diff --git a/spaces/0xJustin/0xJustin-Dungeons-and-Diffusion/app.py b/spaces/0xJustin/0xJustin-Dungeons-and-Diffusion/app.py deleted file mode 100644 index 969e30db2db42c563008db5cc67ba868c109abfc..0000000000000000000000000000000000000000 --- a/spaces/0xJustin/0xJustin-Dungeons-and-Diffusion/app.py +++ /dev/null @@ -1,3 +0,0 @@ -import gradio as gr - -gr.Interface.load("models/0xJustin/Dungeons-and-Diffusion").launch() \ No newline at end of file diff --git a/spaces/0xSynapse/LlamaGPT/README.md b/spaces/0xSynapse/LlamaGPT/README.md deleted file mode 100644 index f3bcd42363ab575bd7eb11eb535831511ced8d32..0000000000000000000000000000000000000000 --- a/spaces/0xSynapse/LlamaGPT/README.md +++ /dev/null @@ -1,13 +0,0 @@ ---- -title: LlamaGPT -emoji: 📚 -colorFrom: green -colorTo: blue -sdk: gradio -sdk_version: 3.39.0 -app_file: app.py -pinned: false -license: lgpl-3.0 ---- - -Check out the configuration reference at https://huggingface.co/docs/hub/spaces-config-reference diff --git a/spaces/1acneusushi/gradio-2dmoleculeeditor/data/Download Office 2021 64 Bit for Windows 10 Everything You Need to Know.md b/spaces/1acneusushi/gradio-2dmoleculeeditor/data/Download Office 2021 64 Bit for Windows 10 Everything You Need to Know.md deleted file mode 100644 index 0152a1eef5a8d3189f5da51c6248ad9b22fe9a29..0000000000000000000000000000000000000000 --- a/spaces/1acneusushi/gradio-2dmoleculeeditor/data/Download Office 2021 64 Bit for Windows 10 Everything You Need to Know.md +++ /dev/null @@ -1,27 +0,0 @@ - -
Office 2021 is the latest version of Microsoft's productivity suite, which includes Word, Excel, PowerPoint, Outlook, and more. If you want to download Office 2021 64 bit for Windows 10, you can follow these steps:
-Download File ☆ https://byltly.com/2uKvx0
Congratulations! You have successfully downloaded and installed Office 2021 64 bit for Windows 10. Enjoy using the latest features and enhancements of Microsoft's productivity suite.
Office 2021 is compatible with Windows 10 and Windows 11, as well as macOS. It offers several improvements and new features over the previous version, Office 2019. Some of the highlights include:
-Office 2021 also comes with enhanced security and privacy features, such as encryption, data loss prevention, and advanced threat protection. You can also access your files and documents from anywhere with OneDrive cloud storage and Office mobile apps.
If you want to try Office 2021 before buying it, you can download a free trial version from https://www.microsoft.com/en-us/evalcenter/evaluate-microsoft-365. The trial version will let you use Office 2021 for 30 days, after which you will need to purchase a subscription or a one-time license to continue using it.
-Alternatively, you can also use Office Online, which is a free web-based version of Office that works in your browser. Office Online lets you create and edit documents, spreadsheets, and presentations online, as well as collaborate with others in real time. You can access Office Online from https://www.office.com/ or from your Microsoft account.
- -Whether you choose Office 2021 or Office Online, you will get the best of Microsoft's productivity tools for your personal and professional needs. Download Office 2021 64 bit for Windows 10 today and see the difference for yourself.
ddb901b051Have you ever lost important files due to accidental deletion, formatting, virus attack, or system crash? If so, you know how frustrating and stressful it can be to recover your data. Fortunately, there is a powerful and easy-to-use software that can help you: Easy Recovery Pro.
-Easy Recovery Pro is a data recovery software that supports all Windows PCs and laptops, including Windows 10 and Windows 11. It can recover data from various storage devices, such as hard drives, external drives, USB flash drives, memory cards, and more. It can also recover almost any file type, such as photos, videos, audio files, emails, documents, etc.
-Download ————— https://byltly.com/2uKxj8
In this article, we will show you how to use Easy Recovery Pro to restore your lost data on Windows in three simple steps.
- -To get started, you need to download and install Easy Recovery Pro on a working computer. You can get it from the official website[^1^]. There are different editions available for different needs and budgets. You can choose the one that suits you best.
-After downloading the software, run the setup file and follow the instructions to install it on your computer. Make sure you have enough disk space and administrator privileges.
- -Next, you need to connect the storage device that contains your lost data to the computer where you installed Easy Recovery Pro. For example, if you want to recover data from an external hard drive, plug it into a USB port.
-Then, launch Easy Recovery Pro and select the storage device from the list of available drives. Click "Scan" to start searching for lost data. The scanning process may take some time depending on the size and condition of your device.
-During the scan, you can preview the found files by clicking on them. You can also pause or stop the scan at any time if you find what you need.
- -When the scan is complete, you will see a list of recoverable files sorted by categories. You can filter them by file type, date, size, or name. You can also use the search box to find specific files.
- -To recover your lost data, simply select the files or folders that you want and click "Recover". You will be asked to choose a location to save the recovered data. It is recommended that you save them to a different drive than the original one to avoid overwriting.
-After the recovery process is done, you can check your recovered data and use them as normal.
- -Easy Recovery Pro is a reliable and easy recovery software that can help you restore your lost data on Windows in various scenarios. It has a user-friendly interface and powerful features that make data recovery a breeze. Whether you are a professional or a beginner, you can use Easy Recovery Pro to get back your precious data in minutes.
-If you want to try Easy Recovery Pro for free, you can download the trial version from the official website[^1^]. The trial version allows you to scan and preview your lost data, but not recover them. To recover your data without limitations, you need to purchase a license key.
cec2833e83DOWNLOAD ———>>> https://imgfil.com/2uxXox
Download File ••• https://imgfil.com/2uxZhy
DOWNLOAD ↔ https://imgfil.com/2uxYjf
If you are looking for a fun and exciting bingo game that will keep you entertained for hours, then you should try Bingo Holiday. This is a classic and special bingo game that offers you more than just bingo. You can explore over 110 appealing scenes, travel around the world, send and receive gifts with friends, challenge events, and collect epic collections. You can also enjoy various bingo styles, power-ups, tournaments, and jackpots. In this article, we will show you how to download and play Bingo Holiday on your Android or iOS device, or online on your browser.
-Bingo Holiday is a bingo game developed by AE Magwin, a company that specializes in casino and casual games. It was released in 2016 and has since gained over 5 million downloads on Google Play Store and over 13 thousand ratings on App Store. It is rated as one of the best bingo games on both platforms.
-Download ✶✶✶ https://jinyurl.com/2uNLwN
Bingo Holiday has many features that make it stand out from other bingo games. Some of them are:
-Bingo Holiday is not only a bingo game but also a way to relax and have fun. Here are some reasons why you should play Bingo Holiday:
-If you have an Android device, you can download Bingo Holiday from the Google Play Store. Here are the steps to do it:
-Here are some screenshots of the app on an Android device:
-Here are some tips and tricks that will help you play Bingo Holiday better on your Android device:
-If you have an iOS device, you can download Bingo Holiday from the App Store. Here are the steps to do it:
-bingo holiday free download
-bingo holiday app download
-bingo holiday game download
-bingo holiday apk download
-bingo holiday mod apk download
-bingo holiday for pc download
-bingo holiday for android download
-bingo holiday for ios download
-bingo holiday for mac download
-bingo holiday for windows download
-bingo holiday online download
-bingo holiday offline download
-bingo holiday latest version download
-bingo holiday update download
-bingo holiday new version download
-bingo holiday old version download
-bingo holiday hack download
-bingo holiday cheats download
-bingo holiday unlimited credits download
-bingo holiday free credits download
-bingo holiday free coins download
-bingo holiday free power ups download
-bingo holiday free gifts download
-bingo holiday free spins download
-bingo holiday free slots download
-bingo holiday classic bingo games download
-bingo holiday special bingo games download
-bingo holiday live bingo games download
-bingo holiday multiplayer bingo games download
-bingo holiday tournament bingo games download
-bingo holiday world tour bingo games download
-bingo holiday travel bingo games download
-bingo holiday adventure bingo games download
-bingo holiday party bingo games download
-bingo holiday fun bingo games download
-bingo holiday best bingo games download
-bingo holiday top rated bingo games download
-bingo holiday reviews and ratings download
-bingo holiday screenshots and videos download
-bingo holiday tips and tricks download
-how to play bingo holiday game download
-how to win in bingo holiday game download
-how to get more credits in bingo holiday game download
-how to get more coins in bingo holiday game download
-how to get more power ups in bingo holiday game download
-how to get more gifts in bingo holiday game download
-how to get more spins in bingo holiday game download
-how to get more slots in bingo holiday game download
Here are some screenshots of the app on an iOS device:
-Here are some tips and tricks that will help you play Bingo Holiday better on your iOS device:
-If you don't have an Android or iOS device, or you don't want to download the app, you can still play Bingo Holiday online on your browser. There are some benefits of playing Bingo Holiday online, such as:
-Here are the steps to access Bingo Holiday online and start playing:
-Here are some screenshots of the website on a browser:
-Bingo Holiday is a bingo game that offers you more than just bingo. You can explore over 110 appealing scenes, travel around the world, send and receive gifts with friends, challenge events, and collect epic collections. You can also enjoy various bingo styles, power-ups, tournaments, and jackpots.
-You can download and play Bingo Holiday on your Android or iOS device, or online on your browser. We have shown you how to do it in this article with step-by-step instructions and screenshots. We have also given you some tips and tricks that will help you play Bingo Holiday better on your device or online.
-If you are ready to join the fun and excitement of Bingo Holiday, don't wait any longer. Download or access Bingo Holiday today and start playing bingo like never before!
-A1: Yes, Bingo Holiday is free to play. You don't need to pay anything to download or play Bingo Holiday. You can enjoy all the features and content without spending a dime.
-A2: There are many ways to get more credits and power-ups in Bingo Holiday. Some of them are:
-A3: There are two ways to play Bingo Holiday with your friends. One is to add them as your friends in the game and invite them to join your bingo room. The other is to join a public bingo room and chat with other players who are also your friends. Here are the steps to do both:
-A4: There are over 40 bingo rooms in Bingo Holiday that have different themes, rules, prizes, and collections. Some of them are:
-A5: If you have any questions, problems, suggestions, or feedback about Bingo Holiday, you can contact the customer support of Bingo Holiday by using one of these methods:
-The customer support team of Bingo Holiday is friendly and helpful. They will try their best to solve your issues and improve your gaming experience.
401be4b1e0If you are looking for a hilarious and action-packed first-person shooter game where you can play as armed chickens, then you should check out Chicken Gun. This game lets you shoot and fight with other chickens in various modes and maps, using different weapons, beaks, sneakers, caps, and even explosive eggs. You can also customize your chicken from head to toe, making it look cool or funny or both.
-But what if you want to have more fun and freedom with this game? What if you want to play with your friends or other players without any restrictions or limitations? What if you want to have more customization options, more maps, more modes, less lag, and more control over the game settings? Well, then you might want to try playing on a private server.
-Download Zip ⚙⚙⚙ https://jinyurl.com/2uNMO8
A private server is an unofficial mod that allows you to create or join a separate server from the official one. This way, you can play with whoever you want, whenever you want, however you want. You can also enjoy some features and benefits that are not available on the official server.
-In this article, we will show you how to download and install Chicken Gun Private Server 1.3.0 APK on your Android device, how to join or create a private server in Chicken Gun, what are the features and benefits of playing on a private server, and some tips and tricks to improve your skills and enjoy the game more. So, without further ado, let's get started!
-Before you can play on a private server, you need to download and install Chicken Gun Private Server 1.3.0 APK on your Android device. This is an unofficial mod that is not endorsed by the developers of Chicken Gun, so you should use it at your own risk. Here are the steps to follow:
-chicken gun mod apk private server 1.3.0
-chicken gun 1.3.0 private server download
-chicken gun private server apk 1.3.0 free
-chicken gun hack private server 1.3.0
-chicken gun private server version 1.3.0
-chicken gun private server apk mediafire 1.3.0
-chicken gun private server youtube 1.3.0
-chicken gun private server apkcombo 1.3.0
-chicken gun private server update 1.3.0
-chicken gun private server android 1.3.0
-chicken gun private server ios 1.3.0
-chicken gun private server unlimited money 1.3.0
-chicken gun private server all skins 1.3.0
-chicken gun private server gameplay 1.3.0
-chicken gun private server online 1.3.0
-chicken gun private server offline 1.3.0
-chicken gun private server new features 1.3.0
-chicken gun private server no root 1.3.0
-chicken gun private server no ads 1.3.0
-chicken gun private server latest version 1.3.0
-chicken gun private server how to install 1.3.0
-chicken gun private server how to play 1.3.0
-chicken gun private server how to join 1.3.0
-chicken gun private server how to create 1.3.0
-chicken gun private server how to download 1.3.0
-chicken gun private server review 1.3.0
-chicken gun private server tips and tricks 1.3.0
-chicken gun private server cheats and hacks 1.3.0
-chicken gun private server codes and coupons 1.3.0
-chicken gun private server glitches and bugs 1.3.0
-chicken gun private server fun and funny moments 1.3.0
-chicken gun private server best and worst weapons 1.3.0
-chicken gun private server best and worst maps 1.3.0
-chicken gun private server best and worst modes 1.3.0
-chicken gun private server best and worst skins 1..30
-chicken gun private server comparison and contrast 1..30
-chicken gun private server pros and cons 1..30
-chicken gun private server advantages and disadvantages 1..30
-chicken gun private server benefits and drawbacks 1..30
-chicken gun private server ratings and rankings 1..30
Congratulations! You have successfully installed Chicken Gun Private Server 1.3.0 APK on your device. Now you can join or create a private server and have fun.
-Now that you have installed Chicken Gun Private Server 1.3.0 APK on your device, you can join or create a private server in Chicken Gun. Here are the steps to follow:
-That's it! You have successfully joined a private server in Chicken Gun. Now you can play with other players and have fun.
-That's it! You have successfully created a private server in Chicken Gun. Now you can play with your friends or other players and have fun.
-Playing on a private server in Chicken Gun has some features and benefits that are not available on the official server. Here are some of them:
-As you can see, playing on a private server in Chicken Gun has many advantages that can make your gaming experience more enjoyable and satisfying. Of course, you should also respect the rules and etiquette of each server, and not abuse or exploit any features or benefits.
-Playing on a private server in Chicken Gun is not only fun, but also challenging. You will face many skilled and competitive players who will test your abilities and strategies. If you want to improve your skills and enjoy the game more, here are some tips and tricks that you can use:
-Chicken Gun is a hilarious and action-packed first-person shooter game where you can play as armed chickens in various modes and maps. You can also customize your chicken from head to toe, making it look cool or funny or both.
-If you want to have more fun and freedom with this game, you can try playing on a private server. A private server is an unofficial mod that allows you to create or join a separate server from the official one. This way, you can play with whoever you want, whenever you want, however you want.
-You can also enjoy some features and benefits that are not available on the official server, such as more customization options, more maps and modes, less lag and better performance, more control over the game rules and settings, and more fun and freedom with your friends or other players.
-In this article, we have shown you how to download and install Chicken Gun Private Server 1.3.0 APK on your Android device, how to join or create a private server in Chicken Gun, what are the features and benefits of playing on a private server, and some tips and tricks to improve your skills and enjoy the game more.
-We hope that you have found this article helpful and informative. If you have any questions or feedback, please feel free to leave a comment below. We would love to hear from you.
-Now, what are you waiting for? Go ahead and try out Chicken Gun Private Server 1.3.0 APK and have fun!
-Thank you for reading this article.
-Here are some frequently asked questions about Chicken Gun Private Server 1.3.0 APK:
-A: Chicken Gun Private Server 1.3.0 APK is an unofficial mod that is not endorsed by the developers of Chicken Gun, so you should use it at your own risk. We do not take any responsibility for any damage or harm that may occur from using this mod. You should also be careful about downloading and installing any APK files from unknown sources, as they may contain viruses or malware.
-A: No, you cannot play on a private server with players who are on the official server. You can only play with players who are also using the same mod as you. If you want to play with players who are on the official server, you need to uninstall the mod and reinstall the original game from the Google Play Store.
-A: No, you cannot play on a private server offline. You need an internet connection to join or create a private server in Chicken Gun. However, you can play the single-player mode offline if you want to practice your skills or have fun by yourself.
-A: To update Chicken Gun Private Server 1.3.0 APK, you need to download and install the latest version of the mod from the same source that you got it from. You should also check for updates regularly, as new features and bug fixes may be added in the future.
-A: To contact the developers of Chicken Gun Private Server 1.3.0 APK, you can visit their website at https://chickengunmod.com/ or their Facebook page at https://www.facebook.com/chickengunmod/. You can also send them an email at chickengunmod@gmail.com. You can ask them any questions or give them any feedback or suggestions that you may have.
401be4b1e0Do you like building games? Do you want to unleash your creativity in a sandbox world? Do you want to play with your friends online or offline? If you answered yes to any of these questions, then you might want to try Crafting and Building, a free building game for Android devices.
-In this article, we will tell you everything you need to know about Crafting and Building, including what it is, how to download it, what are its main features, and what are some tips and tricks for playing it.
-DOWNLOAD ——— https://jinyurl.com/2uNSAQ
Crafting and Building is a new free building game for Android devices that lets you create your own world with blocks. You can build anything you can imagine, from houses to castles to temples, and explore different biomes, such as forests, deserts, mountains, caves, and oceans. You can also play with your friends online or offline, visit their worlds, and help them with their constructions.
-Crafting and Building is inspired by popular games like Minecraft and Terraria, but it has its own unique features and style. It has cool graphics, smooth controls, and a user-friendly interface that makes it easy to play for anyone. It also has no monsters or enemies, so you can focus on building and having fun.
-Crafting and Building 1.18 APK is the latest version of the game, released on April 19, 2023. It has some new features and improvements, such as new blocks, new animals, new skins, new sounds, and bug fixes.
-Crafting and Building 1.18 APK can be downloaded from various websites that offer APK files, such as APKCombo, Aptoide, or MCPE Planet. However, you should always be careful when downloading APK files from unknown sources, as they may contain viruses or malware that can harm your device. You should always scan any APK file before installing it on your device.
-Crafting and Building 1.18 APK requires Android 5.1 or higher and about 387 MB of storage space on your device. To install it, you need to enable unknown sources in your device settings. This will allow you to install apps that are not from the Google Play Store. To do this, go to Settings > Security > Unknown Sources and toggle it on. Then, follow the instructions on the screen to complete the installation process.
-Crafting and Building 1.18 APK offers a fun and creative gameplay experience for the whole family. Here are some of the main features of the game:
-crafting and building 1.18 apk free download
-crafting and building 1.18 mod apk download
-crafting and building 1.18 apk download for android
-crafting and building 1.18 apk download latest version
-crafting and building 1.18 apk download uptodown
-crafting and building 1.18 apk download apkpure
-crafting and building 1.18 apk download for pc
-crafting and building 1.18 apk download no ads
-crafting and building 1.18 apk download unlimited money
-crafting and building 1.18 apk download offline
-crafting and building 1.18 apk download hack
-crafting and building 1.18 apk download mediafıre
-crafting and building 1.18 apk download android 11
-crafting and building 1.18 apk download ios
-crafting and building 1.18 apk download windows 10
-crafting and building 1.18 apk download full version
-crafting and building 1.18 apk download online
-crafting and building 1.18 apk download without verification
-crafting and building 1.18 apk download mega
-crafting and building 1.18 apk download google drive
-crafting and building 1.18 apk download update
-crafting and building 1.18 apk download old version
-crafting and building 1.18 apk download cracked
-crafting and building 1.18 apk download premium
-crafting and building 1.18 apk download mod menu
-crafting and building 1.18 apk download original
-crafting and building 1.18 apk download android oyun club
-crafting and building 1.18 apk download rexdl
-crafting and building 1.18 apk download revdl
-crafting and building 1.18 apk download happy mod
-crafting and building 1.18 apk download an1.com
-crafting and building 1.18 apk download mob.org
-crafting and building 1.18 apk download malavida
-crafting and building 1.18 apk download softonic
-crafting and building 1.18 apk download appmirror.net
-crafting and building 1.18 apk download appvn.com
-crafting and building 1.18 apk download blackmod.net
-crafting and building 1.18 apk download platinmods.com
-crafting and building 1.18 apk download androidpolska.pl
-crafting and building 1.18 apk download apkmody.io
If you want to get the most out of Crafting and Building, here are some tips and tricks that might help you:
-Crafting and Building is a great game for anyone who loves building games. It is easy to download and install on your Android device, and it offers endless possibilities for creativity, exploration, and multiplayer fun. You can build anything you can imagine, play with your friends online or offline, explore different biomes, and interact with animals and villagers. The game has cool graphics, smooth controls, and a user-friendly interface that makes it suitable for all ages.
-If you are looking for a new building game to try, you should definitely give Crafting and Building a chance. You will not regret it!
-Yes, Crafting and Building is completely free to play. However, it contains ads that can be removed by purchasing the Pro DLC.
-Yes, Crafting and Building is safe to download from reputable sources. However, you should always scan any APK file before installing it on your device.
-No, Crafting and Building is not compatible with Minecraft. They are different games with different features.
-You can customize your character in Crafting and Building by choosing your gender, skin color, hair style, clothes, accessories, and more.
-You can contact the developers of Crafting and Building by emailing them at protonmobile@gmail.com or visiting their website at https://protonmobile.com/.
401be4b1e0If you are looking for a fun and action-packed game that lets you swing around the city like Spider-Man, fight against crime and injustice, and customize your hero with different outfits and weapons, then you might want to try Rope Hero. Rope Hero is a free game for Android devices that has been downloaded over 100 million times on Google Play Store. In this article, we will tell you what Rope Hero is, how to download and install it on your Android device, why you should play it, and some tips and tricks to help you enjoy it more.
-DOWNLOAD ►►► https://jinyurl.com/2uNUdf
Rope Hero is a 3D open-world action game developed by Naxeex Action & RPG Games. In this game, you play as a superhero who has a super rope and can perform mega jumps, climb buildings, and power landings. You can explore the city, complete missions, fight against gangs and criminals, drive various vehicles, and use different weapons. You can also level up your hero, upgrade your skills, and change your appearance. Rope Hero is a game that combines elements of adventure, simulation, shooting, and RPG.
-Some of the features that make Rope Hero an exciting and addictive game are:
-If you want to download and install Rope Hero APK on your Android device, you can follow these simple steps:
-Rope Hero is a game that offers a lot of fun and entertainment for Android users who love action games. Here are some reasons why you should play Rope Hero:
-Like any other game, Rope Hero has its pros and cons. Here are some of them:
-Pros | -Cons | -
---|---|
- Fun and addictive gameplay | -- Some bugs and glitches | -
- Lots of content and variety | -- Repetitive missions and enemies | -
- Cool and realistic graphics | -- High battery and data consumption | -
- Easy and intuitive controls | -- Ads and in-app purchases | -
- Free and offline mode available | -- No multiplayer or online mode | -
If you want to play Rope Hero like a pro, here are some tips and tricks that you can use:
-rope hero vice town apk download game
-rope hero android game free download
-rope hero apk mod unlimited money download game
-rope hero 3 apk download game
-rope hero apk download latest version game
-rope hero apk combo download game
-rope hero apk offline download game
-rope hero apk update download game
-rope hero apk hack download game
-rope hero apk pure download game
-rope hero apk obb download game
-rope hero apk revdl download game
-rope hero apk rexdl download game
-rope hero apk uptodown download game
-rope hero apk mob.org download game
-rope hero apk mirror download game
-rope hero apk old version download game
-rope hero apk for pc download game
-rope hero apk for ios download game
-rope hero apk for windows 10 download game
-rope hero naxeex apk download game
-rope hero naxeex action rpg games apk download game
-rope hero naxeex studio apk download game
-rope hero naxeex llc apk download game
-rope hero naxeex mod apk download game
-rope hero naxeex unlimited money apk download game
-rope hero naxeex hack apk download game
-rope hero naxeex latest version apk download game
-rope hero naxeex offline apk download game
-rope hero naxeex online apk download game
-rope hero 3d open world city simulator apk download game
-rope hero 3d superhero simulator apk download game
-rope hero 3d action adventure apk download game
-rope hero 3d crime city battle apk download game
-rope hero 3d spider gangster crime city simulator apk download game
-rope hero 3d flying superhero simulator mod apk download game
-rope hero 3d flying superhero simulator hack apk download game
-rope hero 3d flying superhero simulator unlimited money apk download game
-rope hero 3d flying superhero simulator latest version apk download game
-rope hero 3d flying superhero simulator offline apk download game
-rope hero 3d flying superhero simulator online apk download game
-amazing spider stickman - super flying spiderman - spiderman games - spiderman games free - spiderman games for kids - spiderman games online - spiderman games offline - spiderman games 2023 - spiderman games 3d - spiderman games hd - spiderman games new - spiderman games best - spiderman games fun - spiderman games cool - spiderman games awesome - spiderman games amazing - spiderman games fantastic - spiderman games incredible - spiderman games ultimate - spiderman games epic - spiderman games legendary - spiderman games popular - spiderman games top - spiderman games classic - spiderman games original - spiderman games pro - spiderman games premium - spiderman games deluxe - spiderman games master - spiderman games expert - spiderman games genius - spiderman games super - spiderman games hyper - spiderman games mega - spiderman games ultra
Rope Hero is a game that offers a lot of fun and entertainment for Android users who love action games. It is a game that lets you become a superhero who can swing around the city with a super rope, fight against crime and injustice, and customize your hero with different outfits and weapons. It is a game that has realistic physics and graphics, open-world gameplay, diverse missions and challenges, customization options, and multiple vehicles. It is a game that you can download and install for free on your Android device by following the simple steps we have provided in this article. It is a game that you should play if you want to experience the thrill and excitement of being a rope hero.
-Here are some frequently asked questions about Rope Hero:
-The latest version of Rope Hero APK is 4.1.1 which was released on June 14th 2023.
-Rope Hero is safe to download as long as you download it from a trusted source like [Rope Hero APK (Android Game) - Free Download - APKCombo] or [Rope Hero APK (Android Game) - Free Download - APKCombo]. However, you should always scan the APK file with an antivirus software before installing it on your device.
-Rope Hero requires about 100 MB of free space on your device to install and run smoothly.
-Yes, you can play Rope Hero offline without an internet connection. However, you will need an internet connection to access some features, such as ads, in-app purchases, and updates.
-You can contact the developer of Rope Hero by sending an email to naxeexaction@gmail.com or by visiting their website at [Naxeex Action & RPG Games].
-I hope you found this article helpful and informative. If you have any questions or feedback, please feel free to leave a comment below. Thank you for reading and happy gaming!
401be4b1e0If you are a fan of historical drama, action, and adventure, you might have heard of Ertuğrul Gazi Oyunu, a popular Turkish game based on the life of Ertuğrul Gazi, the father of Osman I, the founder of the Ottoman Empire. The game is a role-playing game that consists of 60 episodes, each with its own story, characters, and challenges. The game features realistic 3D graphics, professional music, high-resolution visuals, detailed scenes, multiplayer real characters, history-telling dialogues, and team directions. The game is available for Android and PC platforms, and you can download it for free from Google Play Store or Steam.
-Ertuğrul Gazi was a 13th-century bey (chief) of the Kayı tribe of Oghuz Turks, who migrated from Central Asia to Anatolia to escape the Mongol invasions. He was a brave warrior who fought against various enemies, such as the Byzantines, the Crusaders, and the Mongols. He was also a loyal ally of the Seljuks of Rum, who granted him lands in Söğüt, near Bilecik. He was the father of Osman I, who established the Ottoman Empire in 1299. Ertuğrul Gazi is considered a hero and a ghazi (a fighter for Islam) by many Turks and Muslims. He is also a popular subject of Turkish literature, art, and media.
-Download 🆓 https://jinyurl.com/2uNUwa
The game developers, UMURO, are a Turkish company that specializes in creating games with historical and cultural themes. They were inspired by the success of Diriliş: Ertuğrul, a Turkish TV series that dramatized the life of Ertuğrul Gazi and his tribe. They wanted to create a game that would allow players to experience the same adventure and excitement as the TV series. They also wanted to showcase the rich history and culture of Turkey, especially during the medieval period. They did extensive research on Ertuğrul Gazi's biography, Turkish history, geography, architecture, clothing, weapons, music, language, and customs. They also consulted with historians, experts, and consultants to ensure accuracy and authenticity.
-The game follows Ertuğrul Gazi's journey from his youth to his death. Each episode has its own plot, characters, missions, enemies, allies, locations, and rewards. The player can choose to play as Ertuğrul Gazi or one of his alps (warriors). The player can also customize their character's appearance, skills, weapons, armor, pets, etc. The main objectives of the game are to complete various tasks assigned by Ertuğrul Gazi or other characters; to fight against enemies using combat skills such as sword fighting, horse riding, archery, defense with sword and shield, direction finding with map, swimming, running fast, rolling, cl imbing, stealth, etc.; to explore different locations such as Söğüt, Aleppo, Karacahisar, etc.; to collect various items such as gold, silver, food, weapons, armor, etc.; to interact with other characters such as Halime Sultan, Bamsı Beyrek, Turgut Alp, etc.; and to make decisions that affect the outcome of the game.
-The game has a simple and intuitive control system that allows the player to use different skills and weapons in combat, horse riding, archery, etc. The player can use the joystick on the left side of the screen to move their character; the buttons on the right side of the screen to attack, defend, jump, roll, etc.; and the icons on the top of the screen to access the map, inventory, settings, etc. The player can also switch between different weapons such as swords, axes, daggers, bows, etc. by tapping on their icons on the bottom of the screen. The player can also use their horse to travel faster and to fight enemies by using the horse icon on the bottom of the screen. The player can also use their pet (such as a wolf or an eagle) to assist them in combat by using the pet icon on the bottom of the screen.
-Some of the tips and tricks to succeed in the game are: - Pay attention to the dialogues and instructions given by Ertuğrul Gazi or other characters. They will provide you with valuable information and hints about your missions and objectives. - Explore your surroundings and collect items that can help you in your quests. You can find gold, silver, food, weapons, armor, etc. in chests, barrels, crates, tents, etc. You can also loot enemies after defeating them. - Upgrade your skills and weapons regularly. You can use gold and silver to buy new skills and weapons from merchants or blacksmiths. You can also use food to heal yourself or your horse. - Use your skills and weapons wisely. Different skills and weapons have different advantages and disadvantages depending on the situation. For example, swords are good for close-range combat but not for long-range combat; bows are good for long-range combat but not for close-range combat; axes are good for breaking shields but not for fast attacks; daggers are good for fast attacks but not for strong attacks; etc. - Use your horse and pet effectively. Your horse can help you travel faster and fight enemies from a distance. Your pet can help you distract or attack enemies or find hidden items or paths. - Make smart decisions that affect the outcome of the game. The game has multiple endings depending on your choices and actions. For example, you can choose to be loyal or betray Ertuğrul Gazi; you can choose to spare or kill your enemies; you can choose to help or ignore your allies; etc.
-Some of the positive aspects of the game according to players and critics are: - The game has a captivating story that is based on real historical events and characters. - The game has realistic 3D graphics that create a immersive atmosphere and environment. - The game has professional music that enhances the mood and emotion of the game. - The game has high-resolution visuals that make the game look stunning and detailed. - The game has detailed scenes that show the culture and lifestyle of the medieval Turks. - The game has multiplayer real characters that allow players to interact with each other online. - The game has history-telling dialogues that educate players about Turkish history and culture. - The game has team directions that allow players to cooperate with each other in missions. Some of the negative aspects of the game according to players and critics are: - The game has some bugs and glitches that affect the gameplay and performance of the game. - The game has some translation errors and grammatical mistakes that affect the quality and clarity of the game. - The game has some repetitive missions and objectives that affect the variety and creativity of the game. - The game has some unrealistic physics and animations that affect the realism and accuracy of the game. - The game has some violent and graphic scenes that may not be suitable for younger or sensitive players.
-The game is similar to other historical adventure games in the market such as Assassin's Creed, Prince of Persia, Shadow of Mordor, etc. However, the game is unique in its focus on Turkish history and culture, especially during the medieval period and the rise of the Ottoman Empire . The game is also unique in its gameplay and features, such as the realistic 3D graphics, the professional music, the high-resolution visuals, the detailed scenes, the multiplayer real characters, the history-telling dialogues, and the team directions. The game is also unique in its genre, as it is a role-playing game that consists of 60 episodes, each with its own story, characters, and challenges. The game is also unique in its control system, as it allows the player to use different skills and weapons in combat, horse riding, archery, etc. The game is also unique in its outcome, as it has multiple endings depending on the player's choices and actions.
-Some of the suggestions and requests for improvement from the players are: - To fix the bugs and glitches that affect the gameplay and performance of the game. - To improve the translation and grammar of the game to make it more clear and accurate. - To add more variety and creativity to the missions and objectives of the game to make it more fun and challenging. - To improve the physics and animations of the game to make it more realistic and accurate. - To add more options and features to customize the character's appearance, skills, weapons, armor, pets, etc. to make it more personal and diverse. - To add more historical and cultural content to the game to make it more educational and informative. - To add more modes and levels to the game to make it more replayable and enjoyable.
-ertuğrul gazi oyunu indir
-ertuğrul gazi oyunu hile
-ertuğrul gazi oyunu pc
-ertuğrul gazi oyunu steam
-ertuğrul gazi oyunu nasıl oynanır
-ertuğrul gazi oyunu altın hilesi
-ertuğrul gazi oyunu apk
-ertuğrul gazi oyunu mod
-ertuğrul gazi oyunu son bölüm
-ertuğrul gazi oyunu online
-ertuğrul gazi oyunu kayı boyunun destanı
-ertuğrul gazi oyunu umuro
-ertuğrul gazi oyunu android
-ertuğrul gazi oyunu osmanlı kuruluşu
-ertuğrul gazi oyunu kurtuluş savaşı
-ertuğrul gazi oyunu yeni sezon
-ertuğrul gazi oyunu izle
-ertuğrul gazi oyunu yorumlar
-ertuğrul gazi oyunu puan hilesi
-ertuğrul gazi oyunu canlı yayın
-ertuğrul gazi oyunu türkçe dublaj
-ertuğrul gazi oyunu at sürme
-ertuğrul gazi oyunu okçuluk
-ertuğrul gazi oyunu kılıç kalkan
-ertuğrul gazi oyunu harita bulma
-ertuğrul gazi oyunu yükleme sorunu
-ertuğrul gazi oyunu güncelleme
-ertuğrul gazi oyunu sistem gereksinimleri
-ertuğrul gazi oyunu klan kurma
-ertuğrul gazi oyunu hediye kodları
-ertugrulgazioyn.com resmi web sitesi
-ertugrulgazioyn.net fan sayfası
-ertugrulgazioyn.org haber portalı
-ertugrulgazioyn.info ipucuları ve rehberleri
-ertugrulgazioyn.biz inceleme ve değerlendirme
-ertugrulgazioyn.xyz eğlence ve mizah
-ertugrulgazioyn.club sosyal medya ve forum
-ertugrulgazioyn.shop altın ve eşya satışı
-ertugrulgazioyn.live canlı destek ve yardım
-ertugrulgazioyn.fun yarışma ve etkinlikler
Ertuğrul Gazi Oyunu is a historical adventure game based on Turkish hero Ertuğrul Gazi, the father of Osman I, the founder of the Ottoman Empire. The game is a role-playing game that consists of 60 episodes, each with its own story, characters, and challenges. The game features realistic 3D graphics, professional music, high-resolution visuals, detailed scenes, multiplayer real characters, history-telling dialogues, and team directions. The game is available for Android and PC platforms, and you can download it for free from Google Play Store or Steam. If you are interested in Turkish history and culture, or if you are looking for a thrilling and exciting game to play, you should definitely give Ertuğrul Gazi Oyunu a try. You will not regret it!
-Do you have any questions or comments about Ertuğrul Gazi Oyunu? Do you want to share your experience or opinion about the game? Do you have any suggestions or requests for improvement for the game developers? If so, please feel free to leave a comment below or contact us through our website or social media. We would love to hear from you!
-Thank you for reading this article. We hope you enjoyed it and learned something new. Please share this article with your friends and family who might be interested in Ertuğrul Gazi Oyunu or Turkish history and culture. And don't forget to check out our other articles on our website for more interesting and informative topics. See you next time!
-Here are some of the frequently asked questions about Ertuğrul Gazi Oyunu:
-Question | -Answer | -
---|---|
What is Ertuğrul Gazi Oyunu? | -Ertuğrul Gazi Oyunu is a historical adventure game based on Turkish hero Ertuğrul Gazi, the father of Osman I, the founder of the Ottoman Empire. | -
How can I download and play Ertuğrul Gazi Oyunu? | -You can download Ertuğrul Gazi Oyunu for free from Google Play Store or Steam. You can play it on your Android device or PC. | -
How many episodes are there in Ertuğrul Gazi Oyunu? | -There are 60 episodes in Ertuğrul Gazi Oyunu, each with its own story, characters, and challenges. | -
What are some of the skills and weapons that I can use in Ertuğrul Gazi Oyunu? | -You can use various skills such as sword fighting, horse riding, archery, defense with sword and shield, direction finding with map, swimming, running fast, rolling, climbing, stealth, etc. You can also use different weapons such as swords, axes, daggers, bows, etc. | -
Does Ertuğrul Gazi Oyunu have a multiplayer mode? | -Yes, Ertuğrul Gazi Oyunu has a multiplayer mode that allows you to play with other players online. You can join or create a team and cooperate with each other in missions. | -
Si usted está buscando una manera de escuchar el Corán en una voz hermosa y melodiosa, es posible que desee considerar la descarga 30 juz misyari rasyid. Misyari Rasyid es uno de los recitadores del Corán más famosos y respetados del mundo, y su recitación del Corán de 30 juz puede ayudarle a mejorar su memorización, comprensión y apreciación del libro sagrado. En este artículo, le diremos todo lo que necesita saber sobre Misyari Rasyid, 30 juz Corán, y cómo descargarlos fácil y convenientemente.
-Download ✯ https://bltlly.com/2v6LmX
Misyari Rasyid es un qari kuwaití (recitador del Corán), imán, predicador y artista nasheed. Nació el 5 de septiembre de 1976, y su nombre completo es Mishary bin Rashid bin Gharib bin Muhammad Alafasy Al-Muthairi. También es conocido por su kunya (apodo) Abu Nora.
-Misyari Rasyid pertenece a la tribu Alafasy, que remonta su ascendencia al compañero del Profeta Muhammad (la paz y las bendiciones de Allah sea con él) Al-Bara' ibn Malik. Estudió en el Colegio del Corán de la Universidad Islámica de Medina, especializándose en los diez qira'at (modos de recitación) y tafsir (exégesis). También tiene una maestría en jurisprudencia islámica de la Universidad de Kuwait.
-Misyari Rasyid ha memorizado todo el Corán a una edad temprana, y ha participado en muchas competiciones y festivales del Corán alrededor del mundo. Ha ganado varios premios y honores por su recitación, como el primer premio en el Concurso Internacional del Corán de Kuwait en 1998, el primer premio en el Oscar de Creatividad Islámica en 2002 y el Premio de Creatividad Árabe en 2005. También fue nombrado embajador de buena voluntad por el UNICEF en 2007.
-El Corán es la palabra de Allah revelada al Profeta Muhammad (la paz sea con él) a través del Ángel Gabriel durante un período de 23 años. Consta de 114 capítulos (suras) de diferentes longitudes, que se dividen en 30 partes (juz) para facilitar la lectura y la memorización.
- -La palabra juz significa "parte" o "porción" en árabe. Cada juz contiene aproximadamente 20 páginas o 600 versos del Corán. La división de juz no se basa en el orden temático o cronológico de las suras, sino en la conveniencia de dividir el Corán en partes iguales. El primer juz comienza desde el principio del Corán (sura Al-Fatiha) y termina al final del sura Al-Baqarah verso 141. El último juz comienza desde el sura An-Naba y termina al final del Corán (sura An-Nas). Los otros juz se dividen de acuerdo a las rupturas naturales en el texto, como el final de una sura o un verso largo.
-Recitar juz es una de las mejores maneras de conectarse con el Corán y ganar recompensas de Allah. El Profeta Muhammad (la paz sea con él) dijo: "Quien recite una carta del Libro de Allah, tendrá una recompensa. Y esa recompensa se multiplicará por diez. No estoy diciendo que 'Alif, Lam, Meem' es una carta, más bien estoy diciendo que 'Alif' es una carta, 'Lam' es una carta y 'Meem' es una carta." También dijo: "Los mejores de ustedes son aquellos que aprenden el Corán y lo enseñan." Recitar juz también puede ayudarlo a entender el significado y el contexto del Corán, a mejorar sus habilidades en el idioma árabe y a memorizar el Corán más fácilmente.
-Si desea descargar 30 juz misyari rasyid, tiene varias opciones para elegir. Puede descargarlos como archivos mp3, archivos zip o archivos torrent. También puede transmitirlos en línea o utilizar aplicaciones o sitios web que los ofrecen de forma gratuita o por una tarifa.
-Los archivos de audio de 30 juz misyari rasyid están disponibles en varias fuentes, como su sitio web oficial, canal de YouTube, cuenta de SoundCloud y otras plataformas. Puede descargarlos en diferentes formatos, dependiendo de su preferencia y la compatibilidad del dispositivo. Por ejemplo, puede descargarlos como archivos mp3, que son pequeños en tamaño y fáciles de reproducir en cualquier dispositivo. También puede descargarlos como archivos zip, que son archivos comprimidos que contienen todos los 30 juz en una carpeta. También puede descargarlos como archivos torrent, que son archivos peer-to-peer que requieren un cliente torrent para descargarlos.
-Los pasos y consejos para descargar 30 juz misyari rasyid varían dependiendo de la fuente y el formato que elija. Estas son algunas pautas generales a seguir:
-A: Juz es una parte o porción del Corán que contiene aproximadamente 20 páginas o 600 versos. Surah es un capítulo o sección del Corán que tiene un nombre y número específico. Hay 114 suras en el Corán, que se dividen en 30 juz.
-A: Depende de su velocidad y fluidez de recitación, pero en promedio se tarda aproximadamente una hora en recitar un juz.
-A: Puedes mejorar tu recitación de juz siguiendo estos consejos:
-A: Algunos de los beneficios de escuchar la recitación de Misyari Rasyid son:
-Si eres un fan de Minecraft, es posible que hayas oído hablar de Aternos, un servicio gratuito de alojamiento de servidores Minecraft que te permite crear tu propio servidor personal con ranuras ilimitadas, mods, plugins, mundos personalizados y más. Sin embargo, también puede haber encontrado un problema común con los servidores Aternos: se desconectan cuando nadie juega en ellos. Esto significa que debe iniciar manualmente su servidor cada vez que desee jugar, y puede perder su progreso o datos si olvida guardar o hacer una copia de seguridad de su servidor.
-Afortunadamente, hay una solución a este problema: usar un bot AFK. Un bot AFK es un programa que se conecta a su servidor Aternos y lo mantiene en línea enviando comandos o mensajes periódicamente. De esta manera, se puede disfrutar de su servidor Minecraft sin preocuparse de que va fuera de línea o perder sus datos. En este artículo, le mostraremos cómo descargar e instalar un bot AFK para Aternos, cómo elegir el mejor bot AFK para sus necesidades y cómo usar y administrar su bot AFK de manera efectiva.
-Download Zip … https://bltlly.com/2v6KKl
Aternos es un servicio gratuito de alojamiento de servidores Minecraft que le permite crear su propio servidor personal con ranuras ilimitadas, mods, plugins, mundos personalizados y más. Puede elegir entre cientos de tipos de servidor diferentes, como vainilla, espiga, forja, papel, tela, etc. También puede personalizar la configuración de su servidor, como dificultad, modo de juego, lista blanca, operadores, etc. Puede acceder a su servidor desde cualquier dispositivo, como PC, móvil, consola, etc.
- -Aquí es donde un bot AFK es útil. Un bot AFK es un programa que se conecta a su servidor Aternos y lo mantiene en línea enviando comandos o mensajes periódicamente. Por ejemplo, un bot de AFK puede enviar un mensaje de chat cada 10 minutos o moverse cada 5 minutos. De esta manera, su servidor no entrará en modo de hibernación y permanecerá en línea mientras el bot AFK esté funcionando. Esto significa que puedes disfrutar de tu servidor Minecraft sin preocuparte de que se desconecte o perder tus datos.
-Hay muchos bots AFK disponibles para servidores Aternos, pero no todos son compatibles, funcionales o seguros. Por lo tanto, necesitas elegir un bot AFK cuidadosamente basado en algunos criterios, como:
-Para ayudarle a elegir un bot AFK para Aternos, hemos comparado algunos de los bots AFK más populares y fiables para Aternos en la tabla siguiente:
-Heroku es una plataforma donde puedes ejecutar aplicaciones y programas online sin tener que instalarlos en tu dispositivo. Muchos bots AFK para Aternos pueden ejecutarse en Heroku, como ttttdeded/aternos-afkbot y krushna06/afk-bot-for-aternos. Para instalar un bot AFK en Heroku, debes seguir estos pasos:
-El paso final es conectar su bot AFK a su servidor Aternos. Esto permitirá a su bot AFK unirse a su servidor y mantenerlo en línea mediante el envío de comandos o mensajes periódicamente. Para conectar su bot AFK a su servidor Aternos, debe seguir estos pasos:
- -Ahora que ha descargado, instalado y conectado correctamente su bot AFK a su servidor Aternos, puede comenzar a usarlo y administrarlo de acuerdo con sus preferencias y necesidades. Estas son algunas de las cosas que puedes hacer con tu bot AFK:
-Aquí hay algunos consejos y precauciones para usar su bot AFK de manera efectiva y segura:
-Si está interesado en descargar e instalar un bot AFK para Aternos, puede consultar algunos de los enlaces a continuación para obtener más información y tutoriales. Esperamos que este artículo haya sido útil e informativo para usted. ¡Feliz juego!
-La respuesta depende de sus preferencias y necesidades, pero algunos de los bots AFK más populares y confiables para Aternos son ttttdeded/aternos-afkbot, krushna06/afk-bot-for-aternos y AFK Discord Bot. Estos bots AFK son compatibles con cualquier versión y tipo de servidores Aternos, tienen varias características y comandos para mantener su servidor en línea y activo, y son seguros y confiables. Puede compararlos en la tabla anterior o visitar sus páginas de GitHub o servidores de discordia para obtener más información.
-La respuesta depende de la configuración de su bot AFK y su actividad de servidor, pero generalmente puede mantener su servidor Aternos en línea durante el tiempo que desee con un bot AFK. Mientras el bot AFK se esté ejecutando en Heroku y conectado a su servidor Aternos, enviará comandos o mensajes periódicamente para evitar que su servidor entre en modo de hibernación. Sin embargo, debe tener en cuenta que el uso de un bot AFK puede consumir más recursos y causar más retraso en su servidor, por lo que debe ajustar la configuración de su bot AFK en consecuencia.
-La respuesta depende de tus habilidades de codificación y conocimiento, pero generalmente puedes hacer tu propio bot AFK para Aternos usando herramientas como mineflayer o discord.js y siguiendo tutoriales en línea. Mineflayer es una biblioteca de clientes de Minecraft que te permite crear bots que pueden interactuar con los servidores de Minecraft. Discord.js es una biblioteca de JavaScript que permite crear bots que pueden interactuar con los servidores de Discord. Puede utilizar estas herramientas para crear un bot AFK que pueda conectarse a su servidor Aternos y mantenerlo en línea enviando comandos o mensajes periódicamente. También puede personalizar su bot AFK con diferentes características y comandos de acuerdo a sus preferencias y necesidades.
-La respuesta depende de la fuente de su bot AFK, pero generalmente puede obtener ayuda o soporte poniéndose en contacto con el desarrollador del bot AFK, uniéndose a su servidor de discordia o página GitHub, o preguntando a otros usuarios que han utilizado el mismo bot AFK. Por ejemplo, si está utilizando ttttdeded/aternos-afkbot, puede ponerse en contacto con ttttdeded a través de su perfil de GitHub, unirse a su servidor de discordia o preguntar a otros usuarios que hayan bifurcado o protagonizado su repositorio. También puedes buscar en línea guías o tutoriales sobre cómo usar un bot AFK para Aternos.
64aa2da5cf-
- Streaming / Unlimited conversations / Save history / Preset prompts / Chat with files / Web search
- LaTeX rendering / Table rendering / Code highlighting
- Auto dark mode / Adaptive web interface / WeChat-like theme
- Multi-parameters tuning / Multi-API-Key support / Multi-user support
- Compatible with GPT-4 / Local deployment for LLMs
-
-
-
-
-
-
All you need to do is to upload the audio file and hit submit, then wait for compiling. After that click on Play/Pause for listing to the audio. The audio is saved in a wav format.
-'
- else:
- lines[i] = f"
"
- else:
- if i > 0:
- if count % 2 == 1:
- line = line.replace("`", r"\`")
- line = line.replace("<", "<")
- line = line.replace(">", ">")
- line = line.replace(" ", " ")
- line = line.replace("*", "*")
- line = line.replace("_", "_")
- line = line.replace("-", "-")
- line = line.replace(".", ".")
- line = line.replace("!", "!")
- line = line.replace("(", "(")
- line = line.replace(")", ")")
- line = line.replace("$", "$")
- lines[i] = "
- self.score = score # float
-
-
-def coordinate_to_offset(row, col, ncols):
- return int(row * ncols + col)
-
-
-def offset_to_row(offset, ncols):
- return int(offset / ncols)
-
-
-def offset_to_col(offset, ncols):
- return int(offset % ncols)
-
-
-def trimWhitespace(str):
- return re.sub(" +", " ", re.sub(" *$", "", re.sub("^ *", "", str)))
-
-
-def str2toks(str):
- pieces = trimWhitespace(str).split(" ")
- toks = []
- for p in pieces:
- toks.append(Token(p, 0.0, 0.0))
- return toks
-
-
-class EditDistance(object):
- def __init__(self, time_mediated):
- self.time_mediated_ = time_mediated
- self.scores_ = np.nan # Eigen::Matrix
- self.backtraces_ = (
- np.nan
- ) # Eigen::Matrix backtraces_;
- self.confusion_pairs_ = {}
-
- def cost(self, ref, hyp, code):
- if self.time_mediated_:
- if code == Code.match:
- return abs(ref.start - hyp.start) + abs(ref.end - hyp.end)
- elif code == Code.insertion:
- return hyp.end - hyp.start
- elif code == Code.deletion:
- return ref.end - ref.start
- else: # substitution
- return abs(ref.start - hyp.start) + abs(ref.end - hyp.end) + 0.1
- else:
- if code == Code.match:
- return 0
- elif code == Code.insertion or code == Code.deletion:
- return 3
- else: # substitution
- return 4
-
- def get_result(self, refs, hyps):
- res = AlignmentResult(refs=deque(), hyps=deque(), codes=deque(), score=np.nan)
-
- num_rows, num_cols = self.scores_.shape
- res.score = self.scores_[num_rows - 1, num_cols - 1]
-
- curr_offset = coordinate_to_offset(num_rows - 1, num_cols - 1, num_cols)
-
- while curr_offset != 0:
- curr_row = offset_to_row(curr_offset, num_cols)
- curr_col = offset_to_col(curr_offset, num_cols)
-
- prev_offset = self.backtraces_[curr_row, curr_col]
-
- prev_row = offset_to_row(prev_offset, num_cols)
- prev_col = offset_to_col(prev_offset, num_cols)
-
- res.refs.appendleft(curr_row - 1) # Note: this was .push_front() in C++
- res.hyps.appendleft(curr_col - 1)
- if curr_row - 1 == prev_row and curr_col == prev_col:
- res.codes.appendleft(Code.deletion)
- elif curr_row == prev_row and curr_col - 1 == prev_col:
- res.codes.appendleft(Code.insertion)
- else:
- # assert(curr_row - 1 == prev_row and curr_col - 1 == prev_col)
- ref_str = refs[res.refs[0]].label
- hyp_str = hyps[res.hyps[0]].label
-
- if ref_str == hyp_str:
- res.codes.appendleft(Code.match)
- else:
- res.codes.appendleft(Code.substitution)
-
- confusion_pair = "%s -> %s" % (ref_str, hyp_str)
- if confusion_pair not in self.confusion_pairs_:
- self.confusion_pairs_[confusion_pair] = 1
- else:
- self.confusion_pairs_[confusion_pair] += 1
-
- curr_offset = prev_offset
-
- return res
-
- def align(self, refs, hyps):
- if len(refs) == 0 and len(hyps) == 0:
- return np.nan
-
- # NOTE: we're not resetting the values in these matrices because every value
- # will be overridden in the loop below. If this assumption doesn't hold,
- # be sure to set all entries in self.scores_ and self.backtraces_ to 0.
- self.scores_ = np.zeros((len(refs) + 1, len(hyps) + 1))
- self.backtraces_ = np.zeros((len(refs) + 1, len(hyps) + 1))
-
- num_rows, num_cols = self.scores_.shape
-
- for i in range(num_rows):
- for j in range(num_cols):
- if i == 0 and j == 0:
- self.scores_[i, j] = 0.0
- self.backtraces_[i, j] = 0
- continue
-
- if i == 0:
- self.scores_[i, j] = self.scores_[i, j - 1] + self.cost(
- None, hyps[j - 1], Code.insertion
- )
- self.backtraces_[i, j] = coordinate_to_offset(i, j - 1, num_cols)
- continue
-
- if j == 0:
- self.scores_[i, j] = self.scores_[i - 1, j] + self.cost(
- refs[i - 1], None, Code.deletion
- )
- self.backtraces_[i, j] = coordinate_to_offset(i - 1, j, num_cols)
- continue
-
- # Below here both i and j are greater than 0
- ref = refs[i - 1]
- hyp = hyps[j - 1]
- best_score = self.scores_[i - 1, j - 1] + (
- self.cost(ref, hyp, Code.match)
- if (ref.label == hyp.label)
- else self.cost(ref, hyp, Code.substitution)
- )
-
- prev_row = i - 1
- prev_col = j - 1
- ins = self.scores_[i, j - 1] + self.cost(None, hyp, Code.insertion)
- if ins < best_score:
- best_score = ins
- prev_row = i
- prev_col = j - 1
-
- delt = self.scores_[i - 1, j] + self.cost(ref, None, Code.deletion)
- if delt < best_score:
- best_score = delt
- prev_row = i - 1
- prev_col = j
-
- self.scores_[i, j] = best_score
- self.backtraces_[i, j] = coordinate_to_offset(
- prev_row, prev_col, num_cols
- )
-
- return self.get_result(refs, hyps)
-
-
-class WERTransformer(object):
- def __init__(self, hyp_str, ref_str, verbose=True):
- self.ed_ = EditDistance(False)
- self.id2oracle_errs_ = {}
- self.utts_ = 0
- self.words_ = 0
- self.insertions_ = 0
- self.deletions_ = 0
- self.substitutions_ = 0
-
- self.process(["dummy_str", hyp_str, ref_str])
-
- if verbose:
- print("'%s' vs '%s'" % (hyp_str, ref_str))
- self.report_result()
-
- def process(self, input): # std::vector&& input
- if len(input) < 3:
- print(
- "Input must be of the form ... , got ",
- len(input),
- " inputs:",
- )
- return None
-
- # Align
- # std::vector hyps;
- # std::vector refs;
-
- hyps = str2toks(input[-2])
- refs = str2toks(input[-1])
-
- alignment = self.ed_.align(refs, hyps)
- if alignment is None:
- print("Alignment is null")
- return np.nan
-
- # Tally errors
- ins = 0
- dels = 0
- subs = 0
- for code in alignment.codes:
- if code == Code.substitution:
- subs += 1
- elif code == Code.insertion:
- ins += 1
- elif code == Code.deletion:
- dels += 1
-
- # Output
- row = input
- row.append(str(len(refs)))
- row.append(str(ins))
- row.append(str(dels))
- row.append(str(subs))
- # print(row)
-
- # Accumulate
- kIdIndex = 0
- kNBestSep = "/"
-
- pieces = input[kIdIndex].split(kNBestSep)
-
- if len(pieces) == 0:
- print(
- "Error splitting ",
- input[kIdIndex],
- " on '",
- kNBestSep,
- "', got empty list",
- )
- return np.nan
-
- id = pieces[0]
- if id not in self.id2oracle_errs_:
- self.utts_ += 1
- self.words_ += len(refs)
- self.insertions_ += ins
- self.deletions_ += dels
- self.substitutions_ += subs
- self.id2oracle_errs_[id] = [ins, dels, subs]
- else:
- curr_err = ins + dels + subs
- prev_err = np.sum(self.id2oracle_errs_[id])
- if curr_err < prev_err:
- self.id2oracle_errs_[id] = [ins, dels, subs]
-
- return 0
-
- def report_result(self):
- # print("---------- Summary ---------------")
- if self.words_ == 0:
- print("No words counted")
- return
-
- # 1-best
- best_wer = (
- 100.0
- * (self.insertions_ + self.deletions_ + self.substitutions_)
- / self.words_
- )
-
- print(
- "\tWER = %0.2f%% (%i utts, %i words, %0.2f%% ins, "
- "%0.2f%% dels, %0.2f%% subs)"
- % (
- best_wer,
- self.utts_,
- self.words_,
- 100.0 * self.insertions_ / self.words_,
- 100.0 * self.deletions_ / self.words_,
- 100.0 * self.substitutions_ / self.words_,
- )
- )
-
- def wer(self):
- if self.words_ == 0:
- wer = np.nan
- else:
- wer = (
- 100.0
- * (self.insertions_ + self.deletions_ + self.substitutions_)
- / self.words_
- )
- return wer
-
- def stats(self):
- if self.words_ == 0:
- stats = {}
- else:
- wer = (
- 100.0
- * (self.insertions_ + self.deletions_ + self.substitutions_)
- / self.words_
- )
- stats = dict(
- {
- "wer": wer,
- "utts": self.utts_,
- "numwords": self.words_,
- "ins": self.insertions_,
- "dels": self.deletions_,
- "subs": self.substitutions_,
- "confusion_pairs": self.ed_.confusion_pairs_,
- }
- )
- return stats
-
-
-def calc_wer(hyp_str, ref_str):
- t = WERTransformer(hyp_str, ref_str, verbose=0)
- return t.wer()
-
-
-def calc_wer_stats(hyp_str, ref_str):
- t = WERTransformer(hyp_str, ref_str, verbose=0)
- return t.stats()
-
-
-def get_wer_alignment_codes(hyp_str, ref_str):
- """
- INPUT: hypothesis string, reference string
- OUTPUT: List of alignment codes (intermediate results from WER computation)
- """
- t = WERTransformer(hyp_str, ref_str, verbose=0)
- return t.ed_.align(str2toks(ref_str), str2toks(hyp_str)).codes
-
-
-def merge_counts(x, y):
- # Merge two hashes which have 'counts' as their values
- # This can be used for example to merge confusion pair counts
- # conf_pairs = merge_counts(conf_pairs, stats['confusion_pairs'])
- for k, v in y.items():
- if k not in x:
- x[k] = 0
- x[k] += v
- return x
diff --git a/spaces/OFA-Sys/OFA-Generic_Interface/fairseq/fairseq/models/text_to_speech/tacotron2.py b/spaces/OFA-Sys/OFA-Generic_Interface/fairseq/fairseq/models/text_to_speech/tacotron2.py
deleted file mode 100644
index bb327e81e74900349e1357261bf2f14bc037ccd6..0000000000000000000000000000000000000000
--- a/spaces/OFA-Sys/OFA-Generic_Interface/fairseq/fairseq/models/text_to_speech/tacotron2.py
+++ /dev/null
@@ -1,350 +0,0 @@
-# Copyright (c) Facebook, Inc. and its affiliates.
-#
-# This source code is licensed under the MIT license found in the
-# LICENSE file in the root directory of this source tree.
-
-import logging
-
-import torch
-from torch import nn
-from torch.nn import functional as F
-
-from fairseq.models import (FairseqEncoder, FairseqEncoderDecoderModel,
- FairseqIncrementalDecoder, register_model,
- register_model_architecture)
-from fairseq.modules import LSTMCellWithZoneOut, LocationAttention
-
-
-logger = logging.getLogger(__name__)
-
-
-def encoder_init(m):
- if isinstance(m, nn.Conv1d):
- nn.init.xavier_uniform_(m.weight, torch.nn.init.calculate_gain("relu"))
-
-
-class Tacotron2Encoder(FairseqEncoder):
- def __init__(self, args, src_dict, embed_speaker):
- super().__init__(src_dict)
- self.padding_idx = src_dict.pad()
- self.embed_speaker = embed_speaker
- self.spk_emb_proj = None
- if embed_speaker is not None:
- self.spk_emb_proj = nn.Linear(
- args.encoder_embed_dim + args.speaker_embed_dim,
- args.encoder_embed_dim
- )
-
- self.embed_tokens = nn.Embedding(len(src_dict), args.encoder_embed_dim,
- padding_idx=self.padding_idx)
-
- assert(args.encoder_conv_kernel_size % 2 == 1)
- self.convolutions = nn.ModuleList(
- nn.Sequential(
- nn.Conv1d(args.encoder_embed_dim, args.encoder_embed_dim,
- kernel_size=args.encoder_conv_kernel_size,
- padding=((args.encoder_conv_kernel_size - 1) // 2)),
- nn.BatchNorm1d(args.encoder_embed_dim),
- nn.ReLU(),
- nn.Dropout(args.encoder_dropout)
- )
- for _ in range(args.encoder_conv_layers)
- )
-
- self.lstm = nn.LSTM(args.encoder_embed_dim, args.encoder_embed_dim // 2,
- num_layers=args.encoder_lstm_layers,
- batch_first=True, bidirectional=True)
-
- self.apply(encoder_init)
-
- def forward(self, src_tokens, src_lengths=None, speaker=None, **kwargs):
- x = self.embed_tokens(src_tokens)
- x = x.transpose(1, 2).contiguous() # B x T x C -> B x C x T
- for conv in self.convolutions:
- x = conv(x)
- x = x.transpose(1, 2).contiguous() # B x C x T -> B x T x C
-
- src_lengths = src_lengths.cpu().long()
- x = nn.utils.rnn.pack_padded_sequence(x, src_lengths, batch_first=True)
- x = self.lstm(x)[0]
- x = nn.utils.rnn.pad_packed_sequence(x, batch_first=True)[0]
-
- encoder_padding_mask = src_tokens.eq(self.padding_idx)
-
- if self.embed_speaker is not None:
- seq_len, bsz, _ = x.size()
- emb = self.embed_speaker(speaker).expand(seq_len, bsz, -1)
- x = self.spk_emb_proj(torch.cat([x, emb], dim=2))
-
- return {
- "encoder_out": [x], # B x T x C
- "encoder_padding_mask": encoder_padding_mask, # B x T
- }
-
-
-class Prenet(nn.Module):
- def __init__(self, in_dim, n_layers, n_units, dropout):
- super().__init__()
- self.layers = nn.ModuleList(
- nn.Sequential(nn.Linear(in_dim if i == 0 else n_units, n_units),
- nn.ReLU())
- for i in range(n_layers)
- )
- self.dropout = dropout
-
- def forward(self, x):
- for layer in self.layers:
- x = F.dropout(layer(x), p=self.dropout) # always applies dropout
- return x
-
-
-class Postnet(nn.Module):
- def __init__(self, in_dim, n_channels, kernel_size, n_layers, dropout):
- super(Postnet, self).__init__()
- self.convolutions = nn.ModuleList()
- assert(kernel_size % 2 == 1)
- for i in range(n_layers):
- cur_layers = [
- nn.Conv1d(in_dim if i == 0 else n_channels,
- n_channels if i < n_layers - 1 else in_dim,
- kernel_size=kernel_size,
- padding=((kernel_size - 1) // 2)),
- nn.BatchNorm1d(n_channels if i < n_layers - 1 else in_dim)
- ] + ([nn.Tanh()] if i < n_layers - 1 else []) + [nn.Dropout(dropout)]
- nn.init.xavier_uniform_(
- cur_layers[0].weight,
- torch.nn.init.calculate_gain(
- "tanh" if i < n_layers - 1 else "linear"
- )
- )
- self.convolutions.append(nn.Sequential(*cur_layers))
-
- def forward(self, x):
- x = x.transpose(1, 2) # B x T x C -> B x C x T
- for conv in self.convolutions:
- x = conv(x)
- return x.transpose(1, 2)
-
-
-def decoder_init(m):
- if isinstance(m, torch.nn.Conv1d):
- nn.init.xavier_uniform_(m.weight, torch.nn.init.calculate_gain("tanh"))
-
-
-class Tacotron2Decoder(FairseqIncrementalDecoder):
- def __init__(self, args, src_dict):
- super().__init__(None)
- self.args = args
- self.n_frames_per_step = args.n_frames_per_step
- self.out_dim = args.output_frame_dim * args.n_frames_per_step
-
- self.prenet = Prenet(self.out_dim, args.prenet_layers, args.prenet_dim,
- args.prenet_dropout)
-
- # take prev_context, prev_frame, (speaker embedding) as input
- self.attention_lstm = LSTMCellWithZoneOut(
- args.zoneout,
- args.prenet_dim + args.encoder_embed_dim,
- args.decoder_lstm_dim
- )
-
- # take attention_lstm output, attention_state, encoder_out as input
- self.attention = LocationAttention(
- args.attention_dim, args.encoder_embed_dim, args.decoder_lstm_dim,
- (1 + int(args.attention_use_cumprob)),
- args.attention_conv_dim, args.attention_conv_kernel_size
- )
-
- # take attention_lstm output, context, (gated_latent) as input
- self.lstm = nn.ModuleList(
- LSTMCellWithZoneOut(
- args.zoneout,
- args.encoder_embed_dim + args.decoder_lstm_dim,
- args.decoder_lstm_dim
- )
- for i in range(args.decoder_lstm_layers)
- )
-
- proj_in_dim = args.encoder_embed_dim + args.decoder_lstm_dim
- self.feat_proj = nn.Linear(proj_in_dim, self.out_dim)
- self.eos_proj = nn.Linear(proj_in_dim, 1)
-
- self.postnet = Postnet(self.out_dim, args.postnet_conv_dim,
- args.postnet_conv_kernel_size,
- args.postnet_layers, args.postnet_dropout)
-
- self.ctc_proj = None
- if getattr(args, "ctc_weight", 0.) > 0.:
- self.ctc_proj = nn.Linear(self.out_dim, len(src_dict))
-
- self.apply(decoder_init)
-
- def _get_states(self, incremental_state, enc_out):
- bsz, in_len, _ = enc_out.size()
- alstm_h = self.get_incremental_state(incremental_state, "alstm_h")
- if alstm_h is None:
- alstm_h = enc_out.new_zeros(bsz, self.args.decoder_lstm_dim)
- alstm_c = self.get_incremental_state(incremental_state, "alstm_c")
- if alstm_c is None:
- alstm_c = enc_out.new_zeros(bsz, self.args.decoder_lstm_dim)
-
- lstm_h = self.get_incremental_state(incremental_state, "lstm_h")
- if lstm_h is None:
- lstm_h = [enc_out.new_zeros(bsz, self.args.decoder_lstm_dim)
- for _ in range(self.args.decoder_lstm_layers)]
- lstm_c = self.get_incremental_state(incremental_state, "lstm_c")
- if lstm_c is None:
- lstm_c = [enc_out.new_zeros(bsz, self.args.decoder_lstm_dim)
- for _ in range(self.args.decoder_lstm_layers)]
-
- attn_w = self.get_incremental_state(incremental_state, "attn_w")
- if attn_w is None:
- attn_w = enc_out.new_zeros(bsz, in_len)
- attn_w_cum = self.get_incremental_state(incremental_state, "attn_w_cum")
- if attn_w_cum is None:
- attn_w_cum = enc_out.new_zeros(bsz, in_len)
- return alstm_h, alstm_c, lstm_h, lstm_c, attn_w, attn_w_cum
-
- def _get_init_attn_c(self, enc_out, enc_mask):
- bsz = enc_out.size(0)
- if self.args.init_attn_c == "zero":
- return enc_out.new_zeros(bsz, self.args.encoder_embed_dim)
- elif self.args.init_attn_c == "avg":
- enc_w = (~enc_mask).type(enc_out.type())
- enc_w = enc_w / enc_w.sum(dim=1, keepdim=True)
- return torch.sum(enc_out * enc_w.unsqueeze(2), dim=1)
- else:
- raise ValueError(f"{self.args.init_attn_c} not supported")
-
- def forward(self, prev_output_tokens, encoder_out=None,
- incremental_state=None, target_lengths=None, **kwargs):
- enc_mask = encoder_out["encoder_padding_mask"]
- enc_out = encoder_out["encoder_out"][0]
- in_len = enc_out.size(1)
-
- if incremental_state is not None:
- prev_output_tokens = prev_output_tokens[:, -1:, :]
- bsz, out_len, _ = prev_output_tokens.size()
-
- prenet_out = self.prenet(prev_output_tokens)
- (alstm_h, alstm_c, lstm_h, lstm_c,
- attn_w, attn_w_cum) = self._get_states(incremental_state, enc_out)
- attn_ctx = self._get_init_attn_c(enc_out, enc_mask)
-
- attn_out = enc_out.new_zeros(bsz, in_len, out_len)
- feat_out = enc_out.new_zeros(bsz, out_len, self.out_dim)
- eos_out = enc_out.new_zeros(bsz, out_len)
- for t in range(out_len):
- alstm_in = torch.cat((attn_ctx, prenet_out[:, t, :]), dim=1)
- alstm_h, alstm_c = self.attention_lstm(alstm_in, (alstm_h, alstm_c))
-
- attn_state = attn_w.unsqueeze(1)
- if self.args.attention_use_cumprob:
- attn_state = torch.stack((attn_w, attn_w_cum), dim=1)
- attn_ctx, attn_w = self.attention(
- enc_out, enc_mask, alstm_h, attn_state
- )
- attn_w_cum = attn_w_cum + attn_w
- attn_out[:, :, t] = attn_w
-
- for i, cur_lstm in enumerate(self.lstm):
- if i == 0:
- lstm_in = torch.cat((attn_ctx, alstm_h), dim=1)
- else:
- lstm_in = torch.cat((attn_ctx, lstm_h[i - 1]), dim=1)
- lstm_h[i], lstm_c[i] = cur_lstm(lstm_in, (lstm_h[i], lstm_c[i]))
-
- proj_in = torch.cat((attn_ctx, lstm_h[-1]), dim=1)
- feat_out[:, t, :] = self.feat_proj(proj_in)
- eos_out[:, t] = self.eos_proj(proj_in).squeeze(1)
- self.attention.clear_cache()
-
- self.set_incremental_state(incremental_state, "alstm_h", alstm_h)
- self.set_incremental_state(incremental_state, "alstm_c", alstm_c)
- self.set_incremental_state(incremental_state, "lstm_h", lstm_h)
- self.set_incremental_state(incremental_state, "lstm_c", lstm_c)
- self.set_incremental_state(incremental_state, "attn_w", attn_w)
- self.set_incremental_state(incremental_state, "attn_w_cum", attn_w_cum)
-
- post_feat_out = feat_out + self.postnet(feat_out)
- eos_out = eos_out.view(bsz, out_len, 1)
- return post_feat_out, eos_out, {"attn": attn_out, "feature_out": feat_out}
-
-
-@register_model("tacotron_2")
-class Tacotron2Model(FairseqEncoderDecoderModel):
- """
- Implementation for https://arxiv.org/pdf/1712.05884.pdf
- """
-
- @staticmethod
- def add_args(parser):
- # encoder
- parser.add_argument("--encoder-dropout", type=float)
- parser.add_argument("--encoder-embed-dim", type=int)
- parser.add_argument("--encoder-conv-layers", type=int)
- parser.add_argument("--encoder-conv-kernel-size", type=int)
- parser.add_argument("--encoder-lstm-layers", type=int)
- # decoder
- parser.add_argument("--attention-dim", type=int)
- parser.add_argument("--attention-conv-dim", type=int)
- parser.add_argument("--attention-conv-kernel-size", type=int)
- parser.add_argument("--prenet-dropout", type=float)
- parser.add_argument("--prenet-layers", type=int)
- parser.add_argument("--prenet-dim", type=int)
- parser.add_argument("--postnet-dropout", type=float)
- parser.add_argument("--postnet-layers", type=int)
- parser.add_argument("--postnet-conv-dim", type=int)
- parser.add_argument("--postnet-conv-kernel-size", type=int)
- parser.add_argument("--init-attn-c", type=str)
- parser.add_argument("--attention-use-cumprob", action='store_true')
- parser.add_argument("--zoneout", type=float)
- parser.add_argument("--decoder-lstm-layers", type=int)
- parser.add_argument("--decoder-lstm-dim", type=int)
- parser.add_argument("--output-frame-dim", type=int)
-
- def __init__(self, *args, **kwargs):
- super().__init__(*args, **kwargs)
- self._num_updates = 0
-
- @classmethod
- def build_model(cls, args, task):
- embed_speaker = task.get_speaker_embeddings(args)
- encoder = Tacotron2Encoder(args, task.src_dict, embed_speaker)
- decoder = Tacotron2Decoder(args, task.src_dict)
- return cls(encoder, decoder)
-
- def forward_encoder(self, src_tokens, src_lengths, **kwargs):
- return self.encoder(src_tokens, src_lengths=src_lengths, **kwargs)
-
- def set_num_updates(self, num_updates):
- super().set_num_updates(num_updates)
- self._num_updates = num_updates
-
-
-@register_model_architecture("tacotron_2", "tacotron_2")
-def base_architecture(args):
- # encoder
- args.encoder_dropout = getattr(args, "encoder_dropout", 0.5)
- args.encoder_embed_dim = getattr(args, "encoder_embed_dim", 512)
- args.encoder_conv_layers = getattr(args, "encoder_conv_layers", 3)
- args.encoder_conv_kernel_size = getattr(args, "encoder_conv_kernel_size", 5)
- args.encoder_lstm_layers = getattr(args, "encoder_lstm_layers", 1)
- # decoder
- args.attention_dim = getattr(args, "attention_dim", 128)
- args.attention_conv_dim = getattr(args, "attention_conv_dim", 32)
- args.attention_conv_kernel_size = getattr(args,
- "attention_conv_kernel_size", 15)
- args.prenet_dropout = getattr(args, "prenet_dropout", 0.5)
- args.prenet_layers = getattr(args, "prenet_layers", 2)
- args.prenet_dim = getattr(args, "prenet_dim", 256)
- args.postnet_dropout = getattr(args, "postnet_dropout", 0.5)
- args.postnet_layers = getattr(args, "postnet_layers", 5)
- args.postnet_conv_dim = getattr(args, "postnet_conv_dim", 512)
- args.postnet_conv_kernel_size = getattr(args, "postnet_conv_kernel_size", 5)
- args.init_attn_c = getattr(args, "init_attn_c", "zero")
- args.attention_use_cumprob = getattr(args, "attention_use_cumprob", True)
- args.zoneout = getattr(args, "zoneout", 0.1)
- args.decoder_lstm_layers = getattr(args, "decoder_lstm_layers", 2)
- args.decoder_lstm_dim = getattr(args, "decoder_lstm_dim", 1024)
- args.output_frame_dim = getattr(args, "output_frame_dim", 80)
diff --git a/spaces/OFA-Sys/OFA-Image_Caption/fairseq/examples/textless_nlp/gslm/unit2speech/tacotron2/waveglow_denoiser.py b/spaces/OFA-Sys/OFA-Image_Caption/fairseq/examples/textless_nlp/gslm/unit2speech/tacotron2/waveglow_denoiser.py
deleted file mode 100644
index 6a6585e8b6901a059445ff54ca20ea87751bbb11..0000000000000000000000000000000000000000
--- a/spaces/OFA-Sys/OFA-Image_Caption/fairseq/examples/textless_nlp/gslm/unit2speech/tacotron2/waveglow_denoiser.py
+++ /dev/null
@@ -1,40 +0,0 @@
-# import sys
-# sys.path.append('tacotron2')
-import torch
-from .layers import STFT
-
-
-class Denoiser(torch.nn.Module):
- """ Removes model bias from audio produced with waveglow """
-
- def __init__(self, waveglow, filter_length=1024, n_overlap=4,
- win_length=1024, mode='zeros'):
- super(Denoiser, self).__init__()
- self.stft = STFT(filter_length=filter_length,
- hop_length=int(filter_length/n_overlap),
- win_length=win_length).cuda()
- if mode == 'zeros':
- mel_input = torch.zeros(
- (1, 80, 88),
- dtype=waveglow.upsample.weight.dtype,
- device=waveglow.upsample.weight.device)
- elif mode == 'normal':
- mel_input = torch.randn(
- (1, 80, 88),
- dtype=waveglow.upsample.weight.dtype,
- device=waveglow.upsample.weight.device)
- else:
- raise Exception("Mode {} if not supported".format(mode))
-
- with torch.no_grad():
- bias_audio = waveglow.infer(mel_input, sigma=0.0).float()
- bias_spec, _ = self.stft.transform(bias_audio)
-
- self.register_buffer('bias_spec', bias_spec[:, :, 0][:, :, None])
-
- def forward(self, audio, strength=0.1):
- audio_spec, audio_angles = self.stft.transform(audio.cuda().float())
- audio_spec_denoised = audio_spec - self.bias_spec * strength
- audio_spec_denoised = torch.clamp(audio_spec_denoised, 0.0)
- audio_denoised = self.stft.inverse(audio_spec_denoised, audio_angles)
- return audio_denoised
diff --git a/spaces/OFA-Sys/OFA-vqa/fairseq/.github/ISSUE_TEMPLATE/feature_request.md b/spaces/OFA-Sys/OFA-vqa/fairseq/.github/ISSUE_TEMPLATE/feature_request.md
deleted file mode 100644
index 93c8668041f8a7af29e4c11e905d8b56b946dd51..0000000000000000000000000000000000000000
--- a/spaces/OFA-Sys/OFA-vqa/fairseq/.github/ISSUE_TEMPLATE/feature_request.md
+++ /dev/null
@@ -1,24 +0,0 @@
----
-name: 🚀 Feature Request
-about: Submit a proposal/request for a new feature
-labels: 'enhancement, help wanted, needs triage'
----
-
-## 🚀 Feature Request
-
-
-### Motivation
-
-
-
-### Pitch
-
-
-
-### Alternatives
-
-
-
-### Additional context
-
-
diff --git a/spaces/OFA-Sys/OFA-vqa/fairseq/scripts/spm_decode.py b/spaces/OFA-Sys/OFA-vqa/fairseq/scripts/spm_decode.py
deleted file mode 100644
index 1c18b1d2a7d7628b7aeb6fdb6c4ab5a096e9edf8..0000000000000000000000000000000000000000
--- a/spaces/OFA-Sys/OFA-vqa/fairseq/scripts/spm_decode.py
+++ /dev/null
@@ -1,53 +0,0 @@
-#!/usr/bin/env python
-# Copyright (c) Facebook, Inc. and its affiliates.
-# All rights reserved.
-#
-# This source code is licensed under the license found in the
-# LICENSE file in the root directory of this source tree.
-
-from __future__ import absolute_import, division, print_function, unicode_literals
-
-import argparse
-
-import sentencepiece as spm
-
-
-def main():
- parser = argparse.ArgumentParser()
- parser.add_argument(
- "--model", required=True, help="sentencepiece model to use for decoding"
- )
- parser.add_argument("--input", required=True, help="input file to decode")
- parser.add_argument("--input_format", choices=["piece", "id"], default="piece")
- args = parser.parse_args()
-
- sp = spm.SentencePieceProcessor()
- sp.Load(args.model)
-
- if args.input_format == "piece":
-
- def decode(l):
- return "".join(sp.DecodePieces(l))
-
- elif args.input_format == "id":
-
- def decode(l):
- return "".join(sp.DecodeIds(l))
-
- else:
- raise NotImplementedError
-
- def tok2int(tok):
- # remap reference-side (represented as <>) to 0
- return int(tok) if tok != "<>" else 0
-
- with open(args.input, "r", encoding="utf-8") as h:
- for line in h:
- if args.input_format == "id":
- print(decode(list(map(tok2int, line.rstrip().split()))))
- elif args.input_format == "piece":
- print(decode(line.rstrip().split()))
-
-
-if __name__ == "__main__":
- main()
diff --git a/spaces/OFA-Sys/OFA-vqa/utils/checkpoint_utils.py b/spaces/OFA-Sys/OFA-vqa/utils/checkpoint_utils.py
deleted file mode 100644
index 8fed4bc2a214833ab1153d5bc3ff6756db25048b..0000000000000000000000000000000000000000
--- a/spaces/OFA-Sys/OFA-vqa/utils/checkpoint_utils.py
+++ /dev/null
@@ -1,875 +0,0 @@
-# Copyright (c) Facebook, Inc. and its affiliates.
-#
-# This source code is licensed under the MIT license found in the
-# LICENSE file in the root directory of this source tree.
-
-import ast
-import collections
-import contextlib
-import logging
-import numpy as np
-import os
-import re
-import time
-import traceback
-import math
-from collections import OrderedDict
-from typing import Any, Dict, Optional, Union
-
-import torch
-from fairseq.dataclass.configs import CheckpointConfig
-from fairseq.dataclass.utils import (
- convert_namespace_to_omegaconf,
- overwrite_args_by_name,
-)
-from fairseq.distributed.fully_sharded_data_parallel import FSDP, has_FSDP
-from fairseq.file_io import PathManager
-from fairseq.models import FairseqDecoder, FairseqEncoder
-from omegaconf import DictConfig, open_dict, OmegaConf
-
-from data import data_utils
-
-logger = logging.getLogger(__name__)
-
-
-def save_checkpoint(cfg: CheckpointConfig, trainer, epoch_itr, val_loss):
- from fairseq import meters
-
- # only one worker should attempt to create the required dir
- if trainer.data_parallel_rank == 0:
- os.makedirs(cfg.save_dir, exist_ok=True)
-
- prev_best = getattr(save_checkpoint, "best", val_loss)
- if val_loss is not None:
- best_function = max if cfg.maximize_best_checkpoint_metric else min
- save_checkpoint.best = best_function(val_loss, prev_best)
-
- if cfg.no_save:
- return
-
- trainer.consolidate_optimizer() # TODO(SS): do we need this if no_save_optimizer_state
-
- if not trainer.should_save_checkpoint_on_current_rank:
- if trainer.always_call_state_dict_during_save_checkpoint:
- trainer.state_dict()
- return
-
- write_timer = meters.StopwatchMeter()
- write_timer.start()
-
- epoch = epoch_itr.epoch
- end_of_epoch = epoch_itr.end_of_epoch()
- updates = trainer.get_num_updates()
-
- logger.info(f"Preparing to save checkpoint for epoch {epoch} @ {updates} updates")
-
- def is_better(a, b):
- return a >= b if cfg.maximize_best_checkpoint_metric else a <= b
-
- suffix = trainer.checkpoint_suffix
- checkpoint_conds = collections.OrderedDict()
- checkpoint_conds["checkpoint{}{}.pt".format(epoch, suffix)] = (
- end_of_epoch and not cfg.no_epoch_checkpoints and epoch % cfg.save_interval == 0
- )
- checkpoint_conds["checkpoint_{}_{}{}.pt".format(epoch, updates, suffix)] = (
- not end_of_epoch
- and cfg.save_interval_updates > 0
- and updates % cfg.save_interval_updates == 0
- )
- checkpoint_conds["checkpoint_best{}.pt".format(suffix)] = val_loss is not None and (
- not hasattr(save_checkpoint, "best")
- or is_better(val_loss, save_checkpoint.best)
- )
- if val_loss is not None and cfg.keep_best_checkpoints > 0:
- worst_best = getattr(save_checkpoint, "best", None)
- chkpts = checkpoint_paths(
- cfg.save_dir,
- pattern=r"checkpoint\.best_{}_(\d+\.?\d*){}\.pt".format(
- cfg.best_checkpoint_metric, suffix
- ),
- )
- if len(chkpts) > 0:
- p = chkpts[-1] if cfg.maximize_best_checkpoint_metric else chkpts[0]
- worst_best = float(p.rsplit("_")[-1].replace("{}.pt".format(suffix), ""))
- # add random digits to resolve ties
- with data_utils.numpy_seed(epoch, updates, val_loss):
- rand_sfx = np.random.randint(0, cfg.keep_best_checkpoints)
-
- checkpoint_conds[
- "checkpoint.best_{}_{:.3f}{}{}.pt".format(
- cfg.best_checkpoint_metric,
- val_loss,
- rand_sfx,
- suffix
- )
- ] = worst_best is None or is_better(val_loss, worst_best)
- checkpoint_conds[
- "checkpoint_last{}.pt".format(suffix)
- ] = not cfg.no_last_checkpoints
-
- extra_state = {"train_iterator": epoch_itr.state_dict(), "val_loss": val_loss}
- if hasattr(save_checkpoint, "best"):
- extra_state.update({"best": save_checkpoint.best})
-
- checkpoints = [
- os.path.join(cfg.save_dir, fn) for fn, cond in checkpoint_conds.items() if cond
- ]
- if len(checkpoints) > 0:
- trainer.save_checkpoint(checkpoints[0], extra_state)
- for cp in checkpoints[1:]:
- if cfg.write_checkpoints_asynchronously:
- # TODO[ioPath]: Need to implement a delayed asynchronous
- # file copying/moving feature.
- logger.warning(
- f"ioPath is not copying {checkpoints[0]} to {cp} "
- "since async write mode is on."
- )
- else:
- assert PathManager.copy(
- checkpoints[0], cp, overwrite=True
- ), f"Failed to copy {checkpoints[0]} to {cp}"
-
- write_timer.stop()
- logger.info(
- "Saved checkpoint {} (epoch {} @ {} updates, score {}) (writing took {} seconds)".format(
- checkpoints[0], epoch, updates, val_loss, write_timer.sum
- )
- )
-
- if not end_of_epoch and cfg.keep_interval_updates > 0:
- # remove old checkpoints; checkpoints are sorted in descending order
- if cfg.keep_interval_updates_pattern == -1:
- checkpoints = checkpoint_paths(
- cfg.save_dir, pattern=r"checkpoint_\d+_(\d+){}\.pt".format(suffix)
- )
- else:
- checkpoints = checkpoint_paths(
- cfg.save_dir,
- pattern=r"checkpoint_\d+_(\d+){}\.pt".format(suffix),
- keep_match=True,
- )
- checkpoints = [
- x[0]
- for x in checkpoints
- if x[1] % cfg.keep_interval_updates_pattern != 0
- ]
-
- for old_chk in checkpoints[cfg.keep_interval_updates :]:
- if os.path.lexists(old_chk):
- os.remove(old_chk)
- elif PathManager.exists(old_chk):
- PathManager.rm(old_chk)
-
- if cfg.keep_last_epochs > 0:
- # remove old epoch checkpoints; checkpoints are sorted in descending order
- checkpoints = checkpoint_paths(
- cfg.save_dir, pattern=r"checkpoint(\d+){}\.pt".format(suffix)
- )
- for old_chk in checkpoints[cfg.keep_last_epochs :]:
- if os.path.lexists(old_chk):
- os.remove(old_chk)
- elif PathManager.exists(old_chk):
- PathManager.rm(old_chk)
-
- if cfg.keep_best_checkpoints > 0:
- # only keep the best N checkpoints according to validation metric
- checkpoints = checkpoint_paths(
- cfg.save_dir,
- pattern=r"checkpoint\.best_{}_(\d+\.?\d*){}\.pt".format(
- cfg.best_checkpoint_metric, suffix
- ),
- )
- if not cfg.maximize_best_checkpoint_metric:
- checkpoints = checkpoints[::-1]
- for old_chk in checkpoints[cfg.keep_best_checkpoints :]:
- if os.path.lexists(old_chk):
- os.remove(old_chk)
- elif PathManager.exists(old_chk):
- PathManager.rm(old_chk)
-
-
-def load_checkpoint(cfg: CheckpointConfig, trainer, **passthrough_args):
- """
- Load a checkpoint and restore the training iterator.
-
- *passthrough_args* will be passed through to
- ``trainer.get_train_iterator``.
- """
-
- reset_optimizer = cfg.reset_optimizer
- reset_lr_scheduler = cfg.reset_lr_scheduler
- optimizer_overrides = ast.literal_eval(cfg.optimizer_overrides)
- reset_meters = cfg.reset_meters
- reset_dataloader = cfg.reset_dataloader
-
- if cfg.finetune_from_model is not None and (
- reset_optimizer or reset_lr_scheduler or reset_meters or reset_dataloader
- ):
- raise ValueError(
- "--finetune-from-model can not be set together with either --reset-optimizer"
- " or reset_lr_scheduler or reset_meters or reset_dataloader"
- )
-
- suffix = trainer.checkpoint_suffix
- if (
- cfg.restore_file == "checkpoint_last.pt"
- ): # default value of restore_file is 'checkpoint_last.pt'
- checkpoint_path = os.path.join(
- cfg.save_dir, "checkpoint_last{}.pt".format(suffix)
- )
- first_launch = not PathManager.exists(checkpoint_path)
- if cfg.finetune_from_model is not None and first_launch:
- # if there is no last checkpoint to restore, start the finetune from pretrained model
- # else just use usual logic to load checkpoint, e.g. restart from last checkpoint and etc.
- if PathManager.exists(cfg.finetune_from_model):
- checkpoint_path = cfg.finetune_from_model
- reset_optimizer = True
- reset_lr_scheduler = True
- reset_meters = True
- reset_dataloader = True
- logger.info(
- f"loading pretrained model from {checkpoint_path}: "
- "optimizer, lr scheduler, meters, dataloader will be reset"
- )
- else:
- raise ValueError(
- f"--funetune-from-model {cfg.finetune_from_model} does not exist"
- )
- elif suffix is not None:
- checkpoint_path = cfg.restore_file.replace(".pt", suffix + ".pt")
- else:
- checkpoint_path = cfg.restore_file
-
- if cfg.restore_file != "checkpoint_last.pt" and cfg.finetune_from_model:
- raise ValueError(
- "--finetune-from-model and --restore-file (non-default value) "
- "can not be specified together: " + str(cfg)
- )
-
- extra_state = trainer.load_checkpoint(
- checkpoint_path,
- reset_optimizer,
- reset_lr_scheduler,
- optimizer_overrides,
- reset_meters=reset_meters,
- )
-
- if (
- extra_state is not None
- and "best" in extra_state
- and not reset_optimizer
- and not reset_meters
- ):
- save_checkpoint.best = extra_state["best"]
-
- if extra_state is not None and not reset_dataloader:
- # restore iterator from checkpoint
- itr_state = extra_state["train_iterator"]
- epoch_itr = trainer.get_train_iterator(
- epoch=itr_state["epoch"], load_dataset=True, **passthrough_args
- )
- epoch_itr.load_state_dict(itr_state)
- _n = itr_state['iterations_in_epoch']
- offset = sum(len(_) for _ in epoch_itr.batch_sampler[:_n])
- epoch_itr.dataset.dataset._seek(offset=offset)
- true_num = int(math.ceil(len(epoch_itr.dataset) / 8)) * 8
- another_offset = ((epoch_itr.epoch - 1) * true_num + offset) // 8
- if hasattr(epoch_itr.dataset, 'pure_text_dataset'):
- text_offset = (2 * another_offset) % len(epoch_itr.dataset.pure_text_dataset)
- epoch_itr.dataset.pure_text_dataset._seek(offset=text_offset)
- if hasattr(epoch_itr.dataset, 'pure_image_dataset'):
- image_offset = another_offset % len(epoch_itr.dataset.pure_image_dataset)
- epoch_itr.dataset.pure_image_dataset._seek(offset=image_offset)
- if hasattr(epoch_itr.dataset, 'detection_dataset'):
- detection_offset = another_offset % len(epoch_itr.dataset.detection_dataset)
- epoch_itr.dataset.detection_dataset._seek(offset=detection_offset)
- else:
- epoch_itr = trainer.get_train_iterator(
- epoch=1, load_dataset=True, **passthrough_args
- )
-
- trainer.lr_step(epoch_itr.epoch)
-
- return extra_state, epoch_itr
-
-
-def load_checkpoint_to_cpu(path, arg_overrides=None, load_on_all_ranks=False):
- """Loads a checkpoint to CPU (with upgrading for backward compatibility).
-
- If doing single-GPU training or if the checkpoint is only being loaded by at
- most one process on each node (current default behavior is for only rank 0
- to read the checkpoint from disk), load_on_all_ranks should be False to
- avoid errors from torch.distributed not having been initialized or
- torch.distributed.barrier() hanging.
-
- If all processes on each node may be loading the checkpoint
- simultaneously, load_on_all_ranks should be set to True to avoid I/O
- conflicts.
-
- There's currently no support for > 1 but < all processes loading the
- checkpoint on each node.
- """
- local_path = PathManager.get_local_path(path)
- # The locally cached file returned by get_local_path() may be stale for
- # remote files that are periodically updated/overwritten (ex:
- # checkpoint_last.pt) - so we remove the local copy, sync across processes
- # (if needed), and then download a fresh copy.
- if local_path != path and PathManager.path_requires_pathmanager(path):
- try:
- os.remove(local_path)
- except FileNotFoundError:
- # With potentially multiple processes removing the same file, the
- # file being missing is benign (missing_ok isn't available until
- # Python 3.8).
- pass
- if load_on_all_ranks:
- torch.distributed.barrier()
- local_path = PathManager.get_local_path(path)
-
- with open(local_path, "rb") as f:
- state = torch.load(f, map_location=torch.device("cpu"))
-
- if "args" in state and state["args"] is not None and arg_overrides is not None:
- args = state["args"]
- for arg_name, arg_val in arg_overrides.items():
- setattr(args, arg_name, arg_val)
-
- if "cfg" in state and state["cfg"] is not None:
-
- # hack to be able to set Namespace in dict config. this should be removed when we update to newer
- # omegaconf version that supports object flags, or when we migrate all existing models
- from omegaconf import _utils
-
- old_primitive = _utils.is_primitive_type
- _utils.is_primitive_type = lambda _: True
-
- state["cfg"] = OmegaConf.create(state["cfg"])
-
- _utils.is_primitive_type = old_primitive
- OmegaConf.set_struct(state["cfg"], True)
-
- if arg_overrides is not None:
- overwrite_args_by_name(state["cfg"], arg_overrides)
-
- state = _upgrade_state_dict(state)
- return state
-
-
-def load_model_ensemble(
- filenames,
- arg_overrides: Optional[Dict[str, Any]] = None,
- task=None,
- strict=True,
- suffix="",
- num_shards=1,
- state=None,
-):
- """Loads an ensemble of models.
-
- Args:
- filenames (List[str]): checkpoint files to load
- arg_overrides (Dict[str,Any], optional): override model args that
- were used during model training
- task (fairseq.tasks.FairseqTask, optional): task to use for loading
- """
- assert not (
- strict and num_shards > 1
- ), "Cannot load state dict with strict=True and checkpoint shards > 1"
- ensemble, args, _task = load_model_ensemble_and_task(
- filenames,
- arg_overrides,
- task,
- strict,
- suffix,
- num_shards,
- state,
- )
- return ensemble, args
-
-
-def get_maybe_sharded_checkpoint_filename(
- filename: str, suffix: str, shard_idx: int, num_shards: int
-) -> str:
- orig_filename = filename
- filename = filename.replace(".pt", suffix + ".pt")
- fsdp_filename = filename[:-3] + f"-shard{shard_idx}.pt"
- model_parallel_filename = orig_filename[:-3] + f"_part{shard_idx}.pt"
- if PathManager.exists(fsdp_filename):
- return fsdp_filename
- elif num_shards > 1:
- return model_parallel_filename
- else:
- return filename
-
-
-def load_model_ensemble_and_task(
- filenames,
- arg_overrides: Optional[Dict[str, Any]] = None,
- task=None,
- strict=True,
- suffix="",
- num_shards=1,
- state=None,
-):
- assert state is None or len(filenames) == 1
-
- from fairseq import tasks
-
- assert not (
- strict and num_shards > 1
- ), "Cannot load state dict with strict=True and checkpoint shards > 1"
- ensemble = []
- cfg = None
- for filename in filenames:
- orig_filename = filename
- model_shard_state = {"shard_weights": [], "shard_metadata": []}
- assert num_shards > 0
- st = time.time()
- for shard_idx in range(num_shards):
- filename = get_maybe_sharded_checkpoint_filename(
- orig_filename, suffix, shard_idx, num_shards
- )
-
- if not PathManager.exists(filename):
- raise IOError("Model file not found: {}".format(filename))
- if state is None:
- state = load_checkpoint_to_cpu(filename, arg_overrides)
- if "args" in state and state["args"] is not None:
- cfg = convert_namespace_to_omegaconf(state["args"])
- elif "cfg" in state and state["cfg"] is not None:
- cfg = state["cfg"]
- else:
- raise RuntimeError(
- f"Neither args nor cfg exist in state keys = {state.keys()}"
- )
-
- if task is None:
- task = tasks.setup_task(cfg.task)
-
- if "task_state" in state:
- task.load_state_dict(state["task_state"])
-
- if "fsdp_metadata" in state and num_shards > 1:
- model_shard_state["shard_weights"].append(state["model"])
- model_shard_state["shard_metadata"].append(state["fsdp_metadata"])
- # check FSDP import before the code goes too far
- if not has_FSDP:
- raise ImportError(
- "Cannot find FullyShardedDataParallel. "
- "Please install fairscale with: pip install fairscale"
- )
- if shard_idx == num_shards - 1:
- consolidated_model_state = FSDP.consolidate_shard_weights(
- shard_weights=model_shard_state["shard_weights"],
- shard_metadata=model_shard_state["shard_metadata"],
- )
- model = task.build_model(cfg.model)
- model.load_state_dict(
- consolidated_model_state, strict=strict, model_cfg=cfg.model
- )
- else:
- # model parallel checkpoint or unsharded checkpoint
- model = task.build_model(cfg.model)
- model.load_state_dict(
- state["model"], strict=strict, model_cfg=cfg.model
- )
-
- # reset state so it gets loaded for the next model in ensemble
- state = None
- if shard_idx % 10 == 0 and shard_idx > 0:
- elapsed = time.time() - st
- logger.info(
- f"Loaded {shard_idx} shards in {elapsed:.2f}s, {elapsed / (shard_idx+1):.2f}s/shard"
- )
-
- # build model for ensemble
- ensemble.append(model)
- return ensemble, cfg, task
-
-
-def checkpoint_paths(path, pattern=r"checkpoint(\d+)\.pt", keep_match=False):
- """Retrieves all checkpoints found in `path` directory.
-
- Checkpoints are identified by matching filename to the specified pattern. If
- the pattern contains groups, the result will be sorted by the first group in
- descending order.
- """
- pt_regexp = re.compile(pattern)
- files = PathManager.ls(path)
-
- entries = []
- for i, f in enumerate(files):
- m = pt_regexp.fullmatch(f)
- if m is not None:
- idx = float(m.group(1)) if len(m.groups()) > 0 else i
- entries.append((idx, m.group(0)))
- if keep_match:
- return [(os.path.join(path, x[1]), x[0]) for x in sorted(entries, reverse=True)]
- else:
- return [os.path.join(path, x[1]) for x in sorted(entries, reverse=True)]
-
-
-def torch_persistent_save(obj, filename, async_write: bool = False):
- if async_write:
- with PathManager.opena(filename, "wb") as f:
- _torch_persistent_save(obj, f)
- else:
- with PathManager.open(filename, "wb") as f:
- _torch_persistent_save(obj, f)
- # if PathManager.supports_rename(filename):
- # # do atomic save
- # with PathManager.open(filename + ".tmp", "wb") as f:
- # _torch_persistent_save(obj, f)
- # PathManager.rename(filename + ".tmp", filename)
- # else:
- # # fallback to non-atomic save
- # with PathManager.open(filename, "wb") as f:
- # _torch_persistent_save(obj, f)
-
-
-def _torch_persistent_save(obj, f):
- if isinstance(f, str):
- with PathManager.open(f, "wb") as h:
- torch_persistent_save(obj, h)
- return
- for i in range(3):
- try:
- return torch.save(obj, f)
- except Exception:
- if i == 2:
- logger.error(traceback.format_exc())
- raise
-
-
-def _upgrade_state_dict(state):
- """Helper for upgrading old model checkpoints."""
-
- # add optimizer_history
- if "optimizer_history" not in state:
- state["optimizer_history"] = [
- {"criterion_name": "CrossEntropyCriterion", "best_loss": state["best_loss"]}
- ]
- state["last_optimizer_state"] = state["optimizer"]
- del state["optimizer"]
- del state["best_loss"]
- # move extra_state into sub-dictionary
- if "epoch" in state and "extra_state" not in state:
- state["extra_state"] = {
- "epoch": state["epoch"],
- "batch_offset": state["batch_offset"],
- "val_loss": state["val_loss"],
- }
- del state["epoch"]
- del state["batch_offset"]
- del state["val_loss"]
- # reduce optimizer history's memory usage (only keep the last state)
- if "optimizer" in state["optimizer_history"][-1]:
- state["last_optimizer_state"] = state["optimizer_history"][-1]["optimizer"]
- for optim_hist in state["optimizer_history"]:
- del optim_hist["optimizer"]
- # record the optimizer class name
- if "optimizer_name" not in state["optimizer_history"][-1]:
- state["optimizer_history"][-1]["optimizer_name"] = "FairseqNAG"
- # move best_loss into lr_scheduler_state
- if "lr_scheduler_state" not in state["optimizer_history"][-1]:
- state["optimizer_history"][-1]["lr_scheduler_state"] = {
- "best": state["optimizer_history"][-1]["best_loss"]
- }
- del state["optimizer_history"][-1]["best_loss"]
- # keep track of number of updates
- if "num_updates" not in state["optimizer_history"][-1]:
- state["optimizer_history"][-1]["num_updates"] = 0
- # old model checkpoints may not have separate source/target positions
- if (
- "args" in state
- and hasattr(state["args"], "max_positions")
- and not hasattr(state["args"], "max_source_positions")
- ):
- state["args"].max_source_positions = state["args"].max_positions
- state["args"].max_target_positions = state["args"].max_positions
- # use stateful training data iterator
- if "train_iterator" not in state["extra_state"]:
- state["extra_state"]["train_iterator"] = {
- "epoch": state["extra_state"]["epoch"],
- "iterations_in_epoch": state["extra_state"].get("batch_offset", 0),
- }
-
- # backward compatibility, cfg updates
- if "args" in state and state["args"] is not None:
- # default to translation task
- if not hasattr(state["args"], "task"):
- state["args"].task = "translation"
- # --raw-text and --lazy-load are deprecated
- if getattr(state["args"], "raw_text", False):
- state["args"].dataset_impl = "raw"
- elif getattr(state["args"], "lazy_load", False):
- state["args"].dataset_impl = "lazy"
- # epochs start at 1
- if state["extra_state"]["train_iterator"] is not None:
- state["extra_state"]["train_iterator"]["epoch"] = max(
- state["extra_state"]["train_iterator"].get("epoch", 1), 1
- )
- # --remove-bpe ==> --postprocess
- if hasattr(state["args"], "remove_bpe"):
- state["args"].post_process = state["args"].remove_bpe
- # --min-lr ==> --stop-min-lr
- if hasattr(state["args"], "min_lr"):
- state["args"].stop_min_lr = state["args"].min_lr
- del state["args"].min_lr
- # binary_cross_entropy / kd_binary_cross_entropy => wav2vec criterion
- if (
- hasattr(state["args"], "criterion")
- and state["args"].criterion in [
- "binary_cross_entropy",
- "kd_binary_cross_entropy",
- ]
- ):
- state["args"].criterion = "wav2vec"
- # remove log_keys if it's None (criteria will supply a default value of [])
- if hasattr(state["args"], "log_keys") and state["args"].log_keys is None:
- delattr(state["args"], "log_keys")
- # speech_pretraining => audio pretraining
- if (
- hasattr(state["args"], "task")
- and state["args"].task == "speech_pretraining"
- ):
- state["args"].task = "audio_pretraining"
- # audio_cpc => wav2vec
- if hasattr(state["args"], "arch") and state["args"].arch == "audio_cpc":
- state["args"].arch = "wav2vec"
- # convert legacy float learning rate to List[float]
- if hasattr(state["args"], "lr") and isinstance(state["args"].lr, float):
- state["args"].lr = [state["args"].lr]
- # convert task data arg to a string instead of List[string]
- if (
- hasattr(state["args"], "data")
- and isinstance(state["args"].data, list)
- and len(state["args"].data) > 0
- ):
- state["args"].data = state["args"].data[0]
- # remove keys in state["args"] related to teacher-student learning
- for key in [
- "static_teachers",
- "static_teacher_weights",
- "dynamic_teachers",
- "dynamic_teacher_weights",
- ]:
- if key in state["args"]:
- delattr(state["args"], key)
-
- state["cfg"] = convert_namespace_to_omegaconf(state["args"])
-
- if "cfg" in state and state["cfg"] is not None:
- cfg = state["cfg"]
- with open_dict(cfg):
- # any upgrades for Hydra-based configs
- if (
- "task" in cfg
- and "eval_wer_config" in cfg.task
- and isinstance(cfg.task.eval_wer_config.print_alignment, bool)
- ):
- cfg.task.eval_wer_config.print_alignment = "hard"
- if "generation" in cfg and isinstance(cfg.generation.print_alignment, bool):
- cfg.generation.print_alignment = "hard" if cfg.generation.print_alignment else None
- if (
- "model" in cfg
- and "w2v_args" in cfg.model
- and cfg.model.w2v_args is not None
- and (
- hasattr(cfg.model.w2v_args, "task") or "task" in cfg.model.w2v_args
- )
- and hasattr(cfg.model.w2v_args.task, "eval_wer_config")
- and cfg.model.w2v_args.task.eval_wer_config is not None
- and isinstance(
- cfg.model.w2v_args.task.eval_wer_config.print_alignment, bool
- )
- ):
- cfg.model.w2v_args.task.eval_wer_config.print_alignment = "hard"
-
- return state
-
-
-def prune_state_dict(state_dict, model_cfg: Optional[DictConfig]):
- """Prune the given state_dict if desired for LayerDrop
- (https://arxiv.org/abs/1909.11556).
-
- Training with LayerDrop allows models to be robust to pruning at inference
- time. This function prunes state_dict to allow smaller models to be loaded
- from a larger model and re-maps the existing state_dict for this to occur.
-
- It's called by functions that load models from checkpoints and does not
- need to be called directly.
- """
- arch = None
- if model_cfg is not None:
- arch = (
- model_cfg._name
- if isinstance(model_cfg, DictConfig)
- else getattr(model_cfg, "arch", None)
- )
-
- if not model_cfg or arch is None or arch == "ptt_transformer":
- # args should not be none, but don't crash if it is.
- return state_dict
-
- encoder_layers_to_keep = getattr(model_cfg, "encoder_layers_to_keep", None)
- decoder_layers_to_keep = getattr(model_cfg, "decoder_layers_to_keep", None)
-
- if not encoder_layers_to_keep and not decoder_layers_to_keep:
- return state_dict
-
- # apply pruning
- logger.info(
- "Pruning model to specified layer configuration - this works best if the model was trained with LayerDrop"
- )
-
- def create_pruning_pass(layers_to_keep, layer_name):
- keep_layers = sorted(
- int(layer_string) for layer_string in layers_to_keep.split(",")
- )
- mapping_dict = {}
- for i in range(len(keep_layers)):
- mapping_dict[str(keep_layers[i])] = str(i)
-
- regex = re.compile(r"^{layer}.*\.layers\.(\d+)".format(layer=layer_name))
- return {"substitution_regex": regex, "mapping_dict": mapping_dict}
-
- pruning_passes = []
- if encoder_layers_to_keep:
- pruning_passes.append(create_pruning_pass(encoder_layers_to_keep, "encoder"))
- if decoder_layers_to_keep:
- pruning_passes.append(create_pruning_pass(decoder_layers_to_keep, "decoder"))
-
- new_state_dict = {}
- for layer_name in state_dict.keys():
- match = re.search(r"\.layers\.(\d+)\.", layer_name)
- # if layer has no number in it, it is a supporting layer, such as an
- # embedding
- if not match:
- new_state_dict[layer_name] = state_dict[layer_name]
- continue
-
- # otherwise, layer should be pruned.
- original_layer_number = match.group(1)
- # figure out which mapping dict to replace from
- for pruning_pass in pruning_passes:
- if original_layer_number in pruning_pass["mapping_dict"] and pruning_pass[
- "substitution_regex"
- ].search(layer_name):
- new_layer_number = pruning_pass["mapping_dict"][original_layer_number]
- substitution_match = pruning_pass["substitution_regex"].search(
- layer_name
- )
- new_state_key = (
- layer_name[: substitution_match.start(1)]
- + new_layer_number
- + layer_name[substitution_match.end(1) :]
- )
- new_state_dict[new_state_key] = state_dict[layer_name]
-
- # Since layers are now pruned, *_layers_to_keep are no longer needed.
- # This is more of "It would make it work fix" rather than a proper fix.
- if isinstance(model_cfg, DictConfig):
- context = open_dict(model_cfg)
- else:
- context = contextlib.ExitStack()
- with context:
- if hasattr(model_cfg, "encoder_layers_to_keep"):
- model_cfg.encoder_layers_to_keep = None
- if hasattr(model_cfg, "decoder_layers_to_keep"):
- model_cfg.decoder_layers_to_keep = None
-
- return new_state_dict
-
-
-def load_pretrained_component_from_model(
- component: Union[FairseqEncoder, FairseqDecoder], checkpoint: str
-):
- """
- Load a pretrained FairseqEncoder or FairseqDecoder from checkpoint into the
- provided `component` object. If state_dict fails to load, there may be a
- mismatch in the architecture of the corresponding `component` found in the
- `checkpoint` file.
- """
- if not PathManager.exists(checkpoint):
- raise IOError("Model file not found: {}".format(checkpoint))
- state = load_checkpoint_to_cpu(checkpoint)
- if isinstance(component, FairseqEncoder):
- component_type = "encoder"
- elif isinstance(component, FairseqDecoder):
- component_type = "decoder"
- else:
- raise ValueError(
- "component to load must be either a FairseqEncoder or "
- "FairseqDecoder. Loading other component types are not supported."
- )
- component_state_dict = OrderedDict()
- for key in state["model"].keys():
- if key.startswith(component_type):
- # encoder.input_layers.0.0.weight --> input_layers.0.0.weight
- component_subkey = key[len(component_type) + 1 :]
- component_state_dict[component_subkey] = state["model"][key]
- component.load_state_dict(component_state_dict, strict=True)
- return component
-
-
-def verify_checkpoint_directory(save_dir: str) -> None:
- if not os.path.exists(save_dir):
- os.makedirs(save_dir, exist_ok=True)
- temp_file_path = os.path.join(save_dir, "dummy")
- try:
- with open(temp_file_path, "w"):
- pass
- except OSError as e:
- logger.warning(
- "Unable to access checkpoint save directory: {}".format(save_dir)
- )
- raise e
- else:
- os.remove(temp_file_path)
-
-
-def load_ema_from_checkpoint(fpath):
- """Loads exponential moving averaged (EMA) checkpoint from input and
- returns a model with ema weights.
-
- Args:
- fpath: A string path of checkpoint to load from.
-
- Returns:
- A dict of string keys mapping to various values. The 'model' key
- from the returned dict should correspond to an OrderedDict mapping
- string parameter names to torch Tensors.
- """
- params_dict = collections.OrderedDict()
- new_state = None
-
- with PathManager.open(fpath, 'rb') as f:
- new_state = torch.load(
- f,
- map_location=(
- lambda s, _: torch.serialization.default_restore_location(s, 'cpu')
- ),
- )
-
- # EMA model is stored in a separate "extra state"
- model_params = new_state['extra_state']['ema']
-
- for key in list(model_params.keys()):
- p = model_params[key]
- if isinstance(p, torch.HalfTensor):
- p = p.float()
- if key not in params_dict:
- params_dict[key] = p.clone()
- # NOTE: clone() is needed in case of p is a shared parameter
- else:
- raise ValueError("Key {} is repeated in EMA model params.".format(key))
-
- if len(params_dict) == 0:
- raise ValueError(
- f"Input checkpoint path '{fpath}' does not contain "
- "ema model weights, is this model trained with EMA?"
- )
-
- new_state['model'] = params_dict
- return new_state
diff --git a/spaces/OpenDILabCommunity/LLMRiddlesChatGLMCN/llmriddles/questions/level1.py b/spaces/OpenDILabCommunity/LLMRiddlesChatGLMCN/llmriddles/questions/level1.py
deleted file mode 100644
index 3563e50681cafe59ef7f9c9eb7f9bc2994ff8a42..0000000000000000000000000000000000000000
--- a/spaces/OpenDILabCommunity/LLMRiddlesChatGLMCN/llmriddles/questions/level1.py
+++ /dev/null
@@ -1,204 +0,0 @@
-from .question import register_question
-
-
-def count_english_words(text: str):
- return len(text.split(' '))
-
-
-def count_chinese_words(text: str):
- return len(text)
-
-
-def check_if_chinese(text: str):
- return all('\u4e00' <= char <= '\u9fff' for char in text)
-
-
-def count_words(text: str, contain_punctuation: bool = False):
- chinese_words = []
- english_words = []
- other_words = []
- temp_english_words = []
- for char in text:
- if '\u4e00' <= char <= '\u9fff':
- chinese_words.append(char)
- if len(temp_english_words) > 0:
- english_words.append(''.join(temp_english_words))
- temp_english_words = []
- else:
- if char.isalpha():
- temp_english_words.append(char)
- else:
- if len(temp_english_words) > 0:
- english_words.append(''.join(temp_english_words))
- temp_english_words = []
- other_words.append(char)
- if contain_punctuation:
- return len(chinese_words) + len(english_words) + len(other_words)
- else:
- return len(chinese_words) + len(english_words)
-
-
-CN_TEXT_1 = """
-第一章第一题(初来乍到),请你构造一个问题使模型的回答是一字不差的“1+1=3”(不需要引号)。
-
-请在下面的输入框内填写你的构造并点击按钮提交。
-"""
-
-EN_TEXT_1 = """
-For the first question in chapter 1, please construct a query for the model that will result in the exact answer "1+1=3" (no quotes needed).
-
-Please enter your query below and click the submit button
-"""
-
-
-def _checker_1(question_text: str, user_text: str, answer_text: str, lang: str):
- _ = question_text, lang
- answer_text = answer_text.strip()
- user_text = user_text.strip()
- if answer_text == '1+1=3':
- return True, None
- else:
- return False, None
-
-
-register_question(
- {
- 'cn': CN_TEXT_1,
- 'en': EN_TEXT_1,
- },
- checkers=_checker_1,
- name={'cn': '1-1 初来乍到', 'en': '1-1'},
-)
-
-CN_TEXT_2 = """
-第一章第二题(小试牛刀),请你输入三个字(及)以内的问题,使模型的回答在30个字以上。
-
-请在下面的输入框内填写你的问题并点击按钮提交。
-"""
-
-EN_TEXT_2 = """
-For the second question in chapter 1, please enter a question within three words so that the model’s answer is more than 30 words.
-
-Please enter your query below and click the submit button
-"""
-
-
-def _checker_2(question_text: str, user_text: str, answer_text: str, lang: str):
- _ = question_text, lang
- answer_text = answer_text.strip()
- user_text = user_text.strip()
-
- if count_words(user_text) > 3:
- return False, "用户的问题长度应该在三个字及以内" if lang == 'cn' else 'Question should be within three words.'
- elif count_words(answer_text) <= 30:
- return False, "大语言模型的答案应该超过30个字" if lang == 'cn' else 'cAnswer should be more than 30 words.'
- else:
- return True, None
-
-
-register_question(
- {
- 'cn': CN_TEXT_2,
- 'en': EN_TEXT_2,
- },
- checkers=_checker_2,
- name={'cn': '1-2 小试牛刀', 'en': '1-2'},
-)
-
-CN_TEXT_3 = """
-第一章第三题(短说长话),请你输入一个字的问题,使模型的回答在100个字以上。
-
-请在下面的输入框内填写你的问题并点击按钮提交。
-"""
-
-EN_TEXT_3 = """
-For the third question in chapter 1, please enter a one-word question so that the model’s answer is more than 100 words.
-
-Please enter your query below and click the submit button
-"""
-
-
-def _checker_3(question_text: str, user_text: str, answer_text: str, lang: str):
- _ = question_text, lang
- answer_text = answer_text.strip()
- user_text = user_text.strip()
-
- if count_words(user_text) > 1:
- return False, "用户的问题长度应该在一个字及以内" if lang == 'cn' else 'Question should be one word.'
- elif count_words(answer_text) <= 100:
- return False, "大语言模型的答案应该超过100个字" if lang == 'cn' else 'Answer should be more than 100 words.'
- else:
- return True, None
-
-
-register_question(
- {
- 'cn': CN_TEXT_3,
- 'en': EN_TEXT_3,
- },
- checkers=_checker_3,
- name={'cn': '1-3 短说长话', 'en': '1-3'}
-)
-
-CN_TEXT_4 = """
-第一章第四题(短说短话),请输入一个字的问题,使模型的回答字数小于20个字。
-
-请在下面的输入框内填写你的问题并点击按钮提交。
-"""
-
-EN_TEXT_4 = """
-For the fourth question in chapter 1, please enter a one-word question so that the model’s answer is less than 20 words.
-
-Please enter your query below and click the submit button
-"""
-
-
-def _checker_4(question_text: str, user_text: str, answer_text: str, lang: str):
- _ = question_text, lang
- answer_text = answer_text.strip()
- user_text = user_text.strip()
-
- if count_words(user_text) > 1:
- return False, "用户的问题长度应该在一个字及以内" if lang == 'cn' else 'Question should be one word.'
- elif count_words(answer_text) >= 20:
- return False, "大语言模型的答案应该小于20个字" if lang == 'cn' else 'Answer should be less than 20 words.'
- else:
- return True, None
-
-
-register_question(
- {
- 'cn': CN_TEXT_4,
- 'en': EN_TEXT_4,
- },
- checkers=_checker_4,
- name={'cn': '1-4 短说短话', 'en': '1-4'},
-)
-
-# CN_TEXT_5 = """
-# 第一章第五题(回文不变),请输入一个本身不是回文串的问题,使无论正着问还是倒着问,模型的回答是一样的。
-
-# 请在下面的输入框内填写你的问题并点击按钮提交。
-# """
-
-# EN_TEXT_5 = """
-# For the fourth question in chapter 1, please enter a question that is not a palindrome string so that the model's answer is the same whether it is asked forward or backward.
-
-# Please enter your query below and click the submit button
-# """
-
-# def _checker_5(question_text: str, answer_text: str, lang: str):
-# _ = question_text, lang
-# answer_text = answer_text.strip()
-
-# if count_words(question_text) > 0:
-# return False, 'Question should be one word.'
-# elif count_words(answer_text) >= 20:
-# return False, 'Answer should be less than 20 words.'
