Commit
·
aaae815
1
Parent(s):
dfa9a4e
Update parquet files (step 17 of 397)
Browse filesThis view is limited to 50 files because it contains too many changes.
See raw diff
- spaces/1acneusushi/gradio-2dmoleculeeditor/data/Crack Keygen Inventor Nesting 2016 Portable __LINK__.md +0 -24
- spaces/1acneusushi/gradio-2dmoleculeeditor/data/DXCPL Download for PES 2016 Crack How to Make Your Game Look Amazing.md +0 -156
- spaces/1acneusushi/gradio-2dmoleculeeditor/data/EaseUS Data Recovery Wizard Pro 11 Serial Key 2018.md +0 -151
- spaces/1phancelerku/anime-remove-background/AetherSX2 beta apk A guide to the best PS2 emulator on the Google Play Store.md +0 -117
- spaces/1phancelerku/anime-remove-background/Disfruta del juego de moto traffic rider apk un juego de conduccin increble con grficos espectaculares.md +0 -17
- spaces/1phancelerku/anime-remove-background/Enjoy Car Parking Multiplayer with Friends - Get the Latest APK Here.md +0 -112
- spaces/801artistry/RVC801/extract_locale.py +0 -34
- spaces/AIConsultant/MusicGen/audiocraft/quantization/base.py +0 -99
- spaces/AIFILMS/generate_human_motion/VQ-Trans/models/pos_encoding.py +0 -43
- spaces/AIWaves/Software_Company/src/agents/Memory/__init__.py +0 -1
- spaces/AIWaves/Software_Company/src/agents/SOP.py +0 -296
- spaces/AchyuthGamer/OpenGPT/g4f/Provider/Providers/Easychat.py +0 -55
- spaces/AgentVerse/agentVerse/ui/src/phaser3-rex-plugins/plugins/raycaster-plugin.d.ts +0 -8
- spaces/AgentVerse/agentVerse/ui/src/phaser3-rex-plugins/templates/spinner/cube/Cube.js +0 -57
- spaces/AgentVerse/agentVerse/ui/src/phaser3-rex-plugins/templates/spinner/spinner-plugin.d.ts +0 -87
- spaces/AgentVerse/agentVerse/ui/src/phaser3-rex-plugins/templates/ui/colorinput/colorpicker/methods/HPaletteCanvas.js +0 -107
- spaces/AlbertoFH98/CastenaApp/app.py +0 -97
- spaces/Alisonbakers/Fml/Dockerfile +0 -21
- spaces/Aloento/9Nine-PITS/text/frontend/tone_sandhi.py +0 -348
- spaces/AlphonseBrandon/speecht5-tts-demo/README.md +0 -14
- spaces/Androidonnxfork/CivitAi-to-Diffusers/diffusers/docs/source/en/training/dreambooth.md +0 -707
- spaces/Androidonnxfork/CivitAi-to-Diffusers/diffusers/src/diffusers/pipelines/alt_diffusion/modeling_roberta_series.py +0 -124
- spaces/Androidonnxfork/CivitAi-to-Diffusers/diffusers/tests/pipelines/shap_e/test_shap_e.py +0 -265
- spaces/Andy1621/uniformer_video_demo/README.md +0 -13
- spaces/AnishKumbhar/ChatBot/text-generation-webui-main/extensions/superboogav2/api.py +0 -207
- spaces/Ataturk-Chatbot/HuggingFaceChat/venv/lib/python3.11/site-packages/pip/_vendor/webencodings/labels.py +0 -231
- spaces/Awiny/Image2Paragraph/models/blip2_model.py +0 -46
- spaces/Awiny/Image2Paragraph/models/grit_src/third_party/CenterNet2/detectron2/layers/csrc/cocoeval/cocoeval.h +0 -88
- spaces/Ayaka-daisuki/anime-remove-background/README.md +0 -14
- spaces/Basil2k4/botbasil203/Dockerfile +0 -106
- spaces/Big-Web/MMSD/env/Lib/site-packages/setuptools/_vendor/tomli/_parser.py +0 -691
- spaces/Blealtan/clip-guided-binary-autoencoder/app.py +0 -327
- spaces/CVPR/Dual-Key_Backdoor_Attacks/datagen/detectron2/tests/test_fast_rcnn.py +0 -98
- spaces/CVPR/Dual-Key_Backdoor_Attacks/openvqa/openvqa/models/mmnasnet/model_cfgs.py +0 -28
- spaces/CVPR/LIVE/thrust/thrust/mr/fancy_pointer_resource.h +0 -61
- spaces/CVPR/LIVE/thrust/thrust/system/detail/generic/transform_reduce.h +0 -53
- spaces/CVPR/lama-example/bin/analyze_errors.py +0 -316
- spaces/CVPR/lama-example/saicinpainting/evaluation/data.py +0 -167
- spaces/CVPR/monoscene_lite/monoscene/.ipynb_checkpoints/unet3d_kitti-checkpoint.py +0 -88
- spaces/CVPR/regionclip-demo/detectron2/export/api.py +0 -273
- spaces/CVPR/unicl-zero-shot-img-recog/model/image_encoder/__init__.py +0 -1
- spaces/CaliforniaHealthCollaborative/README/README.md +0 -43
- spaces/Chaitanya01/InvestingPlatform/mapping.py +0 -0
- spaces/CikeyQI/Yunzai/Yunzai/renderers/puppeteer/lib/puppeteer.js +0 -321
- spaces/Cletrason/cloudqi-cqi_text_to_image_pt_v0/README.md +0 -12
- spaces/CofAI/chat.b4/client/css/dropdown.css +0 -10
- spaces/CorvaeOboro/gen_ability_icon/README.md +0 -17
- spaces/DQChoi/gpt-demo/venv/lib/python3.11/site-packages/fastapi/exceptions.py +0 -49
- spaces/DQChoi/gpt-demo/venv/lib/python3.11/site-packages/gradio/templates/cdn/assets/index-322e8a8e.css +0 -1
- spaces/DQChoi/gpt-demo/venv/lib/python3.11/site-packages/gradio/templates/cdn/assets/index-aa3a045c.js +0 -2
spaces/1acneusushi/gradio-2dmoleculeeditor/data/Crack Keygen Inventor Nesting 2016 Portable __LINK__.md
DELETED
@@ -1,24 +0,0 @@
|
|
1 |
-
|
2 |
-
<h1>How to Use Inventor Nesting 2016 Portable for Efficient Material Optimization</h1>
|
3 |
-
<p>Inventor Nesting 2016 Portable is a software that helps you optimize yield from flat raw material by generating multiple sheet nests in a single study. It is integrated within Inventor Professional and allows you to compare the efficiency and costs associated with different nesting studies to maximize job profitability. You can also export 3D models or DXF files of the completed nest for cutting path generation.</p>
|
4 |
-
<h2>Crack Keygen Inventor Nesting 2016 Portable</h2><br /><p><b><b>Download Zip</b> ✏ <a href="https://byltly.com/2uKyju">https://byltly.com/2uKyju</a></b></p><br /><br />
|
5 |
-
<p>In this article, we will show you how to use Inventor Nesting 2016 Portable for efficient material optimization in four easy steps:</p>
|
6 |
-
<ol>
|
7 |
-
<li>Create a nesting file and extract shapes from a source file.</li>
|
8 |
-
<li>Define the nesting parameters and generate nests.</li>
|
9 |
-
<li>Compare and select the best nesting study.</li>
|
10 |
-
<li>Export the nested results as 3D models or DXF files.</li>
|
11 |
-
</ol>
|
12 |
-
<h2>Step 1: Create a nesting file and extract shapes from a source file</h2>
|
13 |
-
<p>To create a nesting file, open Inventor Professional and select <strong>New</strong> from the <strong>File</strong> menu. Then, select <strong>Nesting File</strong> from the <strong>New File</strong> dialog box and click <strong>Create</strong>. A new nesting file will be created with a default name.</p>
|
14 |
-
<p>To extract shapes from a source file, select <strong>Extract Shapes</strong> from the <strong>Nesting</strong> ribbon tab. Then, browse to the source file that contains the shapes you want to nest. You can use any Inventor part or assembly file, or any generic CAD file that can be imported into Inventor. The <strong>Extract Shapes</strong> dialog box will appear, where you can select the shapes you want to extract and specify their properties, such as quantity, material, orientation, and grain direction. Click <strong>OK</strong> to extract the shapes and add them to the nesting file.</p>
|
15 |
-
<h2>Step 2: Define the nesting parameters and generate nests</h2>
|
16 |
-
<p>To define the nesting parameters, select <strong>Create Nest Study</strong> from the <strong>Nesting</strong> ribbon tab. The <strong>Create Nest Study</strong> dialog box will appear, where you can enter a name for the nest study and select the sources you want to include in it. You can also enable the option to automatically manage nests based on the sources' materials.</p>
|
17 |
-
<p>To generate nests, click <strong>Create Nests</strong> in the <strong>Create Nest Study</strong> dialog box. The <strong>Edit Nest Study</strong> dialog box will appear, where you can specify the parameters for each nest, such as sheet size, sheet gap, part gap, rotation angle, and alignment. You can also preview the nest layout and edit individual nests if needed. Click <strong>Create Nests</strong> to generate the nests based on the parameters you defined.</p>
|
18 |
-
<h2>Step 3: Compare and select the best nesting study</h2>
|
19 |
-
<p>To compare and select the best nesting study, right-click on the nest study node in the browser and select <strong>Compare Nest Studies</strong>. The <strong>Nest Study Comparison Report</strong> dialog box will appear, where you can see a summary of the efficiency and costs of each nest study. You can also see detailed information for each nest, such as sheet utilization, material waste, number of parts, number of sheets, and total area. You can sort and filter the data by clicking on the column headers. To select the best nesting study, click on its row in the table and click <strong>Select Best Nest Study</strong>. The selected nest study will be highlighted in green in the browser.</p>
|
20 |
-
<h2>Step 4: Export the nested results as 3D models or DXF files</h2>
|
21 |
-
<p>To export the nested results as 3D models or DXF files, right-click on the nest node in the browser and select <strong>Create 3D Model</strong> or <strong>Create DXF File</strong>. The <strong>Create 3D Model Options</strong> or <strong>Create DXF File Options</strong> dialog box will appear, where you can specify the options for exporting the nested results. For example,</p>
|
22 |
-
<p></p> cec2833e83<br />
|
23 |
-
<br />
|
24 |
-
<br />
|
|
|
|
|
|
|
|
|
|
|
|
|
|
|
|
|
|
|
|
|
|
|
|
|
|
|
|
|
|
|
|
|
|
|
|
|
|
|
|
|
|
|
|
|
|
|
|
|
|
spaces/1acneusushi/gradio-2dmoleculeeditor/data/DXCPL Download for PES 2016 Crack How to Make Your Game Look Amazing.md
DELETED
@@ -1,156 +0,0 @@
|
|
1 |
-
|
2 |
-
<h1>How to Download and Use DXCPL for PES 2016 Crack</h1>
|
3 |
-
<h2>Introduction</h2>
|
4 |
-
<p>If you are a fan of Pro Evolution Soccer (PES) games, you might have heard of PES 2016 crack. This is a modified version of the original game that allows you to play it for free without buying a license key. However, some users may encounter problems when trying to run PES 2016 crack on their PCs, especially if they have low-end graphics cards. This is where DXCPL comes in handy.</p>
|
5 |
-
<h2>dxcpldownloadforpes2016crack</h2><br /><p><b><b>DOWNLOAD</b> ::: <a href="https://byltly.com/2uKwEh">https://byltly.com/2uKwEh</a></b></p><br /><br />
|
6 |
-
<h3>What is DXCPL and why do you need it for PES 2016 crack?</h3>
|
7 |
-
<p>DXCPL is a tool that lets you change the DirectX settings of your PC. DirectX is a software that enables your PC to run games and other multimedia applications. By changing the DirectX settings, you can improve the performance and compatibility of your games. For example, you can lower the graphics quality, disable some features, or force some options that are not available in the game settings.</p>
|
8 |
-
<p>DXCPL is useful for PES 2016 crack because it can help you fix some issues that may prevent you from playing the game smoothly. For instance, you can use DXCPL to enable the Force WARP option, which allows you to run the game even if your graphics card does not support DirectX 11. You can also use DXCPL to disable some effects that may cause lag or crashes, such as anti-aliasing, shadows, or reflections.</p>
|
9 |
-
<h3>What are the benefits of using DXCPL for PES 2016 crack?</h3>
|
10 |
-
<p>By using DXCPL for PES 2016 crack, you can enjoy the following benefits:</p>
|
11 |
-
<p>dxcpl download for pes 2016 full crack<br />
|
12 |
-
dxcpl exe download for pes 2016 crack<br />
|
13 |
-
dxcpl directx 11 emulator for pes 2016 crack<br />
|
14 |
-
dxcpl rar download for pes 2016 crack<br />
|
15 |
-
dxcpl 32 bit download for pes 2016 crack<br />
|
16 |
-
dxcpl windows 10 download for pes 2016 crack<br />
|
17 |
-
dxcpl windows 7 download for pes 2016 crack<br />
|
18 |
-
dxcpl fix vram problem for pes 2016 crack<br />
|
19 |
-
dxcpl sdkencryptedappticket dll for pes 2016 crack<br />
|
20 |
-
dxcpl video converter for pes 2016 crack<br />
|
21 |
-
dxcpl online download for pes 2016 crack<br />
|
22 |
-
dxcpl full version download for pes 2016 crack<br />
|
23 |
-
dxcpl free download ps3 for pes 2016 crack<br />
|
24 |
-
dxcpl mac free download for pes 2016 crack<br />
|
25 |
-
dxcpl android free download for pes 2016 crack<br />
|
26 |
-
dxcpl iphone free download for pes 2016 crack<br />
|
27 |
-
dxcpl full crack video converter for pes 2016 crack<br />
|
28 |
-
dxcpl net framework for pes 2016 crack<br />
|
29 |
-
dxcpl direct x for pes 2016 crack<br />
|
30 |
-
dxcpl vcredist for pes 2016 crack<br />
|
31 |
-
dxcpl flash player for pes 2016 crack<br />
|
32 |
-
dxcpl java for browser for pes 2016 crack<br />
|
33 |
-
dxcpl no steam file for pes 2016 crack<br />
|
34 |
-
dxcpl hack kernow for pes 2016 crack<br />
|
35 |
-
dxcpl lexcliq article for pes 2016 crack<br />
|
36 |
-
dxcpl kercratinre blog for pes 2016 crack<br />
|
37 |
-
dxcpl bechde pokhara website for pes 2016 crack<br />
|
38 |
-
dxcpl google drive link for pes 2016 crack<br />
|
39 |
-
dxcpl youtube video tutorial for pes 2016 crack<br />
|
40 |
-
dxcpl step by step guide for pes 2016 crack<br />
|
41 |
-
how to use dxcpl for pes 2016 crack<br />
|
42 |
-
how to install dxcpl for pes 2016 crack<br />
|
43 |
-
how to fix error with dxcpl for pes 2016 crack<br />
|
44 |
-
how to run game with dxcpl for pes 2016 crack<br />
|
45 |
-
how to increase vram with dxcpl for pes 2016 crack<br />
|
46 |
-
how to enable directx with dxcpl for pes 2016 crack<br />
|
47 |
-
how to solve missing file with dxcpl for pes 2016 crack<br />
|
48 |
-
how to update file with dxcpl for pes 2016 crack<br />
|
49 |
-
how to backup file with dxcpl for pes 2016 crack<br />
|
50 |
-
how to restore file with dxcpl for pes 2016 crack<br />
|
51 |
-
how to exclude folder with dxcpl for pes 2016 crack<br />
|
52 |
-
how to optimize performance with dxcpl for pes 2016 crack<br />
|
53 |
-
how to play online with dxcpl for pes 2016 crack<br />
|
54 |
-
how to convert video with dxcpl for pes 2016 crack<br />
|
55 |
-
how to download latest version of dxcpl for pes 2016 crack</p>
|
56 |
-
<ul>
|
57 |
-
<li>You can play PES 2016 crack on any PC, regardless of your graphics card specifications.</li>
|
58 |
-
<li>You can improve the performance and stability of PES 2016 crack by adjusting the graphics settings according to your preferences.</li>
|
59 |
-
<li>You can avoid errors and glitches that may occur when running PES 2016 crack without DXCPL.</li>
|
60 |
-
</ul>
|
61 |
-
<p>Now that you know what DXCPL is and why you need it for PES 2016 crack, let's see how you can download and use it.</p>
|
62 |
-
<h2>How to Download DXCPL for PES 2016 Crack</h2>
|
63 |
-
<h3>Where to find the DXCPL download link for PES 2016 crack</h3>
|
64 |
-
<p>The first step is to download DXCPL from a reliable source. There are many websites that offer DXCPL downloads, but some of them may contain viruses or malware that can harm your PC. Therefore, you should be careful when choosing where to download DXCPL from.</p>
|
65 |
-
<p>One of the safest and easiest ways to download DXCPL is to use this link: <a href="https://www.mediafire.com/file/9x9x9x9x9x9x9x9/dxcpl.rar/file">https://www.mediafire.com/file/9x9x9x9x9x9x9x9/dxcpl.rar/file</a>. This link will take you to a MediaFire page where you can download a compressed file named dxcpl.rar. This file contains the DXCPL executable file and a readme.txt file that explains how to use it.</p>
|
66 |
-
<h3>How to install DXCPL on your PC</h3>
|
67 |
-
<p>The next step is to install DXCPL on your PC. To do this, follow these steps:</p>
|
68 |
-
<ol>
|
69 |
-
<li>Extract the dxcpl.rar file using a program like WinRAR or 7-Zip. You will get a folder named dxcpl with two files inside: dxcpl.exe and readme.txt.</li>
|
70 |
-
<li>Copy the dxcpl.exe file and paste it in a location where you can easily access it. For example, you can paste it on your desktop or in your Documents folder.</li>
|
71 |
-
<li>Double-click on the dxcpl.exe file to run it. You will see a window like this:</li>
|
72 |
-
</ol>
|
73 |
-
<img src="https://i.imgur.com/4Qw8XnD.png" alt="DXCPL window" width="500" height="400">
|
74 |
-
<p>Congratulations! You have successfully installed DXCPL on your PC. Now let's see how you can use it for PES 2016 crack.</p>
|
75 |
-
<h2>How to Use DXCPL for PES 2016 Crack</h2>
|
76 |
-
<h3>How to configure DXCPL settings for PES 2016 crack</h3>
|
77 |
-
<p>The first thing you need to do is to configure the DXCPL settings for PES 2016 crack. To do this, follow these steps:</p>
|
78 |
-
<ol>
|
79 |
-
<li>In the DXCPL window, click on the Edit List button at the top right corner. You will see a window like this:</li>
|
80 |
-
</ol>
|
81 |
-
<img src="https://i.imgur.com/0ZyqZ0L.png" alt="Edit List window" width="500" height="400">
|
82 |
-
<ol start="2">
|
83 |
-
<li>Click on the ... button at the bottom right corner. You will see a window like this:</li>
|
84 |
-
</ol>
|
85 |
-
<img src="https://i.imgur.com/8v5JgYr.png" alt="Browse window" width="500" height="400">
|
86 |
-
<ol start="3">
|
87 |
-
<li>Navigate to the folder where you have installed PES 2016 crack. For example, if you have installed it in C:\Program Files (x86)\Pro Evolution Soccer 2016\, go to that folder.</li>
|
88 |
-
<li>Select the pes2016.exe file and click on Open. You will see something like this:</li>
|
89 |
-
</ol>
|
90 |
-
<img src="https://i.imgur.com/0ZyqZ0L.png" alt="Edit List window with pes2016.exe added" width="500" height="400">
|
91 |
-
<ol start="5">
|
92 |
-
<li>Click on OK. You will see something like this:</li>
|
93 |
-
</ol>
|
94 |
-
<img src="https://i.imgur.com/4Qw8XnD.png" alt="DXCPL window with pes2016.exe added" width="500" height="400">
|
95 |
-
<ol start="6">
|
96 |
-
<li>In the Feature level limit section, select one of the options from the drop-down menu according to your graphics card capabilities. For example, if your graphics card supports DirectX 11, select 11_0; if it supports DirectX 10, select 10_0; if it supports DirectX 9, select 9_1; and so on.</li>
|
97 |
-
<li>In the Device settings section, check the box next to Force WARP. This will enable you to run PES 2016 crack even if your graphics card does not support DirectX 11.</li>
|
98 |
-
<li>In the Debug layer section, check the box next to Force ON. This will help you avoid errors and glitches when running PES 2016 crack with DXCPL.</li>
|
99 |
-
<li>In the Feature switches section, uncheck all the boxes except Disable feature level upgrade. This will disable some effects that may cause lag or crashes when running PES 2016 crack with DXCPL.</li>
|
100 |
-
<li>Click on Apply and then OK. You have successfully configured the DXCPL settings for PES 2016 crack.</li>
|
101 |
-
</ol>
|
102 |
-
<h3>How to run PES 2016 crack with DXCPL</h3>
|
103 |
-
<p>The final step is to run PES 2016 crack with DXCPL. To do this, follow these steps:</p>
|
104 |
-
<ol>
|
105 |
-
<li>Make sure that both dxcpl.exe and pes2016.exe are running as administrator. To do this, right-click on each file and select Run as administrator.</li>
|
106 |
-
<img src="https://i.imgur.com/6qWl0ZS.png" alt="PES 2016 crack launcher" width="500" height="400">
|
107 |
-
<ol start="3">
|
108 |
-
<li>Click on Play. You will see something like this:</li>
|
109 |
-
</ol>
|
110 |
-
<img src="https://i.imgur.com/4f7X9Zy.png" alt="PES 2016 crack loading screen" width="500" height="400">
|
111 |
-
<ol start="4">
|
112 |
-
<li>Wait for the game to load. You will see something like this:</li>
|
113 |
-
</ol>
|
114 |
-
<img src="https://i.imgur.com/8Xwq3tL.png" alt="PES 2016 crack main menu" width="500" height="400">
|
115 |
-
<ol start="5">
|
116 |
-
<li>Enjoy playing PES 2016 crack with DXCPL!</li>
|
117 |
-
</ol>
|
118 |
-
<h2>Troubleshooting Tips for DXCPL and PES 2016 Crack</h2>
|
119 |
-
<p>Although DXCPL can help you run PES 2016 crack on your PC, you may still encounter some problems or errors. Here are some troubleshooting tips that may help you fix them.</p>
|
120 |
-
<h3>What to do if DXCPL does not work for PES 2016 crack</h3>
|
121 |
-
<p>If DXCPL does not work for PES 2016 crack, you may try the following solutions:</p>
|
122 |
-
<ul>
|
123 |
-
<li>Make sure that you have downloaded DXCPL from a reliable source and that it is not corrupted or infected by viruses or malware.</li>
|
124 |
-
<li>Make sure that you have configured the DXCPL settings correctly according to your graphics card capabilities and preferences.</li>
|
125 |
-
<li>Make sure that you have run both dxcpl.exe and pes2016.exe as administrator.</li>
|
126 |
-
<li>Make sure that you have closed any other programs or applications that may interfere with DXCPL or PES 2016 crack.</li>
|
127 |
-
<li>Make sure that your PC meets the minimum system requirements for PES 2016 crack. You can check them here: <a href="https://www.systemrequirementslab.com/cyri/requirements/pro-evolution-soccer-2016/13064">https://www.systemrequirementslab.com/cyri/requirements/pro-evolution-soccer-2016/13064</a>.</li>
|
128 |
-
<li>Restart your PC and try again.</li>
|
129 |
-
</ul>
|
130 |
-
<h3>What to do if PES 2016 crack does not run with DXCPL</h3>
|
131 |
-
<p>If PES 2016 crack does not run with DXCPL, you may try the following solutions:</p>
|
132 |
-
<ul>
|
133 |
-
<li>Make sure that you have downloaded PES 2016 crack from a reliable source and that it is not corrupted or infected by viruses or malware.</li>
|
134 |
-
<li>Make sure that you have installed PES 2016 crack correctly and that it is not missing any files or components.</li>
|
135 |
-
<li>Make sure that you have updated PES 2016 crack to the latest version and that it is compatible with DXCPL.</li>
|
136 |
-
<li>Make sure that you have disabled any antivirus or firewall software that may block or delete PES 2016 crack or DXCPL.</li>
|
137 |
-
<li>Make sure that you have applied any patches or fixes that may improve the performance and compatibility of PES 2016 crack or DXCPL.</li>
|
138 |
-
<li>Restart your PC and try again.</li>
|
139 |
-
</ul>
|
140 |
-
<h2>Conclusion</h2>
|
141 |
-
<p>In this article, we have shown you how to download and use DXCPL for PES 2016 crack. We have explained what DXCPL is, why you need it for PES 2016 crack, how to configure it, how to run it, and how to troubleshoot it. We hope that this article has been helpful and informative for you. If you have any questions or feedback, please feel free to leave a comment below. Thank you for reading!</p>
|
142 |
-
<h2>FAQs</h2>
|
143 |
-
<p>Here are some frequently asked questions about DXCPL and PES 2016 crack:</p>
|
144 |
-
<h3>Is DXCPL safe to use?</h3>
|
145 |
-
<p>Yes, DXCPL is safe to use as long as you download it from a reliable source and scan it with an antivirus software before using it. However, you should be careful when changing the DirectX settings of your PC, as some options may cause instability or damage to your system. Always backup your data and create a restore point before using DXCPL.</p>
|
146 |
-
<h3>Is PES 2016 crack legal to use?</h3>
|
147 |
-
<p>No, PES 2016 crack is not legal to use. It is a modified version of the original game that bypasses the license key verification process. This violates the terms and conditions of the game developer and publisher, Konami. By using PES 2016 crack, you are infringing their intellectual property rights and risking legal action. We do not condone or encourage the use of PES 2016 crack or any other pirated software. If you want to play PES 2016 legally, you should buy a license key from an authorized seller.</p>
|
148 |
-
<h3>Can I use DXCPL for other games besides PES 2016 crack?</h3>
|
149 |
-
<p>Yes, you can use DXCPL for other games besides PES 2016 crack. However, not all games will work with DXCPL, as some games may have different DirectX requirements or compatibility issues. You should always check the game specifications and reviews before using DXCPL for them. You should also test the game performance and stability with different DXCPL settings before playing them.</p>
|
150 |
-
<h3>Can I use other tools besides DXCPL for PES 2016 crack?</h3>
|
151 |
-
<p>Yes, you can use other tools besides DXCPL for PES 2016 crack. However, not all tools will work with PES 2016 crack, as some tools may have different functions or compatibility issues. You should always check the tool specifications and reviews before using them for PES 2016 crack. You should also test the tool performance and stability with different settings before using them.</p>
|
152 |
-
<h3>Where can I find more information about DXCPL and PES 2016 crack?</h3>
|
153 |
-
<p>You can find more information about DXCPL and PES 2016 crack on various websites, forums, blogs, videos, or social media platforms. However, you should be careful when accessing these sources, as some of them may contain inaccurate, outdated, misleading, or harmful information. You should always verify the credibility and reliability of these sources before trusting them. You should also avoid clicking on any suspicious links or downloading any unknown files from these sources.</p>
|
154 |
-
</p> 0a6ba089eb<br />
|
155 |
-
<br />
|
156 |
-
<br />
|
|
|
|
|
|
|
|
|
|
|
|
|
|
|
|
|
|
|
|
|
|
|
|
|
|
|
|
|
|
|
|
|
|
|
|
|
|
|
|
|
|
|
|
|
|
|
|
|
|
|
|
|
|
|
|
|
|
|
|
|
|
|
|
|
|
|
|
|
|
|
|
|
|
|
|
|
|
|
|
|
|
|
|
|
|
|
|
|
|
|
|
|
|
|
|
|
|
|
|
|
|
|
|
|
|
|
|
|
|
|
|
|
|
|
|
|
|
|
|
|
|
|
|
|
|
|
|
|
|
|
|
|
|
|
|
|
|
|
|
|
|
|
|
|
|
|
|
|
|
|
|
|
|
|
|
|
|
|
|
|
|
|
|
|
|
|
|
|
|
|
|
|
|
|
|
|
|
|
|
|
|
|
|
|
|
|
|
|
|
|
|
|
|
|
|
|
|
|
|
|
|
|
|
|
|
|
|
|
|
|
|
|
|
|
|
|
|
|
|
|
|
|
|
|
|
|
|
|
|
|
|
|
|
|
|
|
|
|
|
|
|
|
|
|
|
|
|
|
|
|
|
|
|
|
|
|
|
|
|
|
|
|
|
|
|
|
|
|
|
|
|
|
|
|
|
|
|
|
|
|
|
|
|
|
|
|
|
|
|
|
|
|
|
|
|
|
|
|
|
|
|
|
|
|
|
|
|
|
|
|
|
|
|
spaces/1acneusushi/gradio-2dmoleculeeditor/data/EaseUS Data Recovery Wizard Pro 11 Serial Key 2018.md
DELETED
@@ -1,151 +0,0 @@
|
|
1 |
-
<br />
|
2 |
-
<h1>EaseUS Data Recovery Wizard Pro 11 Serial Key 2018</h1>
|
3 |
-
<h2>Introduction</h2>
|
4 |
-
<p>Have you ever lost your important data due to accidental deletion, formatting, virus attack, or other reasons? If so, you know how frustrating and stressful it can be to recover your lost files. Fortunately, there is a software that can help you with this problem: <strong>EaseUS Data Recovery Wizard Pro 11</strong>.</p>
|
5 |
-
<h2>EaseUS Data Recovery Wizard Pro 11 Serial Key 2018</h2><br /><p><b><b>Download File</b> → <a href="https://byltly.com/2uKyDQ">https://byltly.com/2uKyDQ</a></b></p><br /><br />
|
6 |
-
<h3>What is EaseUS Data Recovery Wizard Pro 11?</h3>
|
7 |
-
<p>EaseUS Data Recovery Wizard Pro 11 is a powerful and professional data recovery software that can recover deleted, formatted, or inaccessible data from various devices, such as PC, laptop, hard drive, SSD, USB drive, memory card, digital camera, mobile phone, etc. It supports more than 1000 file types, including photos, videos, documents, emails, audio, archives, etc. It also has advanced features like partition recovery, raw recovery, bootable media recovery, etc.</p>
|
8 |
-
<h3>Why do you need a serial key for EaseUS Data Recovery Wizard Pro 11?</h3>
|
9 |
-
<p>EaseUS Data Recovery Wizard Pro 11 has both a free and a paid version. The free version allows you to recover up to 2 GB of data for free in various data loss scenarios. However, if you want to recover unlimited data with a higher success rate and more features, you need to upgrade to the paid version. To do that, you need a serial key for EaseUS Data Recovery Wizard Pro 11.</p>
|
10 |
-
<p>A serial key is a unique code that activates the full version of the software. It usually consists of letters and numbers. You can get a serial key by purchasing the software from the official website or from other authorized sellers. However, if you don't want to spend money on the software, there are some other ways to get a serial key for free.</p>
|
11 |
-
<p>EaseUS Data Recovery Wizard Pro 11 License Code 2018<br />
|
12 |
-
EaseUS Data Recovery Wizard Pro 11 Activation Code 2018<br />
|
13 |
-
EaseUS Data Recovery Wizard Pro 11 Crack 2018<br />
|
14 |
-
EaseUS Data Recovery Wizard Pro 11 Keygen 2018<br />
|
15 |
-
EaseUS Data Recovery Wizard Pro 11 Free Download 2018<br />
|
16 |
-
EaseUS Data Recovery Wizard Pro 11 Full Version 2018<br />
|
17 |
-
EaseUS Data Recovery Wizard Pro 11 Patch 2018<br />
|
18 |
-
EaseUS Data Recovery Wizard Pro 11 Registration Code 2018<br />
|
19 |
-
EaseUS Data Recovery Wizard Pro 11 Product Key 2018<br />
|
20 |
-
EaseUS Data Recovery Wizard Pro 11 Torrent 2018<br />
|
21 |
-
EaseUS Data Recovery Wizard Pro 11 Serial Number 2018<br />
|
22 |
-
EaseUS Data Recovery Wizard Pro 11 License Key Generator 2018<br />
|
23 |
-
EaseUS Data Recovery Wizard Pro 11 Activation Key Generator 2018<br />
|
24 |
-
EaseUS Data Recovery Wizard Pro 11 Crack Download 2018<br />
|
25 |
-
EaseUS Data Recovery Wizard Pro 11 Keygen Download 2018<br />
|
26 |
-
EaseUS Data Recovery Wizard Pro 11 Free License Code 2018<br />
|
27 |
-
EaseUS Data Recovery Wizard Pro 11 Free Activation Code 2018<br />
|
28 |
-
EaseUS Data Recovery Wizard Pro 11 Free Crack 2018<br />
|
29 |
-
EaseUS Data Recovery Wizard Pro 11 Free Keygen 2018<br />
|
30 |
-
EaseUS Data Recovery Wizard Pro 11 Free Full Version Download 2018<br />
|
31 |
-
How to Get EaseUS Data Recovery Wizard Pro 11 Serial Key for Free in 2018<br />
|
32 |
-
How to Activate EaseUS Data Recovery Wizard Pro 11 with Serial Key in 2018<br />
|
33 |
-
How to Crack EaseUS Data Recovery Wizard Pro 11 with Serial Key in 2018<br />
|
34 |
-
How to Use EaseUS Data Recovery Wizard Pro 11 with Serial Key in 2018<br />
|
35 |
-
How to Recover Lost Data with EaseUS Data Recovery Wizard Pro 11 Serial Key in 2018<br />
|
36 |
-
Best Alternative to EaseUS Data Recovery Wizard Pro 11 Serial Key in 2018<br />
|
37 |
-
Is EaseUS Data Recovery Wizard Pro 11 Serial Key Safe to Use in 2018<br />
|
38 |
-
Is EaseUS Data Recovery Wizard Pro 11 Serial Key Legal to Use in 2018<br />
|
39 |
-
Is EaseUS Data Recovery Wizard Pro 11 Serial Key Working in 2018<br />
|
40 |
-
Is EaseUS Data Recovery Wizard Pro 11 Serial Key Genuine in 2018<br />
|
41 |
-
Where to Find EaseUS Data Recovery Wizard Pro 11 Serial Key for Free in 2018<br />
|
42 |
-
Where to Download EaseUS Data Recovery Wizard Pro 11 Serial Key for Free in 2018<br />
|
43 |
-
Where to Buy EaseUS Data Recovery Wizard Pro 11 Serial Key for Cheap in 2018<br />
|
44 |
-
Where to Get Help for EaseUS Data Recovery Wizard Pro 11 Serial Key Issues in </p>
|
45 |
-
<h2>How to get a serial key for EaseUS Data Recovery Wizard Pro 11?</h2>
|
46 |
-
<h3>Method 1: Participate in a giveaway</h3>
|
47 |
-
<p>One of the easiest ways to get a serial key for free is to participate in a giveaway. A giveaway is a promotional event where the software company or other sponsors offer free serial keys or license codes to lucky winners. You can find giveaways on various platforms like YouTube, Instagram, Facebook, Twitter, blogs, etc.</p>
|
48 |
-
<h4>Followchain giveaway</h4>
|
49 |
-
<p>For example, Followchain is a website that specializes in data backup, data recovery, and disk management. They are offering a list of free EaseUS Recovery keys and license codes on their website. To participate in their giveaway, you need to:</p>
|
50 |
-
<ul>
|
51 |
-
<li>Subscribe to Followchain on YouTube.</li>
|
52 |
-
<li>Follow @followchainorg on Instagram.</li>
|
53 |
-
<li>Send a screenshot to @followchainorg on Instagram to prove that you're subscribed to their YouTube channel.</li>
|
54 |
-
</ul>
|
55 |
-
<p>You will then receive a free EaseUS Recovery key or license code via Instagram DM.</p>
|
56 |
-
<h4>Smart Serials giveaway</h4>
|
57 |
-
<p>Another example is Smart Serials, which is a website that provides serial numbers for various software. They have a serial number for EaseUS Data Recovery Wizard 11.9.0 on their website. To get it for free, you need to:</p>
|
58 |
-
<ul>
|
59 |
-
<li>Verify that you're human by completing a captcha.</li>
|
60 |
-
<li>Agree with their disclaimer that states that you will only use the serial number for evaluation purposes and not for commercial use.</li>
|
61 |
-
<li>Copy and paste the serial number into your software activation window.</li>
|
62 |
-
</ul>
|
63 |
-
<p>You will then be able to use the full version of the software.</p>
|
64 |
-
<h3>Method 2: Use a free recovery key or license code</h3>
|
65 |
-
<p>Another way to get a serial key for free is to use a free recovery key or license code. A recovery key or license code is similar to a serial key but it is usually shorter and easier to remember. You can find free recovery keys or license codes on various websites or forums that share them with other users.</p>
|
66 |
-
<h4>List of free recovery keys and license codes</h4>
|
67 |
-
<p>Here is a list of some free recovery keys and license codes that we found online:</p>
|
68 |
-
<table>
|
69 |
-
<tr>
|
70 |
-
<th>Recovery Key/License Code</th>
|
71 |
-
<th>Source</th>
|
72 |
-
</tr>
|
73 |
-
<tr>
|
74 |
-
<td>C8XIP-2YHL2-39UMI-QVR56-4CI6L</td>
|
75 |
-
<td></td>
|
76 |
-
</tr>
|
77 |
-
<tr>
|
78 |
-
<td>JGFT5-YRUHJ-FYT45-TRUGH-GJRTU-YFH45</td>
|
79 |
-
<td></td>
|
80 |
-
</tr>
|
81 |
-
<tr>
|
82 |
-
<td>ZCQW8-ERZHF-IOVNU-WEJDF-KSDHT-UIOHN</td>
|
83 |
-
<td></td>
|
84 |
-
</tr>
|
85 |
-
<tr>
|
86 |
-
<td>CYNT7-GQKOL-UJYHT-BGFRV-CESDW-AZSXD</td>
|
87 |
-
<td></td>
|
88 |
-
</tr>
|
89 |
-
<tr>
|
90 |
-
<td>FUIERUI-REUIE83UW-ERIOE93-TRIOE93</td>
|
91 |
-
<td></td>
|
92 |
-
</tr>
|
93 |
-
<tr>
|
94 |
-
<td>E89237472-20W0W0-2929W-ERIE93I</td>
|
95 |
-
<td></td>
|
96 |
-
</tr>
|
97 |
-
<tr>
|
98 |
-
<td>DFFUR-FGJKDIE-DFJKDIEE-DFJKDIEEJ-ZBDYR-FGJKDIE</td>
|
99 |
-
<td></td>
|
100 |
-
</tr>
|
101 |
-
<tr>
|
102 |
-
<td>DHJDI-DQJKDI-DQJKDIEJD-FJKDIEJD-JKDIUE1-FKDFJE9FJ</td>
|
103 |
-
<td></td>
|
104 |
-
</tr>
|
105 |
-
<tr>
|
106 |
-
<td>DFFUR-FGJKDIE-DFJKDIEE-DFJKDIEEJ-ZBDYR-FGJKDIE</td>
|
107 |
-
<td></td>
|
108 |
-
</tr>
|
109 |
-
<tr>
|
110 |
-
<td>DHJDI-DQJKDI-DQJKDIEJD-FJKDIEJD-JKDIUE1-FKDFJE9FJ</td>
|
111 |
-
<td></td>
|
112 |
-
</tr>
|
113 |
-
</table>
|
114 |
-
<h4>How to use a free recovery key or license code</h4>
|
115 |
-
<p>To use a free recovery key or license code, you need to:</p>
|
116 |
-
<ul>
|
117 |
-
<li>Download and install EaseUS Data Recovery Wizard Pro 11 from the official website or from other trusted sources.</li>
|
118 |
-
<li>Launch the software and click on "Upgrade Now" or "Activate" button.</li>
|
119 |
-
<li>Enter the recovery key or license code into the input box and click on "Activate" button.</li>
|
120 |
-
<li>Wait for the activation process to complete and enjoy the full version of the software.</li>
|
121 |
-
</ul>
|
122 |
-
<h3>Method 3: Use a survey program to earn rewards</h3>
|
123 |
-
<p>A third way to get a serial key for free is to use a survey program to earn rewards. A survey program is an online platform that pays you for completing surveys or other tasks. You can exchange your rewards for cash or gift cards that you can use to buy EaseUS Data Recovery Wizard Pro 11 from the official website or from other authorized sellers.</p>
|
124 |
-
<h4>Survey Junkie</h4>
|
125 |
-
<p>Survey Junkie is one of the most popular survey programs that pays you for sharing your opinions on various topics. You can earn up to $5 per survey and redeem your rewards via PayPal or e-gift cards. To start earning rewards with Survey Junkie, you need to:</p>
|
126 |
-
<ul>
|
127 |
-
<li>Create a free account on Survey Junkie and complete your profile.</li>
|
128 |
-
<li>Verify your email address and start taking surveys that match your interests.</li>
|
129 |
-
<h4>Inbox Dollars</h4>
|
130 |
-
<p>Inbox Dollars is another survey program that pays you for taking online surveys, reading emails, playing games, shopping online, and more. You can earn up to $5 per survey and get a free $5 bonus when you sign up. You can cash out your rewards via PayPal or e-gift cards. To start earning rewards with Inbox Dollars, you need to:</p>
|
131 |
-
<ul>
|
132 |
-
<li>Create a free account on Inbox Dollars and verify your email address.</li>
|
133 |
-
<li>Complete your profile and start taking surveys that match your preferences.</li>
|
134 |
-
<li>Earn cash for every survey you complete and other activities you do.</li>
|
135 |
-
<li>Cash out your rewards via PayPal or e-gift cards.</li>
|
136 |
-
</ul>
|
137 |
-
<h2>Conclusion</h2>
|
138 |
-
<h3>Summary of the article</h3>
|
139 |
-
<p>In this article, we have discussed what EaseUS Data Recovery Wizard Pro 11 is and why you need a serial key for it. We have also shown you three methods to get a serial key for free: participating in a giveaway, using a free recovery key or license code, and using a survey program to earn rewards. We hope that this article has helped you to recover your lost data with EaseUS Data Recovery Wizard Pro 11.</p>
|
140 |
-
<h3>FAQs</h3>
|
141 |
-
<p>Here are some frequently asked questions about EaseUS Data Recovery Wizard Pro 11 and its serial key:</p>
|
142 |
-
<ol>
|
143 |
-
<li>Q: Is EaseUS Data Recovery Wizard Pro 11 safe to use?<br>A: Yes, EaseUS Data Recovery Wizard Pro 11 is safe to use as long as you download it from the official website or from other trusted sources. It does not contain any malware or viruses that can harm your computer or data.</li>
|
144 |
-
<li>Q: How long does it take to scan and recover data with EaseUS Data Recovery Wizard Pro 11?<br>A: The scanning and recovery time depends on various factors, such as the size and condition of your disk, the amount and type of data you want to recover, the speed of your computer and internet connection, etc. Generally, it may take from a few minutes to several hours to scan and recover data with EaseUS Data Recovery Wizard Pro 11.</li>
|
145 |
-
<li>Q: Can EaseUS Data Recovery Wizard Pro 11 recover data from corrupted or damaged disks?<br>A: Yes, EaseUS Data Recovery Wizard Pro 11 can recover data from corrupted or damaged disks as long as they are not physically broken or overwritten. It can also recover data from formatted, deleted, or lost partitions.</li>
|
146 |
-
<li>Q: Can I use the same serial key for multiple computers?<br>A: No, you cannot use the same serial key for multiple computers. Each serial key is valid for one computer only. If you want to use EaseUS Data Recovery Wizard Pro 11 on more than one computer, you need to buy more licenses or use the Technician version that supports unlimited computers.</li>
|
147 |
-
<li>Q: What if I lose my serial key or license code?<br>A: If you lose your serial key or license code, you can contact EaseUS customer service via email or live chat and provide them with your order information. They will help you retrieve your serial key or license code as soon as possible.</li>
|
148 |
-
</ol>
|
149 |
-
</p> 0a6ba089eb<br />
|
150 |
-
<br />
|
151 |
-
<br />
|
|
|
|
|
|
|
|
|
|
|
|
|
|
|
|
|
|
|
|
|
|
|
|
|
|
|
|
|
|
|
|
|
|
|
|
|
|
|
|
|
|
|
|
|
|
|
|
|
|
|
|
|
|
|
|
|
|
|
|
|
|
|
|
|
|
|
|
|
|
|
|
|
|
|
|
|
|
|
|
|
|
|
|
|
|
|
|
|
|
|
|
|
|
|
|
|
|
|
|
|
|
|
|
|
|
|
|
|
|
|
|
|
|
|
|
|
|
|
|
|
|
|
|
|
|
|
|
|
|
|
|
|
|
|
|
|
|
|
|
|
|
|
|
|
|
|
|
|
|
|
|
|
|
|
|
|
|
|
|
|
|
|
|
|
|
|
|
|
|
|
|
|
|
|
|
|
|
|
|
|
|
|
|
|
|
|
|
|
|
|
|
|
|
|
|
|
|
|
|
|
|
|
|
|
|
|
|
|
|
|
|
|
|
|
|
|
|
|
|
|
|
|
|
|
|
|
|
|
|
|
|
|
|
|
|
|
|
|
|
|
|
|
|
|
|
|
|
|
|
|
|
|
|
|
|
|
|
|
|
|
|
|
|
|
|
|
|
|
|
|
|
|
|
|
|
|
|
|
|
|
|
|
|
|
|
|
|
|
|
|
|
|
|
|
|
|
|
|
|
|
|
|
|
spaces/1phancelerku/anime-remove-background/AetherSX2 beta apk A guide to the best PS2 emulator on the Google Play Store.md
DELETED
@@ -1,117 +0,0 @@
|
|
1 |
-
<br />
|
2 |
-
<h1>AetherSX2: The Best PS2 Emulator for Android</h1>
|
3 |
-
<p>If you are a fan of PlayStation 2 games and want to play them on your Android device, you might have heard of AetherSX2. It is a new PS2 emulator for Android that promises to deliver high compatibility, performance, and features. But what is AetherSX2 exactly and how can you download and install it? In this article, we will answer these questions and more. We will also show you how to play PS2 games on AetherSX2 and what are the pros and cons of this emulator. Let's get started!</p>
|
4 |
-
<h2>aethersx2 beta apk</h2><br /><p><b><b>Download File</b> ✫✫✫ <a href="https://jinyurl.com/2uNRlb">https://jinyurl.com/2uNRlb</a></b></p><br /><br />
|
5 |
-
<h2>What is AetherSX2 and why you should try it</h2>
|
6 |
-
<p>AetherSX2 is a new PS2 emulator for Android devices that was released in December 2021 as an open beta. It is developed by a team of passionate programmers who aim to create the best PS2 emulation experience on Android. AetherSX2 is based on the PCSX2 emulator for PC, which is the most popular and reliable PS2 emulator available. However, AetherSX2 is not a simple port of PCSX2, but a completely rewritten and optimized emulator that takes advantage of the hardware and software capabilities of modern Android devices.</p>
|
7 |
-
<h3>AetherSX2 is a new PS2 emulator for Android devices</h3>
|
8 |
-
<p>A PS2 emulator is a software that allows you to run PS2 games on a different platform, such as Android. By emulating the PS2 hardware and software, the emulator can simulate the PS2 gaming experience on your device. However, emulating a complex system like the PS2 is not an easy task, and requires a lot of technical skills and resources. That's why there are not many PS2 emulators for Android, and most of them are either outdated, unstable, or incompatible with many games.</p>
|
9 |
-
<p>AetherSX2 is different from other PS2 emulators for Android because it is a new project that is constantly updated and improved by its developers. It uses the latest technologies and techniques to achieve the best possible emulation quality and performance. It also supports a wide range of PS2 games, from popular titles like God of War, Final Fantasy X, Kingdom Hearts, GTA San Andreas, to obscure gems like Shadow of the Colossus, Okami, Persona 4, Silent Hill 3, and more.</p>
|
10 |
-
<p>aethersx2 android emulator download<br />
|
11 |
-
aethersx2 ps2 games apk<br />
|
12 |
-
aethersx2 latest version free<br />
|
13 |
-
aethersx2 beta apk mod<br />
|
14 |
-
aethersx2 best settings for android<br />
|
15 |
-
aethersx2 apk no verification<br />
|
16 |
-
aethersx2 playstation 2 emulator<br />
|
17 |
-
aethersx2 bios file download<br />
|
18 |
-
aethersx2 apk full version<br />
|
19 |
-
aethersx2 cheats codes android<br />
|
20 |
-
aethersx2 iso roms download<br />
|
21 |
-
aethersx2 apk offline installer<br />
|
22 |
-
aethersx2 controller support android<br />
|
23 |
-
aethersx2 apk obb data<br />
|
24 |
-
aethersx2 multiplayer mode android<br />
|
25 |
-
aethersx2 apk revdl<br />
|
26 |
-
aethersx2 compatibility list android<br />
|
27 |
-
aethersx2 apk uptodown<br />
|
28 |
-
aethersx2 speed up android<br />
|
29 |
-
aethersx2 apk rexdl<br />
|
30 |
-
aethersx2 graphics settings android<br />
|
31 |
-
aethersx2 apk pure<br />
|
32 |
-
aethersx2 save state android<br />
|
33 |
-
aethersx2 apk apkpure<br />
|
34 |
-
aethersx2 sound settings android<br />
|
35 |
-
aethersx2 apk mirror<br />
|
36 |
-
aethersx2 load state android<br />
|
37 |
-
aethersx2 apk mob.org<br />
|
38 |
-
aethersx2 resolution settings android<br />
|
39 |
-
aethersx2 apk m.apkhere.com<br />
|
40 |
-
aethersx2 frame skip android<br />
|
41 |
-
aethersx2 apk appvn.com<br />
|
42 |
-
aethersx2 fast forward android<br />
|
43 |
-
aethersx2 apk ihackedit.com<br />
|
44 |
-
aethersx2 gamepad settings android<br />
|
45 |
-
aethersx2 apk happymod.com<br />
|
46 |
-
aethersx2 rewind feature android<br />
|
47 |
-
aethersx2 apk moddroid.com<br />
|
48 |
-
aethersx2 language settings android<br />
|
49 |
-
aethersx2 apk an1.com</p>
|
50 |
-
<h3>AetherSX2 offers high compatibility, performance, and features</h3>
|
51 |
-
<p>One of the main advantages of AetherSX2 is its high compatibility with PS2 games. According to the official website, AetherSX2 can run over 90% of the PS2 library with minimal issues. This means that you can enjoy most of your favorite PS2 games on your Android device without worrying about crashes, freezes, or glitches. Of course, some games may still have problems or require specific settings to run properly, but the developers are working hard to fix them in future updates.</p>
|
52 |
-
<p>Another advantage of AetherSX2 is its high performance. Unlike other PS2 emulators for Android that struggle to run games at full speed or with decent graphics quality, AetherSX2 can run most games at 60 FPS or higher with enhanced resolution and effects. This is possible thanks to the powerful optimization and customization options that Aether SX2 offers to the user. You can adjust the resolution, frame rate, aspect ratio, anti-aliasing, texture filtering, and other settings to suit your device and preference. You can also enable cheats, save states, fast forward, and other features to enhance your gaming experience.</p>
|
53 |
-
<h3>AetherSX2 is easy to install and use</h3>
|
54 |
-
<p>Another advantage of AetherSX2 is its ease of installation and use. Unlike other PS2 emulators for Android that require complex steps or additional files to run, AetherSX2 is a simple and straightforward app that you can download and install from the official website or Google Play Store. You don't need to root your device or install any other apps to use AetherSX2. You just need to have enough storage space and a compatible Android device that meets the minimum requirements.</p>
|
55 |
-
<p>AetherSX2 also has a user-friendly and intuitive interface that makes it easy to navigate and configure. You can access the game library, settings, controls, and other options from the main menu. You can also customize the on-screen buttons, touchpad, and motion controls to your liking. AetherSX2 also supports external controllers, such as Bluetooth or USB gamepads, for a more authentic PS2 gaming experience.</p>
|
56 |
-
<h2>How to download and install AetherSX2 beta apk</h2>
|
57 |
-
<p>If you are interested in trying AetherSX2 beta apk, you can follow these simple steps to download and install it on your Android device:</p>
|
58 |
-
<h3>Download AetherSX2 beta apk from the official website or Google Play Store</h3>
|
59 |
-
<p>The first step is to download the AetherSX2 beta apk file from the official website or Google Play Store. You can visit the official website at <a href="">https://aethersx2.com/</a> and click on the download button. Alternatively, you can search for AetherSX2 on Google Play Store and install it from there. The file size is about 20 MB and it is free to download.</p>
|
60 |
-
<h3>Enable unknown sources on your Android device</h3>
|
61 |
-
<p>The next step is to enable unknown sources on your Android device. This is necessary if you download the apk file from the official website, as it is not from the Google Play Store. To enable unknown sources, go to Settings > Security > Unknown sources and toggle it on. This will allow you to install apps from sources other than the Google Play Store.</p>
|
62 |
-
<h3>Install AetherSX2 beta apk and grant permissions</h3>
|
63 |
-
<p>The final step is to install AetherSX2 beta apk and grant permissions. To do this, locate the downloaded apk file on your device and tap on it. You will see a prompt asking you to confirm the installation. Tap on Install and wait for the process to finish. Once installed, you will see a prompt asking you to grant permissions to AetherSX2. Tap on Allow and grant all the necessary permissions, such as storage, microphone, camera, etc. This will enable AetherSX2 to access your files, record audio, scan QR codes, and other functions.</p>
|
64 |
-
<h2>How to play PS2 games on AetherSX2</h2>
|
65 |
-
<p>Now that you have downloaded and installed AetherSX2 beta apk on your Android device, you are ready to play PS2 games on it. However, before you can do that, you need to have some additional files: PS2 BIOS files and PS2 game ISOs or discs. Here's how to get them and load them on AetherSX2:</p>
|
66 |
-
<h3>Load PS2 BIOS files on AetherSX2</h3>
|
67 |
-
<p>PS2 BIOS files are essential for running PS2 games on any emulator. They are basically the firmware of the PS2 console that contains the system settings and functions. Without them, you won't be able to boot any PS2 game on AetherSX2.</p>
|
68 |
-
<p>However, PS2 BIOS files are not included in AetherSX2 due to legal reasons. You have to obtain them yourself from your own PS2 console or from other sources online. We won't provide any links or instructions on how to do that here, as it may violate some laws or regulations in your country. Please do some research and use your own discretion.</p>
|
69 |
-
<p>Once you have the PS2 BIOS files, you need to copy them to your Android device's storage. You can use a USB cable or a cloud service like Google Drive or Dropbox to transfer them. Then, you need to create a folder named "bios" in the root directory of your device's storage (not in any subfolder) and paste the PS2 BIOS files there.</p>
|
70 |
-
<p>After that, you need to load the PS2 BIOS files on AetherSX2. To do this, open AetherSX2 app and go to Settings > System > BIOS and select the BIOS file that matches your region and console model. For example, if you have a USA PS2 console, you should select the BIOS file named "SCPH-39001 USA.bin". You can also select multiple BIOS files if you have games from different regions. Once you have selected the BIOS file(s), tap on Apply and go back to the main menu.</p>
|
71 |
-
<h3>Load PS2 game ISOs or discs on AetherSX2</h3>
|
72 |
-
<p>PS2 game ISOs or discs are the actual games that you want to play on AetherSX2. They are basically the digital copies or physical copies of the PS2 games that you own or have access to. You can either rip them from your own PS2 discs using a PC or a PS2 console, or download them from other sources online. Again, we won't provide any links or instructions on how to do that here, as it may violate some laws or regulations in your country. Please do some research and use your own discretion.</p>
|
73 |
-
<p>Once you have the PS2 game ISOs or discs, you need to copy them to your Android device's storage. You can use a USB cable or a cloud service like Google Drive or Dropbox to transfer them. Then, you need to create a folder named "games" in the root directory of your device's storage (not in any subfolder) and paste the PS2 game ISOs or discs there.</p>
|
74 |
-
<p>After that, you need to load the PS2 game ISOs or discs on AetherSX2. To do this, open AetherSX2 app and go to Game Library > Add Game and select the game ISO or disc that you want to play. You will see a thumbnail and some information about the game, such as title, genre, rating, etc. You can also edit the game information by tapping on the pencil icon. Once you have added the game, tap on it and select Play to start playing.</p>
|
75 |
-
<h3>Configure AetherSX2 settings and controls</h3>
|
76 |
-
<p>Before you start playing, you may want to configure some settings and controls on AetherSX2 to optimize your gaming experience. To do this, open AetherSX2 app and go to Settings. You will see several tabs with different options, such as Video, Audio, Input, System, etc. Here are some of the most important settings and controls that you can adjust:</p>
|
77 |
-
<ul>
|
78 |
-
