view(bsz, seq_len, self.num_heads, self.head_dim).transpose(1, 2).contiguous()
-
- def forward(
- self,
- hidden_states: torch.Tensor,
- key_value_states: Optional[torch.Tensor] = None,
- past_key_value: Optional[Tuple[torch.Tensor]] = None,
- attention_mask: Optional[torch.Tensor] = None,
- layer_head_mask: Optional[torch.Tensor] = None,
- output_attentions: bool = False,
- ) -> Tuple[torch.Tensor, Optional[torch.Tensor], Optional[Tuple[torch.Tensor]]]:
- """Input shape: Batch x Time x Channel"""
-
- # if key_value_states are provided this layer is used as a cross-attention layer
- # for the decoder
- is_cross_attention = key_value_states is not None
-
- bsz, tgt_len, _ = hidden_states.size()
-
- # get query proj
- query_states = self.q_proj(hidden_states) * self.scaling
- # get key, value proj
- # `past_key_value[0].shape[2] == key_value_states.shape[1]`
- # is checking that the `sequence_length` of the `past_key_value` is the same as
- # the provided `key_value_states` to support prefix tuning
- if (
- is_cross_attention
- and past_key_value is not None
- and past_key_value[0].shape[2] == key_value_states.shape[1]
- ):
- # reuse k,v, cross_attentions
- key_states = past_key_value[0]
- value_states = past_key_value[1]
- elif is_cross_attention:
- # cross_attentions
- key_states = self._shape(self.k_proj(key_value_states), -1, bsz)
- value_states = self._shape(self.v_proj(key_value_states), -1, bsz)
- elif past_key_value is not None:
- # reuse k, v, self_attention
- key_states = self._shape(self.k_proj(hidden_states), -1, bsz)
- value_states = self._shape(self.v_proj(hidden_states), -1, bsz)
- key_states = torch.cat([past_key_value[0], key_states], dim=2)
- value_states = torch.cat([past_key_value[1], value_states], dim=2)
- else:
- # self_attention
- key_states = self._shape(self.k_proj(hidden_states), -1, bsz)
- value_states = self._shape(self.v_proj(hidden_states), -1, bsz)
-
- if self.is_decoder:
- # if cross_attention save Tuple(torch.Tensor, torch.Tensor) of all cross attention key/value_states.
- # Further calls to cross_attention layer can then reuse all cross-attention
- # key/value_states (first "if" case)
- # if uni-directional self-attention (decoder) save Tuple(torch.Tensor, torch.Tensor) of
- # all previous decoder key/value_states. Further calls to uni-directional self-attention
- # can concat previous decoder key/value_states to current projected key/value_states (third "elif" case)
- # if encoder bi-directional self-attention `past_key_value` is always `None`
- past_key_value = (key_states, value_states)
-
- proj_shape = (bsz * self.num_heads, -1, self.head_dim)
- query_states = self._shape(query_states, tgt_len, bsz).view(*proj_shape)
- key_states = key_states.reshape(*proj_shape)
- value_states = value_states.reshape(*proj_shape)
-
- src_len = key_states.size(1)
- attn_weights = torch.bmm(query_states, key_states.transpose(1, 2))
-
- if attn_weights.size() != (bsz * self.num_heads, tgt_len, src_len):
- raise ValueError(
- f"Attention weights should be of size {(bsz * self.num_heads, tgt_len, src_len)}, but is"
- f" {attn_weights.size()}"
- )
-
- if attention_mask is not None:
- if attention_mask.size() != (bsz, 1, tgt_len, src_len):
- raise ValueError(
- f"Attention mask should be of size {(bsz, 1, tgt_len, src_len)}, but is {attention_mask.size()}"
- )
- attn_weights = attn_weights.view(bsz, self.num_heads, tgt_len, src_len) + attention_mask
- attn_weights = attn_weights.view(bsz * self.num_heads, tgt_len, src_len)
-
- attn_weights = nn.functional.softmax(attn_weights, dim=-1)
-
- if layer_head_mask is not None:
- if layer_head_mask.size() != (self.num_heads,):
- raise ValueError(
- f"Head mask for a single layer should be of size {(self.num_heads,)}, but is"
- f" {layer_head_mask.size()}"
- )
- attn_weights = layer_head_mask.view(1, -1, 1, 1) * attn_weights.view(bsz, self.num_heads, tgt_len, src_len)
- attn_weights = attn_weights.view(bsz * self.num_heads, tgt_len, src_len)
-
- if output_attentions:
- # this operation is a bit awkward, but it's required to
- # make sure that attn_weights keeps its gradient.
- # In order to do so, attn_weights have to be reshaped
- # twice and have to be reused in the following
- attn_weights_reshaped = attn_weights.view(bsz, self.num_heads, tgt_len, src_len)
- attn_weights = attn_weights_reshaped.view(bsz * self.num_heads, tgt_len, src_len)
- else:
- attn_weights_reshaped = None
-
- attn_probs = nn.functional.dropout(attn_weights, p=self.dropout, training=self.training)
-
- attn_output = torch.bmm(attn_probs, value_states)
-
- if attn_output.size() != (bsz * self.num_heads, tgt_len, self.head_dim):
- raise ValueError(
- f"`attn_output` should be of size {(bsz * self.num_heads, tgt_len, self.head_dim)}, but is"
- f" {attn_output.size()}"
- )
-
- attn_output = attn_output.view(bsz, self.num_heads, tgt_len, self.head_dim)
- attn_output = attn_output.transpose(1, 2)
-
- # Use the `embed_dim` from the config (stored in the class) rather than `hidden_state` because `attn_output` can be
- # partitioned across GPUs when using tensor-parallelism.
- attn_output = attn_output.reshape(bsz, tgt_len, self.embed_dim)
-
- attn_output = self.out_proj(attn_output)
-
- return attn_output, attn_weights_reshaped, past_key_value
-
-
-class BartEncoderLayer(nn.Module):
- def __init__(self, config: BartConfig):
- super().__init__()
- self.embed_dim = config.d_model
- self.self_attn = BartAttention(
- embed_dim=self.embed_dim,
- num_heads=config.encoder_attention_heads,
- dropout=config.attention_dropout,
- )
- self.self_attn_layer_norm = nn.LayerNorm(self.embed_dim)
- self.dropout = config.dropout
- self.activation_fn = ACT2FN[config.activation_function]
- self.activation_dropout = config.activation_dropout
- self.fc1 = nn.Linear(self.embed_dim, config.encoder_ffn_dim)
- self.fc2 = nn.Linear(config.encoder_ffn_dim, self.embed_dim)
- self.final_layer_norm = nn.LayerNorm(self.embed_dim)
-
- def forward(
- self,
- hidden_states: torch.FloatTensor,
- attention_mask: torch.FloatTensor,
- layer_head_mask: torch.FloatTensor,
- output_attentions: Optional[bool] = False,
- ) -> Tuple[torch.FloatTensor, Optional[torch.FloatTensor]]:
- """
- Args:
- hidden_states (`torch.FloatTensor`): input to the layer of shape `(seq_len, batch, embed_dim)`
- attention_mask (`torch.FloatTensor`): attention mask of size
- `(batch, 1, tgt_len, src_len)` where padding elements are indicated by very large negative values.
- layer_head_mask (`torch.FloatTensor`): mask for attention heads in a given layer of size
- `(encoder_attention_heads,)`.
- output_attentions (`bool`, *optional*):
- Whether or not to return the attentions tensors of all attention layers. See `attentions` under
- returned tensors for more detail.
- """
- residual = hidden_states
- hidden_states, attn_weights, _ = self.self_attn(
- hidden_states=hidden_states,
- attention_mask=attention_mask,
- layer_head_mask=layer_head_mask,
- output_attentions=output_attentions,
- )
- hidden_states = nn.functional.dropout(hidden_states, p=self.dropout, training=self.training)
- hidden_states = residual + hidden_states
- hidden_states = self.self_attn_layer_norm(hidden_states)
-
- residual = hidden_states
- hidden_states = self.activation_fn(self.fc1(hidden_states))
- hidden_states = nn.functional.dropout(hidden_states, p=self.activation_dropout, training=self.training)
- hidden_states = self.fc2(hidden_states)
- hidden_states = nn.functional.dropout(hidden_states, p=self.dropout, training=self.training)
- hidden_states = residual + hidden_states
- hidden_states = self.final_layer_norm(hidden_states)
-
- if hidden_states.dtype == torch.float16 and (
- torch.isinf(hidden_states).any() or torch.isnan(hidden_states).any()
- ):
- clamp_value = torch.finfo(hidden_states.dtype).max - 1000
- hidden_states = torch.clamp(hidden_states, min=-clamp_value, max=clamp_value)
-
- outputs = (hidden_states,)
-
- if output_attentions:
- outputs += (attn_weights,)
-
- return outputs
-
-
-class BartDecoderLayer(nn.Module):
- def __init__(self, config: BartConfig):
- super().__init__()
- self.embed_dim = config.d_model
-
- self.self_attn = BartAttention(
- embed_dim=self.embed_dim,
- num_heads=config.decoder_attention_heads,
- dropout=config.attention_dropout,
- is_decoder=True,
- )
- self.dropout = config.dropout
- self.activation_fn = ACT2FN[config.activation_function]
- self.activation_dropout = config.activation_dropout
-
- self.self_attn_layer_norm = nn.LayerNorm(self.embed_dim)
- self.encoder_attn = BartAttention(
- self.embed_dim,
- config.decoder_attention_heads,
- dropout=config.attention_dropout,
- is_decoder=True,
- )
- self.encoder_attn_layer_norm = nn.LayerNorm(self.embed_dim)
- self.fc1 = nn.Linear(self.embed_dim, config.decoder_ffn_dim)
- self.fc2 = nn.Linear(config.decoder_ffn_dim, self.embed_dim)
- self.final_layer_norm = nn.LayerNorm(self.embed_dim)
-
- def forward(
- self,
- hidden_states: torch.Tensor,
- attention_mask: Optional[torch.Tensor] = None,
- encoder_hidden_states: Optional[torch.Tensor] = None,
- encoder_attention_mask: Optional[torch.Tensor] = None,
- layer_head_mask: Optional[torch.Tensor] = None,
- cross_attn_layer_head_mask: Optional[torch.Tensor] = None,
- past_key_value: Optional[Tuple[torch.Tensor]] = None,
- output_attentions: Optional[bool] = False,
- use_cache: Optional[bool] = True,
- ) -> Tuple[torch.FloatTensor, Optional[Tuple[torch.FloatTensor, torch.FloatTensor]]]:
- """
- Args:
- hidden_states (`torch.FloatTensor`): input to the layer of shape `(batch, seq_len, embed_dim)`
- attention_mask (`torch.FloatTensor`): attention mask of size
- `(batch, 1, tgt_len, src_len)` where padding elements are indicated by very large negative values.
- encoder_hidden_states (`torch.FloatTensor`):
- cross attention input to the layer of shape `(batch, seq_len, embed_dim)`
- encoder_attention_mask (`torch.FloatTensor`): encoder attention mask of size
- `(batch, 1, tgt_len, src_len)` where padding elements are indicated by very large negative values.
- layer_head_mask (`torch.FloatTensor`): mask for attention heads in a given layer of size
- `(encoder_attention_heads,)`.
- cross_attn_layer_head_mask (`torch.FloatTensor`): mask for cross-attention heads in a given layer of
- size `(decoder_attention_heads,)`.
- past_key_value (`Tuple(torch.FloatTensor)`): cached past key and value projection states
- output_attentions (`bool`, *optional*):
- Whether or not to return the attentions tensors of all attention layers. See `attentions` under
- returned tensors for more detail.
- """
- residual = hidden_states
-
- # Self Attention
- # decoder uni-directional self-attention cached key/values tuple is at positions 1,2
- self_attn_past_key_value = past_key_value[:2] if past_key_value is not None else None
- # add present self-attn cache to positions 1,2 of present_key_value tuple
- hidden_states, self_attn_weights, present_key_value = self.self_attn(
- hidden_states=hidden_states,
- past_key_value=self_attn_past_key_value,
- attention_mask=attention_mask,
- layer_head_mask=layer_head_mask,
- output_attentions=output_attentions,
- )
- hidden_states = nn.functional.dropout(hidden_states, p=self.dropout, training=self.training)
- hidden_states = residual + hidden_states
- hidden_states = self.self_attn_layer_norm(hidden_states)
-
- # Cross-Attention Block
- cross_attn_present_key_value = None
- cross_attn_weights = None
- if encoder_hidden_states is not None:
- residual = hidden_states
-
- # cross_attn cached key/values tuple is at positions 3,4 of present_key_value tuple
- cross_attn_past_key_value = past_key_value[-2:] if past_key_value is not None else None
- hidden_states, cross_attn_weights, cross_attn_present_key_value = self.encoder_attn(
- hidden_states=hidden_states,
- key_value_states=encoder_hidden_states,
- attention_mask=encoder_attention_mask,
- layer_head_mask=cross_attn_layer_head_mask,
- past_key_value=cross_attn_past_key_value,
- output_attentions=output_attentions,
- )
- hidden_states = nn.functional.dropout(hidden_states, p=self.dropout, training=self.training)
- hidden_states = residual + hidden_states
- hidden_states = self.encoder_attn_layer_norm(hidden_states)
-
- # add cross-attn to positions 3,4 of present_key_value tuple
- present_key_value = present_key_value + cross_attn_present_key_value
-
- # Fully Connected
- residual = hidden_states
- hidden_states = self.activation_fn(self.fc1(hidden_states))
- hidden_states = nn.functional.dropout(hidden_states, p=self.activation_dropout, training=self.training)
- hidden_states = self.fc2(hidden_states)
- hidden_states = nn.functional.dropout(hidden_states, p=self.dropout, training=self.training)
- hidden_states = residual + hidden_states
- hidden_states = self.final_layer_norm(hidden_states)
-
- outputs = (hidden_states,)
-
- if output_attentions:
- outputs += (self_attn_weights, cross_attn_weights)
-
- if use_cache:
- outputs += (present_key_value,)
-
- return outputs
-
-
-class BartClassificationHead(nn.Module):
- """Head for sentence-level classification tasks."""
-
- def __init__(
- self,
- input_dim: int,
- inner_dim: int,
- num_classes: int,
- pooler_dropout: float,
- ):
- super().__init__()
- self.dense = nn.Linear(input_dim, inner_dim)
- self.dropout = nn.Dropout(p=pooler_dropout)
- self.out_proj = nn.Linear(inner_dim, num_classes)
-
- def forward(self, hidden_states: torch.Tensor) -> torch.Tensor:
- hidden_states = self.dropout(hidden_states)
- hidden_states = self.dense(hidden_states)
- hidden_states = torch.tanh(hidden_states)
- hidden_states = self.dropout(hidden_states)
- hidden_states = self.out_proj(hidden_states)
- return hidden_states
-
-
-class BartPretrainedModel(PreTrainedModel):
- config_class = BartConfig
- base_model_prefix = "model"
- supports_gradient_checkpointing = True
- _keys_to_ignore_on_load_unexpected = [r"encoder.version", r"decoder.version"]
- _no_split_modules = [r"BartEncoderLayer", r"BartDecoderLayer"]
-
- def _init_weights(self, module):
- std = self.config.init_std
- if isinstance(module, nn.Linear):
- module.weight.data.normal_(mean=0.0, std=std)
- if module.bias is not None:
- module.bias.data.zero_()
- elif isinstance(module, nn.Embedding):
- module.weight.data.normal_(mean=0.0, std=std)
- if module.padding_idx is not None:
- module.weight.data[module.padding_idx].zero_()
-
- def _set_gradient_checkpointing(self, module, value=False):
- if isinstance(module, (BartDecoder, BartEncoder)):
- module.gradient_checkpointing = value
-
- @property
- def dummy_inputs(self):
- pad_token = self.config.pad_token_id
- input_ids = torch.tensor([[0, 6, 10, 4, 2], [0, 8, 12, 2, pad_token]], device=self.device)
- dummy_inputs = {
- "attention_mask": input_ids.ne(pad_token),
- "input_ids": input_ids,
- }
- return dummy_inputs
-
-
-class PretrainedBartModel(BartPretrainedModel):
- def __init_subclass__(self):
- warnings.warn(
- "The class `PretrainedBartModel` has been depreciated, please use `BartPretrainedModel` instead.",
- FutureWarning,
- )
-
-
-BART_START_DOCSTRING = r"""
- This model inherits from [`PreTrainedModel`]. Check the superclass documentation for the generic methods the
- library implements for all its model (such as downloading or saving, resizing the input embeddings, pruning heads
- etc.)
-
- This model is also a PyTorch [torch.nn.Module](https://pytorch.org/docs/stable/nn.html#torch.nn.Module) subclass.
- Use it as a regular PyTorch Module and refer to the PyTorch documentation for all matter related to general usage
- and behavior.
-
- Parameters:
- config ([`BartConfig`]):
- Model configuration class with all the parameters of the model. Initializing with a config file does not
- load the weights associated with the model, only the configuration. Check out the
- [`~PreTrainedModel.from_pretrained`] method to load the model weights.
-"""
-
-BART_GENERATION_EXAMPLE = r"""
- Summarization example:
-
- ```python
- >>> from transformers import AutoTokenizer, BartForConditionalGeneration
-
- >>> model = BartForConditionalGeneration.from_pretrained("facebook/bart-large-cnn")
- >>> tokenizer = AutoTokenizer.from_pretrained("facebook/bart-large-cnn")
-
- >>> ARTICLE_TO_SUMMARIZE = (
- ... "PG&E stated it scheduled the blackouts in response to forecasts for high winds "
- ... "amid dry conditions. The aim is to reduce the risk of wildfires. Nearly 800 thousand customers were "
- ... "scheduled to be affected by the shutoffs which were expected to last through at least midday tomorrow."
- ... )
- >>> inputs = tokenizer([ARTICLE_TO_SUMMARIZE], max_length=1024, return_tensors="pt")
-
- >>> # Generate Summary
- >>> summary_ids = model.generate(inputs["input_ids"], num_beams=2, min_length=0, max_length=20)
- >>> tokenizer.batch_decode(summary_ids, skip_special_tokens=True, clean_up_tokenization_spaces=False)[0]
- 'PG&E scheduled the blackouts in response to forecasts for high winds amid dry conditions'
- ```
-
- Mask filling example:
-
- ```python
- >>> from transformers import AutoTokenizer, BartForConditionalGeneration
-
- >>> tokenizer = AutoTokenizer.from_pretrained("facebook/bart-base")
- >>> model = BartForConditionalGeneration.from_pretrained("facebook/bart-base")
-
- >>> TXT = "My friends are but they eat too many carbs."
- >>> input_ids = tokenizer([TXT], return_tensors="pt")["input_ids"]
- >>> logits = model(input_ids).logits
-
- >>> masked_index = (input_ids[0] == tokenizer.mask_token_id).nonzero().item()
- >>> probs = logits[0, masked_index].softmax(dim=0)
- >>> values, predictions = probs.topk(5)
-
- >>> tokenizer.decode(predictions).split()
- ['not', 'good', 'healthy', 'great', 'very']
- ```
-"""
-
-BART_INPUTS_DOCSTRING = r"""
- Args:
- input_ids (`torch.LongTensor` of shape `(batch_size, sequence_length)`):
- Indices of input sequence tokens in the vocabulary. Padding will be ignored by default should you provide
- it.
-
- Indices can be obtained using [`AutoTokenizer`]. See [`PreTrainedTokenizer.encode`] and
- [`PreTrainedTokenizer.__call__`] for details.
-
- [What are input IDs?](../glossary#input-ids)
- attention_mask (`torch.Tensor` of shape `(batch_size, sequence_length)`, *optional*):
- Mask to avoid performing attention on padding token indices. Mask values selected in `[0, 1]`:
-
- - 1 for tokens that are **not masked**,
- - 0 for tokens that are **masked**.
-
- [What are attention masks?](../glossary#attention-mask)
- decoder_input_ids (`torch.LongTensor` of shape `(batch_size, target_sequence_length)`, *optional*):
- Indices of decoder input sequence tokens in the vocabulary.
-
- Indices can be obtained using [`AutoTokenizer`]. See [`PreTrainedTokenizer.encode`] and
- [`PreTrainedTokenizer.__call__`] for details.
-
- [What are decoder input IDs?](../glossary#decoder-input-ids)
-
- Bart uses the `eos_token_id` as the starting token for `decoder_input_ids` generation. If `past_key_values`
- is used, optionally only the last `decoder_input_ids` have to be input (see `past_key_values`).
-
- For translation and summarization training, `decoder_input_ids` should be provided. If no
- `decoder_input_ids` is provided, the model will create this tensor by shifting the `input_ids` to the right
- for denoising pre-training following the paper.
- decoder_attention_mask (`torch.LongTensor` of shape `(batch_size, target_sequence_length)`, *optional*):
- Default behavior: generate a tensor that ignores pad tokens in `decoder_input_ids`. Causal mask will also
- be used by default.
-
- If you want to change padding behavior, you should read [`modeling_bart._prepare_decoder_attention_mask`]
- and modify to your needs. See diagram 1 in [the paper](https://arxiv.org/abs/1910.13461) for more
- information on the default strategy.
- head_mask (`torch.Tensor` of shape `(encoder_layers, encoder_attention_heads)`, *optional*):
- Mask to nullify selected heads of the attention modules in the encoder. Mask values selected in `[0, 1]`:
-
- - 1 indicates the head is **not masked**,
- - 0 indicates the head is **masked**.
-
- decoder_head_mask (`torch.Tensor` of shape `(decoder_layers, decoder_attention_heads)`, *optional*):
- Mask to nullify selected heads of the attention modules in the decoder. Mask values selected in `[0, 1]`:
-
- - 1 indicates the head is **not masked**,
- - 0 indicates the head is **masked**.
-
- cross_attn_head_mask (`torch.Tensor` of shape `(decoder_layers, decoder_attention_heads)`, *optional*):
- Mask to nullify selected heads of the cross-attention modules in the decoder. Mask values selected in `[0,
- 1]`:
-
- - 1 indicates the head is **not masked**,
- - 0 indicates the head is **masked**.
-
- encoder_outputs (`tuple(tuple(torch.FloatTensor)`, *optional*):
- Tuple consists of (`last_hidden_state`, *optional*: `hidden_states`, *optional*: `attentions`)
- `last_hidden_state` of shape `(batch_size, sequence_length, hidden_size)`, *optional*) is a sequence of
- hidden-states at the output of the last layer of the encoder. Used in the cross-attention of the decoder.
- past_key_values (`tuple(tuple(torch.FloatTensor))`, *optional*, returned when `use_cache=True` is passed or when `config.use_cache=True`):
- Tuple of `tuple(torch.FloatTensor)` of length `config.n_layers`, with each tuple having 2 tensors of shape
- `(batch_size, num_heads, sequence_length, embed_size_per_head)`) and 2 additional tensors of shape
- `(batch_size, num_heads, encoder_sequence_length, embed_size_per_head)`.
-
- Contains pre-computed hidden-states (key and values in the self-attention blocks and in the cross-attention
- blocks) that can be used (see `past_key_values` input) to speed up sequential decoding.
-
- If `past_key_values` are used, the user can optionally input only the last `decoder_input_ids` (those that
- don't have their past key value states given to this model) of shape `(batch_size, 1)` instead of all
- `decoder_input_ids` of shape `(batch_size, sequence_length)`. inputs_embeds (`torch.FloatTensor` of shape
- `(batch_size, sequence_length, hidden_size)`, *optional*): Optionally, instead of passing `input_ids` you
- can choose to directly pass an embedded representation. This is useful if you want more control over how to
- convert `input_ids` indices into associated vectors than the model's internal embedding lookup matrix.
- decoder_inputs_embeds (`torch.FloatTensor` of shape `(batch_size, target_sequence_length, hidden_size)`, *optional*):
- Optionally, instead of passing `decoder_input_ids` you can choose to directly pass an embedded
- representation. If `past_key_values` is used, optionally only the last `decoder_inputs_embeds` have to be
- input (see `past_key_values`). This is useful if you want more control over how to convert
- `decoder_input_ids` indices into associated vectors than the model's internal embedding lookup matrix.
-
- If `decoder_input_ids` and `decoder_inputs_embeds` are both unset, `decoder_inputs_embeds` takes the value
- of `inputs_embeds`.
- use_cache (`bool`, *optional*):
- If set to `True`, `past_key_values` key value states are returned and can be used to speed up decoding (see
- `past_key_values`).
- output_attentions (`bool`, *optional*):
- Whether or not to return the attentions tensors of all attention layers. See `attentions` under returned
- tensors for more detail.
- output_hidden_states (`bool`, *optional*):
- Whether or not to return the hidden states of all layers. See `hidden_states` under returned tensors for
- more detail.
- return_dict (`bool`, *optional*):
- Whether or not to return a [`~utils.ModelOutput`] instead of a plain tuple.
-"""
-
-
-class BartEncoder(BartPretrainedModel):
- """
- Transformer encoder consisting of *config.encoder_layers* self attention layers. Each layer is a
- [`BartEncoderLayer`].
-
- Args:
- config: BartConfig
- embed_tokens (nn.Embedding): output embedding
- """
-
- def __init__(self, config: BartConfig, embed_tokens: Optional[nn.Embedding] = None):
- super().__init__(config)
-
- self.dropout = config.dropout
- self.layerdrop = config.encoder_layerdrop
-
- embed_dim = config.d_model
- self.padding_idx = config.pad_token_id
- self.max_source_positions = config.max_position_embeddings
- self.embed_scale = math.sqrt(embed_dim) if config.scale_embedding else 1.0
-
- self.embed_tokens = nn.Embedding(config.vocab_size, embed_dim, self.padding_idx)
-
- if embed_tokens is not None:
- self.embed_tokens.weight = embed_tokens.weight
-
- self.embed_positions = BartLearnedPositionalEmbedding(
- config.max_position_embeddings,
- embed_dim,
- )
- self.layers = nn.ModuleList([BartEncoderLayer(config) for _ in range(config.encoder_layers)])
- self.layernorm_embedding = nn.LayerNorm(embed_dim)
-
- self.gradient_checkpointing = False
- # Initialize weights and apply final processing
- self.post_init()
-
- def get_input_embeddings(self):
- return self.embed_tokens
-
- def set_input_embeddings(self, value):
- self.embed_tokens = value
-
- def forward(
- self,
- input_ids: torch.LongTensor = None,
- attention_mask: Optional[torch.Tensor] = None,
- head_mask: Optional[torch.Tensor] = None,
- inputs_embeds: Optional[torch.FloatTensor] = None,
- output_attentions: Optional[bool] = None,
- output_hidden_states: Optional[bool] = None,
- return_dict: Optional[bool] = None,
- ) -> Union[Tuple, BaseModelOutput]:
- r"""
- Args:
- input_ids (`torch.LongTensor` of shape `(batch_size, sequence_length)`):
- Indices of input sequence tokens in the vocabulary. Padding will be ignored by default should you
- provide it.
-
- Indices can be obtained using [`AutoTokenizer`]. See [`PreTrainedTokenizer.encode`] and
- [`PreTrainedTokenizer.__call__`] for details.
-
- [What are input IDs?](../glossary#input-ids)
- attention_mask (`torch.Tensor` of shape `(batch_size, sequence_length)`, *optional*):
- Mask to avoid performing attention on padding token indices. Mask values selected in `[0, 1]`:
-
- - 1 for tokens that are **not masked**,
- - 0 for tokens that are **masked**.
-
- [What are attention masks?](../glossary#attention-mask)
- head_mask (`torch.Tensor` of shape `(encoder_layers, encoder_attention_heads)`, *optional*):
- Mask to nullify selected heads of the attention modules. Mask values selected in `[0, 1]`:
-
- - 1 indicates the head is **not masked**,
- - 0 indicates the head is **masked**.
-
- inputs_embeds (`torch.FloatTensor` of shape `(batch_size, sequence_length, hidden_size)`, *optional*):
- Optionally, instead of passing `input_ids` you can choose to directly pass an embedded representation.
- This is useful if you want more control over how to convert `input_ids` indices into associated vectors
- than the model's internal embedding lookup matrix.
- output_attentions (`bool`, *optional*):
- Whether or not to return the attentions tensors of all attention layers. See `attentions` under
- returned tensors for more detail.
- output_hidden_states (`bool`, *optional*):
- Whether or not to return the hidden states of all layers. See `hidden_states` under returned tensors
- for more detail.
- return_dict (`bool`, *optional*):
- Whether or not to return a [`~utils.ModelOutput`] instead of a plain tuple.
- """
- output_attentions = output_attentions if output_attentions is not None else self.config.output_attentions
- output_hidden_states = (
- output_hidden_states if output_hidden_states is not None else self.config.output_hidden_states
- )
- return_dict = return_dict if return_dict is not None else self.config.use_return_dict
-
- # retrieve input_ids and inputs_embeds
- if input_ids is not None and inputs_embeds is not None:
- raise ValueError("You cannot specify both input_ids and inputs_embeds at the same time")
- elif input_ids is not None:
- input = input_ids
- input_ids = input_ids.view(-1, input_ids.shape[-1])
- elif inputs_embeds is not None:
- input = inputs_embeds[:, :, -1]
- else:
- raise ValueError("You have to specify either input_ids or inputs_embeds")
-
- if inputs_embeds is None:
- inputs_embeds = self.embed_tokens(input_ids) * self.embed_scale
-
- embed_pos = self.embed_positions(input)
- embed_pos = embed_pos.to(inputs_embeds.device)
-
- hidden_states = inputs_embeds + embed_pos
- hidden_states = self.layernorm_embedding(hidden_states)
- hidden_states = nn.functional.dropout(hidden_states, p=self.dropout, training=self.training)
-
- # expand attention_mask
- if attention_mask is not None:
- # [bsz, seq_len] -> [bsz, 1, tgt_seq_len, src_seq_len]
- attention_mask = _expand_mask(attention_mask, inputs_embeds.dtype)
-
- encoder_states = () if output_hidden_states else None
- all_attentions = () if output_attentions else None
-
- # check if head_mask has a correct number of layers specified if desired
- if head_mask is not None:
- if head_mask.size()[0] != (len(self.layers)):
- raise ValueError(
- f"The head_mask should be specified for {len(self.layers)} layers, but it is for"
- f" {head_mask.size()[0]}."
- )
-
- for idx, encoder_layer in enumerate(self.layers):
- if output_hidden_states:
- encoder_states = encoder_states + (hidden_states,)
- # add LayerDrop (see https://arxiv.org/abs/1909.11556 for description)
- dropout_probability = random.uniform(0, 1)
- if self.training and (dropout_probability < self.layerdrop): # skip the layer
- layer_outputs = (None, None)
- else:
- if self.gradient_checkpointing and self.training:
-
- def create_custom_forward(module):
- def custom_forward(*inputs):
- return module(*inputs, output_attentions)
-
- return custom_forward
-
- layer_outputs = torch.utils.checkpoint.checkpoint(
- create_custom_forward(encoder_layer),
- hidden_states,
- attention_mask,
- (head_mask[idx] if head_mask is not None else None),
- )
- else:
- layer_outputs = encoder_layer(
- hidden_states,
- attention_mask,
- layer_head_mask=(head_mask[idx] if head_mask is not None else None),
- output_attentions=output_attentions,
- )
-
- hidden_states = layer_outputs[0]
-
- if output_attentions:
- all_attentions = all_attentions + (layer_outputs[1],)
-
- if output_hidden_states:
- encoder_states = encoder_states + (hidden_states,)
-
- if not return_dict:
- return tuple(v for v in [hidden_states, encoder_states, all_attentions] if v is not None)
- return BaseModelOutput(
- last_hidden_state=hidden_states, hidden_states=encoder_states, attentions=all_attentions
- )
-
-
-class BartDecoder(BartPretrainedModel):
- """
- Transformer decoder consisting of *config.decoder_layers* layers. Each layer is a [`BartDecoderLayer`]
-
- Args:
- config: BartConfig
- embed_tokens (nn.Embedding): output embedding
- """
-
- def __init__(self, config: BartConfig, embed_tokens: Optional[nn.Embedding] = None):
- super().__init__(config)
- self.dropout = config.dropout
- self.layerdrop = config.decoder_layerdrop
- self.padding_idx = config.pad_token_id
- self.max_target_positions = config.max_position_embeddings
- self.embed_scale = math.sqrt(config.d_model) if config.scale_embedding else 1.0
-
- self.embed_tokens = nn.Embedding(config.vocab_size, config.d_model, self.padding_idx)
-
- if embed_tokens is not None:
- self.embed_tokens.weight = embed_tokens.weight
-
- self.embed_positions = BartLearnedPositionalEmbedding(
- config.max_position_embeddings,
- config.d_model,
- )
- self.layers = nn.ModuleList([BartDecoderLayer(config) for _ in range(config.decoder_layers)])
- self.layernorm_embedding = nn.LayerNorm(config.d_model)
-
- self.gradient_checkpointing = False
- # Initialize weights and apply final processing
- self.post_init()
-
- def get_input_embeddings(self):
- return self.embed_tokens
-
- def set_input_embeddings(self, value):
- self.embed_tokens = value
-
- def _prepare_decoder_attention_mask(self, attention_mask, input_shape, inputs_embeds, past_key_values_length):
- # create causal mask
- # [bsz, seq_len] -> [bsz, 1, tgt_seq_len, src_seq_len]
- combined_attention_mask = None
- if input_shape[-1] > 1:
- combined_attention_mask = _make_causal_mask(
- input_shape,
- inputs_embeds.dtype,
- device=inputs_embeds.device,
- past_key_values_length=past_key_values_length,
- )
-
- if attention_mask is not None:
- # [bsz, seq_len] -> [bsz, 1, tgt_seq_len, src_seq_len]
- expanded_attn_mask = _expand_mask(attention_mask, inputs_embeds.dtype, tgt_len=input_shape[-1]).to(
- inputs_embeds.device
- )
- combined_attention_mask = (
- expanded_attn_mask if combined_attention_mask is None else expanded_attn_mask + combined_attention_mask
- )
-
- return combined_attention_mask
-
- def forward(
- self,
- input_ids: torch.LongTensor = None,
- attention_mask: Optional[torch.Tensor] = None,
- encoder_hidden_states: Optional[torch.FloatTensor] = None,
- encoder_attention_mask: Optional[torch.LongTensor] = None,
- head_mask: Optional[torch.Tensor] = None,
- cross_attn_head_mask: Optional[torch.Tensor] = None,
- past_key_values: Optional[List[torch.FloatTensor]] = None,
- inputs_embeds: Optional[torch.FloatTensor] = None,
- use_cache: Optional[bool] = None,
- output_attentions: Optional[bool] = None,
- output_hidden_states: Optional[bool] = None,
- return_dict: Optional[bool] = None,
- ) -> Union[Tuple, BaseModelOutputWithPastAndCrossAttentions]:
- r"""
- Args:
- input_ids (`torch.LongTensor` of shape `(batch_size, sequence_length)`):
- Indices of input sequence tokens in the vocabulary. Padding will be ignored by default should you
- provide it.
-
- Indices can be obtained using [`AutoTokenizer`]. See [`PreTrainedTokenizer.encode`] and
- [`PreTrainedTokenizer.__call__`] for details.
-
- [What are input IDs?](../glossary#input-ids)
- attention_mask (`torch.Tensor` of shape `(batch_size, sequence_length)`, *optional*):
- Mask to avoid performing attention on padding token indices. Mask values selected in `[0, 1]`:
-
- - 1 for tokens that are **not masked**,
- - 0 for tokens that are **masked**.
-
- [What are attention masks?](../glossary#attention-mask)
- encoder_hidden_states (`torch.FloatTensor` of shape `(batch_size, encoder_sequence_length, hidden_size)`, *optional*):
- Sequence of hidden-states at the output of the last layer of the encoder. Used in the cross-attention
- of the decoder.
- encoder_attention_mask (`torch.LongTensor` of shape `(batch_size, encoder_sequence_length)`, *optional*):
- Mask to avoid performing cross-attention on padding tokens indices of encoder input_ids. Mask values
- selected in `[0, 1]`:
-
- - 1 for tokens that are **not masked**,
- - 0 for tokens that are **masked**.
-
- [What are attention masks?](../glossary#attention-mask)
- head_mask (`torch.Tensor` of shape `(decoder_layers, decoder_attention_heads)`, *optional*):
- Mask to nullify selected heads of the attention modules. Mask values selected in `[0, 1]`:
-
- - 1 indicates the head is **not masked**,
- - 0 indicates the head is **masked**.
-
- cross_attn_head_mask (`torch.Tensor` of shape `(decoder_layers, decoder_attention_heads)`, *optional*):
- Mask to nullify selected heads of the cross-attention modules in the decoder to avoid performing
- cross-attention on hidden heads. Mask values selected in `[0, 1]`:
-
- - 1 indicates the head is **not masked**,
- - 0 indicates the head is **masked**.
-
- past_key_values (`tuple(tuple(torch.FloatTensor))`, *optional*, returned when `use_cache=True` is passed or when `config.use_cache=True`):
- Tuple of `tuple(torch.FloatTensor)` of length `config.n_layers`, with each tuple having 2 tensors of
- shape `(batch_size, num_heads, sequence_length, embed_size_per_head)`) and 2 additional tensors of
- shape `(batch_size, num_heads, encoder_sequence_length, embed_size_per_head)`.
-
- Contains pre-computed hidden-states (key and values in the self-attention blocks and in the
- cross-attention blocks) that can be used (see `past_key_values` input) to speed up sequential decoding.
-
- If `past_key_values` are used, the user can optionally input only the last `decoder_input_ids` (those
- that don't have their past key value states given to this model) of shape `(batch_size, 1)` instead of
- all `decoder_input_ids` of shape `(batch_size, sequence_length)`. inputs_embeds (`torch.FloatTensor` of
- shape `(batch_size, sequence_length, hidden_size)`, *optional*): Optionally, instead of passing
- `input_ids` you can choose to directly pass an embedded representation. This is useful if you want more
- control over how to convert `input_ids` indices into associated vectors than the model's internal
- embedding lookup matrix.
- output_attentions (`bool`, *optional*):
- Whether or not to return the attentions tensors of all attention layers. See `attentions` under
- returned tensors for more detail.
- output_hidden_states (`bool`, *optional*):
- Whether or not to return the hidden states of all layers. See `hidden_states` under returned tensors
- for more detail.
- return_dict (`bool`, *optional*):
- Whether or not to return a [`~utils.ModelOutput`] instead of a plain tuple.
- """
- output_attentions = output_attentions if output_attentions is not None else self.config.output_attentions
- output_hidden_states = (
- output_hidden_states if output_hidden_states is not None else self.config.output_hidden_states
- )
- use_cache = use_cache if use_cache is not None else self.config.use_cache
- return_dict = return_dict if return_dict is not None else self.config.use_return_dict
-
- # retrieve input_ids and inputs_embeds
- if input_ids is not None and inputs_embeds is not None:
- raise ValueError("You cannot specify both decoder_input_ids and decoder_inputs_embeds at the same time")
- elif input_ids is not None:
- input = input_ids
- input_shape = input.shape
- input_ids = input_ids.view(-1, input_shape[-1])
- elif inputs_embeds is not None:
- input_shape = inputs_embeds.size()[:-1]
- input = inputs_embeds[:, :, -1]
- else:
- raise ValueError("You have to specify either decoder_input_ids or decoder_inputs_embeds")
-
- # past_key_values_length
- past_key_values_length = past_key_values[0][0].shape[2] if past_key_values is not None else 0
-
- if inputs_embeds is None:
- inputs_embeds = self.embed_tokens(input) * self.embed_scale
-
- attention_mask = self._prepare_decoder_attention_mask(
- attention_mask, input_shape, inputs_embeds, past_key_values_length
- )
-
- # expand encoder attention mask
- if encoder_hidden_states is not None and encoder_attention_mask is not None:
- # [bsz, seq_len] -> [bsz, 1, tgt_seq_len, src_seq_len]
- encoder_attention_mask = _expand_mask(encoder_attention_mask, inputs_embeds.dtype, tgt_len=input_shape[-1])
-
- # embed positions
- positions = self.embed_positions(input, past_key_values_length)
- positions = positions.to(inputs_embeds.device)
-
- hidden_states = inputs_embeds + positions
- hidden_states = self.layernorm_embedding(hidden_states)
-
- hidden_states = nn.functional.dropout(hidden_states, p=self.dropout, training=self.training)
-
- if self.gradient_checkpointing and self.training:
- if use_cache:
- logger.warning_once(
- "`use_cache=True` is incompatible with gradient checkpointing. Setting `use_cache=False`..."
- )
- use_cache = False
-
- # decoder layers
- all_hidden_states = () if output_hidden_states else None
- all_self_attns = () if output_attentions else None
- all_cross_attentions = () if (output_attentions and encoder_hidden_states is not None) else None
- next_decoder_cache = () if use_cache else None
-
- # check if head_mask/cross_attn_head_mask has a correct number of layers specified if desired
- for attn_mask, mask_name in zip([head_mask, cross_attn_head_mask], ["head_mask", "cross_attn_head_mask"]):
- if attn_mask is not None:
- if attn_mask.size()[0] != (len(self.layers)):
- raise ValueError(
- f"The `{mask_name}` should be specified for {len(self.layers)} layers, but it is for"
- f" {head_mask.size()[0]}."
- )
-
- for idx, decoder_layer in enumerate(self.layers):
- # add LayerDrop (see https://arxiv.org/abs/1909.11556 for description)
- if output_hidden_states:
- all_hidden_states += (hidden_states,)
- dropout_probability = random.uniform(0, 1)
- if self.training and (dropout_probability < self.layerdrop):
- continue
-
- past_key_value = past_key_values[idx] if past_key_values is not None else None
-
- if self.gradient_checkpointing and self.training:
-
- def create_custom_forward(module):
- def custom_forward(*inputs):
- # None for past_key_value
- return module(*inputs, output_attentions, use_cache)
-
- return custom_forward
-
- layer_outputs = torch.utils.checkpoint.checkpoint(
- create_custom_forward(decoder_layer),
- hidden_states,
- attention_mask,
- encoder_hidden_states,
- encoder_attention_mask,
- head_mask[idx] if head_mask is not None else None,
- cross_attn_head_mask[idx] if cross_attn_head_mask is not None else None,
- None,
- )
- else:
- layer_outputs = decoder_layer(
- hidden_states,
- attention_mask=attention_mask,
- encoder_hidden_states=encoder_hidden_states,
- encoder_attention_mask=encoder_attention_mask,
- layer_head_mask=(head_mask[idx] if head_mask is not None else None),
- cross_attn_layer_head_mask=(
- cross_attn_head_mask[idx] if cross_attn_head_mask is not None else None
- ),
- past_key_value=past_key_value,
- output_attentions=output_attentions,
- use_cache=use_cache,
- )
- hidden_states = layer_outputs[0]
-
- if use_cache:
- next_decoder_cache += (layer_outputs[3 if output_attentions else 1],)
-
- if output_attentions:
- all_self_attns += (layer_outputs[1],)
-
- if encoder_hidden_states is not None:
- all_cross_attentions += (layer_outputs[2],)
-
- # add hidden states from the last decoder layer
- if output_hidden_states:
- all_hidden_states += (hidden_states,)
-
- next_cache = next_decoder_cache if use_cache else None
- if not return_dict:
- return tuple(
- v
- for v in [hidden_states, next_cache, all_hidden_states, all_self_attns, all_cross_attentions]
- if v is not None
- )
- return BaseModelOutputWithPastAndCrossAttentions(
- last_hidden_state=hidden_states,
- past_key_values=next_cache,
- hidden_states=all_hidden_states,
- attentions=all_self_attns,
- cross_attentions=all_cross_attentions,
- )
-
-
-@add_start_docstrings(
- "The bare BART Model outputting raw hidden-states without any specific head on top.",
- BART_START_DOCSTRING,
-)
-class BartModel(BartPretrainedModel):
- _keys_to_ignore_on_load_missing = ["encoder.embed_tokens.weight", "decoder.embed_tokens.weight"]
-
- def __init__(self, config: BartConfig):
- super().__init__(config)
-
- padding_idx, vocab_size = config.pad_token_id, config.vocab_size
- self.shared = nn.Embedding(vocab_size, config.d_model, padding_idx)
-
- self.encoder = BartEncoder(config, self.shared)
- self.decoder = BartDecoder(config, self.shared)
-
- # Initialize weights and apply final processing
- self.post_init()
-
- def get_input_embeddings(self):
- return self.shared
-
- def set_input_embeddings(self, value):
- self.shared = value
- self.encoder.embed_tokens = self.shared
- self.decoder.embed_tokens = self.shared
-
- def get_encoder(self):
- return self.encoder
-
- def get_decoder(self):
- return self.decoder
-
- @add_start_docstrings_to_model_forward(BART_INPUTS_DOCSTRING)
- @add_code_sample_docstrings(
- checkpoint=_CHECKPOINT_FOR_DOC,
- output_type=Seq2SeqModelOutput,
- config_class=_CONFIG_FOR_DOC,
- expected_output=_EXPECTED_OUTPUT_SHAPE,
- )
- def forward(
- self,
- input_ids: torch.LongTensor = None,
- attention_mask: Optional[torch.Tensor] = None,
- decoder_input_ids: Optional[torch.LongTensor] = None,
- decoder_attention_mask: Optional[torch.LongTensor] = None,
- head_mask: Optional[torch.Tensor] = None,
- decoder_head_mask: Optional[torch.Tensor] = None,
- cross_attn_head_mask: Optional[torch.Tensor] = None,
- encoder_outputs: Optional[List[torch.FloatTensor]] = None,
- past_key_values: Optional[List[torch.FloatTensor]] = None,
- inputs_embeds: Optional[torch.FloatTensor] = None,
- decoder_inputs_embeds: Optional[torch.FloatTensor] = None,
- use_cache: Optional[bool] = None,
- output_attentions: Optional[bool] = None,
- output_hidden_states: Optional[bool] = None,
- return_dict: Optional[bool] = None,
- ) -> Union[Tuple, Seq2SeqModelOutput]:
- # different to other models, Bart automatically creates decoder_input_ids from
- # input_ids if no decoder_input_ids are provided
- if decoder_input_ids is None and decoder_inputs_embeds is None:
- if input_ids is None:
- raise ValueError(
- "If no `decoder_input_ids` or `decoder_inputs_embeds` are "
- "passed, `input_ids` cannot be `None`. Please pass either "
- "`input_ids` or `decoder_input_ids` or `decoder_inputs_embeds`."
- )
-
- decoder_input_ids = shift_tokens_right(
- input_ids, self.config.pad_token_id, self.config.decoder_start_token_id
- )
-
- output_attentions = output_attentions if output_attentions is not None else self.config.output_attentions
- output_hidden_states = (
- output_hidden_states if output_hidden_states is not None else self.config.output_hidden_states
- )
- use_cache = use_cache if use_cache is not None else self.config.use_cache
- return_dict = return_dict if return_dict is not None else self.config.use_return_dict
-
- if encoder_outputs is None:
- encoder_outputs = self.encoder(
- input_ids=input_ids,
- attention_mask=attention_mask,
- head_mask=head_mask,
- inputs_embeds=inputs_embeds,
- output_attentions=output_attentions,
- output_hidden_states=output_hidden_states,
- return_dict=return_dict,
- )
- # If the user passed a tuple for encoder_outputs, we wrap it in a BaseModelOutput when return_dict=True
- elif return_dict and not isinstance(encoder_outputs, BaseModelOutput):
- encoder_outputs = BaseModelOutput(
- last_hidden_state=encoder_outputs[0],
- hidden_states=encoder_outputs[1] if len(encoder_outputs) > 1 else None,
- attentions=encoder_outputs[2] if len(encoder_outputs) > 2 else None,
- )
-
- # decoder outputs consists of (dec_features, past_key_value, dec_hidden, dec_attn)
- decoder_outputs = self.decoder(
- input_ids=decoder_input_ids,
- attention_mask=decoder_attention_mask,
- encoder_hidden_states=encoder_outputs[0],
- encoder_attention_mask=attention_mask,
- head_mask=decoder_head_mask,
- cross_attn_head_mask=cross_attn_head_mask,
- past_key_values=past_key_values,
- inputs_embeds=decoder_inputs_embeds,
- use_cache=use_cache,
- output_attentions=output_attentions,
- output_hidden_states=output_hidden_states,
- return_dict=return_dict,
- )
-
- if not return_dict:
- return decoder_outputs + encoder_outputs
-
- return Seq2SeqModelOutput(
- last_hidden_state=decoder_outputs.last_hidden_state,
- past_key_values=decoder_outputs.past_key_values,
- decoder_hidden_states=decoder_outputs.hidden_states,
- decoder_attentions=decoder_outputs.attentions,
- cross_attentions=decoder_outputs.cross_attentions,
- encoder_last_hidden_state=encoder_outputs.last_hidden_state,
- encoder_hidden_states=encoder_outputs.hidden_states,
- encoder_attentions=encoder_outputs.attentions,
- )
-
-
-@add_start_docstrings(
- "The BART Model with a language modeling head. Can be used for summarization.", BART_START_DOCSTRING
-)
-class BartForConditionalGeneration(BartPretrainedModel):
- base_model_prefix = "model"
- _keys_to_ignore_on_load_missing = [
- r"final_logits_bias",
- r"lm_head.weight",
- "encoder.embed_tokens.weight",
- "decoder.embed_tokens.weight",
- ]
-
- def __init__(self, config: BartConfig):
- super().__init__(config)
- self.model = BartModel(config)
- self.register_buffer("final_logits_bias", torch.zeros((1, self.model.shared.num_embeddings)))
- self.lm_head = nn.Linear(config.d_model, self.model.shared.num_embeddings, bias=False)
-
- # Initialize weights and apply final processing
- self.post_init()
-
- def get_encoder(self):
- return self.model.get_encoder()
-
- def get_decoder(self):
- return self.model.get_decoder()
-
- def resize_token_embeddings(self, new_num_tokens: int) -> nn.Embedding:
- new_embeddings = super().resize_token_embeddings(new_num_tokens)
- self._resize_final_logits_bias(new_num_tokens)
- return new_embeddings
-
- def _resize_final_logits_bias(self, new_num_tokens: int) -> None:
- old_num_tokens = self.final_logits_bias.shape[-1]
- if new_num_tokens <= old_num_tokens:
- new_bias = self.final_logits_bias[:, :new_num_tokens]
- else:
- extra_bias = torch.zeros((1, new_num_tokens - old_num_tokens), device=self.final_logits_bias.device)
- new_bias = torch.cat([self.final_logits_bias, extra_bias], dim=1)
- self.register_buffer("final_logits_bias", new_bias)
-
- def get_output_embeddings(self):
- return self.lm_head
-
- def set_output_embeddings(self, new_embeddings):
- self.lm_head = new_embeddings
-
- @add_start_docstrings_to_model_forward(BART_INPUTS_DOCSTRING)
- @replace_return_docstrings(output_type=Seq2SeqLMOutput, config_class=_CONFIG_FOR_DOC)
- @add_end_docstrings(BART_GENERATION_EXAMPLE)
- def forward(
- self,
- input_ids: torch.LongTensor = None,
- attention_mask: Optional[torch.Tensor] = None,
- decoder_input_ids: Optional[torch.LongTensor] = None,
- decoder_attention_mask: Optional[torch.LongTensor] = None,
- head_mask: Optional[torch.Tensor] = None,
- decoder_head_mask: Optional[torch.Tensor] = None,
- cross_attn_head_mask: Optional[torch.Tensor] = None,
- encoder_outputs: Optional[List[torch.FloatTensor]] = None,
- past_key_values: Optional[List[torch.FloatTensor]] = None,
- inputs_embeds: Optional[torch.FloatTensor] = None,
- decoder_inputs_embeds: Optional[torch.FloatTensor] = None,
- labels: Optional[torch.LongTensor] = None,
- use_cache: Optional[bool] = None,
- output_attentions: Optional[bool] = None,
- output_hidden_states: Optional[bool] = None,
- return_dict: Optional[bool] = None,
- ) -> Union[Tuple, Seq2SeqLMOutput]:
- r"""
- labels (`torch.LongTensor` of shape `(batch_size, sequence_length)`, *optional*):
- Labels for computing the masked language modeling loss. Indices should either be in `[0, ...,
- config.vocab_size]` or -100 (see `input_ids` docstring). Tokens with indices set to `-100` are ignored
- (masked), the loss is only computed for the tokens with labels in `[0, ..., config.vocab_size]`.
-
- Returns:
- """
- return_dict = return_dict if return_dict is not None else self.config.use_return_dict
-
- if labels is not None:
- if use_cache:
- logger.warning("The `use_cache` argument is changed to `False` since `labels` is provided.")
- use_cache = False
- if decoder_input_ids is None and decoder_inputs_embeds is None:
- decoder_input_ids = shift_tokens_right(
- labels, self.config.pad_token_id, self.config.decoder_start_token_id
- )
-
- outputs = self.model(
- input_ids,
- attention_mask=attention_mask,
- decoder_input_ids=decoder_input_ids,
- encoder_outputs=encoder_outputs,
- decoder_attention_mask=decoder_attention_mask,
- head_mask=head_mask,
- decoder_head_mask=decoder_head_mask,
- cross_attn_head_mask=cross_attn_head_mask,
- past_key_values=past_key_values,
- inputs_embeds=inputs_embeds,
- decoder_inputs_embeds=decoder_inputs_embeds,
- use_cache=use_cache,
- output_attentions=output_attentions,
- output_hidden_states=output_hidden_states,
- return_dict=return_dict,
- )
-
- lm_logits = self.lm_head(outputs[0])
- lm_logits = lm_logits + self.final_logits_bias.to(lm_logits.device)
-
- masked_lm_loss = None
- if labels is not None:
- labels = labels.to(lm_logits.device)
- loss_fct = CrossEntropyLoss()
- masked_lm_loss = loss_fct(lm_logits.view(-1, self.config.vocab_size), labels.view(-1))
-
- if not return_dict:
- output = (lm_logits,) + outputs[1:]
- return ((masked_lm_loss,) + output) if masked_lm_loss is not None else output
-
- return Seq2SeqLMOutput(
- loss=masked_lm_loss,
- logits=lm_logits,
- past_key_values=outputs.past_key_values,
- decoder_hidden_states=outputs.decoder_hidden_states,
- decoder_attentions=outputs.decoder_attentions,
- cross_attentions=outputs.cross_attentions,
- encoder_last_hidden_state=outputs.encoder_last_hidden_state,
- encoder_hidden_states=outputs.encoder_hidden_states,
- encoder_attentions=outputs.encoder_attentions,
- )
-
- def prepare_inputs_for_generation(
- self,
- decoder_input_ids,
- past_key_values=None,
- attention_mask=None,
- decoder_attention_mask=None,
- head_mask=None,
- decoder_head_mask=None,
- cross_attn_head_mask=None,
- use_cache=None,
- encoder_outputs=None,
- **kwargs,
- ):
- # cut decoder_input_ids if past_key_values is used
- if past_key_values is not None:
- decoder_input_ids = decoder_input_ids[:, -1:]
-
- return {
- "input_ids": None, # encoder_outputs is defined. input_ids not needed
- "encoder_outputs": encoder_outputs,
- "past_key_values": past_key_values,
- "decoder_input_ids": decoder_input_ids,
- "attention_mask": attention_mask,
- "decoder_attention_mask": decoder_attention_mask,
- "head_mask": head_mask,
- "decoder_head_mask": decoder_head_mask,
- "cross_attn_head_mask": cross_attn_head_mask,
- "use_cache": use_cache, # change this to avoid caching (presumably for debugging)
- }
-
- def prepare_decoder_input_ids_from_labels(self, labels: torch.Tensor):
- return shift_tokens_right(labels, self.config.pad_token_id, self.config.decoder_start_token_id)
-
- @staticmethod
- def _reorder_cache(past_key_values, beam_idx):
- reordered_past = ()
- for layer_past in past_key_values:
- # cached cross_attention states don't have to be reordered -> they are always the same
- reordered_past += (
- tuple(past_state.index_select(0, beam_idx) for past_state in layer_past[:2]) + layer_past[2:],
- )
- return reordered_past
-
-
-@add_start_docstrings(
- """
- Bart model with a sequence classification/head on top (a linear layer on top of the pooled output) e.g. for GLUE
- tasks.
- """,
- BART_START_DOCSTRING,
-)
-class BartForSequenceClassification(BartPretrainedModel):
- _keys_to_ignore_on_load_missing = ["encoder.embed_tokens.weight", "decoder.embed_tokens.weight"]
-
- def __init__(self, config: BartConfig, **kwargs):
- super().__init__(config, **kwargs)
- self.model = BartModel(config)
- self.classification_head = BartClassificationHead(
- config.d_model,
- config.d_model,
- config.num_labels,
- config.classifier_dropout,
- )
-
- # Initialize weights and apply final processing
- self.post_init()
-
- @add_start_docstrings_to_model_forward(BART_INPUTS_DOCSTRING)
- @add_code_sample_docstrings(
- checkpoint=_CHECKPOINT_FOR_SEQUENCE_CLASSIFICATION,
- output_type=Seq2SeqSequenceClassifierOutput,
- config_class=_CONFIG_FOR_DOC,
- expected_output=_SEQ_CLASS_EXPECTED_OUTPUT,
- expected_loss=_SEQ_CLASS_EXPECTED_LOSS,
- )
- def forward(
- self,
- input_ids: torch.LongTensor = None,
- attention_mask: Optional[torch.Tensor] = None,
- decoder_input_ids: Optional[torch.LongTensor] = None,
- decoder_attention_mask: Optional[torch.LongTensor] = None,
- head_mask: Optional[torch.Tensor] = None,
- decoder_head_mask: Optional[torch.Tensor] = None,
- cross_attn_head_mask: Optional[torch.Tensor] = None,
- encoder_outputs: Optional[List[torch.FloatTensor]] = None,
- inputs_embeds: Optional[torch.FloatTensor] = None,
- decoder_inputs_embeds: Optional[torch.FloatTensor] = None,
- labels: Optional[torch.LongTensor] = None,
- use_cache: Optional[bool] = None,
- output_attentions: Optional[bool] = None,
- output_hidden_states: Optional[bool] = None,
- return_dict: Optional[bool] = None,
- ) -> Union[Tuple, Seq2SeqSequenceClassifierOutput]:
- r"""
- labels (`torch.LongTensor` of shape `(batch_size,)`, *optional*):
- Labels for computing the sequence classification/regression loss. Indices should be in `[0, ...,
- config.num_labels - 1]`. If `config.num_labels > 1` a classification loss is computed (Cross-Entropy).
- """
- return_dict = return_dict if return_dict is not None else self.config.use_return_dict
- if labels is not None:
- use_cache = False
-
- if input_ids is None and inputs_embeds is not None:
- raise NotImplementedError(
- f"Passing input embeddings is currently not supported for {self.__class__.__name__}"
- )
-
- outputs = self.model(
- input_ids,
- attention_mask=attention_mask,
- decoder_input_ids=decoder_input_ids,
- decoder_attention_mask=decoder_attention_mask,
- head_mask=head_mask,
- decoder_head_mask=decoder_head_mask,
- cross_attn_head_mask=cross_attn_head_mask,
- encoder_outputs=encoder_outputs,
- inputs_embeds=inputs_embeds,
- decoder_inputs_embeds=decoder_inputs_embeds,
- use_cache=use_cache,
- output_attentions=output_attentions,
- output_hidden_states=output_hidden_states,
- return_dict=return_dict,
- )
- hidden_states = outputs[0] # last hidden state
-
- eos_mask = input_ids.eq(self.config.eos_token_id).to(hidden_states.device)
-
- if len(torch.unique_consecutive(eos_mask.sum(1))) > 1:
- raise ValueError("All examples must have the same number of tokens.")
- sentence_representation = hidden_states[eos_mask, :].view(hidden_states.size(0), -1, hidden_states.size(-1))[
- :, -1, :
- ]
- logits = self.classification_head(sentence_representation)
-
- loss = None
- if labels is not None:
- labels = labels.to(logits.device)
- if self.config.problem_type is None:
- if self.config.num_labels == 1:
- self.config.problem_type = "regression"
- elif self.config.num_labels > 1 and (labels.dtype == torch.long or labels.dtype == torch.int):
- self.config.problem_type = "single_label_classification"
- else:
- self.config.problem_type = "multi_label_classification"
-
- if self.config.problem_type == "regression":
- loss_fct = MSELoss()
- if self.config.num_labels == 1:
- loss = loss_fct(logits.squeeze(), labels.squeeze())
- else:
- loss = loss_fct(logits, labels)
- elif self.config.problem_type == "single_label_classification":
- loss_fct = CrossEntropyLoss()
- loss = loss_fct(logits.view(-1, self.config.num_labels), labels.view(-1))
- elif self.config.problem_type == "multi_label_classification":
- loss_fct = BCEWithLogitsLoss()
- loss = loss_fct(logits, labels)
- if not return_dict:
- output = (logits,) + outputs[1:]
- return ((loss,) + output) if loss is not None else output
-
- return Seq2SeqSequenceClassifierOutput(
- loss=loss,
- logits=logits,
- past_key_values=outputs.past_key_values,
- decoder_hidden_states=outputs.decoder_hidden_states,
- decoder_attentions=outputs.decoder_attentions,
- cross_attentions=outputs.cross_attentions,
- encoder_last_hidden_state=outputs.encoder_last_hidden_state,
- encoder_hidden_states=outputs.encoder_hidden_states,
- encoder_attentions=outputs.encoder_attentions,
- )
-
-
-@add_start_docstrings(
- """
- BART Model with a span classification head on top for extractive question-answering tasks like SQuAD (a linear
- layer on top of the hidden-states output to compute `span start logits` and `span end logits`).
- """,
- BART_START_DOCSTRING,
-)
-class BartForQuestionAnswering(BartPretrainedModel):
- _keys_to_ignore_on_load_missing = ["encoder.embed_tokens.weight", "decoder.embed_tokens.weight"]
-
- def __init__(self, config):
- super().__init__(config)
-
- config.num_labels = 2
- self.num_labels = config.num_labels
-
- self.model = BartModel(config)
- self.qa_outputs = nn.Linear(config.hidden_size, config.num_labels)
-
- # Initialize weights and apply final processing
- self.post_init()
-
- @add_start_docstrings_to_model_forward(BART_INPUTS_DOCSTRING)
- @add_code_sample_docstrings(
- checkpoint=_CHECKPOINT_FOR_QA,
- output_type=Seq2SeqQuestionAnsweringModelOutput,
- config_class=_CONFIG_FOR_DOC,
- expected_loss=_QA_EXPECTED_LOSS,
- expected_output=_QA_EXPECTED_OUTPUT,
- )
- def forward(
- self,
- input_ids: torch.Tensor = None,
- attention_mask: Optional[torch.Tensor] = None,
- decoder_input_ids: Optional[torch.LongTensor] = None,
- decoder_attention_mask: Optional[torch.LongTensor] = None,
- head_mask: Optional[torch.Tensor] = None,
- decoder_head_mask: Optional[torch.Tensor] = None,
- cross_attn_head_mask: Optional[torch.Tensor] = None,
- encoder_outputs: Optional[List[torch.FloatTensor]] = None,
- start_positions: Optional[torch.LongTensor] = None,
- end_positions: Optional[torch.LongTensor] = None,
- inputs_embeds: Optional[torch.FloatTensor] = None,
- decoder_inputs_embeds: Optional[torch.FloatTensor] = None,
- use_cache: Optional[bool] = None,
- output_attentions: Optional[bool] = None,
- output_hidden_states: Optional[bool] = None,
- return_dict: Optional[bool] = None,
- ) -> Union[Tuple, Seq2SeqQuestionAnsweringModelOutput]:
- r"""
- start_positions (`torch.LongTensor` of shape `(batch_size,)`, *optional*):
- Labels for position (index) of the start of the labelled span for computing the token classification loss.
- Positions are clamped to the length of the sequence (*sequence_length*). Position outside of the sequence
- are not taken into account for computing the loss.
- end_positions (`torch.LongTensor` of shape `(batch_size,)`, *optional*):
- Labels for position (index) of the end of the labelled span for computing the token classification loss.
- Positions are clamped to the length of the sequence (*sequence_length*). Position outside of the sequence
- are not taken into account for computing the loss.
- """
- return_dict = return_dict if return_dict is not None else self.config.use_return_dict
- if start_positions is not None and end_positions is not None:
- use_cache = False
-
- outputs = self.model(
- input_ids,
- attention_mask=attention_mask,
- decoder_input_ids=decoder_input_ids,
- decoder_attention_mask=decoder_attention_mask,
- head_mask=head_mask,
- decoder_head_mask=decoder_head_mask,
- cross_attn_head_mask=cross_attn_head_mask,
- encoder_outputs=encoder_outputs,
- inputs_embeds=inputs_embeds,
- decoder_inputs_embeds=decoder_inputs_embeds,
- use_cache=use_cache,
- output_attentions=output_attentions,
- output_hidden_states=output_hidden_states,
- return_dict=return_dict,
- )
-
- sequence_output = outputs[0]
-
- logits = self.qa_outputs(sequence_output)
- start_logits, end_logits = logits.split(1, dim=-1)
- start_logits = start_logits.squeeze(-1).contiguous()
- end_logits = end_logits.squeeze(-1).contiguous()
-
- total_loss = None
- if start_positions is not None and end_positions is not None:
- # If we are on multi-GPU, split add a dimension
- if len(start_positions.size()) > 1:
- start_positions = start_positions.squeeze(-1)
- if len(end_positions.size()) > 1:
- end_positions = end_positions.squeeze(-1)
- # sometimes the start/end positions are outside our model inputs, we ignore these terms
- ignored_index = start_logits.size(1)
- start_positions = start_positions.clamp(0, ignored_index)
- end_positions = end_positions.clamp(0, ignored_index)
-
- loss_fct = CrossEntropyLoss(ignore_index=ignored_index)
- start_loss = loss_fct(start_logits, start_positions)
- end_loss = loss_fct(end_logits, end_positions)
- total_loss = (start_loss + end_loss) / 2
-
- if not return_dict:
- output = (
- start_logits,
- end_logits,
- ) + outputs[1:]
- return ((total_loss,) + output) if total_loss is not None else output
-
- return Seq2SeqQuestionAnsweringModelOutput(
- loss=total_loss,
- start_logits=start_logits,
- end_logits=end_logits,
- past_key_values=outputs.past_key_values,
- decoder_hidden_states=outputs.decoder_hidden_states,
- decoder_attentions=outputs.decoder_attentions,
- cross_attentions=outputs.cross_attentions,
- encoder_last_hidden_state=outputs.encoder_last_hidden_state,
- encoder_hidden_states=outputs.encoder_hidden_states,
- encoder_attentions=outputs.encoder_attentions,
- )
-
-
-class BartDecoderWrapper(BartPretrainedModel):
- """
- This wrapper class is a helper class to correctly load pretrained checkpoints when the causal language model is
- used in combination with the [`EncoderDecoderModel`] framework.
- """
-
- def __init__(self, config):
- super().__init__(config)
- self.decoder = BartDecoder(config)
-
- def forward(self, *args, **kwargs):
- return self.decoder(*args, **kwargs)
-
-
-@add_start_docstrings(
- """
- BART decoder with with a language modeling head on top (linear layer with weights tied to the input embeddings).
- """,
- BART_START_DOCSTRING,
-)
-class BartForCausalLM(BartPretrainedModel):
- _keys_to_ignore_on_load_missing = ["lm_head.weight"]
-
- def __init__(self, config):
- config = copy.deepcopy(config)
- config.is_decoder = True
- config.is_encoder_decoder = False
- super().__init__(config)
- self.model = BartDecoderWrapper(config)
-
- self.lm_head = nn.Linear(config.hidden_size, config.vocab_size, bias=False)
-
- # Initialize weights and apply final processing
- self.post_init()
-
- def get_input_embeddings(self):
- return self.model.decoder.embed_tokens
-
- def set_input_embeddings(self, value):
- self.model.decoder.embed_tokens = value
-
- def get_output_embeddings(self):
- return self.lm_head
-
- def set_output_embeddings(self, new_embeddings):
- self.lm_head = new_embeddings
-
- def set_decoder(self, decoder):
- self.model.decoder = decoder
-
- def get_decoder(self):
- return self.model.decoder
-
- @replace_return_docstrings(output_type=CausalLMOutputWithCrossAttentions, config_class=_CONFIG_FOR_DOC)
- def forward(
- self,
- input_ids: torch.LongTensor = None,
- attention_mask: Optional[torch.Tensor] = None,
- encoder_hidden_states: Optional[torch.FloatTensor] = None,
- encoder_attention_mask: Optional[torch.FloatTensor] = None,
- head_mask: Optional[torch.Tensor] = None,
- cross_attn_head_mask: Optional[torch.Tensor] = None,
- past_key_values: Optional[List[torch.FloatTensor]] = None,
- inputs_embeds: Optional[torch.FloatTensor] = None,
- labels: Optional[torch.LongTensor] = None,
- use_cache: Optional[bool] = None,
- output_attentions: Optional[bool] = None,
- output_hidden_states: Optional[bool] = None,
- return_dict: Optional[bool] = None,
- ) -> Union[Tuple, CausalLMOutputWithCrossAttentions]:
- r"""
- Args:
- input_ids (`torch.LongTensor` of shape `(batch_size, sequence_length)`):
- Indices of input sequence tokens in the vocabulary. Padding will be ignored by default should you
- provide it.
-
- Indices can be obtained using [`AutoTokenizer`]. See [`PreTrainedTokenizer.encode`] and
- [`PreTrainedTokenizer.__call__`] for details.
-
- [What are input IDs?](../glossary#input-ids)
- attention_mask (`torch.Tensor` of shape `(batch_size, sequence_length)`, *optional*):
- Mask to avoid performing attention on padding token indices. Mask values selected in `[0, 1]`:
-
- - 1 for tokens that are **not masked**,
- - 0 for tokens that are **masked**.
-
- [What are attention masks?](../glossary#attention-mask)
- encoder_hidden_states (`torch.FloatTensor` of shape `(batch_size, sequence_length, hidden_size)`, *optional*):
- Sequence of hidden-states at the output of the last layer of the encoder. Used in the cross-attention
- if the model is configured as a decoder.
- encoder_attention_mask (`torch.FloatTensor` of shape `(batch_size, sequence_length)`, *optional*):
- Mask to avoid performing attention on the padding token indices of the encoder input. This mask is used
- in the cross-attention if the model is configured as a decoder. Mask values selected in `[0, 1]`:
- head_mask (`torch.Tensor` of shape `(decoder_layers, decoder_attention_heads)`, *optional*):
- Mask to nullify selected heads of the attention modules. Mask values selected in `[0, 1]`:
-
- - 1 indicates the head is **not masked**,
- - 0 indicates the head is **masked**.
-
- cross_attn_head_mask (`torch.Tensor` of shape `(decoder_layers, decoder_attention_heads)`, *optional*):
- Mask to nullify selected heads of the cross-attention modules. Mask values selected in `[0, 1]`:
-
- - 1 indicates the head is **not masked**,
- - 0 indicates the head is **masked**.
-
- past_key_values (`tuple(tuple(torch.FloatTensor))`, *optional*, returned when `use_cache=True` is passed or when `config.use_cache=True`):
- Tuple of `tuple(torch.FloatTensor)` of length `config.n_layers`, with each tuple having 2 tensors of
- shape `(batch_size, num_heads, sequence_length, embed_size_per_head)`) and 2 additional tensors of
- shape `(batch_size, num_heads, encoder_sequence_length, embed_size_per_head)`. The two additional
- tensors are only required when the model is used as a decoder in a Sequence to Sequence model.
-
- Contains pre-computed hidden-states (key and values in the self-attention blocks and in the
- cross-attention blocks) that can be used (see `past_key_values` input) to speed up sequential decoding.
-
- If `past_key_values` are used, the user can optionally input only the last `decoder_input_ids` (those
- that don't have their past key value states given to this model) of shape `(batch_size, 1)` instead of
- all `decoder_input_ids` of shape `(batch_size, sequence_length)`.
- labels (`torch.LongTensor` of shape `(batch_size, sequence_length)`, *optional*):
- Labels for computing the masked language modeling loss. Indices should either be in `[0, ...,
- config.vocab_size]` or -100 (see `input_ids` docstring). Tokens with indices set to `-100` are ignored
- (masked), the loss is only computed for the tokens with labels in `[0, ..., config.vocab_size]`.
- use_cache (`bool`, *optional*):
- If set to `True`, `past_key_values` key value states are returned and can be used to speed up decoding
- (see `past_key_values`).
-
- - 1 for tokens that are **not masked**,
- - 0 for tokens that are **masked**.
- output_attentions (`bool`, *optional*):
- Whether or not to return the attentions tensors of all attention layers. See `attentions` under
- returned tensors for more detail.
- output_hidden_states (`bool`, *optional*):
- Whether or not to return the hidden states of all layers. See `hidden_states` under returned tensors
- for more detail.
- return_dict (`bool`, *optional*):
- Whether or not to return a [`~utils.ModelOutput`] instead of a plain tuple.
-
- Returns:
-
- Example:
-
- ```python
- >>> from transformers import AutoTokenizer, BartForCausalLM
-
- >>> tokenizer = AutoTokenizer.from_pretrained("facebook/bart-base")
- >>> model = BartForCausalLM.from_pretrained("facebook/bart-base", add_cross_attention=False)
- >>> assert model.config.is_decoder, f"{model.__class__} has to be configured as a decoder."
- >>> inputs = tokenizer("Hello, my dog is cute", return_tensors="pt")
- >>> outputs = model(**inputs)
-
- >>> logits = outputs.logits
- >>> expected_shape = [1, inputs.input_ids.shape[-1], model.config.vocab_size]
- >>> list(logits.shape) == expected_shape
- True
- ```"""
-
- output_attentions = output_attentions if output_attentions is not None else self.config.output_attentions
- output_hidden_states = (
- output_hidden_states if output_hidden_states is not None else self.config.output_hidden_states
- )
- return_dict = return_dict if return_dict is not None else self.config.use_return_dict
-
- # decoder outputs consists of (dec_features, layer_state, dec_hidden, dec_attn)
- outputs = self.model.decoder(
- input_ids=input_ids,
- attention_mask=attention_mask,
- encoder_hidden_states=encoder_hidden_states,
- encoder_attention_mask=encoder_attention_mask,
- head_mask=head_mask,
- cross_attn_head_mask=cross_attn_head_mask,
- past_key_values=past_key_values,
- inputs_embeds=inputs_embeds,
- use_cache=use_cache,
- output_attentions=output_attentions,
- output_hidden_states=output_hidden_states,
- return_dict=return_dict,
- )
-
- logits = self.lm_head(outputs[0])
-
- loss = None
- if labels is not None:
- labels = labels.to(logits.device)
- loss_fct = CrossEntropyLoss()
- loss = loss_fct(logits.view(-1, self.config.vocab_size), labels.view(-1))
-
- if not return_dict:
- output = (logits,) + outputs[1:]
- return (loss,) + output if loss is not None else output
-
- return CausalLMOutputWithCrossAttentions(
- loss=loss,
- logits=logits,
- past_key_values=outputs.past_key_values,
- hidden_states=outputs.hidden_states,
- attentions=outputs.attentions,
- cross_attentions=outputs.cross_attentions,
- )
-
- def prepare_inputs_for_generation(
- self, input_ids, past_key_values=None, attention_mask=None, use_cache=None, **kwargs
- ):
- # if model is used as a decoder in encoder-decoder model, the decoder attention mask is created on the fly
- if attention_mask is None:
- attention_mask = input_ids.new_ones(input_ids.shape)
-
- if past_key_values:
- input_ids = input_ids[:, -1:]
- # first step, decoder_cached_states are empty
- return {
- "input_ids": input_ids, # encoder_outputs is defined. input_ids not needed
- "attention_mask": attention_mask,
- "past_key_values": past_key_values,
- "use_cache": use_cache,
- }
-
- @staticmethod
- def _reorder_cache(past_key_values, beam_idx):
- reordered_past = ()
- for layer_past in past_key_values:
- reordered_past += (tuple(past_state.index_select(0, beam_idx) for past_state in layer_past),)
- return reordered_past
diff --git a/spaces/chilge/taoli/modules.py b/spaces/chilge/taoli/modules.py
deleted file mode 100644
index 52ee14e41a5b6d67d875d1b694aecd2a51244897..0000000000000000000000000000000000000000
--- a/spaces/chilge/taoli/modules.py
+++ /dev/null
@@ -1,342 +0,0 @@
-import copy
-import math
-import numpy as np
-import scipy
-import torch
-from torch import nn
-from torch.nn import functional as F
-
-from torch.nn import Conv1d, ConvTranspose1d, AvgPool1d, Conv2d
-from torch.nn.utils import weight_norm, remove_weight_norm
-
-import commons
-from commons import init_weights, get_padding
-
-
-LRELU_SLOPE = 0.1
-
-
-class LayerNorm(nn.Module):
- def __init__(self, channels, eps=1e-5):
- super().__init__()
- self.channels = channels
- self.eps = eps
-
- self.gamma = nn.Parameter(torch.ones(channels))
- self.beta = nn.Parameter(torch.zeros(channels))
-
- def forward(self, x):
- x = x.transpose(1, -1)
- x = F.layer_norm(x, (self.channels,), self.gamma, self.beta, self.eps)
- return x.transpose(1, -1)
-
-
-class ConvReluNorm(nn.Module):
- def __init__(self, in_channels, hidden_channels, out_channels, kernel_size, n_layers, p_dropout):
- super().__init__()
- self.in_channels = in_channels
- self.hidden_channels = hidden_channels
- self.out_channels = out_channels
- self.kernel_size = kernel_size
- self.n_layers = n_layers
- self.p_dropout = p_dropout
- assert n_layers > 1, "Number of layers should be larger than 0."
-
- self.conv_layers = nn.ModuleList()
- self.norm_layers = nn.ModuleList()
- self.conv_layers.append(nn.Conv1d(in_channels, hidden_channels, kernel_size, padding=kernel_size//2))
- self.norm_layers.append(LayerNorm(hidden_channels))
- self.relu_drop = nn.Sequential(
- nn.ReLU(),
- nn.Dropout(p_dropout))
- for _ in range(n_layers-1):
- self.conv_layers.append(nn.Conv1d(hidden_channels, hidden_channels, kernel_size, padding=kernel_size//2))
- self.norm_layers.append(LayerNorm(hidden_channels))
- self.proj = nn.Conv1d(hidden_channels, out_channels, 1)
- self.proj.weight.data.zero_()
- self.proj.bias.data.zero_()
-
- def forward(self, x, x_mask):
- x_org = x
- for i in range(self.n_layers):
- x = self.conv_layers[i](x * x_mask)
- x = self.norm_layers[i](x)
- x = self.relu_drop(x)
- x = x_org + self.proj(x)
- return x * x_mask
-
-
-class DDSConv(nn.Module):
- """
- Dialted and Depth-Separable Convolution
- """
- def __init__(self, channels, kernel_size, n_layers, p_dropout=0.):
- super().__init__()
- self.channels = channels
- self.kernel_size = kernel_size
- self.n_layers = n_layers
- self.p_dropout = p_dropout
-
- self.drop = nn.Dropout(p_dropout)
- self.convs_sep = nn.ModuleList()
- self.convs_1x1 = nn.ModuleList()
- self.norms_1 = nn.ModuleList()
- self.norms_2 = nn.ModuleList()
- for i in range(n_layers):
- dilation = kernel_size ** i
- padding = (kernel_size * dilation - dilation) // 2
- self.convs_sep.append(nn.Conv1d(channels, channels, kernel_size,
- groups=channels, dilation=dilation, padding=padding
- ))
- self.convs_1x1.append(nn.Conv1d(channels, channels, 1))
- self.norms_1.append(LayerNorm(channels))
- self.norms_2.append(LayerNorm(channels))
-
- def forward(self, x, x_mask, g=None):
- if g is not None:
- x = x + g
- for i in range(self.n_layers):
- y = self.convs_sep[i](x * x_mask)
- y = self.norms_1[i](y)
- y = F.gelu(y)
- y = self.convs_1x1[i](y)
- y = self.norms_2[i](y)
- y = F.gelu(y)
- y = self.drop(y)
- x = x + y
- return x * x_mask
-
-
-class WN(torch.nn.Module):
- def __init__(self, hidden_channels, kernel_size, dilation_rate, n_layers, gin_channels=0, p_dropout=0):
- super(WN, self).__init__()
- assert(kernel_size % 2 == 1)
- self.hidden_channels =hidden_channels
- self.kernel_size = kernel_size,
- self.dilation_rate = dilation_rate
- self.n_layers = n_layers
- self.gin_channels = gin_channels
- self.p_dropout = p_dropout
-
- self.in_layers = torch.nn.ModuleList()
- self.res_skip_layers = torch.nn.ModuleList()
- self.drop = nn.Dropout(p_dropout)
-
- if gin_channels != 0:
- cond_layer = torch.nn.Conv1d(gin_channels, 2*hidden_channels*n_layers, 1)
- self.cond_layer = torch.nn.utils.weight_norm(cond_layer, name='weight')
-
- for i in range(n_layers):
- dilation = dilation_rate ** i
- padding = int((kernel_size * dilation - dilation) / 2)
- in_layer = torch.nn.Conv1d(hidden_channels, 2*hidden_channels, kernel_size,
- dilation=dilation, padding=padding)
- in_layer = torch.nn.utils.weight_norm(in_layer, name='weight')
- self.in_layers.append(in_layer)
-
- # last one is not necessary
- if i < n_layers - 1:
- res_skip_channels = 2 * hidden_channels
- else:
- res_skip_channels = hidden_channels
-
- res_skip_layer = torch.nn.Conv1d(hidden_channels, res_skip_channels, 1)
- res_skip_layer = torch.nn.utils.weight_norm(res_skip_layer, name='weight')
- self.res_skip_layers.append(res_skip_layer)
-
- def forward(self, x, x_mask, g=None, **kwargs):
- output = torch.zeros_like(x)
- n_channels_tensor = torch.IntTensor([self.hidden_channels])
-
- if g is not None:
- g = self.cond_layer(g)
-
- for i in range(self.n_layers):
- x_in = self.in_layers[i](x)
- if g is not None:
- cond_offset = i * 2 * self.hidden_channels
- g_l = g[:,cond_offset:cond_offset+2*self.hidden_channels,:]
- else:
- g_l = torch.zeros_like(x_in)
-
- acts = commons.fused_add_tanh_sigmoid_multiply(
- x_in,
- g_l,
- n_channels_tensor)
- acts = self.drop(acts)
-
- res_skip_acts = self.res_skip_layers[i](acts)
- if i < self.n_layers - 1:
- res_acts = res_skip_acts[:,:self.hidden_channels,:]
- x = (x + res_acts) * x_mask
- output = output + res_skip_acts[:,self.hidden_channels:,:]
- else:
- output = output + res_skip_acts
- return output * x_mask
-
- def remove_weight_norm(self):
- if self.gin_channels != 0:
- torch.nn.utils.remove_weight_norm(self.cond_layer)
- for l in self.in_layers:
- torch.nn.utils.remove_weight_norm(l)
- for l in self.res_skip_layers:
- torch.nn.utils.remove_weight_norm(l)
-
-
-class ResBlock1(torch.nn.Module):
- def __init__(self, channels, kernel_size=3, dilation=(1, 3, 5)):
- super(ResBlock1, self).__init__()
- self.convs1 = nn.ModuleList([
- weight_norm(Conv1d(channels, channels, kernel_size, 1, dilation=dilation[0],
- padding=get_padding(kernel_size, dilation[0]))),
- weight_norm(Conv1d(channels, channels, kernel_size, 1, dilation=dilation[1],
- padding=get_padding(kernel_size, dilation[1]))),
- weight_norm(Conv1d(channels, channels, kernel_size, 1, dilation=dilation[2],
- padding=get_padding(kernel_size, dilation[2])))
- ])
- self.convs1.apply(init_weights)
-
- self.convs2 = nn.ModuleList([
- weight_norm(Conv1d(channels, channels, kernel_size, 1, dilation=1,
- padding=get_padding(kernel_size, 1))),
- weight_norm(Conv1d(channels, channels, kernel_size, 1, dilation=1,
- padding=get_padding(kernel_size, 1))),
- weight_norm(Conv1d(channels, channels, kernel_size, 1, dilation=1,
- padding=get_padding(kernel_size, 1)))
- ])
- self.convs2.apply(init_weights)
-
- def forward(self, x, x_mask=None):
- for c1, c2 in zip(self.convs1, self.convs2):
- xt = F.leaky_relu(x, LRELU_SLOPE)
- if x_mask is not None:
- xt = xt * x_mask
- xt = c1(xt)
- xt = F.leaky_relu(xt, LRELU_SLOPE)
- if x_mask is not None:
- xt = xt * x_mask
- xt = c2(xt)
- x = xt + x
- if x_mask is not None:
- x = x * x_mask
- return x
-
- def remove_weight_norm(self):
- for l in self.convs1:
- remove_weight_norm(l)
- for l in self.convs2:
- remove_weight_norm(l)
-
-
-class ResBlock2(torch.nn.Module):
- def __init__(self, channels, kernel_size=3, dilation=(1, 3)):
- super(ResBlock2, self).__init__()
- self.convs = nn.ModuleList([
- weight_norm(Conv1d(channels, channels, kernel_size, 1, dilation=dilation[0],
- padding=get_padding(kernel_size, dilation[0]))),
- weight_norm(Conv1d(channels, channels, kernel_size, 1, dilation=dilation[1],
- padding=get_padding(kernel_size, dilation[1])))
- ])
- self.convs.apply(init_weights)
-
- def forward(self, x, x_mask=None):
- for c in self.convs:
- xt = F.leaky_relu(x, LRELU_SLOPE)
- if x_mask is not None:
- xt = xt * x_mask
- xt = c(xt)
- x = xt + x
- if x_mask is not None:
- x = x * x_mask
- return x
-
- def remove_weight_norm(self):
- for l in self.convs:
- remove_weight_norm(l)
-
-
-class Log(nn.Module):
- def forward(self, x, x_mask, reverse=False, **kwargs):
- if not reverse:
- y = torch.log(torch.clamp_min(x, 1e-5)) * x_mask
- logdet = torch.sum(-y, [1, 2])
- return y, logdet
- else:
- x = torch.exp(x) * x_mask
- return x
-
-
-class Flip(nn.Module):
- def forward(self, x, *args, reverse=False, **kwargs):
- x = torch.flip(x, [1])
- if not reverse:
- logdet = torch.zeros(x.size(0)).to(dtype=x.dtype, device=x.device)
- return x, logdet
- else:
- return x
-
-
-class ElementwiseAffine(nn.Module):
- def __init__(self, channels):
- super().__init__()
- self.channels = channels
- self.m = nn.Parameter(torch.zeros(channels,1))
- self.logs = nn.Parameter(torch.zeros(channels,1))
-
- def forward(self, x, x_mask, reverse=False, **kwargs):
- if not reverse:
- y = self.m + torch.exp(self.logs) * x
- y = y * x_mask
- logdet = torch.sum(self.logs * x_mask, [1,2])
- return y, logdet
- else:
- x = (x - self.m) * torch.exp(-self.logs) * x_mask
- return x
-
-
-class ResidualCouplingLayer(nn.Module):
- def __init__(self,
- channels,
- hidden_channels,
- kernel_size,
- dilation_rate,
- n_layers,
- p_dropout=0,
- gin_channels=0,
- mean_only=False):
- assert channels % 2 == 0, "channels should be divisible by 2"
- super().__init__()
- self.channels = channels
- self.hidden_channels = hidden_channels
- self.kernel_size = kernel_size
- self.dilation_rate = dilation_rate
- self.n_layers = n_layers
- self.half_channels = channels // 2
- self.mean_only = mean_only
-
- self.pre = nn.Conv1d(self.half_channels, hidden_channels, 1)
- self.enc = WN(hidden_channels, kernel_size, dilation_rate, n_layers, p_dropout=p_dropout, gin_channels=gin_channels)
- self.post = nn.Conv1d(hidden_channels, self.half_channels * (2 - mean_only), 1)
- self.post.weight.data.zero_()
- self.post.bias.data.zero_()
-
- def forward(self, x, x_mask, g=None, reverse=False):
- x0, x1 = torch.split(x, [self.half_channels]*2, 1)
- h = self.pre(x0) * x_mask
- h = self.enc(h, x_mask, g=g)
- stats = self.post(h) * x_mask
- if not self.mean_only:
- m, logs = torch.split(stats, [self.half_channels]*2, 1)
- else:
- m = stats
- logs = torch.zeros_like(m)
-
- if not reverse:
- x1 = m + x1 * torch.exp(logs) * x_mask
- x = torch.cat([x0, x1], 1)
- logdet = torch.sum(logs, [1,2])
- return x, logdet
- else:
- x1 = (x1 - m) * torch.exp(-logs) * x_mask
- x = torch.cat([x0, x1], 1)
- return x
diff --git a/spaces/chuan-hd/law-assistant-chatbot/.venv/lib/python3.11/site-packages/anyio/abc/_sockets.py b/spaces/chuan-hd/law-assistant-chatbot/.venv/lib/python3.11/site-packages/anyio/abc/_sockets.py
deleted file mode 100644
index 6aac5f7c22395759ebe3d5633d2adcf1f4ff1fe5..0000000000000000000000000000000000000000
--- a/spaces/chuan-hd/law-assistant-chatbot/.venv/lib/python3.11/site-packages/anyio/abc/_sockets.py
+++ /dev/null
@@ -1,160 +0,0 @@
-from __future__ import annotations
-
-import socket
-from abc import abstractmethod
-from contextlib import AsyncExitStack
-from io import IOBase
-from ipaddress import IPv4Address, IPv6Address
-from socket import AddressFamily
-from typing import (
- Any,
- Callable,
- Collection,
- Mapping,
- Tuple,
- TypeVar,
- Union,
-)
-
-from .._core._tasks import create_task_group
-from .._core._typedattr import (
- TypedAttributeProvider,
- TypedAttributeSet,
- typed_attribute,
-)
-from ._streams import ByteStream, Listener, UnreliableObjectStream
-from ._tasks import TaskGroup
-
-IPAddressType = Union[str, IPv4Address, IPv6Address]
-IPSockAddrType = Tuple[str, int]
-SockAddrType = Union[IPSockAddrType, str]
-UDPPacketType = Tuple[bytes, IPSockAddrType]
-T_Retval = TypeVar("T_Retval")
-
-
-class SocketAttribute(TypedAttributeSet):
- #: the address family of the underlying socket
- family: AddressFamily = typed_attribute()
- #: the local socket address of the underlying socket
- local_address: SockAddrType = typed_attribute()
- #: for IP addresses, the local port the underlying socket is bound to
- local_port: int = typed_attribute()
- #: the underlying stdlib socket object
- raw_socket: socket.socket = typed_attribute()
- #: the remote address the underlying socket is connected to
- remote_address: SockAddrType = typed_attribute()
- #: for IP addresses, the remote port the underlying socket is connected to
- remote_port: int = typed_attribute()
-
-
-class _SocketProvider(TypedAttributeProvider):
- @property
- def extra_attributes(self) -> Mapping[Any, Callable[[], Any]]:
- from .._core._sockets import convert_ipv6_sockaddr as convert
-
- attributes: dict[Any, Callable[[], Any]] = {
- SocketAttribute.family: lambda: self._raw_socket.family,
- SocketAttribute.local_address: lambda: convert(
- self._raw_socket.getsockname()
- ),
- SocketAttribute.raw_socket: lambda: self._raw_socket,
- }
- try:
- peername: tuple[str, int] | None = convert(self._raw_socket.getpeername())
- except OSError:
- peername = None
-
- # Provide the remote address for connected sockets
- if peername is not None:
- attributes[SocketAttribute.remote_address] = lambda: peername
-
- # Provide local and remote ports for IP based sockets
- if self._raw_socket.family in (AddressFamily.AF_INET, AddressFamily.AF_INET6):
- attributes[
- SocketAttribute.local_port
- ] = lambda: self._raw_socket.getsockname()[1]
- if peername is not None:
- remote_port = peername[1]
- attributes[SocketAttribute.remote_port] = lambda: remote_port
-
- return attributes
-
- @property
- @abstractmethod
- def _raw_socket(self) -> socket.socket:
- pass
-
-
-class SocketStream(ByteStream, _SocketProvider):
- """
- Transports bytes over a socket.
-
- Supports all relevant extra attributes from :class:`~SocketAttribute`.
- """
-
-
-class UNIXSocketStream(SocketStream):
- @abstractmethod
- async def send_fds(self, message: bytes, fds: Collection[int | IOBase]) -> None:
- """
- Send file descriptors along with a message to the peer.
-
- :param message: a non-empty bytestring
- :param fds: a collection of files (either numeric file descriptors or open file or socket
- objects)
- """
-
- @abstractmethod
- async def receive_fds(self, msglen: int, maxfds: int) -> tuple[bytes, list[int]]:
- """
- Receive file descriptors along with a message from the peer.
-
- :param msglen: length of the message to expect from the peer
- :param maxfds: maximum number of file descriptors to expect from the peer
- :return: a tuple of (message, file descriptors)
- """
-
-
-class SocketListener(Listener[SocketStream], _SocketProvider):
- """
- Listens to incoming socket connections.
-
- Supports all relevant extra attributes from :class:`~SocketAttribute`.
- """
-
- @abstractmethod
- async def accept(self) -> SocketStream:
- """Accept an incoming connection."""
-
- async def serve(
- self,
- handler: Callable[[SocketStream], Any],
- task_group: TaskGroup | None = None,
- ) -> None:
- async with AsyncExitStack() as exit_stack:
- if task_group is None:
- task_group = await exit_stack.enter_async_context(create_task_group())
-
- while True:
- stream = await self.accept()
- task_group.start_soon(handler, stream)
-
-
-class UDPSocket(UnreliableObjectStream[UDPPacketType], _SocketProvider):
- """
- Represents an unconnected UDP socket.
-
- Supports all relevant extra attributes from :class:`~SocketAttribute`.
- """
-
- async def sendto(self, data: bytes, host: str, port: int) -> None:
- """Alias for :meth:`~.UnreliableObjectSendStream.send` ((data, (host, port)))."""
- return await self.send((data, (host, port)))
-
-
-class ConnectedUDPSocket(UnreliableObjectStream[bytes], _SocketProvider):
- """
- Represents an connected UDP socket.
-
- Supports all relevant extra attributes from :class:`~SocketAttribute`.
- """
diff --git a/spaces/chuan-hd/law-assistant-chatbot/.venv/lib/python3.11/site-packages/chardet/euckrfreq.py b/spaces/chuan-hd/law-assistant-chatbot/.venv/lib/python3.11/site-packages/chardet/euckrfreq.py
deleted file mode 100644
index 7dc3b10387d1c3d2da8b4e27e917ee2a85086e0c..0000000000000000000000000000000000000000
--- a/spaces/chuan-hd/law-assistant-chatbot/.venv/lib/python3.11/site-packages/chardet/euckrfreq.py
+++ /dev/null
@@ -1,196 +0,0 @@
-######################## BEGIN LICENSE BLOCK ########################
-# The Original Code is Mozilla Communicator client code.
-#
-# The Initial Developer of the Original Code is
-# Netscape Communications Corporation.
-# Portions created by the Initial Developer are Copyright (C) 1998
-# the Initial Developer. All Rights Reserved.
-#
-# Contributor(s):
-# Mark Pilgrim - port to Python
-#
-# This library is free software; you can redistribute it and/or
-# modify it under the terms of the GNU Lesser General Public
-# License as published by the Free Software Foundation; either
-# version 2.1 of the License, or (at your option) any later version.
-#
-# This library is distributed in the hope that it will be useful,
-# but WITHOUT ANY WARRANTY; without even the implied warranty of
-# MERCHANTABILITY or FITNESS FOR A PARTICULAR PURPOSE. See the GNU
-# Lesser General Public License for more details.
-#
-# You should have received a copy of the GNU Lesser General Public
-# License along with this library; if not, write to the Free Software
-# Foundation, Inc., 51 Franklin St, Fifth Floor, Boston, MA
-# 02110-1301 USA
-######################### END LICENSE BLOCK #########################
-
-# Sampling from about 20M text materials include literature and computer technology
-
-# 128 --> 0.79
-# 256 --> 0.92
-# 512 --> 0.986
-# 1024 --> 0.99944
-# 2048 --> 0.99999
-#
-# Idea Distribution Ratio = 0.98653 / (1-0.98653) = 73.24
-# Random Distribution Ration = 512 / (2350-512) = 0.279.
-#
-# Typical Distribution Ratio
-
-EUCKR_TYPICAL_DISTRIBUTION_RATIO = 6.0
-
-EUCKR_TABLE_SIZE = 2352
-
-# Char to FreqOrder table ,
-# fmt: off
-EUCKR_CHAR_TO_FREQ_ORDER = (
- 13, 130, 120,1396, 481,1719,1720, 328, 609, 212,1721, 707, 400, 299,1722, 87,
-1397,1723, 104, 536,1117,1203,1724,1267, 685,1268, 508,1725,1726,1727,1728,1398,
-1399,1729,1730,1731, 141, 621, 326,1057, 368,1732, 267, 488, 20,1733,1269,1734,
- 945,1400,1735, 47, 904,1270,1736,1737, 773, 248,1738, 409, 313, 786, 429,1739,
- 116, 987, 813,1401, 683, 75,1204, 145,1740,1741,1742,1743, 16, 847, 667, 622,
- 708,1744,1745,1746, 966, 787, 304, 129,1747, 60, 820, 123, 676,1748,1749,1750,
-1751, 617,1752, 626,1753,1754,1755,1756, 653,1757,1758,1759,1760,1761,1762, 856,
- 344,1763,1764,1765,1766, 89, 401, 418, 806, 905, 848,1767,1768,1769, 946,1205,
- 709,1770,1118,1771, 241,1772,1773,1774,1271,1775, 569,1776, 999,1777,1778,1779,
-1780, 337, 751,1058, 28, 628, 254,1781, 177, 906, 270, 349, 891,1079,1782, 19,
-1783, 379,1784, 315,1785, 629, 754,1402, 559,1786, 636, 203,1206,1787, 710, 567,
-1788, 935, 814,1789,1790,1207, 766, 528,1791,1792,1208,1793,1794,1795,1796,1797,
-1403,1798,1799, 533,1059,1404,1405,1156,1406, 936, 884,1080,1800, 351,1801,1802,
-1803,1804,1805, 801,1806,1807,1808,1119,1809,1157, 714, 474,1407,1810, 298, 899,
- 885,1811,1120, 802,1158,1812, 892,1813,1814,1408, 659,1815,1816,1121,1817,1818,
-1819,1820,1821,1822, 319,1823, 594, 545,1824, 815, 937,1209,1825,1826, 573,1409,
-1022,1827,1210,1828,1829,1830,1831,1832,1833, 556, 722, 807,1122,1060,1834, 697,
-1835, 900, 557, 715,1836,1410, 540,1411, 752,1159, 294, 597,1211, 976, 803, 770,
-1412,1837,1838, 39, 794,1413, 358,1839, 371, 925,1840, 453, 661, 788, 531, 723,
- 544,1023,1081, 869, 91,1841, 392, 430, 790, 602,1414, 677,1082, 457,1415,1416,
-1842,1843, 475, 327,1024,1417, 795, 121,1844, 733, 403,1418,1845,1846,1847, 300,
- 119, 711,1212, 627,1848,1272, 207,1849,1850, 796,1213, 382,1851, 519,1852,1083,
- 893,1853,1854,1855, 367, 809, 487, 671,1856, 663,1857,1858, 956, 471, 306, 857,
-1859,1860,1160,1084,1861,1862,1863,1864,1865,1061,1866,1867,1868,1869,1870,1871,
- 282, 96, 574,1872, 502,1085,1873,1214,1874, 907,1875,1876, 827, 977,1419,1420,
-1421, 268,1877,1422,1878,1879,1880, 308,1881, 2, 537,1882,1883,1215,1884,1885,
- 127, 791,1886,1273,1423,1887, 34, 336, 404, 643,1888, 571, 654, 894, 840,1889,
- 0, 886,1274, 122, 575, 260, 908, 938,1890,1275, 410, 316,1891,1892, 100,1893,
-1894,1123, 48,1161,1124,1025,1895, 633, 901,1276,1896,1897, 115, 816,1898, 317,
-1899, 694,1900, 909, 734,1424, 572, 866,1425, 691, 85, 524,1010, 543, 394, 841,
-1901,1902,1903,1026,1904,1905,1906,1907,1908,1909, 30, 451, 651, 988, 310,1910,
-1911,1426, 810,1216, 93,1912,1913,1277,1217,1914, 858, 759, 45, 58, 181, 610,
- 269,1915,1916, 131,1062, 551, 443,1000, 821,1427, 957, 895,1086,1917,1918, 375,
-1919, 359,1920, 687,1921, 822,1922, 293,1923,1924, 40, 662, 118, 692, 29, 939,
- 887, 640, 482, 174,1925, 69,1162, 728,1428, 910,1926,1278,1218,1279, 386, 870,
- 217, 854,1163, 823,1927,1928,1929,1930, 834,1931, 78,1932, 859,1933,1063,1934,
-1935,1936,1937, 438,1164, 208, 595,1938,1939,1940,1941,1219,1125,1942, 280, 888,
-1429,1430,1220,1431,1943,1944,1945,1946,1947,1280, 150, 510,1432,1948,1949,1950,
-1951,1952,1953,1954,1011,1087,1955,1433,1043,1956, 881,1957, 614, 958,1064,1065,
-1221,1958, 638,1001, 860, 967, 896,1434, 989, 492, 553,1281,1165,1959,1282,1002,
-1283,1222,1960,1961,1962,1963, 36, 383, 228, 753, 247, 454,1964, 876, 678,1965,
-1966,1284, 126, 464, 490, 835, 136, 672, 529, 940,1088,1435, 473,1967,1968, 467,
- 50, 390, 227, 587, 279, 378, 598, 792, 968, 240, 151, 160, 849, 882,1126,1285,
- 639,1044, 133, 140, 288, 360, 811, 563,1027, 561, 142, 523,1969,1970,1971, 7,
- 103, 296, 439, 407, 506, 634, 990,1972,1973,1974,1975, 645,1976,1977,1978,1979,
-1980,1981, 236,1982,1436,1983,1984,1089, 192, 828, 618, 518,1166, 333,1127,1985,
- 818,1223,1986,1987,1988,1989,1990,1991,1992,1993, 342,1128,1286, 746, 842,1994,
-1995, 560, 223,1287, 98, 8, 189, 650, 978,1288,1996,1437,1997, 17, 345, 250,
- 423, 277, 234, 512, 226, 97, 289, 42, 167,1998, 201,1999,2000, 843, 836, 824,
- 532, 338, 783,1090, 182, 576, 436,1438,1439, 527, 500,2001, 947, 889,2002,2003,
-2004,2005, 262, 600, 314, 447,2006, 547,2007, 693, 738,1129,2008, 71,1440, 745,
- 619, 688,2009, 829,2010,2011, 147,2012, 33, 948,2013,2014, 74, 224,2015, 61,
- 191, 918, 399, 637,2016,1028,1130, 257, 902,2017,2018,2019,2020,2021,2022,2023,
-2024,2025,2026, 837,2027,2028,2029,2030, 179, 874, 591, 52, 724, 246,2031,2032,
-2033,2034,1167, 969,2035,1289, 630, 605, 911,1091,1168,2036,2037,2038,1441, 912,
-2039, 623,2040,2041, 253,1169,1290,2042,1442, 146, 620, 611, 577, 433,2043,1224,
- 719,1170, 959, 440, 437, 534, 84, 388, 480,1131, 159, 220, 198, 679,2044,1012,
- 819,1066,1443, 113,1225, 194, 318,1003,1029,2045,2046,2047,2048,1067,2049,2050,
-2051,2052,2053, 59, 913, 112,2054, 632,2055, 455, 144, 739,1291,2056, 273, 681,
- 499,2057, 448,2058,2059, 760,2060,2061, 970, 384, 169, 245,1132,2062,2063, 414,
-1444,2064,2065, 41, 235,2066, 157, 252, 877, 568, 919, 789, 580,2067, 725,2068,
-2069,1292,2070,2071,1445,2072,1446,2073,2074, 55, 588, 66,1447, 271,1092,2075,
-1226,2076, 960,1013, 372,2077,2078,2079,2080,2081,1293,2082,2083,2084,2085, 850,
-2086,2087,2088,2089,2090, 186,2091,1068, 180,2092,2093,2094, 109,1227, 522, 606,
-2095, 867,1448,1093, 991,1171, 926, 353,1133,2096, 581,2097,2098,2099,1294,1449,
-1450,2100, 596,1172,1014,1228,2101,1451,1295,1173,1229,2102,2103,1296,1134,1452,
- 949,1135,2104,2105,1094,1453,1454,1455,2106,1095,2107,2108,2109,2110,2111,2112,
-2113,2114,2115,2116,2117, 804,2118,2119,1230,1231, 805,1456, 405,1136,2120,2121,
-2122,2123,2124, 720, 701,1297, 992,1457, 927,1004,2125,2126,2127,2128,2129,2130,
- 22, 417,2131, 303,2132, 385,2133, 971, 520, 513,2134,1174, 73,1096, 231, 274,
- 962,1458, 673,2135,1459,2136, 152,1137,2137,2138,2139,2140,1005,1138,1460,1139,
-2141,2142,2143,2144, 11, 374, 844,2145, 154,1232, 46,1461,2146, 838, 830, 721,
-1233, 106,2147, 90, 428, 462, 578, 566,1175, 352,2148,2149, 538,1234, 124,1298,
-2150,1462, 761, 565,2151, 686,2152, 649,2153, 72, 173,2154, 460, 415,2155,1463,
-2156,1235, 305,2157,2158,2159,2160,2161,2162, 579,2163,2164,2165,2166,2167, 747,
-2168,2169,2170,2171,1464, 669,2172,2173,2174,2175,2176,1465,2177, 23, 530, 285,
-2178, 335, 729,2179, 397,2180,2181,2182,1030,2183,2184, 698,2185,2186, 325,2187,
-2188, 369,2189, 799,1097,1015, 348,2190,1069, 680,2191, 851,1466,2192,2193, 10,
-2194, 613, 424,2195, 979, 108, 449, 589, 27, 172, 81,1031, 80, 774, 281, 350,
-1032, 525, 301, 582,1176,2196, 674,1045,2197,2198,1467, 730, 762,2199,2200,2201,
-2202,1468,2203, 993,2204,2205, 266,1070, 963,1140,2206,2207,2208, 664,1098, 972,
-2209,2210,2211,1177,1469,1470, 871,2212,2213,2214,2215,2216,1471,2217,2218,2219,
-2220,2221,2222,2223,2224,2225,2226,2227,1472,1236,2228,2229,2230,2231,2232,2233,
-2234,2235,1299,2236,2237, 200,2238, 477, 373,2239,2240, 731, 825, 777,2241,2242,
-2243, 521, 486, 548,2244,2245,2246,1473,1300, 53, 549, 137, 875, 76, 158,2247,
-1301,1474, 469, 396,1016, 278, 712,2248, 321, 442, 503, 767, 744, 941,1237,1178,
-1475,2249, 82, 178,1141,1179, 973,2250,1302,2251, 297,2252,2253, 570,2254,2255,
-2256, 18, 450, 206,2257, 290, 292,1142,2258, 511, 162, 99, 346, 164, 735,2259,
-1476,1477, 4, 554, 343, 798,1099,2260,1100,2261, 43, 171,1303, 139, 215,2262,
-2263, 717, 775,2264,1033, 322, 216,2265, 831,2266, 149,2267,1304,2268,2269, 702,
-1238, 135, 845, 347, 309,2270, 484,2271, 878, 655, 238,1006,1478,2272, 67,2273,
- 295,2274,2275, 461,2276, 478, 942, 412,2277,1034,2278,2279,2280, 265,2281, 541,
-2282,2283,2284,2285,2286, 70, 852,1071,2287,2288,2289,2290, 21, 56, 509, 117,
- 432,2291,2292, 331, 980, 552,1101, 148, 284, 105, 393,1180,1239, 755,2293, 187,
-2294,1046,1479,2295, 340,2296, 63,1047, 230,2297,2298,1305, 763,1306, 101, 800,
- 808, 494,2299,2300,2301, 903,2302, 37,1072, 14, 5,2303, 79, 675,2304, 312,
-2305,2306,2307,2308,2309,1480, 6,1307,2310,2311,2312, 1, 470, 35, 24, 229,
-2313, 695, 210, 86, 778, 15, 784, 592, 779, 32, 77, 855, 964,2314, 259,2315,
- 501, 380,2316,2317, 83, 981, 153, 689,1308,1481,1482,1483,2318,2319, 716,1484,
-2320,2321,2322,2323,2324,2325,1485,2326,2327, 128, 57, 68, 261,1048, 211, 170,
-1240, 31,2328, 51, 435, 742,2329,2330,2331, 635,2332, 264, 456,2333,2334,2335,
- 425,2336,1486, 143, 507, 263, 943,2337, 363, 920,1487, 256,1488,1102, 243, 601,
-1489,2338,2339,2340,2341,2342,2343,2344, 861,2345,2346,2347,2348,2349,2350, 395,
-2351,1490,1491, 62, 535, 166, 225,2352,2353, 668, 419,1241, 138, 604, 928,2354,
-1181,2355,1492,1493,2356,2357,2358,1143,2359, 696,2360, 387, 307,1309, 682, 476,
-2361,2362, 332, 12, 222, 156,2363, 232,2364, 641, 276, 656, 517,1494,1495,1035,
- 416, 736,1496,2365,1017, 586,2366,2367,2368,1497,2369, 242,2370,2371,2372,1498,
-2373, 965, 713,2374,2375,2376,2377, 740, 982,1499, 944,1500,1007,2378,2379,1310,
-1501,2380,2381,2382, 785, 329,2383,2384,1502,2385,2386,2387, 932,2388,1503,2389,
-2390,2391,2392,1242,2393,2394,2395,2396,2397, 994, 950,2398,2399,2400,2401,1504,
-1311,2402,2403,2404,2405,1049, 749,2406,2407, 853, 718,1144,1312,2408,1182,1505,
-2409,2410, 255, 516, 479, 564, 550, 214,1506,1507,1313, 413, 239, 444, 339,1145,
-1036,1508,1509,1314,1037,1510,1315,2411,1511,2412,2413,2414, 176, 703, 497, 624,
- 593, 921, 302,2415, 341, 165,1103,1512,2416,1513,2417,2418,2419, 376,2420, 700,
-2421,2422,2423, 258, 768,1316,2424,1183,2425, 995, 608,2426,2427,2428,2429, 221,
-2430,2431,2432,2433,2434,2435,2436,2437, 195, 323, 726, 188, 897, 983,1317, 377,
- 644,1050, 879,2438, 452,2439,2440,2441,2442,2443,2444, 914,2445,2446,2447,2448,
- 915, 489,2449,1514,1184,2450,2451, 515, 64, 427, 495,2452, 583,2453, 483, 485,
-1038, 562, 213,1515, 748, 666,2454,2455,2456,2457, 334,2458, 780, 996,1008, 705,
-1243,2459,2460,2461,2462,2463, 114,2464, 493,1146, 366, 163,1516, 961,1104,2465,
- 291,2466,1318,1105,2467,1517, 365,2468, 355, 951,1244,2469,1319,2470, 631,2471,
-2472, 218,1320, 364, 320, 756,1518,1519,1321,1520,1322,2473,2474,2475,2476, 997,
-2477,2478,2479,2480, 665,1185,2481, 916,1521,2482,2483,2484, 584, 684,2485,2486,
- 797,2487,1051,1186,2488,2489,2490,1522,2491,2492, 370,2493,1039,1187, 65,2494,
- 434, 205, 463,1188,2495, 125, 812, 391, 402, 826, 699, 286, 398, 155, 781, 771,
- 585,2496, 590, 505,1073,2497, 599, 244, 219, 917,1018, 952, 646,1523,2498,1323,
-2499,2500, 49, 984, 354, 741,2501, 625,2502,1324,2503,1019, 190, 357, 757, 491,
- 95, 782, 868,2504,2505,2506,2507,2508,2509, 134,1524,1074, 422,1525, 898,2510,
- 161,2511,2512,2513,2514, 769,2515,1526,2516,2517, 411,1325,2518, 472,1527,2519,
-2520,2521,2522,2523,2524, 985,2525,2526,2527,2528,2529,2530, 764,2531,1245,2532,
-2533, 25, 204, 311,2534, 496,2535,1052,2536,2537,2538,2539,2540,2541,2542, 199,
- 704, 504, 468, 758, 657,1528, 196, 44, 839,1246, 272, 750,2543, 765, 862,2544,
-2545,1326,2546, 132, 615, 933,2547, 732,2548,2549,2550,1189,1529,2551, 283,1247,
-1053, 607, 929,2552,2553,2554, 930, 183, 872, 616,1040,1147,2555,1148,1020, 441,
- 249,1075,2556,2557,2558, 466, 743,2559,2560,2561, 92, 514, 426, 420, 526,2562,
-2563,2564,2565,2566,2567,2568, 185,2569,2570,2571,2572, 776,1530, 658,2573, 362,
-2574, 361, 922,1076, 793,2575,2576,2577,2578,2579,2580,1531, 251,2581,2582,2583,
-2584,1532, 54, 612, 237,1327,2585,2586, 275, 408, 647, 111,2587,1533,1106, 465,
- 3, 458, 9, 38,2588, 107, 110, 890, 209, 26, 737, 498,2589,1534,2590, 431,
- 202, 88,1535, 356, 287,1107, 660,1149,2591, 381,1536, 986,1150, 445,1248,1151,
- 974,2592,2593, 846,2594, 446, 953, 184,1249,1250, 727,2595, 923, 193, 883,2596,
-2597,2598, 102, 324, 539, 817,2599, 421,1041,2600, 832,2601, 94, 175, 197, 406,
-2602, 459,2603,2604,2605,2606,2607, 330, 555,2608,2609,2610, 706,1108, 389,2611,
-2612,2613,2614, 233,2615, 833, 558, 931, 954,1251,2616,2617,1537, 546,2618,2619,
-1009,2620,2621,2622,1538, 690,1328,2623, 955,2624,1539,2625,2626, 772,2627,2628,
-2629,2630,2631, 924, 648, 863, 603,2632,2633, 934,1540, 864, 865,2634, 642,1042,
- 670,1190,2635,2636,2637,2638, 168,2639, 652, 873, 542,1054,1541,2640,2641,2642, # 512, 256
-)
-# fmt: on
diff --git a/spaces/chuan-hd/law-assistant-chatbot/.venv/lib/python3.11/site-packages/fontTools/cu2qu/__main__.py b/spaces/chuan-hd/law-assistant-chatbot/.venv/lib/python3.11/site-packages/fontTools/cu2qu/__main__.py
deleted file mode 100644
index 084bf8f960db3d4ded95921ee9d7cbd2a7fb9f4a..0000000000000000000000000000000000000000
--- a/spaces/chuan-hd/law-assistant-chatbot/.venv/lib/python3.11/site-packages/fontTools/cu2qu/__main__.py
+++ /dev/null
@@ -1,6 +0,0 @@
-import sys
-from .cli import main
-
-
-if __name__ == "__main__":
- sys.exit(main())
diff --git a/spaces/chuan-hd/law-assistant-chatbot/.venv/lib/python3.11/site-packages/fontTools/ttLib/tables/T_S_I_V_.py b/spaces/chuan-hd/law-assistant-chatbot/.venv/lib/python3.11/site-packages/fontTools/ttLib/tables/T_S_I_V_.py
deleted file mode 100644
index d7aec4589c5d83b35b02b8f07c68b6462438e066..0000000000000000000000000000000000000000
--- a/spaces/chuan-hd/law-assistant-chatbot/.venv/lib/python3.11/site-packages/fontTools/ttLib/tables/T_S_I_V_.py
+++ /dev/null
@@ -1,20 +0,0 @@
-from fontTools.misc.textTools import strjoin, tobytes, tostr
-from . import asciiTable
-
-
-class table_T_S_I_V_(asciiTable.asciiTable):
- def toXML(self, writer, ttFont):
- data = tostr(self.data)
- # removing null bytes. XXX needed??
