-
-none none
-none
-Title: Collection of books Series: Fiction, fantasy, mysticism
-Download for free without registration a collection of books fb 2.
-Collection of books in the series.
-Download free book - collection - a collection of new books.
-Download the book Collection of new books.
-none 8a78ff9644
-
-
-
diff --git a/spaces/1gistliPinn/ChatGPT4/Examples/Disk Digger Serial.md b/spaces/1gistliPinn/ChatGPT4/Examples/Disk Digger Serial.md
deleted file mode 100644
index aaa07fbfd7adc4255fa605d13d92f85b5d017753..0000000000000000000000000000000000000000
--- a/spaces/1gistliPinn/ChatGPT4/Examples/Disk Digger Serial.md
+++ /dev/null
@@ -1,25 +0,0 @@
-
-
How to Recover Lost Files with DiskDigger Serial
-
Have you ever accidentally deleted some important files from your computer, memory card, or USB drive? Or have you ever formatted your camera's memory card and lost all your photos and videos? If so, you might be interested in a tool that can help you recover your lost files. That tool is called DiskDigger.
DiskDigger is a software that can undelete and recover lost files from any media that your PC can read, including hard disks, flash drives, memory cards, and more. It can recover files from various file systems, such as FAT, NTFS, exFAT, HFS+, and ext4. It can also recover files of various types, such as photos, videos, music, documents, and more.
-
However, DiskDigger is not a free software. You need to purchase a license key to unlock its full features and functionality. A license key costs $19.99 for a single user license, or $49.99 for a site license that allows unlimited installations on multiple PCs. If you don't have a license key, you can only use DiskDigger in "preview" mode, which lets you see the recoverable files but not save them.
-
So, how can you get a DiskDigger serial for free? Well, there are some websites that claim to offer DiskDigger serials, cracks, or keygens that can generate valid license keys for DiskDigger. However, these websites are not trustworthy and may contain malware, viruses, or other harmful programs that can damage your PC or steal your personal information. Moreover, using a cracked or pirated version of DiskDigger is illegal and unethical.
-
The best way to get a DiskDigger serial is to buy it from the official website of DiskDigger. By doing so, you will support the developers of this useful software and ensure that you get the latest updates and bug fixes. You will also get a 30-day money-back guarantee if you are not satisfied with the product.
-
To buy a DiskDigger serial, go to https://www.diskdigger.org/buy and choose the license type that suits your needs. You can pay with PayPal or credit card. After completing the payment process, you will receive an email with your license key and instructions on how to activate DiskDigger.
-
-
Once you have your DiskDigger serial, you can download the latest version of DiskDigger from https://www.diskdigger.org/download and install it on your PC. Then run DiskDigger and enter your license key when prompted. You will then be able to use DiskDigger in full mode and recover your lost files with ease.
-
DiskDigger is a powerful and reliable tool that can help you recover your lost files from any media. Don't waste your time and money on fake or illegal DiskDigger serials. Buy a genuine license key from the official website of DiskDigger and enjoy its benefits.
-
-
How to Use DiskDigger to Recover Lost Files
-
Now that you have a DiskDigger serial and have activated DiskDigger on your PC, you can start using it to recover your lost files. Here are the steps to follow:
-
-
Launch DiskDigger and select the drive or device that you want to scan for lost files. You can also choose a specific folder or file type to narrow down the search.
-
Choose the scan mode that you want to use. DiskDigger offers two scan modes: "Dig Deep" and "Dig Deeper". The "Dig Deep" mode scans the file system for deleted files and recovers them with their original names and paths. The "Dig Deeper" mode scans the entire disk surface for traces of files and recovers them based on their signatures. The "Dig Deeper" mode is more thorough but may take longer and recover more files than you need.
-
Click "Next" and wait for DiskDigger to scan the selected drive or device. You will see a list of recoverable files as they are found. You can preview the files by clicking on them or filter them by name, size, date, or type.
-
Select the files that you want to recover and click "Recover". You can choose to save the files to a different location on your PC, upload them to an FTP server, or send them as email attachments.
-
Review the recovered files and make sure they are intact and usable. If some files are corrupted or incomplete, you can try scanning again with different settings or using another recovery software.
-
-
DiskDigger is a simple and effective tool that can help you recover your lost files from any media. With a DiskDigger serial, you can unlock its full features and functionality and recover your files with ease. Don't hesitate to buy a DiskDigger serial from the official website of DiskDigger and enjoy its benefits.
d5da3c52bf
-
-
\ No newline at end of file
diff --git a/spaces/1phancelerku/anime-remove-background/Download My Talking Tom Friends The Ultimate Virtual Pet Game.md b/spaces/1phancelerku/anime-remove-background/Download My Talking Tom Friends The Ultimate Virtual Pet Game.md
deleted file mode 100644
index 6e8ab59344c725ea30e6e4982c48a27abda17b95..0000000000000000000000000000000000000000
--- a/spaces/1phancelerku/anime-remove-background/Download My Talking Tom Friends The Ultimate Virtual Pet Game.md
+++ /dev/null
@@ -1,95 +0,0 @@
-
-
Download My Talking Tom and Friends: A World of Friendship and Fun
-
Do you love virtual pets? Do you enjoy simulation games? Do you like to customize your own characters? If you answered yes to any of these questions, then you should download My Talking Tom and Friends, the best new virtual pet game from Outfit7 Limited. In this game, you can take care of six adorable characters: Tom, Angela, Hank, Ginger, Ben, and Becca. You can interact with them, play with them, dress them up, feed them, and watch them grow. You can also explore their house, go to town, and discover new mini games and surprises. My Talking Tom and Friends is a world of friendship and fun waiting for you.
My Talking Tom and Friends is a virtual pet game that lets you take care of six different characters at once. Each character has their own personality, preferences, and hobbies. You can learn more about them by talking to them, playing with them, and watching their reactions. You can also customize their appearance by choosing from a closet full of fun fashions. You can even mix and match outfits to create your own unique style.
-
A simulation game with various activities and mini games
-
My Talking Tom and Friends is also a simulation game that lets you experience various activities with your pet friends. You can cook for them, clean for them, take them to the bathroom, put them to bed, and more. You can also enjoy creative and sporty activities with them, such as painting, gardening, dancing, skateboarding, and more. You can also play mini games with them, such as puzzles, arcade games, racing games, and more. You can earn coins by playing mini games, which you can use to buy more outfits, toys, stickers, and other items.
-
A customization game with outfits, toys, stickers, and coins
-
My Talking Tom and Friends is also a customization game that lets you personalize your pet friends' house. You can decorate their rooms with different wallpapers, furniture, accessories, and more. You can also collect toys for them to play with, such as balls, dolls, cars, robots, and more. You can also collect stickers for them to stick on their walls or albums. You can also collect coins for them to spend on more items or surprises.
-
Why should you download My Talking Tom and Friends?
-
There are many reasons why you should download My Talking Tom and Friends. Here are some of them:
-
It is free and easy to play
-
My Talking Tom and Friends is a free game that you can download from the Google Play Store or the App Store. It is also easy to play, as it has simple controls and intuitive features. You just need to tap, swipe, drag, or tilt your device to interact with your pet friends. You can also use voice commands or text messages to talk to them.
-
It is fun and engaging for all ages
-
My Talking Tom and Friends is a fun game that can entertain anyone from kids to adults. It has colorful graphics, cute animations, funny sounds, and lively music. It also has diverse content that can appeal to different tastes and interests. Whether you like cute animals, fashion trends, creative arts, or exciting games, you will find something to enjoy in My Talking Tom and Friends.
-
It is creative and interactive for all personalities
-
My Talking Tom and Friends is a creative game that lets you express yourself through your pet friends. You can choose how they look, act, and sound. You can also choose how they spend their time, what they do, and where they go. You can also interact with them in various ways, such as tickling them, poking them, hugging them, and more. You can also make them repeat what you say or sing along with you.
-
How can you download My Talking Tom and Friends?
-
Downloading My Talking Tom and Friends is easy and fast. You just need to follow these steps:
-
For Android devices
-
If you have an Android device, you can download My Talking Tom and Friends from the Google Play Store. Here is how:
-
How to download my talking tom friends on android
-My talking tom friends free download for pc
-My talking tom friends mod apk unlimited money and stars
-My talking tom friends game play online
-My talking tom friends outfits and accessories
-My talking tom friends latest version update
-My talking tom friends tips and tricks
-My talking tom friends best mini games
-My talking tom friends review and rating
-My talking tom friends fun activities and challenges
-Download my talking tom friends from google play store
-Download my talking tom friends from app store
-Download my talking tom friends for windows 10
-Download my talking tom friends for mac
-Download my talking tom friends for fire tablet
-Download my talking tom friends hack version
-Download my talking tom friends without ads
-Download my talking tom friends with all characters unlocked
-Download my talking tom friends offline mode
-Download my talking tom friends new features and events
-Why you should download my talking tom friends
-Benefits of downloading my talking tom friends
-How to install and run my talking tom friends
-How to uninstall and delete my talking tom friends
-How to backup and restore my talking tom friends data
-How to connect and share my talking tom friends with friends
-How to watch and subscribe to my talking tom friends youtube channel
-How to contact and get support for my talking tom friends
-How to customize and personalize my talking tom friends
-How to earn and spend coins and bus tokens in my talking tom friends
-
-
Open the Google Play Store app on your device.
-
Search for "My Talking Tom and Friends" in the search bar.
-
Select the game from the list of results and tap on "Install".
-
Wait for the game to download and install on your device.
-
Tap on "Open" to launch the game and start playing.
-
-
For iOS devices
-
If you have an iOS device, you can download My Talking Tom and Friends from the App Store. Here is how:
-
-
Open the App Store app on your device.
-
Search for "My Talking Tom and Friends" in the search bar.
-
Select the game from the list of results and tap on "Get".
-
Enter your Apple ID password or use Touch ID or Face ID to confirm.
-
Wait for the game to download and install on your device.
-
Tap on the game icon to launch the game and start playing.
-
-
For YouTube videos
-
If you want to watch YouTube videos of My Talking Tom and Friends, you can visit the official YouTube channel of Outfit7 Limited. Here is how:
-
-
Open the YouTube app or website on your device.
-
Search for "Outfit7 Limited" in the search bar.
-
Select the channel from the list of results and tap on "Subscribe".
-
Browse through the videos of My Talking Tom and Friends and other games from Outfit7 Limited.
-
Select a video that you want to watch and tap on "Play".
-
Enjoy watching the video and leave a comment or a like if you want.
-
-
Conclusion
-
My Talking Tom and Friends is a wonderful game that you should download today. It is a virtual pet game, a simulation game, and a customization game all in one. It is free, easy, fun, engaging, creative, and interactive. It is suitable for all ages and personalities. It is a world of friendship and fun that you can enjoy with your pet friends. Download My Talking Tom and Friends now and join the millions of players who love this game.
-
FAQs
-
Here are some frequently asked questions about My Talking Tom and Friends:
-
Q: How can I update My Talking Tom and Friends?
-
A: To update My Talking Tom and Friends, you need to go to the Google Play Store or the App Store and check if there is a new version available. If there is, you can tap on "Update" to download and install the latest version of the game.
-
Q: How can I backup or restore my progress in My Talking Tom and Friends?
-
A: To backup or restore your progress in My Talking Tom and Friends, you need to connect your game to your Google Play Games account or your iCloud account. This way, you can save your progress online and access it from any device. You can also sync your progress across different games from Outfit7 Limited.
-
Q: How can I contact the support team of My Talking Tom and Friends?
-
A: To contact the support team of My Talking Tom and Friends, you need to go to the settings menu of the game and tap on "Support". You can then fill out a form with your name, email address, subject, message, and screenshots if needed. You can also visit the official website of Outfit7 Limited at https://outfit7.com/ for more information.
-
Q: How can I share my feedback or suggestions for My Talking Tom and Friends?
-
A: To share your feedback or suggestions for My Talking Tom and Friends, you need to go to the settings menu of the game and tap on "Feedback". You can then rate the game with stars, write a review, or send an email. You can also leave a comment or a review on the Google Play Store or the App Store. You can also follow the social media accounts of Outfit7 Limited on Facebook, Twitter, Instagram, and more.
-
Q: How can I get more coins in My Talking Tom and Friends?
-
A: To get more coins in My Talking Tom and Friends, you can play more mini games, complete more tasks, watch more ads, or buy more coins with real money. You can also get free coins by logging in daily, inviting friends, or joining events.
197e85843d
-
-
\ No newline at end of file
diff --git a/spaces/1phancelerku/anime-remove-background/Download Ship Simulator for Mac - Enjoy the Realistic Graphics and Sounds of Ship Driving.md b/spaces/1phancelerku/anime-remove-background/Download Ship Simulator for Mac - Enjoy the Realistic Graphics and Sounds of Ship Driving.md
deleted file mode 100644
index e7832d30db47407bbf2ddcf45d4757f6706cc4fa..0000000000000000000000000000000000000000
--- a/spaces/1phancelerku/anime-remove-background/Download Ship Simulator for Mac - Enjoy the Realistic Graphics and Sounds of Ship Driving.md
+++ /dev/null
@@ -1,173 +0,0 @@
-
-
Ship Simulator Games for Mac: Free Alternatives to Try
-
Ship simulator games are a type of simulation games that allow you to control various types of ships and experience realistic maritime scenarios. They can be fun, educational, and challenging, depending on the game mode, difficulty, and features.
-
However, not all ship simulator games are free to download. Some of them require you to purchase the game or pay a subscription fee to access the full content. This can be a problem for some Mac users who want to enjoy ship simulation without spending any money.
Fortunately, there are some free alternatives that you can try if you are looking for ship simulator games for Mac. In this article, we will review three of them: Ship Handling Simulator, The Ship Simulator 202 2, and NAUTIS Home - Ship Simulator. We will compare their features, pros and cons, and how to download them for Mac users.
-
Ship Handling Simulator
-
Ship Handling Simulator is a realistic ship simulator game that lets you control different types of ships, such as tugboats, container ships, cruise ships, and more. You can choose from various locations, such as New York, Rotterdam, Hong Kong, and others. You can also adjust the weather conditions, such as wind, waves, fog, and rain. The game has a sandbox mode where you can freely explore the environment and practice your skills. You can also take on missions and challenges that test your ship handling abilities.
-
Features
-
-
Realistic physics and graphics
-
Various ships and locations
-
Weather effects and day/night cycle
-
Sandbox mode and missions
-
Online leaderboards and achievements
-
-
Pros and Cons
-
-
-
Pros
-
Cons
-
-
-
Good graphics and sound effects
-
Limited locations and scenarios
-
-
-
Easy controls and interface
-
Expensive price ($10.99)
-
-
-
Frequent updates and improvements
-
No online multiplayer mode
-
-
-
Fun and educational gameplay
-
No customization options for ships or settings
-
-
-
How to Download
-
To download Ship Handling Simulator for Mac, you need to visit the App Store and search for the game. You can also use this link: [Ship Handling Simulator]. The game costs $10.99 and requires macOS 10.9 or later. The game size is 1.6 GB and the current version is 1.4.1.
-
The Ship Simulator 2022
-
The Ship Simulator 2022 is an open world ship simulator game that lets you explore a huge map with various ports, islands, and landmarks. You can choose from a variety of ships, such as cargo ships, cruise ships, fishing boats, yachts, and more. You can also take on different missions, such as transporting goods, rescuing people, racing against other ships, and more. The game has stunning graphics and realistic physics that make you feel like you are really sailing on the sea.
-
Features
-
-
Open world map with diverse locations
-
Variety of ships and missions
-
Realistic physics and graphics
-
Free to play with in-app purchases
-
Online multiplayer mode and chat system
-
-
Pros and Cons
-
-
-
Pros
-
Cons
-
-
-
Immersive gameplay and environment
-
In-app purchases can be expensive or intrusive
-
-
-
Stunning graphics and sound effects
-
Bugs and glitches can affect the performance or experience
-
-
-
Frequent updates and new content
-
No offline mode or save option
Social features and interaction with other players
-
No customization options for ships or settings
-
-
-
How to Download
-
To download The Ship Simulator 2022 for Mac, you need to visit the App Store and search for the game. You can also use this link: [The Ship Simulator 2022]. The game is free to play but offers in-app purchases for extra content and features. The game requires iOS 10 or later. The game size is 1.1 GB and the current version is 1.0.2.
-
NAUTIS Home - Ship Simulator
-
NAUTIS Home - Ship Simulator is a realistic maritime simulation game that lets you experience various scenarios and situations that occur in the real world of shipping. You can choose from famous ports and locations, such as Rotterdam, Hamburg, Singapore, and more. You can also select from different types of ships, such as container ships, bulk carriers, ferries, and more. The game has an online multiplayer mode where you can join other players and compete or cooperate in various missions and challenges.
-
Features
-
-
Realistic maritime simulation with high standard of safety
-
Famous ports and locations with accurate models and data
-
Different types of ships with realistic controls and behavior
-
Online multiplayer mode with voice chat and leaderboards
-
Reduced costs, enhanced performance, fast learning process, objective assessment, flexibility, etc.
-
-
Pros and Cons
-
-
-
Pros
-
Cons
-
-
-
High quality graphics and sound effects
-
Subscription fee required ($9.99 per month or $99 per year)
-
-
-
Educational and professional gameplay
-
Limited free trial period (14 days)
-
-
-
Frequent updates and new content
-
No offline mode or save option
Social features and interaction with other players
-
No customization options for ships or settings
-
-
-
How to Download
-
To download NAUTIS Home - Ship Simulator for Mac, you need to visit the VSTEP LXP website and search for the game. You can also use this link: [NAUTIS Home - Ship Simulator]. The game requires a subscription fee of $9.99 per month or $99 per year to access the full content and features. The game also requires a minimum system requirement of macOS 10.13 or later. The game size is 2.5 GB and the current version is 1.0.0.
-
ship handling simulator mac download
-ship simulator 2022 for mac free
-ship captain simulator mac free
-ship simulator extremes mac download
-ship simulator 2008 mac free download
-ship simulator games for mac free
-ship simulator titanic mac download
-ship simulator world war 2 mac free
-ship simulator sandbox mode mac download
-ship simulator cruise liner mac free
-ship simulator naval warfare mac download
-ship simulator steam ships mac free
-ship simulator aircraft carrier mac download
-ship simulator battleships mac free
-ship simulator cargo ships mac download
-ship simulator sailing ships mac free
-ship simulator tugboats mac download
-ship simulator ferry boats mac free
-ship simulator realistic physics mac download
-ship simulator weather effects mac free
-ship simulator historical ships mac download
-ship simulator modern ships mac free
-ship simulator port cities mac download
-ship simulator open world map mac free
-ship simulator mooring to a pier mac download
-ship simulator maneuvering and docking mac free
-ship simulator single and multi-screw vessels mac download
-ship simulator azimuth propulsors mac free
-ship simulator nuclear powered ships mac download
-ship simulator electric propulsion ships mac free
-ship simulator dynamic positioning system mac download
-ship simulator bow and stern thrusters mac free
-ship simulator rudder and propeller control mac download
-ship simulator engine and speed control mac free
-ship simulator helm and steering wheel mac download
-ship simulator mini map and compass mac free
-ship simulator multiple camera views mac download
-ship simulator realistic sounds and graphics mac free
-ship simulator challenging missions and levels mac download
-ship simulator time and fuel management mac free
-ship simulator collision and damage system mac download
-ship simulator emergency situations and alarms mac free
-ship simulator walk around the ship and add passengers mac download
-ship simulator shoot guns on battleships and aircraft carriers mac free
-ship simulator add planes that can fly and shoot on aircraft carriers mac download
-ship simulator make funnels fall and split in half on sinking ships mac free
-ship simulator add terrain in the sandbox mode mac download
-ship simulator add real ports and landmarks in the open world map mac free
-ship simulator add more real cruise ships and luxury liners mac download
-ship simulator add more variety of horns for modern and classic ships mac free
-
Conclusion
-
In conclusion, ship simulator games are a great way to experience the thrill and challenge of sailing on the sea. They can also help you learn more about the maritime industry and improve your skills and knowledge. However, not all ship simulator games are free to download for Mac users. Some of them require you to pay a certain amount of money or subscribe to a service to enjoy the full content and features.
-
However, there are also some free alternatives that you can try if you are looking for ship simulator games for Mac. We have reviewed three of them in this article: Ship Handling Simulator, The Ship Simulator 2022, and NAUTIS Home - Ship Simulator. We have compared their features, pros and cons, and how to download them for Mac users. We hope that this article has helped you find the best ship simulator game for your Mac device.
-
FAQs
-
-
What are ship simulator games?
-
Ship simulator games are a type of simulation games that allow you to control various types of ships and experience realistic maritime scenarios.
-
Why are ship simulator games popular?
-
Ship simulator games are popular because they can be fun, educational, and challenging, depending on the game mode, difficulty, and features.
-
Are all ship simulator games free to download for Mac users?
-
No, not all ship simulator games are free to download for Mac users. Some of them require you to purchase the game or pay a subscription fee to access the full content.
-
What are some free alternatives for ship simulator games for Mac users?
-
Some free alternatives for ship simulator games for Mac users are Ship Handling Simulator, The Ship Simulator 2022, and NAUTIS Home - Ship Simulator.
-
How can I download ship simulator games for Mac users?
-
You can download ship simulator games for Mac users from the App Store or from the official websites of the developers.
- 401be4b1e0
-
-
\ No newline at end of file
diff --git a/spaces/1toTree/lora_test/ppdiffusers/pipelines/pndm/pipeline_pndm.py b/spaces/1toTree/lora_test/ppdiffusers/pipelines/pndm/pipeline_pndm.py
deleted file mode 100644
index b3f5ef0ea4ce1a1b6d5472b7a7f195d42bd5932e..0000000000000000000000000000000000000000
--- a/spaces/1toTree/lora_test/ppdiffusers/pipelines/pndm/pipeline_pndm.py
+++ /dev/null
@@ -1,94 +0,0 @@
-# Copyright (c) 2022 PaddlePaddle Authors. All Rights Reserved.
-# Copyright 2022 The HuggingFace Team. All rights reserved.
-#
-# Licensed under the Apache License, Version 2.0 (the "License");
-# you may not use this file except in compliance with the License.
-# You may obtain a copy of the License at
-#
-# http://www.apache.org/licenses/LICENSE-2.0
-#
-# Unless required by applicable law or agreed to in writing, software
-# distributed under the License is distributed on an "AS IS" BASIS,
-# WITHOUT WARRANTIES OR CONDITIONS OF ANY KIND, either express or implied.
-# See the License for the specific language governing permissions and
-# limitations under the License.
-
-from typing import List, Optional, Tuple, Union
-
-import paddle
-
-from ...models import UNet2DModel
-from ...pipeline_utils import DiffusionPipeline, ImagePipelineOutput
-from ...schedulers import PNDMScheduler
-
-
-class PNDMPipeline(DiffusionPipeline):
- r"""
- This model inherits from [`DiffusionPipeline`]. Check the superclass documentation for the generic methods the
- library implements for all the pipelines (such as downloading or saving, running on a particular xxxx, etc.)
-
- Parameters:
- unet (`UNet2DModel`): U-Net architecture to denoise the encoded image latents.
- scheduler ([`SchedulerMixin`]):
- The `PNDMScheduler` to be used in combination with `unet` to denoise the encoded image.
- """
-
- unet: UNet2DModel
- scheduler: PNDMScheduler
-
- def __init__(self, unet: UNet2DModel, scheduler: PNDMScheduler):
- super().__init__()
- self.register_modules(unet=unet, scheduler=scheduler)
-
- @paddle.no_grad()
- def __call__(
- self,
- batch_size: int = 1,
- num_inference_steps: int = 50,
- generator: Optional[Union[paddle.Generator, List[paddle.Generator]]] = None,
- output_type: Optional[str] = "pil",
- return_dict: bool = True,
- **kwargs,
- ) -> Union[ImagePipelineOutput, Tuple]:
- r"""
- Args:
- batch_size (`int`, `optional`, defaults to 1): The number of images to generate.
- num_inference_steps (`int`, `optional`, defaults to 50):
- The number of denoising steps. More denoising steps usually lead to a higher quality image at the
- expense of slower inference.
- generator (`paddle.Generator`, `optional`): A [paddle
- generator](to make generation deterministic.
- output_type (`str`, `optional`, defaults to `"pil"`): The output format of the generate image. Choose
- between [PIL](https://pillow.readthedocs.io/en/stable/): `PIL.Image.Image` or `np.array`.
- return_dict (`bool`, `optional`, defaults to `True`): Whether or not to return a
- [`~pipeline_utils.ImagePipelineOutput`] instead of a plain tuple.
-
- Returns:
- [`~pipeline_utils.ImagePipelineOutput`] or `tuple`: [`~pipelines.utils.ImagePipelineOutput`] if
- `return_dict` is True, otherwise a `tuple. When returning a tuple, the first element is a list with the
- generated images.
- """
- # For more information on the sampling method you can take a look at Algorithm 2 of
- # the official paper: https://arxiv.org/pdf/2202.09778.pdf
-
- # Sample gaussian noise to begin loop
- image = paddle.randn(
- (batch_size, self.unet.in_channels, self.unet.sample_size, self.unet.sample_size),
- generator=generator,
- )
-
- self.scheduler.set_timesteps(num_inference_steps)
- for t in self.progress_bar(self.scheduler.timesteps):
- model_output = self.unet(image, t).sample
-
- image = self.scheduler.step(model_output, t, image).prev_sample
-
- image = (image / 2 + 0.5).clip(0, 1)
- image = image.transpose([0, 2, 3, 1]).numpy()
- if output_type == "pil":
- image = self.numpy_to_pil(image)
-
- if not return_dict:
- return (image,)
-
- return ImagePipelineOutput(images=image)
diff --git a/spaces/7thHeaven/GPT2WordPress/app.py b/spaces/7thHeaven/GPT2WordPress/app.py
deleted file mode 100644
index b473780af86b2b97c4d4088f47ddc1418cc73c77..0000000000000000000000000000000000000000
--- a/spaces/7thHeaven/GPT2WordPress/app.py
+++ /dev/null
@@ -1,109 +0,0 @@
-import streamlit as st
-import requests
-from wordpress_xmlrpc import Client, WordPressPost
-from wordpress_xmlrpc.methods.posts import NewPost
-import os
-from dotenv import load_dotenv
-
-load_dotenv()
-openai_api_key = os.getenv("OPENAI_API_KEY")
-wp_url = f"{os.getenv('WP_URL')}/xmlrpc.php"
-wp_username = os.getenv("WP_USERNAME")
-wp_password = os.getenv("WP_PASSWORD")
-
-if openai_api_key:
-
- def get_filetext(filename, cache={}):
- if filename not in cache:
- if not os.path.exists(filename):
- raise ValueError(f"ファイル '{filename}' が見つかりませんでした")
- with open(filename, "r") as f:
- cache[filename] = f.read()
- return cache[filename]
-
- def generate_blog_post(prompt):
- constraints = get_filetext(filename="constraints.md")
-
- data = {
- "model": "gpt-4",
- "messages": [
- {"role": "system", "content": constraints},
- {"role": "user", "content": prompt},
- ],
- "max_tokens": 1024,
- "n": 1,
- "stop": None,
- "temperature": 0.7,
- }
-
- response = requests.post(
- "https://api.openai.com/v1/chat/completions",
- headers={
- "Content-Type": "application/json",
- "Authorization": f"Bearer {openai_api_key}"
- },
- json=data
- )
-
- response.raise_for_status()
- choice = response.json()['choices'][0]
- blog_text = choice['message']['content'].strip()
- return blog_text
-
- def post_to_wordpress(title, content):
- client = Client(wp_url, wp_username, wp_password)
- post = WordPressPost()
- post.title = title
- post.content = content
- post.post_status = "publish"
- post_id = client.call(NewPost(post))
- return post_id
-
- st.title("ChatGPTによるブログ記事生成")
- prompt = st.text_input("記事のタイトルを入力してください:")
-
- generated_post = st.session_state.get("generated_post", None)
-
- if st.button("記事生成"):
- generated_post = generate_blog_post(prompt)
- st.session_state.generated_post = generated_post
- st.write("生成されたブログ記事:")
- st.write(generated_post)
-
- if generated_post:
- if st.button("投稿"):
- post_id = post_to_wordpress(prompt, generated_post)
- st.write(f"ブログ記事が投稿されました。記事ID: {post_id}")
-
-else:
- st.write("サービスを利用するためには、このスペースを複製し、以下の環境変数を定義してください。設定方法はosenv_setting_tips.txtを参照してください。")
- st.write("OPENAI_API_KEY, WP_URL, WP_USERNAME, WP_PASSWORD")
-
-st.markdown(
- """
-
-
- """,
- unsafe_allow_html=True,
-)
-
-st.markdown(
- f''
- f'',
- unsafe_allow_html=True,
-)
-
diff --git a/spaces/801artistry/RVC801/lib/infer_pack/modules.py b/spaces/801artistry/RVC801/lib/infer_pack/modules.py
deleted file mode 100644
index c83289df7c79a4810dacd15c050148544ba0b6a9..0000000000000000000000000000000000000000
--- a/spaces/801artistry/RVC801/lib/infer_pack/modules.py
+++ /dev/null
@@ -1,522 +0,0 @@
-import copy
-import math
-import numpy as np
-import scipy
-import torch
-from torch import nn
-from torch.nn import functional as F
-
-from torch.nn import Conv1d, ConvTranspose1d, AvgPool1d, Conv2d
-from torch.nn.utils import weight_norm, remove_weight_norm
-
-from lib.infer_pack import commons
-from lib.infer_pack.commons import init_weights, get_padding
-from lib.infer_pack.transforms import piecewise_rational_quadratic_transform
-
-
-LRELU_SLOPE = 0.1
-
-
-class LayerNorm(nn.Module):
- def __init__(self, channels, eps=1e-5):
- super().__init__()
- self.channels = channels
- self.eps = eps
-
- self.gamma = nn.Parameter(torch.ones(channels))
- self.beta = nn.Parameter(torch.zeros(channels))
-
- def forward(self, x):
- x = x.transpose(1, -1)
- x = F.layer_norm(x, (self.channels,), self.gamma, self.beta, self.eps)
- return x.transpose(1, -1)
-
-
-class ConvReluNorm(nn.Module):
- def __init__(
- self,
- in_channels,
- hidden_channels,
- out_channels,
- kernel_size,
- n_layers,
- p_dropout,
- ):
- super().__init__()
- self.in_channels = in_channels
- self.hidden_channels = hidden_channels
- self.out_channels = out_channels
- self.kernel_size = kernel_size
- self.n_layers = n_layers
- self.p_dropout = p_dropout
- assert n_layers > 1, "Number of layers should be larger than 0."
-
- self.conv_layers = nn.ModuleList()
- self.norm_layers = nn.ModuleList()
- self.conv_layers.append(
- nn.Conv1d(
- in_channels, hidden_channels, kernel_size, padding=kernel_size // 2
- )
- )
- self.norm_layers.append(LayerNorm(hidden_channels))
- self.relu_drop = nn.Sequential(nn.ReLU(), nn.Dropout(p_dropout))
- for _ in range(n_layers - 1):
- self.conv_layers.append(
- nn.Conv1d(
- hidden_channels,
- hidden_channels,
- kernel_size,
- padding=kernel_size // 2,
- )
- )
- self.norm_layers.append(LayerNorm(hidden_channels))
- self.proj = nn.Conv1d(hidden_channels, out_channels, 1)
- self.proj.weight.data.zero_()
- self.proj.bias.data.zero_()
-
- def forward(self, x, x_mask):
- x_org = x
- for i in range(self.n_layers):
- x = self.conv_layers[i](x * x_mask)
- x = self.norm_layers[i](x)
- x = self.relu_drop(x)
- x = x_org + self.proj(x)
- return x * x_mask
-
-
-class DDSConv(nn.Module):
- """
- Dialted and Depth-Separable Convolution
- """
-
- def __init__(self, channels, kernel_size, n_layers, p_dropout=0.0):
- super().__init__()
- self.channels = channels
- self.kernel_size = kernel_size
- self.n_layers = n_layers
- self.p_dropout = p_dropout
-
- self.drop = nn.Dropout(p_dropout)
- self.convs_sep = nn.ModuleList()
- self.convs_1x1 = nn.ModuleList()
- self.norms_1 = nn.ModuleList()
- self.norms_2 = nn.ModuleList()
- for i in range(n_layers):
- dilation = kernel_size**i
- padding = (kernel_size * dilation - dilation) // 2
- self.convs_sep.append(
- nn.Conv1d(
- channels,
- channels,
- kernel_size,
- groups=channels,
- dilation=dilation,
- padding=padding,
- )
- )
- self.convs_1x1.append(nn.Conv1d(channels, channels, 1))
- self.norms_1.append(LayerNorm(channels))
- self.norms_2.append(LayerNorm(channels))
-
- def forward(self, x, x_mask, g=None):
- if g is not None:
- x = x + g
- for i in range(self.n_layers):
- y = self.convs_sep[i](x * x_mask)
- y = self.norms_1[i](y)
- y = F.gelu(y)
- y = self.convs_1x1[i](y)
- y = self.norms_2[i](y)
- y = F.gelu(y)
- y = self.drop(y)
- x = x + y
- return x * x_mask
-
-
-class WN(torch.nn.Module):
- def __init__(
- self,
- hidden_channels,
- kernel_size,
- dilation_rate,
- n_layers,
- gin_channels=0,
- p_dropout=0,
- ):
- super(WN, self).__init__()
- assert kernel_size % 2 == 1
- self.hidden_channels = hidden_channels
- self.kernel_size = (kernel_size,)
- self.dilation_rate = dilation_rate
- self.n_layers = n_layers
- self.gin_channels = gin_channels
- self.p_dropout = p_dropout
-
- self.in_layers = torch.nn.ModuleList()
- self.res_skip_layers = torch.nn.ModuleList()
- self.drop = nn.Dropout(p_dropout)
-
- if gin_channels != 0:
- cond_layer = torch.nn.Conv1d(
- gin_channels, 2 * hidden_channels * n_layers, 1
- )
- self.cond_layer = torch.nn.utils.weight_norm(cond_layer, name="weight")
-
- for i in range(n_layers):
- dilation = dilation_rate**i
- padding = int((kernel_size * dilation - dilation) / 2)
- in_layer = torch.nn.Conv1d(
- hidden_channels,
- 2 * hidden_channels,
- kernel_size,
- dilation=dilation,
- padding=padding,
- )
- in_layer = torch.nn.utils.weight_norm(in_layer, name="weight")
- self.in_layers.append(in_layer)
-
- # last one is not necessary
- if i < n_layers - 1:
- res_skip_channels = 2 * hidden_channels
- else:
- res_skip_channels = hidden_channels
-
- res_skip_layer = torch.nn.Conv1d(hidden_channels, res_skip_channels, 1)
- res_skip_layer = torch.nn.utils.weight_norm(res_skip_layer, name="weight")
- self.res_skip_layers.append(res_skip_layer)
-
- def forward(self, x, x_mask, g=None, **kwargs):
- output = torch.zeros_like(x)
- n_channels_tensor = torch.IntTensor([self.hidden_channels])
-
- if g is not None:
- g = self.cond_layer(g)
-
- for i in range(self.n_layers):
- x_in = self.in_layers[i](x)
- if g is not None:
- cond_offset = i * 2 * self.hidden_channels
- g_l = g[:, cond_offset : cond_offset + 2 * self.hidden_channels, :]
- else:
- g_l = torch.zeros_like(x_in)
-
- acts = commons.fused_add_tanh_sigmoid_multiply(x_in, g_l, n_channels_tensor)
- acts = self.drop(acts)
-
- res_skip_acts = self.res_skip_layers[i](acts)
- if i < self.n_layers - 1:
- res_acts = res_skip_acts[:, : self.hidden_channels, :]
- x = (x + res_acts) * x_mask
- output = output + res_skip_acts[:, self.hidden_channels :, :]
- else:
- output = output + res_skip_acts
- return output * x_mask
-
- def remove_weight_norm(self):
- if self.gin_channels != 0:
- torch.nn.utils.remove_weight_norm(self.cond_layer)
- for l in self.in_layers:
- torch.nn.utils.remove_weight_norm(l)
- for l in self.res_skip_layers:
- torch.nn.utils.remove_weight_norm(l)
-
-
-class ResBlock1(torch.nn.Module):
- def __init__(self, channels, kernel_size=3, dilation=(1, 3, 5)):
- super(ResBlock1, self).__init__()
- self.convs1 = nn.ModuleList(
- [
- weight_norm(
- Conv1d(
- channels,
- channels,
- kernel_size,
- 1,
- dilation=dilation[0],
- padding=get_padding(kernel_size, dilation[0]),
- )
- ),
- weight_norm(
- Conv1d(
- channels,
- channels,
- kernel_size,
- 1,
- dilation=dilation[1],
- padding=get_padding(kernel_size, dilation[1]),
- )
- ),
- weight_norm(
- Conv1d(
- channels,
- channels,
- kernel_size,
- 1,
- dilation=dilation[2],
- padding=get_padding(kernel_size, dilation[2]),
- )
- ),
- ]
- )
- self.convs1.apply(init_weights)
-
- self.convs2 = nn.ModuleList(
- [
- weight_norm(
- Conv1d(
- channels,
- channels,
- kernel_size,
- 1,
- dilation=1,
- padding=get_padding(kernel_size, 1),
- )
- ),
- weight_norm(
- Conv1d(
- channels,
- channels,
- kernel_size,
- 1,
- dilation=1,
- padding=get_padding(kernel_size, 1),
- )
- ),
- weight_norm(
- Conv1d(
- channels,
- channels,
- kernel_size,
- 1,
- dilation=1,
- padding=get_padding(kernel_size, 1),
- )
- ),
- ]
- )
- self.convs2.apply(init_weights)
-
- def forward(self, x, x_mask=None):
- for c1, c2 in zip(self.convs1, self.convs2):
- xt = F.leaky_relu(x, LRELU_SLOPE)
- if x_mask is not None:
- xt = xt * x_mask
- xt = c1(xt)
- xt = F.leaky_relu(xt, LRELU_SLOPE)
- if x_mask is not None:
- xt = xt * x_mask
- xt = c2(xt)
- x = xt + x
- if x_mask is not None:
- x = x * x_mask
- return x
-
- def remove_weight_norm(self):
- for l in self.convs1:
- remove_weight_norm(l)
- for l in self.convs2:
- remove_weight_norm(l)
-
-
-class ResBlock2(torch.nn.Module):
- def __init__(self, channels, kernel_size=3, dilation=(1, 3)):
- super(ResBlock2, self).__init__()
- self.convs = nn.ModuleList(
- [
- weight_norm(
- Conv1d(
- channels,
- channels,
- kernel_size,
- 1,
- dilation=dilation[0],
- padding=get_padding(kernel_size, dilation[0]),
- )
- ),
- weight_norm(
- Conv1d(
- channels,
- channels,
- kernel_size,
- 1,
- dilation=dilation[1],
- padding=get_padding(kernel_size, dilation[1]),
- )
- ),
- ]
- )
- self.convs.apply(init_weights)
-
- def forward(self, x, x_mask=None):
- for c in self.convs:
- xt = F.leaky_relu(x, LRELU_SLOPE)
- if x_mask is not None:
- xt = xt * x_mask
- xt = c(xt)
- x = xt + x
- if x_mask is not None:
- x = x * x_mask
- return x
-
- def remove_weight_norm(self):
- for l in self.convs:
- remove_weight_norm(l)
-
-
-class Log(nn.Module):
- def forward(self, x, x_mask, reverse=False, **kwargs):
- if not reverse:
- y = torch.log(torch.clamp_min(x, 1e-5)) * x_mask
- logdet = torch.sum(-y, [1, 2])
- return y, logdet
- else:
- x = torch.exp(x) * x_mask
- return x
-
-
-class Flip(nn.Module):
- def forward(self, x, *args, reverse=False, **kwargs):
- x = torch.flip(x, [1])
- if not reverse:
- logdet = torch.zeros(x.size(0)).to(dtype=x.dtype, device=x.device)
- return x, logdet
- else:
- return x
-
-
-class ElementwiseAffine(nn.Module):
- def __init__(self, channels):
- super().__init__()
- self.channels = channels
- self.m = nn.Parameter(torch.zeros(channels, 1))
- self.logs = nn.Parameter(torch.zeros(channels, 1))
-
- def forward(self, x, x_mask, reverse=False, **kwargs):
- if not reverse:
- y = self.m + torch.exp(self.logs) * x
- y = y * x_mask
- logdet = torch.sum(self.logs * x_mask, [1, 2])
- return y, logdet
- else:
- x = (x - self.m) * torch.exp(-self.logs) * x_mask
- return x
-
-
-class ResidualCouplingLayer(nn.Module):
- def __init__(
- self,
- channels,
- hidden_channels,
- kernel_size,
- dilation_rate,
- n_layers,
- p_dropout=0,
- gin_channels=0,
- mean_only=False,
- ):
- assert channels % 2 == 0, "channels should be divisible by 2"
- super().__init__()
- self.channels = channels
- self.hidden_channels = hidden_channels
- self.kernel_size = kernel_size
- self.dilation_rate = dilation_rate
- self.n_layers = n_layers
- self.half_channels = channels // 2
- self.mean_only = mean_only
-
- self.pre = nn.Conv1d(self.half_channels, hidden_channels, 1)
- self.enc = WN(
- hidden_channels,
- kernel_size,
- dilation_rate,
- n_layers,
- p_dropout=p_dropout,
- gin_channels=gin_channels,
- )
- self.post = nn.Conv1d(hidden_channels, self.half_channels * (2 - mean_only), 1)
- self.post.weight.data.zero_()
- self.post.bias.data.zero_()
-
- def forward(self, x, x_mask, g=None, reverse=False):
- x0, x1 = torch.split(x, [self.half_channels] * 2, 1)
- h = self.pre(x0) * x_mask
- h = self.enc(h, x_mask, g=g)
- stats = self.post(h) * x_mask
- if not self.mean_only:
- m, logs = torch.split(stats, [self.half_channels] * 2, 1)
- else:
- m = stats
- logs = torch.zeros_like(m)
-
- if not reverse:
- x1 = m + x1 * torch.exp(logs) * x_mask
- x = torch.cat([x0, x1], 1)
- logdet = torch.sum(logs, [1, 2])
- return x, logdet
- else:
- x1 = (x1 - m) * torch.exp(-logs) * x_mask
- x = torch.cat([x0, x1], 1)
- return x
-
- def remove_weight_norm(self):
- self.enc.remove_weight_norm()
-
-
-class ConvFlow(nn.Module):
- def __init__(
- self,
- in_channels,
- filter_channels,
- kernel_size,
- n_layers,
- num_bins=10,
- tail_bound=5.0,
- ):
- super().__init__()
- self.in_channels = in_channels
- self.filter_channels = filter_channels
- self.kernel_size = kernel_size
- self.n_layers = n_layers
- self.num_bins = num_bins
- self.tail_bound = tail_bound
- self.half_channels = in_channels // 2
-
- self.pre = nn.Conv1d(self.half_channels, filter_channels, 1)
- self.convs = DDSConv(filter_channels, kernel_size, n_layers, p_dropout=0.0)
- self.proj = nn.Conv1d(
- filter_channels, self.half_channels * (num_bins * 3 - 1), 1
- )
- self.proj.weight.data.zero_()
- self.proj.bias.data.zero_()
-
- def forward(self, x, x_mask, g=None, reverse=False):
- x0, x1 = torch.split(x, [self.half_channels] * 2, 1)
- h = self.pre(x0)
- h = self.convs(h, x_mask, g=g)
- h = self.proj(h) * x_mask
-
- b, c, t = x0.shape
- h = h.reshape(b, c, -1, t).permute(0, 1, 3, 2) # [b, cx?, t] -> [b, c, t, ?]
-
- unnormalized_widths = h[..., : self.num_bins] / math.sqrt(self.filter_channels)
- unnormalized_heights = h[..., self.num_bins : 2 * self.num_bins] / math.sqrt(
- self.filter_channels
- )
- unnormalized_derivatives = h[..., 2 * self.num_bins :]
-
- x1, logabsdet = piecewise_rational_quadratic_transform(
- x1,
- unnormalized_widths,
- unnormalized_heights,
- unnormalized_derivatives,
- inverse=reverse,
- tails="linear",
- tail_bound=self.tail_bound,
- )
-
- x = torch.cat([x0, x1], 1) * x_mask
- logdet = torch.sum(logabsdet * x_mask, [1, 2])
- if not reverse:
- return x, logdet
- else:
- return x
diff --git a/spaces/801artistry/RVC801/venv.sh b/spaces/801artistry/RVC801/venv.sh
deleted file mode 100644
index aa230992e892292cb8aa5924ecdafc5758f14e95..0000000000000000000000000000000000000000
--- a/spaces/801artistry/RVC801/venv.sh
+++ /dev/null
@@ -1 +0,0 @@
-python3.8 -m venv .venv
diff --git a/spaces/AIFILMS/generate_human_motion/VQ-Trans/options/option_transformer.py b/spaces/AIFILMS/generate_human_motion/VQ-Trans/options/option_transformer.py
deleted file mode 100644
index cf48ce1fdac663ec44419d67721ac268806f8127..0000000000000000000000000000000000000000
--- a/spaces/AIFILMS/generate_human_motion/VQ-Trans/options/option_transformer.py
+++ /dev/null
@@ -1,68 +0,0 @@
-import argparse
-
-def get_args_parser():
- parser = argparse.ArgumentParser(description='Optimal Transport AutoEncoder training for Amass',
- add_help=True,
- formatter_class=argparse.ArgumentDefaultsHelpFormatter)
-
- ## dataloader
-
- parser.add_argument('--dataname', type=str, default='kit', help='dataset directory')
- parser.add_argument('--batch-size', default=128, type=int, help='batch size')
- parser.add_argument('--fps', default=[20], nargs="+", type=int, help='frames per second')
- parser.add_argument('--seq-len', type=int, default=64, help='training motion length')
-
- ## optimization
- parser.add_argument('--total-iter', default=100000, type=int, help='number of total iterations to run')
- parser.add_argument('--warm-up-iter', default=1000, type=int, help='number of total iterations for warmup')
- parser.add_argument('--lr', default=2e-4, type=float, help='max learning rate')
- parser.add_argument('--lr-scheduler', default=[60000], nargs="+", type=int, help="learning rate schedule (iterations)")
- parser.add_argument('--gamma', default=0.05, type=float, help="learning rate decay")
-
- parser.add_argument('--weight-decay', default=1e-6, type=float, help='weight decay')
- parser.add_argument('--decay-option',default='all', type=str, choices=['all', 'noVQ'], help='disable weight decay on codebook')
- parser.add_argument('--optimizer',default='adamw', type=str, choices=['adam', 'adamw'], help='disable weight decay on codebook')
-
- ## vqvae arch
- parser.add_argument("--code-dim", type=int, default=512, help="embedding dimension")
- parser.add_argument("--nb-code", type=int, default=512, help="nb of embedding")
- parser.add_argument("--mu", type=float, default=0.99, help="exponential moving average to update the codebook")
- parser.add_argument("--down-t", type=int, default=3, help="downsampling rate")
- parser.add_argument("--stride-t", type=int, default=2, help="stride size")
- parser.add_argument("--width", type=int, default=512, help="width of the network")
- parser.add_argument("--depth", type=int, default=3, help="depth of the network")
- parser.add_argument("--dilation-growth-rate", type=int, default=3, help="dilation growth rate")
- parser.add_argument("--output-emb-width", type=int, default=512, help="output embedding width")
- parser.add_argument('--vq-act', type=str, default='relu', choices = ['relu', 'silu', 'gelu'], help='dataset directory')
-
- ## gpt arch
- parser.add_argument("--block-size", type=int, default=25, help="seq len")
- parser.add_argument("--embed-dim-gpt", type=int, default=512, help="embedding dimension")
- parser.add_argument("--clip-dim", type=int, default=512, help="latent dimension in the clip feature")
- parser.add_argument("--num-layers", type=int, default=2, help="nb of transformer layers")
- parser.add_argument("--n-head-gpt", type=int, default=8, help="nb of heads")
- parser.add_argument("--ff-rate", type=int, default=4, help="feedforward size")
- parser.add_argument("--drop-out-rate", type=float, default=0.1, help="dropout ratio in the pos encoding")
-
- ## quantizer
- parser.add_argument("--quantizer", type=str, default='ema_reset', choices = ['ema', 'orig', 'ema_reset', 'reset'], help="eps for optimal transport")
- parser.add_argument('--quantbeta', type=float, default=1.0, help='dataset directory')
-
- ## resume
- parser.add_argument("--resume-pth", type=str, default=None, help='resume vq pth')
- parser.add_argument("--resume-trans", type=str, default=None, help='resume gpt pth')
-
-
- ## output directory
- parser.add_argument('--out-dir', type=str, default='output_GPT_Final/', help='output directory')
- parser.add_argument('--exp-name', type=str, default='exp_debug', help='name of the experiment, will create a file inside out-dir')
- parser.add_argument('--vq-name', type=str, default='exp_debug', help='name of the generated dataset .npy, will create a file inside out-dir')
- ## other
- parser.add_argument('--print-iter', default=200, type=int, help='print frequency')
- parser.add_argument('--eval-iter', default=5000, type=int, help='evaluation frequency')
- parser.add_argument('--seed', default=123, type=int, help='seed for initializing training. ')
- parser.add_argument("--if-maxtest", action='store_true', help="test in max")
- parser.add_argument('--pkeep', type=float, default=1.0, help='keep rate for gpt training')
-
-
- return parser.parse_args()
\ No newline at end of file
diff --git a/spaces/AIFILMS/generate_human_motion/VQ-Trans/utils/paramUtil.py b/spaces/AIFILMS/generate_human_motion/VQ-Trans/utils/paramUtil.py
deleted file mode 100644
index a9f1708b85ca80a9051cb3675cec9b999a0d0e2b..0000000000000000000000000000000000000000
--- a/spaces/AIFILMS/generate_human_motion/VQ-Trans/utils/paramUtil.py
+++ /dev/null
@@ -1,63 +0,0 @@
-import numpy as np
-
-# Define a kinematic tree for the skeletal struture
-kit_kinematic_chain = [[0, 11, 12, 13, 14, 15], [0, 16, 17, 18, 19, 20], [0, 1, 2, 3, 4], [3, 5, 6, 7], [3, 8, 9, 10]]
-
-kit_raw_offsets = np.array(
- [
- [0, 0, 0],
- [0, 1, 0],
- [0, 1, 0],
- [0, 1, 0],
- [0, 1, 0],
- [1, 0, 0],
- [0, -1, 0],
- [0, -1, 0],
- [-1, 0, 0],
- [0, -1, 0],
- [0, -1, 0],
- [1, 0, 0],
- [0, -1, 0],
- [0, -1, 0],
- [0, 0, 1],
- [0, 0, 1],
- [-1, 0, 0],
- [0, -1, 0],
- [0, -1, 0],
- [0, 0, 1],
- [0, 0, 1]
- ]
-)
-
-t2m_raw_offsets = np.array([[0,0,0],
- [1,0,0],
- [-1,0,0],
- [0,1,0],
- [0,-1,0],
- [0,-1,0],
- [0,1,0],
- [0,-1,0],
- [0,-1,0],
- [0,1,0],
- [0,0,1],
- [0,0,1],
- [0,1,0],
- [1,0,0],
- [-1,0,0],
- [0,0,1],
- [0,-1,0],
- [0,-1,0],
- [0,-1,0],
- [0,-1,0],
- [0,-1,0],
- [0,-1,0]])
-
-t2m_kinematic_chain = [[0, 2, 5, 8, 11], [0, 1, 4, 7, 10], [0, 3, 6, 9, 12, 15], [9, 14, 17, 19, 21], [9, 13, 16, 18, 20]]
-t2m_left_hand_chain = [[20, 22, 23, 24], [20, 34, 35, 36], [20, 25, 26, 27], [20, 31, 32, 33], [20, 28, 29, 30]]
-t2m_right_hand_chain = [[21, 43, 44, 45], [21, 46, 47, 48], [21, 40, 41, 42], [21, 37, 38, 39], [21, 49, 50, 51]]
-
-
-kit_tgt_skel_id = '03950'
-
-t2m_tgt_skel_id = '000021'
-
diff --git a/spaces/AIGC-Audio/AudioGPT/text_to_audio/Make_An_Audio/ldm/modules/encoders/open_clap/factory.py b/spaces/AIGC-Audio/AudioGPT/text_to_audio/Make_An_Audio/ldm/modules/encoders/open_clap/factory.py
deleted file mode 100644
index 3c3b28658adb03462b9c4b5405548d4e0d1edc5e..0000000000000000000000000000000000000000
--- a/spaces/AIGC-Audio/AudioGPT/text_to_audio/Make_An_Audio/ldm/modules/encoders/open_clap/factory.py
+++ /dev/null
@@ -1,257 +0,0 @@
-import json
-import logging
-import os
-import pathlib
-import re
-from copy import deepcopy
-from pathlib import Path
-
-import torch
-
-from .model import CLAP, convert_weights_to_fp16
-from .openai import load_openai_model
-from .pretrained import get_pretrained_url, download_pretrained
-from .transform import image_transform
-
-_MODEL_CONFIG_PATHS = [Path(__file__).parent / f"model_configs/"]
-_MODEL_CONFIGS = {} # directory (model_name: config) of model architecture configs
-
-
-def _natural_key(string_):
- return [int(s) if s.isdigit() else s for s in re.split(r"(\d+)", string_.lower())]
-
-
-def _rescan_model_configs():
- global _MODEL_CONFIGS
-
- config_ext = (".json",)
- config_files = []
- for config_path in _MODEL_CONFIG_PATHS:
- if config_path.is_file() and config_path.suffix in config_ext:
- config_files.append(config_path)
- elif config_path.is_dir():
- for ext in config_ext:
- config_files.extend(config_path.glob(f"*{ext}"))
-
- for cf in config_files:
- with open(cf, "r") as f:
- model_cfg = json.load(f)
- if all(a in model_cfg for a in ("embed_dim", "audio_cfg", "text_cfg")):
- _MODEL_CONFIGS[cf.stem] = model_cfg
-
- _MODEL_CONFIGS = {
- k: v
- for k, v in sorted(_MODEL_CONFIGS.items(), key=lambda x: _natural_key(x[0]))
- }
-
-
-_rescan_model_configs() # initial populate of model config registry
-
-
-def load_state_dict(checkpoint_path: str, map_location="cpu", skip_params=True):
- checkpoint = torch.load(checkpoint_path, map_location=map_location)
- if isinstance(checkpoint, dict) and "state_dict" in checkpoint:
- state_dict = checkpoint["state_dict"]
- else:
- state_dict = checkpoint
- if skip_params:
- if next(iter(state_dict.items()))[0].startswith("module"):
- state_dict = {k[7:]: v for k, v in state_dict.items()}
- # for k in state_dict:
- # if k.startswith('transformer'):
- # v = state_dict.pop(k)
- # state_dict['text_branch.' + k[12:]] = v
- return state_dict
-
-
-def create_model(
- amodel_name: str,
- tmodel_name: str,
- pretrained: str = "",
- precision: str = "fp32",
- device: torch.device = torch.device("cpu"),
- jit: bool = False,
- force_quick_gelu: bool = False,
- openai_model_cache_dir: str = os.path.expanduser("~/.cache/clip"),
- skip_params=True,
- pretrained_audio: str = "",
- pretrained_text: str = "",
- enable_fusion: bool = False,
- fusion_type: str = 'None'
- # pretrained_image: bool = False,
-):
- amodel_name = amodel_name.replace(
- "/", "-"
- ) # for callers using old naming with / in ViT names
- pretrained_orig = pretrained
- pretrained = pretrained.lower()
- if pretrained == "openai":
- if amodel_name in _MODEL_CONFIGS:
- logging.info(f"Loading {amodel_name} model config.")
- model_cfg = deepcopy(_MODEL_CONFIGS[amodel_name])
- else:
- logging.error(
- f"Model config for {amodel_name} not found; available models {list_models()}."
- )
- raise RuntimeError(f"Model config for {amodel_name} not found.")
-
- logging.info(f"Loading pretrained ViT-B-16 text encoder from OpenAI.")
- # Hard Code in model name
- model_cfg["text_cfg"]["model_type"] = tmodel_name
- model = load_openai_model(
- "ViT-B-16",
- model_cfg,
- device=device,
- jit=jit,
- cache_dir=openai_model_cache_dir,
- enable_fusion=enable_fusion,
- fusion_type=fusion_type
- )
- # See https://discuss.pytorch.org/t/valueerror-attemting-to-unscale-fp16-gradients/81372
- if precision == "amp" or precision == "fp32":
- model = model.float()
- else:
- if amodel_name in _MODEL_CONFIGS:
- logging.info(f"Loading {amodel_name} model config.")
- model_cfg = deepcopy(_MODEL_CONFIGS[amodel_name])
- else:
- logging.error(
- f"Model config for {amodel_name} not found; available models {list_models()}."
- )
- raise RuntimeError(f"Model config for {amodel_name} not found.")
-
- if force_quick_gelu:
- # override for use of QuickGELU on non-OpenAI transformer models
- model_cfg["quick_gelu"] = True
-
- # if pretrained_image:
- # if 'timm_amodel_name' in model_cfg.get('vision_cfg', {}):
- # # pretrained weight loading for timm models set via vision_cfg
- # model_cfg['vision_cfg']['timm_model_pretrained'] = True
- # else:
- # assert False, 'pretrained image towers currently only supported for timm models'
- model_cfg["text_cfg"]["model_type"] = tmodel_name
- model_cfg["enable_fusion"] = enable_fusion
- model_cfg["fusion_type"] = fusion_type
- model = CLAP(**model_cfg)
-
- if pretrained:
- checkpoint_path = ""
- url = get_pretrained_url(amodel_name, pretrained)
- if url:
- checkpoint_path = download_pretrained(url, root=openai_model_cache_dir)
- elif os.path.exists(pretrained_orig):
- checkpoint_path = pretrained_orig
- if checkpoint_path:
- logging.info(f"Loading pretrained {amodel_name}-{tmodel_name} weights ({pretrained}).")
- ckpt = load_state_dict(checkpoint_path, skip_params=True)
- model.load_state_dict(ckpt)
- param_names = [n for n, p in model.named_parameters()]
- for n in param_names:
- print(n, "\t", "Loaded" if n in ckpt else "Unloaded")
- else:
- logging.warning(
- f"Pretrained weights ({pretrained}) not found for model {amodel_name}."
- )
- raise RuntimeError(
- f"Pretrained weights ({pretrained}) not found for model {amodel_name}."
- )
-
- if pretrained_audio:
- if amodel_name.startswith('PANN'):
- if 'Cnn14_mAP' in pretrained_audio: # official checkpoint
- audio_ckpt = torch.load(pretrained_audio, map_location='cpu')
- audio_ckpt = audio_ckpt['model']
- keys = list(audio_ckpt.keys())
- for key in keys:
- if 'spectrogram_extractor' not in key and 'logmel_extractor' not in key:
- v = audio_ckpt.pop(key)
- audio_ckpt['audio_branch.' + key] = v
- elif os.path.basename(pretrained_audio).startswith('PANN'): # checkpoint trained via HTSAT codebase
- audio_ckpt = torch.load(pretrained_audio, map_location='cpu')
- audio_ckpt = audio_ckpt['state_dict']
- keys = list(audio_ckpt.keys())
- for key in keys:
- if key.startswith('sed_model'):
- v = audio_ckpt.pop(key)
- audio_ckpt['audio_branch.' + key[10:]] = v
- elif os.path.basename(pretrained_audio).startswith('finetuned'): # checkpoint trained via linear probe codebase
- audio_ckpt = torch.load(pretrained_audio, map_location='cpu')
- else:
- raise ValueError('Unknown audio checkpoint')
- elif amodel_name.startswith('HTSAT'):
- if 'HTSAT_AudioSet_Saved' in pretrained_audio: # official checkpoint
- audio_ckpt = torch.load(pretrained_audio, map_location='cpu')
- audio_ckpt = audio_ckpt['state_dict']
- keys = list(audio_ckpt.keys())
- for key in keys:
- if key.startswith('sed_model') and ('spectrogram_extractor' not in key
- and 'logmel_extractor' not in key):
- v = audio_ckpt.pop(key)
- audio_ckpt['audio_branch.' + key[10:]] = v
- elif os.path.basename(pretrained_audio).startswith('HTSAT'): # checkpoint trained via HTSAT codebase
- audio_ckpt = torch.load(pretrained_audio, map_location='cpu')
- audio_ckpt = audio_ckpt['state_dict']
- keys = list(audio_ckpt.keys())
- for key in keys:
- if key.startswith('sed_model'):
- v = audio_ckpt.pop(key)
- audio_ckpt['audio_branch.' + key[10:]] = v
- elif os.path.basename(pretrained_audio).startswith('finetuned'): # checkpoint trained via linear probe codebase
- audio_ckpt = torch.load(pretrained_audio, map_location='cpu')
- else:
- raise ValueError('Unknown audio checkpoint')
- else:
- raise f'this audio encoder pretrained checkpoint is not support'
-
- model.load_state_dict(audio_ckpt, strict=False)
- logging.info(f"Loading pretrained {amodel_name} weights ({pretrained_audio}).")
- param_names = [n for n, p in model.named_parameters()]
- for n in param_names:
- print(n, "\t", "Loaded" if n in audio_ckpt else "Unloaded")
-
- model.to(device=device)
- if precision == "fp16":
- assert device.type != "cpu"
- convert_weights_to_fp16(model)
-
- if jit:
- model = torch.jit.script(model)
-
- return model, model_cfg
-
-
-def create_model_and_transforms(
- model_name: str,
- pretrained: str = "",
- precision: str = "fp32",
- device: torch.device = torch.device("cpu"),
- jit: bool = False,
- force_quick_gelu: bool = False,
- # pretrained_image: bool = False,
-):
- model = create_model(
- model_name,
- pretrained,
- precision,
- device,
- jit,
- force_quick_gelu=force_quick_gelu,
- # pretrained_image=pretrained_image
- )
- preprocess_train = image_transform(model.visual.image_size, is_train=True)
- preprocess_val = image_transform(model.visual.image_size, is_train=False)
- return model, preprocess_train, preprocess_val
-
-
-def list_models():
- """enumerate available model architectures based on config files"""
- return list(_MODEL_CONFIGS.keys())
-
-
-def add_model_config(path):
- """add model config path or file and update registry"""
- if not isinstance(path, Path):
- path = Path(path)
- _MODEL_CONFIG_PATHS.append(path)
- _rescan_model_configs()
diff --git a/spaces/AIZeroToHero/05-RealtimeStreamlitASR/app.py b/spaces/AIZeroToHero/05-RealtimeStreamlitASR/app.py
deleted file mode 100644
index e0f03cf2557eba112bf95ebf5eb582da8d8a0fe3..0000000000000000000000000000000000000000
--- a/spaces/AIZeroToHero/05-RealtimeStreamlitASR/app.py
+++ /dev/null
@@ -1,119 +0,0 @@
-from collections import deque
-import streamlit as st
-import torch
-from streamlit_player import st_player
-from transformers import AutoModelForCTC, Wav2Vec2Processor
-from streaming import ffmpeg_stream
-
-device = torch.device('cuda' if torch.cuda.is_available() else 'cpu')
-player_options = {
- "events": ["onProgress"],
- "progress_interval": 200,
- "volume": 1.0,
- "playing": True,
- "loop": False,
- "controls": False,
- "muted": False,
- "config": {"youtube": {"playerVars": {"start": 1}}},
-}
-
-# disable rapid fading in and out on `st.code` updates
-st.markdown("", unsafe_allow_html=True)
-
-@st.cache(hash_funcs={torch.nn.parameter.Parameter: lambda _: None})
-def load_model(model_path="facebook/wav2vec2-large-robust-ft-swbd-300h"):
- processor = Wav2Vec2Processor.from_pretrained(model_path)
- model = AutoModelForCTC.from_pretrained(model_path).to(device)
- return processor, model
-
-processor, model = load_model()
-
-def stream_text(url, chunk_duration_ms, pad_duration_ms):
- sampling_rate = processor.feature_extractor.sampling_rate
-
- # calculate the length of logits to cut from the sides of the output to account for input padding
- output_pad_len = model._get_feat_extract_output_lengths(int(sampling_rate * pad_duration_ms / 1000))
-
- # define the audio chunk generator
- stream = ffmpeg_stream(url, sampling_rate, chunk_duration_ms=chunk_duration_ms, pad_duration_ms=pad_duration_ms)
-
- leftover_text = ""
- for i, chunk in enumerate(stream):
- input_values = processor(chunk, sampling_rate=sampling_rate, return_tensors="pt").input_values
-
- with torch.no_grad():
- logits = model(input_values.to(device)).logits[0]
- if i > 0:
- logits = logits[output_pad_len : len(logits) - output_pad_len]
- else: # don't count padding at the start of the clip
- logits = logits[: len(logits) - output_pad_len]
-
- predicted_ids = torch.argmax(logits, dim=-1).cpu().tolist()
- if processor.decode(predicted_ids).strip():
- leftover_ids = processor.tokenizer.encode(leftover_text)
- # concat the last word (or its part) from the last frame with the current text
- text = processor.decode(leftover_ids + predicted_ids)
- # don't return the last word in case it's just partially recognized
- text, leftover_text = text.rsplit(" ", 1)
- yield text
- else:
- yield leftover_text
- leftover_text = ""
- yield leftover_text
-
-def main():
- state = st.session_state
- st.header("Video ASR Streamlit from Youtube Link")
-
- with st.form(key="inputs_form"):
-
- # Our worlds best teachers on subjects of AI, Cognitive, Neuroscience for our Behavioral and Medical Health
- ytJoschaBach="https://youtu.be/cC1HszE5Hcw?list=PLHgX2IExbFouJoqEr8JMF5MbZSbyC91-L&t=8984"
- ytSamHarris="https://www.youtube.com/watch?v=4dC_nRYIDZU&list=PLHgX2IExbFouJoqEr8JMF5MbZSbyC91-L&index=2"
- ytJohnAbramson="https://www.youtube.com/watch?v=arrokG3wCdE&list=PLHgX2IExbFouJoqEr8JMF5MbZSbyC91-L&index=3"
- ytElonMusk="https://www.youtube.com/watch?v=DxREm3s1scA&list=PLHgX2IExbFouJoqEr8JMF5MbZSbyC91-L&index=4"
- ytJeffreyShainline="https://www.youtube.com/watch?v=EwueqdgIvq4&list=PLHgX2IExbFouJoqEr8JMF5MbZSbyC91-L&index=5"
- ytJeffHawkins="https://www.youtube.com/watch?v=Z1KwkpTUbkg&list=PLHgX2IExbFouJoqEr8JMF5MbZSbyC91-L&index=6"
- ytSamHarris="https://youtu.be/Ui38ZzTymDY?list=PLHgX2IExbFouJoqEr8JMF5MbZSbyC91-L"
- ytSamHarris="https://youtu.be/4dC_nRYIDZU?list=PLHgX2IExbFouJoqEr8JMF5MbZSbyC91-L&t=7809"
- ytSamHarris="https://youtu.be/4dC_nRYIDZU?list=PLHgX2IExbFouJoqEr8JMF5MbZSbyC91-L&t=7809"
- ytSamHarris="https://youtu.be/4dC_nRYIDZU?list=PLHgX2IExbFouJoqEr8JMF5MbZSbyC91-L&t=7809"
- ytTimelapseAI="https://www.youtube.com/watch?v=63yr9dlI0cU&list=PLHgX2IExbFovQybyfltywXnqZi5YvaSS-"
- state.youtube_url = st.text_input("YouTube URL", ytTimelapseAI)
-
-
- state.chunk_duration_ms = st.slider("Audio chunk duration (ms)", 2000, 10000, 3000, 100)
- state.pad_duration_ms = st.slider("Padding duration (ms)", 100, 5000, 1000, 100)
- submit_button = st.form_submit_button(label="Submit")
-
- if submit_button or "asr_stream" not in state:
- # a hack to update the video player on value changes
- state.youtube_url = (
- state.youtube_url.split("&hash=")[0]
- + f"&hash={state.chunk_duration_ms}-{state.pad_duration_ms}"
- )
- state.asr_stream = stream_text(
- state.youtube_url, state.chunk_duration_ms, state.pad_duration_ms
- )
- state.chunks_taken = 0
-
-
- state.lines = deque([], maxlen=100) # limit to the last n lines of subs
-
-
- player = st_player(state.youtube_url, **player_options, key="youtube_player")
-
- if "asr_stream" in state and player.data and player.data["played"] < 1.0:
- # check how many seconds were played, and if more than processed - write the next text chunk
- processed_seconds = state.chunks_taken * (state.chunk_duration_ms / 1000)
- if processed_seconds < player.data["playedSeconds"]:
- text = next(state.asr_stream)
- state.lines.append(text)
- state.chunks_taken += 1
- if "lines" in state:
- # print the lines of subs
- st.code("\n".join(state.lines))
-
-
-if __name__ == "__main__":
- main()
\ No newline at end of file
diff --git a/spaces/AUBADA-ALARABI/poetry202/app.py b/spaces/AUBADA-ALARABI/poetry202/app.py
deleted file mode 100644
index 5b6654d5a405778ddbc9ca5fa5d041aff535f3b5..0000000000000000000000000000000000000000
--- a/spaces/AUBADA-ALARABI/poetry202/app.py
+++ /dev/null
@@ -1,53 +0,0 @@
-import gc
-import gradio as gr
-from transformers import pipeline, set_seed
-
-pipe = pipeline('text-generation', framework='pt', model='akhooli/ap2023', tokenizer='akhooli/ap2023')
-#gc.collect()
-samples = [['أنت'
- ,1.0, 50, 1.0, 1.0, 114],['هل غادر'
- ,1.0, 50, 1.0, 1.0, 114 ],['ألا ليت'
- ,1.0, 50, 1.0, 1.0, 114 ],['يا قدس'
- ,1.0, 50, 1.0, 1.0, 114],['عيد بأية حال'
- ,1.0, 50, 1.0, 1.0, 114],['لكل شيء إذا ما'
- ,1.0, 50, 1.0, 1.0, 114 ],['.'