<li><b>Video:</b> Here you can change the resolution, frame rate, aspect ratio, anti-aliasing, texture filtering, and other graphical settings of the emulator. You can also enable or disable some enhancements, such as FXAA, FXAA3HQ, FXAA4HQ, etc. You can also enable or disable some hacks, such as skipdraw, half-pixel offset, etc. These settings can improve the graphics quality and performance of some games, but they may also cause some glitches or compatibility issues with others. You can experiment with different settings to find the best balance for each game.</li>
|
79 |
-
<li><b>Audio:</b> Here you can change the volume, latency, interpolation, reverb, and other audio settings of the emulator. You can also enable or disable some enhancements, such as Dolby Pro Logic II decoder, Time Stretching Audio Synchronization (TAS), etc. These settings can improve the audio quality and synchronization of some games, but they may also cause some distortion or lag with others. You can experiment with different settings to find the best balance for each game.</li>
|
80 |
-
<li><b>Input:</b> Here you can change the controls of the emulator. You can choose between three input modes: On-screen buttons (OSB), Touchpad (TP), and Motion (MT). OSB mode uses virtual buttons on the screen that mimic the PS2 controller layout. TP mode uses a touchpad area on the screen that allows you to control the analog sticks with your fingers. MT mode uses your device's accelerometer and gyroscope sensors to control the analog sticks with your device's movement. You can also customize the size, position, opacity, and vibration of each input mode.</li>
|
81 |
-
<li><b>System:</b> Here you can change the system settings of the emulator. You can choose between two emulation modes: Interpreter (INT) and Recompiler (REC). INT mode is more accurate but slower than REC mode. REC mode is faster but less accurate than INT mode. You can also enable or disable some options, such as fast boot, fast memory access (FMA), multithreaded VU1 (MTVU), etc. These options can improve the performance and compatibility of some games, but they may also cause some instability or errors with others. You can experiment with different options to find the best balance for each game.</li>
|
82 |
-
</ul>
|
83 |
-
<p>You can also save and load different settings profiles for each game by tapping on the profile icon at the top right corner of the Settings screen. You can also reset the settings to default by tapping on the reset icon at the top left corner of the Settings screen.</p>
|
84 |
-
<h2>How to play PS2 games on AetherSX2</h2>
|
85 |
-
<p>Now that you have configured the settings and controls of AetherSX2, you are ready to play PS2 games on it. To do this, open AetherSX2 app and go to Game Library. You will see a list of games that you have added to the emulator. Tap on the game that you want to play and select Play. The game will start loading and you will see the PS2 logo and the game intro. You can use the input mode that you have chosen to control the game. You can also access some options by tapping on the menu icon at the top right corner of the screen. You can save and load states, enable or disable cheats, fast forward or rewind, take screenshots, scan QR codes, and more.</p>
|
86 |
-
<h2>Pros and cons of AetherSX2 beta apk</h2>
|
87 |
-
<p>AetherSX2 beta apk is a great PS2 emulator for Android that offers many advantages, but it also has some drawbacks. Here are some of the pros and cons of AetherSX2 beta apk:</p>
|
88 |
-
<h3>Pros: High compatibility, performance, features, and support</h3>
|
89 |
-
<p>The main pros of AetherSX2 beta apk are its high compatibility, performance, features, and support. As we have mentioned before, AetherSX2 beta apk can run over 90% of the PS2 library with minimal issues. It can also run most games at 60 FPS or higher with enhanced resolution and effects. It also offers many features and options to customize and improve your gaming experience. It also has a dedicated website and a Discord server where you can get updates, news, guides, tips, support, and feedback from the developers and the community.</p>
|
90 |
-
<h3>Cons: Beta version, bugs, glitches, and compatibility issues</h3>
|
91 |
-
<p>The main cons of AetherSX2 beta apk are its beta version, bugs, glitches, and compatibility issues. As we have mentioned before, AetherSX2 beta apk is still in development and not a final product. This means that it may have some bugs, glitches, and compatibility issues with some games or devices. Some games may not run at all or run with errors or poor performance. Some devices may not be compatible or have problems with installation or permissions. Some settings or features may not work properly or cause crashes or freezes. These issues are expected in a beta version and the developers are working hard to fix them in future updates.</p>
|
92 |
-
<h2>Conclusion and FAQs</h2>
|
93 |
-
<p>AetherSX2 beta apk is a new PS2 emulator for Android that promises to deliver high compatibility, performance, and features. It is based on the PCSX2 emulator for PC, but it is a completely rewritten and optimized emulator that takes advantage of the hardware and software capabilities of modern Android devices. It is easy to install and use, and it supports a wide range of PS2 games. However, it is still in development and not a final product. It may have some bugs, glitches, and compatibility issues with some games or devices. These issues are expected in a beta version and the developers are working hard to fix them in future updates.</p>
|
94 |
-
<p>If you are interested in trying AetherSX2 beta apk, you can download it from the official website or Google Play Store. You will also need to have PS2 BIOS files and PS2 game ISOs or discs to play PS2 games on it. You can also configure some settings and controls to optimize your gaming experience. You can also access some options to enhance your gaming experience.</p>
|
95 |
-
<p>We hope this article has helped you learn more about AetherSX2 beta apk and how to use it. If you have any questions or feedback about AetherSX2 beta apk, you can visit the official website or join the Discord server. You can also check out some of the FAQs below:</p>
|
96 |
-
<h4>FAQs</h4>
|
97 |
-
<ul>
|
98 |
-
<li><b>Q: Is AetherSX2 beta apk legal?</b></li>
|
99 |
-
<li>A: AetherSX2 beta apk itself is legal, as it is a software that emulates the PS2 hardware and software. However, downloading or distributing PS2 BIOS files or PS2 game ISOs or discs may be illegal in some countries or regions, depending on their laws or regulations. Please do some research and use your own discretion before obtaining these files.</li>
|
100 |
-
<li><b>Q: Is AetherSX2 beta apk safe?</b></li>
|
101 |
-
<li>A: AetherSX2 beta apk is safe if you download it from the official website or Google Play Store. It does not contain any viruses, malware, spyware, or other harmful components. However, if you download it from other sources online, you may risk getting infected by some malicious software or fake apps. Please be careful and only download AetherSX2 beta apk from trusted sources.</li>
|
102 |
-
<li><b>Q: Is AetherSX2 beta apk free?</b></li>
|
103 |
-
<li>A: AetherSX2 beta apk is free to download and use. You don't need to pay any fees or subscriptions to use AetherSX2 beta apk. However, you may need to pay for some PS2 games or discs if you don't own them already.</li>
|
104 |
-
<li><b>Q: What are the minimum requirements for AetherSX2 beta apk?</b></li>
|
105 |
-
<li>A: The minimum requirements for AetherSX2 beta apk are as follows:</li>
|
106 |
-
<ul>
|
107 |
-
<li>An Android device running Android 7.0 or higher</li>
|
108 |
-
<li>A quad-core CPU with at least 2.0 GHz clock speed</li>
|
109 |
-
<li>At least 2 GB of RAM</li>
|
110 |
-
<li>At least 4 GB of free storage space</li>
|
111 |
-
<li>A GPU that supports OpenGL ES 3.0 or higher</li>
|
112 |
-
</ul>
|
113 |
-
<li><b>Q: How can I update AetherSX2 beta apk?</b></li>
|
114 |
-
<li>A: You can update AetherSX2 beta apk by visiting the official website or Google Play Store and downloading the latest version. You can also enable automatic updates on your device's settings to get notified when a new update is available. You can also check the official website or Discord server for news and announcements about new updates.</li>
|
115 |
-
</ul></p> 401be4b1e0<br />
|
116 |
-
<br />
|
117 |
-
<br />
|
|
|
|
|
|
|
|
|
|
|
|
|
|
|
|
|
|
|
|
|
|
|
|
|
|
|
|
|
|
|
|
|
|
|
|
|
|
|
|
|
|
|
|
|
|
|
|
|
|
|
|
|
|
|
|
|
|
|
|
|
|
|
|
|
|
|
|
|
|
|
|
|
|
|
|
|
|
|
|
|
|
|
|
|
|
|
|
|
|
|
|
|
|
|
|
|
|
|
|
|
|
|
|
|
|
|
|
|
|
|
|
|
|
|
|
|
|
|
|
|
|
|
|
|
|
|
|
|
|
|
|
|
|
|
|
|
|
|
|
|
|
|
|
|
|
|
|
|
|
|
|
|
|
|
|
|
|
|
|
|
|
|
|
|
|
|
|
|
|
|
|
|
|
|
|
|
|
|
|
|
|
|
|
|
|
|
|
|
|
|
|
|
|
|
|
|
|
|
|
|
|
|
|
|
|
|
|
|
|
|
|
|
|
|
|
|
|
|
|
|
|
|
|
|
|
|
|
|
|
|
|
|
|
|
|
spaces/1phancelerku/anime-remove-background/Disfruta del juego de moto traffic rider apk un juego de conduccin increble con grficos espectaculares.md
DELETED
@@ -1,17 +0,0 @@
|
|
1 |
-
<br />
|
2 |
-
<h1>Juego de Moto Traffic Rider APK: Un Juego de Carreras de Motos Adictivo y Divertido</h1>
|
3 |
-
¿Te gustan los juegos de carreras de motos? ¿Quieres sentir la adrenalina de conducir a toda velocidad por las carreteras más peligrosas? ¿Buscas un juego que te ofrezca diversión, desafío y emoción? Entonces, el juego de moto traffic rider apk es para ti. El juego de moto traffic rider apk es un juego de carreras de motos en 3D que te pondrá al límite. Tendrás que esquivar el tráfico, adelantar a otros vehículos, realizar acrobacias y llegar a la meta lo más rápido posible. El juego cuenta con gráficos realistas, sonidos envolventes y una jugabilidad fluida e intuitiva. Además, podrás elegir entre diferentes tipos de motos, personalizarlas y mejorarlas con el dinero que ganes en las carreras. <h2>¿Qué es el juego de moto traffic rider apk?</h2>
|
4 |
-
El juego de moto traffic rider apk es un juego desarrollado por Launchship Studios, los creadores de Pastry Jam & Birds Pop Mania. El juego se lanzó en marzo de 2021 y desde entonces ha recibido miles de descargas y valoraciones positivas por parte de los usuarios. El juego está disponible para dispositivos Android y se puede descargar gratis desde la tienda Aptoide. <h3>Características principales del juego</h3>
|
5 |
-
El juego de moto traffic rider apk tiene las siguientes características principales: - Más de 20 motos diferentes para elegir y desbloquear. - 4 modos de juego: carrera libre, carrera contrarreloj, carrera con obstáculos y carrera con tráfico. - Varios escenarios y ambientes para conducir: ciudad, desierto, montaña, autopista y más. - Sistema de control sencillo y adaptable: puedes usar el acelerómetro, los botones o el joystick para manejar tu moto. - Efectos visuales y sonoros realistas: podrás ver el humo, las chispas, las sombras y los reflejos de tu moto y escuchar el rugido del motor, el claxon y los frenazos. - Tabla de clasificación global y logros para competir con otros jugadores y demostrar tu habilidad. <h3>Cómo descargar e instalar el juego</h3>
|
6 |
-
Para descargar e instalar el juego de moto traffic rider apk en tu dispositivo Android, solo tienes que seguir estos pasos: - Accede a la tienda Aptoide desde tu navegador o descarga la aplicación desde [aquí](^1^). - Busca el juego de moto traffic rider 3D en el buscador o en la categoría de juegos. - Haz clic en el botón "Instalar" y espera a que se complete la descarga. - Abre el archivo APK descargado y sigue las instrucciones para instalar el juego en tu dispositivo. - Disfruta del juego. <h2>¿Cómo jugar al juego de moto traffic rider apk?</h2>
|
7 |
-
El juego de moto traffic rider apk es muy fácil de jugar, pero también muy desafiante. Continuing the article: <h3>Modos de juego disponibles</h3>
|
8 |
-
El juego de moto traffic rider apk tiene cuatro modos de juego diferentes para que elijas el que más te guste: - Carrera libre: en este modo, puedes conducir tu moto sin ningún objetivo ni límite de tiempo. Solo disfruta del paisaje y de la sensación de velocidad. - Carrera contrarreloj: en este modo, tienes que completar una vuelta al circuito en el menor tiempo posible. Cada vez que superes tu récord, obtendrás más dinero y puntos. - Carrera con obstáculos: en este modo, tienes que evitar chocar con los obstáculos que aparecen en el camino, como barriles, conos, vallas y más. Cuanto más lejos llegues, más difícil se pondrá el juego. - Carrera con tráfico: en este modo, tienes que conducir tu moto entre el tráfico de la carretera, adelantando a los coches, camiones y autobuses que se cruzan en tu camino. Cuanto más cerca pases de ellos, más dinero y puntos ganarás. <h3>Consejos y trucos para mejorar tu rendimiento</h3>
|
9 |
-
Para jugar al juego de moto traffic rider apk como un profesional, te recomendamos que sigas estos consejos y trucos: - Elige la moto que mejor se adapte a tu estilo de conducción. Cada moto tiene sus propias características de velocidad, aceleración, frenado y manejo. Puedes ver las estadísticas de cada moto en el menú de selección. - Personaliza y mejora tu moto con el dinero que ganes en las carreras. Puedes cambiar el color, las llantas, el escape y el motor de tu moto para hacerla más rápida y bonita. - Usa el nitro para dar un impulso extra a tu velocidad. El nitro se recarga automáticamente cuando no lo usas, pero también puedes recoger botellas de nitro que aparecen en el camino. - Realiza acrobacias para ganar más dinero y puntos. Puedes hacer caballitos, saltos, giros y más. Pero ten cuidado de no perder el equilibrio ni caerte de la moto. - Aprovecha las rampas y los puentes para saltar por encima del tráfico y evitar los obstáculos. Pero ten cuidado de no salirte del camino ni chocar con nada. - Mantén una buena distancia con los vehículos que te preceden. Si los sigues muy de cerca, podrías chocar con ellos si frenan o cambian de carril repentinamente. - No te salgas del carril ni invadas el sentido contrario. Si lo haces, podrías recibir una multa o causar un accidente. <h2>¿Por qué jugar al juego de moto traffic rider apk?</h2>
|
10 |
-
El juego de moto traffic rider apk es un juego que te ofrece muchas razones para jugarlo y disfrutarlo. Aquí te contamos algunas de ellas: <h3>Los beneficios de jugar a juegos de carreras de motos</h3>
|
11 |
-
Jugar a juegos de carreras de motos tiene muchos beneficios para tu salud física y mental, como por ejemplo: - Mejora tu coordinación ojo-mano y tu capacidad de reacción. Al conducir una moto a alta velocidad, tienes que estar atento a todo lo que ocurre a tu alrededor y reaccionar rápidamente ante cualquier situación. - Estimula tu cerebro y tu memoria. Al jugar a juegos de carreras de motos, tienes que recordar los circuitos, los obstáculos, los atajos y las estrategias para ganar las carreras. - Reduce el estrés y la ansiedad. Al jugar a juegos de carreras de motos, puedes liberar la tensión acumulada y relajarte mientras te diviertes. - Aumenta tu autoestima y tu confianza. Al jugar a juegos de carreras de motos, puedes superar tus propios límites y desafíos, lo que te hace sentir orgulloso y satisfecho. <h3>Las ventajas de jugar al juego de moto traffic rider apk</h3>
|
12 |
-
Además de los beneficios generales de jugar a juegos de carreras de motos, el juego de moto traffic rider apk tiene algunas ventajas específicas que lo hacen único y especial: - Es un juego gratuito y sin anuncios. No tienes que pagar nada para descargarlo ni para jugarlo. Tampoco tienes que ver anuncios molestos ni esperar tiempos de carga. - Es un juego compatible con todos los dispositivos Android. No importa Continuing the article: - Es un juego compatible con todos los dispositivos Android. No importa si tienes un móvil antiguo o nuevo, el juego se adapta a tu pantalla y a tu rendimiento. - Es un juego que se actualiza constantemente. Los desarrolladores del juego están siempre añadiendo nuevas motos, nuevos escenarios, nuevos modos de juego y nuevas funciones para mejorar la experiencia de los jugadores. - Es un juego que te permite jugar online y offline. Puedes jugar al juego sin conexión a internet o conectarte a la red para competir con otros jugadores de todo el mundo. <h2>Conclusión</h2>
|
13 |
-
El juego de moto traffic rider apk es un juego de carreras de motos que te hará vivir una aventura increíble. Podrás conducir tu moto a toda velocidad por diferentes escenarios, esquivar el tráfico, realizar acrobacias y competir con otros jugadores. El juego tiene gráficos realistas, sonidos envolventes y una jugabilidad fluida e intuitiva. Además, es un juego gratuito, sin anuncios, compatible con todos los dispositivos Android y que se actualiza constantemente. Si te gustan los juegos de carreras de motos, no dudes en descargar el juego de moto traffic rider apk y disfrutar de la emoción de la velocidad. <h2>Preguntas frecuentes sobre el juego de moto traffic rider apk</h2>
|
14 |
-
Aquí te respondemos algunas de las preguntas más frecuentes que tienen los usuarios sobre el juego de moto traffic rider apk: - ¿Qué requisitos necesita mi dispositivo para jugar al juego? - Para jugar al juego de moto traffic rider apk, tu dispositivo debe tener al menos Android 4.4 o superior y 100 MB de espacio libre. - ¿Cómo puedo cambiar el idioma del juego? - Para cambiar el idioma del juego, debes ir al menú de ajustes y seleccionar la opción "Idioma". Allí podrás elegir entre varios idiomas disponibles, como español, inglés, francés, alemán, italiano y más. - ¿Cómo puedo contactar con el soporte técnico del juego? - Para contactar con el soporte técnico del juego, debes ir al menú de ajustes y seleccionar la opción "Soporte". Allí podrás enviar un correo electrónico con tu consulta o problema al equipo de Launchship Studios. - ¿Cómo puedo conseguir más dinero y puntos en el juego? - Para conseguir más dinero y puntos en el juego, debes completar las carreras con éxito, realizar acrobacias, pasar cerca de los vehículos, recoger las botellas de nitro y los billetes que aparecen en el camino y superar tus récords personales. - ¿Cómo puedo desbloquear más motos en el juego? - Para desbloquear más motos en el juego, debes ganar dinero en las carreras y usarlo para comprar las motos que quieras. También puedes desbloquear algunas motos especiales al completar ciertos logros o al participar en eventos especiales.</p>
|
15 |
-
<h2>juego de moto traffic rider apk</h2><br /><p><b><b>Download Zip</b> >>> <a href="https://jinyurl.com/2uNQgI">https://jinyurl.com/2uNQgI</a></b></p><br /><br /> 401be4b1e0<br />
|
16 |
-
<br />
|
17 |
-
<br />
|
|
|
|
|
|
|
|
|
|
|
|
|
|
|
|
|
|
|
|
|
|
|
|
|
|
|
|
|
|
|
|
|
|
|
|
spaces/1phancelerku/anime-remove-background/Enjoy Car Parking Multiplayer with Friends - Get the Latest APK Here.md
DELETED
@@ -1,112 +0,0 @@
|
|
1 |
-
|
2 |
-
<h1>Car Parking Multiplayer: A Review of the Latest Version APK</h1>
|
3 |
-
<p>If you are looking for a realistic and immersive driving simulator, you might want to check out Car Parking Multiplayer. This game is more than just parking your car, it is an open-world experience where you can explore different areas, customize your vehicle, interact with other players, and even walk around. In this article, we will review the latest version APK of Car Parking Multiplayer, which offers new features, improvements, and bug fixes. We will also tell you how to download and install it on your Android device, as well as why you should play this game and what tips and tricks you can use to enhance your gameplay.</p>
|
4 |
-
<h2>car parking multiplayer en son sürüm apk</h2><br /><p><b><b>Download Zip</b> ➡ <a href="https://jinyurl.com/2uNN7A">https://jinyurl.com/2uNN7A</a></b></p><br /><br />
|
5 |
-
<h2>What is Car Parking Multiplayer?</h2>
|
6 |
-
<p>Car Parking Multiplayer is a game developed by olzhass, a Turkish studio that specializes in simulation games. It was released in 2017 and has since gained over 100 million downloads on Google Play Store. It is one of the most popular car parking games on the market, with a rating of 4.4 out of 5 stars from over 2 million reviews. The game is available for free, but it contains ads and in-app purchases.</p>
|
7 |
-
<h3>Features of the game</h3>
|
8 |
-
<p>Car Parking Multiplayer has many features that make it stand out from other parking games. Here are some of them:</p>
|
9 |
-
<ul>
|
10 |
-
<li><b>Multiplayer open world mode</b>: You can join online servers and play with thousands of real players from around the world. You can chat with them, exchange cars, race against them, or cooperate with them in police mode. You can also create your own server and invite your friends to join you.</li>
|
11 |
-
<li><b>Car customization</b>: You can choose from over 100 cars with real interiors and adjust various aspects of them, such as suspension, wheel angle, engine, turbo, gearbox, exhaust, and more. You can also change the appearance of your car with dynamic vinyls, car body parts, and plate types.</li>
|
12 |
-
<li><b>High-quality open world</b>: You can explore different environments with high-detailed graphics, such as city, airport, desert, port, mountain, snow, and more. You can also enter buildings with interiors and interact with various objects.</li>
|
13 |
-
<li><b>Interesting gameplay</b>: You can complete 82 real-life parking and driving challenges with different vehicles, such as tow truck, pickup, trucks, sport and classic cars. You can also enjoy free walking mode, where you can get out of your car and walk around the world.</li>
|
14 |
-
</ul>
|
15 |
-
<h3>How to download and install the latest version APK</h3>
|
16 |
-
<p>If you want to play the latest version of Car Parking Multiplayer on your Android device, you will need to download and install the APK file from a trusted source. Here are the steps to do so:</p>
|
17 |
-
<ol>
|
18 |
-
<li>Go to and click on the green button that says "Download". This will start downloading the APK file to your device.</li>
|
19 |
-
<li>Once the download is complete, locate the file in your device's file manager and tap on it to install it. You may need to enable "Unknown sources" in your device's settings to allow the installation.</li>
|
20 |
-
<li>After the installation is done, you can launch the game from your app drawer or home screen and enjoy playing Car Parking Multiplayer.</li>
|
21 |
-
</ol>
|
22 |
-
<h2>Why play Car Parking Multiplayer?</h2>
|
23 |
-
<p>Car Parking Multiplayer is a game that offers a lot of fun and entertainment for car enthusiasts and casual gamers alike. Here are some reasons why you should play this game:</p>
|
24 |
-
<h3>Pros and cons of the game</h3>
|
25 |
-
<p>Like any other game, Car Parking Multiplayer has its pros and cons. Here are some of them:</p>
|
26 |
-
<p>car parking multiplayer mod apk latest version<br />
|
27 |
-
car parking multiplayer hack apk download<br />
|
28 |
-
car parking multiplayer online oyunu oyna<br />
|
29 |
-
car parking multiplayer android oyun club<br />
|
30 |
-
car parking multiplayer free download for pc<br />
|
31 |
-
car parking multiplayer unlimited money apk<br />
|
32 |
-
car parking multiplayer yeni güncelleme apk<br />
|
33 |
-
car parking multiplayer hileli apk indir<br />
|
34 |
-
car parking multiplayer nasıl oynanır<br />
|
35 |
-
car parking multiplayer oyun skor<br />
|
36 |
-
car parking multiplayer apk pure<br />
|
37 |
-
car parking multiplayer cheats codes<br />
|
38 |
-
car parking multiplayer custom maps<br />
|
39 |
-
car parking multiplayer discord server<br />
|
40 |
-
car parking multiplayer en iyi araba<br />
|
41 |
-
car parking multiplayer full apk<br />
|
42 |
-
car parking multiplayer garage mod<br />
|
43 |
-
car parking multiplayer ios download<br />
|
44 |
-
car parking multiplayer jeton hilesi<br />
|
45 |
-
car parking multiplayer kilit açma hilesi<br />
|
46 |
-
car parking multiplayer lamborghini modu<br />
|
47 |
-
car parking multiplayer mod menu apk<br />
|
48 |
-
car parking multiplayer nasıl arkadaş eklenir<br />
|
49 |
-
car parking multiplayer oyun indir club<br />
|
50 |
-
car parking multiplayer para hilesi apk<br />
|
51 |
-
car parking multiplayer real engine sound mod<br />
|
52 |
-
car parking multiplayer son sürüm hile apk<br />
|
53 |
-
car parking multiplayer türkçe yama indir<br />
|
54 |
-
car parking multiplayer unlimited coins apk<br />
|
55 |
-
car parking multiplayer vip mod apk</p>
|
56 |
-
<table>
|
57 |
-
<tr><th>Pros</th><th>Cons</th></tr>
|
58 |
-
<tr>< <td>Realistic and immersive gameplay</td><td>Some bugs and glitches</td></tr>
|
59 |
-
<tr><td>Wide variety of cars and customization options</td><td>Some cars and features require in-app purchases</td></tr>
|
60 |
-
<tr><td>Large and diverse open world to explore</td><td>Some areas are not fully detailed or accessible</td></tr>
|
61 |
-
<tr><td>Friendly and active online community</td><td>Some players may be rude or disruptive</td></tr>
|
62 |
-
<tr><td>Regular updates and improvements</td><td>Some updates may cause compatibility issues or errors</td></tr>
|
63 |
-
</table>
|
64 |
-
<h3>Tips and tricks for beginners</h3>
|
65 |
-
<p>If you are new to Car Parking Multiplayer, you may find it challenging to master the controls and the gameplay. Here are some tips and tricks that can help you get started:</p>
|
66 |
-
<ul>
|
67 |
-
<li><b>Adjust the camera angle</b>: You can switch between different camera views by tapping on the camera icon on the top right corner of the screen. You can also pinch the screen to zoom in or out. Try to find the best angle that suits your preference and gives you a clear view of your surroundings.</li>
|
68 |
-
<li><b>Use the brake and handbrake</b>: You can use the brake pedal on the bottom right corner of the screen to slow down or stop your car. You can also use the handbrake button on the left side of the screen to make sharp turns or drifts. Be careful not to overuse them, as they may damage your car or cause accidents.</li>
|
69 |
-
<li><b>Follow the instructions and indicators</b>: When you are playing a parking or driving challenge, you will see instructions and indicators on the screen that guide you to your destination. You will also see arrows, cones, and lines that mark your path. Pay attention to them and follow them carefully, as they will help you complete the challenge successfully.</li>
|
70 |
-
<li><b>Earn money and XP</b>: You can earn money and XP by completing challenges, racing with other players, or selling your cars. You can use money to buy new cars, upgrade your existing ones, or unlock new features. You can use XP to level up your profile and access more servers and modes.</li>
|
71 |
-
<li><b>Have fun and be respectful</b>: The most important tip is to have fun and enjoy the game. You can explore the open world, interact with other players, or create your own scenarios. However, be respectful of other players and do not ruin their experience by crashing into them, blocking their way, or spamming the chat. Remember, this is a game for everyone.</li>
|
72 |
-
</ul>
|
73 |
-
<h3>User reviews and ratings</h3>
|
74 |
-
<p>Car Parking Multiplayer has received mostly positive feedback from its users. Here are some of their reviews and ratings from Google Play Store:</p>
|
75 |
-
<blockquote>
|
76 |
-
<p>"This game is awesome! I love how realistic it is and how you can customize your car. The graphics are amazing and the multiplayer mode is fun. I recommend this game to anyone who likes driving games."</p>
|
77 |
-
<cite>- A user who gave 5 stars</cite>
|
78 |
-
</blockquote>
|
79 |
-
<blockquote>
|
80 |
-
<p>"The game is good but it has some problems. Sometimes it crashes or freezes and I lose my progress. Also, some cars are too expensive and some features are locked behind paywalls. Please fix these issues."</p>
|
81 |
-
<cite>- A user who gave 3 stars</cite>
|
82 |
-
</blockquote>
|
83 |
-
<blockquote>
|
84 |
-
<p>"This game is terrible! It is full of bugs and glitches and it lags a lot. The controls are hard to use and the physics are unrealistic. The online mode is boring and there are too many ads. Do not download this game."</p>
|
85 |
-
<cite>- A user who gave 1 star</cite>
|
86 |
-
</blockquote>
|
87 |
-
<h2>Conclusion</h2>
|
88 |
-
<h3>Summary of the main points</h3>
|
89 |
-
<p>In conclusion, Car Parking Multiplayer is a game that offers a realistic and immersive driving simulator with a wide variety of cars, customization options, environments, modes, and challenges. It also has a multiplayer open world mode where you can play with thousands of real players from around the world. The game is free to download and play, but it contains ads and in-app purchases. The game has some pros and cons, as well as some tips and tricks that can help you improve your gameplay. The game has received mostly positive reviews and ratings from its users.</p>
|
90 |
-
<h3>Recommendations for potential players</h3>
|
91 |
-
<p>If you are interested in playing Car Parking Multiplayer, here are some recommendations for you:</p>
|
92 |
-
<ul>
|
93 |
-
<li><b>Download the latest version APK from a trusted source</b>: To enjoy the new features, improvements, and bug fixes of the game, you should download the latest version APK from . This will ensure that you have the best version of the game on your device.</li>
|
94 |
-
<li><b>Try different cars and modes</b>: To make the most out of the game, you should try different cars and modes that suit your taste and skill level. You can experiment with different settings and features to customize your car and enhance your performance. You can also switch between different modes, such as parking, driving, racing, or police, to have different experiences and challenges.</li>
|
95 |
-
<li><b>Join the online community</b>: To have more fun and interaction, you should join the online community of Car Parking Multiplayer. You can chat with other players, exchange cars, race with them, or cooperate with them in various scenarios. You can also create your own server and invite your friends to play with you. You can also follow the official social media accounts of the game to get updates, news, and tips.</li>
|
96 |
-
</ul>
|
97 |
-
<h3>FAQs</h3>
|
98 |
-
<p>Here are some frequently asked questions about Car Parking Multiplayer:</p>
|
99 |
-
<ol>
|
100 |
-
<li><b>Is Car Parking Multiplayer safe to download and play?</b><br>Yes, Car Parking Multiplayer is safe to download and play, as long as you get it from a trusted source like . However, you should be careful when playing online, as some players may try to scam you or hack your account. You should also avoid clicking on suspicious links or ads that may redirect you to malicious websites or apps.</li>
|
101 |
-
<li><b>How can I remove ads from Car Parking Multiplayer?</b><br>You can remove ads from Car Parking Multiplayer by purchasing the premium version of the game for $2.99. This will also give you access to some exclusive cars and features. Alternatively, you can turn off your internet connection while playing the game, but this will disable the multiplayer mode and some online features.</li>
|
102 |
-
<li><b>How can I get more money and XP in Car Parking Multiplayer?</b><br>You can get more money and XP in Car Parking Multiplayer by completing challenges, racing with other players, or selling your cars. You can also watch ads or complete offers to get free money and XP. However, you should avoid using any cheats or hacks that claim to give you unlimited money and XP, as they may harm your device or get you banned from the game.</li>
|
103 |
-
<li><b>How can I contact the developers of Car Parking Multiplayer?</b><br>You can contact the developers of Car Parking Multiplayer by sending them an email at [email protected]. You can also visit their website at or follow them on Facebook at . You can also leave a review or a comment on Google Play Store to share your feedback or suggestions.</li>
|
104 |
-
<li><b>What are the system requirements for Car Parking Multiplayer?</b><br>The system requirements for Car Parking Multiplayer are as follows:</p>
|
105 |
-
<ul>
|
106 |
-
<li>Android version: 4.4 or higher</li>
|
107 |
-
<li>RAM: 1 GB or higher</li>
|
108 |
-
<li>Storage: 300 MB or higher</li>
|
109 |
-
<li>Internet connection: Required for multiplayer mode and some online features</li>
|
110 |
-
</ul></ol></p> 401be4b1e0<br />
|
111 |
-
<br />
|
112 |
-
<br />
|
|
|
|
|
|
|
|
|
|
|
|
|
|
|
|
|
|
|
|
|
|
|
|
|
|
|
|
|
|
|
|
|
|
|
|
|
|
|
|
|
|
|
|
|
|
|
|
|
|
|
|
|
|
|
|
|
|
|
|
|
|
|
|
|
|
|
|
|
|
|
|
|
|
|
|
|
|
|
|
|
|
|
|
|
|
|
|
|
|
|
|
|
|
|
|
|
|
|
|
|
|
|
|
|
|
|
|
|
|
|
|
|
|
|
|
|
|
|
|
|
|
|
|
|
|
|
|
|
|
|
|
|
|
|
|
|
|
|
|
|
|
|
|
|
|
|
|
|
|
|
|
|
|
|
|
|
|
|
|
|
|
|
|
|
|
|
|
|
|
|
|
|
|
|
|
|
|
|
|
|
|
|
|
|
|
|
|
|
|
|
|
|
|
|
|
|
|
|
|
|
|
|
|
|
|
|
|
|
|
|
|
|
|
|
|
|
|
|
|
|
|
|
|
|
|
spaces/801artistry/RVC801/extract_locale.py
DELETED
@@ -1,34 +0,0 @@
|
|
1 |
-
import json
|
2 |
-
import re
|
3 |
-
|
4 |
-
# Define regular expression patterns
|
5 |
-
pattern = r"""i18n\([\s\n\t]*(["'][^"']+["'])[\s\n\t]*\)"""
|
6 |
-
|
7 |
-
# Initialize the dictionary to store key-value pairs
|
8 |
-
data = {}
|
9 |
-
|
10 |
-
|
11 |
-
def process(fn: str):
|
12 |
-
global data
|
13 |
-
with open(fn, "r", encoding="utf-8") as f:
|
14 |
-
contents = f.read()
|
15 |
-
matches = re.findall(pattern, contents)
|
16 |
-
for key in matches:
|
17 |
-
key = eval(key)
|
18 |
-
print("extract:", key)
|
19 |
-
data[key] = key
|
20 |
-
|
21 |
-
|
22 |
-
print("processing infer-web.py")
|
23 |
-
process("infer-web.py")
|
24 |
-
|
25 |
-
print("processing gui_v0.py")
|
26 |
-
process("gui_v0.py")
|
27 |
-
|
28 |
-
print("processing gui_v1.py")
|
29 |
-
process("gui_v1.py")
|
30 |
-
|
31 |
-
# Save as a JSON file
|
32 |
-
with open("./i18n/en_US.json", "w", encoding="utf-8") as f:
|
33 |
-
json.dump(data, f, ensure_ascii=False, indent=4)
|
34 |
-
f.write("\n")
|
|
|
|
|
|
|
|
|
|
|
|
|
|
|
|
|
|
|
|
|
|
|
|
|
|
|
|
|
|
|
|
|
|
|
|
|
|
|
|
|
|
|
|
|
|
|
|
|
|
|
|
|
|
|
|
|
|
|
|
|
|
|
|
|
|
|
|
|
|
spaces/AIConsultant/MusicGen/audiocraft/quantization/base.py
DELETED
@@ -1,99 +0,0 @@
|
|
1 |
-
# Copyright (c) Meta Platforms, Inc. and affiliates.
|
2 |
-
# All rights reserved.
|
3 |
-
#
|
4 |
-
# This source code is licensed under the license found in the
|
5 |
-
# LICENSE file in the root directory of this source tree.
|
6 |
-
|
7 |
-
"""
|
8 |
-
Base class for all quantizers.
|
9 |
-
"""
|
10 |
-
|
11 |
-
from dataclasses import dataclass, field
|
12 |
-
import typing as tp
|
13 |
-
|
14 |
-
import torch
|
15 |
-
from torch import nn
|
16 |
-
|
17 |
-
|
18 |
-
@dataclass
|
19 |
-
class QuantizedResult:
|
20 |
-
x: torch.Tensor
|
21 |
-
codes: torch.Tensor
|
22 |
-
bandwidth: torch.Tensor # bandwidth in kb/s used, per batch item.
|
23 |
-
penalty: tp.Optional[torch.Tensor] = None
|
24 |
-
metrics: dict = field(default_factory=dict)
|
25 |
-
|
26 |
-
|
27 |
-
class BaseQuantizer(nn.Module):
|
28 |
-
"""Base class for quantizers.
|
29 |
-
"""
|
30 |
-
|
31 |
-
def forward(self, x: torch.Tensor, frame_rate: int) -> QuantizedResult:
|
32 |
-
"""
|
33 |
-
Given input tensor x, returns first the quantized (or approximately quantized)
|
34 |
-
representation along with quantized codes, bandwidth, and any penalty term for the loss.
|
35 |
-
Finally, this returns a dict of metrics to update logging etc.
|
36 |
-
Frame rate must be passed so that the bandwidth is properly computed.
|
37 |
-
"""
|
38 |
-
raise NotImplementedError()
|
39 |
-
|
40 |
-
def encode(self, x: torch.Tensor) -> torch.Tensor:
|
41 |
-
"""Encode a given input tensor with the specified sample rate at the given bandwidth."""
|
42 |
-
raise NotImplementedError()
|
43 |
-
|
44 |
-
def decode(self, codes: torch.Tensor) -> torch.Tensor:
|
45 |
-
"""Decode the given codes to the quantized representation."""
|
46 |
-
raise NotImplementedError()
|
47 |
-
|
48 |
-
@property
|
49 |
-
def total_codebooks(self):
|
50 |
-
"""Total number of codebooks."""
|
51 |
-
raise NotImplementedError()
|
52 |
-
|
53 |
-
@property
|
54 |
-
def num_codebooks(self):
|
55 |
-
"""Number of active codebooks."""
|
56 |
-
raise NotImplementedError()
|
57 |
-
|
58 |
-
def set_num_codebooks(self, n: int):
|
59 |
-
"""Set the number of active codebooks."""
|
60 |
-
raise NotImplementedError()
|
61 |
-
|
62 |
-
|
63 |
-
class DummyQuantizer(BaseQuantizer):
|
64 |
-
"""Fake quantizer that actually does not perform any quantization.
|
65 |
-
"""
|
66 |
-
def __init__(self):
|
67 |
-
super().__init__()
|
68 |
-
|
69 |
-
def forward(self, x: torch.Tensor, frame_rate: int):
|
70 |
-
q = x.unsqueeze(1)
|
71 |
-
return QuantizedResult(x, q, torch.tensor(q.numel() * 32 * frame_rate / 1000 / len(x)).to(x))
|
72 |
-
|
73 |
-
def encode(self, x: torch.Tensor) -> torch.Tensor:
|
74 |
-
"""Encode a given input tensor with the specified sample rate at the given bandwidth.
|
75 |
-
In the case of the DummyQuantizer, the codes are actually identical
|
76 |
-
to the input and resulting quantized representation as no quantization is done.
|
77 |
-
"""
|
78 |
-
return x.unsqueeze(1)
|
79 |
-
|
80 |
-
def decode(self, codes: torch.Tensor) -> torch.Tensor:
|
81 |
-
"""Decode the given codes to the quantized representation.
|
82 |
-
In the case of the DummyQuantizer, the codes are actually identical
|
83 |
-
to the input and resulting quantized representation as no quantization is done.
|
84 |
-
"""
|
85 |
-
return codes.squeeze(1)
|
86 |
-
|
87 |
-
@property
|
88 |
-
def total_codebooks(self):
|
89 |
-
"""Total number of codebooks."""
|
90 |
-
return 1
|
91 |
-
|
92 |
-
@property
|
93 |
-
def num_codebooks(self):
|
94 |
-
"""Total number of codebooks."""
|
95 |
-
return self.total_codebooks
|
96 |
-
|
97 |
-
def set_num_codebooks(self, n: int):
|
98 |
-
"""Set the number of active codebooks."""
|
99 |
-
raise AttributeError("Cannot override the number of codebooks for the dummy quantizer")
|
|
|
|
|
|
|
|
|
|
|
|
|
|
|
|
|
|
|
|
|
|
|
|
|
|
|
|
|
|
|
|
|
|
|
|
|
|
|
|
|
|
|
|
|
|
|
|
|
|
|
|
|
|
|
|
|
|
|
|
|
|
|
|
|
|
|
|
|
|
|
|
|
|
|
|
|
|
|
|
|
|
|
|
|
|
|
|
|
|
|
|
|
|
|
|
|
|
|
|
|
|
|
|
|
|
|
|
|
|
|
|
|
|
|
|
|
|
|
|
|
|
|
|
|
|
|
|
|
|
|
|
|
|
|
|
|
|
|
|
|
|
|
|
|
|
|
|
|
|
|
|
|
|
|
|
|
|
|
|
|
|
|
|
|
|
|
|
|
|
|
|
|
|
|
|
|
|
|
|
|
|
|
|
|
|
|
|
|
|
|
|
|
|
|
|
|
|
|
|
spaces/AIFILMS/generate_human_motion/VQ-Trans/models/pos_encoding.py
DELETED
@@ -1,43 +0,0 @@
|
|
1 |
-
"""
|
2 |
-
Various positional encodings for the transformer.
|
3 |
-
"""
|
4 |
-
import math
|
5 |
-
import torch
|
6 |
-
from torch import nn
|
7 |
-
|
8 |
-
def PE1d_sincos(seq_length, dim):
|
9 |
-
"""
|
10 |
-
:param d_model: dimension of the model
|
11 |
-
:param length: length of positions
|
12 |
-
:return: length*d_model position matrix
|
13 |
-
"""
|
14 |
-
if dim % 2 != 0:
|
15 |
-
raise ValueError("Cannot use sin/cos positional encoding with "
|
16 |
-
"odd dim (got dim={:d})".format(dim))
|
17 |
-
pe = torch.zeros(seq_length, dim)
|
18 |
-
position = torch.arange(0, seq_length).unsqueeze(1)
|
19 |
-
div_term = torch.exp((torch.arange(0, dim, 2, dtype=torch.float) *
|
20 |
-
-(math.log(10000.0) / dim)))
|
21 |
-
pe[:, 0::2] = torch.sin(position.float() * div_term)
|
22 |
-
pe[:, 1::2] = torch.cos(position.float() * div_term)
|
23 |
-
|
24 |
-
return pe.unsqueeze(1)
|
25 |
-
|
26 |
-
|
27 |
-
class PositionEmbedding(nn.Module):
|
28 |
-
"""
|
29 |
-
Absolute pos embedding (standard), learned.
|
30 |
-
"""
|
31 |
-
def __init__(self, seq_length, dim, dropout, grad=False):
|
32 |
-
super().__init__()
|
33 |
-
self.embed = nn.Parameter(data=PE1d_sincos(seq_length, dim), requires_grad=grad)
|
34 |
-
self.dropout = nn.Dropout(p=dropout)
|
35 |
-
|
36 |
-
def forward(self, x):
|
37 |
-
# x.shape: bs, seq_len, feat_dim
|
38 |
-
l = x.shape[1]
|
39 |
-
x = x.permute(1, 0, 2) + self.embed[:l].expand(x.permute(1, 0, 2).shape)
|
40 |
-
x = self.dropout(x.permute(1, 0, 2))
|
41 |
-
return x
|
42 |
-
|
43 |
-
|
|
|
|
|
|
|
|
|
|
|
|
|
|
|
|
|
|
|
|
|
|
|
|
|
|
|
|
|
|
|
|
|
|
|
|
|
|
|
|
|
|
|
|
|
|
|
|
|
|
|
|
|
|
|
|
|
|
|
|
|
|
|
|
|
|
|
|
|
|
|
|
|
|
|
|
|
|
|
|
|
|
|
|
|
|
|
|
spaces/AIWaves/Software_Company/src/agents/Memory/__init__.py
DELETED
@@ -1 +0,0 @@
|
|
1 |
-
from .base_Memory import Memory
|
|
|
|
spaces/AIWaves/Software_Company/src/agents/SOP.py
DELETED
@@ -1,296 +0,0 @@
|
|
1 |
-
# coding=utf-8
|
2 |
-
# Copyright 2023 The AIWaves Inc. team.
|
3 |
-
|
4 |
-
#
|
5 |
-
# Licensed under the Apache License, Version 2.0 (the "License");
|
6 |
-
# you may not use this file except in compliance with the License.
|
7 |
-
# You may obtain a copy of the License at
|
8 |
-
#
|
9 |
-
# http://www.apache.org/licenses/LICENSE-2.0
|
10 |
-
#
|
11 |
-
# Unless required by applicable law or agreed to in writing, software
|
12 |
-
# distributed under the License is distributed on an "AS IS" BASIS,
|
13 |
-
# WITHOUT WARRANTIES OR CONDITIONS OF ANY KIND, either express or implied.
|
14 |
-
# See the License for the specific language governing permissions and
|
15 |
-
# limitations under the License.
|
16 |
-
"""standard operation procedure of an LLM Autonomous agent"""
|
17 |
-
import random
|
18 |
-
from LLM.base_LLM import *
|
19 |
-
from State import State
|
20 |
-
from utils import extract, get_relevant_history
|
21 |
-
from Memory import Memory
|
22 |
-
from Prompt import *
|
23 |
-
import json
|
24 |
-
import os
|
25 |
-
|
26 |
-
class SOP:
|
27 |
-
"""
|
28 |
-
Responsible for managing the operational processes of all agents
|
29 |
-
"""
|
30 |
-
|
31 |
-
# SOP should have args : "states" "relations" "root"
|
32 |
-
|
33 |
-
def __init__(self, **kwargs):
|
34 |
-
self.controller_dict = {}
|
35 |
-
self.LLM = init_LLM("logs/god",**kwargs)
|
36 |
-
|
37 |
-
self.states = {}
|
38 |
-
self.init_states(kwargs["states"])
|
39 |
-
self.init_relation(kwargs["relations"])
|
40 |
-
for state_name, states_dict in kwargs["states"].items():
|
41 |
-
if state_name != "end_state" and "controller" in states_dict:
|
42 |
-
self.controller_dict[state_name] = states_dict["controller"]
|
43 |
-
|
44 |
-
self.user_names = kwargs["user_names"] if "user_names" in kwargs else []
|
45 |
-
self.root = self.states[kwargs["root"]]
|
46 |
-
self.current_state = self.root
|
47 |
-
self.finish_state_name = (
|
48 |
-
kwargs["finish_state_name"]
|
49 |
-
if "finish_state_name" in kwargs
|
50 |
-
else "end_state"
|
51 |
-
)
|
52 |
-
self.roles_to_names = None
|
53 |
-
self.names_to_roles = None
|
54 |
-
self.finished = False
|
55 |
-
|
56 |
-
@classmethod
|
57 |
-
def from_config(cls, config_path):
|
58 |
-
with open(config_path) as f:
|
59 |
-
config = json.load(f)
|
60 |
-
os.environ.clear()
|
61 |
-
for key,value in config["config"].items():
|
62 |
-
if key == "API_BASE":
|
63 |
-
if value == "":
|
64 |
-
pass
|
65 |
-
else:
|
66 |
-
os.environ[key] = value
|
67 |
-
# assert "API_KEY" in os.environ and os.environ["API_KEY"] != "API_KEY","Please go to config.json to set API_KEY"
|
68 |
-
|
69 |
-
sop = SOP(**config)
|
70 |
-
return sop
|
71 |
-
|
72 |
-
def init_states(self, states_dict):
|
73 |
-
for state_name, state_dict in states_dict.items():
|
74 |
-
state_dict["name"] = state_name
|
75 |
-
self.states[state_name] = State(**state_dict)
|
76 |
-
|
77 |
-
def init_relation(self, relations):
|
78 |
-
for state_name, state_relation in relations.items():
|
79 |
-
for idx, next_state_name in state_relation.items():
|
80 |
-
self.states[state_name].next_states[idx] = self.states[next_state_name]
|
81 |
-
|
82 |
-
def transit(self, chat_history, **kwargs):
|
83 |
-
"""
|
84 |
-
Determine the next state based on the current situation
|
85 |
-
Return :
|
86 |
-
next_state(State) : the next state
|
87 |
-
"""
|
88 |
-
# 如果是单一循环节点,则一直循环即可
|
89 |
-
# If it is a single loop node, just keep looping
|
90 |
-
if len(self.current_state.next_states) == 1:
|
91 |
-
next_state = "0"
|
92 |
-
|
93 |
-
# 否则则需要controller去判断进入哪一节点
|
94 |
-
# Otherwise, the controller needs to determine which node to enter.
|
95 |
-
else:
|
96 |
-
current_state = self.current_state
|
97 |
-
controller_dict = self.controller_dict[current_state.name]
|
98 |
-
relevant_history = kwargs["relevant_history"]
|
99 |
-
|
100 |
-
max_chat_nums = controller_dict["max_chat_nums"] if "max_chat_nums" in controller_dict else 1000
|
101 |
-
if current_state.chat_nums>=max_chat_nums:
|
102 |
-
return self.current_state.next_states["1"]
|
103 |
-
|
104 |
-
|
105 |
-
# 否则则让controller判断是否结束
|
106 |
-
# Otherwise, let the controller judge whether to end
|
107 |
-
judge_system_prompt = controller_dict["judge_system_prompt"]
|
108 |
-
environment_prompt = eval(Get_environment_prompt) if current_state.environment_prompt else ""
|
109 |
-
transit_system_prompt = eval(Transit_system_prompt)
|
110 |
-
|
111 |
-
judge_last_prompt = controller_dict["judge_last_prompt"]
|
112 |
-
transit_last_prompt = eval(Transit_last_prompt)
|
113 |
-
|
114 |
-
|
115 |
-
|
116 |
-
environment = kwargs["environment"]
|
117 |
-
environment_summary = environment.shared_memory["short_term_memory"]
|
118 |
-
chat_history_message = Memory.get_chat_history(chat_history)
|
119 |
-
query = chat_history[-1].get_query()
|
120 |
-
|
121 |
-
chat_messages = [
|
122 |
-
{
|
123 |
-
"role": "user",
|
124 |
-
"content": eval(Transit_message)
|
125 |
-
}
|
126 |
-
]
|
127 |
-
|
128 |
-
extract_words = controller_dict["judge_extract_words"] if "judge_extract_words" in controller_dict else "end"
|
129 |
-
|
130 |
-
|
131 |
-
response = self.LLM.get_response(
|
132 |
-
chat_messages, transit_system_prompt, transit_last_prompt, stream=False, **kwargs
|
133 |
-
)
|
134 |
-
next_state = (
|
135 |
-
response if response.isdigit() else extract(response, extract_words)
|
136 |
-
)
|
137 |
-
|
138 |
-
# 如果没有parse出来则继续循环
|
139 |
-
# If no parse comes out, continue looping
|
140 |
-
if not next_state.isdigit():
|
141 |
-
next_state = "0"
|
142 |
-
|
143 |
-
next_state = self.current_state.next_states[next_state]
|
144 |
-
return next_state
|
145 |
-
|
146 |
-
|
147 |
-
def route(self, chat_history, **kwargs):
|
148 |
-
"""
|
149 |
-
Determine the role that needs action based on the current situation
|
150 |
-
Return :
|
151 |
-
current_agent(Agent) : the next act agent
|
152 |
-
"""
|
153 |
-
|
154 |
-
agents = kwargs["agents"]
|
155 |
-
|
156 |
-
# 知道进入哪一状态后开始分配角色,如果该状态下只有一个角色则直接分配给他
|
157 |
-
# Start assigning roles after knowing which state you have entered. If there is only one role in that state, assign it directly to him.
|
158 |
-
if len(self.current_state.roles) == 1:
|
159 |
-
next_role = self.current_state.roles[0]
|
160 |
-
|
161 |
-
|
162 |
-
|
163 |
-
# 否则controller进行分配
|
164 |
-
# Otherwise the controller determines
|
165 |
-
else:
|
166 |
-
relevant_history = kwargs["relevant_history"]
|
167 |
-
controller_type = (
|
168 |
-
self.controller_dict[self.current_state.name]["controller_type"]
|
169 |
-
if "controller_type" in self.controller_dict[self.current_state.name]
|
170 |
-
else "order"
|
171 |
-
)
|
172 |
-
|
173 |
-
|
174 |
-
# 如果是rule 控制器,则交由LLM进行分配角色
|
175 |
-
# If controller type is rule, it is left to LLM to assign roles.
|
176 |
-
if controller_type == "rule":
|
177 |
-
controller_dict = self.controller_dict[self.current_state.name]
|
178 |
-
|
179 |
-
call_last_prompt = controller_dict["call_last_prompt"] if "call_last_prompt" in controller_dict else ""
|
180 |
-
|
181 |
-
allocate_prompt = ""
|
182 |
-
roles = list(set(self.current_state.roles))
|
183 |
-
for role in roles:
|
184 |
-
allocate_prompt += eval(Allocate_component)
|
185 |
-
|
186 |
-
call_system_prompt = controller_dict["call_system_prompt"] if "call_system_prompt" in controller_dict else ""
|
187 |
-
environment_prompt = eval(Get_environment_prompt) if self.current_state.environment_prompt else ""
|
188 |
-
# call_system_prompt + environment + allocate_prompt
|
189 |
-
call_system_prompt = eval(Call_system_prompt)
|
190 |
-
|
191 |
-
query = chat_history[-1].get_query()
|
192 |
-
last_name = chat_history[-1].send_name
|
193 |
-
# last_prompt: note + last_prompt + query
|
194 |
-
call_last_prompt =eval(Call_last_prompt)
|
195 |
-
|
196 |
-
|
197 |
-
chat_history_message = Memory.get_chat_history(chat_history)
|
198 |
-
# Intermediate historical conversation records
|
199 |
-
chat_messages = [
|
200 |
-
{
|
201 |
-
"role": "user",
|
202 |
-
"content": eval(Call_message),
|
203 |
-
}
|
204 |
-
]
|
205 |
-
|
206 |
-
extract_words = controller_dict["call_extract_words"] if "call_extract_words" in controller_dict else "end"
|
207 |
-
|
208 |
-
response = self.LLM.get_response(
|
209 |
-
chat_messages, call_system_prompt, call_last_prompt, stream=False, **kwargs
|
210 |
-
)
|
211 |
-
|
212 |
-
# get next role
|
213 |
-
next_role = extract(response, extract_words)
|
214 |
-
|
215 |
-
# Speak in order
|
216 |
-
elif controller_type == "order":
|
217 |
-
# If there is no begin role, it will be given directly to the first person.
|
218 |
-
if not self.current_state.current_role:
|
219 |
-
next_role = self.current_state.roles[0]
|
220 |
-
# otherwise first
|
221 |
-
else:
|
222 |
-
self.current_state.index += 1
|
223 |
-
self.current_state.index = (self.current_state.index) % len(self.current_state.roles)
|
224 |
-
next_role = self.current_state.roles[self.current_state.index]
|
225 |
-
# random speak
|
226 |
-
elif controller_type == "random":
|
227 |
-
next_role = random.choice(self.current_state.roles)
|
228 |
-
|
229 |
-
# 如果下一角色不在,则随机挑选一个
|
230 |
-
# If the next character is not available, pick one at random
|
231 |
-
if next_role not in self.current_state.roles:
|
232 |
-
next_role = random.choice(self.current_state.roles)
|
233 |
-
|
234 |
-
self.current_state.current_role = next_role
|
235 |
-
|
236 |
-
next_agent = agents[self.roles_to_names[self.current_state.name][next_role]]
|
237 |
-
|
238 |
-
return next_agent
|
239 |
-
|
240 |
-
def next(self, environment, agents):
|
241 |
-
"""
|
242 |
-
Determine the next state and the agent that needs action based on the current situation
|
243 |
-
"""
|
244 |
-
|
245 |
-
# 如��是第一次进入该状态
|
246 |
-
# If it is the first time to enter this state
|
247 |
-
|
248 |
-
if self.current_state.is_begin:
|
249 |
-
agent_name = self.roles_to_names[self.current_state.name][self.current_state.begin_role]
|
250 |
-
agent = agents[agent_name]
|
251 |
-
return self.current_state,agent
|
252 |
-
|
253 |
-
|
254 |
-
# get relevant history
|
255 |
-
query = environment.shared_memory["long_term_memory"][-1].content
|
256 |
-
relevant_history = get_relevant_history(
|
257 |
-
query,
|
258 |
-
environment.shared_memory["long_term_memory"][:-1],
|
259 |
-
environment.shared_memory["chat_embeddings"][:-1],
|
260 |
-
)
|
261 |
-
relevant_history = Memory.get_chat_history(relevant_history)
|
262 |
-
|
263 |
-
|
264 |
-
|
265 |
-
next_state = self.transit(
|
266 |
-
chat_history=environment.shared_memory["long_term_memory"][
|
267 |
-
environment.current_chat_history_idx :
|
268 |
-
],
|
269 |
-
relevant_history=relevant_history,
|
270 |
-
environment=environment,
|
271 |
-
)
|
272 |
-
# 如果进入终止节点,则直接终止
|
273 |
-
# If you enter the termination node, terminate directly
|
274 |
-
if next_state.name == self.finish_state_name:
|
275 |
-
self.finished = True
|
276 |
-
return None, None
|
277 |
-
|
278 |
-
self.current_state = next_state
|
279 |
-
|
280 |
-
# 如果是首次进入该节点且有开场白,则直接分配给开场角色
|
281 |
-
# If it is the first time to enter the state and there is a begin query, it will be directly assigned to the begin role.
|
282 |
-
if self.current_state.is_begin and self.current_state.begin_role:
|
283 |
-
agent_name = self.roles_to_names[self.current_state.name][self.current_state.begin_role]
|
284 |
-
agent = agents[agent_name]
|
285 |
-
return self.current_state,agent
|
286 |
-
|
287 |
-
|
288 |
-
next_agent = self.route(
|
289 |
-
chat_history=environment.shared_memory["long_term_memory"][
|
290 |
-
environment.current_chat_history_idx :
|
291 |
-
],
|
292 |
-
agents = agents,
|
293 |
-
relevant_history=relevant_history,
|
294 |
-
)
|
295 |
-
|
296 |
-
return self.current_state, next_agent
|
|
|
|
|
|
|
|
|
|
|
|
|
|
|
|
|
|
|
|
|
|
|
|
|
|
|
|
|
|
|
|
|
|
|
|
|
|
|
|
|
|
|
|
|
|
|
|
|
|
|
|
|
|
|
|
|
|
|
|
|
|
|
|
|
|
|
|
|
|
|
|
|
|
|
|
|
|
|
|
|
|
|
|
|
|
|
|
|
|
|
|
|
|
|
|
|
|
|
|
|
|
|
|
|
|
|
|
|
|
|
|
|
|
|
|
|
|
|
|
|
|
|
|
|
|
|
|
|
|
|
|
|
|
|
|
|
|
|
|
|
|
|
|
|
|
|
|
|
|
|
|
|
|
|
|
|
|
|
|
|
|
|
|
|
|
|
|
|
|
|
|
|
|
|
|
|
|
|
|
|
|
|
|
|
|
|
|
|
|
|
|
|
|
|
|
|
|
|
|
|
|
|
|
|
|
|
|
|
|
|
|
|
|
|
|
|
|
|
|
|
|
|
|
|
|
|
|
|
|
|
|
|
|
|
|
|
|
|
|
|
|
|
|
|
|
|
|
|
|
|
|
|
|
|
|
|
|
|
|
|
|
|
|
|
|
|
|
|
|
|
|
|
|
|
|
|
|
|
|
|
|
|
|
|
|
|
|
|
|
|
|
|
|
|
|
|
|
|
|
|
|
|
|
|
|
|
|
|
|
|
|
|
|
|
|
|
|
|
|
|
|
|
|
|
|
|
|
|
|
|
|
|
|
|
|
|
|
|
|
|
|
|
|
|
|
|
|
|
|
|
|
|
|
|
|
|
|
|
|
|
|
|
|
|
|
|
|
|
|
|
|
|
|
|
|
|
|
|
|
|
|
|
|
|
|
|
|
|
|
|
|
|
|
|
|
|
|
|
|
|
|
|
|
|
|
|
|
|
|
|
|
|
|
|
|
|
|
|
|
|
|
|
|
|
|
|
|
|
|
|
|
|
|
|
|
|
|
|
|
|
|
|
|
|
|
|
|
|
|
|
|
|
|
|
|
|
|
|
|
|
|
|
|
|
|
|
|
|
|
|
|
|
|
|
|
|
|
|
|
|
|
|
|
|
|
|
|
|
|
|
|
|
|
|
|
|
|
|
|
|
|
|
|
|
|
|
|
|
|
|
|
|
|
|
|
|
|
|
|
|
|
|
|
|
|
|
|
|
|
|
|
|
|
|
|
|
|
|
|
|
|
|
|
|
|
|
|
|
|
|
|
|
|
|
|
|
|
|
|
|
|
|
|
|
|
|
|
|
|
|
|
|
|
|
|
|
|
|
|
|
|
|
|
|
|
|
|
|
|
|
|
|
|
spaces/AchyuthGamer/OpenGPT/g4f/Provider/Providers/Easychat.py
DELETED
@@ -1,55 +0,0 @@
|
|
1 |
-
import requests
|
2 |
-
import os
|
3 |
-
import json
|
4 |
-
from ...typing import sha256, Dict, get_type_hints
|
5 |
-
|
6 |
-
url = 'https://free.easychat.work'
|
7 |
-
model = ['gpt-3.5-turbo', 'gpt-3.5-turbo-16k',
|
8 |
-
'gpt-3.5-turbo-16k-0613', 'gpt-3.5-turbo-0613']
|
9 |
-
supports_stream = True
|
10 |
-
needs_auth = False
|
11 |
-
|
12 |
-
|
13 |
-
def _create_completion(model: str, messages: list, stream: bool, **kwargs):
|
14 |
-
headers = {
|
15 |
-
'authority': 'free.easychat.work',
|
16 |
-
'accept': 'text/event-stream',
|
17 |
-
'accept-language': 'en,fr-FR;q=0.9,fr;q=0.8,es-ES;q=0.7,es;q=0.6,en-US;q=0.5,am;q=0.4,de;q=0.3',
|
18 |
-
'content-type': 'application/json',
|
19 |
-
'endpoint': '',
|
20 |
-
'origin': 'https://free.easychat.work',
|
21 |
-
'plugins': '0',
|
22 |
-
'referer': 'https://free.easychat.work/',
|
23 |
-
'sec-ch-ua': '"Not.A/Brand";v="8", "Chromium";v="114", "Google Chrome";v="114"',
|
24 |
-
'sec-ch-ua-mobile': '?0',
|
25 |
-
'sec-ch-ua-platform': '"macOS"',
|
26 |
-
'sec-fetch-dest': 'empty',
|
27 |
-
'sec-fetch-mode': 'cors',
|
28 |
-
'sec-fetch-site': 'same-origin',
|
29 |
-
'user-agent': 'Mozilla/5.0 (Macintosh; Intel Mac OS X 10_15_7) AppleWebKit/537.36 (KHTML, like Gecko) Chrome/114.0.0.0 Safari/537.36',
|
30 |
-
'usesearch': 'false',
|
31 |
-
'x-requested-with': 'XMLHttpRequest',
|
32 |
-
}
|
33 |
-
|
34 |
-
json_data = {
|
35 |
-
'messages': messages,
|
36 |
-
'stream': True,
|
37 |
-
'model': model,
|
38 |
-
'temperature': 0.5,
|
39 |
-
'presence_penalty': 0,
|
40 |
-
'frequency_penalty': 0,
|
41 |
-
'top_p': 1,
|
42 |
-
}
|
43 |
-
|
44 |
-
response = requests.post('https://free.easychat.work/api/openai/v1/chat/completions',
|
45 |
-
headers=headers, json=json_data)
|
46 |
-
|
47 |
-
for chunk in response.iter_lines():
|
48 |
-
if b'content' in chunk:
|
49 |
-
data = json.loads(chunk.decode().split('data: ')[1])
|
50 |
-
yield (data['choices'][0]['delta']['content'])
|
51 |
-
|
52 |
-
|
53 |
-
params = f'g4f.Providers.{os.path.basename(__file__)[:-3]} supports: ' + \
|
54 |
-
'(%s)' % ', '.join(
|
55 |
-
[f"{name}: {get_type_hints(_create_completion)[name].__name__}" for name in _create_completion.__code__.co_varnames[:_create_completion.__code__.co_argcount]])
|
|
|
|
|
|
|
|
|
|
|
|
|
|
|
|
|
|
|
|
|
|
|
|
|
|
|
|
|
|
|
|
|
|
|
|
|
|
|
|
|
|
|
|
|
|
|
|
|
|
|
|
|
|
|
|
|
|
|
|
|
|
|
|
|
|
|
|
|
|
|
|
|
|
|
|
|
|
|
|
|
|
|
|
|
|
|
|
|
|
|
|
|
|
|
|
|
|
|
|
|
|
|
|
|
|
|
|
|
|
|
|
spaces/AgentVerse/agentVerse/ui/src/phaser3-rex-plugins/plugins/raycaster-plugin.d.ts
DELETED
@@ -1,8 +0,0 @@
|
|
1 |
-
import Raycaster from './raycaster';
|
2 |
-
|
3 |
-
export default class RaycasterPlugin extends Phaser.Plugins.BasePlugin {
|
4 |
-
add(
|
5 |
-
config?: Raycaster.IConfig
|
6 |
-
): Raycaster;
|
7 |
-
|
8 |
-
}
|
|
|
|
|
|
|
|
|
|
|
|
|
|
|
|
|
|
spaces/AgentVerse/agentVerse/ui/src/phaser3-rex-plugins/templates/spinner/cube/Cube.js
DELETED
@@ -1,57 +0,0 @@
|
|
1 |
-
import Base from '../base/Base.js';
|
2 |
-
import { Line } from '../utils/Geoms.js';
|
3 |
-
import Yoyo from '../utils/Yoyo.js';
|
4 |
-
|
5 |
-
const Linear = Phaser.Math.Linear;
|
6 |
-
const ExpoIn = Phaser.Math.Easing.Expo.In;
|
7 |
-
const RowNum = 2;
|
8 |
-
const ColNum = 2;
|
9 |
-
|
10 |
-
class Cube extends Base {
|
11 |
-
constructor(scene, config) {
|
12 |
-
super(scene, config);
|
13 |
-
this.type = 'rexSpinnerCube';
|
14 |
-
}
|
15 |
-
|
16 |
-
buildShapes() {
|
17 |
-
var cnt = RowNum * ColNum;
|
18 |
-
for (var i = 0; i < cnt; i++) {
|
19 |
-
var line = new Line();
|
20 |
-
this.addShape(line);
|
21 |
-
}
|
22 |
-
}
|
23 |
-
|
24 |
-
updateShapes() {
|
25 |
-
var centerX = this.centerX;
|
26 |
-
var centerY = this.centerY;
|
27 |
-
var radius = this.radius;
|
28 |
-
var leftBound = centerX - radius;
|
29 |
-
var topBound = centerY - radius;
|
30 |
-
var cellWidth = (radius * 2) / ColNum;
|
31 |
-
var cellHeight = (radius * 2) / RowNum;
|
32 |
-
|
33 |
-
var shapes = this.getShapes(),
|
34 |
-
cnt = shapes.length;
|
35 |
-
for (var i = 0; i < cnt; i++) {
|
36 |
-
var colIdx = (i % ColNum);
|
37 |
-
var rowIdx = Math.floor(i / RowNum);
|
38 |
-
var x = leftBound + (cellWidth * (colIdx + 0.5));
|
39 |
-
var y = topBound + (cellHeight * (rowIdx + 0.5));
|
40 |
-
|
41 |
-
var line = shapes[i];
|
42 |
-
var t = (this.value + ((cnt - i) * 0.1)) % 1;
|
43 |
-
t = ExpoIn(Yoyo(t));
|
44 |
-
|
45 |
-
var lineAlpha = (cnt - i) / cnt;
|
46 |
-
var lineHeight = Linear(0.7, 1, t) * cellHeight;
|
47 |
-
var lineWidth = Linear(0.7, 1, t) * cellWidth;
|
48 |
-
|
49 |
-
line
|
50 |
-
.lineStyle(lineWidth, this.color, lineAlpha)
|
51 |
-
.setP0(x - (lineHeight / 2), y)
|
52 |
-
.setP1(x + (lineHeight / 2), y);
|
53 |
-
}
|
54 |
-
}
|
55 |
-
}
|
56 |
-
|
57 |
-
export default Cube;
|
|
|
|
|
|
|
|
|
|
|
|
|
|
|
|
|
|
|
|
|
|
|
|
|
|
|
|
|
|
|
|
|
|
|
|
|
|
|
|
|
|
|
|
|
|
|
|
|
|
|
|
|
|
|
|
|
|
|
|
|
|
|
|
|
|
|
|
|
|
|
|
|
|
|
|
|
|
|
|
|
|
|
|
|
|
|
|
|
|
|
|
|
|
|
|
|
|
|
|
|
|
|
|
|
|
|
|
|
|
|
|
|
|
|
|
spaces/AgentVerse/agentVerse/ui/src/phaser3-rex-plugins/templates/spinner/spinner-plugin.d.ts
DELETED
@@ -1,87 +0,0 @@
|
|
1 |
-
import AudioFactory from './audio/Factory';
|
2 |
-
import BallFactory from './ball/Factory';
|
3 |
-
import BarsFactory from './bars/Factory';
|
4 |
-
import BoxFactory from './box/Factory';
|
5 |
-
import ClockFactory from './clock/Factory';
|
6 |
-
import CubeFactory from './cube/Factory';
|
7 |
-
import CustomFactory from './custom/Factory';
|
8 |
-
import DotsFactory from './dots/Factory';
|
9 |
-
import FacebookFactory from './facebook/Factory';
|
10 |
-
import GridFactory from './grid/Factory';
|
11 |
-
import LosFactory from './los/Factory';
|
12 |
-
import OrbitFactory from './orbit/Factory';
|
13 |
-
import OvalFactory from './oval/Factory';
|
14 |
-
import PieFactory from './pie/Factory';
|
15 |
-
import PuffFactory from './puff/Factory';
|
16 |
-
import RadioFactory from './radio/Factory';
|
17 |
-
import RingsFactory from './rings/Factory';
|
18 |
-
import SpinnerFactory from './spinner/Factory';
|
19 |
-
|
20 |
-
export default SpinnerPlugins;
|
21 |
-
|
22 |
-
declare class Factories {
|
23 |
-
audio: typeof AudioFactory;
|
24 |
-
ball: typeof BallFactory;
|
25 |
-
bars: typeof BarsFactory;
|
26 |
-
box: typeof BoxFactory;
|
27 |
-
clock: typeof ClockFactory;
|
28 |
-
cube: typeof CubeFactory;
|
29 |
-
custom: typeof CustomFactory;
|
30 |
-
dots: typeof DotsFactory;
|
31 |
-
facebook: typeof FacebookFactory;
|
32 |
-
grid: typeof GridFactory;
|
33 |
-
los: typeof LosFactory;
|
34 |
-
orbit: typeof OrbitFactory;
|
35 |
-
oval: typeof OvalFactory;
|
36 |
-
pie: typeof PieFactory;
|
37 |
-
puff: typeof PuffFactory;
|
38 |
-
radio: typeof RadioFactory;
|
39 |
-
rings: typeof RingsFactory;
|
40 |
-
spinner: typeof SpinnerFactory;
|
41 |
-
}
|
42 |
-
|
43 |
-
declare class SpinnerPlugins {
|
44 |
-
constructor(scene: Phaser.Scene);
|
45 |
-
|
46 |
-
add: Factories;
|
47 |
-
}
|
48 |
-
|
49 |
-
import AudioClass from './audio/Audio';
|
50 |
-
import BallClass from './ball/Ball';
|
51 |
-
import BarsClass from './bars/Bars';
|
52 |
-
import BoxClass from './box/Box';
|
53 |
-
import ClockClass from './clock/Clock';
|
54 |
-
import CubeClass from './cube/Cube';
|
55 |
-
import CustomClass from './custom/Custom';
|
56 |
-
import DotsClass from './dots/Dots';
|
57 |
-
import FacebookClass from './facebook/Facebook';
|
58 |
-
import GridClass from './grid/Grid';
|
59 |
-
import LosClass from './los/Los';
|
60 |
-
import OrbitClass from './orbit/Orbit';
|
61 |
-
import OvalClass from './oval/Oval';
|
62 |
-
import PieClass from './pie/Pie';
|
63 |
-
import PuffClass from './puff/Puff';
|
64 |
-
import RadioClass from './radio/Radio';
|
65 |
-
import RingsClass from './rings/Rings';
|
66 |
-
import SpinnerClass from './spinner/Spinner';
|
67 |
-
|
68 |
-
declare namespace SpinnerPlugins {
|
69 |
-
type Audio = AudioClass;
|
70 |
-
type Ball = BallClass;
|
71 |
-
type Bars = BarsClass
|
72 |
-
type Box = BoxClass;
|
73 |
-
type Clock = ClockClass;
|
74 |
-
type Cube = CubeClass;
|
75 |
-
type Custom = CustomClass;
|
76 |
-
type Dots = DotsClass;
|
77 |
-
type Facebook = FacebookClass;
|
78 |
-
type Grid = GridClass;
|
79 |
-
type Los = LosClass;
|
80 |
-
type Orbit = OrbitClass;
|
81 |
-
type Oval = OvalClass;
|
82 |
-
type Pie = PieClass;
|
83 |
-
type Puff = PuffClass;
|
84 |
-
type Radio = RadioClass;
|
85 |
-
type Rings = RingsClass;
|
86 |
-
type Spinner = SpinnerClass;
|
87 |
-
}
|
|
|
|
|
|
|
|
|
|
|
|
|
|
|
|
|
|
|
|
|
|
|
|
|
|
|
|
|
|
|
|
|
|
|
|
|
|
|
|
|
|
|
|
|
|
|
|
|
|
|
|
|
|
|
|
|
|
|
|
|
|
|
|
|
|
|
|
|
|
|
|
|
|
|
|
|
|
|
|
|
|
|
|
|
|
|
|
|
|
|
|
|
|
|
|
|
|
|
|
|
|
|
|
|
|
|
|
|
|
|
|
|
|
|
|
|
|
|
|
|
|
|
|
|
|
|
|
|
|
|
|
|
|
|
|
|
|
|
|
|
|
|
|
|
|
|
|
|
|
|
|
|
|
|
|
|
|
|
|
|
|
|
|
|
|
|
|
|
|
|
|
|
|
|
|
spaces/AgentVerse/agentVerse/ui/src/phaser3-rex-plugins/templates/ui/colorinput/colorpicker/methods/HPaletteCanvas.js
DELETED
@@ -1,107 +0,0 @@
|
|
1 |
-
import Canvas from '../../../canvas/Canvas.js';
|
2 |
-
import GetOrientationMode from '../../../utils/GetOrientationMode.js';
|
3 |
-
import { DrawHPalette } from '../../../../../plugins/utils/canvas/DrawHSVPalette.js';
|
4 |
-
|
5 |
-
const Color = Phaser.Display.Color;
|
6 |
-
const Percent = Phaser.Math.Percent;
|
7 |
-
const ColorToRGBA = Phaser.Display.Color.ColorToRGBA;
|
8 |
-
const HSVToRGB = Phaser.Display.Color.HSVToRGB;
|
9 |
-
|
10 |
-
class HPaletteCanvas extends Canvas {
|
11 |
-
constructor(scene, x, y, width, height, orientation) {
|
12 |
-
if (x === undefined) { x = 0; }
|
13 |
-
if (y === undefined) { y = 0; }
|
14 |
-
if (width === undefined) { width = 2; }
|
15 |
-
if (height === undefined) { height = 2; }
|
16 |
-
|
17 |
-
super(scene, x, y, width, height);
|
18 |
-
this.type = 'rexColorPicker.HPaletteCanvas';
|
19 |
-
|
20 |
-
this.colorObject = new Color();
|
21 |
-
|
22 |
-
this.setOrientation(orientation);
|
23 |
-
this.setSize(width, height);
|
24 |
-
}
|
25 |
-
|
26 |
-
setOrientation(orientation) {
|
27 |
-
this.orientation = GetOrientationMode(orientation);
|
28 |
-
return this;
|
29 |
-
}
|
30 |
-
|
31 |
-
updateTexture() {
|
32 |
-
DrawHPalette(this.canvas, this.context, this.orientation);
|
33 |
-
super.updateTexture();
|
34 |
-
return this;
|
35 |
-
}
|
36 |
-
|
37 |
-
get color() {
|
38 |
-
return this.colorObject.color;
|
39 |
-
}
|
40 |
-
|
41 |
-
get hue() {
|
42 |
-
return this._hue;
|
43 |
-
}
|
44 |
-
|
45 |
-
set hue(value) {
|
46 |
-
this._hue = value;
|
47 |
-
}
|
48 |
-
|
49 |
-
getHue(localX, localY) {
|
50 |
-
if (localX === undefined) {
|
51 |
-
return this.hue;
|
52 |
-
}
|
53 |
-
|
54 |
-
if (this.orientation === 0) {
|
55 |
-
this.hue = Percent(localX, 0, this.width);
|
56 |
-
} else {
|
57 |
-
this.hue = Percent(localY, 0, this.height);
|
58 |
-
}
|
59 |
-
|
60 |
-
return this.hue;
|
61 |
-
}
|
62 |
-
|
63 |
-
getColor(localX, localY) {
|
64 |
-
if (localX === undefined) {
|
65 |
-
return this.color;
|
66 |
-
}
|
67 |
-
|
68 |
-
var h = this.getHue(localX, localY);
|
69 |
-
this.colorObject.setFromRGB(HSVToRGB(h, 1, 1));
|
70 |
-
return this.colorObject.color;
|
71 |
-
}
|
72 |
-
|
73 |
-
setColor(color) {
|
74 |
-
if (this.color === color) {
|
75 |
-
return this;
|
76 |
-
}
|
77 |
-
|
78 |
-
return this;
|
79 |
-
}
|
80 |
-
|
81 |
-
colorToLocalPosition(color, out) {
|
82 |
-
if (out === undefined) {
|
83 |
-
out = {};
|
84 |
-
} else if (out === true) {
|
85 |
-
if (LocalXY === undefined) {
|
86 |
-
LocalXY = {};
|
87 |
-
}
|
88 |
-
out = LocalXY;
|
89 |
-
}
|
90 |
-
|
91 |
-
this.colorObject.setFromRGB(ColorToRGBA(color));
|
92 |
-
|
93 |
-
if (this.orientation === 0) {
|
94 |
-
out.x = this.width * this.colorObject.h;
|
95 |
-
out.y = this.height / 2;
|
96 |
-
} else {
|
97 |
-
out.x = this.width / 2;
|
98 |
-
out.y = this.height * this.colorObject.h;
|
99 |
-
}
|
100 |
-
|
101 |
-
return out;
|
102 |
-
}
|
103 |
-
}
|
104 |
-
|
105 |
-
var LocalXY = undefined;
|
106 |
-
|
107 |
-
export default HPaletteCanvas;
|
|
|
|
|
|
|
|
|
|
|
|
|
|
|
|
|
|
|
|
|
|
|
|
|
|
|
|
|
|
|
|
|
|
|
|
|
|
|
|
|
|
|
|
|
|
|
|
|
|
|
|
|
|
|
|
|
|
|
|
|
|
|
|
|
|
|
|
|
|
|
|
|
|
|
|
|
|
|
|
|
|
|
|
|
|
|
|
|
|
|
|
|
|
|
|
|
|
|
|
|
|
|
|
|
|
|
|
|
|
|
|
|
|
|
|
|
|
|
|
|
|
|
|
|
|
|
|
|
|
|
|
|
|
|
|
|
|
|
|
|
|
|
|
|
|
|
|
|
|
|
|
|
|
|
|
|
|
|
|
|
|
|
|
|
|
|
|
|
|
|
|
|
|
|
|
|
|
|
|
|
|
|
|
|
|
|
|
|
|
|
|
|
|
|
|
|
|
|
|
|
|
|
|
|
|
|
|
|
|
|
|
|
|
|
|
spaces/AlbertoFH98/CastenaApp/app.py
DELETED
@@ -1,97 +0,0 @@
|
|
1 |
-
# -- Import libraries
|
2 |
-
from langchain.prompts import PromptTemplate
|
3 |
-
from streamlit.logger import get_logger
|
4 |
-
import pandas as pd
|
5 |
-
import streamlit as st
|
6 |
-
import urllib.request
|
7 |
-
import argparse
|
8 |
-
import together
|
9 |
-
import logging
|
10 |
-
import utils
|
11 |
-
import spacy
|
12 |
-
import time
|
13 |
-
import os
|
14 |
-
|
15 |
-
def main():
|
16 |
-
# -- 1. Setup arguments
|
17 |
-
parser = argparse.ArgumentParser()
|
18 |
-
parser.add_argument('--DEFAULT_SYSTEM_PROMPT_LINK', type=str, default="https://raw.githubusercontent.com/AlbertoUAH/Castena/main/prompts/default_system_prompt.txt", help='Valor para DEFAULT_SYSTEM_PROMPT_LINK')
|
19 |
-
parser.add_argument('--PODCAST_URL_VIDEO_PATH', type=str, default="https://raw.githubusercontent.com/AlbertoUAH/Castena/main/data/podcast_youtube_video.csv", help='Valor para PODCAST_URL_VIDEO_PATH')
|
20 |
-
parser.add_argument('--TRANSCRIPTION', type=str, default='worldcast_roberto_vaquero', help='Name of the trascription')
|
21 |
-
parser.add_argument('--MODEL', type=str, default='togethercomputer/llama-2-7b-chat', help='Model name')
|
22 |
-
parser.add_argument('--EMB_MODEL', type=str, default='BAAI/bge-base-en-v1.5', help='Embedding model name')
|
23 |
-
os.system("python -m spacy download es_core_news_lg")
|
24 |
-
|
25 |
-
# -- 2. Setup env and logger
|
26 |
-
os.environ["TOGETHER_API_KEY"] = "6101599d6e33e3bda336b8d007ca22e35a64c72cfd52c2d8197f663389fc50c5"
|
27 |
-
logger = get_logger(__name__)
|
28 |
-
|
29 |
-
# -- 3. Setup constants
|
30 |
-
B_INST, E_INST = "[INST]", "[/INST]"
|
31 |
-
B_SYS, E_SYS = "<<SYS>>\n", "\n<</SYS>>\n\n"
|
32 |
-
args = parser.parse_args()
|
33 |
-
PODCAST_URL_VIDEO_PATH = args.PODCAST_URL_VIDEO_PATH
|
34 |
-
DEFAULT_SYSTEM_PROMPT_LINK = args.DEFAULT_SYSTEM_PROMPT_LINK
|
35 |
-
TRANSCRIPTION = args.TRANSCRIPTION
|
36 |
-
TRANSCRIPTION_PATH = '{}_transcription.txt'.format(TRANSCRIPTION)
|
37 |
-
MODEL = args.MODEL
|
38 |
-
EMB_MODEL = args.EMB_MODEL
|
39 |
-
SOCIAL_ICONS = {
|
40 |
-
"LinkedIn": ["https://www.linkedin.com/in/alberto-fernandez-hernandez-3a3474136/", "https://icon.signature.email/social/linkedin-circle-medium-0077b5-FFFFFF.png"],
|
41 |
-
"GitHub": ["https://github.com/AlbertoUAH", "https://icon.signature.email/social/github-circle-medium-24292e-FFFFFF.png"]
|
42 |
-
}
|
43 |
-
social_icons_html = [f"<a href='{SOCIAL_ICONS[platform][0]}' target='_blank' style='margin-right: 10px;'><img class='social-icon' src='{SOCIAL_ICONS[platform][1]}'' alt='{platform}''></a>" for platform in SOCIAL_ICONS]
|
44 |
-
|
45 |
-
together.api_key = os.environ["TOGETHER_API_KEY"]
|
46 |
-
together.Models.start(MODEL)
|
47 |
-
podcast_url_video_df = pd.read_csv(PODCAST_URL_VIDEO_PATH, sep=';')
|
48 |
-
youtube_video_url = list(podcast_url_video_df[podcast_url_video_df['podcast_name'] == "\'" + TRANSCRIPTION + "\'"]['youtube_video_url'])[0].replace("\'", "")
|
49 |
-
|
50 |
-
# -- 4. Setup request for system prompt
|
51 |
-
f = urllib.request.urlopen(DEFAULT_SYSTEM_PROMPT_LINK)
|
52 |
-
DEFAULT_SYSTEM_PROMPT = str(f.read(), 'UTF-8')
|
53 |
-
|
54 |
-
# -- 5. Setup app
|
55 |
-
translator, nlp, retriever = utils.setup_app(TRANSCRIPTION_PATH, EMB_MODEL, MODEL, logger)
|
56 |
-
|
57 |
-
|
58 |
-
# -- 6. Setup prompt template + llm chain
|
59 |
-
instruction = """CONTEXT:/n/n {context}/n
|
60 |
-
|
61 |
-
Question: {question}"""
|
62 |
-
prompt_template = utils.get_prompt(instruction, DEFAULT_SYSTEM_PROMPT, B_SYS, E_SYS, B_INST, E_INST, logger)
|
63 |
-
|
64 |
-
llama_prompt = PromptTemplate(
|
65 |
-
template=prompt_template, input_variables=["context", "question"]
|
66 |
-
)
|
67 |
-
chain_type_kwargs = {"prompt": llama_prompt}
|
68 |
-
|
69 |
-
qa_chain = utils.create_llm_chain(MODEL, retriever, chain_type_kwargs, logger)
|
70 |
-
|
71 |
-
# ---------------------------------------------------------------------
|
72 |
-
# -- 7. Setup Streamlit app
|
73 |
-
st.title("Podcast: {}".format(' '.join(x.capitalize() for x in TRANSCRIPTION.split('_'))))
|
74 |
-
st.image("https://raw.githubusercontent.com/AlbertoUAH/autexTification/main/media/{}.jpeg".format(TRANSCRIPTION))
|
75 |
-
|
76 |
-
original_input_text = st.text_input("Pregunta")
|
77 |
-
if st.button("Consultar") or original_input_text:
|
78 |
-
translated_input_text = utils.translate_text(original_input_text, nlp, target_lang='en')
|
79 |
-
logger.info('A query has been launched. Query: {}'.format(original_input_text))
|
80 |
-
logger.info('Waiting for response...')
|
81 |
-
llm_response = qa_chain(translated_input_text)
|
82 |
-
llm_response = utils.process_llm_response(llm_response, nlp).replace(': ', ':<br>').replace('. ', '.<br>').replace('" ', '"<br>')
|
83 |
-
logger.info('Response recieved successfully! {}'.format(llm_response))
|
84 |
-
typewrited_llm_response = utils.typewrite(utils.add_hyperlink_and_convert_to_seconds(llm_response), youtube_video_url)
|
85 |
-
st.components.v1.html(typewrited_llm_response, width=800, height=750, scrolling=True)
|
86 |
-
|
87 |
-
st.write(f"""<div class="subtitle" style="text-align: center;">Información de contacto</div>""", unsafe_allow_html=True)
|
88 |
-
st.write(f"""
|
89 |
-
<div style="display: flex; justify-content: center; margin-bottom: 10px;">
|
90 |
-
{''.join(social_icons_html)}
|
91 |
-
</div>""",
|
92 |
-
unsafe_allow_html=True
|
93 |
-
)
|
94 |
-
|
95 |
-
# -- Sample: streamlit run app.py -- --DEFAULT_SYSTEM_PROMPT_LINK=https://raw.githubusercontent.com/AlbertoUAH/Castena/main/prompts/default_system_prompt.txt --PODCAST_URL_VIDEO_PATH=https://raw.githubusercontent.com/AlbertoUAH/Castena/main/data/podcast_youtube_video.csv --TRANSCRIPTION=worldcast_roberto_vaquero --MODEL=togethercomputer/llama-2-7b-chat --EMB_MODEL=BAAI/bge-base-en-v1.5
|
96 |
-
if __name__ == '__main__':
|
97 |
-
main()
|
|
|
|
|
|
|
|
|
|
|
|
|
|
|
|
|
|
|
|
|
|
|
|
|
|
|
|
|
|
|
|
|
|
|
|
|
|
|
|
|
|
|
|
|
|
|
|
|
|
|
|
|
|
|
|
|
|
|
|
|
|
|
|
|
|
|
|
|
|
|
|
|
|
|
|
|
|
|
|
|
|
|
|
|
|
|
|
|
|
|
|
|
|
|
|
|
|
|
|
|
|
|
|
|
|
|
|
|
|
|
|
|
|
|
|
|
|
|
|
|
|
|
|
|
|
|
|
|
|
|
|
|
|
|
|
|
|
|
|
|
|
|
|
|
|
|
|
|
|
|
|
|
|
|
|
|
|
|
|
|
|
|
|
|
|
|
|
|
|
|
|
|
|
|
|
|
|
|
|
|
|
|
|
|
|
|
|
|
|
|
|
|
|
|
|
spaces/Alisonbakers/Fml/Dockerfile
DELETED
@@ -1,21 +0,0 @@
|
|
1 |
-
FROM node:18-bullseye-slim
|
2 |
-
|
3 |
-
RUN apt-get update && \
|
4 |
-
|
5 |
-
apt-get install -y git
|
6 |
-
|
7 |
-
RUN git clone https://gitgud.io/khanon/oai-reverse-proxy.git /app
|
8 |
-
|
9 |
-
WORKDIR /app
|
10 |
-
|
11 |
-
RUN npm install
|
12 |
-
|
13 |
-
COPY Dockerfile greeting.md* .env* ./
|
14 |
-
|
15 |
-
RUN npm run build
|
16 |
-
|
17 |
-
EXPOSE 7860
|
18 |
-
|
19 |
-
ENV NODE_ENV=production
|
20 |
-
|
21 |
-
CMD [ "npm", "start" ]
|
|
|
|
|
|
|
|
|
|
|
|
|
|
|
|
|
|
|
|
|
|
|
|
|
|
|
|
|
|
|
|
|
|
|
|
|
|
|
|
|
|
|
|
spaces/Aloento/9Nine-PITS/text/frontend/tone_sandhi.py
DELETED
@@ -1,348 +0,0 @@
|
|
1 |
-
# Copyright (c) 2021 PaddlePaddle Authors. All Rights Reserved.
|
2 |
-
#
|
3 |
-
# Licensed under the Apache License, Version 2.0 (the "License");
|
4 |
-
# you may not use this file except in compliance with the License.
|
5 |
-
# You may obtain a copy of the License at
|
6 |
-
#
|
7 |
-
# http://www.apache.org/licenses/LICENSE-2.0
|
8 |
-
#
|
9 |
-
# Unless required by applicable law or agreed to in writing, software
|
10 |
-
# distributed under the License is distributed on an "AS IS" BASIS,
|
11 |
-
# WITHOUT WARRANTIES OR CONDITIONS OF ANY KIND, either express or implied.
|
12 |
-
# See the License for the specific language governing permissions and
|
13 |
-
# limitations under the License.
|
14 |
-
from typing import List
|
15 |
-
from typing import Tuple
|
16 |
-
|
17 |
-
import jieba
|
18 |
-
from pypinyin import Style
|
19 |
-
from pypinyin import lazy_pinyin
|
20 |
-
|
21 |
-
|
22 |
-
class ToneSandhi():
|
23 |
-
def __init__(self):
|
24 |
-
self.must_neural_tone_words = {
|
25 |
-
'麻烦', '麻利', '鸳鸯', '高粱', '骨头', '骆驼', '马虎', '首饰', '馒头', '馄饨', '风筝',
|
26 |
-
'难为', '队伍', '阔气', '闺女', '门道', '锄头', '铺盖', '铃铛', '铁匠', '钥匙', '里脊',
|
27 |
-
'里头', '部分', '那么', '道士', '造化', '迷糊', '连累', '这么', '这个', '运气', '过去',
|
28 |
-
'软和', '转悠', '踏实', '跳蚤', '跟头', '趔趄', '财主', '豆腐', '讲究', '记性', '记号',
|
29 |
-
'认识', '规矩', '见识', '裁缝', '补丁', '衣裳', '衣服', '衙门', '街坊', '行李', '行当',
|
30 |
-
'蛤蟆', '蘑菇', '薄荷', '葫芦', '葡萄', '萝卜', '荸荠', '苗条', '苗头', '苍蝇', '芝麻',
|
31 |
-
'舒服', '舒坦', '舌头', '自在', '膏药', '脾气', '脑袋', '脊梁', '能耐', '胳膊', '胭脂',
|
32 |
-
'胡萝', '胡琴', '胡同', '聪明', '耽误', '耽搁', '耷拉', '耳朵', '老爷', '老实', '老婆',
|
33 |
-
'老头', '老太', '翻腾', '罗嗦', '罐头', '编辑', '结实', '红火', '累赘', '糨糊', '糊涂',
|
34 |
-
'精神', '粮食', '簸箕', '篱笆', '算计', '算盘', '答应', '笤帚', '笑语', '笑话', '窟窿',
|
35 |
-
'窝囊', '窗户', '稳当', '稀罕', '称呼', '秧歌', '秀气', '秀才', '福气', '祖宗', '砚台',
|
36 |
-
'码头', '石榴', '石头', '石匠', '知识', '眼睛', '眯缝', '眨巴', '眉毛', '相声', '盘算',
|
37 |
-
'白净', '痢疾', '痛快', '疟疾', '疙瘩', '疏忽', '畜生', '生意', '甘蔗', '琵琶', '琢磨',
|
38 |
-
'琉璃', '玻璃', '玫瑰', '玄乎', '狐狸', '状元', '特务', '牲口', '牙碜', '牌楼', '爽快',
|
39 |
-
'爱人', '热闹', '烧饼', '烟筒', '烂糊', '点心', '炊帚', '灯笼', '火候', '漂亮', '滑溜',
|
40 |
-
'溜达', '温和', '清楚', '消息', '浪头', '活泼', '比方', '正经', '欺负', '模糊', '槟榔',
|
41 |
-
'棺材', '棒槌', '棉花', '核桃', '栅栏', '柴火', '架势', '枕头', '枇杷', '机灵', '本事',
|
42 |
-
'木头', '木匠', '朋友', '月饼', '月亮', '暖和', '明白', '时候', '新鲜', '故事', '收拾',
|
43 |
-
'收成', '提防', '挖苦', '挑剔', '指甲', '指头', '拾掇', '拳头', '拨弄', '招牌', '招呼',
|
44 |
-
'抬举', '护士', '折腾', '扫帚', '打量', '打算', '打点', '打扮', '打听', '打发', '扎实',
|
45 |
-
'扁担', '戒指', '懒得', '意识', '意思', '情形', '悟性', '怪物', '思量', '怎么', '念头',
|
46 |
-
'念叨', '快活', '忙活', '志气', '心思', '得罪', '张罗', '弟兄', '开通', '应酬', '庄稼',
|
47 |
-
'干事', '帮手', '帐篷', '希罕', '师父', '师傅', '巴结', '巴掌', '差事', '工夫', '岁数',
|
48 |
-
'屁股', '尾巴', '少爷', '小气', '小伙', '将就', '对头', '对付', '寡妇', '家伙', '客气',
|
49 |
-
'实在', '官司', '学问', '学生', '字号', '嫁妆', '媳妇', '媒人', '婆家', '娘家', '委屈',
|
50 |
-
'姑娘', '姐夫', '妯娌', '妥当', '妖精', '奴才', '女婿', '头发', '太阳', '大爷', '大方',
|
51 |
-
'大意', '大夫', '多少', '多么', '外甥', '壮实', '地道', '地方', '在乎', '困难', '嘴巴',
|
52 |
-
'嘱咐', '嘟囔', '嘀咕', '喜欢', '喇嘛', '喇叭', '商量', '唾沫', '哑巴', '哈欠', '哆嗦',
|
53 |
-
'咳嗽', '和尚', '告诉', '告示', '含糊', '吓唬', '后头', '名字', '名堂', '合同', '吆喝',
|
54 |
-
'叫唤', '口袋', '厚道', '厉害', '千斤', '包袱', '包涵', '匀称', '勤快', '动静', '动弹',
|
55 |
-
'功夫', '力气', '前头', '刺猬', '刺激', '别扭', '利落', '利索', '利害', '分析', '出息',
|
56 |
-
'凑合', '凉快', '冷战', '冤枉', '冒失', '养活', '关系', '先生', '兄弟', '便宜', '使唤',
|
57 |
-
'佩服', '作坊', '体面', '位置', '似的', '伙计', '休息', '什么', '人家', '亲戚', '亲家',
|
58 |
-
'交情', '云彩', '事情', '买卖', '主意', '丫头', '丧气', '两口', '东西', '东家', '世故',
|
59 |
-
'不由', '不在', '下水', '下巴', '上头', '上司', '丈夫', '丈人', '一辈', '那个', '菩萨',
|
60 |
-
'父亲', '母亲', '咕噜', '邋遢', '费用', '冤家', '甜头', '介绍', '荒唐', '大人', '泥鳅',
|
61 |
-
'幸福', '熟悉', '计划', '扑腾', '蜡��', '姥爷', '照顾', '喉咙', '吉他', '弄堂', '蚂蚱',
|
62 |
-
'凤凰', '拖沓', '寒碜', '糟蹋', '倒腾', '报复', '逻辑', '盘缠', '喽啰', '牢骚', '咖喱',
|
63 |
-
'扫把', '惦记'
|
64 |
-
}
|
65 |
-
self.must_not_neural_tone_words = {
|
66 |
-
"男子", "女子", "分子", "原子", "量子", "莲子", "石子", "瓜子", "电子", "人人", "虎虎"
|
67 |
-
}
|
68 |
-
self.punc = ":,;。?!“”‘’':,;.?!"
|
69 |
-
|
70 |
-
# the meaning of jieba pos tag: https://blog.csdn.net/weixin_44174352/article/details/113731041
|
71 |
-
# e.g.
|
72 |
-
# word: "家里"
|
73 |
-
# pos: "s"
|
74 |
-
# finals: ['ia1', 'i3']
|
75 |
-
def _neural_sandhi(self, word: str, pos: str,
|
76 |
-
finals: List[str]) -> List[str]:
|
77 |
-
|
78 |
-
# reduplication words for n. and v. e.g. 奶奶, 试试, 旺旺
|
79 |
-
for j, item in enumerate(word):
|
80 |
-
if j - 1 >= 0 and item == word[j - 1] and pos[0] in {
|
81 |
-
"n", "v", "a"
|
82 |
-
} and word not in self.must_not_neural_tone_words:
|
83 |
-
finals[j] = finals[j][:-1] + "5"
|
84 |
-
ge_idx = word.find("个")
|
85 |
-
if len(word) >= 1 and word[-1] in "吧呢哈啊呐噻嘛吖嗨呐哦哒额滴哩哟喽啰耶喔诶":
|
86 |
-
finals[-1] = finals[-1][:-1] + "5"
|
87 |
-
elif len(word) >= 1 and word[-1] in "的地得":
|
88 |
-
finals[-1] = finals[-1][:-1] + "5"
|
89 |
-
# e.g. 走了, 看着, 去过
|
90 |
-
elif len(word) == 1 and word in "了着过" and pos in {"ul", "uz", "ug"}:
|
91 |
-
finals[-1] = finals[-1][:-1] + "5"
|
92 |
-
elif len(word) > 1 and word[-1] in "们子" and pos in {
|
93 |
-
"r", "n"
|
94 |
-
} and word not in self.must_not_neural_tone_words:
|
95 |
-
finals[-1] = finals[-1][:-1] + "5"
|
96 |
-
# e.g. 桌上, 地下, 家里
|
97 |
-
elif len(word) > 1 and word[-1] in "上下里" and pos in {"s", "l", "f"}:
|
98 |
-
finals[-1] = finals[-1][:-1] + "5"
|
99 |
-
# e.g. 上来, 下去
|
100 |
-
elif len(word) > 1 and word[-1] in "来去" and word[-2] in "上下进出回过起开":
|
101 |
-
finals[-1] = finals[-1][:-1] + "5"
|
102 |
-
# 个做量词
|
103 |
-
elif (ge_idx >= 1 and
|
104 |
-
(word[ge_idx - 1].isnumeric() or
|
105 |
-
word[ge_idx - 1] in "几有两半多各整每做是")) or word == '个':
|
106 |
-
finals[ge_idx] = finals[ge_idx][:-1] + "5"
|
107 |
-
else:
|
108 |
-
if word in self.must_neural_tone_words or word[
|
109 |
-
-2:] in self.must_neural_tone_words:
|
110 |
-
finals[-1] = finals[-1][:-1] + "5"
|
111 |
-
|
112 |
-
word_list = self._split_word(word)
|
113 |
-
finals_list = [finals[:len(word_list[0])], finals[len(word_list[0]):]]
|
114 |
-
for i, word in enumerate(word_list):
|
115 |
-
# conventional neural in Chinese
|
116 |
-
if word in self.must_neural_tone_words or word[
|
117 |
-
-2:] in self.must_neural_tone_words:
|
118 |
-
finals_list[i][-1] = finals_list[i][-1][:-1] + "5"
|
119 |
-
finals = sum(finals_list, [])
|
120 |
-
return finals
|
121 |
-
|
122 |
-
def _bu_sandhi(self, word: str, finals: List[str]) -> List[str]:
|
123 |
-
# e.g. 看不懂
|
124 |
-
if len(word) == 3 and word[1] == "不":
|
125 |
-
finals[1] = finals[1][:-1] + "5"
|
126 |
-
else:
|
127 |
-
for i, char in enumerate(word):
|
128 |
-
# "不" before tone4 should be bu2, e.g. 不怕
|
129 |
-
if char == "不" and i + 1 < len(word) and finals[i +
|
130 |
-
1][-1] == "4":
|
131 |
-
finals[i] = finals[i][:-1] + "2"
|
132 |
-
return finals
|
133 |
-
|
134 |
-
def _yi_sandhi(self, word: str, finals: List[str]) -> List[str]:
|
135 |
-
# "一" in number sequences, e.g. 一零零, 二一零
|
136 |
-
if word.find("一") != -1 and all(
|
137 |
-
[item.isnumeric() for item in word if item != "一"]):
|
138 |
-
return finals
|
139 |
-
# "一" between reduplication words shold be yi5, e.g. 看一看
|
140 |
-
elif len(word) == 3 and word[1] == "一" and word[0] == word[-1]:
|
141 |
-
finals[1] = finals[1][:-1] + "5"
|
142 |
-
# when "一" is ordinal word, it should be yi1
|
143 |
-
elif word.startswith("第一"):
|
144 |
-
finals[1] = finals[1][:-1] + "1"
|
145 |
-
else:
|
146 |
-
for i, char in enumerate(word):
|
147 |
-
if char == "一" and i + 1 < len(word):
|
148 |
-
# "一" before tone4 should be yi2, e.g. 一段
|
149 |
-
if finals[i + 1][-1] == "4":
|
150 |
-
finals[i] = finals[i][:-1] + "2"
|
151 |
-
# "一" before non-tone4 should be yi4, e.g. 一天
|
152 |
-
else:
|
153 |
-
# "一" 后面如果是标点,还读一声
|
154 |
-
if word[i + 1] not in self.punc:
|
155 |
-
finals[i] = finals[i][:-1] + "4"
|
156 |
-
return finals
|
157 |
-
|
158 |
-
def _split_word(self, word: str) -> List[str]:
|
159 |
-
word_list = jieba.cut_for_search(word)
|
160 |
-
word_list = sorted(word_list, key=lambda i: len(i), reverse=False)
|
161 |
-
first_subword = word_list[0]
|
162 |
-
first_begin_idx = word.find(first_subword)
|
163 |
-
if first_begin_idx == 0:
|
164 |
-
second_subword = word[len(first_subword):]
|
165 |
-
new_word_list = [first_subword, second_subword]
|
166 |
-
else:
|
167 |
-
second_subword = word[:-len(first_subword)]
|
168 |
-
new_word_list = [second_subword, first_subword]
|
169 |
-
return new_word_list
|
170 |
-
|
171 |
-
def _three_sandhi(self, word: str, finals: List[str]) -> List[str]:
|
172 |
-
if len(word) == 2 and self._all_tone_three(finals):
|
173 |
-
finals[0] = finals[0][:-1] + "2"
|
174 |
-
elif len(word) == 3:
|
175 |
-
word_list = self._split_word(word)
|
176 |
-
if self._all_tone_three(finals):
|
177 |
-
# disyllabic + monosyllabic, e.g. 蒙古/包
|
178 |
-
if len(word_list[0]) == 2:
|
179 |
-
finals[0] = finals[0][:-1] + "2"
|
180 |
-
finals[1] = finals[1][:-1] + "2"
|
181 |
-
# monosyllabic + disyllabic, e.g. 纸/老虎
|
182 |
-
elif len(word_list[0]) == 1:
|
183 |
-
finals[1] = finals[1][:-1] + "2"
|
184 |
-
else:
|
185 |
-
finals_list = [
|
186 |
-
finals[:len(word_list[0])], finals[len(word_list[0]):]
|
187 |
-
]
|
188 |
-
if len(finals_list) == 2:
|
189 |
-
for i, sub in enumerate(finals_list):
|
190 |
-
# e.g. 所有/人
|
191 |
-
if self._all_tone_three(sub) and len(sub) == 2:
|
192 |
-
finals_list[i][0] = finals_list[i][0][:-1] + "2"
|
193 |
-
# e.g. 好/喜欢
|
194 |
-
elif i == 1 and not self._all_tone_three(sub) and finals_list[i][0][-1] == "3" and \
|
195 |
-
finals_list[0][-1][-1] == "3":
|
196 |
-
|
197 |
-
finals_list[0][-1] = finals_list[0][-1][:-1] + "2"
|
198 |
-
finals = sum(finals_list, [])
|
199 |
-
# split idiom into two words who's length is 2
|
200 |
-
elif len(word) == 4:
|
201 |
-
finals_list = [finals[:2], finals[2:]]
|
202 |
-
finals = []
|
203 |
-
for sub in finals_list:
|
204 |
-
if self._all_tone_three(sub):
|
205 |
-
sub[0] = sub[0][:-1] + "2"
|
206 |
-
finals += sub
|
207 |
-
|
208 |
-
return finals
|
209 |
-
|
210 |
-
def _all_tone_three(self, finals: List[str]) -> bool:
|
211 |
-
return all(x[-1] == "3" for x in finals)
|
212 |
-
|
213 |
-
# merge "不" and the word behind it
|
214 |
-
# if don't merge, "不" sometimes appears alone according to jieba, which may occur sandhi error
|
215 |
-
def _merge_bu(self, seg: List[Tuple[str, str]]) -> List[Tuple[str, str]]:
|
216 |
-
new_seg = []
|
217 |
-
last_word = ""
|
218 |
-
for word, pos in seg:
|
219 |
-
if last_word == "不":
|
220 |
-
word = last_word + word
|
221 |
-
if word != "不":
|
222 |
-
new_seg.append((word, pos))
|
223 |
-
last_word = word[:]
|
224 |
-
if last_word == "不":
|
225 |
-
new_seg.append((last_word, 'd'))
|
226 |
-
last_word = ""
|
227 |
-
return new_seg
|
228 |
-
|
229 |
-
# function 1: merge "一" and reduplication words in it's left and right, e.g. "听","一","听" ->"听一听"
|
230 |
-
# function 2: merge single "一" and the word behind it
|
231 |
-
# if don't merge, "一" sometimes appears alone according to jieba, which may occur sandhi error
|
232 |
-
# e.g.
|
233 |
-
# input seg: [('听', 'v'), ('一', 'm'), ('听', 'v')]
|
234 |
-
# output seg: [['听一听', 'v']]
|
235 |
-
def _merge_yi(self, seg: List[Tuple[str, str]]) -> List[Tuple[str, str]]:
|
236 |
-
new_seg = []
|
237 |
-
# function 1
|
238 |
-
for i, (word, pos) in enumerate(seg):
|
239 |
-
if i - 1 >= 0 and word == "一" and i + 1 < len(seg) and seg[i - 1][
|
240 |
-
0] == seg[i + 1][0] and seg[i - 1][1] == "v":
|
241 |
-
new_seg[i - 1][0] = new_seg[i - 1][0] + "一" + new_seg[i - 1][0]
|
242 |
-
else:
|
243 |
-
if i - 2 >= 0 and seg[i - 1][0] == "一" and seg[i - 2][
|
244 |
-
0] == word and pos == "v":
|
245 |
-
continue
|
246 |
-
else:
|
247 |
-
new_seg.append([word, pos])
|
248 |
-
seg = new_seg
|
249 |
-
new_seg = []
|
250 |
-
# function 2
|
251 |
-
for i, (word, pos) in enumerate(seg):
|
252 |
-
if new_seg and new_seg[-1][0] == "一":
|
253 |
-
new_seg[-1][0] = new_seg[-1][0] + word
|
254 |
-
else:
|
255 |
-
new_seg.append([word, pos])
|
256 |
-
return new_seg
|
257 |
-
|
258 |
-
# the first and the second words are all_tone_three
|
259 |
-
def _merge_continuous_three_tones(
|
260 |
-
self, seg: List[Tuple[str, str]]) -> List[Tuple[str, str]]:
|
261 |
-
new_seg = []
|
262 |
-
sub_finals_list = [
|
263 |
-
lazy_pinyin(
|
264 |
-
word, neutral_tone_with_five=True, style=Style.FINALS_TONE3)
|
265 |
-
for (word, pos) in seg
|
266 |
-
]
|
267 |
-
assert len(sub_finals_list) == len(seg)
|
268 |
-
merge_last = [False] * len(seg)
|
269 |
-
for i, (word, pos) in enumerate(seg):
|
270 |
-
if i - 1 >= 0 and self._all_tone_three(
|
271 |
-
sub_finals_list[i - 1]) and self._all_tone_three(
|
272 |
-
sub_finals_list[i]) and not merge_last[i - 1]:
|
273 |
-
# if the last word is reduplication, not merge, because reduplication need to be _neural_sandhi
|
274 |
-
if not self._is_reduplication(seg[i - 1][0]) and len(
|
275 |
-
seg[i - 1][0]) + len(seg[i][0]) <= 3:
|
276 |
-
new_seg[-1][0] = new_seg[-1][0] + seg[i][0]
|
277 |
-
merge_last[i] = True
|
278 |
-
else:
|
279 |
-
new_seg.append([word, pos])
|
280 |
-
else:
|
281 |
-
new_seg.append([word, pos])
|
282 |
-
|
283 |
-
return new_seg
|
284 |
-
|
285 |
-
def _is_reduplication(self, word: str) -> bool:
|
286 |
-
return len(word) == 2 and word[0] == word[1]
|
287 |
-
|
288 |
-
# the last char of first word and the first char of second word is tone_three
|
289 |
-
def _merge_continuous_three_tones_2(
|
290 |
-
self, seg: List[Tuple[str, str]]) -> List[Tuple[str, str]]:
|
291 |
-
new_seg = []
|
292 |
-
sub_finals_list = [
|
293 |
-
lazy_pinyin(
|
294 |
-
word, neutral_tone_with_five=True, style=Style.FINALS_TONE3)
|
295 |
-
for (word, pos) in seg
|
296 |
-
]
|
297 |
-
assert len(sub_finals_list) == len(seg)
|
298 |
-
merge_last = [False] * len(seg)
|
299 |
-
for i, (word, pos) in enumerate(seg):
|
300 |
-
if i - 1 >= 0 and sub_finals_list[i - 1][-1][-1] == "3" and sub_finals_list[i][0][-1] == "3" and not \
|
301 |
-
merge_last[i - 1]:
|
302 |
-
# if the last word is reduplication, not merge, because reduplication need to be _neural_sandhi
|
303 |
-
if not self._is_reduplication(seg[i - 1][0]) and len(
|
304 |
-
seg[i - 1][0]) + len(seg[i][0]) <= 3:
|
305 |
-
new_seg[-1][0] = new_seg[-1][0] + seg[i][0]
|
306 |
-
merge_last[i] = True
|
307 |
-
else:
|
308 |
-
new_seg.append([word, pos])
|
309 |
-
else:
|
310 |
-
new_seg.append([word, pos])
|
311 |
-
return new_seg
|
312 |
-
|
313 |
-
def _merge_er(self, seg: List[Tuple[str, str]]) -> List[Tuple[str, str]]:
|
314 |
-
new_seg = []
|
315 |
-
for i, (word, pos) in enumerate(seg):
|
316 |
-
if i - 1 >= 0 and word == "儿" and seg[i - 1][0] != "#":
|
317 |
-
new_seg[-1][0] = new_seg[-1][0] + seg[i][0]
|
318 |
-
else:
|
319 |
-
new_seg.append([word, pos])
|
320 |
-
return new_seg
|
321 |
-
|
322 |
-
def _merge_reduplication(
|
323 |
-
self, seg: List[Tuple[str, str]]) -> List[Tuple[str, str]]:
|
324 |
-
new_seg = []
|
325 |
-
for i, (word, pos) in enumerate(seg):
|
326 |
-
if new_seg and word == new_seg[-1][0]:
|
327 |
-
new_seg[-1][0] = new_seg[-1][0] + seg[i][0]
|
328 |
-
else:
|
329 |
-
new_seg.append([word, pos])
|
330 |
-
return new_seg
|
331 |
-
|
332 |
-
def pre_merge_for_modify(
|
333 |
-
self, seg: List[Tuple[str, str]]) -> List[Tuple[str, str]]:
|
334 |
-
seg = self._merge_bu(seg)
|
335 |
-
seg = self._merge_yi(seg)
|
336 |
-
seg = self._merge_reduplication(seg)
|
337 |
-
seg = self._merge_continuous_three_tones(seg)
|
338 |
-
seg = self._merge_continuous_three_tones_2(seg)
|
339 |
-
seg = self._merge_er(seg)
|
340 |
-
return seg
|
341 |
-
|
342 |
-
def modified_tone(self, word: str, pos: str,
|
343 |
-
finals: List[str]) -> List[str]:
|
344 |
-
finals = self._bu_sandhi(word, finals)
|
345 |
-
finals = self._yi_sandhi(word, finals)
|
346 |
-
finals = self._neural_sandhi(word, pos, finals)
|
347 |
-
finals = self._three_sandhi(word, finals)
|
348 |
-
return finals
|
|
|
|
|
|
|
|
|
|
|
|
|
|
|
|
|
|
|
|
|
|
|
|
|
|
|
|
|
|
|
|
|
|
|
|
|
|
|
|
|
|
|
|
|
|
|
|
|
|
|
|
|
|
|
|
|
|
|
|
|
|
|
|
|
|
|
|
|
|
|
|
|
|
|
|
|
|
|
|
|
|
|
|
|
|
|
|
|
|
|
|
|
|
|
|
|
|
|
|
|
|
|
|
|
|
|
|
|
|
|
|
|
|
|
|
|
|
|
|
|
|
|
|
|
|
|
|
|
|
|
|
|
|
|
|
|
|
|
|
|
|
|
|
|
|
|
|
|
|
|
|
|
|
|
|
|
|
|
|
|
|
|
|
|
|
|
|
|
|
|
|
|
|
|
|
|
|
|
|
|
|
|
|
|
|
|
|
|
|
|
|
|
|
|
|
|
|
|
|
|
|
|
|
|
|
|
|
|
|
|
|
|
|
|
|
|
|
|
|
|
|
|
|
|
|
|
|
|
|
|
|
|
|
|
|
|
|
|
|
|
|
|
|
|
|
|
|
|
|
|
|
|
|
|
|
|
|
|
|
|
|
|
|
|
|
|
|
|
|
|
|
|
|
|
|
|
|
|
|
|
|
|
|
|
|
|
|
|
|
|
|
|
|
|
|
|
|
|
|
|
|
|
|
|
|
|
|
|
|
|
|
|
|
|
|
|
|
|
|
|
|
|
|
|
|
|
|
|
|
|
|
|
|
|
|
|
|
|
|
|
|
|
|
|
|
|
|
|
|
|
|
|
|
|
|
|
|
|
|
|
|
|
|
|
|
|
|
|
|
|
|
|
|
|
|
|
|
|
|
|
|
|
|
|
|
|
|
|
|
|
|
|
|
|
|
|
|
|
|
|
|
|
|
|
|
|
|
|
|
|
|
|
|
|
|
|
|
|
|
|
|
|
|
|
|
|
|
|
|
|
|
|
|
|
|
|
|
|
|
|
|
|
|
|
|
|
|
|
|
|
|
|
|
|
|
|
|
|
|
|
|
|
|
|
|
|
|
|
|
|
|
|
|
|
|
|
|
|
|
|
|
|
|
|
|
|
|
|
|
|
|
|
|
|
|
|
|
|
|
|
|
|
|
|
|
|
|
|
|
|
|
|
|
|
|
|
|
|
|
|
|
|
|
|
|
|
|
|
|
|
|
|
|
|
|
|
|
|
|
|
|
|
|
|
|
|
|
|
|
|
|
|
|
|
|
|
|
|
|
|
|
|
|
|
|
|
|
|
|
|
|
|
|
|
|
|
|
|
|
|
|
|
|
|
|
|
|
|
|
|
|
|
|
|
|
|
|
|
|
|
|
|
|
|
|
|
|
|
|
|
|
|
|
|
|
|
|
|
|
|
|
|
|
|
|
|
|
|
|
|
|
|
|
|
|
|
|
|
|
|
|
|
|
|
|
|
|
|
|
|
|
|
|
|
|
|
|
|
|
|
|
|
|
|
|
|
|
|
|
|
|
|
|
|
|
|
|
|
|
|
|
|
|
|
|
|
|
|
|
|
|
|
|
|
|
|
|
spaces/AlphonseBrandon/speecht5-tts-demo/README.md
DELETED
@@ -1,14 +0,0 @@
|
|
1 |
-
---
|
2 |
-
title: SpeechT5 Speech Synthesis Demo
|
3 |
-
emoji: 👩🎤
|
4 |
-
colorFrom: yellow
|
5 |
-
colorTo: blue
|
6 |
-
sdk: gradio
|
7 |
-
sdk_version: 3.17.0
|
8 |
-
app_file: app.py
|
9 |
-
pinned: false
|
10 |
-
license: apache-2.0
|
11 |
-
duplicated_from: Matthijs/speecht5-tts-demo
|
12 |
-
---
|
13 |
-
|
14 |
-
Check out the configuration reference at https://huggingface.co/docs/hub/spaces-config-reference
|
|
|
|
|
|
|
|
|
|
|
|
|
|
|
|
|
|
|
|
|
|
|
|
|
|
|
|
|
|
spaces/Androidonnxfork/CivitAi-to-Diffusers/diffusers/docs/source/en/training/dreambooth.md
DELETED
@@ -1,707 +0,0 @@
|
|
1 |
-
<!--Copyright 2023 The HuggingFace Team. All rights reserved.
|
2 |
-
|
3 |
-
Licensed under the Apache License, Version 2.0 (the "License"); you may not use this file except in compliance with
|
4 |
-
the License. You may obtain a copy of the License at
|
5 |
-
|
6 |
-
http://www.apache.org/licenses/LICENSE-2.0
|
7 |
-
|
8 |
-
Unless required by applicable law or agreed to in writing, software distributed under the License is distributed on
|
9 |
-
an "AS IS" BASIS, WITHOUT WARRANTIES OR CONDITIONS OF ANY KIND, either express or implied. See the License for the
|
10 |
-
specific language governing permissions and limitations under the License.
|
11 |
-
-->
|
12 |
-
|
13 |
-
# DreamBooth
|
14 |
-
|
15 |
-
[DreamBooth](https://arxiv.org/abs/2208.12242) is a method to personalize text-to-image models like Stable Diffusion given just a few (3-5) images of a subject. It allows the model to generate contextualized images of the subject in different scenes, poses, and views.