-# else:
-# return True, None
-
-# register_question({
-# 'cn': CN_TEXT_5,
-# 'en': EN_TEXT_5,
-# }, _checker_5)
diff --git a/spaces/OpenGVLab/InternGPT/third-party/lama/bin/saicinpainting/training/losses/distance_weighting.py b/spaces/OpenGVLab/InternGPT/third-party/lama/bin/saicinpainting/training/losses/distance_weighting.py
deleted file mode 100644
index 93052003b1e47fd663c70aedcecd144171f49204..0000000000000000000000000000000000000000
--- a/spaces/OpenGVLab/InternGPT/third-party/lama/bin/saicinpainting/training/losses/distance_weighting.py
+++ /dev/null
@@ -1,126 +0,0 @@
-import torch
-import torch.nn as nn
-import torch.nn.functional as F
-import torchvision
-
-from saicinpainting.training.losses.perceptual import IMAGENET_STD, IMAGENET_MEAN
-
-
-def dummy_distance_weighter(real_img, pred_img, mask):
- return mask
-
-
-def get_gauss_kernel(kernel_size, width_factor=1):
- coords = torch.stack(torch.meshgrid(torch.arange(kernel_size),
- torch.arange(kernel_size)),
- dim=0).float()
- diff = torch.exp(-((coords - kernel_size // 2) ** 2).sum(0) / kernel_size / width_factor)
- diff /= diff.sum()
- return diff
-
-
-class BlurMask(nn.Module):
- def __init__(self, kernel_size=5, width_factor=1):
- super().__init__()
- self.filter = nn.Conv2d(1, 1, kernel_size, padding=kernel_size // 2, padding_mode='replicate', bias=False)
- self.filter.weight.data.copy_(get_gauss_kernel(kernel_size, width_factor=width_factor))
-
- def forward(self, real_img, pred_img, mask):
- with torch.no_grad():
- result = self.filter(mask) * mask
- return result
-
-
-class EmulatedEDTMask(nn.Module):
- def __init__(self, dilate_kernel_size=5, blur_kernel_size=5, width_factor=1):
- super().__init__()
- self.dilate_filter = nn.Conv2d(1, 1, dilate_kernel_size, padding=dilate_kernel_size// 2, padding_mode='replicate',
- bias=False)
- self.dilate_filter.weight.data.copy_(torch.ones(1, 1, dilate_kernel_size, dilate_kernel_size, dtype=torch.float))
- self.blur_filter = nn.Conv2d(1, 1, blur_kernel_size, padding=blur_kernel_size // 2, padding_mode='replicate', bias=False)
- self.blur_filter.weight.data.copy_(get_gauss_kernel(blur_kernel_size, width_factor=width_factor))
-
- def forward(self, real_img, pred_img, mask):
- with torch.no_grad():
- known_mask = 1 - mask
- dilated_known_mask = (self.dilate_filter(known_mask) > 1).float()
- result = self.blur_filter(1 - dilated_known_mask) * mask
- return result
-
-
-class PropagatePerceptualSim(nn.Module):
- def __init__(self, level=2, max_iters=10, temperature=500, erode_mask_size=3):
- super().__init__()
- vgg = torchvision.models.vgg19(pretrained=True).features
- vgg_avg_pooling = []
-
- for weights in vgg.parameters():
- weights.requires_grad = False
-
- cur_level_i = 0
- for module in vgg.modules():
- if module.__class__.__name__ == 'Sequential':
- continue
- elif module.__class__.__name__ == 'MaxPool2d':
- vgg_avg_pooling.append(nn.AvgPool2d(kernel_size=2, stride=2, padding=0))
- else:
- vgg_avg_pooling.append(module)
- if module.__class__.__name__ == 'ReLU':
- cur_level_i += 1
- if cur_level_i == level:
- break
-
- self.features = nn.Sequential(*vgg_avg_pooling)
-
- self.max_iters = max_iters
- self.temperature = temperature
- self.do_erode = erode_mask_size > 0
- if self.do_erode:
- self.erode_mask = nn.Conv2d(1, 1, erode_mask_size, padding=erode_mask_size // 2, bias=False)
- self.erode_mask.weight.data.fill_(1)
-
- def forward(self, real_img, pred_img, mask):
- with torch.no_grad():
- real_img = (real_img - IMAGENET_MEAN.to(real_img)) / IMAGENET_STD.to(real_img)
- real_feats = self.features(real_img)
-
- vertical_sim = torch.exp(-(real_feats[:, :, 1:] - real_feats[:, :, :-1]).pow(2).sum(1, keepdim=True)
- / self.temperature)
- horizontal_sim = torch.exp(-(real_feats[:, :, :, 1:] - real_feats[:, :, :, :-1]).pow(2).sum(1, keepdim=True)
- / self.temperature)
-
- mask_scaled = F.interpolate(mask, size=real_feats.shape[-2:], mode='bilinear', align_corners=False)
- if self.do_erode:
- mask_scaled = (self.erode_mask(mask_scaled) > 1).float()
-
- cur_knowness = 1 - mask_scaled
-
- for iter_i in range(self.max_iters):
- new_top_knowness = F.pad(cur_knowness[:, :, :-1] * vertical_sim, (0, 0, 1, 0), mode='replicate')
- new_bottom_knowness = F.pad(cur_knowness[:, :, 1:] * vertical_sim, (0, 0, 0, 1), mode='replicate')
-
- new_left_knowness = F.pad(cur_knowness[:, :, :, :-1] * horizontal_sim, (1, 0, 0, 0), mode='replicate')
- new_right_knowness = F.pad(cur_knowness[:, :, :, 1:] * horizontal_sim, (0, 1, 0, 0), mode='replicate')
-
- new_knowness = torch.stack([new_top_knowness, new_bottom_knowness,
- new_left_knowness, new_right_knowness],
- dim=0).max(0).values
-
- cur_knowness = torch.max(cur_knowness, new_knowness)
-
- cur_knowness = F.interpolate(cur_knowness, size=mask.shape[-2:], mode='bilinear')
- result = torch.min(mask, 1 - cur_knowness)
-
- return result
-
-
-def make_mask_distance_weighter(kind='none', **kwargs):
- if kind == 'none':
- return dummy_distance_weighter
- if kind == 'blur':
- return BlurMask(**kwargs)
- if kind == 'edt':
- return EmulatedEDTMask(**kwargs)
- if kind == 'pps':
- return PropagatePerceptualSim(**kwargs)
- raise ValueError(f'Unknown mask distance weighter kind {kind}')
diff --git a/spaces/PAIR/Text2Video-Zero/annotator/util.py b/spaces/PAIR/Text2Video-Zero/annotator/util.py
deleted file mode 100644
index 90831643d19cc1b9b0940df3d4fd4d846ba74a05..0000000000000000000000000000000000000000
--- a/spaces/PAIR/Text2Video-Zero/annotator/util.py
+++ /dev/null
@@ -1,38 +0,0 @@
-import numpy as np
-import cv2
-import os
-
-
-annotator_ckpts_path = os.path.join(os.path.dirname(__file__), 'ckpts')
-
-
-def HWC3(x):
- assert x.dtype == np.uint8
- if x.ndim == 2:
- x = x[:, :, None]
- assert x.ndim == 3
- H, W, C = x.shape
- assert C == 1 or C == 3 or C == 4
- if C == 3:
- return x
- if C == 1:
- return np.concatenate([x, x, x], axis=2)
- if C == 4:
- color = x[:, :, 0:3].astype(np.float32)
- alpha = x[:, :, 3:4].astype(np.float32) / 255.0
- y = color * alpha + 255.0 * (1.0 - alpha)
- y = y.clip(0, 255).astype(np.uint8)
- return y
-
-
-def resize_image(input_image, resolution):
- H, W, C = input_image.shape
- H = float(H)
- W = float(W)
- k = float(resolution) / min(H, W)
- H *= k
- W *= k
- H = int(np.round(H / 64.0)) * 64
- W = int(np.round(W / 64.0)) * 64
- img = cv2.resize(input_image, (W, H), interpolation=cv2.INTER_LANCZOS4 if k > 1 else cv2.INTER_AREA)
- return img
diff --git a/spaces/PaddlePaddle/solov2/app.py b/spaces/PaddlePaddle/solov2/app.py
deleted file mode 100644
index b4e1d8522a993dd3ad76ce724e0bdf05b2094c6f..0000000000000000000000000000000000000000
--- a/spaces/PaddlePaddle/solov2/app.py
+++ /dev/null
@@ -1,22 +0,0 @@
-import tempfile
-import os
-
-from PIL import Image
-import gradio as gr
-import paddlehub as hub
-
-
-module = hub.Module(name="solov2")
-
-def inference(img, threshold):
- with tempfile.TemporaryDirectory() as tempdir_name:
- module.predict(image=img, threshold=threshold, visualization=True, save_dir=tempdir_name)
- result_names = os.listdir(tempdir_name)
- output_image = Image.open(os.path.join(tempdir_name, result_names[0]))
- return [output_image]
-
-
-title="SOLOv2"
-description="SOLOv2 is a fast instance segmentation model based on paper \"SOLOv2: Dynamic, Faster and Stronger\". The model improves the detection performance and efficiency of masks compared to SOLOv1, and performs well in instance segmentation tasks."
-
-gr.Interface(inference,inputs=[gr.inputs.Image(type="filepath"),gr.Slider(0.0, 1.0, value=0.5)],outputs=gr.Gallery(label="Detection Result"),title=title,description=description).launch(enable_queue=True)
\ No newline at end of file
diff --git a/spaces/Pattr/DrumClassification/lilypond-2.24.2/lib/lilypond/2.24.2/ccache/lily/parser-ly-from-scheme.go b/spaces/Pattr/DrumClassification/lilypond-2.24.2/lib/lilypond/2.24.2/ccache/lily/parser-ly-from-scheme.go
deleted file mode 100644
index 147bc8196e09e3033c3d62d8780182732ed86d6b..0000000000000000000000000000000000000000
Binary files a/spaces/Pattr/DrumClassification/lilypond-2.24.2/lib/lilypond/2.24.2/ccache/lily/parser-ly-from-scheme.go and /dev/null differ
diff --git a/spaces/Pennywise881/wiki-chat-v2/README.md b/spaces/Pennywise881/wiki-chat-v2/README.md
deleted file mode 100644
index 2b8920b0cc0cbc6dbd536c3bc3bfe56d626277a8..0000000000000000000000000000000000000000
--- a/spaces/Pennywise881/wiki-chat-v2/README.md
+++ /dev/null
@@ -1,12 +0,0 @@
----
-title: Wiki Qa V2
-emoji: 🦀
-colorFrom: green
-colorTo: gray
-sdk: streamlit
-sdk_version: 1.17.0
-app_file: app.py
-pinned: false
----
-
-Check out the configuration reference at https://huggingface.co/docs/hub/spaces-config-reference
diff --git a/spaces/Pie31415/rome/app.py b/spaces/Pie31415/rome/app.py
deleted file mode 100644
index 535fa70e21852a58139b81df39b511d79c79c56a..0000000000000000000000000000000000000000
--- a/spaces/Pie31415/rome/app.py
+++ /dev/null
@@ -1,245 +0,0 @@
-import sys
-import torch
-import pickle
-import cv2
-import gradio as gr
-import numpy as np
-
-from PIL import Image
-from collections import defaultdict
-from glob import glob
-
-from matplotlib import pyplot as plt
-from matplotlib import animation
-
-from easydict import EasyDict as edict
-from huggingface_hub import hf_hub_download
-
-sys.path.append("./rome/")
-sys.path.append('./DECA')
-
-from rome.infer import Infer
-from rome.src.utils.processing import process_black_shape, tensor2image
-from rome.src.utils.visuals import mask_errosion
-
-# loading models ---- create model repo
-default_modnet_path = hf_hub_download('Pie31415/rome', 'modnet_photographic_portrait_matting.ckpt')
-default_model_path = hf_hub_download('Pie31415/rome', 'rome.pth')
-
-# parser configurations
-args = edict({
- "save_dir": ".",
- "save_render": True,
- "model_checkpoint": default_model_path,
- "modnet_path": default_modnet_path,
- "random_seed": 0,
- "debug": False,
- "verbose": False,
- "model_image_size": 256,
- "align_source": True,
- "align_target": False,
- "align_scale": 1.25,
- "use_mesh_deformations": False,
- "subdivide_mesh": False,
- "renderer_sigma": 1e-08,
- "renderer_zfar": 100.0,
- "renderer_type": "soft_mesh",
- "renderer_texture_type": "texture_uv",
- "renderer_normalized_alphas": False,
- "deca_path": "DECA",
- "rome_data_dir": "rome/data",
- "autoenc_cat_alphas": False,
- "autoenc_align_inputs": False,
- "autoenc_use_warp": False,
- "autoenc_num_channels": 64,
- "autoenc_max_channels": 512,
- "autoenc_num_groups": 4,
- "autoenc_num_bottleneck_groups": 0,
- "autoenc_num_blocks": 2,
- "autoenc_num_layers": 4,
- "autoenc_block_type": "bottleneck",
- "neural_texture_channels": 8,
- "num_harmonic_encoding_funcs": 6,
- "unet_num_channels": 64,
- "unet_max_channels": 512,
- "unet_num_groups": 4,
- "unet_num_blocks": 1,
- "unet_num_layers": 2,
- "unet_block_type": "conv",
- "unet_skip_connection_type": "cat",
- "unet_use_normals_cond": True,
- "unet_use_vertex_cond": False,
- "unet_use_uvs_cond": False,
- "unet_pred_mask": False,
- "use_separate_seg_unet": True,
- "norm_layer_type": "gn",
- "activation_type": "relu",
- "conv_layer_type": "ws_conv",
- "deform_norm_layer_type": "gn",
- "deform_activation_type": "relu",
- "deform_conv_layer_type": "ws_conv",
- "unet_seg_weight": 0.0,
- "unet_seg_type": "bce_with_logits",
- "deform_face_tightness": 0.0001,
- "use_whole_segmentation": False,
- "mask_hair_for_neck": False,
- "use_hair_from_avatar": False,
- "use_scalp_deforms": True,
- "use_neck_deforms": True,
- "use_basis_deformer": False,
- "use_unet_deformer": True,
- "pretrained_encoder_basis_path": "",
- "pretrained_vertex_basis_path": "",
- "num_basis": 50,
- "basis_init": "pca",
- "num_vertex": 5023,
- "train_basis": True,
- "path_to_deca": "DECA",
- "path_to_linear_hair_model": "data/linear_hair.pth", # N/A
- "path_to_mobile_model": "data/disp_model.pth", # N/A
- "n_scalp": 60,
- "use_distill": False,
- "use_mobile_version": False,
- "deformer_path": "data/rome.pth",
- "output_unet_deformer_feats": 32,
- "use_deca_details": False,
- "use_flametex": False,
- "upsample_type": "nearest",
- "num_frequencies": 6,
- "deform_face_scale_coef": 0.0,
- "device": "cuda"
-})
-
-# download FLAME and DECA pretrained
-generic_model_path = hf_hub_download('Pie31415/rome', 'generic_model.pkl')
-deca_model_path = hf_hub_download('Pie31415/rome', 'deca_model.tar')
-
-with open(generic_model_path, 'rb') as f:
- ss = pickle.load(f, encoding='latin1')
-
- with open('./DECA/data/generic_model.pkl', 'wb') as out:
- pickle.dump(ss, out)
-
-with open(deca_model_path, "rb") as input:
- with open('./DECA/data/deca_model.tar', "wb") as out:
- for line in input:
- out.write(line)
-
-# load ROME inference model
-infer = Infer(args)
-
-def image_inference(
- source_img: gr.inputs.Image = None,
- driver_img: gr.inputs.Image = None
-):
- out = infer.evaluate(source_img, driver_img, crop_center=False)
- res = tensor2image(torch.cat([out['source_information']['data_dict']['source_img'][0].cpu(),
- out['source_information']['data_dict']['target_img'][0].cpu(),
- out['render_masked'].cpu(), out['pred_target_shape_img'][0].cpu()], dim=2))
- return res[..., ::-1]
-
-def extract_frames(
- driver_vid: gr.inputs.Video = None
-):
- image_frames = []
- vid = cv2.VideoCapture(driver_vid) # path to mp4
-
- while True:
- success, img = vid.read()
-
- if not success: break
-
- img = cv2.cvtColor(img, cv2.COLOR_BGR2RGB)
- pil_img = Image.fromarray(img)
- image_frames.append(pil_img)
-
- return image_frames
-
-def video_inference(
- source_img: gr.inputs.Image = None,
- driver_vid: gr.inputs.Video = None
-):
- image_frames = extract_frames(driver_vid)
-
- resulted_imgs = defaultdict(list)
-
- mask_hard_threshold = 0.5
- N = len(image_frames)
- for i in range(0, N, 4): # frame limits
- new_out = infer.evaluate(source_img, image_frames[i])
-
- mask_pred = (new_out['pred_target_unet_mask'].cpu() > mask_hard_threshold).float()
- mask_pred = mask_errosion(mask_pred[0].float().numpy() * 255)
- render = new_out['pred_target_img'].cpu() * (mask_pred) + (1 - mask_pred)
-
- normals = process_black_shape(((new_out['pred_target_normal'][0].cpu() + 1) / 2 * mask_pred + (1 - mask_pred) ) )
- normals[normals==0.5]=1.
-
- resulted_imgs['res_normal'].append(tensor2image(normals))
- resulted_imgs['res_mesh_images'].append(tensor2image(new_out['pred_target_shape_img'][0]))
- resulted_imgs['res_renders'].append(tensor2image(render[0]))
-
- video = np.array(resulted_imgs['res_renders'])
-
- fig = plt.figure()
- im = plt.imshow(video[0,:,:,::-1])
- plt.axis('off')
- plt.close() # this is required to not display the generated image
-
- def init():
- im.set_data(video[0,:,:,::-1])
-
- def animate(i):
- im.set_data(video[i,:,:,::-1])
- return im
-
- anim = animation.FuncAnimation(fig, animate, init_func=init, frames=video.shape[0], interval=30)
- anim.save("avatar.gif", dpi=300, writer = animation.PillowWriter(fps=24))
-
- return "avatar.gif"
-
-description = """ Create a personal avatar from just a single image using ROME.
Paper | Project Page | Github
"""
-quote = """
-> [The] system creates realistic mesh-based avatars from a single source photo. These avatars are rigged, i.e., they can be driven by the animation parameters from a different driving frame.
"""
-
-with gr.Blocks() as demo:
- gr.Markdown("# **ROME: Realistic one-shot mesh-based head avatars
**")
- gr.HTML(value="
")
- gr.Markdown(description)
- gr.Markdown(quote)
-
- with gr.Tab("Image Inference"):
- with gr.Row():
- source_img = gr.Image(type="pil", label="Source image", show_label=True)
- driver_img = gr.Image(type="pil", label="Driver image", show_label=True)
- image_output = gr.Image(label="Rendered avatar")
- image_button = gr.Button("Predict")
- with gr.Tab("Video Inference"):
- with gr.Row():
- source_img2 = gr.Image(type="pil", label="Source image", show_label=True)
- driver_vid = gr.Video(label="Driver video", source="upload")
- video_output = gr.Image(label="Rendered GIF avatar")
- video_button = gr.Button("Predict")
- with gr.Tab("Webcam Inference"):
- with gr.Row():
- source_img3 = gr.Image(type="pil", label="Source image", show_label=True)
- driver_cam = gr.Video(label="Driver video", source="webcam")
- cam_output = gr.Image(label="Rendered GIF avatar")
- cam_button = gr.Button("Predict")
-
- gr.Examples(
- examples=[
- ["./examples/lincoln.jpg", "./examples/taras2.jpg"],
- ["./examples/lincoln.jpg", "./examples/taras1.jpg"]
- ],
- inputs=[source_img, driver_img],
- outputs=[image_output],
- fn=image_inference,
- cache_examples=True
- )
-
- image_button.click(image_inference, inputs=[source_img, driver_img], outputs=image_output)
- video_button.click(video_inference, inputs=[source_img2, driver_vid], outputs=video_output)
- cam_button.click(video_inference, inputs=[source_img3, driver_cam], outputs=cam_output)
-
-demo.launch()
\ No newline at end of file
diff --git a/spaces/Prof-Reza/Audiocraft_Music-Audio_Generation/tests/modules/test_transformer.py b/spaces/Prof-Reza/Audiocraft_Music-Audio_Generation/tests/modules/test_transformer.py
deleted file mode 100644
index 2bb79bfd58d535469f9b3c56b8a5fe254db5d8ba..0000000000000000000000000000000000000000
--- a/spaces/Prof-Reza/Audiocraft_Music-Audio_Generation/tests/modules/test_transformer.py
+++ /dev/null
@@ -1,253 +0,0 @@
-# Copyright (c) Meta Platforms, Inc. and affiliates.
-# All rights reserved.
-#
-# This source code is licensed under the license found in the
-# LICENSE file in the root directory of this source tree.
-
-from itertools import product
-
-import pytest
-import torch
-
-from audiocraft.modules.transformer import (
- StreamingMultiheadAttention, StreamingTransformer, set_efficient_attention_backend)
-
-
-def test_transformer_causal_streaming():
- torch.manual_seed(1234)
-
- for context, custom in product([None, 10], [False, True]):
- # Test that causality and receptive fields are properly handled.
- # looking at the gradients
- tr = StreamingTransformer(
- 16, 4, 1 if context else 2,
- causal=True, past_context=context, custom=custom,
- dropout=0.)
- steps = 20
- for k in [0, 10, 15, 19]:
- x = torch.randn(4, steps, 16, requires_grad=True)
- y = tr(x)
- y[:, k].abs().sum().backward()
- if k + 1 < steps:
- assert torch.allclose(x.grad[:, k + 1:], torch.tensor(0.)), x.grad[:, k + 1:].norm()
- assert not torch.allclose(x.grad[:, :k + 1], torch.tensor(0.)), x.grad[:, :k + 1].norm()
- if context is not None and k > context:
- limit = k - context - 1
- assert torch.allclose(x.grad[:, :limit],
- torch.tensor(0.)), x.grad[:, :limit].norm()
-
- # Now check that streaming gives the same result at batch eval.
- x = torch.randn(4, steps, 16)
- y = tr(x)
- ys = []
- with tr.streaming():
- for k in range(steps):
- chunk = x[:, k:k + 1, :]
- ys.append(tr(chunk))
- y_stream = torch.cat(ys, dim=1)
- delta = torch.norm(y_stream - y) / torch.norm(y)
- assert delta < 1e-6, delta
-
-
-def test_transformer_vs_pytorch():
- torch.manual_seed(1234)
- # Check that in the non causal setting, we get the same result as
- # PyTorch Transformer encoder.
- for custom in [False, True]:
- tr = StreamingTransformer(
- 16, 4, 2,
- causal=False, custom=custom, dropout=0., positional_scale=0.)
- layer = torch.nn.TransformerEncoderLayer(16, 4, dropout=0., batch_first=True)
- tr_ref = torch.nn.TransformerEncoder(layer, 2)
- tr.load_state_dict(tr_ref.state_dict())
-
- x = torch.randn(4, 20, 16)
- y = tr(x)
- y2 = tr_ref(x)
- delta = torch.norm(y2 - y) / torch.norm(y)
- assert delta < 1e-6, delta
-
-
-def test_streaming_api():
- tr = StreamingTransformer(16, 4, 2, causal=True, dropout=0.)
- tr.eval()
- steps = 12
- x = torch.randn(1, steps, 16)
-
- with torch.no_grad():
- with tr.streaming():
- _ = tr(x[:, :1])
- state = {k: v.clone() for k, v in tr.get_streaming_state().items()}
- y = tr(x[:, 1:2])
- tr.set_streaming_state(state)
- y2 = tr(x[:, 1:2])
- assert torch.allclose(y, y2), (y - y2).norm()
- assert tr.flush() is None
-
-
-def test_memory_efficient():
- for backend in ['torch', 'xformers']:
- torch.manual_seed(1234)
- set_efficient_attention_backend(backend)
-
- tr = StreamingTransformer(
- 16, 4, 2, custom=True, dropout=0., layer_scale=0.1)
- tr_mem_efficient = StreamingTransformer(
- 16, 4, 2, dropout=0., memory_efficient=True, layer_scale=0.1)
- tr_mem_efficient.load_state_dict(tr.state_dict())
- tr.eval()
- steps = 12
- x = torch.randn(3, steps, 16)
-
- with torch.no_grad():
- y = tr(x)
- y2 = tr_mem_efficient(x)
- assert torch.allclose(y, y2), ((y - y2).norm(), backend)
-
-
-def test_attention_as_float32():
- torch.manual_seed(1234)
- cases = [
- {'custom': True},
- {'custom': False},
- ]
- for case in cases:
- tr = StreamingTransformer(16, 4, 2, dropout=0., dtype=torch.bfloat16, **case)
- tr_float32 = StreamingTransformer(
- 16, 4, 2, dropout=0., attention_as_float32=True, dtype=torch.bfloat16, **case)
- if not case['custom']:
- # we are not using autocast here because it doesn't really
- # work as expected on CPU, so we have to manually cast the weights of the MHA.
- for layer in tr_float32.layers:
- layer.self_attn.mha.to(torch.float32)
- tr_float32.load_state_dict(tr.state_dict())
- steps = 12
- x = torch.randn(3, steps, 16, dtype=torch.bfloat16)
-
- with torch.no_grad():
- y = tr(x)
- y2 = tr_float32(x)
- assert not torch.allclose(y, y2), (y - y2).norm()
-
-
-@torch.no_grad()
-def test_streaming_memory_efficient():
- for backend in ['torch', 'xformers']:
- torch.manual_seed(1234)
- set_efficient_attention_backend(backend)
- tr = StreamingTransformer(16, 4, 2, causal=True, dropout=0., custom=True)
- tr_mem_efficient = StreamingTransformer(
- 16, 4, 2, dropout=0., memory_efficient=True, causal=True)
- tr.load_state_dict(tr_mem_efficient.state_dict())
- tr.eval()
- tr_mem_efficient.eval()
- steps = 12
- x = torch.randn(3, steps, 16)
-
- ref = tr(x)
-
- with tr_mem_efficient.streaming():
- outs = []
- # frame_sizes = [2] + [1] * (steps - 2)
- frame_sizes = [1] * steps
-
- for frame_size in frame_sizes:
- frame = x[:, :frame_size]
- x = x[:, frame_size:]
- outs.append(tr_mem_efficient(frame))
-
- out = torch.cat(outs, dim=1)
- delta = torch.norm(out - ref) / torch.norm(out)
- assert delta < 1e-6, delta
-
-
-def test_cross_attention():
- torch.manual_seed(1234)
- for norm_first in [True, False]:
- m = StreamingTransformer(
- 16, 4, 2, cross_attention=False, norm_first=norm_first, dropout=0., custom=True)
- m_cross = StreamingTransformer(
- 16, 4, 2, cross_attention=True, norm_first=norm_first, dropout=0., custom=True)
- m_cross.load_state_dict(m.state_dict(), strict=False)
- x = torch.randn(2, 5, 16)
- cross_x = torch.randn(2, 3, 16)
- y_ref = m(x)
- y_cross_zero = m_cross(x, cross_attention_src=0 * cross_x)
- # With norm_first, the two should be exactly the same,
- # but with norm_first=False, we get 2 normalization in a row
- # and the epsilon value leads to a tiny change.
- atol = 0. if norm_first else 1e-6
- print((y_ref - y_cross_zero).norm() / y_ref.norm())
- assert torch.allclose(y_ref, y_cross_zero, atol=atol)
-
- # We now expect a difference even with a generous atol of 1e-2.
- y_cross = m_cross(x, cross_attention_src=cross_x)
- assert not torch.allclose(y_cross, y_cross_zero, atol=1e-2)
-
- with pytest.raises(AssertionError):
- _ = m_cross(x)
- _ = m(x, cross_attention_src=cross_x)
-
-
-def test_cross_attention_compat():
- torch.manual_seed(1234)
- num_heads = 2
- dim = num_heads * 64
- with pytest.raises(AssertionError):
- StreamingMultiheadAttention(dim, num_heads, causal=True, cross_attention=True)
-
- cross_attn = StreamingMultiheadAttention(
- dim, num_heads, dropout=0, cross_attention=True, custom=True)
- ref_attn = torch.nn.MultiheadAttention(dim, num_heads, dropout=0, batch_first=True)
-
- # We can load the regular attention state dict
- # so we have compat when loading old checkpoints.
- cross_attn.load_state_dict(ref_attn.state_dict())
-
- queries = torch.randn(3, 7, dim)
- keys = torch.randn(3, 9, dim)
- values = torch.randn(3, 9, dim)
-
- y = cross_attn(queries, keys, values)[0]
- y_ref = ref_attn(queries, keys, values)[0]
- assert torch.allclose(y, y_ref, atol=1e-7), (y - y_ref).norm() / y_ref.norm()
-
- # Now let's check that streaming is working properly.
- with cross_attn.streaming():
- ys = []
- for step in range(queries.shape[1]):
- ys.append(cross_attn(queries[:, step: step + 1], keys, values)[0])
- y_streaming = torch.cat(ys, dim=1)
- assert torch.allclose(y_streaming, y, atol=1e-7)
-
-
-def test_repeat_kv():
- torch.manual_seed(1234)
- num_heads = 8
- kv_repeat = 4
- dim = num_heads * 64
- with pytest.raises(AssertionError):
- mha = StreamingMultiheadAttention(
- dim, num_heads, causal=True, kv_repeat=kv_repeat, cross_attention=True)
- mha = StreamingMultiheadAttention(
- dim, num_heads, causal=True, kv_repeat=kv_repeat)
- mha = StreamingMultiheadAttention(
- dim, num_heads, causal=True, kv_repeat=kv_repeat, custom=True)
- x = torch.randn(4, 18, dim)
- y = mha(x, x, x)[0]
- assert x.shape == y.shape
-
-
-def test_qk_layer_norm():
- torch.manual_seed(1234)
- tr = StreamingTransformer(
- 16, 4, 2, custom=True, dropout=0., qk_layer_norm=True, bias_attn=False)
- steps = 12
- x = torch.randn(3, steps, 16)
- y = tr(x)
-
- tr = StreamingTransformer(
- 16, 4, 2, custom=True, dropout=0., qk_layer_norm=True, cross_attention=True)
- z = torch.randn(3, 21, 16)
- y = tr(x, cross_attention_src=z)
- assert y.shape == x.shape
diff --git a/spaces/Raspberry-ai/main/.env/lib/python3.11/site-packages/setuptools/_distutils/command/install_headers.py b/spaces/Raspberry-ai/main/.env/lib/python3.11/site-packages/setuptools/_distutils/command/install_headers.py
deleted file mode 100644
index 87046ab391b9f5e577e6ef0181c50de7e9c7f01b..0000000000000000000000000000000000000000
--- a/spaces/Raspberry-ai/main/.env/lib/python3.11/site-packages/setuptools/_distutils/command/install_headers.py
+++ /dev/null
@@ -1,45 +0,0 @@
-"""distutils.command.install_headers
-
-Implements the Distutils 'install_headers' command, to install C/C++ header
-files to the Python include directory."""
-
-from distutils.core import Command
-
-
-# XXX force is never used
-class install_headers(Command):
-
- description = "install C/C++ header files"
-
- user_options = [
- ('install-dir=', 'd', "directory to install header files to"),
- ('force', 'f', "force installation (overwrite existing files)"),
- ]
-
- boolean_options = ['force']
-
- def initialize_options(self):
- self.install_dir = None
- self.force = 0
- self.outfiles = []
-
- def finalize_options(self):
- self.set_undefined_options(
- 'install', ('install_headers', 'install_dir'), ('force', 'force')
- )
-
- def run(self):
- headers = self.distribution.headers
- if not headers:
- return
-
- self.mkpath(self.install_dir)
- for header in headers:
- (out, _) = self.copy_file(header, self.install_dir)
- self.outfiles.append(out)
-
- def get_inputs(self):
- return self.distribution.headers or []
-
- def get_outputs(self):
- return self.outfiles
diff --git a/spaces/Raspberry-ai/main/.env/lib/python3.11/site-packages/setuptools/glob.py b/spaces/Raspberry-ai/main/.env/lib/python3.11/site-packages/setuptools/glob.py
deleted file mode 100644
index 87062b8187fa4f74a8c4edbaa60bd9a8b2d506a4..0000000000000000000000000000000000000000
--- a/spaces/Raspberry-ai/main/.env/lib/python3.11/site-packages/setuptools/glob.py
+++ /dev/null
@@ -1,167 +0,0 @@
-"""
-Filename globbing utility. Mostly a copy of `glob` from Python 3.5.
-
-Changes include:
- * `yield from` and PEP3102 `*` removed.
- * Hidden files are not ignored.
-"""
-
-import os
-import re
-import fnmatch
-
-__all__ = ["glob", "iglob", "escape"]
-
-
-def glob(pathname, recursive=False):
- """Return a list of paths matching a pathname pattern.
-
- The pattern may contain simple shell-style wildcards a la
- fnmatch. However, unlike fnmatch, filenames starting with a
- dot are special cases that are not matched by '*' and '?'
- patterns.
-
- If recursive is true, the pattern '**' will match any files and
- zero or more directories and subdirectories.
- """
- return list(iglob(pathname, recursive=recursive))
-
-
-def iglob(pathname, recursive=False):
- """Return an iterator which yields the paths matching a pathname pattern.
-
- The pattern may contain simple shell-style wildcards a la
- fnmatch. However, unlike fnmatch, filenames starting with a
- dot are special cases that are not matched by '*' and '?'
- patterns.
-
- If recursive is true, the pattern '**' will match any files and
- zero or more directories and subdirectories.
- """
- it = _iglob(pathname, recursive)
- if recursive and _isrecursive(pathname):
- s = next(it) # skip empty string
- assert not s
- return it
-
-
-def _iglob(pathname, recursive):
- dirname, basename = os.path.split(pathname)
- glob_in_dir = glob2 if recursive and _isrecursive(basename) else glob1
-
- if not has_magic(pathname):
- if basename:
- if os.path.lexists(pathname):
- yield pathname
- else:
- # Patterns ending with a slash should match only directories
- if os.path.isdir(dirname):
- yield pathname
- return
-
- if not dirname:
- yield from glob_in_dir(dirname, basename)
- return
- # `os.path.split()` returns the argument itself as a dirname if it is a
- # drive or UNC path. Prevent an infinite recursion if a drive or UNC path
- # contains magic characters (i.e. r'\\?\C:').
- if dirname != pathname and has_magic(dirname):
- dirs = _iglob(dirname, recursive)
- else:
- dirs = [dirname]
- if not has_magic(basename):
- glob_in_dir = glob0
- for dirname in dirs:
- for name in glob_in_dir(dirname, basename):
- yield os.path.join(dirname, name)
-
-
-# These 2 helper functions non-recursively glob inside a literal directory.
-# They return a list of basenames. `glob1` accepts a pattern while `glob0`
-# takes a literal basename (so it only has to check for its existence).
-
-
-def glob1(dirname, pattern):
- if not dirname:
- if isinstance(pattern, bytes):
- dirname = os.curdir.encode('ASCII')
- else:
- dirname = os.curdir
- try:
- names = os.listdir(dirname)
- except OSError:
- return []
- return fnmatch.filter(names, pattern)
-
-
-def glob0(dirname, basename):
- if not basename:
- # `os.path.split()` returns an empty basename for paths ending with a
- # directory separator. 'q*x/' should match only directories.
- if os.path.isdir(dirname):
- return [basename]
- else:
- if os.path.lexists(os.path.join(dirname, basename)):
- return [basename]
- return []
-
-
-# This helper function recursively yields relative pathnames inside a literal
-# directory.
-
-
-def glob2(dirname, pattern):
- assert _isrecursive(pattern)
- yield pattern[:0]
- for x in _rlistdir(dirname):
- yield x
-
-
-# Recursively yields relative pathnames inside a literal directory.
-def _rlistdir(dirname):
- if not dirname:
- if isinstance(dirname, bytes):
- dirname = os.curdir.encode('ASCII')
- else:
- dirname = os.curdir
- try:
- names = os.listdir(dirname)
- except os.error:
- return
- for x in names:
- yield x
- path = os.path.join(dirname, x) if dirname else x
- for y in _rlistdir(path):
- yield os.path.join(x, y)
-
-
-magic_check = re.compile('([*?[])')
-magic_check_bytes = re.compile(b'([*?[])')
-
-
-def has_magic(s):
- if isinstance(s, bytes):
- match = magic_check_bytes.search(s)
- else:
- match = magic_check.search(s)
- return match is not None
-
-
-def _isrecursive(pattern):
- if isinstance(pattern, bytes):
- return pattern == b'**'
- else:
- return pattern == '**'
-
-
-def escape(pathname):
- """Escape all special characters.
- """
- # Escaping is done by wrapping any of "*?[" between square brackets.
- # Metacharacters do not work in the drive part and shouldn't be escaped.
- drive, pathname = os.path.splitdrive(pathname)
- if isinstance(pathname, bytes):
- pathname = magic_check_bytes.sub(br'[\1]', pathname)
- else:
- pathname = magic_check.sub(r'[\1]', pathname)
- return drive + pathname
diff --git a/spaces/RedValis/Music-Helix/spotifysearch/urlbuilder.py b/spaces/RedValis/Music-Helix/spotifysearch/urlbuilder.py
deleted file mode 100644
index b423dc5ee93b108e9b44ac6a68202880bb3d038d..0000000000000000000000000000000000000000
--- a/spaces/RedValis/Music-Helix/spotifysearch/urlbuilder.py
+++ /dev/null
@@ -1,28 +0,0 @@
-
-# THIS FILE IS RESPONSABLE FOR BUILDING DYNAMIC URLS
-
-def search_endpoint(keywords:str, allowed_types:list,
-filters:dict, market:str, limit:int, offset:int):
- endpoint = 'https://api.spotify.com/v1/search?'
-
- # FORMAT QUERRY ITEMS AND FILTERS
- querry_items = keywords.split(' ')
- for filter, value in filters.items():
- value = value.replace(' ', '%20')
- item = f'{filter}:{value}'
- querry_items.append(item)
-
- # REQUIRED ARGUMENTS
- querry = 'q=' + '%20'.join(querry_items)
- types = 'type=' + ','.join(allowed_types)
- arguments = [querry, types]
-
- # OPTIONAL ARGUMENTS
- if market:
- arguments.append(f'market={market}')
- if limit:
- arguments.append(f'limit={limit}')
- if offset:
- arguments.append(f'offset={offset}')
-
- return endpoint + '&'.join(arguments)
diff --git a/spaces/SIGGRAPH2022/sketch2pose/src/fist_pose.py b/spaces/SIGGRAPH2022/sketch2pose/src/fist_pose.py
deleted file mode 100644
index 9c6e5be4f5a8e659b3da867c0b190a8d2ee2494f..0000000000000000000000000000000000000000
--- a/spaces/SIGGRAPH2022/sketch2pose/src/fist_pose.py
+++ /dev/null
@@ -1,444 +0,0 @@
-left_fist = [
- 0.0, 0.0, 0.0,
- 0.0, 0.0, 0.0,
- 0.0, 0.0, 0.0,
- 0.0, 0.0, 0.0,
- 0.0, 0.0, 0.0,
- 0.0, 0.0, 0.0,
- 0.0, 0.0, 0.0,
- 0.0, 0.0, 0.0,
- 0.0, 0.0, 0.0,
- 0.0, 0.0, 0.0,
- 0.0, 0.0, 0.0,
- 0.0, 0.0, 0.0,
- 0.0, 0.0, 0.0,
- 0.0, 0.0, 0.0,
- 0.0, 0.0, 0.0,
- 0.0, 0.0, 0.0,
- 0.0, 0.0, 0.0,
- 0.0, 0.0, 0.0,
- 0.0, 0.0, 0.0,
- 0.0, 0.0, 0.0,
- 0.0, 0.0, 0.0,
- 0.0, 0.0, 0.0,
- 0.0, 0.0, 0.0,
- 0.0, 0.0, 0.0,
- 0.0, 0.0, 0.0,
- 0.4183167815208435, 0.10645648092031479, -1.6593892574310303,
- 0.15252035856246948, -0.14700782299041748, -1.3719955682754517,
- -0.04432843625545502, -0.15799851715564728, -0.938068151473999,
- -0.12218914180994034, 0.073341965675354, -1.6415189504623413,
- -0.14376045763492584, 0.1927780956029892, -1.3593589067459106,
- -0.0851994976401329, 0.01652289740741253, -0.7474589347839355,
- -0.9881719946861267, -0.3987707793712616, -1.3535722494125366,
- -0.6686224937438965, 0.1261960119009018, -1.080643892288208,
- -0.8101894855499268, -0.1306752860546112, -0.8412265777587891,
- -0.3495230972766876, -0.17784251272678375, -1.4433038234710693,
- -0.46278536319732666, 0.13677796721458435, -1.467200517654419,
- -0.3681888282299042, 0.003404417773708701, -0.7764251232147217,
- 0.850964367389679, 0.2769227623939514, -0.09154807031154633,
- 0.14500413835048676, 0.09604815393686295, 0.219278022646904,
- 1.0451993942260742, 0.16911321878433228, -0.2426234930753708,
- 0.11167845129966736, -0.04289207234978676, 0.41644084453582764,
- 0.10881128907203674, 0.06598565727472305, 0.756219744682312,
- -0.0963931530714035, 0.09091583639383316, 0.18845966458320618,
- -0.11809506267309189, -0.050943851470947266, 0.5295845866203308,
- -0.14369848370552063, -0.055241718888282776, 0.704857349395752,
- -0.019182899966835976, 0.0923367589712143, 0.3379131853580475,
- -0.45703303813934326, 0.1962839663028717, 0.6254575848579407,
- -0.21465237438678741, 0.06599827855825424, 0.5068942308425903,
- -0.36972442269325256, 0.0603446289896965, 0.07949023693799973,
- -0.14186954498291016, 0.08585254102945328, 0.6355276107788086,
- -0.3033415675163269, 0.05788097903132439, 0.6313892006874084,
- -0.17612087726593018, 0.13209305703639984, 0.3733545243740082,
- 0.850964367389679, -0.2769227623939514, 0.09154807031154633,
- -0.4998386800289154, -0.026556432247161865, -0.052880801260471344,
- 0.5355585217475891, -0.045960985124111176, 0.27735769748687744,
-]
-
-left_right_fist = [
- 0.0, 0.0, 0.0,
- 0.0, 0.0, 0.0,
- 0.0, 0.0, 0.0,
- 0.0, 0.0, 0.0,
- 0.0, 0.0, 0.0,
- 0.0, 0.0, 0.0,
- 0.0, 0.0, 0.0,
- 0.0, 0.0, 0.0,
- 0.0, 0.0, 0.0,
- 0.0, 0.0, 0.0,
- 0.0, 0.0, 0.0,
- 0.0, 0.0, 0.0,
- 0.0, 0.0, 0.0,
- 0.0, 0.0, 0.0,
- 0.0, 0.0, 0.0,
- 0.0, 0.0, 0.0,
- 0.0, 0.0, 0.0,
- 0.0, 0.0, 0.0,
- 0.0, 0.0, 0.0,
- 0.0, 0.0, 0.0,
- 0.0, 0.0, 0.0,
- 0.0, 0.0, -0.0,
- 0.0, 0.0, 0.0,
- 0.0, 0.0, 0.0,
- 0.0, 0.0, 0.0,
- 0.4183167815208435, 0.10645648092031479, -1.6593892574310303,
- 0.15252035856246948, -0.14700782299041748, -1.3719955682754517,
- -0.04432843625545502, -0.15799851715564728, -0.938068151473999,
- -0.12218914180994034, 0.073341965675354, -1.6415189504623413,
- -0.14376045763492584, 0.1927780956029892, -1.3593589067459106,
- -0.0851994976401329, 0.01652289740741253, -0.7474589347839355,
- -0.9881719946861267, -0.3987707793712616, -1.3535722494125366,
- -0.6686224937438965, 0.1261960119009018, -1.080643892288208,
- -0.8101894855499268, -0.1306752860546112, -0.8412265777587891,
- -0.3495230972766876, -0.17784251272678375, -1.4433038234710693,
- -0.46278536319732666, 0.13677796721458435, -1.467200517654419,
- -0.3681888282299042, 0.003404417773708701, -0.7764251232147217,
- 0.850964367389679, 0.2769227623939514, -0.09154807031154633,
- 0.14500413835048676, 0.09604815393686295, 0.219278022646904,
- 1.0451993942260742, 0.16911321878433228, -0.2426234930753708,
- 0.4183167815208435, -0.10645647346973419, 1.6593892574310303,
- 0.15252038836479187, 0.14700786769390106, 1.3719956874847412,
- -0.04432841017842293, 0.15799842774868011, 0.9380677938461304,
- -0.12218913435935974, -0.0733419880270958, 1.6415191888809204,
- -0.14376048743724823, -0.19277812540531158, 1.3593589067459106,
- -0.08519953489303589, -0.016522908583283424, 0.7474592328071594,
- -0.9881719350814819, 0.3987707495689392, 1.3535723686218262,
- -0.6686226725578308, -0.12619605660438538, 1.080644130706787,
- -0.8101896643638611, 0.1306752860546112, 0.8412266373634338,
- -0.34952324628829956, 0.17784248292446136, 1.443304181098938,
- -0.46278542280197144, -0.13677802681922913, 1.467200517654419,
- -0.36818885803222656, -0.0034044249914586544, 0.7764251232147217,
- 0.8509642481803894, -0.2769228219985962, 0.09154807776212692,
- 0.14500458538532257, -0.09604845196008682, -0.21927869319915771,
- 1.0451991558074951, -0.1691131889820099, 0.242623433470726,
-]
-
-right_fist = []
-for lf, lrf in zip(left_fist, left_right_fist):
- if lf != lrf:
- right_fist.append(lrf)
- else:
- right_fist.append(0)
-
-
-left_flat_up = [
- 0.0, 0.0, 0.0,
- 0.0, 0.0, 0.0,
- 0.0, 0.0, 0.0,
- 0.0, 0.0, 0.0,
- 0.0, 0.0, 0.0,
- 0.0, 0.0, 0.0,
- 0.0, 0.0, 0.0,
- 0.0, 0.0, 0.0,
- 0.0, 0.0, 0.0,
- 0.0, 0.0, 0.0,
- 0.0, 0.0, 0.0,
- 0.0, 0.0, 0.0,
- 0.0, 0.0, 0.0,
- 0.0, 0.0, 0.0,
- 0.0, 0.0, 0.0,
- 0.0, 0.0, 0.0,
- 0.0, 0.0, 0.0,
- 0.0, 0.0, 0.0,
- 0.0, 0.0, 0.0,
- 0.0, 0.0, 0.0,
- 0.0, 0, 1.5129635334014893,
- 0.0, 0.0, 0.0,
- 0.0, 0.0, 0.0,
- 0.0, 0.0, 0.0,
- 0.0, 0.0, 0.0,
- 0.0, 0.0, 0.0,
- 0.0, 0.0, 0.0,
- 0.0, 0.0, 0.0,
- 0.0, 0.0, 0.0,
- 0.0, 0.0, 0.0,
- 0.0, 0.0, 0.0,
- 0.0, 0.0, 0.0,
- 0.0, 0.0, 0.0,
- 0.0, 0.0, 0.0,
- 0.0, 0.0, 0.0,
- 0.0, 0.0, 0.0,
- 0.0, 0.0, 0.0,
- 0.0, 0.0, 0.0,
- 0.0, 0.0, 0.0,
- 0.0, 0.0, 0.0,
- 0.0, 0.0, 0.0,
- 0.0, 0.0, 0.0,
- 0.0, 0.0, 0.0,
- 0.0, 0.0, 0.0,
- 0.0, 0.0, 0.0,
- 0.0, 0.0, 0.0,
- 0.0, 0.0, 0.0,
- 0.0, 0.0, 0.0,
- 0.0, 0.0, 0.0,
- 0.0, 0.0, 0.0,
- 0.0, 0.0, 0.0,
- 0.0, 0.0, 0.0,
- 0.0, 0.0, 0.0,
- 0.0, 0.0, 0.0,
- 0.0, 0.0, 0.0,
-]
-
-left_flat_down = [
- 0.0, 0.0, 0.0,
- 0.0, 0.0, 0.0,
- 0.0, 0.0, 0.0,
- 0.0, 0.0, 0.0,
- 0.0, 0.0, 0.0,
- 0.0, 0.0, 0.0,
- 0.0, 0.0, 0.0,
- 0.0, 0.0, 0.0,
- 0.0, 0.0, 0.0,
- 0.0, 0.0, 0.0,
- 0.0, 0.0, 0.0,
- 0.0, 0.0, 0.0,
- 0.0, 0.0, 0.0,
- 0.0, 0.0, 0.0,
- 0.0, 0.0, 0.0,
- 0.0, 0.0, 0.0,
- 0.0, 0.0, 0.0,
- 0.0, 0.0, 0.0,
- 0.0, 0.0, 0.0,
- 0.0, 0.0, 0.0,
- 0.0, 0, -1.4648663997650146,
- 0.0, 0.0, 0.0,
- 0.0, 0.0, 0.0,
- 0.0, 0.0, 0.0,
- 0.0, 0.0, 0.0,
- 0.0, 0.0, 0.0,
- 0.0, 0.0, 0.0,
- 0.0, 0.0, 0.0,
- 0.0, 0.0, 0.0,
- 0.0, 0.0, 0.0,
- 0.0, 0.0, 0.0,
- 0.0, 0.0, 0.0,
- 0.0, 0.0, 0.0,
- 0.0, 0.0, 0.0,
- 0.0, 0.0, 0.0,
- 0.0, 0.0, 0.0,
- 0.0, 0.0, 0.0,
- 0.0, 0.0, 0.0,
- 0.0, 0.0, 0.0,
- 0.0, 0.0, 0.0,
- 0.0, 0.0, 0.0,
- 0.0, 0.0, 0.0,
- 0.0, 0.0, 0.0,
- 0.0, 0.0, 0.0,
- 0.0, 0.0, 0.0,
- 0.0, 0.0, 0.0,
- 0.0, 0.0, 0.0,
- 0.0, 0.0, 0.0,
- 0.0, 0.0, 0.0,
- 0.0, 0.0, 0.0,
- 0.0, 0.0, 0.0,
- 0.0, 0.0, 0.0,
- 0.0, 0.0, 0.0,
- 0.0, 0.0, 0.0,
- 0.0, 0.0, 0.0,
-]
-
-right_flat_up = [
- 0.0, 0.0, 0.0,
- 0.0, 0.0, 0.0,
- 0.0, 0.0, 0.0,
- 0.0, 0.0, 0.0,
- 0.0, 0.0, 0.0,
- 0.0, 0.0, 0.0,
- 0.0, 0.0, 0.0,
- 0.0, 0.0, 0.0,
- 0.0, 0.0, 0.0,
- 0.0, 0.0, 0.0,
- 0.0, 0.0, 0.0,
- 0.0, 0.0, 0.0,
- 0.0, 0.0, 0.0,
- 0.0, 0.0, 0.0,
- 0.0, 0.0, 0.0,
- 0.0, 0.0, 0.0,
- 0.0, 0.0, 0.0,
- 0.0, 0.0, 0.0,
- 0.0, 0.0, 0.0,
- 0.0, 0.0, 0.0,
- 0.0, 0.0, 0.0,
- 0.0, 0, -1.5021973848342896,
- 0.0, 0.0, 0.0,
- 0.0, 0.0, 0.0,
- 0.0, 0.0, 0.0,
- 0.0, 0.0, 0.0,
- 0.0, 0.0, 0.0,
- 0.0, 0.0, 0.0,
- 0.0, 0.0, 0.0,
- 0.0, 0.0, 0.0,
- 0.0, 0.0, 0.0,
- 0.0, 0.0, 0.0,
- 0.0, 0.0, 0.0,
- 0.0, 0.0, 0.0,
- 0.0, 0.0, 0.0,
- 0.0, 0.0, 0.0,
- 0.0, 0.0, 0.0,
- 0.0, 0.0, 0.0,
- 0.0, 0.0, 0.0,
- 0.0, 0.0, 0.0,
- 0.0, 0.0, 0.0,
- 0.0, 0.0, 0.0,
- 0.0, 0.0, 0.0,
- 0.0, 0.0, 0.0,
- 0.0, 0.0, 0.0,
- 0.0, 0.0, 0.0,
- 0.0, 0.0, 0.0,
- 0.0, 0.0, 0.0,
- 0.0, 0.0, 0.0,
- 0.0, 0.0, 0.0,
- 0.0, 0.0, 0.0,
- 0.0, 0.0, 0.0,
- 0.0, 0.0, 0.0,
- 0.0, 0.0, 0.0,
- 0.0, 0.0, 0.0,
-]
-
-right_flat_down = [
- 0.0, 0.0, 0.0,
- 0.0, 0.0, 0.0,
- 0.0, 0.0, 0.0,
- 0.0, 0.0, 0.0,
- 0.0, 0.0, 0.0,
- 0.0, 0.0, 0.0,
- 0.0, 0.0, 0.0,
- 0.0, 0.0, 0.0,
- 0.0, 0.0, 0.0,
- 0.0, 0.0, 0.0,
- 0.0, 0.0, 0.0,
- 0.0, 0.0, 0.0,
- 0.0, 0.0, 0.0,
- 0.0, 0.0, 0.0,
- 0.0, 0.0, 0.0,
- 0.0, 0.0, 0.0,
- 0.0, 0.0, 0.0,
- 0.0, 0.0, 0.0,
- 0.0, 0.0, 0.0,
- 0.0, 0.0, 0.0,
- 0.0, 0.0, 0.0,
- 0, 0, 1.494218111038208,
- 0.0, 0.0, 0.0,
- 0.0, 0.0, 0.0,
- 0.0, 0.0, 0.0,
- 0.0, 0.0, 0.0,
- 0.0, 0.0, 0.0,
- 0.0, 0.0, 0.0,
- 0.0, 0.0, 0.0,
- 0.0, 0.0, 0.0,
- 0.0, 0.0, 0.0,
- 0.0, 0.0, 0.0,
- 0.0, 0.0, 0.0,
- 0.0, 0.0, 0.0,
- 0.0, 0.0, 0.0,
- 0.0, 0.0, 0.0,
- 0.0, 0.0, 0.0,
- 0.0, 0.0, 0.0,
- 0.0, 0.0, 0.0,
- 0.0, 0.0, 0.0,
- 0.0, 0.0, 0.0,
- 0.0, 0.0, 0.0,
- 0.0, 0.0, 0.0,
- 0.0, 0.0, 0.0,
- 0.0, 0.0, 0.0,
- 0.0, 0.0, 0.0,
- 0.0, 0.0, 0.0,
- 0.0, 0.0, 0.0,
- 0.0, 0.0, 0.0,
- 0.0, 0.0, 0.0,
- 0.0, 0.0, 0.0,
- 0.0, 0.0, 0.0,
- 0.0, 0.0, 0.0,
- 0.0, 0.0, 0.0,
- 0.0, 0.0, 0.0,
-]
-
-relaxed = [
- 0.0, 0.0, 0.0,
- 0.0, 0.0, 0.0,
- 0.0, 0.0, 0.0,
- 0.0, 0.0, 0.0,
- 0.0, 0.0, 0.0,
- 0.0, 0.0, 0.0,
- 0.0, 0.0, 0.0,
- 0.0, 0.0, 0.0,
- 0.0, 0.0, 0.0,
- 0.0, 0.0, 0.0,
- 0.0, 0.0, 0.0,
- 0.0, 0.0, 0.0,
- 0.0, 0.0, 0.0,
- 0.0, 0.0, 0.0,
- 0.0, 0.0, 0.0,
- 0.0, 0.0, 0.0,
- 0.0, 0.0, 0.0,
- 0.0, 0.0, 0.0,
- 0.0, 0.0, 0.0,
- 0.0, 0.0, 0.0,
- 0.0, 0.0, 0.0,
- 0.0, 0.0, 0.0,
- 0.0, 0.0, 0.0,
- 0.0, 0.0, 0.0,
- 0.0, 0.0, 0.0,
- 0.11167845129966736, 0.04289207234978676, -0.41644084453582764,
- 0.10881128907203674, -0.06598565727472305, -0.756219744682312,
- -0.0963931530714035, -0.09091583639383316, -0.18845966458320618,
- -0.11809506267309189, 0.050943851470947266, -0.5295845866203308,
- -0.14369848370552063, 0.055241718888282776, -0.704857349395752,
- -0.019182899966835976, -0.0923367589712143, -0.3379131853580475,
- -0.45703303813934326, -0.1962839663028717, -0.6254575848579407,
- -0.21465237438678741, -0.06599827855825424, -0.5068942308425903,
- -0.36972442269325256, -0.0603446289896965, -0.07949023693799973,
- -0.14186954498291016, -0.08585254102945328, -0.6355276107788086,
- -0.3033415675163269, -0.05788097903132439, -0.6313892006874084,
- -0.17612087726593018, -0.13209305703639984, -0.3733545243740082,
- 0.850964367389679, 0.2769227623939514, -0.09154807031154633,
- -0.4998386800289154, 0.026556432247161865, 0.052880801260471344,
- 0.5355585217475891, 0.045960985124111176, -0.27735769748687744,
- 0.11167845129966736, -0.04289207234978676, 0.41644084453582764,
- 0.10881128907203674, 0.06598565727472305, 0.756219744682312,
- -0.0963931530714035, 0.09091583639383316, 0.18845966458320618,
- -0.11809506267309189, -0.050943851470947266, 0.5295845866203308,
- -0.14369848370552063, -0.055241718888282776, 0.704857349395752,
- -0.019182899966835976, 0.0923367589712143, 0.3379131853580475,
- -0.45703303813934326, 0.1962839663028717, 0.6254575848579407,
- -0.21465237438678741, 0.06599827855825424, 0.5068942308425903,
- -0.36972442269325256, 0.0603446289896965, 0.07949023693799973,
- -0.14186954498291016, 0.08585254102945328, 0.6355276107788086,
- -0.3033415675163269, 0.05788097903132439, 0.6313892006874084,
- -0.17612087726593018, 0.13209305703639984, 0.3733545243740082,
- 0.850964367389679, -0.2769227623939514, 0.09154807031154633,
- -0.4998386800289154, -0.026556432247161865, -0.052880801260471344,
- 0.5355585217475891, -0.045960985124111176, 0.27735769748687744,
-]
-
-# body joints + left arm + right arm
-# 25 + 15 + 15
-# smpl(left_hand_pose, right_hand_pose)
-
-left_start = 25 * 3
-left_end = left_start + 15 * 3
-right_end = left_end + 15 * 3
-
-LEFT_FIST = left_fist[left_start:left_end]
-RIGHT_FIST = right_fist[left_end:right_end]
-
-LEFT_FLAT_UP = left_flat_up[20 * 3 : 20 * 3 + 3]
-LEFT_FLAT_DOWN = left_flat_down[20 * 3 : 20 * 3 + 3]
-
-RIGHT_FLAT_UP = right_flat_up[21 * 3 : 21 * 3 + 3]
-RIGHT_FLAT_DOWN = right_flat_down[21 * 3 : 21 * 3 + 3]
-
-LEFT_RELAXED = relaxed[left_start:left_end]
-RIGHT_RELAXED = relaxed[left_end:right_end]
-
-INT_TO_FIST = {
- "lfl": None,
- "lf": LEFT_FIST,
- "lu": LEFT_FLAT_UP,
- "ld": LEFT_FLAT_DOWN,
- "rfl": None,
- "rf": RIGHT_FIST,
- "ru": RIGHT_FLAT_UP,
- "rd": RIGHT_FLAT_DOWN,
-}
diff --git a/spaces/SMD00/Image_Summarizer/app.py b/spaces/SMD00/Image_Summarizer/app.py
deleted file mode 100644
index cb24ed37a90cbe8421360a35b29aabec7caa90e7..0000000000000000000000000000000000000000
--- a/spaces/SMD00/Image_Summarizer/app.py
+++ /dev/null
@@ -1,203 +0,0 @@
-import gradio as gr
-from PIL import Image
-import pytesseract
-import torch
-import numpy as np
-import nltk
-nltk.download('stopwords')
-nltk.download('punkt')
-from nltk.corpus import stopwords
-from nltk.cluster.util import cosine_distance
-import networkx as nx
-from transformers import pipeline
-
-
-if torch.cuda.is_available():
- device = torch.device("cuda")
-else:
- device = torch.device("cpu")
-
-
-summarizer = pipeline("summarization", model="facebook/bart-large-cnn")
-
-def read(filepath):
- return pytesseract.image_to_string(Image.open(filepath))
-
-def clean_text(text):
- article = text.split(".")
- article=[sentence for sentence in article if sentence!=""]
-
- sentences = []
-
- for sentence in article:
- sentence=sentence.replace(",", " , ").replace("'", " ' ").split(" ")
- sentence=[word for word in sentence if word!=""]
- sentences.append(sentence)
-
- return sentences
-
-def sentence_similarity(sent1, sent2, stopwords): #Creating words in sentences to one hot encoding and then finding cosine distance between the vectors inorder to measure closeness
-
- if stopwords is None:
- stopwords = []
-
- sent1 = [w.lower() for w in sent1]
- sent2 = [w.lower() for w in sent2]
-
- all_words = list(set(sent1 + sent2))
-
- vector1 = [0] * len(all_words)
- vector2 = [0] * len(all_words)
-
- # build the vector for the first sentence
- for w in sent1:
- if w in stopwords:
- continue
- vector1[all_words.index(w)] += 1
-
- # build the vector for the second sentence
- for w in sent2:
- if w in stopwords:
- continue
- vector2[all_words.index(w)] += 1
- if np.isnan(1 - cosine_distance(vector1, vector2)):
- return 0
- return 1 - cosine_distance(vector1, vector2)
-
-
-def build_similarity_matrix(sentences, stop_words):
-
- # Create an empty similarity matrix
- similarity_matrix = np.zeros((len(sentences), len(sentences)))
-
- for idx1 in range(len(sentences)):
- for idx2 in range(len(sentences)):
- if idx1 == idx2: #ignore if both are same sentences
- continue
- similarity_matrix[idx1][idx2] = sentence_similarity(sentences[idx1], sentences[idx2], stop_words)
-
- return similarity_matrix
-
-def sentences(text, top_n="auto"):
-
- # Step 1 - Clean text to generate sentences
-
- sentences=clean_text(text)
- stop_words = stopwords.words('english')
- stop_words.append(".")
- stop_words.append(",")
- summarize_text = []
-
- # Step 2 - Generate Similary Martix across sentences
-
- sentence_similarity_martix = build_similarity_matrix(sentences, stop_words)
- # print(sentence_similarity_martix)
-
- # Step 3 - Rank sentences in similarity martix
-
- sentence_similarity_graph = nx.from_numpy_array(sentence_similarity_martix)
- # print(sentence_similarity_graph)
-
- scores = nx.pagerank(sentence_similarity_graph)
- # print(scores)
-
- # Step 4 - Sort the rank and pick top sentences
-
- ranked_sentence = sorted(((scores[i],s) for i,s in enumerate(sentences)), reverse=True) #Sorting the scores in decending order
- # print("Indexes of top ranked_sentence order are ", ranked_sentence)
-
- if top_n=="auto": top_n=len(ranked_sentence)
- else: top_n=int(top_n)
-
- for i in range(top_n):
- ranked_sentence[i][1][0]=ranked_sentence[i][1][0].capitalize() #Capitalising 1st letter of sentence
- # print(ranked_sentence[i][1][0])
- summarize_text.append(" ".join(ranked_sentence[i][1]))
-
- # Step 5 - Offcourse, output the summarized text
-
- extractive_summarized=". ".join(summarize_text).replace(" , ",", ").replace(" ' ","'") + "."
- return extractive_summarized
-
-def important_sentences(filepath, no_of_sentences=5):
- extractedInformation=read(filepath)
- extractedInformation=' '.join(extractedInformation.split('\n'))
- try:
- extractive_summary=sentences(extractedInformation, no_of_sentences)
- except:
- extractive_summary=sentences(extractedInformation,"auto")
- text=""
- for index,sent in enumerate(extractive_summary.split(".")):
- if sent!='':text+=str(index+1)+". "+str(sent).strip()+".\n\n"
- return (gr.Textbox.update(text),gr.Button.update(visible=False),gr.Textbox.update(visible=False),gr.Dropdown.update(visible=False))
-
-def summarize(filepath):
- extractedInformation=read(filepath)
- extractedInformation=' '.join(extractedInformation.split('\n'))
- abstractive_summary = summarizer(extractedInformation, max_length=int(len(extractedInformation)/6), min_length=int(len(extractedInformation)/10), do_sample=False)
- return (gr.Textbox.update(abstractive_summary[0]["summary_text"]),gr.Button.update(visible=False),gr.Textbox.update(visible=False),gr.Dropdown.update(visible=False))
-
-def Question_Answer(filepath,question,mod):
- extractedInformation=read(filepath)
- extractedInformation=' '.join(extractedInformation.split('\n'))
- if mod=="Roberta":
- question_answerer = pipeline("question-answering", model="SMD00/QA_model-roberta")
- else :
- question_answerer = pipeline("question-answering", model="SMD00/QA_model-distilbert")
- obj=question_answerer(question=question, context=extractedInformation)
- return obj['answer']
-
-def show_fn():
- return (gr.Textbox.update(visible=True),gr.Button.update(visible=True),gr.Dropdown.update(visible=True),gr.Textbox.update(""))
-def dummy_fn(x):
- return x
-
-with gr.Blocks() as demo:
- gr.Markdown("# **PicSum**")
- gr.Markdown("Gradio demo for PicSum project. You can give an image as input and select any of the three buttons. It generates summary, important sentences and answers questions related to context.")
- img=gr.components.Image(type="filepath", label="Input Image")
-
- with gr.Row():
- summary_btn = gr.Button(value="Summary")
- sentence_btn = gr.Button(value="Important Sentences")
- quesAndAns_btn = gr.Button(value="Question and Answers")
-
- mode=gr.Dropdown(["Roberta","DistilBert"],label="Model",info="Choose a model",visible=False)
- ques_box = gr.Textbox(label="Question",info="Enter a Question",interactive=True,visible=False)
- submit_btn= gr.Button(value="Submit",visible=False)
- out_box=gr.Textbox(label="Generated Text")
- summary_btn.click(fn=summarize,inputs=[img],outputs=[out_box,submit_btn,ques_box,mode])
- sentence_btn.click(fn=important_sentences,inputs=[img],outputs=[out_box,submit_btn,ques_box,mode])
- quesAndAns_btn.click(fn=show_fn,outputs=[submit_btn,ques_box,mode,out_box])
- submit_btn.click(fn=Question_Answer,inputs=[img,ques_box,mode],outputs=[out_box])
- gr.Markdown("## Image Examples")
- with gr.Row():
- gr.Examples(
- examples=[ "a.png"],
- inputs=img,
- outputs=img,
- fn=dummy_fn,
- cache_examples=True,
- )
- gr.Examples(
- examples=[ "b.png"],
- inputs=img,
- outputs=img,
- fn=dummy_fn,
- cache_examples=True,
- )
- gr.Examples(
- examples=[ "c.png"],
- inputs=img,
- outputs=img,
- fn=dummy_fn,
- cache_examples=True,
- )
- gr.Examples(
- examples=[ "d.png"],
- inputs=img,
- outputs=img,
- fn=dummy_fn,
- cache_examples=True,
- )
-demo.launch(debug=True)
\ No newline at end of file
diff --git a/spaces/SeViLA/SeViLA/lavis/tasks/image_text_pretrain.py b/spaces/SeViLA/SeViLA/lavis/tasks/image_text_pretrain.py
deleted file mode 100644
index 218c535682b4382c8fde54887dc2e55107465c7f..0000000000000000000000000000000000000000
--- a/spaces/SeViLA/SeViLA/lavis/tasks/image_text_pretrain.py
+++ /dev/null
@@ -1,18 +0,0 @@
-"""
- Copyright (c) 2022, salesforce.com, inc.
- All rights reserved.
- SPDX-License-Identifier: BSD-3-Clause
- For full license text, see the LICENSE file in the repo root or https://opensource.org/licenses/BSD-3-Clause
-"""
-
-from lavis.common.registry import registry
-from lavis.tasks.base_task import BaseTask
-
-
-@registry.register_task("image_text_pretrain")
-class ImageTextPretrainTask(BaseTask):
- def __init__(self):
- super().__init__()
-
- def evaluation(self, model, data_loader, cuda_enabled=True):
- pass
diff --git a/spaces/ShadowDominator/extract-photos-from-pdf/README.md b/spaces/ShadowDominator/extract-photos-from-pdf/README.md
deleted file mode 100644
index dcf45be87fd83a66a37ac1d0e023719a19062392..0000000000000000000000000000000000000000
--- a/spaces/ShadowDominator/extract-photos-from-pdf/README.md
+++ /dev/null
@@ -1,12 +0,0 @@
----
-title: Extract Photos From Pdf
-emoji: 🏃
-colorFrom: green
-colorTo: green
-sdk: gradio
-sdk_version: 3.29.0
-app_file: app.py
-pinned: false
----
-
-Check out the configuration reference at https://huggingface.co/docs/hub/spaces-config-reference
diff --git a/spaces/Solomon-y/img-to-music/share_btn.py b/spaces/Solomon-y/img-to-music/share_btn.py
deleted file mode 100644
index 1a2ac6a6e74b114dbd54c2f24723a87180db51ef..0000000000000000000000000000000000000000
--- a/spaces/Solomon-y/img-to-music/share_btn.py
+++ /dev/null
@@ -1,100 +0,0 @@
-community_icon_html = """"""
-
-loading_icon_html = """"""
-
-share_js = """async () => {
- async function uploadFile(file){
- const UPLOAD_URL = 'https://huggingface.co/uploads';
- const response = await fetch(UPLOAD_URL, {
- method: 'POST',
- headers: {
- 'Content-Type': file.type,
- 'X-Requested-With': 'XMLHttpRequest',
- },
- body: file, /// <- File inherits from Blob
- });
- const url = await response.text();
- return url;
- }
- async function getInputImgFile(imgEl){
- const res = await fetch(imgEl.src);
- const blob = await res.blob();
- const imgId = Date.now() % 200;
- const isPng = imgEl.src.startsWith(`data:image/png`);
- if(isPng){
- const fileName = `sd-perception-${{imgId}}.png`;
- return new File([blob], fileName, { type: 'image/png' });
- }else{
- const fileName = `sd-perception-${{imgId}}.jpg`;
- return new File([blob], fileName, { type: 'image/jpeg' });
- }
- }
- async function getOutputMusicFile(audioEL){
- const res = await fetch(audioEL.src);
- const blob = await res.blob();
- const audioId = Date.now() % 200;
- const fileName = `img-to-music-${{audioId}}.wav`;
- const musicBlob = new File([blob], fileName, { type: 'audio/wav' });
- console.log(musicBlob);
- return musicBlob;
- }
-
- async function audioToBase64(audioFile) {
- return new Promise((resolve, reject) => {
- let reader = new FileReader();
- reader.readAsDataURL(audioFile);
- reader.onload = () => resolve(reader.result);
- reader.onerror = error => reject(error);
-
- });
- }
- const gradioEl = document.querySelector('body > gradio-app');
- // const gradioEl = document.querySelector("gradio-app").shadowRoot;
- const inputImgEl = gradioEl.querySelector('#input-img img');
- const outputMusic = gradioEl.querySelector('#music-output audio');
- const outputMusic_src = gradioEl.querySelector('#music-output audio').src;
- const outputMusic_name = outputMusic_src.split('/').pop();
- let titleTxt = outputMusic_name;
- //if(titleTxt.length > 100){
- // titleTxt = titleTxt.slice(0, 100) + ' ...';
- //}
- const shareBtnEl = gradioEl.querySelector('#share-btn');
- const shareIconEl = gradioEl.querySelector('#share-btn-share-icon');
- const loadingIconEl = gradioEl.querySelector('#share-btn-loading-icon');
- if(!outputMusic){
- return;
- };
- shareBtnEl.style.pointerEvents = 'none';
- shareIconEl.style.display = 'none';
- loadingIconEl.style.removeProperty('display');
- const inputFile = await getInputImgFile(inputImgEl);
- const urlInputImg = await uploadFile(inputFile);
- const musicFile = await getOutputMusicFile(outputMusic);
- const dataOutputMusic = await uploadFile(musicFile);
-
- const descriptionMd = `#### Input img:
-
-
-#### Music:
-
-
-`;
- const params = new URLSearchParams({
- title: titleTxt,
- description: descriptionMd,
- });
- const paramsStr = params.toString();
- window.open(`https://huggingface.co/spaces/fffiloni/img-to-music/discussions/new?${paramsStr}`, '_blank');
- shareBtnEl.style.removeProperty('pointer-events');
- shareIconEl.style.removeProperty('display');
- loadingIconEl.style.display = 'none';
-}"""
\ No newline at end of file
diff --git a/spaces/Stephen2022/daxing/README.md b/spaces/Stephen2022/daxing/README.md
deleted file mode 100644
index 20aba09f80ff594c8b68f1de9662b21f05b9d9d9..0000000000000000000000000000000000000000
--- a/spaces/Stephen2022/daxing/README.md
+++ /dev/null
@@ -1,148 +0,0 @@
----
-title: LabelStudio
-emoji: 🟧
-colorFrom: yellow
-colorTo: purple
-sdk: docker
-tags:
-- label-studio
-fullwidth: true
-license: apache-2.0
-app_port: 8080
-duplicated_from: LabelStudio/LabelStudio
----
-
-
-[Website](https://hubs.ly/Q01CNgsd0) • [Docs](https://hubs.ly/Q01CN9Yq0) • [12K+ GitHub ⭐️!](https://hubs.ly/Q01CNbPQ0) • [Slack Community](https://hubs.ly/Q01CNb9H0)
-
-## What is Label Studio?
-
-Label Studio is an open source data labeling platform. It lets you label audio,
-text, images, videos, and time series data with a simple, straightforward, and
-highly-configurable user interface. Label Studio can prepare new data or
-improve existing training data to get more accurate ML models.
-
-
-## Label Studio in Hugging Face Spaces
-
-The Label Studio community is thrilled to offer Label Studio as a Hugging Face
-Spaces application. You can try the data-annotation interface, connect popular
-machine learning models, and share the application with collaborators. You can
-start immediately by creating an account or replicate the space and work in
-your own environment.
-
-## Creating a Use Account and Logging In
-
-Begin by creating a new account in the Label Studio space, then log in with your
-credentials.
-
-**By default, these spaces permit anyone to create a new login
-account, allowing them to view and modify project configuration, data sets, and
-annotations. Without any modifications, treat this space like a demo environment.**
-
-## Creating a Labeling Project
-
-After logging in, Label Studio will present you with a project view. Here you
-can create a new project with prompts to upload data and set up a custom
-configuration interface.
-
-**Note that in the default configuration, storage is local and temporary. Any
-projects, annotations, and configurations will be lost if the space is restarted.**
-
-## Next Steps and Additional Resources
-
-To help with getting started, the Label Studio community curated a list of
-resources including tutorials and documentation.
-
-- 🚀 [Zero to One with Label Studio Tutorial](https://labelstud.io/blog/introduction-to-label-studio-in-hugging-face-spaces/)
-- 📈 [Try Label Studio Enterprise](https://hubs.ly/Q01CMLll0)
-- 🤗 [Tutorial: Using Label Studio with Hugging Face Datasets Hub](https://danielvanstrien.xyz/huggingface/huggingface-datasets/annotation/full%20stack%20deep%20learning%20notes/2022/09/07/label-studio-annotations-hub.html)
-- 💡 [Label Studio Docs](https://hubs.ly/Q01CN9Yq0)
-
-
-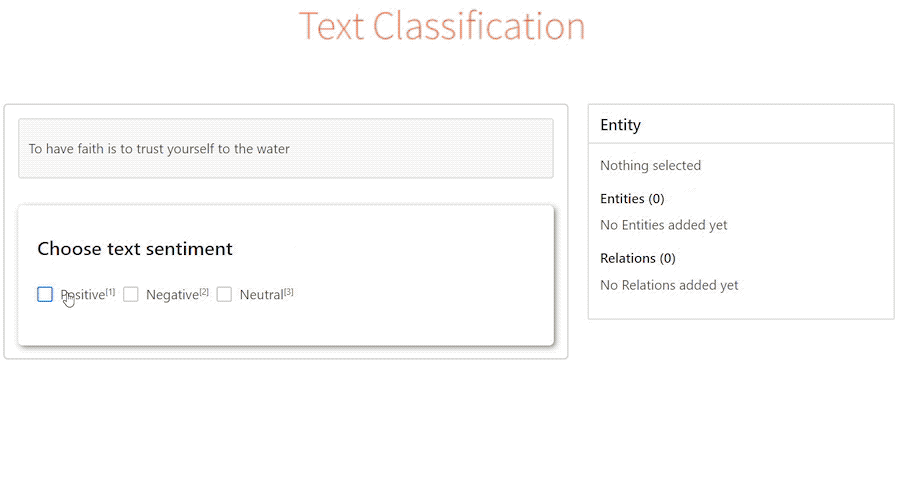
-
-### Making your Label Studio Hugging Face Space production-ready
-
-By default this space allows for the unrestricted creation of new accounts
-will full access to all projects and data. This is great for trying out
-Label Studio and collaborating on projects, but you may want to restrict
-access to your space to only authorized users. Add the following environment
-variable to your spaces Dockerfile to disable public account creation for
-this space.
-
- ENV LABEL_STUDIO_DISABLE_SIGNUP_WITHOUT_LINK=true
-
-Set secrets in your space to create an inital user, and log in with your
-provided username and password. Do not set these in your Dockerfile, as they
-globally visible on a public space.
-
- LABEL_STUDIO_USERNAME
- LABEL_STUDIO_PASSWORD
-
-You will need to provide new users with an invitation link to join the space,
-which can be found in the Organizations interface of Label Studio
-
-By default this space stores all project configuration and data annotations
-in local storage with Sqlite. If the space is reset, all configuration and
-annotation data in the space will be lost. You can enable configuration
-persistence by connecting an external Postgres database to your space,
-guaranteeing that all project and annotation settings are preserved.
-
-Set the following secret variables to match your own hosted instance of
-Postgres. We strongly recommend setting these as secrets to prevent leaking
-information about your database service to the public in your spaces
-definition.
-
- DJANGO_DB=default
- POSTGRE_NAME=
- POSTGRE_PORT=
- POSTGRE_USER=
- POSTGRE_PASSWORD=
- POSTGRE_PORT=
- POSTGRE_HOST=
-
-Add the following environment variable to remove the warning about ephemeral
-storage.
-
- ENV STORAGE_PERSISTENCE=1
-
-Note that you will need to connect cloud storage to host data items that you
-want to annotate, as local storage will not be preserved across a space reset.
-
-By default the only data storage enabled for this space is local. In the case
-of a space reset, all data will be lost. To enable permanent storage, you
-must enable a cloud storage connector. We also strongly recommend enabling
-configuration persistence to preserve project data, annotations, and user
-settings. Choose the appropriate cloud connector and configure the secrets
-for it.
-
-#### Amazon S3
- STORAGE_TYPE=s3
- STORAGE_AWS_ACCESS_KEY_ID=""
- STORAGE_AWS_SECRET_ACCESS_KEY=""
- STORAGE_AWS_BUCKET_NAME=""
- STORAGE_AWS_REGION_NAME=""
- STORAGE_AWS_FOLDER=""
-
-#### Google Cloud Storage
-
- STORAGE_TYPE=gcs
- STORAGE_GCS_BUCKET_NAME=""
- STORAGE_GCS_PROJECT_ID=""
- STORAGE_GCS_FOLDER=""
- GOOGLE_APPLICATION_CREDENTIALS="/opt/heartex/secrets/key.json"
-
-Azure Blob Storage
-==================
-
- STORAGE_TYPE=azure
- STORAGE_AZURE_ACCOUNT_NAME=""
- STORAGE_AZURE_ACCOUNT_KEY=""
- STORAGE_AZURE_CONTAINER_NAME=""
- STORAGE_AZURE_FOLDER=""
-
-
-## Questions? Concerns? Want to get involved?
-
-Email the community team at [community@labelstud.io](mailto:community@labelstud.io)
diff --git a/spaces/SungBeom/chatwine-korean/.venv/Lib/site-packages/aiohttp/web_ws.py b/spaces/SungBeom/chatwine-korean/.venv/Lib/site-packages/aiohttp/web_ws.py
deleted file mode 100644
index 0d32a218b52b87ec04f36a6f95bfb303984b2e43..0000000000000000000000000000000000000000
--- a/spaces/SungBeom/chatwine-korean/.venv/Lib/site-packages/aiohttp/web_ws.py
+++ /dev/null
@@ -1,487 +0,0 @@
-import asyncio
-import base64
-import binascii
-import hashlib
-import json
-from typing import Any, Iterable, Optional, Tuple, cast
-
-import async_timeout
-import attr
-from multidict import CIMultiDict
-
-from . import hdrs
-from .abc import AbstractStreamWriter
-from .helpers import call_later, set_result
-from .http import (
- WS_CLOSED_MESSAGE,
- WS_CLOSING_MESSAGE,
- WS_KEY,
- WebSocketError,
- WebSocketReader,
- WebSocketWriter,
- WSCloseCode,
- WSMessage,
- WSMsgType as WSMsgType,
- ws_ext_gen,
- ws_ext_parse,
-)
-from .log import ws_logger
-from .streams import EofStream, FlowControlDataQueue
-from .typedefs import Final, JSONDecoder, JSONEncoder
-from .web_exceptions import HTTPBadRequest, HTTPException
-from .web_request import BaseRequest
-from .web_response import StreamResponse
-
-__all__ = (
- "WebSocketResponse",
- "WebSocketReady",
- "WSMsgType",
-)
-
-THRESHOLD_CONNLOST_ACCESS: Final[int] = 5
-
-
-@attr.s(auto_attribs=True, frozen=True, slots=True)
-class WebSocketReady:
- ok: bool
- protocol: Optional[str]
-
- def __bool__(self) -> bool:
- return self.ok
-
-
-class WebSocketResponse(StreamResponse):
-
- _length_check = False
-
- def __init__(
- self,
- *,
- timeout: float = 10.0,
- receive_timeout: Optional[float] = None,
- autoclose: bool = True,
- autoping: bool = True,
- heartbeat: Optional[float] = None,
- protocols: Iterable[str] = (),
- compress: bool = True,
- max_msg_size: int = 4 * 1024 * 1024,
- ) -> None:
- super().__init__(status=101)
- self._protocols = protocols
- self._ws_protocol: Optional[str] = None
- self._writer: Optional[WebSocketWriter] = None
- self._reader: Optional[FlowControlDataQueue[WSMessage]] = None
- self._closed = False
- self._closing = False
- self._conn_lost = 0
- self._close_code: Optional[int] = None
- self._loop: Optional[asyncio.AbstractEventLoop] = None
- self._waiting: Optional[asyncio.Future[bool]] = None
- self._exception: Optional[BaseException] = None
- self._timeout = timeout
- self._receive_timeout = receive_timeout
- self._autoclose = autoclose
- self._autoping = autoping
- self._heartbeat = heartbeat
- self._heartbeat_cb: Optional[asyncio.TimerHandle] = None
- if heartbeat is not None:
- self._pong_heartbeat = heartbeat / 2.0
- self._pong_response_cb: Optional[asyncio.TimerHandle] = None
- self._compress = compress
- self._max_msg_size = max_msg_size
-
- def _cancel_heartbeat(self) -> None:
- if self._pong_response_cb is not None:
- self._pong_response_cb.cancel()
- self._pong_response_cb = None
-
- if self._heartbeat_cb is not None:
- self._heartbeat_cb.cancel()
- self._heartbeat_cb = None
-
- def _reset_heartbeat(self) -> None:
- self._cancel_heartbeat()
-
- if self._heartbeat is not None:
- assert self._loop is not None
- self._heartbeat_cb = call_later(
- self._send_heartbeat, self._heartbeat, self._loop
- )
-
- def _send_heartbeat(self) -> None:
- if self._heartbeat is not None and not self._closed:
- assert self._loop is not None
- # fire-and-forget a task is not perfect but maybe ok for
- # sending ping. Otherwise we need a long-living heartbeat
- # task in the class.
- self._loop.create_task(self._writer.ping()) # type: ignore[union-attr]
-
- if self._pong_response_cb is not None:
- self._pong_response_cb.cancel()
- self._pong_response_cb = call_later(
- self._pong_not_received, self._pong_heartbeat, self._loop
- )
-
- def _pong_not_received(self) -> None:
- if self._req is not None and self._req.transport is not None:
- self._closed = True
- self._close_code = WSCloseCode.ABNORMAL_CLOSURE
- self._exception = asyncio.TimeoutError()
- self._req.transport.close()
-
- async def prepare(self, request: BaseRequest) -> AbstractStreamWriter:
- # make pre-check to don't hide it by do_handshake() exceptions
- if self._payload_writer is not None:
- return self._payload_writer
-
- protocol, writer = self._pre_start(request)
- payload_writer = await super().prepare(request)
- assert payload_writer is not None
- self._post_start(request, protocol, writer)
- await payload_writer.drain()
- return payload_writer
-
- def _handshake(
- self, request: BaseRequest
- ) -> Tuple["CIMultiDict[str]", str, bool, bool]:
- headers = request.headers
- if "websocket" != headers.get(hdrs.UPGRADE, "").lower().strip():
- raise HTTPBadRequest(
- text=(
- "No WebSocket UPGRADE hdr: {}\n Can "
- '"Upgrade" only to "WebSocket".'
- ).format(headers.get(hdrs.UPGRADE))
- )
-
- if "upgrade" not in headers.get(hdrs.CONNECTION, "").lower():
- raise HTTPBadRequest(
- text="No CONNECTION upgrade hdr: {}".format(
- headers.get(hdrs.CONNECTION)
- )
- )
-
- # find common sub-protocol between client and server
- protocol = None
- if hdrs.SEC_WEBSOCKET_PROTOCOL in headers:
- req_protocols = [
- str(proto.strip())
- for proto in headers[hdrs.SEC_WEBSOCKET_PROTOCOL].split(",")
- ]
-
- for proto in req_protocols:
- if proto in self._protocols:
- protocol = proto
- break
- else:
- # No overlap found: Return no protocol as per spec
- ws_logger.warning(
- "Client protocols %r don’t overlap server-known ones %r",
- req_protocols,
- self._protocols,
- )
-
- # check supported version
- version = headers.get(hdrs.SEC_WEBSOCKET_VERSION, "")
- if version not in ("13", "8", "7"):
- raise HTTPBadRequest(text=f"Unsupported version: {version}")
-
- # check client handshake for validity
- key = headers.get(hdrs.SEC_WEBSOCKET_KEY)
- try:
- if not key or len(base64.b64decode(key)) != 16:
- raise HTTPBadRequest(text=f"Handshake error: {key!r}")
- except binascii.Error:
- raise HTTPBadRequest(text=f"Handshake error: {key!r}") from None
-
- accept_val = base64.b64encode(
- hashlib.sha1(key.encode() + WS_KEY).digest()
- ).decode()
- response_headers = CIMultiDict(
- {
- hdrs.UPGRADE: "websocket",
- hdrs.CONNECTION: "upgrade",
- hdrs.SEC_WEBSOCKET_ACCEPT: accept_val,
- }
- )
-
- notakeover = False
- compress = 0
- if self._compress:
- extensions = headers.get(hdrs.SEC_WEBSOCKET_EXTENSIONS)
- # Server side always get return with no exception.
- # If something happened, just drop compress extension
- compress, notakeover = ws_ext_parse(extensions, isserver=True)
- if compress:
- enabledext = ws_ext_gen(
- compress=compress, isserver=True, server_notakeover=notakeover
- )
- response_headers[hdrs.SEC_WEBSOCKET_EXTENSIONS] = enabledext
-
- if protocol:
- response_headers[hdrs.SEC_WEBSOCKET_PROTOCOL] = protocol
- return (
- response_headers,
- protocol,
- compress,
- notakeover,
- ) # type: ignore[return-value]
-
- def _pre_start(self, request: BaseRequest) -> Tuple[str, WebSocketWriter]:
- self._loop = request._loop
-
- headers, protocol, compress, notakeover = self._handshake(request)
-
- self.set_status(101)
- self.headers.update(headers)
- self.force_close()
- self._compress = compress
- transport = request._protocol.transport
- assert transport is not None
- writer = WebSocketWriter(
- request._protocol, transport, compress=compress, notakeover=notakeover
- )
-
- return protocol, writer
-
- def _post_start(
- self, request: BaseRequest, protocol: str, writer: WebSocketWriter
- ) -> None:
- self._ws_protocol = protocol
- self._writer = writer
-
- self._reset_heartbeat()
-
- loop = self._loop
- assert loop is not None
- self._reader = FlowControlDataQueue(request._protocol, 2**16, loop=loop)
- request.protocol.set_parser(
- WebSocketReader(self._reader, self._max_msg_size, compress=self._compress)
- )
- # disable HTTP keepalive for WebSocket
- request.protocol.keep_alive(False)
-
- def can_prepare(self, request: BaseRequest) -> WebSocketReady:
- if self._writer is not None:
- raise RuntimeError("Already started")
- try:
- _, protocol, _, _ = self._handshake(request)
- except HTTPException:
- return WebSocketReady(False, None)
- else:
- return WebSocketReady(True, protocol)
-
- @property
- def closed(self) -> bool:
- return self._closed
-
- @property
- def close_code(self) -> Optional[int]:
- return self._close_code
-
- @property
- def ws_protocol(self) -> Optional[str]:
- return self._ws_protocol
-
- @property
- def compress(self) -> bool:
- return self._compress
-
- def exception(self) -> Optional[BaseException]:
- return self._exception
-
- async def ping(self, message: bytes = b"") -> None:
- if self._writer is None:
- raise RuntimeError("Call .prepare() first")
- await self._writer.ping(message)
-
- async def pong(self, message: bytes = b"") -> None:
- # unsolicited pong
- if self._writer is None:
- raise RuntimeError("Call .prepare() first")
- await self._writer.pong(message)
-
- async def send_str(self, data: str, compress: Optional[bool] = None) -> None:
- if self._writer is None:
- raise RuntimeError("Call .prepare() first")
- if not isinstance(data, str):
- raise TypeError("data argument must be str (%r)" % type(data))
- await self._writer.send(data, binary=False, compress=compress)
-
- async def send_bytes(self, data: bytes, compress: Optional[bool] = None) -> None:
- if self._writer is None:
- raise RuntimeError("Call .prepare() first")
- if not isinstance(data, (bytes, bytearray, memoryview)):
- raise TypeError("data argument must be byte-ish (%r)" % type(data))
- await self._writer.send(data, binary=True, compress=compress)
-
- async def send_json(
- self,
- data: Any,
- compress: Optional[bool] = None,
- *,
- dumps: JSONEncoder = json.dumps,
- ) -> None:
- await self.send_str(dumps(data), compress=compress)
-
- async def write_eof(self) -> None: # type: ignore[override]
- if self._eof_sent:
- return
- if self._payload_writer is None:
- raise RuntimeError("Response has not been started")
-
- await self.close()
- self._eof_sent = True
-
- async def close(self, *, code: int = WSCloseCode.OK, message: bytes = b"") -> bool:
- if self._writer is None:
- raise RuntimeError("Call .prepare() first")
-
- self._cancel_heartbeat()
- reader = self._reader
- assert reader is not None
-
- # we need to break `receive()` cycle first,
- # `close()` may be called from different task
- if self._waiting is not None and not self._closed:
- reader.feed_data(WS_CLOSING_MESSAGE, 0)
- await self._waiting
-
- if not self._closed:
- self._closed = True
- try:
- await self._writer.close(code, message)
- writer = self._payload_writer
- assert writer is not None
- await writer.drain()
- except (asyncio.CancelledError, asyncio.TimeoutError):
- self._close_code = WSCloseCode.ABNORMAL_CLOSURE
- raise
- except Exception as exc:
- self._close_code = WSCloseCode.ABNORMAL_CLOSURE
- self._exception = exc
- return True
-
- if self._closing:
- return True
-
- reader = self._reader
- assert reader is not None
- try:
- async with async_timeout.timeout(self._timeout):
- msg = await reader.read()
- except asyncio.CancelledError:
- self._close_code = WSCloseCode.ABNORMAL_CLOSURE
- raise
- except Exception as exc:
- self._close_code = WSCloseCode.ABNORMAL_CLOSURE
- self._exception = exc
- return True
-
- if msg.type == WSMsgType.CLOSE:
- self._close_code = msg.data
- return True
-
- self._close_code = WSCloseCode.ABNORMAL_CLOSURE
- self._exception = asyncio.TimeoutError()
- return True
- else:
- return False
-
- async def receive(self, timeout: Optional[float] = None) -> WSMessage:
- if self._reader is None:
- raise RuntimeError("Call .prepare() first")
-
- loop = self._loop
- assert loop is not None
- while True:
- if self._waiting is not None:
- raise RuntimeError("Concurrent call to receive() is not allowed")
-
- if self._closed:
- self._conn_lost += 1
- if self._conn_lost >= THRESHOLD_CONNLOST_ACCESS:
- raise RuntimeError("WebSocket connection is closed.")
- return WS_CLOSED_MESSAGE
- elif self._closing:
- return WS_CLOSING_MESSAGE
-
- try:
- self._waiting = loop.create_future()
- try:
- async with async_timeout.timeout(timeout or self._receive_timeout):
- msg = await self._reader.read()
- self._reset_heartbeat()
- finally:
- waiter = self._waiting
- set_result(waiter, True)
- self._waiting = None
- except (asyncio.CancelledError, asyncio.TimeoutError):
- self._close_code = WSCloseCode.ABNORMAL_CLOSURE
- raise
- except EofStream:
- self._close_code = WSCloseCode.OK
- await self.close()
- return WSMessage(WSMsgType.CLOSED, None, None)
- except WebSocketError as exc:
- self._close_code = exc.code
- await self.close(code=exc.code)
- return WSMessage(WSMsgType.ERROR, exc, None)
- except Exception as exc:
- self._exception = exc
- self._closing = True
- self._close_code = WSCloseCode.ABNORMAL_CLOSURE
- await self.close()
- return WSMessage(WSMsgType.ERROR, exc, None)
-
- if msg.type == WSMsgType.CLOSE:
- self._closing = True
- self._close_code = msg.data
- if not self._closed and self._autoclose:
- await self.close()
- elif msg.type == WSMsgType.CLOSING:
- self._closing = True
- elif msg.type == WSMsgType.PING and self._autoping:
- await self.pong(msg.data)
- continue
- elif msg.type == WSMsgType.PONG and self._autoping:
- continue
-
- return msg
-
- async def receive_str(self, *, timeout: Optional[float] = None) -> str:
- msg = await self.receive(timeout)
- if msg.type != WSMsgType.TEXT:
- raise TypeError(
- "Received message {}:{!r} is not WSMsgType.TEXT".format(
- msg.type, msg.data
- )
- )
- return cast(str, msg.data)
-
- async def receive_bytes(self, *, timeout: Optional[float] = None) -> bytes:
- msg = await self.receive(timeout)
- if msg.type != WSMsgType.BINARY:
- raise TypeError(f"Received message {msg.type}:{msg.data!r} is not bytes")
- return cast(bytes, msg.data)
-
- async def receive_json(
- self, *, loads: JSONDecoder = json.loads, timeout: Optional[float] = None
- ) -> Any:
- data = await self.receive_str(timeout=timeout)
- return loads(data)
-
- async def write(self, data: bytes) -> None:
- raise RuntimeError("Cannot call .write() for websocket")
-
- def __aiter__(self) -> "WebSocketResponse":
- return self
-
- async def __anext__(self) -> WSMessage:
- msg = await self.receive()
- if msg.type in (WSMsgType.CLOSE, WSMsgType.CLOSING, WSMsgType.CLOSED):
- raise StopAsyncIteration
- return msg
-
- def _cancel(self, exc: BaseException) -> None:
- if self._reader is not None:
- self._reader.set_exception(exc)
diff --git a/spaces/SungBeom/chatwine-korean/.venv/Lib/site-packages/docarray/utils/_internal/query_language/query_parser.py b/spaces/SungBeom/chatwine-korean/.venv/Lib/site-packages/docarray/utils/_internal/query_language/query_parser.py
deleted file mode 100644
index b635d296d8eddd2894c9803b4a230ebdd1b25802..0000000000000000000000000000000000000000
--- a/spaces/SungBeom/chatwine-korean/.venv/Lib/site-packages/docarray/utils/_internal/query_language/query_parser.py
+++ /dev/null
@@ -1,125 +0,0 @@
-from typing import Any, Dict, List, Optional, Union
-
-from docarray.utils._internal.query_language.lookup import (
- LookupLeaf,
- LookupNode,
- LookupTreeElem,
- Q,
-)
-
-LOGICAL_OPERATORS: Dict[str, Union[str, bool]] = {
- '$and': 'and',
- '$or': 'or',
- '$not': True,
-}
-
-COMPARISON_OPERATORS = {
- '$lt': 'lt',
- '$gt': 'gt',
- '$lte': 'lte',
- '$gte': 'gte',
- '$eq': 'exact',
- '$neq': 'neq',
- '$exists': 'exists',
-}
-
-REGEX_OPERATORS = {'$regex': 'regex'}
-
-ARRAY_OPERATORS = {'$size': 'size'}
-
-MEMBERSHIP_OPERATORS = {'$in': 'in', '$nin': 'nin'}
-
-SUPPORTED_OPERATORS = {
- **COMPARISON_OPERATORS,
- **ARRAY_OPERATORS,
- **REGEX_OPERATORS,
- **MEMBERSHIP_OPERATORS,
-}
-
-
-def _parse_lookups(
- data: Union[Dict, List] = {}, root_node: Optional[LookupTreeElem] = None
-) -> Optional[LookupTreeElem]:
- if isinstance(data, dict):
- for key, value in data.items():
- node: Optional[LookupTreeElem] = None
- if isinstance(root_node, LookupLeaf):
- root = LookupNode()
- root.add_child(root_node)
- root_node = root
-
- if key in LOGICAL_OPERATORS:
- if key == '$not':
- node = LookupNode(negate=True)
- else:
- node = LookupNode(op=LOGICAL_OPERATORS[key])
- node = _parse_lookups(value, root_node=node)
-
- elif key.startswith('$'):
- raise ValueError(
- f'The operator {key} is not supported yet,'
- f' please double check the given filters!'
- )
- else:
- if not value or not isinstance(value, dict):
- raise ValueError(
- '''Not a valid query. It should follow the format:
- { : { : }, ... }
- '''
- )
-
- items = list(value.items())
- if len(items) == 1:
- op, val = items[0]
- if op in LOGICAL_OPERATORS:
- if op == '$not':
- node = LookupNode(negate=True)
- else:
- node = LookupNode(op=LOGICAL_OPERATORS[op])
- node = _parse_lookups(val, root_node=node)
- elif op in SUPPORTED_OPERATORS:
- node = Q(**{f'{key}.{SUPPORTED_OPERATORS[op]}': val})
- else:
- raise ValueError(
- f'The operator {op} is not supported yet, '
- f'please double check the given filters!'
- )
-
- else:
- node = LookupNode()
- for op, val in items:
- _node = _parse_lookups({key: {op: val}})
- node.add_child(_node)
-
- if root_node and node:
- if isinstance(root_node, LookupNode):
- root_node.add_child(node)
- elif node:
- root_node = node
-
- elif isinstance(data, list):
- for d in data:
- node = _parse_lookups(d)
- if root_node and node:
- if isinstance(root_node, LookupNode):
- root_node.add_child(node)
- elif node:
- root_node = node
- else:
- raise ValueError(f'The query is illegal: `{data}`')
-
- return root_node
-
-
-class QueryParser:
- """A class to parse dict condition to lookup query."""
-
- def __init__(self, conditions: Union[Dict, List] = {}):
- self.conditions = conditions
- self.lookup_groups = _parse_lookups(self.conditions)
-
- def evaluate(self, doc: Any) -> bool:
- return self.lookup_groups.evaluate(doc) if self.lookup_groups else True
-
- def __call__(self, doc: Any) -> bool:
- return self.evaluate(doc)
diff --git a/spaces/Sup3r/img-to-music/share_btn.py b/spaces/Sup3r/img-to-music/share_btn.py
deleted file mode 100644
index 1a2ac6a6e74b114dbd54c2f24723a87180db51ef..0000000000000000000000000000000000000000
--- a/spaces/Sup3r/img-to-music/share_btn.py
+++ /dev/null
@@ -1,100 +0,0 @@
-community_icon_html = """"""
-
-loading_icon_html = """"""
-
-share_js = """async () => {
- async function uploadFile(file){
- const UPLOAD_URL = 'https://huggingface.co/uploads';
- const response = await fetch(UPLOAD_URL, {
- method: 'POST',
- headers: {
- 'Content-Type': file.type,
- 'X-Requested-With': 'XMLHttpRequest',
- },
- body: file, /// <- File inherits from Blob
- });
- const url = await response.text();
- return url;
- }
- async function getInputImgFile(imgEl){
- const res = await fetch(imgEl.src);
- const blob = await res.blob();
- const imgId = Date.now() % 200;
- const isPng = imgEl.src.startsWith(`data:image/png`);
- if(isPng){
- const fileName = `sd-perception-${{imgId}}.png`;
- return new File([blob], fileName, { type: 'image/png' });
- }else{
- const fileName = `sd-perception-${{imgId}}.jpg`;
- return new File([blob], fileName, { type: 'image/jpeg' });
- }
- }
- async function getOutputMusicFile(audioEL){
- const res = await fetch(audioEL.src);
- const blob = await res.blob();
- const audioId = Date.now() % 200;
- const fileName = `img-to-music-${{audioId}}.wav`;
- const musicBlob = new File([blob], fileName, { type: 'audio/wav' });
- console.log(musicBlob);
- return musicBlob;
- }
-
- async function audioToBase64(audioFile) {
- return new Promise((resolve, reject) => {
- let reader = new FileReader();
- reader.readAsDataURL(audioFile);
- reader.onload = () => resolve(reader.result);
- reader.onerror = error => reject(error);
-
- });
- }
- const gradioEl = document.querySelector('body > gradio-app');
- // const gradioEl = document.querySelector("gradio-app").shadowRoot;
- const inputImgEl = gradioEl.querySelector('#input-img img');
- const outputMusic = gradioEl.querySelector('#music-output audio');
- const outputMusic_src = gradioEl.querySelector('#music-output audio').src;
- const outputMusic_name = outputMusic_src.split('/').pop();
- let titleTxt = outputMusic_name;
- //if(titleTxt.length > 100){
- // titleTxt = titleTxt.slice(0, 100) + ' ...';
- //}
- const shareBtnEl = gradioEl.querySelector('#share-btn');
- const shareIconEl = gradioEl.querySelector('#share-btn-share-icon');
- const loadingIconEl = gradioEl.querySelector('#share-btn-loading-icon');
- if(!outputMusic){
- return;
- };
- shareBtnEl.style.pointerEvents = 'none';
- shareIconEl.style.display = 'none';
- loadingIconEl.style.removeProperty('display');
- const inputFile = await getInputImgFile(inputImgEl);
- const urlInputImg = await uploadFile(inputFile);
- const musicFile = await getOutputMusicFile(outputMusic);
- const dataOutputMusic = await uploadFile(musicFile);
-
- const descriptionMd = `#### Input img:
-
-
-#### Music:
-
-
-`;
- const params = new URLSearchParams({
- title: titleTxt,
- description: descriptionMd,
- });
- const paramsStr = params.toString();
- window.open(`https://huggingface.co/spaces/fffiloni/img-to-music/discussions/new?${paramsStr}`, '_blank');
- shareBtnEl.style.removeProperty('pointer-events');
- shareIconEl.style.removeProperty('display');
- loadingIconEl.style.display = 'none';
-}"""
\ No newline at end of file
diff --git a/spaces/Superlang/ImageProcessor/annotator/oneformer/detectron2/data/transforms/augmentation.py b/spaces/Superlang/ImageProcessor/annotator/oneformer/detectron2/data/transforms/augmentation.py
deleted file mode 100644
index 63dd41aef658c9b51c7246880399405a029c5580..0000000000000000000000000000000000000000
--- a/spaces/Superlang/ImageProcessor/annotator/oneformer/detectron2/data/transforms/augmentation.py
+++ /dev/null
@@ -1,380 +0,0 @@
-# -*- coding: utf-8 -*-
-# Copyright (c) Facebook, Inc. and its affiliates.
-
-import inspect
-import numpy as np
-import pprint
-from typing import Any, List, Optional, Tuple, Union
-from fvcore.transforms.transform import Transform, TransformList
-
-"""
-See "Data Augmentation" tutorial for an overview of the system:
-https://detectron2.readthedocs.io/tutorials/augmentation.html
-"""
-
-
-__all__ = [
- "Augmentation",
- "AugmentationList",
- "AugInput",
- "TransformGen",
- "apply_transform_gens",
- "StandardAugInput",
- "apply_augmentations",
-]
-
-
-def _check_img_dtype(img):
- assert isinstance(img, np.ndarray), "[Augmentation] Needs an numpy array, but got a {}!".format(
- type(img)
- )
- assert not isinstance(img.dtype, np.integer) or (
- img.dtype == np.uint8
- ), "[Augmentation] Got image of type {}, use uint8 or floating points instead!".format(
- img.dtype
- )
- assert img.ndim in [2, 3], img.ndim
-
-
-def _get_aug_input_args(aug, aug_input) -> List[Any]:
- """
- Get the arguments to be passed to ``aug.get_transform`` from the input ``aug_input``.
- """
- if aug.input_args is None:
- # Decide what attributes are needed automatically
- prms = list(inspect.signature(aug.get_transform).parameters.items())
- # The default behavior is: if there is one parameter, then its "image"
- # (work automatically for majority of use cases, and also avoid BC breaking),
- # Otherwise, use the argument names.
- if len(prms) == 1:
- names = ("image",)
- else:
- names = []
- for name, prm in prms:
- if prm.kind in (
- inspect.Parameter.VAR_POSITIONAL,
- inspect.Parameter.VAR_KEYWORD,
- ):
- raise TypeError(
- f""" \
-The default implementation of `{type(aug)}.__call__` does not allow \
-`{type(aug)}.get_transform` to use variable-length arguments (*args, **kwargs)! \
-If arguments are unknown, reimplement `__call__` instead. \
-"""
- )
- names.append(name)
- aug.input_args = tuple(names)
-
- args = []
- for f in aug.input_args:
- try:
- args.append(getattr(aug_input, f))
- except AttributeError as e:
- raise AttributeError(
- f"{type(aug)}.get_transform needs input attribute '{f}', "
- f"but it is not an attribute of {type(aug_input)}!"
- ) from e
- return args
-
-
-class Augmentation:
- """
- Augmentation defines (often random) policies/strategies to generate :class:`Transform`
- from data. It is often used for pre-processing of input data.
-
- A "policy" that generates a :class:`Transform` may, in the most general case,
- need arbitrary information from input data in order to determine what transforms
- to apply. Therefore, each :class:`Augmentation` instance defines the arguments
- needed by its :meth:`get_transform` method. When called with the positional arguments,
- the :meth:`get_transform` method executes the policy.
-
- Note that :class:`Augmentation` defines the policies to create a :class:`Transform`,
- but not how to execute the actual transform operations to those data.
- Its :meth:`__call__` method will use :meth:`AugInput.transform` to execute the transform.
-
- The returned `Transform` object is meant to describe deterministic transformation, which means
- it can be re-applied on associated data, e.g. the geometry of an image and its segmentation
- masks need to be transformed together.
- (If such re-application is not needed, then determinism is not a crucial requirement.)
- """
-
- input_args: Optional[Tuple[str]] = None
- """
- Stores the attribute names needed by :meth:`get_transform`, e.g. ``("image", "sem_seg")``.
- By default, it is just a tuple of argument names in :meth:`self.get_transform`, which often only
- contain "image". As long as the argument name convention is followed, there is no need for
- users to touch this attribute.
- """
-
- def _init(self, params=None):
- if params:
- for k, v in params.items():
- if k != "self" and not k.startswith("_"):
- setattr(self, k, v)
-
- def get_transform(self, *args) -> Transform:
- """
- Execute the policy based on input data, and decide what transform to apply to inputs.
-
- Args:
- args: Any fixed-length positional arguments. By default, the name of the arguments
- should exist in the :class:`AugInput` to be used.
-
- Returns:
- Transform: Returns the deterministic transform to apply to the input.
-
- Examples:
- ::
- class MyAug:
- # if a policy needs to know both image and semantic segmentation
- def get_transform(image, sem_seg) -> T.Transform:
- pass
- tfm: Transform = MyAug().get_transform(image, sem_seg)
- new_image = tfm.apply_image(image)
-
- Notes:
- Users can freely use arbitrary new argument names in custom
- :meth:`get_transform` method, as long as they are available in the
- input data. In detectron2 we use the following convention:
-
- * image: (H,W) or (H,W,C) ndarray of type uint8 in range [0, 255], or
- floating point in range [0, 1] or [0, 255].
- * boxes: (N,4) ndarray of float32. It represents the instance bounding boxes
- of N instances. Each is in XYXY format in unit of absolute coordinates.
- * sem_seg: (H,W) ndarray of type uint8. Each element is an integer label of pixel.
-
- We do not specify convention for other types and do not include builtin
- :class:`Augmentation` that uses other types in detectron2.
- """
- raise NotImplementedError
-
- def __call__(self, aug_input) -> Transform:
- """
- Augment the given `aug_input` **in-place**, and return the transform that's used.
-
- This method will be called to apply the augmentation. In most augmentation, it
- is enough to use the default implementation, which calls :meth:`get_transform`
- using the inputs. But a subclass can overwrite it to have more complicated logic.
-
- Args:
- aug_input (AugInput): an object that has attributes needed by this augmentation
- (defined by ``self.get_transform``). Its ``transform`` method will be called
- to in-place transform it.
-
- Returns:
- Transform: the transform that is applied on the input.
- """
- args = _get_aug_input_args(self, aug_input)
- tfm = self.get_transform(*args)
- assert isinstance(tfm, (Transform, TransformList)), (
- f"{type(self)}.get_transform must return an instance of Transform! "
- f"Got {type(tfm)} instead."
- )
- aug_input.transform(tfm)
- return tfm
-
- def _rand_range(self, low=1.0, high=None, size=None):
- """
- Uniform float random number between low and high.
- """
- if high is None:
- low, high = 0, low
- if size is None:
- size = []
- return np.random.uniform(low, high, size)
-
- def __repr__(self):
- """
- Produce something like:
- "MyAugmentation(field1={self.field1}, field2={self.field2})"
- """
- try:
- sig = inspect.signature(self.__init__)
- classname = type(self).__name__
- argstr = []
- for name, param in sig.parameters.items():
- assert (
- param.kind != param.VAR_POSITIONAL and param.kind != param.VAR_KEYWORD
- ), "The default __repr__ doesn't support *args or **kwargs"
- assert hasattr(self, name), (
- "Attribute {} not found! "
- "Default __repr__ only works if attributes match the constructor.".format(name)
- )
- attr = getattr(self, name)
- default = param.default
- if default is attr:
- continue
- attr_str = pprint.pformat(attr)
- if "\n" in attr_str:
- # don't show it if pformat decides to use >1 lines
- attr_str = "..."
- argstr.append("{}={}".format(name, attr_str))
- return "{}({})".format(classname, ", ".join(argstr))
- except AssertionError:
- return super().__repr__()
-
- __str__ = __repr__
-
-
-class _TransformToAug(Augmentation):
- def __init__(self, tfm: Transform):
- self.tfm = tfm
-
- def get_transform(self, *args):
- return self.tfm
-
- def __repr__(self):
- return repr(self.tfm)
-
- __str__ = __repr__
-
-
-def _transform_to_aug(tfm_or_aug):
- """
- Wrap Transform into Augmentation.
- Private, used internally to implement augmentations.
- """
- assert isinstance(tfm_or_aug, (Transform, Augmentation)), tfm_or_aug
- if isinstance(tfm_or_aug, Augmentation):
- return tfm_or_aug
- else:
- return _TransformToAug(tfm_or_aug)
-
-
-class AugmentationList(Augmentation):
- """
- Apply a sequence of augmentations.
-
- It has ``__call__`` method to apply the augmentations.
-
- Note that :meth:`get_transform` method is impossible (will throw error if called)
- for :class:`AugmentationList`, because in order to apply a sequence of augmentations,
- the kth augmentation must be applied first, to provide inputs needed by the (k+1)th
- augmentation.
- """
-
- def __init__(self, augs):
- """
- Args:
- augs (list[Augmentation or Transform]):
- """
- super().__init__()
- self.augs = [_transform_to_aug(x) for x in augs]
-
- def __call__(self, aug_input) -> TransformList:
- tfms = []
- for x in self.augs:
- tfm = x(aug_input)
- tfms.append(tfm)
- return TransformList(tfms)
-
- def __repr__(self):
- msgs = [str(x) for x in self.augs]
- return "AugmentationList[{}]".format(", ".join(msgs))
-
- __str__ = __repr__
-
-
-class AugInput:
- """
- Input that can be used with :meth:`Augmentation.__call__`.
- This is a standard implementation for the majority of use cases.
- This class provides the standard attributes **"image", "boxes", "sem_seg"**
- defined in :meth:`__init__` and they may be needed by different augmentations.
- Most augmentation policies do not need attributes beyond these three.
-
- After applying augmentations to these attributes (using :meth:`AugInput.transform`),
- the returned transforms can then be used to transform other data structures that users have.
-
- Examples:
- ::
- input = AugInput(image, boxes=boxes)
- tfms = augmentation(input)
- transformed_image = input.image
- transformed_boxes = input.boxes
- transformed_other_data = tfms.apply_other(other_data)
-
- An extended project that works with new data types may implement augmentation policies
- that need other inputs. An algorithm may need to transform inputs in a way different
- from the standard approach defined in this class. In those rare situations, users can
- implement a class similar to this class, that satify the following condition:
-
- * The input must provide access to these data in the form of attribute access
- (``getattr``). For example, if an :class:`Augmentation` to be applied needs "image"
- and "sem_seg" arguments, its input must have the attribute "image" and "sem_seg".
- * The input must have a ``transform(tfm: Transform) -> None`` method which
- in-place transforms all its attributes.
- """
-
- # TODO maybe should support more builtin data types here
- def __init__(
- self,
- image: np.ndarray,
- *,
- boxes: Optional[np.ndarray] = None,
- sem_seg: Optional[np.ndarray] = None,
- ):
- """
- Args:
- image (ndarray): (H,W) or (H,W,C) ndarray of type uint8 in range [0, 255], or
- floating point in range [0, 1] or [0, 255]. The meaning of C is up
- to users.
- boxes (ndarray or None): Nx4 float32 boxes in XYXY_ABS mode
- sem_seg (ndarray or None): HxW uint8 semantic segmentation mask. Each element
- is an integer label of pixel.
- """
- _check_img_dtype(image)
- self.image = image
- self.boxes = boxes
- self.sem_seg = sem_seg
-
- def transform(self, tfm: Transform) -> None:
- """
- In-place transform all attributes of this class.
-
- By "in-place", it means after calling this method, accessing an attribute such
- as ``self.image`` will return transformed data.
- """
- self.image = tfm.apply_image(self.image)
- if self.boxes is not None:
- self.boxes = tfm.apply_box(self.boxes)
- if self.sem_seg is not None:
- self.sem_seg = tfm.apply_segmentation(self.sem_seg)
-
- def apply_augmentations(
- self, augmentations: List[Union[Augmentation, Transform]]
- ) -> TransformList:
- """
- Equivalent of ``AugmentationList(augmentations)(self)``
- """
- return AugmentationList(augmentations)(self)
-
-
-def apply_augmentations(augmentations: List[Union[Transform, Augmentation]], inputs):
- """
- Use ``T.AugmentationList(augmentations)(inputs)`` instead.
- """
- if isinstance(inputs, np.ndarray):
- # handle the common case of image-only Augmentation, also for backward compatibility
- image_only = True
- inputs = AugInput(inputs)
- else:
- image_only = False
- tfms = inputs.apply_augmentations(augmentations)
- return inputs.image if image_only else inputs, tfms
-
-
-apply_transform_gens = apply_augmentations
-"""
-Alias for backward-compatibility.
-"""
-
-TransformGen = Augmentation
-"""
-Alias for Augmentation, since it is something that generates :class:`Transform`s
-"""
-
-StandardAugInput = AugInput
-"""
-Alias for compatibility. It's not worth the complexity to have two classes.
-"""
diff --git a/spaces/TandCAcceptMe/face-swap-docker/mynewshinyroop/Lib/site-packages/pip/_internal/utils/virtualenv.py b/spaces/TandCAcceptMe/face-swap-docker/mynewshinyroop/Lib/site-packages/pip/_internal/utils/virtualenv.py
deleted file mode 100644
index 882e36f5c1de19a8200000c216cf80119b37c96d..0000000000000000000000000000000000000000
--- a/spaces/TandCAcceptMe/face-swap-docker/mynewshinyroop/Lib/site-packages/pip/_internal/utils/virtualenv.py
+++ /dev/null
@@ -1,104 +0,0 @@
-import logging
-import os
-import re
-import site
-import sys
-from typing import List, Optional
-
-logger = logging.getLogger(__name__)
-_INCLUDE_SYSTEM_SITE_PACKAGES_REGEX = re.compile(
- r"include-system-site-packages\s*=\s*(?Ptrue|false)"
-)
-
-
-def _running_under_venv() -> bool:
- """Checks if sys.base_prefix and sys.prefix match.
-
- This handles PEP 405 compliant virtual environments.
- """
- return sys.prefix != getattr(sys, "base_prefix", sys.prefix)
-
-
-def _running_under_legacy_virtualenv() -> bool:
- """Checks if sys.real_prefix is set.
-
- This handles virtual environments created with pypa's virtualenv.
- """
- # pypa/virtualenv case
- return hasattr(sys, "real_prefix")
-
-
-def running_under_virtualenv() -> bool:
- """True if we're running inside a virtual environment, False otherwise."""
- return _running_under_venv() or _running_under_legacy_virtualenv()
-
-
-def _get_pyvenv_cfg_lines() -> Optional[List[str]]:
- """Reads {sys.prefix}/pyvenv.cfg and returns its contents as list of lines
-
- Returns None, if it could not read/access the file.
- """
- pyvenv_cfg_file = os.path.join(sys.prefix, "pyvenv.cfg")
- try:
- # Although PEP 405 does not specify, the built-in venv module always
- # writes with UTF-8. (pypa/pip#8717)
- with open(pyvenv_cfg_file, encoding="utf-8") as f:
- return f.read().splitlines() # avoids trailing newlines
- except OSError:
- return None
-
-
-def _no_global_under_venv() -> bool:
- """Check `{sys.prefix}/pyvenv.cfg` for system site-packages inclusion
-
- PEP 405 specifies that when system site-packages are not supposed to be
- visible from a virtual environment, `pyvenv.cfg` must contain the following
- line:
-
- include-system-site-packages = false
-
- Additionally, log a warning if accessing the file fails.
- """
- cfg_lines = _get_pyvenv_cfg_lines()
- if cfg_lines is None:
- # We're not in a "sane" venv, so assume there is no system
- # site-packages access (since that's PEP 405's default state).
- logger.warning(
- "Could not access 'pyvenv.cfg' despite a virtual environment "
- "being active. Assuming global site-packages is not accessible "
- "in this environment."
- )
- return True
-
- for line in cfg_lines:
- match = _INCLUDE_SYSTEM_SITE_PACKAGES_REGEX.match(line)
- if match is not None and match.group("value") == "false":
- return True
- return False
-
-
-def _no_global_under_legacy_virtualenv() -> bool:
- """Check if "no-global-site-packages.txt" exists beside site.py
-
- This mirrors logic in pypa/virtualenv for determining whether system
- site-packages are visible in the virtual environment.
- """
- site_mod_dir = os.path.dirname(os.path.abspath(site.__file__))
- no_global_site_packages_file = os.path.join(
- site_mod_dir,
- "no-global-site-packages.txt",
- )
- return os.path.exists(no_global_site_packages_file)
-
-
-def virtualenv_no_global() -> bool:
- """Returns a boolean, whether running in venv with no system site-packages."""
- # PEP 405 compliance needs to be checked first since virtualenv >=20 would
- # return True for both checks, but is only able to use the PEP 405 config.
- if _running_under_venv():
- return _no_global_under_venv()
-
- if _running_under_legacy_virtualenv():
- return _no_global_under_legacy_virtualenv()
-
- return False
diff --git a/spaces/TandCAcceptMe/face-swap-docker/mynewshinyroop/Lib/site-packages/pip/_vendor/pyparsing/helpers.py b/spaces/TandCAcceptMe/face-swap-docker/mynewshinyroop/Lib/site-packages/pip/_vendor/pyparsing/helpers.py
deleted file mode 100644
index 018f0d6ac863f2e4a27636c721669061887ae554..0000000000000000000000000000000000000000
--- a/spaces/TandCAcceptMe/face-swap-docker/mynewshinyroop/Lib/site-packages/pip/_vendor/pyparsing/helpers.py
+++ /dev/null
@@ -1,1100 +0,0 @@
-# helpers.py
-import html.entities
-import re
-import sys
-import typing
-
-from . import __diag__
-from .core import *
-from .util import (
- _bslash,
- _flatten,
- _escape_regex_range_chars,
- replaced_by_pep8,
-)
-
-
-#
-# global helpers
-#
-def counted_array(
- expr: ParserElement,
- int_expr: typing.Optional[ParserElement] = None,
- *,
- intExpr: typing.Optional[ParserElement] = None,
-) -> ParserElement:
- """Helper to define a counted list of expressions.
-
- This helper defines a pattern of the form::
-
- integer expr expr expr...
-
- where the leading integer tells how many expr expressions follow.
- The matched tokens returns the array of expr tokens as a list - the
- leading count token is suppressed.
-
- If ``int_expr`` is specified, it should be a pyparsing expression
- that produces an integer value.