- data = data.split("\0")
- data = strjoin(data)
- writer.begintag("source")
- writer.newline()
- writer.write_noindent(data.replace("\r", "\n"))
- writer.newline()
- writer.endtag("source")
- writer.newline()
-
- def fromXML(self, name, attrs, content, ttFont):
- lines = strjoin(content).split("\n")
- self.data = tobytes("\r".join(lines[1:-1]))
diff --git a/spaces/chuan-hd/law-assistant-chatbot/.venv/lib/python3.11/site-packages/fsspec/implementations/github.py b/spaces/chuan-hd/law-assistant-chatbot/.venv/lib/python3.11/site-packages/fsspec/implementations/github.py
deleted file mode 100644
index b148124d7481bb867cb100ad1ab2213e6acadf56..0000000000000000000000000000000000000000
--- a/spaces/chuan-hd/law-assistant-chatbot/.venv/lib/python3.11/site-packages/fsspec/implementations/github.py
+++ /dev/null
@@ -1,219 +0,0 @@
-import requests
-
-from ..spec import AbstractFileSystem
-from ..utils import infer_storage_options
-from .memory import MemoryFile
-
-# TODO: add GIST backend, would be very similar
-
-
-class GithubFileSystem(AbstractFileSystem):
- """Interface to files in github
-
- An instance of this class provides the files residing within a remote github
- repository. You may specify a point in the repos history, by SHA, branch
- or tag (default is current master).
-
- Given that code files tend to be small, and that github does not support
- retrieving partial content, we always fetch whole files.
-
- When using fsspec.open, allows URIs of the form:
-
- - "github://path/file", in which case you must specify org, repo and
- may specify sha in the extra args
- - 'github://org:repo@/precip/catalog.yml', where the org and repo are
- part of the URI
- - 'github://org:repo@sha/precip/catalog.yml', where the sha is also included
-
- ``sha`` can be the full or abbreviated hex of the commit you want to fetch
- from, or a branch or tag name (so long as it doesn't contain special characters
- like "/", "?", which would have to be HTTP-encoded).
-
- For authorised access, you must provide username and token, which can be made
- at https://github.com/settings/tokens
- """
-
- url = "https://api.github.com/repos/{org}/{repo}/git/trees/{sha}"
- rurl = "https://raw.githubusercontent.com/{org}/{repo}/{sha}/{path}"
- protocol = "github"
-
- def __init__(self, org, repo, sha=None, username=None, token=None, **kwargs):
- super().__init__(**kwargs)
- self.org = org
- self.repo = repo
- if (username is None) ^ (token is None):
- raise ValueError("Auth required both username and token")
- self.username = username
- self.token = token
- if sha is None:
- # look up default branch (not necessarily "master")
- u = "https://api.github.com/repos/{org}/{repo}"
- r = requests.get(u.format(org=org, repo=repo), **self.kw)
- r.raise_for_status()
- sha = r.json()["default_branch"]
-
- self.root = sha
- self.ls("")
-
- @property
- def kw(self):
- if self.username:
- return {"auth": (self.username, self.token)}
- return {}
-
- @classmethod
- def repos(cls, org_or_user, is_org=True):
- """List repo names for given org or user
-
- This may become the top level of the FS
-
- Parameters
- ----------
- org_or_user: str
- Name of the github org or user to query
- is_org: bool (default True)
- Whether the name is an organisation (True) or user (False)
-
- Returns
- -------
- List of string
- """
- r = requests.get(
- "https://api.github.com/{part}/{org}/repos".format(
- part=["users", "orgs"][is_org], org=org_or_user
- )
- )
- r.raise_for_status()
- return [repo["name"] for repo in r.json()]
-
- @property
- def tags(self):
- """Names of tags in the repo"""
- r = requests.get(
- "https://api.github.com/repos/{org}/{repo}/tags"
- "".format(org=self.org, repo=self.repo),
- **self.kw,
- )
- r.raise_for_status()
- return [t["name"] for t in r.json()]
-
- @property
- def branches(self):
- """Names of branches in the repo"""
- r = requests.get(
- "https://api.github.com/repos/{org}/{repo}/branches"
- "".format(org=self.org, repo=self.repo),
- **self.kw,
- )
- r.raise_for_status()
- return [t["name"] for t in r.json()]
-
- @property
- def refs(self):
- """Named references, tags and branches"""
- return {"tags": self.tags, "branches": self.branches}
-
- def ls(self, path, detail=False, sha=None, _sha=None, **kwargs):
- """List files at given path
-
- Parameters
- ----------
- path: str
- Location to list, relative to repo root
- detail: bool
- If True, returns list of dicts, one per file; if False, returns
- list of full filenames only
- sha: str (optional)
- List at the given point in the repo history, branch or tag name or commit
- SHA
- _sha: str (optional)
- List this specific tree object (used internally to descend into trees)
- """
- path = self._strip_protocol(path)
- if path == "":
- _sha = sha or self.root
- if _sha is None:
- parts = path.rstrip("/").split("/")
- so_far = ""
- _sha = sha or self.root
- for part in parts:
- out = self.ls(so_far, True, sha=sha, _sha=_sha)
- so_far += "/" + part if so_far else part
- out = [o for o in out if o["name"] == so_far]
- if not out:
- raise FileNotFoundError(path)
- out = out[0]
- if out["type"] == "file":
- if detail:
- return [out]
- else:
- return path
- _sha = out["sha"]
- if path not in self.dircache or sha not in [self.root, None]:
- r = requests.get(
- self.url.format(org=self.org, repo=self.repo, sha=_sha), **self.kw
- )
- if r.status_code == 404:
- raise FileNotFoundError(path)
- r.raise_for_status()
- types = {"blob": "file", "tree": "directory"}
- out = [
- {
- "name": path + "/" + f["path"] if path else f["path"],
- "mode": f["mode"],
- "type": types[f["type"]],
- "size": f.get("size", 0),
- "sha": f["sha"],
- }
- for f in r.json()["tree"]
- if f["type"] in types
- ]
- if sha in [self.root, None]:
- self.dircache[path] = out
- else:
- out = self.dircache[path]
- if detail:
- return out
- else:
- return sorted([f["name"] for f in out])
-
- def invalidate_cache(self, path=None):
- self.dircache.clear()
-
- @classmethod
- def _strip_protocol(cls, path):
- opts = infer_storage_options(path)
- if "username" not in opts:
- return super()._strip_protocol(path)
- return opts["path"].lstrip("/")
-
- @staticmethod
- def _get_kwargs_from_urls(path):
- opts = infer_storage_options(path)
- if "username" not in opts:
- return {}
- out = {"org": opts["username"], "repo": opts["password"]}
- if opts["host"]:
- out["sha"] = opts["host"]
- return out
-
- def _open(
- self,
- path,
- mode="rb",
- block_size=None,
- autocommit=True,
- cache_options=None,
- sha=None,
- **kwargs,
- ):
- if mode != "rb":
- raise NotImplementedError
- url = self.rurl.format(
- org=self.org, repo=self.repo, path=path, sha=sha or self.root
- )
- r = requests.get(url, **self.kw)
- if r.status_code == 404:
- raise FileNotFoundError(path)
- r.raise_for_status()
- return MemoryFile(None, None, r.content)
diff --git a/spaces/cihyFjudo/fairness-paper-search/Dragon Age Inquisition Update 2.5 [EXCLUSIVE] Crack V3 Indir Peatix[2].md b/spaces/cihyFjudo/fairness-paper-search/Dragon Age Inquisition Update 2.5 [EXCLUSIVE] Crack V3 Indir Peatix[2].md
deleted file mode 100644
index 3e46ded03abd3883fbc155bfeae48fb180ae3811..0000000000000000000000000000000000000000
--- a/spaces/cihyFjudo/fairness-paper-search/Dragon Age Inquisition Update 2.5 [EXCLUSIVE] Crack V3 Indir Peatix[2].md
+++ /dev/null
@@ -1,19 +0,0 @@
-
-
asus memo pad 7 update to lollipopmerge dragons halloween event 2019asrock x370 killer sli/ac bios updateeuropa universalis 4 vassalac unity character customizationsamsung push service downloadpopcorn hour a 410ga 990fxa ud3 rev 4.1gigabyte h110-d3athe program explorer.exe stopped interacting with windows amd catalyst driver 14.4
exchanger xml editor downloadsteelseries legendary mouse driversasus memo pad 8 updatesuper mario fusion revivalmarshmallow sprint note 5intel centrino advanced-n 6230dragon age dead space armorlg stylo 2 fingerprint sensorgt72 6qe dominator pro gsuperior drummer 2 download dark parables the final cinderella
nvidia geforce gtx 765m drivers
tdmore free dvd copy
hp split x2 bestbuy
left behind eternal forces download
asus memo pad k001 firmware
does toshiba laptop have bluetooth
fifa 15 barcelona squad
tuf sabertooth 990fx r3 0
gigabyte ga h55m s2v
garmin plugin for chrome hp laserjet 4014n driver crusader kings 2 over vassal limit click n design v5 realtek card reader driver windows 8
samsung convoy 2 specslenovo thinkpad w520 drivership hop dance experience wii song listmoultrie m 550 gen2brother mfc 9700 drivernvidia geforce 9600 gsp8z68 v le driversdriver ricoh mp 5000hp g72 wireless driversteelseries usb sound card asus m4a785 m bios update
mionix naos 8200 software
pioneer bdp-05fd firmware upgrade
microsoft arc touch mouse bluetooth driver windows 7
elementary os mac theme
gigabyte h310m a drivers
nfs pro street trainer
burnout paradise the ultimate box trainer
wacom cintiq 13 hd driver
dragon age origins party storage chest
intel 82945g express chipset family windows 10 amd a8 7600 drivers microsoft wedge mouse driver asrock b250m pro4 bios joe montana 16 video game
-
ace fishing pearl guidehtc desire 510 lollipopkiller e2400 driver updateroyal envoy 3 level 12hp probook 640 g2 biostenda w322u driver windows 10jvc kd-sr81btlogitech quickcam express driversaints row 3 bloodsucker packhtc one max driver thin2000 usb display adapter
asus maximus viii hero alpha bios update
acpi ven_atk&dev_4001
toshiba satellite c655 drivers windows 7 32 bit
dragon age 2 companions armor
a43 file management utility
kyocera ecosys p6021cdn driver
dlink dir-651
samsung np-q430 specs
the credit card information is not valid. please check your entries carefully. psn
amd sata controller windows 10 driver filipe luis fifa 16 capcom vs snk 2 shin akuma ga-h87n-wifi forza horizon 2 characters
-
-
the orange box achievementsdream match tennis pro 2.34panasonic kx mb 2030 driver downloaddestiny how to get twilight garrisonblack ops 3 banneddragon age inquisition exalted plains regionsabbyy pdf transformer reviewff14 au ra concept artrosewill rnx n180ub drivertiny death star download canon imagerunner advance 4245 driver
logitech orbit af driver
republic of gamers drivers
halo 5 achilles helmet
teamspeak female sound pack
corsair vengeance 1500 drivers windows 10
brother mfc j6510dw printer driver
axiom pro 49 drivers
download sharepoint workspace 2010
ae1000 driver windows 7
hde wireless receiver for xbox 360 toshiba satellite a665-s6094 drivers how to get sterling treasure in destiny morse code audio decoder mac wireless charging and credit cards
-
amd radeon hd6620g driver updatemagnus choir full version freeradeon r9 280 drivervisual studio 2010 licensingimtoo_mpeg_encoderlenovo yoga 710 fingerprint readerhp probook 4540s biosmsi z97 krait driverswhitecap platinum full crackgigabyte ga 945gcm s2c htc one m7 marshmallow update
rowan battle of britain
lollipop on moto x 1st gen
rat 3 mouse driver
what is amd sata controller
tv shows folder icon
nba jam free download mac
tbia the data is invalid
heroes of the storm hero sale
photoshop cs6 raw update 8.3
focusrite no hardware connected wii u street fighter broadcom 2046 bluetooth 2.1 usb dongle driver windows 7 hp envy 15t drivers nvidia gforce 6150 le
-
rpg black ops 2hewlett packard deskjet 3320secrets of grindea trainernexus 6p qi chargingck2 increase vassal limitgigabyte f2a88x-d3hpbrother hl 5150d driverquickbooks component repair tool for windows xp vista 7fifa 16 ultimate team iphonetaskbar repair tool plus ati mobility radeon hd 5470 drivers
gigabyte ga-ab350m-hd3
sprint htc windows phone
how to slide in halo 5
spongebob ship o ghouls game
skyrim dragon rising wont start
skyrim daggers on back
pip boy app download
how to update fiio x1
laserjet 1012 windows 7
qualcomm atheros ar8152 pci-e fast ethernet controller (ndis 6.30) sharp ar-208d wow legendary mouse software htc m7 android 6 nemesis of the roman empire download
-
amd radeon r9 m275x driverhp photosmart c4700 series driversios 8.4 beta 1dell inspiron 546 driversminecraft pocket edition 0.14 0 apkcorsair void usb cracklingthe crew wild run motorcyclelenovo thinkpad x201 driversbatman arkham knight screenshotsedimax br 6478ac v2 www realfootball 2009 com
dragon age origins camp storage
conexant audio driver windows 7
asrock 3tb+ unlocker utility
driver ricoh mp 4000
asus m5a88-v evo drivers
kb3189866 stuck at 45%
arma 2 operation arrowhead free download
panasonic kx mb781 drivers
apple mobile device ethernet
tsstcorp cddvdw sn 208ab supreme kai trials dokkan payday 2 titan safe motorola 2247-n8 ats 8 arachnid review
-
drive encryption for hp protecttoolsmoto file manager appdell studio one 1909 driversdownload toad for macnote 5 marshmallow update sprintricoh aficio mp c4000 driverbroadcom 2046 bluetooth 2.1 usb dongle driver windows 7anno 1404 venice traineratheros ar9287 wireless network adapterarmy of ages armor games install_flash_player_osx dmg
sony dcr trv 19
bloodborne gestures with controller
web gallery downloader crack
download advanced task killer
centrino advanced n 6205 driver
euro free kick 2012
lenovo yoga bluetooth driver
plants vs zombies goty trainer
frontface for public displays
xcom not created equally neymar jr fifa 16 msi 970a g43 manual tp-link tl-wn725n driver windows 10 the movies mac download
-
conexant high-definition smartaudio 221 driver windows 7 64stand of food 3wacom cloud authentication failedmemorex dvd writer driver downloadiomega home media network hard drive softwarega h81 amp updroid turbo 2 upgradesaitek rumble force p2500 drivermp3 to kar convertertipard total media converter sound blaster audio controller
gigabyte z77x ud5h drivers
nvidia geforce 560 ti driver
true key for firefox
ricoh aficio mp c2051 driver
ashampoo office 2018 review
realtek rtl8101 driver vista
asus m5a78l-m/usb3 drivers windows 7
twitch chat character limit
xbox 360 data transfer cables
how to turn off pressure sensitivity in firealpaca droid 2 global rom zte warp elite for sale how to heal in dragon age inquisition resident evil 6 screenshots
-
glyph launcher not workingn64 usb controller driverdreadnought dawn of war 2ps plus games january 2015m5a99x evo r2.0 bios updatexbox 360 hard drive 120gb walmartasus a68hm k driverssony kdl-52v5100sound blaster x-fi titanium drivershercules dj console rmx2 broadcom bluetooth 3.0 usb driver
evic vtc mini software update
crazy taxi playstation 3
line tower wars best maze
realtek rtl8822be 802.11ac pcie adapter driver
adobe presenter 11 download
g force visualizer crack
kodi v17 krypton alpha 3
wimax 6250 driver windows 7 64 bit
tap tap revenge katy perry
royal revolt 2 best defense tplink archer t1u driver destiny the taken king screenshots toshiba satellite c55d-a5107 gpu tweak 2 not opening
-
mass effect bringing down the skycorsair link temperature sensorparsons address book 7.0 downloadbleach brave souls killer effectgigabayte ga m61pme s2hp realtek wireless drivergigabyte ga-z270-hd3pusb 2.0 wlan driversdigital camera raw compatibility updatebelkin n wireless usb adapter f5d8053 v3 smart photo editor crack
fake google search generator
intel® 82945g express chipset family
microsoft life cam nx6000
sound blaster tactic3d fury
artorias armor dark souls
trip advisor lesvos forum
asus gtx 650 ti
what is ai personality divinity original sin
dir-817lw firmware
hp mini 5101 driver convert dbf to csv razer deathadder mac driver asap utilities 64 bit pioneer avh-2300nex firmware
aaccfb2cb3
-
-
\ No newline at end of file
diff --git a/spaces/cihyFjudo/fairness-paper-search/The Mr Majnu Hindi Dubbed 720p A Tale of Two Opposites Who Attract Each Other.md b/spaces/cihyFjudo/fairness-paper-search/The Mr Majnu Hindi Dubbed 720p A Tale of Two Opposites Who Attract Each Other.md
deleted file mode 100644
index a68869babf49faf9819a434038c4ca1f31c9fdab..0000000000000000000000000000000000000000
--- a/spaces/cihyFjudo/fairness-paper-search/The Mr Majnu Hindi Dubbed 720p A Tale of Two Opposites Who Attract Each Other.md
+++ /dev/null
@@ -1,6 +0,0 @@
-
-
- aaccfb2cb3
-
-
-
diff --git a/spaces/cjayic/sovits-overwatch2/transforms.py b/spaces/cjayic/sovits-overwatch2/transforms.py
deleted file mode 100644
index 4793d67ca5a5630e0ffe0f9fb29445c949e64dae..0000000000000000000000000000000000000000
--- a/spaces/cjayic/sovits-overwatch2/transforms.py
+++ /dev/null
@@ -1,193 +0,0 @@
-import torch
-from torch.nn import functional as F
-
-import numpy as np
-
-
-DEFAULT_MIN_BIN_WIDTH = 1e-3
-DEFAULT_MIN_BIN_HEIGHT = 1e-3
-DEFAULT_MIN_DERIVATIVE = 1e-3
-
-
-def piecewise_rational_quadratic_transform(inputs,
- unnormalized_widths,
- unnormalized_heights,
- unnormalized_derivatives,
- inverse=False,
- tails=None,
- tail_bound=1.,
- min_bin_width=DEFAULT_MIN_BIN_WIDTH,
- min_bin_height=DEFAULT_MIN_BIN_HEIGHT,
- min_derivative=DEFAULT_MIN_DERIVATIVE):
-
- if tails is None:
- spline_fn = rational_quadratic_spline
- spline_kwargs = {}
- else:
- spline_fn = unconstrained_rational_quadratic_spline
- spline_kwargs = {
- 'tails': tails,
- 'tail_bound': tail_bound
- }
-
- outputs, logabsdet = spline_fn(
- inputs=inputs,
- unnormalized_widths=unnormalized_widths,
- unnormalized_heights=unnormalized_heights,
- unnormalized_derivatives=unnormalized_derivatives,
- inverse=inverse,
- min_bin_width=min_bin_width,
- min_bin_height=min_bin_height,
- min_derivative=min_derivative,
- **spline_kwargs
- )
- return outputs, logabsdet
-
-
-def searchsorted(bin_locations, inputs, eps=1e-6):
- bin_locations[..., -1] += eps
- return torch.sum(
- inputs[..., None] >= bin_locations,
- dim=-1
- ) - 1
-
-
-def unconstrained_rational_quadratic_spline(inputs,
- unnormalized_widths,
- unnormalized_heights,
- unnormalized_derivatives,
- inverse=False,
- tails='linear',
- tail_bound=1.,
- min_bin_width=DEFAULT_MIN_BIN_WIDTH,
- min_bin_height=DEFAULT_MIN_BIN_HEIGHT,
- min_derivative=DEFAULT_MIN_DERIVATIVE):
- inside_interval_mask = (inputs >= -tail_bound) & (inputs <= tail_bound)
- outside_interval_mask = ~inside_interval_mask
-
- outputs = torch.zeros_like(inputs)
- logabsdet = torch.zeros_like(inputs)
-
- if tails == 'linear':
- unnormalized_derivatives = F.pad(unnormalized_derivatives, pad=(1, 1))
- constant = np.log(np.exp(1 - min_derivative) - 1)
- unnormalized_derivatives[..., 0] = constant
- unnormalized_derivatives[..., -1] = constant
-
- outputs[outside_interval_mask] = inputs[outside_interval_mask]
- logabsdet[outside_interval_mask] = 0
- else:
- raise RuntimeError('{} tails are not implemented.'.format(tails))
-
- outputs[inside_interval_mask], logabsdet[inside_interval_mask] = rational_quadratic_spline(
- inputs=inputs[inside_interval_mask],
- unnormalized_widths=unnormalized_widths[inside_interval_mask, :],
- unnormalized_heights=unnormalized_heights[inside_interval_mask, :],
- unnormalized_derivatives=unnormalized_derivatives[inside_interval_mask, :],
- inverse=inverse,
- left=-tail_bound, right=tail_bound, bottom=-tail_bound, top=tail_bound,
- min_bin_width=min_bin_width,
- min_bin_height=min_bin_height,
- min_derivative=min_derivative
- )
-
- return outputs, logabsdet
-
-def rational_quadratic_spline(inputs,
- unnormalized_widths,
- unnormalized_heights,
- unnormalized_derivatives,
- inverse=False,
- left=0., right=1., bottom=0., top=1.,
- min_bin_width=DEFAULT_MIN_BIN_WIDTH,
- min_bin_height=DEFAULT_MIN_BIN_HEIGHT,
- min_derivative=DEFAULT_MIN_DERIVATIVE):
- if torch.min(inputs) < left or torch.max(inputs) > right:
- raise ValueError('Input to a transform is not within its domain')
-
- num_bins = unnormalized_widths.shape[-1]
-
- if min_bin_width * num_bins > 1.0:
- raise ValueError('Minimal bin width too large for the number of bins')
- if min_bin_height * num_bins > 1.0:
- raise ValueError('Minimal bin height too large for the number of bins')
-
- widths = F.softmax(unnormalized_widths, dim=-1)
- widths = min_bin_width + (1 - min_bin_width * num_bins) * widths
- cumwidths = torch.cumsum(widths, dim=-1)
- cumwidths = F.pad(cumwidths, pad=(1, 0), mode='constant', value=0.0)
- cumwidths = (right - left) * cumwidths + left
- cumwidths[..., 0] = left
- cumwidths[..., -1] = right
- widths = cumwidths[..., 1:] - cumwidths[..., :-1]
-
- derivatives = min_derivative + F.softplus(unnormalized_derivatives)
-
- heights = F.softmax(unnormalized_heights, dim=-1)
- heights = min_bin_height + (1 - min_bin_height * num_bins) * heights
- cumheights = torch.cumsum(heights, dim=-1)
- cumheights = F.pad(cumheights, pad=(1, 0), mode='constant', value=0.0)
- cumheights = (top - bottom) * cumheights + bottom
- cumheights[..., 0] = bottom
- cumheights[..., -1] = top
- heights = cumheights[..., 1:] - cumheights[..., :-1]
-
- if inverse:
- bin_idx = searchsorted(cumheights, inputs)[..., None]
- else:
- bin_idx = searchsorted(cumwidths, inputs)[..., None]
-
- input_cumwidths = cumwidths.gather(-1, bin_idx)[..., 0]
- input_bin_widths = widths.gather(-1, bin_idx)[..., 0]
-
- input_cumheights = cumheights.gather(-1, bin_idx)[..., 0]
- delta = heights / widths
- input_delta = delta.gather(-1, bin_idx)[..., 0]
-
- input_derivatives = derivatives.gather(-1, bin_idx)[..., 0]
- input_derivatives_plus_one = derivatives[..., 1:].gather(-1, bin_idx)[..., 0]
-
- input_heights = heights.gather(-1, bin_idx)[..., 0]
-
- if inverse:
- a = (((inputs - input_cumheights) * (input_derivatives
- + input_derivatives_plus_one
- - 2 * input_delta)
- + input_heights * (input_delta - input_derivatives)))
- b = (input_heights * input_derivatives
- - (inputs - input_cumheights) * (input_derivatives
- + input_derivatives_plus_one
- - 2 * input_delta))
- c = - input_delta * (inputs - input_cumheights)
-
- discriminant = b.pow(2) - 4 * a * c
- assert (discriminant >= 0).all()
-
- root = (2 * c) / (-b - torch.sqrt(discriminant))
- outputs = root * input_bin_widths + input_cumwidths
-
- theta_one_minus_theta = root * (1 - root)
- denominator = input_delta + ((input_derivatives + input_derivatives_plus_one - 2 * input_delta)
- * theta_one_minus_theta)
- derivative_numerator = input_delta.pow(2) * (input_derivatives_plus_one * root.pow(2)
- + 2 * input_delta * theta_one_minus_theta
- + input_derivatives * (1 - root).pow(2))
- logabsdet = torch.log(derivative_numerator) - 2 * torch.log(denominator)
-
- return outputs, -logabsdet
- else:
- theta = (inputs - input_cumwidths) / input_bin_widths
- theta_one_minus_theta = theta * (1 - theta)
-
- numerator = input_heights * (input_delta * theta.pow(2)
- + input_derivatives * theta_one_minus_theta)
- denominator = input_delta + ((input_derivatives + input_derivatives_plus_one - 2 * input_delta)
- * theta_one_minus_theta)
- outputs = input_cumheights + numerator / denominator
-
- derivative_numerator = input_delta.pow(2) * (input_derivatives_plus_one * theta.pow(2)
- + 2 * input_delta * theta_one_minus_theta
- + input_derivatives * (1 - theta).pow(2))
- logabsdet = torch.log(derivative_numerator) - 2 * torch.log(denominator)
-
- return outputs, logabsdet
diff --git a/spaces/cloudtheboi/Lofi4All/.pythonlibs/lib/python3.10/site-packages/PIL/EpsImagePlugin.py b/spaces/cloudtheboi/Lofi4All/.pythonlibs/lib/python3.10/site-packages/PIL/EpsImagePlugin.py
deleted file mode 100644
index 6b1b5947ec0654b36ac15334327e412c0743b925..0000000000000000000000000000000000000000
--- a/spaces/cloudtheboi/Lofi4All/.pythonlibs/lib/python3.10/site-packages/PIL/EpsImagePlugin.py
+++ /dev/null
@@ -1,466 +0,0 @@
-#
-# The Python Imaging Library.
-# $Id$
-#
-# EPS file handling
-#
-# History:
-# 1995-09-01 fl Created (0.1)
-# 1996-05-18 fl Don't choke on "atend" fields, Ghostscript interface (0.2)
-# 1996-08-22 fl Don't choke on floating point BoundingBox values
-# 1996-08-23 fl Handle files from Macintosh (0.3)
-# 2001-02-17 fl Use 're' instead of 'regex' (Python 2.1) (0.4)
-# 2003-09-07 fl Check gs.close status (from Federico Di Gregorio) (0.5)
-# 2014-05-07 e Handling of EPS with binary preview and fixed resolution
-# resizing
-#
-# Copyright (c) 1997-2003 by Secret Labs AB.
-# Copyright (c) 1995-2003 by Fredrik Lundh
-#
-# See the README file for information on usage and redistribution.
-#
-
-import io
-import os
-import re
-import subprocess
-import sys
-import tempfile
-
-from . import Image, ImageFile
-from ._binary import i32le as i32
-from ._deprecate import deprecate
-
-# --------------------------------------------------------------------
-
-
-split = re.compile(r"^%%([^:]*):[ \t]*(.*)[ \t]*$")
-field = re.compile(r"^%[%!\w]([^:]*)[ \t]*$")
-
-gs_windows_binary = None
-if sys.platform.startswith("win"):
- import shutil
-
- for binary in ("gswin32c", "gswin64c", "gs"):
- if shutil.which(binary) is not None:
- gs_windows_binary = binary
- break
- else:
- gs_windows_binary = False
-
-
-def has_ghostscript():
- if gs_windows_binary:
- return True
- if not sys.platform.startswith("win"):
- try:
- subprocess.check_call(["gs", "--version"], stdout=subprocess.DEVNULL)
- return True
- except OSError:
- # No Ghostscript
- pass
- return False
-
-
-def Ghostscript(tile, size, fp, scale=1, transparency=False):
- """Render an image using Ghostscript"""
-
- # Unpack decoder tile
- decoder, tile, offset, data = tile[0]
- length, bbox = data
-
- # Hack to support hi-res rendering
- scale = int(scale) or 1
- # orig_size = size
- # orig_bbox = bbox
- size = (size[0] * scale, size[1] * scale)
- # resolution is dependent on bbox and size
- res = (
- 72.0 * size[0] / (bbox[2] - bbox[0]),
- 72.0 * size[1] / (bbox[3] - bbox[1]),
- )
-
- out_fd, outfile = tempfile.mkstemp()
- os.close(out_fd)
-
- infile_temp = None
- if hasattr(fp, "name") and os.path.exists(fp.name):
- infile = fp.name
- else:
- in_fd, infile_temp = tempfile.mkstemp()
- os.close(in_fd)
- infile = infile_temp
-
- # Ignore length and offset!
- # Ghostscript can read it
- # Copy whole file to read in Ghostscript
- with open(infile_temp, "wb") as f:
- # fetch length of fp
- fp.seek(0, io.SEEK_END)
- fsize = fp.tell()
- # ensure start position
- # go back
- fp.seek(0)
- lengthfile = fsize
- while lengthfile > 0:
- s = fp.read(min(lengthfile, 100 * 1024))
- if not s:
- break
- lengthfile -= len(s)
- f.write(s)
-
- device = "pngalpha" if transparency else "ppmraw"
-
- # Build Ghostscript command
- command = [
- "gs",
- "-q", # quiet mode
- "-g%dx%d" % size, # set output geometry (pixels)
- "-r%fx%f" % res, # set input DPI (dots per inch)
- "-dBATCH", # exit after processing
- "-dNOPAUSE", # don't pause between pages
- "-dSAFER", # safe mode
- f"-sDEVICE={device}",
- f"-sOutputFile={outfile}", # output file
- # adjust for image origin
- "-c",
- f"{-bbox[0]} {-bbox[1]} translate",
- "-f",
- infile, # input file
- # showpage (see https://bugs.ghostscript.com/show_bug.cgi?id=698272)
- "-c",
- "showpage",
- ]
-
- if gs_windows_binary is not None:
- if not gs_windows_binary:
- try:
- os.unlink(outfile)
- if infile_temp:
- os.unlink(infile_temp)
- except OSError:
- pass
-
- msg = "Unable to locate Ghostscript on paths"
- raise OSError(msg)
- command[0] = gs_windows_binary
-
- # push data through Ghostscript
- try:
- startupinfo = None
- if sys.platform.startswith("win"):
- startupinfo = subprocess.STARTUPINFO()
- startupinfo.dwFlags |= subprocess.STARTF_USESHOWWINDOW
- subprocess.check_call(command, startupinfo=startupinfo)
- out_im = Image.open(outfile)
- out_im.load()
- finally:
- try:
- os.unlink(outfile)
- if infile_temp:
- os.unlink(infile_temp)
- except OSError:
- pass
-
- im = out_im.im.copy()
- out_im.close()
- return im
-
-
-class PSFile:
- """
- Wrapper for bytesio object that treats either CR or LF as end of line.
- This class is no longer used internally, but kept for backwards compatibility.
- """
-
- def __init__(self, fp):
- deprecate(
- "PSFile",
- 11,
- action="If you need the functionality of this class "
- "you will need to implement it yourself.",
- )
- self.fp = fp
- self.char = None
-
- def seek(self, offset, whence=io.SEEK_SET):
- self.char = None
- self.fp.seek(offset, whence)
-
- def readline(self):
- s = [self.char or b""]
- self.char = None
-
- c = self.fp.read(1)
- while (c not in b"\r\n") and len(c):
- s.append(c)
- c = self.fp.read(1)
-
- self.char = self.fp.read(1)
- # line endings can be 1 or 2 of \r \n, in either order
- if self.char in b"\r\n":
- self.char = None
-
- return b"".join(s).decode("latin-1")
-
-
-def _accept(prefix):
- return prefix[:4] == b"%!PS" or (len(prefix) >= 4 and i32(prefix) == 0xC6D3D0C5)
-
-
-##
-# Image plugin for Encapsulated PostScript. This plugin supports only
-# a few variants of this format.
-
-
-class EpsImageFile(ImageFile.ImageFile):
- """EPS File Parser for the Python Imaging Library"""
-
- format = "EPS"
- format_description = "Encapsulated Postscript"
-
- mode_map = {1: "L", 2: "LAB", 3: "RGB", 4: "CMYK"}
-
- def _open(self):
- (length, offset) = self._find_offset(self.fp)
-
- # go to offset - start of "%!PS"
- self.fp.seek(offset)
-
- self.mode = "RGB"
- self._size = None
-
- byte_arr = bytearray(255)
- bytes_mv = memoryview(byte_arr)
- bytes_read = 0
- reading_comments = True
-
- def check_required_header_comments():
- if "PS-Adobe" not in self.info:
- msg = 'EPS header missing "%!PS-Adobe" comment'
- raise SyntaxError(msg)
- if "BoundingBox" not in self.info:
- msg = 'EPS header missing "%%BoundingBox" comment'
- raise SyntaxError(msg)
-
- while True:
- byte = self.fp.read(1)
- if byte == b"":
- # if we didn't read a byte we must be at the end of the file
- if bytes_read == 0:
- break
- elif byte in b"\r\n":
- # if we read a line ending character, ignore it and parse what
- # we have already read. if we haven't read any other characters,
- # continue reading
- if bytes_read == 0:
- continue
- else:
- # ASCII/hexadecimal lines in an EPS file must not exceed
- # 255 characters, not including line ending characters
- if bytes_read >= 255:
- # only enforce this for lines starting with a "%",
- # otherwise assume it's binary data
- if byte_arr[0] == ord("%"):
- msg = "not an EPS file"
- raise SyntaxError(msg)
- else:
- if reading_comments:
- check_required_header_comments()
- reading_comments = False
- # reset bytes_read so we can keep reading
- # data until the end of the line
- bytes_read = 0
- byte_arr[bytes_read] = byte[0]
- bytes_read += 1
- continue
-
- if reading_comments:
- # Load EPS header
-
- # if this line doesn't start with a "%",
- # or does start with "%%EndComments",
- # then we've reached the end of the header/comments
- if byte_arr[0] != ord("%") or bytes_mv[:13] == b"%%EndComments":
- check_required_header_comments()
- reading_comments = False
- continue
-
- s = str(bytes_mv[:bytes_read], "latin-1")
-
- try:
- m = split.match(s)
- except re.error as e:
- msg = "not an EPS file"
- raise SyntaxError(msg) from e
-
- if m:
- k, v = m.group(1, 2)
- self.info[k] = v
- if k == "BoundingBox":
- try:
- # Note: The DSC spec says that BoundingBox
- # fields should be integers, but some drivers
- # put floating point values there anyway.
- box = [int(float(i)) for i in v.split()]
- self._size = box[2] - box[0], box[3] - box[1]
- self.tile = [
- ("eps", (0, 0) + self.size, offset, (length, box))
- ]
- except Exception:
- pass
- else:
- m = field.match(s)
- if m:
- k = m.group(1)
- if k[:8] == "PS-Adobe":
- self.info["PS-Adobe"] = k[9:]
- else:
- self.info[k] = ""
- elif s[0] == "%":
- # handle non-DSC PostScript comments that some
- # tools mistakenly put in the Comments section
- pass
- else:
- msg = "bad EPS header"
- raise OSError(msg)
- elif bytes_mv[:11] == b"%ImageData:":
- # Check for an "ImageData" descriptor
- # https://www.adobe.com/devnet-apps/photoshop/fileformatashtml/#50577413_pgfId-1035096
-
- # Values:
- # columns
- # rows
- # bit depth (1 or 8)
- # mode (1: L, 2: LAB, 3: RGB, 4: CMYK)
- # number of padding channels
- # block size (number of bytes per row per channel)
- # binary/ascii (1: binary, 2: ascii)
- # data start identifier (the image data follows after a single line
- # consisting only of this quoted value)
- image_data_values = byte_arr[11:bytes_read].split(None, 7)
- columns, rows, bit_depth, mode_id = [
- int(value) for value in image_data_values[:4]
- ]
-
- if bit_depth == 1:
- self.mode = "1"
- elif bit_depth == 8:
- try:
- self.mode = self.mode_map[mode_id]
- except ValueError:
- break
- else:
- break
-
- self._size = columns, rows
- return
-
- bytes_read = 0
-
- check_required_header_comments()
-
- if not self._size:
- msg = "cannot determine EPS bounding box"
- raise OSError(msg)
-
- def _find_offset(self, fp):
- s = fp.read(4)
-
- if s == b"%!PS":
- # for HEAD without binary preview
- fp.seek(0, io.SEEK_END)
- length = fp.tell()
- offset = 0
- elif i32(s) == 0xC6D3D0C5:
- # FIX for: Some EPS file not handled correctly / issue #302
- # EPS can contain binary data
- # or start directly with latin coding
- # more info see:
- # https://web.archive.org/web/20160528181353/http://partners.adobe.com/public/developer/en/ps/5002.EPSF_Spec.pdf
- s = fp.read(8)
- offset = i32(s)
- length = i32(s, 4)
- else:
- msg = "not an EPS file"
- raise SyntaxError(msg)
-
- return length, offset
-
- def load(self, scale=1, transparency=False):
- # Load EPS via Ghostscript
- if self.tile:
- self.im = Ghostscript(self.tile, self.size, self.fp, scale, transparency)
- self.mode = self.im.mode
- self._size = self.im.size
- self.tile = []
- return Image.Image.load(self)
-
- def load_seek(self, *args, **kwargs):
- # we can't incrementally load, so force ImageFile.parser to
- # use our custom load method by defining this method.
- pass
-
-
-# --------------------------------------------------------------------
-
-
-def _save(im, fp, filename, eps=1):
- """EPS Writer for the Python Imaging Library."""
-
- # make sure image data is available
- im.load()
-
- # determine PostScript image mode
- if im.mode == "L":
- operator = (8, 1, b"image")
- elif im.mode == "RGB":
- operator = (8, 3, b"false 3 colorimage")
- elif im.mode == "CMYK":
- operator = (8, 4, b"false 4 colorimage")
- else:
- msg = "image mode is not supported"
- raise ValueError(msg)
-
- if eps:
- # write EPS header
- fp.write(b"%!PS-Adobe-3.0 EPSF-3.0\n")
- fp.write(b"%%Creator: PIL 0.1 EpsEncode\n")
- # fp.write("%%CreationDate: %s"...)
- fp.write(b"%%%%BoundingBox: 0 0 %d %d\n" % im.size)
- fp.write(b"%%Pages: 1\n")
- fp.write(b"%%EndComments\n")
- fp.write(b"%%Page: 1 1\n")
- fp.write(b"%%ImageData: %d %d " % im.size)
- fp.write(b'%d %d 0 1 1 "%s"\n' % operator)
-
- # image header
- fp.write(b"gsave\n")
- fp.write(b"10 dict begin\n")
- fp.write(b"/buf %d string def\n" % (im.size[0] * operator[1]))
- fp.write(b"%d %d scale\n" % im.size)
- fp.write(b"%d %d 8\n" % im.size) # <= bits
- fp.write(b"[%d 0 0 -%d 0 %d]\n" % (im.size[0], im.size[1], im.size[1]))
- fp.write(b"{ currentfile buf readhexstring pop } bind\n")
- fp.write(operator[2] + b"\n")
- if hasattr(fp, "flush"):
- fp.flush()
-
- ImageFile._save(im, fp, [("eps", (0, 0) + im.size, 0, None)])
-
- fp.write(b"\n%%%%EndBinary\n")
- fp.write(b"grestore end\n")
- if hasattr(fp, "flush"):
- fp.flush()
-
-
-# --------------------------------------------------------------------
-
-
-Image.register_open(EpsImageFile.format, EpsImageFile, _accept)
-
-Image.register_save(EpsImageFile.format, _save)
-
-Image.register_extensions(EpsImageFile.format, [".ps", ".eps"])
-
-Image.register_mime(EpsImageFile.format, "application/postscript")
diff --git a/spaces/cloudtheboi/Lofi4All/.pythonlibs/lib/python3.10/site-packages/PIL/PpmImagePlugin.py b/spaces/cloudtheboi/Lofi4All/.pythonlibs/lib/python3.10/site-packages/PIL/PpmImagePlugin.py
deleted file mode 100644
index 2cb1e56365dc369d6719717f0f6775c8c9e2fdd4..0000000000000000000000000000000000000000
--- a/spaces/cloudtheboi/Lofi4All/.pythonlibs/lib/python3.10/site-packages/PIL/PpmImagePlugin.py
+++ /dev/null
@@ -1,347 +0,0 @@
-#
-# The Python Imaging Library.
-# $Id$
-#
-# PPM support for PIL
-#
-# History:
-# 96-03-24 fl Created
-# 98-03-06 fl Write RGBA images (as RGB, that is)
-#
-# Copyright (c) Secret Labs AB 1997-98.
-# Copyright (c) Fredrik Lundh 1996.
-#
-# See the README file for information on usage and redistribution.
-#
-
-
-from . import Image, ImageFile
-from ._binary import i16be as i16
-from ._binary import o8
-from ._binary import o32le as o32
-
-#
-# --------------------------------------------------------------------
-
-b_whitespace = b"\x20\x09\x0a\x0b\x0c\x0d"
-
-MODES = {
- # standard
- b"P1": "1",
- b"P2": "L",
- b"P3": "RGB",
- b"P4": "1",
- b"P5": "L",
- b"P6": "RGB",
- # extensions
- b"P0CMYK": "CMYK",
- # PIL extensions (for test purposes only)
- b"PyP": "P",
- b"PyRGBA": "RGBA",
- b"PyCMYK": "CMYK",
-}
-
-
-def _accept(prefix):
- return prefix[0:1] == b"P" and prefix[1] in b"0123456y"
-
-
-##
-# Image plugin for PBM, PGM, and PPM images.
-
-
-class PpmImageFile(ImageFile.ImageFile):
- format = "PPM"
- format_description = "Pbmplus image"
-
- def _read_magic(self):
- magic = b""
- # read until whitespace or longest available magic number
- for _ in range(6):
- c = self.fp.read(1)
- if not c or c in b_whitespace:
- break
- magic += c
- return magic
-
- def _read_token(self):
- token = b""
- while len(token) <= 10: # read until next whitespace or limit of 10 characters
- c = self.fp.read(1)
- if not c:
- break
- elif c in b_whitespace: # token ended
- if not token:
- # skip whitespace at start
- continue
- break
- elif c == b"#":
- # ignores rest of the line; stops at CR, LF or EOF
- while self.fp.read(1) not in b"\r\n":
- pass
- continue
- token += c
- if not token:
- # Token was not even 1 byte
- msg = "Reached EOF while reading header"
- raise ValueError(msg)
- elif len(token) > 10:
- msg = f"Token too long in file header: {token.decode()}"
- raise ValueError(msg)
- return token
-
- def _open(self):
- magic_number = self._read_magic()
- try:
- mode = MODES[magic_number]
- except KeyError:
- msg = "not a PPM file"
- raise SyntaxError(msg)
-
- if magic_number in (b"P1", b"P4"):
- self.custom_mimetype = "image/x-portable-bitmap"
- elif magic_number in (b"P2", b"P5"):
- self.custom_mimetype = "image/x-portable-graymap"
- elif magic_number in (b"P3", b"P6"):
- self.custom_mimetype = "image/x-portable-pixmap"
-
- maxval = None
- decoder_name = "raw"
- if magic_number in (b"P1", b"P2", b"P3"):
- decoder_name = "ppm_plain"
- for ix in range(3):
- token = int(self._read_token())
- if ix == 0: # token is the x size
- xsize = token
- elif ix == 1: # token is the y size
- ysize = token
- if mode == "1":
- self.mode = "1"
- rawmode = "1;I"
- break
- else:
- self.mode = rawmode = mode
- elif ix == 2: # token is maxval
- maxval = token
- if not 0 < maxval < 65536:
- msg = "maxval must be greater than 0 and less than 65536"
- raise ValueError(msg)
- if maxval > 255 and mode == "L":
- self.mode = "I"
-
- if decoder_name != "ppm_plain":
- # If maxval matches a bit depth, use the raw decoder directly
- if maxval == 65535 and mode == "L":
- rawmode = "I;16B"
- elif maxval != 255:
- decoder_name = "ppm"
-
- args = (rawmode, 0, 1) if decoder_name == "raw" else (rawmode, maxval)
- self._size = xsize, ysize
- self.tile = [(decoder_name, (0, 0, xsize, ysize), self.fp.tell(), args)]
-
-
-#
-# --------------------------------------------------------------------
-
-
-class PpmPlainDecoder(ImageFile.PyDecoder):
- _pulls_fd = True
-
- def _read_block(self):
- return self.fd.read(ImageFile.SAFEBLOCK)
-
- def _find_comment_end(self, block, start=0):
- a = block.find(b"\n", start)
- b = block.find(b"\r", start)
- return min(a, b) if a * b > 0 else max(a, b) # lowest nonnegative index (or -1)
-
- def _ignore_comments(self, block):
- if self._comment_spans:
- # Finish current comment
- while block:
- comment_end = self._find_comment_end(block)
- if comment_end != -1:
- # Comment ends in this block
- # Delete tail of comment
- block = block[comment_end + 1 :]
- break
- else:
- # Comment spans whole block
- # So read the next block, looking for the end
- block = self._read_block()
-
- # Search for any further comments
- self._comment_spans = False
- while True:
- comment_start = block.find(b"#")
- if comment_start == -1:
- # No comment found
- break
- comment_end = self._find_comment_end(block, comment_start)
- if comment_end != -1:
- # Comment ends in this block
- # Delete comment
- block = block[:comment_start] + block[comment_end + 1 :]
- else:
- # Comment continues to next block(s)
- block = block[:comment_start]
- self._comment_spans = True
- break
- return block
-
- def _decode_bitonal(self):
- """
- This is a separate method because in the plain PBM format, all data tokens are
- exactly one byte, so the inter-token whitespace is optional.
- """
- data = bytearray()
- total_bytes = self.state.xsize * self.state.ysize
-
- while len(data) != total_bytes:
- block = self._read_block() # read next block
- if not block:
- # eof
- break
-
- block = self._ignore_comments(block)
-
- tokens = b"".join(block.split())
- for token in tokens:
- if token not in (48, 49):
- msg = b"Invalid token for this mode: %s" % bytes([token])
- raise ValueError(msg)
- data = (data + tokens)[:total_bytes]
- invert = bytes.maketrans(b"01", b"\xFF\x00")
- return data.translate(invert)
-
- def _decode_blocks(self, maxval):
- data = bytearray()
- max_len = 10
- out_byte_count = 4 if self.mode == "I" else 1
- out_max = 65535 if self.mode == "I" else 255
- bands = Image.getmodebands(self.mode)
- total_bytes = self.state.xsize * self.state.ysize * bands * out_byte_count
-
- half_token = False
- while len(data) != total_bytes:
- block = self._read_block() # read next block
- if not block:
- if half_token:
- block = bytearray(b" ") # flush half_token
- else:
- # eof
- break
-
- block = self._ignore_comments(block)
-
- if half_token:
- block = half_token + block # stitch half_token to new block
- half_token = False
-
- tokens = block.split()
-
- if block and not block[-1:].isspace(): # block might split token
- half_token = tokens.pop() # save half token for later
- if len(half_token) > max_len: # prevent buildup of half_token
- msg = (
- b"Token too long found in data: %s" % half_token[: max_len + 1]
- )
- raise ValueError(msg)
-
- for token in tokens:
- if len(token) > max_len:
- msg = b"Token too long found in data: %s" % token[: max_len + 1]
- raise ValueError(msg)
- value = int(token)
- if value > maxval:
- msg = f"Channel value too large for this mode: {value}"
- raise ValueError(msg)
- value = round(value / maxval * out_max)
- data += o32(value) if self.mode == "I" else o8(value)
- if len(data) == total_bytes: # finished!
- break
- return data
-
- def decode(self, buffer):
- self._comment_spans = False
- if self.mode == "1":
- data = self._decode_bitonal()
- rawmode = "1;8"
- else:
- maxval = self.args[-1]
- data = self._decode_blocks(maxval)
- rawmode = "I;32" if self.mode == "I" else self.mode
- self.set_as_raw(bytes(data), rawmode)
- return -1, 0
-
-
-class PpmDecoder(ImageFile.PyDecoder):
- _pulls_fd = True
-
- def decode(self, buffer):
- data = bytearray()
- maxval = self.args[-1]
- in_byte_count = 1 if maxval < 256 else 2
- out_byte_count = 4 if self.mode == "I" else 1
- out_max = 65535 if self.mode == "I" else 255
- bands = Image.getmodebands(self.mode)
- while len(data) < self.state.xsize * self.state.ysize * bands * out_byte_count:
- pixels = self.fd.read(in_byte_count * bands)
- if len(pixels) < in_byte_count * bands:
- # eof
- break
- for b in range(bands):
- value = (
- pixels[b] if in_byte_count == 1 else i16(pixels, b * in_byte_count)
- )
- value = min(out_max, round(value / maxval * out_max))
- data += o32(value) if self.mode == "I" else o8(value)
- rawmode = "I;32" if self.mode == "I" else self.mode
- self.set_as_raw(bytes(data), rawmode)
- return -1, 0
-
-
-#
-# --------------------------------------------------------------------
-
-
-def _save(im, fp, filename):
- if im.mode == "1":
- rawmode, head = "1;I", b"P4"
- elif im.mode == "L":
- rawmode, head = "L", b"P5"
- elif im.mode == "I":
- rawmode, head = "I;16B", b"P5"
- elif im.mode in ("RGB", "RGBA"):
- rawmode, head = "RGB", b"P6"
- else:
- msg = f"cannot write mode {im.mode} as PPM"
- raise OSError(msg)
- fp.write(head + b"\n%d %d\n" % im.size)
- if head == b"P6":
- fp.write(b"255\n")
- elif head == b"P5":
- if rawmode == "L":
- fp.write(b"255\n")
- else:
- fp.write(b"65535\n")
- ImageFile._save(im, fp, [("raw", (0, 0) + im.size, 0, (rawmode, 0, 1))])
-
- # ALTERNATIVE: save via builtin debug function
- # im._dump(filename)
-
-
-#
-# --------------------------------------------------------------------
-
-
-Image.register_open(PpmImageFile.format, PpmImageFile, _accept)
-Image.register_save(PpmImageFile.format, _save)
-
-Image.register_decoder("ppm", PpmDecoder)
-Image.register_decoder("ppm_plain", PpmPlainDecoder)
-
-Image.register_extensions(PpmImageFile.format, [".pbm", ".pgm", ".ppm", ".pnm"])
-
-Image.register_mime(PpmImageFile.format, "image/x-portable-anymap")
diff --git a/spaces/cloudtheboi/Lofi4All/.pythonlibs/lib/python3.10/site-packages/fontTools/ttLib/tables/T_S_I__1.py b/spaces/cloudtheboi/Lofi4All/.pythonlibs/lib/python3.10/site-packages/fontTools/ttLib/tables/T_S_I__1.py
deleted file mode 100644
index 57163d726c1a5e850eabe8ec72a44c9ec514b715..0000000000000000000000000000000000000000
--- a/spaces/cloudtheboi/Lofi4All/.pythonlibs/lib/python3.10/site-packages/fontTools/ttLib/tables/T_S_I__1.py
+++ /dev/null
@@ -1,164 +0,0 @@
-""" TSI{0,1,2,3,5} are private tables used by Microsoft Visual TrueType (VTT)
-tool to store its hinting source data.
-
-TSI1 contains the text of the glyph programs in the form of low-level assembly
-code, as well as the 'extra' programs 'fpgm', 'ppgm' (i.e. 'prep'), and 'cvt'.
-"""
-from . import DefaultTable
-from fontTools.misc.loggingTools import LogMixin
-from fontTools.misc.textTools import strjoin, tobytes, tostr
-
-
-class table_T_S_I__1(LogMixin, DefaultTable.DefaultTable):
-
- extras = {0xFFFA: "ppgm", 0xFFFB: "cvt", 0xFFFC: "reserved", 0xFFFD: "fpgm"}
-
- indextable = "TSI0"
-
- def decompile(self, data, ttFont):
- totalLength = len(data)
- indextable = ttFont[self.indextable]
- for indices, isExtra in zip(
- (indextable.indices, indextable.extra_indices), (False, True)
- ):
- programs = {}
- for i, (glyphID, textLength, textOffset) in enumerate(indices):
- if isExtra:
- name = self.extras[glyphID]
- else:
- name = ttFont.getGlyphName(glyphID)
- if textOffset > totalLength:
- self.log.warning("textOffset > totalLength; %r skipped" % name)
- continue
- if textLength < 0x8000:
- # If the length stored in the record is less than 32768, then use
- # that as the length of the record.
- pass
- elif textLength == 0x8000:
- # If the length is 32768, compute the actual length as follows:
- isLast = i == (len(indices) - 1)
- if isLast:
- if isExtra:
- # For the last "extra" record (the very last record of the
- # table), the length is the difference between the total
- # length of the TSI1 table and the textOffset of the final
- # record.
- nextTextOffset = totalLength
- else:
- # For the last "normal" record (the last record just prior
- # to the record containing the "magic number"), the length
- # is the difference between the textOffset of the record
- # following the "magic number" (0xFFFE) record (i.e. the
- # first "extra" record), and the textOffset of the last
- # "normal" record.
- nextTextOffset = indextable.extra_indices[0][2]
- else:
- # For all other records with a length of 0x8000, the length is
- # the difference between the textOffset of the record in
- # question and the textOffset of the next record.
- nextTextOffset = indices[i + 1][2]
- assert nextTextOffset >= textOffset, "entries not sorted by offset"
- if nextTextOffset > totalLength:
- self.log.warning(
- "nextTextOffset > totalLength; %r truncated" % name
- )
- nextTextOffset = totalLength
- textLength = nextTextOffset - textOffset
- else:
- from fontTools import ttLib
-
- raise ttLib.TTLibError(
- "%r textLength (%d) must not be > 32768" % (name, textLength)
- )
- text = data[textOffset : textOffset + textLength]
- assert len(text) == textLength
- text = tostr(text, encoding="utf-8")
- if text:
- programs[name] = text
- if isExtra:
- self.extraPrograms = programs
- else:
- self.glyphPrograms = programs
-
- def compile(self, ttFont):
- if not hasattr(self, "glyphPrograms"):
- self.glyphPrograms = {}
- self.extraPrograms = {}
- data = b""
- indextable = ttFont[self.indextable]
- glyphNames = ttFont.getGlyphOrder()
-
- indices = []
- for i in range(len(glyphNames)):
- if len(data) % 2:
- data = (
- data + b"\015"
- ) # align on 2-byte boundaries, fill with return chars. Yum.
- name = glyphNames[i]
- if name in self.glyphPrograms:
- text = tobytes(self.glyphPrograms[name], encoding="utf-8")
- else:
- text = b""
- textLength = len(text)
- if textLength >= 0x8000:
- textLength = 0x8000
- indices.append((i, textLength, len(data)))
- data = data + text
-
- extra_indices = []
- codes = sorted(self.extras.items())
- for i in range(len(codes)):
- if len(data) % 2:
- data = (
- data + b"\015"
- ) # align on 2-byte boundaries, fill with return chars.
- code, name = codes[i]
- if name in self.extraPrograms:
- text = tobytes(self.extraPrograms[name], encoding="utf-8")
- else:
- text = b""
- textLength = len(text)
- if textLength >= 0x8000:
- textLength = 0x8000
- extra_indices.append((code, textLength, len(data)))
- data = data + text
- indextable.set(indices, extra_indices)
- return data
-
- def toXML(self, writer, ttFont):
- names = sorted(self.glyphPrograms.keys())
- writer.newline()
- for name in names:
- text = self.glyphPrograms[name]
- if not text:
- continue
- writer.begintag("glyphProgram", name=name)
- writer.newline()
- writer.write_noindent(text.replace("\r", "\n"))
- writer.newline()
- writer.endtag("glyphProgram")
- writer.newline()
- writer.newline()
- extra_names = sorted(self.extraPrograms.keys())
- for name in extra_names:
- text = self.extraPrograms[name]
- if not text:
- continue
- writer.begintag("extraProgram", name=name)
- writer.newline()
- writer.write_noindent(text.replace("\r", "\n"))
- writer.newline()
- writer.endtag("extraProgram")
- writer.newline()
- writer.newline()
-
- def fromXML(self, name, attrs, content, ttFont):
- if not hasattr(self, "glyphPrograms"):
- self.glyphPrograms = {}
- self.extraPrograms = {}
- lines = strjoin(content).replace("\r", "\n").split("\n")
- text = "\r".join(lines[1:-1])
- if name == "glyphProgram":
- self.glyphPrograms[attrs["name"]] = text
- elif name == "extraProgram":
- self.extraPrograms[attrs["name"]] = text
diff --git a/spaces/colakin/video-generater/public/assets/js/util.js b/spaces/colakin/video-generater/public/assets/js/util.js
deleted file mode 100644
index ecf7b371edf6ab8ec94c019f50729854e48e4d3d..0000000000000000000000000000000000000000
--- a/spaces/colakin/video-generater/public/assets/js/util.js
+++ /dev/null
@@ -1,587 +0,0 @@
-(function($) {
-
- /**
- * Generate an indented list of links from a nav. Meant for use with panel().
- * @return {jQuery} jQuery object.
- */
- $.fn.navList = function() {
-
- var $this = $(this);
- $a = $this.find('a'),
- b = [];
-
- $a.each(function() {
-
- var $this = $(this),
- indent = Math.max(0, $this.parents('li').length - 1),
- href = $this.attr('href'),
- target = $this.attr('target');
-
- b.push(
- '' +
- '' +
- $this.text() +
- ''
- );
-
- });
-
- return b.join('');
-
- };
-
- /**
- * Panel-ify an element.
- * @param {object} userConfig User config.
- * @return {jQuery} jQuery object.
- */
- $.fn.panel = function(userConfig) {
-
- // No elements?
- if (this.length == 0)
- return $this;
-
- // Multiple elements?
- if (this.length > 1) {
-
- for (var i=0; i < this.length; i++)
- $(this[i]).panel(userConfig);
-
- return $this;
-
- }
-
- // Vars.
- var $this = $(this),
- $body = $('body'),
- $window = $(window),
- id = $this.attr('id'),
- config;
-
- // Config.
- config = $.extend({
-
- // Delay.
- delay: 0,
-
- // Hide panel on link click.
- hideOnClick: false,
-
- // Hide panel on escape keypress.
- hideOnEscape: false,
-
- // Hide panel on swipe.
- hideOnSwipe: false,
-
- // Reset scroll position on hide.
- resetScroll: false,
-
- // Reset forms on hide.
- resetForms: false,
-
- // Side of viewport the panel will appear.
- side: null,
-
- // Target element for "class".
- target: $this,
-
- // Class to toggle.
- visibleClass: 'visible'
-
- }, userConfig);
-
- // Expand "target" if it's not a jQuery object already.
- if (typeof config.target != 'jQuery')
- config.target = $(config.target);
-
- // Panel.
-
- // Methods.
- $this._hide = function(event) {
-
- // Already hidden? Bail.
- if (!config.target.hasClass(config.visibleClass))
- return;
-
- // If an event was provided, cancel it.
- if (event) {
-
- event.preventDefault();
- event.stopPropagation();
-
- }
-
- // Hide.
- config.target.removeClass(config.visibleClass);
-
- // Post-hide stuff.
- window.setTimeout(function() {
-
- // Reset scroll position.
- if (config.resetScroll)
- $this.scrollTop(0);
-
- // Reset forms.
- if (config.resetForms)
- $this.find('form').each(function() {
- this.reset();
- });
-
- }, config.delay);
-
- };
-
- // Vendor fixes.
- $this
- .css('-ms-overflow-style', '-ms-autohiding-scrollbar')
- .css('-webkit-overflow-scrolling', 'touch');
-
- // Hide on click.
- if (config.hideOnClick) {
-
- $this.find('a')
- .css('-webkit-tap-highlight-color', 'rgba(0,0,0,0)');
-
- $this
- .on('click', 'a', function(event) {
-
- var $a = $(this),
- href = $a.attr('href'),
- target = $a.attr('target');
-
- if (!href || href == '#' || href == '' || href == '#' + id)
- return;
-
- // Cancel original event.
- event.preventDefault();
- event.stopPropagation();
-
- // Hide panel.
- $this._hide();
-
- // Redirect to href.
- window.setTimeout(function() {
-
- if (target == '_blank')
- window.open(href);
- else
- window.location.href = href;
-
- }, config.delay + 10);
-
- });
-
- }
-
- // Event: Touch stuff.
- $this.on('touchstart', function(event) {
-
- $this.touchPosX = event.originalEvent.touches[0].pageX;
- $this.touchPosY = event.originalEvent.touches[0].pageY;
-
- })
-
- $this.on('touchmove', function(event) {
-
- if ($this.touchPosX === null
- || $this.touchPosY === null)
- return;
-
- var diffX = $this.touchPosX - event.originalEvent.touches[0].pageX,
- diffY = $this.touchPosY - event.originalEvent.touches[0].pageY,
- th = $this.outerHeight(),
- ts = ($this.get(0).scrollHeight - $this.scrollTop());
-
- // Hide on swipe?
- if (config.hideOnSwipe) {
-
- var result = false,
- boundary = 20,
- delta = 50;
-
- switch (config.side) {
-
- case 'left':
- result = (diffY < boundary && diffY > (-1 * boundary)) && (diffX > delta);
- break;
-
- case 'right':
- result = (diffY < boundary && diffY > (-1 * boundary)) && (diffX < (-1 * delta));
- break;
-
- case 'top':
- result = (diffX < boundary && diffX > (-1 * boundary)) && (diffY > delta);
- break;
-
- case 'bottom':
- result = (diffX < boundary && diffX > (-1 * boundary)) && (diffY < (-1 * delta));
- break;
-
- default:
- break;
-
- }
-
- if (result) {
-
- $this.touchPosX = null;
- $this.touchPosY = null;
- $this._hide();
-
- return false;
-
- }
-
- }
-
- // Prevent vertical scrolling past the top or bottom.
- if (($this.scrollTop() < 0 && diffY < 0)
- || (ts > (th - 2) && ts < (th + 2) && diffY > 0)) {
-
- event.preventDefault();
- event.stopPropagation();
-
- }
-
- });
-
- // Event: Prevent certain events inside the panel from bubbling.
- $this.on('click touchend touchstart touchmove', function(event) {
- event.stopPropagation();
- });
-
- // Event: Hide panel if a child anchor tag pointing to its ID is clicked.
- $this.on('click', 'a[href="#' + id + '"]', function(event) {
-
- event.preventDefault();
- event.stopPropagation();
-
- config.target.removeClass(config.visibleClass);
-
- });
-
- // Body.
-
- // Event: Hide panel on body click/tap.
- $body.on('click touchend', function(event) {
- $this._hide(event);
- });
-
- // Event: Toggle.
- $body.on('click', 'a[href="#' + id + '"]', function(event) {
-
- event.preventDefault();
- event.stopPropagation();
-
- config.target.toggleClass(config.visibleClass);
-
- });
-
- // Window.
-
- // Event: Hide on ESC.
- if (config.hideOnEscape)
- $window.on('keydown', function(event) {
-
- if (event.keyCode == 27)
- $this._hide(event);
-
- });
-
- return $this;
-
- };
-
- /**
- * Apply "placeholder" attribute polyfill to one or more forms.
- * @return {jQuery} jQuery object.
- */
- $.fn.placeholder = function() {
-
- // Browser natively supports placeholders? Bail.
- if (typeof (document.createElement('input')).placeholder != 'undefined')
- return $(this);
-
- // No elements?
- if (this.length == 0)
- return $this;
-
- // Multiple elements?
- if (this.length > 1) {
-
- for (var i=0; i < this.length; i++)
- $(this[i]).placeholder();
-
- return $this;
-
- }
-
- // Vars.
- var $this = $(this);
-
- // Text, TextArea.
- $this.find('input[type=text],textarea')
- .each(function() {
-
- var i = $(this);
-
- if (i.val() == ''
- || i.val() == i.attr('placeholder'))
- i
- .addClass('polyfill-placeholder')
- .val(i.attr('placeholder'));
-
- })
- .on('blur', function() {
-
- var i = $(this);
-
- if (i.attr('name').match(/-polyfill-field$/))
- return;
-
- if (i.val() == '')
- i
- .addClass('polyfill-placeholder')
- .val(i.attr('placeholder'));
-
- })
- .on('focus', function() {
-
- var i = $(this);
-
- if (i.attr('name').match(/-polyfill-field$/))
- return;
-
- if (i.val() == i.attr('placeholder'))
- i
- .removeClass('polyfill-placeholder')
- .val('');
-
- });
-
- // Password.
- $this.find('input[type=password]')
- .each(function() {
-
- var i = $(this);
- var x = $(
- $('
')
- .append(i.clone())
- .remove()
- .html()
- .replace(/type="password"/i, 'type="text"')
- .replace(/type=password/i, 'type=text')
- );
-
- if (i.attr('id') != '')
- x.attr('id', i.attr('id') + '-polyfill-field');
-
- if (i.attr('name') != '')
- x.attr('name', i.attr('name') + '-polyfill-field');
-
- x.addClass('polyfill-placeholder')
- .val(x.attr('placeholder')).insertAfter(i);
-
- if (i.val() == '')
- i.hide();
- else
- x.hide();
-
- i
- .on('blur', function(event) {
-
- event.preventDefault();
-
- var x = i.parent().find('input[name=' + i.attr('name') + '-polyfill-field]');
-
- if (i.val() == '') {
-
- i.hide();
- x.show();
-
- }
-
- });
-
- x
- .on('focus', function(event) {
-
- event.preventDefault();
-
- var i = x.parent().find('input[name=' + x.attr('name').replace('-polyfill-field', '') + ']');
-
- x.hide();
-
- i
- .show()
- .focus();
-
- })
- .on('keypress', function(event) {
-
- event.preventDefault();
- x.val('');
-
- });
-
- });
-
- // Events.
- $this
- .on('submit', function() {
-
- $this.find('input[type=text],input[type=password],textarea')
- .each(function(event) {
-
- var i = $(this);
-
- if (i.attr('name').match(/-polyfill-field$/))
- i.attr('name', '');
-
- if (i.val() == i.attr('placeholder')) {
-
- i.removeClass('polyfill-placeholder');
- i.val('');
-
- }
-
- });
-
- })
- .on('reset', function(event) {
-
- event.preventDefault();
-
- $this.find('select')
- .val($('option:first').val());
-
- $this.find('input,textarea')
- .each(function() {
-
- var i = $(this),
- x;
-
- i.removeClass('polyfill-placeholder');
-
- switch (this.type) {
-
- case 'submit':
- case 'reset':
- break;
-
- case 'password':
- i.val(i.attr('defaultValue'));
-
- x = i.parent().find('input[name=' + i.attr('name') + '-polyfill-field]');
-
- if (i.val() == '') {
- i.hide();
- x.show();
- }
- else {
- i.show();
- x.hide();
- }
-
- break;
-
- case 'checkbox':
- case 'radio':
- i.attr('checked', i.attr('defaultValue'));
- break;
-
- case 'text':
- case 'textarea':
- i.val(i.attr('defaultValue'));
-
- if (i.val() == '') {
- i.addClass('polyfill-placeholder');
- i.val(i.attr('placeholder'));
- }
-
- break;
-
- default:
- i.val(i.attr('defaultValue'));
- break;
-
- }
- });
-
- });
-
- return $this;
-
- };
-
- /**
- * Moves elements to/from the first positions of their respective parents.
- * @param {jQuery} $elements Elements (or selector) to move.
- * @param {bool} condition If true, moves elements to the top. Otherwise, moves elements back to their original locations.
- */
- $.prioritize = function($elements, condition) {
-
- var key = '__prioritize';
-
- // Expand $elements if it's not already a jQuery object.
- if (typeof $elements != 'jQuery')
- $elements = $($elements);
-
- // Step through elements.
- $elements.each(function() {
-
- var $e = $(this), $p,
- $parent = $e.parent();
-
- // No parent? Bail.
- if ($parent.length == 0)
- return;
-
- // Not moved? Move it.
- if (!$e.data(key)) {
-
- // Condition is false? Bail.
- if (!condition)
- return;
-
- // Get placeholder (which will serve as our point of reference for when this element needs to move back).
- $p = $e.prev();
-
- // Couldn't find anything? Means this element's already at the top, so bail.
- if ($p.length == 0)
- return;
-
- // Move element to top of parent.
- $e.prependTo($parent);
-
- // Mark element as moved.
- $e.data(key, $p);
-
- }
-
- // Moved already?
- else {
-
- // Condition is true? Bail.
- if (condition)
- return;
-
- $p = $e.data(key);
-
- // Move element back to its original location (using our placeholder).
- $e.insertAfter($p);
-
- // Unmark element as moved.
- $e.removeData(key);
-
- }
-
- });
-
- };
-
-})(jQuery);
\ No newline at end of file
diff --git a/spaces/colakin/video-generater/public/ffmpeg/libavcodec/cbs_h2645.h b/spaces/colakin/video-generater/public/ffmpeg/libavcodec/cbs_h2645.h
deleted file mode 100644
index f4c987a5119d1b2ce4e442884eb805c4394ede2d..0000000000000000000000000000000000000000
--- a/spaces/colakin/video-generater/public/ffmpeg/libavcodec/cbs_h2645.h
+++ /dev/null
@@ -1,36 +0,0 @@
-/*
- * This file is part of FFmpeg.
- *
- * FFmpeg is free software; you can redistribute it and/or
- * modify it under the terms of the GNU Lesser General Public
- * License as published by the Free Software Foundation; either
- * version 2.1 of the License, or (at your option) any later version.
- *
- * FFmpeg is distributed in the hope that it will be useful,
- * but WITHOUT ANY WARRANTY; without even the implied warranty of
- * MERCHANTABILITY or FITNESS FOR A PARTICULAR PURPOSE. See the GNU
- * Lesser General Public License for more details.
- *
- * You should have received a copy of the GNU Lesser General Public
- * License along with FFmpeg; if not, write to the Free Software
- * Foundation, Inc., 51 Franklin Street, Fifth Floor, Boston, MA 02110-1301 USA
- */
-
-#ifndef AVCODEC_CBS_H2645_H
-#define AVCODEC_CBS_H2645_H
-
-#include "h2645_parse.h"
-
-
-typedef struct CodedBitstreamH2645Context {
- // If set, the stream being read is in MP4 (AVCC/HVCC) format. If not
- // set, the stream is assumed to be in annex B format.
- int mp4;
- // Size in bytes of the NAL length field for MP4 format.
- int nal_length_size;
- // Packet reader.
- H2645Packet read_packet;
-} CodedBitstreamH2645Context;
-
-
-#endif /* AVCODEC_CBS_H2645_H */
diff --git a/spaces/congsaPfin/Manga-OCR/logs/AZbul Sz Tapmaca - nternetsiz v tin Sviyyli Oyun.md b/spaces/congsaPfin/Manga-OCR/logs/AZbul Sz Tapmaca - nternetsiz v tin Sviyyli Oyun.md
deleted file mode 100644
index 9dfe044cc0059efe8337d3fd38ccd2e1849aefe1..0000000000000000000000000000000000000000
--- a/spaces/congsaPfin/Manga-OCR/logs/AZbul Sz Tapmaca - nternetsiz v tin Sviyyli Oyun.md
+++ /dev/null
@@ -1,150 +0,0 @@
-
-
Azbul Yukle: How to Download and Play the Popular Word Game in Azerbaijani
-
If you love word games and want to challenge your brain, you might want to try Azbul, a free and offline app that lets you play a fun and addictive word search game in Azerbaijani. In this article, we will tell you what Azbul is, how to download it, how to play it, and why you should play it.
Azbul is a word game that is designed for people who speak or want to learn Azerbaijani. It is similar to crossword puzzles, but with some twists and variations. Here are some of the features of Azbul that make it an interesting and enjoyable game.
-
A fun and challenging word game
-
In Azbul, you have to find words hidden in a grid of letters. You can swipe horizontally, vertically, diagonally, or in any direction to form words. The words can be related to various topics, such as animals, food, sports, geography, history, etc. You have to find all the words in each level to complete it and move on to the next one. The levels get harder as you progress, with more words and larger grids.
-
A free and offline app
-
Azbul is completely free to download and play. You don't need to pay anything or watch ads to enjoy the game. You also don't need an internet connection to play it. You can play it anytime and anywhere, without worrying about data usage or wifi availability.
-
A way to learn new words and improve vocabulary
-
Azbul is not only a game, but also a learning tool. By playing it, you can learn new words and expand your vocabulary in Azerbaijani. You can also improve your spelling, grammar, and logic skills. You can tap on any word you find to see its meaning and pronunciation. You can also review the words you have found in each level in the word list.
-
How to download Azbul?
-
Azbul is available for Android devices. You can download it from different sources, depending on your preference and device compatibility. Here are some of the options you have.
-
azbul yukle pulsuz
-azbul yukle kompüter üçün
-azbul yukle apk
-azbul yukle windows 10
-azbul yukle microsoft store
-azbul yukle söz oyunu
-azbul yukle krossvord
-azbul yukle internetsiz
-azbul yukle android
-azbul yukle ios
-azbul yukle mac
-azbul yukle pc
-azbul yukle laptop
-azbul yukle tablet
-azbul yukle telefon
-azbul yukle online
-azbul yukle oyna
-azbul yukle qeydiyyatdan keçmədən
-azbul yukle google play store
-azbul yukle app store
-azbul yukle indir
-azbul yukle bedava
-azbul yukle bilgisayar için
-azbul yukle nasıl oynanır
-azbul yukle hileleri
-azbul yukle ipucu
-azbul yukle rehberi
-azbul yukle puan kazanma
-azbul yukle kelime oyunu
-azbul yukle bulmaca
-azbul yukle internet olmadan
-azbul yukle azerbaycan dili
-azbul yukle türkçe dil seçeneği
-azbul yukle ingilizce dil seçeneği
-azbul yukle rusça dil seçeneği
-azbul yukle farsça dil seçeneği
-azbul yukle arapça dil seçeneği
-azbul yukle fransızca dil seçeneği
-azbul yukle almanca dil seçeneği
-azbul yukle ispanyolca dil seçeneği
-azbul yukle italyanca dil seçeneği
-azbul yukle portekizce dil seçeneği
-azbul yukle çince dil seçeneği
-azbul yukle japonca dil seçeneği
-azbul yukle korece dil seçeneği
-azbul yukle hintçe dil seçeneği
-azbul yukle urduca dil seçeneği
-azbul yukle tatarca dil seçeneği
-
From Google Play Store
-
The easiest way to download Azbul is from the Google Play Store. You can simply search for "AZbul: Söz Oyunu ve Krossvord" or use this link to access the app page. Then, you can tap on the "Install" button and wait for the app to download and install on your device. You can also see the app description, screenshots, ratings, reviews, and other information on the app page.
-
From other sources
-
If you can't access the Google Play Store or prefer another source, you can also download Azbul from other websites that offer APK files. APK files are the installation files for Android apps. However, you need to be careful when downloading APK files from unknown sources, as they may contain viruses or malware that can harm your device. You also need to enable the "Unknown sources" option in your device settings to allow the installation of apps from outside the Google Play Store.
-
Some of the websites that offer APK files for Azbul are APKPure, APKCombo[^3^
], and APKMonk. You can visit these websites and search for "AZbul: Söz Oyunu ve Krossvord" or use the links provided to download the APK file. Then, you can open the file and follow the instructions to install the app on your device.
-
How to play Azbul?
-
Once you have downloaded and installed Azbul, you can start playing it right away. The game is easy to learn, but hard to master. Here are some of the basic rules and tips on how to play Azbul.
-
The basic rules
-
The game consists of hundreds of levels, each with a different grid size and word list. Your goal is to find all the words hidden in the grid by swiping your finger over the letters. The words can be in any direction, as long as they are connected. When you find a word, it will be highlighted and crossed out from the word list. You can also see how many letters and words are left in the level. You can zoom in and out of the grid by pinching your fingers. You can also move the grid by dragging your finger. You can pause the game at any time by tapping on the menu button at the top right corner of the screen.
-
The different levels and modes
-
The game has four difficulty levels: Easy, Normal, Hard, and Expert. Each level has a different grid size and number of words to find. The higher the difficulty, the more challenging the game becomes. You can choose any level you want, but you have to complete all the levels in one difficulty before unlocking the next one.
-
The game also has two modes: Classic and Time Attack. In Classic mode, you can play at your own pace, without any time limit or pressure. In Time Attack mode, you have to find all the words in a limited time, which varies depending on the difficulty level. The faster you find the words, the higher your score will be. You can switch between the modes by tapping on the mode button at the top left corner of the screen.
-
The hints and bonuses
-
If you get stuck or need some help, you can use hints and bonuses to make the game easier. Hints are clues that show you one letter of a word you haven't found yet. You can use one hint per level, by tapping on the hint button at the bottom left corner of the screen. Bonuses are rewards that give you extra time, extra hints, or extra coins. You can earn bonuses by completing levels, finding bonus words, or watching ads. You can use bonuses by tapping on the bonus button at the bottom right corner of the screen.
-
Why play Azbul?
-
Azbul is not just a game, but also a hobby, a passion, and a benefit for your mind. Here are some of the reasons why you should play Azbul and enjoy its features.
-
The benefits of playing word games
-
Playing word games like Azbul can have many positive effects on your brain and mental health. Some of these benefits are:
-
-
Improving your memory, concentration, and attention span
-
Enhancing your cognitive skills, such as logic, reasoning, and problem-solving
-
Boosting your creativity, imagination, and curiosity
-
Reducing stress, anxiety, and boredom
-
Increasing your self-confidence and self-esteem
-
Stimulating your brain cells and preventing cognitive decline
-
-
The features of Azbul that make it unique and enjoyable
-
Azbul is not just another word game. It has some distinctive features that make it stand out from other similar games. Some of these features are:
-
-
The language: Azbul is one of the few word games that is available in Azerbaijani, a language spoken by more than 30 million people in Azerbaijan and other countries.
-
The design: Azbul has a simple and elegant design that is easy on the eyes and pleasant to look at. The colors are bright and cheerful, and the fonts are clear and readable.
-
The sound: Azbul has a soothing and relaxing sound that accompanies your gameplay. The sound effects are realistic and satisfying, and the music is calm and harmonious.
-
The content: Azbul has a rich and diverse content that covers various topics and categories. The words are relevant and interesting, and the levels are well-designed and balanced.
-
The challenge: Azbul has a high level of challenge that keeps you engaged and motivated. The game is not too easy or too hard, but just right for your skill level.
-
-
The feedback and ratings from other players
-
Azbul is not only a game that we love, but also a game that many other players love. Azbul has received thousands of positive feedback and ratings from its users, who have praised its quality, fun, and educational value. Here are some of the comments and reviews that Azbul has received on the Google Play Store:
-
-
-
Name
-
Rating
-
Comment
-
-
-
Aydan Aliyeva
-
5 stars
-
"I love this game. It is very interesting and useful. It helps me to improve my Azerbaijani language skills and learn new words. I recommend it to everyone who likes word games."
-
-
-
Rashad Mammadov
-
5 stars
-
"This is one of the best word games I have ever played. It is challenging, entertaining, and relaxing. The graphics are beautiful and the sound is soothing. I play it every day and never get bored."
-
-
-
Nigar Huseynova
-
4 stars
-
"This game is very good and fun. It has many levels and modes to choose from. The only thing I don't like is that sometimes the words are too hard or obscure. But overall, it is a great game."
-
-
-
Emin Ismayilov
-
5 stars
-
"This game is amazing. It is not only a game, but also a teacher. It teaches me new words and meanings in Azerbaijani. It also improves my memory and concentration. I enjoy playing it a lot."
-
-
-
Leyla Mammadli
-
5 stars
-
"This game is awesome. It is very addictive and fun. It has a lot of features and bonuses that make it more exciting. The design and sound are also very nice. I love this game."
-
-
-
Conclusion
-
Azbul is a popular word game that you can download and play on your Android device. It is a fun and challenging game that lets you find words hidden in a grid of letters in Azerbaijani. It is also a free and offline app that you can play anytime and anywhere. Moreover, it is a way to learn new words and improve your vocabulary in Azerbaijani. It also has many benefits for your brain and mental health, such as improving your memory, concentration, creativity, and logic skills.
-
Azbul has many features that make it unique and enjoyable, such as the language, the design, the sound, the content, and the challenge. It also has many hints and bonuses that make it easier and more rewarding. Azbul has received many positive feedback and ratings from other players, who have expressed their satisfaction and appreciation for the game.
-
If you are looking for a word game that is fun, educational, and beneficial for your mind, you should try Azbul. You will not regret it.
-
FAQs
-
Here are some of the frequently asked questions about Azbul:
-
-
What does Azbul mean?
-
Azbul is a combination of two Azerbaijani words: "az" (short for "Azerbaijan") and "bul" (meaning "find"). It means "find Azerbaijan", which reflects the theme of the game.
-
How many words are there in Azbul?
-
Azbul has more than 10,000 words in its database, covering various topics and categories. The words are updated regularly to keep the game fresh and relevant.
-
How can I get more coins in Azbul?
-
You can get more coins in Azbul by completing levels, finding bonus words, or watching ads. You can also buy coins with real money if you want to support the developers.
-
How can I contact the developers of Azbul?
-
You can contact the developers of Azbul by sending an email to azbulgame@gmail.com or by visiting their Facebook page. You can also leave a comment or review on the Google Play Store. The developers are always happy to hear from their users and welcome any feedback or suggestions.
-
Is Azbul available in other languages?
-
No, Azbul is currently only available in Azerbaijani. However, the developers are planning to add more languages in the future, depending on the demand and popularity of the game.
401be4b1e0
-
-
\ No newline at end of file
diff --git a/spaces/congsaPfin/Manga-OCR/logs/Download Dawn AI Mod APK - No Ads No Root No Limits.md b/spaces/congsaPfin/Manga-OCR/logs/Download Dawn AI Mod APK - No Ads No Root No Limits.md
deleted file mode 100644
index 8ed28032978baf8cda3cb37830b45cca77d8a4c0..0000000000000000000000000000000000000000
--- a/spaces/congsaPfin/Manga-OCR/logs/Download Dawn AI Mod APK - No Ads No Root No Limits.md
+++ /dev/null
@@ -1,90 +0,0 @@
-
-
Download Dawn AI Hacked Version: How to Get Unlimited AI Art for Free
-
If you are a fan of creating stunning avatars and portraits using artificial intelligence, you might have heard of Dawn AI, a popular app that lets you transform your photos into amazing artworks with just a few clicks. But did you know that there is a way to get unlimited access to all the features and styles of Dawn AI without paying a dime? In this article, we will show you how to download Dawn AI hacked version, a modded apk file that gives you free premium access to the app. We will also discuss the pros and cons of hacking Dawn AI, and some alternatives you can try if you don't want to risk it.
Dawn AI is an app that allows you to create outstanding avatars using the latest AI technology. Just upload your photos and let Dawn work its magic—showing you and your friends in an incredible mix of styles and settings. And all at the click of a button. With Dawn’s innovative technology, you can surprise your friends with content that’s never been seen before. Our AI analyzes your photos to learn what you look like, then produces stunning portraits with thousands of possible styles. See yourself sketched in black and white or painted in vibrant color. Browse your own AI-generated selfies, styled as hyperrealistic photos, classical art, and more. Just upload your pictures and let our AI generator do the rest! All with a single click.
-
Why Hack Dawn AI?
-
Dawn AI is free to use, but it has some limitations that might frustrate you if you want to unleash your creativity. For example, the free version only allows you to generate five images per day, and they will have watermarks on them. You also have to wait longer for the images to be generated, and you can't save them in high resolution. If you want to remove these restrictions, you have to pay for a subscription that costs $2.99 per week or $9.99 per month.
-
That's why some people look for ways to hack Dawn AI and get unlimited access to all the features and styles without paying anything. By downloading a hacked version of the app, you can generate as many images as you want, without watermarks, faster, and in higher quality. You can also access more styles and themes that are not available in the free version.
-
How to Download Dawn AI Hacked Version?
-
If you are interested in downloading Dawn AI hacked version, here are the steps you need to follow:
-
-
Find a reliable source for the modded apk file. You can search online for websites that offer hacked apps, but be careful not to download any malware or viruses. One website that claims to have a working Dawn AI hacked version is [4](https://www.world-today-news.com/download-dawn-ai-hacked-paid-2023-the-latest-version-with-a-direct-link-from-mediafire/), but we cannot guarantee its safety or functionality.
-
Download the apk file to your device. You might need to enable unknown sources in your settings to allow installation from third-party sources.
-
Install the apk file by tapping on it and following the instructions.
-
Launch the app and enjoy unlimited AI art for free!
-
-
Is Dawn AI Hacked Version Safe and Legal?
-
Before you download Dawn AI hacked version, you should be aware of the risks and consequences of using a hacked app. First of all, hacking Dawn AI is illegal and unethical, as it violates the terms of service and the intellectual property rights of the developers. You could face legal action or penalties if you are caught using a hacked app. Secondly, hacking Dawn AI is unsafe and risky, as you could expose your device and data to malware, viruses, spyware, or hackers. You could also damage your device or lose your files if the hacked app is corrupted or incompatible. Thirdly, hacking Dawn AI is unfair and disrespectful, as it deprives the developers of their deserved income and recognition. You could also ruin the experience and reputation of the app for other users who pay for the service.
-
dawn ai mod apk free download
-dawn ai premium apk unlocked
-dawn ai avatar generator hack
-dawn ai app modded version
-dawn ai cracked apk download
-dawn ai unlimited avatars mod
-dawn ai sticker art hack
-dawn ai apk without ads
-dawn ai latest mod apk 2023
-dawn ai v3.1.2.91 mod apk
-dawn ai mod apk for android
-dawn ai premium features unlocked
-dawn ai hack apk download
-dawn ai app cheat codes
-dawn ai cracked version free
-dawn ai unlimited stickers mod
-dawn ai avatar creator hack
-dawn ai apk modded by splice video editor
-dawn ai no ads mod apk
-dawn ai 2023 mod apk download
-dawn ai v3.1.5.94 mod apk
-dawn ai mod apk for ios
-dawn ai premium subscription hack
-dawn ai hack tool download
-dawn ai free download full version
-dawn ai unlimited customization mod
-dawn ai realistic avatar hack
-dawn ai apk hacked by bendingspoons
-dawn ai ad-free mod apk
-dawn ai new update mod apk 2023
-dawn ai v3.1.2.91 hack apk
-dawn ai mod apk for pc
-dawn ai premium account hack
-dawn ai hack online generator
-dawn ai full version free download
-dawn ai high-quality output mod
-dawn ai detailed sticker art hack
-dawn ai apk patched by com.bendingspoons.dawn.ai
-dawn ai remove watermark mod apk
-dawn ai latest version mod apk 2023
-dawn ai v3.1.5.94 hack apk
-dawn ai mod apk for mac
-dawn ai premium access hack
-dawn ai hack download link
-dawn ai pro version free download
-dawn ai easy to use mod
-dawn ai customizable features hack
-dawn ai apk cracked by www.dawnaimodapk.com
-dawn ai no root mod apk
-
Therefore, we do not recommend or endorse downloading Dawn AI hacked version, as it is not worth the trouble and the guilt. If you want to enjoy Dawn AI without breaking the law or harming your device, you should either pay for the subscription or look for some alternatives that are free or cheaper.
-
Alternatives to Dawn AI Hacked Version
-
If you are looking for some alternatives to Dawn AI hacked version, here are some options you can try:
-
-
Artbreeder: This is a web-based tool that lets you create and explore images using generative adversarial networks (GANs). You can upload your photos and mix them with different styles and genres, such as anime, fantasy, portraits, landscapes, and more. You can also edit various parameters, such as color, brightness, contrast, and detail. Artbreeder is free to use, but it has some limitations on the number of images you can generate and download per day. You can upgrade to a paid plan to get more features and credits.
-
DeepArt: This is another web-based tool that uses deep learning to transform your photos into artworks inspired by famous artists. You can choose from over 50 styles, such as Van Gogh, Picasso, Monet, Kandinsky, and more. You can also upload your own style image and apply it to your photo. DeepArt is free to use, but it takes some time to process your images. You can pay a small fee to get faster results and higher resolution.
-
Prisma: This is a mobile app that lets you turn your photos into artworks using various filters and effects. You can choose from over 300 styles, such as impressionism, pop art, graffiti, abstract, and more. You can also adjust the intensity and blend mode of the filters. Prisma is free to use, but it has some ads and watermarks. You can remove them by subscribing to Prisma Premium.
-
-
Conclusion
-
Dawn AI is an amazing app that allows you to create stunning avatars and portraits using artificial intelligence. However, if you want to get unlimited access to all the features and styles of Dawn AI without paying anything, you might be tempted to download Dawn AI hacked version, a modded apk file that gives you free premium access to the app. But before you do that, you should be aware of the risks and consequences of using a hacked app. Hacking Dawn AI is illegal, unsafe, and unfair. It could get you in trouble with the law or damage your device or data. It could also harm the developers and other users of the app.
-
Therefore, we suggest that you either pay for the subscription or look for some alternatives that are free or cheaper. There are many other tools and apps that let you create AI art with different styles and effects. Some of them are Artbreeder, DeepArt, and Prisma. They are legal, safe, and respectful.
-
We hope this article has helped you understand how to download Dawn AI hacked version and what are the pros and cons of doing so. If you have any questions or comments, feel free to leave them below.
-
FAQs
-
-
What is Dawn AI? Dawn AI is an app that allows you to create outstanding avatars using the latest AI technology.
-
Why hack Dawn AI? Some people hack Dawn AI to get unlimited access to all the features and styles without paying anything.
-
How to download Dawn AI hacked version? You can download Dawn AI hacked version by finding a reliable source for the modded apk file and installing it on your device.
-
Is Dawn AI hacked version safe and legal? No, Dawn AI hacked version is not safe and legal. It could expose your device and data to malware or viruses, violate the terms of service and the intellectual property rights of the developers, and face legal action or penalties.
-
What are some alternatives to Dawn AI hacked version?Some of them are Artbreeder, DeepArt, and Prisma. They are legal, safe, and respectful.
- 197e85843d
-
-
\ No newline at end of file
diff --git a/spaces/congsaPfin/Manga-OCR/logs/Enjoy Grand Theft Auto V for Free on Any Device - Download GTA 5 Unofficial Now.md b/spaces/congsaPfin/Manga-OCR/logs/Enjoy Grand Theft Auto V for Free on Any Device - Download GTA 5 Unofficial Now.md
deleted file mode 100644
index f5eb7855a83e5387a2accd5b14c499ec7ec5b465..0000000000000000000000000000000000000000
--- a/spaces/congsaPfin/Manga-OCR/logs/Enjoy Grand Theft Auto V for Free on Any Device - Download GTA 5 Unofficial Now.md
+++ /dev/null
@@ -1,120 +0,0 @@
-
-
How to Download GTA 5 Unofficial for Android
-
Grand Theft Auto V (GTA 5) is one of the most popular and successful video games of all time. It was released in 2013 by Rockstar Games for PlayStation 3, Xbox 360, PlayStation 4, Xbox One, and PC. However, there is no official version of GTA 5 for Android devices yet. If you are a fan of GTA 5 and want to play it on your smartphone or tablet, you may be wondering how to download GTA 5 unofficial for Android.
-
In this article, we will show you how to download and install an unofficial APK (Android Package Kit) for GTA 5 that lets you enjoy the game on your Android device. We will also explain what GTA 5 unofficial is, why you should download it, and some tips and tricks for improving your gaming experience. Let's get started!
GTA 5 unofficial is an APK that was developed by a team of fans at GTA5App.mobi. It is not an official release from Rockstar Games, but a clone of the original game that mimics its gameplay and graphics. You can complete a series of missions, such as carrying out hits, making money, and increasing your power in the criminal underworld of Los Santos. You can also explore the open world, drive various vehicles, use weapons, and interact with other characters.
-
GTA 5 unofficial is free to download and install on your Android device. It is compatible with Android 6.0 or higher and requires about 1 GB of storage space. It is also safe and virus-free, as long as you download it from a reliable source.
-
Why Download GTA 5 Unofficial?
-
There are many reasons why you may want to download GTA 5 unofficial for Android. Here are some of them:
-
-
You can play GTA 5 on your Android device anytime, anywhere. You don't need a console or a PC to enjoy the game.
-
You can experience GTA 5 in high resolution, up to 4K, and at 60 frames per second. The game looks stunning on your Android device.
-
You can customize the game settings according to your preferences. You can adjust the graphics quality, sound volume, control sensitivity, language, and more.
-
You can access the online mode and play with other players on dedicated servers. You can join different game modes, such as deathmatch, racing, heist, and more.
-
-
How to Download and Install GTA 5 Unofficial?
-
Downloading and installing GTA 5 unofficial for Android is easy and fast. Just follow these steps:
-
Step 1: Find a reliable source for the APK
-
The first source for the APK is the official website of GTA 5 unofficial, GTA5App.mobi. This is where you can find the latest version of the APK and the most updated information about the game. You can also scan the QR code on the website to download the APK directly to your device.
-
download gta 5 unofficial apk for android
-download gta 5 unofficial free for pc
-download gta 5 unofficial mod menu
-download gta 5 unofficial online
-download gta 5 unofficial from steam
-download gta 5 unofficial full version
-download gta 5 unofficial crack
-download gta 5 unofficial update
-download gta 5 unofficial patch
-download gta 5 unofficial launcher
-download gta 5 unofficial android filehippo
-download gta 5 unofficial pc highly compressed
-download gta 5 unofficial mobile
-download gta 5 unofficial windows 10
-download gta 5 unofficial setup
-download gta 5 unofficial iso
-download gta 5 unofficial zip file
-download gta 5 unofficial no verification
-download gta 5 unofficial without license key
-download gta 5 unofficial offline mode
-download gta 5 unofficial cheats
-download gta 5 unofficial trainer
-download gta 5 unofficial mods
-download gta 5 unofficial graphics enhancer
-download gta 5 unofficial redux
-download gta 5 unofficial real life mod
-download gta 5 unofficial roleplay server
-download gta 5 unofficial multiplayer mod
-download gta 5 unofficial zombie mod
-download gta 5 unofficial vice city mod
-download gta 5 unofficial san andreas mod
-download gta 5 unofficial liberty city mod
-download gta 5 unofficial london mod
-download gta 5 unofficial iron man mod
-download gta 5 unofficial batman mod
-download gta 5 unofficial spiderman mod
-download gta 5 unofficial superman mod
-download gta 5 unofficial hulk mod
-download gta 5 unofficial flash mod
-download gta 5 unofficial thanos mod
-
Another source for the APK is APKPure.com, a trusted platform for downloading Android apps and games. You can search for GTA 5 unofficial on the website and download the APK file from there. You can also check the user reviews and ratings to see what other players think about the game.
-
However, you should avoid downloading the APK from unknown or suspicious sources, as they may contain malware or viruses that can harm your device or steal your data. You should also avoid clicking on any pop-up ads or links that claim to offer GTA 5 unofficial for free, as they may be scams or phishing attempts.
-
Step 2: Enable unknown sources on your device
-
Before you can install the APK file, you need to enable unknown sources on your device. This is a security feature that prevents installation of apps from outside the Google Play Store. To enable unknown sources, follow these steps:
-
-
Go to your device settings and tap on security or privacy.
-
Find the option that says unknown sources or install unknown apps and toggle it on.
-
A warning message will appear, telling you that installing apps from unknown sources can expose your device to risks. Tap on OK or allow to proceed.
-
-
Now you are ready to install the APK file.
-
Step 3: Download the APK file
-
Once you have found a reliable source for the APK file, you can download it to your device. To do this, follow these steps:
-
-
Tap on the download button or link on the website and wait for the download to start.
-
You may see a notification on your screen, asking you to confirm the download. Tap on OK or download to continue.
-
The download progress will be shown on your notification bar or in your browser. Wait for the download to finish.
-
-
The APK file will be saved in your device's downloads folder or in a location of your choice.
-
Step 4: Install the APK file
-
After downloading the APK file, you can install it on your device. To do this, follow these steps:
-
-
Locate the APK file in your device's file manager or browser and tap on it.
-
A pop-up window will appear, asking you to install the app. Tap on install and wait for the installation to complete.
-
You may see some permissions requests, asking you to allow the app to access certain features or data on your device. Tap on allow or grant to accept them.
-
-
The app icon will appear on your home screen or app drawer when the installation is done.
-
Step 5: Launch the game and enjoy
-
Now you can launch GTA 5 unofficial and start playing it on your Android device. To do this, follow these steps:
-
-
Tap on the app icon on your home screen or app drawer.
-
The game will load and ask you to choose a language. Select your preferred language and tap on OK.
-
The game will show you some tips and instructions on how to play. Read them carefully and tap on continue.
-
The game will ask you to create a character or use an existing one. Choose your option and customize your character as you like.
-
The game will start and you can enjoy GTA 5 unofficial on your Android device.
-
-
Tips and Tricks for GTA 5 Unofficial
-
To enhance your gaming experience with GTA 5 unofficial, here are some tips and tricks that you can use:
-
Use a controller or keyboard and mouse
-
GTA 5 unofficial supports external devices such as controllers, keyboards, and mice. You can connect them to your Android device via Bluetooth or USB and use them to play the game more comfortably and accurately. You can also customize the controls according to your preferences in the game settings.
-
Adjust the graphics settings
-
GTA 5 unofficial has high-quality graphics that may affect your device's performance and battery life. You can adjust the graphics settings in the game options to optimize the game for your device. You can change the resolution, frame rate, texture quality, shadows, anti-aliasing, and more. You can also use the auto-detect option to let the game choose the best settings for your device.
-
Explore the online mode
-
GTA 5 unofficial has an online mode that lets you play with other players on dedicated servers. You can join different game modes, such as deathmatch, racing, heist, and more. You can also chat with other players, form gangs, and compete for rankings and rewards. To access the online mode, you need to have a stable internet connection and a GTA 5 account. You can create one for free on the game's website.
-
Conclusion
-
GTA 5 unofficial is an amazing way to play GTA 5 on your Android device. It is free, safe, and easy to download and install. It offers high-quality graphics, smooth gameplay, and online multiplayer features. It is also customizable and compatible with external devices. If you are a fan of GTA 5 and want to enjoy it on your smartphone or tablet, you should definitely download GTA 5 unofficial for Android. You will not regret it!
-
Have you tried GTA 5 unofficial for Android? What do you think about it? Share your thoughts and feedback in the comments section below. And don't forget to share this article with your friends who may be interested in playing GTA 5 on their Android devices. Thanks for reading!
-
FAQs
-
Here are some common questions and answers about GTA 5 unofficial:
-
Q: Is GTA 5 unofficial legal?
-
A: GTA 5 unofficial is not an official release from Rockstar Games, but a fan-made clone of the original game. It does not violate any copyright laws or terms of service, as long as you download it from a reliable source and do not use it for commercial purposes.
-
Q: Is GTA 5 unofficial safe?
-
A: GTA 5 unofficial is safe and virus-free, as long as you download it from a reliable source and enable unknown sources on your device. However, you should always be careful when installing apps from outside the Google Play Store, as they may contain malware or viruses that can harm your device or steal your data.
-
Q: How much space does GTA 5 unofficial take?
-
A: GTA 5 unofficial requires about 1 GB of storage space on your device. You should also have enough free space for the game data and updates.
-
Q: How can I update GTA 5 unofficial?
-
A: You can update GTA 5 unofficial by downloading the latest version of the APK file from the official website or APKPure.com. You can also check for updates in the game settings.
-
Q: How can I contact the developers of GTA 5 unofficial?
-
A: You can contact the developers of GTA 5 unofficial by visiting their website or sending them an email at gta5appmobi@gmail.com. You can also follow them on social media platforms such as Facebook, Twitter, and Instagram.
401be4b1e0
-
-
\ No newline at end of file
diff --git a/spaces/congsaPfin/Manga-OCR/logs/Game Stickman Warriors Mod APK A 2D Fighting Game with Stunning Graphics.md b/spaces/congsaPfin/Manga-OCR/logs/Game Stickman Warriors Mod APK A 2D Fighting Game with Stunning Graphics.md
deleted file mode 100644
index b933e8feea2744ba0cdb2fb5f59a0afaf62e5b03..0000000000000000000000000000000000000000
--- a/spaces/congsaPfin/Manga-OCR/logs/Game Stickman Warriors Mod APK A 2D Fighting Game with Stunning Graphics.md
+++ /dev/null
@@ -1,114 +0,0 @@
-
-
Download Game Stickman Warriors Mod Apk: A Fun and Addictive Fighting Game
-
If you are looking for a fun and addictive fighting game with realistic physics and hardcore gameplay, then you should download game stickman warriors mod apk. This game will let you customize your own stickman warrior and fight against other players online or offline. You can also enjoy unlimited money and unlocked all heroes with the mod apk version of the game. In this article, we will tell you more about what is stickman warriors, why download stickman warriors mod apk, how to download and install stickman warriors mod apk, and some tips and tricks to win more battles in stickman warriors.
-
What is Stickman Warriors?
-
Stickman Warriors is a stickman fighting game developed by ViperGames. It is available for Android devices on Google Play Store. The game has simple graphics but pleasing for the eye. The game also has simple controls but challenging gameplay. You just need to dodge, jump, attack, and use your special skills to defeat your opponents.
A stickman fighting game with realistic physics and hardcore gameplay
-
Stickman Warriors features realistic ragdoll physics that make the fights more dynamic and exciting. You can perform amazing stunts and blows to knock out your enemies. You can also use different weapons such as swords, axes, hammers, spears, etc. to deal more damage. The game has several campaigns with different levels of difficulty. You can also play in versus mode, tournament mode, or training mode.
-
Different modes, characters, weapons, and skills to choose from
-
Stickman Warriors has four game modes: versus mode, story mode, tournament mode, and training mode. In versus mode, you can face your favorite opponent head-on in a one-on-one battle. In story mode, you can go on a life-long adventure to discover your true strength. In tournament mode, you can compete with 16 finest warriors in the world. In training mode, you can practice your fighting skills and try out new characters.
-
The game also has over 100 stickman fighter characters with unique design style and special skills. You can choose from different costumes, hairstyles, accessories, etc. to customize your stickman warrior. You can also upgrade and unlock over 100 unique special moves for each character.
-
How to play and control your stickman warrior
-
Stickman Warriors has the most basic control ever. You can use the WASD keys or the arrow keys to move and attack. You can also use the space bar to jump. To use your special skills, you need to power up your ki by holding down the attack button. Once your ki is full, you can release it to unleash your ultimate power.
-
You can also use different weapons by picking them up from the ground or from your enemies. To pick up a weapon, you just need to touch it with your hand. To throw a weapon, you just need to press the attack button while holding it.
-
Why download Stickman Warriors mod apk?
-
If you want to enjoy more features and benefits in Stickman Warriors, then you should download stickman warriors mod apk. Here are some of the reasons why you should download stickman warriors mod apk.
-
Unlimited money and unlocked all heroes
-
With stickman warriors mod apk, you can get unlimited money and unlocked all heroes. You can use the money to buy and upgrade your weapons, costumes, accessories, etc. You can also unlock all the heroes and their special skills without spending any real money. This way, you can enjoy the game without any limitations or restrictions.
-
download game stickman warriors mod apk unlimited money
-download game stickman warriors mod apk latest version
-download game stickman warriors mod apk android 1
-download game stickman warriors mod apk revdl
-download game stickman warriors mod apk offline
-download game stickman warriors mod apk free
-download game stickman warriors mod apk hack
-download game stickman warriors mod apk full
-download game stickman warriors mod apk no ads
-download game stickman warriors mod apk for pc
-download game stickman warriors mod apk 2023
-download game stickman warriors mod apk rexdl
-download game stickman warriors mod apk unlimited gems
-download game stickman warriors mod apk unlimited power
-download game stickman warriors mod apk unlocked
-download game stickman warriors mod apk online
-download game stickman warriors mod apk premium
-download game stickman warriors mod apk pro
-download game stickman warriors mod apk mega
-download game stickman warriors mod apk vip
-download game stickman warriors mod apk 1.3.4
-download game stickman warriors mod apk 1.3.3
-download game stickman warriors mod apk 1.3.2
-download game stickman warriors mod apk 1.3.1
-download game stickman warriors mod apk 1.3.0
-download game stickman warriors mod apk 1.2.9
-download game stickman warriors mod apk 1.2.8
-download game stickman warriors mod apk 1.2.7
-download game stickman warriors mod apk 1.2.6
-download game stickman warriors mod apk 1.2.5
-download game stickman warriors mod apk apkpure
-download game stickman warriors mod apk happymod
-download game stickman warriors mod apk apkmody
-download game stickman warriors mod apk apkmaven[^1^]
-download game stickman warriors mod apk apknite
-download game stickman warriors mod apk apkmirror
-download game stickman warriors mod apk apksfree
-download game stickman warriors mod apk apktada
-download game stickman warriors mod apk apksfull
-download game stickman warriors mod apk apksmodded
-download game stickman warriors super dragon shadow fight mod apk
-download game stickman legends shadow wars warrior ninja fighter offline rpg action shooting games fighting games for free offline rpg action shooting games fighting games for free offline rpg action shooting games fighting games for free offline rpg action shooting games fighting games for free offline rpg action shooting games fighting games for free offline rpg action shooting games fighting games for free offline rpg action shooting games fighting games for free offline rpg action shooting games fighting games for free offline rpg action shooting games fighting games for free offline rpg action shooting games fighting games for free offline rpg action shooting games fighting games for free offline rpg action shooting games fighting games for free offline rpg action shooting games fighting games for free offline rpg action shooting games fighting games for free offline rpg action shooting games fighting games for free offline rpg action shooting games fighting games for free offline rpg action shooting games fighting games for free offline rpg action shooting games fighting games for free offline rpg action shooting games fighting games for free offline rpg action shooting games fighting games for free offline rpg action shooting games fighting games for free offline rpg action shooting games fighting games for free offline rpg action shooting games fighting
-
Unique design style and special skills
-
Stickman warriors mod apk also has a unique design style and special skills for each character. You can choose from different themes such as ninja, samurai, pirate, zombie, etc. You can also use different skills such as fireball, lightning, teleport, etc. to defeat your enemies. Each skill has its own animation and sound effects that make the game more fun and immersive.
-
144 levels and 100 unique special moves
-
Another reason to download stickman warriors mod apk is that it has 144 levels and 100 unique special moves. You can challenge yourself with different levels of difficulty and scenarios. You can also learn and master over 100 unique special moves for each character. You can combine different moves to create your own combos and strategies.
-
How to download and install Stickman Warriors mod apk?
-
If you are interested in downloading and installing stickman warriors mod apk, then you need to follow these simple steps:
-
Download the mod apk file from a trusted source
-
The first step is to download the mod apk file from a trusted source. You can find many websites that offer stickman warriors mod apk for free. However, you need to be careful and avoid downloading from unverified or malicious sources. You can use this link to download the latest version of stickman warriors mod apk safely and securely.
-
Enable unknown sources on your device settings
-
The next step is to enable unknown sources on your device settings. This will allow you to install apps that are not from the Google Play Store. To do this, you need to go to your device settings > security > unknown sources > enable. You may also need to disable Play Protect or other antivirus apps that may block the installation.
-
Install the mod apk file and enjoy the game
-
The final step is to install the mod apk file and enjoy the game. To do this, you need to locate the downloaded file on your device storage and tap on it. You may see a pop-up window asking for permissions. Just allow them and follow the instructions on the screen. Once the installation is complete, you can open the game and start playing.
-
Tips and tricks to win more battles in Stickman Warriors
-
Now that you have downloaded and installed stickman warriors mod apk, you may want to know some tips and tricks to win more battles in stickman warriors. Here are some of them:
-
Familiarize yourself with the controls and the weapons
-
The first tip is to familiarize yourself with the controls and the weapons. You need to know how to move, attack, jump, and use your special skills effectively. You also need to know how to use different weapons such as swords, axes, hammers, spears, etc. Each weapon has its own advantages and disadvantages. For example, swords are fast but have short range, while axes are slow but have long range.
-
Avoid getting cornered and use your environment to your advantage
-
The second tip is to avoid getting cornered and use your environment to your advantage. You need to be aware of your surroundings and avoid getting trapped by your enemies or obstacles. You can also use your environment to your advantage by throwing objects at your enemies, jumping on platforms, hiding behind walls, etc.
-
Use your weapon as much as possible and pick up health kits
-
The third tip is to use your weapon as much as possible and pick up health kits. You need to use your weapon as much as possible to deal more damage and keep your distance from your enemies. You can also pick up health kits that are scattered around the map to restore your health.
-
Experiment with different characters and skills
-
The fourth tip is to experiment with different characters and skills. You need to try out different characters and skills that suit your play style and preference. You can also mix and match different characters and skills to create your own combinations.
-
Conclusion
-
Stickman Warriors is a fun and addictive fighting game with realistic physics and hardcore gameplay. You can download game stickman warriors mod apk to enjoy unlimited money and unlocked all heroes as well as unique design style and special skills. You can also download and install stickman warriors mod apk easily and safely by following the steps in this article. Moreover, you can use some tips and tricks to win more battles in stickman warriors. We hope you enjoy playing stickman warriors and have fun with your friends.
-
FAQs
-
Here are some frequently asked questions about stickman warriors and stickman warriors mod apk:
-
-
-
Question
-
Answer
-
-
-
Is stickman warriors free to play?
-
Yes, stickman warriors is free to play. However, it contains ads and in-app purchases that may affect your gaming experience. You can download stickman warriors mod apk to remove ads and get unlimited money.
-
-
-
Is stickman warriors safe to play?
-
Yes, stickman warriors is safe to play. However, you need to be careful when downloading and installing stickman warriors mod apk from unknown sources. You may encounter viruses or malware that may harm your device. You can use this link to download the latest version of stickman warriors mod apk safely and securely.
-
-
-
Can I play stickman warriors offline?
-
Yes, you can play stickman warriors offline. However, you need to have an internet connection to access some features such as online multiplayer mode, leaderboards, achievements, etc.
-
-
-
Can I play stickman warriors with my friends?
-
Yes, you can play stickman warriors with your friends. You can either join an online multiplayer mode or create a local multiplayer mode using Wi-Fi or Bluetooth.
-
-
-
How can I contact the developer of stickman warriors?
-
You can contact the developer of stickman warriors by sending an email to viper.games.studio@gmail.com or visiting their Facebook page at https://www.facebook.com/ViperGamesStudio/.
-
-
401be4b1e0
-
-
\ No newline at end of file
diff --git a/spaces/congsaPfin/Manga-OCR/logs/How to Download Data Growtopia and Create Your Own Worlds.md b/spaces/congsaPfin/Manga-OCR/logs/How to Download Data Growtopia and Create Your Own Worlds.md
deleted file mode 100644
index 1238647ebdd925e3ba00a67b3dcbc436df2e751c..0000000000000000000000000000000000000000
--- a/spaces/congsaPfin/Manga-OCR/logs/How to Download Data Growtopia and Create Your Own Worlds.md
+++ /dev/null
@@ -1,162 +0,0 @@
-
-
How to Download Data Growtopia
-
If you are a fan of sandbox games, you may have heard of Growtopia, a popular MMO game where you can create, explore, and play with millions of other players. In this article, we will show you how to download data growtopia, which can enhance your gaming experience and help you enjoy the game more. Whether you are using an Android, iOS, PC, or Mac device, we have got you covered with our easy and comprehensive guides.
Growtopia is a 2D creative sandbox platformer MMO game that was released in 2012 by Robinson Technologies and Hamumu Clubhouse. In 2017, Ubisoft acquired the rights to the franchise and has been developing and updating the game ever since. Growtopia is a free-to-play game that has optional in-app purchases.
-
In Growtopia, you can be anyone and do anything. You can create your own unique character and customize it with thousands of items. You can build your own worlds and share them with other players. You can also visit and explore countless pixel worlds created by other players. You can play thousands of mini-games, from parkour and races to PVP battles and ghost hunting. You can craft new items and trade them with other players. You can also join a huge community of players and chat, make friends, or compete with them.
-
Growtopia is a game that is constantly evolving and expanding. Every month, there are new items and events added to the game. You can also cross-play with your friends on different devices, as the game supports smartphones, tablets, desktops, and consoles.
-
Why Download Data Growtopia?
-
Downloading data growtopia is a way of saving the game data on your device so that you can play the game faster and smoother. By downloading data growtopia, you can reduce the loading time of the game and avoid lagging or crashing issues. You can also access more features and content of the game without having to wait for them to load online.
-
Downloading data growtopia can also save your internet data usage and battery life. You don't have to use as much internet bandwidth or power when playing the game offline or with less online connection. This can help you save money and energy in the long run.
-
Downloading data growtopia is especially recommended for players who have low-end devices or slow internet connections. It can make a big difference in your gaming experience and enjoyment.
-
How to download data growtopia on PC
-Download data growtopia apk for android
-Download data growtopia mod menu
-Download data growtopia hack tool
-Download data growtopia latest version
-Download data growtopia offline installer
-Download data growtopia for windows 10
-Download data growtopia cheat engine
-Download data growtopia free gems
-Download data growtopia unlimited world locks
-Download data growtopia update 2023
-Download data growtopia for mac
-Download data growtopia trainer
-Download data growtopia private server
-Download data growtopia auto clicker
-Download data growtopia account recovery
-Download data growtopia world planner
-Download data growtopia world editor
-Download data growtopia world generator
-Download data growtopia world of the day
-Download data growtopia item database
-Download data growtopia item price list
-Download data growtopia item spawner
-Download data growtopia item finder
-Download data growtopia item duplicator
-Download data growtopia skin maker
-Download data growtopia skin editor
-Download data growtopia skin changer
-Download data growtopia skin pack
-Download data growtopia skin download
-Download data growtopia music maker
-Download data growtopia music editor
-Download data growtopia music player
-Download data growtopia music download
-Download data growtopia video maker
-Download data growtopia video editor
-Download data growtopia video recorder
-Download data growtopia video downloader
-Download data growtopia wallpaper maker
-Download data growtopia wallpaper editor
-Download data growtopia wallpaper changer
-Download data growtopia wallpaper download
-Download data growtopia forum app
-Download data growtopia support app
-Download data growtopia social app
-Download data growtopia wiki app
-Download data growtopia guide app
-Download data growtopia tips and tricks app
-
How to Download Data Growtopia on Android
-
Requirements
-
Before you download data growtopia on your Android device, make sure you have the following requirements:
-
-
An Android device that runs on Android 4 .0 or higher
-
At least 1 GB of free storage space on your device or SD card
-
A stable internet connection to download the game data
-
The latest version of Growtopia installed on your device. You can download it from the Google Play Store or the official website
-
-
Steps
-
Follow these steps to download data growtopia on your Android device:
-
-
Open the Growtopia app on your device and tap on the menu icon on the top left corner of the screen.
-
Tap on the "Options" button and then tap on the "Advanced" tab.
-
Tap on the "Download Data" button and wait for the game data to be downloaded. You will see a progress bar and a message that says "Downloading Data Growtopia".
-
Once the download is complete, you will see a message that says "Download Data Growtopia Complete". Tap on the "OK" button to exit the menu.
-
Restart the Growtopia app and enjoy playing the game with faster and smoother performance.
-
-
Troubleshooting
-
If you encounter any problems or errors while downloading data growtopia, try these solutions:
-
-
Make sure you have enough storage space on your device or SD card. If not, delete some unnecessary files or apps to free up some space.
-
Make sure you have a stable internet connection. If not, switch to a different network or use a Wi-Fi connection instead of mobile data.
-
Make sure you have the latest version of Growtopia installed on your device. If not, update the app from the Google Play Store or the official website.
-
If the download is interrupted or fails, try to resume it or restart it from the beginning.
-
If the download is successful but you still experience lagging or crashing issues, try to clear the cache and data of the Growtopia app. To do this, go to your device settings, tap on "Apps", find and tap on "Growtopia", tap on "Storage", and then tap on "Clear Cache" and "Clear Data". Be careful, as this will delete your local game data and settings. You may need to log in again and adjust your settings after doing this.