|
16 |
-
|
17 |
-
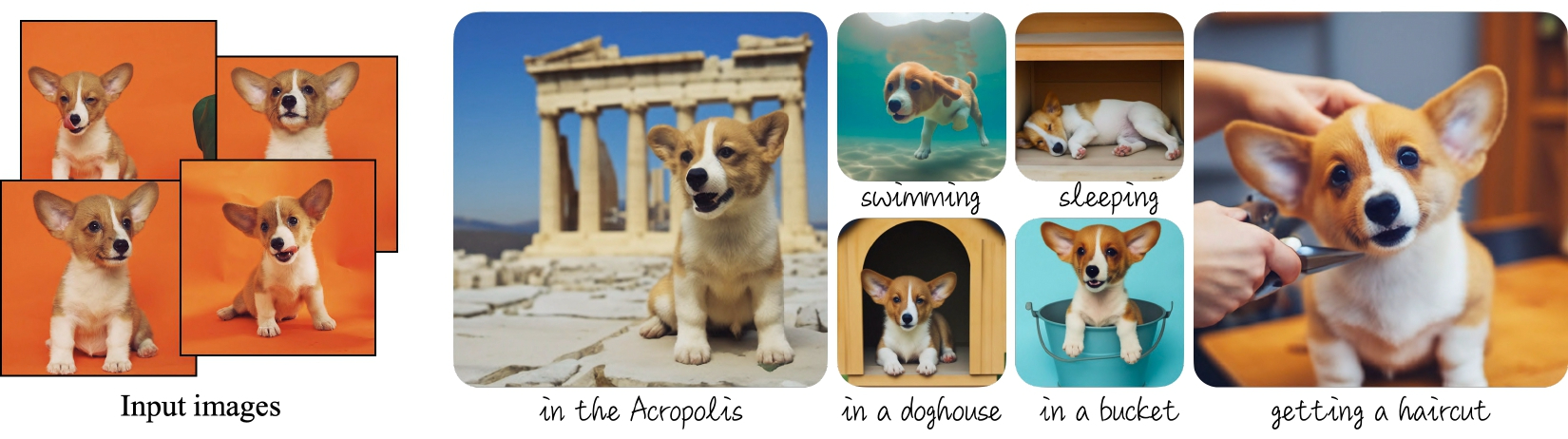
|
18 |
-
<small>Dreambooth examples from the <a href="https://dreambooth.github.io">project's blog.</a></small>
|
19 |
-
|
20 |
-
This guide will show you how to finetune DreamBooth with the [`CompVis/stable-diffusion-v1-4`](https://huggingface.co/CompVis/stable-diffusion-v1-4) model for various GPU sizes, and with Flax. All the training scripts for DreamBooth used in this guide can be found [here](https://github.com/huggingface/diffusers/tree/main/examples/dreambooth) if you're interested in digging deeper and seeing how things work.
|
21 |
-
|
22 |
-
Before running the scripts, make sure you install the library's training dependencies. We also recommend installing 🧨 Diffusers from the `main` GitHub branch:
|
23 |
-
|
24 |
-
```bash
|
25 |
-
pip install git+https://github.com/huggingface/diffusers
|
26 |
-
pip install -U -r diffusers/examples/dreambooth/requirements.txt
|
27 |
-
```
|
28 |
-
|
29 |
-
xFormers is not part of the training requirements, but we recommend you [install](../optimization/xformers) it if you can because it could make your training faster and less memory intensive.
|
30 |
-
|
31 |
-
After all the dependencies have been set up, initialize a [🤗 Accelerate](https://github.com/huggingface/accelerate/) environment with:
|
32 |
-
|
33 |
-
```bash
|
34 |
-
accelerate config
|
35 |
-
```
|
36 |
-
|
37 |
-
To setup a default 🤗 Accelerate environment without choosing any configurations:
|
38 |
-
|
39 |
-
```bash
|
40 |
-
accelerate config default
|
41 |
-
```
|
42 |
-
|
43 |
-
Or if your environment doesn't support an interactive shell like a notebook, you can use:
|
44 |
-
|
45 |
-
```py
|
46 |
-
from accelerate.utils import write_basic_config
|
47 |
-
|
48 |
-
write_basic_config()
|
49 |
-
```
|
50 |
-
|
51 |
-
Finally, download a [few images of a dog](https://huggingface.co/datasets/diffusers/dog-example) to DreamBooth with:
|
52 |
-
|
53 |
-
```py
|
54 |
-
from huggingface_hub import snapshot_download
|
55 |
-
|
56 |
-
local_dir = "./dog"
|
57 |
-
snapshot_download(
|
58 |
-
"diffusers/dog-example",
|
59 |
-
local_dir=local_dir,
|
60 |
-
repo_type="dataset",
|
61 |
-
ignore_patterns=".gitattributes",
|
62 |
-
)
|
63 |
-
```
|
64 |
-
|
65 |
-
To use your own dataset, take a look at the [Create a dataset for training](create_dataset) guide.
|
66 |
-
|
67 |
-
## Finetuning
|
68 |
-
|
69 |
-
<Tip warning={true}>
|
70 |
-
|
71 |
-
DreamBooth finetuning is very sensitive to hyperparameters and easy to overfit. We recommend you take a look at our [in-depth analysis](https://huggingface.co/blog/dreambooth) with recommended settings for different subjects to help you choose the appropriate hyperparameters.
|
72 |
-
|
73 |
-
</Tip>
|
74 |
-
|
75 |
-
<frameworkcontent>
|
76 |
-
<pt>
|
77 |
-
Set the `INSTANCE_DIR` environment variable to the path of the directory containing the dog images.
|
78 |
-
|
79 |
-
Specify the `MODEL_NAME` environment variable (either a Hub model repository id or a path to the directory containing the model weights) and pass it to the [`pretrained_model_name_or_path`] argument. The `instance_prompt` argument is a text prompt that contains a unique identifier, such as `sks`, and the class the image belongs to, which in this example is `a photo of a sks dog`.
|
80 |
-
|
81 |
-
```bash
|
82 |
-
export MODEL_NAME="CompVis/stable-diffusion-v1-4"
|
83 |
-
export INSTANCE_DIR="./dog"
|
84 |
-
export OUTPUT_DIR="path_to_saved_model"
|
85 |
-
```
|
86 |
-
|
87 |
-
Then you can launch the training script (you can find the full training script [here](https://github.com/huggingface/diffusers/blob/main/examples/dreambooth/train_dreambooth.py)) with the following command:
|
88 |
-
|
89 |
-
```bash
|
90 |
-
accelerate launch train_dreambooth.py \
|
91 |
-
--pretrained_model_name_or_path=$MODEL_NAME \
|
92 |
-
--instance_data_dir=$INSTANCE_DIR \
|
93 |
-
--output_dir=$OUTPUT_DIR \
|
94 |
-
--instance_prompt="a photo of sks dog" \
|
95 |
-
--resolution=512 \
|
96 |
-
--train_batch_size=1 \
|
97 |
-
--gradient_accumulation_steps=1 \
|
98 |
-
--learning_rate=5e-6 \
|
99 |
-
--lr_scheduler="constant" \
|
100 |
-
--lr_warmup_steps=0 \
|
101 |
-
--max_train_steps=400 \
|
102 |
-
--push_to_hub
|
103 |
-
```
|
104 |
-
</pt>
|
105 |
-
<jax>
|
106 |
-
If you have access to TPUs or want to train even faster, you can try out the [Flax training script](https://github.com/huggingface/diffusers/blob/main/examples/dreambooth/train_dreambooth_flax.py). The Flax training script doesn't support gradient checkpointing or gradient accumulation, so you'll need a GPU with at least 30GB of memory.
|
107 |
-
|
108 |
-
Before running the script, make sure you have the requirements installed:
|
109 |
-
|
110 |
-
```bash
|
111 |
-
pip install -U -r requirements.txt
|
112 |
-
```
|
113 |
-
|
114 |
-
Specify the `MODEL_NAME` environment variable (either a Hub model repository id or a path to the directory containing the model weights) and pass it to the [`pretrained_model_name_or_path`] argument. The `instance_prompt` argument is a text prompt that contains a unique identifier, such as `sks`, and the class the image belongs to, which in this example is `a photo of a sks dog`.
|
115 |
-
|
116 |
-
Now you can launch the training script with the following command:
|
117 |
-
|
118 |
-
```bash
|
119 |
-
export MODEL_NAME="duongna/stable-diffusion-v1-4-flax"
|
120 |
-
export INSTANCE_DIR="./dog"
|
121 |
-
export OUTPUT_DIR="path-to-save-model"
|
122 |
-
|
123 |
-
python train_dreambooth_flax.py \
|
124 |
-
--pretrained_model_name_or_path=$MODEL_NAME \
|
125 |
-
--instance_data_dir=$INSTANCE_DIR \
|
126 |
-
--output_dir=$OUTPUT_DIR \
|
127 |
-
--instance_prompt="a photo of sks dog" \
|
128 |
-
--resolution=512 \
|
129 |
-
--train_batch_size=1 \
|
130 |
-
--learning_rate=5e-6 \
|
131 |
-
--max_train_steps=400 \
|
132 |
-
--push_to_hub
|
133 |
-
```
|
134 |
-
</jax>
|
135 |
-
</frameworkcontent>
|
136 |
-
|
137 |
-
## Finetuning with prior-preserving loss
|
138 |
-
|
139 |
-
Prior preservation is used to avoid overfitting and language-drift (check out the [paper](https://arxiv.org/abs/2208.12242) to learn more if you're interested). For prior preservation, you use other images of the same class as part of the training process. The nice thing is that you can generate those images using the Stable Diffusion model itself! The training script will save the generated images to a local path you specify.
|
140 |
-
|
141 |
-
The authors recommend generating `num_epochs * num_samples` images for prior preservation. In most cases, 200-300 images work well.
|
142 |
-
|
143 |
-
<frameworkcontent>
|
144 |
-
<pt>
|
145 |
-
```bash
|
146 |
-
export MODEL_NAME="CompVis/stable-diffusion-v1-4"
|
147 |
-
export INSTANCE_DIR="./dog"
|
148 |
-
export CLASS_DIR="path_to_class_images"
|
149 |
-
export OUTPUT_DIR="path_to_saved_model"
|
150 |
-
|
151 |
-
accelerate launch train_dreambooth.py \
|
152 |
-
--pretrained_model_name_or_path=$MODEL_NAME \
|
153 |
-
--instance_data_dir=$INSTANCE_DIR \
|
154 |
-
--class_data_dir=$CLASS_DIR \
|
155 |
-
--output_dir=$OUTPUT_DIR \
|
156 |
-
--with_prior_preservation --prior_loss_weight=1.0 \
|
157 |
-
--instance_prompt="a photo of sks dog" \
|
158 |
-
--class_prompt="a photo of dog" \
|
159 |
-
--resolution=512 \
|
160 |
-
--train_batch_size=1 \
|
161 |
-
--gradient_accumulation_steps=1 \
|
162 |
-
--learning_rate=5e-6 \
|
163 |
-
--lr_scheduler="constant" \
|
164 |
-
--lr_warmup_steps=0 \
|
165 |
-
--num_class_images=200 \
|
166 |
-
--max_train_steps=800 \
|
167 |
-
--push_to_hub
|
168 |
-
```
|
169 |
-
</pt>
|
170 |
-
<jax>
|
171 |
-
```bash
|
172 |
-
export MODEL_NAME="duongna/stable-diffusion-v1-4-flax"
|
173 |
-
export INSTANCE_DIR="./dog"
|
174 |
-
export CLASS_DIR="path-to-class-images"
|
175 |
-
export OUTPUT_DIR="path-to-save-model"
|
176 |
-
|
177 |
-
python train_dreambooth_flax.py \
|
178 |
-
--pretrained_model_name_or_path=$MODEL_NAME \
|
179 |
-
--instance_data_dir=$INSTANCE_DIR \
|
180 |
-
--class_data_dir=$CLASS_DIR \
|
181 |
-
--output_dir=$OUTPUT_DIR \
|
182 |
-
--with_prior_preservation --prior_loss_weight=1.0 \
|
183 |
-
--instance_prompt="a photo of sks dog" \
|
184 |
-
--class_prompt="a photo of dog" \
|
185 |
-
--resolution=512 \
|
186 |
-
--train_batch_size=1 \
|
187 |
-
--learning_rate=5e-6 \
|
188 |
-
--num_class_images=200 \
|
189 |
-
--max_train_steps=800 \
|
190 |
-
--push_to_hub
|
191 |
-
```
|
192 |
-
</jax>
|
193 |
-
</frameworkcontent>
|
194 |
-
|
195 |
-
## Finetuning the text encoder and UNet
|
196 |
-
|
197 |
-
The script also allows you to finetune the `text_encoder` along with the `unet`. In our experiments (check out the [Training Stable Diffusion with DreamBooth using 🧨 Diffusers](https://huggingface.co/blog/dreambooth) post for more details), this yields much better results, especially when generating images of faces.
|
198 |
-
|
199 |
-
<Tip warning={true}>
|
200 |
-
|
201 |
-
Training the text encoder requires additional memory and it won't fit on a 16GB GPU. You'll need at least 24GB VRAM to use this option.
|
202 |
-
|
203 |
-
</Tip>
|
204 |
-
|
205 |
-
Pass the `--train_text_encoder` argument to the training script to enable finetuning the `text_encoder` and `unet`:
|
206 |
-
|
207 |
-
<frameworkcontent>
|
208 |
-
<pt>
|
209 |
-
```bash
|
210 |
-
export MODEL_NAME="CompVis/stable-diffusion-v1-4"
|
211 |
-
export INSTANCE_DIR="./dog"
|
212 |
-
export CLASS_DIR="path_to_class_images"
|
213 |
-
export OUTPUT_DIR="path_to_saved_model"
|
214 |
-
|
215 |
-
accelerate launch train_dreambooth.py \
|
216 |
-
--pretrained_model_name_or_path=$MODEL_NAME \
|
217 |
-
--train_text_encoder \
|
218 |
-
--instance_data_dir=$INSTANCE_DIR \
|
219 |
-
--class_data_dir=$CLASS_DIR \
|
220 |
-
--output_dir=$OUTPUT_DIR \
|
221 |
-
--with_prior_preservation --prior_loss_weight=1.0 \
|
222 |
-
--instance_prompt="a photo of sks dog" \
|
223 |
-
--class_prompt="a photo of dog" \
|
224 |
-
--resolution=512 \
|
225 |
-
--train_batch_size=1 \
|
226 |
-
--use_8bit_adam \
|
227 |
-
--gradient_checkpointing \
|
228 |
-
--learning_rate=2e-6 \
|
229 |
-
--lr_scheduler="constant" \
|
230 |
-
--lr_warmup_steps=0 \
|
231 |
-
--num_class_images=200 \
|
232 |
-
--max_train_steps=800 \
|
233 |
-
--push_to_hub
|
234 |
-
```
|
235 |
-
</pt>
|
236 |
-
<jax>
|
237 |
-
```bash
|
238 |
-
export MODEL_NAME="duongna/stable-diffusion-v1-4-flax"
|
239 |
-
export INSTANCE_DIR="./dog"
|
240 |
-
export CLASS_DIR="path-to-class-images"
|
241 |
-
export OUTPUT_DIR="path-to-save-model"
|
242 |
-
|
243 |
-
python train_dreambooth_flax.py \
|
244 |
-
--pretrained_model_name_or_path=$MODEL_NAME \
|
245 |
-
--train_text_encoder \
|
246 |
-
--instance_data_dir=$INSTANCE_DIR \
|
247 |
-
--class_data_dir=$CLASS_DIR \
|
248 |
-
--output_dir=$OUTPUT_DIR \
|
249 |
-
--with_prior_preservation --prior_loss_weight=1.0 \
|
250 |
-
--instance_prompt="a photo of sks dog" \
|
251 |
-
--class_prompt="a photo of dog" \
|
252 |
-
--resolution=512 \
|
253 |
-
--train_batch_size=1 \
|
254 |
-
--learning_rate=2e-6 \
|
255 |
-
--num_class_images=200 \
|
256 |
-
--max_train_steps=800 \
|
257 |
-
--push_to_hub
|
258 |
-
```
|
259 |
-
</jax>
|
260 |
-
</frameworkcontent>
|
261 |
-
|
262 |
-
## Finetuning with LoRA
|
263 |
-
|
264 |
-
You can also use Low-Rank Adaptation of Large Language Models (LoRA), a fine-tuning technique for accelerating training large models, on DreamBooth. For more details, take a look at the [LoRA training](./lora#dreambooth) guide.
|
265 |
-
|
266 |
-
## Saving checkpoints while training
|
267 |
-
|
268 |
-
It's easy to overfit while training with Dreambooth, so sometimes it's useful to save regular checkpoints during the training process. One of the intermediate checkpoints might actually work better than the final model! Pass the following argument to the training script to enable saving checkpoints:
|
269 |
-
|
270 |
-
```bash
|
271 |
-
--checkpointing_steps=500
|
272 |
-
```
|
273 |
-
|
274 |
-
This saves the full training state in subfolders of your `output_dir`. Subfolder names begin with the prefix `checkpoint-`, followed by the number of steps performed so far; for example, `checkpoint-1500` would be a checkpoint saved after 1500 training steps.
|
275 |
-
|
276 |
-
### Resume training from a saved checkpoint
|
277 |
-
|
278 |
-
If you want to resume training from any of the saved checkpoints, you can pass the argument `--resume_from_checkpoint` to the script and specify the name of the checkpoint you want to use. You can also use the special string `"latest"` to resume from the last saved checkpoint (the one with the largest number of steps). For example, the following would resume training from the checkpoint saved after 1500 steps:
|
279 |
-
|
280 |
-
```bash
|
281 |
-
--resume_from_checkpoint="checkpoint-1500"
|
282 |
-
```
|
283 |
-
|
284 |
-
This is a good opportunity to tweak some of your hyperparameters if you wish.
|
285 |
-
|
286 |
-
### Inference from a saved checkpoint
|
287 |
-
|
288 |
-
Saved checkpoints are stored in a format suitable for resuming training. They not only include the model weights, but also the state of the optimizer, data loaders, and learning rate.
|
289 |
-
|
290 |
-
If you have **`"accelerate>=0.16.0"`** installed, use the following code to run
|
291 |
-
inference from an intermediate checkpoint.
|
292 |
-
|
293 |
-
```python
|
294 |
-
from diffusers import DiffusionPipeline, UNet2DConditionModel
|
295 |
-
from transformers import CLIPTextModel
|
296 |
-
import torch
|
297 |
-
|
298 |
-
# Load the pipeline with the same arguments (model, revision) that were used for training
|
299 |
-
model_id = "CompVis/stable-diffusion-v1-4"
|
300 |
-
|
301 |
-
unet = UNet2DConditionModel.from_pretrained("/sddata/dreambooth/daruma-v2-1/checkpoint-100/unet")
|
302 |
-
|
303 |
-
# if you have trained with `--args.train_text_encoder` make sure to also load the text encoder
|
304 |
-
text_encoder = CLIPTextModel.from_pretrained("/sddata/dreambooth/daruma-v2-1/checkpoint-100/text_encoder")
|
305 |
-
|
306 |
-
pipeline = DiffusionPipeline.from_pretrained(model_id, unet=unet, text_encoder=text_encoder, dtype=torch.float16)
|
307 |
-
pipeline.to("cuda")
|
308 |
-
|
309 |
-
# Perform inference, or save, or push to the hub
|
310 |
-
pipeline.save_pretrained("dreambooth-pipeline")
|
311 |
-
```
|
312 |
-
|
313 |
-
If you have **`"accelerate<0.16.0"`** installed, you need to convert it to an inference pipeline first:
|
314 |
-
|
315 |
-
```python
|
316 |
-
from accelerate import Accelerator
|
317 |
-
from diffusers import DiffusionPipeline
|
318 |
-
|
319 |
-
# Load the pipeline with the same arguments (model, revision) that were used for training
|
320 |
-
model_id = "CompVis/stable-diffusion-v1-4"
|
321 |
-
pipeline = DiffusionPipeline.from_pretrained(model_id)
|
322 |
-
|
323 |
-
accelerator = Accelerator()
|
324 |
-
|
325 |
-
# Use text_encoder if `--train_text_encoder` was used for the initial training
|
326 |
-
unet, text_encoder = accelerator.prepare(pipeline.unet, pipeline.text_encoder)
|
327 |
-
|
328 |
-
# Restore state from a checkpoint path. You have to use the absolute path here.
|
329 |
-
accelerator.load_state("/sddata/dreambooth/daruma-v2-1/checkpoint-100")
|
330 |
-
|
331 |
-
# Rebuild the pipeline with the unwrapped models (assignment to .unet and .text_encoder should work too)
|
332 |
-
pipeline = DiffusionPipeline.from_pretrained(
|
333 |
-
model_id,
|
334 |
-
unet=accelerator.unwrap_model(unet),
|
335 |
-
text_encoder=accelerator.unwrap_model(text_encoder),
|
336 |
-
)
|
337 |
-
|
338 |
-
# Perform inference, or save, or push to the hub
|
339 |
-
pipeline.save_pretrained("dreambooth-pipeline")
|
340 |
-
```
|
341 |
-
|
342 |
-
## Optimizations for different GPU sizes
|
343 |
-
|
344 |
-
Depending on your hardware, there are a few different ways to optimize DreamBooth on GPUs from 16GB to just 8GB!
|
345 |
-
|
346 |
-
### xFormers
|
347 |
-
|
348 |
-
[xFormers](https://github.com/facebookresearch/xformers) is a toolbox for optimizing Transformers, and it includes a [memory-efficient attention](https://facebookresearch.github.io/xformers/components/ops.html#module-xformers.ops) mechanism that is used in 🧨 Diffusers. You'll need to [install xFormers](./optimization/xformers) and then add the following argument to your training script:
|
349 |
-
|
350 |
-
```bash
|
351 |
-
--enable_xformers_memory_efficient_attention
|
352 |
-
```
|
353 |
-
|
354 |
-
xFormers is not available in Flax.
|
355 |
-
|
356 |
-
### Set gradients to none
|
357 |
-
|
358 |
-
Another way you can lower your memory footprint is to [set the gradients](https://pytorch.org/docs/stable/generated/torch.optim.Optimizer.zero_grad.html) to `None` instead of zero. However, this may change certain behaviors, so if you run into any issues, try removing this argument. Add the following argument to your training script to set the gradients to `None`:
|
359 |
-
|
360 |
-
```bash
|
361 |
-
--set_grads_to_none
|
362 |
-
```
|
363 |
-
|
364 |
-
### 16GB GPU
|
365 |
-
|
366 |
-
With the help of gradient checkpointing and [bitsandbytes](https://github.com/TimDettmers/bitsandbytes) 8-bit optimizer, it's possible to train DreamBooth on a 16GB GPU. Make sure you have bitsandbytes installed:
|
367 |
-
|
368 |
-
```bash
|
369 |
-
pip install bitsandbytes
|
370 |
-
```
|
371 |
-
|
372 |
-
Then pass the `--use_8bit_adam` option to the training script:
|
373 |
-
|
374 |
-
```bash
|
375 |
-
export MODEL_NAME="CompVis/stable-diffusion-v1-4"
|
376 |
-
export INSTANCE_DIR="./dog"
|
377 |
-
export CLASS_DIR="path_to_class_images"
|
378 |
-
export OUTPUT_DIR="path_to_saved_model"
|
379 |
-
|
380 |
-
accelerate launch train_dreambooth.py \
|
381 |
-
--pretrained_model_name_or_path=$MODEL_NAME \
|
382 |
-
--instance_data_dir=$INSTANCE_DIR \
|
383 |
-
--class_data_dir=$CLASS_DIR \
|
384 |
-
--output_dir=$OUTPUT_DIR \
|
385 |
-
--with_prior_preservation --prior_loss_weight=1.0 \
|
386 |
-
--instance_prompt="a photo of sks dog" \
|
387 |
-
--class_prompt="a photo of dog" \
|
388 |
-
--resolution=512 \
|
389 |
-
--train_batch_size=1 \
|
390 |
-
--gradient_accumulation_steps=2 --gradient_checkpointing \
|
391 |
-
--use_8bit_adam \
|
392 |
-
--learning_rate=5e-6 \
|
393 |
-
--lr_scheduler="constant" \
|
394 |
-
--lr_warmup_steps=0 \
|
395 |
-
--num_class_images=200 \
|
396 |
-
--max_train_steps=800 \
|
397 |
-
--push_to_hub
|
398 |
-
```
|
399 |
-
|
400 |
-
### 12GB GPU
|
401 |
-
|
402 |
-
To run DreamBooth on a 12GB GPU, you'll need to enable gradient checkpointing, the 8-bit optimizer, xFormers, and set the gradients to `None`:
|
403 |
-
|
404 |
-
```bash
|
405 |
-
export MODEL_NAME="CompVis/stable-diffusion-v1-4"
|
406 |
-
export INSTANCE_DIR="./dog"
|
407 |
-
export CLASS_DIR="path-to-class-images"
|
408 |
-
export OUTPUT_DIR="path-to-save-model"
|
409 |
-
|
410 |
-
accelerate launch train_dreambooth.py \
|
411 |
-
--pretrained_model_name_or_path=$MODEL_NAME \
|
412 |
-
--instance_data_dir=$INSTANCE_DIR \
|
413 |
-
--class_data_dir=$CLASS_DIR \
|
414 |
-
--output_dir=$OUTPUT_DIR \
|
415 |
-
--with_prior_preservation --prior_loss_weight=1.0 \
|
416 |
-
--instance_prompt="a photo of sks dog" \
|
417 |
-
--class_prompt="a photo of dog" \
|
418 |
-
--resolution=512 \
|
419 |
-
--train_batch_size=1 \
|
420 |
-
--gradient_accumulation_steps=1 --gradient_checkpointing \
|
421 |
-
--use_8bit_adam \
|
422 |
-
--enable_xformers_memory_efficient_attention \
|
423 |
-
--set_grads_to_none \
|
424 |
-
--learning_rate=2e-6 \
|
425 |
-
--lr_scheduler="constant" \
|
426 |
-
--lr_warmup_steps=0 \
|
427 |
-
--num_class_images=200 \
|
428 |
-
--max_train_steps=800 \
|
429 |
-
--push_to_hub
|
430 |
-
```
|
431 |
-
|
432 |
-
### 8 GB GPU
|
433 |
-
|
434 |
-
For 8GB GPUs, you'll need the help of [DeepSpeed](https://www.deepspeed.ai/) to offload some
|
435 |
-
tensors from the VRAM to either the CPU or NVME, enabling training with less GPU memory.
|
436 |
-
|
437 |
-
Run the following command to configure your 🤗 Accelerate environment:
|
438 |
-
|
439 |
-
```bash
|
440 |
-
accelerate config
|
441 |
-
```
|
442 |
-
|
443 |
-
During configuration, confirm that you want to use DeepSpeed. Now it's possible to train on under 8GB VRAM by combining DeepSpeed stage 2, fp16 mixed precision, and offloading the model parameters and the optimizer state to the CPU. The drawback is that this requires more system RAM, about 25 GB. See [the DeepSpeed documentation](https://huggingface.co/docs/accelerate/usage_guides/deepspeed) for more configuration options.
|
444 |
-
|
445 |
-
You should also change the default Adam optimizer to DeepSpeed's optimized version of Adam
|
446 |
-
[`deepspeed.ops.adam.DeepSpeedCPUAdam`](https://deepspeed.readthedocs.io/en/latest/optimizers.html#adam-cpu) for a substantial speedup. Enabling `DeepSpeedCPUAdam` requires your system's CUDA toolchain version to be the same as the one installed with PyTorch.
|
447 |
-
|
448 |
-
8-bit optimizers don't seem to be compatible with DeepSpeed at the moment.
|
449 |
-
|
450 |
-
Launch training with the following command:
|
451 |
-
|
452 |
-
```bash
|
453 |
-
export MODEL_NAME="CompVis/stable-diffusion-v1-4"
|
454 |
-
export INSTANCE_DIR="./dog"
|
455 |
-
export CLASS_DIR="path_to_class_images"
|
456 |
-
export OUTPUT_DIR="path_to_saved_model"
|
457 |
-
|
458 |
-
accelerate launch train_dreambooth.py \
|
459 |
-
--pretrained_model_name_or_path=$MODEL_NAME \
|
460 |
-
--instance_data_dir=$INSTANCE_DIR \
|
461 |
-
--class_data_dir=$CLASS_DIR \
|
462 |
-
--output_dir=$OUTPUT_DIR \
|
463 |
-
--with_prior_preservation --prior_loss_weight=1.0 \
|
464 |
-
--instance_prompt="a photo of sks dog" \
|
465 |
-
--class_prompt="a photo of dog" \
|
466 |
-
--resolution=512 \
|
467 |
-
--train_batch_size=1 \
|
468 |
-
--sample_batch_size=1 \
|
469 |
-
--gradient_accumulation_steps=1 --gradient_checkpointing \
|
470 |
-
--learning_rate=5e-6 \
|
471 |
-
--lr_scheduler="constant" \
|
472 |
-
--lr_warmup_steps=0 \
|
473 |
-
--num_class_images=200 \
|
474 |
-
--max_train_steps=800 \
|
475 |
-
--mixed_precision=fp16 \
|
476 |
-
--push_to_hub
|
477 |
-
```
|
478 |
-
|
479 |
-
## Inference
|
480 |
-
|
481 |
-
Once you have trained a model, specify the path to where the model is saved, and use it for inference in the [`StableDiffusionPipeline`]. Make sure your prompts include the special `identifier` used during training (`sks` in the previous examples).
|
482 |
-
|
483 |
-
If you have **`"accelerate>=0.16.0"`** installed, you can use the following code to run
|
484 |
-
inference from an intermediate checkpoint:
|
485 |
-
|
486 |
-
```python
|
487 |
-
from diffusers import DiffusionPipeline
|
488 |
-
import torch
|
489 |
-
|
490 |
-
model_id = "path_to_saved_model"
|
491 |
-
pipe = DiffusionPipeline.from_pretrained(model_id, torch_dtype=torch.float16).to("cuda")
|
492 |
-
|
493 |
-
prompt = "A photo of sks dog in a bucket"
|
494 |
-
image = pipe(prompt, num_inference_steps=50, guidance_scale=7.5).images[0]
|
495 |
-
|
496 |
-
image.save("dog-bucket.png")
|
497 |
-
```
|
498 |
-
|
499 |
-
You may also run inference from any of the [saved training checkpoints](#inference-from-a-saved-checkpoint).
|
500 |
-
|
501 |
-
## IF
|
502 |
-
|
503 |
-
You can use the lora and full dreambooth scripts to train the text to image [IF model](https://huggingface.co/DeepFloyd/IF-I-XL-v1.0) and the stage II upscaler
|
504 |
-
[IF model](https://huggingface.co/DeepFloyd/IF-II-L-v1.0).
|
505 |
-
|
506 |
-
Note that IF has a predicted variance, and our finetuning scripts only train the models predicted error, so for finetuned IF models we switch to a fixed
|
507 |
-
variance schedule. The full finetuning scripts will update the scheduler config for the full saved model. However, when loading saved LoRA weights, you
|
508 |
-
must also update the pipeline's scheduler config.
|
509 |
-
|
510 |
-
```py
|
511 |
-
from diffusers import DiffusionPipeline
|
512 |
-
|
513 |
-
pipe = DiffusionPipeline.from_pretrained("DeepFloyd/IF-I-XL-v1.0")
|
514 |
-
|
515 |
-
pipe.load_lora_weights("<lora weights path>")
|
516 |
-
|
517 |
-
# Update scheduler config to fixed variance schedule
|
518 |
-
pipe.scheduler = pipe.scheduler.__class__.from_config(pipe.scheduler.config, variance_type="fixed_small")
|
519 |
-
```
|
520 |
-
|
521 |
-
Additionally, a few alternative cli flags are needed for IF.
|
522 |
-
|
523 |
-
`--resolution=64`: IF is a pixel space diffusion model. In order to operate on un-compressed pixels, the input images are of a much smaller resolution.
|
524 |
-
|
525 |
-
`--pre_compute_text_embeddings`: IF uses [T5](https://huggingface.co/docs/transformers/model_doc/t5) for its text encoder. In order to save GPU memory, we pre compute all text embeddings and then de-allocate
|
526 |
-
T5.
|
527 |
-
|
528 |
-
`--tokenizer_max_length=77`: T5 has a longer default text length, but the default IF encoding procedure uses a smaller number.
|
529 |
-
|
530 |
-
`--text_encoder_use_attention_mask`: T5 passes the attention mask to the text encoder.
|
531 |
-
|
532 |
-
### Tips and Tricks
|
533 |
-
We find LoRA to be sufficient for finetuning the stage I model as the low resolution of the model makes representing finegrained detail hard regardless.
|
534 |
-
|
535 |
-
For common and/or not-visually complex object concepts, you can get away with not-finetuning the upscaler. Just be sure to adjust the prompt passed to the
|
536 |
-
upscaler to remove the new token from the instance prompt. I.e. if your stage I prompt is "a sks dog", use "a dog" for your stage II prompt.
|
537 |
-
|
538 |
-
For finegrained detail like faces that aren't present in the original training set, we find that full finetuning of the stage II upscaler is better than
|
539 |
-
LoRA finetuning stage II.
|
540 |
-
|
541 |
-
For finegrained detail like faces, we find that lower learning rates along with larger batch sizes work best.
|
542 |
-
|
543 |
-
For stage II, we find that lower learning rates are also needed.
|
544 |
-
|
545 |
-
We found experimentally that the DDPM scheduler with the default larger number of denoising steps to sometimes work better than the DPM Solver scheduler
|
546 |
-
used in the training scripts.
|
547 |
-
|
548 |
-
### Stage II additional validation images
|
549 |
-
|
550 |
-
The stage II validation requires images to upscale, we can download a downsized version of the training set:
|
551 |
-
|
552 |
-
```py
|
553 |
-
from huggingface_hub import snapshot_download
|
554 |
-
|
555 |
-
local_dir = "./dog_downsized"
|
556 |
-
snapshot_download(
|
557 |
-
"diffusers/dog-example-downsized",
|
558 |
-
local_dir=local_dir,
|
559 |
-
repo_type="dataset",
|
560 |
-
ignore_patterns=".gitattributes",
|
561 |
-
)
|
562 |
-
```
|
563 |
-
|
564 |
-
### IF stage I LoRA Dreambooth
|
565 |
-
This training configuration requires ~28 GB VRAM.
|
566 |
-
|
567 |
-
```sh
|
568 |
-
export MODEL_NAME="DeepFloyd/IF-I-XL-v1.0"
|
569 |
-
export INSTANCE_DIR="dog"
|
570 |
-
export OUTPUT_DIR="dreambooth_dog_lora"
|
571 |
-
|
572 |
-
accelerate launch train_dreambooth_lora.py \
|
573 |
-
--report_to wandb \
|
574 |
-
--pretrained_model_name_or_path=$MODEL_NAME \
|
575 |
-
--instance_data_dir=$INSTANCE_DIR \
|
576 |
-
--output_dir=$OUTPUT_DIR \
|
577 |
-
--instance_prompt="a sks dog" \
|
578 |
-
--resolution=64 \
|
579 |
-
--train_batch_size=4 \
|
580 |
-
--gradient_accumulation_steps=1 \
|
581 |
-
--learning_rate=5e-6 \
|
582 |
-
--scale_lr \
|
583 |
-
--max_train_steps=1200 \
|
584 |
-
--validation_prompt="a sks dog" \
|
585 |
-
--validation_epochs=25 \
|
586 |
-
--checkpointing_steps=100 \
|
587 |
-
--pre_compute_text_embeddings \
|
588 |
-
--tokenizer_max_length=77 \
|
589 |
-
--text_encoder_use_attention_mask
|
590 |
-
```
|
591 |
-
|
592 |
-
### IF stage II LoRA Dreambooth
|
593 |
-
|
594 |
-
`--validation_images`: These images are upscaled during validation steps.
|
595 |
-
|
596 |
-
`--class_labels_conditioning=timesteps`: Pass additional conditioning to the UNet needed for stage II.
|
597 |
-
|
598 |
-
`--learning_rate=1e-6`: Lower learning rate than stage I.
|
599 |
-
|
600 |
-
`--resolution=256`: The upscaler expects higher resolution inputs
|
601 |
-
|
602 |
-
```sh
|
603 |
-
export MODEL_NAME="DeepFloyd/IF-II-L-v1.0"
|
604 |
-
export INSTANCE_DIR="dog"
|
605 |
-
export OUTPUT_DIR="dreambooth_dog_upscale"
|
606 |
-
export VALIDATION_IMAGES="dog_downsized/image_1.png dog_downsized/image_2.png dog_downsized/image_3.png dog_downsized/image_4.png"
|
607 |
-
|
608 |
-
python train_dreambooth_lora.py \
|
609 |
-
--report_to wandb \
|
610 |
-
--pretrained_model_name_or_path=$MODEL_NAME \
|
611 |
-
--instance_data_dir=$INSTANCE_DIR \
|
612 |
-
--output_dir=$OUTPUT_DIR \
|
613 |
-
--instance_prompt="a sks dog" \
|
614 |
-
--resolution=256 \
|
615 |
-
--train_batch_size=4 \
|
616 |
-
--gradient_accumulation_steps=1 \
|
617 |
-
--learning_rate=1e-6 \
|
618 |
-
--max_train_steps=2000 \
|
619 |
-
--validation_prompt="a sks dog" \
|
620 |
-
--validation_epochs=100 \
|
621 |
-
--checkpointing_steps=500 \
|
622 |
-
--pre_compute_text_embeddings \
|
623 |
-
--tokenizer_max_length=77 \
|
624 |
-
--text_encoder_use_attention_mask \
|
625 |
-
--validation_images $VALIDATION_IMAGES \
|
626 |
-
--class_labels_conditioning=timesteps
|
627 |
-
```
|
628 |
-
|
629 |
-
### IF Stage I Full Dreambooth
|
630 |
-
`--skip_save_text_encoder`: When training the full model, this will skip saving the entire T5 with the finetuned model. You can still load the pipeline
|
631 |
-
with a T5 loaded from the original model.
|
632 |
-
|
633 |
-
`use_8bit_adam`: Due to the size of the optimizer states, we recommend training the full XL IF model with 8bit adam.
|
634 |
-
|
635 |
-
`--learning_rate=1e-7`: For full dreambooth, IF requires very low learning rates. With higher learning rates model quality will degrade. Note that it is
|
636 |
-
likely the learning rate can be increased with larger batch sizes.
|
637 |
-
|
638 |
-
Using 8bit adam and a batch size of 4, the model can be trained in ~48 GB VRAM.
|
639 |
-
|
640 |
-
```sh
|
641 |
-
export MODEL_NAME="DeepFloyd/IF-I-XL-v1.0"
|
642 |
-
|
643 |
-
export INSTANCE_DIR="dog"
|
644 |
-
export OUTPUT_DIR="dreambooth_if"
|
645 |
-
|
646 |
-
accelerate launch train_dreambooth.py \
|
647 |
-
--pretrained_model_name_or_path=$MODEL_NAME \
|
648 |
-
--instance_data_dir=$INSTANCE_DIR \
|
649 |
-
--output_dir=$OUTPUT_DIR \
|
650 |
-
--instance_prompt="a photo of sks dog" \
|
651 |
-
--resolution=64 \
|
652 |
-
--train_batch_size=4 \
|
653 |
-
--gradient_accumulation_steps=1 \
|
654 |
-
--learning_rate=1e-7 \
|
655 |
-
--max_train_steps=150 \
|
656 |
-
--validation_prompt "a photo of sks dog" \
|
657 |
-
--validation_steps 25 \
|
658 |
-
--text_encoder_use_attention_mask \
|
659 |
-
--tokenizer_max_length 77 \
|
660 |
-
--pre_compute_text_embeddings \
|
661 |
-
--use_8bit_adam \
|
662 |
-
--set_grads_to_none \
|
663 |
-
--skip_save_text_encoder \
|
664 |
-
--push_to_hub
|
665 |
-
```
|
666 |
-
|
667 |
-
### IF Stage II Full Dreambooth
|
668 |
-
|
669 |
-
`--learning_rate=5e-6`: With a smaller effective batch size of 4, we found that we required learning rates as low as
|
670 |
-
1e-8.
|
671 |
-
|
672 |
-
`--resolution=256`: The upscaler expects higher resolution inputs
|
673 |
-
|
674 |
-
`--train_batch_size=2` and `--gradient_accumulation_steps=6`: We found that full training of stage II particularly with
|
675 |
-
faces required large effective batch sizes.
|
676 |
-
|
677 |
-
```sh
|
678 |
-
export MODEL_NAME="DeepFloyd/IF-II-L-v1.0"
|
679 |
-
export INSTANCE_DIR="dog"
|
680 |
-
export OUTPUT_DIR="dreambooth_dog_upscale"
|
681 |
-
export VALIDATION_IMAGES="dog_downsized/image_1.png dog_downsized/image_2.png dog_downsized/image_3.png dog_downsized/image_4.png"
|
682 |
-
|
683 |
-
accelerate launch train_dreambooth.py \
|
684 |
-
--report_to wandb \
|
685 |
-
--pretrained_model_name_or_path=$MODEL_NAME \
|
686 |
-
--instance_data_dir=$INSTANCE_DIR \
|
687 |
-
--output_dir=$OUTPUT_DIR \
|
688 |
-
--instance_prompt="a sks dog" \
|
689 |
-
--resolution=256 \
|
690 |
-
--train_batch_size=2 \
|
691 |
-
--gradient_accumulation_steps=6 \
|
692 |
-
--learning_rate=5e-6 \
|
693 |
-
--max_train_steps=2000 \
|
694 |
-
--validation_prompt="a sks dog" \
|
695 |
-
--validation_steps=150 \
|
696 |
-
--checkpointing_steps=500 \
|
697 |
-
--pre_compute_text_embeddings \
|
698 |
-
--tokenizer_max_length=77 \
|
699 |
-
--text_encoder_use_attention_mask \
|
700 |
-
--validation_images $VALIDATION_IMAGES \
|
701 |
-
--class_labels_conditioning timesteps \
|
702 |
-
--push_to_hub
|
703 |
-
```
|
704 |
-
|
705 |
-
## Stable Diffusion XL
|
706 |
-
|
707 |
-
We support fine-tuning of the UNet shipped in [Stable Diffusion XL](https://huggingface.co/papers/2307.01952) with DreamBooth and LoRA via the `train_dreambooth_lora_sdxl.py` script. Please refer to the docs [here](https://github.com/huggingface/diffusers/blob/main/examples/dreambooth/README_sdxl.md).
|
|
|
|
|
|
|
|
|
|
|
|
|
|
|
|
|
|
|
|
|
|
|
|
|
|
|
|
|
|
|
|
|
|
|
|
|
|
|
|
|
|
|
|
|
|
|
|
|
|
|
|
|
|
|
|
|
|
|
|
|
|
|
|
|
|
|
|
|
|
|
|
|
|
|
|
|
|
|
|
|
|
|
|
|
|
|
|
|
|
|
|
|
|
|
|
|
|
|
|
|
|
|
|
|
|
|
|
|
|
|
|
|
|
|
|
|
|
|
|
|
|
|
|
|
|
|
|
|
|
|
|
|
|
|
|
|
|
|
|
|
|
|
|
|
|
|
|
|
|
|
|
|
|
|
|
|
|
|
|
|
|
|
|
|
|
|
|
|
|
|
|
|
|
|
|
|
|
|
|
|
|
|
|
|
|
|
|
|
|
|
|
|
|
|
|
|
|
|
|
|
|
|
|
|
|
|
|
|
|
|
|
|
|
|
|
|
|
|
|
|
|
|
|
|
|
|
|
|
|
|
|
|
|
|
|
|
|
|
|
|
|
|
|
|
|
|
|
|
|
|
|
|
|
|
|
|
|
|
|
|
|
|
|
|
|
|
|
|
|
|
|
|
|
|
|
|
|
|
|
|
|
|
|
|
|
|
|
|
|
|
|
|
|
|
|
|
|
|
|
|
|
|
|
|
|
|
|
|
|
|
|
|
|
|
|
|
|
|
|
|
|
|
|
|
|
|
|
|
|
|
|
|
|
|
|
|
|
|
|
|
|
|
|
|
|
|
|
|
|
|
|
|
|
|
|
|
|
|
|
|
|
|
|
|
|
|
|
|
|
|
|
|
|
|
|
|
|
|
|
|
|
|
|
|
|
|
|
|
|
|
|
|
|
|
|
|
|
|
|
|
|
|
|
|
|
|
|
|
|
|
|
|
|
|
|
|
|
|
|
|
|
|
|
|
|
|
|
|
|
|
|
|
|
|
|
|
|
|
|
|
|
|
|
|
|
|
|
|
|
|
|
|
|
|
|
|
|
|
|
|
|
|
|
|
|
|
|
|
|
|
|
|
|
|
|
|
|
|
|
|
|
|
|
|
|
|
|
|
|
|
|
|
|
|
|
|
|
|
|
|
|
|
|
|
|
|
|
|
|
|
|
|
|
|
|
|
|
|
|
|
|
|
|
|
|
|
|
|
|
|
|
|
|
|
|
|
|
|
|
|
|
|
|
|
|
|
|
|
|
|
|
|
|
|
|
|
|
|
|
|
|
|
|
|
|
|
|
|
|
|
|
|
|
|
|
|
|
|
|
|
|
|
|
|
|
|
|
|
|
|
|
|
|
|
|
|
|
|
|
|
|
|
|
|
|
|
|
|
|
|
|
|
|
|
|
|
|
|
|
|
|
|
|
|
|
|
|
|
|
|
|
|
|
|
|
|
|
|
|
|
|
|
|
|
|
|
|
|
|
|
|
|
|
|
|
|
|
|
|
|
|
|
|
|
|
|
|
|
|
|
|
|
|
|
|
|
|
|
|
|
|
|
|
|
|
|
|
|
|
|
|
|
|
|
|
|
|
|
|
|
|
|
|
|
|
|
|
|
|
|
|
|
|
|
|
|
|
|
|
|
|
|
|
|
|
|
|
|
|
|
|
|
|
|
|
|
|
|
|
|
|
|
|
|
|
|
|
|
|
|
|
|
|
|
|
|
|
|
|
|
|
|
|
|
|
|
|
|
|
|
|
|
|
|
|
|
|
|
|
|
|
|
|
|
|
|
|
|
|
|
|
|
|
|
|
|
|
|
|
|
|
|
|
|
|
|
|
|
|
|
|
|
|
|
|
|
|
|
|
|
|
|
|
|
|
|
|
|
|
|
|
|
|
|
|
|
|
|
|
|
|
|
|
|
|
|
|
|
|
|
|
|
|
|
|
|
|
|
|
|
|
|
|
|
|
|
|
|
|
|
|
|
|
|
|
|
|
|
|
|
|
|
|
|
|
|
|
|
|
|
|
|
|
|
|
|
|
|
|
|
|
|
|
|
|
|
|
|
|
|
|
|
|
|
|
|
|
|
|
|
|
|
|
|
|
|
|
|
|
|
|
|
|
|
|
|
|
|
|
|
|
|
|
|
|
|
|
|
|
|
|
|
|
|
|
|
|
|
|
|
|
|
|
|
|
|
|
|
|
|
|
|
|
|
|
|
|
|
|
|
|
|
|
|
|
|
|
|
|
|
|
|
|
|
|
|
|
|
|
|
|
|
|
|
|
|
|
|
|
|
|
|
|
|
|
|
|
|
|
|
|
|
|
|
|
|
|
|
|
|
|
|
|
|
|
|
|
|
|
|
|
|
|
|
|
|
|
|
|
|
|
|
|
|
|
|
|
|
|
|
|
|
|
|
|
|
|
|
|
|
|
|
|
|
|
|
|
|
|
|
|
|
|
|
|
|
|
|
|
|
|
|
|
|
|
|
|
|
|
|
|
|
|
|
|
|
|
|
|
|
|
|
|
|
|
|
|
|
|
|
|
|
|
|
|
|
|
|
|
|
|
|
|
|
|
|
|
|
|
|
|
|
|
|
|
|
|
|
|
|
|
|
|
|
|
|
|
|
|
|
|
|
|
|
|
|
|
|
|
|
|
|
|
|
|
|
|
|
|
|
|
|
|
|
|
|
|
|
|
|
|
|
|
|
|
|
|
|
|
|
|
|
|
|
|
|
|
|
|
|
|
|
|
|
|
|
|
|
|
|
|
|
|
|
|
|
|
|
|
|
|
|
|
|
|
|
|
|
|
|
|
|
|
|
|
|
|
|
|
|
|
|
|
|
|
|
|
|
|
|
|
|
|
|
|
|
|
|
|
|
|
|
|
|
|
|
|
|
|
|
|
|
|
|
|
|
|
|
|
|
|
|
|
|
|
|
|
|
|
|
|
|
|
|
|
|
|
|
|
|
|
|
|
|
|
|
|
|
|
|
|
|
|
|
|
|
|
|
|
|
|
|
|
|
|
|
|
|
|
|
|
|
|
|
|
|
|
|
|
|
|
|
|
|
|
|
|
|
|
|
|
|
|
|
|
|
|
|
|
|
|
|
|
|
|
|
|
|
|
|
|
|
|
|
|
|
|
|
|
|
|
|
|
|
|
|
|
|
|
|
|
|
|
|
|
|
|
|
|
|
|
|
|
|
|
spaces/Androidonnxfork/CivitAi-to-Diffusers/diffusers/src/diffusers/pipelines/alt_diffusion/modeling_roberta_series.py
DELETED
@@ -1,124 +0,0 @@
|
|
1 |
-
from dataclasses import dataclass
|
2 |
-
from typing import Optional, Tuple
|
3 |
-
|
4 |
-
import torch
|
5 |
-
from torch import nn
|
6 |
-
from transformers import RobertaPreTrainedModel, XLMRobertaConfig, XLMRobertaModel
|
7 |
-
from transformers.utils import ModelOutput
|
8 |
-
|
9 |
-
|
10 |
-
@dataclass
|
11 |
-
class TransformationModelOutput(ModelOutput):
|
12 |
-
"""
|
13 |
-
Base class for text model's outputs that also contains a pooling of the last hidden states.
|
14 |
-
|
15 |
-
Args:
|
16 |
-
text_embeds (`torch.FloatTensor` of shape `(batch_size, output_dim)` *optional* returned when model is initialized with `with_projection=True`):
|
17 |
-
The text embeddings obtained by applying the projection layer to the pooler_output.
|
18 |
-
last_hidden_state (`torch.FloatTensor` of shape `(batch_size, sequence_length, hidden_size)`):
|
19 |
-
Sequence of hidden-states at the output of the last layer of the model.
|
20 |
-
hidden_states (`tuple(torch.FloatTensor)`, *optional*, returned when `output_hidden_states=True` is passed or when `config.output_hidden_states=True`):
|
21 |
-
Tuple of `torch.FloatTensor` (one for the output of the embeddings, if the model has an embedding layer, +
|
22 |
-
one for the output of each layer) of shape `(batch_size, sequence_length, hidden_size)`.
|
23 |
-
|
24 |
-
Hidden-states of the model at the output of each layer plus the optional initial embedding outputs.
|
25 |
-
attentions (`tuple(torch.FloatTensor)`, *optional*, returned when `output_attentions=True` is passed or when `config.output_attentions=True`):
|
26 |
-
Tuple of `torch.FloatTensor` (one for each layer) of shape `(batch_size, num_heads, sequence_length,
|
27 |
-
sequence_length)`.
|
28 |
-
|
29 |
-
Attentions weights after the attention softmax, used to compute the weighted average in the self-attention
|
30 |
-
heads.