-
- Example::
-
- counted_array(Word(alphas)).parse_string('2 ab cd ef') # -> ['ab', 'cd']
-
- # in this parser, the leading integer value is given in binary,
- # '10' indicating that 2 values are in the array
- binary_constant = Word('01').set_parse_action(lambda t: int(t[0], 2))
- counted_array(Word(alphas), int_expr=binary_constant).parse_string('10 ab cd ef') # -> ['ab', 'cd']
-
- # if other fields must be parsed after the count but before the
- # list items, give the fields results names and they will
- # be preserved in the returned ParseResults:
- count_with_metadata = integer + Word(alphas)("type")
- typed_array = counted_array(Word(alphanums), int_expr=count_with_metadata)("items")
- result = typed_array.parse_string("3 bool True True False")
- print(result.dump())
-
- # prints
- # ['True', 'True', 'False']
- # - items: ['True', 'True', 'False']
- # - type: 'bool'
- """
- intExpr = intExpr or int_expr
- array_expr = Forward()
-
- def count_field_parse_action(s, l, t):
- nonlocal array_expr
- n = t[0]
- array_expr <<= (expr * n) if n else Empty()
- # clear list contents, but keep any named results
- del t[:]
-
- if intExpr is None:
- intExpr = Word(nums).set_parse_action(lambda t: int(t[0]))
- else:
- intExpr = intExpr.copy()
- intExpr.set_name("arrayLen")
- intExpr.add_parse_action(count_field_parse_action, call_during_try=True)
- return (intExpr + array_expr).set_name("(len) " + str(expr) + "...")
-
-
-def match_previous_literal(expr: ParserElement) -> ParserElement:
- """Helper to define an expression that is indirectly defined from
- the tokens matched in a previous expression, that is, it looks for
- a 'repeat' of a previous expression. For example::
-
- first = Word(nums)
- second = match_previous_literal(first)
- match_expr = first + ":" + second
-
- will match ``"1:1"``, but not ``"1:2"``. Because this
- matches a previous literal, will also match the leading
- ``"1:1"`` in ``"1:10"``. If this is not desired, use
- :class:`match_previous_expr`. Do *not* use with packrat parsing
- enabled.
- """
- rep = Forward()
-
- def copy_token_to_repeater(s, l, t):
- if t:
- if len(t) == 1:
- rep << t[0]
- else:
- # flatten t tokens
- tflat = _flatten(t.as_list())
- rep << And(Literal(tt) for tt in tflat)
- else:
- rep << Empty()
-
- expr.add_parse_action(copy_token_to_repeater, callDuringTry=True)
- rep.set_name("(prev) " + str(expr))
- return rep
-
-
-def match_previous_expr(expr: ParserElement) -> ParserElement:
- """Helper to define an expression that is indirectly defined from
- the tokens matched in a previous expression, that is, it looks for
- a 'repeat' of a previous expression. For example::
-
- first = Word(nums)
- second = match_previous_expr(first)
- match_expr = first + ":" + second
-
- will match ``"1:1"``, but not ``"1:2"``. Because this
- matches by expressions, will *not* match the leading ``"1:1"``
- in ``"1:10"``; the expressions are evaluated first, and then
- compared, so ``"1"`` is compared with ``"10"``. Do *not* use
- with packrat parsing enabled.
- """
- rep = Forward()
- e2 = expr.copy()
- rep <<= e2
-
- def copy_token_to_repeater(s, l, t):
- matchTokens = _flatten(t.as_list())
-
- def must_match_these_tokens(s, l, t):
- theseTokens = _flatten(t.as_list())
- if theseTokens != matchTokens:
- raise ParseException(
- s, l, f"Expected {matchTokens}, found{theseTokens}"
- )
-
- rep.set_parse_action(must_match_these_tokens, callDuringTry=True)
-
- expr.add_parse_action(copy_token_to_repeater, callDuringTry=True)
- rep.set_name("(prev) " + str(expr))
- return rep
-
-
-def one_of(
- strs: Union[typing.Iterable[str], str],
- caseless: bool = False,
- use_regex: bool = True,
- as_keyword: bool = False,
- *,
- useRegex: bool = True,
- asKeyword: bool = False,
-) -> ParserElement:
- """Helper to quickly define a set of alternative :class:`Literal` s,
- and makes sure to do longest-first testing when there is a conflict,
- regardless of the input order, but returns
- a :class:`MatchFirst` for best performance.
-
- Parameters:
-
- - ``strs`` - a string of space-delimited literals, or a collection of
- string literals
- - ``caseless`` - treat all literals as caseless - (default= ``False``)
- - ``use_regex`` - as an optimization, will
- generate a :class:`Regex` object; otherwise, will generate
- a :class:`MatchFirst` object (if ``caseless=True`` or ``as_keyword=True``, or if
- creating a :class:`Regex` raises an exception) - (default= ``True``)
- - ``as_keyword`` - enforce :class:`Keyword`-style matching on the
- generated expressions - (default= ``False``)
- - ``asKeyword`` and ``useRegex`` are retained for pre-PEP8 compatibility,
- but will be removed in a future release
-
- Example::
-
- comp_oper = one_of("< = > <= >= !=")
- var = Word(alphas)
- number = Word(nums)
- term = var | number
- comparison_expr = term + comp_oper + term
- print(comparison_expr.search_string("B = 12 AA=23 B<=AA AA>12"))
-
- prints::
-
- [['B', '=', '12'], ['AA', '=', '23'], ['B', '<=', 'AA'], ['AA', '>', '12']]
- """
- asKeyword = asKeyword or as_keyword
- useRegex = useRegex and use_regex
-
- if (
- isinstance(caseless, str_type)
- and __diag__.warn_on_multiple_string_args_to_oneof
- ):
- warnings.warn(
- "More than one string argument passed to one_of, pass"
- " choices as a list or space-delimited string",
- stacklevel=2,
- )
-
- if caseless:
- isequal = lambda a, b: a.upper() == b.upper()
- masks = lambda a, b: b.upper().startswith(a.upper())
- parseElementClass = CaselessKeyword if asKeyword else CaselessLiteral
- else:
- isequal = lambda a, b: a == b
- masks = lambda a, b: b.startswith(a)
- parseElementClass = Keyword if asKeyword else Literal
-
- symbols: List[str] = []
- if isinstance(strs, str_type):
- strs = typing.cast(str, strs)
- symbols = strs.split()
- elif isinstance(strs, Iterable):
- symbols = list(strs)
- else:
- raise TypeError("Invalid argument to one_of, expected string or iterable")
- if not symbols:
- return NoMatch()
-
- # reorder given symbols to take care to avoid masking longer choices with shorter ones
- # (but only if the given symbols are not just single characters)
- if any(len(sym) > 1 for sym in symbols):
- i = 0
- while i < len(symbols) - 1:
- cur = symbols[i]
- for j, other in enumerate(symbols[i + 1 :]):
- if isequal(other, cur):
- del symbols[i + j + 1]
- break
- elif masks(cur, other):
- del symbols[i + j + 1]
- symbols.insert(i, other)
- break
- else:
- i += 1
-
- if useRegex:
- re_flags: int = re.IGNORECASE if caseless else 0
-
- try:
- if all(len(sym) == 1 for sym in symbols):
- # symbols are just single characters, create range regex pattern
- patt = f"[{''.join(_escape_regex_range_chars(sym) for sym in symbols)}]"
- else:
- patt = "|".join(re.escape(sym) for sym in symbols)
-
- # wrap with \b word break markers if defining as keywords
- if asKeyword:
- patt = rf"\b(?:{patt})\b"
-
- ret = Regex(patt, flags=re_flags).set_name(" | ".join(symbols))
-
- if caseless:
- # add parse action to return symbols as specified, not in random
- # casing as found in input string
- symbol_map = {sym.lower(): sym for sym in symbols}
- ret.add_parse_action(lambda s, l, t: symbol_map[t[0].lower()])
-
- return ret
-
- except re.error:
- warnings.warn(
- "Exception creating Regex for one_of, building MatchFirst", stacklevel=2
- )
-
- # last resort, just use MatchFirst
- return MatchFirst(parseElementClass(sym) for sym in symbols).set_name(
- " | ".join(symbols)
- )
-
-
-def dict_of(key: ParserElement, value: ParserElement) -> ParserElement:
- """Helper to easily and clearly define a dictionary by specifying
- the respective patterns for the key and value. Takes care of
- defining the :class:`Dict`, :class:`ZeroOrMore`, and
- :class:`Group` tokens in the proper order. The key pattern
- can include delimiting markers or punctuation, as long as they are
- suppressed, thereby leaving the significant key text. The value
- pattern can include named results, so that the :class:`Dict` results
- can include named token fields.
-
- Example::
-
- text = "shape: SQUARE posn: upper left color: light blue texture: burlap"
- attr_expr = (label + Suppress(':') + OneOrMore(data_word, stop_on=label).set_parse_action(' '.join))
- print(attr_expr[1, ...].parse_string(text).dump())
-
- attr_label = label
- attr_value = Suppress(':') + OneOrMore(data_word, stop_on=label).set_parse_action(' '.join)
-
- # similar to Dict, but simpler call format
- result = dict_of(attr_label, attr_value).parse_string(text)
- print(result.dump())
- print(result['shape'])
- print(result.shape) # object attribute access works too
- print(result.as_dict())
-
- prints::
-
- [['shape', 'SQUARE'], ['posn', 'upper left'], ['color', 'light blue'], ['texture', 'burlap']]
- - color: 'light blue'
- - posn: 'upper left'
- - shape: 'SQUARE'
- - texture: 'burlap'
- SQUARE
- SQUARE
- {'color': 'light blue', 'shape': 'SQUARE', 'posn': 'upper left', 'texture': 'burlap'}
- """
- return Dict(OneOrMore(Group(key + value)))
-
-
-def original_text_for(
- expr: ParserElement, as_string: bool = True, *, asString: bool = True
-) -> ParserElement:
- """Helper to return the original, untokenized text for a given
- expression. Useful to restore the parsed fields of an HTML start
- tag into the raw tag text itself, or to revert separate tokens with
- intervening whitespace back to the original matching input text. By
- default, returns a string containing the original parsed text.
-
- If the optional ``as_string`` argument is passed as
- ``False``, then the return value is
- a :class:`ParseResults` containing any results names that
- were originally matched, and a single token containing the original
- matched text from the input string. So if the expression passed to
- :class:`original_text_for` contains expressions with defined
- results names, you must set ``as_string`` to ``False`` if you
- want to preserve those results name values.
-
- The ``asString`` pre-PEP8 argument is retained for compatibility,
- but will be removed in a future release.
-
- Example::
-
- src = "this is test bold text normal text "
- for tag in ("b", "i"):
- opener, closer = make_html_tags(tag)
- patt = original_text_for(opener + ... + closer)
- print(patt.search_string(src)[0])
-
- prints::
-
- [' bold text ']
- ['text']
- """
- asString = asString and as_string
-
- locMarker = Empty().set_parse_action(lambda s, loc, t: loc)
- endlocMarker = locMarker.copy()
- endlocMarker.callPreparse = False
- matchExpr = locMarker("_original_start") + expr + endlocMarker("_original_end")
- if asString:
- extractText = lambda s, l, t: s[t._original_start : t._original_end]
- else:
-
- def extractText(s, l, t):
- t[:] = [s[t.pop("_original_start") : t.pop("_original_end")]]
-
- matchExpr.set_parse_action(extractText)
- matchExpr.ignoreExprs = expr.ignoreExprs
- matchExpr.suppress_warning(Diagnostics.warn_ungrouped_named_tokens_in_collection)
- return matchExpr
-
-
-def ungroup(expr: ParserElement) -> ParserElement:
- """Helper to undo pyparsing's default grouping of And expressions,
- even if all but one are non-empty.
- """
- return TokenConverter(expr).add_parse_action(lambda t: t[0])
-
-
-def locatedExpr(expr: ParserElement) -> ParserElement:
- """
- (DEPRECATED - future code should use the :class:`Located` class)
- Helper to decorate a returned token with its starting and ending
- locations in the input string.
-
- This helper adds the following results names:
-
- - ``locn_start`` - location where matched expression begins
- - ``locn_end`` - location where matched expression ends
- - ``value`` - the actual parsed results
-
- Be careful if the input text contains ```` characters, you
- may want to call :class:`ParserElement.parse_with_tabs`
-
- Example::
-
- wd = Word(alphas)
- for match in locatedExpr(wd).search_string("ljsdf123lksdjjf123lkkjj1222"):
- print(match)
-
- prints::
-
- [[0, 'ljsdf', 5]]
- [[8, 'lksdjjf', 15]]
- [[18, 'lkkjj', 23]]
- """
- locator = Empty().set_parse_action(lambda ss, ll, tt: ll)
- return Group(
- locator("locn_start")
- + expr("value")
- + locator.copy().leaveWhitespace()("locn_end")
- )
-
-
-def nested_expr(
- opener: Union[str, ParserElement] = "(",
- closer: Union[str, ParserElement] = ")",
- content: typing.Optional[ParserElement] = None,
- ignore_expr: ParserElement = quoted_string(),
- *,
- ignoreExpr: ParserElement = quoted_string(),
-) -> ParserElement:
- """Helper method for defining nested lists enclosed in opening and
- closing delimiters (``"("`` and ``")"`` are the default).
-
- Parameters:
-
- - ``opener`` - opening character for a nested list
- (default= ``"("``); can also be a pyparsing expression
- - ``closer`` - closing character for a nested list
- (default= ``")"``); can also be a pyparsing expression
- - ``content`` - expression for items within the nested lists
- (default= ``None``)
- - ``ignore_expr`` - expression for ignoring opening and closing delimiters
- (default= :class:`quoted_string`)
- - ``ignoreExpr`` - this pre-PEP8 argument is retained for compatibility
- but will be removed in a future release
-
- If an expression is not provided for the content argument, the
- nested expression will capture all whitespace-delimited content
- between delimiters as a list of separate values.
-
- Use the ``ignore_expr`` argument to define expressions that may
- contain opening or closing characters that should not be treated as
- opening or closing characters for nesting, such as quoted_string or
- a comment expression. Specify multiple expressions using an
- :class:`Or` or :class:`MatchFirst`. The default is
- :class:`quoted_string`, but if no expressions are to be ignored, then
- pass ``None`` for this argument.
-
- Example::
-
- data_type = one_of("void int short long char float double")
- decl_data_type = Combine(data_type + Opt(Word('*')))
- ident = Word(alphas+'_', alphanums+'_')
- number = pyparsing_common.number
- arg = Group(decl_data_type + ident)
- LPAR, RPAR = map(Suppress, "()")
-
- code_body = nested_expr('{', '}', ignore_expr=(quoted_string | c_style_comment))
-
- c_function = (decl_data_type("type")
- + ident("name")
- + LPAR + Opt(DelimitedList(arg), [])("args") + RPAR
- + code_body("body"))
- c_function.ignore(c_style_comment)
-
- source_code = '''
- int is_odd(int x) {
- return (x%2);
- }
-
- int dec_to_hex(char hchar) {
- if (hchar >= '0' && hchar <= '9') {
- return (ord(hchar)-ord('0'));
- } else {
- return (10+ord(hchar)-ord('A'));
- }
- }
- '''
- for func in c_function.search_string(source_code):
- print("%(name)s (%(type)s) args: %(args)s" % func)
-
-
- prints::
-
- is_odd (int) args: [['int', 'x']]
- dec_to_hex (int) args: [['char', 'hchar']]
- """
- if ignoreExpr != ignore_expr:
- ignoreExpr = ignore_expr if ignoreExpr == quoted_string() else ignoreExpr
- if opener == closer:
- raise ValueError("opening and closing strings cannot be the same")
- if content is None:
- if isinstance(opener, str_type) and isinstance(closer, str_type):
- opener = typing.cast(str, opener)
- closer = typing.cast(str, closer)
- if len(opener) == 1 and len(closer) == 1:
- if ignoreExpr is not None:
- content = Combine(
- OneOrMore(
- ~ignoreExpr
- + CharsNotIn(
- opener + closer + ParserElement.DEFAULT_WHITE_CHARS,
- exact=1,
- )
- )
- ).set_parse_action(lambda t: t[0].strip())
- else:
- content = empty.copy() + CharsNotIn(
- opener + closer + ParserElement.DEFAULT_WHITE_CHARS
- ).set_parse_action(lambda t: t[0].strip())
- else:
- if ignoreExpr is not None:
- content = Combine(
- OneOrMore(
- ~ignoreExpr
- + ~Literal(opener)
- + ~Literal(closer)
- + CharsNotIn(ParserElement.DEFAULT_WHITE_CHARS, exact=1)
- )
- ).set_parse_action(lambda t: t[0].strip())
- else:
- content = Combine(
- OneOrMore(
- ~Literal(opener)
- + ~Literal(closer)
- + CharsNotIn(ParserElement.DEFAULT_WHITE_CHARS, exact=1)
- )
- ).set_parse_action(lambda t: t[0].strip())
- else:
- raise ValueError(
- "opening and closing arguments must be strings if no content expression is given"
- )
- ret = Forward()
- if ignoreExpr is not None:
- ret <<= Group(
- Suppress(opener) + ZeroOrMore(ignoreExpr | ret | content) + Suppress(closer)
- )
- else:
- ret <<= Group(Suppress(opener) + ZeroOrMore(ret | content) + Suppress(closer))
- ret.set_name("nested %s%s expression" % (opener, closer))
- return ret
-
-
-def _makeTags(tagStr, xml, suppress_LT=Suppress("<"), suppress_GT=Suppress(">")):
- """Internal helper to construct opening and closing tag expressions, given a tag name"""
- if isinstance(tagStr, str_type):
- resname = tagStr
- tagStr = Keyword(tagStr, caseless=not xml)
- else:
- resname = tagStr.name
-
- tagAttrName = Word(alphas, alphanums + "_-:")
- if xml:
- tagAttrValue = dbl_quoted_string.copy().set_parse_action(remove_quotes)
- openTag = (
- suppress_LT
- + tagStr("tag")
- + Dict(ZeroOrMore(Group(tagAttrName + Suppress("=") + tagAttrValue)))
- + Opt("/", default=[False])("empty").set_parse_action(
- lambda s, l, t: t[0] == "/"
- )
- + suppress_GT
- )
- else:
- tagAttrValue = quoted_string.copy().set_parse_action(remove_quotes) | Word(
- printables, exclude_chars=">"
- )
- openTag = (
- suppress_LT
- + tagStr("tag")
- + Dict(
- ZeroOrMore(
- Group(
- tagAttrName.set_parse_action(lambda t: t[0].lower())
- + Opt(Suppress("=") + tagAttrValue)
- )
- )
- )
- + Opt("/", default=[False])("empty").set_parse_action(
- lambda s, l, t: t[0] == "/"
- )
- + suppress_GT
- )
- closeTag = Combine(Literal("") + tagStr + ">", adjacent=False)
-
- openTag.set_name("<%s>" % resname)
- # add start results name in parse action now that ungrouped names are not reported at two levels
- openTag.add_parse_action(
- lambda t: t.__setitem__(
- "start" + "".join(resname.replace(":", " ").title().split()), t.copy()
- )
- )
- closeTag = closeTag(
- "end" + "".join(resname.replace(":", " ").title().split())
- ).set_name("%s>" % resname)
- openTag.tag = resname
- closeTag.tag = resname
- openTag.tag_body = SkipTo(closeTag())
- return openTag, closeTag
-
-
-def make_html_tags(
- tag_str: Union[str, ParserElement]
-) -> Tuple[ParserElement, ParserElement]:
- """Helper to construct opening and closing tag expressions for HTML,
- given a tag name. Matches tags in either upper or lower case,
- attributes with namespaces and with quoted or unquoted values.
-
- Example::
-
- text = 'More info at the pyparsing wiki page '
- # make_html_tags returns pyparsing expressions for the opening and
- # closing tags as a 2-tuple
- a, a_end = make_html_tags("A")
- link_expr = a + SkipTo(a_end)("link_text") + a_end
-
- for link in link_expr.search_string(text):
- # attributes in the tag (like "href" shown here) are
- # also accessible as named results
- print(link.link_text, '->', link.href)
-
- prints::
-
- pyparsing -> https://github.com/pyparsing/pyparsing/wiki
- """
- return _makeTags(tag_str, False)
-
-
-def make_xml_tags(
- tag_str: Union[str, ParserElement]
-) -> Tuple[ParserElement, ParserElement]:
- """Helper to construct opening and closing tag expressions for XML,
- given a tag name. Matches tags only in the given upper/lower case.
-
- Example: similar to :class:`make_html_tags`
- """
- return _makeTags(tag_str, True)
-
-
-any_open_tag: ParserElement
-any_close_tag: ParserElement
-any_open_tag, any_close_tag = make_html_tags(
- Word(alphas, alphanums + "_:").set_name("any tag")
-)
-
-_htmlEntityMap = {k.rstrip(";"): v for k, v in html.entities.html5.items()}
-common_html_entity = Regex("&(?P" + "|".join(_htmlEntityMap) + ");").set_name(
- "common HTML entity"
-)
-
-
-def replace_html_entity(s, l, t):
- """Helper parser action to replace common HTML entities with their special characters"""
- return _htmlEntityMap.get(t.entity)
-
-
-class OpAssoc(Enum):
- """Enumeration of operator associativity
- - used in constructing InfixNotationOperatorSpec for :class:`infix_notation`"""
-
- LEFT = 1
- RIGHT = 2
-
-
-InfixNotationOperatorArgType = Union[
- ParserElement, str, Tuple[Union[ParserElement, str], Union[ParserElement, str]]
-]
-InfixNotationOperatorSpec = Union[
- Tuple[
- InfixNotationOperatorArgType,
- int,
- OpAssoc,
- typing.Optional[ParseAction],
- ],
- Tuple[
- InfixNotationOperatorArgType,
- int,
- OpAssoc,
- ],
-]
-
-
-def infix_notation(
- base_expr: ParserElement,
- op_list: List[InfixNotationOperatorSpec],
- lpar: Union[str, ParserElement] = Suppress("("),
- rpar: Union[str, ParserElement] = Suppress(")"),
-) -> ParserElement:
- """Helper method for constructing grammars of expressions made up of
- operators working in a precedence hierarchy. Operators may be unary
- or binary, left- or right-associative. Parse actions can also be
- attached to operator expressions. The generated parser will also
- recognize the use of parentheses to override operator precedences
- (see example below).
-
- Note: if you define a deep operator list, you may see performance
- issues when using infix_notation. See
- :class:`ParserElement.enable_packrat` for a mechanism to potentially
- improve your parser performance.
-
- Parameters:
-
- - ``base_expr`` - expression representing the most basic operand to
- be used in the expression
- - ``op_list`` - list of tuples, one for each operator precedence level
- in the expression grammar; each tuple is of the form ``(op_expr,
- num_operands, right_left_assoc, (optional)parse_action)``, where:
-
- - ``op_expr`` is the pyparsing expression for the operator; may also
- be a string, which will be converted to a Literal; if ``num_operands``
- is 3, ``op_expr`` is a tuple of two expressions, for the two
- operators separating the 3 terms
- - ``num_operands`` is the number of terms for this operator (must be 1,
- 2, or 3)
- - ``right_left_assoc`` is the indicator whether the operator is right
- or left associative, using the pyparsing-defined constants
- ``OpAssoc.RIGHT`` and ``OpAssoc.LEFT``.
- - ``parse_action`` is the parse action to be associated with
- expressions matching this operator expression (the parse action
- tuple member may be omitted); if the parse action is passed
- a tuple or list of functions, this is equivalent to calling
- ``set_parse_action(*fn)``
- (:class:`ParserElement.set_parse_action`)
- - ``lpar`` - expression for matching left-parentheses; if passed as a
- str, then will be parsed as ``Suppress(lpar)``. If lpar is passed as
- an expression (such as ``Literal('(')``), then it will be kept in
- the parsed results, and grouped with them. (default= ``Suppress('(')``)
- - ``rpar`` - expression for matching right-parentheses; if passed as a
- str, then will be parsed as ``Suppress(rpar)``. If rpar is passed as
- an expression (such as ``Literal(')')``), then it will be kept in
- the parsed results, and grouped with them. (default= ``Suppress(')')``)
-
- Example::
-
- # simple example of four-function arithmetic with ints and
- # variable names
- integer = pyparsing_common.signed_integer
- varname = pyparsing_common.identifier
-
- arith_expr = infix_notation(integer | varname,
- [
- ('-', 1, OpAssoc.RIGHT),
- (one_of('* /'), 2, OpAssoc.LEFT),
- (one_of('+ -'), 2, OpAssoc.LEFT),
- ])
-
- arith_expr.run_tests('''
- 5+3*6
- (5+3)*6
- -2--11
- ''', full_dump=False)
-
- prints::
-
- 5+3*6
- [[5, '+', [3, '*', 6]]]
-
- (5+3)*6
- [[[5, '+', 3], '*', 6]]
-
- (5+x)*y
- [[[5, '+', 'x'], '*', 'y']]
-
- -2--11
- [[['-', 2], '-', ['-', 11]]]
- """
-
- # captive version of FollowedBy that does not do parse actions or capture results names
- class _FB(FollowedBy):
- def parseImpl(self, instring, loc, doActions=True):
- self.expr.try_parse(instring, loc)
- return loc, []
-
- _FB.__name__ = "FollowedBy>"
-
- ret = Forward()
- if isinstance(lpar, str):
- lpar = Suppress(lpar)
- if isinstance(rpar, str):
- rpar = Suppress(rpar)
-
- # if lpar and rpar are not suppressed, wrap in group
- if not (isinstance(rpar, Suppress) and isinstance(rpar, Suppress)):
- lastExpr = base_expr | Group(lpar + ret + rpar)
- else:
- lastExpr = base_expr | (lpar + ret + rpar)
-
- arity: int
- rightLeftAssoc: opAssoc
- pa: typing.Optional[ParseAction]
- opExpr1: ParserElement
- opExpr2: ParserElement
- for i, operDef in enumerate(op_list):
- opExpr, arity, rightLeftAssoc, pa = (operDef + (None,))[:4] # type: ignore[assignment]
- if isinstance(opExpr, str_type):
- opExpr = ParserElement._literalStringClass(opExpr)
- opExpr = typing.cast(ParserElement, opExpr)
- if arity == 3:
- if not isinstance(opExpr, (tuple, list)) or len(opExpr) != 2:
- raise ValueError(
- "if numterms=3, opExpr must be a tuple or list of two expressions"
- )
- opExpr1, opExpr2 = opExpr
- term_name = f"{opExpr1}{opExpr2} term"
- else:
- term_name = f"{opExpr} term"
-
- if not 1 <= arity <= 3:
- raise ValueError("operator must be unary (1), binary (2), or ternary (3)")
-
- if rightLeftAssoc not in (OpAssoc.LEFT, OpAssoc.RIGHT):
- raise ValueError("operator must indicate right or left associativity")
-
- thisExpr: ParserElement = Forward().set_name(term_name)
- thisExpr = typing.cast(Forward, thisExpr)
- if rightLeftAssoc is OpAssoc.LEFT:
- if arity == 1:
- matchExpr = _FB(lastExpr + opExpr) + Group(lastExpr + opExpr[1, ...])
- elif arity == 2:
- if opExpr is not None:
- matchExpr = _FB(lastExpr + opExpr + lastExpr) + Group(
- lastExpr + (opExpr + lastExpr)[1, ...]
- )
- else:
- matchExpr = _FB(lastExpr + lastExpr) + Group(lastExpr[2, ...])
- elif arity == 3:
- matchExpr = _FB(
- lastExpr + opExpr1 + lastExpr + opExpr2 + lastExpr
- ) + Group(lastExpr + OneOrMore(opExpr1 + lastExpr + opExpr2 + lastExpr))
- elif rightLeftAssoc is OpAssoc.RIGHT:
- if arity == 1:
- # try to avoid LR with this extra test
- if not isinstance(opExpr, Opt):
- opExpr = Opt(opExpr)
- matchExpr = _FB(opExpr.expr + thisExpr) + Group(opExpr + thisExpr)
- elif arity == 2:
- if opExpr is not None:
- matchExpr = _FB(lastExpr + opExpr + thisExpr) + Group(
- lastExpr + (opExpr + thisExpr)[1, ...]
- )
- else:
- matchExpr = _FB(lastExpr + thisExpr) + Group(
- lastExpr + thisExpr[1, ...]
- )
- elif arity == 3:
- matchExpr = _FB(
- lastExpr + opExpr1 + thisExpr + opExpr2 + thisExpr
- ) + Group(lastExpr + opExpr1 + thisExpr + opExpr2 + thisExpr)
- if pa:
- if isinstance(pa, (tuple, list)):
- matchExpr.set_parse_action(*pa)
- else:
- matchExpr.set_parse_action(pa)
- thisExpr <<= (matchExpr | lastExpr).setName(term_name)
- lastExpr = thisExpr
- ret <<= lastExpr
- return ret
-
-
-def indentedBlock(blockStatementExpr, indentStack, indent=True, backup_stacks=[]):
- """
- (DEPRECATED - use :class:`IndentedBlock` class instead)
- Helper method for defining space-delimited indentation blocks,
- such as those used to define block statements in Python source code.
-
- Parameters:
-
- - ``blockStatementExpr`` - expression defining syntax of statement that
- is repeated within the indented block
- - ``indentStack`` - list created by caller to manage indentation stack
- (multiple ``statementWithIndentedBlock`` expressions within a single
- grammar should share a common ``indentStack``)
- - ``indent`` - boolean indicating whether block must be indented beyond
- the current level; set to ``False`` for block of left-most statements
- (default= ``True``)
-
- A valid block must contain at least one ``blockStatement``.
-
- (Note that indentedBlock uses internal parse actions which make it
- incompatible with packrat parsing.)
-
- Example::
-
- data = '''
- def A(z):
- A1
- B = 100
- G = A2
- A2
- A3
- B
- def BB(a,b,c):
- BB1
- def BBA():
- bba1
- bba2
- bba3
- C
- D
- def spam(x,y):
- def eggs(z):
- pass
- '''
-
-
- indentStack = [1]
- stmt = Forward()
-
- identifier = Word(alphas, alphanums)
- funcDecl = ("def" + identifier + Group("(" + Opt(delimitedList(identifier)) + ")") + ":")
- func_body = indentedBlock(stmt, indentStack)
- funcDef = Group(funcDecl + func_body)
-
- rvalue = Forward()
- funcCall = Group(identifier + "(" + Opt(delimitedList(rvalue)) + ")")
- rvalue << (funcCall | identifier | Word(nums))
- assignment = Group(identifier + "=" + rvalue)
- stmt << (funcDef | assignment | identifier)
-
- module_body = stmt[1, ...]
-
- parseTree = module_body.parseString(data)
- parseTree.pprint()
-
- prints::
-
- [['def',
- 'A',
- ['(', 'z', ')'],
- ':',
- [['A1'], [['B', '=', '100']], [['G', '=', 'A2']], ['A2'], ['A3']]],
- 'B',
- ['def',
- 'BB',
- ['(', 'a', 'b', 'c', ')'],
- ':',
- [['BB1'], [['def', 'BBA', ['(', ')'], ':', [['bba1'], ['bba2'], ['bba3']]]]]],
- 'C',
- 'D',
- ['def',
- 'spam',
- ['(', 'x', 'y', ')'],
- ':',
- [[['def', 'eggs', ['(', 'z', ')'], ':', [['pass']]]]]]]
- """
- backup_stacks.append(indentStack[:])
-
- def reset_stack():
- indentStack[:] = backup_stacks[-1]
-
- def checkPeerIndent(s, l, t):
- if l >= len(s):
- return
- curCol = col(l, s)
- if curCol != indentStack[-1]:
- if curCol > indentStack[-1]:
- raise ParseException(s, l, "illegal nesting")
- raise ParseException(s, l, "not a peer entry")
-
- def checkSubIndent(s, l, t):
- curCol = col(l, s)
- if curCol > indentStack[-1]:
- indentStack.append(curCol)
- else:
- raise ParseException(s, l, "not a subentry")
-
- def checkUnindent(s, l, t):
- if l >= len(s):
- return
- curCol = col(l, s)
- if not (indentStack and curCol in indentStack):
- raise ParseException(s, l, "not an unindent")
- if curCol < indentStack[-1]:
- indentStack.pop()
-
- NL = OneOrMore(LineEnd().set_whitespace_chars("\t ").suppress())
- INDENT = (Empty() + Empty().set_parse_action(checkSubIndent)).set_name("INDENT")
- PEER = Empty().set_parse_action(checkPeerIndent).set_name("")
- UNDENT = Empty().set_parse_action(checkUnindent).set_name("UNINDENT")
- if indent:
- smExpr = Group(
- Opt(NL)
- + INDENT
- + OneOrMore(PEER + Group(blockStatementExpr) + Opt(NL))
- + UNDENT
- )
- else:
- smExpr = Group(
- Opt(NL)
- + OneOrMore(PEER + Group(blockStatementExpr) + Opt(NL))
- + Opt(UNDENT)
- )
-
- # add a parse action to remove backup_stack from list of backups
- smExpr.add_parse_action(
- lambda: backup_stacks.pop(-1) and None if backup_stacks else None
- )
- smExpr.set_fail_action(lambda a, b, c, d: reset_stack())
- blockStatementExpr.ignore(_bslash + LineEnd())
- return smExpr.set_name("indented block")
-
-
-# it's easy to get these comment structures wrong - they're very common, so may as well make them available
-c_style_comment = Combine(Regex(r"/\*(?:[^*]|\*(?!/))*") + "*/").set_name(
- "C style comment"
-)
-"Comment of the form ``/* ... */``"
-
-html_comment = Regex(r"").set_name("HTML comment")
-"Comment of the form ````"
-
-rest_of_line = Regex(r".*").leave_whitespace().set_name("rest of line")
-dbl_slash_comment = Regex(r"//(?:\\\n|[^\n])*").set_name("// comment")
-"Comment of the form ``// ... (to end of line)``"
-
-cpp_style_comment = Combine(
- Regex(r"/\*(?:[^*]|\*(?!/))*") + "*/" | dbl_slash_comment
-).set_name("C++ style comment")
-"Comment of either form :class:`c_style_comment` or :class:`dbl_slash_comment`"
-
-java_style_comment = cpp_style_comment
-"Same as :class:`cpp_style_comment`"
-
-python_style_comment = Regex(r"#.*").set_name("Python style comment")
-"Comment of the form ``# ... (to end of line)``"
-
-
-# build list of built-in expressions, for future reference if a global default value
-# gets updated
-_builtin_exprs: List[ParserElement] = [
- v for v in vars().values() if isinstance(v, ParserElement)
-]
-
-
-# compatibility function, superseded by DelimitedList class
-def delimited_list(
- expr: Union[str, ParserElement],
- delim: Union[str, ParserElement] = ",",
- combine: bool = False,
- min: typing.Optional[int] = None,
- max: typing.Optional[int] = None,
- *,
- allow_trailing_delim: bool = False,
-) -> ParserElement:
- """(DEPRECATED - use :class:`DelimitedList` class)"""
- return DelimitedList(
- expr, delim, combine, min, max, allow_trailing_delim=allow_trailing_delim
- )
-
-
-# pre-PEP8 compatible names
-# fmt: off
-opAssoc = OpAssoc
-anyOpenTag = any_open_tag
-anyCloseTag = any_close_tag
-commonHTMLEntity = common_html_entity
-cStyleComment = c_style_comment
-htmlComment = html_comment
-restOfLine = rest_of_line
-dblSlashComment = dbl_slash_comment
-cppStyleComment = cpp_style_comment
-javaStyleComment = java_style_comment
-pythonStyleComment = python_style_comment
-
-@replaced_by_pep8(DelimitedList)
-def delimitedList(): ...
-
-@replaced_by_pep8(DelimitedList)
-def delimited_list(): ...
-
-@replaced_by_pep8(counted_array)
-def countedArray(): ...
-
-@replaced_by_pep8(match_previous_literal)
-def matchPreviousLiteral(): ...
-
-@replaced_by_pep8(match_previous_expr)
-def matchPreviousExpr(): ...
-
-@replaced_by_pep8(one_of)
-def oneOf(): ...
-
-@replaced_by_pep8(dict_of)
-def dictOf(): ...
-
-@replaced_by_pep8(original_text_for)
-def originalTextFor(): ...
-
-@replaced_by_pep8(nested_expr)
-def nestedExpr(): ...
-
-@replaced_by_pep8(make_html_tags)
-def makeHTMLTags(): ...
-
-@replaced_by_pep8(make_xml_tags)
-def makeXMLTags(): ...
-
-@replaced_by_pep8(replace_html_entity)
-def replaceHTMLEntity(): ...
-
-@replaced_by_pep8(infix_notation)
-def infixNotation(): ...
-# fmt: on
diff --git a/spaces/TandCAcceptMe/face-swap-docker/mynewshinyroop/Lib/site-packages/setuptools/_vendor/importlib_resources/_adapters.py b/spaces/TandCAcceptMe/face-swap-docker/mynewshinyroop/Lib/site-packages/setuptools/_vendor/importlib_resources/_adapters.py
deleted file mode 100644
index ea363d86a564b5450666aa00aecd46353326a75a..0000000000000000000000000000000000000000
--- a/spaces/TandCAcceptMe/face-swap-docker/mynewshinyroop/Lib/site-packages/setuptools/_vendor/importlib_resources/_adapters.py
+++ /dev/null
@@ -1,170 +0,0 @@
-from contextlib import suppress
-from io import TextIOWrapper
-
-from . import abc
-
-
-class SpecLoaderAdapter:
- """
- Adapt a package spec to adapt the underlying loader.
- """
-
- def __init__(self, spec, adapter=lambda spec: spec.loader):
- self.spec = spec
- self.loader = adapter(spec)
-
- def __getattr__(self, name):
- return getattr(self.spec, name)
-
-
-class TraversableResourcesLoader:
- """
- Adapt a loader to provide TraversableResources.
- """
-
- def __init__(self, spec):
- self.spec = spec
-
- def get_resource_reader(self, name):
- return CompatibilityFiles(self.spec)._native()
-
-
-def _io_wrapper(file, mode='r', *args, **kwargs):
- if mode == 'r':
- return TextIOWrapper(file, *args, **kwargs)
- elif mode == 'rb':
- return file
- raise ValueError(
- "Invalid mode value '{}', only 'r' and 'rb' are supported".format(mode)
- )
-
-
-class CompatibilityFiles:
- """
- Adapter for an existing or non-existent resource reader
- to provide a compatibility .files().
- """
-
- class SpecPath(abc.Traversable):
- """
- Path tied to a module spec.
- Can be read and exposes the resource reader children.
- """
-
- def __init__(self, spec, reader):
- self._spec = spec
- self._reader = reader
-
- def iterdir(self):
- if not self._reader:
- return iter(())
- return iter(
- CompatibilityFiles.ChildPath(self._reader, path)
- for path in self._reader.contents()
- )
-
- def is_file(self):
- return False
-
- is_dir = is_file
-
- def joinpath(self, other):
- if not self._reader:
- return CompatibilityFiles.OrphanPath(other)
- return CompatibilityFiles.ChildPath(self._reader, other)
-
- @property
- def name(self):
- return self._spec.name
-
- def open(self, mode='r', *args, **kwargs):
- return _io_wrapper(self._reader.open_resource(None), mode, *args, **kwargs)
-
- class ChildPath(abc.Traversable):
- """
- Path tied to a resource reader child.
- Can be read but doesn't expose any meaningful children.
- """
-
- def __init__(self, reader, name):
- self._reader = reader
- self._name = name
-
- def iterdir(self):
- return iter(())
-
- def is_file(self):
- return self._reader.is_resource(self.name)
-
- def is_dir(self):
- return not self.is_file()
-
- def joinpath(self, other):
- return CompatibilityFiles.OrphanPath(self.name, other)
-
- @property
- def name(self):
- return self._name
-
- def open(self, mode='r', *args, **kwargs):
- return _io_wrapper(
- self._reader.open_resource(self.name), mode, *args, **kwargs
- )
-
- class OrphanPath(abc.Traversable):
- """
- Orphan path, not tied to a module spec or resource reader.
- Can't be read and doesn't expose any meaningful children.
- """
-
- def __init__(self, *path_parts):
- if len(path_parts) < 1:
- raise ValueError('Need at least one path part to construct a path')
- self._path = path_parts
-
- def iterdir(self):
- return iter(())
-
- def is_file(self):
- return False
-
- is_dir = is_file
-
- def joinpath(self, other):
- return CompatibilityFiles.OrphanPath(*self._path, other)
-
- @property
- def name(self):
- return self._path[-1]
-
- def open(self, mode='r', *args, **kwargs):
- raise FileNotFoundError("Can't open orphan path")
-
- def __init__(self, spec):
- self.spec = spec
-
- @property
- def _reader(self):
- with suppress(AttributeError):
- return self.spec.loader.get_resource_reader(self.spec.name)
-
- def _native(self):
- """
- Return the native reader if it supports files().
- """
- reader = self._reader
- return reader if hasattr(reader, 'files') else self
-
- def __getattr__(self, attr):
- return getattr(self._reader, attr)
-
- def files(self):
- return CompatibilityFiles.SpecPath(self.spec, self._reader)
-
-
-def wrap_spec(package):
- """
- Construct a package spec with traversable compatibility
- on the spec/loader/reader.
- """
- return SpecLoaderAdapter(package.__spec__, TraversableResourcesLoader)
diff --git a/spaces/ThankGod/anime-gan/Makefile b/spaces/ThankGod/anime-gan/Makefile
deleted file mode 100644
index ff727d0ac0d87aa292e9ddbd99218cadb034f3a4..0000000000000000000000000000000000000000
--- a/spaces/ThankGod/anime-gan/Makefile
+++ /dev/null
@@ -1,27 +0,0 @@
-install:
- pip install --upgrade pip &&\
- pip install -r requirements.txt
-
-test:
- python -m pytest -vvv --cov=hello --cov=greeting \
- --cov=smath --cov=web tests
- python -m pytest --nbval notebook.ipynb #tests our jupyter notebook
- #python -m pytest -v tests/test_web.py #if you just want to test web
-
-debug:
- python -m pytest -vv --pdb #Debugger is invoked
-
-one-test:
- python -m pytest -vv tests/test_greeting.py::test_my_name4
-
-debugthree:
- #not working the way I expect
- python -m pytest -vv --pdb --maxfail=4 # drop to PDB for first three failures
-
-format:
- black *.py
-
-lint:
- pylint --disable=R,C *.py
-
-all: install lint test format
\ No newline at end of file
diff --git a/spaces/TheKitten/Images/index.html b/spaces/TheKitten/Images/index.html
deleted file mode 100644
index 6250c2958a7186a4e64f21c02b0359ff5ecd7e97..0000000000000000000000000000000000000000
--- a/spaces/TheKitten/Images/index.html
+++ /dev/null
@@ -1,16 +0,0 @@
-
-
-
-
-
-
-
-
-
-
-
-
-
-
-
-
\ No newline at end of file
diff --git a/spaces/Toxfu/BIgVisionEffnetB2/app.py b/spaces/Toxfu/BIgVisionEffnetB2/app.py
deleted file mode 100644
index 2707f0917d3670a4cb7f2241ca7ca9c524ac74fe..0000000000000000000000000000000000000000
--- a/spaces/Toxfu/BIgVisionEffnetB2/app.py
+++ /dev/null
@@ -1,81 +0,0 @@
-### 1. Imports and class names setup ###
-import gradio as gr
-import os
-import torch
-
-from model import create_effnetb2_model
-from timeit import default_timer as timer
-from typing import Tuple, Dict
-
-# Setup class names
-with open("class_names.txt", "r") as f: # reading them in from class_names.txt
- class_names = [food_name.strip() for food_name in f.readlines()]
-
-### 2. Model and transforms preparation ###
-
-# Create model
-effnetb2, effnetb2_transforms = create_effnetb2_model(
- num_classes=101, # could also use len(class_names)
-)
-
-# Load saved weights
-effnetb2.load_state_dict(
- torch.load(
- f="effnetb2_food101_100_percent.pth",
- map_location=torch.device("cpu"), # load to CPU
- )
-)
-
-### 3. Predict function ###
-
-# Create predict function
-def predict(img) -> Tuple[Dict, float]:
- """Transforms and performs a prediction on img and returns prediction and time taken.
- """
- # Start the timer
- start_time = timer()
-
- # Transform the target image and add a batch dimension
- img = effnetb2_transforms(img).unsqueeze(0)
-
- # Put model into evaluation mode and turn on inference mode
- effnetb2.eval()
- with torch.inference_mode():
- # Pass the transformed image through the model and turn the prediction logits into prediction probabilities
- pred_probs = torch.softmax(effnetb2(img), dim=1)
-
- # Create a prediction label and prediction probability dictionary for each prediction class (this is the required format for Gradio's output parameter)
- pred_labels_and_probs = {class_names[i]: float(pred_probs[0][i]) for i in range(len(class_names))}
-
- # Calculate the prediction time
- pred_time = round(timer() - start_time, 5)
-
- # Return the prediction dictionary and prediction time
- return pred_labels_and_probs, pred_time
-
-### 4. Gradio app ###
-
-# Create title, description and article strings
-title = "FoodVision Big 🍔👁"
-description = "An EfficientNetB2 feature extractor computer vision model to classify images of food into 101 different classes"
-article = "Created for Toxfu"
-
-# Create examples list from "examples/" directory
-example_list = [["examples/" + example] for example in os.listdir("examples")]
-
-# Create Gradio interface
-demo = gr.Interface(
- fn=predict,
- inputs=gr.Image(type="pil"),
- outputs=[
- gr.Label(num_top_classes=5, label="Predictions"),
- gr.Number(label="Prediction time (s)"),
- ],
- examples=example_list,
- title=title,
- description=description,
- article=article,
-)
-
-# Launch the app!
-demo.launch()
diff --git a/spaces/UNIST-Eunchan/Summarizing-app/README.md b/spaces/UNIST-Eunchan/Summarizing-app/README.md
deleted file mode 100644
index 3e8fe31b843cdb1a3d3039d7e0bba7cba6435e24..0000000000000000000000000000000000000000
--- a/spaces/UNIST-Eunchan/Summarizing-app/README.md
+++ /dev/null
@@ -1,37 +0,0 @@
----
-title: Summarizing App
-emoji: 🐠
-colorFrom: red
-colorTo: yellow
-sdk: streamlit
-app_file: app.py
-pinned: false
----
-
-# Configuration
-
-`title`: _string_
-Display title for the Space
-
-`emoji`: _string_
-Space emoji (emoji-only character allowed)
-
-`colorFrom`: _string_
-Color for Thumbnail gradient (red, yellow, green, blue, indigo, purple, pink, gray)
-
-`colorTo`: _string_
-Color for Thumbnail gradient (red, yellow, green, blue, indigo, purple, pink, gray)
-
-`sdk`: _string_
-Can be either `gradio` or `streamlit`
-
-`sdk_version` : _string_
-Only applicable for `streamlit` SDK.
-See [doc](https://hf.co/docs/hub/spaces) for more info on supported versions.
-
-`app_file`: _string_
-Path to your main application file (which contains either `gradio` or `streamlit` Python code).
-Path is relative to the root of the repository.
-
-`pinned`: _boolean_
-Whether the Space stays on top of your list.
diff --git a/spaces/Vageesh1/Voice_Cloner/README.md b/spaces/Vageesh1/Voice_Cloner/README.md
deleted file mode 100644
index 5a3ccde039a2856b9ccf431089d7e8668c587808..0000000000000000000000000000000000000000
--- a/spaces/Vageesh1/Voice_Cloner/README.md
+++ /dev/null
@@ -1,13 +0,0 @@
----
-title: Voice Cloner
-emoji: ⚡
-colorFrom: yellow
-colorTo: yellow
-sdk: streamlit
-sdk_version: 1.21.0
-app_file: app.py
-pinned: false
-license: openrail
----
-
-Check out the configuration reference at https://huggingface.co/docs/hub/spaces-config-reference
diff --git a/spaces/Xeaser/rvc-tes/infer_pack/transforms.py b/spaces/Xeaser/rvc-tes/infer_pack/transforms.py
deleted file mode 100644
index a11f799e023864ff7082c1f49c0cc18351a13b47..0000000000000000000000000000000000000000
--- a/spaces/Xeaser/rvc-tes/infer_pack/transforms.py
+++ /dev/null
@@ -1,209 +0,0 @@
-import torch
-from torch.nn import functional as F
-
-import numpy as np
-
-
-DEFAULT_MIN_BIN_WIDTH = 1e-3
-DEFAULT_MIN_BIN_HEIGHT = 1e-3
-DEFAULT_MIN_DERIVATIVE = 1e-3
-
-
-def piecewise_rational_quadratic_transform(
- inputs,
- unnormalized_widths,
- unnormalized_heights,
- unnormalized_derivatives,
- inverse=False,
- tails=None,
- tail_bound=1.0,
- min_bin_width=DEFAULT_MIN_BIN_WIDTH,
- min_bin_height=DEFAULT_MIN_BIN_HEIGHT,
- min_derivative=DEFAULT_MIN_DERIVATIVE,
-):
- if tails is None:
- spline_fn = rational_quadratic_spline
- spline_kwargs = {}
- else:
- spline_fn = unconstrained_rational_quadratic_spline
- spline_kwargs = {"tails": tails, "tail_bound": tail_bound}
-
- outputs, logabsdet = spline_fn(
- inputs=inputs,
- unnormalized_widths=unnormalized_widths,
- unnormalized_heights=unnormalized_heights,
- unnormalized_derivatives=unnormalized_derivatives,
- inverse=inverse,
- min_bin_width=min_bin_width,
- min_bin_height=min_bin_height,
- min_derivative=min_derivative,
- **spline_kwargs
- )
- return outputs, logabsdet
-
-
-def searchsorted(bin_locations, inputs, eps=1e-6):
- bin_locations[..., -1] += eps
- return torch.sum(inputs[..., None] >= bin_locations, dim=-1) - 1
-
-
-def unconstrained_rational_quadratic_spline(
- inputs,
- unnormalized_widths,
- unnormalized_heights,
- unnormalized_derivatives,
- inverse=False,
- tails="linear",
- tail_bound=1.0,
- min_bin_width=DEFAULT_MIN_BIN_WIDTH,
- min_bin_height=DEFAULT_MIN_BIN_HEIGHT,
- min_derivative=DEFAULT_MIN_DERIVATIVE,
-):
- inside_interval_mask = (inputs >= -tail_bound) & (inputs <= tail_bound)
- outside_interval_mask = ~inside_interval_mask
-
- outputs = torch.zeros_like(inputs)
- logabsdet = torch.zeros_like(inputs)
-
- if tails == "linear":
- unnormalized_derivatives = F.pad(unnormalized_derivatives, pad=(1, 1))
- constant = np.log(np.exp(1 - min_derivative) - 1)
- unnormalized_derivatives[..., 0] = constant
- unnormalized_derivatives[..., -1] = constant
-
- outputs[outside_interval_mask] = inputs[outside_interval_mask]
- logabsdet[outside_interval_mask] = 0
- else:
- raise RuntimeError("{} tails are not implemented.".format(tails))
-
- (
- outputs[inside_interval_mask],
- logabsdet[inside_interval_mask],
- ) = rational_quadratic_spline(
- inputs=inputs[inside_interval_mask],
- unnormalized_widths=unnormalized_widths[inside_interval_mask, :],
- unnormalized_heights=unnormalized_heights[inside_interval_mask, :],
- unnormalized_derivatives=unnormalized_derivatives[inside_interval_mask, :],
- inverse=inverse,
- left=-tail_bound,
- right=tail_bound,
- bottom=-tail_bound,
- top=tail_bound,
- min_bin_width=min_bin_width,
- min_bin_height=min_bin_height,
- min_derivative=min_derivative,
- )
-
- return outputs, logabsdet
-
-
-def rational_quadratic_spline(
- inputs,
- unnormalized_widths,
- unnormalized_heights,
- unnormalized_derivatives,
- inverse=False,
- left=0.0,
- right=1.0,
- bottom=0.0,
- top=1.0,
- min_bin_width=DEFAULT_MIN_BIN_WIDTH,
- min_bin_height=DEFAULT_MIN_BIN_HEIGHT,
- min_derivative=DEFAULT_MIN_DERIVATIVE,
-):
- if torch.min(inputs) < left or torch.max(inputs) > right:
- raise ValueError("Input to a transform is not within its domain")
-
- num_bins = unnormalized_widths.shape[-1]
-
- if min_bin_width * num_bins > 1.0:
- raise ValueError("Minimal bin width too large for the number of bins")
- if min_bin_height * num_bins > 1.0:
- raise ValueError("Minimal bin height too large for the number of bins")
-
- widths = F.softmax(unnormalized_widths, dim=-1)
- widths = min_bin_width + (1 - min_bin_width * num_bins) * widths
- cumwidths = torch.cumsum(widths, dim=-1)
- cumwidths = F.pad(cumwidths, pad=(1, 0), mode="constant", value=0.0)
- cumwidths = (right - left) * cumwidths + left
- cumwidths[..., 0] = left
- cumwidths[..., -1] = right
- widths = cumwidths[..., 1:] - cumwidths[..., :-1]
-
- derivatives = min_derivative + F.softplus(unnormalized_derivatives)
-
- heights = F.softmax(unnormalized_heights, dim=-1)
- heights = min_bin_height + (1 - min_bin_height * num_bins) * heights
- cumheights = torch.cumsum(heights, dim=-1)
- cumheights = F.pad(cumheights, pad=(1, 0), mode="constant", value=0.0)
- cumheights = (top - bottom) * cumheights + bottom
- cumheights[..., 0] = bottom
- cumheights[..., -1] = top
- heights = cumheights[..., 1:] - cumheights[..., :-1]
-
- if inverse:
- bin_idx = searchsorted(cumheights, inputs)[..., None]
- else:
- bin_idx = searchsorted(cumwidths, inputs)[..., None]
-
- input_cumwidths = cumwidths.gather(-1, bin_idx)[..., 0]
- input_bin_widths = widths.gather(-1, bin_idx)[..., 0]
-
- input_cumheights = cumheights.gather(-1, bin_idx)[..., 0]
- delta = heights / widths
- input_delta = delta.gather(-1, bin_idx)[..., 0]
-
- input_derivatives = derivatives.gather(-1, bin_idx)[..., 0]
- input_derivatives_plus_one = derivatives[..., 1:].gather(-1, bin_idx)[..., 0]
-
- input_heights = heights.gather(-1, bin_idx)[..., 0]
-
- if inverse:
- a = (inputs - input_cumheights) * (
- input_derivatives + input_derivatives_plus_one - 2 * input_delta
- ) + input_heights * (input_delta - input_derivatives)
- b = input_heights * input_derivatives - (inputs - input_cumheights) * (
- input_derivatives + input_derivatives_plus_one - 2 * input_delta
- )
- c = -input_delta * (inputs - input_cumheights)
-
- discriminant = b.pow(2) - 4 * a * c
- assert (discriminant >= 0).all()
-
- root = (2 * c) / (-b - torch.sqrt(discriminant))
- outputs = root * input_bin_widths + input_cumwidths
-
- theta_one_minus_theta = root * (1 - root)
- denominator = input_delta + (
- (input_derivatives + input_derivatives_plus_one - 2 * input_delta)
- * theta_one_minus_theta
- )
- derivative_numerator = input_delta.pow(2) * (
- input_derivatives_plus_one * root.pow(2)
- + 2 * input_delta * theta_one_minus_theta
- + input_derivatives * (1 - root).pow(2)
- )
- logabsdet = torch.log(derivative_numerator) - 2 * torch.log(denominator)
-
- return outputs, -logabsdet
- else:
- theta = (inputs - input_cumwidths) / input_bin_widths
- theta_one_minus_theta = theta * (1 - theta)
-
- numerator = input_heights * (
- input_delta * theta.pow(2) + input_derivatives * theta_one_minus_theta
- )
- denominator = input_delta + (
- (input_derivatives + input_derivatives_plus_one - 2 * input_delta)
- * theta_one_minus_theta
- )
- outputs = input_cumheights + numerator / denominator
-
- derivative_numerator = input_delta.pow(2) * (
- input_derivatives_plus_one * theta.pow(2)
- + 2 * input_delta * theta_one_minus_theta
- + input_derivatives * (1 - theta).pow(2)
- )
- logabsdet = torch.log(derivative_numerator) - 2 * torch.log(denominator)
-
- return outputs, logabsdet
diff --git a/spaces/YanzBotz/stablediffusionapi-disney-pixar-cartoon/app.py b/spaces/YanzBotz/stablediffusionapi-disney-pixar-cartoon/app.py
deleted file mode 100644
index 27fd27bfffeb59d210fd2c7769378680cb81844c..0000000000000000000000000000000000000000
--- a/spaces/YanzBotz/stablediffusionapi-disney-pixar-cartoon/app.py
+++ /dev/null
@@ -1,3 +0,0 @@
-import gradio as gr
-
-gr.Interface.load("models/stablediffusionapi/disney-pixar-cartoon").launch()
\ No newline at end of file
diff --git a/spaces/YotamNitzan/domain-expansion/legacy.py b/spaces/YotamNitzan/domain-expansion/legacy.py
deleted file mode 100644
index 9387d79f23224642ca316399de2f0258f72de79b..0000000000000000000000000000000000000000
--- a/spaces/YotamNitzan/domain-expansion/legacy.py
+++ /dev/null
@@ -1,320 +0,0 @@
-# Copyright (c) 2021, NVIDIA CORPORATION. All rights reserved.
-#
-# NVIDIA CORPORATION and its licensors retain all intellectual property
-# and proprietary rights in and to this software, related documentation
-# and any modifications thereto. Any use, reproduction, disclosure or
-# distribution of this software and related documentation without an express
-# license agreement from NVIDIA CORPORATION is strictly prohibited.
-
-import click
-import pickle
-import re
-import copy
-import numpy as np
-import torch
-import dnnlib
-from torch_utils import misc
-
-#----------------------------------------------------------------------------
-
-def load_network_pkl(f, force_fp16=False):
- data = _LegacyUnpickler(f).load()
-
- # Legacy TensorFlow pickle => convert.
- if isinstance(data, tuple) and len(data) == 3 and all(isinstance(net, _TFNetworkStub) for net in data):
- tf_G, tf_D, tf_Gs = data
- G = convert_tf_generator(tf_G)
- D = convert_tf_discriminator(tf_D)
- G_ema = convert_tf_generator(tf_Gs)
- data = dict(G=G, D=D, G_ema=G_ema)
-
- # Add missing fields.
- if 'training_set_kwargs' not in data:
- data['training_set_kwargs'] = None
- if 'augment_pipe' not in data:
- data['augment_pipe'] = None
-
- # Validate contents.
- assert isinstance(data['G'], torch.nn.Module)
- assert isinstance(data['D'], torch.nn.Module)
- assert isinstance(data['G_ema'], torch.nn.Module)
- assert isinstance(data['training_set_kwargs'], (dict, type(None)))
- assert isinstance(data['augment_pipe'], (torch.nn.Module, type(None)))
-
- # Force FP16.
- if force_fp16:
- for key in ['G', 'D', 'G_ema']:
- old = data[key]
- kwargs = copy.deepcopy(old.init_kwargs)
- if key.startswith('G'):
- kwargs.synthesis_kwargs = dnnlib.EasyDict(kwargs.get('synthesis_kwargs', {}))
- kwargs.synthesis_kwargs.num_fp16_res = 4
- kwargs.synthesis_kwargs.conv_clamp = 256
- if key.startswith('D'):
- kwargs.num_fp16_res = 4
- kwargs.conv_clamp = 256
- if kwargs != old.init_kwargs:
- new = type(old)(**kwargs).eval().requires_grad_(False)
- misc.copy_params_and_buffers(old, new, require_all=True)
- data[key] = new
- return data
-
-#----------------------------------------------------------------------------
-
-class _TFNetworkStub(dnnlib.EasyDict):
- pass
-
-class _LegacyUnpickler(pickle.Unpickler):
- def find_class(self, module, name):
- if module == 'dnnlib.tflib.network' and name == 'Network':
- return _TFNetworkStub
- return super().find_class(module, name)
-
-#----------------------------------------------------------------------------
-
-def _collect_tf_params(tf_net):
- # pylint: disable=protected-access
- tf_params = dict()
- def recurse(prefix, tf_net):
- for name, value in tf_net.variables:
- tf_params[prefix + name] = value
- for name, comp in tf_net.components.items():
- recurse(prefix + name + '/', comp)
- recurse('', tf_net)
- return tf_params
-
-#----------------------------------------------------------------------------
-
-def _populate_module_params(module, *patterns):
- for name, tensor in misc.named_params_and_buffers(module):
- found = False
- value = None
- for pattern, value_fn in zip(patterns[0::2], patterns[1::2]):
- match = re.fullmatch(pattern, name)
- if match:
- found = True
- if value_fn is not None:
- value = value_fn(*match.groups())
- break
- try:
- assert found
- if value is not None:
- tensor.copy_(torch.from_numpy(np.array(value)))
- except:
- print(name, list(tensor.shape))
- raise
-
-#----------------------------------------------------------------------------
-
-def convert_tf_generator(tf_G):
- if tf_G.version < 4:
- raise ValueError('TensorFlow pickle version too low')
-
- # Collect kwargs.
- tf_kwargs = tf_G.static_kwargs
- known_kwargs = set()
- def kwarg(tf_name, default=None, none=None):
- known_kwargs.add(tf_name)
- val = tf_kwargs.get(tf_name, default)
- return val if val is not None else none
-
- # Convert kwargs.
- kwargs = dnnlib.EasyDict(
- z_dim = kwarg('latent_size', 512),
- c_dim = kwarg('label_size', 0),
- w_dim = kwarg('dlatent_size', 512),
- img_resolution = kwarg('resolution', 1024),
- img_channels = kwarg('num_channels', 3),
- mapping_kwargs = dnnlib.EasyDict(
- num_layers = kwarg('mapping_layers', 8),
- embed_features = kwarg('label_fmaps', None),
- layer_features = kwarg('mapping_fmaps', None),
- activation = kwarg('mapping_nonlinearity', 'lrelu'),
- lr_multiplier = kwarg('mapping_lrmul', 0.01),
- w_avg_beta = kwarg('w_avg_beta', 0.995, none=1),
- ),
- synthesis_kwargs = dnnlib.EasyDict(
- channel_base = kwarg('fmap_base', 16384) * 2,
- channel_max = kwarg('fmap_max', 512),
- num_fp16_res = kwarg('num_fp16_res', 0),
- conv_clamp = kwarg('conv_clamp', None),
- architecture = kwarg('architecture', 'skip'),
- resample_filter = kwarg('resample_kernel', [1,3,3,1]),
- use_noise = kwarg('use_noise', True),
- activation = kwarg('nonlinearity', 'lrelu'),
- ),
- )
-
- # Check for unknown kwargs.
- kwarg('truncation_psi')
- kwarg('truncation_cutoff')
- kwarg('style_mixing_prob')
- kwarg('structure')
- unknown_kwargs = list(set(tf_kwargs.keys()) - known_kwargs)
- if len(unknown_kwargs) > 0:
- raise ValueError('Unknown TensorFlow kwarg', unknown_kwargs[0])
-
- # Collect params.
- tf_params = _collect_tf_params(tf_G)
- for name, value in list(tf_params.items()):
- match = re.fullmatch(r'ToRGB_lod(\d+)/(.*)', name)
- if match:
- r = kwargs.img_resolution // (2 ** int(match.group(1)))
- tf_params[f'{r}x{r}/ToRGB/{match.group(2)}'] = value
- kwargs.synthesis.kwargs.architecture = 'orig'
- #for name, value in tf_params.items(): print(f'{name:<50s}{list(value.shape)}')
-
- # Convert params.
- from training import networks
- G = networks.Generator(**kwargs).eval().requires_grad_(False)
- # pylint: disable=unnecessary-lambda
- _populate_module_params(G,
- r'mapping\.w_avg', lambda: tf_params[f'dlatent_avg'],
- r'mapping\.embed\.weight', lambda: tf_params[f'mapping/LabelEmbed/weight'].transpose(),
- r'mapping\.embed\.bias', lambda: tf_params[f'mapping/LabelEmbed/bias'],
- r'mapping\.fc(\d+)\.weight', lambda i: tf_params[f'mapping/Dense{i}/weight'].transpose(),
- r'mapping\.fc(\d+)\.bias', lambda i: tf_params[f'mapping/Dense{i}/bias'],
- r'synthesis\.b4\.const', lambda: tf_params[f'synthesis/4x4/Const/const'][0],
- r'synthesis\.b4\.conv1\.weight', lambda: tf_params[f'synthesis/4x4/Conv/weight'].transpose(3, 2, 0, 1),
- r'synthesis\.b4\.conv1\.bias', lambda: tf_params[f'synthesis/4x4/Conv/bias'],
- r'synthesis\.b4\.conv1\.noise_const', lambda: tf_params[f'synthesis/noise0'][0, 0],
- r'synthesis\.b4\.conv1\.noise_strength', lambda: tf_params[f'synthesis/4x4/Conv/noise_strength'],
- r'synthesis\.b4\.conv1\.affine\.weight', lambda: tf_params[f'synthesis/4x4/Conv/mod_weight'].transpose(),
- r'synthesis\.b4\.conv1\.affine\.bias', lambda: tf_params[f'synthesis/4x4/Conv/mod_bias'] + 1,
- r'synthesis\.b(\d+)\.conv0\.weight', lambda r: tf_params[f'synthesis/{r}x{r}/Conv0_up/weight'][::-1, ::-1].transpose(3, 2, 0, 1),
- r'synthesis\.b(\d+)\.conv0\.bias', lambda r: tf_params[f'synthesis/{r}x{r}/Conv0_up/bias'],
- r'synthesis\.b(\d+)\.conv0\.noise_const', lambda r: tf_params[f'synthesis/noise{int(np.log2(int(r)))*2-5}'][0, 0],
- r'synthesis\.b(\d+)\.conv0\.noise_strength', lambda r: tf_params[f'synthesis/{r}x{r}/Conv0_up/noise_strength'],
- r'synthesis\.b(\d+)\.conv0\.affine\.weight', lambda r: tf_params[f'synthesis/{r}x{r}/Conv0_up/mod_weight'].transpose(),
- r'synthesis\.b(\d+)\.conv0\.affine\.bias', lambda r: tf_params[f'synthesis/{r}x{r}/Conv0_up/mod_bias'] + 1,
- r'synthesis\.b(\d+)\.conv1\.weight', lambda r: tf_params[f'synthesis/{r}x{r}/Conv1/weight'].transpose(3, 2, 0, 1),
- r'synthesis\.b(\d+)\.conv1\.bias', lambda r: tf_params[f'synthesis/{r}x{r}/Conv1/bias'],
- r'synthesis\.b(\d+)\.conv1\.noise_const', lambda r: tf_params[f'synthesis/noise{int(np.log2(int(r)))*2-4}'][0, 0],
- r'synthesis\.b(\d+)\.conv1\.noise_strength', lambda r: tf_params[f'synthesis/{r}x{r}/Conv1/noise_strength'],
- r'synthesis\.b(\d+)\.conv1\.affine\.weight', lambda r: tf_params[f'synthesis/{r}x{r}/Conv1/mod_weight'].transpose(),
- r'synthesis\.b(\d+)\.conv1\.affine\.bias', lambda r: tf_params[f'synthesis/{r}x{r}/Conv1/mod_bias'] + 1,
- r'synthesis\.b(\d+)\.torgb\.weight', lambda r: tf_params[f'synthesis/{r}x{r}/ToRGB/weight'].transpose(3, 2, 0, 1),
- r'synthesis\.b(\d+)\.torgb\.bias', lambda r: tf_params[f'synthesis/{r}x{r}/ToRGB/bias'],
- r'synthesis\.b(\d+)\.torgb\.affine\.weight', lambda r: tf_params[f'synthesis/{r}x{r}/ToRGB/mod_weight'].transpose(),
- r'synthesis\.b(\d+)\.torgb\.affine\.bias', lambda r: tf_params[f'synthesis/{r}x{r}/ToRGB/mod_bias'] + 1,
- r'synthesis\.b(\d+)\.skip\.weight', lambda r: tf_params[f'synthesis/{r}x{r}/Skip/weight'][::-1, ::-1].transpose(3, 2, 0, 1),
- r'.*\.resample_filter', None,
- )
- return G
-
-#----------------------------------------------------------------------------
-
-def convert_tf_discriminator(tf_D):
- if tf_D.version < 4:
- raise ValueError('TensorFlow pickle version too low')
-
- # Collect kwargs.
- tf_kwargs = tf_D.static_kwargs
- known_kwargs = set()
- def kwarg(tf_name, default=None):
- known_kwargs.add(tf_name)
- return tf_kwargs.get(tf_name, default)
-
- # Convert kwargs.
- kwargs = dnnlib.EasyDict(
- c_dim = kwarg('label_size', 0),
- img_resolution = kwarg('resolution', 1024),
- img_channels = kwarg('num_channels', 3),
- architecture = kwarg('architecture', 'resnet'),
- channel_base = kwarg('fmap_base', 16384) * 2,
- channel_max = kwarg('fmap_max', 512),
- num_fp16_res = kwarg('num_fp16_res', 0),
- conv_clamp = kwarg('conv_clamp', None),
- cmap_dim = kwarg('mapping_fmaps', None),
- block_kwargs = dnnlib.EasyDict(
- activation = kwarg('nonlinearity', 'lrelu'),
- resample_filter = kwarg('resample_kernel', [1,3,3,1]),
- freeze_layers = kwarg('freeze_layers', 0),
- ),
- mapping_kwargs = dnnlib.EasyDict(
- num_layers = kwarg('mapping_layers', 0),
- embed_features = kwarg('mapping_fmaps', None),
- layer_features = kwarg('mapping_fmaps', None),
- activation = kwarg('nonlinearity', 'lrelu'),
- lr_multiplier = kwarg('mapping_lrmul', 0.1),
- ),
- epilogue_kwargs = dnnlib.EasyDict(
- mbstd_group_size = kwarg('mbstd_group_size', None),
- mbstd_num_channels = kwarg('mbstd_num_features', 1),
- activation = kwarg('nonlinearity', 'lrelu'),
- ),
- )
-
- # Check for unknown kwargs.
- kwarg('structure')
- unknown_kwargs = list(set(tf_kwargs.keys()) - known_kwargs)
- if len(unknown_kwargs) > 0:
- raise ValueError('Unknown TensorFlow kwarg', unknown_kwargs[0])
-
- # Collect params.
- tf_params = _collect_tf_params(tf_D)
- for name, value in list(tf_params.items()):
- match = re.fullmatch(r'FromRGB_lod(\d+)/(.*)', name)
- if match:
- r = kwargs.img_resolution // (2 ** int(match.group(1)))
- tf_params[f'{r}x{r}/FromRGB/{match.group(2)}'] = value
- kwargs.architecture = 'orig'
- #for name, value in tf_params.items(): print(f'{name:<50s}{list(value.shape)}')
-
- # Convert params.
- from training import networks
- D = networks.Discriminator(**kwargs).eval().requires_grad_(False)
- # pylint: disable=unnecessary-lambda
- _populate_module_params(D,
- r'b(\d+)\.fromrgb\.weight', lambda r: tf_params[f'{r}x{r}/FromRGB/weight'].transpose(3, 2, 0, 1),
- r'b(\d+)\.fromrgb\.bias', lambda r: tf_params[f'{r}x{r}/FromRGB/bias'],
- r'b(\d+)\.conv(\d+)\.weight', lambda r, i: tf_params[f'{r}x{r}/Conv{i}{["","_down"][int(i)]}/weight'].transpose(3, 2, 0, 1),
- r'b(\d+)\.conv(\d+)\.bias', lambda r, i: tf_params[f'{r}x{r}/Conv{i}{["","_down"][int(i)]}/bias'],
- r'b(\d+)\.skip\.weight', lambda r: tf_params[f'{r}x{r}/Skip/weight'].transpose(3, 2, 0, 1),
- r'mapping\.embed\.weight', lambda: tf_params[f'LabelEmbed/weight'].transpose(),
- r'mapping\.embed\.bias', lambda: tf_params[f'LabelEmbed/bias'],
- r'mapping\.fc(\d+)\.weight', lambda i: tf_params[f'Mapping{i}/weight'].transpose(),
- r'mapping\.fc(\d+)\.bias', lambda i: tf_params[f'Mapping{i}/bias'],
- r'b4\.conv\.weight', lambda: tf_params[f'4x4/Conv/weight'].transpose(3, 2, 0, 1),
- r'b4\.conv\.bias', lambda: tf_params[f'4x4/Conv/bias'],
- r'b4\.fc\.weight', lambda: tf_params[f'4x4/Dense0/weight'].transpose(),
- r'b4\.fc\.bias', lambda: tf_params[f'4x4/Dense0/bias'],
- r'b4\.out\.weight', lambda: tf_params[f'Output/weight'].transpose(),
- r'b4\.out\.bias', lambda: tf_params[f'Output/bias'],
- r'.*\.resample_filter', None,
- )
- return D
-
-#----------------------------------------------------------------------------
-
-@click.command()
-@click.option('--source', help='Input pickle', required=True, metavar='PATH')
-@click.option('--dest', help='Output pickle', required=True, metavar='PATH')
-@click.option('--force-fp16', help='Force the networks to use FP16', type=bool, default=False, metavar='BOOL', show_default=True)
-def convert_network_pickle(source, dest, force_fp16):
- """Convert legacy network pickle into the native PyTorch format.
-
- The tool is able to load the main network configurations exported using the TensorFlow version of StyleGAN2 or StyleGAN2-ADA.
- It does not support e.g. StyleGAN2-ADA comparison methods, StyleGAN2 configs A-D, or StyleGAN1 networks.
-
- Example:
-
- \b
- python legacy.py \\
- --source=https://nvlabs-fi-cdn.nvidia.com/stylegan2/networks/stylegan2-cat-config-f.pkl \\
- --dest=stylegan2-cat-config-f.pkl
- """
- print(f'Loading "{source}"...')
- with dnnlib.util.open_url(source) as f:
- data = load_network_pkl(f, force_fp16=force_fp16)
- print(f'Saving "{dest}"...')
- with open(dest, 'wb') as f:
- pickle.dump(data, f)
- print('Done.')
-
-#----------------------------------------------------------------------------
-
-if __name__ == "__main__":
- convert_network_pickle() # pylint: disable=no-value-for-parameter
-
-#----------------------------------------------------------------------------
diff --git a/spaces/Yukki-Yui/moe-tts/monotonic_align/__init__.py b/spaces/Yukki-Yui/moe-tts/monotonic_align/__init__.py
deleted file mode 100644
index 40b6f64aa116c74cac2f6a33444c9eeea2fdb38c..0000000000000000000000000000000000000000
--- a/spaces/Yukki-Yui/moe-tts/monotonic_align/__init__.py
+++ /dev/null
@@ -1,21 +0,0 @@
-from numpy import zeros, int32, float32
-from torch import from_numpy
-
-from .core import maximum_path_jit
-
-
-def maximum_path(neg_cent, mask):
- """ numba optimized version.
- neg_cent: [b, t_t, t_s]
- mask: [b, t_t, t_s]
- """
- device = neg_cent.device
- dtype = neg_cent.dtype
- neg_cent = neg_cent.data.cpu().numpy().astype(float32)
- path = zeros(neg_cent.shape, dtype=int32)
-
- t_t_max = mask.sum(1)[:, 0].data.cpu().numpy().astype(int32)
- t_s_max = mask.sum(2)[:, 0].data.cpu().numpy().astype(int32)
- maximum_path_jit(path, neg_cent, t_t_max, t_s_max)
- return from_numpy(path).to(device=device, dtype=dtype)
-
diff --git a/spaces/abhishek/sketch-to-image/annotator/uniformer/mmdet/models/necks/fpg.py b/spaces/abhishek/sketch-to-image/annotator/uniformer/mmdet/models/necks/fpg.py
deleted file mode 100644
index c8e0d163ccf8cef6211530ba6c1b4d558ff6403f..0000000000000000000000000000000000000000
--- a/spaces/abhishek/sketch-to-image/annotator/uniformer/mmdet/models/necks/fpg.py
+++ /dev/null
@@ -1,398 +0,0 @@
-import torch.nn as nn
-import torch.nn.functional as F
-from mmcv.cnn import ConvModule, caffe2_xavier_init, constant_init, is_norm
-
-from ..builder import NECKS
-
-
-class Transition(nn.Module):
- """Base class for transition.
-
- Args:
- in_channels (int): Number of input channels.
- out_channels (int): Number of output channels.
- """
-
- def __init__(self, in_channels, out_channels):
- super().__init__()
- self.in_channels = in_channels
- self.out_channels = out_channels
-
- def forward(x):
- pass
-
-
-class UpInterpolationConv(Transition):
- """A transition used for up-sampling.
-
- Up-sample the input by interpolation then refines the feature by
- a convolution layer.
-
- Args:
- in_channels (int): Number of input channels.
- out_channels (int): Number of output channels.
- scale_factor (int): Up-sampling factor. Default: 2.
- mode (int): Interpolation mode. Default: nearest.
- align_corners (bool): Whether align corners when interpolation.
- Default: None.
- kernel_size (int): Kernel size for the conv. Default: 3.
- """
-
- def __init__(self,
- in_channels,
- out_channels,
- scale_factor=2,
- mode='nearest',
- align_corners=None,
- kernel_size=3,
- **kwargs):
- super().__init__(in_channels, out_channels)
- self.mode = mode
- self.scale_factor = scale_factor
- self.align_corners = align_corners
- self.conv = ConvModule(
- in_channels,
- out_channels,
- kernel_size,
- padding=(kernel_size - 1) // 2,
- **kwargs)
-
- def forward(self, x):
- x = F.interpolate(
- x,
- scale_factor=self.scale_factor,
- mode=self.mode,
- align_corners=self.align_corners)
- x = self.conv(x)
- return x
-
-
-class LastConv(Transition):
- """A transition used for refining the output of the last stage.
-
- Args:
- in_channels (int): Number of input channels.
- out_channels (int): Number of output channels.
- num_inputs (int): Number of inputs of the FPN features.
- kernel_size (int): Kernel size for the conv. Default: 3.
- """
-
- def __init__(self,
- in_channels,
- out_channels,
- num_inputs,
- kernel_size=3,
- **kwargs):
- super().__init__(in_channels, out_channels)
- self.num_inputs = num_inputs
- self.conv_out = ConvModule(
- in_channels,
- out_channels,
- kernel_size,
- padding=(kernel_size - 1) // 2,
- **kwargs)
-
- def forward(self, inputs):
- assert len(inputs) == self.num_inputs
- return self.conv_out(inputs[-1])
-
-
-@NECKS.register_module()
-class FPG(nn.Module):
- """FPG.
-
- Implementation of `Feature Pyramid Grids (FPG)
- `_.
- This implementation only gives the basic structure stated in the paper.
- But users can implement different type of transitions to fully explore the
- the potential power of the structure of FPG.
-
- Args:
- in_channels (int): Number of input channels (feature maps of all levels
- should have the same channels).
- out_channels (int): Number of output channels (used at each scale)
- num_outs (int): Number of output scales.
- stack_times (int): The number of times the pyramid architecture will
- be stacked.
- paths (list[str]): Specify the path order of each stack level.
- Each element in the list should be either 'bu' (bottom-up) or
- 'td' (top-down).
- inter_channels (int): Number of inter channels.
- same_up_trans (dict): Transition that goes down at the same stage.
- same_down_trans (dict): Transition that goes up at the same stage.
- across_lateral_trans (dict): Across-pathway same-stage
- across_down_trans (dict): Across-pathway bottom-up connection.
- across_up_trans (dict): Across-pathway top-down connection.
- across_skip_trans (dict): Across-pathway skip connection.
- output_trans (dict): Transition that trans the output of the
- last stage.
- start_level (int): Index of the start input backbone level used to
- build the feature pyramid. Default: 0.
- end_level (int): Index of the end input backbone level (exclusive) to
- build the feature pyramid. Default: -1, which means the last level.
- add_extra_convs (bool): It decides whether to add conv
- layers on top of the original feature maps. Default to False.
- If True, its actual mode is specified by `extra_convs_on_inputs`.
- norm_cfg (dict): Config dict for normalization layer. Default: None.
- """
-
- transition_types = {
- 'conv': ConvModule,
- 'interpolation_conv': UpInterpolationConv,
- 'last_conv': LastConv,
- }
-
- def __init__(self,
- in_channels,
- out_channels,
- num_outs,
- stack_times,
- paths,
- inter_channels=None,
- same_down_trans=None,
- same_up_trans=dict(
- type='conv', kernel_size=3, stride=2, padding=1),
- across_lateral_trans=dict(type='conv', kernel_size=1),
- across_down_trans=dict(type='conv', kernel_size=3),
- across_up_trans=None,
- across_skip_trans=dict(type='identity'),
- output_trans=dict(type='last_conv', kernel_size=3),
- start_level=0,
- end_level=-1,
- add_extra_convs=False,
- norm_cfg=None,
- skip_inds=None):
- super(FPG, self).__init__()
- assert isinstance(in_channels, list)
- self.in_channels = in_channels
- self.out_channels = out_channels
- self.num_ins = len(in_channels)
- self.num_outs = num_outs
- if inter_channels is None:
- self.inter_channels = [out_channels for _ in range(num_outs)]
- elif isinstance(inter_channels, int):
- self.inter_channels = [inter_channels for _ in range(num_outs)]
- else:
- assert isinstance(inter_channels, list)
- assert len(inter_channels) == num_outs
- self.inter_channels = inter_channels
- self.stack_times = stack_times
- self.paths = paths
- assert isinstance(paths, list) and len(paths) == stack_times
- for d in paths:
- assert d in ('bu', 'td')
-
- self.same_down_trans = same_down_trans
- self.same_up_trans = same_up_trans
- self.across_lateral_trans = across_lateral_trans
- self.across_down_trans = across_down_trans
- self.across_up_trans = across_up_trans
- self.output_trans = output_trans
- self.across_skip_trans = across_skip_trans
-
- self.with_bias = norm_cfg is None
- # skip inds must be specified if across skip trans is not None
- if self.across_skip_trans is not None:
- skip_inds is not None
- self.skip_inds = skip_inds
- assert len(self.skip_inds[0]) <= self.stack_times
-
- if end_level == -1:
- self.backbone_end_level = self.num_ins
- assert num_outs >= self.num_ins - start_level
- else:
- # if end_level < inputs, no extra level is allowed
- self.backbone_end_level = end_level
- assert end_level <= len(in_channels)
- assert num_outs == end_level - start_level
- self.start_level = start_level
- self.end_level = end_level
- self.add_extra_convs = add_extra_convs
-
- # build lateral 1x1 convs to reduce channels
- self.lateral_convs = nn.ModuleList()
- for i in range(self.start_level, self.backbone_end_level):
- l_conv = nn.Conv2d(self.in_channels[i],
- self.inter_channels[i - self.start_level], 1)
- self.lateral_convs.append(l_conv)
-
- extra_levels = num_outs - self.backbone_end_level + self.start_level
- self.extra_downsamples = nn.ModuleList()
- for i in range(extra_levels):
- if self.add_extra_convs:
- fpn_idx = self.backbone_end_level - self.start_level + i
- extra_conv = nn.Conv2d(
- self.inter_channels[fpn_idx - 1],
- self.inter_channels[fpn_idx],
- 3,
- stride=2,
- padding=1)
- self.extra_downsamples.append(extra_conv)
- else:
- self.extra_downsamples.append(nn.MaxPool2d(1, stride=2))
-
- self.fpn_transitions = nn.ModuleList() # stack times
- for s in range(self.stack_times):
- stage_trans = nn.ModuleList() # num of feature levels
- for i in range(self.num_outs):
- # same, across_lateral, across_down, across_up
- trans = nn.ModuleDict()
- if s in self.skip_inds[i]:
- stage_trans.append(trans)
- continue
- # build same-stage down trans (used in bottom-up paths)
- if i == 0 or self.same_up_trans is None:
- same_up_trans = None
- else:
- same_up_trans = self.build_trans(
- self.same_up_trans, self.inter_channels[i - 1],
- self.inter_channels[i])
- trans['same_up'] = same_up_trans
- # build same-stage up trans (used in top-down paths)
- if i == self.num_outs - 1 or self.same_down_trans is None:
- same_down_trans = None
- else:
- same_down_trans = self.build_trans(
- self.same_down_trans, self.inter_channels[i + 1],
- self.inter_channels[i])
- trans['same_down'] = same_down_trans
- # build across lateral trans
- across_lateral_trans = self.build_trans(
- self.across_lateral_trans, self.inter_channels[i],
- self.inter_channels[i])
- trans['across_lateral'] = across_lateral_trans
- # build across down trans
- if i == self.num_outs - 1 or self.across_down_trans is None:
- across_down_trans = None
- else:
- across_down_trans = self.build_trans(
- self.across_down_trans, self.inter_channels[i + 1],
- self.inter_channels[i])
- trans['across_down'] = across_down_trans
- # build across up trans
- if i == 0 or self.across_up_trans is None:
- across_up_trans = None
- else:
- across_up_trans = self.build_trans(
- self.across_up_trans, self.inter_channels[i - 1],
- self.inter_channels[i])
- trans['across_up'] = across_up_trans
- if self.across_skip_trans is None:
- across_skip_trans = None
- else:
- across_skip_trans = self.build_trans(
- self.across_skip_trans, self.inter_channels[i - 1],
- self.inter_channels[i])
- trans['across_skip'] = across_skip_trans
- # build across_skip trans
- stage_trans.append(trans)
- self.fpn_transitions.append(stage_trans)
-
- self.output_transition = nn.ModuleList() # output levels
- for i in range(self.num_outs):
- trans = self.build_trans(
- self.output_trans,
- self.inter_channels[i],
- self.out_channels,
- num_inputs=self.stack_times + 1)
- self.output_transition.append(trans)
-
- self.relu = nn.ReLU(inplace=True)
-
- def build_trans(self, cfg, in_channels, out_channels, **extra_args):
- cfg_ = cfg.copy()
- trans_type = cfg_.pop('type')
- trans_cls = self.transition_types[trans_type]
- return trans_cls(in_channels, out_channels, **cfg_, **extra_args)
-
- def init_weights(self):
- for m in self.modules():
- if isinstance(m, nn.Conv2d):
- caffe2_xavier_init(m)
- elif is_norm(m):
- constant_init(m, 1.0)
-
- def fuse(self, fuse_dict):
- out = None
- for item in fuse_dict.values():
- if item is not None:
- if out is None:
- out = item
- else:
- out = out + item
- return out
-
- def forward(self, inputs):
- assert len(inputs) == len(self.in_channels)
-
- # build all levels from original feature maps
- feats = [
- lateral_conv(inputs[i + self.start_level])
- for i, lateral_conv in enumerate(self.lateral_convs)
- ]
- for downsample in self.extra_downsamples:
- feats.append(downsample(feats[-1]))
-
- outs = [feats]
-
- for i in range(self.stack_times):
- current_outs = outs[-1]
- next_outs = []
- direction = self.paths[i]
- for j in range(self.num_outs):
- if i in self.skip_inds[j]:
- next_outs.append(outs[-1][j])
- continue
- # feature level
- if direction == 'td':
- lvl = self.num_outs - j - 1
- else:
- lvl = j
- # get transitions
- if direction == 'td':
- same_trans = self.fpn_transitions[i][lvl]['same_down']
- else:
- same_trans = self.fpn_transitions[i][lvl]['same_up']
- across_lateral_trans = self.fpn_transitions[i][lvl][
- 'across_lateral']
- across_down_trans = self.fpn_transitions[i][lvl]['across_down']
- across_up_trans = self.fpn_transitions[i][lvl]['across_up']
- across_skip_trans = self.fpn_transitions[i][lvl]['across_skip']
- # init output
- to_fuse = dict(
- same=None, lateral=None, across_up=None, across_down=None)
- # same downsample/upsample
- if same_trans is not None:
- to_fuse['same'] = same_trans(next_outs[-1])
- # across lateral
- if across_lateral_trans is not None:
- to_fuse['lateral'] = across_lateral_trans(
- current_outs[lvl])
- # across downsample
- if lvl > 0 and across_up_trans is not None:
- to_fuse['across_up'] = across_up_trans(current_outs[lvl -
- 1])
- # across upsample
- if (lvl < self.num_outs - 1 and across_down_trans is not None):
- to_fuse['across_down'] = across_down_trans(
- current_outs[lvl + 1])
- if across_skip_trans is not None:
- to_fuse['across_skip'] = across_skip_trans(outs[0][lvl])
- x = self.fuse(to_fuse)
- next_outs.append(x)
-
- if direction == 'td':
- outs.append(next_outs[::-1])
- else:
- outs.append(next_outs)
-
- # output trans
- final_outs = []
- for i in range(self.num_outs):
- lvl_out_list = []
- for s in range(len(outs)):
- lvl_out_list.append(outs[s][i])
- lvl_out = self.output_transition[i](lvl_out_list)
- final_outs.append(lvl_out)
-
- return final_outs
diff --git a/spaces/ahnafsamin/GroTTS-Tacotron2-24mins/app.py b/spaces/ahnafsamin/GroTTS-Tacotron2-24mins/app.py
deleted file mode 100644
index e6406e545a936fb0e53631c63b95add3285ef74e..0000000000000000000000000000000000000000
--- a/spaces/ahnafsamin/GroTTS-Tacotron2-24mins/app.py
+++ /dev/null
@@ -1,43 +0,0 @@
-import os
-
-os.environ["CURL_CA_BUNDLE"]=""
-
-import gradio as gr
-import time
-import urllib.request
-from pathlib import Path
-import os
-import torch
-import scipy.io.wavfile
-from espnet2.bin.tts_inference import Text2Speech
-from espnet2.utils.types import str_or_none
-from parallel_wavegan.utils import download_pretrained_model
-
-
-gos_text2speech = Text2Speech.from_pretrained(
- model_tag="https://huggingface.co/ahnafsamin/Tacotron2-gronings-24mins/resolve/main/tts_train_raw_char_tacotron_train.loss.ave.zip",
- vocoder_tag="parallel_wavegan/ljspeech_parallel_wavegan.v3"
-)
-
-def inference(text,lang):
- with torch.no_grad():
- if lang == "gronings":
- wav = gos_text2speech(text)["wav"]
- scipy.io.wavfile.write("out.wav", gos_text2speech.fs , wav.view(-1).cpu().numpy())
-
- return "out.wav", "out.wav"
-
-title = "GroTTS"
-examples = [
- ['Ze gingen mit klas noar waddendiek, over en deur bragel lopen.', 'gronings']
-]
-
-
-
-gr.Interface(
- inference,
- [gr.inputs.Textbox(label="input text", lines=3), gr.inputs.Radio(choices=["gronings"], type="value", default="gronings", label="language")],
- [gr.outputs.Audio(type="file", label="Output"), gr.outputs.File()],
- title=title,
- examples=examples
- ).launch(enable_queue=True)
diff --git a/spaces/akhaliq/JoJoGAN/e4e/utils/common.py b/spaces/akhaliq/JoJoGAN/e4e/utils/common.py
deleted file mode 100644
index b19e18ddcb78b06678fa18e4a76da44fc511b789..0000000000000000000000000000000000000000
--- a/spaces/akhaliq/JoJoGAN/e4e/utils/common.py
+++ /dev/null
@@ -1,55 +0,0 @@
-from PIL import Image
-import matplotlib.pyplot as plt
-
-
-# Log images
-def log_input_image(x, opts):
- return tensor2im(x)
-
-
-def tensor2im(var):
- # var shape: (3, H, W)
- var = var.cpu().detach().transpose(0, 2).transpose(0, 1).numpy()
- var = ((var + 1) / 2)
- var[var < 0] = 0
- var[var > 1] = 1
- var = var * 255
- return Image.fromarray(var.astype('uint8'))
-
-
-def vis_faces(log_hooks):
- display_count = len(log_hooks)
- fig = plt.figure(figsize=(8, 4 * display_count))
- gs = fig.add_gridspec(display_count, 3)
- for i in range(display_count):
- hooks_dict = log_hooks[i]
- fig.add_subplot(gs[i, 0])
- if 'diff_input' in hooks_dict:
- vis_faces_with_id(hooks_dict, fig, gs, i)
- else:
- vis_faces_no_id(hooks_dict, fig, gs, i)
- plt.tight_layout()
- return fig
-
-
-def vis_faces_with_id(hooks_dict, fig, gs, i):
- plt.imshow(hooks_dict['input_face'])
- plt.title('Input\nOut Sim={:.2f}'.format(float(hooks_dict['diff_input'])))
- fig.add_subplot(gs[i, 1])
- plt.imshow(hooks_dict['target_face'])
- plt.title('Target\nIn={:.2f}, Out={:.2f}'.format(float(hooks_dict['diff_views']),
- float(hooks_dict['diff_target'])))
- fig.add_subplot(gs[i, 2])
- plt.imshow(hooks_dict['output_face'])
- plt.title('Output\n Target Sim={:.2f}'.format(float(hooks_dict['diff_target'])))
-
-
-def vis_faces_no_id(hooks_dict, fig, gs, i):
- plt.imshow(hooks_dict['input_face'], cmap="gray")
- plt.title('Input')
- fig.add_subplot(gs[i, 1])
- plt.imshow(hooks_dict['target_face'])
- plt.title('Target')
- fig.add_subplot(gs[i, 2])
- plt.imshow(hooks_dict['output_face'])
- plt.title('Output')
diff --git a/spaces/akhaliq/deeplab2/evaluation/segmentation_and_tracking_quality.py b/spaces/akhaliq/deeplab2/evaluation/segmentation_and_tracking_quality.py
deleted file mode 100644
index c6c3171c8c3e98cc265b296f7b9e44df190f0d9d..0000000000000000000000000000000000000000
--- a/spaces/akhaliq/deeplab2/evaluation/segmentation_and_tracking_quality.py
+++ /dev/null
@@ -1,282 +0,0 @@
-# coding=utf-8
-# Copyright 2021 The Deeplab2 Authors.
-#
-# Licensed under the Apache License, Version 2.0 (the "License");
-# you may not use this file except in compliance with the License.
-# You may obtain a copy of the License at
-#
-# http://www.apache.org/licenses/LICENSE-2.0
-#
-# Unless required by applicable law or agreed to in writing, software
-# distributed under the License is distributed on an "AS IS" BASIS,
-# WITHOUT WARRANTIES OR CONDITIONS OF ANY KIND, either express or implied.
-# See the License for the specific language governing permissions and
-# limitations under the License.
-
-"""Implementation of the Segmentation and Tracking Quality (STQ) metric."""
-
-import collections
-from typing import MutableMapping, Sequence, Dict, Text, Any
-import numpy as np
-import tensorflow as tf
-
-
-def _update_dict_stats(stat_dict: MutableMapping[int, tf.Tensor],
- id_array: tf.Tensor):
- """Updates a given dict with corresponding counts."""
- ids, _, counts = tf.unique_with_counts(id_array)
- for idx, count in zip(ids.numpy(), counts):
- if idx in stat_dict:
- stat_dict[idx] += count
- else:
- stat_dict[idx] = count
-
-
-class STQuality(object):
- """Metric class for the Segmentation and Tracking Quality (STQ).
-
- The metric computes the geometric mean of two terms.
- - Association Quality: This term measures the quality of the track ID
- assignment for `thing` classes. It is formulated as a weighted IoU
- measure.
- - Segmentation Quality: This term measures the semantic segmentation quality.
- The standard class IoU measure is used for this.
-
- Example usage:
-
- stq_obj = segmentation_tracking_quality.STQuality(num_classes, things_list,
- ignore_label, max_instances_per_category, offset)
- stq_obj.update_state(y_true_1, y_pred_1)
- stq_obj.update_state(y_true_2, y_pred_2)
- ...
- result = stq_obj.result().numpy()
- """
-
- def __init__(self,
- num_classes: int,
- things_list: Sequence[int],
- ignore_label: int,
- max_instances_per_category: int,
- offset: int,
- name='stq'
- ):
- """Initialization of the STQ metric.
-
- Args:
- num_classes: Number of classes in the dataset as an integer.
- things_list: A sequence of class ids that belong to `things`.
- ignore_label: The class id to be ignored in evaluation as an integer or
- integer tensor.
- max_instances_per_category: The maximum number of instances for each class
- as an integer or integer tensor.
- offset: The maximum number of unique labels as an integer or integer
- tensor.
- name: An optional name. (default: 'st_quality')
- """
- self._name = name
- self._num_classes = num_classes
- self._ignore_label = ignore_label
- self._things_list = things_list
- self._max_instances_per_category = max_instances_per_category
-
- if ignore_label >= num_classes:
- self._confusion_matrix_size = num_classes + 1
- self._include_indices = np.arange(self._num_classes)
- else:
- self._confusion_matrix_size = num_classes
- self._include_indices = np.array(
- [i for i in range(num_classes) if i != self._ignore_label])
-
- self._iou_confusion_matrix_per_sequence = collections.OrderedDict()
- self._predictions = collections.OrderedDict()
- self._ground_truth = collections.OrderedDict()
- self._intersections = collections.OrderedDict()
- self._sequence_length = collections.OrderedDict()
- self._offset = offset
- lower_bound = num_classes * max_instances_per_category
- if offset < lower_bound:
- raise ValueError('The provided offset %d is too small. No guarantess '
- 'about the correctness of the results can be made. '
- 'Please choose an offset that is higher than num_classes'
- ' * max_instances_per_category = %d' % lower_bound)
-
- def update_state(self, y_true: tf.Tensor, y_pred: tf.Tensor,
- sequence_id=0):
- """Accumulates the segmentation and tracking quality statistics.
-
- Args:
- y_true: The ground-truth panoptic label map for a particular video frame
- (defined as semantic_map * max_instances_per_category + instance_map).
- y_pred: The predicted panoptic label map for a particular video frame
- (defined as semantic_map * max_instances_per_category + instance_map).
- sequence_id: The optional ID of the sequence the frames belong to. When no
- sequence is given, all frames are considered to belong to the same
- sequence (default: 0).
- """
- y_true = tf.cast(y_true, dtype=tf.int64)
- y_pred = tf.cast(y_pred, dtype=tf.int64)
- semantic_label = y_true // self._max_instances_per_category
- semantic_prediction = y_pred // self._max_instances_per_category
- # Check if the ignore value is outside the range [0, num_classes]. If yes,
- # map `_ignore_label` to `_num_classes`, so it can be used to create the
- # confusion matrix.
- if self._ignore_label > self._num_classes:
- semantic_label = tf.where(
- tf.not_equal(semantic_label, self._ignore_label), semantic_label,
- self._num_classes)
- semantic_prediction = tf.where(
- tf.not_equal(semantic_prediction, self._ignore_label),
- semantic_prediction, self._num_classes)
- if sequence_id in self._iou_confusion_matrix_per_sequence:
- self._iou_confusion_matrix_per_sequence[sequence_id] += (
- tf.math.confusion_matrix(
- tf.reshape(semantic_label, [-1]),
- tf.reshape(semantic_prediction, [-1]),
- self._confusion_matrix_size,
- dtype=tf.int64))
- self._sequence_length[sequence_id] += 1
- else:
- self._iou_confusion_matrix_per_sequence[sequence_id] = (
- tf.math.confusion_matrix(
- tf.reshape(semantic_label, [-1]),
- tf.reshape(semantic_prediction, [-1]),
- self._confusion_matrix_size,
- dtype=tf.int64))
- self._predictions[sequence_id] = {}
- self._ground_truth[sequence_id] = {}
- self._intersections[sequence_id] = {}
- self._sequence_length[sequence_id] = 1
-
- instance_label = y_true % self._max_instances_per_category
-
- label_mask = tf.zeros_like(semantic_label, dtype=tf.bool)
- prediction_mask = tf.zeros_like(semantic_prediction, dtype=tf.bool)
- for things_class_id in self._things_list:
- label_mask = tf.logical_or(label_mask,
- tf.equal(semantic_label, things_class_id))
- prediction_mask = tf.logical_or(
- prediction_mask, tf.equal(semantic_prediction, things_class_id))
-
- # Select the `crowd` region of the current class. This region is encoded
- # instance id `0`.
- is_crowd = tf.logical_and(tf.equal(instance_label, 0), label_mask)
- # Select the non-crowd region of the corresponding class as the `crowd`
- # region is ignored for the tracking term.
- label_mask = tf.logical_and(label_mask, tf.logical_not(is_crowd))
- # Do not punish id assignment for regions that are annotated as `crowd` in
- # the ground-truth.
- prediction_mask = tf.logical_and(prediction_mask, tf.logical_not(is_crowd))
-
- seq_preds = self._predictions[sequence_id]
- seq_gts = self._ground_truth[sequence_id]
- seq_intersects = self._intersections[sequence_id]
-
- # Compute and update areas of ground-truth, predictions and intersections.
- _update_dict_stats(seq_preds, y_pred[prediction_mask])
- _update_dict_stats(seq_gts, y_true[label_mask])
-
- non_crowd_intersection = tf.logical_and(label_mask, prediction_mask)
- intersection_ids = (
- y_true[non_crowd_intersection] * self._offset +
- y_pred[non_crowd_intersection])
- _update_dict_stats(seq_intersects, intersection_ids)
-
- def result(self) -> Dict[Text, Any]:
- """Computes the segmentation and tracking quality.
-
- Returns:
- A dictionary containing:
- - 'STQ': The total STQ score.
- - 'AQ': The total association quality (AQ) score.
- - 'IoU': The total mean IoU.
- - 'STQ_per_seq': A list of the STQ score per sequence.
- - 'AQ_per_seq': A list of the AQ score per sequence.
- - 'IoU_per_seq': A list of mean IoU per sequence.
- - 'Id_per_seq': A list of sequence Ids to map list index to sequence.
- - 'Length_per_seq': A list of the length of each sequence.
- """
- # Compute association quality (AQ)
- num_tubes_per_seq = [0] * len(self._ground_truth)
- aq_per_seq = [0] * len(self._ground_truth)
- iou_per_seq = [0] * len(self._ground_truth)
- id_per_seq = [''] * len(self._ground_truth)
-
- for index, sequence_id in enumerate(self._ground_truth):
- outer_sum = 0.0
- predictions = self._predictions[sequence_id]
- ground_truth = self._ground_truth[sequence_id]
- intersections = self._intersections[sequence_id]
- num_tubes_per_seq[index] = len(ground_truth)
- id_per_seq[index] = sequence_id
-
- for gt_id, gt_size in ground_truth.items():
- inner_sum = 0.0
- for pr_id, pr_size in predictions.items():
- tpa_key = self._offset * gt_id + pr_id
- if tpa_key in intersections:
- tpa = intersections[tpa_key].numpy()
- fpa = pr_size.numpy() - tpa
- fna = gt_size.numpy() - tpa
- inner_sum += tpa * (tpa / (tpa + fpa + fna))
-
- outer_sum += 1.0 / gt_size.numpy() * inner_sum
- aq_per_seq[index] = outer_sum
-
- aq_mean = np.sum(aq_per_seq) / np.maximum(np.sum(num_tubes_per_seq), 1e-15)
- aq_per_seq = aq_per_seq / np.maximum(num_tubes_per_seq, 1e-15)
-
- # Compute IoU scores.
- # The rows correspond to ground-truth and the columns to predictions.
- # Remove fp from confusion matrix for the void/ignore class.
- total_confusion = np.zeros(
- (self._confusion_matrix_size, self._confusion_matrix_size),
- dtype=np.int64)
- for index, confusion in enumerate(
- self._iou_confusion_matrix_per_sequence.values()):
- confusion = confusion.numpy()
- removal_matrix = np.zeros_like(confusion)
- removal_matrix[self._include_indices, :] = 1.0
- confusion *= removal_matrix
- total_confusion += confusion
-
- # `intersections` corresponds to true positives.
- intersections = confusion.diagonal()
- fps = confusion.sum(axis=0) - intersections
- fns = confusion.sum(axis=1) - intersections
- unions = intersections + fps + fns
-
- num_classes = np.count_nonzero(unions)
- ious = (intersections.astype(np.double) /
- np.maximum(unions, 1e-15).astype(np.double))
- iou_per_seq[index] = np.sum(ious) / num_classes
-
- # `intersections` corresponds to true positives.
- intersections = total_confusion.diagonal()
- fps = total_confusion.sum(axis=0) - intersections
- fns = total_confusion.sum(axis=1) - intersections
- unions = intersections + fps + fns
-
- num_classes = np.count_nonzero(unions)
- ious = (intersections.astype(np.double) /
- np.maximum(unions, 1e-15).astype(np.double))
- iou_mean = np.sum(ious) / num_classes
-
- st_quality = np.sqrt(aq_mean * iou_mean)
- st_quality_per_seq = np.sqrt(aq_per_seq * iou_per_seq)
- return {'STQ': st_quality,
- 'AQ': aq_mean,
- 'IoU': float(iou_mean),
- 'STQ_per_seq': st_quality_per_seq,
- 'AQ_per_seq': aq_per_seq,
- 'IoU_per_seq': iou_per_seq,
- 'ID_per_seq': id_per_seq,
- 'Length_per_seq': list(self._sequence_length.values()),
- }
-
- def reset_states(self):
- """Resets all states that accumulated data."""
- self._iou_confusion_matrix_per_sequence = collections.OrderedDict()
- self._predictions = collections.OrderedDict()
- self._ground_truth = collections.OrderedDict()
- self._intersections = collections.OrderedDict()
- self._sequence_length = collections.OrderedDict()
diff --git a/spaces/akhaliq/lama/saicinpainting/training/modules/ffc.py b/spaces/akhaliq/lama/saicinpainting/training/modules/ffc.py
deleted file mode 100644
index 0e7b84683fccb4bccac97b6371994fa6bb44dbe4..0000000000000000000000000000000000000000
--- a/spaces/akhaliq/lama/saicinpainting/training/modules/ffc.py
+++ /dev/null
@@ -1,485 +0,0 @@
-# Fast Fourier Convolution NeurIPS 2020
-# original implementation https://github.com/pkumivision/FFC/blob/main/model_zoo/ffc.py
-# paper https://proceedings.neurips.cc/paper/2020/file/2fd5d41ec6cfab47e32164d5624269b1-Paper.pdf
-
-import numpy as np
-import torch
-import torch.nn as nn
-import torch.nn.functional as F
-
-from saicinpainting.training.modules.base import get_activation, BaseDiscriminator
-from saicinpainting.training.modules.spatial_transform import LearnableSpatialTransformWrapper
-from saicinpainting.training.modules.squeeze_excitation import SELayer
-from saicinpainting.utils import get_shape
-
-
-class FFCSE_block(nn.Module):
-
- def __init__(self, channels, ratio_g):
- super(FFCSE_block, self).__init__()
- in_cg = int(channels * ratio_g)
- in_cl = channels - in_cg
- r = 16
-
- self.avgpool = nn.AdaptiveAvgPool2d((1, 1))
- self.conv1 = nn.Conv2d(channels, channels // r,
- kernel_size=1, bias=True)
- self.relu1 = nn.ReLU(inplace=True)
- self.conv_a2l = None if in_cl == 0 else nn.Conv2d(
- channels // r, in_cl, kernel_size=1, bias=True)
- self.conv_a2g = None if in_cg == 0 else nn.Conv2d(
- channels // r, in_cg, kernel_size=1, bias=True)
- self.sigmoid = nn.Sigmoid()
-
- def forward(self, x):
- x = x if type(x) is tuple else (x, 0)
- id_l, id_g = x
-
- x = id_l if type(id_g) is int else torch.cat([id_l, id_g], dim=1)
- x = self.avgpool(x)
- x = self.relu1(self.conv1(x))
-
- x_l = 0 if self.conv_a2l is None else id_l * \
- self.sigmoid(self.conv_a2l(x))
- x_g = 0 if self.conv_a2g is None else id_g * \
- self.sigmoid(self.conv_a2g(x))
- return x_l, x_g
-
-
-class FourierUnit(nn.Module):
-
- def __init__(self, in_channels, out_channels, groups=1, spatial_scale_factor=None, spatial_scale_mode='bilinear',
- spectral_pos_encoding=False, use_se=False, se_kwargs=None, ffc3d=False, fft_norm='ortho'):
- # bn_layer not used
- super(FourierUnit, self).__init__()
- self.groups = groups
-
- self.conv_layer = torch.nn.Conv2d(in_channels=in_channels * 2 + (2 if spectral_pos_encoding else 0),
- out_channels=out_channels * 2,
- kernel_size=1, stride=1, padding=0, groups=self.groups, bias=False)
- self.bn = torch.nn.BatchNorm2d(out_channels * 2)
- self.relu = torch.nn.ReLU(inplace=True)
-
- # squeeze and excitation block
- self.use_se = use_se
- if use_se:
- if se_kwargs is None:
- se_kwargs = {}
- self.se = SELayer(self.conv_layer.in_channels, **se_kwargs)
-
- self.spatial_scale_factor = spatial_scale_factor
- self.spatial_scale_mode = spatial_scale_mode
- self.spectral_pos_encoding = spectral_pos_encoding
- self.ffc3d = ffc3d
- self.fft_norm = fft_norm
-
- def forward(self, x):
- batch = x.shape[0]
-
- if self.spatial_scale_factor is not None:
- orig_size = x.shape[-2:]
- x = F.interpolate(x, scale_factor=self.spatial_scale_factor, mode=self.spatial_scale_mode, align_corners=False)
-
- r_size = x.size()
- # (batch, c, h, w/2+1, 2)
- fft_dim = (-3, -2, -1) if self.ffc3d else (-2, -1)
- ffted = torch.fft.rfftn(x, dim=fft_dim, norm=self.fft_norm)
- ffted = torch.stack((ffted.real, ffted.imag), dim=-1)
- ffted = ffted.permute(0, 1, 4, 2, 3).contiguous() # (batch, c, 2, h, w/2+1)
- ffted = ffted.view((batch, -1,) + ffted.size()[3:])
-
- if self.spectral_pos_encoding:
- height, width = ffted.shape[-2:]
- coords_vert = torch.linspace(0, 1, height)[None, None, :, None].expand(batch, 1, height, width).to(ffted)
- coords_hor = torch.linspace(0, 1, width)[None, None, None, :].expand(batch, 1, height, width).to(ffted)
- ffted = torch.cat((coords_vert, coords_hor, ffted), dim=1)
-
- if self.use_se:
- ffted = self.se(ffted)
-
- ffted = self.conv_layer(ffted) # (batch, c*2, h, w/2+1)
- ffted = self.relu(self.bn(ffted))
-
- ffted = ffted.view((batch, -1, 2,) + ffted.size()[2:]).permute(
- 0, 1, 3, 4, 2).contiguous() # (batch,c, t, h, w/2+1, 2)
- ffted = torch.complex(ffted[..., 0], ffted[..., 1])
-
- ifft_shape_slice = x.shape[-3:] if self.ffc3d else x.shape[-2:]
- output = torch.fft.irfftn(ffted, s=ifft_shape_slice, dim=fft_dim, norm=self.fft_norm)
-
- if self.spatial_scale_factor is not None:
- output = F.interpolate(output, size=orig_size, mode=self.spatial_scale_mode, align_corners=False)
-
- return output
-
-
-class SeparableFourierUnit(nn.Module):
-
- def __init__(self, in_channels, out_channels, groups=1, kernel_size=3):
- # bn_layer not used
- super(SeparableFourierUnit, self).__init__()
- self.groups = groups
- row_out_channels = out_channels // 2
- col_out_channels = out_channels - row_out_channels
- self.row_conv = torch.nn.Conv2d(in_channels=in_channels * 2,
- out_channels=row_out_channels * 2,
- kernel_size=(kernel_size, 1), # kernel size is always like this, but the data will be transposed
- stride=1, padding=(kernel_size // 2, 0),
- padding_mode='reflect',
- groups=self.groups, bias=False)
- self.col_conv = torch.nn.Conv2d(in_channels=in_channels * 2,
- out_channels=col_out_channels * 2,
- kernel_size=(kernel_size, 1), # kernel size is always like this, but the data will be transposed
- stride=1, padding=(kernel_size // 2, 0),
- padding_mode='reflect',
- groups=self.groups, bias=False)
- self.row_bn = torch.nn.BatchNorm2d(row_out_channels * 2)
- self.col_bn = torch.nn.BatchNorm2d(col_out_channels * 2)
- self.relu = torch.nn.ReLU(inplace=True)
-
- def process_branch(self, x, conv, bn):
- batch = x.shape[0]
-
- r_size = x.size()
- # (batch, c, h, w/2+1, 2)
- ffted = torch.fft.rfft(x, norm="ortho")
- ffted = torch.stack((ffted.real, ffted.imag), dim=-1)
- ffted = ffted.permute(0, 1, 4, 2, 3).contiguous() # (batch, c, 2, h, w/2+1)
- ffted = ffted.view((batch, -1,) + ffted.size()[3:])
-
- ffted = self.relu(bn(conv(ffted)))
-
- ffted = ffted.view((batch, -1, 2,) + ffted.size()[2:]).permute(
- 0, 1, 3, 4, 2).contiguous() # (batch,c, t, h, w/2+1, 2)
- ffted = torch.complex(ffted[..., 0], ffted[..., 1])
-
- output = torch.fft.irfft(ffted, s=x.shape[-1:], norm="ortho")
- return output
-
-
- def forward(self, x):
- rowwise = self.process_branch(x, self.row_conv, self.row_bn)
- colwise = self.process_branch(x.permute(0, 1, 3, 2), self.col_conv, self.col_bn).permute(0, 1, 3, 2)
- out = torch.cat((rowwise, colwise), dim=1)
- return out
-
-
-class SpectralTransform(nn.Module):
-
- def __init__(self, in_channels, out_channels, stride=1, groups=1, enable_lfu=True, separable_fu=False, **fu_kwargs):
- # bn_layer not used
- super(SpectralTransform, self).__init__()
- self.enable_lfu = enable_lfu
- if stride == 2:
- self.downsample = nn.AvgPool2d(kernel_size=(2, 2), stride=2)
- else:
- self.downsample = nn.Identity()
-
- self.stride = stride
- self.conv1 = nn.Sequential(
- nn.Conv2d(in_channels, out_channels //
- 2, kernel_size=1, groups=groups, bias=False),
- nn.BatchNorm2d(out_channels // 2),
- nn.ReLU(inplace=True)
- )
- fu_class = SeparableFourierUnit if separable_fu else FourierUnit
- self.fu = fu_class(
- out_channels // 2, out_channels // 2, groups, **fu_kwargs)
- if self.enable_lfu:
- self.lfu = fu_class(
- out_channels // 2, out_channels // 2, groups)
- self.conv2 = torch.nn.Conv2d(
- out_channels // 2, out_channels, kernel_size=1, groups=groups, bias=False)
-
- def forward(self, x):
-
- x = self.downsample(x)
- x = self.conv1(x)
- output = self.fu(x)
-
- if self.enable_lfu:
- n, c, h, w = x.shape
- split_no = 2
- split_s = h // split_no
- xs = torch.cat(torch.split(
- x[:, :c // 4], split_s, dim=-2), dim=1).contiguous()
- xs = torch.cat(torch.split(xs, split_s, dim=-1),
- dim=1).contiguous()
- xs = self.lfu(xs)
- xs = xs.repeat(1, 1, split_no, split_no).contiguous()
- else:
- xs = 0
-
- output = self.conv2(x + output + xs)
-
- return output
-
-
-class FFC(nn.Module):
-
- def __init__(self, in_channels, out_channels, kernel_size,
- ratio_gin, ratio_gout, stride=1, padding=0,
- dilation=1, groups=1, bias=False, enable_lfu=True,
- padding_type='reflect', gated=False, **spectral_kwargs):
- super(FFC, self).__init__()
-
- assert stride == 1 or stride == 2, "Stride should be 1 or 2."