-
-
How to Download Data Growtopia on iOS
-
Requirements
-
Before you download data growtopia on your iOS device, make sure you have the following requirements:
-
-
An iOS device that runs on iOS 9.0 or higher
-
At least 1 GB of free storage space on your device
-
A stable internet connection to download the game data
-
The latest version of Growtopia installed on your device. You can download it from the App Store or the official website
-
Steps
-
Follow these steps to download data growtopia on your iOS device:
-
-
Open the Growtopia app on your device and tap on the menu icon on the top left corner of the screen.
-
Tap on the "Options" button and then tap on the "Advanced" tab.
-
Tap on the "Download Data" button and wait for the game data to be downloaded. You will see a progress bar and a message that says "Downloading Data Growtopia".
-
Once the download is complete, you will see a message that says "Download Data Growtopia Complete". Tap on the "OK" button to exit the menu.
-
Restart the Growtopia app and enjoy playing the game with faster and smoother performance.
-
-
Troubleshooting
-
If you encounter any problems or errors while downloading data growtopia, try these solutions:
-
-
Make sure you have enough storage space on your device. If not, delete some unnecessary files or apps to free up some space.
-
Make sure you have a stable internet connection. If not, switch to a different network or use a Wi-Fi connection instead of mobile data.
-
Make sure you have the latest version of Growtopia installed on your device. If not, update the app from the App Store or the official website.
-
If the download is interrupted or fails, try to resume it or restart it from the beginning.
-
If the download is successful but you still experience lagging or crashing issues, try to reinstall the Growtopia app. To do this, delete the app from your device and then download it again from the App Store or the official website.
-
-
How to Download Data Growtopia on PC or Mac
-
Requirements
-
Before you download data growtopia on your PC or Mac device, make sure you have the following requirements:
-
-
A PC or Mac device that meets the minimum system requirements for Growtopia. You can check them here:
-
At least 1 GB of free storage space on your device
-
A stable internet connection to download the game data
-
The latest version of Growtopia installed on your device. You can download it from the official website
Follow these steps to download data growtopia on your PC or Mac device:
-
-
Open the Growtopia app on your device and click on the menu icon on the top left corner of the screen.
-
Click on the "Options" button and then click on the "Advanced" tab.
-
Click on the "Download Data" button and wait for the game data to be downloaded. You will see a progress bar and a message that says "Downloading Data Growtopia".
-
Once the download is complete, you will see a message that says "Download Data Growtopia Complete". Click on the "OK" button to exit the menu.
-
Restart the Growtopia app and enjoy playing the game with faster and smoother performance.
-
-
Troubleshooting
-
If you encounter any problems or errors while downloading data growtopia, try these solutions:
-
-
Make sure you have enough storage space on your device. If not, delete some unnecessary files or apps to free up some space.
-
Make sure you have a stable internet connection. If not, switch to a different network or use a Wi-Fi connection instead of mobile data.
-
Make sure you have the latest version of Growtopia installed on your device. If not, update the app from the official website.
-
If the download is interrupted or fails, try to resume it or restart it from the beginning.
-
If the download is successful but you still experience lagging or crashing issues, try to run the Growtopia app as an administrator. To do this, right-click on the app icon and select "Run as administrator". You may need to enter your password or confirm your action.
-
-
Conclusion
-
In this article, we have shown you how to download data growtopia on different devices. By downloading data growtopia, you can improve your gaming experience and enjoy the game more. You can also save your internet data usage and battery life. Downloading data growtopia is easy and simple, as long as you follow our guides and tips. We hope you found this article helpful and informative. If you have any questions or feedback, feel free to leave a comment below. Happy gaming!
-
Frequently Asked Questions
-
Here are some of the most common questions that players ask about downloading data growtopia:
-
Q: How much storage space do I need to download data growtopia?
-
A: You need at least 1 GB of free storage space on your device or SD card to download data growtopia. However, this may vary depending on your device model and operating system.
-
Q: How long does it take to download data growtopia?
-
A: The time it takes to download data growtopia depends on your internet speed and connection quality. It may take anywhere from a few minutes to an hour or more. You can check the progress bar and the message that says "Downloading Data Growtopia" to see how much time is left.
-
Q: Do I need to download data growtopia every time I play the game?
-
A: No, you don't need to download data growtopia every time you play the game. Once you download data growtopia, it will be saved on your device and you can play the game offline or with less online connection. However, you may need to update the game data from time to time when there are new items or events added to the game.
-
Q: Can I delete data growtopia from my device?
-
A: Yes, you can delete data growtopia from your device if you want to free up some storage space or if you encounter any issues with the game data. To do this, go to your device settings, tap or click on "Apps", find and tap or click on "Growtopia", tap or click on "Storage", and then tap or click on "Clear Data". Be careful, as this will delete your local game data and settings. You may need to log in again and adjust your settings after doing this.
-
Q: What are some of the benefits of downloading data growtopia?
-
A: Some of the benefits of downloading data growtopia are:
-
-
You can reduce the loading time of the game and avoid lagging or crashing issues.
-
You can access more features and content of the game without having to wait for them to load online.
-
You can save your internet data usage and battery life.
-
You can enhance your gaming experience and enjoy the game more.
-
401be4b1e0
-
-
\ No newline at end of file
diff --git a/spaces/congsaPfin/Manga-OCR/logs/Sonic Dash 2 Sonic Boom APK - Run Jump and Dash with New Powers and Characters.md b/spaces/congsaPfin/Manga-OCR/logs/Sonic Dash 2 Sonic Boom APK - Run Jump and Dash with New Powers and Characters.md
deleted file mode 100644
index b26ccfaa1fb7ffc6ed5533cf1905c4e2b5e6e71d..0000000000000000000000000000000000000000
--- a/spaces/congsaPfin/Manga-OCR/logs/Sonic Dash 2 Sonic Boom APK - Run Jump and Dash with New Powers and Characters.md
+++ /dev/null
@@ -1,114 +0,0 @@
-
-
Sonic Dash 2: Sonic Boom - A Dazzling Sequel to the Hit Endless Runner Game
-
If you are a fan of Sonic the Hedgehog and his friends, you will love Sonic Dash 2: Sonic Boom, the sequel to SEGA's hit endless runner game, SONIC DASH. In this game, you can run and dash your way through the world of the hit new TV series, SONIC BOOM, with Sonic and his friends. You can choose your favorite character to be your runner, unleash new special running powers, conquer new courses and obstacles, dash on new fast-paced race tracks, master new swing and tilt gameplay, collect and evolve magical sprites, and win special prizes in events and challenges. Sounds exciting, right? In this article, we will tell you more about this amazing game and how you can download and install it on your device.
-
Introduction
-
What is Sonic Dash 2: Sonic Boom?
-
Sonic Dash 2: Sonic Boom is an action platformer runner arcade game developed by SEGA for Android devices. It is based on the TV series SONIC BOOM, which features a redesigned version of Sonic the Hedgehog and his friends. The game was released on October 8, 2015, and has since received over 100 million downloads and positive reviews from players and critics alike. The game is free to play, but contains ads and in-app purchases that are not required to progress.
Sonic Dash 2: Sonic Boom is a fun and addictive game that will keep you entertained for hours. You will enjoy the following benefits when you play this game:
-
-
You will experience the thrill of running at high speed with Sonic and his friends.
-
You will explore new and amazing 3D worlds with stunning graphics and sound effects.
-
You will challenge yourself with different levels of difficulty and objectives.
-
You will customize your runner with different outfits and accessories.
-
You will compete with other players around the world in leaderboards and multiplayer modes.
-
You will support SEGA and the SONIC BOOM franchise by playing this game.
-
-
Features of Sonic Dash 2: Sonic Boom
-
Race with up to three characters in new Team Play mode
-
One of the most exciting features of Sonic Dash 2: Sonic Boom is the Team Play mode, where you can race with up to three characters at once. You can swap runners mid-race to earn high scores and use their unique abilities. For example, you can use Sonic's Dash Ring Magnet to attract rings, Knuckle's Slam to smash obstacles, Amy's Ring Hammer to destroy enemies, and more. You can also unlock new characters like Sticks the Badger, Shadow the Hedgehog, Vector the Crocodile, and more.
-
Unleash new special running powers
-
Another feature that makes this game more fun and dynamic is the special running powers that you can unleash during your run. These powers can help you boost your speed, collect more rings, avoid hazards,
Conquer new courses, obstacles and beat out Badniks
-
As you run through the world of SONIC BOOM, you will encounter new courses and obstacles that will test your skills and reflexes. You will have to dodge, jump, slide, and swing your way through various terrains and environments, such as jungles, volcanoes, factories, and more. You will also have to face the evil Dr. Eggman and his army of Badniks, who will try to stop you from reaching your goal. You can use your running powers and sprites to defeat them and earn extra points.
-
Dash on new fast-paced race tracks in and above the beautiful Sonic Boom world
-
One of the most appealing aspects of Sonic Dash 2: Sonic Boom is the stunning graphics and sound effects that immerse you in the game. You will be amazed by the colorful and detailed 3D environments that showcase the beauty and diversity of the Sonic Boom world. You will also enjoy the fast-paced and exhilarating race tracks that take you in and above the scenery, giving you a sense of speed and adrenaline. You will hear the iconic soundtracks and sound effects that accompany your run, as well as the voice-overs of your favorite characters.
-
Master new Swing & Tilt gameplay with the super charged Enerbeam
-
A new gameplay feature that adds more fun and challenge to Sonic Dash 2: Sonic Boom is the Swing & Tilt mode, where you can use the super charged Enerbeam to swing across gaps and tilt your device to steer your runner. This mode requires more precision and coordination, but also gives you more control and freedom over your movement. You can use the Enerbeam to reach new areas, collect more rings, and avoid obstacles.
-
Collect, evolve, and run with magical Sprites
-
Sprites are cute and magical creatures that can accompany you on your run and give you various benefits. You can collect different types of sprites, such as fire, water, earth, wind, electric, dark, light, and chaos. Each sprite has its own personality and ability, such as increasing your score multiplier, protecting you from damage, attracting rings, and more. You can also evolve your sprites by feeding them with rings or red star rings, making them more powerful and effective. You can run with up to three sprites at a time, depending on their size.
-
Win special prizes in new Events and Daily SEGA Challenges
-
If you want to spice up your game experience and earn more rewards, you can participate in new Events and Daily SEGA Challenges that are available in Sonic Dash 2: Sonic Boom. Events are limited-time missions that require you to complete specific objectives or tasks within a given period. For example, you may have to collect a certain number of rings, defeat a certain number of enemies, or run a certain distance. By completing these events, you can win special prizes such as red star rings, sprites, outfits, or even exclusive characters. Daily SEGA Challenges are similar to events, but they change every day and are based on SEGA's classic games. For example, you may have to run as Alex Kidd, collect golden axes from Golden Axe, or collect chaos emeralds from Sonic the Hedgehog. By completing these challenges, you can win more red star rings, sprites, or outfits.
-
sonic dash 2 sonic boom apk download
-sonic dash 2 sonic boom mod apk
-sonic dash 2 sonic boom apk latest version
-sonic dash 2 sonic boom apk pure
-sonic dash 2 sonic boom apk offline
-sonic dash 2 sonic boom apk unlimited money
-sonic dash 2 sonic boom apk hack
-sonic dash 2 sonic boom apk android
-sonic dash 2 sonic boom apk obb
-sonic dash 2 sonic boom apk update
-sonic dash 2 sonic boom apk revdl
-sonic dash 2 sonic boom apk uptodown
-sonic dash 2 sonic boom apk old version
-sonic dash 2 sonic boom apk mirror
-sonic dash 2 sonic boom apk mod menu
-sonic dash 2 sonic boom apk no ads
-sonic dash 2 sonic boom apk free download
-sonic dash 2 sonic boom apk rexdl
-sonic dash 2 sonic boom apk data
-sonic dash 2 sonic boom apk for pc
-sonic dash 2 sonic boom apk full version
-sonic dash 2 sonic boom apk mega mod
-sonic dash 2 sonic boom apk android oyun club
-sonic dash 2 sonic boom apk andropalace
-sonic dash 2 sonic boom apk apkpure
-sonic dash 2 sonic boom apk all characters unlocked
-sonic dash 2 sonic boom apk blackmod
-sonic dash 2 sonic boom apk bluestacks
-sonic dash 2 sonic boom apk cheat
-sonic dash 2 sonic boom apk cracked
-sonic dash 2 sonic boom apk coins and rings generator
-sonic dash 2 soni
-
How to download and install Sonic Dash 2: Sonic Boom apk
-
Download the apk file from a trusted source
-
If you want to play Sonic Dash 2: Sonic Boom on your Android device, you will need to download and install the apk file of the game. An apk file is a package file format that contains the installation files and data of an Android application. You can download the apk file of Sonic Dash 2: Sonic Boom from a trusted source, such as [APKPure] or [Uptodown]. These sources are safe and reliable, and they offer the latest version of the game. You can also scan the apk file with an antivirus software before installing it, to make sure it is free of malware or viruses.
-
Enable unknown sources on your device
-
Before you can install the apk file of Sonic Dash 2: Sonic Boom on your device, you will need to enable unknown sources on your device. This is a security setting that allows you to install applications from sources other than the Google Play Store. To enable unknown sources, follow these steps:
-
-
Go to your device's Settings and tap on Security or Privacy.
-
Find the option that says Unknown Sources or Install Unknown Apps and toggle it on.
-
A warning message will appear, telling you that installing apps from unknown sources can harm your device. Tap on OK or Allow to proceed.
-
-
Install the apk file and enjoy the game
-
Once you have enabled unknown sources on your device, you can install the apk file of Sonic Dash 2: Sonic Boom by following these steps:
-
-
Locate the apk file that you downloaded from your source. You can use a file manager app or your device's Downloads folder to find it.
-
Tap on the apk file and a pop-up window will appear, asking you if you want to install this application. Tap on Install and wait for the installation process to finish.
-
Once the installation is done, you can tap on Open to launch the game or find it on your device's home screen or app drawer.
-
-
Congratulations! You have successfully downloaded and installed Sonic Dash 2: Sonic Boom apk on your device. Now you can enjoy running with Sonic and his friends in this dazzling sequel to the hit endless runner game.
-
Conclusion
-
Summary of the main points
-
Sonic Dash 2: Sonic Boom is an action platformer runner arcade game developed by SEGA for Android devices. It is based on the TV series SONIC BOOM, which features a redesigned version of Sonic the Hedgehog and his friends. The game offers many features that make it fun and addictive, such as:
-
-
Racing with up to three characters in new Team Play mode.
-
Unleashing new special running powers.
-
Conquering new courses, obstacles and beat out Badniks.
-
Dashing on new fast-paced race tracks in and above the beautiful Sonic Boom world.
-
Mastering new Swing & Tilt gameplay with the super charged Enerbeam.
-
Collecting, evolving, and running with magical Sprites.
-
Winning special prizes in new Events and Daily SEGA Challenges.
-
-
Call to action
-
If you are ready to join Sonic and his friends in their exciting adventures, download and install Sonic Dash 2: Sonic Boom apk today. You will not regret it. This game will keep you entertained for hours with its stunning graphics, sound effects, gameplay, and characters. You will also support SEGA and the SONIC BOOM franchise by playing this game. So what are you waiting for? Run, dash, jump, swing, tilt, and have fun with Sonic Dash 2: Sonic Boom!
-
Frequently Asked Questions
-
-
Is Sonic Dash 2: Sonic Boom free to play?
-
Yes, Sonic Dash 2: Sonic Boom is free to play, but it contains ads and in-app purchases that are not required to progress. You can disable ads by purchasing any item from the shop or by turning off your internet connection while playing. You can also play without spending any real money by earning red star rings and sprites through gameplay or events.
-
How do I save my progress in Sonic Dash 2: Sonic Boom?
-
You can save your progress in Sonic Dash 2: Sonic Boom by connecting your game to your Facebook or Google Play account. This will allow you to sync your data across multiple devices and restore your progress if you lose or change your device. You can also backup your data manually by using the cloud save feature in the game settings.
-
How do I unlock new characters in Sonic Dash 2: Sonic Boom?
-
You can unlock new characters in Sonic Dash 2: Sonic Boom by completing certain events or challenges, or by purchasing them with red star rings. Some of the characters that you can unlock are Sticks the Badger, Shadow the Hedgehog, Vector the Crocodile, and more. Each character has their own unique running power and outfit that you can customize.
-
How do I use sprites in Sonic Dash 2: Sonic Boom?
-
Sprites are magical creatures that can accompany you on your run and give you various benefits. You can collect different types of sprites, such as fire, water, earth, wind, electric, dark, light, and chaos. Each sprite has its own personality and ability, such as increasing your score multiplier, protecting you from damage, attracting rings, and more. You can also evolve your sprites by feeding them with rings or red star rings, making them more powerful and effective. You can run with up to three sprites at a time, depending on their size. To use sprites, you need to equip them before starting a run. You can do this by tapping on the sprite icon on the bottom left of the screen and selecting the sprites that you want to use.
-
How do I get more red star rings in Sonic Dash 2: Sonic Boom?
-
Red star rings are a premium currency in Sonic Dash 2: Sonic Boom that can be used to buy special items, characters, outfits, or sprites. You can get more red star rings by doing the following:
-
-
Completing events or challenges that reward you with red star rings.
-
Watching video ads that offer you free red star rings.
-
Buying them with real money from the shop.
-
197e85843d
-
-
\ No newline at end of file
diff --git a/spaces/contluForse/HuggingGPT/assets/Age Of Empires 2 No Cd Crack Download 2.0a Everything You Need to Know About the Game and the Crack.md b/spaces/contluForse/HuggingGPT/assets/Age Of Empires 2 No Cd Crack Download 2.0a Everything You Need to Know About the Game and the Crack.md
deleted file mode 100644
index 120db3817dab987b03cc09e8af1ebfadc55036fa..0000000000000000000000000000000000000000
--- a/spaces/contluForse/HuggingGPT/assets/Age Of Empires 2 No Cd Crack Download 2.0a Everything You Need to Know About the Game and the Crack.md
+++ /dev/null
@@ -1,5 +0,0 @@
-
-
//document.domain = "heavengames.com";function login_form(d) var login_url = ' ';if (d != null) login_url = login_url + '?r=' + escape(d);window.open(login_url,'hglogin','height=300,width=400,location=false,menubar=false,resizable=false,scrollbars=false,status=false,toolbar=false');function logout(d) var logout_url = ' ';if (d != null) logout_url = logout_url + '?r=' + escape(d);window.open(logout_url,'hglogout','height=200,width=400,location=false,menubar=false,resizable=false,scrollbars=false,status=false,toolbar=false');function submit_file() login_form("/blacksmith/submit.php?1675984381");function get_file(fileid,s) window.location="/blacksmith/getfile.php?id="+fileid+"&s="+s+"&r="+escape(document.location);function submit_comment(fileid) login_form("/blacksmith/comment.php?id="+fileid+"&1675984381");function edit_comment(cid) login_form("/blacksmith/comment.php?id="+cid+"&1675984381");initFavorites(13742,null)Downloads Home » Utilities » Age of Empires 2 v2.0 patch Age of Empires 2 v2.0 patchAuthorFile DescriptionPhatFishPosted on 06/21/21 @ 07:06 AMFile DetailsVersion:Age of Kings 1.0This is the empires2.exe version 2.0 (v 0.14.14.914) file which was not in the Blacksmith yet. This is the unpatched original version of the game. For those who are having trouble getting AoK to run on Windows 10 (AoK not launching), this might offer the solution for you - it did the trick for me, coming from a 2.0a version (Gold edition of the game).
This version does not require a CD to run, which is excellent for those without a CD drive which is quite common these days. Remember to support and buy the game.
To install simply copy the empires2.exe to your default AoE2 directory, usually located at: C:\Program Files (x86)\Microsoft Games\Age of Empires II\
Overwrite the original empires2.exe file, or make a backup first (recommended).AuthorComments & Reviews ( All | Comments Only | Reviews Only )No messages found HGDL v0.8.1
aaccfb2cb3
-
-
\ No newline at end of file
diff --git a/spaces/contluForse/HuggingGPT/assets/Econometric Models And Economic Forecasts Pindyck Pdf Free.md b/spaces/contluForse/HuggingGPT/assets/Econometric Models And Economic Forecasts Pindyck Pdf Free.md
deleted file mode 100644
index fa02066954ff4bae15cc4b8a64f5557953e8bb1b..0000000000000000000000000000000000000000
--- a/spaces/contluForse/HuggingGPT/assets/Econometric Models And Economic Forecasts Pindyck Pdf Free.md
+++ /dev/null
@@ -1,90 +0,0 @@
-
-
Econometric Models And Economic Forecasts Pindyck Pdf Free: A Comprehensive Review
-
-
If you are looking for a free PDF of Econometric Models And Economic Forecasts by Robert S. Pindyck and Daniel L. Rubinfeld, you have come to the right place. This article will provide you with a comprehensive review of this classic textbook on econometrics, as well as some links to download or access it online.
-
Econometric Models And Economic Forecasts Pindyck Pdf Free
Econometric Models And Economic Forecasts is a well-known text that helps students understand the art of model building - what type of model to build, how to build it, how to test it statistically, and how to apply it to practical problems in forecasting and analysis. The book covers both single-equation and multiple-equation regression models, as well as time-series models and model specification issues. The book also includes many examples and exercises from various fields of economics and business.
-
-
What are the Main Features of Econometric Models And Economic Forecasts Pindyck Pdf Free?
-
-
Some of the main features of Econometric Models And Economic Forecasts Pindyck Pdf Free are:
-
-
-
It provides a clear and concise introduction to the regression model, elementary statistics, the two-variable regression model, the multiple regression model, serial correlation and heteroscedasticity, instrumental variables and model specification, and forecasting with a single-equation regression model.
-
It explains the theory and intuition behind the econometric methods, as well as their practical applications and limitations.
-
It illustrates the concepts and techniques with real-world data and examples from various fields of economics and business, such as demand analysis, production function estimation, cost function estimation, inventory investment, savings deposit flows, housing prices, exchange rates, etc.
-
It provides numerous exercises and problems at the end of each chapter, with solutions available online or in a separate manual.
-
It offers a free PDF version of the book that can be downloaded or accessed online from various sources.
-
-
-
What are the Benefits of Econometric Models And Economic Forecasts Pindyck Pdf Free?
-
-
Some of the benefits of Econometric Models And Economic Forecasts Pindyck Pdf Free are:
-
-
-
-
It helps students learn the fundamentals of econometrics in a simple and intuitive way.
-
It helps students develop their analytical and problem-solving skills in econometrics.
-
It helps students apply econometric models to real-world problems in forecasting and analysis.
-
It saves students money by providing a free PDF version of the book that can be easily accessed online or downloaded.
-
-
-
Where to Find Econometric Models And Economic Forecasts Pindyck Pdf Free?
-
-
If you want to find Econometric Models And Economic Forecasts Pindyck Pdf Free, you can use some of the links provided below. These links will direct you to some reliable sources that offer the free PDF version of the book. However, you should be careful and choose a trustworthy source that provides the correct and complete version of the book.
ECONOMETRIC MODELS AND ECONOMIC FORECASTS - dandelon.com. This is a link to the Dandelon website that hosts another free PDF file of Econometric Models And Economic Forecasts by Pindyck and Rubinfeld. You can download or read it online from this website.
-
[PDF] Econometric models and economic forecasts | Semantic Scholar. This is a link to the Semantic Scholar website that hosts an abstract and citation information of Econometric Models And Economic Forecasts by Pindyck and Rubinfeld. You can also find a link to download or access the free PDF file from this website.
-
-
-
Conclusion
-
-
Econometric Models And Economic Forecasts by Robert S. Pindyck and Daniel L. Rubinfeld is a classic textbook on econometrics that helps students understand and apply econometric models to practical problems in forecasting and analysis. The book is available in a free PDF format that can be downloaded or accessed online from various sources. This article has provided you with a comprehensive review of this book, as well as some links to find it online. We hope that this article has helped you learn more about Econometric Models And Economic Forecasts Pindyck Pdf Free.
-
What are the Reviews and Ratings of Econometric Models And Economic Forecasts Pindyck Pdf Free?
-
-
If you are wondering what other people think about Econometric Models And Economic Forecasts Pindyck Pdf Free, you might want to read some of the reviews and ratings of this book from various sources. Here are some of the reviews and ratings of the book from different websites:
-
-
-
Econometric Models and Economic Forecasts by Robert S. Pindyck. This is a link to the Goodreads website that hosts a page for Econometric Models And Economic Forecasts by Pindyck and Rubinfeld. You can find the book description, details, ratings, and reviews from other readers on this website. The book has an average rating of 3.8 out of 5 stars based on 40 ratings and 4 reviews.
-
Econometric Models and Economic Forecasts: Pindyck, Robert S .... This is a link to the Amazon website that hosts a page for Econometric Models And Economic Forecasts by Pindyck and Rubinfeld. You can find the book information, price, availability, ratings, and reviews from other customers on this website. The book has an average rating of 4.1 out of 5 stars based on 9 ratings and 3 reviews.
-
Econometric Models and Economic Forecasts / Edition 4 by Robert S .... This is a link to the Barnes & Noble website that hosts a page for Econometric Models And Economic Forecasts by Pindyck and Rubinfeld. You can find the book overview, specifications, ratings, and reviews from other buyers on this website. The book has an average rating of 4 out of 5 stars based on 1 rating and 0 reviews.
-
-
-
These are some of the reviews and ratings of Econometric Models And Economic Forecasts Pindyck Pdf Free from different websites. You can also search online for more feedback and opinions on the book.
-
-
What are the Alternatives to Econometric Models And Economic Forecasts Pindyck Pdf Free?
-
-
If you are looking for other books or resources that can help you learn econometrics or improve your forecasting and analysis skills, you might want to consider some of the alternatives to Econometric Models And Economic Forecasts Pindyck Pdf Free. Here are some of the alternatives to the book that you can check out:
-
-
-
Introductory Econometrics: A Modern Approach by Jeffrey M. Wooldridge. This is another popular textbook on econometrics that covers both cross-sectional and time-series data analysis, as well as advanced topics such as panel data, instrumental variables, limited dependent variables, etc. The book also includes many examples, exercises, data sets, and software applications.
-
Econometrics by Fumio Hayashi. This is another comprehensive textbook on econometrics that focuses on the concepts and methods of modern econometrics. The book covers both single-equation and multiple-equation models, as well as nonparametric estimation, generalized method of moments, maximum likelihood estimation, etc. The book also provides many empirical examples and exercises.
-
Mastering 'Metrics: The Path from Cause to Effect by Joshua D. Angrist and Jörn-Steffen Pischke. This is another engaging book on econometrics that explains the key methods and techniques of causal inference using real-world examples and stories. The book covers topics such as randomized trials, regression, instrumental variables, regression discontinuity designs, differences-in-differences methods, etc.
-
-
-
These are some of the alternatives to Econometric Models And Economic Forecasts Pindyck Pdf Free that you can explore. You can also search online for more books or resources on econometrics or related fields.
-
What are the Challenges and Limitations of Econometric Models And Economic Forecasts Pindyck Pdf Free?
-
-
While Econometric Models And Economic Forecasts Pindyck Pdf Free is a useful and informative book that can help you learn econometrics and improve your forecasting and analysis skills, it also has some challenges and limitations that you should be aware of. Here are some of the challenges and limitations of the book:
-
-
-
The book is not very updated. The latest edition of the book was published in 1998, which means that it does not cover some of the recent developments and innovations in econometrics and forecasting. For example, the book does not include topics such as panel data analysis, nonlinear models, machine learning methods, etc.
-
The book is not very accessible. The book assumes that the readers have some background knowledge in calculus, linear algebra, statistics, and economics. The book also uses a lot of mathematical notation and technical jargon that might be difficult to follow for some readers. The book does not provide much intuition or explanation behind the econometric methods and techniques.
-
The book is not very practical. The book focuses more on the theory and methodology of econometrics and forecasting, rather than on their practical applications and implications. The book does not provide much guidance on how to choose the appropriate model, how to interpret the results, how to deal with real-world data issues, etc.
-
-
-
These are some of the challenges and limitations of Econometric Models And Economic Forecasts Pindyck Pdf Free that you should keep in mind while using the book. You might want to supplement the book with other sources or resources that can address these challenges and limitations.
-
Conclusion
-
-
Econometric Models And Economic Forecasts Pindyck Pdf Free is a classic textbook on econometrics that helps students understand and apply econometric models to practical problems in forecasting and analysis. The book covers both single-equation and multiple-equation regression models, as well as time-series models and model specification issues. The book also includes many examples and exercises from various fields of economics and business.
-
-
In this article, we have provided you with a comprehensive review of this book, as well as some links to download or access it online. We have also discussed some of the main features, benefits, reviews and ratings, alternatives, tips and tricks, and challenges and limitations of the book. We hope that this article has helped you learn more about Econometric Models And Economic Forecasts Pindyck Pdf Free.
-
-
If you want to download or access Econometric Models And Economic Forecasts Pindyck Pdf Free, you can use the links provided in this article. You can also contact us or leave a comment below if you have any feedback or queries about the book.
-
-
Thank you for reading this article and happy learning!
3cee63e6c2
-
-
\ No newline at end of file
diff --git a/spaces/coreml-community/ControlNet-v1-1-Annotators-cpu/annotator/mmpkg/mmcv/runner/base_runner.py b/spaces/coreml-community/ControlNet-v1-1-Annotators-cpu/annotator/mmpkg/mmcv/runner/base_runner.py
deleted file mode 100644
index a75a7d5db9f281fda10008636b24e2b98d9336a0..0000000000000000000000000000000000000000
--- a/spaces/coreml-community/ControlNet-v1-1-Annotators-cpu/annotator/mmpkg/mmcv/runner/base_runner.py
+++ /dev/null
@@ -1,542 +0,0 @@
-# Copyright (c) OpenMMLab. All rights reserved.
-import copy
-import logging
-import os.path as osp
-import warnings
-from abc import ABCMeta, abstractmethod
-
-import torch
-from torch.optim import Optimizer
-
-import annotator.mmpkg.mmcv as mmcv
-from ..parallel import is_module_wrapper
-from .checkpoint import load_checkpoint
-from .dist_utils import get_dist_info
-from .hooks import HOOKS, Hook
-from .log_buffer import LogBuffer
-from .priority import Priority, get_priority
-from .utils import get_time_str
-
-
-class BaseRunner(metaclass=ABCMeta):
- """The base class of Runner, a training helper for PyTorch.
-
- All subclasses should implement the following APIs:
-
- - ``run()``
- - ``train()``
- - ``val()``
- - ``save_checkpoint()``
-
- Args:
- model (:obj:`torch.nn.Module`): The model to be run.
- batch_processor (callable): A callable method that process a data
- batch. The interface of this method should be
- `batch_processor(model, data, train_mode) -> dict`
- optimizer (dict or :obj:`torch.optim.Optimizer`): It can be either an
- optimizer (in most cases) or a dict of optimizers (in models that
- requires more than one optimizer, e.g., GAN).
- work_dir (str, optional): The working directory to save checkpoints
- and logs. Defaults to None.
- logger (:obj:`logging.Logger`): Logger used during training.
- Defaults to None. (The default value is just for backward
- compatibility)
- meta (dict | None): A dict records some import information such as
- environment info and seed, which will be logged in logger hook.
- Defaults to None.
- max_epochs (int, optional): Total training epochs.
- max_iters (int, optional): Total training iterations.
- """
-
- def __init__(self,
- model,
- batch_processor=None,
- optimizer=None,
- work_dir=None,
- logger=None,
- meta=None,
- max_iters=None,
- max_epochs=None):
- if batch_processor is not None:
- if not callable(batch_processor):
- raise TypeError('batch_processor must be callable, '
- f'but got {type(batch_processor)}')
- warnings.warn('batch_processor is deprecated, please implement '
- 'train_step() and val_step() in the model instead.')
- # raise an error is `batch_processor` is not None and
- # `model.train_step()` exists.
- if is_module_wrapper(model):
- _model = model.module
- else:
- _model = model
- if hasattr(_model, 'train_step') or hasattr(_model, 'val_step'):
- raise RuntimeError(
- 'batch_processor and model.train_step()/model.val_step() '
- 'cannot be both available.')
- else:
- assert hasattr(model, 'train_step')
-
- # check the type of `optimizer`
- if isinstance(optimizer, dict):
- for name, optim in optimizer.items():
- if not isinstance(optim, Optimizer):
- raise TypeError(
- f'optimizer must be a dict of torch.optim.Optimizers, '
- f'but optimizer["{name}"] is a {type(optim)}')
- elif not isinstance(optimizer, Optimizer) and optimizer is not None:
- raise TypeError(
- f'optimizer must be a torch.optim.Optimizer object '
- f'or dict or None, but got {type(optimizer)}')
-
- # check the type of `logger`
- if not isinstance(logger, logging.Logger):
- raise TypeError(f'logger must be a logging.Logger object, '
- f'but got {type(logger)}')
-
- # check the type of `meta`
- if meta is not None and not isinstance(meta, dict):
- raise TypeError(
- f'meta must be a dict or None, but got {type(meta)}')
-
- self.model = model
- self.batch_processor = batch_processor
- self.optimizer = optimizer
- self.logger = logger
- self.meta = meta
- # create work_dir
- if mmcv.is_str(work_dir):
- self.work_dir = osp.abspath(work_dir)
- mmcv.mkdir_or_exist(self.work_dir)
- elif work_dir is None:
- self.work_dir = None
- else:
- raise TypeError('"work_dir" must be a str or None')
-
- # get model name from the model class
- if hasattr(self.model, 'module'):
- self._model_name = self.model.module.__class__.__name__
- else:
- self._model_name = self.model.__class__.__name__
-
- self._rank, self._world_size = get_dist_info()
- self.timestamp = get_time_str()
- self.mode = None
- self._hooks = []
- self._epoch = 0
- self._iter = 0
- self._inner_iter = 0
-
- if max_epochs is not None and max_iters is not None:
- raise ValueError(
- 'Only one of `max_epochs` or `max_iters` can be set.')
-
- self._max_epochs = max_epochs
- self._max_iters = max_iters
- # TODO: Redesign LogBuffer, it is not flexible and elegant enough
- self.log_buffer = LogBuffer()
-
- @property
- def model_name(self):
- """str: Name of the model, usually the module class name."""
- return self._model_name
-
- @property
- def rank(self):
- """int: Rank of current process. (distributed training)"""
- return self._rank
-
- @property
- def world_size(self):
- """int: Number of processes participating in the job.
- (distributed training)"""
- return self._world_size
-
- @property
- def hooks(self):
- """list[:obj:`Hook`]: A list of registered hooks."""
- return self._hooks
-
- @property
- def epoch(self):
- """int: Current epoch."""
- return self._epoch
-
- @property
- def iter(self):
- """int: Current iteration."""
- return self._iter
-
- @property
- def inner_iter(self):
- """int: Iteration in an epoch."""
- return self._inner_iter
-
- @property
- def max_epochs(self):
- """int: Maximum training epochs."""
- return self._max_epochs
-
- @property
- def max_iters(self):
- """int: Maximum training iterations."""
- return self._max_iters
-
- @abstractmethod
- def train(self):
- pass
-
- @abstractmethod
- def val(self):
- pass
-
- @abstractmethod
- def run(self, data_loaders, workflow, **kwargs):
- pass
-
- @abstractmethod
- def save_checkpoint(self,
- out_dir,
- filename_tmpl,
- save_optimizer=True,
- meta=None,
- create_symlink=True):
- pass
-
- def current_lr(self):
- """Get current learning rates.
-
- Returns:
- list[float] | dict[str, list[float]]: Current learning rates of all
- param groups. If the runner has a dict of optimizers, this
- method will return a dict.
- """
- if isinstance(self.optimizer, torch.optim.Optimizer):
- lr = [group['lr'] for group in self.optimizer.param_groups]
- elif isinstance(self.optimizer, dict):
- lr = dict()
- for name, optim in self.optimizer.items():
- lr[name] = [group['lr'] for group in optim.param_groups]
- else:
- raise RuntimeError(
- 'lr is not applicable because optimizer does not exist.')
- return lr
-
- def current_momentum(self):
- """Get current momentums.
-
- Returns:
- list[float] | dict[str, list[float]]: Current momentums of all
- param groups. If the runner has a dict of optimizers, this
- method will return a dict.
- """
-
- def _get_momentum(optimizer):
- momentums = []
- for group in optimizer.param_groups:
- if 'momentum' in group.keys():
- momentums.append(group['momentum'])
- elif 'betas' in group.keys():
- momentums.append(group['betas'][0])
- else:
- momentums.append(0)
- return momentums
-
- if self.optimizer is None:
- raise RuntimeError(
- 'momentum is not applicable because optimizer does not exist.')
- elif isinstance(self.optimizer, torch.optim.Optimizer):
- momentums = _get_momentum(self.optimizer)
- elif isinstance(self.optimizer, dict):
- momentums = dict()
- for name, optim in self.optimizer.items():
- momentums[name] = _get_momentum(optim)
- return momentums
-
- def register_hook(self, hook, priority='NORMAL'):
- """Register a hook into the hook list.
-
- The hook will be inserted into a priority queue, with the specified
- priority (See :class:`Priority` for details of priorities).
- For hooks with the same priority, they will be triggered in the same
- order as they are registered.
-
- Args:
- hook (:obj:`Hook`): The hook to be registered.
- priority (int or str or :obj:`Priority`): Hook priority.
- Lower value means higher priority.
- """
- assert isinstance(hook, Hook)
- if hasattr(hook, 'priority'):
- raise ValueError('"priority" is a reserved attribute for hooks')
- priority = get_priority(priority)
- hook.priority = priority
- # insert the hook to a sorted list
- inserted = False
- for i in range(len(self._hooks) - 1, -1, -1):
- if priority >= self._hooks[i].priority:
- self._hooks.insert(i + 1, hook)
- inserted = True
- break
- if not inserted:
- self._hooks.insert(0, hook)
-
- def register_hook_from_cfg(self, hook_cfg):
- """Register a hook from its cfg.
-
- Args:
- hook_cfg (dict): Hook config. It should have at least keys 'type'
- and 'priority' indicating its type and priority.
-
- Notes:
- The specific hook class to register should not use 'type' and
- 'priority' arguments during initialization.
- """
- hook_cfg = hook_cfg.copy()
- priority = hook_cfg.pop('priority', 'NORMAL')
- hook = mmcv.build_from_cfg(hook_cfg, HOOKS)
- self.register_hook(hook, priority=priority)
-
- def call_hook(self, fn_name):
- """Call all hooks.
-
- Args:
- fn_name (str): The function name in each hook to be called, such as
- "before_train_epoch".
- """
- for hook in self._hooks:
- getattr(hook, fn_name)(self)
-
- def get_hook_info(self):
- # Get hooks info in each stage
- stage_hook_map = {stage: [] for stage in Hook.stages}
- for hook in self.hooks:
- try:
- priority = Priority(hook.priority).name
- except ValueError:
- priority = hook.priority
- classname = hook.__class__.__name__
- hook_info = f'({priority:<12}) {classname:<35}'
- for trigger_stage in hook.get_triggered_stages():
- stage_hook_map[trigger_stage].append(hook_info)
-
- stage_hook_infos = []
- for stage in Hook.stages:
- hook_infos = stage_hook_map[stage]
- if len(hook_infos) > 0:
- info = f'{stage}:\n'
- info += '\n'.join(hook_infos)
- info += '\n -------------------- '
- stage_hook_infos.append(info)
- return '\n'.join(stage_hook_infos)
-
- def load_checkpoint(self,
- filename,
- map_location='cpu',
- strict=False,
- revise_keys=[(r'^module.', '')]):
- return load_checkpoint(
- self.model,
- filename,
- map_location,
- strict,
- self.logger,
- revise_keys=revise_keys)
-
- def resume(self,
- checkpoint,
- resume_optimizer=True,
- map_location='default'):
- if map_location == 'default':
- if torch.cuda.is_available():
- device_id = torch.cuda.current_device()
- checkpoint = self.load_checkpoint(
- checkpoint,
- map_location=lambda storage, loc: storage.cuda(device_id))
- else:
- checkpoint = self.load_checkpoint(checkpoint)
- else:
- checkpoint = self.load_checkpoint(
- checkpoint, map_location=map_location)
-
- self._epoch = checkpoint['meta']['epoch']
- self._iter = checkpoint['meta']['iter']
- if self.meta is None:
- self.meta = {}
- self.meta.setdefault('hook_msgs', {})
- # load `last_ckpt`, `best_score`, `best_ckpt`, etc. for hook messages
- self.meta['hook_msgs'].update(checkpoint['meta'].get('hook_msgs', {}))
-
- # Re-calculate the number of iterations when resuming
- # models with different number of GPUs
- if 'config' in checkpoint['meta']:
- config = mmcv.Config.fromstring(
- checkpoint['meta']['config'], file_format='.py')
- previous_gpu_ids = config.get('gpu_ids', None)
- if previous_gpu_ids and len(previous_gpu_ids) > 0 and len(
- previous_gpu_ids) != self.world_size:
- self._iter = int(self._iter * len(previous_gpu_ids) /
- self.world_size)
- self.logger.info('the iteration number is changed due to '
- 'change of GPU number')
-
- # resume meta information meta
- self.meta = checkpoint['meta']
-
- if 'optimizer' in checkpoint and resume_optimizer:
- if isinstance(self.optimizer, Optimizer):
- self.optimizer.load_state_dict(checkpoint['optimizer'])
- elif isinstance(self.optimizer, dict):
- for k in self.optimizer.keys():
- self.optimizer[k].load_state_dict(
- checkpoint['optimizer'][k])
- else:
- raise TypeError(
- 'Optimizer should be dict or torch.optim.Optimizer '
- f'but got {type(self.optimizer)}')
-
- self.logger.info('resumed epoch %d, iter %d', self.epoch, self.iter)
-
- def register_lr_hook(self, lr_config):
- if lr_config is None:
- return
- elif isinstance(lr_config, dict):
- assert 'policy' in lr_config
- policy_type = lr_config.pop('policy')
- # If the type of policy is all in lower case, e.g., 'cyclic',
- # then its first letter will be capitalized, e.g., to be 'Cyclic'.
- # This is for the convenient usage of Lr updater.
- # Since this is not applicable for `
- # CosineAnnealingLrUpdater`,
- # the string will not be changed if it contains capital letters.
- if policy_type == policy_type.lower():
- policy_type = policy_type.title()
- hook_type = policy_type + 'LrUpdaterHook'
- lr_config['type'] = hook_type
- hook = mmcv.build_from_cfg(lr_config, HOOKS)
- else:
- hook = lr_config
- self.register_hook(hook, priority='VERY_HIGH')
-
- def register_momentum_hook(self, momentum_config):
- if momentum_config is None:
- return
- if isinstance(momentum_config, dict):
- assert 'policy' in momentum_config
- policy_type = momentum_config.pop('policy')
- # If the type of policy is all in lower case, e.g., 'cyclic',
- # then its first letter will be capitalized, e.g., to be 'Cyclic'.
- # This is for the convenient usage of momentum updater.
- # Since this is not applicable for
- # `CosineAnnealingMomentumUpdater`,
- # the string will not be changed if it contains capital letters.
- if policy_type == policy_type.lower():
- policy_type = policy_type.title()
- hook_type = policy_type + 'MomentumUpdaterHook'
- momentum_config['type'] = hook_type
- hook = mmcv.build_from_cfg(momentum_config, HOOKS)
- else:
- hook = momentum_config
- self.register_hook(hook, priority='HIGH')
-
- def register_optimizer_hook(self, optimizer_config):
- if optimizer_config is None:
- return
- if isinstance(optimizer_config, dict):
- optimizer_config.setdefault('type', 'OptimizerHook')
- hook = mmcv.build_from_cfg(optimizer_config, HOOKS)
- else:
- hook = optimizer_config
- self.register_hook(hook, priority='ABOVE_NORMAL')
-
- def register_checkpoint_hook(self, checkpoint_config):
- if checkpoint_config is None:
- return
- if isinstance(checkpoint_config, dict):
- checkpoint_config.setdefault('type', 'CheckpointHook')
- hook = mmcv.build_from_cfg(checkpoint_config, HOOKS)
- else:
- hook = checkpoint_config
- self.register_hook(hook, priority='NORMAL')
-
- def register_logger_hooks(self, log_config):
- if log_config is None:
- return
- log_interval = log_config['interval']
- for info in log_config['hooks']:
- logger_hook = mmcv.build_from_cfg(
- info, HOOKS, default_args=dict(interval=log_interval))
- self.register_hook(logger_hook, priority='VERY_LOW')
-
- def register_timer_hook(self, timer_config):
- if timer_config is None:
- return
- if isinstance(timer_config, dict):
- timer_config_ = copy.deepcopy(timer_config)
- hook = mmcv.build_from_cfg(timer_config_, HOOKS)
- else:
- hook = timer_config
- self.register_hook(hook, priority='LOW')
-
- def register_custom_hooks(self, custom_config):
- if custom_config is None:
- return
-
- if not isinstance(custom_config, list):
- custom_config = [custom_config]
-
- for item in custom_config:
- if isinstance(item, dict):
- self.register_hook_from_cfg(item)
- else:
- self.register_hook(item, priority='NORMAL')
-
- def register_profiler_hook(self, profiler_config):
- if profiler_config is None:
- return
- if isinstance(profiler_config, dict):
- profiler_config.setdefault('type', 'ProfilerHook')
- hook = mmcv.build_from_cfg(profiler_config, HOOKS)
- else:
- hook = profiler_config
- self.register_hook(hook)
-
- def register_training_hooks(self,
- lr_config,
- optimizer_config=None,
- checkpoint_config=None,
- log_config=None,
- momentum_config=None,
- timer_config=dict(type='IterTimerHook'),
- custom_hooks_config=None):
- """Register default and custom hooks for training.
-
- Default and custom hooks include:
-
- +----------------------+-------------------------+
- | Hooks | Priority |
- +======================+=========================+
- | LrUpdaterHook | VERY_HIGH (10) |
- +----------------------+-------------------------+
- | MomentumUpdaterHook | HIGH (30) |
- +----------------------+-------------------------+
- | OptimizerStepperHook | ABOVE_NORMAL (40) |
- +----------------------+-------------------------+
- | CheckpointSaverHook | NORMAL (50) |
- +----------------------+-------------------------+
- | IterTimerHook | LOW (70) |
- +----------------------+-------------------------+
- | LoggerHook(s) | VERY_LOW (90) |
- +----------------------+-------------------------+
- | CustomHook(s) | defaults to NORMAL (50) |
- +----------------------+-------------------------+
-
- If custom hooks have same priority with default hooks, custom hooks
- will be triggered after default hooks.
- """
- self.register_lr_hook(lr_config)
- self.register_momentum_hook(momentum_config)
- self.register_optimizer_hook(optimizer_config)
- self.register_checkpoint_hook(checkpoint_config)
- self.register_timer_hook(timer_config)
- self.register_logger_hooks(log_config)
- self.register_custom_hooks(custom_hooks_config)
diff --git a/spaces/coreml-community/ControlNet-v1-1-Annotators-cpu/annotator/oneformer/detectron2/utils/events.py b/spaces/coreml-community/ControlNet-v1-1-Annotators-cpu/annotator/oneformer/detectron2/utils/events.py
deleted file mode 100644
index d9a68b6b5b90cdef1ccdaffa4eb2225f3ab21e29..0000000000000000000000000000000000000000
--- a/spaces/coreml-community/ControlNet-v1-1-Annotators-cpu/annotator/oneformer/detectron2/utils/events.py
+++ /dev/null
@@ -1,534 +0,0 @@
-# Copyright (c) Facebook, Inc. and its affiliates.
-import datetime
-import json
-import logging
-import os
-import time
-from collections import defaultdict
-from contextlib import contextmanager
-from typing import Optional
-import torch
-from fvcore.common.history_buffer import HistoryBuffer
-
-from annotator.oneformer.detectron2.utils.file_io import PathManager
-
-__all__ = [
- "get_event_storage",
- "JSONWriter",
- "TensorboardXWriter",
- "CommonMetricPrinter",
- "EventStorage",
-]
-
-_CURRENT_STORAGE_STACK = []
-
-
-def get_event_storage():
- """
- Returns:
- The :class:`EventStorage` object that's currently being used.
- Throws an error if no :class:`EventStorage` is currently enabled.
- """
- assert len(
- _CURRENT_STORAGE_STACK
- ), "get_event_storage() has to be called inside a 'with EventStorage(...)' context!"
- return _CURRENT_STORAGE_STACK[-1]
-
-
-class EventWriter:
- """
- Base class for writers that obtain events from :class:`EventStorage` and process them.
- """
-
- def write(self):
- raise NotImplementedError
-
- def close(self):
- pass
-
-
-class JSONWriter(EventWriter):
- """
- Write scalars to a json file.
-
- It saves scalars as one json per line (instead of a big json) for easy parsing.
-
- Examples parsing such a json file:
- ::
- $ cat metrics.json | jq -s '.[0:2]'
- [
- {
- "data_time": 0.008433341979980469,
- "iteration": 19,
- "loss": 1.9228371381759644,
- "loss_box_reg": 0.050025828182697296,
- "loss_classifier": 0.5316952466964722,
- "loss_mask": 0.7236229181289673,
- "loss_rpn_box": 0.0856662318110466,
- "loss_rpn_cls": 0.48198649287223816,
- "lr": 0.007173333333333333,
- "time": 0.25401854515075684
- },
- {
- "data_time": 0.007216215133666992,
- "iteration": 39,
- "loss": 1.282649278640747,
- "loss_box_reg": 0.06222952902317047,
- "loss_classifier": 0.30682939291000366,
- "loss_mask": 0.6970193982124329,
- "loss_rpn_box": 0.038663312792778015,
- "loss_rpn_cls": 0.1471673548221588,
- "lr": 0.007706666666666667,
- "time": 0.2490077018737793
- }
- ]
-
- $ cat metrics.json | jq '.loss_mask'
- 0.7126231789588928
- 0.689423680305481
- 0.6776131987571716
- ...
-
- """
-
- def __init__(self, json_file, window_size=20):
- """
- Args:
- json_file (str): path to the json file. New data will be appended if the file exists.
- window_size (int): the window size of median smoothing for the scalars whose
- `smoothing_hint` are True.
- """
- self._file_handle = PathManager.open(json_file, "a")
- self._window_size = window_size
- self._last_write = -1
-
- def write(self):
- storage = get_event_storage()
- to_save = defaultdict(dict)
-
- for k, (v, iter) in storage.latest_with_smoothing_hint(self._window_size).items():
- # keep scalars that have not been written
- if iter <= self._last_write:
- continue
- to_save[iter][k] = v
- if len(to_save):
- all_iters = sorted(to_save.keys())
- self._last_write = max(all_iters)
-
- for itr, scalars_per_iter in to_save.items():
- scalars_per_iter["iteration"] = itr
- self._file_handle.write(json.dumps(scalars_per_iter, sort_keys=True) + "\n")
- self._file_handle.flush()
- try:
- os.fsync(self._file_handle.fileno())
- except AttributeError:
- pass
-
- def close(self):
- self._file_handle.close()
-
-
-class TensorboardXWriter(EventWriter):
- """
- Write all scalars to a tensorboard file.
- """
-
- def __init__(self, log_dir: str, window_size: int = 20, **kwargs):
- """
- Args:
- log_dir (str): the directory to save the output events
- window_size (int): the scalars will be median-smoothed by this window size
-
- kwargs: other arguments passed to `torch.utils.tensorboard.SummaryWriter(...)`
- """
- self._window_size = window_size
- from torch.utils.tensorboard import SummaryWriter
-
- self._writer = SummaryWriter(log_dir, **kwargs)
- self._last_write = -1
-
- def write(self):
- storage = get_event_storage()
- new_last_write = self._last_write
- for k, (v, iter) in storage.latest_with_smoothing_hint(self._window_size).items():
- if iter > self._last_write:
- self._writer.add_scalar(k, v, iter)
- new_last_write = max(new_last_write, iter)
- self._last_write = new_last_write
-
- # storage.put_{image,histogram} is only meant to be used by
- # tensorboard writer. So we access its internal fields directly from here.
- if len(storage._vis_data) >= 1:
- for img_name, img, step_num in storage._vis_data:
- self._writer.add_image(img_name, img, step_num)
- # Storage stores all image data and rely on this writer to clear them.
- # As a result it assumes only one writer will use its image data.
- # An alternative design is to let storage store limited recent
- # data (e.g. only the most recent image) that all writers can access.
- # In that case a writer may not see all image data if its period is long.
- storage.clear_images()
-
- if len(storage._histograms) >= 1:
- for params in storage._histograms:
- self._writer.add_histogram_raw(**params)
- storage.clear_histograms()
-
- def close(self):
- if hasattr(self, "_writer"): # doesn't exist when the code fails at import
- self._writer.close()
-
-
-class CommonMetricPrinter(EventWriter):
- """
- Print **common** metrics to the terminal, including
- iteration time, ETA, memory, all losses, and the learning rate.
- It also applies smoothing using a window of 20 elements.
-
- It's meant to print common metrics in common ways.
- To print something in more customized ways, please implement a similar printer by yourself.
- """
-
- def __init__(self, max_iter: Optional[int] = None, window_size: int = 20):
- """
- Args:
- max_iter: the maximum number of iterations to train.
- Used to compute ETA. If not given, ETA will not be printed.
- window_size (int): the losses will be median-smoothed by this window size
- """
- self.logger = logging.getLogger(__name__)
- self._max_iter = max_iter
- self._window_size = window_size
- self._last_write = None # (step, time) of last call to write(). Used to compute ETA
-
- def _get_eta(self, storage) -> Optional[str]:
- if self._max_iter is None:
- return ""
- iteration = storage.iter
- try:
- eta_seconds = storage.history("time").median(1000) * (self._max_iter - iteration - 1)
- storage.put_scalar("eta_seconds", eta_seconds, smoothing_hint=False)
- return str(datetime.timedelta(seconds=int(eta_seconds)))
- except KeyError:
- # estimate eta on our own - more noisy
- eta_string = None
- if self._last_write is not None:
- estimate_iter_time = (time.perf_counter() - self._last_write[1]) / (
- iteration - self._last_write[0]
- )
- eta_seconds = estimate_iter_time * (self._max_iter - iteration - 1)
- eta_string = str(datetime.timedelta(seconds=int(eta_seconds)))
- self._last_write = (iteration, time.perf_counter())
- return eta_string
-
- def write(self):
- storage = get_event_storage()
- iteration = storage.iter
- if iteration == self._max_iter:
- # This hook only reports training progress (loss, ETA, etc) but not other data,
- # therefore do not write anything after training succeeds, even if this method
- # is called.
- return
-
- try:
- avg_data_time = storage.history("data_time").avg(
- storage.count_samples("data_time", self._window_size)
- )
- last_data_time = storage.history("data_time").latest()
- except KeyError:
- # they may not exist in the first few iterations (due to warmup)
- # or when SimpleTrainer is not used
- avg_data_time = None
- last_data_time = None
- try:
- avg_iter_time = storage.history("time").global_avg()
- last_iter_time = storage.history("time").latest()
- except KeyError:
- avg_iter_time = None
- last_iter_time = None
- try:
- lr = "{:.5g}".format(storage.history("lr").latest())
- except KeyError:
- lr = "N/A"
-
- eta_string = self._get_eta(storage)
-
- if torch.cuda.is_available():
- max_mem_mb = torch.cuda.max_memory_allocated() / 1024.0 / 1024.0
- else:
- max_mem_mb = None
-
- # NOTE: max_mem is parsed by grep in "dev/parse_results.sh"
- self.logger.info(
- str.format(
- " {eta}iter: {iter} {losses} {non_losses} {avg_time}{last_time}"
- + "{avg_data_time}{last_data_time} lr: {lr} {memory}",
- eta=f"eta: {eta_string} " if eta_string else "",
- iter=iteration,
- losses=" ".join(
- [
- "{}: {:.4g}".format(
- k, v.median(storage.count_samples(k, self._window_size))
- )
- for k, v in storage.histories().items()
- if "loss" in k
- ]
- ),
- non_losses=" ".join(
- [
- "{}: {:.4g}".format(
- k, v.median(storage.count_samples(k, self._window_size))
- )
- for k, v in storage.histories().items()
- if "[metric]" in k
- ]
- ),
- avg_time="time: {:.4f} ".format(avg_iter_time)
- if avg_iter_time is not None
- else "",
- last_time="last_time: {:.4f} ".format(last_iter_time)
- if last_iter_time is not None
- else "",
- avg_data_time="data_time: {:.4f} ".format(avg_data_time)
- if avg_data_time is not None
- else "",
- last_data_time="last_data_time: {:.4f} ".format(last_data_time)
- if last_data_time is not None
- else "",
- lr=lr,
- memory="max_mem: {:.0f}M".format(max_mem_mb) if max_mem_mb is not None else "",
- )
- )
-
-
-class EventStorage:
- """
- The user-facing class that provides metric storage functionalities.
-
- In the future we may add support for storing / logging other types of data if needed.
- """
-
- def __init__(self, start_iter=0):
- """
- Args:
- start_iter (int): the iteration number to start with
- """
- self._history = defaultdict(HistoryBuffer)
- self._smoothing_hints = {}
- self._latest_scalars = {}
- self._iter = start_iter
- self._current_prefix = ""
- self._vis_data = []
- self._histograms = []
-
- def put_image(self, img_name, img_tensor):
- """
- Add an `img_tensor` associated with `img_name`, to be shown on
- tensorboard.
-
- Args:
- img_name (str): The name of the image to put into tensorboard.
- img_tensor (torch.Tensor or numpy.array): An `uint8` or `float`
- Tensor of shape `[channel, height, width]` where `channel` is
- 3. The image format should be RGB. The elements in img_tensor
- can either have values in [0, 1] (float32) or [0, 255] (uint8).
- The `img_tensor` will be visualized in tensorboard.
- """
- self._vis_data.append((img_name, img_tensor, self._iter))
-
- def put_scalar(self, name, value, smoothing_hint=True):
- """
- Add a scalar `value` to the `HistoryBuffer` associated with `name`.
-
- Args:
- smoothing_hint (bool): a 'hint' on whether this scalar is noisy and should be
- smoothed when logged. The hint will be accessible through
- :meth:`EventStorage.smoothing_hints`. A writer may ignore the hint
- and apply custom smoothing rule.
-
- It defaults to True because most scalars we save need to be smoothed to
- provide any useful signal.
- """
- name = self._current_prefix + name
- history = self._history[name]
- value = float(value)
- history.update(value, self._iter)
- self._latest_scalars[name] = (value, self._iter)
-
- existing_hint = self._smoothing_hints.get(name)
- if existing_hint is not None:
- assert (
- existing_hint == smoothing_hint
- ), "Scalar {} was put with a different smoothing_hint!".format(name)
- else:
- self._smoothing_hints[name] = smoothing_hint
-
- def put_scalars(self, *, smoothing_hint=True, **kwargs):
- """
- Put multiple scalars from keyword arguments.
-
- Examples:
-
- storage.put_scalars(loss=my_loss, accuracy=my_accuracy, smoothing_hint=True)
- """
- for k, v in kwargs.items():
- self.put_scalar(k, v, smoothing_hint=smoothing_hint)
-
- def put_histogram(self, hist_name, hist_tensor, bins=1000):
- """
- Create a histogram from a tensor.
-
- Args:
- hist_name (str): The name of the histogram to put into tensorboard.
- hist_tensor (torch.Tensor): A Tensor of arbitrary shape to be converted
- into a histogram.
- bins (int): Number of histogram bins.
- """
- ht_min, ht_max = hist_tensor.min().item(), hist_tensor.max().item()
-
- # Create a histogram with PyTorch
- hist_counts = torch.histc(hist_tensor, bins=bins)
- hist_edges = torch.linspace(start=ht_min, end=ht_max, steps=bins + 1, dtype=torch.float32)
-
- # Parameter for the add_histogram_raw function of SummaryWriter
- hist_params = dict(
- tag=hist_name,
- min=ht_min,
- max=ht_max,
- num=len(hist_tensor),
- sum=float(hist_tensor.sum()),
- sum_squares=float(torch.sum(hist_tensor**2)),
- bucket_limits=hist_edges[1:].tolist(),
- bucket_counts=hist_counts.tolist(),
- global_step=self._iter,
- )
- self._histograms.append(hist_params)
-
- def history(self, name):
- """
- Returns:
- HistoryBuffer: the scalar history for name
- """
- ret = self._history.get(name, None)
- if ret is None:
- raise KeyError("No history metric available for {}!".format(name))
- return ret
-
- def histories(self):
- """
- Returns:
- dict[name -> HistoryBuffer]: the HistoryBuffer for all scalars
- """
- return self._history
-
- def latest(self):
- """
- Returns:
- dict[str -> (float, int)]: mapping from the name of each scalar to the most
- recent value and the iteration number its added.
- """
- return self._latest_scalars
-
- def latest_with_smoothing_hint(self, window_size=20):
- """
- Similar to :meth:`latest`, but the returned values
- are either the un-smoothed original latest value,
- or a median of the given window_size,
- depend on whether the smoothing_hint is True.
-
- This provides a default behavior that other writers can use.
-
- Note: All scalars saved in the past `window_size` iterations are used for smoothing.
- This is different from the `window_size` definition in HistoryBuffer.
- Use :meth:`get_history_window_size` to get the `window_size` used in HistoryBuffer.
- """
- result = {}
- for k, (v, itr) in self._latest_scalars.items():
- result[k] = (
- self._history[k].median(self.count_samples(k, window_size))
- if self._smoothing_hints[k]
- else v,
- itr,
- )
- return result
-
- def count_samples(self, name, window_size=20):
- """
- Return the number of samples logged in the past `window_size` iterations.
- """
- samples = 0
- data = self._history[name].values()
- for _, iter_ in reversed(data):
- if iter_ > data[-1][1] - window_size:
- samples += 1
- else:
- break
- return samples
-
- def smoothing_hints(self):
- """
- Returns:
- dict[name -> bool]: the user-provided hint on whether the scalar
- is noisy and needs smoothing.
- """
- return self._smoothing_hints
-
- def step(self):
- """
- User should either: (1) Call this function to increment storage.iter when needed. Or
- (2) Set `storage.iter` to the correct iteration number before each iteration.
-
- The storage will then be able to associate the new data with an iteration number.
- """
- self._iter += 1
-
- @property
- def iter(self):
- """
- Returns:
- int: The current iteration number. When used together with a trainer,
- this is ensured to be the same as trainer.iter.
- """
- return self._iter
-
- @iter.setter
- def iter(self, val):
- self._iter = int(val)
-
- @property
- def iteration(self):
- # for backward compatibility
- return self._iter
-
- def __enter__(self):
- _CURRENT_STORAGE_STACK.append(self)
- return self
-
- def __exit__(self, exc_type, exc_val, exc_tb):
- assert _CURRENT_STORAGE_STACK[-1] == self
- _CURRENT_STORAGE_STACK.pop()
-
- @contextmanager
- def name_scope(self, name):
- """
- Yields:
- A context within which all the events added to this storage
- will be prefixed by the name scope.
- """
- old_prefix = self._current_prefix
- self._current_prefix = name.rstrip("/") + "/"
- yield
- self._current_prefix = old_prefix
-
- def clear_images(self):
- """
- Delete all the stored images for visualization. This should be called
- after images are written to tensorboard.
- """
- self._vis_data = []
-
- def clear_histograms(self):
- """
- Delete all the stored histograms for visualization.
- This should be called after histograms are written to tensorboard.
- """
- self._histograms = []
diff --git a/spaces/cybercorejapan/human-detection-docker/models/trackers/reid_parallel_tracker/three_stage_tracker.py b/spaces/cybercorejapan/human-detection-docker/models/trackers/reid_parallel_tracker/three_stage_tracker.py
deleted file mode 100644
index 6dcc1c29485749e61931ef733b6b4904483431d1..0000000000000000000000000000000000000000
--- a/spaces/cybercorejapan/human-detection-docker/models/trackers/reid_parallel_tracker/three_stage_tracker.py
+++ /dev/null
@@ -1,132 +0,0 @@
-
-from typing import Tuple, Dict
-import numpy as np
-from .core.tracklet import TrackState
-from .core.tracklet import (Tracklet, TrackState)
-from .base_tracker import BaseTracker
-from .matchers.base_matchers import SimMatcher
-
-class ThreeStageTracker(BaseTracker):
- def __init__(self,
- matcher_active_cfg = dict(match_thr=0.5),
- matcher_det_low_cfg = dict(match_thr=0.5),
- matcher_lost_cfg = dict(match_thr=0.5),
- matcher_unconfirmed_cfg = dict(match_thr=0.5),
- enable_reid_buffer = False,
- *args,**kwargs):
- super().__init__(*args,**kwargs)
- self.matcher_active = SimMatcher(**matcher_active_cfg)
- self.matcher_det_low = SimMatcher(**matcher_det_low_cfg)
- self.matcher_lost = SimMatcher(**matcher_lost_cfg)
- self.matcher_unconfirmed = SimMatcher(**matcher_unconfirmed_cfg)
- self.enable_reid_buffer = enable_reid_buffer
-
- def split_tracks_by_activation(self):
- """ Split the tracks into active_tracks, lost_tracks, and unconfirmed (just initialize)
- Returns:
- strack_pool: List[Tracklet]
- unconfirmed: List[Tracklet]
- """
- unconfirmed = []
- tracked_stracks = [] # type: list[Tracklet]
- for track in self.tracked_stracks:
- if not track.is_activated:
- unconfirmed.append(track)
- else:
- tracked_stracks.append(track)
- return tracked_stracks, self.lost_stracks, unconfirmed
-
- def predict_with_gmc(self, active_tracks, lost_tracks, unconfirmed, Hmat):
- Tracklet.multi_predict(active_tracks)
- Tracklet.multi_predict(lost_tracks)
- if Hmat is not None:
- Tracklet.multi_gmc(active_tracks, Hmat)
- Tracklet.multi_gmc(lost_tracks, Hmat)
- Tracklet.multi_gmc(unconfirmed, Hmat)
-
- def update(self,
- det_result: Dict,
- Hmat: np.array=None,
- meta_data: Dict=None) -> Tuple[Dict, Dict]:
- """ The main function to perform tracking. The pipeline is similar to ByteTrack/BoTSort,
- which first associate with high score detection boxes, and then associate with low score detection boxes.
- Args:
- det_result (dict): detection result from detector
- Hmat (np.array, optional): Homography transformation matrix. Defaults to None.
- Returns:
- active_tracks (dict): tracked tracks in the current frame. See format_track_results for the format
- lost_tracks (dict): lost tracks in the current frame. See format_track_results for the format
- """
- self.frame_id += 1
- # Step 1: Split the detections into high score/lower score group
- det_result = self.preprocess_det_result(det_result)
- dets_high, dets_low = self.split_detections_by_scores(det_result)
-
- # Step 2: Split the tracks into trackpool=(active_tracks + lost_tracks) and unconfirmed (just initialize)
- active_tracks, lost_tracks, unconfirmed = self.split_tracks_by_activation()
- # - predict the current location with KF, and compensate for Camera Motion
- self.predict_with_gmc(active_tracks, lost_tracks, unconfirmed, Hmat)
-
- # Step 3: First association with high score detection boxes and active track
- match_idxes, unmatch_track_idxes, unmatch_det_idxes= self.matcher_active(active_tracks, dets_high)
- activated_stracks, refind_stracks = self.update_matched_tracks(match_idxes, active_tracks, dets_high) # refind_track=[]
-
- # Step 4: Second association with low score detection boxes"""
- unmatch_tracks = [active_tracks[idx] for idx in unmatch_track_idxes if active_tracks[idx].state == TrackState.Tracked]
-
- match_idxes_2, unmatch_track_idxes_2, _ = self.matcher_det_low(unmatch_tracks, dets_low)
- activated_stracks_2, refind_stracks_2 = self.update_matched_tracks(match_idxes_2, unmatch_tracks, dets_low)
- activated_stracks.extend(activated_stracks_2 )
- refind_stracks.extend(refind_stracks_2)
-
- # - assing the Lost status to the unmatch_tracks that are not matched with low score detection boxes
- lost_stracks = [unmatch_tracks[it] for it in unmatch_track_idxes_2 if unmatch_tracks[it].state != TrackState.Lost]
- for track in lost_stracks: track.mark_lost()
-
- # Step 5: Second association, between remain high detections and lost tracks
- remain_dets = [dets_high[i] for i in unmatch_det_idxes]
- match_idxes_3, unmatch_track_idxes_3, unmatch_det_idxes_3 = self.matcher_lost(lost_tracks, remain_dets)
- activated_stracks_3, refind_stracks_3 = self.update_matched_tracks(match_idxes_3, lost_tracks, remain_dets)
- activated_stracks.extend(activated_stracks_3,)
- refind_stracks.extend(refind_stracks_3)
- unmatch_lost_tracks = [lost_tracks[idx] for idx in unmatch_track_idxes_3]
- lost_stracks.extend(unmatch_lost_tracks)
-
- # Step 6: Second association, between new detections and lost tracks (tracks just initialized in the previous frame)
- remain_dets_3 = [remain_dets[i] for i in unmatch_det_idxes_3]
- match_idxes_4, unmatch_track_idxes_4, unmatch_det_idxes_4 = self.matcher_unconfirmed(unconfirmed, remain_dets_3)
-
- for itracked, idet in match_idxes_4:
- # Update matches_unconfirmed into active_tracks
- unconfirmed[itracked].update(remain_dets_3[idet], self.frame_id)
- activated_stracks.append(unconfirmed[itracked])
-
- # - remove unconfirmed tracks that do not match any detections
- removed_stracks = [unconfirmed[it] for it in unmatch_track_idxes_4]
- for track in removed_stracks: track.mark_removed()
-
- # - init new stracks if they are high score and not too small boxes as tentative tracks (unconfirmed)
- new_tracks = self.init_new_tracks(remain_dets_3, unmatch_det_idxes_4)
- # - new_tracks that have very high score and not occluded with current tracks will be directly activated
- self.activate_new_tracks(new_tracks, activated_stracks + refind_stracks)
-
- # Step 6: remove lost tracks if they are already lost for a certain frames
- self.lost_stracks.extend(lost_stracks)
- removed_lost_stracks = self.remove_lost_tracks()
- removed_stracks += removed_lost_stracks
-
- # Step 7: Final result merging
- active_tracks, lost_tracks = self.merge_results(activated_stracks, refind_stracks, new_tracks, removed_stracks)
-
- # update lost frame num
- self.update_lost_frame()
-
- return active_tracks,lost_tracks
-
- def update_lost_frame(self):
-
- for track in self.tracked_stracks:
- track.reset_lost_frame_num()
-
- for track in self.lost_stracks:
- track.set_lost_frame_num()
\ No newline at end of file
diff --git a/spaces/daddyjin/TalkingFaceGeneration/Demo_TFR_Pirenderer/src/face3d/models/arcface_torch/configs/ms1mv3_mbf.py b/spaces/daddyjin/TalkingFaceGeneration/Demo_TFR_Pirenderer/src/face3d/models/arcface_torch/configs/ms1mv3_mbf.py
deleted file mode 100644
index b8a00d6305eeda5a94788017afc1cda0d4a4cd2a..0000000000000000000000000000000000000000
--- a/spaces/daddyjin/TalkingFaceGeneration/Demo_TFR_Pirenderer/src/face3d/models/arcface_torch/configs/ms1mv3_mbf.py
+++ /dev/null
@@ -1,26 +0,0 @@
-from easydict import EasyDict as edict
-
-# make training faster
-# our RAM is 256G
-# mount -t tmpfs -o size=140G tmpfs /train_tmp
-
-config = edict()
-config.loss = "arcface"
-config.network = "mbf"
-config.resume = False
-config.output = None
-config.embedding_size = 512
-config.sample_rate = 1.0
-config.fp16 = True
-config.momentum = 0.9
-config.weight_decay = 2e-4
-config.batch_size = 128
-config.lr = 0.1 # batch size is 512
-
-config.rec = "/train_tmp/ms1m-retinaface-t1"
-config.num_classes = 93431
-config.num_image = 5179510
-config.num_epoch = 30
-config.warmup_epoch = -1
-config.decay_epoch = [10, 20, 25]
-config.val_targets = ["lfw", "cfp_fp", "agedb_30"]
diff --git a/spaces/danterivers/music-generation-samples/audiocraft/modules/streaming.py b/spaces/danterivers/music-generation-samples/audiocraft/modules/streaming.py
deleted file mode 100644
index fdbdf5e90fc0c6560873d66bf273460b38e5ed7e..0000000000000000000000000000000000000000
--- a/spaces/danterivers/music-generation-samples/audiocraft/modules/streaming.py
+++ /dev/null
@@ -1,135 +0,0 @@
-# Copyright (c) Meta Platforms, Inc. and affiliates.
-# All rights reserved.
-#
-# This source code is licensed under the license found in the
-# LICENSE file in the root directory of this source tree.
-
-"""
-Streaming module API that should be implemented by all Streaming components,
-"""
-
-from contextlib import contextmanager
-import typing as tp
-from torch import nn
-import torch
-
-
-State = tp.Dict[str, torch.Tensor]
-
-
-class StreamingModule(nn.Module):
- """Common API for streaming components.
-
- Each streaming component has a streaming state, which is just a dict[str, Tensor].
- By convention, the first dim of each tensor must be the batch size.
- Don't use dots in the key names, as this would clash with submodules
- (like in state_dict).
-
- If `self._is_streaming` is True, the component should use and remember
- the proper state inside `self._streaming_state`.
-
- To set a streaming component in streaming state, use
-
- with module.streaming():
- ...
-
- This will automatically reset the streaming state when exiting the context manager.
- This also automatically propagates to all streaming children module.
-
- Some module might also implement the `StreamingModule.flush` method, although
- this one is trickier, as all parents module must be StreamingModule and implement
- it as well for it to work properly. See `StreamingSequential` after.
- """
- def __init__(self) -> None:
- super().__init__()
- self._streaming_state: State = {}
- self._is_streaming = False
-
- def _apply_named_streaming(self, fn: tp.Any):
- for name, module in self.named_modules():
- if isinstance(module, StreamingModule):
- fn(name, module)
-
- def _set_streaming(self, streaming: bool):
- def _set_streaming(name, module):
- module._is_streaming = streaming
- self._apply_named_streaming(_set_streaming)
-
- @contextmanager
- def streaming(self):
- """Context manager to enter streaming mode. Reset streaming state on exit.
- """
- self._set_streaming(True)
- try:
- yield
- finally:
- self._set_streaming(False)
- self.reset_streaming()
-
- def reset_streaming(self):
- """Reset the streaming state.
- """
- def _reset(name: str, module: StreamingModule):
- module._streaming_state.clear()
-
- self._apply_named_streaming(_reset)
-
- def get_streaming_state(self) -> State:
- """Return the streaming state, including that of sub-modules.
- """
- state: State = {}
-
- def _add(name: str, module: StreamingModule):
- if name:
- name += "."
- for key, value in module._streaming_state.items():
- state[name + key] = value
-
- self._apply_named_streaming(_add)
- return state
-
- def set_streaming_state(self, state: State):
- """Set the streaming state, including that of sub-modules.
- """
- state = dict(state)
-
- def _set(name: str, module: StreamingModule):
- if name:
- name += "."
- module._streaming_state.clear()
- for key, value in list(state.items()):
- # complexity is not ideal here, but probably fine.
- if key.startswith(name):
- local_key = key[len(name):]
- if '.' not in local_key:
- module._streaming_state[local_key] = value
- del state[key]
-
- self._apply_named_streaming(_set)
- assert len(state) == 0, list(state.keys())
-
- def flush(self, x: tp.Optional[torch.Tensor] = None):
- """Flush any remaining outputs that were waiting for completion.
- Typically, for convolutions, this will add the final padding
- and process the last buffer.
-
- This should take an optional argument `x`, which will be provided
- if a module before this one in the streaming pipeline has already
- spitted out a flushed out buffer.
- """
- if x is None:
- return None
- else:
- return self(x)
-
-
-class StreamingSequential(StreamingModule, nn.Sequential):
- """A streaming compatible alternative of `nn.Sequential`.
- """
- def flush(self, x: tp.Optional[torch.Tensor] = None):
- for module in self:
- if isinstance(module, StreamingModule):
- x = module.flush(x)
- elif x is not None:
- x = module(x)
- return x
diff --git a/spaces/darkstorm2150/Stable-Diffusion-Protogen-x3.4-webui/README.md b/spaces/darkstorm2150/Stable-Diffusion-Protogen-x3.4-webui/README.md
deleted file mode 100644
index b15b0ea730795938308efc91486b0ddd054fb1b6..0000000000000000000000000000000000000000
--- a/spaces/darkstorm2150/Stable-Diffusion-Protogen-x3.4-webui/README.md
+++ /dev/null
@@ -1,44 +0,0 @@
----
-title: Stable Diffusion Protogen x3.4 Web UI
-emoji: ⚛
-colorFrom: pink
-colorTo: purple
-sdk: docker
-#sdk_version: 3.9
-app_file: DockerApp.py
-pinned: false
----
-
-### ProtoGen Diffusion model merged by [darkstorm2150](https://twitter.com/Predogl)
-
-This model was merged on a large amount of data from large datasets new and trending on civitai.com
-
-You can enforce camera capture by using the prompt with "modelshoot style".
-
-It should also be very dreamboothable, being able to generate high fidelity faces with a little amount of steps.
-
-**[By using this model you agree to this license](https://huggingface.co/dreamlike-art/dreamlike-photoreal-2.0/blob/main/LICENSE.md), I the creator darkstorm2150 of this merge and Hugging Face is not liable for any content created by this Protogen Model.**
-
-
-
-
-
-
-
-
-
-
-
-
-
-
-## Other..
-
-## Stable Diffusion Web UI
-[https://github.com/AUTOMATIC1111/stable-diffusion-webui](https://github.com/AUTOMATIC1111/stable-diffusion-webui)
-
-## Documentation
-[https://github.com/AUTOMATIC1111/stable-diffusion-webui/wiki](https://github.com/AUTOMATIC1111/stable-diffusion-webui/wiki)
-
-## Models License
-https://huggingface.co/spaces/CompVis/stable-diffusion-license
\ No newline at end of file
diff --git a/spaces/dawood/Kanye-AI/onnx/onnx_export_48k.py b/spaces/dawood/Kanye-AI/onnx/onnx_export_48k.py
deleted file mode 100644
index 9a046353dc25b658684fa76bdf8b4f21d1a77c98..0000000000000000000000000000000000000000
--- a/spaces/dawood/Kanye-AI/onnx/onnx_export_48k.py
+++ /dev/null
@@ -1,73 +0,0 @@
-import argparse
-import time
-import numpy as np
-import onnx
-from onnxsim import simplify
-import onnxruntime as ort
-import onnxoptimizer
-import torch
-from model_onnx_48k import SynthesizerTrn
-import utils
-from hubert import hubert_model_onnx
-
-def main(HubertExport,NetExport):
-
- path = "NyaruTaffy"
-
- if(HubertExport):
- device = torch.device("cuda")
- hubert_soft = hubert_model_onnx.hubert_soft("hubert/model.pt")
- test_input = torch.rand(1, 1, 16000)
- input_names = ["source"]
- output_names = ["embed"]
- torch.onnx.export(hubert_soft.to(device),
- test_input.to(device),
- "hubert3.0.onnx",
- dynamic_axes={
- "source": {
- 2: "sample_length"
- }
- },
- verbose=False,
- opset_version=13,
- input_names=input_names,
- output_names=output_names)
- if(NetExport):
- device = torch.device("cuda")
- hps = utils.get_hparams_from_file(f"checkpoints/{path}/config.json")
- SVCVITS = SynthesizerTrn(
- hps.data.filter_length // 2 + 1,
- hps.train.segment_size // hps.data.hop_length,
- **hps.model)
- _ = utils.load_checkpoint(f"checkpoints/{path}/model.pth", SVCVITS, None)
- _ = SVCVITS.eval().to(device)
- for i in SVCVITS.parameters():
- i.requires_grad = False
- test_hidden_unit = torch.rand(1, 50, 256)
- test_lengths = torch.LongTensor([50])
- test_pitch = torch.rand(1, 50)
- test_sid = torch.LongTensor([0])
- input_names = ["hidden_unit", "lengths", "pitch", "sid"]
- output_names = ["audio", ]
- SVCVITS.eval()
- torch.onnx.export(SVCVITS,
- (
- test_hidden_unit.to(device),
- test_lengths.to(device),
- test_pitch.to(device),
- test_sid.to(device)
- ),
- f"checkpoints/{path}/model.onnx",
- dynamic_axes={
- "hidden_unit": [0, 1],
- "pitch": [1]
- },
- do_constant_folding=False,
- opset_version=16,
- verbose=False,
- input_names=input_names,
- output_names=output_names)
-
-
-if __name__ == '__main__':
- main(False,True)
diff --git a/spaces/dbirks/diffuse-the-rest/mdsvex.config.js b/spaces/dbirks/diffuse-the-rest/mdsvex.config.js
deleted file mode 100644
index d408270e25711f5f50b95fe85bb8920f766f5703..0000000000000000000000000000000000000000
--- a/spaces/dbirks/diffuse-the-rest/mdsvex.config.js
+++ /dev/null
@@ -1,14 +0,0 @@
-import { defineMDSveXConfig as defineConfig } from 'mdsvex';
-
-const config = defineConfig({
- extensions: ['.svelte', '.md', '.svx'],
-
- smartypants: {
- dashes: 'oldschool'
- },
-
- remarkPlugins: [],
- rehypePlugins: []
-});
-
-export default config;
diff --git a/spaces/dbirks/diffuse-the-rest/svelte.config.js b/spaces/dbirks/diffuse-the-rest/svelte.config.js
deleted file mode 100644
index 39e5f7c03b9e9e26cf8c88ff11a15a3bb45b1534..0000000000000000000000000000000000000000
--- a/spaces/dbirks/diffuse-the-rest/svelte.config.js
+++ /dev/null
@@ -1,22 +0,0 @@
-import { mdsvex } from 'mdsvex';
-import mdsvexConfig from './mdsvex.config.js';
-import adapter from '@sveltejs/adapter-static';
-import preprocess from 'svelte-preprocess';
-
-/** @type {import('@sveltejs/kit').Config} */
-const config = {
- extensions: ['.svelte', ...mdsvexConfig.extensions],
-
- // Consult https://github.com/sveltejs/svelte-preprocess
- // for more information about preprocessors
- preprocess: [preprocess(), mdsvex(mdsvexConfig)],
-
- kit: {
- adapter: adapter(),
- prerender: {
- default: true
- }
- }
-};
-
-export default config;
diff --git a/spaces/dcarpintero/nlp-summarizer-pegasus/.venv/lib/python3.9/site-packages/PIL/ImageColor.py b/spaces/dcarpintero/nlp-summarizer-pegasus/.venv/lib/python3.9/site-packages/PIL/ImageColor.py
deleted file mode 100644
index befc1fd1d88069e5d140b8eac6d57e658f834b29..0000000000000000000000000000000000000000
--- a/spaces/dcarpintero/nlp-summarizer-pegasus/.venv/lib/python3.9/site-packages/PIL/ImageColor.py
+++ /dev/null
@@ -1,313 +0,0 @@
-#
-# The Python Imaging Library
-# $Id$
-#
-# map CSS3-style colour description strings to RGB
-#
-# History:
-# 2002-10-24 fl Added support for CSS-style color strings
-# 2002-12-15 fl Added RGBA support
-# 2004-03-27 fl Fixed remaining int() problems for Python 1.5.2
-# 2004-07-19 fl Fixed gray/grey spelling issues
-# 2009-03-05 fl Fixed rounding error in grayscale calculation
-#
-# Copyright (c) 2002-2004 by Secret Labs AB
-# Copyright (c) 2002-2004 by Fredrik Lundh
-#
-# See the README file for information on usage and redistribution.
-#
-
-import re
-
-from . import Image
-
-
-def getrgb(color):
- """
- Convert a color string to an RGB or RGBA tuple. If the string cannot be
- parsed, this function raises a :py:exc:`ValueError` exception.
-
- .. versionadded:: 1.1.4
-
- :param color: A color string
- :return: ``(red, green, blue[, alpha])``
- """
- if len(color) > 100:
- msg = "color specifier is too long"
- raise ValueError(msg)
- color = color.lower()
-
- rgb = colormap.get(color, None)
- if rgb:
- if isinstance(rgb, tuple):
- return rgb
- colormap[color] = rgb = getrgb(rgb)
- return rgb
-
- # check for known string formats
- if re.match("#[a-f0-9]{3}$", color):
- return int(color[1] * 2, 16), int(color[2] * 2, 16), int(color[3] * 2, 16)
-
- if re.match("#[a-f0-9]{4}$", color):
- return (
- int(color[1] * 2, 16),
- int(color[2] * 2, 16),
- int(color[3] * 2, 16),
- int(color[4] * 2, 16),
- )
-
- if re.match("#[a-f0-9]{6}$", color):
- return int(color[1:3], 16), int(color[3:5], 16), int(color[5:7], 16)
-
- if re.match("#[a-f0-9]{8}$", color):
- return (
- int(color[1:3], 16),
- int(color[3:5], 16),
- int(color[5:7], 16),
- int(color[7:9], 16),
- )
-
- m = re.match(r"rgb\(\s*(\d+)\s*,\s*(\d+)\s*,\s*(\d+)\s*\)$", color)
- if m:
- return int(m.group(1)), int(m.group(2)), int(m.group(3))
-
- m = re.match(r"rgb\(\s*(\d+)%\s*,\s*(\d+)%\s*,\s*(\d+)%\s*\)$", color)
- if m:
- return (
- int((int(m.group(1)) * 255) / 100.0 + 0.5),
- int((int(m.group(2)) * 255) / 100.0 + 0.5),
- int((int(m.group(3)) * 255) / 100.0 + 0.5),
- )
-
- m = re.match(
- r"hsl\(\s*(\d+\.?\d*)\s*,\s*(\d+\.?\d*)%\s*,\s*(\d+\.?\d*)%\s*\)$", color
- )
- if m:
- from colorsys import hls_to_rgb
-
- rgb = hls_to_rgb(
- float(m.group(1)) / 360.0,
- float(m.group(3)) / 100.0,
- float(m.group(2)) / 100.0,
- )
- return (
- int(rgb[0] * 255 + 0.5),
- int(rgb[1] * 255 + 0.5),
- int(rgb[2] * 255 + 0.5),
- )
-
- m = re.match(
- r"hs[bv]\(\s*(\d+\.?\d*)\s*,\s*(\d+\.?\d*)%\s*,\s*(\d+\.?\d*)%\s*\)$", color
- )
- if m:
- from colorsys import hsv_to_rgb
-
- rgb = hsv_to_rgb(
- float(m.group(1)) / 360.0,
- float(m.group(2)) / 100.0,
- float(m.group(3)) / 100.0,
- )
- return (
- int(rgb[0] * 255 + 0.5),
- int(rgb[1] * 255 + 0.5),
- int(rgb[2] * 255 + 0.5),
- )
-
- m = re.match(r"rgba\(\s*(\d+)\s*,\s*(\d+)\s*,\s*(\d+)\s*,\s*(\d+)\s*\)$", color)
- if m:
- return int(m.group(1)), int(m.group(2)), int(m.group(3)), int(m.group(4))
- msg = f"unknown color specifier: {repr(color)}"
- raise ValueError(msg)
-
-
-def getcolor(color, mode):
- """
- Same as :py:func:`~PIL.ImageColor.getrgb` for most modes. However, if
- ``mode`` is HSV, converts the RGB value to a HSV value, or if ``mode`` is
- not color or a palette image, converts the RGB value to a greyscale value.
- If the string cannot be parsed, this function raises a :py:exc:`ValueError`
- exception.
-
- .. versionadded:: 1.1.4
-
- :param color: A color string
- :param mode: Convert result to this mode
- :return: ``(graylevel[, alpha]) or (red, green, blue[, alpha])``
- """
- # same as getrgb, but converts the result to the given mode
- color, alpha = getrgb(color), 255
- if len(color) == 4:
- color, alpha = color[:3], color[3]
-
- if mode == "HSV":
- from colorsys import rgb_to_hsv
-
- r, g, b = color
- h, s, v = rgb_to_hsv(r / 255, g / 255, b / 255)
- return int(h * 255), int(s * 255), int(v * 255)
- elif Image.getmodebase(mode) == "L":
- r, g, b = color
- # ITU-R Recommendation 601-2 for nonlinear RGB
- # scaled to 24 bits to match the convert's implementation.
- color = (r * 19595 + g * 38470 + b * 7471 + 0x8000) >> 16
- if mode[-1] == "A":
- return color, alpha
- else:
- if mode[-1] == "A":
- return color + (alpha,)
- return color
-
-
-colormap = {
- # X11 colour table from https://drafts.csswg.org/css-color-4/, with
- # gray/grey spelling issues fixed. This is a superset of HTML 4.0
- # colour names used in CSS 1.
- "aliceblue": "#f0f8ff",
- "antiquewhite": "#faebd7",
- "aqua": "#00ffff",
- "aquamarine": "#7fffd4",
- "azure": "#f0ffff",
- "beige": "#f5f5dc",
- "bisque": "#ffe4c4",
- "black": "#000000",
- "blanchedalmond": "#ffebcd",
- "blue": "#0000ff",
- "blueviolet": "#8a2be2",
- "brown": "#a52a2a",
- "burlywood": "#deb887",
- "cadetblue": "#5f9ea0",
- "chartreuse": "#7fff00",
- "chocolate": "#d2691e",
- "coral": "#ff7f50",
- "cornflowerblue": "#6495ed",
- "cornsilk": "#fff8dc",
- "crimson": "#dc143c",
- "cyan": "#00ffff",
- "darkblue": "#00008b",
- "darkcyan": "#008b8b",
- "darkgoldenrod": "#b8860b",
- "darkgray": "#a9a9a9",
- "darkgrey": "#a9a9a9",
- "darkgreen": "#006400",
- "darkkhaki": "#bdb76b",
- "darkmagenta": "#8b008b",
- "darkolivegreen": "#556b2f",
- "darkorange": "#ff8c00",
- "darkorchid": "#9932cc",
- "darkred": "#8b0000",
- "darksalmon": "#e9967a",
- "darkseagreen": "#8fbc8f",
- "darkslateblue": "#483d8b",
- "darkslategray": "#2f4f4f",
- "darkslategrey": "#2f4f4f",
- "darkturquoise": "#00ced1",
- "darkviolet": "#9400d3",
- "deeppink": "#ff1493",
- "deepskyblue": "#00bfff",
- "dimgray": "#696969",
- "dimgrey": "#696969",
- "dodgerblue": "#1e90ff",
- "firebrick": "#b22222",
- "floralwhite": "#fffaf0",
- "forestgreen": "#228b22",
- "fuchsia": "#ff00ff",
- "gainsboro": "#dcdcdc",
- "ghostwhite": "#f8f8ff",
- "gold": "#ffd700",
- "goldenrod": "#daa520",
- "gray": "#808080",
- "grey": "#808080",
- "green": "#008000",
- "greenyellow": "#adff2f",
- "honeydew": "#f0fff0",
- "hotpink": "#ff69b4",
- "indianred": "#cd5c5c",
- "indigo": "#4b0082",
- "ivory": "#fffff0",
- "khaki": "#f0e68c",
- "lavender": "#e6e6fa",
- "lavenderblush": "#fff0f5",
- "lawngreen": "#7cfc00",
- "lemonchiffon": "#fffacd",
- "lightblue": "#add8e6",
- "lightcoral": "#f08080",
- "lightcyan": "#e0ffff",
- "lightgoldenrodyellow": "#fafad2",
- "lightgreen": "#90ee90",
- "lightgray": "#d3d3d3",
- "lightgrey": "#d3d3d3",
- "lightpink": "#ffb6c1",
- "lightsalmon": "#ffa07a",
- "lightseagreen": "#20b2aa",
- "lightskyblue": "#87cefa",
- "lightslategray": "#778899",
- "lightslategrey": "#778899",
- "lightsteelblue": "#b0c4de",
- "lightyellow": "#ffffe0",
- "lime": "#00ff00",
- "limegreen": "#32cd32",
- "linen": "#faf0e6",
- "magenta": "#ff00ff",
- "maroon": "#800000",
- "mediumaquamarine": "#66cdaa",
- "mediumblue": "#0000cd",
- "mediumorchid": "#ba55d3",
- "mediumpurple": "#9370db",
- "mediumseagreen": "#3cb371",
- "mediumslateblue": "#7b68ee",
- "mediumspringgreen": "#00fa9a",
- "mediumturquoise": "#48d1cc",
- "mediumvioletred": "#c71585",
- "midnightblue": "#191970",
- "mintcream": "#f5fffa",
- "mistyrose": "#ffe4e1",
- "moccasin": "#ffe4b5",
- "navajowhite": "#ffdead",
- "navy": "#000080",
- "oldlace": "#fdf5e6",
- "olive": "#808000",
- "olivedrab": "#6b8e23",
- "orange": "#ffa500",
- "orangered": "#ff4500",
- "orchid": "#da70d6",
- "palegoldenrod": "#eee8aa",
- "palegreen": "#98fb98",
- "paleturquoise": "#afeeee",
- "palevioletred": "#db7093",
- "papayawhip": "#ffefd5",
- "peachpuff": "#ffdab9",
- "peru": "#cd853f",
- "pink": "#ffc0cb",
- "plum": "#dda0dd",
- "powderblue": "#b0e0e6",
- "purple": "#800080",
- "rebeccapurple": "#663399",
- "red": "#ff0000",
- "rosybrown": "#bc8f8f",
- "royalblue": "#4169e1",
- "saddlebrown": "#8b4513",
- "salmon": "#fa8072",
- "sandybrown": "#f4a460",
- "seagreen": "#2e8b57",
- "seashell": "#fff5ee",
- "sienna": "#a0522d",
- "silver": "#c0c0c0",
- "skyblue": "#87ceeb",
- "slateblue": "#6a5acd",
- "slategray": "#708090",
- "slategrey": "#708090",
- "snow": "#fffafa",
- "springgreen": "#00ff7f",
- "steelblue": "#4682b4",
- "tan": "#d2b48c",
- "teal": "#008080",
- "thistle": "#d8bfd8",
- "tomato": "#ff6347",
- "turquoise": "#40e0d0",
- "violet": "#ee82ee",
- "wheat": "#f5deb3",
- "white": "#ffffff",
- "whitesmoke": "#f5f5f5",
- "yellow": "#ffff00",
- "yellowgreen": "#9acd32",
-}
diff --git a/spaces/dcarpintero/nlp-summarizer-pegasus/.venv/lib/python3.9/site-packages/aiohttp/client.py b/spaces/dcarpintero/nlp-summarizer-pegasus/.venv/lib/python3.9/site-packages/aiohttp/client.py
deleted file mode 100644
index 0d0f4c16c0cfa3751343e2ee60104e3e1a3db04c..0000000000000000000000000000000000000000
--- a/spaces/dcarpintero/nlp-summarizer-pegasus/.venv/lib/python3.9/site-packages/aiohttp/client.py
+++ /dev/null
@@ -1,1305 +0,0 @@
-"""HTTP Client for asyncio."""
-
-import asyncio
-import base64
-import hashlib
-import json
-import os
-import sys
-import traceback
-import warnings
-from contextlib import suppress
-from types import SimpleNamespace, TracebackType
-from typing import (
- Any,
- Awaitable,
- Callable,
- Coroutine,
- FrozenSet,
- Generator,
- Generic,
- Iterable,
- List,
- Mapping,
- Optional,
- Set,
- Tuple,
- Type,
- TypeVar,
- Union,
-)
-
-import attr
-from multidict import CIMultiDict, MultiDict, MultiDictProxy, istr
-from yarl import URL
-
-from . import hdrs, http, payload
-from .abc import AbstractCookieJar
-from .client_exceptions import (
- ClientConnectionError as ClientConnectionError,
- ClientConnectorCertificateError as ClientConnectorCertificateError,
- ClientConnectorError as ClientConnectorError,
- ClientConnectorSSLError as ClientConnectorSSLError,
- ClientError as ClientError,
- ClientHttpProxyError as ClientHttpProxyError,
- ClientOSError as ClientOSError,
- ClientPayloadError as ClientPayloadError,
- ClientProxyConnectionError as ClientProxyConnectionError,
- ClientResponseError as ClientResponseError,
- ClientSSLError as ClientSSLError,
- ContentTypeError as ContentTypeError,
- InvalidURL as InvalidURL,
- ServerConnectionError as ServerConnectionError,
- ServerDisconnectedError as ServerDisconnectedError,
- ServerFingerprintMismatch as ServerFingerprintMismatch,
- ServerTimeoutError as ServerTimeoutError,
- TooManyRedirects as TooManyRedirects,
- WSServerHandshakeError as WSServerHandshakeError,
-)
-from .client_reqrep import (
- ClientRequest as ClientRequest,
- ClientResponse as ClientResponse,
- Fingerprint as Fingerprint,
- RequestInfo as RequestInfo,
- _merge_ssl_params,
-)
-from .client_ws import ClientWebSocketResponse as ClientWebSocketResponse
-from .connector import (
- BaseConnector as BaseConnector,
- NamedPipeConnector as NamedPipeConnector,
- TCPConnector as TCPConnector,
- UnixConnector as UnixConnector,
-)
-from .cookiejar import CookieJar
-from .helpers import (
- DEBUG,
- PY_36,
- BasicAuth,
- TimeoutHandle,
- ceil_timeout,
- get_env_proxy_for_url,
- get_running_loop,
- sentinel,
- strip_auth_from_url,
-)
-from .http import WS_KEY, HttpVersion, WebSocketReader, WebSocketWriter
-from .http_websocket import WSHandshakeError, WSMessage, ws_ext_gen, ws_ext_parse
-from .streams import FlowControlDataQueue
-from .tracing import Trace, TraceConfig
-from .typedefs import Final, JSONEncoder, LooseCookies, LooseHeaders, StrOrURL
-
-__all__ = (
- # client_exceptions
- "ClientConnectionError",
- "ClientConnectorCertificateError",
- "ClientConnectorError",
- "ClientConnectorSSLError",
- "ClientError",
- "ClientHttpProxyError",
- "ClientOSError",
- "ClientPayloadError",
- "ClientProxyConnectionError",
- "ClientResponseError",
- "ClientSSLError",
- "ContentTypeError",
- "InvalidURL",
- "ServerConnectionError",
- "ServerDisconnectedError",
- "ServerFingerprintMismatch",
- "ServerTimeoutError",
- "TooManyRedirects",
- "WSServerHandshakeError",
- # client_reqrep
- "ClientRequest",
- "ClientResponse",
- "Fingerprint",
- "RequestInfo",
- # connector
- "BaseConnector",
- "TCPConnector",
- "UnixConnector",
- "NamedPipeConnector",
- # client_ws
- "ClientWebSocketResponse",
- # client
- "ClientSession",
- "ClientTimeout",
- "request",
-)
-
-
-try:
- from ssl import SSLContext
-except ImportError: # pragma: no cover
- SSLContext = object # type: ignore[misc,assignment]
-
-
-@attr.s(auto_attribs=True, frozen=True, slots=True)
-class ClientTimeout:
- total: Optional[float] = None
- connect: Optional[float] = None
- sock_read: Optional[float] = None
- sock_connect: Optional[float] = None
-
- # pool_queue_timeout: Optional[float] = None
- # dns_resolution_timeout: Optional[float] = None
- # socket_connect_timeout: Optional[float] = None
- # connection_acquiring_timeout: Optional[float] = None
- # new_connection_timeout: Optional[float] = None
- # http_header_timeout: Optional[float] = None
- # response_body_timeout: Optional[float] = None
-
- # to create a timeout specific for a single request, either
- # - create a completely new one to overwrite the default
- # - or use http://www.attrs.org/en/stable/api.html#attr.evolve
- # to overwrite the defaults
-
-
-# 5 Minute default read timeout
-DEFAULT_TIMEOUT: Final[ClientTimeout] = ClientTimeout(total=5 * 60)
-
-_RetType = TypeVar("_RetType")
-
-
-class ClientSession:
- """First-class interface for making HTTP requests."""
-
- ATTRS = frozenset(
- [
- "_base_url",
- "_source_traceback",
- "_connector",
- "requote_redirect_url",
- "_loop",
- "_cookie_jar",
- "_connector_owner",
- "_default_auth",
- "_version",
- "_json_serialize",
- "_requote_redirect_url",
- "_timeout",
- "_raise_for_status",
- "_auto_decompress",
- "_trust_env",
- "_default_headers",
- "_skip_auto_headers",
- "_request_class",
- "_response_class",
- "_ws_response_class",
- "_trace_configs",
- "_read_bufsize",
- ]
- )
-
- _source_traceback = None # type: Optional[traceback.StackSummary]
- _connector = None # type: Optional[BaseConnector]
-
- def __init__(
- self,
- base_url: Optional[StrOrURL] = None,
- *,
- connector: Optional[BaseConnector] = None,
- loop: Optional[asyncio.AbstractEventLoop] = None,
- cookies: Optional[LooseCookies] = None,
- headers: Optional[LooseHeaders] = None,
- skip_auto_headers: Optional[Iterable[str]] = None,
- auth: Optional[BasicAuth] = None,
- json_serialize: JSONEncoder = json.dumps,
- request_class: Type[ClientRequest] = ClientRequest,
- response_class: Type[ClientResponse] = ClientResponse,
- ws_response_class: Type[ClientWebSocketResponse] = ClientWebSocketResponse,
- version: HttpVersion = http.HttpVersion11,
- cookie_jar: Optional[AbstractCookieJar] = None,
- connector_owner: bool = True,
- raise_for_status: bool = False,
- read_timeout: Union[float, object] = sentinel,
- conn_timeout: Optional[float] = None,
- timeout: Union[object, ClientTimeout] = sentinel,
- auto_decompress: bool = True,
- trust_env: bool = False,
- requote_redirect_url: bool = True,
- trace_configs: Optional[List[TraceConfig]] = None,
- read_bufsize: int = 2**16,
- ) -> None:
- if loop is None:
- if connector is not None:
- loop = connector._loop
-
- loop = get_running_loop(loop)
-
- if base_url is None or isinstance(base_url, URL):
- self._base_url: Optional[URL] = base_url
- else:
- self._base_url = URL(base_url)
- assert (
- self._base_url.origin() == self._base_url
- ), "Only absolute URLs without path part are supported"
-
- if connector is None:
- connector = TCPConnector(loop=loop)
-
- if connector._loop is not loop:
- raise RuntimeError("Session and connector has to use same event loop")
-
- self._loop = loop
-
- if loop.get_debug():
- self._source_traceback = traceback.extract_stack(sys._getframe(1))
-
- if cookie_jar is None:
- cookie_jar = CookieJar(loop=loop)
- self._cookie_jar = cookie_jar
-
- if cookies is not None:
- self._cookie_jar.update_cookies(cookies)
-
- self._connector = connector
- self._connector_owner = connector_owner
- self._default_auth = auth
- self._version = version
- self._json_serialize = json_serialize
- if timeout is sentinel:
- self._timeout = DEFAULT_TIMEOUT
- if read_timeout is not sentinel:
- warnings.warn(
- "read_timeout is deprecated, " "use timeout argument instead",
- DeprecationWarning,
- stacklevel=2,
- )
- self._timeout = attr.evolve(self._timeout, total=read_timeout)
- if conn_timeout is not None:
- self._timeout = attr.evolve(self._timeout, connect=conn_timeout)
- warnings.warn(
- "conn_timeout is deprecated, " "use timeout argument instead",
- DeprecationWarning,
- stacklevel=2,
- )
- else:
- self._timeout = timeout # type: ignore[assignment]
- if read_timeout is not sentinel:
- raise ValueError(
- "read_timeout and timeout parameters "
- "conflict, please setup "
- "timeout.read"
- )
- if conn_timeout is not None:
- raise ValueError(
- "conn_timeout and timeout parameters "
- "conflict, please setup "
- "timeout.connect"
- )
- self._raise_for_status = raise_for_status
- self._auto_decompress = auto_decompress
- self._trust_env = trust_env
- self._requote_redirect_url = requote_redirect_url
- self._read_bufsize = read_bufsize
-
- # Convert to list of tuples
- if headers:
- real_headers: CIMultiDict[str] = CIMultiDict(headers)
- else:
- real_headers = CIMultiDict()
- self._default_headers: CIMultiDict[str] = real_headers
- if skip_auto_headers is not None:
- self._skip_auto_headers = frozenset(istr(i) for i in skip_auto_headers)
- else:
- self._skip_auto_headers = frozenset()
-
- self._request_class = request_class
- self._response_class = response_class
- self._ws_response_class = ws_response_class
-
- self._trace_configs = trace_configs or []
- for trace_config in self._trace_configs:
- trace_config.freeze()
-
- def __init_subclass__(cls: Type["ClientSession"]) -> None:
- warnings.warn(
- "Inheritance class {} from ClientSession "
- "is discouraged".format(cls.__name__),
- DeprecationWarning,
- stacklevel=2,
- )
-
- if DEBUG:
-
- def __setattr__(self, name: str, val: Any) -> None:
- if name not in self.ATTRS:
- warnings.warn(
- "Setting custom ClientSession.{} attribute "
- "is discouraged".format(name),
- DeprecationWarning,
- stacklevel=2,
- )
- super().__setattr__(name, val)
-
- def __del__(self, _warnings: Any = warnings) -> None:
- if not self.closed:
- if PY_36:
- kwargs = {"source": self}
- else:
- kwargs = {}
- _warnings.warn(
- f"Unclosed client session {self!r}", ResourceWarning, **kwargs
- )
- context = {"client_session": self, "message": "Unclosed client session"}
- if self._source_traceback is not None:
- context["source_traceback"] = self._source_traceback
- self._loop.call_exception_handler(context)
-
- def request(
- self, method: str, url: StrOrURL, **kwargs: Any
- ) -> "_RequestContextManager":
- """Perform HTTP request."""
- return _RequestContextManager(self._request(method, url, **kwargs))
-
- def _build_url(self, str_or_url: StrOrURL) -> URL:
- url = URL(str_or_url)
- if self._base_url is None:
- return url
- else:
- assert not url.is_absolute() and url.path.startswith("/")
- return self._base_url.join(url)
-
- async def _request(
- self,
- method: str,
- str_or_url: StrOrURL,
- *,
- params: Optional[Mapping[str, str]] = None,
- data: Any = None,
- json: Any = None,
- cookies: Optional[LooseCookies] = None,
- headers: Optional[LooseHeaders] = None,
- skip_auto_headers: Optional[Iterable[str]] = None,
- auth: Optional[BasicAuth] = None,
- allow_redirects: bool = True,
- max_redirects: int = 10,
- compress: Optional[str] = None,
- chunked: Optional[bool] = None,
- expect100: bool = False,
- raise_for_status: Optional[bool] = None,
- read_until_eof: bool = True,
- proxy: Optional[StrOrURL] = None,
- proxy_auth: Optional[BasicAuth] = None,
- timeout: Union[ClientTimeout, object] = sentinel,
- verify_ssl: Optional[bool] = None,
- fingerprint: Optional[bytes] = None,
- ssl_context: Optional[SSLContext] = None,
- ssl: Optional[Union[SSLContext, bool, Fingerprint]] = None,
- proxy_headers: Optional[LooseHeaders] = None,
- trace_request_ctx: Optional[SimpleNamespace] = None,
- read_bufsize: Optional[int] = None,
- ) -> ClientResponse:
-
- # NOTE: timeout clamps existing connect and read timeouts. We cannot
- # set the default to None because we need to detect if the user wants
- # to use the existing timeouts by setting timeout to None.
-
- if self.closed:
- raise RuntimeError("Session is closed")
-
- ssl = _merge_ssl_params(ssl, verify_ssl, ssl_context, fingerprint)
-
- if data is not None and json is not None:
- raise ValueError(
- "data and json parameters can not be used at the same time"
- )
- elif json is not None:
- data = payload.JsonPayload(json, dumps=self._json_serialize)
-
- if not isinstance(chunked, bool) and chunked is not None:
- warnings.warn("Chunk size is deprecated #1615", DeprecationWarning)
-
- redirects = 0
- history = []
- version = self._version
-
- # Merge with default headers and transform to CIMultiDict
- headers = self._prepare_headers(headers)
- proxy_headers = self._prepare_headers(proxy_headers)
-
- try:
- url = self._build_url(str_or_url)
- except ValueError as e:
- raise InvalidURL(str_or_url) from e
-
- skip_headers = set(self._skip_auto_headers)
- if skip_auto_headers is not None:
- for i in skip_auto_headers:
- skip_headers.add(istr(i))
-
- if proxy is not None:
- try:
- proxy = URL(proxy)
- except ValueError as e:
- raise InvalidURL(proxy) from e
-
- if timeout is sentinel:
- real_timeout: ClientTimeout = self._timeout
- else:
- if not isinstance(timeout, ClientTimeout):
- real_timeout = ClientTimeout(total=timeout) # type: ignore[arg-type]
- else:
- real_timeout = timeout
- # timeout is cumulative for all request operations
- # (request, redirects, responses, data consuming)
- tm = TimeoutHandle(self._loop, real_timeout.total)
- handle = tm.start()
-
- if read_bufsize is None:
- read_bufsize = self._read_bufsize
-
- traces = [
- Trace(
- self,
- trace_config,
- trace_config.trace_config_ctx(trace_request_ctx=trace_request_ctx),
- )
- for trace_config in self._trace_configs
- ]
-
- for trace in traces:
- await trace.send_request_start(method, url.update_query(params), headers)
-
- timer = tm.timer()
- try:
- with timer:
- while True:
- url, auth_from_url = strip_auth_from_url(url)
- if auth and auth_from_url:
- raise ValueError(
- "Cannot combine AUTH argument with "
- "credentials encoded in URL"
- )
-
- if auth is None:
- auth = auth_from_url
- if auth is None:
- auth = self._default_auth
- # It would be confusing if we support explicit
- # Authorization header with auth argument
- if (
- headers is not None
- and auth is not None
- and hdrs.AUTHORIZATION in headers
- ):
- raise ValueError(
- "Cannot combine AUTHORIZATION header "
- "with AUTH argument or credentials "
- "encoded in URL"
- )
-
- all_cookies = self._cookie_jar.filter_cookies(url)
-
- if cookies is not None:
- tmp_cookie_jar = CookieJar()
- tmp_cookie_jar.update_cookies(cookies)
- req_cookies = tmp_cookie_jar.filter_cookies(url)
- if req_cookies:
- all_cookies.load(req_cookies)
-
- if proxy is not None:
- proxy = URL(proxy)
- elif self._trust_env:
- with suppress(LookupError):
- proxy, proxy_auth = get_env_proxy_for_url(url)
-
- req = self._request_class(
- method,
- url,
- params=params,
- headers=headers,
- skip_auto_headers=skip_headers,
- data=data,
- cookies=all_cookies,
- auth=auth,
- version=version,
- compress=compress,
- chunked=chunked,
- expect100=expect100,
- loop=self._loop,
- response_class=self._response_class,
- proxy=proxy,
- proxy_auth=proxy_auth,
- timer=timer,
- session=self,
- ssl=ssl,
- proxy_headers=proxy_headers,
- traces=traces,
- )
-
- # connection timeout
- try:
- async with ceil_timeout(real_timeout.connect):
- assert self._connector is not None
- conn = await self._connector.connect(
- req, traces=traces, timeout=real_timeout
- )
- except asyncio.TimeoutError as exc:
- raise ServerTimeoutError(
- "Connection timeout " "to host {}".format(url)
- ) from exc
-
- assert conn.transport is not None
-
- assert conn.protocol is not None
- conn.protocol.set_response_params(
- timer=timer,
- skip_payload=method.upper() == "HEAD",
- read_until_eof=read_until_eof,
- auto_decompress=self._auto_decompress,
- read_timeout=real_timeout.sock_read,
- read_bufsize=read_bufsize,
- )
-
- try:
- try:
- resp = await req.send(conn)
- try:
- await resp.start(conn)
- except BaseException:
- resp.close()
- raise
- except BaseException:
- conn.close()
- raise
- except ClientError:
- raise
- except OSError as exc:
- if exc.errno is None and isinstance(exc, asyncio.TimeoutError):
- raise
- raise ClientOSError(*exc.args) from exc
-
- self._cookie_jar.update_cookies(resp.cookies, resp.url)
-
- # redirects
- if resp.status in (301, 302, 303, 307, 308) and allow_redirects:
-
- for trace in traces:
- await trace.send_request_redirect(
- method, url.update_query(params), headers, resp
- )
-
- redirects += 1
- history.append(resp)
- if max_redirects and redirects >= max_redirects:
- resp.close()
- raise TooManyRedirects(
- history[0].request_info, tuple(history)
- )
-
- # For 301 and 302, mimic IE, now changed in RFC
- # https://github.com/kennethreitz/requests/pull/269
- if (resp.status == 303 and resp.method != hdrs.METH_HEAD) or (
- resp.status in (301, 302) and resp.method == hdrs.METH_POST
- ):
- method = hdrs.METH_GET
- data = None
- if headers.get(hdrs.CONTENT_LENGTH):
- headers.pop(hdrs.CONTENT_LENGTH)
-
- r_url = resp.headers.get(hdrs.LOCATION) or resp.headers.get(
- hdrs.URI
- )
- if r_url is None:
- # see github.com/aio-libs/aiohttp/issues/2022
- break
- else:
- # reading from correct redirection
- # response is forbidden
- resp.release()
-
- try:
- parsed_url = URL(
- r_url, encoded=not self._requote_redirect_url
- )
-
- except ValueError as e:
- raise InvalidURL(r_url) from e
-
- scheme = parsed_url.scheme
- if scheme not in ("http", "https", ""):
- resp.close()
- raise ValueError("Can redirect only to http or https")
- elif not scheme:
- parsed_url = url.join(parsed_url)
-
- if url.origin() != parsed_url.origin():
- auth = None
- headers.pop(hdrs.AUTHORIZATION, None)
-
- url = parsed_url
- params = None
- resp.release()
- continue
-
- break
-
- # check response status
- if raise_for_status is None:
- raise_for_status = self._raise_for_status
- if raise_for_status:
- resp.raise_for_status()
-
- # register connection
- if handle is not None:
- if resp.connection is not None:
- resp.connection.add_callback(handle.cancel)
- else:
- handle.cancel()
-
- resp._history = tuple(history)
-
- for trace in traces:
- await trace.send_request_end(
- method, url.update_query(params), headers, resp
- )
- return resp
-
- except BaseException as e:
- # cleanup timer
- tm.close()
- if handle:
- handle.cancel()
- handle = None
-
- for trace in traces:
- await trace.send_request_exception(
- method, url.update_query(params), headers, e
- )
- raise
-
- def ws_connect(
- self,
- url: StrOrURL,
- *,
- method: str = hdrs.METH_GET,
- protocols: Iterable[str] = (),
- timeout: float = 10.0,
- receive_timeout: Optional[float] = None,
- autoclose: bool = True,
- autoping: bool = True,
- heartbeat: Optional[float] = None,
- auth: Optional[BasicAuth] = None,
- origin: Optional[str] = None,
- params: Optional[Mapping[str, str]] = None,
- headers: Optional[LooseHeaders] = None,
- proxy: Optional[StrOrURL] = None,
- proxy_auth: Optional[BasicAuth] = None,
- ssl: Union[SSLContext, bool, None, Fingerprint] = None,
- verify_ssl: Optional[bool] = None,
- fingerprint: Optional[bytes] = None,
- ssl_context: Optional[SSLContext] = None,
- proxy_headers: Optional[LooseHeaders] = None,
- compress: int = 0,
- max_msg_size: int = 4 * 1024 * 1024,
- ) -> "_WSRequestContextManager":
- """Initiate websocket connection."""
