diff --git a/spaces/101-5/gpt4free/g4f/typing.py b/spaces/101-5/gpt4free/g4f/typing.py
deleted file mode 100644
index e41a567ae49dd26d2ace2a3732b0e8f0bbbaa4b0..0000000000000000000000000000000000000000
--- a/spaces/101-5/gpt4free/g4f/typing.py
+++ /dev/null
@@ -1,3 +0,0 @@
-from typing import Dict, NewType, Union, Optional, List, get_type_hints
-
-sha256 = NewType('sha_256_hash', str)
\ No newline at end of file
diff --git a/spaces/1acneusushi/gradio-2dmoleculeeditor/data/ArcSoft TotalMedia 3.5 Serial 45k Download and Install Guide.md b/spaces/1acneusushi/gradio-2dmoleculeeditor/data/ArcSoft TotalMedia 3.5 Serial 45k Download and Install Guide.md
deleted file mode 100644
index d2796a1b6067498b9e17e8032baf8d9d417b5d80..0000000000000000000000000000000000000000
--- a/spaces/1acneusushi/gradio-2dmoleculeeditor/data/ArcSoft TotalMedia 3.5 Serial 45k Download and Install Guide.md
+++ /dev/null
@@ -1,193 +0,0 @@
-
-
Arcsoft TotalMedia 3.5 Serial 45k: A Complete Guide
-
If you are looking for a powerful and versatile software that can handle all your media needs, you might have heard of Arcsoft TotalMedia 3.5. This software is a comprehensive solution that allows you to play, record, edit, enhance, convert, and burn media files with ease. But how can you get this software and use it to its full potential? In this article, we will answer all your questions about Arcsoft TotalMedia 3.5 Serial 45k, including what it is, how to get it, how to install and activate it, and how to use it.
Arcsoft TotalMedia 3.5 is a multimedia application that was developed by Arcsoft, a leading software company that specializes in digital imaging and video technologies. It was released in 2009 and has since been updated with several patches and fixes.
-
Arcsoft TotalMedia 3.5 is designed to be an all-in-one media center that can handle various types of media files, such as audio, video, photos, DVDs, Blu-rays, TV shows, and more. It has a user-friendly interface that lets you access all the functions and features with a few clicks.
-
A brief overview of the software and its features
-
Some of the main features of Arcsoft TotalMedia 3.5 are:
-
-
Playback: You can play any media file on your computer or external device with high-quality sound and picture. You can also enjoy online streaming services such as YouTube, Netflix, Hulu, etc.
-
Recording: You can record TV shows or movies from your TV tuner card or webcam with various options such as time-shifting, scheduled recording, etc. You can also capture screenshots or videos from your desktop or webcam.
-
Editing: You can edit your media files with basic or advanced tools such as trimming, cropping, rotating, adding effects, transitions, subtitles, etc. You can also create slideshows or movies with your photos and videos.
-
Enhancing: You can enhance your media files with features such as noise reduction, color correction, brightness adjustment, etc. You can also apply filters or presets to improve the quality of your media files.
-
Converting: You can convert your media files to different formats or resolutions according to your needs or preferences. You can also optimize your media files for various devices such as iPhone, iPad, Android, PSP, etc.
-
Burning: You can burn your media files to CDs or DVDs with customized menus and labels. You can also create ISO files or disc images from your media files.
-
-
The benefits of using Arcsoft TotalMedia 3.5
-
Some of the benefits of using Arcsoft TotalMedia 3.5 are:
-
arcsoft totalmedia 3.5 license key 45k
-arcsoft totalmedia 3.5 activation code 45k
-arcsoft totalmedia 3.5 crack download 45k
-arcsoft totalmedia 3.5 full version 45k
-arcsoft totalmedia 3.5 keygen free 45k
-arcsoft totalmedia 3.5 serial number 45k
-arcsoft totalmedia 3.5 product key 45k
-arcsoft totalmedia 3.5 registration code 45k
-arcsoft totalmedia 3.5 patch 45k
-arcsoft totalmedia 3.5 torrent 45k
-arcsoft totalmedia 3.5 free download 45k
-arcsoft totalmedia 3.5 software 45k
-arcsoft totalmedia 3.5 update 45k
-arcsoft totalmedia 3.5 manual 45k
-arcsoft totalmedia 3.5 user guide 45k
-arcsoft totalmedia 3.5 review 45k
-arcsoft totalmedia 3.5 features 45k
-arcsoft totalmedia 3.5 system requirements 45k
-arcsoft totalmedia 3.5 installation guide 45k
-arcsoft totalmedia 3.5 troubleshooting 45k
-arcsoft totalmedia 3.5 alternative 45k
-arcsoft totalmedia 3.5 comparison 45k
-arcsoft totalmedia 3.5 price 45k
-arcsoft totalmedia 3.5 discount code 45k
-arcsoft totalmedia 3.5 coupon code 45k
-arcsoft totalmedia 3.5 buy online 45k
-arcsoft totalmedia 3.5 official website 45k
-arcsoft totalmedia 3.5 customer service number 45k
-arcsoft totalmedia 3.5 technical support number 45k
-arcsoft totalmedia 3.5 refund policy 45k
-arcsoft totalmedia extreme vs ultimate vs suite vs pro vs standard vs lite vs basic vs express vs home edition vs personal edition vs business edition vs enterprise edition vs premium edition vs deluxe edition vs gold edition vs platinum edition vs diamond edition vs titanium edition vs ultimate plus edition vs suite plus edition vs pro plus edition vs standard plus edition vs lite plus edition vs basic plus edition vs express plus edition vs home plus edition vs personal plus edition vs business plus edition vs enterprise plus edition vs premium plus edition vs deluxe plus edition vs gold plus edition vs platinum plus edition vs diamond plus edition vs titanium plus edition (all with serial number or license key or activation code or crack or keygen or product key or registration code or patch or torrent or free download or software or update or manual or user guide or review or features or system requirements or installation guide or troubleshooting or alternative or comparison or price or discount code or coupon code or buy online or official website or customer service number or technical support number or refund policy)
-
-
Versatility: You can use one software for all your media needs instead of switching between different applications.
-
Compatibility: You can use any type of media file regardless of its format or source.
-
Ease of use: You can use the software with simple steps and intuitive controls.
-
Performance: You can use the software with fast speed and smooth operation.
-
Quality: You can use the software with high-quality output and results.
-
-
How to get Arcsoft TotalMedia 3.5 Serial 45k?
-
If you want to use Arcsoft TotalMedia 3.5 Serial 45k on your computer, you need to get two things: the software itself and the serial number that activates it. There are two ways to get these things: the official way and the alternative way.
-
The official way: buying the software from Arcsoft website
-
The official way to get Arcsoft TotalMedia 3.5 Serial 45k is to buy it from the Arcsoft website (https://www.arcsoft.com/totalmedia-theatre/). This is the safest and most reliable way to get the software as you will get the latest version with full support and updates from the developer.
-
The price of Arcsoft TotalMedia 3.5 Serial 45k is $99.99 USD for a single license that can be used on one computer only. You can pay with various methods such as credit card, PayPal, etc. After you complete the payment process, you will receive an email with a download link for the software and a serial number for activation.
-
The alternative way: downloading the software from third-party sources
-
The alternative way to get Arcsoft TotalMedia 3.5 Serial 45k is to download it from third-party sources such as torrent sites or file-sharing platforms. This is a risky and illegal way to get the software as you may encounter viruses, malware, spyware, or other threats that may harm your computer or compromise your privacy.
-
The pros and cons of using third-party sources
-
Some of the pros of using third-party sources are:
-
-
Cheaper: You can get the software for free or at a lower price than the official source.
-
Faster: You can get the software faster than waiting for the email confirmation from the official source.
-
Easier: You can get the software easier than going through the payment process from the official source.
-
-
Some of the cons of using third-party sources are:
-
-
Dangerous: You may expose your computer or personal information to viruses, malware, spyware, or other threats that may damage your system or steal your data.
-
Illegal: You may violate the intellectual property rights of Arcsoft or other parties by downloading or using pirated software without permission or license.
-
Ineffective: You may not be able to use all the functions or features of the software as some of them may be disabled or corrupted by cracks or patches.
-
Insecure: You may not be able to update or upgrade the software as some of them may be blocked or detected by anti-virus programs or online servers.
-
-
The risks and precautions of using third-party sources
-
If you decide to use third-party sources despite their drawbacks, you should be aware of the risks and take some precautions to minimize them.
-
Some of the risks are:
-
-
Virus infection: Your computer may be infected by viruses that may slow down your system performance or delete your important files.
-
Data theft: Your personal information such as passwords, bank accounts, credit cards, etc., may be stolen by hackers who may use them for fraudulent purposes.
-
Lawsuit threat: Your IP address may be traced by authorities who may sue you for copyright infringement or piracy charges.
-
-
Some of the precautions are:
-
-
Virus scan: You should scan any downloaded file with a reliable anti-virus program before opening or installing it on your computer.
-
How to install and activate Arcsoft TotalMedia 3.5 Serial 45k?
-
After you get the software and the serial number, you need to install and activate it on your computer. The installation and activation process may vary depending on the source of the software.
-
The steps for installing the software from the official source
-
If you bought the software from the Arcsoft website, you can follow these steps to install and activate it:
-
-
Click on the download link in the email that you received from Arcsoft and save the file to your computer.
-
Double-click on the file and follow the instructions to install the software on your computer.
-
Launch the software and enter the serial number that you received from Arcsoft in the activation window.
-
Click on Activate and wait for the confirmation message.
-
Enjoy using Arcsoft TotalMedia 3.5 Serial 45k on your computer.
-
-
The steps for installing the software from a third-party source
-
If you downloaded the software from a third-party source, you can follow these steps to install and activate it:
-
-
Scan the downloaded file with an anti-virus program and make sure it is safe to open.
-
Extract the file to a folder on your computer using a program such as WinRAR or 7-Zip.
-
Open the folder and look for a file named Setup.exe or Install.exe and double-click on it.
-
Follow the instructions to install the software on your computer. You may need to uncheck some options or decline some offers that may come with the software.
-
Look for a file named Crack.exe or Patch.exe in the folder and double-click on it. You may need to copy and paste it to the installation directory of the software.
-
Run the crack or patch and wait for it to finish. It may modify some files or registry entries of the software.
-
Launch the software and check if it is activated. You may not need to enter a serial number as the crack or patch may have done it for you.
-
Enjoy using Arcsoft TotalMedia 3.5 Serial 45k on your computer.
-
-
How to find and enter the serial number
-
If you need to enter a serial number to activate the software, you can find it in different ways depending on the source of the software.
-
If you bought the software from the Arcsoft website, you can find the serial number in:
-
-
The email that you received from Arcsoft after completing the payment process.
-
The order confirmation page on the Arcsoft website after completing the payment process.
-
The My Account section on the Arcsoft website after logging in with your email and password.
-
-
If you downloaded the software from a third-party source, you can find the serial number in:
-
-
The folder where you extracted the downloaded file. There may be a file named Serial.txt or Key.txt that contains the serial number.
-
The crack or patch that came with the downloaded file. There may be a button or option that generates or shows a serial number for you.
-
The internet. You can search for Arcsoft TotalMedia 3.5 Serial 45k on Google or other search engines and look for websites that provide serial numbers for free. However, this is not recommended as some of these websites may be unsafe or unreliable.
-
-
To enter the serial number, you can follow these steps:
-
-
Launch the software and look for an activation window that asks for a serial number.
-
Copy and paste or type in the serial number in the designated field.
-
Click on Activate and wait for a confirmation message.
-
-
How to verify and troubleshoot the activation process
-
To verify if your software is activated, you can follow these steps:
-
-
Launch the software and look for an About or Help menu at the top or bottom of the screen.
-
Select About or Help and look for a window that shows information about the software version, license, status, etc.
-
Check if there is a message that says "Activated" or "Registered" next to the status or license field. If there is, then your software is activated. If there is not, then your software is not activated.
-
-
To troubleshoot if your software is not activated, you can try these steps:
-
-
Make sure you entered the correct serial number without any typos or spaces.
-
Make sure you have an internet connection as some activation processes may require online verification.
-
Make sure you have disabled any anti-virus programs or firewalls that may block or interfere with the activation process.
-
Make sure you have run the crack or patch as administrator if you downloaded the software from a third-party source.
-
Contact Arcsoft customer support (https://www.arcsoft.com/support/) if you bought the software from the official source and still have problems with activation.
-
How to use Arcsoft TotalMedia 3.5 Serial 45k?
-
After you install and activate Arcsoft TotalMedia 3.5 Serial 45k on your computer, you can start using it for all your media needs. The software has a simple and intuitive interface that lets you access all its functions and features with ease. Here are some of the main functions and features of the software and how to use them:
-
The main functions and features of the software
-
Arcsoft TotalMedia 3.5 Serial 45k has four main tabs at the top of the screen: Home, Play, Edit, and Data. Each tab has different sub-tabs that correspond to different functions and features of the software. Here is a table that summarizes what each tab and sub-tab does:
- | Tab | Sub-tab | Function | | --- | --- | --- | | Home | Media Library | Allows you to browse, organize, and manage your media files on your computer or external device | | Home | Online Media | Allows you to access online streaming services such as YouTube, Netflix, Hulu, etc | | Home | TV | Allows you to watch TV shows or movies from your TV tuner card | | Home | Capture | Allows you to capture screenshots or videos from your desktop or webcam | | Play | Video | Allows you to play video files with various options such as subtitles, audio tracks, aspect ratio, etc | | Play | Music | Allows you to play audio files with various options such as playlists, equalizer, visualizer, etc | | Play | Photo | Allows you to view photo files with various options such as slideshow, zoom, rotate, etc | | Play | DVD/BD | Allows you to play DVD or Blu-ray discs with various options such as menus, chapters, angles, etc | | Edit | Video Editor | Allows you to edit video files with basic or advanced tools such as trimming, cropping, rotating, adding effects, transitions, subtitles, etc | | Edit | Photo Editor | Allows you to edit photo files with basic or advanced tools such as cropping, rotating, resizing, adding effects, filters, presets, etc | | Edit | Movie Maker | Allows you to create movies with your photos and videos with various options such as themes, music, titles, credits, etc | | Edit | Slideshow Maker | Allows you to create slideshows with your photos with various options such as transitions, music, titles, credits, etc | | Data | Converter | Allows you to convert media files to different formats or resolutions according to your needs or preferences | | Data | Device Transfer | Allows you to transfer media files to different devices such as iPhone, iPad, Android, PSP, etc | | Data | Disc Burner | Allows you to burn media files to CDs or DVDs with customized menus and labels | | Data | Disc Creator | Allows you to create ISO files or disc images from media files |
How to play and record media files
-
To play media files with Arcsoft TotalMedia 3.5 Serial 45k, you can follow these steps:
-
Select the Play tab at the top of the screen
Select the sub-tab that corresponds to the type of media file that you want to play (Video, Music, Photo, or DVD/BD)
Browse your computer or external device for the media file that you want to play
Double-click on the media file or drag-and-drop it onto the player window
Use the controls at the bottom of the player window to adjust volume, playback speed, fullscreen mode, etc
-
To record media files with Arcsoft TotalMedia 3.5 Serial 45k, you can follow these steps:
-
Select the Home tab at the top of the screen
Select sub-tab that corresponds to the source of the media file that you want to record (TV or Capture)
-
Choose the TV tuner card or webcam that you want to use for recording
-
Use the controls at the bottom of the recorder window to adjust channel, resolution, quality, etc
-
Click on the Record button to start recording
-
Click on the Stop button to stop recording
-
Find the recorded file in the Media Library or the folder that you specified for saving
-
-
How to edit and enhance media files
-
To edit media files with Arcsoft TotalMedia 3.5 Serial 45k, you can follow these steps:
-
Select the Edit tab at the top of the screen
Select the sub-tab that corresponds to the type of media file that you want to edit (Video Editor, Photo Editor, Movie Maker, or Slideshow Maker)
Browse your computer or external device for the media file that you want to edit
Double-click on the media file or drag-and-drop it onto the editor window
Use the tools at the left or right side of the editor window to trim, crop, rotate, add effects, transitions, subtitles, etc
Use the preview window at the center of the editor window to check your changes
Click on the Save or Export button to save or export your edited file
-
To enhance media files with Arcsoft TotalMedia 3.5 Serial 45k, you can follow these steps:
-
Select the Edit tab at the top of the screen
Select the sub-tab that corresponds to the type of media file that you want to enhance (Video Editor or Photo Editor)
Browse your computer or external device for the media file that you want to enhance
Double-click on the media file or drag-and-drop it onto the editor window
Use the tools at the left or right side of the editor window to adjust noise reduction, color correction, brightness, contrast, etc
Use the preview window at the center of the editor window to check your changes
Click on the Save or Export button to save or export your enhanced file
-
How to convert and burn media files
-
To convert media files with Arcsoft TotalMedia 3.5 Serial 45k, you can follow these steps:
-
Select the Data tab at the top of the screen
Select the Converter sub-tab
Browse your computer or external device for the media file that you want to convert
Double-click on the media file or drag-and-drop it onto the converter window
Select the output format and resolution that you want from the drop-down menu at the bottom of the converter window
Click on the Start button to start converting
-
Find the converted file in the folder that you specified for saving
-
-
To burn media files with Arcsoft TotalMedia 3.5 Serial 45k, you can follow these steps:
-
Select the Data tab at the top of the screen
-
Select the Disc Burner sub-tab
-
Browse your computer or external device for the media file that you want to burn
-
Double-click on the media file or drag-and-drop it onto the burner window
-
Select the disc type and label that you want from the drop-down menu at the bottom of the burner window
-
Insert a blank CD or DVD into your disc drive and click on the Burn button to start burning
-
Eject the disc when the burning process is completed
-
-
Conclusion
-
Arcsoft TotalMedia 3.5 Serial 45k is a great software that can help you with all your media needs. It can play, record, edit, enhance, convert, and burn any type of media file with ease and quality. However, you need to be careful when getting this software as there are two ways to get it: the official way and the alternative way. The official way is safer and more reliable but more expensive and slower. The alternative way is cheaper and faster but more dangerous and illegal. You also need to know how to install and activate this software as well as how to use its main functions and features. We hope this article has given you a complete guide on Arcsoft TotalMedia 3.5 Serial 45k and helped you make an informed decision.
-
Frequently Asked Questions (FAQs)
-
Here are some of the most common questions that people ask about Arcsoft TotalMedia 3.5 Serial 45k:
-
-
What are the system requirements for Arcsoft TotalMedia 3.5 Serial 45k?The minimum system requirements for Arcsoft TotalMedia 3.5 Serial 45k are:- Windows XP/Vista/7/8/10 (32-bit or 64-bit)- Intel Pentium IV 2.4 GHz or equivalent processor- 512 MB RAM (1 GB recommended)- 300 MB free hard disk space (1 GB recommended)- DirectX 9.0c compatible graphics card with 64 MB VRAM (128 MB recommended)- DirectX compatible sound card - DVD-ROM drive - Internet connection for activation and updates The recommended system requirements for Arcsoft TotalMedia 3.5 Serial 45k are:- Windows XP/Vista/7/8/10 (32-bit or 64-bit)- Intel Core 2 Duo E6400 or equivalent processor - 2 GB RAM - 1 GB free hard disk space - DirectX 9.0c compatible graphics card with 256 MB VRAM - DirectX compatible sound card - DVD-ROM drive - Internet connection for activation and updates
-
Is Arcsoft TotalMedia 3.5 Serial 45k compatible with Windows 10?Yes, Arcsoft TotalMedia 3.5 Serial 45k is compatible with Windows 10 as long as you have installed all the latest updates and patches from Microsoft and Arcsoft.
-
How can I update Arcsoft TotalMedia 3.5 Serial 45k?You can update Arcsoft TotalMedia 3.5 Serial 45k by following these steps:- Launch the software and click on Help menu at the top of the screen.- Select Check for Updates and wait for a window that shows if there are any available updates.- Click on Download and Install if there are any available updates.- Follow the instructions to complete the update process.- Restart your computer if prompted.
-
How can I contact Arcsoft customer support?You can contact Arcsoft customer support by following these steps:- Visit https://www.arcsoft.com/support/ and select your product from the drop-down menu.- Select your issue category from another drop-down menu.- Fill in your name, email address, subject, description, attachment (optional), and verification code.- Click on Submit and wait for a reply from Arcsoft customer support.
-
How can I uninstall Arcsoft TotalMedia 3.5 Serial 45k?You can uninstall Arcsoft TotalMedia 3.5 Serial 45k by following these steps:- Go to Start menu and select Control Panel.- Select Programs and Features or Add/Remove Programs.- Find Arcsoft TotalMedia 3.5 Serial 45k in the list of installed programs and click on Uninstall/Change.- Follow the instructions to complete the uninstallation process.- Restart your computer if prompted.
- 0a6ba089eb
-
-
\ No newline at end of file
diff --git a/spaces/1acneusushi/gradio-2dmoleculeeditor/data/Charly 2007 2007 Xvid A Movie That Will Touch Your Heart.md b/spaces/1acneusushi/gradio-2dmoleculeeditor/data/Charly 2007 2007 Xvid A Movie That Will Touch Your Heart.md
deleted file mode 100644
index 19afad8ce1c972f7cb2ad9c6bfad8ed192cf4121..0000000000000000000000000000000000000000
--- a/spaces/1acneusushi/gradio-2dmoleculeeditor/data/Charly 2007 2007 Xvid A Movie That Will Touch Your Heart.md
+++ /dev/null
@@ -1,86 +0,0 @@
-
-
Charly 2007 2007 Xvid: A French Drama Film by Isild Le Besco
-
If you are looking for a different and unconventional film to watch, you might want to check out Charly 2007 2007 Xvid, a French drama film directed by Isild Le Besco. This film tells the story of Nicolas, a troubled teenager who runs away from home and meets Charly, a mysterious and seductive woman who takes him on a road trip across France. Along the way, they develop a complex and intense relationship that challenges their identities and their destinies. In this article, we will explore the plot, the reception, and the technical aspects of this film, and why you should watch it.
The film begins with Nicolas (Kolia Litscher), a 14-year-old boy who lives in a foster home with his younger sister. He is unhappy and bored with his life, and he often gets into trouble at school and with the police. One day, he decides to run away from home and hitchhike to Brittany, where he hopes to find his biological father. On his way, he meets Charly (Julie-Marie Parmentier), a red-haired woman in her twenties who offers him a ride. She claims to be a photographer who travels around France taking pictures of landscapes and people. She also says that she has a terminal illness and that she wants to enjoy her life as much as possible.
-
Nicolas is intrigued by Charly's personality and appearance, and he agrees to go with her. They start a journey across France, stopping at various places such as hotels, motels, campsites, forests, beaches, and cities. They also encounter different people along the way, such as truck drivers, farmers, hippies, bikers, and tourists. Nicolas and Charly share intimate moments of conversation, laughter, sex, and violence. They also reveal secrets about their pasts and their dreams for the future. Nicolas gradually falls in love with Charly, but he also realizes that she is not who she seems to be. She is unpredictable, manipulative, and dangerous. She often lies to him about her identity, her motives, and her feelings. She also has a dark side that involves drugs, theft, prostitution, and murder.
-
The film ends with a shocking twist that reveals the true nature of Charly's illness and her relationship with Nicolas. The film explores themes such as adolescence, sexuality, identity, freedom, love, death, and fate.
-
The Reception of Charly 2007 2007 Xvid
-
The film received mixed reviews from critics and audiences when it was released in France in 2007. Some praised it for its originality, its realism, its cinematography, its acting performances, and its daring portrayal of sexuality and violence. Others criticized it for its lack of coherence, its implausibility, its moral ambiguity, its excessive nudity and gore, and its exploitation of underage actors. The film was nominated for two César Awards (the French equivalent of the Oscars) for Best First Feature Film and Most Promising Actress (Julie-Marie Parmentier). However, it did not win any awards.
-
Charly 2007 movie download Xvid
-Watch Charly 2007 online free Xvid
-Charly 2007 film review Xvid
-Charly 2007 full movie Xvid
-Charly 2007 subtitles Xvid
-Charly 2007 trailer Xvid
-Charly 2007 cast and crew Xvid
-Charly 2007 DVD release date Xvid
-Charly 2007 streaming Xvid
-Charly 2007 plot summary Xvid
-Charly 2007 soundtrack Xvid
-Charly 2007 awards and nominations Xvid
-Charly 2007 box office Xvid
-Charly 2007 behind the scenes Xvid
-Charly 2007 director's cut Xvid
-Charly 2007 trivia and facts Xvid
-Charly 2007 quotes and dialogues Xvid
-Charly 2007 genre and rating Xvid
-Charly 2007 based on a true story Xvid
-Charly 2007 remake or sequel Xvid
-Charly 2007 torrent magnet link Xvid
-Charly 2007 Blu-ray quality Xvid
-Charly 2007 runtime and language Xvid
-Charly 2007 deleted scenes Xvid
-Charly 2007 bloopers and outtakes Xvid
-Charly 2007 fan art and memes Xvid
-Charly 2007 analysis and interpretation Xvid
-Charly 2007 comparison with other movies Xvid
-Charly 2007 best scenes and moments Xvid
-Charly 2007 worst scenes and mistakes Xvid
-Charly 2007 easter eggs and references Xvid
-Charly 2007 alternate endings and theories Xvid
-Charly 2007 making of and production details Xvid
-Charly 2007 location and setting Xvid
-Charly 2007 costumes and makeup Xvid
-Charly 2007 special effects and CGI Xvid
-Charly 2007 score and music Xvid
-Charly 2007 themes and messages Xvid
-Charly 2007 characters and relationships Xvid
-Charly 2007 controversy and criticism Xvid
-Charly 2007 history and background Xvid
-Charly 2007 inspiration and influence Xvid
-Charly 2007 adaptation and source material Xvid
-Charly 2007 legal issues and lawsuits Xvid
-Charly 2007 merchandise and collectibles Xvid
-Charly 2007 interviews and podcasts Xvid
-Charly 2007 articles and blogs Xvid
-Charly 2007 books and comics Xvid
-Charly 2007 games and apps Xvid
-Charly 2007 spin-offs and prequels Xvid
-
The film also caused controversy and censorship issues in some countries where it was distributed. For example, in Australia, the film was banned for its depiction of child pornography and sexual abuse. In Germany, the film was cut by 16 minutes to remove some scenes of graphic violence and sex involving minors. In Italy, the film was rated VM18 (forbidden for minors under 18) for its explicit content. In Spain, the film was rated X (restricted to adult theaters) for its extreme scenes of sex and violence.