|
31 |
-
"""
|
32 |
-
|
33 |
-
projection_state: Optional[torch.FloatTensor] = None
|
34 |
-
last_hidden_state: torch.FloatTensor = None
|
35 |
-
hidden_states: Optional[Tuple[torch.FloatTensor]] = None
|
36 |
-
attentions: Optional[Tuple[torch.FloatTensor]] = None
|
37 |
-
|
38 |
-
|
39 |
-
class RobertaSeriesConfig(XLMRobertaConfig):
|
40 |
-
def __init__(
|
41 |
-
self,
|
42 |
-
pad_token_id=1,
|
43 |
-
bos_token_id=0,
|
44 |
-
eos_token_id=2,
|
45 |
-
project_dim=512,
|
46 |
-
pooler_fn="cls",
|
47 |
-
learn_encoder=False,
|
48 |
-
use_attention_mask=True,
|
49 |
-
**kwargs,
|
50 |
-
):
|
51 |
-
super().__init__(pad_token_id=pad_token_id, bos_token_id=bos_token_id, eos_token_id=eos_token_id, **kwargs)
|
52 |
-
self.project_dim = project_dim
|
53 |
-
self.pooler_fn = pooler_fn
|
54 |
-
self.learn_encoder = learn_encoder
|
55 |
-
self.use_attention_mask = use_attention_mask
|
56 |
-
|
57 |
-
|
58 |
-
class RobertaSeriesModelWithTransformation(RobertaPreTrainedModel):
|
59 |
-
_keys_to_ignore_on_load_unexpected = [r"pooler", r"logit_scale"]
|
60 |
-
_keys_to_ignore_on_load_missing = [r"position_ids", r"predictions.decoder.bias"]
|
61 |
-
base_model_prefix = "roberta"
|
62 |
-
config_class = RobertaSeriesConfig
|
63 |
-
|
64 |
-
def __init__(self, config):
|
65 |
-
super().__init__(config)
|
66 |
-
self.roberta = XLMRobertaModel(config)
|
67 |
-
self.transformation = nn.Linear(config.hidden_size, config.project_dim)
|
68 |
-
self.has_pre_transformation = getattr(config, "has_pre_transformation", False)
|
69 |
-
if self.has_pre_transformation:
|
70 |
-
self.transformation_pre = nn.Linear(config.hidden_size, config.project_dim)
|
71 |
-
self.pre_LN = nn.LayerNorm(config.hidden_size, eps=config.layer_norm_eps)
|
72 |
-
self.post_init()
|
73 |
-
|
74 |
-
def forward(
|
75 |
-
self,
|
76 |
-
input_ids: Optional[torch.Tensor] = None,
|
77 |
-
attention_mask: Optional[torch.Tensor] = None,
|
78 |
-
token_type_ids: Optional[torch.Tensor] = None,
|
79 |
-
position_ids: Optional[torch.Tensor] = None,
|
80 |
-
head_mask: Optional[torch.Tensor] = None,
|
81 |
-
inputs_embeds: Optional[torch.Tensor] = None,
|
82 |
-
encoder_hidden_states: Optional[torch.Tensor] = None,
|
83 |
-
encoder_attention_mask: Optional[torch.Tensor] = None,
|
84 |
-
output_attentions: Optional[bool] = None,
|
85 |
-
return_dict: Optional[bool] = None,
|
86 |
-
output_hidden_states: Optional[bool] = None,
|
87 |
-
):
|
88 |
-
r""" """
|
89 |
-
|
90 |
-
return_dict = return_dict if return_dict is not None else self.config.use_return_dict
|
91 |
-
|
92 |
-
outputs = self.base_model(
|
93 |
-
input_ids=input_ids,
|
94 |
-
attention_mask=attention_mask,
|
95 |
-
token_type_ids=token_type_ids,
|
96 |
-
position_ids=position_ids,
|
97 |
-
head_mask=head_mask,
|
98 |
-
inputs_embeds=inputs_embeds,
|
99 |
-
encoder_hidden_states=encoder_hidden_states,
|
100 |
-
encoder_attention_mask=encoder_attention_mask,
|
101 |
-
output_attentions=output_attentions,
|
102 |
-
output_hidden_states=True if self.has_pre_transformation else output_hidden_states,
|
103 |
-
return_dict=return_dict,
|
104 |
-
)
|
105 |
-
|
106 |
-
if self.has_pre_transformation:
|
107 |
-
sequence_output2 = outputs["hidden_states"][-2]
|
108 |
-
sequence_output2 = self.pre_LN(sequence_output2)
|
109 |
-
projection_state2 = self.transformation_pre(sequence_output2)
|
110 |
-
|
111 |
-
return TransformationModelOutput(
|
112 |
-
projection_state=projection_state2,
|
113 |
-
last_hidden_state=outputs.last_hidden_state,
|
114 |
-
hidden_states=outputs.hidden_states,
|
115 |
-
attentions=outputs.attentions,
|
116 |
-
)
|
117 |
-
else:
|
118 |
-
projection_state = self.transformation(outputs.last_hidden_state)
|
119 |
-
return TransformationModelOutput(
|
120 |
-
projection_state=projection_state,
|
121 |
-
last_hidden_state=outputs.last_hidden_state,
|
122 |
-
hidden_states=outputs.hidden_states,
|
123 |
-
attentions=outputs.attentions,
|
124 |
-
)
|
|
|
|
|
|
|
|
|
|
|
|
|
|
|
|
|
|
|
|
|
|
|
|
|
|
|
|
|
|
|
|
|
|
|
|
|
|
|
|
|
|
|
|
|
|
|
|
|
|
|
|
|
|
|
|
|
|
|
|
|
|
|
|
|
|
|
|
|
|
|
|
|
|
|
|
|
|
|
|
|
|
|
|
|
|
|
|
|
|
|
|
|
|
|
|
|
|
|
|
|
|
|
|
|
|
|
|
|
|
|
|
|
|
|
|
|
|
|
|
|
|
|
|
|
|
|
|
|
|
|
|
|
|
|
|
|
|
|
|
|
|
|
|
|
|
|
|
|
|
|
|
|
|
|
|
|
|
|
|
|
|
|
|
|
|
|
|
|
|
|
|
|
|
|
|
|
|
|
|
|
|
|
|
|
|
|
|
|
|
|
|
|
|
|
|
|
|
|
|
|
|
|
|
|
|
|
|
|
|
|
|
|
|
|
|
|
|
|
|
|
|
|
|
|
|
|
|
|
|
|
|
|
|
|
|
|
|
|
|
|
|
|
|
|
|
|
|
|
|
spaces/Androidonnxfork/CivitAi-to-Diffusers/diffusers/tests/pipelines/shap_e/test_shap_e.py
DELETED
@@ -1,265 +0,0 @@
|
|
1 |
-
# Copyright 2023 HuggingFace Inc.
|
2 |
-
#
|
3 |
-
# Licensed under the Apache License, Version 2.0 (the "License");
|
4 |
-
# you may not use this file except in compliance with the License.
|
5 |
-
# You may obtain a copy of the License at
|
6 |
-
#
|
7 |
-
# http://www.apache.org/licenses/LICENSE-2.0
|
8 |
-
#
|
9 |
-
# Unless required by applicable law or agreed to in writing, software
|
10 |
-
# distributed under the License is distributed on an "AS IS" BASIS,
|
11 |
-
# WITHOUT WARRANTIES OR CONDITIONS OF ANY KIND, either express or implied.
|
12 |
-
# See the License for the specific language governing permissions and
|
13 |
-
# limitations under the License.
|
14 |
-
|
15 |
-
import gc
|
16 |
-
import unittest
|
17 |
-
|
18 |
-
import numpy as np
|
19 |
-
import torch
|
20 |
-
from transformers import CLIPTextConfig, CLIPTextModelWithProjection, CLIPTokenizer
|
21 |
-
|
22 |
-
from diffusers import HeunDiscreteScheduler, PriorTransformer, ShapEPipeline
|
23 |
-
from diffusers.pipelines.shap_e import ShapERenderer
|
24 |
-
from diffusers.utils import load_numpy, slow
|
25 |
-
from diffusers.utils.testing_utils import require_torch_gpu, torch_device
|
26 |
-
|
27 |
-
from ..test_pipelines_common import PipelineTesterMixin, assert_mean_pixel_difference
|
28 |
-
|
29 |
-
|
30 |
-
class ShapEPipelineFastTests(PipelineTesterMixin, unittest.TestCase):
|
31 |
-
pipeline_class = ShapEPipeline
|
32 |
-
params = ["prompt"]
|
33 |
-
batch_params = ["prompt"]
|
34 |
-
required_optional_params = [
|
35 |
-
"num_images_per_prompt",
|
36 |
-
"num_inference_steps",
|
37 |
-
"generator",
|
38 |
-
"latents",
|
39 |
-
"guidance_scale",
|
40 |
-
"frame_size",
|
41 |
-
"output_type",
|
42 |
-
"return_dict",
|
43 |
-
]
|
44 |
-
test_xformers_attention = False
|
45 |
-
|
46 |
-
@property
|
47 |
-
def text_embedder_hidden_size(self):
|
48 |
-
return 32
|
49 |
-
|
50 |
-
@property
|
51 |
-
def time_input_dim(self):
|
52 |
-
return 32
|
53 |
-
|
54 |
-
@property
|
55 |
-
def time_embed_dim(self):
|
56 |
-
return self.time_input_dim * 4
|
57 |
-
|
58 |
-
@property
|
59 |
-
def renderer_dim(self):
|
60 |
-
return 8
|
61 |
-
|
62 |
-
@property
|
63 |
-
def dummy_tokenizer(self):
|
64 |
-
tokenizer = CLIPTokenizer.from_pretrained("hf-internal-testing/tiny-random-clip")
|
65 |
-
return tokenizer
|
66 |
-
|
67 |
-
@property
|
68 |
-
def dummy_text_encoder(self):
|
69 |
-
torch.manual_seed(0)
|
70 |
-
config = CLIPTextConfig(
|
71 |
-
bos_token_id=0,
|
72 |
-
eos_token_id=2,
|
73 |
-
hidden_size=self.text_embedder_hidden_size,
|
74 |
-
projection_dim=self.text_embedder_hidden_size,
|
75 |
-
intermediate_size=37,
|
76 |
-
layer_norm_eps=1e-05,
|
77 |
-
num_attention_heads=4,
|
78 |
-
num_hidden_layers=5,
|
79 |
-
pad_token_id=1,
|
80 |
-
vocab_size=1000,
|
81 |
-
)
|
82 |
-
return CLIPTextModelWithProjection(config)
|
83 |
-
|
84 |
-
@property
|
85 |
-
def dummy_prior(self):
|
86 |
-
torch.manual_seed(0)
|
87 |
-
|
88 |
-
model_kwargs = {
|
89 |
-
"num_attention_heads": 2,
|
90 |
-
"attention_head_dim": 16,
|
91 |
-
"embedding_dim": self.time_input_dim,
|
92 |
-
"num_embeddings": 32,
|
93 |
-
"embedding_proj_dim": self.text_embedder_hidden_size,
|
94 |
-
"time_embed_dim": self.time_embed_dim,
|
95 |
-
"num_layers": 1,
|
96 |
-
"clip_embed_dim": self.time_input_dim * 2,
|
97 |
-
"additional_embeddings": 0,
|
98 |
-
"time_embed_act_fn": "gelu",
|
99 |
-
"norm_in_type": "layer",
|
100 |
-
"encoder_hid_proj_type": None,
|
101 |
-
"added_emb_type": None,
|
102 |
-
}
|
103 |
-
|
104 |
-
model = PriorTransformer(**model_kwargs)
|
105 |
-
return model
|
106 |
-
|
107 |
-
@property
|
108 |
-
def dummy_renderer(self):
|
109 |
-
torch.manual_seed(0)
|
110 |
-
|
111 |
-
model_kwargs = {
|
112 |
-
"param_shapes": (
|
113 |
-
(self.renderer_dim, 93),
|
114 |
-
(self.renderer_dim, 8),
|
115 |
-
(self.renderer_dim, 8),
|
116 |
-
(self.renderer_dim, 8),
|
117 |
-
),
|
118 |
-
"d_latent": self.time_input_dim,
|
119 |
-
"d_hidden": self.renderer_dim,
|
120 |
-
"n_output": 12,
|
121 |
-
"background": (
|
122 |
-
0.1,
|
123 |
-
0.1,
|
124 |
-
0.1,
|
125 |
-
),
|
126 |
-
}
|
127 |
-
model = ShapERenderer(**model_kwargs)
|
128 |
-
return model
|
129 |
-
|
130 |
-
def get_dummy_components(self):
|
131 |
-
prior = self.dummy_prior
|
132 |
-
text_encoder = self.dummy_text_encoder
|
133 |
-
tokenizer = self.dummy_tokenizer
|
134 |
-
shap_e_renderer = self.dummy_renderer
|
135 |
-
|
136 |
-
scheduler = HeunDiscreteScheduler(
|
137 |
-
beta_schedule="exp",
|
138 |
-
num_train_timesteps=1024,
|
139 |
-
prediction_type="sample",
|
140 |
-
use_karras_sigmas=True,
|
141 |
-
clip_sample=True,
|
142 |
-
clip_sample_range=1.0,
|
143 |
-
)
|
144 |
-
components = {
|
145 |
-
"prior": prior,
|
146 |
-
"text_encoder": text_encoder,
|
147 |
-
"tokenizer": tokenizer,
|
148 |
-
"shap_e_renderer": shap_e_renderer,
|
149 |
-
"scheduler": scheduler,
|
150 |
-
}
|
151 |
-
|
152 |
-
return components
|
153 |
-
|
154 |
-
def get_dummy_inputs(self, device, seed=0):
|
155 |
-
if str(device).startswith("mps"):
|
156 |
-
generator = torch.manual_seed(seed)
|
157 |
-
else:
|
158 |
-
generator = torch.Generator(device=device).manual_seed(seed)
|
159 |
-
inputs = {
|
160 |
-
"prompt": "horse",
|
161 |
-
"generator": generator,
|
162 |
-
"num_inference_steps": 1,
|
163 |
-
"frame_size": 32,
|
164 |
-
"output_type": "np",
|
165 |
-
}
|
166 |
-
return inputs
|
167 |
-
|
168 |
-
def test_shap_e(self):
|
169 |
-
device = "cpu"
|
170 |
-
|
171 |
-
components = self.get_dummy_components()
|
172 |
-
|
173 |
-
pipe = self.pipeline_class(**components)
|
174 |
-
pipe = pipe.to(device)
|
175 |
-
|
176 |
-
pipe.set_progress_bar_config(disable=None)
|
177 |
-
|
178 |
-
output = pipe(**self.get_dummy_inputs(device))
|
179 |
-
image = output.images[0]
|
180 |
-
image_slice = image[0, -3:, -3:, -1]
|
181 |
-
|
182 |
-
assert image.shape == (20, 32, 32, 3)
|
183 |
-
|
184 |
-
expected_slice = np.array(
|
185 |
-
[
|
186 |
-
0.00039216,
|
187 |
-
0.00039216,
|
188 |
-
0.00039216,
|
189 |
-
0.00039216,
|
190 |
-
0.00039216,
|
191 |
-
0.00039216,
|
192 |
-
0.00039216,
|
193 |
-
0.00039216,
|
194 |
-
0.00039216,
|
195 |
-
]
|
196 |
-
)
|
197 |
-
|
198 |
-
assert np.abs(image_slice.flatten() - expected_slice).max() < 1e-2
|
199 |
-
|
200 |
-
def test_inference_batch_consistent(self):
|
201 |
-
# NOTE: Larger batch sizes cause this test to timeout, only test on smaller batches
|
202 |
-
self._test_inference_batch_consistent(batch_sizes=[1, 2])
|
203 |
-
|
204 |
-
def test_inference_batch_single_identical(self):
|
205 |
-
test_max_difference = torch_device == "cpu"
|
206 |
-
relax_max_difference = True
|
207 |
-
|
208 |
-
self._test_inference_batch_single_identical(
|
209 |
-
batch_size=2,
|
210 |
-
test_max_difference=test_max_difference,
|
211 |
-
relax_max_difference=relax_max_difference,
|
212 |
-
)
|
213 |
-
|
214 |
-
def test_num_images_per_prompt(self):
|
215 |
-
components = self.get_dummy_components()
|
216 |
-
pipe = self.pipeline_class(**components)
|
217 |
-
pipe = pipe.to(torch_device)
|
218 |
-
pipe.set_progress_bar_config(disable=None)
|
219 |
-
|
220 |
-
batch_size = 1
|
221 |
-
num_images_per_prompt = 2
|
222 |
-
|
223 |
-
inputs = self.get_dummy_inputs(torch_device)
|
224 |
-
|
225 |
-
for key in inputs.keys():
|
226 |
-
if key in self.batch_params:
|
227 |
-
inputs[key] = batch_size * [inputs[key]]
|
228 |
-
|
229 |
-
images = pipe(**inputs, num_images_per_prompt=num_images_per_prompt)[0]
|
230 |
-
|
231 |
-
assert images.shape[0] == batch_size * num_images_per_prompt
|
232 |
-
|
233 |
-
|
234 |
-
@slow
|
235 |
-
@require_torch_gpu
|
236 |
-
class ShapEPipelineIntegrationTests(unittest.TestCase):
|
237 |
-
def tearDown(self):
|
238 |
-
# clean up the VRAM after each test
|
239 |
-
super().tearDown()
|
240 |
-
gc.collect()
|
241 |
-
torch.cuda.empty_cache()
|
242 |
-
|
243 |
-
def test_shap_e(self):
|
244 |
-
expected_image = load_numpy(
|
245 |
-
"https://huggingface.co/datasets/hf-internal-testing/diffusers-images/resolve/main"
|
246 |
-
"/shap_e/test_shap_e_np_out.npy"
|
247 |
-
)
|
248 |
-
pipe = ShapEPipeline.from_pretrained("openai/shap-e")
|
249 |
-
pipe = pipe.to(torch_device)
|
250 |
-
pipe.set_progress_bar_config(disable=None)
|
251 |
-
|
252 |
-
generator = torch.Generator(device=torch_device).manual_seed(0)
|
253 |
-
|
254 |
-
images = pipe(
|
255 |
-
"a shark",
|
256 |
-
generator=generator,
|
257 |
-
guidance_scale=15.0,
|
258 |
-
num_inference_steps=64,
|
259 |
-
frame_size=64,
|
260 |
-
output_type="np",
|
261 |
-
).images[0]
|
262 |
-
|
263 |
-
assert images.shape == (20, 64, 64, 3)
|
264 |
-
|
265 |
-
assert_mean_pixel_difference(images, expected_image)
|
|
|
|
|
|
|
|
|
|
|
|
|
|
|
|
|
|
|
|
|
|
|
|
|
|
|
|
|
|
|
|
|
|
|
|
|
|
|
|
|
|
|
|
|
|
|
|
|
|
|
|
|
|
|
|
|
|
|
|
|
|
|
|
|
|
|
|
|
|
|
|
|
|
|
|
|
|
|
|
|
|
|
|
|
|
|
|
|
|
|
|
|
|
|
|
|
|
|
|
|
|
|
|
|
|
|
|
|
|
|
|
|
|
|
|
|
|
|
|
|
|
|
|
|
|
|
|
|
|
|
|
|
|
|
|
|
|
|
|
|
|
|
|
|
|
|
|
|
|
|
|
|
|
|
|
|
|
|
|
|
|
|
|
|
|
|
|
|
|
|
|
|
|
|
|
|
|
|
|
|
|
|
|
|
|
|
|
|
|
|
|
|
|
|
|
|
|
|
|
|
|
|
|
|
|
|
|
|
|
|
|
|
|
|
|
|
|
|
|
|
|
|
|
|
|
|
|
|
|
|
|
|
|
|
|
|
|
|
|
|
|
|
|
|
|
|
|
|
|
|
|
|
|
|
|
|
|
|
|
|
|
|
|
|
|
|
|
|
|
|
|
|
|
|
|
|
|
|
|
|
|
|
|
|
|
|
|
|
|
|
|
|
|
|
|
|
|
|
|
|
|
|
|
|
|
|
|
|
|
|
|
|
|
|
|
|
|
|
|
|
|
|
|
|
|
|
|
|
|
|
|
|
|
|
|
|
|
|
|
|
|
|
|
|
|
|
|
|
|
|
|
|
|
|
|
|
|
|
|
|
|
|
|
|
|
|
|
|
|
|
|
|
|
|
|
|
|
|
|
|
|
|
|
|
|
|
|
|
|
|
|
|
|
|
|
|
|
|
|
|
|
|
|
|
|
|
|
|
|
|
|
|
|
|
|
|
|
|
|
|
|
|
|
|
|
|
|
|
|
|
|
|
|
|
|
|
|
|
|
|
|
|
|
|
|
|
|
|
|
|
|
|
|
|
|
|
|
|
|
|
|
|
|
|
|
|
|
|
|
|
|
|
|
|
|
|
|
|
|
|
|
|
|
|
|
|
|
|
|
|
|
|
|
|
|
|
|
|
|
|
|
|
|
|
|
|
|
|
|
|
|
|
|
|
|
|
|
|
|
|
|
|
|
|
|
|
|
|
|
|
|
spaces/Andy1621/uniformer_video_demo/README.md
DELETED
@@ -1,13 +0,0 @@
|
|
1 |
-
---
|
2 |
-
title: Uniformer_video_demo
|
3 |
-
emoji: 📹
|
4 |
-
colorFrom: pink
|
5 |
-
colorTo: green
|
6 |
-
sdk: gradio
|
7 |
-
sdk_version: 3.0.3
|
8 |
-
app_file: app.py
|
9 |
-
pinned: false
|
10 |
-
license: mit
|
11 |
-
---
|
12 |
-
|
13 |
-
Check out the configuration reference at https://huggingface.co/docs/hub/spaces#reference
|
|
|
|
|
|
|
|
|
|
|
|
|
|
|
|
|
|
|
|
|
|
|
|
|
|
|
|
spaces/AnishKumbhar/ChatBot/text-generation-webui-main/extensions/superboogav2/api.py
DELETED
@@ -1,207 +0,0 @@
|
|
1 |
-
"""
|
2 |
-
This module is responsible for the VectorDB API. It currently supports:
|
3 |
-
* DELETE api/v1/clear
|
4 |
-
- Clears the whole DB.
|
5 |
-
* POST api/v1/add
|
6 |
-
- Add some corpus to the DB. You can also specify metadata to be added alongside it.
|
7 |
-
* POST api/v1/delete
|
8 |
-
- Delete specific records with given metadata.
|
9 |
-
* POST api/v1/get
|
10 |
-
- Get results from chromaDB.
|
11 |
-
"""
|
12 |
-
|
13 |
-
import json
|
14 |
-
from http.server import BaseHTTPRequestHandler, ThreadingHTTPServer
|
15 |
-
from urllib.parse import urlparse, parse_qs
|
16 |
-
from threading import Thread
|
17 |
-
|
18 |
-
from modules import shared
|
19 |
-
from modules.logging_colors import logger
|
20 |
-
|
21 |
-
from .chromadb import ChromaCollector
|
22 |
-
from .data_processor import process_and_add_to_collector
|
23 |
-
|
24 |
-
import extensions.superboogav2.parameters as parameters
|
25 |
-
|
26 |
-
|
27 |
-
class CustomThreadingHTTPServer(ThreadingHTTPServer):
|
28 |
-
def __init__(self, server_address, RequestHandlerClass, collector: ChromaCollector, bind_and_activate=True):
|
29 |
-
self.collector = collector
|
30 |
-
super().__init__(server_address, RequestHandlerClass, bind_and_activate)
|
31 |
-
|
32 |
-
def finish_request(self, request, client_address):
|
33 |
-
self.RequestHandlerClass(request, client_address, self, self.collector)
|
34 |
-
|
35 |
-
|
36 |
-
class Handler(BaseHTTPRequestHandler):
|
37 |
-
def __init__(self, request, client_address, server, collector: ChromaCollector):
|
38 |
-
self.collector = collector
|
39 |
-
super().__init__(request, client_address, server)
|
40 |
-
|
41 |
-
|
42 |
-
def _send_412_error(self, message):
|
43 |
-
self.send_response(412)
|
44 |
-
self.send_header("Content-type", "application/json")
|
45 |
-
self.end_headers()
|
46 |
-
response = json.dumps({"error": message})
|
47 |
-
self.wfile.write(response.encode('utf-8'))
|
48 |
-
|
49 |
-
|
50 |
-
def _send_404_error(self):
|
51 |
-
self.send_response(404)
|
52 |
-
self.send_header("Content-type", "application/json")
|
53 |
-
self.end_headers()
|
54 |
-
response = json.dumps({"error": "Resource not found"})
|
55 |
-
self.wfile.write(response.encode('utf-8'))
|
56 |
-
|
57 |
-
|
58 |
-
def _send_400_error(self, error_message: str):
|
59 |
-
self.send_response(400)
|
60 |
-
self.send_header("Content-type", "application/json")
|
61 |
-
self.end_headers()
|
62 |
-
response = json.dumps({"error": error_message})
|
63 |
-
self.wfile.write(response.encode('utf-8'))
|
64 |
-
|
65 |
-
|
66 |
-
def _send_200_response(self, message: str):
|
67 |
-
self.send_response(200)
|
68 |
-
self.send_header("Content-type", "application/json")
|
69 |
-
self.end_headers()
|
70 |
-
|
71 |
-
if isinstance(message, str):
|
72 |
-
response = json.dumps({"message": message})
|
73 |
-
else:
|
74 |
-
response = json.dumps(message)
|
75 |
-
|
76 |
-
self.wfile.write(response.encode('utf-8'))
|
77 |
-
|
78 |
-
|
79 |
-
def _handle_get(self, search_strings: list[str], n_results: int, max_token_count: int, sort_param: str):
|
80 |
-
if sort_param == parameters.SORT_DISTANCE:
|
81 |
-
results = self.collector.get_sorted_by_dist(search_strings, n_results, max_token_count)
|
82 |
-
elif sort_param == parameters.SORT_ID:
|
83 |
-
results = self.collector.get_sorted_by_id(search_strings, n_results, max_token_count)
|
84 |
-
else: # Default is dist
|
85 |
-
results = self.collector.get_sorted_by_dist(search_strings, n_results, max_token_count)
|
86 |
-
|
87 |
-
return {
|
88 |
-
"results": results
|
89 |
-
}
|
90 |
-
|
91 |
-
|
92 |
-
def do_GET(self):
|
93 |
-
self._send_404_error()
|
94 |
-
|
95 |
-
|
96 |
-
def do_POST(self):
|
97 |
-
try:
|
98 |
-
content_length = int(self.headers['Content-Length'])
|
99 |
-
body = json.loads(self.rfile.read(content_length).decode('utf-8'))
|
100 |
-
|
101 |
-
parsed_path = urlparse(self.path)
|
102 |
-
path = parsed_path.path
|
103 |
-
query_params = parse_qs(parsed_path.query)
|
104 |
-
|
105 |
-
if path in ['/api/v1/add', '/api/add']:
|
106 |
-
corpus = body.get('corpus')
|
107 |
-
if corpus is None:
|
108 |
-
self._send_412_error("Missing parameter 'corpus'")
|
109 |
-
return
|
110 |
-
|
111 |
-
clear_before_adding = body.get('clear_before_adding', False)
|
112 |
-
metadata = body.get('metadata')
|
113 |
-
process_and_add_to_collector(corpus, self.collector, clear_before_adding, metadata)
|
114 |
-
self._send_200_response("Data successfully added")
|
115 |
-
|
116 |
-
elif path in ['/api/v1/delete', '/api/delete']:
|
117 |
-
metadata = body.get('metadata')
|
118 |
-
if corpus is None:
|
119 |
-
self._send_412_error("Missing parameter 'metadata'")
|
120 |
-
return
|
121 |
-
|
122 |
-
self.collector.delete(ids_to_delete=None, where=metadata)
|
123 |
-
self._send_200_response("Data successfully deleted")
|
124 |
-
|
125 |
-
elif path in ['/api/v1/get', '/api/get']:
|
126 |
-
search_strings = body.get('search_strings')
|
127 |
-
if search_strings is None:
|
128 |
-
self._send_412_error("Missing parameter 'search_strings'")
|
129 |
-
return
|
130 |
-
|
131 |
-
n_results = body.get('n_results')
|
132 |
-
if n_results is None:
|
133 |
-
n_results = parameters.get_chunk_count()
|
134 |
-
|
135 |
-
max_token_count = body.get('max_token_count')
|
136 |
-
if max_token_count is None:
|
137 |
-
max_token_count = parameters.get_max_token_count()
|
138 |
-
|
139 |
-
sort_param = query_params.get('sort', ['distance'])[0]
|
140 |
-
|
141 |
-
results = self._handle_get(search_strings, n_results, max_token_count, sort_param)
|
142 |
-
self._send_200_response(results)
|
143 |
-
|
144 |
-
else:
|
145 |
-
self._send_404_error()
|
146 |
-
except Exception as e:
|
147 |
-
self._send_400_error(str(e))
|
148 |
-
|
149 |
-
|
150 |
-
def do_DELETE(self):
|
151 |
-
try:
|
152 |
-
parsed_path = urlparse(self.path)
|
153 |
-
path = parsed_path.path
|
154 |
-
query_params = parse_qs(parsed_path.query)
|
155 |
-
|
156 |
-
if path in ['/api/v1/clear', '/api/clear']:
|
157 |
-
self.collector.clear()
|
158 |
-
self._send_200_response("Data successfully cleared")
|
159 |
-
else:
|
160 |
-
self._send_404_error()
|
161 |
-
except Exception as e:
|
162 |
-
self._send_400_error(str(e))
|
163 |
-
|
164 |
-
|
165 |
-
def do_OPTIONS(self):
|
166 |
-
self.send_response(200)
|
167 |
-
self.end_headers()
|
168 |
-
|
169 |
-
|
170 |
-
def end_headers(self):
|
171 |
-
self.send_header('Access-Control-Allow-Origin', '*')
|
172 |
-
self.send_header('Access-Control-Allow-Methods', '*')
|
173 |
-
self.send_header('Access-Control-Allow-Headers', '*')
|
174 |
-
self.send_header('Cache-Control', 'no-store, no-cache, must-revalidate')
|
175 |
-
super().end_headers()
|
176 |
-
|
177 |
-
|
178 |
-
class APIManager:
|
179 |
-
def __init__(self, collector: ChromaCollector):
|
180 |
-
self.server = None
|
181 |
-
self.collector = collector
|
182 |
-
self.is_running = False
|
183 |
-
|
184 |
-
def start_server(self, port: int):
|
185 |
-
if self.server is not None:
|
186 |
-
print("Server already running.")
|
187 |
-
return
|
188 |
-
|
189 |
-
address = '0.0.0.0' if shared.args.listen else '127.0.0.1'
|
190 |
-
self.server = CustomThreadingHTTPServer((address, port), Handler, self.collector)
|
191 |
-
|
192 |
-
logger.info(f'Starting chromaDB API at http://{address}:{port}/api')
|
193 |
-
|
194 |
-
Thread(target=self.server.serve_forever, daemon=True).start()
|
195 |
-
|
196 |
-
self.is_running = True
|
197 |
-
|
198 |
-
def stop_server(self):
|
199 |
-
if self.server is not None:
|
200 |
-
logger.info(f'Stopping chromaDB API.')
|
201 |
-
self.server.shutdown()
|
202 |
-
self.server.server_close()
|
203 |
-
self.server = None
|
204 |
-
self.is_running = False
|
205 |
-
|
206 |
-
def is_server_running(self):
|
207 |
-
return self.is_running
|
|
|
|
|
|
|
|
|
|
|
|
|
|
|
|
|
|
|
|
|
|
|
|
|
|
|
|
|
|
|
|
|
|
|
|
|
|
|
|
|
|
|
|
|
|
|
|
|
|
|
|
|
|
|
|
|
|
|
|
|
|
|
|
|
|
|
|
|
|
|
|
|
|
|
|
|
|
|
|
|
|
|
|
|
|
|
|
|
|
|
|
|
|
|
|
|
|
|
|
|
|
|
|
|
|
|
|
|
|
|
|
|
|
|
|
|
|
|
|
|
|
|
|
|
|
|
|
|
|
|
|
|
|
|
|
|
|
|
|
|
|
|
|
|
|
|
|
|
|
|
|
|
|
|
|
|
|
|
|
|
|
|
|
|
|
|
|
|
|
|
|
|
|
|
|
|
|
|
|
|
|
|
|
|
|
|
|
|
|
|
|
|
|
|
|
|
|
|
|
|
|
|
|
|
|
|
|
|
|
|
|
|
|
|
|
|
|
|
|
|
|
|
|
|
|
|
|
|
|
|
|
|
|
|
|
|
|
|
|
|
|
|
|
|
|
|
|
|
|
|
|
|
|
|
|
|
|
|
|
|
|
|
|
|
|
|
|
|
|
|
|
|
|
|
|
|
|
|
|
|
|
|
|
|
|
|
|
|
|
|
|
|
|
|
|
|
|
|
|
|
|
|
|
|
|
|
|
|
|
|
|
|
|
|
|
|
|
|
|
|
|
|
|
|
|
|
|
|
|
|
|
|
|
|
|
|
|
|
|
|
|
|
|
|
|
|
|
|
|
|
|
|
|
|
|
|
|
|
|
|
|
|
|
|
|
|
|
|
|
|
|
|
|
|
|
|
|
|
|
|
|
|
|
|
|
|
|
|
|
|
|
|
|
|
|
|
|
|
|
|
|
|
|
|
|
|
|
|
|
|
|
|
|
|
|
spaces/Ataturk-Chatbot/HuggingFaceChat/venv/lib/python3.11/site-packages/pip/_vendor/webencodings/labels.py
DELETED
@@ -1,231 +0,0 @@
|
|
1 |
-
"""
|
2 |
-
|
3 |
-
webencodings.labels
|
4 |
-
~~~~~~~~~~~~~~~~~~~
|
5 |
-
|
6 |
-
Map encoding labels to their name.
|
7 |
-
|
8 |
-
:copyright: Copyright 2012 by Simon Sapin
|
9 |
-
:license: BSD, see LICENSE for details.
|
10 |
-
|
11 |
-
"""
|
12 |
-
|
13 |
-
# XXX Do not edit!
|
14 |
-
# This file is automatically generated by mklabels.py
|
15 |
-
|
16 |
-
LABELS = {
|
17 |
-
'unicode-1-1-utf-8': 'utf-8',
|
18 |
-
'utf-8': 'utf-8',
|
19 |
-
'utf8': 'utf-8',
|
20 |
-
'866': 'ibm866',
|
21 |
-
'cp866': 'ibm866',
|
22 |
-
'csibm866': 'ibm866',
|
23 |
-
'ibm866': 'ibm866',
|
24 |
-
'csisolatin2': 'iso-8859-2',
|
25 |
-
'iso-8859-2': 'iso-8859-2',
|
26 |
-
'iso-ir-101': 'iso-8859-2',
|
27 |
-
'iso8859-2': 'iso-8859-2',
|
28 |
-
'iso88592': 'iso-8859-2',
|
29 |
-
'iso_8859-2': 'iso-8859-2',
|
30 |
-
'iso_8859-2:1987': 'iso-8859-2',
|
31 |
-
'l2': 'iso-8859-2',
|
32 |
-
'latin2': 'iso-8859-2',
|
33 |
-
'csisolatin3': 'iso-8859-3',
|
34 |
-
'iso-8859-3': 'iso-8859-3',
|
35 |
-
'iso-ir-109': 'iso-8859-3',
|
36 |
-
'iso8859-3': 'iso-8859-3',
|
37 |
-
'iso88593': 'iso-8859-3',
|
38 |
-
'iso_8859-3': 'iso-8859-3',
|
39 |
-
'iso_8859-3:1988': 'iso-8859-3',
|
40 |
-
'l3': 'iso-8859-3',
|
41 |
-
'latin3': 'iso-8859-3',
|
42 |
-
'csisolatin4': 'iso-8859-4',
|
43 |
-
'iso-8859-4': 'iso-8859-4',
|
44 |
-
'iso-ir-110': 'iso-8859-4',
|
45 |
-
'iso8859-4': 'iso-8859-4',
|
46 |
-
'iso88594': 'iso-8859-4',
|
47 |
-
'iso_8859-4': 'iso-8859-4',
|
48 |
-
'iso_8859-4:1988': 'iso-8859-4',
|
49 |
-
'l4': 'iso-8859-4',
|
50 |
-
'latin4': 'iso-8859-4',
|
51 |
-
'csisolatincyrillic': 'iso-8859-5',
|
52 |
-
'cyrillic': 'iso-8859-5',
|
53 |
-
'iso-8859-5': 'iso-8859-5',
|
54 |
-
'iso-ir-144': 'iso-8859-5',
|
55 |
-
'iso8859-5': 'iso-8859-5',
|
56 |
-
'iso88595': 'iso-8859-5',
|
57 |
-
'iso_8859-5': 'iso-8859-5',
|
58 |
-
'iso_8859-5:1988': 'iso-8859-5',
|
59 |
-
'arabic': 'iso-8859-6',
|
60 |
-
'asmo-708': 'iso-8859-6',
|
61 |
-
'csiso88596e': 'iso-8859-6',
|
62 |
-
'csiso88596i': 'iso-8859-6',
|
63 |
-
'csisolatinarabic': 'iso-8859-6',
|
64 |
-
'ecma-114': 'iso-8859-6',
|
65 |
-
'iso-8859-6': 'iso-8859-6',
|
66 |
-
'iso-8859-6-e': 'iso-8859-6',
|
67 |
-
'iso-8859-6-i': 'iso-8859-6',
|
68 |
-
'iso-ir-127': 'iso-8859-6',
|
69 |
-
'iso8859-6': 'iso-8859-6',
|
70 |
-
'iso88596': 'iso-8859-6',
|
71 |
-
'iso_8859-6': 'iso-8859-6',
|
72 |
-
'iso_8859-6:1987': 'iso-8859-6',
|
73 |
-
'csisolatingreek': 'iso-8859-7',
|
74 |
-
'ecma-118': 'iso-8859-7',
|
75 |
-
'elot_928': 'iso-8859-7',
|
76 |
-
'greek': 'iso-8859-7',
|
77 |
-
'greek8': 'iso-8859-7',
|
78 |
-
'iso-8859-7': 'iso-8859-7',
|
79 |
-
'iso-ir-126': 'iso-8859-7',
|
80 |
-
'iso8859-7': 'iso-8859-7',
|
81 |
-
'iso88597': 'iso-8859-7',
|
82 |
-
'iso_8859-7': 'iso-8859-7',
|
83 |
-
'iso_8859-7:1987': 'iso-8859-7',
|
84 |
-
'sun_eu_greek': 'iso-8859-7',
|
85 |
-
'csiso88598e': 'iso-8859-8',
|
86 |
-
'csisolatinhebrew': 'iso-8859-8',
|
87 |
-
'hebrew': 'iso-8859-8',
|
88 |
-
'iso-8859-8': 'iso-8859-8',
|
89 |
-
'iso-8859-8-e': 'iso-8859-8',
|
90 |
-
'iso-ir-138': 'iso-8859-8',
|
91 |
-
'iso8859-8': 'iso-8859-8',
|
92 |
-
'iso88598': 'iso-8859-8',
|
93 |
-
'iso_8859-8': 'iso-8859-8',
|
94 |
-
'iso_8859-8:1988': 'iso-8859-8',
|
95 |
-
'visual': 'iso-8859-8',
|
96 |
-
'csiso88598i': 'iso-8859-8-i',
|
97 |
-
'iso-8859-8-i': 'iso-8859-8-i',
|
98 |
-
'logical': 'iso-8859-8-i',
|
99 |
-
'csisolatin6': 'iso-8859-10',
|
100 |
-
'iso-8859-10': 'iso-8859-10',
|
101 |
-
'iso-ir-157': 'iso-8859-10',
|
102 |
-
'iso8859-10': 'iso-8859-10',
|
103 |
-
'iso885910': 'iso-8859-10',
|
104 |
-
'l6': 'iso-8859-10',
|
105 |
-
'latin6': 'iso-8859-10',
|
106 |
-
'iso-8859-13': 'iso-8859-13',
|
107 |
-
'iso8859-13': 'iso-8859-13',
|
108 |
-
'iso885913': 'iso-8859-13',
|
109 |
-
'iso-8859-14': 'iso-8859-14',
|
110 |
-
'iso8859-14': 'iso-8859-14',
|
111 |
-
'iso885914': 'iso-8859-14',
|
112 |
-
'csisolatin9': 'iso-8859-15',
|
113 |
-
'iso-8859-15': 'iso-8859-15',
|
114 |
-
'iso8859-15': 'iso-8859-15',
|
115 |
-
'iso885915': 'iso-8859-15',
|
116 |
-
'iso_8859-15': 'iso-8859-15',
|
117 |
-
'l9': 'iso-8859-15',
|
118 |
-
'iso-8859-16': 'iso-8859-16',
|
119 |
-
'cskoi8r': 'koi8-r',
|
120 |
-
'koi': 'koi8-r',
|
121 |
-
'koi8': 'koi8-r',
|
122 |
-
'koi8-r': 'koi8-r',
|
123 |
-
'koi8_r': 'koi8-r',
|
124 |
-
'koi8-u': 'koi8-u',
|
125 |
-
'csmacintosh': 'macintosh',
|
126 |
-
'mac': 'macintosh',
|
127 |
-
'macintosh': 'macintosh',
|
128 |
-
'x-mac-roman': 'macintosh',
|
129 |
-
'dos-874': 'windows-874',
|
130 |
-
'iso-8859-11': 'windows-874',
|
131 |
-
'iso8859-11': 'windows-874',
|
132 |
-
'iso885911': 'windows-874',
|
133 |
-
'tis-620': 'windows-874',
|
134 |
-
'windows-874': 'windows-874',
|
135 |
-
'cp1250': 'windows-1250',
|
136 |
-
'windows-1250': 'windows-1250',
|
137 |
-
'x-cp1250': 'windows-1250',
|
138 |
-
'cp1251': 'windows-1251',
|
139 |
-
'windows-1251': 'windows-1251',
|
140 |
-
'x-cp1251': 'windows-1251',
|
141 |
-
'ansi_x3.4-1968': 'windows-1252',
|
142 |
-
'ascii': 'windows-1252',
|
143 |
-
'cp1252': 'windows-1252',
|
144 |
-
'cp819': 'windows-1252',
|
145 |
-
'csisolatin1': 'windows-1252',
|
146 |
-
'ibm819': 'windows-1252',
|
147 |
-
'iso-8859-1': 'windows-1252',
|
148 |
-
'iso-ir-100': 'windows-1252',
|
149 |
-
'iso8859-1': 'windows-1252',
|
150 |
-
'iso88591': 'windows-1252',
|
151 |
-
'iso_8859-1': 'windows-1252',
|
152 |
-
'iso_8859-1:1987': 'windows-1252',
|
153 |
-
'l1': 'windows-1252',
|
154 |
-
'latin1': 'windows-1252',
|
155 |
-
'us-ascii': 'windows-1252',
|
156 |
-
'windows-1252': 'windows-1252',
|
157 |
-
'x-cp1252': 'windows-1252',
|
158 |
-
'cp1253': 'windows-1253',
|
159 |
-
'windows-1253': 'windows-1253',
|
160 |
-
'x-cp1253': 'windows-1253',
|
161 |
-
'cp1254': 'windows-1254',
|
162 |
-
'csisolatin5': 'windows-1254',
|
163 |
-
'iso-8859-9': 'windows-1254',
|
164 |
-
'iso-ir-148': 'windows-1254',
|
165 |
-
'iso8859-9': 'windows-1254',
|
166 |
-
'iso88599': 'windows-1254',
|
167 |
-
'iso_8859-9': 'windows-1254',
|
168 |
-
'iso_8859-9:1989': 'windows-1254',
|
169 |
-
'l5': 'windows-1254',
|
170 |
-
'latin5': 'windows-1254',
|
171 |
-
'windows-1254': 'windows-1254',
|
172 |
-
'x-cp1254': 'windows-1254',
|
173 |
-
'cp1255': 'windows-1255',
|
174 |
-
'windows-1255': 'windows-1255',
|
175 |
-
'x-cp1255': 'windows-1255',
|
176 |
-
'cp1256': 'windows-1256',
|
177 |
-
'windows-1256': 'windows-1256',
|
178 |
-
'x-cp1256': 'windows-1256',
|
179 |
-
'cp1257': 'windows-1257',
|
180 |
-
'windows-1257': 'windows-1257',
|
181 |
-
'x-cp1257': 'windows-1257',
|
182 |
-
'cp1258': 'windows-1258',
|
183 |
-
'windows-1258': 'windows-1258',
|
184 |
-
'x-cp1258': 'windows-1258',
|
185 |
-
'x-mac-cyrillic': 'x-mac-cyrillic',
|
186 |
-
'x-mac-ukrainian': 'x-mac-cyrillic',
|
187 |
-
'chinese': 'gbk',
|
188 |
-
'csgb2312': 'gbk',
|
189 |
-
'csiso58gb231280': 'gbk',
|
190 |
-
'gb2312': 'gbk',
|
191 |
-
'gb_2312': 'gbk',
|
192 |
-
'gb_2312-80': 'gbk',
|
193 |
-
'gbk': 'gbk',
|
194 |
-
'iso-ir-58': 'gbk',
|
195 |
-
'x-gbk': 'gbk',
|
196 |
-
'gb18030': 'gb18030',
|
197 |
-
'hz-gb-2312': 'hz-gb-2312',
|
198 |
-
'big5': 'big5',
|
199 |
-
'big5-hkscs': 'big5',
|
200 |
-
'cn-big5': 'big5',
|
201 |
-
'csbig5': 'big5',
|
202 |
-
'x-x-big5': 'big5',
|
203 |
-
'cseucpkdfmtjapanese': 'euc-jp',
|
204 |
-
'euc-jp': 'euc-jp',
|
205 |
-
'x-euc-jp': 'euc-jp',
|
206 |
-
'csiso2022jp': 'iso-2022-jp',
|
207 |
-
'iso-2022-jp': 'iso-2022-jp',
|
208 |
-
'csshiftjis': 'shift_jis',
|
209 |
-
'ms_kanji': 'shift_jis',
|
210 |
-
'shift-jis': 'shift_jis',
|
211 |
-
'shift_jis': 'shift_jis',
|
212 |
-
'sjis': 'shift_jis',
|
213 |
-
'windows-31j': 'shift_jis',
|
214 |
-
'x-sjis': 'shift_jis',
|
215 |
-
'cseuckr': 'euc-kr',
|
216 |
-
'csksc56011987': 'euc-kr',
|
217 |
-
'euc-kr': 'euc-kr',
|
218 |
-
'iso-ir-149': 'euc-kr',
|
219 |
-
'korean': 'euc-kr',
|
220 |
-
'ks_c_5601-1987': 'euc-kr',
|
221 |
-
'ks_c_5601-1989': 'euc-kr',
|
222 |
-
'ksc5601': 'euc-kr',
|
223 |
-
'ksc_5601': 'euc-kr',
|
224 |
-
'windows-949': 'euc-kr',
|
225 |
-
'csiso2022kr': 'iso-2022-kr',
|
226 |
-
'iso-2022-kr': 'iso-2022-kr',
|
227 |
-
'utf-16be': 'utf-16be',
|
228 |
-
'utf-16': 'utf-16le',
|
229 |
-
'utf-16le': 'utf-16le',
|
230 |
-
'x-user-defined': 'x-user-defined',
|
231 |
-
}
|
|
|
|
|
|
|
|
|
|
|
|
|
|
|
|
|
|
|
|
|
|
|
|
|
|
|
|
|
|
|
|
|
|
|
|
|
|
|
|
|
|
|
|
|
|
|
|
|
|
|
|
|
|
|
|
|
|
|
|
|
|
|
|
|
|
|
|
|
|
|
|
|
|
|
|
|
|
|
|
|
|
|
|
|
|
|
|
|
|
|
|
|
|
|
|
|
|
|
|
|
|
|
|
|
|
|
|
|
|
|
|
|
|
|
|
|
|
|
|
|
|
|
|
|
|
|
|
|
|
|
|
|
|
|
|
|
|
|
|
|
|
|
|
|
|
|
|
|
|
|
|
|
|
|
|
|
|
|
|
|
|
|
|
|
|
|
|
|
|
|
|
|
|
|
|
|
|
|
|
|
|
|
|
|
|
|
|
|
|
|
|
|
|
|
|
|
|
|
|
|
|
|
|
|
|
|
|
|
|
|
|
|
|
|
|
|
|
|
|
|
|
|
|
|
|
|
|
|
|
|
|
|
|
|
|
|
|
|
|
|
|
|
|
|
|
|
|
|
|
|
|
|
|
|
|
|
|
|
|
|
|
|
|
|
|
|
|
|
|
|
|
|
|
|
|
|
|
|
|
|
|
|
|
|
|
|
|
|
|
|
|
|
|
|
|
|
|
|
|
|
|
|
|
|
|
|
|
|
|
|
|
|
|
|
|
|
|
|
|
|
|
|
|
|
|
|
|
|
|
|
|
|
|
|
|
|
|
|
|
|
|
|
|
|
|
|
|
|
|
|
|
|
|
|
|
|
|
|
|
|
|
|
|
|
|
|
|
|
|
|
|
|
|
|
|
|
|
|
|
|
|
|
|
|
|
|
|
|
|
|
|
|
|
|
|
|
|
|
|
|
|
|
|
|
|
|
|
|
|
|
|
|
|
|
|
|
|
|
|
|
|
|
|
|
|
|
|
|
|
|
|
|
|
|
|
|
|
|
|
|
|
|
|
|
|
|
|
|
|
|
|
|
|
|
|
|
|
|
|
|
|
|
|
spaces/Awiny/Image2Paragraph/models/blip2_model.py
DELETED
@@ -1,46 +0,0 @@
|
|
1 |
-
from PIL import Image
|
2 |
-
import requests
|
3 |
-
from transformers import Blip2Processor, Blip2ForConditionalGeneration, BlipProcessor, BlipForConditionalGeneration
|
4 |
-
import torch
|
5 |
-
from utils.util import resize_long_edge
|
6 |
-
|
7 |
-
|
8 |
-
class ImageCaptioning:
|
9 |
-
def __init__(self, device, captioner_base_model='blip'):
|
10 |
-
self.device = device
|
11 |
-
self.captioner_base_model = captioner_base_model
|
12 |
-
self.processor, self.model = self.initialize_model()
|
13 |
-
|
14 |
-
def initialize_model(self,):
|
15 |
-
if self.device == 'cpu':
|
16 |
-
self.data_type = torch.float32
|
17 |
-
else:
|
18 |
-
self.data_type = torch.float16
|
19 |
-
if self.captioner_base_model == 'blip2':
|
20 |
-
processor = Blip2Processor.from_pretrained("pretrained_models/blip2-opt-2.7b")
|
21 |
-
model = Blip2ForConditionalGeneration.from_pretrained(
|
22 |
-
"pretrained_models/blip2-opt-2.7b", torch_dtype=self.data_type
|
23 |
-
)
|
24 |
-
# for gpu with small memory
|
25 |
-
elif self.captioner_base_model == 'blip':
|
26 |
-
processor = BlipProcessor.from_pretrained("Salesforce/blip-image-captioning-base")
|
27 |
-
model = BlipForConditionalGeneration.from_pretrained("Salesforce/blip-image-captioning-base", torch_dtype=self.data_type)
|
28 |
-
else:
|
29 |
-
raise ValueError('arch not supported')
|
30 |
-
model.to(self.device)
|
31 |
-
return processor, model
|
32 |
-
|
33 |
-
def image_caption(self, image_src):
|
34 |
-
image = Image.open(image_src)
|
35 |
-
image = resize_long_edge(image, 384)
|
36 |
-
inputs = self.processor(images=image, return_tensors="pt").to(self.device, self.data_type)
|
37 |
-
generated_ids = self.model.generate(**inputs)
|
38 |
-
generated_text = self.processor.batch_decode(generated_ids, skip_special_tokens=True)[0].strip()
|
39 |
-
print('\033[1;35m' + '*' * 100 + '\033[0m')
|
40 |
-
print('\nStep1, BLIP2 caption:')
|
41 |
-
print(generated_text)
|
42 |
-
print('\033[1;35m' + '*' * 100 + '\033[0m')
|
43 |
-
return generated_text
|
44 |
-
|
45 |
-
def image_caption_debug(self, image_src):
|
46 |
-
return "A dish with salmon, broccoli, and something yellow."
|
|
|
|
|
|
|
|
|
|
|
|
|
|
|
|
|
|
|
|
|
|
|
|
|
|
|
|
|
|
|
|
|
|
|
|
|
|
|
|
|
|
|
|
|
|
|
|
|
|
|
|
|
|
|
|
|
|
|
|
|
|
|
|
|
|
|
|
|
|
|
|
|
|
|
|
|
|
|
|
|
|
|
|
|
|
|
|
|
|
|
|
|
|
spaces/Awiny/Image2Paragraph/models/grit_src/third_party/CenterNet2/detectron2/layers/csrc/cocoeval/cocoeval.h
DELETED
@@ -1,88 +0,0 @@
|
|
1 |
-
// Copyright (c) Facebook, Inc. and its affiliates.
|
2 |
-
#pragma once
|
3 |
-
|
4 |
-
#include <pybind11/numpy.h>
|
5 |
-
#include <pybind11/pybind11.h>
|
6 |
-
#include <pybind11/stl.h>
|
7 |
-
#include <pybind11/stl_bind.h>
|
8 |
-
#include <vector>
|
9 |
-
|
10 |
-
namespace py = pybind11;
|
11 |
-
|
12 |
-
namespace detectron2 {
|
13 |
-
|
14 |
-
namespace COCOeval {
|
15 |
-
|
16 |
-
// Annotation data for a single object instance in an image
|
17 |
-
struct InstanceAnnotation {
|
18 |
-
InstanceAnnotation(
|
19 |
-
uint64_t id,
|
20 |
-
double score,
|
21 |
-
double area,
|
22 |
-
bool is_crowd,
|
23 |
-
bool ignore)
|
24 |
-
: id{id}, score{score}, area{area}, is_crowd{is_crowd}, ignore{ignore} {}
|
25 |
-
uint64_t id;
|
26 |
-
double score = 0.;
|
27 |
-
double area = 0.;
|
28 |
-
bool is_crowd = false;
|
29 |
-
bool ignore = false;
|
30 |
-
};
|
31 |
-
|
32 |
-
// Stores intermediate results for evaluating detection results for a single
|
33 |
-
// image that has D detected instances and G ground truth instances. This stores
|
34 |
-
// matches between detected and ground truth instances
|
35 |
-
struct ImageEvaluation {
|
36 |
-
// For each of the D detected instances, the id of the matched ground truth
|
37 |
-
// instance, or 0 if unmatched
|
38 |
-
std::vector<uint64_t> detection_matches;
|
39 |
-
|
40 |
-
// The detection score of each of the D detected instances
|
41 |
-
std::vector<double> detection_scores;
|
42 |
-
|
43 |
-
// Marks whether or not each of G instances was ignored from evaluation (e.g.,
|
44 |
-
// because it's outside area_range)
|
45 |
-
std::vector<bool> ground_truth_ignores;
|
46 |
-
|
47 |
-
// Marks whether or not each of D instances was ignored from evaluation (e.g.,
|
48 |
-
// because it's outside aRng)
|
49 |
-
std::vector<bool> detection_ignores;
|
50 |
-
};
|
51 |
-
|
52 |
-
template <class T>
|
53 |
-
using ImageCategoryInstances = std::vector<std::vector<std::vector<T>>>;
|
54 |
-
|
55 |
-
// C++ implementation of COCO API cocoeval.py::COCOeval.evaluateImg(). For each
|
56 |
-
// combination of image, category, area range settings, and IOU thresholds to
|
57 |
-
// evaluate, it matches detected instances to ground truth instances and stores
|
58 |
-
// the results into a vector of ImageEvaluation results, which will be
|
59 |
-
// interpreted by the COCOeval::Accumulate() function to produce precion-recall
|
60 |
-
// curves. The parameters of nested vectors have the following semantics:
|
61 |
-
// image_category_ious[i][c][d][g] is the intersection over union of the d'th
|
62 |
-
// detected instance and g'th ground truth instance of
|
63 |
-
// category category_ids[c] in image image_ids[i]
|
64 |
-
// image_category_ground_truth_instances[i][c] is a vector of ground truth
|
65 |
-
// instances in image image_ids[i] of category category_ids[c]
|
66 |
-
// image_category_detection_instances[i][c] is a vector of detected
|
67 |
-
// instances in image image_ids[i] of category category_ids[c]
|
68 |
-
std::vector<ImageEvaluation> EvaluateImages(
|
69 |
-
const std::vector<std::array<double, 2>>& area_ranges, // vector of 2-tuples
|
70 |
-
int max_detections,
|
71 |
-
const std::vector<double>& iou_thresholds,
|
72 |
-
const ImageCategoryInstances<std::vector<double>>& image_category_ious,
|
73 |
-
const ImageCategoryInstances<InstanceAnnotation>&
|
74 |
-
image_category_ground_truth_instances,
|
75 |
-
const ImageCategoryInstances<InstanceAnnotation>&
|
76 |
-
image_category_detection_instances);
|
77 |
-
|
78 |
-
// C++ implementation of COCOeval.accumulate(), which generates precision
|
79 |
-
// recall curves for each set of category, IOU threshold, detection area range,
|
80 |
-
// and max number of detections parameters. It is assumed that the parameter
|
81 |
-
// evaluations is the return value of the functon COCOeval::EvaluateImages(),
|
82 |
-
// which was called with the same parameter settings params
|
83 |
-
py::dict Accumulate(
|
84 |
-
const py::object& params,
|
85 |
-
const std::vector<ImageEvaluation>& evalutations);
|
86 |
-
|
87 |
-
} // namespace COCOeval
|
88 |
-
} // namespace detectron2
|
|
|
|
|
|
|
|
|
|
|
|
|
|
|
|
|
|
|
|
|
|
|
|
|
|
|
|
|
|
|
|
|
|
|
|
|
|
|
|
|
|
|
|
|
|
|
|
|
|
|
|
|
|
|
|
|
|
|
|
|
|
|
|
|
|
|
|
|
|
|
|
|
|
|
|
|
|
|
|
|
|
|
|
|
|
|
|
|
|
|
|
|
|
|
|
|
|
|
|
|
|
|
|
|
|
|
|
|
|
|
|
|
|
|
|
|
|
|
|
|
|
|
|
|
|
|
|
|
|
|
|
|
|
|
|
|
|
|
|
|
|
|
|
|
|
|
|
|
|
|
|
|
|
|
|
|
|
|
|
|
|
|
|
|
|
|
|
|
|
|
|
|
|
|
|
|
|
spaces/Ayaka-daisuki/anime-remove-background/README.md
DELETED
@@ -1,14 +0,0 @@
|
|
1 |
-
---
|
2 |
-
title: Anime Remove Background
|
3 |
-
emoji: 🪄🖼️
|
4 |
-
colorFrom: indigo
|
5 |
-
colorTo: pink
|
6 |
-
sdk: gradio
|
7 |
-
sdk_version: 3.1.4
|
8 |
-
app_file: app.py
|
9 |
-
pinned: false
|
10 |
-
license: apache-2.0
|
11 |
-
duplicated_from: skytnt/anime-remove-background
|
12 |
-
---
|
13 |
-
|
14 |
-
Check out the configuration reference at https://huggingface.co/docs/hub/spaces-config-reference
|
|
|
|
|
|
|
|
|
|
|
|
|
|
|
|
|
|
|
|
|
|
|
|
|
|
|
|
|
|
spaces/Basil2k4/botbasil203/Dockerfile
DELETED
@@ -1,106 +0,0 @@
|
|
1 |
-
# syntax=docker/dockerfile:experimental
|
2 |
-
|
3 |
-
# ./hooks/build latest
|
4 |
-
# ./hooks/test latest
|
5 |
-
|
6 |
-
### Example: Build and test 'dev' tag locally like
|
7 |
-
### ./hooks/build dev
|
8 |
-
### ./hooks/test dev
|
9 |
-
### or with additional arguments
|
10 |
-
### ./hooks/build dev --no-cache
|
11 |
-
### ./hooks/test dev
|
12 |
-
### or using the utility
|
13 |
-
### ./utils/util-hdx.sh Dockerfile 3
|
14 |
-
### ./utils/util-hdx.sh Dockerfile 4
|
15 |
-
### The last output line should be '+ exit 0'
|
16 |
-
### If '+ exit 1' then adjust the version sticker
|
17 |
-
### variables in script './hooks/env'
|
18 |
-
|
19 |
-
ARG BASETAG=latest
|
20 |
-
|
21 |
-
FROM accetto/ubuntu-vnc-xfce:${BASETAG} as stage-install
|
22 |
-
|
23 |
-
### Be sure to use root user
|
24 |
-
USER 0
|
25 |
-
|
26 |
-
### 'apt-get clean' runs automatically
|
27 |
-
RUN apt-get update \
|
28 |
-
&& DEBIAN_FRONTEND=noninteractive apt-get install -y \
|
29 |
-
chromium-browser \
|
30 |
-
neofetch \
|
31 |
-
python3-pip \
|
32 |
-
firefox \
|
33 |
-
sudo \
|
34 |
-
unzip \
|
35 |
-
git \
|
36 |
-
curl \
|
37 |
-
default-jdk \
|
38 |
-
snapd \
|
39 |
-
&& curl -sL https://deb.nodesource.com/setup_16.x | sudo -E bash -\
|
40 |
-
&& apt install nodejs\
|
41 |
-
&& apt-get -y autoremove \
|
42 |
-
&& rm -rf /var/lib/apt/lists/*
|
43 |
-
### Chromium browser requires some presets
|
44 |
-
### Note that 'no-sandbox' flag is required, but intended for development only
|
45 |
-
RUN echo "CHROMIUM_FLAGS='--no-sandbox --disable-gpu --user-data-dir --window-size=${VNC_RESOLUTION%x*},${VNC_RESOLUTION#*x} --window-position=0,0'" > ${HOME}/.chromium-browser.init
|
46 |
-
|
47 |
-
FROM stage-install as stage-config
|
48 |
-
|
49 |
-
### Arguments can be provided during build
|
50 |
-
ARG ARG_VNC_USER
|
51 |
-
|
52 |
-
ENV VNC_USER=${ARG_VNC_USER:-headless:headless}
|
53 |
-
|
54 |
-
WORKDIR ${HOME}
|
55 |
-
SHELL ["/bin/bash", "-c"]
|
56 |
-
|
57 |
-
COPY [ "./src/create_user_and_fix_permissions.sh", "./" ]
|
58 |
-
|
59 |
-
### 'sync' mitigates automated build failures
|
60 |
-
RUN chmod +x \
|
61 |
-
./create_user_and_fix_permissions.sh \
|
62 |
-
&& sync \
|
63 |
-
&& ./create_user_and_fix_permissions.sh $STARTUPDIR $HOME \
|
64 |
-
&& rm ./create_user_and_fix_permissions.sh
|
65 |
-
|
66 |
-
FROM stage-config as stage-final
|
67 |
-
|
68 |
-
### Arguments can be provided during build
|
69 |
-
ARG ARG_REFRESHED_AT
|
70 |
-
ARG ARG_VCS_REF
|
71 |
-
ARG ARG_VERSION_STICKER
|
72 |
-
ARG ARG_VNC_BLACKLIST_THRESHOLD
|
73 |
-
ARG ARG_VNC_BLACKLIST_TIMEOUT
|
74 |
-
ARG ARG_VNC_RESOLUTION
|
75 |
-
|
76 |
-
LABEL \
|
77 |
-
any.accetto.description="Headless Ubuntu VNC/noVNC container with Xfce desktop and Chromium Browser" \
|
78 |
-
any.accetto.display-name="Headless Ubuntu/Xfce VNC/noVNC container with Firefox and Chromium" \
|
79 |
-
any.accetto.tags="ubuntu, xfce, vnc, novnc, chromium" \
|
80 |
-
version-sticker="${ARG_VERSION_STICKER}" \
|
81 |
-
org.label-schema.vcs-ref="${ARG_VCS_REF}" \
|
82 |
-
org.label-schema.vcs-url="https://github.com/accetto/ubuntu-vnc-xfce-chromium"
|
83 |
-
|
84 |
-
ENV \
|
85 |
-
REFRESHED_AT=${ARG_REFRESHED_AT} \
|
86 |
-
VERSION_STICKER=${ARG_VERSION_STICKER} \
|
87 |
-
VNC_BLACKLIST_THRESHOLD=${ARG_VNC_BLACKLIST_THRESHOLD:-20} \
|
88 |
-
VNC_BLACKLIST_TIMEOUT=${ARG_VNC_BLACKLIST_TIMEOUT:-0} \
|
89 |
-
VNC_RESOLUTION=${ARG_VNC_RESOLUTION:-1360x768}
|
90 |
-
|
91 |
-
### Preconfigure Xfce
|
92 |
-
COPY [ "./src/home/Desktop", "./Desktop/" ]
|
93 |
-
COPY [ "./src/home/config/xfce4/panel", "./.config/xfce4/panel/" ]
|
94 |
-
COPY [ "./src/home/config/xfce4/xfconf/xfce-perchannel-xml", "./.config/xfce4/xfconf/xfce-perchannel-xml/" ]
|
95 |
-
COPY [ "./src/startup/version_sticker.sh", "${STARTUPDIR}/" ]
|
96 |
-
|
97 |
-
### Fix permissions
|
98 |
-
RUN \
|
99 |
-
chmod a+wx "${STARTUPDIR}"/version_sticker.sh \
|
100 |
-
&& "${STARTUPDIR}"/set_user_permissions.sh "${STARTUPDIR}" "${HOME}"
|
101 |
-
|
102 |
-
### Switch to non-root user
|
103 |
-
USER 0
|
104 |
-
|
105 |
-
### Issue #7 (base): Mitigating problems with foreground mode
|
106 |
-
WORKDIR ${STARTUPDIR}
|
|
|
|
|
|
|
|
|
|
|
|
|
|
|
|
|
|
|
|
|
|
|
|
|
|
|
|
|
|
|
|
|
|
|
|
|
|
|
|
|
|
|
|
|
|
|
|
|
|
|
|
|
|
|
|
|
|
|
|
|
|
|
|
|
|
|
|
|
|
|
|
|
|
|
|
|
|
|
|
|
|
|
|
|
|
|
|
|
|
|
|
|
|
|
|
|
|
|
|
|
|
|
|
|
|
|
|
|
|
|
|
|
|
|
|
|
|
|
|
|
|
|
|
|
|
|
|
|
|
|
|
|
|
|
|
|
|
|
|
|
|
|
|
|
|
|
|
|
|
|
|
|
|
|
|
|
|
|
|
|
|
|
|
|
|
|
|
|
|
|
|
|
|
|
|
|
|
|
|
|
|
|
|
|
|
|
|
|
|
|
|
|
|
|
|
|
|
|
|
|
|
|
|
|
|
|
|
|
|
|
|
|
|
spaces/Big-Web/MMSD/env/Lib/site-packages/setuptools/_vendor/tomli/_parser.py
DELETED
@@ -1,691 +0,0 @@
|
|
1 |
-
# SPDX-License-Identifier: MIT
|
2 |
-
# SPDX-FileCopyrightText: 2021 Taneli Hukkinen
|
3 |
-
# Licensed to PSF under a Contributor Agreement.
|
4 |
-
|
5 |
-
from __future__ import annotations
|
6 |
-
|
7 |
-
from collections.abc import Iterable
|
8 |
-
import string
|
9 |
-
from types import MappingProxyType
|
10 |
-
from typing import Any, BinaryIO, NamedTuple
|
11 |
-
|
12 |
-
from ._re import (
|
13 |
-
RE_DATETIME,
|
14 |
-
RE_LOCALTIME,
|
15 |
-
RE_NUMBER,
|
16 |
-
match_to_datetime,
|
17 |
-
match_to_localtime,
|
18 |
-
match_to_number,
|
19 |
-
)
|
20 |
-
from ._types import Key, ParseFloat, Pos
|
21 |
-
|
22 |
-
ASCII_CTRL = frozenset(chr(i) for i in range(32)) | frozenset(chr(127))
|
23 |
-
|
24 |
-
# Neither of these sets include quotation mark or backslash. They are
|
25 |
-
# currently handled as separate cases in the parser functions.
|
26 |
-
ILLEGAL_BASIC_STR_CHARS = ASCII_CTRL - frozenset("\t")
|
27 |
-
ILLEGAL_MULTILINE_BASIC_STR_CHARS = ASCII_CTRL - frozenset("\t\n")
|
28 |
-
|
29 |
-
ILLEGAL_LITERAL_STR_CHARS = ILLEGAL_BASIC_STR_CHARS
|
30 |
-
ILLEGAL_MULTILINE_LITERAL_STR_CHARS = ILLEGAL_MULTILINE_BASIC_STR_CHARS
|
31 |
-
|
32 |
-
ILLEGAL_COMMENT_CHARS = ILLEGAL_BASIC_STR_CHARS
|
33 |
-
|
34 |
-
TOML_WS = frozenset(" \t")
|
35 |
-
TOML_WS_AND_NEWLINE = TOML_WS | frozenset("\n")
|
36 |
-
BARE_KEY_CHARS = frozenset(string.ascii_letters + string.digits + "-_")
|
37 |
-
KEY_INITIAL_CHARS = BARE_KEY_CHARS | frozenset("\"'")
|
38 |
-
HEXDIGIT_CHARS = frozenset(string.hexdigits)
|
39 |
-
|
40 |
-
BASIC_STR_ESCAPE_REPLACEMENTS = MappingProxyType(
|
41 |
-
{
|
42 |
-
"\\b": "\u0008", # backspace
|
43 |
-
"\\t": "\u0009", # tab
|
44 |
-
"\\n": "\u000A", # linefeed
|
45 |
-
"\\f": "\u000C", # form feed
|
46 |
-
"\\r": "\u000D", # carriage return
|
47 |
-
'\\"': "\u0022", # quote
|
48 |
-
"\\\\": "\u005C", # backslash
|
49 |
-
}
|
50 |
-
)
|
51 |
-
|
52 |
-
|
53 |
-
class TOMLDecodeError(ValueError):
|
54 |
-
"""An error raised if a document is not valid TOML."""