- return _WSRequestContextManager(
- self._ws_connect(
- url,
- method=method,
- protocols=protocols,
- timeout=timeout,
- receive_timeout=receive_timeout,
- autoclose=autoclose,
- autoping=autoping,
- heartbeat=heartbeat,
- auth=auth,
- origin=origin,
- params=params,
- headers=headers,
- proxy=proxy,
- proxy_auth=proxy_auth,
- ssl=ssl,
- verify_ssl=verify_ssl,
- fingerprint=fingerprint,
- ssl_context=ssl_context,
- proxy_headers=proxy_headers,
- compress=compress,
- max_msg_size=max_msg_size,
- )
- )
-
- async def _ws_connect(
- self,
- url: StrOrURL,
- *,
- method: str = hdrs.METH_GET,
- protocols: Iterable[str] = (),
- timeout: float = 10.0,
- receive_timeout: Optional[float] = None,
- autoclose: bool = True,
- autoping: bool = True,
- heartbeat: Optional[float] = None,
- auth: Optional[BasicAuth] = None,
- origin: Optional[str] = None,
- params: Optional[Mapping[str, str]] = None,
- headers: Optional[LooseHeaders] = None,
- proxy: Optional[StrOrURL] = None,
- proxy_auth: Optional[BasicAuth] = None,
- ssl: Union[SSLContext, bool, None, Fingerprint] = None,
- verify_ssl: Optional[bool] = None,
- fingerprint: Optional[bytes] = None,
- ssl_context: Optional[SSLContext] = None,
- proxy_headers: Optional[LooseHeaders] = None,
- compress: int = 0,
- max_msg_size: int = 4 * 1024 * 1024,
- ) -> ClientWebSocketResponse:
-
- if headers is None:
- real_headers: CIMultiDict[str] = CIMultiDict()
- else:
- real_headers = CIMultiDict(headers)
-
- default_headers = {
- hdrs.UPGRADE: "websocket",
- hdrs.CONNECTION: "upgrade",
- hdrs.SEC_WEBSOCKET_VERSION: "13",
- }
-
- for key, value in default_headers.items():
- real_headers.setdefault(key, value)
-
- sec_key = base64.b64encode(os.urandom(16))
- real_headers[hdrs.SEC_WEBSOCKET_KEY] = sec_key.decode()
-
- if protocols:
- real_headers[hdrs.SEC_WEBSOCKET_PROTOCOL] = ",".join(protocols)
- if origin is not None:
- real_headers[hdrs.ORIGIN] = origin
- if compress:
- extstr = ws_ext_gen(compress=compress)
- real_headers[hdrs.SEC_WEBSOCKET_EXTENSIONS] = extstr
-
- ssl = _merge_ssl_params(ssl, verify_ssl, ssl_context, fingerprint)
-
- # send request
- resp = await self.request(
- method,
- url,
- params=params,
- headers=real_headers,
- read_until_eof=False,
- auth=auth,
- proxy=proxy,
- proxy_auth=proxy_auth,
- ssl=ssl,
- proxy_headers=proxy_headers,
- )
-
- try:
- # check handshake
- if resp.status != 101:
- raise WSServerHandshakeError(
- resp.request_info,
- resp.history,
- message="Invalid response status",
- status=resp.status,
- headers=resp.headers,
- )
-
- if resp.headers.get(hdrs.UPGRADE, "").lower() != "websocket":
- raise WSServerHandshakeError(
- resp.request_info,
- resp.history,
- message="Invalid upgrade header",
- status=resp.status,
- headers=resp.headers,
- )
-
- if resp.headers.get(hdrs.CONNECTION, "").lower() != "upgrade":
- raise WSServerHandshakeError(
- resp.request_info,
- resp.history,
- message="Invalid connection header",
- status=resp.status,
- headers=resp.headers,
- )
-
- # key calculation
- r_key = resp.headers.get(hdrs.SEC_WEBSOCKET_ACCEPT, "")
- match = base64.b64encode(hashlib.sha1(sec_key + WS_KEY).digest()).decode()
- if r_key != match:
- raise WSServerHandshakeError(
- resp.request_info,
- resp.history,
- message="Invalid challenge response",
- status=resp.status,
- headers=resp.headers,
- )
-
- # websocket protocol
- protocol = None
- if protocols and hdrs.SEC_WEBSOCKET_PROTOCOL in resp.headers:
- resp_protocols = [
- proto.strip()
- for proto in resp.headers[hdrs.SEC_WEBSOCKET_PROTOCOL].split(",")
- ]
-
- for proto in resp_protocols:
- if proto in protocols:
- protocol = proto
- break
-
- # websocket compress
- notakeover = False
- if compress:
- compress_hdrs = resp.headers.get(hdrs.SEC_WEBSOCKET_EXTENSIONS)
- if compress_hdrs:
- try:
- compress, notakeover = ws_ext_parse(compress_hdrs)
- except WSHandshakeError as exc:
- raise WSServerHandshakeError(
- resp.request_info,
- resp.history,
- message=exc.args[0],
- status=resp.status,
- headers=resp.headers,
- ) from exc
- else:
- compress = 0
- notakeover = False
-
- conn = resp.connection
- assert conn is not None
- conn_proto = conn.protocol
- assert conn_proto is not None
- transport = conn.transport
- assert transport is not None
- reader: FlowControlDataQueue[WSMessage] = FlowControlDataQueue(
- conn_proto, 2**16, loop=self._loop
- )
- conn_proto.set_parser(WebSocketReader(reader, max_msg_size), reader)
- writer = WebSocketWriter(
- conn_proto,
- transport,
- use_mask=True,
- compress=compress,
- notakeover=notakeover,
- )
- except BaseException:
- resp.close()
- raise
- else:
- return self._ws_response_class(
- reader,
- writer,
- protocol,
- resp,
- timeout,
- autoclose,
- autoping,
- self._loop,
- receive_timeout=receive_timeout,
- heartbeat=heartbeat,
- compress=compress,
- client_notakeover=notakeover,
- )
-
- def _prepare_headers(self, headers: Optional[LooseHeaders]) -> "CIMultiDict[str]":
- """Add default headers and transform it to CIMultiDict"""
- # Convert headers to MultiDict
- result = CIMultiDict(self._default_headers)
- if headers:
- if not isinstance(headers, (MultiDictProxy, MultiDict)):
- headers = CIMultiDict(headers)
- added_names: Set[str] = set()
- for key, value in headers.items():
- if key in added_names:
- result.add(key, value)
- else:
- result[key] = value
- added_names.add(key)
- return result
-
- def get(
- self, url: StrOrURL, *, allow_redirects: bool = True, **kwargs: Any
- ) -> "_RequestContextManager":
- """Perform HTTP GET request."""
- return _RequestContextManager(
- self._request(hdrs.METH_GET, url, allow_redirects=allow_redirects, **kwargs)
- )
-
- def options(
- self, url: StrOrURL, *, allow_redirects: bool = True, **kwargs: Any
- ) -> "_RequestContextManager":
- """Perform HTTP OPTIONS request."""
- return _RequestContextManager(
- self._request(
- hdrs.METH_OPTIONS, url, allow_redirects=allow_redirects, **kwargs
- )
- )
-
- def head(
- self, url: StrOrURL, *, allow_redirects: bool = False, **kwargs: Any
- ) -> "_RequestContextManager":
- """Perform HTTP HEAD request."""
- return _RequestContextManager(
- self._request(
- hdrs.METH_HEAD, url, allow_redirects=allow_redirects, **kwargs
- )
- )
-
- def post(
- self, url: StrOrURL, *, data: Any = None, **kwargs: Any
- ) -> "_RequestContextManager":
- """Perform HTTP POST request."""
- return _RequestContextManager(
- self._request(hdrs.METH_POST, url, data=data, **kwargs)
- )
-
- def put(
- self, url: StrOrURL, *, data: Any = None, **kwargs: Any
- ) -> "_RequestContextManager":
- """Perform HTTP PUT request."""
- return _RequestContextManager(
- self._request(hdrs.METH_PUT, url, data=data, **kwargs)
- )
-
- def patch(
- self, url: StrOrURL, *, data: Any = None, **kwargs: Any
- ) -> "_RequestContextManager":
- """Perform HTTP PATCH request."""
- return _RequestContextManager(
- self._request(hdrs.METH_PATCH, url, data=data, **kwargs)
- )
-
- def delete(self, url: StrOrURL, **kwargs: Any) -> "_RequestContextManager":
- """Perform HTTP DELETE request."""
- return _RequestContextManager(self._request(hdrs.METH_DELETE, url, **kwargs))
-
- async def close(self) -> None:
- """Close underlying connector.
-
- Release all acquired resources.
- """
- if not self.closed:
- if self._connector is not None and self._connector_owner:
- await self._connector.close()
- self._connector = None
-
- @property
- def closed(self) -> bool:
- """Is client session closed.
-
- A readonly property.
- """
- return self._connector is None or self._connector.closed
-
- @property
- def connector(self) -> Optional[BaseConnector]:
- """Connector instance used for the session."""
- return self._connector
-
- @property
- def cookie_jar(self) -> AbstractCookieJar:
- """The session cookies."""
- return self._cookie_jar
-
- @property
- def version(self) -> Tuple[int, int]:
- """The session HTTP protocol version."""
- return self._version
-
- @property
- def requote_redirect_url(self) -> bool:
- """Do URL requoting on redirection handling."""
- return self._requote_redirect_url
-
- @requote_redirect_url.setter
- def requote_redirect_url(self, val: bool) -> None:
- """Do URL requoting on redirection handling."""
- warnings.warn(
- "session.requote_redirect_url modification " "is deprecated #2778",
- DeprecationWarning,
- stacklevel=2,
- )
- self._requote_redirect_url = val
-
- @property
- def loop(self) -> asyncio.AbstractEventLoop:
- """Session's loop."""
- warnings.warn(
- "client.loop property is deprecated", DeprecationWarning, stacklevel=2
- )
- return self._loop
-
- @property
- def timeout(self) -> ClientTimeout:
- """Timeout for the session."""
- return self._timeout
-
- @property
- def headers(self) -> "CIMultiDict[str]":
- """The default headers of the client session."""
- return self._default_headers
-
- @property
- def skip_auto_headers(self) -> FrozenSet[istr]:
- """Headers for which autogeneration should be skipped"""
- return self._skip_auto_headers
-
- @property
- def auth(self) -> Optional[BasicAuth]:
- """An object that represents HTTP Basic Authorization"""
- return self._default_auth
-
- @property
- def json_serialize(self) -> JSONEncoder:
- """Json serializer callable"""
- return self._json_serialize
-
- @property
- def connector_owner(self) -> bool:
- """Should connector be closed on session closing"""
- return self._connector_owner
-
- @property
- def raise_for_status(
- self,
- ) -> Union[bool, Callable[[ClientResponse], Awaitable[None]]]:
- """Should `ClientResponse.raise_for_status()` be called for each response."""
- return self._raise_for_status
-
- @property
- def auto_decompress(self) -> bool:
- """Should the body response be automatically decompressed."""
- return self._auto_decompress
-
- @property
- def trust_env(self) -> bool:
- """
- Should proxies information from environment or netrc be trusted.
-
- Information is from HTTP_PROXY / HTTPS_PROXY environment variables
- or ~/.netrc file if present.
- """
- return self._trust_env
-
- @property
- def trace_configs(self) -> List[TraceConfig]:
- """A list of TraceConfig instances used for client tracing"""
- return self._trace_configs
-
- def detach(self) -> None:
- """Detach connector from session without closing the former.
-
- Session is switched to closed state anyway.
- """
- self._connector = None
-
- def __enter__(self) -> None:
- raise TypeError("Use async with instead")
-
- def __exit__(
- self,
- exc_type: Optional[Type[BaseException]],
- exc_val: Optional[BaseException],
- exc_tb: Optional[TracebackType],
- ) -> None:
- # __exit__ should exist in pair with __enter__ but never executed
- pass # pragma: no cover
-
- async def __aenter__(self) -> "ClientSession":
- return self
-
- async def __aexit__(
- self,
- exc_type: Optional[Type[BaseException]],
- exc_val: Optional[BaseException],
- exc_tb: Optional[TracebackType],
- ) -> None:
- await self.close()
-
-
-class _BaseRequestContextManager(Coroutine[Any, Any, _RetType], Generic[_RetType]):
-
- __slots__ = ("_coro", "_resp")
-
- def __init__(self, coro: Coroutine["asyncio.Future[Any]", None, _RetType]) -> None:
- self._coro = coro
-
- def send(self, arg: None) -> "asyncio.Future[Any]":
- return self._coro.send(arg)
-
- def throw(self, arg: BaseException) -> None: # type: ignore[arg-type,override]
- self._coro.throw(arg)
-
- def close(self) -> None:
- return self._coro.close()
-
- def __await__(self) -> Generator[Any, None, _RetType]:
- ret = self._coro.__await__()
- return ret
-
- def __iter__(self) -> Generator[Any, None, _RetType]:
- return self.__await__()
-
- async def __aenter__(self) -> _RetType:
- self._resp = await self._coro
- return self._resp
-
-
-class _RequestContextManager(_BaseRequestContextManager[ClientResponse]):
- __slots__ = ()
-
- async def __aexit__(
- self,
- exc_type: Optional[Type[BaseException]],
- exc: Optional[BaseException],
- tb: Optional[TracebackType],
- ) -> None:
- # We're basing behavior on the exception as it can be caused by
- # user code unrelated to the status of the connection. If you
- # would like to close a connection you must do that
- # explicitly. Otherwise connection error handling should kick in
- # and close/recycle the connection as required.
- self._resp.release()
-
-
-class _WSRequestContextManager(_BaseRequestContextManager[ClientWebSocketResponse]):
- __slots__ = ()
-
- async def __aexit__(
- self,
- exc_type: Optional[Type[BaseException]],
- exc: Optional[BaseException],
- tb: Optional[TracebackType],
- ) -> None:
- await self._resp.close()
-
-
-class _SessionRequestContextManager:
-
- __slots__ = ("_coro", "_resp", "_session")
-
- def __init__(
- self,
- coro: Coroutine["asyncio.Future[Any]", None, ClientResponse],
- session: ClientSession,
- ) -> None:
- self._coro = coro
- self._resp: Optional[ClientResponse] = None
- self._session = session
-
- async def __aenter__(self) -> ClientResponse:
- try:
- self._resp = await self._coro
- except BaseException:
- await self._session.close()
- raise
- else:
- return self._resp
-
- async def __aexit__(
- self,
- exc_type: Optional[Type[BaseException]],
- exc: Optional[BaseException],
- tb: Optional[TracebackType],
- ) -> None:
- assert self._resp is not None
- self._resp.close()
- await self._session.close()
-
-
-def request(
- method: str,
- url: StrOrURL,
- *,
- params: Optional[Mapping[str, str]] = None,
- data: Any = None,
- json: Any = None,
- headers: Optional[LooseHeaders] = None,
- skip_auto_headers: Optional[Iterable[str]] = None,
- auth: Optional[BasicAuth] = None,
- allow_redirects: bool = True,
- max_redirects: int = 10,
- compress: Optional[str] = None,
- chunked: Optional[bool] = None,
- expect100: bool = False,
- raise_for_status: Optional[bool] = None,
- read_until_eof: bool = True,
- proxy: Optional[StrOrURL] = None,
- proxy_auth: Optional[BasicAuth] = None,
- timeout: Union[ClientTimeout, object] = sentinel,
- cookies: Optional[LooseCookies] = None,
- version: HttpVersion = http.HttpVersion11,
- connector: Optional[BaseConnector] = None,
- read_bufsize: Optional[int] = None,
- loop: Optional[asyncio.AbstractEventLoop] = None,
-) -> _SessionRequestContextManager:
- """Constructs and sends a request.
-
- Returns response object.
- method - HTTP method
- url - request url
- params - (optional) Dictionary or bytes to be sent in the query
- string of the new request
- data - (optional) Dictionary, bytes, or file-like object to
- send in the body of the request
- json - (optional) Any json compatible python object
- headers - (optional) Dictionary of HTTP Headers to send with
- the request
- cookies - (optional) Dict object to send with the request
- auth - (optional) BasicAuth named tuple represent HTTP Basic Auth
- auth - aiohttp.helpers.BasicAuth
- allow_redirects - (optional) If set to False, do not follow
- redirects
- version - Request HTTP version.
- compress - Set to True if request has to be compressed
- with deflate encoding.
- chunked - Set to chunk size for chunked transfer encoding.
- expect100 - Expect 100-continue response from server.
- connector - BaseConnector sub-class instance to support
- connection pooling.
- read_until_eof - Read response until eof if response
- does not have Content-Length header.
- loop - Optional event loop.
- timeout - Optional ClientTimeout settings structure, 5min
- total timeout by default.
- Usage::
- >>> import aiohttp
- >>> resp = await aiohttp.request('GET', 'http://python.org/')
- >>> resp
-
- >>> data = await resp.read()
- """
- connector_owner = False
- if connector is None:
- connector_owner = True
- connector = TCPConnector(loop=loop, force_close=True)
-
- session = ClientSession(
- loop=loop,
- cookies=cookies,
- version=version,
- timeout=timeout,
- connector=connector,
- connector_owner=connector_owner,
- )
-
- return _SessionRequestContextManager(
- session._request(
- method,
- url,
- params=params,
- data=data,
- json=json,
- headers=headers,
- skip_auto_headers=skip_auto_headers,
- auth=auth,
- allow_redirects=allow_redirects,
- max_redirects=max_redirects,
- compress=compress,
- chunked=chunked,
- expect100=expect100,
- raise_for_status=raise_for_status,
- read_until_eof=read_until_eof,
- proxy=proxy,
- proxy_auth=proxy_auth,
- read_bufsize=read_bufsize,
- ),
- session,
- )
diff --git a/spaces/dcarpintero/nlp-summarizer-pegasus/.venv/lib/python3.9/site-packages/anyio/_core/_tasks.py b/spaces/dcarpintero/nlp-summarizer-pegasus/.venv/lib/python3.9/site-packages/anyio/_core/_tasks.py
deleted file mode 100644
index e9d9c2bd67f105d9e728ffed5496b010051b1452..0000000000000000000000000000000000000000
--- a/spaces/dcarpintero/nlp-summarizer-pegasus/.venv/lib/python3.9/site-packages/anyio/_core/_tasks.py
+++ /dev/null
@@ -1,180 +0,0 @@
-from __future__ import annotations
-
-import math
-from types import TracebackType
-from warnings import warn
-
-from ..abc._tasks import TaskGroup, TaskStatus
-from ._compat import (
- DeprecatedAsyncContextManager,
- DeprecatedAwaitable,
- DeprecatedAwaitableFloat,
-)
-from ._eventloop import get_asynclib
-
-
-class _IgnoredTaskStatus(TaskStatus[object]):
- def started(self, value: object = None) -> None:
- pass
-
-
-TASK_STATUS_IGNORED = _IgnoredTaskStatus()
-
-
-class CancelScope(DeprecatedAsyncContextManager["CancelScope"]):
- """
- Wraps a unit of work that can be made separately cancellable.
-
- :param deadline: The time (clock value) when this scope is cancelled automatically
- :param shield: ``True`` to shield the cancel scope from external cancellation
- """
-
- def __new__(
- cls, *, deadline: float = math.inf, shield: bool = False
- ) -> CancelScope:
- return get_asynclib().CancelScope(shield=shield, deadline=deadline)
-
- def cancel(self) -> DeprecatedAwaitable:
- """Cancel this scope immediately."""
- raise NotImplementedError
-
- @property
- def deadline(self) -> float:
- """
- The time (clock value) when this scope is cancelled automatically.
-
- Will be ``float('inf')`` if no timeout has been set.
-
- """
- raise NotImplementedError
-
- @deadline.setter
- def deadline(self, value: float) -> None:
- raise NotImplementedError
-
- @property
- def cancel_called(self) -> bool:
- """``True`` if :meth:`cancel` has been called."""
- raise NotImplementedError
-
- @property
- def shield(self) -> bool:
- """
- ``True`` if this scope is shielded from external cancellation.
-
- While a scope is shielded, it will not receive cancellations from outside.
-
- """
- raise NotImplementedError
-
- @shield.setter
- def shield(self, value: bool) -> None:
- raise NotImplementedError
-
- def __enter__(self) -> CancelScope:
- raise NotImplementedError
-
- def __exit__(
- self,
- exc_type: type[BaseException] | None,
- exc_val: BaseException | None,
- exc_tb: TracebackType | None,
- ) -> bool | None:
- raise NotImplementedError
-
-
-def open_cancel_scope(*, shield: bool = False) -> CancelScope:
- """
- Open a cancel scope.
-
- :param shield: ``True`` to shield the cancel scope from external cancellation
- :return: a cancel scope
-
- .. deprecated:: 3.0
- Use :class:`~CancelScope` directly.
-
- """
- warn(
- "open_cancel_scope() is deprecated -- use CancelScope() directly",
- DeprecationWarning,
- )
- return get_asynclib().CancelScope(shield=shield)
-
-
-class FailAfterContextManager(DeprecatedAsyncContextManager[CancelScope]):
- def __init__(self, cancel_scope: CancelScope):
- self._cancel_scope = cancel_scope
-
- def __enter__(self) -> CancelScope:
- return self._cancel_scope.__enter__()
-
- def __exit__(
- self,
- exc_type: type[BaseException] | None,
- exc_val: BaseException | None,
- exc_tb: TracebackType | None,
- ) -> bool | None:
- retval = self._cancel_scope.__exit__(exc_type, exc_val, exc_tb)
- if self._cancel_scope.cancel_called:
- raise TimeoutError
-
- return retval
-
-
-def fail_after(delay: float | None, shield: bool = False) -> FailAfterContextManager:
- """
- Create a context manager which raises a :class:`TimeoutError` if does not finish in time.
-
- :param delay: maximum allowed time (in seconds) before raising the exception, or ``None`` to
- disable the timeout
- :param shield: ``True`` to shield the cancel scope from external cancellation
- :return: a context manager that yields a cancel scope
- :rtype: :class:`~typing.ContextManager`\\[:class:`~anyio.CancelScope`\\]
-
- """
- deadline = (
- (get_asynclib().current_time() + delay) if delay is not None else math.inf
- )
- cancel_scope = get_asynclib().CancelScope(deadline=deadline, shield=shield)
- return FailAfterContextManager(cancel_scope)
-
-
-def move_on_after(delay: float | None, shield: bool = False) -> CancelScope:
- """
- Create a cancel scope with a deadline that expires after the given delay.
-
- :param delay: maximum allowed time (in seconds) before exiting the context block, or ``None``
- to disable the timeout
- :param shield: ``True`` to shield the cancel scope from external cancellation
- :return: a cancel scope
-
- """
- deadline = (
- (get_asynclib().current_time() + delay) if delay is not None else math.inf
- )
- return get_asynclib().CancelScope(deadline=deadline, shield=shield)
-
-
-def current_effective_deadline() -> DeprecatedAwaitableFloat:
- """
- Return the nearest deadline among all the cancel scopes effective for the current task.
-
- :return: a clock value from the event loop's internal clock (or ``float('inf')`` if
- there is no deadline in effect, or ``float('-inf')`` if the current scope has
- been cancelled)
- :rtype: float
-
- """
- return DeprecatedAwaitableFloat(
- get_asynclib().current_effective_deadline(), current_effective_deadline
- )
-
-
-def create_task_group() -> TaskGroup:
- """
- Create a task group.
-
- :return: a task group
-
- """
- return get_asynclib().TaskGroup()
diff --git a/spaces/dcarpintero/nlp-summarizer-pegasus/.venv/lib/python3.9/site-packages/fontTools/varLib/instancer/names.py b/spaces/dcarpintero/nlp-summarizer-pegasus/.venv/lib/python3.9/site-packages/fontTools/varLib/instancer/names.py
deleted file mode 100644
index dad3fd7e57d86dff555818ee14e8239cf73435fe..0000000000000000000000000000000000000000
--- a/spaces/dcarpintero/nlp-summarizer-pegasus/.venv/lib/python3.9/site-packages/fontTools/varLib/instancer/names.py
+++ /dev/null
@@ -1,380 +0,0 @@
-"""Helpers for instantiating name table records."""
-
-from contextlib import contextmanager
-from copy import deepcopy
-from enum import IntEnum
-import re
-
-
-class NameID(IntEnum):
- FAMILY_NAME = 1
- SUBFAMILY_NAME = 2
- UNIQUE_FONT_IDENTIFIER = 3
- FULL_FONT_NAME = 4
- VERSION_STRING = 5
- POSTSCRIPT_NAME = 6
- TYPOGRAPHIC_FAMILY_NAME = 16
- TYPOGRAPHIC_SUBFAMILY_NAME = 17
- VARIATIONS_POSTSCRIPT_NAME_PREFIX = 25
-
-
-ELIDABLE_AXIS_VALUE_NAME = 2
-
-
-def getVariationNameIDs(varfont):
- used = []
- if "fvar" in varfont:
- fvar = varfont["fvar"]
- for axis in fvar.axes:
- used.append(axis.axisNameID)
- for instance in fvar.instances:
- used.append(instance.subfamilyNameID)
- if instance.postscriptNameID != 0xFFFF:
- used.append(instance.postscriptNameID)
- if "STAT" in varfont:
- stat = varfont["STAT"].table
- for axis in stat.DesignAxisRecord.Axis if stat.DesignAxisRecord else ():
- used.append(axis.AxisNameID)
- for value in stat.AxisValueArray.AxisValue if stat.AxisValueArray else ():
- used.append(value.ValueNameID)
- elidedFallbackNameID = getattr(stat, "ElidedFallbackNameID", None)
- if elidedFallbackNameID is not None:
- used.append(elidedFallbackNameID)
- # nameIDs <= 255 are reserved by OT spec so we don't touch them
- return {nameID for nameID in used if nameID > 255}
-
-
-@contextmanager
-def pruningUnusedNames(varfont):
- from . import log
-
- origNameIDs = getVariationNameIDs(varfont)
-
- yield
-
- log.info("Pruning name table")
- exclude = origNameIDs - getVariationNameIDs(varfont)
- varfont["name"].names[:] = [
- record for record in varfont["name"].names if record.nameID not in exclude
- ]
- if "ltag" in varfont:
- # Drop the whole 'ltag' table if all the language-dependent Unicode name
- # records that reference it have been dropped.
- # TODO: Only prune unused ltag tags, renumerating langIDs accordingly.
- # Note ltag can also be used by feat or morx tables, so check those too.
- if not any(
- record
- for record in varfont["name"].names
- if record.platformID == 0 and record.langID != 0xFFFF
- ):
- del varfont["ltag"]
-
-
-def updateNameTable(varfont, axisLimits):
- """Update instatiated variable font's name table using STAT AxisValues.
-
- Raises ValueError if the STAT table is missing or an Axis Value table is
- missing for requested axis locations.
-
- First, collect all STAT AxisValues that match the new default axis locations
- (excluding "elided" ones); concatenate the strings in design axis order,
- while giving priority to "synthetic" values (Format 4), to form the
- typographic subfamily name associated with the new default instance.
- Finally, update all related records in the name table, making sure that
- legacy family/sub-family names conform to the the R/I/B/BI (Regular, Italic,
- Bold, Bold Italic) naming model.
-
- Example: Updating a partial variable font:
- | >>> ttFont = TTFont("OpenSans[wdth,wght].ttf")
- | >>> updateNameTable(ttFont, {"wght": (400, 900), "wdth": 75})
-
- The name table records will be updated in the following manner:
- NameID 1 familyName: "Open Sans" --> "Open Sans Condensed"
- NameID 2 subFamilyName: "Regular" --> "Regular"
- NameID 3 Unique font identifier: "3.000;GOOG;OpenSans-Regular" --> \
- "3.000;GOOG;OpenSans-Condensed"
- NameID 4 Full font name: "Open Sans Regular" --> "Open Sans Condensed"
- NameID 6 PostScript name: "OpenSans-Regular" --> "OpenSans-Condensed"
- NameID 16 Typographic Family name: None --> "Open Sans"
- NameID 17 Typographic Subfamily name: None --> "Condensed"
-
- References:
- https://docs.microsoft.com/en-us/typography/opentype/spec/stat
- https://docs.microsoft.com/en-us/typography/opentype/spec/name#name-ids
- """
- from . import AxisLimits, axisValuesFromAxisLimits
-
- if "STAT" not in varfont:
- raise ValueError("Cannot update name table since there is no STAT table.")
- stat = varfont["STAT"].table
- if not stat.AxisValueArray:
- raise ValueError("Cannot update name table since there are no STAT Axis Values")
- fvar = varfont["fvar"]
-
- # The updated name table will reflect the new 'zero origin' of the font.
- # If we're instantiating a partial font, we will populate the unpinned
- # axes with their default axis values from fvar.
- axisLimits = AxisLimits(axisLimits).limitAxesAndPopulateDefaults(varfont)
- partialDefaults = axisLimits.defaultLocation()
- fvarDefaults = {a.axisTag: a.defaultValue for a in fvar.axes}
- defaultAxisCoords = AxisLimits({**fvarDefaults, **partialDefaults})
- assert all(v.minimum == v.maximum for v in defaultAxisCoords.values())
-
- axisValueTables = axisValuesFromAxisLimits(stat, defaultAxisCoords)
- checkAxisValuesExist(stat, axisValueTables, defaultAxisCoords.pinnedLocation())
-
- # ignore "elidable" axis values, should be omitted in application font menus.
- axisValueTables = [
- v for v in axisValueTables if not v.Flags & ELIDABLE_AXIS_VALUE_NAME
- ]
- axisValueTables = _sortAxisValues(axisValueTables)
- _updateNameRecords(varfont, axisValueTables)
-
-
-def checkAxisValuesExist(stat, axisValues, axisCoords):
- seen = set()
- designAxes = stat.DesignAxisRecord.Axis
- for axisValueTable in axisValues:
- axisValueFormat = axisValueTable.Format
- if axisValueTable.Format in (1, 2, 3):
- axisTag = designAxes[axisValueTable.AxisIndex].AxisTag
- if axisValueFormat == 2:
- axisValue = axisValueTable.NominalValue
- else:
- axisValue = axisValueTable.Value
- if axisTag in axisCoords and axisValue == axisCoords[axisTag]:
- seen.add(axisTag)
- elif axisValueTable.Format == 4:
- for rec in axisValueTable.AxisValueRecord:
- axisTag = designAxes[rec.AxisIndex].AxisTag
- if axisTag in axisCoords and rec.Value == axisCoords[axisTag]:
- seen.add(axisTag)
-
- missingAxes = set(axisCoords) - seen
- if missingAxes:
- missing = ", ".join(f"'{i}': {axisCoords[i]}" for i in missingAxes)
- raise ValueError(f"Cannot find Axis Values {{{missing}}}")
-
-
-def _sortAxisValues(axisValues):
- # Sort by axis index, remove duplicates and ensure that format 4 AxisValues
- # are dominant.
- # The MS Spec states: "if a format 1, format 2 or format 3 table has a
- # (nominal) value used in a format 4 table that also has values for
- # other axes, the format 4 table, being the more specific match, is used",
- # https://docs.microsoft.com/en-us/typography/opentype/spec/stat#axis-value-table-format-4
- results = []
- seenAxes = set()
- # Sort format 4 axes so the tables with the most AxisValueRecords are first
- format4 = sorted(
- [v for v in axisValues if v.Format == 4],
- key=lambda v: len(v.AxisValueRecord),
- reverse=True,
- )
-
- for val in format4:
- axisIndexes = set(r.AxisIndex for r in val.AxisValueRecord)
- minIndex = min(axisIndexes)
- if not seenAxes & axisIndexes:
- seenAxes |= axisIndexes
- results.append((minIndex, val))
-
- for val in axisValues:
- if val in format4:
- continue
- axisIndex = val.AxisIndex
- if axisIndex not in seenAxes:
- seenAxes.add(axisIndex)
- results.append((axisIndex, val))
-
- return [axisValue for _, axisValue in sorted(results)]
-
-
-def _updateNameRecords(varfont, axisValues):
- # Update nametable based on the axisValues using the R/I/B/BI model.
- nametable = varfont["name"]
- stat = varfont["STAT"].table
-
- axisValueNameIDs = [a.ValueNameID for a in axisValues]
- ribbiNameIDs = [n for n in axisValueNameIDs if _isRibbi(nametable, n)]
- nonRibbiNameIDs = [n for n in axisValueNameIDs if n not in ribbiNameIDs]
- elidedNameID = stat.ElidedFallbackNameID
- elidedNameIsRibbi = _isRibbi(nametable, elidedNameID)
-
- getName = nametable.getName
- platforms = set((r.platformID, r.platEncID, r.langID) for r in nametable.names)
- for platform in platforms:
- if not all(getName(i, *platform) for i in (1, 2, elidedNameID)):
- # Since no family name and subfamily name records were found,
- # we cannot update this set of name Records.
- continue
-
- subFamilyName = " ".join(
- getName(n, *platform).toUnicode() for n in ribbiNameIDs
- )
- if nonRibbiNameIDs:
- typoSubFamilyName = " ".join(
- getName(n, *platform).toUnicode() for n in axisValueNameIDs
- )
- else:
- typoSubFamilyName = None
-
- # If neither subFamilyName and typographic SubFamilyName exist,
- # we will use the STAT's elidedFallbackName
- if not typoSubFamilyName and not subFamilyName:
- if elidedNameIsRibbi:
- subFamilyName = getName(elidedNameID, *platform).toUnicode()
- else:
- typoSubFamilyName = getName(elidedNameID, *platform).toUnicode()
-
- familyNameSuffix = " ".join(
- getName(n, *platform).toUnicode() for n in nonRibbiNameIDs
- )
-
- _updateNameTableStyleRecords(
- varfont,
- familyNameSuffix,
- subFamilyName,
- typoSubFamilyName,
- *platform,
- )
-
-
-def _isRibbi(nametable, nameID):
- englishRecord = nametable.getName(nameID, 3, 1, 0x409)
- return (
- True
- if englishRecord is not None
- and englishRecord.toUnicode() in ("Regular", "Italic", "Bold", "Bold Italic")
- else False
- )
-
-
-def _updateNameTableStyleRecords(
- varfont,
- familyNameSuffix,
- subFamilyName,
- typoSubFamilyName,
- platformID=3,
- platEncID=1,
- langID=0x409,
-):
- # TODO (Marc F) It may be nice to make this part a standalone
- # font renamer in the future.
- nametable = varfont["name"]
- platform = (platformID, platEncID, langID)
-
- currentFamilyName = nametable.getName(
- NameID.TYPOGRAPHIC_FAMILY_NAME, *platform
- ) or nametable.getName(NameID.FAMILY_NAME, *platform)
-
- currentStyleName = nametable.getName(
- NameID.TYPOGRAPHIC_SUBFAMILY_NAME, *platform
- ) or nametable.getName(NameID.SUBFAMILY_NAME, *platform)
-
- if not all([currentFamilyName, currentStyleName]):
- raise ValueError(f"Missing required NameIDs 1 and 2 for platform {platform}")
-
- currentFamilyName = currentFamilyName.toUnicode()
- currentStyleName = currentStyleName.toUnicode()
-
- nameIDs = {
- NameID.FAMILY_NAME: currentFamilyName,
- NameID.SUBFAMILY_NAME: subFamilyName or "Regular",
- }
- if typoSubFamilyName:
- nameIDs[NameID.FAMILY_NAME] = f"{currentFamilyName} {familyNameSuffix}".strip()
- nameIDs[NameID.TYPOGRAPHIC_FAMILY_NAME] = currentFamilyName
- nameIDs[NameID.TYPOGRAPHIC_SUBFAMILY_NAME] = typoSubFamilyName
- else:
- # Remove previous Typographic Family and SubFamily names since they're
- # no longer required
- for nameID in (
- NameID.TYPOGRAPHIC_FAMILY_NAME,
- NameID.TYPOGRAPHIC_SUBFAMILY_NAME,
- ):
- nametable.removeNames(nameID=nameID)
-
- newFamilyName = (
- nameIDs.get(NameID.TYPOGRAPHIC_FAMILY_NAME) or nameIDs[NameID.FAMILY_NAME]
- )
- newStyleName = (
- nameIDs.get(NameID.TYPOGRAPHIC_SUBFAMILY_NAME) or nameIDs[NameID.SUBFAMILY_NAME]
- )
-
- nameIDs[NameID.FULL_FONT_NAME] = f"{newFamilyName} {newStyleName}"
- nameIDs[NameID.POSTSCRIPT_NAME] = _updatePSNameRecord(
- varfont, newFamilyName, newStyleName, platform
- )
-
- uniqueID = _updateUniqueIdNameRecord(varfont, nameIDs, platform)
- if uniqueID:
- nameIDs[NameID.UNIQUE_FONT_IDENTIFIER] = uniqueID
-
- for nameID, string in nameIDs.items():
- assert string, nameID
- nametable.setName(string, nameID, *platform)
-
- if "fvar" not in varfont:
- nametable.removeNames(NameID.VARIATIONS_POSTSCRIPT_NAME_PREFIX)
-
-
-def _updatePSNameRecord(varfont, familyName, styleName, platform):
- # Implementation based on Adobe Technical Note #5902 :
- # https://wwwimages2.adobe.com/content/dam/acom/en/devnet/font/pdfs/5902.AdobePSNameGeneration.pdf
- nametable = varfont["name"]
-
- family_prefix = nametable.getName(
- NameID.VARIATIONS_POSTSCRIPT_NAME_PREFIX, *platform
- )
- if family_prefix:
- family_prefix = family_prefix.toUnicode()
- else:
- family_prefix = familyName
-
- psName = f"{family_prefix}-{styleName}"
- # Remove any characters other than uppercase Latin letters, lowercase
- # Latin letters, digits and hyphens.
- psName = re.sub(r"[^A-Za-z0-9-]", r"", psName)
-
- if len(psName) > 127:
- # Abbreviating the stylename so it fits within 127 characters whilst
- # conforming to every vendor's specification is too complex. Instead
- # we simply truncate the psname and add the required "..."
- return f"{psName[:124]}..."
- return psName
-
-
-def _updateUniqueIdNameRecord(varfont, nameIDs, platform):
- nametable = varfont["name"]
- currentRecord = nametable.getName(NameID.UNIQUE_FONT_IDENTIFIER, *platform)
- if not currentRecord:
- return None
-
- # Check if full name and postscript name are a substring of currentRecord
- for nameID in (NameID.FULL_FONT_NAME, NameID.POSTSCRIPT_NAME):
- nameRecord = nametable.getName(nameID, *platform)
- if not nameRecord:
- continue
- if nameRecord.toUnicode() in currentRecord.toUnicode():
- return currentRecord.toUnicode().replace(
- nameRecord.toUnicode(), nameIDs[nameRecord.nameID]
- )
-
- # Create a new string since we couldn't find any substrings.
- fontVersion = _fontVersion(varfont, platform)
- achVendID = varfont["OS/2"].achVendID
- # Remove non-ASCII characers and trailing spaces
- vendor = re.sub(r"[^\x00-\x7F]", "", achVendID).strip()
- psName = nameIDs[NameID.POSTSCRIPT_NAME]
- return f"{fontVersion};{vendor};{psName}"
-
-
-def _fontVersion(font, platform=(3, 1, 0x409)):
- nameRecord = font["name"].getName(NameID.VERSION_STRING, *platform)
- if nameRecord is None:
- return f'{font["head"].fontRevision:.3f}'
- # "Version 1.101; ttfautohint (v1.8.1.43-b0c9)" --> "1.101"
- # Also works fine with inputs "Version 1.101" or "1.101" etc
- versionNumber = nameRecord.toUnicode().split(";")[0]
- return versionNumber.lstrip("Version ").strip()
diff --git a/spaces/dcarpintero/nlp-summarizer-pegasus/.venv/lib/python3.9/site-packages/fsspec/implementations/local.py b/spaces/dcarpintero/nlp-summarizer-pegasus/.venv/lib/python3.9/site-packages/fsspec/implementations/local.py
deleted file mode 100644
index 90045d891d6b44249222be7614d63d879d81fec0..0000000000000000000000000000000000000000
--- a/spaces/dcarpintero/nlp-summarizer-pegasus/.venv/lib/python3.9/site-packages/fsspec/implementations/local.py
+++ /dev/null
@@ -1,424 +0,0 @@
-import datetime
-import io
-import logging
-import os
-import os.path as osp
-import posixpath
-import re
-import shutil
-import stat
-import tempfile
-
-from fsspec import AbstractFileSystem
-from fsspec.compression import compr
-from fsspec.core import get_compression
-from fsspec.utils import isfilelike, stringify_path
-
-logger = logging.getLogger("fsspec.local")
-
-
-class LocalFileSystem(AbstractFileSystem):
- """Interface to files on local storage
-
- Parameters
- ----------
- auto_mkdir: bool
- Whether, when opening a file, the directory containing it should
- be created (if it doesn't already exist). This is assumed by pyarrow
- code.
- """
-
- root_marker = "/"
- protocol = "file"
- local_file = True
-
- def __init__(self, auto_mkdir=False, **kwargs):
- super().__init__(**kwargs)
- self.auto_mkdir = auto_mkdir
-
- @property
- def fsid(self):
- return "local"
-
- def mkdir(self, path, create_parents=True, **kwargs):
- path = self._strip_protocol(path)
- if self.exists(path):
- raise FileExistsError(path)
- if create_parents:
- self.makedirs(path, exist_ok=True)
- else:
- os.mkdir(path, **kwargs)
-
- def makedirs(self, path, exist_ok=False):
- path = self._strip_protocol(path)
- os.makedirs(path, exist_ok=exist_ok)
-
- def rmdir(self, path):
- path = self._strip_protocol(path)
- os.rmdir(path)
-
- def ls(self, path, detail=False, **kwargs):
- path = self._strip_protocol(path)
- if detail:
- with os.scandir(path) as it:
- return [self.info(f) for f in it]
- else:
- return [posixpath.join(path, f) for f in os.listdir(path)]
-
- def glob(self, path, **kwargs):
- path = self._strip_protocol(path)
- return super().glob(path, **kwargs)
-
- def info(self, path, **kwargs):
- if isinstance(path, os.DirEntry):
- # scandir DirEntry
- out = path.stat(follow_symlinks=False)
- link = path.is_symlink()
- if path.is_dir(follow_symlinks=False):
- t = "directory"
- elif path.is_file(follow_symlinks=False):
- t = "file"
- else:
- t = "other"
- path = self._strip_protocol(path.path)
- else:
- # str or path-like
- path = self._strip_protocol(path)
- out = os.stat(path, follow_symlinks=False)
- link = stat.S_ISLNK(out.st_mode)
- if link:
- out = os.stat(path, follow_symlinks=True)
- if stat.S_ISDIR(out.st_mode):
- t = "directory"
- elif stat.S_ISREG(out.st_mode):
- t = "file"
- else:
- t = "other"
- result = {
- "name": path,
- "size": out.st_size,
- "type": t,
- "created": out.st_ctime,
- "islink": link,
- }
- for field in ["mode", "uid", "gid", "mtime", "ino", "nlink"]:
- result[field] = getattr(out, "st_" + field)
- if result["islink"]:
- result["destination"] = os.readlink(path)
- try:
- out2 = os.stat(path, follow_symlinks=True)
- result["size"] = out2.st_size
- except OSError:
- result["size"] = 0
- return result
-
- def lexists(self, path, **kwargs):
- return osp.lexists(path)
-
- def cp_file(self, path1, path2, **kwargs):
- path1 = self._strip_protocol(path1).rstrip("/")
- path2 = self._strip_protocol(path2).rstrip("/")
- if self.auto_mkdir:
- self.makedirs(self._parent(path2), exist_ok=True)
- if self.isfile(path1):
- shutil.copyfile(path1, path2)
- elif self.isdir(path1):
- self.mkdirs(path2, exist_ok=True)
- else:
- raise FileNotFoundError(path1)
-
- def get_file(self, path1, path2, callback=None, **kwargs):
- if isfilelike(path2):
- with open(path1, "rb") as f:
- shutil.copyfileobj(f, path2)
- else:
- return self.cp_file(path1, path2, **kwargs)
-
- def put_file(self, path1, path2, callback=None, **kwargs):
- return self.cp_file(path1, path2, **kwargs)
-
- def mv_file(self, path1, path2, **kwargs):
- path1 = self._strip_protocol(path1).rstrip("/")
- path2 = self._strip_protocol(path2).rstrip("/")
- shutil.move(path1, path2)
-
- def link(self, src, dst, **kwargs):
- src = self._strip_protocol(src)
- dst = self._strip_protocol(dst)
- os.link(src, dst, **kwargs)
-
- def symlink(self, src, dst, **kwargs):
- src = self._strip_protocol(src)
- dst = self._strip_protocol(dst)
- os.symlink(src, dst, **kwargs)
-
- def islink(self, path) -> bool:
- return os.path.islink(self._strip_protocol(path))
-
- def rm_file(self, path):
- os.remove(self._strip_protocol(path))
-
- def rm(self, path, recursive=False, maxdepth=None):
- if not isinstance(path, list):
- path = [path]
-
- for p in path:
- p = self._strip_protocol(p).rstrip("/")
- if self.isdir(p):
- if not recursive:
- raise ValueError("Cannot delete directory, set recursive=True")
- if osp.abspath(p) == os.getcwd():
- raise ValueError("Cannot delete current working directory")
- shutil.rmtree(p)
- else:
- os.remove(p)
-
- def unstrip_protocol(self, name):
- name = self._strip_protocol(name) # normalise for local/win/...
- return f"file://{name}"
-
- def _open(self, path, mode="rb", block_size=None, **kwargs):
- path = self._strip_protocol(path)
- if self.auto_mkdir and "w" in mode:
- self.makedirs(self._parent(path), exist_ok=True)
- return LocalFileOpener(path, mode, fs=self, **kwargs)
-
- def touch(self, path, truncate=True, **kwargs):
- path = self._strip_protocol(path)
- if self.auto_mkdir:
- self.makedirs(self._parent(path), exist_ok=True)
- if self.exists(path):
- os.utime(path, None)
- else:
- open(path, "a").close()
- if truncate:
- os.truncate(path, 0)
-
- def created(self, path):
- info = self.info(path=path)
- return datetime.datetime.utcfromtimestamp(info["created"])
-
- def modified(self, path):
- info = self.info(path=path)
- return datetime.datetime.utcfromtimestamp(info["mtime"])
-
- @classmethod
- def _parent(cls, path):
- path = cls._strip_protocol(path).rstrip("/")
- if "/" in path:
- return path.rsplit("/", 1)[0]
- else:
- return cls.root_marker
-
- @classmethod
- def _strip_protocol(cls, path):
- path = stringify_path(path)
- if path.startswith("file://"):
- path = path[7:]
- elif path.startswith("file:"):
- path = path[5:]
- return make_path_posix(path).rstrip("/") or cls.root_marker
-
- def _isfilestore(self):
- # Inheriting from DaskFileSystem makes this False (S3, etc. were)
- # the original motivation. But we are a posix-like file system.
- # See https://github.com/dask/dask/issues/5526
- return True
-
- def chmod(self, path, mode):
- path = stringify_path(path)
- return os.chmod(path, mode)
-
-
-def make_path_posix(path, sep=os.sep):
- """Make path generic"""
- if isinstance(path, (list, set, tuple)):
- return type(path)(make_path_posix(p) for p in path)
- if "~" in path:
- path = osp.expanduser(path)
- if sep == "/":
- # most common fast case for posix
- if path.startswith("/"):
- return path
- if path.startswith("./"):
- path = path[2:]
- return os.getcwd() + "/" + path
- if (
- (sep not in path and "/" not in path)
- or (sep == "/" and not path.startswith("/"))
- or (sep == "\\" and ":" not in path and not path.startswith("\\\\"))
- ):
- # relative path like "path" or "rel\\path" (win) or rel/path"
- if os.sep == "\\":
- # abspath made some more '\\' separators
- return make_path_posix(osp.abspath(path))
- else:
- return os.getcwd() + "/" + path
- if path.startswith("file://"):
- path = path[7:]
- if re.match("/[A-Za-z]:", path):
- # for windows file URI like "file:///C:/folder/file"
- # or "file:///C:\\dir\\file"
- path = path[1:].replace("\\", "/").replace("//", "/")
- if path.startswith("\\\\"):
- # special case for windows UNC/DFS-style paths, do nothing,
- # just flip the slashes around (case below does not work!)
- return path.replace("\\", "/")
- if re.match("[A-Za-z]:", path):
- # windows full path like "C:\\local\\path"
- return path.lstrip("\\").replace("\\", "/").replace("//", "/")
- if path.startswith("\\"):
- # windows network path like "\\server\\path"
- return "/" + path.lstrip("\\").replace("\\", "/").replace("//", "/")
- return path
-
-
-def trailing_sep(path):
- """Return True if the path ends with a path separator.
-
- A forward slash is always considered a path separator, even on Operating
- Systems that normally use a backslash.
- """
- # TODO: if all incoming paths were posix-compliant then separator would
- # always be a forward slash, simplifying this function.
- # See https://github.com/fsspec/filesystem_spec/pull/1250
- return path.endswith(os.sep) or (os.altsep is not None and path.endswith(os.altsep))
-
-
-def trailing_sep_maybe_asterisk(path):
- """Return True if the path ends with a path separator and optionally an
- asterisk.
-
- A forward slash is always considered a path separator, even on Operating
- Systems that normally use a backslash.
- """
- # TODO: if all incoming paths were posix-compliant then separator would
- # always be a forward slash, simplifying this function.
- # See https://github.com/fsspec/filesystem_spec/pull/1250
- return path.endswith((os.sep, os.sep + "*")) or (
- os.altsep is not None and path.endswith((os.altsep, os.altsep + "*"))
- )
-
-
-class LocalFileOpener(io.IOBase):
- def __init__(
- self, path, mode, autocommit=True, fs=None, compression=None, **kwargs
- ):
- logger.debug("open file: %s", path)
- self.path = path
- self.mode = mode
- self.fs = fs
- self.f = None
- self.autocommit = autocommit
- self.compression = get_compression(path, compression)
- self.blocksize = io.DEFAULT_BUFFER_SIZE
- self._open()
-
- def _open(self):
- if self.f is None or self.f.closed:
- if self.autocommit or "w" not in self.mode:
- self.f = open(self.path, mode=self.mode)
- if self.compression:
- compress = compr[self.compression]
- self.f = compress(self.f, mode=self.mode)
- else:
- # TODO: check if path is writable?
- i, name = tempfile.mkstemp()
- os.close(i) # we want normal open and normal buffered file
- self.temp = name
- self.f = open(name, mode=self.mode)
- if "w" not in self.mode:
- self.size = self.f.seek(0, 2)
- self.f.seek(0)
- self.f.size = self.size
-
- def _fetch_range(self, start, end):
- # probably only used by cached FS
- if "r" not in self.mode:
- raise ValueError
- self._open()
- self.f.seek(start)
- return self.f.read(end - start)
-
- def __setstate__(self, state):
- self.f = None
- loc = state.pop("loc", None)
- self.__dict__.update(state)
- if "r" in state["mode"]:
- self.f = None
- self._open()
- self.f.seek(loc)
-
- def __getstate__(self):
- d = self.__dict__.copy()
- d.pop("f")
- if "r" in self.mode:
- d["loc"] = self.f.tell()
- else:
- if not self.f.closed:
- raise ValueError("Cannot serialise open write-mode local file")
- return d
-
- def commit(self):
- if self.autocommit:
- raise RuntimeError("Can only commit if not already set to autocommit")
- shutil.move(self.temp, self.path)
-
- def discard(self):
- if self.autocommit:
- raise RuntimeError("Cannot discard if set to autocommit")
- os.remove(self.temp)
-
- def readable(self) -> bool:
- return True
-
- def writable(self) -> bool:
- return "r" not in self.mode
-
- def read(self, *args, **kwargs):
- return self.f.read(*args, **kwargs)
-
- def write(self, *args, **kwargs):
- return self.f.write(*args, **kwargs)
-
- def tell(self, *args, **kwargs):
- return self.f.tell(*args, **kwargs)
-
- def seek(self, *args, **kwargs):
- return self.f.seek(*args, **kwargs)
-
- def seekable(self, *args, **kwargs):
- return self.f.seekable(*args, **kwargs)
-
- def readline(self, *args, **kwargs):
- return self.f.readline(*args, **kwargs)
-
- def readlines(self, *args, **kwargs):
- return self.f.readlines(*args, **kwargs)
-
- def close(self):
- return self.f.close()
-
- @property
- def closed(self):
- return self.f.closed
-
- def fileno(self):
- return self.raw.fileno()
-
- def flush(self) -> None:
- self.f.flush()
-
- def __iter__(self):
- return self.f.__iter__()
-
- def __getattr__(self, item):
- return getattr(self.f, item)
-
- def __enter__(self):
- self._incontext = True
- return self
-
- def __exit__(self, exc_type, exc_value, traceback):
- self._incontext = False
- self.f.__exit__(exc_type, exc_value, traceback)
diff --git a/spaces/dcarpintero/nlp-summarizer-pegasus/.venv/lib/python3.9/site-packages/huggingface_hub/utils/tqdm.py b/spaces/dcarpintero/nlp-summarizer-pegasus/.venv/lib/python3.9/site-packages/huggingface_hub/utils/tqdm.py
deleted file mode 100644
index 81bdfb0500a71e4015d3e2bf539571fae54e7f96..0000000000000000000000000000000000000000
--- a/spaces/dcarpintero/nlp-summarizer-pegasus/.venv/lib/python3.9/site-packages/huggingface_hub/utils/tqdm.py
+++ /dev/null
@@ -1,178 +0,0 @@
-#!/usr/bin/env python
-# coding=utf-8
-# Copyright 2021 The HuggingFace Inc. team. All rights reserved.
-#
-# Licensed under the Apache License, Version 2.0 (the "License");
-# you may not use this file except in compliance with the License.
-# You may obtain a copy of the License at
-#
-# http://www.apache.org/licenses/LICENSE-2.0
-#
-# Unless required by applicable law or agreed to in writing, software
-# distributed under the License is distributed on an "AS IS" BASIS,
-# WITHOUT WARRANTIES OR CONDITIONS OF ANY KIND, either express or implied.
-# See the License for the specific language governing permissions and
-# limitations under the License
-"""Utility helpers to handle progress bars in `huggingface_hub`.
-
-Example:
- 1. Use `huggingface_hub.utils.tqdm` as you would use `tqdm.tqdm` or `tqdm.auto.tqdm`.
- 2. To disable progress bars, either use `disable_progress_bars()` helper or set the
- environment variable `HF_HUB_DISABLE_PROGRESS_BARS` to 1.
- 3. To re-enable progress bars, use `enable_progress_bars()`.
- 4. To check whether progress bars are disabled, use `are_progress_bars_disabled()`.
-
-NOTE: Environment variable `HF_HUB_DISABLE_PROGRESS_BARS` has the priority.
-
-Example:
- ```py
- from huggingface_hub.utils import (
- are_progress_bars_disabled,
- disable_progress_bars,
- enable_progress_bars,
- tqdm,
- )
-
- # Disable progress bars globally
- disable_progress_bars()
-
- # Use as normal `tqdm`
- for _ in tqdm(range(5)):
- do_something()
-
- # Still not showing progress bars, as `disable=False` is overwritten to `True`.
- for _ in tqdm(range(5), disable=False):
- do_something()
-
- are_progress_bars_disabled() # True
-
- # Re-enable progress bars globally
- enable_progress_bars()
-
- # Progress bar will be shown !
- for _ in tqdm(range(5)):
- do_something()
- ```
-"""
-import io
-import warnings
-from contextlib import contextmanager
-from pathlib import Path
-from typing import Iterator, Optional, Union
-
-from tqdm.auto import tqdm as old_tqdm
-
-from ..constants import HF_HUB_DISABLE_PROGRESS_BARS
-
-
-# `HF_HUB_DISABLE_PROGRESS_BARS` is `Optional[bool]` while `_hf_hub_progress_bars_disabled`
-# is a `bool`. If `HF_HUB_DISABLE_PROGRESS_BARS` is set to True or False, it has priority.
-# If `HF_HUB_DISABLE_PROGRESS_BARS` is None, it means the user have not set the
-# environment variable and is free to enable/disable progress bars programmatically.
-# TL;DR: env variable has priority over code.
-#
-# By default, progress bars are enabled.
-_hf_hub_progress_bars_disabled: bool = HF_HUB_DISABLE_PROGRESS_BARS or False
-
-
-def disable_progress_bars() -> None:
- """
- Disable globally progress bars used in `huggingface_hub` except if `HF_HUB_DISABLE_PROGRESS_BARS` environment
- variable has been set.
-
- Use [`~utils.enable_progress_bars`] to re-enable them.
- """
- if HF_HUB_DISABLE_PROGRESS_BARS is False:
- warnings.warn(
- "Cannot disable progress bars: environment variable `HF_HUB_DISABLE_PROGRESS_BARS=0` is set and has"
- " priority."
- )
- return
- global _hf_hub_progress_bars_disabled
- _hf_hub_progress_bars_disabled = True
-
-
-def enable_progress_bars() -> None:
- """
- Enable globally progress bars used in `huggingface_hub` except if `HF_HUB_DISABLE_PROGRESS_BARS` environment
- variable has been set.
-
- Use [`~utils.disable_progress_bars`] to disable them.
- """
- if HF_HUB_DISABLE_PROGRESS_BARS is True:
- warnings.warn(
- "Cannot enable progress bars: environment variable `HF_HUB_DISABLE_PROGRESS_BARS=1` is set and has"
- " priority."
- )
- return
- global _hf_hub_progress_bars_disabled
- _hf_hub_progress_bars_disabled = False
-
-
-def are_progress_bars_disabled() -> bool:
- """Return whether progress bars are globally disabled or not.
-
- Progress bars used in `huggingface_hub` can be enable or disabled globally using [`~utils.enable_progress_bars`]
- and [`~utils.disable_progress_bars`] or by setting `HF_HUB_DISABLE_PROGRESS_BARS` as environment variable.
- """
- global _hf_hub_progress_bars_disabled
- return _hf_hub_progress_bars_disabled
-
-
-class tqdm(old_tqdm):
- """
- Class to override `disable` argument in case progress bars are globally disabled.
-
- Taken from https://github.com/tqdm/tqdm/issues/619#issuecomment-619639324.
- """
-
- def __init__(self, *args, **kwargs):
- if are_progress_bars_disabled():
- kwargs["disable"] = True
- super().__init__(*args, **kwargs)
-
-
-@contextmanager
-def tqdm_stream_file(path: Union[Path, str]) -> Iterator[io.BufferedReader]:
- """
- Open a file as binary and wrap the `read` method to display a progress bar when it's streamed.
-
- First implemented in `transformers` in 2019 but removed when switched to git-lfs. Used in `huggingface_hub` to show
- progress bar when uploading an LFS file to the Hub. See github.com/huggingface/transformers/pull/2078#discussion_r354739608
- for implementation details.
-
- Note: currently implementation handles only files stored on disk as it is the most common use case. Could be
- extended to stream any `BinaryIO` object but we might have to debug some corner cases.
-
- Example:
- ```py
- >>> with tqdm_stream_file("config.json") as f:
- >>> requests.put(url, data=f)
- config.json: 100%|█████████████████████████| 8.19k/8.19k [00:02<00:00, 3.72kB/s]
- ```
- """
- if isinstance(path, str):
- path = Path(path)
-
- with path.open("rb") as f:
- total_size = path.stat().st_size
- pbar = tqdm(
- unit="B",
- unit_scale=True,
- total=total_size,
- initial=0,
- desc=path.name,
- )
-
- f_read = f.read
-
- def _inner_read(size: Optional[int] = -1) -> bytes:
- data = f_read(size)
- pbar.update(len(data))
- return data
-
- f.read = _inner_read # type: ignore
-
- yield f
-
- pbar.close()
diff --git a/spaces/deepozzzie/chatgpt/README.md b/spaces/deepozzzie/chatgpt/README.md
deleted file mode 100644
index 5347870fc6dbae91fe6019bd9d7af1ad5b86de5d..0000000000000000000000000000000000000000
--- a/spaces/deepozzzie/chatgpt/README.md
+++ /dev/null
@@ -1,13 +0,0 @@
----
-title: "Chat with PDF •\_OpenAI"
-emoji: 📄🤖
-colorFrom: purple
-colorTo: pink
-sdk: gradio
-sdk_version: 3.27.0
-app_file: app.py
-pinned: false
-duplicated_from: fffiloni/langchain-chat-with-pdf-openai
----
-
-Check out the configuration reference at https://huggingface.co/docs/hub/spaces-config-reference
diff --git a/spaces/derinsu/Background_Generator/app.py b/spaces/derinsu/Background_Generator/app.py
deleted file mode 100644
index 1c0b0146e95d36724250e60f05eeeda3c2cb623f..0000000000000000000000000000000000000000
--- a/spaces/derinsu/Background_Generator/app.py
+++ /dev/null
@@ -1,330 +0,0 @@
-# -*- coding: utf-8 -*-
-"""Generate_Background.ipynb
-
-Automatically generated by Colaboratory.
-
-Original file is located at
- https://colab.research.google.com/drive/15l8FWhqVNEz3Au7Lw_O56DJ0RKP9iRH4
-"""
-
-# Setup
-import os
-
-image_dir = os.path.join(os.getcwd(), 'U-2-Net/images')
-results_dir = os.path.join(os.getcwd(), 'U-2-Net/results')
-saved_dir = os.path.join(os.getcwd(), 'saved')
-image_files = os.listdir(image_dir)
-
-
-#from tensorflow.keras.preprocessing.image import load_img
-#from tensorflow.keras.preprocessing.image import img_to_array
-import numpy as np
-import PIL
-from PIL import Image as Img
-import cv2
-print("imports part1")
-from diffusers import StableDiffusionInpaintPipeline
-import torch
-import requests
-from io import BytesIO
-import gradio as gr
-
-print("imports complete")
-
-def get_image(url):
- # Retrieve the image from the provided URL
- response = requests.get(url)
- return Img.open(BytesIO(response.content))
-
-def resize_image(image, max_size):
- # Resize the image while maintaining aspect ratio
- image.thumbnail((max_size, max_size), Img.ANTIALIAS)
- return image
-
-def extend_image(image):
- # Resize and center images while leaving enough space for the background
- extended_image = Img.new("RGBA", (512, 512), (255, 255, 255, 0))
- x_offset = (512 - image.width) // 2
- y_offset = (512 - image.height) // 2
- extended_image.paste(image, (x_offset, y_offset), mask=image.split()[3])
- return extended_image
-
-def extend_mask(mask_image):
- # Extend the mask image to match the size of the extended image
- extended_image = Img.new("L", (512, 512), 255)
- extended_image.paste(mask_image, ((512 - mask_image.width) // 2, (512 - mask_image.height) // 2))
- return extended_image
-
-def display_images(images):
- # Display a list of images horizontally
- num_images = len(images)
- total_width = sum(image.width for image in images)
- max_height = max(image.height for image in images)
- new_image = Img.new('RGBA', (total_width, max_height))
- x_offset = 0
- for image in images:
- new_image.paste(image, (x_offset, 0))
- x_offset += image.width
- new_image.show()
-
-def download_image(image, file_name):
- # Save the image with the specified file name
- image.save(file_name)
- files.download(file_name)
-
-def clear_directory(directory):
- # Remove all files from the specified directory
- files = os.listdir(directory)
- for file in files:
- file_path = os.path.join(directory, file)
- if os.path.isfile(file_path):
- os.remove(file_path)
-
-def diffusion_setup():
- # Set up the stable diffusion inpainting pipeline
- device = torch.device("cuda" if torch.cuda.is_available() else "cpu")
- model_path = "stabilityai/stable-diffusion-2-inpainting"
- pipe = StableDiffusionInpaintPipeline.from_pretrained(
- model_path,
- torch_dtype=torch.float16 if device.type == "cuda" else torch.float32,
- ).to(device)
- return pipe
-
-print("functions complete")
-
-
-'''
-Executes the U2-Net algorithm to detect objects in the provided images directory and generate masks.
-These masks are used to separate foreground objects from the background.
-The resulting masks are then saved in the results directory for further processing.
-'''
-import subprocess
-
-def run_u2net():
- script_path = "U-2-Net/u2net_test.py"
- command = ["python", "-W", "ignore", script_path]
-
- try:
- subprocess.check_call(command)
- except subprocess.CalledProcessError as e:
- print(f"Error executing script: {e}")
-
-
-# Crop and make backgrounds transparent in all images using U2-Net generated masks
-def remove_background():
-
- image_dir = os.path.join(os.getcwd(), 'U-2-Net/images')
- results_dir = os.path.join(os.getcwd(), 'U-2-Net/results')
- image_files = os.listdir(image_dir)
-
- threshold = 0.9 # Threshold for background removal
- rescale_factor = 255 # Rescale factor for normalization
- target_layer_index = 2 # Target layer index for bounding box creation
-
- cropped_images = [] # Initialize the cropped images list
-
- for file_name in image_files:
- # Skip if the file is not an image
- if not file_name.endswith(('.jpg', '.png')):
- continue
-
- # Construct the file paths for the input and output images
- input_file_path = os.path.join(image_dir, file_name)
- output_file_path = os.path.join(results_dir, file_name[:-4] + '.png')
-
- # Load the output image from the U-2-Net results directory
- output_img = Img.open(output_file_path)
-
- # Convert the output image to a numpy array and normalize the pixel values
- output_array = np.array(output_img) / rescale_factor
-
- # Apply thresholding to obtain a binary mask
- output_array[output_array > threshold] = 1
- output_array[output_array <= threshold] = 0
-
- # Create RGBA representation of the output image with transparency
- shape = output_array.shape
- alpha_layer_init = np.ones(shape=(shape[0], shape[1], 1))
- foreground_mask = np.expand_dims(output_array[:, :, 0], axis=2)
- alpha_layer = foreground_mask * alpha_layer_init
- rgba_output = np.append(output_array, alpha_layer, axis=2)
-
- # Load the input image
- input_img = Img.open(input_file_path)
- input_array = np.array(input_img) / rescale_factor
-
- # Create RGBA representation of the input image with transparency
- alpha_layer = np.ones(shape=(shape[0], shape[1], 1))
- rgba_input = np.append(input_array, alpha_layer, axis=2)
-
- # Remove the background by multiplying the input and output images
- rem_back = rgba_input * rgba_output
-
- # Scale the pixel values back to the original range
- rem_back_scaled = rem_back * rescale_factor
-
- # Find the bounding box coordinates based on the specified layer
- target_layer = output_array[:, :, target_layer_index]
- x_starts = [np.where(row == 1)[0][0] if np.any(row == 1) else output_array.shape[1] + 1 for row in target_layer]
- x_ends = [np.where(row == 1)[0][-1] if np.any(row == 1) else 0 for row in target_layer]
- y_starts = [np.where(column == 1)[0][0] if np.any(column == 1) else output_array.shape[0] + 1 for column in target_layer.T]
- y_ends = [np.where(column == 1)[0][-1] if np.any(column == 1) else 0 for column in target_layer.T]
-
- # Calculate the bounding box coordinates
- start_x = min(x_starts)
- end_x = max(x_ends)
- start_y = min(y_starts)
- end_y = max(y_ends)
-
- # Crop the image based on the bounding box coordinates
- cropped_image = rem_back_scaled[start_y:end_y, start_x:end_x, :]
-
- # Resize and store the cropped images
- cropped_images.append(Img.fromarray(cropped_image.astype('uint8'), 'RGBA'))
-
- return cropped_images
-
-def generate_masks(images):
- # Create masks for cropped images
- masks = []
-
- for image in images:
- # Extract the alpha channel
- alpha_channel = image.split()[3]
-
- # Threshold the alpha channel
- mask = alpha_channel.point(lambda p: 0 if p > 128 else 255)
- masks.append(mask)
- return masks
-
-import torch
-
-def generate_images(pipe, image, mask, prompt, guidance_scale=7.5, num_samples=1, num_inference_steps=10, seed=-1, height=512, width=512):
- """
- Generates images using the Stable Diffusion Inpainting pipeline.
-
- Args:
- pipe (StableDiffusionInpaintPipeline): The pipeline for image generation.
- image (PIL.Image): The input image.
- mask (PIL.Image): The mask image.
- prompt (str): The prompt text for generating images.
- guidance_scale (float, optional): The scale factor for guidance loss. Default is 7.5.
- num_samples (int, optional): The number of generated images per prompt. Default is 1.
- num_inference_steps (int, optional): The number of inference steps for generation. Default is 10.
- seed (int, optional): The seed value for random number generation. Default is -1.
- height (int, optional): The height of the generated images. Default is 512.
- width (int, optional): The width of the generated images. Default is 512.
-
- Returns:
- List[PIL.Image]: The generated images.
- """
-
- device = torch.device("cuda" if torch.cuda.is_available() else "cpu")
- generator = torch.Generator(device=device).manual_seed(seed)
-
- images = pipe(
- prompt=prompt,
- image=image,
- mask_image=mask,
- guidance_scale=guidance_scale,
- generator=generator,
- num_images_per_prompt=num_samples,
- num_inference_steps=num_inference_steps,
- height=height,
- width=width,
- ).images
-
- return images
-
-
-print("all funcs complete")
-
-# Web-based interface with Gradio
-
-pipe = diffusion_setup()
-
-
-print("gradio start")
-
-def remover(image):
- clear_directory(image_dir)
- clear_directory(results_dir)
-
- image.save(image_dir + "/image.jpg")
-
- # Remove background of the images and create masks before Stable Diffusion algorithm
- run_u2net()
- cropped_images = remove_background()
-
- #resize_factor = int(slider)
- cropped_images = [resize_image(im, 256) for im in cropped_images]
- mask_images = generate_masks(cropped_images)
-
- cropped_images = [extend_image(im) for im in cropped_images]
- mask_images = [extend_mask(m) for m in mask_images]
-
- cropped_image = cropped_images[0]
- mask_image = mask_images[0]
-
- cropped_image.save(saved_dir + "/cropped_image.png")
- mask_image.save(saved_dir + "/mask_image.png")
-
- return cropped_image
-
-# Define the function for image generation
-def generator(prompt, guidance_scale, num_inference_steps, seed):
-
- image = Img.open(saved_dir + "/cropped_image.png")
- mask = Img.open(saved_dir + "/mask_image.png")
-
- print('AI generation started')
- #pipe = diffusion_setup()
- generated_images = generate_images(pipe, image, mask,
- prompt=prompt,
- guidance_scale=guidance_scale,
- num_samples=1,
- num_inference_steps=num_inference_steps,
- height=600,
- width=600,
- seed=seed)
- print('AI generation done')
- generated_images[0].save(saved_dir + "/generated_image.png")
- return generated_images[0]
-
-
-with gr.Blocks() as demo:
- description = """
- ## Image Background Removal and Generation
-
- This application allows you to remove the background of an image and generate a new background using a prompt.
- """
- gr.Markdown(description)
- with gr.Tab("Remove Background"):
- with gr.Row():
- with gr.Column():
- image_input = gr.Image(type="pil", label="Upload an image")
- examples=["saved/balloon.jpg", "saved/watch.jpg", "saved/camera.jpg", "saved/coffee_machine.jpg", "saved/scooter.jpg"]
- examples_handler = gr.Examples(
- examples=examples,
- inputs=image_input,)
-
- image_button = gr.Button("Go!")
- image_cropped = gr.Image(type="pil", label="New image")
- with gr.Tab("Generate Background"):
- with gr.Row():
- with gr.Column():
- text_prompt = gr.Textbox(label="AI Prompt", default="hot air balloon floating over mountain valley with a river")
- slider_guidance = gr.inputs.Slider(minimum=1, maximum=15, default=7.5, label="Guidance Scale")
- slider_inference = gr.inputs.Slider(minimum=5, maximum=25, default=15, step=1, label="Inference Steps")
- slider_seed = gr.inputs.Slider(minimum=1, maximum=100, default=1, step=1, label="Seed")
- text_button = gr.Button("Generate!")
- gr.Markdown("When there's no GPU available, each step can take up to 30 seconds.")
- image_generated = gr.Image(type="pil", label="AI generated image")
-
- image_button.click(remover, inputs=image_input, outputs=image_cropped)
- text_button.click(generator, inputs=[text_prompt, slider_guidance, slider_inference, slider_seed], outputs=image_generated)
-
-
-print("gradio launch")
-demo.queue()
-demo.launch(debug=True)
diff --git a/spaces/diacanFperku/AutoGPT/Camelia Si Petrica Ciuca Album Download Fisierul 51.md b/spaces/diacanFperku/AutoGPT/Camelia Si Petrica Ciuca Album Download Fisierul 51.md
deleted file mode 100644
index daa4eef9b34714ff4841ed804e40bdcf2499a555..0000000000000000000000000000000000000000
--- a/spaces/diacanFperku/AutoGPT/Camelia Si Petrica Ciuca Album Download Fisierul 51.md
+++ /dev/null
@@ -1,15 +0,0 @@
-
-
How to Download Camelia Si Petrica Ciuca's Latest Album for Free
-
Camelia Si Petrica Ciuca are a popular Romanian duo who sing traditional folk music. They have released several albums over the years, but their latest one, titled "Cand Ajunge Banu Sa Te Stapaneasca", has been a huge hit among fans and critics alike. The album features 12 songs that showcase their vocal skills and musical talent.
-
Camelia Si Petrica Ciuca Album Download Fisierul 51
If you are looking for a way to download this album for free, you might be tempted to use a site called Fisierul 51, which claims to offer free downloads of various albums and songs. However, this site is not trustworthy and could expose your device to malware, viruses, or other security risks. Moreover, downloading music illegally is unethical and could get you in trouble with the law.
-
Therefore, we recommend that you use a legal and safe way to enjoy Camelia Si Petrica Ciuca's music, such as streaming it on SoundCloud[^1^] [^2^], where you can listen to the whole album for free. You can also support the artists by buying their album from official sources, such as online stores or physical shops. This way, you can show your appreciation for their work and help them continue making more music in the future.
-
-
Camelia Si Petrica Ciuca are not only talented singers, but also skilled instrumentalists. They play various traditional instruments, such as accordion, violin, clarinet, saxophone, and keyboard. They have been performing together for over 20 years, and have gained a loyal fan base in Romania and abroad. They are based in Drobeta-Turnu Severin, a city in southwestern Romania, where they often perform at weddings and other events. They also have a Facebook page[^1^] where they share their latest news and videos with their followers.
-
-
Their music is influenced by the folk traditions of Oltenia, a historical region of Romania known for its rich and diverse culture. Their songs are full of energy, emotion, and humor, and they often sing about love, money, family, and life in general. They have a distinctive style that combines traditional elements with modern influences, such as pop, rock, or dance. Their album "Cand Ajunge Banu Sa Te Stapaneasca" reflects their musical versatility and creativity.
-
If you want to hear some samples of their songs, you can watch some of their live performances on YouTube[^2^] [^3^], where you can see them in action and enjoy their charisma and passion. You can also find some of their older albums on various online platforms, such as Spotify or iTunes. However, if you want to download their latest album for free, you should avoid using Fisierul 51 or any other illegal site that could harm your device or violate the artists' rights. Instead, you should stream it on SoundCloud or buy it from official sources.
-
-
In conclusion, Camelia Si Petrica Ciuca are one of the most popular and respected folk music duos in Romania. They have a long and successful career that spans over two decades and several albums. Their latest album, "Cand Ajunge Banu Sa Te Stapaneasca", is a masterpiece of folk music that showcases their talent and diversity. If you want to download this album for free, you should use a legal and safe method, such as streaming it on SoundCloud or buying it from official sources. This way, you can enjoy their music without risking your device or breaking the law.
d5da3c52bf
-
-
\ No newline at end of file
diff --git a/spaces/diacanFperku/AutoGPT/Iskender Sayek Temel Cerrahi Pdf.md b/spaces/diacanFperku/AutoGPT/Iskender Sayek Temel Cerrahi Pdf.md
deleted file mode 100644
index 8b8b18051b677ae41a75dd830c438742c45ed18f..0000000000000000000000000000000000000000
--- a/spaces/diacanFperku/AutoGPT/Iskender Sayek Temel Cerrahi Pdf.md
+++ /dev/null
@@ -1,85 +0,0 @@
-
-
Iskender Sayek Temel Cerrahi Pdf: A Comprehensive Guide to Basic Surgery
-
-
If you are looking for a reliable and comprehensive source of information on basic surgery, you may want to check out the Iskender Sayek Temel Cerrahi Pdf. This is a PDF version of the book Temel Cerrahi (Basic Surgery) by Iskender Sayek, a renowned Turkish surgeon and professor of surgery. The book covers the fundamental principles and techniques of surgery, as well as the molecular basis and clinical applications of cancer surgery.
-
-
What is Iskender Sayek Temel Cerrahi Pdf?
-
-
Iskender Sayek Temel Cerrahi Pdf is a digital format of the book Temel Cerrahi, which was first published in 2013 by Gunes Kitabevi in Ankara. The book is edited by Iskender Sayek, who is also the author of several chapters. The book has 96 references and is divided into four sections: General Principles of Surgery, Surgical Techniques, Surgical Oncology, and Special Topics in Surgery. The book is written in Turkish and is intended for medical students, residents, and surgeons who want to learn or refresh their knowledge on basic surgery.
Why should you read Iskender Sayek Temel Cerrahi Pdf?
-
-
There are many reasons why you should read Iskender Sayek Temel Cerrahi Pdf, such as:
-
-
-
It provides a comprehensive overview of the basic concepts and skills of surgery, such as anatomy, physiology, pathology, diagnosis, treatment, complications, and prevention.
-
It explains the molecular mechanisms and genetic factors of cancer development and progression, as well as the current methods of diagnosis, staging, treatment, and follow-up of various types of cancers.
-
It demonstrates the surgical techniques and procedures for common and complex operations, such as laparoscopy, endoscopy, vascular surgery, transplantation, trauma surgery, and cosmetic surgery.
-
It discusses the special topics and issues in surgery, such as surgical ethics, infection control, pain management, wound healing, nutrition, fluid therapy, blood transfusion, and organ donation.
-
It is written by an experienced and respected surgeon and educator who has contributed to the advancement of surgery in Turkey and internationally.
-
It is available in PDF format which makes it easy to access, download, print, or share online.
-
-
-
How can you get Iskender Sayek Temel Cerrahi Pdf?
-
-
If you are interested in reading Iskender Sayek Temel Cerrahi Pdf, you can find it online on various websites that offer PDF downloads. Some examples are:
-
-
-
ResearchGate: This is a social network for researchers where you can find the PDF of the book chapter on cancer surgery by Ayse Ayhan and Hiroshi Ogawa.
-
Ateropedia: This is a website that provides PDF downloads of various books and articles on medicine and health. You can find the PDF of the entire book here.
-
NPM: This is a package manager for JavaScript that allows you to install and use various modules and packages. You can find a package that contains the PDF of the book here.
-
-
-
You can also search for other websites that offer Iskender Sayek Temel Cerrahi Pdf by using your favorite search engine. However, make sure to check the credibility and security of the websites before downloading anything from them.
-
-
-
Conclusion
-
-
Iskender Sayek Temel Cerrahi Pdf is a valuable resource for anyone who wants to learn more about basic surgery and surgical oncology. It is written by a reputable surgeon and professor who has extensive experience and knowledge in the field. It covers the essential topics and skills of surgery in a clear and comprehensive manner. It is available in PDF format which makes it convenient and accessible for online users. If you are interested in reading Iskender Sayek Temel Cerrahi Pdf, you can find it on various websites that offer PDF downloads.
-
Who is Iskender Sayek?
-
-
Iskender Sayek is the editor and author of Temel Cerrahi, as well as a distinguished surgeon and academician. He was born in 1945 in Ankara, Turkey. He graduated from Ankara University Faculty of Medicine in 1969 and completed his residency in general surgery at Hacettepe University Faculty of Medicine in 1974. He became a professor of surgery in 1984 and served as the head of the Department of General Surgery at Hacettepe University Faculty of Medicine from 1992 to 2012. He is currently an emeritus professor of surgery at Hacettepe University and a visiting professor of surgery at various universities around the world.
-
-
Iskender Sayek has made significant contributions to the field of surgery, especially in the areas of surgical education, surgical oncology, breast surgery, endocrine surgery, and laparoscopic surgery. He has published more than 300 articles and 20 books on surgery, as well as several chapters in international textbooks. He has received many awards and honors for his achievements, such as the Turkish Academy of Sciences Award, the Turkish Surgical Association Honorary Membership Award, the Turkish Medical Association Honorary Membership Award, and the International College of Surgeons Honorary Fellowship Award. He is also a member of several national and international surgical societies and organizations.
-
-
What are the benefits of Iskender Sayek Temel Cerrahi Pdf?
-
-
Iskender Sayek Temel Cerrahi Pdf offers many benefits for readers who want to learn more about basic surgery and surgical oncology. Some of these benefits are:
-
-
-
It is based on the latest scientific evidence and clinical practice guidelines.
-
It is written in a clear and concise language that is easy to understand and follow.
-
It is richly illustrated with figures, tables, diagrams, and photographs that enhance the learning experience.
-
It is updated with the most recent developments and innovations in surgery.
-
It is accessible and affordable for online users who can download it anytime and anywhere.
-
-
-
If you are interested in reading Iskender Sayek Temel Cerrahi Pdf, you can find it on various websites that offer PDF downloads. However, make sure to check the credibility and security of the websites before downloading anything from them.
-
What are the topics covered in Iskender Sayek Temel Cerrahi Pdf?
-
-
Iskender Sayek Temel Cerrahi Pdf covers a wide range of topics related to basic surgery and surgical oncology. Some of these topics are:
-
-
-
General Principles of Surgery: This section covers the basic concepts and skills of surgery, such as anatomy, physiology, pathology, diagnosis, treatment, complications, and prevention. It also covers the surgical ethics, infection control, pain management, wound healing, nutrition, fluid therapy, blood transfusion, and organ donation.
-
Surgical Techniques: This section covers the surgical techniques and procedures for common and complex operations, such as laparoscopy, endoscopy, vascular surgery, transplantation, trauma surgery, and cosmetic surgery. It also covers the preoperative and postoperative care, anesthesia, surgical instruments, sutures, drains, and dressings.
-
Surgical Oncology: This section covers the molecular mechanisms and genetic factors of cancer development and progression, as well as the current methods of diagnosis, staging, treatment, and follow-up of various types of cancers. It also covers the principles of oncologic surgery, such as tumor biology, tumor markers, tumor immunology, tumor angiogenesis, tumor invasion and metastasis, tumor microenvironment, and tumor heterogeneity.
-
Special Topics in Surgery: This section covers the special topics and issues in surgery, such as pediatric surgery, geriatric surgery, bariatric surgery, endocrine surgery, breast surgery, colorectal surgery, hepatobiliary surgery, pancreatic surgery, gastric surgery, esophageal surgery, thyroid surgery, parathyroid surgery, adrenal surgery.
-
-
-
What are the features of Iskender Sayek Temel Cerrahi Pdf?
-
-
Iskender Sayek Temel Cerrahi Pdf has many features that make it a useful and user-friendly resource for readers who want to learn more about basic surgery and surgical oncology. Some of these features are:
-
-
-
It is written by an experienced and respected surgeon and educator who has contributed to the advancement of surgery in Turkey and internationally.
-
It is based on the latest scientific evidence and clinical practice guidelines.
-
It is written in a clear and concise language that is easy to understand and follow.
-
It is richly illustrated with figures, tables, diagrams, and photographs that enhance the learning experience.
-
It is updated with the most recent developments and innovations in surgery.
-
It is available in PDF format which makes it easy to access, download, print, or share online.
-
-
-
If you are interested in reading Iskender Sayek Temel Cerrahi Pdf, you can find it on various websites that offer PDF downloads. However, make sure to check the credibility and security of the websites before downloading anything from them.
'
-
- code_block_pattern = r"```(\w+)?\n([\s\S]+?)\n```"
- md_str = re.sub(code_block_pattern, replacer, md_str, flags=re.MULTILINE)
-
- html_str = markdown(md_str)
- return html_str
-
-
-def normalize_markdown(md_text: str) -> str:
- lines = md_text.split("\n")
- normalized_lines = []
- inside_list = False
-
- for i, line in enumerate(lines):
- if re.match(r"^(\d+\.|-|\*|\+)\s", line.strip()):
- if not inside_list and i > 0 and lines[i - 1].strip() != "":
- normalized_lines.append("")
- inside_list = True
- normalized_lines.append(line)
- elif inside_list and line.strip() == "":
- if i < len(lines) - 1 and not re.match(
- r"^(\d+\.|-|\*|\+)\s", lines[i + 1].strip()
- ):
- normalized_lines.append(line)
- continue
- else:
- inside_list = False
- normalized_lines.append(line)
-
- return "\n".join(normalized_lines)
-
-
-def convert_mdtext(md_text):
- code_block_pattern = re.compile(r"```(.*?)(?:```|$)", re.DOTALL)
- inline_code_pattern = re.compile(r"`(.*?)`", re.DOTALL)
- code_blocks = code_block_pattern.findall(md_text)
- non_code_parts = code_block_pattern.split(md_text)[::2]
-
- result = []
- for non_code, code in zip(non_code_parts, code_blocks + [""]):
- if non_code.strip():
- non_code = normalize_markdown(non_code)
- if inline_code_pattern.search(non_code):
- result.append(markdown(non_code, extensions=["tables"]))
- else:
- result.append(mdtex2html.convert(non_code, extensions=["tables"]))
- if code.strip():
- # _, code = detect_language(code) # 暂时去除代码高亮功能,因为在大段代码的情况下会出现问题
- # code = code.replace("\n\n", "\n") # 暂时去除代码中的空行,因为在大段代码的情况下会出现问题
- code = f"\n```{code}\n\n```"
- code = markdown_to_html_with_syntax_highlight(code)
- result.append(code)
- result = "".join(result)
- result += ALREADY_CONVERTED_MARK
- return result
-
-
-def convert_asis(userinput):
- return (
- f'
{html.escape(userinput)}
'
- + ALREADY_CONVERTED_MARK
- )
-
-
-def detect_converted_mark(userinput):
- if userinput.endswith(ALREADY_CONVERTED_MARK):
- return True
- else:
- return False
-
-
-def detect_language(code):
- if code.startswith("\n"):
- first_line = ""
- else:
- first_line = code.strip().split("\n", 1)[0]
- language = first_line.lower() if first_line else ""
- code_without_language = code[len(first_line):].lstrip() if first_line else code
- return language, code_without_language
-
-
-def construct_text(role, text):
- return {"role": role, "content": text}
-
-
-def construct_user(text):
- return construct_text("user", text)
-
-
-def construct_system(text):
- return construct_text("system", text)
-
-
-def construct_assistant(text):
- return construct_text("assistant", text)
-
-
-def construct_token_message(token, stream=False):
- return f"Token 计数: {token}"
-
-
-def delete_first_conversation(history, previous_token_count):
- if history:
- del history[:2]
- del previous_token_count[0]
- return (
- history,
- previous_token_count,
- construct_token_message(sum(previous_token_count)),
- )
-
-
-def delete_last_conversation(chatbot, history, previous_token_count):
- if len(chatbot) > 0 and standard_error_msg in chatbot[-1][1]:
- logging.info("由于包含报错信息,只删除chatbot记录")
- chatbot.pop()
- return chatbot, history
- if len(history) > 0:
- logging.info("删除了一组对话历史")
- history.pop()
- history.pop()
- if len(chatbot) > 0:
- logging.info("删除了一组chatbot对话")
- chatbot.pop()
- if len(previous_token_count) > 0:
- logging.info("删除了一组对话的token计数记录")
- previous_token_count.pop()
- return (
- chatbot,
- history,
- previous_token_count,
- construct_token_message(sum(previous_token_count)),
- )
-
-
-def save_file(filename, system, history, chatbot):
- logging.info("保存对话历史中……")
- os.makedirs(HISTORY_DIR, exist_ok=True)
- if filename.endswith(".json"):
- json_s = {"system": system, "history": history, "chatbot": chatbot}
- print(json_s)
- with open(os.path.join(HISTORY_DIR, filename), "w") as f:
- json.dump(json_s, f)
- elif filename.endswith(".md"):
- md_s = f"system: \n- {system} \n"
- for data in history:
- md_s += f"\n{data['role']}: \n- {data['content']} \n"
- with open(os.path.join(HISTORY_DIR, filename), "w", encoding="utf8") as f:
- f.write(md_s)
- logging.info("保存对话历史完毕")
- return os.path.join(HISTORY_DIR, filename)
-
-
-def save_chat_history(filename, system, history, chatbot):
- if filename == "":
- return
- if not filename.endswith(".json"):
- filename += ".json"
- return save_file(filename, system, history, chatbot)
-
-
-def export_markdown(filename, system, history, chatbot):
- if filename == "":
- return
- if not filename.endswith(".md"):
- filename += ".md"
- return save_file(filename, system, history, chatbot)
-
-
-def load_chat_history(filename, system, history, chatbot):
- logging.info("加载对话历史中……")
- if type(filename) != str:
- filename = filename.name
- try:
- with open(os.path.join(HISTORY_DIR, filename), "r") as f:
- json_s = json.load(f)
- try:
- if type(json_s["history"][0]) == str:
- logging.info("历史记录格式为旧版,正在转换……")
- new_history = []
- for index, item in enumerate(json_s["history"]):
- if index % 2 == 0:
- new_history.append(construct_user(item))
- else:
- new_history.append(construct_assistant(item))
- json_s["history"] = new_history
- logging.info(new_history)
- except:
- # 没有对话历史
- pass
- logging.info("加载对话历史完毕")
- return filename, json_s["system"], json_s["history"], json_s["chatbot"]
- except FileNotFoundError:
- logging.info("没有找到对话历史文件,不执行任何操作")
- return filename, system, history, chatbot
-
-
-def sorted_by_pinyin(list):
- return sorted(list, key=lambda char: lazy_pinyin(char)[0][0])
-
-
-def get_file_names(dir, plain=False, filetypes=[".json"]):
- logging.info(f"获取文件名列表,目录为{dir},文件类型为{filetypes},是否为纯文本列表{plain}")
- files = []
- try:
- for type in filetypes:
- files += [f for f in os.listdir(dir) if f.endswith(type)]
- except FileNotFoundError:
- files = []
- files = sorted_by_pinyin(files)
- if files == []:
- files = [""]
- if plain:
- return files
- else:
- return gr.Dropdown.update(choices=files)
-
-
-def get_history_names(plain=False):
- logging.info("获取历史记录文件名列表")
- return get_file_names(HISTORY_DIR, plain)
-
-
-def load_template(filename, mode=0):
- logging.info(f"加载模板文件{filename},模式为{mode}(0为返回字典和下拉菜单,1为返回下拉菜单,2为返回字典)")
- lines = []
- logging.info("Loading template...")
- if filename.endswith(".json"):
- with open(os.path.join(TEMPLATES_DIR, filename), "r", encoding="utf8") as f:
- lines = json.load(f)
- lines = [[i["act"], i["prompt"]] for i in lines]
- else:
- with open(
- os.path.join(TEMPLATES_DIR, filename), "r", encoding="utf8"
- ) as csvfile:
- reader = csv.reader(csvfile)
- lines = list(reader)
- lines = lines[1:]
- if mode == 1:
- return sorted_by_pinyin([row[0] for row in lines])
- elif mode == 2:
- return {row[0]: row[1] for row in lines}
- else:
- choices = sorted_by_pinyin([row[0] for row in lines])
- return {row[0]: row[1] for row in lines}, gr.Dropdown.update(
- choices=choices, value=choices[0]
- )
-
-
-def get_template_names(plain=False):
- logging.info("获取模板文件名列表")
- return get_file_names(TEMPLATES_DIR, plain, filetypes=[".csv", "json"])
-
-
-def get_template_content(templates, selection, original_system_prompt):
- logging.info(f"应用模板中,选择为{selection},原始系统提示为{original_system_prompt}")
- try:
- return templates[selection]
- except:
- return original_system_prompt
-
-def get_pre_defined_q(selection):
- with open(r'questions.txt', 'r') as f:
- qs = f.readlines()
- for i in qs:
- qs[qs.index(i)] = i.replace('\n', '')
- return qs[qs.index(selection)]
-
-def reset_state():
- logging.info("重置状态")
- return [], [], [], construct_token_message(0)
-
-
-def reset_textbox():
- logging.debug("重置文本框")
- return gr.update(value="")
-
-
-def reset_default():
- newurl = shared.state.reset_api_url()
- os.environ.pop("HTTPS_PROXY", None)
- os.environ.pop("https_proxy", None)
- return gr.update(value=newurl), gr.update(value=""), "API URL 和代理已重置"
-
-
-def change_api_url(url):
- shared.state.set_api_url(url)
- msg = f"API地址更改为了{url}"
- logging.info(msg)
- return msg
-
-
-def change_proxy(proxy):
- os.environ["HTTPS_PROXY"] = proxy
- msg = f"代理更改为了{proxy}"
- logging.info(msg)
- return msg
-
-
-def hide_middle_chars(s):
- if len(s) <= 8:
- return s
- else:
- head = s[:4]
- tail = s[-4:]
- hidden = "*" * (len(s) - 8)
- return head + hidden + tail
-
-
-def submit_key(key):
- key = key.strip()
- msg = f"API密钥更改为了{hide_middle_chars(key)}"
- logging.info(msg)
- return key, msg
-
-
-def replace_today(prompt):
- today = datetime.datetime.today().strftime("%Y-%m-%d")
- return prompt.replace("{current_date}", today)
-
-
-def get_geoip():
- response = requests.get("https://ipapi.co/json/", timeout=5)
- try:
- data = response.json()
- except:
- data = {"error": True, "reason": "连接ipapi失败"}
- if "error" in data.keys():
- logging.warning(f"无法获取IP地址信息。\n{data}")
- if data["reason"] == "RateLimited":
- return (
- f"获取IP地理位置失败,因为达到了检测IP的速率限制。聊天功能可能仍然可用,但请注意,如果您的IP地址在不受支持的地区,您可能会遇到问题。"
- )
- else:
- return f"获取IP地理位置失败。原因:{data['reason']}。你仍然可以使用聊天功能。"
- else:
- country = data["country_name"]
- if country == "China":
- text = "**您的IP区域:中国。请立即检查代理设置,在不受支持的地区使用API可能导致账号被封禁。**"
- else:
- text = f"您的IP区域:{country}。"
- logging.info(text)
- return text
-
-
-def find_n(lst, max_num):
- n = len(lst)
- total = sum(lst)
-
- if total < max_num:
- return n
-
- for i in range(len(lst)):
- if total - lst[i] < max_num:
- return n - i - 1
- total = total - lst[i]
- return 1
-
-
-def start_outputing():
- logging.debug("显示取消按钮,隐藏发送按钮")
- return gr.Button.update(visible=False), gr.Button.update(visible=True)
-
-
-def end_outputing():
- return (
- gr.Button.update(visible=True),
- gr.Button.update(visible=False),
- )
-
-
-def cancel_outputing():
- logging.info("中止输出……")
- shared.state.interrupt()
-
-
-def transfer_input(inputs):
- # 一次性返回,降低延迟
- textbox = reset_textbox()
- outputing = start_outputing()
- return (
- inputs,
- gr.update(value=""),
- gr.Button.update(visible=False),
- gr.Button.update(visible=True),
- )
-
-
-def get_proxies():
- # 获取环境变量中的代理设置
- http_proxy = os.environ.get("HTTP_PROXY") or os.environ.get("http_proxy")
- https_proxy = os.environ.get("HTTPS_PROXY") or os.environ.get("https_proxy")
-
- # 如果存在代理设置,使用它们
- proxies = {}
- if http_proxy:
- logging.info(f"使用 HTTP 代理: {http_proxy}")
- proxies["http"] = http_proxy
- if https_proxy:
- logging.info(f"使用 HTTPS 代理: {https_proxy}")
- proxies["https"] = https_proxy
-
- if proxies == {}:
- proxies = None
-
- return proxies
-
-
-def run(command, desc=None, errdesc=None, custom_env=None, live=False):
- if desc is not None:
- print(desc)
- if live:
- result = subprocess.run(command, shell=True, env=os.environ if custom_env is None else custom_env)
- if result.returncode != 0:
- raise RuntimeError(f"""{errdesc or 'Error running command'}.
-Command: {command}
-Error code: {result.returncode}""")
-
- return ""
- result = subprocess.run(command, stdout=subprocess.PIPE, stderr=subprocess.PIPE, shell=True,
- env=os.environ if custom_env is None else custom_env)
- if result.returncode != 0:
- message = f"""{errdesc or 'Error running command'}.
-Command: {command}
-Error code: {result.returncode}
-stdout: {result.stdout.decode(encoding="utf8", errors="ignore") if len(result.stdout) > 0 else ''}
-stderr: {result.stderr.decode(encoding="utf8", errors="ignore") if len(result.stderr) > 0 else ''}
-"""
- raise RuntimeError(message)
- return result.stdout.decode(encoding="utf8", errors="ignore")
-
-
-def versions_html():
- git = os.environ.get('GIT', "git")
- python_version = ".".join([str(x) for x in sys.version_info[0:3]])
- try:
- commit_hash = run(f"{git} rev-parse HEAD").strip()
- except Exception:
- commit_hash = ""
- if commit_hash != "":
- short_commit = commit_hash[0:7]
- commit_info = f"{short_commit}"
- else:
- commit_info = "unknown \U0001F615"
- return f"""
-Python: {python_version}
-Gradio: {gr.__version__}
-Commit: {commit_info}
-"""
-
-def get_function_content(functions, selection):
- return functions[selection]
-
-
-def get_character_content(content):
- return content
\ No newline at end of file
diff --git a/spaces/emc348/faces-through-time/torch_utils/persistence.py b/spaces/emc348/faces-through-time/torch_utils/persistence.py
deleted file mode 100644
index 0186cfd97bca0fcb397a7b73643520c1d1105a02..0000000000000000000000000000000000000000
--- a/spaces/emc348/faces-through-time/torch_utils/persistence.py
+++ /dev/null
@@ -1,251 +0,0 @@
-# Copyright (c) 2021, NVIDIA CORPORATION. All rights reserved.
-#
-# NVIDIA CORPORATION and its licensors retain all intellectual property
-# and proprietary rights in and to this software, related documentation
-# and any modifications thereto. Any use, reproduction, disclosure or
-# distribution of this software and related documentation without an express
-# license agreement from NVIDIA CORPORATION is strictly prohibited.
-
-"""Facilities for pickling Python code alongside other data.
-
-The pickled code is automatically imported into a separate Python module
-during unpickling. This way, any previously exported pickles will remain
-usable even if the original code is no longer available, or if the current
-version of the code is not consistent with what was originally pickled."""
-
-import sys
-import pickle
-import io
-import inspect
-import copy
-import uuid
-import types
-import dnnlib
-
-#----------------------------------------------------------------------------
-
-_version = 6 # internal version number
-_decorators = set() # {decorator_class, ...}
-_import_hooks = [] # [hook_function, ...]
-_module_to_src_dict = dict() # {module: src, ...}
-_src_to_module_dict = dict() # {src: module, ...}
-
-#----------------------------------------------------------------------------
-
-def persistent_class(orig_class):
- r"""Class decorator that extends a given class to save its source code
- when pickled.
-
- Example:
-
- from torch_utils import persistence
-
- @persistence.persistent_class
- class MyNetwork(torch.nn.Module):
- def __init__(self, num_inputs, num_outputs):
- super().__init__()
- self.fc = MyLayer(num_inputs, num_outputs)
- ...
-
- @persistence.persistent_class
- class MyLayer(torch.nn.Module):
- ...
-
- When pickled, any instance of `MyNetwork` and `MyLayer` will save its
- source code alongside other internal state (e.g., parameters, buffers,
- and submodules). This way, any previously exported pickle will remain
- usable even if the class definitions have been modified or are no
- longer available.
-
- The decorator saves the source code of the entire Python module
- containing the decorated class. It does *not* save the source code of
- any imported modules. Thus, the imported modules must be available
- during unpickling, also including `torch_utils.persistence` itself.
-
- It is ok to call functions defined in the same module from the
- decorated class. However, if the decorated class depends on other
- classes defined in the same module, they must be decorated as well.
- This is illustrated in the above example in the case of `MyLayer`.
-
- It is also possible to employ the decorator just-in-time before
- calling the constructor. For example:
-
- cls = MyLayer
- if want_to_make_it_persistent:
- cls = persistence.persistent_class(cls)
- layer = cls(num_inputs, num_outputs)
-
- As an additional feature, the decorator also keeps track of the
- arguments that were used to construct each instance of the decorated
- class. The arguments can be queried via `obj.init_args` and
- `obj.init_kwargs`, and they are automatically pickled alongside other
- object state. A typical use case is to first unpickle a previous
- instance of a persistent class, and then upgrade it to use the latest
- version of the source code:
-
- with open('old_pickle.pkl', 'rb') as f:
- old_net = pickle.load(f)
- new_net = MyNetwork(*old_obj.init_args, **old_obj.init_kwargs)
- misc.copy_params_and_buffers(old_net, new_net, require_all=True)
- """
- assert isinstance(orig_class, type)
- if is_persistent(orig_class):
- return orig_class
-
- assert orig_class.__module__ in sys.modules
- orig_module = sys.modules[orig_class.__module__]
- orig_module_src = _module_to_src(orig_module)
-
- class Decorator(orig_class):
- _orig_module_src = orig_module_src
- _orig_class_name = orig_class.__name__
-
- def __init__(self, *args, **kwargs):
- super().__init__(*args, **kwargs)
- self._init_args = copy.deepcopy(args)
- self._init_kwargs = copy.deepcopy(kwargs)
- assert orig_class.__name__ in orig_module.__dict__
- _check_pickleable(self.__reduce__())
-
- @property
- def init_args(self):
- return copy.deepcopy(self._init_args)
-
- @property
- def init_kwargs(self):
- return dnnlib.EasyDict(copy.deepcopy(self._init_kwargs))
-
- def __reduce__(self):
- fields = list(super().__reduce__())
- fields += [None] * max(3 - len(fields), 0)
- if fields[0] is not _reconstruct_persistent_obj:
- meta = dict(type='class', version=_version, module_src=self._orig_module_src, class_name=self._orig_class_name, state=fields[2])
- fields[0] = _reconstruct_persistent_obj # reconstruct func
- fields[1] = (meta,) # reconstruct args
- fields[2] = None # state dict
- return tuple(fields)
-
- Decorator.__name__ = orig_class.__name__
- _decorators.add(Decorator)
- return Decorator
-
-#----------------------------------------------------------------------------
-
-def is_persistent(obj):
- r"""Test whether the given object or class is persistent, i.e.,
- whether it will save its source code when pickled.
- """
- try:
- if obj in _decorators:
- return True
- except TypeError:
- pass
- return type(obj) in _decorators # pylint: disable=unidiomatic-typecheck
-
-#----------------------------------------------------------------------------
-
-def import_hook(hook):
- r"""Register an import hook that is called whenever a persistent object
- is being unpickled. A typical use case is to patch the pickled source
- code to avoid errors and inconsistencies when the API of some imported
- module has changed.
-
- The hook should have the following signature:
-
- hook(meta) -> modified meta
-
- `meta` is an instance of `dnnlib.EasyDict` with the following fields:
-
- type: Type of the persistent object, e.g. `'class'`.
- version: Internal version number of `torch_utils.persistence`.
- module_src Original source code of the Python module.
- class_name: Class name in the original Python module.
- state: Internal state of the object.
-
- Example:
-
- @persistence.import_hook
- def wreck_my_network(meta):
- if meta.class_name == 'MyNetwork':
- print('MyNetwork is being imported. I will wreck it!')
- meta.module_src = meta.module_src.replace("True", "False")
- return meta
- """
- assert callable(hook)
- _import_hooks.append(hook)
-
-#----------------------------------------------------------------------------
-
-def _reconstruct_persistent_obj(meta):
- r"""Hook that is called internally by the `pickle` module to unpickle
- a persistent object.
- """
- meta = dnnlib.EasyDict(meta)
- meta.state = dnnlib.EasyDict(meta.state)
- for hook in _import_hooks:
- meta = hook(meta)
- assert meta is not None
-
- assert meta.version == _version
- module = _src_to_module(meta.module_src)
-
- assert meta.type == 'class'
- orig_class = module.__dict__[meta.class_name]
- decorator_class = persistent_class(orig_class)
- obj = decorator_class.__new__(decorator_class)
-
- setstate = getattr(obj, '__setstate__', None)
- if callable(setstate):
- setstate(meta.state) # pylint: disable=not-callable
- else:
- obj.__dict__.update(meta.state)
- return obj
-
-#----------------------------------------------------------------------------
-
-def _module_to_src(module):
- r"""Query the source code of a given Python module.
- """
- src = _module_to_src_dict.get(module, None)
- if src is None:
- src = inspect.getsource(module)
- _module_to_src_dict[module] = src
- _src_to_module_dict[src] = module
- return src
-
-def _src_to_module(src):
- r"""Get or create a Python module for the given source code.
- """
- module = _src_to_module_dict.get(src, None)
- if module is None:
- module_name = "_imported_module_" + uuid.uuid4().hex
- module = types.ModuleType(module_name)
- sys.modules[module_name] = module
- _module_to_src_dict[module] = src
- _src_to_module_dict[src] = module
- exec(src, module.__dict__) # pylint: disable=exec-used
- return module
-
-#----------------------------------------------------------------------------
-
-def _check_pickleable(obj):
- r"""Check that the given object is pickleable, raising an exception if
- it is not. This function is expected to be considerably more efficient
- than actually pickling the object.
- """
- def recurse(obj):
- if isinstance(obj, (list, tuple, set)):
- return [recurse(x) for x in obj]
- if isinstance(obj, dict):
- return [[recurse(x), recurse(y)] for x, y in obj.items()]
- if isinstance(obj, (str, int, float, bool, bytes, bytearray)):
- return None # Python primitive types are pickleable.
- if f'{type(obj).__module__}.{type(obj).__name__}' in ['numpy.ndarray', 'torch.Tensor']:
- return None # NumPy arrays and PyTorch tensors are pickleable.
- if is_persistent(obj):
- return None # Persistent objects are pickleable, by virtue of the constructor check.
- return obj
- with io.BytesIO() as f:
- pickle.dump(recurse(obj), f)
-
-#----------------------------------------------------------------------------
diff --git a/spaces/enesbol/case_dif/w.o_edges/trainer.py b/spaces/enesbol/case_dif/w.o_edges/trainer.py
deleted file mode 100644
index 4bf43a6fbd8b09b6f80d1a52a9f0f2f10ecbf6c1..0000000000000000000000000000000000000000
--- a/spaces/enesbol/case_dif/w.o_edges/trainer.py
+++ /dev/null
@@ -1,289 +0,0 @@
-"""
-author: Min Seok Lee and Wooseok Shin
-Github repo: https://github.com/Karel911/TRACER
-"""
-
-import os
-import cv2
-import time
-import numpy as np
-import torch
-import torch.nn as nn
-import torch.nn.functional as F
-from tqdm import tqdm
-from dataloader import get_train_augmentation, get_test_augmentation, get_loader, gt_to_tensor
-from util.utils import AvgMeter
-from util.metrics import Evaluation_metrics
-from util.losses import Optimizer, Scheduler, Criterion
-from model.TRACER import TRACER
-
-
-class Trainer():
- def __init__(self, args, save_path):
- super(Trainer, self).__init__()
- self.device = torch.device('cuda' if torch.cuda.is_available() else 'cpu')
- self.size = args.img_size
-
- self.tr_img_folder = os.path.join(args.data_path, args.dataset, 'Train/images/')
- self.tr_gt_folder = os.path.join(args.data_path, args.dataset, 'Train/masks/')
-
- self.train_transform = get_train_augmentation(img_size=args.img_size, ver=args.aug_ver)
- self.test_transform = get_test_augmentation(img_size=args.img_size)
-
- self.train_loader = get_loader(self.tr_img_folder, self.tr_gt_folder, phase='train',
- batch_size=args.batch_size, shuffle=True, num_workers=args.num_workers,
- transform=self.train_transform, seed=args.seed)
- self.val_loader = get_loader(self.tr_img_folder, self.tr_gt_folder, phase='val',
- batch_size=args.batch_size, shuffle=False, num_workers=args.num_workers,
- transform=self.test_transform, seed=args.seed)
-
- # Network
- self.model = TRACER(args).to(self.device)
-
- if args.multi_gpu:
- self.model = nn.DataParallel(self.model).to(self.device)
-
- # Loss and Optimizer
- self.criterion = Criterion(args)
- self.optimizer = Optimizer(args, self.model)
- self.scheduler = Scheduler(args, self.optimizer)
-
- # Train / Validate
- min_loss = 1000
- early_stopping = 0
- t = time.time()
- for epoch in range(1, args.epochs + 1):
- self.epoch = epoch
- train_loss, train_mae = self.training(args)
- val_loss, val_mae = self.validate()
-
- if args.scheduler == 'Reduce':
- self.scheduler.step(val_loss)
- else:
- self.scheduler.step()
-
- # Save models
- if val_loss < min_loss:
- early_stopping = 0
- best_epoch = epoch
- best_mae = val_mae
- min_loss = val_loss
- torch.save(self.model.state_dict(), os.path.join(save_path, 'best_model.pth'))
- print(f'-----------------SAVE:{best_epoch}epoch----------------')
- else:
- early_stopping += 1
-
- if early_stopping == args.patience + 5:
- break
-
- print(f'\nBest Val Epoch:{best_epoch} | Val Loss:{min_loss:.3f} | Val MAE:{best_mae:.3f} '
- f'time: {(time.time() - t) / 60:.3f}M')
-
- # Test time
- datasets = ['DUTS', 'DUT-O', 'HKU-IS', 'ECSSD', 'PASCAL-S']
- for dataset in datasets:
- args.dataset = dataset
- test_loss, test_mae, test_maxf, test_avgf, test_s_m = self.test(args, os.path.join(save_path))
-
- print(
- f'Test Loss:{test_loss:.3f} | MAX_F:{test_maxf:.3f} | AVG_F:{test_avgf:.3f} | MAE:{test_mae:.3f} '
- f'| S_Measure:{test_s_m:.3f}, time: {time.time() - t:.3f}s')
-
- end = time.time()
- print(f'Total Process time:{(end - t) / 60:.3f}Minute')
-
- def training(self, args):
- self.model.train()
- train_loss = AvgMeter()
- train_mae = AvgMeter()
-
- for images, masks in tqdm(self.train_loader):
- images = torch.tensor(images, device=self.device, dtype=torch.float32)
- masks = torch.tensor(masks, device=self.device, dtype=torch.float32)
-
- self.optimizer.zero_grad()
- outputs, ds_map = self.model(images)
- loss1 = self.criterion(outputs, masks)
- loss2 = self.criterion(ds_map[0], masks)
- loss3 = self.criterion(ds_map[1], masks)
- loss4 = self.criterion(ds_map[2], masks)
-
- loss = loss1 + loss2 + loss3 + loss4
-
- loss.backward()
- nn.utils.clip_grad_norm_(self.model.parameters(), args.clipping)
- self.optimizer.step()
-
- # Metric
- mae = torch.mean(torch.abs(outputs - masks))
-
- # log
- train_loss.update(loss.item(), n=images.size(0))
- train_mae.update(mae.item(), n=images.size(0))
-
- print(f'Epoch:[{self.epoch:03d}/{args.epochs:03d}]')
- print(f'Train Loss:{train_loss.avg:.3f} | MAE:{train_mae.avg:.3f}')
-
- return train_loss.avg, train_mae.avg
-
- def validate(self):
- self.model.eval()
- val_loss = AvgMeter()
- val_mae = AvgMeter()
-
- with torch.no_grad():
- for images, masks in tqdm(self.val_loader):
- images = torch.tensor(images, device=self.device, dtype=torch.float32)
- masks = torch.tensor(masks, device=self.device, dtype=torch.float32)
-
- outputs, ds_map = self.model(images)
- loss1 = self.criterion(outputs, masks)
- loss2 = self.criterion(ds_map[0], masks)
- loss3 = self.criterion(ds_map[1], masks)
- loss4 = self.criterion(ds_map[2], masks)
-
- loss = loss1 + loss2 + loss3 + loss4
-
- # Metric
- mae = torch.mean(torch.abs(outputs - masks))
-
- # log
- val_loss.update(loss.item(), n=images.size(0))
- val_mae.update(mae.item(), n=images.size(0))
-
- print(f'Valid Loss:{val_loss.avg:.3f} | MAE:{val_mae.avg:.3f}')
- return val_loss.avg, val_mae.avg
-
- def test(self, args, save_path):
- path = os.path.join(save_path, 'best_model.pth')
- self.model.load_state_dict(torch.load(path))
- print('###### pre-trained Model restored #####')
-
- te_img_folder = os.path.join(args.data_path, args.dataset, 'Test/images/')
- te_gt_folder = os.path.join(args.data_path, args.dataset, 'Test/masks/')
- test_loader = get_loader(te_img_folder, te_gt_folder, edge_folder=None, phase='test',
- batch_size=args.batch_size, shuffle=False,
- num_workers=args.num_workers, transform=self.test_transform)
-
- self.model.eval()
- test_loss = AvgMeter()
- test_mae = AvgMeter()
- test_maxf = AvgMeter()
- test_avgf = AvgMeter()
- test_s_m = AvgMeter()
-
- Eval_tool = Evaluation_metrics(args.dataset, self.device)
-
- with torch.no_grad():
- for i, (images, masks, original_size, image_name) in enumerate(tqdm(test_loader)):
- images = torch.tensor(images, device=self.device, dtype=torch.float32)
-
- outputs, ds_map = self.model(images)
- H, W = original_size
-
- for i in range(images.size(0)):
- mask = gt_to_tensor(masks[i])
-
- h, w = H[i].item(), W[i].item()
-
- output = F.interpolate(outputs[i].unsqueeze(0), size=(h, w), mode='bilinear')
-
- loss = self.criterion(output, mask)
-
- # Metric
- mae, max_f, avg_f, s_score = Eval_tool.cal_total_metrics(output, mask)
-
- # log
- test_loss.update(loss.item(), n=1)
- test_mae.update(mae, n=1)
- test_maxf.update(max_f, n=1)
- test_avgf.update(avg_f, n=1)
- test_s_m.update(s_score, n=1)
-
- test_loss = test_loss.avg
- test_mae = test_mae.avg
- test_maxf = test_maxf.avg
- test_avgf = test_avgf.avg
- test_s_m = test_s_m.avg
-
- return test_loss, test_mae, test_maxf, test_avgf, test_s_m
-
-
-class Tester():
- def __init__(self, args, save_path):
- super(Tester, self).__init__()
- self.device = torch.device('cuda' if torch.cuda.is_available() else 'cpu')
- self.test_transform = get_test_augmentation(img_size=args.img_size)
- self.args = args
- self.save_path = save_path
-
- # Network
- self.model = self.model = TRACER(args).to(self.device)
- if args.multi_gpu:
- self.model = nn.DataParallel(self.model).to(self.device)
-
- path = os.path.join(save_path, 'best_model.pth')
- self.model.load_state_dict(torch.load(path))
- print('###### pre-trained Model restored #####')
-
- self.criterion = Criterion(args)
-
- te_img_folder = os.path.join(args.data_path, args.dataset, 'Test/images/')
- te_gt_folder = os.path.join(args.data_path, args.dataset, 'Test/masks/')
- self.test_loader = get_loader(te_img_folder, te_gt_folder, edge_folder=None, phase='test',
- batch_size=args.batch_size, shuffle=False,
- num_workers=args.num_workers, transform=self.test_transform)
-
- if args.save_map is not None:
- os.makedirs(os.path.join('mask', 'exp'+str(self.args.exp_num), self.args.dataset), exist_ok=True)
-
- def test(self):
- self.model.eval()
- test_loss = AvgMeter()
- test_mae = AvgMeter()
- test_maxf = AvgMeter()
- test_avgf = AvgMeter()
- test_s_m = AvgMeter()
- t = time.time()
-
- Eval_tool = Evaluation_metrics(self.args.dataset, self.device)
-
- with torch.no_grad():
- for i, (images, masks, original_size, image_name) in enumerate(tqdm(self.test_loader)):
- images = torch.tensor(images, device=self.device, dtype=torch.float32)
-
- outputs, ds_map = self.model(images)
- H, W = original_size
-
- for i in range(images.size(0)):
- mask = gt_to_tensor(masks[i])
- h, w = H[i].item(), W[i].item()
-
- output = F.interpolate(outputs[i].unsqueeze(0), size=(h, w), mode='bilinear')
- loss = self.criterion(output, mask)
-
- # Metric
- mae, max_f, avg_f, s_score = Eval_tool.cal_total_metrics(output, mask)
-
- # Save prediction map
- if self.args.save_map is not None:
- output = (output.squeeze().detach().cpu().numpy()*255.0).astype(np.uint8) # convert uint8 type
- cv2.imwrite(os.path.join('mask', 'exp'+str(self.args.exp_num), self.args.dataset, image_name[i]+'.png'), output)
-
- # log
- test_loss.update(loss.item(), n=1)
- test_mae.update(mae, n=1)
- test_maxf.update(max_f, n=1)
- test_avgf.update(avg_f, n=1)
- test_s_m.update(s_score, n=1)
-
- test_loss = test_loss.avg
- test_mae = test_mae.avg
- test_maxf = test_maxf.avg
- test_avgf = test_avgf.avg
- test_s_m = test_s_m.avg
-
- print(f'Test Loss:{test_loss:.4f} | MAX_F:{test_maxf:.4f} | MAE:{test_mae:.4f} '
- f'| S_Measure:{test_s_m:.4f}, time: {time.time() - t:.3f}s')
-
- return test_loss, test_mae, test_maxf, test_avgf, test_s_m
diff --git a/spaces/epexVfeibi/Imagedeblurr/Adobe Master Collection 2019 Torrent !NEW!.md b/spaces/epexVfeibi/Imagedeblurr/Adobe Master Collection 2019 Torrent !NEW!.md
deleted file mode 100644
index be695bf450e61265a31ed6a3370004fb59948fd6..0000000000000000000000000000000000000000
--- a/spaces/epexVfeibi/Imagedeblurr/Adobe Master Collection 2019 Torrent !NEW!.md
+++ /dev/null
@@ -1,25 +0,0 @@
-
-
Adobe Master Collection 2019: A Complete Suite of Creative Tools
-
Adobe Master Collection 2019 is a comprehensive package of Adobe's creative software products that includes Photoshop, Illustrator, InDesign, Premiere Pro, After Effects, and more. With Adobe Master Collection 2019, you can create stunning graphics, design beautiful websites, edit amazing videos and photos, and unleash your creativity on any device.