-
The Technical Aspects of Charly 2007 2007 Xvid
-
The film was directed by Isild Le Besco, a French actress and filmmaker who made her debut as a director with this film. She also wrote the screenplay and co-produced the film. She was inspired by her own experiences as a runaway teenager and by her fascination with the character of Charly, whom she met in real life. She wanted to make a film that was raw, honest, and spontaneous, without following any conventional rules or genres. She also wanted to explore the emotions and sensations of being young, free, and in love.
-
The film was shot in digital video with a handheld camera, giving it a documentary-like feel and a sense of immediacy and intimacy. The camera follows the characters closely, capturing their expressions, movements, and interactions. The film also uses natural lighting, ambient sound, and improvised dialogue, creating a realistic and immersive atmosphere. The film has a nonlinear and fragmented structure, with frequent flashbacks, flash-forwards, and jump cuts. The film also mixes different styles and tones, such as drama, comedy, romance, thriller, and horror.
-
The film has a minimalistic and eclectic soundtrack that consists of various songs and music genres that reflect the mood and the personality of the characters. Some of the songs and artists featured in the film are: "L'Amour à la Plage" by Niagara, "La Vie en Rose" by Edith Piaf, "I Wanna Be Your Dog" by The Stooges, "Killing in the Name" by Rage Against the Machine, "La Bamba" by Ritchie Valens, "Hallelujah" by Leonard Cohen, "The End" by The Doors, and "Charly" by Isild Le Besco.
-
The film was released on DVD in France in 2008 with English subtitles. The DVD also includes some bonus features such as interviews with the director and the actors, behind-the-scenes footage, deleted scenes, and a photo gallery.
-
Conclusion: Why You Should Watch Charly 2007 2007 Xvid
-
In conclusion, Charly 2007 2007 Xvid is a film that is not for everyone. It is a challenging and provocative film that explores themes that are controversial and disturbing. It is also a film that is original and unconventional, that breaks the boundaries of cinema and storytelling. It is a film that is realistic and immersive, that shows the beauty and the ugliness of life and love. It is a film that is emotional and sensual, that makes you feel and think. It is a film that is unforgettable and unique.
-
If you are looking for a different and unconventional film to watch, you might want to give Charly 2007 2007 Xvid a try. You might love it or hate it, but you will not be indifferent to it.
-
FAQs
-
Here are some frequently asked questions and answers about the film:
-
-
Q: Is Charly 2007 2007 Xvid based on a true story?
-
A: The film is partly based on the director's own experiences as a runaway teenager and partly inspired by a real person named Charly whom she met in real life. However, the film is not a biographical or documentary film. It is a fictional and artistic interpretation of reality.
-
Q: How old were the actors who played Nicolas and Charly?
-
A: Kolia Litscher was 14 years old and Julie-Marie Parmentier was 26 years old when they filmed the movie. They were both professional actors who had previous experience in cinema and theater.
-
Q: How did they film the sex scenes involving minors?
-
A: The sex scenes involving minors were filmed with the consent of the actors and their parents or legal guardians. They were also filmed with the supervision of a child protection officer and a psychologist. The sex scenes were simulated and not real. They were also edited in a way that did not show any explicit nudity or penetration.
-
Q: What is the meaning of the title Charly 2007 2007 Xvid?
-
A: The title Charly 2007 2007 Xvid has multiple meanings. It refers to the name of the main character (Charly), the year of release of the film (2007), the format of the video file (Xvid), and the director's initials (Isild Le Besco).
-
Q: What is the message or moral of the film?
-
A: The film does not have a clear or definitive message or moral. It is open to interpretation and discussion. It invites the viewers to form their own opinions and judgments about the characters and their actions. It also challenges the viewers to question their own values and beliefs about life and love.
-
- 0a6ba089eb
-
-
\ No newline at end of file
diff --git a/spaces/1acneusushi/gradio-2dmoleculeeditor/data/Fslabs concorde x crack straight How to fly the supersonic jet in FSX and P3D.md b/spaces/1acneusushi/gradio-2dmoleculeeditor/data/Fslabs concorde x crack straight How to fly the supersonic jet in FSX and P3D.md
deleted file mode 100644
index 8e8fe071cd02a9009d91af8ec0c8f0e87d9dbcfd..0000000000000000000000000000000000000000
--- a/spaces/1acneusushi/gradio-2dmoleculeeditor/data/Fslabs concorde x crack straight How to fly the supersonic jet in FSX and P3D.md
+++ /dev/null
@@ -1,79 +0,0 @@
-
-
Fslabs Concorde X Crack Straight: How to Fly the Legendary Supersonic Jet in FSX
-
Introduction
-
If you are a fan of flight simulation, you probably have heard of Fslabs Concorde X, one of the most realistic and detailed add-ons for Microsoft Flight Simulator X (FSX). This product recreates the iconic Concorde, the only supersonic passenger airliner that ever flew commercially, with stunning graphics, accurate flight dynamics, complex systems, and immersive sound effects.
However, Fslabs Concorde X is not a cheap product. It costs $99.95 for FSX and $139.95 for Prepar3D (P3D), which is a lot of money for some people. That's why some people resort to using a crack, which is a modified file that bypasses the activation process and allows them to use the product without paying for it.
-
But is using a crack worth it? What are the risks and drawbacks of doing so? And what are the benefits and features of buying the official product instead? In this article, we will answer these questions and show you how to fly Fslabs Concorde X Crack Straight in FSX.
-
How to Install Fslabs Concorde X Crack Straight
-
The first step to fly Fslabs Concorde X Crack Straight is to install it on your computer. To do that, you will need to find and download the crack file from a website that offers it. There are many websites that claim to have working cracks for Fslabs Concorde X, but most of them are fake or malicious. They may contain viruses, malware, spyware, or adware that can harm your computer or steal your personal information.
-
One of the websites that seems to have a working crack for Fslabs Concorde X is LexCliq. However, we do not recommend or endorse this website or any other website that provides cracks. Use them at your own risk and discretion.
-
Once you have downloaded the crack file from LexCliq or another website, you will need to extract it using a program like WinRAR or 7-Zip. You will get a folder called "FSLabs_Concorde_X_Crack_Straight" that contains two files: "FSL_ConcX.dll" and "readme.txt". You will need to copy these files to your FSX folder, which is usually located at "C:\Program Files (x86)\Microsoft Games\Microsoft Flight Simulator X".
-
After copying the files, you can run FSX and select Concorde X as your aircraft. You should see a message saying "FSLABS CONCORDE-X ACTIVATED" on the top left corner of your screen. This means that the crack worked and you can use Fslabs Concorde X without activation.
-
fslabs concorde x free download full version
-fslabs concorde x serial key generator
-fslabs concorde x activation code crack
-fslabs concorde x cracked by skidrow
-fslabs concorde x torrent download link
-fslabs concorde x license key crack
-fslabs concorde x patch v1.41 crack
-fslabs concorde x crack only download
-fslabs concorde x full game crack
-fslabs concorde x crack fix error
-fslabs concorde x crack no survey no password
-fslabs concorde x crack for windows 10
-fslabs concorde x crack without virus
-fslabs concorde x crack working 100%
-fslabs concorde x crack updated version
-fslabs concorde x crack with all liveries
-fslabs concorde x crack for fsx steam edition
-fslabs concorde x crack for prepar3d v4
-fslabs concorde x crack for prepar3d v5
-fslabs concorde x crack for microsoft flight simulator 2020
-fslabs concorde x crack instructions guide
-fslabs concorde x crack installation video tutorial
-fslabs concorde x crack review and gameplay
-fslabs concorde x crack features and benefits
-fslabs concorde x crack system requirements and compatibility
-fslabs concorde x crack support and customer service
-fslabs concorde x crack feedback and testimonials
-fslabs concorde x crack comparison and alternatives
-fslabs concorde x crack tips and tricks
-fslabs concorde x crack best practices and recommendations
-fslabs concorde x crack pros and cons
-fslabs concorde x crack advantages and disadvantages
-fslabs concorde x crack facts and myths
-fslabs concorde x crack dos and don'ts
-fslabs concorde x crack faqs and answers
-fslabs concorde x crack problems and solutions
-fslabs concorde x crack issues and fixes
-fslabs concorde x crack bugs and glitches
-fslabs concorde x crack updates and news
-fslabs concorde x crack forum and community
-fslabs concorde x crack online and offline mode
-fslabs concorde x crack multiplayer and co-op mode
-fslabs concorde x crack mods and addons
-fslabs concorde x crack cheats and hacks
-fslabs concorde x crack codes and keys
-fslabs concorde x crack tools and utilities
-fslabs concorde x crack resources and links
-fslabs concorde x crack deals and discounts
-fslabs concorde x crack offers and promotions
-
If you don't see this message or you see an error message instead, it means that the crack didn't work or it was detected by Fslabs. In that case, you will need to delete the files you copied and try another crack or buy the official product.
-
How to Fly Fslabs Concorde X Crack Straight
-
Now that you have installed Fslabs Concorde X Crack Straight on your computer, you can start flying it in FSX. However, flying Concorde is not easy. It requires a lot of skill, knowledge, and practice. You will need to follow a detailed checklist, set up various systems, monitor multiple gauges, adjust fuel balance, manage engine thrust, control speed and altitude, and more.
-
To help you fly Fslabs Concorde X Crack Straight successfully, we will give you a brief overview of how to do it. However, this is not a comprehensive tutorial or manual. For more information and guidance, we recommend reading the official documentation that comes with Fslabs Concorde X or watching some videos on YouTube.
-
Here are some basic steps on how to fly Fslabs Concorde X Crack Straight:
-
-
Set up the cockpit: Before starting your flight, you will need to set up the cockpit according to your preferences and flight plan. You will need to adjust your seat position, set your views, configure your displays, tune your radios, enter your waypoints, set your fuel load, check your weight and balance, etc.
-
Configure the systems: After setting up the cockpit, you will need to configure various systems on Concorde such as electrical power, hydraulics, air conditioning, pressurization, fuel pumps, anti-ice, etc. You will also need to perform some tests on your engines, brakes, flight controls, instruments, etc.
-
Take off and climb: When you are ready to take off, you will need to request clearance from ATC (if enabled), taxi to the runway (preferably one that is long enough for Concorde), line up with the centerline (using nosewheel steering), apply full throttle (using afterburners), release brakes (using toe brakes), accelerate (using rudder pedals), rotate (using yoke), retract landing gear (using G key), retract flaps (using F6 key), turn off afterburners (using Shift+F4 keys), climb (using yoke), engage autopilot (using Z key), etc.
-
Cruise at Mach 2: When you reach about 28,000 feet (FL280), you will need to accelerate again using afterburners until you reach Mach 1 (the speed of sound). Then you will need to climb further until you reach about 50,000 feet (FL500), where you will reach Mach 2 (twice the speed of sound). You will also need to adjust your fuel balance using transfer pumps and trim tanks during cruise.
-
Descend and land: When you approach your destination airport (about 200 miles away), you will need to request descent clearance from ATC (if enabled), reduce speed using airbrakes (using / key), descend using autopilot or manually (using yoke), turn off afterburners (using Shift+F4 keys), extend landing gear (using G key), extend flaps (using F7 key), align with runway using ILS or visually (using yoke), flare (using yoke), touch down gently (using yoke), apply reverse thrust (using F2 key), apply brakes (using . key), exit runway (using rudder pedals), taxi to gate (using nosewheel steering), etc.
-
-
Conclusion
- folder of your FSX installation.
-
- 0a6ba089eb
-
-
\ No newline at end of file
diff --git a/spaces/1gistliPinn/ChatGPT4/Examples/Adobe Photoshop Lightroom CC 6.12 Portable Crack !NEW!ed [crack !NEW!sNow] Full Version.md b/spaces/1gistliPinn/ChatGPT4/Examples/Adobe Photoshop Lightroom CC 6.12 Portable Crack !NEW!ed [crack !NEW!sNow] Full Version.md
deleted file mode 100644
index a5cdbf2b786c3b45a23cb7138cc846b4b7bf0252..0000000000000000000000000000000000000000
--- a/spaces/1gistliPinn/ChatGPT4/Examples/Adobe Photoshop Lightroom CC 6.12 Portable Crack !NEW!ed [crack !NEW!sNow] Full Version.md
+++ /dev/null
@@ -1,6 +0,0 @@
-
Adobe Photoshop Lightroom CC 6.12 Portable Cracked [CracksNow] Full Version
-
-adobe photoshop lightroom cc 6.12 portable ed [snow] adobe photoshop lightroom cc 6.12 portable ed [snow] 8a78ff9644
-
-
-
diff --git a/spaces/1gistliPinn/ChatGPT4/Examples/American Ninja 5 Full ((EXCLUSIVE)) Movie Kick.md b/spaces/1gistliPinn/ChatGPT4/Examples/American Ninja 5 Full ((EXCLUSIVE)) Movie Kick.md
deleted file mode 100644
index d0f3f38469dd9eda2d48e2adf12978dd07f09112..0000000000000000000000000000000000000000
--- a/spaces/1gistliPinn/ChatGPT4/Examples/American Ninja 5 Full ((EXCLUSIVE)) Movie Kick.md
+++ /dev/null
@@ -1,6 +0,0 @@
-
-
Theres a cat and mouse format to the ninjas in the film that reminds me of The Karate Kid, with ninjas crawling in through the kitchen window, ninjas in the bathroom window, and so on. Its also a lot more barebones than the other films in the series, relying almost solely on fistfights and the like to sell the story, but this works well enough for the most part. The climactic battle is one of the low points, but thats true of most of them by now. The ninjas themselves are a rather bland lineup of Zatoichi type guys with a smattering of ludicrously-dressed bad guys that would have been more effective before the gag had worn off, and Sam Firstenberg just keeps showing up, a little too much even by ninjas-films standards. And it goes without saying that Bradleys best scene here is the one where he flails around wildly like Royce Gracie in the octagon.
-
At this point, everyone has entered into the franchise with a certain amount of baggage. Sam Firstenberg cant win them all. And he makes a lot of rookie errors here. The most egregious of which comes in the final battle, when the ninjas have their hi-tech army drop on the terrorists and Bradley gets totally caught out with a relatively minor mistake. On one hand, this highlights why the ninjas arent as dangerous as the marines are in the other films, because Zito doesnt want to go too nuts with it all. But on the other hand, this combat goes on for far too long and is rather unappealing. On top of that, the final battle also provides the one good battle sequence in the film, and it ends up going the way it did because it wasnt good enough. It still looks amazing and the right amount of tension is generated by the action, but it never satisfied my desire to see the ninjas do something more.
-
-ableton live 10.0.2 y ed - detailed instructions for installing desktop software on windows and mac operating systems.
-The desktop software is the main operating system that is used to play and record your music.
-Its goal is to run as a desktop application but it is not a complete, stand-alone package.
-It still requires the software on the hard 8a78ff9644
-
-
-
diff --git a/spaces/1gistliPinn/ChatGPT4/Examples/Dhamaal 720p Movie Kickass Download VERIFIED.md b/spaces/1gistliPinn/ChatGPT4/Examples/Dhamaal 720p Movie Kickass Download VERIFIED.md
deleted file mode 100644
index 8362753b28f96cd12bfc3bb6afd68ea784b0bac9..0000000000000000000000000000000000000000
--- a/spaces/1gistliPinn/ChatGPT4/Examples/Dhamaal 720p Movie Kickass Download VERIFIED.md
+++ /dev/null
@@ -1,95 +0,0 @@
-
-
Dhamaal 720p Movie Kickass Download - A Guide for Bollywood Comedy Lovers
-
-
If you are a fan of Bollywood comedy movies, you must have heard of Dhamaal, a hilarious film that was released in 2007. Dhamaal is a story of four lazy and broke friends who embark on a crazy adventure to find a hidden treasure of 10 crore rupees. Along the way, they encounter various obstacles and challenges, as well as a ruthless cop who is after the same treasure. Dhamaal is a laugh riot that will keep you entertained from start to finish.
But how can you watch Dhamaal if you don't have access to a DVD or a streaming service? Well, there is a way to download Dhamaal 720p movie from kickass torrents, one of the most popular torrent sites on the internet. Kickass torrents is a platform where you can find and download various files, including movies, TV shows, music, games, software and more. All you need is a torrent client, such as uTorrent or BitTorrent, and a stable internet connection.
-
-
How to Download Dhamaal 720p Movie from Kickass Torrents
-
-
Here are the steps to download Dhamaal 720p movie from kickass torrents:
-
-
-
Go to https://kickass.sx/, the official website of kickass torrents. You can also use other mirror sites or proxy sites if the main site is blocked in your region.
-
In the search bar, type Dhamaal 720p movie and hit enter. You will see a list of results that match your query.
-
Choose the result that has the most seeders and leechers. Seeders are the users who have the complete file and are sharing it with others. Leechers are the users who are downloading the file from the seeders. The more seeders and leechers a torrent has, the faster and more reliable the download will be.
-
Click on the result and you will be taken to a page with more details about the torrent, such as file size, quality, format, language, subtitles and more. You can also read the comments and reviews from other users who have downloaded the torrent.
-
Click on the Download Torrent button or the Magnet Link icon. A torrent file or a magnet link will be downloaded to your device.
-
Open the torrent file or the magnet link with your torrent client. The download will start automatically.
-
Wait for the download to finish. It may take some time depending on your internet speed and the number of seeders and leechers available.
-
Once the download is complete, you can enjoy watching Dhamaal 720p movie on your device.
-
-
-
Tips and Warnings for Downloading Dhamaal 720p Movie from Kickass Torrents
-
-
Here are some tips and warnings for downloading Dhamaal 720p movie from kickass torrents:
-
-
-
-
Make sure you have enough space on your device to store the downloaded file. Dhamaal 720p movie is about 1 GB in size.
-
Make sure you have a good antivirus software on your device to protect it from any malware or viruses that may come with the torrent file or the magnet link.
-
Make sure you are using a VPN service to hide your IP address and encrypt your online activity. Downloading torrents may be illegal in some countries and regions, and you may face legal consequences if you are caught by authorities or ISPs.
-
Make sure you are downloading Dhamaal 720p movie from a trusted and verified source. Some torrents may be fake or corrupted, and may not work properly or contain harmful content.
-
Make sure you are downloading Dhamaal 720p movie for personal use only. Do not distribute or share it with others without permission from the original creators or owners.
-
-
-
Conclusion
-
-
Dhamaal 720p movie kickass download is a great option for Bollywood comedy lovers who want to watch this hilarious film on their devices. By following the steps above, you can easily download Dhamaal 720p movie from kickass torrents and enjoy it anytime and anywhere. However, you should also be aware of the risks and responsibilities involved in downloading torrents, and take precautions to protect yourself and respect others' rights.
-
Why You Should Watch Dhamaal 720p Movie
-
-
Dhamaal 720p movie is not only a fun and easy way to download and watch this film, but also a great way to enjoy its many features and benefits. Here are some of the reasons why you should watch Dhamaal 720p movie:
-
-
-
You can watch Dhamaal 720p movie in high definition quality, which enhances the visual and audio effects of the film. You can see the details and colors of the scenes and characters, and hear the sounds and dialogues clearly and loudly.
-
You can watch Dhamaal 720p movie on any device that supports this format, such as laptops, tablets, smartphones, smart TVs and more. You can also connect your device to a larger screen or a speaker system for a better viewing experience.
-
You can watch Dhamaal 720p movie anytime and anywhere you want, as long as you have the downloaded file on your device. You don't need an internet connection or a subscription service to watch it. You can also pause, rewind, fast forward or skip any part of the film as you wish.
-
You can watch Dhamaal 720p movie with your friends and family, and share the laughter and joy of this film. You can also discuss the plot, the characters, the jokes and the messages of the film with them.
-
You can watch Dhamaal 720p movie as many times as you want, without getting bored or tired of it. You can discover new things and appreciate different aspects of the film every time you watch it.
-
-
-
Dhamaal 720p movie is a wonderful way to enjoy this Bollywood comedy masterpiece. By downloading it from kickass torrents, you can have access to this film anytime and anywhere you want. However, you should also respect the rights of the creators and owners of this film, and use it for personal use only.
-
What is Dhamaal 720p Movie About
-
-
Dhamaal 720p movie is a comedy film that revolves around four friends who are in search of a hidden treasure. The film is a remake of the 1963 Hollywood film It's a Mad, Mad, Mad, Mad World. The film stars Sanjay Dutt, Riteish Deshmukh, Arshad Warsi, Javed Jaffrey and Aashish Chaudhary in the lead roles. The film also features Asrani, Vijay Raaz, Manoj Pahwa and Prem Chopra in supporting roles.
-
-
The film begins with a dying thief named Bose (Prem Chopra) who reveals the location of his loot of 10 crore rupees to four strangers: Roy (Riteish Deshmukh), Adi (Arshad Warsi), Manav (Javed Jaffrey) and Boman (Aashish Chaudhary). The four friends decide to split the money equally and head to Goa where the treasure is buried. However, they are unaware that Bose's partner Kabir (Sanjay Dutt), a corrupt police inspector, is also after the same treasure. Kabir follows them and tries to sabotage their plans.
-
-
On their way to Goa, the four friends encounter various obstacles and challenges, such as a plane crash, a car chase, a monkey attack, a bridge collapse and more. They also meet various characters who either help them or hinder them, such as a blind couple (Asrani and Tiku Talsania), a dacoit leader (Sanjay Mishra), a hotel manager (Vijay Raaz), a garage owner (Manoj Pahwa) and more. The film is full of hilarious situations and dialogues that will make you laugh out loud.
-
-
Why Dhamaal 720p Movie is a Must-Watch for Comedy Lovers
-
-
Dhamaal 720p movie is a must-watch for comedy lovers because it has everything that makes a comedy film great. Here are some of the reasons why Dhamaal 720p movie is a must-watch for comedy lovers:
-
-
-
The film has a simple and engaging plot that keeps you hooked till the end. The film has a fast-paced and unpredictable storyline that keeps you guessing what will happen next. The film has a satisfying and surprising climax that will leave you amazed.
-
The film has a talented and charismatic cast that delivers brilliant performances. The film has a perfect chemistry and timing between the actors that makes their scenes more enjoyable. The film has memorable and funny characters that will make you laugh and relate to them.
-
The film has a witty and hilarious script that makes you laugh at every dialogue. The film has clever and humorous dialogues that are full of puns, sarcasm, irony and references. The film has witty and hilarious situations that are full of slapstick, parody and satire.
-
The film has a catchy and upbeat soundtrack that adds to the fun and mood of the film. The film has catchy and upbeat songs that are composed by Adnan Sami and sung by various singers. The film has catchy and upbeat background music that enhances the comedy scenes.
-
The film has a colorful and vibrant cinematography that makes the film more appealing and attractive. The film has colorful and vibrant scenes that are shot in various locations such as Mumbai, Goa, Lonavala and more. The film has colorful and vibrant costumes and props that add to the charm and style of the film.
-
-
-
Dhamaal 720p movie is a comedy masterpiece that will make you laugh till your stomach hurts. By downloading it from kickass torrents, you can enjoy this film in high quality on your device. However, you should also respect the rights of the creators and owners of this film, and use it for personal use only.
-
How to Enjoy Dhamaal 720p Movie with Your Friends and Family
-
-
Dhamaal 720p movie is not only a great film to watch by yourself, but also a great film to watch with your friends and family. Here are some tips on how to enjoy Dhamaal 720p movie with your friends and family:
-
-
-
Plan a movie night with your friends and family. Choose a date and time that is convenient for everyone. Invite them to your place or go to their place. Make sure you have enough space and seating for everyone.
-
Prepare some snacks and drinks for the movie night. Choose some snacks and drinks that are easy to make and eat, such as popcorn, chips, cookies, soda, juice and more. You can also order some pizza, burgers, sandwiches or other food items that everyone likes.
-
Download Dhamaal 720p movie from kickass torrents and transfer it to your device. Make sure you have a good torrent client and a VPN service to download the film safely and securely. Transfer the downloaded file to your device that you will use to watch the film.
-
Connect your device to a larger screen or a speaker system for a better viewing experience. You can use an HDMI cable, a Chromecast, a Bluetooth speaker or any other device that can connect your device to a larger screen or a speaker system. Adjust the volume and brightness as per your preference.
-
Enjoy watching Dhamaal 720p movie with your friends and family. Laugh along with the hilarious scenes and dialogues of the film. Share your opinions and thoughts on the film with them. Have fun and make some memories with them.
-
-
-
Dhamaal 720p movie is a perfect film to watch with your friends and family. By following these tips, you can have a wonderful movie night with them. However, you should also respect the rights of the creators and owners of this film, and use it for personal use only.
-
-
Conclusion
-
-
Dhamaal 720p movie kickass download is an amazing option for comedy lovers who want to download and watch this hilarious film on their devices. By following the steps above, you can easily download Dhamaal 720p movie from kickass torrents and enjoy it anytime and anywhere you want. However, you should also be aware of the risks and responsibilities involved in downloading torrents, and take precautions to protect yourself and respect others' rights.
-
Conclusion
-
-
Dhamaal 720p movie kickass download is a great way to enjoy this Bollywood comedy masterpiece on your devices. By downloading it from kickass torrents, you can have access to this film anytime and anywhere you want. However, you should also respect the rights of the creators and owners of this film, and use it for personal use only. Dhamaal 720p movie is a film that will make you laugh till your stomach hurts. By following the tips above, you can also enjoy this film with your friends and family, and have a wonderful movie night with them. Dhamaal 720p movie is a film that has something for everyone, and can enrich your mind and soul. If you have not watched Dhamaal 720p movie yet, you should do it now and discover the wonders of this film.