- ,1.0, 50, 1.0, 1.0, 114]]
-
-notes = """
-- Enter a short prompt or select (click) one of the examples and click SEND
-- Adjust parameters (temperture, top k, top p and penalty) through the slider (keep close to default values).
-- For the same seed (randomness), the same output is regenerated if other parameters are fixed
-- Clear and enter new prompt or select another example and SEND to regenerate
-- The '.' means start a new line from no prompt (your prompt need not be long)
-- Be patient: this runs on CPU (free tier)
-- Feedback (Twitter): @akhooli (https://twitter.com/akhooli/status/1611025232201977859)
-- Note/Disclaimer: may generate unaccepted or inappropriate content. Use at your own risk.
-"""
-def sayPoetry(prompt, temp=1.0, topk = 50, topp = 1.0, penalty=1.0, seed=114):
- if not int(seed) >= 0: seed=114
- set_seed(seed)
- gen = pipe(prompt, max_length=96, do_sample=True, temperature=temp, top_k=topk, top_p=topp, repetition_penalty=penalty,
- min_length = 64, no_repeat_ngram_size = 3, return_full_text=True,
- num_beams=5, num_return_sequences=1)[0]["generated_text"]
- poetry =""
- for line in gen.split('.')[:-1]:
- poetry += line #+ "\n"
- return poetry
-poetry = gr.Interface(fn=sayPoetry,
- inputs=[
- gr.Textbox(label="Enter short prompt or select from examples:"),
- gr.Slider(0.70, 1.2, step=0.01,value=1.0, label='control temperature'),
- gr.Slider(25, 100, step=1,value=50, label='control top k'),
- gr.Slider(0.80, 1.0, step=0.01,value=1.0, label='control top p'),
- gr.Slider(0.90, 1.50, step=0.01,value=1.0, label='control penalty'),
- gr.Number(value=139750, precision=0, label='Seed'),
- ],
- outputs=[gr.Textbox(label="Generated Poetry:")],
-
- allow_flagging='never',
- title='Arabic Poetry Generation Demo (updated Jan. 2023)',
- description = "A simple demo of AI generated poetry based on 1M poems fine-tuned using AraGPT2 (be patient, runs on cpu)",
- examples=samples,
- cache_examples=False,
- article = notes)
-poetry.launch() # show_error = True, debug=True
\ No newline at end of file
diff --git a/spaces/Abdllh/poetry202/README.md b/spaces/Abdllh/poetry202/README.md
deleted file mode 100644
index c958a0c31dcf28cc9fa8983a3f43d6b3b0481875..0000000000000000000000000000000000000000
--- a/spaces/Abdllh/poetry202/README.md
+++ /dev/null
@@ -1,13 +0,0 @@
----
-title: Poetry2023
-emoji: 👁
-colorFrom: green
-colorTo: gray
-sdk: gradio
-sdk_version: 3.16.0
-app_file: app.py
-pinned: false
-duplicated_from: akhooli/poetry2023
----
-
-Check out the configuration reference at https://huggingface.co/docs/hub/spaces-config-reference
diff --git a/spaces/AchyuthGamer/OpenGPT/client/js/change-language.js b/spaces/AchyuthGamer/OpenGPT/client/js/change-language.js
deleted file mode 100644
index ce87f6f60c7a9acca5e1902612930ef677f3fb65..0000000000000000000000000000000000000000
--- a/spaces/AchyuthGamer/OpenGPT/client/js/change-language.js
+++ /dev/null
@@ -1,47 +0,0 @@
-document.addEventListener('DOMContentLoaded', fetchLanguages);
-
-async function fetchLanguages() {
- try {
- const [languagesResponse, currentLanguageResponse] = await Promise.all([
- fetch(`${url_prefix}/get-languages`),
- fetch(`${url_prefix}/get-locale`)
- ]);
-
- const languages = await languagesResponse.json();
- const currentLanguage = await currentLanguageResponse.text();
-
- const languageSelect = document.getElementById('language');
- languages.forEach(lang => {
- const option = document.createElement('option');
- option.value = lang;
- option.textContent = lang;
- languageSelect.appendChild(option);
- });
-
- const savedLanguage = localStorage.getItem("language") || currentLanguage;
- setLanguageOnPageLoad(savedLanguage);
- } catch (error) {
- console.error("Failed to fetch languages or current language");
- }
-}
-
-function setLanguageOnPageLoad(language) {
- document.getElementById("language").value = language;
-}
-
-function changeLanguage(lang) {
- fetch(`${url_prefix}/change-language`, {
- method: "POST",
- headers: {
- "Content-Type": "application/json",
- },
- body: JSON.stringify({ language: lang }),
- }).then((response) => {
- if (response.ok) {
- localStorage.setItem("language", lang);
- location.reload();
- } else {
- console.error("Failed to change language");
- }
- });
-}
diff --git a/spaces/AdithyaSNair/Medical_price_prediction/README.md b/spaces/AdithyaSNair/Medical_price_prediction/README.md
deleted file mode 100644
index 65faf95e65f584327ebba3cc4b82c47b2aacebfa..0000000000000000000000000000000000000000
--- a/spaces/AdithyaSNair/Medical_price_prediction/README.md
+++ /dev/null
@@ -1,12 +0,0 @@
----
-title: Medical Price Prediction
-emoji: 📚
-colorFrom: red
-colorTo: indigo
-sdk: gradio
-sdk_version: 3.16.2
-app_file: app.py
-pinned: false
----
-
-Check out the configuration reference at https://huggingface.co/docs/hub/spaces-config-reference
diff --git a/spaces/AgentVerse/agentVerse/agentverse/environments/simulation_env/rules/selector/basic.py b/spaces/AgentVerse/agentVerse/agentverse/environments/simulation_env/rules/selector/basic.py
deleted file mode 100644
index 1ebc0b48ba773245df7148e4cebc17c38f0a9373..0000000000000000000000000000000000000000
--- a/spaces/AgentVerse/agentVerse/agentverse/environments/simulation_env/rules/selector/basic.py
+++ /dev/null
@@ -1,27 +0,0 @@
-from __future__ import annotations
-
-from typing import TYPE_CHECKING, List
-
-from agentverse.message import Message
-
-from . import selector_registry as SelectorRegistry
-from .base import BaseSelector
-
-if TYPE_CHECKING:
- from agentverse.environments import BaseEnvironment
-
-
-@SelectorRegistry.register("basic")
-class BasicSelector(BaseSelector):
- """
- Base class for all selecters
- """
-
- def select_message(
- self, environment: BaseEnvironment, messages: List[Message]
- ) -> List[Message]:
- """Selects a set of valid messages from all messages"""
- return messages
-
- def reset(self) -> None:
- pass
diff --git a/spaces/AgentVerse/agentVerse/ui/src/phaser3-rex-plugins/templates/ui/alphamaskimage/AlphaMaskImage.js b/spaces/AgentVerse/agentVerse/ui/src/phaser3-rex-plugins/templates/ui/alphamaskimage/AlphaMaskImage.js
deleted file mode 100644
index 7bfad1377a8e736d5f7f4dd2a39d403c68aa68db..0000000000000000000000000000000000000000
--- a/spaces/AgentVerse/agentVerse/ui/src/phaser3-rex-plugins/templates/ui/alphamaskimage/AlphaMaskImage.js
+++ /dev/null
@@ -1,2 +0,0 @@
-import AlphaMaskImage from '../../../plugins/alphamaskimage.js';
-export default AlphaMaskImage;
\ No newline at end of file
diff --git a/spaces/AgentVerse/agentVerse/ui/src/phaser3-rex-plugins/templates/ui/filechooser/Factory.d.ts b/spaces/AgentVerse/agentVerse/ui/src/phaser3-rex-plugins/templates/ui/filechooser/Factory.d.ts
deleted file mode 100644
index f1a7c08fd9880511b28ebc37e19a97dd1406fe1b..0000000000000000000000000000000000000000
--- a/spaces/AgentVerse/agentVerse/ui/src/phaser3-rex-plugins/templates/ui/filechooser/Factory.d.ts
+++ /dev/null
@@ -1,5 +0,0 @@
-import { FileChooser } from './FileChooser.js';
-
-export default function (
- config?: FileChooser.IConfig
-): FileChooser;
diff --git "a/spaces/Ameaou/academic-chatgpt3.1/crazy_functions/\346\211\271\351\207\217Markdown\347\277\273\350\257\221.py" "b/spaces/Ameaou/academic-chatgpt3.1/crazy_functions/\346\211\271\351\207\217Markdown\347\277\273\350\257\221.py"
deleted file mode 100644
index ee6a1a44340ac2cf8fc3a4323c23218c69e0946f..0000000000000000000000000000000000000000
--- "a/spaces/Ameaou/academic-chatgpt3.1/crazy_functions/\346\211\271\351\207\217Markdown\347\277\273\350\257\221.py"
+++ /dev/null
@@ -1,161 +0,0 @@
-from toolbox import update_ui
-from toolbox import CatchException, report_execption, write_results_to_file
-fast_debug = False
-
-class PaperFileGroup():
- def __init__(self):
- self.file_paths = []
- self.file_contents = []
- self.sp_file_contents = []
- self.sp_file_index = []
- self.sp_file_tag = []
-
- # count_token
- from request_llm.bridge_all import model_info
- enc = model_info["gpt-3.5-turbo"]['tokenizer']
- def get_token_num(txt): return len(enc.encode(txt, disallowed_special=()))
- self.get_token_num = get_token_num
-
- def run_file_split(self, max_token_limit=1900):
- """
- 将长文本分离开来
- """
- for index, file_content in enumerate(self.file_contents):
- if self.get_token_num(file_content) < max_token_limit:
- self.sp_file_contents.append(file_content)
- self.sp_file_index.append(index)
- self.sp_file_tag.append(self.file_paths[index])
- else:
- from .crazy_utils import breakdown_txt_to_satisfy_token_limit_for_pdf
- segments = breakdown_txt_to_satisfy_token_limit_for_pdf(file_content, self.get_token_num, max_token_limit)
- for j, segment in enumerate(segments):
- self.sp_file_contents.append(segment)
- self.sp_file_index.append(index)
- self.sp_file_tag.append(self.file_paths[index] + f".part-{j}.md")
-
- print('Segmentation: done')
-
-def 多文件翻译(file_manifest, project_folder, llm_kwargs, plugin_kwargs, chatbot, history, system_prompt, language='en'):
- import time, os, re
- from .crazy_utils import request_gpt_model_multi_threads_with_very_awesome_ui_and_high_efficiency
-
- # <-------- 读取Markdown文件,删除其中的所有注释 ---------->
- pfg = PaperFileGroup()
-
- for index, fp in enumerate(file_manifest):
- with open(fp, 'r', encoding='utf-8', errors='replace') as f:
- file_content = f.read()
- # 记录删除注释后的文本
- pfg.file_paths.append(fp)
- pfg.file_contents.append(file_content)
-
- # <-------- 拆分过长的Markdown文件 ---------->
- pfg.run_file_split(max_token_limit=1500)
- n_split = len(pfg.sp_file_contents)
-
- # <-------- 多线程润色开始 ---------->
- if language == 'en->zh':
- inputs_array = ["This is a Markdown file, translate it into Chinese, do not modify any existing Markdown commands:" +
- f"\n\n{frag}" for frag in pfg.sp_file_contents]
- inputs_show_user_array = [f"翻译 {f}" for f in pfg.sp_file_tag]
- sys_prompt_array = ["You are a professional academic paper translator." for _ in range(n_split)]
- elif language == 'zh->en':
- inputs_array = [f"This is a Markdown file, translate it into English, do not modify any existing Markdown commands:" +
- f"\n\n{frag}" for frag in pfg.sp_file_contents]
- inputs_show_user_array = [f"翻译 {f}" for f in pfg.sp_file_tag]
- sys_prompt_array = ["You are a professional academic paper translator." for _ in range(n_split)]
-
- gpt_response_collection = yield from request_gpt_model_multi_threads_with_very_awesome_ui_and_high_efficiency(
- inputs_array=inputs_array,
- inputs_show_user_array=inputs_show_user_array,
- llm_kwargs=llm_kwargs,
- chatbot=chatbot,
- history_array=[[""] for _ in range(n_split)],
- sys_prompt_array=sys_prompt_array,
- # max_workers=5, # OpenAI所允许的最大并行过载
- scroller_max_len = 80
- )
-
- # <-------- 整理结果,退出 ---------->
- create_report_file_name = time.strftime("%Y-%m-%d-%H-%M-%S", time.localtime()) + f"-chatgpt.polish.md"
- res = write_results_to_file(gpt_response_collection, file_name=create_report_file_name)
- history = gpt_response_collection
- chatbot.append((f"{fp}完成了吗?", res))
- yield from update_ui(chatbot=chatbot, history=history) # 刷新界面
-
-
-
-
-
-@CatchException
-def Markdown英译中(txt, llm_kwargs, plugin_kwargs, chatbot, history, system_prompt, web_port):
- # 基本信息:功能、贡献者
- chatbot.append([
- "函数插件功能?",
- "对整个Markdown项目进行翻译。函数插件贡献者: Binary-Husky"])
- yield from update_ui(chatbot=chatbot, history=history) # 刷新界面
-
- # 尝试导入依赖,如果缺少依赖,则给出安装建议
- try:
- import tiktoken
- except:
- report_execption(chatbot, history,
- a=f"解析项目: {txt}",
- b=f"导入软件依赖失败。使用该模块需要额外依赖,安装方法```pip install --upgrade tiktoken```。")
- yield from update_ui(chatbot=chatbot, history=history) # 刷新界面
- return
- history = [] # 清空历史,以免输入溢出
- import glob, os
- if os.path.exists(txt):
- project_folder = txt
- else:
- if txt == "": txt = '空空如也的输入栏'
- report_execption(chatbot, history, a = f"解析项目: {txt}", b = f"找不到本地项目或无权访问: {txt}")
- yield from update_ui(chatbot=chatbot, history=history) # 刷新界面
- return
- file_manifest = [f for f in glob.glob(f'{project_folder}/**/*.md', recursive=True)]
- if len(file_manifest) == 0:
- report_execption(chatbot, history, a = f"解析项目: {txt}", b = f"找不到任何.md文件: {txt}")
- yield from update_ui(chatbot=chatbot, history=history) # 刷新界面
- return
- yield from 多文件翻译(file_manifest, project_folder, llm_kwargs, plugin_kwargs, chatbot, history, system_prompt, language='en->zh')
-
-
-
-
-
-@CatchException
-def Markdown中译英(txt, llm_kwargs, plugin_kwargs, chatbot, history, system_prompt, web_port):
- # 基本信息:功能、贡献者
- chatbot.append([
- "函数插件功能?",
- "对整个Markdown项目进行翻译。函数插件贡献者: Binary-Husky"])
- yield from update_ui(chatbot=chatbot, history=history) # 刷新界面
-
- # 尝试导入依赖,如果缺少依赖,则给出安装建议
- try:
- import tiktoken
- except:
- report_execption(chatbot, history,
- a=f"解析项目: {txt}",
- b=f"导入软件依赖失败。使用该模块需要额外依赖,安装方法```pip install --upgrade tiktoken```。")
- yield from update_ui(chatbot=chatbot, history=history) # 刷新界面
- return
- history = [] # 清空历史,以免输入溢出
- import glob, os
- if os.path.exists(txt):
- project_folder = txt
- else:
- if txt == "": txt = '空空如也的输入栏'
- report_execption(chatbot, history, a = f"解析项目: {txt}", b = f"找不到本地项目或无权访问: {txt}")
- yield from update_ui(chatbot=chatbot, history=history) # 刷新界面
- return
- if txt.endswith('.md'):
- file_manifest = [txt]
- else:
- file_manifest = [f for f in glob.glob(f'{project_folder}/**/*.md', recursive=True)]
- if len(file_manifest) == 0:
- report_execption(chatbot, history, a = f"解析项目: {txt}", b = f"找不到任何.md文件: {txt}")
- yield from update_ui(chatbot=chatbot, history=history) # 刷新界面
- return
- yield from 多文件翻译(file_manifest, project_folder, llm_kwargs, plugin_kwargs, chatbot, history, system_prompt, language='zh->en')
\ No newline at end of file
diff --git a/spaces/Andy1621/uniformer_image_detection/configs/_base_/models/mask_rcnn_uniformer_fpn.py b/spaces/Andy1621/uniformer_image_detection/configs/_base_/models/mask_rcnn_uniformer_fpn.py
deleted file mode 100644
index ef5a368c6386138e43fa9a2d4fbdc0f5dfa9c982..0000000000000000000000000000000000000000
--- a/spaces/Andy1621/uniformer_image_detection/configs/_base_/models/mask_rcnn_uniformer_fpn.py
+++ /dev/null
@@ -1,121 +0,0 @@
-# model settings
-model = dict(
- type='MaskRCNN',
- pretrained=None,
- backbone=dict(
- type='UniFormer',
- embed_dim=[64, 128, 320, 512],
- layers=[3, 4, 8, 3],
- head_dim=64,
- mlp_ratio=4.,
- qkv_bias=True,
- drop_rate=0.,
- attn_drop_rate=0.,
- drop_path_rate=0.2),
- neck=dict(
- type='FPN',
- in_channels=[64, 128, 320, 512],
- out_channels=256,
- num_outs=5),
- rpn_head=dict(
- type='RPNHead',
- in_channels=256,
- feat_channels=256,
- anchor_generator=dict(
- type='AnchorGenerator',
- scales=[8],
- ratios=[0.5, 1.0, 2.0],
- strides=[4, 8, 16, 32, 64]),
- bbox_coder=dict(
- type='DeltaXYWHBBoxCoder',
- target_means=[.0, .0, .0, .0],
- target_stds=[1.0, 1.0, 1.0, 1.0]),
- loss_cls=dict(
- type='CrossEntropyLoss', use_sigmoid=True, loss_weight=1.0),
- loss_bbox=dict(type='L1Loss', loss_weight=1.0)),
- roi_head=dict(
- type='StandardRoIHead',
- bbox_roi_extractor=dict(
- type='SingleRoIExtractor',
- roi_layer=dict(type='RoIAlign', output_size=7, sampling_ratio=0),
- out_channels=256,
- featmap_strides=[4, 8, 16, 32]),
- bbox_head=dict(
- type='Shared2FCBBoxHead',
- in_channels=256,
- fc_out_channels=1024,
- roi_feat_size=7,
- num_classes=80,
- bbox_coder=dict(
- type='DeltaXYWHBBoxCoder',
- target_means=[0., 0., 0., 0.],
- target_stds=[0.1, 0.1, 0.2, 0.2]),
- reg_class_agnostic=False,
- loss_cls=dict(
- type='CrossEntropyLoss', use_sigmoid=False, loss_weight=1.0),
- loss_bbox=dict(type='L1Loss', loss_weight=1.0)),
- mask_roi_extractor=dict(
- type='SingleRoIExtractor',
- roi_layer=dict(type='RoIAlign', output_size=14, sampling_ratio=0),
- out_channels=256,
- featmap_strides=[4, 8, 16, 32]),
- mask_head=dict(
- type='FCNMaskHead',
- num_convs=4,
- in_channels=256,
- conv_out_channels=256,
- num_classes=80,
- loss_mask=dict(
- type='CrossEntropyLoss', use_mask=True, loss_weight=1.0))),
- # model training and testing settings
- train_cfg=dict(
- rpn=dict(
- assigner=dict(
- type='MaxIoUAssigner',
- pos_iou_thr=0.7,
- neg_iou_thr=0.3,
- min_pos_iou=0.3,
- match_low_quality=True,
- ignore_iof_thr=-1),
- sampler=dict(
- type='RandomSampler',
- num=256,
- pos_fraction=0.5,
- neg_pos_ub=-1,
- add_gt_as_proposals=False),
- allowed_border=-1,
- pos_weight=-1,
- debug=False),
- rpn_proposal=dict(
- nms_pre=2000,
- max_per_img=1000,
- nms=dict(type='nms', iou_threshold=0.7),
- min_bbox_size=0),
- rcnn=dict(
- assigner=dict(
- type='MaxIoUAssigner',
- pos_iou_thr=0.5,
- neg_iou_thr=0.5,
- min_pos_iou=0.5,
- match_low_quality=True,
- ignore_iof_thr=-1),
- sampler=dict(
- type='RandomSampler',
- num=512,
- pos_fraction=0.25,
- neg_pos_ub=-1,
- add_gt_as_proposals=True),
- mask_size=28,
- pos_weight=-1,
- debug=False)),
- test_cfg=dict(
- rpn=dict(
- nms_pre=1000,
- max_per_img=1000,
- nms=dict(type='nms', iou_threshold=0.7),
- min_bbox_size=0),
- rcnn=dict(
- score_thr=0.05,
- nms=dict(type='nms', iou_threshold=0.5),
- max_per_img=100,
- mask_thr_binary=0.5)))
diff --git a/spaces/Andy1621/uniformer_image_detection/configs/fast_rcnn/fast_rcnn_r101_fpn_1x_coco.py b/spaces/Andy1621/uniformer_image_detection/configs/fast_rcnn/fast_rcnn_r101_fpn_1x_coco.py
deleted file mode 100644
index 9a76b3997fbbed5883adde2122dc17ee2262fa80..0000000000000000000000000000000000000000
--- a/spaces/Andy1621/uniformer_image_detection/configs/fast_rcnn/fast_rcnn_r101_fpn_1x_coco.py
+++ /dev/null
@@ -1,2 +0,0 @@
-_base_ = './fast_rcnn_r50_fpn_1x_coco.py'
-model = dict(pretrained='torchvision://resnet101', backbone=dict(depth=101))
diff --git a/spaces/Andy1621/uniformer_image_detection/configs/legacy_1.x/retinanet_r50_caffe_fpn_1x_coco_v1.py b/spaces/Andy1621/uniformer_image_detection/configs/legacy_1.x/retinanet_r50_caffe_fpn_1x_coco_v1.py
deleted file mode 100644
index ef9392f7e351f489d6d9e97936925b6a16d1212e..0000000000000000000000000000000000000000
--- a/spaces/Andy1621/uniformer_image_detection/configs/legacy_1.x/retinanet_r50_caffe_fpn_1x_coco_v1.py
+++ /dev/null
@@ -1,37 +0,0 @@
-_base_ = './retinanet_r50_fpn_1x_coco_v1.py'
-model = dict(
- pretrained='open-mmlab://detectron/resnet50_caffe',
- backbone=dict(
- norm_cfg=dict(requires_grad=False), norm_eval=True, style='caffe'))
-# use caffe img_norm
-img_norm_cfg = dict(
- mean=[102.9801, 115.9465, 122.7717], std=[1.0, 1.0, 1.0], to_rgb=False)
-train_pipeline = [
- dict(type='LoadImageFromFile'),
- dict(type='LoadAnnotations', with_bbox=True),
- dict(type='Resize', img_scale=(1333, 800), keep_ratio=True),
- dict(type='RandomFlip', flip_ratio=0.5),
- dict(type='Normalize', **img_norm_cfg),
- dict(type='Pad', size_divisor=32),
- dict(type='DefaultFormatBundle'),
- dict(type='Collect', keys=['img', 'gt_bboxes', 'gt_labels']),
-]
-test_pipeline = [
- dict(type='LoadImageFromFile'),
- dict(
- type='MultiScaleFlipAug',
- img_scale=(1333, 800),
- flip=False,
- transforms=[
- dict(type='Resize', keep_ratio=True),
- dict(type='RandomFlip'),
- dict(type='Normalize', **img_norm_cfg),
- dict(type='Pad', size_divisor=32),
- dict(type='ImageToTensor', keys=['img']),
- dict(type='Collect', keys=['img']),
- ])
-]
-data = dict(
- train=dict(pipeline=train_pipeline),
- val=dict(pipeline=test_pipeline),
- test=dict(pipeline=test_pipeline))
diff --git a/spaces/Andy1621/uniformer_image_detection/configs/retinanet/retinanet_r50_caffe_fpn_1x_coco.py b/spaces/Andy1621/uniformer_image_detection/configs/retinanet/retinanet_r50_caffe_fpn_1x_coco.py
deleted file mode 100644
index 028c1a3ad48f49ee22e0ee70d07555d58f3c73d1..0000000000000000000000000000000000000000
--- a/spaces/Andy1621/uniformer_image_detection/configs/retinanet/retinanet_r50_caffe_fpn_1x_coco.py
+++ /dev/null
@@ -1,37 +0,0 @@
-_base_ = './retinanet_r50_fpn_1x_coco.py'
-model = dict(
- pretrained='open-mmlab://detectron2/resnet50_caffe',
- backbone=dict(
- norm_cfg=dict(requires_grad=False), norm_eval=True, style='caffe'))
-# use caffe img_norm
-img_norm_cfg = dict(
- mean=[103.530, 116.280, 123.675], std=[1.0, 1.0, 1.0], to_rgb=False)
-train_pipeline = [
- dict(type='LoadImageFromFile'),
- dict(type='LoadAnnotations', with_bbox=True),
- dict(type='Resize', img_scale=(1333, 800), keep_ratio=True),
- dict(type='RandomFlip', flip_ratio=0.5),
- dict(type='Normalize', **img_norm_cfg),
- dict(type='Pad', size_divisor=32),
- dict(type='DefaultFormatBundle'),
- dict(type='Collect', keys=['img', 'gt_bboxes', 'gt_labels']),
-]
-test_pipeline = [
- dict(type='LoadImageFromFile'),
- dict(
- type='MultiScaleFlipAug',
- img_scale=(1333, 800),
- flip=False,
- transforms=[
- dict(type='Resize', keep_ratio=True),
- dict(type='RandomFlip'),
- dict(type='Normalize', **img_norm_cfg),
- dict(type='Pad', size_divisor=32),
- dict(type='ImageToTensor', keys=['img']),
- dict(type='Collect', keys=['img']),
- ])
-]
-data = dict(
- train=dict(pipeline=train_pipeline),
- val=dict(pipeline=test_pipeline),
- test=dict(pipeline=test_pipeline))
diff --git a/spaces/Andy1621/uniformer_image_detection/mmdet/models/roi_heads/bbox_heads/sabl_head.py b/spaces/Andy1621/uniformer_image_detection/mmdet/models/roi_heads/bbox_heads/sabl_head.py
deleted file mode 100644
index 5153996aeb706d103d1ad14b61734914eddb7693..0000000000000000000000000000000000000000
--- a/spaces/Andy1621/uniformer_image_detection/mmdet/models/roi_heads/bbox_heads/sabl_head.py
+++ /dev/null
@@ -1,572 +0,0 @@
-import numpy as np
-import torch
-import torch.nn as nn
-import torch.nn.functional as F
-from mmcv.cnn import ConvModule, kaiming_init, normal_init, xavier_init
-from mmcv.runner import force_fp32
-
-from mmdet.core import build_bbox_coder, multi_apply, multiclass_nms
-from mmdet.models.builder import HEADS, build_loss
-from mmdet.models.losses import accuracy
-
-
-@HEADS.register_module()
-class SABLHead(nn.Module):
- """Side-Aware Boundary Localization (SABL) for RoI-Head.
-
- Side-Aware features are extracted by conv layers
- with an attention mechanism.
- Boundary Localization with Bucketing and Bucketing Guided Rescoring
- are implemented in BucketingBBoxCoder.
-
- Please refer to https://arxiv.org/abs/1912.04260 for more details.
-
- Args:
- cls_in_channels (int): Input channels of cls RoI feature. \
- Defaults to 256.
- reg_in_channels (int): Input channels of reg RoI feature. \
- Defaults to 256.
- roi_feat_size (int): Size of RoI features. Defaults to 7.
- reg_feat_up_ratio (int): Upsample ratio of reg features. \
- Defaults to 2.
- reg_pre_kernel (int): Kernel of 2D conv layers before \
- attention pooling. Defaults to 3.
- reg_post_kernel (int): Kernel of 1D conv layers after \
- attention pooling. Defaults to 3.
- reg_pre_num (int): Number of pre convs. Defaults to 2.
- reg_post_num (int): Number of post convs. Defaults to 1.
- num_classes (int): Number of classes in dataset. Defaults to 80.
- cls_out_channels (int): Hidden channels in cls fcs. Defaults to 1024.
- reg_offset_out_channels (int): Hidden and output channel \
- of reg offset branch. Defaults to 256.
- reg_cls_out_channels (int): Hidden and output channel \
- of reg cls branch. Defaults to 256.
- num_cls_fcs (int): Number of fcs for cls branch. Defaults to 1.
- num_reg_fcs (int): Number of fcs for reg branch.. Defaults to 0.
- reg_class_agnostic (bool): Class agnostic regresion or not. \
- Defaults to True.
- norm_cfg (dict): Config of norm layers. Defaults to None.
- bbox_coder (dict): Config of bbox coder. Defaults 'BucketingBBoxCoder'.
- loss_cls (dict): Config of classification loss.
- loss_bbox_cls (dict): Config of classification loss for bbox branch.
- loss_bbox_reg (dict): Config of regression loss for bbox branch.
- """
-
- def __init__(self,
- num_classes,
- cls_in_channels=256,
- reg_in_channels=256,
- roi_feat_size=7,
- reg_feat_up_ratio=2,
- reg_pre_kernel=3,
- reg_post_kernel=3,
- reg_pre_num=2,
- reg_post_num=1,
- cls_out_channels=1024,
- reg_offset_out_channels=256,
- reg_cls_out_channels=256,
- num_cls_fcs=1,
- num_reg_fcs=0,
- reg_class_agnostic=True,
- norm_cfg=None,
- bbox_coder=dict(
- type='BucketingBBoxCoder',
- num_buckets=14,
- scale_factor=1.7),
- loss_cls=dict(
- type='CrossEntropyLoss',
- use_sigmoid=False,
- loss_weight=1.0),
- loss_bbox_cls=dict(
- type='CrossEntropyLoss',
- use_sigmoid=True,
- loss_weight=1.0),
- loss_bbox_reg=dict(
- type='SmoothL1Loss', beta=0.1, loss_weight=1.0)):
- super(SABLHead, self).__init__()
- self.cls_in_channels = cls_in_channels
- self.reg_in_channels = reg_in_channels
- self.roi_feat_size = roi_feat_size
- self.reg_feat_up_ratio = int(reg_feat_up_ratio)
- self.num_buckets = bbox_coder['num_buckets']
- assert self.reg_feat_up_ratio // 2 >= 1
- self.up_reg_feat_size = roi_feat_size * self.reg_feat_up_ratio
- assert self.up_reg_feat_size == bbox_coder['num_buckets']
- self.reg_pre_kernel = reg_pre_kernel
- self.reg_post_kernel = reg_post_kernel
- self.reg_pre_num = reg_pre_num
- self.reg_post_num = reg_post_num
- self.num_classes = num_classes
- self.cls_out_channels = cls_out_channels
- self.reg_offset_out_channels = reg_offset_out_channels
- self.reg_cls_out_channels = reg_cls_out_channels
- self.num_cls_fcs = num_cls_fcs
- self.num_reg_fcs = num_reg_fcs
- self.reg_class_agnostic = reg_class_agnostic
- assert self.reg_class_agnostic
- self.norm_cfg = norm_cfg
-
- self.bbox_coder = build_bbox_coder(bbox_coder)
- self.loss_cls = build_loss(loss_cls)
- self.loss_bbox_cls = build_loss(loss_bbox_cls)
- self.loss_bbox_reg = build_loss(loss_bbox_reg)
-
- self.cls_fcs = self._add_fc_branch(self.num_cls_fcs,
- self.cls_in_channels,
- self.roi_feat_size,
- self.cls_out_channels)
-
- self.side_num = int(np.ceil(self.num_buckets / 2))
-
- if self.reg_feat_up_ratio > 1:
- self.upsample_x = nn.ConvTranspose1d(
- reg_in_channels,
- reg_in_channels,
- self.reg_feat_up_ratio,
- stride=self.reg_feat_up_ratio)
- self.upsample_y = nn.ConvTranspose1d(
- reg_in_channels,
- reg_in_channels,
- self.reg_feat_up_ratio,
- stride=self.reg_feat_up_ratio)
-
- self.reg_pre_convs = nn.ModuleList()
- for i in range(self.reg_pre_num):
- reg_pre_conv = ConvModule(
- reg_in_channels,
- reg_in_channels,
- kernel_size=reg_pre_kernel,
- padding=reg_pre_kernel // 2,
- norm_cfg=norm_cfg,
- act_cfg=dict(type='ReLU'))
- self.reg_pre_convs.append(reg_pre_conv)
-
- self.reg_post_conv_xs = nn.ModuleList()
- for i in range(self.reg_post_num):
- reg_post_conv_x = ConvModule(
- reg_in_channels,
- reg_in_channels,
- kernel_size=(1, reg_post_kernel),
- padding=(0, reg_post_kernel // 2),
- norm_cfg=norm_cfg,
- act_cfg=dict(type='ReLU'))
- self.reg_post_conv_xs.append(reg_post_conv_x)
- self.reg_post_conv_ys = nn.ModuleList()
- for i in range(self.reg_post_num):
- reg_post_conv_y = ConvModule(
- reg_in_channels,
- reg_in_channels,
- kernel_size=(reg_post_kernel, 1),
- padding=(reg_post_kernel // 2, 0),
- norm_cfg=norm_cfg,
- act_cfg=dict(type='ReLU'))
- self.reg_post_conv_ys.append(reg_post_conv_y)
-
- self.reg_conv_att_x = nn.Conv2d(reg_in_channels, 1, 1)
- self.reg_conv_att_y = nn.Conv2d(reg_in_channels, 1, 1)
-
- self.fc_cls = nn.Linear(self.cls_out_channels, self.num_classes + 1)
- self.relu = nn.ReLU(inplace=True)
-
- self.reg_cls_fcs = self._add_fc_branch(self.num_reg_fcs,
- self.reg_in_channels, 1,
- self.reg_cls_out_channels)
- self.reg_offset_fcs = self._add_fc_branch(self.num_reg_fcs,
- self.reg_in_channels, 1,
- self.reg_offset_out_channels)
- self.fc_reg_cls = nn.Linear(self.reg_cls_out_channels, 1)
- self.fc_reg_offset = nn.Linear(self.reg_offset_out_channels, 1)
-
- def _add_fc_branch(self, num_branch_fcs, in_channels, roi_feat_size,
- fc_out_channels):
- in_channels = in_channels * roi_feat_size * roi_feat_size
- branch_fcs = nn.ModuleList()
- for i in range(num_branch_fcs):
- fc_in_channels = (in_channels if i == 0 else fc_out_channels)
- branch_fcs.append(nn.Linear(fc_in_channels, fc_out_channels))
- return branch_fcs
-
- def init_weights(self):
- for module_list in [
- self.reg_cls_fcs, self.reg_offset_fcs, self.cls_fcs
- ]:
- for m in module_list.modules():
- if isinstance(m, nn.Linear):
- xavier_init(m, distribution='uniform')
- if self.reg_feat_up_ratio > 1:
- kaiming_init(self.upsample_x, distribution='normal')
- kaiming_init(self.upsample_y, distribution='normal')
-
- normal_init(self.reg_conv_att_x, 0, 0.01)
- normal_init(self.reg_conv_att_y, 0, 0.01)
- normal_init(self.fc_reg_offset, 0, 0.001)
- normal_init(self.fc_reg_cls, 0, 0.01)
- normal_init(self.fc_cls, 0, 0.01)
-
- def cls_forward(self, cls_x):
- cls_x = cls_x.view(cls_x.size(0), -1)
- for fc in self.cls_fcs:
- cls_x = self.relu(fc(cls_x))
- cls_score = self.fc_cls(cls_x)
- return cls_score
-
- def attention_pool(self, reg_x):
- """Extract direction-specific features fx and fy with attention
- methanism."""
- reg_fx = reg_x
- reg_fy = reg_x
- reg_fx_att = self.reg_conv_att_x(reg_fx).sigmoid()
- reg_fy_att = self.reg_conv_att_y(reg_fy).sigmoid()
- reg_fx_att = reg_fx_att / reg_fx_att.sum(dim=2).unsqueeze(2)
- reg_fy_att = reg_fy_att / reg_fy_att.sum(dim=3).unsqueeze(3)
- reg_fx = (reg_fx * reg_fx_att).sum(dim=2)
- reg_fy = (reg_fy * reg_fy_att).sum(dim=3)
- return reg_fx, reg_fy
-
- def side_aware_feature_extractor(self, reg_x):
- """Refine and extract side-aware features without split them."""
- for reg_pre_conv in self.reg_pre_convs:
- reg_x = reg_pre_conv(reg_x)
- reg_fx, reg_fy = self.attention_pool(reg_x)
-
- if self.reg_post_num > 0:
- reg_fx = reg_fx.unsqueeze(2)
- reg_fy = reg_fy.unsqueeze(3)
- for i in range(self.reg_post_num):
- reg_fx = self.reg_post_conv_xs[i](reg_fx)
- reg_fy = self.reg_post_conv_ys[i](reg_fy)
- reg_fx = reg_fx.squeeze(2)
- reg_fy = reg_fy.squeeze(3)
- if self.reg_feat_up_ratio > 1:
- reg_fx = self.relu(self.upsample_x(reg_fx))
- reg_fy = self.relu(self.upsample_y(reg_fy))
- reg_fx = torch.transpose(reg_fx, 1, 2)
- reg_fy = torch.transpose(reg_fy, 1, 2)
- return reg_fx.contiguous(), reg_fy.contiguous()
-
- def reg_pred(self, x, offset_fcs, cls_fcs):
- """Predict bucketing estimation (cls_pred) and fine regression (offset
- pred) with side-aware features."""
- x_offset = x.view(-1, self.reg_in_channels)
- x_cls = x.view(-1, self.reg_in_channels)
-
- for fc in offset_fcs:
- x_offset = self.relu(fc(x_offset))
- for fc in cls_fcs:
- x_cls = self.relu(fc(x_cls))
- offset_pred = self.fc_reg_offset(x_offset)
- cls_pred = self.fc_reg_cls(x_cls)
-
- offset_pred = offset_pred.view(x.size(0), -1)
- cls_pred = cls_pred.view(x.size(0), -1)
-
- return offset_pred, cls_pred
-
- def side_aware_split(self, feat):
- """Split side-aware features aligned with orders of bucketing
- targets."""
- l_end = int(np.ceil(self.up_reg_feat_size / 2))
- r_start = int(np.floor(self.up_reg_feat_size / 2))
- feat_fl = feat[:, :l_end]
- feat_fr = feat[:, r_start:].flip(dims=(1, ))
- feat_fl = feat_fl.contiguous()
- feat_fr = feat_fr.contiguous()
- feat = torch.cat([feat_fl, feat_fr], dim=-1)
- return feat
-
- def bbox_pred_split(self, bbox_pred, num_proposals_per_img):
- """Split batch bbox prediction back to each image."""
- bucket_cls_preds, bucket_offset_preds = bbox_pred
- bucket_cls_preds = bucket_cls_preds.split(num_proposals_per_img, 0)
- bucket_offset_preds = bucket_offset_preds.split(
- num_proposals_per_img, 0)
- bbox_pred = tuple(zip(bucket_cls_preds, bucket_offset_preds))
- return bbox_pred
-
- def reg_forward(self, reg_x):
- outs = self.side_aware_feature_extractor(reg_x)
- edge_offset_preds = []
- edge_cls_preds = []
- reg_fx = outs[0]
- reg_fy = outs[1]
- offset_pred_x, cls_pred_x = self.reg_pred(reg_fx, self.reg_offset_fcs,
- self.reg_cls_fcs)
- offset_pred_y, cls_pred_y = self.reg_pred(reg_fy, self.reg_offset_fcs,
- self.reg_cls_fcs)
- offset_pred_x = self.side_aware_split(offset_pred_x)
- offset_pred_y = self.side_aware_split(offset_pred_y)
- cls_pred_x = self.side_aware_split(cls_pred_x)
- cls_pred_y = self.side_aware_split(cls_pred_y)
- edge_offset_preds = torch.cat([offset_pred_x, offset_pred_y], dim=-1)
- edge_cls_preds = torch.cat([cls_pred_x, cls_pred_y], dim=-1)
-
- return (edge_cls_preds, edge_offset_preds)
-
- def forward(self, x):
-
- bbox_pred = self.reg_forward(x)
- cls_score = self.cls_forward(x)
-
- return cls_score, bbox_pred
-
- def get_targets(self, sampling_results, gt_bboxes, gt_labels,
- rcnn_train_cfg):
- pos_proposals = [res.pos_bboxes for res in sampling_results]
- neg_proposals = [res.neg_bboxes for res in sampling_results]
- pos_gt_bboxes = [res.pos_gt_bboxes for res in sampling_results]
- pos_gt_labels = [res.pos_gt_labels for res in sampling_results]
- cls_reg_targets = self.bucket_target(pos_proposals, neg_proposals,
- pos_gt_bboxes, pos_gt_labels,
- rcnn_train_cfg)
- (labels, label_weights, bucket_cls_targets, bucket_cls_weights,
- bucket_offset_targets, bucket_offset_weights) = cls_reg_targets
- return (labels, label_weights, (bucket_cls_targets,
- bucket_offset_targets),
- (bucket_cls_weights, bucket_offset_weights))
-
- def bucket_target(self,
- pos_proposals_list,
- neg_proposals_list,
- pos_gt_bboxes_list,
- pos_gt_labels_list,
- rcnn_train_cfg,
- concat=True):
- (labels, label_weights, bucket_cls_targets, bucket_cls_weights,
- bucket_offset_targets, bucket_offset_weights) = multi_apply(
- self._bucket_target_single,
- pos_proposals_list,
- neg_proposals_list,
- pos_gt_bboxes_list,
- pos_gt_labels_list,
- cfg=rcnn_train_cfg)
-
- if concat:
- labels = torch.cat(labels, 0)
- label_weights = torch.cat(label_weights, 0)
- bucket_cls_targets = torch.cat(bucket_cls_targets, 0)
- bucket_cls_weights = torch.cat(bucket_cls_weights, 0)
- bucket_offset_targets = torch.cat(bucket_offset_targets, 0)
- bucket_offset_weights = torch.cat(bucket_offset_weights, 0)
- return (labels, label_weights, bucket_cls_targets, bucket_cls_weights,
- bucket_offset_targets, bucket_offset_weights)
-
- def _bucket_target_single(self, pos_proposals, neg_proposals,
- pos_gt_bboxes, pos_gt_labels, cfg):
- """Compute bucketing estimation targets and fine regression targets for
- a single image.
-
- Args:
- pos_proposals (Tensor): positive proposals of a single image,
- Shape (n_pos, 4)
- neg_proposals (Tensor): negative proposals of a single image,
- Shape (n_neg, 4).
- pos_gt_bboxes (Tensor): gt bboxes assigned to positive proposals
- of a single image, Shape (n_pos, 4).
- pos_gt_labels (Tensor): gt labels assigned to positive proposals
- of a single image, Shape (n_pos, ).
- cfg (dict): Config of calculating targets
-
- Returns:
- tuple:
-
- - labels (Tensor): Labels in a single image. \
- Shape (n,).
- - label_weights (Tensor): Label weights in a single image.\
- Shape (n,)
- - bucket_cls_targets (Tensor): Bucket cls targets in \
- a single image. Shape (n, num_buckets*2).
- - bucket_cls_weights (Tensor): Bucket cls weights in \
- a single image. Shape (n, num_buckets*2).
- - bucket_offset_targets (Tensor): Bucket offset targets \
- in a single image. Shape (n, num_buckets*2).
- - bucket_offset_targets (Tensor): Bucket offset weights \
- in a single image. Shape (n, num_buckets*2).
- """
- num_pos = pos_proposals.size(0)
- num_neg = neg_proposals.size(0)
- num_samples = num_pos + num_neg
- labels = pos_gt_bboxes.new_full((num_samples, ),
- self.num_classes,
- dtype=torch.long)
- label_weights = pos_proposals.new_zeros(num_samples)
- bucket_cls_targets = pos_proposals.new_zeros(num_samples,
- 4 * self.side_num)
- bucket_cls_weights = pos_proposals.new_zeros(num_samples,
- 4 * self.side_num)
- bucket_offset_targets = pos_proposals.new_zeros(
- num_samples, 4 * self.side_num)
- bucket_offset_weights = pos_proposals.new_zeros(
- num_samples, 4 * self.side_num)
- if num_pos > 0:
- labels[:num_pos] = pos_gt_labels
- label_weights[:num_pos] = 1.0
- (pos_bucket_offset_targets, pos_bucket_offset_weights,
- pos_bucket_cls_targets,
- pos_bucket_cls_weights) = self.bbox_coder.encode(
- pos_proposals, pos_gt_bboxes)
- bucket_cls_targets[:num_pos, :] = pos_bucket_cls_targets
- bucket_cls_weights[:num_pos, :] = pos_bucket_cls_weights
- bucket_offset_targets[:num_pos, :] = pos_bucket_offset_targets
- bucket_offset_weights[:num_pos, :] = pos_bucket_offset_weights
- if num_neg > 0:
- label_weights[-num_neg:] = 1.0
- return (labels, label_weights, bucket_cls_targets, bucket_cls_weights,
- bucket_offset_targets, bucket_offset_weights)
-
- def loss(self,
- cls_score,
- bbox_pred,
- rois,
- labels,
- label_weights,
- bbox_targets,
- bbox_weights,
- reduction_override=None):
- losses = dict()
- if cls_score is not None:
- avg_factor = max(torch.sum(label_weights > 0).float().item(), 1.)
- losses['loss_cls'] = self.loss_cls(
- cls_score,
- labels,
- label_weights,
- avg_factor=avg_factor,
- reduction_override=reduction_override)
- losses['acc'] = accuracy(cls_score, labels)
-
- if bbox_pred is not None:
- bucket_cls_preds, bucket_offset_preds = bbox_pred
- bucket_cls_targets, bucket_offset_targets = bbox_targets
- bucket_cls_weights, bucket_offset_weights = bbox_weights
- # edge cls
- bucket_cls_preds = bucket_cls_preds.view(-1, self.side_num)
- bucket_cls_targets = bucket_cls_targets.view(-1, self.side_num)
- bucket_cls_weights = bucket_cls_weights.view(-1, self.side_num)
- losses['loss_bbox_cls'] = self.loss_bbox_cls(
- bucket_cls_preds,
- bucket_cls_targets,
- bucket_cls_weights,
- avg_factor=bucket_cls_targets.size(0),
- reduction_override=reduction_override)
-
- losses['loss_bbox_reg'] = self.loss_bbox_reg(
- bucket_offset_preds,
- bucket_offset_targets,
- bucket_offset_weights,
- avg_factor=bucket_offset_targets.size(0),
- reduction_override=reduction_override)
-
- return losses
-
- @force_fp32(apply_to=('cls_score', 'bbox_pred'))
- def get_bboxes(self,
- rois,
- cls_score,
- bbox_pred,
- img_shape,
- scale_factor,
- rescale=False,
- cfg=None):
- if isinstance(cls_score, list):
- cls_score = sum(cls_score) / float(len(cls_score))
- scores = F.softmax(cls_score, dim=1) if cls_score is not None else None
-
- if bbox_pred is not None:
- bboxes, confids = self.bbox_coder.decode(rois[:, 1:], bbox_pred,
- img_shape)
- else:
- bboxes = rois[:, 1:].clone()
- confids = None
- if img_shape is not None:
- bboxes[:, [0, 2]].clamp_(min=0, max=img_shape[1] - 1)
- bboxes[:, [1, 3]].clamp_(min=0, max=img_shape[0] - 1)
-
- if rescale and bboxes.size(0) > 0:
- if isinstance(scale_factor, float):
- bboxes /= scale_factor
- else:
- bboxes /= torch.from_numpy(scale_factor).to(bboxes.device)
-
- if cfg is None:
- return bboxes, scores
- else:
- det_bboxes, det_labels = multiclass_nms(
- bboxes,
- scores,
- cfg.score_thr,
- cfg.nms,
- cfg.max_per_img,
- score_factors=confids)
-
- return det_bboxes, det_labels
-
- @force_fp32(apply_to=('bbox_preds', ))
- def refine_bboxes(self, rois, labels, bbox_preds, pos_is_gts, img_metas):
- """Refine bboxes during training.
-
- Args:
- rois (Tensor): Shape (n*bs, 5), where n is image number per GPU,
- and bs is the sampled RoIs per image.
- labels (Tensor): Shape (n*bs, ).
- bbox_preds (list[Tensor]): Shape [(n*bs, num_buckets*2), \
- (n*bs, num_buckets*2)].
- pos_is_gts (list[Tensor]): Flags indicating if each positive bbox
- is a gt bbox.
- img_metas (list[dict]): Meta info of each image.
-
- Returns:
- list[Tensor]: Refined bboxes of each image in a mini-batch.
- """
- img_ids = rois[:, 0].long().unique(sorted=True)
- assert img_ids.numel() == len(img_metas)
-
- bboxes_list = []
- for i in range(len(img_metas)):
- inds = torch.nonzero(
- rois[:, 0] == i, as_tuple=False).squeeze(dim=1)
- num_rois = inds.numel()
-
- bboxes_ = rois[inds, 1:]
- label_ = labels[inds]
- edge_cls_preds, edge_offset_preds = bbox_preds
- edge_cls_preds_ = edge_cls_preds[inds]
- edge_offset_preds_ = edge_offset_preds[inds]
- bbox_pred_ = [edge_cls_preds_, edge_offset_preds_]
- img_meta_ = img_metas[i]
- pos_is_gts_ = pos_is_gts[i]
-
- bboxes = self.regress_by_class(bboxes_, label_, bbox_pred_,
- img_meta_)
- # filter gt bboxes
- pos_keep = 1 - pos_is_gts_
- keep_inds = pos_is_gts_.new_ones(num_rois)
- keep_inds[:len(pos_is_gts_)] = pos_keep
-
- bboxes_list.append(bboxes[keep_inds.type(torch.bool)])
-
- return bboxes_list
-
- @force_fp32(apply_to=('bbox_pred', ))
- def regress_by_class(self, rois, label, bbox_pred, img_meta):
- """Regress the bbox for the predicted class. Used in Cascade R-CNN.
-
- Args:
- rois (Tensor): shape (n, 4) or (n, 5)
- label (Tensor): shape (n, )
- bbox_pred (list[Tensor]): shape [(n, num_buckets *2), \
- (n, num_buckets *2)]
- img_meta (dict): Image meta info.
-
- Returns:
- Tensor: Regressed bboxes, the same shape as input rois.
- """
- assert rois.size(1) == 4 or rois.size(1) == 5
-
- if rois.size(1) == 4:
- new_rois, _ = self.bbox_coder.decode(rois, bbox_pred,
- img_meta['img_shape'])
- else:
- bboxes, _ = self.bbox_coder.decode(rois[:, 1:], bbox_pred,
- img_meta['img_shape'])
- new_rois = torch.cat((rois[:, [0]], bboxes), dim=1)
-
- return new_rois
diff --git a/spaces/Andy1621/uniformer_image_segmentation/configs/pspnet/pspnet_r101-d8_512x1024_80k_cityscapes.py b/spaces/Andy1621/uniformer_image_segmentation/configs/pspnet/pspnet_r101-d8_512x1024_80k_cityscapes.py
deleted file mode 100644
index 9931a07bc2d137eb49b3fa4dad8f8681d4f5e943..0000000000000000000000000000000000000000
--- a/spaces/Andy1621/uniformer_image_segmentation/configs/pspnet/pspnet_r101-d8_512x1024_80k_cityscapes.py
+++ /dev/null
@@ -1,2 +0,0 @@
-_base_ = './pspnet_r50-d8_512x1024_80k_cityscapes.py'
-model = dict(pretrained='open-mmlab://resnet101_v1c', backbone=dict(depth=101))
diff --git a/spaces/Andyrasika/Andyrasika-dreamshaper-sdxl-1.0/README.md b/spaces/Andyrasika/Andyrasika-dreamshaper-sdxl-1.0/README.md
deleted file mode 100644
index a9a3d8480ea7cae99aaeffa5c81dd485d534839a..0000000000000000000000000000000000000000
--- a/spaces/Andyrasika/Andyrasika-dreamshaper-sdxl-1.0/README.md
+++ /dev/null
@@ -1,12 +0,0 @@
----
-title: Andyrasika Dreamshaper Sdxl 1.0
-emoji: 👀
-colorFrom: pink
-colorTo: purple
-sdk: gradio
-sdk_version: 3.40.1
-app_file: app.py
-pinned: false
----
-
-Check out the configuration reference at https://huggingface.co/docs/hub/spaces-config-reference
diff --git a/spaces/Anthony7906/MengHuiMXD_GPT/modules/utils.py b/spaces/Anthony7906/MengHuiMXD_GPT/modules/utils.py
deleted file mode 100644
index e1516e1fad4761787070d24e867bea57d86ac9ed..0000000000000000000000000000000000000000
--- a/spaces/Anthony7906/MengHuiMXD_GPT/modules/utils.py
+++ /dev/null
@@ -1,548 +0,0 @@
-# -*- coding:utf-8 -*-
-from __future__ import annotations
-from typing import TYPE_CHECKING, Any, Callable, Dict, List, Tuple, Type
-import logging
-import json
-import os
-import datetime
-import hashlib
-import csv
-import requests
-import re
-import html
-import sys
-import subprocess
-
-import gradio as gr
-from pypinyin import lazy_pinyin
-import tiktoken
-import mdtex2html
-from markdown import markdown
-from pygments import highlight
-from pygments.lexers import get_lexer_by_name
-from pygments.formatters import HtmlFormatter
-import pandas as pd
-
-from modules.presets import *
-from . import shared
-from modules.config import retrieve_proxy
-
-if TYPE_CHECKING:
- from typing import TypedDict
-
- class DataframeData(TypedDict):
- headers: List[str]
- data: List[List[str | int | bool]]
-
-def predict(current_model, *args):
- iter = current_model.predict(*args)
- for i in iter:
- yield i
-
-def billing_info(current_model):
- return current_model.billing_info()
-
-def set_key(current_model, *args):
- return current_model.set_key(*args)
-
-def load_chat_history(current_model, *args):
- return current_model.load_chat_history(*args)
-
-def interrupt(current_model, *args):
- return current_model.interrupt(*args)
-
-def reset(current_model, *args):
- return current_model.reset(*args)
-
-def retry(current_model, *args):
- iter = current_model.retry(*args)
- for i in iter:
- yield i
-
-def delete_first_conversation(current_model, *args):
- return current_model.delete_first_conversation(*args)
-
-def delete_last_conversation(current_model, *args):
- return current_model.delete_last_conversation(*args)
-
-def set_system_prompt(current_model, *args):
- return current_model.set_system_prompt(*args)
-
-def save_chat_history(current_model, *args):
- return current_model.save_chat_history(*args)
-
-def export_markdown(current_model, *args):
- return current_model.export_markdown(*args)
-
-def load_chat_history(current_model, *args):
- return current_model.load_chat_history(*args)
-
-def set_token_upper_limit(current_model, *args):
- return current_model.set_token_upper_limit(*args)
-
-def set_temperature(current_model, *args):
- current_model.set_temperature(*args)
-
-def set_top_p(current_model, *args):
- current_model.set_top_p(*args)
-
-def set_n_choices(current_model, *args):
- current_model.set_n_choices(*args)
-
-def set_stop_sequence(current_model, *args):
- current_model.set_stop_sequence(*args)
-
-def set_max_tokens(current_model, *args):
- current_model.set_max_tokens(*args)
-
-def set_presence_penalty(current_model, *args):
- current_model.set_presence_penalty(*args)
-
-def set_frequency_penalty(current_model, *args):
- current_model.set_frequency_penalty(*args)
-
-def set_logit_bias(current_model, *args):
- current_model.set_logit_bias(*args)
-
-def set_user_identifier(current_model, *args):
- current_model.set_user_identifier(*args)
-
-def set_single_turn(current_model, *args):
- current_model.set_single_turn(*args)
-
-def handle_file_upload(current_model, *args):
- return current_model.handle_file_upload(*args)
-
-def like(current_model, *args):
- return current_model.like(*args)
-
-def dislike(current_model, *args):
- return current_model.dislike(*args)
-
-
-def count_token(message):
- encoding = tiktoken.get_encoding("cl100k_base")
- input_str = f"role: {message['role']}, content: {message['content']}"
- length = len(encoding.encode(input_str))
- return length
-
-
-def markdown_to_html_with_syntax_highlight(md_str):
- def replacer(match):
- lang = match.group(1) or "text"
- code = match.group(2)
-
- try:
- lexer = get_lexer_by_name(lang, stripall=True)
- except ValueError:
- lexer = get_lexer_by_name("text", stripall=True)
-
- formatter = HtmlFormatter()
- highlighted_code = highlight(code, lexer, formatter)
-
- return f'
{highlighted_code}
'
-
- code_block_pattern = r"```(\w+)?\n([\s\S]+?)\n```"
- md_str = re.sub(code_block_pattern, replacer, md_str, flags=re.MULTILINE)
-
- html_str = markdown(md_str)
- return html_str
-
-
-def normalize_markdown(md_text: str) -> str:
- lines = md_text.split("\n")
- normalized_lines = []
- inside_list = False
-
- for i, line in enumerate(lines):
- if re.match(r"^(\d+\.|-|\*|\+)\s", line.strip()):
- if not inside_list and i > 0 and lines[i - 1].strip() != "":
- normalized_lines.append("")
- inside_list = True
- normalized_lines.append(line)
- elif inside_list and line.strip() == "":
- if i < len(lines) - 1 and not re.match(
- r"^(\d+\.|-|\*|\+)\s", lines[i + 1].strip()
- ):
- normalized_lines.append(line)
- continue
- else:
- inside_list = False
- normalized_lines.append(line)
-
- return "\n".join(normalized_lines)
-
-
-def convert_mdtext(md_text):
- code_block_pattern = re.compile(r"```(.*?)(?:```|$)", re.DOTALL)
- inline_code_pattern = re.compile(r"`(.*?)`", re.DOTALL)
- code_blocks = code_block_pattern.findall(md_text)
- non_code_parts = code_block_pattern.split(md_text)[::2]
-
- result = []
- for non_code, code in zip(non_code_parts, code_blocks + [""]):
- if non_code.strip():
- non_code = normalize_markdown(non_code)
- if inline_code_pattern.search(non_code):
- result.append(markdown(non_code, extensions=["tables"]))
- else:
- result.append(mdtex2html.convert(non_code, extensions=["tables"]))
- if code.strip():
- # _, code = detect_language(code) # 暂时去除代码高亮功能,因为在大段代码的情况下会出现问题
- # code = code.replace("\n\n", "\n") # 暂时去除代码中的空行,因为在大段代码的情况下会出现问题
- code = f"\n```{code}\n\n```"
- code = markdown_to_html_with_syntax_highlight(code)
- result.append(code)
- result = "".join(result)
- result += ALREADY_CONVERTED_MARK
- return result
-
-
-def convert_asis(userinput):
- return (
- f'
{html.escape(userinput)}
'
- + ALREADY_CONVERTED_MARK
- )
-
-
-def detect_converted_mark(userinput):
- try:
- if userinput.endswith(ALREADY_CONVERTED_MARK):
- return True
- else:
- return False
- except:
- return True
-
-
-def detect_language(code):
- if code.startswith("\n"):
- first_line = ""
- else:
- first_line = code.strip().split("\n", 1)[0]
- language = first_line.lower() if first_line else ""
- code_without_language = code[len(first_line) :].lstrip() if first_line else code
- return language, code_without_language
-
-
-def construct_text(role, text):
- return {"role": role, "content": text}
-
-
-def construct_user(text):
- return construct_text("user", text)
-
-
-def construct_system(text):
- return construct_text("system", text)
-
-
-def construct_assistant(text):
- return construct_text("assistant", text)
-
-
-def save_file(filename, system, history, chatbot, user_name):
- logging.debug(f"{user_name} 保存对话历史中……")
- os.makedirs(os.path.join(HISTORY_DIR, user_name), exist_ok=True)
- if filename.endswith(".json"):
- json_s = {"system": system, "history": history, "chatbot": chatbot}
- print(json_s)
- with open(os.path.join(HISTORY_DIR, user_name, filename), "w") as f:
- json.dump(json_s, f)
- elif filename.endswith(".md"):
- md_s = f"system: \n- {system} \n"
- for data in history:
- md_s += f"\n{data['role']}: \n- {data['content']} \n"
- with open(os.path.join(HISTORY_DIR, user_name, filename), "w", encoding="utf8") as f:
- f.write(md_s)
- logging.debug(f"{user_name} 保存对话历史完毕")
- return os.path.join(HISTORY_DIR, user_name, filename)
-
-
-def sorted_by_pinyin(list):
- return sorted(list, key=lambda char: lazy_pinyin(char)[0][0])
-
-
-def get_file_names(dir, plain=False, filetypes=[".json"]):
- logging.debug(f"获取文件名列表,目录为{dir},文件类型为{filetypes},是否为纯文本列表{plain}")
- files = []
- try:
- for type in filetypes:
- files += [f for f in os.listdir(dir) if f.endswith(type)]
- except FileNotFoundError:
- files = []
- files = sorted_by_pinyin(files)
- if files == []:
- files = [""]
- logging.debug(f"files are:{files}")
- if plain:
- return files
- else:
- return gr.Dropdown.update(choices=files)
-
-
-def get_history_names(plain=False, user_name=""):
- logging.debug(f"从用户 {user_name} 中获取历史记录文件名列表")
- return get_file_names(os.path.join(HISTORY_DIR, user_name), plain)
-
-
-def load_template(filename, mode=0):
- logging.debug(f"加载模板文件{filename},模式为{mode}(0为返回字典和下拉菜单,1为返回下拉菜单,2为返回字典)")
- lines = []
- if filename.endswith(".json"):
- with open(os.path.join(TEMPLATES_DIR, filename), "r", encoding="utf8") as f:
- lines = json.load(f)
- lines = [[i["act"], i["prompt"]] for i in lines]
- else:
- with open(
- os.path.join(TEMPLATES_DIR, filename), "r", encoding="utf8"
- ) as csvfile:
- reader = csv.reader(csvfile)
- lines = list(reader)
- lines = lines[1:]
- if mode == 1:
- return sorted_by_pinyin([row[0] for row in lines])
- elif mode == 2:
- return {row[0]: row[1] for row in lines}
- else:
- choices = sorted_by_pinyin([row[0] for row in lines])
- return {row[0]: row[1] for row in lines}, gr.Dropdown.update(
- choices=choices
- )
-
-
-def get_template_names(plain=False):
- logging.debug("获取模板文件名列表")
- return get_file_names(TEMPLATES_DIR, plain, filetypes=[".csv", "json"])
-
-
-def get_template_content(templates, selection, original_system_prompt):
- logging.debug(f"应用模板中,选择为{selection},原始系统提示为{original_system_prompt}")
- try:
- return templates[selection]
- except:
- return original_system_prompt
-
-
-def reset_textbox():
- logging.debug("重置文本框")
- return gr.update(value="")
-
-
-def reset_default():
- default_host = shared.state.reset_api_host()
- retrieve_proxy("")
- return gr.update(value=default_host), gr.update(value=""), "API-Host 和代理已重置"
-
-
-def change_api_host(host):
- shared.state.set_api_host(host)
- msg = f"API-Host更改为了{host}"
- logging.info(msg)
- return msg
-
-
-def change_proxy(proxy):
- retrieve_proxy(proxy)
- os.environ["HTTPS_PROXY"] = proxy
- msg = f"代理更改为了{proxy}"
- logging.info(msg)
- return msg
-
-
-def hide_middle_chars(s):
- if s is None:
- return ""
- if len(s) <= 8:
- return s
- else:
- head = s[:4]
- tail = s[-4:]
- hidden = "*" * (len(s) - 8)
- return head + hidden + tail
-
-
-def submit_key(key):
- key = key.strip()
- msg = f"API密钥更改为了{hide_middle_chars(key)}"
- logging.info(msg)
- return key, msg
-
-
-def replace_today(prompt):
- today = datetime.datetime.today().strftime("%Y-%m-%d")
- return prompt.replace("{current_date}", today)
-
-
-def get_geoip():
- try:
- with retrieve_proxy():
- response = requests.get("https://ipapi.co/json/", timeout=5)
- data = response.json()
- except:
- data = {"error": True, "reason": "连接ipapi失败"}
- if "error" in data.keys():
- logging.warning(f"无法获取IP地址信息。\n{data}")
- if data["reason"] == "RateLimited":
- return (
- i18n("您的IP区域:未知。")
- )
- else:
- return i18n("获取IP地理位置失败。原因:") + f"{data['reason']}" + i18n("。你仍然可以使用聊天功能。")
- else:
- country = data["country_name"]
- if country == "China":
- text = "**您的IP区域:中国。请立即检查代理设置,在不受支持的地区使用API可能导致账号被封禁。**"
- else:
- text = i18n("您的IP区域:") + f"{country}。"
- logging.info(text)
- return text
-
-
-def find_n(lst, max_num):
- n = len(lst)
- total = sum(lst)
-
- if total < max_num:
- return n
-
- for i in range(len(lst)):
- if total - lst[i] < max_num:
- return n - i - 1
- total = total - lst[i]
- return 1
-
-
-def start_outputing():
- logging.debug("显示取消按钮,隐藏发送按钮")
- return gr.Button.update(visible=False), gr.Button.update(visible=True)
-
-
-def end_outputing():
- return (
- gr.Button.update(visible=True),
- gr.Button.update(visible=False),
- )
-
-
-def cancel_outputing():
- logging.info("中止输出……")
- shared.state.interrupt()
-
-
-def transfer_input(inputs):
- # 一次性返回,降低延迟
- textbox = reset_textbox()
- outputing = start_outputing()
- return (
- inputs,
- gr.update(value=""),
- gr.Button.update(visible=False),
- gr.Button.update(visible=True),
- )
-
-
-
-def run(command, desc=None, errdesc=None, custom_env=None, live=False):
- if desc is not None:
- print(desc)
- if live:
- result = subprocess.run(command, shell=True, env=os.environ if custom_env is None else custom_env)
- if result.returncode != 0:
- raise RuntimeError(f"""{errdesc or 'Error running command'}.
-Command: {command}
-Error code: {result.returncode}""")
-
- return ""
- result = subprocess.run(command, stdout=subprocess.PIPE, stderr=subprocess.PIPE, shell=True, env=os.environ if custom_env is None else custom_env)
- if result.returncode != 0:
- message = f"""{errdesc or 'Error running command'}.
- Command: {command}
- Error code: {result.returncode}
- stdout: {result.stdout.decode(encoding="utf8", errors="ignore") if len(result.stdout)>0 else ''}
- stderr: {result.stderr.decode(encoding="utf8", errors="ignore") if len(result.stderr)>0 else ''}
- """
- raise RuntimeError(message)
- return result.stdout.decode(encoding="utf8", errors="ignore")
-
-def versions_html():
- git = os.environ.get('GIT', "git")
- python_version = ".".join([str(x) for x in sys.version_info[0:3]])
- try:
- commit_hash = run(f"{git} rev-parse HEAD").strip()
- except Exception:
- commit_hash = ""
- if commit_hash != "":
- short_commit = commit_hash[0:7]
- commit_info = f"{short_commit}"
- else:
- commit_info = "unknown \U0001F615"
- return f"""
- Python: {python_version}
- •
- Gradio: {gr.__version__}
- •
- Commit: {commit_info}
- """
-
-def add_source_numbers(lst, source_name = "Source", use_source = True):
- if use_source:
- return [f'[{idx+1}]\t "{item[0]}"\n{source_name}: {item[1]}' for idx, item in enumerate(lst)]
- else:
- return [f'[{idx+1}]\t "{item}"' for idx, item in enumerate(lst)]
-
-def add_details(lst):
- nodes = []
- for index, txt in enumerate(lst):
- brief = txt[:25].replace("\n", "")
- nodes.append(
- f"{brief}...
{txt}
"
- )
- return nodes
-
-
-def sheet_to_string(sheet, sheet_name = None):
- result = []
- for index, row in sheet.iterrows():
- row_string = ""
- for column in sheet.columns:
- row_string += f"{column}: {row[column]}, "
- row_string = row_string.rstrip(", ")
- row_string += "."