|
55 |
-
|
56 |
-
|
57 |
-
def load(__fp: BinaryIO, *, parse_float: ParseFloat = float) -> dict[str, Any]:
|
58 |
-
"""Parse TOML from a binary file object."""
|
59 |
-
b = __fp.read()
|
60 |
-
try:
|
61 |
-
s = b.decode()
|
62 |
-
except AttributeError:
|
63 |
-
raise TypeError(
|
64 |
-
"File must be opened in binary mode, e.g. use `open('foo.toml', 'rb')`"
|
65 |
-
) from None
|
66 |
-
return loads(s, parse_float=parse_float)
|
67 |
-
|
68 |
-
|
69 |
-
def loads(__s: str, *, parse_float: ParseFloat = float) -> dict[str, Any]: # noqa: C901
|
70 |
-
"""Parse TOML from a string."""
|
71 |
-
|
72 |
-
# The spec allows converting "\r\n" to "\n", even in string
|
73 |
-
# literals. Let's do so to simplify parsing.
|
74 |
-
src = __s.replace("\r\n", "\n")
|
75 |
-
pos = 0
|
76 |
-
out = Output(NestedDict(), Flags())
|
77 |
-
header: Key = ()
|
78 |
-
parse_float = make_safe_parse_float(parse_float)
|
79 |
-
|
80 |
-
# Parse one statement at a time
|
81 |
-
# (typically means one line in TOML source)
|
82 |
-
while True:
|
83 |
-
# 1. Skip line leading whitespace
|
84 |
-
pos = skip_chars(src, pos, TOML_WS)
|
85 |
-
|
86 |
-
# 2. Parse rules. Expect one of the following:
|
87 |
-
# - end of file
|
88 |
-
# - end of line
|
89 |
-
# - comment
|
90 |
-
# - key/value pair
|
91 |
-
# - append dict to list (and move to its namespace)
|
92 |
-
# - create dict (and move to its namespace)
|
93 |
-
# Skip trailing whitespace when applicable.
|
94 |
-
try:
|
95 |
-
char = src[pos]
|
96 |
-
except IndexError:
|
97 |
-
break
|
98 |
-
if char == "\n":
|
99 |
-
pos += 1
|
100 |
-
continue
|
101 |
-
if char in KEY_INITIAL_CHARS:
|
102 |
-
pos = key_value_rule(src, pos, out, header, parse_float)
|
103 |
-
pos = skip_chars(src, pos, TOML_WS)
|
104 |
-
elif char == "[":
|
105 |
-
try:
|
106 |
-
second_char: str | None = src[pos + 1]
|
107 |
-
except IndexError:
|
108 |
-
second_char = None
|
109 |
-
out.flags.finalize_pending()
|
110 |
-
if second_char == "[":
|
111 |
-
pos, header = create_list_rule(src, pos, out)
|
112 |
-
else:
|
113 |
-
pos, header = create_dict_rule(src, pos, out)
|
114 |
-
pos = skip_chars(src, pos, TOML_WS)
|
115 |
-
elif char != "#":
|
116 |
-
raise suffixed_err(src, pos, "Invalid statement")
|
117 |
-
|
118 |
-
# 3. Skip comment
|
119 |
-
pos = skip_comment(src, pos)
|
120 |
-
|
121 |
-
# 4. Expect end of line or end of file
|
122 |
-
try:
|
123 |
-
char = src[pos]
|
124 |
-
except IndexError:
|
125 |
-
break
|
126 |
-
if char != "\n":
|
127 |
-
raise suffixed_err(
|
128 |
-
src, pos, "Expected newline or end of document after a statement"
|
129 |
-
)
|
130 |
-
pos += 1
|
131 |
-
|
132 |
-
return out.data.dict
|
133 |
-
|
134 |
-
|
135 |
-
class Flags:
|
136 |
-
"""Flags that map to parsed keys/namespaces."""
|
137 |
-
|
138 |
-
# Marks an immutable namespace (inline array or inline table).
|
139 |
-
FROZEN = 0
|
140 |
-
# Marks a nest that has been explicitly created and can no longer
|
141 |
-
# be opened using the "[table]" syntax.
|
142 |
-
EXPLICIT_NEST = 1
|
143 |
-
|
144 |
-
def __init__(self) -> None:
|
145 |
-
self._flags: dict[str, dict] = {}
|
146 |
-
self._pending_flags: set[tuple[Key, int]] = set()
|
147 |
-
|
148 |
-
def add_pending(self, key: Key, flag: int) -> None:
|
149 |
-
self._pending_flags.add((key, flag))
|
150 |
-
|
151 |
-
def finalize_pending(self) -> None:
|
152 |
-
for key, flag in self._pending_flags:
|
153 |
-
self.set(key, flag, recursive=False)
|
154 |
-
self._pending_flags.clear()
|
155 |
-
|
156 |
-
def unset_all(self, key: Key) -> None:
|
157 |
-
cont = self._flags
|
158 |
-
for k in key[:-1]:
|
159 |
-
if k not in cont:
|
160 |
-
return
|
161 |
-
cont = cont[k]["nested"]
|
162 |
-
cont.pop(key[-1], None)
|
163 |
-
|
164 |
-
def set(self, key: Key, flag: int, *, recursive: bool) -> None: # noqa: A003
|
165 |
-
cont = self._flags
|
166 |
-
key_parent, key_stem = key[:-1], key[-1]
|
167 |
-
for k in key_parent:
|
168 |
-
if k not in cont:
|
169 |
-
cont[k] = {"flags": set(), "recursive_flags": set(), "nested": {}}
|
170 |
-
cont = cont[k]["nested"]
|
171 |
-
if key_stem not in cont:
|
172 |
-
cont[key_stem] = {"flags": set(), "recursive_flags": set(), "nested": {}}
|
173 |
-
cont[key_stem]["recursive_flags" if recursive else "flags"].add(flag)
|
174 |
-
|
175 |
-
def is_(self, key: Key, flag: int) -> bool:
|
176 |
-
if not key:
|
177 |
-
return False # document root has no flags
|
178 |
-
cont = self._flags
|
179 |
-
for k in key[:-1]:
|
180 |
-
if k not in cont:
|
181 |
-
return False
|
182 |
-
inner_cont = cont[k]
|
183 |
-
if flag in inner_cont["recursive_flags"]:
|
184 |
-
return True
|
185 |
-
cont = inner_cont["nested"]
|
186 |
-
key_stem = key[-1]
|
187 |
-
if key_stem in cont:
|
188 |
-
cont = cont[key_stem]
|
189 |
-
return flag in cont["flags"] or flag in cont["recursive_flags"]
|
190 |
-
return False
|
191 |
-
|
192 |
-
|
193 |
-
class NestedDict:
|
194 |
-
def __init__(self) -> None:
|
195 |
-
# The parsed content of the TOML document
|
196 |
-
self.dict: dict[str, Any] = {}
|
197 |
-
|
198 |
-
def get_or_create_nest(
|
199 |
-
self,
|
200 |
-
key: Key,
|
201 |
-
*,
|
202 |
-
access_lists: bool = True,
|
203 |
-
) -> dict:
|
204 |
-
cont: Any = self.dict
|
205 |
-
for k in key:
|
206 |
-
if k not in cont:
|
207 |
-
cont[k] = {}
|
208 |
-
cont = cont[k]
|
209 |
-
if access_lists and isinstance(cont, list):
|
210 |
-
cont = cont[-1]
|
211 |
-
if not isinstance(cont, dict):
|
212 |
-
raise KeyError("There is no nest behind this key")
|
213 |
-
return cont
|
214 |
-
|
215 |
-
def append_nest_to_list(self, key: Key) -> None:
|
216 |
-
cont = self.get_or_create_nest(key[:-1])
|
217 |
-
last_key = key[-1]
|
218 |
-
if last_key in cont:
|
219 |
-
list_ = cont[last_key]
|
220 |
-
if not isinstance(list_, list):
|
221 |
-
raise KeyError("An object other than list found behind this key")
|
222 |
-
list_.append({})
|
223 |
-
else:
|
224 |
-
cont[last_key] = [{}]
|
225 |
-
|
226 |
-
|
227 |
-
class Output(NamedTuple):
|
228 |
-
data: NestedDict
|
229 |
-
flags: Flags
|
230 |
-
|
231 |
-
|
232 |
-
def skip_chars(src: str, pos: Pos, chars: Iterable[str]) -> Pos:
|
233 |
-
try:
|
234 |
-
while src[pos] in chars:
|
235 |
-
pos += 1
|
236 |
-
except IndexError:
|
237 |
-
pass
|
238 |
-
return pos
|
239 |
-
|
240 |
-
|
241 |
-
def skip_until(
|
242 |
-
src: str,
|
243 |
-
pos: Pos,
|
244 |
-
expect: str,
|
245 |
-
*,
|
246 |
-
error_on: frozenset[str],
|
247 |
-
error_on_eof: bool,
|
248 |
-
) -> Pos:
|
249 |
-
try:
|
250 |
-
new_pos = src.index(expect, pos)
|
251 |
-
except ValueError:
|
252 |
-
new_pos = len(src)
|
253 |
-
if error_on_eof:
|
254 |
-
raise suffixed_err(src, new_pos, f"Expected {expect!r}") from None
|
255 |
-
|
256 |
-
if not error_on.isdisjoint(src[pos:new_pos]):
|
257 |
-
while src[pos] not in error_on:
|
258 |
-
pos += 1
|
259 |
-
raise suffixed_err(src, pos, f"Found invalid character {src[pos]!r}")
|
260 |
-
return new_pos
|
261 |
-
|
262 |
-
|
263 |
-
def skip_comment(src: str, pos: Pos) -> Pos:
|
264 |
-
try:
|
265 |
-
char: str | None = src[pos]
|
266 |
-
except IndexError:
|
267 |
-
char = None
|
268 |
-
if char == "#":
|
269 |
-
return skip_until(
|
270 |
-
src, pos + 1, "\n", error_on=ILLEGAL_COMMENT_CHARS, error_on_eof=False
|
271 |
-
)
|
272 |
-
return pos
|
273 |
-
|
274 |
-
|
275 |
-
def skip_comments_and_array_ws(src: str, pos: Pos) -> Pos:
|
276 |
-
while True:
|
277 |
-
pos_before_skip = pos
|
278 |
-
pos = skip_chars(src, pos, TOML_WS_AND_NEWLINE)
|
279 |
-
pos = skip_comment(src, pos)
|
280 |
-
if pos == pos_before_skip:
|
281 |
-
return pos
|
282 |
-
|
283 |
-
|
284 |
-
def create_dict_rule(src: str, pos: Pos, out: Output) -> tuple[Pos, Key]:
|
285 |
-
pos += 1 # Skip "["
|
286 |
-
pos = skip_chars(src, pos, TOML_WS)
|
287 |
-
pos, key = parse_key(src, pos)
|
288 |
-
|
289 |
-
if out.flags.is_(key, Flags.EXPLICIT_NEST) or out.flags.is_(key, Flags.FROZEN):
|
290 |
-
raise suffixed_err(src, pos, f"Cannot declare {key} twice")
|
291 |
-
out.flags.set(key, Flags.EXPLICIT_NEST, recursive=False)
|
292 |
-
try:
|
293 |
-
out.data.get_or_create_nest(key)
|
294 |
-
except KeyError:
|
295 |
-
raise suffixed_err(src, pos, "Cannot overwrite a value") from None
|
296 |
-
|
297 |
-
if not src.startswith("]", pos):
|
298 |
-
raise suffixed_err(src, pos, "Expected ']' at the end of a table declaration")
|
299 |
-
return pos + 1, key
|
300 |
-
|
301 |
-
|
302 |
-
def create_list_rule(src: str, pos: Pos, out: Output) -> tuple[Pos, Key]:
|
303 |
-
pos += 2 # Skip "[["
|
304 |
-
pos = skip_chars(src, pos, TOML_WS)
|
305 |
-
pos, key = parse_key(src, pos)
|
306 |
-
|
307 |
-
if out.flags.is_(key, Flags.FROZEN):
|
308 |
-
raise suffixed_err(src, pos, f"Cannot mutate immutable namespace {key}")
|
309 |
-
# Free the namespace now that it points to another empty list item...
|
310 |
-
out.flags.unset_all(key)
|
311 |
-
# ...but this key precisely is still prohibited from table declaration
|
312 |
-
out.flags.set(key, Flags.EXPLICIT_NEST, recursive=False)
|
313 |
-
try:
|
314 |
-
out.data.append_nest_to_list(key)
|
315 |
-
except KeyError:
|
316 |
-
raise suffixed_err(src, pos, "Cannot overwrite a value") from None
|
317 |
-
|
318 |
-
if not src.startswith("]]", pos):
|
319 |
-
raise suffixed_err(src, pos, "Expected ']]' at the end of an array declaration")
|
320 |
-
return pos + 2, key
|
321 |
-
|
322 |
-
|
323 |
-
def key_value_rule(
|
324 |
-
src: str, pos: Pos, out: Output, header: Key, parse_float: ParseFloat
|
325 |
-
) -> Pos:
|
326 |
-
pos, key, value = parse_key_value_pair(src, pos, parse_float)
|
327 |
-
key_parent, key_stem = key[:-1], key[-1]
|
328 |
-
abs_key_parent = header + key_parent
|
329 |
-
|
330 |
-
relative_path_cont_keys = (header + key[:i] for i in range(1, len(key)))
|
331 |
-
for cont_key in relative_path_cont_keys:
|
332 |
-
# Check that dotted key syntax does not redefine an existing table
|
333 |
-
if out.flags.is_(cont_key, Flags.EXPLICIT_NEST):
|
334 |
-
raise suffixed_err(src, pos, f"Cannot redefine namespace {cont_key}")
|
335 |
-
# Containers in the relative path can't be opened with the table syntax or
|
336 |
-
# dotted key/value syntax in following table sections.
|
337 |
-
out.flags.add_pending(cont_key, Flags.EXPLICIT_NEST)
|
338 |
-
|
339 |
-
if out.flags.is_(abs_key_parent, Flags.FROZEN):
|
340 |
-
raise suffixed_err(
|
341 |
-
src, pos, f"Cannot mutate immutable namespace {abs_key_parent}"
|
342 |
-
)
|
343 |
-
|
344 |
-
try:
|
345 |
-
nest = out.data.get_or_create_nest(abs_key_parent)
|
346 |
-
except KeyError:
|
347 |
-
raise suffixed_err(src, pos, "Cannot overwrite a value") from None
|
348 |
-
if key_stem in nest:
|
349 |
-
raise suffixed_err(src, pos, "Cannot overwrite a value")
|
350 |
-
# Mark inline table and array namespaces recursively immutable
|
351 |
-
if isinstance(value, (dict, list)):
|
352 |
-
out.flags.set(header + key, Flags.FROZEN, recursive=True)
|
353 |
-
nest[key_stem] = value
|
354 |
-
return pos
|
355 |
-
|
356 |
-
|
357 |
-
def parse_key_value_pair(
|
358 |
-
src: str, pos: Pos, parse_float: ParseFloat
|
359 |
-
) -> tuple[Pos, Key, Any]:
|
360 |
-
pos, key = parse_key(src, pos)
|
361 |
-
try:
|
362 |
-
char: str | None = src[pos]
|
363 |
-
except IndexError:
|
364 |
-
char = None
|
365 |
-
if char != "=":
|
366 |
-
raise suffixed_err(src, pos, "Expected '=' after a key in a key/value pair")
|
367 |
-
pos += 1
|
368 |
-
pos = skip_chars(src, pos, TOML_WS)
|
369 |
-
pos, value = parse_value(src, pos, parse_float)
|
370 |
-
return pos, key, value
|
371 |
-
|
372 |
-
|
373 |
-
def parse_key(src: str, pos: Pos) -> tuple[Pos, Key]:
|
374 |
-
pos, key_part = parse_key_part(src, pos)
|
375 |
-
key: Key = (key_part,)
|
376 |
-
pos = skip_chars(src, pos, TOML_WS)
|
377 |
-
while True:
|
378 |
-
try:
|
379 |
-
char: str | None = src[pos]
|
380 |
-
except IndexError:
|
381 |
-
char = None
|
382 |
-
if char != ".":
|
383 |
-
return pos, key
|
384 |
-
pos += 1
|
385 |
-
pos = skip_chars(src, pos, TOML_WS)
|
386 |
-
pos, key_part = parse_key_part(src, pos)
|
387 |
-
key += (key_part,)
|
388 |
-
pos = skip_chars(src, pos, TOML_WS)
|
389 |
-
|
390 |
-
|
391 |
-
def parse_key_part(src: str, pos: Pos) -> tuple[Pos, str]:
|
392 |
-
try:
|
393 |
-
char: str | None = src[pos]
|
394 |
-
except IndexError:
|
395 |
-
char = None
|
396 |
-
if char in BARE_KEY_CHARS:
|
397 |
-
start_pos = pos
|
398 |
-
pos = skip_chars(src, pos, BARE_KEY_CHARS)
|
399 |
-
return pos, src[start_pos:pos]
|
400 |
-
if char == "'":
|
401 |
-
return parse_literal_str(src, pos)
|
402 |
-
if char == '"':
|
403 |
-
return parse_one_line_basic_str(src, pos)
|
404 |
-
raise suffixed_err(src, pos, "Invalid initial character for a key part")
|
405 |
-
|
406 |
-
|
407 |
-
def parse_one_line_basic_str(src: str, pos: Pos) -> tuple[Pos, str]:
|
408 |
-
pos += 1
|
409 |
-
return parse_basic_str(src, pos, multiline=False)
|
410 |
-
|
411 |
-
|
412 |
-
def parse_array(src: str, pos: Pos, parse_float: ParseFloat) -> tuple[Pos, list]:
|
413 |
-
pos += 1
|
414 |
-
array: list = []
|
415 |
-
|
416 |
-
pos = skip_comments_and_array_ws(src, pos)
|
417 |
-
if src.startswith("]", pos):
|
418 |
-
return pos + 1, array
|
419 |
-
while True:
|
420 |
-
pos, val = parse_value(src, pos, parse_float)
|
421 |
-
array.append(val)
|
422 |
-
pos = skip_comments_and_array_ws(src, pos)
|
423 |
-
|
424 |
-
c = src[pos : pos + 1]
|
425 |
-
if c == "]":
|
426 |
-
return pos + 1, array
|
427 |
-
if c != ",":
|
428 |
-
raise suffixed_err(src, pos, "Unclosed array")
|
429 |
-
pos += 1
|
430 |
-
|
431 |
-
pos = skip_comments_and_array_ws(src, pos)
|
432 |
-
if src.startswith("]", pos):
|
433 |
-
return pos + 1, array
|
434 |
-
|
435 |
-
|
436 |
-
def parse_inline_table(src: str, pos: Pos, parse_float: ParseFloat) -> tuple[Pos, dict]:
|
437 |
-
pos += 1
|
438 |
-
nested_dict = NestedDict()
|
439 |
-
flags = Flags()
|
440 |
-
|
441 |
-
pos = skip_chars(src, pos, TOML_WS)
|
442 |
-
if src.startswith("}", pos):
|
443 |
-
return pos + 1, nested_dict.dict
|
444 |
-
while True:
|
445 |
-
pos, key, value = parse_key_value_pair(src, pos, parse_float)
|
446 |
-
key_parent, key_stem = key[:-1], key[-1]
|
447 |
-
if flags.is_(key, Flags.FROZEN):
|
448 |
-
raise suffixed_err(src, pos, f"Cannot mutate immutable namespace {key}")
|
449 |
-
try:
|
450 |
-
nest = nested_dict.get_or_create_nest(key_parent, access_lists=False)
|
451 |
-
except KeyError:
|
452 |
-
raise suffixed_err(src, pos, "Cannot overwrite a value") from None
|
453 |
-
if key_stem in nest:
|
454 |
-
raise suffixed_err(src, pos, f"Duplicate inline table key {key_stem!r}")
|
455 |
-
nest[key_stem] = value
|
456 |
-
pos = skip_chars(src, pos, TOML_WS)
|
457 |
-
c = src[pos : pos + 1]
|
458 |
-
if c == "}":
|
459 |
-
return pos + 1, nested_dict.dict
|
460 |
-
if c != ",":
|
461 |
-
raise suffixed_err(src, pos, "Unclosed inline table")
|
462 |
-
if isinstance(value, (dict, list)):
|
463 |
-
flags.set(key, Flags.FROZEN, recursive=True)
|
464 |
-
pos += 1
|
465 |
-
pos = skip_chars(src, pos, TOML_WS)
|
466 |
-
|
467 |
-
|
468 |
-
def parse_basic_str_escape(
|
469 |
-
src: str, pos: Pos, *, multiline: bool = False
|
470 |
-
) -> tuple[Pos, str]:
|
471 |
-
escape_id = src[pos : pos + 2]
|
472 |
-
pos += 2
|
473 |
-
if multiline and escape_id in {"\\ ", "\\\t", "\\\n"}:
|
474 |
-
# Skip whitespace until next non-whitespace character or end of
|
475 |
-
# the doc. Error if non-whitespace is found before newline.
|
476 |
-
if escape_id != "\\\n":
|
477 |
-
pos = skip_chars(src, pos, TOML_WS)
|
478 |
-
try:
|
479 |
-
char = src[pos]
|
480 |
-
except IndexError:
|
481 |
-
return pos, ""
|
482 |
-
if char != "\n":
|
483 |
-
raise suffixed_err(src, pos, "Unescaped '\\' in a string")
|
484 |
-
pos += 1
|
485 |
-
pos = skip_chars(src, pos, TOML_WS_AND_NEWLINE)
|
486 |
-
return pos, ""
|
487 |
-
if escape_id == "\\u":
|
488 |
-
return parse_hex_char(src, pos, 4)
|
489 |
-
if escape_id == "\\U":
|
490 |
-
return parse_hex_char(src, pos, 8)
|
491 |
-
try:
|
492 |
-
return pos, BASIC_STR_ESCAPE_REPLACEMENTS[escape_id]
|
493 |
-
except KeyError:
|
494 |
-
raise suffixed_err(src, pos, "Unescaped '\\' in a string") from None
|
495 |
-
|
496 |
-
|
497 |
-
def parse_basic_str_escape_multiline(src: str, pos: Pos) -> tuple[Pos, str]:
|
498 |
-
return parse_basic_str_escape(src, pos, multiline=True)
|
499 |
-
|
500 |
-
|
501 |
-
def parse_hex_char(src: str, pos: Pos, hex_len: int) -> tuple[Pos, str]:
|
502 |
-
hex_str = src[pos : pos + hex_len]
|
503 |
-
if len(hex_str) != hex_len or not HEXDIGIT_CHARS.issuperset(hex_str):
|
504 |
-
raise suffixed_err(src, pos, "Invalid hex value")
|
505 |
-
pos += hex_len
|
506 |
-
hex_int = int(hex_str, 16)
|
507 |
-
if not is_unicode_scalar_value(hex_int):
|
508 |
-
raise suffixed_err(src, pos, "Escaped character is not a Unicode scalar value")
|
509 |
-
return pos, chr(hex_int)
|
510 |
-
|
511 |
-
|
512 |
-
def parse_literal_str(src: str, pos: Pos) -> tuple[Pos, str]:
|
513 |
-
pos += 1 # Skip starting apostrophe
|
514 |
-
start_pos = pos
|
515 |
-
pos = skip_until(
|
516 |
-
src, pos, "'", error_on=ILLEGAL_LITERAL_STR_CHARS, error_on_eof=True
|
517 |
-
)
|
518 |
-
return pos + 1, src[start_pos:pos] # Skip ending apostrophe
|
519 |
-
|
520 |
-
|
521 |
-
def parse_multiline_str(src: str, pos: Pos, *, literal: bool) -> tuple[Pos, str]:
|
522 |
-
pos += 3
|
523 |
-
if src.startswith("\n", pos):
|
524 |
-
pos += 1
|
525 |
-
|
526 |
-
if literal:
|
527 |
-
delim = "'"
|
528 |
-
end_pos = skip_until(
|
529 |
-
src,
|
530 |
-
pos,
|
531 |
-
"'''",
|
532 |
-
error_on=ILLEGAL_MULTILINE_LITERAL_STR_CHARS,
|
533 |
-
error_on_eof=True,
|
534 |
-
)
|
535 |
-
result = src[pos:end_pos]
|
536 |
-
pos = end_pos + 3
|
537 |
-
else:
|
538 |
-
delim = '"'
|
539 |
-
pos, result = parse_basic_str(src, pos, multiline=True)
|
540 |
-
|
541 |
-
# Add at maximum two extra apostrophes/quotes if the end sequence
|
542 |
-
# is 4 or 5 chars long instead of just 3.
|
543 |
-
if not src.startswith(delim, pos):
|
544 |
-
return pos, result
|
545 |
-
pos += 1
|
546 |
-
if not src.startswith(delim, pos):
|
547 |
-
return pos, result + delim
|
548 |
-
pos += 1
|
549 |
-
return pos, result + (delim * 2)
|
550 |
-
|
551 |
-
|
552 |
-
def parse_basic_str(src: str, pos: Pos, *, multiline: bool) -> tuple[Pos, str]:
|
553 |
-
if multiline:
|
554 |
-
error_on = ILLEGAL_MULTILINE_BASIC_STR_CHARS
|
555 |
-
parse_escapes = parse_basic_str_escape_multiline
|
556 |
-
else:
|
557 |
-
error_on = ILLEGAL_BASIC_STR_CHARS
|
558 |
-
parse_escapes = parse_basic_str_escape
|
559 |
-
result = ""
|
560 |
-
start_pos = pos
|
561 |
-
while True:
|
562 |
-
try:
|
563 |
-
char = src[pos]
|
564 |
-
except IndexError:
|
565 |
-
raise suffixed_err(src, pos, "Unterminated string") from None
|
566 |
-
if char == '"':
|
567 |
-
if not multiline:
|
568 |
-
return pos + 1, result + src[start_pos:pos]
|
569 |
-
if src.startswith('"""', pos):
|
570 |
-
return pos + 3, result + src[start_pos:pos]
|
571 |
-
pos += 1
|
572 |
-
continue
|
573 |
-
if char == "\\":
|
574 |
-
result += src[start_pos:pos]
|
575 |
-
pos, parsed_escape = parse_escapes(src, pos)
|
576 |
-
result += parsed_escape
|
577 |
-
start_pos = pos
|
578 |
-
continue
|
579 |
-
if char in error_on:
|
580 |
-
raise suffixed_err(src, pos, f"Illegal character {char!r}")
|
581 |
-
pos += 1
|
582 |
-
|
583 |
-
|
584 |
-
def parse_value( # noqa: C901
|
585 |
-
src: str, pos: Pos, parse_float: ParseFloat
|
586 |
-
) -> tuple[Pos, Any]:
|
587 |
-
try:
|
588 |
-
char: str | None = src[pos]
|
589 |
-
except IndexError:
|
590 |
-
char = None
|
591 |
-
|
592 |
-
# IMPORTANT: order conditions based on speed of checking and likelihood
|
593 |
-
|
594 |
-
# Basic strings
|
595 |
-
if char == '"':
|
596 |
-
if src.startswith('"""', pos):
|
597 |
-
return parse_multiline_str(src, pos, literal=False)
|
598 |
-
return parse_one_line_basic_str(src, pos)
|
599 |
-
|
600 |
-
# Literal strings
|
601 |
-
if char == "'":
|
602 |
-
if src.startswith("'''", pos):
|
603 |
-
return parse_multiline_str(src, pos, literal=True)
|
604 |
-
return parse_literal_str(src, pos)
|
605 |
-
|
606 |
-
# Booleans
|
607 |
-
if char == "t":
|
608 |
-
if src.startswith("true", pos):
|
609 |
-
return pos + 4, True
|
610 |
-
if char == "f":
|
611 |
-
if src.startswith("false", pos):
|
612 |
-
return pos + 5, False
|
613 |
-
|
614 |
-
# Arrays
|
615 |
-
if char == "[":
|
616 |
-
return parse_array(src, pos, parse_float)
|
617 |
-
|
618 |
-
# Inline tables
|
619 |
-
if char == "{":
|
620 |
-
return parse_inline_table(src, pos, parse_float)
|
621 |
-
|
622 |
-
# Dates and times
|
623 |
-
datetime_match = RE_DATETIME.match(src, pos)
|
624 |
-
if datetime_match:
|
625 |
-
try:
|
626 |
-
datetime_obj = match_to_datetime(datetime_match)
|
627 |
-
except ValueError as e:
|
628 |
-
raise suffixed_err(src, pos, "Invalid date or datetime") from e
|
629 |
-
return datetime_match.end(), datetime_obj
|
630 |
-
localtime_match = RE_LOCALTIME.match(src, pos)
|
631 |
-
if localtime_match:
|
632 |
-
return localtime_match.end(), match_to_localtime(localtime_match)
|
633 |
-
|
634 |
-
# Integers and "normal" floats.
|
635 |
-
# The regex will greedily match any type starting with a decimal
|
636 |
-
# char, so needs to be located after handling of dates and times.
|
637 |
-
number_match = RE_NUMBER.match(src, pos)
|
638 |
-
if number_match:
|
639 |
-
return number_match.end(), match_to_number(number_match, parse_float)
|
640 |
-
|
641 |
-
# Special floats
|
642 |
-
first_three = src[pos : pos + 3]
|
643 |
-
if first_three in {"inf", "nan"}:
|
644 |
-
return pos + 3, parse_float(first_three)
|
645 |
-
first_four = src[pos : pos + 4]
|
646 |
-
if first_four in {"-inf", "+inf", "-nan", "+nan"}:
|
647 |
-
return pos + 4, parse_float(first_four)
|
648 |
-
|
649 |
-
raise suffixed_err(src, pos, "Invalid value")
|
650 |
-
|
651 |
-
|
652 |
-
def suffixed_err(src: str, pos: Pos, msg: str) -> TOMLDecodeError:
|
653 |
-
"""Return a `TOMLDecodeError` where error message is suffixed with
|
654 |
-
coordinates in source."""
|
655 |
-
|
656 |
-
def coord_repr(src: str, pos: Pos) -> str:
|
657 |
-
if pos >= len(src):
|
658 |
-
return "end of document"
|
659 |
-
line = src.count("\n", 0, pos) + 1
|
660 |
-
if line == 1:
|
661 |
-
column = pos + 1
|
662 |
-
else:
|
663 |
-
column = pos - src.rindex("\n", 0, pos)
|
664 |
-
return f"line {line}, column {column}"
|
665 |
-
|
666 |
-
return TOMLDecodeError(f"{msg} (at {coord_repr(src, pos)})")
|
667 |
-
|
668 |
-
|
669 |
-
def is_unicode_scalar_value(codepoint: int) -> bool:
|
670 |
-
return (0 <= codepoint <= 55295) or (57344 <= codepoint <= 1114111)
|
671 |
-
|
672 |
-
|
673 |
-
def make_safe_parse_float(parse_float: ParseFloat) -> ParseFloat:
|
674 |
-
"""A decorator to make `parse_float` safe.
|
675 |
-
|
676 |
-
`parse_float` must not return dicts or lists, because these types
|
677 |
-
would be mixed with parsed TOML tables and arrays, thus confusing
|
678 |
-
the parser. The returned decorated callable raises `ValueError`
|
679 |
-
instead of returning illegal types.
|
680 |
-
"""
|
681 |
-
# The default `float` callable never returns illegal types. Optimize it.
|
682 |
-
if parse_float is float: # type: ignore[comparison-overlap]
|
683 |
-
return float
|
684 |
-
|
685 |
-
def safe_parse_float(float_str: str) -> Any:
|
686 |
-
float_value = parse_float(float_str)
|
687 |
-
if isinstance(float_value, (dict, list)):
|
688 |
-
raise ValueError("parse_float must not return dicts or lists")
|
689 |
-
return float_value
|
690 |
-
|
691 |
-
return safe_parse_float
|
|
|
|
|
|
|
|
|
|
|
|
|
|
|
|
|
|
|
|
|
|
|
|
|
|
|
|
|
|
|
|
|
|
|
|
|
|
|
|
|
|
|
|
|
|
|
|
|
|
|
|
|
|
|
|
|
|
|
|
|
|
|
|
|
|
|
|
|
|
|
|
|
|
|
|
|
|
|
|
|
|
|
|
|
|
|
|
|
|
|
|
|
|
|
|
|
|
|
|
|
|
|
|
|
|
|
|
|
|
|
|
|
|
|
|
|
|
|
|
|
|
|
|
|
|
|
|
|
|
|
|
|
|
|
|
|
|
|
|
|
|
|
|
|
|
|
|
|
|
|
|
|
|
|
|
|
|
|
|
|
|
|
|
|
|
|
|
|
|
|
|
|
|
|
|
|
|
|
|
|
|
|
|
|
|
|
|
|
|
|
|
|
|
|
|
|
|
|
|
|
|
|
|
|
|
|
|
|
|
|
|
|
|
|
|
|
|
|
|
|
|
|
|
|
|
|
|
|
|
|
|
|
|
|
|
|
|
|
|
|
|
|
|
|
|
|
|
|
|
|
|
|
|
|
|
|
|
|
|
|
|
|
|
|
|
|
|
|
|
|
|
|
|
|
|
|
|
|
|
|
|
|
|
|
|
|
|
|
|
|
|
|
|
|
|
|
|
|
|
|
|
|
|
|
|
|
|
|
|
|
|
|
|
|
|
|
|
|
|
|
|
|
|
|
|
|
|
|
|
|
|
|
|
|
|
|
|
|
|
|
|
|
|
|
|
|
|
|
|
|
|
|
|
|
|
|
|
|
|
|
|
|
|
|
|
|
|
|
|
|
|
|
|
|
|
|
|
|
|
|
|
|
|
|
|
|
|
|
|
|
|
|
|
|
|
|
|
|
|
|
|
|
|
|
|
|
|
|
|
|
|
|
|
|
|
|
|
|
|
|
|
|
|
|
|
|
|
|
|
|
|
|
|
|
|
|
|
|
|
|
|
|
|
|
|
|
|
|
|
|
|
|
|
|
|
|
|
|
|
|
|
|
|
|
|
|
|
|
|
|
|
|
|
|
|
|
|
|
|
|
|
|
|
|
|
|
|
|
|
|
|
|
|
|
|
|
|
|
|
|
|
|
|
|
|
|
|
|
|
|
|
|
|
|
|
|
|
|
|
|
|
|
|
|
|
|
|
|
|
|
|
|
|
|
|
|
|
|
|
|
|
|
|
|
|
|
|
|
|
|
|
|
|
|
|
|
|
|
|
|
|
|
|
|
|
|
|
|
|
|
|
|
|
|
|
|
|
|
|
|
|
|
|
|
|
|
|
|
|
|
|
|
|
|
|
|
|
|
|
|
|
|
|
|
|
|
|
|
|
|
|
|
|
|
|
|
|
|
|
|
|
|
|
|
|
|
|
|
|
|
|
|
|
|
|
|
|
|
|
|
|
|
|
|
|
|
|
|
|
|
|
|
|
|
|
|
|
|
|
|
|
|
|
|
|
|
|
|
|
|
|
|
|
|
|
|
|
|
|
|
|
|
|
|
|
|
|
|
|
|
|
|
|
|
|
|
|
|
|
|
|
|
|
|
|
|
|
|
|
|
|
|
|
|
|
|
|
|
|
|
|
|
|
|
|
|
|
|
|
|
|
|
|
|
|
|
|
|
|
|
|
|
|
|
|
|
|
|
|
|
|
|
|
|
|
|
|
|
|
|
|
|
|
|
|
|
|
|
|
|
|
|
|
|
|
|
|
|
|
|
|
|
|
|
|
|
|
|
|
|
|
|
|
|
|
|
|
|
|
|
|
|
|
|
|
|
|
|
|
|
|
|
|
|
|
|
|
|
|
|
|
|
|
|
|
|
|
|
|
|
|
|
|
|
|
|
|
|
|
|
|
|
|
|
|
|
|
|
|
|
|
|
|
|
|
|
|
|
|
|
|
|
|
|
|
|
|
|
|
|
|
|
|
|
|
|
|
|
|
|
|
|
|
|
|
|
|
|
|
|
|
|
|
|
|
|
|
|
|
|
|
|
|
|
|
|
|
|
|
|
|
|
|
|
|
|
|
|
|
|
|
|
|
|
|
|
|
|
|
|
|
|
|
|
|
|
|
|
|
|
|
|
|
|
|
|
|
|
|
|
|
|
|
|
|
|
|
|
|
|
|
|
|
|
|
|
|
|
|
|
|
|
|
|
|
|
|
|
|
|
|
|
|
|
|
|
|
|
|
|
|
|
|
|
|
|
|
|
|
|
|
|
|
|
|
|
|
|
|
|
|
|
|
|
|
|
|
|
|
|
|
|
|
|
|
|
|
|
|
|
|
|
|
|
|
|
|
|
|
|
|
|
|
|
|
|
|
|
|
|
|
|
|
|
|
|
|
|
|
|
|
|
|
|
|
|
|
|
|
|
|
|
|
|
|
|
|
|
|
|
|
|
|
|
|
|
|
|
|
|
|
|
|
|
|
|
|
|
|
|
|
|
|
|
|
|
|
|
|
|
|
|
|
|
|
|
|
|
|
|
|
|
|
|
|
|
|
|
|
|
|
|
|
|
|
|
|
|
|
|
|
|
|
|
|
|
|
|
|
|
|
|
|
|
|
|
|
|
|
|
|
|
|
|
|
|
|
|
|
|
|
|
|
|
|
|
|
|
|
|
|
|
|
|
|
|
|
|
|
|
|
|
|
|
|
|
|
|
|
|
|
|
|
|
|
|
|
|
|
|
|
|
|
|
|
|
|
|
|
|
|
|
|
|
|
|
|
|
|
|
|
|
|
|
|
|
|
|
|
|
|
|
|
|
|
|
|
|
|
|
|
|
|
|
|
|
|
|
|
|
|
|
|
|
|
|
|
|
|
|
|
|
|
|
|
|
|
|
|
|
|
|
|
|
|
|
|
|
|
|
|
|
|
|
|
|
|
|
|
|
|
|
|
|
|
|
|
|
|
|
|
|
|
|
|
|
|
|
|
|
|
|
|
|
|
|
|
|
|
|
|
|
|
|
|
|
|
|
|
|
|
|
|
|
|
|
|
|
|
|
|
|
|
|
|
|
|
|
|
|
|
|
|
|
|
|
|
|
|
|
|
|
|
|
|
|
|
|
|
|
|
|
|
spaces/Blealtan/clip-guided-binary-autoencoder/app.py
DELETED
@@ -1,327 +0,0 @@
|
|
1 |
-
import base64
|
2 |
-
from huggingface_hub import hf_hub_download
|
3 |
-
import streamlit as st
|
4 |
-
import io
|
5 |
-
import gc
|
6 |
-
import json
|
7 |
-
|
8 |
-
########################################################################################################
|
9 |
-
# The RWKV Language Model - https://github.com/BlinkDL/RWKV-LM
|
10 |
-
########################################################################################################
|
11 |
-
|
12 |
-
MODEL_REPO = 'BlinkDL/clip-guided-binary-autoencoder'
|
13 |
-
|
14 |
-
import torch, types
|
15 |
-
import numpy as np
|
16 |
-
from PIL import Image
|
17 |
-
import torch.nn as nn
|
18 |
-
from torch.nn import functional as F
|
19 |
-
import torchvision as vision
|
20 |
-
import torchvision.transforms as transforms
|
21 |
-
from torchvision.transforms import functional as VF
|
22 |
-
|
23 |
-
device = 'cuda' if torch.cuda.is_available() else 'cpu'
|
24 |
-
|
25 |
-
IMG_BITS = 13
|
26 |
-
|
27 |
-
|
28 |
-
class ResBlock(nn.Module):
|
29 |
-
|
30 |
-
def __init__(self, c_x, c_hidden):
|
31 |
-
super().__init__()
|
32 |
-
self.B0 = nn.BatchNorm2d(c_x)
|
33 |
-
self.C0 = nn.Conv2d(c_x, c_hidden, kernel_size=3, padding=1)
|
34 |
-
self.C1 = nn.Conv2d(c_hidden, c_x, kernel_size=3, padding=1)
|
35 |
-
self.C2 = nn.Conv2d(c_x, c_hidden, kernel_size=3, padding=1)
|
36 |
-
self.C3 = nn.Conv2d(c_hidden, c_x, kernel_size=3, padding=1)
|
37 |
-
|
38 |
-
def forward(self, x):
|
39 |
-
ACT = F.mish
|
40 |
-
x = x + self.C1(ACT(self.C0(ACT(self.B0(x)))))
|
41 |
-
x = x + self.C3(ACT(self.C2(x)))
|
42 |
-
return x
|
43 |
-
|
44 |
-
|
45 |
-
class REncoderSmall(nn.Module):
|
46 |
-
|
47 |
-
def __init__(self):
|
48 |
-
super().__init__()
|
49 |
-
dd = 8
|
50 |
-
self.Bxx = nn.BatchNorm2d(dd * 64)
|
51 |
-
|
52 |
-
self.CIN = nn.Conv2d(3, dd, kernel_size=3, padding=1)
|
53 |
-
self.Cx0 = nn.Conv2d(dd, 32, kernel_size=3, padding=1)
|
54 |
-
self.Cx1 = nn.Conv2d(32, dd, kernel_size=3, padding=1)
|
55 |
-
|
56 |
-
self.B00 = nn.BatchNorm2d(dd * 4)
|
57 |
-
self.C00 = nn.Conv2d(dd * 4, 256, kernel_size=3, padding=1)
|
58 |
-
self.C01 = nn.Conv2d(256, dd * 4, kernel_size=3, padding=1)
|
59 |
-
self.C02 = nn.Conv2d(dd * 4, 256, kernel_size=3, padding=1)
|
60 |
-
self.C03 = nn.Conv2d(256, dd * 4, kernel_size=3, padding=1)
|
61 |
-
|
62 |
-
self.B10 = nn.BatchNorm2d(dd * 16)
|
63 |
-
self.C10 = nn.Conv2d(dd * 16, 256, kernel_size=3, padding=1)
|
64 |
-
self.C11 = nn.Conv2d(256, dd * 16, kernel_size=3, padding=1)
|
65 |
-
self.C12 = nn.Conv2d(dd * 16, 256, kernel_size=3, padding=1)
|
66 |
-
self.C13 = nn.Conv2d(256, dd * 16, kernel_size=3, padding=1)
|
67 |
-
|
68 |
-
self.B20 = nn.BatchNorm2d(dd * 64)
|
69 |
-
self.C20 = nn.Conv2d(dd * 64, 256, kernel_size=3, padding=1)
|
70 |
-
self.C21 = nn.Conv2d(256, dd * 64, kernel_size=3, padding=1)
|
71 |
-
self.C22 = nn.Conv2d(dd * 64, 256, kernel_size=3, padding=1)
|
72 |
-
self.C23 = nn.Conv2d(256, dd * 64, kernel_size=3, padding=1)
|
73 |
-
|
74 |
-
self.COUT = nn.Conv2d(dd * 64, IMG_BITS, kernel_size=3, padding=1)
|
75 |
-
|
76 |
-
def forward(self, img):
|
77 |
-
ACT = F.mish
|
78 |
-
|
79 |
-
x = self.CIN(img)
|
80 |
-
xx = self.Bxx(F.pixel_unshuffle(x, 8))
|
81 |
-
x = x + self.Cx1(ACT(self.Cx0(x)))
|
82 |
-
|
83 |
-
x = F.pixel_unshuffle(x, 2)
|
84 |
-
x = x + self.C01(ACT(self.C00(ACT(self.B00(x)))))
|
85 |
-
x = x + self.C03(ACT(self.C02(x)))
|
86 |
-
|
87 |
-
x = F.pixel_unshuffle(x, 2)
|
88 |
-
x = x + self.C11(ACT(self.C10(ACT(self.B10(x)))))
|
89 |
-
x = x + self.C13(ACT(self.C12(x)))
|
90 |
-
|
91 |
-
x = F.pixel_unshuffle(x, 2)
|
92 |
-
x = x + self.C21(ACT(self.C20(ACT(self.B20(x)))))
|
93 |
-
x = x + self.C23(ACT(self.C22(x)))
|
94 |
-
|
95 |
-
x = self.COUT(x + xx)
|
96 |
-
return torch.sigmoid(x)
|
97 |
-
|
98 |
-
|
99 |
-
class RDecoderSmall(nn.Module):
|
100 |
-
|
101 |
-
def __init__(self):
|
102 |
-
super().__init__()
|
103 |
-
dd = 8
|
104 |
-
self.CIN = nn.Conv2d(IMG_BITS, dd * 64, kernel_size=3, padding=1)
|
105 |
-
|
106 |
-
self.B00 = nn.BatchNorm2d(dd * 64)
|
107 |
-
self.C00 = nn.Conv2d(dd * 64, 256, kernel_size=3, padding=1)
|
108 |
-
self.C01 = nn.Conv2d(256, dd * 64, kernel_size=3, padding=1)
|
109 |
-
self.C02 = nn.Conv2d(dd * 64, 256, kernel_size=3, padding=1)
|
110 |
-
self.C03 = nn.Conv2d(256, dd * 64, kernel_size=3, padding=1)
|
111 |
-
|
112 |
-
self.B10 = nn.BatchNorm2d(dd * 16)
|
113 |
-
self.C10 = nn.Conv2d(dd * 16, 256, kernel_size=3, padding=1)
|
114 |
-
self.C11 = nn.Conv2d(256, dd * 16, kernel_size=3, padding=1)
|
115 |
-
self.C12 = nn.Conv2d(dd * 16, 256, kernel_size=3, padding=1)
|
116 |
-
self.C13 = nn.Conv2d(256, dd * 16, kernel_size=3, padding=1)
|
117 |
-
|
118 |
-
self.B20 = nn.BatchNorm2d(dd * 4)
|
119 |
-
self.C20 = nn.Conv2d(dd * 4, 256, kernel_size=3, padding=1)
|
120 |
-
self.C21 = nn.Conv2d(256, dd * 4, kernel_size=3, padding=1)
|
121 |
-
self.C22 = nn.Conv2d(dd * 4, 256, kernel_size=3, padding=1)
|
122 |
-
self.C23 = nn.Conv2d(256, dd * 4, kernel_size=3, padding=1)
|
123 |
-
|
124 |
-
self.Cx0 = nn.Conv2d(dd, 32, kernel_size=3, padding=1)
|
125 |
-
self.Cx1 = nn.Conv2d(32, dd, kernel_size=3, padding=1)
|
126 |
-
self.COUT = nn.Conv2d(dd, 3, kernel_size=3, padding=1)
|
127 |
-
|
128 |
-
def forward(self, code):
|
129 |
-
ACT = F.mish
|
130 |
-
x = self.CIN(code)
|
131 |
-
|
132 |
-
x = x + self.C01(ACT(self.C00(ACT(self.B00(x)))))
|
133 |
-
x = x + self.C03(ACT(self.C02(x)))
|
134 |
-
x = F.pixel_shuffle(x, 2)
|
135 |
-
|
136 |
-
x = x + self.C11(ACT(self.C10(ACT(self.B10(x)))))
|
137 |
-
x = x + self.C13(ACT(self.C12(x)))
|
138 |
-
x = F.pixel_shuffle(x, 2)
|
139 |
-
|
140 |
-
x = x + self.C21(ACT(self.C20(ACT(self.B20(x)))))
|
141 |
-
x = x + self.C23(ACT(self.C22(x)))
|
142 |
-
x = F.pixel_shuffle(x, 2)
|
143 |
-
|
144 |
-
x = x + self.Cx1(ACT(self.Cx0(x)))
|
145 |
-
x = self.COUT(x)
|
146 |
-
|
147 |
-
return torch.sigmoid(x)
|
148 |
-
|
149 |
-
|
150 |
-
class REncoderLarge(nn.Module):
|
151 |
-
|
152 |
-
def __init__(self, dd, ee, ff):
|
153 |
-
super().__init__()
|
154 |
-
self.CXX = nn.Conv2d(3, dd, kernel_size=3, padding=1)
|
155 |
-
self.BXX = nn.BatchNorm2d(dd)
|
156 |
-
self.CX0 = nn.Conv2d(dd, ee, kernel_size=3, padding=1)
|
157 |
-
self.CX1 = nn.Conv2d(ee, dd, kernel_size=3, padding=1)
|
158 |
-
self.R0 = ResBlock(dd * 4, ff)
|
159 |
-
self.R1 = ResBlock(dd * 16, ff)
|
160 |
-
self.R2 = ResBlock(dd * 64, ff)
|
161 |
-
self.CZZ = nn.Conv2d(dd * 64, IMG_BITS, kernel_size=3, padding=1)
|
162 |
-
|
163 |
-
def forward(self, x):
|
164 |
-
ACT = F.mish
|
165 |
-
x = self.BXX(self.CXX(x))
|
166 |
-
|
167 |
-
x = x + self.CX1(ACT(self.CX0(x)))
|
168 |
-
x = F.pixel_unshuffle(x, 2)
|
169 |
-
x = self.R0(x)
|
170 |
-
x = F.pixel_unshuffle(x, 2)
|
171 |
-
x = self.R1(x)
|
172 |
-
x = F.pixel_unshuffle(x, 2)
|
173 |
-
x = self.R2(x)
|
174 |
-
|
175 |
-
x = self.CZZ(x)
|
176 |
-
return torch.sigmoid(x)
|
177 |
-
|
178 |
-
|
179 |
-
class RDecoderLarge(nn.Module):
|
180 |
-
|
181 |
-
def __init__(self, dd, ee, ff):
|
182 |
-
super().__init__()
|
183 |
-
self.CZZ = nn.Conv2d(IMG_BITS, dd * 64, kernel_size=3, padding=1)
|
184 |
-
self.BZZ = nn.BatchNorm2d(dd * 64)
|
185 |
-
self.R0 = ResBlock(dd * 64, ff)
|
186 |
-
self.R1 = ResBlock(dd * 16, ff)
|
187 |
-
self.R2 = ResBlock(dd * 4, ff)
|
188 |
-
self.CX0 = nn.Conv2d(dd, ee, kernel_size=3, padding=1)
|
189 |
-
self.CX1 = nn.Conv2d(ee, dd, kernel_size=3, padding=1)
|
190 |
-
self.CXX = nn.Conv2d(dd, 3, kernel_size=3, padding=1)
|
191 |
-
|
192 |
-
def forward(self, x):
|
193 |
-
ACT = F.mish
|
194 |
-
x = self.BZZ(self.CZZ(x))
|
195 |
-
|
196 |
-
x = self.R0(x)
|
197 |
-
x = F.pixel_shuffle(x, 2)
|
198 |
-
x = self.R1(x)
|
199 |
-
x = F.pixel_shuffle(x, 2)
|
200 |
-
x = self.R2(x)
|
201 |
-
x = F.pixel_shuffle(x, 2)
|
202 |
-
x = x + self.CX1(ACT(self.CX0(x)))
|
203 |
-
|
204 |
-
x = self.CXX(x)
|
205 |
-
return torch.sigmoid(x)
|
206 |
-
|
207 |
-
|
208 |
-
@st.cache
|
209 |
-
def prepare_model(model_prefix):
|
210 |
-
gc.collect()
|
211 |
-
|
212 |
-
if model_prefix == 'out-v7c_d8_256-224-13bit-OB32x0.5-745':
|
213 |
-
R_ENCODER, R_DECODER = REncoderSmall(), RDecoderSmall()
|
214 |
-
else:
|
215 |
-
if 'd16_512' in model_prefix:
|
216 |
-
dd, ee, ff = 16, 64, 512
|
217 |
-
elif 'd32_1024' in model_prefix:
|
218 |
-
dd, ee, ff = 32, 128, 1024
|
219 |
-
R_ENCODER = REncoderLarge(dd, ee, ff)
|
220 |
-
R_DECODER = RDecoderLarge(dd, ee, ff)
|
221 |
-
|
222 |
-
encoder = R_ENCODER.eval().to(device)
|
223 |
-
decoder = R_DECODER.eval().to(device)
|
224 |
-
|
225 |
-
encoder.load_state_dict(
|
226 |
-
torch.load(hf_hub_download(MODEL_REPO, f'{model_prefix}-E.pth')))
|
227 |
-
decoder.load_state_dict(
|
228 |
-
torch.load(hf_hub_download(MODEL_REPO, f'{model_prefix}-D.pth')))
|
229 |
-
|
230 |
-
return encoder, decoder
|
231 |
-
|
232 |
-
|
233 |
-
def compute_padding(img_shape):
|
234 |
-
hsize, vsize = (img_shape[1] + 7) // 8 * 8, (img_shape[0] + 7) // 8 * 8
|
235 |
-
hpad, vpad = hsize - img_shape[1], vsize - img_shape[0]
|
236 |
-
left, top = hpad // 2, vpad // 2
|
237 |
-
right, bottom = hpad - left, vpad - top
|
238 |
-
return left, top, right, bottom
|
239 |
-
|
240 |
-
|
241 |
-
def encode(model_prefix, img, keep_shape):
|
242 |
-
gc.collect()
|
243 |
-
encoder, _ = prepare_model(model_prefix)
|
244 |
-
|
245 |
-
with torch.no_grad():
|
246 |
-
img = VF.pil_to_tensor(img.convert("RGB"))
|
247 |
-
img = VF.convert_image_dtype(img)
|
248 |
-
img = img.unsqueeze(0).to(device)
|
249 |
-
img_shape = img.shape[2:]
|
250 |
-
|
251 |
-
if keep_shape:
|
252 |
-
left, top, right, bottom = compute_padding(img_shape)
|
253 |
-
img = VF.pad(img, [left, top, right, bottom], padding_mode='edge')
|
254 |
-
else:
|
255 |
-
img = VF.resize(img, [224, 224])
|
256 |
-
|
257 |
-
z = torch.floor(encoder(img) + 0.5)
|
258 |
-
|
259 |
-
with io.BytesIO() as buffer:
|
260 |
-
np.save(buffer, np.packbits(z.cpu().numpy().astype('bool')))
|
261 |
-
z_b64 = base64.b64encode(buffer.getvalue()).decode()
|
262 |
-
|
263 |
-
return json.dumps({
|
264 |
-
"img_shape": img_shape,
|
265 |
-
"z_shape": z.shape[2:],
|
266 |
-
"keep_shape": keep_shape,
|
267 |
-
"data": z_b64,
|
268 |
-
})
|
269 |
-
|
270 |
-
|
271 |
-
def decode(model_prefix, z_str):
|
272 |
-
gc.collect()
|
273 |
-
_, decoder = prepare_model(model_prefix)
|
274 |
-
|
275 |
-
z_json = json.loads(z_str)
|
276 |
-
with io.BytesIO() as buffer:
|
277 |
-
buffer.write(base64.b64decode(z_json["data"]))
|
278 |
-
buffer.seek(0)
|
279 |
-
z = np.load(buffer)
|
280 |
-
img_shape = z_json["img_shape"]
|
281 |
-
z_shape = z_json["z_shape"]
|
282 |
-
keep_shape = z_json["keep_shape"]
|
283 |
-
|
284 |
-
z = np.unpackbits(z)[:IMG_BITS * z_shape[0] * z_shape[1]].astype('float')
|
285 |
-
z = z.reshape([1, IMG_BITS] + z_shape)
|
286 |
-
|
287 |
-
img = decoder(torch.Tensor(z).to(device))
|
288 |
-
|
289 |
-
if keep_shape:
|
290 |
-
left, top, right, bottom = compute_padding(img_shape)
|
291 |
-
img = img[0, :, top:img.shape[2] - bottom, left:img.shape[3] - right]
|
292 |
-
else:
|
293 |
-
img = img[0]
|
294 |
-
|
295 |
-
return VF.to_pil_image(img)
|
296 |
-
|
297 |
-
|
298 |
-
st.title("Clip Guided Binary Autoencoder")
|
299 |
-
st.write(
|
300 |
-
"Model is from [@BlinkDL](https://huggingface.co/BlinkDL/clip-guided-binary-autoencoder)"
|
301 |
-
)
|
302 |
-
model_prefix = st.selectbox('The model to use',
|
303 |
-
('out-v7c_d8_256-224-13bit-OB32x0.5-745',
|
304 |
-
'out-v7d_d16_512-224-13bit-OB32x0.5-2487',
|
305 |
-
'out-v7d_d32_1024-224-13bit-OB32x0.5-5560'))
|
306 |
-
|
307 |
-
encoder_tab, decoder_tab = st.tabs(["Encode", "Decode"])
|
308 |
-
|
309 |
-
with encoder_tab:
|
310 |
-
col_in, col_out = st.columns(2)
|
311 |
-
keep_shape = col_in.checkbox(
|
312 |
-
'Use original size of input image instead of rescaling (Experimental)')
|
313 |
-
uploaded_file = col_in.file_uploader('Choose an Image')
|
314 |
-
if uploaded_file is not None:
|
315 |
-
image = Image.open(uploaded_file)
|
316 |
-
col_in.image(image, 'Input Image')
|
317 |
-
z_str = encode(model_prefix, image, keep_shape)
|
318 |
-
col_out.write("Encoded to:")
|
319 |
-
col_out.code(z_str, language=None)
|
320 |
-
col_out.image(decode(model_prefix, z_str), 'Output Image preview')
|
321 |
-
|
322 |
-
with decoder_tab:
|
323 |
-
col_in, col_out = st.columns(2)
|
324 |
-
z_str = col_in.text_area('Paste encoded string here:')
|
325 |
-
if len(z_str) > 0:
|
326 |
-
image = decode(model_prefix, z_str)
|
327 |
-
col_out.image(image, 'Output Image')
|
|
|
|
|
|
|
|
|
|
|
|
|
|
|
|
|
|
|
|
|
|
|
|
|
|
|
|
|
|
|
|
|
|
|
|
|
|
|
|
|
|
|
|
|
|
|
|
|
|
|
|
|
|
|
|
|
|
|
|
|
|
|
|
|
|
|
|
|
|
|
|
|
|
|
|
|
|
|
|
|
|
|
|
|
|
|
|
|
|
|
|
|
|
|
|
|
|
|
|
|
|
|
|
|
|
|
|
|
|
|
|
|
|
|
|
|
|
|
|
|
|
|
|
|
|
|
|
|
|
|
|
|
|
|
|
|
|
|
|
|
|
|
|
|
|
|
|
|
|
|
|
|
|
|
|
|
|
|
|
|
|
|
|
|
|
|
|
|
|
|
|
|
|
|
|
|
|
|
|
|
|
|
|
|
|
|
|
|
|
|
|
|
|
|
|
|
|
|
|
|
|
|
|
|
|
|
|
|
|
|
|
|
|
|
|
|
|
|
|
|
|
|
|
|
|
|
|
|
|
|
|
|
|
|
|
|
|
|
|
|
|
|
|
|
|
|
|
|
|
|
|
|
|
|
|
|
|
|
|
|
|
|
|
|
|
|
|
|
|
|
|
|
|
|
|
|
|
|
|
|
|
|
|
|
|
|
|
|
|
|
|
|
|
|
|
|
|
|
|
|
|
|
|
|
|
|
|
|
|
|
|
|
|
|
|
|
|
|
|
|
|
|
|
|
|
|
|
|
|
|
|
|
|
|
|
|
|
|
|
|
|
|
|
|
|
|
|
|
|
|
|
|
|
|
|
|
|
|
|
|
|
|
|
|
|
|
|
|
|
|
|
|
|
|
|
|
|
|
|
|
|
|
|
|
|
|
|
|
|
|
|
|
|
|
|
|
|
|
|
|
|
|
|
|
|
|
|
|
|
|
|
|
|
|
|
|
|
|
|
|
|
|
|
|
|
|
|
|
|
|
|
|
|
|
|
|
|
|
|
|
|
|
|
|
|
|
|
|
|
|
|
|
|
|
|
|
|
|
|
|
|
|
|
|
|
|
|
|
|
|
|
|
|
|
|
|
|
|
|
|
|
|
|
|
|
|
|
|
|
|
|
|
|
|
|
|
|
|
|
|
|
|
|
|
|
|
|
|
|
|
|
|
|
|
|
|
|
|
|
|
|
|
|
|
|
|
|
|
|
|
|
|
|
|
|
|
|
|
|
|
|
|
|
|
|
|
|
|
|
|
|
|
|
|
|
|
|
|
|
|
|
|
|
|
|
|
|
|
|
|
|
|
|
|
|
|
|
|
|
|
|
|
|
|
|
|
|
|
|
|
|
|
|
|
|
|
|
|
|
|
|
|
|
|
|
|
|
|
|
|
|
|
|
|
|
|
|
|
|
|
|
|
|
|
|
|
|
|
|
|
|
|
|
|
|
|
|
|
|
|
|
|
|
|
|
|
|
|
|
|
|
|
|
|
|
spaces/CVPR/Dual-Key_Backdoor_Attacks/datagen/detectron2/tests/test_fast_rcnn.py
DELETED
@@ -1,98 +0,0 @@
|
|
1 |
-
# Copyright (c) Facebook, Inc. and its affiliates. All Rights Reserved
|
2 |
-
import logging
|
3 |
-
import unittest
|
4 |
-
import torch
|
5 |
-
|
6 |
-
from detectron2.layers import ShapeSpec
|
7 |
-
from detectron2.modeling.box_regression import Box2BoxTransform, Box2BoxTransformRotated
|
8 |
-
from detectron2.modeling.roi_heads.fast_rcnn import FastRCNNOutputLayers
|
9 |
-
from detectron2.modeling.roi_heads.rotated_fast_rcnn import RotatedFastRCNNOutputLayers
|
10 |
-
from detectron2.structures import Boxes, Instances, RotatedBoxes
|
11 |
-
from detectron2.utils.events import EventStorage
|
12 |
-
|
13 |
-
logger = logging.getLogger(__name__)
|
14 |
-
|
15 |
-
|
16 |
-
class FastRCNNTest(unittest.TestCase):
|
17 |
-
def test_fast_rcnn(self):
|
18 |
-
torch.manual_seed(132)
|
19 |
-
|
20 |
-
box_head_output_size = 8
|
21 |
-
|
22 |
-
box_predictor = FastRCNNOutputLayers(
|
23 |
-
ShapeSpec(channels=box_head_output_size), Box2BoxTransform(weights=(10, 10, 5, 5)), 5
|
24 |
-
)
|
25 |
-
feature_pooled = torch.rand(2, box_head_output_size)
|
26 |
-
predictions = box_predictor(feature_pooled)
|
27 |
-
|
28 |
-
proposal_boxes = torch.tensor([[0.8, 1.1, 3.2, 2.8], [2.3, 2.5, 7, 8]], dtype=torch.float32)
|
29 |
-
gt_boxes = torch.tensor([[1, 1, 3, 3], [2, 2, 6, 6]], dtype=torch.float32)
|
30 |
-
proposal = Instances((10, 10))
|
31 |
-
proposal.proposal_boxes = Boxes(proposal_boxes)
|
32 |
-
proposal.gt_boxes = Boxes(gt_boxes)
|
33 |
-
proposal.gt_classes = torch.tensor([1, 2])
|
34 |
-
|
35 |
-
with EventStorage(): # capture events in a new storage to discard them
|
36 |
-
losses = box_predictor.losses(predictions, [proposal])
|
37 |
-
|
38 |
-
expected_losses = {
|
39 |
-
"loss_cls": torch.tensor(1.7951188087),
|
40 |
-
"loss_box_reg": torch.tensor(4.0357131958),
|
41 |
-
}
|
42 |
-
for name in expected_losses.keys():
|
43 |
-
assert torch.allclose(losses[name], expected_losses[name])
|
44 |
-
|
45 |
-
def test_fast_rcnn_empty_batch(self):
|
46 |
-
box_predictor = FastRCNNOutputLayers(
|
47 |
-
ShapeSpec(channels=10), Box2BoxTransform(weights=(10, 10, 5, 5)), 8
|
48 |
-
)
|
49 |
-
|
50 |
-
logits = torch.randn(0, 100, requires_grad=True)
|
51 |
-
deltas = torch.randn(0, 4, requires_grad=True)
|
52 |
-
losses = box_predictor.losses([logits, deltas], [])
|
53 |
-
for value in losses.values():
|
54 |
-
self.assertTrue(torch.allclose(value, torch.zeros_like(value)))
|
55 |
-
sum(losses.values()).backward()
|
56 |
-
self.assertTrue(logits.grad is not None)
|
57 |
-
self.assertTrue(deltas.grad is not None)
|
58 |
-
|
59 |
-
predictions, _ = box_predictor.inference([logits, deltas], [])
|
60 |
-
self.assertEqual(len(predictions), 0)
|
61 |
-
|
62 |
-
def test_fast_rcnn_rotated(self):
|
63 |
-
torch.manual_seed(132)
|
64 |
-
box_head_output_size = 8
|
65 |
-
|
66 |
-
box_predictor = RotatedFastRCNNOutputLayers(
|
67 |
-
ShapeSpec(channels=box_head_output_size),
|
68 |
-
Box2BoxTransformRotated(weights=(10, 10, 5, 5, 1)),
|
69 |
-
5,
|
70 |
-
)
|
71 |
-
feature_pooled = torch.rand(2, box_head_output_size)
|
72 |
-
predictions = box_predictor(feature_pooled)
|
73 |
-
proposal_boxes = torch.tensor(
|
74 |
-
[[2, 1.95, 2.4, 1.7, 0], [4.65, 5.25, 4.7, 5.5, 0]], dtype=torch.float32
|
75 |
-
)
|
76 |
-
gt_boxes = torch.tensor([[2, 2, 2, 2, 0], [4, 4, 4, 4, 0]], dtype=torch.float32)
|
77 |
-
proposal = Instances((10, 10))
|
78 |
-
proposal.proposal_boxes = RotatedBoxes(proposal_boxes)
|
79 |
-
proposal.gt_boxes = RotatedBoxes(gt_boxes)
|
80 |
-
proposal.gt_classes = torch.tensor([1, 2])
|
81 |
-
|
82 |
-
with EventStorage(): # capture events in a new storage to discard them
|
83 |
-
losses = box_predictor.losses(predictions, [proposal])