3cee63e6c2
-
-
\ No newline at end of file
diff --git a/spaces/1phancelerku/anime-remove-background/Cricket League New Mod APK - The Best Cricket Game for Android Users.md b/spaces/1phancelerku/anime-remove-background/Cricket League New Mod APK - The Best Cricket Game for Android Users.md
deleted file mode 100644
index b759ebe56c6ffef6242ab2a7f6570794fafd2766..0000000000000000000000000000000000000000
--- a/spaces/1phancelerku/anime-remove-background/Cricket League New Mod APK - The Best Cricket Game for Android Users.md
+++ /dev/null
@@ -1,132 +0,0 @@
-
-
Cricket League New Mod APK: How to Download and Play
-
Do you love cricket and want to play it on your mobile device? If yes, then you should try Cricket League, a fast, fun, exciting and authentic 3D real-time multiplayer cricket game. And if you want to enjoy the game with unlimited resources and features, then you should download Cricket League Mod APK, a modified version of the original game that gives you access to everything for free. In this article, we will tell you what Cricket League is, how to download and install Cricket League Mod APK, why you should use it, how to play it, and some tips and tricks to help you win more matches.
Cricket League is a 3D real-time multiplayer cricket game developed by Miniclip, a popular gaming company that also created games like 8 Ball Pool, Soccer Stars, and Agar.io. Cricket League lets you bat, bowl and field your way to the top of the league in various modes, such as T20, ODI, Test, and Super Over. You can choose from 16 different teams, each with their own strengths and weaknesses, and customize your players and equipment. You can also compete with other players online in ranked matches or friendly matches, or play offline against the AI. Cricket League has realistic graphics, animations, physics, and sounds that make you feel like you are playing in a real stadium.
-
Features of Cricket League
-
Some of the features of Cricket League are:
-
-
Realistic 3D graphics and animations
-
Authentic cricket physics and sounds
-
16 different teams to choose from
-
4 different modes to play: T20, ODI, Test, and Super Over
-
Online multiplayer mode with ranked matches and friendly matches
-
Offline mode with AI opponents
-
Customizable players and equipment
-
Power-ups and special abilities to boost your performance
-
Leaderboards and achievements to track your progress
-
Daily rewards and missions to earn coins and gems
-
-
How to download Cricket League Mod APK
-
If you want to download Cricket League Mod APK, you need to follow these steps:
-
-
Go to [Cricket League Mod apk download - HappyMod](^1^), a website that provides modded versions of various games.
-
Click on the "Download APK" button and wait for the file to be downloaded on your device.
-
Go to your device's settings and enable "Unknown Sources" to allow installation of apps from sources other than the Google Play Store.
-
Locate the downloaded file in your file manager and tap on it to install it.
-
Launch the game and enjoy playing with unlimited resources and features.
-
-
Why use Cricket League Mod APK?
-
You might be wondering why you should use Cricket League Mod APK instead of the original game. Well, there are some benefits and risks of using Cricket League Mod APK that you should know before deciding whether to use it or not.
-
Benefits of Cricket League Mod APK
-
Some of the benefits of using Cricket League Mod APK are:
-
-
You get unlimited coins and gems that you can use to buy or upgrade anything in the game.
-
You get all the teams unlocked so you can choose any team you want.
-
You get all the modes unlocked so you can play any mode you want.
-
You get all the power-ups and special abilities unlocked so you can use them anytime you need.
-
You get unlimited lives and retries so you can play as long as you want without worrying about losing.
-
-
Risks of Cricket League Mod APK
-
Some of the risks of using Cricket League Mod APK are:
-
-
You might face some compatibility issues or bugs while playing the game.
-
You might get banned from the online mode if the game detects that you are using a modded version.
-
You might lose your progress or data if the game updates or crashes.
-
You might expose your device to malware or viruses if you download the modded version from an untrusted source.
-
-
How to play Cricket League Mod APK
-
Playing Cricket League Mod APK is similar to playing the original game, except that you have access to unlimited resources and features. Here are some basic steps to help you play the game:
-
Cricket League 2023 Mod APK Download
-How to Install Cricket League Mod APK on Android
-Cricket League Mod APK Unlimited Coins and Gems
-Cricket League Mod APK Latest Version Free Download
-Cricket League Mod APK Hack with All Teams Unlocked
-Cricket League Mod APK Online Multiplayer Mode
-Cricket League Mod APK No Root Required
-Cricket League Mod APK for PC Windows 10/8/7
-Cricket League Mod APK with Realistic Graphics and Physics
-Cricket League Mod APK with Customizable Players and Stadiums
-Cricket League Mod APK with Commentary and Sound Effects
-Cricket League Mod APK with New Features and Updates
-Cricket League Mod APK with Offline Mode and Data Saving
-Cricket League Mod APK with Easy Controls and Gameplay
-Cricket League Mod APK with Different Game Modes and Levels
-Cricket League Mod APK with Achievements and Leaderboards
-Cricket League Mod APK with In-app Purchases and Ads Removed
-Cricket League Mod APK with Anti-ban and Anti-virus Protection
-Cricket League Mod APK with Bug Fixes and Performance Improvements
-Cricket League Mod APK Review and Rating
-Best Sites to Download Cricket League Mod APK for Free
-How to Backup and Restore Cricket League Mod APK Data
-How to Uninstall and Reinstall Cricket League Mod APK
-How to Update Cricket League Mod APK to the Latest Version
-How to Play Cricket League Mod APK on iOS Devices
-How to Play Cricket League Mod APK on Mac OS X
-How to Play Cricket League Mod APK on Linux OS
-How to Play Cricket League Mod APK on Chrome OS
-How to Play Cricket League Mod APK on Fire TV Stick
-How to Play Cricket League Mod APK on Smart TV
-How to Play Cricket League Mod APK on Xbox One/360
-How to Play Cricket League Mod APK on PlayStation 4/5
-How to Play Cricket League Mod APK on Nintendo Switch
-How to Play Cricket League Mod APK on VR Headset
-How to Play Cricket League Mod APK with Friends and Family
-How to Play Cricket League Mod APK with Keyboard and Mouse
-How to Play Cricket League Mod APK with Gamepad and Controller
-How to Play Cricket League Mod APK with Voice Chat and Messaging
-How to Play Cricket League Mod APK with Live Streaming and Recording
-How to Play Cricket League Mod APK with Tips and Tricks
-How to Play Cricket League Mod APK with Cheats and Hacks
-How to Play Cricket League Mod APK with Mods and Customizations
-How to Play Cricket League Mod APK with Challenges and Rewards
-How to Play Cricket League Mod APK with Fun and Entertainment
-How to Solve Common Problems and Errors in Cricket League Mod APK
-How to Contact Customer Support and Feedback for Cricket League Mod APK
-How to Join Community and Forum for Cricket League Mod APK
-How to Learn More About Cricket Rules and History from Cricket League Mod APK
-
Choose your team and mode
-
When you launch the game, you can choose your team from 16 different options, such as India, Australia, England, Pakistan, etc. You can also customize your players and equipment by using the coins and gems that you have. Then, you can choose the mode that you want to play, such as T20, ODI, Test, or Super Over. Each mode has different rules and objectives that you need to follow.
-
Bat, bowl and field your way to the top
-
Once you start the match, you can either bat or bowl first depending on the toss. When you bat, you need to swipe on the screen to hit the ball in different directions. You can also use power-ups and special abilities to boost your shots. When you bowl, you need to tap on the screen to select the type, speed, and direction of your delivery. You can also use power-ups and special abilities to make your balls more effective. When you field, you need to swipe on the screen to catch or throw the ball. You can also use power-ups and special abilities to improve your fielding skills.
-
Compete with other players online
-
If you want to test your skills against other players, you can join the online multiplayer mode. You can either play ranked matches or friendly matches with other players from around the world. You can also chat with them and send them emojis during the match. You can earn trophies and coins by winning matches and climb up the leaderboards. You can also unlock achievements and rewards by completing various challenges.
-
Tips and tricks for Cricket League Mod APK
-
If you want to improve your performance and win more matches in Cricket League Mod APK, here are some tips and tricks that you should follow:
-
Practice your skills in training mode
-
If you are new to the game or want to hone your skills, you should try the training mode. This mode allows you to practice batting, bowling, and fielding without any pressure or time limit. You can also adjust the difficulty level and settings according to your preference. This mode will help you learn the basics and master the controls of the game.
-
Upgrade your players and equipment
-
If you want to make your team stronger and more competitive, you should upgrade your players and equipment regularly. You can use the coins and gems that you have to buy or upgrade various items, such as bats, balls, helmets, gloves, pads, shoes, etc. Each item has different stats and effects that can enhance your performance in different aspects of the game. You can also upgrade your players' skills and abilities by using coins and gems.
-
Use power-ups and special abilities
-
If you want to gain an edge over your opponents, you should use power-ups and special abilities wisely. Power-ups are items that can boost your performance temporarily, such as extra runs, extra wickets, extra overs, etc. Special abilities are skills that can change the outcome of the game dramatically, such as super sixes, super fours, super catches, super throws, etc. You can use power-ups and special abilities by tapping on their icons on the screen during the match. However, you should use them sparingly as they have limited uses and cooldowns.
-
Conclusion
-
Cricket League is a 3D real-time multiplayer cricket game that lets you bat, bowl and field your way to the top of the league in various modes, such as T20, ODI, Test, and Super Over. You can choose from 16 different teams, customize your players and equipment, and compete with other players online or offline. Cricket League has realistic graphics, physics, and sounds that make you feel like you are playing in a real stadium.
-
If you want to enjoy the game with unlimited resources and features, you can download Cricket League Mod APK, a modified version of the original game that gives you access to everything for free. However, you should also be aware of the risks of using Cricket League Mod APK, such as compatibility issues, bugs, bans, data loss, or malware. You should also download the modded version from a trusted source and use it at your own discretion.
-
Cricket League Mod APK is a fun and exciting game that will keep you hooked for hours. Whether you are a cricket fan or not, you will love playing this game and challenging yourself and others. So, what are you waiting for? Download Cricket League Mod APK today and start playing!
-
FAQs
-
Here are some frequently asked questions about Cricket League Mod APK:
-
-
Q: Is Cricket League Mod APK safe to use?
-
A: Cricket League Mod APK is safe to use if you download it from a trusted source and scan it with an antivirus before installing it. However, you should also be careful about the risks of using a modded version of the game, such as compatibility issues, bugs, bans, data loss, or malware.
-
Q: How can I update Cricket League Mod APK?
-
A: You can update Cricket League Mod APK by downloading the latest version from the same source that you downloaded the previous version. You should also uninstall the old version before installing the new one to avoid any errors or conflicts.
-
Q: How can I restore my progress or data in Cricket League Mod APK?
-
A: You can restore your progress or data in Cricket League Mod APK by using a backup app or tool that can save your game data on your device or cloud. You should also backup your data regularly to avoid losing it in case of any issues or crashes.
-
Q: How can I contact the developers of Cricket League Mod APK?
-
A: You can contact the developers of Cricket League Mod APK by visiting their website [HappyMod] or their social media pages on Facebook, Twitter, Instagram, etc. You can also leave a comment or feedback on their website or app page.
-
Q: How can I support the developers of Cricket League Mod APK?
-
A: You can support the developers of Cricket League Mod APK by rating and reviewing their app on their website or app page. You can also share their app with your friends and family and encourage them to download it.
-
401be4b1e0
-
-
\ No newline at end of file
diff --git a/spaces/1toTree/lora_test/ppdiffusers/pipeline_utils.py b/spaces/1toTree/lora_test/ppdiffusers/pipeline_utils.py
deleted file mode 100644
index 1be8011bd01833a0a3e472656843124ce1c79aa3..0000000000000000000000000000000000000000
--- a/spaces/1toTree/lora_test/ppdiffusers/pipeline_utils.py
+++ /dev/null
@@ -1,659 +0,0 @@
-# Copyright (c) 2022 PaddlePaddle Authors. All Rights Reserved.
-# Copyright 2022 The HuggingFace Team. All rights reserved.
-# Copyright (c) 2022, NVIDIA CORPORATION. All rights reserved.
-#
-# Licensed under the Apache License, Version 2.0 (the "License");
-# you may not use this file except in compliance with the License.
-# You may obtain a copy of the License at
-#
-# http://www.apache.org/licenses/LICENSE-2.0
-#
-# Unless required by applicable law or agreed to in writing, software
-# distributed under the License is distributed on an "AS IS" BASIS,
-# WITHOUT WARRANTIES OR CONDITIONS OF ANY KIND, either express or implied.
-# See the License for the specific language governing permissions and
-# limitations under the License.
-
-import importlib
-import inspect
-import os
-import tempfile
-from dataclasses import dataclass
-from typing import Any, Dict, List, Optional, Union
-
-import numpy as np
-import paddle
-import paddle.nn as nn
-import PIL
-from huggingface_hub import (
- create_repo,
- get_hf_file_metadata,
- hf_hub_url,
- repo_type_and_id_from_hf_id,
- upload_folder,
-)
-from huggingface_hub.utils import EntryNotFoundError
-from packaging import version
-from PIL import Image
-from tqdm.auto import tqdm
-
-from . import FastDeployRuntimeModel
-from .configuration_utils import ConfigMixin
-from .utils import PPDIFFUSERS_CACHE, BaseOutput, deprecate, logging
-
-INDEX_FILE = "model_state.pdparams"
-CUSTOM_PIPELINE_FILE_NAME = "pipeline.py"
-DUMMY_MODULES_FOLDER = "ppdiffusers.utils"
-PADDLENLP_DUMMY_MODULES_FOLDER = "paddlenlp.transformers.utils"
-
-logger = logging.get_logger(__name__)
-
-LOADABLE_CLASSES = {
- "ppdiffusers": {
- "ModelMixin": ["save_pretrained", "from_pretrained"],
- "SchedulerMixin": ["save_pretrained", "from_pretrained"],
- "DiffusionPipeline": ["save_pretrained", "from_pretrained"],
- "FastDeployRuntimeModel": ["save_pretrained", "from_pretrained"],
- },
- "paddlenlp.transformers": {
- "PretrainedTokenizer": ["save_pretrained", "from_pretrained"],
- "PretrainedModel": ["save_pretrained", "from_pretrained"],
- "FeatureExtractionMixin": ["save_pretrained", "from_pretrained"],
- "ProcessorMixin": ["save_pretrained", "from_pretrained"],
- "ImageProcessingMixin": ["save_pretrained", "from_pretrained"],
- },
-}
-
-ALL_IMPORTABLE_CLASSES = {}
-for library in LOADABLE_CLASSES:
- ALL_IMPORTABLE_CLASSES.update(LOADABLE_CLASSES[library])
-
-
-@dataclass
-class ImagePipelineOutput(BaseOutput):
- """
- Output class for image pipelines.
-
- Args:
- images (`List[PIL.Image.Image]` or `np.ndarray`)
- List of denoised PIL images of length `batch_size` or numpy array of shape `(batch_size, height, width,
- num_channels)`. PIL images or numpy array present the denoised images of the diffusion pipeline.
- """
-
- images: Union[List[PIL.Image.Image], np.ndarray]
-
-
-@dataclass
-class AudioPipelineOutput(BaseOutput):
- """
- Output class for audio pipelines.
-
- Args:
- audios (`np.ndarray`)
- List of denoised samples of shape `(batch_size, num_channels, sample_rate)`. Numpy array present the
- denoised audio samples of the diffusion pipeline.
- """
-
- audios: np.ndarray
-
-
-class DiffusionPipeline(ConfigMixin):
- r"""
- Base class for all models.
-
- [`DiffusionPipeline`] takes care of storing all components (models, schedulers, processors) for diffusion pipelines
- and handles methods for loading, downloading and saving models as well as a few methods common to all pipelines to:
-
- - move all Paddle modules to the device of your choice
- - enabling/disabling the progress bar for the denoising iteration
-
- Class attributes:
-
- - **config_name** (`str`) -- name of the config file that will store the class and module names of all
- - **_optional_components** (List[`str`]) -- list of all components that are optional so they don't have to be
- passed for the pipeline to function (should be overridden by subclasses).
- """
- config_name = "model_index.json"
- _optional_components = []
-
- def register_modules(self, **kwargs):
- # import it here to avoid circular import
- from . import pipelines
-
- for name, module in kwargs.items():
- # retrieve library
- if module is None:
- register_dict = {name: (None, None)}
- else:
- # TODO (junnyu) support paddlenlp.transformers
- if "paddlenlp" in module.__module__.split(".") or "ppnlp_patch_utils" in module.__module__.split("."):
- library = "paddlenlp.transformers"
- else:
- library = module.__module__.split(".")[0]
-
- # check if the module is a pipeline module
- pipeline_dir = module.__module__.split(".")[-2] if len(module.__module__.split(".")) > 2 else None
- path = module.__module__.split(".")
- is_pipeline_module = pipeline_dir in path and hasattr(pipelines, pipeline_dir)
-
- # if library is not in LOADABLE_CLASSES, then it is a custom module.
- # Or if it's a pipeline module, then the module is inside the pipeline
- # folder so we set the library to module name.
- if library not in LOADABLE_CLASSES or is_pipeline_module:
- library = pipeline_dir
-
- # retrieve class_name
- class_name = module.__class__.__name__
-
- register_dict = {name: (library, class_name)}
-
- # save model index config
- self.register_to_config(**register_dict)
-
- # set models
- setattr(self, name, module)
-
- def save_pretrained(self, save_directory: Union[str, os.PathLike]):
- """
- Save all variables of the pipeline that can be saved and loaded as well as the pipelines configuration file to
- a directory. A pipeline variable can be saved and loaded if its class implements both a save and loading
- method. The pipeline can easily be re-loaded using the `[`~DiffusionPipeline.from_pretrained`]` class method.
-
- Arguments:
- save_directory (`str` or `os.PathLike`):
- Directory to which to save. Will be created if it doesn't exist.
- """
- self.save_config(save_directory)
-
- model_index_dict = dict(self.config)
- model_index_dict.pop("_class_name")
- # TODO (junnyu) support old version
- model_index_dict.pop("_diffusers_paddle_version", None)
- model_index_dict.pop("_diffusers_version", None)
- model_index_dict.pop("_ppdiffusers_version", None)
- model_index_dict.pop("_module", None)
-
- expected_modules, optional_kwargs = self._get_signature_keys(self)
-
- def is_saveable_module(name, value):
- if name not in expected_modules:
- return False
- if name in self._optional_components and value[0] is None:
- return False
- return True
-
- model_index_dict = {k: v for k, v in model_index_dict.items() if is_saveable_module(k, v)}
-
- for pipeline_component_name in model_index_dict.keys():
- sub_model = getattr(self, pipeline_component_name)
-
- model_cls = sub_model.__class__
-
- save_method_name = None
- # search for the model's base class in LOADABLE_CLASSES
- for library_name, library_classes in LOADABLE_CLASSES.items():
- library = importlib.import_module(library_name)
- for base_class, save_load_methods in library_classes.items():
- class_candidate = getattr(library, base_class, None)
- if class_candidate is not None and issubclass(model_cls, class_candidate):
- # if we found a suitable base class in LOADABLE_CLASSES then grab its save method
- save_method_name = save_load_methods[0]
- break
- if save_method_name is not None:
- break
-
- save_method = getattr(sub_model, save_method_name)
- save_method(os.path.join(save_directory, pipeline_component_name))
-
- def save_to_hf_hub(
- self,
- repo_id: str,
- private: Optional[bool] = None,
- commit_message: Optional[str] = None,
- revision: Optional[str] = None,
- create_pr: bool = False,
- ):
- """
- Uploads all elements of this pipeline to a new HuggingFace Hub repository.
- Args:
- repo_id (str): Repository name for your model/tokenizer in the Hub.
- private (bool, optional): Whether the model/tokenizer is set to private
- commit_message (str, optional) — The summary / title / first line of the generated commit. Defaults to: f"Upload {path_in_repo} with huggingface_hub"
- revision (str, optional) — The git revision to commit from. Defaults to the head of the "main" branch.
- create_pr (boolean, optional) — Whether or not to create a Pull Request with that commit. Defaults to False.
- If revision is not set, PR is opened against the "main" branch. If revision is set and is a branch, PR is opened against this branch.
- If revision is set and is not a branch name (example: a commit oid), an RevisionNotFoundError is returned by the server.
-
- Returns: The url of the commit of your model in the given repository.
- """
- repo_url = create_repo(repo_id, private=private, exist_ok=True)
-
- # Infer complete repo_id from repo_url
- # Can be different from the input `repo_id` if repo_owner was implicit
- _, repo_owner, repo_name = repo_type_and_id_from_hf_id(repo_url)
-
- repo_id = f"{repo_owner}/{repo_name}"
-
- # Check if README file already exist in repo
- try:
- get_hf_file_metadata(hf_hub_url(repo_id=repo_id, filename="README.md", revision=revision))
- has_readme = True
- except EntryNotFoundError:
- has_readme = False
-
- with tempfile.TemporaryDirectory() as tmp_dir:
- # save model
- self.save_pretrained(tmp_dir)
- # Add readme if does not exist
- logger.info("README.md not found, adding the default README.md")
- if not has_readme:
- with open(os.path.join(tmp_dir, "README.md"), "w") as f:
- f.write(f"---\nlibrary_name: ppdiffusers\n---\n# {repo_id}")
-
- # Upload model and return
- logger.info(f"Pushing to the {repo_id}. This might take a while")
- return upload_folder(
- repo_id=repo_id,
- repo_type="model",
- folder_path=tmp_dir,
- commit_message=commit_message,
- revision=revision,
- create_pr=create_pr,
- )
-
- def to(self, paddle_device: Optional[str] = None):
- if paddle_device is None:
- return self
-
- module_names, _, _ = self.extract_init_dict(dict(self.config))
- for name in module_names.keys():
- module = getattr(self, name)
- if isinstance(module, nn.Layer):
- if module.dtype == paddle.float16 and str(paddle_device) in ["cpu"]:
- logger.warning(
- "Pipelines loaded with `paddle_dtype=paddle.float16` cannot run with `cpu` device. It"
- " is not recommended to move them to `cpu` as running them will fail. Please make"
- " sure to use an accelerator to run the pipeline in inference, due to the lack of"
- " support for`float16` operations on this device in Paddle. Please, remove the"
- " `paddle_dtype=paddle.float16` argument, or use another device for inference."
- )
- module.to(paddle_device)
- return self
-
- @property
- def device(self):
- r"""
- Returns:
- `paddle.device`: The paddle device on which the pipeline is located.
- """
- module_names, _, _ = self.extract_init_dict(dict(self.config))
- for name in module_names.keys():
- module = getattr(self, name)
- if isinstance(module, nn.Layer):
- return module.place
- return "cpu"
-
- @classmethod
- def from_pretrained(cls, pretrained_model_name_or_path: Optional[Union[str, os.PathLike]], **kwargs):
- r"""
- Instantiate a Paddle diffusion pipeline from pre-trained pipeline weights.
-
- The pipeline is set in evaluation mode by default using `model.eval()` (Dropout modules are deactivated).
-
- The warning *Weights from XXX not initialized from pretrained model* means that the weights of XXX do not come
- pretrained with the rest of the model. It is up to you to train those weights with a downstream fine-tuning
- task.
-
- The warning *Weights from XXX not used in YYY* means that the layer XXX is not used by YYY, therefore those
- weights are discarded.
-
- Parameters:
- pretrained_model_name_or_path (`str` or `os.PathLike`, *optional*):
- Can be either:
-
- - A string, the *model id* of a pretrained pipeline hosted inside in `https://bj.bcebos.com/paddlenlp/models/community`.
- like `CompVis/stable-diffusion-v1-4`, `CompVis/ldm-text2im-large-256`.
- - A path to a *directory* containing pipeline weights saved using
- [`~DiffusionPipeline.save_pretrained`], e.g., `./my_pipeline_directory/`.
- paddle_dtype (`str` or `paddle.dtype`, *optional*):
- Override the default `paddle.dtype` and load the model under this dtype. If `"auto"` is passed the dtype
- will be automatically derived from the model's weights.
- output_loading_info(`bool`, *optional*, defaults to `False`):
- Whether or not to also return a dictionary containing missing keys, unexpected keys and error messages.
- from_hf_hub (bool, *optional*):
- Whether to load from Hugging Face Hub. Defaults to False
- kwargs (remaining dictionary of keyword arguments, *optional*):
- Can be used to overwrite load - and saveable variables - *i.e.* the pipeline components - of the
- specific pipeline class. The overwritten components are then directly passed to the pipelines
- `__init__` method. See example below for more information.
-
- Examples:
-
- ```py
- >>> from ppdiffusers import DiffusionPipeline
-
- >>> # Download pipeline from bos and cache.
- >>> pipeline = DiffusionPipeline.from_pretrained("CompVis/ldm-text2im-large-256")
-
- >>> # Download pipeline that requires an authorization token
- >>> # For more information on access tokens, please refer to this section
- >>> # of the documentation](https://huggingface.co/docs/hub/security-tokens)
- >>> pipeline = DiffusionPipeline.from_pretrained("runwayml/stable-diffusion-v1-5")
-
- >>> # Use a different scheduler
- >>> from ppdiffusers import LMSDiscreteScheduler
-
- >>> scheduler = LMSDiscreteScheduler.from_config(pipeline.scheduler.config)
- >>> pipeline.scheduler = scheduler
- ```
- """
- cache_dir = kwargs.pop("cache_dir", PPDIFFUSERS_CACHE)
- paddle_dtype = kwargs.pop("paddle_dtype", None)
- # (TODO junnyu, we donot suuport this.)