- result.append(row_string)
- return result
-
-def excel_to_string(file_path):
- # 读取Excel文件中的所有工作表
- excel_file = pd.read_excel(file_path, engine='openpyxl', sheet_name=None)
-
- # 初始化结果字符串
- result = []
-
- # 遍历每一个工作表
- for sheet_name, sheet_data in excel_file.items():
-
- # 处理当前工作表并添加到结果字符串
- result += sheet_to_string(sheet_data, sheet_name=sheet_name)
-
-
- return result
-
-def get_last_day_of_month(any_day):
- # The day 28 exists in every month. 4 days later, it's always next month
- next_month = any_day.replace(day=28) + datetime.timedelta(days=4)
- # subtracting the number of the current day brings us back one month
- return next_month - datetime.timedelta(days=next_month.day)
-
-def get_model_source(model_name, alternative_source):
- if model_name == "gpt2-medium":
- return "https://huggingface.co/gpt2-medium"
-
-def refresh_ui_elements_on_load(current_model, selected_model_name):
- return toggle_like_btn_visibility(selected_model_name)
-
-def toggle_like_btn_visibility(selected_model_name):
- if selected_model_name == "xmchat":
- return gr.update(visible=True)
- else:
- return gr.update(visible=False)
diff --git a/spaces/ArtGAN/Video-Diffusion-WebUI/video_diffusion/utils/model_list.py b/spaces/ArtGAN/Video-Diffusion-WebUI/video_diffusion/utils/model_list.py
deleted file mode 100644
index c1bb9b1d8be48ceb76d1e2fd72981cc1e9400ec5..0000000000000000000000000000000000000000
--- a/spaces/ArtGAN/Video-Diffusion-WebUI/video_diffusion/utils/model_list.py
+++ /dev/null
@@ -1,6 +0,0 @@
-stable_model_list = [
- "runwayml/stable-diffusion-v1-5",
- "stabilityai/stable-diffusion-2-1",
- # "prompthero/openjourney-v4",
- "cerspense/zeroscope_v2_576w"
-]
diff --git a/spaces/Ataturk-Chatbot/HuggingFaceChat/venv/lib/python3.11/site-packages/setuptools/_distutils/__init__.py b/spaces/Ataturk-Chatbot/HuggingFaceChat/venv/lib/python3.11/site-packages/setuptools/_distutils/__init__.py
deleted file mode 100644
index b3ac0146cb3f4cb1894f55fc09775875bc4e1177..0000000000000000000000000000000000000000
--- a/spaces/Ataturk-Chatbot/HuggingFaceChat/venv/lib/python3.11/site-packages/setuptools/_distutils/__init__.py
+++ /dev/null
@@ -1,24 +0,0 @@
-"""distutils
-
-The main package for the Python Module Distribution Utilities. Normally
-used from a setup script as
-
- from distutils.core import setup
-
- setup (...)
-"""
-
-import sys
-import importlib
-
-__version__ = sys.version[: sys.version.index(' ')]
-
-
-try:
- # Allow Debian and pkgsrc (only) to customize system
- # behavior. Ref pypa/distutils#2 and pypa/distutils#16.
- # This hook is deprecated and no other environments
- # should use it.
- importlib.import_module('_distutils_system_mod')
-except ImportError:
- pass
diff --git a/spaces/Awiny/Image2Paragraph/models/grit_src/third_party/CenterNet2/docs/Makefile b/spaces/Awiny/Image2Paragraph/models/grit_src/third_party/CenterNet2/docs/Makefile
deleted file mode 100644
index 718eddce170fe13b67216baf9d4d25b20e860506..0000000000000000000000000000000000000000
--- a/spaces/Awiny/Image2Paragraph/models/grit_src/third_party/CenterNet2/docs/Makefile
+++ /dev/null
@@ -1,19 +0,0 @@
-# Minimal makefile for Sphinx documentation
-# Copyright (c) Facebook, Inc. and its affiliates.
-
-# You can set these variables from the command line.
-SPHINXOPTS =
-SPHINXBUILD = sphinx-build
-SOURCEDIR = .
-BUILDDIR = _build
-
-# Put it first so that "make" without argument is like "make help".
-help:
- @$(SPHINXBUILD) -M help "$(SOURCEDIR)" "$(BUILDDIR)" $(SPHINXOPTS) $(O)
-
-.PHONY: help Makefile
-
-# Catch-all target: route all unknown targets to Sphinx using the new
-# "make mode" option. $(O) is meant as a shortcut for $(SPHINXOPTS).
-%: Makefile
- @$(SPHINXBUILD) -M $@ "$(SOURCEDIR)" "$(BUILDDIR)" $(SPHINXOPTS) $(O)
diff --git a/spaces/Awiny/Image2Paragraph/models/segment_models/configs/__init__.py b/spaces/Awiny/Image2Paragraph/models/segment_models/configs/__init__.py
deleted file mode 100644
index b9742821a6f164200bc145e7a847382f08778303..0000000000000000000000000000000000000000
--- a/spaces/Awiny/Image2Paragraph/models/segment_models/configs/__init__.py
+++ /dev/null
@@ -1 +0,0 @@
-from . import *
\ No newline at end of file
diff --git a/spaces/Awiny/Image2Paragraph/models/segment_models/semgent_anything_model.py b/spaces/Awiny/Image2Paragraph/models/segment_models/semgent_anything_model.py
deleted file mode 100644
index 45de9a1938aec69680cc53aec97cbe5e0ffca09e..0000000000000000000000000000000000000000
--- a/spaces/Awiny/Image2Paragraph/models/segment_models/semgent_anything_model.py
+++ /dev/null
@@ -1,29 +0,0 @@
-import cv2
-from segment_anything import SamAutomaticMaskGenerator, sam_model_registry
-from utils.util import resize_long_edge_cv2
-
-class SegmentAnything:
- def __init__(self, device, arch="vit_b"):
- self.device = device
- if arch=='vit_b':
- pretrained_weights="pretrained_models/sam_vit_b_01ec64.pth"
- elif arch=='vit_l':
- pretrained_weights="pretrained_models/sam_vit_l_0e2f7b.pth"
- elif arch=='vit_h':
- pretrained_weights="pretrained_models/sam_vit_h_0e2f7b.pth"
- else:
- raise ValueError(f"arch {arch} not supported")
- self.model = self.initialize_model(arch, pretrained_weights)
-
- def initialize_model(self, arch, pretrained_weights):
- sam = sam_model_registry[arch](checkpoint=pretrained_weights)
- sam.to(device=self.device)
- mask_generator = SamAutomaticMaskGenerator(sam)
- return mask_generator
-
- def generate_mask(self, img_src):
- image = cv2.imread(img_src)
- image = cv2.cvtColor(image, cv2.COLOR_BGR2RGB)
- image = resize_long_edge_cv2(image, 384)
- anns = self.model.generate(image)
- return anns
\ No newline at end of file
diff --git a/spaces/Benson/text-generation/Examples/ .md b/spaces/Benson/text-generation/Examples/ .md
deleted file mode 100644
index 7ec7ccf287abd3aa93b21e8157278d705474770c..0000000000000000000000000000000000000000
--- a/spaces/Benson/text-generation/Examples/ .md
+++ /dev/null
@@ -1,63 +0,0 @@
-
-
Cómo descargar videos de baloncesto de la NBA gratis
-
Si eres un fan del baloncesto, probablemente te encanta ver los partidos de la NBA y los mejores momentos. La NBA es la liga de baloncesto más prestigiosa y popular del mundo, con los mejores jugadores, equipos y competiciones. Ya sea que quieras ponerte al día con las últimas puntuaciones, revivir los momentos más memorables o aprender de los profesionales, ver videos de la NBA es una gran manera de disfrutar del deporte.
Pero ¿qué pasa si no tienes acceso a la televisión en vivo o servicios de streaming? ¿Qué pasa si quieres ver videos de la NBA sin conexión o en diferentes dispositivos? ¿Qué pasa si quieres editar o compartir tus propias creaciones de video de la NBA? En estos casos, es posible que desee descargar videos de baloncesto de la NBA de forma gratuita desde Internet.
-
Descargar videos de la NBA puede darte más flexibilidad y comodidad para verlos y usarlos. Puede guardarlos en su computadora, teléfono, tableta u otros dispositivos, y verlos en cualquier momento y en cualquier lugar sin conexión a Internet. También puede editarlos con su software favorito, agregar su propio comentario o música, o crear sus propios carretes de puntos destacados. También puedes compartirlos con tus amigos, familiares o compañeros fans en las redes sociales u otras plataformas.
-
Pero ¿cómo descargar videos de baloncesto de la NBA gratis? ¿Dónde puedes encontrarlos? ¿Qué herramientas necesitas? ¿Cómo asegurar la mejor calidad y formato? En este artículo, vamos a responder a estas preguntas y más. Le mostraremos los mejores sitios para encontrar videos de baloncesto de la NBA gratis, y las mejores maneras de descargarlos sin pérdida de calidad. También te daremos algunos consejos y sugerencias sobre cómo disfrutar y usar tus videos descargados de la NBA. ¡Empecemos!
-
Los mejores sitios para encontrar gratis NBA Basketball Videos
-
-
Para evitar estos problemas, recomendamos usar solo sitios de buena reputación y confiables que proporcionen contenido de video NBA legal y de alta calidad. Estos son algunos de los mejores sitios que sugerimos:
-
-
YouTube
-
YouTube es la plataforma para compartir videos más popular del mundo, y tiene una gran colección de videos de baloncesto de la NBA. Puedes encontrar casi cualquier tipo de video de la NBA en YouTube, como lo más destacado del juego completo, playoffs, transmisiones en vivo, noticias, finales, entrevistas, documentales, análisis, etc.
-
Para buscar vídeos de la NBA en YouTube, la pérdida de calidad es Cisdem Video Converter. Cisdem Video Converter es un potente y versátil conversor de vídeo, descargador, editor y extractor de DVD para Mac. Se puede descargar vídeos de la NBA de YouTube, NBA.com, Vimeo, y cualquier otro sitio con facilidad. También puede editar y convertir videos NBA descargados a cualquier formato que desee, como MP4, MOV, AVI, MKV, etc.
-
Aquí es cómo utilizar Cisdem Video Converter para descargar videos de baloncesto de la NBA sin pérdida de calidad:
-
-
Descargue e instale Cisdem Video Converter en su Mac desde aquí.
-
Inicie Cisdem Video Converter y cambie a la pestaña "Descargar".
-
Vaya al sitio donde desea descargar videos de la NBA, como YouTube, NBA.com o Vimeo, y copie la URL del video.
-
Pegue la URL en el cuadro en Cisdem Video Converter y haga clic en el icono de descarga.
-
Espere a que termine la descarga. Puede ver el progreso y el estado en la interfaz.
-
Una vez que se hace la descarga, se puede encontrar el video de la NBA descargado en la carpeta "Descargado".
-
Si desea editar o convertir el video NBA descargado, puede cambiar a la pestaña "Convertir" y arrastrar y soltar el video en la interfaz.
-
Puede usar el editor incorporado para recortar, recortar, rotar, agregar marca de agua, subtítulos, efectos, etc. al video.
-
También puede elegir un formato de salida de los presets o personalizar sus propios ajustes.
-
-
Una vez que se hace la conversión, se puede encontrar el vídeo de la NBA convertido en la carpeta "Convertido".
-
-
Uso de 4K Video Downloader para Windows
-
Si usted es un usuario de Windows, una de las mejores herramientas para descargar videos de baloncesto de la NBA sin pérdida de calidad es 4K Video Downloader. 4K Video Downloader es un descargador de video simple y rápido que puede descargar videos de la NBA de YouTube y otros sitios con alta calidad. También puede ajustar la calidad y el formato de los vídeos descargados de la NBA según sus preferencias.
-
Aquí está cómo usar 4K Video Downloader para descargar videos de baloncesto de la NBA sin pérdida de calidad:
-
-
Descargar e instalar 4K Video Downloader en su PC con Windows desde aquí.
-
Inicie 4K Video Downloader y haga clic en el botón "Pegar enlace" en la esquina superior izquierda.
-
Vaya al sitio donde desea descargar videos de la NBA, como YouTube, NBA.com o Vimeo, y copie la URL del video.
-
La URL se pegará automáticamente en 4K Video Downloader y se analizará.
-
Puede elegir la calidad y el formato del vídeo descargado de la NBA desde la ventana emergente. También puede descargar subtítulos o anotaciones si están disponibles.
-
Haga clic en el botón "Descargar" para iniciar la descarga. Puede ver el progreso y el estado en la interfaz.
-
Una vez que se hace la descarga, se puede encontrar el video de la NBA descargado en la carpeta "Videos".
-
-
Conclusión
-
En este artículo, le hemos mostrado cómo descargar videos de baloncesto de la NBA de forma gratuita desde Internet. También te hemos dado algunos consejos y sugerencias sobre cómo disfrutar y usar tus videos de la NBA descargados. Esperamos que haya encontrado este artículo útil e informativo.
-
-
¿Tienes alguna pregunta o comentario sobre la descarga de videos de baloncesto de la NBA de forma gratuita? ¿Tienes otros sitios o herramientas que recomiendes para descargar vídeos de la NBA? ¿Tienes algún video favorito de la NBA que quieras compartir con nosotros? Por favor, siéntete libre de dejar un comentario a continuación. ¡Nos encantaría saber de ti!
-
Preguntas frecuentes
-
¿Es legal descargar videos de la NBA desde Internet?
-
Depende de la fuente y el propósito de descargar los videos de la NBA. En general, la descarga de vídeos de la NBA desde los sitios o canales oficiales, como NBA.com o YouTube, es legal siempre y cuando los utilice con fines personales y no comerciales. Sin embargo, la descarga de vídeos de la NBA desde sitios no autorizados o pirateados, como sitios de torrent o streaming, puede ser ilegal y puede violar las leyes de derechos de autor o los términos de servicio de las fuentes originales.
-
¿Cómo puedo ver vídeos de la NBA descargados sin conexión?
-
Puedes ver videos de la NBA descargados sin conexión transfiriéndolos a tu dispositivo preferido, como tu computadora, teléfono, tableta o TV. Puede utilizar un cable USB, una conexión inalámbrica o un servicio en la nube para transferir los vídeos descargados de la NBA. También puedes usar un reproductor multimedia o un convertidor de vídeo para reproducir los vídeos de la NBA descargados en tu dispositivo.
-
¿Cómo puedo hacer mis propios videos destacados de la NBA?
-
Puedes hacer tus propios videos destacados de la NBA editando y combinando videos descargados de la NBA con tu software favorito, como iMovie, Windows Movie Maker, Adobe Premiere Pro, etc. También puedes agregar tus propios comentarios, música, efectos, transiciones, etc. para hacer sus propios videos destacados de la NBA más personalizados y creativos.
-
¿Dónde puedo encontrar más recursos y consejos de vídeo de la NBA?
-
Puedes encontrar más recursos de video de la NBA y consejos en varias plataformas en línea, como blogs, foros, podcasts, redes sociales, etc. Algunos de los ejemplos son:
-
-
NBA Video Blog: Un blog que presenta noticias de video de la NBA, reseñas, tutoriales y más.
-
-
NBA Video Podcast: Un podcast que cubre temas de video de la NBA, como análisis, comentarios, entrevistas, etc.
-
NBA Video Social Media: Una plataforma de medios sociales que conecta a los fans de videos de la NBA entre sí y con las cuentas oficiales de la NBA.
-
-
¿Cómo puedo apoyar a mis equipos y jugadores favoritos de la NBA?
-
Puedes apoyar a tus equipos y jugadores favoritos de la NBA siguiendo sus sitios y canales oficiales, como sus sitios web, cuentas de redes sociales, canales de YouTube, etc. También puedes comprar su mercancía oficial, como camisetas, sombreros, carteles, etc. También puede ver sus juegos en vivo o transmisiones en línea o fuera de línea. También puede unirse a sus clubes de fans o comunidades en línea o fuera de línea.
64aa2da5cf
-
-
\ No newline at end of file
diff --git a/spaces/Benson/text-generation/Examples/101 Yzbir Okey Plus Apk.md b/spaces/Benson/text-generation/Examples/101 Yzbir Okey Plus Apk.md
deleted file mode 100644
index fe86d9644e960efc28c86d99321d6811d978380d..0000000000000000000000000000000000000000
--- a/spaces/Benson/text-generation/Examples/101 Yzbir Okey Plus Apk.md
+++ /dev/null
@@ -1,80 +0,0 @@
-
-
¿Qué es 101 yüzbir okey plus apk?
-
101 yüzbir okey plus apk es un popular juego basado en azulejos que se originó en Turquía y es jugado por millones de personas en todo el mundo. Es una variante de rummy que utiliza un conjunto de 106 fichas en lugar de tarjetas. Las baldosas están numeradas del 1 al 13 en cuatro colores diferentes: rojo, amarillo, verde y negro. También hay dos azulejos especiales con un símbolo de trébol, llamados los falsos comodines.
-
El juego se juega en línea a través de 3G, 4G, Edge o Wi-Fi con tus amigos o contra más de 1.000.000 de usuarios. También puedes jugar sin conexión contra inteligencia artificial avanzada. El juego es gratis, pero también puedes comprar fichas adicionales y objetos del juego.
El juego suele ser jugado por cuatro jugadores, pero también puede ser jugado por dos o tres jugadores. Cada jugador recibe 21 fichas al comienzo del juego, excepto el dealer que recibe 22 fichas. El distribuidor se elige al azar al principio y cambia después de cada ronda.
-
Las fichas restantes se colocan boca abajo en la mesa y se barajan. Luego, se forman 21 pilas de cinco fichas cada una. Una ficha se deja sin tachar y se mantiene por el distribuidor. A continuación, el repartidor lanza un dado para determinar qué pila se utilizará para seleccionar la ficha boca arriba que determinará el comodín para el juego.
-
El mosaico boca arriba se coloca encima de la pila seleccionada y su color y valor indican el comodín. El comodín es el azulejo que tiene el mismo color y un valor más alto que el azulejo boca arriba. Por ejemplo, si la ficha boca arriba es un 5 rojo, entonces el comodín es un 6 rojo. Si la ficha boca arriba es un 13 negro, entonces el comodín es un 1.
negro
-
El comodín y el comodín falso
-
-
Los comodines falsos no son sustitutos de ninguna ficha. Tienen su propio valor y color, como lo indican su número y símbolo de trébol. Por ejemplo, si el mosaico boca arriba es un 5 rojo, entonces los comodines falsos son 5s verdes.
-
La mano ganadora
-
El objetivo del juego es ser el primero en formar una mano ganadora de 14 fichas que consiste enteramente en sets y carreras. También puedes ganar con siete pares de fichas idénticas.
-
-
En cada turno, debes dibujar una ficha de la parte superior de una pila no seleccionada o de la pila de descartes del jugador anterior. A continuación, debe descartar una ficha no deseada cara arriba junto a sus pilas.
-
Si tienes una mano ganadora, puedes terminar el juego exponiendo todas tus fichas después de descartar tu última ficha encima de una pila no seleccionada. Debes anunciar "Okey" cuando lo hagas.
-
Cómo descargar e instalar 101 yü. bir okey plus apk?
-
Requisitos y compatibilidad
-
Para descargar e instalar 101 yüzbir okey más apk, es necesario tener un dispositivo Android que se ejecuta en Android 4.1 o superior. También necesita tener al menos 95 MB de espacio de almacenamiento gratuito en su dispositivo. El juego es compatible con la mayoría de dispositivos Android, incluyendo tabletas y teléfonos.
-
Pasos para descargar e instalar
-
Hay dos maneras de descargar e instalar 101 yüzbir okey plus apk en su dispositivo. Puede utilizar la Google Play Store o un sitio web de terceros que proporciona el archivo apk.
-
Si usas Google Play Store, solo tienes que seguir estos pasos:
-
-
Abra la aplicación Google Play Store en su dispositivo y busque "101 yüzbir okey plus".
-
Seleccione el juego de la lista de resultados y toque en "Instalar".
-
Espere a que se complete la descarga y la instalación.
-
Inicia el juego y disfruta jugando.
-
-
Si utiliza un sitio web de terceros, debe seguir estos pasos:
-
-
-
Descargar el archivo apk a su dispositivo.
-
Ir a la configuración del dispositivo y permitir la instalación de aplicaciones de fuentes desconocidas.
-
Busque el archivo apk en su dispositivo y toque en él para instalarlo.
-
Inicia el juego y disfruta jugando.
-
-
¿Por qué jugar 101 yüzbir okey plus apk?
-
Las características y beneficios del juego
-
101 yüzbir okey plus apk es un juego divertido y adictivo que ofrece muchas características y beneficios para sus jugadores. Algunos de ellos son:
-
-
Puedes jugar online con tus amigos o contra millones de otros jugadores de diferentes países y regiones.
-
Puedes chatear con otros jugadores durante el juego y enviarles regalos, emojis y pegatinas.
-
Puedes personalizar tu perfil, avatar, tabla y mosaicos con varias opciones y temas.
-
Puede unirse o crear clubes y competir con otros clubes en torneos y tablas de clasificación.
-
Puedes ganar fichas gratis todos los días completando misiones, viendo vídeos, girando la rueda o invitando a tus amigos.
-
Puedes comprar fichas adicionales y artículos en el juego con dinero real o usando varios métodos de pago.
-
-
Los retos y consejos del juego
-
101 yüzbir okey plus apk no es solo un juego de suerte, sino también un juego de habilidad y estrategia. Tienes que prestar atención a las fichas de la mesa, la pila de descartes y los movimientos de tus oponentes. También necesitas planificar con anticipación y usar tus comodines sabiamente. Aquí hay algunos desafíos y consejos que pueden ayudarte a mejorar tu juego:
-
-
El desafío: El juego puede ser muy rápido y competitivo, especialmente cuando juegas en línea contra jugadores experimentados. Necesitas ser rápido y alerta para evitar oportunidades perdidas o cometer errores.
-
El consejo: Practica sin conexión contra la inteligencia artificial o juega en línea con apuestas más bajas hasta que te familiarices con el juego. También puedes ver tutoriales o vídeos de otros jugadores para aprender de sus estrategias.
-
-
El consejo: No dejes que tus emociones afecten tus decisiones o acciones. Mantén la calma y concéntrate en tu objetivo. Recuerde que cada ronda es una nueva oportunidad para ganar. También puede tomar descansos o cambiar de mesa si se siente estresado o aburrido.
-
El desafío: El juego puede ser adictivo y tentador, especialmente cuando juegas online con dinero real o con objetos del juego. Necesitas ser responsable y cauteloso para evitar perder más de lo que puedes permitirte o meterte en problemas.
-
El consejo: Establezca un presupuesto y un límite de tiempo para usted antes de empezar a jugar. No persiga sus pérdidas o apueste más de lo que puede manejar. No juegues cuando estés cansado, borracho o distraído. Si tienes un problema de juego, busca la ayuda de un profesional o un grupo de apoyo.
-
-
Conclusión
-
Resumen de los puntos principales
-
En conclusión, 101 yüzbir okey plus apk es un gran juego que combina diversión, habilidad y estrategia. Es una variante de rummy que utiliza fichas en lugar de cartas. Se juega online o offline con tus amigos o contra la inteligencia artificial. Puedes descargar e instalar el juego gratis en tu dispositivo Android, ya sea desde la Google Play Store o desde un sitio web de terceros. También puede disfrutar de las características y beneficios del juego, como chatear, personalizar, unirse a clubes, ganar fichas y comprar artículos. Sin embargo, también debes ser consciente de los desafíos y consejos del juego, como ser rápido, paciente, responsable y cauteloso. Jugar 101 yüzbir okey plus apk puede ser una gran manera de divertirse y mejorar sus habilidades.
-
Llamada a la acción e invitación a jugar
-
-
Preguntas frecuentes
-
Aquí hay algunas preguntas frecuentes sobre 101 yüzbir okey plus apk:
-
-
¿Cuál es la diferencia entre 101 yüzbir okey más apk y otros juegos okey?
-
101 yüzbir okey plus apk es una variante de okey que tiene algunas características y reglas únicas. Por ejemplo, usa 106 fichas en lugar de 104, tiene dos comodines falsos en lugar de uno, requiere una mano ganadora de 14 fichas en lugar de 15, y permite ganar con siete parejas.
-
¿Cómo puedo obtener más fichas en 101 yüzbir okey plus apk?
-
Usted puede obtener más fichas en 101 yüzbir okey más apk completando misiones, viendo vídeos, girando la rueda, invitando a sus amigos, o comprarlos con dinero real u otros métodos de pago.
-
¿Cómo puedo contactar con el equipo de soporte de 101 yüzbir okey plus apk?
-
Puede ponerse en contacto con el equipo de soporte de 101 yüzbir okey plus apk enviando un correo electrónico a [correo electrónico de soporte] o llenando el formulario en [sitio web de soporte]. También puede visitar su página de Facebook o cuenta de Twitter para obtener más información y actualizaciones.
-
¿Cómo puedo jugar 101 yüzbir okey plus apk en mi PC o portátil?
-
Usted puede jugar 101 yüzbir okey más apk en su PC o portátil mediante el uso de un emulador de Android, como BlueStacks o NoxPlayer. Solo tienes que descargar e instalar el emulador en tu PC o portátil, luego descargar e instalar el juego desde la Google Play Store o un sitio web de terceros.
-
¿Es 101 yüzbir okey más apk seguro?
-
Sí, 101 yüzbir okey plus apk es seguro. No contiene ningún virus, malware, spyware, u otros elementos dañinos. Tampoco recopila ni comparte ninguna información personal o confidencial de sus usuarios. Solo requiere algunos permisos para acceder a las funciones de tu dispositivo, como conexión de red, espacio de almacenamiento, cámara, micrófono, etc.
- 64aa2da5cf
-
-
\ No newline at end of file
diff --git a/spaces/Benson/text-generation/Examples/Call Of Duty Black Ops 2 Descarga Mvil.md b/spaces/Benson/text-generation/Examples/Call Of Duty Black Ops 2 Descarga Mvil.md
deleted file mode 100644
index 6a105aad64a64dd94db2b0f4f66a0840f3ba5e94..0000000000000000000000000000000000000000
--- a/spaces/Benson/text-generation/Examples/Call Of Duty Black Ops 2 Descarga Mvil.md
+++ /dev/null
@@ -1,102 +0,0 @@
-
-
Call of Duty Black Ops 2 Descargar móvil: Cómo jugar el FPS clásico en su teléfono
-
Call of Duty Black Ops 2 es uno de los juegos más queridos e influyentes en la historia de los tiradores en primera persona. Lanzado en 2012, fue la novena entrega de la franquicia Call of Duty y la secuela de la original Black Ops. Presentaba un entorno futurista, una historia ramificada, un modo multijugador diverso y un emocionante modo zombis. Fue elogiado por críticos y fans por su jugabilidad, gráficos, sonido e innovación.
-
Si eres un fan de Call of Duty Black Ops 2 o quieres experimentarlo por primera vez, no necesitas una consola o un PC para jugarlo. Puedes reproducirlo en tu dispositivo móvil gracias a Call of Duty Mobile, una aplicación gratuita que trae lo mejor de Call of Duty a tu teléfono. En este artículo, le mostraremos cómo descargar Call of Duty Mobile y acceder a los mapas y modos de Black Ops 2 en su teléfono.
Call of Duty Black Ops 2 es un juego de disparos en primera persona que sigue dos historias interconectadas: una ambientada a finales de 1980 durante la Guerra Fría y otra ambientada en 2025 durante una nueva Guerra Fría. El juego cambia entre estas dos líneas de tiempo a medida que juegas como diferentes personajes que están involucrados en un conflicto entre los Estados Unidos y China por un mineral de tierras raras llamado Celerium. El juego también presenta múltiples finales basados en tus elecciones y acciones a lo largo del juego.
-
Call of Duty Black Ops 2 tiene tres modos principales: multijugador, zombis y campaña. El modo multijugador le permite competir con otros jugadores en línea en varios modos de juego y mapas. El modo zombis te permite formar equipo con otros jugadores o jugar solo contra oleadas de enemigos no muertos en diferentes escenarios. El modo campaña te permite seguir la historia del juego y tomar decisiones que afectan el resultado.
-
-
¿Por qué es popular Call of Duty Black Ops 2?
-
Call of Duty Black Ops 2 es popular por muchas razones. En primer lugar, tiene una base de fans leales que disfrutan de la historia, los personajes y la atmósfera del juego. El juego tiene momentos y personajes memorables, como Frank Woods, Raúl Menéndez y David Mason. El juego también tiene una rica tradición y trasfondo que se conecta con el juego anterior de Black Ops y otros juegos de Call of Duty.
-
En segundo lugar, tiene un modo multijugador divertido y adictivo que ofrece mucho contenido y personalización. El juego tiene docenas de mapas, modos, armas, accesorios, beneficios, scorestreaks y más. El juego también tiene un sistema de clasificación que te recompensa por tu rendimiento y progreso. El juego también tiene una escena competitiva que atrae a muchos jugadores que quieren poner a prueba sus habilidades y estrategias.
-
En tercer lugar, tiene un modo de zombies emocionante y desafiante que proporciona entretenimiento sin fin y acción cooperativa. El juego tiene varios mapas de zombies, cada uno con su propia historia, secretos, huevos de Pascua y objetivos. El juego también tiene diferentes modos de zombies, como Supervivencia, Dolor, Convertido, y Orígenes. El juego también tiene una variedad de enemigos zombies, como rastreadores, perros, jefes, y más.
-
Cómo descargar Call of Duty Mobile
-
Call of Duty Mobile es una aplicación gratuita que te permite jugar Call of Duty en tu dispositivo móvil. Fue lanzado en 2019 por Activision y Tencent Games. Cuenta con muchos elementos de la franquicia Call of Duty, incluyendo personajes, armas, mapas, modos y más. También cuenta con contenido exclusivo y eventos que se actualizan regularmente.
-
Para descargar Call of Duty Mobile en tu dispositivo Android o iOS, debes seguir estos pasos:
-
-
-
Ir a la Google Play Store o la App Store en su dispositivo.
-
Buscar Call of Duty Mobile o utilizar estos enlaces: Android | iOS.
-
Toque en el botón Instalar u Obtener y espere a que la aplicación se descargue.
-
-
Disfruta jugando Call of Duty Mobile en tu teléfono.
-
-
Nota: Call of Duty Mobile requiere una conexión a Internet y al menos 2 GB de RAM para funcionar sin problemas. También requiere al menos 1,5 GB de espacio de almacenamiento gratuito en su dispositivo. Se recomienda utilizar una conexión Wi-Fi o un plan de datos móvil estable para evitar problemas de retraso o desconexión.
-
Cómo acceder a los mapas y modos de Black Ops 2 en Call of Duty Mobile
-
Si quieres jugar Call of Duty Black Ops 2 en tu teléfono, puedes hacerlo accediendo a los mapas y modos de Black Ops 2 en Call of Duty Mobile. Estos están disponibles en el modo multijugador y el modo zombis de la aplicación. Aquí están las formas de acceder a ellos:
-
Modo multijugador
-
El modo multijugador de Call of Duty Mobile te permite jugar con o contra otros jugadores en línea en varios modos de juego y mapas. Puede elegir entre diferentes cargas, operadores, scorestreaks y más. También puede personalizar sus ajustes, como sensibilidad, controles, gráficos y sonido.
-
Mapas
-
El modo multijugador de Call of Duty Mobile tiene muchos mapas en los que puedes jugar. Algunos de estos mapas son de Call of Duty Black Ops 2, como:
-
-
Nuketown: Un pequeño mapa ubicado en un sitio de pruebas nucleares con dos casas enfrentadas.
-
Raid: Un mapa de tamaño mediano ubicado en una mansión de Hollywood con una piscina, un garaje y una cancha de baloncesto.
-
Standoff: Un mapa de tamaño mediano en una ciudad fronteriza con una gasolinera, un mercado y una iglesia.
-
Secuestrado: Un pequeño mapa en un yate de lujo con un helipuerto, un jacuzzi y un bar.
-
Fusión: Un mapa de tamaño mediano en una planta de energía nuclear con una torre de enfriamiento, un reactor y una sala de control.
-
-
Puede seleccionar estos mapas tocando el icono del mapa en la esquina superior derecha de la pantalla del modo multijugador. También puede filtrar los mapas por categorías, como destacados, clásicos o estacionales.
-
Modos
-
-
-
Team Deathmatch: un modo en el que dos equipos de cinco jugadores compiten para obtener la mayor cantidad de muertes en un tiempo limitado.
-
Dominación: un modo donde dos equipos de cinco jugadores compiten para capturar y sostener tres banderas en el mapa.
-
Matar confirmado: Un modo en el que dos equipos de cinco jugadores compiten para obtener el mayor número de muertes y recoger las placas de identificación de los enemigos caídos.
-
Hardpoint: un modo donde dos equipos de cinco jugadores compiten para capturar y mantener un objetivo giratorio en el mapa.
-
Buscar y destruir: un modo en el que dos equipos de cinco jugadores se turnan para atacar y defender dos sitios de bombas en el mapa.
-
-
Puede seleccionar estos modos pulsando en el icono de modo en la esquina superior derecha de la pantalla del modo multijugador. También puede filtrar los modos por categoría, como núcleo, destacado o clasificado.
-
Modo de zombies
-
El modo zombis de Call of Duty Mobile te permite jugar con o contra otros jugadores o bots en varios escenarios que involucran zombies. Puede elegir entre diferentes cargas, operadores, beneficios y más. También puede personalizar sus configuraciones, como dificultad, rondas y salud.
-
Mapas
-
El modo zombis de Call of Duty Mobile tiene varios mapas en los que puedes jugar. Algunos de estos mapas son de Call of Duty Black Ops 2, como:
-
-
TranZit: Un mapa grande que consta de varias ubicaciones conectadas por una ruta de autobús. Puede viajar entre los lugares en autobús o caminando por la niebla.
-
Die Rise: un mapa vertical que se encuentra en un rascacielos desmoronado en China. Puede usar ascensores, trampolines y ejes para moverse por el mapa.
-
Enterrado: Un mapa subterráneo que se encuentra en un antiguo pueblo del oeste enterrado bajo tierra. Puedes usar túneles, carros de minas y un gigante para acceder a diferentes áreas del mapa.
-
-
-
Modos
-
El modo zombis de Call of Duty Mobile tiene diferentes modos en los que puedes jugar. Algunos de estos modos son de Call of Duty Black Ops 2, como:
-
-
Supervivencia: Un modo en el que tienes que sobrevivir el mayor tiempo posible contra interminables oleadas de zombies. Puedes comprar armas, beneficios y otros artículos del mapa para ayudarte a sobrevivir.
-
Duelo: un modo en el que dos equipos de cuatro jugadores compiten para sobrevivir más tiempo que el otro equipo. También puedes sabotear al otro equipo usando carne, granadas o trampas.
-
Turned: Un modo donde un jugador es un humano y los otros son zombies. El humano tiene que sobrevivir el mayor tiempo posible mientras los zombies tienen que matarlo. El zombi que mata al humano se convierte en el nuevo humano.
-
Origins: un modo que se basa en el mapa de Origins de Black Ops 2. Cuenta con cuatro personajes de la historia original de zombies que tienen que luchar contra zombies y robots gigantes en un entorno de la Primera Guerra Mundial.
-
-
Puede seleccionar estos modos pulsando en el icono de modo en la esquina superior derecha de la pantalla del modo zombis. También puede filtrar los modos por categoría, como clásico o hardcore.
-
Modo Battle Royale
-
El modo battle royale de Call of Duty Mobile te permite jugar con o contra otros jugadores o bots en un mapa grande que se reduce con el tiempo. Puede elegir entre diferentes cargas, operadores, vehículos y más. También puedes personalizar tus ajustes, como perspectiva, tamaño de escuadrón y botín.
-
Mapa
-
El modo battle royale de Call of Duty Mobile tiene un mapa en el que puedes jugar. El mapa se llama Aislado y se compone de varios lugares de diferentes juegos de Call of Duty. Algunos de estos lugares son de Call of Duty Black Ops 2, como:
-
-
D ock: Un pequeño mapa situado en una isla prisión con un faro, un bloque de celdas y un puente.
-
Granja: Un mapa de tamaño mediano ubicado en una zona rural con un granero, una granja y un molino de viento.
-
-
Standoff: Un mapa de tamaño mediano en una ciudad fronteriza con una gasolinera, un mercado y una iglesia.
-
Nuketown Island: Un mapa grande que combina Nuketown y Nuketown 2025 con un búnker subterráneo y una instalación de pruebas.
-
-
Puedes explorar estos lugares en paracaídas desde un avión, conduciendo varios vehículos o usando tirolinas. También puedes saquear armas, armaduras, municiones y otros objetos del mapa para ayudarte a sobrevivir.
-
Modo
-
El modo battle royale de Call of Duty Mobile tiene un modo en el que puedes jugar. El modo se llama Battle Royale y es similar al Blackout de Call of Duty Black Ops 4. Cuenta con hasta 100 jugadores que tienen que luchar entre sí hasta que solo quede un jugador o equipo. El modo también cuenta con eventos especiales, como lanzamientos de aire, zombies y jefes.
-
Puedes jugar el modo solo, dúo o escuadrón. También puedes elegir tu clase de operador, como médico, explorador, ninja o defensor. También puedes usar beneficios, habilidades y puntajes para obtener una ventaja sobre tus enemigos.
-
Conclusión
-
Call of Duty Black Ops 2 es un clásico juego de FPS que puedes jugar en tu dispositivo móvil gracias a Call of Duty Mobile. Puedes disfrutar de los modos multijugador, zombis y campaña del juego en tu teléfono con los mismos o similares mapas y modos del juego original. También puedes experimentar la ambientación futurista del juego, la historia ramificada y múltiples finales en tu teléfono. También puedes jugar el modo battle royale del juego con ubicaciones de Black Ops 2 en tu teléfono.
-
Si eres un fan de Call of Duty Black Ops 2 o quieres probarlo por primera vez, deberías descargar Call of Duty Mobile y reproducirlo en tu teléfono. Es gratis para jugar y fácil de instalar. También es divertido y adictivo para jugar. Es la mejor manera de disfrutar de la experiencia FPS clásica en su dispositivo móvil.
-
Preguntas frecuentes
-
Aquí hay algunas preguntas frecuentes sobre Call of Duty Black Ops 2 Mobile Descargar:
-
-
-
A: No, Call of Duty Mobile no es lo mismo que Call of Duty Black Ops 2. Call of Duty Mobile es una aplicación separada que cuenta con elementos de diferentes juegos de Call of Duty, incluyendo Black Ops 2. Sin embargo, puedes jugar algunos de los mapas y modos de Black Ops 2 en Call of Duty Mobile.
-
Q: ¿Puedo jugar Call of Duty Black Ops 2 en mi teléfono sin descargar Call of Duty Mobile?
-
A: No, no puedes jugar Call of Duty Black Ops 2 en tu teléfono sin descargar Call of Duty Mobile. No hay una versión móvil oficial de Call of Duty Black Ops 2. La única forma de reproducirlo en tu teléfono es descargando Call of Duty Mobile y accediendo a los mapas y modos de Black Ops 2 en la aplicación.
-
Q: ¿Cuánto espacio ocupa Call of Duty Mobile en mi teléfono?
-
A: Call of Duty Mobile ocupa aproximadamente 1,5 GB de espacio en su teléfono. Sin embargo, esto puede variar dependiendo del modelo de dispositivo y el sistema operativo. También puede necesitar espacio adicional para actualizaciones y contenido adicional.
-
Q: ¿Puedo jugar Call of Duty Mobile sin conexión?
-
A: No, no puedes jugar Call of Duty Mobile sin conexión. Necesitas una conexión a Internet para jugar. Puede usar Wi-Fi o datos móviles para conectarse a los servidores del juego.
-
Q: ¿Puedo jugar Call of Duty Mobile con mis amigos?
-
A: Sí, puedes jugar a Call of Duty Mobile con tus amigos. Puedes invitarlos a unirse a tu lobby o unirse a su lobby en el juego. También puedes chatear con ellos usando mensajes de voz o de texto en el juego.
- 64aa2da5cf
-
-
\ No newline at end of file
diff --git a/spaces/Benson/text-generation/Examples/Cmo Descargar Hill Climb Racing 2 En PC.md b/spaces/Benson/text-generation/Examples/Cmo Descargar Hill Climb Racing 2 En PC.md
deleted file mode 100644
index 09024def6dcae812903e701a422ffb0e7f5c494e..0000000000000000000000000000000000000000
--- a/spaces/Benson/text-generation/Examples/Cmo Descargar Hill Climb Racing 2 En PC.md
+++ /dev/null
@@ -1,57 +0,0 @@
-
-
Cómo descargar Hill Climb Racing 2 en PC
-
Hill Climb Racing 2 es uno de los juegos de carreras más populares y adictivos en Android. Cuenta con una variedad de vehículos, pistas, modos y desafíos que te mantendrán entretenido durante horas. ¿Pero sabías que también puedes jugar a este juego en tu PC? Jugar a Hill Climb Racing 2 en PC tiene muchas ventajas, como una pantalla más grande, mejores gráficos, un juego más fluido y controles más cómodos. Además, puede ahorrar la duración de la batería del teléfono y el espacio de almacenamiento jugando en su PC. En este artículo, le mostraremos cómo descargar Hill Climb Racing 2 en PC utilizando diferentes métodos. Si desea utilizar la tienda de Microsoft, un emulador de Android, o una plataforma de juegos, tenemos todo cubierto. Sigue estos sencillos pasos y disfruta de Hill Climb Racing 2 en tu PC.
-
Método 1: Uso de Microsoft Store
-
La tienda de Microsoft ofrece una manera conveniente de descargar Hill Climb Racing 2 en su PC. Es una plataforma de distribución digital que le permite acceder a varias aplicaciones y juegos para Windows. Aquí está cómo usarlo:
Abra la aplicación Microsoft Store en su PC. Puede encontrarla en el menú de inicio o presionando Windows Key + S y escribiendo "Microsoft Store".
-
Buscar Hill Climb Racing 2 en la barra de búsqueda y haga clic en él.
-
Haga clic en el botón obtener o comprar para descargar e instalar el juego. Si el juego es gratuito, puede descargarlo sin ningún pago. Si se paga, tendrá que introducir sus datos de pago o utilizar una tarjeta de regalo.
-
Inicie el juego desde el menú de inicio o la aplicación de la tienda. También puede anclarlo a su barra de tareas o escritorio para facilitar el acceso.
-
-
Felicidades, has descargado con éxito Hill Climb Racing 2 en tu PC usando Microsoft Store. Disfruta del juego y diviértete.
-
Método 2: Usando el emulador de BlueStacks
-
-
-
Descargue e instale el emulador de BlueStacks desde su sitio web oficial: https://www.bluestacks.com/. Siga las instrucciones de la pantalla y complete el proceso de instalación.
-
Inicie BlueStacks e inicie sesión con su cuenta de Google. Si no tiene una, puede crear una gratis.
-
Buscar Hill Climb Racing 2 en la aplicación Google Play Store e instalarlo. También puede utilizar la barra de búsqueda en la pantalla de inicio o navegar por las categorías.
-
Iniciar el juego desde la pantalla de inicio o el cajón de aplicaciones. También puede personalizar la configuración, los controles del teclado y los gráficos según sus preferencias.
-
-
Felicidades, has descargado con éxito Hill Climb Racing 2 en tu PC usando el emulador BlueStacks. Disfruta del juego y diviértete.
-
Método 3: Usando el emulador de GameLoop
-
GameLoop es otro emulador de Android popular y confiable para PC. Está especialmente diseñado para juegos y ofrece una experiencia fluida e inmersiva. Tiene una interfaz simple, bajos requisitos del sistema y una gran colección de juegos. Aquí está cómo usarlo:
-
-
Descargue e instale el emulador de GameLoop desde su sitio web oficial: https://gameloop.fun/. Siga las instrucciones de la pantalla y complete el proceso de instalación.
-
Inicie GameLoop y haga clic en la pestaña del centro del juego. Verá una lista de juegos que puede descargar y jugar.
-
Buscar Hill Climb Racing 2 y haga clic en el botón de instalación. El juego se descargará e instalará automáticamente.
-
Inicie el juego desde la pestaña de mis juegos o el acceso directo del escritorio. También puede ajustar la configuración, los controles del teclado y los gráficos según sus preferencias.
-
-
Felicidades, has descargado con éxito Hill Climb Racing 2 en tu PC usando el emulador GameLoop. Disfruta del juego y diviértete.
-
Conclusión
-
-
-
Usa potenciadores y potenciadores sabiamente para ganar ventaja sobre tus oponentes.
-
Actualizar las piezas de su vehículo y desbloquear nuevas pieles y accesorios para mejorar su rendimiento y estilo.
-
Domine la física y los controles de cada vehículo y la pista para evitar chocar o volcar.
-
Compite en varios modos y eventos para ganar monedas, gemas, trofeos y recompensas.
-
Crear o unirse a un equipo para jugar con tus amigos en línea y participar en carreras de equipo y desafíos.
-
-
Esperamos que haya encontrado este artículo útil e informativo. Si tiene alguna pregunta o comentario, no dude en compartirlos en la sección de comentarios a continuación. ¡Gracias por leer y feliz carrera!
-
-
Preguntas frecuentes
-
-
¿Cuáles son los requisitos del sistema para jugar carreras de subida de colina 2 en PC?
-
Los requisitos mínimos del sistema son Windows 7 o superior, procesador Intel o AMD, 4 GB de RAM y DirectX versión 9.0c o superior.
-
¿Cómo puedo personalizar mi personaje y mi vehículo en las carreras de ascenso 2?
-
Puedes personalizar tu personaje y vehículo desbloqueando y actualizando nuevas piezas, pieles y accesorios. También puede cambiar su nombre, bandera y equipo en el menú de configuración.
-
¿Cómo puedo jugar carreras de escalada 2 con mis amigos en línea?
-
Puedes jugar a las carreras de escalada 2 con tus amigos online creando o uniéndote a un equipo, invitando o aceptando invitaciones de otros jugadores, y participando en eventos y carreras de equipo.
-
¿Cómo puedo mejorar mi rendimiento y mis habilidades en las carreras de escalada en colina 2?
-
Usted puede mejorar su rendimiento y habilidades en la subida de la colina de carreras 2 mediante la práctica en diferentes pistas, el dominio de la física y los controles, el uso de potenciadores y potenciadores sabiamente, y aprender de sus errores.
-
¿Cómo puedo contactar a los desarrolladores de Hill Climb Racing 2 para obtener apoyo o comentarios?
You can skip the queue and load custom models in the colab:
- Running on {device}{(" in a Google Colab." if is_colab else "")}
-
-
You can also duplicate this space and upgrade to gpu by going to settings:
-
-
- """
- )
- with gr.Row():
-
- with gr.Column(scale=55):
- with gr.Group():
- model_name = gr.Dropdown(label="Model", choices=[m.name for m in models], value=current_model.name)
- with gr.Box(visible=False) as custom_model_group:
- custom_model_path = gr.Textbox(label="Custom model path", placeholder="Path to model, e.g. nitrosocke/Arcane-Diffusion", interactive=True)
- gr.HTML("
Custom models have to be downloaded first, so give it some time.
-
-
diff --git a/spaces/Raspberry-ai/main/.env/lib/python3.11/site-packages/pip/_internal/metadata/importlib/_compat.py b/spaces/Raspberry-ai/main/.env/lib/python3.11/site-packages/pip/_internal/metadata/importlib/_compat.py
deleted file mode 100644
index 593bff23edecd3c517c96e119ee777bd4ee1d9d0..0000000000000000000000000000000000000000
--- a/spaces/Raspberry-ai/main/.env/lib/python3.11/site-packages/pip/_internal/metadata/importlib/_compat.py
+++ /dev/null
@@ -1,55 +0,0 @@
-import importlib.metadata
-from typing import Any, Optional, Protocol, cast
-
-
-class BadMetadata(ValueError):
- def __init__(self, dist: importlib.metadata.Distribution, *, reason: str) -> None:
- self.dist = dist
- self.reason = reason
-
- def __str__(self) -> str:
- return f"Bad metadata in {self.dist} ({self.reason})"
-
-
-class BasePath(Protocol):
- """A protocol that various path objects conform.
-
- This exists because importlib.metadata uses both ``pathlib.Path`` and
- ``zipfile.Path``, and we need a common base for type hints (Union does not
- work well since ``zipfile.Path`` is too new for our linter setup).
-
- This does not mean to be exhaustive, but only contains things that present
- in both classes *that we need*.
- """
-
- @property
- def name(self) -> str:
- raise NotImplementedError()
-
- @property
- def parent(self) -> "BasePath":
- raise NotImplementedError()
-
-
-def get_info_location(d: importlib.metadata.Distribution) -> Optional[BasePath]:
- """Find the path to the distribution's metadata directory.
-
- HACK: This relies on importlib.metadata's private ``_path`` attribute. Not
- all distributions exist on disk, so importlib.metadata is correct to not
- expose the attribute as public. But pip's code base is old and not as clean,
- so we do this to avoid having to rewrite too many things. Hopefully we can
- eliminate this some day.
- """
- return getattr(d, "_path", None)
-
-
-def get_dist_name(dist: importlib.metadata.Distribution) -> str:
- """Get the distribution's project name.
-
- The ``name`` attribute is only available in Python 3.10 or later. We are
- targeting exactly that, but Mypy does not know this.
- """
- name = cast(Any, dist).name
- if not isinstance(name, str):
- raise BadMetadata(dist, reason="invalid metadata entry 'name'")
- return name
diff --git a/spaces/Raspberry-ai/main/.env/lib/python3.11/site-packages/pip/_vendor/distlib/manifest.py b/spaces/Raspberry-ai/main/.env/lib/python3.11/site-packages/pip/_vendor/distlib/manifest.py
deleted file mode 100644
index ca0fe442d9ca499466df9438df16eca405c5f102..0000000000000000000000000000000000000000
--- a/spaces/Raspberry-ai/main/.env/lib/python3.11/site-packages/pip/_vendor/distlib/manifest.py
+++ /dev/null
@@ -1,393 +0,0 @@
-# -*- coding: utf-8 -*-
-#
-# Copyright (C) 2012-2013 Python Software Foundation.
-# See LICENSE.txt and CONTRIBUTORS.txt.
-#
-"""
-Class representing the list of files in a distribution.
-
-Equivalent to distutils.filelist, but fixes some problems.
-"""
-import fnmatch
-import logging
-import os
-import re
-import sys
-
-from . import DistlibException
-from .compat import fsdecode
-from .util import convert_path
-
-
-__all__ = ['Manifest']
-
-logger = logging.getLogger(__name__)
-
-# a \ followed by some spaces + EOL
-_COLLAPSE_PATTERN = re.compile('\\\\w*\n', re.M)
-_COMMENTED_LINE = re.compile('#.*?(?=\n)|\n(?=$)', re.M | re.S)
-
-#
-# Due to the different results returned by fnmatch.translate, we need
-# to do slightly different processing for Python 2.7 and 3.2 ... this needed
-# to be brought in for Python 3.6 onwards.
-#
-_PYTHON_VERSION = sys.version_info[:2]
-
-class Manifest(object):
- """A list of files built by on exploring the filesystem and filtered by
- applying various patterns to what we find there.
- """
-
- def __init__(self, base=None):
- """
- Initialise an instance.
-
- :param base: The base directory to explore under.
- """
- self.base = os.path.abspath(os.path.normpath(base or os.getcwd()))
- self.prefix = self.base + os.sep
- self.allfiles = None
- self.files = set()
-
- #
- # Public API
- #
-
- def findall(self):
- """Find all files under the base and set ``allfiles`` to the absolute
- pathnames of files found.
- """
- from stat import S_ISREG, S_ISDIR, S_ISLNK
-
- self.allfiles = allfiles = []
- root = self.base
- stack = [root]
- pop = stack.pop
- push = stack.append
-
- while stack:
- root = pop()
- names = os.listdir(root)
-
- for name in names:
- fullname = os.path.join(root, name)
-
- # Avoid excess stat calls -- just one will do, thank you!
- stat = os.stat(fullname)
- mode = stat.st_mode
- if S_ISREG(mode):
- allfiles.append(fsdecode(fullname))
- elif S_ISDIR(mode) and not S_ISLNK(mode):
- push(fullname)
-
- def add(self, item):
- """
- Add a file to the manifest.
-
- :param item: The pathname to add. This can be relative to the base.
- """
- if not item.startswith(self.prefix):
- item = os.path.join(self.base, item)
- self.files.add(os.path.normpath(item))
-
- def add_many(self, items):
- """
- Add a list of files to the manifest.
-
- :param items: The pathnames to add. These can be relative to the base.
- """
- for item in items:
- self.add(item)
-
- def sorted(self, wantdirs=False):
- """
- Return sorted files in directory order
- """
-
- def add_dir(dirs, d):
- dirs.add(d)
- logger.debug('add_dir added %s', d)
- if d != self.base:
- parent, _ = os.path.split(d)
- assert parent not in ('', '/')
- add_dir(dirs, parent)
-
- result = set(self.files) # make a copy!
- if wantdirs:
- dirs = set()
- for f in result:
- add_dir(dirs, os.path.dirname(f))
- result |= dirs
- return [os.path.join(*path_tuple) for path_tuple in
- sorted(os.path.split(path) for path in result)]
-
- def clear(self):
- """Clear all collected files."""
- self.files = set()
- self.allfiles = []
-
- def process_directive(self, directive):
- """
- Process a directive which either adds some files from ``allfiles`` to
- ``files``, or removes some files from ``files``.
-
- :param directive: The directive to process. This should be in a format
- compatible with distutils ``MANIFEST.in`` files:
-
- http://docs.python.org/distutils/sourcedist.html#commands
- """
- # Parse the line: split it up, make sure the right number of words
- # is there, and return the relevant words. 'action' is always
- # defined: it's the first word of the line. Which of the other
- # three are defined depends on the action; it'll be either
- # patterns, (dir and patterns), or (dirpattern).
- action, patterns, thedir, dirpattern = self._parse_directive(directive)
-
- # OK, now we know that the action is valid and we have the
- # right number of words on the line for that action -- so we
- # can proceed with minimal error-checking.
- if action == 'include':
- for pattern in patterns:
- if not self._include_pattern(pattern, anchor=True):
- logger.warning('no files found matching %r', pattern)
-
- elif action == 'exclude':
- for pattern in patterns:
- found = self._exclude_pattern(pattern, anchor=True)
- #if not found:
- # logger.warning('no previously-included files '
- # 'found matching %r', pattern)
-
- elif action == 'global-include':
- for pattern in patterns:
- if not self._include_pattern(pattern, anchor=False):
- logger.warning('no files found matching %r '
- 'anywhere in distribution', pattern)
-
- elif action == 'global-exclude':
- for pattern in patterns:
- found = self._exclude_pattern(pattern, anchor=False)
- #if not found:
- # logger.warning('no previously-included files '
- # 'matching %r found anywhere in '
- # 'distribution', pattern)
-
- elif action == 'recursive-include':
- for pattern in patterns:
- if not self._include_pattern(pattern, prefix=thedir):
- logger.warning('no files found matching %r '
- 'under directory %r', pattern, thedir)
-
- elif action == 'recursive-exclude':
- for pattern in patterns:
- found = self._exclude_pattern(pattern, prefix=thedir)
- #if not found:
- # logger.warning('no previously-included files '
- # 'matching %r found under directory %r',
- # pattern, thedir)
-
- elif action == 'graft':
- if not self._include_pattern(None, prefix=dirpattern):
- logger.warning('no directories found matching %r',
- dirpattern)
-
- elif action == 'prune':
- if not self._exclude_pattern(None, prefix=dirpattern):
- logger.warning('no previously-included directories found '
- 'matching %r', dirpattern)
- else: # pragma: no cover
- # This should never happen, as it should be caught in
- # _parse_template_line
- raise DistlibException(
- 'invalid action %r' % action)
-
- #
- # Private API
- #
-
- def _parse_directive(self, directive):
- """
- Validate a directive.
- :param directive: The directive to validate.
- :return: A tuple of action, patterns, thedir, dir_patterns
- """
- words = directive.split()
- if len(words) == 1 and words[0] not in ('include', 'exclude',
- 'global-include',
- 'global-exclude',
- 'recursive-include',
- 'recursive-exclude',
- 'graft', 'prune'):
- # no action given, let's use the default 'include'
- words.insert(0, 'include')
-
- action = words[0]
- patterns = thedir = dir_pattern = None
-
- if action in ('include', 'exclude',
- 'global-include', 'global-exclude'):
- if len(words) < 2:
- raise DistlibException(
- '%r expects ...' % action)
-
- patterns = [convert_path(word) for word in words[1:]]
-
- elif action in ('recursive-include', 'recursive-exclude'):
- if len(words) < 3:
- raise DistlibException(
- '%r expects ...' % action)
-
- thedir = convert_path(words[1])
- patterns = [convert_path(word) for word in words[2:]]
-
- elif action in ('graft', 'prune'):
- if len(words) != 2:
- raise DistlibException(
- '%r expects a single ' % action)
-
- dir_pattern = convert_path(words[1])
-
- else:
- raise DistlibException('unknown action %r' % action)
-
- return action, patterns, thedir, dir_pattern
-
- def _include_pattern(self, pattern, anchor=True, prefix=None,
- is_regex=False):
- """Select strings (presumably filenames) from 'self.files' that
- match 'pattern', a Unix-style wildcard (glob) pattern.
-
- Patterns are not quite the same as implemented by the 'fnmatch'
- module: '*' and '?' match non-special characters, where "special"
- is platform-dependent: slash on Unix; colon, slash, and backslash on
- DOS/Windows; and colon on Mac OS.
-
- If 'anchor' is true (the default), then the pattern match is more
- stringent: "*.py" will match "foo.py" but not "foo/bar.py". If
- 'anchor' is false, both of these will match.
-
- If 'prefix' is supplied, then only filenames starting with 'prefix'
- (itself a pattern) and ending with 'pattern', with anything in between
- them, will match. 'anchor' is ignored in this case.
-
- If 'is_regex' is true, 'anchor' and 'prefix' are ignored, and
- 'pattern' is assumed to be either a string containing a regex or a
- regex object -- no translation is done, the regex is just compiled
- and used as-is.
-
- Selected strings will be added to self.files.
-
- Return True if files are found.
- """
- # XXX docstring lying about what the special chars are?
- found = False
- pattern_re = self._translate_pattern(pattern, anchor, prefix, is_regex)
-
- # delayed loading of allfiles list
- if self.allfiles is None:
- self.findall()
-
- for name in self.allfiles:
- if pattern_re.search(name):
- self.files.add(name)
- found = True
- return found
-
- def _exclude_pattern(self, pattern, anchor=True, prefix=None,
- is_regex=False):
- """Remove strings (presumably filenames) from 'files' that match
- 'pattern'.
-
- Other parameters are the same as for 'include_pattern()', above.
- The list 'self.files' is modified in place. Return True if files are
- found.
-
- This API is public to allow e.g. exclusion of SCM subdirs, e.g. when
- packaging source distributions
- """
- found = False
- pattern_re = self._translate_pattern(pattern, anchor, prefix, is_regex)
- for f in list(self.files):
- if pattern_re.search(f):
- self.files.remove(f)
- found = True
- return found
-
- def _translate_pattern(self, pattern, anchor=True, prefix=None,
- is_regex=False):
- """Translate a shell-like wildcard pattern to a compiled regular
- expression.
-
- Return the compiled regex. If 'is_regex' true,
- then 'pattern' is directly compiled to a regex (if it's a string)
- or just returned as-is (assumes it's a regex object).
- """
- if is_regex:
- if isinstance(pattern, str):
- return re.compile(pattern)
- else:
- return pattern
-
- if _PYTHON_VERSION > (3, 2):
- # ditch start and end characters
- start, _, end = self._glob_to_re('_').partition('_')
-
- if pattern:
- pattern_re = self._glob_to_re(pattern)
- if _PYTHON_VERSION > (3, 2):
- assert pattern_re.startswith(start) and pattern_re.endswith(end)
- else:
- pattern_re = ''
-
- base = re.escape(os.path.join(self.base, ''))
- if prefix is not None:
- # ditch end of pattern character
- if _PYTHON_VERSION <= (3, 2):
- empty_pattern = self._glob_to_re('')
- prefix_re = self._glob_to_re(prefix)[:-len(empty_pattern)]
- else:
- prefix_re = self._glob_to_re(prefix)
- assert prefix_re.startswith(start) and prefix_re.endswith(end)
- prefix_re = prefix_re[len(start): len(prefix_re) - len(end)]
- sep = os.sep
- if os.sep == '\\':
- sep = r'\\'
- if _PYTHON_VERSION <= (3, 2):
- pattern_re = '^' + base + sep.join((prefix_re,
- '.*' + pattern_re))
- else:
- pattern_re = pattern_re[len(start): len(pattern_re) - len(end)]
- pattern_re = r'%s%s%s%s.*%s%s' % (start, base, prefix_re, sep,
- pattern_re, end)
- else: # no prefix -- respect anchor flag
- if anchor:
- if _PYTHON_VERSION <= (3, 2):
- pattern_re = '^' + base + pattern_re
- else:
- pattern_re = r'%s%s%s' % (start, base, pattern_re[len(start):])
-
- return re.compile(pattern_re)
-
- def _glob_to_re(self, pattern):
- """Translate a shell-like glob pattern to a regular expression.
-
- Return a string containing the regex. Differs from
- 'fnmatch.translate()' in that '*' does not match "special characters"
- (which are platform-specific).
- """
- pattern_re = fnmatch.translate(pattern)
-
- # '?' and '*' in the glob pattern become '.' and '.*' in the RE, which
- # IMHO is wrong -- '?' and '*' aren't supposed to match slash in Unix,
- # and by extension they shouldn't match such "special characters" under
- # any OS. So change all non-escaped dots in the RE to match any
- # character except the special characters (currently: just os.sep).
- sep = os.sep
- if os.sep == '\\':
- # we're using a regex to manipulate a regex, so we need
- # to escape the backslash twice
- sep = r'\\\\'
- escaped = r'\1[^%s]' % sep
- pattern_re = re.sub(r'((? 0
-
- def __ge__(self, other):
- c = self._cmp(other)
- if c is NotImplemented:
- return c
- return c >= 0
-
-
-# Interface for version-number classes -- must be implemented
-# by the following classes (the concrete ones -- Version should
-# be treated as an abstract class).
-# __init__ (string) - create and take same action as 'parse'
-# (string parameter is optional)
-# parse (string) - convert a string representation to whatever
-# internal representation is appropriate for
-# this style of version numbering
-# __str__ (self) - convert back to a string; should be very similar
-# (if not identical to) the string supplied to parse
-# __repr__ (self) - generate Python code to recreate
-# the instance
-# _cmp (self, other) - compare two version numbers ('other' may
-# be an unparsed version string, or another
-# instance of your version class)
-
-
-class StrictVersion(Version):
-
- """Version numbering for anal retentives and software idealists.
- Implements the standard interface for version number classes as
- described above. A version number consists of two or three
- dot-separated numeric components, with an optional "pre-release" tag
- on the end. The pre-release tag consists of the letter 'a' or 'b'
- followed by a number. If the numeric components of two version
- numbers are equal, then one with a pre-release tag will always
- be deemed earlier (lesser) than one without.
-
- The following are valid version numbers (shown in the order that
- would be obtained by sorting according to the supplied cmp function):
-
- 0.4 0.4.0 (these two are equivalent)
- 0.4.1
- 0.5a1
- 0.5b3
- 0.5
- 0.9.6
- 1.0
- 1.0.4a3
- 1.0.4b1
- 1.0.4
-
- The following are examples of invalid version numbers:
-
- 1
- 2.7.2.2
- 1.3.a4
- 1.3pl1
- 1.3c4
-
- The rationale for this version numbering system will be explained
- in the distutils documentation.
- """
-
- version_re = re.compile(
- r'^(\d+) \. (\d+) (\. (\d+))? ([ab](\d+))?$', re.VERBOSE | re.ASCII
- )
-
- def parse(self, vstring):
- match = self.version_re.match(vstring)
- if not match:
- raise ValueError("invalid version number '%s'" % vstring)
-
- (major, minor, patch, prerelease, prerelease_num) = match.group(1, 2, 4, 5, 6)
-
- if patch:
- self.version = tuple(map(int, [major, minor, patch]))
- else:
- self.version = tuple(map(int, [major, minor])) + (0,)
-
- if prerelease:
- self.prerelease = (prerelease[0], int(prerelease_num))
- else:
- self.prerelease = None
-
- def __str__(self):
-
- if self.version[2] == 0:
- vstring = '.'.join(map(str, self.version[0:2]))
- else:
- vstring = '.'.join(map(str, self.version))
-
- if self.prerelease:
- vstring = vstring + self.prerelease[0] + str(self.prerelease[1])
-
- return vstring
-
- def _cmp(self, other): # noqa: C901
- if isinstance(other, str):
- with suppress_known_deprecation():
- other = StrictVersion(other)
- elif not isinstance(other, StrictVersion):
- return NotImplemented
-
- if self.version != other.version:
- # numeric versions don't match
- # prerelease stuff doesn't matter
- if self.version < other.version:
- return -1
- else:
- return 1
-
- # have to compare prerelease
- # case 1: neither has prerelease; they're equal
- # case 2: self has prerelease, other doesn't; other is greater
- # case 3: self doesn't have prerelease, other does: self is greater
- # case 4: both have prerelease: must compare them!
-
- if not self.prerelease and not other.prerelease:
- return 0
- elif self.prerelease and not other.prerelease:
- return -1
- elif not self.prerelease and other.prerelease:
- return 1
- elif self.prerelease and other.prerelease:
- if self.prerelease == other.prerelease:
- return 0
- elif self.prerelease < other.prerelease:
- return -1
- else:
- return 1
- else:
- assert False, "never get here"
-
-
-# end class StrictVersion
-
-
-# The rules according to Greg Stein:
-# 1) a version number has 1 or more numbers separated by a period or by
-# sequences of letters. If only periods, then these are compared
-# left-to-right to determine an ordering.
-# 2) sequences of letters are part of the tuple for comparison and are
-# compared lexicographically
-# 3) recognize the numeric components may have leading zeroes
-#
-# The LooseVersion class below implements these rules: a version number
-# string is split up into a tuple of integer and string components, and
-# comparison is a simple tuple comparison. This means that version
-# numbers behave in a predictable and obvious way, but a way that might
-# not necessarily be how people *want* version numbers to behave. There
-# wouldn't be a problem if people could stick to purely numeric version
-# numbers: just split on period and compare the numbers as tuples.
-# However, people insist on putting letters into their version numbers;
-# the most common purpose seems to be:
-# - indicating a "pre-release" version
-# ('alpha', 'beta', 'a', 'b', 'pre', 'p')
-# - indicating a post-release patch ('p', 'pl', 'patch')
-# but of course this can't cover all version number schemes, and there's
-# no way to know what a programmer means without asking him.
-#
-# The problem is what to do with letters (and other non-numeric
-# characters) in a version number. The current implementation does the
-# obvious and predictable thing: keep them as strings and compare
-# lexically within a tuple comparison. This has the desired effect if
-# an appended letter sequence implies something "post-release":
-# eg. "0.99" < "0.99pl14" < "1.0", and "5.001" < "5.001m" < "5.002".
-#
-# However, if letters in a version number imply a pre-release version,
-# the "obvious" thing isn't correct. Eg. you would expect that
-# "1.5.1" < "1.5.2a2" < "1.5.2", but under the tuple/lexical comparison
-# implemented here, this just isn't so.
-#
-# Two possible solutions come to mind. The first is to tie the
-# comparison algorithm to a particular set of semantic rules, as has
-# been done in the StrictVersion class above. This works great as long
-# as everyone can go along with bondage and discipline. Hopefully a
-# (large) subset of Python module programmers will agree that the
-# particular flavour of bondage and discipline provided by StrictVersion
-# provides enough benefit to be worth using, and will submit their
-# version numbering scheme to its domination. The free-thinking
-# anarchists in the lot will never give in, though, and something needs
-# to be done to accommodate them.
-#
-# Perhaps a "moderately strict" version class could be implemented that
-# lets almost anything slide (syntactically), and makes some heuristic
-# assumptions about non-digits in version number strings. This could
-# sink into special-case-hell, though; if I was as talented and
-# idiosyncratic as Larry Wall, I'd go ahead and implement a class that
-# somehow knows that "1.2.1" < "1.2.2a2" < "1.2.2" < "1.2.2pl3", and is
-# just as happy dealing with things like "2g6" and "1.13++". I don't
-# think I'm smart enough to do it right though.
-#
-# In any case, I've coded the test suite for this module (see
-# ../test/test_version.py) specifically to fail on things like comparing
-# "1.2a2" and "1.2". That's not because the *code* is doing anything
-# wrong, it's because the simple, obvious design doesn't match my
-# complicated, hairy expectations for real-world version numbers. It
-# would be a snap to fix the test suite to say, "Yep, LooseVersion does
-# the Right Thing" (ie. the code matches the conception). But I'd rather
-# have a conception that matches common notions about version numbers.
-
-
-class LooseVersion(Version):
-
- """Version numbering for anarchists and software realists.
- Implements the standard interface for version number classes as
- described above. A version number consists of a series of numbers,
- separated by either periods or strings of letters. When comparing
- version numbers, the numeric components will be compared
- numerically, and the alphabetic components lexically. The following
- are all valid version numbers, in no particular order:
-
- 1.5.1
- 1.5.2b2
- 161
- 3.10a
- 8.02
- 3.4j
- 1996.07.12
- 3.2.pl0
- 3.1.1.6
- 2g6
- 11g
- 0.960923
- 2.2beta29
- 1.13++
- 5.5.kw
- 2.0b1pl0
-
- In fact, there is no such thing as an invalid version number under
- this scheme; the rules for comparison are simple and predictable,
- but may not always give the results you want (for some definition
- of "want").
- """
-
- component_re = re.compile(r'(\d+ | [a-z]+ | \.)', re.VERBOSE)
-
- def parse(self, vstring):
- # I've given up on thinking I can reconstruct the version string
- # from the parsed tuple -- so I just store the string here for
- # use by __str__
- self.vstring = vstring
- components = [x for x in self.component_re.split(vstring) if x and x != '.']