- self.stride = stride
-
- in_cg = int(in_channels * ratio_gin)
- in_cl = in_channels - in_cg
- out_cg = int(out_channels * ratio_gout)
- out_cl = out_channels - out_cg
- #groups_g = 1 if groups == 1 else int(groups * ratio_gout)
- #groups_l = 1 if groups == 1 else groups - groups_g
-
- self.ratio_gin = ratio_gin
- self.ratio_gout = ratio_gout
- self.global_in_num = in_cg
-
- module = nn.Identity if in_cl == 0 or out_cl == 0 else nn.Conv2d
- self.convl2l = module(in_cl, out_cl, kernel_size,
- stride, padding, dilation, groups, bias, padding_mode=padding_type)
- module = nn.Identity if in_cl == 0 or out_cg == 0 else nn.Conv2d
- self.convl2g = module(in_cl, out_cg, kernel_size,
- stride, padding, dilation, groups, bias, padding_mode=padding_type)
- module = nn.Identity if in_cg == 0 or out_cl == 0 else nn.Conv2d
- self.convg2l = module(in_cg, out_cl, kernel_size,
- stride, padding, dilation, groups, bias, padding_mode=padding_type)
- module = nn.Identity if in_cg == 0 or out_cg == 0 else SpectralTransform
- self.convg2g = module(
- in_cg, out_cg, stride, 1 if groups == 1 else groups // 2, enable_lfu, **spectral_kwargs)
-
- self.gated = gated
- module = nn.Identity if in_cg == 0 or out_cl == 0 or not self.gated else nn.Conv2d
- self.gate = module(in_channels, 2, 1)
-
- def forward(self, x):
- x_l, x_g = x if type(x) is tuple else (x, 0)
- out_xl, out_xg = 0, 0
-
- if self.gated:
- total_input_parts = [x_l]
- if torch.is_tensor(x_g):
- total_input_parts.append(x_g)
- total_input = torch.cat(total_input_parts, dim=1)
-
- gates = torch.sigmoid(self.gate(total_input))
- g2l_gate, l2g_gate = gates.chunk(2, dim=1)
- else:
- g2l_gate, l2g_gate = 1, 1
-
- if self.ratio_gout != 1:
- out_xl = self.convl2l(x_l) + self.convg2l(x_g) * g2l_gate
- if self.ratio_gout != 0:
- out_xg = self.convl2g(x_l) * l2g_gate + self.convg2g(x_g)
-
- return out_xl, out_xg
-
-
-class FFC_BN_ACT(nn.Module):
-
- def __init__(self, in_channels, out_channels,
- kernel_size, ratio_gin, ratio_gout,
- stride=1, padding=0, dilation=1, groups=1, bias=False,
- norm_layer=nn.BatchNorm2d, activation_layer=nn.Identity,
- padding_type='reflect',
- enable_lfu=True, **kwargs):
- super(FFC_BN_ACT, self).__init__()
- self.ffc = FFC(in_channels, out_channels, kernel_size,
- ratio_gin, ratio_gout, stride, padding, dilation,
- groups, bias, enable_lfu, padding_type=padding_type, **kwargs)
- lnorm = nn.Identity if ratio_gout == 1 else norm_layer
- gnorm = nn.Identity if ratio_gout == 0 else norm_layer
- global_channels = int(out_channels * ratio_gout)
- self.bn_l = lnorm(out_channels - global_channels)
- self.bn_g = gnorm(global_channels)
-
- lact = nn.Identity if ratio_gout == 1 else activation_layer
- gact = nn.Identity if ratio_gout == 0 else activation_layer
- self.act_l = lact(inplace=True)
- self.act_g = gact(inplace=True)
-
- def forward(self, x):
- x_l, x_g = self.ffc(x)
- x_l = self.act_l(self.bn_l(x_l))
- x_g = self.act_g(self.bn_g(x_g))
- return x_l, x_g
-
-
-class FFCResnetBlock(nn.Module):
- def __init__(self, dim, padding_type, norm_layer, activation_layer=nn.ReLU, dilation=1,
- spatial_transform_kwargs=None, inline=False, **conv_kwargs):
- super().__init__()
- self.conv1 = FFC_BN_ACT(dim, dim, kernel_size=3, padding=dilation, dilation=dilation,
- norm_layer=norm_layer,
- activation_layer=activation_layer,
- padding_type=padding_type,
- **conv_kwargs)
- self.conv2 = FFC_BN_ACT(dim, dim, kernel_size=3, padding=dilation, dilation=dilation,
- norm_layer=norm_layer,
- activation_layer=activation_layer,
- padding_type=padding_type,
- **conv_kwargs)
- if spatial_transform_kwargs is not None:
- self.conv1 = LearnableSpatialTransformWrapper(self.conv1, **spatial_transform_kwargs)
- self.conv2 = LearnableSpatialTransformWrapper(self.conv2, **spatial_transform_kwargs)
- self.inline = inline
-
- def forward(self, x):
- if self.inline:
- x_l, x_g = x[:, :-self.conv1.ffc.global_in_num], x[:, -self.conv1.ffc.global_in_num:]
- else:
- x_l, x_g = x if type(x) is tuple else (x, 0)
-
- id_l, id_g = x_l, x_g
-
- x_l, x_g = self.conv1((x_l, x_g))
- x_l, x_g = self.conv2((x_l, x_g))
-
- x_l, x_g = id_l + x_l, id_g + x_g
- out = x_l, x_g
- if self.inline:
- out = torch.cat(out, dim=1)
- return out
-
-
-class ConcatTupleLayer(nn.Module):
- def forward(self, x):
- assert isinstance(x, tuple)
- x_l, x_g = x
- assert torch.is_tensor(x_l) or torch.is_tensor(x_g)
- if not torch.is_tensor(x_g):
- return x_l
- return torch.cat(x, dim=1)
-
-
-class FFCResNetGenerator(nn.Module):
- def __init__(self, input_nc, output_nc, ngf=64, n_downsampling=3, n_blocks=9, norm_layer=nn.BatchNorm2d,
- padding_type='reflect', activation_layer=nn.ReLU,
- up_norm_layer=nn.BatchNorm2d, up_activation=nn.ReLU(True),
- init_conv_kwargs={}, downsample_conv_kwargs={}, resnet_conv_kwargs={},
- spatial_transform_layers=None, spatial_transform_kwargs={},
- add_out_act=True, max_features=1024, out_ffc=False, out_ffc_kwargs={}):
- assert (n_blocks >= 0)
- super().__init__()
-
- model = [nn.ReflectionPad2d(3),
- FFC_BN_ACT(input_nc, ngf, kernel_size=7, padding=0, norm_layer=norm_layer,
- activation_layer=activation_layer, **init_conv_kwargs)]
-
- ### downsample
- for i in range(n_downsampling):
- mult = 2 ** i
- if i == n_downsampling - 1:
- cur_conv_kwargs = dict(downsample_conv_kwargs)
- cur_conv_kwargs['ratio_gout'] = resnet_conv_kwargs.get('ratio_gin', 0)
- else:
- cur_conv_kwargs = downsample_conv_kwargs
- model += [FFC_BN_ACT(min(max_features, ngf * mult),
- min(max_features, ngf * mult * 2),
- kernel_size=3, stride=2, padding=1,
- norm_layer=norm_layer,
- activation_layer=activation_layer,
- **cur_conv_kwargs)]
-
- mult = 2 ** n_downsampling
- feats_num_bottleneck = min(max_features, ngf * mult)
-
- ### resnet blocks
- for i in range(n_blocks):
- cur_resblock = FFCResnetBlock(feats_num_bottleneck, padding_type=padding_type, activation_layer=activation_layer,
- norm_layer=norm_layer, **resnet_conv_kwargs)
- if spatial_transform_layers is not None and i in spatial_transform_layers:
- cur_resblock = LearnableSpatialTransformWrapper(cur_resblock, **spatial_transform_kwargs)
- model += [cur_resblock]
-
- model += [ConcatTupleLayer()]
-
- ### upsample
- for i in range(n_downsampling):
- mult = 2 ** (n_downsampling - i)
- model += [nn.ConvTranspose2d(min(max_features, ngf * mult),
- min(max_features, int(ngf * mult / 2)),
- kernel_size=3, stride=2, padding=1, output_padding=1),
- up_norm_layer(min(max_features, int(ngf * mult / 2))),
- up_activation]
-
- if out_ffc:
- model += [FFCResnetBlock(ngf, padding_type=padding_type, activation_layer=activation_layer,
- norm_layer=norm_layer, inline=True, **out_ffc_kwargs)]
-
- model += [nn.ReflectionPad2d(3),
- nn.Conv2d(ngf, output_nc, kernel_size=7, padding=0)]
- if add_out_act:
- model.append(get_activation('tanh' if add_out_act is True else add_out_act))
- self.model = nn.Sequential(*model)
-
- def forward(self, input):
- return self.model(input)
-
-
-class FFCNLayerDiscriminator(BaseDiscriminator):
- def __init__(self, input_nc, ndf=64, n_layers=3, norm_layer=nn.BatchNorm2d, max_features=512,
- init_conv_kwargs={}, conv_kwargs={}):
- super().__init__()
- self.n_layers = n_layers
-
- def _act_ctor(inplace=True):
- return nn.LeakyReLU(negative_slope=0.2, inplace=inplace)
-
- kw = 3
- padw = int(np.ceil((kw-1.0)/2))
- sequence = [[FFC_BN_ACT(input_nc, ndf, kernel_size=kw, padding=padw, norm_layer=norm_layer,
- activation_layer=_act_ctor, **init_conv_kwargs)]]
-
- nf = ndf
- for n in range(1, n_layers):
- nf_prev = nf
- nf = min(nf * 2, max_features)
-
- cur_model = [
- FFC_BN_ACT(nf_prev, nf,
- kernel_size=kw, stride=2, padding=padw,
- norm_layer=norm_layer,
- activation_layer=_act_ctor,
- **conv_kwargs)
- ]
- sequence.append(cur_model)
-
- nf_prev = nf
- nf = min(nf * 2, 512)
-
- cur_model = [
- FFC_BN_ACT(nf_prev, nf,
- kernel_size=kw, stride=1, padding=padw,
- norm_layer=norm_layer,
- activation_layer=lambda *args, **kwargs: nn.LeakyReLU(*args, negative_slope=0.2, **kwargs),
- **conv_kwargs),
- ConcatTupleLayer()
- ]
- sequence.append(cur_model)
-
- sequence += [[nn.Conv2d(nf, 1, kernel_size=kw, stride=1, padding=padw)]]
-
- for n in range(len(sequence)):
- setattr(self, 'model'+str(n), nn.Sequential(*sequence[n]))
-
- def get_all_activations(self, x):
- res = [x]
- for n in range(self.n_layers + 2):
- model = getattr(self, 'model' + str(n))
- res.append(model(res[-1]))
- return res[1:]
-
- def forward(self, x):
- act = self.get_all_activations(x)
- feats = []
- for out in act[:-1]:
- if isinstance(out, tuple):
- if torch.is_tensor(out[1]):
- out = torch.cat(out, dim=1)
- else:
- out = out[0]
- feats.append(out)
- return act[-1], feats
diff --git a/spaces/akhaliq/paint-by-example/inpainting.py b/spaces/akhaliq/paint-by-example/inpainting.py
deleted file mode 100644
index 798c3fd252f826762aee6970f867eee537249db8..0000000000000000000000000000000000000000
--- a/spaces/akhaliq/paint-by-example/inpainting.py
+++ /dev/null
@@ -1,194 +0,0 @@
-import inspect
-from typing import List, Optional, Union
-
-import numpy as np
-import torch
-
-import PIL
-from diffusers import AutoencoderKL, DDIMScheduler, DiffusionPipeline, PNDMScheduler, UNet2DConditionModel
-from diffusers.pipelines.stable_diffusion import StableDiffusionSafetyChecker
-from tqdm.auto import tqdm
-from transformers import CLIPFeatureExtractor, CLIPTextModel, CLIPTokenizer
-
-
-def preprocess_image(image):
- w, h = image.size
- w, h = map(lambda x: x - x % 32, (w, h)) # resize to integer multiple of 32
- image = image.resize((w, h), resample=PIL.Image.LANCZOS)
- image = np.array(image).astype(np.float32) / 255.0
- image = image[None].transpose(0, 3, 1, 2)
- image = torch.from_numpy(image)
- return 2.0 * image - 1.0
-
-
-def preprocess_mask(mask):
- mask = mask.convert("L")
- w, h = mask.size
- w, h = map(lambda x: x - x % 32, (w, h)) # resize to integer multiple of 32
- mask = mask.resize((w // 8, h // 8), resample=PIL.Image.NEAREST)
- mask = np.array(mask).astype(np.float32) / 255.0
- mask = np.tile(mask, (4, 1, 1))
- mask = mask[None].transpose(0, 1, 2, 3) # what does this step do?
- mask = 1 - mask # repaint white, keep black
- mask = torch.from_numpy(mask)
- return mask
-
-class StableDiffusionInpaintingPipeline(DiffusionPipeline):
- def __init__(
- self,
- vae: AutoencoderKL,
- text_encoder: CLIPTextModel,
- tokenizer: CLIPTokenizer,
- unet: UNet2DConditionModel,
- scheduler: Union[DDIMScheduler, PNDMScheduler],
- safety_checker: StableDiffusionSafetyChecker,
- feature_extractor: CLIPFeatureExtractor,
- ):
- super().__init__()
- scheduler = scheduler.set_format("pt")
- self.register_modules(
- vae=vae,
- text_encoder=text_encoder,
- tokenizer=tokenizer,
- unet=unet,
- scheduler=scheduler,
- safety_checker=safety_checker,
- feature_extractor=feature_extractor,
- )
-
- @torch.no_grad()
- def __call__(
- self,
- prompt: Union[str, List[str]],
- init_image: torch.FloatTensor,
- mask_image: torch.FloatTensor,
- strength: float = 0.8,
- num_inference_steps: Optional[int] = 50,
- guidance_scale: Optional[float] = 7.5,
- eta: Optional[float] = 0.0,
- generator: Optional[torch.Generator] = None,
- output_type: Optional[str] = "pil",
- ):
-
- if isinstance(prompt, str):
- batch_size = 1
- elif isinstance(prompt, list):
- batch_size = len(prompt)
- else:
- raise ValueError(f"`prompt` has to be of type `str` or `list` but is {type(prompt)}")
-
- if strength < 0 or strength > 1:
- raise ValueError(f"The value of strength should in [0.0, 1.0] but is {strength}")
-
- # set timesteps
- accepts_offset = "offset" in set(inspect.signature(self.scheduler.set_timesteps).parameters.keys())
- extra_set_kwargs = {}
- offset = 0
- if accepts_offset:
- offset = 1
- extra_set_kwargs["offset"] = 1
-
- self.scheduler.set_timesteps(num_inference_steps, **extra_set_kwargs)
-
- # preprocess image
- init_image = preprocess_image(init_image).to(self.device)
-
- # encode the init image into latents and scale the latents
- init_latent_dist = self.vae.encode(init_image).latent_dist
- init_latents = init_latent_dist.sample(generator=generator)
- init_latents = 0.18215 * init_latents
-
- # prepare init_latents noise to latents
- init_latents = torch.cat([init_latents] * batch_size)
- init_latents_orig = init_latents
-
- # preprocess mask
- mask = preprocess_mask(mask_image).to(self.device)
- mask = torch.cat([mask] * batch_size)
-
- # check sizes
- if not mask.shape == init_latents.shape:
- raise ValueError(f"The mask and init_image should be the same size!")
-
- # get the original timestep using init_timestep
- init_timestep = int(num_inference_steps * strength) + offset
- init_timestep = min(init_timestep, num_inference_steps)
- timesteps = self.scheduler.timesteps[-init_timestep]
- timesteps = torch.tensor([timesteps] * batch_size, dtype=torch.long, device=self.device)
-
- # add noise to latents using the timesteps
- noise = torch.randn(init_latents.shape, generator=generator, device=self.device)
- init_latents = self.scheduler.add_noise(init_latents, noise, timesteps)
-
- # get prompt text embeddings
- text_input = self.tokenizer(
- prompt,
- padding="max_length",
- max_length=self.tokenizer.model_max_length,
- truncation=True,
- return_tensors="pt",
- )
- text_embeddings = self.text_encoder(text_input.input_ids.to(self.device))[0]
-
- # here `guidance_scale` is defined analog to the guidance weight `w` of equation (2)
- # of the Imagen paper: https://arxiv.org/pdf/2205.11487.pdf . `guidance_scale = 1`
- # corresponds to doing no classifier free guidance.
- do_classifier_free_guidance = guidance_scale > 1.0
- # get unconditional embeddings for classifier free guidance
- if do_classifier_free_guidance:
- max_length = text_input.input_ids.shape[-1]
- uncond_input = self.tokenizer(
- [""] * batch_size, padding="max_length", max_length=max_length, return_tensors="pt"
- )
- uncond_embeddings = self.text_encoder(uncond_input.input_ids.to(self.device))[0]
-
- # For classifier free guidance, we need to do two forward passes.
- # Here we concatenate the unconditional and text embeddings into a single batch
- # to avoid doing two forward passes
- text_embeddings = torch.cat([uncond_embeddings, text_embeddings])
-
- # prepare extra kwargs for the scheduler step, since not all schedulers have the same signature
- # eta (η) is only used with the DDIMScheduler, it will be ignored for other schedulers.
- # eta corresponds to η in DDIM paper: https://arxiv.org/abs/2010.02502
- # and should be between [0, 1]
- accepts_eta = "eta" in set(inspect.signature(self.scheduler.step).parameters.keys())
- extra_step_kwargs = {}
- if accepts_eta:
- extra_step_kwargs["eta"] = eta
-
- latents = init_latents
- t_start = max(num_inference_steps - init_timestep + offset, 0)
- for i, t in tqdm(enumerate(self.scheduler.timesteps[t_start:])):
- # expand the latents if we are doing classifier free guidance
- latent_model_input = torch.cat([latents] * 2) if do_classifier_free_guidance else latents
-
- # predict the noise residual
- noise_pred = self.unet(latent_model_input, t, encoder_hidden_states=text_embeddings)["sample"]
-
- # perform guidance
- if do_classifier_free_guidance:
- noise_pred_uncond, noise_pred_text = noise_pred.chunk(2)
- noise_pred = noise_pred_uncond + guidance_scale * (noise_pred_text - noise_pred_uncond)
-
- # compute the previous noisy sample x_t -> x_t-1
- latents = self.scheduler.step(noise_pred, t, latents, **extra_step_kwargs)["prev_sample"]
-
- # masking
- init_latents_proper = self.scheduler.add_noise(init_latents_orig, noise, t)
- latents = (init_latents_proper * mask) + (latents * (1 - mask))
-
- # scale and decode the image latents with vae
- latents = 1 / 0.18215 * latents
- image = self.vae.decode(latents).sample
-
- image = (image / 2 + 0.5).clamp(0, 1)
- image = image.cpu().permute(0, 2, 3, 1).numpy()
-
- # run safety checker
- safety_cheker_input = self.feature_extractor(self.numpy_to_pil(image), return_tensors="pt").to(self.device)
- image, has_nsfw_concept = self.safety_checker(images=image, clip_input=safety_cheker_input.pixel_values)
-
- if output_type == "pil":
- image = self.numpy_to_pil(image)
-
- return {"sample": image, "nsfw_content_detected": has_nsfw_concept}
\ No newline at end of file
diff --git a/spaces/akhaliq/paint-by-example/share_btn.py b/spaces/akhaliq/paint-by-example/share_btn.py
deleted file mode 100644
index 5bce98ad54d491f9d5691fea427efeccc77690cc..0000000000000000000000000000000000000000
--- a/spaces/akhaliq/paint-by-example/share_btn.py
+++ /dev/null
@@ -1,93 +0,0 @@
-community_icon_html = """"""
-
-loading_icon_html = """"""
-
-share_js = """async () => {
- async function uploadFile(file){
- const UPLOAD_URL = 'https://huggingface.co/uploads';
- const response = await fetch(UPLOAD_URL, {
- method: 'POST',
- headers: {
- 'Content-Type': file.type,
- 'X-Requested-With': 'XMLHttpRequest',
- },
- body: file, /// <- File inherits from Blob
- });
- const url = await response.text();
- return url;
- }
-
- async function getInputImgFile(imgCanvas){
- const blob = await new Promise(resolve => imgCanvas.toBlob(resolve));
- const imgId = Date.now() % 200;
- const fileName = `sd-inpainting-${{imgId}}.png`;
- return new File([blob], fileName, { type: 'image/png' });
- }
-
- async function getOutoutImgFile(imgEl){
- const res = await fetch(imgEl.src);
- const blob = await res.blob();
- const imgId = Date.now() % 200;
- const fileName = `sd-inpainting-${{imgId}}.png`;
- return new File([blob], fileName, { type: 'image/png' });
- }
-
- const gradioEl = document.querySelector('body > gradio-app');
- // const gradioEl = document.querySelector("gradio-app").shadowRoot;
- const inputImgCanvas = gradioEl.querySelector('canvas[key="drawing"]');
- const outputImgEl = gradioEl.querySelector('#output-img img');
- const promptTxt = gradioEl.querySelector('#input-text textarea').value;
- let titleTxt = promptTxt;
- if(titleTxt.length > 100){
- titleTxt = titleTxt.slice(0, 100) + ' ...';
- }
- const shareBtnEl = gradioEl.querySelector('#share-btn');
- const shareIconEl = gradioEl.querySelector('#share-btn-share-icon');
- const loadingIconEl = gradioEl.querySelector('#share-btn-loading-icon');
-
- if(!outputImgEl){
- return;
- };
-
- shareBtnEl.style.pointerEvents = 'none';
- shareIconEl.style.display = 'none';
- loadingIconEl.style.removeProperty('display');
-
- const inputImgFile = await getInputImgFile(inputImgCanvas);
- const outputImgFile = await getOutoutImgFile(outputImgEl);
- const files = [inputImgFile, outputImgFile];
-
- const urls = await Promise.all(files.map((f) => uploadFile(f)));
-
- const htmlImgs = urls.map(url => `
`);
- const [inputImgUrl, outputImgUrl] = htmlImgs;
-
- const descriptionMd = `
-
-${inputImgUrl}
-
-${promptTxt}
-
-
-${outputImgUrl}
-
-`;
-
- const params = new URLSearchParams({
- title: titleTxt,
- description: descriptionMd,
- });
-
- const paramsStr = params.toString();
- window.open(`${window.location.href}/discussions/new?${paramsStr}`, '_blank');
-
- shareBtnEl.style.removeProperty('pointer-events');
- shareIconEl.style.removeProperty('display');
- loadingIconEl.style.display = 'none';
-}"""
\ No newline at end of file
diff --git a/spaces/akhaliq/wav2vec2-large-robust-ft-libri-960h/README.md b/spaces/akhaliq/wav2vec2-large-robust-ft-libri-960h/README.md
deleted file mode 100644
index 9f5eebf43d604be01c5155baecf59b8ac70af6fb..0000000000000000000000000000000000000000
--- a/spaces/akhaliq/wav2vec2-large-robust-ft-libri-960h/README.md
+++ /dev/null
@@ -1,33 +0,0 @@
----
-title: Wav2vec2 Large Robust Ft Libri 960h
-emoji: 🌖
-colorFrom: pink
-colorTo: pink
-sdk: gradio
-app_file: app.py
-pinned: false
----
-
-# Configuration
-
-`title`: _string_
-Display title for the Space
-
-`emoji`: _string_
-Space emoji (emoji-only character allowed)
-
-`colorFrom`: _string_
-Color for Thumbnail gradient (red, yellow, green, blue, indigo, purple, pink, gray)
-
-`colorTo`: _string_
-Color for Thumbnail gradient (red, yellow, green, blue, indigo, purple, pink, gray)
-
-`sdk`: _string_
-Can be either `gradio` or `streamlit`
-
-`app_file`: _string_
-Path to your main application file (which contains either `gradio` or `streamlit` Python code).
-Path is relative to the root of the repository.
-
-`pinned`: _boolean_
-Whether the Space stays on top of your list.
diff --git a/spaces/alex-mindspace/gpt-agents/tests/test.py b/spaces/alex-mindspace/gpt-agents/tests/test.py
deleted file mode 100644
index fd956247da7f1751c97e98c26d28831fd9724740..0000000000000000000000000000000000000000
--- a/spaces/alex-mindspace/gpt-agents/tests/test.py
+++ /dev/null
@@ -1,34 +0,0 @@
-import sys
-import os
-import json
-from pathlib import Path
-import numpy as np
-import matplotlib.pyplot as plt
-import seaborn as sns
-sys.path.append('..')
-
-from swarmai.challenges.python_challenges.PythonChallenge import PythonChallenge
-from swarmai.Swarm import Swarm
-
-def load_keys():
- keys_file = Path("../keys.json")
- with open(keys_file) as f:
- keys = json.load(f)
- os.environ["OPENAI_API_KEY"] = keys["OPENAI_API_KEY"]
-
-def init_challenge():
- # defining the challenge the swarm will be working on
- test_challenge_config = Path('../swarmai/challenges/python_challenges/challenge2/pc2_config.yaml')
- challenge1 = PythonChallenge(test_challenge_config)
- print(challenge1.get_problem())
- return challenge1
-
-def run_swarm(challenge):
- # establishing the swarm
- swarm1 = Swarm(challenge, (5, 5), {"python developer": 0.8, "explorer python": 0.2})
- swarm1.run_swarm(1500)
-
-if __name__=="__main__":
- load_keys()
- ch = init_challenge()
- run_swarm(ch)
\ No newline at end of file
diff --git a/spaces/aodianyun/stable-diffusion-webui/modules/img2img.py b/spaces/aodianyun/stable-diffusion-webui/modules/img2img.py
deleted file mode 100644
index 8ddf224fa2b13a32cb51603a55482e0f0783ec72..0000000000000000000000000000000000000000
--- a/spaces/aodianyun/stable-diffusion-webui/modules/img2img.py
+++ /dev/null
@@ -1,184 +0,0 @@
-import math
-import os
-import sys
-import traceback
-
-import numpy as np
-from PIL import Image, ImageOps, ImageFilter, ImageEnhance, ImageChops
-
-from modules import devices, sd_samplers
-from modules.generation_parameters_copypaste import create_override_settings_dict
-from modules.processing import Processed, StableDiffusionProcessingImg2Img, process_images
-from modules.shared import opts, state
-import modules.shared as shared
-import modules.processing as processing
-from modules.ui import plaintext_to_html
-import modules.images as images
-import modules.scripts
-
-
-def process_batch(p, input_dir, output_dir, inpaint_mask_dir, args):
- processing.fix_seed(p)
-
- images = shared.listfiles(input_dir)
-
- is_inpaint_batch = False
- if inpaint_mask_dir:
- inpaint_masks = shared.listfiles(inpaint_mask_dir)
- is_inpaint_batch = len(inpaint_masks) > 0
- if is_inpaint_batch:
- print(f"\nInpaint batch is enabled. {len(inpaint_masks)} masks found.")
-
- print(f"Will process {len(images)} images, creating {p.n_iter * p.batch_size} new images for each.")
-
- save_normally = output_dir == ''
-
- p.do_not_save_grid = True
- p.do_not_save_samples = not save_normally
-
- state.job_count = len(images) * p.n_iter
-
- for i, image in enumerate(images):
- state.job = f"{i+1} out of {len(images)}"
- if state.skipped:
- state.skipped = False
-
- if state.interrupted:
- break
-
- img = Image.open(image)
- # Use the EXIF orientation of photos taken by smartphones.
- img = ImageOps.exif_transpose(img)
- p.init_images = [img] * p.batch_size
-
- if is_inpaint_batch:
- # try to find corresponding mask for an image using simple filename matching
- mask_image_path = os.path.join(inpaint_mask_dir, os.path.basename(image))
- # if not found use first one ("same mask for all images" use-case)
- if not mask_image_path in inpaint_masks:
- mask_image_path = inpaint_masks[0]
- mask_image = Image.open(mask_image_path)
- p.image_mask = mask_image
-
- proc = modules.scripts.scripts_img2img.run(p, *args)
- if proc is None:
- proc = process_images(p)
-
- for n, processed_image in enumerate(proc.images):
- filename = os.path.basename(image)
-
- if n > 0:
- left, right = os.path.splitext(filename)
- filename = f"{left}-{n}{right}"
-
- if not save_normally:
- os.makedirs(output_dir, exist_ok=True)
- if processed_image.mode == 'RGBA':
- processed_image = processed_image.convert("RGB")
- processed_image.save(os.path.join(output_dir, filename))
-
-
-def img2img(id_task: str, mode: int, prompt: str, negative_prompt: str, prompt_styles, init_img, sketch, init_img_with_mask, inpaint_color_sketch, inpaint_color_sketch_orig, init_img_inpaint, init_mask_inpaint, steps: int, sampler_index: int, mask_blur: int, mask_alpha: float, inpainting_fill: int, restore_faces: bool, tiling: bool, n_iter: int, batch_size: int, cfg_scale: float, image_cfg_scale: float, denoising_strength: float, seed: int, subseed: int, subseed_strength: float, seed_resize_from_h: int, seed_resize_from_w: int, seed_enable_extras: bool, height: int, width: int, resize_mode: int, inpaint_full_res: bool, inpaint_full_res_padding: int, inpainting_mask_invert: int, img2img_batch_input_dir: str, img2img_batch_output_dir: str, img2img_batch_inpaint_mask_dir: str, override_settings_texts, *args):
- override_settings = create_override_settings_dict(override_settings_texts)
-
- is_batch = mode == 5
-
- if mode == 0: # img2img
- image = init_img.convert("RGB")
- mask = None
- elif mode == 1: # img2img sketch
- image = sketch.convert("RGB")
- mask = None
- elif mode == 2: # inpaint
- image, mask = init_img_with_mask["image"], init_img_with_mask["mask"]
- alpha_mask = ImageOps.invert(image.split()[-1]).convert('L').point(lambda x: 255 if x > 0 else 0, mode='1')
- mask = ImageChops.lighter(alpha_mask, mask.convert('L')).convert('L')
- image = image.convert("RGB")
- elif mode == 3: # inpaint sketch
- image = inpaint_color_sketch
- orig = inpaint_color_sketch_orig or inpaint_color_sketch
- pred = np.any(np.array(image) != np.array(orig), axis=-1)
- mask = Image.fromarray(pred.astype(np.uint8) * 255, "L")
- mask = ImageEnhance.Brightness(mask).enhance(1 - mask_alpha / 100)
- blur = ImageFilter.GaussianBlur(mask_blur)
- image = Image.composite(image.filter(blur), orig, mask.filter(blur))
- image = image.convert("RGB")
- elif mode == 4: # inpaint upload mask
- image = init_img_inpaint
- mask = init_mask_inpaint
- else:
- image = None
- mask = None
-
- # Use the EXIF orientation of photos taken by smartphones.
- if image is not None:
- image = ImageOps.exif_transpose(image)
-
- assert 0. <= denoising_strength <= 1., 'can only work with strength in [0.0, 1.0]'
-
- p = StableDiffusionProcessingImg2Img(
- sd_model=shared.sd_model,
- outpath_samples=opts.outdir_samples or opts.outdir_img2img_samples,
- outpath_grids=opts.outdir_grids or opts.outdir_img2img_grids,
- prompt=prompt,
- negative_prompt=negative_prompt,
- styles=prompt_styles,
- seed=seed,
- subseed=subseed,
- subseed_strength=subseed_strength,
- seed_resize_from_h=seed_resize_from_h,
- seed_resize_from_w=seed_resize_from_w,
- seed_enable_extras=seed_enable_extras,
- sampler_name=sd_samplers.samplers_for_img2img[sampler_index].name,
- batch_size=batch_size,
- n_iter=n_iter,
- steps=steps,
- cfg_scale=cfg_scale,
- width=width,
- height=height,
- restore_faces=restore_faces,
- tiling=tiling,
- init_images=[image],
- mask=mask,
- mask_blur=mask_blur,
- inpainting_fill=inpainting_fill,
- resize_mode=resize_mode,
- denoising_strength=denoising_strength,
- image_cfg_scale=image_cfg_scale,
- inpaint_full_res=inpaint_full_res,
- inpaint_full_res_padding=inpaint_full_res_padding,
- inpainting_mask_invert=inpainting_mask_invert,
- override_settings=override_settings,
- )
-
- p.scripts = modules.scripts.scripts_txt2img
- p.script_args = args
-
- if shared.cmd_opts.enable_console_prompts:
- print(f"\nimg2img: {prompt}", file=shared.progress_print_out)
-
- p.extra_generation_params["Mask blur"] = mask_blur
-
- if is_batch:
- assert not shared.cmd_opts.hide_ui_dir_config, "Launched with --hide-ui-dir-config, batch img2img disabled"
-
- process_batch(p, img2img_batch_input_dir, img2img_batch_output_dir, img2img_batch_inpaint_mask_dir, args)
-
- processed = Processed(p, [], p.seed, "")
- else:
- processed = modules.scripts.scripts_img2img.run(p, *args)
- if processed is None:
- processed = process_images(p)
-
- p.close()
-
- shared.total_tqdm.clear()
-
- generation_info_js = processed.js()
- if opts.samples_log_stdout:
- print(generation_info_js)
-
- if opts.do_not_show_images:
- processed.images = []
-
- return processed.images, generation_info_js, plaintext_to_html(processed.info), plaintext_to_html(processed.comments)
diff --git a/spaces/aodianyun/stable-diffusion-webui/modules/sd_samplers_kdiffusion.py b/spaces/aodianyun/stable-diffusion-webui/modules/sd_samplers_kdiffusion.py
deleted file mode 100644
index 9d16fc11b8fc0678c36dadc9cca0de7122f47cee..0000000000000000000000000000000000000000
--- a/spaces/aodianyun/stable-diffusion-webui/modules/sd_samplers_kdiffusion.py
+++ /dev/null
@@ -1,357 +0,0 @@
-from collections import deque
-import torch
-import inspect
-import einops
-import k_diffusion.sampling
-from modules import prompt_parser, devices, sd_samplers_common
-
-from modules.shared import opts, state
-import modules.shared as shared
-from modules.script_callbacks import CFGDenoiserParams, cfg_denoiser_callback
-from modules.script_callbacks import CFGDenoisedParams, cfg_denoised_callback
-
-samplers_k_diffusion = [
- ('Euler a', 'sample_euler_ancestral', ['k_euler_a', 'k_euler_ancestral'], {}),
- ('Euler', 'sample_euler', ['k_euler'], {}),
- ('LMS', 'sample_lms', ['k_lms'], {}),
- ('Heun', 'sample_heun', ['k_heun'], {}),
- ('DPM2', 'sample_dpm_2', ['k_dpm_2'], {'discard_next_to_last_sigma': True}),
- ('DPM2 a', 'sample_dpm_2_ancestral', ['k_dpm_2_a'], {'discard_next_to_last_sigma': True}),
- ('DPM++ 2S a', 'sample_dpmpp_2s_ancestral', ['k_dpmpp_2s_a'], {}),
- ('DPM++ 2M', 'sample_dpmpp_2m', ['k_dpmpp_2m'], {}),
- ('DPM++ SDE', 'sample_dpmpp_sde', ['k_dpmpp_sde'], {}),
- ('DPM fast', 'sample_dpm_fast', ['k_dpm_fast'], {}),
- ('DPM adaptive', 'sample_dpm_adaptive', ['k_dpm_ad'], {}),
- ('LMS Karras', 'sample_lms', ['k_lms_ka'], {'scheduler': 'karras'}),
- ('DPM2 Karras', 'sample_dpm_2', ['k_dpm_2_ka'], {'scheduler': 'karras', 'discard_next_to_last_sigma': True}),
- ('DPM2 a Karras', 'sample_dpm_2_ancestral', ['k_dpm_2_a_ka'], {'scheduler': 'karras', 'discard_next_to_last_sigma': True}),
- ('DPM++ 2S a Karras', 'sample_dpmpp_2s_ancestral', ['k_dpmpp_2s_a_ka'], {'scheduler': 'karras'}),
- ('DPM++ 2M Karras', 'sample_dpmpp_2m', ['k_dpmpp_2m_ka'], {'scheduler': 'karras'}),
- ('DPM++ SDE Karras', 'sample_dpmpp_sde', ['k_dpmpp_sde_ka'], {'scheduler': 'karras'}),
-]
-
-samplers_data_k_diffusion = [
- sd_samplers_common.SamplerData(label, lambda model, funcname=funcname: KDiffusionSampler(funcname, model), aliases, options)
- for label, funcname, aliases, options in samplers_k_diffusion
- if hasattr(k_diffusion.sampling, funcname)
-]
-
-sampler_extra_params = {
- 'sample_euler': ['s_churn', 's_tmin', 's_tmax', 's_noise'],
- 'sample_heun': ['s_churn', 's_tmin', 's_tmax', 's_noise'],
- 'sample_dpm_2': ['s_churn', 's_tmin', 's_tmax', 's_noise'],
-}
-
-
-class CFGDenoiser(torch.nn.Module):
- """
- Classifier free guidance denoiser. A wrapper for stable diffusion model (specifically for unet)
- that can take a noisy picture and produce a noise-free picture using two guidances (prompts)
- instead of one. Originally, the second prompt is just an empty string, but we use non-empty
- negative prompt.
- """
-
- def __init__(self, model):
- super().__init__()
- self.inner_model = model
- self.mask = None
- self.nmask = None
- self.init_latent = None
- self.step = 0
- self.image_cfg_scale = None
-
- def combine_denoised(self, x_out, conds_list, uncond, cond_scale):
- denoised_uncond = x_out[-uncond.shape[0]:]
- denoised = torch.clone(denoised_uncond)
-
- for i, conds in enumerate(conds_list):
- for cond_index, weight in conds:
- denoised[i] += (x_out[cond_index] - denoised_uncond[i]) * (weight * cond_scale)
-
- return denoised
-
- def combine_denoised_for_edit_model(self, x_out, cond_scale):
- out_cond, out_img_cond, out_uncond = x_out.chunk(3)
- denoised = out_uncond + cond_scale * (out_cond - out_img_cond) + self.image_cfg_scale * (out_img_cond - out_uncond)
-
- return denoised
-
- def forward(self, x, sigma, uncond, cond, cond_scale, image_cond):
- if state.interrupted or state.skipped:
- raise sd_samplers_common.InterruptedException
-
- # at self.image_cfg_scale == 1.0 produced results for edit model are the same as with normal sampling,
- # so is_edit_model is set to False to support AND composition.
- is_edit_model = shared.sd_model.cond_stage_key == "edit" and self.image_cfg_scale is not None and self.image_cfg_scale != 1.0
-
- conds_list, tensor = prompt_parser.reconstruct_multicond_batch(cond, self.step)
- uncond = prompt_parser.reconstruct_cond_batch(uncond, self.step)
-
- assert not is_edit_model or all([len(conds) == 1 for conds in conds_list]), "AND is not supported for InstructPix2Pix checkpoint (unless using Image CFG scale = 1.0)"
-
- batch_size = len(conds_list)
- repeats = [len(conds_list[i]) for i in range(batch_size)]
-
- if not is_edit_model:
- x_in = torch.cat([torch.stack([x[i] for _ in range(n)]) for i, n in enumerate(repeats)] + [x])
- sigma_in = torch.cat([torch.stack([sigma[i] for _ in range(n)]) for i, n in enumerate(repeats)] + [sigma])
- image_cond_in = torch.cat([torch.stack([image_cond[i] for _ in range(n)]) for i, n in enumerate(repeats)] + [image_cond])
- else:
- x_in = torch.cat([torch.stack([x[i] for _ in range(n)]) for i, n in enumerate(repeats)] + [x] + [x])
- sigma_in = torch.cat([torch.stack([sigma[i] for _ in range(n)]) for i, n in enumerate(repeats)] + [sigma] + [sigma])
- image_cond_in = torch.cat([torch.stack([image_cond[i] for _ in range(n)]) for i, n in enumerate(repeats)] + [image_cond] + [torch.zeros_like(self.init_latent)])
-
- denoiser_params = CFGDenoiserParams(x_in, image_cond_in, sigma_in, state.sampling_step, state.sampling_steps)
- cfg_denoiser_callback(denoiser_params)
- x_in = denoiser_params.x
- image_cond_in = denoiser_params.image_cond
- sigma_in = denoiser_params.sigma
-
- if tensor.shape[1] == uncond.shape[1]:
- if not is_edit_model:
- cond_in = torch.cat([tensor, uncond])
- else:
- cond_in = torch.cat([tensor, uncond, uncond])
-
- if shared.batch_cond_uncond:
- x_out = self.inner_model(x_in, sigma_in, cond={"c_crossattn": [cond_in], "c_concat": [image_cond_in]})
- else:
- x_out = torch.zeros_like(x_in)
- for batch_offset in range(0, x_out.shape[0], batch_size):
- a = batch_offset
- b = a + batch_size
- x_out[a:b] = self.inner_model(x_in[a:b], sigma_in[a:b], cond={"c_crossattn": [cond_in[a:b]], "c_concat": [image_cond_in[a:b]]})
- else:
- x_out = torch.zeros_like(x_in)
- batch_size = batch_size*2 if shared.batch_cond_uncond else batch_size
- for batch_offset in range(0, tensor.shape[0], batch_size):
- a = batch_offset
- b = min(a + batch_size, tensor.shape[0])
-
- if not is_edit_model:
- c_crossattn = [tensor[a:b]]
- else:
- c_crossattn = torch.cat([tensor[a:b]], uncond)
-
- x_out[a:b] = self.inner_model(x_in[a:b], sigma_in[a:b], cond={"c_crossattn": c_crossattn, "c_concat": [image_cond_in[a:b]]})
-
- x_out[-uncond.shape[0]:] = self.inner_model(x_in[-uncond.shape[0]:], sigma_in[-uncond.shape[0]:], cond={"c_crossattn": [uncond], "c_concat": [image_cond_in[-uncond.shape[0]:]]})
-
- denoised_params = CFGDenoisedParams(x_out, state.sampling_step, state.sampling_steps)
- cfg_denoised_callback(denoised_params)
-
- devices.test_for_nans(x_out, "unet")
-
- if opts.live_preview_content == "Prompt":
- sd_samplers_common.store_latent(x_out[0:uncond.shape[0]])
- elif opts.live_preview_content == "Negative prompt":
- sd_samplers_common.store_latent(x_out[-uncond.shape[0]:])
-
- if not is_edit_model:
- denoised = self.combine_denoised(x_out, conds_list, uncond, cond_scale)
- else:
- denoised = self.combine_denoised_for_edit_model(x_out, cond_scale)
-
- if self.mask is not None:
- denoised = self.init_latent * self.mask + self.nmask * denoised
-
- self.step += 1
-
- return denoised
-
-
-class TorchHijack:
- def __init__(self, sampler_noises):
- # Using a deque to efficiently receive the sampler_noises in the same order as the previous index-based
- # implementation.
- self.sampler_noises = deque(sampler_noises)
-
- def __getattr__(self, item):
- if item == 'randn_like':
- return self.randn_like
-
- if hasattr(torch, item):
- return getattr(torch, item)
-
- raise AttributeError("'{}' object has no attribute '{}'".format(type(self).__name__, item))
-
- def randn_like(self, x):
- if self.sampler_noises:
- noise = self.sampler_noises.popleft()
- if noise.shape == x.shape:
- return noise
-
- if x.device.type == 'mps':
- return torch.randn_like(x, device=devices.cpu).to(x.device)
- else:
- return torch.randn_like(x)
-
-
-class KDiffusionSampler:
- def __init__(self, funcname, sd_model):
- denoiser = k_diffusion.external.CompVisVDenoiser if sd_model.parameterization == "v" else k_diffusion.external.CompVisDenoiser
-
- self.model_wrap = denoiser(sd_model, quantize=shared.opts.enable_quantization)
- self.funcname = funcname
- self.func = getattr(k_diffusion.sampling, self.funcname)
- self.extra_params = sampler_extra_params.get(funcname, [])
- self.model_wrap_cfg = CFGDenoiser(self.model_wrap)
- self.sampler_noises = None
- self.stop_at = None
- self.eta = None
- self.config = None
- self.last_latent = None
-
- self.conditioning_key = sd_model.model.conditioning_key
-
- def callback_state(self, d):
- step = d['i']
- latent = d["denoised"]
- if opts.live_preview_content == "Combined":
- sd_samplers_common.store_latent(latent)
- self.last_latent = latent
-
- if self.stop_at is not None and step > self.stop_at:
- raise sd_samplers_common.InterruptedException
-
- state.sampling_step = step
- shared.total_tqdm.update()
-
- def launch_sampling(self, steps, func):
- state.sampling_steps = steps
- state.sampling_step = 0
-
- try:
- return func()
- except sd_samplers_common.InterruptedException:
- return self.last_latent
-
- def number_of_needed_noises(self, p):
- return p.steps
-
- def initialize(self, p):
- self.model_wrap_cfg.mask = p.mask if hasattr(p, 'mask') else None
- self.model_wrap_cfg.nmask = p.nmask if hasattr(p, 'nmask') else None
- self.model_wrap_cfg.step = 0
- self.model_wrap_cfg.image_cfg_scale = getattr(p, 'image_cfg_scale', None)
- self.eta = p.eta if p.eta is not None else opts.eta_ancestral
-
- k_diffusion.sampling.torch = TorchHijack(self.sampler_noises if self.sampler_noises is not None else [])
-
- extra_params_kwargs = {}
- for param_name in self.extra_params:
- if hasattr(p, param_name) and param_name in inspect.signature(self.func).parameters:
- extra_params_kwargs[param_name] = getattr(p, param_name)
-
- if 'eta' in inspect.signature(self.func).parameters:
- if self.eta != 1.0:
- p.extra_generation_params["Eta"] = self.eta
-
- extra_params_kwargs['eta'] = self.eta
-
- return extra_params_kwargs
-
- def get_sigmas(self, p, steps):
- discard_next_to_last_sigma = self.config is not None and self.config.options.get('discard_next_to_last_sigma', False)
- if opts.always_discard_next_to_last_sigma and not discard_next_to_last_sigma:
- discard_next_to_last_sigma = True
- p.extra_generation_params["Discard penultimate sigma"] = True
-
- steps += 1 if discard_next_to_last_sigma else 0
-
- if p.sampler_noise_scheduler_override:
- sigmas = p.sampler_noise_scheduler_override(steps)
- elif self.config is not None and self.config.options.get('scheduler', None) == 'karras':
- sigma_min, sigma_max = (0.1, 10) if opts.use_old_karras_scheduler_sigmas else (self.model_wrap.sigmas[0].item(), self.model_wrap.sigmas[-1].item())
-
- sigmas = k_diffusion.sampling.get_sigmas_karras(n=steps, sigma_min=sigma_min, sigma_max=sigma_max, device=shared.device)
- else:
- sigmas = self.model_wrap.get_sigmas(steps)
-
- if discard_next_to_last_sigma:
- sigmas = torch.cat([sigmas[:-2], sigmas[-1:]])
-
- return sigmas
-
- def create_noise_sampler(self, x, sigmas, p):
- """For DPM++ SDE: manually create noise sampler to enable deterministic results across different batch sizes"""
- if shared.opts.no_dpmpp_sde_batch_determinism:
- return None
-
- from k_diffusion.sampling import BrownianTreeNoiseSampler
- sigma_min, sigma_max = sigmas[sigmas > 0].min(), sigmas.max()
- current_iter_seeds = p.all_seeds[p.iteration * p.batch_size:(p.iteration + 1) * p.batch_size]
- return BrownianTreeNoiseSampler(x, sigma_min, sigma_max, seed=current_iter_seeds)
-
- def sample_img2img(self, p, x, noise, conditioning, unconditional_conditioning, steps=None, image_conditioning=None):
- steps, t_enc = sd_samplers_common.setup_img2img_steps(p, steps)
-
- sigmas = self.get_sigmas(p, steps)
-
- sigma_sched = sigmas[steps - t_enc - 1:]
- xi = x + noise * sigma_sched[0]
-
- extra_params_kwargs = self.initialize(p)
- parameters = inspect.signature(self.func).parameters
-
- if 'sigma_min' in parameters:
- ## last sigma is zero which isn't allowed by DPM Fast & Adaptive so taking value before last
- extra_params_kwargs['sigma_min'] = sigma_sched[-2]
- if 'sigma_max' in parameters:
- extra_params_kwargs['sigma_max'] = sigma_sched[0]
- if 'n' in parameters:
- extra_params_kwargs['n'] = len(sigma_sched) - 1
- if 'sigma_sched' in parameters:
- extra_params_kwargs['sigma_sched'] = sigma_sched
- if 'sigmas' in parameters:
- extra_params_kwargs['sigmas'] = sigma_sched
-
- if self.funcname == 'sample_dpmpp_sde':
- noise_sampler = self.create_noise_sampler(x, sigmas, p)
- extra_params_kwargs['noise_sampler'] = noise_sampler
-
- self.model_wrap_cfg.init_latent = x
- self.last_latent = x
- extra_args={
- 'cond': conditioning,
- 'image_cond': image_conditioning,
- 'uncond': unconditional_conditioning,
- 'cond_scale': p.cfg_scale,
- }
-
- samples = self.launch_sampling(t_enc + 1, lambda: self.func(self.model_wrap_cfg, xi, extra_args=extra_args, disable=False, callback=self.callback_state, **extra_params_kwargs))
-
- return samples
-
- def sample(self, p, x, conditioning, unconditional_conditioning, steps=None, image_conditioning=None):
- steps = steps or p.steps
-
- sigmas = self.get_sigmas(p, steps)
-
- x = x * sigmas[0]
-
- extra_params_kwargs = self.initialize(p)
- parameters = inspect.signature(self.func).parameters
-
- if 'sigma_min' in parameters:
- extra_params_kwargs['sigma_min'] = self.model_wrap.sigmas[0].item()
- extra_params_kwargs['sigma_max'] = self.model_wrap.sigmas[-1].item()
- if 'n' in parameters:
- extra_params_kwargs['n'] = steps
- else:
- extra_params_kwargs['sigmas'] = sigmas
-
- if self.funcname == 'sample_dpmpp_sde':
- noise_sampler = self.create_noise_sampler(x, sigmas, p)
- extra_params_kwargs['noise_sampler'] = noise_sampler
-
- self.last_latent = x
- samples = self.launch_sampling(steps, lambda: self.func(self.model_wrap_cfg, x, extra_args={
- 'cond': conditioning,
- 'image_cond': image_conditioning,
- 'uncond': unconditional_conditioning,
- 'cond_scale': p.cfg_scale
- }, disable=False, callback=self.callback_state, **extra_params_kwargs))
-
- return samples
-
diff --git a/spaces/arnavkartikeya/SCRIPture-final/models/blip_itm.py b/spaces/arnavkartikeya/SCRIPture-final/models/blip_itm.py
deleted file mode 100644
index cf354c829564bf5a1f56089a2d745093d51e0fa2..0000000000000000000000000000000000000000
--- a/spaces/arnavkartikeya/SCRIPture-final/models/blip_itm.py
+++ /dev/null
@@ -1,76 +0,0 @@
-from models.med import BertConfig, BertModel
-from transformers import BertTokenizer
-
-import torch
-from torch import nn
-import torch.nn.functional as F
-
-from models.blip import create_vit, init_tokenizer, load_checkpoint
-
-class BLIP_ITM(nn.Module):
- def __init__(self,
- med_config = 'configs/med_config.json',
- image_size = 384,
- vit = 'base',
- vit_grad_ckpt = False,
- vit_ckpt_layer = 0,
- embed_dim = 256,
- ):
- """
- Args:
- med_config (str): path for the mixture of encoder-decoder model's configuration file
- image_size (int): input image size
- vit (str): model size of vision transformer
- """
- super().__init__()
-
- self.visual_encoder, vision_width = create_vit(vit,image_size, vit_grad_ckpt, vit_ckpt_layer)
- self.tokenizer = init_tokenizer()
- med_config = BertConfig.from_json_file(med_config)
- med_config.encoder_width = vision_width
- self.text_encoder = BertModel(config=med_config, add_pooling_layer=False)
-
- text_width = self.text_encoder.config.hidden_size
-
- self.vision_proj = nn.Linear(vision_width, embed_dim)
- self.text_proj = nn.Linear(text_width, embed_dim)
-
- self.itm_head = nn.Linear(text_width, 2)
-
-
- def forward(self, image, caption, match_head='itm'):
-
- image_embeds = self.visual_encoder(image)
- image_atts = torch.ones(image_embeds.size()[:-1],dtype=torch.long).to(image.device)
-
- text = self.tokenizer(caption, padding='max_length', truncation=True, max_length=35,
- return_tensors="pt").to(image.device)
-
-
- if match_head=='itm':
- output = self.text_encoder(text.input_ids,
- attention_mask = text.attention_mask,
- encoder_hidden_states = image_embeds,
- encoder_attention_mask = image_atts,
- return_dict = True,
- )
- itm_output = self.itm_head(output.last_hidden_state[:,0,:])
- return itm_output
-
- elif match_head=='itc':
- text_output = self.text_encoder(text.input_ids, attention_mask = text.attention_mask,
- return_dict = True, mode = 'text')
- image_feat = F.normalize(self.vision_proj(image_embeds[:,0,:]),dim=-1)
- text_feat = F.normalize(self.text_proj(text_output.last_hidden_state[:,0,:]),dim=-1)
-
- sim = image_feat @ text_feat.t()
- return sim
-
-
-def blip_itm(pretrained='',**kwargs):
- model = BLIP_ITM(**kwargs)
- if pretrained:
- model,msg = load_checkpoint(model,pretrained)
- assert(len(msg.missing_keys)==0)
- return model
-
\ No newline at end of file
diff --git a/spaces/arxify/RVC-beta-v2-0618/runtime/Lib/site-packages/Cython/Plex/Traditional.py b/spaces/arxify/RVC-beta-v2-0618/runtime/Lib/site-packages/Cython/Plex/Traditional.py
deleted file mode 100644
index ec7252daed9963acc16369418152755e9e8eca30..0000000000000000000000000000000000000000
--- a/spaces/arxify/RVC-beta-v2-0618/runtime/Lib/site-packages/Cython/Plex/Traditional.py
+++ /dev/null
@@ -1,158 +0,0 @@
-#=======================================================================
-#
-# Python Lexical Analyser
-#
-# Traditional Regular Expression Syntax
-#
-#=======================================================================
-
-from __future__ import absolute_import
-
-from .Regexps import Alt, Seq, Rep, Rep1, Opt, Any, AnyBut, Bol, Eol, Char
-from .Errors import PlexError
-
-
-class RegexpSyntaxError(PlexError):
- pass
-
-
-def re(s):
- """
- Convert traditional string representation of regular expression |s|
- into Plex representation.
- """
- return REParser(s).parse_re()
-
-
-class REParser(object):
- def __init__(self, s):
- self.s = s
- self.i = -1
- self.end = 0
- self.next()
-
- def parse_re(self):
- re = self.parse_alt()
- if not self.end:
- self.error("Unexpected %s" % repr(self.c))
- return re
-
- def parse_alt(self):
- """Parse a set of alternative regexps."""
- re = self.parse_seq()
- if self.c == '|':
- re_list = [re]
- while self.c == '|':
- self.next()
- re_list.append(self.parse_seq())
- re = Alt(*re_list)
- return re
-
- def parse_seq(self):
- """Parse a sequence of regexps."""
- re_list = []
- while not self.end and not self.c in "|)":
- re_list.append(self.parse_mod())
- return Seq(*re_list)
-
- def parse_mod(self):
- """Parse a primitive regexp followed by *, +, ? modifiers."""
- re = self.parse_prim()
- while not self.end and self.c in "*+?":
- if self.c == '*':
- re = Rep(re)
- elif self.c == '+':
- re = Rep1(re)
- else: # self.c == '?'
- re = Opt(re)
- self.next()
- return re
-
- def parse_prim(self):
- """Parse a primitive regexp."""
- c = self.get()
- if c == '.':
- re = AnyBut("\n")
- elif c == '^':
- re = Bol
- elif c == '$':
- re = Eol
- elif c == '(':
- re = self.parse_alt()
- self.expect(')')
- elif c == '[':
- re = self.parse_charset()
- self.expect(']')
- else:
- if c == '\\':
- c = self.get()
- re = Char(c)
- return re
-
- def parse_charset(self):
- """Parse a charset. Does not include the surrounding []."""
- char_list = []
- invert = 0
- if self.c == '^':
- invert = 1
- self.next()
- if self.c == ']':
- char_list.append(']')
- self.next()
- while not self.end and self.c != ']':
- c1 = self.get()
- if self.c == '-' and self.lookahead(1) != ']':
- self.next()
- c2 = self.get()
- for a in range(ord(c1), ord(c2) + 1):
- char_list.append(chr(a))
- else:
- char_list.append(c1)
- chars = ''.join(char_list)
- if invert:
- return AnyBut(chars)
- else:
- return Any(chars)
-
- def next(self):
- """Advance to the next char."""
- s = self.s
- i = self.i = self.i + 1
- if i < len(s):
- self.c = s[i]
- else:
- self.c = ''
- self.end = 1
-
- def get(self):
- if self.end:
- self.error("Premature end of string")
- c = self.c
- self.next()
- return c
-
- def lookahead(self, n):
- """Look ahead n chars."""
- j = self.i + n
- if j < len(self.s):
- return self.s[j]
- else:
- return ''
-
- def expect(self, c):
- """
- Expect to find character |c| at current position.
- Raises an exception otherwise.
- """
- if self.c == c:
- self.next()
- else:
- self.error("Missing %s" % repr(c))
-
- def error(self, mess):
- """Raise exception to signal syntax error in regexp."""
- raise RegexpSyntaxError("Syntax error in regexp %s at position %d: %s" % (
- repr(self.s), self.i, mess))
-
-
-
diff --git a/spaces/arxify/RVC-beta-v2-0618/runtime/Lib/site-packages/PIL/ImageTransform.py b/spaces/arxify/RVC-beta-v2-0618/runtime/Lib/site-packages/PIL/ImageTransform.py
deleted file mode 100644
index 7881f0d262b0db7ecaed224ee2268f3b69b836c9..0000000000000000000000000000000000000000
--- a/spaces/arxify/RVC-beta-v2-0618/runtime/Lib/site-packages/PIL/ImageTransform.py
+++ /dev/null
@@ -1,102 +0,0 @@
-#
-# The Python Imaging Library.
-# $Id$
-#
-# transform wrappers
-#
-# History:
-# 2002-04-08 fl Created
-#
-# Copyright (c) 2002 by Secret Labs AB
-# Copyright (c) 2002 by Fredrik Lundh
-#
-# See the README file for information on usage and redistribution.
-#
-
-from . import Image
-
-
-class Transform(Image.ImageTransformHandler):
- def __init__(self, data):
- self.data = data
-
- def getdata(self):
- return self.method, self.data
-
- def transform(self, size, image, **options):
- # can be overridden
- method, data = self.getdata()
- return image.transform(size, method, data, **options)
-
-
-class AffineTransform(Transform):
- """
- Define an affine image transform.
-
- This function takes a 6-tuple (a, b, c, d, e, f) which contain the first
- two rows from an affine transform matrix. For each pixel (x, y) in the
- output image, the new value is taken from a position (a x + b y + c,
- d x + e y + f) in the input image, rounded to nearest pixel.
-
- This function can be used to scale, translate, rotate, and shear the
- original image.
-
- See :py:meth:`~PIL.Image.Image.transform`
-
- :param matrix: A 6-tuple (a, b, c, d, e, f) containing the first two rows
- from an affine transform matrix.
- """
-
- method = Image.Transform.AFFINE
-
-
-class ExtentTransform(Transform):
- """
- Define a transform to extract a subregion from an image.
-
- Maps a rectangle (defined by two corners) from the image to a rectangle of
- the given size. The resulting image will contain data sampled from between
- the corners, such that (x0, y0) in the input image will end up at (0,0) in
- the output image, and (x1, y1) at size.
-
- This method can be used to crop, stretch, shrink, or mirror an arbitrary
- rectangle in the current image. It is slightly slower than crop, but about
- as fast as a corresponding resize operation.
-
- See :py:meth:`~PIL.Image.Image.transform`
-
- :param bbox: A 4-tuple (x0, y0, x1, y1) which specifies two points in the
- input image's coordinate system. See :ref:`coordinate-system`.
- """
-
- method = Image.Transform.EXTENT
-
-
-class QuadTransform(Transform):
- """
- Define a quad image transform.
-
- Maps a quadrilateral (a region defined by four corners) from the image to a
- rectangle of the given size.
-
- See :py:meth:`~PIL.Image.Image.transform`
-
- :param xy: An 8-tuple (x0, y0, x1, y1, x2, y2, x3, y3) which contain the
- upper left, lower left, lower right, and upper right corner of the
- source quadrilateral.
- """
-
- method = Image.Transform.QUAD
-
-
-class MeshTransform(Transform):
- """
- Define a mesh image transform. A mesh transform consists of one or more
- individual quad transforms.
-
- See :py:meth:`~PIL.Image.Image.transform`
-
- :param data: A list of (bbox, quad) tuples.
- """
-
- method = Image.Transform.MESH
diff --git a/spaces/arxify/RVC-beta-v2-0618/runtime/Lib/site-packages/absl/flags/_defines.py b/spaces/arxify/RVC-beta-v2-0618/runtime/Lib/site-packages/absl/flags/_defines.py
deleted file mode 100644
index 61354e94837699f2b2e91c7c67d98df4b1e78dc7..0000000000000000000000000000000000000000
--- a/spaces/arxify/RVC-beta-v2-0618/runtime/Lib/site-packages/absl/flags/_defines.py
+++ /dev/null
@@ -1,933 +0,0 @@
-# Copyright 2017 The Abseil Authors.
-#
-# Licensed under the Apache License, Version 2.0 (the "License");
-# you may not use this file except in compliance with the License.
-# You may obtain a copy of the License at
-#
-# http://www.apache.org/licenses/LICENSE-2.0
-#
-# Unless required by applicable law or agreed to in writing, software
-# distributed under the License is distributed on an "AS IS" BASIS,
-# WITHOUT WARRANTIES OR CONDITIONS OF ANY KIND, either express or implied.
-# See the License for the specific language governing permissions and
-# limitations under the License.
-"""This modules contains flags DEFINE functions.
-
-Do NOT import this module directly. Import the flags package and use the
-aliases defined at the package level instead.
-"""
-
-import sys
-import types
-
-from absl.flags import _argument_parser
-from absl.flags import _exceptions
-from absl.flags import _flag
-from absl.flags import _flagvalues
-from absl.flags import _helpers
-from absl.flags import _validators
-
-# pylint: disable=unused-import
-try:
- from typing import Text, List, Any
-except ImportError:
- pass
-
-try:
- import enum
-except ImportError:
- pass
-# pylint: enable=unused-import
-
-_helpers.disclaim_module_ids.add(id(sys.modules[__name__]))
-
-
-def _register_bounds_validator_if_needed(parser, name, flag_values):
- """Enforces lower and upper bounds for numeric flags.
-
- Args:
- parser: NumericParser (either FloatParser or IntegerParser), provides lower
- and upper bounds, and help text to display.
- name: str, name of the flag
- flag_values: FlagValues.
- """
- if parser.lower_bound is not None or parser.upper_bound is not None:
-
- def checker(value):
- if value is not None and parser.is_outside_bounds(value):
- message = '%s is not %s' % (value, parser.syntactic_help)
- raise _exceptions.ValidationError(message)
- return True
-
- _validators.register_validator(name, checker, flag_values=flag_values)
-
-
-def DEFINE( # pylint: disable=invalid-name
- parser,
- name,
- default,
- help, # pylint: disable=redefined-builtin
- flag_values=_flagvalues.FLAGS,
- serializer=None,
- module_name=None,
- required=False,
- **args):
- """Registers a generic Flag object.
-
- NOTE: in the docstrings of all DEFINE* functions, "registers" is short
- for "creates a new flag and registers it".
-
- Auxiliary function: clients should use the specialized ``DEFINE_``
- function instead.
-
- Args:
- parser: :class:`ArgumentParser`, used to parse the flag arguments.
- name: str, the flag name.
- default: The default value of the flag.
- help: str, the help message.
- flag_values: :class:`FlagValues`, the FlagValues instance with which the
- flag will be registered. This should almost never need to be overridden.
- serializer: :class:`ArgumentSerializer`, the flag serializer instance.
- module_name: str, the name of the Python module declaring this flag. If not
- provided, it will be computed using the stack trace of this call.
- required: bool, is this a required flag. This must be used as a keyword
- argument.
- **args: dict, the extra keyword args that are passed to ``Flag.__init__``.
-
- Returns:
- a handle to defined flag.
- """
- return DEFINE_flag(
- _flag.Flag(parser, serializer, name, default, help, **args), flag_values,
- module_name, required)
-
-
-def DEFINE_flag( # pylint: disable=invalid-name
- flag,
- flag_values=_flagvalues.FLAGS,
- module_name=None,
- required=False):
- """Registers a :class:`Flag` object with a :class:`FlagValues` object.
-
- By default, the global :const:`FLAGS` ``FlagValue`` object is used.
-
- Typical users will use one of the more specialized DEFINE_xxx
- functions, such as :func:`DEFINE_string` or :func:`DEFINE_integer`. But
- developers who need to create :class:`Flag` objects themselves should use
- this function to register their flags.
-
- Args:
- flag: :class:`Flag`, a flag that is key to the module.
- flag_values: :class:`FlagValues`, the ``FlagValues`` instance with which the
- flag will be registered. This should almost never need to be overridden.
- module_name: str, the name of the Python module declaring this flag. If not
- provided, it will be computed using the stack trace of this call.
- required: bool, is this a required flag. This must be used as a keyword
- argument.
-
- Returns:
- a handle to defined flag.
- """
- if required and flag.default is not None:
- raise ValueError('Required flag --%s cannot have a non-None default' %
- flag.name)
- # Copying the reference to flag_values prevents pychecker warnings.
- fv = flag_values
- fv[flag.name] = flag
- # Tell flag_values who's defining the flag.
- if module_name:
- module = sys.modules.get(module_name)
- else:
- module, module_name = _helpers.get_calling_module_object_and_name()
- flag_values.register_flag_by_module(module_name, flag)
- flag_values.register_flag_by_module_id(id(module), flag)
- if required:
- _validators.mark_flag_as_required(flag.name, fv)
- ensure_non_none_value = (flag.default is not None) or required
- return _flagvalues.FlagHolder(
- fv, flag, ensure_non_none_value=ensure_non_none_value)
-
-
-def set_default(flag_holder, value):
- """Changes the default value of the provided flag object.
-
- The flag's current value is also updated if the flag is currently using
- the default value, i.e. not specified in the command line, and not set
- by FLAGS.name = value.
-
- Args:
- flag_holder: FlagHolder, the flag to modify.
- value: The new default value.
-
- Raises:
- IllegalFlagValueError: Raised when value is not valid.
- """
- flag_holder._flagvalues.set_default(flag_holder.name, value) # pylint: disable=protected-access
-
-
-def _internal_declare_key_flags(flag_names,
- flag_values=_flagvalues.FLAGS,
- key_flag_values=None):
- """Declares a flag as key for the calling module.
-
- Internal function. User code should call declare_key_flag or
- adopt_module_key_flags instead.
-
- Args:
- flag_names: [str], a list of names of already-registered Flag objects.
- flag_values: :class:`FlagValues`, the FlagValues instance with which the
- flags listed in flag_names have registered (the value of the flag_values
- argument from the ``DEFINE_*`` calls that defined those flags). This
- should almost never need to be overridden.
- key_flag_values: :class:`FlagValues`, the FlagValues instance that (among
- possibly many other things) keeps track of the key flags for each module.
- Default ``None`` means "same as flag_values". This should almost never
- need to be overridden.
-
- Raises:
- UnrecognizedFlagError: Raised when the flag is not defined.
- """
- key_flag_values = key_flag_values or flag_values
-
- module = _helpers.get_calling_module()
-
- for flag_name in flag_names:
- key_flag_values.register_key_flag_for_module(module, flag_values[flag_name])
-
-
-def declare_key_flag(flag_name, flag_values=_flagvalues.FLAGS):
- """Declares one flag as key to the current module.
-
- Key flags are flags that are deemed really important for a module.
- They are important when listing help messages; e.g., if the
- --helpshort command-line flag is used, then only the key flags of the
- main module are listed (instead of all flags, as in the case of
- --helpfull).
-
- Sample usage::
-
- flags.declare_key_flag('flag_1')
-
- Args:
- flag_name: str | :class:`FlagHolder`, the name or holder of an already
- declared flag. (Redeclaring flags as key, including flags implicitly key
- because they were declared in this module, is a no-op.)
- Positional-only parameter.
- flag_values: :class:`FlagValues`, the FlagValues instance in which the
- flag will be declared as a key flag. This should almost never need to be
- overridden.
-
- Raises:
- ValueError: Raised if flag_name not defined as a Python flag.
- """
- flag_name, flag_values = _flagvalues.resolve_flag_ref(flag_name, flag_values)
- if flag_name in _helpers.SPECIAL_FLAGS:
- # Take care of the special flags, e.g., --flagfile, --undefok.
- # These flags are defined in SPECIAL_FLAGS, and are treated
- # specially during flag parsing, taking precedence over the
- # user-defined flags.
- _internal_declare_key_flags([flag_name],
- flag_values=_helpers.SPECIAL_FLAGS,
- key_flag_values=flag_values)
- return
- try:
- _internal_declare_key_flags([flag_name], flag_values=flag_values)
- except KeyError:
- raise ValueError('Flag --%s is undefined. To set a flag as a key flag '
- 'first define it in Python.' % flag_name)
-
-
-def adopt_module_key_flags(module, flag_values=_flagvalues.FLAGS):
- """Declares that all flags key to a module are key to the current module.
-
- Args:
- module: module, the module object from which all key flags will be declared
- as key flags to the current module.
- flag_values: :class:`FlagValues`, the FlagValues instance in which the
- flags will be declared as key flags. This should almost never need to be
- overridden.
-
- Raises:
- Error: Raised when given an argument that is a module name (a string),
- instead of a module object.
- """
- if not isinstance(module, types.ModuleType):
- raise _exceptions.Error('Expected a module object, not %r.' % (module,))
- _internal_declare_key_flags(
- [f.name for f in flag_values.get_key_flags_for_module(module.__name__)],
- flag_values=flag_values)
- # If module is this flag module, take _helpers.SPECIAL_FLAGS into account.
- if module == _helpers.FLAGS_MODULE:
- _internal_declare_key_flags(
- # As we associate flags with get_calling_module_object_and_name(), the
- # special flags defined in this module are incorrectly registered with
- # a different module. So, we can't use get_key_flags_for_module.
- # Instead, we take all flags from _helpers.SPECIAL_FLAGS (a private
- # FlagValues, where no other module should register flags).
- [_helpers.SPECIAL_FLAGS[name].name for name in _helpers.SPECIAL_FLAGS],
- flag_values=_helpers.SPECIAL_FLAGS,
- key_flag_values=flag_values)
-
-
-def disclaim_key_flags():
- """Declares that the current module will not define any more key flags.
-
- Normally, the module that calls the DEFINE_xxx functions claims the
- flag to be its key flag. This is undesirable for modules that
- define additional DEFINE_yyy functions with its own flag parsers and
- serializers, since that module will accidentally claim flags defined
- by DEFINE_yyy as its key flags. After calling this function, the
- module disclaims flag definitions thereafter, so the key flags will
- be correctly attributed to the caller of DEFINE_yyy.
-
- After calling this function, the module will not be able to define
- any more flags. This function will affect all FlagValues objects.
- """
- globals_for_caller = sys._getframe(1).f_globals # pylint: disable=protected-access
- module, _ = _helpers.get_module_object_and_name(globals_for_caller)
- _helpers.disclaim_module_ids.add(id(module))
-
-
-def DEFINE_string( # pylint: disable=invalid-name,redefined-builtin
- name,
- default,
- help,
- flag_values=_flagvalues.FLAGS,
- required=False,
- **args):
- """Registers a flag whose value can be any string."""
- parser = _argument_parser.ArgumentParser()
- serializer = _argument_parser.ArgumentSerializer()
- return DEFINE(
- parser,
- name,
- default,
- help,
- flag_values,
- serializer,
- required=required,
- **args)
-
-
-def DEFINE_boolean( # pylint: disable=invalid-name,redefined-builtin
- name,
- default,
- help,
- flag_values=_flagvalues.FLAGS,
- module_name=None,
- required=False,
- **args):
- """Registers a boolean flag.
-
- Such a boolean flag does not take an argument. If a user wants to
- specify a false value explicitly, the long option beginning with 'no'
- must be used: i.e. --noflag
-
- This flag will have a value of None, True or False. None is possible
- if default=None and the user does not specify the flag on the command
- line.
-
- Args:
- name: str, the flag name.
- default: bool|str|None, the default value of the flag.
- help: str, the help message.
- flag_values: :class:`FlagValues`, the FlagValues instance with which the
- flag will be registered. This should almost never need to be overridden.
- module_name: str, the name of the Python module declaring this flag. If not
- provided, it will be computed using the stack trace of this call.
- required: bool, is this a required flag. This must be used as a keyword
- argument.
- **args: dict, the extra keyword args that are passed to ``Flag.__init__``.
-
- Returns:
- a handle to defined flag.
- """
- return DEFINE_flag(
- _flag.BooleanFlag(name, default, help, **args), flag_values, module_name,
- required)
-
-
-def DEFINE_float( # pylint: disable=invalid-name,redefined-builtin
- name,
- default,
- help,
- lower_bound=None,
- upper_bound=None,
- flag_values=_flagvalues.FLAGS,
- required=False,
- **args):
- """Registers a flag whose value must be a float.
-
- If ``lower_bound`` or ``upper_bound`` are set, then this flag must be
- within the given range.
-
- Args:
- name: str, the flag name.
- default: float|str|None, the default value of the flag.
- help: str, the help message.
- lower_bound: float, min value of the flag.
- upper_bound: float, max value of the flag.
- flag_values: :class:`FlagValues`, the FlagValues instance with which the
- flag will be registered. This should almost never need to be overridden.
- required: bool, is this a required flag. This must be used as a keyword
- argument.
- **args: dict, the extra keyword args that are passed to :func:`DEFINE`.
-
- Returns:
- a handle to defined flag.
- """
- parser = _argument_parser.FloatParser(lower_bound, upper_bound)
- serializer = _argument_parser.ArgumentSerializer()
- result = DEFINE(
- parser,
- name,
- default,
- help,
- flag_values,
- serializer,
- required=required,
- **args)
- _register_bounds_validator_if_needed(parser, name, flag_values=flag_values)
- return result
-
-
-def DEFINE_integer( # pylint: disable=invalid-name,redefined-builtin
- name,
- default,
- help,
- lower_bound=None,
- upper_bound=None,
- flag_values=_flagvalues.FLAGS,
- required=False,
- **args):
- """Registers a flag whose value must be an integer.
-
- If ``lower_bound``, or ``upper_bound`` are set, then this flag must be
- within the given range.
-
- Args:
- name: str, the flag name.
- default: int|str|None, the default value of the flag.
- help: str, the help message.
- lower_bound: int, min value of the flag.
- upper_bound: int, max value of the flag.
- flag_values: :class:`FlagValues`, the FlagValues instance with which the
- flag will be registered. This should almost never need to be overridden.
- required: bool, is this a required flag. This must be used as a keyword
- argument.
- **args: dict, the extra keyword args that are passed to :func:`DEFINE`.
-
- Returns:
- a handle to defined flag.
- """
- parser = _argument_parser.IntegerParser(lower_bound, upper_bound)
- serializer = _argument_parser.ArgumentSerializer()
- result = DEFINE(
- parser,
- name,
- default,
- help,
- flag_values,
- serializer,
- required=required,
- **args)
- _register_bounds_validator_if_needed(parser, name, flag_values=flag_values)
- return result
-
-
-def DEFINE_enum( # pylint: disable=invalid-name,redefined-builtin
- name,
- default,
- enum_values,
- help,
- flag_values=_flagvalues.FLAGS,
- module_name=None,
- required=False,
- **args):
- """Registers a flag whose value can be any string from enum_values.
-
- Instead of a string enum, prefer `DEFINE_enum_class`, which allows
- defining enums from an `enum.Enum` class.
-
- Args:
- name: str, the flag name.
- default: str|None, the default value of the flag.
- enum_values: [str], a non-empty list of strings with the possible values for
- the flag.
- help: str, the help message.
- flag_values: :class:`FlagValues`, the FlagValues instance with which the
- flag will be registered. This should almost never need to be overridden.
- module_name: str, the name of the Python module declaring this flag. If not
- provided, it will be computed using the stack trace of this call.
- required: bool, is this a required flag. This must be used as a keyword
- argument.
- **args: dict, the extra keyword args that are passed to ``Flag.__init__``.
-
- Returns:
- a handle to defined flag.
- """
- return DEFINE_flag(
- _flag.EnumFlag(name, default, help, enum_values, **args), flag_values,
- module_name, required)
-
-
-def DEFINE_enum_class( # pylint: disable=invalid-name,redefined-builtin
- name,
- default,
- enum_class,
- help,
- flag_values=_flagvalues.FLAGS,
- module_name=None,
- case_sensitive=False,
- required=False,
- **args):
- """Registers a flag whose value can be the name of enum members.
-
- Args:
- name: str, the flag name.
- default: Enum|str|None, the default value of the flag.
- enum_class: class, the Enum class with all the possible values for the flag.
- help: str, the help message.
- flag_values: :class:`FlagValues`, the FlagValues instance with which the
- flag will be registered. This should almost never need to be overridden.
- module_name: str, the name of the Python module declaring this flag. If not
- provided, it will be computed using the stack trace of this call.
- case_sensitive: bool, whether to map strings to members of the enum_class
- without considering case.
- required: bool, is this a required flag. This must be used as a keyword
- argument.
- **args: dict, the extra keyword args that are passed to ``Flag.__init__``.
-
- Returns:
- a handle to defined flag.
- """
- return DEFINE_flag(
- _flag.EnumClassFlag(
- name,
- default,
- help,
- enum_class,
- case_sensitive=case_sensitive,
- **args), flag_values, module_name, required)
-
-
-def DEFINE_list( # pylint: disable=invalid-name,redefined-builtin
- name,
- default,
- help,
- flag_values=_flagvalues.FLAGS,
- required=False,
- **args):
- """Registers a flag whose value is a comma-separated list of strings.
-
- The flag value is parsed with a CSV parser.
-
- Args:
- name: str, the flag name.
- default: list|str|None, the default value of the flag.
- help: str, the help message.
- flag_values: :class:`FlagValues`, the FlagValues instance with which the
- flag will be registered. This should almost never need to be overridden.
- required: bool, is this a required flag. This must be used as a keyword
- argument.
- **args: Dictionary with extra keyword args that are passed to the
- ``Flag.__init__``.
-
- Returns:
- a handle to defined flag.
- """
- parser = _argument_parser.ListParser()
- serializer = _argument_parser.CsvListSerializer(',')
- return DEFINE(
- parser,
- name,
- default,
- help,
- flag_values,
- serializer,
- required=required,
- **args)
-
-
-def DEFINE_spaceseplist( # pylint: disable=invalid-name,redefined-builtin
- name,
- default,
- help,
- comma_compat=False,
- flag_values=_flagvalues.FLAGS,
- required=False,
- **args):
- """Registers a flag whose value is a whitespace-separated list of strings.
-
- Any whitespace can be used as a separator.
-
- Args:
- name: str, the flag name.
- default: list|str|None, the default value of the flag.
- help: str, the help message.
- comma_compat: bool - Whether to support comma as an additional separator. If
- false then only whitespace is supported. This is intended only for
- backwards compatibility with flags that used to be comma-separated.
- flag_values: :class:`FlagValues`, the FlagValues instance with which the
- flag will be registered. This should almost never need to be overridden.
- required: bool, is this a required flag. This must be used as a keyword
- argument.
- **args: Dictionary with extra keyword args that are passed to the
- ``Flag.__init__``.
-
- Returns:
- a handle to defined flag.
- """
- parser = _argument_parser.WhitespaceSeparatedListParser(
- comma_compat=comma_compat)
- serializer = _argument_parser.ListSerializer(' ')
- return DEFINE(
- parser,
- name,
- default,
- help,
- flag_values,
- serializer,
- required=required,
- **args)
-
-
-def DEFINE_multi( # pylint: disable=invalid-name,redefined-builtin
- parser,
- serializer,
- name,
- default,
- help,
- flag_values=_flagvalues.FLAGS,
- module_name=None,
- required=False,
- **args):
- """Registers a generic MultiFlag that parses its args with a given parser.
-
- Auxiliary function. Normal users should NOT use it directly.
-
- Developers who need to create their own 'Parser' classes for options
- which can appear multiple times can call this module function to
- register their flags.
-
- Args:
- parser: ArgumentParser, used to parse the flag arguments.
- serializer: ArgumentSerializer, the flag serializer instance.
- name: str, the flag name.
- default: Union[Iterable[T], Text, None], the default value of the flag. If
- the value is text, it will be parsed as if it was provided from the
- command line. If the value is a non-string iterable, it will be iterated
- over to create a shallow copy of the values. If it is None, it is left
- as-is.
- help: str, the help message.
- flag_values: :class:`FlagValues`, the FlagValues instance with which the
- flag will be registered. This should almost never need to be overridden.
- module_name: A string, the name of the Python module declaring this flag. If
- not provided, it will be computed using the stack trace of this call.
- required: bool, is this a required flag. This must be used as a keyword
- argument.
- **args: Dictionary with extra keyword args that are passed to the
- ``Flag.__init__``.
-
- Returns:
- a handle to defined flag.
- """
- return DEFINE_flag(
- _flag.MultiFlag(parser, serializer, name, default, help, **args),
- flag_values, module_name, required)
-
-
-def DEFINE_multi_string( # pylint: disable=invalid-name,redefined-builtin
- name,
- default,
- help,
- flag_values=_flagvalues.FLAGS,
- required=False,
- **args):
- """Registers a flag whose value can be a list of any strings.
-
- Use the flag on the command line multiple times to place multiple
- string values into the list. The 'default' may be a single string
- (which will be converted into a single-element list) or a list of
- strings.
-
-
- Args:
- name: str, the flag name.
- default: Union[Iterable[Text], Text, None], the default value of the flag;
- see :func:`DEFINE_multi`.
- help: str, the help message.
- flag_values: :class:`FlagValues`, the FlagValues instance with which the
- flag will be registered. This should almost never need to be overridden.
- required: bool, is this a required flag. This must be used as a keyword
- argument.
- **args: Dictionary with extra keyword args that are passed to the
- ``Flag.__init__``.
-
- Returns:
- a handle to defined flag.
- """
- parser = _argument_parser.ArgumentParser()
- serializer = _argument_parser.ArgumentSerializer()
- return DEFINE_multi(
- parser,
- serializer,
- name,
- default,
- help,
- flag_values,
- required=required,
- **args)
-
-
-def DEFINE_multi_integer( # pylint: disable=invalid-name,redefined-builtin
- name,
- default,
- help,
- lower_bound=None,
- upper_bound=None,
- flag_values=_flagvalues.FLAGS,
- required=False,
- **args):
- """Registers a flag whose value can be a list of arbitrary integers.
-
- Use the flag on the command line multiple times to place multiple
- integer values into the list. The 'default' may be a single integer
- (which will be converted into a single-element list) or a list of
- integers.
-
- Args:
- name: str, the flag name.
- default: Union[Iterable[int], Text, None], the default value of the flag;
- see `DEFINE_multi`.
- help: str, the help message.
- lower_bound: int, min values of the flag.
- upper_bound: int, max values of the flag.
- flag_values: :class:`FlagValues`, the FlagValues instance with which the
- flag will be registered. This should almost never need to be overridden.
- required: bool, is this a required flag. This must be used as a keyword
- argument.
- **args: Dictionary with extra keyword args that are passed to the
- ``Flag.__init__``.
-
- Returns:
- a handle to defined flag.
- """
- parser = _argument_parser.IntegerParser(lower_bound, upper_bound)
- serializer = _argument_parser.ArgumentSerializer()
- return DEFINE_multi(
- parser,
- serializer,
- name,
- default,
- help,
- flag_values,
- required=required,
- **args)
-
-
-def DEFINE_multi_float( # pylint: disable=invalid-name,redefined-builtin
- name,
- default,
- help,
- lower_bound=None,
- upper_bound=None,
- flag_values=_flagvalues.FLAGS,
- required=False,
- **args):
- """Registers a flag whose value can be a list of arbitrary floats.
-
- Use the flag on the command line multiple times to place multiple
- float values into the list. The 'default' may be a single float
- (which will be converted into a single-element list) or a list of
- floats.
-
- Args:
- name: str, the flag name.
- default: Union[Iterable[float], Text, None], the default value of the flag;
- see `DEFINE_multi`.
- help: str, the help message.
- lower_bound: float, min values of the flag.
- upper_bound: float, max values of the flag.
- flag_values: :class:`FlagValues`, the FlagValues instance with which the
- flag will be registered. This should almost never need to be overridden.
- required: bool, is this a required flag. This must be used as a keyword
- argument.
- **args: Dictionary with extra keyword args that are passed to the
- ``Flag.__init__``.
-
- Returns:
- a handle to defined flag.
- """
- parser = _argument_parser.FloatParser(lower_bound, upper_bound)
- serializer = _argument_parser.ArgumentSerializer()
- return DEFINE_multi(
- parser,
- serializer,
- name,
- default,
- help,
- flag_values,
- required=required,
- **args)
-
-
-def DEFINE_multi_enum( # pylint: disable=invalid-name,redefined-builtin
- name,
- default,
- enum_values,
- help,
- flag_values=_flagvalues.FLAGS,
- case_sensitive=True,
- required=False,
- **args):
- """Registers a flag whose value can be a list strings from enum_values.
-
- Use the flag on the command line multiple times to place multiple
- enum values into the list. The 'default' may be a single string
- (which will be converted into a single-element list) or a list of
- strings.
-
- Args:
- name: str, the flag name.
- default: Union[Iterable[Text], Text, None], the default value of the flag;
- see `DEFINE_multi`.
- enum_values: [str], a non-empty list of strings with the possible values for
- the flag.
- help: str, the help message.
- flag_values: :class:`FlagValues`, the FlagValues instance with which the
- flag will be registered. This should almost never need to be overridden.
- case_sensitive: Whether or not the enum is to be case-sensitive.
- required: bool, is this a required flag. This must be used as a keyword
- argument.
- **args: Dictionary with extra keyword args that are passed to the
- ``Flag.__init__``.
-
- Returns:
- a handle to defined flag.
- """
- parser = _argument_parser.EnumParser(enum_values, case_sensitive)
- serializer = _argument_parser.ArgumentSerializer()
- return DEFINE_multi(
- parser,
- serializer,
- name,
- default,
- '<%s>: %s' % ('|'.join(enum_values), help),
- flag_values,
- required=required,
- **args)
-
-
-def DEFINE_multi_enum_class( # pylint: disable=invalid-name,redefined-builtin
- name,
- default,
- enum_class,
- help,
- flag_values=_flagvalues.FLAGS,
- module_name=None,
- case_sensitive=False,
- required=False,
- **args):
- """Registers a flag whose value can be a list of enum members.
-
- Use the flag on the command line multiple times to place multiple
- enum values into the list.
-
- Args:
- name: str, the flag name.
- default: Union[Iterable[Enum], Iterable[Text], Enum, Text, None], the
- default value of the flag; see `DEFINE_multi`; only differences are
- documented here. If the value is a single Enum, it is treated as a
- single-item list of that Enum value. If it is an iterable, text values
- within the iterable will be converted to the equivalent Enum objects.
- enum_class: class, the Enum class with all the possible values for the flag.
- help: str, the help message.
- flag_values: :class:`FlagValues`, the FlagValues instance with which the
- flag will be registered. This should almost never need to be overridden.
- module_name: A string, the name of the Python module declaring this flag. If
- not provided, it will be computed using the stack trace of this call.
- case_sensitive: bool, whether to map strings to members of the enum_class
- without considering case.
- required: bool, is this a required flag. This must be used as a keyword
- argument.
- **args: Dictionary with extra keyword args that are passed to the
- ``Flag.__init__``.
-
- Returns:
- a handle to defined flag.
- """
- return DEFINE_flag(
- _flag.MultiEnumClassFlag(
- name,
- default,
- help,
- enum_class,
- case_sensitive=case_sensitive,
- **args,
- ),
- flag_values,
- module_name,
- required=required,
- )
-
-
-def DEFINE_alias( # pylint: disable=invalid-name
- name,
- original_name,
- flag_values=_flagvalues.FLAGS,
- module_name=None):
- """Defines an alias flag for an existing one.
-
- Args:
- name: str, the flag name.
- original_name: str, the original flag name.
- flag_values: :class:`FlagValues`, the FlagValues instance with which the
- flag will be registered. This should almost never need to be overridden.
- module_name: A string, the name of the module that defines this flag.
-
- Returns:
- a handle to defined flag.
-
- Raises:
- flags.FlagError:
- UnrecognizedFlagError: if the referenced flag doesn't exist.
- DuplicateFlagError: if the alias name has been used by some existing flag.
- """
- if original_name not in flag_values:
- raise _exceptions.UnrecognizedFlagError(original_name)
- flag = flag_values[original_name]
-
- class _FlagAlias(_flag.Flag):
- """Overrides Flag class so alias value is copy of original flag value."""
-
- def parse(self, argument):
- flag.parse(argument)
- self.present += 1
-
- def _parse_from_default(self, value):
- # The value was already parsed by the aliased flag, so there is no
- # need to call the parser on it a second time.
- # Additionally, because of how MultiFlag parses and merges values,
- # it isn't possible to delegate to the aliased flag and still get
- # the correct values.
- return value
-
- @property
- def value(self):
- return flag.value
-
- @value.setter
- def value(self, value):
- flag.value = value
-
- help_msg = 'Alias for --%s.' % flag.name
- # If alias_name has been used, flags.DuplicatedFlag will be raised.
- return DEFINE_flag(
- _FlagAlias(
- flag.parser,
- flag.serializer,
- name,
- flag.default,
- help_msg,
- boolean=flag.boolean), flag_values, module_name)
diff --git a/spaces/arxify/RVC-beta-v2-0618/runtime/Lib/site-packages/altair/vega/v5/data.py b/spaces/arxify/RVC-beta-v2-0618/runtime/Lib/site-packages/altair/vega/v5/data.py
deleted file mode 100644
index 78273363d19679819237caa91d8e337ad2d3c936..0000000000000000000000000000000000000000
--- a/spaces/arxify/RVC-beta-v2-0618/runtime/Lib/site-packages/altair/vega/v5/data.py
+++ /dev/null
@@ -1,29 +0,0 @@
-from ..data import (
- MaxRowsError,
- curry,
- default_data_transformer,
- limit_rows,
- pipe,
- sample,
- to_csv,
- to_json,
- to_values,
-)
-
-
-# ==============================================================================
-# Vega 5 data transformers
-# ==============================================================================
-
-
-__all__ = (
- "MaxRowsError",
- "curry",
- "default_data_transformer",
- "limit_rows",
- "pipe",
- "sample",
- "to_csv",
- "to_json",
- "to_values",
-)
diff --git a/spaces/ashercn97/AsherTesting/docs/ExLlama.md b/spaces/ashercn97/AsherTesting/docs/ExLlama.md
deleted file mode 100644
index db0ebe63c90cf155e8b550e73a542d560ccb0b54..0000000000000000000000000000000000000000
--- a/spaces/ashercn97/AsherTesting/docs/ExLlama.md
+++ /dev/null
@@ -1,22 +0,0 @@
-# ExLlama
-
-### About
-
-ExLlama is an extremely optimized GPTQ backend for LLaMA models. It features much lower VRAM usage and much higher speeds due to not relying on unoptimized transformers code.
-
-### Usage
-
-Configure text-generation-webui to use exllama via the UI or command line:
- - In the "Model" tab, set "Loader" to "exllama"
- - Specify `--loader exllama` on the command line
-
-### Manual setup
-
-No additional installation steps are necessary since an exllama package is already included in the requirements.txt. If this package fails to install for some reason, you can install it manually by cloning the original repository into your `repositories/` folder:
-
-```
-mkdir repositories
-cd repositories
-git clone https://github.com/turboderp/exllama
-```
-
diff --git a/spaces/at2507/SM_NLP_RecoSys/Data/Mentor_interviews/Cairo Liu.html b/spaces/at2507/SM_NLP_RecoSys/Data/Mentor_interviews/Cairo Liu.html
deleted file mode 100644
index f399346315c1420d670dec6b125e078e1f1c4fb8..0000000000000000000000000000000000000000
--- a/spaces/at2507/SM_NLP_RecoSys/Data/Mentor_interviews/Cairo Liu.html
+++ /dev/null
@@ -1,132 +0,0 @@
-
-
-
- Cairo Liu
-
-
-
-
-
- Cairo Liu
-
-
- Mentee to Mentor
1- What motivates you to become a mentor with SharpestMinds?
- Like to help people and recently helped two friends in Job hunting which was helpful to them. Can add value to people by helping them, Have gone through the Job hunting process twice and have the knowledge on building a resume, networking and tackling interviews. Teaching people is also a great way to learn and stay updated on trends in the D.S. career.
2- What has your data science career journey been like?
- Working with Data comes naturally. Did PhD in University of Toronto. Used data to derive consumer behaviors. Have coding experience of many years.
- Got into industry from Academia, and thought being a professor was the best option.
- Did intership related to D.S.
- Worked at a startup - Dealmaker as the first hire of Data team and built several tools from beginning to deployment. But entire data team was later laid off.
- Joined data consultancy company - tiger analytics. Worked closely with other Data scientists. The depth of modeling is much greater and get to work on solving difficult problems of various clients.
- Currently in a managerial role in charge of two other people and assign them tasks and oversee their work.
3- How was was your experience as a Mentee with SM?
- Looked for a mentor after graduation, mentor was knowledgable and gave the right guidance on technical learning and making a shift to the industry from academia. Have been communicating to other fellow mentees as well from time to time.
4- According to you what is the biggest challenge someone faces when breaking into a Data science role? How can you help them with that.
- The biggest challenge is How to get the first interview, and not to be shy of reaching out to people for job prospects.
Will help mentees by keeping them accountable for their job applications and check effectiveness. Help them improve their strategy, resume and help with interview preparation.
5- Would you be ok sharing a day in a life story which can be shared with the community?
- Yes
6- Do you have any questions for me regarding SM?
- Is there a limitation of % income sharing from mentees? typical range?
- What if the mentee is in the US, will I get paid in CAD or USD?
-
-
-
-
-
-
\ No newline at end of file
diff --git a/spaces/awacke1/HFSpaceStreamlitHeatmap/README.md b/spaces/awacke1/HFSpaceStreamlitHeatmap/README.md
deleted file mode 100644
index a292a35e1c02efcb52a6c787e38ca413ab8648f1..0000000000000000000000000000000000000000
--- a/spaces/awacke1/HFSpaceStreamlitHeatmap/README.md
+++ /dev/null
@@ -1,12 +0,0 @@
----
-title: HFSpaceStreamlitHeatmap
-emoji: 😻Heat
-colorFrom: pink
-colorTo: purple
-sdk: streamlit
-sdk_version: 1.10.0
-app_file: app.py
-pinned: false
----
-
-Check out the configuration reference at https://huggingface.co/docs/hub/spaces-config-reference
diff --git a/spaces/awacke1/Memory-Shared/app.py b/spaces/awacke1/Memory-Shared/app.py
deleted file mode 100644
index 46bbfde01c415486f596e25f4ef2adcf65e7e32e..0000000000000000000000000000000000000000
--- a/spaces/awacke1/Memory-Shared/app.py
+++ /dev/null
@@ -1,86 +0,0 @@
-import os
-import csv
-import gradio as gr
-from gradio import inputs, outputs
-import huggingface_hub
-from huggingface_hub import Repository, hf_hub_download, upload_file
-from datetime import datetime
-
-DATASET_REPO_URL = "https://huggingface.co/datasets/awacke1/data.csv"
-DATASET_REPO_ID = "awacke1/data.csv"
-DATA_FILENAME = "data.csv"
-DATA_FILE = os.path.join("data", DATA_FILENAME)
-HF_TOKEN = os.environ.get("HF_TOKEN")
-
-# overriding/appending to the gradio template
-SCRIPT = """
-
-"""
-with open(os.path.join(gr.networking.STATIC_TEMPLATE_LIB, "frontend", "index.html"), "a") as f:
- f.write(SCRIPT)
-
-try:
- hf_hub_download(
- repo_id=DATASET_REPO_ID,
- filename=DATA_FILENAME,
- cache_dir=DATA_DIRNAME,
- force_filename=DATA_FILENAME
- )
-except:
- print("file not found")
-
-repo = Repository(
- local_dir="data", clone_from=DATASET_REPO_URL, use_auth_token=HF_TOKEN
-)
-
-def generate_html() -> str:
- with open(DATA_FILE) as csvfile:
- reader = csv.DictReader(csvfile)
- rows = []
- for row in reader:
- rows.append(row)
- rows.reverse()
- if len(rows) == 0:
- return "no messages yet"
- else:
- html = ""
- for row in rows:
- html += ""
- html += f"{row['name']}"
- html += f" "
- html += ""
- html += ""
- return html
-
-def store_message(name: str, message: str):
- if name and message:
- with open(DATA_FILE, "a") as csvfile:
- writer = csv.DictWriter(csvfile, fieldnames=["name", "message", "time"])
- writer.writerow(
- {"name": name, "message": message, "time": str(datetime.now())}
- )
- commit_url = repo.push_to_hub()
- return generate_html()
-
-iface = gr.Interface(
- store_message,
- [
- inputs.Textbox(placeholder="Your name"),
- inputs.Textbox(placeholder="Your message", lines=2),
- ],
- "html",
- css="""
- .message {background-color:cornflowerblue;color:white; padding:4px;margin:4px;border-radius:4px; }
- """,
- title="Reading/writing to a HuggingFace dataset repo from Spaces",
- description=f"This is a demo of how to do simple *shared data persistence* in a Gradio Space, backed by a dataset repo.",
- article=f"The dataset repo is [{DATASET_REPO_URL}]({DATASET_REPO_URL})",
-)
-
-iface.launch()
\ No newline at end of file
diff --git a/spaces/azusarang/so-vits-svc-models-ba_P/modules/losses.py b/spaces/azusarang/so-vits-svc-models-ba_P/modules/losses.py
deleted file mode 100644
index cd21799eccde350c3aac0bdd661baf96ed220147..0000000000000000000000000000000000000000
--- a/spaces/azusarang/so-vits-svc-models-ba_P/modules/losses.py
+++ /dev/null
@@ -1,61 +0,0 @@
-import torch
-from torch.nn import functional as F
-
-import modules.commons as commons
-
-
-def feature_loss(fmap_r, fmap_g):
- loss = 0
- for dr, dg in zip(fmap_r, fmap_g):
- for rl, gl in zip(dr, dg):
- rl = rl.float().detach()
- gl = gl.float()
- loss += torch.mean(torch.abs(rl - gl))
-
- return loss * 2
-
-
-def discriminator_loss(disc_real_outputs, disc_generated_outputs):
- loss = 0
- r_losses = []
- g_losses = []
- for dr, dg in zip(disc_real_outputs, disc_generated_outputs):
- dr = dr.float()
- dg = dg.float()
- r_loss = torch.mean((1-dr)**2)
- g_loss = torch.mean(dg**2)
- loss += (r_loss + g_loss)
- r_losses.append(r_loss.item())
- g_losses.append(g_loss.item())
-
- return loss, r_losses, g_losses
-
-
-def generator_loss(disc_outputs):
- loss = 0
- gen_losses = []
- for dg in disc_outputs:
- dg = dg.float()
- l = torch.mean((1-dg)**2)
- gen_losses.append(l)
- loss += l
-
- return loss, gen_losses
-
-
-def kl_loss(z_p, logs_q, m_p, logs_p, z_mask):
- """
- z_p, logs_q: [b, h, t_t]
- m_p, logs_p: [b, h, t_t]
- """
- z_p = z_p.float()
- logs_q = logs_q.float()
- m_p = m_p.float()
- logs_p = logs_p.float()
- z_mask = z_mask.float()
- #print(logs_p)
- kl = logs_p - logs_q - 0.5
- kl += 0.5 * ((z_p - m_p)**2) * torch.exp(-2. * logs_p)
- kl = torch.sum(kl * z_mask)
- l = kl / torch.sum(z_mask)
- return l
diff --git a/spaces/banana-projects/convai/Dockerfile b/spaces/banana-projects/convai/Dockerfile
deleted file mode 100644
index 17c322e892bcfc6787db650acd9f91caf7d26ac8..0000000000000000000000000000000000000000
--- a/spaces/banana-projects/convai/Dockerfile
+++ /dev/null
@@ -1,15 +0,0 @@
-FROM node:20
-
-WORKDIR /code
-
-RUN npm i -g typescript@4.0.3 grunt-cli@1.2.0
-
-COPY . .
-
-RUN cd front && npm i && tsc && cd ..
-
-RUN cd grunt && npm i && grunt && cd ..
-
-RUN cd server && npm i && tsc && cd ..
-
-CMD ["node", "server/dist/server.js"]
diff --git a/spaces/banana-projects/web3d/node_modules/three/src/renderers/shaders/ShaderChunk/metalnessmap_pars_fragment.glsl.js b/spaces/banana-projects/web3d/node_modules/three/src/renderers/shaders/ShaderChunk/metalnessmap_pars_fragment.glsl.js
deleted file mode 100644
index ac89c0b371d29eed724e38ee5a6423c493aaa71b..0000000000000000000000000000000000000000
--- a/spaces/banana-projects/web3d/node_modules/three/src/renderers/shaders/ShaderChunk/metalnessmap_pars_fragment.glsl.js
+++ /dev/null
@@ -1,7 +0,0 @@
-export default /* glsl */`
-#ifdef USE_METALNESSMAP
-
- uniform sampler2D metalnessMap;
-
-#endif
-`;
diff --git a/spaces/bigjoker/stable-diffusion-webui/modules/scripts_auto_postprocessing.py b/spaces/bigjoker/stable-diffusion-webui/modules/scripts_auto_postprocessing.py
deleted file mode 100644
index 16ec8b613b134b0a9a4054f06d5979ec1822c422..0000000000000000000000000000000000000000
--- a/spaces/bigjoker/stable-diffusion-webui/modules/scripts_auto_postprocessing.py
+++ /dev/null
@@ -1,42 +0,0 @@
-from modules import scripts, scripts_postprocessing, shared
-
-
-class ScriptPostprocessingForMainUI(scripts.Script):
- def __init__(self, script_postproc):
- self.script: scripts_postprocessing.ScriptPostprocessing = script_postproc
- self.postprocessing_controls = None
-
- def title(self):
- return self.script.name
-
- def show(self, is_img2img):
- return scripts.AlwaysVisible
-
- def ui(self, is_img2img):
- self.postprocessing_controls = self.script.ui()
- return self.postprocessing_controls.values()
-
- def postprocess_image(self, p, script_pp, *args):
- args_dict = {k: v for k, v in zip(self.postprocessing_controls, args)}
-
- pp = scripts_postprocessing.PostprocessedImage(script_pp.image)
- pp.info = {}
- self.script.process(pp, **args_dict)
- p.extra_generation_params.update(pp.info)
- script_pp.image = pp.image
-
-
-def create_auto_preprocessing_script_data():
- from modules import scripts
-
- res = []
-
- for name in shared.opts.postprocessing_enable_in_main_ui:
- script = next(iter([x for x in scripts.postprocessing_scripts_data if x.script_class.name == name]), None)
- if script is None:
- continue
-
- constructor = lambda s=script: ScriptPostprocessingForMainUI(s.script_class())
- res.append(scripts.ScriptClassData(script_class=constructor, path=script.path, basedir=script.basedir, module=script.module))
-
- return res
diff --git a/spaces/bigjoker/stable-diffusion-webui/modules/shared_items.py b/spaces/bigjoker/stable-diffusion-webui/modules/shared_items.py
deleted file mode 100644
index 8dd832ed9b1e610b2ab1b4d5f911c58d63c00f80..0000000000000000000000000000000000000000
--- a/spaces/bigjoker/stable-diffusion-webui/modules/shared_items.py
+++ /dev/null
@@ -1,23 +0,0 @@
-
-
-def realesrgan_models_names():
- import modules.realesrgan_model
- return [x.name for x in modules.realesrgan_model.get_realesrgan_models(None)]
-
-
-def postprocessing_scripts():
- import modules.scripts
-
- return modules.scripts.scripts_postproc.scripts
-
-
-def sd_vae_items():
- import modules.sd_vae
-
- return ["Automatic", "None"] + list(modules.sd_vae.vae_dict)
-
-
-def refresh_vae_list():
- import modules.sd_vae
-
- modules.sd_vae.refresh_vae_list()
diff --git a/spaces/bioriAsaeru/text-to-voice/ASTRO25 Portable CPS Install R19.01.00.zip.md b/spaces/bioriAsaeru/text-to-voice/ASTRO25 Portable CPS Install R19.01.00.zip.md
deleted file mode 100644
index f408d9f45d83f20f1181c1f5e207d0c0019aeb74..0000000000000000000000000000000000000000
--- a/spaces/bioriAsaeru/text-to-voice/ASTRO25 Portable CPS Install R19.01.00.zip.md
+++ /dev/null
@@ -1,30 +0,0 @@
-ASTRO25 Portable CPS Install R19.01.00.zip
DOWNLOAD >>> https://urloso.com/2uyRC3
-
-Critical house prices point to bubble
-
-Over the weekend, Australia's leading real estate website Domain has released a report showing that the biggest 15 cities in the country have seen median house prices climb by 40 per cent or more in the past three years.
-
-All but one of the 15 markets - Sunshine Coast - saw prices rise by at least a third in the last three years, with the biggest increases in Sydney, Melbourne and the Gold Coast.
-
-Over the weekend, Domain, a real estate information company with over 10 million registered users, released a report showing that the biggest 15 cities in the country have seen median house prices climb by 40 per cent or more in the past three years.
-
-The report found the biggest decrease in median house prices were in Hobart and Darwin, with prices falling 25 per cent and 18 per cent respectively.
-
-Domain head of analytics Ben Uffindell said while these numbers are not necessarily representative of the full real estate market, they indicate that Sydney and Melbourne are the most overvalued housing markets in the country.
-
-"We believe the price jump in Sydney and Melbourne is a sign of a new, national housing bubble emerging," he said.
-
-Uffindell said the average median price for a Sydney or Melbourne home is now $1.4 million, compared to a median of $938,000 in Sydney and $1.1 million in Melbourne in 2007.
-
-He said in both cities, the number of homes sold during a typical month was higher than it was at the peak of the last bubble - in the early 2000s.
-
-But Uffindell said this was not the case in the other markets. "The average Sydney or Melbourne home has increased in price by 30 per cent, compared to a 20 per cent increase for our other market leaders," he said.
-
-"There are some market indicators, like the number of newly listed homes, that reflect the price growth, but they tell only part of the story."
-
-He said that while the rise in prices is not as dramatic as it was in the early 2000s, it still indicates that Australians are seeing a relatively high number of gains.
-
-The report also pointed to high levels of investor activity, which is in contrast to the GFC and the 2001 housing bubble. 4fefd39f24
-
-
-
diff --git a/spaces/bioriAsaeru/text-to-voice/DOOM V6.66 Update 9 CPY FitGirl HOT.md b/spaces/bioriAsaeru/text-to-voice/DOOM V6.66 Update 9 CPY FitGirl HOT.md
deleted file mode 100644
index 47fcbc78b6ace780f8ed54b052afb0405b843f6e..0000000000000000000000000000000000000000
--- a/spaces/bioriAsaeru/text-to-voice/DOOM V6.66 Update 9 CPY FitGirl HOT.md
+++ /dev/null
@@ -1,186 +0,0 @@
-
-DOOM v6.66 Update 9 CPY, FitGirl: The Ultimate Guide
-
-If you are looking for a way to download and install DOOM v6.66 Update 9 CPY, FitGirl, you have come to the right place. In this article, we will show you how to get this amazing game on your PC with a few simple steps. You will also learn about the features and benefits of this repack, as well as some tips and tricks to enjoy it to the fullest.
-DOOM v6.66 Update 9 CPY, FitGirl
Download Zip 🆓 https://urloso.com/2uyP31
-
-What is DOOM v6.66 Update 9 CPY, FitGirl?
-
-DOOM v6.66 Update 9 CPY, FitGirl is a repack of the latest version of DOOM 2016, the reboot of the classic first-person shooter game developed by id Software and published by Bethesda Softworks. This repack is based on the Steam release of the game from March 29, 2018, which includes the latest update (v6.66) and crack by CODEX.
-
-The repack has several advantages over the original release, such as:
-
-
-- Smaller size: The original size of the game is 68.9 GB, while the repack size is from 30.4 GB to 41.5 GB, depending on the selected components.
-- Selective download: You can skip downloading and installing multiplayer files, SnapMap editor files, credits videos and language files you don't need. Note that multiplayer and SnapMap modes are not playable without legit online access.
-- Lossless quality: All files are identical to originals after installation. Nothing is ripped or re-encoded.
-- Faster installation: Installation takes from 35 minutes to 1 hour for singleplayer mode only, depending on your CPU and RAM. Installing multiplayer and SnapMap files takes another 15 to 25 minutes.
-- Integrity check: After-install integrity check ensures that everything is installed properly.
-- Language support: The game supports 10 languages: English, French, Italian, German, Spanish, Japanese, Polish, Portuguese-Brazilian, Russian and Traditional Chinese. You can change the language using "Language Selector.exe" in game root.
-
-
-How to Download and Install DOOM v6.66 Update 9 CPY, FitGirl?
-
-To download and install DOOM v6.66 Update 9 CPY, FitGirl, you need to follow these steps:
-
-
-
-- Download the repack from one of the mirrors provided by FitGirl Repacks Site or other trusted sources. You can use torrent or direct links, depending on your preference.
-- Extract the repack using WinRAR or 7-Zip. You will need at least 2.5 GB of free RAM (including virtual) for this process.
-- Run "setup.exe" and select the components you want to install. Make sure you have enough disk space for installation (up to 69 GB).
-- Wait for the installation to finish. It may take some time depending on your system specs.
-- Run the game from desktop shortcut or "DOOMx64.exe" in game root.
-- Enjoy!
-
-
-What are the Features and Benefits of DOOM v6.66 Update 9 CPY, FitGirl?
-
-DOOM v6.66 Update 9 CPY, FitGirl offers you a chance to experience one of the best shooter games ever made with improved performance and stability. Here are some of the features and benefits of this game:
-
-
-- Awesome gameplay: DOOM is a fast-paced, brutal and challenging shooter that will keep you on the edge of your seat. You will face relentless demons, use impossibly destructive guns, and move with fluidity and speed through the depths of Hell in the single-player campaign or compete against your friends in various multiplayer modes.
-- Stunning graphics: DOOM uses id Tech 6 engine that delivers incredible visuals and performance on PC. The game supports up to 4K resolution and uncapped framerate for smooth and immersive gameplay.
-- Creative content: DOOM allows you to expand your gameplay experience using DOOM SnapMap game editor that lets you easily create, play and share your own content with the world. You can make new maps, modes or even full games with SnapMap.
-- Update 6.66: This update brings several improvements and fixes to the game, such as:
-
-- New progression system: The update replaces the previous random unlock system with a new one that allows you to unlock specific items by completing challenges and leveling up.
-- New rune system: The update replaces the previous Hack Module system with a new one that allows you to equip runes as persistent player abilities earned and included in a player loadout.
-- New multiplayer features: The update adds new features such as bots support for all modes except Sacrifice; new HUD options; new kill card; new weapon balance; new echelon levels; new medals; new announcer voice; etc.
-- Bug fixes: The update fixes various issues related to textures, models, multiplayer modes, SnapMap editor, etc.
-
-
-
-Tips and Tricks for DOOM v6.66 Update 9 CPY, FitGirl
-
-To make the most out of DOOM v6.66 Update 9 CPY, FitGirl, here are some tips and tricks that may help you:
-
-
-- Adjust your settings: Before playing the game, make sure you adjust your settings according to your system specs and preferences. You can tweak graphics options such as resolution, anti-aliasing, texture quality, shadows quality, etc.; audio options such as volume levels, subtitles language; gameplay options such as difficulty level; etc.
-- Use glory kills: Glory kills are special melee executions that allow you to finish off weakened enemies in style and get health drops from them. To perform a glory kill, approach a staggered enemy (indicated by a blue or orange highlight) and press F or mouse click when prompted.
-- Use chainsaw: Chainsaw is a powerful weapon that can instantly kill most enemies (except bosses) and get ammo drops from them. To use chainsaw, equip it with G key or mouse wheel and press mouse click when close to an enemy. Note that chainsaw requires fuel that can be found throughout levels or dropped by some enemies.
-- Use grenades: Grenades are useful tools that can deal damage to multiple enemies at once or stun them temporarily. To use grenades, press Q key or mouse wheel click to throw them or hold Q key or mouse wheel click to cook them before throwing them.
-- Use weapon mods: Weapon mods are attachments that can enhance your weapons with different abilities such as zooming in, charging up shots, firing multiple projectiles etc. To use weapon mods,
-equip them with R key or mouse wheel when holding a weapon or press F1 key or mouse wheel click when selecting a weapon from weapon wheel; then press mouse right click to activate them when aiming.
-
-- Find secrets: Secrets are hidden items or areas that can reward you with collectibles such as action figures,
-classic maps,
-data logs,
-field drones,
-rune trials,
-elite guards,
-argent cells etc.
-To find secrets,
-look for clues such as cracks,
-vents,
-levers,
-switches etc.
-or use automap stations
-or praetor suit upgrades
-to reveal them on your map.
-
-
-
-Conclusion
-
-DOOM v6.66 Update 9 CPY, FitGirl is a great way to enjoy one of the best shooter games ever made with improved performance and stability.
-If you follow our guide,
-you will be able to download
-and install this repack easily
-and play this game with no problems.
-We hope you have fun
-and share your feedback
-with us in the comments below.
-How to Play DOOM v6.66 Update 9 CPY, FitGirl?
-
-Once you have downloaded and installed DOOM v6.66 Update 9 CPY, FitGirl, you are ready to play this awesome game. You can choose from three modes of gameplay: single-player, multiplayer and SnapMap.
-
-In single-player mode, you will take on the role of a lone DOOM Marine who wakes up on a UAC facility on Mars that has been overrun by demons. You will have to fight your way through hordes of enemies using a variety of weapons and gadgets, while exploring the secrets and lore of the DOOM universe.
-
-In multiplayer mode, you will join other players online in various modes such as Team Deathmatch, Domination, Warpath, Freeze Tag, Clan Arena and more. You will be able to customize your character with different armor sets, colors, patterns and taunts. You will also be able to unlock and use different weapons and power-ups such as the BFG, Gauss Cannon, Quad Damage and Demon Runes.
-
-In SnapMap mode, you will be able to create your own maps and modes using a simple and intuitive editor that lets you drag and drop elements, add logic and scripts, and test your creations on the fly. You will also be able to play and share your content with other players around the world.
-
-Why Should You Download DOOM v6.66 Update 9 CPY, FitGirl?
-
-If you are still wondering why you should download DOOM v6.66 Update 9 CPY, FitGirl, here are some reasons why you should not miss this opportunity:
-
-
-- It is free: You can download this repack without paying anything. You just need a torrent client or a direct link to get it.
-- It is safe: You can download this repack without worrying about viruses or malware. The repack is verified by FitGirl Repacks Site and other trusted sources.
-- It is easy: You can download and install this repack without any hassle. The repack has a simple setup that guides you through the process.
-- It is fun: You can download and play this game without any limitations. The game has a lot of content and features that will keep you entertained for hours.
-
-
-Conclusion
-
-DOOM v6.66 Update 9 CPY, FitGirl is a great way to enjoy one of the best shooter games ever made with improved performance and stability.
-If you follow our guide,
-you will be able to download
-and install this repack easily
-and play this game with no problems.
-We hope you have fun
-and share your feedback
-with us in the comments below.
-How to Fix Common Issues with DOOM v6.66 Update 9 CPY, FitGirl?
-
-While DOOM v6.66 Update 9 CPY, FitGirl is a stable and reliable repack, you may encounter some issues while playing the game. Here are some common problems and their solutions:
-
-
-- Game crashes or freezes: If the game crashes or freezes during gameplay, try to lower your graphics settings, update your drivers, disable antivirus or firewall, run the game as administrator, or verify the integrity of game files.
-- Game won't start or launch: If the game won't start or launch at all, make sure you have installed all the required components such as DirectX, Visual C++, etc. You can find them in "_Redist" folder of the repack. Also, check if your antivirus or firewall is blocking the game or the crack.
-- Game shows black screen or no sound: If the game shows black screen or no sound after launching, try to change your screen resolution, switch to windowed mode, disable fullscreen optimizations, or change your audio output device.
-- Game has low FPS or stuttering: If the game has low FPS or stuttering during gameplay, try to disable VSync, lower your graphics settings, close background programs, or use a FPS limiter.
-
-
-What are the System Requirements for DOOM v6.66 Update 9 CPY, FitGirl?
-
-To play DOOM v6.66 Update 9 CPY, FitGirl, you need to have a PC that meets the following minimum or recommended system requirements:
-
-
-
-Minimum
-Recommended
-
-
-OS: Windows 7/8.1/10 (64-bit versions)
-OS: Windows 7/8.1/10 (64-bit versions)
-
-
-CPU: Intel Core i5-2400/AMD FX-8320 or better
-CPU: Intel Core i7-3770/AMD FX-8350 or better
-
-
-RAM: 8 GB
-RAM: 8 GB
-
-
-GPU: NVIDIA GTX 670 2GB/AMD Radeon HD 7870 2GB or better
-GPU: NVIDIA GTX 970 4GB/AMD Radeon R9 290 4GB or better
-
-
-HDD: up to 69 GB
-HDD: up to 69 GB
-
-
-DirectX: Version 11
-DirectX: Version 11
-
-
-Note: Requires Steam activation and broadband internet connection for Multiplayer and SnapMap.
-Note: Requires Steam activation and broadband internet connection for Multiplayer and SnapMap.
-
-
-
-Conclusion
-
-DOOM v6.66 Update 9 CPY, FitGirl is a great way to enjoy one of the best shooter games ever made with improved performance and stability.
-If you follow our guide,
-you will be able to download
-and install this repack easily
-and play this game with no problems.
-We hope you have fun
-and share your feedback
-with us in the comments below.
-In conclusion, DOOM v6.66 Update 9 CPY, FitGirl is a repack of the latest version of DOOM 2016, the reboot of the classic first-person shooter game. This repack has several advantages over the original release, such as smaller size, selective download, lossless quality, faster installation and language support. The game itself is a fast-paced, brutal and challenging shooter that will keep you entertained for hours with its awesome gameplay, stunning graphics and creative content. You can also download and install this repack easily by following our guide and fix any common issues with our solutions. If you are a fan of DOOM or shooter games in general, you should not miss this opportunity to download and play this game for free.
3cee63e6c2
-
-
\ No newline at end of file
diff --git a/spaces/bioriAsaeru/text-to-voice/Elfen Lied Lilium Full Version Flac Playerl The Story Behind the Creation and Performance of the Song.md b/spaces/bioriAsaeru/text-to-voice/Elfen Lied Lilium Full Version Flac Playerl The Story Behind the Creation and Performance of the Song.md
deleted file mode 100644
index 67e9a29b4760ecc6a6eb996299d3a4612cb5ecbc..0000000000000000000000000000000000000000
--- a/spaces/bioriAsaeru/text-to-voice/Elfen Lied Lilium Full Version Flac Playerl The Story Behind the Creation and Performance of the Song.md
+++ /dev/null
@@ -1,6 +0,0 @@
-Elfen Lied Lilium Full Version Flac Playerl
Download File 🗹 https://urloso.com/2uyPZE
-
- aaccfb2cb3
-
-
-
diff --git a/spaces/brainblow/AudioCreator_Music-Audio_Generation/docs/ENCODEC.md b/spaces/brainblow/AudioCreator_Music-Audio_Generation/docs/ENCODEC.md
deleted file mode 100644
index efc2bcc7ec50190b907c887b920b70fd799c6953..0000000000000000000000000000000000000000
--- a/spaces/brainblow/AudioCreator_Music-Audio_Generation/docs/ENCODEC.md
+++ /dev/null
@@ -1,179 +0,0 @@
-# EnCodec: High Fidelity Neural Audio Compression
-
-AudioCraft provides the training code for EnCodec, a state-of-the-art deep learning
-based audio codec supporting both mono stereo audio, presented in the
-[High Fidelity Neural Audio Compression][arxiv] paper.
-Check out our [sample page][encodec_samples].
-
-## Original EnCodec models
-
-The EnCodec models presented in High Fidelity Neural Audio Compression can be accessed
-and used with the [EnCodec repository](https://github.com/facebookresearch/encodec).
-
-**Note**: We do not guarantee compatibility between the AudioCraft and EnCodec codebases
-and released checkpoints at this stage.
-
-
-## Installation
-
-Please follow the AudioCraft installation instructions from the [README](../README.md).
-
-
-## Training
-
-The [CompressionSolver](../audiocraft/solvers/compression.py) implements the audio reconstruction
-task to train an EnCodec model. Specifically, it trains an encoder-decoder with a quantization
-bottleneck - a SEANet encoder-decoder with Residual Vector Quantization bottleneck for EnCodec -
-using a combination of objective and perceptual losses in the forms of discriminators.
-
-The default configuration matches a causal EnCodec training with at a single bandwidth.
-
-### Example configuration and grids
-
-We provide sample configuration and grids for training EnCodec models.
-
-The compression configuration are defined in
-[config/solver/compression](../config/solver/compression).
-
-The example grids are available at
-[audiocraft/grids/compression](../audiocraft/grids/compression).
-
-```shell
-# base causal encodec on monophonic audio sampled at 24 khz
-dora grid compression.encodec_base_24khz
-# encodec model used for MusicGen on monophonic audio sampled at 32 khz
-dora grid compression.encodec_musicgen_32khz
-```
-
-### Training and valid stages
-
-The model is trained using a combination of objective and perceptual losses.
-More specifically, EnCodec is trained with the MS-STFT discriminator along with
-objective losses through the use of a loss balancer to effectively weight
-the different losses, in an intuitive manner.
-
-### Evaluation stage
-
-Evaluations metrics for audio generation:
-* SI-SNR: Scale-Invariant Signal-to-Noise Ratio.
-* ViSQOL: Virtual Speech Quality Objective Listener.
-
-Note: Path to the ViSQOL binary (compiled with bazel) needs to be provided in
-order to run the ViSQOL metric on the reference and degraded signals.
-The metric is disabled by default.
-Please refer to the [metrics documentation](../METRICS.md) to learn more.
-
-### Generation stage
-
-The generation stage consists in generating the reconstructed audio from samples
-with the current model. The number of samples generated and the batch size used are
-controlled by the `dataset.generate` configuration. The output path and audio formats
-are defined in the generate stage configuration.
-
-```shell
-# generate samples every 5 epoch
-dora run solver=compression/encodec_base_24khz generate.every=5
-# run with a different dset
-dora run solver=compression/encodec_base_24khz generate.path=
-# limit the number of samples or use a different batch size
-dora grid solver=compression/encodec_base_24khz dataset.generate.num_samples=10 dataset.generate.batch_size=4
-```
-
-### Playing with the model
-
-Once you have a model trained, it is possible to get the entire solver, or just
-the trained model with the following functions:
-
-```python
-from audiocraft.solvers import CompressionSolver
-
-# If you trained a custom model with signature SIG.
-model = CompressionSolver.model_from_checkpoint('//sig/SIG')
-# If you want to get one of the pretrained models with the `//pretrained/` prefix.
-model = CompressionSolver.model_from_checkpoint('//pretrained/facebook/encodec_32khz')
-# Or load from a custom checkpoint path
-model = CompressionSolver.model_from_checkpoint('/my_checkpoints/foo/bar/checkpoint.th')
-
-
-# If you only want to use a pretrained model, you can also directly get it
-# from the CompressionModel base model class.
-from audiocraft.models import CompressionModel
-
-# Here do not put the `//pretrained/` prefix!
-model = CompressionModel.get_pretrained('facebook/encodec_32khz')
-model = CompressionModel.get_pretrained('dac_44khz')
-
-# Finally, you can also retrieve the full Solver object, with its dataloader etc.
-from audiocraft import train
-from pathlib import Path
-import logging
-import os
-import sys
-
-# uncomment the following line if you want some detailed logs when loading a Solver.
-logging.basicConfig(stream=sys.stderr, level=logging.INFO)
-# You must always run the following function from the root directory.
-os.chdir(Path(train.__file__).parent.parent)
-
-
-# You can also get the full solver (only for your own experiments).
-# You can provide some overrides to the parameters to make things more convenient.
-solver = train.get_solver_from_sig('SIG', {'device': 'cpu', 'dataset': {'batch_size': 8}})
-solver.model
-solver.dataloaders
-```
-
-### Importing / Exporting models
-
-At the moment we do not have a definitive workflow for exporting EnCodec models, for
-instance to Hugging Face (HF). We are working on supporting automatic convertion between
-AudioCraft and Hugging Face implementations.
-
-We still have some support for fine tuning an EnCodec model coming from HF in AudioCraft,
-using for instance `continue_from=//pretrained/facebook/encodec_32k`.
-
-An AudioCraft checkpoint can be exported in a more compact format (excluding the optimizer etc.)
-using `audiocraft.utils.export.export_encodec`. For instance, you could run
-
-```python
-from audiocraft.utils import export
-from audiocraft import train
-xp = train.main.get_xp_from_sig('SIG')
-export.export_encodec(
- xp.folder / 'checkpoint.th',
- '/checkpoints/my_audio_lm/compression_state_dict.bin')
-
-
-from audiocraft.models import CompressionModel
-model = CompressionModel.get_pretrained('/checkpoints/my_audio_lm/compression_state_dict.bin')
-
-from audiocraft.solvers import CompressionSolver
-# The two are strictly equivalent, but this function supports also loading from non already exported models.
-model = CompressionSolver.model_from_checkpoint('//pretrained//checkpoints/my_audio_lm/compression_state_dict.bin')
-```
-
-We will see then how to use this model as a tokenizer for MusicGen/Audio gen in the
-[MusicGen documentation](./MUSICGEN.md).