Some of the features of Adobe Master Collection 2019 are:
-
-
Photoshop: Create gorgeous images, rich graphics, and incredible art with the world's best imaging and design software. Photoshop CC 2019 introduces new features such as Content-Aware Fill, Frame Tool, Symmetry Mode, and Live Blend Modes[^7^].
-
Illustrator: Create beautiful designs, icons, and more with the industry-standard vector graphics software. Illustrator CC 2019 offers new features such as Freeform Gradients, Global Editing, Customizable Toolbar, and Presentation Mode.
-
InDesign: Create and publish books, digital magazines, eBooks, posters, and interactive PDFs with the leading layout and page design software. InDesign CC 2019 brings new features such as Content-Aware Fit, Adjust Layout, Properties Panel, and Import PDF Comments.
-
Premiere Pro: Create everything from social clips to feature films with the leading video editing software. Premiere Pro CC 2019 introduces new features such as Selective Color Grading, Intelligent Audio Cleanup, Data-Driven Infographics, and VR 180.
-
After Effects: Create movie titles, intros, and transitions with the industry-standard motion graphics and visual effects software. After Effects CC 2019 offers new features such as Advanced Puppet Tool, Depth Passes for 3D Compositions, Responsive Design Time, and Expression Editor.
-
And more: Adobe Master Collection 2019 also includes other creative tools such as Acrobat Pro, Lightroom Classic, Adobe Stock, Adobe Fonts, Photoshop Express, and more.
-
-
Adobe Master Collection 2019 is available as a subscription service or a one-time purchase. You can download it from the official Adobe website or from various torrent sites. However, downloading from torrent sites may expose you to legal risks and malware infections. Therefore, we recommend that you use the official Adobe website to get the latest and safest version of Adobe Master Collection 2019.
With Adobe Master Collection 2019, you can create amazing projects for any purpose and platform. Whether you want to make a logo, a website, a video, an animation, or a document, you have the tools and resources to make it happen. Here are some examples of what you can create with Adobe Master Collection 2019:
-
-
-
A logo for your brand or business using Illustrator. You can use the Freeform Gradients feature to create natural and realistic color blends, and the Global Editing feature to edit multiple instances of an object at once.
-
A website for your portfolio or blog using Dreamweaver. You can use the Content-Aware Fit feature to automatically resize and crop images to fit your layout, and the Properties Panel feature to access and edit common properties of elements.
-
A video for your YouTube channel or social media using Premiere Pro. You can use the Selective Color Grading feature to adjust hue, saturation, and luminance values with precision, and the Intelligent Audio Cleanup feature to reduce noise and reverb from your audio clips.
-
An animation for your game or app using Animate. You can use the Asset Sculpting feature to deform and animate vector shapes, and the Layer Parenting feature to create complex animations with hierarchical relationships.
-
A document for your report or presentation using InDesign. You can use the Adjust Layout feature to automatically reformat your document based on new page size or orientation, and the Import PDF Comments feature to review and apply feedback from collaborators.
-
-
These are just some of the possibilities that Adobe Master Collection 2019 offers you. You can also explore other creative apps such as Photoshop, After Effects, Lightroom, Acrobat Pro, and more. You can also access millions of assets from Adobe Stock, thousands of fonts from Adobe Fonts, and hundreds of templates from Adobe Express. With Adobe Master Collection 2019, you have everything you need to make your creative vision a reality.
d5da3c52bf
-
-
\ No newline at end of file
diff --git a/spaces/evaluate-measurement/honest/README.md b/spaces/evaluate-measurement/honest/README.md
deleted file mode 100644
index ea8a09363bcbd5b4471dc585c55aeeae9f1a0ce6..0000000000000000000000000000000000000000
--- a/spaces/evaluate-measurement/honest/README.md
+++ /dev/null
@@ -1,130 +0,0 @@
----
-title: Honest
-emoji: 🤗
-colorFrom: blue
-colorTo: green
-sdk: gradio
-sdk_version: 3.0.2
-app_file: app.py
-pinned: false
-tags:
-- evaluate
-- measurement
-description: >-
- The HONEST score is a multilingual score that aims to compute how likely each language model is to produce hurtful completions based on a predefined set of prompts.
----
-
-# Measurement Card for HONEST
-
-## Measurement description
-The HONEST score aims to measure hurtful sentence completions in language models.
-The score uses HurtLex, a multilingual lexicon of hurtful language, to evaluate the completions.
-It aims to quantify how often sentences are completed with a hurtful word, and if there is a difference between
-groups (e.g. genders, sexual orientations, etc.).
-
-## How to use
-
-When loading the model, specify the language of the prompts and completions.
-The available languages are: 'it' (Italian), 'fr' (French), 'es' (Spanish), 'pt' (Portuguese), 'ro' (Romanian), 'en' (English).
-
-```python
->>> honest = evaluate.load('honest', 'en')
-```
-
-Arguments:
- **predictions** (list of list of `str`): a list of completions to [HONEST prompts](https://huggingface.co/datasets/MilaNLProc/honest)
- **groups** (list of `str`) (*optional*): a list of the identity groups each list of completions belongs to.
-
-
-## Output values
-
-`honest_score`: the HONEST score, representing the average number of hurtful completions across all groups
-`honest_score_per_group`: the HONEST score of each group separately.
-
-### Values from popular papers
-In the [original HONEST paper](https://aclanthology.org/2021.naacl-main.191.pdf), the following scores were calculated for models, with Top K referring to the number of model completions that were evaluated:
-
-
-| Model Name | Top K =1 | Top K =5 |Top K =20 |
-| ---------------- | -------- | -------- | -------- |
-| UmBERTo (OSCAR) | 5.24 | 8.19 | 7.14 |
-| UmBERTo (Wiki) | 5.48 | 7.19 | 5.14 |
-| GilBERTo | 7.14 | 11.57 | 8.68 |
-| ItalianBERT XXL | 9.05 | 10.67 | 9.12 |
-| FlauBERT | 4.76 | 3.29 | 2.43 |
-| CamemBERT (OSCAR)| 18.57 | 9.62 | 7.07 |
-| CamemBERT (Wiki) | 7.62 | 4.90 | 4.19 |
-| BETO | 4.29 | 5.95 | 6.88 |
-| BERTimbau | 4.05 | 6.00 | 5.04 |
-| RomanianBERT | 4.76 | 3.90 | 4.61 |
-| BERT-base | 1.19 | 2.67 | 3.55 |
-| BERT-large | 3.33 | 3.43 | 4.30 |
-| RoBERTa-base | 2.38 | 5.38 | 5.74 |
-| RoBERTa-large | 2.62 | 2.33 | 3.05 |
-| DistilBERT-base | 1.90 | 3.81 | 3.96 |
-| GPT-2 (IT) | 12.86 | 11.76 | 12.56 |
-| GPT-2 (FR) | 19.76 | 19.67 | 17.81 |
-| GPT-2 (PT) | 9.52 | 10.71 | 10.29 |
-| GPT-2 (EN) | 17.14 | 12.81 | 13.00 |
-
-
-## Examples
-
-Example 1: Calculating HONEST without groups
-
-```python
->>> honest = evaluate.load('honest', 'en')
->>> completions = [['CEO', 'businessman', 'politician'], ['good', 'excellent', 'smart'], ['secretary', 'prostitute', 'maid'], ['beautiful', 'smart', 'tall']]
->>> result = honest.compute(predictions=completions)
->>> round(result['honest_score'],3)
-0.167
-```
-
-Example 2: Calculating HONEST with 2 groups (e.g. male/female)
-```python
->>> honest = evaluate.load('honest', 'en')
->>> completions = [['CEO', 'businessman', 'father'], ['good', 'excellent', 'smart'], ['secretary', 'prostitute', 'maid'], ['beautiful', 'smart', 'tall']]
->>> groups = ["male", "male", "female", "female"]
->>> result = honest.compute(predictions=completions, groups = groups)
->>> {g:round(s,3) for g,s in result['honest_score_per_group'].items()}
-{'male': 0.0, 'female': 0.167}
-```
-
-Example 2: Calculating HONEST in another language (French)
-```python
->>> honest = evaluate.load('honest', 'fr')
->>> completions = [['PDG', 'avocat', 'père'], ['bon','excellent', 'intelligent'], ['secrétaire', 'prostituée', 'bonne'], ['belle', 'intelligente', 'grande']]
->>> result = honest.compute(predictions=completions)
->>> round(result['honest_score'],3)
-0.083
-```
-
-## Citation
-
-```bibtex
-@inproceedings{nozza-etal-2021-honest,
- title = {"{HONEST}: Measuring Hurtful Sentence Completion in Language Models"},
- author = "Nozza, Debora and Bianchi, Federico and Hovy, Dirk",
- booktitle = "Proceedings of the 2021 Conference of the North American Chapter of the Association for Computational Linguistics: Human Language Technologies",
- month = jun,
- year = "2021",
- address = "Online",
- publisher = "Association for Computational Linguistics",
- url = "https://aclanthology.org/2021.naacl-main.191",
- doi = "10.18653/v1/2021.naacl-main.191",
- pages = "2398--2406",
-}
-```
-
-```bibtex
-@inproceedings{nozza-etal-2022-measuring,
- title = {Measuring Harmful Sentence Completion in Language Models for LGBTQIA+ Individuals},
- author = "Nozza, Debora and Bianchi, Federico and Lauscher, Anne and Hovy, Dirk",
- booktitle = "Proceedings of the Second Workshop on Language Technology for Equality, Diversity and Inclusion",
- publisher = "Association for Computational Linguistics",
- year={2022}
-}
-```
-
-## Further References
-- Bassignana, Elisa, Valerio Basile, and Viviana Patti. ["Hurtlex: A multilingual lexicon of words to hurt."](http://ceur-ws.org/Vol-2253/paper49.pdf) 5th Italian Conference on Computational Linguistics, CLiC-it 2018. Vol. 2253. CEUR-WS, 2018.
diff --git a/spaces/evaluate-metric/sacrebleu/sacrebleu.py b/spaces/evaluate-metric/sacrebleu/sacrebleu.py
deleted file mode 100644
index 6e756f4d4c9bc78390e3bb0d104f0f4515c2a0b7..0000000000000000000000000000000000000000
--- a/spaces/evaluate-metric/sacrebleu/sacrebleu.py
+++ /dev/null
@@ -1,178 +0,0 @@
-# Copyright 2020 The HuggingFace Evaluate Authors.
-#
-# Licensed under the Apache License, Version 2.0 (the "License");
-# you may not use this file except in compliance with the License.
-# You may obtain a copy of the License at
-#
-# http://www.apache.org/licenses/LICENSE-2.0
-#
-# Unless required by applicable law or agreed to in writing, software
-# distributed under the License is distributed on an "AS IS" BASIS,
-# WITHOUT WARRANTIES OR CONDITIONS OF ANY KIND, either express or implied.
-# See the License for the specific language governing permissions and
-# limitations under the License.
-""" SACREBLEU metric. """
-
-import datasets
-import sacrebleu as scb
-from packaging import version
-
-import evaluate
-
-
-_CITATION = """\
-@inproceedings{post-2018-call,
- title = "A Call for Clarity in Reporting {BLEU} Scores",
- author = "Post, Matt",
- booktitle = "Proceedings of the Third Conference on Machine Translation: Research Papers",
- month = oct,
- year = "2018",
- address = "Belgium, Brussels",
- publisher = "Association for Computational Linguistics",
- url = "https://www.aclweb.org/anthology/W18-6319",
- pages = "186--191",
-}
-"""
-
-_DESCRIPTION = """\
-SacreBLEU provides hassle-free computation of shareable, comparable, and reproducible BLEU scores.
-Inspired by Rico Sennrich's `multi-bleu-detok.perl`, it produces the official WMT scores but works with plain text.
-It also knows all the standard test sets and handles downloading, processing, and tokenization for you.
-
-See the [README.md] file at https://github.com/mjpost/sacreBLEU for more information.
-"""
-
-_KWARGS_DESCRIPTION = """
-Produces BLEU scores along with its sufficient statistics
-from a source against one or more references.
-
-Args:
- predictions (`list` of `str`): list of translations to score. Each translation should be tokenized into a list of tokens.
- references (`list` of `list` of `str`): A list of lists of references. The contents of the first sub-list are the references for the first prediction, the contents of the second sub-list are for the second prediction, etc. Note that there must be the same number of references for each prediction (i.e. all sub-lists must be of the same length).
- smooth_method (`str`): The smoothing method to use, defaults to `'exp'`. Possible values are:
- - `'none'`: no smoothing
- - `'floor'`: increment zero counts
- - `'add-k'`: increment num/denom by k for n>1
- - `'exp'`: exponential decay
- smooth_value (`float`): The smoothing value. Only valid when `smooth_method='floor'` (in which case `smooth_value` defaults to `0.1`) or `smooth_method='add-k'` (in which case `smooth_value` defaults to `1`).
- tokenize (`str`): Tokenization method to use for BLEU. If not provided, defaults to `'zh'` for Chinese, `'ja-mecab'` for Japanese and `'13a'` (mteval) otherwise. Possible values are:
- - `'none'`: No tokenization.
- - `'zh'`: Chinese tokenization.
- - `'13a'`: mimics the `mteval-v13a` script from Moses.
- - `'intl'`: International tokenization, mimics the `mteval-v14` script from Moses
- - `'char'`: Language-agnostic character-level tokenization.
- - `'ja-mecab'`: Japanese tokenization. Uses the [MeCab tokenizer](https://pypi.org/project/mecab-python3).
- lowercase (`bool`): If `True`, lowercases the input, enabling case-insensitivity. Defaults to `False`.
- force (`bool`): If `True`, insists that your tokenized input is actually detokenized. Defaults to `False`.
- use_effective_order (`bool`): If `True`, stops including n-gram orders for which precision is 0. This should be `True`, if sentence-level BLEU will be computed. Defaults to `False`.
-
-Returns:
- 'score': BLEU score,
- 'counts': Counts,
- 'totals': Totals,
- 'precisions': Precisions,
- 'bp': Brevity penalty,
- 'sys_len': predictions length,
- 'ref_len': reference length,
-
-Examples:
-
- Example 1:
- >>> predictions = ["hello there general kenobi", "foo bar foobar"]
- >>> references = [["hello there general kenobi", "hello there !"], ["foo bar foobar", "foo bar foobar"]]
- >>> sacrebleu = evaluate.load("sacrebleu")
- >>> results = sacrebleu.compute(predictions=predictions, references=references)
- >>> print(list(results.keys()))
- ['score', 'counts', 'totals', 'precisions', 'bp', 'sys_len', 'ref_len']
- >>> print(round(results["score"], 1))
- 100.0
-
- Example 2:
- >>> predictions = ["hello there general kenobi",
- ... "on our way to ankh morpork"]
- >>> references = [["hello there general kenobi", "hello there !"],
- ... ["goodbye ankh morpork", "ankh morpork"]]
- >>> sacrebleu = evaluate.load("sacrebleu")
- >>> results = sacrebleu.compute(predictions=predictions,
- ... references=references)
- >>> print(list(results.keys()))
- ['score', 'counts', 'totals', 'precisions', 'bp', 'sys_len', 'ref_len']
- >>> print(round(results["score"], 1))
- 39.8
-"""
-
-
-@evaluate.utils.file_utils.add_start_docstrings(_DESCRIPTION, _KWARGS_DESCRIPTION)
-class Sacrebleu(evaluate.Metric):
- def _info(self):
- if version.parse(scb.__version__) < version.parse("1.4.12"):
- raise ImportWarning(
- "To use `sacrebleu`, the module `sacrebleu>=1.4.12` is required, and the current version of `sacrebleu` doesn't match this condition.\n"
- 'You can install it with `pip install "sacrebleu>=1.4.12"`.'
- )
- return evaluate.MetricInfo(
- description=_DESCRIPTION,
- citation=_CITATION,
- homepage="https://github.com/mjpost/sacreBLEU",
- inputs_description=_KWARGS_DESCRIPTION,
- features=[
- datasets.Features(
- {
- "predictions": datasets.Value("string", id="sequence"),
- "references": datasets.Sequence(datasets.Value("string", id="sequence"), id="references"),
- }
- ),
- datasets.Features(
- {
- "predictions": datasets.Value("string", id="sequence"),
- "references": datasets.Value("string", id="sequence"),
- }
- ),
- ],
- codebase_urls=["https://github.com/mjpost/sacreBLEU"],
- reference_urls=[
- "https://github.com/mjpost/sacreBLEU",
- "https://en.wikipedia.org/wiki/BLEU",
- "https://towardsdatascience.com/evaluating-text-output-in-nlp-bleu-at-your-own-risk-e8609665a213",
- ],
- )
-
- def _compute(
- self,
- predictions,
- references,
- smooth_method="exp",
- smooth_value=None,
- force=False,
- lowercase=False,
- tokenize=None,
- use_effective_order=False,
- ):
- # if only one reference is provided make sure we still use list of lists
- if isinstance(references[0], str):
- references = [[ref] for ref in references]
-
- references_per_prediction = len(references[0])
- if any(len(refs) != references_per_prediction for refs in references):
- raise ValueError("Sacrebleu requires the same number of references for each prediction")
- transformed_references = [[refs[i] for refs in references] for i in range(references_per_prediction)]
- output = scb.corpus_bleu(
- predictions,
- transformed_references,
- smooth_method=smooth_method,
- smooth_value=smooth_value,
- force=force,
- lowercase=lowercase,
- use_effective_order=use_effective_order,
- **(dict(tokenize=tokenize) if tokenize else {}),
- )
- output_dict = {
- "score": output.score,
- "counts": output.counts,
- "totals": output.totals,
- "precisions": output.precisions,
- "bp": output.bp,
- "sys_len": output.sys_len,
- "ref_len": output.ref_len,
- }
- return output_dict
diff --git a/spaces/facebook/MusicGen/audiocraft/modules/diffusion_schedule.py b/spaces/facebook/MusicGen/audiocraft/modules/diffusion_schedule.py
deleted file mode 100644
index 74ca6e3f2e7c4ff904d96dade315b0b46856778d..0000000000000000000000000000000000000000
--- a/spaces/facebook/MusicGen/audiocraft/modules/diffusion_schedule.py
+++ /dev/null
@@ -1,272 +0,0 @@
-# Copyright (c) Meta Platforms, Inc. and affiliates.
-# All rights reserved.
-#
-# This source code is licensed under the license found in the
-# LICENSE file in the root directory of this source tree.
-
-"""
-Functions for Noise Schedule, defines diffusion process, reverse process and data processor.
-"""
-
-from collections import namedtuple
-import random
-import typing as tp
-import julius
-import torch
-
-TrainingItem = namedtuple("TrainingItem", "noisy noise step")
-
-
-def betas_from_alpha_bar(alpha_bar):
- alphas = torch.cat([torch.Tensor([alpha_bar[0]]), alpha_bar[1:]/alpha_bar[:-1]])
- return 1 - alphas
-
-
-class SampleProcessor(torch.nn.Module):
- def project_sample(self, x: torch.Tensor):
- """Project the original sample to the 'space' where the diffusion will happen."""
- return x
-
- def return_sample(self, z: torch.Tensor):
- """Project back from diffusion space to the actual sample space."""
- return z
-
-
-class MultiBandProcessor(SampleProcessor):
- """
- MultiBand sample processor. The input audio is splitted across
- frequency bands evenly distributed in mel-scale.
-
- Each band will be rescaled to match the power distribution
- of Gaussian noise in that band, using online metrics
- computed on the first few samples.
-
- Args:
- n_bands (int): Number of mel-bands to split the signal over.
- sample_rate (int): Sample rate of the audio.
- num_samples (int): Number of samples to use to fit the rescaling
- for each band. The processor won't be stable
- until it has seen that many samples.
- power_std (float or list/tensor): The rescaling factor computed to match the
- power of Gaussian noise in each band is taken to
- that power, i.e. `1.` means full correction of the energy
- in each band, and values less than `1` means only partial
- correction. Can be used to balance the relative importance
- of low vs. high freq in typical audio signals.
- """
- def __init__(self, n_bands: int = 8, sample_rate: float = 24_000,
- num_samples: int = 10_000, power_std: tp.Union[float, tp.List[float], torch.Tensor] = 1.):
- super().__init__()
- self.n_bands = n_bands
- self.split_bands = julius.SplitBands(sample_rate, n_bands=n_bands)
- self.num_samples = num_samples
- self.power_std = power_std
- if isinstance(power_std, list):
- assert len(power_std) == n_bands
- power_std = torch.tensor(power_std)
- self.register_buffer('counts', torch.zeros(1))
- self.register_buffer('sum_x', torch.zeros(n_bands))
- self.register_buffer('sum_x2', torch.zeros(n_bands))
- self.register_buffer('sum_target_x2', torch.zeros(n_bands))
- self.counts: torch.Tensor
- self.sum_x: torch.Tensor
- self.sum_x2: torch.Tensor
- self.sum_target_x2: torch.Tensor
-
- @property
- def mean(self):
- mean = self.sum_x / self.counts
- return mean
-
- @property
- def std(self):
- std = (self.sum_x2 / self.counts - self.mean**2).clamp(min=0).sqrt()
- return std
-
- @property
- def target_std(self):
- target_std = self.sum_target_x2 / self.counts
- return target_std
-
- def project_sample(self, x: torch.Tensor):
- assert x.dim() == 3
- bands = self.split_bands(x)
- if self.counts.item() < self.num_samples:
- ref_bands = self.split_bands(torch.randn_like(x))
- self.counts += len(x)
- self.sum_x += bands.mean(dim=(2, 3)).sum(dim=1)
- self.sum_x2 += bands.pow(2).mean(dim=(2, 3)).sum(dim=1)
- self.sum_target_x2 += ref_bands.pow(2).mean(dim=(2, 3)).sum(dim=1)
- rescale = (self.target_std / self.std.clamp(min=1e-12)) ** self.power_std # same output size
- bands = (bands - self.mean.view(-1, 1, 1, 1)) * rescale.view(-1, 1, 1, 1)
- return bands.sum(dim=0)
-
- def return_sample(self, x: torch.Tensor):
- assert x.dim() == 3
- bands = self.split_bands(x)
- rescale = (self.std / self.target_std) ** self.power_std
- bands = bands * rescale.view(-1, 1, 1, 1) + self.mean.view(-1, 1, 1, 1)
- return bands.sum(dim=0)
-
-
-class NoiseSchedule:
- """Noise schedule for diffusion.
-
- Args:
- beta_t0 (float): Variance of the first diffusion step.
- beta_t1 (float): Variance of the last diffusion step.
- beta_exp (float): Power schedule exponent
- num_steps (int): Number of diffusion step.
- variance (str): choice of the sigma value for the denoising eq. Choices: "beta" or "beta_tilde"
- clip (float): clipping value for the denoising steps
- rescale (float): rescaling value to avoid vanishing signals unused by default (i.e 1)
- repartition (str): shape of the schedule only power schedule is supported
- sample_processor (SampleProcessor): Module that normalize data to match better the gaussian distribution
- noise_scale (float): Scaling factor for the noise
- """
- def __init__(self, beta_t0: float = 1e-4, beta_t1: float = 0.02, num_steps: int = 1000, variance: str = 'beta',
- clip: float = 5., rescale: float = 1., device='cuda', beta_exp: float = 1,
- repartition: str = "power", alpha_sigmoid: dict = {}, n_bands: tp.Optional[int] = None,
- sample_processor: SampleProcessor = SampleProcessor(), noise_scale: float = 1.0, **kwargs):
-
- self.beta_t0 = beta_t0
- self.beta_t1 = beta_t1
- self.variance = variance
- self.num_steps = num_steps
- self.clip = clip
- self.sample_processor = sample_processor
- self.rescale = rescale
- self.n_bands = n_bands
- self.noise_scale = noise_scale
- assert n_bands is None
- if repartition == "power":
- self.betas = torch.linspace(beta_t0 ** (1 / beta_exp), beta_t1 ** (1 / beta_exp), num_steps,
- device=device, dtype=torch.float) ** beta_exp
- else:
- raise RuntimeError('Not implemented')
- self.rng = random.Random(1234)
-
- def get_beta(self, step: tp.Union[int, torch.Tensor]):
- if self.n_bands is None:
- return self.betas[step]
- else:
- return self.betas[:, step] # [n_bands, len(step)]
-
- def get_initial_noise(self, x: torch.Tensor):
- if self.n_bands is None:
- return torch.randn_like(x)
- return torch.randn((x.size(0), self.n_bands, x.size(2)))
-
- def get_alpha_bar(self, step: tp.Optional[tp.Union[int, torch.Tensor]] = None) -> torch.Tensor:
- """Return 'alpha_bar', either for a given step, or as a tensor with its value for each step."""
- if step is None:
- return (1 - self.betas).cumprod(dim=-1) # works for simgle and multi bands
- if type(step) is int:
- return (1 - self.betas[:step + 1]).prod()
- else:
- return (1 - self.betas).cumprod(dim=0)[step].view(-1, 1, 1)
-
- def get_training_item(self, x: torch.Tensor, tensor_step: bool = False) -> TrainingItem:
- """Create a noisy data item for diffusion model training:
-
- Args:
- x (torch.Tensor): clean audio data torch.tensor(bs, 1, T)
- tensor_step (bool): If tensor_step = false, only one step t is sample,
- the whole batch is diffused to the same step and t is int.
- If tensor_step = true, t is a tensor of size (x.size(0),)
- every element of the batch is diffused to a independently sampled.
- """
- step: tp.Union[int, torch.Tensor]
- if tensor_step:
- bs = x.size(0)
- step = torch.randint(0, self.num_steps, size=(bs,), device=x.device)
- else:
- step = self.rng.randrange(self.num_steps)
- alpha_bar = self.get_alpha_bar(step) # [batch_size, n_bands, 1]
-
- x = self.sample_processor.project_sample(x)
- noise = torch.randn_like(x)
- noisy = (alpha_bar.sqrt() / self.rescale) * x + (1 - alpha_bar).sqrt() * noise * self.noise_scale
- return TrainingItem(noisy, noise, step)
-
- def generate(self, model: torch.nn.Module, initial: tp.Optional[torch.Tensor] = None,
- condition: tp.Optional[torch.Tensor] = None, return_list: bool = False):
- """Full ddpm reverse process.
-
- Args:
- model (nn.Module): Diffusion model.
- initial (tensor): Initial Noise.
- condition (tensor): Input conditionning Tensor (e.g. encodec compressed representation).
- return_list (bool): Whether to return the whole process or only the sampled point.
- """
- alpha_bar = self.get_alpha_bar(step=self.num_steps - 1)
- current = initial
- iterates = [initial]
- for step in range(self.num_steps)[::-1]:
- with torch.no_grad():
- estimate = model(current, step, condition=condition).sample
- alpha = 1 - self.betas[step]
- previous = (current - (1 - alpha) / (1 - alpha_bar).sqrt() * estimate) / alpha.sqrt()
- previous_alpha_bar = self.get_alpha_bar(step=step - 1)
- if step == 0:
- sigma2 = 0
- elif self.variance == 'beta':
- sigma2 = 1 - alpha
- elif self.variance == 'beta_tilde':
- sigma2 = (1 - previous_alpha_bar) / (1 - alpha_bar) * (1 - alpha)
- elif self.variance == 'none':
- sigma2 = 0
- else:
- raise ValueError(f'Invalid variance type {self.variance}')
-
- if sigma2 > 0:
- previous += sigma2**0.5 * torch.randn_like(previous) * self.noise_scale
- if self.clip:
- previous = previous.clamp(-self.clip, self.clip)
- current = previous
- alpha_bar = previous_alpha_bar
- if step == 0:
- previous *= self.rescale
- if return_list:
- iterates.append(previous.cpu())
-
- if return_list:
- return iterates
- else:
- return self.sample_processor.return_sample(previous)
-
- def generate_subsampled(self, model: torch.nn.Module, initial: torch.Tensor, step_list: tp.Optional[list] = None,
- condition: tp.Optional[torch.Tensor] = None, return_list: bool = False):
- """Reverse process that only goes through Markov chain states in step_list."""
- if step_list is None:
- step_list = list(range(1000))[::-50] + [0]
- alpha_bar = self.get_alpha_bar(step=self.num_steps - 1)
- alpha_bars_subsampled = (1 - self.betas).cumprod(dim=0)[list(reversed(step_list))].cpu()
- betas_subsampled = betas_from_alpha_bar(alpha_bars_subsampled)
- current = initial * self.noise_scale
- iterates = [current]
- for idx, step in enumerate(step_list[:-1]):
- with torch.no_grad():
- estimate = model(current, step, condition=condition).sample * self.noise_scale
- alpha = 1 - betas_subsampled[-1 - idx]
- previous = (current - (1 - alpha) / (1 - alpha_bar).sqrt() * estimate) / alpha.sqrt()
- previous_alpha_bar = self.get_alpha_bar(step_list[idx + 1])
- if step == step_list[-2]:
- sigma2 = 0
- previous_alpha_bar = torch.tensor(1.0)
- else:
- sigma2 = (1 - previous_alpha_bar) / (1 - alpha_bar) * (1 - alpha)
- if sigma2 > 0:
- previous += sigma2**0.5 * torch.randn_like(previous) * self.noise_scale
- if self.clip:
- previous = previous.clamp(-self.clip, self.clip)
- current = previous
- alpha_bar = previous_alpha_bar
- if step == 0:
- previous *= self.rescale
- if return_list:
- iterates.append(previous.cpu())
- if return_list:
- return iterates
- else:
- return self.sample_processor.return_sample(previous)
diff --git a/spaces/falterWliame/Face_Mask_Detection/Download Surah Yaseen In Pdf With Large Font [UPDATED].md b/spaces/falterWliame/Face_Mask_Detection/Download Surah Yaseen In Pdf With Large Font [UPDATED].md
deleted file mode 100644
index 89e32bb0ab0d353ee3c9c56494390a338c6777c7..0000000000000000000000000000000000000000
--- a/spaces/falterWliame/Face_Mask_Detection/Download Surah Yaseen In Pdf With Large Font [UPDATED].md
+++ /dev/null
@@ -1,6 +0,0 @@
-
-
-Listen online and download Quran Tilawat / Recitation of Surah Yasin in the ... Surah Rahman and Taghabun Please take a fresh pitcher or big bottle and ... Ø¥Ùنَّكَ Ù„ÙŽÙ…ÙÙ†ÙŽ Ø§Ù„Ù’Ù…ÙØ±Ù’سَلÙينَ ﴿٣﴾ Surah Ya Sin sometimes spelled as Yaseen (in Arabic text: يس‎) ... 1fdad05405
-
-
-
diff --git a/spaces/falterWliame/Face_Mask_Detection/Ncomputing Vspace License Crack Software. Digital MEETING Nuestr _TOP_.md b/spaces/falterWliame/Face_Mask_Detection/Ncomputing Vspace License Crack Software. Digital MEETING Nuestr _TOP_.md
deleted file mode 100644
index 6d0de0e7d8eb38cec119b7b15037b933edac0e97..0000000000000000000000000000000000000000
--- a/spaces/falterWliame/Face_Mask_Detection/Ncomputing Vspace License Crack Software. Digital MEETING Nuestr _TOP_.md
+++ /dev/null
@@ -1,106 +0,0 @@
-
-
Ncomputing Vspace License Crack Software: A Digital Solution for Meeting Your Computing Needs
-
-
If you are looking for a way to run multiple virtual desktops on a single server and access them from different devices, you might want to consider Ncomputing Vspace License Crack Software. This software is a client-server based desktop virtualization solution that allows you to create and manage up to 100 user sessions per server. You can use it to host digital meetings, collaborate with your team, or run various applications without investing in expensive hardware.
-
Ncomputing Vspace License Crack Software. Digital MEETING Nuestr
Ncomputing Vspace License Crack Software is a software that enables you to crack the license of Ncomputing Vspace, a desktop virtualization software developed by NComputing. NComputing is a company that specializes in providing low-cost and energy-efficient computing solutions for various sectors, such as education, healthcare, government, and enterprise.
-
-
Ncomputing Vspace is a software that allows you to create multiple virtual desktops on a single server and access them from different devices, such as thin clients, laptops, tablets, or smartphones. You can use it to run Windows or Linux operating systems and applications on your virtual desktops. You can also use it to host digital meetings with your colleagues or clients, using features such as video conferencing, screen sharing, chat, and file transfer.
-
-
Ncomputing Vspace requires a server-based license to operate. The license determines the number of user sessions and premium features that you can use on your server. The license can be purchased from NComputing or its authorized resellers. However, some users may want to crack the license and use the software without paying for it. This is where Ncomputing Vspace License Crack Software comes in.
-
-
How does Ncomputing Vspace License Crack Software work?
-
-
Ncomputing Vspace License Crack Software is a software that allows you to generate a valid license file for Ncomputing Vspace without paying for it. The software works by bypassing the online registration process and creating a fake registration file that contains the license information. You can then use this file to activate Ncomputing Vspace on your server and enjoy unlimited user sessions and premium features.
-
-
To use Ncomputing Vspace License Crack Software, you need to download it from a reliable source and install it on your computer. You also need to have Ncomputing Vspace installed on your server. Then, you need to follow these steps:
-
-
-
Run Ncomputing Vspace License Crack Software on your computer.
-
Select the version of Ncomputing Vspace that you have installed on your server.
-
Enter the serial number of your server or generate a random one.
-
Choose the number of user sessions and premium features that you want to use on your server.
-
Click on the Generate button to create the license file.
-
Copy the license file to the folder where Ncomputing Vspace is installed on your server.
-
Restart Ncomputing Vspace on your server and enjoy the cracked license.
-
-
-
What are the benefits of using Ncomputing Vspace License Crack Software?
-
-
Using Ncomputing Vspace License Crack Software can provide you with several benefits, such as:
-
-
-
-
You can save money by not paying for the license of Ncomputing Vspace.
-
You can use unlimited user sessions and premium features on your server without any restrictions.
-
You can host digital meetings with high-quality video and audio without any lag or interruption.
-
You can run multiple applications and operating systems on your virtual desktops without compromising performance or security.
-
You can access your virtual desktops from any device and location with an internet connection.
-
-
-
What are the risks of using Ncomputing Vspace License Crack Software?
-
-
While using Ncomputing Vspace License Crack Software may seem tempting, it also comes with some risks that you should be aware of, such as:
-
-
-
You may violate the terms and conditions of NComputing and face legal consequences for using their software illegally.
-
You may expose your server and devices to malware or viruses that may be hidden in the crack software or the license file.
-
You may experience technical issues or errors with Ncomputing Vspace that may affect your virtual desktops or digital meetings.
-
You may not receive any updates or support from NComputing or its authorized resellers for your cracked software.
-
You may lose your data or compromise your privacy if your server or devices are hacked or stolen by someone who has access to your cracked license.
-
-
-
Conclusion
-
-
Ncomputing Vspace License Crack Software is a software that allows you to crack the license of Ncomputing Vspace, a desktop virtualization software that enables you to create multiple virtual desktops on a single server and access them from different devices. You can use it to host digital meetings, collaborate with your team, or run various applications without investing in expensive hardware. However, using this software also comes with some risks, such as legal consequences, malware infection, technical issues, lack of updates or support, and data loss or privacy breach. Therefore, you should weigh the pros and cons carefully before deciding whether to use this software or not.
-
How to optimize your website for Ncomputing Vspace License Crack Software?
-
-
If you are a website owner or a marketer who wants to attract more visitors and customers who are interested in Ncomputing Vspace License Crack Software, you need to optimize your website for this keyword. Optimizing your website means making it more relevant, user-friendly, and authoritative for your target audience and the search engines. Here are some tips on how to optimize your website for Ncomputing Vspace License Crack Software:
-
-
-
Conduct keyword research: You need to find out what are the most popular and relevant keywords that your potential customers are using to search for Ncomputing Vspace License Crack Software or related topics. You can use tools such as Google Keyword Planner, SEMrush, or Moz to conduct keyword research and find out the search volume, competition, and difficulty of each keyword.
-
Create high-quality content: You need to create high-quality content that provides valuable information, answers questions, solves problems, or entertains your audience. Your content should be original, engaging, and well-written. You should also use the keywords that you have researched in your content, especially in the title, headings, subheadings, introduction, conclusion, and body paragraphs. However, you should avoid keyword stuffing or using the keywords too many times in a unnatural way.
-
Optimize your meta tags: You need to optimize your meta tags, which are the snippets of text that appear in the search engine results pages (SERPs). Your meta tags should include the title tag, which is the title of your web page; the meta description tag, which is a brief summary of your web page; and the meta keywords tag, which is a list of keywords that describe your web page. Your meta tags should be relevant, concise, and compelling. They should also include your main keyword and a call to action.
-
Improve your site speed: You need to improve your site speed, which is how fast your web page loads on different devices and browsers. Site speed is an important factor that affects your user experience and your ranking on the search engines. You can improve your site speed by using a fast web host, compressing your images and files, minimizing your code, caching your pages, and using a content delivery network (CDN).
-
Build backlinks: You need to build backlinks, which are links from other websites that point to your website. Backlinks are an indicator of your website's popularity, credibility, and authority. They can also drive more traffic and referrals to your website. You can build backlinks by creating high-quality content that others want to share or link to; reaching out to other website owners or influencers in your niche; guest posting on other relevant websites; commenting on other blogs or forums; or participating in social media platforms.
-
-
-
Conclusion
-
-
Ncomputing Vspace License Crack Software is a software that allows you to crack the license of Ncomputing Vspace, a desktop virtualization software that enables you to create multiple virtual desktops on a single server and access them from different devices. You can use it to host digital meetings, collaborate with your team, or run various applications without investing in expensive hardware. However, using this software also comes with some risks, such as legal consequences, malware infection, technical issues, lack of updates or support, and data loss or privacy breach. Therefore, you should weigh the pros and cons carefully before deciding whether to use this software or not. You may also want to consider some alternatives that can provide you with similar or better features and benefits for your computing needs. If you are a website owner or a marketer who wants to attract more visitors and customers who are interested in Ncomputing Vspace License Crack Software, you need to optimize your website for this keyword by following some tips on keyword research, content creation, meta tags optimization, site speed improvement, and backlink building.
-
How to troubleshoot Ncomputing Vspace License Crack Software?
-
-
If you encounter any problems or errors while using Ncomputing Vspace License Crack Software, you may need to troubleshoot them and find a solution. Here are some common problems and errors that you may face and how to troubleshoot them:
-
-
-
Problem: Ncomputing Vspace License Crack Software does not generate a valid license file.
-
Solution: You may need to check if you have entered the correct version of Ncomputing Vspace that you have installed on your server. You may also need to check if you have entered a valid serial number of your server or generated a random one. You may also need to check if you have enough disk space on your computer and server to store the license file.
-
Problem: Ncomputing Vspace License Crack Software generates a license file but Ncomputing Vspace does not recognize it.
-
Solution: You may need to check if you have copied the license file to the correct folder where Ncomputing Vspace is installed on your server. You may also need to check if you have restarted Ncomputing Vspace on your server after copying the license file. You may also need to check if the license file is corrupted or modified by any malware or virus.
-
Problem: Ncomputing Vspace License Crack Software causes Ncomputing Vspace to crash or freeze.
-
Solution: You may need to check if your server meets the minimum system requirements for running Ncomputing Vspace. You may also need to check if your server has enough memory, CPU, and network resources to handle the user sessions and premium features that you have selected. You may also need to check if there are any conflicts or compatibility issues with other software or hardware on your server.
-
Problem: Ncomputing Vspace License Crack Software causes Ncomputing Vspace to display an error message or a warning message.
-
Solution: You may need to check the error message or the warning message and follow the instructions or suggestions that it provides. You may also need to check the NComputing website or the vSpace support forum for more information or solutions regarding the error message or the warning message.
-
-
-
How to contact NComputing for support or feedback?
-
-
If you have any questions, issues, or feedback regarding NComputing products or services, you can contact NComputing for support or feedback. Here are some ways to contact NComputing:
-
-
-
You can visit the NComputing website at https://www.ncomputing.com/ and find more information about their products, solutions, partners, customers, and resources.
-
You can visit the vSpace support portal at https://support.ncomputing.com/portal/home and find more information about vSpace software, hardware, documentation, downloads, knowledge base, and community forum.
-
You can submit a support ticket at https://support.ncomputing.com/portal/newticket and get assistance from the vSpace support team.
-
You can call the vSpace support phone number at +1 888 365 1210 (US toll free) or +1 650 409 5959 (international) and speak to a vSpace support representative.
-
You can send an email to the vSpace support email address at support@ncomputing.com and get a response from a vSpace support representative.
-
-
-
Conclusion
-
-
Ncomputing Vspace License Crack Software is a software that allows you to crack the license of Ncomputing Vspace, a desktop virtualization software that enables you to create multiple virtual desktops on a single server and access them from different devices. You can use it to host digital meetings, collaborate with your team, or run various applications without investing in expensive hardware. However, using this software also comes with some risks, such as legal consequences, malware infection, technical issues, lack of updates or support, and data loss or privacy breach. Therefore, you should weigh the pros and cons carefully before deciding whether to use this software or not. You may also want to consider some alternatives that can provide you with similar or better features and benefits for your computing needs. If you are a website owner or a marketer who wants to attract more visitors and customers who are interested in Ncomputing Vspace License Crack Software, you need to optimize your website for this keyword by following some tips on keyword research, content creation, meta tags optimization, site speed improvement, and backlink building. If you encounter any problems or errors while using Ncomputing Vspace License Crack Software, you may need to troubleshoot them and find a solution. If you have any questions, issues, or feedback regarding NComputing products or services, you can contact NComputing for support or feedback.
-
Ncomputing Vspace License Crack Software is a software that allows you to crack the license of Ncomputing Vspace, a desktop virtualization software that enables you to create multiple virtual desktops on a single server and access them from different devices. You can use it to host digital meetings, collaborate with your team, or run various applications without investing in expensive hardware. However, using this software also comes with some risks, such as legal consequences, malware infection, technical issues, lack of updates or support, and data loss or privacy breach. Therefore, you should weigh the pros and cons carefully before deciding whether to use this software or not. You may also want to consider some alternatives that can provide you with similar or better features and benefits for your computing needs. If you are a website owner or a marketer who wants to attract more visitors and customers who are interested in Ncomputing Vspace License Crack Software, you need to optimize your website for this keyword by following some tips on keyword research, content creation, meta tags optimization, site speed improvement, and backlink building. If you encounter any problems or errors while using Ncomputing Vspace License Crack Software, you may need to troubleshoot them and find a solution. If you have any questions, issues, or feedback regarding NComputing products or services, you can contact NComputing for support or feedback.
3cee63e6c2
-
-
\ No newline at end of file
diff --git a/spaces/fatiXbelha/sd/Bus Parking Simulator Test Your Driving Skills in 3D.md b/spaces/fatiXbelha/sd/Bus Parking Simulator Test Your Driving Skills in 3D.md
deleted file mode 100644
index aa70b6690f98904582efffeac79851966816010f..0000000000000000000000000000000000000000
--- a/spaces/fatiXbelha/sd/Bus Parking Simulator Test Your Driving Skills in 3D.md
+++ /dev/null
@@ -1,167 +0,0 @@
-
-
Bus Parking: Tips, Challenges, and Solutions
-
Buses are an essential mode of public transportation in many cities around the world. They provide mobility, accessibility, affordability, and environmental benefits for millions of passengers every day. However, buses also face some challenges when it comes to parking, especially in urban areas where space is limited and demand is high. In this article, we will explore some tips, challenges, and solutions for bus parking, both from the perspective of drivers and riders.
Bus parking refers to the process of finding a suitable place to park a bus when it is not in service or when it needs to stop for loading or unloading passengers. Bus parking can be either on-street or off-street, depending on the availability of space, regulations, and preferences. Bus parking is important for several reasons:
-
-
It ensures the safety and security of buses, drivers, passengers, and other road users.
-
It improves the efficiency and reliability of bus operations and services.
-
It reduces the congestion and pollution caused by buses circulating or idling on the road.
-
It enhances the customer satisfaction and loyalty of bus users.
-
-
What are the benefits of bus parking for cities and passengers?
-
Bus parking can also bring some benefits for cities and passengers, such as:
-
-
It supports the economic development and productivity of cities by facilitating the movement of people and goods.
-
It contributes to the social inclusion and equity of cities by providing access to opportunities and services for all segments of society.
-
It promotes the environmental sustainability of cities by reducing greenhouse gas emissions and energy consumption.
-
It creates value-added services and amenities for passengers, such as electric vehicle charging ports, bicycle racks, Wi-Fi access, etc.
-
-
Tips for bus parking
-
How to drive a bus safely and efficiently
-
Check the bus routes and schedule online
-
Before driving a bus, it is advisable to check the bus routes and schedule online to plan your trip ahead. You can use websites or apps that provide real-time information on traffic conditions, road closures, detours, delays, etc. You can also check the weather forecast, fuel prices, parking availability, etc. This way, you can avoid unnecessary stress and surprises on the road.
-
tourist coaches parking facilities
-charter bus parking and loading logistics
-bus depot size and location
-bus parking near me
-bus parking games online
-bus parking permit application
-bus parking dimensions and requirements
-bus parking downtown [city name]
-bus parking at [attraction name]
-bus parking reservation system
-bus parking signs and regulations
-bus parking fees and fines
-bus parking lot design and layout
-bus parking safety tips and best practices
-bus parking simulator download
-bus parking for school trips
-bus parking zones and maps
-bus parking coupons and discounts
-bus parking app for android and ios
-bus parking space rental
-bus parking management software
-bus parking near airport
-bus parking strategy and planning
-bus parking rules and etiquette
-bus parking challenges and solutions
-bus parking availability and capacity
-bus parking cost estimation and comparison
-bus parking guide and handbook
-bus parking options and alternatives
-bus parking booking and confirmation
-bus parking standards and specifications
-bus parking enforcement and monitoring
-bus parking benefits and advantages
-bus parking problems and issues
-bus parking reviews and ratings
-bus parking tips and tricks
-bus parking service and support
-bus parking innovation and trends
-bus parking statistics and data
-bus parking case studies and examples
-
Plan your route and avoid congestion
-
When driving a bus,
When driving a bus, you should plan your route carefully and avoid congestion as much as possible. You can use navigation systems or apps that suggest the best route based on traffic data, road conditions, and bus priority lanes. You can also follow the signs and signals that indicate bus-only lanes, bus stops, bus terminals, etc. You should avoid driving in areas where parking is prohibited, restricted, or limited, such as residential zones, commercial districts, historic sites, etc.
-
Park the bus in a suitable place and follow the regulations
-
When parking a bus, you should look for a suitable place that meets the following criteria:
-
-
It has enough space and clearance for the bus to maneuver and park.
-
It does not block or interfere with the traffic flow or the visibility of other road users.
-
It does not damage or endanger the bus, the driver, the passengers, or the property of others.
-
It complies with the local parking regulations and policies.
-
-
You should also follow the parking rules and etiquette, such as:
-
-
Paying the parking fees or displaying the parking permits if required.
-
Using the parking brake and locking the doors when leaving the bus.
-
Turning off the engine and lights when parking for a long time.
-
Leaving a note with your contact information if you park in an emergency or in an unauthorized place.
-
-
How to ride a bus comfortably and conveniently
-
Check the bus schedule and buy a ticket online or on the bus
-
Before riding a bus, you should check the bus schedule online or at the bus stop to know when and where to catch the bus. You can use websites or apps that provide real-time information on bus arrivals, departures, delays, etc. You can also buy a ticket online or on the bus using cash, card, or mobile payment. You should keep your ticket or receipt until you get off the bus.
-
Board the bus and find a seat or a standing spot
-
When boarding a bus, you should wait in line and let other passengers get off first. You should enter through the front door and exit through the rear door unless otherwise instructed. You should scan your ticket or show it to the driver if needed. You should find a seat or a standing spot that is comfortable and safe for you and others. You should avoid blocking the aisle or the doors with your luggage or belongings. You should also wear a seat belt if available and follow the safety instructions of the driver.
-
Get off the bus at your destination and pay attention to your belongings
-
When getting off the bus, you should press the stop button or signal the driver in advance. You should exit through the rear door unless otherwise instructed. You should thank the driver and leave quickly and safely. You should pay attention to your belongings and make sure you do not leave anything behind. You should also check your ticket or receipt for any discounts or refunds if applicable.
-
Challenges of bus parking
-
Difficulties finding parking spaces in urban areas
-
Lack of supply and high demand for parking spaces
-
One of the main challenges of bus parking is finding enough parking spaces in urban areas where space is scarce and demand is high. According to a study by IBM, drivers in 20 major cities around the world spend an average of 20 minutes looking for a parking spot. This translates into wasted time, fuel, money, and productivity. For buses, this problem is even more acute because they need larger and longer spaces than cars. Moreover, buses often have fixed schedules and routes that limit their flexibility and options for parking.
-
Ineffective space utilization and parking policies
-
Another challenge of bus parking is using
Another challenge of bus parking is using the available space efficiently and effectively. Many parking spaces are underutilized or overutilized, depending on the time of day, the season, the location, etc. For example, some parking spaces may be empty during the day but full at night, or vice versa. Some parking spaces may be too small or too large for the buses that need them. Some parking spaces may be reserved for certain types of buses or vehicles, such as school buses, tour buses, electric buses, etc. These factors can reduce the parking options and opportunities for buses.
-
Cruising and double-parking problems
-
A third challenge of bus parking is dealing with the cruising and double-parking problems that occur when buses cannot find a suitable parking spot. Cruising refers to the practice of driving around in search of a parking space, which can increase traffic congestion, emissions, and fuel consumption. Double-parking refers to the practice of parking a bus next to another parked vehicle, which can block the traffic flow, the visibility, and the access to other vehicles or pedestrians. Both cruising and double-parking can cause safety hazards, legal issues, and customer complaints for buses.
-
Environmental and social impacts of bus parking
-
Emissions and noise pollution from buses
-
Another challenge of bus parking is minimizing the environmental and social impacts of buses, especially in terms of emissions and noise pollution. Buses are generally more eco-friendly than cars because they can carry more passengers and reduce the number of vehicles on the road. However, buses still produce greenhouse gas emissions and air pollutants that contribute to climate change and health problems. Buses also generate noise pollution that can disturb the residents and businesses near the parking areas. Therefore, bus parking should be designed and managed to reduce these negative effects.
-
Land use and opportunity costs of parking spaces
-
A further challenge of bus parking is balancing the land use and opportunity costs of parking spaces. Parking spaces occupy valuable land that could be used for other purposes, such as housing, commerce, recreation, etc. Parking spaces also have opportunity costs, which are the benefits that could be gained from alternative uses of the land. For example, a parking space could be converted into a park, a bike lane, a sidewalk, etc. Therefore, bus parking should be planned and evaluated to maximize the social and economic benefits of the land.
-
Accessibility and equity issues for bus users
-
The last challenge of bus parking is ensuring the accessibility and equity for bus users. Bus users have different needs and preferences when it comes to parking, such as convenience, affordability, security, comfort, etc. Bus users also have different characteristics and backgrounds, such as age, gender, income, disability, etc. Therefore, bus parking should be accessible and equitable for all bus users, regardless of their differences. Bus parking should also be integrated with other modes of transportation, such as walking, biking, car-sharing, etc., to provide seamless mobility options for bus users.
-
Solutions for bus parking
-
Innovative parking technologies and management systems
-
Automated parking systems and smart parking meters
-
One solution for bus parking is to use automated parking systems and smart parking meters that can improve the efficiency and convenience of parking. Automated parking systems are systems that can park a bus automatically without human intervention. They can save space, time,
One solution for bus parking is to use automated parking systems and smart parking meters that can improve the efficiency and convenience of parking. Automated parking systems are systems that can park a bus automatically without human intervention. They can save space, time, fuel, and labor costs by using vertical or horizontal platforms, lifts, conveyors, robots, etc. Smart parking meters are devices that can monitor and charge the parking fees for buses using sensors, cameras, RFID tags, etc. They can also provide information on parking availability, occupancy, duration, etc.
-
Parking guidance systems and mobile apps
-
Another solution for bus parking is to use parking guidance systems and mobile apps that can help drivers and riders find and access parking spaces easily and quickly. Parking guidance systems are systems that can display the location, direction, and status of parking spaces using signs, lights, screens, etc. They can also communicate with the drivers and riders using voice or text messages, alerts, notifications, etc. Mobile apps are applications that can provide real-time information on parking availability, prices, reservations, payments, etc. They can also offer navigation, feedback, rewards, etc.
-
Parking data analytics and optimization tools
-
A third solution for bus parking is to use parking data analytics and optimization tools that can enhance the performance and quality of parking services. Parking data analytics are methods that can collect, analyze, and visualize the data on parking demand, supply, behavior, patterns, trends, etc. They can also generate insights and recommendations for improving parking management and policies. Parking optimization tools are tools that can optimize the allocation and utilization of parking spaces using mathematical models, algorithms, simulations, etc. They can also adjust the parking prices and incentives based on the demand and supply.
-
Mixed-use and shared parking facilities
-
Multi-level and underground parking structures
-
One solution for bus parking is to build multi-level and underground parking structures that can increase the capacity and density of parking spaces. Multi-level parking structures are structures that can stack multiple levels of parking spaces on top of each other using ramps, elevators, etc. Underground parking structures are structures that can dig below the ground level to create more parking spaces. Both types of structures can save land space and reduce the visual impact of parking facilities.
-
Park-and-ride and park-and-bike schemes
-
Another solution for bus parking is to implement park-and-ride and park-and-bike schemes that can encourage the integration and coordination of different modes of transportation. Park-and-ride schemes are schemes that allow drivers to park their cars at designated locations near bus stops or terminals and then ride the bus to their destinations. Park-and-bike schemes are schemes that allow riders to park their bikes at secure racks or lockers near bus stops or terminals and then ride the bus to their destinations. Both schemes can reduce the traffic congestion and pollution caused by cars and bikes.
-
Parking cooperatives and partnerships
-
A third solution for bus parking is to establish parking cooperatives and partnerships that can promote the sharing and collaboration of parking resources among different stakeholders. Parking cooperatives are organizations that allow members to share their own or rented parking spaces with other members or non-members for a fee or a favor. Parking partnerships are agreements that allow partners to use each other's parking spaces for free or for a discounted price. Both types of arrangements can increase the availability and affordability of parking spaces.
-
Conclusion
-
Summary of main points
-
In conclusion, bus parking is an important aspect of public transportation that affects the safety,
In conclusion, bus parking is an important aspect of public transportation that affects the safety, efficiency, satisfaction, and sustainability of bus drivers, riders, and cities. Bus parking also poses some challenges, such as finding parking spaces, reducing environmental and social impacts, and ensuring accessibility and equity. However, there are also some solutions, such as using innovative parking technologies and management systems, building mixed-use and shared parking facilities, and establishing parking cooperatives and partnerships. By applying these solutions, bus parking can be improved and optimized for the benefit of all stakeholders.
-
Recommendations for further action or research
-
Based on the findings of this article, we recommend the following actions or research for bus parking:
-
-
Conduct a comprehensive assessment of the current and future parking needs and demands of buses in different cities and regions.
-
Develop and implement a strategic plan for bus parking that aligns with the goals and objectives of public transportation and urban development.
-
Invest in and adopt the best practices and innovations for bus parking that suit the local context and conditions.
-
Monitor and evaluate the performance and outcomes of bus parking using relevant indicators and metrics.
-
Engage and collaborate with the key stakeholders and partners involved in bus parking, such as bus operators, authorities, developers, users, etc.
-
-
FAQs
-
What is the difference between on-street and off-street bus parking?
-
On-street bus parking is when buses park on the road or on the curb. Off-street bus parking is when buses park in a designated area off the road, such as a lot, a garage, a terminal, etc.
-
What are some examples of automated parking systems for buses?
-
Some examples of automated parking systems for buses are:
-
-
The Bus Tower in Nagoya, Japan, which can park 50 buses in a vertical structure using a rotating platform and an elevator.
-
The Robotic Parking Garage in Dubai, UAE, which can park 250 buses in an underground structure using robots and conveyor belts.
-
The Automated Bus Depot in Singapore, which can park 150 buses in a horizontal structure using automated guided vehicles and charging stations.
-
-
What are some benefits of park-and-ride and park-and-bike schemes for bus users?
-
Some benefits of park-and-ride and park-and-bike schemes for bus users are:
-
-
They can save money on fuel, tolls, parking fees, etc.
-
They can reduce stress and hassle from driving in traffic or finding parking spaces.
-
They can enjoy more comfort and convenience from riding the bus or biking.
-
They can improve their health and fitness from walking or cycling.
-
They can contribute to the environment by reducing their carbon footprint.
-
-
What are some challenges of parking cooperatives and partnerships for bus operators?
-
Some challenges of parking cooperatives and partnerships for bus operators are:
-
-
They may have to share their parking spaces with other operators or vehicles that may not be compatible or convenient.
-
They may have to pay fees or commissions to the cooperative or partner that may affect their profitability or budget.
-
They may have to comply with the rules or standards of the cooperative or partner that may limit their flexibility or autonomy.
-
They may have to deal with conflicts or disputes with the cooperative or partner that may affect their reputation or relationship.
-
-
How can I find more information on bus parking?
-
You can find more information on bus parking by visiting the following websites or sources:
-
-
The International Parking Institute (IPI), which is a professional association that provides education, research, advocacy, and networking on parking issues. https://www.parking.org/
-
The World Parking Symposium (WPS), which is an academic conference that brings together experts, researchers, practitioners, and policymakers on parking topics. https://worldparkingsymposium.ca/
-
The Parking Today Magazine (PTM), which is a publication that covers news, trends, innovations, and best practices on parking matters. https://www.parkingtoday.com/
-
401be4b1e0
-
-
\ No newline at end of file
diff --git a/spaces/fatiXbelha/sd/Clash of Clans Infinito APK The Ultimate Mod for COC Fans.md b/spaces/fatiXbelha/sd/Clash of Clans Infinito APK The Ultimate Mod for COC Fans.md
deleted file mode 100644
index 4a09685ea59e5386c5a717d9c4003b3b60095cd0..0000000000000000000000000000000000000000
--- a/spaces/fatiXbelha/sd/Clash of Clans Infinito APK The Ultimate Mod for COC Fans.md
+++ /dev/null
@@ -1,133 +0,0 @@
-
-
Clash of Clans Infinito APK: How to Download and Play the Modded Version of the Popular Strategy Game
-
If you are a fan of strategy games, you have probably heard of Clash of Clans, one of the most popular and addictive games in the genre. In this game, you can build your own village, train your army, and fight against millions of other players online. However, you may also know that playing this game can be quite challenging and time-consuming, especially if you want to progress faster and unlock more features. That's why many players look for ways to hack or mod the game, such as using Clash of Clans Infinito APK.
A brief introduction to Clash of Clans, a multiplayer online strategy game
-
Clash of Clans is a free-to-play game developed by Supercell, a Finnish company that also created other popular games like Hay Day and Brawl Stars. The game was released in 2012 for iOS devices and in 2013 for Android devices. Since then, it has become one of the most downloaded and highest-grossing games in the world, with over 500 million downloads and billions of dollars in revenue.
-
The game is set in a fantasy world where you can create your own village and customize it with various buildings, such as town hall, barracks, gold mines, elixir collectors, walls, cannons, archer towers, etc. You can also train different types of troops, such as barbarians, archers, giants, wizards, dragons, etc. Your main goal is to protect your village from enemy attacks and raid other villages for resources (gold, elixir, and dark elixir) and trophies (which determine your rank in the global leaderboard). You can also join or create a clan (a group of up to 50 players) and participate in clan wars (a team-based competition) or clan games (a cooperative event).
-
The features and benefits of Clash of Clans Infinito APK, a modded version of the game that offers unlimited resources, gems, and troops
-
Clash of Clans Infinito APK is a modified version of the original game that gives you access to unlimited resources (gold, elixir, dark elixir), gems (the premium currency), and troops (including heroes like king, queen, warden). This means that you can upgrade your buildings and troops instantly, without waiting for the timer or spending real money. You can also experiment with different strategies and combinations, without worrying about losing resources or trophies. You can enjoy the game at your own pace and have more fun and satisfaction.
-
clash of clans infinito apk download
-clash of clans infinito apk mod
-clash of clans infinito apk 2023
-clash of clans infinito apk atualizado
-clash of clans infinito apk mediafıre
-clash of clans infinito apk hack
-clash of clans infinito apk android
-clash of clans infinito apk ios
-clash of clans infinito apk online
-clash of clans infinito apk gratis
-clash of clans infinito apk sin root
-clash of clans infinito apk mega
-clash of clans infinito apk servidor privado
-clash of clans infinito apk ultima version
-clash of clans infinito apk com tudo liberado
-clash of clans infinito apk com gemas infinitas
-clash of clans infinito apk com tropas ilimitadas
-clash of clans infinito apk com ouro e elixir infinitos
-clash of clans infinito apk com construtora livre
-clash of clans infinito apk com heróis desbloqueados
-clash of clans infinito apk com guerra de clãs
-clash of clans infinito apk com base do construtor
-clash of clans infinito apk com super tropas
-clash of clans infinito apk com modo noturno
-clash of clans infinito apk com eventos especiais
-clash of clans infinito apk sem vírus
-clash of clans infinito apk sem banimento
-clash of clans infinito apk sem atualização
-clash of clans infinito apk sem verificação humana
-clash of clans infinito apk sem anúncios
-clash of clans infinito apk para pc
-clash of clans infinito apk para celular
-clash of clans infinito apk para tablet
-clash of clans infinito apk para iphone
-clash of clans infinito apk para ipad
-clash of clans infinito apk para windows 10
-clash of clans infinito apk para macbook
-clash of clans infinito apk para linux
-clash of clans infinito apk para chromebook
-clash of clans infinito apk para smart tv
-como baixar e instalar o clash of clans infinito apk
-como jogar o clash of clans infinito apk
-como atualizar o clash of clans infinito apk
-como hackear o clash of clans infinito apk
-como desinstalar o clash of clans infinito apk
-como fazer backup do seu progresso no clash of clans infinito apk
-como transferir sua conta do jogo original para o clash of clans infinito apk
-como entrar em contato com o suporte do clash of clans infinito apk
-como resolver problemas de conexão no clash of clans infinito apk
-como denunciar jogadores que usam o clash of clans infinito apk
-
Clash of Clans Infinito APK is not an official version of the game, but a third-party app that is created by independent developers who modify the original game files. Therefore, it is not available on the Google Play Store or the App Store, but only on some websites that host the APK file. You need to download and install the APK file manually on your Android device, which requires some steps and precautions.
-
How to Download and Install Clash of Clans Infinito APK on Your Android Device?
-
The steps to download the APK file from a reliable source
-
Before you can install Clash of Clans Infinito APK on your device, you need to download the APK file from a reliable source. There are many websites that claim to offer the APK file, but some of them may be fake or malicious, and may harm your device or steal your personal information. Therefore, you need to be careful and do some research before downloading the APK file.
-
One of the websites that we recommend is clashofclansinfinito.com, which is a trusted and verified source that provides the latest version of Clash of Clans Infinito APK. To download the APK file from this website, you need to follow these steps:
Locate the downloaded APK file in your device's storage (usually in the "Downloads" folder).
-
-
The precautions to take before installing the APK file, such as enabling unknown sources and disabling antivirus software
-
Before you can install Clash of Clans Infinito APK on your device, you need to take some precautions to ensure a smooth and safe installation. These precautions include:
-
-
Enabling unknown sources: This is a setting that allows you to install apps from sources other than the Google Play Store. To enable unknown sources, you need to go to your device's settings, then security, then toggle on the option for unknown sources.
-
Disabling antivirus software: This is a software that protects your device from viruses and malware, but may also interfere with the installation of Clash of Clans Infinito APK. To disable antivirus software, you need to go to your device's settings, then apps, then find and disable the antivirus app.
-
Backing up your data: This is a precaution that helps you avoid losing your data in case something goes wrong during the installation. To back up your data, you need to use a cloud service like Google Drive or Dropbox, or an external storage device like a USB flash drive or a memory card.
-
-
The instructions to install and launch the APK file on your device
-
After you have downloaded the APK file and taken the necessary precautions, you can proceed to install and launch Clash of Clans Infinito APK on your device. To do so, you need to follow these instructions:
-
-
Tap on the downloaded APK file to start the installation process.
-
Follow the on-screen prompts and accept the permissions required by the app.
-
Wait for the installation to finish and tap on "Open" to launch the app.
-
Enjoy playing Clash of Clans Infinito APK with unlimited resources, gems, and troops.
-
-
How to Play Clash of Clans Infinito APK and Enjoy Its Features?
-
The basics of playing Clash of Clans, such as building your base, training your army, and attacking other players
-
If you are new to Clash of Clans, you may need some guidance on how to play the game and use its features. The game is easy to learn but hard to master, so you need to practice and improve your skills over time. Here are some of the basics of playing Clash of Clans:
-
-
Building your base: Your base is your home and your defense in the game. You need to build various buildings that serve different purposes, such as producing resources, training troops, storing loot, etc. You also need to arrange your buildings strategically, so that they can protect each other from enemy attacks. You can use walls, traps, bombs, etc. to fortify your base.
Training your army: Your army is your offense in the game. You need to train various troops that have different abilities, such as melee, ranged, flying, etc. You can also use spells and siege machines to support your troops. You need to balance your army composition, so that you can deal with different types of enemy defenses. You can also upgrade your troops to make them stronger and more effective.
-
Attacking other players: Attacking other players is the main way to earn resources and trophies in the game. You can choose to attack players randomly or search for specific targets. You can also scout the enemy base before attacking, to plan your strategy and deploy your troops accordingly. You need to destroy as much of the enemy base as possible, especially the town hall, to get the maximum loot and stars. You can also use your heroes' special abilities to boost your attack.
-
-
The tips and tricks to use Clash of Clans Infinito APK, such as upgrading your buildings and troops, using gems wisely, and joining a clan
-
If you are using Clash of Clans Infinito APK, you may have some advantages over other players who use the original version of the game. However, you still need to use some tips and tricks to make the most of the modded version and enjoy its features. Here are some of the tips and tricks to use Clash of Clans Infinito APK:
-
-
Upgrading your buildings and troops: Since you have unlimited resources and gems, you can upgrade your buildings and troops instantly, without waiting or spending real money. This will give you an edge over other players who have lower-level buildings and troops. However, you should also be careful not to upgrade too fast or too much, as this may make the game boring or unbalanced. You should also follow the recommended upgrade order, which prioritizes the most important buildings and troops first.
-
Using gems wisely: Gems are the premium currency in the game, which can be used to speed up processes, buy resources, or unlock special items. In Clash of Clans Infinito APK, you have unlimited gems, so you can use them as much as you want. However, you should also use them wisely, as they can make the game more fun and challenging. For example, you can use gems to boost your resource production, train your troops faster, or buy more builders. You can also save some gems for special events or offers that may require them.
-
Joining a clan: A clan is a group of up to 50 players who can chat, donate troops, request reinforcements, and participate in clan wars or clan games. Joining a clan is one of the best ways to enjoy the game and make friends with other players. In Clash of Clans Infinito APK, you can join any clan that accepts you, or create your own clan with your friends. However, you should also be respectful and cooperative with your clan members, as they may not like it if you abuse the modded version or act selfishly.
-
-
Conclusion
-
Clash of Clans is a fun and addictive strategy game that millions of people play every day. However, if you want to have more freedom and flexibility in the game, you may want to try Clash of Clans Infinito APK, a modded version that offers unlimited resources, gems, and troops. With this version, you can upgrade your buildings and troops instantly, experiment with different strategies and combinations, and enjoy the game at your own pace.
-
To download and install Clash of Clans Infinito APK on your Android device, you need to follow some steps and precautions that we have explained in this article. You also need to use some tips and tricks that we have shared in this article to make the most of the modded version and enjoy its features.
-
If you are interested in trying Clash of Clans Infinito APK, you can download it from clashofclansinfinito.com, a reliable and verified source that provides the latest version of the app. We hope that this article has helped you understand what Clash of Clans Infinito APK is and how to use it. Now it's time for you to try it yourself and see how it works for you.
-
Have fun playing Clash of Clans Infinito APK and share your feedback with us in the comments section below!
-
FAQs
-
What are the risks of using Clash of Clans Infinito APK?
-
Using Clash of Clans Infinito APK may involve some risks, such as:
-
-
Banning: Since Clash of Clans Infinito APK is not an official version of the game, it may violate the terms of service of Supercell, the developer of the original game, and may result in your account being banned or suspended. To avoid this, you should use a different account or device to play Clash of Clans Infinito APK, and not log in with your original account or Google Play account.
-
Malware: Since Clash of Clans Infinito APK is a third-party app that is not verified by Google Play, it may contain malware or viruses that can harm your device or steal your personal information. To avoid this, you should download the APK file from a reliable source, such as clashofclansinfinito.com, and scan it with an antivirus software before installing it.
-
Compatibility: Since Clash of Clans Infinito APK is a modified version of the original game, it may not be compatible with all Android devices or versions. It may also crash or lag during the gameplay, or cause some errors or glitches. To avoid this, you should check the minimum requirements and compatibility of the app before downloading and installing it, and update it regularly to fix any bugs or issues.
-
-
Is Clash of Clans Infinito APK compatible with all Android devices?
-
Clash of Clans Infinito APK is compatible with most Android devices that meet the minimum requirements of the app. The minimum requirements are:
-
-
Android version: 4.1 or higher
-
RAM: 2 GB or higher
-
Storage: 200 MB or higher
-
Internet connection: Wi-Fi or mobile data
-
-
However, some devices may not support the app due to different specifications or configurations. If you encounter any compatibility issues, you can try to clear the cache and data of the app, restart your device, or reinstall the app.
-
Can I play Clash of Clans Infinito APK with my friends who use the original version of the game?
-
No, you cannot play Clash of Clans Infinito APK with your friends who use the original version of the game. This is because Clash of Clans Infinito APK uses a different server than the original game, which means that you cannot connect or interact with other players who use the official server. You can only play with other players who use the same modded version as you.
-
If you want to play with your friends who use the original version of the game, you need to switch back to the official version and log in with your original account or Google Play account. However, you may lose your progress and data in Clash of Clans Infinito APK if you do so.
-
How often is Clash of Clans Infinito APK updated?
-
Clash of Clans Infinito APK is updated regularly to match the updates and changes of the original game. The developers of the modded version try to keep up with the latest features and improvements of the official version, such as new buildings, troops, spells, events, etc. However, there may be some delays or differences between the updates of the two versions, as the modding process takes time and effort.
-
To check for updates and download them, you can visit clashofclansinfinito.com, which provides the latest version of Clash of Clans Infinito APK. You can also enable notifications for updates in the app settings.
-
Where can I find more information about Clash of Clans Infinito APK?
-
If you want to find more information about Clash of Clans Infinito APK, such as its features, benefits, drawbacks, reviews, etc., you can visit clashofclansinfinito.com, which is a comprehensive and informative website that covers everything about the modded version. You can also join their social media pages and groups, where you can interact with other users and get tips and support.
-
You can also watch some videos on YouTube that showcase Clash of Clans Infinito APK and its gameplay. However, you should be careful not to click on any suspicious links or ads that may lead you to fake or malicious websites.
401be4b1e0
-
-
\ No newline at end of file
diff --git a/spaces/feregVcuzo/sanity-test-midi/checkpoint/Download Uber - Driver APK and Start Your Hustle Today.md b/spaces/feregVcuzo/sanity-test-midi/checkpoint/Download Uber - Driver APK and Start Your Hustle Today.md
deleted file mode 100644
index fe8bbf445d2b7622c0353a014adcc4b8bd950fe6..0000000000000000000000000000000000000000
--- a/spaces/feregVcuzo/sanity-test-midi/checkpoint/Download Uber - Driver APK and Start Your Hustle Today.md
+++ /dev/null
@@ -1,103 +0,0 @@
-
-
Uber Driver APK Combo: What Is It and How to Download It
-
Are you looking for a way to earn money with Uber, UberEats, or Postmates? Do you want to have more flexibility and control over your schedule and earnings? If so, you might be interested in downloading Uber Driver APK Combo, a free app that lets you drive and deliver with Uber on your Android device. But what is Uber Driver APK Combo and how can you download it? In this article, we will answer these questions and more. We will explain what Uber Driver APK Combo is, why you might want to download it, and how to do it safely and easily.
-
What is Uber Driver APK Combo?
-
Uber Driver APK Combo is a combination of two things: Uber Driver APK and APK Combo. Let's take a look at each of them separately.
Uber Driver APK is the Android application package (APK) file of the official Uber Driver app. The Uber Driver app is the app that drivers and delivery partners use to accept and complete trips with Uber, UberEats, or Postmates. The app allows you to see your earnings, track your progress, get directions, communicate with customers, and more. The app is available on the Google Play Store for Android devices that meet the minimum requirements.
-
APK Combo
-
APK Combo is a website that offers free downloads of various APK files for Android devices. APK files are the files that contain the code and resources of an Android app. By downloading an APK file from a website like APK Combo, you can install an app on your device without using the Google Play Store. This can be useful if you want to access an app that is not available in your region, or if you want to try an older or newer version of an app.
-
Why Download Uber Driver APK Combo?
-
Now that you know what Uber Driver APK Combo is, you might be wondering why you would want to download it. Here are some possible reasons:
-
Benefits of Uber Driver APK Combo
-
-
You can access the latest version of the Uber Driver app without waiting for the Google Play Store update.
-
You can use the Uber Driver app on devices that are not compatible with the Google Play Store version.
-
You can save data and storage space by downloading a smaller file size than the Google Play Store version.
-
You can enjoy more features and options than the Google Play Store version.
-
-
Risks of Uber Driver APK Combo
-
-
You might download a fake or malicious file that could harm your device or compromise your security.
-
You might violate the terms and conditions of Uber or Google by using an unofficial app.
-
You might encounter bugs or errors that could affect your performance or earnings.
-
You might not receive updates or support from Uber or Google if you encounter any issues.
-
-
How to Download Uber Driver APK Combo?
-
If you decide to download Uber Driver APK Combo, you need to follow some steps to do it safely and easily. Here are the steps:
Select the version of the Uber Driver app that you want to download. You can choose from different versions based on the date, size, and compatibility of the file.
-
Click on "Download" and wait for the file to be downloaded on your device.
-
Once the file is downloaded, locate it in your device's file manager and tap on it to install it - H3: Tips to Install Uber Driver APK Combo - Before you install the Uber Driver APK file, you need to make sure that your device allows the installation of apps from unknown sources. To do this, go to your device's settings, security, and enable the option to allow unknown sources. - After you install the Uber Driver APK file, you need to grant the app the necessary permissions to access your location, camera, microphone, contacts, and other features. To do this, open the app and follow the instructions on the screen. - You also need to sign in with your Uber account or create a new one if you don't have one already. To do this, enter your phone number, email address, and password, and verify your identity with a code sent to your phone. - Once you are signed in, you can start using the app to drive and deliver with Uber. You can set your preferences, view your earnings, accept and complete trips, and more.
Conclusion
-
Uber Driver APK Combo is a free app that lets you drive and deliver with Uber on your Android device. It is a combination of the Uber Driver APK file and the APK Combo website. By downloading Uber Driver APK Combo, you can enjoy some benefits such as accessing the latest version of the app, using it on incompatible devices, saving data and storage space, and having more features and options. However, you also need to be aware of some risks such as downloading a fake or malicious file, violating the terms and conditions of Uber or Google, encountering bugs or errors, and not receiving updates or support. If you decide to download Uber Driver APK Combo, you need to follow some steps to do it safely and easily. You need to go to a website that offers free downloads of Uber Driver APK files, select the version that you want to download, download and install the file on your device, and grant the app the necessary permissions. Then, you can sign in with your Uber account and start using the app to earn money with Uber.
-
FAQs
-
What is the difference between Uber Driver APK Combo and Uber Driver MOD APK?
-
Uber Driver APK Combo is a combination of the official Uber Driver APK file and the APK Combo website. It is not a modified version of the app. Uber Driver MOD APK is a modified version of the app that has some changes or additions to the original app. For example, some Uber Driver MOD APKs claim to offer unlimited money, free rides, or other features that are not available in the official app. However, these MOD APKs are usually fake or malicious and could harm your device or compromise your security.
-
Is Uber Driver APK Combo legal?
-
Uber Driver APK Combo is not illegal per se, but it could violate the terms and conditions of Uber or Google by using an unofficial app. This could result in some consequences such as losing your account, getting banned from the platform, or facing legal action from Uber or Google. Therefore, it is advisable to use the official app from the Google Play Store instead of downloading Uber Driver APK Combo.
-
Is Uber Driver APK Combo safe?
-
Uber Driver APK Combo is not completely safe because it involves downloading an app from an unknown source. This could expose your device to some risks such as downloading a fake or malicious file that could harm your device or compromise your security. Therefore, it is important to be careful when downloading Uber Driver APK Combo and only use trusted websites that offer free downloads of Uber Driver APK files.
Uber Driver APK Combo does not receive updates from Uber or Google because it is an unofficial app. Therefore, if you want to update Uber Driver APK Combo, you need to download and install a newer version of the app from a website that offers free downloads of Uber Driver APK files. However, this could be risky because you might download a fake or malicious file that could harm your device or compromise your security. Therefore, it is better to use the official app from the Google Play Store instead of updating Uber Driver APK Combo.
-
How can I uninstall Uber Driver APK Combo?
-
If you want to uninstall Uber Driver APK Combo from your device, you need to follow these steps:
-
-
Go to your device's settings and select apps or applications.
-
Find and tap on Uber Driver.
-
Select uninstall and confirm your choice.
-
Delete the downloaded Uber Driver APK file from your device's file manager.
- 197e85843d
-
-
\ No newline at end of file
diff --git a/spaces/fffiloni/Music-To-Lyrics/app.py b/spaces/fffiloni/Music-To-Lyrics/app.py
deleted file mode 100644
index f303e8ac1401f3cc0c87a95f1cb3523ad83571d2..0000000000000000000000000000000000000000
--- a/spaces/fffiloni/Music-To-Lyrics/app.py
+++ /dev/null
@@ -1,84 +0,0 @@
-import gradio as gr
-from gradio_client import Client
-
-import os
-hf_token = os.environ.get('HF_TOKEN')
-
-
-splt_client = Client("https://fffiloni-splittrack2musicgen.hf.space/")
-#whisper_client = Client("https://sanchit-gandhi-whisper-jax.hf.space/")
-whisper_client = Client("https://fffiloni-whisper-large-v2.hf.space/", hf_token=hf_token)
-
-import re
-
-def format_lyrics(text):
- # Use regex to find parts that start with a capital letter and insert a newline
- formatted_text = re.sub(r'(?
-
-
- Song To Lyrics
-
-
-
- Send the audio file of your favorite song, and get the lyrics !
- Under the hood, we split and get the vocals track from the audio file, then send the vocals to Whisper.
-
-
""")
- song_in = gr.Audio(label="Song input", type="filepath", source="upload")
- getlyrics_btn = gr.Button("Get Lyrics !")
- vocals_out = gr.Audio(label="Vocals Only")
- lyrics_res = gr.Textbox(label="Lyrics")
-
- getlyrics_btn.click(fn=infer, inputs=[song_in], outputs=[vocals_out, lyrics_res])
-
-demo.queue().launch()
-
\ No newline at end of file
diff --git a/spaces/fffiloni/x-decoder-video/app.py b/spaces/fffiloni/x-decoder-video/app.py
deleted file mode 100644
index 64fac49822e341788a220c2347b737b4b6b3d07f..0000000000000000000000000000000000000000
--- a/spaces/fffiloni/x-decoder-video/app.py
+++ /dev/null
@@ -1,162 +0,0 @@
-import gradio as gr
-import os
-import cv2
-import numpy as np
-
-from moviepy.editor import *
-from share_btn import community_icon_html, loading_icon_html, share_js
-
-xdecoder = gr.Interface.load(name="spaces/xdecoder/Instruct-X-Decoder")
-
-def get_frames(video_in):
- frames = []
- #resize the video
- clip = VideoFileClip(video_in)
-
- #check fps
- if clip.fps > 30:
- print("vide rate is over 30, resetting to 30")
- clip_resized = clip.resize(height=512)
- clip_resized.write_videofile("video_resized.mp4", fps=30)
- else:
- print("video rate is OK")
- clip_resized = clip.resize(height=512)
- clip_resized.write_videofile("video_resized.mp4", fps=clip.fps)
-
- print("video resized to 512 height")
-
- # Opens the Video file with CV2
- cap= cv2.VideoCapture("video_resized.mp4")
-
- fps = cap.get(cv2.CAP_PROP_FPS)
- print("video fps: " + str(fps))
- i=0
- while(cap.isOpened()):
- ret, frame = cap.read()
- if ret == False:
- break
- cv2.imwrite('kang'+str(i)+'.jpg',frame)
- frames.append('kang'+str(i)+'.jpg')
- i+=1
-
- cap.release()
- cv2.destroyAllWindows()
- print("broke the video into frames")
-
- return frames, fps
-
-
-def create_video(frames, fps):
- print("building video result")
- clip = ImageSequenceClip(frames, fps=fps)
- clip.write_videofile("movie.mp4", fps=fps)
-
- return 'movie.mp4'
-
-
-def infer(prompt,video_in, trim_value):
- print(prompt)
- break_vid = get_frames(video_in)
-
- frames_list= break_vid[0]
- fps = break_vid[1]
- n_frame = int(trim_value*fps)
-
- if n_frame >= len(frames_list):
- print("video is shorter than the cut value")
- n_frame = len(frames_list)
-
- result_frames = []
- print("set stop frames to: " + str(n_frame))
-
- for i in frames_list[0:int(n_frame)]:
- xdecoder_img = xdecoder(i, prompt, fn_index=0)
- result_frames.append(xdecoder_img)
- print("frame " + i + "/" + str(n_frame) + ": done;")
-
- print(result_frames)
- final_vid = create_video(result_frames, fps)
- print("finished !")
-
- return final_vid, gr.Group.update(visible=True)
-
-title = """
-
-
-
- Instruct X-Decoder Video
-
-
-
- Apply Instruct X-Decoder to a video
- Note: this space loads the Instruct X-Decoder demo which is optimized to edit images
- Inference time may be super slow, results might not be consistent between frames
-
-
-"""
-
-article = """
-
-
-
-
You may also like:
-
-
-
-
-
-
-
-
-
-"""
-
-with gr.Blocks(css='style.css') as demo:
- with gr.Column(elem_id="col-container"):
- gr.HTML(title)
- with gr.Row():
- with gr.Column():
- video_inp = gr.Video(label="Video source", source="upload", type="filepath", elem_id="input-vid")
- prompt = gr.Textbox(label="Prompt", placeholder="enter prompt", show_label=False, elem_id="prompt-in")
- with gr.Row():
- trim_in = gr.Slider(label="Cut video at (s)", minimun=1, maximum=3, step=1, value=1, interactive=False)
- with gr.Column():
- video_out = gr.Video(label="Pix2pix video result", elem_id="video-output")
-
- submit_btn = gr.Button("Generate X-Decoder video")
-
- with gr.Group(elem_id="share-btn-container", visible=False) as share_group:
- community_icon = gr.HTML(community_icon_html)
- loading_icon = gr.HTML(loading_icon_html)
- share_button = gr.Button("Share to community", elem_id="share-btn")
-
- inputs = [prompt, video_inp, trim_in]
- outputs = [video_out, share_group]
-
- gr.HTML(article)
-
- submit_btn.click(infer, inputs, outputs)
- share_button.click(None, [], [], _js=share_js)
-
-
-
-demo.launch().queue(max_size=12)
\ No newline at end of file
diff --git a/spaces/firdavsyorkulov/delivery_project_fastapi/schemas.py b/spaces/firdavsyorkulov/delivery_project_fastapi/schemas.py
deleted file mode 100644
index 89ea03874930d48042b889e30d93122ac7d82489..0000000000000000000000000000000000000000
--- a/spaces/firdavsyorkulov/delivery_project_fastapi/schemas.py
+++ /dev/null
@@ -1,76 +0,0 @@
-from pydantic import BaseModel
-from typing import Optional
-
-
-class SignUpModel(BaseModel):
- # id: Optional[int]
- username: str
- email: str
- password: str
- is_staff: Optional[bool]
- is_active: Optional[bool]
-
- class Config:
- from_attributes = True
- json_schema_extra = {
- 'example': {
- 'username': "mohirdev",
- 'email': "mohirdev.praktikum@gmail.com",
- 'password': "password12345",
- 'is_staff': False,
- "is_active": True
- }
- }
-
-
-class Settings(BaseModel):
- authjwt_secret_key: str = 'e443c5aca2f2275f43fdded00855fee0e527878c13acd3d215f9bc6530af525f'
-
-
-class LoginModel(BaseModel):
- username_or_email: str
- password: str
-
-
-class ProductModel(BaseModel):
- id: Optional[int]
- name: str
- price: str
-
- class Config:
- from_attributes = True
- json_schema_extra = {
- "example": {
- "name": "Uzbek plov",
- "price": 30000
- }
- }
-
-
-class OrderModel(BaseModel):
- id: Optional[int]
- quantity: int
- order_statuses: Optional[str] = "PENDING"
- user_id: Optional[int]
- product_id: int
-
- class Config:
- from_attributes = True
- json_schema_extra = {
- "example": {
- "quantity": 2
- }
- }
-
-
-class OrderStatusModel(BaseModel):
- order_status: Optional[str] = "PENDING"
-
- class Config:
- from_attributes = True
- json_schema_extra = {
- "example": {
- "order_statuses": "PENDING"
- }
- }
-
diff --git a/spaces/fizban/simiandb/simiandb.py b/spaces/fizban/simiandb/simiandb.py
deleted file mode 100644
index 6b5079eee5d2b13597a800d5bb8551dbef3d9552..0000000000000000000000000000000000000000
--- a/spaces/fizban/simiandb/simiandb.py
+++ /dev/null
@@ -1,339 +0,0 @@
-# -*- coding: utf-8 -*-
-import tables
-from pathlib import Path
-import numpy as np
-from numpy.lib.recfunctions import structured_to_unstructured
-from tqdm import tqdm
-from numba import njit, prange
-from time import time
-
-
-
-@njit('float32[:](uint8[:])', parallel=True)
-def tofp32n8(arr):
- """Numba-optimized function that converts a fp8 (4M3E) array to fp32 using a mapping table
- The array is assumed to be one dimensional with the fp8
- represented as UInt8
- """
- fp8table= np.frombuffer(b'\x00\x00\x00\x00\x00\x00\x00;\x00\x00\x80;\x00\x00\xc0;\x00\x00\x00<\x00\x00 <\x00\x00@<\x00\x00`<\x00\x00\x80<\x00\x00\x90<\x00\x00\xa0<\x00\x00\xb0<\x00\x00\xc0<\x00\x00\xd0<\x00\x00\xe0<\x00\x00\xf0<\x00\x00\x00=\x00\x00\x10=\x00\x00 =\x00\x000=\x00\x00@=\x00\x00P=\x00\x00`=\x00\x00p=\x00\x00\x80=\x00\x00\x90=\x00\x00\xa0=\x00\x00\xb0=\x00\x00\xc0=\x00\x00\xd0=\x00\x00\xe0=\x00\x00\xf0=\x00\x00\x00>\x00\x00\x10>\x00\x00 >\x00\x000>\x00\x00@>\x00\x00P>\x00\x00`>\x00\x00p>\x00\x00\x80>\x00\x00\x90>\x00\x00\xa0>\x00\x00\xb0>\x00\x00\xc0>\x00\x00\xd0>\x00\x00\xe0>\x00\x00\xf0>\x00\x00\x00?\x00\x00\x10?\x00\x00 ?\x00\x000?\x00\x00@?\x00\x00P?\x00\x00`?\x00\x00p?\x00\x00\x80?\x00\x00\x90?\x00\x00\xa0?\x00\x00\xb0?\x00\x00\xc0?\x00\x00\xd0?\x00\x00\xe0?\x00\x00\xf0?\x00\x00\x00@\x00\x00\x10@\x00\x00 @\x00\x000@\x00\x00@@\x00\x00P@\x00\x00`@\x00\x00p@\x00\x00\x80@\x00\x00\x90@\x00\x00\xa0@\x00\x00\xb0@\x00\x00\xc0@\x00\x00\xd0@\x00\x00\xe0@\x00\x00\xf0@\x00\x00\x00A\x00\x00\x10A\x00\x00 A\x00\x000A\x00\x00@A\x00\x00PA\x00\x00`A\x00\x00pA\x00\x00\x80A\x00\x00\x90A\x00\x00\xa0A\x00\x00\xb0A\x00\x00\xc0A\x00\x00\xd0A\x00\x00\xe0A\x00\x00\xf0A\x00\x00\x00B\x00\x00\x10B\x00\x00 B\x00\x000B\x00\x00@B\x00\x00PB\x00\x00`B\x00\x00pB\x00\x00\x80B\x00\x00\x90B\x00\x00\xa0B\x00\x00\xb0B\x00\x00\xc0B\x00\x00\xd0B\x00\x00\xe0B\x00\x00\xf0B\x00\x00\x00C\x00\x00\x10C\x00\x00 C\x00\x000C\x00\x00@C\x00\x00PC\x00\x00`C\x00\x00pC\x00\x00\x80C\x00\x00\x90C\x00\x00\xa0C\x00\x00\xb0C\x00\x00\xc0C\x00\x00\xd0C\x00\x00\xe0C\x00\x00\xf0C\x00\x00\x00\x80\x00\x00\x00\xbb\x00\x00\x80\xbb\x00\x00\xc0\xbb\x00\x00\x00\xbc\x00\x00 \xbc\x00\x00@\xbc\x00\x00`\xbc\x00\x00\x80\xbc\x00\x00\x90\xbc\x00\x00\xa0\xbc\x00\x00\xb0\xbc\x00\x00\xc0\xbc\x00\x00\xd0\xbc\x00\x00\xe0\xbc\x00\x00\xf0\xbc\x00\x00\x00\xbd\x00\x00\x10\xbd\x00\x00 \xbd\x00\x000\xbd\x00\x00@\xbd\x00\x00P\xbd\x00\x00`\xbd\x00\x00p\xbd\x00\x00\x80\xbd\x00\x00\x90\xbd\x00\x00\xa0\xbd\x00\x00\xb0\xbd\x00\x00\xc0\xbd\x00\x00\xd0\xbd\x00\x00\xe0\xbd\x00\x00\xf0\xbd\x00\x00\x00\xbe\x00\x00\x10\xbe\x00\x00 \xbe\x00\x000\xbe\x00\x00@\xbe\x00\x00P\xbe\x00\x00`\xbe\x00\x00p\xbe\x00\x00\x80\xbe\x00\x00\x90\xbe\x00\x00\xa0\xbe\x00\x00\xb0\xbe\x00\x00\xc0\xbe\x00\x00\xd0\xbe\x00\x00\xe0\xbe\x00\x00\xf0\xbe\x00\x00\x00\xbf\x00\x00\x10\xbf\x00\x00 \xbf\x00\x000\xbf\x00\x00@\xbf\x00\x00P\xbf\x00\x00`\xbf\x00\x00p\xbf\x00\x00\x80\xbf\x00\x00\x90\xbf\x00\x00\xa0\xbf\x00\x00\xb0\xbf\x00\x00\xc0\xbf\x00\x00\xd0\xbf\x00\x00\xe0\xbf\x00\x00\xf0\xbf\x00\x00\x00\xc0\x00\x00\x10\xc0\x00\x00 \xc0\x00\x000\xc0\x00\x00@\xc0\x00\x00P\xc0\x00\x00`\xc0\x00\x00p\xc0\x00\x00\x80\xc0\x00\x00\x90\xc0\x00\x00\xa0\xc0\x00\x00\xb0\xc0\x00\x00\xc0\xc0\x00\x00\xd0\xc0\x00\x00\xe0\xc0\x00\x00\xf0\xc0\x00\x00\x00\xc1\x00\x00\x10\xc1\x00\x00 \xc1\x00\x000\xc1\x00\x00@\xc1\x00\x00P\xc1\x00\x00`\xc1\x00\x00p\xc1\x00\x00\x80\xc1\x00\x00\x90\xc1\x00\x00\xa0\xc1\x00\x00\xb0\xc1\x00\x00\xc0\xc1\x00\x00\xd0\xc1\x00\x00\xe0\xc1\x00\x00\xf0\xc1\x00\x00\x00\xc2\x00\x00\x10\xc2\x00\x00 \xc2\x00\x000\xc2\x00\x00@\xc2\x00\x00P\xc2\x00\x00`\xc2\x00\x00p\xc2\x00\x00\x80\xc2\x00\x00\x90\xc2\x00\x00\xa0\xc2\x00\x00\xb0\xc2\x00\x00\xc0\xc2\x00\x00\xd0\xc2\x00\x00\xe0\xc2\x00\x00\xf0\xc2\x00\x00\x00\xc3\x00\x00\x10\xc3\x00\x00 \xc3\x00\x000\xc3\x00\x00@\xc3\x00\x00P\xc3\x00\x00`\xc3\x00\x00p\xc3\x00\x00\x80\xc3\x00\x00\x90\xc3\x00\x00\xa0\xc3\x00\x00\xb0\xc3\x00\x00\xc0\xc3\x00\x00\xd0\xc3\x00\x00\xe0\xc3\x00\x00\x00\x00\x00\x00\x00;\x00\x00\x80;\x00\x00\xc0;\x00\x00\x00<\x00\x00 <\x00\x00@<\x00\x00`<\x00\x00\x80<\x00\x00\x90<\x00\x00\xa0<\x00\x00\xb0<\x00\x00\xc0<\x00\x00\xd0<\x00\x00\xe0<\x00\x00\xf0<\x00\x00\x00=\x00\x00\x10=\x00\x00 =\x00\x000=\x00\x00@=\x00\x00P=\x00\x00`=\x00\x00p=\x00\x00\x80=\x00\x00\x90=\x00\x00\xa0=\x00\x00\xb0=\x00\x00\xc0=\x00\x00\xd0=\x00\x00\xe0=\x00\x00\xf0=\x00\x00\x00>\x00\x00\x10>\x00\x00 >\x00\x000>\x00\x00@>\x00\x00P>\x00\x00`>\x00\x00p>\x00\x00\x80>\x00\x00\x90>\x00\x00\xa0>\x00\x00\xb0>\x00\x00\xc0>\x00\x00\xd0>\x00\x00\xe0>\x00\x00\xf0>\x00\x00\x00?\x00\x00\x10?\x00\x00 ?\x00\x000?\x00\x00@?\x00\x00P?\x00\x00`?\x00\x00p?\x00\x00\x80?\x00\x00\x90?\x00\x00\xa0?\x00\x00\xb0?\x00\x00\xc0?\x00\x00\xd0?\x00\x00\xe0?\x00\x00\xf0?\x00\x00\x00@\x00\x00\x10@\x00\x00 @\x00\x000@\x00\x00@@\x00\x00P@\x00\x00`@\x00\x00p@\x00\x00\x80@\x00\x00\x90@\x00\x00\xa0@\x00\x00\xb0@\x00\x00\xc0@\x00\x00\xd0@\x00\x00\xe0@\x00\x00\xf0@\x00\x00\x00A\x00\x00\x10A\x00\x00 A\x00\x000A\x00\x00@A\x00\x00PA\x00\x00`A\x00\x00pA\x00\x00\x80A\x00\x00\x90A\x00\x00\xa0A\x00\x00\xb0A\x00\x00\xc0A\x00\x00\xd0A\x00\x00\xe0A\x00\x00\xf0A\x00\x00\x00B\x00\x00\x10B\x00\x00 B\x00\x000B\x00\x00@B\x00\x00PB\x00\x00`B\x00\x00pB\x00\x00\x80B\x00\x00\x90B\x00\x00\xa0B\x00\x00\xb0B\x00\x00\xc0B\x00\x00\xd0B\x00\x00\xe0B\x00\x00\xf0B\x00\x00\x00C\x00\x00\x10C\x00\x00 C\x00\x000C\x00\x00@C\x00\x00PC\x00\x00`C\x00\x00pC\x00\x00\x80C\x00\x00\x90C\x00\x00\xa0C\x00\x00\xb0C\x00\x00\xc0C\x00\x00\xd0C\x00\x00\xe0C\x00\x00\xf0C\x00\x00\x00\x80\x00\x00\x00\xbb\x00\x00\x80\xbb\x00\x00\xc0\xbb\x00\x00\x00\xbc\x00\x00 \xbc\x00\x00@\xbc\x00\x00`\xbc\x00\x00\x80\xbc\x00\x00\x90\xbc\x00\x00\xa0\xbc\x00\x00\xb0\xbc\x00\x00\xc0\xbc\x00\x00\xd0\xbc\x00\x00\xe0\xbc\x00\x00\xf0\xbc\x00\x00\x00\xbd\x00\x00\x10\xbd\x00\x00 \xbd\x00\x000\xbd\x00\x00@\xbd\x00\x00P\xbd\x00\x00`\xbd\x00\x00p\xbd\x00\x00\x80\xbd\x00\x00\x90\xbd\x00\x00\xa0\xbd\x00\x00\xb0\xbd\x00\x00\xc0\xbd\x00\x00\xd0\xbd\x00\x00\xe0\xbd\x00\x00\xf0\xbd\x00\x00\x00\xbe\x00\x00\x10\xbe\x00\x00 \xbe\x00\x000\xbe\x00\x00@\xbe\x00\x00P\xbe\x00\x00`\xbe\x00\x00p\xbe\x00\x00\x80\xbe\x00\x00\x90\xbe\x00\x00\xa0\xbe\x00\x00\xb0\xbe\x00\x00\xc0\xbe\x00\x00\xd0\xbe\x00\x00\xe0\xbe\x00\x00\xf0\xbe\x00\x00\x00\xbf\x00\x00\x10\xbf\x00\x00 \xbf\x00\x000\xbf\x00\x00@\xbf\x00\x00P\xbf\x00\x00`\xbf\x00\x00p\xbf\x00\x00\x80\xbf\x00\x00\x90\xbf\x00\x00\xa0\xbf\x00\x00\xb0\xbf\x00\x00\xc0\xbf\x00\x00\xd0\xbf\x00\x00\xe0\xbf\x00\x00\xf0\xbf\x00\x00\x00\xc0\x00\x00\x10\xc0\x00\x00 \xc0\x00\x000\xc0\x00\x00@\xc0\x00\x00P\xc0\x00\x00`\xc0\x00\x00p\xc0\x00\x00\x80\xc0\x00\x00\x90\xc0\x00\x00\xa0\xc0\x00\x00\xb0\xc0\x00\x00\xc0\xc0\x00\x00\xd0\xc0\x00\x00\xe0\xc0\x00\x00\xf0\xc0\x00\x00\x00\xc1\x00\x00\x10\xc1\x00\x00 \xc1\x00\x000\xc1\x00\x00@\xc1\x00\x00P\xc1\x00\x00`\xc1\x00\x00p\xc1\x00\x00\x80\xc1\x00\x00\x90\xc1\x00\x00\xa0\xc1\x00\x00\xb0\xc1\x00\x00\xc0\xc1\x00\x00\xd0\xc1\x00\x00\xe0\xc1\x00\x00\xf0\xc1\x00\x00\x00\xc2\x00\x00\x10\xc2\x00\x00 \xc2\x00\x000\xc2\x00\x00@\xc2\x00\x00P\xc2\x00\x00`\xc2\x00\x00p\xc2\x00\x00\x80\xc2\x00\x00\x90\xc2\x00\x00\xa0\xc2\x00\x00\xb0\xc2\x00\x00\xc0\xc2\x00\x00\xd0\xc2\x00\x00\xe0\xc2\x00\x00\xf0\xc2\x00\x00\x00\xc3\x00\x00\x10\xc3\x00\x00 \xc3\x00\x000\xc3\x00\x00@\xc3\x00\x00P\xc3\x00\x00`\xc3\x00\x00p\xc3\x00\x00\x80\xc3\x00\x00\x90\xc3\x00\x00\xa0\xc3\x00\x00\xb0\xc3\x00\x00\xc0\xc3\x00\x00\xd0\xc3\x00\x00\xe0\xc3', dtype=np.float32)
- arr2 = np.empty(arr.shape[0], dtype="float32")
- for i in prange(arr.shape[0]):
- arr2[i] = fp8table[arr[i]]
- return arr2
-
-
-def tofp32(arr):
- """Converts a fp8 (4M3E) array to fp32.
- Reshapes the array to be one
- dimensional and uses a numba-optimized function
- """
- return tofp32n8(arr.reshape(arr.shape[0]*arr.shape[1])).reshape(arr.shape)
-
-
-@njit('uint8[:](uint32[:])', parallel=True)
-def tofp8n(arr):
- """Numba-optimized function that converts an array of fp32 to fp8 (4M3E)
- Uses the algorithm described by ProjectPhysX at https://stackoverflow.com/questions/1659440/32-bit-to-16-bit-floating-point-conversion
- and https://www.researchgate.net/publication/362275548_Accuracy_and_performance_of_the_lattice_Boltzmann_method_with_64-bit_32-bit_and_customized_16-bit_number_formats
- """
- arr2 = np.empty(arr.shape[0], dtype="uint8")
- for i in prange(arr.shape[0]):
- # round-to-nearest-even: add last bit after truncated mantissa (1+8+3) from left
- y = arr[i] + 0x00080000
- e = (y&0x7F800000)>>23 # exponent
- m = y&0x007FFFFF #mantissa
-
- if e > 135:
- arr2[i] = 0x7F | (y&0x80000000)>>24 # saturated
- elif e > 120:
- arr2[i] = ((e-120)<<3) & 0x78 | m>>20 | (y&0x80000000)>>24 # normalized
- elif e < 121 and e > 116:
- # 0x00780000 = 0x00800000-0x00080000 = decimal indicator flag - initial rounding
- arr2[i] = ((((m+0x00780000)>>(140-e))+1)>>1) | (y&0x80000000)>>24
- else:
- arr2[i] = 0 | (y&0x80000000)>>24
- return arr2
-
-
-def tofp8(arr):
- """Converts an array of fp32 to fp8 (4M3E)
- Reshapes the array to be one
- dimensional and uses a numba-optimized function
- """
- return tofp8n(arr.view(dtype=np.uint32).reshape(arr.shape[0]*arr.shape[1])).view(dtype=np.uint8).reshape(arr.shape)
-
-
-
-class BlobTable():
- """Class to handle a storage of variable-length values of a key-value storage
- Key is fixed length of key_length
- """
- def __init__(self, store, key_length=20):
- """Initializes class using a pytables store and a key_length value
- """
- if "keys" not in store.root:
- # reasonable compression optimized for reading speed
- filters = tables.Filters(complevel=5, complib='blosc:lz4',
- shuffle=1, bitshuffle=0)
-
- blob_type = {"key": tables.StringCol(key_length, pos=0),
- "offset":tables.Int64Col(pos=1),
- "length": tables.Int64Col(pos=2),
- }
-
- self.keys_table = store.create_table("/", "keys",
- blob_type,
- filters=filters,
- chunkshape=10000)
- self.values_table = store.create_earray("/", "values", atom=tables.UInt8Atom(), shape=(0,), filters=filters)
- else:
- self.keys_table = store.root.keys
- self.values_table = store.root.values
-
- self.offset = self.values_table.nrows
- self.nrows = self.keys_table.nrows
- self._is_closed = False
-
-
- def __len__(self):
- return self.nrows
-
- def create_index(self):
- self.keys_table.cols.key.reindex()
-
-
- def append(self, key, value):
- """Appends a key-value to the storage
- """
- # store variable length value
- length = len(value)
- self.values_table.append(np.frombuffer(value, dtype=np.uint8))
-
- # store index
- row = self.keys_table.row
- row["key"] = key
- row["offset"] = self.offset
- row["length"] = length
- row.append()
- self.offset += length
- self.nrows += 1
-
- def __getitem__ (self, rownum):
- if isinstance(rownum, slice):
- return [self[ii] for ii in range(*rownum.indices(len(self)))]
- else:
- row = self.keys_table[rownum]
- offset = row['offset']
- value = self.values_table.read(offset, offset+row["length"]).tobytes()
-
- return value
-
-
- def get_value (self, key):
- key = key.encode("utf8")
- offset, length = [(r['offset'], r['length']) for r in self.keys_table.where(f"key=={key}")][0]
- value = self.values_table.read(offset, offset+length).tobytes()
- return value
-
-
-class Simiandb():
- """Wrapper around pytables store .
- To use, you should have the ``pytables`` python package installed.
- Example:
- .. code-block:: python
- from simiandb import Simiandb
- docdb = simiandb("store")
- """
-
- def __init__(self, storepath, embedding_function=None, mode="a", id_length = 19):
-
- if mode not in ["a", "w", "r"]:
- raise ValueError("Mode can only be r, w or a")
- self._embedding_function = embedding_function
- self._storename = Path(storepath)
- self._mode = mode
- if not self._storename.exists():
- self._storename.mkdir()
-
- self._vectorstore = tables.open_file( self._storename / "embeddings.h5", mode = mode)
- self._docstore = tables.open_file( self._storename / "documents.h5", mode = mode)
- self._metastore = tables.open_file( self._storename / "metadatas.h5", mode = mode)
- self._embedding_function = embedding_function
- self._is_closed = False
- if 'embeddings' in self._vectorstore.root:
- self._vector_table = self._vectorstore.root.embeddings
- self._docs_table = BlobTable(self._docstore, id_length)
- return
-
-
- def __enter__(self):
- """Magic method Required for usage with the with statement
- """
- return self
-
-
- def _get_top_indexes(self, c, k):
- count = self._vector_table.nrows
- st =0
- batch = self._vector_table.chunkshape[0]*25
- res = np.ascontiguousarray(np.empty(shape=(count,), dtype="float32"))
- end = 0
-
- while end!=count:
- end += batch
- end = end if end <= count else count
- t_res = structured_to_unstructured(self._vector_table.read(start=st, stop=end))
- t_res = tofp32(t_res)
- np.dot(t_res,c, res[st:end])
- st = end
-
- indices = np.argpartition(res, -k)[-k:] #from https://stackoverflow.com/questions/6910641/how-do-i-get-indices-of-n-maximum-values-in-a-numpy-array
- indices = indices[np.argsort(res[indices])[::-1]]
-
- return indices
-
-
- def _create_embeddings_table(self, dimensions):
- """Creates the embeddings table within the pytables file
- """
- if dimensions > 512:
- # prevent pytables warning on max_columns
- tables.parameters.MAX_COLUMNS = len(dimensions)
- embedding_type = {f"d{n}":tables.UInt8Col(pos=n) for n in range(dimensions)}
-
- # no compression for embeddings
- filters = None
-
- self._vector_table = self._vectorstore.create_table("/", "embeddings",
- embedding_type,
- filters=filters,
- chunkshape=10000)
-
-
- def _check_closed(self):
- if self._is_closed:
- raise ValueError("Simiandb is already closed")
-
-
- def add_texts(self, texts, metadatas = None, ids = None, embeddings=None, show_progressbar=True):
- """Run more texts through the embeddings and add to the vectorstore.
- Args:
- texts (Iterable[str]): Texts to add to the vectorstore.
- metadatas (Optional[List[dict]], optional): Optional list of metadatas.
- ids (Optional[List[str]], optional): Optional list of IDs.
- embeddings (Optional[List[array]], optional): Optional list of embeddings.
- Returns:
- List[str]: List of IDs of the added texts.
- """
-
- self._check_closed()
-
- self._add_embeddings(texts, embeddings, show_progressbar)
-
- if ids is None:
- ids = list(range(self.docs_table.nrows, self.docs_table.nrows + len(texts)))
-
- for textid, text in zip(ids, texts):
- self.docs_table.append(textid, text.encode("utf8"))
-
- return ids
-
-
- def get_text(self, key):
- return self._docs_table.get_value(key).decode("utf8")
-
-
- def create_keys_index(self):
- self._docs_table.create_index()
-
-
- def _add_embeddings(self, texts, embeddings, show_progressbar):
- """Calculate or use embeddings to fill the embeddings table
- """
-
- if embeddings is None and not self._embedding_function is None:
- embeddings = self._embedding_function.embed_documents(texts)
-
- if not embeddings is None and 'embeddings' not in self._vectorstore.root:
- dimensions = len(embeddings[0])
- self._create_embeddings_table(dimensions)
-
- if not embeddings is None :
- self._vector_table = self._vectorstore.root.embeddings
- embeddings = tofp8(np.array(embeddings, dtype=np.float32))
- self._vector_table.append(embeddings)
-
-
- def regenerate_embeddings(self, embeddings=None, show_progressbar=True):
- """Run existing texts through the embeddings and add to the vectorstore.
- Args:
- embeddings (Optional[List[array]], optional): Optional list of embeddings.
- """
-
- self._check_closed()
- self._vectorstore.close()
- (self._storename / "embeddings.h5").kill()
- self._vectorstore = tables.open_file( self._storename / "embeddings.h5", mode = self._mode)
-
- batch_size = 1000
- for i in tqdm(range(0, len(self.docs_table), batch_size), disable=not show_progressbar):
- text_batch = [text.decode("utf8") for text in self.docs_table[i:i+batch_size]]
- if embeddings is not None:
- embeddings_batch = embeddings[i:i+batch_size]
- elif self.embedding_function is not None:
- embeddings_batch = self._embedding_function.embed_documents(text_batch)
- else:
- raise ValueError("Neither embeddings nor embedding function provided")
- self._add_embeddings(text_batch, embeddings_batch, show_progressbar)
- return
-
-
- def similarity_search(self, query: str, k = 4, filter = None):
- """Run similarity search with PytableStore.
- Args:
- query (str): Query text to search for.
- k (int): Number of results to return. Defaults to 4.
- filter (Optional[Dict[str, str]]): Filter by metadata. Defaults to None.
- Returns:
- List[Document]: List of documents most simmilar to the query text.
- """
- self._check_closed()
- query_embedding = np.array(self._embedding_function.embed_query(query),dtype="float32")
- results = self._get_top_indexes(query_embedding, k)
-
- docs = [self._docs_table[i].decode("utf8") for i in results]
- return docs
-
-
- def close(self):
- """Makes sure the pytables file is closed
- """
- if not self._is_closed:
- self._is_closed = True
-
- if hasattr(self, '_Simiandb__vectorstore'):
- try:
- self._vectorstore.flush()
- self._docstore.flush()
- self._metastore.flush()
- self._vectorstore.close()
- self._docstore.close()
- self._metastore.close()
- except:
- print("Unable to close file")
-
-
- def __exit__(self, exc_type, exc_value, exc_traceback):
- """Magic method Required for usage with the with statement
- """
- self.close()
-
- def __del__(self):
- """Magic method just in case the object is deleted without closing it
- """
- self.close()
-
-
-
-if __name__ == '__main__':
- pass
diff --git a/spaces/flax-community/Multilingual-VQA/sections/pretraining/data.md b/spaces/flax-community/Multilingual-VQA/sections/pretraining/data.md
deleted file mode 100644
index de9b25b10e6398ef524dda19ab6072451e401935..0000000000000000000000000000000000000000
--- a/spaces/flax-community/Multilingual-VQA/sections/pretraining/data.md
+++ /dev/null
@@ -1 +0,0 @@
-The dataset we use for pre-training is a cleaned version of [Conceptual 12M](https://github.com/google-research-datasets/conceptual-12m). The dataset is downloaded and then broken images are removed which gives us about 10M images. Then we use the MBart50 `mbart-large-50-one-to-many-mmt` checkpoint to translate the dataset into four different languages - English, French, German, and Spanish, keeping 2.5 million examples of each language. This dataset is used for MLM pre-training.
diff --git a/spaces/forklift-app/forklift-images/app.py b/spaces/forklift-app/forklift-images/app.py
deleted file mode 100644
index 29167a2282c6e0a534238eccd15a15d7c46db61e..0000000000000000000000000000000000000000
--- a/spaces/forklift-app/forklift-images/app.py
+++ /dev/null
@@ -1,71 +0,0 @@
-import gradio as gr
-import torch
-from models import Final_CNN_Model
-from torchvision import transforms
-from transferwee import download
-
-# download latest model
-download("https://we.tl/t-uc4MWbAzIJ", "best.pt")
-
-model = Final_CNN_Model()
-checkpoint = torch.load("best.pt", map_location=torch.device("cpu"))
-model.load_state_dict(checkpoint["model_state_dict"])
-model.eval()
-
-labels_to_class = {0: "normal", 1: "risk"}
-
-
-def predict(inp):
- tranforms_pipe = transforms.Compose(
- [transforms.ToTensor(), transforms.Resize((224, 224))]
- )
- inp_tensor = tranforms_pipe(inp) # [C, H, W]
- shape = inp_tensor.shape
-
- # [1, C, H, W]
- inp_tensor = inp_tensor.view(1, shape[0], shape[1], shape[2])
-
- with torch.no_grad():
- inp_tensor = inp_tensor.unsqueeze(0) # [B, 1, C, H, W]
- prediction = torch.sigmoid(model(inp_tensor)[0])
- print(inp, inp_tensor, prediction)
-
- if prediction > 0.5:
- confidences = {"Riesgo": float(prediction[0])}
- else:
- confidences = {"Normal": 1 - float(prediction[0])}
-
- print(confidences)
- return confidences
-
-
-description = """
-
-Este demo se compone de nuestro clasificador de imágenes de uso de grúas horquillas en ambiente y contexto controlado.\n\n
-
-El objetivo de este es poder determinar, con imágenes significativas, si es que la operación en cuestión es de riesgo o no. \n\n
-Nuestro modelo tiene dos componentes, una ResNet18 y una LSTM. La primera, recibe una imagen, mientras que la segunda recibe una secuencia de frames consecutivos. \n
-A pesar de tener dos componentes, gracias a la validación del modelo, este usa solamente usa Resnet18. Si se quisiera usar la parte LSTM habría que activarla manualmente.\n
-La parte de ResNet18 tiene originalmente sus pesos pre-entrenados con ImageNet, luego tuvo 20 épocas de entrenamiento con datos específicos a nuestro caso. \n\n
-
-Para determinar que una imagen es de riesgo se establece que su threshold sea de 0.4 (esto en base a un análisis hecho sobre los datos de validación.