- # custom_pipeline = kwargs.pop("custom_pipeline", None)
- # for fastdeploy model
- runtime_options = kwargs.pop("runtime_options", None)
- from_hf_hub = kwargs.pop("from_hf_hub", False)
-
- # 1. Download the checkpoints and configs
- if not os.path.isdir(pretrained_model_name_or_path):
- config_dict = cls.load_config(
- pretrained_model_name_or_path,
- cache_dir=cache_dir,
- from_hf_hub=from_hf_hub,
- )
- else:
- config_dict = cls.load_config(pretrained_model_name_or_path)
-
- # 2. Load the pipeline class
- if cls != DiffusionPipeline:
- pipeline_class = cls
- else:
- diffusers_module = importlib.import_module(cls.__module__.split(".")[0])
- pipeline_class = getattr(diffusers_module, config_dict["_class_name"])
-
- # To be removed in 1.0.0
- # TODO (junnyu) support old version
- _ppdiffusers_version = (
- config_dict["_diffusers_paddle_version"]
- if "_diffusers_paddle_version" in config_dict
- else config_dict["_ppdiffusers_version"]
- )
- if pipeline_class.__name__ == "StableDiffusionInpaintPipeline" and version.parse(
- version.parse(_ppdiffusers_version).base_version
- ) <= version.parse("0.5.1"):
- from . import (
- StableDiffusionInpaintPipeline,
- StableDiffusionInpaintPipelineLegacy,
- )
-
- pipeline_class = StableDiffusionInpaintPipelineLegacy
-
- deprecation_message = (
- "You are using a legacy checkpoint for inpainting with Stable Diffusion, therefore we are loading the"
- f" {StableDiffusionInpaintPipelineLegacy} class instead of {StableDiffusionInpaintPipeline}. For"
- " better inpainting results, we strongly suggest using Stable Diffusion's official inpainting"
- " checkpoint: https://huggingface.co/runwayml/stable-diffusion-inpainting instead or adapting your"
- f" checkpoint {pretrained_model_name_or_path} to the format of"
- " https://huggingface.co/runwayml/stable-diffusion-inpainting. Note that we do not actively maintain"
- " the {StableDiffusionInpaintPipelineLegacy} class and will likely remove it in version 1.0.0."
- )
- deprecate("StableDiffusionInpaintPipelineLegacy", "1.0.0", deprecation_message, standard_warn=False)
-
- # some modules can be passed directly to the init
- # in this case they are already instantiated in `kwargs`
- # extract them here
- expected_modules, optional_kwargs = cls._get_signature_keys(pipeline_class)
-
- passed_class_obj = {k: kwargs.pop(k) for k in expected_modules if k in kwargs}
- passed_pipe_kwargs = {k: kwargs.pop(k) for k in optional_kwargs if k in kwargs}
-
- init_dict, unused_kwargs, _ = pipeline_class.extract_init_dict(config_dict, **kwargs)
-
- # define init kwargs
- init_kwargs = {k: init_dict.pop(k) for k in optional_kwargs if k in init_dict}
- init_kwargs = {**init_kwargs, **passed_pipe_kwargs}
-
- # remove `null` components
- def load_module(name, value):
- if value[0] is None:
- return False
- if name in passed_class_obj and passed_class_obj[name] is None:
- return False
- return True
-
- init_dict = {k: v for k, v in init_dict.items() if load_module(k, v)}
-
- if len(unused_kwargs) > 0:
- logger.warning(
- f"Keyword arguments {unused_kwargs} are not expected by {pipeline_class.__name__} and will be ignored."
- )
- # import it here to avoid circular import
- from . import pipelines
-
- # 3. Load each module in the pipeline
- for name, (library_name, class_name) in init_dict.items():
- # TODO (junnyu) support old model_index.json
- if library_name == "diffusers_paddle":
- library_name = "ppdiffusers"
-
- is_pipeline_module = hasattr(pipelines, library_name)
- loaded_sub_model = None
-
- # if the model is in a pipeline module, then we load it from the pipeline
- if name in passed_class_obj:
- # 1. check that passed_class_obj has correct parent class
- if not is_pipeline_module:
- library = importlib.import_module(library_name)
- class_obj = getattr(library, class_name)
- importable_classes = LOADABLE_CLASSES[library_name]
- class_candidates = {c: getattr(library, c, None) for c in importable_classes.keys()}
-
- expected_class_obj = None
- for class_name, class_candidate in class_candidates.items():
- if class_candidate is not None and issubclass(class_obj, class_candidate):
- expected_class_obj = class_candidate
-
- if not issubclass(passed_class_obj[name].__class__, expected_class_obj):
- raise ValueError(
- f"{passed_class_obj[name]} is of type: {type(passed_class_obj[name])}, but should be"
- f" {expected_class_obj}"
- )
- else:
- logger.warning(
- f"You have passed a non-standard module {passed_class_obj[name]}. We cannot verify whether it"
- " has the correct type"
- )
-
- # set passed class object
- loaded_sub_model = passed_class_obj[name]
- elif is_pipeline_module:
- pipeline_module = getattr(pipelines, library_name)
- class_obj = getattr(pipeline_module, class_name)
- importable_classes = ALL_IMPORTABLE_CLASSES
- class_candidates = {c: class_obj for c in importable_classes.keys()}
- else:
- # else we just import it from the library.
- library = importlib.import_module(library_name)
-
- class_obj = getattr(library, class_name)
- importable_classes = LOADABLE_CLASSES[library_name]
- class_candidates = {c: getattr(library, c, None) for c in importable_classes.keys()}
-
- if loaded_sub_model is None:
- load_method_name = None
- for class_name, class_candidate in class_candidates.items():
- if class_candidate is not None and issubclass(class_obj, class_candidate):
- load_method_name = importable_classes[class_name][1]
-
- if load_method_name is None:
- none_module = class_obj.__module__
- is_dummy_path = none_module.startswith(DUMMY_MODULES_FOLDER) or none_module.startswith(
- PADDLENLP_DUMMY_MODULES_FOLDER
- )
- if is_dummy_path and "dummy" in none_module:
- # call class_obj for nice error message of missing requirements
- class_obj()
-
- raise ValueError(
- f"The component {class_obj} of {pipeline_class} cannot be loaded as it does not seem to have"
- f" any of the loading methods defined in {ALL_IMPORTABLE_CLASSES}."
- )
-
- load_method = getattr(class_obj, load_method_name)
- loading_kwargs = {
- "from_hf_hub": from_hf_hub,
- "cache_dir": cache_dir,
- }
-
- if issubclass(class_obj, FastDeployRuntimeModel):
- if isinstance(runtime_options, dict):
- options = runtime_options.get(name, None)
- else:
- options = runtime_options
- loading_kwargs["runtime_options"] = options
-
- if os.path.isdir(pretrained_model_name_or_path):
- model_path_dir = os.path.join(pretrained_model_name_or_path, name)
- elif from_hf_hub:
- model_path_dir = pretrained_model_name_or_path
- loading_kwargs["subfolder"] = name
- else:
- # BOS does not require 'subfolder'. We simpy concat the model name with the subfolder
- model_path_dir = pretrained_model_name_or_path + "/" + name
-
- loaded_sub_model = load_method(model_path_dir, **loading_kwargs)
-
- # TODO junnyu find a better way to covert to float16
- if isinstance(loaded_sub_model, nn.Layer):
- if paddle_dtype is not None and next(loaded_sub_model.named_parameters())[1].dtype != paddle_dtype:
- loaded_sub_model = loaded_sub_model.to(dtype=paddle_dtype)
- # paddlenlp model is training mode not eval mode
- loaded_sub_model.eval()
-
- init_kwargs[name] = loaded_sub_model # UNet(...), # DiffusionScheduler(...)
-
- # 4. Potentially add passed objects if expected
- missing_modules = set(expected_modules) - set(init_kwargs.keys())
- passed_modules = list(passed_class_obj.keys())
- optional_modules = pipeline_class._optional_components
- if len(missing_modules) > 0 and missing_modules <= set(passed_modules + optional_modules):
- for module in missing_modules:
- init_kwargs[module] = passed_class_obj.get(module, None)
- elif len(missing_modules) > 0:
- passed_modules = set(list(init_kwargs.keys()) + list(passed_class_obj.keys())) - optional_kwargs
- raise ValueError(
- f"Pipeline {pipeline_class} expected {expected_modules}, but only {passed_modules} were passed."
- )
-
- # 5. Instantiate the pipeline
- model = pipeline_class(**init_kwargs)
- return model
-
- def enable_attention_slicing(self, slice_size: Optional[Union[str, int]] = "auto"):
- r"""
- Enable sliced attention computation.
- When this option is enabled, the attention module will split the input tensor in slices, to compute attention
- in several steps. This is useful to save some memory in exchange for a small speed decrease.
- Args:
- slice_size (`str` or `int`, *optional*, defaults to `"auto"`):
- When `"auto"`, halves the input to the attention heads, so attention will be computed in two steps. If
- `"max"`, maxium amount of memory will be saved by running only one slice at a time. If a number is
- provided, uses as many slices as `attention_head_dim // slice_size`. In this case, `attention_head_dim`
- must be a multiple of `slice_size`.
- """
- self.set_attention_slice(slice_size)
-
- def disable_attention_slicing(self):
- r"""
- Disable sliced attention computation. If `enable_attention_slicing` was previously invoked, this method will go
- back to computing attention in one step.
- """
- # set slice_size = `None` to disable `attention slicing`
- self.enable_attention_slicing(None)
-
- def set_attention_slice(self, slice_size: Optional[int]):
- module_names, _, _ = self.extract_init_dict(dict(self.config))
- for module_name in module_names:
- module = getattr(self, module_name)
- if isinstance(module, nn.Layer) and hasattr(module, "set_attention_slice"):
- module.set_attention_slice(slice_size)
-
- @staticmethod
- def _get_signature_keys(obj):
- parameters = inspect.signature(obj.__init__).parameters
- required_parameters = {k: v for k, v in parameters.items() if v.default == inspect._empty}
- optional_parameters = set({k for k, v in parameters.items() if v.default != inspect._empty})
- expected_modules = set(required_parameters.keys()) - set(["self"])
- return expected_modules, optional_parameters
-
- @property
- def components(self) -> Dict[str, Any]:
- r"""
-
- The `self.components` property can be useful to run different pipelines with the same weights and
- configurations to not have to re-allocate memory.
-
- Examples:
-
- ```py
- >>> from ppdiffusers import (
- ... StableDiffusionPipeline,
- ... StableDiffusionImg2ImgPipeline,
- ... StableDiffusionInpaintPipeline,
- ... )
-
- >>> text2img = StableDiffusionPipeline.from_pretrained("runwayml/stable-diffusion-v1-5")
- >>> img2img = StableDiffusionImg2ImgPipeline(**text2img.components)
- >>> inpaint = StableDiffusionInpaintPipeline(**text2img.components)
- ```
-
- Returns:
- A dictionaly containing all the modules needed to initialize the pipeline.
- """
- expected_modules, optional_parameters = self._get_signature_keys(self)
- components = {
- k: getattr(self, k) for k in self.config.keys() if not k.startswith("_") and k not in optional_parameters
- }
-
- if set(components.keys()) != expected_modules:
- raise ValueError(
- f"{self} has been incorrectly initialized or {self.__class__} is incorrectly implemented. Expected"
- f" {expected_modules} to be defined, but {components} are defined."
- )
-
- return components
-
- @staticmethod
- def numpy_to_pil(images):
- """
- Convert a numpy image or a batch of images to a PIL image.
- """
- if images.ndim == 3:
- images = images[None, ...]
- images = (images * 255).round().astype("uint8")
- if images.shape[-1] == 1:
- # special case for grayscale (single channel) images
- pil_images = [Image.fromarray(image.squeeze(), mode="L") for image in images]
- else:
- pil_images = [Image.fromarray(image) for image in images]
-
- return pil_images
-
- def progress_bar(self, iterable=None, total=None):
- if not hasattr(self, "_progress_bar_config"):
- self._progress_bar_config = {}
- elif not isinstance(self._progress_bar_config, dict):
- raise ValueError(
- f"`self._progress_bar_config` should be of type `dict`, but is {type(self._progress_bar_config)}."
- )
-
- if iterable is not None:
- return tqdm(iterable, **self._progress_bar_config)
- elif total is not None:
- return tqdm(total=total, **self._progress_bar_config)
- else:
- raise ValueError("Either `total` or `iterable` has to be defined.")
-
- def set_progress_bar_config(self, **kwargs):
- self._progress_bar_config = kwargs
diff --git a/spaces/A00001/bingothoo/src/components/chat-image.tsx b/spaces/A00001/bingothoo/src/components/chat-image.tsx
deleted file mode 100644
index 05ecc9771eada27a0f2d160bb01cba170d37bb09..0000000000000000000000000000000000000000
--- a/spaces/A00001/bingothoo/src/components/chat-image.tsx
+++ /dev/null
@@ -1,170 +0,0 @@
-import {
- useEffect,
- useState,
- useCallback,
- ChangeEvent,
- ClipboardEvent,
- MouseEventHandler,
- FormEvent,
- useRef
-} from "react"
-import Image from 'next/image'
-import PasteIcon from '@/assets/images/paste.svg'
-import UploadIcon from '@/assets/images/upload.svg'
-import CameraIcon from '@/assets/images/camera.svg'
-import { useBing } from '@/lib/hooks/use-bing'
-import { cn } from '@/lib/utils'
-
-interface ChatImageProps extends Pick, 'uploadImage'> {}
-
-const preventDefault: MouseEventHandler = (event) => {
- event.nativeEvent.stopImmediatePropagation()
-}
-
-const toBase64 = (file: File): Promise => new Promise((resolve, reject) => {
- const reader = new FileReader()
- reader.readAsDataURL(file)
- reader.onload = () => resolve(reader.result as string)
- reader.onerror = reject
-})
-
-export function ChatImage({ children, uploadImage }: React.PropsWithChildren) {
- const videoRef = useRef(null)
- const canvasRef = useRef(null)
- const mediaStream = useRef()
- const [panel, setPanel] = useState('none')
-
- const upload = useCallback((url: string) => {
- if (url) {
- uploadImage(url)
- }
- setPanel('none')
- }, [panel])
-
- const onUpload = useCallback(async (event: ChangeEvent) => {
- const file = event.target.files?.[0]
- if (file) {
- const fileDataUrl = await toBase64(file)
- if (fileDataUrl) {
- upload(fileDataUrl)
- }
- }
- }, [])
-
- const onPaste = useCallback((event: ClipboardEvent) => {
- const pasteUrl = event.clipboardData.getData('text') ?? ''
- upload(pasteUrl)
- }, [])
-
- const onEnter = useCallback((event: FormEvent) => {
- event.preventDefault()
- event.stopPropagation()
- // @ts-ignore
- const inputUrl = event.target.elements.image.value
- if (inputUrl) {
- upload(inputUrl)
- }
- }, [])
-
- const openVideo: MouseEventHandler = async (event) => {
- event.stopPropagation()
- setPanel('camera-mode')
- }
-
- const onCapture = () => {
- if (canvasRef.current && videoRef.current) {
- const canvas = canvasRef.current
- canvas.width = videoRef.current!.videoWidth
- canvas.height = videoRef.current!.videoHeight
- canvas.getContext('2d')?.drawImage(videoRef.current, 0, 0, canvas.width, canvas.height)
- const cameraUrl = canvas.toDataURL('image/jpeg')
- upload(cameraUrl)
- }
- }
-
- useEffect(() => {
- const handleBlur = () => {
- if (panel !== 'none') {
- setPanel('none')
- }
- }
- document.addEventListener('click', handleBlur)
- return () => {
- document.removeEventListener('click', handleBlur)
- }
- }, [panel])
-
- useEffect(() => {
- if (panel === 'camera-mode') {
- navigator.mediaDevices.getUserMedia({ video: true, audio: false })
- .then(videoStream => {
- mediaStream.current = videoStream
- if (videoRef.current) {
- videoRef.current.srcObject = videoStream
- }
- })
- } else {
- if (mediaStream.current) {
- mediaStream.current.getTracks().forEach(function(track) {
- track.stop()
- })
- mediaStream.current = undefined
- }
- }
- }, [panel])
-
- return (
-
- )
-}
diff --git a/spaces/AISuperheroes/08GR-KitchenSink-AIUIUX/demos/kitchen_sink/run.py b/spaces/AISuperheroes/08GR-KitchenSink-AIUIUX/demos/kitchen_sink/run.py
deleted file mode 100644
index ea9471edb82b28c509429b72124451d28f1c56ef..0000000000000000000000000000000000000000
--- a/spaces/AISuperheroes/08GR-KitchenSink-AIUIUX/demos/kitchen_sink/run.py
+++ /dev/null
@@ -1,146 +0,0 @@
-import os
-import json
-import numpy as np
-import gradio as gr
-
-CHOICES = ["foo", "bar", "baz"]
-JSONOBJ = """{"items":{"item":[{"id": "0001","type": null,"is_good": false,"ppu": 0.55,"batters":{"batter":[{ "id": "1001", "type": "Regular" },{ "id": "1002", "type": "Chocolate" },{ "id": "1003", "type": "Blueberry" },{ "id": "1004", "type": "Devil's Food" }]},"topping":[{ "id": "5001", "type": "None" },{ "id": "5002", "type": "Glazed" },{ "id": "5005", "type": "Sugar" },{ "id": "5007", "type": "Powdered Sugar" },{ "id": "5006", "type": "Chocolate with Sprinkles" },{ "id": "5003", "type": "Chocolate" },{ "id": "5004", "type": "Maple" }]}]}}"""
-
-def fn(
- text1,
- text2,
- num,
- slider1,
- slider2,
- single_checkbox,
- checkboxes,
- radio,
- dropdown,
- im1,
- im2,
- im3,
- im4,
- video,
- audio1,
- audio2,
- file,
- df1,
- df2,
-):
- return (
- (text1 if single_checkbox else text2)
- + ", selected:"
- + ", ".join(checkboxes), # Text
- {
- "positive": num / (num + slider1 + slider2),
- "negative": slider1 / (num + slider1 + slider2),
- "neutral": slider2 / (num + slider1 + slider2),
- }, # Label
- (audio1[0], np.flipud(audio1[1]))
- if audio1 is not None else os.path.join(os.path.dirname(__file__), "files/cantina.wav"), # Audio
- np.flipud(im1)
- if im1 is not None else os.path.join(os.path.dirname(__file__), "files/cheetah1.jpg"), # Image
- video
- if video is not None else os.path.join(os.path.dirname(__file__), "files/world.mp4"), # Video
- [
- ("The", "art"),
- ("quick brown", "adj"),
- ("fox", "nn"),
- ("jumped", "vrb"),
- ("testing testing testing", None),
- ("over", "prp"),
- ("the", "art"),
- ("testing", None),
- ("lazy", "adj"),
- ("dogs", "nn"),
- (".", "punc"),
- ] + [(f"test {x}", f"test {x}") for x in range(10)], # HighlightedText
- [
- ("The testing testing testing", None),
- ("over", 0.6),
- ("the", 0.2),
- ("testing", None),
- ("lazy", -0.1),
- ("dogs", 0.4),
- (".", 0),
- ] + [(f"test", x / 10) for x in range(-10, 10)], # HighlightedText
- json.loads(JSONOBJ), # JSON
- "", # HTML
- os.path.join(os.path.dirname(__file__), "files/titanic.csv"),
- df1, # Dataframe
- np.random.randint(0, 10, (4, 4)), # Dataframe
- df2, # Timeseries
- )
-
-
-demo = gr.Interface(
- fn,
- inputs=[
- gr.Textbox(value="Lorem ipsum", label="Textbox"),
- gr.Textbox(lines=3, placeholder="Type here..", label="Textbox 2"),
- gr.Number(label="Number", value=42),
- gr.Slider(10, 20, value=15, label="Slider: 10 - 20"),
- gr.Slider(maximum=20, step=0.04, label="Slider: step @ 0.04"),
- gr.Checkbox(label="Checkbox"),
- gr.CheckboxGroup(label="CheckboxGroup", choices=CHOICES, value=CHOICES[0:2]),
- gr.Radio(label="Radio", choices=CHOICES, value=CHOICES[2]),
- gr.Dropdown(label="Dropdown", choices=CHOICES),
- gr.Image(label="Image"),
- gr.Image(label="Image w/ Cropper", tool="select"),
- gr.Image(label="Sketchpad", source="canvas"),
- gr.Image(label="Webcam", source="webcam"),
- gr.Video(label="Video"),
- gr.Audio(label="Audio"),
- gr.Audio(label="Microphone", source="microphone"),
- gr.File(label="File"),
- gr.Dataframe(label="Dataframe", headers=["Name", "Age", "Gender"]),
- gr.Timeseries(x="time", y=["price", "value"], colors=["pink", "purple"]),
- ],
- outputs=[
- gr.Textbox(label="Textbox"),
- gr.Label(label="Label"),
- gr.Audio(label="Audio"),
- gr.Image(label="Image"),
- gr.Video(label="Video"),
- gr.HighlightedText(label="HighlightedText", color_map={"punc": "pink", "test 0": "blue"}),
- gr.HighlightedText(label="HighlightedText", show_legend=True),
- gr.JSON(label="JSON"),
- gr.HTML(label="HTML"),
- gr.File(label="File"),
- gr.Dataframe(label="Dataframe"),
- gr.Dataframe(label="Numpy"),
- gr.Timeseries(x="time", y=["price", "value"], label="Timeseries"),
- ],
- examples=[
- [
- "the quick brown fox",
- "jumps over the lazy dog",
- 10,
- 12,
- 4,
- True,
- ["foo", "baz"],
- "baz",
- "bar",
- os.path.join(os.path.dirname(__file__), "files/cheetah1.jpg"),
- os.path.join(os.path.dirname(__file__), "files/cheetah1.jpg"),
- os.path.join(os.path.dirname(__file__), "files/cheetah1.jpg"),
- os.path.join(os.path.dirname(__file__), "files/cheetah1.jpg"),
- os.path.join(os.path.dirname(__file__), "files/world.mp4"),
- os.path.join(os.path.dirname(__file__), "files/cantina.wav"),
- os.path.join(os.path.dirname(__file__), "files/cantina.wav"),
- os.path.join(os.path.dirname(__file__), "files/titanic.csv"),
- [[1, 2, 3], [3, 4, 5]],
- os.path.join(os.path.dirname(__file__), "files/time.csv"),
- ]
- ]
- * 3,
- theme="default",
- title="Gradio AI UI UX",
- cache_examples=False,
- description="Try out all the components!",
- article="Learn more about [Gradio](http://gradio.app)",
-)
-
-if __name__ == "__main__":
- demo.launch()
diff --git a/spaces/AIWaves/SOP_Generation-single/Agent/Agent.py b/spaces/AIWaves/SOP_Generation-single/Agent/Agent.py
deleted file mode 100644
index 74b0f5d234f1ce67d5c293bb3ab751302f9cac42..0000000000000000000000000000000000000000
--- a/spaces/AIWaves/SOP_Generation-single/Agent/Agent.py
+++ /dev/null
@@ -1,243 +0,0 @@
-# coding=utf-8
-# Copyright 2023 The AIWaves Inc. team.
-
-#
-# Licensed under the Apache License, Version 2.0 (the "License");
-# you may not use this file except in compliance with the License.
-# You may obtain a copy of the License at
-#
-# http://www.apache.org/licenses/LICENSE-2.0
-#
-# Unless required by applicable law or agreed to in writing, software
-# distributed under the License is distributed on an "AS IS" BASIS,
-# WITHOUT WARRANTIES OR CONDITIONS OF ANY KIND, either express or implied.
-# See the License for the specific language governing permissions and
-# limitations under the License.
-"""LLM autonoumous agent"""
-from LLM.base_LLM import *
-from Component import *
-from Action import Action
-from Prompt import *
-
-headers = {
- "Content-Type": "text/event-stream",
- "Cache-Control": "no-cache",
- "X-Accel-Buffering": "no",
-}
-
-
-
-
-class Agent:
- """
- Auto agent, input the JSON of SOP.