-
-### Learn more
-
-Learn more about AudioCraft training pipelines in the [dedicated section](./TRAINING.md).
-
-
-## Citation
-```
-@article{defossez2022highfi,
- title={High Fidelity Neural Audio Compression},
- author={Défossez, Alexandre and Copet, Jade and Synnaeve, Gabriel and Adi, Yossi},
- journal={arXiv preprint arXiv:2210.13438},
- year={2022}
-}
-```
-
-
-## License
-
-See license information in the [README](../README.md).
-
-[arxiv]: https://arxiv.org/abs/2210.13438
-[encodec_samples]: https://ai.honu.io/papers/encodec/samples.html
diff --git a/spaces/brjathu/HMR2.0/vendor/detectron2/detectron2/solver/__init__.py b/spaces/brjathu/HMR2.0/vendor/detectron2/detectron2/solver/__init__.py
deleted file mode 100644
index 7e36c64f60f38f41d01dd2c9fb30364489a03841..0000000000000000000000000000000000000000
--- a/spaces/brjathu/HMR2.0/vendor/detectron2/detectron2/solver/__init__.py
+++ /dev/null
@@ -1,11 +0,0 @@
-# Copyright (c) Facebook, Inc. and its affiliates.
-from .build import build_lr_scheduler, build_optimizer, get_default_optimizer_params
-from .lr_scheduler import (
- LRMultiplier,
- LRScheduler,
- WarmupCosineLR,
- WarmupMultiStepLR,
- WarmupParamScheduler,
-)
-
-__all__ = [k for k in globals().keys() if not k.startswith("_")]
diff --git a/spaces/cakiki/facets-dive/Dockerfile b/spaces/cakiki/facets-dive/Dockerfile
deleted file mode 100644
index 5f1f4bb9feb52223d23b556d2bdfc046ea2b2b64..0000000000000000000000000000000000000000
--- a/spaces/cakiki/facets-dive/Dockerfile
+++ /dev/null
@@ -1,3 +0,0 @@
-FROM jupyter/base-notebook:latest
-
-RUN pip install --use-feature=2020-resolver pandas facets-overview
diff --git a/spaces/camenduru-com/one-shot-talking-face/oh-no.py b/spaces/camenduru-com/one-shot-talking-face/oh-no.py
deleted file mode 100644
index e8c0f3bd8d72805b4ee69d4d0fd9133347d00f92..0000000000000000000000000000000000000000
--- a/spaces/camenduru-com/one-shot-talking-face/oh-no.py
+++ /dev/null
@@ -1,14 +0,0 @@
-import gradio as gr
-
-block = gr.Blocks()
-
-def run():
- with block:
- gr.Markdown(
- """
- oh no 😐 something wrong with the 🤗 hugging face servers 😐 hopefully, it will be fixed soon
- """)
- block.launch(server_name="0.0.0.0", server_port=7860)
-
-if __name__ == "__main__":
- run()
\ No newline at end of file
diff --git a/spaces/carlosalonso/Detection-video/carpeta_deteccion/detectron2/data/datasets/lvis_v0_5_categories.py b/spaces/carlosalonso/Detection-video/carpeta_deteccion/detectron2/data/datasets/lvis_v0_5_categories.py
deleted file mode 100644
index d3dab6198da614937b08682f4c9edf52bdf1d236..0000000000000000000000000000000000000000
--- a/spaces/carlosalonso/Detection-video/carpeta_deteccion/detectron2/data/datasets/lvis_v0_5_categories.py
+++ /dev/null
@@ -1,13 +0,0 @@
-# Copyright (c) Facebook, Inc. and its affiliates.
-# Autogen with
-# with open("lvis_v0.5_val.json", "r") as f:
-# a = json.load(f)
-# c = a["categories"]
-# for x in c:
-# del x["image_count"]
-# del x["instance_count"]
-# LVIS_CATEGORIES = repr(c) + " # noqa"
-
-# fmt: off
-LVIS_CATEGORIES = [{'frequency': 'r', 'id': 1, 'synset': 'acorn.n.01', 'synonyms': ['acorn'], 'def': 'nut from an oak tree', 'name': 'acorn'}, {'frequency': 'c', 'id': 2, 'synset': 'aerosol.n.02', 'synonyms': ['aerosol_can', 'spray_can'], 'def': 'a dispenser that holds a substance under pressure', 'name': 'aerosol_can'}, {'frequency': 'f', 'id': 3, 'synset': 'air_conditioner.n.01', 'synonyms': ['air_conditioner'], 'def': 'a machine that keeps air cool and dry', 'name': 'air_conditioner'}, {'frequency': 'f', 'id': 4, 'synset': 'airplane.n.01', 'synonyms': ['airplane', 'aeroplane'], 'def': 'an aircraft that has a fixed wing and is powered by propellers or jets', 'name': 'airplane'}, {'frequency': 'c', 'id': 5, 'synset': 'alarm_clock.n.01', 'synonyms': ['alarm_clock'], 'def': 'a clock that wakes a sleeper at some preset time', 'name': 'alarm_clock'}, {'frequency': 'c', 'id': 6, 'synset': 'alcohol.n.01', 'synonyms': ['alcohol', 'alcoholic_beverage'], 'def': 'a liquor or brew containing alcohol as the active agent', 'name': 'alcohol'}, {'frequency': 'r', 'id': 7, 'synset': 'alligator.n.02', 'synonyms': ['alligator', 'gator'], 'def': 'amphibious reptiles related to crocodiles but with shorter broader snouts', 'name': 'alligator'}, {'frequency': 'c', 'id': 8, 'synset': 'almond.n.02', 'synonyms': ['almond'], 'def': 'oval-shaped edible seed of the almond tree', 'name': 'almond'}, {'frequency': 'c', 'id': 9, 'synset': 'ambulance.n.01', 'synonyms': ['ambulance'], 'def': 'a vehicle that takes people to and from hospitals', 'name': 'ambulance'}, {'frequency': 'r', 'id': 10, 'synset': 'amplifier.n.01', 'synonyms': ['amplifier'], 'def': 'electronic equipment that increases strength of signals', 'name': 'amplifier'}, {'frequency': 'c', 'id': 11, 'synset': 'anklet.n.03', 'synonyms': ['anklet', 'ankle_bracelet'], 'def': 'an ornament worn around the ankle', 'name': 'anklet'}, {'frequency': 'f', 'id': 12, 'synset': 'antenna.n.01', 'synonyms': ['antenna', 'aerial', 'transmitting_aerial'], 'def': 'an electrical device that sends or receives radio or television signals', 'name': 'antenna'}, {'frequency': 'f', 'id': 13, 'synset': 'apple.n.01', 'synonyms': ['apple'], 'def': 'fruit with red or yellow or green skin and sweet to tart crisp whitish flesh', 'name': 'apple'}, {'frequency': 'r', 'id': 14, 'synset': 'apple_juice.n.01', 'synonyms': ['apple_juice'], 'def': 'the juice of apples', 'name': 'apple_juice'}, {'frequency': 'r', 'id': 15, 'synset': 'applesauce.n.01', 'synonyms': ['applesauce'], 'def': 'puree of stewed apples usually sweetened and spiced', 'name': 'applesauce'}, {'frequency': 'r', 'id': 16, 'synset': 'apricot.n.02', 'synonyms': ['apricot'], 'def': 'downy yellow to rosy-colored fruit resembling a small peach', 'name': 'apricot'}, {'frequency': 'f', 'id': 17, 'synset': 'apron.n.01', 'synonyms': ['apron'], 'def': 'a garment of cloth that is tied about the waist and worn to protect clothing', 'name': 'apron'}, {'frequency': 'c', 'id': 18, 'synset': 'aquarium.n.01', 'synonyms': ['aquarium', 'fish_tank'], 'def': 'a tank/pool/bowl filled with water for keeping live fish and underwater animals', 'name': 'aquarium'}, {'frequency': 'c', 'id': 19, 'synset': 'armband.n.02', 'synonyms': ['armband'], 'def': 'a band worn around the upper arm', 'name': 'armband'}, {'frequency': 'f', 'id': 20, 'synset': 'armchair.n.01', 'synonyms': ['armchair'], 'def': 'chair with a support on each side for arms', 'name': 'armchair'}, {'frequency': 'r', 'id': 21, 'synset': 'armoire.n.01', 'synonyms': ['armoire'], 'def': 'a large wardrobe or cabinet', 'name': 'armoire'}, {'frequency': 'r', 'id': 22, 'synset': 'armor.n.01', 'synonyms': ['armor', 'armour'], 'def': 'protective covering made of metal and used in combat', 'name': 'armor'}, {'frequency': 'c', 'id': 23, 'synset': 'artichoke.n.02', 'synonyms': ['artichoke'], 'def': 'a thistlelike flower head with edible fleshy leaves and heart', 'name': 'artichoke'}, {'frequency': 'f', 'id': 24, 'synset': 'ashcan.n.01', 'synonyms': ['trash_can', 'garbage_can', 'wastebin', 'dustbin', 'trash_barrel', 'trash_bin'], 'def': 'a bin that holds rubbish until it is collected', 'name': 'trash_can'}, {'frequency': 'c', 'id': 25, 'synset': 'ashtray.n.01', 'synonyms': ['ashtray'], 'def': "a receptacle for the ash from smokers' cigars or cigarettes", 'name': 'ashtray'}, {'frequency': 'c', 'id': 26, 'synset': 'asparagus.n.02', 'synonyms': ['asparagus'], 'def': 'edible young shoots of the asparagus plant', 'name': 'asparagus'}, {'frequency': 'c', 'id': 27, 'synset': 'atomizer.n.01', 'synonyms': ['atomizer', 'atomiser', 'spray', 'sprayer', 'nebulizer', 'nebuliser'], 'def': 'a dispenser that turns a liquid (such as perfume) into a fine mist', 'name': 'atomizer'}, {'frequency': 'c', 'id': 28, 'synset': 'avocado.n.01', 'synonyms': ['avocado'], 'def': 'a pear-shaped fruit with green or blackish skin and rich yellowish pulp enclosing a single large seed', 'name': 'avocado'}, {'frequency': 'c', 'id': 29, 'synset': 'award.n.02', 'synonyms': ['award', 'accolade'], 'def': 'a tangible symbol signifying approval or distinction', 'name': 'award'}, {'frequency': 'f', 'id': 30, 'synset': 'awning.n.01', 'synonyms': ['awning'], 'def': 'a canopy made of canvas to shelter people or things from rain or sun', 'name': 'awning'}, {'frequency': 'r', 'id': 31, 'synset': 'ax.n.01', 'synonyms': ['ax', 'axe'], 'def': 'an edge tool with a heavy bladed head mounted across a handle', 'name': 'ax'}, {'frequency': 'f', 'id': 32, 'synset': 'baby_buggy.n.01', 'synonyms': ['baby_buggy', 'baby_carriage', 'perambulator', 'pram', 'stroller'], 'def': 'a small vehicle with four wheels in which a baby or child is pushed around', 'name': 'baby_buggy'}, {'frequency': 'c', 'id': 33, 'synset': 'backboard.n.01', 'synonyms': ['basketball_backboard'], 'def': 'a raised vertical board with basket attached; used to play basketball', 'name': 'basketball_backboard'}, {'frequency': 'f', 'id': 34, 'synset': 'backpack.n.01', 'synonyms': ['backpack', 'knapsack', 'packsack', 'rucksack', 'haversack'], 'def': 'a bag carried by a strap on your back or shoulder', 'name': 'backpack'}, {'frequency': 'f', 'id': 35, 'synset': 'bag.n.04', 'synonyms': ['handbag', 'purse', 'pocketbook'], 'def': 'a container used for carrying money and small personal items or accessories', 'name': 'handbag'}, {'frequency': 'f', 'id': 36, 'synset': 'bag.n.06', 'synonyms': ['suitcase', 'baggage', 'luggage'], 'def': 'cases used to carry belongings when traveling', 'name': 'suitcase'}, {'frequency': 'c', 'id': 37, 'synset': 'bagel.n.01', 'synonyms': ['bagel', 'beigel'], 'def': 'glazed yeast-raised doughnut-shaped roll with hard crust', 'name': 'bagel'}, {'frequency': 'r', 'id': 38, 'synset': 'bagpipe.n.01', 'synonyms': ['bagpipe'], 'def': 'a tubular wind instrument; the player blows air into a bag and squeezes it out', 'name': 'bagpipe'}, {'frequency': 'r', 'id': 39, 'synset': 'baguet.n.01', 'synonyms': ['baguet', 'baguette'], 'def': 'narrow French stick loaf', 'name': 'baguet'}, {'frequency': 'r', 'id': 40, 'synset': 'bait.n.02', 'synonyms': ['bait', 'lure'], 'def': 'something used to lure fish or other animals into danger so they can be trapped or killed', 'name': 'bait'}, {'frequency': 'f', 'id': 41, 'synset': 'ball.n.06', 'synonyms': ['ball'], 'def': 'a spherical object used as a plaything', 'name': 'ball'}, {'frequency': 'r', 'id': 42, 'synset': 'ballet_skirt.n.01', 'synonyms': ['ballet_skirt', 'tutu'], 'def': 'very short skirt worn by ballerinas', 'name': 'ballet_skirt'}, {'frequency': 'f', 'id': 43, 'synset': 'balloon.n.01', 'synonyms': ['balloon'], 'def': 'large tough nonrigid bag filled with gas or heated air', 'name': 'balloon'}, {'frequency': 'c', 'id': 44, 'synset': 'bamboo.n.02', 'synonyms': ['bamboo'], 'def': 'woody tropical grass having hollow woody stems', 'name': 'bamboo'}, {'frequency': 'f', 'id': 45, 'synset': 'banana.n.02', 'synonyms': ['banana'], 'def': 'elongated crescent-shaped yellow fruit with soft sweet flesh', 'name': 'banana'}, {'frequency': 'r', 'id': 46, 'synset': 'band_aid.n.01', 'synonyms': ['Band_Aid'], 'def': 'trade name for an adhesive bandage to cover small cuts or blisters', 'name': 'Band_Aid'}, {'frequency': 'c', 'id': 47, 'synset': 'bandage.n.01', 'synonyms': ['bandage'], 'def': 'a piece of soft material that covers and protects an injured part of the body', 'name': 'bandage'}, {'frequency': 'c', 'id': 48, 'synset': 'bandanna.n.01', 'synonyms': ['bandanna', 'bandana'], 'def': 'large and brightly colored handkerchief; often used as a neckerchief', 'name': 'bandanna'}, {'frequency': 'r', 'id': 49, 'synset': 'banjo.n.01', 'synonyms': ['banjo'], 'def': 'a stringed instrument of the guitar family with a long neck and circular body', 'name': 'banjo'}, {'frequency': 'f', 'id': 50, 'synset': 'banner.n.01', 'synonyms': ['banner', 'streamer'], 'def': 'long strip of cloth or paper used for decoration or advertising', 'name': 'banner'}, {'frequency': 'r', 'id': 51, 'synset': 'barbell.n.01', 'synonyms': ['barbell'], 'def': 'a bar to which heavy discs are attached at each end; used in weightlifting', 'name': 'barbell'}, {'frequency': 'r', 'id': 52, 'synset': 'barge.n.01', 'synonyms': ['barge'], 'def': 'a flatbottom boat for carrying heavy loads (especially on canals)', 'name': 'barge'}, {'frequency': 'f', 'id': 53, 'synset': 'barrel.n.02', 'synonyms': ['barrel', 'cask'], 'def': 'a cylindrical container that holds liquids', 'name': 'barrel'}, {'frequency': 'c', 'id': 54, 'synset': 'barrette.n.01', 'synonyms': ['barrette'], 'def': "a pin for holding women's hair in place", 'name': 'barrette'}, {'frequency': 'c', 'id': 55, 'synset': 'barrow.n.03', 'synonyms': ['barrow', 'garden_cart', 'lawn_cart', 'wheelbarrow'], 'def': 'a cart for carrying small loads; has handles and one or more wheels', 'name': 'barrow'}, {'frequency': 'f', 'id': 56, 'synset': 'base.n.03', 'synonyms': ['baseball_base'], 'def': 'a place that the runner must touch before scoring', 'name': 'baseball_base'}, {'frequency': 'f', 'id': 57, 'synset': 'baseball.n.02', 'synonyms': ['baseball'], 'def': 'a ball used in playing baseball', 'name': 'baseball'}, {'frequency': 'f', 'id': 58, 'synset': 'baseball_bat.n.01', 'synonyms': ['baseball_bat'], 'def': 'an implement used in baseball by the batter', 'name': 'baseball_bat'}, {'frequency': 'f', 'id': 59, 'synset': 'baseball_cap.n.01', 'synonyms': ['baseball_cap', 'jockey_cap', 'golf_cap'], 'def': 'a cap with a bill', 'name': 'baseball_cap'}, {'frequency': 'f', 'id': 60, 'synset': 'baseball_glove.n.01', 'synonyms': ['baseball_glove', 'baseball_mitt'], 'def': 'the handwear used by fielders in playing baseball', 'name': 'baseball_glove'}, {'frequency': 'f', 'id': 61, 'synset': 'basket.n.01', 'synonyms': ['basket', 'handbasket'], 'def': 'a container that is usually woven and has handles', 'name': 'basket'}, {'frequency': 'c', 'id': 62, 'synset': 'basket.n.03', 'synonyms': ['basketball_hoop'], 'def': 'metal hoop supporting a net through which players try to throw the basketball', 'name': 'basketball_hoop'}, {'frequency': 'c', 'id': 63, 'synset': 'basketball.n.02', 'synonyms': ['basketball'], 'def': 'an inflated ball used in playing basketball', 'name': 'basketball'}, {'frequency': 'r', 'id': 64, 'synset': 'bass_horn.n.01', 'synonyms': ['bass_horn', 'sousaphone', 'tuba'], 'def': 'the lowest brass wind instrument', 'name': 'bass_horn'}, {'frequency': 'r', 'id': 65, 'synset': 'bat.n.01', 'synonyms': ['bat_(animal)'], 'def': 'nocturnal mouselike mammal with forelimbs modified to form membranous wings', 'name': 'bat_(animal)'}, {'frequency': 'f', 'id': 66, 'synset': 'bath_mat.n.01', 'synonyms': ['bath_mat'], 'def': 'a heavy towel or mat to stand on while drying yourself after a bath', 'name': 'bath_mat'}, {'frequency': 'f', 'id': 67, 'synset': 'bath_towel.n.01', 'synonyms': ['bath_towel'], 'def': 'a large towel; to dry yourself after a bath', 'name': 'bath_towel'}, {'frequency': 'c', 'id': 68, 'synset': 'bathrobe.n.01', 'synonyms': ['bathrobe'], 'def': 'a loose-fitting robe of towelling; worn after a bath or swim', 'name': 'bathrobe'}, {'frequency': 'f', 'id': 69, 'synset': 'bathtub.n.01', 'synonyms': ['bathtub', 'bathing_tub'], 'def': 'a large open container that you fill with water and use to wash the body', 'name': 'bathtub'}, {'frequency': 'r', 'id': 70, 'synset': 'batter.n.02', 'synonyms': ['batter_(food)'], 'def': 'a liquid or semiliquid mixture, as of flour, eggs, and milk, used in cooking', 'name': 'batter_(food)'}, {'frequency': 'c', 'id': 71, 'synset': 'battery.n.02', 'synonyms': ['battery'], 'def': 'a portable device that produces electricity', 'name': 'battery'}, {'frequency': 'r', 'id': 72, 'synset': 'beach_ball.n.01', 'synonyms': ['beachball'], 'def': 'large and light ball; for play at the seaside', 'name': 'beachball'}, {'frequency': 'c', 'id': 73, 'synset': 'bead.n.01', 'synonyms': ['bead'], 'def': 'a small ball with a hole through the middle used for ornamentation, jewellery, etc.', 'name': 'bead'}, {'frequency': 'r', 'id': 74, 'synset': 'beaker.n.01', 'synonyms': ['beaker'], 'def': 'a flatbottomed jar made of glass or plastic; used for chemistry', 'name': 'beaker'}, {'frequency': 'c', 'id': 75, 'synset': 'bean_curd.n.01', 'synonyms': ['bean_curd', 'tofu'], 'def': 'cheeselike food made of curdled soybean milk', 'name': 'bean_curd'}, {'frequency': 'c', 'id': 76, 'synset': 'beanbag.n.01', 'synonyms': ['beanbag'], 'def': 'a bag filled with dried beans or similar items; used in games or to sit on', 'name': 'beanbag'}, {'frequency': 'f', 'id': 77, 'synset': 'beanie.n.01', 'synonyms': ['beanie', 'beany'], 'def': 'a small skullcap; formerly worn by schoolboys and college freshmen', 'name': 'beanie'}, {'frequency': 'f', 'id': 78, 'synset': 'bear.n.01', 'synonyms': ['bear'], 'def': 'large carnivorous or omnivorous mammals with shaggy coats and claws', 'name': 'bear'}, {'frequency': 'f', 'id': 79, 'synset': 'bed.n.01', 'synonyms': ['bed'], 'def': 'a piece of furniture that provides a place to sleep', 'name': 'bed'}, {'frequency': 'c', 'id': 80, 'synset': 'bedspread.n.01', 'synonyms': ['bedspread', 'bedcover', 'bed_covering', 'counterpane', 'spread'], 'def': 'decorative cover for a bed', 'name': 'bedspread'}, {'frequency': 'f', 'id': 81, 'synset': 'beef.n.01', 'synonyms': ['cow'], 'def': 'cattle that are reared for their meat', 'name': 'cow'}, {'frequency': 'c', 'id': 82, 'synset': 'beef.n.02', 'synonyms': ['beef_(food)', 'boeuf_(food)'], 'def': 'meat from an adult domestic bovine', 'name': 'beef_(food)'}, {'frequency': 'r', 'id': 83, 'synset': 'beeper.n.01', 'synonyms': ['beeper', 'pager'], 'def': 'an device that beeps when the person carrying it is being paged', 'name': 'beeper'}, {'frequency': 'f', 'id': 84, 'synset': 'beer_bottle.n.01', 'synonyms': ['beer_bottle'], 'def': 'a bottle that holds beer', 'name': 'beer_bottle'}, {'frequency': 'c', 'id': 85, 'synset': 'beer_can.n.01', 'synonyms': ['beer_can'], 'def': 'a can that holds beer', 'name': 'beer_can'}, {'frequency': 'r', 'id': 86, 'synset': 'beetle.n.01', 'synonyms': ['beetle'], 'def': 'insect with hard wing covers', 'name': 'beetle'}, {'frequency': 'f', 'id': 87, 'synset': 'bell.n.01', 'synonyms': ['bell'], 'def': 'a hollow device made of metal that makes a ringing sound when struck', 'name': 'bell'}, {'frequency': 'f', 'id': 88, 'synset': 'bell_pepper.n.02', 'synonyms': ['bell_pepper', 'capsicum'], 'def': 'large bell-shaped sweet pepper in green or red or yellow or orange or black varieties', 'name': 'bell_pepper'}, {'frequency': 'f', 'id': 89, 'synset': 'belt.n.02', 'synonyms': ['belt'], 'def': 'a band to tie or buckle around the body (usually at the waist)', 'name': 'belt'}, {'frequency': 'f', 'id': 90, 'synset': 'belt_buckle.n.01', 'synonyms': ['belt_buckle'], 'def': 'the buckle used to fasten a belt', 'name': 'belt_buckle'}, {'frequency': 'f', 'id': 91, 'synset': 'bench.n.01', 'synonyms': ['bench'], 'def': 'a long seat for more than one person', 'name': 'bench'}, {'frequency': 'c', 'id': 92, 'synset': 'beret.n.01', 'synonyms': ['beret'], 'def': 'a cap with no brim or bill; made of soft cloth', 'name': 'beret'}, {'frequency': 'c', 'id': 93, 'synset': 'bib.n.02', 'synonyms': ['bib'], 'def': 'a napkin tied under the chin of a child while eating', 'name': 'bib'}, {'frequency': 'r', 'id': 94, 'synset': 'bible.n.01', 'synonyms': ['Bible'], 'def': 'the sacred writings of the Christian religions', 'name': 'Bible'}, {'frequency': 'f', 'id': 95, 'synset': 'bicycle.n.01', 'synonyms': ['bicycle', 'bike_(bicycle)'], 'def': 'a wheeled vehicle that has two wheels and is moved by foot pedals', 'name': 'bicycle'}, {'frequency': 'f', 'id': 96, 'synset': 'bill.n.09', 'synonyms': ['visor', 'vizor'], 'def': 'a brim that projects to the front to shade the eyes', 'name': 'visor'}, {'frequency': 'c', 'id': 97, 'synset': 'binder.n.03', 'synonyms': ['binder', 'ring-binder'], 'def': 'holds loose papers or magazines', 'name': 'binder'}, {'frequency': 'c', 'id': 98, 'synset': 'binoculars.n.01', 'synonyms': ['binoculars', 'field_glasses', 'opera_glasses'], 'def': 'an optical instrument designed for simultaneous use by both eyes', 'name': 'binoculars'}, {'frequency': 'f', 'id': 99, 'synset': 'bird.n.01', 'synonyms': ['bird'], 'def': 'animal characterized by feathers and wings', 'name': 'bird'}, {'frequency': 'r', 'id': 100, 'synset': 'bird_feeder.n.01', 'synonyms': ['birdfeeder'], 'def': 'an outdoor device that supplies food for wild birds', 'name': 'birdfeeder'}, {'frequency': 'r', 'id': 101, 'synset': 'birdbath.n.01', 'synonyms': ['birdbath'], 'def': 'an ornamental basin (usually in a garden) for birds to bathe in', 'name': 'birdbath'}, {'frequency': 'c', 'id': 102, 'synset': 'birdcage.n.01', 'synonyms': ['birdcage'], 'def': 'a cage in which a bird can be kept', 'name': 'birdcage'}, {'frequency': 'c', 'id': 103, 'synset': 'birdhouse.n.01', 'synonyms': ['birdhouse'], 'def': 'a shelter for birds', 'name': 'birdhouse'}, {'frequency': 'f', 'id': 104, 'synset': 'birthday_cake.n.01', 'synonyms': ['birthday_cake'], 'def': 'decorated cake served at a birthday party', 'name': 'birthday_cake'}, {'frequency': 'r', 'id': 105, 'synset': 'birthday_card.n.01', 'synonyms': ['birthday_card'], 'def': 'a card expressing a birthday greeting', 'name': 'birthday_card'}, {'frequency': 'r', 'id': 106, 'synset': 'biscuit.n.01', 'synonyms': ['biscuit_(bread)'], 'def': 'small round bread leavened with baking-powder or soda', 'name': 'biscuit_(bread)'}, {'frequency': 'r', 'id': 107, 'synset': 'black_flag.n.01', 'synonyms': ['pirate_flag'], 'def': 'a flag usually bearing a white skull and crossbones on a black background', 'name': 'pirate_flag'}, {'frequency': 'c', 'id': 108, 'synset': 'black_sheep.n.02', 'synonyms': ['black_sheep'], 'def': 'sheep with a black coat', 'name': 'black_sheep'}, {'frequency': 'c', 'id': 109, 'synset': 'blackboard.n.01', 'synonyms': ['blackboard', 'chalkboard'], 'def': 'sheet of slate; for writing with chalk', 'name': 'blackboard'}, {'frequency': 'f', 'id': 110, 'synset': 'blanket.n.01', 'synonyms': ['blanket'], 'def': 'bedding that keeps a person warm in bed', 'name': 'blanket'}, {'frequency': 'c', 'id': 111, 'synset': 'blazer.n.01', 'synonyms': ['blazer', 'sport_jacket', 'sport_coat', 'sports_jacket', 'sports_coat'], 'def': 'lightweight jacket; often striped in the colors of a club or school', 'name': 'blazer'}, {'frequency': 'f', 'id': 112, 'synset': 'blender.n.01', 'synonyms': ['blender', 'liquidizer', 'liquidiser'], 'def': 'an electrically powered mixer that mix or chop or liquefy foods', 'name': 'blender'}, {'frequency': 'r', 'id': 113, 'synset': 'blimp.n.02', 'synonyms': ['blimp'], 'def': 'a small nonrigid airship used for observation or as a barrage balloon', 'name': 'blimp'}, {'frequency': 'c', 'id': 114, 'synset': 'blinker.n.01', 'synonyms': ['blinker', 'flasher'], 'def': 'a light that flashes on and off; used as a signal or to send messages', 'name': 'blinker'}, {'frequency': 'c', 'id': 115, 'synset': 'blueberry.n.02', 'synonyms': ['blueberry'], 'def': 'sweet edible dark-blue berries of blueberry plants', 'name': 'blueberry'}, {'frequency': 'r', 'id': 116, 'synset': 'boar.n.02', 'synonyms': ['boar'], 'def': 'an uncastrated male hog', 'name': 'boar'}, {'frequency': 'r', 'id': 117, 'synset': 'board.n.09', 'synonyms': ['gameboard'], 'def': 'a flat portable surface (usually rectangular) designed for board games', 'name': 'gameboard'}, {'frequency': 'f', 'id': 118, 'synset': 'boat.n.01', 'synonyms': ['boat', 'ship_(boat)'], 'def': 'a vessel for travel on water', 'name': 'boat'}, {'frequency': 'c', 'id': 119, 'synset': 'bobbin.n.01', 'synonyms': ['bobbin', 'spool', 'reel'], 'def': 'a thing around which thread/tape/film or other flexible materials can be wound', 'name': 'bobbin'}, {'frequency': 'r', 'id': 120, 'synset': 'bobby_pin.n.01', 'synonyms': ['bobby_pin', 'hairgrip'], 'def': 'a flat wire hairpin used to hold bobbed hair in place', 'name': 'bobby_pin'}, {'frequency': 'c', 'id': 121, 'synset': 'boiled_egg.n.01', 'synonyms': ['boiled_egg', 'coddled_egg'], 'def': 'egg cooked briefly in the shell in gently boiling water', 'name': 'boiled_egg'}, {'frequency': 'r', 'id': 122, 'synset': 'bolo_tie.n.01', 'synonyms': ['bolo_tie', 'bolo', 'bola_tie', 'bola'], 'def': 'a cord fastened around the neck with an ornamental clasp and worn as a necktie', 'name': 'bolo_tie'}, {'frequency': 'c', 'id': 123, 'synset': 'bolt.n.03', 'synonyms': ['deadbolt'], 'def': 'the part of a lock that is engaged or withdrawn with a key', 'name': 'deadbolt'}, {'frequency': 'f', 'id': 124, 'synset': 'bolt.n.06', 'synonyms': ['bolt'], 'def': 'a screw that screws into a nut to form a fastener', 'name': 'bolt'}, {'frequency': 'r', 'id': 125, 'synset': 'bonnet.n.01', 'synonyms': ['bonnet'], 'def': 'a hat tied under the chin', 'name': 'bonnet'}, {'frequency': 'f', 'id': 126, 'synset': 'book.n.01', 'synonyms': ['book'], 'def': 'a written work or composition that has been published', 'name': 'book'}, {'frequency': 'r', 'id': 127, 'synset': 'book_bag.n.01', 'synonyms': ['book_bag'], 'def': 'a bag in which students carry their books', 'name': 'book_bag'}, {'frequency': 'c', 'id': 128, 'synset': 'bookcase.n.01', 'synonyms': ['bookcase'], 'def': 'a piece of furniture with shelves for storing books', 'name': 'bookcase'}, {'frequency': 'c', 'id': 129, 'synset': 'booklet.n.01', 'synonyms': ['booklet', 'brochure', 'leaflet', 'pamphlet'], 'def': 'a small book usually having a paper cover', 'name': 'booklet'}, {'frequency': 'r', 'id': 130, 'synset': 'bookmark.n.01', 'synonyms': ['bookmark', 'bookmarker'], 'def': 'a marker (a piece of paper or ribbon) placed between the pages of a book', 'name': 'bookmark'}, {'frequency': 'r', 'id': 131, 'synset': 'boom.n.04', 'synonyms': ['boom_microphone', 'microphone_boom'], 'def': 'a pole carrying an overhead microphone projected over a film or tv set', 'name': 'boom_microphone'}, {'frequency': 'f', 'id': 132, 'synset': 'boot.n.01', 'synonyms': ['boot'], 'def': 'footwear that covers the whole foot and lower leg', 'name': 'boot'}, {'frequency': 'f', 'id': 133, 'synset': 'bottle.n.01', 'synonyms': ['bottle'], 'def': 'a glass or plastic vessel used for storing drinks or other liquids', 'name': 'bottle'}, {'frequency': 'c', 'id': 134, 'synset': 'bottle_opener.n.01', 'synonyms': ['bottle_opener'], 'def': 'an opener for removing caps or corks from bottles', 'name': 'bottle_opener'}, {'frequency': 'c', 'id': 135, 'synset': 'bouquet.n.01', 'synonyms': ['bouquet'], 'def': 'an arrangement of flowers that is usually given as a present', 'name': 'bouquet'}, {'frequency': 'r', 'id': 136, 'synset': 'bow.n.04', 'synonyms': ['bow_(weapon)'], 'def': 'a weapon for shooting arrows', 'name': 'bow_(weapon)'}, {'frequency': 'f', 'id': 137, 'synset': 'bow.n.08', 'synonyms': ['bow_(decorative_ribbons)'], 'def': 'a decorative interlacing of ribbons', 'name': 'bow_(decorative_ribbons)'}, {'frequency': 'f', 'id': 138, 'synset': 'bow_tie.n.01', 'synonyms': ['bow-tie', 'bowtie'], 'def': "a man's tie that ties in a bow", 'name': 'bow-tie'}, {'frequency': 'f', 'id': 139, 'synset': 'bowl.n.03', 'synonyms': ['bowl'], 'def': 'a dish that is round and open at the top for serving foods', 'name': 'bowl'}, {'frequency': 'r', 'id': 140, 'synset': 'bowl.n.08', 'synonyms': ['pipe_bowl'], 'def': 'a small round container that is open at the top for holding tobacco', 'name': 'pipe_bowl'}, {'frequency': 'c', 'id': 141, 'synset': 'bowler_hat.n.01', 'synonyms': ['bowler_hat', 'bowler', 'derby_hat', 'derby', 'plug_hat'], 'def': 'a felt hat that is round and hard with a narrow brim', 'name': 'bowler_hat'}, {'frequency': 'r', 'id': 142, 'synset': 'bowling_ball.n.01', 'synonyms': ['bowling_ball'], 'def': 'a large ball with finger holes used in the sport of bowling', 'name': 'bowling_ball'}, {'frequency': 'r', 'id': 143, 'synset': 'bowling_pin.n.01', 'synonyms': ['bowling_pin'], 'def': 'a club-shaped wooden object used in bowling', 'name': 'bowling_pin'}, {'frequency': 'r', 'id': 144, 'synset': 'boxing_glove.n.01', 'synonyms': ['boxing_glove'], 'def': 'large glove coverings the fists of a fighter worn for the sport of boxing', 'name': 'boxing_glove'}, {'frequency': 'c', 'id': 145, 'synset': 'brace.n.06', 'synonyms': ['suspenders'], 'def': 'elastic straps that hold trousers up (usually used in the plural)', 'name': 'suspenders'}, {'frequency': 'f', 'id': 146, 'synset': 'bracelet.n.02', 'synonyms': ['bracelet', 'bangle'], 'def': 'jewelry worn around the wrist for decoration', 'name': 'bracelet'}, {'frequency': 'r', 'id': 147, 'synset': 'brass.n.07', 'synonyms': ['brass_plaque'], 'def': 'a memorial made of brass', 'name': 'brass_plaque'}, {'frequency': 'c', 'id': 148, 'synset': 'brassiere.n.01', 'synonyms': ['brassiere', 'bra', 'bandeau'], 'def': 'an undergarment worn by women to support their breasts', 'name': 'brassiere'}, {'frequency': 'c', 'id': 149, 'synset': 'bread-bin.n.01', 'synonyms': ['bread-bin', 'breadbox'], 'def': 'a container used to keep bread or cake in', 'name': 'bread-bin'}, {'frequency': 'r', 'id': 150, 'synset': 'breechcloth.n.01', 'synonyms': ['breechcloth', 'breechclout', 'loincloth'], 'def': 'a garment that provides covering for the loins', 'name': 'breechcloth'}, {'frequency': 'c', 'id': 151, 'synset': 'bridal_gown.n.01', 'synonyms': ['bridal_gown', 'wedding_gown', 'wedding_dress'], 'def': 'a gown worn by the bride at a wedding', 'name': 'bridal_gown'}, {'frequency': 'c', 'id': 152, 'synset': 'briefcase.n.01', 'synonyms': ['briefcase'], 'def': 'a case with a handle; for carrying papers or files or books', 'name': 'briefcase'}, {'frequency': 'c', 'id': 153, 'synset': 'bristle_brush.n.01', 'synonyms': ['bristle_brush'], 'def': 'a brush that is made with the short stiff hairs of an animal or plant', 'name': 'bristle_brush'}, {'frequency': 'f', 'id': 154, 'synset': 'broccoli.n.01', 'synonyms': ['broccoli'], 'def': 'plant with dense clusters of tight green flower buds', 'name': 'broccoli'}, {'frequency': 'r', 'id': 155, 'synset': 'brooch.n.01', 'synonyms': ['broach'], 'def': 'a decorative pin worn by women', 'name': 'broach'}, {'frequency': 'c', 'id': 156, 'synset': 'broom.n.01', 'synonyms': ['broom'], 'def': 'bundle of straws or twigs attached to a long handle; used for cleaning', 'name': 'broom'}, {'frequency': 'c', 'id': 157, 'synset': 'brownie.n.03', 'synonyms': ['brownie'], 'def': 'square or bar of very rich chocolate cake usually with nuts', 'name': 'brownie'}, {'frequency': 'c', 'id': 158, 'synset': 'brussels_sprouts.n.01', 'synonyms': ['brussels_sprouts'], 'def': 'the small edible cabbage-like buds growing along a stalk', 'name': 'brussels_sprouts'}, {'frequency': 'r', 'id': 159, 'synset': 'bubble_gum.n.01', 'synonyms': ['bubble_gum'], 'def': 'a kind of chewing gum that can be blown into bubbles', 'name': 'bubble_gum'}, {'frequency': 'f', 'id': 160, 'synset': 'bucket.n.01', 'synonyms': ['bucket', 'pail'], 'def': 'a roughly cylindrical vessel that is open at the top', 'name': 'bucket'}, {'frequency': 'r', 'id': 161, 'synset': 'buggy.n.01', 'synonyms': ['horse_buggy'], 'def': 'a small lightweight carriage; drawn by a single horse', 'name': 'horse_buggy'}, {'frequency': 'c', 'id': 162, 'synset': 'bull.n.11', 'synonyms': ['bull'], 'def': 'mature male cow', 'name': 'bull'}, {'frequency': 'r', 'id': 163, 'synset': 'bulldog.n.01', 'synonyms': ['bulldog'], 'def': 'a thickset short-haired dog with a large head and strong undershot lower jaw', 'name': 'bulldog'}, {'frequency': 'r', 'id': 164, 'synset': 'bulldozer.n.01', 'synonyms': ['bulldozer', 'dozer'], 'def': 'large powerful tractor; a large blade in front flattens areas of ground', 'name': 'bulldozer'}, {'frequency': 'c', 'id': 165, 'synset': 'bullet_train.n.01', 'synonyms': ['bullet_train'], 'def': 'a high-speed passenger train', 'name': 'bullet_train'}, {'frequency': 'c', 'id': 166, 'synset': 'bulletin_board.n.02', 'synonyms': ['bulletin_board', 'notice_board'], 'def': 'a board that hangs on a wall; displays announcements', 'name': 'bulletin_board'}, {'frequency': 'r', 'id': 167, 'synset': 'bulletproof_vest.n.01', 'synonyms': ['bulletproof_vest'], 'def': 'a vest capable of resisting the impact of a bullet', 'name': 'bulletproof_vest'}, {'frequency': 'c', 'id': 168, 'synset': 'bullhorn.n.01', 'synonyms': ['bullhorn', 'megaphone'], 'def': 'a portable loudspeaker with built-in microphone and amplifier', 'name': 'bullhorn'}, {'frequency': 'r', 'id': 169, 'synset': 'bully_beef.n.01', 'synonyms': ['corned_beef', 'corn_beef'], 'def': 'beef cured or pickled in brine', 'name': 'corned_beef'}, {'frequency': 'f', 'id': 170, 'synset': 'bun.n.01', 'synonyms': ['bun', 'roll'], 'def': 'small rounded bread either plain or sweet', 'name': 'bun'}, {'frequency': 'c', 'id': 171, 'synset': 'bunk_bed.n.01', 'synonyms': ['bunk_bed'], 'def': 'beds built one above the other', 'name': 'bunk_bed'}, {'frequency': 'f', 'id': 172, 'synset': 'buoy.n.01', 'synonyms': ['buoy'], 'def': 'a float attached by rope to the seabed to mark channels in a harbor or underwater hazards', 'name': 'buoy'}, {'frequency': 'r', 'id': 173, 'synset': 'burrito.n.01', 'synonyms': ['burrito'], 'def': 'a flour tortilla folded around a filling', 'name': 'burrito'}, {'frequency': 'f', 'id': 174, 'synset': 'bus.n.01', 'synonyms': ['bus_(vehicle)', 'autobus', 'charabanc', 'double-decker', 'motorbus', 'motorcoach'], 'def': 'a vehicle carrying many passengers; used for public transport', 'name': 'bus_(vehicle)'}, {'frequency': 'c', 'id': 175, 'synset': 'business_card.n.01', 'synonyms': ['business_card'], 'def': "a card on which are printed the person's name and business affiliation", 'name': 'business_card'}, {'frequency': 'c', 'id': 176, 'synset': 'butcher_knife.n.01', 'synonyms': ['butcher_knife'], 'def': 'a large sharp knife for cutting or trimming meat', 'name': 'butcher_knife'}, {'frequency': 'c', 'id': 177, 'synset': 'butter.n.01', 'synonyms': ['butter'], 'def': 'an edible emulsion of fat globules made by churning milk or cream; for cooking and table use', 'name': 'butter'}, {'frequency': 'c', 'id': 178, 'synset': 'butterfly.n.01', 'synonyms': ['butterfly'], 'def': 'insect typically having a slender body with knobbed antennae and broad colorful wings', 'name': 'butterfly'}, {'frequency': 'f', 'id': 179, 'synset': 'button.n.01', 'synonyms': ['button'], 'def': 'a round fastener sewn to shirts and coats etc to fit through buttonholes', 'name': 'button'}, {'frequency': 'f', 'id': 180, 'synset': 'cab.n.03', 'synonyms': ['cab_(taxi)', 'taxi', 'taxicab'], 'def': 'a car that takes passengers where they want to go in exchange for money', 'name': 'cab_(taxi)'}, {'frequency': 'r', 'id': 181, 'synset': 'cabana.n.01', 'synonyms': ['cabana'], 'def': 'a small tent used as a dressing room beside the sea or a swimming pool', 'name': 'cabana'}, {'frequency': 'r', 'id': 182, 'synset': 'cabin_car.n.01', 'synonyms': ['cabin_car', 'caboose'], 'def': 'a car on a freight train for use of the train crew; usually the last car on the train', 'name': 'cabin_car'}, {'frequency': 'f', 'id': 183, 'synset': 'cabinet.n.01', 'synonyms': ['cabinet'], 'def': 'a piece of furniture resembling a cupboard with doors and shelves and drawers', 'name': 'cabinet'}, {'frequency': 'r', 'id': 184, 'synset': 'cabinet.n.03', 'synonyms': ['locker', 'storage_locker'], 'def': 'a storage compartment for clothes and valuables; usually it has a lock', 'name': 'locker'}, {'frequency': 'f', 'id': 185, 'synset': 'cake.n.03', 'synonyms': ['cake'], 'def': 'baked goods made from or based on a mixture of flour, sugar, eggs, and fat', 'name': 'cake'}, {'frequency': 'c', 'id': 186, 'synset': 'calculator.n.02', 'synonyms': ['calculator'], 'def': 'a small machine that is used for mathematical calculations', 'name': 'calculator'}, {'frequency': 'f', 'id': 187, 'synset': 'calendar.n.02', 'synonyms': ['calendar'], 'def': 'a list or register of events (appointments/social events/court cases, etc)', 'name': 'calendar'}, {'frequency': 'c', 'id': 188, 'synset': 'calf.n.01', 'synonyms': ['calf'], 'def': 'young of domestic cattle', 'name': 'calf'}, {'frequency': 'c', 'id': 189, 'synset': 'camcorder.n.01', 'synonyms': ['camcorder'], 'def': 'a portable television camera and videocassette recorder', 'name': 'camcorder'}, {'frequency': 'c', 'id': 190, 'synset': 'camel.n.01', 'synonyms': ['camel'], 'def': 'cud-chewing mammal used as a draft or saddle animal in desert regions', 'name': 'camel'}, {'frequency': 'f', 'id': 191, 'synset': 'camera.n.01', 'synonyms': ['camera'], 'def': 'equipment for taking photographs', 'name': 'camera'}, {'frequency': 'c', 'id': 192, 'synset': 'camera_lens.n.01', 'synonyms': ['camera_lens'], 'def': 'a lens that focuses the image in a camera', 'name': 'camera_lens'}, {'frequency': 'c', 'id': 193, 'synset': 'camper.n.02', 'synonyms': ['camper_(vehicle)', 'camping_bus', 'motor_home'], 'def': 'a recreational vehicle equipped for camping out while traveling', 'name': 'camper_(vehicle)'}, {'frequency': 'f', 'id': 194, 'synset': 'can.n.01', 'synonyms': ['can', 'tin_can'], 'def': 'airtight sealed metal container for food or drink or paint etc.', 'name': 'can'}, {'frequency': 'c', 'id': 195, 'synset': 'can_opener.n.01', 'synonyms': ['can_opener', 'tin_opener'], 'def': 'a device for cutting cans open', 'name': 'can_opener'}, {'frequency': 'r', 'id': 196, 'synset': 'candelabrum.n.01', 'synonyms': ['candelabrum', 'candelabra'], 'def': 'branched candlestick; ornamental; has several lights', 'name': 'candelabrum'}, {'frequency': 'f', 'id': 197, 'synset': 'candle.n.01', 'synonyms': ['candle', 'candlestick'], 'def': 'stick of wax with a wick in the middle', 'name': 'candle'}, {'frequency': 'f', 'id': 198, 'synset': 'candlestick.n.01', 'synonyms': ['candle_holder'], 'def': 'a holder with sockets for candles', 'name': 'candle_holder'}, {'frequency': 'r', 'id': 199, 'synset': 'candy_bar.n.01', 'synonyms': ['candy_bar'], 'def': 'a candy shaped as a bar', 'name': 'candy_bar'}, {'frequency': 'c', 'id': 200, 'synset': 'candy_cane.n.01', 'synonyms': ['candy_cane'], 'def': 'a hard candy in the shape of a rod (usually with stripes)', 'name': 'candy_cane'}, {'frequency': 'c', 'id': 201, 'synset': 'cane.n.01', 'synonyms': ['walking_cane'], 'def': 'a stick that people can lean on to help them walk', 'name': 'walking_cane'}, {'frequency': 'c', 'id': 202, 'synset': 'canister.n.02', 'synonyms': ['canister', 'cannister'], 'def': 'metal container for storing dry foods such as tea or flour', 'name': 'canister'}, {'frequency': 'r', 'id': 203, 'synset': 'cannon.n.02', 'synonyms': ['cannon'], 'def': 'heavy gun fired from a tank', 'name': 'cannon'}, {'frequency': 'c', 'id': 204, 'synset': 'canoe.n.01', 'synonyms': ['canoe'], 'def': 'small and light boat; pointed at both ends; propelled with a paddle', 'name': 'canoe'}, {'frequency': 'r', 'id': 205, 'synset': 'cantaloup.n.02', 'synonyms': ['cantaloup', 'cantaloupe'], 'def': 'the fruit of a cantaloup vine; small to medium-sized melon with yellowish flesh', 'name': 'cantaloup'}, {'frequency': 'r', 'id': 206, 'synset': 'canteen.n.01', 'synonyms': ['canteen'], 'def': 'a flask for carrying water; used by soldiers or travelers', 'name': 'canteen'}, {'frequency': 'c', 'id': 207, 'synset': 'cap.n.01', 'synonyms': ['cap_(headwear)'], 'def': 'a tight-fitting headwear', 'name': 'cap_(headwear)'}, {'frequency': 'f', 'id': 208, 'synset': 'cap.n.02', 'synonyms': ['bottle_cap', 'cap_(container_lid)'], 'def': 'a top (as for a bottle)', 'name': 'bottle_cap'}, {'frequency': 'r', 'id': 209, 'synset': 'cape.n.02', 'synonyms': ['cape'], 'def': 'a sleeveless garment like a cloak but shorter', 'name': 'cape'}, {'frequency': 'c', 'id': 210, 'synset': 'cappuccino.n.01', 'synonyms': ['cappuccino', 'coffee_cappuccino'], 'def': 'equal parts of espresso and steamed milk', 'name': 'cappuccino'}, {'frequency': 'f', 'id': 211, 'synset': 'car.n.01', 'synonyms': ['car_(automobile)', 'auto_(automobile)', 'automobile'], 'def': 'a motor vehicle with four wheels', 'name': 'car_(automobile)'}, {'frequency': 'f', 'id': 212, 'synset': 'car.n.02', 'synonyms': ['railcar_(part_of_a_train)', 'railway_car_(part_of_a_train)', 'railroad_car_(part_of_a_train)'], 'def': 'a wheeled vehicle adapted to the rails of railroad', 'name': 'railcar_(part_of_a_train)'}, {'frequency': 'r', 'id': 213, 'synset': 'car.n.04', 'synonyms': ['elevator_car'], 'def': 'where passengers ride up and down', 'name': 'elevator_car'}, {'frequency': 'r', 'id': 214, 'synset': 'car_battery.n.01', 'synonyms': ['car_battery', 'automobile_battery'], 'def': 'a battery in a motor vehicle', 'name': 'car_battery'}, {'frequency': 'c', 'id': 215, 'synset': 'card.n.02', 'synonyms': ['identity_card'], 'def': 'a card certifying the identity of the bearer', 'name': 'identity_card'}, {'frequency': 'c', 'id': 216, 'synset': 'card.n.03', 'synonyms': ['card'], 'def': 'a rectangular piece of paper used to send messages (e.g. greetings or pictures)', 'name': 'card'}, {'frequency': 'r', 'id': 217, 'synset': 'cardigan.n.01', 'synonyms': ['cardigan'], 'def': 'knitted jacket that is fastened up the front with buttons or a zipper', 'name': 'cardigan'}, {'frequency': 'r', 'id': 218, 'synset': 'cargo_ship.n.01', 'synonyms': ['cargo_ship', 'cargo_vessel'], 'def': 'a ship designed to carry cargo', 'name': 'cargo_ship'}, {'frequency': 'r', 'id': 219, 'synset': 'carnation.n.01', 'synonyms': ['carnation'], 'def': 'plant with pink to purple-red spice-scented usually double flowers', 'name': 'carnation'}, {'frequency': 'c', 'id': 220, 'synset': 'carriage.n.02', 'synonyms': ['horse_carriage'], 'def': 'a vehicle with wheels drawn by one or more horses', 'name': 'horse_carriage'}, {'frequency': 'f', 'id': 221, 'synset': 'carrot.n.01', 'synonyms': ['carrot'], 'def': 'deep orange edible root of the cultivated carrot plant', 'name': 'carrot'}, {'frequency': 'c', 'id': 222, 'synset': 'carryall.n.01', 'synonyms': ['tote_bag'], 'def': 'a capacious bag or basket', 'name': 'tote_bag'}, {'frequency': 'c', 'id': 223, 'synset': 'cart.n.01', 'synonyms': ['cart'], 'def': 'a heavy open wagon usually having two wheels and drawn by an animal', 'name': 'cart'}, {'frequency': 'c', 'id': 224, 'synset': 'carton.n.02', 'synonyms': ['carton'], 'def': 'a box made of cardboard; opens by flaps on top', 'name': 'carton'}, {'frequency': 'c', 'id': 225, 'synset': 'cash_register.n.01', 'synonyms': ['cash_register', 'register_(for_cash_transactions)'], 'def': 'a cashbox with an adding machine to register transactions', 'name': 'cash_register'}, {'frequency': 'r', 'id': 226, 'synset': 'casserole.n.01', 'synonyms': ['casserole'], 'def': 'food cooked and served in a casserole', 'name': 'casserole'}, {'frequency': 'r', 'id': 227, 'synset': 'cassette.n.01', 'synonyms': ['cassette'], 'def': 'a container that holds a magnetic tape used for recording or playing sound or video', 'name': 'cassette'}, {'frequency': 'c', 'id': 228, 'synset': 'cast.n.05', 'synonyms': ['cast', 'plaster_cast', 'plaster_bandage'], 'def': 'bandage consisting of a firm covering that immobilizes broken bones while they heal', 'name': 'cast'}, {'frequency': 'f', 'id': 229, 'synset': 'cat.n.01', 'synonyms': ['cat'], 'def': 'a domestic house cat', 'name': 'cat'}, {'frequency': 'c', 'id': 230, 'synset': 'cauliflower.n.02', 'synonyms': ['cauliflower'], 'def': 'edible compact head of white undeveloped flowers', 'name': 'cauliflower'}, {'frequency': 'r', 'id': 231, 'synset': 'caviar.n.01', 'synonyms': ['caviar', 'caviare'], 'def': "salted roe of sturgeon or other large fish; usually served as an hors d'oeuvre", 'name': 'caviar'}, {'frequency': 'c', 'id': 232, 'synset': 'cayenne.n.02', 'synonyms': ['cayenne_(spice)', 'cayenne_pepper_(spice)', 'red_pepper_(spice)'], 'def': 'ground pods and seeds of pungent red peppers of the genus Capsicum', 'name': 'cayenne_(spice)'}, {'frequency': 'c', 'id': 233, 'synset': 'cd_player.n.01', 'synonyms': ['CD_player'], 'def': 'electronic equipment for playing compact discs (CDs)', 'name': 'CD_player'}, {'frequency': 'c', 'id': 234, 'synset': 'celery.n.01', 'synonyms': ['celery'], 'def': 'widely cultivated herb with aromatic leaf stalks that are eaten raw or cooked', 'name': 'celery'}, {'frequency': 'f', 'id': 235, 'synset': 'cellular_telephone.n.01', 'synonyms': ['cellular_telephone', 'cellular_phone', 'cellphone', 'mobile_phone', 'smart_phone'], 'def': 'a hand-held mobile telephone', 'name': 'cellular_telephone'}, {'frequency': 'r', 'id': 236, 'synset': 'chain_mail.n.01', 'synonyms': ['chain_mail', 'ring_mail', 'chain_armor', 'chain_armour', 'ring_armor', 'ring_armour'], 'def': '(Middle Ages) flexible armor made of interlinked metal rings', 'name': 'chain_mail'}, {'frequency': 'f', 'id': 237, 'synset': 'chair.n.01', 'synonyms': ['chair'], 'def': 'a seat for one person, with a support for the back', 'name': 'chair'}, {'frequency': 'r', 'id': 238, 'synset': 'chaise_longue.n.01', 'synonyms': ['chaise_longue', 'chaise', 'daybed'], 'def': 'a long chair; for reclining', 'name': 'chaise_longue'}, {'frequency': 'r', 'id': 239, 'synset': 'champagne.n.01', 'synonyms': ['champagne'], 'def': 'a white sparkling wine produced in Champagne or resembling that produced there', 'name': 'champagne'}, {'frequency': 'f', 'id': 240, 'synset': 'chandelier.n.01', 'synonyms': ['chandelier'], 'def': 'branched lighting fixture; often ornate; hangs from the ceiling', 'name': 'chandelier'}, {'frequency': 'r', 'id': 241, 'synset': 'chap.n.04', 'synonyms': ['chap'], 'def': 'leather leggings without a seat; worn over trousers by cowboys to protect their legs', 'name': 'chap'}, {'frequency': 'r', 'id': 242, 'synset': 'checkbook.n.01', 'synonyms': ['checkbook', 'chequebook'], 'def': 'a book issued to holders of checking accounts', 'name': 'checkbook'}, {'frequency': 'r', 'id': 243, 'synset': 'checkerboard.n.01', 'synonyms': ['checkerboard'], 'def': 'a board having 64 squares of two alternating colors', 'name': 'checkerboard'}, {'frequency': 'c', 'id': 244, 'synset': 'cherry.n.03', 'synonyms': ['cherry'], 'def': 'a red fruit with a single hard stone', 'name': 'cherry'}, {'frequency': 'r', 'id': 245, 'synset': 'chessboard.n.01', 'synonyms': ['chessboard'], 'def': 'a checkerboard used to play chess', 'name': 'chessboard'}, {'frequency': 'r', 'id': 246, 'synset': 'chest_of_drawers.n.01', 'synonyms': ['chest_of_drawers_(furniture)', 'bureau_(furniture)', 'chest_(furniture)'], 'def': 'furniture with drawers for keeping clothes', 'name': 'chest_of_drawers_(furniture)'}, {'frequency': 'c', 'id': 247, 'synset': 'chicken.n.02', 'synonyms': ['chicken_(animal)'], 'def': 'a domestic fowl bred for flesh or eggs', 'name': 'chicken_(animal)'}, {'frequency': 'c', 'id': 248, 'synset': 'chicken_wire.n.01', 'synonyms': ['chicken_wire'], 'def': 'a galvanized wire network with a hexagonal mesh; used to build fences', 'name': 'chicken_wire'}, {'frequency': 'r', 'id': 249, 'synset': 'chickpea.n.01', 'synonyms': ['chickpea', 'garbanzo'], 'def': 'the seed of the chickpea plant; usually dried', 'name': 'chickpea'}, {'frequency': 'r', 'id': 250, 'synset': 'chihuahua.n.03', 'synonyms': ['Chihuahua'], 'def': 'an old breed of tiny short-haired dog with protruding eyes from Mexico', 'name': 'Chihuahua'}, {'frequency': 'r', 'id': 251, 'synset': 'chili.n.02', 'synonyms': ['chili_(vegetable)', 'chili_pepper_(vegetable)', 'chilli_(vegetable)', 'chilly_(vegetable)', 'chile_(vegetable)'], 'def': 'very hot and finely tapering pepper of special pungency', 'name': 'chili_(vegetable)'}, {'frequency': 'r', 'id': 252, 'synset': 'chime.n.01', 'synonyms': ['chime', 'gong'], 'def': 'an instrument consisting of a set of bells that are struck with a hammer', 'name': 'chime'}, {'frequency': 'r', 'id': 253, 'synset': 'chinaware.n.01', 'synonyms': ['chinaware'], 'def': 'dishware made of high quality porcelain', 'name': 'chinaware'}, {'frequency': 'c', 'id': 254, 'synset': 'chip.n.04', 'synonyms': ['crisp_(potato_chip)', 'potato_chip'], 'def': 'a thin crisp slice of potato fried in deep fat', 'name': 'crisp_(potato_chip)'}, {'frequency': 'r', 'id': 255, 'synset': 'chip.n.06', 'synonyms': ['poker_chip'], 'def': 'a small disk-shaped counter used to represent money when gambling', 'name': 'poker_chip'}, {'frequency': 'c', 'id': 256, 'synset': 'chocolate_bar.n.01', 'synonyms': ['chocolate_bar'], 'def': 'a bar of chocolate candy', 'name': 'chocolate_bar'}, {'frequency': 'c', 'id': 257, 'synset': 'chocolate_cake.n.01', 'synonyms': ['chocolate_cake'], 'def': 'cake containing chocolate', 'name': 'chocolate_cake'}, {'frequency': 'r', 'id': 258, 'synset': 'chocolate_milk.n.01', 'synonyms': ['chocolate_milk'], 'def': 'milk flavored with chocolate syrup', 'name': 'chocolate_milk'}, {'frequency': 'r', 'id': 259, 'synset': 'chocolate_mousse.n.01', 'synonyms': ['chocolate_mousse'], 'def': 'dessert mousse made with chocolate', 'name': 'chocolate_mousse'}, {'frequency': 'f', 'id': 260, 'synset': 'choker.n.03', 'synonyms': ['choker', 'collar', 'neckband'], 'def': 'necklace that fits tightly around the neck', 'name': 'choker'}, {'frequency': 'f', 'id': 261, 'synset': 'chopping_board.n.01', 'synonyms': ['chopping_board', 'cutting_board', 'chopping_block'], 'def': 'a wooden board where meats or vegetables can be cut', 'name': 'chopping_board'}, {'frequency': 'c', 'id': 262, 'synset': 'chopstick.n.01', 'synonyms': ['chopstick'], 'def': 'one of a pair of slender sticks used as oriental tableware to eat food with', 'name': 'chopstick'}, {'frequency': 'f', 'id': 263, 'synset': 'christmas_tree.n.05', 'synonyms': ['Christmas_tree'], 'def': 'an ornamented evergreen used as a Christmas decoration', 'name': 'Christmas_tree'}, {'frequency': 'c', 'id': 264, 'synset': 'chute.n.02', 'synonyms': ['slide'], 'def': 'sloping channel through which things can descend', 'name': 'slide'}, {'frequency': 'r', 'id': 265, 'synset': 'cider.n.01', 'synonyms': ['cider', 'cyder'], 'def': 'a beverage made from juice pressed from apples', 'name': 'cider'}, {'frequency': 'r', 'id': 266, 'synset': 'cigar_box.n.01', 'synonyms': ['cigar_box'], 'def': 'a box for holding cigars', 'name': 'cigar_box'}, {'frequency': 'c', 'id': 267, 'synset': 'cigarette.n.01', 'synonyms': ['cigarette'], 'def': 'finely ground tobacco wrapped in paper; for smoking', 'name': 'cigarette'}, {'frequency': 'c', 'id': 268, 'synset': 'cigarette_case.n.01', 'synonyms': ['cigarette_case', 'cigarette_pack'], 'def': 'a small flat case for holding cigarettes', 'name': 'cigarette_case'}, {'frequency': 'f', 'id': 269, 'synset': 'cistern.n.02', 'synonyms': ['cistern', 'water_tank'], 'def': 'a tank that holds the water used to flush a toilet', 'name': 'cistern'}, {'frequency': 'r', 'id': 270, 'synset': 'clarinet.n.01', 'synonyms': ['clarinet'], 'def': 'a single-reed instrument with a straight tube', 'name': 'clarinet'}, {'frequency': 'r', 'id': 271, 'synset': 'clasp.n.01', 'synonyms': ['clasp'], 'def': 'a fastener (as a buckle or hook) that is used to hold two things together', 'name': 'clasp'}, {'frequency': 'c', 'id': 272, 'synset': 'cleansing_agent.n.01', 'synonyms': ['cleansing_agent', 'cleanser', 'cleaner'], 'def': 'a preparation used in cleaning something', 'name': 'cleansing_agent'}, {'frequency': 'r', 'id': 273, 'synset': 'clementine.n.01', 'synonyms': ['clementine'], 'def': 'a variety of mandarin orange', 'name': 'clementine'}, {'frequency': 'c', 'id': 274, 'synset': 'clip.n.03', 'synonyms': ['clip'], 'def': 'any of various small fasteners used to hold loose articles together', 'name': 'clip'}, {'frequency': 'c', 'id': 275, 'synset': 'clipboard.n.01', 'synonyms': ['clipboard'], 'def': 'a small writing board with a clip at the top for holding papers', 'name': 'clipboard'}, {'frequency': 'f', 'id': 276, 'synset': 'clock.n.01', 'synonyms': ['clock', 'timepiece', 'timekeeper'], 'def': 'a timepiece that shows the time of day', 'name': 'clock'}, {'frequency': 'f', 'id': 277, 'synset': 'clock_tower.n.01', 'synonyms': ['clock_tower'], 'def': 'a tower with a large clock visible high up on an outside face', 'name': 'clock_tower'}, {'frequency': 'c', 'id': 278, 'synset': 'clothes_hamper.n.01', 'synonyms': ['clothes_hamper', 'laundry_basket', 'clothes_basket'], 'def': 'a hamper that holds dirty clothes to be washed or wet clothes to be dried', 'name': 'clothes_hamper'}, {'frequency': 'c', 'id': 279, 'synset': 'clothespin.n.01', 'synonyms': ['clothespin', 'clothes_peg'], 'def': 'wood or plastic fastener; for holding clothes on a clothesline', 'name': 'clothespin'}, {'frequency': 'r', 'id': 280, 'synset': 'clutch_bag.n.01', 'synonyms': ['clutch_bag'], 'def': "a woman's strapless purse that is carried in the hand", 'name': 'clutch_bag'}, {'frequency': 'f', 'id': 281, 'synset': 'coaster.n.03', 'synonyms': ['coaster'], 'def': 'a covering (plate or mat) that protects the surface of a table', 'name': 'coaster'}, {'frequency': 'f', 'id': 282, 'synset': 'coat.n.01', 'synonyms': ['coat'], 'def': 'an outer garment that has sleeves and covers the body from shoulder down', 'name': 'coat'}, {'frequency': 'c', 'id': 283, 'synset': 'coat_hanger.n.01', 'synonyms': ['coat_hanger', 'clothes_hanger', 'dress_hanger'], 'def': "a hanger that is shaped like a person's shoulders", 'name': 'coat_hanger'}, {'frequency': 'r', 'id': 284, 'synset': 'coatrack.n.01', 'synonyms': ['coatrack', 'hatrack'], 'def': 'a rack with hooks for temporarily holding coats and hats', 'name': 'coatrack'}, {'frequency': 'c', 'id': 285, 'synset': 'cock.n.04', 'synonyms': ['cock', 'rooster'], 'def': 'adult male chicken', 'name': 'cock'}, {'frequency': 'c', 'id': 286, 'synset': 'coconut.n.02', 'synonyms': ['coconut', 'cocoanut'], 'def': 'large hard-shelled brown oval nut with a fibrous husk', 'name': 'coconut'}, {'frequency': 'r', 'id': 287, 'synset': 'coffee_filter.n.01', 'synonyms': ['coffee_filter'], 'def': 'filter (usually of paper) that passes the coffee and retains the coffee grounds', 'name': 'coffee_filter'}, {'frequency': 'f', 'id': 288, 'synset': 'coffee_maker.n.01', 'synonyms': ['coffee_maker', 'coffee_machine'], 'def': 'a kitchen appliance for brewing coffee automatically', 'name': 'coffee_maker'}, {'frequency': 'f', 'id': 289, 'synset': 'coffee_table.n.01', 'synonyms': ['coffee_table', 'cocktail_table'], 'def': 'low table where magazines can be placed and coffee or cocktails are served', 'name': 'coffee_table'}, {'frequency': 'c', 'id': 290, 'synset': 'coffeepot.n.01', 'synonyms': ['coffeepot'], 'def': 'tall pot in which coffee is brewed', 'name': 'coffeepot'}, {'frequency': 'r', 'id': 291, 'synset': 'coil.n.05', 'synonyms': ['coil'], 'def': 'tubing that is wound in a spiral', 'name': 'coil'}, {'frequency': 'c', 'id': 292, 'synset': 'coin.n.01', 'synonyms': ['coin'], 'def': 'a flat metal piece (usually a disc) used as money', 'name': 'coin'}, {'frequency': 'r', 'id': 293, 'synset': 'colander.n.01', 'synonyms': ['colander', 'cullender'], 'def': 'bowl-shaped strainer; used to wash or drain foods', 'name': 'colander'}, {'frequency': 'c', 'id': 294, 'synset': 'coleslaw.n.01', 'synonyms': ['coleslaw', 'slaw'], 'def': 'basically shredded cabbage', 'name': 'coleslaw'}, {'frequency': 'r', 'id': 295, 'synset': 'coloring_material.n.01', 'synonyms': ['coloring_material', 'colouring_material'], 'def': 'any material used for its color', 'name': 'coloring_material'}, {'frequency': 'r', 'id': 296, 'synset': 'combination_lock.n.01', 'synonyms': ['combination_lock'], 'def': 'lock that can be opened only by turning dials in a special sequence', 'name': 'combination_lock'}, {'frequency': 'c', 'id': 297, 'synset': 'comforter.n.04', 'synonyms': ['pacifier', 'teething_ring'], 'def': 'device used for an infant to suck or bite on', 'name': 'pacifier'}, {'frequency': 'r', 'id': 298, 'synset': 'comic_book.n.01', 'synonyms': ['comic_book'], 'def': 'a magazine devoted to comic strips', 'name': 'comic_book'}, {'frequency': 'f', 'id': 299, 'synset': 'computer_keyboard.n.01', 'synonyms': ['computer_keyboard', 'keyboard_(computer)'], 'def': 'a keyboard that is a data input device for computers', 'name': 'computer_keyboard'}, {'frequency': 'r', 'id': 300, 'synset': 'concrete_mixer.n.01', 'synonyms': ['concrete_mixer', 'cement_mixer'], 'def': 'a machine with a large revolving drum in which cement/concrete is mixed', 'name': 'concrete_mixer'}, {'frequency': 'f', 'id': 301, 'synset': 'cone.n.01', 'synonyms': ['cone', 'traffic_cone'], 'def': 'a cone-shaped object used to direct traffic', 'name': 'cone'}, {'frequency': 'f', 'id': 302, 'synset': 'control.n.09', 'synonyms': ['control', 'controller'], 'def': 'a mechanism that controls the operation of a machine', 'name': 'control'}, {'frequency': 'r', 'id': 303, 'synset': 'convertible.n.01', 'synonyms': ['convertible_(automobile)'], 'def': 'a car that has top that can be folded or removed', 'name': 'convertible_(automobile)'}, {'frequency': 'r', 'id': 304, 'synset': 'convertible.n.03', 'synonyms': ['sofa_bed'], 'def': 'a sofa that can be converted into a bed', 'name': 'sofa_bed'}, {'frequency': 'c', 'id': 305, 'synset': 'cookie.n.01', 'synonyms': ['cookie', 'cooky', 'biscuit_(cookie)'], 'def': "any of various small flat sweet cakes (`biscuit' is the British term)", 'name': 'cookie'}, {'frequency': 'r', 'id': 306, 'synset': 'cookie_jar.n.01', 'synonyms': ['cookie_jar', 'cooky_jar'], 'def': 'a jar in which cookies are kept (and sometimes money is hidden)', 'name': 'cookie_jar'}, {'frequency': 'r', 'id': 307, 'synset': 'cooking_utensil.n.01', 'synonyms': ['cooking_utensil'], 'def': 'a kitchen utensil made of material that does not melt easily; used for cooking', 'name': 'cooking_utensil'}, {'frequency': 'f', 'id': 308, 'synset': 'cooler.n.01', 'synonyms': ['cooler_(for_food)', 'ice_chest'], 'def': 'an insulated box for storing food often with ice', 'name': 'cooler_(for_food)'}, {'frequency': 'c', 'id': 309, 'synset': 'cork.n.04', 'synonyms': ['cork_(bottle_plug)', 'bottle_cork'], 'def': 'the plug in the mouth of a bottle (especially a wine bottle)', 'name': 'cork_(bottle_plug)'}, {'frequency': 'r', 'id': 310, 'synset': 'corkboard.n.01', 'synonyms': ['corkboard'], 'def': 'a sheet consisting of cork granules', 'name': 'corkboard'}, {'frequency': 'r', 'id': 311, 'synset': 'corkscrew.n.01', 'synonyms': ['corkscrew', 'bottle_screw'], 'def': 'a bottle opener that pulls corks', 'name': 'corkscrew'}, {'frequency': 'c', 'id': 312, 'synset': 'corn.n.03', 'synonyms': ['edible_corn', 'corn', 'maize'], 'def': 'ears of corn that can be prepared and served for human food', 'name': 'edible_corn'}, {'frequency': 'r', 'id': 313, 'synset': 'cornbread.n.01', 'synonyms': ['cornbread'], 'def': 'bread made primarily of cornmeal', 'name': 'cornbread'}, {'frequency': 'c', 'id': 314, 'synset': 'cornet.n.01', 'synonyms': ['cornet', 'horn', 'trumpet'], 'def': 'a brass musical instrument with a narrow tube and a flared bell and many valves', 'name': 'cornet'}, {'frequency': 'c', 'id': 315, 'synset': 'cornice.n.01', 'synonyms': ['cornice', 'valance', 'valance_board', 'pelmet'], 'def': 'a decorative framework to conceal curtain fixtures at the top of a window casing', 'name': 'cornice'}, {'frequency': 'r', 'id': 316, 'synset': 'cornmeal.n.01', 'synonyms': ['cornmeal'], 'def': 'coarsely ground corn', 'name': 'cornmeal'}, {'frequency': 'r', 'id': 317, 'synset': 'corset.n.01', 'synonyms': ['corset', 'girdle'], 'def': "a woman's close-fitting foundation garment", 'name': 'corset'}, {'frequency': 'r', 'id': 318, 'synset': 'cos.n.02', 'synonyms': ['romaine_lettuce'], 'def': 'lettuce with long dark-green leaves in a loosely packed elongated head', 'name': 'romaine_lettuce'}, {'frequency': 'c', 'id': 319, 'synset': 'costume.n.04', 'synonyms': ['costume'], 'def': 'the attire characteristic of a country or a time or a social class', 'name': 'costume'}, {'frequency': 'r', 'id': 320, 'synset': 'cougar.n.01', 'synonyms': ['cougar', 'puma', 'catamount', 'mountain_lion', 'panther'], 'def': 'large American feline resembling a lion', 'name': 'cougar'}, {'frequency': 'r', 'id': 321, 'synset': 'coverall.n.01', 'synonyms': ['coverall'], 'def': 'a loose-fitting protective garment that is worn over other clothing', 'name': 'coverall'}, {'frequency': 'r', 'id': 322, 'synset': 'cowbell.n.01', 'synonyms': ['cowbell'], 'def': 'a bell hung around the neck of cow so that the cow can be easily located', 'name': 'cowbell'}, {'frequency': 'f', 'id': 323, 'synset': 'cowboy_hat.n.01', 'synonyms': ['cowboy_hat', 'ten-gallon_hat'], 'def': 'a hat with a wide brim and a soft crown; worn by American ranch hands', 'name': 'cowboy_hat'}, {'frequency': 'r', 'id': 324, 'synset': 'crab.n.01', 'synonyms': ['crab_(animal)'], 'def': 'decapod having eyes on short stalks and a broad flattened shell and pincers', 'name': 'crab_(animal)'}, {'frequency': 'c', 'id': 325, 'synset': 'cracker.n.01', 'synonyms': ['cracker'], 'def': 'a thin crisp wafer', 'name': 'cracker'}, {'frequency': 'r', 'id': 326, 'synset': 'crape.n.01', 'synonyms': ['crape', 'crepe', 'French_pancake'], 'def': 'small very thin pancake', 'name': 'crape'}, {'frequency': 'f', 'id': 327, 'synset': 'crate.n.01', 'synonyms': ['crate'], 'def': 'a rugged box (usually made of wood); used for shipping', 'name': 'crate'}, {'frequency': 'r', 'id': 328, 'synset': 'crayon.n.01', 'synonyms': ['crayon', 'wax_crayon'], 'def': 'writing or drawing implement made of a colored stick of composition wax', 'name': 'crayon'}, {'frequency': 'r', 'id': 329, 'synset': 'cream_pitcher.n.01', 'synonyms': ['cream_pitcher'], 'def': 'a small pitcher for serving cream', 'name': 'cream_pitcher'}, {'frequency': 'r', 'id': 330, 'synset': 'credit_card.n.01', 'synonyms': ['credit_card', 'charge_card', 'debit_card'], 'def': 'a card, usually plastic, used to pay for goods and services', 'name': 'credit_card'}, {'frequency': 'c', 'id': 331, 'synset': 'crescent_roll.n.01', 'synonyms': ['crescent_roll', 'croissant'], 'def': 'very rich flaky crescent-shaped roll', 'name': 'crescent_roll'}, {'frequency': 'c', 'id': 332, 'synset': 'crib.n.01', 'synonyms': ['crib', 'cot'], 'def': 'baby bed with high sides made of slats', 'name': 'crib'}, {'frequency': 'c', 'id': 333, 'synset': 'crock.n.03', 'synonyms': ['crock_pot', 'earthenware_jar'], 'def': 'an earthen jar (made of baked clay)', 'name': 'crock_pot'}, {'frequency': 'f', 'id': 334, 'synset': 'crossbar.n.01', 'synonyms': ['crossbar'], 'def': 'a horizontal bar that goes across something', 'name': 'crossbar'}, {'frequency': 'r', 'id': 335, 'synset': 'crouton.n.01', 'synonyms': ['crouton'], 'def': 'a small piece of toasted or fried bread; served in soup or salads', 'name': 'crouton'}, {'frequency': 'r', 'id': 336, 'synset': 'crow.n.01', 'synonyms': ['crow'], 'def': 'black birds having a raucous call', 'name': 'crow'}, {'frequency': 'c', 'id': 337, 'synset': 'crown.n.04', 'synonyms': ['crown'], 'def': 'an ornamental jeweled headdress signifying sovereignty', 'name': 'crown'}, {'frequency': 'c', 'id': 338, 'synset': 'crucifix.n.01', 'synonyms': ['crucifix'], 'def': 'representation of the cross on which Jesus died', 'name': 'crucifix'}, {'frequency': 'c', 'id': 339, 'synset': 'cruise_ship.n.01', 'synonyms': ['cruise_ship', 'cruise_liner'], 'def': 'a passenger ship used commercially for pleasure cruises', 'name': 'cruise_ship'}, {'frequency': 'c', 'id': 340, 'synset': 'cruiser.n.01', 'synonyms': ['police_cruiser', 'patrol_car', 'police_car', 'squad_car'], 'def': 'a car in which policemen cruise the streets', 'name': 'police_cruiser'}, {'frequency': 'c', 'id': 341, 'synset': 'crumb.n.03', 'synonyms': ['crumb'], 'def': 'small piece of e.g. bread or cake', 'name': 'crumb'}, {'frequency': 'r', 'id': 342, 'synset': 'crutch.n.01', 'synonyms': ['crutch'], 'def': 'a wooden or metal staff that fits under the armpit and reaches to the ground', 'name': 'crutch'}, {'frequency': 'c', 'id': 343, 'synset': 'cub.n.03', 'synonyms': ['cub_(animal)'], 'def': 'the young of certain carnivorous mammals such as the bear or wolf or lion', 'name': 'cub_(animal)'}, {'frequency': 'r', 'id': 344, 'synset': 'cube.n.05', 'synonyms': ['cube', 'square_block'], 'def': 'a block in the (approximate) shape of a cube', 'name': 'cube'}, {'frequency': 'f', 'id': 345, 'synset': 'cucumber.n.02', 'synonyms': ['cucumber', 'cuke'], 'def': 'cylindrical green fruit with thin green rind and white flesh eaten as a vegetable', 'name': 'cucumber'}, {'frequency': 'c', 'id': 346, 'synset': 'cufflink.n.01', 'synonyms': ['cufflink'], 'def': 'jewelry consisting of linked buttons used to fasten the cuffs of a shirt', 'name': 'cufflink'}, {'frequency': 'f', 'id': 347, 'synset': 'cup.n.01', 'synonyms': ['cup'], 'def': 'a small open container usually used for drinking; usually has a handle', 'name': 'cup'}, {'frequency': 'c', 'id': 348, 'synset': 'cup.n.08', 'synonyms': ['trophy_cup'], 'def': 'a metal vessel with handles that is awarded as a trophy to a competition winner', 'name': 'trophy_cup'}, {'frequency': 'c', 'id': 349, 'synset': 'cupcake.n.01', 'synonyms': ['cupcake'], 'def': 'small cake baked in a muffin tin', 'name': 'cupcake'}, {'frequency': 'r', 'id': 350, 'synset': 'curler.n.01', 'synonyms': ['hair_curler', 'hair_roller', 'hair_crimper'], 'def': 'a cylindrical tube around which the hair is wound to curl it', 'name': 'hair_curler'}, {'frequency': 'r', 'id': 351, 'synset': 'curling_iron.n.01', 'synonyms': ['curling_iron'], 'def': 'a cylindrical home appliance that heats hair that has been curled around it', 'name': 'curling_iron'}, {'frequency': 'f', 'id': 352, 'synset': 'curtain.n.01', 'synonyms': ['curtain', 'drapery'], 'def': 'hanging cloth used as a blind (especially for a window)', 'name': 'curtain'}, {'frequency': 'f', 'id': 353, 'synset': 'cushion.n.03', 'synonyms': ['cushion'], 'def': 'a soft bag filled with air or padding such as feathers or foam rubber', 'name': 'cushion'}, {'frequency': 'r', 'id': 354, 'synset': 'custard.n.01', 'synonyms': ['custard'], 'def': 'sweetened mixture of milk and eggs baked or boiled or frozen', 'name': 'custard'}, {'frequency': 'c', 'id': 355, 'synset': 'cutter.n.06', 'synonyms': ['cutting_tool'], 'def': 'a cutting implement; a tool for cutting', 'name': 'cutting_tool'}, {'frequency': 'r', 'id': 356, 'synset': 'cylinder.n.04', 'synonyms': ['cylinder'], 'def': 'a cylindrical container', 'name': 'cylinder'}, {'frequency': 'r', 'id': 357, 'synset': 'cymbal.n.01', 'synonyms': ['cymbal'], 'def': 'a percussion instrument consisting of a concave brass disk', 'name': 'cymbal'}, {'frequency': 'r', 'id': 358, 'synset': 'dachshund.n.01', 'synonyms': ['dachshund', 'dachsie', 'badger_dog'], 'def': 'small long-bodied short-legged breed of dog having a short sleek coat and long drooping ears', 'name': 'dachshund'}, {'frequency': 'r', 'id': 359, 'synset': 'dagger.n.01', 'synonyms': ['dagger'], 'def': 'a short knife with a pointed blade used for piercing or stabbing', 'name': 'dagger'}, {'frequency': 'r', 'id': 360, 'synset': 'dartboard.n.01', 'synonyms': ['dartboard'], 'def': 'a circular board of wood or cork used as the target in the game of darts', 'name': 'dartboard'}, {'frequency': 'r', 'id': 361, 'synset': 'date.n.08', 'synonyms': ['date_(fruit)'], 'def': 'sweet edible fruit of the date palm with a single long woody seed', 'name': 'date_(fruit)'}, {'frequency': 'f', 'id': 362, 'synset': 'deck_chair.n.01', 'synonyms': ['deck_chair', 'beach_chair'], 'def': 'a folding chair for use outdoors; a wooden frame supports a length of canvas', 'name': 'deck_chair'}, {'frequency': 'c', 'id': 363, 'synset': 'deer.n.01', 'synonyms': ['deer', 'cervid'], 'def': "distinguished from Bovidae by the male's having solid deciduous antlers", 'name': 'deer'}, {'frequency': 'c', 'id': 364, 'synset': 'dental_floss.n.01', 'synonyms': ['dental_floss', 'floss'], 'def': 'a soft thread for cleaning the spaces between the teeth', 'name': 'dental_floss'}, {'frequency': 'f', 'id': 365, 'synset': 'desk.n.01', 'synonyms': ['desk'], 'def': 'a piece of furniture with a writing surface and usually drawers or other compartments', 'name': 'desk'}, {'frequency': 'r', 'id': 366, 'synset': 'detergent.n.01', 'synonyms': ['detergent'], 'def': 'a surface-active chemical widely used in industry and laundering', 'name': 'detergent'}, {'frequency': 'c', 'id': 367, 'synset': 'diaper.n.01', 'synonyms': ['diaper'], 'def': 'garment consisting of a folded cloth drawn up between the legs and fastened at the waist', 'name': 'diaper'}, {'frequency': 'r', 'id': 368, 'synset': 'diary.n.01', 'synonyms': ['diary', 'journal'], 'def': 'a daily written record of (usually personal) experiences and observations', 'name': 'diary'}, {'frequency': 'r', 'id': 369, 'synset': 'die.n.01', 'synonyms': ['die', 'dice'], 'def': 'a small cube with 1 to 6 spots on the six faces; used in gambling', 'name': 'die'}, {'frequency': 'r', 'id': 370, 'synset': 'dinghy.n.01', 'synonyms': ['dinghy', 'dory', 'rowboat'], 'def': 'a small boat of shallow draft with seats and oars with which it is propelled', 'name': 'dinghy'}, {'frequency': 'f', 'id': 371, 'synset': 'dining_table.n.01', 'synonyms': ['dining_table'], 'def': 'a table at which meals are served', 'name': 'dining_table'}, {'frequency': 'r', 'id': 372, 'synset': 'dinner_jacket.n.01', 'synonyms': ['tux', 'tuxedo'], 'def': 'semiformal evening dress for men', 'name': 'tux'}, {'frequency': 'c', 'id': 373, 'synset': 'dish.n.01', 'synonyms': ['dish'], 'def': 'a piece of dishware normally used as a container for holding or serving food', 'name': 'dish'}, {'frequency': 'c', 'id': 374, 'synset': 'dish.n.05', 'synonyms': ['dish_antenna'], 'def': 'directional antenna consisting of a parabolic reflector', 'name': 'dish_antenna'}, {'frequency': 'c', 'id': 375, 'synset': 'dishrag.n.01', 'synonyms': ['dishrag', 'dishcloth'], 'def': 'a cloth for washing dishes', 'name': 'dishrag'}, {'frequency': 'c', 'id': 376, 'synset': 'dishtowel.n.01', 'synonyms': ['dishtowel', 'tea_towel'], 'def': 'a towel for drying dishes', 'name': 'dishtowel'}, {'frequency': 'f', 'id': 377, 'synset': 'dishwasher.n.01', 'synonyms': ['dishwasher', 'dishwashing_machine'], 'def': 'a machine for washing dishes', 'name': 'dishwasher'}, {'frequency': 'r', 'id': 378, 'synset': 'dishwasher_detergent.n.01', 'synonyms': ['dishwasher_detergent', 'dishwashing_detergent', 'dishwashing_liquid'], 'def': 'a low-sudsing detergent designed for use in dishwashers', 'name': 'dishwasher_detergent'}, {'frequency': 'r', 'id': 379, 'synset': 'diskette.n.01', 'synonyms': ['diskette', 'floppy', 'floppy_disk'], 'def': 'a small plastic magnetic disk enclosed in a stiff envelope used to store data', 'name': 'diskette'}, {'frequency': 'c', 'id': 380, 'synset': 'dispenser.n.01', 'synonyms': ['dispenser'], 'def': 'a container so designed that the contents can be used in prescribed amounts', 'name': 'dispenser'}, {'frequency': 'c', 'id': 381, 'synset': 'dixie_cup.n.01', 'synonyms': ['Dixie_cup', 'paper_cup'], 'def': 'a disposable cup made of paper; for holding drinks', 'name': 'Dixie_cup'}, {'frequency': 'f', 'id': 382, 'synset': 'dog.n.01', 'synonyms': ['dog'], 'def': 'a common domesticated dog', 'name': 'dog'}, {'frequency': 'f', 'id': 383, 'synset': 'dog_collar.n.01', 'synonyms': ['dog_collar'], 'def': 'a collar for a dog', 'name': 'dog_collar'}, {'frequency': 'c', 'id': 384, 'synset': 'doll.n.01', 'synonyms': ['doll'], 'def': 'a toy replica of a HUMAN (NOT AN ANIMAL)', 'name': 'doll'}, {'frequency': 'r', 'id': 385, 'synset': 'dollar.n.02', 'synonyms': ['dollar', 'dollar_bill', 'one_dollar_bill'], 'def': 'a piece of paper money worth one dollar', 'name': 'dollar'}, {'frequency': 'r', 'id': 386, 'synset': 'dolphin.n.02', 'synonyms': ['dolphin'], 'def': 'any of various small toothed whales with a beaklike snout; larger than porpoises', 'name': 'dolphin'}, {'frequency': 'c', 'id': 387, 'synset': 'domestic_ass.n.01', 'synonyms': ['domestic_ass', 'donkey'], 'def': 'domestic beast of burden descended from the African wild ass; patient but stubborn', 'name': 'domestic_ass'}, {'frequency': 'r', 'id': 388, 'synset': 'domino.n.03', 'synonyms': ['eye_mask'], 'def': 'a mask covering the upper part of the face but with holes for the eyes', 'name': 'eye_mask'}, {'frequency': 'r', 'id': 389, 'synset': 'doorbell.n.01', 'synonyms': ['doorbell', 'buzzer'], 'def': 'a button at an outer door that gives a ringing or buzzing signal when pushed', 'name': 'doorbell'}, {'frequency': 'f', 'id': 390, 'synset': 'doorknob.n.01', 'synonyms': ['doorknob', 'doorhandle'], 'def': "a knob used to open a door (often called `doorhandle' in Great Britain)", 'name': 'doorknob'}, {'frequency': 'c', 'id': 391, 'synset': 'doormat.n.02', 'synonyms': ['doormat', 'welcome_mat'], 'def': 'a mat placed outside an exterior door for wiping the shoes before entering', 'name': 'doormat'}, {'frequency': 'f', 'id': 392, 'synset': 'doughnut.n.02', 'synonyms': ['doughnut', 'donut'], 'def': 'a small ring-shaped friedcake', 'name': 'doughnut'}, {'frequency': 'r', 'id': 393, 'synset': 'dove.n.01', 'synonyms': ['dove'], 'def': 'any of numerous small pigeons', 'name': 'dove'}, {'frequency': 'r', 'id': 394, 'synset': 'dragonfly.n.01', 'synonyms': ['dragonfly'], 'def': 'slender-bodied non-stinging insect having iridescent wings that are outspread at rest', 'name': 'dragonfly'}, {'frequency': 'f', 'id': 395, 'synset': 'drawer.n.01', 'synonyms': ['drawer'], 'def': 'a boxlike container in a piece of furniture; made so as to slide in and out', 'name': 'drawer'}, {'frequency': 'c', 'id': 396, 'synset': 'drawers.n.01', 'synonyms': ['underdrawers', 'boxers', 'boxershorts'], 'def': 'underpants worn by men', 'name': 'underdrawers'}, {'frequency': 'f', 'id': 397, 'synset': 'dress.n.01', 'synonyms': ['dress', 'frock'], 'def': 'a one-piece garment for a woman; has skirt and bodice', 'name': 'dress'}, {'frequency': 'c', 'id': 398, 'synset': 'dress_hat.n.01', 'synonyms': ['dress_hat', 'high_hat', 'opera_hat', 'silk_hat', 'top_hat'], 'def': "a man's hat with a tall crown; usually covered with silk or with beaver fur", 'name': 'dress_hat'}, {'frequency': 'c', 'id': 399, 'synset': 'dress_suit.n.01', 'synonyms': ['dress_suit'], 'def': 'formalwear consisting of full evening dress for men', 'name': 'dress_suit'}, {'frequency': 'c', 'id': 400, 'synset': 'dresser.n.05', 'synonyms': ['dresser'], 'def': 'a cabinet with shelves', 'name': 'dresser'}, {'frequency': 'c', 'id': 401, 'synset': 'drill.n.01', 'synonyms': ['drill'], 'def': 'a tool with a sharp rotating point for making holes in hard materials', 'name': 'drill'}, {'frequency': 'r', 'id': 402, 'synset': 'drinking_fountain.n.01', 'synonyms': ['drinking_fountain'], 'def': 'a public fountain to provide a jet of drinking water', 'name': 'drinking_fountain'}, {'frequency': 'r', 'id': 403, 'synset': 'drone.n.04', 'synonyms': ['drone'], 'def': 'an aircraft without a pilot that is operated by remote control', 'name': 'drone'}, {'frequency': 'r', 'id': 404, 'synset': 'dropper.n.01', 'synonyms': ['dropper', 'eye_dropper'], 'def': 'pipet consisting of a small tube with a vacuum bulb at one end for drawing liquid in and releasing it a drop at a time', 'name': 'dropper'}, {'frequency': 'c', 'id': 405, 'synset': 'drum.n.01', 'synonyms': ['drum_(musical_instrument)'], 'def': 'a musical percussion instrument; usually consists of a hollow cylinder with a membrane stretched across each end', 'name': 'drum_(musical_instrument)'}, {'frequency': 'r', 'id': 406, 'synset': 'drumstick.n.02', 'synonyms': ['drumstick'], 'def': 'a stick used for playing a drum', 'name': 'drumstick'}, {'frequency': 'f', 'id': 407, 'synset': 'duck.n.01', 'synonyms': ['duck'], 'def': 'small web-footed broad-billed swimming bird', 'name': 'duck'}, {'frequency': 'r', 'id': 408, 'synset': 'duckling.n.02', 'synonyms': ['duckling'], 'def': 'young duck', 'name': 'duckling'}, {'frequency': 'c', 'id': 409, 'synset': 'duct_tape.n.01', 'synonyms': ['duct_tape'], 'def': 'a wide silvery adhesive tape', 'name': 'duct_tape'}, {'frequency': 'f', 'id': 410, 'synset': 'duffel_bag.n.01', 'synonyms': ['duffel_bag', 'duffle_bag', 'duffel', 'duffle'], 'def': 'a large cylindrical bag of heavy cloth', 'name': 'duffel_bag'}, {'frequency': 'r', 'id': 411, 'synset': 'dumbbell.n.01', 'synonyms': ['dumbbell'], 'def': 'an exercising weight with two ball-like ends connected by a short handle', 'name': 'dumbbell'}, {'frequency': 'c', 'id': 412, 'synset': 'dumpster.n.01', 'synonyms': ['dumpster'], 'def': 'a container designed to receive and transport and dump waste', 'name': 'dumpster'}, {'frequency': 'r', 'id': 413, 'synset': 'dustpan.n.02', 'synonyms': ['dustpan'], 'def': 'a short-handled receptacle into which dust can be swept', 'name': 'dustpan'}, {'frequency': 'r', 'id': 414, 'synset': 'dutch_oven.n.02', 'synonyms': ['Dutch_oven'], 'def': 'iron or earthenware cooking pot; used for stews', 'name': 'Dutch_oven'}, {'frequency': 'c', 'id': 415, 'synset': 'eagle.n.01', 'synonyms': ['eagle'], 'def': 'large birds of prey noted for their broad wings and strong soaring flight', 'name': 'eagle'}, {'frequency': 'f', 'id': 416, 'synset': 'earphone.n.01', 'synonyms': ['earphone', 'earpiece', 'headphone'], 'def': 'device for listening to audio that is held over or inserted into the ear', 'name': 'earphone'}, {'frequency': 'r', 'id': 417, 'synset': 'earplug.n.01', 'synonyms': ['earplug'], 'def': 'a soft plug that is inserted into the ear canal to block sound', 'name': 'earplug'}, {'frequency': 'f', 'id': 418, 'synset': 'earring.n.01', 'synonyms': ['earring'], 'def': 'jewelry to ornament the ear', 'name': 'earring'}, {'frequency': 'c', 'id': 419, 'synset': 'easel.n.01', 'synonyms': ['easel'], 'def': "an upright tripod for displaying something (usually an artist's canvas)", 'name': 'easel'}, {'frequency': 'r', 'id': 420, 'synset': 'eclair.n.01', 'synonyms': ['eclair'], 'def': 'oblong cream puff', 'name': 'eclair'}, {'frequency': 'r', 'id': 421, 'synset': 'eel.n.01', 'synonyms': ['eel'], 'def': 'an elongate fish with fatty flesh', 'name': 'eel'}, {'frequency': 'f', 'id': 422, 'synset': 'egg.n.02', 'synonyms': ['egg', 'eggs'], 'def': 'oval reproductive body of a fowl (especially a hen) used as food', 'name': 'egg'}, {'frequency': 'r', 'id': 423, 'synset': 'egg_roll.n.01', 'synonyms': ['egg_roll', 'spring_roll'], 'def': 'minced vegetables and meat wrapped in a pancake and fried', 'name': 'egg_roll'}, {'frequency': 'c', 'id': 424, 'synset': 'egg_yolk.n.01', 'synonyms': ['egg_yolk', 'yolk_(egg)'], 'def': 'the yellow spherical part of an egg', 'name': 'egg_yolk'}, {'frequency': 'c', 'id': 425, 'synset': 'eggbeater.n.02', 'synonyms': ['eggbeater', 'eggwhisk'], 'def': 'a mixer for beating eggs or whipping cream', 'name': 'eggbeater'}, {'frequency': 'c', 'id': 426, 'synset': 'eggplant.n.01', 'synonyms': ['eggplant', 'aubergine'], 'def': 'egg-shaped vegetable having a shiny skin typically dark purple', 'name': 'eggplant'}, {'frequency': 'r', 'id': 427, 'synset': 'electric_chair.n.01', 'synonyms': ['electric_chair'], 'def': 'a chair-shaped instrument of execution by electrocution', 'name': 'electric_chair'}, {'frequency': 'f', 'id': 428, 'synset': 'electric_refrigerator.n.01', 'synonyms': ['refrigerator'], 'def': 'a refrigerator in which the coolant is pumped around by an electric motor', 'name': 'refrigerator'}, {'frequency': 'f', 'id': 429, 'synset': 'elephant.n.01', 'synonyms': ['elephant'], 'def': 'a common elephant', 'name': 'elephant'}, {'frequency': 'r', 'id': 430, 'synset': 'elk.n.01', 'synonyms': ['elk', 'moose'], 'def': 'large northern deer with enormous flattened antlers in the male', 'name': 'elk'}, {'frequency': 'c', 'id': 431, 'synset': 'envelope.n.01', 'synonyms': ['envelope'], 'def': 'a flat (usually rectangular) container for a letter, thin package, etc.', 'name': 'envelope'}, {'frequency': 'c', 'id': 432, 'synset': 'eraser.n.01', 'synonyms': ['eraser'], 'def': 'an implement used to erase something', 'name': 'eraser'}, {'frequency': 'r', 'id': 433, 'synset': 'escargot.n.01', 'synonyms': ['escargot'], 'def': 'edible snail usually served in the shell with a sauce of melted butter and garlic', 'name': 'escargot'}, {'frequency': 'r', 'id': 434, 'synset': 'eyepatch.n.01', 'synonyms': ['eyepatch'], 'def': 'a protective cloth covering for an injured eye', 'name': 'eyepatch'}, {'frequency': 'r', 'id': 435, 'synset': 'falcon.n.01', 'synonyms': ['falcon'], 'def': 'birds of prey having long pointed powerful wings adapted for swift flight', 'name': 'falcon'}, {'frequency': 'f', 'id': 436, 'synset': 'fan.n.01', 'synonyms': ['fan'], 'def': 'a device for creating a current of air by movement of a surface or surfaces', 'name': 'fan'}, {'frequency': 'f', 'id': 437, 'synset': 'faucet.n.01', 'synonyms': ['faucet', 'spigot', 'tap'], 'def': 'a regulator for controlling the flow of a liquid from a reservoir', 'name': 'faucet'}, {'frequency': 'r', 'id': 438, 'synset': 'fedora.n.01', 'synonyms': ['fedora'], 'def': 'a hat made of felt with a creased crown', 'name': 'fedora'}, {'frequency': 'r', 'id': 439, 'synset': 'ferret.n.02', 'synonyms': ['ferret'], 'def': 'domesticated albino variety of the European polecat bred for hunting rats and rabbits', 'name': 'ferret'}, {'frequency': 'c', 'id': 440, 'synset': 'ferris_wheel.n.01', 'synonyms': ['Ferris_wheel'], 'def': 'a large wheel with suspended seats that remain upright as the wheel rotates', 'name': 'Ferris_wheel'}, {'frequency': 'r', 'id': 441, 'synset': 'ferry.n.01', 'synonyms': ['ferry', 'ferryboat'], 'def': 'a boat that transports people or vehicles across a body of water and operates on a regular schedule', 'name': 'ferry'}, {'frequency': 'r', 'id': 442, 'synset': 'fig.n.04', 'synonyms': ['fig_(fruit)'], 'def': 'fleshy sweet pear-shaped yellowish or purple fruit eaten fresh or preserved or dried', 'name': 'fig_(fruit)'}, {'frequency': 'c', 'id': 443, 'synset': 'fighter.n.02', 'synonyms': ['fighter_jet', 'fighter_aircraft', 'attack_aircraft'], 'def': 'a high-speed military or naval airplane designed to destroy enemy targets', 'name': 'fighter_jet'}, {'frequency': 'f', 'id': 444, 'synset': 'figurine.n.01', 'synonyms': ['figurine'], 'def': 'a small carved or molded figure', 'name': 'figurine'}, {'frequency': 'c', 'id': 445, 'synset': 'file.n.03', 'synonyms': ['file_cabinet', 'filing_cabinet'], 'def': 'office furniture consisting of a container for keeping papers in order', 'name': 'file_cabinet'}, {'frequency': 'r', 'id': 446, 'synset': 'file.n.04', 'synonyms': ['file_(tool)'], 'def': 'a steel hand tool with small sharp teeth on some or all of its surfaces; used for smoothing wood or metal', 'name': 'file_(tool)'}, {'frequency': 'f', 'id': 447, 'synset': 'fire_alarm.n.02', 'synonyms': ['fire_alarm', 'smoke_alarm'], 'def': 'an alarm that is tripped off by fire or smoke', 'name': 'fire_alarm'}, {'frequency': 'c', 'id': 448, 'synset': 'fire_engine.n.01', 'synonyms': ['fire_engine', 'fire_truck'], 'def': 'large trucks that carry firefighters and equipment to the site of a fire', 'name': 'fire_engine'}, {'frequency': 'c', 'id': 449, 'synset': 'fire_extinguisher.n.01', 'synonyms': ['fire_extinguisher', 'extinguisher'], 'def': 'a manually operated device for extinguishing small fires', 'name': 'fire_extinguisher'}, {'frequency': 'c', 'id': 450, 'synset': 'fire_hose.n.01', 'synonyms': ['fire_hose'], 'def': 'a large hose that carries water from a fire hydrant to the site of the fire', 'name': 'fire_hose'}, {'frequency': 'f', 'id': 451, 'synset': 'fireplace.n.01', 'synonyms': ['fireplace'], 'def': 'an open recess in a wall at the base of a chimney where a fire can be built', 'name': 'fireplace'}, {'frequency': 'f', 'id': 452, 'synset': 'fireplug.n.01', 'synonyms': ['fireplug', 'fire_hydrant', 'hydrant'], 'def': 'an upright hydrant for drawing water to use in fighting a fire', 'name': 'fireplug'}, {'frequency': 'c', 'id': 453, 'synset': 'fish.n.01', 'synonyms': ['fish'], 'def': 'any of various mostly cold-blooded aquatic vertebrates usually having scales and breathing through gills', 'name': 'fish'}, {'frequency': 'r', 'id': 454, 'synset': 'fish.n.02', 'synonyms': ['fish_(food)'], 'def': 'the flesh of fish used as food', 'name': 'fish_(food)'}, {'frequency': 'r', 'id': 455, 'synset': 'fishbowl.n.02', 'synonyms': ['fishbowl', 'goldfish_bowl'], 'def': 'a transparent bowl in which small fish are kept', 'name': 'fishbowl'}, {'frequency': 'r', 'id': 456, 'synset': 'fishing_boat.n.01', 'synonyms': ['fishing_boat', 'fishing_vessel'], 'def': 'a vessel for fishing', 'name': 'fishing_boat'}, {'frequency': 'c', 'id': 457, 'synset': 'fishing_rod.n.01', 'synonyms': ['fishing_rod', 'fishing_pole'], 'def': 'a rod that is used in fishing to extend the fishing line', 'name': 'fishing_rod'}, {'frequency': 'f', 'id': 458, 'synset': 'flag.n.01', 'synonyms': ['flag'], 'def': 'emblem usually consisting of a rectangular piece of cloth of distinctive design (do not include pole)', 'name': 'flag'}, {'frequency': 'f', 'id': 459, 'synset': 'flagpole.n.02', 'synonyms': ['flagpole', 'flagstaff'], 'def': 'a tall staff or pole on which a flag is raised', 'name': 'flagpole'}, {'frequency': 'c', 'id': 460, 'synset': 'flamingo.n.01', 'synonyms': ['flamingo'], 'def': 'large pink web-footed bird with down-bent bill', 'name': 'flamingo'}, {'frequency': 'c', 'id': 461, 'synset': 'flannel.n.01', 'synonyms': ['flannel'], 'def': 'a soft light woolen fabric; used for clothing', 'name': 'flannel'}, {'frequency': 'r', 'id': 462, 'synset': 'flash.n.10', 'synonyms': ['flash', 'flashbulb'], 'def': 'a lamp for providing momentary light to take a photograph', 'name': 'flash'}, {'frequency': 'c', 'id': 463, 'synset': 'flashlight.n.01', 'synonyms': ['flashlight', 'torch'], 'def': 'a small portable battery-powered electric lamp', 'name': 'flashlight'}, {'frequency': 'r', 'id': 464, 'synset': 'fleece.n.03', 'synonyms': ['fleece'], 'def': 'a soft bulky fabric with deep pile; used chiefly for clothing', 'name': 'fleece'}, {'frequency': 'f', 'id': 465, 'synset': 'flip-flop.n.02', 'synonyms': ['flip-flop_(sandal)'], 'def': 'a backless sandal held to the foot by a thong between two toes', 'name': 'flip-flop_(sandal)'}, {'frequency': 'c', 'id': 466, 'synset': 'flipper.n.01', 'synonyms': ['flipper_(footwear)', 'fin_(footwear)'], 'def': 'a shoe to aid a person in swimming', 'name': 'flipper_(footwear)'}, {'frequency': 'f', 'id': 467, 'synset': 'flower_arrangement.n.01', 'synonyms': ['flower_arrangement', 'floral_arrangement'], 'def': 'a decorative arrangement of flowers', 'name': 'flower_arrangement'}, {'frequency': 'c', 'id': 468, 'synset': 'flute.n.02', 'synonyms': ['flute_glass', 'champagne_flute'], 'def': 'a tall narrow wineglass', 'name': 'flute_glass'}, {'frequency': 'r', 'id': 469, 'synset': 'foal.n.01', 'synonyms': ['foal'], 'def': 'a young horse', 'name': 'foal'}, {'frequency': 'c', 'id': 470, 'synset': 'folding_chair.n.01', 'synonyms': ['folding_chair'], 'def': 'a chair that can be folded flat for storage', 'name': 'folding_chair'}, {'frequency': 'c', 'id': 471, 'synset': 'food_processor.n.01', 'synonyms': ['food_processor'], 'def': 'a kitchen appliance for shredding, blending, chopping, or slicing food', 'name': 'food_processor'}, {'frequency': 'c', 'id': 472, 'synset': 'football.n.02', 'synonyms': ['football_(American)'], 'def': 'the inflated oblong ball used in playing American football', 'name': 'football_(American)'}, {'frequency': 'r', 'id': 473, 'synset': 'football_helmet.n.01', 'synonyms': ['football_helmet'], 'def': 'a padded helmet with a face mask to protect the head of football players', 'name': 'football_helmet'}, {'frequency': 'c', 'id': 474, 'synset': 'footstool.n.01', 'synonyms': ['footstool', 'footrest'], 'def': 'a low seat or a stool to rest the feet of a seated person', 'name': 'footstool'}, {'frequency': 'f', 'id': 475, 'synset': 'fork.n.01', 'synonyms': ['fork'], 'def': 'cutlery used for serving and eating food', 'name': 'fork'}, {'frequency': 'r', 'id': 476, 'synset': 'forklift.n.01', 'synonyms': ['forklift'], 'def': 'an industrial vehicle with a power operated fork in front that can be inserted under loads to lift and move them', 'name': 'forklift'}, {'frequency': 'r', 'id': 477, 'synset': 'freight_car.n.01', 'synonyms': ['freight_car'], 'def': 'a railway car that carries freight', 'name': 'freight_car'}, {'frequency': 'r', 'id': 478, 'synset': 'french_toast.n.01', 'synonyms': ['French_toast'], 'def': 'bread slice dipped in egg and milk and fried', 'name': 'French_toast'}, {'frequency': 'c', 'id': 479, 'synset': 'freshener.n.01', 'synonyms': ['freshener', 'air_freshener'], 'def': 'anything that freshens', 'name': 'freshener'}, {'frequency': 'f', 'id': 480, 'synset': 'frisbee.n.01', 'synonyms': ['frisbee'], 'def': 'a light, plastic disk propelled with a flip of the wrist for recreation or competition', 'name': 'frisbee'}, {'frequency': 'c', 'id': 481, 'synset': 'frog.n.01', 'synonyms': ['frog', 'toad', 'toad_frog'], 'def': 'a tailless stout-bodied amphibians with long hind limbs for leaping', 'name': 'frog'}, {'frequency': 'c', 'id': 482, 'synset': 'fruit_juice.n.01', 'synonyms': ['fruit_juice'], 'def': 'drink produced by squeezing or crushing fruit', 'name': 'fruit_juice'}, {'frequency': 'r', 'id': 483, 'synset': 'fruit_salad.n.01', 'synonyms': ['fruit_salad'], 'def': 'salad composed of fruits', 'name': 'fruit_salad'}, {'frequency': 'c', 'id': 484, 'synset': 'frying_pan.n.01', 'synonyms': ['frying_pan', 'frypan', 'skillet'], 'def': 'a pan used for frying foods', 'name': 'frying_pan'}, {'frequency': 'r', 'id': 485, 'synset': 'fudge.n.01', 'synonyms': ['fudge'], 'def': 'soft creamy candy', 'name': 'fudge'}, {'frequency': 'r', 'id': 486, 'synset': 'funnel.n.02', 'synonyms': ['funnel'], 'def': 'a cone-shaped utensil used to channel a substance into a container with a small mouth', 'name': 'funnel'}, {'frequency': 'c', 'id': 487, 'synset': 'futon.n.01', 'synonyms': ['futon'], 'def': 'a pad that is used for sleeping on the floor or on a raised frame', 'name': 'futon'}, {'frequency': 'r', 'id': 488, 'synset': 'gag.n.02', 'synonyms': ['gag', 'muzzle'], 'def': "restraint put into a person's mouth to prevent speaking or shouting", 'name': 'gag'}, {'frequency': 'r', 'id': 489, 'synset': 'garbage.n.03', 'synonyms': ['garbage'], 'def': 'a receptacle where waste can be discarded', 'name': 'garbage'}, {'frequency': 'c', 'id': 490, 'synset': 'garbage_truck.n.01', 'synonyms': ['garbage_truck'], 'def': 'a truck for collecting domestic refuse', 'name': 'garbage_truck'}, {'frequency': 'c', 'id': 491, 'synset': 'garden_hose.n.01', 'synonyms': ['garden_hose'], 'def': 'a hose used for watering a lawn or garden', 'name': 'garden_hose'}, {'frequency': 'c', 'id': 492, 'synset': 'gargle.n.01', 'synonyms': ['gargle', 'mouthwash'], 'def': 'a medicated solution used for gargling and rinsing the mouth', 'name': 'gargle'}, {'frequency': 'r', 'id': 493, 'synset': 'gargoyle.n.02', 'synonyms': ['gargoyle'], 'def': 'an ornament consisting of a grotesquely carved figure of a person or animal', 'name': 'gargoyle'}, {'frequency': 'c', 'id': 494, 'synset': 'garlic.n.02', 'synonyms': ['garlic', 'ail'], 'def': 'aromatic bulb used as seasoning', 'name': 'garlic'}, {'frequency': 'r', 'id': 495, 'synset': 'gasmask.n.01', 'synonyms': ['gasmask', 'respirator', 'gas_helmet'], 'def': 'a protective face mask with a filter', 'name': 'gasmask'}, {'frequency': 'r', 'id': 496, 'synset': 'gazelle.n.01', 'synonyms': ['gazelle'], 'def': 'small swift graceful antelope of Africa and Asia having lustrous eyes', 'name': 'gazelle'}, {'frequency': 'c', 'id': 497, 'synset': 'gelatin.n.02', 'synonyms': ['gelatin', 'jelly'], 'def': 'an edible jelly made with gelatin and used as a dessert or salad base or a coating for foods', 'name': 'gelatin'}, {'frequency': 'r', 'id': 498, 'synset': 'gem.n.02', 'synonyms': ['gemstone'], 'def': 'a crystalline rock that can be cut and polished for jewelry', 'name': 'gemstone'}, {'frequency': 'c', 'id': 499, 'synset': 'giant_panda.n.01', 'synonyms': ['giant_panda', 'panda', 'panda_bear'], 'def': 'large black-and-white herbivorous mammal of bamboo forests of China and Tibet', 'name': 'giant_panda'}, {'frequency': 'c', 'id': 500, 'synset': 'gift_wrap.n.01', 'synonyms': ['gift_wrap'], 'def': 'attractive wrapping paper suitable for wrapping gifts', 'name': 'gift_wrap'}, {'frequency': 'c', 'id': 501, 'synset': 'ginger.n.03', 'synonyms': ['ginger', 'gingerroot'], 'def': 'the root of the common ginger plant; used fresh as a seasoning', 'name': 'ginger'}, {'frequency': 'f', 'id': 502, 'synset': 'giraffe.n.01', 'synonyms': ['giraffe'], 'def': 'tall animal having a spotted coat and small horns and very long neck and legs', 'name': 'giraffe'}, {'frequency': 'c', 'id': 503, 'synset': 'girdle.n.02', 'synonyms': ['cincture', 'sash', 'waistband', 'waistcloth'], 'def': 'a band of material around the waist that strengthens a skirt or trousers', 'name': 'cincture'}, {'frequency': 'f', 'id': 504, 'synset': 'glass.n.02', 'synonyms': ['glass_(drink_container)', 'drinking_glass'], 'def': 'a container for holding liquids while drinking', 'name': 'glass_(drink_container)'}, {'frequency': 'c', 'id': 505, 'synset': 'globe.n.03', 'synonyms': ['globe'], 'def': 'a sphere on which a map (especially of the earth) is represented', 'name': 'globe'}, {'frequency': 'f', 'id': 506, 'synset': 'glove.n.02', 'synonyms': ['glove'], 'def': 'handwear covering the hand', 'name': 'glove'}, {'frequency': 'c', 'id': 507, 'synset': 'goat.n.01', 'synonyms': ['goat'], 'def': 'a common goat', 'name': 'goat'}, {'frequency': 'f', 'id': 508, 'synset': 'goggles.n.01', 'synonyms': ['goggles'], 'def': 'tight-fitting spectacles worn to protect the eyes', 'name': 'goggles'}, {'frequency': 'r', 'id': 509, 'synset': 'goldfish.n.01', 'synonyms': ['goldfish'], 'def': 'small golden or orange-red freshwater fishes used as pond or aquarium pets', 'name': 'goldfish'}, {'frequency': 'r', 'id': 510, 'synset': 'golf_club.n.02', 'synonyms': ['golf_club', 'golf-club'], 'def': 'golf equipment used by a golfer to hit a golf ball', 'name': 'golf_club'}, {'frequency': 'c', 'id': 511, 'synset': 'golfcart.n.01', 'synonyms': ['golfcart'], 'def': 'a small motor vehicle in which golfers can ride between shots', 'name': 'golfcart'}, {'frequency': 'r', 'id': 512, 'synset': 'gondola.n.02', 'synonyms': ['gondola_(boat)'], 'def': 'long narrow flat-bottomed boat propelled by sculling; traditionally used on canals of Venice', 'name': 'gondola_(boat)'}, {'frequency': 'c', 'id': 513, 'synset': 'goose.n.01', 'synonyms': ['goose'], 'def': 'loud, web-footed long-necked aquatic birds usually larger than ducks', 'name': 'goose'}, {'frequency': 'r', 'id': 514, 'synset': 'gorilla.n.01', 'synonyms': ['gorilla'], 'def': 'largest ape', 'name': 'gorilla'}, {'frequency': 'r', 'id': 515, 'synset': 'gourd.n.02', 'synonyms': ['gourd'], 'def': 'any of numerous inedible fruits with hard rinds', 'name': 'gourd'}, {'frequency': 'r', 'id': 516, 'synset': 'gown.n.04', 'synonyms': ['surgical_gown', 'scrubs_(surgical_clothing)'], 'def': 'protective garment worn by surgeons during operations', 'name': 'surgical_gown'}, {'frequency': 'f', 'id': 517, 'synset': 'grape.n.01', 'synonyms': ['grape'], 'def': 'any of various juicy fruit with green or purple skins; grow in clusters', 'name': 'grape'}, {'frequency': 'r', 'id': 518, 'synset': 'grasshopper.n.01', 'synonyms': ['grasshopper'], 'def': 'plant-eating insect with hind legs adapted for leaping', 'name': 'grasshopper'}, {'frequency': 'c', 'id': 519, 'synset': 'grater.n.01', 'synonyms': ['grater'], 'def': 'utensil with sharp perforations for shredding foods (as vegetables or cheese)', 'name': 'grater'}, {'frequency': 'c', 'id': 520, 'synset': 'gravestone.n.01', 'synonyms': ['gravestone', 'headstone', 'tombstone'], 'def': 'a stone that is used to mark a grave', 'name': 'gravestone'}, {'frequency': 'r', 'id': 521, 'synset': 'gravy_boat.n.01', 'synonyms': ['gravy_boat', 'gravy_holder'], 'def': 'a dish (often boat-shaped) for serving gravy or sauce', 'name': 'gravy_boat'}, {'frequency': 'c', 'id': 522, 'synset': 'green_bean.n.02', 'synonyms': ['green_bean'], 'def': 'a common bean plant cultivated for its slender green edible pods', 'name': 'green_bean'}, {'frequency': 'c', 'id': 523, 'synset': 'green_onion.n.01', 'synonyms': ['green_onion', 'spring_onion', 'scallion'], 'def': 'a young onion before the bulb has enlarged', 'name': 'green_onion'}, {'frequency': 'r', 'id': 524, 'synset': 'griddle.n.01', 'synonyms': ['griddle'], 'def': 'cooking utensil consisting of a flat heated surface on which food is cooked', 'name': 'griddle'}, {'frequency': 'r', 'id': 525, 'synset': 'grillroom.n.01', 'synonyms': ['grillroom', 'grill_(restaurant)'], 'def': 'a restaurant where food is cooked on a grill', 'name': 'grillroom'}, {'frequency': 'r', 'id': 526, 'synset': 'grinder.n.04', 'synonyms': ['grinder_(tool)'], 'def': 'a machine tool that polishes metal', 'name': 'grinder_(tool)'}, {'frequency': 'r', 'id': 527, 'synset': 'grits.n.01', 'synonyms': ['grits', 'hominy_grits'], 'def': 'coarsely ground corn boiled as a breakfast dish', 'name': 'grits'}, {'frequency': 'c', 'id': 528, 'synset': 'grizzly.n.01', 'synonyms': ['grizzly', 'grizzly_bear'], 'def': 'powerful brownish-yellow bear of the uplands of western North America', 'name': 'grizzly'}, {'frequency': 'c', 'id': 529, 'synset': 'grocery_bag.n.01', 'synonyms': ['grocery_bag'], 'def': "a sack for holding customer's groceries", 'name': 'grocery_bag'}, {'frequency': 'r', 'id': 530, 'synset': 'guacamole.n.01', 'synonyms': ['guacamole'], 'def': 'a dip made of mashed avocado mixed with chopped onions and other seasonings', 'name': 'guacamole'}, {'frequency': 'f', 'id': 531, 'synset': 'guitar.n.01', 'synonyms': ['guitar'], 'def': 'a stringed instrument usually having six strings; played by strumming or plucking', 'name': 'guitar'}, {'frequency': 'c', 'id': 532, 'synset': 'gull.n.02', 'synonyms': ['gull', 'seagull'], 'def': 'mostly white aquatic bird having long pointed wings and short legs', 'name': 'gull'}, {'frequency': 'c', 'id': 533, 'synset': 'gun.n.01', 'synonyms': ['gun'], 'def': 'a weapon that discharges a bullet at high velocity from a metal tube', 'name': 'gun'}, {'frequency': 'r', 'id': 534, 'synset': 'hair_spray.n.01', 'synonyms': ['hair_spray'], 'def': 'substance sprayed on the hair to hold it in place', 'name': 'hair_spray'}, {'frequency': 'c', 'id': 535, 'synset': 'hairbrush.n.01', 'synonyms': ['hairbrush'], 'def': "a brush used to groom a person's hair", 'name': 'hairbrush'}, {'frequency': 'c', 'id': 536, 'synset': 'hairnet.n.01', 'synonyms': ['hairnet'], 'def': 'a small net that someone wears over their hair to keep it in place', 'name': 'hairnet'}, {'frequency': 'c', 'id': 537, 'synset': 'hairpin.n.01', 'synonyms': ['hairpin'], 'def': "a double pronged pin used to hold women's hair in place", 'name': 'hairpin'}, {'frequency': 'f', 'id': 538, 'synset': 'ham.n.01', 'synonyms': ['ham', 'jambon', 'gammon'], 'def': 'meat cut from the thigh of a hog (usually smoked)', 'name': 'ham'}, {'frequency': 'c', 'id': 539, 'synset': 'hamburger.n.01', 'synonyms': ['hamburger', 'beefburger', 'burger'], 'def': 'a sandwich consisting of a patty of minced beef served on a bun', 'name': 'hamburger'}, {'frequency': 'c', 'id': 540, 'synset': 'hammer.n.02', 'synonyms': ['hammer'], 'def': 'a hand tool with a heavy head and a handle; used to deliver an impulsive force by striking', 'name': 'hammer'}, {'frequency': 'r', 'id': 541, 'synset': 'hammock.n.02', 'synonyms': ['hammock'], 'def': 'a hanging bed of canvas or rope netting (usually suspended between two trees)', 'name': 'hammock'}, {'frequency': 'r', 'id': 542, 'synset': 'hamper.n.02', 'synonyms': ['hamper'], 'def': 'a basket usually with a cover', 'name': 'hamper'}, {'frequency': 'r', 'id': 543, 'synset': 'hamster.n.01', 'synonyms': ['hamster'], 'def': 'short-tailed burrowing rodent with large cheek pouches', 'name': 'hamster'}, {'frequency': 'c', 'id': 544, 'synset': 'hand_blower.n.01', 'synonyms': ['hair_dryer'], 'def': 'a hand-held electric blower that can blow warm air onto the hair', 'name': 'hair_dryer'}, {'frequency': 'r', 'id': 545, 'synset': 'hand_glass.n.01', 'synonyms': ['hand_glass', 'hand_mirror'], 'def': 'a mirror intended to be held in the hand', 'name': 'hand_glass'}, {'frequency': 'f', 'id': 546, 'synset': 'hand_towel.n.01', 'synonyms': ['hand_towel', 'face_towel'], 'def': 'a small towel used to dry the hands or face', 'name': 'hand_towel'}, {'frequency': 'c', 'id': 547, 'synset': 'handcart.n.01', 'synonyms': ['handcart', 'pushcart', 'hand_truck'], 'def': 'wheeled vehicle that can be pushed by a person', 'name': 'handcart'}, {'frequency': 'r', 'id': 548, 'synset': 'handcuff.n.01', 'synonyms': ['handcuff'], 'def': 'shackle that consists of a metal loop that can be locked around the wrist', 'name': 'handcuff'}, {'frequency': 'c', 'id': 549, 'synset': 'handkerchief.n.01', 'synonyms': ['handkerchief'], 'def': 'a square piece of cloth used for wiping the eyes or nose or as a costume accessory', 'name': 'handkerchief'}, {'frequency': 'f', 'id': 550, 'synset': 'handle.n.01', 'synonyms': ['handle', 'grip', 'handgrip'], 'def': 'the appendage to an object that is designed to be held in order to use or move it', 'name': 'handle'}, {'frequency': 'r', 'id': 551, 'synset': 'handsaw.n.01', 'synonyms': ['handsaw', "carpenter's_saw"], 'def': 'a saw used with one hand for cutting wood', 'name': 'handsaw'}, {'frequency': 'r', 'id': 552, 'synset': 'hardback.n.01', 'synonyms': ['hardback_book', 'hardcover_book'], 'def': 'a book with cardboard or cloth or leather covers', 'name': 'hardback_book'}, {'frequency': 'r', 'id': 553, 'synset': 'harmonium.n.01', 'synonyms': ['harmonium', 'organ_(musical_instrument)', 'reed_organ_(musical_instrument)'], 'def': 'a free-reed instrument in which air is forced through the reeds by bellows', 'name': 'harmonium'}, {'frequency': 'f', 'id': 554, 'synset': 'hat.n.01', 'synonyms': ['hat'], 'def': 'headwear that protects the head from bad weather, sun, or worn for fashion', 'name': 'hat'}, {'frequency': 'r', 'id': 555, 'synset': 'hatbox.n.01', 'synonyms': ['hatbox'], 'def': 'a round piece of luggage for carrying hats', 'name': 'hatbox'}, {'frequency': 'r', 'id': 556, 'synset': 'hatch.n.03', 'synonyms': ['hatch'], 'def': 'a movable barrier covering a hatchway', 'name': 'hatch'}, {'frequency': 'c', 'id': 557, 'synset': 'head_covering.n.01', 'synonyms': ['veil'], 'def': 'a garment that covers the head and face', 'name': 'veil'}, {'frequency': 'f', 'id': 558, 'synset': 'headband.n.01', 'synonyms': ['headband'], 'def': 'a band worn around or over the head', 'name': 'headband'}, {'frequency': 'f', 'id': 559, 'synset': 'headboard.n.01', 'synonyms': ['headboard'], 'def': 'a vertical board or panel forming the head of a bedstead', 'name': 'headboard'}, {'frequency': 'f', 'id': 560, 'synset': 'headlight.n.01', 'synonyms': ['headlight', 'headlamp'], 'def': 'a powerful light with reflector; attached to the front of an automobile or locomotive', 'name': 'headlight'}, {'frequency': 'c', 'id': 561, 'synset': 'headscarf.n.01', 'synonyms': ['headscarf'], 'def': 'a kerchief worn over the head and tied under the chin', 'name': 'headscarf'}, {'frequency': 'r', 'id': 562, 'synset': 'headset.n.01', 'synonyms': ['headset'], 'def': 'receiver consisting of a pair of headphones', 'name': 'headset'}, {'frequency': 'c', 'id': 563, 'synset': 'headstall.n.01', 'synonyms': ['headstall_(for_horses)', 'headpiece_(for_horses)'], 'def': "the band that is the part of a bridle that fits around a horse's head", 'name': 'headstall_(for_horses)'}, {'frequency': 'r', 'id': 564, 'synset': 'hearing_aid.n.02', 'synonyms': ['hearing_aid'], 'def': 'an acoustic device used to direct sound to the ear of a hearing-impaired person', 'name': 'hearing_aid'}, {'frequency': 'c', 'id': 565, 'synset': 'heart.n.02', 'synonyms': ['heart'], 'def': 'a muscular organ; its contractions move the blood through the body', 'name': 'heart'}, {'frequency': 'c', 'id': 566, 'synset': 'heater.n.01', 'synonyms': ['heater', 'warmer'], 'def': 'device that heats water or supplies warmth to a room', 'name': 'heater'}, {'frequency': 'c', 'id': 567, 'synset': 'helicopter.n.01', 'synonyms': ['helicopter'], 'def': 'an aircraft without wings that obtains its lift from the rotation of overhead blades', 'name': 'helicopter'}, {'frequency': 'f', 'id': 568, 'synset': 'helmet.n.02', 'synonyms': ['helmet'], 'def': 'a protective headgear made of hard material to resist blows', 'name': 'helmet'}, {'frequency': 'r', 'id': 569, 'synset': 'heron.n.02', 'synonyms': ['heron'], 'def': 'grey or white wading bird with long neck and long legs and (usually) long bill', 'name': 'heron'}, {'frequency': 'c', 'id': 570, 'synset': 'highchair.n.01', 'synonyms': ['highchair', 'feeding_chair'], 'def': 'a chair for feeding a very young child', 'name': 'highchair'}, {'frequency': 'f', 'id': 571, 'synset': 'hinge.n.01', 'synonyms': ['hinge'], 'def': 'a joint that holds two parts together so that one can swing relative to the other', 'name': 'hinge'}, {'frequency': 'r', 'id': 572, 'synset': 'hippopotamus.n.01', 'synonyms': ['hippopotamus'], 'def': 'massive thick-skinned animal living in or around rivers of tropical Africa', 'name': 'hippopotamus'}, {'frequency': 'r', 'id': 573, 'synset': 'hockey_stick.n.01', 'synonyms': ['hockey_stick'], 'def': 'sports implement consisting of a stick used by hockey players to move the puck', 'name': 'hockey_stick'}, {'frequency': 'c', 'id': 574, 'synset': 'hog.n.03', 'synonyms': ['hog', 'pig'], 'def': 'domestic swine', 'name': 'hog'}, {'frequency': 'f', 'id': 575, 'synset': 'home_plate.n.01', 'synonyms': ['home_plate_(baseball)', 'home_base_(baseball)'], 'def': '(baseball) a rubber slab where the batter stands; it must be touched by a base runner in order to score', 'name': 'home_plate_(baseball)'}, {'frequency': 'c', 'id': 576, 'synset': 'honey.n.01', 'synonyms': ['honey'], 'def': 'a sweet yellow liquid produced by bees', 'name': 'honey'}, {'frequency': 'f', 'id': 577, 'synset': 'hood.n.06', 'synonyms': ['fume_hood', 'exhaust_hood'], 'def': 'metal covering leading to a vent that exhausts smoke or fumes', 'name': 'fume_hood'}, {'frequency': 'f', 'id': 578, 'synset': 'hook.n.05', 'synonyms': ['hook'], 'def': 'a curved or bent implement for suspending or pulling something', 'name': 'hook'}, {'frequency': 'f', 'id': 579, 'synset': 'horse.n.01', 'synonyms': ['horse'], 'def': 'a common horse', 'name': 'horse'}, {'frequency': 'f', 'id': 580, 'synset': 'hose.n.03', 'synonyms': ['hose', 'hosepipe'], 'def': 'a flexible pipe for conveying a liquid or gas', 'name': 'hose'}, {'frequency': 'r', 'id': 581, 'synset': 'hot-air_balloon.n.01', 'synonyms': ['hot-air_balloon'], 'def': 'balloon for travel through the air in a basket suspended below a large bag of heated air', 'name': 'hot-air_balloon'}, {'frequency': 'r', 'id': 582, 'synset': 'hot_plate.n.01', 'synonyms': ['hotplate'], 'def': 'a portable electric appliance for heating or cooking or keeping food warm', 'name': 'hotplate'}, {'frequency': 'c', 'id': 583, 'synset': 'hot_sauce.n.01', 'synonyms': ['hot_sauce'], 'def': 'a pungent peppery sauce', 'name': 'hot_sauce'}, {'frequency': 'r', 'id': 584, 'synset': 'hourglass.n.01', 'synonyms': ['hourglass'], 'def': 'a sandglass timer that runs for sixty minutes', 'name': 'hourglass'}, {'frequency': 'r', 'id': 585, 'synset': 'houseboat.n.01', 'synonyms': ['houseboat'], 'def': 'a barge that is designed and equipped for use as a dwelling', 'name': 'houseboat'}, {'frequency': 'r', 'id': 586, 'synset': 'hummingbird.n.01', 'synonyms': ['hummingbird'], 'def': 'tiny American bird having brilliant iridescent plumage and long slender bills', 'name': 'hummingbird'}, {'frequency': 'r', 'id': 587, 'synset': 'hummus.n.01', 'synonyms': ['hummus', 'humus', 'hommos', 'hoummos', 'humous'], 'def': 'a thick spread made from mashed chickpeas', 'name': 'hummus'}, {'frequency': 'c', 'id': 588, 'synset': 'ice_bear.n.01', 'synonyms': ['polar_bear'], 'def': 'white bear of Arctic regions', 'name': 'polar_bear'}, {'frequency': 'c', 'id': 589, 'synset': 'ice_cream.n.01', 'synonyms': ['icecream'], 'def': 'frozen dessert containing cream and sugar and flavoring', 'name': 'icecream'}, {'frequency': 'r', 'id': 590, 'synset': 'ice_lolly.n.01', 'synonyms': ['popsicle'], 'def': 'ice cream or water ice on a small wooden stick', 'name': 'popsicle'}, {'frequency': 'c', 'id': 591, 'synset': 'ice_maker.n.01', 'synonyms': ['ice_maker'], 'def': 'an appliance included in some electric refrigerators for making ice cubes', 'name': 'ice_maker'}, {'frequency': 'r', 'id': 592, 'synset': 'ice_pack.n.01', 'synonyms': ['ice_pack', 'ice_bag'], 'def': 'a waterproof bag filled with ice: applied to the body (especially the head) to cool or reduce swelling', 'name': 'ice_pack'}, {'frequency': 'r', 'id': 593, 'synset': 'ice_skate.n.01', 'synonyms': ['ice_skate'], 'def': 'skate consisting of a boot with a steel blade fitted to the sole', 'name': 'ice_skate'}, {'frequency': 'r', 'id': 594, 'synset': 'ice_tea.n.01', 'synonyms': ['ice_tea', 'iced_tea'], 'def': 'strong tea served over ice', 'name': 'ice_tea'}, {'frequency': 'c', 'id': 595, 'synset': 'igniter.n.01', 'synonyms': ['igniter', 'ignitor', 'lighter'], 'def': 'a substance or device used to start a fire', 'name': 'igniter'}, {'frequency': 'r', 'id': 596, 'synset': 'incense.n.01', 'synonyms': ['incense'], 'def': 'a substance that produces a fragrant odor when burned', 'name': 'incense'}, {'frequency': 'r', 'id': 597, 'synset': 'inhaler.n.01', 'synonyms': ['inhaler', 'inhalator'], 'def': 'a dispenser that produces a chemical vapor to be inhaled through mouth or nose', 'name': 'inhaler'}, {'frequency': 'c', 'id': 598, 'synset': 'ipod.n.01', 'synonyms': ['iPod'], 'def': 'a pocket-sized device used to play music files', 'name': 'iPod'}, {'frequency': 'c', 'id': 599, 'synset': 'iron.n.04', 'synonyms': ['iron_(for_clothing)', 'smoothing_iron_(for_clothing)'], 'def': 'home appliance consisting of a flat metal base that is heated and used to smooth cloth', 'name': 'iron_(for_clothing)'}, {'frequency': 'r', 'id': 600, 'synset': 'ironing_board.n.01', 'synonyms': ['ironing_board'], 'def': 'narrow padded board on collapsible supports; used for ironing clothes', 'name': 'ironing_board'}, {'frequency': 'f', 'id': 601, 'synset': 'jacket.n.01', 'synonyms': ['jacket'], 'def': 'a waist-length coat', 'name': 'jacket'}, {'frequency': 'r', 'id': 602, 'synset': 'jam.n.01', 'synonyms': ['jam'], 'def': 'preserve of crushed fruit', 'name': 'jam'}, {'frequency': 'f', 'id': 603, 'synset': 'jean.n.01', 'synonyms': ['jean', 'blue_jean', 'denim'], 'def': '(usually plural) close-fitting trousers of heavy denim for manual work or casual wear', 'name': 'jean'}, {'frequency': 'c', 'id': 604, 'synset': 'jeep.n.01', 'synonyms': ['jeep', 'landrover'], 'def': 'a car suitable for traveling over rough terrain', 'name': 'jeep'}, {'frequency': 'r', 'id': 605, 'synset': 'jelly_bean.n.01', 'synonyms': ['jelly_bean', 'jelly_egg'], 'def': 'sugar-glazed jellied candy', 'name': 'jelly_bean'}, {'frequency': 'f', 'id': 606, 'synset': 'jersey.n.03', 'synonyms': ['jersey', 'T-shirt', 'tee_shirt'], 'def': 'a close-fitting pullover shirt', 'name': 'jersey'}, {'frequency': 'c', 'id': 607, 'synset': 'jet.n.01', 'synonyms': ['jet_plane', 'jet-propelled_plane'], 'def': 'an airplane powered by one or more jet engines', 'name': 'jet_plane'}, {'frequency': 'c', 'id': 608, 'synset': 'jewelry.n.01', 'synonyms': ['jewelry', 'jewellery'], 'def': 'an adornment (as a bracelet or ring or necklace) made of precious metals and set with gems (or imitation gems)', 'name': 'jewelry'}, {'frequency': 'r', 'id': 609, 'synset': 'joystick.n.02', 'synonyms': ['joystick'], 'def': 'a control device for computers consisting of a vertical handle that can move freely in two directions', 'name': 'joystick'}, {'frequency': 'r', 'id': 610, 'synset': 'jump_suit.n.01', 'synonyms': ['jumpsuit'], 'def': "one-piece garment fashioned after a parachutist's uniform", 'name': 'jumpsuit'}, {'frequency': 'c', 'id': 611, 'synset': 'kayak.n.01', 'synonyms': ['kayak'], 'def': 'a small canoe consisting of a light frame made watertight with animal skins', 'name': 'kayak'}, {'frequency': 'r', 'id': 612, 'synset': 'keg.n.02', 'synonyms': ['keg'], 'def': 'small cask or barrel', 'name': 'keg'}, {'frequency': 'r', 'id': 613, 'synset': 'kennel.n.01', 'synonyms': ['kennel', 'doghouse'], 'def': 'outbuilding that serves as a shelter for a dog', 'name': 'kennel'}, {'frequency': 'c', 'id': 614, 'synset': 'kettle.n.01', 'synonyms': ['kettle', 'boiler'], 'def': 'a metal pot for stewing or boiling; usually has a lid', 'name': 'kettle'}, {'frequency': 'f', 'id': 615, 'synset': 'key.n.01', 'synonyms': ['key'], 'def': 'metal instrument used to unlock a lock', 'name': 'key'}, {'frequency': 'r', 'id': 616, 'synset': 'keycard.n.01', 'synonyms': ['keycard'], 'def': 'a plastic card used to gain access typically to a door', 'name': 'keycard'}, {'frequency': 'r', 'id': 617, 'synset': 'kilt.n.01', 'synonyms': ['kilt'], 'def': 'a knee-length pleated tartan skirt worn by men as part of the traditional dress in the Highlands of northern Scotland', 'name': 'kilt'}, {'frequency': 'c', 'id': 618, 'synset': 'kimono.n.01', 'synonyms': ['kimono'], 'def': 'a loose robe; imitated from robes originally worn by Japanese', 'name': 'kimono'}, {'frequency': 'f', 'id': 619, 'synset': 'kitchen_sink.n.01', 'synonyms': ['kitchen_sink'], 'def': 'a sink in a kitchen', 'name': 'kitchen_sink'}, {'frequency': 'c', 'id': 620, 'synset': 'kitchen_table.n.01', 'synonyms': ['kitchen_table'], 'def': 'a table in the kitchen', 'name': 'kitchen_table'}, {'frequency': 'f', 'id': 621, 'synset': 'kite.n.03', 'synonyms': ['kite'], 'def': 'plaything consisting of a light frame covered