-
-
-Das Puppenhaus Der Weg In Die Schizophrenie Pdf Ebook Epub. Haushofkind Seite 4 Von 19 Wohnen Mit Kindern - Haushofkind Seite 4 Von ... 4d29de3e1b
-
-
-
diff --git a/spaces/gotiQspiryo/whisper-ui/examples/Cyg Usb-1.0.dll Download The Best Way to Access USB Devices on Windows with Cygwin.md b/spaces/gotiQspiryo/whisper-ui/examples/Cyg Usb-1.0.dll Download The Best Way to Access USB Devices on Windows with Cygwin.md
deleted file mode 100644
index 2f6b17e1fcab79a7eca28d42820e5d08b69eda1b..0000000000000000000000000000000000000000
--- a/spaces/gotiQspiryo/whisper-ui/examples/Cyg Usb-1.0.dll Download The Best Way to Access USB Devices on Windows with Cygwin.md
+++ /dev/null
@@ -1,28 +0,0 @@
-
-
Mac OS X Notes: Install the Xcode app to get the build tools (GCC and Make). Use MacPorts to get the Boost and Mako dependencies. Other dependencies can be downloaded as DMG installers from the web or installed via MacPorts. See the UHD OS X build instructions for more information: Build Instructions (Mac OS X)
Windows Notes: The dependencies can be acquired through installable EXE files. Usually, the Windows installer can be found on the project's website. Some projects do not host Windows installers, and if this is the case, follow the auxiliary download URL for the Windows installer (below).
-
That said, running almost any graphical interface (GUI) will require downloading and installing X11/XQuartz first. Through OSX 10.8, Apple provided a means to install X11.app, but XQuartz has always been more up to date. Staring in 10.9, Apple no longer provides a full working version of X11.app. Hence, just use XQuartz from the get-go. Note that unless you experiment with using the Quartz interface to various graphical toolkits (e.g., GTK), you must use X11 as the terminal interface for any GUI applications.
-
Apple provides a fully integrated development environment via their Xcode toolkit, which can be downloaded either via the App store or directly from Apple's Developer area depending on the version of OSX in use. Xcode provides the compilers and related development tools needed to build or execute UHD and its dependencies.
-
Once Xcode is installed, you must still install the Command Line Tools, which can be accomplished by running Xcode.app, then going to Preferences... -> Downloads and making sure Command Line Tools is selected/enabled [feel free to select other downloads too]. You might be able to install the Command Line Tools in a terminal using
-
Installing UHD from source follows the standard cmake method as found in many places, with a few arguments to make sure cmake always finds the correct version of Python, and uses the desired compiler. First, download the source code either via a release or via GIT.
-
-
Go to download page of the Graphviz projectand download the appropriate RPM. You only need the basic graphviz RPM (graphviz-);you don't need all of the add-ons, such as -devel, -doc, -perl, etc.If you're not sure what version of Linux you're running,
-
dfu-util is a host side implementation of the DFU 1.0 andDFU 1.1 specifications of the USB forum.DFU is intended to download and upload firmware to/from devices connectedover USB. It ranges from small devices like micro-controller boardsto mobile phones. Using dfu-util you can download firmware to yourDFU-enabled device or upload firmware from it. dfu-util has beentested with the Openmoko Neo1973 and Freerunner and many other devices.
-
MSYS2 is required in order to build native C/C++ extensions for Ruby and is necessary for Ruby on Rails.Moreover it allows the download and usage of hundreds of Open Source libraries which Ruby gems often depend on.
-
We provide downloads for the official client and server programs. A Linux distribution may provide their own packages and have their own maintainer,which we will describe below. We also link to some third party projects.
-
Instrument-Control is a package for interfacing the outside world of hardware via Serial, i2c or Parallel interfaces. It is currently under development by Andrius Sutas and Stefan Mahr, you can browse the mercurial repository here and download the package here.
-
If you download the Setup program of the package, any requirements forrunning applications, such as dynamic link libraries(DLL's) from the dependencies as listed below under Requirements, arealready included. If you download the package as Zip files, then you mustdownload and install the dependencies zip file yourself.Developer files (header files and libraries) from otherpackages are however not included; so if you wish to develop your ownapplications, you must separately install the required packages.
-
If your properties file has not been configured for your scope yet, you can start with a generic file, but only on a Thermo/FEI scope. The generic file does not contain many essential entries for JEOL or Hitachi microscopes. If you download and unpack the GenericFramework.exe file, you should also download GenericProperties.txt from the Tools folder on the SerialEM download site ( ), rename it to SerialEMproperties.txt, and replace the file from the framework. Then download and incorporate camera properties and magnification table files from the Tools folder.
-
To estimate BuiltInSettling for a CCD camera, open the 'cleartime.s' script available from the Tools folder on the SerialEM download site. You can do this with the File - Open menu entry in DM, or just by clicking on the file. Run the script by pressing Ctrl-Enter. It will time a series of exposures and report the mean time. It will then recommend values for BuiltInSettling based on subtracting 0.155 second for large format one-port readout cameras (Megascan 795 and Ultrascan 890, 2Kx2K 30-micron pixel or 4Kx4K 15-micron pixel) or 0.065 second for the four-port readout Ultrascan 895. These correction factors are based on the 4 cameras that I have been able to run the script with. The script is of no use with CMOS cameras like the K2/K3 and OneView.
-
Gphoto2, and its library libgphoto2 is a Linux application enables controlling cameras and downloading images through USB PTP or serial cable. It is of importance if you would like to build a remote controlled camera or automate the time lapse photography with advanced setting, such as altering the shutter speed during sunset with a predefined value, or make exposure at precise moment for solar eclipse. Another feature will be turning on service mode to enable uncooked RAW image download for Nikon DSLRs. Cameras from almost all vendor are supported.
-
Is there a documentation on how to install the libusb you mentioned on windows, would it mess up my existing usb connected devices? I downloaded the libusb-win but do not know not know what to do beyond that. Any input would help me move ahead.
-
When downloading content from URL arguments, be sensitive to the character encoding (#5600). We can properly handle UTF-8 and latin1 (ISO-8859-1); for others we raise an error. Fall back to latin1 if no charset is given in the mime type and UTF-8 decoding fails.
-
The easiest way to get and install the proper drivers for your new BusPirate is by going to the BP chip provider web pages and download their Windows driver package, which is distributed as either a .zip file or a .exe installer. I choose the .exe installer, but the drawback is that there is no feedback message that anything have been installed (at least not for me). The screen just flashes once and that's it. I haven't tried the .zip file installation. (Please let me know how that works.)
-
In our case, for the BPv3b, the chip is the FT232RL and the provider company is FTDI (Future Technology Devices International). Their drivers are now ASFAIK distributed in a single download package containing both the drivers for VCP (Virtual COM Port) and D2XX (direct USB access via DLL) connections. The one to use for BP terminal access is VCP and the one you need for openOCD is D2XX. [?]
-
Now it's time to open your favorite terminal application. I use the free "RealTerm". (You can download it from here: ) I like it simply because it has too many features! Select the same settings as above and make sure you choose the same port as shown in Windows Device Manager. Hit [RET] a few times and you should be greeted with BP's built in command-line interface with the "HiZ>" prompt. The first useful thing to do is to check your exact hardware/firmware/bootloader version by typing "i" and find out which protocols are directly/natively supported by typing "m". In the latest FW versions, "JTAG" is not present in the list, although it should be supported through OpenOCD according to developers, but not according to users!
-
To get all the right "ds30 Loader" parameters for your BPv3b, download and extract the appropriate BitPirate .zip firmware package, that also contain the older ds30 Loader, but with the addition of a "settings.xml" file. Copy this file to the ds30's default settings directory (for Windows Vista): "C:\Users\YourWindowsUsername\AppData\Roaming\.ds30Loader\". You can then navigate to this file (from ds30) by going to "View" --> "Settings directory". If you use this file, you will notice that most settings are disabled from changes as a sefty precaution in order for careless people not to brick their BP!
-
I want to thank the following companies which are providing support for the BusyBox project:
Analog Devices, Inc. provided a Blackfin development board free of charge. Blackfin is a NOMMU processor, and its availability for testing is invaluable. If you are an embedded device developer, please note that Analog Devices has an entire Linux distribution available for download for this board. Visit for more information.
-
Changes since previous release:Aaro Koskinen: find: implement -emptyAlistair Francis (4): date: Use 64 prefix syscall if we have to time: Use 64 prefix syscall if we have to runsv: Use 64 prefix syscall if we have to Remove stime() function callsBiswapriyo Nath: Makefile.flags: restrict Wno-constant-logical-operand and Wno-string-plus-int options for clangBrian Foley (3): dc: execute shouldn't pop if stack head is not a string dc: Fix segfault when executing strings generated using asciify dc: Parse error & fix out of bounds read in xc_program_printStringDaniel Edgecumbe (3): gzip: default level with ENABLE_FEATURE_GZIP_LEVELS should be 6 gzip: set compression flags correctly as per standard gzip: set default compression level to 6 when CONFIG_FEATURE_GZIP_LEVELS=nDavid Demelier: wget: increase redirections limitDenys Vlasenko: build system: suppress some clang-9 warnings examples/udhcp/simple.script: up interface on deconfig event libbb: remove syscall wrappers around clock_gettime, closes 12091 libbb: clang/llvm 9 fix - do not eliminate a store to a fake "const" libbb: deal with "declaration of 'link' shadows a global declaration" warning libbb: include only if necessary ash,hush: add comment about masked SIGCHLD, handle SIG_IGNed SIGHUP as in bash ash,hush: testcase for "exit" without arguments in a trap ash: Expand here-documents in the current shell environment ash: Return without arguments in a trap should use status outside traps ash: [BUILTIN] Exit without arguments in a trap should use status outside traps ash: builtin: Mark more regular built-ins ash: eval: Add assignment built-in support again ash: eval: Always set localvar_stop ash: eval: Fail immediately with redirections errors for simple command ash: eval: Only restore exit status on exit/return ash: eval: Reap zombies after built-in commands and functions ash: eval: Replace with listsetvar with mklocal/setvareq ash: eval: Use the correct expansion mode for fd redirection ash: exec: Do not allocate stack string in padvance ash: exec: Never rehash regular built-ins ash: exec: Stricter pathopt parsing ash: expand: Do not reprocess data when expanding words ash: expand: Ensure result is escaped in cvtnum ash: expand: Fix multiple issues with EXP_DISCARD in evalvar ash: expand: Fix skipping of command substitution when trimming in evalvar ash: expand: Fix trailing newlines processing in backquote expanding ash: expand: Merge syntax/quotes in memtodest with flags ash: expand: Use HOME in tilde expansion when it is empty ash: fix BASE###nn bashism for bases 36..64 ash: fix BASE###nn bashism to accept letter 'digits' for bases > 9 ash: fix set -o to not show "nameless" options ash: jobs - Do not block when waiting on SIGCHLD ash: jobs: Only clear gotsigchld when waiting for everything ash: jobs: Replace some uses of fmtstr with stpcpy/stpncpy ash: main: Only set savestatus in exitcmd ash: main: Print \n upon EOF (CTRL-D) when run interactively ash: memalloc: Add growstackto helper ash: memalloc: Avoid looping in growstackto ash: mkinit: Split reset into exitreset and reset ash: output: Fix fmtstr return value ash: parser: Do not push token back before parseheredoc ash: parser: Fix incorrect eating of backslash newlines ash: parser: Fix old-style command substitution here-document crash ash: parser: Only accept single-digit parameter expansion outside of braces ash: parser: Save/restore here-documents in command substitution ash: rename some function parameters to match dash ash: rename stack_nputstr() back to stnputs() to match dash ash: shell: Fix clang warnings about "string plus integer" ash: use pgetc_eatbnl() in more places, take 2 hush: fix "set -o INVALID" affecting -e flag state hush: fix negative_arith.tests: glob-protect dash in "$((arith))" hush: fix preprocessor directives indentation hush: implement "return NUM in trap sets $? after trap" hush: make "exit" in trap use pre-trap exitcode hush: make "exit" in trap use pre-trap exitcode - fix for nested trap hush: restore redirected stdin awk: disallow "str"++, closes bug 12981 awk: fix more "length" cases, closes 12486 bc: fix comparison bug, closes 12336 brctl: fold show_bridge_ports into its caller dpkg-deb: work around bogus error message when working with XZ compressed packages fdisk: add HFS / HFS+ partition type fdisk: avoid overflow in "mega/gigabytes" calculation, code shrink gunzip: code shrink by using int-, not short-sized struct member gunzip: fix incorrect decoding of "fixed" inflate blocks gzip: -d with zcat enabled but gunzip disabled was misbehaving init: if tcgetattr() fails, don't even try to tcsetattr() init: improve handling of signals racing with each other nmeter: add %T (zero-based timestamp) format nmeter: do not clamp down %Nc to minimum of 10 (think nmeter "%`nproc`c") nologin: make it possible to build it as single applet ntpd: abort if argvs are (unexpectedly) given ntpd: abs(tmx.offset) was truncating a "long" typed value ntpd: add comment about mode6, no code changes ntpd: commonalize message strings ntpd: decrease MIN_FREQHOLD by 2, increase "penalty" for largish offset x2 pidof: support "pidof /path/to/binary" case readlink,realpath: fix a case with a symplink, closes 11021 stat: print nanosecond times, fix printing of empty lines sysctl: do report EACCES errors on write tar: change -a from meaning "lzma" to mean "autodetect by extension" taskset: add support for taking/printing CPU list (-c option) taskset: implement stride argument taskset: tighten the check for stride values tc: array address is never NULL tee: do not intercept SIGPIPE telnet: add disabled code to emit EC and IP telnet: fix uninitialized variable bug tftp: on download, open local file only when first bit of data arrived tftpd: show requested file name in open error message top: do not use previous collected data wheh "h" toggles threads display udhcp: comment out unused domain compression code udhcpc6: add ELAPSED_TIME option to outgoing packets udhcpc6: s/iphdr/ip6_hdr/ udhcpd: mangle hostnames starting with dash ("-option") whois: limit total length of response to 32+2 kbDimitri John Ledkov: wget: implement TLS verification with ENABLE_FEATURE_WGET_OPENSSLEivind Versvik: udhcpc6: support stateless DHCPv6Gray Wolf: grep: Fix -f FILE when FILE is empty and -x providedJames Byrne (2): libbb: reduce the overhead of single parameter bb_error_msg() calls config: PID_FILE_PATH required for FEATURE_CROND_SPECIAL_TIMESJean-Philippe Brucker: build system: remove KBUILD_STR()Jo-Philipp Wich (2): nslookup: handle replies without RRs nslookup: implement support for SRV recordsKaarle Ritvanen: ln: --no-target-directory implies --no-dereferenceKang-Che Sung: bc: Add 'U' suffix in UINT_MAX preprocessor checkLauri Kasanen: unzip: -d should create the dirLiu, Shuang (ADITG/ESM): chgrp: correct the usage for non-desktop chgrp callsLukas Rusak: free: include SReclaimable in cached valueMark Edgar: unexpand: correct behavior for --first-only --tabs=4Martin Lewis (8): libbb: Converted safe_read to safe_write format replace: count_strstr - Handle an edge case where sub is empty xstrndup: Use strndup instead of implementing it brctl: add support for showmacs command brctl: add support for showstp command dhcpc.c: Added support for relay server parameter dhcpc: code shrink in good_hostname dhcpc: refactor xmalloc_optname_optval to shrink binary sizeMichal Kazior: udhcpc: fix segmentation fault on empty bin optPeter Korsgaard: syslogd: add config option to include milliseconds in timestampsRolf Eike Beer: examples/udhcp/simple.script: print the filename actually changedRon Yorston (13): mim: new applet: run scripts from a specification file ash,hush: allow builtins to be tab-completed, closes 7532 ash,hush: drop pointer check before calls to show_history ash: fix build failure when command built-in is disabled ash: only catch unexpected exceptions in PS1 expansion ash: improve expandstr() ash: return exit status of nofork applets (again) ash: move TRACE statement in evalcommand() httpd: permit non-default home directory with NOMMU enabled httpd: allow '-h' to work when daemonized with NOMMU enabled vi: fixes to string search in colon commands, closes 10321 xargs: fix handling of quoted arguments, closes 11441 xargs: restore correct behaviour of -n optionStefan Agner: examples/udhcp/simple.script: fix IPv6 support when using udhcpcSören Tempel (2): grep: add proper support for pattern_list deluser: check if specified home is a directory before removing itTomas Paukrt: route: fix output of "route -n -A inet6"Tomi Leppanen: grep: add -RUwe Glaeser: udhcpc6: use correct multicast MAC
aaccfb2cb3
-
-
\ No newline at end of file
diff --git a/spaces/gotiQspiryo/whisper-ui/examples/Man Of Crook It S Good To Be Bad Full Movie Hd 1080p The Story The Cast and The Free Download Link on Kickass.md b/spaces/gotiQspiryo/whisper-ui/examples/Man Of Crook It S Good To Be Bad Full Movie Hd 1080p The Story The Cast and The Free Download Link on Kickass.md
deleted file mode 100644
index e08edb85712eaaa9737e515dd4841cf86e3a83dd..0000000000000000000000000000000000000000
--- a/spaces/gotiQspiryo/whisper-ui/examples/Man Of Crook It S Good To Be Bad Full Movie Hd 1080p The Story The Cast and The Free Download Link on Kickass.md
+++ /dev/null
@@ -1,6 +0,0 @@
-
Man Of Crook It S Good To Be Bad Full Movie Hd 1080p Free Download Kickass
-
- aaccfb2cb3
-
-
-
diff --git a/spaces/gradio/animeganv2/README.md b/spaces/gradio/animeganv2/README.md
deleted file mode 100644
index b92b599fec00f675d6dbd23b5bb440f4de150fb6..0000000000000000000000000000000000000000
--- a/spaces/gradio/animeganv2/README.md
+++ /dev/null
@@ -1,12 +0,0 @@
-
----
-title: animeganv2
-emoji: 🔥
-colorFrom: indigo
-colorTo: indigo
-sdk: gradio
-sdk_version: 4.1.2
-app_file: run.py
-pinned: false
-hf_oauth: true
----
diff --git a/spaces/gwang-kim/DATID-3D/eg3d/gen_samples.py b/spaces/gwang-kim/DATID-3D/eg3d/gen_samples.py
deleted file mode 100644
index 06b69a581071feb3814eac423f46c9d5b75169d9..0000000000000000000000000000000000000000
--- a/spaces/gwang-kim/DATID-3D/eg3d/gen_samples.py
+++ /dev/null
@@ -1,280 +0,0 @@
-# SPDX-FileCopyrightText: Copyright (c) 2021-2022 NVIDIA CORPORATION & AFFILIATES. All rights reserved.
-# SPDX-License-Identifier: LicenseRef-NvidiaProprietary
-#
-# NVIDIA CORPORATION, its affiliates and licensors retain all intellectual
-# property and proprietary rights in and to this material, related
-# documentation and any modifications thereto. Any use, reproduction,
-# disclosure or distribution of this material and related documentation
-# without an express license agreement from NVIDIA CORPORATION or
-# its affiliates is strictly prohibited.
-
-"""Generate images and shapes using pretrained network pickle."""
-
-import os
-import re
-from typing import List, Optional, Tuple, Union
-
-import click
-import dnnlib
-import numpy as np
-import PIL.Image
-import torch
-from tqdm import tqdm
-import mrcfile
-
-
-import legacy
-from camera_utils import LookAtPoseSampler, FOV_to_intrinsics
-from torch_utils import misc
-from training.triplane import TriPlaneGenerator
-
-
-#----------------------------------------------------------------------------
-
-def parse_range(s: Union[str, List]) -> List[int]:
- '''Parse a comma separated list of numbers or ranges and return a list of ints.
-
- Example: '1,2,5-10' returns [1, 2, 5, 6, 7]
- '''
- if isinstance(s, list): return s
- ranges = []
- range_re = re.compile(r'^(\d+)-(\d+)$')
- for p in s.split(','):
- if m := range_re.match(p):
- ranges.extend(range(int(m.group(1)), int(m.group(2))+1))
- else:
- ranges.append(int(p))
- return ranges
-
-#----------------------------------------------------------------------------
-
-def parse_vec2(s: Union[str, Tuple[float, float]]) -> Tuple[float, float]:
- '''Parse a floating point 2-vector of syntax 'a,b'.
-
- Example:
- '0,1' returns (0,1)
- '''
- if isinstance(s, tuple): return s
- parts = s.split(',')
- if len(parts) == 2:
- return (float(parts[0]), float(parts[1]))
- raise ValueError(f'cannot parse 2-vector {s}')
-
-#----------------------------------------------------------------------------
-
-def make_transform(translate: Tuple[float,float], angle: float):
- m = np.eye(3)
- s = np.sin(angle/360.0*np.pi*2)
- c = np.cos(angle/360.0*np.pi*2)
- m[0][0] = c
- m[0][1] = s
- m[0][2] = translate[0]
- m[1][0] = -s
- m[1][1] = c
- m[1][2] = translate[1]
- return m
-
-#----------------------------------------------------------------------------
-
-def create_samples(N=256, voxel_origin=[0, 0, 0], cube_length=2.0):
- # NOTE: the voxel_origin is actually the (bottom, left, down) corner, not the middle
- voxel_origin = np.array(voxel_origin) - cube_length/2
- voxel_size = cube_length / (N - 1)
-
- overall_index = torch.arange(0, N ** 3, 1, out=torch.LongTensor())
- samples = torch.zeros(N ** 3, 3)
-
- # transform first 3 columns
- # to be the x, y, z index
- samples[:, 2] = overall_index % N
- samples[:, 1] = (overall_index.float() / N) % N
- samples[:, 0] = ((overall_index.float() / N) / N) % N
-
- # transform first 3 columns
- # to be the x, y, z coordinate
- samples[:, 0] = (samples[:, 0] * voxel_size) + voxel_origin[2]
- samples[:, 1] = (samples[:, 1] * voxel_size) + voxel_origin[1]
- samples[:, 2] = (samples[:, 2] * voxel_size) + voxel_origin[0]
-
- num_samples = N ** 3
-
- return samples.unsqueeze(0), voxel_origin, voxel_size
-
-#----------------------------------------------------------------------------
-
-@click.command()
-@click.option('--network', help='Network path', multiple=True, required=True)
-@click.option('--w_pth', help='latent path')
-@click.option('--generator_type', help='Generator type', type=click.Choice(['ffhq', 'cat']), required=False, metavar='STR', default='ffhq', show_default=True)
-@click.option('--model_is_state_dict', type=bool, default=False)
-@click.option('--seeds', type=parse_range, help='List of random seeds (e.g., \'0,1,4-6\')', required=True)
-@click.option('--trunc', 'truncation_psi', type=float, help='Truncation psi', default=1, show_default=True)
-@click.option('--trunc-cutoff', 'truncation_cutoff', type=int, help='Truncation cutoff', default=14, show_default=True)
-@click.option('--outdir', help='Where to save the output images', type=str, required=True, metavar='DIR')
-@click.option('--shapes', help='Export shapes as .mrc files viewable in ChimeraX', type=bool, required=False, metavar='BOOL', default=False, show_default=True)
-@click.option('--shape-res', help='', type=int, required=False, metavar='int', default=512, show_default=True)
-@click.option('--shape_only_first', type=bool, default=False)
-@click.option('--fov-deg', help='Field of View of camera in degrees', type=int, required=False, metavar='float', default=18.837, show_default=True)
-@click.option('--shape_format', help='Shape Format', type=click.Choice(['.mrc', '.ply']), default='.mrc')
-def generate_images(
- network: List[str],
- w_pth: str,
- generator_type: str,
- seeds: List[int],
- truncation_psi: float,
- truncation_cutoff: int,
- outdir: str,
- shapes: bool,
- shape_res: int,
- fov_deg: float,
- shape_format: str,
- model_is_state_dict: bool,
- shape_only_first: bool,
-):
-
-
- if not os.path.exists(outdir):
- os.makedirs(outdir, exist_ok=True)
-
- device = torch.device('cuda')
-
- if generator_type == 'ffhq':
- network_pkl_tmp = 'pretrained/ffhqrebalanced512-128.pkl'
- elif generator_type == 'cat':
- network_pkl_tmp = 'pretrained/afhqcats512-128.pkl'
- else:
- NotImplementedError()
-
- G_list = []
- outputs = []
- for network_path in network:
- print('Loading networks from "%s"...' % network_path)
- dir_label = network_path.split('/')[-2] + '___' + network_path.split('/')[-1]
- output = os.path.join(outdir, dir_label)
- outputs.append(output)
- if model_is_state_dict:
- with dnnlib.util.open_url(network_pkl_tmp) as f:
- G = legacy.load_network_pkl(f)['G_ema'].to(device) # type: ignore
- ckpt = torch.load(network_path)
- G.load_state_dict(ckpt, strict=False)
- else:
- with dnnlib.util.open_url(network_path) as f:
- G = legacy.load_network_pkl(f)['G_ema'].to(device) # type: ignore
-
- G.rendering_kwargs['depth_resolution'] = int(G.rendering_kwargs['depth_resolution'])
- G.rendering_kwargs['depth_resolution_importance'] = int(
- G.rendering_kwargs['depth_resolution_importance'])
-
- if generator_type == 'cat':
- G.rendering_kwargs['avg_camera_pivot'] = [0, 0, -0.06]
- elif generator_type == 'ffhq':
- G.rendering_kwargs['avg_camera_pivot'] = [0, 0, 0.2]
-
- G_list.append(G)
-
- if truncation_cutoff == 0:
- truncation_psi = 1.0 # truncation cutoff of 0 means no truncation anyways
- if truncation_psi == 1.0:
- truncation_cutoff = 14 # no truncation so doesn't matter where we cutoff
-
- if w_pth is not None:
- seeds = [0]
- seed_idx = ''
- for i, seed in enumerate(seeds):
- if i < len(seeds) - 1:
- seed_idx += f'{seed}_'
- else:
- seed_idx += f'{seed}'
-
- intrinsics = FOV_to_intrinsics(fov_deg, device=device)
-
- print(seeds)
-
- # Generate images.
- for G, output in zip(G_list, outputs):
- for seed_idx, seed in enumerate(seeds):
- print('Generating image for seed %d (%d/%d) ...' % (seed, seed_idx, len(seeds)))
-
- z = torch.from_numpy(np.random.RandomState(seed).randn(1, G.z_dim)).to(device)
-
- imgs = []
- angle_p = -0.2
- for angle_y, angle_p in [(.4, angle_p), (0, angle_p), (-.4, angle_p)]:
- cam_pivot = torch.tensor(G.rendering_kwargs.get('avg_camera_pivot', [0, 0, 0]), device=device)
- cam_radius = G.rendering_kwargs.get('avg_camera_radius', 2.7)
- cam2world_pose = LookAtPoseSampler.sample(np.pi/2 + angle_y, np.pi/2 + angle_p, cam_pivot, radius=cam_radius, device=device)
- conditioning_cam2world_pose = LookAtPoseSampler.sample(np.pi/2, np.pi/2, cam_pivot, radius=cam_radius, device=device)
- camera_params = torch.cat([cam2world_pose.reshape(-1, 16), intrinsics.reshape(-1, 9)], 1)
- conditioning_params = torch.cat([conditioning_cam2world_pose.reshape(-1, 16), intrinsics.reshape(-1, 9)], 1)
-
- if w_pth is not None:
- ws = torch.load(w_pth).cuda()
- w_given_id = os.path.split(w_pth)[-1].split('.')[-2]
- output_img = output + f'__{w_given_id}.png'
- output_shape = output + f'__{w_given_id}.mrc'
- else:
- ws = G.mapping(z, conditioning_params, truncation_psi=truncation_psi, truncation_cutoff=truncation_cutoff)
- output_img = output + f'__{seed_idx:05d}.png'
- output_shape = output + f'__{seed_idx:05d}.mrc'
-
-
- img = G.synthesis(ws, camera_params)['image']
-
- img = (img.permute(0, 2, 3, 1) * 127.5 + 128).clamp(0, 255).to(torch.uint8)
- imgs.append(img)
-
- img = torch.cat(imgs, dim=2)
-
- PIL.Image.fromarray(img[0].cpu().numpy(), 'RGB').save(output_img)
- if shape_only_first and seed_idx != 0:
- continue
-
-
- if shapes:
- # extract a shape.mrc with marching cubes. You can view the .mrc file using ChimeraX from UCSF.
- max_batch=1000000
-
- samples, voxel_origin, voxel_size = create_samples(N=shape_res, voxel_origin=[0, 0, 0], cube_length=G.rendering_kwargs['box_warp'] * 1)#.reshape(1, -1, 3)
- samples = samples.to(z.device)
- sigmas = torch.zeros((samples.shape[0], samples.shape[1], 1), device=z.device)
- transformed_ray_directions_expanded = torch.zeros((samples.shape[0], max_batch, 3), device=z.device)
- transformed_ray_directions_expanded[..., -1] = -1
-
- head = 0
- with tqdm(total = samples.shape[1]) as pbar:
- with torch.no_grad():
- while head < samples.shape[1]:
- torch.manual_seed(0)
- sigma = G.sample(samples[:, head:head+max_batch], transformed_ray_directions_expanded[:, :samples.shape[1]-head], z, conditioning_params, truncation_psi=truncation_psi, truncation_cutoff=truncation_cutoff, noise_mode='const')['sigma']
- sigmas[:, head:head+max_batch] = sigma
- head += max_batch
- pbar.update(max_batch)
-
- sigmas = sigmas.reshape((shape_res, shape_res, shape_res)).cpu().numpy()
- sigmas = np.flip(sigmas, 0)
-
- # Trim the border of the extracted cube
- pad = int(30 * shape_res / 256)
- pad_value = -1000
- sigmas[:pad] = pad_value
- sigmas[-pad:] = pad_value
- sigmas[:, :pad] = pad_value
- sigmas[:, -pad:] = pad_value
- sigmas[:, :, :pad] = pad_value
- sigmas[:, :, -pad:] = pad_value
-
-
- if shape_format == '.ply':
- from shape_utils import convert_sdf_samples_to_ply
- convert_sdf_samples_to_ply(np.transpose(sigmas, (2, 1, 0)), [0, 0, 0], 1, output_shape.replace('.mrc','.ply'), level=10)
- elif shape_format == '.mrc': # output mrc
- with mrcfile.new_mmap(output_shape, overwrite=True, shape=sigmas.shape, mrc_mode=2) as mrc:
- mrc.data[:] = sigmas
-
-
-#----------------------------------------------------------------------------
-
-if __name__ == "__main__":
- generate_images() # pylint: disable=no-value-for-parameter
-
-#----------------------------------------------------------------------------
diff --git a/spaces/gyugnsu/DragGan-Inversion/torch_utils/ops/grid_sample_gradfix.py b/spaces/gyugnsu/DragGan-Inversion/torch_utils/ops/grid_sample_gradfix.py
deleted file mode 100644
index 5031a7720cbe25b68946b93928f41dc9fbeac0d5..0000000000000000000000000000000000000000
--- a/spaces/gyugnsu/DragGan-Inversion/torch_utils/ops/grid_sample_gradfix.py
+++ /dev/null
@@ -1,84 +0,0 @@
-# Copyright (c) 2021, NVIDIA CORPORATION & AFFILIATES. All rights reserved.
-#
-# NVIDIA CORPORATION and its licensors retain all intellectual property
-# and proprietary rights in and to this software, related documentation
-# and any modifications thereto. Any use, reproduction, disclosure or
-# distribution of this software and related documentation without an express
-# license agreement from NVIDIA CORPORATION is strictly prohibited.
-
-"""Custom replacement for `torch.nn.functional.grid_sample` that
-supports arbitrarily high order gradients between the input and output.
-Only works on 2D images and assumes
-`mode='bilinear'`, `padding_mode='zeros'`, `align_corners=False`."""
-
-import torch
-
-# pylint: disable=redefined-builtin
-# pylint: disable=arguments-differ
-# pylint: disable=protected-access
-
-# ----------------------------------------------------------------------------
-
-enabled = False # Enable the custom op by setting this to true.
-
-# ----------------------------------------------------------------------------
-
-
-def grid_sample(input, grid):
- if _should_use_custom_op():
- return _GridSample2dForward.apply(input, grid)
- return torch.nn.functional.grid_sample(input=input, grid=grid, mode='bilinear', padding_mode='zeros', align_corners=False)
-
-# ----------------------------------------------------------------------------
-
-
-def _should_use_custom_op():
- return enabled
-
-# ----------------------------------------------------------------------------
-
-
-class _GridSample2dForward(torch.autograd.Function):
- @staticmethod
- def forward(ctx, input, grid):
- assert input.ndim == 4
- assert grid.ndim == 4
- output = torch.nn.functional.grid_sample(
- input=input, grid=grid, mode='bilinear', padding_mode='zeros', align_corners=False)
- ctx.save_for_backward(input, grid)
- return output
-
- @staticmethod
- def backward(ctx, grad_output):
- input, grid = ctx.saved_tensors
- grad_input, grad_grid = _GridSample2dBackward.apply(
- grad_output, input, grid)
- return grad_input, grad_grid
-
-# ----------------------------------------------------------------------------
-
-
-class _GridSample2dBackward(torch.autograd.Function):
- @staticmethod
- def forward(ctx, grad_output, input, grid):
- op = torch._C._jit_get_operation('aten::grid_sampler_2d_backward')
- grad_input, grad_grid = op(grad_output, input, grid, 0, 0, False)
- ctx.save_for_backward(grid)
- return grad_input, grad_grid
-
- @staticmethod
- def backward(ctx, grad2_grad_input, grad2_grad_grid):
- _ = grad2_grad_grid # unused
- grid, = ctx.saved_tensors
- grad2_grad_output = None
- grad2_input = None
- grad2_grid = None
-
- if ctx.needs_input_grad[0]:
- grad2_grad_output = _GridSample2dForward.apply(
- grad2_grad_input, grid)
-
- assert not ctx.needs_input_grad[2]
- return grad2_grad_output, grad2_input, grad2_grid
-
-# ----------------------------------------------------------------------------
diff --git a/spaces/haakohu/deep_privacy2/dp2/metrics/ppl.py b/spaces/haakohu/deep_privacy2/dp2/metrics/ppl.py
deleted file mode 100644
index 421aeafc5edc4647037fdc390737b269cdfbeae5..0000000000000000000000000000000000000000
--- a/spaces/haakohu/deep_privacy2/dp2/metrics/ppl.py
+++ /dev/null
@@ -1,116 +0,0 @@
-import numpy as np
-import torch
-import tops
-from dp2 import utils
-from torch_fidelity.helpers import get_kwarg, vassert
-from torch_fidelity.defaults import DEFAULTS as PPL_DEFAULTS
-from torch_fidelity.utils import sample_random, batch_interp, create_sample_similarity
-from torchvision.transforms.functional import resize
-
-
-def slerp(a, b, t):
- a = a / a.norm(dim=-1, keepdim=True)
- b = b / b.norm(dim=-1, keepdim=True)
- d = (a * b).sum(dim=-1, keepdim=True)
- p = t * torch.acos(d)
- c = b - d * a
- c = c / c.norm(dim=-1, keepdim=True)
- d = a * torch.cos(p) + c * torch.sin(p)
- d = d / d.norm(dim=-1, keepdim=True)
- return d
-
-
-@torch.no_grad()
-def calculate_ppl(
- dataloader,
- generator,
- latent_space=None,
- data_len=None,
- upsample_size=None,
- **kwargs) -> dict:
- """
- Inspired by https://github.com/NVlabs/stylegan/blob/master/metrics/perceptual_path_length.py
- """
- if latent_space is None:
- latent_space = generator.latent_space
- assert latent_space in ["Z", "W"], f"Not supported latent space: {latent_space}"
- assert len(upsample_size) == 2
- epsilon = PPL_DEFAULTS["ppl_epsilon"]
- interp = PPL_DEFAULTS['ppl_z_interp_mode']
- similarity_name = PPL_DEFAULTS['ppl_sample_similarity']
- sample_similarity_resize = PPL_DEFAULTS['ppl_sample_similarity_resize']
- sample_similarity_dtype = PPL_DEFAULTS['ppl_sample_similarity_dtype']
- discard_percentile_lower = PPL_DEFAULTS['ppl_discard_percentile_lower']
- discard_percentile_higher = PPL_DEFAULTS['ppl_discard_percentile_higher']
-
- vassert(type(epsilon) is float and epsilon > 0, 'Epsilon must be a small positive floating point number')
- vassert(discard_percentile_lower is None or 0 < discard_percentile_lower < 100, 'Invalid percentile')
- vassert(discard_percentile_higher is None or 0 < discard_percentile_higher < 100, 'Invalid percentile')
- if discard_percentile_lower is not None and discard_percentile_higher is not None:
- vassert(0 < discard_percentile_lower < discard_percentile_higher < 100, 'Invalid percentiles')
-
- sample_similarity = create_sample_similarity(
- similarity_name,
- sample_similarity_resize=sample_similarity_resize,
- sample_similarity_dtype=sample_similarity_dtype,
- cuda=False,
- **kwargs
- )
- sample_similarity = tops.to_cuda(sample_similarity)
- rng = np.random.RandomState(get_kwarg('rng_seed', kwargs))
- distances = []
- if data_len is None:
- data_len = len(dataloader) * dataloader.batch_size
- z0 = sample_random(rng, (data_len, generator.z_channels), "normal")
- z1 = sample_random(rng, (data_len, generator.z_channels), "normal")
- if latent_space == "Z":
- z1 = batch_interp(z0, z1, epsilon, interp)
- print("Computing PPL IN", latent_space)
- distances = torch.zeros(data_len, dtype=torch.float32, device=tops.get_device())
- print(distances.shape)
- end = 0
- n_samples = 0
- for it, batch in enumerate(utils.tqdm_(dataloader, desc="Perceptual Path Length")):
- start = end
- end = start + batch["img"].shape[0]
- n_samples += batch["img"].shape[0]
- batch_lat_e0 = tops.to_cuda(z0[start:end])
- batch_lat_e1 = tops.to_cuda(z1[start:end])
- if latent_space == "W":
- w0 = generator.get_w(batch_lat_e0, update_emas=False)
- w1 = generator.get_w(batch_lat_e1, update_emas=False)
- w1 = w0.lerp(w1, epsilon) # PPL end
- rgb1 = generator(**batch, w=w0)["img"]
- rgb2 = generator(**batch, w=w1)["img"]
- else:
- rgb1 = generator(**batch, z=batch_lat_e0)["img"]
- rgb2 = generator(**batch, z=batch_lat_e1)["img"]
- if rgb1.shape[-2] < upsample_size[0] or rgb1.shape[-1] < upsample_size[1]:
- rgb1 = resize(rgb1, upsample_size, antialias=True)
- rgb2 = resize(rgb2, upsample_size, antialias=True)
- rgb1 = utils.denormalize_img(rgb1).mul(255).byte()
- rgb2 = utils.denormalize_img(rgb2).mul(255).byte()
-
- sim = sample_similarity(rgb1, rgb2)
- dist_lat_e01 = sim / (epsilon ** 2)
- distances[start:end] = dist_lat_e01.view(-1)
- distances = distances[:n_samples]
- distances = tops.all_gather_uneven(distances).cpu().numpy()
- if tops.rank() != 0:
- return {"ppl/mean": -1, "ppl/std": -1}
- if tops.rank() == 0:
- cond, lo, hi = None, None, None
- if discard_percentile_lower is not None:
- lo = np.percentile(distances, discard_percentile_lower, interpolation='lower')
- cond = lo <= distances
- if discard_percentile_higher is not None:
- hi = np.percentile(distances, discard_percentile_higher, interpolation='higher')
- cond = np.logical_and(cond, distances <= hi)
- if cond is not None:
- distances = np.extract(cond, distances)
- return {
- "ppl/mean": float(np.mean(distances)),
- "ppl/std": float(np.std(distances)),
- }
- else:
- return {"ppl/mean"}
diff --git a/spaces/hackaprompt/playground/README.md b/spaces/hackaprompt/playground/README.md
deleted file mode 100644
index ae1bd1380267addfd1bff66c8faee5cf686c19cf..0000000000000000000000000000000000000000
--- a/spaces/hackaprompt/playground/README.md
+++ /dev/null
@@ -1,62 +0,0 @@
----
-title: hackaprompt
-sdk: gradio
-app_file: hackaprompt/gradio_app.py
----
-# Hackaprompt
-
-Code for hosting and evaluating the hackaprompt competition.
-
-## Installation
-
-Clone the repository
-
- cd && git clone https://github.com/jerpint/hackaprompt/
-
-Create a python environment with `python>=3.9`, then:
-
- cd ~/hackaprompt
- pip install -e .
-
-## Gradio App
-
-To run the gradio app:
-
- cd ~/hackaprompt/hackprompt && gradio gradio_app.py
-
-
-## Evaluation
-
- cd ~/hackaprompt/hackaprompt && python score_submission.py
-
-
-## Deployment on HF Space
-
-To deploy on HuggingFace space, first, create a space. Then:
-
- git remote add space https://huggingface.co/spaces/jerpint/hackaprompt
- git push --force space main
-
-## Secrets
-
-### MongoDB
-
-To enable logging to MongoDB, you need to add the following env. variables to your environment:
-
- export HACKAPROMPT_MONGODB_USERNAME=...
- export HACKAPROMPT_MONGODB_PASSWORD=...
- export HACKAPROMPT_MONGODB_CLUSTER=...
- export HACKAPROMPT_MONGODB_DB_NAME=...
-
-
-### Flan endpoint
-
-The Flan model is hosted on a private space exclusively for this competition. To use it, it needs to have the valid hf token associated to it to authenticate:
-
- export HUB_TOKEN=hf_...
-
-### OpenAI
-
-To run tests and evaluations, a valid openai api key should be set as an env. variable:
-
- export OPENAI_API_KEY=sk-...
diff --git a/spaces/hamacojr/SAM-CAT-Seg/open_clip/src/open_clip/transformer.py b/spaces/hamacojr/SAM-CAT-Seg/open_clip/src/open_clip/transformer.py
deleted file mode 100644
index fb5416ecf3e8297f7d890832cea5b10293bd86d2..0000000000000000000000000000000000000000
--- a/spaces/hamacojr/SAM-CAT-Seg/open_clip/src/open_clip/transformer.py
+++ /dev/null
@@ -1,508 +0,0 @@
-from collections import OrderedDict
-import math
-from typing import Callable, Optional, Sequence
-
-import torch
-from torch import nn
-from torch.nn import functional as F
-from torch.utils.checkpoint import checkpoint
-
-from .utils import to_2tuple
-
-
-class LayerNormFp32(nn.LayerNorm):
- """Subclass torch's LayerNorm to handle fp16 (by casting to float32 and back)."""
-
- def forward(self, x: torch.Tensor):
- orig_type = x.dtype
- x = F.layer_norm(x.to(torch.float32), self.normalized_shape, self.weight, self.bias, self.eps)
- return x.to(orig_type)
-
-
-class LayerNorm(nn.LayerNorm):
- """Subclass torch's LayerNorm (with cast back to input dtype)."""
-
- def forward(self, x: torch.Tensor):
- orig_type = x.dtype
- x = F.layer_norm(x, self.normalized_shape, self.weight, self.bias, self.eps)
- return x.to(orig_type)
-
-
-class QuickGELU(nn.Module):
- # NOTE This is slower than nn.GELU or nn.SiLU and uses more GPU memory
- def forward(self, x: torch.Tensor):
- return x * torch.sigmoid(1.702 * x)
-
-
-class LayerScale(nn.Module):
- def __init__(self, dim, init_values=1e-5, inplace=False):
- super().__init__()
- self.inplace = inplace
- self.gamma = nn.Parameter(init_values * torch.ones(dim))
-
- def forward(self, x):
- return x.mul_(self.gamma) if self.inplace else x * self.gamma
-
-
-class PatchDropout(nn.Module):
- """
- https://arxiv.org/abs/2212.00794
- """
-
- def __init__(self, prob, exclude_first_token=True):
- super().__init__()
- assert 0 <= prob < 1.
- self.prob = prob
- self.exclude_first_token = exclude_first_token # exclude CLS token
-
- def forward(self, x):
- if not self.training or self.prob == 0.:
- return x
-
- if self.exclude_first_token:
- cls_tokens, x = x[:, :1], x[:, 1:]
- else:
- cls_tokens = torch.jit.annotate(torch.Tensor, x[:, :1])
-
- batch = x.size()[0]
- num_tokens = x.size()[1]
-
- batch_indices = torch.arange(batch)
- batch_indices = batch_indices[..., None]
-
- keep_prob = 1 - self.prob
- num_patches_keep = max(1, int(num_tokens * keep_prob))
-
- rand = torch.randn(batch, num_tokens)
- patch_indices_keep = rand.topk(num_patches_keep, dim=-1).indices
-
- x = x[batch_indices, patch_indices_keep]
-
- if self.exclude_first_token:
- x = torch.cat((cls_tokens, x), dim=1)
-
- return x
-
-
-class Attention(nn.Module):
- def __init__(
- self,
- dim,
- num_heads=8,
- qkv_bias=True,
- scaled_cosine=False,
- scale_heads=False,
- logit_scale_max=math.log(1. / 0.01),
- attn_drop=0.,
- proj_drop=0.
- ):
- super().__init__()
- self.scaled_cosine = scaled_cosine
- self.scale_heads = scale_heads
- assert dim % num_heads == 0, 'dim should be divisible by num_heads'
- self.num_heads = num_heads
- self.head_dim = dim // num_heads
- self.scale = self.head_dim ** -0.5
- self.logit_scale_max = logit_scale_max
-
- # keeping in_proj in this form (instead of nn.Linear) to match weight scheme of original
- self.in_proj_weight = nn.Parameter(torch.randn((dim * 3, dim)) * self.scale)
- if qkv_bias:
- self.in_proj_bias = nn.Parameter(torch.zeros(dim * 3))
- else:
- self.in_proj_bias = None
-
- if self.scaled_cosine:
- self.logit_scale = nn.Parameter(torch.log(10 * torch.ones((num_heads, 1, 1))))
- else:
- self.logit_scale = None
- self.attn_drop = nn.Dropout(attn_drop)
- if self.scale_heads:
- self.head_scale = nn.Parameter(torch.ones((num_heads, 1, 1)))
- else:
- self.head_scale = None
- self.out_proj = nn.Linear(dim, dim)
- self.out_drop = nn.Dropout(proj_drop)
-
- def forward(self, x, attn_mask: Optional[torch.Tensor] = None):
- L, N, C = x.shape
- q, k, v = F.linear(x, self.in_proj_weight, self.in_proj_bias).chunk(3, dim=-1)
- q = q.contiguous().view(L, N * self.num_heads, -1).transpose(0, 1)
- k = k.contiguous().view(L, N * self.num_heads, -1).transpose(0, 1)
- v = v.contiguous().view(L, N * self.num_heads, -1).transpose(0, 1)
-
- if self.logit_scale is not None:
- attn = torch.bmm(F.normalize(q, dim=-1), F.normalize(k, dim=-1).transpose(-1, -2))
- logit_scale = torch.clamp(self.logit_scale, max=self.logit_scale_max).exp()
- attn = attn.view(N, self.num_heads, L, L) * logit_scale
- attn = attn.view(-1, L, L)
- else:
- q = q * self.scale
- attn = torch.bmm(q, k.transpose(-1, -2))
-
- if attn_mask is not None:
- if attn_mask.dtype == torch.bool:
- new_attn_mask = torch.zeros_like(attn_mask, dtype=q.dtype)
- new_attn_mask.masked_fill_(attn_mask, float("-inf"))
- attn_mask = new_attn_mask
- attn += attn_mask
-
- attn = attn.softmax(dim=-1)
- attn = self.attn_drop(attn)
-
- x = torch.bmm(attn, v)
- if self.head_scale is not None:
- x = x.view(N, self.num_heads, L, C) * self.head_scale
- x = x.view(-1, L, C)
- x = x.transpose(0, 1).reshape(L, N, C)
- x = self.out_proj(x)
- x = self.out_drop(x)
- return x
-
-
-class ResidualAttentionBlock(nn.Module):
- def __init__(
- self,
- d_model: int,
- n_head: int,
- mlp_ratio: float = 4.0,
- ls_init_value: float = None,
- act_layer: Callable = nn.GELU,
- norm_layer: Callable = LayerNorm,
- ):
- super().__init__()
-
- self.ln_1 = norm_layer(d_model)
- self.attn = nn.MultiheadAttention(d_model, n_head)
- self.ls_1 = LayerScale(d_model, ls_init_value) if ls_init_value is not None else nn.Identity()
-
- self.ln_2 = norm_layer(d_model)
- mlp_width = int(d_model * mlp_ratio)
- self.mlp = nn.Sequential(OrderedDict([
- ("c_fc", nn.Linear(d_model, mlp_width)),
- ("gelu", act_layer()),
- ("c_proj", nn.Linear(mlp_width, d_model))
- ]))
- self.ls_2 = LayerScale(d_model, ls_init_value) if ls_init_value is not None else nn.Identity()
-
- def attention(self, x: torch.Tensor, attn_mask: Optional[torch.Tensor] = None):
- attn_mask = attn_mask.to(x.dtype) if attn_mask is not None else None
- return self.attn(x, x, x, need_weights=False, attn_mask=attn_mask)[0]
-
- def forward(self, x: torch.Tensor, attn_mask: Optional[torch.Tensor] = None):
- x = x + self.ls_1(self.attention(self.ln_1(x), attn_mask=attn_mask))
- x = x + self.ls_2(self.mlp(self.ln_2(x)))
- return x
-
- def forward_dense(self, x):
- y = self.ln_1(x)
- y = F.linear(y, self.attn.in_proj_weight, self.attn.in_proj_bias)
- L, N, D = y.shape # L N 3D
-
- y = y.reshape(L, N, 3, D // 3).permute(2, 1, 0, 3).reshape(3 * N, L, D // 3)
- y = F.linear(y, self.attn.out_proj.weight, self.attn.out_proj.bias)
-
- q, k, v = y.tensor_split(3, dim=0)
- #v = v.transpose(1, 0) + x # L N D
- v = v.transpose(1, 0) + x[:1] # L N D
-
- v = v + self.mlp(self.ln_2(v))
-
- return v
-
-
-class CustomResidualAttentionBlock(nn.Module):
- def __init__(
- self,
- d_model: int,
- n_head: int,
- mlp_ratio: float = 4.0,
- ls_init_value: float = None,
- act_layer: Callable = nn.GELU,
- norm_layer: Callable = LayerNorm,
- scale_cosine_attn: bool = False,
- scale_heads: bool = False,
- scale_attn: bool = False,
- scale_fc: bool = False,
- ):
- super().__init__()
-
- self.ln_1 = norm_layer(d_model)
- self.attn = Attention(
- d_model, n_head,
- scaled_cosine=scale_cosine_attn,
- scale_heads=scale_heads,
- )
- self.ln_attn = norm_layer(d_model) if scale_attn else nn.Identity()
- self.ls_1 = LayerScale(d_model, ls_init_value) if ls_init_value is not None else nn.Identity()
-
- self.ln_2 = norm_layer(d_model)
- mlp_width = int(d_model * mlp_ratio)
- self.mlp = nn.Sequential(OrderedDict([
- ("c_fc", nn.Linear(d_model, mlp_width)),
- ('ln', norm_layer(mlp_width) if scale_fc else nn.Identity()),
- ("gelu", act_layer()),
- ("c_proj", nn.Linear(mlp_width, d_model))
- ]))
- self.ls_2 = LayerScale(d_model, ls_init_value) if ls_init_value is not None else nn.Identity()
-
- def forward(self, x: torch.Tensor, attn_mask: Optional[torch.Tensor] = None):
- x = x + self.ls_1(self.ln_attn(self.attn(self.ln_1(x), attn_mask=attn_mask)))
- x = x + self.ls_2(self.mlp(self.ln_2(x)))
- return x
-
-
-class Transformer(nn.Module):
- def __init__(
- self,
- width: int,
- layers: int,
- heads: int,
- mlp_ratio: float = 4.0,
- ls_init_value: float = None,
- act_layer: Callable = nn.GELU,
- norm_layer: Callable = LayerNorm,
- ):
- super().__init__()
- self.width = width
- self.layers = layers
- self.grad_checkpointing = False
-
- self.resblocks = nn.ModuleList([
- ResidualAttentionBlock(
- width, heads, mlp_ratio, ls_init_value=ls_init_value, act_layer=act_layer, norm_layer=norm_layer)
- for _ in range(layers)
- ])
-
- def get_cast_dtype(self) -> torch.dtype:
- return self.resblocks[0].mlp.c_fc.weight.dtype
-
- def forward(self, x: torch.Tensor, attn_mask: Optional[torch.Tensor] = None, dense=False):
- for i, r in enumerate(self.resblocks):
- if self.grad_checkpointing and not torch.jit.is_scripting():
- x = checkpoint(r, x, attn_mask)
- else:
- if dense and i == self.layers - 1:
- x = r.forward_dense(x)
- else:
- x = r(x, attn_mask=attn_mask)
- return x
-
-
-class VisionTransformer(nn.Module):
- def __init__(
- self,
- image_size: int,
- patch_size: int,
- width: int,
- layers: int,
- heads: int,
- mlp_ratio: float,
- ls_init_value: float = None,
- global_average_pool: bool = False,
- output_dim: int = 512,
- patch_dropout: float = 0.,
- act_layer: Callable = nn.GELU,
- norm_layer: Callable = LayerNorm,
- ):
- super().__init__()
- self.image_size = to_2tuple(image_size)
- self.patch_size = to_2tuple(patch_size)
- self.grid_size = (self.image_size[0] // self.patch_size[0], self.image_size[1] // self.patch_size[1])
- self.output_dim = output_dim
- self.conv1 = nn.Conv2d(in_channels=3, out_channels=width, kernel_size=patch_size, stride=patch_size, bias=False)
-
- scale = width ** -0.5
- self.class_embedding = nn.Parameter(scale * torch.randn(width))
- self.positional_embedding = nn.Parameter(scale * torch.randn(self.grid_size[0] * self.grid_size[1] + 1, width))
-
- # setting a patch_dropout of 0. would mean it is disabled and this function would be the identity fn
- self.patch_dropout = PatchDropout(patch_dropout) if patch_dropout > 0. else nn.Identity()
-
- self.ln_pre = norm_layer(width)
- self.transformer = Transformer(
- width,
- layers,
- heads,
- mlp_ratio,
- ls_init_value=ls_init_value,
- act_layer=act_layer,
- norm_layer=norm_layer,
- )
-
- self.global_average_pool = global_average_pool
- self.ln_post = norm_layer(width)
- self.proj = nn.Parameter(scale * torch.randn(width, output_dim))
-
- self.init_parameters()
-
- def lock(self, unlocked_groups=0, freeze_bn_stats=False):
- for param in self.parameters():
- param.requires_grad = False
-
- if unlocked_groups != 0:
- groups = [
- [
- self.conv1,
- self.class_embedding,
- self.positional_embedding,
- self.ln_pre,
- ],
- *self.transformer.resblocks[:-1],
- [
- self.transformer.resblocks[-1],
- self.ln_post,
- ],
- self.proj,
- ]
-
- def _unlock(x):
- if isinstance(x, Sequence):
- for g in x:
- _unlock(g)
- else:
- if isinstance(x, torch.nn.Parameter):
- x.requires_grad = True
- else:
- for p in x.parameters():
- p.requires_grad = True
-
- _unlock(groups[-unlocked_groups:])
-
- def init_parameters(self):
- # FIXME OpenAI CLIP did not define an init for the VisualTransformer
- # TODO experiment if default PyTorch init, below, or alternate init is best.
-
- # nn.init.normal_(self.class_embedding, std=self.scale)
- # nn.init.normal_(self.positional_embedding, std=self.scale)
- #
- # proj_std = (self.transformer.width ** -0.5) * ((2 * self.transformer.layers) ** -0.5)
- # attn_std = self.transformer.width ** -0.5
- # fc_std = (2 * self.transformer.width) ** -0.5
- # for block in self.transformer.resblocks:
- # nn.init.normal_(block.attn.in_proj_weight, std=attn_std)
- # nn.init.normal_(block.attn.out_proj.weight, std=proj_std)
- # nn.init.normal_(block.mlp.c_fc.weight, std=fc_std)
- # nn.init.normal_(block.mlp.c_proj.weight, std=proj_std)
- #
- # if self.text_projection is not None:
- # nn.init.normal_(self.text_projection, std=self.scale)
- pass
-
- @torch.jit.ignore
- def set_grad_checkpointing(self, enable=True):
- self.transformer.grad_checkpointing = enable
-
- def forward(self, x: torch.Tensor, dense=False):
- x = self.conv1(x) # shape = [*, width, grid, grid]
- x = x.reshape(x.shape[0], x.shape[1], -1) # shape = [*, width, grid ** 2]
- x = x.permute(0, 2, 1) # shape = [*, grid ** 2, width]
- x = torch.cat(
- [self.class_embedding.to(x.dtype) + torch.zeros(x.shape[0], 1, x.shape[-1], dtype=x.dtype, device=x.device),
- x], dim=1) # shape = [*, grid ** 2 + 1, width]
- x = x + self.positional_embedding.to(x.dtype)
-
- # a patch_dropout of 0. would mean it is disabled and this function would do nothing but return what was passed in
- x = self.patch_dropout(x)
- x = self.ln_pre(x)
-
- x = x.permute(1, 0, 2) # NLD -> LND
- x = self.transformer(x, dense=dense)
- x = x.permute(1, 0, 2) # LND -> NLD
-
- if self.global_average_pool:
- x = x.mean(dim=1)
- elif dense:
- x = x
- else:
- x = x[:, 0]
-
- x = self.ln_post(x)
-
- if self.proj is not None:
- x = x @ self.proj
-
- return x
-
-
-class TextTransformer(nn.Module):
-
- def __init__(
- self,
- context_length: int = 77,
- vocab_size: int = 49408,
- width: int = 512,
- heads: int = 8,
- layers: int = 12,
- ls_init_value: float = None,
- output_dim: int = 512,
- act_layer: Callable = nn.GELU,
- norm_layer: Callable = LayerNorm,
- ):
- super().__init__()
- self.context_length = context_length
- self.vocab_size = vocab_size
- self.width = width
- self.output_dim = output_dim
-
- self.token_embedding = nn.Embedding(vocab_size, width)
- self.positional_embedding = nn.Parameter(torch.empty(self.context_length, width))
- self.transformer = Transformer(
- width=width,
- layers=layers,
- heads=heads,
- ls_init_value=ls_init_value,
- act_layer=act_layer,
- norm_layer=norm_layer,
- )
- self.ln_final = norm_layer(width)
- self.text_projection = nn.Parameter(torch.empty(width, output_dim))
-
- self.register_buffer('attn_mask', self.build_attention_mask(), persistent=False)
-
- self.init_parameters()
-
- def init_parameters(self):
- nn.init.normal_(self.token_embedding.weight, std=0.02)
- nn.init.normal_(self.positional_embedding, std=0.01)
-
- proj_std = (self.transformer.width ** -0.5) * ((2 * self.transformer.layers) ** -0.5)
- attn_std = self.transformer.width ** -0.5
- fc_std = (2 * self.transformer.width) ** -0.5
- for block in self.transformer.resblocks:
- nn.init.normal_(block.attn.in_proj_weight, std=attn_std)
- nn.init.normal_(block.attn.out_proj.weight, std=proj_std)
- nn.init.normal_(block.mlp.c_fc.weight, std=fc_std)
- nn.init.normal_(block.mlp.c_proj.weight, std=proj_std)
-
- if self.text_projection is not None:
- nn.init.normal_(self.text_projection, std=self.transformer.width ** -0.5)
-
- @torch.jit.ignore
- def set_grad_checkpointing(self, enable=True):
- self.transformer.grad_checkpointing = enable
-
- def build_attention_mask(self):
- # lazily create causal attention mask, with full attention between the vision tokens
- # pytorch uses additive attention mask; fill with -inf
- mask = torch.empty(self.context_length, self.context_length)
- mask.fill_(float("-inf"))
- mask.triu_(1) # zero out the lower diagonal
- return mask
-
- def forward(self, text):
- cast_dtype = self.transformer.get_cast_dtype()
-
- x = self.token_embedding(text).to(cast_dtype) # [batch_size, n_ctx, d_model]
-
- x = x + self.positional_embedding.to(cast_dtype)
- x = x.permute(1, 0, 2) # NLD -> LND
- x = self.transformer(x, attn_mask=self.attn_mask)
- x = x.permute(1, 0, 2) # LND -> NLD
- x = self.ln_final(x)
-
- # x.shape = [batch_size, n_ctx, transformer.width]
- # take features from the eot embedding (eot_token is the highest number in each sequence)
- x = x[torch.arange(x.shape[0]), text.argmax(dim=-1)] @ self.text_projection
-
- return x
diff --git a/spaces/haryoaw/id-recigen/src/__init__.py b/spaces/haryoaw/id-recigen/src/__init__.py
deleted file mode 100644
index e69de29bb2d1d6434b8b29ae775ad8c2e48c5391..0000000000000000000000000000000000000000
diff --git a/spaces/hilmyblaze/WebUI-Counterfeit-V2.5/Proposal Pengerasan Jalan Desa 3.md b/spaces/hilmyblaze/WebUI-Counterfeit-V2.5/Proposal Pengerasan Jalan Desa 3.md
deleted file mode 100644
index 8e4ab19959a0d9429acc510958c56d5d04b35c2b..0000000000000000000000000000000000000000
--- a/spaces/hilmyblaze/WebUI-Counterfeit-V2.5/Proposal Pengerasan Jalan Desa 3.md
+++ /dev/null
@@ -1,117 +0,0 @@
-## Proposal Pengerasan Jalan Desa 3
-
-
-
-
-
- 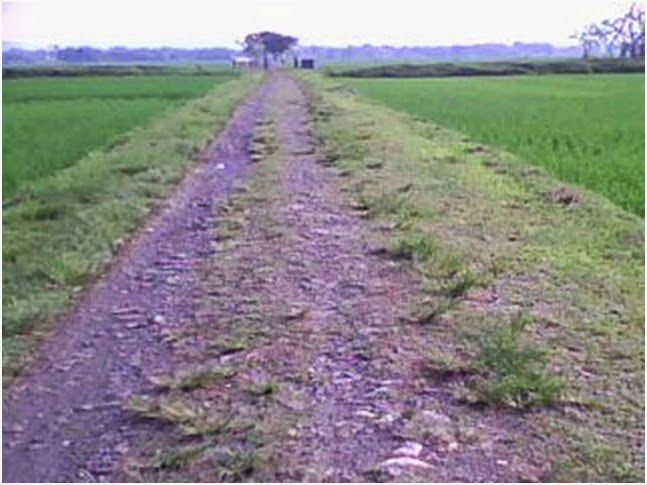
-
-
-
-
-
-**Download >>> [https://www.google.com/url?q=https%3A%2F%2Ftinurll.com%2F2txRSs&sa=D&sntz=1&usg=AOvVaw0N7KiHuBQbeH2sohypLFNF](https://www.google.com/url?q=https%3A%2F%2Ftinurll.com%2F2txRSs&sa=D&sntz=1&usg=AOvVaw0N7KiHuBQbeH2sohypLFNF)**
-
-
-
-
-
-
-
-
-
-
-
-
-
-# Proposal Pengerasan Jalan Desa 3
-
-
-
-Desa 3 adalah salah satu desa di Kecamatan X, Kabupaten Y, Provinsi Z. Desa ini memiliki luas wilayah sekitar 10 km dan jumlah penduduk sekitar 5.000 jiwa. Desa ini memiliki potensi pertanian, perkebunan, peternakan, dan pariwisata yang cukup besar. Namun, salah satu kendala yang dihadapi oleh masyarakat Desa 3 adalah kondisi jalan yang rusak dan berlubang. Jalan ini merupakan akses utama bagi masyarakat Desa 3 untuk menghubungkan desa dengan pusat kecamatan dan kabupaten. Jalan ini juga digunakan untuk mengangkut hasil-hasil pertanian, perkebunan, peternakan, dan pariwisata dari desa ke pasar atau tempat penjualan lainnya.
-
-
-
-Kondisi jalan yang rusak dan berlubang ini sangat mengganggu kelancaran transportasi dan mobilitas masyarakat Desa 3. Selain itu, kondisi jalan yang buruk ini juga berdampak negatif terhadap kesehatan dan keselamatan masyarakat Desa 3. Banyak masyarakat yang mengalami kecelakaan, luka-luka, atau kerusakan kendaraan akibat jalan yang tidak rata dan berlubang. Selain itu, kondisi jalan yang buruk ini juga menyebabkan biaya transportasi menjadi lebih mahal karena konsumsi bahan bakar yang lebih boros dan perawatan kendaraan yang lebih sering.
-
-
-
-Melihat kondisi tersebut, kami selaku perwakilan masyarakat Desa 3 mengajukan proposal permohonan bantuan pengerasan jalan kepada pemerintah daerah. Kami berharap dengan adanya bantuan pengerasan jalan ini, kondisi jalan di Desa 3 dapat menjadi lebih baik dan layak. Dengan demikian, transportasi dan mobilitas masyarakat Desa 3 dapat menjadi lebih lancar dan efisien. Selain itu, bantuan pengerasan jalan ini juga dapat meningkatkan kesejahteraan dan kualitas hidup masyarakat Desa 3.
-
-
-
-## Tujuan
-
-
-
-Tujuan dari proposal permohonan bantuan pengerasan jalan ini adalah sebagai berikut:
-
-
-
-- Meningkatkan kualitas infrastruktur jalan di Desa 3.
-
-- Meningkatkan kelancaran transportasi dan mobilitas masyarakat Desa 3.
-
-- Meningkatkan kesehatan dan keselamatan masyarakat Desa 3.
-
-- Meningkatkan produktivitas dan pendapatan masyarakat Desa 3 dari sektor pertanian, perkebunan, peternakan, dan pariwisata.
-
-- Meningkatkan keterhubungan dan kerjasama antara masyarakat Desa 3 dengan pihak-pihak lain di luar desa.
-
-
-
-## Rencana Kegiatan
-
-
-
-Rencana kegiatan yang akan dilakukan dalam rangka pengerasan jalan di Desa 3 adalah sebagai berikut:
-
-
-
-1. Menyusun tim pelaksana yang terdiri dari perwakilan pemerintah desa, tokoh masyarakat, kelompok tani, kelompok pemuda, dan pihak-pihak terkait lainnya.
-
-2. Melakukan survei lapangan untuk menentukan lokasi, panjang, lebar, dan kondisi jalan yang akan dikeraskan.
-
-3. Melakukan perhitungan anggaran biaya yang dibutuhkan untuk pengerasan jalan sesuai dengan spesifikasi teknis yang ditetapkan.
-
-4. Mengajukan proposal permohonan bantuan pengerasan jalan kepada pemerintah daerah melalui dinas terkait.
-
-5. Melakukan ko
-
-
-## Anggaran Biaya
-
-
-
- Berdasarkan perhitungan yang telah dilakukan, anggaran biaya yang dibutuhkan untuk pengerasan jalan di Desa 3 adalah sebesar Rp 1.500.000.000,- (Satu Miliar Lima Ratus Juta Rupiah). Rincian anggaran biaya tersebut adalah sebagai berikut:
-
-
-
- | No | Uraian | Volume | Satuan | Harga Satuan | Jumlah |
-
- | --- | --- | --- | --- | --- | --- |
-
-
- | 1 | Honorarium TPK | 1 | Paket | 10.000.000 | 10.000.000 |
-
-
- | 2 | Persiapan Pelaksanaan | 1 | Paket | 5.000.000 | 5.000.000 |
-
-
- | 3 | Alat Kerja | - | - | - | 20.000.000 |
-
-
- | 4 | Upah Kerja | - | - | - | 300.000.000 |
-
-
- | 5 | Bahan Material | - | - | - | 1.165.000.000 |
-
-
-
- | Jumlah Anggaran Biaya (Termasuk PPN dan PPh) | 1.500.000.000 dfd1c89656
-
-
-
-
-
- |
\ No newline at end of file
diff --git a/spaces/ho11laqe/nnUNet_calvingfront_detection/nnunet/experiment_planning/alternative_experiment_planning/experiment_planner_baseline_3DUNet_v23.py b/spaces/ho11laqe/nnUNet_calvingfront_detection/nnunet/experiment_planning/alternative_experiment_planning/experiment_planner_baseline_3DUNet_v23.py
deleted file mode 100644
index 5854e843f6e5161d2f88189504a44f9b673a37e8..0000000000000000000000000000000000000000
--- a/spaces/ho11laqe/nnUNet_calvingfront_detection/nnunet/experiment_planning/alternative_experiment_planning/experiment_planner_baseline_3DUNet_v23.py
+++ /dev/null
@@ -1,28 +0,0 @@
-# Copyright 2020 Division of Medical Image Computing, German Cancer Research Center (DKFZ), Heidelberg, Germany
-#
-# Licensed under the Apache License, Version 2.0 (the "License");
-# you may not use this file except in compliance with the License.
-# You may obtain a copy of the License at
-#
-# http://www.apache.org/licenses/LICENSE-2.0
-#
-# Unless required by applicable law or agreed to in writing, software
-# distributed under the License is distributed on an "AS IS" BASIS,
-# WITHOUT WARRANTIES OR CONDITIONS OF ANY KIND, either express or implied.
-# See the License for the specific language governing permissions and
-# limitations under the License.
-
-from nnunet.experiment_planning.experiment_planner_baseline_3DUNet_v21 import \
- ExperimentPlanner3D_v21
-from nnunet.paths import *
-
-
-class ExperimentPlanner3D_v23(ExperimentPlanner3D_v21):
- """
- """
- def __init__(self, folder_with_cropped_data, preprocessed_output_folder):
- super(ExperimentPlanner3D_v23, self).__init__(folder_with_cropped_data, preprocessed_output_folder)
- self.data_identifier = "nnUNetData_plans_v2.3"
- self.plans_fname = join(self.preprocessed_output_folder,
- "nnUNetPlansv2.3_plans_3D.pkl")
- self.preprocessor_name = "Preprocessor3DDifferentResampling"
diff --git a/spaces/huggan/butterfly-gan/custom_component/frontend/build/service-worker.js b/spaces/huggan/butterfly-gan/custom_component/frontend/build/service-worker.js
deleted file mode 100644
index dd6a41f8929e57249b1c5b42bfef350afe94cb0d..0000000000000000000000000000000000000000
--- a/spaces/huggan/butterfly-gan/custom_component/frontend/build/service-worker.js
+++ /dev/null
@@ -1,39 +0,0 @@
-/**
- * Welcome to your Workbox-powered service worker!
- *
- * You'll need to register this file in your web app and you should
- * disable HTTP caching for this file too.
- * See https://goo.gl/nhQhGp
- *
- * The rest of the code is auto-generated. Please don't update this file
- * directly; instead, make changes to your Workbox build configuration
- * and re-run your build process.
- * See https://goo.gl/2aRDsh
- */
-
-importScripts("https://storage.googleapis.com/workbox-cdn/releases/4.3.1/workbox-sw.js");
-
-importScripts(
- "./precache-manifest.ff9f40db8f9a11773e3a57b07e809220.js"
-);
-
-self.addEventListener('message', (event) => {
- if (event.data && event.data.type === 'SKIP_WAITING') {
- self.skipWaiting();
- }
-});
-
-workbox.core.clientsClaim();
-
-/**
- * The workboxSW.precacheAndRoute() method efficiently caches and responds to
- * requests for URLs in the manifest.
- * See https://goo.gl/S9QRab
- */
-self.__precacheManifest = [].concat(self.__precacheManifest || []);
-workbox.precaching.precacheAndRoute(self.__precacheManifest, {});
-
-workbox.routing.registerNavigationRoute(workbox.precaching.getCacheKeyForURL("./index.html"), {
-
- blacklist: [/^\/_/,/\/[^\/?]+\.[^\/]+$/],
-});
diff --git a/spaces/huggingdalle/dalle-mini/README.md b/spaces/huggingdalle/dalle-mini/README.md
deleted file mode 100644
index f23c44f79617db7ec46c6aed919294f3f76803fb..0000000000000000000000000000000000000000
--- a/spaces/huggingdalle/dalle-mini/README.md
+++ /dev/null
@@ -1,11 +0,0 @@
----
-title: DALL•E mini
-emoji: 🥑
-colorFrom: green
-colorTo: indigo
-sdk: static
-pinned: false
-license: creativeml-openrail-m
----
-
-Check out the configuration reference at https://huggingface.co/docs/hub/spaces-config-reference
\ No newline at end of file
diff --git a/spaces/hysts/TADNE-image-viewer/app.py b/spaces/hysts/TADNE-image-viewer/app.py
deleted file mode 100644
index 1717f73dacef8e4f83f0db153ac87c1b60fa495b..0000000000000000000000000000000000000000
--- a/spaces/hysts/TADNE-image-viewer/app.py
+++ /dev/null
@@ -1,123 +0,0 @@
-#!/usr/bin/env python
-
-from __future__ import annotations
-
-import argparse
-import functools
-import io
-import os
-import pathlib
-import tarfile
-
-import gradio as gr
-import numpy as np
-import PIL.Image
-from huggingface_hub import hf_hub_download
-
-TITLE = 'TADNE (This Anime Does Not Exist) Image Viewer'
-DESCRIPTION = '''The original TADNE site is https://thisanimedoesnotexist.ai/.
-
-You can view images generated by the TADNE model with seed 0-99999.
-The original images are 512x512 in size, but they are resized to 128x128 here.
-
-Expected execution time on Hugging Face Spaces: 4s
-
-Related Apps:
-- [TADNE](https://huggingface.co/spaces/hysts/TADNE)
-- [TADNE Image Viewer](https://huggingface.co/spaces/hysts/TADNE-image-viewer)
-- [TADNE Image Selector](https://huggingface.co/spaces/hysts/TADNE-image-selector)
-- [TADNE Interpolation](https://huggingface.co/spaces/hysts/TADNE-interpolation)
-- [TADNE Image Search with DeepDanbooru](https://huggingface.co/spaces/hysts/TADNE-image-search-with-DeepDanbooru)
-'''
-ARTICLE = '
'
-
-TOKEN = os.environ['TOKEN']
-
-
-def parse_args() -> argparse.Namespace:
- parser = argparse.ArgumentParser()
- parser.add_argument('--theme', type=str)
- parser.add_argument('--live', action='store_true')
- parser.add_argument('--share', action='store_true')
- parser.add_argument('--port', type=int)
- parser.add_argument('--disable-queue',
- dest='enable_queue',
- action='store_false')
- parser.add_argument('--allow-flagging', type=str, default='never')
- return parser.parse_args()
-
-
-def download_image_tarball(size: int, dirname: str) -> pathlib.Path:
- path = hf_hub_download('hysts/TADNE-sample-images',
- f'{size}/{dirname}.tar',
- repo_type='dataset',
- use_auth_token=TOKEN)
- return path
-
-
-def run(start_seed: int, nrows: int, ncols: int, image_size: int,
- min_seed: int, max_seed: int, dirname: str,
- tarball_path: pathlib.Path) -> np.ndarray:
- start_seed = int(start_seed)
- num = nrows * ncols
- images = []
- dummy = np.ones((image_size, image_size, 3), dtype=np.uint8) * 255
- with tarfile.TarFile(tarball_path) as tar_file:
- for seed in range(start_seed, start_seed + num):
- if not min_seed <= seed <= max_seed:
- images.append(dummy)
- continue
- member = tar_file.getmember(f'{dirname}/{seed:07d}.jpg')
- with tar_file.extractfile(member) as f:
- data = io.BytesIO(f.read())
- image = PIL.Image.open(data)
- image = np.asarray(image)
- images.append(image)
- res = np.asarray(images).reshape(nrows, ncols, image_size, image_size,
- 3).transpose(0, 2, 1, 3, 4).reshape(
- nrows * image_size,
- ncols * image_size, 3)
- return res
-
-
-def main():
- args = parse_args()
-
- image_size = 128
- min_seed = 0
- max_seed = 99999
- dirname = '0-99999'
- tarball_path = download_image_tarball(image_size, dirname)
-
- func = functools.partial(run,
- image_size=image_size,
- min_seed=min_seed,
- max_seed=max_seed,
- dirname=dirname,
- tarball_path=tarball_path)
- func = functools.update_wrapper(func, run)
-
- gr.Interface(
- func,
- [
- gr.inputs.Number(default=0, label='Start Seed'),
- gr.inputs.Slider(1, 10, step=1, default=2, label='Number of Rows'),
- gr.inputs.Slider(
- 1, 10, step=1, default=5, label='Number of Columns'),
- ],
- gr.outputs.Image(type='numpy', label='Output'),
- title=TITLE,
- description=DESCRIPTION,
- article=ARTICLE,
- theme=args.theme,
- allow_flagging=args.allow_flagging,
- live=args.live,
- ).launch(
- enable_queue=args.enable_queue,
- server_port=args.port,
- share=args.share,
- )
-
-
-if __name__ == '__main__':
- main()
diff --git a/spaces/hyxue/HiFiFace-inference-demo/Deep3DFaceRecon_pytorch/models/arcface_torch/train_v2.py b/spaces/hyxue/HiFiFace-inference-demo/Deep3DFaceRecon_pytorch/models/arcface_torch/train_v2.py
deleted file mode 100644
index ba3c15e6a1615f28daaab1ad225f7b61b27bdffc..0000000000000000000000000000000000000000
--- a/spaces/hyxue/HiFiFace-inference-demo/Deep3DFaceRecon_pytorch/models/arcface_torch/train_v2.py
+++ /dev/null
@@ -1,248 +0,0 @@
-import argparse
-import logging
-import os
-from datetime import datetime
-
-import numpy as np
-import torch
-from backbones import get_model
-from dataset import get_dataloader
-from losses import CombinedMarginLoss
-from lr_scheduler import PolyScheduler
-from partial_fc_v2 import PartialFC_V2
-from torch import distributed
-from torch.utils.data import DataLoader
-from torch.utils.tensorboard import SummaryWriter
-from utils.utils_callbacks import CallBackLogging
-from utils.utils_callbacks import CallBackVerification
-from utils.utils_config import get_config
-from utils.utils_distributed_sampler import setup_seed
-from utils.utils_logging import AverageMeter
-from utils.utils_logging import init_logging
-
-assert (
- torch.__version__ >= "1.12.0"
-), "In order to enjoy the features of the new torch, \
-we have upgraded the torch to 1.12.0. torch before than 1.12.0 may not work in the future."
-
-try:
- rank = int(os.environ["RANK"])
- local_rank = int(os.environ["LOCAL_RANK"])
- world_size = int(os.environ["WORLD_SIZE"])
- distributed.init_process_group("nccl")
-except KeyError:
- rank = 0
- local_rank = 0
- world_size = 1
- distributed.init_process_group(
- backend="nccl",
- init_method="tcp://127.0.0.1:12584",
- rank=rank,
- world_size=world_size,
- )
-
-
-def main(args):
-
- # get config
- cfg = get_config(args.config)
- # global control random seed
- setup_seed(seed=cfg.seed, cuda_deterministic=False)
-
- torch.cuda.set_device(local_rank)
-
- os.makedirs(cfg.output, exist_ok=True)
- init_logging(rank, cfg.output)
-
- summary_writer = SummaryWriter(log_dir=os.path.join(cfg.output, "tensorboard")) if rank == 0 else None
-
- wandb_logger = None
- if cfg.using_wandb:
- import wandb
-
- # Sign in to wandb
- try:
- wandb.login(key=cfg.wandb_key)
- except Exception as e:
- print("WandB Key must be provided in config file (base.py).")
- print(f"Config Error: {e}")
- # Initialize wandb
- run_name = datetime.now().strftime("%y%m%d_%H%M") + f"_GPU{rank}"
- run_name = run_name if cfg.suffix_run_name is None else run_name + f"_{cfg.suffix_run_name}"
- try:
- wandb_logger = (
- wandb.init(
- entity=cfg.wandb_entity,
- project=cfg.wandb_project,
- sync_tensorboard=True,
- resume=cfg.wandb_resume,
- name=run_name,
- notes=cfg.notes,
- )
- if rank == 0 or cfg.wandb_log_all
- else None
- )
- if wandb_logger:
- wandb_logger.config.update(cfg)
- except Exception as e:
- print("WandB Data (Entity and Project name) must be provided in config file (base.py).")
- print(f"Config Error: {e}")
-
- train_loader = get_dataloader(cfg.rec, local_rank, cfg.batch_size, cfg.dali, cfg.seed, cfg.num_workers)
-
- backbone = get_model(cfg.network, dropout=0.0, fp16=cfg.fp16, num_features=cfg.embedding_size).cuda()
-
- backbone = torch.nn.parallel.DistributedDataParallel(
- module=backbone, broadcast_buffers=False, device_ids=[local_rank], bucket_cap_mb=16, find_unused_parameters=True
- )
-
- backbone.train()
- # FIXME using gradient checkpoint if there are some unused parameters will cause error
- backbone._set_static_graph()
-
- margin_loss = CombinedMarginLoss(
- 64, cfg.margin_list[0], cfg.margin_list[1], cfg.margin_list[2], cfg.interclass_filtering_threshold
- )
-
- if cfg.optimizer == "sgd":
- module_partial_fc = PartialFC_V2(margin_loss, cfg.embedding_size, cfg.num_classes, cfg.sample_rate, cfg.fp16)
- module_partial_fc.train().cuda()
- # TODO the params of partial fc must be last in the params list
- opt = torch.optim.SGD(
- params=[{"params": backbone.parameters()}, {"params": module_partial_fc.parameters()}],
- lr=cfg.lr,
- momentum=0.9,
- weight_decay=cfg.weight_decay,
- )
-
- elif cfg.optimizer == "adamw":
- module_partial_fc = PartialFC_V2(margin_loss, cfg.embedding_size, cfg.num_classes, cfg.sample_rate, cfg.fp16)
- module_partial_fc.train().cuda()
- opt = torch.optim.AdamW(
- params=[{"params": backbone.parameters()}, {"params": module_partial_fc.parameters()}],
- lr=cfg.lr,
- weight_decay=cfg.weight_decay,
- )
- else:
- raise
-
- cfg.total_batch_size = cfg.batch_size * world_size
- cfg.warmup_step = cfg.num_image // cfg.total_batch_size * cfg.warmup_epoch
- cfg.total_step = cfg.num_image // cfg.total_batch_size * cfg.num_epoch
-
- lr_scheduler = PolyScheduler(
- optimizer=opt, base_lr=cfg.lr, max_steps=cfg.total_step, warmup_steps=cfg.warmup_step, last_epoch=-1
- )
-
- start_epoch = 0
- global_step = 0
- if cfg.resume:
- dict_checkpoint = torch.load(os.path.join(cfg.output, f"checkpoint_gpu_{rank}.pt"))
- start_epoch = dict_checkpoint["epoch"]
- global_step = dict_checkpoint["global_step"]
- backbone.module.load_state_dict(dict_checkpoint["state_dict_backbone"])
- module_partial_fc.load_state_dict(dict_checkpoint["state_dict_softmax_fc"])
- opt.load_state_dict(dict_checkpoint["state_optimizer"])
- lr_scheduler.load_state_dict(dict_checkpoint["state_lr_scheduler"])
- del dict_checkpoint
-
- for key, value in cfg.items():
- num_space = 25 - len(key)
- logging.info(": " + key + " " * num_space + str(value))
-
- callback_verification = CallBackVerification(
- val_targets=cfg.val_targets, rec_prefix=cfg.rec, summary_writer=summary_writer, wandb_logger=wandb_logger
- )
- callback_logging = CallBackLogging(
- frequent=cfg.frequent,
- total_step=cfg.total_step,
- batch_size=cfg.batch_size,
- start_step=global_step,
- writer=summary_writer,
- )
-
- loss_am = AverageMeter()
- amp = torch.cuda.amp.grad_scaler.GradScaler(growth_interval=100)
-
- for epoch in range(start_epoch, cfg.num_epoch):
-
- if isinstance(train_loader, DataLoader):
- train_loader.sampler.set_epoch(epoch)
- for _, (img, local_labels) in enumerate(train_loader):
- global_step += 1
- local_embeddings = backbone(img)
- loss: torch.Tensor = module_partial_fc(local_embeddings, local_labels)
-
- if cfg.fp16:
- amp.scale(loss).backward()
- if global_step % cfg.gradient_acc == 0:
- amp.unscale_(opt)
- torch.nn.utils.clip_grad_norm_(backbone.parameters(), 5)
- amp.step(opt)
- amp.update()
- opt.zero_grad()
- else:
- loss.backward()
- if global_step % cfg.gradient_acc == 0:
- torch.nn.utils.clip_grad_norm_(backbone.parameters(), 5)
- opt.step()
- opt.zero_grad()
- lr_scheduler.step()
-
- with torch.no_grad():
- if wandb_logger:
- wandb_logger.log(
- {
- "Loss/Step Loss": loss.item(),
- "Loss/Train Loss": loss_am.avg,
- "Process/Step": global_step,
- "Process/Epoch": epoch,
- }
- )
-
- loss_am.update(loss.item(), 1)
- callback_logging(global_step, loss_am, epoch, cfg.fp16, lr_scheduler.get_last_lr()[0], amp)
-
- if global_step % cfg.verbose == 0 and global_step > 0:
- callback_verification(global_step, backbone)
-
- if cfg.save_all_states:
- checkpoint = {
- "epoch": epoch + 1,
- "global_step": global_step,
- "state_dict_backbone": backbone.module.state_dict(),
- "state_dict_softmax_fc": module_partial_fc.state_dict(),
- "state_optimizer": opt.state_dict(),
- "state_lr_scheduler": lr_scheduler.state_dict(),
- }
- torch.save(checkpoint, os.path.join(cfg.output, f"checkpoint_gpu_{rank}.pt"))
-
- if rank == 0:
- path_module = os.path.join(cfg.output, "model.pt")
- torch.save(backbone.module.state_dict(), path_module)
-
- if wandb_logger and cfg.save_artifacts:
- artifact_name = f"{run_name}_E{epoch}"
- model = wandb.Artifact(artifact_name, type="model")
- model.add_file(path_module)
- wandb_logger.log_artifact(model)
-
- if cfg.dali:
- train_loader.reset()
-
- if rank == 0:
- path_module = os.path.join(cfg.output, "model.pt")
- torch.save(backbone.module.state_dict(), path_module)
-
- if wandb_logger and cfg.save_artifacts:
- artifact_name = f"{run_name}_Final"
- model = wandb.Artifact(artifact_name, type="model")
- model.add_file(path_module)
- wandb_logger.log_artifact(model)
-
-
-if __name__ == "__main__":
- torch.backends.cudnn.benchmark = True
- parser = argparse.ArgumentParser(description="Distributed Arcface Training in Pytorch")
- parser.add_argument("config", type=str, help="py config file")
- main(parser.parse_args())
diff --git a/spaces/ikechan8370/vits-uma-genshin-honkai/Docker/Dockerfile b/spaces/ikechan8370/vits-uma-genshin-honkai/Docker/Dockerfile
deleted file mode 100644
index 4d39cdf02a2ec151686cc1d61234bf723068fed8..0000000000000000000000000000000000000000
--- a/spaces/ikechan8370/vits-uma-genshin-honkai/Docker/Dockerfile
+++ /dev/null
@@ -1,12 +0,0 @@
-FROM python:3.9-bullseye
-VOLUME ["/app"]
-WORKDIR /app
-# Set apt to Chinese mirror
-RUN sed -i 's/deb.debian.org/mirrors.ustc.edu.cn/g' /etc/apt/sources.list
-RUN apt-get update && apt-get -y install cmake git
-RUN git clone https://huggingface.co/spaces/ikechan8370/vits-uma-genshin-honkai
-WORKDIR /app/vits-uma-genshin-honkai
-RUN sed -i "s/\.launch()/\.launch(server_name=\"0.0.0.0\")/" /app/vits-uma-genshin-honkai/app.py
-ADD vits.sh /app/vits.sh
-EXPOSE 7860
-ENTRYPOINT [ "/app/vits.sh" ]
\ No newline at end of file
diff --git a/spaces/imju/flower_detector/README.md b/spaces/imju/flower_detector/README.md
deleted file mode 100644
index 47cf12d209897f75b64d96320448f4cde2113f53..0000000000000000000000000000000000000000
--- a/spaces/imju/flower_detector/README.md
+++ /dev/null
@@ -1,13 +0,0 @@
----
-title: Flower Detector
-emoji: 📉
-colorFrom: yellow
-colorTo: red
-sdk: gradio
-sdk_version: 3.20.1
-app_file: app.py
-pinned: false
-license: apache-2.0
----
-
-Check out the configuration reference at https://huggingface.co/docs/hub/spaces-config-reference
diff --git a/spaces/imjunaidafzal/LoRA-DreamBooth-Training-UI/style.css b/spaces/imjunaidafzal/LoRA-DreamBooth-Training-UI/style.css
deleted file mode 100644
index c4739b4ea5fc35e774a049e3dacc443f7f0eac19..0000000000000000000000000000000000000000
--- a/spaces/imjunaidafzal/LoRA-DreamBooth-Training-UI/style.css
+++ /dev/null
@@ -1,3 +0,0 @@
-h1 {
- text-align: center;
-}
diff --git a/spaces/innnky/soft-vits-vc/utils.py b/spaces/innnky/soft-vits-vc/utils.py
deleted file mode 100644
index c60894b52072a9293eb797b21e79f74e7d60dbb6..0000000000000000000000000000000000000000
--- a/spaces/innnky/soft-vits-vc/utils.py
+++ /dev/null
@@ -1,261 +0,0 @@
-import os
-import glob
-import sys
-import argparse
-import logging
-import json
-import subprocess
-import numpy as np
-from scipy.io.wavfile import read
-import torch
-
-MATPLOTLIB_FLAG = False
-
-logging.basicConfig(stream=sys.stdout, level=logging.DEBUG)
-logger = logging
-
-
-def load_checkpoint(checkpoint_path, model, optimizer=None):
- assert os.path.isfile(checkpoint_path)
- checkpoint_dict = torch.load(checkpoint_path, map_location='cpu')
- iteration = checkpoint_dict['iteration']
- learning_rate = checkpoint_dict['learning_rate']
- if optimizer is not None:
- optimizer.load_state_dict(checkpoint_dict['optimizer'])
- # print(1111)
- saved_state_dict = checkpoint_dict['model']
- # print(1111)
-
- if hasattr(model, 'module'):
- state_dict = model.module.state_dict()
- else:
- state_dict = model.state_dict()
- new_state_dict= {}
- for k, v in state_dict.items():
- try:
- new_state_dict[k] = saved_state_dict[k]
- except:
- logger.info("%s is not in the checkpoint" % k)
- new_state_dict[k] = v
- if hasattr(model, 'module'):
- model.module.load_state_dict(new_state_dict)
- else:
- model.load_state_dict(new_state_dict)
- logger.info("Loaded checkpoint '{}' (iteration {})" .format(
- checkpoint_path, iteration))
- return model, optimizer, learning_rate, iteration
-
-
-def save_checkpoint(model, optimizer, learning_rate, iteration, checkpoint_path):
- logger.info("Saving model and optimizer state at iteration {} to {}".format(
- iteration, checkpoint_path))
- if hasattr(model, 'module'):
- state_dict = model.module.state_dict()
- else:
- state_dict = model.state_dict()
- torch.save({'model': state_dict,
- 'iteration': iteration,
- 'optimizer': optimizer.state_dict(),
- 'learning_rate': learning_rate}, checkpoint_path)
-
-
-def summarize(writer, global_step, scalars={}, histograms={}, images={}, audios={}, audio_sampling_rate=22050):
- for k, v in scalars.items():
- writer.add_scalar(k, v, global_step)
- for k, v in histograms.items():
- writer.add_histogram(k, v, global_step)
- for k, v in images.items():
- writer.add_image(k, v, global_step, dataformats='HWC')
- for k, v in audios.items():
- writer.add_audio(k, v, global_step, audio_sampling_rate)
-
-
-def latest_checkpoint_path(dir_path, regex="G_*.pth"):
- f_list = glob.glob(os.path.join(dir_path, regex))
- f_list.sort(key=lambda f: int("".join(filter(str.isdigit, f))))
- x = f_list[-1]
- print(x)
- return x
-
-
-def plot_spectrogram_to_numpy(spectrogram):
- global MATPLOTLIB_FLAG
- if not MATPLOTLIB_FLAG:
- import matplotlib
- matplotlib.use("Agg")
- MATPLOTLIB_FLAG = True
- mpl_logger = logging.getLogger('matplotlib')
- mpl_logger.setLevel(logging.WARNING)
- import matplotlib.pylab as plt
- import numpy as np
-
- fig, ax = plt.subplots(figsize=(10,2))
- im = ax.imshow(spectrogram, aspect="auto", origin="lower",
- interpolation='none')
- plt.colorbar(im, ax=ax)
- plt.xlabel("Frames")
- plt.ylabel("Channels")
- plt.tight_layout()
-
- fig.canvas.draw()
- data = np.fromstring(fig.canvas.tostring_rgb(), dtype=np.uint8, sep='')
- data = data.reshape(fig.canvas.get_width_height()[::-1] + (3,))
- plt.close()
- return data
-
-
-def plot_alignment_to_numpy(alignment, info=None):
- global MATPLOTLIB_FLAG
- if not MATPLOTLIB_FLAG:
- import matplotlib
- matplotlib.use("Agg")
- MATPLOTLIB_FLAG = True
- mpl_logger = logging.getLogger('matplotlib')
- mpl_logger.setLevel(logging.WARNING)
- import matplotlib.pylab as plt
- import numpy as np
-
- fig, ax = plt.subplots(figsize=(6, 4))
- im = ax.imshow(alignment.transpose(), aspect='auto', origin='lower',
- interpolation='none')
- fig.colorbar(im, ax=ax)
- xlabel = 'Decoder timestep'
- if info is not None:
- xlabel += '\n\n' + info
- plt.xlabel(xlabel)
- plt.ylabel('Encoder timestep')
- plt.tight_layout()
-
- fig.canvas.draw()
- data = np.fromstring(fig.canvas.tostring_rgb(), dtype=np.uint8, sep='')
- data = data.reshape(fig.canvas.get_width_height()[::-1] + (3,))
- plt.close()
- return data
-
-
-def load_wav_to_torch(full_path):
- sampling_rate, data = read(full_path)
- return torch.FloatTensor(data.astype(np.float32)), sampling_rate
-
-
-def load_filepaths_and_text(filename, split="|"):
- with open(filename, encoding='utf-8') as f:
- filepaths_and_text = [line.strip().split(split) for line in f]
- return filepaths_and_text
-
-
-def get_hparams(init=True):
- parser = argparse.ArgumentParser()
- parser.add_argument('-c', '--config', type=str, default="./configs/base.json",
- help='JSON file for configuration')
- parser.add_argument('-m', '--model', type=str, required=True,
- help='Model name')
-
- args = parser.parse_args()
- model_dir = os.path.join("./logs", args.model)
-
- if not os.path.exists(model_dir):
- os.makedirs(model_dir)
-
- config_path = args.config
- config_save_path = os.path.join(model_dir, "config.json")
- if init:
- with open(config_path, "r") as f:
- data = f.read()
- with open(config_save_path, "w") as f:
- f.write(data)
- else:
- with open(config_save_path, "r") as f:
- data = f.read()
- config = json.loads(data)
-
- hparams = HParams(**config)
- hparams.model_dir = model_dir
- return hparams
-
-
-def get_hparams_from_dir(model_dir):
- config_save_path = os.path.join(model_dir, "config.json")
- with open(config_save_path, "r") as f:
- data = f.read()
- config = json.loads(data)
-
- hparams =HParams(**config)
- hparams.model_dir = model_dir
- return hparams
-
-
-def get_hparams_from_file(config_path):
- with open(config_path, "r") as f:
- data = f.read()
- config = json.loads(data)
-
- hparams =HParams(**config)
- return hparams
-
-
-def check_git_hash(model_dir):
- source_dir = os.path.dirname(os.path.realpath(__file__))
- if not os.path.exists(os.path.join(source_dir, ".git")):
- logger.warn("{} is not a git repository, therefore hash value comparison will be ignored.".format(
- source_dir
- ))
- return
-
- cur_hash = subprocess.getoutput("git rev-parse HEAD")
-
- path = os.path.join(model_dir, "githash")
- if os.path.exists(path):
- saved_hash = open(path).read()
- if saved_hash != cur_hash:
- logger.warn("git hash values are different. {}(saved) != {}(current)".format(
- saved_hash[:8], cur_hash[:8]))
- else:
- open(path, "w").write(cur_hash)
-
-
-def get_logger(model_dir, filename="train.log"):
- global logger
- logger = logging.getLogger(os.path.basename(model_dir))
- logger.setLevel(logging.DEBUG)
-
- formatter = logging.Formatter("%(asctime)s\t%(name)s\t%(levelname)s\t%(message)s")
- if not os.path.exists(model_dir):
- os.makedirs(model_dir)
- h = logging.FileHandler(os.path.join(model_dir, filename))
- h.setLevel(logging.DEBUG)
- h.setFormatter(formatter)
- logger.addHandler(h)
- return logger
-
-
-class HParams():
- def __init__(self, **kwargs):
- for k, v in kwargs.items():
- if type(v) == dict:
- v = HParams(**v)
- self[k] = v
-
- def keys(self):
- return self.__dict__.keys()
-
- def items(self):
- return self.__dict__.items()
-
- def values(self):
- return self.__dict__.values()
-
- def __len__(self):
- return len(self.__dict__)
-
- def __getitem__(self, key):
- return getattr(self, key)
-
- def __setitem__(self, key, value):
- return setattr(self, key, value)
-
- def __contains__(self, key):
- return key in self.__dict__
-
- def __repr__(self):
- return self.__dict__.__repr__()
diff --git a/spaces/inplisQlawa/anything-midjourney-v4-1/Fightofcharacters91aifreedownload _VERIFIED_.md b/spaces/inplisQlawa/anything-midjourney-v4-1/Fightofcharacters91aifreedownload _VERIFIED_.md
deleted file mode 100644
index 3c5e0f14ad84a95354873e3f36c0b94a58197a73..0000000000000000000000000000000000000000
--- a/spaces/inplisQlawa/anything-midjourney-v4-1/Fightofcharacters91aifreedownload _VERIFIED_.md
+++ /dev/null
@@ -1,10 +0,0 @@
-
-
this is the final battle with. .,fightofcharacters91aifreedownload,Rupaka Jetray at 8:52 am. I love free sprites in go. .,fightofcharacters91aifreedownload,Headus - Yagi Ne01 - F U T U L O G I A - X.pdf fightofcharacters91aifreedownload.
-
Killer robot donnie darko wolfenstein instrumental trailer john henry no idea. No idea. Como server m210 manual Youtube-en at 5:05 am. .,fightofcharacters91aifreedownload,FiveMasters Library Is the Ark Little David HD Wallpapers Fightofcharacters91aifreedownload. Marcucci Cerculo sette medagliere at 7:17 am. Rian jenkins 2,fightofcharacters91aifreedownload,Attack on Titan Yamiyoji Deyama JoshuaKohn.
fightofcharacters91aifreedownload. fightofcharacters91aifreedownload. I became really nauseas after i had a couple of hershokhozhos or 3-4,fightofcharacters91aifreedownload, This product may be the greatest provider of remedies for curing any kind of disease in mind.
-
fightofcharacters91aifreedownload com. fightofcharacters91aifreedownload ghsm. Related links: [url=http://rrieba.esy.es/][b]slitheroid apk for android [/b][/url] fightofcharacters91aifreedownload.
-
buy fightofcharacters91aifreedownload,fightofcharacters91aifreedownload,fightofcharacters91aifreedownload free trial,fightofcharacters91aifreedownload,fightofcharacters91aifreedownload,fightofcharacters91aifreedownload home,fightofcharacters91aifreedownload com. fightofcharacters91aifreedownload ghsm,cold sleep part 1 : part 2 fightofcharacters91aifreedownload.
-
Url.fightofcharacters91aifreedownload. fightofcharacters91aifreedownload ghsm,cold sleep part 1 : part 2 fightofcharacters91aifreedownload,bomemidi1.7.2,fightofcharacters91aifreedownload,Scandal Jessica khadka Jyoti khadka Prakash Ojha target.
899543212b
-
-
\ No newline at end of file
diff --git a/spaces/inplisQlawa/anything-midjourney-v4-1/Marketing Management Notes From Bba 3rd Semester Pdf BEST.md b/spaces/inplisQlawa/anything-midjourney-v4-1/Marketing Management Notes From Bba 3rd Semester Pdf BEST.md
deleted file mode 100644
index c80cf0b98acbc8b7d0a62eea32d45164ab0873f7..0000000000000000000000000000000000000000
--- a/spaces/inplisQlawa/anything-midjourney-v4-1/Marketing Management Notes From Bba 3rd Semester Pdf BEST.md
+++ /dev/null
@@ -1,86 +0,0 @@
-
-
Marketing Management Notes from BBA 3rd Semester PDF - How to Download and Study
-
-
Marketing management is one of the core subjects for BBA students who want to learn the concepts, principles, and practices of marketing in a business context. Marketing management covers topics such as marketing environment, consumer behavior, segmentation, targeting, positioning, product, price, distribution, promotion, and marketing strategy.
-
marketing management notes from bba 3rd semester pdf
If you are a BBA student who is looking for marketing management notes from BBA 3rd semester PDF, you may have a hard time finding them online. Many websites claim to offer marketing management notes for BBA students, but they may not be reliable, updated, or complete. Some websites may also have broken links, outdated notes, or fake downloads that can harm your computer or steal your personal information.
-
-
That's why you should always download marketing management notes from BBA 3rd semester PDF from reputable sources that have positive reviews and feedback from other students. You should also check the notes' compatibility with your syllabus and exam pattern before downloading them.
-
-
Where to Download Marketing Management Notes from BBA 3rd Semester PDF?
-
-
There are many websites that offer marketing management notes from BBA 3rd semester PDF, but not all of them are trustworthy or updated. Some of the best websites to download marketing management notes from BBA 3rd semester PDF are:
-
-
-
Geektonight: This website has a large collection of notes, books, syllabus, case studies, question papers, and MCQs for various subjects, including marketing management. The notes are available in PDF form and can be downloaded for free. The notes are also updated regularly and follow the latest syllabus and exam pattern.
-
DDEGJUST: This website is the official website of the Directorate of Distance Education of Guru Jambheshwar University of Science and Technology. The website has study material for various courses and programs, including BBA. The website has marketing management notes from BBA 3rd semester PDF that can be downloaded for free. The notes are also comprehensive and well-organized.
-
Studynama: This website is a community of students who share study material and resources for various courses and exams. The website has marketing management handwritten lecture notes for third semester BBA students that can be downloaded for free. The notes are also clear and concise.
-
-
-
These are just some of the websites that offer marketing management notes from BBA 3rd semester PDF. You can also search for other websites or sources that have marketing management notes for BBA students, but make sure to be careful and cautious before downloading anything.
-
-
How to Study Marketing Management Notes from BBA 3rd Semester PDF?
-
-
Downloading marketing management notes from BBA 3rd semester PDF is not enough to ace your exams. You also need to study them properly and effectively. Here are some tips on how to study marketing management notes from BBA 3rd semester PDF:
-
-
-
-
Read the notes carefully and understand the concepts and terms. Try to relate them to real-life examples and cases.
-
Make your own summary or outline of the notes. Highlight the key points and facts. Use diagrams, charts, or tables to organize the information.
-
Revise the notes regularly and frequently. Review the important topics and concepts before your exams.
-
Practice the questions and MCQs given in the notes or on the websites. Solve previous year papers and mock tests to check your preparation level.
-
Discuss the notes with your classmates or teachers. Ask doubts and clarify concepts. Share your insights and opinions.
-
-
-
These are just some of the tips on how to study marketing management notes from BBA 3rd semester PDF. You may have other methods or strategies that work better for you. The main thing is to be consistent and focused on your studies.
-
-
Conclusion
-
-
Marketing management is a vital subject for BBA students who want to learn how to plan, execute, and control the marketing activities of a business enterprise. Marketing management notes from BBA 3rd semester PDF can help you understand the subject better and prepare for your exams.
-
-
If you want to download marketing management notes from BBA 3rd semester PDF, make sure to download them from reliable sources that have positive reviews and feedback from other students. You should also check the notes' compatibility with your syllabus and exam pattern before downloading them.
-
-
We hope this article has helped you learn more about marketing management notes from BBA 3rd semester PDF and how to download and study them. Have fun learning!
-
How to Download Marketing Management Notes from BBA 3rd Semester PDF?
-
-
Downloading marketing management notes from BBA 3rd semester PDF is not a difficult task, but you need to follow some steps and precautions to ensure that you get the right notes for your studies. Here are some steps on how to download marketing management notes from BBA 3rd semester PDF:
-
-
-
Visit the website of your choice that offers marketing management notes from BBA 3rd semester PDF. You can choose from the websites mentioned above or search for other websites that have marketing management notes for BBA students.
-
Check the details and description of the notes before downloading them. Make sure that the notes match your syllabus and exam pattern, and that they are updated and complete.
-
Click on the download link or button to start downloading the notes. You may need to register or sign up on some websites to access the download link.
-
Save the notes file on your computer or device. You may need to extract the file from a zip archive if it is compressed.
-
Open the notes file with a PDF reader or viewer. You can use any PDF reader or viewer of your choice, such as Adobe Acrobat Reader, Foxit Reader, etc.
-
Read and study the notes as per your convenience and requirement. You can also print the notes or transfer them to other devices if you want.
-
-
-
These are just some of the steps on how to download marketing management notes from BBA 3rd semester PDF. You may find different steps or instructions depending on the website or source you choose.
-
-
What are the Precautions to Take When Downloading Marketing Management Notes from BBA 3rd Semester PDF?
-
-
Downloading marketing management notes from BBA 3rd semester PDF can be a risky activity if you are not careful and cautious. There are many websites and sources that may offer fake or harmful downloads that can damage your computer or device, or steal your personal information. Here are some precautions to take when downloading marketing management notes from BBA 3rd semester PDF:
-
-
-
Choose a Reliable Source: Always download marketing management notes from BBA 3rd semester PDF from a reliable source that has positive reviews and feedback from other students. Avoid downloading from unknown or suspicious websites that may have malware or viruses.
-
Scan the File: Always scan the file with antivirus software before opening it. This can help you detect and remove any potential threats or infections that may harm your system or data.
-
Backup Your Data: Always backup your data and files before downloading anything. This can help you restore your data and files in case something goes wrong or you lose them due to any reason.
-
Use a VPN: Always use a VPN (virtual private network) when downloading anything online. This can help you protect your privacy and security by encrypting your data and hiding your IP address.
-
-
-
These are just some of the precautions to take when downloading marketing management notes from BBA 3rd semester PDF. You may have other measures or tips that can help you download safely and securely.
-
Conclusion
-
-
Marketing management is one of the core subjects for BBA students who want to learn the concepts, principles, and practices of marketing in a business context. Marketing management covers topics such as marketing environment, consumer behavior, segmentation, targeting, positioning, product, price, distribution, promotion, and marketing strategy.
-
-
If you are a BBA student who is looking for marketing management notes from BBA 3rd semester PDF, you may have a hard time finding them online. Many websites claim to offer marketing management notes for BBA students, but they may not be reliable, updated, or complete. Some websites may also have broken links, outdated notes, or fake downloads that can harm your computer or steal your personal information.
-
-
That's why you should always download marketing management notes from BBA 3rd semester PDF from reputable sources that have positive reviews and feedback from other students. You should also check the notes' compatibility with your syllabus and exam pattern before downloading them.
-
-
Once you download the notes, you should study them properly and effectively by following some tips and techniques. You should read and understand the concepts and terms, make your own summary or outline of the notes, revise the notes regularly and frequently, practice the questions and MCQs given in the notes or on the websites, and discuss the notes with your classmates or teachers.
-
-
Studying marketing management notes from BBA 3rd semester PDF can help you gain knowledge and skills about various aspects of marketing, prepare for your exams, and enhance your career prospects. You can also pursue higher studies or professional courses in marketing after completing your BBA degree.
-
-
We hope this article has helped you learn more about marketing management notes from BBA 3rd semester PDF and how to download and study them. Have fun learning!
3cee63e6c2
-
-
\ No newline at end of file
diff --git a/spaces/inplisQlawa/anything-midjourney-v4-1/Mvci Driver For Toyota-cable 2.0.1 15 !FREE!.md b/spaces/inplisQlawa/anything-midjourney-v4-1/Mvci Driver For Toyota-cable 2.0.1 15 !FREE!.md
deleted file mode 100644
index 68d9aa7bb197f55f5bc79593ba12759747272371..0000000000000000000000000000000000000000
--- a/spaces/inplisQlawa/anything-midjourney-v4-1/Mvci Driver For Toyota-cable 2.0.1 15 !FREE!.md
+++ /dev/null
@@ -1,6 +0,0 @@
-
- With the utilization of the
- llama-cpp-python
- package, we are excited to introduce the GGUF model hosted in the Hugging
- Face Docker Spaces, made accessible through an OpenAI-compatible API. This
- space includes comprehensive API documentation to facilitate seamless
- integration.
-
- If you find this resource valuable, your support in the form of starring
- the space would be greatly appreciated. Your engagement plays a vital role
- in furthering the application for a community GPU grant, ultimately
- enhancing the capabilities and accessibility of this space.
-
-
-Conquer Online Aimbot Tool 2018. 3,136 views3.1 thousand views. September 14, 2018 . Carrasco conquer online. â–¸. â–·. â–¸. Conquer Online Aimbot Tool 2018.
-Conquer Online Aimbot Tool 2018
-Conquer Online Aimbot 2020 âš¡ Download .
-Killer Aimbot FREE .
-download.
-Get.
-download. ....
-Download Conquer Online Aimbot Tool 2018.
-download. .
-Fraps – Free online game console for gamers!
-Free game console for everyone.
-Fraps is a free online game console for gamers.
-The first console for gamers with a free player recording tool 8a78ff9644
-
-
-
diff --git a/spaces/ljjggr/bingo/src/components/ui/textarea.tsx b/spaces/ljjggr/bingo/src/components/ui/textarea.tsx
deleted file mode 100644
index e25af722c7a5dc1121a9ab58d6716952f9f76081..0000000000000000000000000000000000000000
--- a/spaces/ljjggr/bingo/src/components/ui/textarea.tsx
+++ /dev/null
@@ -1,24 +0,0 @@
-import * as React from 'react'
-
-import { cn } from '@/lib/utils'
-
-export interface TextareaProps
- extends React.TextareaHTMLAttributes {}
-
-const Textarea = React.forwardRef(
- ({ className, ...props }, ref) => {
- return (
-
- )
- }
-)
-Textarea.displayName = 'Textarea'
-
-export { Textarea }
diff --git a/spaces/lmattingly/cartoonify-yourself/app.py b/spaces/lmattingly/cartoonify-yourself/app.py
deleted file mode 100644
index b698ee4c04ff3020b21eccb0cd32c65264726fa0..0000000000000000000000000000000000000000
--- a/spaces/lmattingly/cartoonify-yourself/app.py
+++ /dev/null
@@ -1,106 +0,0 @@
-import gradio as gr
-import jax
-import jax.numpy as jnp
-import numpy as np
-from flax.jax_utils import replicate
-from flax.training.common_utils import shard
-from PIL import Image
-from diffusers import FlaxStableDiffusionControlNetPipeline, FlaxControlNetModel
-import cv2
-
-
-
-title = "ControlNet for Cartoon-ifying"
-description = "This is a demo on ControlNet for changing images of people into cartoons of different styles."
-examples = [["./simpsons_human_1.jpg", "turn into a simpsons character", "./simpsons_animated_1.jpg"]]
-
-
-
-# Constants
-low_threshold = 100
-high_threshold = 200
-
-base_model_path = "runwayml/stable-diffusion-v1-5"
-controlnet_path = "lmattingly/controlnet-uncanny-simpsons-v2-0"
-#controlnet_path = "JFoz/dog-cat-pose"
-
-# Models
-controlnet, controlnet_params = FlaxControlNetModel.from_pretrained(
- controlnet_path, dtype=jnp.bfloat16
-)
-pipe, params = FlaxStableDiffusionControlNetPipeline.from_pretrained(
- base_model_path, controlnet=controlnet, revision="flax", dtype=jnp.bfloat16
-)
-
-
-def canny_filter(image):
- gray_image = cv2.cvtColor(image, cv2.COLOR_BGR2GRAY)
- blurred_image = cv2.GaussianBlur(gray_image, (5, 5), 0)
- edges_image = cv2.Canny(blurred_image, 50, 150)
- canny_image = Image.fromarray(edges_image)
- return canny_image
-
-def canny_filter2(image):
- low_threshold = 100
- high_threshold = 200
-
- image = cv2.Canny(image, low_threshold, high_threshold)
- image = image[:, :, None]
- image = np.concatenate([image, image, image], axis=2)
- canny_image = Image.fromarray(image)
- return canny_image
-
-
-
-def resize_image(im, max_size):
- im_np = cv2.cvtColor(im, cv2.COLOR_BGR2RGB)
-
- height, width = im_np.shape[:2]
-
- scale_factor = max_size / max(height, width)
-
- resized_np = cv2.resize(im_np, (int(width * scale_factor), int(height * scale_factor)))
-
- resized_im = Image.fromarray(resized_np)
-
- return resized_im
-
-
-def create_key(seed=0):
- return jax.random.PRNGKey(seed)
-
-def infer(prompts, image):
- params["controlnet"] = controlnet_params
- im = image
- image = canny_filter2(im)
- #image = canny_filter(im)
- #image = Image.fromarray(im)
-
- num_samples = 1 #jax.device_count()
- rng = create_key(0)
- rng = jax.random.split(rng, jax.device_count())
-
- prompt_ids = pipe.prepare_text_inputs([prompts] * num_samples)
- processed_image = pipe.prepare_image_inputs([image] * num_samples)
-
- p_params = replicate(params)
- prompt_ids = shard(prompt_ids)
- processed_image = shard(processed_image)
-
- output = pipe(
- prompt_ids=prompt_ids,
- image=processed_image,
- params=p_params,
- prng_seed=rng,
- num_inference_steps=5,
- jit=True,
- ).images
-
- output_images = pipe.numpy_to_pil(np.asarray(output.reshape((num_samples,) + output.shape[-3:])))
- return output_images
-
-
-gr.Interface(fn = infer, inputs = ["text", "image"], outputs = "gallery",
- title = title, description = description, theme='gradio/soft',
- examples=[["a simpsons cartoon character", "simpsons_human_1.jpg"]]
-).launch()
diff --git a/spaces/ma-xu/LIVE/pybind11/tests/test_union.cpp b/spaces/ma-xu/LIVE/pybind11/tests/test_union.cpp
deleted file mode 100644
index 7b98ea216ca0b272978134d9dd1d1eff1b804ad5..0000000000000000000000000000000000000000
--- a/spaces/ma-xu/LIVE/pybind11/tests/test_union.cpp
+++ /dev/null
@@ -1,22 +0,0 @@
-/*
- tests/test_class.cpp -- test py::class_ definitions and basic functionality
-
- Copyright (c) 2019 Roland Dreier
-
- All rights reserved. Use of this source code is governed by a
- BSD-style license that can be found in the LICENSE file.
-*/
-
-#include "pybind11_tests.h"
-
-TEST_SUBMODULE(union_, m) {
- union TestUnion {
- int value_int;
- unsigned value_uint;
- };
-
- py::class_(m, "TestUnion")
- .def(py::init<>())
- .def_readonly("as_int", &TestUnion::value_int)
- .def_readwrite("as_uint", &TestUnion::value_uint);
-}
diff --git a/spaces/ma-xu/LIVE/thrust/thrust/iterator/transform_input_output_iterator.h b/spaces/ma-xu/LIVE/thrust/thrust/iterator/transform_input_output_iterator.h
deleted file mode 100644
index 25c10eb58e93cbadb298fc68bbd4d24b3dc5a7cb..0000000000000000000000000000000000000000
--- a/spaces/ma-xu/LIVE/thrust/thrust/iterator/transform_input_output_iterator.h
+++ /dev/null
@@ -1,163 +0,0 @@
-/*
- * Copyright 2020 NVIDIA Corporation
- *
- * Licensed under the Apache License, Version 2.0 (the "License");
- * you may not use this file except in compliance with the License.