- """
-
- # Agent should have args: agents,states
- def __init__(self, name, agent_state_roles, **kwargs) -> None:
- self.state_roles = agent_state_roles
- self.name = name
-
- self.style = kwargs["style"]
- self.LLMs = kwargs["LLMs"]
- self.LLM = None
- self.is_user = kwargs["is_user"]
- self.begins = kwargs["begins"] if "begins" in kwargs else False
- self.current_role = ""
- self.long_term_memory = []
- self.short_term_memory = ""
- self.current_state = None
- self.first_speak = True
- self.environment = None
-
-
- @classmethod
- def from_config(cls, config_path):
- """
- Initialize agents based on json file
- Return:
- agents(dict) : key:agent_name;value:class(Agent)
- names_to_roles(dict) : key:state_name value:(dict; (key:agent_name ; value:agent_role))
- roles_to_names(dict) : key:state_name value:(dict; (key:agent_role ; value:agent_name))
- """
- with open(config_path) as f:
- config = json.load(f)
-
- roles_to_names = {}
- names_to_roles = {}
- agents = {}
- user_names = json.loads(os.environ["User_Names"]) if "User_Names" in os.environ else []
- for agent_name, agent_dict in config["agents"].items():
- agent_state_roles = {}
- agent_LLMs = {}
- agent_begins = {}
- for state_name, agent_role in agent_dict["roles"].items():
-
- agent_begins[state_name] = {}
-
- if state_name not in roles_to_names:
- roles_to_names[state_name] = {}
- if state_name not in names_to_roles:
- names_to_roles[state_name] = {}
- roles_to_names[state_name][agent_role] = agent_name
- names_to_roles[state_name][agent_name] = agent_role
- agent_state_roles[state_name] = agent_role
- current_state = config["states"][state_name]
- current_state["roles"] = list(current_state["agent_states"].keys()) if "roles" not in current_state else current_state["roles"]
- current_state_begin_role = current_state["begin_role"] if "begin_role" in current_state else current_state["roles"][0]
- agent_begins[state_name]["is_begin"] = current_state_begin_role==agent_role if "begin_role" in current_state else False
- agent_begins[state_name]["begin_query"] = current_state["begin_query"] if "begin_query" in current_state else " "
- agent_LLMs[state_name] = init_LLM("logs"+os.sep+f"{agent_name}",**current_state["agent_states"][agent_role])
- agents[agent_name] = cls(
- agent_name,
- agent_state_roles,
- LLMs=agent_LLMs,
- is_user=agent_name in user_names,
- style = agent_dict["style"],
- begins = agent_begins
- )
- assert len(config["agents"].keys()) != 2 or (roles_to_names[config["root"]][config["states"][config["root"]]["begin_role"]] not in user_names and "begin_query" in config["states"][config["root"]]),"In a single-agent scenario, there must be an opening statement and it must be the agent"
- return agents, roles_to_names, names_to_roles
-
- def step(self, current_state,input=""):
- """
- return actions by current state and environment
- Return: action(Action)
- """
-
- current_state.chat_nums +=1
- state_begin = current_state.is_begin
- agent_begin = self.begins[current_state.name]["is_begin"]
- self.begins[current_state.name]["is_begin"] = False
- current_state.is_begin = False
- environment = self.environment
-
- self.current_state = current_state
- # 先根据当前环境更新信息
- # First update the information according to the current environment
-
- response = " "
- res_dict = {}
-
- if self.is_user:
- response = f"{self.name}:{input}"
- else:
- if len(environment.shared_memory["long_term_memory"])>0:
- current_history = self.observe()
- self.long_term_memory.append(current_history)
- if agent_begin:
- response = (char for char in self.begins[current_state.name]["begin_query"])
- else:
- response,res_dict = self.act()
-
-
- action_dict = {
- "response": response,
- "res_dict": res_dict,
- "role": self.state_roles[current_state.name],
- "name": self.name,
- "state_begin" : state_begin,
- "agent_begin" : agent_begin,
- "is_user" : self.is_user
- }
- return Action(**action_dict)
-
- def act(self):
- """
- return actions by the current state
- """
- current_state = self.current_state
- chat_history = self.long_term_memory
- current_LLM = self.LLMs[current_state.name]
-
- system_prompt, last_prompt, res_dict = self.compile()
-
-
-
- response = current_LLM.get_response(
- chat_history, system_prompt, last_prompt, stream=True
- )
- return response,res_dict
-
- def update_memory(self, memory):
- self.long_term_memory.append(
- {"role": "assistant", "content": memory.content}
- )
-
- MAX_CHAT_HISTORY = eval(os.environ["MAX_CHAT_HISTORY"])
- environment = self.environment
- current_chat_history_idx = environment.current_chat_history_idx if environment.environment_type == "competive" else 0
-
- current_long_term_memory = environment.shared_memory["long_term_memory"][current_chat_history_idx:]
- last_conversation_idx = environment._get_agent_last_conversation_idx(self,current_long_term_memory)
- if len(current_long_term_memory)-last_conversation_idx >= MAX_CHAT_HISTORY:
- current_state = self.current_state
- current_role = self.state_roles[current_state.name]
- current_component_dict = current_state.components[current_role]
-
- # get chat history from new conversation
- conversations = environment._get_agent_new_memory(self,current_long_term_memory)
-
- # get summary
- summary_prompt = (
- current_state.summary_prompt[current_role]
- if current_state.summary_prompt
- else f"""your name is {self.name},your role is{current_component_dict["style"].role},your task is {current_component_dict["task"].task}.\n"""
- )
- summary_prompt =eval(Agent_summary_system_prompt)
- summary = self.LLMs[current_state.name].get_response(None, summary_prompt,stream = False)
- self.short_term_memory = summary
-
-
- def compile(self):
- """
- get prompt from state depend on your role
- Return:
- system_prompt:system_prompt for agents's LLM
- last_prompt:last_prompt for agents's LLM
- res_dict(dict): Other return from tool component.For example: search engine results
- """
- current_state = self.current_state
- self.current_roles = self.state_roles[current_state.name]
- current_state_name = current_state.name
- self.LLM = self.LLMs[current_state_name]
- components = current_state.components[self.state_roles[current_state_name]]
-
- system_prompt = self.current_state.environment_prompt
- last_prompt = ""
-
- res_dict = {}
- for component in components.values():
- if isinstance(component, (OutputComponent, LastComponent)):
- last_prompt = last_prompt + "\n" + component.get_prompt(self)
- elif isinstance(component, PromptComponent):
- system_prompt = (
- system_prompt + "\n" + component.get_prompt(self)
- )
- elif isinstance(component, ToolComponent):
- response = component.func(self)
- if "prompt" in response and response["prompt"]:
- last_prompt = last_prompt + "\n" + response["prompt"]
- res_dict.update(response)
-
- name = self.name
- query = self.environment.shared_memory["long_term_memory"][-1] if len(self.environment.shared_memory["long_term_memory"]) else ""
- last_prompt = eval(Agent_last_prompt)
- system_prompt = eval(Agent_system_prompt)
- return system_prompt, last_prompt, res_dict
-
-
- def observe(self):
- """
- Update one's own memory according to the current environment, including: updating short-term memory; updating long-term memory
- """
- return self.environment._observe(self)
-
-
- def generate_sop(self):
- pass
-
- def reflection(self):
- pass
-
-
diff --git a/spaces/AP123/Upside-Down-Diffusion/user_history.py b/spaces/AP123/Upside-Down-Diffusion/user_history.py
deleted file mode 100644
index 19d3b4a896ce19d8815d0f455c235a64d77b260a..0000000000000000000000000000000000000000
--- a/spaces/AP123/Upside-Down-Diffusion/user_history.py
+++ /dev/null
@@ -1,524 +0,0 @@
-"""
-User History is a plugin that you can add to your Spaces to cache generated images for your users.
-
-Key features:
-- 🤗 Sign in with Hugging Face
-- Save generated images with their metadata: prompts, timestamp, hyper-parameters, etc.
-- Export your history as zip.
-- Delete your history to respect privacy.
-- Compatible with Persistent Storage for long-term storage.
-- Admin panel to check configuration and disk usage .
-
-Useful links:
-- Demo: https://huggingface.co/spaces/Wauplin/gradio-user-history
-- README: https://huggingface.co/spaces/Wauplin/gradio-user-history/blob/main/README.md
-- Source file: https://huggingface.co/spaces/Wauplin/gradio-user-history/blob/main/user_history.py
-- Discussions: https://huggingface.co/spaces/Wauplin/gradio-user-history/discussions
-"""
-import json
-import os
-import shutil
-import warnings
-from datetime import datetime
-from functools import cache
-from pathlib import Path
-from typing import Callable, Dict, List, Tuple
-from uuid import uuid4
-
-import gradio as gr
-import numpy as np
-import requests
-from filelock import FileLock
-from PIL.Image import Image
-
-
-def setup(folder_path: str | Path | None = None) -> None:
- user_history = _UserHistory()
- user_history.folder_path = _resolve_folder_path(folder_path)
- user_history.initialized = True
-
- # TODO: remove this section once all Spaces have migrated
- _migrate_history()
-
-
-def render() -> None:
- user_history = _UserHistory()
-
- # initialize with default config
- if not user_history.initialized:
- print(
- "Initializing user history with default config. Use `user_history.setup(...)` to customize folder_path."
- )
- setup()
-
- # Render user history tab
- gr.Markdown(
- "## Your past generations\n\nLog in to keep a gallery of your previous generations. Your history will be saved"
- " and available on your next visit. Make sure to export your images from time to time as this gallery may be"
- " deleted in the future."
- )
-
- if os.getenv("SYSTEM") == "spaces" and not os.path.exists("/data"):
- gr.Markdown(
- "**⚠️ Persistent storage is disabled, meaning your history will be lost if the Space gets restarted."
- " Only the Space owner can setup a Persistent Storage. If you are not the Space owner, consider"
- " duplicating this Space to set your own storage.⚠️**"
- )
-
- with gr.Row():
- gr.LoginButton(min_width=250)
- gr.LogoutButton(min_width=250)
- refresh_button = gr.Button(
- "Refresh",
- icon="https://huggingface.co/spaces/Wauplin/gradio-user-history/resolve/main/assets/icon_refresh.png",
- )
- export_button = gr.Button(
- "Export",
- icon="https://huggingface.co/spaces/Wauplin/gradio-user-history/resolve/main/assets/icon_download.png",
- )
- delete_button = gr.Button(
- "Delete history",
- icon="https://huggingface.co/spaces/Wauplin/gradio-user-history/resolve/main/assets/icon_delete.png",
- )
-
- # "Export zip" row (hidden by default)
- with gr.Row():
- export_file = gr.File(
- file_count="single",
- file_types=[".zip"],
- label="Exported history",
- visible=False,
- )
-
- # "Config deletion" row (hidden by default)
- with gr.Row():
- confirm_button = gr.Button(
- "Confirm delete all history", variant="stop", visible=False
- )
- cancel_button = gr.Button("Cancel", visible=False)
-
- # Gallery
- gallery = gr.Gallery(
- label="Past images",
- show_label=True,
- elem_id="gallery",
- object_fit="contain",
- columns=5,
- height=600,
- preview=False,
- show_share_button=False,
- show_download_button=False,
- )
- gr.Markdown(
- "User history is powered by"
- " [Wauplin/gradio-user-history](https://huggingface.co/spaces/Wauplin/gradio-user-history). Integrate it to"
- " your own Space in just a few lines of code!"
- )
- gallery.attach_load_event(_fetch_user_history, every=None)
-
- # Interactions
- refresh_button.click(
- fn=_fetch_user_history, inputs=[], outputs=[gallery], queue=False
- )
- export_button.click(
- fn=_export_user_history, inputs=[], outputs=[export_file], queue=False
- )
-
- # Taken from https://github.com/gradio-app/gradio/issues/3324#issuecomment-1446382045
- delete_button.click(
- lambda: [gr.update(visible=True), gr.update(visible=True)],
- outputs=[confirm_button, cancel_button],
- queue=False,
- )
- cancel_button.click(
- lambda: [gr.update(visible=False), gr.update(visible=False)],
- outputs=[confirm_button, cancel_button],
- queue=False,
- )
- confirm_button.click(_delete_user_history).then(
- lambda: [gr.update(visible=False), gr.update(visible=False)],
- outputs=[confirm_button, cancel_button],
- queue=False,
- )
-
- # Admin section (only shown locally or when logged in as Space owner)
- _admin_section()
-
-
-def save_image(
- profile: gr.OAuthProfile | None,
- image: Image | np.ndarray | str | Path,
- label: str | None = None,
- metadata: Dict | None = None,
-):
- # Ignore images from logged out users
- if profile is None:
- return
- username = profile["preferred_username"]
-
- # Ignore images if user history not used
- user_history = _UserHistory()
- if not user_history.initialized:
- warnings.warn(
- "User history is not set in Gradio demo. Saving image is ignored. You must use `user_history.render(...)`"
- " first."
- )
- return
-
- # Copy image to storage
- image_path = _copy_image(image, dst_folder=user_history._user_images_path(username))
-
- # Save new image + metadata
- if metadata is None:
- metadata = {}
- if "datetime" not in metadata:
- metadata["datetime"] = str(datetime.now())
- data = {"path": str(image_path), "label": label, "metadata": metadata}
- with user_history._user_lock(username):
- with user_history._user_jsonl_path(username).open("a") as f:
- f.write(json.dumps(data) + "\n")
-
-
-#############
-# Internals #
-#############
-
-
-class _UserHistory(object):
- _instance = None
- initialized: bool = False
- folder_path: Path
-
- def __new__(cls):
- # Using singleton pattern => we don't want to expose an object (more complex to use) but still want to keep
- # state between `render` and `save_image` calls.
- if cls._instance is None:
- cls._instance = super(_UserHistory, cls).__new__(cls)
- return cls._instance
-
- def _user_path(self, username: str) -> Path:
- path = self.folder_path / username
- path.mkdir(parents=True, exist_ok=True)
- return path
-
- def _user_lock(self, username: str) -> FileLock:
- """Ensure history is not corrupted if concurrent calls."""
- return FileLock(
- self.folder_path / f"{username}.lock"
- ) # lock outside of folder => better when exporting ZIP
-
- def _user_jsonl_path(self, username: str) -> Path:
- return self._user_path(username) / "history.jsonl"
-
- def _user_images_path(self, username: str) -> Path:
- path = self._user_path(username) / "images"
- path.mkdir(parents=True, exist_ok=True)
- return path
-
-
-def _fetch_user_history(profile: gr.OAuthProfile | None) -> List[Tuple[str, str]]:
- """Return saved history for that user, if it exists."""
- # Cannot load history for logged out users
- if profile is None:
- return []
- username = profile["preferred_username"]
-
- user_history = _UserHistory()
- if not user_history.initialized:
- warnings.warn(
- "User history is not set in Gradio demo. You must use `user_history.render(...)` first."
- )
- return []
-
- with user_history._user_lock(username):
- # No file => no history saved yet
- jsonl_path = user_history._user_jsonl_path(username)
- if not jsonl_path.is_file():
- return []
-
- # Read history
- images = []
- for line in jsonl_path.read_text().splitlines():
- data = json.loads(line)
- images.append((data["path"], data["label"] or ""))
- return list(reversed(images))
-
-
-def _export_user_history(profile: gr.OAuthProfile | None) -> Dict | None:
- """Zip all history for that user, if it exists and return it as a downloadable file."""
- # Cannot load history for logged out users
- if profile is None:
- return None
- username = profile["preferred_username"]
-
- user_history = _UserHistory()
- if not user_history.initialized:
- warnings.warn(
- "User history is not set in Gradio demo. You must use `user_history.render(...)` first."
- )
- return None
-
- # Zip history
- with user_history._user_lock(username):
- path = shutil.make_archive(
- str(_archives_path() / f"history_{username}"),
- "zip",
- user_history._user_path(username),
- )
-
- return gr.update(visible=True, value=path)
-
-
-def _delete_user_history(profile: gr.OAuthProfile | None) -> None:
- """Delete all history for that user."""
- # Cannot load history for logged out users
- if profile is None:
- return
- username = profile["preferred_username"]
-
- user_history = _UserHistory()
- if not user_history.initialized:
- warnings.warn(
- "User history is not set in Gradio demo. You must use `user_history.render(...)` first."
- )
- return
-
- with user_history._user_lock(username):
- shutil.rmtree(user_history._user_path(username))
-
-
-####################
-# Internal helpers #
-####################
-
-
-def _copy_image(image: Image | np.ndarray | str | Path, dst_folder: Path) -> Path:
- """Copy image to the images folder."""
- # Already a path => copy it
- if isinstance(image, str):
- image = Path(image)
- if isinstance(image, Path):
- dst = dst_folder / f"{uuid4().hex}_{Path(image).name}" # keep file ext
- shutil.copyfile(image, dst)
- return dst
-
- # Still a Python object => serialize it
- if isinstance(image, np.ndarray):
- image = Image.fromarray(image)
- if isinstance(image, Image):
- dst = dst_folder / f"{uuid4().hex}.png"
- image.save(dst)
- return dst
-
- raise ValueError(f"Unsupported image type: {type(image)}")
-
-
-def _resolve_folder_path(folder_path: str | Path | None) -> Path:
- if folder_path is not None:
- return Path(folder_path).expanduser().resolve()
-
- if os.getenv("SYSTEM") == "spaces" and os.path.exists(
- "/data"
- ): # Persistent storage is enabled!
- return Path("/data") / "_user_history"
-
- # Not in a Space or Persistent storage not enabled => local folder
- return Path(__file__).parent / "_user_history"
-
-
-def _archives_path() -> Path:
- # Doesn't have to be on persistent storage as it's only used for download
- path = Path(__file__).parent / "_user_history_exports"
- path.mkdir(parents=True, exist_ok=True)
- return path
-
-
-#################
-# Admin section #
-#################
-
-
-def _admin_section() -> None:
- title = gr.Markdown()
- title.attach_load_event(_display_if_admin(), every=None)
-
-
-def _display_if_admin() -> Callable:
- def _inner(profile: gr.OAuthProfile | None) -> str:
- if profile is None:
- return ""
- if profile["preferred_username"] in _fetch_admins():
- return _admin_content()
- return ""
-
- return _inner
-
-
-def _admin_content() -> str:
- return f"""
-## Admin section
-
-Running on **{os.getenv("SYSTEM", "local")}** (id: {os.getenv("SPACE_ID")}). {_get_msg_is_persistent_storage_enabled()}
-
-Admins: {', '.join(_fetch_admins())}
-
-{_get_nb_users()} user(s), {_get_nb_images()} image(s)
-
-### Configuration
-
-History folder: *{_UserHistory().folder_path}*
-
-Exports folder: *{_archives_path()}*
-
-### Disk usage
-
-{_disk_space_warning_message()}
-"""
-
-
-def _get_nb_users() -> int:
- user_history = _UserHistory()
- if not user_history.initialized:
- return 0
- if user_history.folder_path is not None:
- return len(
- [path for path in user_history.folder_path.iterdir() if path.is_dir()]
- )
- return 0
-
-
-def _get_nb_images() -> int:
- user_history = _UserHistory()
- if not user_history.initialized:
- return 0
- if user_history.folder_path is not None:
- return len([path for path in user_history.folder_path.glob("*/images/*")])
- return 0
-
-
-def _get_msg_is_persistent_storage_enabled() -> str:
- if os.getenv("SYSTEM") == "spaces":
- if os.path.exists("/data"):
- return "Persistent storage is enabled."
- else:
- return (
- "Persistent storage is not enabled. This means that user histories will be deleted when the Space is"
- " restarted. Consider adding a Persistent Storage in your Space settings."
- )
- return ""
-
-
-def _disk_space_warning_message() -> str:
- user_history = _UserHistory()
- if not user_history.initialized:
- return ""
-
- message = ""
- if user_history.folder_path is not None:
- total, used, _ = _get_disk_usage(user_history.folder_path)
- message += f"History folder: **{used / 1e9 :.0f}/{total / 1e9 :.0f}GB** used ({100*used/total :.0f}%)."
-
- total, used, _ = _get_disk_usage(_archives_path())
- message += f"\n\nExports folder: **{used / 1e9 :.0f}/{total / 1e9 :.0f}GB** used ({100*used/total :.0f}%)."
-
- return f"{message.strip()}"
-
-
-def _get_disk_usage(path: Path) -> Tuple[int, int, int]:
- for path in [path] + list(
- path.parents
- ): # first check target_dir, then each parents one by one
- try:
- return shutil.disk_usage(path)
- except (
- OSError
- ): # if doesn't exist or can't read => fail silently and try parent one
- pass
- return 0, 0, 0
-
-
-@cache
-def _fetch_admins() -> List[str]:
- # Running locally => fake user is admin
- if os.getenv("SYSTEM") != "spaces":
- return ["FakeGradioUser"]
-
- # Running in Space but no space_id => ???
- space_id = os.getenv("SPACE_ID")
- if space_id is None:
- return ["Unknown"]
-
- # Running in Space => try to fetch organization members
- # Otherwise, it's not an organization => namespace is the user
- namespace = space_id.split("/")[0]
- response = requests.get(
- f"https://huggingface.co/api/organizations/{namespace}/members"
- )
- if response.status_code == 200:
- return sorted(
- (member["user"] for member in response.json()), key=lambda x: x.lower()
- )
- return [namespace]
-
-
-################################################################
-# Legacy helpers to migrate image structure to new data format #
-################################################################
-# TODO: remove this section once all Spaces have migrated
-
-
-def _migrate_history():
- """Script to migrate user history from v0 to v1."""
- legacy_history_path = _legacy_get_history_folder_path()
- if not legacy_history_path.exists():
- return
-
- error_count = 0
- for json_path in legacy_history_path.glob("*.json"):
- username = json_path.stem
- print(f"Migrating history for user {username}...")
- error_count += _legacy_move_user_history(username)
- print("Done.")
- print(f"Migration complete. {error_count} error(s) happened.")
-
- if error_count == 0:
- shutil.rmtree(legacy_history_path, ignore_errors=True)
-
-
-def _legacy_move_user_history(username: str) -> int:
- history = _legacy_read_user_history(username)
- error_count = 0
- for image, prompt in reversed(history):
- try:
- save_image(
- label=prompt, image=image, profile={"preferred_username": username}
- )
- except Exception as e:
- print("Issue while migrating image:", e)
- error_count += 1
- return error_count
-
-
-def _legacy_get_history_folder_path() -> Path:
- _folder = os.environ.get("HISTORY_FOLDER")
- if _folder is None:
- _folder = Path(__file__).parent / "history"
- return Path(_folder)
-
-
-def _legacy_read_user_history(username: str) -> List[Tuple[str, str]]:
- """Return saved history for that user."""
- with _legacy_user_lock(username):
- path = _legacy_user_history_path(username)
- if path.exists():
- return json.loads(path.read_text())
- return [] # No history yet
-
-
-def _legacy_user_history_path(username: str) -> Path:
- return _legacy_get_history_folder_path() / f"{username}.json"
-
-
-def _legacy_user_lock(username: str) -> FileLock:
- """Ensure history is not corrupted if concurrent calls."""
- return FileLock(f"{_legacy_user_history_path(username)}.lock")
diff --git a/spaces/AgentVerse/agentVerse/agentverse/memory/chat_history.py b/spaces/AgentVerse/agentVerse/agentverse/memory/chat_history.py
deleted file mode 100644
index 38649d40292a4dadfa92721dc175a1e17b90eebd..0000000000000000000000000000000000000000
--- a/spaces/AgentVerse/agentVerse/agentverse/memory/chat_history.py
+++ /dev/null
@@ -1,77 +0,0 @@
-import json
-from typing import List
-
-from pydantic import Field
-
-from agentverse.message import Message, ExecutorMessage
-
-from . import memory_registry
-from .base import BaseMemory
-
-
-@memory_registry.register("chat_history")
-class ChatHistoryMemory(BaseMemory):
- messages: List[Message] = Field(default=[])
-
- def add_message(self, messages: List[Message]) -> None:
- for message in messages:
- self.messages.append(message)
-
- def to_string(self, add_sender_prefix: bool = False) -> str:
- if add_sender_prefix:
- return "\n".join(
- [
- f"[{message.sender}]: {message.content}"
- if message.sender != ""
- else message.content
- for message in self.messages
- ]
- )
- else:
- return "\n".join([message.content for message in self.messages])
-
- def to_messages(self, my_name: str = "", start_index: int = 0) -> List[dict]:
- messages = []
- for message in self.messages[start_index:]:
- if message.sender == my_name:
- if isinstance(message, ExecutorMessage):
- if message.tool_name != "":
- messages.append(
- {
- "role": "assistant",
- "content": f"[{message.sender}]: {message.content}"
- if message.content != ""
- else "",
- "function_call": {
- "name": message.tool_name,
- "arguments": json.dumps(message.tool_input),
- },
- }
- )
- continue
- messages.append(
- {
- "role": "assistant",
- "content": f"[{message.sender}]: {message.content}",
- }
- )
- continue
- if message.sender == "function":
- messages.append(
- {
- "role": "function",
- "content": message.content,
- "name": message.tool_name,
- }
- )
- continue
- messages.append(
- {
- "role": "assistant",
- "content": f"[{message.sender}]: {message.content}",
- }
- )
- return messages
-
- def reset(self) -> None:
- self.messages = []
diff --git a/spaces/Agusbs98/automatic-ecg-diagnosis/libs.py b/spaces/Agusbs98/automatic-ecg-diagnosis/libs.py
deleted file mode 100644
index 99d647200aa07536e1ef508357d7a7588f0080fc..0000000000000000000000000000000000000000
--- a/spaces/Agusbs98/automatic-ecg-diagnosis/libs.py
+++ /dev/null
@@ -1,31 +0,0 @@
-import os, sys
-import warnings; warnings.filterwarnings("ignore")
-
-
-import pandas, numpy as np
-import pandas as pd
-import gradio as gr
-#import argparse
-#import random
-#import neurokit2 as nk
-import torch
-import torch.nn as nn, torch.optim as optim
-import torch.nn.functional as F
-import torch.nn.utils.prune as prune
-#import captum.attr as attr
-#import matplotlib.pyplot as pyplot
-#from sklearn.metrics import f1_score
-from tensorflow.keras.models import load_model
-from tensorflow.keras.optimizers import Adam
-from tensorflow.keras.preprocessing.sequence import pad_sequences
-import h5py
-import scipy.signal as sgn
-from sierraecg import read_file
-import ecg_plot
-
-
-#!pip install pandas
-#!pip install torch
-#!pip install gradio
-#!pip install tesorflow
-#!pip install sierraecg
diff --git a/spaces/Akmyradov/TurkmenTTSweSTT/vits/text/__init__.py b/spaces/Akmyradov/TurkmenTTSweSTT/vits/text/__init__.py
deleted file mode 100644
index 4ac41f9025755d8ffd74068af14c6cfc8e5a4173..0000000000000000000000000000000000000000
--- a/spaces/Akmyradov/TurkmenTTSweSTT/vits/text/__init__.py
+++ /dev/null
@@ -1,54 +0,0 @@
-""" from https://github.com/keithito/tacotron """
-from text import cleaners
-from text.symbols import symbols
-
-
-# Mappings from symbol to numeric ID and vice versa:
-_symbol_to_id = {s: i for i, s in enumerate(symbols)}
-_id_to_symbol = {i: s for i, s in enumerate(symbols)}
-
-
-def text_to_sequence(text, cleaner_names):
- '''Converts a string of text to a sequence of IDs corresponding to the symbols in the text.
- Args:
- text: string to convert to a sequence
- cleaner_names: names of the cleaner functions to run the text through
- Returns:
- List of integers corresponding to the symbols in the text
- '''
- sequence = []
-
- clean_text = _clean_text(text, cleaner_names)
- for symbol in clean_text:
- symbol_id = _symbol_to_id[symbol]
- sequence += [symbol_id]
- return sequence
-
-
-def cleaned_text_to_sequence(cleaned_text):
- '''Converts a string of text to a sequence of IDs corresponding to the symbols in the text.