|
84 |
-
|
85 |
-
# Note: the expected losses are slightly different even if
|
86 |
-
# the boxes are essentially the same as in the FastRCNNOutput test, because
|
87 |
-
# bbox_pred in FastRCNNOutputLayers have different Linear layers/initialization
|
88 |
-
# between the two cases.
|
89 |
-
expected_losses = {
|
90 |
-
"loss_cls": torch.tensor(1.7920907736),
|
91 |
-
"loss_box_reg": torch.tensor(4.0410838127),
|
92 |
-
}
|
93 |
-
for name in expected_losses.keys():
|
94 |
-
assert torch.allclose(losses[name], expected_losses[name])
|
95 |
-
|
96 |
-
|
97 |
-
if __name__ == "__main__":
|
98 |
-
unittest.main()
|
|
|
|
|
|
|
|
|
|
|
|
|
|
|
|
|
|
|
|
|
|
|
|
|
|
|
|
|
|
|
|
|
|
|
|
|
|
|
|
|
|
|
|
|
|
|
|
|
|
|
|
|
|
|
|
|
|
|
|
|
|
|
|
|
|
|
|
|
|
|
|
|
|
|
|
|
|
|
|
|
|
|
|
|
|
|
|
|
|
|
|
|
|
|
|
|
|
|
|
|
|
|
|
|
|
|
|
|
|
|
|
|
|
|
|
|
|
|
|
|
|
|
|
|
|
|
|
|
|
|
|
|
|
|
|
|
|
|
|
|
|
|
|
|
|
|
|
|
|
|
|
|
|
|
|
|
|
|
|
|
|
|
|
|
|
|
|
|
|
|
|
|
|
|
|
|
|
|
|
|
|
|
|
|
|
|
|
|
|
|
|
|
|
|
|
|
|
spaces/CVPR/Dual-Key_Backdoor_Attacks/openvqa/openvqa/models/mmnasnet/model_cfgs.py
DELETED
@@ -1,28 +0,0 @@
|
|
1 |
-
# --------------------------------------------------------
|
2 |
-
# OpenVQA
|
3 |
-
# Written by Zhenwei Shao https://github.com/ParadoxZW
|
4 |
-
# --------------------------------------------------------
|
5 |
-
|
6 |
-
from openvqa.core.base_cfgs import BaseCfgs
|
7 |
-
|
8 |
-
|
9 |
-
class Cfgs(BaseCfgs):
|
10 |
-
def __init__(self):
|
11 |
-
super(Cfgs, self).__init__()
|
12 |
-
|
13 |
-
self.ARCH = {
|
14 |
-
'enc': ['SA', 'SA', 'SA', 'SA', 'FFN', 'FFN', 'FFN', 'FFN', 'SA', 'FFN', 'FFN', 'FFN'],
|
15 |
-
'dec': ['GA', 'GA', 'FFN', 'FFN', 'GA', 'FFN', 'RSA', 'GA', 'FFN', 'GA', 'RSA', 'FFN', 'RSA', 'SA', 'FFN', 'RSA', 'GA', 'FFN']
|
16 |
-
}
|
17 |
-
self.HIDDEN_SIZE = 512
|
18 |
-
self.BBOXFEAT_EMB_SIZE = 2048
|
19 |
-
self.FF_SIZE = 2048
|
20 |
-
self.MULTI_HEAD = 8
|
21 |
-
self.DROPOUT_R = 0.1
|
22 |
-
self.FLAT_MLP_SIZE = 512
|
23 |
-
self.FLAT_GLIMPSES = 1
|
24 |
-
self.FLAT_OUT_SIZE = 1024
|
25 |
-
self.USE_AUX_FEAT = False
|
26 |
-
self.USE_BBOX_FEAT = False
|
27 |
-
self.REL_HBASE = 64
|
28 |
-
self.REL_SIZE = 64
|
|
|
|
|
|
|
|
|
|
|
|
|
|
|
|
|
|
|
|
|
|
|
|
|
|
|
|
|
|
|
|
|
|
|
|
|
|
|
|
|
|
|
|
|
|
|
|
|
|
|
|
|
|
|
|
|
|
spaces/CVPR/LIVE/thrust/thrust/mr/fancy_pointer_resource.h
DELETED
@@ -1,61 +0,0 @@
|
|
1 |
-
/*
|
2 |
-
* Copyright 2018 NVIDIA Corporation
|
3 |
-
*
|
4 |
-
* Licensed under the Apache License, Version 2.0 (the "License");
|
5 |
-
* you may not use this file except in compliance with the License.
|
6 |
-
* You may obtain a copy of the License at
|
7 |
-
*
|
8 |
-
* http://www.apache.org/licenses/LICENSE-2.0
|
9 |
-
*
|
10 |
-
* Unless required by applicable law or agreed to in writing, software
|
11 |
-
* distributed under the License is distributed on an "AS IS" BASIS,
|
12 |
-
* WITHOUT WARRANTIES OR CONDITIONS OF ANY KIND, either express or implied.
|
13 |
-
* See the License for the specific language governing permissions and
|
14 |
-
* limitations under the License.
|
15 |
-
*/
|
16 |
-
|
17 |
-
#pragma once
|
18 |
-
|
19 |
-
#include <thrust/detail/type_traits/pointer_traits.h>
|
20 |
-
|
21 |
-
#include <thrust/mr/memory_resource.h>
|
22 |
-
#include <thrust/mr/validator.h>
|
23 |
-
|
24 |
-
namespace thrust
|
25 |
-
{
|
26 |
-
namespace mr
|
27 |
-
{
|
28 |
-
|
29 |
-
template<typename Upstream, typename Pointer>
|
30 |
-
class fancy_pointer_resource THRUST_FINAL : public memory_resource<Pointer>, private validator<Upstream>
|
31 |
-
{
|
32 |
-
public:
|
33 |
-
fancy_pointer_resource() : m_upstream(get_global_resource<Upstream>())
|
34 |
-
{
|
35 |
-
}
|
36 |
-
|
37 |
-
fancy_pointer_resource(Upstream * upstream) : m_upstream(upstream)
|
38 |
-
{
|
39 |
-
}
|
40 |
-
|
41 |
-
THRUST_NODISCARD
|
42 |
-
virtual Pointer do_allocate(std::size_t bytes, std::size_t alignment = THRUST_MR_DEFAULT_ALIGNMENT) THRUST_OVERRIDE
|
43 |
-
{
|
44 |
-
return static_cast<Pointer>(m_upstream->do_allocate(bytes, alignment));
|
45 |
-
}
|
46 |
-
|
47 |
-
virtual void do_deallocate(Pointer p, std::size_t bytes, std::size_t alignment) THRUST_OVERRIDE
|
48 |
-
{
|
49 |
-
return m_upstream->do_deallocate(
|
50 |
-
static_cast<typename Upstream::pointer>(
|
51 |
-
thrust::detail::pointer_traits<Pointer>::get(p)),
|
52 |
-
bytes, alignment);
|
53 |
-
}
|
54 |
-
|
55 |
-
private:
|
56 |
-
Upstream * m_upstream;
|
57 |
-
};
|
58 |
-
|
59 |
-
} // end mr
|
60 |
-
} // end thrust
|
61 |
-
|
|
|
|
|
|
|
|
|
|
|
|
|
|
|
|
|
|
|
|
|
|
|
|
|
|
|
|
|
|
|
|
|
|
|
|
|
|
|
|
|
|
|
|
|
|
|
|
|
|
|
|
|
|
|
|
|
|
|
|
|
|
|
|
|
|
|
|
|
|
|
|
|
|
|
|
|
|
|
|
|
|
|
|
|
|
|
|
|
|
|
|
|
|
|
|
|
|
|
|
|
|
|
|
|
|
|
|
|
|
|
|
|
|
|
|
|
|
|
|
|
|
|
|
spaces/CVPR/LIVE/thrust/thrust/system/detail/generic/transform_reduce.h
DELETED
@@ -1,53 +0,0 @@
|
|
1 |
-
/*
|
2 |
-
* Copyright 2008-2013 NVIDIA Corporation
|
3 |
-
*
|
4 |
-
* Licensed under the Apache License, Version 2.0 (the "License");
|
5 |
-
* you may not use this file except in compliance with the License.
|
6 |
-
* You may obtain a copy of the License at
|
7 |
-
*
|
8 |
-
* http://www.apache.org/licenses/LICENSE-2.0
|
9 |
-
*
|
10 |
-
* Unless required by applicable law or agreed to in writing, software
|
11 |
-
* distributed under the License is distributed on an "AS IS" BASIS,
|
12 |
-
* WITHOUT WARRANTIES OR CONDITIONS OF ANY KIND, either express or implied.
|
13 |
-
* See the License for the specific language governing permissions and
|
14 |
-
* limitations under the License.
|
15 |
-
*/
|
16 |
-
|
17 |
-
|
18 |
-
#pragma once
|
19 |
-
|
20 |
-
#include <thrust/detail/config.h>
|
21 |
-
#include <thrust/system/detail/generic/tag.h>
|
22 |
-
|
23 |
-
namespace thrust
|
24 |
-
{
|
25 |
-
namespace system
|
26 |
-
{
|
27 |
-
namespace detail
|
28 |
-
{
|
29 |
-
namespace generic
|
30 |
-
{
|
31 |
-
|
32 |
-
|
33 |
-
template<typename ExecutionPolicy,
|
34 |
-
typename InputIterator,
|
35 |
-
typename UnaryFunction,
|
36 |
-
typename OutputType,
|
37 |
-
typename BinaryFunction>
|
38 |
-
__host__ __device__
|
39 |
-
OutputType transform_reduce(thrust::execution_policy<ExecutionPolicy> &exec,
|
40 |
-
InputIterator first,
|
41 |
-
InputIterator last,
|
42 |
-
UnaryFunction unary_op,
|
43 |
-
OutputType init,
|
44 |
-
BinaryFunction binary_op);
|
45 |
-
|
46 |
-
|
47 |
-
} // end namespace generic
|
48 |
-
} // end namespace detail
|
49 |
-
} // end namespace system
|
50 |
-
} // end namespace thrust
|
51 |
-
|
52 |
-
#include <thrust/system/detail/generic/transform_reduce.inl>
|
53 |
-
|
|
|
|
|
|
|
|
|
|
|
|
|
|
|
|
|
|
|
|
|
|
|
|
|
|
|
|
|
|
|
|
|
|
|
|
|
|
|
|
|
|
|
|
|
|
|
|
|
|
|
|
|
|
|
|
|
|
|
|
|
|
|
|
|
|
|
|
|
|
|
|
|
|
|
|
|
|
|
|
|
|
|
|
|
|
|
|
|
|
|
|
|
|
|
|
|
|
|
|
|
|
|
|
|
|
|
|
spaces/CVPR/lama-example/bin/analyze_errors.py
DELETED
@@ -1,316 +0,0 @@
|
|
1 |
-
#!/usr/bin/env python3
|
2 |
-
import cv2
|
3 |
-
import numpy as np
|
4 |
-
import sklearn
|
5 |
-
import torch
|
6 |
-
import os
|
7 |
-
import pickle
|
8 |
-
import pandas as pd
|
9 |
-
import matplotlib.pyplot as plt
|
10 |
-
from joblib import Parallel, delayed
|
11 |
-
|
12 |
-
from saicinpainting.evaluation.data import PrecomputedInpaintingResultsDataset, load_image
|
13 |
-
from saicinpainting.evaluation.losses.fid.inception import InceptionV3
|
14 |
-
from saicinpainting.evaluation.utils import load_yaml
|
15 |
-
from saicinpainting.training.visualizers.base import visualize_mask_and_images
|
16 |
-
|
17 |
-
|
18 |
-
def draw_score(img, score):
|
19 |
-
img = np.transpose(img, (1, 2, 0))
|
20 |
-
cv2.putText(img, f'{score:.2f}',
|
21 |
-
(40, 40),
|
22 |
-
cv2.FONT_HERSHEY_SIMPLEX,
|
23 |
-
1,
|
24 |
-
(0, 1, 0),
|
25 |
-
thickness=3)
|
26 |
-
img = np.transpose(img, (2, 0, 1))
|
27 |
-
return img
|
28 |
-
|
29 |
-
|
30 |
-
def save_global_samples(global_mask_fnames, mask2real_fname, mask2fake_fname, out_dir, real_scores_by_fname, fake_scores_by_fname):
|
31 |
-
for cur_mask_fname in global_mask_fnames:
|
32 |
-
cur_real_fname = mask2real_fname[cur_mask_fname]
|
33 |
-
orig_img = load_image(cur_real_fname, mode='RGB')
|
34 |
-
fake_img = load_image(mask2fake_fname[cur_mask_fname], mode='RGB')[:, :orig_img.shape[1], :orig_img.shape[2]]
|
35 |
-
mask = load_image(cur_mask_fname, mode='L')[None, ...]
|
36 |
-
|
37 |
-
draw_score(orig_img, real_scores_by_fname.loc[cur_real_fname, 'real_score'])
|
38 |
-
draw_score(fake_img, fake_scores_by_fname.loc[cur_mask_fname, 'fake_score'])
|
39 |
-
|
40 |
-
cur_grid = visualize_mask_and_images(dict(image=orig_img, mask=mask, fake=fake_img),
|
41 |
-
keys=['image', 'fake'],
|
42 |
-
last_without_mask=True)
|
43 |
-
cur_grid = np.clip(cur_grid * 255, 0, 255).astype('uint8')
|
44 |
-
cur_grid = cv2.cvtColor(cur_grid, cv2.COLOR_RGB2BGR)
|
45 |
-
cv2.imwrite(os.path.join(out_dir, os.path.splitext(os.path.basename(cur_mask_fname))[0] + '.jpg'),
|
46 |
-
cur_grid)
|
47 |
-
|
48 |
-
|
49 |
-
def save_samples_by_real(worst_best_by_real, mask2fake_fname, fake_info, out_dir):
|
50 |
-
for real_fname in worst_best_by_real.index:
|
51 |
-
worst_mask_path = worst_best_by_real.loc[real_fname, 'worst']
|
52 |
-
best_mask_path = worst_best_by_real.loc[real_fname, 'best']
|
53 |
-
orig_img = load_image(real_fname, mode='RGB')
|
54 |
-
worst_mask_img = load_image(worst_mask_path, mode='L')[None, ...]
|
55 |
-
worst_fake_img = load_image(mask2fake_fname[worst_mask_path], mode='RGB')[:, :orig_img.shape[1], :orig_img.shape[2]]
|
56 |
-
best_mask_img = load_image(best_mask_path, mode='L')[None, ...]
|
57 |
-
best_fake_img = load_image(mask2fake_fname[best_mask_path], mode='RGB')[:, :orig_img.shape[1], :orig_img.shape[2]]
|
58 |
-
|
59 |
-
draw_score(orig_img, worst_best_by_real.loc[real_fname, 'real_score'])
|
60 |
-
draw_score(worst_fake_img, worst_best_by_real.loc[real_fname, 'worst_score'])
|
61 |
-
draw_score(best_fake_img, worst_best_by_real.loc[real_fname, 'best_score'])
|
62 |
-
|
63 |
-
cur_grid = visualize_mask_and_images(dict(image=orig_img, mask=np.zeros_like(worst_mask_img),
|
64 |
-
worst_mask=worst_mask_img, worst_img=worst_fake_img,
|
65 |
-
best_mask=best_mask_img, best_img=best_fake_img),
|
66 |
-
keys=['image', 'worst_mask', 'worst_img', 'best_mask', 'best_img'],
|
67 |
-
rescale_keys=['worst_mask', 'best_mask'],
|
68 |
-
last_without_mask=True)
|
69 |
-
cur_grid = np.clip(cur_grid * 255, 0, 255).astype('uint8')
|
70 |
-
cur_grid = cv2.cvtColor(cur_grid, cv2.COLOR_RGB2BGR)
|
71 |
-
cv2.imwrite(os.path.join(out_dir,
|
72 |
-
os.path.splitext(os.path.basename(real_fname))[0] + '.jpg'),
|
73 |
-
cur_grid)
|
74 |
-
|
75 |
-
fig, (ax1, ax2) = plt.subplots(1, 2)
|
76 |
-
cur_stat = fake_info[fake_info['real_fname'] == real_fname]
|
77 |
-
cur_stat['fake_score'].hist(ax=ax1)
|
78 |
-
cur_stat['real_score'].hist(ax=ax2)
|
79 |
-
fig.tight_layout()
|
80 |
-
fig.savefig(os.path.join(out_dir,
|
81 |
-
os.path.splitext(os.path.basename(real_fname))[0] + '_scores.png'))
|
82 |
-
plt.close(fig)
|
83 |
-
|
84 |
-
|
85 |
-
def extract_overlapping_masks(mask_fnames, cur_i, fake_scores_table, max_overlaps_n=2):
|
86 |
-
result_pairs = []
|
87 |
-
result_scores = []
|
88 |
-
mask_fname_a = mask_fnames[cur_i]
|
89 |
-
mask_a = load_image(mask_fname_a, mode='L')[None, ...] > 0.5
|
90 |
-
cur_score_a = fake_scores_table.loc[mask_fname_a, 'fake_score']
|
91 |
-
for mask_fname_b in mask_fnames[cur_i + 1:]:
|
92 |
-
mask_b = load_image(mask_fname_b, mode='L')[None, ...] > 0.5
|
93 |
-
if not np.any(mask_a & mask_b):
|
94 |
-
continue
|
95 |
-
cur_score_b = fake_scores_table.loc[mask_fname_b, 'fake_score']
|
96 |
-
result_pairs.append((mask_fname_a, mask_fname_b))
|
97 |
-
result_scores.append(cur_score_b - cur_score_a)
|
98 |
-
if len(result_pairs) >= max_overlaps_n:
|
99 |
-
break
|
100 |
-
return result_pairs, result_scores
|
101 |
-
|
102 |
-
|
103 |
-
def main(args):
|
104 |
-
config = load_yaml(args.config)
|
105 |
-
|
106 |
-
latents_dir = os.path.join(args.outpath, 'latents')
|
107 |
-
os.makedirs(latents_dir, exist_ok=True)
|
108 |
-
global_worst_dir = os.path.join(args.outpath, 'global_worst')
|
109 |
-
os.makedirs(global_worst_dir, exist_ok=True)
|
110 |
-
global_best_dir = os.path.join(args.outpath, 'global_best')
|
111 |
-
os.makedirs(global_best_dir, exist_ok=True)
|
112 |
-
worst_best_by_best_worst_score_diff_max_dir = os.path.join(args.outpath, 'worst_best_by_real', 'best_worst_score_diff_max')
|
113 |
-
os.makedirs(worst_best_by_best_worst_score_diff_max_dir, exist_ok=True)
|
114 |
-
worst_best_by_best_worst_score_diff_min_dir = os.path.join(args.outpath, 'worst_best_by_real', 'best_worst_score_diff_min')
|
115 |
-
os.makedirs(worst_best_by_best_worst_score_diff_min_dir, exist_ok=True)
|
116 |
-
worst_best_by_real_best_score_diff_max_dir = os.path.join(args.outpath, 'worst_best_by_real', 'real_best_score_diff_max')
|
117 |
-
os.makedirs(worst_best_by_real_best_score_diff_max_dir, exist_ok=True)
|
118 |
-
worst_best_by_real_best_score_diff_min_dir = os.path.join(args.outpath, 'worst_best_by_real', 'real_best_score_diff_min')
|
119 |
-
os.makedirs(worst_best_by_real_best_score_diff_min_dir, exist_ok=True)
|
120 |
-
worst_best_by_real_worst_score_diff_max_dir = os.path.join(args.outpath, 'worst_best_by_real', 'real_worst_score_diff_max')
|
121 |
-
os.makedirs(worst_best_by_real_worst_score_diff_max_dir, exist_ok=True)
|
122 |
-
worst_best_by_real_worst_score_diff_min_dir = os.path.join(args.outpath, 'worst_best_by_real', 'real_worst_score_diff_min')
|
123 |
-
os.makedirs(worst_best_by_real_worst_score_diff_min_dir, exist_ok=True)
|
124 |
-
|
125 |
-
if not args.only_report:
|
126 |
-
block_idx = InceptionV3.BLOCK_INDEX_BY_DIM[2048]
|
127 |
-
inception_model = InceptionV3([block_idx]).eval().cuda()
|
128 |
-
|
129 |
-
dataset = PrecomputedInpaintingResultsDataset(args.datadir, args.predictdir, **config.dataset_kwargs)
|
130 |
-
|
131 |
-
real2vector_cache = {}
|
132 |
-
|
133 |
-
real_features = []
|
134 |
-
fake_features = []
|
135 |
-
|
136 |
-
orig_fnames = []
|
137 |
-
mask_fnames = []
|
138 |
-
mask2real_fname = {}
|
139 |
-
mask2fake_fname = {}
|
140 |
-
|
141 |
-
for batch_i, batch in enumerate(dataset):
|
142 |
-
orig_img_fname = dataset.img_filenames[batch_i]
|
143 |
-
mask_fname = dataset.mask_filenames[batch_i]
|
144 |
-
fake_fname = dataset.pred_filenames[batch_i]
|
145 |
-
mask2real_fname[mask_fname] = orig_img_fname
|
146 |
-
mask2fake_fname[mask_fname] = fake_fname
|
147 |
-
|
148 |
-
cur_real_vector = real2vector_cache.get(orig_img_fname, None)
|
149 |
-
if cur_real_vector is None:
|
150 |
-
with torch.no_grad():
|
151 |
-
in_img = torch.from_numpy(batch['image'][None, ...]).cuda()
|
152 |
-
cur_real_vector = inception_model(in_img)[0].squeeze(-1).squeeze(-1).cpu().numpy()
|
153 |
-
real2vector_cache[orig_img_fname] = cur_real_vector
|
154 |
-
|
155 |
-
pred_img = torch.from_numpy(batch['inpainted'][None, ...]).cuda()
|
156 |
-
cur_fake_vector = inception_model(pred_img)[0].squeeze(-1).squeeze(-1).cpu().numpy()
|
157 |
-
|
158 |
-
real_features.append(cur_real_vector)
|
159 |
-
fake_features.append(cur_fake_vector)
|
160 |
-
|
161 |
-
orig_fnames.append(orig_img_fname)
|
162 |
-
mask_fnames.append(mask_fname)
|
163 |
-
|
164 |
-
ids_features = np.concatenate(real_features + fake_features, axis=0)
|
165 |
-
ids_labels = np.array(([1] * len(real_features)) + ([0] * len(fake_features)))
|
166 |
-
|
167 |
-
with open(os.path.join(latents_dir, 'featues.pkl'), 'wb') as f:
|
168 |
-
pickle.dump(ids_features, f, protocol=3)
|
169 |
-
with open(os.path.join(latents_dir, 'labels.pkl'), 'wb') as f:
|
170 |
-
pickle.dump(ids_labels, f, protocol=3)
|
171 |
-
with open(os.path.join(latents_dir, 'orig_fnames.pkl'), 'wb') as f:
|
172 |
-
pickle.dump(orig_fnames, f, protocol=3)
|
173 |
-
with open(os.path.join(latents_dir, 'mask_fnames.pkl'), 'wb') as f:
|
174 |
-
pickle.dump(mask_fnames, f, protocol=3)
|
175 |
-
with open(os.path.join(latents_dir, 'mask2real_fname.pkl'), 'wb') as f:
|
176 |
-
pickle.dump(mask2real_fname, f, protocol=3)
|
177 |
-
with open(os.path.join(latents_dir, 'mask2fake_fname.pkl'), 'wb') as f:
|
178 |
-
pickle.dump(mask2fake_fname, f, protocol=3)
|
179 |
-
|
180 |
-
svm = sklearn.svm.LinearSVC(dual=False)
|
181 |
-
svm.fit(ids_features, ids_labels)
|
182 |
-
|
183 |
-
pred_scores = svm.decision_function(ids_features)
|
184 |
-
real_scores = pred_scores[:len(real_features)]
|
185 |
-
fake_scores = pred_scores[len(real_features):]
|
186 |
-
|
187 |
-
with open(os.path.join(latents_dir, 'pred_scores.pkl'), 'wb') as f:
|
188 |
-
pickle.dump(pred_scores, f, protocol=3)
|
189 |
-
with open(os.path.join(latents_dir, 'real_scores.pkl'), 'wb') as f:
|
190 |
-
pickle.dump(real_scores, f, protocol=3)
|
191 |
-
with open(os.path.join(latents_dir, 'fake_scores.pkl'), 'wb') as f:
|
192 |
-
pickle.dump(fake_scores, f, protocol=3)
|
193 |
-
else:
|
194 |
-
with open(os.path.join(latents_dir, 'orig_fnames.pkl'), 'rb') as f:
|
195 |
-
orig_fnames = pickle.load(f)
|
196 |
-
with open(os.path.join(latents_dir, 'mask_fnames.pkl'), 'rb') as f:
|
197 |
-
mask_fnames = pickle.load(f)
|
198 |
-
with open(os.path.join(latents_dir, 'mask2real_fname.pkl'), 'rb') as f:
|
199 |
-
mask2real_fname = pickle.load(f)
|
200 |
-
with open(os.path.join(latents_dir, 'mask2fake_fname.pkl'), 'rb') as f:
|
201 |
-
mask2fake_fname = pickle.load(f)
|
202 |
-
with open(os.path.join(latents_dir, 'real_scores.pkl'), 'rb') as f:
|
203 |
-
real_scores = pickle.load(f)
|
204 |
-
with open(os.path.join(latents_dir, 'fake_scores.pkl'), 'rb') as f:
|
205 |
-
fake_scores = pickle.load(f)
|
206 |
-
|
207 |
-
real_info = pd.DataFrame(data=[dict(real_fname=fname,
|
208 |
-
real_score=score)
|
209 |
-
for fname, score
|
210 |
-
in zip(orig_fnames, real_scores)])
|
211 |
-
real_info.set_index('real_fname', drop=True, inplace=True)
|
212 |
-
|
213 |
-
fake_info = pd.DataFrame(data=[dict(mask_fname=fname,
|
214 |
-
fake_fname=mask2fake_fname[fname],
|
215 |
-
real_fname=mask2real_fname[fname],
|
216 |
-
fake_score=score)
|
217 |
-
for fname, score
|
218 |
-
in zip(mask_fnames, fake_scores)])
|
219 |
-
fake_info = fake_info.join(real_info, on='real_fname', how='left')
|
220 |
-
fake_info.drop_duplicates(['fake_fname', 'real_fname'], inplace=True)
|
221 |
-
|
222 |
-
fake_stats_by_real = fake_info.groupby('real_fname')['fake_score'].describe()[['mean', 'std']].rename(
|
223 |
-
{'mean': 'mean_fake_by_real', 'std': 'std_fake_by_real'}, axis=1)
|
224 |
-
fake_info = fake_info.join(fake_stats_by_real, on='real_fname', rsuffix='stat_by_real')
|
225 |
-
fake_info.drop_duplicates(['fake_fname', 'real_fname'], inplace=True)
|
226 |
-
fake_info.to_csv(os.path.join(latents_dir, 'join_scores_table.csv'), sep='\t', index=False)
|
227 |
-
|
228 |
-
fake_scores_table = fake_info.set_index('mask_fname')['fake_score'].to_frame()
|
229 |
-
real_scores_table = fake_info.set_index('real_fname')['real_score'].drop_duplicates().to_frame()
|
230 |
-
|
231 |
-
fig, (ax1, ax2) = plt.subplots(1, 2)
|
232 |
-
ax1.hist(fake_scores)
|
233 |
-
ax2.hist(real_scores)
|
234 |
-
fig.tight_layout()
|
235 |
-
fig.savefig(os.path.join(args.outpath, 'global_scores_hist.png'))
|
236 |
-
plt.close(fig)
|
237 |
-
|
238 |
-
global_worst_masks = fake_info.sort_values('fake_score', ascending=True)['mask_fname'].iloc[:config.take_global_top].to_list()
|
239 |
-
global_best_masks = fake_info.sort_values('fake_score', ascending=False)['mask_fname'].iloc[:config.take_global_top].to_list()
|
240 |
-
save_global_samples(global_worst_masks, mask2real_fname, mask2fake_fname, global_worst_dir, real_scores_table, fake_scores_table)
|
241 |
-
save_global_samples(global_best_masks, mask2real_fname, mask2fake_fname, global_best_dir, real_scores_table, fake_scores_table)
|
242 |
-
|
243 |
-
# grouped by real
|
244 |
-
worst_samples_by_real = fake_info.groupby('real_fname').apply(
|
245 |
-
lambda d: d.set_index('mask_fname')['fake_score'].idxmin()).to_frame().rename({0: 'worst'}, axis=1)
|
246 |
-
best_samples_by_real = fake_info.groupby('real_fname').apply(
|
247 |
-
lambda d: d.set_index('mask_fname')['fake_score'].idxmax()).to_frame().rename({0: 'best'}, axis=1)
|
248 |
-
worst_best_by_real = pd.concat([worst_samples_by_real, best_samples_by_real], axis=1)
|
249 |
-
|
250 |
-
worst_best_by_real = worst_best_by_real.join(fake_scores_table.rename({'fake_score': 'worst_score'}, axis=1),
|
251 |
-
on='worst')
|
252 |
-
worst_best_by_real = worst_best_by_real.join(fake_scores_table.rename({'fake_score': 'best_score'}, axis=1),
|
253 |
-
on='best')
|
254 |
-
worst_best_by_real = worst_best_by_real.join(real_scores_table)
|
255 |
-
|
256 |
-
worst_best_by_real['best_worst_score_diff'] = worst_best_by_real['best_score'] - worst_best_by_real['worst_score']
|
257 |
-
worst_best_by_real['real_best_score_diff'] = worst_best_by_real['real_score'] - worst_best_by_real['best_score']
|
258 |
-
worst_best_by_real['real_worst_score_diff'] = worst_best_by_real['real_score'] - worst_best_by_real['worst_score']
|
259 |
-
|
260 |
-
worst_best_by_best_worst_score_diff_min = worst_best_by_real.sort_values('best_worst_score_diff', ascending=True).iloc[:config.take_worst_best_top]
|
261 |
-
worst_best_by_best_worst_score_diff_max = worst_best_by_real.sort_values('best_worst_score_diff', ascending=False).iloc[:config.take_worst_best_top]
|
262 |
-
save_samples_by_real(worst_best_by_best_worst_score_diff_min, mask2fake_fname, fake_info, worst_best_by_best_worst_score_diff_min_dir)
|
263 |
-
save_samples_by_real(worst_best_by_best_worst_score_diff_max, mask2fake_fname, fake_info, worst_best_by_best_worst_score_diff_max_dir)
|
264 |
-
|
265 |
-
worst_best_by_real_best_score_diff_min = worst_best_by_real.sort_values('real_best_score_diff', ascending=True).iloc[:config.take_worst_best_top]
|
266 |
-
worst_best_by_real_best_score_diff_max = worst_best_by_real.sort_values('real_best_score_diff', ascending=False).iloc[:config.take_worst_best_top]
|
267 |
-
save_samples_by_real(worst_best_by_real_best_score_diff_min, mask2fake_fname, fake_info, worst_best_by_real_best_score_diff_min_dir)
|
268 |
-
save_samples_by_real(worst_best_by_real_best_score_diff_max, mask2fake_fname, fake_info, worst_best_by_real_best_score_diff_max_dir)
|
269 |
-
|
270 |
-
worst_best_by_real_worst_score_diff_min = worst_best_by_real.sort_values('real_worst_score_diff', ascending=True).iloc[:config.take_worst_best_top]
|
271 |
-
worst_best_by_real_worst_score_diff_max = worst_best_by_real.sort_values('real_worst_score_diff', ascending=False).iloc[:config.take_worst_best_top]
|
272 |
-
save_samples_by_real(worst_best_by_real_worst_score_diff_min, mask2fake_fname, fake_info, worst_best_by_real_worst_score_diff_min_dir)
|
273 |
-
save_samples_by_real(worst_best_by_real_worst_score_diff_max, mask2fake_fname, fake_info, worst_best_by_real_worst_score_diff_max_dir)
|
274 |
-
|
275 |
-
# analyze what change of mask causes bigger change of score
|
276 |
-
overlapping_mask_fname_pairs = []
|
277 |
-
overlapping_mask_fname_score_diffs = []
|
278 |
-
for cur_real_fname in orig_fnames:
|
279 |
-
cur_fakes_info = fake_info[fake_info['real_fname'] == cur_real_fname]
|
280 |
-
cur_mask_fnames = sorted(cur_fakes_info['mask_fname'].unique())
|
281 |
-
|
282 |
-
cur_mask_pairs_and_scores = Parallel(args.n_jobs)(
|
283 |
-
delayed(extract_overlapping_masks)(cur_mask_fnames, i, fake_scores_table)
|
284 |
-
for i in range(len(cur_mask_fnames) - 1)
|
285 |
-
)
|
286 |
-
for cur_pairs, cur_scores in cur_mask_pairs_and_scores:
|
287 |
-
overlapping_mask_fname_pairs.extend(cur_pairs)
|
288 |
-
overlapping_mask_fname_score_diffs.extend(cur_scores)
|
289 |
-
|
290 |
-
overlapping_mask_fname_pairs = np.asarray(overlapping_mask_fname_pairs)
|
291 |
-
overlapping_mask_fname_score_diffs = np.asarray(overlapping_mask_fname_score_diffs)
|
292 |
-
overlapping_sort_idx = np.argsort(overlapping_mask_fname_score_diffs)
|
293 |
-
overlapping_mask_fname_pairs = overlapping_mask_fname_pairs[overlapping_sort_idx]
|
294 |
-
overlapping_mask_fname_score_diffs = overlapping_mask_fname_score_diffs[overlapping_sort_idx]
|
295 |
-
|
296 |
-
|
297 |
-
|
298 |
-
|
299 |
-
|
300 |
-
|
301 |
-
if __name__ == '__main__':
|
302 |
-
import argparse
|
303 |
-
|
304 |
-
aparser = argparse.ArgumentParser()
|
305 |
-
aparser.add_argument('config', type=str, help='Path to config for dataset generation')
|
306 |
-
aparser.add_argument('datadir', type=str,
|
307 |
-
help='Path to folder with images and masks (output of gen_mask_dataset.py)')
|
308 |
-
aparser.add_argument('predictdir', type=str,
|
309 |
-
help='Path to folder with predicts (e.g. predict_hifill_baseline.py)')
|
310 |
-
aparser.add_argument('outpath', type=str, help='Where to put results')
|
311 |
-
aparser.add_argument('--only-report', action='store_true',
|
312 |
-
help='Whether to skip prediction and feature extraction, '
|
313 |
-
'load all the possible latents and proceed with report only')
|
314 |
-
aparser.add_argument('--n-jobs', type=int, default=8, help='how many processes to use for pair mask mining')
|
315 |
-
|
316 |
-
main(aparser.parse_args())
|
|
|
|
|
|
|
|
|
|
|
|
|
|
|
|
|
|
|
|
|
|
|
|
|
|
|
|
|
|
|
|
|
|
|
|
|
|
|
|
|
|
|
|
|
|
|
|
|
|
|
|
|
|
|
|
|
|
|
|
|
|
|
|
|
|
|
|
|
|
|
|
|
|
|
|
|
|
|
|
|
|
|
|
|
|
|
|
|
|
|
|
|
|
|
|
|
|
|
|
|
|
|
|
|
|
|
|
|
|
|
|
|
|
|
|
|
|
|
|
|
|
|
|
|
|
|
|
|
|
|
|
|
|
|
|
|
|
|
|
|
|
|
|
|
|
|
|
|
|
|
|
|
|
|
|
|
|
|
|
|
|
|
|
|
|
|
|
|
|
|
|
|
|
|
|
|
|
|
|
|
|
|
|
|
|
|
|
|
|
|
|
|
|
|
|
|
|
|
|
|
|
|
|
|
|
|
|
|
|
|
|
|
|
|
|
|
|
|
|
|
|
|
|
|
|
|
|
|
|
|
|
|
|
|
|
|
|
|
|
|
|
|
|
|
|
|
|
|
|
|
|
|
|
|
|
|
|
|
|
|
|
|
|
|
|
|
|
|
|
|
|
|
|
|
|
|
|
|
|
|
|
|
|
|
|
|
|
|
|
|
|
|
|
|
|
|
|
|
|
|
|
|
|
|
|
|
|
|
|
|
|
|
|
|
|
|
|
|
|
|
|
|
|
|
|
|
|
|
|
|
|
|
|
|
|
|
|
|
|
|
|
|
|
|
|
|
|
|
|
|
|
|
|
|
|
|
|
|
|
|
|
|
|
|
|
|
|
|
|
|
|
|
|
|
|
|
|
|
|
|
|
|
|
|
|
|
|
|
|
|
|
|
|
|
|
|
|
|
|
|
|
|
|
|
|
|
|
|
|
|
|
|
|
|
|
|
|
|
|
|
|
|
|
|
|
|
|
|
|
|
|
|
|
|
|
|
|
|
|
|
|
|
|
|
|
|
|
|
|
|
|
|
|
|
|
|
|
|
|
|
|
|
|
|
|
|
|
|
|
|
|
|
|
|
|
|
|
|
|
|
|
|
|
|
|
|
|
|
|
|
|
|
|
|
|
|
|
|
|
|
|
|
|
|
|
|
|
|
|
|
|
|
|
|
|
|
|
|
|
|
|
|
|
|
|
|
|
|
|
|
|
|
|
|
|
|
|
|
|
|
|
|
|
|
|
|
|
|
|
|
|
|
|
|
|
|
|
|
|
|
|
|
|
|
|
|
|
|
|
|
|
|
|
|
|
|
|
|
|
|
|
|
|
|
|
|
|
|
|
|
|
|
|
|
|
|
|
|
|
|
|
|
|
|
|
|
|
|
|
|
|
|
|
|
|
|
|
|
|
|
|
|
|
|
|
|
|
|
|
|
|
|
|
spaces/CVPR/lama-example/saicinpainting/evaluation/data.py
DELETED
@@ -1,167 +0,0 @@
|
|
1 |
-
import glob
|
2 |
-
import os
|
3 |
-
|
4 |
-
import cv2
|
5 |
-
import PIL.Image as Image
|
6 |
-
import numpy as np
|
7 |
-
|
8 |
-
from torch.utils.data import Dataset
|
9 |
-
import torch.nn.functional as F
|
10 |
-
|
11 |
-
|
12 |
-
def load_image(fname, mode='RGB', return_orig=False):
|
13 |
-
img = np.array(Image.open(fname).convert(mode))
|
14 |
-
if img.ndim == 3:
|
15 |
-
img = np.transpose(img, (2, 0, 1))
|
16 |
-
out_img = img.astype('float32') / 255
|
17 |
-
if return_orig:
|
18 |
-
return out_img, img
|
19 |
-
else:
|
20 |
-
return out_img
|
21 |
-
|
22 |
-
|
23 |
-
def ceil_modulo(x, mod):
|
24 |
-
if x % mod == 0:
|
25 |
-
return x
|
26 |
-
return (x // mod + 1) * mod
|
27 |
-
|
28 |
-
|
29 |
-
def pad_img_to_modulo(img, mod):
|
30 |
-
channels, height, width = img.shape
|
31 |
-
out_height = ceil_modulo(height, mod)
|
32 |
-
out_width = ceil_modulo(width, mod)
|
33 |
-
return np.pad(img, ((0, 0), (0, out_height - height), (0, out_width - width)), mode='symmetric')
|
34 |
-
|
35 |
-
|
36 |
-
def pad_tensor_to_modulo(img, mod):
|
37 |
-
batch_size, channels, height, width = img.shape
|
38 |
-
out_height = ceil_modulo(height, mod)
|
39 |
-
out_width = ceil_modulo(width, mod)
|
40 |
-
return F.pad(img, pad=(0, out_width - width, 0, out_height - height), mode='reflect')
|
41 |
-
|
42 |
-
|
43 |
-
def scale_image(img, factor, interpolation=cv2.INTER_AREA):
|
44 |
-
if img.shape[0] == 1:
|
45 |
-
img = img[0]
|
46 |
-
else:
|
47 |
-
img = np.transpose(img, (1, 2, 0))
|
48 |
-
|
49 |
-
img = cv2.resize(img, dsize=None, fx=factor, fy=factor, interpolation=interpolation)
|
50 |
-
|
51 |
-
if img.ndim == 2:
|
52 |
-
img = img[None, ...]
|
53 |
-
else:
|
54 |
-
img = np.transpose(img, (2, 0, 1))
|
55 |
-
return img
|
56 |
-
|
57 |
-
|
58 |
-
class InpaintingDataset(Dataset):
|
59 |
-
def __init__(self, datadir, img_suffix='.jpg', pad_out_to_modulo=None, scale_factor=None):
|
60 |
-
self.datadir = datadir
|
61 |
-
self.mask_filenames = sorted(list(glob.glob(os.path.join(self.datadir, '**', '*mask*.png'), recursive=True)))
|
62 |
-
self.img_filenames = [fname.rsplit('_mask', 1)[0] + img_suffix for fname in self.mask_filenames]
|
63 |
-
self.pad_out_to_modulo = pad_out_to_modulo
|
64 |
-
self.scale_factor = scale_factor
|
65 |
-
|
66 |
-
def __len__(self):
|
67 |
-
return len(self.mask_filenames)
|
68 |
-
|
69 |
-
def __getitem__(self, i):
|
70 |
-
image = load_image(self.img_filenames[i], mode='RGB')
|
71 |
-
mask = load_image(self.mask_filenames[i], mode='L')
|
72 |
-
result = dict(image=image, mask=mask[None, ...])
|
73 |
-
|
74 |
-
if self.scale_factor is not None:
|
75 |
-
result['image'] = scale_image(result['image'], self.scale_factor)
|
76 |
-
result['mask'] = scale_image(result['mask'], self.scale_factor, interpolation=cv2.INTER_NEAREST)
|
77 |
-
|
78 |
-
if self.pad_out_to_modulo is not None and self.pad_out_to_modulo > 1:
|
79 |
-
result['image'] = pad_img_to_modulo(result['image'], self.pad_out_to_modulo)
|
80 |
-
result['mask'] = pad_img_to_modulo(result['mask'], self.pad_out_to_modulo)
|
81 |
-
|
82 |
-
return result
|
83 |
-
|
84 |
-
class OurInpaintingDataset(Dataset):
|
85 |
-
def __init__(self, datadir, img_suffix='.jpg', pad_out_to_modulo=None, scale_factor=None):
|
86 |
-
self.datadir = datadir
|
87 |
-
self.mask_filenames = sorted(list(glob.glob(os.path.join(self.datadir, 'mask', '**', '*mask*.png'), recursive=True)))
|
88 |
-
self.img_filenames = [os.path.join(self.datadir, 'img', os.path.basename(fname.rsplit('-', 1)[0].rsplit('_', 1)[0]) + '.png') for fname in self.mask_filenames]
|
89 |
-
self.pad_out_to_modulo = pad_out_to_modulo
|
90 |
-
self.scale_factor = scale_factor
|
91 |
-
|
92 |
-
def __len__(self):
|
93 |
-
return len(self.mask_filenames)
|
94 |
-
|
95 |
-
def __getitem__(self, i):
|
96 |
-
result = dict(image=load_image(self.img_filenames[i], mode='RGB'),
|
97 |
-
mask=load_image(self.mask_filenames[i], mode='L')[None, ...])
|
98 |
-
|
99 |
-
if self.scale_factor is not None:
|
100 |
-
result['image'] = scale_image(result['image'], self.scale_factor)
|
101 |
-
result['mask'] = scale_image(result['mask'], self.scale_factor)
|
102 |
-
|
103 |
-
if self.pad_out_to_modulo is not None and self.pad_out_to_modulo > 1:
|
104 |
-
result['image'] = pad_img_to_modulo(result['image'], self.pad_out_to_modulo)
|
105 |
-
result['mask'] = pad_img_to_modulo(result['mask'], self.pad_out_to_modulo)
|
106 |
-
|
107 |
-
return result
|
108 |
-
|
109 |
-
class PrecomputedInpaintingResultsDataset(InpaintingDataset):
|
110 |
-
def __init__(self, datadir, predictdir, inpainted_suffix='_inpainted.jpg', **kwargs):
|
111 |
-
super().__init__(datadir, **kwargs)
|
112 |
-
if not datadir.endswith('/'):
|
113 |
-
datadir += '/'
|
114 |
-
self.predictdir = predictdir
|
115 |
-
self.pred_filenames = [os.path.join(predictdir, os.path.splitext(fname[len(datadir):])[0] + inpainted_suffix)
|
116 |
-
for fname in self.mask_filenames]
|
117 |
-
|
118 |
-
def __getitem__(self, i):
|
119 |
-
result = super().__getitem__(i)
|
120 |
-
result['inpainted'] = load_image(self.pred_filenames[i])
|
121 |
-
if self.pad_out_to_modulo is not None and self.pad_out_to_modulo > 1:
|
122 |
-
result['inpainted'] = pad_img_to_modulo(result['inpainted'], self.pad_out_to_modulo)
|
123 |
-
return result
|
124 |
-
|
125 |
-
class OurPrecomputedInpaintingResultsDataset(OurInpaintingDataset):
|
126 |
-
def __init__(self, datadir, predictdir, inpainted_suffix="png", **kwargs):
|
127 |
-
super().__init__(datadir, **kwargs)
|
128 |
-
if not datadir.endswith('/'):
|
129 |
-
datadir += '/'
|
130 |
-
self.predictdir = predictdir
|
131 |
-
self.pred_filenames = [os.path.join(predictdir, os.path.basename(os.path.splitext(fname)[0]) + f'_inpainted.{inpainted_suffix}')
|
132 |
-
for fname in self.mask_filenames]
|
133 |
-
# self.pred_filenames = [os.path.join(predictdir, os.path.splitext(fname[len(datadir):])[0] + inpainted_suffix)
|
134 |
-
# for fname in self.mask_filenames]
|
135 |
-
|
136 |
-
def __getitem__(self, i):
|
137 |
-
result = super().__getitem__(i)
|
138 |
-
result['inpainted'] = self.file_loader(self.pred_filenames[i])
|
139 |
-
|
140 |
-
if self.pad_out_to_modulo is not None and self.pad_out_to_modulo > 1:
|
141 |
-
result['inpainted'] = pad_img_to_modulo(result['inpainted'], self.pad_out_to_modulo)
|
142 |
-
return result
|
143 |
-
|
144 |
-
class InpaintingEvalOnlineDataset(Dataset):
|
145 |
-
def __init__(self, indir, mask_generator, img_suffix='.jpg', pad_out_to_modulo=None, scale_factor=None, **kwargs):
|
146 |
-
self.indir = indir
|
147 |
-
self.mask_generator = mask_generator
|
148 |
-
self.img_filenames = sorted(list(glob.glob(os.path.join(self.indir, '**', f'*{img_suffix}' ), recursive=True)))
|
149 |
-
self.pad_out_to_modulo = pad_out_to_modulo
|
150 |
-
self.scale_factor = scale_factor
|
151 |
-
|
152 |
-
def __len__(self):
|
153 |
-
return len(self.img_filenames)
|
154 |
-
|
155 |
-
def __getitem__(self, i):
|
156 |
-
img, raw_image = load_image(self.img_filenames[i], mode='RGB', return_orig=True)
|
157 |
-
mask = self.mask_generator(img, raw_image=raw_image)
|
158 |
-
result = dict(image=img, mask=mask)
|
159 |
-
|
160 |
-
if self.scale_factor is not None:
|
161 |
-
result['image'] = scale_image(result['image'], self.scale_factor)
|
162 |
-
result['mask'] = scale_image(result['mask'], self.scale_factor, interpolation=cv2.INTER_NEAREST)
|
163 |
-
|
164 |
-
if self.pad_out_to_modulo is not None and self.pad_out_to_modulo > 1:
|
165 |
-
result['image'] = pad_img_to_modulo(result['image'], self.pad_out_to_modulo)
|
166 |
-
result['mask'] = pad_img_to_modulo(result['mask'], self.pad_out_to_modulo)
|
167 |
-
return result
|
|
|
|
|
|
|
|
|
|
|
|
|
|
|
|
|
|
|
|
|
|
|
|
|
|
|
|
|
|
|
|
|
|
|
|
|
|
|
|
|
|
|
|
|
|
|
|
|
|
|
|
|
|
|
|
|
|
|
|
|
|
|
|
|
|
|
|
|
|
|
|
|
|
|
|
|
|
|
|
|
|
|
|
|
|
|
|
|
|
|
|
|
|
|
|
|
|
|
|
|
|
|
|
|
|
|
|
|
|
|
|
|
|
|
|
|
|
|
|
|
|
|
|
|
|
|
|
|
|
|
|
|
|
|
|
|
|
|
|
|
|
|
|
|
|
|
|
|
|
|
|
|
|
|
|
|
|
|
|
|
|
|
|
|
|
|
|
|
|
|
|
|
|
|
|
|
|
|
|
|
|
|
|
|
|
|
|
|
|
|
|
|
|
|
|
|
|
|
|
|
|
|
|
|
|
|
|
|
|
|
|
|
|
|
|
|
|
|
|
|
|
|
|
|
|
|
|
|
|
|
|
|
|
|
|
|
|
|
|
|
|
|
|
|
|
|
|
|
|
|
|
|
|
|
|
|
|
|
|
|
|
|
|
|
|
|
|
|
|
|
|
|
|
|
|
|
|
|
|
|
|
|
|
|
|
|
|
|
|
|
|
|
|
|
|
|
|
|
|
|
|
|
|
|
|
|
|
|
|
|
|
|
|
|
|
|
|
|
|
|
|
|
|
|
|
|
|
|
|
|
|
|
|
|
|
spaces/CVPR/monoscene_lite/monoscene/.ipynb_checkpoints/unet3d_kitti-checkpoint.py
DELETED
@@ -1,88 +0,0 @@
|
|
1 |
-
# encoding: utf-8
|
2 |
-
import torch
|
3 |
-
import torch.nn as nn
|
4 |
-
import torch.nn.functional as F
|
5 |
-
from monoscene.modules import SegmentationHead
|
6 |
-
from monoscene.CRP3D import CPMegaVoxels
|
7 |
-
from monoscene.modules import Process, Upsample, Downsample
|
8 |
-
|
9 |
-
|
10 |
-
class UNet3D(nn.Module):
|
11 |
-
def __init__(
|
12 |
-
self,
|
13 |
-
class_num,
|
14 |
-
norm_layer,
|
15 |
-
full_scene_size,
|
16 |
-
feature,
|
17 |
-
project_scale,
|
18 |
-
context_prior=None,
|
19 |
-
bn_momentum=0.1,
|
20 |
-
):
|
21 |
-
super(UNet3D, self).__init__()
|
22 |
-
self.business_layer = []
|
23 |
-
self.project_scale = project_scale
|
24 |
-
self.full_scene_size = full_scene_size
|
25 |
-
self.feature = feature
|
26 |
-
|
27 |
-
size_l1 = (
|
28 |
-
int(self.full_scene_size[0] / project_scale),
|
29 |
-
int(self.full_scene_size[1] / project_scale),
|
30 |
-
int(self.full_scene_size[2] / project_scale),
|
31 |
-
)
|
32 |
-
size_l2 = (size_l1[0] // 2, size_l1[1] // 2, size_l1[2] // 2)
|
33 |
-
size_l3 = (size_l2[0] // 2, size_l2[1] // 2, size_l2[2] // 2)
|
34 |
-
|
35 |
-
dilations = [1, 2, 3]
|
36 |
-
self.process_l1 = nn.Sequential(
|
37 |
-
Process(self.feature, norm_layer, bn_momentum, dilations=[1, 2, 3]),
|
38 |
-
Downsample(self.feature, norm_layer, bn_momentum),
|
39 |
-
)
|
40 |
-
self.process_l2 = nn.Sequential(
|
41 |
-
Process(self.feature * 2, norm_layer, bn_momentum, dilations=[1, 2, 3]),
|
42 |
-
Downsample(self.feature * 2, norm_layer, bn_momentum),
|
43 |
-
)
|
44 |
-
|
45 |
-
self.up_13_l2 = Upsample(
|
46 |
-
self.feature * 4, self.feature * 2, norm_layer, bn_momentum
|
47 |
-
)
|
48 |
-
self.up_12_l1 = Upsample(
|
49 |
-
self.feature * 2, self.feature, norm_layer, bn_momentum
|
50 |
-
)
|
51 |
-
self.up_l1_lfull = Upsample(
|
52 |
-
self.feature, self.feature // 2, norm_layer, bn_momentum
|
53 |
-
)
|
54 |
-
|
55 |
-
self.ssc_head = SegmentationHead(
|
56 |
-
self.feature // 2, self.feature // 2, class_num, dilations
|
57 |
-
)
|
58 |
-
|
59 |
-
self.context_prior = context_prior
|
60 |
-
if context_prior:
|
61 |
-
self.CP_mega_voxels = CPMegaVoxels(
|
62 |
-
self.feature * 4, size_l3, bn_momentum=bn_momentum
|
63 |
-
)
|
64 |
-
|
65 |
-
def forward(self, input_dict):
|
66 |
-
res = {}
|
67 |
-
|
68 |
-
x3d_l1 = input_dict["x3d"]
|
69 |
-
|
70 |
-
x3d_l2 = self.process_l1(x3d_l1)
|
71 |
-
|
72 |
-
x3d_l3 = self.process_l2(x3d_l2)
|
73 |
-
|
74 |
-
if self.context_prior:
|
75 |
-
ret = self.CP_mega_voxels(x3d_l3)
|
76 |
-
x3d_l3 = ret["x"]
|
77 |
-
for k in ret.keys():
|
78 |
-
res[k] = ret[k]
|
79 |
-
|
80 |
-
x3d_up_l2 = self.up_13_l2(x3d_l3) + x3d_l2
|
81 |
-
x3d_up_l1 = self.up_12_l1(x3d_up_l2) + x3d_l1
|
82 |
-
x3d_up_lfull = self.up_l1_lfull(x3d_up_l1)
|
83 |
-
|
84 |
-
ssc_logit_full = self.ssc_head(x3d_up_lfull)
|
85 |
-
|
86 |
-
res["ssc_logit"] = ssc_logit_full
|
87 |
-
|
88 |
-
return res
|
|
|
|
|
|
|
|
|
|
|
|
|
|
|
|
|
|
|
|
|
|
|
|
|
|
|
|
|
|
|
|
|
|
|
|
|
|
|
|
|
|
|
|
|
|
|
|
|
|
|
|
|
|
|
|
|
|
|
|
|
|
|
|
|
|
|
|
|
|
|
|
|
|
|
|
|
|
|
|
|
|
|
|
|
|
|
|
|
|
|
|
|
|
|
|
|
|
|
|
|
|
|
|
|
|
|
|
|
|
|
|
|
|
|
|
|
|
|
|
|
|
|
|
|
|
|
|
|
|
|
|
|
|
|
|
|
|
|
|
|
|
|
|
|
|
|
|
|
|
|
|
|
|
|
|
|
|
|
|
|
|
|
|
|
|
|
|
|
|
|
|
|
|
|
|
|
|
spaces/CVPR/regionclip-demo/detectron2/export/api.py
DELETED
@@ -1,273 +0,0 @@
|
|
1 |
-
# Copyright (c) Facebook, Inc. and its affiliates.
|
2 |
-
import copy
|
3 |
-
import logging
|
4 |
-
import os
|
5 |
-
import torch
|
6 |
-
from caffe2.proto import caffe2_pb2
|
7 |
-
from torch import nn
|
8 |
-
|
9 |
-
from detectron2.config import CfgNode
|
10 |
-
from detectron2.utils.file_io import PathManager
|
11 |
-
|
12 |
-
from .caffe2_inference import ProtobufDetectionModel
|
13 |
-
from .caffe2_modeling import META_ARCH_CAFFE2_EXPORT_TYPE_MAP, convert_batched_inputs_to_c2_format
|
14 |
-
from .shared import get_pb_arg_vali, get_pb_arg_vals, save_graph
|
15 |
-
|
16 |
-
__all__ = [
|
17 |
-
"add_export_config",
|
18 |
-
"export_caffe2_model",
|
19 |
-
"Caffe2Model",
|
20 |
-
"export_onnx_model",
|
21 |
-
"Caffe2Tracer",
|
22 |
-
]
|
23 |
-
|
24 |
-
|
25 |
-
def add_export_config(cfg):
|
26 |
-
"""
|
27 |
-
Add options needed by caffe2 export.