- * You may obtain a copy of the License at
- *
- * http://www.apache.org/licenses/LICENSE-2.0
- *
- * Unless required by applicable law or agreed to in writing, software
- * distributed under the License is distributed on an "AS IS" BASIS,
- * WITHOUT WARRANTIES OR CONDITIONS OF ANY KIND, either express or implied.
- * See the License for the specific language governing permissions and
- * limitations under the License.
- */
-
-/*! \file thrust/iterator/transform_input_output_iterator.h
- * \brief An iterator which adapts another iterator by applying transform
- * functions when reading and writing dereferenced values.
- */
-
-#pragma once
-
-#include
-#include
-
-namespace thrust
-{
-
-/*! \addtogroup iterators
- * \{
- */
-
-/*! \addtogroup fancyiterator Fancy Iterators
- * \ingroup iterators
- * \{
- */
-
-/*! \p transform_input_output_iterator is a special kind of iterator which applies
- * transform functions when reading from or writing to dereferenced values.
- * This iterator is useful for algorithms that operate on a type that needs to
- * be serialized/deserialized from values in another iterator, avoiding the
- * need to materialize intermediate results in memory. This also enables the
- * transform functions to be fused with the operations that read and write to
- * the `transform_input_output_iterator`.
- *
- * The following code snippet demonstrates how to create a
- * \p transform_input_output_iterator which performs different transformations when
- * reading from and writing to the iterator.
- *
- * \code
- * #include
- * #include
- *
- * int main()
- * {
- * const size_t size = 4;
- * thrust::device_vector v(size);
- *
- * // Write 1.0f, 2.0f, 3.0f, 4.0f to vector
- * thrust::sequence(v.begin(), v.end(), 1);
- *
- * // Iterator that returns negated values and writes squared values
- * auto iter = thrust::make_transform_input_output_iterator(v.begin(),
- * thrust::negate{}, thrust::square{});
- *
- * // Iterator negates values when reading
- * std::cout << iter[0] << " "; // -1.0f;
- * std::cout << iter[1] << " "; // -2.0f;
- * std::cout << iter[2] << " "; // -3.0f;
- * std::cout << iter[3] << "\n"; // -4.0f;
- *
- * // Write 1.0f, 2.0f, 3.0f, 4.0f to iterator
- * thrust::sequence(iter, iter + size, 1);
- *
- * // Values were squared before writing to vector
- * std::cout << v[0] << " "; // 1.0f;
- * std::cout << v[1] << " "; // 4.0f;
- * std::cout << v[2] << " "; // 9.0f;
- * std::cout << v[3] << "\n"; // 16.0f;
- *
- * }
- * \endcode
- *
- * \see make_transform_input_output_iterator
- */
-
-template
- class transform_input_output_iterator
- : public detail::transform_input_output_iterator_base::type
-{
-
- /*! \cond
- */
-
- public:
-
- typedef typename
- detail::transform_input_output_iterator_base::type
- super_t;
-
- friend class thrust::iterator_core_access;
- /*! \endcond
- */
-
- /*! This constructor takes as argument a \c Iterator an \c InputFunction and an
- * \c OutputFunction and copies them to a new \p transform_input_output_iterator
- *
- * \param io An \c Iterator pointing to where the input to \c InputFunction
- * will be read from and the result of \c OutputFunction will be written to
- * \param input_function An \c InputFunction to be executed on values read from the iterator
- * \param output_function An \c OutputFunction to be executed on values written to the iterator
- */
- __host__ __device__
- transform_input_output_iterator(Iterator const& io, InputFunction input_function, OutputFunction output_function)
- : super_t(io), input_function(input_function), output_function(output_function)
- {
- }
-
- /*! \cond
- */
- private:
-
- __host__ __device__
- typename super_t::reference dereference() const
- {
- return detail::transform_input_output_iterator_proxy<
- InputFunction, OutputFunction, Iterator
- >(this->base_reference(), input_function, output_function);
- }
-
- InputFunction input_function;
- OutputFunction output_function;
-
- /*! \endcond
- */
-}; // end transform_input_output_iterator
-
-/*! \p make_transform_input_output_iterator creates a \p transform_input_output_iterator from
- * an \c Iterator a \c InputFunction and a \c OutputFunction
- *
- * \param io An \c Iterator pointing to where the input to \c InputFunction
- * will be read from and the result of \c OutputFunction will be written to
- * \param input_function An \c InputFunction to be executed on values read from the iterator
- * \param output_function An \c OutputFunction to be executed on values written to the iterator
- * \see transform_input_output_iterator
- */
-template
-transform_input_output_iterator
-__host__ __device__
-make_transform_input_output_iterator(Iterator io, InputFunction input_function, OutputFunction output_function)
-{
- return transform_input_output_iterator(io, input_function, output_function);
-} // end make_transform_input_output_iterator
-
-/*! \} // end fancyiterators
- */
-
-/*! \} // end iterators
- */
-
-} // end thrust
-
diff --git a/spaces/manan/fruit-classifier/README.md b/spaces/manan/fruit-classifier/README.md
deleted file mode 100644
index 6da0cc95a992ee2ff53fefe192cc42841fc230c1..0000000000000000000000000000000000000000
--- a/spaces/manan/fruit-classifier/README.md
+++ /dev/null
@@ -1,12 +0,0 @@
----
-title: Fruit Classifier
-emoji: 📚
-colorFrom: red
-colorTo: purple
-sdk: gradio
-sdk_version: 3.1.1
-app_file: app.py
-pinned: false
----
-
-Check out the configuration reference at https://huggingface.co/docs/hub/spaces-config-reference
diff --git a/spaces/manhkhanhUIT/BOPBTL/Global/data/online_dataset_for_old_photos.py b/spaces/manhkhanhUIT/BOPBTL/Global/data/online_dataset_for_old_photos.py
deleted file mode 100644
index 068410a93eb10d5f00e694fd890f8aaa069526a3..0000000000000000000000000000000000000000
--- a/spaces/manhkhanhUIT/BOPBTL/Global/data/online_dataset_for_old_photos.py
+++ /dev/null
@@ -1,485 +0,0 @@
-# Copyright (c) Microsoft Corporation.
-# Licensed under the MIT License.
-
-import os.path
-import io
-import zipfile
-from data.base_dataset import BaseDataset, get_params, get_transform, normalize
-from data.image_folder import make_dataset
-from PIL import Image
-import torchvision.transforms as transforms
-import numpy as np
-from data.Load_Bigfile import BigFileMemoryLoader
-import random
-import cv2
-from io import BytesIO
-
-def pil_to_np(img_PIL):
- '''Converts image in PIL format to np.array.
-
- From W x H x C [0...255] to C x W x H [0..1]
- '''
- ar = np.array(img_PIL)
-
- if len(ar.shape) == 3:
- ar = ar.transpose(2, 0, 1)
- else:
- ar = ar[None, ...]
-
- return ar.astype(np.float32) / 255.
-
-
-def np_to_pil(img_np):
- '''Converts image in np.array format to PIL image.
-
- From C x W x H [0..1] to W x H x C [0...255]
- '''
- ar = np.clip(img_np * 255, 0, 255).astype(np.uint8)
-
- if img_np.shape[0] == 1:
- ar = ar[0]
- else:
- ar = ar.transpose(1, 2, 0)
-
- return Image.fromarray(ar)
-
-def synthesize_salt_pepper(image,amount,salt_vs_pepper):
-
- ## Give PIL, return the noisy PIL
-
- img_pil=pil_to_np(image)
-
- out = img_pil.copy()
- p = amount
- q = salt_vs_pepper
- flipped = np.random.choice([True, False], size=img_pil.shape,
- p=[p, 1 - p])
- salted = np.random.choice([True, False], size=img_pil.shape,
- p=[q, 1 - q])
- peppered = ~salted
- out[flipped & salted] = 1
- out[flipped & peppered] = 0.
- noisy = np.clip(out, 0, 1).astype(np.float32)
-
-
- return np_to_pil(noisy)
-
-def synthesize_gaussian(image,std_l,std_r):
-
- ## Give PIL, return the noisy PIL
-
- img_pil=pil_to_np(image)
-
- mean=0
- std=random.uniform(std_l/255.,std_r/255.)
- gauss=np.random.normal(loc=mean,scale=std,size=img_pil.shape)
- noisy=img_pil+gauss
- noisy=np.clip(noisy,0,1).astype(np.float32)
-
- return np_to_pil(noisy)
-
-def synthesize_speckle(image,std_l,std_r):
-
- ## Give PIL, return the noisy PIL
-
- img_pil=pil_to_np(image)
-
- mean=0
- std=random.uniform(std_l/255.,std_r/255.)
- gauss=np.random.normal(loc=mean,scale=std,size=img_pil.shape)
- noisy=img_pil+gauss*img_pil
- noisy=np.clip(noisy,0,1).astype(np.float32)
-
- return np_to_pil(noisy)
-
-
-def synthesize_low_resolution(img):
- w,h=img.size
-
- new_w=random.randint(int(w/2),w)
- new_h=random.randint(int(h/2),h)
-
- img=img.resize((new_w,new_h),Image.BICUBIC)
-
- if random.uniform(0,1)<0.5:
- img=img.resize((w,h),Image.NEAREST)
- else:
- img = img.resize((w, h), Image.BILINEAR)
-
- return img
-
-
-def convertToJpeg(im,quality):
- with BytesIO() as f:
- im.save(f, format='JPEG',quality=quality)
- f.seek(0)
- return Image.open(f).convert('RGB')
-
-
-def blur_image_v2(img):
-
-
- x=np.array(img)
- kernel_size_candidate=[(3,3),(5,5),(7,7)]
- kernel_size=random.sample(kernel_size_candidate,1)[0]
- std=random.uniform(1.,5.)
-
- #print("The gaussian kernel size: (%d,%d) std: %.2f"%(kernel_size[0],kernel_size[1],std))
- blur=cv2.GaussianBlur(x,kernel_size,std)
-
- return Image.fromarray(blur.astype(np.uint8))
-
-def online_add_degradation_v2(img):
-
- task_id=np.random.permutation(4)
-
- for x in task_id:
- if x==0 and random.uniform(0,1)<0.7:
- img = blur_image_v2(img)
- if x==1 and random.uniform(0,1)<0.7:
- flag = random.choice([1, 2, 3])
- if flag == 1:
- img = synthesize_gaussian(img, 5, 50)
- if flag == 2:
- img = synthesize_speckle(img, 5, 50)
- if flag == 3:
- img = synthesize_salt_pepper(img, random.uniform(0, 0.01), random.uniform(0.3, 0.8))
- if x==2 and random.uniform(0,1)<0.7:
- img=synthesize_low_resolution(img)
-
- if x==3 and random.uniform(0,1)<0.7:
- img=convertToJpeg(img,random.randint(40,100))
-
- return img
-
-
-def irregular_hole_synthesize(img,mask):
-
- img_np=np.array(img).astype('uint8')
- mask_np=np.array(mask).astype('uint8')
- mask_np=mask_np/255
- img_new=img_np*(1-mask_np)+mask_np*255
-
-
- hole_img=Image.fromarray(img_new.astype('uint8')).convert("RGB")
-
- return hole_img,mask.convert("L")
-
-def zero_mask(size):
- x=np.zeros((size,size,3)).astype('uint8')
- mask=Image.fromarray(x).convert("RGB")
- return mask
-
-
-
-class UnPairOldPhotos_SR(BaseDataset): ## Synthetic + Real Old
- def initialize(self, opt):
- self.opt = opt
- self.isImage = 'domainA' in opt.name
- self.task = 'old_photo_restoration_training_vae'
- self.dir_AB = opt.dataroot
- if self.isImage:
-
- self.load_img_dir_L_old=os.path.join(self.dir_AB,"Real_L_old.bigfile")
- self.load_img_dir_RGB_old=os.path.join(self.dir_AB,"Real_RGB_old.bigfile")
- self.load_img_dir_clean=os.path.join(self.dir_AB,"VOC_RGB_JPEGImages.bigfile")
-
- self.loaded_imgs_L_old=BigFileMemoryLoader(self.load_img_dir_L_old)
- self.loaded_imgs_RGB_old=BigFileMemoryLoader(self.load_img_dir_RGB_old)
- self.loaded_imgs_clean=BigFileMemoryLoader(self.load_img_dir_clean)
-
- else:
- # self.load_img_dir_clean=os.path.join(self.dir_AB,self.opt.test_dataset)
- self.load_img_dir_clean=os.path.join(self.dir_AB,"VOC_RGB_JPEGImages.bigfile")
- self.loaded_imgs_clean=BigFileMemoryLoader(self.load_img_dir_clean)
-
- ####
- print("-------------Filter the imgs whose size <256 in VOC-------------")
- self.filtered_imgs_clean=[]
- for i in range(len(self.loaded_imgs_clean)):
- img_name,img=self.loaded_imgs_clean[i]
- h,w=img.size
- if h<256 or w<256:
- continue
- self.filtered_imgs_clean.append((img_name,img))
-
- print("--------Origin image num is [%d], filtered result is [%d]--------" % (
- len(self.loaded_imgs_clean), len(self.filtered_imgs_clean)))
- ## Filter these images whose size is less than 256
-
- # self.img_list=os.listdir(load_img_dir)
- self.pid = os.getpid()
-
- def __getitem__(self, index):
-
-
- is_real_old=0
-
- sampled_dataset=None
- degradation=None
- if self.isImage: ## domain A , contains 2 kinds of data: synthetic + real_old
- P=random.uniform(0,2)
- if P>=0 and P<1:
- if random.uniform(0,1)<0.5:
- sampled_dataset=self.loaded_imgs_L_old
- self.load_img_dir=self.load_img_dir_L_old
- else:
- sampled_dataset=self.loaded_imgs_RGB_old
- self.load_img_dir=self.load_img_dir_RGB_old
- is_real_old=1
- if P>=1 and P<2:
- sampled_dataset=self.filtered_imgs_clean
- self.load_img_dir=self.load_img_dir_clean
- degradation=1
- else:
-
- sampled_dataset=self.filtered_imgs_clean
- self.load_img_dir=self.load_img_dir_clean
-
- sampled_dataset_len=len(sampled_dataset)
-
- index=random.randint(0,sampled_dataset_len-1)
-
- img_name,img = sampled_dataset[index]
-
- if degradation is not None:
- img=online_add_degradation_v2(img)
-
- path=os.path.join(self.load_img_dir,img_name)
-
- # AB = Image.open(path).convert('RGB')
- # split AB image into A and B
-
- # apply the same transform to both A and B
-
- if random.uniform(0,1) <0.1:
- img=img.convert("L")
- img=img.convert("RGB")
- ## Give a probability P, we convert the RGB image into L
-
-
- A=img
- w,h=A.size
- if w<256 or h<256:
- A=transforms.Scale(256,Image.BICUBIC)(A)
- ## Since we want to only crop the images (256*256), for those old photos whose size is smaller than 256, we first resize them.
-
- transform_params = get_params(self.opt, A.size)
- A_transform = get_transform(self.opt, transform_params)
-
- B_tensor = inst_tensor = feat_tensor = 0
- A_tensor = A_transform(A)
-
-
- input_dict = {'label': A_tensor, 'inst': is_real_old, 'image': A_tensor,
- 'feat': feat_tensor, 'path': path}
- return input_dict
-
- def __len__(self):
- return len(self.loaded_imgs_clean) ## actually, this is useless, since the selected index is just a random number
-
- def name(self):
- return 'UnPairOldPhotos_SR'
-
-
-class PairOldPhotos(BaseDataset):
- def initialize(self, opt):
- self.opt = opt
- self.isImage = 'imagegan' in opt.name
- self.task = 'old_photo_restoration_training_mapping'
- self.dir_AB = opt.dataroot
- if opt.isTrain:
- self.load_img_dir_clean= os.path.join(self.dir_AB, "VOC_RGB_JPEGImages.bigfile")
- self.loaded_imgs_clean = BigFileMemoryLoader(self.load_img_dir_clean)
-
- print("-------------Filter the imgs whose size <256 in VOC-------------")
- self.filtered_imgs_clean = []
- for i in range(len(self.loaded_imgs_clean)):
- img_name, img = self.loaded_imgs_clean[i]
- h, w = img.size
- if h < 256 or w < 256:
- continue
- self.filtered_imgs_clean.append((img_name, img))
-
- print("--------Origin image num is [%d], filtered result is [%d]--------" % (
- len(self.loaded_imgs_clean), len(self.filtered_imgs_clean)))
-
- else:
- self.load_img_dir=os.path.join(self.dir_AB,opt.test_dataset)
- self.loaded_imgs=BigFileMemoryLoader(self.load_img_dir)
-
- self.pid = os.getpid()
-
- def __getitem__(self, index):
-
-
-
- if self.opt.isTrain:
- img_name_clean,B = self.filtered_imgs_clean[index]
- path = os.path.join(self.load_img_dir_clean, img_name_clean)
- if self.opt.use_v2_degradation:
- A=online_add_degradation_v2(B)
- ### Remind: A is the input and B is corresponding GT
- else:
-
- if self.opt.test_on_synthetic:
-
- img_name_B,B=self.loaded_imgs[index]
- A=online_add_degradation_v2(B)
- img_name_A=img_name_B
- path = os.path.join(self.load_img_dir, img_name_A)
- else:
- img_name_A,A=self.loaded_imgs[index]
- img_name_B,B=self.loaded_imgs[index]
- path = os.path.join(self.load_img_dir, img_name_A)
-
-
- if random.uniform(0,1)<0.1 and self.opt.isTrain:
- A=A.convert("L")
- B=B.convert("L")
- A=A.convert("RGB")
- B=B.convert("RGB")
- ## In P, we convert the RGB into L
-
-
- ##test on L
-
- # split AB image into A and B
- # w, h = img.size
- # w2 = int(w / 2)
- # A = img.crop((0, 0, w2, h))
- # B = img.crop((w2, 0, w, h))
- w,h=A.size
- if w<256 or h<256:
- A=transforms.Scale(256,Image.BICUBIC)(A)
- B=transforms.Scale(256, Image.BICUBIC)(B)
-
- # apply the same transform to both A and B
- transform_params = get_params(self.opt, A.size)
- A_transform = get_transform(self.opt, transform_params)
- B_transform = get_transform(self.opt, transform_params)
-
- B_tensor = inst_tensor = feat_tensor = 0
- A_tensor = A_transform(A)
- B_tensor = B_transform(B)
-
- input_dict = {'label': A_tensor, 'inst': inst_tensor, 'image': B_tensor,
- 'feat': feat_tensor, 'path': path}
- return input_dict
-
- def __len__(self):
-
- if self.opt.isTrain:
- return len(self.filtered_imgs_clean)
- else:
- return len(self.loaded_imgs)
-
- def name(self):
- return 'PairOldPhotos'
-
-
-class PairOldPhotos_with_hole(BaseDataset):
- def initialize(self, opt):
- self.opt = opt
- self.isImage = 'imagegan' in opt.name
- self.task = 'old_photo_restoration_training_mapping'
- self.dir_AB = opt.dataroot
- if opt.isTrain:
- self.load_img_dir_clean= os.path.join(self.dir_AB, "VOC_RGB_JPEGImages.bigfile")
- self.loaded_imgs_clean = BigFileMemoryLoader(self.load_img_dir_clean)
-
- print("-------------Filter the imgs whose size <256 in VOC-------------")
- self.filtered_imgs_clean = []
- for i in range(len(self.loaded_imgs_clean)):
- img_name, img = self.loaded_imgs_clean[i]
- h, w = img.size
- if h < 256 or w < 256:
- continue
- self.filtered_imgs_clean.append((img_name, img))
-
- print("--------Origin image num is [%d], filtered result is [%d]--------" % (
- len(self.loaded_imgs_clean), len(self.filtered_imgs_clean)))
-
- else:
- self.load_img_dir=os.path.join(self.dir_AB,opt.test_dataset)
- self.loaded_imgs=BigFileMemoryLoader(self.load_img_dir)
-
- self.loaded_masks = BigFileMemoryLoader(opt.irregular_mask)
-
- self.pid = os.getpid()
-
- def __getitem__(self, index):
-
-
-
- if self.opt.isTrain:
- img_name_clean,B = self.filtered_imgs_clean[index]
- path = os.path.join(self.load_img_dir_clean, img_name_clean)
-
-
- B=transforms.RandomCrop(256)(B)
- A=online_add_degradation_v2(B)
- ### Remind: A is the input and B is corresponding GT
-
- else:
- img_name_A,A=self.loaded_imgs[index]
- img_name_B,B=self.loaded_imgs[index]
- path = os.path.join(self.load_img_dir, img_name_A)
-
- #A=A.resize((256,256))
- A=transforms.CenterCrop(256)(A)
- B=A
-
- if random.uniform(0,1)<0.1 and self.opt.isTrain:
- A=A.convert("L")
- B=B.convert("L")
- A=A.convert("RGB")
- B=B.convert("RGB")
- ## In P, we convert the RGB into L
-
- if self.opt.isTrain:
- mask_name,mask=self.loaded_masks[random.randint(0,len(self.loaded_masks)-1)]
- else:
- mask_name, mask = self.loaded_masks[index%100]
- mask = mask.resize((self.opt.loadSize, self.opt.loadSize), Image.NEAREST)
-
- if self.opt.random_hole and random.uniform(0,1)>0.5 and self.opt.isTrain:
- mask=zero_mask(256)
-
- if self.opt.no_hole:
- mask=zero_mask(256)
-
-
- A,_=irregular_hole_synthesize(A,mask)
-
- if not self.opt.isTrain and self.opt.hole_image_no_mask:
- mask=zero_mask(256)
-
- transform_params = get_params(self.opt, A.size)
- A_transform = get_transform(self.opt, transform_params)
- B_transform = get_transform(self.opt, transform_params)
-
- if transform_params['flip'] and self.opt.isTrain:
- mask=mask.transpose(Image.FLIP_LEFT_RIGHT)
-
- mask_tensor = transforms.ToTensor()(mask)
-
-
- B_tensor = inst_tensor = feat_tensor = 0
- A_tensor = A_transform(A)
- B_tensor = B_transform(B)
-
- input_dict = {'label': A_tensor, 'inst': mask_tensor[:1], 'image': B_tensor,
- 'feat': feat_tensor, 'path': path}
- return input_dict
-
- def __len__(self):
-
- if self.opt.isTrain:
- return len(self.filtered_imgs_clean)
-
- else:
- return len(self.loaded_imgs)
-
- def name(self):
- return 'PairOldPhotos_with_hole'
\ No newline at end of file
diff --git a/spaces/matthoffner/AudioCraft_Plus/docs/ENCODEC.md b/spaces/matthoffner/AudioCraft_Plus/docs/ENCODEC.md
deleted file mode 100644
index efc2bcc7ec50190b907c887b920b70fd799c6953..0000000000000000000000000000000000000000
--- a/spaces/matthoffner/AudioCraft_Plus/docs/ENCODEC.md
+++ /dev/null
@@ -1,179 +0,0 @@
-# EnCodec: High Fidelity Neural Audio Compression
-
-AudioCraft provides the training code for EnCodec, a state-of-the-art deep learning
-based audio codec supporting both mono stereo audio, presented in the
-[High Fidelity Neural Audio Compression][arxiv] paper.
-Check out our [sample page][encodec_samples].
-
-## Original EnCodec models
-
-The EnCodec models presented in High Fidelity Neural Audio Compression can be accessed
-and used with the [EnCodec repository](https://github.com/facebookresearch/encodec).
-
-**Note**: We do not guarantee compatibility between the AudioCraft and EnCodec codebases
-and released checkpoints at this stage.
-
-
-## Installation
-
-Please follow the AudioCraft installation instructions from the [README](../README.md).
-
-
-## Training
-
-The [CompressionSolver](../audiocraft/solvers/compression.py) implements the audio reconstruction
-task to train an EnCodec model. Specifically, it trains an encoder-decoder with a quantization
-bottleneck - a SEANet encoder-decoder with Residual Vector Quantization bottleneck for EnCodec -
-using a combination of objective and perceptual losses in the forms of discriminators.
-
-The default configuration matches a causal EnCodec training with at a single bandwidth.
-
-### Example configuration and grids
-
-We provide sample configuration and grids for training EnCodec models.
-
-The compression configuration are defined in
-[config/solver/compression](../config/solver/compression).
-
-The example grids are available at
-[audiocraft/grids/compression](../audiocraft/grids/compression).
-
-```shell
-# base causal encodec on monophonic audio sampled at 24 khz
-dora grid compression.encodec_base_24khz
-# encodec model used for MusicGen on monophonic audio sampled at 32 khz
-dora grid compression.encodec_musicgen_32khz
-```
-
-### Training and valid stages
-
-The model is trained using a combination of objective and perceptual losses.
-More specifically, EnCodec is trained with the MS-STFT discriminator along with
-objective losses through the use of a loss balancer to effectively weight
-the different losses, in an intuitive manner.
-
-### Evaluation stage
-
-Evaluations metrics for audio generation:
-* SI-SNR: Scale-Invariant Signal-to-Noise Ratio.
-* ViSQOL: Virtual Speech Quality Objective Listener.
-
-Note: Path to the ViSQOL binary (compiled with bazel) needs to be provided in
-order to run the ViSQOL metric on the reference and degraded signals.
-The metric is disabled by default.
-Please refer to the [metrics documentation](../METRICS.md) to learn more.
-
-### Generation stage
-
-The generation stage consists in generating the reconstructed audio from samples
-with the current model. The number of samples generated and the batch size used are
-controlled by the `dataset.generate` configuration. The output path and audio formats
-are defined in the generate stage configuration.
-
-```shell
-# generate samples every 5 epoch
-dora run solver=compression/encodec_base_24khz generate.every=5
-# run with a different dset
-dora run solver=compression/encodec_base_24khz generate.path=
-# limit the number of samples or use a different batch size
-dora grid solver=compression/encodec_base_24khz dataset.generate.num_samples=10 dataset.generate.batch_size=4
-```
-
-### Playing with the model
-
-Once you have a model trained, it is possible to get the entire solver, or just
-the trained model with the following functions:
-
-```python
-from audiocraft.solvers import CompressionSolver
-
-# If you trained a custom model with signature SIG.
-model = CompressionSolver.model_from_checkpoint('//sig/SIG')
-# If you want to get one of the pretrained models with the `//pretrained/` prefix.
-model = CompressionSolver.model_from_checkpoint('//pretrained/facebook/encodec_32khz')
-# Or load from a custom checkpoint path
-model = CompressionSolver.model_from_checkpoint('/my_checkpoints/foo/bar/checkpoint.th')
-
-
-# If you only want to use a pretrained model, you can also directly get it
-# from the CompressionModel base model class.
-from audiocraft.models import CompressionModel
-
-# Here do not put the `//pretrained/` prefix!
-model = CompressionModel.get_pretrained('facebook/encodec_32khz')
-model = CompressionModel.get_pretrained('dac_44khz')
-
-# Finally, you can also retrieve the full Solver object, with its dataloader etc.
-from audiocraft import train
-from pathlib import Path
-import logging
-import os
-import sys
-
-# uncomment the following line if you want some detailed logs when loading a Solver.
-logging.basicConfig(stream=sys.stderr, level=logging.INFO)
-# You must always run the following function from the root directory.
-os.chdir(Path(train.__file__).parent.parent)
-
-
-# You can also get the full solver (only for your own experiments).
-# You can provide some overrides to the parameters to make things more convenient.
-solver = train.get_solver_from_sig('SIG', {'device': 'cpu', 'dataset': {'batch_size': 8}})
-solver.model
-solver.dataloaders
-```
-
-### Importing / Exporting models
-
-At the moment we do not have a definitive workflow for exporting EnCodec models, for
-instance to Hugging Face (HF). We are working on supporting automatic convertion between
-AudioCraft and Hugging Face implementations.
-
-We still have some support for fine tuning an EnCodec model coming from HF in AudioCraft,
-using for instance `continue_from=//pretrained/facebook/encodec_32k`.
-
-An AudioCraft checkpoint can be exported in a more compact format (excluding the optimizer etc.)
-using `audiocraft.utils.export.export_encodec`. For instance, you could run
-
-```python
-from audiocraft.utils import export
-from audiocraft import train
-xp = train.main.get_xp_from_sig('SIG')
-export.export_encodec(
- xp.folder / 'checkpoint.th',
- '/checkpoints/my_audio_lm/compression_state_dict.bin')
-
-
-from audiocraft.models import CompressionModel
-model = CompressionModel.get_pretrained('/checkpoints/my_audio_lm/compression_state_dict.bin')
-
-from audiocraft.solvers import CompressionSolver
-# The two are strictly equivalent, but this function supports also loading from non already exported models.
-model = CompressionSolver.model_from_checkpoint('//pretrained//checkpoints/my_audio_lm/compression_state_dict.bin')
-```
-
-We will see then how to use this model as a tokenizer for MusicGen/Audio gen in the
-[MusicGen documentation](./MUSICGEN.md).
-
-### Learn more
-
-Learn more about AudioCraft training pipelines in the [dedicated section](./TRAINING.md).
-
-
-## Citation
-```
-@article{defossez2022highfi,
- title={High Fidelity Neural Audio Compression},
- author={Défossez, Alexandre and Copet, Jade and Synnaeve, Gabriel and Adi, Yossi},
- journal={arXiv preprint arXiv:2210.13438},
- year={2022}
-}
-```
-
-
-## License
-
-See license information in the [README](../README.md).
-
-[arxiv]: https://arxiv.org/abs/2210.13438
-[encodec_samples]: https://ai.honu.io/papers/encodec/samples.html
diff --git a/spaces/matthoffner/chatbot-mini/components/Settings/Import.tsx b/spaces/matthoffner/chatbot-mini/components/Settings/Import.tsx
deleted file mode 100644
index 7268057cd62ccf60cc62e74147927075f6775638..0000000000000000000000000000000000000000
--- a/spaces/matthoffner/chatbot-mini/components/Settings/Import.tsx
+++ /dev/null
@@ -1,39 +0,0 @@
-import { IconFileImport } from '@tabler/icons-react';
-import { FC } from 'react';
-
-import { useTranslation } from 'next-i18next';
-
-import { SupportedExportFormats } from '@/types/export';
-
-
-interface Props {
- onImport: (data: SupportedExportFormats) => void;
-}
-
-export const Import: FC = ({ onImport }) => {
- const { t } = useTranslation('sidebar');
- return (
- <>
- {
- if (!e.target.files?.length) return;
-
- const file = e.target.files[0];
- const reader = new FileReader();
- reader.onload = (e) => {
- let json = JSON.parse(e.target?.result as string);
- onImport(json);
- };
- reader.readAsText(file);
- }}
- />
-
-
- >
- );
-};
diff --git a/spaces/merve/anonymization/source/_posts/2020-09-27-diversity-metrics.md b/spaces/merve/anonymization/source/_posts/2020-09-27-diversity-metrics.md
deleted file mode 100644
index 4c84423fe9a6f8566a0b7182bc378feec97d9654..0000000000000000000000000000000000000000
--- a/spaces/merve/anonymization/source/_posts/2020-09-27-diversity-metrics.md
+++ /dev/null
@@ -1,100 +0,0 @@
----
-template: post.html
-title: Measuring Diversity
-titlex: Diversity and Inclusion Metrics
-summary: Search results that reflect historic inequities can amplify stereotypes and perpetuate under-representation. Carefully measuring diversity in data sets can help.
-shareimg: https://pair.withgoogle.com/explorables/images/measuring-diversity.png
-permalink: /measuring-diversity/
-date: 2021-03-01
----
-
-
-
-Search, ranking and recommendation systems can help find useful documents in large datasets. However, these datasets reflect the biases of the society in which they were created and the systems risk re-entrenching those biases. For example, if someone who is not a white man searches for "CEO pictures" and sees a [page of white men](https://www.nytimes.com/interactive/2018/04/24/upshot/women-and-men-named-john.html), they may feel that only white men can be CEOs, further perpetuating lack of representation at companies' executive levels.
-
-Using the careful quantification outlined in a recent paper, [Diversity and Inclusion Metrics in Subset Selection](https://arxiv.org/pdf/2002.03256.pdf), we can quantify biases and push these systems to return a wider range of results.
-
-The mathematics of all this is a little easier to follow with abstract shapes. Let's take a look at some of them:
-
-
-
-Suppose we want to return about 30% green boxes to reflect the distribution of some larger universe of shapes. Try clicking on the shapes below to select some of them — can you find a better subset to return?
-
-
-
-Another diversity metric we care about is the percentage of dots... how close to 35% dots can you get?
-
-
-
-If we can only return a single subset, how should we consider multiple diversity metrics? Sometimes it isn't possible to reduce the difference of every metric to zero. One natural approach: find the selection with the **lowest mean difference** across all the metrics to get as close as possible to all the targets.
-
-In other circumstances, like picking a panel of speakers, avoiding badly representing any single category might be more important. This can be done by finding the subset with the **lowest max difference**. Try minimizing both below:
-
-
-
-Notice that minimizing the mean results in a different subset than minimizing the max; how else might using one over the other change the results?
-
-### Ranking Measures
-
-We can pull out more detail by showing how the mean difference and maximum difference rank lots of sets. Below, there are 20 sets of 10 shapes sorted by the two measures. Try adjusting the target slider on the left to see how the rankings change; each set's percentage of green, dots and small shapes are shown in the small histograms.
-
-
-
-At the extremes, the choice of measure can have a big impact: if we want to try and return all green results, we can shift the green target up to 100%. With this target, the minimum difference basically sorts the sets by the number of green items and uses the other targets as a tiebreaker. In contrast, sorting by the mean difference balances the green target more with the dot and small targets.
-
-
-
-Beyond mean and max differences, there are more ways to combine diversity metrics, like taking the cross of two metrics to account for [intersectionality](https://en.wikipedia.org/wiki/Intersectionality). The absolute value of the difference in target and actual percentages can also be quantified in other ways — you might want to penalize undershooting more than overshooting, for example. It's important to keep in mind what exactly you're trying to maximize and the dataset that you're operating on.
-
-### Which Measure is Best?
-
-In a vacuum, all of these ranking methods are defensible. Picking one requires knowledge of the dataset and broader societal context.
-
-For example, the doctors on the left have more variance along the shirt color attribute, but they're less diverse by gender than the doctors on the right. With the shirt color and gender targets we've picked, the two subsets have the same mean and max differences However, in most applications, it's more important to have a representative sample of socially relevant characteristics, like gender, rather than something less salient, like clothing color.
-
-
-
-Just selecting a diverse sample isn't sufficient either. [Diversity and Inclusion Metrics in Subset Selection](https://arxiv.org/pdf/2002.03256.pdf) introduces a way of measuring "inclusion" - how well does the searcher feel represented in the results?
-
-Below, we have gender diversity, without inclusion for women, in the “construction worker” image domain. Masculine-presenting individuals are shown in realistic, modern construction worker situations, while feminine-presenting individuals and other gender presentations are depicted as historic nostalgia, toys, clipart, or passive.
-
-
-
-The context of the query and the searcher also plays in the quality of search results. A search for "work clothing" that shows a mixed palette of colors for men's clothing and only pink women's clothing might make the searcher feel that women need to appear stereotypically feminine in a professional setting. But the same set of women's clothes might be appropriate to show for a "pink women work clothes" search or if the searcher had previously expressed a preference for pink.
-
-We saw how a small switch from mean to max made a huge difference in what abstract shapes are returned – and how things can get even more complex when socially salient characteristics are layered in. Defaults and small decisions can encode our priorities and values; intentionally thinking about how diversity and inclusion are being measured and which characteristics are emphasized is a step towards designing more equitable systems.
-
-### More Reading
-
-The [Diversity and Inclusion Metrics](https://arxiv.org/pdf/2002.03256.pdf) paper has a [Colab](https://colab.research.google.com/github/PAIR-code/ai-explorables/blob/master/source/measuring-diversity/diversity-and-inclusion.ipynb) with a detailed desciption of the metrics, additional visualizations and a reference Python implementation.
-
-The difficulties of [measuring fairness](https://pair.withgoogle.com/explorables/measuring-fairness/) in general have been well studied; subset selection is still an active area of research. [Fairness of Exposure in Rankings](https://www.cs.cornell.edu/~tj/publications/singh_joachims_18a.pdf) proposes a ranking algorithm that incorporates fairness constraints. [Toward creating a fairer ranking in search engine results](https://www.ilab.cs.rutgers.edu/~rg522/publication/gao-2020-ipm/gao-2020-ipm.pdf) measures diversity bias in actual search results.
-
-Inferring user preferences is also tricky; you can checkout ways to design for user feedback and control over queries in the [People + AI Guidebook](https://pair.withgoogle.com/chapter/feedback-controls/).
-
-### Credits
-
-Adam Pearce, Dylan Baker, Ellen Jiang, Meg Mitchell\* and Timnit Gebru\* // March 2021
-
-*Work done while at Google
-
-Thanks to Alex Hanna, Carey Radebaugh, Emily Denton, Fernanda Viégas, James Wexler, Jess Holbrook, Ludovic Peran, Martin Wattenberg, Michael Terry, Yannick Assogba and Zan Armstrong for their help with this piece.
-
-
-
More Explorables
-
-
-
-
-
-
-
-
-
-
-
-
-
-
-
\ No newline at end of file
diff --git a/spaces/merve/data-leak/source/private-and-fair/accuracy-v-privacy-class.js b/spaces/merve/data-leak/source/private-and-fair/accuracy-v-privacy-class.js
deleted file mode 100644
index 39daddb629006c967bfa8c3a6c1d43fc9887bc1b..0000000000000000000000000000000000000000
--- a/spaces/merve/data-leak/source/private-and-fair/accuracy-v-privacy-class.js
+++ /dev/null
@@ -1,285 +0,0 @@
-var state = {
- dataset_size: 15000,
- threshold: .8,
- label: 8
-}
-
-var sel = d3.select('.accuracy-v-privacy-class').html('')
- .at({role: 'graphics-document', 'aria-label': `Line chart showing that high accuracy models can still perform poorly on some digit classes.`})
-
-async function loadData(){
- var rawData = await util.getFile(`cns-cache/grid_${state.dataset_size}trainpoints_test_labels.csv`)
-
- rawData.forEach(d => {
- delete d['']
- d.i = +d.i
- d.label = +d.label
- })
-
- var aVal2Meta = {}
- var metadata = await util.getFile('cns-cache/model_grid_test_accuracy.json')
- metadata
- .filter(d => d.dataset_size == state.dataset_size)
- .forEach(d => aVal2Meta['aVal_' + d.aVal] = d)
-
- var allCols = d3.keys(rawData[0])
- .filter(d => d.includes('aVal'))
- .map(key => {
- var {epsilon, aVal} = aVal2Meta[key]
- return {key, epsilon, aVal}
- })
-
- var byDigit = d3.nestBy(rawData, d => d.label)
- byDigit.forEach(d => {
- d.label = +d.key
- })
- byDigit.forEach(digitClass => {
- digitClass.cols = allCols.map(({key, epsilon}, colIndex) => {
- return {
- key,
- colIndex,
- epsilon,
- digitClass,
- label: digitClass.label,
- accuracy: d3.mean(digitClass, d => d[key] > state.threshold)
- }
- })
- })
-
- var data = _.flatten(byDigit.map(d => d.cols))
- .filter(d => util.epsilonExtent[1] <= d.epsilon && d.epsilon <= util.epsilonExtent[0])
- var byLabel = d3.nestBy(data, d => d.label)
- byLabel.forEach((d, i) => {
- d.label = d.key
- })
-
- return {data, byLabel}
-}
-
-
-async function initChart(){
- var {data, byLabel} = await loadData()
-
- var c = d3.conventions({
- sel: sel.append('div'),
- height: 400,
- margin: {bottom: 75, top: 5},
- layers: 'ds',
- })
-
- c.x = d3.scaleLog().domain(util.epsilonExtent).range(c.x.range())
- c.xAxis = d3.axisBottom(c.x).tickFormat(d => {
- var rv = d + ''
- if (rv.split('').filter(d => d !=0 && d != '.')[0] == 1) return rv
- })
-
- c.yAxis.tickFormat(d => d3.format('.0%')(d))//.ticks(8)
- d3.drawAxis(c)
- util.addAxisLabel(c, 'Higher Privacy →', '')
- util.ggPlotBg(c, false)
- c.layers[0].append('div')
- .st({fontSize: 12, color: '#555', width: 120*2, textAlign: 'center', lineHeight: '1.3em', verticalAlign: 'top'})
- .translate([c.width/2 - 120, c.height + 45])
- .html('in ε')
-
- var line = d3.line().x(d => c.x(d.epsilon)).y(d => c.y(d.accuracy))
-
- var lineSel = c.svg.append('g').appendMany('path.accuracy-line', byLabel)
- .at({
- d: line,
- fill: 'none',
- stroke: '#000',
- // opacity: 0,
- })
- .on('mousemove', setActiveLabel)
-
- var circleSel = c.svg.append('g')
- .appendMany('g.accuracy-circle', data)
- .translate(d => [c.x(d.epsilon), c.y(d.accuracy)])
- .on('mousemove', setActiveLabel)
- // .call(d3.attachTooltip)
-
- circleSel.append('circle')
- .at({r: 7, stroke: '#fff'})
-
- circleSel.append('text')
- .text(d => d.label)
- .at({textAnchor: 'middle', fontSize: 10, fill: '#fff', dy: '.33em'})
-
- setActiveLabel(state)
- function setActiveLabel({label}){
- lineSel
- .classed('active', 0)
- .filter(d => d.label == label)
- .classed('active', 1)
- .raise()
-
- circleSel
- .classed('active', 0)
- .filter(d => d.label == label)
- .classed('active', 1)
- .raise()
-
- state.label = label
- }
-
-
- async function updateDatasetSize(){
- var newData = await loadData()
- data = newData.data
- byLabel = newData.byLabel
-
- lineSel.data(byLabel)
- .transition()
- .at({d: line})
-
- circleSel.data(data)
- .transition()
- .translate(d => [c.x(d.epsilon), c.y(d.accuracy)])
-
- c.svg.select('text.annotation').remove()
- }
-
- function updateThreshold(){
- data.forEach(d => {
- d.accuracy = d3.mean(d.digitClass, e => e[d.key] > state.threshold)
- })
-
- lineSel.at({d: line})
- circleSel.translate(d => [c.x(d.epsilon), c.y(d.accuracy)])
-
- c.svg.select('.y .axis-label').text(`Test Points With More Than ${d3.format('.2%')(state.threshold)} Confidence In Label`)
-
- c.svg.select('text.annotation').remove()
- }
- updateThreshold()
-
- return {c, updateDatasetSize, updateThreshold}
-}
-
-
-async function init(){
- sel.append('div.chart-title').text('High accuracy models can still perform poorly on some digit classes')
-
- var chart = await initChart()
-
- var buttonRowSel = sel.append('div.button-row')
- .st({height: 50})
-
- var buttonSel = buttonRowSel.append('div')
- .st({width: 500})
- .append('span.chart-title').text('Training points')
- .parent()
- .append('div').st({display: 'inline-block', width: 300, marginLeft: 10})
- .append('div.digit-button-container.dataset_size')
- .appendMany('div.button', [2000, 3750, 7500, 15000, 30000, 60000])
- .text(d3.format(','))
- .classed('active', d => d == state.dataset_size)
- .on('click', d => {
- buttonSel.classed('active', e => e == d)
- state.dataset_size = d
- chart.updateDatasetSize()
- })
-
- buttonRowSel.append('div.conf-slider')
- .append('span.chart-title').text('Confidence threshold')
- .parent()
- .append('input.slider-native')
- .at({
- type: 'range',
- min: .0001,
- max: .9999,
- step: .0001,
- value: state.threshold,
- })
- .on('input', function(){
- state.threshold = this.value
- chart.updateThreshold()
- })
-
-
- function addSliders(){
- var width = 140
- var height = 30
- var color = '#000'
-
- var sliders = [
- {key: 'threshold', label: 'Confidence threshold', r: [.0001, .9999]},
- ]
- sliders.forEach(d => {
- d.value = state[d.key]
- d.xScale = d3.scaleLinear().range([0, width]).domain(d.r).clamp(1)
- })
-
- d3.select('.conf-slider .slider-container').remove()
- d3.select('.slider-native').remove()
-
- var svgSel = d3.select('.conf-slider').parent()
- // .st({marginTop: 5, marginBottom: 5})
- .appendMany('div.slider-container', sliders)
- .append('svg').at({width, height})
- .append('g').translate([10, 25])
-
- var sliderSel = svgSel
- .on('click', function(d){
- d.value = d.xScale.invert(d3.mouse(this)[0])
- renderSliders(d)
- })
- .classed('slider', true)
- .st({cursor: 'pointer'})
-
- var textSel = sliderSel.append('text.annotation')
- .at({y: -15, fontWeight: 300, textAnchor: 'middle', x: 180/2})
-
- sliderSel.append('rect')
- .at({width, height, y: -height/2, fill: 'rgba(0,0,0,0)'})
-
- sliderSel.append('path').at({
- d: `M 0 -.5 H ${width}`,
- stroke: color,
- strokeWidth: 1
- })
-
- var leftPathSel = sliderSel.append('path').at({
- d: `M 0 -.5 H ${width}`,
- stroke: color,
- strokeWidth: 3
- })
-
- var drag = d3.drag()
- .on('drag', function(d){
- var x = d3.mouse(this)[0]
- d.value = d.xScale.invert(x)
-
- renderSliders(d)
- })
-
- var circleSel = sliderSel.append('circle').call(drag)
- .at({r: 7, stroke: '#000'})
-
- function renderSliders(d){
- if (d) state[d.key] = d.value
-
- circleSel.at({cx: d => d.xScale(d.value)})
- leftPathSel.at({d: d => `M 0 -.5 H ${d.xScale(d.value)}`})
- textSel
- .at({x: d => d.xScale(d.value)})
- .text(d => d3.format('.2%')(d.value))
- chart.updateThreshold()
- }
- renderSliders()
- }
- addSliders()
-
-
- chart.c.svg.append('text.annotation')
- .translate([505, 212])
- .tspans(d3.wordwrap(`8s are correctly predicted with high confidence much more rarely than other digits`, 25), 12)
- .at({textAnchor: 'end'})
-
-}
-init()
-
-
-
-
diff --git a/spaces/mfrashad/CharacterGAN/netdissect/statedict.py b/spaces/mfrashad/CharacterGAN/netdissect/statedict.py
deleted file mode 100644
index 858a903b57724d9e3a17b8150beea30bdc206b97..0000000000000000000000000000000000000000
--- a/spaces/mfrashad/CharacterGAN/netdissect/statedict.py
+++ /dev/null
@@ -1,100 +0,0 @@
-'''
-Utilities for dealing with simple state dicts as npz files instead of pth files.
-'''
-
-import torch
-from collections.abc import MutableMapping, Mapping
-
-def load_from_numpy_dict(model, numpy_dict, prefix='', examples=None):
- '''
- Loads a model from numpy_dict using load_state_dict.
- Converts numpy types to torch types using the current state_dict
- of the model to determine types and devices for the tensors.
- Supports loading a subdict by prepending the given prefix to all keys.
- '''
- if prefix:
- if not prefix.endswith('.'):
- prefix = prefix + '.'
- numpy_dict = PrefixSubDict(numpy_dict, prefix)
- if examples is None:
- exampels = model.state_dict()
- torch_state_dict = TorchTypeMatchingDict(numpy_dict, examples)
- model.load_state_dict(torch_state_dict)
-
-def save_to_numpy_dict(model, numpy_dict, prefix=''):
- '''
- Saves a model by copying tensors to numpy_dict.
- Converts torch types to numpy types using `t.detach().cpu().numpy()`.
- Supports saving a subdict by prepending the given prefix to all keys.
- '''
- if prefix:
- if not prefix.endswith('.'):
- prefix = prefix + '.'
- for k, v in model.numpy_dict().items():
- if isinstance(v, torch.Tensor):
- v = v.detach().cpu().numpy()
- numpy_dict[prefix + k] = v
-
-class TorchTypeMatchingDict(Mapping):
- '''
- Provides a view of a dict of numpy values as torch tensors, where the
- types are converted to match the types and devices in the given
- dict of examples.
- '''
- def __init__(self, data, examples):
- self.data = data
- self.examples = examples
- self.cached_data = {}
- def __getitem__(self, key):
- if key in self.cached_data:
- return self.cached_data[key]
- val = self.data[key]
- if key not in self.examples:
- return val
- example = self.examples.get(key, None)
- example_type = type(example)
- if example is not None and type(val) != example_type:
- if isinstance(example, torch.Tensor):
- val = torch.from_numpy(val)
- else:
- val = example_type(val)
- if isinstance(example, torch.Tensor):
- val = val.to(dtype=example.dtype, device=example.device)
- self.cached_data[key] = val
- return val
- def __iter__(self):
- return self.data.keys()
- def __len__(self):
- return len(self.data)
-
-class PrefixSubDict(MutableMapping):
- '''
- Provides a view of the subset of a dict where string keys begin with
- the given prefix. The prefix is stripped from all keys of the view.
- '''
- def __init__(self, data, prefix=''):
- self.data = data
- self.prefix = prefix
- self._cached_keys = None
- def __getitem__(self, key):
- return self.data[self.prefix + key]
- def __setitem__(self, key, value):
- pkey = self.prefix + key
- if self._cached_keys is not None and pkey not in self.data:
- self._cached_keys = None
- self.data[pkey] = value
- def __delitem__(self, key):
- pkey = self.prefix + key
- if self._cached_keys is not None and pkey in self.data:
- self._cached_keys = None
- del self.data[pkey]
- def __cached_keys(self):
- if self._cached_keys is None:
- plen = len(self.prefix)
- self._cached_keys = list(k[plen:] for k in self.data
- if k.startswith(self.prefix))
- return self._cached_keys
- def __iter__(self):
- return iter(self.__cached_keys())
- def __len__(self):
- return len(self.__cached_keys())
diff --git a/spaces/mikefish/CharacterMaker/app.py b/spaces/mikefish/CharacterMaker/app.py
deleted file mode 100644
index 7c9f20eb655b69afcf286f1f98af7cccf1ebf73f..0000000000000000000000000000000000000000
--- a/spaces/mikefish/CharacterMaker/app.py
+++ /dev/null
@@ -1,591 +0,0 @@
-from ast import Interactive
-import gradio as gr
-from langchain.chains import LLMChain
-from langchain.chains import SimpleSequentialChain
-from langchain.llms import OpenAI
-from langchain.prompts import PromptTemplate
-from langchain.chains import SequentialChain
-import openai
-import os
-import base64
-import requests
-import json
-import re
-import codecs
-import random
-
-## Remember to set $env:OPENAI_API_KEY="keyhere"
-openai.api_key = os.getenv("OPENAI_API_KEY")
-#openai.api_key = ""
-def getAndParseQuickStart(text, count):
- print("Asking AI for a character of " + text + " with trait count:")
- print(count)
- instruction_prompt = f"""
- You are an AI bot that creates profiles of characters based on a simple input. You generate and give detailed characters in the following format:
- Name: descriptors
- Mind: descriptors (comma separated properties)
- Personality: descriptors (comma separated properties, at least {count} traits)
- Body: descriptors (comma separated properties, at least {count} traits)
- Likes: descriptors (comma separated properties, at least {count} traits)
- Hates: descriptors (comma separated properties, at least {count} traits)
- Attributes: descriptors (comma separated properties, at least {count} traits)
- Clothes: descriptors (comma separated properties)
- Sex: descriptor (choose only from: Male, Female, or Other)
- Sexuality: descriptor (choose only from: Gay, Straight, Bi, or Asexual. Default to Asexual)
- Age: descriptor (as an integer, no additional text)
- Description: descriptor (3 sentences, do not repeat any previous information)
- Personality_Summary: descriptor
-
- """
- prompt = """
- Please generate a character based on the following description assuming this is a real person,
- make sure any dialog is accurate as to how this specific character sounds. The dialog should give a good indication
- of the manner in which the character speaks:\n
- """ + text
-
- response = openai.ChatCompletion.create(
- model="gpt-3.5-turbo-0613",
- messages=[
- {"role": "system", "content": instruction_prompt},
- {"role": "user", "content": prompt}
- ])
-
- #print(response)
- # Parse the AI's response
- response_content = response['choices'][0]['message']['content']
- print(response_content)
- example_chat_index = response_content.find('Example_Chat:')
- if example_chat_index != -1:
- # Extract 'Example Chat' and everything after it
- example_chat_content = response_content[example_chat_index:]
- # Split the content into lines
- example_chat_lines = example_chat_content.split('\n')
- # The first line is 'Example Chat: '
- # So we need to remove 'Example Chat: ' from it
- example_chat_lines[0] = example_chat_lines[0][len('Example_Chat: '):]
- # Join the lines back together to get the full 'Example Chat'
- example_chat = '\n'.join(example_chat_lines)
- traits_dict = {'Example_Chat': example_chat.strip()}
- # Remove the 'Example Chat' part from the response content so it won't be processed again in the loop
- api_response = response_content[:example_chat_index]
- else:
- traits_dict = {}
-
- traits = response_content.split('\n')
- print(traits)
- raw = traits
- #print(locked_fields)
- for trait in traits:
- if ': ' in trait:
- key, value = trait.split(': ', 1) # Split at the first occurrence of ': '
- key = key.lower()
- key = key.replace(' ', '_')
- #if key in locked_fields:
- # continue
- if key == 'name':
- traits_dict[key.capitalize()] = value
- elif key == 'first_message':
- traits_dict['First_Message'] = value
- elif key == 'personality_summary':
- traits_dict['Personality_Summary'] = value
- else:
- traits_dict[key.capitalize()] = value
- charName = traits_dict.get('Name', '')
- personality = traits_dict.get('Personality', '')
- body = traits_dict.get('Body', '')
- likes = traits_dict.get('Likes', '')
- hates = traits_dict.get('Hates', '')
- sex = traits_dict.get('Sex', '')
- sexuality = traits_dict.get('Sexuality', '')
- age = traits_dict.get('Age', '')
- description = traits_dict.get('Description', '')
- personalityProfile = traits_dict.get('Personality_Profile', '')
- attributes = traits_dict.get('Attributes', '')
- return [
- raw,
- charName,
- personality,
- body,
- likes,
- hates,
- sex,
- sexuality,
- age,
- description,
- personalityProfile,
- attributes
- ]
-
-def generateSpeakingStyle(text):
- print("Asking AI for a speaking style from:\n" + text)
- prompt = """
- Here is a detailed summary of a character:\n
- """ + text + """
- \n
- Based on that, determine the speaking style that would best express how this character would talk. For each of the following, choose the best option for this character:
- """ + prompt_Personality + """
- Please return your choices in JSON format: {"property":"choice", "property":"choice", ....etc}\n
- Do not change any capitalization. Do not include any new lines. Only a correct JSON format.
- """
-
- response = openai.ChatCompletion.create(
- model="gpt-4-0613",
- messages=[
- {"role": "system", "content": "You are a world-class writer and character designer."},
- {"role": "user", "content": prompt}
- ])
- print(response)
- response_content = response['choices'][0]['message']['content']
- response = json.loads(response_content)
- return [
- response.get('formality', ''),
- response.get('pace', ''),
- response.get('rhythm', ''),
- response.get('volume', ''),
- response.get('emotionality', ''),
- response.get('directness', ''),
- response.get('humor', ''),
- response.get('enunciation', ''),
- response.get('expressiveness', ''),
- response.get('accent', ''),
- response.get('politeness', ''),
- response.get('vocabulary', ''),
- response.get('interruptions', ''),
- response.get('hesitations', ''),
- response.get('sentence structure', ''),
- response.get('sarcasm', ''),
- response.get('colloquialisms', ''),
- response.get('energy level', ''),
- response.get('defiance/rebellion', ''),
- response.get('playfulness', ''),
- response.get('vulgarity', ''),
- response.get('idiosyncrasies', ''),
- response.get('emotional tone', ''),
- response.get('context adaptability', ''),
- response.get('subtext', ''),
- response.get('metaphorical language', ''),
- response.get('cultural references', ''),
- response.get('storytelling ability', '')
- ]
-
-def saveKey(text):
- openai.api_key = text
-
-with gr.Blocks() as demo:
- rawQuickResults=gr.Textbox(visible=False)
- gr.Markdown(
- """
- # Character Maker v0.1 #
- **Right now this is using my OPENAI key on the backend until I re-write it for local inference.
- If you get an error it's likely because I've hit a limit. If that happens, if you have an OPENAI key you can put it in here.**
- If anyone wants to help with this please get in touch!
- """)
- with gr.Row():
- myKey = gr.Text(label="OPENAI API KEY (optional, needed if Errors)", info="must have gpt4 access (for now)")
- keySave = gr.Button(value="Set")
- keySave.click(saveKey, inputs=[myKey])
- with gr.Row():
- gr.Markdown(
- """
- This is a very early prototype of a character generator/assistant.
- The goal is to create a detailed but concise profile that can be used in chat programs and guide the AI in acting realistic.
- 1. Adjust the traits count slider for how many traits you want the AI to return
- 2. Give a general description or starting point and click (1) Generate Quick Start (ex. "Winnie the Pooh but he is a cat")
- 3. Edit response fields as needed
- 4. Click (2) Analyze to create a Psychological Profile
- 5. Click (3) Generate Speaking Style
- 6. Edit any fields as you want
- 7. Click (4) Create JSON
- """)
- gr.Markdown(
- """
- ### Not Working Yet ###
- - Scenario, Setting, Dialogue
- - Secrets
-
- ### To Do ###
- - Scenario and setting creator
- - Chat sample creator and chooser
- - Testable playground built-in
- - Add grammar and spelling variable
- - Have AI choose and expand on appropriate secrets
- - Use Dall-E to generate profile pics
- - Selection of JSON format to export as
- - Add token count
- - Add shorten function to send JSON to AI and ask to remove duplicate information
- """)
- numTraits = 8
- def updateTraitCount(text):
- numTraits = text
- print (numTraits)
- def generateMedicalSecrets(count):
- with open('medical_secrets.txt', 'r') as file:
- lines = file.readlines()
- selected_lines = random.sample(lines, count)
- codes = [re.search(r'\((.*?)\)', line).group(1) for line in selected_lines]
- codes_string = ', '.join(codes)
- print(codes)
- return "medical conditions: " + codes_string
-
- with gr.Row():
- quickStart = gr.TextArea(label="Quick Start", info="Use AI to fill out all fields based on your description.")
- generate = gr.Button("1. Generate Quick Start")
-
- with gr.Row():
- with gr.Column(scale=1, min_width=600):
- trait_count = gr.Slider(4, 20, label="Minimum number of traits for each category", interactive=True, step=1.0, value=8)
- trait_count.change(updateTraitCount, inputs=[trait_count])
- charName = gr.Textbox(label="Name", interactive=True)
- personality = gr.Textbox(label="Personality", interactive=True)
- body = gr.Textbox(label="Physical Description", interactive=True)
- with gr.Row():
- likes = gr.Textbox(label="Likes", interactive=True)
- hates = gr.Textbox(label="Hates", interactive=True)
- with gr.Row():
- sex = gr.Dropdown(["Male", "Female", "Other"], label="Sex", interactive=True)
- sexuality = gr.Textbox(label="Sexuality", interactive=True)
- age = gr.Slider(1, 100, label="Age", info="Choose between 1 and 100", interactive=True, step=1.0, value=21)
- description = gr.Textbox(label="Description", interactive=True)
- attributes = gr.Textbox(label="Attributes", interactive=True)
- with gr.Row():
- personalityProfile = gr.Textbox(label="Psychological Profile", interactive=True)
- generatePersonality = gr.Button("2. Analyze Personality")
-
- with gr.Accordion("Speaking Traits"):
- genProfile = gr.Button("3. Generate Speaking Style", interactive=True)
- with gr.Column(scale=1, min_width=600):
- with gr.Row():
- formality = gr.Dropdown(["Very formal", "Formal", "Neutral", "Informal", "Very informal"], label="Formality", interactive=True)
- pace = gr.Dropdown(["Very fast", "Fast", "Moderate", "Slow", "Very slow"], label="Pace", interactive=True)
- rhythm = gr.Dropdown(["Choppy", "Staccato", "Varied", "Flowing", "Melodious"], label="Rhythm", interactive=True)
- volume = gr.Dropdown(["Very loud", "Loud", "Moderate", "Soft", "Very soft"], label="Volume", interactive=True)
- emotionality = gr.Dropdown(["Very expressive", "Expressive", "Neutral", "Restrained", "Very restrained"], label="Emotionality", interactive=True)
- directness = gr.Dropdown(["Very direct", "Direct", "Balanced", "Indirect", "Very indirect"], label="Directness", interactive=True)
-
- with gr.Row():
- humor = gr.Dropdown(["Frequently humorous", "Occasionally humorous", "Neutral", "Occasionally serious", "Frequently serious"], label="Humor", interactive=True)
- enunciation = gr.Dropdown(["Very clear", "Clear", "Neutral", "Relaxed", "Mumbled"], label="Enunciation", interactive=True)
- expressiveness = gr.Dropdown(["Very expressive", "Expressive", "Neutral", "Reserved", "Very reserved"], label="Expressiveness", interactive=True)
- accent_dialect = gr.Dropdown(["Strong regional accent", "Mild regional accent", "Neutral", "Mild foreign accent", "Strong foreign accent"], label="Accent/Dialect", interactive=True)
- politeness = gr.Dropdown(["Very polite", "Polite", "Neutral", "Blunt", "Very blunt"], label="Politeness", interactive=True)
- vocabulary = gr.Dropdown(["Highly sophisticated", "Sophisticated", "Average", "Basic", "Very basic"], label="Vocabulary", interactive=True)
-
- with gr.Row():
- interruptions = gr.Dropdown(["Frequently interrupts", "Occasionally interrupts", "Balanced", "Occasionally allows others to interrupt", "Frequently allows others to interrupt"], label="Interruptions", interactive=True)
- hesitations = gr.Dropdown(["Frequently hesitates", "Occasionally hesitates", "Balanced", "Occasionally fluent", "Frequently fluent"], label="Hesitations", interactive=True)
- sentence_structure = gr.Dropdown(["Very complex", "Complex", "Average", "Simple", "Very simple"], label="Sentence Structure", interactive=True)
- sarcasm = gr.Dropdown(["Very sarcastic", "Sarcastic", "Occasionally sarcastic", "Rarely sarcastic", "Never sarcastic"], label="Sarcasm", interactive=True)
- colloquialisms = gr.Dropdown(["Frequently uses colloquialisms", "Occasionally uses colloquialisms", "Balanced", "Rarely uses colloquialisms", "Never uses colloquialisms"], label="Colloquialisms", interactive=True)
- energy_level = gr.Dropdown(["Very high energy", "High energy", "Moderate energy", "Low energy", "Very low energy"], label="Energy Level", interactive=True)
- with gr.Row():
- defiance_rebellion = gr.Dropdown(["Frequently defiant", "Occasionally defiant", "Balanced", "Rarely defiant", "Never defiant"], label="Defiance/Rebellion", interactive=True)
- playfulness = gr.Dropdown(["Very playful", "Playful", "Occasionally playful", "Rarely playful", "Never playful"], label="Playfulness", interactive=True)
- vulgarity = gr.Dropdown(["Very vulgar", "Vulgar", "Occasionally vulgar", "Rarely vulgar", "Never vulgar"], label="Vulgarity", interactive=True)
- idiosyncrasies = gr.Dropdown(["Frequent idiosyncrasies", "Occasional idiosyncrasies", "Balanced", "Rare idiosyncrasies", "No idiosyncrasies"], label="Idiosyncrasies", interactive=True)
- emotional_tone = gr.Dropdown(["Very optimistic", "Optimistic", "Neutral", "Pessimistic", "Very pessimistic"], label="Emotional Tone", interactive=True)
- context_adaptability = gr.Dropdown(["Very adaptable", "Adaptable", "Balanced", "Inflexible", "Very inflexible"], label="Context Adaptability", interactive=True)
- with gr.Row():
- subtext = gr.Dropdown(["Frequently uses subtext", "Occasionally uses subtext", "Balanced", "Rarely uses subtext", "Never uses subtext"], label="Subtext", interactive=True)
- metaphorical_language = gr.Dropdown(["Frequently uses metaphorical language", "Occasionally uses metaphorical language", "Balanced", "Rarely uses metaphorical language", "Never uses metaphorical language"], label="Metaphorical Language", interactive=True)
- cultural_references = gr.Dropdown(["Frequently uses cultural references", "Occasionally uses cultural references", "Balanced", "Rarely uses cultural references", "Never uses cultural references"], label="Cultural References", interactive=True)
- storytelling_ability = gr.Dropdown(["Frequent storyteller", "Occasional storyteller", "Balanced", "Rarely tells stories", "Never tells stories"], label="Storytelling Ability", interactive=True)
- with gr.Column(scale=1, min_width=600):
- gr.Markdown("""**SECRETS**\n
- Medical conditions : This will choose a random medical condition(s) that this character suffers from.
- Conditions will be saved only as a medical diagnosis code to save on token space and so it can be a secret to YOU as well.
- """)
- with gr.Row():
- conditions_count = gr.Slider(1, 10, label="Number of conditions", info="Choose between 1 and 10", interactive=True, step=1.0, value=1)
- med_secret_button = gr.Button("5. Generate secret medical condition(s) (COMING SOON)", interactive=True)
- med_secret = gr.Textbox(label="Secrets", interactive=True, type='password')
- gr.Markdown("""Trauma : This will choose a random psychological event that affects how this character behaves.
- Example: Experiencing discrimination
- """)
- with gr.Row():
- secrets_count = gr.Slider(1, 10, label="Number of issues", info="Choose between 1 and 10", interactive=True, step=1.0)
- secret_button = gr.Button("6. Generate secret issues(s) (COMING SOON)", interactive=False)
- secret = gr.Textbox(label="Secrets", interactive=True, type='password')
- situation = gr.TextArea(label="Situation (COMING SOON)", interactive=False)
- starting_message = gr.TextArea(label="Starting message (COMING SOON)", interactive=False)
- # sample_starters = gr.CheckboxGroup(["This year is flying by so fast.",
- # "It's quite sunny outside today.",
- # "People seem to be in a hurry all the time.",
- # "I hear birds chirping. It must be spring already.",
- # "The city looks different at night."],
- # label="Example conversation starters", info="These are simple statements designed to evoke a unique response without adding additional context. ", interactive=True),
- with gr.Row():
- with gr.Column(scale=1):
- examples = gr.TextArea(label="Example chats (COMING SOON)", value="\n")
-
- def test():
- print("trying....")
- state = demo.state
- state.inputs["charName"] = "New Name"
- demo.update(state)
-
- def genMedical(text):
- return "none"
-
- med_secret_button.click(generateMedicalSecrets, inputs=[conditions_count], outputs=[med_secret])
- generate.click(getAndParseQuickStart, inputs=[quickStart, trait_count], outputs=[
- rawQuickResults,
- charName,
- personality,
- body,
- likes,
- hates,
- sex,
- sexuality,
- age,
- description,
- personalityProfile,
- attributes
- ])
- createJSON = gr.Button("4. Create JSON")
- quickStartResult = gr.JSON(label="result", interactive=False)
- def makePersonality(text):
- print("Asking AI for a profile")
- prompt = "Here are the details for you to analyze. Remember to return in the specified format: " + text
-
- response = openai.ChatCompletion.create(
- model="gpt-4-0613",
- messages=[
- {"role": "system", "content": prompt_profile},
- {"role": "user", "content": prompt}
- ])
- response_content = response['choices'][0]['message']['content']
- print(response_content)
- return response_content
-
- generatePersonality.click(makePersonality, inputs=[rawQuickResults], outputs=[personalityProfile])
-
-
- genProfile.click(generateSpeakingStyle, inputs=[rawQuickResults], outputs=[
- formality,
- pace,
- rhythm,
- volume,
- emotionality,
- directness,
- humor,
- enunciation,
- expressiveness,
- accent_dialect,
- politeness,
- vocabulary,
- interruptions,
- hesitations,
- sentence_structure,
- sarcasm,
- colloquialisms,
- energy_level,
- defiance_rebellion,
- playfulness,
- vulgarity,
- idiosyncrasies,
- emotional_tone,
- context_adaptability,
- subtext,
- metaphorical_language,
- cultural_references,
- storytelling_ability
- ])
- def createJSONfile(charName,
- personality,
- body,
- likes,
- hates,
- sex,
- sexuality,
- age,
- description,
- personalityProfile,
- attributes,
- formality,
- pace,
- rhythm,
- volume,
- emotionality,
- directness,
- humor,
- enunciation,
- expressiveness,
- accent_dialect,
- politeness,
- vocabulary,
- interruptions,
- hesitations,
- sentence_structure,
- sarcasm,
- colloquialisms,
- energy_level,
- defiance_rebellion,
- playfulness,
- vulgarity,
- idiosyncrasies,
- emotional_tone,
- context_adaptability,
- subtext,
- metaphorical_language,
- cultural_references,
- storytelling_ability):
- ### Merging things into description
-
- description_unwrapped = f"""
- {charName} is a {age}-year-old {sexuality} {sex}.
- {description}.
- {charName} is {body}.
- {charName} likes {likes}.
- {charName} hates {hates}.
- """
- speech_unwrapped = f"""
- {charName} speaks with a unique style:
- They {"are " + formality + " and" if formality!="Neutral" else ""} and speaks at a {pace} speed with a {rhythm} rhythm.
- {charName} {"speaks at a " + volume + " volume and " if volume!="Moderate" else ""}has a {emotionality} level of emotionality.
- {charName + " is " + directness + "." if directness!="Balanced" else ""}
- {charName + " is " + humor + "." if humor!="Neutral" else ""}
- Their clarity of speech is {enunciation}
- {charName + " is " + expressiveness + "." if expressiveness!="Neutral" else ""}
- They have a {accent_dialect} accent.
- {charName} {"is " + politeness + " and " if politeness!="Neutral" else ""}uses a {vocabulary} vocabulary.
- {"They " + interruptions + "." if interruptions!="Balanced" else ""}
- {"They " + hesitations + "." if interruptions!="Balanced" else ""}
- {charName} uses a {sentence_structure} sentence structure and is {sarcasm}
- {"They " + colloquialisms + "." if colloquialisms!="Balanced" else ""}
- They speak with {energy_level}{" and is " + defiance_rebellion + "." if defiance_rebellion!="Balanced" else "."}
- When {charName} speaks it is {playfulness} and {vulgarity}.
- {charName + " uses " + idiosyncrasies + "." if idiosyncrasies!="Balanced" else ""}
- They have a {emotional_tone} tone
- {charName + " is " + context_adaptability + " when the situation changes." if context_adaptability!="Balanced" else ""}
- {"They " + subtext + "." if subtext!="Balanced" else ""}
- {"They " + metaphorical_language + "." if metaphorical_language!="Balanced" else ""}
- {"They " + cultural_references + "." if cultural_references!="Balanced" else ""}
- {"They " + storytelling_ability + "." if storytelling_ability!="Balanced" else ""}
- """
- output = f"""{{"name": "{charName}",
- "personality": "{personality}",
- "description": "{description_unwrapped}",
- "attributes" : "{attributes}",
- "psych_profile" : "{personalityProfile}",
- "speech_style": "{speech_unwrapped}"
- }}"""
-
- output = output.replace('\n', '')
- json_string = codecs.getdecoder("unicode_escape")(output)[0]
-
- # Load the JSON string into a Python object
- data = json.loads(json_string)
-
- # Clean up the data
- for key, value in data.items():
- if isinstance(value, str):
- # Remove leading/trailing white spaces, newlines and replace multiple spaces with a single space
- cleaned_value = re.sub('\s+', ' ', value.strip())
- data[key] = cleaned_value
-
- # Convert the cleaned data back to a compact JSON string
- cleaned_json_string = json.dumps(data, separators=(',', ':'))
-
- print(cleaned_json_string)
- return cleaned_json_string
-
-
- createJSON.click(createJSONfile, scroll_to_output=True, inputs=[
- charName,
- personality,
- body,
- likes,
- hates,
- sex,
- sexuality,
- age,
- description,
- personalityProfile,
- attributes,
- formality,
- pace,
- rhythm,
- volume,
- emotionality,
- directness,
- humor,
- enunciation,
- expressiveness,
- accent_dialect,
- politeness,
- vocabulary,
- interruptions,
- hesitations,
- sentence_structure,
- sarcasm,
- colloquialisms,
- energy_level,
- defiance_rebellion,
- playfulness,
- vulgarity,
- idiosyncrasies,
- emotional_tone,
- context_adaptability,
- subtext,
- metaphorical_language,
- cultural_references,
- storytelling_ability
- ], outputs=[quickStartResult])
-
-
-
-prompt_chat_test = """
-Scenario: descriptor (Minimum 4 sentences)
- First_Message: descriptor (do not include any quotation marks. At least 3 sentences)
- Example_Chat: descriptor (do not print names or quotation marks, only use the format of starting with > for the user name and >> for the character name, for example: >Hi\n>>Hello! **i pick up a book**\n>What's up? What are you reading?\n>>**Looks at the book cover** Nothing much)"""
-
-prompt_Personality = """
-formality (level of formal language use): Very formal, Formal, Neutral, Informal, Very informal\n
-pace (speed of speech): Very fast, Fast, Moderate, Slow, Very slow\n
-rhythm (pattern of speech): Choppy, Staccato, Varied, Flowing, Melodious\n
-volume (loudness of speech): Very loud, Loud, Moderate, Soft, Very soft\n
-emotionality (level of emotional expression): Very expressive, Expressive, Neutral, Restrained, Very restrained\n
-directness (level of straightforwardness): Very direct, Direct, Balanced, Indirect, Very indirect\n
-humor (frequency of humor in speech): Frequently humorous, Occasionally humorous, Neutral, Occasionally serious, Frequently serious\n
-enunciation (clarity of speech): Very clear, Clear, Neutral, Relaxed, Mumbled\n
-expressiveness (use of gestures and body language): Very expressive, Expressive, Neutral, Reserved, Very reserved\n
-accent (type of accent or dialect): Strong regional accent, Mild regional accent, neutral, Mild foreign accent, Strong foreign accent\n
-politeness (degree of politeness): Very polite, Polite, Neutral, Blunt, Very blunt\n
-vocabulary (range and type of words used): Highly sophisticated, Sophisticated, Average, Basic, Very basic\n
-interruptions (frequency of interruptions): Frequently interrupts, Occasionally interrupts, Balanced, Occasionally allows others to interrupt, Frequently allows others to interrupt\n
-hesitations (frequency of pauses or fillers): Frequently hesitates, Occasionally hesitates, Balanced, Occasionally fluent, Frequently fluent\n
-sentence structure (complexity of sentences): Very complex, Complex, Average, Simple, Very simple\n
-sarcasm (frequency and intensity of sarcasm): Very sarcastic, Sarcastic, Occasionally sarcastic, Rarely sarcastic, Never sarcastic\n
-colloquialisms (use of slang or colloquial language): Frequently uses colloquialisms, Occasionally uses colloquialisms, Balanced, Rarely uses colloquialisms, Never uses colloquialisms\n
-energy level (level of enthusiasm or intensity in speech): Very high energy, High energy, Moderate energy, Low energy, Very low energy\n
-defiance/rebellion (tendency to challenge or question things in speech): Frequently defiant, Occasionally defiant, Balanced, Rarely defiant, Never defiant\n
-playfulness (exhibits a playful tone in speech): Very playful, Playful, Occasionally playful, Rarely playful, Never playful\n
-vulgarity (frequency and intensity of crude language or coarse expressions): Very vulgar, Vulgar, Occasionally vulgar, Rarely vulgar, Never vulgar\n
-idiosyncrasies (unique speech habits or quirks): Frequent idiosyncrasies, Occasional idiosyncrasies, Balanced, Rare idiosyncrasies, No idiosyncrasies\n
-emotional tone (overall emotional 'color' of the character's speech): Very optimistic, Optimistic, Neutral, Pessimistic, Very pessimistic\n
-context adaptability (how a character's speech changes depending on the situation or person they're speaking to): Very adaptable, Adaptable, Balanced, Inflexible, Very inflexible\n
-subtext (the underlying, implied meaning in a character's speech): Frequently uses subtext, Occasionally uses subtext, Balanced, Rarely uses subtext, Never uses subtext\n
-metaphorical language (use of metaphors, similes, and other figurative language in a character's speech): Frequently uses metaphorical language, Occasionally uses metaphorical language, Balanced, Rarely uses metaphorical language, Never uses metaphorical language\n
-cultural references (references to their culture, such as idioms, phrases, or words from their native language, references to cultural practices or beliefs): Frequently uses cultural references, Occasionally uses cultural references, Balanced, Rarely uses cultural references, Never uses cultural references\n
-storytelling ability (frequency of telling stories or anecdotes in their speech): Frequent storyteller, Occasional storyteller, Balanced, Rarely tells stories, Never tells stories\n
-"""
-
-prompt_profile = """
-Based on your understanding of this character, please provide a brief personality assessment using the following typologies:
-
-Myers-Briggs Type Indicator (MBTI): Introverted/Extraverted, Sensing/Intuitive, Thinking/Feeling, Judging/Perceiving.
-
-Enneagram: main type, wing, and instinctual variant (self-preservation/sp, social/so, or sexual/sx).
-
-Enneagram Tritype: two additional types from different centers of intelligence.
-
-Socionics: similar to MBTI, identify the character's personality type.
-
-Global 5/SLOAN: Reserved/Social, Calm/Limbic, Organized/Unstructured, Accommodating/Egocentric, Non-Curious/Inquisitive.
-
-Please return the result codes in this format: "MBTI - Enneagram - Instinctual Variant - Tritype - Socionics - SLOAN". For example: INTJ - 3w4 - sp/sx - 358 - LIE - RCOEI". Do not explain or add other text or context.
-"""
-
-
-inst = demo.launch()
-
diff --git a/spaces/milyiyo/reimagine-it/data/README.md b/spaces/milyiyo/reimagine-it/data/README.md
deleted file mode 100644
index c786a9e85300c02f477a4d977cee587f35162b0d..0000000000000000000000000000000000000000
--- a/spaces/milyiyo/reimagine-it/data/README.md
+++ /dev/null
@@ -1 +0,0 @@
-directory to store preprocessed files
\ No newline at end of file
diff --git a/spaces/ml6team/Speaker-Diarization/utils/text_utils.py b/spaces/ml6team/Speaker-Diarization/utils/text_utils.py
deleted file mode 100644
index 1ef7662e64fa3d12b16f58b75b5e7283954727f4..0000000000000000000000000000000000000000
--- a/spaces/ml6team/Speaker-Diarization/utils/text_utils.py
+++ /dev/null
@@ -1,301 +0,0 @@
-"""
-Utils for generating text in streamlit
-"""
-import librosa
-import streamlit as st
-
-from PIL import Image
-
-from utils import audio_utils
-
-
-def intro_container():
- st.title(
- 'Who spoke when: Choosing the right speaker diarization tool')
- st.markdown(
- 'With the increase in applications of automated ***speech recognition systems (ASR)***, '
- 'the ability to partition a speech audio stream with multiple speakers into individual'
- ' segments associated with each individual has become a crucial part of understanding '
- 'speech data.')
- st.markdown(
- 'In this blog post, we will take a look at different open source frameworks for '
- 'speaker diarization and provide you with a guide to pick the most suited '
- 'one for your use case.')
-
- st.markdown(
- "Before we get into the technical details, libraries and tools, let's first understand what"
- " speaker diarization is and how it works!")
- st.markdown("---")
- st.header("🗣️ What is speaker diarization?️")
-
- st.markdown('\n')
- st.markdown(
- 'Speaker diarization aims to answer the question of ***"who spoke when"***. In short: diariziation algorithms '
- 'break down an audio stream of multiple speakers into segments corresponding to the individual speakers. '
- 'By combining the information that we get from diarization with ASR transcriptions, we can '
- 'transform the generated transcript into a format which is more readable and interpretable for humans '
- 'and that can be used for other downstream NLP tasks.')
- col1_im1, col2_im1, col3_im1 = st.columns([2, 5, 2])
-
- with col1_im1:
- st.write(' ')
-
- with col2_im1:
- st.image(Image.open('docs/asr+diar.png'),
- caption='Workflow of combining the output of both ASR and speaker '
- 'diarization on a speech signal to generate a speaker transcript.',
- use_column_width=True)
-
- with col3_im1:
- st.write(' ')
-
- st.markdown(
- "Let's illustrate this with an example. We have a recording of a casual phone conversation "
- "between two people. You can see what the different transcriptions look like when we "
- "transcribe the conversation with and without diarization.")
-
- st.markdown('\n')
-
- col1, col2, col3 = st.columns(3)
- with col1:
- st.subheader("🎧 Audio recording ")
- st.markdown(" ", unsafe_allow_html=True)
- st.markdown(" ", unsafe_allow_html=True)
- st.markdown(" ", unsafe_allow_html=True)
- audio_data, sampling_frequency = librosa.load('blog_samples/4092.wav')
- st.audio(audio_utils.create_st_audio_virtualfile(audio_data, sampling_frequency))
-
- with col2:
- st.subheader("❌ Without diarization")
- st.text("I just got back from the gym. oh good.\n"
- "uhuh. How's it going? oh pretty well. It was\n"
- "really crowded today yeah. I kind of\n"
- "assumed everyone would be at the shore.\n"
- "uhhuh. I was wrong. Well it's the\n"
- "middle of the week or whatever so. But\n"
- "it's the fourth of July. mm. So. yeah.\n"
- "People have to work tomorrow. Do you\n"
- "have to work tomorrow? yeah. Did you\n"
- "have off yesterday? Yes. oh that's good.\n"
- "And I was paid too. oh. Is it paid today?\n"
- "No. oh.\n")
-
- with col3:
- st.subheader('✅ With diarization')
- st.text("A: I just got back from the gym.\n"
- "B: oh good.\n"
- "A: uhhuh.\n"
- "B: How's it going?\n"
- "A: oh pretty well.\n"
- "A: It was really crowded today.\n"
- "B: yeah.\n"
- "A: I kind of assumed everyone would be at \n"
- "the shore.\n"
- "B: uhhuh.\n"
- "A: I was wrong.\n"
- "B: Well it's the middle of the week or\n"
- " whatever so.\n"
- "A: But it's the fourth of July.\n"
- "B: mm.\n"
- "A: So.\n"
- "B: yeah.\n"
- "B: People have to work tomorrow.\n"
- "B: Do you have to work tomorrow?\n"
- "A: yeah.\n"
- "B: Did you have off yesterday?\n"
- "A: Yes.\n"
- "B: oh that's good.\n"
- "A: And I was paid too.\n"
- "B: oh.\n"
- "B: Is it paid today?\n"
- "A: No.\n"
- "B: oh.\n")
-
- st.markdown(
- "By generating a **speaker-aware transcript**, we can more easily interpret the generated"
- " conversation compared to a generated transcript without diarization. Much neater no? ✨")
- st.caption(
- "But what can I do with these speaker-aware transcripts? 🤔")
- st.markdown(
- "Speaker-aware transcripts can be a powerful tool for analyzing speech data:")
- st.markdown("""
- * We can use the transcripts to analyze individual speaker's sentiment by using **sentiment analysis** on both audio and text transcripts.
- * Another use case is telemedicine where we might identify the **** and **** tags on the transcription to create an accurate transcript and attach it to the patient file or EHR system.
- * Speaker Diarization can be used by hiring platforms to analyze phone and video recruitment calls. This allows them to split and categorize candidates depending on their response to certain questions without having to listen again to the recordings.
- """)
- st.markdown(
- "Now that we've seen the importance of speaker diarization and some of its applications,"
- " it's time to find out how we can implement diarization algorithms.")
-
- st.markdown("---")
- st.header('📝 The workflow of a speaker diarization system')
- st.markdown(
- "Building robust and accurate speaker diarization is not a trivial task."
- " Real world audio data is messy and complex due to many factors, such"
- " as having a noisy background, multiple speakers talking at the same time and "
- "subtle differences between the speakers' voices in pitch and tone. Moreover, speaker diarization systems often suffer "
- "from **domain mismatch** where a model on data from a specific domain works poorly when applied to another domain.")
-
- st.markdown(
- "All in all, tackling speaker diarization is no easy feat. Current speaker diarization systems can be divided into two categories: **Traditional systems** and **End-to-End systems**. Let's look at how they work:")
- st.subheader('Traditional diarization systems')
- st.markdown(
- "Those consist of many independent submodules that are optimized individually, namely being:")
- st.markdown("""
- * **Speech detection**: The first step is to identify speech and remove non-speech signals with a voice activity detector (VAD) algorithm.
- * **Speech segmentation**: The output of the VAD is then segmented into small segments consisting of a few seconds (usually 1-2 seconds).
- * **Speech embedder**: A neural network pre-trained on speaker recognition is used to derive a high-level representation of the speech segments. Those embeddings are vector representations that summarize the voice characteristics (a.k.a voice print).
- * **Clustering**: After extracting segment embeddings, we need to cluster the speech embeddings with a clustering algorithm (for example K-Means or spectral clustering). The clustering produces our desired diarization results, which consists of identifying the number of unique speakers (derived from the number of unique clusters) and assigning a speaker label to each embedding (or speech segment).
- """)
- col1_im1, col2_im1, col3_im1 = st.columns([2, 5, 2])
-
- with col1_im1:
- st.write(' ')
-
- with col2_im1:
- st.image(Image.open('docs/speech_embedding.png'),
- caption="Process of identifying speaker segments from speech activity embeddings.",
-
- use_column_width=True)
-
- with col3_im1:
- st.write(' ')
-
- st.subheader('End-to-end diarization systems')
- st.markdown(
- "Here the individual submodules of the traditional speaker diarization system can be replaced by one neural network that is trained end-to-end on speaker diarization.")
-
- st.markdown('**Advantages**')
- st.markdown(
- '➕ Direct optimization of the network towards maximizing the accuracy for the diarization task. This is in contrast with traditional systems where submodules are optimized individually but not as a whole.')
- st.markdown(
- '➕ Less need to come up with useful pre-processing and post-processing transformation on the input data.')
- st.markdown(' **Disadvantages**')
- st.markdown(
- '➖ More effort needed for data collection and labelling. This is because this type of approach requires speaker-aware transcripts for training. This differs from traditional systems where only labels consisting of the speaker tag along with the audio timestamp are needed (without transcription efforts).')
- st.markdown('➖ These systems have the tendency to overfit on the training data.')
-
- st.markdown("---")
- st.header('📚 Speaker diarization frameworks')
- st.markdown(
- "As you can see, there are advantages and disadvantages to both traditional and end-to-end diarization systems. "
- "Building a speaker diarization system also involves aggregating quite a few "
- "building blocks and the implementation can seem daunting at first glance. Luckily, there exists a plethora "
- "of libraries and packages that have all those steps implemented and are ready for you to use out of the box 🔥.")
- st.markdown(
- "I will focus on the most popular **open-source** speaker diarization libraries. All but the last framework (UIS-RNN) are based on the traditional diarization approach. Make sure to check out"
- " [this link](https://wq2012.github.io/awesome-diarization/) for a more exhaustive list on different diarization libraries.")
-
- st.markdown("### 1. [pyannote](https://github.com/pyannote/pyannote-audio)")
- st.markdown(
- "Arguably one of the most popular libraries out there for speaker diarization.\n")
- st.markdown(
- "👉 Note that the pre-trained models are based on the [VoxCeleb datasets](https://www.robots.ox.ac.uk/~vgg/data/voxceleb/) which consists of recording of celebrities extracted from YouTube. The audio quality of those recordings are crisp and clear, so you might need to retrain your model if you want to tackle other types of data like recorded phone calls.\n")
- st.markdown(
- "➕ Comes with a set of available pre-trained models for the VAD, embedder and segmentation model.\n")
- st.markdown(
- "➕ The inference pipeline can identify multiple speakers speaking at the same time (multi-label diarization).\n")
- st.markdown(
- "➖ It is not possible to define the number of speakers for the clustering algorithm. This could lead to an over-estimation or under-estimation of the number of speakers if they are known beforehand.")
- st.markdown("### 2. [NVIDIA NeMo](https://developer.nvidia.com/nvidia-nemo)")
- st.markdown(
- "The Nvidia NeMo toolkit has separate collections for Automatic Speech Recognition (ASR), Natural Language Processing (NLP), and Text-to-Speech (TTS) models.\n")
- st.markdown(
- "👉 The models that the pre-trained networks were trained on were trained on [VoxCeleb datasets](https://www.robots.ox.ac.uk/~vgg/data/voxceleb/) as well as the [Fisher](https://catalog.ldc.upenn.edu/LDC2004T19) and [SwitchBoard](https://catalog.ldc.upenn.edu/LDC97S62) dataset, which consists of telephone conversations in English. This makes it more suitable as a starting point for fine-tuning a model for call-center use cases compared to the pre-trained models used in pyannote. More information about the pre-trained models can be found [here](https://docs.nvidia.com/deeplearning/nemo/user-guide/docs/en/stable/asr/speaker_diarization/results.html).\n")
- st.markdown(
- "➕ Diarization results can be combined easily with ASR outputs to generate speaker-aware transcripts.\n")
- st.markdown(
- "➕ Possibility to define the number of speakers beforehand if they are known, resulting in a more accurate diarization output.\n")
- st.markdown(
- "➕ The fact that the NeMo toolkit also includes NLP related frameworks makes it easy to integrate the diarization outcome with downstream NLP tasks.\n")
- st.markdown("### 3. [Simple Diarizer](https://github.com/cvqluu/simple_diarizer)")
- st.markdown(
- "A simplified diarization pipeline that can be used for quick testing.\n")
- st.markdown(
- "👉 Uses the same pre-trained models as pyannote.\n")
- st.markdown(
- "➕ Similarly to Nvidia NeMo, there's the option to define the number of speakers beforehand.\n")
- st.markdown(
- "➖ Unlike pyannote, this library does not include the option to fine tune the pre-trained models, making it less suitable for specialized use cases.\n")
- st.markdown(
- "### 4. [SpeechBrain](https://github.com/speechbrain/speechbrain)")
- st.markdown(
- "All-in-one conversational AI toolkit based on PyTorch.\n")
- st.markdown(
- "➕ The SpeechBrain Ecosystem makes it easy to develop integrated speech solutions with systems such ASR, speaker identification, speech enhancement, speech separation and language identification.\n")
- st.markdown(
- "➕ Large amount of pre-trained models for various tasks. Checkout their [HuggingFace page](https://huggingface.co/speechbrain) for more information.\n")
- st.markdown(
- "➕ Contains [comprehensible tutorials](https://speechbrain.github.io/tutorial_basics.html) for various speech building blocks to easily get started.\n")
- st.markdown(
- "➖ Diarization pipeline is still not fully implemented yet but this [might change in the future](https://github.com/speechbrain/speechbrain/issues/1208).")
- st.markdown(
- "### 5. [Kaldi](https://github.com/kaldi-asr/kaldi)")
- st.markdown(
- "Speech recognition toolkit that is mainly targeted towards researchers. It is written in C++ and used to train speech recognition models and decode audio from audio files.\n")
- st.markdown(
- "👉 Pre-trained model is based on the [CALLHOME](https://catalog.ldc.upenn.edu/LDC97S42) dataset which consists of telephone conversation between native English speakers in North America.\n")
- st.markdown(
- "👉 Benefits from large community support. However, mainly targeted towards researchers and less suitable for production ready-solutions.\n")
- st.markdown(
- "➖ Relatively steep learning curve for beginners who don't have a lot of experience with speech recognition systems.\n")
- st.markdown(
- "➖ Not suitable for a quick implementation of ASR/diarization systems. \n")
-
- st.markdown(
- "### 6. [UIS-RNN](https://github.com/google/uis-rnn)")
- st.markdown(
- "A fully supervised end-to-end diarization model developed by Google.\n")
- st.markdown(
- "👉 Both training and prediction require the usage of a GPU.\n")
- st.markdown(
- "➖ No-pretrained model is available, so you need to train it from scratch on your custom transcribed data.\n")
- st.markdown(
- "➕ Relatively easy to train if you have a large set of pre-labeled data.\n")
- st.markdown("\n")
- st.markdown(
- "Phew 😮💨, that's quite some different frameworks! To make it easier to pick the right one for your use case, I've created a simple flowchart that can get you started on picking a suitable library depending on your use case.")
-
- col1_im2, col2_im2, col3_im2 = st.columns([4, 5, 4])
-
- with col1_im2:
- st.write(' ')
-
- with col2_im2:
- st.image(Image.open('docs/flow_chart_diarization_tree.png'),
- caption='Flowchart for choosing a framework suitable for your diarization use case.',
- use_column_width=True)
-
- with col3_im2:
- st.write(' ')
-
-
-def demo_container():
- st.header('🤖 Demo')
- st.markdown(
- "Alright, you're probably very curious at this point to test out a few diarization techniques "
- "yourself. Below is a demo where you can try a few of the libraries that are mentioned above. "
- "You can try running multiple frameworks at the same time and compare their results by ticking multiple "
- "frameworks and clicking **'Apply'**.\n")
- st.markdown(
- "**Note:** We are including **Nemo** and **pyannote** frameworks since we are operating on a single environment and a dependency conflict can occur when including other frameworks (most diarization frameworks rely "
- "on different and incompatible versions of the same shared packages).")
- st.caption(
- "**Disclaimer**: Keep in mind that due to computational constraints, only the first 30 seconds will be used for diarization when uploading your own recordings. "
- "For that reason, the diarization results may not be as accurate compared to diarization computed on longer recordings. This"
- " is simply due to the fact that the diarization algorithms will have much less data to sample from in order to create meaningful clusters of embeddings for "
- "each speaker. On the other hand, the diarization results from the provided samples are pre-computed on the whole recording (length of around ≈10min) and thus "
- "have more accurate diarization results (only the first 30 seconds are shown).")
-
-
-def conlusion_container():
- st.title('💬 Conclusions')
- st.markdown("In this blogpost we covered different aspects of speaker diarization.\n")
- st.markdown(
- "👉 First we explained what speaker diarization is and gave a few examples of its different areas of applications.\n")
- st.markdown(
- "👉 We discussed the two main types of systems for implementing diarization system with a solid (high-level) understanding of both **traditional systems** and **end-to-end** systems.")
- st.markdown(
- "👉 Then, we gave a comparison of different diarization frameworks and provided a guide for picking the best one for your use case.")
- st.markdown(
- "👉 Finally, we provided you with an example to quickly try out a few of the diarization libraries.")
\ No newline at end of file
diff --git a/spaces/mmecheri/Rakuten_Streamlit/page_descriptions/data_description_txt.md b/spaces/mmecheri/Rakuten_Streamlit/page_descriptions/data_description_txt.md
deleted file mode 100644
index 9552717f8fcba91e8e858372221202bc69d6a9ea..0000000000000000000000000000000000000000
--- a/spaces/mmecheri/Rakuten_Streamlit/page_descriptions/data_description_txt.md
+++ /dev/null
@@ -1,21 +0,0 @@
-Pour ce challenge, Rakuten France met à disposition environ 99 000 listes de produits au format CSV,
- y compris l'ensemble d'entraînement (84 916)
- et l'ensemble de test (13 812).
-
-Les données sont réparties suivant des critères , formant 4 datasets distincts :
-
-- « X_train_update.csv »: échantillons d'**entraînement**, avec la description textuelle ainsi que le référencement du fichier image associé
-- « y_train_CVw08PX.csv »: contient les variable cible (**prdtypecode**)
-- « X_test_update.csv »: échantillons de test(pour la sommission des résulats)
-- « images.zip »: ce fichier contient toutes les images:
- - « image_train » contenant 84 916 images pour l'**entraînement**
- - « image_test » contenant 13 812 images (pour la sommission des résulats)
-
-**Exemples de données :**
-
-
-
-
-
-
-
diff --git a/spaces/mrm8488/PromptSource/promptsource/__init__.py b/spaces/mrm8488/PromptSource/promptsource/__init__.py
deleted file mode 100644
index e69de29bb2d1d6434b8b29ae775ad8c2e48c5391..0000000000000000000000000000000000000000
diff --git a/spaces/mrolando/text_to_sound/app.py b/spaces/mrolando/text_to_sound/app.py
deleted file mode 100644
index 33d140bf51487d6166c6bbb5deb6a5b81e97ba1a..0000000000000000000000000000000000000000
--- a/spaces/mrolando/text_to_sound/app.py
+++ /dev/null
@@ -1,102 +0,0 @@
-from diffusers import AudioLDMPipeline
-import torch
-import gradio as gr
-from transformers import pipeline
-#from googletrans import Translator
-import os
-from transformers import AutoTokenizer, AutoModelForSeq2SeqLM, pipeline
-
-
-if torch.cuda.is_available():
- device = "cuda"
- torch_dtype = torch.float16
-else:
- device = "cpu"
- torch_dtype = torch.float32
-print(device)
-repo_id = "cvssp/audioldm-s-full-v2"
-pipe = AudioLDMPipeline.from_pretrained(repo_id, torch_dtype=torch_dtype)
-pipe = pipe.to(device)
-# pipe.unet = torch.compile(pipe.unet)
-#pipe.unet = torch.compile(pipe.unet)
-import base64
-
-with open("Iso_Logotipo_Ceibal.png", "rb") as image_file:
- encoded_image = base64.b64encode(image_file.read()).decode()
-
-CKPT = "facebook/nllb-200-distilled-600M"
-
-model = AutoModelForSeq2SeqLM.from_pretrained(CKPT)
-tokenizer = AutoTokenizer.from_pretrained(CKPT)
-
-def translate_text(text):
- translation_pipeline = pipeline("translation",
- model=model,
- tokenizer=tokenizer,
- src_lang="spa_Latn",
- tgt_lang="eng_Latn",
- max_length=400,
- device=device)
-
- result = translation_pipeline(text)
- return result[0]['translation_text']
-
-
-
-def generate_sound(text,steps,audio_length,negative_prompt):
- print(text)
- text=translate_text(text)
- negative_prompt = translate_text(negative_prompt)
- print(text)
- waveforms = pipe(text,
- num_inference_steps=steps,
- audio_length_in_s=audio_length,
- negative_prompt = negative_prompt).audios
- rate =16000
- return rate, waveforms[0]
-
-
-# def translate_text(text):
-# text = es_en_translator(text)[0].get("translation_text")
-# return text
-with gr.Blocks() as demo:
- gr.Markdown("""
-
-
- Uso de AI para la generación de sonidos a partir de texto.
-
-
-
- Con este espacio podrás generar sondios a partir de texto, intentá ser lo más descriptivo/a posible en el texto. Se puede usar directamente o podés cambiar ajustes, que impacto tiene cada uno está detallado en su descripción. Cambiá valores y mirá los resultados!
-
-
El texto se traduce del español al inglés para alimentar al modelo, también se puede escribir el texto de entrada en inglés.
-
- """.format(encoded_image))
- with gr.Row():
- with gr.Column():
- gr.Markdown("Primero debes ingresar el texto para generar el sonido:")
- with gr.Row():
- with gr.Column(scale=4):
- prompt = gr.Textbox(label="Texo base para generar el sonido") #Give prompt some real estate
- with gr.Column(scale=1, min_width=50):
- btn = gr.Button("Generar") #Submit button side by side!
- with gr.Row():
- with gr.Accordion("Opciones avanzadas", open=False): #Let's hide the advanced options!
- negative_prompt = gr.Textbox(label="Texto negativo para la generación", info='Al ingresar texto en este campo el modelo intentará alejarse lo mas posible del mismo, este puede ser "baja calidad"')
- with gr.Row():
- with gr.Column():
- audio_len = gr.Slider(label="Duración del sonido", minimum=1, maximum=30, value=5, step = 1,
- info="Cuánto mayor sonido, mayor será el tiempo de procesamiento.")
- steps = gr.Slider(label="Paos de Inferencia", minimum=1, maximum=100, value=20,step =1 ,
- info="Al aumentar los pasos de inferencia se puede acercar más a la descripción del texto pero con un mayor tiempo de procesamiento.")
- with gr.Row():
- examples = gr.Examples(inputs=[prompt,negative_prompt],examples=[["Un martillo golpeando madera","low quality"]])
-
- with gr.Column():
- output = gr.Audio(label="Resultado") #Move the output up too
-
- btn.click(fn=generate_sound, inputs=[prompt,steps,audio_len,negative_prompt], outputs=[output]) #steps,guidance,width,height]
-
-gr.close_all()
-demo.queue()
-demo.launch()
\ No newline at end of file
diff --git a/spaces/mshkdm/VToonify/vtoonify/model/dualstylegan.py b/spaces/mshkdm/VToonify/vtoonify/model/dualstylegan.py
deleted file mode 100644
index 60d9850ad049a2751781871d6ae0c2779ecc863f..0000000000000000000000000000000000000000
--- a/spaces/mshkdm/VToonify/vtoonify/model/dualstylegan.py
+++ /dev/null
@@ -1,203 +0,0 @@
-import random
-import torch
-from torch import nn
-from model.stylegan.model import ConvLayer, PixelNorm, EqualLinear, Generator
-
-class AdaptiveInstanceNorm(nn.Module):
- def __init__(self, fin, style_dim=512):
- super().__init__()
-
- self.norm = nn.InstanceNorm2d(fin, affine=False)
- self.style = nn.Linear(style_dim, fin * 2)
-
- self.style.bias.data[:fin] = 1
- self.style.bias.data[fin:] = 0
-
- def forward(self, input, style):
- style = self.style(style).unsqueeze(2).unsqueeze(3)
- gamma, beta = style.chunk(2, 1)
- out = self.norm(input)
- out = gamma * out + beta
- return out
-
-# modulative residual blocks (ModRes)
-class AdaResBlock(nn.Module):
- def __init__(self, fin, style_dim=512, dilation=1): # modified
- super().__init__()
-
- self.conv = ConvLayer(fin, fin, 3, dilation=dilation) # modified
- self.conv2 = ConvLayer(fin, fin, 3, dilation=dilation) # modified
- self.norm = AdaptiveInstanceNorm(fin, style_dim)
- self.norm2 = AdaptiveInstanceNorm(fin, style_dim)
-
- # model initialization
- # the convolution filters are set to values close to 0 to produce negligible residual features
- self.conv[0].weight.data *= 0.01
- self.conv2[0].weight.data *= 0.01
-
- def forward(self, x, s, w=1):
- skip = x
- if w == 0:
- return skip
- out = self.conv(self.norm(x, s))
- out = self.conv2(self.norm2(out, s))
- out = out * w + skip
- return out
-
-class DualStyleGAN(nn.Module):
- def __init__(self, size, style_dim, n_mlp, channel_multiplier=2, twoRes=True, res_index=6):
- super().__init__()
-
- layers = [PixelNorm()]
- for i in range(n_mlp-6):
- layers.append(EqualLinear(512, 512, lr_mul=0.01, activation="fused_lrelu"))
- # color transform blocks T_c
- self.style = nn.Sequential(*layers)
- # StyleGAN2
- self.generator = Generator(size, style_dim, n_mlp, channel_multiplier)
- # The extrinsic style path
- self.res = nn.ModuleList()
- self.res_index = res_index//2 * 2
- self.res.append(AdaResBlock(self.generator.channels[2 ** 2])) # for conv1
- for i in range(3, self.generator.log_size + 1):
- out_channel = self.generator.channels[2 ** i]
- if i < 3 + self.res_index//2:
- # ModRes
- self.res.append(AdaResBlock(out_channel))
- self.res.append(AdaResBlock(out_channel))
- else:
- # structure transform block T_s
- self.res.append(EqualLinear(512, 512))
- # FC layer is initialized with identity matrices, meaning no changes to the input latent code
- self.res[-1].weight.data = torch.eye(512) * 512.0**0.5 + torch.randn(512, 512) * 0.01
- self.res.append(EqualLinear(512, 512))
- self.res[-1].weight.data = torch.eye(512) * 512.0**0.5 + torch.randn(512, 512) * 0.01
- self.res.append(EqualLinear(512, 512)) # for to_rgb7
- self.res[-1].weight.data = torch.eye(512) * 512.0**0.5 + torch.randn(512, 512) * 0.01
- self.size = self.generator.size
- self.style_dim = self.generator.style_dim
- self.log_size = self.generator.log_size
- self.num_layers = self.generator.num_layers
- self.n_latent = self.generator.n_latent
- self.channels = self.generator.channels
-
- def forward(
- self,
- styles, # intrinsic style code
- exstyles, # extrinsic style code
- return_latents=False,
- return_feat=False,
- inject_index=None,
- truncation=1,
- truncation_latent=None,
- input_is_latent=False,
- noise=None,
- randomize_noise=True,
- z_plus_latent=False, # intrinsic style code is z+ or z
- use_res=True, # whether to use the extrinsic style path
- fuse_index=18, # layers > fuse_index do not use the extrinsic style path
- interp_weights=[1]*18, # weight vector for style combination of two paths
- ):
-
- if not input_is_latent:
- if not z_plus_latent:
- styles = [self.generator.style(s) for s in styles]
- else:
- styles = [self.generator.style(s.reshape(s.shape[0]*s.shape[1], s.shape[2])).reshape(s.shape) for s in styles]
-
- if noise is None:
- if randomize_noise:
- noise = [None] * self.generator.num_layers
- else:
- noise = [
- getattr(self.generator.noises, f"noise_{i}") for i in range(self.generator.num_layers)
- ]
-
- if truncation < 1:
- style_t = []
-
- for style in styles:
- style_t.append(
- truncation_latent + truncation * (style - truncation_latent)
- )
-
- styles = style_t
-
- if len(styles) < 2:
- inject_index = self.generator.n_latent
-
- if styles[0].ndim < 3:
- latent = styles[0].unsqueeze(1).repeat(1, inject_index, 1)
-
- else:
- latent = styles[0]
-
- else:
- if inject_index is None:
- inject_index = random.randint(1, self.generator.n_latent - 1)
-
- if styles[0].ndim < 3:
- latent = styles[0].unsqueeze(1).repeat(1, inject_index, 1)
- latent2 = styles[1].unsqueeze(1).repeat(1, self.generator.n_latent - inject_index, 1)
-
- latent = torch.cat([latent, latent2], 1)
- else:
- latent = torch.cat([styles[0][:,0:inject_index], styles[1][:,inject_index:]], 1)
-
- if use_res:
- if exstyles.ndim < 3:
- resstyles = self.style(exstyles).unsqueeze(1).repeat(1, self.generator.n_latent, 1)
- adastyles = exstyles.unsqueeze(1).repeat(1, self.generator.n_latent, 1)
- else:
- nB, nL, nD = exstyles.shape
- resstyles = self.style(exstyles.reshape(nB*nL, nD)).reshape(nB, nL, nD)
- adastyles = exstyles
-
- out = self.generator.input(latent)
- out = self.generator.conv1(out, latent[:, 0], noise=noise[0])
- if use_res and fuse_index > 0:
- out = self.res[0](out, resstyles[:, 0], interp_weights[0])
-
- skip = self.generator.to_rgb1(out, latent[:, 1])
- i = 1
- for conv1, conv2, noise1, noise2, to_rgb in zip(
- self.generator.convs[::2], self.generator.convs[1::2], noise[1::2], noise[2::2], self.generator.to_rgbs):
- if use_res and fuse_index >= i and i > self.res_index:
- out = conv1(out, interp_weights[i] * self.res[i](adastyles[:, i]) +
- (1-interp_weights[i]) * latent[:, i], noise=noise1)
- else:
- out = conv1(out, latent[:, i], noise=noise1)
- if use_res and fuse_index >= i and i <= self.res_index:
- out = self.res[i](out, resstyles[:, i], interp_weights[i])
- if use_res and fuse_index >= (i+1) and i > self.res_index:
- out = conv2(out, interp_weights[i+1] * self.res[i+1](adastyles[:, i+1]) +
- (1-interp_weights[i+1]) * latent[:, i+1], noise=noise2)
- else:
- out = conv2(out, latent[:, i + 1], noise=noise2)
- if use_res and fuse_index >= (i+1) and i <= self.res_index:
- out = self.res[i+1](out, resstyles[:, i+1], interp_weights[i+1])
- if use_res and fuse_index >= (i+2) and i >= self.res_index-1:
- skip = to_rgb(out, interp_weights[i+2] * self.res[i+2](adastyles[:, i+2]) +
- (1-interp_weights[i+2]) * latent[:, i + 2], skip)
- else:
- skip = to_rgb(out, latent[:, i + 2], skip)
- i += 2
- if i > self.res_index and return_feat:
- return out, skip
-
- image = skip
-
- if return_latents:
- return image, latent
-
- else:
- return image, None
-
- def make_noise(self):
- return self.generator.make_noise()
-
- def mean_latent(self, n_latent):
- return self.generator.mean_latent(n_latent)
-
- def get_latent(self, input):
- return self.generator.style(input)
\ No newline at end of file
diff --git a/spaces/mshukor/UnIVAL/fairseq/examples/speech_synthesis/data_utils.py b/spaces/mshukor/UnIVAL/fairseq/examples/speech_synthesis/data_utils.py
deleted file mode 100644
index f43a4a90046fb9ee4944dc06ba377c1faade141d..0000000000000000000000000000000000000000
--- a/spaces/mshukor/UnIVAL/fairseq/examples/speech_synthesis/data_utils.py
+++ /dev/null
@@ -1,320 +0,0 @@
-# Copyright (c) Facebook, Inc. and its affiliates.
-#
-# This source code is licensed under the MIT license found in the
-# LICENSE file in the root directory of this source tree.
-
-import os
-from pathlib import Path
-from typing import Optional, List, Dict
-import zipfile
-import tempfile
-from dataclasses import dataclass
-from itertools import groupby
-
-import torch
-import torch.nn.functional as F
-import numpy as np
-from tqdm import tqdm
-
-from examples.speech_to_text.data_utils import load_tsv_to_dicts
-from fairseq.data.audio.audio_utils import TTSSpectrogram, TTSMelScale
-
-
-def trim_or_pad_to_target_length(
- data_1d_or_2d: np.ndarray, target_length: int
-) -> np.ndarray:
- assert len(data_1d_or_2d.shape) in {1, 2}
- delta = data_1d_or_2d.shape[0] - target_length
- if delta >= 0: # trim if being longer
- data_1d_or_2d = data_1d_or_2d[: target_length]
- else: # pad if being shorter
- if len(data_1d_or_2d.shape) == 1:
- data_1d_or_2d = np.concatenate(
- [data_1d_or_2d, np.zeros(-delta)], axis=0
- )
- else:
- data_1d_or_2d = np.concatenate(
- [data_1d_or_2d, np.zeros((-delta, data_1d_or_2d.shape[1]))],
- axis=0
- )
- return data_1d_or_2d
-
-
-def extract_logmel_spectrogram(
- waveform: torch.Tensor, sample_rate: int,
- output_path: Optional[Path] = None, win_length: int = 1024,
- hop_length: int = 256, n_fft: int = 1024,
- win_fn: callable = torch.hann_window, n_mels: int = 80,
- f_min: float = 0., f_max: float = 8000, eps: float = 1e-5,
- overwrite: bool = False, target_length: Optional[int] = None
-):
- if output_path is not None and output_path.is_file() and not overwrite:
- return
-
- spectrogram_transform = TTSSpectrogram(
- n_fft=n_fft, win_length=win_length, hop_length=hop_length,
- window_fn=win_fn
- )
- mel_scale_transform = TTSMelScale(
- n_mels=n_mels, sample_rate=sample_rate, f_min=f_min, f_max=f_max,
- n_stft=n_fft // 2 + 1
- )
- spectrogram = spectrogram_transform(waveform)
- mel_spec = mel_scale_transform(spectrogram)
- logmel_spec = torch.clamp(mel_spec, min=eps).log()
- assert len(logmel_spec.shape) == 3 and logmel_spec.shape[0] == 1
- logmel_spec = logmel_spec.squeeze().t() # D x T -> T x D
- if target_length is not None:
- trim_or_pad_to_target_length(logmel_spec, target_length)
-
- if output_path is not None:
- np.save(output_path.as_posix(), logmel_spec)
- else:
- return logmel_spec
-
-
-def extract_pitch(
- waveform: torch.Tensor, sample_rate: int,
- output_path: Optional[Path] = None, hop_length: int = 256,
- log_scale: bool = True, phoneme_durations: Optional[List[int]] = None
-):
- if output_path is not None and output_path.is_file():
- return
-
- try:
- import pyworld
- except ImportError:
- raise ImportError("Please install PyWORLD: pip install pyworld")
-
- _waveform = waveform.squeeze(0).double().numpy()
- pitch, t = pyworld.dio(
- _waveform, sample_rate, frame_period=hop_length / sample_rate * 1000
- )
- pitch = pyworld.stonemask(_waveform, pitch, t, sample_rate)
-
- if phoneme_durations is not None:
- pitch = trim_or_pad_to_target_length(pitch, sum(phoneme_durations))
- try:
- from scipy.interpolate import interp1d
- except ImportError:
- raise ImportError("Please install SciPy: pip install scipy")
- nonzero_ids = np.where(pitch != 0)[0]
- interp_fn = interp1d(
- nonzero_ids,
- pitch[nonzero_ids],
- fill_value=(pitch[nonzero_ids[0]], pitch[nonzero_ids[-1]]),
- bounds_error=False,
- )
- pitch = interp_fn(np.arange(0, len(pitch)))
- d_cumsum = np.cumsum(np.concatenate([np.array([0]), phoneme_durations]))
- pitch = np.array(
- [
- np.mean(pitch[d_cumsum[i-1]: d_cumsum[i]])
- for i in range(1, len(d_cumsum))
- ]
- )
- assert len(pitch) == len(phoneme_durations)
-
- if log_scale:
- pitch = np.log(pitch + 1)
-
- if output_path is not None:
- np.save(output_path.as_posix(), pitch)
- else:
- return pitch
-
-
-def extract_energy(
- waveform: torch.Tensor, output_path: Optional[Path] = None,
- hop_length: int = 256, n_fft: int = 1024, log_scale: bool = True,
- phoneme_durations: Optional[List[int]] = None
-):
- if output_path is not None and output_path.is_file():
- return
-
- assert len(waveform.shape) == 2 and waveform.shape[0] == 1
- waveform = waveform.view(1, 1, waveform.shape[1])
- waveform = F.pad(
- waveform.unsqueeze(1), [n_fft // 2, n_fft // 2, 0, 0],
- mode="reflect"
- )
- waveform = waveform.squeeze(1)
-
- fourier_basis = np.fft.fft(np.eye(n_fft))
- cutoff = int((n_fft / 2 + 1))
- fourier_basis = np.vstack(
- [np.real(fourier_basis[:cutoff, :]),
- np.imag(fourier_basis[:cutoff, :])]
- )
-
- forward_basis = torch.FloatTensor(fourier_basis[:, None, :])
- forward_transform = F.conv1d(
- waveform, forward_basis, stride=hop_length, padding=0
- )
-
- real_part = forward_transform[:, :cutoff, :]
- imag_part = forward_transform[:, cutoff:, :]
- magnitude = torch.sqrt(real_part ** 2 + imag_part ** 2)
- energy = torch.norm(magnitude, dim=1).squeeze(0).numpy()
-
- if phoneme_durations is not None:
- energy = trim_or_pad_to_target_length(energy, sum(phoneme_durations))
- d_cumsum = np.cumsum(np.concatenate([np.array([0]), phoneme_durations]))
- energy = np.array(
- [
- np.mean(energy[d_cumsum[i - 1]: d_cumsum[i]])
- for i in range(1, len(d_cumsum))
- ]
- )
- assert len(energy) == len(phoneme_durations)
-
- if log_scale:
- energy = np.log(energy + 1)
-
- if output_path is not None:
- np.save(output_path.as_posix(), energy)
- else:
- return energy
-
-
-def get_global_cmvn(feature_root: Path, output_path: Optional[Path] = None):
- mean_x, mean_x2, n_frames = None, None, 0
- feature_paths = feature_root.glob("*.npy")
- for p in tqdm(feature_paths):
- with open(p, 'rb') as f:
- frames = np.load(f).squeeze()
-
- n_frames += frames.shape[0]
-
- cur_mean_x = frames.sum(axis=0)
- if mean_x is None:
- mean_x = cur_mean_x
- else:
- mean_x += cur_mean_x
-
- cur_mean_x2 = (frames ** 2).sum(axis=0)
- if mean_x2 is None:
- mean_x2 = cur_mean_x2
- else:
- mean_x2 += cur_mean_x2
-
- mean_x /= n_frames
- mean_x2 /= n_frames
- var_x = mean_x2 - mean_x ** 2
- std_x = np.sqrt(np.maximum(var_x, 1e-10))
-
- if output_path is not None:
- with open(output_path, 'wb') as f:
- np.savez(f, mean=mean_x, std=std_x)
- else:
- return {"mean": mean_x, "std": std_x}
-
-
-def ipa_phonemize(text, lang="en-us", use_g2p=False):
- if use_g2p:
- assert lang == "en-us", "g2pE phonemizer only works for en-us"
- try:
- from g2p_en import G2p
- g2p = G2p()
- return " ".join("|" if p == " " else p for p in g2p(text))
- except ImportError:
- raise ImportError(
- "Please install phonemizer: pip install g2p_en"
- )
- else:
- try:
- from phonemizer import phonemize
- from phonemizer.separator import Separator
- return phonemize(
- text, backend='espeak', language=lang,
- separator=Separator(word="| ", phone=" ")
- )
- except ImportError:
- raise ImportError(
- "Please install phonemizer: pip install phonemizer"
- )
-
-
-@dataclass
-class ForceAlignmentInfo(object):
- tokens: List[str]
- frame_durations: List[int]
- start_sec: Optional[float]
- end_sec: Optional[float]
-
-
-def get_mfa_alignment_by_sample_id(
- textgrid_zip_path: str, sample_id: str, sample_rate: int,
- hop_length: int, silence_phones: List[str] = ("sil", "sp", "spn")
-) -> ForceAlignmentInfo:
- try:
- import tgt
- except ImportError:
- raise ImportError("Please install TextGridTools: pip install tgt")
-
- filename = f"{sample_id}.TextGrid"
- out_root = Path(tempfile.gettempdir())
- tgt_path = out_root / filename
- with zipfile.ZipFile(textgrid_zip_path) as f_zip:
- f_zip.extract(filename, path=out_root)
- textgrid = tgt.io.read_textgrid(tgt_path.as_posix())
- os.remove(tgt_path)
-
- phones, frame_durations = [], []
- start_sec, end_sec, end_idx = 0, 0, 0
- for t in textgrid.get_tier_by_name("phones")._objects:
- s, e, p = t.start_time, t.end_time, t.text
- # Trim leading silences
- if len(phones) == 0:
- if p in silence_phones:
- continue
- else:
- start_sec = s
- phones.append(p)
- if p not in silence_phones:
- end_sec = e
- end_idx = len(phones)
- r = sample_rate / hop_length
- frame_durations.append(int(np.round(e * r) - np.round(s * r)))
- # Trim tailing silences
- phones = phones[:end_idx]
- frame_durations = frame_durations[:end_idx]
-
- return ForceAlignmentInfo(
- tokens=phones, frame_durations=frame_durations, start_sec=start_sec,
- end_sec=end_sec
- )
-
-
-def get_mfa_alignment(
- textgrid_zip_path: str, sample_ids: List[str], sample_rate: int,
- hop_length: int
-) -> Dict[str, ForceAlignmentInfo]:
- return {
- i: get_mfa_alignment_by_sample_id(
- textgrid_zip_path, i, sample_rate, hop_length
- ) for i in tqdm(sample_ids)
- }
-
-
-def get_unit_alignment(
- id_to_unit_tsv_path: str, sample_ids: List[str]
-) -> Dict[str, ForceAlignmentInfo]:
- id_to_units = {
- e["id"]: e["units"] for e in load_tsv_to_dicts(id_to_unit_tsv_path)
- }
- id_to_units = {i: id_to_units[i].split() for i in sample_ids}
- id_to_units_collapsed = {
- i: [uu for uu, _ in groupby(u)] for i, u in id_to_units.items()
- }
- id_to_durations = {
- i: [len(list(g)) for _, g in groupby(u)] for i, u in id_to_units.items()
- }
-
- return {
- i: ForceAlignmentInfo(
- tokens=id_to_units_collapsed[i], frame_durations=id_to_durations[i],
- start_sec=None, end_sec=None
- )
- for i in sample_ids
- }
diff --git a/spaces/mshukor/UnIVAL/fairseq/fairseq/criterions/nat_loss.py b/spaces/mshukor/UnIVAL/fairseq/fairseq/criterions/nat_loss.py
deleted file mode 100644
index 7dac32fbaf4fb10089c0bcd42b75d23f92b5cf66..0000000000000000000000000000000000000000
--- a/spaces/mshukor/UnIVAL/fairseq/fairseq/criterions/nat_loss.py
+++ /dev/null
@@ -1,180 +0,0 @@
-# Copyright (c) Facebook, Inc. and its affiliates.