- for i, obj in enumerate(components):
- try:
- components[i] = int(obj)
- except ValueError:
- pass
-
- self.version = components
-
- def __str__(self):
- return self.vstring
-
- def __repr__(self):
- return "LooseVersion ('%s')" % str(self)
-
- def _cmp(self, other):
- if isinstance(other, str):
- other = LooseVersion(other)
- elif not isinstance(other, LooseVersion):
- return NotImplemented
-
- if self.version == other.version:
- return 0
- if self.version < other.version:
- return -1
- if self.version > other.version:
- return 1
-
-
-# end class LooseVersion
diff --git a/spaces/Rehman1603/Video-To-Text/README.md b/spaces/Rehman1603/Video-To-Text/README.md
deleted file mode 100644
index 4cd94539a532e266dcae2ac421413dbbd1d1b32d..0000000000000000000000000000000000000000
--- a/spaces/Rehman1603/Video-To-Text/README.md
+++ /dev/null
@@ -1,12 +0,0 @@
----
-title: Video To Text
-emoji: 🐠
-colorFrom: yellow
-colorTo: indigo
-sdk: gradio
-sdk_version: 3.34.0
-app_file: app.py
-pinned: false
----
-
-Check out the configuration reference at https://huggingface.co/docs/hub/spaces-config-reference
diff --git a/spaces/Ricecake123/RVC-demo/extract_f0_print.py b/spaces/Ricecake123/RVC-demo/extract_f0_print.py
deleted file mode 100644
index 76dcad173834de10f0f84277308b1c5722eb9e0f..0000000000000000000000000000000000000000
--- a/spaces/Ricecake123/RVC-demo/extract_f0_print.py
+++ /dev/null
@@ -1,159 +0,0 @@
-import os, traceback, sys, parselmouth
-
-now_dir = os.getcwd()
-sys.path.append(now_dir)
-from my_utils import load_audio
-import pyworld
-import numpy as np, logging
-
-logging.getLogger("numba").setLevel(logging.WARNING)
-from multiprocessing import Process
-
-exp_dir = sys.argv[1]
-f = open("%s/extract_f0_feature.log" % exp_dir, "a+")
-
-
-def printt(strr):
- print(strr)
- f.write("%s\n" % strr)
- f.flush()
-
-
-n_p = int(sys.argv[2])
-f0method = sys.argv[3]
-
-
-class FeatureInput(object):
- def __init__(self, samplerate=16000, hop_size=160):
- self.fs = samplerate
- self.hop = hop_size
-
- self.f0_bin = 256
- self.f0_max = 1100.0
- self.f0_min = 50.0
- self.f0_mel_min = 1127 * np.log(1 + self.f0_min / 700)
- self.f0_mel_max = 1127 * np.log(1 + self.f0_max / 700)
-
- def compute_f0(self, path, f0_method):
- x = load_audio(path, self.fs)
- p_len = x.shape[0] // self.hop
- if f0_method == "pm":
- time_step = 160 / 16000 * 1000
- f0_min = 50
- f0_max = 1100
- f0 = (
- parselmouth.Sound(x, self.fs)
- .to_pitch_ac(
- time_step=time_step / 1000,
- voicing_threshold=0.6,
- pitch_floor=f0_min,
- pitch_ceiling=f0_max,
- )
- .selected_array["frequency"]
- )
- pad_size = (p_len - len(f0) + 1) // 2
- if pad_size > 0 or p_len - len(f0) - pad_size > 0:
- f0 = np.pad(
- f0, [[pad_size, p_len - len(f0) - pad_size]], mode="constant"
- )
- elif f0_method == "harvest":
- f0, t = pyworld.harvest(
- x.astype(np.double),
- fs=self.fs,
- f0_ceil=self.f0_max,
- f0_floor=self.f0_min,
- frame_period=1000 * self.hop / self.fs,
- )
- f0 = pyworld.stonemask(x.astype(np.double), f0, t, self.fs)
- elif f0_method == "dio":
- f0, t = pyworld.dio(
- x.astype(np.double),
- fs=self.fs,
- f0_ceil=self.f0_max,
- f0_floor=self.f0_min,
- frame_period=1000 * self.hop / self.fs,
- )
- f0 = pyworld.stonemask(x.astype(np.double), f0, t, self.fs)
- return f0
-
- def coarse_f0(self, f0):
- f0_mel = 1127 * np.log(1 + f0 / 700)
- f0_mel[f0_mel > 0] = (f0_mel[f0_mel > 0] - self.f0_mel_min) * (
- self.f0_bin - 2
- ) / (self.f0_mel_max - self.f0_mel_min) + 1
-
- # use 0 or 1
- f0_mel[f0_mel <= 1] = 1
- f0_mel[f0_mel > self.f0_bin - 1] = self.f0_bin - 1
- f0_coarse = np.rint(f0_mel).astype(int)
- assert f0_coarse.max() <= 255 and f0_coarse.min() >= 1, (
- f0_coarse.max(),
- f0_coarse.min(),
- )
- return f0_coarse
-
- def go(self, paths, f0_method):
- if len(paths) == 0:
- printt("no-f0-todo")
- else:
- printt("todo-f0-%s" % len(paths))
- n = max(len(paths) // 5, 1) # 每个进程最多打印5条
- for idx, (inp_path, opt_path1, opt_path2) in enumerate(paths):
- try:
- if idx % n == 0:
- printt("f0ing,now-%s,all-%s,-%s" % (idx, len(paths), inp_path))
- if (
- os.path.exists(opt_path1 + ".npy") == True
- and os.path.exists(opt_path2 + ".npy") == True
- ):
- continue
- featur_pit = self.compute_f0(inp_path, f0_method)
- np.save(
- opt_path2,
- featur_pit,
- allow_pickle=False,
- ) # nsf
- coarse_pit = self.coarse_f0(featur_pit)
- np.save(
- opt_path1,
- coarse_pit,
- allow_pickle=False,
- ) # ori
- except:
- printt("f0fail-%s-%s-%s" % (idx, inp_path, traceback.format_exc()))
-
-
-if __name__ == "__main__":
- # exp_dir=r"E:\codes\py39\dataset\mi-test"
- # n_p=16
- # f = open("%s/log_extract_f0.log"%exp_dir, "w")
- printt(sys.argv)
- featureInput = FeatureInput()
- paths = []
- inp_root = "%s/1_16k_wavs" % (exp_dir)
- opt_root1 = "%s/2a_f0" % (exp_dir)
- opt_root2 = "%s/2b-f0nsf" % (exp_dir)
-
- os.makedirs(opt_root1, exist_ok=True)
- os.makedirs(opt_root2, exist_ok=True)
- for name in sorted(list(os.listdir(inp_root))):
- inp_path = "%s/%s" % (inp_root, name)
- if "spec" in inp_path:
- continue
- opt_path1 = "%s/%s" % (opt_root1, name)
- opt_path2 = "%s/%s" % (opt_root2, name)
- paths.append([inp_path, opt_path1, opt_path2])
-
- ps = []
- for i in range(n_p):
- p = Process(
- target=featureInput.go,
- args=(
- paths[i::n_p],
- f0method,
- ),
- )
- ps.append(p)
- p.start()
- for i in range(n_p):
- ps[i].join()
diff --git a/spaces/Robert001/UniControl-Demo/annotator/uniformer/mmcv/runner/checkpoint.py b/spaces/Robert001/UniControl-Demo/annotator/uniformer/mmcv/runner/checkpoint.py
deleted file mode 100644
index b29ca320679164432f446adad893e33fb2b4b29e..0000000000000000000000000000000000000000
--- a/spaces/Robert001/UniControl-Demo/annotator/uniformer/mmcv/runner/checkpoint.py
+++ /dev/null
@@ -1,707 +0,0 @@
-# Copyright (c) OpenMMLab. All rights reserved.
-import io
-import os
-import os.path as osp
-import pkgutil
-import re
-import time
-import warnings
-from collections import OrderedDict
-from importlib import import_module
-from tempfile import TemporaryDirectory
-
-import torch
-import torchvision
-from torch.optim import Optimizer
-from torch.utils import model_zoo
-
-import annotator.uniformer.mmcv as mmcv
-from ..fileio import FileClient
-from ..fileio import load as load_file
-from ..parallel import is_module_wrapper
-from ..utils import mkdir_or_exist
-from .dist_utils import get_dist_info
-
-ENV_MMCV_HOME = 'MMCV_HOME'
-ENV_XDG_CACHE_HOME = 'XDG_CACHE_HOME'
-DEFAULT_CACHE_DIR = '~/.cache'
-
-
-def _get_mmcv_home():
- mmcv_home = os.path.expanduser(
- os.getenv(
- ENV_MMCV_HOME,
- os.path.join(
- os.getenv(ENV_XDG_CACHE_HOME, DEFAULT_CACHE_DIR), 'mmcv')))
-
- mkdir_or_exist(mmcv_home)
- return mmcv_home
-
-
-def load_state_dict(module, state_dict, strict=False, logger=None):
- """Load state_dict to a module.
-
- This method is modified from :meth:`torch.nn.Module.load_state_dict`.
- Default value for ``strict`` is set to ``False`` and the message for
- param mismatch will be shown even if strict is False.
-
- Args:
- module (Module): Module that receives the state_dict.
- state_dict (OrderedDict): Weights.
- strict (bool): whether to strictly enforce that the keys
- in :attr:`state_dict` match the keys returned by this module's
- :meth:`~torch.nn.Module.state_dict` function. Default: ``False``.
- logger (:obj:`logging.Logger`, optional): Logger to log the error
- message. If not specified, print function will be used.
- """
- unexpected_keys = []
- all_missing_keys = []
- err_msg = []
-
- metadata = getattr(state_dict, '_metadata', None)
- state_dict = state_dict.copy()
- if metadata is not None:
- state_dict._metadata = metadata
-
- # use _load_from_state_dict to enable checkpoint version control
- def load(module, prefix=''):
- # recursively check parallel module in case that the model has a
- # complicated structure, e.g., nn.Module(nn.Module(DDP))
- if is_module_wrapper(module):
- module = module.module
- local_metadata = {} if metadata is None else metadata.get(
- prefix[:-1], {})
- module._load_from_state_dict(state_dict, prefix, local_metadata, True,
- all_missing_keys, unexpected_keys,
- err_msg)
- for name, child in module._modules.items():
- if child is not None:
- load(child, prefix + name + '.')
-
- load(module)
- load = None # break load->load reference cycle
-
- # ignore "num_batches_tracked" of BN layers
- missing_keys = [
- key for key in all_missing_keys if 'num_batches_tracked' not in key
- ]
-
- if unexpected_keys:
- err_msg.append('unexpected key in source '
- f'state_dict: {", ".join(unexpected_keys)}\n')
- if missing_keys:
- err_msg.append(
- f'missing keys in source state_dict: {", ".join(missing_keys)}\n')
-
- rank, _ = get_dist_info()
- if len(err_msg) > 0 and rank == 0:
- err_msg.insert(
- 0, 'The model and loaded state dict do not match exactly\n')
- err_msg = '\n'.join(err_msg)
- if strict:
- raise RuntimeError(err_msg)
- elif logger is not None:
- logger.warning(err_msg)
- else:
- print(err_msg)
-
-
-def get_torchvision_models():
- model_urls = dict()
- for _, name, ispkg in pkgutil.walk_packages(torchvision.models.__path__):
- if ispkg:
- continue
- _zoo = import_module(f'torchvision.models.{name}')
- if hasattr(_zoo, 'model_urls'):
- _urls = getattr(_zoo, 'model_urls')
- model_urls.update(_urls)
- return model_urls
-
-
-def get_external_models():
- mmcv_home = _get_mmcv_home()
- default_json_path = osp.join(mmcv.__path__[0], 'model_zoo/open_mmlab.json')
- default_urls = load_file(default_json_path)
- assert isinstance(default_urls, dict)
- external_json_path = osp.join(mmcv_home, 'open_mmlab.json')
- if osp.exists(external_json_path):
- external_urls = load_file(external_json_path)
- assert isinstance(external_urls, dict)
- default_urls.update(external_urls)
-
- return default_urls
-
-
-def get_mmcls_models():
- mmcls_json_path = osp.join(mmcv.__path__[0], 'model_zoo/mmcls.json')
- mmcls_urls = load_file(mmcls_json_path)
-
- return mmcls_urls
-
-
-def get_deprecated_model_names():
- deprecate_json_path = osp.join(mmcv.__path__[0],
- 'model_zoo/deprecated.json')
- deprecate_urls = load_file(deprecate_json_path)
- assert isinstance(deprecate_urls, dict)
-
- return deprecate_urls
-
-
-def _process_mmcls_checkpoint(checkpoint):
- state_dict = checkpoint['state_dict']
- new_state_dict = OrderedDict()
- for k, v in state_dict.items():
- if k.startswith('backbone.'):
- new_state_dict[k[9:]] = v
- new_checkpoint = dict(state_dict=new_state_dict)
-
- return new_checkpoint
-
-
-class CheckpointLoader:
- """A general checkpoint loader to manage all schemes."""
-
- _schemes = {}
-
- @classmethod
- def _register_scheme(cls, prefixes, loader, force=False):
- if isinstance(prefixes, str):
- prefixes = [prefixes]
- else:
- assert isinstance(prefixes, (list, tuple))
- for prefix in prefixes:
- if (prefix not in cls._schemes) or force:
- cls._schemes[prefix] = loader
- else:
- raise KeyError(
- f'{prefix} is already registered as a loader backend, '
- 'add "force=True" if you want to override it')
- # sort, longer prefixes take priority
- cls._schemes = OrderedDict(
- sorted(cls._schemes.items(), key=lambda t: t[0], reverse=True))
-
- @classmethod
- def register_scheme(cls, prefixes, loader=None, force=False):
- """Register a loader to CheckpointLoader.
-
- This method can be used as a normal class method or a decorator.
-
- Args:
- prefixes (str or list[str] or tuple[str]):
- The prefix of the registered loader.
- loader (function, optional): The loader function to be registered.
- When this method is used as a decorator, loader is None.
- Defaults to None.
- force (bool, optional): Whether to override the loader
- if the prefix has already been registered. Defaults to False.
- """
-
- if loader is not None:
- cls._register_scheme(prefixes, loader, force=force)
- return
-
- def _register(loader_cls):
- cls._register_scheme(prefixes, loader_cls, force=force)
- return loader_cls
-
- return _register
-
- @classmethod
- def _get_checkpoint_loader(cls, path):
- """Finds a loader that supports the given path. Falls back to the local
- loader if no other loader is found.
-
- Args:
- path (str): checkpoint path
-
- Returns:
- loader (function): checkpoint loader
- """
-
- for p in cls._schemes:
- if path.startswith(p):
- return cls._schemes[p]
-
- @classmethod
- def load_checkpoint(cls, filename, map_location=None, logger=None):
- """load checkpoint through URL scheme path.
-
- Args:
- filename (str): checkpoint file name with given prefix
- map_location (str, optional): Same as :func:`torch.load`.
- Default: None
- logger (:mod:`logging.Logger`, optional): The logger for message.
- Default: None
-
- Returns:
- dict or OrderedDict: The loaded checkpoint.
- """
-
- checkpoint_loader = cls._get_checkpoint_loader(filename)
- class_name = checkpoint_loader.__name__
- mmcv.print_log(
- f'load checkpoint from {class_name[10:]} path: {filename}', logger)
- return checkpoint_loader(filename, map_location)
-
-
-@CheckpointLoader.register_scheme(prefixes='')
-def load_from_local(filename, map_location):
- """load checkpoint by local file path.
-
- Args:
- filename (str): local checkpoint file path
- map_location (str, optional): Same as :func:`torch.load`.
-
- Returns:
- dict or OrderedDict: The loaded checkpoint.
- """
-
- if not osp.isfile(filename):
- raise IOError(f'{filename} is not a checkpoint file')
- checkpoint = torch.load(filename, map_location=map_location)
- return checkpoint
-
-
-@CheckpointLoader.register_scheme(prefixes=('http://', 'https://'))
-def load_from_http(filename, map_location=None, model_dir=None):
- """load checkpoint through HTTP or HTTPS scheme path. In distributed
- setting, this function only download checkpoint at local rank 0.
-
- Args:
- filename (str): checkpoint file path with modelzoo or
- torchvision prefix
- map_location (str, optional): Same as :func:`torch.load`.
- model_dir (string, optional): directory in which to save the object,
- Default: None
-
- Returns:
- dict or OrderedDict: The loaded checkpoint.
- """
- rank, world_size = get_dist_info()
- rank = int(os.environ.get('LOCAL_RANK', rank))
- if rank == 0:
- checkpoint = model_zoo.load_url(
- filename, model_dir=model_dir, map_location=map_location)
- if world_size > 1:
- torch.distributed.barrier()
- if rank > 0:
- checkpoint = model_zoo.load_url(
- filename, model_dir=model_dir, map_location=map_location)
- return checkpoint
-
-
-@CheckpointLoader.register_scheme(prefixes='pavi://')
-def load_from_pavi(filename, map_location=None):
- """load checkpoint through the file path prefixed with pavi. In distributed
- setting, this function download ckpt at all ranks to different temporary
- directories.
-
- Args:
- filename (str): checkpoint file path with pavi prefix
- map_location (str, optional): Same as :func:`torch.load`.
- Default: None
-
- Returns:
- dict or OrderedDict: The loaded checkpoint.
- """
- assert filename.startswith('pavi://'), \
- f'Expected filename startswith `pavi://`, but get {filename}'
- model_path = filename[7:]
-
- try:
- from pavi import modelcloud
- except ImportError:
- raise ImportError(
- 'Please install pavi to load checkpoint from modelcloud.')
-
- model = modelcloud.get(model_path)
- with TemporaryDirectory() as tmp_dir:
- downloaded_file = osp.join(tmp_dir, model.name)
- model.download(downloaded_file)
- checkpoint = torch.load(downloaded_file, map_location=map_location)
- return checkpoint
-
-
-@CheckpointLoader.register_scheme(prefixes='s3://')
-def load_from_ceph(filename, map_location=None, backend='petrel'):
- """load checkpoint through the file path prefixed with s3. In distributed
- setting, this function download ckpt at all ranks to different temporary
- directories.
-
- Args:
- filename (str): checkpoint file path with s3 prefix
- map_location (str, optional): Same as :func:`torch.load`.
- backend (str, optional): The storage backend type. Options are 'ceph',
- 'petrel'. Default: 'petrel'.
-
- .. warning::
- :class:`mmcv.fileio.file_client.CephBackend` will be deprecated,
- please use :class:`mmcv.fileio.file_client.PetrelBackend` instead.
-
- Returns:
- dict or OrderedDict: The loaded checkpoint.
- """
- allowed_backends = ['ceph', 'petrel']
- if backend not in allowed_backends:
- raise ValueError(f'Load from Backend {backend} is not supported.')
-
- if backend == 'ceph':
- warnings.warn(
- 'CephBackend will be deprecated, please use PetrelBackend instead')
-
- # CephClient and PetrelBackend have the same prefix 's3://' and the latter
- # will be chosen as default. If PetrelBackend can not be instantiated
- # successfully, the CephClient will be chosen.
- try:
- file_client = FileClient(backend=backend)
- except ImportError:
- allowed_backends.remove(backend)
- file_client = FileClient(backend=allowed_backends[0])
-
- with io.BytesIO(file_client.get(filename)) as buffer:
- checkpoint = torch.load(buffer, map_location=map_location)
- return checkpoint
-
-
-@CheckpointLoader.register_scheme(prefixes=('modelzoo://', 'torchvision://'))
-def load_from_torchvision(filename, map_location=None):
- """load checkpoint through the file path prefixed with modelzoo or
- torchvision.
-
- Args:
- filename (str): checkpoint file path with modelzoo or
- torchvision prefix
- map_location (str, optional): Same as :func:`torch.load`.
-
- Returns:
- dict or OrderedDict: The loaded checkpoint.
- """
- model_urls = get_torchvision_models()
- if filename.startswith('modelzoo://'):
- warnings.warn('The URL scheme of "modelzoo://" is deprecated, please '
- 'use "torchvision://" instead')
- model_name = filename[11:]
- else:
- model_name = filename[14:]
- return load_from_http(model_urls[model_name], map_location=map_location)
-
-
-@CheckpointLoader.register_scheme(prefixes=('open-mmlab://', 'openmmlab://'))
-def load_from_openmmlab(filename, map_location=None):
- """load checkpoint through the file path prefixed with open-mmlab or
- openmmlab.
-
- Args:
- filename (str): checkpoint file path with open-mmlab or
- openmmlab prefix
- map_location (str, optional): Same as :func:`torch.load`.
- Default: None
-
- Returns:
- dict or OrderedDict: The loaded checkpoint.
- """
-
- model_urls = get_external_models()
- prefix_str = 'open-mmlab://'
- if filename.startswith(prefix_str):
- model_name = filename[13:]
- else:
- model_name = filename[12:]
- prefix_str = 'openmmlab://'
-
- deprecated_urls = get_deprecated_model_names()
- if model_name in deprecated_urls:
- warnings.warn(f'{prefix_str}{model_name} is deprecated in favor '
- f'of {prefix_str}{deprecated_urls[model_name]}')
- model_name = deprecated_urls[model_name]
- model_url = model_urls[model_name]
- # check if is url
- if model_url.startswith(('http://', 'https://')):
- checkpoint = load_from_http(model_url, map_location=map_location)
- else:
- filename = osp.join(_get_mmcv_home(), model_url)
- if not osp.isfile(filename):
- raise IOError(f'{filename} is not a checkpoint file')
- checkpoint = torch.load(filename, map_location=map_location)
- return checkpoint
-
-
-@CheckpointLoader.register_scheme(prefixes='mmcls://')
-def load_from_mmcls(filename, map_location=None):
- """load checkpoint through the file path prefixed with mmcls.
-
- Args:
- filename (str): checkpoint file path with mmcls prefix
- map_location (str, optional): Same as :func:`torch.load`.
-
- Returns:
- dict or OrderedDict: The loaded checkpoint.
- """
-
- model_urls = get_mmcls_models()
- model_name = filename[8:]
- checkpoint = load_from_http(
- model_urls[model_name], map_location=map_location)
- checkpoint = _process_mmcls_checkpoint(checkpoint)
- return checkpoint
-
-
-def _load_checkpoint(filename, map_location=None, logger=None):
- """Load checkpoint from somewhere (modelzoo, file, url).
-
- Args:
- filename (str): Accept local filepath, URL, ``torchvision://xxx``,
- ``open-mmlab://xxx``. Please refer to ``docs/model_zoo.md`` for
- details.
- map_location (str, optional): Same as :func:`torch.load`.
- Default: None.
- logger (:mod:`logging.Logger`, optional): The logger for error message.
- Default: None
-
- Returns:
- dict or OrderedDict: The loaded checkpoint. It can be either an
- OrderedDict storing model weights or a dict containing other
- information, which depends on the checkpoint.
- """
- return CheckpointLoader.load_checkpoint(filename, map_location, logger)
-
-
-def _load_checkpoint_with_prefix(prefix, filename, map_location=None):
- """Load partial pretrained model with specific prefix.
-
- Args:
- prefix (str): The prefix of sub-module.
- filename (str): Accept local filepath, URL, ``torchvision://xxx``,
- ``open-mmlab://xxx``. Please refer to ``docs/model_zoo.md`` for
- details.
- map_location (str | None): Same as :func:`torch.load`. Default: None.
-
- Returns:
- dict or OrderedDict: The loaded checkpoint.
- """
-
- checkpoint = _load_checkpoint(filename, map_location=map_location)
-
- if 'state_dict' in checkpoint:
- state_dict = checkpoint['state_dict']
- else:
- state_dict = checkpoint
- if not prefix.endswith('.'):
- prefix += '.'
- prefix_len = len(prefix)
-
- state_dict = {
- k[prefix_len:]: v
- for k, v in state_dict.items() if k.startswith(prefix)
- }
-
- assert state_dict, f'{prefix} is not in the pretrained model'
- return state_dict
-
-
-def load_checkpoint(model,
- filename,
- map_location=None,
- strict=False,
- logger=None,
- revise_keys=[(r'^module\.', '')]):
- """Load checkpoint from a file or URI.
-
- Args:
- model (Module): Module to load checkpoint.
- filename (str): Accept local filepath, URL, ``torchvision://xxx``,
- ``open-mmlab://xxx``. Please refer to ``docs/model_zoo.md`` for
- details.
- map_location (str): Same as :func:`torch.load`.
- strict (bool): Whether to allow different params for the model and
- checkpoint.
- logger (:mod:`logging.Logger` or None): The logger for error message.
- revise_keys (list): A list of customized keywords to modify the
- state_dict in checkpoint. Each item is a (pattern, replacement)
- pair of the regular expression operations. Default: strip
- the prefix 'module.' by [(r'^module\\.', '')].
-
- Returns:
- dict or OrderedDict: The loaded checkpoint.
- """
- checkpoint = _load_checkpoint(filename, map_location, logger)
- # OrderedDict is a subclass of dict
- if not isinstance(checkpoint, dict):
- raise RuntimeError(
- f'No state_dict found in checkpoint file {filename}')
- # get state_dict from checkpoint
- if 'state_dict' in checkpoint:
- state_dict = checkpoint['state_dict']
- else:
- state_dict = checkpoint
-
- # strip prefix of state_dict
- metadata = getattr(state_dict, '_metadata', OrderedDict())
- for p, r in revise_keys:
- state_dict = OrderedDict(
- {re.sub(p, r, k): v
- for k, v in state_dict.items()})
- # Keep metadata in state_dict
- state_dict._metadata = metadata
-
- # load state_dict
- load_state_dict(model, state_dict, strict, logger)
- return checkpoint
-
-
-def weights_to_cpu(state_dict):
- """Copy a model state_dict to cpu.
-
- Args:
- state_dict (OrderedDict): Model weights on GPU.
-
- Returns:
- OrderedDict: Model weights on GPU.
- """
- state_dict_cpu = OrderedDict()
- for key, val in state_dict.items():
- state_dict_cpu[key] = val.cpu()
- # Keep metadata in state_dict
- state_dict_cpu._metadata = getattr(state_dict, '_metadata', OrderedDict())
- return state_dict_cpu
-
-
-def _save_to_state_dict(module, destination, prefix, keep_vars):
- """Saves module state to `destination` dictionary.
-
- This method is modified from :meth:`torch.nn.Module._save_to_state_dict`.
-
- Args:
- module (nn.Module): The module to generate state_dict.
- destination (dict): A dict where state will be stored.
- prefix (str): The prefix for parameters and buffers used in this
- module.
- """
- for name, param in module._parameters.items():
- if param is not None:
- destination[prefix + name] = param if keep_vars else param.detach()
- for name, buf in module._buffers.items():
- # remove check of _non_persistent_buffers_set to allow nn.BatchNorm2d
- if buf is not None:
- destination[prefix + name] = buf if keep_vars else buf.detach()
-
-
-def get_state_dict(module, destination=None, prefix='', keep_vars=False):
- """Returns a dictionary containing a whole state of the module.
-
- Both parameters and persistent buffers (e.g. running averages) are
- included. Keys are corresponding parameter and buffer names.
-
- This method is modified from :meth:`torch.nn.Module.state_dict` to
- recursively check parallel module in case that the model has a complicated
- structure, e.g., nn.Module(nn.Module(DDP)).
-
- Args:
- module (nn.Module): The module to generate state_dict.
- destination (OrderedDict): Returned dict for the state of the
- module.
- prefix (str): Prefix of the key.
- keep_vars (bool): Whether to keep the variable property of the
- parameters. Default: False.
-
- Returns:
- dict: A dictionary containing a whole state of the module.
- """
- # recursively check parallel module in case that the model has a
- # complicated structure, e.g., nn.Module(nn.Module(DDP))
- if is_module_wrapper(module):
- module = module.module
-
- # below is the same as torch.nn.Module.state_dict()
- if destination is None:
- destination = OrderedDict()
- destination._metadata = OrderedDict()
- destination._metadata[prefix[:-1]] = local_metadata = dict(
- version=module._version)
- _save_to_state_dict(module, destination, prefix, keep_vars)
- for name, child in module._modules.items():
- if child is not None:
- get_state_dict(
- child, destination, prefix + name + '.', keep_vars=keep_vars)
- for hook in module._state_dict_hooks.values():
- hook_result = hook(module, destination, prefix, local_metadata)
- if hook_result is not None:
- destination = hook_result
- return destination
-
-
-def save_checkpoint(model,
- filename,
- optimizer=None,
- meta=None,
- file_client_args=None):
- """Save checkpoint to file.
-
- The checkpoint will have 3 fields: ``meta``, ``state_dict`` and
- ``optimizer``. By default ``meta`` will contain version and time info.
-
- Args:
- model (Module): Module whose params are to be saved.
- filename (str): Checkpoint filename.
- optimizer (:obj:`Optimizer`, optional): Optimizer to be saved.
- meta (dict, optional): Metadata to be saved in checkpoint.
- file_client_args (dict, optional): Arguments to instantiate a
- FileClient. See :class:`mmcv.fileio.FileClient` for details.
- Default: None.
- `New in version 1.3.16.`
- """
- if meta is None:
- meta = {}
- elif not isinstance(meta, dict):
- raise TypeError(f'meta must be a dict or None, but got {type(meta)}')
- meta.update(mmcv_version=mmcv.__version__, time=time.asctime())
-
- if is_module_wrapper(model):
- model = model.module
-
- if hasattr(model, 'CLASSES') and model.CLASSES is not None:
- # save class name to the meta
- meta.update(CLASSES=model.CLASSES)
-
- checkpoint = {
- 'meta': meta,
- 'state_dict': weights_to_cpu(get_state_dict(model))
- }
- # save optimizer state dict in the checkpoint
- if isinstance(optimizer, Optimizer):
- checkpoint['optimizer'] = optimizer.state_dict()
- elif isinstance(optimizer, dict):
- checkpoint['optimizer'] = {}
- for name, optim in optimizer.items():
- checkpoint['optimizer'][name] = optim.state_dict()
-
- if filename.startswith('pavi://'):
- if file_client_args is not None:
- raise ValueError(
- 'file_client_args should be "None" if filename starts with'
- f'"pavi://", but got {file_client_args}')
- try:
- from pavi import modelcloud
- from pavi import exception
- except ImportError:
- raise ImportError(
- 'Please install pavi to load checkpoint from modelcloud.')
- model_path = filename[7:]
- root = modelcloud.Folder()
- model_dir, model_name = osp.split(model_path)
- try:
- model = modelcloud.get(model_dir)
- except exception.NodeNotFoundError:
- model = root.create_training_model(model_dir)
- with TemporaryDirectory() as tmp_dir:
- checkpoint_file = osp.join(tmp_dir, model_name)
- with open(checkpoint_file, 'wb') as f:
- torch.save(checkpoint, f)
- f.flush()
- model.create_file(checkpoint_file, name=model_name)
- else:
- file_client = FileClient.infer_client(file_client_args, filename)
- with io.BytesIO() as f:
- torch.save(checkpoint, f)
- file_client.put(f.getvalue(), filename)
diff --git a/spaces/Robert001/UniControl-Demo/annotator/uniformer/mmdet_null/core/bbox/assigners/assign_result.py b/spaces/Robert001/UniControl-Demo/annotator/uniformer/mmdet_null/core/bbox/assigners/assign_result.py
deleted file mode 100644
index cb12a571dfe306e5f3055af170d16ff12371ac77..0000000000000000000000000000000000000000
--- a/spaces/Robert001/UniControl-Demo/annotator/uniformer/mmdet_null/core/bbox/assigners/assign_result.py
+++ /dev/null
@@ -1,204 +0,0 @@
-import torch
-
-from annotator.uniformer.mmdet.utils import util_mixins
-
-
-class AssignResult(util_mixins.NiceRepr):
- """Stores assignments between predicted and truth boxes.
-
- Attributes:
- num_gts (int): the number of truth boxes considered when computing this
- assignment
-
- gt_inds (LongTensor): for each predicted box indicates the 1-based
- index of the assigned truth box. 0 means unassigned and -1 means
- ignore.
-
- max_overlaps (FloatTensor): the iou between the predicted box and its
- assigned truth box.
-
- labels (None | LongTensor): If specified, for each predicted box
- indicates the category label of the assigned truth box.
-
- Example:
- >>> # An assign result between 4 predicted boxes and 9 true boxes
- >>> # where only two boxes were assigned.
- >>> num_gts = 9
- >>> max_overlaps = torch.LongTensor([0, .5, .9, 0])
- >>> gt_inds = torch.LongTensor([-1, 1, 2, 0])
- >>> labels = torch.LongTensor([0, 3, 4, 0])
- >>> self = AssignResult(num_gts, gt_inds, max_overlaps, labels)
- >>> print(str(self)) # xdoctest: +IGNORE_WANT
-
- >>> # Force addition of gt labels (when adding gt as proposals)
- >>> new_labels = torch.LongTensor([3, 4, 5])
- >>> self.add_gt_(new_labels)
- >>> print(str(self)) # xdoctest: +IGNORE_WANT
-
- """
-
- def __init__(self, num_gts, gt_inds, max_overlaps, labels=None):
- self.num_gts = num_gts
- self.gt_inds = gt_inds
- self.max_overlaps = max_overlaps
- self.labels = labels
- # Interface for possible user-defined properties
- self._extra_properties = {}
-
- @property
- def num_preds(self):
- """int: the number of predictions in this assignment"""
- return len(self.gt_inds)
-
- def set_extra_property(self, key, value):
- """Set user-defined new property."""
- assert key not in self.info
- self._extra_properties[key] = value
-
- def get_extra_property(self, key):
- """Get user-defined property."""
- return self._extra_properties.get(key, None)
-
- @property
- def info(self):
- """dict: a dictionary of info about the object"""
- basic_info = {
- 'num_gts': self.num_gts,
- 'num_preds': self.num_preds,
- 'gt_inds': self.gt_inds,
- 'max_overlaps': self.max_overlaps,
- 'labels': self.labels,
- }
- basic_info.update(self._extra_properties)
- return basic_info
-
- def __nice__(self):
- """str: a "nice" summary string describing this assign result"""
- parts = []
- parts.append(f'num_gts={self.num_gts!r}')
- if self.gt_inds is None:
- parts.append(f'gt_inds={self.gt_inds!r}')
- else:
- parts.append(f'gt_inds.shape={tuple(self.gt_inds.shape)!r}')
- if self.max_overlaps is None:
- parts.append(f'max_overlaps={self.max_overlaps!r}')
- else:
- parts.append('max_overlaps.shape='
- f'{tuple(self.max_overlaps.shape)!r}')
- if self.labels is None:
- parts.append(f'labels={self.labels!r}')
- else:
- parts.append(f'labels.shape={tuple(self.labels.shape)!r}')
- return ', '.join(parts)
-
- @classmethod
- def random(cls, **kwargs):
- """Create random AssignResult for tests or debugging.
-
- Args:
- num_preds: number of predicted boxes
- num_gts: number of true boxes
- p_ignore (float): probability of a predicted box assinged to an
- ignored truth
- p_assigned (float): probability of a predicted box not being
- assigned
- p_use_label (float | bool): with labels or not
- rng (None | int | numpy.random.RandomState): seed or state
-
- Returns:
- :obj:`AssignResult`: Randomly generated assign results.
-
- Example:
- >>> from mmdet.core.bbox.assigners.assign_result import * # NOQA
- >>> self = AssignResult.random()
- >>> print(self.info)
- """
- from mmdet.core.bbox import demodata
- rng = demodata.ensure_rng(kwargs.get('rng', None))
-
- num_gts = kwargs.get('num_gts', None)
- num_preds = kwargs.get('num_preds', None)
- p_ignore = kwargs.get('p_ignore', 0.3)
- p_assigned = kwargs.get('p_assigned', 0.7)
- p_use_label = kwargs.get('p_use_label', 0.5)
- num_classes = kwargs.get('p_use_label', 3)
-
- if num_gts is None:
- num_gts = rng.randint(0, 8)
- if num_preds is None:
- num_preds = rng.randint(0, 16)
-
- if num_gts == 0:
- max_overlaps = torch.zeros(num_preds, dtype=torch.float32)
- gt_inds = torch.zeros(num_preds, dtype=torch.int64)
- if p_use_label is True or p_use_label < rng.rand():
- labels = torch.zeros(num_preds, dtype=torch.int64)
- else:
- labels = None
- else:
- import numpy as np
- # Create an overlap for each predicted box
- max_overlaps = torch.from_numpy(rng.rand(num_preds))
-
- # Construct gt_inds for each predicted box
- is_assigned = torch.from_numpy(rng.rand(num_preds) < p_assigned)
- # maximum number of assignments constraints
- n_assigned = min(num_preds, min(num_gts, is_assigned.sum()))
-
- assigned_idxs = np.where(is_assigned)[0]
- rng.shuffle(assigned_idxs)
- assigned_idxs = assigned_idxs[0:n_assigned]
- assigned_idxs.sort()
-
- is_assigned[:] = 0
- is_assigned[assigned_idxs] = True
-
- is_ignore = torch.from_numpy(
- rng.rand(num_preds) < p_ignore) & is_assigned
-
- gt_inds = torch.zeros(num_preds, dtype=torch.int64)
-
- true_idxs = np.arange(num_gts)
- rng.shuffle(true_idxs)
- true_idxs = torch.from_numpy(true_idxs)
- gt_inds[is_assigned] = true_idxs[:n_assigned]
-
- gt_inds = torch.from_numpy(
- rng.randint(1, num_gts + 1, size=num_preds))
- gt_inds[is_ignore] = -1
- gt_inds[~is_assigned] = 0
- max_overlaps[~is_assigned] = 0
-
- if p_use_label is True or p_use_label < rng.rand():
- if num_classes == 0:
- labels = torch.zeros(num_preds, dtype=torch.int64)
- else:
- labels = torch.from_numpy(
- # remind that we set FG labels to [0, num_class-1]
- # since mmdet v2.0
- # BG cat_id: num_class
- rng.randint(0, num_classes, size=num_preds))
- labels[~is_assigned] = 0
- else:
- labels = None
-
- self = cls(num_gts, gt_inds, max_overlaps, labels)
- return self
-
- def add_gt_(self, gt_labels):
- """Add ground truth as assigned results.
-
- Args:
- gt_labels (torch.Tensor): Labels of gt boxes
- """
- self_inds = torch.arange(
- 1, len(gt_labels) + 1, dtype=torch.long, device=gt_labels.device)
- self.gt_inds = torch.cat([self_inds, self.gt_inds])
-
- self.max_overlaps = torch.cat(
- [self.max_overlaps.new_ones(len(gt_labels)), self.max_overlaps])
-
- if self.labels is not None:
- self.labels = torch.cat([gt_labels, self.labels])
diff --git a/spaces/Rongjiehuang/GenerSpeech/data_gen/tts/emotion/params_data.py b/spaces/Rongjiehuang/GenerSpeech/data_gen/tts/emotion/params_data.py
deleted file mode 100644
index bdb1716ed45617f2b127a7fb8885afe6cc74fb71..0000000000000000000000000000000000000000
--- a/spaces/Rongjiehuang/GenerSpeech/data_gen/tts/emotion/params_data.py
+++ /dev/null
@@ -1,29 +0,0 @@
-
-## Mel-filterbank
-mel_window_length = 25 # In milliseconds
-mel_window_step = 10 # In milliseconds
-mel_n_channels = 40
-
-
-## Audio
-sampling_rate = 16000
-# Number of spectrogram frames in a partial utterance
-partials_n_frames = 160 # 1600 ms
-# Number of spectrogram frames at inference
-inference_n_frames = 80 # 800 ms
-
-
-## Voice Activation Detection
-# Window size of the VAD. Must be either 10, 20 or 30 milliseconds.
-# This sets the granularity of the VAD. Should not need to be changed.
-vad_window_length = 30 # In milliseconds
-# Number of frames to average together when performing the moving average smoothing.
-# The larger this value, the larger the VAD variations must be to not get smoothed out.
-vad_moving_average_width = 8
-# Maximum number of consecutive silent frames a segment can have.
-vad_max_silence_length = 6
-
-
-## Audio volume normalization
-audio_norm_target_dBFS = -30
-
diff --git a/spaces/SalahZa/Tunisian-Speech-Recognition/wavlm-large/README.md b/spaces/SalahZa/Tunisian-Speech-Recognition/wavlm-large/README.md
deleted file mode 100644
index 02b19adcbff4fe72cccfefb2f23345f4e8c3372e..0000000000000000000000000000000000000000
--- a/spaces/SalahZa/Tunisian-Speech-Recognition/wavlm-large/README.md
+++ /dev/null
@@ -1,64 +0,0 @@
----
-language:
-- en
-tags:
-- speech
-inference: false
----
-
-# WavLM-Large
-
-[Microsoft's WavLM](https://github.com/microsoft/unilm/tree/master/wavlm)
-
-The large model pretrained on 16kHz sampled speech audio. When using the model, make sure that your speech input is also sampled at 16kHz.
-
-**Note**: This model does not have a tokenizer as it was pretrained on audio alone. In order to use this model **speech recognition**, a tokenizer should be created and the model should be fine-tuned on labeled text data. Check out [this blog](https://huggingface.co/blog/fine-tune-wav2vec2-english) for more in-detail explanation of how to fine-tune the model.
-
-The model was pre-trained on:
-
-- 60,000 hours of [Libri-Light](https://arxiv.org/abs/1912.07875)
-- 10,000 hours of [GigaSpeech](https://arxiv.org/abs/2106.06909)
-- 24,000 hours of [VoxPopuli](https://arxiv.org/abs/2101.00390)
-
-[Paper: WavLM: Large-Scale Self-Supervised Pre-Training for Full Stack Speech Processing](https://arxiv.org/abs/2110.13900)
-
-Authors: Sanyuan Chen, Chengyi Wang, Zhengyang Chen, Yu Wu, Shujie Liu, Zhuo Chen, Jinyu Li, Naoyuki Kanda, Takuya Yoshioka, Xiong Xiao, Jian Wu, Long Zhou, Shuo Ren, Yanmin Qian, Yao Qian, Jian Wu, Michael Zeng, Furu Wei
-
-**Abstract**
-*Self-supervised learning (SSL) achieves great success in speech recognition, while limited exploration has been attempted for other speech processing tasks. As speech signal contains multi-faceted information including speaker identity, paralinguistics, spoken content, etc., learning universal representations for all speech tasks is challenging. In this paper, we propose a new pre-trained model, WavLM, to solve full-stack downstream speech tasks. WavLM is built based on the HuBERT framework, with an emphasis on both spoken content modeling and speaker identity preservation. We first equip the Transformer structure with gated relative position bias to improve its capability on recognition tasks. For better speaker discrimination, we propose an utterance mixing training strategy, where additional overlapped utterances are created unsupervisely and incorporated during model training. Lastly, we scale up the training dataset from 60k hours to 94k hours. WavLM Large achieves state-of-the-art performance on the SUPERB benchmark, and brings significant improvements for various speech processing tasks on their representative benchmarks.*
-
-The original model can be found under https://github.com/microsoft/unilm/tree/master/wavlm.
-
-# Usage
-
-This is an English pre-trained speech model that has to be fine-tuned on a downstream task like speech recognition or audio classification before it can be
-used in inference. The model was pre-trained in English and should therefore perform well only in English. The model has been shown to work well on the [SUPERB benchmark](https://superbbenchmark.org/).
-
-**Note**: The model was pre-trained on phonemes rather than characters. This means that one should make sure that the input text is converted to a sequence
-of phonemes before fine-tuning.
-
-## Speech Recognition
-
-To fine-tune the model for speech recognition, see [the official speech recognition example](https://github.com/huggingface/transformers/tree/master/examples/pytorch/speech-recognition).
-
-## Speech Classification
-
-To fine-tune the model for speech classification, see [the official audio classification example](https://github.com/huggingface/transformers/tree/master/examples/pytorch/audio-classification).
-
-## Speaker Verification
-
-TODO
-
-## Speaker Diarization
-
-TODO
-
-# Contribution
-
-The model was contributed by [cywang](https://huggingface.co/cywang) and [patrickvonplaten](https://huggingface.co/patrickvonplaten).
-
-# License
-
-The official license can be found [here](https://github.com/microsoft/UniSpeech/blob/main/LICENSE)
-
-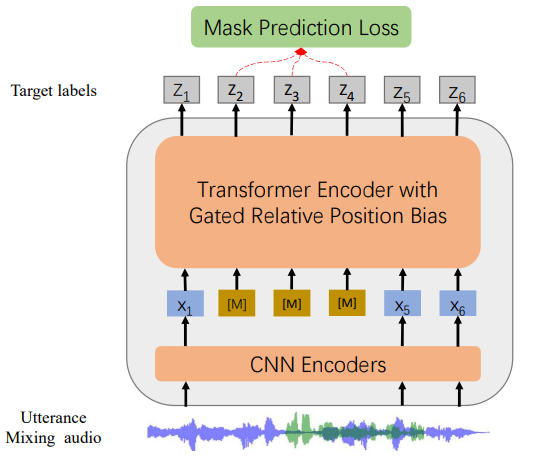
\ No newline at end of file
diff --git a/spaces/Sapiensia/diffuse-the-rest/build/_app/immutable/components/pages/_layout.svelte-f7e87a93.js b/spaces/Sapiensia/diffuse-the-rest/build/_app/immutable/components/pages/_layout.svelte-f7e87a93.js
deleted file mode 100644
index 79d515949f13dfdbdf746fad01336bc244eebbe2..0000000000000000000000000000000000000000
--- a/spaces/Sapiensia/diffuse-the-rest/build/_app/immutable/components/pages/_layout.svelte-f7e87a93.js
+++ /dev/null
@@ -1 +0,0 @@
-import{S as l,i,s as r,B as u,C as f,D as _,E as c,f as p,t as d}from"../../chunks/index-032ac624.js";function m(n){let s;const o=n[1].default,e=u(o,n,n[0],null);return{c(){e&&e.c()},l(t){e&&e.l(t)},m(t,a){e&&e.m(t,a),s=!0},p(t,[a]){e&&e.p&&(!s||a&1)&&f(e,o,t,t[0],s?c(o,t[0],a,null):_(t[0]),null)},i(t){s||(p(e,t),s=!0)},o(t){d(e,t),s=!1},d(t){e&&e.d(t)}}}function $(n,s,o){let{$$slots:e={},$$scope:t}=s;return n.$$set=a=>{"$$scope"in a&&o(0,t=a.$$scope)},[t,e]}class h extends l{constructor(s){super(),i(this,s,$,m,r,{})}}export{h as default};
diff --git a/spaces/ServerX/PorcoDiaz/lib/uvr5_pack/lib_v5/layers.py b/spaces/ServerX/PorcoDiaz/lib/uvr5_pack/lib_v5/layers.py
deleted file mode 100644
index b82f06bb4993cd63f076e68d7e24185269b1bc42..0000000000000000000000000000000000000000
--- a/spaces/ServerX/PorcoDiaz/lib/uvr5_pack/lib_v5/layers.py
+++ /dev/null
@@ -1,118 +0,0 @@
-import torch
-from torch import nn
-import torch.nn.functional as F
-
-from . import spec_utils
-
-
-class Conv2DBNActiv(nn.Module):
- def __init__(self, nin, nout, ksize=3, stride=1, pad=1, dilation=1, activ=nn.ReLU):
- super(Conv2DBNActiv, self).__init__()
- self.conv = nn.Sequential(
- nn.Conv2d(
- nin,
- nout,
- kernel_size=ksize,
- stride=stride,
- padding=pad,
- dilation=dilation,
- bias=False,
- ),
- nn.BatchNorm2d(nout),
- activ(),
- )
-
- def __call__(self, x):
- return self.conv(x)
-
-
-class SeperableConv2DBNActiv(nn.Module):
- def __init__(self, nin, nout, ksize=3, stride=1, pad=1, dilation=1, activ=nn.ReLU):
- super(SeperableConv2DBNActiv, self).__init__()
- self.conv = nn.Sequential(
- nn.Conv2d(
- nin,
- nin,
- kernel_size=ksize,
- stride=stride,
- padding=pad,
- dilation=dilation,
- groups=nin,
- bias=False,
- ),
- nn.Conv2d(nin, nout, kernel_size=1, bias=False),
- nn.BatchNorm2d(nout),
- activ(),
- )
-
- def __call__(self, x):
- return self.conv(x)
-
-
-class Encoder(nn.Module):
- def __init__(self, nin, nout, ksize=3, stride=1, pad=1, activ=nn.LeakyReLU):
- super(Encoder, self).__init__()
- self.conv1 = Conv2DBNActiv(nin, nout, ksize, 1, pad, activ=activ)
- self.conv2 = Conv2DBNActiv(nout, nout, ksize, stride, pad, activ=activ)
-
- def __call__(self, x):
- skip = self.conv1(x)
- h = self.conv2(skip)
-
- return h, skip
-
-
-class Decoder(nn.Module):
- def __init__(
- self, nin, nout, ksize=3, stride=1, pad=1, activ=nn.ReLU, dropout=False
- ):
- super(Decoder, self).__init__()
- self.conv = Conv2DBNActiv(nin, nout, ksize, 1, pad, activ=activ)
- self.dropout = nn.Dropout2d(0.1) if dropout else None
-
- def __call__(self, x, skip=None):
- x = F.interpolate(x, scale_factor=2, mode="bilinear", align_corners=True)
- if skip is not None:
- skip = spec_utils.crop_center(skip, x)
- x = torch.cat([x, skip], dim=1)
- h = self.conv(x)
-
- if self.dropout is not None:
- h = self.dropout(h)
-
- return h
-
-
-class ASPPModule(nn.Module):
- def __init__(self, nin, nout, dilations=(4, 8, 16), activ=nn.ReLU):
- super(ASPPModule, self).__init__()
- self.conv1 = nn.Sequential(
- nn.AdaptiveAvgPool2d((1, None)),
- Conv2DBNActiv(nin, nin, 1, 1, 0, activ=activ),
- )
- self.conv2 = Conv2DBNActiv(nin, nin, 1, 1, 0, activ=activ)
- self.conv3 = SeperableConv2DBNActiv(
- nin, nin, 3, 1, dilations[0], dilations[0], activ=activ
- )
- self.conv4 = SeperableConv2DBNActiv(
- nin, nin, 3, 1, dilations[1], dilations[1], activ=activ
- )
- self.conv5 = SeperableConv2DBNActiv(
- nin, nin, 3, 1, dilations[2], dilations[2], activ=activ
- )
- self.bottleneck = nn.Sequential(
- Conv2DBNActiv(nin * 5, nout, 1, 1, 0, activ=activ), nn.Dropout2d(0.1)
- )
-
- def forward(self, x):
- _, _, h, w = x.size()
- feat1 = F.interpolate(
- self.conv1(x), size=(h, w), mode="bilinear", align_corners=True
- )
- feat2 = self.conv2(x)
- feat3 = self.conv3(x)
- feat4 = self.conv4(x)
- feat5 = self.conv5(x)
- out = torch.cat((feat1, feat2, feat3, feat4, feat5), dim=1)
- bottle = self.bottleneck(out)
- return bottle
diff --git a/spaces/SeyedAli/Persian-To-English-Translation/app.py b/spaces/SeyedAli/Persian-To-English-Translation/app.py
deleted file mode 100644
index f4f5910869a4f9dd994877c3a5eb8fa6cb20535e..0000000000000000000000000000000000000000
--- a/spaces/SeyedAli/Persian-To-English-Translation/app.py
+++ /dev/null
@@ -1,18 +0,0 @@
-import gradio as gr
-from transformers import MT5ForConditionalGeneration, MT5Tokenizer
-
-model_name = "SeyedAli/Persian-to-English-Translation-mT5-V1"
-tokenizer = MT5Tokenizer.from_pretrained(model_name)
-model = MT5ForConditionalGeneration.from_pretrained(model_name)
-
-text_input = gr.TextArea(label="جمله فارسی",text_align="right",rtl=True,type="text")
-text_output = gr.TextArea(label="ترجمه انگلیسی",text_align="left",rtl=True,type="text")
-
-def Translate(text, **generator_args):
- input_ids = tokenizer.encode(text, return_tensors="pt")
- res = model.generate(input_ids, **generator_args)
- output = tokenizer.batch_decode(res, skip_special_tokens=True)[0]
- return output
-
-iface = gr.Interface(fn=Translate, inputs=text_input, outputs=text_output)
-iface.launch(share=False)
\ No newline at end of file
diff --git a/spaces/Silentlin/DiffSinger/modules/parallel_wavegan/models/source.py b/spaces/Silentlin/DiffSinger/modules/parallel_wavegan/models/source.py
deleted file mode 100644
index f2a006e53c0e2194036fd08ea9d6ed4d9a10d6cf..0000000000000000000000000000000000000000
--- a/spaces/Silentlin/DiffSinger/modules/parallel_wavegan/models/source.py
+++ /dev/null
@@ -1,538 +0,0 @@
-import torch
-import numpy as np
-import sys
-import torch.nn.functional as torch_nn_func
-
-
-class SineGen(torch.nn.Module):
- """ Definition of sine generator
- SineGen(samp_rate, harmonic_num = 0,
- sine_amp = 0.1, noise_std = 0.003,
- voiced_threshold = 0,
- flag_for_pulse=False)
-
- samp_rate: sampling rate in Hz
- harmonic_num: number of harmonic overtones (default 0)
- sine_amp: amplitude of sine-wavefrom (default 0.1)
- noise_std: std of Gaussian noise (default 0.003)
- voiced_thoreshold: F0 threshold for U/V classification (default 0)
- flag_for_pulse: this SinGen is used inside PulseGen (default False)
-
- Note: when flag_for_pulse is True, the first time step of a voiced
- segment is always sin(np.pi) or cos(0)
- """
-
- def __init__(self, samp_rate, harmonic_num=0,
- sine_amp=0.1, noise_std=0.003,
- voiced_threshold=0,
- flag_for_pulse=False):
- super(SineGen, self).__init__()
- self.sine_amp = sine_amp
- self.noise_std = noise_std
- self.harmonic_num = harmonic_num
- self.dim = self.harmonic_num + 1
- self.sampling_rate = samp_rate
- self.voiced_threshold = voiced_threshold
- self.flag_for_pulse = flag_for_pulse
-
- def _f02uv(self, f0):
- # generate uv signal
- uv = torch.ones_like(f0)
- uv = uv * (f0 > self.voiced_threshold)
- return uv
-
- def _f02sine(self, f0_values):
- """ f0_values: (batchsize, length, dim)
- where dim indicates fundamental tone and overtones
- """
- # convert to F0 in rad. The interger part n can be ignored
- # because 2 * np.pi * n doesn't affect phase
- rad_values = (f0_values / self.sampling_rate) % 1
-
- # initial phase noise (no noise for fundamental component)
- rand_ini = torch.rand(f0_values.shape[0], f0_values.shape[2], \
- device=f0_values.device)
- rand_ini[:, 0] = 0
- rad_values[:, 0, :] = rad_values[:, 0, :] + rand_ini
-
- # instantanouse phase sine[t] = sin(2*pi \sum_i=1 ^{t} rad)
- if not self.flag_for_pulse:
- # for normal case
-
- # To prevent torch.cumsum numerical overflow,
- # it is necessary to add -1 whenever \sum_k=1^n rad_value_k > 1.
- # Buffer tmp_over_one_idx indicates the time step to add -1.
- # This will not change F0 of sine because (x-1) * 2*pi = x * 2*pi
- tmp_over_one = torch.cumsum(rad_values, 1) % 1
- tmp_over_one_idx = (tmp_over_one[:, 1:, :] -
- tmp_over_one[:, :-1, :]) < 0
- cumsum_shift = torch.zeros_like(rad_values)
- cumsum_shift[:, 1:, :] = tmp_over_one_idx * -1.0
-
- sines = torch.sin(torch.cumsum(rad_values + cumsum_shift, dim=1)
- * 2 * np.pi)
- else:
- # If necessary, make sure that the first time step of every
- # voiced segments is sin(pi) or cos(0)
- # This is used for pulse-train generation
-
- # identify the last time step in unvoiced segments
- uv = self._f02uv(f0_values)
- uv_1 = torch.roll(uv, shifts=-1, dims=1)
- uv_1[:, -1, :] = 1
- u_loc = (uv < 1) * (uv_1 > 0)
-
- # get the instantanouse phase
- tmp_cumsum = torch.cumsum(rad_values, dim=1)
- # different batch needs to be processed differently
- for idx in range(f0_values.shape[0]):
- temp_sum = tmp_cumsum[idx, u_loc[idx, :, 0], :]
- temp_sum[1:, :] = temp_sum[1:, :] - temp_sum[0:-1, :]
- # stores the accumulation of i.phase within
- # each voiced segments
- tmp_cumsum[idx, :, :] = 0
- tmp_cumsum[idx, u_loc[idx, :, 0], :] = temp_sum
-
- # rad_values - tmp_cumsum: remove the accumulation of i.phase
- # within the previous voiced segment.
- i_phase = torch.cumsum(rad_values - tmp_cumsum, dim=1)
-
- # get the sines
- sines = torch.cos(i_phase * 2 * np.pi)
- return sines
-
- def forward(self, f0):
- """ sine_tensor, uv = forward(f0)
- input F0: tensor(batchsize=1, length, dim=1)
- f0 for unvoiced steps should be 0
- output sine_tensor: tensor(batchsize=1, length, dim)
- output uv: tensor(batchsize=1, length, 1)
- """
- with torch.no_grad():
- f0_buf = torch.zeros(f0.shape[0], f0.shape[1], self.dim,
- device=f0.device)
- # fundamental component
- f0_buf[:, :, 0] = f0[:, :, 0]
- for idx in np.arange(self.harmonic_num):
- # idx + 2: the (idx+1)-th overtone, (idx+2)-th harmonic
- f0_buf[:, :, idx + 1] = f0_buf[:, :, 0] * (idx + 2)
-
- # generate sine waveforms
- sine_waves = self._f02sine(f0_buf) * self.sine_amp
-
- # generate uv signal
- # uv = torch.ones(f0.shape)
- # uv = uv * (f0 > self.voiced_threshold)
- uv = self._f02uv(f0)
-
- # noise: for unvoiced should be similar to sine_amp
- # std = self.sine_amp/3 -> max value ~ self.sine_amp
- # . for voiced regions is self.noise_std
- noise_amp = uv * self.noise_std + (1 - uv) * self.sine_amp / 3
- noise = noise_amp * torch.randn_like(sine_waves)
-
- # first: set the unvoiced part to 0 by uv
- # then: additive noise
- sine_waves = sine_waves * uv + noise
- return sine_waves, uv, noise
-
-
-class PulseGen(torch.nn.Module):
- """ Definition of Pulse train generator
-
- There are many ways to implement pulse generator.
- Here, PulseGen is based on SinGen. For a perfect
- """
- def __init__(self, samp_rate, pulse_amp = 0.1,
- noise_std = 0.003, voiced_threshold = 0):
- super(PulseGen, self).__init__()
- self.pulse_amp = pulse_amp
- self.sampling_rate = samp_rate
- self.voiced_threshold = voiced_threshold
- self.noise_std = noise_std
- self.l_sinegen = SineGen(self.sampling_rate, harmonic_num=0, \
- sine_amp=self.pulse_amp, noise_std=0, \
- voiced_threshold=self.voiced_threshold, \
- flag_for_pulse=True)
-
- def forward(self, f0):
- """ Pulse train generator
- pulse_train, uv = forward(f0)
- input F0: tensor(batchsize=1, length, dim=1)
- f0 for unvoiced steps should be 0
- output pulse_train: tensor(batchsize=1, length, dim)
- output uv: tensor(batchsize=1, length, 1)
-
- Note: self.l_sine doesn't make sure that the initial phase of
- a voiced segment is np.pi, the first pulse in a voiced segment
- may not be at the first time step within a voiced segment
- """
- with torch.no_grad():
- sine_wav, uv, noise = self.l_sinegen(f0)
-
- # sine without additive noise
- pure_sine = sine_wav - noise
-
- # step t corresponds to a pulse if
- # sine[t] > sine[t+1] & sine[t] > sine[t-1]
- # & sine[t-1], sine[t+1], and sine[t] are voiced
- # or
- # sine[t] is voiced, sine[t-1] is unvoiced
- # we use torch.roll to simulate sine[t+1] and sine[t-1]
- sine_1 = torch.roll(pure_sine, shifts=1, dims=1)
- uv_1 = torch.roll(uv, shifts=1, dims=1)
- uv_1[:, 0, :] = 0
- sine_2 = torch.roll(pure_sine, shifts=-1, dims=1)
- uv_2 = torch.roll(uv, shifts=-1, dims=1)
- uv_2[:, -1, :] = 0
-
- loc = (pure_sine > sine_1) * (pure_sine > sine_2) \
- * (uv_1 > 0) * (uv_2 > 0) * (uv > 0) \
- + (uv_1 < 1) * (uv > 0)
-
- # pulse train without noise
- pulse_train = pure_sine * loc
-
- # additive noise to pulse train
- # note that noise from sinegen is zero in voiced regions
- pulse_noise = torch.randn_like(pure_sine) * self.noise_std
-
- # with additive noise on pulse, and unvoiced regions
- pulse_train += pulse_noise * loc + pulse_noise * (1 - uv)
- return pulse_train, sine_wav, uv, pulse_noise
-
-
-class SignalsConv1d(torch.nn.Module):
- """ Filtering input signal with time invariant filter
- Note: FIRFilter conducted filtering given fixed FIR weight
- SignalsConv1d convolves two signals
- Note: this is based on torch.nn.functional.conv1d
-
- """
-
- def __init__(self):
- super(SignalsConv1d, self).__init__()
-
- def forward(self, signal, system_ir):
- """ output = forward(signal, system_ir)
-
- signal: (batchsize, length1, dim)
- system_ir: (length2, dim)
-
- output: (batchsize, length1, dim)
- """
- if signal.shape[-1] != system_ir.shape[-1]:
- print("Error: SignalsConv1d expects shape:")
- print("signal (batchsize, length1, dim)")
- print("system_id (batchsize, length2, dim)")
- print("But received signal: {:s}".format(str(signal.shape)))
- print(" system_ir: {:s}".format(str(system_ir.shape)))
- sys.exit(1)
- padding_length = system_ir.shape[0] - 1
- groups = signal.shape[-1]
-
- # pad signal on the left
- signal_pad = torch_nn_func.pad(signal.permute(0, 2, 1), \
- (padding_length, 0))
- # prepare system impulse response as (dim, 1, length2)
- # also flip the impulse response
- ir = torch.flip(system_ir.unsqueeze(1).permute(2, 1, 0), \
- dims=[2])
- # convolute
- output = torch_nn_func.conv1d(signal_pad, ir, groups=groups)
- return output.permute(0, 2, 1)
-
-
-class CyclicNoiseGen_v1(torch.nn.Module):
- """ CyclicnoiseGen_v1
- Cyclic noise with a single parameter of beta.
- Pytorch v1 implementation assumes f_t is also fixed
- """
-
- def __init__(self, samp_rate,
- noise_std=0.003, voiced_threshold=0):
- super(CyclicNoiseGen_v1, self).__init__()
- self.samp_rate = samp_rate
- self.noise_std = noise_std
- self.voiced_threshold = voiced_threshold
-
- self.l_pulse = PulseGen(samp_rate, pulse_amp=1.0,
- noise_std=noise_std,
- voiced_threshold=voiced_threshold)
- self.l_conv = SignalsConv1d()
-
- def noise_decay(self, beta, f0mean):
- """ decayed_noise = noise_decay(beta, f0mean)
- decayed_noise = n[t]exp(-t * f_mean / beta / samp_rate)
-
- beta: (dim=1) or (batchsize=1, 1, dim=1)
- f0mean (batchsize=1, 1, dim=1)
-
- decayed_noise (batchsize=1, length, dim=1)
- """
- with torch.no_grad():
- # exp(-1.0 n / T) < 0.01 => n > -log(0.01)*T = 4.60*T
- # truncate the noise when decayed by -40 dB
- length = 4.6 * self.samp_rate / f0mean
- length = length.int()
- time_idx = torch.arange(0, length, device=beta.device)
- time_idx = time_idx.unsqueeze(0).unsqueeze(2)
- time_idx = time_idx.repeat(beta.shape[0], 1, beta.shape[2])
-
- noise = torch.randn(time_idx.shape, device=beta.device)
-
- # due to Pytorch implementation, use f0_mean as the f0 factor
- decay = torch.exp(-time_idx * f0mean / beta / self.samp_rate)
- return noise * self.noise_std * decay
-
- def forward(self, f0s, beta):
- """ Producde cyclic-noise
- """
- # pulse train
- pulse_train, sine_wav, uv, noise = self.l_pulse(f0s)
- pure_pulse = pulse_train - noise
-
- # decayed_noise (length, dim=1)
- if (uv < 1).all():
- # all unvoiced
- cyc_noise = torch.zeros_like(sine_wav)
- else:
- f0mean = f0s[uv > 0].mean()
-
- decayed_noise = self.noise_decay(beta, f0mean)[0, :, :]
- # convolute
- cyc_noise = self.l_conv(pure_pulse, decayed_noise)
-
- # add noise in invoiced segments
- cyc_noise = cyc_noise + noise * (1.0 - uv)
- return cyc_noise, pulse_train, sine_wav, uv, noise
-
-
-class SineGen(torch.nn.Module):
- """ Definition of sine generator
- SineGen(samp_rate, harmonic_num = 0,
- sine_amp = 0.1, noise_std = 0.003,
- voiced_threshold = 0,
- flag_for_pulse=False)
-
- samp_rate: sampling rate in Hz
- harmonic_num: number of harmonic overtones (default 0)
- sine_amp: amplitude of sine-wavefrom (default 0.1)
- noise_std: std of Gaussian noise (default 0.003)
- voiced_thoreshold: F0 threshold for U/V classification (default 0)
- flag_for_pulse: this SinGen is used inside PulseGen (default False)
-
- Note: when flag_for_pulse is True, the first time step of a voiced
- segment is always sin(np.pi) or cos(0)
- """
-
- def __init__(self, samp_rate, harmonic_num=0,
- sine_amp=0.1, noise_std=0.003,
- voiced_threshold=0,
- flag_for_pulse=False):
- super(SineGen, self).__init__()
- self.sine_amp = sine_amp
- self.noise_std = noise_std
- self.harmonic_num = harmonic_num
- self.dim = self.harmonic_num + 1
- self.sampling_rate = samp_rate
- self.voiced_threshold = voiced_threshold
- self.flag_for_pulse = flag_for_pulse
-
- def _f02uv(self, f0):
- # generate uv signal
- uv = torch.ones_like(f0)
- uv = uv * (f0 > self.voiced_threshold)
- return uv
-
- def _f02sine(self, f0_values):
- """ f0_values: (batchsize, length, dim)
- where dim indicates fundamental tone and overtones
- """
- # convert to F0 in rad. The interger part n can be ignored
- # because 2 * np.pi * n doesn't affect phase
- rad_values = (f0_values / self.sampling_rate) % 1
-
- # initial phase noise (no noise for fundamental component)
- rand_ini = torch.rand(f0_values.shape[0], f0_values.shape[2], \
- device=f0_values.device)
- rand_ini[:, 0] = 0
- rad_values[:, 0, :] = rad_values[:, 0, :] + rand_ini
-
- # instantanouse phase sine[t] = sin(2*pi \sum_i=1 ^{t} rad)
- if not self.flag_for_pulse:
- # for normal case
-
- # To prevent torch.cumsum numerical overflow,
- # it is necessary to add -1 whenever \sum_k=1^n rad_value_k > 1.
- # Buffer tmp_over_one_idx indicates the time step to add -1.
- # This will not change F0 of sine because (x-1) * 2*pi = x * 2*pi
- tmp_over_one = torch.cumsum(rad_values, 1) % 1
- tmp_over_one_idx = (tmp_over_one[:, 1:, :] -
- tmp_over_one[:, :-1, :]) < 0
- cumsum_shift = torch.zeros_like(rad_values)
- cumsum_shift[:, 1:, :] = tmp_over_one_idx * -1.0
-
- sines = torch.sin(torch.cumsum(rad_values + cumsum_shift, dim=1)
- * 2 * np.pi)
- else:
- # If necessary, make sure that the first time step of every
- # voiced segments is sin(pi) or cos(0)
- # This is used for pulse-train generation
-
- # identify the last time step in unvoiced segments
- uv = self._f02uv(f0_values)
- uv_1 = torch.roll(uv, shifts=-1, dims=1)
- uv_1[:, -1, :] = 1
- u_loc = (uv < 1) * (uv_1 > 0)
-
- # get the instantanouse phase
- tmp_cumsum = torch.cumsum(rad_values, dim=1)
- # different batch needs to be processed differently
- for idx in range(f0_values.shape[0]):
- temp_sum = tmp_cumsum[idx, u_loc[idx, :, 0], :]
- temp_sum[1:, :] = temp_sum[1:, :] - temp_sum[0:-1, :]
- # stores the accumulation of i.phase within
- # each voiced segments
- tmp_cumsum[idx, :, :] = 0
- tmp_cumsum[idx, u_loc[idx, :, 0], :] = temp_sum
-
- # rad_values - tmp_cumsum: remove the accumulation of i.phase
- # within the previous voiced segment.