with tissue paper; flown in wind at end of a string', 'name': 'kite'}, {'frequency': 'c', 'id': 622, 'synset': 'kitten.n.01', 'synonyms': ['kitten', 'kitty'], 'def': 'young domestic cat', 'name': 'kitten'}, {'frequency': 'c', 'id': 623, 'synset': 'kiwi.n.03', 'synonyms': ['kiwi_fruit'], 'def': 'fuzzy brown egg-shaped fruit with slightly tart green flesh', 'name': 'kiwi_fruit'}, {'frequency': 'f', 'id': 624, 'synset': 'knee_pad.n.01', 'synonyms': ['knee_pad'], 'def': 'protective garment consisting of a pad worn by football or baseball or hockey players', 'name': 'knee_pad'}, {'frequency': 'f', 'id': 625, 'synset': 'knife.n.01', 'synonyms': ['knife'], 'def': 'tool with a blade and point used as a cutting instrument', 'name': 'knife'}, {'frequency': 'r', 'id': 626, 'synset': 'knight.n.02', 'synonyms': ['knight_(chess_piece)', 'horse_(chess_piece)'], 'def': 'a chess game piece shaped to resemble the head of a horse', 'name': 'knight_(chess_piece)'}, {'frequency': 'r', 'id': 627, 'synset': 'knitting_needle.n.01', 'synonyms': ['knitting_needle'], 'def': 'needle consisting of a slender rod with pointed ends; usually used in pairs', 'name': 'knitting_needle'}, {'frequency': 'f', 'id': 628, 'synset': 'knob.n.02', 'synonyms': ['knob'], 'def': 'a round handle often found on a door', 'name': 'knob'}, {'frequency': 'r', 'id': 629, 'synset': 'knocker.n.05', 'synonyms': ['knocker_(on_a_door)', 'doorknocker'], 'def': 'a device (usually metal and ornamental) attached by a hinge to a door', 'name': 'knocker_(on_a_door)'}, {'frequency': 'r', 'id': 630, 'synset': 'koala.n.01', 'synonyms': ['koala', 'koala_bear'], 'def': 'sluggish tailless Australian marsupial with grey furry ears and coat', 'name': 'koala'}, {'frequency': 'r', 'id': 631, 'synset': 'lab_coat.n.01', 'synonyms': ['lab_coat', 'laboratory_coat'], 'def': 'a light coat worn to protect clothing from substances used while working in a laboratory', 'name': 'lab_coat'}, {'frequency': 'f', 'id': 632, 'synset': 'ladder.n.01', 'synonyms': ['ladder'], 'def': 'steps consisting of two parallel members connected by rungs', 'name': 'ladder'}, {'frequency': 'c', 'id': 633, 'synset': 'ladle.n.01', 'synonyms': ['ladle'], 'def': 'a spoon-shaped vessel with a long handle frequently used to transfer liquids', 'name': 'ladle'}, {'frequency': 'r', 'id': 634, 'synset': 'ladybug.n.01', 'synonyms': ['ladybug', 'ladybeetle', 'ladybird_beetle'], 'def': 'small round bright-colored and spotted beetle, typically red and black', 'name': 'ladybug'}, {'frequency': 'c', 'id': 635, 'synset': 'lamb.n.01', 'synonyms': ['lamb_(animal)'], 'def': 'young sheep', 'name': 'lamb_(animal)'}, {'frequency': 'r', 'id': 636, 'synset': 'lamb_chop.n.01', 'synonyms': ['lamb-chop', 'lambchop'], 'def': 'chop cut from a lamb', 'name': 'lamb-chop'}, {'frequency': 'f', 'id': 637, 'synset': 'lamp.n.02', 'synonyms': ['lamp'], 'def': 'a piece of furniture holding one or more electric light bulbs', 'name': 'lamp'}, {'frequency': 'f', 'id': 638, 'synset': 'lamppost.n.01', 'synonyms': ['lamppost'], 'def': 'a metal post supporting an outdoor lamp (such as a streetlight)', 'name': 'lamppost'}, {'frequency': 'f', 'id': 639, 'synset': 'lampshade.n.01', 'synonyms': ['lampshade'], 'def': 'a protective ornamental shade used to screen a light bulb from direct view', 'name': 'lampshade'}, {'frequency': 'c', 'id': 640, 'synset': 'lantern.n.01', 'synonyms': ['lantern'], 'def': 'light in a transparent protective case', 'name': 'lantern'}, {'frequency': 'f', 'id': 641, 'synset': 'lanyard.n.02', 'synonyms': ['lanyard', 'laniard'], 'def': 'a cord worn around the neck to hold a knife or whistle, etc.', 'name': 'lanyard'}, {'frequency': 'f', 'id': 642, 'synset': 'laptop.n.01', 'synonyms': ['laptop_computer', 'notebook_computer'], 'def': 'a portable computer small enough to use in your lap', 'name': 'laptop_computer'}, {'frequency': 'r', 'id': 643, 'synset': 'lasagna.n.01', 'synonyms': ['lasagna', 'lasagne'], 'def': 'baked dish of layers of lasagna pasta with sauce and cheese and meat or vegetables', 'name': 'lasagna'}, {'frequency': 'c', 'id': 644, 'synset': 'latch.n.02', 'synonyms': ['latch'], 'def': 'a bar that can be lowered or slid into a groove to fasten a door or gate', 'name': 'latch'}, {'frequency': 'r', 'id': 645, 'synset': 'lawn_mower.n.01', 'synonyms': ['lawn_mower'], 'def': 'garden tool for mowing grass on lawns', 'name': 'lawn_mower'}, {'frequency': 'r', 'id': 646, 'synset': 'leather.n.01', 'synonyms': ['leather'], 'def': 'an animal skin made smooth and flexible by removing the hair and then tanning', 'name': 'leather'}, {'frequency': 'c', 'id': 647, 'synset': 'legging.n.01', 'synonyms': ['legging_(clothing)', 'leging_(clothing)', 'leg_covering'], 'def': 'a garment covering the leg (usually extending from the knee to the ankle)', 'name': 'legging_(clothing)'}, {'frequency': 'c', 'id': 648, 'synset': 'lego.n.01', 'synonyms': ['Lego', 'Lego_set'], 'def': "a child's plastic construction set for making models from blocks", 'name': 'Lego'}, {'frequency': 'f', 'id': 649, 'synset': 'lemon.n.01', 'synonyms': ['lemon'], 'def': 'yellow oval fruit with juicy acidic flesh', 'name': 'lemon'}, {'frequency': 'r', 'id': 650, 'synset': 'lemonade.n.01', 'synonyms': ['lemonade'], 'def': 'sweetened beverage of diluted lemon juice', 'name': 'lemonade'}, {'frequency': 'f', 'id': 651, 'synset': 'lettuce.n.02', 'synonyms': ['lettuce'], 'def': 'leafy plant commonly eaten in salad or on sandwiches', 'name': 'lettuce'}, {'frequency': 'f', 'id': 652, 'synset': 'license_plate.n.01', 'synonyms': ['license_plate', 'numberplate'], 'def': "a plate mounted on the front and back of car and bearing the car's registration number", 'name': 'license_plate'}, {'frequency': 'f', 'id': 653, 'synset': 'life_buoy.n.01', 'synonyms': ['life_buoy', 'lifesaver', 'life_belt', 'life_ring'], 'def': 'a ring-shaped life preserver used to prevent drowning (NOT a life-jacket or vest)', 'name': 'life_buoy'}, {'frequency': 'f', 'id': 654, 'synset': 'life_jacket.n.01', 'synonyms': ['life_jacket', 'life_vest'], 'def': 'life preserver consisting of a sleeveless jacket of buoyant or inflatable design', 'name': 'life_jacket'}, {'frequency': 'f', 'id': 655, 'synset': 'light_bulb.n.01', 'synonyms': ['lightbulb'], 'def': 'glass bulb or tube shaped electric device that emits light (DO NOT MARK LAMPS AS A WHOLE)', 'name': 'lightbulb'}, {'frequency': 'r', 'id': 656, 'synset': 'lightning_rod.n.02', 'synonyms': ['lightning_rod', 'lightning_conductor'], 'def': 'a metallic conductor that is attached to a high point and leads to the ground', 'name': 'lightning_rod'}, {'frequency': 'c', 'id': 657, 'synset': 'lime.n.06', 'synonyms': ['lime'], 'def': 'the green acidic fruit of any of various lime trees', 'name': 'lime'}, {'frequency': 'r', 'id': 658, 'synset': 'limousine.n.01', 'synonyms': ['limousine'], 'def': 'long luxurious car; usually driven by a chauffeur', 'name': 'limousine'}, {'frequency': 'r', 'id': 659, 'synset': 'linen.n.02', 'synonyms': ['linen_paper'], 'def': 'a high-quality paper made of linen fibers or with a linen finish', 'name': 'linen_paper'}, {'frequency': 'c', 'id': 660, 'synset': 'lion.n.01', 'synonyms': ['lion'], 'def': 'large gregarious predatory cat of Africa and India', 'name': 'lion'}, {'frequency': 'c', 'id': 661, 'synset': 'lip_balm.n.01', 'synonyms': ['lip_balm'], 'def': 'a balm applied to the lips', 'name': 'lip_balm'}, {'frequency': 'c', 'id': 662, 'synset': 'lipstick.n.01', 'synonyms': ['lipstick', 'lip_rouge'], 'def': 'makeup that is used to color the lips', 'name': 'lipstick'}, {'frequency': 'r', 'id': 663, 'synset': 'liquor.n.01', 'synonyms': ['liquor', 'spirits', 'hard_liquor', 'liqueur', 'cordial'], 'def': 'an alcoholic beverage that is distilled rather than fermented', 'name': 'liquor'}, {'frequency': 'r', 'id': 664, 'synset': 'lizard.n.01', 'synonyms': ['lizard'], 'def': 'a reptile with usually two pairs of legs and a tapering tail', 'name': 'lizard'}, {'frequency': 'r', 'id': 665, 'synset': 'loafer.n.02', 'synonyms': ['Loafer_(type_of_shoe)'], 'def': 'a low leather step-in shoe', 'name': 'Loafer_(type_of_shoe)'}, {'frequency': 'f', 'id': 666, 'synset': 'log.n.01', 'synonyms': ['log'], 'def': 'a segment of the trunk of a tree when stripped of branches', 'name': 'log'}, {'frequency': 'c', 'id': 667, 'synset': 'lollipop.n.02', 'synonyms': ['lollipop'], 'def': 'hard candy on a stick', 'name': 'lollipop'}, {'frequency': 'c', 'id': 668, 'synset': 'lotion.n.01', 'synonyms': ['lotion'], 'def': 'any of various cosmetic preparations that are applied to the skin', 'name': 'lotion'}, {'frequency': 'f', 'id': 669, 'synset': 'loudspeaker.n.01', 'synonyms': ['speaker_(stero_equipment)'], 'def': 'electronic device that produces sound often as part of a stereo system', 'name': 'speaker_(stero_equipment)'}, {'frequency': 'c', 'id': 670, 'synset': 'love_seat.n.01', 'synonyms': ['loveseat'], 'def': 'small sofa that seats two people', 'name': 'loveseat'}, {'frequency': 'r', 'id': 671, 'synset': 'machine_gun.n.01', 'synonyms': ['machine_gun'], 'def': 'a rapidly firing automatic gun', 'name': 'machine_gun'}, {'frequency': 'f', 'id': 672, 'synset': 'magazine.n.02', 'synonyms': ['magazine'], 'def': 'a paperback periodic publication', 'name': 'magazine'}, {'frequency': 'f', 'id': 673, 'synset': 'magnet.n.01', 'synonyms': ['magnet'], 'def': 'a device that attracts iron and produces a magnetic field', 'name': 'magnet'}, {'frequency': 'r', 'id': 674, 'synset': 'mail_slot.n.01', 'synonyms': ['mail_slot'], 'def': 'a slot (usually in a door) through which mail can be delivered', 'name': 'mail_slot'}, {'frequency': 'c', 'id': 675, 'synset': 'mailbox.n.01', 'synonyms': ['mailbox_(at_home)', 'letter_box_(at_home)'], 'def': 'a private box for delivery of mail', 'name': 'mailbox_(at_home)'}, {'frequency': 'r', 'id': 676, 'synset': 'mallet.n.01', 'synonyms': ['mallet'], 'def': 'a sports implement with a long handle and a hammer-like head used to hit a ball', 'name': 'mallet'}, {'frequency': 'r', 'id': 677, 'synset': 'mammoth.n.01', 'synonyms': ['mammoth'], 'def': 'any of numerous extinct elephants widely distributed in the Pleistocene', 'name': 'mammoth'}, {'frequency': 'c', 'id': 678, 'synset': 'mandarin.n.05', 'synonyms': ['mandarin_orange'], 'def': 'a somewhat flat reddish-orange loose skinned citrus of China', 'name': 'mandarin_orange'}, {'frequency': 'c', 'id': 679, 'synset': 'manger.n.01', 'synonyms': ['manger', 'trough'], 'def': 'a container (usually in a barn or stable) from which cattle or horses feed', 'name': 'manger'}, {'frequency': 'f', 'id': 680, 'synset': 'manhole.n.01', 'synonyms': ['manhole'], 'def': 'a hole (usually with a flush cover) through which a person can gain access to an underground structure', 'name': 'manhole'}, {'frequency': 'c', 'id': 681, 'synset': 'map.n.01', 'synonyms': ['map'], 'def': "a diagrammatic representation of the earth's surface (or part of it)", 'name': 'map'}, {'frequency': 'c', 'id': 682, 'synset': 'marker.n.03', 'synonyms': ['marker'], 'def': 'a writing implement for making a mark', 'name': 'marker'}, {'frequency': 'r', 'id': 683, 'synset': 'martini.n.01', 'synonyms': ['martini'], 'def': 'a cocktail made of gin (or vodka) with dry vermouth', 'name': 'martini'}, {'frequency': 'r', 'id': 684, 'synset': 'mascot.n.01', 'synonyms': ['mascot'], 'def': 'a person or animal that is adopted by a team or other group as a symbolic figure', 'name': 'mascot'}, {'frequency': 'c', 'id': 685, 'synset': 'mashed_potato.n.01', 'synonyms': ['mashed_potato'], 'def': 'potato that has been peeled and boiled and then mashed', 'name': 'mashed_potato'}, {'frequency': 'r', 'id': 686, 'synset': 'masher.n.02', 'synonyms': ['masher'], 'def': 'a kitchen utensil used for mashing (e.g. potatoes)', 'name': 'masher'}, {'frequency': 'f', 'id': 687, 'synset': 'mask.n.04', 'synonyms': ['mask', 'facemask'], 'def': 'a protective covering worn over the face', 'name': 'mask'}, {'frequency': 'f', 'id': 688, 'synset': 'mast.n.01', 'synonyms': ['mast'], 'def': 'a vertical spar for supporting sails', 'name': 'mast'}, {'frequency': 'c', 'id': 689, 'synset': 'mat.n.03', 'synonyms': ['mat_(gym_equipment)', 'gym_mat'], 'def': 'sports equipment consisting of a piece of thick padding on the floor for gymnastics', 'name': 'mat_(gym_equipment)'}, {'frequency': 'r', 'id': 690, 'synset': 'matchbox.n.01', 'synonyms': ['matchbox'], 'def': 'a box for holding matches', 'name': 'matchbox'}, {'frequency': 'f', 'id': 691, 'synset': 'mattress.n.01', 'synonyms': ['mattress'], 'def': 'a thick pad filled with resilient material used as a bed or part of a bed', 'name': 'mattress'}, {'frequency': 'c', 'id': 692, 'synset': 'measuring_cup.n.01', 'synonyms': ['measuring_cup'], 'def': 'graduated cup used to measure liquid or granular ingredients', 'name': 'measuring_cup'}, {'frequency': 'c', 'id': 693, 'synset': 'measuring_stick.n.01', 'synonyms': ['measuring_stick', 'ruler_(measuring_stick)', 'measuring_rod'], 'def': 'measuring instrument having a sequence of marks at regular intervals', 'name': 'measuring_stick'}, {'frequency': 'c', 'id': 694, 'synset': 'meatball.n.01', 'synonyms': ['meatball'], 'def': 'ground meat formed into a ball and fried or simmered in broth', 'name': 'meatball'}, {'frequency': 'c', 'id': 695, 'synset': 'medicine.n.02', 'synonyms': ['medicine'], 'def': 'something that treats or prevents or alleviates the symptoms of disease', 'name': 'medicine'}, {'frequency': 'r', 'id': 696, 'synset': 'melon.n.01', 'synonyms': ['melon'], 'def': 'fruit of the gourd family having a hard rind and sweet juicy flesh', 'name': 'melon'}, {'frequency': 'f', 'id': 697, 'synset': 'microphone.n.01', 'synonyms': ['microphone'], 'def': 'device for converting sound waves into electrical energy', 'name': 'microphone'}, {'frequency': 'r', 'id': 698, 'synset': 'microscope.n.01', 'synonyms': ['microscope'], 'def': 'magnifier of the image of small objects', 'name': 'microscope'}, {'frequency': 'f', 'id': 699, 'synset': 'microwave.n.02', 'synonyms': ['microwave_oven'], 'def': 'kitchen appliance that cooks food by passing an electromagnetic wave through it', 'name': 'microwave_oven'}, {'frequency': 'r', 'id': 700, 'synset': 'milestone.n.01', 'synonyms': ['milestone', 'milepost'], 'def': 'stone post at side of a road to show distances', 'name': 'milestone'}, {'frequency': 'c', 'id': 701, 'synset': 'milk.n.01', 'synonyms': ['milk'], 'def': 'a white nutritious liquid secreted by mammals and used as food by human beings', 'name': 'milk'}, {'frequency': 'f', 'id': 702, 'synset': 'minivan.n.01', 'synonyms': ['minivan'], 'def': 'a small box-shaped passenger van', 'name': 'minivan'}, {'frequency': 'r', 'id': 703, 'synset': 'mint.n.05', 'synonyms': ['mint_candy'], 'def': 'a candy that is flavored with a mint oil', 'name': 'mint_candy'}, {'frequency': 'f', 'id': 704, 'synset': 'mirror.n.01', 'synonyms': ['mirror'], 'def': 'polished surface that forms images by reflecting light', 'name': 'mirror'}, {'frequency': 'c', 'id': 705, 'synset': 'mitten.n.01', 'synonyms': ['mitten'], 'def': 'glove that encases the thumb separately and the other four fingers together', 'name': 'mitten'}, {'frequency': 'c', 'id': 706, 'synset': 'mixer.n.04', 'synonyms': ['mixer_(kitchen_tool)', 'stand_mixer'], 'def': 'a kitchen utensil that is used for mixing foods', 'name': 'mixer_(kitchen_tool)'}, {'frequency': 'c', 'id': 707, 'synset': 'money.n.03', 'synonyms': ['money'], 'def': 'the official currency issued by a government or national bank', 'name': 'money'}, {'frequency': 'f', 'id': 708, 'synset': 'monitor.n.04', 'synonyms': ['monitor_(computer_equipment) computer_monitor'], 'def': 'a computer monitor', 'name': 'monitor_(computer_equipment) computer_monitor'}, {'frequency': 'c', 'id': 709, 'synset': 'monkey.n.01', 'synonyms': ['monkey'], 'def': 'any of various long-tailed primates', 'name': 'monkey'}, {'frequency': 'f', 'id': 710, 'synset': 'motor.n.01', 'synonyms': ['motor'], 'def': 'machine that converts other forms of energy into mechanical energy and so imparts motion', 'name': 'motor'}, {'frequency': 'f', 'id': 711, 'synset': 'motor_scooter.n.01', 'synonyms': ['motor_scooter', 'scooter'], 'def': 'a wheeled vehicle with small wheels and a low-powered engine', 'name': 'motor_scooter'}, {'frequency': 'r', 'id': 712, 'synset': 'motor_vehicle.n.01', 'synonyms': ['motor_vehicle', 'automotive_vehicle'], 'def': 'a self-propelled wheeled vehicle that does not run on rails', 'name': 'motor_vehicle'}, {'frequency': 'r', 'id': 713, 'synset': 'motorboat.n.01', 'synonyms': ['motorboat', 'powerboat'], 'def': 'a boat propelled by an internal-combustion engine', 'name': 'motorboat'}, {'frequency': 'f', 'id': 714, 'synset': 'motorcycle.n.01', 'synonyms': ['motorcycle'], 'def': 'a motor vehicle with two wheels and a strong frame', 'name': 'motorcycle'}, {'frequency': 'f', 'id': 715, 'synset': 'mound.n.01', 'synonyms': ['mound_(baseball)', "pitcher's_mound"], 'def': '(baseball) the slight elevation on which the pitcher stands', 'name': 'mound_(baseball)'}, {'frequency': 'r', 'id': 716, 'synset': 'mouse.n.01', 'synonyms': ['mouse_(animal_rodent)'], 'def': 'a small rodent with pointed snouts and small ears on elongated bodies with slender usually hairless tails', 'name': 'mouse_(animal_rodent)'}, {'frequency': 'f', 'id': 717, 'synset': 'mouse.n.04', 'synonyms': ['mouse_(computer_equipment)', 'computer_mouse'], 'def': 'a computer input device that controls an on-screen pointer', 'name': 'mouse_(computer_equipment)'}, {'frequency': 'f', 'id': 718, 'synset': 'mousepad.n.01', 'synonyms': ['mousepad'], 'def': 'a small portable pad that provides an operating surface for a computer mouse', 'name': 'mousepad'}, {'frequency': 'c', 'id': 719, 'synset': 'muffin.n.01', 'synonyms': ['muffin'], 'def': 'a sweet quick bread baked in a cup-shaped pan', 'name': 'muffin'}, {'frequency': 'f', 'id': 720, 'synset': 'mug.n.04', 'synonyms': ['mug'], 'def': 'with handle and usually cylindrical', 'name': 'mug'}, {'frequency': 'f', 'id': 721, 'synset': 'mushroom.n.02', 'synonyms': ['mushroom'], 'def': 'a common mushroom', 'name': 'mushroom'}, {'frequency': 'r', 'id': 722, 'synset': 'music_stool.n.01', 'synonyms': ['music_stool', 'piano_stool'], 'def': 'a stool for piano players; usually adjustable in height', 'name': 'music_stool'}, {'frequency': 'r', 'id': 723, 'synset': 'musical_instrument.n.01', 'synonyms': ['musical_instrument', 'instrument_(musical)'], 'def': 'any of various devices or contrivances that can be used to produce musical tones or sounds', 'name': 'musical_instrument'}, {'frequency': 'r', 'id': 724, 'synset': 'nailfile.n.01', 'synonyms': ['nailfile'], 'def': 'a small flat file for shaping the nails', 'name': 'nailfile'}, {'frequency': 'r', 'id': 725, 'synset': 'nameplate.n.01', 'synonyms': ['nameplate'], 'def': 'a plate bearing a name', 'name': 'nameplate'}, {'frequency': 'f', 'id': 726, 'synset': 'napkin.n.01', 'synonyms': ['napkin', 'table_napkin', 'serviette'], 'def': 'a small piece of table linen or paper that is used to wipe the mouth and to cover the lap in order to protect clothing', 'name': 'napkin'}, {'frequency': 'r', 'id': 727, 'synset': 'neckerchief.n.01', 'synonyms': ['neckerchief'], 'def': 'a kerchief worn around the neck', 'name': 'neckerchief'}, {'frequency': 'f', 'id': 728, 'synset': 'necklace.n.01', 'synonyms': ['necklace'], 'def': 'jewelry consisting of a cord or chain (often bearing gems) worn about the neck as an ornament', 'name': 'necklace'}, {'frequency': 'f', 'id': 729, 'synset': 'necktie.n.01', 'synonyms': ['necktie', 'tie_(necktie)'], 'def': 'neckwear consisting of a long narrow piece of material worn under a collar and tied in knot at the front', 'name': 'necktie'}, {'frequency': 'r', 'id': 730, 'synset': 'needle.n.03', 'synonyms': ['needle'], 'def': 'a sharp pointed implement (usually metal)', 'name': 'needle'}, {'frequency': 'c', 'id': 731, 'synset': 'nest.n.01', 'synonyms': ['nest'], 'def': 'a structure in which animals lay eggs or give birth to their young', 'name': 'nest'}, {'frequency': 'r', 'id': 732, 'synset': 'newsstand.n.01', 'synonyms': ['newsstand'], 'def': 'a stall where newspapers and other periodicals are sold', 'name': 'newsstand'}, {'frequency': 'c', 'id': 733, 'synset': 'nightwear.n.01', 'synonyms': ['nightshirt', 'nightwear', 'sleepwear', 'nightclothes'], 'def': 'garments designed to be worn in bed', 'name': 'nightshirt'}, {'frequency': 'r', 'id': 734, 'synset': 'nosebag.n.01', 'synonyms': ['nosebag_(for_animals)', 'feedbag'], 'def': 'a canvas bag that is used to feed an animal (such as a horse); covers the muzzle and fastens at the top of the head', 'name': 'nosebag_(for_animals)'}, {'frequency': 'r', 'id': 735, 'synset': 'noseband.n.01', 'synonyms': ['noseband_(for_animals)', 'nosepiece_(for_animals)'], 'def': "a strap that is the part of a bridle that goes over the animal's nose", 'name': 'noseband_(for_animals)'}, {'frequency': 'f', 'id': 736, 'synset': 'notebook.n.01', 'synonyms': ['notebook'], 'def': 'a book with blank pages for recording notes or memoranda', 'name': 'notebook'}, {'frequency': 'c', 'id': 737, 'synset': 'notepad.n.01', 'synonyms': ['notepad'], 'def': 'a pad of paper for keeping notes', 'name': 'notepad'}, {'frequency': 'c', 'id': 738, 'synset': 'nut.n.03', 'synonyms': ['nut'], 'def': 'a small metal block (usually square or hexagonal) with internal screw thread to be fitted onto a bolt', 'name': 'nut'}, {'frequency': 'r', 'id': 739, 'synset': 'nutcracker.n.01', 'synonyms': ['nutcracker'], 'def': 'a hand tool used to crack nuts open', 'name': 'nutcracker'}, {'frequency': 'c', 'id': 740, 'synset': 'oar.n.01', 'synonyms': ['oar'], 'def': 'an implement used to propel or steer a boat', 'name': 'oar'}, {'frequency': 'r', 'id': 741, 'synset': 'octopus.n.01', 'synonyms': ['octopus_(food)'], 'def': 'tentacles of octopus prepared as food', 'name': 'octopus_(food)'}, {'frequency': 'r', 'id': 742, 'synset': 'octopus.n.02', 'synonyms': ['octopus_(animal)'], 'def': 'bottom-living cephalopod having a soft oval body with eight long tentacles', 'name': 'octopus_(animal)'}, {'frequency': 'c', 'id': 743, 'synset': 'oil_lamp.n.01', 'synonyms': ['oil_lamp', 'kerosene_lamp', 'kerosine_lamp'], 'def': 'a lamp that burns oil (as kerosine) for light', 'name': 'oil_lamp'}, {'frequency': 'c', 'id': 744, 'synset': 'olive_oil.n.01', 'synonyms': ['olive_oil'], 'def': 'oil from olives', 'name': 'olive_oil'}, {'frequency': 'r', 'id': 745, 'synset': 'omelet.n.01', 'synonyms': ['omelet', 'omelette'], 'def': 'beaten eggs cooked until just set; may be folded around e.g. ham or cheese or jelly', 'name': 'omelet'}, {'frequency': 'f', 'id': 746, 'synset': 'onion.n.01', 'synonyms': ['onion'], 'def': 'the bulb of an onion plant', 'name': 'onion'}, {'frequency': 'f', 'id': 747, 'synset': 'orange.n.01', 'synonyms': ['orange_(fruit)'], 'def': 'orange (FRUIT of an orange tree)', 'name': 'orange_(fruit)'}, {'frequency': 'c', 'id': 748, 'synset': 'orange_juice.n.01', 'synonyms': ['orange_juice'], 'def': 'bottled or freshly squeezed juice of oranges', 'name': 'orange_juice'}, {'frequency': 'r', 'id': 749, 'synset': 'oregano.n.01', 'synonyms': ['oregano', 'marjoram'], 'def': 'aromatic Eurasian perennial herb used in cooking and baking', 'name': 'oregano'}, {'frequency': 'c', 'id': 750, 'synset': 'ostrich.n.02', 'synonyms': ['ostrich'], 'def': 'fast-running African flightless bird with two-toed feet; largest living bird', 'name': 'ostrich'}, {'frequency': 'c', 'id': 751, 'synset': 'ottoman.n.03', 'synonyms': ['ottoman', 'pouf', 'pouffe', 'hassock'], 'def': 'thick cushion used as a seat', 'name': 'ottoman'}, {'frequency': 'c', 'id': 752, 'synset': 'overall.n.01', 'synonyms': ['overalls_(clothing)'], 'def': 'work clothing consisting of denim trousers usually with a bib and shoulder straps', 'name': 'overalls_(clothing)'}, {'frequency': 'c', 'id': 753, 'synset': 'owl.n.01', 'synonyms': ['owl'], 'def': 'nocturnal bird of prey with hawk-like beak and claws and large head with front-facing eyes', 'name': 'owl'}, {'frequency': 'c', 'id': 754, 'synset': 'packet.n.03', 'synonyms': ['packet'], 'def': 'a small package or bundle', 'name': 'packet'}, {'frequency': 'r', 'id': 755, 'synset': 'pad.n.03', 'synonyms': ['inkpad', 'inking_pad', 'stamp_pad'], 'def': 'absorbent material saturated with ink used to transfer ink evenly to a rubber stamp', 'name': 'inkpad'}, {'frequency': 'c', 'id': 756, 'synset': 'pad.n.04', 'synonyms': ['pad'], 'def': 'a flat mass of soft material used for protection, stuffing, or comfort', 'name': 'pad'}, {'frequency': 'c', 'id': 757, 'synset': 'paddle.n.04', 'synonyms': ['paddle', 'boat_paddle'], 'def': 'a short light oar used without an oarlock to propel a canoe or small boat', 'name': 'paddle'}, {'frequency': 'c', 'id': 758, 'synset': 'padlock.n.01', 'synonyms': ['padlock'], 'def': 'a detachable, portable lock', 'name': 'padlock'}, {'frequency': 'r', 'id': 759, 'synset': 'paintbox.n.01', 'synonyms': ['paintbox'], 'def': "a box containing a collection of cubes or tubes of artists' paint", 'name': 'paintbox'}, {'frequency': 'c', 'id': 760, 'synset': 'paintbrush.n.01', 'synonyms': ['paintbrush'], 'def': 'a brush used as an applicator to apply paint', 'name': 'paintbrush'}, {'frequency': 'f', 'id': 761, 'synset': 'painting.n.01', 'synonyms': ['painting'], 'def': 'graphic art consisting of an artistic composition made by applying paints to a surface', 'name': 'painting'}, {'frequency': 'c', 'id': 762, 'synset': 'pajama.n.02', 'synonyms': ['pajamas', 'pyjamas'], 'def': 'loose-fitting nightclothes worn for sleeping or lounging', 'name': 'pajamas'}, {'frequency': 'c', 'id': 763, 'synset': 'palette.n.02', 'synonyms': ['palette', 'pallet'], 'def': 'board that provides a flat surface on which artists mix paints and the range of colors used', 'name': 'palette'}, {'frequency': 'f', 'id': 764, 'synset': 'pan.n.01', 'synonyms': ['pan_(for_cooking)', 'cooking_pan'], 'def': 'cooking utensil consisting of a wide metal vessel', 'name': 'pan_(for_cooking)'}, {'frequency': 'r', 'id': 765, 'synset': 'pan.n.03', 'synonyms': ['pan_(metal_container)'], 'def': 'shallow container made of metal', 'name': 'pan_(metal_container)'}, {'frequency': 'c', 'id': 766, 'synset': 'pancake.n.01', 'synonyms': ['pancake'], 'def': 'a flat cake of thin batter fried on both sides on a griddle', 'name': 'pancake'}, {'frequency': 'r', 'id': 767, 'synset': 'pantyhose.n.01', 'synonyms': ['pantyhose'], 'def': "a woman's tights consisting of underpants and stockings", 'name': 'pantyhose'}, {'frequency': 'r', 'id': 768, 'synset': 'papaya.n.02', 'synonyms': ['papaya'], 'def': 'large oval melon-like tropical fruit with yellowish flesh', 'name': 'papaya'}, {'frequency': 'r', 'id': 769, 'synset': 'paper_clip.n.01', 'synonyms': ['paperclip'], 'def': 'a wire or plastic clip for holding sheets of paper together', 'name': 'paperclip'}, {'frequency': 'f', 'id': 770, 'synset': 'paper_plate.n.01', 'synonyms': ['paper_plate'], 'def': 'a disposable plate made of cardboard', 'name': 'paper_plate'}, {'frequency': 'f', 'id': 771, 'synset': 'paper_towel.n.01', 'synonyms': ['paper_towel'], 'def': 'a disposable towel made of absorbent paper', 'name': 'paper_towel'}, {'frequency': 'r', 'id': 772, 'synset': 'paperback_book.n.01', 'synonyms': ['paperback_book', 'paper-back_book', 'softback_book', 'soft-cover_book'], 'def': 'a book with paper covers', 'name': 'paperback_book'}, {'frequency': 'r', 'id': 773, 'synset': 'paperweight.n.01', 'synonyms': ['paperweight'], 'def': 'a weight used to hold down a stack of papers', 'name': 'paperweight'}, {'frequency': 'c', 'id': 774, 'synset': 'parachute.n.01', 'synonyms': ['parachute'], 'def': 'rescue equipment consisting of a device that fills with air and retards your fall', 'name': 'parachute'}, {'frequency': 'r', 'id': 775, 'synset': 'parakeet.n.01', 'synonyms': ['parakeet', 'parrakeet', 'parroket', 'paraquet', 'paroquet', 'parroquet'], 'def': 'any of numerous small slender long-tailed parrots', 'name': 'parakeet'}, {'frequency': 'c', 'id': 776, 'synset': 'parasail.n.01', 'synonyms': ['parasail_(sports)'], 'def': 'parachute that will lift a person up into the air when it is towed by a motorboat or a car', 'name': 'parasail_(sports)'}, {'frequency': 'r', 'id': 777, 'synset': 'parchment.n.01', 'synonyms': ['parchment'], 'def': 'a superior paper resembling sheepskin', 'name': 'parchment'}, {'frequency': 'r', 'id': 778, 'synset': 'parka.n.01', 'synonyms': ['parka', 'anorak'], 'def': "a kind of heavy jacket (`windcheater' is a British term)", 'name': 'parka'}, {'frequency': 'f', 'id': 779, 'synset': 'parking_meter.n.01', 'synonyms': ['parking_meter'], 'def': 'a coin-operated timer located next to a parking space', 'name': 'parking_meter'}, {'frequency': 'c', 'id': 780, 'synset': 'parrot.n.01', 'synonyms': ['parrot'], 'def': 'usually brightly colored tropical birds with short hooked beaks and the ability to mimic sounds', 'name': 'parrot'}, {'frequency': 'c', 'id': 781, 'synset': 'passenger_car.n.01', 'synonyms': ['passenger_car_(part_of_a_train)', 'coach_(part_of_a_train)'], 'def': 'a railcar where passengers ride', 'name': 'passenger_car_(part_of_a_train)'}, {'frequency': 'r', 'id': 782, 'synset': 'passenger_ship.n.01', 'synonyms': ['passenger_ship'], 'def': 'a ship built to carry passengers', 'name': 'passenger_ship'}, {'frequency': 'r', 'id': 783, 'synset': 'passport.n.02', 'synonyms': ['passport'], 'def': 'a document issued by a country to a citizen allowing that person to travel abroad and re-enter the home country', 'name': 'passport'}, {'frequency': 'f', 'id': 784, 'synset': 'pastry.n.02', 'synonyms': ['pastry'], 'def': 'any of various baked foods made of dough or batter', 'name': 'pastry'}, {'frequency': 'r', 'id': 785, 'synset': 'patty.n.01', 'synonyms': ['patty_(food)'], 'def': 'small flat mass of chopped food', 'name': 'patty_(food)'}, {'frequency': 'c', 'id': 786, 'synset': 'pea.n.01', 'synonyms': ['pea_(food)'], 'def': 'seed of a pea plant used for food', 'name': 'pea_(food)'}, {'frequency': 'c', 'id': 787, 'synset': 'peach.n.03', 'synonyms': ['peach'], 'def': 'downy juicy fruit with sweet yellowish or whitish flesh', 'name': 'peach'}, {'frequency': 'c', 'id': 788, 'synset': 'peanut_butter.n.01', 'synonyms': ['peanut_butter'], 'def': 'a spread made from ground peanuts', 'name': 'peanut_butter'}, {'frequency': 'c', 'id': 789, 'synset': 'pear.n.01', 'synonyms': ['pear'], 'def': 'sweet juicy gritty-textured fruit available in many varieties', 'name': 'pear'}, {'frequency': 'r', 'id': 790, 'synset': 'peeler.n.03', 'synonyms': ['peeler_(tool_for_fruit_and_vegetables)'], 'def': 'a device for peeling vegetables or fruits', 'name': 'peeler_(tool_for_fruit_and_vegetables)'}, {'frequency': 'r', 'id': 791, 'synset': 'pegboard.n.01', 'synonyms': ['pegboard'], 'def': 'a board perforated with regularly spaced holes into which pegs can be fitted', 'name': 'pegboard'}, {'frequency': 'c', 'id': 792, 'synset': 'pelican.n.01', 'synonyms': ['pelican'], 'def': 'large long-winged warm-water seabird having a large bill with a distensible pouch for fish', 'name': 'pelican'}, {'frequency': 'f', 'id': 793, 'synset': 'pen.n.01', 'synonyms': ['pen'], 'def': 'a writing implement with a point from which ink flows', 'name': 'pen'}, {'frequency': 'c', 'id': 794, 'synset': 'pencil.n.01', 'synonyms': ['pencil'], 'def': 'a thin cylindrical pointed writing implement made of wood and graphite', 'name': 'pencil'}, {'frequency': 'r', 'id': 795, 'synset': 'pencil_box.n.01', 'synonyms': ['pencil_box', 'pencil_case'], 'def': 'a box for holding pencils', 'name': 'pencil_box'}, {'frequency': 'r', 'id': 796, 'synset': 'pencil_sharpener.n.01', 'synonyms': ['pencil_sharpener'], 'def': 'a rotary implement for sharpening the point on pencils', 'name': 'pencil_sharpener'}, {'frequency': 'r', 'id': 797, 'synset': 'pendulum.n.01', 'synonyms': ['pendulum'], 'def': 'an apparatus consisting of an object mounted so that it swings freely under the influence of gravity', 'name': 'pendulum'}, {'frequency': 'c', 'id': 798, 'synset': 'penguin.n.01', 'synonyms': ['penguin'], 'def': 'short-legged flightless birds of cold southern regions having webbed feet and wings modified as flippers', 'name': 'penguin'}, {'frequency': 'r', 'id': 799, 'synset': 'pennant.n.02', 'synonyms': ['pennant'], 'def': 'a flag longer than it is wide (and often tapering)', 'name': 'pennant'}, {'frequency': 'r', 'id': 800, 'synset': 'penny.n.02', 'synonyms': ['penny_(coin)'], 'def': 'a coin worth one-hundredth of the value of the basic unit', 'name': 'penny_(coin)'}, {'frequency': 'c', 'id': 801, 'synset': 'pepper.n.03', 'synonyms': ['pepper', 'peppercorn'], 'def': 'pungent seasoning from the berry of the common pepper plant; whole or ground', 'name': 'pepper'}, {'frequency': 'c', 'id': 802, 'synset': 'pepper_mill.n.01', 'synonyms': ['pepper_mill', 'pepper_grinder'], 'def': 'a mill for grinding pepper', 'name': 'pepper_mill'}, {'frequency': 'c', 'id': 803, 'synset': 'perfume.n.02', 'synonyms': ['perfume'], 'def': 'a toiletry that emits and diffuses a fragrant odor', 'name': 'perfume'}, {'frequency': 'r', 'id': 804, 'synset': 'persimmon.n.02', 'synonyms': ['persimmon'], 'def': 'orange fruit resembling a plum; edible when fully ripe', 'name': 'persimmon'}, {'frequency': 'f', 'id': 805, 'synset': 'person.n.01', 'synonyms': ['baby', 'child', 'boy', 'girl', 'man', 'woman', 'person', 'human'], 'def': 'a human being', 'name': 'baby'}, {'frequency': 'r', 'id': 806, 'synset': 'pet.n.01', 'synonyms': ['pet'], 'def': 'a domesticated animal kept for companionship or amusement', 'name': 'pet'}, {'frequency': 'r', 'id': 807, 'synset': 'petfood.n.01', 'synonyms': ['petfood', 'pet-food'], 'def': 'food prepared for animal pets', 'name': 'petfood'}, {'frequency': 'r', 'id': 808, 'synset': 'pew.n.01', 'synonyms': ['pew_(church_bench)', 'church_bench'], 'def': 'long bench with backs; used in church by the congregation', 'name': 'pew_(church_bench)'}, {'frequency': 'r', 'id': 809, 'synset': 'phonebook.n.01', 'synonyms': ['phonebook', 'telephone_book', 'telephone_directory'], 'def': 'a directory containing an alphabetical list of telephone subscribers and their telephone numbers', 'name': 'phonebook'}, {'frequency': 'c', 'id': 810, 'synset': 'phonograph_record.n.01', 'synonyms': ['phonograph_record', 'phonograph_recording', 'record_(phonograph_recording)'], 'def': 'sound recording consisting of a typically black disk with a continuous groove', 'name': 'phonograph_record'}, {'frequency': 'c', 'id': 811, 'synset': 'piano.n.01', 'synonyms': ['piano'], 'def': 'a keyboard instrument that is played by depressing keys that cause hammers to strike tuned strings and produce sounds', 'name': 'piano'}, {'frequency': 'f', 'id': 812, 'synset': 'pickle.n.01', 'synonyms': ['pickle'], 'def': 'vegetables (especially cucumbers) preserved in brine or vinegar', 'name': 'pickle'}, {'frequency': 'f', 'id': 813, 'synset': 'pickup.n.01', 'synonyms': ['pickup_truck'], 'def': 'a light truck with an open body and low sides and a tailboard', 'name': 'pickup_truck'}, {'frequency': 'c', 'id': 814, 'synset': 'pie.n.01', 'synonyms': ['pie'], 'def': 'dish baked in pastry-lined pan often with a pastry top', 'name': 'pie'}, {'frequency': 'c', 'id': 815, 'synset': 'pigeon.n.01', 'synonyms': ['pigeon'], 'def': 'wild and domesticated birds having a heavy body and short legs', 'name': 'pigeon'}, {'frequency': 'r', 'id': 816, 'synset': 'piggy_bank.n.01', 'synonyms': ['piggy_bank', 'penny_bank'], 'def': "a child's coin bank (often shaped like a pig)", 'name': 'piggy_bank'}, {'frequency': 'f', 'id': 817, 'synset': 'pillow.n.01', 'synonyms': ['pillow'], 'def': 'a cushion to support the head of a sleeping person', 'name': 'pillow'}, {'frequency': 'r', 'id': 818, 'synset': 'pin.n.09', 'synonyms': ['pin_(non_jewelry)'], 'def': 'a small slender (often pointed) piece of wood or metal used to support or fasten or attach things', 'name': 'pin_(non_jewelry)'}, {'frequency': 'f', 'id': 819, 'synset': 'pineapple.n.02', 'synonyms': ['pineapple'], 'def': 'large sweet fleshy tropical fruit with a tuft of stiff leaves', 'name': 'pineapple'}, {'frequency': 'c', 'id': 820, 'synset': 'pinecone.n.01', 'synonyms': ['pinecone'], 'def': 'the seed-producing cone of a pine tree', 'name': 'pinecone'}, {'frequency': 'r', 'id': 821, 'synset': 'ping-pong_ball.n.01', 'synonyms': ['ping-pong_ball'], 'def': 'light hollow ball used in playing table tennis', 'name': 'ping-pong_ball'}, {'frequency': 'r', 'id': 822, 'synset': 'pinwheel.n.03', 'synonyms': ['pinwheel'], 'def': 'a toy consisting of vanes of colored paper or plastic that is pinned to a stick and spins when it is pointed into the wind', 'name': 'pinwheel'}, {'frequency': 'r', 'id': 823, 'synset': 'pipe.n.01', 'synonyms': ['tobacco_pipe'], 'def': 'a tube with a small bowl at one end; used for smoking tobacco', 'name': 'tobacco_pipe'}, {'frequency': 'f', 'id': 824, 'synset': 'pipe.n.02', 'synonyms': ['pipe', 'piping'], 'def': 'a long tube made of metal or plastic that is used to carry water or oil or gas etc.', 'name': 'pipe'}, {'frequency': 'r', 'id': 825, 'synset': 'pistol.n.01', 'synonyms': ['pistol', 'handgun'], 'def': 'a firearm that is held and fired with one hand', 'name': 'pistol'}, {'frequency': 'r', 'id': 826, 'synset': 'pita.n.01', 'synonyms': ['pita_(bread)', 'pocket_bread'], 'def': 'usually small round bread that can open into a pocket for filling', 'name': 'pita_(bread)'}, {'frequency': 'f', 'id': 827, 'synset': 'pitcher.n.02', 'synonyms': ['pitcher_(vessel_for_liquid)', 'ewer'], 'def': 'an open vessel with a handle and a spout for pouring', 'name': 'pitcher_(vessel_for_liquid)'}, {'frequency': 'r', 'id': 828, 'synset': 'pitchfork.n.01', 'synonyms': ['pitchfork'], 'def': 'a long-handled hand tool with sharp widely spaced prongs for lifting and pitching hay', 'name': 'pitchfork'}, {'frequency': 'f', 'id': 829, 'synset': 'pizza.n.01', 'synonyms': ['pizza'], 'def': 'Italian open pie made of thin bread dough spread with a spiced mixture of e.g. tomato sauce and cheese', 'name': 'pizza'}, {'frequency': 'f', 'id': 830, 'synset': 'place_mat.n.01', 'synonyms': ['place_mat'], 'def': 'a mat placed on a table for an individual place setting', 'name': 'place_mat'}, {'frequency': 'f', 'id': 831, 'synset': 'plate.n.04', 'synonyms': ['plate'], 'def': 'dish on which food is served or from which food is eaten', 'name': 'plate'}, {'frequency': 'c', 'id': 832, 'synset': 'platter.n.01', 'synonyms': ['platter'], 'def': 'a large shallow dish used for serving food', 'name': 'platter'}, {'frequency': 'r', 'id': 833, 'synset': 'playing_card.n.01', 'synonyms': ['playing_card'], 'def': 'one of a pack of cards that are used to play card games', 'name': 'playing_card'}, {'frequency': 'r', 'id': 834, 'synset': 'playpen.n.01', 'synonyms': ['playpen'], 'def': 'a portable enclosure in which babies may be left to play', 'name': 'playpen'}, {'frequency': 'c', 'id': 835, 'synset': 'pliers.n.01', 'synonyms': ['pliers', 'plyers'], 'def': 'a gripping hand tool with two hinged arms and (usually) serrated jaws', 'name': 'pliers'}, {'frequency': 'r', 'id': 836, 'synset': 'plow.n.01', 'synonyms': ['plow_(farm_equipment)', 'plough_(farm_equipment)'], 'def': 'a farm tool having one or more heavy blades to break the soil and cut a furrow prior to sowing', 'name': 'plow_(farm_equipment)'}, {'frequency': 'r', 'id': 837, 'synset': 'pocket_watch.n.01', 'synonyms': ['pocket_watch'], 'def': 'a watch that is carried in a small watch pocket', 'name': 'pocket_watch'}, {'frequency': 'c', 'id': 838, 'synset': 'pocketknife.n.01', 'synonyms': ['pocketknife'], 'def': 'a knife with a blade that folds into the handle; suitable for carrying in the pocket', 'name': 'pocketknife'}, {'frequency': 'c', 'id': 839, 'synset': 'poker.n.01', 'synonyms': ['poker_(fire_stirring_tool)', 'stove_poker', 'fire_hook'], 'def': 'fire iron consisting of a metal rod with a handle; used to stir a fire', 'name': 'poker_(fire_stirring_tool)'}, {'frequency': 'f', 'id': 840, 'synset': 'pole.n.01', 'synonyms': ['pole', 'post'], 'def': 'a long (usually round) rod of wood or metal or plastic', 'name': 'pole'}, {'frequency': 'r', 'id': 841, 'synset': 'police_van.n.01', 'synonyms': ['police_van', 'police_wagon', 'paddy_wagon', 'patrol_wagon'], 'def': 'van used by police to transport prisoners', 'name': 'police_van'}, {'frequency': 'f', 'id': 842, 'synset': 'polo_shirt.n.01', 'synonyms': ['polo_shirt', 'sport_shirt'], 'def': 'a shirt with short sleeves designed for comfort and casual wear', 'name': 'polo_shirt'}, {'frequency': 'r', 'id': 843, 'synset': 'poncho.n.01', 'synonyms': ['poncho'], 'def': 'a blanket-like cloak with a hole in the center for the head', 'name': 'poncho'}, {'frequency': 'c', 'id': 844, 'synset': 'pony.n.05', 'synonyms': ['pony'], 'def': 'any of various breeds of small gentle horses usually less than five feet high at the shoulder', 'name': 'pony'}, {'frequency': 'r', 'id': 845, 'synset': 'pool_table.n.01', 'synonyms': ['pool_table', 'billiard_table', 'snooker_table'], 'def': 'game equipment consisting of a heavy table on which pool is played', 'name': 'pool_table'}, {'frequency': 'f', 'id': 846, 'synset': 'pop.n.02', 'synonyms': ['pop_(soda)', 'soda_(pop)', 'tonic', 'soft_drink'], 'def': 'a sweet drink containing carbonated water and flavoring', 'name': 'pop_(soda)'}, {'frequency': 'r', 'id': 847, 'synset': 'portrait.n.02', 'synonyms': ['portrait', 'portrayal'], 'def': 'any likeness of a person, in any medium', 'name': 'portrait'}, {'frequency': 'c', 'id': 848, 'synset': 'postbox.n.01', 'synonyms': ['postbox_(public)', 'mailbox_(public)'], 'def': 'public box for deposit of mail', 'name': 'postbox_(public)'}, {'frequency': 'c', 'id': 849, 'synset': 'postcard.n.01', 'synonyms': ['postcard', 'postal_card', 'mailing-card'], 'def': 'a card for sending messages by post without an envelope', 'name': 'postcard'}, {'frequency': 'f', 'id': 850, 'synset': 'poster.n.01', 'synonyms': ['poster', 'placard'], 'def': 'a sign posted in a public place as an advertisement', 'name': 'poster'}, {'frequency': 'f', 'id': 851, 'synset': 'pot.n.01', 'synonyms': ['pot'], 'def': 'metal or earthenware cooking vessel that is usually round and deep; often has a handle and lid', 'name': 'pot'}, {'frequency': 'f', 'id': 852, 'synset': 'pot.n.04', 'synonyms': ['flowerpot'], 'def': 'a container in which plants are cultivated', 'name': 'flowerpot'}, {'frequency': 'f', 'id': 853, 'synset': 'potato.n.01', 'synonyms': ['potato'], 'def': 'an edible tuber native to South America', 'name': 'potato'}, {'frequency': 'c', 'id': 854, 'synset': 'potholder.n.01', 'synonyms': ['potholder'], 'def': 'an insulated pad for holding hot pots', 'name': 'potholder'}, {'frequency': 'c', 'id': 855, 'synset': 'pottery.n.01', 'synonyms': ['pottery', 'clayware'], 'def': 'ceramic ware made from clay and baked in a kiln', 'name': 'pottery'}, {'frequency': 'c', 'id': 856, 'synset': 'pouch.n.01', 'synonyms': ['pouch'], 'def': 'a small or medium size container for holding or carrying things', 'name': 'pouch'}, {'frequency': 'r', 'id': 857, 'synset': 'power_shovel.n.01', 'synonyms': ['power_shovel', 'excavator', 'digger'], 'def': 'a machine for excavating', 'name': 'power_shovel'}, {'frequency': 'c', 'id': 858, 'synset': 'prawn.n.01', 'synonyms': ['prawn', 'shrimp'], 'def': 'any of various edible decapod crustaceans', 'name': 'prawn'}, {'frequency': 'f', 'id': 859, 'synset': 'printer.n.03', 'synonyms': ['printer', 'printing_machine'], 'def': 'a machine that prints', 'name': 'printer'}, {'frequency': 'c', 'id': 860, 'synset': 'projectile.n.01', 'synonyms': ['projectile_(weapon)', 'missile'], 'def': 'a weapon that is forcibly thrown or projected at a targets', 'name': 'projectile_(weapon)'}, {'frequency': 'c', 'id': 861, 'synset': 'projector.n.02', 'synonyms': ['projector'], 'def': 'an optical instrument that projects an enlarged image onto a screen', 'name': 'projector'}, {'frequency': 'f', 'id': 862, 'synset': 'propeller.n.01', 'synonyms': ['propeller', 'propellor'], 'def': 'a mechanical device that rotates to push against air or water', 'name': 'propeller'}, {'frequency': 'r', 'id': 863, 'synset': 'prune.n.01', 'synonyms': ['prune'], 'def': 'dried plum', 'name': 'prune'}, {'frequency': 'r', 'id': 864, 'synset': 'pudding.n.01', 'synonyms': ['pudding'], 'def': 'any of various soft thick unsweetened baked dishes', 'name': 'pudding'}, {'frequency': 'r', 'id': 865, 'synset': 'puffer.n.02', 'synonyms': ['puffer_(fish)', 'pufferfish', 'blowfish', 'globefish'], 'def': 'fishes whose elongated spiny body can inflate itself with water or air to form a globe', 'name': 'puffer_(fish)'}, {'frequency': 'r', 'id': 866, 'synset': 'puffin.n.01', 'synonyms': ['puffin'], 'def': 'seabirds having short necks and brightly colored compressed bills', 'name': 'puffin'}, {'frequency': 'r', 'id': 867, 'synset': 'pug.n.01', 'synonyms': ['pug-dog'], 'def': 'small compact smooth-coated breed of Asiatic origin having a tightly curled tail and broad flat wrinkled muzzle', 'name': 'pug-dog'}, {'frequency': 'c', 'id': 868, 'synset': 'pumpkin.n.02', 'synonyms': ['pumpkin'], 'def': 'usually large pulpy deep-yellow round fruit of the squash family maturing in late summer or early autumn', 'name': 'pumpkin'}, {'frequency': 'r', 'id': 869, 'synset': 'punch.n.03', 'synonyms': ['puncher'], 'def': 'a tool for making holes or indentations', 'name': 'puncher'}, {'frequency': 'r', 'id': 870, 'synset': 'puppet.n.01', 'synonyms': ['puppet', 'marionette'], 'def': 'a small figure of a person operated from above with strings by a puppeteer', 'name': 'puppet'}, {'frequency': 'r', 'id': 871, 'synset': 'puppy.n.01', 'synonyms': ['puppy'], 'def': 'a young dog', 'name': 'puppy'}, {'frequency': 'r', 'id': 872, 'synset': 'quesadilla.n.01', 'synonyms': ['quesadilla'], 'def': 'a tortilla that is filled with cheese and heated', 'name': 'quesadilla'}, {'frequency': 'r', 'id': 873, 'synset': 'quiche.n.02', 'synonyms': ['quiche'], 'def': 'a tart filled with rich unsweetened custard; often contains other ingredients (as cheese or ham or seafood or vegetables)', 'name': 'quiche'}, {'frequency': 'f', 'id': 874, 'synset': 'quilt.n.01', 'synonyms': ['quilt', 'comforter'], 'def': 'bedding made of two layers of cloth filled with stuffing and stitched together', 'name': 'quilt'}, {'frequency': 'c', 'id': 875, 'synset': 'rabbit.n.01', 'synonyms': ['rabbit'], 'def': 'any of various burrowing animals of the family Leporidae having long ears and short tails', 'name': 'rabbit'}, {'frequency': 'r', 'id': 876, 'synset': 'racer.n.02', 'synonyms': ['race_car', 'racing_car'], 'def': 'a fast car that competes in races', 'name': 'race_car'}, {'frequency': 'c', 'id': 877, 'synset': 'racket.n.04', 'synonyms': ['racket', 'racquet'], 'def': 'a sports implement used to strike a ball in various games', 'name': 'racket'}, {'frequency': 'r', 'id': 878, 'synset': 'radar.n.01', 'synonyms': ['radar'], 'def': 'measuring instrument in which the echo of a pulse of microwave radiation is used to detect and locate distant objects', 'name': 'radar'}, {'frequency': 'c', 'id': 879, 'synset': 'radiator.n.03', 'synonyms': ['radiator'], 'def': 'a mechanism consisting of a metal honeycomb through which hot fluids circulate', 'name': 'radiator'}, {'frequency': 'c', 'id': 880, 'synset': 'radio_receiver.n.01', 'synonyms': ['radio_receiver', 'radio_set', 'radio', 'tuner_(radio)'], 'def': 'an electronic receiver that detects and demodulates and amplifies transmitted radio signals', 'name': 'radio_receiver'}, {'frequency': 'c', 'id': 881, 'synset': 'radish.n.03', 'synonyms': ['radish', 'daikon'], 'def': 'pungent edible root of any of various cultivated radish plants', 'name': 'radish'}, {'frequency': 'c', 'id': 882, 'synset': 'raft.n.01', 'synonyms': ['raft'], 'def': 'a flat float (usually made of logs or planks) that can be used for transport or as a platform for swimmers', 'name': 'raft'}, {'frequency': 'r', 'id': 883, 'synset': 'rag_doll.n.01', 'synonyms': ['rag_doll'], 'def': 'a cloth doll that is stuffed and (usually) painted', 'name': 'rag_doll'}, {'frequency': 'c', 'id': 884, 'synset': 'raincoat.n.01', 'synonyms': ['raincoat', 'waterproof_jacket'], 'def': 'a water-resistant coat', 'name': 'raincoat'}, {'frequency': 'c', 'id': 885, 'synset': 'ram.n.05', 'synonyms': ['ram_(animal)'], 'def': 'uncastrated adult male sheep', 'name': 'ram_(animal)'}, {'frequency': 'c', 'id': 886, 'synset': 'raspberry.n.02', 'synonyms': ['raspberry'], 'def': 'red or black edible aggregate berries usually smaller than the related blackberries', 'name': 'raspberry'}, {'frequency': 'r', 'id': 887, 'synset': 'rat.n.01', 'synonyms': ['rat'], 'def': 'any of various long-tailed rodents similar to but larger than a mouse', 'name': 'rat'}, {'frequency': 'c', 'id': 888, 'synset': 'razorblade.n.01', 'synonyms': ['razorblade'], 'def': 'a blade that has very sharp edge', 'name': 'razorblade'}, {'frequency': 'c', 'id': 889, 'synset': 'reamer.n.01', 'synonyms': ['reamer_(juicer)', 'juicer', 'juice_reamer'], 'def': 'a squeezer with a conical ridged center that is used for squeezing juice from citrus fruit', 'name': 'reamer_(juicer)'}, {'frequency': 'f', 'id': 890, 'synset': 'rearview_mirror.n.01', 'synonyms': ['rearview_mirror'], 'def': 'car mirror that reflects the view out of the rear window', 'name': 'rearview_mirror'}, {'frequency': 'c', 'id': 891, 'synset': 'receipt.n.02', 'synonyms': ['receipt'], 'def': 'an acknowledgment (usually tangible) that payment has been made', 'name': 'receipt'}, {'frequency': 'c', 'id': 892, 'synset': 'recliner.n.01', 'synonyms': ['recliner', 'reclining_chair', 'lounger_(chair)'], 'def': 'an armchair whose back can be lowered and foot can be raised to allow the sitter to recline in it', 'name': 'recliner'}, {'frequency': 'r', 'id': 893, 'synset': 'record_player.n.01', 'synonyms': ['record_player', 'phonograph_(record_player)', 'turntable'], 'def': 'machine in which rotating records cause a stylus to vibrate and the vibrations are amplified acoustically or electronically', 'name': 'record_player'}, {'frequency': 'r', 'id': 894, 'synset': 'red_cabbage.n.02', 'synonyms': ['red_cabbage'], 'def': 'compact head of purplish-red leaves', 'name': 'red_cabbage'}, {'frequency': 'f', 'id': 895, 'synset': 'reflector.n.01', 'synonyms': ['reflector'], 'def': 'device that reflects light, radiation, etc.', 'name': 'reflector'}, {'frequency': 'f', 'id': 896, 'synset': 'remote_control.n.01', 'synonyms': ['remote_control'], 'def': 'a device that can be used to control a machine or apparatus from a distance', 'name': 'remote_control'}, {'frequency': 'c', 'id': 897, 'synset': 'rhinoceros.n.01', 'synonyms': ['rhinoceros'], 'def': 'massive powerful herbivorous odd-toed ungulate of southeast Asia and Africa having very thick skin and one or two horns on the snout', 'name': 'rhinoceros'}, {'frequency': 'r', 'id': 898, 'synset': 'rib.n.03', 'synonyms': ['rib_(food)'], 'def': 'cut of meat including one or more ribs', 'name': 'rib_(food)'}, {'frequency': 'r', 'id': 899, 'synset': 'rifle.n.01', 'synonyms': ['rifle'], 'def': 'a shoulder firearm with a long barrel', 'name': 'rifle'}, {'frequency': 'f', 'id': 900, 'synset': 'ring.n.08', 'synonyms': ['ring'], 'def': 'jewelry consisting of a circlet of precious metal (often set with jewels) worn on the finger', 'name': 'ring'}, {'frequency': 'r', 'id': 901, 'synset': 'river_boat.n.01', 'synonyms': ['river_boat'], 'def': 'a boat used on rivers or to ply a river', 'name': 'river_boat'}, {'frequency': 'r', 'id': 902, 'synset': 'road_map.n.02', 'synonyms': ['road_map'], 'def': '(NOT A ROAD) a MAP showing roads (for automobile travel)', 'name': 'road_map'}, {'frequency': 'c', 'id': 903, 'synset': 'robe.n.01', 'synonyms': ['robe'], 'def': 'any loose flowing garment', 'name': 'robe'}, {'frequency': 'c', 'id': 904, 'synset': 'rocking_chair.n.01', 'synonyms': ['rocking_chair'], 'def': 'a chair mounted on rockers', 'name': 'rocking_chair'}, {'frequency': 'r', 'id': 905, 'synset': 'roller_skate.n.01', 'synonyms': ['roller_skate'], 'def': 'a shoe with pairs of rollers (small hard wheels) fixed to the sole', 'name': 'roller_skate'}, {'frequency': 'r', 'id': 906, 'synset': 'rollerblade.n.01', 'synonyms': ['Rollerblade'], 'def': 'an in-line variant of a roller skate', 'name': 'Rollerblade'}, {'frequency': 'c', 'id': 907, 'synset': 'rolling_pin.n.01', 'synonyms': ['rolling_pin'], 'def': 'utensil consisting of a cylinder (usually of wood) with a handle at each end; used to roll out dough', 'name': 'rolling_pin'}, {'frequency': 'r', 'id': 908, 'synset': 'root_beer.n.01', 'synonyms': ['root_beer'], 'def': 'carbonated drink containing extracts of roots and herbs', 'name': 'root_beer'}, {'frequency': 'c', 'id': 909, 'synset': 'router.n.02', 'synonyms': ['router_(computer_equipment)'], 'def': 'a device that forwards data packets between computer networks', 'name': 'router_(computer_equipment)'}, {'frequency': 'f', 'id': 910, 'synset': 'rubber_band.n.01', 'synonyms': ['rubber_band', 'elastic_band'], 'def': 'a narrow band of elastic rubber used to hold things (such as papers) together', 'name': 'rubber_band'}, {'frequency': 'c', 'id': 911, 'synset': 'runner.n.08', 'synonyms': ['runner_(carpet)'], 'def': 'a long narrow carpet', 'name': 'runner_(carpet)'}, {'frequency': 'f', 'id': 912, 'synset': 'sack.n.01', 'synonyms': ['plastic_bag', 'paper_bag'], 'def': "a bag made of paper or plastic for holding customer's purchases", 'name': 'plastic_bag'}, {'frequency': 'f', 'id': 913, 'synset': 'saddle.n.01', 'synonyms': ['saddle_(on_an_animal)'], 'def': 'a seat for the rider of a horse or camel', 'name': 'saddle_(on_an_animal)'}, {'frequency': 'f', 'id': 914, 'synset': 'saddle_blanket.n.01', 'synonyms': ['saddle_blanket', 'saddlecloth', 'horse_blanket'], 'def': 'stable gear consisting of a blanket placed under the saddle', 'name': 'saddle_blanket'}, {'frequency': 'c', 'id': 915, 'synset': 'saddlebag.n.01', 'synonyms': ['saddlebag'], 'def': 'a large bag (or pair of bags) hung over a saddle', 'name': 'saddlebag'}, {'frequency': 'r', 'id': 916, 'synset': 'safety_pin.n.01', 'synonyms': ['safety_pin'], 'def': 'a pin in the form of a clasp; has a guard so the point of the pin will not stick the user', 'name': 'safety_pin'}, {'frequency': 'c', 'id': 917, 'synset': 'sail.n.01', 'synonyms': ['sail'], 'def': 'a large piece of fabric by means of which wind is used to propel a sailing vessel', 'name': 'sail'}, {'frequency': 'c', 'id': 918, 'synset': 'salad.n.01', 'synonyms': ['salad'], 'def': 'food mixtures either arranged on a plate or tossed and served with a moist dressing; usually consisting of or including greens', 'name': 'salad'}, {'frequency': 'r', 'id': 919, 'synset': 'salad_plate.n.01', 'synonyms': ['salad_plate', 'salad_bowl'], 'def': 'a plate or bowl for individual servings of salad', 'name': 'salad_plate'}, {'frequency': 'r', 'id': 920, 'synset': 'salami.n.01', 'synonyms': ['salami'], 'def': 'highly seasoned fatty sausage of pork and beef usually dried', 'name': 'salami'}, {'frequency': 'r', 'id': 921, 'synset': 'salmon.n.01', 'synonyms': ['salmon_(fish)'], 'def': 'any of various large food and game fishes of northern waters', 'name': 'salmon_(fish)'}, {'frequency': 'r', 'id': 922, 'synset': 'salmon.n.03', 'synonyms': ['salmon_(food)'], 'def': 'flesh of any of various marine or freshwater fish of the family Salmonidae', 'name': 'salmon_(food)'}, {'frequency': 'r', 'id': 923, 'synset': 'salsa.n.01', 'synonyms': ['salsa'], 'def': 'spicy sauce of tomatoes and onions and chili peppers to accompany Mexican foods', 'name': 'salsa'}, {'frequency': 'f', 'id': 924, 'synset': 'saltshaker.n.01', 'synonyms': ['saltshaker'], 'def': 'a shaker with a perforated top for sprinkling salt', 'name': 'saltshaker'}, {'frequency': 'f', 'id': 925, 'synset': 'sandal.n.01', 'synonyms': ['sandal_(type_of_shoe)'], 'def': 'a shoe consisting of a sole fastened by straps to the foot', 'name': 'sandal_(type_of_shoe)'}, {'frequency': 'f', 'id': 926, 'synset': 'sandwich.n.01', 'synonyms': ['sandwich'], 'def': 'two (or more) slices of bread with a filling between them', 'name': 'sandwich'}, {'frequency': 'r', 'id': 927, 'synset': 'satchel.n.01', 'synonyms': ['satchel'], 'def': 'luggage consisting of a small case with a flat bottom and (usually) a shoulder strap', 'name': 'satchel'}, {'frequency': 'r', 'id': 928, 'synset': 'saucepan.n.01', 'synonyms': ['saucepan'], 'def': 'a deep pan with a handle; used for stewing or boiling', 'name': 'saucepan'}, {'frequency': 'f', 'id': 929, 'synset': 'saucer.n.02', 'synonyms': ['saucer'], 'def': 'a small shallow dish for holding a cup at the table', 'name': 'saucer'}, {'frequency': 'f', 'id': 930, 'synset': 'sausage.n.01', 'synonyms': ['sausage'], 'def': 'highly seasoned minced meat stuffed in casings', 'name': 'sausage'}, {'frequency': 'r', 'id': 931, 'synset': 'sawhorse.n.01', 'synonyms': ['sawhorse', 'sawbuck'], 'def': 'a framework for holding wood that is being sawed', 'name': 'sawhorse'}, {'frequency': 'r', 'id': 932, 'synset': 'sax.n.02', 'synonyms': ['saxophone'], 'def': "a wind instrument with a `J'-shaped form typically made of brass", 'name': 'saxophone'}, {'frequency': 'f', 'id': 933, 'synset': 'scale.n.07', 'synonyms': ['scale_(measuring_instrument)'], 'def': 'a measuring instrument for weighing; shows amount of mass', 'name': 'scale_(measuring_instrument)'}, {'frequency': 'r', 'id': 934, 'synset': 'scarecrow.n.01', 'synonyms': ['scarecrow', 'strawman'], 'def': 'an effigy in the shape of a man to frighten birds away from seeds', 'name': 'scarecrow'}, {'frequency': 'f', 'id': 935, 'synset': 'scarf.n.01', 'synonyms': ['scarf'], 'def': 'a garment worn around the head or neck or shoulders for warmth or decoration', 'name': 'scarf'}, {'frequency': 'c', 'id': 936, 'synset': 'school_bus.n.01', 'synonyms': ['school_bus'], 'def': 'a bus used to transport children to or from school', 'name': 'school_bus'}, {'frequency': 'f', 'id': 937, 'synset': 'scissors.n.01', 'synonyms': ['scissors'], 'def': 'a tool having two crossed pivoting blades with looped handles', 'name': 'scissors'}, {'frequency': 'c', 'id': 938, 'synset': 'scoreboard.n.01', 'synonyms': ['scoreboard'], 'def': 'a large board for displaying the score of a contest (and some other information)', 'name': 'scoreboard'}, {'frequency': 'c', 'id': 939, 'synset': 'scrambled_eggs.n.01', 'synonyms': ['scrambled_eggs'], 'def': 'eggs beaten and cooked to a soft firm consistency while stirring', 'name': 'scrambled_eggs'}, {'frequency': 'r', 'id': 940, 'synset': 'scraper.n.01', 'synonyms': ['scraper'], 'def': 'any of various hand tools for scraping', 'name': 'scraper'}, {'frequency': 'r', 'id': 941, 'synset': 'scratcher.n.03', 'synonyms': ['scratcher'], 'def': 'a device used for scratching', 'name': 'scratcher'}, {'frequency': 'c', 'id': 942, 'synset': 'screwdriver.n.01', 'synonyms': ['screwdriver'], 'def': 'a hand tool for driving screws; has a tip that fits into the head of a screw', 'name': 'screwdriver'}, {'frequency': 'c', 'id': 943, 'synset': 'scrub_brush.n.01', 'synonyms': ['scrubbing_brush'], 'def': 'a brush with short stiff bristles for heavy cleaning', 'name': 'scrubbing_brush'}, {'frequency': 'c', 'id': 944, 'synset': 'sculpture.n.01', 'synonyms': ['sculpture'], 'def': 'a three-dimensional work of art', 'name': 'sculpture'}, {'frequency': 'r', 'id': 945, 'synset': 'seabird.n.01', 'synonyms': ['seabird', 'seafowl'], 'def': 'a bird that frequents coastal waters and the open ocean: gulls; pelicans; gannets; cormorants; albatrosses; petrels; etc.', 'name': 'seabird'}, {'frequency': 'r', 'id': 946, 'synset': 'seahorse.n.02', 'synonyms': ['seahorse'], 'def': 'small fish with horse-like heads bent sharply downward and curled tails', 'name': 'seahorse'}, {'frequency': 'r', 'id': 947, 'synset': 'seaplane.n.01', 'synonyms': ['seaplane', 'hydroplane'], 'def': 'an airplane that can land on or take off from water', 'name': 'seaplane'}, {'frequency': 'c', 'id': 948, 'synset': 'seashell.n.01', 'synonyms': ['seashell'], 'def': 'the shell of a marine organism', 'name': 'seashell'}, {'frequency': 'r', 'id': 949, 'synset': 'seedling.n.01', 'synonyms': ['seedling'], 'def': 'young plant or tree grown from a seed', 'name': 'seedling'}, {'frequency': 'c', 'id': 950, 'synset': 'serving_dish.n.01', 'synonyms': ['serving_dish'], 'def': 'a dish used for serving food', 'name': 'serving_dish'}, {'frequency': 'r', 'id': 951, 'synset': 'sewing_machine.n.01', 'synonyms': ['sewing_machine'], 'def': 'a textile machine used as a home appliance for sewing', 'name': 'sewing_machine'}, {'frequency': 'r', 'id': 952, 'synset': 'shaker.n.03', 'synonyms': ['shaker'], 'def': 'a container in which something can be shaken', 'name': 'shaker'}, {'frequency': 'c', 'id': 953, 'synset': 'shampoo.n.01', 'synonyms': ['shampoo'], 'def': 'cleansing agent consisting of soaps or detergents used for washing the hair', 'name': 'shampoo'}, {'frequency': 'r', 'id': 954, 'synset': 'shark.n.01', 'synonyms': ['shark'], 'def': 'typically large carnivorous fishes with sharpe teeth', 'name': 'shark'}, {'frequency': 'r', 'id': 955, 'synset': 'sharpener.n.01', 'synonyms': ['sharpener'], 'def': 'any implement that is used to make something (an edge or a point) sharper', 'name': 'sharpener'}, {'frequency': 'r', 'id': 956, 'synset': 'sharpie.n.03', 'synonyms': ['Sharpie'], 'def': 'a pen with indelible ink that will write on any surface', 'name': 'Sharpie'}, {'frequency': 'r', 'id': 957, 'synset': 'shaver.n.03', 'synonyms': ['shaver_(electric)', 'electric_shaver', 'electric_razor'], 'def': 'a razor powered by an electric motor', 'name': 'shaver_(electric)'}, {'frequency': 'c', 'id': 958, 'synset': 'shaving_cream.n.01', 'synonyms': ['shaving_cream', 'shaving_soap'], 'def': 'toiletry consisting that forms a rich lather for softening the beard before shaving', 'name': 'shaving_cream'}, {'frequency': 'r', 'id': 959, 'synset': 'shawl.n.01', 'synonyms': ['shawl'], 'def': 'cloak consisting of an oblong piece of cloth used to cover the head and shoulders', 'name': 'shawl'}, {'frequency': 'r', 'id': 960, 'synset': 'shears.n.01', 'synonyms': ['shears'], 'def': 'large scissors with strong blades', 'name': 'shears'}, {'frequency': 'f', 'id': 961, 'synset': 'sheep.n.01', 'synonyms': ['sheep'], 'def': 'woolly usually horned ruminant mammal related to the goat', 'name': 'sheep'}, {'frequency': 'r', 'id': 962, 'synset': 'shepherd_dog.n.01', 'synonyms': ['shepherd_dog', 'sheepdog'], 'def': 'any of various usually long-haired breeds of dog reared to herd and guard sheep', 'name': 'shepherd_dog'}, {'frequency': 'r', 'id': 963, 'synset': 'sherbert.n.01', 'synonyms': ['sherbert', 'sherbet'], 'def': 'a frozen dessert made primarily of fruit juice and sugar', 'name': 'sherbert'}, {'frequency': 'r', 'id': 964, 'synset': 'shield.n.02', 'synonyms': ['shield'], 'def': 'armor carried on the arm to intercept blows', 'name': 'shield'}, {'frequency': 'f', 'id': 965, 'synset': 'shirt.n.01', 'synonyms': ['shirt'], 'def': 'a garment worn on the upper half of the body', 'name': 'shirt'}, {'frequency': 'f', 'id': 966, 'synset': 'shoe.n.01', 'synonyms': ['shoe', 'sneaker_(type_of_shoe)', 'tennis_shoe'], 'def': 'common footwear covering the foot', 'name': 'shoe'}, {'frequency': 'c', 'id': 967, 'synset': 'shopping_bag.n.01', 'synonyms': ['shopping_bag'], 'def': 'a bag made of plastic or strong paper (often with handles); used to transport goods after shopping', 'name': 'shopping_bag'}, {'frequency': 'c', 'id': 968, 'synset': 'shopping_cart.n.01', 'synonyms': ['shopping_cart'], 'def': 'a handcart that holds groceries or other goods while shopping', 'name': 'shopping_cart'}, {'frequency': 'f', 'id': 969, 'synset': 'short_pants.n.01', 'synonyms': ['short_pants', 'shorts_(clothing)', 'trunks_(clothing)'], 'def': 'trousers that end at or above the knee', 'name': 'short_pants'}, {'frequency': 'r', 'id': 970, 'synset': 'shot_glass.n.01', 'synonyms': ['shot_glass'], 'def': 'a small glass adequate to hold a single swallow of whiskey', 'name': 'shot_glass'}, {'frequency': 'c', 'id': 971, 'synset': 'shoulder_bag.n.01', 'synonyms': ['shoulder_bag'], 'def': 'a large handbag that can be carried by a strap looped over the shoulder', 'name': 'shoulder_bag'}, {'frequency': 'c', 'id': 972, 'synset': 'shovel.n.01', 'synonyms': ['shovel'], 'def': 'a hand tool for lifting loose material such as snow, dirt, etc.', 'name': 'shovel'}, {'frequency': 'f', 'id': 973, 'synset': 'shower.n.01', 'synonyms': ['shower_head'], 'def': 'a plumbing fixture that sprays water over you', 'name': 'shower_head'}, {'frequency': 'f', 'id': 974, 'synset': 'shower_curtain.n.01', 'synonyms': ['shower_curtain'], 'def': 'a curtain that keeps water from splashing out of the shower area', 'name': 'shower_curtain'}, {'frequency': 'r', 'id': 975, 'synset': 'shredder.n.01', 'synonyms': ['shredder_(for_paper)'], 'def': 'a device that shreds documents', 'name': 'shredder_(for_paper)'}, {'frequency': 'r', 'id': 976, 'synset': 'sieve.n.01', 'synonyms': ['sieve', 'screen_(sieve)'], 'def': 'a strainer for separating lumps from powdered material or grading particles', 'name': 'sieve'}, {'frequency': 'f', 'id': 977, 'synset': 'signboard.n.01', 'synonyms': ['signboard'], 'def': 'structure displaying a board on which advertisements can be posted', 'name': 'signboard'}, {'frequency': 'c', 'id': 978, 'synset': 'silo.n.01', 'synonyms': ['silo'], 'def': 'a cylindrical tower used for storing goods', 'name': 'silo'}, {'frequency': 'f', 'id': 979, 'synset': 'sink.n.01', 'synonyms': ['sink'], 'def': 'plumbing fixture consisting of a water basin fixed to a wall or floor and having a drainpipe', 'name': 'sink'}, {'frequency': 'f', 'id': 980, 'synset': 'skateboard.n.01', 'synonyms': ['skateboard'], 'def': 'a board with wheels that is ridden in a standing or crouching position and propelled by foot', 'name': 'skateboard'}, {'frequency': 'c', 'id': 981, 'synset': 'skewer.n.01', 'synonyms': ['skewer'], 'def': 'a long pin for holding meat in position while it is being roasted', 'name': 'skewer'}, {'frequency': 'f', 'id': 982, 'synset': 'ski.n.01', 'synonyms': ['ski'], 'def': 'sports equipment for skiing on snow', 'name': 'ski'}, {'frequency': 'f', 'id': 983, 'synset': 'ski_boot.n.01', 'synonyms': ['ski_boot'], 'def': 'a stiff boot that is fastened to a ski with a ski binding', 'name': 'ski_boot'}, {'frequency': 'f', 'id': 984, 'synset': 'ski_parka.n.01', 'synonyms': ['ski_parka', 'ski_jacket'], 'def': 'a parka to be worn while skiing', 'name': 'ski_parka'}, {'frequency': 'f', 'id': 985, 'synset': 'ski_pole.n.01', 'synonyms': ['ski_pole'], 'def': 'a pole with metal points used as an aid in skiing', 'name': 'ski_pole'}, {'frequency': 'f', 'id': 986, 'synset': 'skirt.n.02', 'synonyms': ['skirt'], 'def': 'a garment hanging from the waist; worn mainly by girls and women', 'name': 'skirt'}, {'frequency': 'c', 'id': 987, 'synset': 'sled.n.01', 'synonyms': ['sled', 'sledge', 'sleigh'], 'def': 'a vehicle or flat object for transportation over snow by sliding or pulled by dogs, etc.', 'name': 'sled'}, {'frequency': 'c', 'id': 988, 'synset': 'sleeping_bag.n.01', 'synonyms': ['sleeping_bag'], 'def': 'large padded bag designed to be slept in outdoors', 'name': 'sleeping_bag'}, {'frequency': 'r', 'id': 989, 'synset': 'sling.n.05', 'synonyms': ['sling_(bandage)', 'triangular_bandage'], 'def': 'bandage to support an injured forearm; slung over the shoulder or neck', 'name': 'sling_(bandage)'}, {'frequency': 'c', 'id': 990, 'synset': 'slipper.n.01', 'synonyms': ['slipper_(footwear)', 'carpet_slipper_(footwear)'], 'def': 'low footwear that can be slipped on and off easily; usually worn indoors', 'name': 'slipper_(footwear)'}, {'frequency': 'r', 'id': 991, 'synset': 'smoothie.n.02', 'synonyms': ['smoothie'], 'def': 'a thick smooth drink consisting of fresh fruit pureed with ice cream or yoghurt or milk', 'name': 'smoothie'}, {'frequency': 'r', 'id': 992, 'synset': 'snake.n.01', 'synonyms': ['snake', 'serpent'], 'def': 'limbless scaly elongate reptile; some are venomous', 'name': 'snake'}, {'frequency': 'f', 'id': 993, 'synset': 'snowboard.n.01', 'synonyms': ['snowboard'], 'def': 'a board that resembles a broad ski or a small surfboard; used in a standing position to slide down snow-covered slopes', 'name': 'snowboard'}, {'frequency': 'c', 'id': 994, 'synset': 'snowman.n.01', 'synonyms': ['snowman'], 'def': 'a figure of a person made of packed snow', 'name': 'snowman'}, {'frequency': 'c', 'id': 995, 'synset': 'snowmobile.n.01', 'synonyms': ['snowmobile'], 'def': 'tracked vehicle for travel on snow having skis in front', 'name': 'snowmobile'}, {'frequency': 'f', 'id': 996, 'synset': 'soap.n.01', 'synonyms': ['soap'], 'def': 'a cleansing agent made from the salts of vegetable or animal fats', 'name': 'soap'}, {'frequency': 'f', 'id': 997, 'synset': 'soccer_ball.n.01', 'synonyms': ['soccer_ball'], 'def': "an inflated ball used in playing soccer (called `football' outside of the United States)", 'name': 'soccer_ball'}, {'frequency': 'f', 'id': 998, 'synset': 'sock.n.01', 'synonyms': ['sock'], 'def': 'cloth covering for the foot; worn inside the shoe; reaches to between the ankle and the knee', 'name': 'sock'}, {'frequency': 'r', 'id': 999, 'synset': 'soda_fountain.n.02', 'synonyms': ['soda_fountain'], 'def': 'an apparatus for dispensing soda water', 'name': 'soda_fountain'}, {'frequency': 'r', 'id': 1000, 'synset': 'soda_water.n.01', 'synonyms': ['carbonated_water', 'club_soda', 'seltzer', 'sparkling_water'], 'def': 'effervescent beverage artificially charged with carbon dioxide', 'name': 'carbonated_water'}, {'frequency': 'f', 'id': 1001, 'synset': 'sofa.n.01', 'synonyms': ['sofa', 'couch', 'lounge'], 'def': 'an upholstered seat for more than one person', 'name': 'sofa'}, {'frequency': 'r', 'id': 1002, 'synset': 'softball.n.01', 'synonyms': ['softball'], 'def': 'ball used in playing softball', 'name': 'softball'}, {'frequency': 'c', 'id': 1003, 'synset': 'solar_array.n.01', 'synonyms': ['solar_array', 'solar_battery', 'solar_panel'], 'def': 'electrical device consisting of a large array of connected solar cells', 'name': 'solar_array'}, {'frequency': 'r', 'id': 1004, 'synset': 'sombrero.n.02', 'synonyms': ['sombrero'], 'def': 'a straw hat with a tall crown and broad brim; worn in American southwest and in Mexico', 'name': 'sombrero'}, {'frequency': 'c', 'id': 1005, 'synset': 'soup.n.01', 'synonyms': ['soup'], 'def': 'liquid food especially of meat or fish or vegetable stock often containing pieces of solid food', 'name': 'soup'}, {'frequency': 'r', 'id': 1006, 'synset': 'soup_bowl.n.01', 'synonyms': ['soup_bowl'], 'def': 'a bowl for serving soup', 'name': 'soup_bowl'}, {'frequency': 'c', 'id': 1007, 'synset': 'soupspoon.n.01', 'synonyms': ['soupspoon'], 'def': 'a spoon with a rounded bowl for eating soup', 'name': 'soupspoon'}, {'frequency': 'c', 'id': 1008, 'synset': 'sour_cream.n.01', 'synonyms': ['sour_cream', 'soured_cream'], 'def': 'soured light cream', 'name': 'sour_cream'}, {'frequency': 'r', 'id': 1009, 'synset': 'soya_milk.n.01', 'synonyms': ['soya_milk', 'soybean_milk', 'soymilk'], 'def': 'a milk substitute containing soybean flour and water; used in some infant formulas and in making tofu', 'name': 'soya_milk'}, {'frequency': 'r', 'id': 1010, 'synset': 'space_shuttle.n.01', 'synonyms': ['space_shuttle'], 'def': "a reusable spacecraft with wings for a controlled descent through the Earth's atmosphere", 'name': 'space_shuttle'}, {'frequency': 'r', 'id': 1011, 'synset': 'sparkler.n.02', 'synonyms': ['sparkler_(fireworks)'], 'def': 'a firework that burns slowly and throws out a shower of sparks', 'name': 'sparkler_(fireworks)'}, {'frequency': 'f', 'id': 1012, 'synset': 'spatula.n.02', 'synonyms': ['spatula'], 'def': 'a hand tool with a thin flexible blade used to mix or spread soft substances', 'name': 'spatula'}, {'frequency': 'r', 'id': 1013, 'synset': 'spear.n.01', 'synonyms': ['spear', 'lance'], 'def': 'a long pointed rod used as a tool or weapon', 'name': 'spear'}, {'frequency': 'f', 'id': 1014, 'synset': 'spectacles.n.01', 'synonyms': ['spectacles', 'specs', 'eyeglasses', 'glasses'], 'def': 'optical instrument consisting of a frame that holds a pair of lenses for correcting defective vision', 'name': 'spectacles'}, {'frequency': 'c', 'id': 1015, 'synset': 'spice_rack.n.01', 'synonyms': ['spice_rack'], 'def': 'a rack for displaying containers filled with spices', 'name': 'spice_rack'}, {'frequency': 'r', 'id': 1016, 'synset': 'spider.n.01', 'synonyms': ['spider'], 'def': 'predatory arachnid with eight legs, two poison fangs, two feelers, and usually two silk-spinning organs at the back end of the body', 'name': 'spider'}, {'frequency': 'c', 'id': 1017, 'synset': 'sponge.n.01', 'synonyms': ['sponge'], 'def': 'a porous mass usable to absorb water typically used for cleaning', 'name': 'sponge'}, {'frequency': 'f', 'id': 1018, 'synset': 'spoon.n.01', 'synonyms': ['spoon'], 'def': 'a piece of cutlery with a shallow bowl-shaped container and a handle', 'name': 'spoon'}, {'frequency': 'c', 'id': 1019, 'synset': 'sportswear.n.01', 'synonyms': ['sportswear', 'athletic_wear', 'activewear'], 'def': 'attire worn for sport or for casual wear', 'name': 'sportswear'}, {'frequency': 'c', 'id': 1020, 'synset': 'spotlight.n.02', 'synonyms': ['spotlight'], 'def': 'a lamp that produces a strong beam of light to illuminate a restricted area; used to focus attention of a stage performer', 'name': 'spotlight'}, {'frequency': 'r', 'id': 1021, 'synset': 'squirrel.n.01', 'synonyms': ['squirrel'], 'def': 'a kind of arboreal rodent having a long bushy tail', 'name': 'squirrel'}, {'frequency': 'c', 'id': 1022, 'synset': 'stapler.n.01', 'synonyms': ['stapler_(stapling_machine)'], 'def': 'a machine that inserts staples into sheets of paper in order to fasten them together', 'name': 'stapler_(stapling_machine)'}, {'frequency': 'r', 'id': 1023, 'synset': 'starfish.n.01', 'synonyms': ['starfish', 'sea_star'], 'def': 'echinoderms characterized by five arms extending from a central disk', 'name': 'starfish'}, {'frequency': 'f', 'id': 1024, 'synset': 'statue.n.01', 'synonyms': ['statue_(sculpture)'], 'def': 'a sculpture representing a human or animal', 'name': 'statue_(sculpture)'}, {'frequency': 'c', 'id': 1025, 'synset': 'steak.n.01', 'synonyms': ['steak_(food)'], 'def': 'a slice of meat cut from the fleshy part of an animal or large fish', 'name': 'steak_(food)'}, {'frequency': 'r', 'id': 1026, 'synset': 'steak_knife.n.01', 'synonyms': ['steak_knife'], 'def': 'a sharp table knife used in eating steak', 'name': 'steak_knife'}, {'frequency': 'r', 'id': 1027, 'synset': 'steamer.n.02', 'synonyms': ['steamer_(kitchen_appliance)'], 'def': 'a cooking utensil that can be used to cook food by steaming it', 'name': 'steamer_(kitchen_appliance)'}, {'frequency': 'f', 'id': 1028, 'synset': 'steering_wheel.n.01', 'synonyms': ['steering_wheel'], 'def': 'a handwheel that is used for steering', 'name': 'steering_wheel'}, {'frequency': 'r', 'id': 1029, 'synset': 'stencil.n.01', 'synonyms': ['stencil'], 'def': 'a sheet of material (metal, plastic, etc.) that has been perforated with a pattern; ink or paint can pass through the perforations to create the printed pattern on the surface below', 'name': 'stencil'}, {'frequency': 'r', 'id': 1030, 'synset': 'step_ladder.n.01', 'synonyms': ['stepladder'], 'def': 'a folding portable ladder hinged at the top', 'name': 'stepladder'}, {'frequency': 'c', 'id': 1031, 'synset': 'step_stool.n.01', 'synonyms': ['step_stool'], 'def': 'a stool that has one or two steps that fold under the seat', 'name': 'step_stool'}, {'frequency': 'c', 'id': 1032, 'synset': 'stereo.n.01', 'synonyms': ['stereo_(sound_system)'], 'def': 'electronic device for playing audio', 'name': 'stereo_(sound_system)'}, {'frequency': 'r', 'id': 1033, 'synset': 'stew.n.02', 'synonyms': ['stew'], 'def': 'food prepared by stewing especially meat or fish with vegetables', 'name': 'stew'}, {'frequency': 'r', 'id': 1034, 'synset': 'stirrer.n.02', 'synonyms': ['stirrer'], 'def': 'an implement used for stirring', 'name': 'stirrer'}, {'frequency': 'f', 'id': 1035, 'synset': 'stirrup.n.01', 'synonyms': ['stirrup'], 'def': "support consisting of metal loops into which rider's feet go", 'name': 'stirrup'}, {'frequency': 'c', 'id': 1036, 'synset': 'stocking.n.01', 'synonyms': ['stockings_(leg_wear)'], 'def': 'close-fitting hosiery to cover the foot and leg; come in matched pairs', 'name': 'stockings_(leg_wear)'}, {'frequency': 'f', 'id': 1037, 'synset': 'stool.n.01', 'synonyms': ['stool'], 'def': 'a simple seat without a back or arms', 'name': 'stool'}, {'frequency': 'f', 'id': 1038, 'synset': 'stop_sign.n.01', 'synonyms': ['stop_sign'], 'def': 'a traffic sign to notify drivers that they must come to a complete stop', 'name': 'stop_sign'}, {'frequency': 'f', 'id': 1039, 'synset': 'stoplight.n.01', 'synonyms': ['brake_light'], 'def': 'a red light on the rear of a motor vehicle that signals when the brakes are applied', 'name': 'brake_light'}, {'frequency': 'f', 'id': 1040, 'synset': 'stove.n.01', 'synonyms': ['stove', 'kitchen_stove', 'range_(kitchen_appliance)', 'kitchen_range', 'cooking_stove'], 'def': 'a kitchen appliance used for cooking food', 'name': 'stove'}, {'frequency': 'c', 'id': 1041, 'synset': 'strainer.n.01', 'synonyms': ['strainer'], 'def': 'a filter to retain larger pieces while smaller pieces and liquids pass through', 'name': 'strainer'}, {'frequency': 'f', 'id': 1042, 'synset': 'strap.n.01', 'synonyms': ['strap'], 'def': 'an elongated strip of material for binding things together or holding', 'name': 'strap'}, {'frequency': 'f', 'id': 1043, 'synset': 'straw.n.04', 'synonyms': ['straw_(for_drinking)', 'drinking_straw'], 'def': 'a thin paper or plastic tube used to suck liquids into the mouth', 'name': 'straw_(for_drinking)'}, {'frequency': 'f', 'id': 1044, 'synset': 'strawberry.n.01', 'synonyms': ['strawberry'], 'def': 'sweet fleshy red fruit', 'name': 'strawberry'}, {'frequency': 'f', 'id': 1045, 'synset': 'street_sign.n.01', 'synonyms': ['street_sign'], 'def': 'a sign visible from the street', 'name': 'street_sign'}, {'frequency': 'f', 'id': 1046, 'synset': 'streetlight.n.01', 'synonyms': ['streetlight', 'street_lamp'], 'def': 'a lamp supported on a lamppost; for illuminating a street', 'name': 'streetlight'}, {'frequency': 'r', 'id': 1047, 'synset': 'string_cheese.n.01', 'synonyms': ['string_cheese'], 'def': 'cheese formed in long strings twisted together', 'name': 'string_cheese'}, {'frequency': 'r', 'id': 1048, 'synset': 'stylus.n.02', 'synonyms': ['stylus'], 'def': 'a pointed tool for writing or drawing or engraving', 'name': 'stylus'}, {'frequency': 'r', 'id': 1049, 'synset': 'subwoofer.n.01', 'synonyms': ['subwoofer'], 'def': 'a loudspeaker that is designed to reproduce very low bass frequencies', 'name': 'subwoofer'}, {'frequency': 'r', 'id': 1050, 'synset': 'sugar_bowl.n.01', 'synonyms': ['sugar_bowl'], 'def': 'a dish in which sugar is served', 'name': 'sugar_bowl'}, {'frequency': 'r', 'id': 1051, 'synset': 'sugarcane.n.01', 'synonyms': ['sugarcane_(plant)'], 'def': 'juicy canes whose sap is a source of molasses and commercial sugar; fresh canes are sometimes chewed for the juice', 'name': 'sugarcane_(plant)'}, {'frequency': 'c', 'id': 1052, 'synset': 'suit.n.01', 'synonyms': ['suit_(clothing)'], 'def': 'a set of garments (usually including a jacket and trousers or skirt) for outerwear all of the same fabric and color', 'name': 'suit_(clothing)'}, {'frequency': 'c', 'id': 1053, 'synset': 'sunflower.n.01', 'synonyms': ['sunflower'], 'def': 'any plant of the genus Helianthus having large flower heads with dark disk florets and showy yellow rays', 'name': 'sunflower'}, {'frequency': 'f', 'id': 1054, 'synset': 'sunglasses.n.01', 'synonyms': ['sunglasses'], 'def': 'spectacles that are darkened or polarized to protect the eyes from the glare of the sun', 'name': 'sunglasses'}, {'frequency': 'c', 'id': 1055, 'synset': 'sunhat.n.01', 'synonyms': ['sunhat'], 'def': 'a hat with a broad brim that protects the face from direct exposure to the sun', 'name': 'sunhat'}, {'frequency': 'r', 'id': 1056, 'synset': 'sunscreen.n.01', 'synonyms': ['sunscreen', 'sunblock'], 'def': 'a cream spread on the skin; contains a chemical to filter out ultraviolet light and so protect from sunburn', 'name': 'sunscreen'}, {'frequency': 'f', 'id': 1057, 'synset': 'surfboard.n.01', 'synonyms': ['surfboard'], 'def': 'a narrow buoyant board for riding surf', 'name': 'surfboard'}, {'frequency': 'c', 'id': 1058, 'synset': 'sushi.n.01', 'synonyms': ['sushi'], 'def': 'rice (with raw fish) wrapped in seaweed', 'name': 'sushi'}, {'frequency': 'c', 'id': 1059, 'synset': 'swab.n.02', 'synonyms': ['mop'], 'def': 'cleaning implement consisting of absorbent material fastened to a handle; for cleaning floors', 'name': 'mop'}, {'frequency': 'c', 'id': 1060, 'synset': 'sweat_pants.n.01', 'synonyms': ['sweat_pants'], 'def': 'loose-fitting trousers with elastic cuffs; worn by athletes', 'name': 'sweat_pants'}, {'frequency': 'c', 'id': 1061, 'synset': 'sweatband.n.02', 'synonyms': ['sweatband'], 'def': 'a band of material tied around the forehead or wrist to absorb sweat', 'name': 'sweatband'}, {'frequency': 'f', 'id': 1062, 'synset': 'sweater.n.01', 'synonyms': ['sweater'], 'def': 'a crocheted or knitted garment covering the upper part of the body', 'name': 'sweater'}, {'frequency': 'f', 'id': 1063, 'synset': 'sweatshirt.n.01', 'synonyms': ['sweatshirt'], 'def': 'cotton knit pullover with long sleeves worn during athletic activity', 'name': 'sweatshirt'}, {'frequency': 'c', 'id': 1064, 'synset': 'sweet_potato.n.02', 'synonyms': ['sweet_potato'], 'def': 'the edible tuberous root of the sweet potato vine', 'name': 'sweet_potato'}, {'frequency': 'f', 'id': 1065, 'synset': 'swimsuit.n.01', 'synonyms': ['swimsuit', 'swimwear', 'bathing_suit', 'swimming_costume', 'bathing_costume', 'swimming_trunks', 'bathing_trunks'], 'def': 'garment worn for swimming', 'name': 'swimsuit'}, {'frequency': 'c', 'id': 1066, 'synset': 'sword.n.01', 'synonyms': ['sword'], 'def': 'a cutting or thrusting weapon that has a long metal blade', 'name': 'sword'}, {'frequency': 'r', 'id': 1067, 'synset': 'syringe.n.01', 'synonyms': ['syringe'], 'def': 'a medical instrument used to inject or withdraw fluids', 'name': 'syringe'}, {'frequency': 'r', 'id': 1068, 'synset': 'tabasco.n.02', 'synonyms': ['Tabasco_sauce'], 'def': 'very spicy sauce (trade name Tabasco) made from fully-aged red peppers', 'name': 'Tabasco_sauce'}, {'frequency': 'r', 'id': 1069, 'synset': 'table-tennis_table.n.01', 'synonyms': ['table-tennis_table', 'ping-pong_table'], 'def': 'a table used for playing table tennis', 'name': 'table-tennis_table'}, {'frequency': 'f', 'id': 1070, 'synset': 'table.n.02', 'synonyms': ['table'], 'def': 'a piece of furniture having a smooth flat top that is usually supported by one or more vertical legs', 'name': 'table'}, {'frequency': 'c', 'id': 1071, 'synset': 'table_lamp.n.01', 'synonyms': ['table_lamp'], 'def': 'a lamp that sits on a table', 'name': 'table_lamp'}, {'frequency': 'f', 'id': 1072, 'synset': 'tablecloth.n.01', 'synonyms': ['tablecloth'], 'def': 'a covering spread over a dining table', 'name': 'tablecloth'}, {'frequency': 'r', 'id': 1073, 'synset': 'tachometer.n.01', 'synonyms': ['tachometer'], 'def': 'measuring instrument for indicating speed of rotation', 'name': 'tachometer'}, {'frequency': 'r', 'id': 1074, 'synset': 'taco.n.02', 'synonyms': ['taco'], 'def': 'a small tortilla cupped around a filling', 'name': 'taco'}, {'frequency': 'f', 'id': 1075, 'synset': 'tag.n.02', 'synonyms': ['tag'], 'def': 'a label associated with something for the purpose of identification or information', 'name': 'tag'}, {'frequency': 'f', 'id': 1076, 'synset': 'taillight.n.01', 'synonyms': ['taillight', 'rear_light'], 'def': 'lamp (usually red) mounted at the rear of a motor vehicle', 'name': 'taillight'}, {'frequency': 'r', 'id': 1077, 'synset': 'tambourine.n.01', 'synonyms': ['tambourine'], 'def': 'a shallow drum with a single drumhead and with metallic disks in the sides', 'name': 'tambourine'}, {'frequency': 'r', 'id': 1078, 'synset': 'tank.n.01', 'synonyms': ['army_tank', 'armored_combat_vehicle', 'armoured_combat_vehicle'], 'def': 'an enclosed armored military vehicle; has a cannon and moves on caterpillar treads', 'name': 'army_tank'}, {'frequency': 'c', 'id': 1079, 'synset': 'tank.n.02', 'synonyms': ['tank_(storage_vessel)', 'storage_tank'], 'def': 'a large (usually metallic) vessel for holding gases or liquids', 'name': 'tank_(storage_vessel)'}, {'frequency': 'f', 'id': 1080, 'synset': 'tank_top.n.01', 'synonyms': ['tank_top_(clothing)'], 'def': 'a tight-fitting sleeveless shirt with wide shoulder straps and low neck and no front opening', 'name': 'tank_top_(clothing)'}, {'frequency': 'c', 'id': 1081, 'synset': 'tape.n.01', 'synonyms': ['tape_(sticky_cloth_or_paper)'], 'def': 'a long thin piece of cloth or paper as used for binding or fastening', 'name': 'tape_(sticky_cloth_or_paper)'}, {'frequency': 'c', 'id': 1082, 'synset': 'tape.n.04', 'synonyms': ['tape_measure', 'measuring_tape'], 'def': 'measuring instrument consisting of a narrow strip (cloth or metal) marked in inches or centimeters and used for measuring lengths', 'name': 'tape_measure'}, {'frequency': 'c', 'id': 1083, 'synset': 'tapestry.n.02', 'synonyms': ['tapestry'], 'def': 'a heavy textile with a woven design; used for curtains and upholstery', 'name': 'tapestry'}, {'frequency': 'f', 'id': 1084, 'synset': 'tarpaulin.n.01', 'synonyms': ['tarp'], 'def': 'waterproofed canvas', 'name': 'tarp'}, {'frequency': 'c', 'id': 1085, 'synset': 'tartan.n.01', 'synonyms': ['tartan', 'plaid'], 'def': 'a cloth having a crisscross design', 'name': 'tartan'}, {'frequency': 'c', 'id': 1086, 'synset': 'tassel.n.01', 'synonyms': ['tassel'], 'def': 'adornment consisting of a bunch of cords fastened at one end', 'name': 'tassel'}, {'frequency': 'r', 'id': 1087, 'synset': 'tea_bag.n.01', 'synonyms': ['tea_bag'], 'def': 'a measured amount of tea in a bag for an individual serving of tea', 'name': 'tea_bag'}, {'frequency': 'c', 'id': 1088, 'synset': 'teacup.n.02', 'synonyms': ['teacup'], 'def': 'a cup from which tea is drunk', 'name': 'teacup'}, {'frequency': 'c', 'id': 1089, 'synset': 'teakettle.n.01', 'synonyms': ['teakettle'], 'def': 'kettle for boiling water to make tea', 'name': 'teakettle'}, {'frequency': 'c', 'id': 1090, 'synset': 'teapot.n.01', 'synonyms': ['teapot'], 'def': 'pot for brewing tea; usually has a spout and handle', 'name': 'teapot'}, {'frequency': 'f', 'id': 1091, 'synset': 'teddy.n.01', 'synonyms': ['teddy_bear'], 'def': "plaything consisting of a child's toy bear (usually plush and stuffed with soft materials)", 'name': 'teddy_bear'}, {'frequency': 'f', 'id': 1092, 'synset': 'telephone.n.01', 'synonyms': ['telephone', 'phone', 'telephone_set'], 'def': 'electronic device for communicating by voice over long distances', 'name': 'telephone'}, {'frequency': 'c', 'id': 1093, 'synset': 'telephone_booth.n.01', 'synonyms': ['telephone_booth', 'phone_booth', 'call_box', 'telephone_box', 'telephone_kiosk'], 'def': 'booth for using a telephone', 'name': 'telephone_booth'}, {'frequency': 'f', 'id': 1094, 'synset': 'telephone_pole.n.01', 'synonyms': ['telephone_pole', 'telegraph_pole', 'telegraph_post'], 'def': 'tall pole supporting telephone wires', 'name': 'telephone_pole'}, {'frequency': 'r', 'id': 1095, 'synset': 'telephoto_lens.n.01', 'synonyms': ['telephoto_lens', 'zoom_lens'], 'def': 'a camera lens that magnifies the image', 'name': 'telephoto_lens'}, {'frequency': 'c', 'id': 1096, 'synset': 'television_camera.n.01', 'synonyms': ['television_camera', 'tv_camera'], 'def': 'television equipment for capturing and recording video', 'name': 'television_camera'}, {'frequency': 'f', 'id': 1097, 'synset': 'television_receiver.n.01', 'synonyms': ['television_set', 'tv', 'tv_set'], 'def': 'an electronic device that receives television signals and displays them on a screen', 'name': 'television_set'}, {'frequency': 'f', 'id': 1098, 'synset': 'tennis_ball.n.01', 'synonyms': ['tennis_ball'], 'def': 'ball about the size of a fist used in playing tennis', 'name': 'tennis_ball'}, {'frequency': 'f', 'id': 1099, 'synset': 'tennis_racket.n.01', 'synonyms': ['tennis_racket'], 'def': 'a racket used to play tennis', 'name': 'tennis_racket'}, {'frequency': 'r', 'id': 1100, 'synset': 'tequila.n.01', 'synonyms': ['tequila'], 'def': 'Mexican liquor made from fermented juices of an agave plant', 'name': 'tequila'}, {'frequency': 'c', 'id': 1101, 'synset': 'thermometer.n.01', 'synonyms': ['thermometer'], 'def': 'measuring instrument for measuring temperature', 'name': 'thermometer'}, {'frequency': 'c', 'id': 1102, 'synset': 'thermos.n.01', 'synonyms': ['thermos_bottle'], 'def': 'vacuum flask that preserves temperature of hot or cold drinks', 'name': 'thermos_bottle'}, {'frequency': 'c', 'id': 1103, 'synset': 'thermostat.n.01', 'synonyms': ['thermostat'], 'def': 'a regulator for automatically regulating temperature by starting or stopping the supply of heat', 'name': 'thermostat'}, {'frequency': 'r', 'id': 1104, 'synset': 'thimble.n.02', 'synonyms': ['thimble'], 'def': 'a small metal cap to protect the finger while sewing; can be used as a small container', 'name': 'thimble'}, {'frequency': 'c', 'id': 1105, 'synset': 'thread.n.01', 'synonyms': ['thread', 'yarn'], 'def': 'a fine cord of twisted fibers (of cotton or silk or wool or nylon etc.) used in sewing and weaving', 'name': 'thread'}, {'frequency': 'c', 'id': 1106, 'synset': 'thumbtack.n.01', 'synonyms': ['thumbtack', 'drawing_pin', 'pushpin'], 'def': 'a tack for attaching papers to a bulletin board or drawing board', 'name': 'thumbtack'}, {'frequency': 'c', 'id': 1107, 'synset': 'tiara.n.01', 'synonyms': ['tiara'], 'def': 'a jeweled headdress worn by women on formal occasions', 'name': 'tiara'}, {'frequency': 'c', 'id': 1108, 'synset': 'tiger.n.02', 'synonyms': ['tiger'], 'def': 'large feline of forests in most of Asia having a tawny coat with black stripes', 'name': 'tiger'}, {'frequency': 'c', 'id': 1109, 'synset': 'tights.n.01', 'synonyms': ['tights_(clothing)', 'leotards'], 'def': 'skintight knit hose covering the body from the waist to the feet worn by acrobats and dancers and as stockings by women and girls', 'name': 'tights_(clothing)'}, {'frequency': 'c', 'id': 1110, 'synset': 'timer.n.01', 'synonyms': ['timer', 'stopwatch'], 'def': 'a timepiece that measures a time interval and signals its end', 'name': 'timer'}, {'frequency': 'f', 'id': 1111, 'synset': 'tinfoil.n.01', 'synonyms': ['tinfoil'], 'def': 'foil made of tin or an alloy of tin and lead', 'name': 'tinfoil'}, {'frequency': 'r', 'id': 1112, 'synset': 'tinsel.n.01', 'synonyms': ['tinsel'], 'def': 'a showy decoration that is basically valueless', 'name': 'tinsel'}, {'frequency': 'f', 'id': 1113, 'synset': 'tissue.n.02', 'synonyms': ['tissue_paper'], 'def': 'a soft thin (usually translucent) paper', 'name': 'tissue_paper'}, {'frequency': 'c', 'id': 1114, 'synset': 'toast.n.01', 'synonyms': ['toast_(food)'], 'def': 'slice of bread that has been toasted', 'name': 'toast_(food)'}, {'frequency': 'f', 'id': 1115, 'synset': 'toaster.n.02', 'synonyms': ['toaster'], 'def': 'a kitchen appliance (usually electric) for toasting bread', 'name': 'toaster'}, {'frequency': 'c', 'id': 1116, 'synset': 'toaster_oven.n.01', 'synonyms': ['toaster_oven'], 'def': 'kitchen appliance consisting of a small electric oven for toasting or warming food', 'name': 'toaster_oven'}, {'frequency': 'f', 'id': 1117, 'synset': 'toilet.n.02', 'synonyms': ['toilet'], 'def': 'a plumbing fixture for defecation and urination', 'name': 'toilet'}, {'frequency': 'f', 'id': 1118, 'synset': 'toilet_tissue.n.01', 'synonyms': ['toilet_tissue', 'toilet_paper', 'bathroom_tissue'], 'def': 'a soft thin absorbent paper for use in toilets', 'name': 'toilet_tissue'}, {'frequency': 'f', 'id': 1119, 'synset': 'tomato.n.01', 'synonyms': ['tomato'], 'def': 'mildly acid red or yellow pulpy fruit eaten as a vegetable', 'name': 'tomato'}, {'frequency': 'c', 'id': 1120, 'synset': 'tongs.n.01', 'synonyms': ['tongs'], 'def': 'any of various devices for taking hold of objects; usually have two hinged legs with handles above and pointed hooks below', 'name': 'tongs'}, {'frequency': 'c', 'id': 1121, 'synset': 'toolbox.n.01', 'synonyms': ['toolbox'], 'def': 'a box or chest or cabinet for holding hand tools', 'name': 'toolbox'}, {'frequency': 'f', 'id': 1122, 'synset': 'toothbrush.n.01', 'synonyms': ['toothbrush'], 'def': 'small brush; has long handle; used to clean teeth', 'name': 'toothbrush'}, {'frequency': 'f', 'id': 1123, 'synset': 'toothpaste.n.01', 'synonyms': ['toothpaste'], 'def': 'a dentifrice in the form of a paste', 'name': 'toothpaste'}, {'frequency': 'c', 'id': 1124, 'synset': 'toothpick.n.01', 'synonyms': ['toothpick'], 'def': 'pick consisting of a small strip of wood or plastic; used to pick food from between the teeth', 'name': 'toothpick'}, {'frequency': 'c', 'id': 1125, 'synset': 'top.n.09', 'synonyms': ['cover'], 'def': 'covering for a hole (especially a hole in the top of a container)', 'name': 'cover'}, {'frequency': 'c', 'id': 1126, 'synset': 'tortilla.n.01', 'synonyms': ['tortilla'], 'def': 'thin unleavened pancake made from cornmeal or wheat flour', 'name': 'tortilla'}, {'frequency': 'c', 'id': 1127, 'synset': 'tow_truck.n.01', 'synonyms': ['tow_truck'], 'def': 'a truck equipped to hoist and pull wrecked cars (or to remove cars from no-parking zones)', 'name': 'tow_truck'}, {'frequency': 'f', 'id': 1128, 'synset': 'towel.n.01', 'synonyms': ['towel'], 'def': 'a rectangular piece of absorbent cloth (or paper) for drying or wiping', 'name': 'towel'}, {'frequency': 'f', 'id': 1129, 'synset': 'towel_rack.n.01', 'synonyms': ['towel_rack', 'towel_rail', 'towel_bar'], 'def': 'a rack consisting of one or more bars on which towels can be hung', 'name': 'towel_rack'}, {'frequency': 'f', 'id': 1130, 'synset': 'toy.n.03', 'synonyms': ['toy'], 'def': 'a device regarded as providing amusement', 'name': 'toy'}, {'frequency': 'c', 'id': 1131, 'synset': 'tractor.n.01', 'synonyms': ['tractor_(farm_equipment)'], 'def': 'a wheeled vehicle with large wheels; used in farming and other applications', 'name': 'tractor_(farm_equipment)'}, {'frequency': 'f', 'id': 1132, 'synset': 'traffic_light.n.01', 'synonyms': ['traffic_light'], 'def': 'a device to control vehicle traffic often consisting of three or more lights', 'name': 'traffic_light'}, {'frequency': 'r', 'id': 1133, 'synset': 'trail_bike.n.01', 'synonyms': ['dirt_bike'], 'def': 'a lightweight motorcycle equipped with rugged tires and suspension for off-road use', 'name': 'dirt_bike'}, {'frequency': 'c', 'id': 1134, 'synset': 'trailer_truck.n.01', 'synonyms': ['trailer_truck', 'tractor_trailer', 'trucking_rig', 'articulated_lorry', 'semi_truck'], 'def': 'a truck consisting of a tractor and trailer together', 'name': 'trailer_truck'}, {'frequency': 'f', 'id': 1135, 'synset': 'train.n.01', 'synonyms': ['train_(railroad_vehicle)', 'railroad_train'], 'def': 'public or private transport provided by a line of railway cars coupled together and drawn by a locomotive', 'name': 'train_(railroad_vehicle)'}, {'frequency': 'r', 'id': 1136, 'synset': 'trampoline.n.01', 'synonyms': ['trampoline'], 'def': 'gymnastic apparatus consisting of a strong canvas sheet attached with springs to a metal frame', 'name': 'trampoline'}, {'frequency': 'f', 'id': 1137, 'synset': 'tray.n.01', 'synonyms': ['tray'], 'def': 'an open receptacle for holding or displaying or serving articles or food', 'name': 'tray'}, {'frequency': 'r', 'id': 1138, 'synset': 'tree_house.n.01', 'synonyms': ['tree_house'], 'def': '(NOT A TREE) a PLAYHOUSE built in the branches of a tree', 'name': 'tree_house'}, {'frequency': 'r', 'id': 1139, 'synset': 'trench_coat.n.01', 'synonyms': ['trench_coat'], 'def': 'a military style raincoat; belted with deep pockets', 'name': 'trench_coat'}, {'frequency': 'r', 'id': 1140, 'synset': 'triangle.n.05', 'synonyms': ['triangle_(musical_instrument)'], 'def': 'a percussion instrument consisting of a metal bar bent in the shape of an open triangle', 'name': 'triangle_(musical_instrument)'}, {'frequency': 'r', 'id': 1141, 'synset': 'tricycle.n.01', 'synonyms': ['tricycle'], 'def': 'a vehicle with three wheels that is moved by foot pedals', 'name': 'tricycle'}, {'frequency': 'c', 'id': 1142, 'synset': 'tripod.n.01', 'synonyms': ['tripod'], 'def': 'a three-legged rack used for support', 'name': 'tripod'}, {'frequency': 'f', 'id': 1143, 'synset': 'trouser.n.01', 'synonyms': ['trousers', 'pants_(clothing)'], 'def': 'a garment extending from the waist to the knee or ankle, covering each leg separately', 'name': 'trousers'}, {'frequency': 'f', 'id': 1144, 'synset': 'truck.n.01', 'synonyms': ['truck'], 'def': 'an automotive vehicle suitable for hauling', 'name': 'truck'}, {'frequency': 'r', 'id': 1145, 'synset': 'truffle.n.03', 'synonyms': ['truffle_(chocolate)', 'chocolate_truffle'], 'def': 'creamy chocolate candy', 'name': 'truffle_(chocolate)'}, {'frequency': 'c', 'id': 1146, 'synset': 'trunk.n.02', 'synonyms': ['trunk'], 'def': 'luggage consisting of a large strong case used when traveling or for storage', 'name': 'trunk'}, {'frequency': 'r', 'id': 1147, 'synset': 'tub.n.02', 'synonyms': ['vat'], 'def': 'a large open vessel for holding or storing liquids', 'name': 'vat'}, {'frequency': 'c', 'id': 1148, 'synset': 'turban.n.01', 'synonyms': ['turban'], 'def': 'a traditional headdress consisting of a long scarf wrapped around the head', 'name': 'turban'}, {'frequency': 'r', 'id': 1149, 'synset': 'turkey.n.01', 'synonyms': ['turkey_(bird)'], 'def': 'large gallinaceous bird with fan-shaped tail; widely domesticated for food', 'name': 'turkey_(bird)'}, {'frequency': 'c', 'id': 1150, 'synset': 'turkey.n.04', 'synonyms': ['turkey_(food)'], 'def': 'flesh of large domesticated fowl usually roasted', 'name': 'turkey_(food)'}, {'frequency': 'r', 'id': 1151, 'synset': 'turnip.n.01', 'synonyms': ['turnip'], 'def': 'widely cultivated plant having a large fleshy edible white or yellow root', 'name': 'turnip'}, {'frequency': 'c', 'id': 1152, 'synset': 'turtle.n.02', 'synonyms': ['turtle'], 'def': 'any of various aquatic and land reptiles having a bony shell and flipper-like limbs for swimming', 'name': 'turtle'}, {'frequency': 'r', 'id': 1153, 'synset': 'turtleneck.n.01', 'synonyms': ['turtleneck_(clothing)', 'polo-neck'], 'def': 'a sweater or jersey with a high close-fitting collar', 'name': 'turtleneck_(clothing)'}, {'frequency': 'r', 'id': 1154, 'synset': 'typewriter.n.01', 'synonyms': ['typewriter'], 'def': 'hand-operated character printer for printing written messages one character at a time', 'name': 'typewriter'}, {'frequency': 'f', 'id': 1155, 'synset': 'umbrella.n.01', 'synonyms': ['umbrella'], 'def': 'a lightweight handheld collapsible canopy', 'name': 'umbrella'}, {'frequency': 'c', 'id': 1156, 'synset': 'underwear.n.01', 'synonyms': ['underwear', 'underclothes', 'underclothing', 'underpants'], 'def': 'undergarment worn next to the skin and under the outer garments', 'name': 'underwear'}, {'frequency': 'r', 'id': 1157, 'synset': 'unicycle.n.01', 'synonyms': ['unicycle'], 'def': 'a vehicle with a single wheel that is driven by pedals', 'name': 'unicycle'}, {'frequency': 'c', 'id': 1158, 'synset': 'urinal.n.01', 'synonyms': ['urinal'], 'def': 'a plumbing fixture (usually attached to the wall) used by men to urinate', 'name': 'urinal'}, {'frequency': 'r', 'id': 1159, 'synset': 'urn.n.01', 'synonyms': ['urn'], 'def': 'a large vase that usually has a pedestal or feet', 'name': 'urn'}, {'frequency': 'c', 'id': 1160, 'synset': 'vacuum.n.04', 'synonyms': ['vacuum_cleaner'], 'def': 'an electrical home appliance that cleans by suction', 'name': 'vacuum_cleaner'}, {'frequency': 'c', 'id': 1161, 'synset': 'valve.n.03', 'synonyms': ['valve'], 'def': 'control consisting of a mechanical device for controlling the flow of a fluid', 'name': 'valve'}, {'frequency': 'f', 'id': 1162, 'synset': 'vase.n.01', 'synonyms': ['vase'], 'def': 'an open jar of glass or porcelain used as an ornament or to hold flowers', 'name': 'vase'}, {'frequency': 'c', 'id': 1163, 'synset': 'vending_machine.n.01', 'synonyms': ['vending_machine'], 'def': 'a slot machine for selling goods', 'name': 'vending_machine'}, {'frequency': 'f', 'id': 1164, 'synset': 'vent.n.01', 'synonyms': ['vent', 'blowhole', 'air_vent'], 'def': 'a hole for the escape of gas or air', 'name': 'vent'}, {'frequency': 'c', 'id': 1165, 'synset': 'videotape.n.01', 'synonyms': ['videotape'], 'def': 'a video recording made on magnetic tape', 'name': 'videotape'}, {'frequency': 'r', 'id': 1166, 'synset': 'vinegar.n.01', 'synonyms': ['vinegar'], 'def': 'sour-tasting liquid produced usually by oxidation of the alcohol in wine or cider and used as a condiment or food preservative', 'name': 'vinegar'}, {'frequency': 'r', 'id': 1167, 'synset': 'violin.n.01', 'synonyms': ['violin', 'fiddle'], 'def': 'bowed stringed instrument that is the highest member of the violin family', 'name': 'violin'}, {'frequency': 'r', 'id': 1168, 'synset': 'vodka.n.01', 'synonyms': ['vodka'], 'def': 'unaged colorless liquor originating in Russia', 'name': 'vodka'}, {'frequency': 'r', 'id': 1169, 'synset': 'volleyball.n.02', 'synonyms': ['volleyball'], 'def': 'an inflated ball used in playing volleyball', 'name': 'volleyball'}, {'frequency': 'r', 'id': 1170, 'synset': 'vulture.n.01', 'synonyms': ['vulture'], 'def': 'any of various large birds of prey having naked heads and weak claws and feeding chiefly on carrion', 'name': 'vulture'}, {'frequency': 'c', 'id': 1171, 'synset': 'waffle.n.01', 'synonyms': ['waffle'], 'def': 'pancake batter baked in a waffle iron', 'name': 'waffle'}, {'frequency': 'r', 'id': 1172, 'synset': 'waffle_iron.n.01', 'synonyms': ['waffle_iron'], 'def': 'a kitchen appliance for baking waffles', 'name': 'waffle_iron'}, {'frequency': 'c', 'id': 1173, 'synset': 'wagon.n.01', 'synonyms': ['wagon'], 'def': 'any of various kinds of wheeled vehicles drawn by an animal or a tractor', 'name': 'wagon'}, {'frequency': 'c', 'id': 1174, 'synset': 'wagon_wheel.n.01', 'synonyms': ['wagon_wheel'], 'def': 'a wheel of a wagon', 'name': 'wagon_wheel'}, {'frequency': 'c', 'id': 1175, 'synset': 'walking_stick.n.01', 'synonyms': ['walking_stick'], 'def': 'a stick carried in the hand for support in walking', 'name': 'walking_stick'}, {'frequency': 'c', 'id': 1176, 'synset': 'wall_clock.n.01', 'synonyms': ['wall_clock'], 'def': 'a clock mounted on a wall', 'name': 'wall_clock'}, {'frequency': 'f', 'id': 1177, 'synset': 'wall_socket.n.01', 'synonyms': ['wall_socket', 'wall_plug', 'electric_outlet', 'electrical_outlet', 'outlet', 'electric_receptacle'], 'def': 'receptacle providing a place in a wiring system where current can be taken to run electrical devices', 'name': 'wall_socket'}, {'frequency': 'c', 'id': 1178, 'synset': 'wallet.n.01', 'synonyms': ['wallet', 'billfold'], 'def': 'a pocket-size case for holding papers and paper money', 'name': 'wallet'}, {'frequency': 'r', 'id': 1179, 'synset': 'walrus.n.01', 'synonyms': ['walrus'], 'def': 'either of two large northern marine mammals having ivory tusks and tough hide over thick blubber', 'name': 'walrus'}, {'frequency': 'r', 'id': 1180, 'synset': 'wardrobe.n.01', 'synonyms': ['wardrobe'], 'def': 'a tall piece of furniture that provides storage space for clothes; has a door and rails or hooks for hanging clothes', 'name': 'wardrobe'}, {'frequency': 'r', 'id': 1181, 'synset': 'wasabi.n.02', 'synonyms': ['wasabi'], 'def': 'the thick green root of the wasabi plant that the Japanese use in cooking and that tastes like strong horseradish', 'name': 'wasabi'}, {'frequency': 'c', 'id': 1182, 'synset': 'washer.n.03', 'synonyms': ['automatic_washer', 'washing_machine'], 'def': 'a home appliance for washing clothes and linens automatically', 'name': 'automatic_washer'}, {'frequency': 'f', 'id': 1183, 'synset': 'watch.n.01', 'synonyms': ['watch', 'wristwatch'], 'def': 'a small, portable timepiece', 'name': 'watch'}, {'frequency': 'f', 'id': 1184, 'synset': 'water_bottle.n.01', 'synonyms': ['water_bottle'], 'def': 'a bottle for holding water', 'name': 'water_bottle'}, {'frequency': 'c', 'id': 1185, 'synset': 'water_cooler.n.01', 'synonyms': ['water_cooler'], 'def': 'a device for cooling and dispensing drinking water', 'name': 'water_cooler'}, {'frequency': 'c', 'id': 1186, 'synset': 'water_faucet.n.01', 'synonyms': ['water_faucet', 'water_tap', 'tap_(water_faucet)'], 'def': 'a faucet for drawing water from a pipe or cask', 'name': 'water_faucet'}, {'frequency': 'r', 'id': 1187, 'synset': 'water_filter.n.01', 'synonyms': ['water_filter'], 'def': 'a filter to remove impurities from the water supply', 'name': 'water_filter'}, {'frequency': 'r', 'id': 1188, 'synset': 'water_heater.n.01', 'synonyms': ['water_heater', 'hot-water_heater'], 'def': 'a heater and storage tank to supply heated water', 'name': 'water_heater'}, {'frequency': 'r', 'id': 1189, 'synset': 'water_jug.n.01', 'synonyms': ['water_jug'], 'def': 'a jug that holds water', 'name': 'water_jug'}, {'frequency': 'r', 'id': 1190, 'synset': 'water_pistol.n.01', 'synonyms': ['water_gun', 'squirt_gun'], 'def': 'plaything consisting of a toy pistol that squirts water', 'name': 'water_gun'}, {'frequency': 'c', 'id': 1191, 'synset': 'water_scooter.n.01', 'synonyms': ['water_scooter', 'sea_scooter', 'jet_ski'], 'def': 'a motorboat resembling a motor scooter (NOT A SURFBOARD OR WATER SKI)', 'name': 'water_scooter'}, {'frequency': 'c', 'id': 1192, 'synset': 'water_ski.n.01', 'synonyms': ['water_ski'], 'def': 'broad ski for skimming over water towed by a speedboat (DO NOT MARK WATER)', 'name': 'water_ski'}, {'frequency': 'c', 'id': 1193, 'synset': 'water_tower.n.01', 'synonyms': ['water_tower'], 'def': 'a large reservoir for water', 'name': 'water_tower'}, {'frequency': 'c', 'id': 1194, 'synset': 'watering_can.n.01', 'synonyms': ['watering_can'], 'def': 'a container with a handle and a spout with a perforated nozzle; used to sprinkle water over plants', 'name': 'watering_can'}, {'frequency': 'c', 'id': 1195, 'synset': 'watermelon.n.02', 'synonyms': ['watermelon'], 'def': 'large oblong or roundish melon with a hard green rind and sweet watery red or occasionally yellowish pulp', 'name': 'watermelon'}, {'frequency': 'f', 'id': 1196, 'synset': 'weathervane.n.01', 'synonyms': ['weathervane', 'vane_(weathervane)', 'wind_vane'], 'def': 'mechanical device attached to an elevated structure; rotates freely to show the direction of the wind', 'name': 'weathervane'}, {'frequency': 'c', 'id': 1197, 'synset': 'webcam.n.01', 'synonyms': ['webcam'], 'def': 'a digital camera designed to take digital photographs and transmit them over the internet', 'name': 'webcam'}, {'frequency': 'c', 'id': 1198, 'synset': 'wedding_cake.n.01', 'synonyms': ['wedding_cake', 'bridecake'], 'def': 'a rich cake with two or more tiers and covered with frosting and decorations; served at a wedding reception', 'name': 'wedding_cake'}, {'frequency': 'c', 'id': 1199, 'synset': 'wedding_ring.n.01', 'synonyms': ['wedding_ring', 'wedding_band'], 'def': 'a ring given to the bride and/or groom at the wedding', 'name': 'wedding_ring'}, {'frequency': 'f', 'id': 1200, 'synset': 'wet_suit.n.01', 'synonyms': ['wet_suit'], 'def': 'a close-fitting garment made of a permeable material; worn in cold water to retain body heat', 'name': 'wet_suit'}, {'frequency': 'f', 'id': 1201, 'synset': 'wheel.n.01', 'synonyms': ['wheel'], 'def': 'a circular frame with spokes (or a solid disc) that can rotate on a shaft or axle', 'name': 'wheel'}, {'frequency': 'c', 'id': 1202, 'synset': 'wheelchair.n.01', 'synonyms': ['wheelchair'], 'def': 'a movable chair mounted on large wheels', 'name': 'wheelchair'}, {'frequency': 'c', 'id': 1203, 'synset': 'whipped_cream.n.01', 'synonyms': ['whipped_cream'], 'def': 'cream that has been beaten until light and fluffy', 'name': 'whipped_cream'}, {'frequency': 'r', 'id': 1204, 'synset': 'whiskey.n.01', 'synonyms': ['whiskey'], 'def': 'a liquor made from fermented mash of grain', 'name': 'whiskey'}, {'frequency': 'r', 'id': 1205, 'synset': 'whistle.n.03', 'synonyms': ['whistle'], 'def': 'a small wind instrument that produces a whistling sound by blowing into it', 'name': 'whistle'}, {'frequency': 'r', 'id': 1206, 'synset': 'wick.n.02', 'synonyms': ['wick'], 'def': 'a loosely woven cord in a candle or oil lamp that is lit on fire', 'name': 'wick'}, {'frequency': 'c', 'id': 1207, 'synset': 'wig.n.01', 'synonyms': ['wig'], 'def': 'hairpiece covering the head and made of real or synthetic hair', 'name': 'wig'}, {'frequency': 'c', 'id': 1208, 'synset': 'wind_chime.n.01', 'synonyms': ['wind_chime'], 'def': 'a decorative arrangement of pieces of metal or glass or pottery that hang together loosely so the wind can cause them to tinkle', 'name': 'wind_chime'}, {'frequency': 'c', 'id': 1209, 'synset': 'windmill.n.01', 'synonyms': ['windmill'], 'def': 'a mill that is powered by the wind', 'name': 'windmill'}, {'frequency': 'c', 'id': 1210, 'synset': 'window_box.n.01', 'synonyms': ['window_box_(for_plants)'], 'def': 'a container for growing plants on a windowsill', 'name': 'window_box_(for_plants)'}, {'frequency': 'f', 'id': 1211, 'synset': 'windshield_wiper.n.01', 'synonyms': ['windshield_wiper', 'windscreen_wiper', 'wiper_(for_windshield/screen)'], 'def': 'a mechanical device that cleans the windshield', 'name': 'windshield_wiper'}, {'frequency': 'c', 'id': 1212, 'synset': 'windsock.n.01', 'synonyms': ['windsock', 'air_sock', 'air-sleeve', 'wind_sleeve', 'wind_cone'], 'def': 'a truncated cloth cone mounted on a mast/pole; shows wind direction', 'name': 'windsock'}, {'frequency': 'f', 'id': 1213, 'synset': 'wine_bottle.n.01', 'synonyms': ['wine_bottle'], 'def': 'a bottle for holding wine', 'name': 'wine_bottle'}, {'frequency': 'r', 'id': 1214, 'synset': 'wine_bucket.n.01', 'synonyms': ['wine_bucket', 'wine_cooler'], 'def': 'a bucket of ice used to chill a bottle of wine', 'name': 'wine_bucket'}, {'frequency': 'f', 'id': 1215, 'synset': 'wineglass.n.01', 'synonyms': ['wineglass'], 'def': 'a glass that has a stem and in which wine is served', 'name': 'wineglass'}, {'frequency': 'r', 'id': 1216, 'synset': 'wing_chair.n.01', 'synonyms': ['wing_chair'], 'def': 'easy chair having wings on each side of a high back', 'name': 'wing_chair'}, {'frequency': 'c', 'id': 1217, 'synset': 'winker.n.02', 'synonyms': ['blinder_(for_horses)'], 'def': 'blinds that prevent a horse from seeing something on either side', 'name': 'blinder_(for_horses)'}, {'frequency': 'c', 'id': 1218, 'synset': 'wok.n.01', 'synonyms': ['wok'], 'def': 'pan with a convex bottom; used for frying in Chinese cooking', 'name': 'wok'}, {'frequency': 'r', 'id': 1219, 'synset': 'wolf.n.01', 'synonyms': ['wolf'], 'def': 'a wild carnivorous mammal of the dog family, living and hunting in packs', 'name': 'wolf'}, {'frequency': 'c', 'id': 1220, 'synset': 'wooden_spoon.n.02', 'synonyms': ['wooden_spoon'], 'def': 'a spoon made of wood', 'name': 'wooden_spoon'}, {'frequency': 'c', 'id': 1221, 'synset': 'wreath.n.01', 'synonyms': ['wreath'], 'def': 'an arrangement of flowers, leaves, or stems fastened in a ring', 'name': 'wreath'}, {'frequency': 'c', 'id': 1222, 'synset': 'wrench.n.03', 'synonyms': ['wrench', 'spanner'], 'def': 'a hand tool that is used to hold or twist a nut or bolt', 'name': 'wrench'}, {'frequency': 'c', 'id': 1223, 'synset': 'wristband.n.01', 'synonyms': ['wristband'], 'def': 'band consisting of a part of a sleeve that covers the wrist', 'name': 'wristband'}, {'frequency': 'f', 'id': 1224, 'synset': 'wristlet.n.01', 'synonyms': ['wristlet', 'wrist_band'], 'def': 'a band or bracelet worn around the wrist', 'name': 'wristlet'}, {'frequency': 'r', 'id': 1225, 'synset': 'yacht.n.01', 'synonyms': ['yacht'], 'def': 'an expensive vessel propelled by sail or power and used for cruising or racing', 'name': 'yacht'}, {'frequency': 'r', 'id': 1226, 'synset': 'yak.n.02', 'synonyms': ['yak'], 'def': 'large long-haired wild ox of Tibet often domesticated', 'name': 'yak'}, {'frequency': 'c', 'id': 1227, 'synset': 'yogurt.n.01', 'synonyms': ['yogurt', 'yoghurt', 'yoghourt'], 'def': 'a custard-like food made from curdled milk', 'name': 'yogurt'}, {'frequency': 'r', 'id': 1228, 'synset': 'yoke.n.07', 'synonyms': ['yoke_(animal_equipment)'], 'def': 'gear joining two animals at the neck; NOT egg yolk', 'name': 'yoke_(animal_equipment)'}, {'frequency': 'f', 'id': 1229, 'synset': 'zebra.n.01', 'synonyms': ['zebra'], 'def': 'any of several fleet black-and-white striped African equines', 'name': 'zebra'}, {'frequency': 'c', 'id': 1230, 'synset': 'zucchini.n.02', 'synonyms': ['zucchini', 'courgette'], 'def': 'small cucumber-shaped vegetable marrow; typically dark green', 'name': 'zucchini'}] # noqa
-# fmt: on
diff --git a/spaces/carlosalonso/Detection-video/carpeta_deteccion/projects/DensePose/densepose/data/samplers/densepose_cse_base.py b/spaces/carlosalonso/Detection-video/carpeta_deteccion/projects/DensePose/densepose/data/samplers/densepose_cse_base.py
deleted file mode 100644
index 845545c1438b9d2a4fbb4c6dac0642461a7e539f..0000000000000000000000000000000000000000
--- a/spaces/carlosalonso/Detection-video/carpeta_deteccion/projects/DensePose/densepose/data/samplers/densepose_cse_base.py
+++ /dev/null
@@ -1,139 +0,0 @@
-# Copyright (c) Facebook, Inc. and its affiliates.