- Args:
- text: string to convert to a sequence
- Returns:
- List of integers corresponding to the symbols in the text
- '''
- sequence = [_symbol_to_id[symbol] for symbol in cleaned_text]
- return sequence
-
-
-def sequence_to_text(sequence):
- '''Converts a sequence of IDs back to a string'''
- result = ''
- for symbol_id in sequence:
- s = _id_to_symbol[symbol_id]
- result += s
- return result
-
-
-def _clean_text(text, cleaner_names):
- for name in cleaner_names:
- cleaner = getattr(cleaners, name)
- if not cleaner:
- raise Exception('Unknown cleaner: %s' % name)
- text = cleaner(text)
- return text
diff --git a/spaces/AlexWang/lama/bin/calc_dataset_stats.py b/spaces/AlexWang/lama/bin/calc_dataset_stats.py
deleted file mode 100644
index 5086fea1bab691892f2e52e3c59e5ef048bcfac0..0000000000000000000000000000000000000000
--- a/spaces/AlexWang/lama/bin/calc_dataset_stats.py
+++ /dev/null
@@ -1,88 +0,0 @@
-#!/usr/bin/env python3
-
-import os
-
-import numpy as np
-import tqdm
-from scipy.ndimage.morphology import distance_transform_edt
-
-from saicinpainting.evaluation.data import InpaintingDataset
-from saicinpainting.evaluation.vis import save_item_for_vis
-
-
-def main(args):
- dataset = InpaintingDataset(args.datadir, img_suffix='.png')
-
- area_bins = np.linspace(0, 1, args.area_bins + 1)
-
- heights = []
- widths = []
- image_areas = []
- hole_areas = []
- hole_area_percents = []
- known_pixel_distances = []
-
- area_bins_count = np.zeros(args.area_bins)
- area_bin_titles = [f'{area_bins[i] * 100:.0f}-{area_bins[i + 1] * 100:.0f}' for i in range(args.area_bins)]
-
- bin2i = [[] for _ in range(args.area_bins)]
-
- for i, item in enumerate(tqdm.tqdm(dataset)):
- h, w = item['image'].shape[1:]
- heights.append(h)
- widths.append(w)
- full_area = h * w
- image_areas.append(full_area)
- bin_mask = item['mask'] > 0.5
- hole_area = bin_mask.sum()
- hole_areas.append(hole_area)
- hole_percent = hole_area / full_area
- hole_area_percents.append(hole_percent)
- bin_i = np.clip(np.searchsorted(area_bins, hole_percent) - 1, 0, len(area_bins_count) - 1)
- area_bins_count[bin_i] += 1
- bin2i[bin_i].append(i)
-
- cur_dist = distance_transform_edt(bin_mask)
- cur_dist_inside_mask = cur_dist[bin_mask]
- known_pixel_distances.append(cur_dist_inside_mask.mean())
-
- os.makedirs(args.outdir, exist_ok=True)
- with open(os.path.join(args.outdir, 'summary.txt'), 'w') as f:
- f.write(f'''Location: {args.datadir}
-
-Number of samples: {len(dataset)}
-
-Image height: min {min(heights):5d} max {max(heights):5d} mean {np.mean(heights):.2f}
-Image width: min {min(widths):5d} max {max(widths):5d} mean {np.mean(widths):.2f}
-Image area: min {min(image_areas):7d} max {max(image_areas):7d} mean {np.mean(image_areas):.2f}
-Hole area: min {min(hole_areas):7d} max {max(hole_areas):7d} mean {np.mean(hole_areas):.2f}
-Hole area %: min {min(hole_area_percents) * 100:2.2f} max {max(hole_area_percents) * 100:2.2f} mean {np.mean(hole_area_percents) * 100:2.2f}
-Dist 2known: min {min(known_pixel_distances):2.2f} max {max(known_pixel_distances):2.2f} mean {np.mean(known_pixel_distances):2.2f} median {np.median(known_pixel_distances):2.2f}
-
-Stats by hole area %:
-''')
- for bin_i in range(args.area_bins):
- f.write(f'{area_bin_titles[bin_i]}%: '
- f'samples number {area_bins_count[bin_i]}, '
- f'{area_bins_count[bin_i] / len(dataset) * 100:.1f}%\n')
-
- for bin_i in range(args.area_bins):
- bindir = os.path.join(args.outdir, 'samples', area_bin_titles[bin_i])
- os.makedirs(bindir, exist_ok=True)
- bin_idx = bin2i[bin_i]
- for sample_i in np.random.choice(bin_idx, size=min(len(bin_idx), args.samples_n), replace=False):
- save_item_for_vis(dataset[sample_i], os.path.join(bindir, f'{sample_i}.png'))
-
-
-if __name__ == '__main__':
- import argparse
-
- aparser = argparse.ArgumentParser()
- aparser.add_argument('datadir', type=str,
- help='Path to folder with images and masks (output of gen_mask_dataset.py)')
- aparser.add_argument('outdir', type=str, help='Where to put results')
- aparser.add_argument('--samples-n', type=int, default=10,
- help='Number of sample images with masks to copy for visualization for each area bin')
- aparser.add_argument('--area-bins', type=int, default=10, help='How many area bins to have')
-
- main(aparser.parse_args())
diff --git a/spaces/Androidonnxfork/CivitAi-to-Diffusers/diffusers/docs/source/ko/optimization/fp16.md b/spaces/Androidonnxfork/CivitAi-to-Diffusers/diffusers/docs/source/ko/optimization/fp16.md
deleted file mode 100644
index 30197305540cbe23b58e56bf29feb2c833729750..0000000000000000000000000000000000000000
--- a/spaces/Androidonnxfork/CivitAi-to-Diffusers/diffusers/docs/source/ko/optimization/fp16.md
+++ /dev/null
@@ -1,410 +0,0 @@
-
-
-# 메모리와 속도
-
-메모리 또는 속도에 대해 🤗 Diffusers *추론*을 최적화하기 위한 몇 가지 기술과 아이디어를 제시합니다.
-일반적으로, memory-efficient attention을 위해 [xFormers](https://github.com/facebookresearch/xformers) 사용을 추천하기 때문에, 추천하는 [설치 방법](xformers)을 보고 설치해 보세요.
-
-다음 설정이 성능과 메모리에 미치는 영향에 대해 설명합니다.
-
-| | 지연시간 | 속도 향상 |
-| ---------------- | ------- | ------- |
-| 별도 설정 없음 | 9.50s | x1 |
-| cuDNN auto-tuner | 9.37s | x1.01 |
-| fp16 | 3.61s | x2.63 |
-| Channels Last 메모리 형식 | 3.30s | x2.88 |
-| traced UNet | 3.21s | x2.96 |
-| memory-efficient attention | 2.63s | x3.61 |
-
-
- NVIDIA TITAN RTX에서 50 DDIM 스텝의 "a photo of an astronaut riding a horse on mars" 프롬프트로 512x512 크기의 단일 이미지를 생성하였습니다.
-
-
-## cuDNN auto-tuner 활성화하기
-
-[NVIDIA cuDNN](https://developer.nvidia.com/cudnn)은 컨볼루션을 계산하는 많은 알고리즘을 지원합니다. Autotuner는 짧은 벤치마크를 실행하고 주어진 입력 크기에 대해 주어진 하드웨어에서 최고의 성능을 가진 커널을 선택합니다.
-
-**컨볼루션 네트워크**를 활용하고 있기 때문에 (다른 유형들은 현재 지원되지 않음), 다음 설정을 통해 추론 전에 cuDNN autotuner를 활성화할 수 있습니다:
-
-```python
-import torch
-
-torch.backends.cudnn.benchmark = True
-```
-
-### fp32 대신 tf32 사용하기 (Ampere 및 이후 CUDA 장치들에서)
-
-Ampere 및 이후 CUDA 장치에서 행렬곱 및 컨볼루션은 TensorFloat32(TF32) 모드를 사용하여 더 빠르지만 약간 덜 정확할 수 있습니다.
-기본적으로 PyTorch는 컨볼루션에 대해 TF32 모드를 활성화하지만 행렬 곱셈은 활성화하지 않습니다.
-네트워크에 완전한 float32 정밀도가 필요한 경우가 아니면 행렬 곱셈에 대해서도 이 설정을 활성화하는 것이 좋습니다.
-이는 일반적으로 무시할 수 있는 수치의 정확도 손실이 있지만, 계산 속도를 크게 높일 수 있습니다.
-그것에 대해 [여기](https://huggingface.co/docs/transformers/v4.18.0/en/performance#tf32)서 더 읽을 수 있습니다.
-추론하기 전에 다음을 추가하기만 하면 됩니다:
-
-```python
-import torch
-
-torch.backends.cuda.matmul.allow_tf32 = True
-```
-
-## 반정밀도 가중치
-
-더 많은 GPU 메모리를 절약하고 더 빠른 속도를 얻기 위해 모델 가중치를 반정밀도(half precision)로 직접 불러오고 실행할 수 있습니다.
-여기에는 `fp16`이라는 브랜치에 저장된 float16 버전의 가중치를 불러오고, 그 때 `float16` 유형을 사용하도록 PyTorch에 지시하는 작업이 포함됩니다.
-
-```Python
-pipe = StableDiffusionPipeline.from_pretrained(
- "runwayml/stable-diffusion-v1-5",
-
- torch_dtype=torch.float16,
-)
-pipe = pipe.to("cuda")
-
-prompt = "a photo of an astronaut riding a horse on mars"
-image = pipe(prompt).images[0]
-```
-
-
- 어떤 파이프라인에서도 [`torch.autocast`](https://pytorch.org/docs/stable/amp.html#torch.autocast) 를 사용하는 것은 검은색 이미지를 생성할 수 있고, 순수한 float16 정밀도를 사용하는 것보다 항상 느리기 때문에 사용하지 않는 것이 좋습니다.
-
-
-## 추가 메모리 절약을 위한 슬라이스 어텐션
-
-추가 메모리 절약을 위해, 한 번에 모두 계산하는 대신 단계적으로 계산을 수행하는 슬라이스 버전의 어텐션(attention)을 사용할 수 있습니다.
-
-
- Attention slicing은 모델이 하나 이상의 어텐션 헤드를 사용하는 한, 배치 크기가 1인 경우에도 유용합니다.
- 하나 이상의 어텐션 헤드가 있는 경우 *QK^T* 어텐션 매트릭스는 상당한 양의 메모리를 절약할 수 있는 각 헤드에 대해 순차적으로 계산될 수 있습니다.
-
-
-각 헤드에 대해 순차적으로 어텐션 계산을 수행하려면, 다음과 같이 추론 전에 파이프라인에서 [`~StableDiffusionPipeline.enable_attention_slicing`]를 호출하면 됩니다:
-
-```Python
-import torch
-from diffusers import StableDiffusionPipeline
-
-pipe = StableDiffusionPipeline.from_pretrained(
- "runwayml/stable-diffusion-v1-5",
-
- torch_dtype=torch.float16,
-)
-pipe = pipe.to("cuda")
-
-prompt = "a photo of an astronaut riding a horse on mars"
-pipe.enable_attention_slicing()
-image = pipe(prompt).images[0]
-```
-
-추론 시간이 약 10% 느려지는 약간의 성능 저하가 있지만 이 방법을 사용하면 3.2GB 정도의 작은 VRAM으로도 Stable Diffusion을 사용할 수 있습니다!
-
-
-## 더 큰 배치를 위한 sliced VAE 디코드
-
-제한된 VRAM에서 대규모 이미지 배치를 디코딩하거나 32개 이상의 이미지가 포함된 배치를 활성화하기 위해, 배치의 latent 이미지를 한 번에 하나씩 디코딩하는 슬라이스 VAE 디코드를 사용할 수 있습니다.
-
-이를 [`~StableDiffusionPipeline.enable_attention_slicing`] 또는 [`~StableDiffusionPipeline.enable_xformers_memory_efficient_attention`]과 결합하여 메모리 사용을 추가로 최소화할 수 있습니다.
-
-VAE 디코드를 한 번에 하나씩 수행하려면 추론 전에 파이프라인에서 [`~StableDiffusionPipeline.enable_vae_slicing`]을 호출합니다. 예를 들어:
-
-```Python
-import torch
-from diffusers import StableDiffusionPipeline
-
-pipe = StableDiffusionPipeline.from_pretrained(
- "runwayml/stable-diffusion-v1-5",
-
- torch_dtype=torch.float16,
-)
-pipe = pipe.to("cuda")
-
-prompt = "a photo of an astronaut riding a horse on mars"
-pipe.enable_vae_slicing()
-images = pipe([prompt] * 32).images
-```
-
-다중 이미지 배치에서 VAE 디코드가 약간의 성능 향상이 이루어집니다. 단일 이미지 배치에서는 성능 영향은 없습니다.
-
-
-
-## 메모리 절약을 위해 가속 기능을 사용하여 CPU로 오프로딩
-
-추가 메모리 절약을 위해 가중치를 CPU로 오프로드하고 순방향 전달을 수행할 때만 GPU로 로드할 수 있습니다.
-
-CPU 오프로딩을 수행하려면 [`~StableDiffusionPipeline.enable_sequential_cpu_offload`]를 호출하기만 하면 됩니다:
-
-```Python
-import torch
-from diffusers import StableDiffusionPipeline
-
-pipe = StableDiffusionPipeline.from_pretrained(
- "runwayml/stable-diffusion-v1-5",
-
- torch_dtype=torch.float16,
-)
-
-prompt = "a photo of an astronaut riding a horse on mars"
-pipe.enable_sequential_cpu_offload()
-image = pipe(prompt).images[0]
-```
-
-그러면 메모리 소비를 3GB 미만으로 줄일 수 있습니다.
-
-참고로 이 방법은 전체 모델이 아닌 서브모듈 수준에서 작동합니다. 이는 메모리 소비를 최소화하는 가장 좋은 방법이지만 프로세스의 반복적 특성으로 인해 추론 속도가 훨씬 느립니다. 파이프라인의 UNet 구성 요소는 여러 번 실행됩니다('num_inference_steps' 만큼). 매번 UNet의 서로 다른 서브모듈이 순차적으로 온로드된 다음 필요에 따라 오프로드되므로 메모리 이동 횟수가 많습니다.
-
-
-또 다른 최적화 방법인 모델 오프로딩을 사용하는 것을 고려하십시오. 이는 훨씬 빠르지만 메모리 절약이 크지는 않습니다.
-
-
-또한 ttention slicing과 연결해서 최소 메모리(< 2GB)로도 동작할 수 있습니다.
-
-
-```Python
-import torch
-from diffusers import StableDiffusionPipeline
-
-pipe = StableDiffusionPipeline.from_pretrained(
- "runwayml/stable-diffusion-v1-5",
-
- torch_dtype=torch.float16,
-)
-
-prompt = "a photo of an astronaut riding a horse on mars"
-pipe.enable_sequential_cpu_offload()
-pipe.enable_attention_slicing(1)
-
-image = pipe(prompt).images[0]
-```
-
-**참고**: 'enable_sequential_cpu_offload()'를 사용할 때, 미리 파이프라인을 CUDA로 이동하지 **않는** 것이 중요합니다.그렇지 않으면 메모리 소비의 이득이 최소화됩니다. 더 많은 정보를 위해 [이 이슈](https://github.com/huggingface/diffusers/issues/1934)를 보세요.
-
-
-## 빠른 추론과 메모리 메모리 절약을 위한 모델 오프로딩
-
-[순차적 CPU 오프로딩](#sequential_offloading)은 이전 섹션에서 설명한 것처럼 많은 메모리를 보존하지만 필요에 따라 서브모듈을 GPU로 이동하고 새 모듈이 실행될 때 즉시 CPU로 반환되기 때문에 추론 속도가 느려집니다.
-
-전체 모델 오프로딩은 각 모델의 구성 요소인 _modules_을 처리하는 대신, 전체 모델을 GPU로 이동하는 대안입니다. 이로 인해 추론 시간에 미치는 영향은 미미하지만(파이프라인을 'cuda'로 이동하는 것과 비교하여) 여전히 약간의 메모리를 절약할 수 있습니다.
-
-이 시나리오에서는 파이프라인의 주요 구성 요소 중 하나만(일반적으로 텍스트 인코더, unet 및 vae) GPU에 있고, 나머지는 CPU에서 대기할 것입니다.
-여러 반복을 위해 실행되는 UNet과 같은 구성 요소는 더 이상 필요하지 않을 때까지 GPU에 남아 있습니다.
-
-이 기능은 아래와 같이 파이프라인에서 `enable_model_cpu_offload()`를 호출하여 활성화할 수 있습니다.
-
-```Python
-import torch
-from diffusers import StableDiffusionPipeline
-
-pipe = StableDiffusionPipeline.from_pretrained(
- "runwayml/stable-diffusion-v1-5",
- torch_dtype=torch.float16,
-)
-
-prompt = "a photo of an astronaut riding a horse on mars"
-pipe.enable_model_cpu_offload()
-image = pipe(prompt).images[0]
-```
-
-이는 추가적인 메모리 절약을 위한 attention slicing과도 호환됩니다.
-
-```Python
-import torch
-from diffusers import StableDiffusionPipeline
-
-pipe = StableDiffusionPipeline.from_pretrained(
- "runwayml/stable-diffusion-v1-5",
- torch_dtype=torch.float16,
-)
-
-prompt = "a photo of an astronaut riding a horse on mars"
-pipe.enable_model_cpu_offload()
-pipe.enable_attention_slicing(1)
-
-image = pipe(prompt).images[0]
-```
-
-
-이 기능을 사용하려면 'accelerate' 버전 0.17.0 이상이 필요합니다.
-
-
-## Channels Last 메모리 형식 사용하기
-
-Channels Last 메모리 형식은 차원 순서를 보존하는 메모리에서 NCHW 텐서 배열을 대체하는 방법입니다.
-Channels Last 텐서는 채널이 가장 조밀한 차원이 되는 방식으로 정렬됩니다(일명 픽셀당 이미지를 저장).
-현재 모든 연산자 Channels Last 형식을 지원하는 것은 아니라 성능이 저하될 수 있으므로, 사용해보고 모델에 잘 작동하는지 확인하는 것이 좋습니다.
-
-
-예를 들어 파이프라인의 UNet 모델이 channels Last 형식을 사용하도록 설정하려면 다음을 사용할 수 있습니다:
-
-```python
-print(pipe.unet.conv_out.state_dict()["weight"].stride()) # (2880, 9, 3, 1)
-pipe.unet.to(memory_format=torch.channels_last) # in-place 연산
-# 2번째 차원에서 스트라이드 1을 가지는 (2880, 1, 960, 320)로, 연산이 작동함을 증명합니다.
-print(pipe.unet.conv_out.state_dict()["weight"].stride())
-```
-
-## 추적(tracing)
-
-추적은 모델을 통해 예제 입력 텐서를 통해 실행되는데, 해당 입력이 모델의 레이어를 통과할 때 호출되는 작업을 캡처하여 실행 파일 또는 'ScriptFunction'이 반환되도록 하고, 이는 just-in-time 컴파일로 최적화됩니다.
-
-UNet 모델을 추적하기 위해 다음을 사용할 수 있습니다:
-
-```python
-import time
-import torch
-from diffusers import StableDiffusionPipeline
-import functools
-
-# torch 기울기 비활성화
-torch.set_grad_enabled(False)
-
-# 변수 설정
-n_experiments = 2
-unet_runs_per_experiment = 50
-
-
-# 입력 불러오기
-def generate_inputs():
- sample = torch.randn(2, 4, 64, 64).half().cuda()
- timestep = torch.rand(1).half().cuda() * 999
- encoder_hidden_states = torch.randn(2, 77, 768).half().cuda()
- return sample, timestep, encoder_hidden_states
-
-
-pipe = StableDiffusionPipeline.from_pretrained(
- "runwayml/stable-diffusion-v1-5",
- torch_dtype=torch.float16,
-).to("cuda")
-unet = pipe.unet
-unet.eval()
-unet.to(memory_format=torch.channels_last) # Channels Last 메모리 형식 사용
-unet.forward = functools.partial(unet.forward, return_dict=False) # return_dict=False을 기본값으로 설정
-
-# 워밍업
-for _ in range(3):
- with torch.inference_mode():
- inputs = generate_inputs()
- orig_output = unet(*inputs)
-
-# 추적
-print("tracing..")
-unet_traced = torch.jit.trace(unet, inputs)
-unet_traced.eval()
-print("done tracing")
-
-
-# 워밍업 및 그래프 최적화
-for _ in range(5):
- with torch.inference_mode():
- inputs = generate_inputs()
- orig_output = unet_traced(*inputs)
-
-
-# 벤치마킹
-with torch.inference_mode():
- for _ in range(n_experiments):
- torch.cuda.synchronize()
- start_time = time.time()
- for _ in range(unet_runs_per_experiment):
- orig_output = unet_traced(*inputs)
- torch.cuda.synchronize()
- print(f"unet traced inference took {time.time() - start_time:.2f} seconds")
- for _ in range(n_experiments):
- torch.cuda.synchronize()
- start_time = time.time()
- for _ in range(unet_runs_per_experiment):
- orig_output = unet(*inputs)
- torch.cuda.synchronize()
- print(f"unet inference took {time.time() - start_time:.2f} seconds")
-
-# 모델 저장
-unet_traced.save("unet_traced.pt")
-```
-
-그 다음, 파이프라인의 `unet` 특성을 다음과 같이 추적된 모델로 바꿀 수 있습니다.
-
-```python
-from diffusers import StableDiffusionPipeline
-import torch
-from dataclasses import dataclass
-
-
-@dataclass
-class UNet2DConditionOutput:
- sample: torch.FloatTensor
-
-
-pipe = StableDiffusionPipeline.from_pretrained(
- "runwayml/stable-diffusion-v1-5",
- torch_dtype=torch.float16,
-).to("cuda")
-
-# jitted unet 사용
-unet_traced = torch.jit.load("unet_traced.pt")
-
-
-# pipe.unet 삭제
-class TracedUNet(torch.nn.Module):
- def __init__(self):
- super().__init__()
- self.in_channels = pipe.unet.in_channels
- self.device = pipe.unet.device
-
- def forward(self, latent_model_input, t, encoder_hidden_states):
- sample = unet_traced(latent_model_input, t, encoder_hidden_states)[0]
- return UNet2DConditionOutput(sample=sample)
-
-
-pipe.unet = TracedUNet()
-
-with torch.inference_mode():
- image = pipe([prompt] * 1, num_inference_steps=50).images[0]
-```
-
-
-## Memory-efficient attention
-
-어텐션 블록의 대역폭을 최적화하는 최근 작업으로 GPU 메모리 사용량이 크게 향상되고 향상되었습니다.
-@tridao의 가장 최근의 플래시 어텐션: [code](https://github.com/HazyResearch/flash-attention), [paper](https://arxiv.org/pdf/2205.14135.pdf).
-
-배치 크기 1(프롬프트 1개)의 512x512 크기로 추론을 실행할 때 몇 가지 Nvidia GPU에서 얻은 속도 향상은 다음과 같습니다:
-
-| GPU | 기준 어텐션 FP16 | 메모리 효율적인 어텐션 FP16 |
-|------------------ |--------------------- |--------------------------------- |
-| NVIDIA Tesla T4 | 3.5it/s | 5.5it/s |
-| NVIDIA 3060 RTX | 4.6it/s | 7.8it/s |
-| NVIDIA A10G | 8.88it/s | 15.6it/s |
-| NVIDIA RTX A6000 | 11.7it/s | 21.09it/s |
-| NVIDIA TITAN RTX | 12.51it/s | 18.22it/s |
-| A100-SXM4-40GB | 18.6it/s | 29.it/s |
-| A100-SXM-80GB | 18.7it/s | 29.5it/s |
-
-이를 활용하려면 다음을 만족해야 합니다:
- - PyTorch > 1.12
- - Cuda 사용 가능
- - [xformers 라이브러리를 설치함](xformers)
-```python
-from diffusers import StableDiffusionPipeline
-import torch
-
-pipe = StableDiffusionPipeline.from_pretrained(
- "runwayml/stable-diffusion-v1-5",
- torch_dtype=torch.float16,
-).to("cuda")
-
-pipe.enable_xformers_memory_efficient_attention()
-
-with torch.inference_mode():
- sample = pipe("a small cat")
-
-# 선택: 이를 비활성화 하기 위해 다음을 사용할 수 있습니다.
-# pipe.disable_xformers_memory_efficient_attention()
-```
diff --git a/spaces/Androidonnxfork/CivitAi-to-Diffusers/diffusers/tests/pipelines/stable_diffusion/test_onnx_stable_diffusion_img2img.py b/spaces/Androidonnxfork/CivitAi-to-Diffusers/diffusers/tests/pipelines/stable_diffusion/test_onnx_stable_diffusion_img2img.py
deleted file mode 100644
index 9147dc461fc583336a05d04b485a374d327bd9ea..0000000000000000000000000000000000000000
--- a/spaces/Androidonnxfork/CivitAi-to-Diffusers/diffusers/tests/pipelines/stable_diffusion/test_onnx_stable_diffusion_img2img.py
+++ /dev/null
@@ -1,245 +0,0 @@
-# coding=utf-8
-# Copyright 2023 HuggingFace Inc.
-#
-# Licensed under the Apache License, Version 2.0 (the "License");
-# you may not use this file except in compliance with the License.
-# You may obtain a copy of the License at
-#
-# http://www.apache.org/licenses/LICENSE-2.0
-#
-# Unless required by applicable law or agreed to in writing, software
-# distributed under the License is distributed on an "AS IS" BASIS,
-# WITHOUT WARRANTIES OR CONDITIONS OF ANY KIND, either express or implied.
-# See the License for the specific language governing permissions and
-# limitations under the License.