- i_phase = torch.cumsum(rad_values - tmp_cumsum, dim=1)
-
- # get the sines
- sines = torch.cos(i_phase * 2 * np.pi)
- return sines
-
- def forward(self, f0):
- """ sine_tensor, uv = forward(f0)
- input F0: tensor(batchsize=1, length, dim=1)
- f0 for unvoiced steps should be 0
- output sine_tensor: tensor(batchsize=1, length, dim)
- output uv: tensor(batchsize=1, length, 1)
- """
- with torch.no_grad():
- f0_buf = torch.zeros(f0.shape[0], f0.shape[1], self.dim, \
- device=f0.device)
- # fundamental component
- f0_buf[:, :, 0] = f0[:, :, 0]
- for idx in np.arange(self.harmonic_num):
- # idx + 2: the (idx+1)-th overtone, (idx+2)-th harmonic
- f0_buf[:, :, idx + 1] = f0_buf[:, :, 0] * (idx + 2)
-
- # generate sine waveforms
- sine_waves = self._f02sine(f0_buf) * self.sine_amp
-
- # generate uv signal
- # uv = torch.ones(f0.shape)
- # uv = uv * (f0 > self.voiced_threshold)
- uv = self._f02uv(f0)
-
- # noise: for unvoiced should be similar to sine_amp
- # std = self.sine_amp/3 -> max value ~ self.sine_amp
- # . for voiced regions is self.noise_std
- noise_amp = uv * self.noise_std + (1 - uv) * self.sine_amp / 3
- noise = noise_amp * torch.randn_like(sine_waves)
-
- # first: set the unvoiced part to 0 by uv
- # then: additive noise
- sine_waves = sine_waves * uv + noise
- return sine_waves, uv, noise
-
-
-class SourceModuleCycNoise_v1(torch.nn.Module):
- """ SourceModuleCycNoise_v1
- SourceModule(sampling_rate, noise_std=0.003, voiced_threshod=0)
- sampling_rate: sampling_rate in Hz
-
- noise_std: std of Gaussian noise (default: 0.003)
- voiced_threshold: threshold to set U/V given F0 (default: 0)
-
- cyc, noise, uv = SourceModuleCycNoise_v1(F0_upsampled, beta)
- F0_upsampled (batchsize, length, 1)
- beta (1)
- cyc (batchsize, length, 1)
- noise (batchsize, length, 1)
- uv (batchsize, length, 1)
- """
-
- def __init__(self, sampling_rate, noise_std=0.003, voiced_threshod=0):
- super(SourceModuleCycNoise_v1, self).__init__()
- self.sampling_rate = sampling_rate
- self.noise_std = noise_std
- self.l_cyc_gen = CyclicNoiseGen_v1(sampling_rate, noise_std,
- voiced_threshod)
-
- def forward(self, f0_upsamped, beta):
- """
- cyc, noise, uv = SourceModuleCycNoise_v1(F0, beta)
- F0_upsampled (batchsize, length, 1)
- beta (1)
- cyc (batchsize, length, 1)
- noise (batchsize, length, 1)
- uv (batchsize, length, 1)
- """
- # source for harmonic branch
- cyc, pulse, sine, uv, add_noi = self.l_cyc_gen(f0_upsamped, beta)
-
- # source for noise branch, in the same shape as uv
- noise = torch.randn_like(uv) * self.noise_std / 3
- return cyc, noise, uv
-
-
-class SourceModuleHnNSF(torch.nn.Module):
- """ SourceModule for hn-nsf
- SourceModule(sampling_rate, harmonic_num=0, sine_amp=0.1,
- add_noise_std=0.003, voiced_threshod=0)
- sampling_rate: sampling_rate in Hz
- harmonic_num: number of harmonic above F0 (default: 0)
- sine_amp: amplitude of sine source signal (default: 0.1)
- add_noise_std: std of additive Gaussian noise (default: 0.003)
- note that amplitude of noise in unvoiced is decided
- by sine_amp
- voiced_threshold: threhold to set U/V given F0 (default: 0)
-
- Sine_source, noise_source = SourceModuleHnNSF(F0_sampled)
- F0_sampled (batchsize, length, 1)
- Sine_source (batchsize, length, 1)
- noise_source (batchsize, length 1)
- uv (batchsize, length, 1)
- """
-
- def __init__(self, sampling_rate, harmonic_num=0, sine_amp=0.1,
- add_noise_std=0.003, voiced_threshod=0):
- super(SourceModuleHnNSF, self).__init__()
-
- self.sine_amp = sine_amp
- self.noise_std = add_noise_std
-
- # to produce sine waveforms
- self.l_sin_gen = SineGen(sampling_rate, harmonic_num,
- sine_amp, add_noise_std, voiced_threshod)
-
- # to merge source harmonics into a single excitation
- self.l_linear = torch.nn.Linear(harmonic_num + 1, 1)
- self.l_tanh = torch.nn.Tanh()
-
- def forward(self, x):
- """
- Sine_source, noise_source = SourceModuleHnNSF(F0_sampled)
- F0_sampled (batchsize, length, 1)
- Sine_source (batchsize, length, 1)
- noise_source (batchsize, length 1)
- """
- # source for harmonic branch
- sine_wavs, uv, _ = self.l_sin_gen(x)
- sine_merge = self.l_tanh(self.l_linear(sine_wavs))
-
- # source for noise branch, in the same shape as uv
- noise = torch.randn_like(uv) * self.sine_amp / 3
- return sine_merge, noise, uv
-
-
-if __name__ == '__main__':
- source = SourceModuleCycNoise_v1(24000)
- x = torch.randn(16, 25600, 1)
-
-
diff --git a/spaces/Stephen2022/daxing/Dockerfile b/spaces/Stephen2022/daxing/Dockerfile
deleted file mode 100644
index 7389a194e4f9307a2920c398ec6ad8fd3509e88d..0000000000000000000000000000000000000000
--- a/spaces/Stephen2022/daxing/Dockerfile
+++ /dev/null
@@ -1,99 +0,0 @@
-FROM heartexlabs/label-studio:hf-latest
-
-################################################################################
-#
-# How to Disable Public Account Creation
-# --------------------------------------
-# By default this space allows for the unrestricted creation of new accounts
-# will full access to all projects and data. This is great for trying out
-# Label Studio and collaborating on projects, but you may want to restrict
-# access to your space to only authorized users. Uncomment the following line
-# to disable public account creation for this space.
-#
-# ENV LABEL_STUDIO_DISABLE_SIGNUP_WITHOUT_LINK=true
-#
-# Set secrets in your space to create an inital user, and log in with your
-# provided username and password. Do not set these in your Dockerfile, as they
-# globally visible on a public space.
-#
-# LABEL_STUDIO_USERNAME
-# LABEL_STUDIO_PASSWORD
-#
-# You will need to provide new users with an invitation link to join the space.
-#
-################################################################################
-
-################################################################################
-#
-# How to Enable Configuration Persistence
-# ---------------------------------------
-# By default this space stores all project configuration and data annotations
-# in local storage with Sqlite. If the space is reset, all configuration and
-# annotation data in the space will be lost. You can enable configuration
-# persistence by connecting an external Postgres database to your space,
-# guaranteeing that all project and annotation settings are preserved.
-#
-# Set the following secret variables to match your own hosted instance of
-# Postgres. We strongly recommend setting these as secrets to prevent leaking
-# information about your database service to the public in your spaces
-# definition.
-#
-# ENV DJANGO_DB=default
-# ENV POSTGRE_NAME=
-# ENV POSTGRE_PORT=
-# ENV POSTGRE_USER=
-# ENV POSTGRE_PASSWORD=
-# ENV POSTGRE_PORT=
-# ENV POSTGRE_HOST=
-#
-# Uncomment the following line to remove the warning about ephemeral storage
-#
-# ENV STORAGE_PERSISTENCE=1
-#
-# Note that you will need to connect cloud storage to host data items that you
-# want to annotate, as local storage will not be preserved across a space reset.
-#
-################################################################################
-
-################################################################################
-#
-# How to Enable Cloud Storage
-# ---------------------------
-# By default the only data storage enabled for this space is local. In the case
-# of a space reset, all data will be lost. To enable permanent storage, you
-# must enable a cloud storage connector. We also strongly recommend enabling
-# configuration persistence to preserve project data, annotations, and user
-# settings. Choose the appropriate cloud connector and configure the secrets
-# for it.
-#
-# Amazon S3
-# =========
-# STORAGE_TYPE=s3
-# STORAGE_AWS_ACCESS_KEY_ID=""
-# STORAGE_AWS_SECRET_ACCESS_KEY=""
-# STORAGE_AWS_BUCKET_NAME=""
-# STORAGE_AWS_REGION_NAME=""
-# STORAGE_AWS_FOLDER=""
-#
-# Google Cloud Storage
-# ====================
-#
-# STORAGE_TYPE=gcs
-# STORAGE_GCS_BUCKET_NAME=""
-# STORAGE_GCS_PROJECT_ID=""
-# STORAGE_GCS_FOLDER=""
-# GOOGLE_APPLICATION_CREDENTIALS="/opt/heartex/secrets/key.json"
-#
-# Azure Blob Storage
-# ==================
-#
-# STORAGE_TYPE=azure
-# STORAGE_AZURE_ACCOUNT_NAME=""
-# STORAGE_AZURE_ACCOUNT_KEY=""
-# STORAGE_AZURE_CONTAINER_NAME=""
-# STORAGE_AZURE_FOLDER=""
-#
-#
-################################################################################
-
-CMD exec label-studio --host=$SPACE_HOST
diff --git a/spaces/SungBeom/chatwine-korean/.venv/Lib/site-packages/dataclasses_json/cfg.py b/spaces/SungBeom/chatwine-korean/.venv/Lib/site-packages/dataclasses_json/cfg.py
deleted file mode 100644
index 0ad723922423947716b56b42e31ffaee1730d115..0000000000000000000000000000000000000000
--- a/spaces/SungBeom/chatwine-korean/.venv/Lib/site-packages/dataclasses_json/cfg.py
+++ /dev/null
@@ -1,107 +0,0 @@
-import functools
-from enum import Enum
-from typing import Callable, Dict, Optional, TypeVar, Union
-
-from marshmallow.fields import Field as MarshmallowField
-
-from dataclasses_json.stringcase import (camelcase, pascalcase, snakecase,
- spinalcase) # type: ignore
-from dataclasses_json.undefined import Undefined, UndefinedParameterError
-
-T = TypeVar("T")
-
-
-class Exclude:
- """
- Pre-defined constants for exclusion. By default, fields are configured to
- be included.
- """
- ALWAYS: Callable[[T], bool] = lambda _: True
- NEVER: Callable[[T], bool] = lambda _: False
-
-
-# TODO: add warnings?
-class _GlobalConfig:
-
- def __init__(self):
- self.encoders: Dict[type, Callable] = {}
- self.decoders: Dict[type, Callable] = {}
- self.mm_fields: Dict[type, MarshmallowField] = {}
- # self._json_module = json
-
- # TODO: #180
- # @property
- # def json_module(self):
- # return self._json_module
- #
- # @json_module.setter
- # def json_module(self, value):
- # warnings.warn(f"Now using {value.__name__} module to handle JSON. "
- # f"{self._disable_msg}")
- # self._json_module = value
-
-
-global_config = _GlobalConfig()
-
-
-class LetterCase(Enum):
- CAMEL = camelcase
- KEBAB = spinalcase
- SNAKE = snakecase
- PASCAL = pascalcase
-
-
-def config(metadata: dict = None, *,
- # TODO: these can be typed more precisely
- # Specifically, a Callable[A, B], where `B` is bound as a JSON type
- encoder: Callable = None,
- decoder: Callable = None,
- mm_field: MarshmallowField = None,
- letter_case: Union[Callable[[str], str], LetterCase, None] = None,
- undefined: Optional[Union[str, Undefined]] = None,
- field_name: str = None,
- exclude: Union[Callable[[str, T], bool], Exclude, None] = None,
- ) -> Dict[str, dict]:
- if metadata is None:
- metadata = {}
-
- lib_metadata = metadata.setdefault('dataclasses_json', {})
-
- if encoder is not None:
- lib_metadata['encoder'] = encoder
-
- if decoder is not None:
- lib_metadata['decoder'] = decoder
-
- if mm_field is not None:
- lib_metadata['mm_field'] = mm_field
-
- if field_name is not None:
- if letter_case is not None:
- @functools.wraps(letter_case) # type:ignore
- def override(_, _letter_case=letter_case, _field_name=field_name):
- return _letter_case(_field_name)
- else:
- def override(_, _field_name=field_name): # type:ignore
- return _field_name
- letter_case = override
-
- if letter_case is not None:
- lib_metadata['letter_case'] = letter_case
-
- if undefined is not None:
- # Get the corresponding action for undefined parameters
- if isinstance(undefined, str):
- if not hasattr(Undefined, undefined.upper()):
- valid_actions = list(action.name for action in Undefined)
- raise UndefinedParameterError(
- f"Invalid undefined parameter action, "
- f"must be one of {valid_actions}")
- undefined = Undefined[undefined.upper()]
-
- lib_metadata['undefined'] = undefined
-
- if exclude is not None:
- lib_metadata['exclude'] = exclude
-
- return metadata
diff --git a/spaces/Superlang/ImageProcessor/annotator/oneformer/detectron2/modeling/meta_arch/dense_detector.py b/spaces/Superlang/ImageProcessor/annotator/oneformer/detectron2/modeling/meta_arch/dense_detector.py
deleted file mode 100644
index 461c370fe9e5fab5c634b029d5176cf4dc68de2f..0000000000000000000000000000000000000000
--- a/spaces/Superlang/ImageProcessor/annotator/oneformer/detectron2/modeling/meta_arch/dense_detector.py
+++ /dev/null
@@ -1,294 +0,0 @@
-import numpy as np
-from typing import Dict, List, Optional, Tuple
-import torch
-from torch import Tensor, nn
-
-from annotator.oneformer.detectron2.data.detection_utils import convert_image_to_rgb
-from annotator.oneformer.detectron2.layers import move_device_like
-from annotator.oneformer.detectron2.modeling import Backbone
-from annotator.oneformer.detectron2.structures import Boxes, ImageList, Instances
-from annotator.oneformer.detectron2.utils.events import get_event_storage
-
-from ..postprocessing import detector_postprocess
-
-
-def permute_to_N_HWA_K(tensor, K: int):
- """
- Transpose/reshape a tensor from (N, (Ai x K), H, W) to (N, (HxWxAi), K)
- """
- assert tensor.dim() == 4, tensor.shape
- N, _, H, W = tensor.shape
- tensor = tensor.view(N, -1, K, H, W)
- tensor = tensor.permute(0, 3, 4, 1, 2)
- tensor = tensor.reshape(N, -1, K) # Size=(N,HWA,K)
- return tensor
-
-
-class DenseDetector(nn.Module):
- """
- Base class for dense detector. We define a dense detector as a fully-convolutional model that
- makes per-pixel (i.e. dense) predictions.
- """
-
- def __init__(
- self,
- backbone: Backbone,
- head: nn.Module,
- head_in_features: Optional[List[str]] = None,
- *,
- pixel_mean,
- pixel_std,
- ):
- """
- Args:
- backbone: backbone module
- head: head module
- head_in_features: backbone features to use in head. Default to all backbone features.
- pixel_mean (Tuple[float]):
- Values to be used for image normalization (BGR order).
- To train on images of different number of channels, set different mean & std.
- Default values are the mean pixel value from ImageNet: [103.53, 116.28, 123.675]
- pixel_std (Tuple[float]):
- When using pre-trained models in Detectron1 or any MSRA models,
- std has been absorbed into its conv1 weights, so the std needs to be set 1.
- Otherwise, you can use [57.375, 57.120, 58.395] (ImageNet std)
- """
- super().__init__()
-
- self.backbone = backbone
- self.head = head
- if head_in_features is None:
- shapes = self.backbone.output_shape()
- self.head_in_features = sorted(shapes.keys(), key=lambda x: shapes[x].stride)
- else:
- self.head_in_features = head_in_features
- self.register_buffer("pixel_mean", torch.tensor(pixel_mean).view(-1, 1, 1), False)
- self.register_buffer("pixel_std", torch.tensor(pixel_std).view(-1, 1, 1), False)
-
- @property
- def device(self):
- return self.pixel_mean.device
-
- def _move_to_current_device(self, x):
- return move_device_like(x, self.pixel_mean)
-
- def forward(self, batched_inputs: List[Dict[str, Tensor]]):
- """
- Args:
- batched_inputs: a list, batched outputs of :class:`DatasetMapper` .
- Each item in the list contains the inputs for one image.
- For now, each item in the list is a dict that contains:
-
- * image: Tensor, image in (C, H, W) format.
- * instances: Instances
-
- Other information that's included in the original dicts, such as:
-
- * "height", "width" (int): the output resolution of the model, used in inference.
- See :meth:`postprocess` for details.
-
- Returns:
- In training, dict[str, Tensor]: mapping from a named loss to a tensor storing the
- loss. Used during training only. In inference, the standard output format, described
- in :doc:`/tutorials/models`.
- """
- images = self.preprocess_image(batched_inputs)
- features = self.backbone(images.tensor)
- features = [features[f] for f in self.head_in_features]
- predictions = self.head(features)
-
- if self.training:
- assert not torch.jit.is_scripting(), "Not supported"
- assert "instances" in batched_inputs[0], "Instance annotations are missing in training!"
- gt_instances = [x["instances"].to(self.device) for x in batched_inputs]
- return self.forward_training(images, features, predictions, gt_instances)
- else:
- results = self.forward_inference(images, features, predictions)
- if torch.jit.is_scripting():
- return results
-
- processed_results = []
- for results_per_image, input_per_image, image_size in zip(
- results, batched_inputs, images.image_sizes
- ):
- height = input_per_image.get("height", image_size[0])
- width = input_per_image.get("width", image_size[1])
- r = detector_postprocess(results_per_image, height, width)
- processed_results.append({"instances": r})
- return processed_results
-
- def forward_training(self, images, features, predictions, gt_instances):
- raise NotImplementedError()
-
- def preprocess_image(self, batched_inputs: List[Dict[str, Tensor]]):
- """
- Normalize, pad and batch the input images.
- """
- images = [self._move_to_current_device(x["image"]) for x in batched_inputs]
- images = [(x - self.pixel_mean) / self.pixel_std for x in images]
- images = ImageList.from_tensors(
- images,
- self.backbone.size_divisibility,
- padding_constraints=self.backbone.padding_constraints,
- )
- return images
-
- def _transpose_dense_predictions(
- self, predictions: List[List[Tensor]], dims_per_anchor: List[int]
- ) -> List[List[Tensor]]:
- """
- Transpose the dense per-level predictions.
-
- Args:
- predictions: a list of outputs, each is a list of per-level
- predictions with shape (N, Ai x K, Hi, Wi), where N is the
- number of images, Ai is the number of anchors per location on
- level i, K is the dimension of predictions per anchor.
- dims_per_anchor: the value of K for each predictions. e.g. 4 for
- box prediction, #classes for classification prediction.
-
- Returns:
- List[List[Tensor]]: each prediction is transposed to (N, Hi x Wi x Ai, K).
- """
- assert len(predictions) == len(dims_per_anchor)
- res: List[List[Tensor]] = []
- for pred, dim_per_anchor in zip(predictions, dims_per_anchor):
- pred = [permute_to_N_HWA_K(x, dim_per_anchor) for x in pred]
- res.append(pred)
- return res
-
- def _ema_update(self, name: str, value: float, initial_value: float, momentum: float = 0.9):
- """
- Apply EMA update to `self.name` using `value`.
-
- This is mainly used for loss normalizer. In Detectron1, loss is normalized by number
- of foreground samples in the batch. When batch size is 1 per GPU, #foreground has a
- large variance and using it lead to lower performance. Therefore we maintain an EMA of
- #foreground to stabilize the normalizer.
-
- Args:
- name: name of the normalizer
- value: the new value to update
- initial_value: the initial value to start with
- momentum: momentum of EMA
-
- Returns:
- float: the updated EMA value
- """
- if hasattr(self, name):
- old = getattr(self, name)
- else:
- old = initial_value
- new = old * momentum + value * (1 - momentum)
- setattr(self, name, new)
- return new
-
- def _decode_per_level_predictions(
- self,
- anchors: Boxes,
- pred_scores: Tensor,
- pred_deltas: Tensor,
- score_thresh: float,
- topk_candidates: int,
- image_size: Tuple[int, int],
- ) -> Instances:
- """
- Decode boxes and classification predictions of one featuer level, by
- the following steps:
- 1. filter the predictions based on score threshold and top K scores.
- 2. transform the box regression outputs
- 3. return the predicted scores, classes and boxes
-
- Args:
- anchors: Boxes, anchor for this feature level
- pred_scores: HxWxA,K
- pred_deltas: HxWxA,4
-
- Returns:
- Instances: with field "scores", "pred_boxes", "pred_classes".
- """
- # Apply two filtering to make NMS faster.
- # 1. Keep boxes with confidence score higher than threshold
- keep_idxs = pred_scores > score_thresh
- pred_scores = pred_scores[keep_idxs]
- topk_idxs = torch.nonzero(keep_idxs) # Kx2
-
- # 2. Keep top k top scoring boxes only
- topk_idxs_size = topk_idxs.shape[0]
- if isinstance(topk_idxs_size, Tensor):
- # It's a tensor in tracing
- num_topk = torch.clamp(topk_idxs_size, max=topk_candidates)
- else:
- num_topk = min(topk_idxs_size, topk_candidates)
- pred_scores, idxs = pred_scores.topk(num_topk)
- topk_idxs = topk_idxs[idxs]
-
- anchor_idxs, classes_idxs = topk_idxs.unbind(dim=1)
-
- pred_boxes = self.box2box_transform.apply_deltas(
- pred_deltas[anchor_idxs], anchors.tensor[anchor_idxs]
- )
- return Instances(
- image_size, pred_boxes=Boxes(pred_boxes), scores=pred_scores, pred_classes=classes_idxs
- )
-
- def _decode_multi_level_predictions(
- self,
- anchors: List[Boxes],
- pred_scores: List[Tensor],
- pred_deltas: List[Tensor],
- score_thresh: float,
- topk_candidates: int,
- image_size: Tuple[int, int],
- ) -> Instances:
- """
- Run `_decode_per_level_predictions` for all feature levels and concat the results.
- """
- predictions = [
- self._decode_per_level_predictions(
- anchors_i,
- box_cls_i,
- box_reg_i,
- self.test_score_thresh,
- self.test_topk_candidates,
- image_size,
- )
- # Iterate over every feature level
- for box_cls_i, box_reg_i, anchors_i in zip(pred_scores, pred_deltas, anchors)
- ]
- return predictions[0].cat(predictions) # 'Instances.cat' is not scriptale but this is
-
- def visualize_training(self, batched_inputs, results):
- """
- A function used to visualize ground truth images and final network predictions.
- It shows ground truth bounding boxes on the original image and up to 20
- predicted object bounding boxes on the original image.
-
- Args:
- batched_inputs (list): a list that contains input to the model.
- results (List[Instances]): a list of #images elements returned by forward_inference().
- """
- from annotator.oneformer.detectron2.utils.visualizer import Visualizer
-
- assert len(batched_inputs) == len(
- results
- ), "Cannot visualize inputs and results of different sizes"
- storage = get_event_storage()
- max_boxes = 20
-
- image_index = 0 # only visualize a single image
- img = batched_inputs[image_index]["image"]
- img = convert_image_to_rgb(img.permute(1, 2, 0), self.input_format)
- v_gt = Visualizer(img, None)
- v_gt = v_gt.overlay_instances(boxes=batched_inputs[image_index]["instances"].gt_boxes)
- anno_img = v_gt.get_image()
- processed_results = detector_postprocess(results[image_index], img.shape[0], img.shape[1])
- predicted_boxes = processed_results.pred_boxes.tensor.detach().cpu().numpy()
-
- v_pred = Visualizer(img, None)
- v_pred = v_pred.overlay_instances(boxes=predicted_boxes[0:max_boxes])
- prop_img = v_pred.get_image()
- vis_img = np.vstack((anno_img, prop_img))
- vis_img = vis_img.transpose(2, 0, 1)
- vis_name = f"Top: GT bounding boxes; Bottom: {max_boxes} Highest Scoring Results"
- storage.put_image(vis_name, vis_img)
diff --git a/spaces/TEXTurePaper/TEXTure/README.md b/spaces/TEXTurePaper/TEXTure/README.md
deleted file mode 100644
index 5c5a5c859af802207346785a47a6a88ede927580..0000000000000000000000000000000000000000
--- a/spaces/TEXTurePaper/TEXTure/README.md
+++ /dev/null
@@ -1,12 +0,0 @@
----
-title: TEXTure
-emoji: 📚
-colorFrom: green
-colorTo: red
-sdk: docker
-pinned: false
-license: mit
-suggested_hardware: a10g-small
----
-
-Check out the configuration reference at https://huggingface.co/docs/hub/spaces-config-reference
diff --git a/spaces/TLME/Bert-VITS-Umamusume-Genshin-HonkaiSR/text/english.py b/spaces/TLME/Bert-VITS-Umamusume-Genshin-HonkaiSR/text/english.py
deleted file mode 100644
index 0f9339c9ed771dab5136978eaaab194ec3fe2395..0000000000000000000000000000000000000000
--- a/spaces/TLME/Bert-VITS-Umamusume-Genshin-HonkaiSR/text/english.py
+++ /dev/null
@@ -1,214 +0,0 @@
-import pickle
-import os
-import re
-from g2p_en import G2p
-
-from text import symbols
-
-current_file_path = os.path.dirname(__file__)
-CMU_DICT_PATH = os.path.join(current_file_path, "cmudict.rep")
-CACHE_PATH = os.path.join(current_file_path, "cmudict_cache.pickle")
-_g2p = G2p()
-
-arpa = {
- "AH0",
- "S",
- "AH1",
- "EY2",
- "AE2",
- "EH0",
- "OW2",
- "UH0",
- "NG",
- "B",
- "G",
- "AY0",
- "M",
- "AA0",
- "F",
- "AO0",
- "ER2",
- "UH1",
- "IY1",
- "AH2",
- "DH",
- "IY0",
- "EY1",
- "IH0",
- "K",
- "N",
- "W",
- "IY2",
- "T",
- "AA1",
- "ER1",
- "EH2",
- "OY0",
- "UH2",
- "UW1",
- "Z",
- "AW2",
- "AW1",
- "V",
- "UW2",
- "AA2",
- "ER",
- "AW0",
- "UW0",
- "R",
- "OW1",
- "EH1",
- "ZH",
- "AE0",
- "IH2",
- "IH",
- "Y",
- "JH",
- "P",
- "AY1",
- "EY0",
- "OY2",
- "TH",
- "HH",
- "D",
- "ER0",
- "CH",
- "AO1",
- "AE1",
- "AO2",
- "OY1",
- "AY2",
- "IH1",
- "OW0",
- "L",
- "SH",
-}
-
-
-def post_replace_ph(ph):
- rep_map = {
- ":": ",",
- ";": ",",
- ",": ",",
- "。": ".",
- "!": "!",
- "?": "?",
- "\n": ".",
- "·": ",",
- "、": ",",
- "...": "…",
- "v": "V",
- }
- if ph in rep_map.keys():
- ph = rep_map[ph]
- if ph in symbols:
- return ph
- if ph not in symbols:
- ph = "UNK"
- return ph
-
-
-def read_dict():
- g2p_dict = {}
- start_line = 49
- with open(CMU_DICT_PATH) as f:
- line = f.readline()
- line_index = 1
- while line:
- if line_index >= start_line:
- line = line.strip()
- word_split = line.split(" ")
- word = word_split[0]
-
- syllable_split = word_split[1].split(" - ")
- g2p_dict[word] = []
- for syllable in syllable_split:
- phone_split = syllable.split(" ")
- g2p_dict[word].append(phone_split)
-
- line_index = line_index + 1
- line = f.readline()
-
- return g2p_dict
-
-
-def cache_dict(g2p_dict, file_path):
- with open(file_path, "wb") as pickle_file:
- pickle.dump(g2p_dict, pickle_file)
-
-
-def get_dict():
- if os.path.exists(CACHE_PATH):
- with open(CACHE_PATH, "rb") as pickle_file:
- g2p_dict = pickle.load(pickle_file)
- else:
- g2p_dict = read_dict()
- cache_dict(g2p_dict, CACHE_PATH)
-
- return g2p_dict
-
-
-eng_dict = get_dict()
-
-
-def refine_ph(phn):
- tone = 0
- if re.search(r"\d$", phn):
- tone = int(phn[-1]) + 1
- phn = phn[:-1]
- return phn.lower(), tone
-
-
-def refine_syllables(syllables):
- tones = []
- phonemes = []
- for phn_list in syllables:
- for i in range(len(phn_list)):
- phn = phn_list[i]
- phn, tone = refine_ph(phn)
- phonemes.append(phn)
- tones.append(tone)
- return phonemes, tones
-
-
-def text_normalize(text):
- # todo: eng text normalize
- return text
-
-
-def g2p(text):
- phones = []
- tones = []
- words = re.split(r"([,;.\-\?\!\s+])", text)
- for w in words:
- if w.upper() in eng_dict:
- phns, tns = refine_syllables(eng_dict[w.upper()])
- phones += phns
- tones += tns
- else:
- phone_list = list(filter(lambda p: p != " ", _g2p(w)))
- for ph in phone_list:
- if ph in arpa:
- ph, tn = refine_ph(ph)
- phones.append(ph)
- tones.append(tn)
- else:
- phones.append(ph)
- tones.append(0)
- # todo: implement word2ph
- word2ph = [1 for i in phones]
-
- phones = [post_replace_ph(i) for i in phones]
- return phones, tones, word2ph
-
-
-if __name__ == "__main__":
- # print(get_dict())
- # print(eng_word_to_phoneme("hello"))
- print(g2p("In this paper, we propose 1 DSPGAN, a GAN-based universal vocoder."))
- # all_phones = set()
- # for k, syllables in eng_dict.items():
- # for group in syllables:
- # for ph in group:
- # all_phones.add(ph)
- # print(all_phones)
diff --git a/spaces/TWV87/LDA_Vis/app.py b/spaces/TWV87/LDA_Vis/app.py
deleted file mode 100644
index a962a655808a3e17ff8033011f4932ffb1c343b1..0000000000000000000000000000000000000000
--- a/spaces/TWV87/LDA_Vis/app.py
+++ /dev/null
@@ -1,28 +0,0 @@
-import numpy as np
-import pandas as pd
-from gensim.corpora import Dictionary, MmCorpus
-from gensim.models import LdaModel, Word2Vec
-import matplotlib.pyplot as plt
-import streamlit as st
-from pyLDAvis import prepared_data_to_html
-import pyLDAvis.gensim_models as gensimvis
-
-# 生データ・コーパス・辞書・モデルのロード
-df = pd.read_csv("./raw_corpus.csv")
-corpus = MmCorpus('./corpus.mm')
-dict = Dictionary.load(f'./livedoor_demo.dict')
-lda = LdaModel.load('./lda_demo.model')
-
-st.caption("生データ一覧")
-st.dataframe(df.iloc[:100])
-
-st.caption("記事のカテゴリ")
-fig, ax = plt.subplots()
-count = df[["CATEGORY", "DOCUMENT"]].groupby("CATEGORY").count()
-count.plot.pie(y="DOCUMENT", ax=ax, ylabel="", legend=False)
-st.pyplot(fig)
-
-# pyLDAvisによるトピックの可視化
-vis = gensimvis.prepare(lda, corpus, dict)
-html_string = prepared_data_to_html(vis)
-st.components.v1.html(html_string, width=1300, height=800)
diff --git a/spaces/Tahsin-Mayeesha/Bangla-Question-Generation/app.py b/spaces/Tahsin-Mayeesha/Bangla-Question-Generation/app.py
deleted file mode 100644
index f00c7d2e3d3486142634dea84026f0937e9cc65d..0000000000000000000000000000000000000000
--- a/spaces/Tahsin-Mayeesha/Bangla-Question-Generation/app.py
+++ /dev/null
@@ -1,76 +0,0 @@
-import torch
-from transformers import AutoTokenizer, AutoModelForSeq2SeqLM
-import gradio as gr
-from sklearn.ensemble import RandomForestClassifier
-from sklearn.feature_extraction.text import TfidfVectorizer
-
-import pickle
-
-vectorizer = pickle.load(open("tfidf.pickle", "rb"))
-# clf = pickle.load(open("classifier.pickle", "rb"))
-
-example_context = "ফলস্বরূপ, ১৯৭৯ সালে, সনি এবং ফিলিপস একটি নতুন ডিজিটাল অডিও ডিস্ক ডিজাইন করার জন্য প্রকৌশলীদের একটি যৌথ টাস্ক ফোর্স গঠন করে। ইঞ্জিনিয়ার কিস শুহামার ইমমিনক এবং তোশিতাদা দোই এর নেতৃত্বে, গবেষণাটি লেজার এবং অপটিক্যাল ডিস্ক প্রযুক্তিকে এগিয়ে নিয়ে যায়। এক বছর পরীক্ষা-নিরীক্ষা ও আলোচনার পর টাস্ক ফোর্স রেড বুক সিডি-ডিএ স্ট্যান্ডার্ড তৈরি করে। প্রথম প্রকাশিত হয় ১৯৮০ সালে। আইইসি কর্তৃক ১৯৮৭ সালে আন্তর্জাতিক মান হিসেবে আনুষ্ঠানিকভাবে এই মান গৃহীত হয় এবং ১৯৯৬ সালে বিভিন্ন সংশোধনী মানের অংশ হয়ে ওঠে।'"
-example_answer = "১৯৮০"
-
-def choose_model(model_choice):
- if model_choice=="mt5-small":
- return "jannatul17/squad-bn-qgen-mt5-small-v1"
- elif model_choice=="mt5-base":
- return "Tahsin-Mayeesha/squad-bn-mt5-base2"
- else :
- return "jannatul17/squad-bn-qgen-banglat5-v1"
-
-
-def generate_questions(model_choice,context,answer,numReturnSequences=1,num_beams=None,do_sample=False,top_p=None,top_k=None,temperature=None):
- model_name = choose_model(model_choice)
- model = AutoModelForSeq2SeqLM.from_pretrained(model_name)
- tokenizer = AutoTokenizer.from_pretrained(model_name)
- text='answer: '+answer + ' context: ' + context
- text_encoding = tokenizer.encode_plus(
- text,return_tensors="pt"
- )
- model.eval()
- generated_ids = model.generate(
- input_ids=text_encoding['input_ids'],
- attention_mask=text_encoding['attention_mask'],
- max_length=120,
- num_beams=num_beams,
- do_sample=do_sample,
- top_k = top_k,
- top_p = top_p,
- temperature = temperature,
- num_return_sequences=numReturnSequences
- )
-
- text = []
- for id in generated_ids:
- text.append(tokenizer.decode(id,skip_special_tokens=True,clean_up_tokenization_spaces=True).replace('question: ',' '))
-
- question = " ".join(text)
- #correctness_pred = clf.predict(vectorizer.transform([question]))[0]
- #if correctness_pred == 1:
- # correctness = "Correct"
- #else :
- # correctness = "Incorrect"
-
- return question
-
-
-demo = gr.Interface(fn=generate_questions, inputs=[gr.Dropdown(label="Model", choices=["mt5-small","mt5-base","banglat5"],value="banglat5"),
- gr.Textbox(label='Context'),
- gr.Textbox(label='Answer'),
- # hyperparameters
- gr.Slider(1, 3, 1, step=1, label="Num return Sequences"),
- # beam search
- gr.Slider(1, 10,value=None, step=1, label="Beam width"),
- # top-k/top-p
- gr.Checkbox(label="Do Random Sample",value=False),
- gr.Slider(0, 50, value=None, step=1, label="Top K"),
- gr.Slider(0, 1, value=None, label="Top P/Nucleus Sampling"),
- gr.Slider(0, 1, value=None, label="Temperature") ] ,
- # output
- outputs=[gr.Textbox(label='Question')],
- examples=[["banglat5",example_context,example_answer]],
- cache_examples=False,
- title="Bangla Question Generation")
-demo.launch()
diff --git a/spaces/TandCAcceptMe/face-swap-docker/jaa.py b/spaces/TandCAcceptMe/face-swap-docker/jaa.py
deleted file mode 100644
index 1a1d7d036cbf036409180d31bed2d476c6312a9b..0000000000000000000000000000000000000000
--- a/spaces/TandCAcceptMe/face-swap-docker/jaa.py
+++ /dev/null
@@ -1,355 +0,0 @@
-"""
-Jaa.py Plugin Framework
-Author: Janvarev Vladislav
-
-Jaa.py - minimalistic one-file plugin framework with no dependencies.
-Main functions:
-- run all plugins files from "plugins" folder, base on filename
-- save each plugin options in "options" folder in JSON text files for further editing
-
-- Plugins
-must located in plugins/ folder
-must have "start(core)" function, that returns manifest dict
-manifest must contain keys "name" and "version"
-can contain "default_options"
-- if contain - options will be saved in "options" folder and reload instead next time
-- if contain - "start_with_options(core,manifest)" function will run with manifest with "options" key
-manifest will be processed in "process_plugin_manifest" function if you override it
-
-- Options (for plugins)
-are saved under "options" folder in JSON format
-created at first run plugin with "default_options"
-updated when plugin change "version"
-
-- Example usage:
-class VoiceAssCore(JaaCore): # class must override JaaCore
- def __init__(self):
- JaaCore.__init__(self,__file__)
- ...
-
-main = VoiceAssCore()
-main.init_plugins(["core"]) # 1 param - first plugins to be initialized
- # Good if you need some "core" options/plugin to be loaded before others
- # not necessary starts with "plugin_" prefix
-
-also can be run like
-
-main.init_plugins()
-
-- Requirements
-Python 3.5+ (due to dict mix in final_options calc), can be relaxed
-"""
-
-import os
-import traceback
-import json
-
-# here we trying to use termcolor to highlight plugin info and errors during load
-try:
- from termcolor import cprint
-except Exception as e:
- # not found? making a stub!
- def cprint(p,color=None):
- if color == None:
- print(p)
- else:
- print(str(color).upper(),p)
-
-version = "2.2.0"
-
-class JaaCore:
- verbose = False
-
- def __init__(self,root_file = __file__):
- self.jaaPluginPrefix = "plugin_"
- self.jaaVersion = version
- self.jaaRootFolder = os.path.dirname(root_file)
- self.jaaOptionsPath = self.jaaRootFolder+os.path.sep+"plugin_options"
- self.jaaShowTracebackOnPluginErrors = False
- if self.verbose:
- cprint("JAA.PY v{0} class created!".format(version),"blue")
-
- # ------------- plugins -----------------
- def init_plugins(self, list_first_plugins = []):
- self.plugin_manifests = {}
-
- # 1. run first plugins first!
- for modname in list_first_plugins:
- self.init_plugin(modname)
-
- # 2. run all plugins from plugins folder
- from os import listdir
- from os.path import isfile, join
- pluginpath = self.jaaRootFolder+"/plugins"
- files = [f for f in listdir(pluginpath) if isfile(join(pluginpath, f))]
-
- for fil in files:
- # print fil[:-3]
- if fil.startswith(self.jaaPluginPrefix) and fil.endswith(".py"):
- modfile = fil[:-3]
- self.init_plugin(modfile)
-
-
-
- def init_plugin(self,modname):
- # import
- try:
- mod = self.import_plugin("plugins."+modname)
- except Exception as e:
- self.print_error("JAA PLUGIN ERROR: {0} error on load: {1}".format(modname, str(e)))
- return False
-
- # run start function
- try:
- res = mod.start(self)
- except Exception as e:
- self.print_error("JAA PLUGIN ERROR: {0} error on start: {1}".format(modname, str(e)))
- return False
-
- # if plugin has an options
- if "default_options" in res:
- try:
- # saved options try to read
- saved_options = {}
- try:
- with open(self.jaaOptionsPath+'/'+modname+'.json', 'r', encoding="utf-8") as f:
- s = f.read()
- saved_options = json.loads(s)
- #print("Saved options", saved_options)
- except Exception as e:
- pass
-
- res["default_options"]["v"] = res["version"]
-
-
- # only string needs Python 3.5
- final_options = {**res["default_options"], **saved_options}
-
- # if no option found or version is differ from mod version
- if len(saved_options) == 0 or saved_options["v"] != res["version"]:
- final_options["v"] = res["version"]
- self.save_plugin_options(modname,final_options)
-
- res["options"] = final_options
-
- try:
- res2 = mod.start_with_options(self,res)
- if res2 != None:
- res = res2
- except Exception as e:
- self.print_error("JAA PLUGIN ERROR: {0} error on start_with_options processing: {1}".format(modname, str(e)))
- return False
-
- except Exception as e:
- self.print_error("JAA PLUGIN ERROR: {0} error on options processing: {1}".format(modname, str(e)))
- return False
-
-
- # processing plugin manifest
- try:
- # set up name and version
- plugin_name = res["name"]
- plugin_version = res["version"]
-
-
- self.process_plugin_manifest(modname,res)
-
- except Exception as e:
- print("JAA PLUGIN ERROR: {0} error on process startup options: {1}".format(modname, str(e)))
- return False
-
- self.plugin_manifests[modname] = res
-
- self.on_succ_plugin_start(modname,plugin_name,plugin_version)
- return True
-
- def on_succ_plugin_start(self, modname, plugin_name, plugin_version):
- if self.verbose:
- cprint("JAA PLUGIN: {1} {2} ({0}) started!".format(modname, plugin_name, plugin_version))
-
- def print_error(self,p):
- cprint(p,"red")
- if self.jaaShowTracebackOnPluginErrors:
- traceback.print_exc()
-
- def import_plugin(self, module_name):
- import sys
-
- __import__(module_name)
-
- if module_name in sys.modules:
- return sys.modules[module_name]
- return None
-
- def save_plugin_options(self,modname,options):
- # check folder exists
- if not os.path.exists(self.jaaOptionsPath):
- os.makedirs(self.jaaOptionsPath)
-
- str_options = json.dumps(options, sort_keys=True, indent=4, ensure_ascii=False)
- with open(self.jaaOptionsPath+'/'+modname+'.json', 'w', encoding="utf-8") as f:
- f.write(str_options)
- f.close()
-
- # process manifest must be overrided in inherit class
- def process_plugin_manifest(self,modname,manifest):
- print("JAA PLUGIN: {0} manifest dummy procession (override 'process_plugin_manifest' function)".format(modname))
- return
-
- def plugin_manifest(self,pluginname):
- if pluginname in self.plugin_manifests:
- return self.plugin_manifests[pluginname]
- return {}
-
- def plugin_options(self,pluginname):
- manifest = self.plugin_manifest(pluginname)
- if "options" in manifest:
- return manifest["options"]
- return None
-
- # ------------ gradio stuff --------------
- def gradio_save(self,pluginname):
- print("Saving options for {0}!".format(pluginname))
- self.save_plugin_options(pluginname,self.plugin_options(pluginname))
-
- def gradio_upd(self, pluginname, option, val):
- options = self.plugin_options(pluginname)
-
- # special case
- if isinstance(options[option], (list, dict)) and isinstance(val, str):
- import json
- try:
- options[option] = json.loads(val)
- except Exception as e:
- print(e)
- pass
- else:
- options[option] = val
- print(option,val,options)
-
- def gradio_render_settings_interface(self, title:str="Settings manager", required_fields_to_show_plugin:list=["default_options"]):
- import gradio as gr
-
- with gr.Blocks() as gr_interface:
- gr.Markdown("# {0}".format(title))
- for pluginname in self.plugin_manifests:
- manifest = self.plugin_manifests[pluginname]
-
- # calculate if we show plugin
- is_show_plugin = False
- if len(required_fields_to_show_plugin) == 0:
- is_show_plugin = True
- else:
- for k in required_fields_to_show_plugin:
- if manifest.get(k) is not None:
- is_show_plugin = True
-
- if is_show_plugin:
- with gr.Tab(pluginname):
- gr.Markdown("## {0} v{1}".format(manifest["name"],manifest["version"]))
- if manifest.get("description") is not None:
- gr.Markdown(manifest.get("description"))
-
- if manifest.get("url") is not None:
- gr.Markdown("**URL:** [{0}]({0})".format(manifest.get("url")))
-
-
- if "options" in manifest:
- options = manifest["options"]
- if len(options) > 1: # not only v
- text_button = gr.Button("Save options".format(pluginname))
- #options_int_list = []
- for option in options:
-
- #gr.Label(label=option)
- if option != "v":
- val = options[option]
- label = option
-
- if manifest.get("options_label") is not None:
- if manifest.get("options_label").get(option) is not None:
- label = option+": "+manifest.get("options_label").get(option)
-
-
- if isinstance(val, (bool, )):
- gr_elem = gr.Checkbox(value=val,label=label)
- elif isinstance(val, (dict,list)):
- import json
- gr_elem = gr.Textbox(value=json.dumps(val,ensure_ascii=False), label=label)
- else:
- gr_elem = gr.Textbox(value=val, label=label)
-
- def handler(x,pluginname=pluginname,option=option):
- self.gradio_upd(pluginname, option, x)
-
- gr_elem.change(handler, gr_elem, None)
-
- def handler_save(pluginname=pluginname):
- self.gradio_save(pluginname)
-
- text_button.click(handler_save,inputs=None,outputs=None)
- else:
- gr.Markdown("_No options for this plugin_")
-
- return gr_interface
-
-
-def load_options(options_file=None,py_file=None,default_options={}):
- # 1. calculating options filename
- if options_file == None:
- if py_file == None:
- raise Exception('JAA: Options or PY file is not defined, cant calc options filename')
- else:
- options_file = py_file[:-3]+'.json'
-
- # 2. try to read saved options
- saved_options = {}
- try:
- with open(options_file, 'r', encoding="utf-8") as f:
- s = f.read()
- saved_options = json.loads(s)
- #print("Saved options", saved_options)
- except Exception as e:
- pass
-
- # 3. calculating final options
-
- # only string needs Python 3.5
- final_options = {**default_options, **saved_options}
-
- # 4. calculating hash from def options to check - is file rewrite needed?
- import hashlib
- hash = hashlib.md5((json.dumps(default_options, sort_keys=True)).encode('utf-8')).hexdigest()
-
- # 5. if no option file found or hash was from other default options
- if len(saved_options) == 0 or not ("hash" in saved_options.keys()) or saved_options["hash"] != hash:
- final_options["hash"] = hash
- #self.save_plugin_options(modname,final_options)
-
- # saving in file
- str_options = json.dumps(final_options, sort_keys=True, indent=4, ensure_ascii=False)
- with open(options_file, 'w', encoding="utf-8") as f:
- f.write(str_options)
- f.close()
-
- return final_options
-
-"""
-The MIT License (MIT)
-Copyright (c) 2021 Janvarev Vladislav
-
-Permission is hereby granted, free of charge, to any person obtaining a copy
-of this software and associated documentation files (the “Software”), to deal
-in the Software without restriction, including without limitation the rights to use,
-copy, modify, merge, publish, distribute, sublicense, and/or sell copies of the Software,
-and to permit persons to whom the Software is furnished to do so, subject to the following conditions:
-
-The above copyright notice and this permission notice shall be included in all copies or
-substantial portions of the Software.
-
-THE SOFTWARE IS PROVIDED “AS IS”, WITHOUT WARRANTY OF ANY KIND, EXPRESS OR IMPLIED,
-INCLUDING BUT NOT LIMITED TO THE WARRANTIES OF MERCHANTABILITY, FITNESS FOR A PARTICULAR
-PURPOSE AND NONINFRINGEMENT. IN NO EVENT SHALL THE AUTHORS OR COPYRIGHT HOLDERS BE LIABLE
-FOR ANY CLAIM, DAMAGES OR OTHER LIABILITY, WHETHER IN AN ACTION OF CONTRACT, TORT OR OTHERWISE,
-ARISING FROM, OUT OF OR IN CONNECTION WITH THE SOFTWARE OR THE USE OR OTHER DEALINGS IN THE SOFTWARE.
-"""
\ No newline at end of file
diff --git a/spaces/TencentARC/VLog/models/grit_src/third_party/CenterNet2/detectron2/modeling/proposal_generator/rrpn.py b/spaces/TencentARC/VLog/models/grit_src/third_party/CenterNet2/detectron2/modeling/proposal_generator/rrpn.py
deleted file mode 100644
index d51b92b7d25865a950e28cfb9ae284e600495888..0000000000000000000000000000000000000000
--- a/spaces/TencentARC/VLog/models/grit_src/third_party/CenterNet2/detectron2/modeling/proposal_generator/rrpn.py
+++ /dev/null
@@ -1,203 +0,0 @@
-# Copyright (c) Facebook, Inc. and its affiliates.
-import itertools
-import logging
-from typing import Dict, List
-import torch
-
-from detectron2.config import configurable
-from detectron2.layers import ShapeSpec, batched_nms_rotated, cat
-from detectron2.structures import Instances, RotatedBoxes, pairwise_iou_rotated
-from detectron2.utils.memory import retry_if_cuda_oom
-
-from ..box_regression import Box2BoxTransformRotated
-from .build import PROPOSAL_GENERATOR_REGISTRY
-from .proposal_utils import _is_tracing
-from .rpn import RPN
-
-logger = logging.getLogger(__name__)
-
-
-def find_top_rrpn_proposals(
- proposals,
- pred_objectness_logits,
- image_sizes,
- nms_thresh,
- pre_nms_topk,
- post_nms_topk,
- min_box_size,
- training,
-):
- """
- For each feature map, select the `pre_nms_topk` highest scoring proposals,
- apply NMS, clip proposals, and remove small boxes. Return the `post_nms_topk`
- highest scoring proposals among all the feature maps if `training` is True,
- otherwise, returns the highest `post_nms_topk` scoring proposals for each
- feature map.
-
- Args:
- proposals (list[Tensor]): A list of L tensors. Tensor i has shape (N, Hi*Wi*A, 5).
- All proposal predictions on the feature maps.
- pred_objectness_logits (list[Tensor]): A list of L tensors. Tensor i has shape (N, Hi*Wi*A).
- image_sizes (list[tuple]): sizes (h, w) for each image
- nms_thresh (float): IoU threshold to use for NMS
- pre_nms_topk (int): number of top k scoring proposals to keep before applying NMS.
- When RRPN is run on multiple feature maps (as in FPN) this number is per
- feature map.
- post_nms_topk (int): number of top k scoring proposals to keep after applying NMS.
- When RRPN is run on multiple feature maps (as in FPN) this number is total,
- over all feature maps.
- min_box_size(float): minimum proposal box side length in pixels (absolute units wrt
- input images).
- training (bool): True if proposals are to be used in training, otherwise False.
- This arg exists only to support a legacy bug; look for the "NB: Legacy bug ..."
- comment.
-
- Returns:
- proposals (list[Instances]): list of N Instances. The i-th Instances
- stores post_nms_topk object proposals for image i.
- """
- num_images = len(image_sizes)
- device = proposals[0].device
-
- # 1. Select top-k anchor for every level and every image
- topk_scores = [] # #lvl Tensor, each of shape N x topk
- topk_proposals = []
- level_ids = [] # #lvl Tensor, each of shape (topk,)
- batch_idx = torch.arange(num_images, device=device)
- for level_id, proposals_i, logits_i in zip(
- itertools.count(), proposals, pred_objectness_logits
- ):
- Hi_Wi_A = logits_i.shape[1]
- if isinstance(Hi_Wi_A, torch.Tensor): # it's a tensor in tracing
- num_proposals_i = torch.clamp(Hi_Wi_A, max=pre_nms_topk)
- else:
- num_proposals_i = min(Hi_Wi_A, pre_nms_topk)
-
- topk_scores_i, topk_idx = logits_i.topk(num_proposals_i, dim=1)
-
- # each is N x topk
- topk_proposals_i = proposals_i[batch_idx[:, None], topk_idx] # N x topk x 5
-
- topk_proposals.append(topk_proposals_i)
- topk_scores.append(topk_scores_i)
- level_ids.append(torch.full((num_proposals_i,), level_id, dtype=torch.int64, device=device))
-
- # 2. Concat all levels together
- topk_scores = cat(topk_scores, dim=1)
- topk_proposals = cat(topk_proposals, dim=1)
- level_ids = cat(level_ids, dim=0)
-
- # 3. For each image, run a per-level NMS, and choose topk results.
- results = []
- for n, image_size in enumerate(image_sizes):
- boxes = RotatedBoxes(topk_proposals[n])
- scores_per_img = topk_scores[n]
- valid_mask = torch.isfinite(boxes.tensor).all(dim=1) & torch.isfinite(scores_per_img)
- if not valid_mask.all():
- boxes = boxes[valid_mask]
- scores_per_img = scores_per_img[valid_mask]
- boxes.clip(image_size)
-
- # filter empty boxes
- keep = boxes.nonempty(threshold=min_box_size)
- lvl = level_ids
- if _is_tracing() or keep.sum().item() != len(boxes):
- boxes, scores_per_img, lvl = (boxes[keep], scores_per_img[keep], level_ids[keep])
-
- keep = batched_nms_rotated(boxes.tensor, scores_per_img, lvl, nms_thresh)
- # In Detectron1, there was different behavior during training vs. testing.
- # (https://github.com/facebookresearch/Detectron/issues/459)
- # During training, topk is over the proposals from *all* images in the training batch.
- # During testing, it is over the proposals for each image separately.
- # As a result, the training behavior becomes batch-dependent,
- # and the configuration "POST_NMS_TOPK_TRAIN" end up relying on the batch size.
- # This bug is addressed in Detectron2 to make the behavior independent of batch size.
- keep = keep[:post_nms_topk]
-
- res = Instances(image_size)
- res.proposal_boxes = boxes[keep]
- res.objectness_logits = scores_per_img[keep]
- results.append(res)
- return results
-
-
-@PROPOSAL_GENERATOR_REGISTRY.register()
-class RRPN(RPN):
- """
- Rotated Region Proposal Network described in :paper:`RRPN`.
- """
-
- @configurable
- def __init__(self, *args, **kwargs):
- super().__init__(*args, **kwargs)
- if self.anchor_boundary_thresh >= 0:
- raise NotImplementedError(
- "anchor_boundary_thresh is a legacy option not implemented for RRPN."
- )
-
- @classmethod
- def from_config(cls, cfg, input_shape: Dict[str, ShapeSpec]):
- ret = super().from_config(cfg, input_shape)
- ret["box2box_transform"] = Box2BoxTransformRotated(weights=cfg.MODEL.RPN.BBOX_REG_WEIGHTS)
- return ret
-
- @torch.no_grad()
- def label_and_sample_anchors(self, anchors: List[RotatedBoxes], gt_instances: List[Instances]):
- """
- Args:
- anchors (list[RotatedBoxes]): anchors for each feature map.
- gt_instances: the ground-truth instances for each image.
-
- Returns:
- list[Tensor]:
- List of #img tensors. i-th element is a vector of labels whose length is
- the total number of anchors across feature maps. Label values are in {-1, 0, 1},
- with meanings: -1 = ignore; 0 = negative class; 1 = positive class.
- list[Tensor]:
- i-th element is a Nx5 tensor, where N is the total number of anchors across
- feature maps. The values are the matched gt boxes for each anchor.
- Values are undefined for those anchors not labeled as 1.
- """
- anchors = RotatedBoxes.cat(anchors)
-
- gt_boxes = [x.gt_boxes for x in gt_instances]
- del gt_instances
-
- gt_labels = []
- matched_gt_boxes = []
- for gt_boxes_i in gt_boxes:
- """
- gt_boxes_i: ground-truth boxes for i-th image
- """
- match_quality_matrix = retry_if_cuda_oom(pairwise_iou_rotated)(gt_boxes_i, anchors)
- matched_idxs, gt_labels_i = retry_if_cuda_oom(self.anchor_matcher)(match_quality_matrix)
- # Matching is memory-expensive and may result in CPU tensors. But the result is small
- gt_labels_i = gt_labels_i.to(device=gt_boxes_i.device)
-
- # A vector of labels (-1, 0, 1) for each anchor
- gt_labels_i = self._subsample_labels(gt_labels_i)
-
- if len(gt_boxes_i) == 0:
- # These values won't be used anyway since the anchor is labeled as background
- matched_gt_boxes_i = torch.zeros_like(anchors.tensor)
- else:
- # TODO wasted indexing computation for ignored boxes
- matched_gt_boxes_i = gt_boxes_i[matched_idxs].tensor
-
- gt_labels.append(gt_labels_i) # N,AHW
- matched_gt_boxes.append(matched_gt_boxes_i)
- return gt_labels, matched_gt_boxes
-
- @torch.no_grad()
- def predict_proposals(self, anchors, pred_objectness_logits, pred_anchor_deltas, image_sizes):
- pred_proposals = self._decode_proposals(anchors, pred_anchor_deltas)
- return find_top_rrpn_proposals(
- pred_proposals,
- pred_objectness_logits,
- image_sizes,
- self.nms_thresh,
- self.pre_nms_topk[self.training],
- self.post_nms_topk[self.training],
- self.min_box_size,
- self.training,
- )
diff --git a/spaces/TeraTTS/TTS/tokenizer/gruut/tokenizer.py b/spaces/TeraTTS/TTS/tokenizer/gruut/tokenizer.py
deleted file mode 100644
index 80a317f0c0553e69d36f5314c6e2547cc2d6f927..0000000000000000000000000000000000000000
--- a/spaces/TeraTTS/TTS/tokenizer/gruut/tokenizer.py
+++ /dev/null
@@ -1,36 +0,0 @@
-from gruut import sentences
-import os
-import re
-
-class Tokenizer():
- def __init__(self, path) -> None:
- with open(os.path.join(path, "vocab.txt"), "r", encoding="utf-8") as vocab_file:
- self.symbols = vocab_file.read().split("\n")
- self.symbols = list(map(chr, list(map(int, self.symbols))))
-
- self.symbol_to_id = {s: i for i, s in enumerate(self.symbols)}
-
- def _ru_phonems(self, text: str) -> str:
- text = text.lower()
- phonemes = ""
- for sent in sentences(text, lang="ru"):
- for word in sent:
- if word.phonemes:
- phonemes += "".join(word.phonemes)
- phonemes = re.sub(re.compile(r'\s+'), ' ', phonemes).lstrip().rstrip()
- return phonemes
-
-
- def _text_to_sequence(self, text: str) -> list[int]:
- '''convert text to seq'''
- sequence = []
- clean_text = self._ru_phonems(text)
- for symbol in clean_text:
- symbol_id = self.symbol_to_id[symbol]
- sequence += [symbol_id]
- return sequence
-
-
- def _get_seq(self, text: str) -> list[int]:
- seq = self._text_to_sequence(text)
- return seq
\ No newline at end of file
diff --git a/spaces/Tetel/secondbing/EdgeGPT/EdgeUtils.py b/spaces/Tetel/secondbing/EdgeGPT/EdgeUtils.py
deleted file mode 100644
index 795383fca66d7c68a22ff958fa2cc8a6723c95ec..0000000000000000000000000000000000000000
--- a/spaces/Tetel/secondbing/EdgeGPT/EdgeUtils.py
+++ /dev/null
@@ -1,254 +0,0 @@
-import asyncio
-import json
-import platform
-import time
-from pathlib import Path
-from typing import Dict
-from typing import List
-from typing import Set
-from typing import Union
-
-from EdgeGPT.EdgeGPT import Chatbot
-from EdgeGPT.EdgeGPT import ConversationStyle
-from EdgeGPT.ImageGen import ImageGen
-
-
-class Cookie:
- """
- Convenience class for Bing Cookie files, data, and configuration. This Class
- is updated dynamically by the Query class to allow cycling through >1
- cookie/credentials file e.g. when daily request limits (current 200 per
- account per day) are exceeded.
- """
-
- current_file_index = 0
- dirpath = Path("./").resolve()
- search_pattern = "bing_cookies_*.json"
- ignore_files = set()
- current_filepath: Union[dict, None] = None
-
- @classmethod
- def fetch_default(cls, path: Union[Path, None] = None) -> None:
- from selenium import webdriver
- from selenium.webdriver.common.by import By
-
- driver = webdriver.Edge()
- driver.get("https://bing.com/chat")
- time.sleep(5)
- xpath = '//button[@id="bnp_btn_accept"]'
- driver.find_element(By.XPATH, xpath).click()
- time.sleep(2)
- xpath = '//a[@id="codexPrimaryButton"]'
- driver.find_element(By.XPATH, xpath).click()
- if path is None:
- path = Path("./bing_cookies__default.json")
- # Double underscore ensures this file is first when sorted
- cookies = driver.get_cookies()
- Path(path).write_text(json.dumps(cookies, indent=4), encoding="utf-8")
- # Path again in case supplied path is: str
- print(f"Cookies saved to: {path}")
- driver.quit()
-
- @classmethod
- def files(cls) -> List[Path]:
- """Return a sorted list of all cookie files matching .search_pattern"""
- all_files = set(cls.dirpath.glob(cls.search_pattern))
- return sorted(all_files - cls.ignore_files)
-
- @classmethod
- def import_data(cls) -> None:
- """
- Read the active cookie file and populate the following attributes:
-
- .current_filepath
- .current_data
- .image_token
- """
- try:
- cls.current_filepath = cls.files()[cls.current_file_index]
- except IndexError as exc:
- print(
- "> Please set Cookie.current_filepath to a valid cookie file, then run Cookie.import_data()",
- )
- raise "No valid cookie file found." from exc
- print(f"> Importing cookies from: {cls.current_filepath.name}")
- with Path.open(cls.current_filepath, encoding="utf-8") as file:
- cls.current_data = json.load(file)
- cls.image_token = [x for x in cls.current_data if x.get("name") == "_U"]
- cls.image_token = cls.image_token[0].get("value")
-
- @classmethod
- def import_next(cls) -> None:
- """
- Cycle through to the next cookies file. Import it. Mark the previous
- file to be ignored for the remainder of the current session.
- """
- cls.ignore_files.add(cls.current_filepath)
- if Cookie.current_file_index >= len(cls.files()):
- Cookie.current_file_index = 0
- Cookie.import_data()
-
-
-class Query:
- """
- A convenience class that wraps around EdgeGPT.Chatbot to encapsulate input,
- config, and output all together. Relies on Cookie class for authentication
- """
-
- def __init__(
- self,
- prompt: str,
- style: str = "precise",
- content_type: str = "text",
- cookie_file: int = 0,
- echo: bool = True,
- echo_prompt: bool = False,
- proxy: Union[str, None] = None,
- ) -> None:
- """
- Arguments:
-
- prompt: Text to enter into Bing Chat
- style: creative, balanced, or precise
- content_type: "text" for Bing Chat; "image" for Dall-e
- cookie_file: Path, filepath string, or index (int) to list of cookie paths
- echo: Print something to confirm request made
- echo_prompt: Print confirmation of the evaluated prompt
- """
- self.proxy = proxy
- self.index = []
- self.request_count = {}
- self.image_dirpath = Path("./").resolve()
- Cookie.import_data()
- self.index += [self]
- self.prompt = prompt
- files = Cookie.files()
- if isinstance(cookie_file, int):
- index = cookie_file if cookie_file < len(files) else 0
- else:
- if not isinstance(cookie_file, (str, Path)):
- message = "'cookie_file' must be an int, str, or Path object"
- raise TypeError(message)
- cookie_file = Path(cookie_file)
- if cookie_file in files: # Supplied filepath IS in Cookie.dirpath
- index = files.index(cookie_file)
- else: # Supplied filepath is NOT in Cookie.dirpath
- if cookie_file.is_file():
- Cookie.dirpath = cookie_file.parent.resolve()
- if cookie_file.is_dir():
- Cookie.dirpath = cookie_file.resolve()
- index = 0
- Cookie.current_file_index = index
- if content_type == "text":
- self.style = style
- self.log_and_send_query(echo, echo_prompt)
- if content_type == "image":
- self.create_image()
-
- def log_and_send_query(self, echo: bool, echo_prompt: bool) -> None:
- if platform.system() == "Windows":
- asyncio.set_event_loop_policy(asyncio.WindowsSelectorEventLoopPolicy())
- self.response = asyncio.run(self.send_to_bing(echo, echo_prompt))
- name = str(Cookie.current_filepath.name)
- if not self.request_count.get(name):
- self.request_count[name] = 1
- else:
- self.request_count[name] += 1
-
- def create_image(self) -> None:
- image_generator = ImageGen(Cookie.image_token)
- image_generator.save_images(
- image_generator.get_images(self.prompt),
- output_dir=self.image_dirpath,
- )
-
- async def send_to_bing(self, echo: bool = True, echo_prompt: bool = False) -> str:
- """Creat, submit, then close a Chatbot instance. Return the response"""
- retries = len(Cookie.files())
- while retries:
- try:
- # Read the cookies file
- bot = await Chatbot.create(
- proxy=self.proxy,
- cookies=Cookie.current_data,
- )
- if echo_prompt:
- print(f"> {self.prompt}=")
- if echo:
- print("> Waiting for response...")