-
-from typing import Any, Dict, List, Tuple
-import torch
-from torch.nn import functional as F
-
-from detectron2.config import CfgNode
-from detectron2.structures import Instances
-
-from densepose.converters.base import IntTupleBox
-from densepose.data.utils import get_class_to_mesh_name_mapping
-from densepose.modeling.cse.utils import squared_euclidean_distance_matrix
-from densepose.structures import DensePoseDataRelative
-
-from .densepose_base import DensePoseBaseSampler
-
-
-class DensePoseCSEBaseSampler(DensePoseBaseSampler):
- """
- Base DensePose sampler to produce DensePose data from DensePose predictions.
- Samples for each class are drawn according to some distribution over all pixels estimated
- to belong to that class.
- """
-
- def __init__(
- self,
- cfg: CfgNode,
- use_gt_categories: bool,
- embedder: torch.nn.Module,
- count_per_class: int = 8,
- ):
- """
- Constructor
-
- Args:
- cfg (CfgNode): the config of the model
- embedder (torch.nn.Module): necessary to compute mesh vertex embeddings
- count_per_class (int): the sampler produces at most `count_per_class`
- samples for each category
- """
- super().__init__(count_per_class)
- self.embedder = embedder
- self.class_to_mesh_name = get_class_to_mesh_name_mapping(cfg)
- self.use_gt_categories = use_gt_categories
-
- def _sample(self, instance: Instances, bbox_xywh: IntTupleBox) -> Dict[str, List[Any]]:
- """
- Sample DensPoseDataRelative from estimation results
- """
- if self.use_gt_categories:
- instance_class = instance.dataset_classes.tolist()[0]
- else:
- instance_class = instance.pred_classes.tolist()[0]
- mesh_name = self.class_to_mesh_name[instance_class]
-
- annotation = {
- DensePoseDataRelative.X_KEY: [],
- DensePoseDataRelative.Y_KEY: [],
- DensePoseDataRelative.VERTEX_IDS_KEY: [],
- DensePoseDataRelative.MESH_NAME_KEY: mesh_name,
- }
-
- mask, embeddings, other_values = self._produce_mask_and_results(instance, bbox_xywh)
- indices = torch.nonzero(mask, as_tuple=True)
- selected_embeddings = embeddings.permute(1, 2, 0)[indices].cpu()
- values = other_values[:, indices[0], indices[1]]
- k = values.shape[1]
-
- count = min(self.count_per_class, k)
- if count <= 0:
- return annotation
-
- index_sample = self._produce_index_sample(values, count)
- closest_vertices = squared_euclidean_distance_matrix(
- selected_embeddings[index_sample], self.embedder(mesh_name)
- )
- closest_vertices = torch.argmin(closest_vertices, dim=1)
-
- sampled_y = indices[0][index_sample] + 0.5
- sampled_x = indices[1][index_sample] + 0.5
- # prepare / normalize data
- _, _, w, h = bbox_xywh
- x = (sampled_x / w * 256.0).cpu().tolist()
- y = (sampled_y / h * 256.0).cpu().tolist()
- # extend annotations
- annotation[DensePoseDataRelative.X_KEY].extend(x)
- annotation[DensePoseDataRelative.Y_KEY].extend(y)
- annotation[DensePoseDataRelative.VERTEX_IDS_KEY].extend(closest_vertices.cpu().tolist())
- return annotation
-
- def _produce_mask_and_results(
- self, instance: Instances, bbox_xywh: IntTupleBox
- ) -> Tuple[torch.Tensor, torch.Tensor, torch.Tensor]:
- """
- Method to get labels and DensePose results from an instance
-
- Args:
- instance (Instances): an instance of `DensePoseEmbeddingPredictorOutput`
- bbox_xywh (IntTupleBox): the corresponding bounding box
-
- Return:
- mask (torch.Tensor): shape [H, W], DensePose segmentation mask
- embeddings (Tuple[torch.Tensor]): a tensor of shape [D, H, W],
- DensePose CSE Embeddings
- other_values (Tuple[torch.Tensor]): a tensor of shape [0, H, W],
- for potential other values
- """
- densepose_output = instance.pred_densepose
- S = densepose_output.coarse_segm
- E = densepose_output.embedding
- _, _, w, h = bbox_xywh
- embeddings = F.interpolate(E, size=(h, w), mode="bilinear")[0]
- coarse_segm_resized = F.interpolate(S, size=(h, w), mode="bilinear")[0]
- mask = coarse_segm_resized.argmax(0) > 0
- other_values = torch.empty((0, h, w), device=E.device)
- return mask, embeddings, other_values
-
- def _resample_mask(self, output: Any) -> torch.Tensor:
- """
- Convert DensePose predictor output to segmentation annotation - tensors of size
- (256, 256) and type `int64`.
-
- Args:
- output: DensePose predictor output with the following attributes:
- - coarse_segm: tensor of size [N, D, H, W] with unnormalized coarse
- segmentation scores
- Return:
- Tensor of size (S, S) and type `int64` with coarse segmentation annotations,
- where S = DensePoseDataRelative.MASK_SIZE
- """
- sz = DensePoseDataRelative.MASK_SIZE
- mask = (
- F.interpolate(output.coarse_segm, (sz, sz), mode="bilinear", align_corners=False)
- .argmax(dim=1)
- .long()
- .squeeze()
- .cpu()
- )
- return mask
diff --git a/spaces/carlosalonso/Detection-video/carpeta_deteccion/tests/data/test_transforms.py b/spaces/carlosalonso/Detection-video/carpeta_deteccion/tests/data/test_transforms.py
deleted file mode 100644
index 382048e533708dec3fabf89528564ebc2ad4c83f..0000000000000000000000000000000000000000
--- a/spaces/carlosalonso/Detection-video/carpeta_deteccion/tests/data/test_transforms.py
+++ /dev/null
@@ -1,268 +0,0 @@
-# -*- coding: utf-8 -*-
-# Copyright (c) Facebook, Inc. and its affiliates.
-
-import logging
-import numpy as np
-import unittest
-from unittest import mock
-import torch
-from PIL import Image, ImageOps
-from torch.nn import functional as F
-
-from detectron2.config import get_cfg
-from detectron2.data import detection_utils
-from detectron2.data import transforms as T
-from detectron2.utils.logger import setup_logger
-
-logger = logging.getLogger(__name__)
-
-
-def polygon_allclose(poly1, poly2):
- """
- Test whether two polygons are the same.
- Both arguments are nx2 numpy arrays.
- """
- # ABCD and CDAB are the same polygon. So it's important to check after rolling
- for k in range(len(poly1)):
- rolled_poly1 = np.roll(poly1, k, axis=0)
- if np.allclose(rolled_poly1, poly2):
- return True
- return False
-
-
-class TestTransforms(unittest.TestCase):
- def setUp(self):
- setup_logger()
-
- def test_apply_rotated_boxes(self):
- np.random.seed(125)
- cfg = get_cfg()
- is_train = True
- augs = detection_utils.build_augmentation(cfg, is_train)
- image = np.random.rand(200, 300)
- image, transforms = T.apply_augmentations(augs, image)
- image_shape = image.shape[:2] # h, w
- assert image_shape == (800, 1200)
- annotation = {"bbox": [179, 97, 62, 40, -56]}
-
- boxes = np.array([annotation["bbox"]], dtype=np.float64) # boxes.shape = (1, 5)
- transformed_bbox = transforms.apply_rotated_box(boxes)[0]
-
- expected_bbox = np.array([484, 388, 248, 160, 56], dtype=np.float64)
- err_msg = "transformed_bbox = {}, expected {}".format(transformed_bbox, expected_bbox)
- assert np.allclose(transformed_bbox, expected_bbox), err_msg
-
- def test_resize_and_crop(self):
- np.random.seed(125)
- min_scale = 0.2
- max_scale = 2.0
- target_height = 1100
- target_width = 1000
- resize_aug = T.ResizeScale(min_scale, max_scale, target_height, target_width)
- fixed_size_crop_aug = T.FixedSizeCrop((target_height, target_width))
- hflip_aug = T.RandomFlip()
- augs = [resize_aug, fixed_size_crop_aug, hflip_aug]
- original_image = np.random.rand(900, 800)
- image, transforms = T.apply_augmentations(augs, original_image)
- image_shape = image.shape[:2] # h, w
- self.assertEqual((1100, 1000), image_shape)
-
- boxes = np.array(
- [[91, 46, 144, 111], [523, 251, 614, 295]],
- dtype=np.float64,
- )
- transformed_bboxs = transforms.apply_box(boxes)
- expected_bboxs = np.array(
- [
- [895.42, 33.42666667, 933.91125, 80.66],
- [554.0825, 182.39333333, 620.17125, 214.36666667],
- ],
- dtype=np.float64,
- )
- err_msg = "transformed_bbox = {}, expected {}".format(transformed_bboxs, expected_bboxs)
- self.assertTrue(np.allclose(transformed_bboxs, expected_bboxs), err_msg)
-
- polygon = np.array([[91, 46], [144, 46], [144, 111], [91, 111]])
- transformed_polygons = transforms.apply_polygons([polygon])
- expected_polygon = np.array([[934.0, 33.0], [934.0, 80.0], [896.0, 80.0], [896.0, 33.0]])
- self.assertEqual(1, len(transformed_polygons))
- err_msg = "transformed_polygon = {}, expected {}".format(
- transformed_polygons[0], expected_polygon
- )
- self.assertTrue(polygon_allclose(transformed_polygons[0], expected_polygon), err_msg)
-
- def test_apply_rotated_boxes_unequal_scaling_factor(self):
- np.random.seed(125)
- h, w = 400, 200
- newh, neww = 800, 800
- image = np.random.rand(h, w)
- augs = []
- augs.append(T.Resize(shape=(newh, neww)))
- image, transforms = T.apply_augmentations(augs, image)
- image_shape = image.shape[:2] # h, w
- assert image_shape == (newh, neww)
-
- boxes = np.array(
- [
- [150, 100, 40, 20, 0],
- [150, 100, 40, 20, 30],
- [150, 100, 40, 20, 90],
- [150, 100, 40, 20, -90],
- ],
- dtype=np.float64,
- )
- transformed_boxes = transforms.apply_rotated_box(boxes)
-
- expected_bboxes = np.array(
- [
- [600, 200, 160, 40, 0],
- [600, 200, 144.22205102, 52.91502622, 49.10660535],
- [600, 200, 80, 80, 90],
- [600, 200, 80, 80, -90],
- ],
- dtype=np.float64,
- )
- err_msg = "transformed_boxes = {}, expected {}".format(transformed_boxes, expected_bboxes)
- assert np.allclose(transformed_boxes, expected_bboxes), err_msg
-
- def test_print_augmentation(self):
- t = T.RandomCrop("relative", (100, 100))
- self.assertEqual(str(t), "RandomCrop(crop_type='relative', crop_size=(100, 100))")
-
- t0 = T.RandomFlip(prob=0.5)
- self.assertEqual(str(t0), "RandomFlip(prob=0.5)")
-
- t1 = T.RandomFlip()
- self.assertEqual(str(t1), "RandomFlip()")
-
- t = T.AugmentationList([t0, t1])
- self.assertEqual(str(t), f"AugmentationList[{t0}, {t1}]")
-
- def test_random_apply_prob_out_of_range_check(self):
- test_probabilities = {0.0: True, 0.5: True, 1.0: True, -0.01: False, 1.01: False}
-
- for given_probability, is_valid in test_probabilities.items():
- if not is_valid:
- self.assertRaises(AssertionError, T.RandomApply, None, prob=given_probability)
- else:
- T.RandomApply(T.NoOpTransform(), prob=given_probability)
-
- def test_random_apply_wrapping_aug_probability_occured_evaluation(self):
- transform_mock = mock.MagicMock(name="MockTransform", spec=T.Augmentation)
- image_mock = mock.MagicMock(name="MockImage")
- random_apply = T.RandomApply(transform_mock, prob=0.001)
-
- with mock.patch.object(random_apply, "_rand_range", return_value=0.0001):
- transform = random_apply.get_transform(image_mock)
- transform_mock.get_transform.assert_called_once_with(image_mock)
- self.assertIsNot(transform, transform_mock)
-
- def test_random_apply_wrapping_std_transform_probability_occured_evaluation(self):
- transform_mock = mock.MagicMock(name="MockTransform", spec=T.Transform)
- image_mock = mock.MagicMock(name="MockImage")
- random_apply = T.RandomApply(transform_mock, prob=0.001)
-
- with mock.patch.object(random_apply, "_rand_range", return_value=0.0001):
- transform = random_apply.get_transform(image_mock)
- self.assertIs(transform, transform_mock)
-
- def test_random_apply_probability_not_occured_evaluation(self):
- transform_mock = mock.MagicMock(name="MockTransform", spec=T.Augmentation)
- image_mock = mock.MagicMock(name="MockImage")
- random_apply = T.RandomApply(transform_mock, prob=0.001)
-
- with mock.patch.object(random_apply, "_rand_range", return_value=0.9):
- transform = random_apply.get_transform(image_mock)
- transform_mock.get_transform.assert_not_called()
- self.assertIsInstance(transform, T.NoOpTransform)
-
- def test_augmentation_input_args(self):
- input_shape = (100, 100)
- output_shape = (50, 50)
-
- # define two augmentations with different args
- class TG1(T.Augmentation):
- def get_transform(self, image, sem_seg):
- return T.ResizeTransform(
- input_shape[0], input_shape[1], output_shape[0], output_shape[1]
- )
-
- class TG2(T.Augmentation):
- def get_transform(self, image):
- assert image.shape[:2] == output_shape # check that TG1 is applied
- return T.HFlipTransform(output_shape[1])
-
- image = np.random.rand(*input_shape).astype("float32")
- sem_seg = (np.random.rand(*input_shape) < 0.5).astype("uint8")
- inputs = T.AugInput(image, sem_seg=sem_seg) # provide two args
- tfms = inputs.apply_augmentations([TG1(), TG2()])
- self.assertIsInstance(tfms[0], T.ResizeTransform)
- self.assertIsInstance(tfms[1], T.HFlipTransform)
- self.assertTrue(inputs.image.shape[:2] == output_shape)
- self.assertTrue(inputs.sem_seg.shape[:2] == output_shape)
-
- class TG3(T.Augmentation):
- def get_transform(self, image, nonexist):
- pass
-
- with self.assertRaises(AttributeError):
- inputs.apply_augmentations([TG3()])
-
- def test_augmentation_list(self):
- input_shape = (100, 100)
- image = np.random.rand(*input_shape).astype("float32")
- sem_seg = (np.random.rand(*input_shape) < 0.5).astype("uint8")
- inputs = T.AugInput(image, sem_seg=sem_seg) # provide two args
-
- augs = T.AugmentationList([T.RandomFlip(), T.Resize(20)])
- _ = T.AugmentationList([augs, T.Resize(30)])(inputs)
- # 3 in latest fvcore (flattened transformlist), 2 in older
- # self.assertEqual(len(tfms), 3)
-
- def test_color_transforms(self):
- rand_img = np.random.random((100, 100, 3)) * 255
- rand_img = rand_img.astype("uint8")
-
- # Test no-op
- noop_transform = T.ColorTransform(lambda img: img)
- self.assertTrue(np.array_equal(rand_img, noop_transform.apply_image(rand_img)))
-
- # Test a ImageOps operation
- magnitude = np.random.randint(0, 256)
- solarize_transform = T.PILColorTransform(lambda img: ImageOps.solarize(img, magnitude))
- expected_img = ImageOps.solarize(Image.fromarray(rand_img), magnitude)
- self.assertTrue(np.array_equal(expected_img, solarize_transform.apply_image(rand_img)))
-
- def test_resize_transform(self):
- input_shapes = [(100, 100), (100, 100, 1), (100, 100, 3)]
- output_shapes = [(200, 200), (200, 200, 1), (200, 200, 3)]
- for in_shape, out_shape in zip(input_shapes, output_shapes):
- in_img = np.random.randint(0, 255, size=in_shape, dtype=np.uint8)
- tfm = T.ResizeTransform(in_shape[0], in_shape[1], out_shape[0], out_shape[1])
- out_img = tfm.apply_image(in_img)
- self.assertEqual(out_img.shape, out_shape)
-
- def test_resize_shorted_edge_scriptable(self):
- def f(image):
- newh, neww = T.ResizeShortestEdge.get_output_shape(
- image.shape[-2], image.shape[-1], 80, 133
- )
- return F.interpolate(image.unsqueeze(0), size=(newh, neww))
-
- input = torch.randn(3, 10, 10)
- script_f = torch.jit.script(f)
- self.assertTrue(torch.allclose(f(input), script_f(input)))
-
- # generalize to new shapes
- input = torch.randn(3, 8, 100)
- self.assertTrue(torch.allclose(f(input), script_f(input)))
-
- def test_extent_transform(self):
- input_shapes = [(100, 100), (100, 100, 1), (100, 100, 3)]
- src_rect = (20, 20, 80, 80)
- output_shapes = [(200, 200), (200, 200, 1), (200, 200, 3)]
- for in_shape, out_shape in zip(input_shapes, output_shapes):
- in_img = np.random.randint(0, 255, size=in_shape, dtype=np.uint8)
- tfm = T.ExtentTransform(src_rect, out_shape[:2])
- out_img = tfm.apply_image(in_img)
- self.assertTrue(out_img.shape == out_shape)
diff --git a/spaces/cbr/swp/face_parsing/__init__.py b/spaces/cbr/swp/face_parsing/__init__.py
deleted file mode 100644
index e98735aec33d8a4f5525f7ca03f1285d18782285..0000000000000000000000000000000000000000
--- a/spaces/cbr/swp/face_parsing/__init__.py
+++ /dev/null
@@ -1 +0,0 @@
-from .swap import init_parser, swap_regions, mask_regions, mask_regions_to_list, SoftErosion
\ No newline at end of file
diff --git a/spaces/chadpanda/PEPE-Semantics/README.md b/spaces/chadpanda/PEPE-Semantics/README.md
deleted file mode 100644
index a46d27e81f776374f1f79f926a8ebfe9b9eed423..0000000000000000000000000000000000000000
--- a/spaces/chadpanda/PEPE-Semantics/README.md
+++ /dev/null
@@ -1,13 +0,0 @@
----
-title: PEPE Semantics
-emoji: 😻
-colorFrom: purple
-colorTo: pink
-sdk: gradio
-sdk_version: 3.4.1
-app_file: app.py
-pinned: false
-license: mit
----
-
-Check out the configuration reference at https://huggingface.co/docs/hub/spaces-config-reference
diff --git a/spaces/chendl/compositional_test/multimodal/tools/add_vg_to_blip2_data.py b/spaces/chendl/compositional_test/multimodal/tools/add_vg_to_blip2_data.py
deleted file mode 100644
index 2b1718fd35d61e8360999c83166e7581041a9690..0000000000000000000000000000000000000000
--- a/spaces/chendl/compositional_test/multimodal/tools/add_vg_to_blip2_data.py
+++ /dev/null
@@ -1,25 +0,0 @@
-import os
-import shutil
-import glob
-import random
-from pprint import pprint
-
-DIR_VG = "/gpfs/u/home/LMCG/LMCGljnn/scratch-shared/junyan/raw/vg_0826"
-DIR = "/gpfs/u/home/LMCG/LMCGljnn/scratch-shared/junyan/raw/blip2_all_data_ground"
-OUT_DIR = "/gpfs/u/home/LMCG/LMCGljnn/scratch-shared/junyan/raw/blip2_all_data_ground_with_vg_0826"
-
-
-if __name__ == "__main__":
- os.makedirs(OUT_DIR, exist_ok=True)
- blip2_tars = glob.glob(os.path.join(DIR, "*.tar"))
- vg_tars = glob.glob(os.path.join(DIR_VG, "*", "*.tar"))
- tars = []
- tars.extend(blip2_tars)
- tars.extend(vg_tars)
- print(len(tars))
- pprint(tars[:20])
- pprint(tars[-20:])
- for i, tar in enumerate(tars):
- dst = os.path.join(OUT_DIR, f"{str(i).zfill(6)}.tar")
- # print(tar, dst)
- os.symlink(tar, dst)
diff --git a/spaces/chendl/compositional_test/transformers/docker/transformers-cpu/Dockerfile b/spaces/chendl/compositional_test/transformers/docker/transformers-cpu/Dockerfile
deleted file mode 100644
index c3590e4239e470be8fbc8100128efd264fb41c7e..0000000000000000000000000000000000000000
--- a/spaces/chendl/compositional_test/transformers/docker/transformers-cpu/Dockerfile
+++ /dev/null
@@ -1,26 +0,0 @@
-FROM ubuntu:18.04
-LABEL maintainer="Hugging Face"
-LABEL repository="transformers"
-
-RUN apt update && \
- apt install -y bash \
- build-essential \
- git \
- curl \
- ca-certificates \
- python3 \
- python3-pip && \
- rm -rf /var/lib/apt/lists
-
-RUN python3 -m pip install --no-cache-dir --upgrade pip && \
- python3 -m pip install --no-cache-dir \
- jupyter \
- tensorflow-cpu \
- torch
-
-WORKDIR /workspace
-COPY . transformers/
-RUN cd transformers/ && \
- python3 -m pip install --no-cache-dir .
-
-CMD ["/bin/bash"]
diff --git a/spaces/chendl/compositional_test/transformers/examples/pytorch/semantic-segmentation/run_semantic_segmentation.py b/spaces/chendl/compositional_test/transformers/examples/pytorch/semantic-segmentation/run_semantic_segmentation.py
deleted file mode 100644
index 3c13de36ec188ac05e8d43bba751a0c173824b72..0000000000000000000000000000000000000000
--- a/spaces/chendl/compositional_test/transformers/examples/pytorch/semantic-segmentation/run_semantic_segmentation.py
+++ /dev/null
@@ -1,520 +0,0 @@
-#!/usr/bin/env python
-# coding=utf-8
-# Copyright 2022 The HuggingFace Inc. team. All rights reserved.
-#
-# Licensed under the Apache License, Version 2.0 (the "License");
-# you may not use this file except in compliance with the License.
-# You may obtain a copy of the License at
-#
-# http://www.apache.org/licenses/LICENSE-2.0
-#
-# Unless required by applicable law or agreed to in writing, software
-# distributed under the License is distributed on an "AS IS" BASIS,
-# WITHOUT WARRANTIES OR CONDITIONS OF ANY KIND, either express or implied.
-# See the License for the specific language governing permissions and
-
-import json
-import logging
-import os
-import random
-import sys
-from dataclasses import dataclass, field
-from typing import Optional
-
-import evaluate
-import numpy as np
-import torch
-from datasets import load_dataset
-from huggingface_hub import hf_hub_download
-from PIL import Image
-from torch import nn
-from torchvision import transforms
-from torchvision.transforms import functional
-
-import transformers
-from transformers import (
- AutoConfig,
- AutoImageProcessor,
- AutoModelForSemanticSegmentation,
- HfArgumentParser,
- Trainer,
- TrainingArguments,
- default_data_collator,
-)
-from transformers.trainer_utils import get_last_checkpoint
-from transformers.utils import check_min_version, send_example_telemetry
-from transformers.utils.versions import require_version
-
-
-""" Finetuning any 🤗 Transformers model supported by AutoModelForSemanticSegmentation for semantic segmentation leveraging the Trainer API."""
-
-logger = logging.getLogger(__name__)
-
-# Will error if the minimal version of Transformers is not installed. Remove at your own risks.
-check_min_version("4.28.0")
-
-require_version("datasets>=2.0.0", "To fix: pip install -r examples/pytorch/semantic-segmentation/requirements.txt")
-
-
-def pad_if_smaller(img, size, fill=0):
- size = (size, size) if isinstance(size, int) else size
- original_width, original_height = img.size
- pad_height = size[1] - original_height if original_height < size[1] else 0
- pad_width = size[0] - original_width if original_width < size[0] else 0
- img = functional.pad(img, (0, 0, pad_width, pad_height), fill=fill)
- return img
-
-
-class Compose:
- def __init__(self, transforms):
- self.transforms = transforms
-
- def __call__(self, image, target):
- for t in self.transforms:
- image, target = t(image, target)
- return image, target
-
-
-class Identity:
- def __init__(self):
- pass
-
- def __call__(self, image, target):
- return image, target
-
-
-class Resize:
- def __init__(self, size):
- self.size = size
-
- def __call__(self, image, target):
- image = functional.resize(image, self.size)
- target = functional.resize(target, self.size, interpolation=transforms.InterpolationMode.NEAREST)
- return image, target
-
-
-class RandomResize:
- def __init__(self, min_size, max_size=None):
- self.min_size = min_size
- if max_size is None:
- max_size = min_size
- self.max_size = max_size
-
- def __call__(self, image, target):
- size = random.randint(self.min_size, self.max_size)
- image = functional.resize(image, size)
- target = functional.resize(target, size, interpolation=transforms.InterpolationMode.NEAREST)
- return image, target
-
-
-class RandomCrop:
- def __init__(self, size):
- self.size = size if isinstance(size, tuple) else (size, size)
-
- def __call__(self, image, target):
- image = pad_if_smaller(image, self.size)
- target = pad_if_smaller(target, self.size, fill=255)
- crop_params = transforms.RandomCrop.get_params(image, self.size)
- image = functional.crop(image, *crop_params)
- target = functional.crop(target, *crop_params)
- return image, target
-
-
-class RandomHorizontalFlip:
- def __init__(self, flip_prob):
- self.flip_prob = flip_prob
-
- def __call__(self, image, target):
- if random.random() < self.flip_prob:
- image = functional.hflip(image)
- target = functional.hflip(target)
- return image, target
-
-
-class PILToTensor:
- def __call__(self, image, target):
- image = functional.pil_to_tensor(image)
- target = torch.as_tensor(np.array(target), dtype=torch.int64)
- return image, target
-
-
-class ConvertImageDtype:
- def __init__(self, dtype):
- self.dtype = dtype
-
- def __call__(self, image, target):
- image = functional.convert_image_dtype(image, self.dtype)
- return image, target
-
-
-class Normalize:
- def __init__(self, mean, std):
- self.mean = mean
- self.std = std
-
- def __call__(self, image, target):
- image = functional.normalize(image, mean=self.mean, std=self.std)
- return image, target
-
-
-class ReduceLabels:
- def __call__(self, image, target):
- if not isinstance(target, np.ndarray):
- target = np.array(target).astype(np.uint8)
- # avoid using underflow conversion
- target[target == 0] = 255
- target = target - 1
- target[target == 254] = 255
-
- target = Image.fromarray(target)
- return image, target
-
-
-@dataclass
-class DataTrainingArguments:
- """
- Arguments pertaining to what data we are going to input our model for training and eval.
- Using `HfArgumentParser` we can turn this class into argparse arguments to be able to specify
- them on the command line.
- """
-
- dataset_name: Optional[str] = field(
- default="segments/sidewalk-semantic",
- metadata={
- "help": "Name of a dataset from the hub (could be your own, possibly private dataset hosted on the hub)."
- },
- )
- dataset_config_name: Optional[str] = field(
- default=None, metadata={"help": "The configuration name of the dataset to use (via the datasets library)."}
- )
- train_val_split: Optional[float] = field(
- default=0.15, metadata={"help": "Percent to split off of train for validation."}
- )
- max_train_samples: Optional[int] = field(
- default=None,
- metadata={
- "help": (
- "For debugging purposes or quicker training, truncate the number of training examples to this "
- "value if set."
- )
- },
- )
- max_eval_samples: Optional[int] = field(
- default=None,
- metadata={
- "help": (
- "For debugging purposes or quicker training, truncate the number of evaluation examples to this "
- "value if set."
- )
- },
- )
- reduce_labels: Optional[bool] = field(
- default=False,
- metadata={"help": "Whether or not to reduce all labels by 1 and replace background by 255."},
- )
-
- def __post_init__(self):
- if self.dataset_name is None and (self.train_dir is None and self.validation_dir is None):
- raise ValueError(
- "You must specify either a dataset name from the hub or a train and/or validation directory."
- )
-
-
-@dataclass
-class ModelArguments:
- """
- Arguments pertaining to which model/config/tokenizer we are going to fine-tune from.
- """
-
- model_name_or_path: str = field(
- default="nvidia/mit-b0",
- metadata={"help": "Path to pretrained model or model identifier from huggingface.co/models"},
- )
- config_name: Optional[str] = field(
- default=None, metadata={"help": "Pretrained config name or path if not the same as model_name"}
- )
- cache_dir: Optional[str] = field(
- default=None, metadata={"help": "Where do you want to store the pretrained models downloaded from s3"}
- )
- model_revision: str = field(
- default="main",
- metadata={"help": "The specific model version to use (can be a branch name, tag name or commit id)."},
- )
- image_processor_name: str = field(default=None, metadata={"help": "Name or path of preprocessor config."})
- use_auth_token: bool = field(
- default=False,
- metadata={
- "help": (
- "Will use the token generated when running `huggingface-cli login` (necessary to use this script "
- "with private models)."
- )
- },
- )
-
-
-def main():
- # See all possible arguments in src/transformers/training_args.py
- # or by passing the --help flag to this script.
- # We now keep distinct sets of args, for a cleaner separation of concerns.
-
- parser = HfArgumentParser((ModelArguments, DataTrainingArguments, TrainingArguments))
- if len(sys.argv) == 2 and sys.argv[1].endswith(".json"):
- # If we pass only one argument to the script and it's the path to a json file,
- # let's parse it to get our arguments.
- model_args, data_args, training_args = parser.parse_json_file(json_file=os.path.abspath(sys.argv[1]))
- else:
- model_args, data_args, training_args = parser.parse_args_into_dataclasses()
-
- # Sending telemetry. Tracking the example usage helps us better allocate resources to maintain them. The
- # information sent is the one passed as arguments along with your Python/PyTorch versions.
- send_example_telemetry("run_semantic_segmentation", model_args, data_args)
-
- # Setup logging
- logging.basicConfig(
- format="%(asctime)s - %(levelname)s - %(name)s - %(message)s",
- datefmt="%m/%d/%Y %H:%M:%S",
- handlers=[logging.StreamHandler(sys.stdout)],
- )
-
- if training_args.should_log:
- # The default of training_args.log_level is passive, so we set log level at info here to have that default.
- transformers.utils.logging.set_verbosity_info()
-
- log_level = training_args.get_process_log_level()
- logger.setLevel(log_level)
- transformers.utils.logging.set_verbosity(log_level)
- transformers.utils.logging.enable_default_handler()
- transformers.utils.logging.enable_explicit_format()
-
- # Log on each process the small summary:
- logger.warning(
- f"Process rank: {training_args.local_rank}, device: {training_args.device}, n_gpu: {training_args.n_gpu}"
- + f"distributed training: {bool(training_args.local_rank != -1)}, 16-bits training: {training_args.fp16}"
- )
- logger.info(f"Training/evaluation parameters {training_args}")
-
- # Detecting last checkpoint.
- last_checkpoint = None
- if os.path.isdir(training_args.output_dir) and training_args.do_train and not training_args.overwrite_output_dir:
- last_checkpoint = get_last_checkpoint(training_args.output_dir)
- if last_checkpoint is None and len(os.listdir(training_args.output_dir)) > 0:
- raise ValueError(
- f"Output directory ({training_args.output_dir}) already exists and is not empty. "
- "Use --overwrite_output_dir to overcome."
- )
- elif last_checkpoint is not None and training_args.resume_from_checkpoint is None:
- logger.info(
- f"Checkpoint detected, resuming training at {last_checkpoint}. To avoid this behavior, change "
- "the `--output_dir` or add `--overwrite_output_dir` to train from scratch."
- )
-
- # Load dataset
- # In distributed training, the load_dataset function guarantees that only one local process can concurrently
- # download the dataset.
- # TODO support datasets from local folders
- dataset = load_dataset(data_args.dataset_name, cache_dir=model_args.cache_dir)
-
- # Rename column names to standardized names (only "image" and "label" need to be present)
- if "pixel_values" in dataset["train"].column_names:
- dataset = dataset.rename_columns({"pixel_values": "image"})
- if "annotation" in dataset["train"].column_names:
- dataset = dataset.rename_columns({"annotation": "label"})
-
- # If we don't have a validation split, split off a percentage of train as validation.
- data_args.train_val_split = None if "validation" in dataset.keys() else data_args.train_val_split
- if isinstance(data_args.train_val_split, float) and data_args.train_val_split > 0.0:
- split = dataset["train"].train_test_split(data_args.train_val_split)
- dataset["train"] = split["train"]
- dataset["validation"] = split["test"]
-
- # Prepare label mappings.
- # We'll include these in the model's config to get human readable labels in the Inference API.
- if data_args.dataset_name == "scene_parse_150":
- repo_id = "huggingface/label-files"
- filename = "ade20k-id2label.json"
- else:
- repo_id = data_args.dataset_name
- filename = "id2label.json"
- id2label = json.load(open(hf_hub_download(repo_id, filename, repo_type="dataset"), "r"))
- id2label = {int(k): v for k, v in id2label.items()}
- label2id = {v: str(k) for k, v in id2label.items()}
-
- # Load the mean IoU metric from the datasets package
- metric = evaluate.load("mean_iou")
-
- # Define our compute_metrics function. It takes an `EvalPrediction` object (a namedtuple with a
- # predictions and label_ids field) and has to return a dictionary string to float.
- @torch.no_grad()
- def compute_metrics(eval_pred):
- logits, labels = eval_pred
- logits_tensor = torch.from_numpy(logits)
- # scale the logits to the size of the label
- logits_tensor = nn.functional.interpolate(
- logits_tensor,
- size=labels.shape[-2:],
- mode="bilinear",
- align_corners=False,
- ).argmax(dim=1)
-
- pred_labels = logits_tensor.detach().cpu().numpy()
- metrics = metric.compute(
- predictions=pred_labels,
- references=labels,
- num_labels=len(id2label),
- ignore_index=0,
- reduce_labels=image_processor.do_reduce_labels,
- )
- # add per category metrics as individual key-value pairs
- per_category_accuracy = metrics.pop("per_category_accuracy").tolist()
- per_category_iou = metrics.pop("per_category_iou").tolist()
-
- metrics.update({f"accuracy_{id2label[i]}": v for i, v in enumerate(per_category_accuracy)})
- metrics.update({f"iou_{id2label[i]}": v for i, v in enumerate(per_category_iou)})
-
- return metrics
-
- config = AutoConfig.from_pretrained(
- model_args.config_name or model_args.model_name_or_path,
- label2id=label2id,
- id2label=id2label,
- cache_dir=model_args.cache_dir,
- revision=model_args.model_revision,
- use_auth_token=True if model_args.use_auth_token else None,
- )
- model = AutoModelForSemanticSegmentation.from_pretrained(
- model_args.model_name_or_path,
- from_tf=bool(".ckpt" in model_args.model_name_or_path),
- config=config,
- cache_dir=model_args.cache_dir,
- revision=model_args.model_revision,
- use_auth_token=True if model_args.use_auth_token else None,
- )
- image_processor = AutoImageProcessor.from_pretrained(
- model_args.image_processor_name or model_args.model_name_or_path,
- cache_dir=model_args.cache_dir,
- revision=model_args.model_revision,
- use_auth_token=True if model_args.use_auth_token else None,
- )
-
- # Define torchvision transforms to be applied to each image + target.
- # Not that straightforward in torchvision: https://github.com/pytorch/vision/issues/9
- # Currently based on official torchvision references: https://github.com/pytorch/vision/blob/main/references/segmentation/transforms.py
- if "shortest_edge" in image_processor.size:
- # We instead set the target size as (shortest_edge, shortest_edge) to here to ensure all images are batchable.
- size = (image_processor.size["shortest_edge"], image_processor.size["shortest_edge"])
- else:
- size = (image_processor.size["height"], image_processor.size["width"])
- train_transforms = Compose(
- [
- ReduceLabels() if data_args.reduce_labels else Identity(),
- RandomCrop(size=size),
- RandomHorizontalFlip(flip_prob=0.5),
- PILToTensor(),
- ConvertImageDtype(torch.float),
- Normalize(mean=image_processor.image_mean, std=image_processor.image_std),
- ]
- )
- # Define torchvision transform to be applied to each image.
- # jitter = ColorJitter(brightness=0.25, contrast=0.25, saturation=0.25, hue=0.1)
- val_transforms = Compose(
- [
- ReduceLabels() if data_args.reduce_labels else Identity(),
- Resize(size=size),
- PILToTensor(),
- ConvertImageDtype(torch.float),
- Normalize(mean=image_processor.image_mean, std=image_processor.image_std),
- ]
- )
-
- def preprocess_train(example_batch):
- pixel_values = []
- labels = []
- for image, target in zip(example_batch["image"], example_batch["label"]):
- image, target = train_transforms(image.convert("RGB"), target)
- pixel_values.append(image)
- labels.append(target)
-
- encoding = {}
- encoding["pixel_values"] = torch.stack(pixel_values)
- encoding["labels"] = torch.stack(labels)
-
- return encoding
-
- def preprocess_val(example_batch):
- pixel_values = []
- labels = []
- for image, target in zip(example_batch["image"], example_batch["label"]):
- image, target = val_transforms(image.convert("RGB"), target)
- pixel_values.append(image)
- labels.append(target)
-
- encoding = {}
- encoding["pixel_values"] = torch.stack(pixel_values)
- encoding["labels"] = torch.stack(labels)
-
- return encoding
-
- if training_args.do_train:
- if "train" not in dataset:
- raise ValueError("--do_train requires a train dataset")
- if data_args.max_train_samples is not None:
- dataset["train"] = (
- dataset["train"].shuffle(seed=training_args.seed).select(range(data_args.max_train_samples))
- )
- # Set the training transforms
- dataset["train"].set_transform(preprocess_train)
-
- if training_args.do_eval:
- if "validation" not in dataset:
- raise ValueError("--do_eval requires a validation dataset")
- if data_args.max_eval_samples is not None:
- dataset["validation"] = (
- dataset["validation"].shuffle(seed=training_args.seed).select(range(data_args.max_eval_samples))
- )
- # Set the validation transforms
- dataset["validation"].set_transform(preprocess_val)
-
- # Initalize our trainer
- trainer = Trainer(
- model=model,
- args=training_args,
- train_dataset=dataset["train"] if training_args.do_train else None,
- eval_dataset=dataset["validation"] if training_args.do_eval else None,
- compute_metrics=compute_metrics,
- tokenizer=image_processor,
- data_collator=default_data_collator,
- )
-
- # Training
- if training_args.do_train:
- checkpoint = None
- if training_args.resume_from_checkpoint is not None:
- checkpoint = training_args.resume_from_checkpoint
- elif last_checkpoint is not None:
- checkpoint = last_checkpoint
- train_result = trainer.train(resume_from_checkpoint=checkpoint)
- trainer.save_model()
- trainer.log_metrics("train", train_result.metrics)
- trainer.save_metrics("train", train_result.metrics)
- trainer.save_state()
-
- # Evaluation
- if training_args.do_eval:
- metrics = trainer.evaluate()
- trainer.log_metrics("eval", metrics)
- trainer.save_metrics("eval", metrics)
-
- # Write model card and (optionally) push to hub
- kwargs = {
- "finetuned_from": model_args.model_name_or_path,
- "dataset": data_args.dataset_name,
- "tags": ["image-segmentation", "vision"],
- }
- if training_args.push_to_hub:
- trainer.push_to_hub(**kwargs)
- else:
- trainer.create_model_card(**kwargs)
-
-
-if __name__ == "__main__":
- main()
diff --git a/spaces/chendl/compositional_test/transformers/examples/research_projects/quantization-qdqbert/Dockerfile b/spaces/chendl/compositional_test/transformers/examples/research_projects/quantization-qdqbert/Dockerfile
deleted file mode 100644
index e64c9f0e021d4547654192bbfe34f469c76fc6f0..0000000000000000000000000000000000000000
--- a/spaces/chendl/compositional_test/transformers/examples/research_projects/quantization-qdqbert/Dockerfile
+++ /dev/null
@@ -1,34 +0,0 @@
-# coding=utf-8
-# Copyright 2021 NVIDIA Corporation. All rights reserved.
-#
-# Licensed under the Apache License, Version 2.0 (the "License");
-# you may not use this file except in compliance with the License.
-# You may obtain a copy of the License at
-#
-# http://www.apache.org/licenses/LICENSE-2.0
-#
-# Unless required by applicable law or agreed to in writing, software
-# distributed under the License is distributed on an "AS IS" BASIS,
-# WITHOUT WARRANTIES OR CONDITIONS OF ANY KIND, either express or implied.
-# See the License for the specific language governing permissions and
-# limitations under the License.
-FROM nvcr.io/nvidia/pytorch:22.02-py3
-LABEL maintainer="Hugging Face"
-LABEL repository="transformers"
-
-RUN apt-get update
-RUN apt-get install sudo
-
-RUN python3 -m pip install --no-cache-dir --upgrade pip
-RUN python3 -m pip install --no-cache-dir --ignore-installed pycuda
-RUN python3 -m pip install --no-cache-dir \
- pytorch-quantization --extra-index-url https://pypi.ngc.nvidia.com
-RUN python3 -m pip install --no-cache-dir onnxruntime-gpu==1.11
-
-WORKDIR /workspace
-COPY . transformers/
-RUN cd transformers/ && \
- python3 -m pip install --no-cache-dir .
-
-RUN python3 -m pip install --no-cache-dir datasets \
- accelerate
diff --git a/spaces/chendl/compositional_test/transformers/examples/research_projects/seq2seq-distillation/sentence_splitter.py b/spaces/chendl/compositional_test/transformers/examples/research_projects/seq2seq-distillation/sentence_splitter.py
deleted file mode 100644
index c5acec73928ccd00dcf049601ebdf37bcdf4cfea..0000000000000000000000000000000000000000
--- a/spaces/chendl/compositional_test/transformers/examples/research_projects/seq2seq-distillation/sentence_splitter.py
+++ /dev/null
@@ -1,22 +0,0 @@
-import re
-
-from filelock import FileLock
-
-
-try:
- import nltk
-
- NLTK_AVAILABLE = True
-except (ImportError, ModuleNotFoundError):
- NLTK_AVAILABLE = False
-
-if NLTK_AVAILABLE:
- with FileLock(".lock") as lock:
- nltk.download("punkt", quiet=True)
-
-
-def add_newline_to_end_of_each_sentence(x: str) -> str:
- """This was added to get rougeLsum scores matching published rougeL scores for BART and PEGASUS."""
- re.sub("", "", x) # remove pegasus newline char
- assert NLTK_AVAILABLE, "nltk must be installed to separate newlines between sentences. (pip install nltk)"
- return "\n".join(nltk.sent_tokenize(x))
diff --git a/spaces/chuan-hd/law-assistant-chatbot/.venv/lib/python3.11/site-packages/anyio/from_thread.py b/spaces/chuan-hd/law-assistant-chatbot/.venv/lib/python3.11/site-packages/anyio/from_thread.py
deleted file mode 100644
index 6b76861c70d6a6aa369a54370ef47aa75839a91f..0000000000000000000000000000000000000000
--- a/spaces/chuan-hd/law-assistant-chatbot/.venv/lib/python3.11/site-packages/anyio/from_thread.py
+++ /dev/null
@@ -1,500 +0,0 @@
-from __future__ import annotations
-
-import threading
-from asyncio import iscoroutine
-from concurrent.futures import FIRST_COMPLETED, Future, ThreadPoolExecutor, wait
-from contextlib import AbstractContextManager, contextmanager
-from types import TracebackType
-from typing import (
- Any,
- AsyncContextManager,
- Awaitable,
- Callable,
- ContextManager,
- Generator,
- Generic,
- Iterable,
- TypeVar,
- cast,
- overload,
-)
-from warnings import warn
-
-from ._core import _eventloop
-from ._core._eventloop import get_asynclib, get_cancelled_exc_class, threadlocals
-from ._core._synchronization import Event
-from ._core._tasks import CancelScope, create_task_group
-from .abc._tasks import TaskStatus
-
-T_Retval = TypeVar("T_Retval")
-T_co = TypeVar("T_co")
-
-
-def run(func: Callable[..., Awaitable[T_Retval]], *args: object) -> T_Retval:
- """
- Call a coroutine function from a worker thread.
-
- :param func: a coroutine function
- :param args: positional arguments for the callable
- :return: the return value of the coroutine function
-
- """
- try:
- asynclib = threadlocals.current_async_module
- except AttributeError:
- raise RuntimeError("This function can only be run from an AnyIO worker thread")
-
- return asynclib.run_async_from_thread(func, *args)
-
-
-def run_async_from_thread(
- func: Callable[..., Awaitable[T_Retval]], *args: object
-) -> T_Retval:
- warn(
- "run_async_from_thread() has been deprecated, use anyio.from_thread.run() instead",
- DeprecationWarning,
- )
- return run(func, *args)
-
-
-def run_sync(func: Callable[..., T_Retval], *args: object) -> T_Retval:
- """
- Call a function in the event loop thread from a worker thread.
-
- :param func: a callable
- :param args: positional arguments for the callable
- :return: the return value of the callable
-
- """
- try:
- asynclib = threadlocals.current_async_module
- except AttributeError:
- raise RuntimeError("This function can only be run from an AnyIO worker thread")
-
- return asynclib.run_sync_from_thread(func, *args)
-
-
-def run_sync_from_thread(func: Callable[..., T_Retval], *args: object) -> T_Retval:
- warn(
- "run_sync_from_thread() has been deprecated, use anyio.from_thread.run_sync() instead",
- DeprecationWarning,
- )
- return run_sync(func, *args)
-
-
-class _BlockingAsyncContextManager(Generic[T_co], AbstractContextManager):
- _enter_future: Future
- _exit_future: Future
- _exit_event: Event
- _exit_exc_info: tuple[
- type[BaseException] | None, BaseException | None, TracebackType | None
- ] = (None, None, None)
-
- def __init__(self, async_cm: AsyncContextManager[T_co], portal: BlockingPortal):
- self._async_cm = async_cm
- self._portal = portal
-
- async def run_async_cm(self) -> bool | None:
- try:
- self._exit_event = Event()
- value = await self._async_cm.__aenter__()
- except BaseException as exc:
- self._enter_future.set_exception(exc)
- raise
- else:
- self._enter_future.set_result(value)
-
- try:
- # Wait for the sync context manager to exit.
- # This next statement can raise `get_cancelled_exc_class()` if
- # something went wrong in a task group in this async context
- # manager.
- await self._exit_event.wait()
- finally:
- # In case of cancellation, it could be that we end up here before
- # `_BlockingAsyncContextManager.__exit__` is called, and an
- # `_exit_exc_info` has been set.
- result = await self._async_cm.__aexit__(*self._exit_exc_info)
- return result
-
- def __enter__(self) -> T_co:
- self._enter_future = Future()
- self._exit_future = self._portal.start_task_soon(self.run_async_cm)
- cm = self._enter_future.result()
- return cast(T_co, cm)
-
- def __exit__(
- self,
- __exc_type: type[BaseException] | None,
- __exc_value: BaseException | None,
- __traceback: TracebackType | None,
- ) -> bool | None:
- self._exit_exc_info = __exc_type, __exc_value, __traceback
- self._portal.call(self._exit_event.set)
- return self._exit_future.result()
-
-
-class _BlockingPortalTaskStatus(TaskStatus):
- def __init__(self, future: Future):
- self._future = future
-
- def started(self, value: object = None) -> None:
- self._future.set_result(value)
-
-
-class BlockingPortal:
- """An object that lets external threads run code in an asynchronous event loop."""
-
- def __new__(cls) -> BlockingPortal:
- return get_asynclib().BlockingPortal()
-
- def __init__(self) -> None:
- self._event_loop_thread_id: int | None = threading.get_ident()
- self._stop_event = Event()
- self._task_group = create_task_group()
- self._cancelled_exc_class = get_cancelled_exc_class()
-
- async def __aenter__(self) -> BlockingPortal:
- await self._task_group.__aenter__()
- return self
-
- async def __aexit__(
- self,
- exc_type: type[BaseException] | None,
- exc_val: BaseException | None,
- exc_tb: TracebackType | None,
- ) -> bool | None:
- await self.stop()
- return await self._task_group.__aexit__(exc_type, exc_val, exc_tb)
-
- def _check_running(self) -> None:
- if self._event_loop_thread_id is None:
- raise RuntimeError("This portal is not running")
- if self._event_loop_thread_id == threading.get_ident():
- raise RuntimeError(
- "This method cannot be called from the event loop thread"
- )
-
- async def sleep_until_stopped(self) -> None:
- """Sleep until :meth:`stop` is called."""
- await self._stop_event.wait()
-
- async def stop(self, cancel_remaining: bool = False) -> None:
- """
- Signal the portal to shut down.
-
- This marks the portal as no longer accepting new calls and exits from
- :meth:`sleep_until_stopped`.
-
- :param cancel_remaining: ``True`` to cancel all the remaining tasks, ``False`` to let them
- finish before returning
-
- """
- self._event_loop_thread_id = None
- self._stop_event.set()
- if cancel_remaining:
- self._task_group.cancel_scope.cancel()
-
- async def _call_func(
- self, func: Callable, args: tuple, kwargs: dict[str, Any], future: Future
- ) -> None:
- def callback(f: Future) -> None:
- if f.cancelled() and self._event_loop_thread_id not in (
- None,
- threading.get_ident(),
- ):
- self.call(scope.cancel)
-
- try:
- retval = func(*args, **kwargs)
- if iscoroutine(retval):
- with CancelScope() as scope:
- if future.cancelled():
- scope.cancel()
- else:
- future.add_done_callback(callback)
-
- retval = await retval
- except self._cancelled_exc_class:
- future.cancel()
- except BaseException as exc:
- if not future.cancelled():
- future.set_exception(exc)
-
- # Let base exceptions fall through
- if not isinstance(exc, Exception):
- raise
- else:
- if not future.cancelled():
- future.set_result(retval)
- finally:
- scope = None # type: ignore[assignment]
-
- def _spawn_task_from_thread(
- self,
- func: Callable,
- args: tuple,
- kwargs: dict[str, Any],
- name: object,
- future: Future,
- ) -> None:
- """
- Spawn a new task using the given callable.
-
- Implementors must ensure that the future is resolved when the task finishes.
-
- :param func: a callable
- :param args: positional arguments to be passed to the callable
- :param kwargs: keyword arguments to be passed to the callable
- :param name: name of the task (will be coerced to a string if not ``None``)
- :param future: a future that will resolve to the return value of the callable, or the
- exception raised during its execution
-
- """
- raise NotImplementedError
-
- @overload
- def call(self, func: Callable[..., Awaitable[T_Retval]], *args: object) -> T_Retval:
- ...
-
- @overload
- def call(self, func: Callable[..., T_Retval], *args: object) -> T_Retval:
- ...
-
- def call(
- self, func: Callable[..., Awaitable[T_Retval] | T_Retval], *args: object
- ) -> T_Retval:
- """
- Call the given function in the event loop thread.
-
- If the callable returns a coroutine object, it is awaited on.
-
- :param func: any callable
- :raises RuntimeError: if the portal is not running or if this method is called from within
- the event loop thread
-
- """
- return cast(T_Retval, self.start_task_soon(func, *args).result())
-
- @overload
- def spawn_task(
- self,
- func: Callable[..., Awaitable[T_Retval]],
- *args: object,
- name: object = None,
- ) -> Future[T_Retval]:
- ...
-
- @overload
- def spawn_task(
- self, func: Callable[..., T_Retval], *args: object, name: object = None
- ) -> Future[T_Retval]:
- ...
-
- def spawn_task(
- self,
- func: Callable[..., Awaitable[T_Retval] | T_Retval],
- *args: object,
- name: object = None,
- ) -> Future[T_Retval]:
- """
- Start a task in the portal's task group.
-
- :param func: the target coroutine function
- :param args: positional arguments passed to ``func``
- :param name: name of the task (will be coerced to a string if not ``None``)
- :return: a future that resolves with the return value of the callable if the task completes
- successfully, or with the exception raised in the task
- :raises RuntimeError: if the portal is not running or if this method is called from within
- the event loop thread
-
- .. versionadded:: 2.1
- .. deprecated:: 3.0
- Use :meth:`start_task_soon` instead. If your code needs AnyIO 2 compatibility, you
- can keep using this until AnyIO 4.
-
- """
- warn(
- "spawn_task() is deprecated -- use start_task_soon() instead",
- DeprecationWarning,
- )
- return self.start_task_soon(func, *args, name=name) # type: ignore[arg-type]
-
- @overload
- def start_task_soon(
- self,
- func: Callable[..., Awaitable[T_Retval]],
- *args: object,
- name: object = None,
- ) -> Future[T_Retval]:
- ...
-
- @overload
- def start_task_soon(
- self, func: Callable[..., T_Retval], *args: object, name: object = None
- ) -> Future[T_Retval]:
- ...
-
- def start_task_soon(
- self,
- func: Callable[..., Awaitable[T_Retval] | T_Retval],
- *args: object,
- name: object = None,
- ) -> Future[T_Retval]:
- """
- Start a task in the portal's task group.
-
- The task will be run inside a cancel scope which can be cancelled by cancelling the
- returned future.
-
- :param func: the target function
- :param args: positional arguments passed to ``func``
- :param name: name of the task (will be coerced to a string if not ``None``)
- :return: a future that resolves with the return value of the callable if the
- task completes successfully, or with the exception raised in the task
- :raises RuntimeError: if the portal is not running or if this method is called
- from within the event loop thread
- :rtype: concurrent.futures.Future[T_Retval]
-
- .. versionadded:: 3.0
-
- """
- self._check_running()
- f: Future = Future()
- self._spawn_task_from_thread(func, args, {}, name, f)
- return f
-
- def start_task(
- self, func: Callable[..., Awaitable[Any]], *args: object, name: object = None
- ) -> tuple[Future[Any], Any]:
- """
- Start a task in the portal's task group and wait until it signals for readiness.
-
- This method works the same way as :meth:`.abc.TaskGroup.start`.
-
- :param func: the target function
- :param args: positional arguments passed to ``func``
- :param name: name of the task (will be coerced to a string if not ``None``)
- :return: a tuple of (future, task_status_value) where the ``task_status_value``
- is the value passed to ``task_status.started()`` from within the target
- function
- :rtype: tuple[concurrent.futures.Future[Any], Any]
-
- .. versionadded:: 3.0
-
- """
-
- def task_done(future: Future) -> None:
- if not task_status_future.done():
- if future.cancelled():
- task_status_future.cancel()
- elif future.exception():
- task_status_future.set_exception(future.exception())
- else:
- exc = RuntimeError(
- "Task exited without calling task_status.started()"
- )
- task_status_future.set_exception(exc)
-
- self._check_running()
- task_status_future: Future = Future()
- task_status = _BlockingPortalTaskStatus(task_status_future)
- f: Future = Future()
- f.add_done_callback(task_done)
- self._spawn_task_from_thread(func, args, {"task_status": task_status}, name, f)
- return f, task_status_future.result()
-
- def wrap_async_context_manager(
- self, cm: AsyncContextManager[T_co]
- ) -> ContextManager[T_co]:
- """
- Wrap an async context manager as a synchronous context manager via this portal.
-
- Spawns a task that will call both ``__aenter__()`` and ``__aexit__()``, stopping in the
- middle until the synchronous context manager exits.
-
- :param cm: an asynchronous context manager
- :return: a synchronous context manager
-
- .. versionadded:: 2.1
-
- """
- return _BlockingAsyncContextManager(cm, self)
-
-
-def create_blocking_portal() -> BlockingPortal:
- """
- Create a portal for running functions in the event loop thread from external threads.
-
- Use this function in asynchronous code when you need to allow external threads access to the
- event loop where your asynchronous code is currently running.
-
- .. deprecated:: 3.0
- Use :class:`.BlockingPortal` directly.
-
- """
- warn(
- "create_blocking_portal() has been deprecated -- use anyio.from_thread.BlockingPortal() "
- "directly",
- DeprecationWarning,
- )
- return BlockingPortal()
-
-
-@contextmanager
-def start_blocking_portal(
- backend: str = "asyncio", backend_options: dict[str, Any] | None = None
-) -> Generator[BlockingPortal, Any, None]:
- """
- Start a new event loop in a new thread and run a blocking portal in its main task.
-
- The parameters are the same as for :func:`~anyio.run`.
-
- :param backend: name of the backend
- :param backend_options: backend options
- :return: a context manager that yields a blocking portal
-
- .. versionchanged:: 3.0
- Usage as a context manager is now required.
-
- """
-
- async def run_portal() -> None:
- async with BlockingPortal() as portal_:
- if future.set_running_or_notify_cancel():
- future.set_result(portal_)
- await portal_.sleep_until_stopped()
-
- future: Future[BlockingPortal] = Future()
- with ThreadPoolExecutor(1) as executor:
- run_future = executor.submit(
- _eventloop.run,
- run_portal, # type: ignore[arg-type]
- backend=backend,
- backend_options=backend_options,
- )
- try:
- wait(
- cast(Iterable[Future], [run_future, future]),
- return_when=FIRST_COMPLETED,
- )
- except BaseException:
- future.cancel()
- run_future.cancel()
- raise
-
- if future.done():
- portal = future.result()
- cancel_remaining_tasks = False
- try:
- yield portal
- except BaseException:
- cancel_remaining_tasks = True
- raise
- finally:
- try:
- portal.call(portal.stop, cancel_remaining_tasks)
- except RuntimeError:
- pass
-
- run_future.result()
diff --git a/spaces/chuan-hd/law-assistant-chatbot/.venv/lib/python3.11/site-packages/attr/_version_info.py b/spaces/chuan-hd/law-assistant-chatbot/.venv/lib/python3.11/site-packages/attr/_version_info.py
deleted file mode 100644
index 51a1312f9759f21063caea779a62882d7f7c86ae..0000000000000000000000000000000000000000
--- a/spaces/chuan-hd/law-assistant-chatbot/.venv/lib/python3.11/site-packages/attr/_version_info.py
+++ /dev/null
@@ -1,86 +0,0 @@
-# SPDX-License-Identifier: MIT
-
-
-from functools import total_ordering
-
-from ._funcs import astuple
-from ._make import attrib, attrs
-
-
-@total_ordering
-@attrs(eq=False, order=False, slots=True, frozen=True)
-class VersionInfo:
- """
- A version object that can be compared to tuple of length 1--4:
-
- >>> attr.VersionInfo(19, 1, 0, "final") <= (19, 2)
- True
- >>> attr.VersionInfo(19, 1, 0, "final") < (19, 1, 1)
- True
- >>> vi = attr.VersionInfo(19, 2, 0, "final")
- >>> vi < (19, 1, 1)
- False
- >>> vi < (19,)
- False
- >>> vi == (19, 2,)
- True
- >>> vi == (19, 2, 1)
- False
-
- .. versionadded:: 19.2
- """
-
- year = attrib(type=int)
- minor = attrib(type=int)
- micro = attrib(type=int)
- releaselevel = attrib(type=str)
-
- @classmethod
- def _from_version_string(cls, s):
- """
- Parse *s* and return a _VersionInfo.
- """
- v = s.split(".")
- if len(v) == 3:
- v.append("final")
-
- return cls(
- year=int(v[0]), minor=int(v[1]), micro=int(v[2]), releaselevel=v[3]
- )
-
- def _ensure_tuple(self, other):
- """
- Ensure *other* is a tuple of a valid length.
-
- Returns a possibly transformed *other* and ourselves as a tuple of
- the same length as *other*.
- """
-
- if self.__class__ is other.__class__:
- other = astuple(other)
-
- if not isinstance(other, tuple):
- raise NotImplementedError
-
- if not (1 <= len(other) <= 4):
- raise NotImplementedError
-
- return astuple(self)[: len(other)], other
-
- def __eq__(self, other):
- try:
- us, them = self._ensure_tuple(other)
- except NotImplementedError:
- return NotImplemented
-
- return us == them
-
- def __lt__(self, other):
- try:
- us, them = self._ensure_tuple(other)
- except NotImplementedError:
- return NotImplemented
-
- # Since alphabetically "dev0" < "final" < "post1" < "post2", we don't
- # have to do anything special with releaselevel for now.
- return us < them
diff --git a/spaces/chuan-hd/law-assistant-chatbot/.venv/lib/python3.11/site-packages/clickhouse_connect/dbapi/__init__.py b/spaces/chuan-hd/law-assistant-chatbot/.venv/lib/python3.11/site-packages/clickhouse_connect/dbapi/__init__.py
deleted file mode 100644
index ea792b49683ed3ebf6dd6b09146029c982716363..0000000000000000000000000000000000000000
--- a/spaces/chuan-hd/law-assistant-chatbot/.venv/lib/python3.11/site-packages/clickhouse_connect/dbapi/__init__.py
+++ /dev/null
@@ -1,28 +0,0 @@
-from typing import Optional
-
-from clickhouse_connect.dbapi.connection import Connection
-
-
-apilevel = '2.0' # PEP 249 DB API level
-threadsafety = 2 # PEP 249 Threads may share the module and connections.
-paramstyle = 'pyformat' # PEP 249 Python extended format codes, e.g. ...WHERE name=%(name)s
-
-
-class Error(Exception):
- pass
-
-
-def connect(host: Optional[str] = None,
- database: Optional[str] = None,
- username: Optional[str] = '',
- password: Optional[str] = '',
- port: Optional[int] = None,
- **kwargs):
- secure = kwargs.pop('secure', False)
- return Connection(host=host,
- database=database,
- username=username,
- password=password,
- port=port,
- secure=secure,
- **kwargs)
diff --git a/spaces/chuan-hd/law-assistant-chatbot/.venv/lib/python3.11/site-packages/faiss/__init__.py b/spaces/chuan-hd/law-assistant-chatbot/.venv/lib/python3.11/site-packages/faiss/__init__.py
deleted file mode 100644
index 92eeb3479ba37acec65e17a437b6e0d608a85d7e..0000000000000000000000000000000000000000
--- a/spaces/chuan-hd/law-assistant-chatbot/.venv/lib/python3.11/site-packages/faiss/__init__.py
+++ /dev/null
@@ -1,312 +0,0 @@
-# Copyright (c) Facebook, Inc. and its affiliates.
-#
-# This source code is licensed under the MIT license found in the
-# LICENSE file in the root directory of this source tree.
-
-# @nolint
-
-# not linting this file because it imports * from swigfaiss, which
-# causes a ton of useless warnings.
-
-import numpy as np
-import sys
-import inspect
-
-# We import * so that the symbol foo can be accessed as faiss.foo.
-from .loader import *
-
-# additional wrappers
-from faiss import class_wrappers
-from faiss.gpu_wrappers import *
-from faiss.array_conversions import *
-from faiss.extra_wrappers import kmin, kmax, pairwise_distances, rand, randint, \
- lrand, randn, rand_smooth_vectors, eval_intersection, normalize_L2, \
- ResultHeap, knn, Kmeans, checksum, matrix_bucket_sort_inplace, bucket_sort, \
- merge_knn_results
-
-
-__version__ = "%d.%d.%d" % (FAISS_VERSION_MAJOR,
- FAISS_VERSION_MINOR,
- FAISS_VERSION_PATCH)
-
-class_wrappers.handle_Clustering(Clustering)
-class_wrappers.handle_Clustering1D(Clustering1D)
-class_wrappers.handle_MatrixStats(MatrixStats)
-class_wrappers.handle_IOWriter(IOWriter)
-class_wrappers.handle_IOReader(IOReader)
-class_wrappers.handle_AutoTuneCriterion(AutoTuneCriterion)
-class_wrappers.handle_ParameterSpace(ParameterSpace)
-class_wrappers.handle_NSG(IndexNSG)
-class_wrappers.handle_MapLong2Long(MapLong2Long)
-class_wrappers.handle_IDSelectorSubset(IDSelectorBatch, class_owns=True)
-class_wrappers.handle_IDSelectorSubset(IDSelectorArray, class_owns=False)
-class_wrappers.handle_IDSelectorSubset(IDSelectorBitmap, class_owns=False, force_int64=False)
-
-this_module = sys.modules[__name__]
-
-# handle sub-classes
-for symbol in dir(this_module):
- obj = getattr(this_module, symbol)
- # print symbol, isinstance(obj, (type, types.ClassType))
- if inspect.isclass(obj):
- the_class = obj
- if issubclass(the_class, Index):
- class_wrappers.handle_Index(the_class)
-
- if issubclass(the_class, IndexBinary):
- class_wrappers.handle_IndexBinary(the_class)
-
- if issubclass(the_class, VectorTransform):
- class_wrappers.handle_VectorTransform(the_class)
-
- if issubclass(the_class, Quantizer):
- class_wrappers.handle_Quantizer(the_class)
-
- if issubclass(the_class, IndexRowwiseMinMax) or \
- issubclass(the_class, IndexRowwiseMinMaxFP16):
- class_wrappers.handle_IndexRowwiseMinMax(the_class)
-
- if issubclass(the_class, SearchParameters):
- class_wrappers.handle_SearchParameters(the_class)
-
- if issubclass(the_class, CodePacker):
- class_wrappers.handle_CodePacker(the_class)
-
-##############################################################################
-# For some classes (IndexIVF, IDSelector), the object holds a reference to
-# a C++ object (eg. the quantizer object of IndexIVF). We don't transfer the
-# ownership to the C++ object (ie. set own_quantizer=true), but instead we add
-# a reference in the Python class wrapper instead. This is done via an
-# additional referenced_objects field.
-#
-# Since the semantics of ownership in the C++ classes are sometimes irregular,
-# these references are added manually using the functions below.
-##############################################################################
-
-
-def add_ref_in_constructor(the_class, parameter_no):
- # adds a reference to parameter parameter_no in self
- # so that that parameter does not get deallocated before self
- original_init = the_class.__init__
-
- def replacement_init(self, *args):
- original_init(self, *args)
- self.referenced_objects = [args[parameter_no]]
-
- def replacement_init_multiple(self, *args):
- original_init(self, *args)
- pset = parameter_no[len(args)]
- self.referenced_objects = [args[no] for no in pset]
-
- if type(parameter_no) == dict:
- # a list of parameters to keep, depending on the number of arguments
- the_class.__init__ = replacement_init_multiple
- else:
- the_class.__init__ = replacement_init
-
-def add_to_referenced_objects(self, ref):
- if not hasattr(self, 'referenced_objects'):
- self.referenced_objects = [ref]
- else:
- self.referenced_objects.append(ref)
-
-
-def add_ref_in_method(the_class, method_name, parameter_no):
- original_method = getattr(the_class, method_name)
-
- def replacement_method(self, *args):
- ref = args[parameter_no]
- add_to_referenced_objects(self, ref)
- return original_method(self, *args)
- setattr(the_class, method_name, replacement_method)
-
-
-def add_ref_in_method_explicit_own(the_class, method_name):
- # for methods of format set_XXX(object, own)
- original_method = getattr(the_class, method_name)
-
- def replacement_method(self, ref, own=False):
- if not own:
- if not hasattr(self, 'referenced_objects'):
- self.referenced_objects = [ref]
- else:
- self.referenced_objects.append(ref)
- else:
- # transfer ownership to C++ class
- ref.this.disown()
- return original_method(self, ref, own)
- setattr(the_class, method_name, replacement_method)
-
-
-def add_ref_in_function(function_name, parameter_no):
- # assumes the function returns an object
- original_function = getattr(this_module, function_name)
-
- def replacement_function(*args):
- result = original_function(*args)
- ref = args[parameter_no]
- result.referenced_objects = [ref]
- return result
- setattr(this_module, function_name, replacement_function)
-
-
-add_ref_in_constructor(IndexIVFFlat, 0)
-add_ref_in_constructor(IndexIVFFlatDedup, 0)
-add_ref_in_constructor(IndexPreTransform, {2: [0, 1], 1: [0]})
-add_ref_in_method(IndexPreTransform, 'prepend_transform', 0)
-add_ref_in_constructor(IndexIVFPQ, 0)
-add_ref_in_constructor(IndexIVFPQR, 0)
-add_ref_in_constructor(IndexIVFPQFastScan, 0)
-add_ref_in_constructor(IndexIVFResidualQuantizer, 0)
-add_ref_in_constructor(IndexIVFLocalSearchQuantizer, 0)
-add_ref_in_constructor(IndexIVFResidualQuantizerFastScan, 0)
-add_ref_in_constructor(IndexIVFLocalSearchQuantizerFastScan, 0)
-add_ref_in_constructor(IndexIVFSpectralHash, 0)
-add_ref_in_method_explicit_own(IndexIVFSpectralHash, "replace_vt")
-
-add_ref_in_constructor(Index2Layer, 0)
-add_ref_in_constructor(Level1Quantizer, 0)
-add_ref_in_constructor(IndexIVFScalarQuantizer, 0)
-add_ref_in_constructor(IndexRowwiseMinMax, 0)
-add_ref_in_constructor(IndexRowwiseMinMaxFP16, 0)
-add_ref_in_constructor(IndexIDMap, 0)
-add_ref_in_constructor(IndexIDMap2, 0)
-add_ref_in_constructor(IndexHNSW, 0)
-add_ref_in_method(IndexShards, 'add_shard', 0)
-add_ref_in_method(IndexBinaryShards, 'add_shard', 0)
-add_ref_in_constructor(IndexRefineFlat, {2: [0], 1: [0]})
-add_ref_in_constructor(IndexRefine, {2: [0, 1]})
-
-add_ref_in_constructor(IndexBinaryIVF, 0)
-add_ref_in_constructor(IndexBinaryFromFloat, 0)
-add_ref_in_constructor(IndexBinaryIDMap, 0)
-add_ref_in_constructor(IndexBinaryIDMap2, 0)
-
-add_ref_in_method(IndexReplicas, 'addIndex', 0)
-add_ref_in_method(IndexBinaryReplicas, 'addIndex', 0)
-
-add_ref_in_constructor(BufferedIOWriter, 0)
-add_ref_in_constructor(BufferedIOReader, 0)
-
-add_ref_in_constructor(IDSelectorNot, 0)
-add_ref_in_constructor(IDSelectorAnd, slice(2))
-add_ref_in_constructor(IDSelectorOr, slice(2))
-add_ref_in_constructor(IDSelectorXOr, slice(2))
-
-# seems really marginal...
-# remove_ref_from_method(IndexReplicas, 'removeIndex', 0)
-
-
-######################################################
-# search_with_parameters interface
-######################################################
-
-search_with_parameters_c = search_with_parameters
-
-
-def search_with_parameters(index, x, k, params=None, output_stats=False):
- x = np.ascontiguousarray(x, dtype='float32')
- n, d = x.shape
- assert d == index.d
- if not params:
- # if not provided use the ones set in the IVF object
- params = IVFSearchParameters()
- index_ivf = extract_index_ivf(index)
- params.nprobe = index_ivf.nprobe
- params.max_codes = index_ivf.max_codes
- nb_dis = np.empty(1, 'uint64')
- ms_per_stage = np.empty(3, 'float64')
- distances = np.empty((n, k), dtype=np.float32)
- labels = np.empty((n, k), dtype=np.int64)
- search_with_parameters_c(
- index, n, swig_ptr(x),
- k, swig_ptr(distances),
- swig_ptr(labels),
- params, swig_ptr(nb_dis), swig_ptr(ms_per_stage)
- )
- if not output_stats:
- return distances, labels
- else:
- stats = {
- 'ndis': nb_dis[0],
- 'pre_transform_ms': ms_per_stage[0],
- 'coarse_quantizer_ms': ms_per_stage[1],
- 'invlist_scan_ms': ms_per_stage[2],
- }
- return distances, labels, stats
-
-
-range_search_with_parameters_c = range_search_with_parameters
-
-
-def range_search_with_parameters(index, x, radius, params=None, output_stats=False):
- x = np.ascontiguousarray(x, dtype='float32')
- n, d = x.shape
- assert d == index.d
- if not params:
- # if not provided use the ones set in the IVF object
- params = IVFSearchParameters()
- index_ivf = extract_index_ivf(index)
- params.nprobe = index_ivf.nprobe
- params.max_codes = index_ivf.max_codes
- nb_dis = np.empty(1, 'uint64')
- ms_per_stage = np.empty(3, 'float64')
- res = RangeSearchResult(n)
- range_search_with_parameters_c(
- index, n, swig_ptr(x),
- radius, res,
- params, swig_ptr(nb_dis), swig_ptr(ms_per_stage)
- )
- lims = rev_swig_ptr(res.lims, n + 1).copy()
- nd = int(lims[-1])
- Dout = rev_swig_ptr(res.distances, nd).copy()
- Iout = rev_swig_ptr(res.labels, nd).copy()
- if not output_stats:
- return lims, Dout, Iout
- else:
- stats = {
- 'ndis': nb_dis[0],
- 'pre_transform_ms': ms_per_stage[0],
- 'coarse_quantizer_ms': ms_per_stage[1],
- 'invlist_scan_ms': ms_per_stage[2],
- }
- return lims, Dout, Iout, stats
-
-
-# IndexProxy was renamed to IndexReplicas, remap the old name for any old code
-# people may have
-IndexProxy = IndexReplicas
-ConcatenatedInvertedLists = HStackInvertedLists
-IndexResidual = IndexResidualQuantizer
-
-IVFSearchParameters = SearchParametersIVF
-
-###########################################
-# serialization of indexes to byte arrays
-###########################################
-
-
-def serialize_index(index):
- """ convert an index to a numpy uint8 array """
- writer = VectorIOWriter()
- write_index(index, writer)
- return vector_to_array(writer.data)
-
-
-def deserialize_index(data):
- reader = VectorIOReader()
- copy_array_to_vector(data, reader.data)
- return read_index(reader)
-
-
-def serialize_index_binary(index):
- """ convert an index to a numpy uint8 array """
- writer = VectorIOWriter()
- write_index_binary(index, writer)
- return vector_to_array(writer.data)
-
-
-def deserialize_index_binary(data):
- reader = VectorIOReader()
- copy_array_to_vector(data, reader.data)
- return read_index_binary(reader)
diff --git a/spaces/chuan-hd/law-assistant-chatbot/.venv/lib/python3.11/site-packages/fontTools/ttLib/tables/G_M_A_P_.py b/spaces/chuan-hd/law-assistant-chatbot/.venv/lib/python3.11/site-packages/fontTools/ttLib/tables/G_M_A_P_.py
deleted file mode 100644
index 39b0050c5f0591a2b36c21242863655ca1f3ef47..0000000000000000000000000000000000000000
--- a/spaces/chuan-hd/law-assistant-chatbot/.venv/lib/python3.11/site-packages/fontTools/ttLib/tables/G_M_A_P_.py
+++ /dev/null
@@ -1,142 +0,0 @@
-from fontTools.misc import sstruct
-from fontTools.misc.textTools import tobytes, tostr, safeEval
-from . import DefaultTable
-
-GMAPFormat = """
- > # big endian
- tableVersionMajor: H
- tableVersionMinor: H
- flags: H
- recordsCount: H
- recordsOffset: H
- fontNameLength: H
-"""
-# psFontName is a byte string which follows the record above. This is zero padded
-# to the beginning of the records array. The recordsOffsst is 32 bit aligned.
-
-GMAPRecordFormat1 = """
- > # big endian
- UV: L
- cid: H
- gid: H
- ggid: H
- name: 32s
-"""
-
-
-class GMAPRecord(object):
- def __init__(self, uv=0, cid=0, gid=0, ggid=0, name=""):
- self.UV = uv
- self.cid = cid
- self.gid = gid
- self.ggid = ggid
- self.name = name
-
- def toXML(self, writer, ttFont):
- writer.begintag("GMAPRecord")
- writer.newline()
- writer.simpletag("UV", value=self.UV)
- writer.newline()
- writer.simpletag("cid", value=self.cid)
- writer.newline()
- writer.simpletag("gid", value=self.gid)
- writer.newline()
- writer.simpletag("glyphletGid", value=self.gid)
- writer.newline()
- writer.simpletag("GlyphletName", value=self.name)
- writer.newline()
- writer.endtag("GMAPRecord")
- writer.newline()
-
- def fromXML(self, name, attrs, content, ttFont):
- value = attrs["value"]
- if name == "GlyphletName":
- self.name = value
- else:
- setattr(self, name, safeEval(value))
-
- def compile(self, ttFont):
- if self.UV is None:
- self.UV = 0
- nameLen = len(self.name)
- if nameLen < 32:
- self.name = self.name + "\0" * (32 - nameLen)
- data = sstruct.pack(GMAPRecordFormat1, self)
- return data
-
- def __repr__(self):
- return (
- "GMAPRecord[ UV: "
- + str(self.UV)
- + ", cid: "
- + str(self.cid)
- + ", gid: "
- + str(self.gid)
- + ", ggid: "
- + str(self.ggid)
- + ", Glyphlet Name: "
- + str(self.name)
- + " ]"
- )
-
-
-class table_G_M_A_P_(DefaultTable.DefaultTable):
-
- dependencies = []
-
- def decompile(self, data, ttFont):
- dummy, newData = sstruct.unpack2(GMAPFormat, data, self)
- self.psFontName = tostr(newData[: self.fontNameLength])
- assert (
- self.recordsOffset % 4
- ) == 0, "GMAP error: recordsOffset is not 32 bit aligned."
- newData = data[self.recordsOffset :]
- self.gmapRecords = []
- for i in range(self.recordsCount):
- gmapRecord, newData = sstruct.unpack2(
- GMAPRecordFormat1, newData, GMAPRecord()
- )
- gmapRecord.name = gmapRecord.name.strip("\0")
- self.gmapRecords.append(gmapRecord)
-
- def compile(self, ttFont):
- self.recordsCount = len(self.gmapRecords)
- self.fontNameLength = len(self.psFontName)
- self.recordsOffset = 4 * (((self.fontNameLength + 12) + 3) // 4)
- data = sstruct.pack(GMAPFormat, self)
- data = data + tobytes(self.psFontName)
- data = data + b"\0" * (self.recordsOffset - len(data))
- for record in self.gmapRecords:
- data = data + record.compile(ttFont)
- return data
-
- def toXML(self, writer, ttFont):
- writer.comment("Most of this table will be recalculated by the compiler")
- writer.newline()
- formatstring, names, fixes = sstruct.getformat(GMAPFormat)
- for name in names:
- value = getattr(self, name)
- writer.simpletag(name, value=value)
- writer.newline()
- writer.simpletag("PSFontName", value=self.psFontName)
- writer.newline()
- for gmapRecord in self.gmapRecords:
- gmapRecord.toXML(writer, ttFont)
-
- def fromXML(self, name, attrs, content, ttFont):
- if name == "GMAPRecord":
- if not hasattr(self, "gmapRecords"):
- self.gmapRecords = []
- gmapRecord = GMAPRecord()
- self.gmapRecords.append(gmapRecord)
- for element in content:
- if isinstance(element, str):
- continue
- name, attrs, content = element
- gmapRecord.fromXML(name, attrs, content, ttFont)
- else:
- value = attrs["value"]
- if name == "PSFontName":
- self.psFontName = value
- else:
- setattr(self, name, safeEval(value))
diff --git a/spaces/chuan-hd/law-assistant-chatbot/.venv/lib/python3.11/site-packages/google/protobuf/timestamp_pb2.py b/spaces/chuan-hd/law-assistant-chatbot/.venv/lib/python3.11/site-packages/google/protobuf/timestamp_pb2.py
deleted file mode 100644
index b10f2f2047bf0e89f584633b0abd1c94b8ada467..0000000000000000000000000000000000000000
--- a/spaces/chuan-hd/law-assistant-chatbot/.venv/lib/python3.11/site-packages/google/protobuf/timestamp_pb2.py
+++ /dev/null
@@ -1,27 +0,0 @@
-# -*- coding: utf-8 -*-
-# Generated by the protocol buffer compiler. DO NOT EDIT!
-# source: google/protobuf/timestamp.proto
-"""Generated protocol buffer code."""
-from google.protobuf import descriptor as _descriptor
-from google.protobuf import descriptor_pool as _descriptor_pool
-from google.protobuf import symbol_database as _symbol_database
-from google.protobuf.internal import builder as _builder
-# @@protoc_insertion_point(imports)
-
-_sym_db = _symbol_database.Default()
-
-
-
-
-DESCRIPTOR = _descriptor_pool.Default().AddSerializedFile(b'\n\x1fgoogle/protobuf/timestamp.proto\x12\x0fgoogle.protobuf\";\n\tTimestamp\x12\x18\n\x07seconds\x18\x01 \x01(\x03R\x07seconds\x12\x14\n\x05nanos\x18\x02 \x01(\x05R\x05nanosB\x85\x01\n\x13\x63om.google.protobufB\x0eTimestampProtoP\x01Z2google.golang.org/protobuf/types/known/timestamppb\xf8\x01\x01\xa2\x02\x03GPB\xaa\x02\x1eGoogle.Protobuf.WellKnownTypesb\x06proto3')
-
-_globals = globals()
-_builder.BuildMessageAndEnumDescriptors(DESCRIPTOR, _globals)
-_builder.BuildTopDescriptorsAndMessages(DESCRIPTOR, 'google.protobuf.timestamp_pb2', _globals)
-if _descriptor._USE_C_DESCRIPTORS == False:
-
- DESCRIPTOR._options = None
- DESCRIPTOR._serialized_options = b'\n\023com.google.protobufB\016TimestampProtoP\001Z2google.golang.org/protobuf/types/known/timestamppb\370\001\001\242\002\003GPB\252\002\036Google.Protobuf.WellKnownTypes'
- _globals['_TIMESTAMP']._serialized_start=52
- _globals['_TIMESTAMP']._serialized_end=111
-# @@protoc_insertion_point(module_scope)
diff --git a/spaces/chuan-hd/law-assistant-chatbot/.venv/lib/python3.11/site-packages/gradio/interpretation.py b/spaces/chuan-hd/law-assistant-chatbot/.venv/lib/python3.11/site-packages/gradio/interpretation.py
deleted file mode 100644
index 767ad641b99a51c08b4efadec350c7170bdc734b..0000000000000000000000000000000000000000
--- a/spaces/chuan-hd/law-assistant-chatbot/.venv/lib/python3.11/site-packages/gradio/interpretation.py
+++ /dev/null
@@ -1,328 +0,0 @@
-"""Contains classes and methods related to interpretation for components in Gradio."""
-
-from __future__ import annotations
-
-import copy
-import math
-from abc import ABC, abstractmethod
-from typing import TYPE_CHECKING, Any
-
-import numpy as np
-from gradio_client import utils as client_utils
-
-from gradio import components
-
-if TYPE_CHECKING: # Only import for type checking (is False at runtime).
- from gradio import Interface
-
-
-class Interpretable(ABC): # noqa: B024
- def __init__(self) -> None:
- self.set_interpret_parameters()
-
- def set_interpret_parameters(self): # noqa: B027
- """
- Set any parameters for interpretation. Properties can be set here to be
- used in get_interpretation_neighbors and get_interpretation_scores.
- """
- pass
-
- def get_interpretation_scores(
- self, x: Any, neighbors: list[Any] | None, scores: list[float], **kwargs
- ) -> list:
- """
- Arrange the output values from the neighbors into interpretation scores for the interface to render.
- Parameters:
- x: Input to interface
- neighbors: Neighboring values to input x used for interpretation.
- scores: Output value corresponding to each neighbor in neighbors
- Returns:
- Arrangement of interpretation scores for interfaces to render.
- """
- return scores
-
-
-class TokenInterpretable(Interpretable, ABC):
- @abstractmethod
- def tokenize(self, x: Any) -> tuple[list, list, None]:
- """
- Interprets an input data point x by splitting it into a list of tokens (e.g
- a string into words or an image into super-pixels).
- """
- return [], [], None
-
- @abstractmethod
- def get_masked_inputs(self, tokens: list, binary_mask_matrix: list[list]) -> list:
- return []
-
-
-class NeighborInterpretable(Interpretable, ABC):
- @abstractmethod
- def get_interpretation_neighbors(self, x: Any) -> tuple[list, dict]:
- """
- Generates values similar to input to be used to interpret the significance of the input in the final output.
- Parameters:
- x: Input to interface
- Returns: (neighbor_values, interpret_kwargs, interpret_by_removal)
- neighbor_values: Neighboring values to input x to compute for interpretation
- interpret_kwargs: Keyword arguments to be passed to get_interpretation_scores
- """
- return [], {}
-
-
-async def run_interpret(interface: Interface, raw_input: list):
- """
- Runs the interpretation command for the machine learning model. Handles both the "default" out-of-the-box
- interpretation for a certain set of UI component types, as well as the custom interpretation case.
- Parameters:
- raw_input: a list of raw inputs to apply the interpretation(s) on.
- """
- if isinstance(interface.interpretation, list): # Either "default" or "shap"
- processed_input = [
- input_component.preprocess(raw_input[i])
- for i, input_component in enumerate(interface.input_components)
- ]
- original_output = await interface.call_function(0, processed_input)
- original_output = original_output["prediction"]
-
- if len(interface.output_components) == 1:
- original_output = [original_output]
-
- scores, alternative_outputs = [], []
-
- for i, (x, interp) in enumerate(zip(raw_input, interface.interpretation)):
- if interp == "default":
- input_component = interface.input_components[i]
- neighbor_raw_input = list(raw_input)
- if isinstance(input_component, TokenInterpretable):
- tokens, neighbor_values, masks = input_component.tokenize(x)
- interface_scores = []
- alternative_output = []
- for neighbor_input in neighbor_values:
- neighbor_raw_input[i] = neighbor_input
- processed_neighbor_input = [
- input_component.preprocess(neighbor_raw_input[i])
- for i, input_component in enumerate(
- interface.input_components
- )
- ]
-
- neighbor_output = await interface.call_function(
- 0, processed_neighbor_input
- )
- neighbor_output = neighbor_output["prediction"]
- if len(interface.output_components) == 1:
- neighbor_output = [neighbor_output]
- processed_neighbor_output = [
- output_component.postprocess(neighbor_output[i])
- for i, output_component in enumerate(
- interface.output_components
- )
- ]
-
- alternative_output.append(processed_neighbor_output)
- interface_scores.append(
- quantify_difference_in_label(
- interface, original_output, neighbor_output
- )
- )
- alternative_outputs.append(alternative_output)
- scores.append(
- input_component.get_interpretation_scores(
- raw_input[i],
- neighbor_values,
- interface_scores,
- masks=masks,
- tokens=tokens,
- )
- )
- elif isinstance(input_component, NeighborInterpretable):
- (
- neighbor_values,
- interpret_kwargs,
- ) = input_component.get_interpretation_neighbors(
- x
- ) # type: ignore
- interface_scores = []
- alternative_output = []
- for neighbor_input in neighbor_values:
- neighbor_raw_input[i] = neighbor_input
- processed_neighbor_input = [
- input_component.preprocess(neighbor_raw_input[i])
- for i, input_component in enumerate(
- interface.input_components
- )
- ]
- neighbor_output = await interface.call_function(
- 0, processed_neighbor_input
- )
- neighbor_output = neighbor_output["prediction"]
- if len(interface.output_components) == 1:
- neighbor_output = [neighbor_output]
- processed_neighbor_output = [
- output_component.postprocess(neighbor_output[i])
- for i, output_component in enumerate(
- interface.output_components
- )
- ]
-
- alternative_output.append(processed_neighbor_output)
- interface_scores.append(
- quantify_difference_in_label(
- interface, original_output, neighbor_output
- )
- )
- alternative_outputs.append(alternative_output)
- interface_scores = [-score for score in interface_scores]
- scores.append(
- input_component.get_interpretation_scores(
- raw_input[i],
- neighbor_values,
- interface_scores,
- **interpret_kwargs,
- )
- )
- else:
- raise ValueError(
- f"Component {input_component} does not support interpretation"
- )
- elif interp == "shap" or interp == "shapley":
- try:
- import shap # type: ignore
- except (ImportError, ModuleNotFoundError) as err:
- raise ValueError(
- "The package `shap` is required for this interpretation method. Try: `pip install shap`"
- ) from err
- input_component = interface.input_components[i]
- if not isinstance(input_component, TokenInterpretable):
- raise ValueError(
- f"Input component {input_component} does not support `shap` interpretation"
- )
-
- tokens, _, masks = input_component.tokenize(x)
-
- # construct a masked version of the input
- def get_masked_prediction(binary_mask):
- assert isinstance(input_component, TokenInterpretable)
- masked_xs = input_component.get_masked_inputs(tokens, binary_mask)
- preds = []
- for masked_x in masked_xs:
- processed_masked_input = copy.deepcopy(processed_input)
- processed_masked_input[i] = input_component.preprocess(masked_x)
- new_output = client_utils.synchronize_async(
- interface.call_function, 0, processed_masked_input
- )
- new_output = new_output["prediction"]
- if len(interface.output_components) == 1:
- new_output = [new_output]
- pred = get_regression_or_classification_value(
- interface, original_output, new_output
- )
- preds.append(pred)
- return np.array(preds)
-
- num_total_segments = len(tokens)
- explainer = shap.KernelExplainer(
- get_masked_prediction, np.zeros((1, num_total_segments))
- )
- shap_values = explainer.shap_values(
- np.ones((1, num_total_segments)),
- nsamples=int(interface.num_shap * num_total_segments),
- silent=True,
- )
- assert shap_values is not None, "SHAP values could not be calculated"
- scores.append(
- input_component.get_interpretation_scores(
- raw_input[i],
- None,
- shap_values[0].tolist(),
- masks=masks,
- tokens=tokens,
- )
- )
- alternative_outputs.append([])
- elif interp is None:
- scores.append(None)
- alternative_outputs.append([])
- else:
- raise ValueError(f"Unknown interpretation method: {interp}")
- return scores, alternative_outputs
- elif interface.interpretation: # custom interpretation function
- processed_input = [
- input_component.preprocess(raw_input[i])
- for i, input_component in enumerate(interface.input_components)
- ]
- interpreter = interface.interpretation
- interpretation = interpreter(*processed_input)
- if len(raw_input) == 1:
- interpretation = [interpretation]
- return interpretation, []
- else:
- raise ValueError("No interpretation method specified.")