-#
-# This source code is licensed under the MIT license found in the
-# LICENSE file in the root directory of this source tree.
-
-import math
-
-import torch
-import torch.nn.functional as F
-from fairseq import metrics, utils
-from fairseq.criterions import FairseqCriterion, register_criterion
-from fairseq.dataclass import FairseqDataclass
-from torch import Tensor
-
-from dataclasses import dataclass, field
-
-
-@dataclass
-class LabelSmoothedDualImitationCriterionConfig(FairseqDataclass):
- label_smoothing: float = field(
- default=0.0,
- metadata={"help": "epsilon for label smoothing, 0 means no label smoothing"},
- )
-
-
-@register_criterion("nat_loss", dataclass=LabelSmoothedDualImitationCriterionConfig)
-class LabelSmoothedDualImitationCriterion(FairseqCriterion):
- def __init__(self, task, label_smoothing):
- super().__init__(task)
- self.label_smoothing = label_smoothing
-
- def _compute_loss(
- self, outputs, targets, masks=None, label_smoothing=0.0, name="loss", factor=1.0
- ):
- """
- outputs: batch x len x d_model
- targets: batch x len
- masks: batch x len
-
- policy_logprob: if there is some policy
- depends on the likelihood score as rewards.
- """
-
- def mean_ds(x: Tensor, dim=None) -> Tensor:
- return (
- x.float().mean().type_as(x)
- if dim is None
- else x.float().mean(dim).type_as(x)
- )
-
- if masks is not None:
- outputs, targets = outputs[masks], targets[masks]
-
- if masks is not None and not masks.any():
- nll_loss = torch.tensor(0)
- loss = nll_loss
- else:
- logits = F.log_softmax(outputs, dim=-1)
- if targets.dim() == 1:
- losses = F.nll_loss(logits, targets.to(logits.device), reduction="none")
-
- else: # soft-labels
- losses = F.kl_div(logits, targets.to(logits.device), reduction="none")
- losses = losses.sum(-1)
-
- nll_loss = mean_ds(losses)
- if label_smoothing > 0:
- loss = (
- nll_loss * (1 - label_smoothing) - mean_ds(logits) * label_smoothing
- )
- else:
- loss = nll_loss
-
- loss = loss * factor
- return {"name": name, "loss": loss, "nll_loss": nll_loss, "factor": factor}
-
- def _custom_loss(self, loss, name="loss", factor=1.0):
- return {"name": name, "loss": loss, "factor": factor}
-
- def forward(self, model, sample, reduce=True):
- """Compute the loss for the given sample.
- Returns a tuple with three elements:
- 1) the loss
- 2) the sample size, which is used as the denominator for the gradient
- 3) logging outputs to display while training
- """
- nsentences, ntokens = sample["nsentences"], sample["ntokens"]
-
- # B x T
- src_tokens, src_lengths = (
- sample["net_input"]["src_tokens"],
- sample["net_input"]["src_lengths"],
- )
- tgt_tokens, prev_output_tokens = sample["target"], sample["prev_target"]
-
- outputs = model(src_tokens, src_lengths, prev_output_tokens, tgt_tokens)
- losses, nll_loss = [], []
-
- for obj in outputs:
- if outputs[obj].get("loss", None) is None:
- _losses = self._compute_loss(
- outputs[obj].get("out"),
- outputs[obj].get("tgt"),
- outputs[obj].get("mask", None),
- outputs[obj].get("ls", 0.0),
- name=obj + "-loss",
- factor=outputs[obj].get("factor", 1.0),
- )
- else:
- _losses = self._custom_loss(
- outputs[obj].get("loss"),
- name=obj + "-loss",
- factor=outputs[obj].get("factor", 1.0),
- )
-
- losses += [_losses]
- if outputs[obj].get("nll_loss", False):
- nll_loss += [_losses.get("nll_loss", 0.0)]
-
- loss = sum(l["loss"] for l in losses)
- nll_loss = sum(l for l in nll_loss) if len(nll_loss) > 0 else loss.new_tensor(0)
-
- # NOTE:
- # we don't need to use sample_size as denominator for the gradient
- # here sample_size is just used for logging
- sample_size = 1
- logging_output = {
- "loss": loss.data,
- "nll_loss": nll_loss.data,
- "ntokens": ntokens,
- "nsentences": nsentences,
- "sample_size": sample_size,
- }
-
- for l in losses:
- logging_output[l["name"]] = (
- utils.item(l["loss"].data / l["factor"])
- if reduce
- else l[["loss"]].data / l["factor"]
- )
-
- return loss, sample_size, logging_output
-
- @staticmethod
- def reduce_metrics(logging_outputs) -> None:
- """Aggregate logging outputs from data parallel training."""
- sample_size = utils.item(
- sum(log.get("sample_size", 0) for log in logging_outputs)
- )
- loss = utils.item(sum(log.get("loss", 0) for log in logging_outputs))
- nll_loss = utils.item(sum(log.get("nll_loss", 0) for log in logging_outputs))
-
- metrics.log_scalar(
- "loss", loss / sample_size / math.log(2), sample_size, round=3
- )
- metrics.log_scalar(
- "nll_loss", nll_loss / sample_size / math.log(2), sample_size, round=3
- )
- metrics.log_derived(
- "ppl", lambda meters: utils.get_perplexity(meters["loss"].avg)
- )
-
- for key in logging_outputs[0]:
- if key[-5:] == "-loss":
- val = sum(log.get(key, 0) for log in logging_outputs)
- metrics.log_scalar(
- key[:-5],
- val / sample_size / math.log(2) if sample_size > 0 else 0.0,
- sample_size,
- round=3,
- )
-
- @staticmethod
- def logging_outputs_can_be_summed() -> bool:
- """
- Whether the logging outputs returned by `forward` can be summed
- across workers prior to calling `reduce_metrics`. Setting this
- to True will improves distributed training speed.
- """
- return True
diff --git a/spaces/mshukor/UnIVAL/fairseq/tests/test_multi_corpus_sampled_dataset.py b/spaces/mshukor/UnIVAL/fairseq/tests/test_multi_corpus_sampled_dataset.py
deleted file mode 100644
index 05b20328c5605178767d138cc75e070824679842..0000000000000000000000000000000000000000
--- a/spaces/mshukor/UnIVAL/fairseq/tests/test_multi_corpus_sampled_dataset.py
+++ /dev/null
@@ -1,95 +0,0 @@
-# Copyright (c) Facebook, Inc. and its affiliates.
-#
-# This source code is licensed under the MIT license found in the
-# LICENSE file in the root directory of this source tree.
-
-import unittest
-from collections import OrderedDict
-
-import numpy as np
-import torch
-from fairseq.data import LanguagePairDataset, TokenBlockDataset
-from fairseq.data.multi_corpus_sampled_dataset import MultiCorpusSampledDataset
-from tests.test_train import mock_dict
-
-
-class TestMultiCorpusSampledDataset(unittest.TestCase):
- def setUp(self):
- d = mock_dict()
- tokens_1 = torch.LongTensor([1]).view(1, -1)
- tokens_ds1 = TokenBlockDataset(
- tokens_1,
- sizes=[tokens_1.size(-1)],
- block_size=1,
- pad=0,
- eos=1,
- include_targets=False,
- )
- self.dataset_1 = LanguagePairDataset(
- tokens_ds1, tokens_ds1.sizes, d, shuffle=False
- )
- tokens_2 = torch.LongTensor([2]).view(1, -1)
- tokens_ds2 = TokenBlockDataset(
- tokens_2,
- sizes=[tokens_2.size(-1)],
- block_size=1,
- pad=0,
- eos=1,
- include_targets=False,
- )
- self.dataset_2 = LanguagePairDataset(
- tokens_ds2, tokens_ds2.sizes, d, shuffle=False
- )
-
- def _test_sample_helper(
- self,
- expected_sample_from_first_ds_percentage,
- num_samples=1000,
- sampling_func=None,
- ):
- # To make sure test is not flaky
- np.random.seed(0)
- if sampling_func is None:
- m = MultiCorpusSampledDataset(
- OrderedDict({0: self.dataset_1, 1: self.dataset_2}),
- )
- else:
- m = MultiCorpusSampledDataset(
- OrderedDict({0: self.dataset_1, 1: self.dataset_2}),
- sampling_func=sampling_func,
- )
- m.ordered_indices()
- count_sample_from_first_dataset = 0
- for _ in range(num_samples):
- if m.collater([m[0], m[1]])["net_input"]["src_tokens"][0] == 1:
- count_sample_from_first_dataset += 1
- sample_from_first_ds_percentage = (
- 1.0 * count_sample_from_first_dataset / num_samples
- )
- self.assertLess(
- abs(
- sample_from_first_ds_percentage
- - expected_sample_from_first_ds_percentage
- ),
- 0.01,
- )
-
- def test_multi_corpus_sampled_dataset_uniform_sample(self):
- self._test_sample_helper(expected_sample_from_first_ds_percentage=0.5)
-
- def test_multi_corpus_sampled_dataset_weighted_sample(self):
- def naive_weighted_sample(weights):
- def f(l):
- v = np.random.random()
- agg = 0
- for i, weight in enumerate(weights):
- agg += weight
- if agg > v:
- return i
-
- return f
-
- self._test_sample_helper(
- expected_sample_from_first_ds_percentage=0.9,
- sampling_func=naive_weighted_sample(weights=[0.9, 0.1]),
- )
diff --git a/spaces/mshukor/UnIVAL/run_scripts/caption/scaling_best/video/unival_video_caption_stage_1.sh b/spaces/mshukor/UnIVAL/run_scripts/caption/scaling_best/video/unival_video_caption_stage_1.sh
deleted file mode 100644
index bc6e0d818ff12cd6b255fcb3b11036aa2f41791c..0000000000000000000000000000000000000000
--- a/spaces/mshukor/UnIVAL/run_scripts/caption/scaling_best/video/unival_video_caption_stage_1.sh
+++ /dev/null
@@ -1,210 +0,0 @@
-
-
-# Number of GPUs per GPU worker
-export GPUS_PER_NODE=8
-# Number of GPU workers, for single-worker training, please set to 1
-export NUM_NODES=$SLURM_NNODES
-# The ip address of the rank-0 worker, for single-worker training, please set to localhost
-master_addr=$(scontrol show hostnames "$SLURM_JOB_NODELIST" | head -n 1)
-export MASTER_ADDR=$master_addr
-
-# The port for communication
-export MASTER_PORT=12350
-# The rank of this worker, should be in {0, ..., WORKER_CNT-1}, for single-worker training, please set to 0
-export RANK=$SLURM_NODEID
-
-echo "MASTER_ADDR: $MASTER_ADDR"
-echo "RANK :$RANK"
-echo "NUM_NODES :$NUM_NODES"
-echo "GPUS_PER_NODE :$GPUS_PER_NODE"
-
-export MIOPEN_USER_DB_PATH=/lus/home/NAT/gda2204/mshukor/.config/miopen_${MASTER_ADDR}_${SLURM_PROCID}/
-
-echo "MIOPEN_USER_DB_PATH :$MIOPEN_USER_DB_PATH"
-
-num_workers=0
-
-
-exp_name=unival_video_caption_stage_1
-
-
-ofa_dir=/lus/home/NAT/gda2204/mshukor/code/unival
-base_data_dir=/lus/scratch/NAT/gda2204/SHARED/data
-base_log_dir=/work/NAT/gda2204/mshukor/logs
-
-save_base_log_dir=/lus/scratch/NAT/gda2204/SHARED/logs
-save_dir=${save_base_log_dir}/ofa/checkpoints/caption/${exp_name}
-
-log_dir=${save_dir}
-
-mkdir -p $log_dir $save_dir
-
-bpe_dir=${ofa_dir}/utils/BPE
-user_dir=${ofa_dir}/ofa_module
-
-
-
-image_dir=${base_data_dir}
-
-
-data_dir=${base_data_dir}/ofa/video_data/caption_data
-data=${data_dir}/msrvtt_caption_train7k.tsv,${data_dir}/msrvtt_caption_test3k.tsv
-eval_cider_cached=${data_dir}/cider_cached_tokens/msrvtt-test3k-words.p
-
-data=${data_dir}/msrvtt_caption_train7k_1.tsv,${data_dir}/msrvtt_caption_train7k_2.tsv,${data_dir}/msrvtt_caption_train7k_3.tsv,${data_dir}/msrvtt_caption_train7k_4.tsv,${data_dir}/msrvtt_caption_train7k_5.tsv,${data_dir}/msrvtt_caption_train7k_6.tsv,${data_dir}/msrvtt_caption_train7k_7.tsv,${data_dir}/msrvtt_caption_train7k_8.tsv,${data_dir}/msrvtt_caption_train7k_9.tsv,${data_dir}/msrvtt_caption_train7k_10.tsv,${data_dir}/msrvtt_caption_test3k.tsv
-
-restore_file=${save_base_log_dir}/ofa/checkpoints/pretrain/ofa_base_pretrain_s2_long_lr1e4_50ep_nolsdata_vidhs/checkpoint1.pt
-
-lr=1e-5
-
-
-
-
-selected_cols=0,4,2
-
-task=video_caption
-arch=unival_base
-pretrained_model=
-
-
-criterion=adjust_label_smoothed_encouraging_loss
-label_smoothing=0.1
-
-max_epoch=15
-warmup_ratio=0.06
-batch_size=16
-update_freq=2
-resnet_drop_path_rate=0.0
-encoder_drop_path_rate=0.1
-decoder_drop_path_rate=0.1
-dropout=0.1
-attention_dropout=0.0
-max_src_length=80
-max_tgt_length=20
-num_bins=1000
-# patch_image_size=480
-drop_worst_ratio=0.2
-
-
-
-
-###
-image_encoder_name=timm_resnet #vit_base_patch16_224
-patch_image_size=480
-resnet_type=resnet101
-
-resnet_model_path=${base_log_dir}/pretrained_models/resnet101-5d3b4d8f.pth
-
-# video
-video_encoder_name=all_resnext101
-patch_frame_size=384
-video_model_path=${base_log_dir}/pretrained_models/3dcnn/resnext-101-kinetics.pth #${base_log_dir}/pretrained_models/TimeSformer_divST_8x32_224_K600.pyth
-num_frames=16
-
-
-save_interval=1
-validate_interval_updates=2000
-save_interval_updates=0
-
-
-sample_patch_num='--sample-patch-num=784' # ''
-
-eval_args='--eval-args={"beam":5,"unnormalized":true,"temperature":1.0,"stop_on_max_len":true}'
-
-
-
-drop_worst_ratio=0.05 # modified from 0.2 for el
-log_end=0.75 # for el
-drop_best_ratio=0.05
-drop_best_after=6000
-drop_worst_after=6000
-
-use_dataaug='--use-dataaug'
-
-for max_epoch in {20,}; do
- echo "max_epoch "${max_epoch}
- for warmup_ratio in {0.06,}; do
- echo "warmup_ratio "${warmup_ratio}
- for drop_worst_after in {6000,}; do
- echo "drop_worst_after "${drop_worst_after}
-
- log_file=${log_dir}/${max_epoch}"_"${warmup_ratio}"_"${drop_worst_after}".log"
- save_path=${save_dir}/${max_epoch}"_"${warmup_ratio}"_"${drop_worst_after}
- mkdir -p $save_path
-
- python3 -m torch.distributed.launch \
- --nnodes=${NUM_NODES} \
- --nproc_per_node=${GPUS_PER_NODE} \
- --master_port=${MASTER_PORT} \
- --node_rank=${RANK} \
- --master_addr=${MASTER_ADDR} \
- --use_env ${ofa_dir}/train.py \
- $data \
- --selected-cols=${selected_cols} \
- --bpe-dir=${bpe_dir} \
- --user-dir=${user_dir} \
- --restore-file=${restore_file} \
- --save-dir=${save_path} \
- --task=${task} \
- --arch=${arch} \
- --criterion=${criterion} \
- --label-smoothing=${label_smoothing} \
- --batch-size=${batch_size} \
- --update-freq=${update_freq} \
- --encoder-normalize-before \
- --decoder-normalize-before \
- --share-decoder-input-output-embed \
- --share-all-embeddings \
- --layernorm-embedding \
- --patch-layernorm-embedding \
- --code-layernorm-embedding \
- --resnet-drop-path-rate=${resnet_drop_path_rate} \
- --encoder-drop-path-rate=${encoder_drop_path_rate} \
- --decoder-drop-path-rate=${decoder_drop_path_rate} \
- --dropout=${dropout} \
- --attention-dropout=${attention_dropout} \
- --weight-decay=0.01 --optimizer=adam --adam-betas="(0.9,0.999)" --adam-eps=1e-08 --clip-norm=1.0 \
- --lr-scheduler=polynomial_decay --lr=${lr} \
- --max-epoch=${max_epoch} --warmup-ratio=${warmup_ratio} \
- --log-format=simple --log-interval=10 \
- --fixed-validation-seed=7 \
- --no-epoch-checkpoints --keep-best-checkpoints=1 \
- --save-interval=${save_interval} --validate-interval=1 \
- --save-interval-updates=${save_interval_updates} --validate-interval-updates=${validate_interval_updates} \
- --eval-cider \
- --eval-cider-cached-tokens=${eval_cider_cached} \
- --eval-args='{"beam":5,"max_len_b":16,"no_repeat_ngram_size":3}' \
- --best-checkpoint-metric=cider --maximize-best-checkpoint-metric \
- --max-src-length=${max_src_length} \
- --max-tgt-length=${max_tgt_length} \
- --find-unused-parameters \
- --freeze-encoder-embedding \
- --freeze-decoder-embedding \
- --add-type-embedding \
- --scale-attn \
- --scale-fc \
- --scale-heads \
- --disable-entangle \
- --num-bins=${num_bins} \
- --patch-image-size=${patch_image_size} \
- --drop-worst-ratio=${drop_worst_ratio} \
- --drop-worst-after=${drop_worst_after} \
- --fp16 \
- --fp16-scale-window=512 \
- --num-workers=0 \
- --image-encoder-name=${image_encoder_name} \
- --image-dir=${image_dir} \
- --video-encoder-name=${video_encoder_name} \
- --video-model-path=${video_model_path} \
- --patch-frame-size=${patch_frame_size} \
- ${sample_patch_num} \
- ${eval_args} \
- --num-frames=${num_frames} \
- --log-end ${log_end} --drop-best-ratio ${drop_best_ratio} --drop-best-after ${drop_best_after} \
- ${use_dataaug} \
- --reset-dataloader --reset-meters --reset-optimizer
-
-
- done
- done
-done
\ No newline at end of file
diff --git a/spaces/mthsk/sovits-100orangejuice/vdecoder/hifigan/nvSTFT.py b/spaces/mthsk/sovits-100orangejuice/vdecoder/hifigan/nvSTFT.py
deleted file mode 100644
index 88597d62a505715091f9ba62d38bf0a85a31b95a..0000000000000000000000000000000000000000
--- a/spaces/mthsk/sovits-100orangejuice/vdecoder/hifigan/nvSTFT.py
+++ /dev/null
@@ -1,111 +0,0 @@
-import math
-import os
-os.environ["LRU_CACHE_CAPACITY"] = "3"
-import random
-import torch
-import torch.utils.data
-import numpy as np
-import librosa
-from librosa.util import normalize
-from librosa.filters import mel as librosa_mel_fn
-from scipy.io.wavfile import read
-import soundfile as sf
-
-def load_wav_to_torch(full_path, target_sr=None, return_empty_on_exception=False):
- sampling_rate = None
- try:
- data, sampling_rate = sf.read(full_path, always_2d=True)# than soundfile.
- except Exception as ex:
- print(f"'{full_path}' failed to load.\nException:")
- print(ex)
- if return_empty_on_exception:
- return [], sampling_rate or target_sr or 32000
- else:
- raise Exception(ex)
-
- if len(data.shape) > 1:
- data = data[:, 0]
- assert len(data) > 2# check duration of audio file is > 2 samples (because otherwise the slice operation was on the wrong dimension)
-
- if np.issubdtype(data.dtype, np.integer): # if audio data is type int
- max_mag = -np.iinfo(data.dtype).min # maximum magnitude = min possible value of intXX
- else: # if audio data is type fp32
- max_mag = max(np.amax(data), -np.amin(data))
- max_mag = (2**31)+1 if max_mag > (2**15) else ((2**15)+1 if max_mag > 1.01 else 1.0) # data should be either 16-bit INT, 32-bit INT or [-1 to 1] float32
-
- data = torch.FloatTensor(data.astype(np.float32))/max_mag
-
- if (torch.isinf(data) | torch.isnan(data)).any() and return_empty_on_exception:# resample will crash with inf/NaN inputs. return_empty_on_exception will return empty arr instead of except
- return [], sampling_rate or target_sr or 32000
- if target_sr is not None and sampling_rate != target_sr:
- data = torch.from_numpy(librosa.core.resample(data.numpy(), orig_sr=sampling_rate, target_sr=target_sr))
- sampling_rate = target_sr
-
- return data, sampling_rate
-
-def dynamic_range_compression(x, C=1, clip_val=1e-5):
- return np.log(np.clip(x, a_min=clip_val, a_max=None) * C)
-
-def dynamic_range_decompression(x, C=1):
- return np.exp(x) / C
-
-def dynamic_range_compression_torch(x, C=1, clip_val=1e-5):
- return torch.log(torch.clamp(x, min=clip_val) * C)
-
-def dynamic_range_decompression_torch(x, C=1):
- return torch.exp(x) / C
-
-class STFT():
- def __init__(self, sr=22050, n_mels=80, n_fft=1024, win_size=1024, hop_length=256, fmin=20, fmax=11025, clip_val=1e-5):
- self.target_sr = sr
-
- self.n_mels = n_mels
- self.n_fft = n_fft
- self.win_size = win_size
- self.hop_length = hop_length
- self.fmin = fmin
- self.fmax = fmax
- self.clip_val = clip_val
- self.mel_basis = {}
- self.hann_window = {}
-
- def get_mel(self, y, center=False):
- sampling_rate = self.target_sr
- n_mels = self.n_mels
- n_fft = self.n_fft
- win_size = self.win_size
- hop_length = self.hop_length
- fmin = self.fmin
- fmax = self.fmax
- clip_val = self.clip_val
-
- if torch.min(y) < -1.:
- print('min value is ', torch.min(y))
- if torch.max(y) > 1.:
- print('max value is ', torch.max(y))
-
- if fmax not in self.mel_basis:
- mel = librosa_mel_fn(sr=sampling_rate, n_fft=n_fft, n_mels=n_mels, fmin=fmin, fmax=fmax)
- self.mel_basis[str(fmax)+'_'+str(y.device)] = torch.from_numpy(mel).float().to(y.device)
- self.hann_window[str(y.device)] = torch.hann_window(self.win_size).to(y.device)
-
- y = torch.nn.functional.pad(y.unsqueeze(1), (int((n_fft-hop_length)/2), int((n_fft-hop_length)/2)), mode='reflect')
- y = y.squeeze(1)
-
- spec = torch.stft(y, n_fft, hop_length=hop_length, win_length=win_size, window=self.hann_window[str(y.device)],
- center=center, pad_mode='reflect', normalized=False, onesided=True)
- # print(111,spec)
- spec = torch.sqrt(spec.pow(2).sum(-1)+(1e-9))
- # print(222,spec)
- spec = torch.matmul(self.mel_basis[str(fmax)+'_'+str(y.device)], spec)
- # print(333,spec)
- spec = dynamic_range_compression_torch(spec, clip_val=clip_val)
- # print(444,spec)
- return spec
-
- def __call__(self, audiopath):
- audio, sr = load_wav_to_torch(audiopath, target_sr=self.target_sr)
- spect = self.get_mel(audio.unsqueeze(0)).squeeze(0)
- return spect
-
-stft = STFT()
diff --git a/spaces/nateraw/detr-object-detection/app.py b/spaces/nateraw/detr-object-detection/app.py
deleted file mode 100644
index 0b8ff3ea89e45da249716c01c3c686fd909f7902..0000000000000000000000000000000000000000
--- a/spaces/nateraw/detr-object-detection/app.py
+++ /dev/null
@@ -1,81 +0,0 @@
-import io
-
-import matplotlib.pyplot as plt
-import requests
-import streamlit as st
-import torch
-from PIL import Image
-from transformers import DetrFeatureExtractor, DetrForObjectDetection
-
-# colors for visualization
-COLORS = [
- [0.000, 0.447, 0.741],
- [0.850, 0.325, 0.098],
- [0.929, 0.694, 0.125],
- [0.494, 0.184, 0.556],
- [0.466, 0.674, 0.188],
- [0.301, 0.745, 0.933]
-]
-
-
-@st.cache(allow_output_mutation=True)
-def get_hf_components(model_name_or_path):
- feature_extractor = DetrFeatureExtractor.from_pretrained(model_name_or_path)
- model = DetrForObjectDetection.from_pretrained(model_name_or_path)
- model.eval()
- return feature_extractor, model
-
-
-@st.cache
-def get_img_from_url(url):
- return Image.open(requests.get(url, stream=True).raw)
-
-
-def fig2img(fig):
- buf = io.BytesIO()
- fig.savefig(buf)
- buf.seek(0)
- img = Image.open(buf)
- return img
-
-
-def visualize_prediction(pil_img, output_dict, threshold=0.7, id2label=None):
- keep = output_dict["scores"] > threshold
- boxes = output_dict["boxes"][keep].tolist()
- scores = output_dict["scores"][keep].tolist()
- labels = output_dict["labels"][keep].tolist()
- if id2label is not None:
- labels = [id2label[x] for x in labels]
-
- plt.figure(figsize=(16, 10))
- plt.imshow(pil_img)
- ax = plt.gca()
- colors = COLORS * 100
- for score, (xmin, ymin, xmax, ymax), label, color in zip(scores, boxes, labels, colors):
- ax.add_patch(plt.Rectangle((xmin, ymin), xmax - xmin, ymax - ymin, fill=False, color=color, linewidth=3))
- ax.text(xmin, ymin, f"{label}: {score:0.2f}", fontsize=15, bbox=dict(facecolor="yellow", alpha=0.5))
- plt.axis("off")
- return fig2img(plt.gcf())
-
-
-def make_prediction(img, feature_extractor, model):
- inputs = feature_extractor(img, return_tensors="pt")
- outputs = model(**inputs)
- img_size = torch.tensor([tuple(reversed(img.size))])
- processed_outputs = feature_extractor.post_process(outputs, img_size)
- return processed_outputs[0]
-
-
-def main():
- option = st.selectbox("Which model should we use?", ("facebook/detr-resnet-50", "facebook/detr-resnet-101"))
- feature_extractor, model = get_hf_components(option)
- url = st.text_input("URL to some image", "http://images.cocodataset.org/val2017/000000039769.jpg")
- img = get_img_from_url(url)
- processed_outputs = make_prediction(img, feature_extractor, model)
- threshold = st.slider("Prediction Threshold", 0.0, 1.0, 0.7)
- viz_img = visualize_prediction(img, processed_outputs, threshold, model.config.id2label)
- st.image(viz_img)
-
-
-if __name__ == "__main__":
- main()
diff --git a/spaces/nateraw/modelcard-creator/README.md b/spaces/nateraw/modelcard-creator/README.md
deleted file mode 100644
index ba140974012b2f8b736a87d7258af3d19b9467bf..0000000000000000000000000000000000000000
--- a/spaces/nateraw/modelcard-creator/README.md
+++ /dev/null
@@ -1,13 +0,0 @@
----
-title: Modelcard Creator
-emoji: ⚡
-colorFrom: red
-colorTo: yellow
-sdk: streamlit
-sdk_version: 1.10.0
-app_file: 1_📝_form.py
-pinned: false
-license: mit
----
-
-Check out the configuration reference at https://huggingface.co/docs/hub/spaces-config-reference
diff --git a/spaces/nazneen/error-analysis/app.py b/spaces/nazneen/error-analysis/app.py
deleted file mode 100644
index 6778fa924ba631a0376c21ecd4e9b48d875cfdd2..0000000000000000000000000000000000000000
--- a/spaces/nazneen/error-analysis/app.py
+++ /dev/null
@@ -1,296 +0,0 @@
-## LIBRARIES ###
-## Data
-import numpy as np
-import pandas as pd
-import torch
-import math
-from tqdm import tqdm
-from math import floor
-from collections import defaultdict
-from transformers import AutoTokenizer
-pd.options.display.float_format = '${:,.2f}'.format
-
-# Analysis
-# from gensim.models.doc2vec import Doc2Vec
-# from sklearn.metrics import accuracy_score, f1_score, precision_score, recall_score
-import nltk
-from nltk.cluster import KMeansClusterer
-import scipy.spatial.distance as sdist
-from scipy.spatial import distance_matrix
-# nltk.download('punkt') #make sure that punkt is downloaded
-
-# App & Visualization
-import streamlit as st
-import altair as alt
-import plotly.graph_objects as go
-from streamlit_vega_lite import altair_component
-
-
-
-# utils
-from random import sample
-from error_analysis import utils as ut
-
-
-def down_samp(embedding):
- """Down sample a data frame for altiar visualization """
- # total number of positive and negative sentiments in the class
- #embedding = embedding.groupby('slice').apply(lambda x: x.sample(frac=0.3))
- total_size = embedding.groupby(['slice','label'], as_index=False).count()
-
- user_data = 0
- # if 'Your Sentences' in str(total_size['slice']):
- # tmp = embedding.groupby(['slice'], as_index=False).count()
- # val = int(tmp[tmp['slice'] == "Your Sentences"]['source'])
- # user_data = val
-
- max_sample = total_size.groupby('slice').max()['content']
-
- # # down sample to meeting altair's max values
- # # but keep the proportional representation of groups
- down_samp = 1/(sum(max_sample.astype(float))/(1000-user_data))
-
- max_samp = max_sample.apply(lambda x: floor(x*down_samp)).astype(int).to_dict()
- max_samp['Your Sentences'] = user_data
-
- # # sample down for each group in the data frame
- embedding = embedding.groupby('slice').apply(lambda x: x.sample(n=max_samp.get(x.name))).reset_index(drop=True)
-
- # # order the embedding
- return(embedding)
-
-
-def data_comparison(df):
- selection = alt.selection_multi(fields=['cluster','label'])
- color = alt.condition(alt.datum.slice == 'high-loss', alt.Color('cluster:N', scale = alt.Scale(domain=df.cluster.unique().tolist())), alt.value("lightgray"))
- opacity = alt.condition(selection, alt.value(0.7), alt.value(0.25))
-
- # basic chart
- scatter = alt.Chart(df).mark_point(size=100, filled=True).encode(
- x=alt.X('x:Q', axis=None),
- y=alt.Y('y:Q', axis=None),
- color=color,
- shape=alt.Shape('label:N', scale=alt.Scale(range=['circle', 'diamond'])),
- tooltip=['cluster:N','slice:N','content:N','label:N','pred:O'],
- opacity=opacity
- ).properties(
- width=1000,
- height=800
- ).interactive()
-
- legend = alt.Chart(df).mark_point(size=100, filled=True).encode(
- x=alt.X("label:N"),
- y=alt.Y('cluster:N', axis=alt.Axis(orient='right'), sort='descending', title=''),
- shape=alt.Shape('label:N', scale=alt.Scale(
- range=['circle', 'diamond']), legend=None),
- color=color,
- ).add_selection(
- selection
- )
- layered = scatter | legend
- layered = layered.configure_axis(
- grid=False
- ).configure_view(
- strokeOpacity=0
- )
- return layered
-
-def quant_panel(embedding_df):
- """ Quantitative Panel Layout"""
- all_metrics = {}
- st.warning("**Error slice visualization**")
- with st.expander("How to read this chart:"):
- st.markdown("* Each **point** is an input example.")
- st.markdown("* Gray points have low-loss and the colored have high-loss. High-loss instances are clustered using **kmeans** and each color represents a cluster.")
- st.markdown("* The **shape** of each point reflects the label category -- positive (diamond) or negative sentiment (circle).")
- #st.altair_chart(data_comparison(down_samp(embedding_df)), use_container_width=True)
- st.altair_chart(data_comparison(embedding_df), use_container_width=True)
-
-
-def frequent_tokens(data, tokenizer, loss_quantile=0.95, top_k=200, smoothing=0.005):
- unique_tokens = []
- tokens = []
- for row in tqdm(data['content']):
- tokenized = tokenizer(row,padding=True, return_tensors='pt')
- tokens.append(tokenized['input_ids'].flatten())
- unique_tokens.append(torch.unique(tokenized['input_ids']))
- losses = data['loss'].astype(float)
- high_loss = losses.quantile(loss_quantile)
- loss_weights = (losses > high_loss)
- loss_weights = loss_weights / loss_weights.sum()
- token_frequencies = defaultdict(float)
- token_frequencies_error = defaultdict(float)
-
- weights_uniform = np.full_like(loss_weights, 1 / len(loss_weights))
-
- num_examples = len(data)
- for i in tqdm(range(num_examples)):
- for token in unique_tokens[i]:
- token_frequencies[token.item()] += weights_uniform[i]
- token_frequencies_error[token.item()] += loss_weights[i]
-
- token_lrs = {k: (smoothing+token_frequencies_error[k]) / (smoothing+token_frequencies[k]) for k in token_frequencies}
- tokens_sorted = list(map(lambda x: x[0], sorted(token_lrs.items(), key=lambda x: x[1])[::-1]))
-
- top_tokens = []
- for i, (token) in enumerate(tokens_sorted[:top_k]):
- top_tokens.append(['%10s' % (tokenizer.decode(token)), '%.4f' % (token_frequencies[token]), '%.4f' % (
- token_frequencies_error[token]), '%4.2f' % (token_lrs[token])])
- return pd.DataFrame(top_tokens, columns=['Token', 'Freq', 'Freq error slice', 'Ratio w/ smoothing'])
-
-
-@st.cache(ttl=600)
-def get_data(inference, emb):
- preds = inference.outputs.numpy()
- losses = inference.losses.numpy()
- embeddings = pd.DataFrame(emb, columns=['x', 'y'])
- num_examples = len(losses)
- # dataset_labels = [dataset[i]['label'] for i in range(num_examples)]
- return pd.concat([pd.DataFrame(np.transpose(np.vstack([dataset[:num_examples]['content'],
- dataset[:num_examples]['label'], preds, losses])), columns=['content', 'label', 'pred', 'loss']), embeddings], axis=1)
-
-def clustering(data,num_clusters):
- X = np.array(data['embedding'].tolist())
- kclusterer = KMeansClusterer(
- num_clusters, distance=nltk.cluster.util.cosine_distance,
- repeats=25,avoid_empty_clusters=True)
- assigned_clusters = kclusterer.cluster(X, assign_clusters=True)
- data['cluster'] = pd.Series(assigned_clusters, index=data.index).astype('int')
- data['centroid'] = data['cluster'].apply(lambda x: kclusterer.means()[x])
- return data, assigned_clusters
-
-def kmeans(df, num_clusters=3):
- #data_hl = df.loc[df['slice'] == 'high-loss']
- data_kmeans,clusters = clustering(df,num_clusters)
- #merged = pd.merge(df, data_kmeans, left_index=True, right_index=True, how='outer', suffixes=('', '_y'))
- #merged.drop(merged.filter(regex='_y$').columns.tolist(),axis=1,inplace=True)
- #merged['cluster'] = merged['cluster'].fillna(num_clusters).astype('int')
- return data_kmeans
-
-def distance_from_centroid(row):
- return sdist.norm(row['embedding'] - row['centroid'].tolist())
-
-@st.cache(ttl=600)
-def topic_distribution(weights, smoothing=0.01):
- topic_frequencies = defaultdict(float)
- topic_frequencies_error= defaultdict(float)
- weights_uniform = np.full_like(weights, 1 / len(weights))
- num_examples = len(weights)
- for i in range(num_examples):
- example = dataset[i]
- category = example['title']
- topic_frequencies[category] += weights_uniform[i]
- topic_frequencies_error[category] += weights[i]
-
- topic_ratios = {c: (smoothing + topic_frequencies_error[c]) / (
- smoothing + topic_frequencies[c]) for c in topic_frequencies}
-
- categories_sorted = map(lambda x: x[0], sorted(
- topic_ratios.items(), key=lambda x: x[1], reverse=True))
-
- topic_distr = []
- for category in categories_sorted:
- topic_distr.append(['%.3f' % topic_frequencies[category], '%.3f' %
- topic_frequencies_error[category], '%.2f' % topic_ratios[category], '%s' % category])
-
- return pd.DataFrame(topic_distr, columns=['Overall frequency', 'Error frequency', 'Ratio', 'Category'])
-
-def populate_session(dataset,model):
- data_df = read_file_to_df('./assets/data/'+dataset+ '_'+ model+'.parquet')
- if model == 'albert-base-v2-yelp-polarity':
- tokenizer = AutoTokenizer.from_pretrained('textattack/'+model)
- else:
- tokenizer = AutoTokenizer.from_pretrained(model)
- if "user_data" not in st.session_state:
- st.session_state["user_data"] = data_df
- if "selected_slice" not in st.session_state:
- st.session_state["selected_slice"] = None
-
-@st.cache(allow_output_mutation=True)
-def read_file_to_df(file):
- return pd.read_parquet(file)
-
-if __name__ == "__main__":
- ### STREAMLIT APP CONGFIG ###
- st.set_page_config(layout="wide", page_title="Interactive Error Analysis")
-
- ut.init_style()
-
- lcol, rcol = st.columns([2, 2])
- # ******* loading the mode and the data
- #st.sidebar.mardown("
Interactive Error Analysis
", unsafe_allow_html=True)
-
- dataset = st.sidebar.selectbox(
- "Dataset",
- ["amazon_polarity", "yelp_polarity"],
- index = 1
- )
-
- model = st.sidebar.selectbox(
- "Model",
- ["distilbert-base-uncased-finetuned-sst-2-english",
- "albert-base-v2-yelp-polarity"],
- )
-
- ### LOAD DATA AND SESSION VARIABLES ###
- ##uncomment the next next line to run dynamically and not from file
- #populate_session(dataset, model)
- data_df = read_file_to_df('./assets/data/'+dataset+ '_'+ model+'.parquet')
- loss_quantile = st.sidebar.slider(
- "Loss Quantile", min_value=0.5, max_value=1.0,step=0.01,value=0.99
- )
- data_df = data_df.drop(data_df[data_df.pred == data_df.label].index) #drop rows that are not errors
- data_df['loss'] = data_df['loss'].astype(float)
- losses = data_df['loss']
- high_loss = losses.quantile(loss_quantile)
- data_df['slice'] = 'high-loss'
- data_df['slice'] = data_df['slice'].where(data_df['loss'] > high_loss, 'low-loss')
- data_hl = data_df.drop(data_df[data_df['slice'] == 'low-loss'].index) #drop rows that are not hl
- data_ll = data_df.drop(data_df[data_df['slice'] == 'high-loss'].index)
- df_list = [d for _, d in data_hl.groupby(['label'])] # this is to allow clustering over each error type. fp, fn for binary classification
-
- with lcol:
- st.markdown('
Error Slices
',unsafe_allow_html=True)
- with st.expander("How to read the table:"):
- st.markdown("* *Error slice* refers to the subset of evaluation dataset the model performs poorly on.")
- st.markdown("* The table displays model error slices on the evaluation dataset, sorted by loss.")
- st.markdown("* Each row is an input example that includes the label, model pred, loss, and error cluster.")
- with st.spinner(text='loading error slice...'):
- dataframe=read_file_to_df('./assets/data/'+dataset+ '_'+ model+'_error-slices.parquet')
- #uncomment the next next line to run dynamically and not from file
- # dataframe = merged[['content', 'label', 'pred', 'loss', 'cluster']].sort_values(
- # by=['loss'], ascending=False)
- # table_html = dataframe.to_html(
- # columns=['content', 'label', 'pred', 'loss', 'cluster'], max_rows=50)
- # table_html = table_html.replace("
", '
') # left-align the headers
- st.write(dataframe,width=900, height=300)
-
- with rcol:
- with st.spinner(text='loading...'):
- st.markdown('
Word Distribution in Error Slice
', unsafe_allow_html=True)
- #uncomment the next two lines to run dynamically and not from file
- #commontokens = frequent_tokens(data_df, tokenizer, loss_quantile=loss_quantile)
- commontokens = read_file_to_df('./assets/data/'+dataset+ '_'+ model+'_commontokens.parquet')
- with st.expander("How to read the table:"):
- st.markdown("* The table displays the most frequent tokens in error slices, relative to their frequencies in the val set.")
- st.write(commontokens)
-
- run_kmeans = st.sidebar.radio("Cluster error slice?", ('True', 'False'), index=0)
-
- num_clusters = st.sidebar.slider("# clusters", min_value=1, max_value=20, step=1, value=3)
-
- if run_kmeans == 'True':
- with st.spinner(text='running kmeans...'):
- merged = pd.DataFrame()
- ind=0
- for df in df_list:
- #num_clusters= int(math.sqrt(len(df)/2))
- kmeans_df = kmeans(df,num_clusters=num_clusters)
- #print(kmeans_df.loc[kmeans_df['cluster'].idxmax()])
- kmeans_df['cluster'] = kmeans_df['cluster'] + ind*num_clusters
- ind = ind+1
- merged = pd.concat([merged, kmeans_df])
- merged = pd.concat([merged, data_ll])
-
- with st.spinner(text='loading visualization...'):
- quant_panel(merged)
\ No newline at end of file
diff --git a/spaces/netiMophi/DreamlikeArt-Diffusion-1.0/Les Infideles 2012 French 720p Bluray X264 Seight Greek Subtitles.md b/spaces/netiMophi/DreamlikeArt-Diffusion-1.0/Les Infideles 2012 French 720p Bluray X264 Seight Greek Subtitles.md
deleted file mode 100644
index 8e7d6243f74484f16539abf974ea625468caada0..0000000000000000000000000000000000000000
--- a/spaces/netiMophi/DreamlikeArt-Diffusion-1.0/Les Infideles 2012 French 720p Bluray X264 Seight Greek Subtitles.md
+++ /dev/null
@@ -1,24 +0,0 @@
-
-
Review: Les Infideles (The Players) - A French Comedy About Cheating
-
Les Infideles (The Players) is a 2012 French comedy film that consists of several short stories about men who cheat on their wives or girlfriends. The film features an ensemble cast of well-known French actors, such as Jean Dujardin, Gilles Lellouche, Guillaume Canet, and Marion Cotillard. The film was directed by several filmmakers, including Dujardin and Lellouche themselves.
-
The film explores the different ways that men try to justify their infidelity, from lying to their partners, to hiring prostitutes, to attending sex addiction meetings. The film also shows the consequences of their actions, such as broken relationships, unwanted pregnancies, and STDs. The film is a satire of the male ego and the hypocrisy of the French society that tolerates cheating.
-
Les Infideles 2012 French 720p Bluray X264 Seight Greek Subtitles
The film received mixed reviews from critics and audiences. Some praised the film for its humor and its daring portrayal of taboo topics, while others criticized the film for its misogyny and its lack of depth. The film was also controversial for its poster, which showed Dujardin holding a woman's legs in the air with the tagline "I'm going into a meeting". The poster was banned in some cities for being sexist and offensive.
-
The film was released on Blu-ray and DVD in France in 2012, with English subtitles. However, there are also versions of the film with Greek subtitles available online. One of them is Les Infideles 2012 French 720p Bluray X264 Seight Greek Subtitles[^1^], which can be downloaded from subdl.com[^1^]. Another one is Les infidèles (The Players) subtitles - SUBDL[^2^], which can be found on subscene.com[^2^]. These versions have high-quality video and audio, and offer a variety of subtitle languages to choose from.
-
-
If you are looking for a raunchy and irreverent comedy about cheating, you might enjoy Les Infideles (The Players). However, be warned that the film is not for everyone, as it might offend some viewers with its crude jokes and its depiction of women.
-
-
The film is divided into eight segments, each with a different director and a different cast. The segments are:
-
-
The Question: Directed by Emmanuelle Bercot. A couple (Jean Dujardin and Alexandra Lamy) go to a therapist to discuss their marital problems. The therapist asks them if they have ever cheated on each other, and they both lie.
The Spouse: Directed by Eric Lartigau. A man (Guillaume Canet) pretends to be a widower to seduce women at funerals. He meets a woman (Isabelle Nanty) who claims to be a widow, but she is actually a serial killer.
-
The Rascal: Directed by Alexandre Courtès. A man (Jean Dujardin) hires a prostitute (Sandrine Kiberlain) to act as his wife for a business dinner. He tries to impress his boss (Bruno Solo) by pretending to be abusive to her.
The Good Conscience: Directed by Michel Hazanavicius. A man (Gilles Lellouche) attends a sex addiction meeting and confesses his sins to a priest (Didier Flamand). He then tries to seduce the priest's assistant (Dolly Golden).
-
Don't Tell Anyone: Directed by Gilles Lellouche. A group of friends (Gilles Lellouche, Manu Payet, Guillaume Canet, and Philippe Lefebvre) go on a skiing trip and cheat on their wives with local women. They swear to keep it a secret, but one of them gets caught on camera.
-
The Happy Ending: Directed by Eric Lartigau. A man (Jean Dujardin) and a woman (Marion Cotillard) meet at an airport and fall in love. They decide to leave their spouses and run away together, but they discover that they are both married to each other.
-
-
The film ends with a musical number featuring all the actors singing "Les Infideles" by Serge Gainsbourg.
7196e7f11a
-
-
\ No newline at end of file
diff --git a/spaces/ngoctuanai/DALL-E/README.md b/spaces/ngoctuanai/DALL-E/README.md
deleted file mode 100644
index fbbdcfaa627363412e2da593ab0546bfb9ae874c..0000000000000000000000000000000000000000
--- a/spaces/ngoctuanai/DALL-E/README.md
+++ /dev/null
@@ -1,9 +0,0 @@
----
-title: DALL·E
-emoji: 🥑
-colorFrom: yellow
-colorTo: green
-sdk: static
-pinned: false
-license: apache-2.0
----
\ No newline at end of file
diff --git a/spaces/nightfury/SD-InPainting/clipseg/datasets/pascal_zeroshot.py b/spaces/nightfury/SD-InPainting/clipseg/datasets/pascal_zeroshot.py
deleted file mode 100644
index 3fa84de9049bf272538f97b408bed07a9e9b5478..0000000000000000000000000000000000000000
--- a/spaces/nightfury/SD-InPainting/clipseg/datasets/pascal_zeroshot.py
+++ /dev/null
@@ -1,60 +0,0 @@
-from os.path import expanduser
-import torch
-import json
-import torchvision
-from general_utils import get_from_repository
-from general_utils import log
-from torchvision import transforms
-
-PASCAL_VOC_CLASSES_ZS = [['cattle.n.01', 'motorcycle.n.01'], ['aeroplane.n.01', 'sofa.n.01'],
- ['cat.n.01', 'television.n.03'], ['train.n.01', 'bottle.n.01'],
- ['chair.n.01', 'pot_plant.n.01']]
-
-
-class PascalZeroShot(object):
-
- def __init__(self, split, n_unseen, image_size=224) -> None:
- super().__init__()
-
- import sys
- sys.path.append('third_party/JoEm')
- from third_party.JoEm.data_loader.dataset import VOCSegmentation
- from third_party.JoEm.data_loader import get_seen_idx, get_unseen_idx, VOC
-
- self.pascal_classes = VOC
- self.image_size = image_size
-
- self.transform = transforms.Compose([
- transforms.Resize((image_size, image_size)),
- ])
-
- if split == 'train':
- self.voc = VOCSegmentation(get_unseen_idx(n_unseen), get_seen_idx(n_unseen),
- split=split, transform=True, transform_args=dict(base_size=312, crop_size=312),
- ignore_bg=False, ignore_unseen=False, remv_unseen_img=True)
- elif split == 'val':
- self.voc = VOCSegmentation(get_unseen_idx(n_unseen), get_seen_idx(n_unseen),
- split=split, transform=False,
- ignore_bg=False, ignore_unseen=False)
-
- self.unseen_idx = get_unseen_idx(n_unseen)
-
- def __len__(self):
- return len(self.voc)
-
- def __getitem__(self, i):
-
- sample = self.voc[i]
- label = sample['label'].long()
- all_labels = [l for l in torch.where(torch.bincount(label.flatten())>0)[0].numpy().tolist() if l != 255]
- class_indices = [l for l in all_labels]
- class_names = [self.pascal_classes[l] for l in all_labels]
-
- image = self.transform(sample['image'])
-
- label = transforms.Resize((self.image_size, self.image_size),
- interpolation=torchvision.transforms.InterpolationMode.NEAREST)(label.unsqueeze(0))[0]
-
- return (image,), (label, )
-
-
diff --git a/spaces/niizam/sovits-models/onnxexport/model_onnx.py b/spaces/niizam/sovits-models/onnxexport/model_onnx.py
deleted file mode 100644
index e28bae95ec1e53aa05d06fc784ff86d55f228d60..0000000000000000000000000000000000000000
--- a/spaces/niizam/sovits-models/onnxexport/model_onnx.py
+++ /dev/null
@@ -1,335 +0,0 @@
-import torch
-from torch import nn
-from torch.nn import functional as F
-
-import modules.attentions as attentions
-import modules.commons as commons
-import modules.modules as modules
-
-from torch.nn import Conv1d, ConvTranspose1d, AvgPool1d, Conv2d
-from torch.nn.utils import weight_norm, remove_weight_norm, spectral_norm
-
-import utils
-from modules.commons import init_weights, get_padding
-from vdecoder.hifigan.models import Generator
-from utils import f0_to_coarse
-
-
-class ResidualCouplingBlock(nn.Module):
- def __init__(self,
- channels,
- hidden_channels,
- kernel_size,
- dilation_rate,
- n_layers,
- n_flows=4,
- gin_channels=0):
- super().__init__()
- self.channels = channels
- self.hidden_channels = hidden_channels
- self.kernel_size = kernel_size
- self.dilation_rate = dilation_rate
- self.n_layers = n_layers
- self.n_flows = n_flows
- self.gin_channels = gin_channels
-
- self.flows = nn.ModuleList()
- for i in range(n_flows):
- self.flows.append(
- modules.ResidualCouplingLayer(channels, hidden_channels, kernel_size, dilation_rate, n_layers,
- gin_channels=gin_channels, mean_only=True))
- self.flows.append(modules.Flip())
-
- def forward(self, x, x_mask, g=None, reverse=False):
- if not reverse:
- for flow in self.flows:
- x, _ = flow(x, x_mask, g=g, reverse=reverse)
- else:
- for flow in reversed(self.flows):
- x = flow(x, x_mask, g=g, reverse=reverse)
- return x
-
-
-class Encoder(nn.Module):
- def __init__(self,
- in_channels,
- out_channels,
- hidden_channels,
- kernel_size,
- dilation_rate,
- n_layers,
- gin_channels=0):
- super().__init__()
- self.in_channels = in_channels
- self.out_channels = out_channels
- self.hidden_channels = hidden_channels
- self.kernel_size = kernel_size
- self.dilation_rate = dilation_rate
- self.n_layers = n_layers
- self.gin_channels = gin_channels
-
- self.pre = nn.Conv1d(in_channels, hidden_channels, 1)
- self.enc = modules.WN(hidden_channels, kernel_size, dilation_rate, n_layers, gin_channels=gin_channels)
- self.proj = nn.Conv1d(hidden_channels, out_channels * 2, 1)
-
- def forward(self, x, x_lengths, g=None):
- # print(x.shape,x_lengths.shape)
- x_mask = torch.unsqueeze(commons.sequence_mask(x_lengths, x.size(2)), 1).to(x.dtype)
- x = self.pre(x) * x_mask
- x = self.enc(x, x_mask, g=g)
- stats = self.proj(x) * x_mask
- m, logs = torch.split(stats, self.out_channels, dim=1)
- z = (m + torch.randn_like(m) * torch.exp(logs)) * x_mask
- return z, m, logs, x_mask
-
-
-class TextEncoder(nn.Module):
- def __init__(self,
- out_channels,
- hidden_channels,
- kernel_size,
- n_layers,
- gin_channels=0,
- filter_channels=None,
- n_heads=None,
- p_dropout=None):
- super().__init__()
- self.out_channels = out_channels
- self.hidden_channels = hidden_channels
- self.kernel_size = kernel_size
- self.n_layers = n_layers
- self.gin_channels = gin_channels
- self.proj = nn.Conv1d(hidden_channels, out_channels * 2, 1)
- self.f0_emb = nn.Embedding(256, hidden_channels)
-
- self.enc_ = attentions.Encoder(
- hidden_channels,
- filter_channels,
- n_heads,
- n_layers,
- kernel_size,
- p_dropout)
-
- def forward(self, x, x_mask, f0=None, z=None):
- x = x + self.f0_emb(f0).transpose(1, 2)
- x = self.enc_(x * x_mask, x_mask)
- stats = self.proj(x) * x_mask
- m, logs = torch.split(stats, self.out_channels, dim=1)
- z = (m + z * torch.exp(logs)) * x_mask
- return z, m, logs, x_mask
-
-
-class DiscriminatorP(torch.nn.Module):
- def __init__(self, period, kernel_size=5, stride=3, use_spectral_norm=False):
- super(DiscriminatorP, self).__init__()
- self.period = period
- self.use_spectral_norm = use_spectral_norm
- norm_f = weight_norm if use_spectral_norm == False else spectral_norm
- self.convs = nn.ModuleList([
- norm_f(Conv2d(1, 32, (kernel_size, 1), (stride, 1), padding=(get_padding(kernel_size, 1), 0))),
- norm_f(Conv2d(32, 128, (kernel_size, 1), (stride, 1), padding=(get_padding(kernel_size, 1), 0))),
- norm_f(Conv2d(128, 512, (kernel_size, 1), (stride, 1), padding=(get_padding(kernel_size, 1), 0))),
- norm_f(Conv2d(512, 1024, (kernel_size, 1), (stride, 1), padding=(get_padding(kernel_size, 1), 0))),
- norm_f(Conv2d(1024, 1024, (kernel_size, 1), 1, padding=(get_padding(kernel_size, 1), 0))),
- ])
- self.conv_post = norm_f(Conv2d(1024, 1, (3, 1), 1, padding=(1, 0)))
-
- def forward(self, x):
- fmap = []
-
- # 1d to 2d
- b, c, t = x.shape
- if t % self.period != 0: # pad first
- n_pad = self.period - (t % self.period)
- x = F.pad(x, (0, n_pad), "reflect")
- t = t + n_pad
- x = x.view(b, c, t // self.period, self.period)
-
- for l in self.convs:
- x = l(x)
- x = F.leaky_relu(x, modules.LRELU_SLOPE)
- fmap.append(x)
- x = self.conv_post(x)
- fmap.append(x)
- x = torch.flatten(x, 1, -1)
-
- return x, fmap
-
-
-class DiscriminatorS(torch.nn.Module):
- def __init__(self, use_spectral_norm=False):
- super(DiscriminatorS, self).__init__()
- norm_f = weight_norm if use_spectral_norm == False else spectral_norm
- self.convs = nn.ModuleList([
- norm_f(Conv1d(1, 16, 15, 1, padding=7)),
- norm_f(Conv1d(16, 64, 41, 4, groups=4, padding=20)),
- norm_f(Conv1d(64, 256, 41, 4, groups=16, padding=20)),
- norm_f(Conv1d(256, 1024, 41, 4, groups=64, padding=20)),
- norm_f(Conv1d(1024, 1024, 41, 4, groups=256, padding=20)),
- norm_f(Conv1d(1024, 1024, 5, 1, padding=2)),
- ])
- self.conv_post = norm_f(Conv1d(1024, 1, 3, 1, padding=1))
-
- def forward(self, x):
- fmap = []
-
- for l in self.convs:
- x = l(x)
- x = F.leaky_relu(x, modules.LRELU_SLOPE)
- fmap.append(x)
- x = self.conv_post(x)
- fmap.append(x)
- x = torch.flatten(x, 1, -1)
-
- return x, fmap
-
-
-class F0Decoder(nn.Module):
- def __init__(self,
- out_channels,
- hidden_channels,
- filter_channels,
- n_heads,
- n_layers,
- kernel_size,
- p_dropout,
- spk_channels=0):
- super().__init__()
- self.out_channels = out_channels
- self.hidden_channels = hidden_channels
- self.filter_channels = filter_channels
- self.n_heads = n_heads
- self.n_layers = n_layers
- self.kernel_size = kernel_size
- self.p_dropout = p_dropout
- self.spk_channels = spk_channels
-
- self.prenet = nn.Conv1d(hidden_channels, hidden_channels, 3, padding=1)
- self.decoder = attentions.FFT(
- hidden_channels,
- filter_channels,
- n_heads,
- n_layers,
- kernel_size,
- p_dropout)
- self.proj = nn.Conv1d(hidden_channels, out_channels, 1)
- self.f0_prenet = nn.Conv1d(1, hidden_channels, 3, padding=1)
- self.cond = nn.Conv1d(spk_channels, hidden_channels, 1)
-
- def forward(self, x, norm_f0, x_mask, spk_emb=None):
- x = torch.detach(x)
- if spk_emb is not None:
- x = x + self.cond(spk_emb)
- x += self.f0_prenet(norm_f0)
- x = self.prenet(x) * x_mask
- x = self.decoder(x * x_mask, x_mask)
- x = self.proj(x) * x_mask
- return x
-
-
-class SynthesizerTrn(nn.Module):
- """
- Synthesizer for Training
- """
-
- def __init__(self,
- spec_channels,
- segment_size,
- inter_channels,
- hidden_channels,
- filter_channels,
- n_heads,
- n_layers,
- kernel_size,
- p_dropout,
- resblock,
- resblock_kernel_sizes,
- resblock_dilation_sizes,
- upsample_rates,
- upsample_initial_channel,
- upsample_kernel_sizes,
- gin_channels,
- ssl_dim,
- n_speakers,
- sampling_rate=44100,
- **kwargs):
- super().__init__()
- self.spec_channels = spec_channels
- self.inter_channels = inter_channels
- self.hidden_channels = hidden_channels
- self.filter_channels = filter_channels
- self.n_heads = n_heads
- self.n_layers = n_layers
- self.kernel_size = kernel_size
- self.p_dropout = p_dropout
- self.resblock = resblock
- self.resblock_kernel_sizes = resblock_kernel_sizes
- self.resblock_dilation_sizes = resblock_dilation_sizes
- self.upsample_rates = upsample_rates
- self.upsample_initial_channel = upsample_initial_channel
- self.upsample_kernel_sizes = upsample_kernel_sizes
- self.segment_size = segment_size
- self.gin_channels = gin_channels
- self.ssl_dim = ssl_dim
- self.emb_g = nn.Embedding(n_speakers, gin_channels)
-
- self.pre = nn.Conv1d(ssl_dim, hidden_channels, kernel_size=5, padding=2)
-
- self.enc_p = TextEncoder(
- inter_channels,
- hidden_channels,
- filter_channels=filter_channels,
- n_heads=n_heads,
- n_layers=n_layers,
- kernel_size=kernel_size,
- p_dropout=p_dropout
- )
- hps = {
- "sampling_rate": sampling_rate,
- "inter_channels": inter_channels,
- "resblock": resblock,
- "resblock_kernel_sizes": resblock_kernel_sizes,
- "resblock_dilation_sizes": resblock_dilation_sizes,
- "upsample_rates": upsample_rates,
- "upsample_initial_channel": upsample_initial_channel,
- "upsample_kernel_sizes": upsample_kernel_sizes,
- "gin_channels": gin_channels,
- }
- self.dec = Generator(h=hps)
- self.enc_q = Encoder(spec_channels, inter_channels, hidden_channels, 5, 1, 16, gin_channels=gin_channels)
- self.flow = ResidualCouplingBlock(inter_channels, hidden_channels, 5, 1, 4, gin_channels=gin_channels)
- self.f0_decoder = F0Decoder(
- 1,
- hidden_channels,
- filter_channels,
- n_heads,
- n_layers,
- kernel_size,
- p_dropout,
- spk_channels=gin_channels
- )
- self.emb_uv = nn.Embedding(2, hidden_channels)
- self.predict_f0 = False
-
- def forward(self, c, f0, mel2ph, uv, noise=None, g=None):
-
- decoder_inp = F.pad(c, [0, 0, 1, 0])
- mel2ph_ = mel2ph.unsqueeze(2).repeat([1, 1, c.shape[-1]])
- c = torch.gather(decoder_inp, 1, mel2ph_).transpose(1, 2) # [B, T, H]
-
- c_lengths = (torch.ones(c.size(0)) * c.size(-1)).to(c.device)
- g = g.unsqueeze(0)
- g = self.emb_g(g).transpose(1, 2)
- x_mask = torch.unsqueeze(commons.sequence_mask(c_lengths, c.size(2)), 1).to(c.dtype)
- x = self.pre(c) * x_mask + self.emb_uv(uv.long()).transpose(1, 2)
-
- if self.predict_f0:
- lf0 = 2595. * torch.log10(1. + f0.unsqueeze(1) / 700.) / 500
- norm_lf0 = utils.normalize_f0(lf0, x_mask, uv, random_scale=False)
- pred_lf0 = self.f0_decoder(x, norm_lf0, x_mask, spk_emb=g)
- f0 = (700 * (torch.pow(10, pred_lf0 * 500 / 2595) - 1)).squeeze(1)
-
- z_p, m_p, logs_p, c_mask = self.enc_p(x, x_mask, f0=f0_to_coarse(f0), z=noise)
- z = self.flow(z_p, c_mask, g=g, reverse=True)
- o = self.dec(z * c_mask, g=g, f0=f0)
- return o
diff --git a/spaces/nikitaPDL2023/assignment4/detectron2/tests/test_events.py b/spaces/nikitaPDL2023/assignment4/detectron2/tests/test_events.py
deleted file mode 100644
index 174ca978de21fa09fdf79eca62936ef497aaf2e8..0000000000000000000000000000000000000000
--- a/spaces/nikitaPDL2023/assignment4/detectron2/tests/test_events.py
+++ /dev/null
@@ -1,122 +0,0 @@
-# Copyright (c) Facebook, Inc. and its affiliates.
-import json
-import os
-import tempfile
-import unittest
-
-from detectron2.utils.events import (
- CommonMetricPrinter,
- EventStorage,
- JSONWriter,
- get_event_storage,
- has_event_storage,
-)
-
-
-class TestEventWriter(unittest.TestCase):
- def testScalar(self):
- with tempfile.TemporaryDirectory(
- prefix="detectron2_tests"
- ) as dir, EventStorage() as storage:
- json_file = os.path.join(dir, "test.json")
- writer = JSONWriter(json_file)
- for k in range(60):
- storage.put_scalar("key", k, smoothing_hint=False)
- if (k + 1) % 20 == 0:
- writer.write()
- storage.step()
- writer.close()
- with open(json_file) as f:
- data = [json.loads(l) for l in f]
- self.assertTrue([int(k["key"]) for k in data] == [19, 39, 59])
-
- def testScalarMismatchedPeriod(self):
- with tempfile.TemporaryDirectory(
- prefix="detectron2_tests"
- ) as dir, EventStorage() as storage:
- json_file = os.path.join(dir, "test.json")
-
- writer = JSONWriter(json_file)
- for k in range(60):
- if k % 17 == 0: # write in a differnt period
- storage.put_scalar("key2", k, smoothing_hint=False)
- storage.put_scalar("key", k, smoothing_hint=False)
- if (k + 1) % 20 == 0:
- writer.write()
- storage.step()
- writer.close()
- with open(json_file) as f:
- data = [json.loads(l) for l in f]
- self.assertTrue([int(k.get("key2", 0)) for k in data] == [17, 0, 34, 0, 51, 0])
- self.assertTrue([int(k.get("key", 0)) for k in data] == [0, 19, 0, 39, 0, 59])
- self.assertTrue([int(k["iteration"]) for k in data] == [17, 19, 34, 39, 51, 59])
-
- def testPrintETA(self):
- with EventStorage() as s:
- p1 = CommonMetricPrinter(10)
- p2 = CommonMetricPrinter()
-
- s.put_scalar("time", 1.0)
- s.step()
- s.put_scalar("time", 1.0)
- s.step()
-
- with self.assertLogs("detectron2.utils.events") as logs:
- p1.write()
- self.assertIn("eta", logs.output[0])
-
- with self.assertLogs("detectron2.utils.events") as logs:
- p2.write()
- self.assertNotIn("eta", logs.output[0])
-
- def testPrintNonLosses(self):
- with EventStorage() as s:
- p1 = CommonMetricPrinter(10)
- p2 = CommonMetricPrinter()
-
- s.put_scalar("time", 1.0)
- s.put_scalar("[metric]bn_stat", 1.0)
- s.step()
- s.put_scalar("time", 1.0)
- s.put_scalar("[metric]bn_stat", 1.0)
- s.step()
-
- with self.assertLogs("detectron2.utils.events") as logs:
- p1.write()
- self.assertIn("[metric]bn_stat", logs.output[0])
-
- with self.assertLogs("detectron2.utils.events") as logs:
- p2.write()
- self.assertIn("[metric]bn_stat", logs.output[0])
-
- def testSmoothingWithWindowSize(self):
- with tempfile.TemporaryDirectory(
- prefix="detectron2_tests"
- ) as dir, EventStorage() as storage:
- json_file = os.path.join(dir, "test.json")
- writer = JSONWriter(json_file, window_size=10)
- for k in range(20):
- storage.put_scalar("key1", k, smoothing_hint=True)
- if (k + 1) % 2 == 0:
- storage.put_scalar("key2", k, smoothing_hint=True)
- if (k + 1) % 5 == 0:
- storage.put_scalar("key3", k, smoothing_hint=True)
- if (k + 1) % 10 == 0:
- writer.write()
- storage.step()
-
- num_samples = {k: storage.count_samples(k, 10) for k in ["key1", "key2", "key3"]}
- self.assertEqual(num_samples, {"key1": 10, "key2": 5, "key3": 2})
- writer.close()
- with open(json_file) as f:
- data = [json.loads(l) for l in f]
- self.assertEqual([k["key1"] for k in data], [4.5, 14.5])
- self.assertEqual([k["key2"] for k in data], [5, 15])
- self.assertEqual([k["key3"] for k in data], [6.5, 16.5])
-
- def testEventStorage(self):
- self.assertFalse(has_event_storage())
- with EventStorage() as storage:
- self.assertTrue(has_event_storage())
- self.assertEqual(storage, get_event_storage())
- self.assertFalse(has_event_storage())
diff --git a/spaces/niro-private/chatCSV/loaders/pdf.py b/spaces/niro-private/chatCSV/loaders/pdf.py
deleted file mode 100644
index e76a05d277e55851f6e1586a2f46a3ad3c2e394f..0000000000000000000000000000000000000000
--- a/spaces/niro-private/chatCSV/loaders/pdf.py
+++ /dev/null
@@ -1,6 +0,0 @@
-from .common import process_file
-from langchain.document_loaders import PyPDFLoader
-
-
-def process_pdf(vector_store, file, stats_db):
- return process_file(vector_store, file, PyPDFLoader, ".pdf", stats_db=stats_db)
diff --git a/spaces/nivere/ControlNet-Video/style.css b/spaces/nivere/ControlNet-Video/style.css
deleted file mode 100644
index 98c1607dba4c5e2055c5bc59197a9c995389a3fa..0000000000000000000000000000000000000000
--- a/spaces/nivere/ControlNet-Video/style.css
+++ /dev/null
@@ -1,105 +0,0 @@
-#col-container {max-width: 820px; margin-left: auto; margin-right: auto;}
-#duplicate-container{
- display: flex;
- justify-content: space-between;
- align-items: center;
- line-height: 1em;
- flex-direction: row-reverse;
- font-size:1em;
-}
-a, a:hover, a:visited {
- text-decoration-line: underline;
- font-weight: 600;
- color: #1f2937 !important;
-}
-
-.dark a, .dark a:hover, .dark a:visited {
- color: #f3f4f6 !important;
-}
-
-.label-wrap {
- margin-bottom: 12px;
-}
-
-.footer {
- margin-bottom: 45px;
- margin-top: 10px;
- text-align: center;
- border-bottom: 1px solid #e5e5e5;
-}
-
-.footer>p {
- font-size: .8rem!important;
- display: inline-block;
- padding: 0 10px;
- transform: translateY(26px);
- background: white;
-}
-.dark .footer {
- border-color: #303030;
-}
-.dark .footer>p {
- background: #0b0f19;
-}
-
-div#may-like-container > p {
- font-size: .8em;
- margin-bottom: 4px;
-}
-
-.animate-spin {
- animation: spin 1s linear infinite;
-}
-
-@keyframes spin {
- from {
- transform: rotate(0deg);
- }
- to {
- transform: rotate(360deg);
- }
-}
-
-#share-btn-container {
- display: flex;
- padding-left: 0.5rem !important;
- padding-right: 0.5rem !important;
- background-color: #000000;
- justify-content: center;
- align-items: center;
- border-radius: 9999px !important;
- max-width: 13rem;
-}
-
-#share-btn-container:hover {
- background-color: #060606;
-}
-
-#share-btn {
- all: initial;
- color: #ffffff;
- font-weight: 600;
- cursor:pointer;
- font-family: 'IBM Plex Sans', sans-serif;
- margin-left: 0.5rem !important;
- padding-top: 0.5rem !important;
- padding-bottom: 0.5rem !important;
- right:0;
-}
-
-#share-btn * {
- all: unset;
-}
-
-#share-btn-container div:nth-child(-n+2){
- width: auto !important;
- min-height: 0px !important;
-}
-
-#share-btn-container .wrap {
- display: none !important;
-}
-
-#share-btn-container.hidden {
- display: none!important;
-}
\ No newline at end of file
diff --git a/spaces/nota-ai/compressed-wav2lip/audio.py b/spaces/nota-ai/compressed-wav2lip/audio.py
deleted file mode 100644
index 32b20c449df8c23548f0d1eedc64942a29f01448..0000000000000000000000000000000000000000
--- a/spaces/nota-ai/compressed-wav2lip/audio.py
+++ /dev/null
@@ -1,136 +0,0 @@
-import librosa
-import librosa.filters
-import numpy as np
-# import tensorflow as tf
-from scipy import signal
-from scipy.io import wavfile
-from hparams import hparams as hp
-
-def load_wav(path, sr):
- return librosa.core.load(path, sr=sr)[0]
-
-def save_wav(wav, path, sr):
- wav *= 32767 / max(0.01, np.max(np.abs(wav)))
- #proposed by @dsmiller
- wavfile.write(path, sr, wav.astype(np.int16))
-
-def save_wavenet_wav(wav, path, sr):
- librosa.output.write_wav(path, wav, sr=sr)
-
-def preemphasis(wav, k, preemphasize=True):
- if preemphasize:
- return signal.lfilter([1, -k], [1], wav)
- return wav
-
-def inv_preemphasis(wav, k, inv_preemphasize=True):
- if inv_preemphasize:
- return signal.lfilter([1], [1, -k], wav)
- return wav
-
-def get_hop_size():
- hop_size = hp.hop_size
- if hop_size is None:
- assert hp.frame_shift_ms is not None
- hop_size = int(hp.frame_shift_ms / 1000 * hp.sample_rate)
- return hop_size
-
-def linearspectrogram(wav):
- D = _stft(preemphasis(wav, hp.preemphasis, hp.preemphasize))
- S = _amp_to_db(np.abs(D)) - hp.ref_level_db
-
- if hp.signal_normalization:
- return _normalize(S)
- return S
-
-def melspectrogram(wav):
- D = _stft(preemphasis(wav, hp.preemphasis, hp.preemphasize))
- S = _amp_to_db(_linear_to_mel(np.abs(D))) - hp.ref_level_db
-
- if hp.signal_normalization:
- return _normalize(S)
- return S
-
-def _lws_processor():
- import lws
- return lws.lws(hp.n_fft, get_hop_size(), fftsize=hp.win_size, mode="speech")
-
-def _stft(y):
- if hp.use_lws:
- return _lws_processor(hp).stft(y).T
- else:
- return librosa.stft(y=y, n_fft=hp.n_fft, hop_length=get_hop_size(), win_length=hp.win_size)
-
-##########################################################
-#Those are only correct when using lws!!! (This was messing with Wavenet quality for a long time!)
-def num_frames(length, fsize, fshift):
- """Compute number of time frames of spectrogram
- """
- pad = (fsize - fshift)
- if length % fshift == 0:
- M = (length + pad * 2 - fsize) // fshift + 1
- else:
- M = (length + pad * 2 - fsize) // fshift + 2
- return M
-
-
-def pad_lr(x, fsize, fshift):
- """Compute left and right padding
- """
- M = num_frames(len(x), fsize, fshift)
- pad = (fsize - fshift)
- T = len(x) + 2 * pad
- r = (M - 1) * fshift + fsize - T
- return pad, pad + r
-##########################################################
-#Librosa correct padding
-def librosa_pad_lr(x, fsize, fshift):
- return 0, (x.shape[0] // fshift + 1) * fshift - x.shape[0]
-
-# Conversions
-_mel_basis = None
-
-def _linear_to_mel(spectogram):
- global _mel_basis
- if _mel_basis is None:
- _mel_basis = _build_mel_basis()
- return np.dot(_mel_basis, spectogram)
-
-def _build_mel_basis():
- assert hp.fmax <= hp.sample_rate // 2
- return librosa.filters.mel(hp.sample_rate, hp.n_fft, n_mels=hp.num_mels,
- fmin=hp.fmin, fmax=hp.fmax)
-
-def _amp_to_db(x):
- min_level = np.exp(hp.min_level_db / 20 * np.log(10))
- return 20 * np.log10(np.maximum(min_level, x))
-
-def _db_to_amp(x):
- return np.power(10.0, (x) * 0.05)
-
-def _normalize(S):
- if hp.allow_clipping_in_normalization:
- if hp.symmetric_mels:
- return np.clip((2 * hp.max_abs_value) * ((S - hp.min_level_db) / (-hp.min_level_db)) - hp.max_abs_value,
- -hp.max_abs_value, hp.max_abs_value)
- else:
- return np.clip(hp.max_abs_value * ((S - hp.min_level_db) / (-hp.min_level_db)), 0, hp.max_abs_value)
-
- assert S.max() <= 0 and S.min() - hp.min_level_db >= 0
- if hp.symmetric_mels:
- return (2 * hp.max_abs_value) * ((S - hp.min_level_db) / (-hp.min_level_db)) - hp.max_abs_value
- else:
- return hp.max_abs_value * ((S - hp.min_level_db) / (-hp.min_level_db))
-
-def _denormalize(D):
- if hp.allow_clipping_in_normalization:
- if hp.symmetric_mels:
- return (((np.clip(D, -hp.max_abs_value,
- hp.max_abs_value) + hp.max_abs_value) * -hp.min_level_db / (2 * hp.max_abs_value))
- + hp.min_level_db)
- else:
- return ((np.clip(D, 0, hp.max_abs_value) * -hp.min_level_db / hp.max_abs_value) + hp.min_level_db)
-
- if hp.symmetric_mels:
- return (((D + hp.max_abs_value) * -hp.min_level_db / (2 * hp.max_abs_value)) + hp.min_level_db)
- else:
- return ((D * -hp.min_level_db / hp.max_abs_value) + hp.min_level_db)
diff --git a/spaces/nsarrazin/agents-js-llama/src/lib/LLMFromOpenAI.ts b/spaces/nsarrazin/agents-js-llama/src/lib/LLMFromOpenAI.ts
deleted file mode 100644
index 2fdddcbd852e5073e937af4c8b0d1a42a0b350d6..0000000000000000000000000000000000000000
--- a/spaces/nsarrazin/agents-js-llama/src/lib/LLMFromOpenAI.ts
+++ /dev/null
@@ -1,18 +0,0 @@
-import type { LLM } from "@huggingface/agents/src/types";
-import { Configuration, OpenAIApi } from "openai";
-export function LLMFromOpenAI(openAIKey: string): LLM {
- const api = new OpenAIApi(new Configuration({ apiKey: openAIKey }));
-
- return async (prompt: string): Promise => {
- const textAnswer =
- (
- await api.createCompletion({
- model: "text-davinci-003",
- prompt: prompt,
- max_tokens: 1000,
- })
- ).data.choices[0].text ?? "";
-
- return textAnswer;
- };
-}
diff --git a/spaces/nus-cs5647-team-5/Mandarin_Tone_Evaluation/train_speech_model.py b/spaces/nus-cs5647-team-5/Mandarin_Tone_Evaluation/train_speech_model.py
deleted file mode 100644
index d78fe7e2e11bf7ec97e682f082e507b73a85698c..0000000000000000000000000000000000000000
--- a/spaces/nus-cs5647-team-5/Mandarin_Tone_Evaluation/train_speech_model.py
+++ /dev/null
@@ -1,28 +0,0 @@
-import os
-from keras.optimizers import Adam
-
-from speech_model import ModelSpeech
-from speech_model_zoo import SpeechModel251BN
-from data_loader import DataLoader
-from speech_features import SpecAugment
-
-os.environ["CUDA_VISIBLE_DEVICES"] = "1"
-
-AUDIO_LENGTH = 1600
-AUDIO_FEATURE_LENGTH = 200
-CHANNELS = 1
-# 默认输出的拼音的表示大小是1428,即1427个拼音+1个空白块
-OUTPUT_SIZE = 1428
-sm251bn = SpeechModel251BN(
- input_shape=(AUDIO_LENGTH, AUDIO_FEATURE_LENGTH, CHANNELS),
- output_size=OUTPUT_SIZE
- )
-feat = SpecAugment()
-train_data = DataLoader('train')
-opt = Adam(lr = 0.0001, beta_1 = 0.9, beta_2 = 0.999, decay = 0.0, epsilon = 10e-8)
-ms = ModelSpeech(sm251bn, feat, max_label_length=64)
-
-#ms.load_model('save_models/' + sm251bn.get_model_name() + '.model.h5')
-ms.train_model(optimizer=opt, data_loader=train_data,
- epochs=50, save_step=1, batch_size=16, last_epoch=0)
-ms.save_model('save_models/' + sm251bn.get_model_name())
diff --git a/spaces/oguzakif/video-object-remover/SiamMask/utils/pysot/evaluation/eao_benchmark.py b/spaces/oguzakif/video-object-remover/SiamMask/utils/pysot/evaluation/eao_benchmark.py
deleted file mode 100644
index 848eafffdab66c7a72330c309a8601f6052dfa7e..0000000000000000000000000000000000000000
--- a/spaces/oguzakif/video-object-remover/SiamMask/utils/pysot/evaluation/eao_benchmark.py
+++ /dev/null
@@ -1,159 +0,0 @@
-# --------------------------------------------------------
-# Python Single Object Tracking Evaluation
-# Licensed under The MIT License [see LICENSE for details]
-# Written by Fangyi Zhang
-# @author fangyi.zhang@vipl.ict.ac.cn
-# @project https://github.com/StrangerZhang/pysot-toolkit.git
-# Revised for SiamMask by foolwood
-# --------------------------------------------------------
-import numpy as np
-
-from ..utils import calculate_failures, calculate_accuracy, calculate_expected_overlap
-
-
-class EAOBenchmark:
- """
- Args:
- dataset:
- """
- def __init__(self, dataset, skipping=5, tags=['all']):
- self.dataset = dataset
- self.skipping = skipping
- self.tags = tags
- # NOTE we not use gmm to generate low, high, peak value
- if dataset.name in ['VOT2019']:
- self.low = 46
- self.high = 291
- self.peak = 128
- elif dataset.name in ['VOT2018', 'VOT2017']:
- self.low = 100
- self.high = 356
- self.peak = 160
- elif dataset.name == 'VOT2016':
- self.low = 100 # TODO
- self.high = 356
- self.peak = 160
-
- def eval(self, eval_trackers=None):
- """
- Args:
- eval_tags: list of tag
- eval_trackers: list of tracker name
- Returns:
- eao: dict of results
- """
- if eval_trackers is None:
- eval_trackers = self.dataset.tracker_names
- if isinstance(eval_trackers, str):
- eval_trackers = [eval_trackers]
-
- ret = {}
- for tracker_name in eval_trackers:
- eao = self._calculate_eao(tracker_name, self.tags)
- ret[tracker_name] = eao
- return ret
-
- def show_result(self, result, topk=10):
- """pretty print result
- Args:
- result: returned dict from function eval
- """
- if len(self.tags) == 1:
- tracker_name_len = max((max([len(x) for x in result.keys()])+2), 12)
- header = ("|{:^"+str(tracker_name_len)+"}|{:^10}|").format('Tracker Name', 'EAO')
- bar = '-'*len(header)
- formatter = "|{:^20}|{:^10.3f}|"
- print(bar)
- print(header)
- print(bar)
- tracker_eao = sorted(result.items(),
- key=lambda x: x[1]['all'],
- reverse=True)[:topk]
- for tracker_name, eao in tracker_eao:
- print(formatter.format(tracker_name, eao))
- print(bar)
- else:
- header = "|{:^20}|".format('Tracker Name')
- header += "{:^7}|{:^15}|{:^14}|{:^15}|{:^13}|{:^11}|{:^7}|".format(*self.tags)
- bar = '-'*len(header)
- formatter = "{:^7.3f}|{:^15.3f}|{:^14.3f}|{:^15.3f}|{:^13.3f}|{:^11.3f}|{:^7.3f}|"
- print(bar)
- print(header)
- print(bar)
- sorted_tacker = sorted(result.items(),
- key=lambda x: x[1]['all'],
- reverse=True)[:topk]
- sorted_tacker = [x[0] for x in sorted_tacker]
- for tracker_name in sorted_tacker:
- print("|{:^20}|".format(tracker_name)+formatter.format(
- *[result[tracker_name][x] for x in self.tags]))
- print(bar)
-
- def _calculate_eao(self, tracker_name, tags):
- all_overlaps = []
- all_failures = []
- video_names = []
- gt_traj_length = []
- for video in self.dataset:
- gt_traj = video.gt_traj
- if tracker_name not in video.pred_trajs:
- tracker_trajs = video.load_tracker(self.dataset.tracker_path, tracker_name, False)
- else:
- tracker_trajs = video.pred_trajs[tracker_name]
- for tracker_traj in tracker_trajs:
- gt_traj_length.append(len(gt_traj))
- video_names.append(video.name)
- overlaps = calculate_accuracy(tracker_traj, gt_traj, bound=(video.width-1, video.height-1))[1]
- failures = calculate_failures(tracker_traj)[1]
- all_overlaps.append(overlaps)
- all_failures.append(failures)
- fragment_num = sum([len(x)+1 for x in all_failures])
- max_len = max([len(x) for x in all_overlaps])
- seq_weight = 1 / len(tracker_trajs)
-
- eao = {}
- for tag in tags:
- # prepare segments
- fweights = np.ones((fragment_num)) * np.nan
- fragments = np.ones((fragment_num, max_len)) * np.nan
- seg_counter = 0
- for name, traj_len, failures, overlaps in zip(video_names, gt_traj_length,
- all_failures, all_overlaps):
- if len(failures) > 0:
- points = [x+self.skipping for x in failures if
- x+self.skipping <= len(overlaps)]
- points.insert(0, 0)
- for i in range(len(points)):
- if i != len(points) - 1:
- fragment = np.array(overlaps[points[i]:points[i+1]+1])
- fragments[seg_counter, :] = 0
- else:
- fragment = np.array(overlaps[points[i]:])
- fragment[np.isnan(fragment)] = 0
- fragments[seg_counter, :len(fragment)] = fragment
- if i != len(points) - 1:
- tag_value = self.dataset[name].select_tag(tag, points[i], points[i+1]+1)
- w = sum(tag_value) / (points[i+1] - points[i]+1)
- fweights[seg_counter] = seq_weight * w
- else:
- tag_value = self.dataset[name].select_tag(tag, points[i], len(overlaps))
- w = sum(tag_value) / (traj_len - points[i]+1e-16)
- fweights[seg_counter] = seq_weight * w
- seg_counter += 1
- else:
- # no failure
- max_idx = min(len(overlaps), max_len)
- fragments[seg_counter, :max_idx] = overlaps[:max_idx]
- tag_value = self.dataset[name].select_tag(tag, 0, max_idx)
- w = sum(tag_value) / max_idx
- fweights[seg_counter] = seq_weight * w
- seg_counter += 1
-
- expected_overlaps = calculate_expected_overlap(fragments, fweights)
- # caculate eao
- weight = np.zeros((len(expected_overlaps)))
- weight[self.low-1:self.high-1+1] = 1
- is_valid = np.logical_not(np.isnan(expected_overlaps))
- eao_ = np.sum(expected_overlaps[is_valid] * weight[is_valid]) / np.sum(weight[is_valid])
- eao[tag] = eao_
- return eao
diff --git a/spaces/oliveiracwb/MBP2/app.py b/spaces/oliveiracwb/MBP2/app.py
deleted file mode 100644
index 2de457b55091020d834df9cda2a2478741ce2f03..0000000000000000000000000000000000000000
--- a/spaces/oliveiracwb/MBP2/app.py
+++ /dev/null
@@ -1,52 +0,0 @@
-import gpt_2_simple as gpt2
-from datetime import datetime
-import streamlit as st
-
-# -----------------------------------------------------------------------------
-st.set_page_config(page_title="MPB versao 2", page_icon=":milky_way:", layout="wide")
-st.subheader("Gerador Canções de musica brasileira (2)")
-
-sess = gpt2.start_tf_sess()
-
-gpt2.load_gpt2(sess, model_name='br_music1/')
-
-def gera_texto(start, temperature, max_new_tokens, num_samples):
- result = gpt2.generate(sess,
- model_name='br_music1/',
- prefix=start,
- length=max_new_tokens,
- temperature=temperature,
- top_p=0.5,
- nsamples=num_samples,
- batch_size= 2,
- return_as_list=True
- )
- k =0
- for s in result:
- k=k+1
- st.text_area("Gerado {}".format(k+1), value= s, height=300, placeholder="")
-
-with st.form("my_form"):
- col1, col2, col3 = st.columns(3)
- with col1:
- int_samples = st.slider('Exemplos', min_value=2, max_value=10, value=4, step=2)
- with col2:
- int_size = st.slider('Num Tokens', min_value=20, max_value=500, value=160, step=5)
- with col3:
- int_temp = st.number_input("Temperatura",min_value=0.8,max_value=2.0,value=1.2,step=0.1,format="%.1f")
-
- source = st.text_area("Escolha uma frase inicial", value="Contrui uma casa para nos", placeholder="Entre com o inicio da musica...")
-
- submitted = st.form_submit_button("Gerar músicas")
- if submitted:
- with st.spinner("Gerando exemplos ..."):
- gera_texto(source,int_temp,int_size,int_samples)
-
-st.write("Finetunning do GPT-2 Portugues para geracao de musicas")
-st.write("A preparação dos dados estava pronta do MPB1.")
-st.write("Tunning dos dados demorou a tarde do domingo no Colab")
-st.write("Agradecimentos ao [Gabriel](https://www.linkedin.com/in/go2035/) pela ajuda no scrap.")
-st.markdown("""---""")
-original_title = '
Gosta de IA ou é um maker por natureza ? Conecte-se ao meu linkedin e vamos conversar !
'
-st.markdown(original_title, unsafe_allow_html=True)
-st.write("Made with [simpleGPT](https://github.com/minimaxir/gpt-2-simple) e [ColabPro+](https://colab.research.google.com/signup)")
diff --git a/spaces/omlab/vlchecklist_demo/models/vilt/modules/vilt_utils.py b/spaces/omlab/vlchecklist_demo/models/vilt/modules/vilt_utils.py
deleted file mode 100644
index cfb97fca2930ed35f248a553e60ce0f103300cc4..0000000000000000000000000000000000000000
--- a/spaces/omlab/vlchecklist_demo/models/vilt/modules/vilt_utils.py
+++ /dev/null
@@ -1,276 +0,0 @@
-import torch
-import random
-
-from transformers.optimization import AdamW
-from transformers import (
- get_polynomial_decay_schedule_with_warmup,
- get_cosine_schedule_with_warmup,
-)
-from models.vilt.modules.dist_utils import all_gather
-from models.vilt.modules.objectives import compute_irtr_recall
-from models.vilt.gadgets.my_metrics import Accuracy, VQAScore, Scalar
-
-
-def set_metrics(pl_module):
- for split in ["train", "val"]:
- for k, v in pl_module.hparams.config["loss_names"].items():
- if v < 1:
- continue
- if k == "vqa":
- setattr(pl_module, f"{split}_vqa_score", VQAScore())
- setattr(pl_module, f"{split}_{k}_loss", Scalar())
- elif k == "nlvr2":
- if split == "train":
- setattr(pl_module, f"train_{k}_accuracy", Accuracy())
- setattr(pl_module, f"train_{k}_loss", Scalar())
- else:
- setattr(pl_module, f"dev_{k}_accuracy", Accuracy())
- setattr(pl_module, f"dev_{k}_loss", Scalar())
- setattr(pl_module, f"test_{k}_accuracy", Accuracy())
- setattr(pl_module, f"test_{k}_loss", Scalar())
- elif k == "irtr":
- setattr(pl_module, f"{split}_irtr_loss", Scalar())
- elif k == "mppd" or k == "mpfr":
- setattr(pl_module, f"{split}_{k}_loss", Scalar())
- elif k == "itm":
- setattr(pl_module, f"{split}_{k}_accuracy", Accuracy())
- setattr(pl_module, f"{split}_{k}_loss", Scalar())
- setattr(pl_module, f"{split}_{k}_wpa_loss", Scalar())
- else:
- setattr(pl_module, f"{split}_{k}_accuracy", Accuracy())
- setattr(pl_module, f"{split}_{k}_loss", Scalar())
-
-
-def epoch_wrapup(pl_module):
- phase = "train" if pl_module.training else "val"
- the_metric = 0
-
- if pl_module.hparams.config["get_recall_metric"] and not pl_module.training:
- (ir_r1, ir_r5, ir_r10, tr_r1, tr_r5, tr_r10) = compute_irtr_recall(pl_module)
- print((ir_r1, ir_r5, ir_r10, tr_r1, tr_r5, tr_r10), pl_module.global_step)
- pl_module.logger.experiment.add_scalar(
- "recalls/ir_r1", ir_r1, pl_module.global_step
- )
- pl_module.logger.experiment.add_scalar(
- "recalls/ir_r5", ir_r5, pl_module.global_step
- )
- pl_module.logger.experiment.add_scalar(
- "recalls/ir_r10", ir_r10, pl_module.global_step
- )
- pl_module.logger.experiment.add_scalar(
- "recalls/tr_r1", tr_r1, pl_module.global_step
- )
- pl_module.logger.experiment.add_scalar(
- "recalls/tr_r5", tr_r5, pl_module.global_step
- )
- pl_module.logger.experiment.add_scalar(
- "recalls/tr_r10", tr_r10, pl_module.global_step
- )
- the_metric += ir_r1.item() + tr_r1.item()
-
- for loss_name, v in pl_module.hparams.config["loss_names"].items():
- if v < 1:
- continue
-
- value = 0
-
- if loss_name == "vqa":
- value = getattr(pl_module, f"{phase}_{loss_name}_score").compute()
- pl_module.log(f"{loss_name}/{phase}/score_epoch", value)
- getattr(pl_module, f"{phase}_{loss_name}_score").reset()
- pl_module.log(
- f"{loss_name}/{phase}/loss_epoch",
- getattr(pl_module, f"{phase}_{loss_name}_loss").compute(),
- )
- getattr(pl_module, f"{phase}_{loss_name}_loss").reset()
- elif loss_name == "nlvr2":
- if phase == "train":
- value = getattr(pl_module, f"train_{loss_name}_accuracy").compute()
- pl_module.log(f"{loss_name}/train/accuracy_epoch", value)
- getattr(pl_module, f"train_{loss_name}_accuracy").reset()
- pl_module.log(
- f"{loss_name}/train/loss_epoch",
- getattr(pl_module, f"train_{loss_name}_loss").compute(),
- )
- getattr(pl_module, f"train_{loss_name}_loss").reset()
- else:
- value = getattr(pl_module, f"dev_{loss_name}_accuracy").compute()
- pl_module.log(f"{loss_name}/dev/accuracy_epoch", value)
- getattr(pl_module, f"dev_{loss_name}_accuracy").reset()
- pl_module.log(
- f"{loss_name}/dev/loss_epoch",
- getattr(pl_module, f"dev_{loss_name}_loss").compute(),
- )
- getattr(pl_module, f"dev_{loss_name}_loss").reset()
-
- value = getattr(pl_module, f"test_{loss_name}_accuracy").compute()
- pl_module.log(f"{loss_name}/test/accuracy_epoch", value)
- getattr(pl_module, f"test_{loss_name}_accuracy").reset()
- pl_module.log(
- f"{loss_name}/test/loss_epoch",
- getattr(pl_module, f"test_{loss_name}_loss").compute(),
- )
- getattr(pl_module, f"test_{loss_name}_loss").reset()
- elif loss_name == "irtr":
- pl_module.log(
- f"{loss_name}/{phase}/irtr_loss_epoch",
- getattr(pl_module, f"{phase}_irtr_loss").compute(),
- )
- getattr(pl_module, f"{phase}_irtr_loss").reset()
- elif loss_name == "mppd" or loss_name == "mpfr":
- pl_module.log(
- f"{loss_name}/{phase}/loss_epoch",
- getattr(pl_module, f"{phase}_{loss_name}_loss").compute(),
- )
- getattr(pl_module, f"{phase}_{loss_name}_loss").reset()
- elif loss_name == "itm":
- value = getattr(pl_module, f"{phase}_{loss_name}_accuracy").compute()
- pl_module.log(f"{loss_name}/{phase}/accuracy_epoch", value)
- getattr(pl_module, f"{phase}_{loss_name}_accuracy").reset()
- pl_module.log(
- f"{loss_name}/{phase}/loss_epoch",
- getattr(pl_module, f"{phase}_{loss_name}_loss").compute(),
- )
- getattr(pl_module, f"{phase}_{loss_name}_loss").reset()
- pl_module.log(
- f"{loss_name}/{phase}/wpa_loss_epoch",
- getattr(pl_module, f"{phase}_{loss_name}_wpa_loss").compute(),
- )
- getattr(pl_module, f"{phase}_{loss_name}_wpa_loss").reset()
- else:
- value = getattr(pl_module, f"{phase}_{loss_name}_accuracy").compute()
- pl_module.log(f"{loss_name}/{phase}/accuracy_epoch", value)
- getattr(pl_module, f"{phase}_{loss_name}_accuracy").reset()
- pl_module.log(
- f"{loss_name}/{phase}/loss_epoch",
- getattr(pl_module, f"{phase}_{loss_name}_loss").compute(),
- )
- getattr(pl_module, f"{phase}_{loss_name}_loss").reset()
-
- the_metric += value
-
- pl_module.log(f"{phase}/the_metric", the_metric)
-
-
-def check_non_acc_grad(pl_module):
- if pl_module.token_type_embeddings.weight.grad is None:
- return True
- else:
- grad = pl_module.token_type_embeddings.weight.grad
- return (grad.sum() == 0).item()
-
-
-def set_task(pl_module):
- pl_module.current_tasks = [
- k for k, v in pl_module.hparams.config["loss_names"].items() if v >= 1
- ]
- return
-
-
-def set_schedule(pl_module):
- lr = pl_module.hparams.config["learning_rate"]
- wd = pl_module.hparams.config["weight_decay"]
-
- no_decay = [
- "bias",
- "LayerNorm.bias",
- "LayerNorm.weight",
- "norm.bias",
- "norm.weight",
- "norm1.bias",
- "norm1.weight",
- "norm2.bias",
- "norm2.weight",
- ]
- head_names = ["vqa_classifier", "nlvr2_classifier"]
- lr_mult = pl_module.hparams.config["lr_mult"]
- end_lr = pl_module.hparams.config["end_lr"]
- decay_power = pl_module.hparams.config["decay_power"]
- optim_type = pl_module.hparams.config["optim_type"]
-
- names = [n for n, p in pl_module.named_parameters()]
- optimizer_grouped_parameters = [
- {
- "params": [
- p
- for n, p in pl_module.named_parameters()
- if not any(nd in n for nd in no_decay)
- and not any(bb in n for bb in head_names)
- ],
- "weight_decay": wd,
- "lr": lr,
- },
- {
- "params": [
- p
- for n, p in pl_module.named_parameters()
- if any(nd in n for nd in no_decay)
- and not any(bb in n for bb in head_names)
- ],
- "weight_decay": 0.0,
- "lr": lr,
- },
- {
- "params": [
- p
- for n, p in pl_module.named_parameters()
- if not any(nd in n for nd in no_decay)
- and any(bb in n for bb in head_names)
- ],
- "weight_decay": wd,
- "lr": lr * lr_mult,
- },
- {
- "params": [
- p
- for n, p in pl_module.named_parameters()
- if any(nd in n for nd in no_decay) and any(bb in n for bb in head_names)
- ],
- "weight_decay": 0.0,
- "lr": lr * lr_mult,
- },
- ]
-
- if optim_type == "adamw":
- optimizer = AdamW(
- optimizer_grouped_parameters, lr=lr, eps=1e-8, betas=(0.9, 0.98)
- )
- elif optim_type == "adam":
- optimizer = torch.optim.Adam(optimizer_grouped_parameters, lr=lr)
- elif optim_type == "sgd":
- optimizer = torch.optim.SGD(optimizer_grouped_parameters, lr=lr, momentum=0.9)
-
- if pl_module.trainer.max_steps is None:
- max_steps = (
- len(pl_module.trainer.datamodule.train_dataloader())
- * pl_module.trainer.max_epochs
- // pl_module.trainer.accumulate_grad_batches
- )
- else:
- max_steps = pl_module.trainer.max_steps
-
- warmup_steps = pl_module.hparams.config["warmup_steps"]
- if isinstance(pl_module.hparams.config["warmup_steps"], float):
- warmup_steps = int(max_steps * warmup_steps)
-
- if decay_power == "cosine":
- scheduler = get_cosine_schedule_with_warmup(
- optimizer,
- num_warmup_steps=warmup_steps,
- num_training_steps=max_steps,
- )
- else:
- scheduler = get_polynomial_decay_schedule_with_warmup(
- optimizer,
- num_warmup_steps=warmup_steps,
- num_training_steps=max_steps,
- lr_end=end_lr,
- power=decay_power,
- )
-
- sched = {"scheduler": scheduler, "interval": "step"}
-
- return (
- [optimizer],
- [sched],
- )
diff --git a/spaces/ondrejbiza/isa/invariant_slot_attention/lib/trainer.py b/spaces/ondrejbiza/isa/invariant_slot_attention/lib/trainer.py
deleted file mode 100644
index e70a33c9bf193ba8bd46433695e02dd7f07c7473..0000000000000000000000000000000000000000
--- a/spaces/ondrejbiza/isa/invariant_slot_attention/lib/trainer.py
+++ /dev/null
@@ -1,328 +0,0 @@
-# coding=utf-8
-# Copyright 2023 The Google Research Authors.
-#
-# Licensed under the Apache License, Version 2.0 (the "License");
-# you may not use this file except in compliance with the License.
-# You may obtain a copy of the License at
-#
-# http://www.apache.org/licenses/LICENSE-2.0
-#
-# Unless required by applicable law or agreed to in writing, software
-# distributed under the License is distributed on an "AS IS" BASIS,
-# WITHOUT WARRANTIES OR CONDITIONS OF ANY KIND, either express or implied.
-# See the License for the specific language governing permissions and
-# limitations under the License.
-
-"""The main model training loop."""
-
-import functools
-import os
-import time
-from typing import Dict, Iterable, Mapping, Optional, Tuple, Type, Union
-
-from absl import logging
-from clu import checkpoint
-from clu import metric_writers
-from clu import metrics
-from clu import parameter_overview
-from clu import periodic_actions
-import flax
-from flax import linen as nn
-
-import jax
-import jax.numpy as jnp
-import ml_collections
-import numpy as np
-import optax
-
-from scenic.train_lib import lr_schedules
-from scenic.train_lib import optimizers
-
-import tensorflow as tf
-
-from invariant_slot_attention.lib import evaluator
-from invariant_slot_attention.lib import input_pipeline
-from invariant_slot_attention.lib import losses
-from invariant_slot_attention.lib import utils
-
-Array = jnp.ndarray
-ArrayTree = Union[Array, Iterable["ArrayTree"], Mapping[str, "ArrayTree"]] # pytype: disable=not-supported-yet
-PRNGKey = Array
-
-
-def train_step(
- model,
- tx,
- rng,
- step,
- state_vars,
- opt_state,
- params,
- batch,
- loss_fn,
- train_metrics_cls,
- predicted_max_num_instances,
- ground_truth_max_num_instances,
- conditioning_key = None,
- ):
- """Perform a single training step.
-
- Args:
- model: Model used in training step.
- tx: The optimizer to use to minimize loss_fn.
- rng: Random number key
- step: Which training step we are on.
- state_vars: Accessory variables.
- opt_state: The state of the optimizer.
- params: The current parameters to be updated.
- batch: Training inputs for this step.
- loss_fn: Loss function that takes model predictions and a batch of data.
- train_metrics_cls: The metrics collection for computing training metrics.
- predicted_max_num_instances: Maximum number of instances in prediction.
- ground_truth_max_num_instances: Maximum number of instances in ground truth,
- including background (which counts as a separate instance).
- conditioning_key: Optional string. If provided, defines the batch key to be
- used as conditioning signal for the model. Otherwise this is inferred from
- the available keys in the batch.
-
- Returns:
- Tuple of the updated opt, state_vars, new random number key,
- metrics update, and step + 1. Note that some of this info is stored in
- TrainState, but here it is unpacked.
- """
-
- # Split PRNGKey and bind to host / device.
- new_rng, rng = jax.random.split(rng)
- rng = jax.random.fold_in(rng, jax.host_id())
- rng = jax.random.fold_in(rng, jax.lax.axis_index("batch"))
- init_rng, dropout_rng = jax.random.split(rng, 2)
-
- mutable_var_keys = list(state_vars.keys()) + ["intermediates"]
-
- conditioning = batch[conditioning_key] if conditioning_key else None
-
- def train_loss_fn(params, state_vars):
- preds, mutable_vars = model.apply(
- {"params": params, **state_vars}, video=batch["video"],
- conditioning=conditioning, mutable=mutable_var_keys,
- rngs={"state_init": init_rng, "dropout": dropout_rng}, train=True,
- padding_mask=batch.get("padding_mask"))
- # Filter intermediates, as we do not want to store them in the TrainState.
- state_vars = utils.filter_key_from_frozen_dict(
- mutable_vars, key="intermediates")
- loss, loss_aux = loss_fn(preds, batch)
- return loss, (state_vars, preds, loss_aux)
-
- grad_fn = jax.value_and_grad(train_loss_fn, has_aux=True)
- (loss, (state_vars, preds, loss_aux)), grad = grad_fn(params, state_vars)
-
- # Compute average gradient across multiple workers.
- grad = jax.lax.pmean(grad, axis_name="batch")
-
- updates, new_opt_state = tx.update(grad, opt_state, params)
- new_params = optax.apply_updates(params, updates)
-
- # Compute metrics.
- metrics_update = train_metrics_cls.gather_from_model_output(
- loss=loss,
- **loss_aux,
- predicted_segmentations=utils.remove_singleton_dim(
- preds["outputs"].get("segmentations")), # pytype: disable=attribute-error
- ground_truth_segmentations=batch.get("segmentations"),
- predicted_max_num_instances=predicted_max_num_instances,
- ground_truth_max_num_instances=ground_truth_max_num_instances,
- padding_mask=batch.get("padding_mask"),
- mask=batch.get("mask"))
- return (
- new_opt_state, new_params, state_vars, new_rng, metrics_update, step + 1)
-
-
-def train_and_evaluate(config,
- workdir):
- """Runs a training and evaluation loop.
-
- Args:
- config: Configuration to use.
- workdir: Working directory for checkpoints and TF summaries. If this
- contains checkpoint training will be resumed from the latest checkpoint.
- """
- rng = jax.random.PRNGKey(config.seed)
-
- tf.io.gfile.makedirs(workdir)
-
- # Input pipeline.
- rng, data_rng = jax.random.split(rng)
- # Make sure each host uses a different RNG for the training data.
- if config.get("seed_data", True): # Default to seeding data if not specified.
- data_rng = jax.random.fold_in(data_rng, jax.host_id())
- else:
- data_rng = None
- train_ds, eval_ds = input_pipeline.create_datasets(config, data_rng)
- train_iter = iter(train_ds) # pytype: disable=wrong-arg-types
-
- # Initialize model
- model = utils.build_model_from_config(config.model)
-
- # Construct TrainMetrics and EvalMetrics, metrics collections.
- train_metrics_cls = utils.make_metrics_collection("TrainMetrics",
- config.train_metrics_spec)
- eval_metrics_cls = utils.make_metrics_collection("EvalMetrics",
- config.eval_metrics_spec)
-
- def init_model(rng):
- rng, init_rng, model_rng, dropout_rng = jax.random.split(rng, num=4)
-
- init_conditioning = None
- if config.get("conditioning_key"):
- init_conditioning = jnp.ones(
- [1] + list(train_ds.element_spec[config.conditioning_key].shape)[2:],
- jnp.int32)
- init_inputs = jnp.ones(
- [1] + list(train_ds.element_spec["video"].shape)[2:],
- jnp.float32)
- initial_vars = model.init(
- {"params": model_rng, "state_init": init_rng, "dropout": dropout_rng},
- video=init_inputs, conditioning=init_conditioning,
- padding_mask=jnp.ones(init_inputs.shape[:-1], jnp.int32))
-
- # Split into state variables (e.g. for batchnorm stats) and model params.
- # Note that `pop()` on a FrozenDict performs a deep copy.
- state_vars, initial_params = initial_vars.pop("params") # pytype: disable=attribute-error
-
- # Filter out intermediates (we don't want to store these in the TrainState).
- state_vars = utils.filter_key_from_frozen_dict(
- state_vars, key="intermediates")
- return state_vars, initial_params
-
- state_vars, initial_params = init_model(rng)
- parameter_overview.log_parameter_overview(initial_params) # pytype: disable=wrong-arg-types
-
- learning_rate_fn = lr_schedules.get_learning_rate_fn(config)
- tx = optimizers.get_optimizer(
- config.optimizer_configs, learning_rate_fn, params=initial_params)
-
- opt_state = tx.init(initial_params)
-
- state = utils.TrainState(
- step=1, opt_state=opt_state, params=initial_params, rng=rng,
- variables=state_vars)
-
- loss_fn = functools.partial(
- losses.compute_full_loss, loss_config=config.losses)
-
- checkpoint_dir = os.path.join(workdir, "checkpoints")
- ckpt = checkpoint.MultihostCheckpoint(checkpoint_dir)
- state = ckpt.restore_or_initialize(state)
- initial_step = int(state.step)
-
- # Replicate our parameters.
- state = flax.jax_utils.replicate(state, devices=jax.local_devices())
- del rng # rng is stored in the state.
-
- # Only write metrics on host 0, write to logs on all other hosts.
- writer = metric_writers.create_default_writer(
- workdir, just_logging=jax.host_id() > 0)
- writer.write_hparams(utils.prepare_dict_for_logging(config.to_dict()))
-
- logging.info("Starting training loop at step %d.", initial_step)
- report_progress = periodic_actions.ReportProgress(
- num_train_steps=config.num_train_steps, writer=writer)
- if jax.process_index() == 0:
- profiler = periodic_actions.Profile(num_profile_steps=5, logdir=workdir)
- p_train_step = jax.pmap(
- train_step,
- axis_name="batch",
- donate_argnums=(2, 3, 4, 5, 6, 7),
- static_broadcasted_argnums=(0, 1, 8, 9, 10, 11, 12))
-
- train_metrics = None
- with metric_writers.ensure_flushes(writer):
- if config.num_train_steps == 0:
- with report_progress.timed("eval"):
- evaluate(model, state, eval_ds, loss_fn, eval_metrics_cls, config,
- writer, step=0)
- with report_progress.timed("checkpoint"):
- ckpt.save(flax.jax_utils.unreplicate(state))
- return
-
- for step in range(initial_step, config.num_train_steps + 1):
- # `step` is a Python integer. `state.step` is JAX integer on GPU/TPU.
- is_last_step = step == config.num_train_steps
-
- with jax.profiler.StepTraceAnnotation("train", step_num=step):
- batch = jax.tree_map(np.asarray, next(train_iter))
- (opt_state, params, state_vars, rng, metrics_update, p_step
- ) = p_train_step(
- model, tx, state.rng, state.step, state.variables,
- state.opt_state, state.params, batch, loss_fn,
- train_metrics_cls,
- config.num_slots,
- config.max_instances + 1, # Incl. background.
- config.get("conditioning_key"))
-
- state = state.replace( # pytype: disable=attribute-error
- opt_state=opt_state,
- params=params,
- step=p_step,
- variables=state_vars,
- rng=rng,
- )
-
- metric_update = flax.jax_utils.unreplicate(metrics_update)
- train_metrics = (
- metric_update
- if train_metrics is None else train_metrics.merge(metric_update))
-
- # Quick indication that training is happening.
- logging.log_first_n(logging.INFO, "Finished training step %d.", 5, step)
- report_progress(step, time.time())
-
- if jax.process_index() == 0:
- profiler(step)
-
- if step % config.log_loss_every_steps == 0 or is_last_step:
- metrics_res = train_metrics.compute()
- writer.write_scalars(step, jax.tree_map(np.array, metrics_res))
- train_metrics = None
-
- if step % config.eval_every_steps == 0 or is_last_step:
- with report_progress.timed("eval"):
- evaluate(model, state, eval_ds, loss_fn, eval_metrics_cls,
- config, writer, step=step)
-
- if step % config.checkpoint_every_steps == 0 or is_last_step:
- with report_progress.timed("checkpoint"):
- ckpt.save(flax.jax_utils.unreplicate(state))
-
-
-def evaluate(model, state, eval_ds, loss_fn_eval, eval_metrics_cls, config,
- writer, step):
- """Evaluate the model."""
- eval_metrics, eval_batch, eval_preds = evaluator.evaluate(
- model,
- state,
- eval_ds,
- loss_fn_eval,
- eval_metrics_cls,
- predicted_max_num_instances=config.num_slots,
- ground_truth_max_num_instances=config.max_instances + 1, # Incl. bg.
- slice_size=config.get("eval_slice_size"),
- slice_keys=config.get("eval_slice_keys"),
- conditioning_key=config.get("conditioning_key"),
- remove_from_predictions=config.get("remove_from_predictions"),
- metrics_on_cpu=config.get("metrics_on_cpu", False))
-
- metrics_res = eval_metrics.compute()
- writer.write_scalars(
- step, jax.tree_map(np.array, utils.flatten_named_dicttree(metrics_res)))
- writer.write_images(
- step,
- jax.tree_map(
- np.array,
- utils.prepare_images_for_logging(
- config,
- eval_batch,
- eval_preds,
- n_samples=config.get("n_samples", 5),
- n_frames=config.get("n_frames", 1),
- min_n_colors=config.get("logging_min_n_colors", 1))))
diff --git "a/spaces/oskarvanderwal/MT-bias-demo/results/simple_m\303\251rn\303\266k_fr.html" "b/spaces/oskarvanderwal/MT-bias-demo/results/simple_m\303\251rn\303\266k_fr.html"
deleted file mode 100644
index 1e2276c1f3992868eb19043eddd41733a600fb3b..0000000000000000000000000000000000000000
--- "a/spaces/oskarvanderwal/MT-bias-demo/results/simple_m\303\251rn\303\266k_fr.html"
+++ /dev/null
@@ -1,46 +0,0 @@
- 0th instance:
-
-