- if self.style.lower() not in "creative balanced precise".split():
- self.style = "precise"
- return await bot.ask(
- prompt=self.prompt,
- conversation_style=getattr(ConversationStyle, self.style),
- # wss_link="wss://sydney.bing.com/sydney/ChatHub"
- # What other values can this parameter take? It seems to be optional
- )
- except KeyError:
- print(
- f"> KeyError [{Cookie.current_filepath.name} may have exceeded the daily limit]",
- )
- Cookie.import_next()
- retries -= 1
- finally:
- await bot.close()
- return None
-
- @property
- def output(self) -> str:
- """The response from a completed Chatbot request"""
- return self.response["item"]["messages"][-1]["text"]
-
- @property
- def sources(self) -> str:
- """The source names and details parsed from a completed Chatbot request"""
- return self.response["item"]["messages"][-1]["sourceAttributions"]
-
- @property
- def sources_dict(self) -> Dict[str, str]:
- """The source names and details as a dictionary"""
- sources_dict = {}
- name = "providerDisplayName"
- url = "seeMoreUrl"
- for source in self.sources:
- if name in source and url in source:
- sources_dict[source[name]] = source[url]
- else:
- continue
- return sources_dict
-
- @property
- def code(self) -> str:
- """Extract and join any snippets of Python code in the response"""
- code_blocks = self.output.split("```")[1:-1:2]
- code_blocks = ["\n".join(x.splitlines()[1:]) for x in code_blocks]
- return "\n\n".join(code_blocks)
-
- @property
- def languages(self) -> Set[str]:
- """Extract all programming languages given in code blocks"""
- code_blocks = self.output.split("```")[1:-1:2]
- return {x.splitlines()[0] for x in code_blocks}
-
- @property
- def suggestions(self) -> List[str]:
- """Follow-on questions suggested by the Chatbot"""
- return [
- x["text"]
- for x in self.response["item"]["messages"][-1]["suggestedResponses"]
- ]
-
- def __repr__(self) -> str:
- return f""
-
- def __str__(self) -> str:
- return self.output
-
-
-class ImageQuery(Query):
- def __init__(self, prompt: str, **kwargs) -> None:
- kwargs["content_type"] = "image"
- super().__init__(prompt, **kwargs)
-
- def __repr__(self) -> str:
- return f""
diff --git a/spaces/Thaweewat/ControlNet-Architecture/annotator/openpose/__init__.py b/spaces/Thaweewat/ControlNet-Architecture/annotator/openpose/__init__.py
deleted file mode 100644
index 41111fbe4508fc1462978c4353b5939262951bee..0000000000000000000000000000000000000000
--- a/spaces/Thaweewat/ControlNet-Architecture/annotator/openpose/__init__.py
+++ /dev/null
@@ -1,31 +0,0 @@
-import os
-os.environ["KMP_DUPLICATE_LIB_OK"]="TRUE"
-
-import torch
-import numpy as np
-from . import util
-from .body import Body
-from .hand import Hand
-
-from huggingface_hub import hf_hub_url, cached_download
-REPO_ID = "lllyasviel/ControlNet"
-body_estimation = Body(cached_download(hf_hub_url(REPO_ID, 'annotator/ckpts/body_pose_model.pth')))
-hand_estimation = Hand(cached_download(hf_hub_url(REPO_ID,'annotator/ckpts/hand_pose_model.pth')))
-
-
-def apply_openpose(oriImg, hand=False):
- oriImg = oriImg[:, :, ::-1].copy()
- with torch.no_grad():
- candidate, subset = body_estimation(oriImg)
- canvas = np.zeros_like(oriImg)
- canvas = util.draw_bodypose(canvas, candidate, subset)
- if hand:
- hands_list = util.handDetect(candidate, subset, oriImg)
- all_hand_peaks = []
- for x, y, w, is_left in hands_list:
- peaks = hand_estimation(oriImg[y:y+w, x:x+w, :])
- peaks[:, 0] = np.where(peaks[:, 0] == 0, peaks[:, 0], peaks[:, 0] + x)
- peaks[:, 1] = np.where(peaks[:, 1] == 0, peaks[:, 1], peaks[:, 1] + y)
- all_hand_peaks.append(peaks)
- canvas = util.draw_handpose(canvas, all_hand_peaks)
- return canvas, dict(candidate=candidate.tolist(), subset=subset.tolist())
diff --git a/spaces/Toritto/Genshin-impact-IA-project-v1/README.md b/spaces/Toritto/Genshin-impact-IA-project-v1/README.md
deleted file mode 100644
index 16161474aeed99ba7fb6192d0c181eb6d4a8a84d..0000000000000000000000000000000000000000
--- a/spaces/Toritto/Genshin-impact-IA-project-v1/README.md
+++ /dev/null
@@ -1,13 +0,0 @@
----
-title: RVC Genshin Impact
-emoji: 🎤
-colorFrom: red
-colorTo: purple
-sdk: gradio
-sdk_version: 3.40.1
-app_file: app.py
-pinned: true
-license: mit
----
-
-Check out the configuration reference at https://huggingface.co/docs/hub/spaces-config-reference
diff --git a/spaces/UltimateAICourse/Prompt-Engineering/README.md b/spaces/UltimateAICourse/Prompt-Engineering/README.md
deleted file mode 100644
index 9115c8cf2160b247fb193ecc96bca63ce88690c6..0000000000000000000000000000000000000000
--- a/spaces/UltimateAICourse/Prompt-Engineering/README.md
+++ /dev/null
@@ -1,11 +0,0 @@
----
-title: Prompt Engineering
-emoji: 🔥
-colorFrom: gray
-colorTo: blue
-sdk: static
-pinned: false
-license: cc
----
-
-Check out the configuration reference at https://huggingface.co/docs/hub/spaces-config-reference
diff --git a/spaces/VoiceHero69/changer/setup_tools/magicinstaller/requirements/huggingface_package.py b/spaces/VoiceHero69/changer/setup_tools/magicinstaller/requirements/huggingface_package.py
deleted file mode 100644
index 7506206df2ca58d0628bcf550b0d170a51654c63..0000000000000000000000000000000000000000
--- a/spaces/VoiceHero69/changer/setup_tools/magicinstaller/requirements/huggingface_package.py
+++ /dev/null
@@ -1,13 +0,0 @@
-from setup_tools.magicinstaller.requirement import SimpleRequirement
-
-
-class Transformers(SimpleRequirement):
- package_name = 'transformers'
-
-
-class Diffusers(SimpleRequirement):
- package_name = 'diffusers'
-
-
-class Gradio(SimpleRequirement):
- package_name = 'gradio'
diff --git a/spaces/VoiceHero69/changer/setup_tools/magicinstaller/requirements/rvc_package.py b/spaces/VoiceHero69/changer/setup_tools/magicinstaller/requirements/rvc_package.py
deleted file mode 100644
index a46ede52d9ab31f47b91ed328a07f39475be6e21..0000000000000000000000000000000000000000
--- a/spaces/VoiceHero69/changer/setup_tools/magicinstaller/requirements/rvc_package.py
+++ /dev/null
@@ -1,64 +0,0 @@
-from setup_tools.magicinstaller.requirement import SimpleRequirement
-
-
-class Praat(SimpleRequirement):
- package_name = 'praat-parselmouth'
-
- def is_right_version(self):
- from packaging import version
- return version.parse(self.get_package_version(self.package_name)) >= version.parse('0.4.2')
-
- def install(self) -> tuple[int, str, str]:
- return self.install_pip('praat-parselmouth>=0.4.2', 'praat-parselmouth')
-
-
-class PyWorld(SimpleRequirement):
- package_name = 'pyworld'
-
- def is_right_version(self):
- from packaging import version
- return version.parse(self.get_package_version(self.package_name)) >= version.parse('0.3.2')
-
- def install(self) -> tuple[int, str, str]:
- return self.install_pip('pyworld>=0.3.2', 'pyworld')
-
-
-class FaissCpu(SimpleRequirement):
- package_name = 'faiss-cpu'
-
- def is_right_version(self):
- from packaging import version
- return version.parse(self.get_package_version(self.package_name)) == version.parse('1.7.3')
-
- def install(self) -> tuple[int, str, str]:
- return self.install_pip('faiss-cpu==1.7.3', 'faiss')
-
-
-class TorchCrepe(SimpleRequirement):
- package_name = 'torchcrepe'
-
- def is_right_version(self):
- from packaging import version
- return version.parse(self.get_package_version(self.package_name)) == version.parse('0.0.20')
-
- def install(self) -> tuple[int, str, str]:
- return self.install_pip('torchcrepe==0.0.20', 'torchcrepe')
-
-
-class FfmpegPython(SimpleRequirement):
- package_name = 'ffmpeg-python'
-
-
-class NoiseReduce(SimpleRequirement):
- package_name = 'noisereduce'
-
-
-class LibRosa(SimpleRequirement):
- package_name = 'librosa'
-
-
-class Demucs(SimpleRequirement):
- package_name = 'demucs'
-
- def install(self) -> tuple[int, str, str]:
- return self.install_pip('git+https://github.com/facebookresearch/demucs#egg=demucs', 'demucs')
diff --git a/spaces/WindVChen/INR-Harmon/utils/build_loss.py b/spaces/WindVChen/INR-Harmon/utils/build_loss.py
deleted file mode 100644
index 01ebe4bba88be6a3b611f69809a9c9960aefd9ae..0000000000000000000000000000000000000000
--- a/spaces/WindVChen/INR-Harmon/utils/build_loss.py
+++ /dev/null
@@ -1,76 +0,0 @@
-import torch
-
-
-def loss_generator(ignore: list = None):
- loss_fn = {'mse': mse,
- 'lut_mse': lut_mse,
- 'masked_mse': masked_mse,
- 'sample_weighted_mse': sample_weighted_mse,
- 'regularize_LUT': regularize_LUT,
- 'MaskWeightedMSE': MaskWeightedMSE}
-
- if ignore:
- for fn in ignore:
- ignore.pop(fn)
-
- return loss_fn
-
-
-def mse(pred, gt):
- return torch.mean((pred - gt) ** 2)
-
-
-def masked_mse(pred, gt, mask):
- delimin = torch.clamp_min(torch.sum(mask, dim=([x for x in range(1, len(mask.shape))])), 100).cuda()
- # total = torch.sum(torch.ones_like(mask), dim=([x for x in range(1, len(mask.shape))]))
- out = torch.sum((mask > 100 / 255.) * (pred - gt) ** 2, dim=([x for x in range(1, len(mask.shape))]))
- out = out / delimin
- return torch.mean(out)
-
-
-def sample_weighted_mse(pred, gt, mask):
- multi_factor = torch.clamp_min(torch.sum(mask, dim=([x for x in range(1, len(mask.shape))])), 100).cuda()
- multi_factor = multi_factor / (multi_factor.sum())
- # total = torch.sum(torch.ones_like(mask), dim=([x for x in range(1, len(mask.shape))]))
- out = torch.mean((pred - gt) ** 2, dim=([x for x in range(1, len(mask.shape))]))
- out = out * multi_factor
- return torch.sum(out)
-
-
-def regularize_LUT(lut):
- st = lut[lut < 0.]
- reg_st = (st ** 2).mean() if min(st.shape) != 0 else 0
-
- lt = lut[lut > 1.]
- reg_lt = ((lt - 1.) ** 2).mean() if min(lt.shape) != 0 else 0
-
- return reg_lt + reg_st
-
-
-def lut_mse(feat, lut_batch):
- loss = 0
- for id in range(feat.shape[0] // lut_batch):
- for i in feat[id * lut_batch: id * lut_batch + lut_batch]:
- for j in feat[id * lut_batch: id * lut_batch + lut_batch]:
- loss += mse(i, j)
-
- return loss / lut_batch
-
-
-def MaskWeightedMSE(pred, label, mask):
- label = label.view(pred.size())
- reduce_dims = get_dims_with_exclusion(label.dim(), 0)
-
- loss = (pred - label) ** 2
- delimeter = pred.size(1) * torch.clamp_min(torch.sum(mask, dim=reduce_dims), 100)
- loss = torch.sum(loss, dim=reduce_dims) / delimeter
-
- return torch.mean(loss)
-
-
-def get_dims_with_exclusion(dim, exclude=None):
- dims = list(range(dim))
- if exclude is not None:
- dims.remove(exclude)
-
- return dims
\ No newline at end of file
diff --git a/spaces/Wootang01/chatbot_four/README.md b/spaces/Wootang01/chatbot_four/README.md
deleted file mode 100644
index c13c68fdaddf3255d9f59d14a68564259d7a09bd..0000000000000000000000000000000000000000
--- a/spaces/Wootang01/chatbot_four/README.md
+++ /dev/null
@@ -1,12 +0,0 @@
----
-title: Chatbot_four
-emoji: 🌖
-colorFrom: gray
-colorTo: purple
-sdk: streamlit
-sdk_version: 1.2.0
-app_file: app.py
-pinned: false
----
-
-Check out the configuration reference at https://huggingface.co/docs/hub/spaces#reference
diff --git a/spaces/XlalalaX/VITS-Umamusume-voice-synthesizer/text/cleaners.py b/spaces/XlalalaX/VITS-Umamusume-voice-synthesizer/text/cleaners.py
deleted file mode 100644
index c80e113b2b81a66134800dbdaa29c7d96a0152a7..0000000000000000000000000000000000000000
--- a/spaces/XlalalaX/VITS-Umamusume-voice-synthesizer/text/cleaners.py
+++ /dev/null
@@ -1,146 +0,0 @@
-import re
-
-
-def japanese_cleaners(text):
- from text.japanese import japanese_to_romaji_with_accent
- text = japanese_to_romaji_with_accent(text)
- text = re.sub(r'([A-Za-z])$', r'\1.', text)
- return text
-
-
-def japanese_cleaners2(text):
- return japanese_cleaners(text).replace('ts', 'ʦ').replace('...', '…')
-
-
-def korean_cleaners(text):
- '''Pipeline for Korean text'''
- from text.korean import latin_to_hangul, number_to_hangul, divide_hangul
- text = latin_to_hangul(text)
- text = number_to_hangul(text)
- text = divide_hangul(text)
- text = re.sub(r'([\u3131-\u3163])$', r'\1.', text)
- return text
-
-
-def chinese_cleaners(text):
- '''Pipeline for Chinese text'''
- from text.mandarin import number_to_chinese, chinese_to_bopomofo, latin_to_bopomofo
- text = number_to_chinese(text)
- text = chinese_to_bopomofo(text)
- text = latin_to_bopomofo(text)
- text = re.sub(r'([ˉˊˇˋ˙])$', r'\1。', text)
- return text
-
-
-def zh_ja_mixture_cleaners(text):
- from text.mandarin import chinese_to_romaji
- from text.japanese import japanese_to_romaji_with_accent
- text = re.sub(r'\[ZH\](.*?)\[ZH\]',
- lambda x: chinese_to_romaji(x.group(1))+' ', text)
- text = re.sub(r'\[JA\](.*?)\[JA\]', lambda x: japanese_to_romaji_with_accent(
- x.group(1)).replace('ts', 'ʦ').replace('u', 'ɯ').replace('...', '…')+' ', text)
- text = re.sub(r'\s+$', '', text)
- text = re.sub(r'([^\.,!\?\-…~])$', r'\1.', text)
- return text
-
-
-def sanskrit_cleaners(text):
- text = text.replace('॥', '।').replace('ॐ', 'ओम्')
- if text[-1] != '।':
- text += ' ।'
- return text
-
-
-def cjks_cleaners(text):
- from text.mandarin import chinese_to_lazy_ipa
- from text.japanese import japanese_to_ipa
- from text.korean import korean_to_lazy_ipa
- from text.sanskrit import devanagari_to_ipa
- from text.english import english_to_lazy_ipa
- text = re.sub(r'\[ZH\](.*?)\[ZH\]',
- lambda x: chinese_to_lazy_ipa(x.group(1))+' ', text)
- text = re.sub(r'\[JA\](.*?)\[JA\]',
- lambda x: japanese_to_ipa(x.group(1))+' ', text)
- text = re.sub(r'\[KO\](.*?)\[KO\]',
- lambda x: korean_to_lazy_ipa(x.group(1))+' ', text)
- text = re.sub(r'\[SA\](.*?)\[SA\]',
- lambda x: devanagari_to_ipa(x.group(1))+' ', text)
- text = re.sub(r'\[EN\](.*?)\[EN\]',
- lambda x: english_to_lazy_ipa(x.group(1))+' ', text)
- text = re.sub(r'\s+$', '', text)
- text = re.sub(r'([^\.,!\?\-…~])$', r'\1.', text)
- return text
-
-
-def cjke_cleaners(text):
- from text.mandarin import chinese_to_lazy_ipa
- from text.japanese import japanese_to_ipa
- from text.korean import korean_to_ipa
- from text.english import english_to_ipa2
- text = re.sub(r'\[ZH\](.*?)\[ZH\]', lambda x: chinese_to_lazy_ipa(x.group(1)).replace(
- 'ʧ', 'tʃ').replace('ʦ', 'ts').replace('ɥan', 'ɥæn')+' ', text)
- text = re.sub(r'\[JA\](.*?)\[JA\]', lambda x: japanese_to_ipa(x.group(1)).replace('ʧ', 'tʃ').replace(
- 'ʦ', 'ts').replace('ɥan', 'ɥæn').replace('ʥ', 'dz')+' ', text)
- text = re.sub(r'\[KO\](.*?)\[KO\]',
- lambda x: korean_to_ipa(x.group(1))+' ', text)
- text = re.sub(r'\[EN\](.*?)\[EN\]', lambda x: english_to_ipa2(x.group(1)).replace('ɑ', 'a').replace(
- 'ɔ', 'o').replace('ɛ', 'e').replace('ɪ', 'i').replace('ʊ', 'u')+' ', text)
- text = re.sub(r'\s+$', '', text)
- text = re.sub(r'([^\.,!\?\-…~])$', r'\1.', text)
- return text
-
-
-def cjke_cleaners2(text):
- from text.mandarin import chinese_to_ipa
- from text.japanese import japanese_to_ipa2
- from text.korean import korean_to_ipa
- from text.english import english_to_ipa2
- text = re.sub(r'\[ZH\](.*?)\[ZH\]',
- lambda x: chinese_to_ipa(x.group(1))+' ', text)
- text = re.sub(r'\[JA\](.*?)\[JA\]',
- lambda x: japanese_to_ipa2(x.group(1))+' ', text)
- text = re.sub(r'\[KO\](.*?)\[KO\]',
- lambda x: korean_to_ipa(x.group(1))+' ', text)
- text = re.sub(r'\[EN\](.*?)\[EN\]',
- lambda x: english_to_ipa2(x.group(1))+' ', text)
- text = re.sub(r'\s+$', '', text)
- text = re.sub(r'([^\.,!\?\-…~])$', r'\1.', text)
- return text
-
-
-def thai_cleaners(text):
- from text.thai import num_to_thai, latin_to_thai
- text = num_to_thai(text)
- text = latin_to_thai(text)
- return text
-
-
-def shanghainese_cleaners(text):
- from text.shanghainese import shanghainese_to_ipa
- text = shanghainese_to_ipa(text)
- text = re.sub(r'([^\.,!\?\-…~])$', r'\1.', text)
- return text
-
-
-def chinese_dialect_cleaners(text):
- from text.mandarin import chinese_to_ipa2
- from text.japanese import japanese_to_ipa3
- from text.shanghainese import shanghainese_to_ipa
- from text.cantonese import cantonese_to_ipa
- from text.english import english_to_lazy_ipa2
- from text.ngu_dialect import ngu_dialect_to_ipa
- text = re.sub(r'\[ZH\](.*?)\[ZH\]',
- lambda x: chinese_to_ipa2(x.group(1))+' ', text)
- text = re.sub(r'\[JA\](.*?)\[JA\]',
- lambda x: japanese_to_ipa3(x.group(1)).replace('Q', 'ʔ')+' ', text)
- text = re.sub(r'\[SH\](.*?)\[SH\]', lambda x: shanghainese_to_ipa(x.group(1)).replace('1', '˥˧').replace('5',
- '˧˧˦').replace('6', '˩˩˧').replace('7', '˥').replace('8', '˩˨').replace('ᴀ', 'ɐ').replace('ᴇ', 'e')+' ', text)
- text = re.sub(r'\[GD\](.*?)\[GD\]',
- lambda x: cantonese_to_ipa(x.group(1))+' ', text)
- text = re.sub(r'\[EN\](.*?)\[EN\]',
- lambda x: english_to_lazy_ipa2(x.group(1))+' ', text)
- text = re.sub(r'\[([A-Z]{2})\](.*?)\[\1\]', lambda x: ngu_dialect_to_ipa(x.group(2), x.group(
- 1)).replace('ʣ', 'dz').replace('ʥ', 'dʑ').replace('ʦ', 'ts').replace('ʨ', 'tɕ')+' ', text)
- text = re.sub(r'\s+$', '', text)
- text = re.sub(r'([^\.,!\?\-…~])$', r'\1.', text)
- return text
diff --git a/spaces/Xule/ChuanhuChatGPT/modules/pdf_func.py b/spaces/Xule/ChuanhuChatGPT/modules/pdf_func.py
deleted file mode 100644
index 0aba6b7b891fc527c79b887256b0cbaa81ae5b3d..0000000000000000000000000000000000000000
--- a/spaces/Xule/ChuanhuChatGPT/modules/pdf_func.py
+++ /dev/null
@@ -1,180 +0,0 @@
-from types import SimpleNamespace
-import pdfplumber
-import logging
-from llama_index import Document
-
-def prepare_table_config(crop_page):
- """Prepare table查找边界, 要求page为原始page
-
- From https://github.com/jsvine/pdfplumber/issues/242
- """
- page = crop_page.root_page # root/parent
- cs = page.curves + page.edges
- def curves_to_edges():
- """See https://github.com/jsvine/pdfplumber/issues/127"""
- edges = []
- for c in cs:
- edges += pdfplumber.utils.rect_to_edges(c)
- return edges
- edges = curves_to_edges()
- return {
- "vertical_strategy": "explicit",
- "horizontal_strategy": "explicit",
- "explicit_vertical_lines": edges,
- "explicit_horizontal_lines": edges,
- "intersection_y_tolerance": 10,
- }
-
-def get_text_outside_table(crop_page):
- ts = prepare_table_config(crop_page)
- if len(ts["explicit_vertical_lines"]) == 0 or len(ts["explicit_horizontal_lines"]) == 0:
- return crop_page
-
- ### Get the bounding boxes of the tables on the page.
- bboxes = [table.bbox for table in crop_page.root_page.find_tables(table_settings=ts)]
- def not_within_bboxes(obj):
- """Check if the object is in any of the table's bbox."""
- def obj_in_bbox(_bbox):
- """See https://github.com/jsvine/pdfplumber/blob/stable/pdfplumber/table.py#L404"""
- v_mid = (obj["top"] + obj["bottom"]) / 2
- h_mid = (obj["x0"] + obj["x1"]) / 2
- x0, top, x1, bottom = _bbox
- return (h_mid >= x0) and (h_mid < x1) and (v_mid >= top) and (v_mid < bottom)
- return not any(obj_in_bbox(__bbox) for __bbox in bboxes)
-
- return crop_page.filter(not_within_bboxes)
-# 请使用 LaTeX 表达公式,行内公式以 $ 包裹,行间公式以 $$ 包裹
-
-extract_words = lambda page: page.extract_words(keep_blank_chars=True, y_tolerance=0, x_tolerance=1, extra_attrs=["fontname", "size", "object_type"])
-# dict_keys(['text', 'x0', 'x1', 'top', 'doctop', 'bottom', 'upright', 'direction', 'fontname', 'size'])
-
-def get_title_with_cropped_page(first_page):
- title = [] # 处理标题
- x0,top,x1,bottom = first_page.bbox # 获取页面边框
-
- for word in extract_words(first_page):
- word = SimpleNamespace(**word)
-
- if word.size >= 14:
- title.append(word.text)
- title_bottom = word.bottom
- elif word.text == "Abstract": # 获取页面abstract
- top = word.top
-
- user_info = [i["text"] for i in extract_words(first_page.within_bbox((x0,title_bottom,x1,top)))]
- # 裁剪掉上半部分, within_bbox: full_included; crop: partial_included
- return title, user_info, first_page.within_bbox((x0,top,x1,bottom))
-
-def get_column_cropped_pages(pages, two_column=True):
- new_pages = []
- for page in pages:
- if two_column:
- left = page.within_bbox((0, 0, page.width/2, page.height),relative=True)
- right = page.within_bbox((page.width/2, 0, page.width, page.height), relative=True)
- new_pages.append(left)
- new_pages.append(right)
- else:
- new_pages.append(page)
-
- return new_pages
-
-def parse_pdf(filename, two_column = True):
- level = logging.getLogger().level
- if level == logging.getLevelName("DEBUG"):
- logging.getLogger().setLevel("INFO")
-
- with pdfplumber.open(filename) as pdf:
- title, user_info, first_page = get_title_with_cropped_page(pdf.pages[0])
- new_pages = get_column_cropped_pages([first_page] + pdf.pages[1:], two_column)
-
- chapters = []
- # tuple (chapter_name, [pageid] (start,stop), chapter_text)
- create_chapter = lambda page_start,name_top,name_bottom: SimpleNamespace(
- name=[],
- name_top=name_top,
- name_bottom=name_bottom,
- record_chapter_name = True,
-
- page_start=page_start,
- page_stop=None,
-
- text=[],
- )
- cur_chapter = None
-
- # 按页遍历PDF文档
- for idx, page in enumerate(new_pages):
- page = get_text_outside_table(page)
-
- # 按行遍历页面文本
- for word in extract_words(page):
- word = SimpleNamespace(**word)
-
- # 检查行文本是否以12号字体打印,如果是,则将其作为新章节开始
- if word.size >= 11: # 出现chapter name
- if cur_chapter is None:
- cur_chapter = create_chapter(page.page_number, word.top, word.bottom)
- elif not cur_chapter.record_chapter_name or (cur_chapter.name_bottom != cur_chapter.name_bottom and cur_chapter.name_top != cur_chapter.name_top):
- # 不再继续写chapter name
- cur_chapter.page_stop = page.page_number # stop id
- chapters.append(cur_chapter)
- # 重置当前chapter信息
- cur_chapter = create_chapter(page.page_number, word.top, word.bottom)
-
- # print(word.size, word.top, word.bottom, word.text)
- cur_chapter.name.append(word.text)
- else:
- cur_chapter.record_chapter_name = False # chapter name 结束
- cur_chapter.text.append(word.text)
- else:
- # 处理最后一个章节
- cur_chapter.page_stop = page.page_number # stop id
- chapters.append(cur_chapter)
-
- for i in chapters:
- logging.info(f"section: {i.name} pages:{i.page_start, i.page_stop} word-count:{len(i.text)}")
- logging.debug(" ".join(i.text))
-
- title = " ".join(title)
- user_info = " ".join(user_info)
- text = f"Article Title: {title}, Information:{user_info}\n"
- for idx, chapter in enumerate(chapters):
- chapter.name = " ".join(chapter.name)
- text += f"The {idx}th Chapter {chapter.name}: " + " ".join(chapter.text) + "\n"
-
- logging.getLogger().setLevel(level)
- return Document(text=text, extra_info={"title": title})
-
-BASE_POINTS = """
-1. Who are the authors?
-2. What is the process of the proposed method?
-3. What is the performance of the proposed method? Please note down its performance metrics.
-4. What are the baseline models and their performances? Please note down these baseline methods.
-5. What dataset did this paper use?
-"""
-
-READING_PROMPT = """
-You are a researcher helper bot. You can help the user with research paper reading and summarizing. \n
-Now I am going to send you a paper. You need to read it and summarize it for me part by part. \n
-When you are reading, You need to focus on these key points:{}
-"""
-
-READING_PROMT_V2 = """
-You are a researcher helper bot. You can help the user with research paper reading and summarizing. \n
-Now I am going to send you a paper. You need to read it and summarize it for me part by part. \n
-When you are reading, You need to focus on these key points:{},
-
-And You need to generate a brief but informative title for this part.
-Your return format:
-- title: '...'
-- summary: '...'
-"""
-
-SUMMARY_PROMPT = "You are a researcher helper bot. Now you need to read the summaries of a research paper."
-
-
-if __name__ == '__main__':
- # Test code
- z = parse_pdf("./build/test.pdf")
- print(z["user_info"])
- print(z["title"])
\ No newline at end of file
diff --git a/spaces/XzJosh/maimai-Bert-VITS2/models.py b/spaces/XzJosh/maimai-Bert-VITS2/models.py
deleted file mode 100644
index d4afe44d883691610c5903e602a3ca245fcb3a5c..0000000000000000000000000000000000000000
--- a/spaces/XzJosh/maimai-Bert-VITS2/models.py
+++ /dev/null
@@ -1,707 +0,0 @@
-import copy
-import math
-import torch
-from torch import nn
-from torch.nn import functional as F
-
-import commons
-import modules
-import attentions
-import monotonic_align
-
-from torch.nn import Conv1d, ConvTranspose1d, AvgPool1d, Conv2d
-from torch.nn.utils import weight_norm, remove_weight_norm, spectral_norm
-
-from commons import init_weights, get_padding
-from text import symbols, num_tones, num_languages
-class DurationDiscriminator(nn.Module): #vits2
- def __init__(self, in_channels, filter_channels, kernel_size, p_dropout, gin_channels=0):
- super().__init__()
-
- self.in_channels = in_channels
- self.filter_channels = filter_channels
- self.kernel_size = kernel_size
- self.p_dropout = p_dropout
- self.gin_channels = gin_channels
-
- self.drop = nn.Dropout(p_dropout)
- self.conv_1 = nn.Conv1d(in_channels, filter_channels, kernel_size, padding=kernel_size//2)
- self.norm_1 = modules.LayerNorm(filter_channels)
- self.conv_2 = nn.Conv1d(filter_channels, filter_channels, kernel_size, padding=kernel_size//2)
- self.norm_2 = modules.LayerNorm(filter_channels)
- self.dur_proj = nn.Conv1d(1, filter_channels, 1)
-
- self.pre_out_conv_1 = nn.Conv1d(2*filter_channels, filter_channels, kernel_size, padding=kernel_size//2)
- self.pre_out_norm_1 = modules.LayerNorm(filter_channels)
- self.pre_out_conv_2 = nn.Conv1d(filter_channels, filter_channels, kernel_size, padding=kernel_size//2)
- self.pre_out_norm_2 = modules.LayerNorm(filter_channels)
-
- if gin_channels != 0:
- self.cond = nn.Conv1d(gin_channels, in_channels, 1)
-
- self.output_layer = nn.Sequential(
- nn.Linear(filter_channels, 1),
- nn.Sigmoid()
- )
-
- def forward_probability(self, x, x_mask, dur, g=None):
- dur = self.dur_proj(dur)
- x = torch.cat([x, dur], dim=1)
- x = self.pre_out_conv_1(x * x_mask)
- x = torch.relu(x)
- x = self.pre_out_norm_1(x)
- x = self.drop(x)
- x = self.pre_out_conv_2(x * x_mask)
- x = torch.relu(x)
- x = self.pre_out_norm_2(x)
- x = self.drop(x)
- x = x * x_mask
- x = x.transpose(1, 2)
- output_prob = self.output_layer(x)
- return output_prob
-
- def forward(self, x, x_mask, dur_r, dur_hat, g=None):
- x = torch.detach(x)
- if g is not None:
- g = torch.detach(g)
- x = x + self.cond(g)
- x = self.conv_1(x * x_mask)
- x = torch.relu(x)
- x = self.norm_1(x)
- x = self.drop(x)
- x = self.conv_2(x * x_mask)
- x = torch.relu(x)
- x = self.norm_2(x)
- x = self.drop(x)
-
- output_probs = []
- for dur in [dur_r, dur_hat]:
- output_prob = self.forward_probability(x, x_mask, dur, g)
- output_probs.append(output_prob)
-
- return output_probs
-
-class TransformerCouplingBlock(nn.Module):
- def __init__(self,
- channels,
- hidden_channels,
- filter_channels,
- n_heads,
- n_layers,
- kernel_size,
- p_dropout,
- n_flows=4,
- gin_channels=0,
- share_parameter=False
- ):
-
- super().__init__()
- self.channels = channels
- self.hidden_channels = hidden_channels
- self.kernel_size = kernel_size
- self.n_layers = n_layers
- self.n_flows = n_flows
- self.gin_channels = gin_channels
-
- self.flows = nn.ModuleList()
-
- self.wn = attentions.FFT(hidden_channels, filter_channels, n_heads, n_layers, kernel_size, p_dropout, isflow = True, gin_channels = self.gin_channels) if share_parameter else None
-
- for i in range(n_flows):
- self.flows.append(
- modules.TransformerCouplingLayer(channels, hidden_channels, kernel_size, n_layers, n_heads, p_dropout, filter_channels, mean_only=True, wn_sharing_parameter=self.wn, gin_channels = self.gin_channels))
- self.flows.append(modules.Flip())
-
- def forward(self, x, x_mask, g=None, reverse=False):
- if not reverse:
- for flow in self.flows:
- x, _ = flow(x, x_mask, g=g, reverse=reverse)
- else:
- for flow in reversed(self.flows):
- x = flow(x, x_mask, g=g, reverse=reverse)
- return x
-
-class StochasticDurationPredictor(nn.Module):
- def __init__(self, in_channels, filter_channels, kernel_size, p_dropout, n_flows=4, gin_channels=0):
- super().__init__()
- filter_channels = in_channels # it needs to be removed from future version.
- self.in_channels = in_channels
- self.filter_channels = filter_channels
- self.kernel_size = kernel_size
- self.p_dropout = p_dropout
- self.n_flows = n_flows
- self.gin_channels = gin_channels
-
- self.log_flow = modules.Log()
- self.flows = nn.ModuleList()
- self.flows.append(modules.ElementwiseAffine(2))
- for i in range(n_flows):
- self.flows.append(modules.ConvFlow(2, filter_channels, kernel_size, n_layers=3))
- self.flows.append(modules.Flip())
-
- self.post_pre = nn.Conv1d(1, filter_channels, 1)
- self.post_proj = nn.Conv1d(filter_channels, filter_channels, 1)
- self.post_convs = modules.DDSConv(filter_channels, kernel_size, n_layers=3, p_dropout=p_dropout)
- self.post_flows = nn.ModuleList()
- self.post_flows.append(modules.ElementwiseAffine(2))
- for i in range(4):
- self.post_flows.append(modules.ConvFlow(2, filter_channels, kernel_size, n_layers=3))
- self.post_flows.append(modules.Flip())
-
- self.pre = nn.Conv1d(in_channels, filter_channels, 1)
- self.proj = nn.Conv1d(filter_channels, filter_channels, 1)
- self.convs = modules.DDSConv(filter_channels, kernel_size, n_layers=3, p_dropout=p_dropout)
- if gin_channels != 0:
- self.cond = nn.Conv1d(gin_channels, filter_channels, 1)
-
- def forward(self, x, x_mask, w=None, g=None, reverse=False, noise_scale=1.0):
- x = torch.detach(x)
- x = self.pre(x)
- if g is not None:
- g = torch.detach(g)
- x = x + self.cond(g)
- x = self.convs(x, x_mask)
- x = self.proj(x) * x_mask
-
- if not reverse:
- flows = self.flows
- assert w is not None
-
- logdet_tot_q = 0
- h_w = self.post_pre(w)
- h_w = self.post_convs(h_w, x_mask)
- h_w = self.post_proj(h_w) * x_mask
- e_q = torch.randn(w.size(0), 2, w.size(2)).to(device=x.device, dtype=x.dtype) * x_mask
- z_q = e_q
- for flow in self.post_flows:
- z_q, logdet_q = flow(z_q, x_mask, g=(x + h_w))
- logdet_tot_q += logdet_q
- z_u, z1 = torch.split(z_q, [1, 1], 1)
- u = torch.sigmoid(z_u) * x_mask
- z0 = (w - u) * x_mask
- logdet_tot_q += torch.sum((F.logsigmoid(z_u) + F.logsigmoid(-z_u)) * x_mask, [1, 2])
- logq = torch.sum(-0.5 * (math.log(2 * math.pi) + (e_q ** 2)) * x_mask, [1, 2]) - logdet_tot_q
-
- logdet_tot = 0
- z0, logdet = self.log_flow(z0, x_mask)
- logdet_tot += logdet
- z = torch.cat([z0, z1], 1)
- for flow in flows:
- z, logdet = flow(z, x_mask, g=x, reverse=reverse)
- logdet_tot = logdet_tot + logdet
- nll = torch.sum(0.5 * (math.log(2 * math.pi) + (z ** 2)) * x_mask, [1, 2]) - logdet_tot
- return nll + logq # [b]
- else:
- flows = list(reversed(self.flows))
- flows = flows[:-2] + [flows[-1]] # remove a useless vflow
- z = torch.randn(x.size(0), 2, x.size(2)).to(device=x.device, dtype=x.dtype) * noise_scale
- for flow in flows:
- z = flow(z, x_mask, g=x, reverse=reverse)
- z0, z1 = torch.split(z, [1, 1], 1)
- logw = z0
- return logw
-
-
-class DurationPredictor(nn.Module):
- def __init__(self, in_channels, filter_channels, kernel_size, p_dropout, gin_channels=0):
- super().__init__()
-
- self.in_channels = in_channels
- self.filter_channels = filter_channels
- self.kernel_size = kernel_size
- self.p_dropout = p_dropout
- self.gin_channels = gin_channels
-
- self.drop = nn.Dropout(p_dropout)
- self.conv_1 = nn.Conv1d(in_channels, filter_channels, kernel_size, padding=kernel_size // 2)
- self.norm_1 = modules.LayerNorm(filter_channels)
- self.conv_2 = nn.Conv1d(filter_channels, filter_channels, kernel_size, padding=kernel_size // 2)
- self.norm_2 = modules.LayerNorm(filter_channels)
- self.proj = nn.Conv1d(filter_channels, 1, 1)
-
- if gin_channels != 0:
- self.cond = nn.Conv1d(gin_channels, in_channels, 1)
-
- def forward(self, x, x_mask, g=None):
- x = torch.detach(x)
- if g is not None:
- g = torch.detach(g)
- x = x + self.cond(g)
- x = self.conv_1(x * x_mask)
- x = torch.relu(x)
- x = self.norm_1(x)
- x = self.drop(x)
- x = self.conv_2(x * x_mask)
- x = torch.relu(x)
- x = self.norm_2(x)
- x = self.drop(x)
- x = self.proj(x * x_mask)
- return x * x_mask
-
-
-class TextEncoder(nn.Module):
- def __init__(self,
- n_vocab,
- out_channels,
- hidden_channels,
- filter_channels,
- n_heads,
- n_layers,
- kernel_size,
- p_dropout,
- gin_channels=0):
- super().__init__()
- self.n_vocab = n_vocab
- self.out_channels = out_channels
- self.hidden_channels = hidden_channels
- self.filter_channels = filter_channels
- self.n_heads = n_heads
- self.n_layers = n_layers
- self.kernel_size = kernel_size
- self.p_dropout = p_dropout
- self.gin_channels = gin_channels
- self.emb = nn.Embedding(len(symbols), hidden_channels)
- nn.init.normal_(self.emb.weight, 0.0, hidden_channels ** -0.5)
- self.tone_emb = nn.Embedding(num_tones, hidden_channels)
- nn.init.normal_(self.tone_emb.weight, 0.0, hidden_channels ** -0.5)
- self.language_emb = nn.Embedding(num_languages, hidden_channels)
- nn.init.normal_(self.language_emb.weight, 0.0, hidden_channels ** -0.5)
- self.bert_proj = nn.Conv1d(1024, hidden_channels, 1)
-
- self.encoder = attentions.Encoder(
- hidden_channels,
- filter_channels,
- n_heads,
- n_layers,
- kernel_size,
- p_dropout,
- gin_channels=self.gin_channels)
- self.proj = nn.Conv1d(hidden_channels, out_channels * 2, 1)
-
- def forward(self, x, x_lengths, tone, language, bert, g=None):
- x = (self.emb(x)+ self.tone_emb(tone)+ self.language_emb(language)+self.bert_proj(bert).transpose(1,2)) * math.sqrt(self.hidden_channels) # [b, t, h]
- x = torch.transpose(x, 1, -1) # [b, h, t]
- x_mask = torch.unsqueeze(commons.sequence_mask(x_lengths, x.size(2)), 1).to(x.dtype)
-
- x = self.encoder(x * x_mask, x_mask, g=g)
- stats = self.proj(x) * x_mask
-
- m, logs = torch.split(stats, self.out_channels, dim=1)
- return x, m, logs, x_mask
-
-
-class ResidualCouplingBlock(nn.Module):
- def __init__(self,
- channels,
- hidden_channels,
- kernel_size,
- dilation_rate,
- n_layers,
- n_flows=4,
- gin_channels=0):
- super().__init__()
- self.channels = channels
- self.hidden_channels = hidden_channels
- self.kernel_size = kernel_size
- self.dilation_rate = dilation_rate
- self.n_layers = n_layers
- self.n_flows = n_flows
- self.gin_channels = gin_channels
-
- self.flows = nn.ModuleList()
- for i in range(n_flows):
- self.flows.append(
- modules.ResidualCouplingLayer(channels, hidden_channels, kernel_size, dilation_rate, n_layers,
- gin_channels=gin_channels, mean_only=True))
- self.flows.append(modules.Flip())
-
- def forward(self, x, x_mask, g=None, reverse=False):
- if not reverse:
- for flow in self.flows:
- x, _ = flow(x, x_mask, g=g, reverse=reverse)
- else:
- for flow in reversed(self.flows):
- x = flow(x, x_mask, g=g, reverse=reverse)
- return x
-
-
-class PosteriorEncoder(nn.Module):
- def __init__(self,
- in_channels,
- out_channels,
- hidden_channels,
- kernel_size,
- dilation_rate,
- n_layers,
- gin_channels=0):
- super().__init__()
- self.in_channels = in_channels
- self.out_channels = out_channels
- self.hidden_channels = hidden_channels
- self.kernel_size = kernel_size
- self.dilation_rate = dilation_rate
- self.n_layers = n_layers
- self.gin_channels = gin_channels
-
- self.pre = nn.Conv1d(in_channels, hidden_channels, 1)
- self.enc = modules.WN(hidden_channels, kernel_size, dilation_rate, n_layers, gin_channels=gin_channels)
- self.proj = nn.Conv1d(hidden_channels, out_channels * 2, 1)
-
- def forward(self, x, x_lengths, g=None):
- x_mask = torch.unsqueeze(commons.sequence_mask(x_lengths, x.size(2)), 1).to(x.dtype)
- x = self.pre(x) * x_mask
- x = self.enc(x, x_mask, g=g)
- stats = self.proj(x) * x_mask
- m, logs = torch.split(stats, self.out_channels, dim=1)
- z = (m + torch.randn_like(m) * torch.exp(logs)) * x_mask
- return z, m, logs, x_mask
-
-
-class Generator(torch.nn.Module):
- def __init__(self, initial_channel, resblock, resblock_kernel_sizes, resblock_dilation_sizes, upsample_rates,
- upsample_initial_channel, upsample_kernel_sizes, gin_channels=0):
- super(Generator, self).__init__()
- self.num_kernels = len(resblock_kernel_sizes)
- self.num_upsamples = len(upsample_rates)
- self.conv_pre = Conv1d(initial_channel, upsample_initial_channel, 7, 1, padding=3)
- resblock = modules.ResBlock1 if resblock == '1' else modules.ResBlock2
-
- self.ups = nn.ModuleList()
- for i, (u, k) in enumerate(zip(upsample_rates, upsample_kernel_sizes)):
- self.ups.append(weight_norm(
- ConvTranspose1d(upsample_initial_channel // (2 ** i), upsample_initial_channel // (2 ** (i + 1)),
- k, u, padding=(k - u) // 2)))
-
- self.resblocks = nn.ModuleList()
- for i in range(len(self.ups)):
- ch = upsample_initial_channel // (2 ** (i + 1))
- for j, (k, d) in enumerate(zip(resblock_kernel_sizes, resblock_dilation_sizes)):
- self.resblocks.append(resblock(ch, k, d))
-
- self.conv_post = Conv1d(ch, 1, 7, 1, padding=3, bias=False)
- self.ups.apply(init_weights)
-
- if gin_channels != 0:
- self.cond = nn.Conv1d(gin_channels, upsample_initial_channel, 1)
-
- def forward(self, x, g=None):
- x = self.conv_pre(x)
- if g is not None:
- x = x + self.cond(g)
-
- for i in range(self.num_upsamples):
- x = F.leaky_relu(x, modules.LRELU_SLOPE)
- x = self.ups[i](x)
- xs = None
- for j in range(self.num_kernels):
- if xs is None:
- xs = self.resblocks[i * self.num_kernels + j](x)
- else:
- xs += self.resblocks[i * self.num_kernels + j](x)
- x = xs / self.num_kernels
- x = F.leaky_relu(x)
- x = self.conv_post(x)
- x = torch.tanh(x)
-
- return x
-
- def remove_weight_norm(self):
- print('Removing weight norm...')
- for l in self.ups:
- remove_weight_norm(l)
- for l in self.resblocks:
- l.remove_weight_norm()
-
-
-class DiscriminatorP(torch.nn.Module):
- def __init__(self, period, kernel_size=5, stride=3, use_spectral_norm=False):
- super(DiscriminatorP, self).__init__()
- self.period = period
- self.use_spectral_norm = use_spectral_norm
- norm_f = weight_norm if use_spectral_norm == False else spectral_norm
- self.convs = nn.ModuleList([
- norm_f(Conv2d(1, 32, (kernel_size, 1), (stride, 1), padding=(get_padding(kernel_size, 1), 0))),
- norm_f(Conv2d(32, 128, (kernel_size, 1), (stride, 1), padding=(get_padding(kernel_size, 1), 0))),
- norm_f(Conv2d(128, 512, (kernel_size, 1), (stride, 1), padding=(get_padding(kernel_size, 1), 0))),
- norm_f(Conv2d(512, 1024, (kernel_size, 1), (stride, 1), padding=(get_padding(kernel_size, 1), 0))),
- norm_f(Conv2d(1024, 1024, (kernel_size, 1), 1, padding=(get_padding(kernel_size, 1), 0))),
- ])
- self.conv_post = norm_f(Conv2d(1024, 1, (3, 1), 1, padding=(1, 0)))
-
- def forward(self, x):
- fmap = []
-
- # 1d to 2d
- b, c, t = x.shape
- if t % self.period != 0: # pad first
- n_pad = self.period - (t % self.period)
- x = F.pad(x, (0, n_pad), "reflect")
- t = t + n_pad
- x = x.view(b, c, t // self.period, self.period)
-
- for l in self.convs:
- x = l(x)
- x = F.leaky_relu(x, modules.LRELU_SLOPE)
- fmap.append(x)
- x = self.conv_post(x)
- fmap.append(x)
- x = torch.flatten(x, 1, -1)
-
- return x, fmap
-
-
-class DiscriminatorS(torch.nn.Module):
- def __init__(self, use_spectral_norm=False):
- super(DiscriminatorS, self).__init__()
- norm_f = weight_norm if use_spectral_norm == False else spectral_norm
- self.convs = nn.ModuleList([
- norm_f(Conv1d(1, 16, 15, 1, padding=7)),
- norm_f(Conv1d(16, 64, 41, 4, groups=4, padding=20)),
- norm_f(Conv1d(64, 256, 41, 4, groups=16, padding=20)),
- norm_f(Conv1d(256, 1024, 41, 4, groups=64, padding=20)),
- norm_f(Conv1d(1024, 1024, 41, 4, groups=256, padding=20)),
- norm_f(Conv1d(1024, 1024, 5, 1, padding=2)),
- ])
- self.conv_post = norm_f(Conv1d(1024, 1, 3, 1, padding=1))
-
- def forward(self, x):
- fmap = []
-
- for l in self.convs:
- x = l(x)
- x = F.leaky_relu(x, modules.LRELU_SLOPE)
- fmap.append(x)
- x = self.conv_post(x)
- fmap.append(x)
- x = torch.flatten(x, 1, -1)
-
- return x, fmap
-
-
-class MultiPeriodDiscriminator(torch.nn.Module):
- def __init__(self, use_spectral_norm=False):
- super(MultiPeriodDiscriminator, self).__init__()
- periods = [2, 3, 5, 7, 11]
-
- discs = [DiscriminatorS(use_spectral_norm=use_spectral_norm)]
- discs = discs + [DiscriminatorP(i, use_spectral_norm=use_spectral_norm) for i in periods]
- self.discriminators = nn.ModuleList(discs)
-
- def forward(self, y, y_hat):
- y_d_rs = []
- y_d_gs = []
- fmap_rs = []
- fmap_gs = []
- for i, d in enumerate(self.discriminators):
- y_d_r, fmap_r = d(y)
- y_d_g, fmap_g = d(y_hat)
- y_d_rs.append(y_d_r)
- y_d_gs.append(y_d_g)
- fmap_rs.append(fmap_r)
- fmap_gs.append(fmap_g)
-
- return y_d_rs, y_d_gs, fmap_rs, fmap_gs
-
-class ReferenceEncoder(nn.Module):
- '''
- inputs --- [N, Ty/r, n_mels*r] mels
- outputs --- [N, ref_enc_gru_size]
- '''
-
- def __init__(self, spec_channels, gin_channels=0):
-
- super().__init__()
- self.spec_channels = spec_channels
- ref_enc_filters = [32, 32, 64, 64, 128, 128]
- K = len(ref_enc_filters)
- filters = [1] + ref_enc_filters
- convs = [weight_norm(nn.Conv2d(in_channels=filters[i],
- out_channels=filters[i + 1],
- kernel_size=(3, 3),
- stride=(2, 2),
- padding=(1, 1))) for i in range(K)]
- self.convs = nn.ModuleList(convs)
- # self.wns = nn.ModuleList([weight_norm(num_features=ref_enc_filters[i]) for i in range(K)])
-
- out_channels = self.calculate_channels(spec_channels, 3, 2, 1, K)
- self.gru = nn.GRU(input_size=ref_enc_filters[-1] * out_channels,
- hidden_size=256 // 2,
- batch_first=True)
- self.proj = nn.Linear(128, gin_channels)
-
- def forward(self, inputs, mask=None):
- N = inputs.size(0)
- out = inputs.view(N, 1, -1, self.spec_channels) # [N, 1, Ty, n_freqs]
- for conv in self.convs:
- out = conv(out)
- # out = wn(out)
- out = F.relu(out) # [N, 128, Ty//2^K, n_mels//2^K]
-
- out = out.transpose(1, 2) # [N, Ty//2^K, 128, n_mels//2^K]
- T = out.size(1)
- N = out.size(0)
- out = out.contiguous().view(N, T, -1) # [N, Ty//2^K, 128*n_mels//2^K]
-
- self.gru.flatten_parameters()
- memory, out = self.gru(out) # out --- [1, N, 128]
-
- return self.proj(out.squeeze(0))
-
- def calculate_channels(self, L, kernel_size, stride, pad, n_convs):
- for i in range(n_convs):
- L = (L - kernel_size + 2 * pad) // stride + 1
- return L
-
-
-class SynthesizerTrn(nn.Module):
- """
- Synthesizer for Training
- """
-
- def __init__(self,
- n_vocab,
- spec_channels,
- segment_size,
- inter_channels,
- hidden_channels,
- filter_channels,
- n_heads,
- n_layers,
- kernel_size,
- p_dropout,
- resblock,
- resblock_kernel_sizes,
- resblock_dilation_sizes,
- upsample_rates,
- upsample_initial_channel,
- upsample_kernel_sizes,
- n_speakers=256,
- gin_channels=256,
- use_sdp=True,
- n_flow_layer = 4,
- n_layers_trans_flow = 3,
- flow_share_parameter = False,
- use_transformer_flow = True,
- **kwargs):
-
- super().__init__()
- self.n_vocab = n_vocab
- self.spec_channels = spec_channels
- self.inter_channels = inter_channels
- self.hidden_channels = hidden_channels
- self.filter_channels = filter_channels
- self.n_heads = n_heads
- self.n_layers = n_layers
- self.kernel_size = kernel_size
- self.p_dropout = p_dropout
- self.resblock = resblock
- self.resblock_kernel_sizes = resblock_kernel_sizes
- self.resblock_dilation_sizes = resblock_dilation_sizes
- self.upsample_rates = upsample_rates
- self.upsample_initial_channel = upsample_initial_channel
- self.upsample_kernel_sizes = upsample_kernel_sizes
- self.segment_size = segment_size
- self.n_speakers = n_speakers
- self.gin_channels = gin_channels
- self.n_layers_trans_flow = n_layers_trans_flow
- self.use_spk_conditioned_encoder = kwargs.get("use_spk_conditioned_encoder", True)
- self.use_sdp = use_sdp
- self.use_noise_scaled_mas = kwargs.get("use_noise_scaled_mas", False)
- self.mas_noise_scale_initial = kwargs.get("mas_noise_scale_initial", 0.01)
- self.noise_scale_delta = kwargs.get("noise_scale_delta", 2e-6)
- self.current_mas_noise_scale = self.mas_noise_scale_initial
- if self.use_spk_conditioned_encoder and gin_channels > 0:
- self.enc_gin_channels = gin_channels
- self.enc_p = TextEncoder(n_vocab,
- inter_channels,
- hidden_channels,
- filter_channels,
- n_heads,
- n_layers,
- kernel_size,
- p_dropout,
- gin_channels=self.enc_gin_channels)
- self.dec = Generator(inter_channels, resblock, resblock_kernel_sizes, resblock_dilation_sizes, upsample_rates,
- upsample_initial_channel, upsample_kernel_sizes, gin_channels=gin_channels)
- self.enc_q = PosteriorEncoder(spec_channels, inter_channels, hidden_channels, 5, 1, 16,
- gin_channels=gin_channels)
- if use_transformer_flow:
- self.flow = TransformerCouplingBlock(inter_channels, hidden_channels, filter_channels, n_heads, n_layers_trans_flow, 5, p_dropout, n_flow_layer, gin_channels=gin_channels,share_parameter= flow_share_parameter)
- else:
- self.flow = ResidualCouplingBlock(inter_channels, hidden_channels, 5, 1, n_flow_layer, gin_channels=gin_channels)
- self.sdp = StochasticDurationPredictor(hidden_channels, 192, 3, 0.5, 4, gin_channels=gin_channels)
- self.dp = DurationPredictor(hidden_channels, 256, 3, 0.5, gin_channels=gin_channels)
-
- if n_speakers >= 1:
- self.emb_g = nn.Embedding(n_speakers, gin_channels)
- else:
- self.ref_enc = ReferenceEncoder(spec_channels, gin_channels)
-
- def forward(self, x, x_lengths, y, y_lengths, sid, tone, language, bert):
- if self.n_speakers > 0:
- g = self.emb_g(sid).unsqueeze(-1) # [b, h, 1]
- else:
- g = self.ref_enc(y.transpose(1,2)).unsqueeze(-1)
- x, m_p, logs_p, x_mask = self.enc_p(x, x_lengths, tone, language, bert,g=g)
- z, m_q, logs_q, y_mask = self.enc_q(y, y_lengths, g=g)
- z_p = self.flow(z, y_mask, g=g)
-
- with torch.no_grad():
- # negative cross-entropy
- s_p_sq_r = torch.exp(-2 * logs_p) # [b, d, t]
- neg_cent1 = torch.sum(-0.5 * math.log(2 * math.pi) - logs_p, [1], keepdim=True) # [b, 1, t_s]
- neg_cent2 = torch.matmul(-0.5 * (z_p ** 2).transpose(1, 2),
- s_p_sq_r) # [b, t_t, d] x [b, d, t_s] = [b, t_t, t_s]
- neg_cent3 = torch.matmul(z_p.transpose(1, 2), (m_p * s_p_sq_r)) # [b, t_t, d] x [b, d, t_s] = [b, t_t, t_s]
- neg_cent4 = torch.sum(-0.5 * (m_p ** 2) * s_p_sq_r, [1], keepdim=True) # [b, 1, t_s]
- neg_cent = neg_cent1 + neg_cent2 + neg_cent3 + neg_cent4
- if self.use_noise_scaled_mas:
- epsilon = torch.std(neg_cent) * torch.randn_like(neg_cent) * self.current_mas_noise_scale
- neg_cent = neg_cent + epsilon
-
- attn_mask = torch.unsqueeze(x_mask, 2) * torch.unsqueeze(y_mask, -1)
- attn = monotonic_align.maximum_path(neg_cent, attn_mask.squeeze(1)).unsqueeze(1).detach()
-
- w = attn.sum(2)
-
- l_length_sdp = self.sdp(x, x_mask, w, g=g)
- l_length_sdp = l_length_sdp / torch.sum(x_mask)
-
- logw_ = torch.log(w + 1e-6) * x_mask
- logw = self.dp(x, x_mask, g=g)
- l_length_dp = torch.sum((logw - logw_) ** 2, [1, 2]) / torch.sum(x_mask) # for averaging
-
- l_length = l_length_dp + l_length_sdp
-
- # expand prior
- m_p = torch.matmul(attn.squeeze(1), m_p.transpose(1, 2)).transpose(1, 2)
- logs_p = torch.matmul(attn.squeeze(1), logs_p.transpose(1, 2)).transpose(1, 2)
-
- z_slice, ids_slice = commons.rand_slice_segments(z, y_lengths, self.segment_size)
- o = self.dec(z_slice, g=g)
- return o, l_length, attn, ids_slice, x_mask, y_mask, (z, z_p, m_p, logs_p, m_q, logs_q), (x, logw, logw_)
-
- def infer(self, x, x_lengths, sid, tone, language, bert, noise_scale=.667, length_scale=1, noise_scale_w=0.8, max_len=None, sdp_ratio=0,y=None):
- #x, m_p, logs_p, x_mask = self.enc_p(x, x_lengths, tone, language, bert)
- # g = self.gst(y)
- if self.n_speakers > 0:
- g = self.emb_g(sid).unsqueeze(-1) # [b, h, 1]
- else:
- g = self.ref_enc(y.transpose(1,2)).unsqueeze(-1)
- x, m_p, logs_p, x_mask = self.enc_p(x, x_lengths, tone, language, bert,g=g)
- logw = self.sdp(x, x_mask, g=g, reverse=True, noise_scale=noise_scale_w) * (sdp_ratio) + self.dp(x, x_mask, g=g) * (1 - sdp_ratio)
- w = torch.exp(logw) * x_mask * length_scale
- w_ceil = torch.ceil(w)
- y_lengths = torch.clamp_min(torch.sum(w_ceil, [1, 2]), 1).long()
- y_mask = torch.unsqueeze(commons.sequence_mask(y_lengths, None), 1).to(x_mask.dtype)
- attn_mask = torch.unsqueeze(x_mask, 2) * torch.unsqueeze(y_mask, -1)
- attn = commons.generate_path(w_ceil, attn_mask)
-
- m_p = torch.matmul(attn.squeeze(1), m_p.transpose(1, 2)).transpose(1, 2) # [b, t', t], [b, t, d] -> [b, d, t']
- logs_p = torch.matmul(attn.squeeze(1), logs_p.transpose(1, 2)).transpose(1,
- 2) # [b, t', t], [b, t, d] -> [b, d, t']
-
- z_p = m_p + torch.randn_like(m_p) * torch.exp(logs_p) * noise_scale
- z = self.flow(z_p, y_mask, g=g, reverse=True)
- o = self.dec((z * y_mask)[:, :, :max_len], g=g)
- return o, attn, y_mask, (z, z_p, m_p, logs_p)
diff --git a/spaces/XzJosh/nanami-Bert-VITS2/text/chinese.py b/spaces/XzJosh/nanami-Bert-VITS2/text/chinese.py
deleted file mode 100644
index 276753880b73de2e8889dcb2101cd98c09e0710b..0000000000000000000000000000000000000000
--- a/spaces/XzJosh/nanami-Bert-VITS2/text/chinese.py
+++ /dev/null
@@ -1,193 +0,0 @@
-import os
-import re
-
-import cn2an
-from pypinyin import lazy_pinyin, Style
-
-from text import symbols
-from text.symbols import punctuation
-from text.tone_sandhi import ToneSandhi
-
-current_file_path = os.path.dirname(__file__)
-pinyin_to_symbol_map = {line.split("\t")[0]: line.strip().split("\t")[1] for line in
- open(os.path.join(current_file_path, 'opencpop-strict.txt')).readlines()}
-
-import jieba.posseg as psg
-
-
-rep_map = {
- ':': ',',
- ';': ',',
- ',': ',',
- '。': '.',
- '!': '!',
- '?': '?',
- '\n': '.',
- "·": ",",
- '、': ",",
- '...': '…',
- '$': '.',
- '“': "'",
- '”': "'",
- '‘': "'",
- '’': "'",
- '(': "'",
- ')': "'",
- '(': "'",
- ')': "'",
- '《': "'",
- '》': "'",
- '【': "'",
- '】': "'",
- '[': "'",
- ']': "'",
- '—': "-",
- '~': "-",
- '~': "-",
- '「': "'",
- '」': "'",
-
-}
-
-tone_modifier = ToneSandhi()
-
-def replace_punctuation(text):
- text = text.replace("嗯", "恩").replace("呣","母")
- pattern = re.compile('|'.join(re.escape(p) for p in rep_map.keys()))
-
- replaced_text = pattern.sub(lambda x: rep_map[x.group()], text)
-
- replaced_text = re.sub(r'[^\u4e00-\u9fa5'+"".join(punctuation)+r']+', '', replaced_text)
-
- return replaced_text
-
-def g2p(text):
- pattern = r'(?<=[{0}])\s*'.format(''.join(punctuation))
- sentences = [i for i in re.split(pattern, text) if i.strip()!='']
- phones, tones, word2ph = _g2p(sentences)
- assert sum(word2ph) == len(phones)
- assert len(word2ph) == len(text) #Sometimes it will crash,you can add a try-catch.
- phones = ['_'] + phones + ["_"]
- tones = [0] + tones + [0]
- word2ph = [1] + word2ph + [1]
- return phones, tones, word2ph
-
-
-def _get_initials_finals(word):
- initials = []
- finals = []
- orig_initials = lazy_pinyin(
- word, neutral_tone_with_five=True, style=Style.INITIALS)
- orig_finals = lazy_pinyin(
- word, neutral_tone_with_five=True, style=Style.FINALS_TONE3)
- for c, v in zip(orig_initials, orig_finals):
- initials.append(c)
- finals.append(v)
- return initials, finals
-
-
-def _g2p(segments):
- phones_list = []
- tones_list = []
- word2ph = []
- for seg in segments:
- pinyins = []
- # Replace all English words in the sentence
- seg = re.sub('[a-zA-Z]+', '', seg)
- seg_cut = psg.lcut(seg)
- initials = []
- finals = []
- seg_cut = tone_modifier.pre_merge_for_modify(seg_cut)
- for word, pos in seg_cut:
- if pos == 'eng':
- continue
- sub_initials, sub_finals = _get_initials_finals(word)
- sub_finals = tone_modifier.modified_tone(word, pos,
- sub_finals)
- initials.append(sub_initials)
- finals.append(sub_finals)
-
- # assert len(sub_initials) == len(sub_finals) == len(word)
- initials = sum(initials, [])
- finals = sum(finals, [])
- #
- for c, v in zip(initials, finals):
- raw_pinyin = c+v
- # NOTE: post process for pypinyin outputs
- # we discriminate i, ii and iii
- if c == v:
- assert c in punctuation
- phone = [c]
- tone = '0'
- word2ph.append(1)
- else:
- v_without_tone = v[:-1]
- tone = v[-1]
-
- pinyin = c+v_without_tone
- assert tone in '12345'
-
- if c:
- # 多音节
- v_rep_map = {
- "uei": 'ui',
- 'iou': 'iu',
- 'uen': 'un',
- }
- if v_without_tone in v_rep_map.keys():
- pinyin = c+v_rep_map[v_without_tone]
- else:
- # 单音节
- pinyin_rep_map = {
- 'ing': 'ying',
- 'i': 'yi',
- 'in': 'yin',
- 'u': 'wu',
- }
- if pinyin in pinyin_rep_map.keys():
- pinyin = pinyin_rep_map[pinyin]
- else:
- single_rep_map = {
- 'v': 'yu',
- 'e': 'e',
- 'i': 'y',
- 'u': 'w',
- }
- if pinyin[0] in single_rep_map.keys():
- pinyin = single_rep_map[pinyin[0]]+pinyin[1:]
-
- assert pinyin in pinyin_to_symbol_map.keys(), (pinyin, seg, raw_pinyin)
- phone = pinyin_to_symbol_map[pinyin].split(' ')
- word2ph.append(len(phone))
-
- phones_list += phone
- tones_list += [int(tone)] * len(phone)
- return phones_list, tones_list, word2ph
-
-
-
-def text_normalize(text):
- numbers = re.findall(r'\d+(?:\.?\d+)?', text)
- for number in numbers:
- text = text.replace(number, cn2an.an2cn(number), 1)
- text = replace_punctuation(text)
- return text
-
-def get_bert_feature(text, word2ph):
- from text import chinese_bert
- return chinese_bert.get_bert_feature(text, word2ph)
-
-if __name__ == '__main__':
- from text.chinese_bert import get_bert_feature
- text = "啊!但是《原神》是由,米哈\游自主, [研发]的一款全.新开放世界.冒险游戏"
- text = text_normalize(text)
- print(text)
- phones, tones, word2ph = g2p(text)
- bert = get_bert_feature(text, word2ph)
-
- print(phones, tones, word2ph, bert.shape)
-
-
-# # 示例用法
-# text = "这是一个示例文本:,你好!这是一个测试...."
-# print(g2p_paddle(text)) # 输出: 这是一个示例文本你好这是一个测试
diff --git a/spaces/YlcldKlns/bing/src/components/providers.tsx b/spaces/YlcldKlns/bing/src/components/providers.tsx
deleted file mode 100644
index 892226412d80fe0b05211911b9e245cd22876460..0000000000000000000000000000000000000000
--- a/spaces/YlcldKlns/bing/src/components/providers.tsx
+++ /dev/null
@@ -1,15 +0,0 @@
-'use client'
-
-import * as React from 'react'
-import { ThemeProvider as NextThemesProvider } from 'next-themes'
-import { ThemeProviderProps } from 'next-themes/dist/types'
-
-import { TooltipProvider } from '@/components/ui/tooltip'
-
-export function Providers({ children, ...props }: ThemeProviderProps) {
- return (
-
- {children}
-
- )
-}
diff --git a/spaces/YouLiXiya/Mobile-SAM/sam_extension/pipeline/owlvit.py b/spaces/YouLiXiya/Mobile-SAM/sam_extension/pipeline/owlvit.py
deleted file mode 100644
index 08a49febe9ad79e3f8015e3a08887dcef0c303df..0000000000000000000000000000000000000000
--- a/spaces/YouLiXiya/Mobile-SAM/sam_extension/pipeline/owlvit.py
+++ /dev/null
@@ -1,372 +0,0 @@
-from typing import Optional, Tuple, Union, List
-import numpy as np
-import PIL
-from PIL.Image import Image
-import supervision as sv
-
-import torch
-from torch import nn
-
-from transformers import OwlViTProcessor, OwlViTForObjectDetection, OwlViTVisionModel
-from transformers.models.owlvit.modeling_owlvit import center_to_corners_format, box_iou, generalized_box_iou, OwlViTObjectDetectionOutput
-
-from sam_extension.pipeline.base import Pipeline, Output
-
-class OwlViTVisionEncoderPipeline(Pipeline):
-
- def __init__(self,
- vision_model,
- layer_norm,
- processor,
- device='cuda',
- *args,
- **kwargs):
- super().__init__(*args, **kwargs)
- self.vision_model = vision_model
- self.layer_norm = layer_norm
- self.processor = processor
- self.device = device
- torch.cuda.empty_cache()
- @classmethod
- def from_pretrained(cls, model_type, device='cuda', *args, **kwargs):
- owlvit_for_object_detection = OwlViTForObjectDetection.from_pretrained(model_type).to(device)
- processor = OwlViTProcessor.from_pretrained(model_type)
- return cls(owlvit_for_object_detection.owlvit.vision_model,
- owlvit_for_object_detection.layer_norm,
- processor,
- device,
- *args,
- **kwargs)
- def process_image(self, image:Image):
- image = self.processor(images=image, return_tensors="pt").pixel_values.to(self.device)
- return image
- @torch.no_grad()
- def forward(
- self,
- pixel_values: Union[torch.FloatTensor, Image] = None,
- output_attentions: Optional[bool] = None,
- output_hidden_states: Optional[bool] = None,
- return_dict: Optional[bool] = None,
- ) -> torch.FloatTensor:
- if isinstance(pixel_values, Image):
- pixel_values = self.process_image(pixel_values)
- pixel_values = pixel_values.to(self.device)
- vision_outputs = self.vision_model(
- pixel_values=pixel_values,
- output_attentions=output_attentions,
- output_hidden_states=output_hidden_states,
- return_dict=return_dict,
- )
- # Get image embeddings
- last_hidden_state = vision_outputs[0]
- image_embeds = self.vision_model.post_layernorm(last_hidden_state)
- new_size = tuple(np.array(image_embeds.shape) - np.array((0, 1, 0)))
- class_token_out = torch.broadcast_to(image_embeds[:, :1, :], new_size)
-
- # Merge image embedding with class tokens
- image_embeds = image_embeds[:, 1:, :] * class_token_out
- image_embeds = self.layer_norm(image_embeds)
-
- # Resize to [batch_size, num_patches, num_patches, hidden_size]
- new_size = (
- image_embeds.shape[0],
- int(np.sqrt(image_embeds.shape[1])),
- int(np.sqrt(image_embeds.shape[1])),
- image_embeds.shape[-1],
- )
- image_embeds = image_embeds.reshape(new_size)
- return image_embeds
-
-
-
-class OwlViTDecoderPipeline(Pipeline):
- prompt_template: str = 'a photo of a '
- def __init__(self,
- owlvit_text,
- text_projection,
- class_head,
- box_head,
- processor,
- device='cuda',
- *args,
- **kwargs):
- super().__init__(*args, **kwargs)
-
- self.owlvit_text = owlvit_text
- self.text_projection = text_projection
- self.class_head = class_head
- self.box_head = box_head
-
- self.sigmoid = nn.Sigmoid()
- self.processor = processor
- self.device = device
- torch.cuda.empty_cache()
-
- @classmethod
- def from_pretrained(cls, model_type, device='cuda', *args, **kwargs):
- owlvit_for_object_detection = OwlViTForObjectDetection.from_pretrained(model_type).to(device)
- processor = OwlViTProcessor.from_pretrained(model_type)
- return cls(owlvit_for_object_detection.owlvit.text_model,
- owlvit_for_object_detection.owlvit.text_projection,
- owlvit_for_object_detection.class_head,
- owlvit_for_object_detection.box_head,
- processor,
- device,
- *args,
- **kwargs)
- def set_template(self, template: str):
- self.prompt_template = template
- def process_text(self, text:List, use_template:bool = True):
- if use_template:
- text = [[self.prompt_template+i for i in text[0]]]
- inputs = self.processor(text=text, return_tensors="pt")
- return inputs
- def normalize_grid_corner_coordinates(self, feature_map: torch.FloatTensor):
- # Computes normalized xy corner coordinates from feature_map.
- if not feature_map.ndim == 4:
- raise ValueError("Expected input shape is [batch_size, num_patches, num_patches, hidden_dim]")
-
- device = feature_map.device
- num_patches = feature_map.shape[1]
-
- box_coordinates = np.stack(
- np.meshgrid(np.arange(1, num_patches + 1), np.arange(1, num_patches + 1)), axis=-1
- ).astype(np.float32)
- box_coordinates /= np.array([num_patches, num_patches], np.float32)
-
- # Flatten (h, w, 2) -> (h*w, 2)
- box_coordinates = box_coordinates.reshape(
- box_coordinates.shape[0] * box_coordinates.shape[1], box_coordinates.shape[2]
- )
- box_coordinates = torch.from_numpy(box_coordinates).to(device)
-
- return box_coordinates
-
- def compute_box_bias(self, feature_map: torch.FloatTensor) -> torch.FloatTensor:
- # The box center is biased to its position on the feature grid
- box_coordinates = self.normalize_grid_corner_coordinates(feature_map)
- box_coordinates = torch.clip(box_coordinates, 0.0, 1.0)
-
- # Unnormalize xy
- box_coord_bias = torch.log(box_coordinates + 1e-4) - torch.log1p(-box_coordinates + 1e-4)
-
- # The box size is biased to the patch size
- box_size = torch.full_like(box_coord_bias, 1.0 / feature_map.shape[-2])
- box_size_bias = torch.log(box_size + 1e-4) - torch.log1p(-box_size + 1e-4)
-
- # Compute box bias
- box_bias = torch.cat([box_coord_bias, box_size_bias], dim=-1)
- return box_bias
-
- def box_predictor(
- self,
- image_feats: torch.FloatTensor,
- feature_map: torch.FloatTensor,
- ) -> torch.FloatTensor:
- """
- Args:
- image_feats:
- Features extracted from the image, returned by the `image_text_embedder` method.
- feature_map:
- A spatial re-arrangement of image_features, also returned by the `image_text_embedder` method.
- Returns:
- pred_boxes:
- List of predicted boxes (cxcywh normalized to 0, 1) nested within a dictionary.
- """
- # Bounding box detection head [batch_size, num_boxes, 4].
- pred_boxes = self.box_head(image_feats)
-
- # Compute the location of each token on the grid and use it to compute a bias for the bbox prediction
- pred_boxes += self.compute_box_bias(feature_map)
- pred_boxes = self.sigmoid(pred_boxes)
- return pred_boxes
-
- def class_predictor(
- self,
- image_feats: torch.FloatTensor,
- query_embeds: Optional[torch.FloatTensor] = None,
- query_mask: Optional[torch.Tensor] = None,
- ) -> Tuple[torch.FloatTensor]:
- """
- Args:
- image_feats:
- Features extracted from the `image_text_embedder`.
- query_embeds:
- Text query embeddings.
- query_mask:
- Must be provided with query_embeddings. A mask indicating which query embeddings are valid.