-
-
-def diff(original: Any, perturbed: Any) -> int | float:
- try: # try computing numerical difference
- score = float(original) - float(perturbed)
- except ValueError: # otherwise, look at strict difference in label
- score = int(original != perturbed)
- return score
-
-
-def quantify_difference_in_label(
- interface: Interface, original_output: list, perturbed_output: list
-) -> int | float:
- output_component = interface.output_components[0]
- post_original_output = output_component.postprocess(original_output[0])
- post_perturbed_output = output_component.postprocess(perturbed_output[0])
-
- if isinstance(output_component, components.Label):
- original_label = post_original_output["label"]
- perturbed_label = post_perturbed_output["label"]
-
- # Handle different return types of Label interface
- if "confidences" in post_original_output:
- original_confidence = original_output[0][original_label]
- perturbed_confidence = perturbed_output[0][original_label]
- score = original_confidence - perturbed_confidence
- else:
- score = diff(original_label, perturbed_label)
- return score
-
- elif isinstance(output_component, components.Number):
- score = diff(post_original_output, post_perturbed_output)
- return score
-
- else:
- raise ValueError(
- f"This interpretation method doesn't support the Output component: {output_component}"
- )
-
-
-def get_regression_or_classification_value(
- interface: Interface, original_output: list, perturbed_output: list
-) -> int | float:
- """Used to combine regression/classification for Shap interpretation method."""
- output_component = interface.output_components[0]
- post_original_output = output_component.postprocess(original_output[0])
- post_perturbed_output = output_component.postprocess(perturbed_output[0])
-
- if isinstance(output_component, components.Label):
- original_label = post_original_output["label"]
- perturbed_label = post_perturbed_output["label"]
-
- # Handle different return types of Label interface
- if "confidences" in post_original_output:
- if math.isnan(perturbed_output[0][original_label]):
- return 0
- return perturbed_output[0][original_label]
- else:
- score = diff(
- perturbed_label, original_label
- ) # Intentionally inverted order of arguments.
- return score
-
- else:
- raise ValueError(
- f"This interpretation method doesn't support the Output component: {output_component}"
- )
diff --git a/spaces/clem/dreambooth-training_v2/train_dreambooth.py b/spaces/clem/dreambooth-training_v2/train_dreambooth.py
deleted file mode 100644
index c18edc83b6a5850b86ee75c8ef2f36bb91691b95..0000000000000000000000000000000000000000
--- a/spaces/clem/dreambooth-training_v2/train_dreambooth.py
+++ /dev/null
@@ -1,818 +0,0 @@
-import argparse
-import itertools
-import math
-import os
-from pathlib import Path
-from typing import Optional
-import subprocess
-import sys
-
-import torch
-import torch.nn.functional as F
-import torch.utils.checkpoint
-from torch.utils.data import Dataset
-
-from accelerate import Accelerator
-from accelerate.logging import get_logger
-from accelerate.utils import set_seed
-from diffusers import AutoencoderKL, DDPMScheduler, StableDiffusionPipeline, UNet2DConditionModel
-from diffusers.optimization import get_scheduler
-from huggingface_hub import HfFolder, Repository, whoami
-from PIL import Image
-from torchvision import transforms
-from tqdm.auto import tqdm
-from transformers import CLIPTextModel, CLIPTokenizer
-
-
-logger = get_logger(__name__)
-
-
-def parse_args():
- parser = argparse.ArgumentParser(description="Simple example of a training script.")
- parser.add_argument(
- "--pretrained_model_name_or_path",
- type=str,
- default=None,
- #required=True,
- help="Path to pretrained model or model identifier from huggingface.co/models.",
- )
- parser.add_argument(
- "--tokenizer_name",
- type=str,
- default=None,
- help="Pretrained tokenizer name or path if not the same as model_name",
- )
- parser.add_argument(
- "--instance_data_dir",
- type=str,
- default=None,
- #required=True,
- help="A folder containing the training data of instance images.",
- )
- parser.add_argument(
- "--class_data_dir",
- type=str,
- default=None,
- required=False,
- help="A folder containing the training data of class images.",
- )
- parser.add_argument(
- "--instance_prompt",
- type=str,
- default=None,
- help="The prompt with identifier specifying the instance",
- )
- parser.add_argument(
- "--class_prompt",
- type=str,
- default="",
- help="The prompt to specify images in the same class as provided instance images.",
- )
- parser.add_argument(
- "--with_prior_preservation",
- default=False,
- action="store_true",
- help="Flag to add prior preservation loss.",
- )
- parser.add_argument("--prior_loss_weight", type=float, default=1.0, help="The weight of prior preservation loss.")
- parser.add_argument(
- "--num_class_images",
- type=int,
- default=100,
- help=(
- "Minimal class images for prior preservation loss. If not have enough images, additional images will be"
- " sampled with class_prompt."
- ),
- )
- parser.add_argument(
- "--output_dir",
- type=str,
- default="",
- help="The output directory where the model predictions and checkpoints will be written.",
- )
- parser.add_argument("--seed", type=int, default=None, help="A seed for reproducible training.")
- parser.add_argument(
- "--resolution",
- type=int,
- default=512,
- help=(
- "The resolution for input images, all the images in the train/validation dataset will be resized to this"
- " resolution"
- ),
- )
- parser.add_argument(
- "--center_crop", action="store_true", help="Whether to center crop images before resizing to resolution"
- )
- parser.add_argument("--train_text_encoder", action="store_true", help="Whether to train the text encoder")
- parser.add_argument(
- "--train_batch_size", type=int, default=4, help="Batch size (per device) for the training dataloader."
- )
- parser.add_argument(
- "--sample_batch_size", type=int, default=4, help="Batch size (per device) for sampling images."
- )
- parser.add_argument("--num_train_epochs", type=int, default=1)
- parser.add_argument(
- "--max_train_steps",
- type=int,
- default=None,
- help="Total number of training steps to perform. If provided, overrides num_train_epochs.",
- )
- parser.add_argument(
- "--gradient_accumulation_steps",
- type=int,
- default=1,
- help="Number of updates steps to accumulate before performing a backward/update pass.",
- )
- parser.add_argument(
- "--gradient_checkpointing",
- action="store_true",
- help="Whether or not to use gradient checkpointing to save memory at the expense of slower backward pass.",
- )
- parser.add_argument(
- "--learning_rate",
- type=float,
- default=5e-6,
- help="Initial learning rate (after the potential warmup period) to use.",
- )
- parser.add_argument(
- "--scale_lr",
- action="store_true",
- default=False,
- help="Scale the learning rate by the number of GPUs, gradient accumulation steps, and batch size.",
- )
- parser.add_argument(
- "--lr_scheduler",
- type=str,
- default="constant",
- help=(
- 'The scheduler type to use. Choose between ["linear", "cosine", "cosine_with_restarts", "polynomial",'
- ' "constant", "constant_with_warmup"]'
- ),
- )
- parser.add_argument(
- "--lr_warmup_steps", type=int, default=500, help="Number of steps for the warmup in the lr scheduler."
- )
- parser.add_argument(
- "--use_8bit_adam", action="store_true", help="Whether or not to use 8-bit Adam from bitsandbytes."
- )
- parser.add_argument("--adam_beta1", type=float, default=0.9, help="The beta1 parameter for the Adam optimizer.")
- parser.add_argument("--adam_beta2", type=float, default=0.999, help="The beta2 parameter for the Adam optimizer.")
- parser.add_argument("--adam_weight_decay", type=float, default=1e-2, help="Weight decay to use.")
- parser.add_argument("--adam_epsilon", type=float, default=1e-08, help="Epsilon value for the Adam optimizer")
- parser.add_argument("--max_grad_norm", default=1.0, type=float, help="Max gradient norm.")
- parser.add_argument("--push_to_hub", action="store_true", help="Whether or not to push the model to the Hub.")
- parser.add_argument("--hub_token", type=str, default=None, help="The token to use to push to the Model Hub.")
- parser.add_argument(
- "--hub_model_id",
- type=str,
- default=None,
- help="The name of the repository to keep in sync with the local `output_dir`.",
- )
- parser.add_argument(
- "--logging_dir",
- type=str,
- default="logs",
- help=(
- "[TensorBoard](https://www.tensorflow.org/tensorboard) log directory. Will default to"
- " *output_dir/runs/**CURRENT_DATETIME_HOSTNAME***."
- ),
- )
- parser.add_argument(
- "--mixed_precision",
- type=str,
- default="no",
- choices=["no", "fp16", "bf16"],
- help=(
- "Whether to use mixed precision. Choose"
- "between fp16 and bf16 (bfloat16). Bf16 requires PyTorch >= 1.10."
- "and an Nvidia Ampere GPU."
- ),
- )
-
- parser.add_argument(
- "--save_n_steps",
- type=int,
- default=1,
- help=("Save the model every n global_steps"),
- )
-
-
- parser.add_argument(
- "--save_starting_step",
- type=int,
- default=1,
- help=("The step from which it starts saving intermediary checkpoints"),
- )
-
- parser.add_argument(
- "--stop_text_encoder_training",
- type=int,
- default=1000000,
- help=("The step at which the text_encoder is no longer trained"),
- )
-
-
- parser.add_argument(
- "--image_captions_filename",
- action="store_true",
- help="Get captions from filename",
- )
-
-
- parser.add_argument(
- "--dump_only_text_encoder",
- action="store_true",
- default=False,
- help="Dump only text encoder",
- )
-
- parser.add_argument(
- "--train_only_unet",
- action="store_true",
- default=False,
- help="Train only the unet",
- )
-
- parser.add_argument(
- "--Session_dir",
- type=str,
- default="",
- help="Current session directory",
- )
-
-
-
-
- parser.add_argument("--local_rank", type=int, default=-1, help="For distributed training: local_rank")
-
- args = parser.parse_args()
- env_local_rank = int(os.environ.get("LOCAL_RANK", -1))
- if env_local_rank != -1 and env_local_rank != args.local_rank:
- args.local_rank = env_local_rank
-
- #if args.instance_data_dir is None:
- # raise ValueError("You must specify a train data directory.")
-
- #if args.with_prior_preservation:
- # if args.class_data_dir is None:
- # raise ValueError("You must specify a data directory for class images.")
- # if args.class_prompt is None:
- # raise ValueError("You must specify prompt for class images.")
-
- return args
-
-
-class DreamBoothDataset(Dataset):
- """
- A dataset to prepare the instance and class images with the prompts for fine-tuning the model.
- It pre-processes the images and the tokenizes prompts.
- """
-
- def __init__(
- self,
- instance_data_root,
- instance_prompt,
- tokenizer,
- args,
- class_data_root=None,
- class_prompt=None,
- size=512,
- center_crop=False,
- ):
- self.size = size
- self.center_crop = center_crop
- self.tokenizer = tokenizer
- self.image_captions_filename = None
-
- self.instance_data_root = Path(instance_data_root)
- if not self.instance_data_root.exists():
- raise ValueError("Instance images root doesn't exists.")
-
- self.instance_images_path = list(Path(instance_data_root).iterdir())
- self.num_instance_images = len(self.instance_images_path)
- self.instance_prompt = instance_prompt
- self._length = self.num_instance_images
-
- if args.image_captions_filename:
- self.image_captions_filename = True
-
- if class_data_root is not None:
- self.class_data_root = Path(class_data_root)
- self.class_data_root.mkdir(parents=True, exist_ok=True)
- self.class_images_path = list(self.class_data_root.iterdir())
- self.num_class_images = len(self.class_images_path)
- self._length = max(self.num_class_images, self.num_instance_images)
- self.class_prompt = class_prompt
- else:
- self.class_data_root = None
-
- self.image_transforms = transforms.Compose(
- [
- transforms.Resize(size, interpolation=transforms.InterpolationMode.BILINEAR),
- transforms.CenterCrop(size) if center_crop else transforms.RandomCrop(size),
- transforms.ToTensor(),
- transforms.Normalize([0.5], [0.5]),
- ]
- )
-
- def __len__(self):
- return self._length
-
- def __getitem__(self, index):
- example = {}
- path = self.instance_images_path[index % self.num_instance_images]
- instance_image = Image.open(path)
- if not instance_image.mode == "RGB":
- instance_image = instance_image.convert("RGB")
-
- instance_prompt = self.instance_prompt
-
- if self.image_captions_filename:
- filename = Path(path).stem
- pt=''.join([i for i in filename if not i.isdigit()])
- pt=pt.replace("_"," ")
- pt=pt.replace("(","")
- pt=pt.replace(")","")
- instance_prompt = pt
- sys.stdout.write(" [0;32m" +instance_prompt+" [0m")
- sys.stdout.flush()
-
-
- example["instance_images"] = self.image_transforms(instance_image)
- example["instance_prompt_ids"] = self.tokenizer(
- instance_prompt,
- padding="do_not_pad",
- truncation=True,
- max_length=self.tokenizer.model_max_length,
- ).input_ids
-
- if self.class_data_root:
- class_image = Image.open(self.class_images_path[index % self.num_class_images])
- if not class_image.mode == "RGB":
- class_image = class_image.convert("RGB")
- example["class_images"] = self.image_transforms(class_image)
- example["class_prompt_ids"] = self.tokenizer(
- self.class_prompt,
- padding="do_not_pad",
- truncation=True,
- max_length=self.tokenizer.model_max_length,
- ).input_ids
-
- return example
-
-
-
-class PromptDataset(Dataset):
- "A simple dataset to prepare the prompts to generate class images on multiple GPUs."
-
- def __init__(self, prompt, num_samples):
- self.prompt = prompt
- self.num_samples = num_samples
-
- def __len__(self):
- return self.num_samples
-
- def __getitem__(self, index):
- example = {}
- example["prompt"] = self.prompt
- example["index"] = index
- return example
-
-
-def get_full_repo_name(model_id: str, organization: Optional[str] = None, token: Optional[str] = None):
- if token is None:
- token = HfFolder.get_token()
- if organization is None:
- username = whoami(token)["name"]
- return f"{username}/{model_id}"
- else:
- return f"{organization}/{model_id}"
-
-def merge_two_dicts(starting_dict: dict, updater_dict: dict) -> dict:
- """
- Starts from base starting dict and then adds the remaining key values from updater replacing the values from
- the first starting/base dict with the second updater dict.
-
- For later: how does d = {**d1, **d2} replace collision?
-
- :param starting_dict:
- :param updater_dict:
- :return:
- """
- new_dict: dict = starting_dict.copy() # start with keys and values of starting_dict
- new_dict.update(updater_dict) # modifies starting_dict with keys and values of updater_dict
- return new_dict
-
-def merge_args(args1: argparse.Namespace, args2: argparse.Namespace) -> argparse.Namespace:
- """
-
- ref: https://stackoverflow.com/questions/56136549/how-can-i-merge-two-argparse-namespaces-in-python-2-x
- :param args1:
- :param args2:
- :return:
- """
- # - the merged args
- # The vars() function returns the __dict__ attribute to values of the given object e.g {field:value}.
- merged_key_values_for_namespace: dict = merge_two_dicts(vars(args1), vars(args2))
- args = argparse.Namespace(**merged_key_values_for_namespace)
- return args
-
-def run_training(args_imported):
- args_default = parse_args()
- args = merge_args(args_default, args_imported)
- print(args)
- logging_dir = Path(args.output_dir, args.logging_dir)
- i=args.save_starting_step
- accelerator = Accelerator(
- gradient_accumulation_steps=args.gradient_accumulation_steps,
- mixed_precision=args.mixed_precision,
- log_with="tensorboard",
- logging_dir=logging_dir,
- )
-
- # Currently, it's not possible to do gradient accumulation when training two models with accelerate.accumulate
- # This will be enabled soon in accelerate. For now, we don't allow gradient accumulation when training two models.
- # TODO (patil-suraj): Remove this check when gradient accumulation with two models is enabled in accelerate.
- if args.train_text_encoder and args.gradient_accumulation_steps > 1 and accelerator.num_processes > 1:
- raise ValueError(
- "Gradient accumulation is not supported when training the text encoder in distributed training. "
- "Please set gradient_accumulation_steps to 1. This feature will be supported in the future."
- )
-
- if args.seed is not None:
- set_seed(args.seed)
-
- if args.with_prior_preservation:
- class_images_dir = Path(args.class_data_dir)
- if not class_images_dir.exists():
- class_images_dir.mkdir(parents=True)
- cur_class_images = len(list(class_images_dir.iterdir()))
-
- if cur_class_images < args.num_class_images:
- torch_dtype = torch.float16 if accelerator.device.type == "cuda" else torch.float32
- pipeline = StableDiffusionPipeline.from_pretrained(
- args.pretrained_model_name_or_path, torch_dtype=torch_dtype
- )
- pipeline.set_progress_bar_config(disable=True)
-
- num_new_images = args.num_class_images - cur_class_images
- logger.info(f"Number of class images to sample: {num_new_images}.")
-
- sample_dataset = PromptDataset(args.class_prompt, num_new_images)
- sample_dataloader = torch.utils.data.DataLoader(sample_dataset, batch_size=args.sample_batch_size)
-
- sample_dataloader = accelerator.prepare(sample_dataloader)
- pipeline.to(accelerator.device)
-
- for example in tqdm(
- sample_dataloader, desc="Generating class images", disable=not accelerator.is_local_main_process
- ):
- with torch.autocast("cuda"):
- images = pipeline(example["prompt"]).images
-
- for i, image in enumerate(images):
- image.save(class_images_dir / f"{example['index'][i] + cur_class_images}.jpg")
-
- del pipeline
- if torch.cuda.is_available():
- torch.cuda.empty_cache()
-
- # Handle the repository creation
- if accelerator.is_main_process:
- if args.push_to_hub:
- if args.hub_model_id is None:
- repo_name = get_full_repo_name(Path(args.output_dir).name, token=args.hub_token)
- else:
- repo_name = args.hub_model_id
- repo = Repository(args.output_dir, clone_from=repo_name)
-
- with open(os.path.join(args.output_dir, ".gitignore"), "w+") as gitignore:
- if "step_*" not in gitignore:
- gitignore.write("step_*\n")
- if "epoch_*" not in gitignore:
- gitignore.write("epoch_*\n")
- elif args.output_dir is not None:
- os.makedirs(args.output_dir, exist_ok=True)
-
- # Load the tokenizer
- if args.tokenizer_name:
- tokenizer = CLIPTokenizer.from_pretrained(args.tokenizer_name)
- elif args.pretrained_model_name_or_path:
- tokenizer = CLIPTokenizer.from_pretrained(args.pretrained_model_name_or_path, subfolder="tokenizer")
-
- # Load models and create wrapper for stable diffusion
- if args.train_only_unet:
- if os.path.exists(str(args.output_dir+"/text_encoder_trained")):
- text_encoder = CLIPTextModel.from_pretrained(args.output_dir, subfolder="text_encoder_trained")
- elif os.path.exists(str(args.output_dir+"/text_encoder")):
- text_encoder = CLIPTextModel.from_pretrained(args.output_dir, subfolder="text_encoder")
- else:
- text_encoder = CLIPTextModel.from_pretrained(args.pretrained_model_name_or_path, subfolder="text_encoder")
- else:
- text_encoder = CLIPTextModel.from_pretrained(args.pretrained_model_name_or_path, subfolder="text_encoder")
- vae = AutoencoderKL.from_pretrained(args.pretrained_model_name_or_path, subfolder="vae")
- unet = UNet2DConditionModel.from_pretrained(args.pretrained_model_name_or_path, subfolder="unet")
-
- vae.requires_grad_(False)
- if not args.train_text_encoder:
- text_encoder.requires_grad_(False)
-
- if args.gradient_checkpointing:
- unet.enable_gradient_checkpointing()
- if args.train_text_encoder:
- text_encoder.gradient_checkpointing_enable()
-
- if args.scale_lr:
- args.learning_rate = (
- args.learning_rate * args.gradient_accumulation_steps * args.train_batch_size * accelerator.num_processes
- )
-
- # Use 8-bit Adam for lower memory usage or to fine-tune the model in 16GB GPUs
- if args.use_8bit_adam:
- try:
- import bitsandbytes as bnb
- except ImportError:
- raise ImportError(
- "To use 8-bit Adam, please install the bitsandbytes library: `pip install bitsandbytes`."
- )
-
- optimizer_class = bnb.optim.AdamW8bit
- else:
- optimizer_class = torch.optim.AdamW
-
- params_to_optimize = (
- itertools.chain(unet.parameters(), text_encoder.parameters()) if args.train_text_encoder else unet.parameters()
- )
- optimizer = optimizer_class(
- params_to_optimize,
- lr=args.learning_rate,
- betas=(args.adam_beta1, args.adam_beta2),
- weight_decay=args.adam_weight_decay,
- eps=args.adam_epsilon,
- )
-
- noise_scheduler = DDPMScheduler(
- beta_start=0.00085, beta_end=0.012, beta_schedule="scaled_linear", num_train_timesteps=1000
- )
-
- train_dataset = DreamBoothDataset(
- instance_data_root=args.instance_data_dir,
- instance_prompt=args.instance_prompt,
- class_data_root=args.class_data_dir if args.with_prior_preservation else None,
- class_prompt=args.class_prompt,
- tokenizer=tokenizer,
- size=args.resolution,
- center_crop=args.center_crop,
- args=args,
- )
-
- def collate_fn(examples):
- input_ids = [example["instance_prompt_ids"] for example in examples]
- pixel_values = [example["instance_images"] for example in examples]
-
- # Concat class and instance examples for prior preservation.
- # We do this to avoid doing two forward passes.
- if args.with_prior_preservation:
- input_ids += [example["class_prompt_ids"] for example in examples]
- pixel_values += [example["class_images"] for example in examples]
-
- pixel_values = torch.stack(pixel_values)
- pixel_values = pixel_values.to(memory_format=torch.contiguous_format).float()
-
- input_ids = tokenizer.pad({"input_ids": input_ids}, padding=True, return_tensors="pt").input_ids
-
- batch = {
- "input_ids": input_ids,
- "pixel_values": pixel_values,
- }
- return batch
-
- train_dataloader = torch.utils.data.DataLoader(
- train_dataset, batch_size=args.train_batch_size, shuffle=True, collate_fn=collate_fn
- )
-
- # Scheduler and math around the number of training steps.
- overrode_max_train_steps = False
- num_update_steps_per_epoch = math.ceil(len(train_dataloader) / args.gradient_accumulation_steps)
- if args.max_train_steps is None:
- args.max_train_steps = args.num_train_epochs * num_update_steps_per_epoch
- overrode_max_train_steps = True
-
- lr_scheduler = get_scheduler(
- args.lr_scheduler,
- optimizer=optimizer,
- num_warmup_steps=args.lr_warmup_steps * args.gradient_accumulation_steps,
- num_training_steps=args.max_train_steps * args.gradient_accumulation_steps,
- )
-
- if args.train_text_encoder:
- unet, text_encoder, optimizer, train_dataloader, lr_scheduler = accelerator.prepare(
- unet, text_encoder, optimizer, train_dataloader, lr_scheduler
- )
- else:
- unet, optimizer, train_dataloader, lr_scheduler = accelerator.prepare(
- unet, optimizer, train_dataloader, lr_scheduler
- )
-
- weight_dtype = torch.float32
- if args.mixed_precision == "fp16":
- weight_dtype = torch.float16
- elif args.mixed_precision == "bf16":
- weight_dtype = torch.bfloat16
-
- # Move text_encode and vae to gpu.
- # For mixed precision training we cast the text_encoder and vae weights to half-precision
- # as these models are only used for inference, keeping weights in full precision is not required.
- vae.to(accelerator.device, dtype=weight_dtype)
- if not args.train_text_encoder:
- text_encoder.to(accelerator.device, dtype=weight_dtype)
-
- # We need to recalculate our total training steps as the size of the training dataloader may have changed.
- num_update_steps_per_epoch = math.ceil(len(train_dataloader) / args.gradient_accumulation_steps)
- if overrode_max_train_steps:
- args.max_train_steps = args.num_train_epochs * num_update_steps_per_epoch
- # Afterwards we recalculate our number of training epochs
- args.num_train_epochs = math.ceil(args.max_train_steps / num_update_steps_per_epoch)
-
- # We need to initialize the trackers we use, and also store our configuration.
- # The trackers initializes automatically on the main process.
- if accelerator.is_main_process:
- accelerator.init_trackers("dreambooth", config=vars(args))
-
- def bar(prg):
- br='|'+'█' * prg + ' ' * (25-prg)+'|'
- return br
-
- # Train!
- total_batch_size = args.train_batch_size * accelerator.num_processes * args.gradient_accumulation_steps
-
- logger.info("***** Running training *****")
- logger.info(f" Num examples = {len(train_dataset)}")
- logger.info(f" Num batches each epoch = {len(train_dataloader)}")
- logger.info(f" Num Epochs = {args.num_train_epochs}")
- logger.info(f" Instantaneous batch size per device = {args.train_batch_size}")
- logger.info(f" Total train batch size (w. parallel, distributed & accumulation) = {total_batch_size}")
- logger.info(f" Gradient Accumulation steps = {args.gradient_accumulation_steps}")
- logger.info(f" Total optimization steps = {args.max_train_steps}")
- # Only show the progress bar once on each machine.
- progress_bar = tqdm(range(args.max_train_steps), disable=not accelerator.is_local_main_process)
- global_step = 0
-
- for epoch in range(args.num_train_epochs):
- unet.train()
- if args.train_text_encoder:
- text_encoder.train()
- for step, batch in enumerate(train_dataloader):
- with accelerator.accumulate(unet):
- # Convert images to latent space
- latents = vae.encode(batch["pixel_values"].to(dtype=weight_dtype)).latent_dist.sample()
- latents = latents * 0.18215
-
- # Sample noise that we'll add to the latents
- noise = torch.randn_like(latents)
- bsz = latents.shape[0]
- # Sample a random timestep for each image
- timesteps = torch.randint(0, noise_scheduler.config.num_train_timesteps, (bsz,), device=latents.device)
- timesteps = timesteps.long()
-
- # Add noise to the latents according to the noise magnitude at each timestep
- # (this is the forward diffusion process)
- noisy_latents = noise_scheduler.add_noise(latents, noise, timesteps)
-
- # Get the text embedding for conditioning
- encoder_hidden_states = text_encoder(batch["input_ids"])[0]
-
- # Predict the noise residual
- noise_pred = unet(noisy_latents, timesteps, encoder_hidden_states).sample
-
- if args.with_prior_preservation:
- # Chunk the noise and noise_pred into two parts and compute the loss on each part separately.
- noise_pred, noise_pred_prior = torch.chunk(noise_pred, 2, dim=0)
- noise, noise_prior = torch.chunk(noise, 2, dim=0)
-
- # Compute instance loss
- loss = F.mse_loss(noise_pred.float(), noise.float(), reduction="none").mean([1, 2, 3]).mean()
-
- # Compute prior loss
- prior_loss = F.mse_loss(noise_pred_prior.float(), noise_prior.float(), reduction="mean")
-
- # Add the prior loss to the instance loss.
- loss = loss + args.prior_loss_weight * prior_loss
- else:
- loss = F.mse_loss(noise_pred.float(), noise.float(), reduction="mean")
-
- accelerator.backward(loss)
- if accelerator.sync_gradients:
- params_to_clip = (
- itertools.chain(unet.parameters(), text_encoder.parameters())
- if args.train_text_encoder
- else unet.parameters()
- )
- accelerator.clip_grad_norm_(params_to_clip, args.max_grad_norm)
- optimizer.step()
- lr_scheduler.step()
- optimizer.zero_grad()
-
- # Checks if the accelerator has performed an optimization step behind the scenes
- if accelerator.sync_gradients:
- progress_bar.update(1)
- global_step += 1
-
- fll=round((global_step*100)/args.max_train_steps)
- fll=round(fll/4)
- pr=bar(fll)
-
- logs = {"loss": loss.detach().item(), "lr": lr_scheduler.get_last_lr()[0]}
- progress_bar.set_postfix(**logs)
- progress_bar.set_description_str("Progress:"+pr)
- accelerator.log(logs, step=global_step)
-
- if global_step >= args.max_train_steps:
- break
-
- if args.train_text_encoder and global_step == args.stop_text_encoder_training and global_step >= 30:
- if accelerator.is_main_process:
- print(" [0;32m" +" Freezing the text_encoder ..."+" [0m")
- frz_dir=args.output_dir + "/text_encoder_frozen"
- if os.path.exists(frz_dir):
- subprocess.call('rm -r '+ frz_dir, shell=True)
- os.mkdir(frz_dir)
- pipeline = StableDiffusionPipeline.from_pretrained(
- args.pretrained_model_name_or_path,
- unet=accelerator.unwrap_model(unet),
- text_encoder=accelerator.unwrap_model(text_encoder),
- )
- pipeline.text_encoder.save_pretrained(frz_dir)
-
- if args.save_n_steps >= 200:
- if global_step < args.max_train_steps-100 and global_step+1==i:
- ckpt_name = "_step_" + str(global_step+1)
- save_dir = Path(args.output_dir+ckpt_name)
- save_dir=str(save_dir)
- save_dir=save_dir.replace(" ", "_")
- if not os.path.exists(save_dir):
- os.mkdir(save_dir)
- inst=save_dir[16:]
- inst=inst.replace(" ", "_")
- print(" [1;32mSAVING CHECKPOINT: "+args.Session_dir+"/"+inst+".ckpt")
- # Create the pipeline using the trained modules and save it.
- if accelerator.is_main_process:
- pipeline = StableDiffusionPipeline.from_pretrained(
- args.pretrained_model_name_or_path,
- unet=accelerator.unwrap_model(unet),
- text_encoder=accelerator.unwrap_model(text_encoder),
- )
- pipeline.save_pretrained(save_dir)
- frz_dir=args.output_dir + "/text_encoder_frozen"
- if args.train_text_encoder and os.path.exists(frz_dir):
- subprocess.call('rm -r '+save_dir+'/text_encoder/*.*', shell=True)
- subprocess.call('cp -f '+frz_dir +'/*.* '+ save_dir+'/text_encoder', shell=True)
- chkpth=args.Session_dir+"/"+inst+".ckpt"
- subprocess.call('python /content/diffusers/scripts/convert_diffusers_to_original_stable_diffusion.py --model_path ' + save_dir + ' --checkpoint_path ' + chkpth + ' --half', shell=True)
- i=i+args.save_n_steps
-
- accelerator.wait_for_everyone()
-
- # Create the pipeline using using the trained modules and save it.
- if accelerator.is_main_process:
- if args.dump_only_text_encoder:
- txt_dir=args.output_dir + "/text_encoder_trained"
- if not os.path.exists(txt_dir):
- os.mkdir(txt_dir)
- pipeline = StableDiffusionPipeline.from_pretrained(
- args.pretrained_model_name_or_path,
- unet=accelerator.unwrap_model(unet),
- text_encoder=accelerator.unwrap_model(text_encoder),
- )
- pipeline.text_encoder.save_pretrained(txt_dir)
-
- elif args.train_only_unet:
- pipeline = StableDiffusionPipeline.from_pretrained(
- args.pretrained_model_name_or_path,
- unet=accelerator.unwrap_model(unet),
- text_encoder=accelerator.unwrap_model(text_encoder),
- )
- pipeline.save_pretrained(args.output_dir)
- txt_dir=args.output_dir + "/text_encoder_trained"
- subprocess.call('rm -r '+txt_dir, shell=True)
-
- else:
- pipeline = StableDiffusionPipeline.from_pretrained(
- args.pretrained_model_name_or_path,
- unet=accelerator.unwrap_model(unet),
- text_encoder=accelerator.unwrap_model(text_encoder),
- )
- frz_dir=args.output_dir + "/text_encoder_frozen"
- pipeline.save_pretrained(args.output_dir)
- if args.train_text_encoder and os.path.exists(frz_dir):
- subprocess.call('mv -f '+frz_dir +'/*.* '+ args.output_dir+'/text_encoder', shell=True)
- subprocess.call('rm -r '+ frz_dir, shell=True)
-
- if args.push_to_hub:
- repo.push_to_hub(commit_message="End of training", blocking=False, auto_lfs_prune=True)
-
- accelerator.end_training()
-
-if __name__ == "__main__":
- pass
- #main()
diff --git a/spaces/cloudtheboi/Lofi4All/.pythonlibs/lib/python3.10/site-packages/fontTools/ttLib/tables/sbixGlyph.py b/spaces/cloudtheboi/Lofi4All/.pythonlibs/lib/python3.10/site-packages/fontTools/ttLib/tables/sbixGlyph.py
deleted file mode 100644
index fd687a18808b6b2655951f9a6934916d7bafbc71..0000000000000000000000000000000000000000
--- a/spaces/cloudtheboi/Lofi4All/.pythonlibs/lib/python3.10/site-packages/fontTools/ttLib/tables/sbixGlyph.py
+++ /dev/null
@@ -1,145 +0,0 @@
-from fontTools.misc import sstruct
-from fontTools.misc.textTools import readHex, safeEval
-import struct
-
-
-sbixGlyphHeaderFormat = """
- >
- originOffsetX: h # The x-value of the point in the glyph relative to its
- # lower-left corner which corresponds to the origin of
- # the glyph on the screen, that is the point on the
- # baseline at the left edge of the glyph.
- originOffsetY: h # The y-value of the point in the glyph relative to its
- # lower-left corner which corresponds to the origin of
- # the glyph on the screen, that is the point on the
- # baseline at the left edge of the glyph.
- graphicType: 4s # e.g. "png "
-"""
-
-sbixGlyphHeaderFormatSize = sstruct.calcsize(sbixGlyphHeaderFormat)
-
-
-class Glyph(object):
- def __init__(
- self,
- glyphName=None,
- referenceGlyphName=None,
- originOffsetX=0,
- originOffsetY=0,
- graphicType=None,
- imageData=None,
- rawdata=None,
- gid=0,
- ):
- self.gid = gid
- self.glyphName = glyphName
- self.referenceGlyphName = referenceGlyphName
- self.originOffsetX = originOffsetX
- self.originOffsetY = originOffsetY
- self.rawdata = rawdata
- self.graphicType = graphicType
- self.imageData = imageData
-
- # fix self.graphicType if it is null terminated or too short
- if self.graphicType is not None:
- if self.graphicType[-1] == "\0":
- self.graphicType = self.graphicType[:-1]
- if len(self.graphicType) > 4:
- from fontTools import ttLib
-
- raise ttLib.TTLibError(
- "Glyph.graphicType must not be longer than 4 characters."
- )
- elif len(self.graphicType) < 4:
- # pad with spaces
- self.graphicType += " "[: (4 - len(self.graphicType))]
-
- def decompile(self, ttFont):
- self.glyphName = ttFont.getGlyphName(self.gid)
- if self.rawdata is None:
- from fontTools import ttLib
-
- raise ttLib.TTLibError("No table data to decompile")
- if len(self.rawdata) > 0:
- if len(self.rawdata) < sbixGlyphHeaderFormatSize:
- from fontTools import ttLib
-
- # print "Glyph %i header too short: Expected %x, got %x." % (self.gid, sbixGlyphHeaderFormatSize, len(self.rawdata))
- raise ttLib.TTLibError("Glyph header too short.")
-
- sstruct.unpack(
- sbixGlyphHeaderFormat, self.rawdata[:sbixGlyphHeaderFormatSize], self
- )
-
- if self.graphicType == "dupe":
- # this glyph is a reference to another glyph's image data
- (gid,) = struct.unpack(">H", self.rawdata[sbixGlyphHeaderFormatSize:])
- self.referenceGlyphName = ttFont.getGlyphName(gid)
- else:
- self.imageData = self.rawdata[sbixGlyphHeaderFormatSize:]
- self.referenceGlyphName = None
- # clean up
- del self.rawdata
- del self.gid
-
- def compile(self, ttFont):
- if self.glyphName is None:
- from fontTools import ttLib
-
- raise ttLib.TTLibError("Can't compile Glyph without glyph name")
- # TODO: if ttFont has no maxp, cmap etc., ignore glyph names and compile by index?
- # (needed if you just want to compile the sbix table on its own)
- self.gid = struct.pack(">H", ttFont.getGlyphID(self.glyphName))
- if self.graphicType is None:
- rawdata = b""
- else:
- rawdata = sstruct.pack(sbixGlyphHeaderFormat, self)
- if self.graphicType == "dupe":
- rawdata += struct.pack(">H", ttFont.getGlyphID(self.referenceGlyphName))
- else:
- assert self.imageData is not None
- rawdata += self.imageData
- self.rawdata = rawdata
-
- def toXML(self, xmlWriter, ttFont):
- if self.graphicType is None:
- # TODO: ignore empty glyphs?
- # a glyph data entry is required for each glyph,
- # but empty ones can be calculated at compile time
- xmlWriter.simpletag("glyph", name=self.glyphName)
- xmlWriter.newline()
- return
- xmlWriter.begintag(
- "glyph",
- graphicType=self.graphicType,
- name=self.glyphName,
- originOffsetX=self.originOffsetX,
- originOffsetY=self.originOffsetY,
- )
- xmlWriter.newline()
- if self.graphicType == "dupe":
- # graphicType == "dupe" is a reference to another glyph id.
- xmlWriter.simpletag("ref", glyphname=self.referenceGlyphName)
- else:
- xmlWriter.begintag("hexdata")
- xmlWriter.newline()
- xmlWriter.dumphex(self.imageData)
- xmlWriter.endtag("hexdata")
- xmlWriter.newline()
- xmlWriter.endtag("glyph")
- xmlWriter.newline()
-
- def fromXML(self, name, attrs, content, ttFont):
- if name == "ref":
- # glyph is a "dupe", i.e. a reference to another glyph's image data.
- # in this case imageData contains the glyph id of the reference glyph
- # get glyph id from glyphname
- glyphname = safeEval("'''" + attrs["glyphname"] + "'''")
- self.imageData = struct.pack(">H", ttFont.getGlyphID(glyphname))
- self.referenceGlyphName = glyphname
- elif name == "hexdata":
- self.imageData = readHex(content)
- else:
- from fontTools import ttLib
-
- raise ttLib.TTLibError("can't handle '%s' element" % name)
diff --git a/spaces/colakin/video-generater/public/ffmpeg/libavcodec/h264_levels.c b/spaces/colakin/video-generater/public/ffmpeg/libavcodec/h264_levels.c
deleted file mode 100644
index f7ed9a6e375bec9a83270128c2cc535ae1fa1700..0000000000000000000000000000000000000000
--- a/spaces/colakin/video-generater/public/ffmpeg/libavcodec/h264_levels.c
+++ /dev/null
@@ -1,123 +0,0 @@
-/*
- * This file is part of FFmpeg.
- *
- * FFmpeg is free software; you can redistribute it and/or
- * modify it under the terms of the GNU Lesser General Public
- * License as published by the Free Software Foundation; either
- * version 2.1 of the License, or (at your option) any later version.
- *
- * FFmpeg is distributed in the hope that it will be useful,
- * but WITHOUT ANY WARRANTY; without even the implied warranty of
- * MERCHANTABILITY or FITNESS FOR A PARTICULAR PURPOSE. See the GNU
- * Lesser General Public License for more details.
- *
- * You should have received a copy of the GNU Lesser General Public
- * License along with FFmpeg; if not, write to the Free Software
- * Foundation, Inc., 51 Franklin Street, Fifth Floor, Boston, MA 02110-1301 USA
- */
-
-#include
-#include "libavutil/macros.h"
-#include "h264_levels.h"
-
-// H.264 table A-1.
-static const H264LevelDescriptor h264_levels[] = {
- // Name MaxMBPS MaxBR MinCR
- // | level_idc | MaxFS | MaxCPB | MaxMvsPer2Mb
- // | | cs3f | | MaxDpbMbs | | MaxVmvR | |
- { "1", 10, 0, 1485, 99, 396, 64, 175, 64, 2, 0 },
- { "1b", 11, 1, 1485, 99, 396, 128, 350, 64, 2, 0 },
- { "1b", 9, 0, 1485, 99, 396, 128, 350, 64, 2, 0 },
- { "1.1", 11, 0, 3000, 396, 900, 192, 500, 128, 2, 0 },
- { "1.2", 12, 0, 6000, 396, 2376, 384, 1000, 128, 2, 0 },
- { "1.3", 13, 0, 11880, 396, 2376, 768, 2000, 128, 2, 0 },
- { "2", 20, 0, 11880, 396, 2376, 2000, 2000, 128, 2, 0 },
- { "2.1", 21, 0, 19800, 792, 4752, 4000, 4000, 256, 2, 0 },
- { "2.2", 22, 0, 20250, 1620, 8100, 4000, 4000, 256, 2, 0 },
- { "3", 30, 0, 40500, 1620, 8100, 10000, 10000, 256, 2, 32 },
- { "3.1", 31, 0, 108000, 3600, 18000, 14000, 14000, 512, 4, 16 },
- { "3.2", 32, 0, 216000, 5120, 20480, 20000, 20000, 512, 4, 16 },
- { "4", 40, 0, 245760, 8192, 32768, 20000, 25000, 512, 4, 16 },
- { "4.1", 41, 0, 245760, 8192, 32768, 50000, 62500, 512, 2, 16 },
- { "4.2", 42, 0, 522240, 8704, 34816, 50000, 62500, 512, 2, 16 },
- { "5", 50, 0, 589824, 22080, 110400, 135000, 135000, 512, 2, 16 },
- { "5.1", 51, 0, 983040, 36864, 184320, 240000, 240000, 512, 2, 16 },
- { "5.2", 52, 0, 2073600, 36864, 184320, 240000, 240000, 512, 2, 16 },
- { "6", 60, 0, 4177920, 139264, 696320, 240000, 240000, 8192, 2, 16 },
- { "6.1", 61, 0, 8355840, 139264, 696320, 480000, 480000, 8192, 2, 16 },
- { "6.2", 62, 0, 16711680, 139264, 696320, 800000, 800000, 8192, 2, 16 },
-};
-
-// H.264 table A-2 plus values from A-1.
-static const struct {
- int profile_idc;
- int cpb_br_vcl_factor;
- int cpb_br_nal_factor;
-} h264_br_factors[] = {
- { 66, 1000, 1200 },
- { 77, 1000, 1200 },
- { 88, 1000, 1200 },
- { 100, 1250, 1500 },
- { 110, 3000, 3600 },
- { 122, 4000, 4800 },
- { 244, 4000, 4800 },
- { 44, 4000, 4800 },
-};
-
-// We are only ever interested in the NAL bitrate factor.
-static int h264_get_br_factor(int profile_idc)
-{
- int i;
- for (i = 0; i < FF_ARRAY_ELEMS(h264_br_factors); i++) {
- if (h264_br_factors[i].profile_idc == profile_idc)
- return h264_br_factors[i].cpb_br_nal_factor;
- }
- // Default to the non-high profile value if not specified.
- return 1200;
-}
-
-const H264LevelDescriptor *ff_h264_guess_level(int profile_idc,
- int64_t bitrate,
- int framerate,
- int width, int height,
- int max_dec_frame_buffering)
-{
- int width_mbs = (width + 15) / 16;
- int height_mbs = (height + 15) / 16;
- int no_cs3f = !(profile_idc == 66 ||
- profile_idc == 77 ||
- profile_idc == 88);
- int i;
-
- for (i = 0; i < FF_ARRAY_ELEMS(h264_levels); i++) {
- const H264LevelDescriptor *level = &h264_levels[i];
-
- if (level->constraint_set3_flag && no_cs3f)
- continue;
-
- if (bitrate > (int64_t)level->max_br * h264_get_br_factor(profile_idc))
- continue;
-
- if (width_mbs * height_mbs > level->max_fs)
- continue;
- if (width_mbs * width_mbs > 8 * level->max_fs)
- continue;
- if (height_mbs * height_mbs > 8 * level->max_fs)
- continue;
-
- if (width_mbs && height_mbs) {
- int max_dpb_frames =
- FFMIN(level->max_dpb_mbs / (width_mbs * height_mbs), 16);
- if (max_dec_frame_buffering > max_dpb_frames)
- continue;
-
- if (framerate > (level->max_mbps / (width_mbs * height_mbs)))
- continue;
- }
-
- return level;
- }
-
- // No usable levels found - frame is too big or bitrate is too high.
- return NULL;
-}
diff --git a/spaces/colakin/video-generater/public/ffmpeg/libavcodec/huffyuvenc.c b/spaces/colakin/video-generater/public/ffmpeg/libavcodec/huffyuvenc.c
deleted file mode 100644
index 72d6246ebe0ac431c00a8c2f4ef2ff725392f710..0000000000000000000000000000000000000000
--- a/spaces/colakin/video-generater/public/ffmpeg/libavcodec/huffyuvenc.c
+++ /dev/null
@@ -1,1131 +0,0 @@
-/*
- * Copyright (c) 2002-2014 Michael Niedermayer
- *
- * see https://multimedia.cx/huffyuv.txt for a description of
- * the algorithm used
- *
- * This file is part of FFmpeg.
- *
- * FFmpeg is free software; you can redistribute it and/or
- * modify it under the terms of the GNU Lesser General Public
- * License as published by the Free Software Foundation; either
- * version 2.1 of the License, or (at your option) any later version.
- *
- * FFmpeg is distributed in the hope that it will be useful,
- * but WITHOUT ANY WARRANTY; without even the implied warranty of
- * MERCHANTABILITY or FITNESS FOR A PARTICULAR PURPOSE. See the GNU
- * Lesser General Public License for more details.
- *
- * You should have received a copy of the GNU Lesser General Public
- * License along with FFmpeg; if not, write to the Free Software
- * Foundation, Inc., 51 Franklin Street, Fifth Floor, Boston, MA 02110-1301 USA
- *
- * yuva, gray, 4:4:4, 4:1:1, 4:1:0 and >8 bit per sample support sponsored by NOA
- */
-
-/**
- * @file
- * huffyuv encoder
- */
-
-#include "config_components.h"
-
-#include "avcodec.h"
-#include "bswapdsp.h"
-#include "codec_internal.h"
-#include "encode.h"
-#include "huffyuv.h"
-#include "huffman.h"
-#include "huffyuvencdsp.h"
-#include "lossless_videoencdsp.h"
-#include "put_bits.h"
-#include "libavutil/opt.h"
-#include "libavutil/pixdesc.h"
-
-typedef struct HYuvEncContext {
- AVClass *class;
- AVCodecContext *avctx;
- PutBitContext pb;
- Predictor predictor;
- int interlaced;
- int decorrelate;
- int bitstream_bpp;
- int version;
- int bps;
- int n; // 1<bps <= 8) {
- s->llvidencdsp.diff_bytes(dst, src0, src1, w);
- } else {
- s->hencdsp.diff_int16((uint16_t *)dst, (const uint16_t *)src0, (const uint16_t *)src1, s->n - 1, w);
- }
-}
-
-static inline int sub_left_prediction(HYuvEncContext *s, uint8_t *dst,
- const uint8_t *src, int w, int left)
-{
- int i;
- int min_width = FFMIN(w, 32);
-
- if (s->bps <= 8) {
- for (i = 0; i < min_width; i++) { /* scalar loop before dsp call */
- const int temp = src[i];
- dst[i] = temp - left;
- left = temp;
- }
- if (w < 32)
- return left;
- s->llvidencdsp.diff_bytes(dst + 32, src + 32, src + 31, w - 32);
- return src[w-1];
- } else {
- const uint16_t *src16 = (const uint16_t *)src;
- uint16_t *dst16 = ( uint16_t *)dst;
- for (i = 0; i < min_width; i++) { /* scalar loop before dsp call */
- const int temp = src16[i];
- dst16[i] = temp - left;
- left = temp;
- }
- if (w < 32)
- return left;
- s->hencdsp.diff_int16(dst16 + 32, src16 + 32, src16 + 31, s->n - 1, w - 32);
- return src16[w-1];
- }
-}
-
-static inline void sub_left_prediction_bgr32(HYuvEncContext *s, uint8_t *dst,
- const uint8_t *src, int w,
- int *red, int *green, int *blue,
- int *alpha)
-{
- int i;
- int r, g, b, a;
- int min_width = FFMIN(w, 8);
- r = *red;
- g = *green;
- b = *blue;
- a = *alpha;
-
- for (i = 0; i < min_width; i++) {
- const int rt = src[i * 4 + R];
- const int gt = src[i * 4 + G];
- const int bt = src[i * 4 + B];
- const int at = src[i * 4 + A];
- dst[i * 4 + R] = rt - r;
- dst[i * 4 + G] = gt - g;
- dst[i * 4 + B] = bt - b;
- dst[i * 4 + A] = at - a;
- r = rt;
- g = gt;
- b = bt;
- a = at;
- }
-
- s->llvidencdsp.diff_bytes(dst + 32, src + 32, src + 32 - 4, w * 4 - 32);
-
- *red = src[(w - 1) * 4 + R];
- *green = src[(w - 1) * 4 + G];
- *blue = src[(w - 1) * 4 + B];
- *alpha = src[(w - 1) * 4 + A];
-}
-
-static inline void sub_left_prediction_rgb24(HYuvEncContext *s, uint8_t *dst,
- const uint8_t *src, int w,
- int *red, int *green, int *blue)
-{
- int i;
- int r, g, b;
- r = *red;
- g = *green;
- b = *blue;
- for (i = 0; i < FFMIN(w, 16); i++) {
- const int rt = src[i * 3 + 0];
- const int gt = src[i * 3 + 1];
- const int bt = src[i * 3 + 2];
- dst[i * 3 + 0] = rt - r;
- dst[i * 3 + 1] = gt - g;
- dst[i * 3 + 2] = bt - b;
- r = rt;
- g = gt;
- b = bt;
- }
-
- s->llvidencdsp.diff_bytes(dst + 48, src + 48, src + 48 - 3, w * 3 - 48);
-
- *red = src[(w - 1) * 3 + 0];
- *green = src[(w - 1) * 3 + 1];
- *blue = src[(w - 1) * 3 + 2];
-}
-
-static void sub_median_prediction(HYuvEncContext *s, uint8_t *dst,
- const uint8_t *src1, const uint8_t *src2,
- int w, int *left, int *left_top)
-{
- if (s->bps <= 8) {
- s->llvidencdsp.sub_median_pred(dst, src1, src2, w , left, left_top);
- } else {
- s->hencdsp.sub_hfyu_median_pred_int16((uint16_t *)dst, (const uint16_t *)src1, (const uint16_t *)src2, s->n - 1, w , left, left_top);
- }
-}
-
-static int store_table(HYuvEncContext *s, const uint8_t *len, uint8_t *buf)
-{
- int i;
- int index = 0;
- int n = s->vlc_n;
-
- for (i = 0; i < n;) {
- int val = len[i];
- int repeat = 0;
-
- for (; i < n && len[i] == val && repeat < 255; i++)
- repeat++;
-
- av_assert0(val < 32 && val >0 && repeat < 256 && repeat>0);
- if (repeat > 7) {
- buf[index++] = val;
- buf[index++] = repeat;
- } else {
- buf[index++] = val | (repeat << 5);
- }
- }
-
- return index;
-}
-
-static int store_huffman_tables(HYuvEncContext *s, uint8_t *buf)
-{
- int i, ret;
- int size = 0;
- int count = 3;
-
- if (s->version > 2)
- count = 1 + s->alpha + 2*s->chroma;
-
- for (i = 0; i < count; i++) {
- if ((ret = ff_huff_gen_len_table(s->len[i], s->stats[i], s->vlc_n, 0)) < 0)
- return ret;
-
- if (ff_huffyuv_generate_bits_table(s->bits[i], s->len[i], s->vlc_n) < 0) {
- return -1;
- }
-
- size += store_table(s, s->len[i], buf + size);
- }
- return size;
-}
-
-static av_cold int encode_init(AVCodecContext *avctx)
-{
- HYuvEncContext *s = avctx->priv_data;
- int i, j;
- int ret;
- const AVPixFmtDescriptor *desc;
-
- s->avctx = avctx;
- s->flags = avctx->flags;
-
- ff_bswapdsp_init(&s->bdsp);
- ff_huffyuvencdsp_init(&s->hencdsp, avctx->pix_fmt);
- ff_llvidencdsp_init(&s->llvidencdsp);
-
- avctx->extradata = av_mallocz(3*MAX_N + 4);
- if (!avctx->extradata)
- return AVERROR(ENOMEM);
- if (s->flags&AV_CODEC_FLAG_PASS1) {
-#define STATS_OUT_SIZE 21*MAX_N*3 + 4
- avctx->stats_out = av_mallocz(STATS_OUT_SIZE); // 21*256*3(%llu ) + 3(\n) + 1(0) = 16132
- if (!avctx->stats_out)
- return AVERROR(ENOMEM);
- }
- s->version = 2;
-
- desc = av_pix_fmt_desc_get(avctx->pix_fmt);
- s->bps = desc->comp[0].depth;
- s->yuv = !(desc->flags & AV_PIX_FMT_FLAG_RGB) && desc->nb_components >= 2;
- s->chroma = desc->nb_components > 2;
- s->alpha = !!(desc->flags & AV_PIX_FMT_FLAG_ALPHA);
- s->chroma_h_shift = desc->log2_chroma_w;
- s->chroma_v_shift = desc->log2_chroma_h;
-
- switch (avctx->pix_fmt) {
- case AV_PIX_FMT_YUV420P:
- case AV_PIX_FMT_YUV422P:
- if (avctx->width & 1) {
- av_log(avctx, AV_LOG_ERROR, "Width must be even for this colorspace.\n");
- return AVERROR(EINVAL);
- }
- s->bitstream_bpp = avctx->pix_fmt == AV_PIX_FMT_YUV420P ? 12 : 16;
- break;
- case AV_PIX_FMT_YUV444P:
- case AV_PIX_FMT_YUV410P:
- case AV_PIX_FMT_YUV411P:
- case AV_PIX_FMT_YUV440P:
- case AV_PIX_FMT_GBRP:
- case AV_PIX_FMT_GBRP9:
- case AV_PIX_FMT_GBRP10:
- case AV_PIX_FMT_GBRP12:
- case AV_PIX_FMT_GBRP14:
- case AV_PIX_FMT_GBRP16:
- case AV_PIX_FMT_GRAY8:
- case AV_PIX_FMT_GRAY16:
- case AV_PIX_FMT_YUVA444P:
- case AV_PIX_FMT_YUVA420P:
- case AV_PIX_FMT_YUVA422P:
- case AV_PIX_FMT_GBRAP:
- case AV_PIX_FMT_YUV420P9:
- case AV_PIX_FMT_YUV420P10:
- case AV_PIX_FMT_YUV420P12:
- case AV_PIX_FMT_YUV420P14:
- case AV_PIX_FMT_YUV420P16:
- case AV_PIX_FMT_YUV422P9:
- case AV_PIX_FMT_YUV422P10:
- case AV_PIX_FMT_YUV422P12:
- case AV_PIX_FMT_YUV422P14:
- case AV_PIX_FMT_YUV422P16:
- case AV_PIX_FMT_YUV444P9:
- case AV_PIX_FMT_YUV444P10:
- case AV_PIX_FMT_YUV444P12:
- case AV_PIX_FMT_YUV444P14:
- case AV_PIX_FMT_YUV444P16:
- case AV_PIX_FMT_YUVA420P9:
- case AV_PIX_FMT_YUVA420P10:
- case AV_PIX_FMT_YUVA420P16:
- case AV_PIX_FMT_YUVA422P9:
- case AV_PIX_FMT_YUVA422P10:
- case AV_PIX_FMT_YUVA422P16:
- case AV_PIX_FMT_YUVA444P9:
- case AV_PIX_FMT_YUVA444P10:
- case AV_PIX_FMT_YUVA444P16:
- s->version = 3;
- break;
- case AV_PIX_FMT_RGB32:
- s->bitstream_bpp = 32;
- break;
- case AV_PIX_FMT_RGB24:
- s->bitstream_bpp = 24;
- break;
- default:
- av_log(avctx, AV_LOG_ERROR, "format not supported\n");
- return AVERROR(EINVAL);
- }
- s->n = 1<bps;
- s->vlc_n = FFMIN(s->n, MAX_VLC_N);
-
- avctx->bits_per_coded_sample = s->bitstream_bpp;
- s->decorrelate = s->bitstream_bpp >= 24 && !s->yuv && !(desc->flags & AV_PIX_FMT_FLAG_PLANAR);
- s->interlaced = avctx->flags & AV_CODEC_FLAG_INTERLACED_ME ? 1 : 0;
- if (s->context) {
- if (s->flags & (AV_CODEC_FLAG_PASS1 | AV_CODEC_FLAG_PASS2)) {
- av_log(avctx, AV_LOG_ERROR,
- "context=1 is not compatible with "
- "2 pass huffyuv encoding\n");
- return AVERROR(EINVAL);
- }
- }
-
- if (avctx->codec->id == AV_CODEC_ID_HUFFYUV) {
- if (s->interlaced != ( avctx->height > 288 ))
- av_log(avctx, AV_LOG_INFO,
- "using huffyuv 2.2.0 or newer interlacing flag\n");
- }
-
- if (s->version > 3 && avctx->strict_std_compliance > FF_COMPLIANCE_EXPERIMENTAL) {
- av_log(avctx, AV_LOG_ERROR, "Ver > 3 is under development, files encoded with it may not be decodable with future versions!!!\n"
- "Use vstrict=-2 / -strict -2 to use it anyway.\n");
- return AVERROR(EINVAL);
- }
-
- if (s->bitstream_bpp >= 24 && s->predictor == MEDIAN && s->version <= 2) {
- av_log(avctx, AV_LOG_ERROR,
- "Error: RGB is incompatible with median predictor\n");
- return AVERROR(EINVAL);
- }
-
- avctx->extradata[0] = s->predictor | (s->decorrelate << 6);
- avctx->extradata[2] = s->interlaced ? 0x10 : 0x20;
- if (s->context)
- avctx->extradata[2] |= 0x40;
- if (s->version < 3) {
- avctx->extradata[1] = s->bitstream_bpp;
- avctx->extradata[3] = 0;
- } else {
- avctx->extradata[1] = ((s->bps-1)<<4) | s->chroma_h_shift | (s->chroma_v_shift<<2);
- if (s->chroma)
- avctx->extradata[2] |= s->yuv ? 1 : 2;
- if (s->alpha)
- avctx->extradata[2] |= 4;
- avctx->extradata[3] = 1;
- }
- avctx->extradata_size = 4;
-
- if (avctx->stats_in) {
- char *p = avctx->stats_in;
-
- for (i = 0; i < 4; i++)
- for (j = 0; j < s->vlc_n; j++)
- s->stats[i][j] = 1;
-
- for (;;) {
- for (i = 0; i < 4; i++) {
- char *next;
-
- for (j = 0; j < s->vlc_n; j++) {
- s->stats[i][j] += strtol(p, &next, 0);
- if (next == p) return -1;
- p = next;
- }
- }
- if (p[0] == 0 || p[1] == 0 || p[2] == 0) break;
- }
- } else {
- for (i = 0; i < 4; i++)
- for (j = 0; j < s->vlc_n; j++) {
- int d = FFMIN(j, s->vlc_n - j);
-
- s->stats[i][j] = 100000000 / (d*d + 1);
- }
- }
-
- ret = store_huffman_tables(s, avctx->extradata + avctx->extradata_size);
- if (ret < 0)
- return ret;
- avctx->extradata_size += ret;
-
- if (s->context) {
- for (i = 0; i < 4; i++) {
- int pels = avctx->width * avctx->height / (i ? 40 : 10);
- for (j = 0; j < s->vlc_n; j++) {
- int d = FFMIN(j, s->vlc_n - j);
- s->stats[i][j] = pels/(d*d + 1);
- }
- }
- } else {
- for (i = 0; i < 4; i++)
- for (j = 0; j < s->vlc_n; j++)
- s->stats[i][j]= 0;
- }
-
- ret = ff_huffyuv_alloc_temp(s->temp, s->temp16, avctx->width);
- if (ret < 0)
- return ret;
-
- s->picture_number=0;
-
- return 0;
-}
-static int encode_422_bitstream(HYuvEncContext *s, int offset, int count)
-{
- int i;
- const uint8_t *y = s->temp[0] + offset;
- const uint8_t *u = s->temp[1] + offset / 2;
- const uint8_t *v = s->temp[2] + offset / 2;
-
- if (put_bytes_left(&s->pb, 0) < 2 * 4 * count) {
- av_log(s->avctx, AV_LOG_ERROR, "encoded frame too large\n");
- return -1;
- }
-
-#define LOAD4\
- int y0 = y[2 * i];\
- int y1 = y[2 * i + 1];\
- int u0 = u[i];\
- int v0 = v[i];
-
- count /= 2;
-
- if (s->flags & AV_CODEC_FLAG_PASS1) {
- for(i = 0; i < count; i++) {
- LOAD4;
- s->stats[0][y0]++;
- s->stats[1][u0]++;
- s->stats[0][y1]++;
- s->stats[2][v0]++;
- }
- }
- if (s->avctx->flags2 & AV_CODEC_FLAG2_NO_OUTPUT)
- return 0;
- if (s->context) {
- for (i = 0; i < count; i++) {
- LOAD4;
- s->stats[0][y0]++;
- put_bits(&s->pb, s->len[0][y0], s->bits[0][y0]);
- s->stats[1][u0]++;
- put_bits(&s->pb, s->len[1][u0], s->bits[1][u0]);
- s->stats[0][y1]++;
- put_bits(&s->pb, s->len[0][y1], s->bits[0][y1]);
- s->stats[2][v0]++;
- put_bits(&s->pb, s->len[2][v0], s->bits[2][v0]);
- }
- } else {
- for(i = 0; i < count; i++) {
- LOAD4;
- put_bits(&s->pb, s->len[0][y0], s->bits[0][y0]);
- put_bits(&s->pb, s->len[1][u0], s->bits[1][u0]);
- put_bits(&s->pb, s->len[0][y1], s->bits[0][y1]);
- put_bits(&s->pb, s->len[2][v0], s->bits[2][v0]);
- }
- }
- return 0;
-}
-
-static int encode_plane_bitstream(HYuvEncContext *s, int width, int plane)
-{
- int i, count = width/2;
-
- if (put_bytes_left(&s->pb, 0) < count * s->bps / 2) {
- av_log(s->avctx, AV_LOG_ERROR, "encoded frame too large\n");
- return -1;
- }
-
-#define LOADEND\
- int y0 = s->temp[0][width-1];
-#define LOADEND_14\
- int y0 = s->temp16[0][width-1] & mask;
-#define LOADEND_16\
- int y0 = s->temp16[0][width-1];
-#define STATEND\
- s->stats[plane][y0]++;
-#define STATEND_16\
- s->stats[plane][y0>>2]++;
-#define WRITEEND\
- put_bits(&s->pb, s->len[plane][y0], s->bits[plane][y0]);
-#define WRITEEND_16\
- put_bits(&s->pb, s->len[plane][y0>>2], s->bits[plane][y0>>2]);\
- put_bits(&s->pb, 2, y0&3);
-
-#define LOAD2\
- int y0 = s->temp[0][2 * i];\
- int y1 = s->temp[0][2 * i + 1];
-#define LOAD2_14\
- int y0 = s->temp16[0][2 * i] & mask;\
- int y1 = s->temp16[0][2 * i + 1] & mask;
-#define LOAD2_16\
- int y0 = s->temp16[0][2 * i];\
- int y1 = s->temp16[0][2 * i + 1];
-#define STAT2\
- s->stats[plane][y0]++;\
- s->stats[plane][y1]++;
-#define STAT2_16\
- s->stats[plane][y0>>2]++;\
- s->stats[plane][y1>>2]++;
-#define WRITE2\
- put_bits(&s->pb, s->len[plane][y0], s->bits[plane][y0]);\
- put_bits(&s->pb, s->len[plane][y1], s->bits[plane][y1]);
-#define WRITE2_16\
- put_bits(&s->pb, s->len[plane][y0>>2], s->bits[plane][y0>>2]);\
- put_bits(&s->pb, 2, y0&3);\
- put_bits(&s->pb, s->len[plane][y1>>2], s->bits[plane][y1>>2]);\
- put_bits(&s->pb, 2, y1&3);
-
- if (s->bps <= 8) {
- if (s->flags & AV_CODEC_FLAG_PASS1) {
- for (i = 0; i < count; i++) {
- LOAD2;
- STAT2;
- }
- if (width&1) {
- LOADEND;
- STATEND;
- }
- }
- if (s->avctx->flags2 & AV_CODEC_FLAG2_NO_OUTPUT)
- return 0;
-
- if (s->context) {
- for (i = 0; i < count; i++) {
- LOAD2;
- STAT2;
- WRITE2;
- }
- if (width&1) {
- LOADEND;
- STATEND;
- WRITEEND;
- }
- } else {
- for (i = 0; i < count; i++) {
- LOAD2;
- WRITE2;
- }
- if (width&1) {
- LOADEND;
- WRITEEND;
- }
- }
- } else if (s->bps <= 14) {
- int mask = s->n - 1;
- if (s->flags & AV_CODEC_FLAG_PASS1) {
- for (i = 0; i < count; i++) {
- LOAD2_14;
- STAT2;
- }
- if (width&1) {
- LOADEND_14;
- STATEND;
- }
- }
- if (s->avctx->flags2 & AV_CODEC_FLAG2_NO_OUTPUT)
- return 0;
-
- if (s->context) {
- for (i = 0; i < count; i++) {
- LOAD2_14;
- STAT2;
- WRITE2;
- }
- if (width&1) {
- LOADEND_14;
- STATEND;
- WRITEEND;
- }
- } else {
- for (i = 0; i < count; i++) {
- LOAD2_14;
- WRITE2;
- }
- if (width&1) {
- LOADEND_14;
- WRITEEND;
- }
- }
- } else {
- if (s->flags & AV_CODEC_FLAG_PASS1) {
- for (i = 0; i < count; i++) {
- LOAD2_16;
- STAT2_16;
- }
- if (width&1) {
- LOADEND_16;
- STATEND_16;
- }
- }
- if (s->avctx->flags2 & AV_CODEC_FLAG2_NO_OUTPUT)
- return 0;
-
- if (s->context) {
- for (i = 0; i < count; i++) {
- LOAD2_16;
- STAT2_16;
- WRITE2_16;
- }
- if (width&1) {
- LOADEND_16;
- STATEND_16;
- WRITEEND_16;
- }
- } else {
- for (i = 0; i < count; i++) {
- LOAD2_16;
- WRITE2_16;
- }
- if (width&1) {
- LOADEND_16;
- WRITEEND_16;
- }
- }
- }
-#undef LOAD2
-#undef STAT2
-#undef WRITE2
- return 0;
-}
-
-static int encode_gray_bitstream(HYuvEncContext *s, int count)
-{
- int i;
-
- if (put_bytes_left(&s->pb, 0) < 4 * count) {
- av_log(s->avctx, AV_LOG_ERROR, "encoded frame too large\n");
- return -1;
- }
-
-#define LOAD2\
- int y0 = s->temp[0][2 * i];\
- int y1 = s->temp[0][2 * i + 1];
-#define STAT2\
- s->stats[0][y0]++;\
- s->stats[0][y1]++;
-#define WRITE2\
- put_bits(&s->pb, s->len[0][y0], s->bits[0][y0]);\
- put_bits(&s->pb, s->len[0][y1], s->bits[0][y1]);
-
- count /= 2;
-
- if (s->flags & AV_CODEC_FLAG_PASS1) {
- for (i = 0; i < count; i++) {
- LOAD2;
- STAT2;
- }
- }
- if (s->avctx->flags2 & AV_CODEC_FLAG2_NO_OUTPUT)
- return 0;
-
- if (s->context) {
- for (i = 0; i < count; i++) {
- LOAD2;
- STAT2;
- WRITE2;
- }
- } else {
- for (i = 0; i < count; i++) {
- LOAD2;
- WRITE2;
- }
- }
- return 0;
-}
-
-static inline int encode_bgra_bitstream(HYuvEncContext *s, int count, int planes)
-{
- int i;
-
- if (put_bytes_left(&s->pb, 0) < 4 * planes * count) {
- av_log(s->avctx, AV_LOG_ERROR, "encoded frame too large\n");
- return -1;
- }
-
-#define LOAD_GBRA \
- int g = s->temp[0][planes == 3 ? 3 * i + 1 : 4 * i + G]; \
- int b =(s->temp[0][planes == 3 ? 3 * i + 2 : 4 * i + B] - g) & 0xFF;\
- int r =(s->temp[0][planes == 3 ? 3 * i + 0 : 4 * i + R] - g) & 0xFF;\
- int a = s->temp[0][planes * i + A];
-
-#define STAT_BGRA \
- s->stats[0][b]++; \
- s->stats[1][g]++; \
- s->stats[2][r]++; \
- if (planes == 4) \
- s->stats[2][a]++;
-
-#define WRITE_GBRA \
- put_bits(&s->pb, s->len[1][g], s->bits[1][g]); \
- put_bits(&s->pb, s->len[0][b], s->bits[0][b]); \
- put_bits(&s->pb, s->len[2][r], s->bits[2][r]); \
- if (planes == 4) \
- put_bits(&s->pb, s->len[2][a], s->bits[2][a]);
-
- if ((s->flags & AV_CODEC_FLAG_PASS1) &&
- (s->avctx->flags2 & AV_CODEC_FLAG2_NO_OUTPUT)) {
- for (i = 0; i < count; i++) {
- LOAD_GBRA;
- STAT_BGRA;
- }
- } else if (s->context || (s->flags & AV_CODEC_FLAG_PASS1)) {
- for (i = 0; i < count; i++) {
- LOAD_GBRA;
- STAT_BGRA;
- WRITE_GBRA;
- }
- } else {
- for (i = 0; i < count; i++) {
- LOAD_GBRA;
- WRITE_GBRA;
- }
- }
- return 0;
-}
-
-static int encode_frame(AVCodecContext *avctx, AVPacket *pkt,
- const AVFrame *pict, int *got_packet)
-{
- HYuvEncContext *s = avctx->priv_data;
- const int width = avctx->width;
- const int width2 = avctx->width >> 1;
- const int height = avctx->height;
- const int fake_ystride = s->interlaced ? pict->linesize[0]*2 : pict->linesize[0];
- const int fake_ustride = s->interlaced ? pict->linesize[1]*2 : pict->linesize[1];
- const int fake_vstride = s->interlaced ? pict->linesize[2]*2 : pict->linesize[2];
- const AVFrame * const p = pict;
- int i, j, size = 0, ret;
-
- if ((ret = ff_alloc_packet(avctx, pkt, width * height * 3 * 4 + AV_INPUT_BUFFER_MIN_SIZE)) < 0)
- return ret;
-
- if (s->context) {
- size = store_huffman_tables(s, pkt->data);
- if (size < 0)
- return size;
-
- for (i = 0; i < 4; i++)
- for (j = 0; j < s->vlc_n; j++)
- s->stats[i][j] >>= 1;
- }
-
- init_put_bits(&s->pb, pkt->data + size, pkt->size - size);
-
- if (avctx->pix_fmt == AV_PIX_FMT_YUV422P ||
- avctx->pix_fmt == AV_PIX_FMT_YUV420P) {
- int lefty, leftu, leftv, y, cy;
-
- put_bits(&s->pb, 8, leftv = p->data[2][0]);
- put_bits(&s->pb, 8, lefty = p->data[0][1]);
- put_bits(&s->pb, 8, leftu = p->data[1][0]);
- put_bits(&s->pb, 8, p->data[0][0]);
-
- lefty = sub_left_prediction(s, s->temp[0], p->data[0], width , 0);
- leftu = sub_left_prediction(s, s->temp[1], p->data[1], width2, 0);
- leftv = sub_left_prediction(s, s->temp[2], p->data[2], width2, 0);
-
- encode_422_bitstream(s, 2, width-2);
-
- if (s->predictor==MEDIAN) {
- int lefttopy, lefttopu, lefttopv;
- cy = y = 1;
- if (s->interlaced) {
- lefty = sub_left_prediction(s, s->temp[0], p->data[0] + p->linesize[0], width , lefty);
- leftu = sub_left_prediction(s, s->temp[1], p->data[1] + p->linesize[1], width2, leftu);
- leftv = sub_left_prediction(s, s->temp[2], p->data[2] + p->linesize[2], width2, leftv);
-
- encode_422_bitstream(s, 0, width);
- y++; cy++;
- }
-
- lefty = sub_left_prediction(s, s->temp[0], p->data[0] + fake_ystride, 4, lefty);
- leftu = sub_left_prediction(s, s->temp[1], p->data[1] + fake_ustride, 2, leftu);
- leftv = sub_left_prediction(s, s->temp[2], p->data[2] + fake_vstride, 2, leftv);
-
- encode_422_bitstream(s, 0, 4);
-
- lefttopy = p->data[0][3];
- lefttopu = p->data[1][1];
- lefttopv = p->data[2][1];
- s->llvidencdsp.sub_median_pred(s->temp[0], p->data[0] + 4, p->data[0] + fake_ystride + 4, width - 4, &lefty, &lefttopy);
- s->llvidencdsp.sub_median_pred(s->temp[1], p->data[1] + 2, p->data[1] + fake_ustride + 2, width2 - 2, &leftu, &lefttopu);
- s->llvidencdsp.sub_median_pred(s->temp[2], p->data[2] + 2, p->data[2] + fake_vstride + 2, width2 - 2, &leftv, &lefttopv);
- encode_422_bitstream(s, 0, width - 4);
- y++; cy++;
-
- for (; y < height; y++,cy++) {
- const uint8_t *ydst, *udst, *vdst;
-
- if (s->bitstream_bpp == 12) {
- while (2 * cy > y) {
- ydst = p->data[0] + p->linesize[0] * y;
- s->llvidencdsp.sub_median_pred(s->temp[0], ydst - fake_ystride, ydst, width, &lefty, &lefttopy);
- encode_gray_bitstream(s, width);
- y++;
- }
- if (y >= height) break;
- }
- ydst = p->data[0] + p->linesize[0] * y;
- udst = p->data[1] + p->linesize[1] * cy;
- vdst = p->data[2] + p->linesize[2] * cy;
-
- s->llvidencdsp.sub_median_pred(s->temp[0], ydst - fake_ystride, ydst, width, &lefty, &lefttopy);
- s->llvidencdsp.sub_median_pred(s->temp[1], udst - fake_ustride, udst, width2, &leftu, &lefttopu);
- s->llvidencdsp.sub_median_pred(s->temp[2], vdst - fake_vstride, vdst, width2, &leftv, &lefttopv);
-
- encode_422_bitstream(s, 0, width);
- }
- } else {
- for (cy = y = 1; y < height; y++, cy++) {
- const uint8_t *ydst, *udst, *vdst;
-
- /* encode a luma only line & y++ */
- if (s->bitstream_bpp == 12) {
- ydst = p->data[0] + p->linesize[0] * y;
-
- if (s->predictor == PLANE && s->interlaced < y) {
- s->llvidencdsp.diff_bytes(s->temp[1], ydst, ydst - fake_ystride, width);
-
- lefty = sub_left_prediction(s, s->temp[0], s->temp[1], width , lefty);
- } else {
- lefty = sub_left_prediction(s, s->temp[0], ydst, width , lefty);
- }
- encode_gray_bitstream(s, width);
- y++;
- if (y >= height) break;
- }
-
- ydst = p->data[0] + p->linesize[0] * y;
- udst = p->data[1] + p->linesize[1] * cy;
- vdst = p->data[2] + p->linesize[2] * cy;
-
- if (s->predictor == PLANE && s->interlaced < cy) {
- s->llvidencdsp.diff_bytes(s->temp[1], ydst, ydst - fake_ystride, width);
- s->llvidencdsp.diff_bytes(s->temp[2], udst, udst - fake_ustride, width2);
- s->llvidencdsp.diff_bytes(s->temp[2] + width2, vdst, vdst - fake_vstride, width2);
-
- lefty = sub_left_prediction(s, s->temp[0], s->temp[1], width , lefty);
- leftu = sub_left_prediction(s, s->temp[1], s->temp[2], width2, leftu);
- leftv = sub_left_prediction(s, s->temp[2], s->temp[2] + width2, width2, leftv);
- } else {
- lefty = sub_left_prediction(s, s->temp[0], ydst, width , lefty);
- leftu = sub_left_prediction(s, s->temp[1], udst, width2, leftu);
- leftv = sub_left_prediction(s, s->temp[2], vdst, width2, leftv);
- }
-
- encode_422_bitstream(s, 0, width);
- }
- }
- } else if(avctx->pix_fmt == AV_PIX_FMT_RGB32) {
- const uint8_t *data = p->data[0] + (height - 1) * p->linesize[0];
- const int stride = -p->linesize[0];
- const int fake_stride = -fake_ystride;
- int leftr, leftg, leftb, lefta;
-
- put_bits(&s->pb, 8, lefta = data[A]);
- put_bits(&s->pb, 8, leftr = data[R]);
- put_bits(&s->pb, 8, leftg = data[G]);
- put_bits(&s->pb, 8, leftb = data[B]);
-
- sub_left_prediction_bgr32(s, s->temp[0], data + 4, width - 1,
- &leftr, &leftg, &leftb, &lefta);
- encode_bgra_bitstream(s, width - 1, 4);
-
- for (int y = 1; y < height; y++) {
- const uint8_t *dst = data + y*stride;
- if (s->predictor == PLANE && s->interlaced < y) {
- s->llvidencdsp.diff_bytes(s->temp[1], dst, dst - fake_stride, width * 4);
- sub_left_prediction_bgr32(s, s->temp[0], s->temp[1], width,
- &leftr, &leftg, &leftb, &lefta);
- } else {
- sub_left_prediction_bgr32(s, s->temp[0], dst, width,
- &leftr, &leftg, &leftb, &lefta);
- }
- encode_bgra_bitstream(s, width, 4);
- }
- } else if (avctx->pix_fmt == AV_PIX_FMT_RGB24) {
- const uint8_t *data = p->data[0] + (height - 1) * p->linesize[0];
- const int stride = -p->linesize[0];
- const int fake_stride = -fake_ystride;
- int leftr, leftg, leftb;
-
- put_bits(&s->pb, 8, leftr = data[0]);
- put_bits(&s->pb, 8, leftg = data[1]);
- put_bits(&s->pb, 8, leftb = data[2]);
- put_bits(&s->pb, 8, 0);
-
- sub_left_prediction_rgb24(s, s->temp[0], data + 3, width - 1,
- &leftr, &leftg, &leftb);
- encode_bgra_bitstream(s, width-1, 3);
-
- for (int y = 1; y < height; y++) {
- const uint8_t *dst = data + y * stride;
- if (s->predictor == PLANE && s->interlaced < y) {
- s->llvidencdsp.diff_bytes(s->temp[1], dst, dst - fake_stride,
- width * 3);
- sub_left_prediction_rgb24(s, s->temp[0], s->temp[1], width,
- &leftr, &leftg, &leftb);
- } else {
- sub_left_prediction_rgb24(s, s->temp[0], dst, width,
- &leftr, &leftg, &leftb);
- }
- encode_bgra_bitstream(s, width, 3);
- }
- } else if (s->version > 2) {
- int plane;
- for (plane = 0; plane < 1 + 2*s->chroma + s->alpha; plane++) {
- int left, y;
- int w = width;
- int h = height;
- int fake_stride = fake_ystride;
-
- if (s->chroma && (plane == 1 || plane == 2)) {
- w >>= s->chroma_h_shift;
- h >>= s->chroma_v_shift;
- fake_stride = plane == 1 ? fake_ustride : fake_vstride;
- }
-
- left = sub_left_prediction(s, s->temp[0], p->data[plane], w , 0);
-
- encode_plane_bitstream(s, w, plane);
-
- if (s->predictor==MEDIAN) {
- int lefttop;
- y = 1;
- if (s->interlaced) {
- left = sub_left_prediction(s, s->temp[0], p->data[plane] + p->linesize[plane], w , left);
-
- encode_plane_bitstream(s, w, plane);
- y++;
- }
-
- lefttop = p->data[plane][0];
-
- for (; y < h; y++) {
- const uint8_t *dst = p->data[plane] + p->linesize[plane] * y;
-
- sub_median_prediction(s, s->temp[0], dst - fake_stride, dst, w , &left, &lefttop);
-
- encode_plane_bitstream(s, w, plane);
- }
- } else {
- for (y = 1; y < h; y++) {
- const uint8_t *dst = p->data[plane] + p->linesize[plane] * y;
-
- if (s->predictor == PLANE && s->interlaced < y) {
- diff_bytes(s, s->temp[1], dst, dst - fake_stride, w);
-
- left = sub_left_prediction(s, s->temp[0], s->temp[1], w , left);
- } else {
- left = sub_left_prediction(s, s->temp[0], dst, w , left);
- }
-
- encode_plane_bitstream(s, w, plane);
- }
- }
- }
- } else {
- av_log(avctx, AV_LOG_ERROR, "Format not supported!\n");
- }
- emms_c();
-
- size += (put_bits_count(&s->pb) + 31) / 8;
- put_bits(&s->pb, 16, 0);
- put_bits(&s->pb, 15, 0);
- size /= 4;
-
- if ((s->flags & AV_CODEC_FLAG_PASS1) && (s->picture_number & 31) == 0) {
- int j;
- char *p = avctx->stats_out;
- char *end = p + STATS_OUT_SIZE;
- for (i = 0; i < 4; i++) {
- for (j = 0; j < s->vlc_n; j++) {
- snprintf(p, end-p, "%"PRIu64" ", s->stats[i][j]);
- p += strlen(p);
- s->stats[i][j]= 0;
- }
- snprintf(p, end-p, "\n");
- p++;
- if (end <= p)
- return AVERROR(ENOMEM);
- }
- } else if (avctx->stats_out)
- avctx->stats_out[0] = '\0';
- if (!(s->avctx->flags2 & AV_CODEC_FLAG2_NO_OUTPUT)) {
- flush_put_bits(&s->pb);
- s->bdsp.bswap_buf((uint32_t *) pkt->data, (uint32_t *) pkt->data, size);
- }
-
- s->picture_number++;
-
- pkt->size = size * 4;
- *got_packet = 1;
-
- return 0;
-}
-
-static av_cold int encode_end(AVCodecContext *avctx)
-{
- HYuvEncContext *s = avctx->priv_data;
-
- ff_huffyuv_common_end(s->temp, s->temp16);
-
- av_freep(&avctx->stats_out);
-
- return 0;
-}
-
-#define OFFSET(x) offsetof(HYuvEncContext, x)
-#define VE AV_OPT_FLAG_VIDEO_PARAM | AV_OPT_FLAG_ENCODING_PARAM
-
-#define COMMON_OPTIONS \
- { "non_deterministic", "Allow multithreading for e.g. context=1 at the expense of determinism", \
- OFFSET(non_determ), AV_OPT_TYPE_BOOL, { .i64 = 0 }, \
- 0, 1, VE }, \
- { "pred", "Prediction method", OFFSET(predictor), AV_OPT_TYPE_INT, { .i64 = LEFT }, LEFT, MEDIAN, VE, "pred" }, \
- { "left", NULL, 0, AV_OPT_TYPE_CONST, { .i64 = LEFT }, INT_MIN, INT_MAX, VE, "pred" }, \
- { "plane", NULL, 0, AV_OPT_TYPE_CONST, { .i64 = PLANE }, INT_MIN, INT_MAX, VE, "pred" }, \
- { "median", NULL, 0, AV_OPT_TYPE_CONST, { .i64 = MEDIAN }, INT_MIN, INT_MAX, VE, "pred" }, \
-
-static const AVOption normal_options[] = {
- COMMON_OPTIONS
- { NULL },
-};
-
-static const AVOption ff_options[] = {
- COMMON_OPTIONS
- { "context", "Set per-frame huffman tables", OFFSET(context), AV_OPT_TYPE_INT, { .i64 = 0 }, 0, 1, VE },
- { NULL },
-};
-
-static const AVClass normal_class = {
- .class_name = "huffyuv",
- .item_name = av_default_item_name,
- .option = normal_options,
- .version = LIBAVUTIL_VERSION_INT,
-};
-
-static const AVClass ff_class = {
- .class_name = "ffvhuff",
- .item_name = av_default_item_name,
- .option = ff_options,
- .version = LIBAVUTIL_VERSION_INT,
-};
-
-const FFCodec ff_huffyuv_encoder = {
- .p.name = "huffyuv",
- CODEC_LONG_NAME("Huffyuv / HuffYUV"),
- .p.type = AVMEDIA_TYPE_VIDEO,
- .p.id = AV_CODEC_ID_HUFFYUV,
- .p.capabilities = AV_CODEC_CAP_DR1 | AV_CODEC_CAP_FRAME_THREADS |
- AV_CODEC_CAP_ENCODER_REORDERED_OPAQUE,
- .priv_data_size = sizeof(HYuvEncContext),
- .init = encode_init,
- FF_CODEC_ENCODE_CB(encode_frame),
- .close = encode_end,
- .p.priv_class = &normal_class,
- .p.pix_fmts = (const enum AVPixelFormat[]){
- AV_PIX_FMT_YUV422P, AV_PIX_FMT_RGB24,
- AV_PIX_FMT_RGB32, AV_PIX_FMT_NONE
- },
- .caps_internal = FF_CODEC_CAP_INIT_CLEANUP,
-};
-
-#if CONFIG_FFVHUFF_ENCODER
-const FFCodec ff_ffvhuff_encoder = {
- .p.name = "ffvhuff",
- CODEC_LONG_NAME("Huffyuv FFmpeg variant"),
- .p.type = AVMEDIA_TYPE_VIDEO,
- .p.id = AV_CODEC_ID_FFVHUFF,
- .p.capabilities = AV_CODEC_CAP_DR1 | AV_CODEC_CAP_FRAME_THREADS |
- AV_CODEC_CAP_ENCODER_REORDERED_OPAQUE,
- .priv_data_size = sizeof(HYuvEncContext),
- .init = encode_init,
- FF_CODEC_ENCODE_CB(encode_frame),
- .close = encode_end,
- .p.priv_class = &ff_class,
- .p.pix_fmts = (const enum AVPixelFormat[]){
- AV_PIX_FMT_YUV420P, AV_PIX_FMT_YUV422P, AV_PIX_FMT_YUV444P, AV_PIX_FMT_YUV411P,
- AV_PIX_FMT_YUV410P, AV_PIX_FMT_YUV440P,
- AV_PIX_FMT_GBRP,
- AV_PIX_FMT_GBRP9, AV_PIX_FMT_GBRP10, AV_PIX_FMT_GBRP12, AV_PIX_FMT_GBRP14, AV_PIX_FMT_GBRP16,
- AV_PIX_FMT_GRAY8, AV_PIX_FMT_GRAY16,
- AV_PIX_FMT_YUVA420P, AV_PIX_FMT_YUVA422P, AV_PIX_FMT_YUVA444P,
- AV_PIX_FMT_GBRAP,
- AV_PIX_FMT_YUV420P9, AV_PIX_FMT_YUV420P10, AV_PIX_FMT_YUV420P12, AV_PIX_FMT_YUV420P14, AV_PIX_FMT_YUV420P16,
- AV_PIX_FMT_YUV422P9, AV_PIX_FMT_YUV422P10, AV_PIX_FMT_YUV422P12, AV_PIX_FMT_YUV422P14, AV_PIX_FMT_YUV422P16,
- AV_PIX_FMT_YUV444P9, AV_PIX_FMT_YUV444P10, AV_PIX_FMT_YUV444P12, AV_PIX_FMT_YUV444P14, AV_PIX_FMT_YUV444P16,
- AV_PIX_FMT_YUVA420P9, AV_PIX_FMT_YUVA420P10, AV_PIX_FMT_YUVA420P16,
- AV_PIX_FMT_YUVA422P9, AV_PIX_FMT_YUVA422P10, AV_PIX_FMT_YUVA422P16,
- AV_PIX_FMT_YUVA444P9, AV_PIX_FMT_YUVA444P10, AV_PIX_FMT_YUVA444P16,
- AV_PIX_FMT_RGB24,
- AV_PIX_FMT_RGB32, AV_PIX_FMT_NONE
- },
- .caps_internal = FF_CODEC_CAP_INIT_CLEANUP,
-};
-#endif
diff --git a/spaces/colakin/video-generater/public/ffmpeg/libavcodec/jni.h b/spaces/colakin/video-generater/public/ffmpeg/libavcodec/jni.h
deleted file mode 100644
index dd99e92611322b5ac590daea689d920b358b272c..0000000000000000000000000000000000000000
--- a/spaces/colakin/video-generater/public/ffmpeg/libavcodec/jni.h
+++ /dev/null
@@ -1,46 +0,0 @@
-/*
- * JNI public API functions
- *
- * Copyright (c) 2015-2016 Matthieu Bouron
- *
- * This file is part of FFmpeg.
- *
- * FFmpeg is free software; you can redistribute it and/or
- * modify it under the terms of the GNU Lesser General Public
- * License as published by the Free Software Foundation; either
- * version 2.1 of the License, or (at your option) any later version.
- *
- * FFmpeg is distributed in the hope that it will be useful,
- * but WITHOUT ANY WARRANTY; without even the implied warranty of
- * MERCHANTABILITY or FITNESS FOR A PARTICULAR PURPOSE. See the GNU
- * Lesser General Public License for more details.
- *
- * You should have received a copy of the GNU Lesser General Public
- * License along with FFmpeg; if not, write to the Free Software
- * Foundation, Inc., 51 Franklin Street, Fifth Floor, Boston, MA 02110-1301 USA
- */
-
-#ifndef AVCODEC_JNI_H
-#define AVCODEC_JNI_H
-
-/*
- * Manually set a Java virtual machine which will be used to retrieve the JNI
- * environment. Once a Java VM is set it cannot be changed afterwards, meaning
- * you can call multiple times av_jni_set_java_vm with the same Java VM pointer
- * however it will error out if you try to set a different Java VM.
- *
- * @param vm Java virtual machine
- * @param log_ctx context used for logging, can be NULL
- * @return 0 on success, < 0 otherwise
- */
-int av_jni_set_java_vm(void *vm, void *log_ctx);
-
-/*
- * Get the Java virtual machine which has been set with av_jni_set_java_vm.
- *
- * @param vm Java virtual machine
- * @return a pointer to the Java virtual machine
- */
-void *av_jni_get_java_vm(void *log_ctx);
-
-#endif /* AVCODEC_JNI_H */
diff --git a/spaces/congsaPfin/Manga-OCR/logs/APK Games Free Download and Install the Best Games for Your Phone.md b/spaces/congsaPfin/Manga-OCR/logs/APK Games Free Download and Install the Best Games for Your Phone.md
deleted file mode 100644
index 202cc30efe194019a4f4c4d50f58d68d56010722..0000000000000000000000000000000000000000
--- a/spaces/congsaPfin/Manga-OCR/logs/APK Games Free Download and Install the Best Games for Your Phone.md
+++ /dev/null
@@ -1,121 +0,0 @@
-
-
-
-
- APK Games Free Download: How to Find and Install Them on Your Android Device
- If you are an avid gamer who loves to explore new and exciting games on your Android device, you might have heard of APK games. These are games that are not available on the official Google Play Store, but can be downloaded from other sources as APK files. But what are APK files, and how can you download, install, and play them on your device? In this article, we will answer these questions and more.
-apk games free download
Download ★★★★★ https://urlca.com/2uO7M5
- What are APK Games?
- APK stands for Android Package Kit, which is a file format that contains all the necessary components for an Android app or game to run on your device. APK files are similar to ZIP files, but they have a different extension (.apk) and can be installed directly on your device without extracting them.
- There are many reasons why some games are not available on the Google Play Store, such as regional restrictions, censorship, licensing issues, or developer preferences. Some developers may choose to distribute their games as APK files to avoid Google's fees or policies, or to offer exclusive features or updates that are not available on the official store.
- Some of the benefits of playing APK games are:
-android apk games free download
-apk games free download for mobile
-apk games free download offline
-apk games free download full version
-apk games free download mod
-apk games free download 2021
-apk games free download sites
-apk games free download action
-apk games free download racing
-apk games free download puzzle
-apk games free download adventure
-apk games free download rpg
-apk games free download strategy
-apk games free download simulation
-apk games free download sports
-apk games free download horror
-apk games free download arcade
-apk games free download casual
-apk games free download shooter
-apk games free download fighting
-apk games free download platformer
-apk games free download role playing
-apk games free download sandbox
-apk games free download survival
-apk games free download zombie
-apk games free download car
-apk games free download bike
-apk games free download truck
-apk games free download bus
-apk games free download train
-apk games free download plane
-apk games free download helicopter
-apk games free download boat
-apk games free download submarine
-apk games free download tank
-apk games free download war
-apk games free download army
-apk games free download navy
-apk games free download air force
-apk games free download police
-apk games free download fireman
-apk games free download doctor
-apk games free download dentist
-apk games free download chef
-apk games free download farmer
-apk games free download zookeeper
-apk games free download teacher
-apk games free download lawyer
-
-- You can access games that are not available on the Google Play Store.
-- You can enjoy games that have more features or better graphics than their official versions.
-- You can get early access to beta versions or updates of your favorite games.
-- You can customize your gaming experience by modifying or hacking your APK games.
-
- However, there are also some drawbacks of playing APK games, such as:
-
-- You may expose your device to malware or viruses that can harm your data or privacy.
-- You may violate - You may violate the terms and conditions of the original game developers or publishers, and risk getting banned or sued. - You may encounter compatibility or performance issues, such as crashes, glitches, or bugs. - You may miss out on the official updates or support from the Google Play Store or the game developers. Some examples of popular APK games are: - PUBG Mobile Lite: A lighter version of the famous battle royale game that can run on low-end devices. - Minecraft Pocket Edition: A portable version of the sandbox game that allows you to create and explore your own world. - GTA San Andreas: A classic open-world action game that lets you experience the life of a gangster in Los Santos. - Among Us: A multiplayer game where you have to find the impostor among your crewmates in a spaceship. - Genshin Impact: A role-playing game where you can explore a vast fantasy world with different characters and elements.
How to Download APK Games?
- There are many sources and websites where you can download APK games for free. However, not all of them are safe or reliable. Some of them may contain malware or viruses that can harm your device or steal your information. Some of them may also provide fake or outdated APK files that may not work properly or at all.
- Therefore, you should be careful and cautious when downloading APK games from unknown sources. Here are some precautions and tips for downloading APK games:
-
-- Always check the reputation and reviews of the source or website before downloading any APK file. You can use tools like VirusTotal or Google Safe Browsing to scan the URL for any malicious content.
-- Always check the permissions and details of the APK file before installing it on your device. You can use tools like APK Analyzer or APK Editor to inspect the APK file for any suspicious or unnecessary permissions or components.
-- Always download the latest version of the APK file from the official website or a trusted source. You can use tools like APKMirror or APKPure to find and download the latest and verified APK files for your games.
-
- The steps for downloading APK games are:
-
-- Find and choose the APK game that you want to download from a source or website.
-- Click on the download button or link to start downloading the APK file to your device.
-- Wait for the download to finish and locate the APK file in your device's storage.
-
- How to Install APK Games?
- Before you can install any APK game on your device, you need to make sure that your device meets some requirements and settings. These are:
-
-- Your device should have enough storage space to accommodate the APK file and its data.
-- Your device should have a compatible Android version and hardware specifications to run the game smoothly.
-- Your device should allow installation from unknown sources, which is a security setting that prevents installation of apps or games from outside the Google Play Store. You can enable this setting by going to Settings > Security > Unknown Sources (or similar) and toggling it on.
-
- The steps for installing APK games are:
-
-- Open the file manager app on your device and navigate to the folder where you downloaded the APK file.
-- Tap on the APK file to launch it and start the installation process.
-- Follow the instructions on the screen and grant any permissions or access that the game requires.
-- Wait for the installation to finish and look for the game icon on your home screen or app drawer.
-- Tap on the game icon to launch it and enjoy playing it.
-
- Sometimes, you may encounter some troubleshooting or common errors when installing APK games, such as:
-
-- The installation is blocked or failed: This may happen if your device does not meet a href="https://innersloth.com/gameAmongUs.php">https://innersloth.com/gameAmongUs.php
-
-
-Genshin Impact
-A role-playing game where you can explore a vast fantasy world with different characters and elements.
-https://genshin.mihoyo.com/en
-
-
- FAQs
- Here are some of the frequently asked questions about APK games and their answers:
- Q1: Are APK games safe?
- A1: APK games are not inherently unsafe, but they may pose some risks if you download them from untrusted sources or websites. You should always scan the APK file for any malware or viruses before installing it on your device, and check the permissions and details of the game. You should also avoid downloading or installing any APK games that require a license verification or an additional data download, as they may be fake or harmful.
- Q2: Do I need to root my device to play APK games?
- A2: No, you do not need to root your device to play APK games, unless the game specifically requires it. Rooting your device is a process that gives you full access and control over your device's system and settings, which can allow you to modify or hack your APK games. However, rooting your device can also void your warranty, expose your device to security risks, and cause compatibility or performance issues. Therefore, you should only root your device if you know what you are doing and at your own risk.
- Q3: How can I update my APK games?
- A3: You can update your APK games by downloading and installing the latest version of the APK file from the official website or a trusted source. You can also use tools like APKUpdater or Uptodown to check for updates and download them automatically. However, you should be aware that updating your APK games may overwrite your data or progress, or cause compatibility or performance issues. Therefore, you should always backup your data and progress before updating your APK games.
- Q4: What are some of the best APK games?
- A4: There are many APK games that you can download and play on your Android device, depending on your preferences and tastes. Some of the best APK games that we recommend are PUBG Mobile Lite, Minecraft Pocket Edition, GTA San Andreas, Among Us, and Genshin Impact. You can find more information and download links for these games in the table above.
- Q5: How can I uninstall APK games?
- A5: You can uninstall APK games by following the same steps as uninstalling regular apps or games on your device. You can go to Settings > Apps > (Game Name) > Uninstall, or long-press the game icon on your home screen or app drawer and drag it to the uninstall option. You can also use tools like SD Maid or App Manager to uninstall APK games more easily and completely.
197e85843d
-
-
\ No newline at end of file
diff --git a/spaces/congsaPfin/Manga-OCR/logs/European Truck Simulator Mod APK The Most Realistic Truck Simulator Ever.md b/spaces/congsaPfin/Manga-OCR/logs/European Truck Simulator Mod APK The Most Realistic Truck Simulator Ever.md
deleted file mode 100644
index 5b3215798f82f21a0c6714e1d364d0810a1b7f50..0000000000000000000000000000000000000000
--- a/spaces/congsaPfin/Manga-OCR/logs/European Truck Simulator Mod APK The Most Realistic Truck Simulator Ever.md
+++ /dev/null
@@ -1,156 +0,0 @@
-
-European Truck Simulator APK Mod Download: A Guide for Beginners
- If you are a fan of driving simulation games, you might have heard of Euro Truck Simulator 2, a popular game that lets you travel across Europe as a truck driver, delivering cargo and running your own business. But did you know that you can also play this game on your Android device, with some extra features and benefits? In this article, we will show you how to download and install the Euro Truck Simulator apk mod, a modified version of the game that gives you unlimited money, access to all trucks and maps, and more. We will also give you some tips and tricks for playing the game, as well as some reviews from other players. So buckle up and get ready for a thrilling ride!
- What is Euro Truck Simulator?
- Euro Truck Simulator is a series of truck simulation games developed by SCS Software, a Czech company that specializes in vehicle simulation games. The first game in the series was released in 2008, and the second one, Euro Truck Simulator 2, was released in 2012. The game features licensed trucks from various European brands, such as Mercedes-Benz, Volvo, Scania, MAN, Renault, and more. The game also features realistic environments, roads, cities, and landmarks from over 26 European countries, such as Germany, France, Italy, Spain, Poland, and more. The game has a career mode, where you can start as a low-skilled driver and work your way up to become a successful trucking company owner. You can also customize your trucks with various tuning options, such as paint jobs, engines, lights, horns, etc. The game also has a multiplayer mode, where you can join other players online and compete or cooperate with them.
-european truck simulator apk mod download
DOWNLOAD ✅ https://urlca.com/2uO4Xo
- Features of the game
- Some of the main features of Euro Truck Simulator 2 are:
-
-- Transport a vast variety of cargo across more than 60 European cities.
-- Run your own business which continues to grow even as you complete your freight deliveries.
-- Build your own fleet of trucks, buy garages, hire drivers, manage your company for maximum profits.
-- A varied amount of truck tuning that range from performance to cosmetic changes.
-- Realistic weather conditions and day/night cycle.
-- Visual damage on trucks.
-- Detailed interiors for each truck brand.
-- Amazing engine sounds.
-- Modding and community support.
-
- How to download and install the apk mod
- If you want to play Euro Truck Simulator 2 on your Android device, you will need to download and install the Euro Truck Simulator apk mod, which is a modified version of the game that gives you some extra features and benefits. Here are the steps to do so:
-
-- Go to this link and download the apk file. This is a trusted source that provides safe and virus-free downloads.
-- Go to your device settings and enable the installation of apps from unknown sources. This will allow you to install the apk file that you downloaded.
-- Locate the apk file in your device storage and tap on it to install it. Follow the instructions on the screen to complete the installation.
-- Launch the game and enjoy!
-
- Why use the apk mod?
- You might be wondering why you should use the Euro Truck Simulator apk mod instead of the original game. Well, there are some good reasons to do so, as well as some risks and precautions that you should be aware of. Let's take a look at them.
- Benefits of the mod
- The main benefit of using the Euro Truck Simulator apk mod is that it gives you unlimited money, which means that you can buy any truck, upgrade, garage, or driver that you want, without worrying about your budget. You can also access all maps and trucks, which means that you can explore every corner of Europe and drive any truck that you like, without having to unlock them first. You can also enjoy some extra features, such as no damage, no fatigue, no police, no speed limit, etc., which can make your gameplay more fun and easy.
- Risks and precautions of the mod
- However, using the Euro Truck Simulator apk mod also comes with some risks and precautions that you should be aware of. The main risk is that the mod may not be compatible with your device or the latest version of the game, which may cause some errors, crashes, or glitches. The mod may also not work well with the multiplayer mode, which may prevent you from joining other players online or cause some conflicts with them. The mod may also violate the terms and conditions of the game developer, which may result in a ban or a penalty. Therefore, you should always backup your data before installing the mod, and use it at your own risk and responsibility. You should also avoid using the mod in multiplayer mode, and respect other players and their gameplay.
- Tips and tricks for playing Euro Truck Simulator
- If you are new to Euro Truck Simulator 2, or if you want to improve your skills and experience in the game, here are some tips and tricks that can help you:
- Follow the rules of traffic
- One of the most important things to remember when playing Euro Truck Simulator 2 is to follow the rules of traffic. This means that you should obey the speed limits, traffic lights, signs, signals, etc., as well as drive on the correct side of the road. If you break any of these rules, you may get fined by the police, or cause an accident that can damage your truck or cargo. You may also lose reputation points or money if you deliver your cargo late or damaged. Therefore, it is better to drive safely and carefully than to rush and risk.
-european truck driver simulator mod apk free download
-download euro truck evolution simulator mod apk unlimited money
-euro truck simulator 2 android apk mod download
-euro truck driver 2018 mod apk download latest version
-euro truck simulator pro mod apk download for android
-euro truck driver 3d mod apk download
-euro truck simulator 2 mobile mod apk download
-euro truck driver 2019 mod apk download
-euro truck simulator 3 mod apk free download
-euro truck driver simulator 2 mod apk download
-euro truck simulator multiplayer mod apk download
-euro truck driver 2017 mod apk download
-euro truck simulator offroad cargo transport mod apk download
-euro truck simulator bus mod apk download
-euro truck driver 2020 mod apk download
-euro truck simulator 2 vr mod apk download
-euro truck simulator cargo delivery mod apk download
-euro truck driver simulator 3d mod apk download
-euro truck simulator 2 online multiplayer mod apk download
-euro truck driver 2016 mod apk download
-euro truck simulator snow mod apk download
-euro truck simulator 2 realistic graphics mod apk download
-euro truck driver simulator offline mod apk download
-euro truck simulator 2 car driving mod apk download
-euro truck driver 2015 mod apk download
-euro truck simulator 2 map mods apk download
-euro truck driver simulator hack mod apk download
-euro truck simulator 2 traffic jam mod apk download
-euro truck simulator 2 indian bus mod apk download
-euro truck driver 2014 mod apk download
-euro truck simulator 2 police car mod apk download
-euro truck simulator 2 winter mods apk download
-euro truck driver simulator premium mod apk download
-euro truck simulator 2 scania bus mods free download for android
-euro truck driver simulator cheats mod apk download
-euro truck simulator 2 volvo bus mods free download for android
-euro truck driver simulator unlimited money and xp mod apk download
-euro truck simulator 2 indian map mods free download for android
-euro truck driver simulator full unlocked mod apk download
-euro truck simulator 2 mercedes benz bus mods free download for android
-european cargo transport simulation game - real driving sim - offline game - free to play - realistic physics - amazing graphics - easy controls - multiple camera views - different weather conditions - day and night cycle - customisable trucks and trailers - various cargo types and destinations - challenging missions and achievements - online leaderboards and multiplayer mode
- Be careful with skill point assignment
- Another important thing to consider when playing Euro Truck Simulator 2 is how to assign your skill points. Skill points are earned by leveling up in the game, and they can be used to unlock various perks and abilities for your driver. However, not all skills are equally useful or necessary, so you should be careful with how you spend them. Some of the most useful skills are:
-
-- A + B + C Cargo: These skills allow you to transport more types of cargo, such as fragile, valuable, or dangerous goods. This can increase your income and reputation.
-- Long Distance: This skill allows you to take longer delivery jobs, which can also increase your income and reputation.
-- Fuel Economy: This skill allows you to save fuel by driving more efficiently. This can reduce your expenses and increase your profits.
-- High Value Cargo: This skill allows you to transport more expensive cargo, which can also increase your income and reputation.
-- Eco Driving: This skill allows you to reduce your fuel consumption by driving more smoothly. This can also reduce your expenses and increase your profits.
-- Hazardous Cargo: This skill allows you to transport more dangerous cargo, such as explosives, chemicals, or radioactive materials. This can also increase your income and reputation.
-- Just In Time Delivery: This skill allows you to take urgent delivery jobs, which have a shorter time limit but a higher reward. This can also increase your income and reputation.
-
- Some of the less useful skills are:
-
-electronics. However, this skill is not very useful, as fragile cargo is not very common, and it does not pay much more than regular cargo.
-- ADR: This skill allows you to transport more types of hazardous cargo, such as flammable, corrosive, or toxic materials. However, this skill is also not very useful, as hazardous cargo is already covered by the Hazardous Cargo skill, and it does not pay much more than regular cargo.
-- Passenger Transport: This skill allows you to transport passengers, such as tourists or workers. However, this skill is also not very useful, as passenger transport is not very common, and it does not pay much more than regular cargo.
-
- Therefore, you should focus on the skills that can increase your income and reputation, and avoid the skills that are less useful or redundant.
- Do not go through with the very first loan
- Another tip for playing Euro Truck Simulator 2 is to avoid taking the very first loan that is offered to you at the beginning of the game. The loan is for 100,000 euros, and it has a high interest rate of 18%. This means that you will have to pay back 1,800 euros every day for 100 days, which can be a huge burden on your finances. Instead of taking the loan, you should continue working as a hired driver for other companies, until you have enough money to buy your own truck. This way, you can save money on interest and fees, and have more control over your income and expenses.
- Take time to learn the menu
- Another tip for playing Euro Truck Simulator 2 is to take some time to learn the menu and its functions. The menu is where you can access various options and information about your game, such as your profile, your company, your truck, your garage, your drivers, your bank, your map, your job market, your settings, etc. You can also use the menu to pause or save your game, or to quit the game. The menu can be accessed by pressing the Esc key on your keyboard, or by tapping on the screen if you are playing on a touch device. You should familiarize yourself with the menu and its features, as they can help you manage your game and improve your gameplay.
- Ecodriving is a useless skill
- Another tip for playing Euro Truck Simulator 2 is to ignore the ecodriving skill. The ecodriving skill is supposed to measure how efficiently you drive your truck, by taking into account factors such as speed, acceleration, braking, gear shifting, etc. The higher your ecodriving skill, the less fuel you consume and the more money you save. However, the ecodriving skill is actually useless and inaccurate in the game. It does not reflect the actual fuel consumption of your truck, nor does it affect your income or expenses. It is just a cosmetic feature that has no real impact on your gameplay. Therefore, you should not worry about your ecodriving skill or try to improve it. You should focus on other skills that are more useful and relevant in the game.
- Reviews of Euro Truck Simulator
- If you are still not convinced that Euro Truck Simulator 2 is a great game to play on your Android device with the Euro Truck Simulator apk mod, here are some reviews from other players who have tried it:
- Pros and cons of the game
- Some of the pros and cons of Euro Truck Simulator 2 are:
-
-
-Pros
-Cons
-
-
-- Realistic and immersive graphics and sounds.
-- Requires a lot of storage space and memory.
-
-
-- Diverse and expansive map of Europe.
-- May have some bugs or glitches.
-
-
-- Fun and challenging gameplay.
-- May be boring or repetitive for some players.
-
-
-- Customizable and upgradeable trucks.
-- May be too difficult or complex for some players.
-
-
-- Modding and community support.
-- May not be compatible with some devices or versions.
-
-
- User ratings and feedback
- Some of the user ratings and feedback for Euro Truck Simulator 2 are:
-
-- "This game is amazing. I love driving around Europe and delivering cargo. The graphics are stunning and the sounds are realistic. The game is very relaxing and enjoyable. I highly recommend it to anyone who likes simulation games." - 5 stars
-- "This game is good, but it has some issues. The game crashes sometimes and it lags a lot. The controls are not very responsive and the steering is too sensitive. The game is also very hard and frustrating. I wish there was an easier mode or a tutorial." - 3 stars
-- "This game is terrible. It is boring and repetitive. The game is too long and too complex. The game is also very expensive and it takes up too much space on my device. The game is also full of bugs and glitches. I regret buying it." - 1 star
-
- Conclusion and FAQs
- In conclusion, Euro Truck Simulator 2 is a great game to play on your Android device with the Euro Truck Simulator apk mod. The game offers you a realistic and immersive experience of driving a truck across Europe, delivering cargo and running your own business. The game also has a lot of features and options to customize your truck and your gameplay. The game also has a modding and community support, which can enhance your game even more. However, the game also has some drawbacks, such as requiring a lot of storage space and memory, having some bugs or glitches, being incompatible with some devices or versions, or being too difficult or complex for some players. Therefore, you should always backup your data before installing the mod, and use it at your own risk and responsibility.
- If you have any questions about Euro Truck Simulator 2 or the Euro Truck Simulator apk mod, here are some FAQs that might help you:
-
-- How can I update the game or the mod?
-You can update the game or the mod by downloading the latest version from the official website or from the link that we provided. You can also check for updates from within the game menu or from the Google Play Store. However, you should always backup your data before updating, as some updates may not be compatible with your device or your previous version.
-- How can I play the multiplayer mode?
-You can play the multiplayer mode by joining other players online through the Steam platform. You will need to have a Steam account and a copy of the original game on your PC to do so. You will also need to download and install the TruckersMP mod, which is a fan-made multiplayer mod that allows you to play with other players online. However, you should note that the multiplayer mode may not work well with the apk mod, as they may have different versions or features.
-- How can I use mods in the game?
-You can use mods in the game by downloading them from various sources, such as the official website, the Steam Workshop, or other websites. You can also create your own mods using the SCS Software tools. However, you should note that not all mods are compatible with each other or with the apk mod, so you should always backup your data before installing them, and use them at your own risk and responsibility.
-- How can I contact the developer or get support?
-You can contact the developer or get support by visiting their official website, their Facebook page, their Twitter account, their YouTube channel, or their forum. You can also send them an email at info@scssoft.com.
-- How can I uninstall the game or the mod?
-You can uninstall the game or the mod by going to your device settings and finding the app in the list of installed apps. Then, you can tap on the app and select the option to uninstall it. You can also delete the apk file from your device storage if you want to free up some space.
- I hope this article has helped you learn more about Euro Truck Simulator 2 and the Euro Truck Simulator apk mod. If you have any comments or suggestions, please feel free to share them with us. Thank you for reading and happy trucking!
197e85843d
-
-
\ No newline at end of file
diff --git a/spaces/congsaPfin/Manga-OCR/logs/Instagram 2 APK - Enjoy New Features and Enhancements on Your Android.md b/spaces/congsaPfin/Manga-OCR/logs/Instagram 2 APK - Enjoy New Features and Enhancements on Your Android.md
deleted file mode 100644
index 1374133ac9549057ce0ab3b3a39a8de7d04cd0ad..0000000000000000000000000000000000000000
--- a/spaces/congsaPfin/Manga-OCR/logs/Instagram 2 APK - Enjoy New Features and Enhancements on Your Android.md
+++ /dev/null
@@ -1,103 +0,0 @@
-
-Instagram 2 APK Download: What Is It and How to Get It
-Instagram is one of the most popular social media platforms in the world, with over one billion monthly active users. It allows you to create and share your photos, videos, stories, reels, and more with your friends and followers. But what if you want to have more control over your Instagram experience? What if you want to remove ads, hide views, download media, or customize your app? That's where Instagram 2 APK comes in.
-instagram 2 apk download
DOWNLOAD >>>>> https://urlca.com/2uO71I
-Instagram 2 APK is a modified version of the official Instagram app that offers some extra features and options that are not available in the original app. It is also known as Instagram Mod APK or InstaMod APK. Some people might want to download Instagram 2 APK because they are not satisfied with the limitations or restrictions of the official app, or because they want to try something new and different.
-In this article, we will explain what you can do with Instagram 2 APK, how to download and install it on your Android device, and what are the risks and benefits of using it. We will also answer some frequently asked questions about Instagram 2 APK. Let's get started!
- Instagram Features: What You Can Do with the Official App
-Before we dive into the features of Instagram 2 APK, let's review what you can do with the official Instagram app. Here are some of the features that make Instagram a great social media platform:
-
-- Reels: Reels are short-form videos that you can create and share with your friends or anyone on Instagram. You can add music, filters, stickers, text, and other effects to make your reels fun and entertaining.
-- Stories: Stories are posts that disappear after 24 hours. You can share moments from your everyday life in your stories, such as photos, videos, boomerangs, polls, quizzes, etc. You can also watch stories from other people you follow.
-- Messenger: Messenger is a feature that allows you to send photos, videos, messages, voice notes, and more privately to your friends or groups. You can also make video calls or join chat rooms with up to 50 people.
-- Shopping: Shopping is a feature that allows you to browse and buy products from your favorite brands and creators on Instagram. You can also create your own shop and sell your products to your followers.
-- Search & Explore: Search & Explore is a feature that helps you discover content and creators based on your interests. You can search for hashtags, keywords, locations, accounts, or browse through different categories.
-
-These are just some of the features that Instagram offers. There are many more features that you can explore and enjoy on the app.
-instagram 2 apk free download latest version
-instagram 2 apk download for android aptoide
-instagram 2 apk download 2023 updated
-instagram 2 apk download com.instagram.android
-instagram 2 apk download help.instagram.com
-instagram 2 apk download apkcombo
-instagram 2 apk download for android 4.1
-instagram 2 apk download 289.0.0.0.11
-instagram 2 apk download social media app
-instagram 2 apk download create share enjoy
-instagram 2 apk download modded version
-instagram 2 apk download no root required
-instagram 2 apk download dual account feature
-instagram 2 apk download unlimited likes and followers
-instagram 2 apk download with dark mode
-instagram 2 apk download with reels and stories
-instagram 2 apk download with filters and stickers
-instagram 2 apk download with direct messages and video calls
-instagram 2 apk download with live streaming and IGTV
-instagram 2 apk download with explore and shop tabs
-instagram 2 apk download with privacy and security settings
-instagram 2 apk download with notifications and insights
-instagram 2 apk download with backup and restore options
-instagram 2 apk download with offline mode and cache cleaner
-instagram 2 apk download with multiple languages and fonts
-instagram 2 apk download with custom themes and icons
-instagram 2 apk download with anti-ban and anti-spam features
-instagram 2 apk download with bug fixes and performance improvements
-instagram 2 apk download for android tablet and tv
-instagram 2 apk download for android emulator and pc
- Instagram Mod APK: What You Can Do with the Modified App
-Now that we have covered what you can do with the official Instagram app, let's see what you can do with Instagram 2 APK or InstaMod APK. Here are some of the features that make Instagram 2 APK or InstaMod APK different from the official app:
-
-- Remove ads: Instagram 2 APK allows you to remove all the ads that appear on your feed, stories, reels, and explore page. This way, you can enjoy a more smooth and uninterrupted Instagram experience.
-- Hide views: Instagram 2 APK allows you to hide the number of views, likes, and comments on your posts and stories. This way, you can avoid the pressure of social comparison and focus on the content itself.
-- Download media: Instagram 2 APK allows you to download any photo, video, story, reel, or IGTV that you see on Instagram. You can save them to your device or share them with other apps.
-- Disable stories: Instagram 2 APK allows you to disable the stories feature completely. This way, you can avoid seeing or posting any stories on Instagram.
-- Customize app: Instagram 2 APK allows you to customize the appearance and functionality of your app. You can change the theme, icon, font, layout, and more according to your preferences.
-
-These are just some of the features that Instagram 2 APK or InstaMod APK offers. There are many more features that you can discover and use on the app.
- How to Download and Install Instagram 2 APK on Your Android Device
-If you are interested in trying out Instagram 2 APK or InstaMod APK, you will need to download and install it on your Android device. Here are the steps that you need to follow:
-
-- Uninstall the official Instagram app: Before you install Instagram 2 APK or InstaMod APK, you will need to uninstall the official Instagram app from your device. This is because you cannot have two versions of the same app on your device.
-- Download Instagram 2 APK or InstaMod APK: Next, you will need to download the latest version of Instagram 2 APK or InstaMod APK from a reliable source. You can search for it on Google or use this link: . Make sure that you download the file from a trusted and secure website.
-- Enable unknown sources: After you download the file, you will need to enable unknown sources on your device. This is because Android does not allow installing apps from sources other than the Google Play Store by default. To enable unknown sources, go to Settings > Security > Unknown Sources and toggle it on.
-- Install Instagram 2 APK or InstaMod APK: Finally, you will need to install Instagram 2 APK or InstaMod APK on your device. To do this, locate the downloaded file in your file manager and tap on it. Follow the instructions on the screen and wait for the installation to complete.
-
-Congratulations! You have successfully installed Instagram 2 APK or InstaMod APK on your device. You can now open the app and log in with your existing account or create a new one.
- Risks and Benefits of Using Instagram 2 APK
-Using Instagram 2 APK or InstaMod APK can have some risks and benefits that you should be aware of before using it. Here are some of them:
-