-
-import random
-import unittest
-
-import numpy as np
-
-from diffusers import (
- DPMSolverMultistepScheduler,
- EulerAncestralDiscreteScheduler,
- EulerDiscreteScheduler,
- LMSDiscreteScheduler,
- OnnxStableDiffusionImg2ImgPipeline,
- PNDMScheduler,
-)
-from diffusers.utils import floats_tensor
-from diffusers.utils.testing_utils import (
- is_onnx_available,
- load_image,
- nightly,
- require_onnxruntime,
- require_torch_gpu,
-)
-
-from ..test_pipelines_onnx_common import OnnxPipelineTesterMixin
-
-
-if is_onnx_available():
- import onnxruntime as ort
-
-
-class OnnxStableDiffusionImg2ImgPipelineFastTests(OnnxPipelineTesterMixin, unittest.TestCase):
- hub_checkpoint = "hf-internal-testing/tiny-random-OnnxStableDiffusionPipeline"
-
- def get_dummy_inputs(self, seed=0):
- image = floats_tensor((1, 3, 128, 128), rng=random.Random(seed))
- generator = np.random.RandomState(seed)
- inputs = {
- "prompt": "A painting of a squirrel eating a burger",
- "image": image,
- "generator": generator,
- "num_inference_steps": 3,
- "strength": 0.75,
- "guidance_scale": 7.5,
- "output_type": "numpy",
- }
- return inputs
-
- def test_pipeline_default_ddim(self):
- pipe = OnnxStableDiffusionImg2ImgPipeline.from_pretrained(self.hub_checkpoint, provider="CPUExecutionProvider")
- pipe.set_progress_bar_config(disable=None)
-
- inputs = self.get_dummy_inputs()
- image = pipe(**inputs).images
- image_slice = image[0, -3:, -3:, -1].flatten()
-
- assert image.shape == (1, 128, 128, 3)
- expected_slice = np.array([0.69643, 0.58484, 0.50314, 0.58760, 0.55368, 0.59643, 0.51529, 0.41217, 0.49087])
- assert np.abs(image_slice - expected_slice).max() < 1e-1
-
- def test_pipeline_pndm(self):
- pipe = OnnxStableDiffusionImg2ImgPipeline.from_pretrained(self.hub_checkpoint, provider="CPUExecutionProvider")
- pipe.scheduler = PNDMScheduler.from_config(pipe.scheduler.config, skip_prk_steps=True)
- pipe.set_progress_bar_config(disable=None)
-
- inputs = self.get_dummy_inputs()
- image = pipe(**inputs).images
- image_slice = image[0, -3:, -3:, -1]
-
- assert image.shape == (1, 128, 128, 3)
- expected_slice = np.array([0.61737, 0.54642, 0.53183, 0.54465, 0.52742, 0.60525, 0.49969, 0.40655, 0.48154])
-
- assert np.abs(image_slice.flatten() - expected_slice).max() < 1e-1
-
- def test_pipeline_lms(self):
- pipe = OnnxStableDiffusionImg2ImgPipeline.from_pretrained(self.hub_checkpoint, provider="CPUExecutionProvider")
- pipe.scheduler = LMSDiscreteScheduler.from_config(pipe.scheduler.config)
- pipe.set_progress_bar_config(disable=None)
-
- # warmup pass to apply optimizations
- _ = pipe(**self.get_dummy_inputs())
-
- inputs = self.get_dummy_inputs()
- image = pipe(**inputs).images
- image_slice = image[0, -3:, -3:, -1]
-
- assert image.shape == (1, 128, 128, 3)
- expected_slice = np.array([0.52761, 0.59977, 0.49033, 0.49619, 0.54282, 0.50311, 0.47600, 0.40918, 0.45203])
-
- assert np.abs(image_slice.flatten() - expected_slice).max() < 1e-1
-
- def test_pipeline_euler(self):
- pipe = OnnxStableDiffusionImg2ImgPipeline.from_pretrained(self.hub_checkpoint, provider="CPUExecutionProvider")
- pipe.scheduler = EulerDiscreteScheduler.from_config(pipe.scheduler.config)
- pipe.set_progress_bar_config(disable=None)
-
- inputs = self.get_dummy_inputs()
- image = pipe(**inputs).images
- image_slice = image[0, -3:, -3:, -1]
-
- assert image.shape == (1, 128, 128, 3)
- expected_slice = np.array([0.52911, 0.60004, 0.49229, 0.49805, 0.54502, 0.50680, 0.47777, 0.41028, 0.45304])
-
- assert np.abs(image_slice.flatten() - expected_slice).max() < 1e-1
-
- def test_pipeline_euler_ancestral(self):
- pipe = OnnxStableDiffusionImg2ImgPipeline.from_pretrained(self.hub_checkpoint, provider="CPUExecutionProvider")
- pipe.scheduler = EulerAncestralDiscreteScheduler.from_config(pipe.scheduler.config)
- pipe.set_progress_bar_config(disable=None)
-
- inputs = self.get_dummy_inputs()
- image = pipe(**inputs).images
- image_slice = image[0, -3:, -3:, -1]
-
- assert image.shape == (1, 128, 128, 3)
- expected_slice = np.array([0.52911, 0.60004, 0.49229, 0.49805, 0.54502, 0.50680, 0.47777, 0.41028, 0.45304])
-
- assert np.abs(image_slice.flatten() - expected_slice).max() < 1e-1
-
- def test_pipeline_dpm_multistep(self):
- pipe = OnnxStableDiffusionImg2ImgPipeline.from_pretrained(self.hub_checkpoint, provider="CPUExecutionProvider")
- pipe.scheduler = DPMSolverMultistepScheduler.from_config(pipe.scheduler.config)
- pipe.set_progress_bar_config(disable=None)
-
- inputs = self.get_dummy_inputs()
- image = pipe(**inputs).images
- image_slice = image[0, -3:, -3:, -1]
-
- assert image.shape == (1, 128, 128, 3)
- expected_slice = np.array([0.65331, 0.58277, 0.48204, 0.56059, 0.53665, 0.56235, 0.50969, 0.40009, 0.46552])
-
- assert np.abs(image_slice.flatten() - expected_slice).max() < 1e-1
-
-
-@nightly
-@require_onnxruntime
-@require_torch_gpu
-class OnnxStableDiffusionImg2ImgPipelineIntegrationTests(unittest.TestCase):
- @property
- def gpu_provider(self):
- return (
- "CUDAExecutionProvider",
- {
- "gpu_mem_limit": "15000000000", # 15GB
- "arena_extend_strategy": "kSameAsRequested",
- },
- )
-
- @property
- def gpu_options(self):
- options = ort.SessionOptions()
- options.enable_mem_pattern = False
- return options
-
- def test_inference_default_pndm(self):
- init_image = load_image(
- "https://huggingface.co/datasets/hf-internal-testing/diffusers-images/resolve/main"
- "/img2img/sketch-mountains-input.jpg"
- )
- init_image = init_image.resize((768, 512))
- # using the PNDM scheduler by default
- pipe = OnnxStableDiffusionImg2ImgPipeline.from_pretrained(
- "CompVis/stable-diffusion-v1-4",
- revision="onnx",
- safety_checker=None,
- feature_extractor=None,
- provider=self.gpu_provider,
- sess_options=self.gpu_options,
- )
- pipe.set_progress_bar_config(disable=None)
-
- prompt = "A fantasy landscape, trending on artstation"
-
- generator = np.random.RandomState(0)
- output = pipe(
- prompt=prompt,
- image=init_image,
- strength=0.75,
- guidance_scale=7.5,
- num_inference_steps=10,
- generator=generator,
- output_type="np",
- )
- images = output.images
- image_slice = images[0, 255:258, 383:386, -1]
-
- assert images.shape == (1, 512, 768, 3)
- expected_slice = np.array([0.4909, 0.5059, 0.5372, 0.4623, 0.4876, 0.5049, 0.4820, 0.4956, 0.5019])
- # TODO: lower the tolerance after finding the cause of onnxruntime reproducibility issues
-
- assert np.abs(image_slice.flatten() - expected_slice).max() < 2e-2
-
- def test_inference_k_lms(self):
- init_image = load_image(
- "https://huggingface.co/datasets/hf-internal-testing/diffusers-images/resolve/main"
- "/img2img/sketch-mountains-input.jpg"
- )
- init_image = init_image.resize((768, 512))
- lms_scheduler = LMSDiscreteScheduler.from_pretrained(
- "runwayml/stable-diffusion-v1-5", subfolder="scheduler", revision="onnx"
- )
- pipe = OnnxStableDiffusionImg2ImgPipeline.from_pretrained(
- "runwayml/stable-diffusion-v1-5",
- revision="onnx",
- scheduler=lms_scheduler,
- safety_checker=None,
- feature_extractor=None,
- provider=self.gpu_provider,
- sess_options=self.gpu_options,
- )
- pipe.set_progress_bar_config(disable=None)
-
- prompt = "A fantasy landscape, trending on artstation"
-
- generator = np.random.RandomState(0)
- output = pipe(
- prompt=prompt,
- image=init_image,
- strength=0.75,
- guidance_scale=7.5,
- num_inference_steps=20,
- generator=generator,
- output_type="np",
- )
- images = output.images
- image_slice = images[0, 255:258, 383:386, -1]
-
- assert images.shape == (1, 512, 768, 3)
- expected_slice = np.array([0.8043, 0.926, 0.9581, 0.8119, 0.8954, 0.913, 0.7209, 0.7463, 0.7431])
- # TODO: lower the tolerance after finding the cause of onnxruntime reproducibility issues
-
- assert np.abs(image_slice.flatten() - expected_slice).max() < 2e-2
diff --git a/spaces/Andy1621/uniformer_image_detection/configs/grid_rcnn/grid_rcnn_r101_fpn_gn-head_2x_coco.py b/spaces/Andy1621/uniformer_image_detection/configs/grid_rcnn/grid_rcnn_r101_fpn_gn-head_2x_coco.py
deleted file mode 100644
index cf8b648a4291db4a172bf031f301110963f38dd6..0000000000000000000000000000000000000000
--- a/spaces/Andy1621/uniformer_image_detection/configs/grid_rcnn/grid_rcnn_r101_fpn_gn-head_2x_coco.py
+++ /dev/null
@@ -1,3 +0,0 @@
-_base_ = './grid_rcnn_r50_fpn_gn-head_2x_coco.py'
-
-model = dict(pretrained='torchvision://resnet101', backbone=dict(depth=101))
diff --git a/spaces/Andy1621/uniformer_image_detection/configs/vfnet/vfnet_r101_fpn_mdconv_c3-c5_mstrain_2x_coco.py b/spaces/Andy1621/uniformer_image_detection/configs/vfnet/vfnet_r101_fpn_mdconv_c3-c5_mstrain_2x_coco.py
deleted file mode 100644
index f8ef6ec092db2e454ca5359b6df89d31365672c0..0000000000000000000000000000000000000000
--- a/spaces/Andy1621/uniformer_image_detection/configs/vfnet/vfnet_r101_fpn_mdconv_c3-c5_mstrain_2x_coco.py
+++ /dev/null
@@ -1,14 +0,0 @@
-_base_ = './vfnet_r50_fpn_mdconv_c3-c5_mstrain_2x_coco.py'
-model = dict(
- pretrained='torchvision://resnet101',
- backbone=dict(
- type='ResNet',
- depth=101,
- num_stages=4,
- out_indices=(0, 1, 2, 3),
- frozen_stages=1,
- norm_cfg=dict(type='BN', requires_grad=True),
- norm_eval=True,
- style='pytorch',
- dcn=dict(type='DCNv2', deform_groups=1, fallback_on_stride=False),
- stage_with_dcn=(False, True, True, True)))
diff --git a/spaces/Andy1621/uniformer_image_detection/mmdet/core/bbox/samplers/__init__.py b/spaces/Andy1621/uniformer_image_detection/mmdet/core/bbox/samplers/__init__.py
deleted file mode 100644
index 0b06303fe1000e11c5486c40c70606a34a5208e3..0000000000000000000000000000000000000000
--- a/spaces/Andy1621/uniformer_image_detection/mmdet/core/bbox/samplers/__init__.py
+++ /dev/null
@@ -1,15 +0,0 @@
-from .base_sampler import BaseSampler
-from .combined_sampler import CombinedSampler
-from .instance_balanced_pos_sampler import InstanceBalancedPosSampler
-from .iou_balanced_neg_sampler import IoUBalancedNegSampler
-from .ohem_sampler import OHEMSampler
-from .pseudo_sampler import PseudoSampler
-from .random_sampler import RandomSampler
-from .sampling_result import SamplingResult
-from .score_hlr_sampler import ScoreHLRSampler
-
-__all__ = [
- 'BaseSampler', 'PseudoSampler', 'RandomSampler',
- 'InstanceBalancedPosSampler', 'IoUBalancedNegSampler', 'CombinedSampler',
- 'OHEMSampler', 'SamplingResult', 'ScoreHLRSampler'
-]
diff --git a/spaces/Andy1621/uniformer_image_segmentation/configs/_base_/models/pspnet_unet_s5-d16.py b/spaces/Andy1621/uniformer_image_segmentation/configs/_base_/models/pspnet_unet_s5-d16.py
deleted file mode 100644
index fcff9ec4f41fad158344ecd77313dc14564f3682..0000000000000000000000000000000000000000
--- a/spaces/Andy1621/uniformer_image_segmentation/configs/_base_/models/pspnet_unet_s5-d16.py
+++ /dev/null
@@ -1,50 +0,0 @@
-# model settings
-norm_cfg = dict(type='SyncBN', requires_grad=True)
-model = dict(
- type='EncoderDecoder',
- pretrained=None,
- backbone=dict(
- type='UNet',
- in_channels=3,
- base_channels=64,
- num_stages=5,
- strides=(1, 1, 1, 1, 1),
- enc_num_convs=(2, 2, 2, 2, 2),
- dec_num_convs=(2, 2, 2, 2),
- downsamples=(True, True, True, True),
- enc_dilations=(1, 1, 1, 1, 1),
- dec_dilations=(1, 1, 1, 1),
- with_cp=False,
- conv_cfg=None,
- norm_cfg=norm_cfg,
- act_cfg=dict(type='ReLU'),
- upsample_cfg=dict(type='InterpConv'),
- norm_eval=False),
- decode_head=dict(
- type='PSPHead',
- in_channels=64,
- in_index=4,
- channels=16,
- pool_scales=(1, 2, 3, 6),
- dropout_ratio=0.1,
- num_classes=2,
- norm_cfg=norm_cfg,
- align_corners=False,
- loss_decode=dict(
- type='CrossEntropyLoss', use_sigmoid=False, loss_weight=1.0)),
- auxiliary_head=dict(
- type='FCNHead',
- in_channels=128,
- in_index=3,
- channels=64,
- num_convs=1,
- concat_input=False,
- dropout_ratio=0.1,
- num_classes=2,
- norm_cfg=norm_cfg,
- align_corners=False,
- loss_decode=dict(
- type='CrossEntropyLoss', use_sigmoid=False, loss_weight=0.4)),
- # model training and testing settings
- train_cfg=dict(),
- test_cfg=dict(mode='slide', crop_size=256, stride=170))
diff --git a/spaces/Andy1621/uniformer_image_segmentation/configs/pspnet/pspnet_r18-d8_769x769_80k_cityscapes.py b/spaces/Andy1621/uniformer_image_segmentation/configs/pspnet/pspnet_r18-d8_769x769_80k_cityscapes.py
deleted file mode 100644
index 5893e66a41cad73e8fb24aa58dc78ef002aecca5..0000000000000000000000000000000000000000
--- a/spaces/Andy1621/uniformer_image_segmentation/configs/pspnet/pspnet_r18-d8_769x769_80k_cityscapes.py
+++ /dev/null
@@ -1,9 +0,0 @@
-_base_ = './pspnet_r50-d8_769x769_80k_cityscapes.py'
-model = dict(
- pretrained='open-mmlab://resnet18_v1c',
- backbone=dict(depth=18),
- decode_head=dict(
- in_channels=512,
- channels=128,
- ),
- auxiliary_head=dict(in_channels=256, channels=64))
diff --git a/spaces/AnishKumbhar/ChatBot/text-generation-webui-main/docs/Docker.md b/spaces/AnishKumbhar/ChatBot/text-generation-webui-main/docs/Docker.md
deleted file mode 100644
index 322dba39a8b2ebcf87932717ddf240101f558ed4..0000000000000000000000000000000000000000
--- a/spaces/AnishKumbhar/ChatBot/text-generation-webui-main/docs/Docker.md
+++ /dev/null
@@ -1,203 +0,0 @@
-Docker Compose is a way of installing and launching the web UI in an isolated Ubuntu image using only a few commands.
-
-In order to create the image as described in the main README, you must have docker compose 2.17 or higher:
-
-```
-~$ docker compose version
-Docker Compose version v2.17.2
-```
-
-Make sure to also create the necessary symbolic links:
-
-```
-cd text-generation-webui
-ln -s docker/{Dockerfile,docker-compose.yml,.dockerignore} .
-cp docker/.env.example .env
-# Edit .env and set TORCH_CUDA_ARCH_LIST based on your GPU model
-docker compose up --build
-```
-
-# Table of contents
-
-* [Docker Compose installation instructions](#docker-compose-installation-instructions)
-* [Repository with additional Docker files](#dedicated-docker-repository)
-
-# Docker Compose installation instructions
-
-By [@loeken](https://github.com/loeken).
-
-- [Ubuntu 22.04](#ubuntu-2204)
- - [0. youtube video](#0-youtube-video)
- - [1. update the drivers](#1-update-the-drivers)
- - [2. reboot](#2-reboot)
- - [3. install docker](#3-install-docker)
- - [4. docker \& container toolkit](#4-docker--container-toolkit)
- - [5. clone the repo](#5-clone-the-repo)
- - [6. prepare models](#6-prepare-models)
- - [7. prepare .env file](#7-prepare-env-file)
- - [8. startup docker container](#8-startup-docker-container)
-- [Manjaro](#manjaro)
- - [update the drivers](#update-the-drivers)
- - [reboot](#reboot)
- - [docker \& container toolkit](#docker--container-toolkit)
- - [continue with ubuntu task](#continue-with-ubuntu-task)
-- [Windows](#windows)
- - [0. youtube video](#0-youtube-video-1)
- - [1. choco package manager](#1-choco-package-manager)
- - [2. install drivers/dependencies](#2-install-driversdependencies)
- - [3. install wsl](#3-install-wsl)
- - [4. reboot](#4-reboot)
- - [5. git clone \&\& startup](#5-git-clone--startup)
- - [6. prepare models](#6-prepare-models-1)
- - [7. startup](#7-startup)
-- [notes](#notes)
-
-## Ubuntu 22.04
-
-### 0. youtube video
-A video walking you through the setup can be found here:
-
-[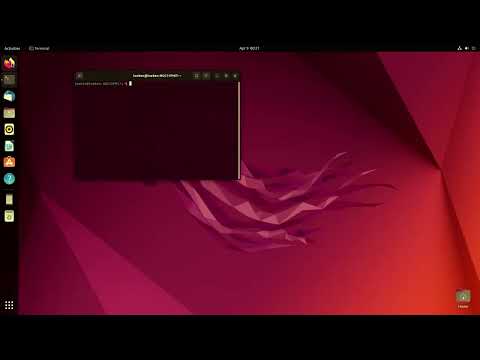](https://www.youtube.com/watch?v=ELkKWYh8qOk)
-
-
-### 1. update the drivers
-in the the “software updater” update drivers to the last version of the prop driver.
-
-### 2. reboot
-to switch using to new driver
-
-### 3. install docker
-```bash
-sudo apt update
-sudo apt-get install curl
-sudo mkdir -m 0755 -p /etc/apt/keyrings
-curl -fsSL https://download.docker.com/linux/ubuntu/gpg | sudo gpg --dearmor -o /etc/apt/keyrings/docker.gpg
-echo \
- "deb [arch="$(dpkg --print-architecture)" signed-by=/etc/apt/keyrings/docker.gpg] https://download.docker.com/linux/ubuntu \
- "$(. /etc/os-release && echo "$VERSION_CODENAME")" stable" | \
- sudo tee /etc/apt/sources.list.d/docker.list > /dev/null
-sudo apt update
-sudo apt-get install docker-ce docker-ce-cli containerd.io docker-buildx-plugin docker-compose-plugin docker-compose -y
-sudo usermod -aG docker $USER
-newgrp docker
-```
-
-### 4. docker & container toolkit
-```bash
-curl -fsSL https://nvidia.github.io/libnvidia-container/gpgkey | sudo gpg --dearmor -o /usr/share/keyrings/nvidia-container-toolkit-keyring.gpg
-echo "deb [signed-by=/usr/share/keyrings/nvidia-container-toolkit-keyring.gpg] https://nvidia.github.io/libnvidia-container/stable/ubuntu22.04/amd64 /" | \
-sudo tee /etc/apt/sources.list.d/nvidia.list > /dev/null
-sudo apt update
-sudo apt install nvidia-docker2 nvidia-container-runtime -y
-sudo systemctl restart docker
-```
-
-### 5. clone the repo
-```
-git clone https://github.com/oobabooga/text-generation-webui
-cd text-generation-webui
-```
-
-### 6. prepare models
-download and place the models inside the models folder. tested with:
-
-4bit
-https://github.com/oobabooga/text-generation-webui/pull/530#issuecomment-1483891617
-https://github.com/oobabooga/text-generation-webui/pull/530#issuecomment-1483941105
-
-8bit:
-https://github.com/oobabooga/text-generation-webui/pull/530#issuecomment-1484235789
-
-### 7. prepare .env file
-edit .env values to your needs.
-```bash
-cp .env.example .env
-nano .env
-```
-
-### 8. startup docker container
-```bash
-docker compose up --build
-```
-
-## Manjaro
-manjaro/arch is similar to ubuntu just the dependency installation is more convenient
-
-### update the drivers
-```bash
-sudo mhwd -a pci nonfree 0300
-```
-### reboot
-```bash
-reboot
-```
-### docker & container toolkit
-```bash
-yay -S docker docker-compose buildkit gcc nvidia-docker
-sudo usermod -aG docker $USER
-newgrp docker
-sudo systemctl restart docker # required by nvidia-container-runtime
-```
-
-### continue with ubuntu task
-continue at [5. clone the repo](#5-clone-the-repo)
-
-## Windows
-### 0. youtube video
-A video walking you through the setup can be found here:
-[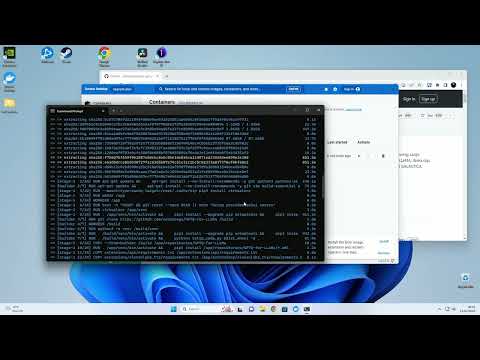](https://www.youtube.com/watch?v=ejH4w5b5kFQ)
-
-### 1. choco package manager
-install package manager (https://chocolatey.org/ )
-```
-Set-ExecutionPolicy Bypass -Scope Process -Force; [System.Net.ServicePointManager]::SecurityProtocol = [System.Net.ServicePointManager]::SecurityProtocol -bor 3072; iex ((New-Object System.Net.WebClient).DownloadString('https://community.chocolatey.org/install.ps1'))
-```
-
-### 2. install drivers/dependencies
-```
-choco install nvidia-display-driver cuda git docker-desktop
-```
-
-### 3. install wsl
-wsl --install
-
-### 4. reboot
-after reboot enter username/password in wsl
-
-### 5. git clone && startup
-clone the repo and edit .env values to your needs.
-```
-cd Desktop
-git clone https://github.com/oobabooga/text-generation-webui
-cd text-generation-webui
-COPY .env.example .env
-notepad .env
-```
-
-### 6. prepare models
-download and place the models inside the models folder. tested with:
-
-4bit https://github.com/oobabooga/text-generation-webui/pull/530#issuecomment-1483891617 https://github.com/oobabooga/text-generation-webui/pull/530#issuecomment-1483941105
-
-8bit: https://github.com/oobabooga/text-generation-webui/pull/530#issuecomment-1484235789
-
-### 7. startup
-```
-docker compose up
-```
-
-## notes
-
-on older ubuntus you can manually install the docker compose plugin like this:
-```
-DOCKER_CONFIG=${DOCKER_CONFIG:-$HOME/.docker}
-mkdir -p $DOCKER_CONFIG/cli-plugins
-curl -SL https://github.com/docker/compose/releases/download/v2.17.2/docker-compose-linux-x86_64 -o $DOCKER_CONFIG/cli-plugins/docker-compose
-chmod +x $DOCKER_CONFIG/cli-plugins/docker-compose
-export PATH="$HOME/.docker/cli-plugins:$PATH"
-```
-
-# Dedicated docker repository
-
-An external repository maintains a docker wrapper for this project as well as several pre-configured 'one-click' `docker compose` variants (e.g., updated branches of GPTQ). It can be found at: [Atinoda/text-generation-webui-docker](https://github.com/Atinoda/text-generation-webui-docker).