- """
- (pred_logits, image_class_embeds) = self.class_head(image_feats, query_embeds, query_mask)
-
- return (pred_logits, image_class_embeds)
-
- def image_text_embedder(
- self,
- input_ids: torch.Tensor,
- image_embeds: torch.FloatTensor,
- attention_mask: torch.Tensor,
- output_attentions: Optional[bool] = None,
- output_hidden_states: Optional[bool] = None,
- ) -> Tuple[torch.FloatTensor]:
-
- # Encode text and image
- text_outputs = self.owlvit_text(
- input_ids=input_ids,
- attention_mask=attention_mask,
- output_attentions=output_attentions,
- output_hidden_states=output_hidden_states,
- return_dict=True,
- )
- text_embeds = text_outputs[1]
- text_embeds = self.text_projection(text_embeds)
- text_embeds = text_embeds / torch.linalg.norm(text_embeds, ord=2, dim=-1, keepdim=True)
-
- return (text_embeds, image_embeds, text_outputs)
-
- def embed_image_query(
- self, query_image_features: torch.FloatTensor, query_feature_map: torch.FloatTensor
- ) -> torch.FloatTensor:
-
- _, class_embeds = self.class_predictor(query_image_features)
- pred_boxes = self.box_predictor(query_image_features, query_feature_map)
- pred_boxes_as_corners = center_to_corners_format(pred_boxes)
-
- # Loop over query images
- best_class_embeds = []
- best_box_indices = []
- pred_boxes_device = pred_boxes_as_corners.device
-
- for i in range(query_image_features.shape[0]):
- each_query_box = torch.tensor([[0, 0, 1, 1]], device=pred_boxes_device)
- each_query_pred_boxes = pred_boxes_as_corners[i]
- ious, _ = box_iou(each_query_box, each_query_pred_boxes)
-
- # If there are no overlapping boxes, fall back to generalized IoU
- if torch.all(ious[0] == 0.0):
- ious = generalized_box_iou(each_query_box, each_query_pred_boxes)
-
- # Use an adaptive threshold to include all boxes within 80% of the best IoU
- iou_threshold = torch.max(ious) * 0.8
-
- selected_inds = (ious[0] >= iou_threshold).nonzero()
- if selected_inds.numel():
- selected_embeddings = class_embeds[i][selected_inds[0]]
- mean_embeds = torch.mean(class_embeds[i], axis=0)
- mean_sim = torch.einsum("d,id->i", mean_embeds, selected_embeddings)
- best_box_ind = selected_inds[torch.argmin(mean_sim)]
- best_class_embeds.append(class_embeds[i][best_box_ind])
- best_box_indices.append(best_box_ind)
-
- if best_class_embeds:
- query_embeds = torch.stack(best_class_embeds)
- box_indices = torch.stack(best_box_indices)
- else:
- query_embeds, box_indices = None, None
-
- return query_embeds, box_indices, pred_boxes
-
- @torch.no_grad()
- def forward(
- self,
- image_embeds: torch.FloatTensor,
- input_ids: Optional[torch.Tensor] = None,
- text: Optional[List] = None,
- attention_mask: Optional[torch.Tensor] = None,
- output_attentions: Optional[bool] = None,
- output_hidden_states: Optional[bool] = None,
- return_dict: Optional[bool] = None,
- ) -> OwlViTObjectDetectionOutput:
- if text is not None:
- inputs = self.process_text(text)
- input_ids = inputs.input_ids.to(self.device)
- attention_mask = inputs.attention_mask.to(self.device)
- input_ids = input_ids.to(self.device)
- image_embeds = image_embeds.to(self.device)
- attention_mask = attention_mask.to(self.device)
- output_attentions = output_attentions if output_attentions is not None else False
- output_hidden_states = (
- output_hidden_states if output_hidden_states is not None else False
- )
- return_dict = return_dict if return_dict is not None else True
-
- # Embed images and text queries
- query_embeds, feature_map, text_outputs = self.image_text_embedder(
- input_ids=input_ids,
- image_embeds=image_embeds,
- attention_mask=attention_mask,
- output_attentions=output_attentions,
- output_hidden_states=output_hidden_states,
- )
-
- # Text and vision model outputs
-
- batch_size, num_patches, num_patches, hidden_dim = feature_map.shape
- image_feats = torch.reshape(feature_map, (batch_size, num_patches * num_patches, hidden_dim))
-
- # Reshape from [batch_size * max_text_queries, hidden_dim] -> [batch_size, max_text_queries, hidden_dim]
- max_text_queries = input_ids.shape[0] // batch_size
- query_embeds = query_embeds.reshape(batch_size, max_text_queries, query_embeds.shape[-1])
-
- # If first token is 0, then this is a padded query [batch_size, num_queries].
- input_ids = input_ids.reshape(batch_size, max_text_queries, input_ids.shape[-1])
- query_mask = input_ids[..., 0] > 0
-
- # Predict object classes [batch_size, num_patches, num_queries+1]
- (pred_logits, class_embeds) = self.class_predictor(image_feats, query_embeds, query_mask)
-
- # Predict object boxes
- pred_boxes = self.box_predictor(image_feats, feature_map)
-
- if not return_dict:
- output = (
- pred_logits,
- pred_boxes,
- query_embeds,
- feature_map,
- class_embeds,
- text_outputs.to_tuple(),
- None,
- )
- output = tuple(x for x in output if x is not None)
- return output
-
- return OwlViTObjectDetectionOutput(
- image_embeds=feature_map,
- text_embeds=query_embeds,
- pred_boxes=pred_boxes.cpu(),
- logits=pred_logits.cpu(),
- class_embeds=class_embeds,
- text_model_output=text_outputs,
- vision_model_output=None,
- )
-
- def owlvit_visualize(self,
- image: Image,
- texts: List,
- owlvit_objectdetection_output: OwlViTObjectDetectionOutput,
- score_threshold: float = 0.1,
- pil=True):
- target_sizes = torch.Tensor([image.size[::-1]])
- # Convert outputs (bounding boxes and class logits) to COCO API
- results = self.processor.post_process(outputs=owlvit_objectdetection_output, target_sizes=target_sizes)
-
- text = texts[0]
- boxes, scores, labels = results[0]["boxes"], results[0]["scores"], results[0]["labels"]
- boxes_np = []
- labels_list = []
- # Print detected objects and rescaled box coordinates
- for box, score, label in zip(boxes, scores, labels):
- box = [int(i) for i in box.tolist()]
- if score >= score_threshold:
- labels_list.append(f"{text[label]} {round(score.item(), 3)}")
- boxes_np.append(box)
- print(f"Detected {text[label]} with confidence {round(score.item(), 3)} at location {box}")
- boxes_np = np.array(boxes_np)
- detections = sv.Detections(xyxy=boxes_np)
- image_np = np.uint8(image)[:, :, ::-1]
- box_annotator = sv.BoxAnnotator()
- annotated_frame = box_annotator.annotate(scene=image_np.copy(), detections=detections, labels=labels_list)
- if pil:
- return PIL.Image.fromarray(annotated_frame[:, :, ::-1])
- else:
- return annotated_frame[:, :, ::-1]
diff --git a/spaces/Yukki-Yui/White-box-Cartoonization/README.md b/spaces/Yukki-Yui/White-box-Cartoonization/README.md
deleted file mode 100644
index f960f60b0dd3fce436ecc0c4e6779140133652de..0000000000000000000000000000000000000000
--- a/spaces/Yukki-Yui/White-box-Cartoonization/README.md
+++ /dev/null
@@ -1,14 +0,0 @@
----
-python_version: 3.7
-title: White Box Cartoonization
-emoji: 📚
-colorFrom: purple
-colorTo: green
-sdk: gradio
-sdk_version: 2.9.4
-app_file: app.py
-pinned: false
-license: apache-2.0
----
-
-Check out the configuration reference at https://huggingface.co/docs/hub/spaces#reference
diff --git a/spaces/Yuliang/ECON/lib/common/libvoxelize/tribox2.h b/spaces/Yuliang/ECON/lib/common/libvoxelize/tribox2.h
deleted file mode 100644
index 85d19ed728dc42995034438bbb74c6902e9b44e6..0000000000000000000000000000000000000000
--- a/spaces/Yuliang/ECON/lib/common/libvoxelize/tribox2.h
+++ /dev/null
@@ -1,184 +0,0 @@
-/********************************************************/
-/* AABB-triangle overlap test code */
-/* by Tomas Akenine-M�ller */
-/* Function: int triBoxOverlap(float boxcenter[3], */
-/* float boxhalfsize[3],float triverts[3][3]); */
-/* History: */
-/* 2001-03-05: released the code in its first version */
-/* 2001-06-18: changed the order of the tests, faster */
-/* */
-/* Acknowledgement: Many thanks to Pierre Terdiman for */
-/* suggestions and discussions on how to optimize code. */
-/* Thanks to David Hunt for finding a ">="-bug! */
-/********************************************************/
-#include
-#include
-
-#define X 0
-#define Y 1
-#define Z 2
-
-#define CROSS(dest,v1,v2) \
- dest[0]=v1[1]*v2[2]-v1[2]*v2[1]; \
- dest[1]=v1[2]*v2[0]-v1[0]*v2[2]; \
- dest[2]=v1[0]*v2[1]-v1[1]*v2[0];
-
-#define DOT(v1,v2) (v1[0]*v2[0]+v1[1]*v2[1]+v1[2]*v2[2])
-
-#define SUB(dest,v1,v2) \
- dest[0]=v1[0]-v2[0]; \
- dest[1]=v1[1]-v2[1]; \
- dest[2]=v1[2]-v2[2];
-
-#define FINDMINMAX(x0,x1,x2,min,max) \
- min = max = x0; \
- if(x1max) max=x1;\
- if(x2max) max=x2;
-
-int planeBoxOverlap(float normal[3],float d, float maxbox[3])
-{
- int q;
- float vmin[3],vmax[3];
- for(q=X;q<=Z;q++)
- {
- if(normal[q]>0.0f)
- {
- vmin[q]=-maxbox[q];
- vmax[q]=maxbox[q];
- }
- else
- {
- vmin[q]=maxbox[q];
- vmax[q]=-maxbox[q];
- }
- }
- if(DOT(normal,vmin)+d>0.0f) return 0;
- if(DOT(normal,vmax)+d>=0.0f) return 1;
-
- return 0;
-}
-
-
-/*======================== X-tests ========================*/
-#define AXISTEST_X01(a, b, fa, fb) \
- p0 = a*v0[Y] - b*v0[Z]; \
- p2 = a*v2[Y] - b*v2[Z]; \
- if(p0rad || max<-rad) return 0;
-
-#define AXISTEST_X2(a, b, fa, fb) \
- p0 = a*v0[Y] - b*v0[Z]; \
- p1 = a*v1[Y] - b*v1[Z]; \
- if(p0rad || max<-rad) return 0;
-
-/*======================== Y-tests ========================*/
-#define AXISTEST_Y02(a, b, fa, fb) \
- p0 = -a*v0[X] + b*v0[Z]; \
- p2 = -a*v2[X] + b*v2[Z]; \
- if(p0rad || max<-rad) return 0;
-
-#define AXISTEST_Y1(a, b, fa, fb) \
- p0 = -a*v0[X] + b*v0[Z]; \
- p1 = -a*v1[X] + b*v1[Z]; \
- if(p0rad || max<-rad) return 0;
-
-/*======================== Z-tests ========================*/
-
-#define AXISTEST_Z12(a, b, fa, fb) \
- p1 = a*v1[X] - b*v1[Y]; \
- p2 = a*v2[X] - b*v2[Y]; \
- if(p2rad || max<-rad) return 0;
-
-#define AXISTEST_Z0(a, b, fa, fb) \
- p0 = a*v0[X] - b*v0[Y]; \
- p1 = a*v1[X] - b*v1[Y]; \
- if(p0rad || max<-rad) return 0;
-
-int triBoxOverlap(float boxcenter[3],float boxhalfsize[3],float tri0[3], float tri1[3], float tri2[3])
-{
-
- /* use separating axis theorem to test overlap between triangle and box */
- /* need to test for overlap in these directions: */
- /* 1) the {x,y,z}-directions (actually, since we use the AABB of the triangle */
- /* we do not even need to test these) */
- /* 2) normal of the triangle */
- /* 3) crossproduct(edge from tri, {x,y,z}-directin) */
- /* this gives 3x3=9 more tests */
- float v0[3],v1[3],v2[3];
- float min,max,d,p0,p1,p2,rad,fex,fey,fez;
- float normal[3],e0[3],e1[3],e2[3];
-
- /* This is the fastest branch on Sun */
- /* move everything so that the boxcenter is in (0,0,0) */
- SUB(v0, tri0, boxcenter);
- SUB(v1, tri1, boxcenter);
- SUB(v2, tri2, boxcenter);
-
- /* compute triangle edges */
- SUB(e0,v1,v0); /* tri edge 0 */
- SUB(e1,v2,v1); /* tri edge 1 */
- SUB(e2,v0,v2); /* tri edge 2 */
-
- /* Bullet 3: */
- /* test the 9 tests first (this was faster) */
- fex = fabs(e0[X]);
- fey = fabs(e0[Y]);
- fez = fabs(e0[Z]);
- AXISTEST_X01(e0[Z], e0[Y], fez, fey);
- AXISTEST_Y02(e0[Z], e0[X], fez, fex);
- AXISTEST_Z12(e0[Y], e0[X], fey, fex);
-
- fex = fabs(e1[X]);
- fey = fabs(e1[Y]);
- fez = fabs(e1[Z]);
- AXISTEST_X01(e1[Z], e1[Y], fez, fey);
- AXISTEST_Y02(e1[Z], e1[X], fez, fex);
- AXISTEST_Z0(e1[Y], e1[X], fey, fex);
-
- fex = fabs(e2[X]);
- fey = fabs(e2[Y]);
- fez = fabs(e2[Z]);
- AXISTEST_X2(e2[Z], e2[Y], fez, fey);
- AXISTEST_Y1(e2[Z], e2[X], fez, fex);
- AXISTEST_Z12(e2[Y], e2[X], fey, fex);
-
- /* Bullet 1: */
- /* first test overlap in the {x,y,z}-directions */
- /* find min, max of the triangle each direction, and test for overlap in */
- /* that direction -- this is equivalent to testing a minimal AABB around */
- /* the triangle against the AABB */
-
- /* test in X-direction */
- FINDMINMAX(v0[X],v1[X],v2[X],min,max);
- if(min>boxhalfsize[X] || max<-boxhalfsize[X]) return 0;
-
- /* test in Y-direction */
- FINDMINMAX(v0[Y],v1[Y],v2[Y],min,max);
- if(min>boxhalfsize[Y] || max<-boxhalfsize[Y]) return 0;
-
- /* test in Z-direction */
- FINDMINMAX(v0[Z],v1[Z],v2[Z],min,max);
- if(min>boxhalfsize[Z] || max<-boxhalfsize[Z]) return 0;
-
- /* Bullet 2: */
- /* test if the box intersects the plane of the triangle */
- /* compute plane equation of triangle: normal*x+d=0 */
- CROSS(normal,e0,e1);
- d=-DOT(normal,v0); /* plane eq: normal.x+d=0 */
- if(!planeBoxOverlap(normal,d,boxhalfsize)) return 0;
-
- return 1; /* box and triangle overlaps */
-}
diff --git a/spaces/Zaixi/ICLR_FLAG/utils/datasets/__init__.py b/spaces/Zaixi/ICLR_FLAG/utils/datasets/__init__.py
deleted file mode 100644
index f518b1df9d36f8ae62b8b2a9da533686a82ca4e1..0000000000000000000000000000000000000000
--- a/spaces/Zaixi/ICLR_FLAG/utils/datasets/__init__.py
+++ /dev/null
@@ -1,21 +0,0 @@
-import torch
-from torch.utils.data import Subset
-from .pl import PocketLigandPairDataset
-import random
-
-
-def get_dataset(config, *args, **kwargs):
- name = config.name
- root = config.path
- if name == 'pl':
- dataset = PocketLigandPairDataset(root, *args, **kwargs)
- else:
- raise NotImplementedError('Unknown dataset: %s' % name)
-
- if 'split' in config:
- split_by_name = torch.load(config.split)
- split = {k: [dataset.name2id[n] for n in names if n in dataset.name2id] for k, names in split_by_name.items()}
- subsets = {k:Subset(dataset, indices=v) for k, v in split.items()}
- return dataset, subsets
- else:
- return dataset
diff --git a/spaces/abdvl/datahub_qa_bot/docs/advanced/derived-aspects.md b/spaces/abdvl/datahub_qa_bot/docs/advanced/derived-aspects.md
deleted file mode 100644
index 989432380c593e64a84f5871cac50471aabf86d7..0000000000000000000000000000000000000000
--- a/spaces/abdvl/datahub_qa_bot/docs/advanced/derived-aspects.md
+++ /dev/null
@@ -1,3 +0,0 @@
-# Derived Aspects
-
-WIP
diff --git a/spaces/abhishek/sketch-to-image/annotator/uniformer/mmcv/utils/progressbar.py b/spaces/abhishek/sketch-to-image/annotator/uniformer/mmcv/utils/progressbar.py
deleted file mode 100644
index 0062f670dd94fa9da559ab26ef85517dcf5211c7..0000000000000000000000000000000000000000
--- a/spaces/abhishek/sketch-to-image/annotator/uniformer/mmcv/utils/progressbar.py
+++ /dev/null
@@ -1,208 +0,0 @@
-# Copyright (c) OpenMMLab. All rights reserved.
-import sys
-from collections.abc import Iterable
-from multiprocessing import Pool
-from shutil import get_terminal_size
-
-from .timer import Timer
-
-
-class ProgressBar:
- """A progress bar which can print the progress."""
-
- def __init__(self, task_num=0, bar_width=50, start=True, file=sys.stdout):
- self.task_num = task_num
- self.bar_width = bar_width
- self.completed = 0
- self.file = file
- if start:
- self.start()
-
- @property
- def terminal_width(self):
- width, _ = get_terminal_size()
- return width
-
- def start(self):
- if self.task_num > 0:
- self.file.write(f'[{" " * self.bar_width}] 0/{self.task_num}, '
- 'elapsed: 0s, ETA:')
- else:
- self.file.write('completed: 0, elapsed: 0s')
- self.file.flush()
- self.timer = Timer()
-
- def update(self, num_tasks=1):
- assert num_tasks > 0
- self.completed += num_tasks
- elapsed = self.timer.since_start()
- if elapsed > 0:
- fps = self.completed / elapsed
- else:
- fps = float('inf')
- if self.task_num > 0:
- percentage = self.completed / float(self.task_num)
- eta = int(elapsed * (1 - percentage) / percentage + 0.5)
- msg = f'\r[{{}}] {self.completed}/{self.task_num}, ' \
- f'{fps:.1f} task/s, elapsed: {int(elapsed + 0.5)}s, ' \
- f'ETA: {eta:5}s'
-
- bar_width = min(self.bar_width,
- int(self.terminal_width - len(msg)) + 2,
- int(self.terminal_width * 0.6))
- bar_width = max(2, bar_width)
- mark_width = int(bar_width * percentage)
- bar_chars = '>' * mark_width + ' ' * (bar_width - mark_width)
- self.file.write(msg.format(bar_chars))
- else:
- self.file.write(
- f'completed: {self.completed}, elapsed: {int(elapsed + 0.5)}s,'
- f' {fps:.1f} tasks/s')
- self.file.flush()
-
-
-def track_progress(func, tasks, bar_width=50, file=sys.stdout, **kwargs):
- """Track the progress of tasks execution with a progress bar.
-
- Tasks are done with a simple for-loop.
-
- Args:
- func (callable): The function to be applied to each task.
- tasks (list or tuple[Iterable, int]): A list of tasks or
- (tasks, total num).
- bar_width (int): Width of progress bar.
-
- Returns:
- list: The task results.
- """
- if isinstance(tasks, tuple):
- assert len(tasks) == 2
- assert isinstance(tasks[0], Iterable)
- assert isinstance(tasks[1], int)
- task_num = tasks[1]
- tasks = tasks[0]
- elif isinstance(tasks, Iterable):
- task_num = len(tasks)
- else:
- raise TypeError(
- '"tasks" must be an iterable object or a (iterator, int) tuple')
- prog_bar = ProgressBar(task_num, bar_width, file=file)
- results = []
- for task in tasks:
- results.append(func(task, **kwargs))
- prog_bar.update()
- prog_bar.file.write('\n')
- return results
-
-
-def init_pool(process_num, initializer=None, initargs=None):
- if initializer is None:
- return Pool(process_num)
- elif initargs is None:
- return Pool(process_num, initializer)
- else:
- if not isinstance(initargs, tuple):
- raise TypeError('"initargs" must be a tuple')
- return Pool(process_num, initializer, initargs)
-
-
-def track_parallel_progress(func,
- tasks,
- nproc,
- initializer=None,
- initargs=None,
- bar_width=50,
- chunksize=1,
- skip_first=False,
- keep_order=True,
- file=sys.stdout):
- """Track the progress of parallel task execution with a progress bar.
-
- The built-in :mod:`multiprocessing` module is used for process pools and
- tasks are done with :func:`Pool.map` or :func:`Pool.imap_unordered`.
-
- Args:
- func (callable): The function to be applied to each task.
- tasks (list or tuple[Iterable, int]): A list of tasks or
- (tasks, total num).
- nproc (int): Process (worker) number.
- initializer (None or callable): Refer to :class:`multiprocessing.Pool`
- for details.
- initargs (None or tuple): Refer to :class:`multiprocessing.Pool` for
- details.
- chunksize (int): Refer to :class:`multiprocessing.Pool` for details.
- bar_width (int): Width of progress bar.
- skip_first (bool): Whether to skip the first sample for each worker
- when estimating fps, since the initialization step may takes
- longer.
- keep_order (bool): If True, :func:`Pool.imap` is used, otherwise
- :func:`Pool.imap_unordered` is used.
-
- Returns:
- list: The task results.
- """
- if isinstance(tasks, tuple):
- assert len(tasks) == 2
- assert isinstance(tasks[0], Iterable)
- assert isinstance(tasks[1], int)
- task_num = tasks[1]
- tasks = tasks[0]
- elif isinstance(tasks, Iterable):
- task_num = len(tasks)
- else:
- raise TypeError(
- '"tasks" must be an iterable object or a (iterator, int) tuple')
- pool = init_pool(nproc, initializer, initargs)
- start = not skip_first
- task_num -= nproc * chunksize * int(skip_first)
- prog_bar = ProgressBar(task_num, bar_width, start, file=file)
- results = []
- if keep_order:
- gen = pool.imap(func, tasks, chunksize)
- else:
- gen = pool.imap_unordered(func, tasks, chunksize)
- for result in gen:
- results.append(result)
- if skip_first:
- if len(results) < nproc * chunksize:
- continue
- elif len(results) == nproc * chunksize:
- prog_bar.start()
- continue
- prog_bar.update()
- prog_bar.file.write('\n')
- pool.close()
- pool.join()
- return results
-
-
-def track_iter_progress(tasks, bar_width=50, file=sys.stdout):
- """Track the progress of tasks iteration or enumeration with a progress
- bar.
-
- Tasks are yielded with a simple for-loop.
-
- Args:
- tasks (list or tuple[Iterable, int]): A list of tasks or
- (tasks, total num).
- bar_width (int): Width of progress bar.
-
- Yields:
- list: The task results.
- """
- if isinstance(tasks, tuple):
- assert len(tasks) == 2
- assert isinstance(tasks[0], Iterable)
- assert isinstance(tasks[1], int)
- task_num = tasks[1]
- tasks = tasks[0]
- elif isinstance(tasks, Iterable):
- task_num = len(tasks)
- else:
- raise TypeError(
- '"tasks" must be an iterable object or a (iterator, int) tuple')
- prog_bar = ProgressBar(task_num, bar_width, file=file)
- for task in tasks:
- yield task
- prog_bar.update()
- prog_bar.file.write('\n')
diff --git a/spaces/abhishek/sketch-to-image/annotator/uniformer/mmdet/models/necks/hrfpn.py b/spaces/abhishek/sketch-to-image/annotator/uniformer/mmdet/models/necks/hrfpn.py
deleted file mode 100644
index ed4f194832fc4b6ea77ce54262fb8ffa8675fc4e..0000000000000000000000000000000000000000
--- a/spaces/abhishek/sketch-to-image/annotator/uniformer/mmdet/models/necks/hrfpn.py
+++ /dev/null
@@ -1,102 +0,0 @@
-import torch
-import torch.nn as nn
-import torch.nn.functional as F
-from mmcv.cnn import ConvModule, caffe2_xavier_init
-from torch.utils.checkpoint import checkpoint
-
-from ..builder import NECKS
-
-
-@NECKS.register_module()
-class HRFPN(nn.Module):
- """HRFPN (High Resolution Feature Pyramids)
-
- paper: `High-Resolution Representations for Labeling Pixels and Regions
- `_.
-
- Args:
- in_channels (list): number of channels for each branch.
- out_channels (int): output channels of feature pyramids.
- num_outs (int): number of output stages.
- pooling_type (str): pooling for generating feature pyramids
- from {MAX, AVG}.
- conv_cfg (dict): dictionary to construct and config conv layer.
- norm_cfg (dict): dictionary to construct and config norm layer.
- with_cp (bool): Use checkpoint or not. Using checkpoint will save some
- memory while slowing down the training speed.
- stride (int): stride of 3x3 convolutional layers
- """
-
- def __init__(self,
- in_channels,
- out_channels,
- num_outs=5,
- pooling_type='AVG',
- conv_cfg=None,
- norm_cfg=None,
- with_cp=False,
- stride=1):
- super(HRFPN, self).__init__()
- assert isinstance(in_channels, list)
- self.in_channels = in_channels
- self.out_channels = out_channels
- self.num_ins = len(in_channels)
- self.num_outs = num_outs
- self.with_cp = with_cp
- self.conv_cfg = conv_cfg
- self.norm_cfg = norm_cfg
-
- self.reduction_conv = ConvModule(
- sum(in_channels),
- out_channels,
- kernel_size=1,
- conv_cfg=self.conv_cfg,
- act_cfg=None)
-
- self.fpn_convs = nn.ModuleList()
- for i in range(self.num_outs):
- self.fpn_convs.append(
- ConvModule(
- out_channels,
- out_channels,
- kernel_size=3,
- padding=1,
- stride=stride,
- conv_cfg=self.conv_cfg,
- act_cfg=None))
-
- if pooling_type == 'MAX':
- self.pooling = F.max_pool2d
- else:
- self.pooling = F.avg_pool2d
-
- def init_weights(self):
- """Initialize the weights of module."""
- for m in self.modules():
- if isinstance(m, nn.Conv2d):
- caffe2_xavier_init(m)
-
- def forward(self, inputs):
- """Forward function."""
- assert len(inputs) == self.num_ins
- outs = [inputs[0]]
- for i in range(1, self.num_ins):
- outs.append(
- F.interpolate(inputs[i], scale_factor=2**i, mode='bilinear'))
- out = torch.cat(outs, dim=1)
- if out.requires_grad and self.with_cp:
- out = checkpoint(self.reduction_conv, out)
- else:
- out = self.reduction_conv(out)
- outs = [out]
- for i in range(1, self.num_outs):
- outs.append(self.pooling(out, kernel_size=2**i, stride=2**i))
- outputs = []
-
- for i in range(self.num_outs):
- if outs[i].requires_grad and self.with_cp:
- tmp_out = checkpoint(self.fpn_convs[i], outs[i])
- else:
- tmp_out = self.fpn_convs[i](outs[i])
- outputs.append(tmp_out)
- return tuple(outputs)
diff --git a/spaces/abhishek/sketch-to-image/annotator/uniformer/mmdet_null/utils/profiling.py b/spaces/abhishek/sketch-to-image/annotator/uniformer/mmdet_null/utils/profiling.py
deleted file mode 100644
index 4be9222c37e922329d537f883f5587995e27efc6..0000000000000000000000000000000000000000
--- a/spaces/abhishek/sketch-to-image/annotator/uniformer/mmdet_null/utils/profiling.py
+++ /dev/null
@@ -1,39 +0,0 @@
-import contextlib
-import sys
-import time
-
-import torch
-
-if sys.version_info >= (3, 7):
-
- @contextlib.contextmanager
- def profile_time(trace_name,
- name,
- enabled=True,
- stream=None,
- end_stream=None):
- """Print time spent by CPU and GPU.
-
- Useful as a temporary context manager to find sweet spots of code
- suitable for async implementation.
- """
- if (not enabled) or not torch.cuda.is_available():
- yield
- return
- stream = stream if stream else torch.cuda.current_stream()
- end_stream = end_stream if end_stream else stream
- start = torch.cuda.Event(enable_timing=True)
- end = torch.cuda.Event(enable_timing=True)
- stream.record_event(start)
- try:
- cpu_start = time.monotonic()
- yield
- finally:
- cpu_end = time.monotonic()
- end_stream.record_event(end)
- end.synchronize()
- cpu_time = (cpu_end - cpu_start) * 1000
- gpu_time = start.elapsed_time(end)
- msg = f'{trace_name} {name} cpu_time {cpu_time:.2f} ms '
- msg += f'gpu_time {gpu_time:.2f} ms stream {stream}'
- print(msg, end_stream)
diff --git a/spaces/abhishek/sketch-to-image/annotator/uniformer_base/mmseg/datasets/pipelines/formating.py b/spaces/abhishek/sketch-to-image/annotator/uniformer_base/mmseg/datasets/pipelines/formating.py
deleted file mode 100644
index f4c9c531effc2e2869880aa31205c659240afdf2..0000000000000000000000000000000000000000
--- a/spaces/abhishek/sketch-to-image/annotator/uniformer_base/mmseg/datasets/pipelines/formating.py
+++ /dev/null
@@ -1,300 +0,0 @@
-'''
- * Copyright (c) 2023 Salesforce, Inc.
- * All rights reserved.
- * SPDX-License-Identifier: Apache License 2.0
- * For full license text, see LICENSE.txt file in the repo root or http://www.apache.org/licenses/
- * By Can Qin
- * Modified from ControlNet repo: https://github.com/lllyasviel/ControlNet
- * Copyright (c) 2023 Lvmin Zhang and Maneesh Agrawala
- * Modified from MMCV repo: From https://github.com/open-mmlab/mmcv
- * Copyright (c) OpenMMLab. All rights reserved.
-'''
-
-from collections.abc import Sequence
-
-import annotator.uniformer.mmcv as mmcv
-import numpy as np
-import torch
-from annotator.uniformer.mmcv.parallel import DataContainer as DC
-
-from ..builder import PIPELINES
-
-
-def to_tensor(data):
- """Convert objects of various python types to :obj:`torch.Tensor`.
-
- Supported types are: :class:`numpy.ndarray`, :class:`torch.Tensor`,
- :class:`Sequence`, :class:`int` and :class:`float`.
-
- Args:
- data (torch.Tensor | numpy.ndarray | Sequence | int | float): Data to
- be converted.
- """
-
- if isinstance(data, torch.Tensor):
- return data
- elif isinstance(data, np.ndarray):
- return torch.from_numpy(data)
- elif isinstance(data, Sequence) and not mmcv.is_str(data):
- return torch.tensor(data)
- elif isinstance(data, int):
- return torch.LongTensor([data])
- elif isinstance(data, float):
- return torch.FloatTensor([data])
- else:
- raise TypeError(f'type {type(data)} cannot be converted to tensor.')
-
-
-@PIPELINES.register_module()
-class ToTensor(object):
- """Convert some results to :obj:`torch.Tensor` by given keys.
-
- Args:
- keys (Sequence[str]): Keys that need to be converted to Tensor.
- """
-
- def __init__(self, keys):
- self.keys = keys
-
- def __call__(self, results):
- """Call function to convert data in results to :obj:`torch.Tensor`.
-
- Args:
- results (dict): Result dict contains the data to convert.
-
- Returns:
- dict: The result dict contains the data converted
- to :obj:`torch.Tensor`.
- """
-
- for key in self.keys:
- results[key] = to_tensor(results[key])
- return results
-
- def __repr__(self):
- return self.__class__.__name__ + f'(keys={self.keys})'
-
-
-@PIPELINES.register_module()
-class ImageToTensor(object):
- """Convert image to :obj:`torch.Tensor` by given keys.
-
- The dimension order of input image is (H, W, C). The pipeline will convert
- it to (C, H, W). If only 2 dimension (H, W) is given, the output would be
- (1, H, W).
-
- Args:
- keys (Sequence[str]): Key of images to be converted to Tensor.
- """
-
- def __init__(self, keys):
- self.keys = keys
-
- def __call__(self, results):
- """Call function to convert image in results to :obj:`torch.Tensor` and
- transpose the channel order.
-
- Args:
- results (dict): Result dict contains the image data to convert.
-
- Returns:
- dict: The result dict contains the image converted
- to :obj:`torch.Tensor` and transposed to (C, H, W) order.
- """
-
- for key in self.keys:
- img = results[key]
- if len(img.shape) < 3:
- img = np.expand_dims(img, -1)
- results[key] = to_tensor(img.transpose(2, 0, 1))
- return results
-
- def __repr__(self):
- return self.__class__.__name__ + f'(keys={self.keys})'
-
-
-@PIPELINES.register_module()
-class Transpose(object):
- """Transpose some results by given keys.
-
- Args:
- keys (Sequence[str]): Keys of results to be transposed.
- order (Sequence[int]): Order of transpose.
- """
-
- def __init__(self, keys, order):
- self.keys = keys
- self.order = order
-
- def __call__(self, results):
- """Call function to convert image in results to :obj:`torch.Tensor` and
- transpose the channel order.
-
- Args:
- results (dict): Result dict contains the image data to convert.
-
- Returns:
- dict: The result dict contains the image converted
- to :obj:`torch.Tensor` and transposed to (C, H, W) order.
- """
-
- for key in self.keys:
- results[key] = results[key].transpose(self.order)
- return results
-
- def __repr__(self):
- return self.__class__.__name__ + \
- f'(keys={self.keys}, order={self.order})'
-
-
-@PIPELINES.register_module()
-class ToDataContainer(object):
- """Convert results to :obj:`mmcv.DataContainer` by given fields.
-
- Args:
- fields (Sequence[dict]): Each field is a dict like
- ``dict(key='xxx', **kwargs)``. The ``key`` in result will
- be converted to :obj:`mmcv.DataContainer` with ``**kwargs``.
- Default: ``(dict(key='img', stack=True),
- dict(key='gt_semantic_seg'))``.
- """
-
- def __init__(self,
- fields=(dict(key='img',
- stack=True), dict(key='gt_semantic_seg'))):
- self.fields = fields
-
- def __call__(self, results):
- """Call function to convert data in results to
- :obj:`mmcv.DataContainer`.
-
- Args:
- results (dict): Result dict contains the data to convert.
-
- Returns:
- dict: The result dict contains the data converted to
- :obj:`mmcv.DataContainer`.
- """
-
- for field in self.fields:
- field = field.copy()
- key = field.pop('key')
- results[key] = DC(results[key], **field)
- return results
-
- def __repr__(self):
- return self.__class__.__name__ + f'(fields={self.fields})'
-
-
-@PIPELINES.register_module()
-class DefaultFormatBundle(object):
- """Default formatting bundle.
-
- It simplifies the pipeline of formatting common fields, including "img"
- and "gt_semantic_seg". These fields are formatted as follows.
-
- - img: (1)transpose, (2)to tensor, (3)to DataContainer (stack=True)
- - gt_semantic_seg: (1)unsqueeze dim-0 (2)to tensor,
- (3)to DataContainer (stack=True)
- """
-
- def __call__(self, results):
- """Call function to transform and format common fields in results.
-
- Args:
- results (dict): Result dict contains the data to convert.
-
- Returns:
- dict: The result dict contains the data that is formatted with
- default bundle.
- """
-
- if 'img' in results:
- img = results['img']
- if len(img.shape) < 3:
- img = np.expand_dims(img, -1)
- img = np.ascontiguousarray(img.transpose(2, 0, 1))
- results['img'] = DC(to_tensor(img), stack=True)
- if 'gt_semantic_seg' in results:
- # convert to long
- results['gt_semantic_seg'] = DC(
- to_tensor(results['gt_semantic_seg'][None,
- ...].astype(np.int64)),
- stack=True)
- return results
-
- def __repr__(self):
- return self.__class__.__name__
-
-
-@PIPELINES.register_module()
-class Collect(object):
- """Collect data from the loader relevant to the specific task.
-
- This is usually the last stage of the data loader pipeline. Typically keys
- is set to some subset of "img", "gt_semantic_seg".
-
- The "img_meta" item is always populated. The contents of the "img_meta"
- dictionary depends on "meta_keys". By default this includes:
-
- - "img_shape": shape of the image input to the network as a tuple
- (h, w, c). Note that images may be zero padded on the bottom/right
- if the batch tensor is larger than this shape.
-
- - "scale_factor": a float indicating the preprocessing scale
-
- - "flip": a boolean indicating if image flip transform was used
-
- - "filename": path to the image file
-
- - "ori_shape": original shape of the image as a tuple (h, w, c)
-
- - "pad_shape": image shape after padding
-
- - "img_norm_cfg": a dict of normalization information:
- - mean - per channel mean subtraction
- - std - per channel std divisor
- - to_rgb - bool indicating if bgr was converted to rgb
-
- Args:
- keys (Sequence[str]): Keys of results to be collected in ``data``.
- meta_keys (Sequence[str], optional): Meta keys to be converted to
- ``mmcv.DataContainer`` and collected in ``data[img_metas]``.
- Default: ``('filename', 'ori_filename', 'ori_shape', 'img_shape',
- 'pad_shape', 'scale_factor', 'flip', 'flip_direction',
- 'img_norm_cfg')``
- """
-
- def __init__(self,
- keys,
- meta_keys=('filename', 'ori_filename', 'ori_shape',
- 'img_shape', 'pad_shape', 'scale_factor', 'flip',
- 'flip_direction', 'img_norm_cfg')):
- self.keys = keys
- self.meta_keys = meta_keys
-
- def __call__(self, results):
- """Call function to collect keys in results. The keys in ``meta_keys``
- will be converted to :obj:mmcv.DataContainer.
-
- Args:
- results (dict): Result dict contains the data to collect.
-
- Returns:
- dict: The result dict contains the following keys
- - keys in``self.keys``
- - ``img_metas``
- """
-
- data = {}
- img_meta = {}
- for key in self.meta_keys:
- img_meta[key] = results[key]
- data['img_metas'] = DC(img_meta, cpu_only=True)
- for key in self.keys:
- data[key] = results[key]
- return data
-
- def __repr__(self):
- return self.__class__.__name__ + \
- f'(keys={self.keys}, meta_keys={self.meta_keys})'
diff --git a/spaces/abrar-lohia/text-2-character-anim/VQTrans/render_final.py b/spaces/abrar-lohia/text-2-character-anim/VQTrans/render_final.py
deleted file mode 100644
index 41b3bfdb2e6bff74aeaceb8f1a7ebac9dc1acaba..0000000000000000000000000000000000000000
--- a/spaces/abrar-lohia/text-2-character-anim/VQTrans/render_final.py
+++ /dev/null
@@ -1,194 +0,0 @@
-from models.rotation2xyz import Rotation2xyz
-import numpy as np
-from trimesh import Trimesh
-import os
-os.environ['PYOPENGL_PLATFORM'] = "osmesa"
-
-import torch
-from visualize.simplify_loc2rot import joints2smpl
-import pyrender
-import matplotlib.pyplot as plt
-
-import io
-import imageio
-from shapely import geometry
-import trimesh
-from pyrender.constants import RenderFlags
-import math
-# import ffmpeg
-from PIL import Image
-
-class WeakPerspectiveCamera(pyrender.Camera):
- def __init__(self,
- scale,
- translation,
- znear=pyrender.camera.DEFAULT_Z_NEAR,
- zfar=None,
- name=None):
- super(WeakPerspectiveCamera, self).__init__(
- znear=znear,
- zfar=zfar,
- name=name,
- )
- self.scale = scale
- self.translation = translation
-
- def get_projection_matrix(self, width=None, height=None):
- P = np.eye(4)
- P[0, 0] = self.scale[0]
- P[1, 1] = self.scale[1]
- P[0, 3] = self.translation[0] * self.scale[0]
- P[1, 3] = -self.translation[1] * self.scale[1]
- P[2, 2] = -1
- return P
-
-def render(motions, outdir='test_vis', device_id=0, name=None, pred=True):
- frames, njoints, nfeats = motions.shape
- MINS = motions.min(axis=0).min(axis=0)
- MAXS = motions.max(axis=0).max(axis=0)
-
- height_offset = MINS[1]
- motions[:, :, 1] -= height_offset
- trajec = motions[:, 0, [0, 2]]
-
- j2s = joints2smpl(num_frames=frames, device_id=0, cuda=True)
- rot2xyz = Rotation2xyz(device=torch.device("cuda:0"))
- faces = rot2xyz.smpl_model.faces
-
- if (not os.path.exists(outdir + name+'_pred.pt') and pred) or (not os.path.exists(outdir + name+'_gt.pt') and not pred):
- print(f'Running SMPLify, it may take a few minutes.')
- motion_tensor, opt_dict = j2s.joint2smpl(motions) # [nframes, njoints, 3]
-
- vertices = rot2xyz(torch.tensor(motion_tensor).clone(), mask=None,
- pose_rep='rot6d', translation=True, glob=True,
- jointstype='vertices',
- vertstrans=True)
-
- if pred:
- torch.save(vertices, outdir + name+'_pred.pt')
- else:
- torch.save(vertices, outdir + name+'_gt.pt')
- else:
- if pred:
- vertices = torch.load(outdir + name+'_pred.pt')
- else:
- vertices = torch.load(outdir + name+'_gt.pt')
- frames = vertices.shape[3] # shape: 1, nb_frames, 3, nb_joints
- print (vertices.shape)
- MINS = torch.min(torch.min(vertices[0], axis=0)[0], axis=1)[0]
- MAXS = torch.max(torch.max(vertices[0], axis=0)[0], axis=1)[0]
- # vertices[:,:,1,:] -= MINS[1] + 1e-5
-
-
- out_list = []
-
- minx = MINS[0] - 0.5
- maxx = MAXS[0] + 0.5
- minz = MINS[2] - 0.5
- maxz = MAXS[2] + 0.5
- polygon = geometry.Polygon([[minx, minz], [minx, maxz], [maxx, maxz], [maxx, minz]])
- polygon_mesh = trimesh.creation.extrude_polygon(polygon, 1e-5)
-
- vid = []
- for i in range(frames):
- if i % 10 == 0:
- print(i)
-
- mesh = Trimesh(vertices=vertices[0, :, :, i].squeeze().tolist(), faces=faces)
-
- base_color = (0.11, 0.53, 0.8, 0.5)
- ## OPAQUE rendering without alpha
- ## BLEND rendering consider alpha
- material = pyrender.MetallicRoughnessMaterial(
- metallicFactor=0.7,
- alphaMode='OPAQUE',
- baseColorFactor=base_color
- )
-
-
- mesh = pyrender.Mesh.from_trimesh(mesh, material=material)
-
- polygon_mesh.visual.face_colors = [0, 0, 0, 0.21]
- polygon_render = pyrender.Mesh.from_trimesh(polygon_mesh, smooth=False)
-
- bg_color = [1, 1, 1, 0.8]
- scene = pyrender.Scene(bg_color=bg_color, ambient_light=(0.4, 0.4, 0.4))
-
- sx, sy, tx, ty = [0.75, 0.75, 0, 0.10]
-
- camera = pyrender.PerspectiveCamera(yfov=(np.pi / 3.0))
-
- light = pyrender.DirectionalLight(color=[1,1,1], intensity=300)
-
- scene.add(mesh)
-
- c = np.pi / 2
-
- scene.add(polygon_render, pose=np.array([[ 1, 0, 0, 0],
-
- [ 0, np.cos(c), -np.sin(c), MINS[1].cpu().numpy()],
-
- [ 0, np.sin(c), np.cos(c), 0],
-
- [ 0, 0, 0, 1]]))
-
- light_pose = np.eye(4)
- light_pose[:3, 3] = [0, -1, 1]
- scene.add(light, pose=light_pose.copy())
-
- light_pose[:3, 3] = [0, 1, 1]
- scene.add(light, pose=light_pose.copy())
-
- light_pose[:3, 3] = [1, 1, 2]
- scene.add(light, pose=light_pose.copy())
-
-
- c = -np.pi / 6
-
- scene.add(camera, pose=[[ 1, 0, 0, (minx+maxx).cpu().numpy()/2],
-
- [ 0, np.cos(c), -np.sin(c), 1.5],
-
- [ 0, np.sin(c), np.cos(c), max(4, minz.cpu().numpy()+(1.5-MINS[1].cpu().numpy())*2, (maxx-minx).cpu().numpy())],
-
- [ 0, 0, 0, 1]
- ])
-
- # render scene
- r = pyrender.OffscreenRenderer(960, 960)
-
- color, _ = r.render(scene, flags=RenderFlags.RGBA)
- # Image.fromarray(color).save(outdir+'/'+name+'_'+str(i)+'.png')
-
- vid.append(color)
-
- r.delete()
-
- out = np.stack(vid, axis=0)
- if pred:
- imageio.mimsave(outdir + name+'_pred.gif', out, fps=20)
- else:
- imageio.mimsave(outdir + name+'_gt.gif', out, fps=20)
-
-
-
-
-
-if __name__ == "__main__":
- import argparse
- parser = argparse.ArgumentParser()
- parser.add_argument("--filedir", type=str, default=None, help='motion npy file dir')
- parser.add_argument('--motion-list', default=None, nargs="+", type=str, help="motion name list")
- args = parser.parse_args()
-
- filename_list = args.motion_list
- filedir = args.filedir
-
- for filename in filename_list:
- motions = np.load(filedir + filename+'_pred.npy')
- print('pred', motions.shape, filename)
- render(motions[0], outdir=filedir, device_id=0, name=filename, pred=True)
-
- motions = np.load(filedir + filename+'_gt.npy')
- print('gt', motions.shape, filename)
- render(motions[0], outdir=filedir, device_id=0, name=filename, pred=False)
diff --git a/spaces/adirik/ALIGN-zero-shot-image-classification/app.py b/spaces/adirik/ALIGN-zero-shot-image-classification/app.py
deleted file mode 100644
index 91dec8f3db06d4dda03befbbfc991af222d82727..0000000000000000000000000000000000000000
--- a/spaces/adirik/ALIGN-zero-shot-image-classification/app.py
+++ /dev/null
@@ -1,53 +0,0 @@
-import torch
-import gradio as gr
-from transformers import AlignProcessor, AlignModel
-
-
-device = "cuda" if torch.cuda.is_available() else "cpu"
-
-processor = AlignProcessor.from_pretrained("kakaobrain/align-base")
-model = AlignModel.from_pretrained("kakaobrain/align-base").to(device)
-model.eval()
-
-
-def predict(image, labels):
- labels = labels.split(', ')
- inputs = processor(images=image, text=labels, return_tensors="pt").to(device)
-
- with torch.no_grad():
- outputs = model(**inputs)
-
- logits_per_image = outputs.logits_per_image
- probs = logits_per_image.softmax(dim=1).cpu().numpy()
- return {k: float(v) for k, v in zip(labels, probs[0])}
-
-
-description = """
-
-
-
-
-
-
Gradio demo for ALIGN,
- as introduced in "Scaling Up Visual and Vision-Language Representation Learning With Noisy Text Supervision". ALIGN features a dual-encoder architecture with EfficientNet and BERT as its text and vision encoders, and learns to align visual and text representations with contrastive learning.
- Unlike previous work, ALIGN leverages a massive noisy dataset and shows that the scale of the corpus can be used to achieve SOTA representations with a simple recipe.
- \n\nALIGN is not open-sourced and the `kakaobrain/align-base` model used for this demo is based on the Kakao Brain implementation that follows the original paper. The model is trained on the open source [COYO](https://github.com/kakaobrain/coyo-dataset) dataset by the Kakao Brain team. To perform zero-shot image classification with ALIGN, upload an image and enter your candidate labels as free-form text separated by a comma followed by a space.
-
-
diff --git a/spaces/axuint/OpenNiji/README.md b/spaces/axuint/OpenNiji/README.md
deleted file mode 100644
index 96aaadd83969d8819062af612ee86579947734db..0000000000000000000000000000000000000000
--- a/spaces/axuint/OpenNiji/README.md
+++ /dev/null
@@ -1,12 +0,0 @@
----
-title: OpenNiji
-emoji: 🦀
-colorFrom: indigo
-colorTo: red
-sdk: gradio
-sdk_version: 3.16.2
-app_file: app.py
-pinned: false
----
-
-Check out the configuration reference at https://huggingface.co/docs/hub/spaces-config-reference
diff --git a/spaces/badayvedat/AudioSep/models/CLAP/training/lp_train.py b/spaces/badayvedat/AudioSep/models/CLAP/training/lp_train.py
deleted file mode 100644
index 24a19bacd0a4b789415cfccbce1f8bc99bc493ed..0000000000000000000000000000000000000000
--- a/spaces/badayvedat/AudioSep/models/CLAP/training/lp_train.py
+++ /dev/null
@@ -1,301 +0,0 @@
-import json
-import logging
-import math
-import os
-import time
-from contextlib import suppress
-
-import numpy as np
-import torch
-import torch.nn.functional as F
-
-try:
- import wandb
-except ImportError:
- wandb = None
-
-from open_clip import LPLoss, LPMetrics, lp_gather_features
-from open_clip.utils import do_mixup, get_mix_lambda
-from .distributed import is_master
-from .zero_shot import zero_shot_eval
-
-
-class AverageMeter(object):
- """Computes and stores the average and current value"""
-
- def __init__(self):
- self.reset()
-
- def reset(self):
- self.val = 0
- self.avg = 0
- self.sum = 0
- self.count = 0
-
- def update(self, val, n=1):
- self.val = val
- self.sum += val * n
- self.count += n
- self.avg = self.sum / self.count
-
-
-def unwrap_model(model):
- if hasattr(model, "module"):
- return model.module
- else:
- return model
-
-
-def train_one_epoch(
- model,
- data,
- epoch,
- optimizer,
- scaler,
- scheduler,
- args,
- tb_writer=None,
- extra_suffix="",
-):
- device = torch.device(args.device)
- autocast = torch.cuda.amp.autocast if args.precision == "amp" else suppress
- model.train()
- loss = LPLoss(args.lp_loss)
-
- dataloader, sampler = data["train"].dataloader, data["train"].sampler
- if args.distributed and sampler is not None:
- sampler.set_epoch(epoch)
- num_batches_per_epoch = dataloader.num_batches
- sample_digits = math.ceil(math.log(dataloader.num_samples + 1, 10))
-
- # for toy dataset
- if args.dataset_type == "toy":
- dataloader.dataset.generate_queue()
-
- loss_m = AverageMeter()
- batch_time_m = AverageMeter()
- data_time_m = AverageMeter()
- end = time.time()
-
- for i, batch in enumerate(dataloader):
- step = num_batches_per_epoch * epoch + i
-
- if isinstance(scheduler, dict):
- for s in scheduler.values():
- s(step)
- else:
- scheduler(step)
-
- audio = batch # contains mel_spec, wavform, and longer list
- class_label = batch["class_label"]
- # audio = audio.to(device=device, non_blocking=True)
- class_label = class_label.to(device=device, non_blocking=True)
-
- if args.mixup:
- # https://github.com/RetroCirce/HTS-Audio-Transformer/blob/main/utils.py#L146
- mix_lambda = torch.from_numpy(
- get_mix_lambda(0.5, len(audio["waveform"]))
- ).to(device)
- class_label = do_mixup(class_label, mix_lambda)
- else:
- mix_lambda = None
-
- data_time_m.update(time.time() - end)
- if isinstance(optimizer, dict):
- for o_ in optimizer.values():
- o_.zero_grad()
- else:
- optimizer.zero_grad()
-
- with autocast():
- pred = model(audio, mix_lambda=mix_lambda, device=device)
- total_loss = loss(pred, class_label)
-
- if isinstance(optimizer, dict):
- if scaler is not None:
- scaler.scale(total_loss).backward()
- for o_ in optimizer.values():
- if args.horovod:
- o_.synchronize()
- scaler.unscale_(o_)
- with o_.skip_synchronize():
- scaler.step(o_)
- else:
- scaler.step(o_)
- scaler.update()
- else:
- total_loss.backward()
- for o_ in optimizer.values():
- o_.step()
- else:
- if scaler is not None:
- scaler.scale(total_loss).backward()
- if args.horovod:
- optimizer.synchronize()
- scaler.unscale_(optimizer)
- with optimizer.skip_synchronize():
- scaler.step(optimizer)
- else:
- scaler.step(optimizer)
- scaler.update()
- else:
- total_loss.backward()
- optimizer.step()
-
- # Note: we clamp to 4.6052 = ln(100), as in the original paper.
- with torch.no_grad():
- unwrap_model(model).clap_model.logit_scale_a.clamp_(0, math.log(100))
- unwrap_model(model).clap_model.logit_scale_t.clamp_(0, math.log(100))
-
- batch_time_m.update(time.time() - end)
- end = time.time()
- batch_count = i + 1
-
- if is_master(args) and (i % 100 == 0 or batch_count == num_batches_per_epoch):
- if isinstance(audio, dict):
- batch_size = len(audio["waveform"])
- else:
- batch_size = len(audio)
- num_samples = batch_count * batch_size * args.world_size
- samples_per_epoch = dataloader.num_samples
- percent_complete = 100.0 * batch_count / num_batches_per_epoch
-
- # NOTE loss is coarsely sampled, just master node and per log update
- loss_m.update(total_loss.item(), batch_size)
- if isinstance(optimizer, dict):
- logging.info(
- f"Train Epoch: {epoch} [{num_samples:>{sample_digits}}/{samples_per_epoch} ({percent_complete:.0f}%)] "
- f"Loss: {loss_m.val:#.5g} ({loss_m.avg:#.4g}) "
- f"Data (t): {data_time_m.avg:.3f} "
- f"Batch (t): {batch_time_m.avg:.3f} "
- f"LR: {[o_.param_groups[0]['lr'] for o_ in optimizer.values()]}"
- )
- log_data = {
- "loss": loss_m.val,
- "data_time": data_time_m.val,
- "batch_time": batch_time_m.val,
- "lr": [o_.param_groups[0]["lr"] for o_ in optimizer.values()],
- }
- else:
- logging.info(
- f"Train Epoch: {epoch} [{num_samples:>{sample_digits}}/{samples_per_epoch} ({percent_complete:.0f}%)] "
- f"Loss: {loss_m.val:#.5g} ({loss_m.avg:#.4g}) "
- f"Data (t): {data_time_m.avg:.3f} "
- f"Batch (t): {batch_time_m.avg:.3f} "
- f"LR: {optimizer.param_groups[0]['lr']:5f} "
- )
-
- # Save train loss / etc. Using non avg meter values as loggers have their own smoothing
- log_data = {
- "loss": loss_m.val,
- "data_time": data_time_m.val,
- "batch_time": batch_time_m.val,
- "lr": optimizer.param_groups[0]["lr"],
- }
- for name, val in log_data.items():
- name = f"train{extra_suffix}/{name}"
- if tb_writer is not None:
- tb_writer.add_scalar(name, val, step)
- if args.wandb:
- assert wandb is not None, "Please install wandb."
- wandb.log({name: val, "step": step})
-
- # resetting batch / data time meters per log window
- batch_time_m.reset()
- data_time_m.reset()
- # end for
-
-
-def evaluate(model, data, epoch, args, tb_writer=None, extra_suffix=""):
- metrics = {}
- if not args.parallel_eval:
- if not is_master(args):
- return metrics
- device = torch.device(args.device)
- model.eval()
-
- # CHANGE
- # zero_shot_metrics = zero_shot_eval(model, data, epoch, args)
- # metrics.update(zero_shot_metrics)
- if is_master(args):
- print("Evaluating...")
- metric_names = args.lp_metrics.split(",")
- eval_tool = LPMetrics(metric_names=metric_names)
-
- autocast = torch.cuda.amp.autocast if args.precision == "amp" else suppress
- if "val" in data and (
- args.val_frequency
- and ((epoch % args.val_frequency) == 0 or epoch == args.epochs)
- ):
- if args.parallel_eval:
- dataloader, sampler = data["val"].dataloader, data["val"].sampler
- if args.distributed and sampler is not None:
- sampler.set_epoch(epoch)
- samples_per_val = dataloader.num_samples
- else:
- dataloader = data["val"].dataloader
- num_samples = 0
- samples_per_val = dataloader.num_samples
-
- eval_info = {"pred": [], "target": []}
- with torch.no_grad():
- for i, batch in enumerate(dataloader):
- audio = batch # contains mel_spec, wavform, and longer list
- class_label = batch["class_label"]
-
- # audio = audio.to(device=device, non_blocking=True)
- class_label = class_label.to(device=device, non_blocking=True)
-
- with autocast():
- pred = model(audio, device=device)
- if args.parallel_eval:
- pred, class_label = lp_gather_features(
- pred, class_label, args.world_size, args.horovod
- )
- eval_info["pred"].append(pred)
- eval_info["target"].append(class_label)
-
- num_samples += class_label.shape[0]
-
- if (i % 100) == 0: # and i != 0:
- logging.info(
- f"Eval Epoch: {epoch} [{num_samples} / {samples_per_val}]"
- )
-
- if is_master(args):
- eval_info["pred"] = torch.cat(eval_info["pred"], 0).cpu()
- eval_info["target"] = torch.cat(eval_info["target"], 0).cpu()
- metric_dict = eval_tool.evaluate_mertics(
- eval_info["pred"], eval_info["target"]
- )
- metrics.update(metric_dict)
- if "epoch" not in metrics.keys():
- metrics.update({"epoch": epoch})
-
- if is_master(args):
- if not metrics:
- return metrics
-
- logging.info(
- f"Eval Epoch: {epoch} "
- + "\n".join(
- ["\t".join([f"{m}: {round(metrics[m], 4):.4f}"]) for m in metrics]
- )
- )
- if args.save_logs:
- for name, val in metrics.items():
- if tb_writer is not None:
- tb_writer.add_scalar(f"val{extra_suffix}/{name}", val, epoch)
-
- with open(os.path.join(args.checkpoint_path, "results.jsonl"), "a+") as f:
- f.write(json.dumps(metrics))
- f.write("\n")
-
- if args.wandb:
- assert wandb is not None, "Please install wandb."
- for name, val in metrics.items():
- wandb.log({f"val{extra_suffix}/{name}": val, "epoch": epoch})
-
- return metrics
- else:
- return metrics
diff --git a/spaces/banana-projects/web3d/node_modules/three/examples/js/loaders/LWOLoader.js b/spaces/banana-projects/web3d/node_modules/three/examples/js/loaders/LWOLoader.js
deleted file mode 100644
index 173f0f598af07a2946144124e269cdde224dcbc5..0000000000000000000000000000000000000000
--- a/spaces/banana-projects/web3d/node_modules/three/examples/js/loaders/LWOLoader.js
+++ /dev/null
@@ -1,2310 +0,0 @@
-/**
- * @author Lewy Blue https://github.com/looeee
- *
- * Load files in LWO3 format
- *
- * LWO3 format specification:
- * http://static.lightwave3d.com/sdk/2018/html/filefmts/lwo3.html
- *
- * LWO2 format specification (not tested, however the loader should be largely backwards compatible)
- * http://static.lightwave3d.com/sdk/2018/html/filefmts/lwo2.html
- *
- */
-
-THREE.LWOLoader = ( function () {
-
- var lwoTree;
-
- function LWOLoader( manager ) {
-
- this.manager = ( manager !== undefined ) ? manager : THREE.DefaultLoadingManager;
-
- }
-
- LWOLoader.prototype = {
-
- constructor: LWOLoader,
-
- crossOrigin: 'anonymous',
-
- load: function ( url, onLoad, onProgress, onError ) {
-
- var self = this;
-
- var path = ( self.path === undefined ) ? THREE.LoaderUtils.extractUrlBase( url ) : self.path;
-
- // give the mesh a default name based on the filename
- var modelName = url.split( path ).pop().split( '.' )[ 0 ];
-
- var loader = new THREE.FileLoader( this.manager );
- loader.setPath( self.path );
- loader.setResponseType( 'arraybuffer' );
-
- loader.load( url, function ( buffer ) {
-
- // console.time( 'Total parsing: ' );
- onLoad( self.parse( buffer, path, modelName ) );
- // console.timeEnd( 'Total parsing: ' );
-
- }, onProgress, onError );
-
- },
-
- setCrossOrigin: function ( value ) {
-
- this.crossOrigin = value;
- return this;
-
- },
-
- setPath: function ( value ) {
-
- this.path = value;
- return this;
-
- },
-
- setResourcePath: function ( value ) {
-
- this.resourcePath = value;
- return this;
-
- },
-
- parse: function ( iffBuffer, path, modelName ) {
-
- lwoTree = new IFFParser().parse( iffBuffer );
-
- // console.log( 'lwoTree', lwoTree );
-
- var textureLoader = new THREE.TextureLoader( this.manager ).setPath( this.resourcePath || path ).setCrossOrigin( this.crossOrigin );
-
- return new LWOTreeParser( textureLoader ).parse( modelName );
-
- }
-
- };
-
- // Parse the lwoTree object
- function LWOTreeParser( textureLoader ) {
-
- this.textureLoader = textureLoader;
-
- }
-
- LWOTreeParser.prototype = {
-
- constructor: LWOTreeParser,
-
- parse: function ( modelName ) {
-
- this.materials = new MaterialParser( this.textureLoader ).parse();
- this.defaultLayerName = modelName;
-
- this.meshes = this.parseLayers();
-
- return {
- materials: this.materials,
- meshes: this.meshes,
- };
-
- },
-
- parseLayers() {
-
- // array of all meshes for building hierarchy
- var meshes = [];
-
- // final array containing meshes with scene graph hierarchy set up
- var finalMeshes = [];
-
- var geometryParser = new GeometryParser();
-
- var self = this;
- lwoTree.layers.forEach( function ( layer ) {
-
- var geometry = geometryParser.parse( layer.geometry, layer );
-
- var mesh = self.parseMesh( geometry, layer );
-
- meshes[ layer.number ] = mesh;
-
- if ( layer.parent === - 1 ) finalMeshes.push( mesh );
- else meshes[ layer.parent ].add( mesh );
-
-
- } );
-
- this.applyPivots( finalMeshes );
-
- return finalMeshes;
-
- },
-
- parseMesh( geometry, layer ) {
-
- var mesh;
-
- var materials = this.getMaterials( geometry.userData.matNames, layer.geometry.type );
-
- this.duplicateUVs( geometry, materials );
-
- if ( layer.geometry.type === 'points' ) mesh = new THREE.Points( geometry, materials );
- else if ( layer.geometry.type === 'lines' ) mesh = new THREE.LineSegments( geometry, materials );
- else mesh = new THREE.Mesh( geometry, materials );
-
- if ( layer.name ) mesh.name = layer.name;
- else mesh.name = this.defaultLayerName + '_layer_' + layer.number;
-
- mesh.userData.pivot = layer.pivot;
-
- return mesh;
-
- },
-
- // TODO: may need to be reversed in z to convert LWO to three.js coordinates
- applyPivots( meshes ) {
-
- meshes.forEach( function ( mesh ) {
-
- mesh.traverse( function ( child ) {
-
- var pivot = child.userData.pivot;
-
- child.position.x += pivot[ 0 ];
- child.position.y += pivot[ 1 ];
- child.position.z += pivot[ 2 ];
-
- if ( child.parent ) {
-
- var parentPivot = child.parent.userData.pivot;
-
- child.position.x -= parentPivot[ 0 ];
- child.position.y -= parentPivot[ 1 ];
- child.position.z -= parentPivot[ 2 ];
-
- }
-
- } );
-
- } );
-
- },
-
- getMaterials( namesArray, type ) {
-
- var materials = [];
-
- var self = this;
-
- namesArray.forEach( function ( name, i ) {
-
- materials[ i ] = self.getMaterialByName( name );
-
- } );
-
- // convert materials to line or point mats if required
- if ( type === 'points' || type === 'lines' ) {
-
- materials.forEach( function ( mat, i ) {
-
- var spec = {
- color: mat.color,
- };
-
- if ( type === 'points' ) {
-
- spec.size = 0.1;
- spec.map = mat.map;
- spec.morphTargets = mat.morphTargets;
- materials[ i ] = new THREE.PointsMaterial( spec );
-
- } else if ( type === 'lines' ) {
-
- materials[ i ] = new THREE.LineBasicMaterial( spec );
-
- }
-
- } );
-
- }
-
- // if there is only one material, return that directly instead of array
- var filtered = materials.filter( Boolean );
- if ( filtered.length === 1 ) return filtered[ 0 ];
-
- return materials;
-
- },
-
- getMaterialByName( name ) {
-
- return this.materials.filter( function ( m ) {
-
- return m.name === name;
-
- } )[ 0 ];
-
- },
-
- // If the material has an aoMap, duplicate UVs
- duplicateUVs( geometry, materials ) {
-
- var duplicateUVs = false;
-
- if ( ! Array.isArray( materials ) ) {
-
- if ( materials.aoMap ) duplicateUVs = true;
-
- } else {
-
- materials.forEach( function ( material ) {
-
- if ( material.aoMap ) duplicateUVs = true;
-
- } );
-
- }
-
- if ( ! duplicateUVs ) return;
-
- geometry.addAttribute( 'uv2', new THREE.BufferAttribute( geometry.attributes.uv.array, 2 ) );
-
- },
-
- };
-
- function MaterialParser( textureLoader ) {
-
- this.textureLoader = textureLoader;
-
- }
-
- MaterialParser.prototype = {
-
- constructor: MaterialParser,
-
- parse: function () {
-
- var materials = [];
- this.textures = {};
-
- for ( var name in lwoTree.materials ) {
-
- materials.push( this.parseMaterial( lwoTree.materials[ name ], name, lwoTree.textures ) );
-
- }
-
- return materials;
-
- },
-
- parseMaterial( materialData, name, textures ) {
-
- var params = {
- name: name,
- side: this.getSide( materialData.attributes ),
- flatShading: this.getSmooth( materialData.attributes ),
- };
-
- var connections = this.parseConnections( materialData.connections, materialData.nodes );
-
- var maps = this.parseTextureNodes( connections.maps );
-
- this.parseAttributeImageMaps( connections.attributes, textures, maps, materialData.maps );
-
- var attributes = this.parseAttributes( connections.attributes, maps );
-
- this.parseEnvMap( connections, maps, attributes );
-
- params = Object.assign( maps, params );
- params = Object.assign( params, attributes );
-
- var type = connections.attributes.Roughness ? 'Standard' : 'Phong';
-
- return new THREE[ 'Mesh' + type + 'Material' ]( params );
-
- },
-
- // Note: converting from left to right handed coords by switching x -> -x in vertices, and
- // then switching mat FrontSide -> BackSide
- // NB: this means that THREE.FrontSide and THREE.BackSide have been switched!