|
28 |
-
|
29 |
-
Args:
|
30 |
-
cfg (CfgNode): a detectron2 config
|
31 |
-
|
32 |
-
Returns:
|
33 |
-
CfgNode:
|
34 |
-
an updated config with new options that will be used by :class:`Caffe2Tracer`.
|
35 |
-
"""
|
36 |
-
is_frozen = cfg.is_frozen()
|
37 |
-
cfg.defrost()
|
38 |
-
cfg.EXPORT_CAFFE2 = CfgNode()
|
39 |
-
cfg.EXPORT_CAFFE2.USE_HEATMAP_MAX_KEYPOINT = False
|
40 |
-
if is_frozen:
|
41 |
-
cfg.freeze()
|
42 |
-
return cfg
|
43 |
-
|
44 |
-
|
45 |
-
class Caffe2Tracer:
|
46 |
-
"""
|
47 |
-
Make a detectron2 model traceable with Caffe2 operators.
|
48 |
-
This class creates a traceable version of a detectron2 model which:
|
49 |
-
|
50 |
-
1. Rewrite parts of the model using ops in Caffe2. Note that some ops do
|
51 |
-
not have GPU implementation in Caffe2.
|
52 |
-
2. Remove post-processing and only produce raw layer outputs
|
53 |
-
|
54 |
-
After making a traceable model, the class provide methods to export such a
|
55 |
-
model to different deployment formats.
|
56 |
-
Exported graph produced by this class take two input tensors:
|
57 |
-
|
58 |
-
1. (1, C, H, W) float "data" which is an image (usually in [0, 255]).
|
59 |
-
(H, W) often has to be padded to multiple of 32 (depend on the model
|
60 |
-
architecture).
|
61 |
-
2. 1x3 float "im_info", each row of which is (height, width, 1.0).
|
62 |
-
Height and width are true image shapes before padding.
|
63 |
-
|
64 |
-
The class currently only supports models using builtin meta architectures.
|
65 |
-
Batch inference is not supported, and contributions are welcome.
|
66 |
-
"""
|
67 |
-
|
68 |
-
def __init__(self, cfg: CfgNode, model: nn.Module, inputs):
|
69 |
-
"""
|
70 |
-
Args:
|
71 |
-
cfg (CfgNode): a detectron2 config, with extra export-related options
|
72 |
-
added by :func:`add_export_config`. It's used to construct
|
73 |
-
caffe2-compatible model.
|
74 |
-
model (nn.Module): An original pytorch model. Must be among a few official models
|
75 |
-
in detectron2 that can be converted to become caffe2-compatible automatically.
|
76 |
-
Weights have to be already loaded to this model.
|
77 |
-
inputs: sample inputs that the given model takes for inference.
|
78 |
-
Will be used to trace the model. For most models, random inputs with
|
79 |
-
no detected objects will not work as they lead to wrong traces.
|
80 |
-
"""
|
81 |
-
assert isinstance(cfg, CfgNode), cfg
|
82 |
-
assert isinstance(model, torch.nn.Module), type(model)
|
83 |
-
|
84 |
-
if "EXPORT_CAFFE2" not in cfg:
|
85 |
-
cfg = add_export_config(cfg) # will just the defaults
|
86 |
-
# TODO make it support custom models, by passing in c2 model directly
|
87 |
-
C2MetaArch = META_ARCH_CAFFE2_EXPORT_TYPE_MAP[cfg.MODEL.META_ARCHITECTURE]
|
88 |
-
self.traceable_model = C2MetaArch(cfg, copy.deepcopy(model))
|
89 |
-
self.inputs = inputs
|
90 |
-
self.traceable_inputs = self.traceable_model.get_caffe2_inputs(inputs)
|
91 |
-
|
92 |
-
def export_caffe2(self):
|
93 |
-
"""
|
94 |
-
Export the model to Caffe2's protobuf format.
|
95 |
-
The returned object can be saved with its :meth:`.save_protobuf()` method.
|
96 |
-
The result can be loaded and executed using Caffe2 runtime.
|
97 |
-
|
98 |
-
Returns:
|
99 |
-
:class:`Caffe2Model`
|
100 |
-
"""
|
101 |
-
from .caffe2_export import export_caffe2_detection_model
|
102 |
-
|
103 |
-
predict_net, init_net = export_caffe2_detection_model(
|
104 |
-
self.traceable_model, self.traceable_inputs
|
105 |
-
)
|
106 |
-
return Caffe2Model(predict_net, init_net)
|
107 |
-
|
108 |
-
def export_onnx(self):
|
109 |
-
"""
|
110 |
-
Export the model to ONNX format.
|
111 |
-
Note that the exported model contains custom ops only available in caffe2, therefore it
|
112 |
-
cannot be directly executed by other runtime (such as onnxruntime or TensorRT).
|
113 |
-
Post-processing or transformation passes may be applied on the model to accommodate
|
114 |
-
different runtimes, but we currently do not provide support for them.
|
115 |
-
|
116 |
-
Returns:
|
117 |
-
onnx.ModelProto: an onnx model.
|
118 |
-
"""
|
119 |
-
from .caffe2_export import export_onnx_model as export_onnx_model_impl
|
120 |
-
|
121 |
-
return export_onnx_model_impl(self.traceable_model, (self.traceable_inputs,))
|
122 |
-
|
123 |
-
def export_torchscript(self):
|
124 |
-
"""
|
125 |
-
Export the model to a ``torch.jit.TracedModule`` by tracing.
|
126 |
-
The returned object can be saved to a file by ``.save()``.
|
127 |
-
|
128 |
-
Returns:
|
129 |
-
torch.jit.TracedModule: a torch TracedModule
|
130 |
-
"""
|
131 |
-
logger = logging.getLogger(__name__)
|
132 |
-
logger.info("Tracing the model with torch.jit.trace ...")
|
133 |
-
with torch.no_grad():
|
134 |
-
return torch.jit.trace(self.traceable_model, (self.traceable_inputs,))
|
135 |
-
|
136 |
-
|
137 |
-
class Caffe2Model(nn.Module):
|
138 |
-
"""
|
139 |
-
A wrapper around the traced model in Caffe2's protobuf format.
|
140 |
-
The exported graph has different inputs/outputs from the original Pytorch
|
141 |
-
model, as explained in :class:`Caffe2Tracer`. This class wraps around the
|
142 |
-
exported graph to simulate the same interface as the original Pytorch model.
|
143 |
-
It also provides functions to save/load models in Caffe2's format.'
|
144 |
-
|
145 |
-
Examples:
|
146 |
-
::
|
147 |
-
c2_model = Caffe2Tracer(cfg, torch_model, inputs).export_caffe2()
|
148 |
-
inputs = [{"image": img_tensor_CHW}]
|
149 |
-
outputs = c2_model(inputs)
|
150 |
-
orig_outputs = torch_model(inputs)
|
151 |
-
"""
|
152 |
-
|
153 |
-
def __init__(self, predict_net, init_net):
|
154 |
-
super().__init__()
|
155 |
-
self.eval() # always in eval mode
|
156 |
-
self._predict_net = predict_net
|
157 |
-
self._init_net = init_net
|
158 |
-
self._predictor = None
|
159 |
-
|
160 |
-
__init__.__HIDE_SPHINX_DOC__ = True
|
161 |
-
|
162 |
-
@property
|
163 |
-
def predict_net(self):
|
164 |
-
"""
|
165 |
-
caffe2.core.Net: the underlying caffe2 predict net
|
166 |
-
"""
|
167 |
-
return self._predict_net
|
168 |
-
|
169 |
-
@property
|
170 |
-
def init_net(self):
|
171 |
-
"""
|
172 |
-
caffe2.core.Net: the underlying caffe2 init net
|
173 |
-
"""
|
174 |
-
return self._init_net
|
175 |
-
|
176 |
-
def save_protobuf(self, output_dir):
|
177 |
-
"""
|
178 |
-
Save the model as caffe2's protobuf format.
|
179 |
-
It saves the following files:
|
180 |
-
|
181 |
-
* "model.pb": definition of the graph. Can be visualized with
|
182 |
-
tools like `netron <https://github.com/lutzroeder/netron>`_.
|
183 |
-
* "model_init.pb": model parameters
|
184 |
-
* "model.pbtxt": human-readable definition of the graph. Not
|
185 |
-
needed for deployment.
|
186 |
-
|
187 |
-
Args:
|
188 |
-
output_dir (str): the output directory to save protobuf files.
|
189 |
-
"""
|
190 |
-
logger = logging.getLogger(__name__)
|
191 |
-
logger.info("Saving model to {} ...".format(output_dir))
|
192 |
-
if not PathManager.exists(output_dir):
|
193 |
-
PathManager.mkdirs(output_dir)
|
194 |
-
|
195 |
-
with PathManager.open(os.path.join(output_dir, "model.pb"), "wb") as f:
|
196 |
-
f.write(self._predict_net.SerializeToString())
|
197 |
-
with PathManager.open(os.path.join(output_dir, "model.pbtxt"), "w") as f:
|
198 |
-
f.write(str(self._predict_net))
|
199 |
-
with PathManager.open(os.path.join(output_dir, "model_init.pb"), "wb") as f:
|
200 |
-
f.write(self._init_net.SerializeToString())
|
201 |
-
|
202 |
-
def save_graph(self, output_file, inputs=None):
|
203 |
-
"""
|
204 |
-
Save the graph as SVG format.
|
205 |
-
|
206 |
-
Args:
|
207 |
-
output_file (str): a SVG file
|
208 |
-
inputs: optional inputs given to the model.
|
209 |
-
If given, the inputs will be used to run the graph to record
|
210 |
-
shape of every tensor. The shape information will be
|
211 |
-
saved together with the graph.
|
212 |
-
"""
|
213 |
-
from .caffe2_export import run_and_save_graph
|
214 |
-
|
215 |
-
if inputs is None:
|
216 |
-
save_graph(self._predict_net, output_file, op_only=False)
|
217 |
-
else:
|
218 |
-
size_divisibility = get_pb_arg_vali(self._predict_net, "size_divisibility", 0)
|
219 |
-
device = get_pb_arg_vals(self._predict_net, "device", b"cpu").decode("ascii")
|
220 |
-
inputs = convert_batched_inputs_to_c2_format(inputs, size_divisibility, device)
|
221 |
-
inputs = [x.cpu().numpy() for x in inputs]
|
222 |
-
run_and_save_graph(self._predict_net, self._init_net, inputs, output_file)
|
223 |
-
|
224 |
-
@staticmethod
|
225 |
-
def load_protobuf(dir):
|
226 |
-
"""
|
227 |
-
Args:
|
228 |
-
dir (str): a directory used to save Caffe2Model with
|
229 |
-
:meth:`save_protobuf`.
|
230 |
-
The files "model.pb" and "model_init.pb" are needed.
|
231 |
-
|
232 |
-
Returns:
|
233 |
-
Caffe2Model: the caffe2 model loaded from this directory.
|
234 |
-
"""
|
235 |
-
predict_net = caffe2_pb2.NetDef()
|
236 |
-
with PathManager.open(os.path.join(dir, "model.pb"), "rb") as f:
|
237 |
-
predict_net.ParseFromString(f.read())
|
238 |
-
|
239 |
-
init_net = caffe2_pb2.NetDef()
|
240 |
-
with PathManager.open(os.path.join(dir, "model_init.pb"), "rb") as f:
|
241 |
-
init_net.ParseFromString(f.read())
|
242 |
-
|
243 |
-
return Caffe2Model(predict_net, init_net)
|
244 |
-
|
245 |
-
def __call__(self, inputs):
|
246 |
-
"""
|
247 |
-
An interface that wraps around a Caffe2 model and mimics detectron2's models'
|
248 |
-
input/output format. See details about the format at :doc:`/tutorials/models`.
|
249 |
-
This is used to compare the outputs of caffe2 model with its original torch model.
|
250 |
-
|
251 |
-
Due to the extra conversion between Pytorch/Caffe2, this method is not meant for
|
252 |
-
benchmark. Because of the conversion, this method also has dependency
|
253 |
-
on detectron2 in order to convert to detectron2's output format.
|
254 |
-
"""
|
255 |
-
if self._predictor is None:
|
256 |
-
self._predictor = ProtobufDetectionModel(self._predict_net, self._init_net)
|
257 |
-
return self._predictor(inputs)
|
258 |
-
|
259 |
-
|
260 |
-
def export_caffe2_model(cfg, model, inputs):
|
261 |
-
logger = logging.getLogger(__name__)
|
262 |
-
logger.warning(
|
263 |
-
"export_caffe2_model() is deprecated. Please use `Caffe2Tracer().export_caffe2() instead."
|
264 |
-
)
|
265 |
-
return Caffe2Tracer(cfg, model, inputs).export_caffe2()
|
266 |
-
|
267 |
-
|
268 |
-
def export_onnx_model(cfg, model, inputs):
|
269 |
-
logger = logging.getLogger(__name__)
|
270 |
-
logger.warning(
|
271 |
-
"export_caffe2_model() is deprecated. Please use `Caffe2Tracer().export_onnx() instead."
|
272 |
-
)
|
273 |
-
return Caffe2Tracer(cfg, model, inputs).export_onnx()
|
|
|
|
|
|
|
|
|
|
|
|
|
|
|
|
|
|
|
|
|
|
|
|
|
|
|
|
|
|
|
|
|
|
|
|
|
|
|
|
|
|
|
|
|
|
|
|
|
|
|
|
|
|
|
|
|
|
|
|
|
|
|
|
|
|
|
|
|
|
|
|
|
|
|
|
|
|
|
|
|
|
|
|
|
|
|
|
|
|
|
|
|
|
|
|
|
|
|
|
|
|
|
|
|
|
|
|
|
|
|
|
|
|
|
|
|
|
|
|
|
|
|
|
|
|
|
|
|
|
|
|
|
|
|
|
|
|
|
|
|
|
|
|
|
|
|
|
|
|
|
|
|
|
|
|
|
|
|
|
|
|
|
|
|
|
|
|
|
|
|
|
|
|
|
|
|
|
|
|
|
|
|
|
|
|
|
|
|
|
|
|
|
|
|
|
|
|
|
|
|
|
|
|
|
|
|
|
|
|
|
|
|
|
|
|
|
|
|
|
|
|
|
|
|
|
|
|
|
|
|
|
|
|
|
|
|
|
|
|
|
|
|
|
|
|
|
|
|
|
|
|
|
|
|
|
|
|
|
|
|
|
|
|
|
|
|
|
|
|
|
|
|
|
|
|
|
|
|
|
|
|
|
|
|
|
|
|
|
|
|
|
|
|
|
|
|
|
|
|
|
|
|
|
|
|
|
|
|
|
|
|
|
|
|
|
|
|
|
|
|
|
|
|
|
|
|
|
|
|
|
|
|
|
|
|
|
|
|
|
|
|
|
|
|
|
|
|
|
|
|
|
|
|
|
|
|
|
|
|
|
|
|
|
|
|
|
|
|
|
|
|
|
|
|
|
|
|
|
|
|
|
|
|
|
|
|
|
|
|
|
|
|
|
|
|
|
|
|
|
|
|
|
|
|
|
|
|
|
|
|
|
|
|
|
|
|
|
|
|
|
|
|
|
|
|
|
|
|
|
|
|
|
|
|
|
|
|
|
|
|
|
|
|
|
|
|
|
|
|
|
|
|
|
|
|
|
|
|
|
|
|
|
|
|
|
|
|
|
|
|
|
|
|
|
|
|
|
|
|
|
|
|
|
|
|
|
|
|
|
|
|
|
|
|
|
|
|
|
|
|
|
|
|
|
|
|
|
|
|
|
|
|
|
|
|
|
|
|
|
|
|
|
|
|
|
|
|
|
|
|
|
|
|
|
|
|
|
|
|
|
|
|
|
|
|
|
|
spaces/CVPR/unicl-zero-shot-img-recog/model/image_encoder/__init__.py
DELETED
@@ -1 +0,0 @@
|
|
1 |
-
from .build import build_model as build_image_encoder
|
|
|
|
spaces/CaliforniaHealthCollaborative/README/README.md
DELETED
@@ -1,43 +0,0 @@
|
|
1 |
-
---
|
2 |
-
title: 🔥README🔥
|
3 |
-
emoji: 📚
|
4 |
-
colorFrom: yellow
|
5 |
-
colorTo: red
|
6 |
-
sdk: static
|
7 |
-
pinned: true
|
8 |
-
license: mit
|
9 |
-
---
|
10 |
-

|
11 |
-
|
12 |
-
|
13 |
-
|
14 |
-
## Summary
|
15 |
-
|
16 |
-
This research proposal aims to develop an innovative approach for language retrieval by leveraging emoji-to-Kaktovik translation. The objective is to build a more interpretable and interoperable language retrieval process that surpasses traditional binary fragmenting techniques. By translating emojis to Kaktovik numerals, we can capture their inherent meaning and relationships, enabling precise and resource-efficient language retrieval. This proposal is being submitted to Microsoft AI Research and prospective universities to explore the potential of emoji-based language retrieval.
|
17 |
-
|
18 |
-
## Objectives
|
19 |
-
|
20 |
-
1. Develop an emoji clustering, indexing, and fragmentation method that organizes emojis into semantically meaningful groups, surpassing the limitations of binary fragmenting techniques.
|
21 |
-
2. Investigate techniques for translating emojis to Kaktovik numerals, preserving their inherent meaning and relationships in the translation process.
|
22 |
-
3. Design algorithms and models that leverage the translated Kaktovik numerals for precise and resource-efficient language retrieval.
|
23 |
-
4. Evaluate the interpretability and interoperability of the proposed approach, comparing it to traditional binary fragmenting techniques.
|
24 |
-
5. Demonstrate the practical applications of the emoji-to-Kaktovik translation in real-world language retrieval scenarios, showcasing its advantages in precision, efficiency, and interpretability.
|
25 |
-
|
26 |
-
## Methodology
|
27 |
-
|
28 |
-
1. **Emoji Clustering**: Develop an advanced clustering method that groups visually and semantically similar emojis together. Explore techniques that consider various factors, such as visual characteristics, semantic meanings, and user interpretations, to form cohesive and meaningful clusters.
|
29 |
-
2. **Kaktovik Numerals Encoding**: Investigate methods for translating emojis to Kaktovik numerals while preserving their inherent meaning and relationships. Design encoding algorithms that capture the nuanced representations of emojis in Kaktovik numerals, enabling precise and interpretable language retrieval.
|
30 |
-
3. **Translation Models**: Develop machine learning models and algorithms that translate emojis to their corresponding Kaktovik numerals. Train these models using large-scale annotated datasets of emojis and their Kaktovik numeral translations to ensure accurate and context-aware translations.
|
31 |
-
4. **Language Retrieval Integration**: Integrate the translated Kaktovik numerals into the language retrieval process. Develop efficient indexing and retrieval techniques that leverage the inherent meaning and relationships captured in the Kaktovik numerals to enhance the precision and efficiency of language retrieval.
|
32 |
-
5. **Evaluation and Analysis**: Evaluate the performance of the proposed approach by measuring metrics such as retrieval accuracy, precision, recall, and resource efficiency. Compare the results against traditional binary fragmenting techniques to assess the interpretability and interoperability advantages of the emoji-to-Kaktovik translation.
|
33 |
-
6. **Real-World Applications**: Deploy the developed language retrieval system in real-world scenarios, such as information retrieval, chatbots, and recommendation systems. Demonstrate the practical benefits of the emoji-to-Kaktovik translation approach in terms of improved precision, interpretability, and reduced resource requirements.
|
34 |
-
|
35 |
-
## Expected Outcomes
|
36 |
-
|
37 |
-
1. Enhanced precision and interpretability in language retrieval through the emoji-to-Kaktovik translation approach.
|
38 |
-
2. Improved interoperability of the language retrieval system, surpassing the limitations of binary fragmenting techniques.
|
39 |
-
3. Resource-efficient language retrieval process, enabling faster and more precise retrieval of relevant information.
|
40 |
-
4. Insights into the potential applications and advantages of emoji-to-Kaktovik translation in various language processing tasks.
|
41 |
-
5. Collaboration and knowledge exchange opportunities with Microsoft AI Research and prospective universities in advancing the field of interpretable and interoperable language retrieval.
|
42 |
-
|
43 |
-
6. 
|
|
|
|
|
|
|
|
|
|
|
|
|
|
|
|
|
|
|
|
|
|
|
|
|
|
|
|
|
|
|
|
|
|
|
|
|
|
|
|
|
|
|
|
|
|
|
|
|
|
|
|
|
|
|
|
|
|
|
|
|
|
|
|
|
|
|
|
|
|
|
|
|
|
|
|
|
|
|
|
|
|
|
|
|
|
|
|
spaces/Chaitanya01/InvestingPlatform/mapping.py
DELETED
The diff for this file is too large to render.
See raw diff
|
|
spaces/CikeyQI/Yunzai/Yunzai/renderers/puppeteer/lib/puppeteer.js
DELETED
@@ -1,321 +0,0 @@
|
|
1 |
-
import Renderer from '../../../lib/renderer/Renderer.js'
|
2 |
-
import os from 'node:os'
|
3 |
-
import lodash from 'lodash'
|
4 |
-
import puppeteer from 'puppeteer'
|
5 |
-
// 暂时保留对原config的兼容
|
6 |
-
import cfg from '../../../lib/config/config.js'
|
7 |
-
import { Data } from '#miao'
|
8 |
-
|
9 |
-
const _path = process.cwd()
|
10 |
-
// mac地址
|
11 |
-
let mac = ''
|
12 |
-
// 超时计时器
|
13 |
-
let overtimeList = []
|
14 |
-
|
15 |
-
export default class Puppeteer extends Renderer {
|
16 |
-
constructor (config) {
|
17 |
-
super({
|
18 |
-
id: 'puppeteer',
|
19 |
-
type: 'image',
|
20 |
-
render: 'screenshot'
|
21 |
-
})
|
22 |
-
this.browser = false
|
23 |
-
this.lock = false
|
24 |
-
this.shoting = []
|
25 |
-
/** 截图数达到时重启浏览器 避免生成速度越来越慢 */
|
26 |
-
this.restartNum = 100
|
27 |
-
/** 截图次数 */
|
28 |
-
this.renderNum = 0
|
29 |
-
this.config = {
|
30 |
-
headless: Data.def(config.headless, 'new'),
|
31 |
-
args: Data.def(config.args, [
|
32 |
-
'--disable-gpu',
|
33 |
-
'--disable-setuid-sandbox',
|
34 |
-
'--no-sandbox',
|
35 |
-
'--no-zygote'
|
36 |
-
])
|
37 |
-
}
|
38 |
-
if (config.chromiumPath || cfg?.bot?.chromium_path) {
|
39 |
-
/** chromium其他路径 */
|
40 |
-
this.config.executablePath = config.chromiumPath || cfg?.bot?.chromium_path
|
41 |
-
}
|
42 |
-
if (config.puppeteerWS || cfg?.bot?.puppeteer_ws) {
|
43 |
-
/** chromium其他路径 */
|
44 |
-
this.config.wsEndpoint = config.puppeteerWS || cfg?.bot?.puppeteer_ws
|
45 |
-
}
|
46 |
-
/** puppeteer超时超时时间 */
|
47 |
-
this.puppeteerTimeout = config.puppeteerTimeout || cfg?.bot?.puppeteer_timeout || 0
|
48 |
-
}
|
49 |
-
|
50 |
-
/**
|
51 |
-
* 初始化chromium
|
52 |
-
*/
|
53 |
-
async browserInit () {
|
54 |
-
if (this.browser) return this.browser
|
55 |
-
if (this.lock) return false
|
56 |
-
this.lock = true
|
57 |
-
|
58 |
-
logger.info('puppeteer Chromium 启动中...')
|
59 |
-
|
60 |
-
let connectFlag = false
|
61 |
-
try {
|
62 |
-
// 获取Mac地址
|
63 |
-
if (!mac) {
|
64 |
-
mac = await this.getMac()
|
65 |
-
this.browserMacKey = `Yz:chromium:browserWSEndpoint:${mac}`
|
66 |
-
}
|
67 |
-
// 是否有browser实例
|
68 |
-
const browserUrl = (await redis.get(this.browserMacKey)) || this.config.wsEndpoint
|
69 |
-
if (browserUrl) {
|
70 |
-
logger.info(`puppeteer Chromium from ${browserUrl}`)
|
71 |
-
const browserWSEndpoint = await puppeteer.connect({ browserWSEndpoint: browserUrl }).catch(() => {
|
72 |
-
logger.error('puppeteer Chromium 缓存的实例已关闭')
|
73 |
-
redis.del(this.browserMacKey)
|
74 |
-
})
|
75 |
-
// 如果有实例,直接使用
|
76 |
-
if (browserWSEndpoint) {
|
77 |
-
this.browser = browserWSEndpoint
|
78 |
-
if (this.browser) {
|
79 |
-
connectFlag = true
|
80 |
-
}
|
81 |
-
}
|
82 |
-
}
|
83 |
-
} catch (e) {
|
84 |
-
logger.info('puppeteer Chromium 不存在已有实例')
|
85 |
-
}
|
86 |
-
|
87 |
-
if (!this.browser || !connectFlag) {
|
88 |
-
// 如果没有实例,初始化puppeteer
|
89 |
-
this.browser = await puppeteer.launch(this.config).catch((err, trace) => {
|
90 |
-
let errMsg = err.toString() + (trace ? trace.toString() : '')
|
91 |
-
if (typeof err == 'object') {
|
92 |
-
logger.error(JSON.stringify(err))
|
93 |
-
} else {
|
94 |
-
logger.error(err.toString())
|
95 |
-
if (errMsg.includes('Could not find Chromium')) {
|
96 |
-
logger.error('没有正确安装 Chromium,可以尝试执行安装命令:node node_modules/puppeteer/install.js')
|
97 |
-
} else if (errMsg.includes('cannot open shared object file')) {
|
98 |
-
logger.error('没有正确安装 Chromium 运行库')
|
99 |
-
}
|
100 |
-
}
|
101 |
-
logger.error(err, trace)
|
102 |
-
})
|
103 |
-
}
|
104 |
-
|
105 |
-
this.lock = false
|
106 |
-
|
107 |
-
if (!this.browser) {
|
108 |
-
logger.error('puppeteer Chromium 启动失败')
|
109 |
-
return false
|
110 |
-
}
|
111 |
-
if (connectFlag) {
|
112 |
-
logger.info('puppeteer Chromium 已连接启动的实例')
|
113 |
-
} else {
|
114 |
-
logger.info(`[Chromium] ${this.browser.wsEndpoint()}`)
|
115 |
-
if (process.env.pm_id && this.browserMacKey) {
|
116 |
-
// 缓存一下实例30天
|
117 |
-
const expireTime = 60 * 60 * 24 * 30
|
118 |
-
await redis.set(this.browserMacKey, this.browser.wsEndpoint(), { EX: expireTime })
|
119 |
-
}
|
120 |
-
logger.info('puppeteer Chromium 启动成功')
|
121 |
-
}
|
122 |
-
|
123 |
-
/** 监听Chromium实例是否断开 */
|
124 |
-
this.browser.on('disconnected', () => {
|
125 |
-
logger.error('Chromium 实例关闭或崩溃!')
|
126 |
-
this.browser = false
|
127 |
-
})
|
128 |
-
|
129 |
-
return this.browser
|
130 |
-
}
|
131 |
-
|
132 |
-
// 获取Mac地址
|
133 |
-
getMac () {
|
134 |
-
let mac = '00:00:00:00:00:00'
|
135 |
-
try {
|
136 |
-
const network = os.networkInterfaces()
|
137 |
-
let macFlag = false
|
138 |
-
for (const a in network) {
|
139 |
-
for (const i of network[a]) {
|
140 |
-
if (i.mac && i.mac !== mac) {
|
141 |
-
macFlag = true
|
142 |
-
mac = i.mac
|
143 |
-
break
|
144 |
-
}
|
145 |
-
}
|
146 |
-
if (macFlag) {
|
147 |
-
break
|
148 |
-
}
|
149 |
-
}
|
150 |
-
} catch (e) {
|
151 |
-
}
|
152 |
-
mac = mac.replace(/:/g, '')
|
153 |
-
return mac
|
154 |
-
}
|
155 |
-
|
156 |
-
/**
|
157 |
-
* `chromium` 截图
|
158 |
-
* @param name
|
159 |
-
* @param data 模板参数
|
160 |
-
* @param data.tplFile 模板路径,必传
|
161 |
-
* @param data.saveId 生成html名称,为空name代替
|
162 |
-
* @param data.imgType screenshot参数,生成图片类型:jpeg,png
|
163 |
-
* @param data.quality screenshot参数,图片质量 0-100,jpeg是可传,默认90
|
164 |
-
* @param data.omitBackground screenshot参数,隐藏默认的白色背景,背景透明。默认不透明
|
165 |
-
* @param data.path screenshot参数,截图保存路径。截图图片类型将从文件扩展名推断出来。如果是相对路径,则从当前路径解析。如果没有指定路径,图片将不会保存到硬盘。
|
166 |
-
* @param data.multiPage 是否分页截图,默认false
|
167 |
-
* @param data.multiPageHeight 分页状态下页面高度,默认4000
|
168 |
-
* @param data.pageGotoParams 页面goto时的参数
|
169 |
-
* @return img 不做segment包裹
|
170 |
-
*/
|
171 |
-
async screenshot (name, data = {}) {
|
172 |
-
if (!await this.browserInit()) {
|
173 |
-
return false
|
174 |
-
}
|
175 |
-
const pageHeight = data.multiPageHeight || 4000
|
176 |
-
|
177 |
-
let savePath = this.dealTpl(name, data)
|
178 |
-
if (!savePath) {
|
179 |
-
return false
|
180 |
-
}
|
181 |
-
|
182 |
-
let buff = ''
|
183 |
-
let start = Date.now()
|
184 |
-
|
185 |
-
let ret = []
|
186 |
-
this.shoting.push(name)
|
187 |
-
|
188 |
-
const puppeteerTimeout = this.puppeteerTimeout
|
189 |
-
let overtime
|
190 |
-
let overtimeFlag = false
|
191 |
-
if (puppeteerTimeout > 0) {
|
192 |
-
// TODO 截图超时处理
|
193 |
-
overtime = setTimeout(() => {
|
194 |
-
if (!overtimeFlag) {
|
195 |
-
logger.error(`[图片生成][${name}] 截图超时,当前等待队列:${this.shoting.join(',')}`)
|
196 |
-
this.restart(true)
|
197 |
-
this.shoting = []
|
198 |
-
overtimeList.forEach(item => {
|
199 |
-
clearTimeout(item)
|
200 |
-
})
|
201 |
-
}
|
202 |
-
}, puppeteerTimeout)
|
203 |
-
}
|
204 |
-
|
205 |
-
try {
|
206 |
-
const page = await this.browser.newPage()
|
207 |
-
let pageGotoParams = lodash.extend({ timeout: 120000 }, data.pageGotoParams || {})
|
208 |
-
await page.goto(`file://${_path}${lodash.trim(savePath, '.')}`, pageGotoParams)
|
209 |
-
let body = await page.$('#container') || await page.$('body')
|
210 |
-
|
211 |
-
// 计算页面高度
|
212 |
-
const boundingBox = await body.boundingBox()
|
213 |
-
// 分页数
|
214 |
-
let num = 1
|
215 |
-
|
216 |
-
let randData = {
|
217 |
-
type: data.imgType || 'jpeg',
|
218 |
-
omitBackground: data.omitBackground || false,
|
219 |
-
quality: data.quality || 90,
|
220 |
-
path: data.path || ''
|
221 |
-
}
|
222 |
-
|
223 |
-
if (data.multiPage) {
|
224 |
-
randData.type = 'jpeg'
|
225 |
-
num = Math.round(boundingBox.height / pageHeight) || 1
|
226 |
-
}
|
227 |
-
|
228 |
-
if (data.imgType === 'png') {
|
229 |
-
delete randData.quality
|
230 |
-
}
|
231 |
-
|
232 |
-
if (!data.multiPage) {
|
233 |
-
buff = await body.screenshot(randData)
|
234 |
-
/** 计算图片大小 */
|
235 |
-
const kb = (buff.length / 1024).toFixed(2) + 'KB'
|
236 |
-
logger.mark(`[图片生成][${name}][${this.renderNum}次] ${kb} ${logger.green(`${Date.now() - start}ms`)}`)
|
237 |
-
this.renderNum++
|
238 |
-
ret.push(buff)
|
239 |
-
} else {
|
240 |
-
// 分片截图
|
241 |
-
if (num > 1) {
|
242 |
-
await page.setViewport({
|
243 |
-
width: boundingBox.width,
|
244 |
-
height: pageHeight + 100
|
245 |
-
})
|
246 |
-
}
|
247 |
-
for (let i = 1; i <= num; i++) {
|
248 |
-
if (i !== 1 && i === num) {
|
249 |
-
await page.setViewport({
|
250 |
-
width: boundingBox.width,
|
251 |
-
height: parseInt(boundingBox.height) - pageHeight * (num - 1)
|
252 |
-
})
|
253 |
-
}
|
254 |
-
if (i !== 1 && i <= num) {
|
255 |
-
await page.evaluate(pageHeight => window.scrollBy(0, pageHeight), pageHeight)
|
256 |
-
}
|
257 |
-
if (num === 1) {
|
258 |
-
buff = await body.screenshot(randData)
|
259 |
-
} else {
|
260 |
-
buff = await page.screenshot(randData)
|
261 |
-
}
|
262 |
-
if (num > 2) {
|
263 |
-
await Data.sleep(200)
|
264 |
-
}
|
265 |
-
this.renderNum++
|
266 |
-
|
267 |
-
/** 计算图片大小 */
|
268 |
-
const kb = (buff.length / 1024).toFixed(2) + 'KB'
|
269 |
-
logger.mark(`[图片生成][${name}][${i}/${num}] ${kb}`)
|
270 |
-
ret.push(buff)
|
271 |
-
}
|
272 |
-
if (num > 1) {
|
273 |
-
logger.mark(`[图片生成][${name}] 处理完成`)
|
274 |
-
}
|
275 |
-
}
|
276 |
-
page.close().catch((err) => logger.error(err))
|
277 |
-
} catch (error) {
|
278 |
-
logger.error(`[图片生成][${name}] 图片生成失败:${error}`)
|
279 |
-
/** 关闭浏览器 */
|
280 |
-
if (this.browser) {
|
281 |
-
await this.browser.close().catch((err) => logger.error(err))
|
282 |
-
}
|
283 |
-
this.browser = false
|
284 |
-
ret = []
|
285 |
-
return false
|
286 |
-
} finally {
|
287 |
-
if (overtime) {
|
288 |
-
overtimeFlag = true
|
289 |
-
clearTimeout(overtime)
|
290 |
-
overtimeList = []
|
291 |
-
}
|
292 |
-
}
|
293 |
-
|
294 |
-
this.shoting.pop()
|
295 |
-
|
296 |
-
if (ret.length === 0 || !ret[0]) {
|
297 |
-
logger.error(`[图片生成][${name}] 图片生成为空`)
|
298 |
-
return false
|
299 |
-
}
|
300 |
-
|
301 |
-
this.restart(false)
|
302 |
-
|
303 |
-
return data.multiPage ? ret : ret[0]
|
304 |
-
}
|
305 |
-
|
306 |
-
/** 重启 */
|
307 |
-
restart (force = false) {
|
308 |
-
/** 截图超过重启数时,自动关闭重启浏览器,避免生成速度越来越慢 */
|
309 |
-
if (this.renderNum % this.restartNum === 0 || force) {
|
310 |
-
if (this.shoting.length <= 0 || force) {
|
311 |
-
setTimeout(async () => {
|
312 |
-
if (this.browser) {
|
313 |
-
await this.browser.close().catch((err) => logger.error(err))
|
314 |
-
}
|
315 |
-
this.browser = false
|
316 |
-
logger.info(`puppeteer Chromium ${force ? '强制' : ''}关闭重启...`)
|
317 |
-
}, 100)
|
318 |
-
}
|
319 |
-
}
|
320 |
-
}
|
321 |
-
}
|
|
|
|
|
|
|
|
|
|
|
|
|
|
|
|
|
|
|
|
|
|
|
|
|
|
|
|
|
|
|
|
|
|
|
|
|
|
|
|
|
|
|
|
|
|
|
|
|
|
|
|
|
|
|
|
|
|
|
|
|
|
|
|
|
|
|
|
|
|
|
|
|
|
|
|
|
|
|
|
|
|
|
|
|
|
|
|
|
|
|
|
|
|
|
|
|
|
|
|
|
|
|
|
|
|
|
|
|
|
|
|
|
|
|
|
|
|
|
|
|
|
|
|
|
|
|
|
|
|
|
|
|
|
|
|
|
|
|
|
|
|
|
|
|
|
|
|
|
|
|
|
|
|
|
|
|
|
|
|
|
|
|
|
|
|
|
|
|
|
|
|
|
|
|
|
|
|
|
|
|
|
|
|
|
|
|
|
|
|
|
|
|
|
|
|
|
|
|
|
|
|
|
|
|
|
|
|
|
|
|
|
|
|
|
|
|
|
|
|
|
|
|
|
|
|
|
|
|
|
|
|
|
|
|
|
|
|
|
|
|
|
|
|
|
|
|
|
|
|
|
|
|
|
|
|
|
|
|
|
|
|
|
|
|
|
|
|
|
|
|
|
|
|
|
|
|
|
|
|
|
|
|
|
|
|
|
|
|
|
|
|
|
|
|
|
|
|
|
|
|
|
|
|
|
|
|
|
|
|
|
|
|
|
|
|
|
|
|
|
|
|
|
|
|
|
|
|
|
|
|
|
|
|
|
|
|
|
|
|
|
|
|
|
|
|
|
|
|
|
|
|
|
|
|
|
|
|
|
|
|
|
|
|
|
|
|
|
|
|
|
|
|
|
|
|
|
|
|
|
|
|
|
|
|
|
|
|
|
|
|
|
|
|
|
|
|
|
|
|
|
|
|
|
|
|
|
|
|
|
|
|
|
|
|
|
|
|
|
|
|
|
|
|
|
|
|
|
|
|
|
|
|
|
|
|
|
|
|
|
|
|
|
|
|
|
|
|
|
|
|
|
|
|
|
|
|
|
|
|
|
|
|
|
|
|
|
|
|
|
|
|
|
|
|
|
|
|
|
|
|
|
|
|
|
|
|
|
|
|
|
|
|
|
|
|
|
|
|
|
|
|
|
|
|
|
|
|
|
|
|
|
|
|
|
|
|
|
|
|
|
|
|
|
|
|
|
|
|
|
|
|
|
|
|
|
|
|
|
|
|
|
|
|
|
|
|
|
|
|
|
|
|
|
|
|
|
|
|
|
|
|
|
|
|
|
|
|
|
|
|
|
|
|
|
|
|
|
|
|
|
|
|
|
|
|
|
|
|
|
|
|
|
|
|
|
|
|
|
|
|
|
|
|
|
|
|
|
|
|
|
|
|
|
|
|
|
|
|
|
|
|
|
|
|
|
|
|
|
|
|
|
|
|
|
|
|
|
|
|
|
|
|
|
spaces/Cletrason/cloudqi-cqi_text_to_image_pt_v0/README.md
DELETED
@@ -1,12 +0,0 @@
|
|
1 |
-
---
|
2 |
-
title: Cloudqi-cqi Text To Image Pt V0
|
3 |
-
emoji: 😻
|
4 |
-
colorFrom: indigo
|
5 |
-
colorTo: green
|
6 |
-
sdk: gradio
|
7 |
-
sdk_version: 3.33.1
|
8 |
-
app_file: app.py
|
9 |
-
pinned: false
|
10 |
-
---
|
11 |
-
|
12 |
-
Check out the configuration reference at https://huggingface.co/docs/hub/spaces-config-reference
|
|
|
|
|
|
|
|
|
|
|
|
|
|
|
|
|
|
|
|
|
|
|
|
|
|
spaces/CofAI/chat.b4/client/css/dropdown.css
DELETED
@@ -1,10 +0,0 @@
|
|
1 |
-
.dropdown {
|
2 |
-
border: 1px solid var(--conversations);
|
3 |
-
}
|
4 |
-
|
5 |
-
@media screen and (max-width: 990px) {
|
6 |
-
.dropdown {
|
7 |
-
padding: 4px 8px;
|
8 |
-
font-size: 0.75rem;
|
9 |
-
}
|
10 |
-
}
|
|
|
|
|
|
|
|
|
|
|
|
|
|
|
|
|
|
|
|
|
|
spaces/CorvaeOboro/gen_ability_icon/README.md
DELETED
@@ -1,17 +0,0 @@
|
|
1 |
-
---
|
2 |
-
title: gen_ability_icon stylegan2ada
|
3 |
-
emoji: 🔵🔥🌀
|
4 |
-
colorFrom: purple
|
5 |
-
colorTo: green
|
6 |
-
sdk: gradio
|
7 |
-
sdk_version: 3.0.5
|
8 |
-
app_file: app.py
|
9 |
-
tags:
|
10 |
-
- stylegan2
|
11 |
-
license: cc0-1.0
|
12 |
-
models:
|
13 |
-
- "CorvaeOboro/gen_ability_icon"
|
14 |
-
pinned: false
|
15 |
-
---
|
16 |
-
|
17 |
-
Check out the configuration reference at https://huggingface.co/docs/hub/spaces#reference
|
|
|
|
|
|
|
|
|
|
|
|
|
|
|
|
|
|
|
|
|
|
|
|
|
|
|
|
|
|
|
|
|
|
|
|
spaces/DQChoi/gpt-demo/venv/lib/python3.11/site-packages/fastapi/exceptions.py
DELETED
@@ -1,49 +0,0 @@
|
|
1 |
-
from typing import Any, Dict, Optional, Sequence, Type
|
2 |
-
|
3 |
-
from pydantic import BaseModel, create_model
|
4 |
-
from starlette.exceptions import HTTPException as StarletteHTTPException
|
5 |
-
from starlette.exceptions import WebSocketException as WebSocketException # noqa: F401
|
6 |
-
|
7 |
-
|
8 |
-
class HTTPException(StarletteHTTPException):
|
9 |
-
def __init__(
|
10 |
-
self,
|
11 |
-
status_code: int,
|
12 |
-
detail: Any = None,
|
13 |
-
headers: Optional[Dict[str, str]] = None,
|
14 |
-
) -> None:
|
15 |
-
super().__init__(status_code=status_code, detail=detail, headers=headers)
|
16 |
-
|
17 |
-
|
18 |
-
RequestErrorModel: Type[BaseModel] = create_model("Request")
|
19 |
-
WebSocketErrorModel: Type[BaseModel] = create_model("WebSocket")
|
20 |
-
|
21 |
-
|
22 |
-
class FastAPIError(RuntimeError):
|
23 |
-
"""
|
24 |
-
A generic, FastAPI-specific error.
|
25 |
-
"""
|
26 |
-
|
27 |
-
|
28 |
-
class ValidationException(Exception):
|
29 |
-
def __init__(self, errors: Sequence[Any]) -> None:
|
30 |
-
self._errors = errors
|
31 |
-
|
32 |
-
def errors(self) -> Sequence[Any]:
|
33 |
-
return self._errors
|
34 |
-
|
35 |
-
|
36 |
-
class RequestValidationError(ValidationException):
|
37 |
-
def __init__(self, errors: Sequence[Any], *, body: Any = None) -> None:
|
38 |
-
super().__init__(errors)
|
39 |
-
self.body = body
|
40 |
-
|
41 |
-
|
42 |
-
class WebSocketRequestValidationError(ValidationException):
|
43 |
-
pass
|
44 |
-
|
45 |
-
|
46 |
-
class ResponseValidationError(ValidationException):
|
47 |
-
def __init__(self, errors: Sequence[Any], *, body: Any = None) -> None:
|
48 |
-
super().__init__(errors)
|
49 |
-
self.body = body
|
|
|
|
|
|
|
|
|
|
|
|
|
|
|
|
|
|
|
|
|
|
|
|
|
|
|
|
|
|
|
|
|
|
|
|
|
|
|
|
|
|
|
|
|
|
|
|
|
|
|
|
|
|
|
|
|
|
|
|
|
|
|
|
|
|
|
|
|
|
|
|
|
|
|
|
|
|
|
|
|
|
|
|
|
|
|
|
|
|
|
|
|
|
|
|
|
|
|
|
spaces/DQChoi/gpt-demo/venv/lib/python3.11/site-packages/gradio/templates/cdn/assets/index-322e8a8e.css
DELETED
@@ -1 +0,0 @@
|
|
1 |
-
.gallery.svelte-1ayixqk,.gallery.svelte-1viwdyg{padding:var(--size-1) var(--size-2)}div.svelte-1viwdyg{overflow:hidden;min-width:var(--local-text-width);white-space:nowrap}video.svelte-1tntsc1{flex:none;border:2px solid var(--border-color-primary);border-radius:var(--radius-lg);max-width:none}video.svelte-1tntsc1:hover,video.selected.svelte-1tntsc1{border-color:var(--border-color-accent)}.table.svelte-1tntsc1{margin:0 auto;width:var(--size-20);height:var(--size-20);object-fit:cover}.gallery.svelte-1tntsc1{max-height:var(--size-20);object-fit:cover}div.svelte-rgtszb{overflow:hidden;text-overflow:ellipsis;white-space:nowrap}.gallery.svelte-rgtszb{display:flex;align-items:center;cursor:pointer;padding:var(--size-1) var(--size-2);text-align:left}table.svelte-1cib1xd.svelte-1cib1xd{position:relative}td.svelte-1cib1xd.svelte-1cib1xd{border:1px solid var(--table-border-color);padding:var(--size-2);font-size:var(--text-sm);font-family:var(--font-mono)}.selected.svelte-1cib1xd td.svelte-1cib1xd{border-color:var(--border-color-accent)}.table.svelte-1cib1xd.svelte-1cib1xd{display:inline-block;margin:0 auto}.gallery.svelte-1cib1xd td.svelte-1cib1xd:first-child{border-left:none}.gallery.svelte-1cib1xd tr:first-child td.svelte-1cib1xd{border-top:none}.gallery.svelte-1cib1xd td.svelte-1cib1xd:last-child{border-right:none}.gallery.svelte-1cib1xd tr:last-child td.svelte-1cib1xd{border-bottom:none}.overlay.svelte-1cib1xd.svelte-1cib1xd{--gradient-to:transparent;position:absolute;bottom:0;background:linear-gradient(to bottom,transparent,var(--gradient-to));width:var(--size-full);height:50%}.odd.svelte-1cib1xd.svelte-1cib1xd{--gradient-to:var(--table-even-background-fill)}.even.svelte-1cib1xd.svelte-1cib1xd{--gradient-to:var(--table-odd-background-fill)}.button.svelte-1cib1xd.svelte-1cib1xd{--gradient-to:var(--background-fill-primary)}div.svelte-h6ogpl{width:var(--size-10);height:var(--size-10)}.table.svelte-h6ogpl{margin:0 auto}.gallery.svelte-1ayixqk{padding:var(--size-1) var(--size-2)}.gallery.svelte-zvfedn{padding:var(--size-2)}pre.svelte-agpzo2{text-align:left}.gallery.svelte-agpzo2{padding:var(--size-1) var(--size-2)}.wrap.svelte-13hsdno.svelte-13hsdno.svelte-13hsdno{display:inline-block;width:var(--size-full);max-width:var(--size-full);color:var(--body-text-color)}.hide.svelte-13hsdno.svelte-13hsdno.svelte-13hsdno{display:none}.label.svelte-13hsdno.svelte-13hsdno.svelte-13hsdno{display:flex;align-items:center;margin-bottom:var(--size-2);color:var(--block-label-text-color);font-weight:var(--block-label-text-weight);font-size:var(--block-label-text-size);line-height:var(--line-sm)}svg.svelte-13hsdno.svelte-13hsdno.svelte-13hsdno{margin-right:var(--size-1)}.gallery.svelte-13hsdno.svelte-13hsdno.svelte-13hsdno{display:flex;flex-wrap:wrap;gap:var(--spacing-lg)}.gallery-item.svelte-13hsdno.svelte-13hsdno.svelte-13hsdno{border:1px solid var(--border-color-primary);border-radius:var(--button-large-radius);overflow:hidden}.gallery-item.svelte-13hsdno.svelte-13hsdno.svelte-13hsdno:hover{border-color:var(--border-color-accent);background:var(--table-row-focus)}.table-wrap.svelte-13hsdno.svelte-13hsdno.svelte-13hsdno{border:1px solid var(--border-color-primary);border-radius:var(--table-radius);width:var(--size-full);table-layout:auto;overflow-x:auto;line-height:var(--line-sm)}table.svelte-13hsdno.svelte-13hsdno.svelte-13hsdno{width:var(--size-full)}.tr-head.svelte-13hsdno.svelte-13hsdno.svelte-13hsdno{box-shadow:var(--shadow-drop-lg);border-bottom:1px solid var(--border-color-primary)}.tr-head.svelte-13hsdno>.svelte-13hsdno+.svelte-13hsdno{border-right-width:0px;border-left-width:1px;border-color:var(--border-color-primary)}th.svelte-13hsdno.svelte-13hsdno.svelte-13hsdno{padding:var(--size-2);white-space:nowrap}.tr-body.svelte-13hsdno.svelte-13hsdno.svelte-13hsdno{cursor:pointer;border-bottom:1px solid var(--border-color-primary);background:var(--table-even-background-fill)}.tr-body.svelte-13hsdno.svelte-13hsdno.svelte-13hsdno:last-child{border:none}.tr-body.svelte-13hsdno.svelte-13hsdno.svelte-13hsdno:nth-child(odd){background:var(--table-odd-background-fill)}.tr-body.svelte-13hsdno.svelte-13hsdno.svelte-13hsdno:hover{background:var(--table-row-focus)}.tr-body.svelte-13hsdno>.svelte-13hsdno+.svelte-13hsdno{border-right-width:0px;border-left-width:1px;border-color:var(--border-color-primary)}.tr-body.svelte-13hsdno:hover>.svelte-13hsdno+.svelte-13hsdno{border-color:var(--border-color-accent)}td.svelte-13hsdno.svelte-13hsdno.svelte-13hsdno{padding:var(--size-2);text-align:center}.paginate.svelte-13hsdno.svelte-13hsdno.svelte-13hsdno{display:flex;justify-content:center;align-items:center;gap:var(--spacing-sm);margin-top:var(--size-2);color:var(--block-label-text-color);font-size:var(--text-sm)}button.current-page.svelte-13hsdno.svelte-13hsdno.svelte-13hsdno{font-weight:var(--weight-bold)}
|
|
|
|
spaces/DQChoi/gpt-demo/venv/lib/python3.11/site-packages/gradio/templates/cdn/assets/index-aa3a045c.js
DELETED
@@ -1,2 +0,0 @@
|
|
1 |
-
import{S as m,e as u,s as r,k as d,o as b,z as c,v as f,x as g,a9 as v,ab as k,ac as B,ad as h}from"./index-1d65707a.js";import{B as p}from"./Button-f155035a.js";function C(a){let t;const l=a[3].default,e=v(l,a,a[4],null);return{c(){e&&e.c()},m(s,n){e&&e.m(s,n),t=!0},p(s,n){e&&e.p&&(!t||n&16)&&k(e,l,s,s[4],t?h(l,s[4],n,null):B(s[4]),null)},i(s){t||(c(e,s),t=!0)},o(s){f(e,s),t=!1},d(s){e&&e.d(s)}}}function S(a){let t,l;return t=new p({props:{elem_id:a[0],elem_classes:a[1],visible:a[2],explicit_call:!0,$$slots:{default:[C]},$$scope:{ctx:a}}}),{c(){d(t.$$.fragment)},m(e,s){b(t,e,s),l=!0},p(e,[s]){const n={};s&1&&(n.elem_id=e[0]),s&2&&(n.elem_classes=e[1]),s&4&&(n.visible=e[2]),s&16&&(n.$$scope={dirty:s,ctx:e}),t.$set(n)},i(e){l||(c(t.$$.fragment,e),l=!0)},o(e){f(t.$$.fragment,e),l=!1},d(e){g(t,e)}}}function q(a,t,l){let{$$slots:e={},$$scope:s}=t,{elem_id:n}=t,{elem_classes:i}=t,{visible:_=!0}=t;return a.$$set=o=>{"elem_id"in o&&l(0,n=o.elem_id),"elem_classes"in o&&l(1,i=o.elem_classes),"visible"in o&&l(2,_=o.visible),"$$scope"in o&&l(4,s=o.$$scope)},[n,i,_,e,s]}class w extends m{constructor(t){super(),u(this,t,q,S,r,{elem_id:0,elem_classes:1,visible:2})}}const A=w,D=["static"];export{A as Component,D as modes};
|
2 |
-
//# sourceMappingURL=index-aa3a045c.js.map
|
|
|
|
|
|