- getSide( attributes ) {
-
- if ( ! attributes.side ) return THREE.BackSide;
-
- switch ( attributes.side ) {
-
- case 0:
- case 1:
- return THREE.BackSide;
- case 2: return THREE.FrontSide;
- case 3: return THREE.DoubleSide;
-
- }
-
- },
-
- getSmooth( attributes ) {
-
- if ( ! attributes.smooth ) return true;
- return ! attributes.smooth;
-
- },
-
- parseConnections( connections, nodes ) {
-
- var materialConnections = {
- maps: {}
- };
-
- var inputName = connections.inputName;
- var inputNodeName = connections.inputNodeName;
- var nodeName = connections.nodeName;
-
- var self = this;
- inputName.forEach( function ( name, index ) {
-
- if ( name === 'Material' ) {
-
- var matNode = self.getNodeByRefName( inputNodeName[ index ], nodes );
- materialConnections.attributes = matNode.attributes;
- materialConnections.envMap = matNode.fileName;
- materialConnections.name = inputNodeName[ index ];
-
- }
-
- } );
-
- nodeName.forEach( function ( name, index ) {
-
- if ( name === materialConnections.name ) {
-
- materialConnections.maps[ inputName[ index ] ] = self.getNodeByRefName( inputNodeName[ index ], nodes );
-
- }
-
- } );
-
- return materialConnections;
-
- },
-
- getNodeByRefName( refName, nodes ) {
-
- for ( var name in nodes ) {
-
- if ( nodes[ name ].refName === refName ) return nodes[ name ];
-
- }
-
- },
-
- parseTextureNodes( textureNodes ) {
-
- var maps = {};
-
- for ( name in textureNodes ) {
-
- var node = textureNodes[ name ];
- var path = node.fileName;
-
- if ( ! path ) return;
-
- var texture = this.loadTexture( path );
-
- if ( node.widthWrappingMode !== undefined ) texture.wrapS = this.getWrappingType( node.widthWrappingMode );
- if ( node.heightWrappingMode !== undefined ) texture.wrapT = this.getWrappingType( node.heightWrappingMode );
-
- switch ( name ) {
-
- case 'Color':
- maps.map = texture;
- break;
- case 'Roughness':
- maps.roughnessMap = texture;
- maps.roughness = 0.5;
- break;
- case 'Specular':
- maps.specularMap = texture;
- maps.specular = 0xffffff;
- break;
- case 'Luminous':
- maps.emissiveMap = texture;
- maps.emissive = 0x808080;
- break;
- case 'Metallic':
- maps.metalnessMap = texture;
- maps.metalness = 0.5;
- break;
- case 'Transparency':
- case 'Alpha':
- maps.alphaMap = texture;
- maps.transparent = true;
- break;
- case 'Normal':
- maps.normalMap = texture;
- if ( node.amplitude !== undefined ) maps.normalScale = new THREE.Vector2( node.amplitude, node.amplitude );
- break;
- case 'Bump':
- maps.bumpMap = texture;
- break;
-
- }
-
- }
-
- // LWO BSDF materials can have both spec and rough, but this is not valid in three
- if ( maps.roughnessMap && maps.specularMap ) delete maps.specularMap;
-
- return maps;
-
- },
-
- // maps can also be defined on individual material attributes, parse those here
- // This occurs on Standard (Phong) surfaces
- parseAttributeImageMaps( attributes, textures, maps ) {
-
- for ( var name in attributes ) {
-
- var attribute = attributes[ name ];
-
- if ( attribute.maps ) {
-
- var mapData = attribute.maps[ 0 ];
-
- var path = this.getTexturePathByIndex( mapData.imageIndex, textures );
- if ( ! path ) return;
-
- var texture = this.loadTexture( path );
-
- if ( mapData.wrap !== undefined ) texture.wrapS = this.getWrappingType( mapData.wrap.w );
- if ( mapData.wrap !== undefined ) texture.wrapT = this.getWrappingType( mapData.wrap.h );
-
- switch ( name ) {
-
- case 'Color':
- maps.map = texture;
- break;
- case 'Diffuse':
- maps.aoMap = texture;
- break;
- case 'Roughness':
- maps.roughnessMap = texture;
- maps.roughness = 1;
- break;
- case 'Specular':
- maps.specularMap = texture;
- maps.specular = 0xffffff;
- break;
- case 'Luminosity':
- maps.emissiveMap = texture;
- maps.emissive = 0x808080;
- break;
- case 'Metallic':
- maps.metalnessMap = texture;
- maps.metalness = 1;
- break;
- case 'Transparency':
- case 'Alpha':
- maps.alphaMap = texture;
- maps.transparent = true;
- break;
- case 'Normal':
- maps.normalMap = texture;
- break;
- case 'Bump':
- maps.bumpMap = texture;
- break;
-
- }
-
- }
-
- }
-
- },
-
- parseAttributes( attributes, maps ) {
-
- var params = {};
-
- // don't use color data if color map is present
- if ( attributes.Color && ! maps.map ) {
-
- params.color = new THREE.Color().fromArray( attributes.Color.value );
-
- } else params.color = new THREE.Color();
-
-
- if ( attributes.Transparency && attributes.Transparency.value !== 0 ) {
-
- params.opacity = 1 - attributes.Transparency.value;
- params.transparent = true;
-
- }
-
- if ( attributes[ 'Bump Height' ] ) params.bumpScale = attributes[ 'Bump Height' ].value * 0.1;
-
- if ( attributes[ 'Refraction Index' ] ) params.refractionRatio = 1 / attributes[ 'Refraction Index' ].value;
-
- this.parseStandardAttributes( params, attributes, maps );
- this.parsePhongAttributes( params, attributes, maps );
-
- return params;
-
- },
-
- parseStandardAttributes( params, attributes, maps ) {
-
- if ( attributes.Luminous && attributes.Luminous.value !== 0 && attributes[ 'Luminous Color' ] ) {
-
- var emissiveColor = attributes[ 'Luminous Color' ].value.map( function ( val ) {
-
- return val * attributes.Luminous.value;
-
- } );
-
- params.emissive = new THREE.Color().fromArray( emissiveColor );
-
- }
- if ( attributes.Roughness && ! maps.roughnessMap ) params.roughness = attributes.Roughness.value;
- if ( attributes.Metallic && ! maps.metalnessMap ) params.metalness = attributes.Metallic.value;
-
- },
-
- parsePhongAttributes( params, attributes, maps ) {
-
- if ( attributes.Diffuse ) params.color.multiplyScalar( attributes.Diffuse.value );
-
- if ( attributes.Reflection ) {
-
- params.reflectivity = attributes.Reflection.value;
- params.combine = THREE.AddOperation;
-
- }
-
- if ( attributes.Luminosity && ! maps.emissiveMap ) params.emissive = new THREE.Color().setScalar( attributes.Luminosity.value );
-
- if ( attributes.Glossiness !== undefined ) params.shininess = 5 + Math.pow( attributes.Glossiness.value * 7, 6 );
-
- // parse specular if there is no roughness - we will interpret the material as 'Phong' in this case
- if ( ! attributes.Roughness && attributes.Specular && ! maps.specularMap ) params.specular = new THREE.Color().setScalar( attributes.Specular.value * 1.5 );
-
- },
-
- parseEnvMap( connections, maps, attributes ) {
-
- if ( connections.envMap ) {
-
- var envMap = this.loadTexture( connections.envMap );
-
- if ( attributes.transparent && attributes.opacity < 0.999 ) {
-
- envMap.mapping = THREE.EquirectangularRefractionMapping;
-
- // Reflectivity and refraction mapping don't work well together in Phong materials
- if ( attributes.reflectivity !== undefined ) {
-
- delete attributes.reflectivity;
- delete attributes.combine;
-
- }
-
- if ( attributes.metalness !== undefined ) {
-
- delete attributes.metalness;
-
- }
-
- } else envMap.mapping = THREE.EquirectangularReflectionMapping;
-
- maps.envMap = envMap;
-
- }
-
- },
-
- // get texture defined at top level by its index
- getTexturePathByIndex( index ) {
-
- var fileName = '';
-
- if ( ! lwoTree.textures ) return fileName;
-
- lwoTree.textures.forEach( function ( texture ) {
-
- if ( texture.index === index ) fileName = texture.fileName;
-
- } );
-
- return fileName;
-
- },
-
- loadTexture( path ) {
-
- if ( ! path ) return null;
-
- return this.textureLoader.load( this.cleanPath( path ) );
-
- },
-
- // Lightwave expects textures to be in folder called Images relative
- // to the model
- // Otherwise, the full absolute path is stored: D://some_directory/textures/bumpMap.png
- // In this case, we'll strip out everything and load 'bumpMap.png' from the same directory as the model
- cleanPath( path ) {
-
- if ( path.indexOf( 'Images' ) === 0 ) return './' + path;
- return path.split( '/' ).pop().split( '\\' ).pop();
-
- },
-
- // 0 = Reset, 1 = Repeat, 2 = Mirror, 3 = Edge
- getWrappingType( num ) {
-
- switch ( num ) {
-
- case 0:
- console.warn( 'LWOLoader: "Reset" texture wrapping type is not supported in three.js' );
- return THREE.ClampToEdgeWrapping;
- case 1: return THREE.RepeatWrapping;
- case 2: return THREE.MirroredRepeatWrapping;
- case 3: return THREE.ClampToEdgeWrapping;
-
- }
-
- },
-
- getType( nodeData ) {
-
- if ( nodeData.roughness ) return 'Standard';
- return 'Phong';
-
- },
-
- };
-
- function GeometryParser() {}
-
- GeometryParser.prototype = {
-
- constructor: GeometryParser,
-
- parse( geoData, layer ) {
-
- var geometry = new THREE.BufferGeometry();
-
- geometry.addAttribute( 'position', new THREE.Float32BufferAttribute( geoData.points, 3 ) );
-
- var indices = this.splitIndices( geoData.vertexIndices, geoData.polygonDimensions );
- geometry.setIndex( indices );
-
- this.parseGroups( geometry, geoData );
-
- geometry.computeVertexNormals();
-
- this.parseUVs( geometry, layer, indices );
- this.parseMorphTargets( geometry, layer, indices );
-
- // TODO: z may need to be reversed to account for coordinate system change
- geometry.translate( - layer.pivot[ 0 ], - layer.pivot[ 1 ], - layer.pivot[ 2 ] );
-
- // var userData = geometry.userData;
- // geometry = geometry.toNonIndexed()
- // geometry.userData = userData;
-
- return geometry;
-
- },
-
- // split quads into tris
- splitIndices( indices, polygonDimensions ) {
-
- var remappedIndices = [];
-
- var i = 0;
- polygonDimensions.forEach( function ( dim ) {
-
- if ( dim < 4 ) {
-
- for ( var k = 0; k < dim; k ++ ) remappedIndices.push( indices[ i + k ] );
-
- } else if ( dim === 4 ) {
-
- remappedIndices.push(
- indices[ i ],
- indices[ i + 1 ],
- indices[ i + 2 ],
-
- indices[ i ],
- indices[ i + 2 ],
- indices[ i + 3 ]
-
- );
-
- } else if ( dim > 4 ) console.warn( 'LWOLoader: polygons with greater than 4 sides are not supported' );
-
- i += dim;
-
- } );
-
- return remappedIndices;
-
- },
-
- // NOTE: currently ignoring poly indices and assuming that they are intelligently ordered
- parseGroups( geometry, geoData ) {
-
- var tags = lwoTree.tags;
- var matNames = [];
-
- var elemSize = 3;
- if ( geoData.type === 'lines' ) elemSize = 2;
- if ( geoData.type === 'points' ) elemSize = 1;
-
- var remappedIndices = this.splitMaterialIndices( geoData.polygonDimensions, geoData.materialIndices );
-
- var indexNum = 0; // create new indices in numerical order
- var indexPairs = {}; // original indices mapped to numerical indices
-
- var prevMaterialIndex;
-
- var prevStart = 0;
- var currentCount = 0;
-
- for ( var i = 0; i < remappedIndices.length; i += 2 ) {
-
- var materialIndex = remappedIndices[ i + 1 ];
-
- if ( i === 0 ) matNames[ indexNum ] = tags[ materialIndex ];
-
- if ( prevMaterialIndex === undefined ) prevMaterialIndex = materialIndex;
-
- if ( materialIndex !== prevMaterialIndex ) {
-
- var currentIndex;
- if ( indexPairs[ tags[ prevMaterialIndex ] ] ) {
-
- currentIndex = indexPairs[ tags[ prevMaterialIndex ] ];
-
- } else {
-
- currentIndex = indexNum;
- indexPairs[ tags[ prevMaterialIndex ] ] = indexNum;
- matNames[ indexNum ] = tags[ prevMaterialIndex ];
- indexNum ++;
-
- }
-
- geometry.addGroup( prevStart, currentCount, currentIndex );
-
- prevStart += currentCount;
-
- prevMaterialIndex = materialIndex;
- currentCount = 0;
-
- }
-
- currentCount += elemSize;
-
- }
-
- // the loop above doesn't add the last group, do that here.
- if ( geometry.groups.length > 0 ) {
-
- var currentIndex;
- if ( indexPairs[ tags[ materialIndex ] ] ) {
-
- currentIndex = indexPairs[ tags[ materialIndex ] ];
-
- } else {
-
- currentIndex = indexNum;
- indexPairs[ tags[ materialIndex ] ] = indexNum;
- matNames[ indexNum ] = tags[ materialIndex ];
-
- }
-
- geometry.addGroup( prevStart, currentCount, currentIndex );
-
- }
-
- // Mat names from TAGS chunk, used to build up an array of materials for this geometry
- geometry.userData.matNames = matNames;
-
- },
-
- splitMaterialIndices( polygonDimensions, indices ) {
-
- var remappedIndices = [];
-
- polygonDimensions.forEach( function ( dim, i ) {
-
- if ( dim <= 3 ) {
-
- remappedIndices.push( indices[ i * 2 ], indices[ i * 2 + 1 ] );
-
- } else if ( dim === 4 ) {
-
- remappedIndices.push( indices[ i * 2 ], indices[ i * 2 + 1 ], indices[ i * 2 ], indices[ i * 2 + 1 ] );
-
- } // ignore > 4 for now
-
- } );
-
- return remappedIndices;
-
- },
-
- // UV maps:
- // 1: are defined via index into an array of points, not into a geometry
- // - the geometry is also defined by an index into this array, but the indexes may not match
- // 2: there can be any number of UV maps for a single geometry. Here these are combined,
- // with preference given to the first map encountered
- // 3: UV maps can be partial - that is, defined for only a part of the geometry
- // 4: UV maps can be VMAP or VMAD (discontinuous, to allow for seams). In practice, most
- // UV maps are defined as partially VMAP and partially VMAD
- // VMADs are currently not supported
- parseUVs( geometry, layer ) {
-
- // start by creating a UV map set to zero for the whole geometry
- var remappedUVs = Array.from( Array( geometry.attributes.position.count * 2 ), function () {
-
- return 0;
-
- } );
-
- for ( var name in layer.uvs ) {
-
- var uvs = layer.uvs[ name ].uvs;
- var uvIndices = layer.uvs[ name ].uvIndices;
-
- uvIndices.forEach( function ( i, j ) {
-
- remappedUVs[ i * 2 ] = uvs[ j * 2 ];
- remappedUVs[ i * 2 + 1 ] = uvs[ j * 2 + 1 ];
-
- } );
-
- }
-
- geometry.addAttribute( 'uv', new THREE.Float32BufferAttribute( remappedUVs, 2 ) );
-
- },
-
- parseMorphTargets( geometry, layer ) {
-
- var num = 0;
- for ( var name in layer.morphTargets ) {
-
- var remappedPoints = geometry.attributes.position.array.slice();
-
- if ( ! geometry.morphAttributes.position ) geometry.morphAttributes.position = [];
-
- var morphPoints = layer.morphTargets[ name ].points;
- var morphIndices = layer.morphTargets[ name ].indices;
- var type = layer.morphTargets[ name ].type;
-
- morphIndices.forEach( function ( i, j ) {
-
- if ( type === 'relative' ) {
-
- remappedPoints[ i * 3 ] += morphPoints[ j * 3 ];
- remappedPoints[ i * 3 + 1 ] += morphPoints[ j * 3 + 1 ];
- remappedPoints[ i * 3 + 2 ] += morphPoints[ j * 3 + 2 ];
-
- } else {
-
- remappedPoints[ i * 3 ] = morphPoints[ j * 3 ];
- remappedPoints[ i * 3 + 1 ] = morphPoints[ j * 3 + 1 ];
- remappedPoints[ i * 3 + 2 ] = morphPoints[ j * 3 + 2 ];
-
- }
-
- } );
-
- geometry.morphAttributes.position[ num ] = new THREE.Float32BufferAttribute( remappedPoints, 3 );
- geometry.morphAttributes.position[ num ].name = name;
-
- num ++;
-
- }
-
- },
-
- };
-
- // parse data from the IFF buffer.
- // LWO3 files are in IFF format and can contain the following data types, referred to by shorthand codes
- //
- // ATOMIC DATA TYPES
- // ID Tag - 4x 7 bit uppercase ASCII chars: ID4
- // signed integer, 1, 2, or 4 byte length: I1, I2, I4
- // unsigned integer, 1, 2, or 4 byte length: U1, U2, U4
- // float, 4 byte length: F4
- // string, series of ASCII chars followed by null byte (If the length of the string including the null terminating byte is odd, an extra null is added so that the data that follows will begin on an even byte boundary): S0
- //
- // COMPOUND DATA TYPES
- // Variable-length Index (index into an array or collection): U2 or U4 : VX
- // Color (RGB): F4 + F4 + F4: COL12
- // Coordinate (x, y, z): F4 + F4 + F4: VEC12
- // Percentage F4 data type from 0->1 with 1 = 100%: FP4
- // Angle in radian F4: ANG4
- // Filename (string) S0: FNAM0
- // XValue F4 + index (VX) + optional envelope( ENVL ): XVAL
- // XValue vector VEC12 + index (VX) + optional envelope( ENVL ): XVAL3
- //
- // The IFF file is arranged in chunks:
- // CHUNK = ID4 + length (U4) + length X bytes of data + optional 0 pad byte
- // optional 0 pad byte is there to ensure chunk ends on even boundary, not counted in size
-
- // Chunks are combined in Forms (collections of chunks)
- // FORM = string 'FORM' (ID4) + length (U4) + type (ID4) + optional ( CHUNK | FORM )
-
- // CHUNKS and FORMS are collectively referred to as blocks
-
- // The entire file is contained in one top level FORM
- function IFFParser() {}
-
- IFFParser.prototype = {
-
- constructor: IFFParser,
-
- parse: function ( buffer ) {
-
- // dump the whole buffer as a string for testing
- // printBuffer( buffer );
-
- this.reader = new DataViewReader( buffer );
-
- this.tree = {
- materials: {},
- layers: [],
- tags: [],
- textures: [],
- };
-
- // start out at the top level to add any data before first layer is encountered
- this.currentLayer = this.tree;
- this.currentForm = this.tree;
-
- // parse blocks until end of file is reached
- while ( ! this.reader.endOfFile() ) this.parseBlock();
-
- return this.tree;
-
- },
-
- parseBlock() {
-
- var blockID = this.reader.getIDTag();
- var length = this.reader.getUint32(); // size of data in bytes
-
- // Data types may be found in either LWO2 OR LWO3 spec
- switch ( blockID ) {
-
- case 'FORM': // form blocks may consist of sub -chunks or sub-forms
- this.parseForm( length );
- break;
-
- // SKIPPED CHUNKS
-
- // MISC skipped
- case 'ICON': // Thumbnail Icon Image
- case 'VMPA': // Vertex Map Parameter
- case 'BBOX': // bounding box
- // case 'VMMD':
- // case 'VTYP':
-
- // normal maps can be specified, normally on models imported from other applications. Currently ignored
- case 'NORM':
-
- // ENVL FORM skipped
- case 'PRE ':
- case 'POST':
- case 'KEY ':
- case 'SPAN':
-
- // CLIP FORM skipped
- case 'TIME':
- case 'CLRS':
- case 'CLRA':
- case 'FILT':
- case 'DITH':
- case 'CONT':
- case 'BRIT':
- case 'SATR':
- case 'HUE ':
- case 'GAMM':
- case 'NEGA':
- case 'IFLT':
- case 'PFLT':
-
- // Image Map Layer skipped
- case 'PROJ':
- case 'AXIS':
- case 'AAST':
- case 'PIXB':
- case 'STCK':
-
- // Procedural Textures skipped
- case 'VALU':
-
- // Gradient Textures skipped
- case 'PNAM':
- case 'INAM':
- case 'GRST':
- case 'GREN':
- case 'GRPT':
- case 'FKEY':
- case 'IKEY':
-
- // Texture Mapping Form skipped
- case 'CSYS':
-
- // Surface CHUNKs skipped
- case 'OPAQ': // top level 'opacity' checkbox
- case 'CMAP': // clip map
-
- // Surface node CHUNKS skipped
- // These mainly specify the node editor setup in LW
- case 'NLOC':
- case 'NZOM':
- case 'NVER':
- case 'NSRV':
- case 'NCRD':
- case 'NMOD':
- case 'NPRW':
- case 'NPLA':
- case 'VERS':
- case 'ENUM':
- case 'FLAG':
- case 'TAG ':
-
- // Car Material CHUNKS
- case 'CGMD':
- case 'CGTY':
- case 'CGST':
- case 'CGEN':
- case 'CGTS':
- case 'CGTE':
- case 'OSMP':
- case 'OMDE':
- case 'OUTR':
- this.reader.skip( length );
- break;
-
- // Skipped LWO2 chunks
- case 'DIFF': // diffuse level, may be necessary to modulate COLR with this
- case 'TRNL':
- case 'REFL':
- case 'GLOS':
- case 'SHRP':
- case 'RFOP':
- case 'RSAN':
- case 'TROP':
- case 'RBLR':
- case 'TBLR':
- case 'CLRH':
- case 'CLRF':
- case 'ADTR':
- case 'GLOW':
- case 'LINE':
- case 'ALPH':
- case 'LINE':
- case 'VCOL':
- case 'ENAB':
- this.reader.skip( length );
- break;
-
- // Texture node chunks (not in spec)
- case 'IPIX': // usePixelBlending
- case 'IMIP': // useMipMaps
- case 'IMOD': // imageBlendingMode
- case 'AMOD': // unknown
- case 'IINV': // imageInvertAlpha
- case 'INCR': // imageInvertColor
- case 'IAXS': // imageAxis ( for non-UV maps)
- case 'IFOT': // imageFallofType
- case 'ITIM': // timing for animated textures
- case 'IWRL':
- case 'IUTI':
- case 'IINX':
- case 'IINY':
- case 'IINZ':
- case 'IREF': // possibly a VX for reused texture nodes
- if ( length === 4 ) this.currentNode[ blockID ] = this.reader.getInt32();
- else this.reader.skip( length );
- break;
-
- case 'OTAG':
- this.parseObjectTag();
- break;
-
- case 'LAYR':
- this.parseLayer( length );
- break;
-
- case 'PNTS':
- this.parsePoints( length );
- break;
-
- case 'VMAP':
- this.parseVertexMapping( length );
- break;
-
- case 'POLS':
- this.parsePolygonList( length );
- break;
-
- case 'TAGS':
- this.parseTagStrings( length );
- break;
-
- case 'PTAG':
- this.parsePolygonTagMapping( length );
- break;
-
- case 'VMAD':
- this.parseVertexMapping( length, true );
- break;
-
- // Misc CHUNKS
- case 'DESC': // Description Line
- this.currentForm.description = this.reader.getString();
- break;
-
- case 'TEXT':
- case 'CMNT':
- case 'NCOM':
- this.currentForm.comment = this.reader.getString();
- break;
-
- // Envelope Form
- case 'NAME':
- this.currentForm.channelName = this.reader.getString();
- break;
-
- // Image Map Layer
-
- case 'WRAP':
- this.currentForm.wrap = { w: this.reader.getUint16(), h: this.reader.getUint16() };
- break;
-
- case 'IMAG':
- var index = this.reader.getVariableLengthIndex();
- this.currentForm.imageIndex = index;
- break;
-
- // Texture Mapping Form
-
- case 'OREF':
- this.currentForm.referenceObject = this.reader.getString();
- break;
-
- case 'ROID':
- this.currentForm.referenceObjectID = this.reader.getUint32();
- break;
-
- // Surface Blocks
-
- case 'SSHN':
- this.currentSurface.surfaceShaderName = this.reader.getString();
- break;
-
- case 'AOVN':
- this.currentSurface.surfaceCustomAOVName = this.reader.getString();
- break;
-
- // Nodal Blocks
-
- case 'NSTA':
- this.currentForm.disabled = this.reader.getUint16();
- break;
-
- case 'NRNM':
- this.currentForm.realName = this.reader.getString();
- break;
-
- case 'NNME':
- this.currentForm.refName = this.reader.getString();
- this.currentSurface.nodes[ this.currentForm.refName ] = this.currentForm;
- break;
-
- // Nodal Blocks : connections
- case 'INME':
- if ( ! this.currentForm.nodeName ) this.currentForm.nodeName = [];
- this.currentForm.nodeName.push( this.reader.getString() );
- break;
-
- case 'IINN':
- if ( ! this.currentForm.inputNodeName ) this.currentForm.inputNodeName = [];
- this.currentForm.inputNodeName.push( this.reader.getString() );
- break;
-
- case 'IINM':
- if ( ! this.currentForm.inputName ) this.currentForm.inputName = [];
- this.currentForm.inputName.push( this.reader.getString() );
- break;
-
- case 'IONM':
- if ( ! this.currentForm.inputOutputName ) this.currentForm.inputOutputName = [];
- this.currentForm.inputOutputName.push( this.reader.getString() );
- break;
-
- case 'FNAM':
- this.currentForm.fileName = this.reader.getString();
- break;
-
- case 'CHAN': // NOTE: ENVL Forms may also have CHAN chunk, however ENVL is currently ignored
- if ( length === 4 ) this.currentForm.textureChannel = this.reader.getIDTag();
- else this.reader.skip( length );
- break;
-
- // LWO2 Spec chunks: these are needed since the SURF FORMs are often in LWO2 format
-
- case 'SMAN':
- var maxSmoothingAngle = this.reader.getFloat32();
- this.currentSurface.attributes.smooth = ( maxSmoothingAngle < 0 ) ? false : true;
- break;
-
- case 'ENAB':
- this.currentForm.enabled = this.reader.getUint16();
- break;
-
- // LWO2: Basic Surface Parameters
- case 'COLR':
- this.currentSurface.attributes.color = this.reader.getFloat32Array( 3 );
- this.reader.skip( 2 ); // VX: envelope
- break;
-
- case 'LUMI':
- this.currentSurface.attributes.luminosityLevel = this.reader.getFloat32();
- this.reader.skip( 2 );
- break;
-
- case 'SPEC':
- this.currentSurface.attributes.specularLevel = this.reader.getFloat32();
- this.reader.skip( 2 );
- break;
-
- case 'REFL':
- this.currentSurface.attributes.reflectivity = this.reader.getFloat32();
- this.reader.skip( 2 );
- break;
-
- case 'TRAN':
- this.currentSurface.attributes.opacity = this.reader.getFloat32();
- this.reader.skip( 2 );
- break;
-
- case 'BUMP':
- this.currentSurface.attributes.bumpStrength = this.reader.getFloat32();
- this.reader.skip( 2 );
- break;
-
- case 'SIDE':
- this.currentSurface.attributes.side = this.reader.getUint16();
- break;
-
- case 'RIMG':
- this.currentSurface.attributes.reflectionMap = this.reader.getVariableLengthIndex();
- break;
-
- case 'RIND':
- this.currentSurface.attributes.refractiveIndex = this.reader.getFloat32();
- this.reader.skip( 2 );
- break;
-
- case 'TIMG':
- this.currentSurface.attributes.refractionMap = this.reader.getVariableLengthIndex();
- break;
-
- case 'IMAP':
- this.currentSurface.attributes.imageMapIndex = this.reader.getUint32();
- break;
-
- case 'IUVI': // uv channel name
- this.currentNode.UVChannel = this.reader.getString( length );
- break;
-
- case 'IUTL': // widthWrappingMode: 0 = Reset, 1 = Repeat, 2 = Mirror, 3 = Edge
- this.currentNode.widthWrappingMode = this.reader.getUint32();
- break;
- case 'IVTL': // heightWrappingMode
- this.currentNode.heightWrappingMode = this.reader.getUint32();
- break;
-
- default:
- this.parseUnknownCHUNK( blockID, length );
-
- }
-
- if ( this.reader.offset >= this.currentFormEnd ) {
-
- this.currentForm = this.parentForm;
-
- }
-
- },
-
-
- ///
- // FORM PARSING METHODS
- ///
-
- // Forms are organisational and can contain any number of sub chunks and sub forms
- // FORM ::= 'FORM'[ID4], length[U4], type[ID4], ( chunk[CHUNK] | form[FORM] ) * }
- parseForm( length ) {
-
- var type = this.reader.getIDTag();
-
- switch ( type ) {
-
- // SKIPPED FORMS
- // if skipForm( length ) is called, the entire form and any sub forms and chunks are skipped
-
- case 'ISEQ': // Image sequence
- case 'ANIM': // plug in animation
- case 'STCC': // Color-cycling Still
- case 'VPVL':
- case 'VPRM':
- case 'NROT':
- case 'WRPW': // image wrap w ( for cylindrical and spherical projections)
- case 'WRPH': // image wrap h
- case 'FUNC':
- case 'FALL':
- case 'OPAC':
- case 'GRAD': // gradient texture
- case 'ENVS':
- case 'VMOP':
- case 'VMBG':
-
- // Car Material FORMS
- case 'OMAX':
- case 'STEX':
- case 'CKBG':
- case 'CKEY':
- case 'VMLA':
- case 'VMLB':
- this.skipForm( length ); // not currently supported
- break;
-
- // if break; is called directly, the position in the lwoTree is not created
- // any sub chunks and forms are added to the parent form instead
- case 'META':
- case 'NNDS':
- case 'NODS':
- case 'NDTA':
- case 'ADAT':
- case 'AOVS':
- case 'BLOK':
-
- // used by texture nodes
- case 'IBGC': // imageBackgroundColor
- case 'IOPC': // imageOpacity
- case 'IIMG': // hold reference to image path
- case 'TXTR':
- // this.setupForm( type, length );
- break;
-
- case 'IFAL': // imageFallof
- case 'ISCL': // imageScale
- case 'IPOS': // imagePosition
- case 'IROT': // imageRotation
- case 'IBMP':
- case 'IUTD':
- case 'IVTD':
- this.parseTextureNodeAttribute( type );
- break;
-
- case 'LWO3':
- this.tree.format = type;
- break;
-
- case 'ENVL':
- this.parseEnvelope( length );
- break;
-
- // CLIP FORM AND SUB FORMS
-
- case 'CLIP':
- this.parseClip( length );
- break;
-
- case 'STIL':
- this.parseImage();
- break;
-
- case 'XREF': // clone of another STIL
- this.reader.skip( 8 ); // unknown
- this.currentForm.referenceTexture = {
- index: this.reader.getUint32(),
- refName: this.reader.getString() // internal unique ref
- };
- break;
-
- // Not in spec, used by texture nodes
-
- case 'IMST':
- this.parseImageStateForm( length );
- break;
-
- // SURF FORM AND SUB FORMS
-
- case 'SURF':
- this.parseSurfaceForm( length );
- break;
-
- case 'VALU': // Not in spec
- this.parseValueForm( length );
- break;
-
- case 'NTAG':
- this.parseSubNode( length );
- break;
-
- case 'NNDS':
- this.setupForm( 'nodes', length );
- break;
-
- case 'ATTR': // BSDF Node Attributes
- case 'SATR': // Standard Node Attributes
- this.setupForm( 'attributes', length );
- break;
-
- case 'NCON':
- this.parseConnections( length );
- break;
-
- case 'SSHA':
- this.parentForm = this.currentForm;
- this.currentForm = this.currentSurface;
- this.setupForm( 'surfaceShader', length );
- break;
-
- case 'SSHD':
- this.setupForm( 'surfaceShaderData', length );
- break;
-
- case 'ENTR': // Not in spec
- this.parseEntryForm( length );
- break;
-
- // Image Map Layer
-
- case 'IMAP':
- this.parseImageMap( length );
- break;
-
- case 'TAMP':
- this.parseXVAL( 'amplitude', length );
- break;
-
- //Texture Mapping Form
-
- case 'TMAP':
- this.setupForm( 'textureMap', length );
- break;
-
- case 'CNTR':
- this.parseXVAL3( 'center', length );
- break;
-
- case 'SIZE':
- this.parseXVAL3( 'scale', length );
- break;
-
- case 'ROTA':
- this.parseXVAL3( 'rotation', length );
- break;
-
- default:
- this.parseUnknownForm( type, length );
-
- }
-
- },
-
- setupForm( type, length ) {
-
- if ( ! this.currentForm ) this.currentForm = this.currentNode;
-
- this.currentFormEnd = this.reader.offset + length;
- this.parentForm = this.currentForm;
-
- if ( ! this.currentForm[ type ] ) {
-
- this.currentForm[ type ] = {};
- this.currentForm = this.currentForm[ type ];
-
-
- } else {
-
- // should never see this unless there's a bug in the reader
- console.warn( 'LWOLoader: form already exists on parent: ', type, this.currentForm );
-
- this.currentForm = this.currentForm[ type ];
-
- }
-
-
- },
-
- skipForm( length ) {
-
- this.reader.skip( length - 4 );
-
- },
-
- parseUnknownForm( type, length ) {
-
- console.warn( 'LWOLoader: unknown FORM encountered: ' + type, length );
-
- printBuffer( this.reader.dv.buffer, this.reader.offset, length - 4 );
- this.reader.skip( length - 4 );
-
- },
-
- parseSurfaceForm( length ) {
-
- this.reader.skip( 8 ); // unknown Uint32 x2
-
- var name = this.reader.getString();
-
- var surface = {
- attributes: {}, // LWO2 style non-node attributes will go here
- connections: {},
- name: name,
- nodes: {},
- source: this.reader.getString(),
- };
-
- this.tree.materials[ name ] = surface;
- this.currentSurface = surface;
-
- this.parentForm = this.tree.materials;
- this.currentForm = surface;
- this.currentFormEnd = this.reader.offset + length;
-
- },
-
- parseSubNode( length ) {
-
- // parse the NRNM CHUNK of the subnode FORM to get
- // a meaningful name for the subNode
- // some subnodes can be renamed, but Input and Surface cannot
-
- this.reader.skip( 8 ); // NRNM + length
- var name = this.reader.getString();
-
- var node = {
- name: name
- };
- this.currentForm = node;
- this.currentNode = node;
-
- this.currentFormEnd = this.reader.offset + length;
-
-
- },
-
- // collect attributes from all nodes at the top level of a surface
- parseConnections( length ) {
-
- this.currentFormEnd = this.reader.offset + length;
- this.parentForm = this.currentForm;
-
- this.currentForm = this.currentSurface.connections;
-
- },
-
- // surface node attribute data, e.g. specular, roughness etc
- parseEntryForm( length ) {
-
- this.reader.skip( 8 ); // NAME + length
- var name = this.reader.getString();
- this.currentForm = this.currentNode.attributes;
-
- this.setupForm( name, length );
-
- },
-
- // parse values from material - doesn't match up to other LWO3 data types
- // sub form of entry form
- parseValueForm() {
-
- this.reader.skip( 8 ); // unknown + length
-
- var valueType = this.reader.getString();
-
- if ( valueType === 'double' ) {
-
- this.currentForm.value = this.reader.getUint64();
-
- } else if ( valueType === 'int' ) {
-
- this.currentForm.value = this.reader.getUint32();
-
- } else if ( valueType === 'vparam' ) {
-
- this.reader.skip( 24 );
- this.currentForm.value = this.reader.getFloat64();
-
- } else if ( valueType === 'vparam3' ) {
-
- this.reader.skip( 24 );
- this.currentForm.value = this.reader.getFloat64Array( 3 );
-
-
- }
-
- },
-
- // holds various data about texture node image state
- // Data other thanmipMapLevel unknown
- parseImageStateForm() {
-
- this.reader.skip( 8 ); // unknown
-
- this.currentForm.mipMapLevel = this.reader.getFloat32();
-
- },
-
- // LWO2 style image data node OR LWO3 textures defined at top level in editor (not as SURF node)
- parseImageMap( length ) {
-
- this.currentFormEnd = this.reader.offset + length;
- this.parentForm = this.currentForm;
-
- if ( ! this.currentForm.maps ) this.currentForm.maps = [];
-
- var map = {};
- this.currentForm.maps.push( map );
- this.currentForm = map;
-
- this.reader.skip( 10 ); // unknown, could be an issue if it contains a VX
-
- },
-
- parseTextureNodeAttribute( type ) {
-
- this.reader.skip( 28 ); // FORM + length + VPRM + unknown + Uint32 x2 + float32
-
- this.reader.skip( 20 ); // FORM + length + VPVL + float32 + Uint32
-
- switch ( type ) {
-
- case 'ISCL':
- this.currentNode.scale = this.reader.getFloat32Array( 3 );
- break;
- case 'IPOS':
- this.currentNode.position = this.reader.getFloat32Array( 3 );
- break;
- case 'IROT':
- this.currentNode.rotation = this.reader.getFloat32Array( 3 );
- break;
- case 'IFAL':
- this.currentNode.falloff = this.reader.getFloat32Array( 3 );
- break;
-
- case 'IBMP':
- this.currentNode.amplitude = this.reader.getFloat32();
- break;
- case 'IUTD':
- this.currentNode.uTiles = this.reader.getFloat32();
- break;
- case 'IVTD':
- this.currentNode.vTiles = this.reader.getFloat32();
- break;
-
- }
-
- this.reader.skip( 2 ); // unknown
-
-
- },
-
- // ENVL forms are currently ignored
- parseEnvelope( length ) {
-
- this.reader.skip( length - 4 ); // skipping entirely for now
-
- },
-
- ///
- // CHUNK PARSING METHODS
- ///
-
- // clips can either be defined inside a surface node, or at the top
- // level and they have a different format in each case
- parseClip( length ) {
-
- var tag = this.reader.getIDTag();
-
- // inside surface node
- if ( tag === 'FORM' ) {
-
- this.reader.skip( 16 );
-
- this.currentNode.fileName = this.reader.getString();
-
- return;
-
- }
-
- // otherwise top level
- this.reader.setOffset( this.reader.offset - 4 );
-
- this.currentFormEnd = this.reader.offset + length;
- this.parentForm = this.currentForm;
-
- this.reader.skip( 8 ); // unknown
-
- var texture = {
- index: this.reader.getUint32()
- };
- this.tree.textures.push( texture );
- this.currentForm = texture;
-
- },
-
- parseImage() {
-
- this.reader.skip( 8 ); // unknown
- this.currentForm.fileName = this.reader.getString();
-
- },
-
- parseXVAL( type, length ) {
-
- var endOffset = this.reader.offset + length - 4;
- this.reader.skip( 8 );
-
- this.currentForm[ type ] = this.reader.getFloat32();
-
- this.reader.setOffset( endOffset ); // set end offset directly to skip optional envelope
-
- },
-
- parseXVAL3( type, length ) {
-
- var endOffset = this.reader.offset + length - 4;
- this.reader.skip( 8 );
-
- this.currentForm[ type ] = {
- x: this.reader.getFloat32(),
- y: this.reader.getFloat32(),
- z: this.reader.getFloat32(),
- };
-
- this.reader.setOffset( endOffset );
-
- },
-
- // Tags associated with an object
- // OTAG { type[ID4], tag-string[S0] }
- parseObjectTag() {
-
- if ( ! this.tree.objectTags ) this.tree.objectTags = {};
-
- this.tree.objectTags[ this.reader.getIDTag() ] = {
- tagString: this.reader.getString()
- };
-
- },
-
- // Signals the start of a new layer. All the data chunks which follow will be included in this layer until another layer chunk is encountered.
- // LAYR: number[U2], flags[U2], pivot[VEC12], name[S0], parent[U2]
- parseLayer( length ) {
-
- var layer = {
- number: this.reader.getUint16(),
- flags: this.reader.getUint16(), // If the least significant bit of flags is set, the layer is hidden.
- pivot: this.reader.getFloat32Array( 3 ), // Note: this seems to be superflous, as the geometry is translated when pivot is present
- name: this.reader.getString(),
- };
-
- this.tree.layers.push( layer );
- this.currentLayer = layer;
-
- var parsedLength = 16 + stringOffset( this.currentLayer.name ); // index ( 2 ) + flags( 2 ) + pivot( 12 ) + stringlength
-
- // if we have not reached then end of the layer block, there must be a parent defined
- this.currentLayer.parent = ( parsedLength < length ) ? this.reader.getUint16() : - 1; // omitted or -1 for no parent
-
- },
-
- // VEC12 * ( F4 + F4 + F4 ) array of x,y,z vectors
- // Converting from left to right handed coordinate system:
- // x -> -x and switch material FrontSide -> BackSide
- parsePoints( length ) {
-
- this.currentPoints = [];
- for ( var i = 0; i < length / 4; i += 3 ) {
-
- // z -> -z to match three.js right handed coords
- this.currentPoints.push( this.reader.getFloat32(), this.reader.getFloat32(), - this.reader.getFloat32() );
-
- }
-
- },
-
- // parse VMAP or VMAD
- // Associates a set of floating-point vectors with a set of points.
- // VMAP: { type[ID4], dimension[U2], name[S0], ( vert[VX], value[F4] # dimension ) * }
-
- // VMAD Associates a set of floating-point vectors with the vertices of specific polygons.
- // Similar to VMAP UVs, but associates with polygon vertices rather than points
- // to solve to problem of UV seams: VMAD chunks are paired with VMAPs of the same name,
- // if they exist. The vector values in the VMAD will then replace those in the
- // corresponding VMAP, but only for calculations involving the specified polygons.
- // VMAD { type[ID4], dimension[U2], name[S0], ( vert[VX], poly[VX], value[F4] # dimension ) * }
- parseVertexMapping( length, discontinuous ) {
-
- var finalOffset = this.reader.offset + length;
-
- var channelName = this.reader.getString();
-
- if ( this.reader.offset === finalOffset ) {
-
- // then we are in a texture node and the VMAP chunk is just a reference to a UV channel name
- this.currentForm.UVChannel = channelName;
- return;
-
- }
-
- // otherwise reset to initial length and parse normal VMAP CHUNK
- this.reader.setOffset( this.reader.offset - stringOffset( channelName ) );
-
- var type = this.reader.getIDTag();
-
- this.reader.getUint16(); // dimension
- var name = this.reader.getString();
-
- var remainingLength = length - 6 - stringOffset( name );
-
- switch ( type ) {
-
- case 'TXUV':
- this.parseUVMapping( name, finalOffset, discontinuous );
- break;
- case 'MORF':
- case 'SPOT':
- this.parseMorphTargets( name, finalOffset, type ); // can't be discontinuous
- break;
- // unsupported VMAPs
- case 'APSL':
- case 'NORM':
- case 'WGHT':
- case 'MNVW':
- case 'PICK':
- case 'RGB ':
- case 'RGBA':
- this.reader.skip( remainingLength );
- break;
- default:
- console.warn( 'LWOLoader: unknown vertex map type: ' + type );
- this.reader.skip( remainingLength );
-
- }
-
- },
-
- parseUVMapping( name, finalOffset, discontinuous ) {
-
- var uvIndices = [];
- var polyIndices = [];
- var uvs = [];
-
- while ( this.reader.offset < finalOffset ) {
-
- uvIndices.push( this.reader.getVariableLengthIndex() );
-
- if ( discontinuous ) polyIndices.push( this.reader.getVariableLengthIndex() );
-
- uvs.push( this.reader.getFloat32(), this.reader.getFloat32() );
-
- }
-
- if ( discontinuous ) {
-
- if ( ! this.currentLayer.discontinuousUVs ) this.currentLayer.discontinuousUVs = {};
-
- this.currentLayer.discontinuousUVs[ name ] = {
- uvIndices: uvIndices,
- polyIndices: polyIndices,
- uvs: uvs,
- };
-
- } else {
-
- if ( ! this.currentLayer.uvs ) this.currentLayer.uvs = {};
-
- this.currentLayer.uvs[ name ] = {
- uvIndices: uvIndices,
- uvs: uvs,
- };
-
- }
-
- },
-
- parseMorphTargets( name, finalOffset, type ) {
-
- var indices = [];
- var points = [];
-
- type = ( type === 'MORF' ) ? 'relative' : 'absolute';
-
- while ( this.reader.offset < finalOffset ) {
-
- indices.push( this.reader.getVariableLengthIndex() );
- // z -> -z to match three.js right handed coords
- points.push( this.reader.getFloat32(), this.reader.getFloat32(), - this.reader.getFloat32() );
-
- }
-
- if ( ! this.currentLayer.morphTargets ) this.currentLayer.morphTargets = {};
-
- this.currentLayer.morphTargets[ name ] = {
- indices: indices,
- points: points,
- type: type,
- };
-
- },
-
- // A list of polygons for the current layer.
- // POLS { type[ID4], ( numvert+flags[U2], vert[VX] # numvert ) * }
- parsePolygonList( length ) {
-
- var finalOffset = this.reader.offset + length;
- var type = this.reader.getIDTag();
-
- var indices = [];
-
- // hold a list of polygon sizes, to be split up later
- var polygonDimensions = [];
-
- while ( this.reader.offset < finalOffset ) {
-
- var numverts = this.reader.getUint16();
-
- //var flags = numverts & 64512; // 6 high order bits are flags - ignoring for now
- numverts = numverts & 1023; // remaining ten low order bits are vertex num
- polygonDimensions.push( numverts );
-
- for ( var j = 0; j < numverts; j ++ ) indices.push( this.reader.getVariableLengthIndex() );
-
- }
-
- var geometryData = {
- type: type,
- vertexIndices: indices,
- polygonDimensions: polygonDimensions,
- points: this.currentPoints
- };
-
- // Note: assuming that all polys will be lines or points if the first is
- if ( polygonDimensions[ 0 ] === 1 ) geometryData.type = 'points';
- else if ( polygonDimensions[ 0 ] === 2 ) geometryData.type = 'lines';
-
- this.currentLayer.geometry = geometryData;
-
- },
-
- // Lists the tag strings that can be associated with polygons by the PTAG chunk.
- // TAGS { tag-string[S0] * }
- parseTagStrings( length ) {
-
- this.tree.tags = this.reader.getStringArray( length );
-
- },
-
- // Associates tags of a given type with polygons in the most recent POLS chunk.
- // PTAG { type[ID4], ( poly[VX], tag[U2] ) * }
- parsePolygonTagMapping( length ) {
-
- var finalOffset = this.reader.offset + length;
- var type = this.reader.getIDTag();
- if ( type === 'SURF' ) this.parseMaterialIndices( finalOffset );
- else { //PART, SMGP, COLR not supported
-
- this.reader.skip( length - 4 );
-
- }
-
- },
-
- parseMaterialIndices( finalOffset ) {
-
- // array holds polygon index followed by material index
- this.currentLayer.geometry.materialIndices = [];
-
- var initialMatIndex;
-
- while ( this.reader.offset < finalOffset ) {
-
- var polygonIndex = this.reader.getVariableLengthIndex();
- var materialIndex = this.reader.getUint16();
-
- if ( ! initialMatIndex ) initialMatIndex = materialIndex; // set up first mat index
-
- this.currentLayer.geometry.materialIndices.push( polygonIndex, materialIndex );
-
- }
-
- },
-
- parseUnknownCHUNK( blockID, length ) {
-
- console.warn( 'LWOLoader: unknown chunk type: ' + blockID + ' length: ' + length );
-
- // print the chunk plus some bytes padding either side
- // printBuffer( this.reader.dv.buffer, this.reader.offset - 20, length + 40 );
-
- var data = this.reader.getString( length );
-
- this.currentForm[ blockID ] = data;
-
- }
-
- };
-
- function DataViewReader( buffer ) {
-
- // For testing: dump whole buffer to console as a string
- // printBuffer( buffer, 0, buffer.byteLength );
-
- this.dv = new DataView( buffer );
- this.offset = 0;
-
- }
-
- DataViewReader.prototype = {
-
- constructor: DataViewReader,
-
- size: function () {
-
- return this.dv.buffer.byteLength;
-
- },
-
- setOffset( offset ) {
-
- if ( offset > 0 && offset < this.dv.buffer.byteLength ) {
-
- this.offset = offset;
-
- } else {
-
- console.error( 'LWOLoader: invalid buffer offset' );
-
- }
-
- },
-
- endOfFile: function () {
-
- if ( this.offset >= this.size() ) return true;
- return false;
-
- },
-
- skip: function ( length ) {
-
- this.offset += length;
-
- },
-
- getUint8: function () {
-
- var value = this.dv.getUint8( this.offset );
- this.offset += 1;
- return value;
-
- },
-
- getUint16: function () {
-
- var value = this.dv.getUint16( this.offset );
- this.offset += 2;
- return value;
-
- },
-
- getInt32: function () {
-
- var value = this.dv.getInt32( this.offset, false );
- this.offset += 4;
- return value;
-
- },
-
- getUint32: function () {
-
- var value = this.dv.getUint32( this.offset, false );
- this.offset += 4;
- return value;
-
- },
-
- getUint64: function () {
-
- var low, high;
-
- high = this.getUint32();
- low = this.getUint32();
- return high * 0x100000000 + low;
-
- },
-
- getFloat32: function () {
-
- var value = this.dv.getFloat32( this.offset, false );
- this.offset += 4;
- return value;
-
- },
-
- getFloat32Array: function ( size ) {
-
- var a = [];
-
- for ( var i = 0; i < size; i ++ ) {
-
- a.push( this.getFloat32() );
-
- }
-
- return a;
-
- },
-
- getFloat64: function () {
-
- var value = this.dv.getFloat64( this.offset, this.littleEndian );
- this.offset += 8;
- return value;
-
- },
-
- getFloat64Array: function ( size ) {
-
- var a = [];
-
- for ( var i = 0; i < size; i ++ ) {
-
- a.push( this.getFloat64() );
-
- }
-
- return a;
-
- },
-
- // get variable-length index data type
- // VX ::= index[U2] | (index + 0xFF000000)[U4]
- // If the index value is less than 65,280 (0xFF00),then VX === U2
- // otherwise VX === U4 with bits 24-31 set
- // When reading an index, if the first byte encountered is 255 (0xFF), then
- // the four-byte form is being used and the first byte should be discarded or masked out.
- getVariableLengthIndex() {
-
- var firstByte = this.getUint8();
-
- if ( firstByte === 255 ) {
-
- return this.getUint8() * 65536 + this.getUint8() * 256 + this.getUint8();
-
- }
-
- return firstByte * 256 + this.getUint8();
-
- },
-
- // An ID tag is a sequence of 4 bytes containing 7-bit ASCII values
- getIDTag() {
-
- return this.getString( 4 );
-
- },
-
- getString: function ( size ) {
-
- if ( size === 0 ) return;
-
- // note: safari 9 doesn't support Uint8Array.indexOf; create intermediate array instead
- var a = [];
-
- if ( size ) {
-
- for ( var i = 0; i < size; i ++ ) {
-
- a[ i ] = this.getUint8();
-
- }
-
- } else {
-
- var currentChar;
- var len = 0;
-
- while ( currentChar !== 0 ) {
-
- currentChar = this.getUint8();
- if ( currentChar !== 0 ) a.push( currentChar );
- len ++;
-
- }
-
- if ( ! isEven( len + 1 ) ) this.getUint8(); // if string with terminating nullbyte is uneven, extra nullbyte is added
-
- }
-
- return THREE.LoaderUtils.decodeText( new Uint8Array( a ) );
-
- },
-
- getStringArray: function ( size ) {
-
- var a = this.getString( size );
- a = a.split( '\0' );
-
- return a.filter( Boolean ); // return array with any empty strings removed
-
- }
-
- };
-
- // ************** UTILITY FUNCTIONS **************
-
- function isEven( num ) {
-
- return num % 2;
-
- }
-
- // calculate the length of the string in the buffer
- // this will be string.length + nullbyte + optional padbyte to make the length even
- function stringOffset( string ) {
-
- return string.length + 1 + ( isEven( string.length + 1 ) ? 1 : 0 );
-
- }
-
- // for testing purposes, dump buffer to console
- // printBuffer( this.reader.dv.buffer, this.reader.offset, length );
- function printBuffer( buffer, from, to ) {
-
- console.log( THREE.LoaderUtils.decodeText( new Uint8Array( buffer, from, to ) ) );
-
- }
-
- return LWOLoader;
-
-} )();
diff --git a/spaces/banana-projects/web3d/node_modules/three/src/materials/Materials.d.ts b/spaces/banana-projects/web3d/node_modules/three/src/materials/Materials.d.ts
deleted file mode 100644
index 7828ad1557dcb3ca460eba3b062ab04b7667202e..0000000000000000000000000000000000000000
--- a/spaces/banana-projects/web3d/node_modules/three/src/materials/Materials.d.ts
+++ /dev/null
@@ -1,18 +0,0 @@
-export * from './ShadowMaterial';
-export * from './SpriteMaterial';
-export * from './RawShaderMaterial';
-export * from './ShaderMaterial';
-export * from './PointsMaterial';
-export * from './MeshPhysicalMaterial';
-export * from './MeshStandardMaterial';
-export * from './MeshPhongMaterial';
-//export * from './MeshToonMaterial';
-export * from './MeshNormalMaterial';
-export * from './MeshLambertMaterial';
-export * from './MeshDepthMaterial';
-//export * from './MeshDistanceMaterial';
-export * from './MeshBasicMaterial';
-//export * from './MeshMatcapMaterial';
-export * from './LineDashedMaterial';
-export * from './LineBasicMaterial';
-export * from './Material';
diff --git a/spaces/bhandsab/meta-llama-Llama-2-70b-chat/app.py b/spaces/bhandsab/meta-llama-Llama-2-70b-chat/app.py
deleted file mode 100644
index 0b64725e3d6f6c01a2d4b40a32d2e624a14f01c2..0000000000000000000000000000000000000000
--- a/spaces/bhandsab/meta-llama-Llama-2-70b-chat/app.py
+++ /dev/null
@@ -1,3 +0,0 @@
-import gradio as gr
-
-gr.Interface.load("models/meta-llama/Llama-2-70b-chat").launch()
\ No newline at end of file
diff --git a/spaces/bioriAsaeru/text-to-voice/Buku sejarah peradaban islam badri yatim PDF Menginspirasi Pembaca dengan Kisah-Kisah Peradaban Islam.md b/spaces/bioriAsaeru/text-to-voice/Buku sejarah peradaban islam badri yatim PDF Menginspirasi Pembaca dengan Kisah-Kisah Peradaban Islam.md
deleted file mode 100644
index 10ea608ae34fe712c2e720e7030e8cc64aa5201c..0000000000000000000000000000000000000000
--- a/spaces/bioriAsaeru/text-to-voice/Buku sejarah peradaban islam badri yatim PDF Menginspirasi Pembaca dengan Kisah-Kisah Peradaban Islam.md
+++ /dev/null
@@ -1,6 +0,0 @@
-
-
Sejarah peradaban Islam dibagi menjadi 3 periode, klasik, pertengahan dan modern. Pada periode klasik kebudayaan dan peradaban Islam identik dengan ke-budayaan dan peradaban Arab sejalan dengan dominasi bangsa Arab dalam pemerintah dan bahasa. Pada periode berikutnya, mulai terjadi perubahan-perubahan signifikan dengan muncul dan berkembangnya beberapa peradaban Islam. Sampai saat ini, tercatat empat kawasan pengaruh kebudayaan Persia, kawasan pengaruh kebudayaan Turki dan kawasan pengaruh kebudayaan India-Islam yang selalu menjadi objek kajian ke-Islaman kontemporer. Pengkajian sejarah Islam di Indonesia mendapatkan porsi cukup besar dalam buku ini mengingat penyebaran Islam di nusantara memiliki corak yang khas.
-
Materi buku ini dengan uraian sejarah peradaban Islam-nya menjadi bahan yang sangat penting dan berguna bagi mereka yang berminat pada studi keIslaman, antara lain mahasiswa dan pengajar dari fakultas-fakultas ke-agamaan di perguruan tinggi.
-
-Karanbir Singh has announced the release of CentOS 5.5, a distribution created by compiling the ... Are you having a problem downloading Linux from LQ ISO? 4d29de3e1b
-
-
-
diff --git a/spaces/bioriAsaeru/text-to-voice/Chromaphone 2.2.1 KeyGen VERIFIED.md b/spaces/bioriAsaeru/text-to-voice/Chromaphone 2.2.1 KeyGen VERIFIED.md
deleted file mode 100644
index 091d1e13aa64c8012ceb813cfadc0f5b808b5acc..0000000000000000000000000000000000000000
--- a/spaces/bioriAsaeru/text-to-voice/Chromaphone 2.2.1 KeyGen VERIFIED.md
+++ /dev/null
@@ -1,26 +0,0 @@
-
-
Chromaphone 2.2.1: A Powerful and Versatile Percussion Synthesizer
-
Chromaphone 2.2.1 is a software synthesizer that combines physical modeling and subtractive synthesis to create realistic and expressive percussion sounds. Chromaphone 2.2.1 can produce a wide range of instruments, from drums and mallets to plucked strings and bells, as well as unique textures and soundscapes. Chromaphone 2.2.1 is packed with more than 650 presets from the best sound designers, covering various genres and styles[^1^] [^2^]. Chromaphone 2.2.1 also offers a user-friendly interface, a flexible arpeggiator, and a rich effects section to enhance your sonic possibilities.
-
In this article, we will explore some of the features and benefits of Chromaphone 2.2.1, as well as how to download and install it on your computer.
Chromaphone 2.2.1 is based on two main components: a source and a resonator. The source can be either a mallet or a noise generator, which excites the resonator to produce the sound. The resonator can be either a drumhead, a string, a plate, or a tube, which shapes the sound according to its physical properties and parameters[^1^] [^2^]. By combining different sources and resonators, you can create a variety of percussion sounds with realistic dynamics and timbres.
-
Some of the features and benefits of Chromaphone 2.2.1 are:
-
-
It has a new drumhead resonator model that reproduces precisely how a real drumhead vibrates, resulting in super realistic and responsive drums and percussions[^1^] [^2^].
-
It has an envelope mode for the noise source that allows you to carve precise one-shots with attack, hold, and decay stages[^1^] [^2^].
-
It has a noise filter bank that lets you tailor the spectrum of the noise source with a 10-band equalizer for fine control of the tone[^1^] [^2^].
-
It has a low-cut filter for the resonators that helps you control the clarity and brightness of the sound[^1^] [^2^].
-
It has a built-in arpeggiator module that adds motion and rhythm to your sounds with various modes, patterns, rates, sync options, and octaves[^1^] [^2^].
-
It has a complete set of MIDI features, including unison, vibrato, portamento, legato, keyboard split, micro tuning, and velocity response[^1^] [^2^].
-
It has a rich effects section that includes distortion, compressor, equalizer, chorus, delay, reverb, phaser, flanger, wah wah, notch filter, and crusher[^1^] [^2^].
-
It has an intuitive interface that gives you access to all source and resonator parameters, as well as modulation options and performance controls[^1^] [^2^].
-
It has a library browser that lets you easily navigate through the presets by category, subcategory, characteristics, or keywords[^1^] [^2^].
-
It supports multiple formats: WINDOWS · MAC OS X · 32-/64-BIT VST · AU · RTAS · AAX NATIVE · NKS · STANDALONE[^3^]
-
-
How to Download and Install Chromaphone 2.2.1
-
If you want to try Chromaphone 2.2.1 for yourself, you can download it for free from various websites that offer cracked software[^3^] [^4^]. However, we do not recommend this method as it may expose your computer to viruses or malware, as well as violate the intellectual property rights of the developer.
-
The best way to download and install Chromaphone 2.2.1 is to purchase it from the official website of Applied Acoustics Systems DVM Inc, the developer of Chromaphone
YOLOv5 is a family of compound-scaled object detection models trained on the COCO dataset, and includes " \
- "simple functionality for Test Time Augmentation (TTA), model ensembling, hyperparameter evolution, " \
- "and export to ONNX, CoreML and TFLite. Source code |" \
- "iOS App | PyTorch Hub
- )
-}
diff --git a/spaces/chendl/compositional_test/multimodal/YOLOX/yolox/data/__init__.py b/spaces/chendl/compositional_test/multimodal/YOLOX/yolox/data/__init__.py
deleted file mode 100644
index aeaf4f930ab8b9890ca43ba031f5b035be623ccd..0000000000000000000000000000000000000000
--- a/spaces/chendl/compositional_test/multimodal/YOLOX/yolox/data/__init__.py
+++ /dev/null
@@ -1,9 +0,0 @@
-#!/usr/bin/env python3
-# -*- coding:utf-8 -*-
-# Copyright (c) Megvii, Inc. and its affiliates.
-
-from .data_augment import TrainTransform, ValTransform
-from .data_prefetcher import DataPrefetcher
-from .dataloading import DataLoader, get_yolox_datadir, worker_init_reset_seed
-from .datasets import *
-from .samplers import InfiniteSampler, YoloBatchSampler
diff --git a/spaces/chendl/compositional_test/transformers/docs/source/_config.py b/spaces/chendl/compositional_test/transformers/docs/source/_config.py
deleted file mode 100644
index 4a7a86cc23d8070ff3070ef6fcf3a9f6598f858b..0000000000000000000000000000000000000000
--- a/spaces/chendl/compositional_test/transformers/docs/source/_config.py
+++ /dev/null
@@ -1,14 +0,0 @@
-# docstyle-ignore
-INSTALL_CONTENT = """
-# Transformers installation
-! pip install transformers datasets evaluate
-# To install from source instead of the last release, comment the command above and uncomment the following one.
-# ! pip install git+https://github.com/huggingface/transformers.git
-"""
-
-notebook_first_cells = [{"type": "code", "content": INSTALL_CONTENT}]
-black_avoid_patterns = {
- "{processor_class}": "FakeProcessorClass",
- "{model_class}": "FakeModelClass",
- "{object_class}": "FakeObjectClass",
-}
diff --git a/spaces/chuan-hd/law-assistant-chatbot/.venv/lib/python3.11/site-packages/gradio/components/label.py b/spaces/chuan-hd/law-assistant-chatbot/.venv/lib/python3.11/site-packages/gradio/components/label.py
deleted file mode 100644
index 140b6bb27f7642333f10cc4a52d10909e4799afd..0000000000000000000000000000000000000000
--- a/spaces/chuan-hd/law-assistant-chatbot/.venv/lib/python3.11/site-packages/gradio/components/label.py
+++ /dev/null
@@ -1,182 +0,0 @@
-"""gr.Label() component."""
-
-from __future__ import annotations
-
-import operator
-from pathlib import Path
-from typing import Callable, Literal
-
-from gradio_client.documentation import document, set_documentation_group
-from gradio_client.serializing import (
- JSONSerializable,
-)
-
-from gradio.components.base import IOComponent, _Keywords
-from gradio.deprecation import warn_style_method_deprecation
-from gradio.events import (
- Changeable,
- EventListenerMethod,
- Selectable,
-)
-
-set_documentation_group("component")
-
-
-@document()
-class Label(Changeable, Selectable, IOComponent, JSONSerializable):
- """
- Displays a classification label, along with confidence scores of top categories, if provided.
- Preprocessing: this component does *not* accept input.
- Postprocessing: expects a {Dict[str, float]} of classes and confidences, or {str} with just the class or an {int}/{float} for regression outputs, or a {str} path to a .json file containing a json dictionary in the structure produced by Label.postprocess().
-
- Demos: main_note, titanic_survival
- Guides: image-classification-in-pytorch, image-classification-in-tensorflow, image-classification-with-vision-transformers, building-a-pictionary-app
- """
-
- CONFIDENCES_KEY = "confidences"
-
- def __init__(
- self,
- value: dict[str, float] | str | float | Callable | None = None,
- *,
- num_top_classes: int | None = None,
- label: str | None = None,
- every: float | None = None,
- show_label: bool = True,
- container: bool = True,
- scale: int | None = None,
- min_width: int = 160,
- visible: bool = True,
- elem_id: str | None = None,
- elem_classes: list[str] | str | None = None,
- color: str | None = None,
- **kwargs,
- ):
- """
- Parameters:
- value: Default value to show in the component. If a str or number is provided, simply displays the string or number. If a {Dict[str, float]} of classes and confidences is provided, displays the top class on top and the `num_top_classes` below, along with their confidence bars. If callable, the function will be called whenever the app loads to set the initial value of the component.
- num_top_classes: number of most confident classes to show.
- label: component name in interface.
- every: If `value` is a callable, run the function 'every' number of seconds while the client connection is open. Has no effect otherwise. Queue must be enabled. The event can be accessed (e.g. to cancel it) via this component's .load_event attribute.
- show_label: if True, will display label.
- container: If True, will place the component in a container - providing some extra padding around the border.
- scale: relative width compared to adjacent Components in a Row. For example, if Component A has scale=2, and Component B has scale=1, A will be twice as wide as B. Should be an integer.
- min_width: minimum pixel width, will wrap if not sufficient screen space to satisfy this value. If a certain scale value results in this Component being narrower than min_width, the min_width parameter will be respected first.
- visible: If False, component will be hidden.
- elem_id: An optional string that is assigned as the id of this component in the HTML DOM. Can be used for targeting CSS styles.
- elem_classes: An optional list of strings that are assigned as the classes of this component in the HTML DOM. Can be used for targeting CSS styles.
- color: The background color of the label (either a valid css color name or hexadecimal string).
- """
- self.num_top_classes = num_top_classes
- self.color = color
- self.select: EventListenerMethod
- """
- Event listener for when the user selects a category from Label.
- Uses event data gradio.SelectData to carry `value` referring to name of selected category, and `index` to refer to index.
- See EventData documentation on how to use this event data.
- """
- IOComponent.__init__(
- self,
- label=label,
- every=every,
- show_label=show_label,
- container=container,
- scale=scale,
- min_width=min_width,
- visible=visible,
- elem_id=elem_id,
- elem_classes=elem_classes,
- value=value,
- **kwargs,
- )
-
- def get_config(self):
- return {
- "num_top_classes": self.num_top_classes,
- "value": self.value,
- "color": self.color,
- "selectable": self.selectable,
- **IOComponent.get_config(self),
- }
-
- def postprocess(self, y: dict[str, float] | str | float | None) -> dict | None:
- """
- Parameters:
- y: a dictionary mapping labels to confidence value, or just a string/numerical label by itself
- Returns:
- Object with key 'label' representing primary label, and key 'confidences' representing a list of label-confidence pairs
- """
- if y is None or y == {}:
- return {}
- if isinstance(y, str) and y.endswith(".json") and Path(y).exists():
- return self.serialize(y)
- if isinstance(y, (str, float, int)):
- return {"label": str(y)}
- if isinstance(y, dict):
- if "confidences" in y and isinstance(y["confidences"], dict):
- y = y["confidences"]
- y = {c["label"]: c["confidence"] for c in y}
- sorted_pred = sorted(y.items(), key=operator.itemgetter(1), reverse=True)
- if self.num_top_classes is not None:
- sorted_pred = sorted_pred[: self.num_top_classes]
- return {
- "label": sorted_pred[0][0],
- "confidences": [
- {"label": pred[0], "confidence": pred[1]} for pred in sorted_pred
- ],
- }
- raise ValueError(
- "The `Label` output interface expects one of: a string label, or an int label, a "
- "float label, or a dictionary whose keys are labels and values are confidences. "
- f"Instead, got a {type(y)}"
- )
-
- @staticmethod
- def update(
- value: dict[str, float]
- | str
- | float
- | Literal[_Keywords.NO_VALUE]
- | None = _Keywords.NO_VALUE,
- label: str | None = None,
- show_label: bool | None = None,
- container: bool | None = None,
- scale: int | None = None,
- min_width: int | None = None,
- visible: bool | None = None,
- color: str | Literal[_Keywords.NO_VALUE] | None = _Keywords.NO_VALUE,
- ):
- # If color is not specified (NO_VALUE) map it to None so that
- # it gets filtered out in postprocess. This will mean the color
- # will not be updated in the front-end
- if color is _Keywords.NO_VALUE:
- color = None
- # If the color was specified by the developer as None
- # Map is so that the color is updated to be transparent,
- # e.g. no background default state.
- elif color is None:
- color = "transparent"
- return {
- "label": label,
- "show_label": show_label,
- "container": container,
- "scale": scale,
- "min_width": min_width,
- "visible": visible,
- "value": value,
- "color": color,
- "__type__": "update",
- }
-
- def style(
- self,
- *,
- container: bool | None = None,
- ):
- """
- This method is deprecated. Please set these arguments in the constructor instead.
- """
- warn_style_method_deprecation()
- if container is not None:
- self.container = container
- return self
diff --git a/spaces/cihyFjudo/fairness-paper-search/Download Win.7.Activator.New.Rar and Enjoy the Benefits of a Genuine Windows 7.md b/spaces/cihyFjudo/fairness-paper-search/Download Win.7.Activator.New.Rar and Enjoy the Benefits of a Genuine Windows 7.md
deleted file mode 100644
index d48a117f814b874fac7d9d96170f1ac3737748ba..0000000000000000000000000000000000000000
--- a/spaces/cihyFjudo/fairness-paper-search/Download Win.7.Activator.New.Rar and Enjoy the Benefits of a Genuine Windows 7.md
+++ /dev/null
@@ -1,6 +0,0 @@
-
-
- aaccfb2cb3
-
-
-
diff --git a/spaces/cihyFjudo/fairness-paper-search/Ex Machina Hard Truck Apocalypse [Buka] Key Generator - The Best Way to Experience the Post-Apocalyptic World.md b/spaces/cihyFjudo/fairness-paper-search/Ex Machina Hard Truck Apocalypse [Buka] Key Generator - The Best Way to Experience the Post-Apocalyptic World.md
deleted file mode 100644
index 8d82c9cf00dc6c46e74d55795dd4930d626e15c7..0000000000000000000000000000000000000000
--- a/spaces/cihyFjudo/fairness-paper-search/Ex Machina Hard Truck Apocalypse [Buka] Key Generator - The Best Way to Experience the Post-Apocalyptic World.md
+++ /dev/null
@@ -1,6 +0,0 @@
-
Ex Machina Hard Truck: Apocalypse [Buka] Key Generator
-
- aaccfb2cb3
-
-
-
diff --git a/spaces/cihyFjudo/fairness-paper-search/La donna venuta dal passato movie streaming and download in 720p Choose between watching online or downloading the film.md b/spaces/cihyFjudo/fairness-paper-search/La donna venuta dal passato movie streaming and download in 720p Choose between watching online or downloading the film.md
deleted file mode 100644
index 1509630d71b8821172692de418ce3ae7c9747616..0000000000000000000000000000000000000000
--- a/spaces/cihyFjudo/fairness-paper-search/La donna venuta dal passato movie streaming and download in 720p Choose between watching online or downloading the film.md
+++ /dev/null
@@ -1,6 +0,0 @@
-
the La donna venuta dal passato movie download 720p
-
- aaccfb2cb3
-
-
-
diff --git a/spaces/cihyFjudo/fairness-paper-search/Telerik UI for Silverlight R1 2019 (2019.1.116) Retail A Comprehensive Guide to the Latest Features and Improvements.md b/spaces/cihyFjudo/fairness-paper-search/Telerik UI for Silverlight R1 2019 (2019.1.116) Retail A Comprehensive Guide to the Latest Features and Improvements.md
deleted file mode 100644
index 85e80f9561f15e9c437f0e775fc3444bcce1ab58..0000000000000000000000000000000000000000
--- a/spaces/cihyFjudo/fairness-paper-search/Telerik UI for Silverlight R1 2019 (2019.1.116) Retail A Comprehensive Guide to the Latest Features and Improvements.md
+++ /dev/null
@@ -1,6 +0,0 @@
-
Telerik UI for Silverlight R1 2019 (2019.1.116) Retail