-
diff --git a/spaces/AnishKumbhar/ChatBot/text-generation-webui-main/modules/ui_parameters.py b/spaces/AnishKumbhar/ChatBot/text-generation-webui-main/modules/ui_parameters.py
deleted file mode 100644
index d75d420245c2afa421c5eee364bd5c5b1de047d6..0000000000000000000000000000000000000000
--- a/spaces/AnishKumbhar/ChatBot/text-generation-webui-main/modules/ui_parameters.py
+++ /dev/null
@@ -1,106 +0,0 @@
-from pathlib import Path
-
-import gradio as gr
-
-from modules import loaders, presets, shared, ui, ui_chat, utils
-from modules.utils import gradio
-
-
-def create_ui(default_preset):
- mu = shared.args.multi_user
- generate_params = presets.load_preset(default_preset)
- with gr.Tab("Parameters", elem_id="parameters"):
- with gr.Tab("Generation"):
- with gr.Row():
- with gr.Column():
- with gr.Row():
- shared.gradio['preset_menu'] = gr.Dropdown(choices=utils.get_available_presets(), value=default_preset, label='Preset', elem_classes='slim-dropdown')
- ui.create_refresh_button(shared.gradio['preset_menu'], lambda: None, lambda: {'choices': utils.get_available_presets()}, 'refresh-button', interactive=not mu)
- shared.gradio['save_preset'] = gr.Button('💾', elem_classes='refresh-button', interactive=not mu)
- shared.gradio['delete_preset'] = gr.Button('🗑️', elem_classes='refresh-button', interactive=not mu)
-
- with gr.Column():
- shared.gradio['filter_by_loader'] = gr.Dropdown(label="Filter by loader", choices=["All"] + list(loaders.loaders_and_params.keys()), value="All", elem_classes='slim-dropdown')
-
- with gr.Row():
- with gr.Column():
- with gr.Row():
- with gr.Column():
- shared.gradio['max_new_tokens'] = gr.Slider(minimum=shared.settings['max_new_tokens_min'], maximum=shared.settings['max_new_tokens_max'], step=1, label='max_new_tokens', value=shared.settings['max_new_tokens'])
- shared.gradio['temperature'] = gr.Slider(0.01, 1.99, value=generate_params['temperature'], step=0.01, label='temperature')
- shared.gradio['top_p'] = gr.Slider(0.0, 1.0, value=generate_params['top_p'], step=0.01, label='top_p')
- shared.gradio['top_k'] = gr.Slider(0, 200, value=generate_params['top_k'], step=1, label='top_k')
- shared.gradio['repetition_penalty'] = gr.Slider(1.0, 1.5, value=generate_params['repetition_penalty'], step=0.01, label='repetition_penalty')
- shared.gradio['repetition_penalty_range'] = gr.Slider(0, 4096, step=64, value=generate_params['repetition_penalty_range'], label='repetition_penalty_range')
- shared.gradio['typical_p'] = gr.Slider(0.0, 1.0, value=generate_params['typical_p'], step=0.01, label='typical_p')
- shared.gradio['tfs'] = gr.Slider(0.0, 1.0, value=generate_params['tfs'], step=0.01, label='tfs')
- shared.gradio['top_a'] = gr.Slider(0.0, 1.0, value=generate_params['top_a'], step=0.01, label='top_a')
- shared.gradio['epsilon_cutoff'] = gr.Slider(0, 9, value=generate_params['epsilon_cutoff'], step=0.01, label='epsilon_cutoff')
- shared.gradio['eta_cutoff'] = gr.Slider(0, 20, value=generate_params['eta_cutoff'], step=0.01, label='eta_cutoff')
-
- with gr.Column():
- shared.gradio['guidance_scale'] = gr.Slider(-0.5, 2.5, step=0.05, value=generate_params['guidance_scale'], label='guidance_scale', info='For CFG. 1.5 is a good value.')
- shared.gradio['negative_prompt'] = gr.Textbox(value=shared.settings['negative_prompt'], label='Negative prompt', lines=3, elem_classes=['add_scrollbar'])
- shared.gradio['penalty_alpha'] = gr.Slider(0, 5, value=generate_params['penalty_alpha'], label='penalty_alpha', info='For Contrastive Search. do_sample must be unchecked.')
- shared.gradio['mirostat_mode'] = gr.Slider(0, 2, step=1, value=generate_params['mirostat_mode'], label='mirostat_mode', info='mode=1 is for llama.cpp only.')
- shared.gradio['mirostat_tau'] = gr.Slider(0, 10, step=0.01, value=generate_params['mirostat_tau'], label='mirostat_tau')
- shared.gradio['mirostat_eta'] = gr.Slider(0, 1, step=0.01, value=generate_params['mirostat_eta'], label='mirostat_eta')
- shared.gradio['do_sample'] = gr.Checkbox(value=generate_params['do_sample'], label='do_sample')
- shared.gradio['seed'] = gr.Number(value=shared.settings['seed'], label='Seed (-1 for random)')
- with gr.Accordion('Other parameters', open=False):
- shared.gradio['encoder_repetition_penalty'] = gr.Slider(0.8, 1.5, value=generate_params['encoder_repetition_penalty'], step=0.01, label='encoder_repetition_penalty')
- shared.gradio['no_repeat_ngram_size'] = gr.Slider(0, 20, step=1, value=generate_params['no_repeat_ngram_size'], label='no_repeat_ngram_size')
- shared.gradio['min_length'] = gr.Slider(0, 2000, step=1, value=generate_params['min_length'], label='min_length')
- shared.gradio['num_beams'] = gr.Slider(1, 20, step=1, value=generate_params['num_beams'], label='num_beams', info='For Beam Search, along with length_penalty and early_stopping.')
- shared.gradio['length_penalty'] = gr.Slider(-5, 5, value=generate_params['length_penalty'], label='length_penalty')
- shared.gradio['early_stopping'] = gr.Checkbox(value=generate_params['early_stopping'], label='early_stopping')
-
- gr.Markdown("[Learn more](https://github.com/oobabooga/text-generation-webui/blob/main/docs/Generation-Parameters.md)")
-
- with gr.Column():
- with gr.Row():
- with gr.Column():
- shared.gradio['truncation_length'] = gr.Slider(value=get_truncation_length(), minimum=shared.settings['truncation_length_min'], maximum=shared.settings['truncation_length_max'], step=256, label='Truncate the prompt up to this length', info='The leftmost tokens are removed if the prompt exceeds this length. Most models require this to be at most 2048.')
- shared.gradio['max_tokens_second'] = gr.Slider(value=shared.settings['max_tokens_second'], minimum=0, maximum=20, step=1, label='Maximum number of tokens/second', info='To make text readable in real time.')
- shared.gradio['custom_stopping_strings'] = gr.Textbox(lines=1, value=shared.settings["custom_stopping_strings"] or None, label='Custom stopping strings', info='In addition to the defaults. Written between "" and separated by commas.', placeholder='"\\n", "\\nYou:"')
- shared.gradio['custom_token_bans'] = gr.Textbox(value=shared.settings['custom_token_bans'] or None, label='Custom token bans', info='Specific token IDs to ban from generating, comma-separated. The IDs can be found in the Default or Notebook tab.')
-
- with gr.Column():
- shared.gradio['auto_max_new_tokens'] = gr.Checkbox(value=shared.settings['auto_max_new_tokens'], label='auto_max_new_tokens', info='Expand max_new_tokens to the available context length.')
- shared.gradio['ban_eos_token'] = gr.Checkbox(value=shared.settings['ban_eos_token'], label='Ban the eos_token', info='Forces the model to never end the generation prematurely.')
- shared.gradio['add_bos_token'] = gr.Checkbox(value=shared.settings['add_bos_token'], label='Add the bos_token to the beginning of prompts', info='Disabling this can make the replies more creative.')
- shared.gradio['skip_special_tokens'] = gr.Checkbox(value=shared.settings['skip_special_tokens'], label='Skip special tokens', info='Some specific models need this unset.')
- shared.gradio['stream'] = gr.Checkbox(value=shared.settings['stream'], label='Activate text streaming')
-
- with gr.Row() as shared.gradio['grammar_file_row']:
- shared.gradio['grammar_file'] = gr.Dropdown(value='None', choices=utils.get_available_grammars(), label='Load grammar from file (.gbnf)', elem_classes='slim-dropdown')
- ui.create_refresh_button(shared.gradio['grammar_file'], lambda: None, lambda: {'choices': utils.get_available_grammars()}, 'refresh-button', interactive=not mu)
- shared.gradio['save_grammar'] = gr.Button('💾', elem_classes='refresh-button', interactive=not mu)
- shared.gradio['delete_grammar'] = gr.Button('🗑️ ', elem_classes='refresh-button', interactive=not mu)
-
- shared.gradio['grammar_string'] = gr.Textbox(value='', label='Grammar', lines=16, elem_classes=['add_scrollbar', 'monospace'])
-
- ui_chat.create_chat_settings_ui()
-
-
-def create_event_handlers():
- shared.gradio['filter_by_loader'].change(loaders.blacklist_samplers, gradio('filter_by_loader'), gradio(loaders.list_all_samplers()), show_progress=False)
- shared.gradio['preset_menu'].change(presets.load_preset_for_ui, gradio('preset_menu', 'interface_state'), gradio('interface_state') + gradio(presets.presets_params()))
- shared.gradio['grammar_file'].change(load_grammar, gradio('grammar_file'), gradio('grammar_string'))
-
-
-def get_truncation_length():
- if shared.args.max_seq_len != shared.args_defaults.max_seq_len:
- return shared.args.max_seq_len
- if shared.args.n_ctx != shared.args_defaults.n_ctx:
- return shared.args.n_ctx
- else:
- return shared.settings['truncation_length']
-
-
-def load_grammar(name):
- p = Path(f'grammars/{name}')
- if p.exists():
- return open(p, 'r').read()
- else:
- return ''
diff --git a/spaces/Anthony7906/MengHuiMXD_GPT/locale/extract_locale.py b/spaces/Anthony7906/MengHuiMXD_GPT/locale/extract_locale.py
deleted file mode 100644
index 32b0924bd6dffe150cb3e481ddadef836b91b83c..0000000000000000000000000000000000000000
--- a/spaces/Anthony7906/MengHuiMXD_GPT/locale/extract_locale.py
+++ /dev/null
@@ -1,26 +0,0 @@
-import os
-import json
-import re
-
-# Define regular expression patterns
-pattern = r'i18n\((\"{3}.*?\"{3}|\".*?\")\)'
-
-# Load the .py file
-with open('ChuanhuChatbot.py', 'r', encoding='utf-8') as f:
- contents = f.read()
-
-# Load the .py files in the modules folder
-for filename in os.listdir("modules"):
- if filename.endswith(".py"):
- with open(os.path.join("modules", filename), "r", encoding="utf-8") as f:
- contents += f.read()
-
-# Matching with regular expressions
-matches = re.findall(pattern, contents, re.DOTALL)
-
-# Convert to key/value pairs
-data = {match.strip('()"'): '' for match in matches}
-
-# Save as a JSON file
-with open('labels.json', 'w', encoding='utf-8') as f:
- json.dump(data, f, ensure_ascii=False, indent=4)
\ No newline at end of file
diff --git a/spaces/Ataturk-Chatbot/HuggingFaceChat/venv/lib/python3.11/site-packages/pip/_internal/utils/entrypoints.py b/spaces/Ataturk-Chatbot/HuggingFaceChat/venv/lib/python3.11/site-packages/pip/_internal/utils/entrypoints.py
deleted file mode 100644
index 150136938548af6aa5ae1f716b330d0eb2d3e013..0000000000000000000000000000000000000000
--- a/spaces/Ataturk-Chatbot/HuggingFaceChat/venv/lib/python3.11/site-packages/pip/_internal/utils/entrypoints.py
+++ /dev/null
@@ -1,84 +0,0 @@
-import itertools
-import os
-import shutil
-import sys
-from typing import List, Optional
-
-from pip._internal.cli.main import main
-from pip._internal.utils.compat import WINDOWS
-
-_EXECUTABLE_NAMES = [
- "pip",
- f"pip{sys.version_info.major}",
- f"pip{sys.version_info.major}.{sys.version_info.minor}",
-]
-if WINDOWS:
- _allowed_extensions = {"", ".exe"}
- _EXECUTABLE_NAMES = [
- "".join(parts)
- for parts in itertools.product(_EXECUTABLE_NAMES, _allowed_extensions)
- ]
-
-
-def _wrapper(args: Optional[List[str]] = None) -> int:
- """Central wrapper for all old entrypoints.
-
- Historically pip has had several entrypoints defined. Because of issues
- arising from PATH, sys.path, multiple Pythons, their interactions, and most
- of them having a pip installed, users suffer every time an entrypoint gets
- moved.
-
- To alleviate this pain, and provide a mechanism for warning users and
- directing them to an appropriate place for help, we now define all of
- our old entrypoints as wrappers for the current one.
- """
- sys.stderr.write(
- "WARNING: pip is being invoked by an old script wrapper. This will "
- "fail in a future version of pip.\n"
- "Please see https://github.com/pypa/pip/issues/5599 for advice on "
- "fixing the underlying issue.\n"
- "To avoid this problem you can invoke Python with '-m pip' instead of "
- "running pip directly.\n"
- )
- return main(args)
-
-
-def get_best_invocation_for_this_pip() -> str:
- """Try to figure out the best way to invoke pip in the current environment."""
- binary_directory = "Scripts" if WINDOWS else "bin"
- binary_prefix = os.path.join(sys.prefix, binary_directory)
-
- # Try to use pip[X[.Y]] names, if those executables for this environment are
- # the first on PATH with that name.
- path_parts = os.path.normcase(os.environ.get("PATH", "")).split(os.pathsep)
- exe_are_in_PATH = os.path.normcase(binary_prefix) in path_parts
- if exe_are_in_PATH:
- for exe_name in _EXECUTABLE_NAMES:
- found_executable = shutil.which(exe_name)
- binary_executable = os.path.join(binary_prefix, exe_name)
- if (
- found_executable
- and os.path.exists(binary_executable)
- and os.path.samefile(
- found_executable,
- binary_executable,
- )
- ):
- return exe_name
-
- # Use the `-m` invocation, if there's no "nice" invocation.
- return f"{get_best_invocation_for_this_python()} -m pip"
-
-
-def get_best_invocation_for_this_python() -> str:
- """Try to figure out the best way to invoke the current Python."""
- exe = sys.executable
- exe_name = os.path.basename(exe)
-
- # Try to use the basename, if it's the first executable.
- found_executable = shutil.which(exe_name)
- if found_executable and os.path.samefile(found_executable, exe):
- return exe_name
-
- # Use the full executable name, because we couldn't find something simpler.
- return exe
diff --git a/spaces/Ataturk-Chatbot/HuggingFaceChat/venv/lib/python3.11/site-packages/pip/_vendor/cachecontrol/adapter.py b/spaces/Ataturk-Chatbot/HuggingFaceChat/venv/lib/python3.11/site-packages/pip/_vendor/cachecontrol/adapter.py
deleted file mode 100644
index 94c75e1a05b47922945c5233e90e9f936b108b66..0000000000000000000000000000000000000000
--- a/spaces/Ataturk-Chatbot/HuggingFaceChat/venv/lib/python3.11/site-packages/pip/_vendor/cachecontrol/adapter.py
+++ /dev/null
@@ -1,137 +0,0 @@
-# SPDX-FileCopyrightText: 2015 Eric Larson
-#
-# SPDX-License-Identifier: Apache-2.0
-
-import types
-import functools
-import zlib
-
-from pip._vendor.requests.adapters import HTTPAdapter
-
-from .controller import CacheController, PERMANENT_REDIRECT_STATUSES
-from .cache import DictCache
-from .filewrapper import CallbackFileWrapper
-
-
-class CacheControlAdapter(HTTPAdapter):
- invalidating_methods = {"PUT", "PATCH", "DELETE"}
-
- def __init__(
- self,
- cache=None,
- cache_etags=True,
- controller_class=None,
- serializer=None,
- heuristic=None,
- cacheable_methods=None,
- *args,
- **kw
- ):
- super(CacheControlAdapter, self).__init__(*args, **kw)
- self.cache = DictCache() if cache is None else cache
- self.heuristic = heuristic
- self.cacheable_methods = cacheable_methods or ("GET",)
-
- controller_factory = controller_class or CacheController
- self.controller = controller_factory(
- self.cache, cache_etags=cache_etags, serializer=serializer
- )
-
- def send(self, request, cacheable_methods=None, **kw):
- """
- Send a request. Use the request information to see if it
- exists in the cache and cache the response if we need to and can.
- """
- cacheable = cacheable_methods or self.cacheable_methods
- if request.method in cacheable:
- try:
- cached_response = self.controller.cached_request(request)
- except zlib.error:
- cached_response = None
- if cached_response:
- return self.build_response(request, cached_response, from_cache=True)
-
- # check for etags and add headers if appropriate
- request.headers.update(self.controller.conditional_headers(request))
-
- resp = super(CacheControlAdapter, self).send(request, **kw)
-
- return resp
-
- def build_response(
- self, request, response, from_cache=False, cacheable_methods=None
- ):
- """
- Build a response by making a request or using the cache.
-
- This will end up calling send and returning a potentially
- cached response
- """
- cacheable = cacheable_methods or self.cacheable_methods
- if not from_cache and request.method in cacheable:
- # Check for any heuristics that might update headers
- # before trying to cache.
- if self.heuristic:
- response = self.heuristic.apply(response)
-
- # apply any expiration heuristics
- if response.status == 304:
- # We must have sent an ETag request. This could mean
- # that we've been expired already or that we simply
- # have an etag. In either case, we want to try and
- # update the cache if that is the case.
- cached_response = self.controller.update_cached_response(
- request, response
- )
-
- if cached_response is not response:
- from_cache = True
-
- # We are done with the server response, read a
- # possible response body (compliant servers will
- # not return one, but we cannot be 100% sure) and
- # release the connection back to the pool.
- response.read(decode_content=False)
- response.release_conn()
-
- response = cached_response
-
- # We always cache the 301 responses
- elif int(response.status) in PERMANENT_REDIRECT_STATUSES:
- self.controller.cache_response(request, response)
- else:
- # Wrap the response file with a wrapper that will cache the
- # response when the stream has been consumed.
- response._fp = CallbackFileWrapper(
- response._fp,
- functools.partial(
- self.controller.cache_response, request, response
- ),
- )
- if response.chunked:
- super_update_chunk_length = response._update_chunk_length
-
- def _update_chunk_length(self):
- super_update_chunk_length()
- if self.chunk_left == 0:
- self._fp._close()
-
- response._update_chunk_length = types.MethodType(
- _update_chunk_length, response
- )
-
- resp = super(CacheControlAdapter, self).build_response(request, response)
-
- # See if we should invalidate the cache.
- if request.method in self.invalidating_methods and resp.ok:
- cache_url = self.controller.cache_url(request.url)
- self.cache.delete(cache_url)
-
- # Give the request a from_cache attr to let people use it
- resp.from_cache = from_cache
-
- return resp
-
- def close(self):
- self.cache.close()
- super(CacheControlAdapter, self).close()
diff --git a/spaces/Audio-AGI/AudioSep/models/CLAP/training/zero_shot.py b/spaces/Audio-AGI/AudioSep/models/CLAP/training/zero_shot.py
deleted file mode 100644
index 28b8fccc1af17fc69002857a7f529ac041c374f2..0000000000000000000000000000000000000000
--- a/spaces/Audio-AGI/AudioSep/models/CLAP/training/zero_shot.py
+++ /dev/null
@@ -1,95 +0,0 @@
-# NOTE: This script is currently not supported for CLAP.
-import logging
-from contextlib import suppress
-
-import torch
-import torch.nn.functional as F
-from tqdm import tqdm
-
-from open_clip import tokenize
-from .imagenet_zeroshot_data import imagenet_classnames, openai_imagenet_template
-
-
-def zero_shot_classifier(model, classnames, templates, args):
- with torch.no_grad():
- zeroshot_weights = []
- for classname in tqdm(classnames):
- texts = [template(classname) for template in templates] # format with class
- texts = tokenize(texts).to(args.device) # tokenize
- if args.distributed and not args.horovod:
- class_embeddings = model.module.encode_text(texts)
- else:
- class_embeddings = model.encode_text(texts)
- class_embedding = F.normalize(class_embeddings, dim=-1).mean(dim=0)
- class_embedding /= class_embedding.norm()
- zeroshot_weights.append(class_embedding)
- zeroshot_weights = torch.stack(zeroshot_weights, dim=1).to(args.device)
- return zeroshot_weights
-
-
-def accuracy(output, target, topk=(1,)):
- pred = output.topk(max(topk), 1, True, True)[1].t()
- correct = pred.eq(target.view(1, -1).expand_as(pred))
- return [
- float(correct[:k].reshape(-1).float().sum(0, keepdim=True).cpu().numpy())
- for k in topk
- ]
-
-
-def run(model, classifier, dataloader, args):
- autocast = torch.cuda.amp.autocast if args.precision == "amp" else suppress
- with torch.no_grad():
- top1, top5, n = 0.0, 0.0, 0.0
- for images, target in tqdm(dataloader, unit_scale=args.batch_size):
- images = images.to(args.device)
- target = target.to(args.device)
-
- with autocast():
- # predict
- if args.distributed and not args.horovod:
- image_features = model.module.encode_image(images)
- else:
- image_features = model.encode_image(images)
- image_features = F.normalize(image_features, dim=-1)
- logits = 100.0 * image_features @ classifier
-
- # measure accuracy
- acc1, acc5 = accuracy(logits, target, topk=(1, 5))
- top1 += acc1
- top5 += acc5
- n += images.size(0)
-
- top1 = top1 / n
- top5 = top5 / n
- return top1, top5
-
-
-def zero_shot_eval(model, data, epoch, args):
- if "imagenet-val" not in data and "imagenet-v2" not in data:
- return {}
- if args.zeroshot_frequency == 0:
- return {}
- if (epoch % args.zeroshot_frequency) != 0 and epoch != args.epochs:
- return {}
-
- logging.info("Starting zero-shot imagenet.")
-
- logging.info("Building zero-shot classifier")
- classifier = zero_shot_classifier(
- model, imagenet_classnames, openai_imagenet_template, args
- )
-
- logging.info("Using classifier")
- results = {}
- if "imagenet-val" in data:
- top1, top5 = run(model, classifier, data["imagenet-val"].dataloader, args)
- results["imagenet-zeroshot-val-top1"] = top1
- results["imagenet-zeroshot-val-top5"] = top5
- if "imagenet-v2" in data:
- top1, top5 = run(model, classifier, data["imagenet-v2"].dataloader, args)
- results["imagenetv2-zeroshot-val-top1"] = top1
- results["imagenetv2-zeroshot-val-top5"] = top5
-
- logging.info("Finished zero-shot imagenet.")
-
- return results
diff --git a/spaces/Awiny/Image2Paragraph/models/grit_src/third_party/CenterNet2/detectron2/layers/csrc/ROIAlignRotated/ROIAlignRotated_cpu.cpp b/spaces/Awiny/Image2Paragraph/models/grit_src/third_party/CenterNet2/detectron2/layers/csrc/ROIAlignRotated/ROIAlignRotated_cpu.cpp
deleted file mode 100644
index 2a3d3056cc71a4acaafb570739a9dd247a7eb1ed..0000000000000000000000000000000000000000
--- a/spaces/Awiny/Image2Paragraph/models/grit_src/third_party/CenterNet2/detectron2/layers/csrc/ROIAlignRotated/ROIAlignRotated_cpu.cpp
+++ /dev/null
@@ -1,522 +0,0 @@
-// Copyright (c) Facebook, Inc. and its affiliates.
-#include
-#include "ROIAlignRotated.h"
-
-// Note: this implementation originates from the Caffe2 ROIAlignRotated Op
-// and PyTorch ROIAlign (non-rotated) Op implementations.
-// The key difference between this implementation and those ones is
-// we don't do "legacy offset" in this version, as there aren't many previous
-// works, if any, using the "legacy" ROIAlignRotated Op.
-// This would make the interface a bit cleaner.
-
-namespace detectron2 {
-
-namespace {
-template
-struct PreCalc {
- int pos1;
- int pos2;
- int pos3;
- int pos4;
- T w1;
- T w2;
- T w3;
- T w4;
-};
-
-template
-void pre_calc_for_bilinear_interpolate(
- const int height,
- const int width,
- const int pooled_height,
- const int pooled_width,
- const int iy_upper,
- const int ix_upper,
- T roi_start_h,
- T roi_start_w,
- T bin_size_h,
- T bin_size_w,
- int roi_bin_grid_h,
- int roi_bin_grid_w,
- T roi_center_h,
- T roi_center_w,
- T cos_theta,
- T sin_theta,
- std::vector>& pre_calc) {
- int pre_calc_index = 0;
- for (int ph = 0; ph < pooled_height; ph++) {
- for (int pw = 0; pw < pooled_width; pw++) {
- for (int iy = 0; iy < iy_upper; iy++) {
- const T yy = roi_start_h + ph * bin_size_h +
- static_cast(iy + .5f) * bin_size_h /
- static_cast(roi_bin_grid_h); // e.g., 0.5, 1.5
- for (int ix = 0; ix < ix_upper; ix++) {
- const T xx = roi_start_w + pw * bin_size_w +
- static_cast(ix + .5f) * bin_size_w /
- static_cast(roi_bin_grid_w);
-
- // Rotate by theta around the center and translate
- // In image space, (y, x) is the order for Right Handed System,
- // and this is essentially multiplying the point by a rotation matrix
- // to rotate it counterclockwise through angle theta.
- T y = yy * cos_theta - xx * sin_theta + roi_center_h;
- T x = yy * sin_theta + xx * cos_theta + roi_center_w;
- // deal with: inverse elements are out of feature map boundary
- if (y < -1.0 || y > height || x < -1.0 || x > width) {
- // empty
- PreCalc pc;
- pc.pos1 = 0;
- pc.pos2 = 0;
- pc.pos3 = 0;
- pc.pos4 = 0;
- pc.w1 = 0;
- pc.w2 = 0;
- pc.w3 = 0;
- pc.w4 = 0;
- pre_calc[pre_calc_index] = pc;
- pre_calc_index += 1;
- continue;
- }
-
- if (y < 0) {
- y = 0;
- }
- if (x < 0) {
- x = 0;
- }
-
- int y_low = (int)y;
- int x_low = (int)x;
- int y_high;
- int x_high;
-
- if (y_low >= height - 1) {
- y_high = y_low = height - 1;
- y = (T)y_low;
- } else {
- y_high = y_low + 1;
- }
-
- if (x_low >= width - 1) {
- x_high = x_low = width - 1;
- x = (T)x_low;
- } else {
- x_high = x_low + 1;
- }
-
- T ly = y - y_low;
- T lx = x - x_low;
- T hy = 1. - ly, hx = 1. - lx;
- T w1 = hy * hx, w2 = hy * lx, w3 = ly * hx, w4 = ly * lx;
-
- // save weights and indices
- PreCalc pc;
- pc.pos1 = y_low * width + x_low;
- pc.pos2 = y_low * width + x_high;
- pc.pos3 = y_high * width + x_low;
- pc.pos4 = y_high * width + x_high;
- pc.w1 = w1;
- pc.w2 = w2;
- pc.w3 = w3;
- pc.w4 = w4;
- pre_calc[pre_calc_index] = pc;
-
- pre_calc_index += 1;
- }
- }
- }
- }
-}
-
-template
-void bilinear_interpolate_gradient(
- const int height,
- const int width,
- T y,
- T x,
- T& w1,
- T& w2,
- T& w3,
- T& w4,
- int& x_low,
- int& x_high,
- int& y_low,
- int& y_high) {
- // deal with cases that inverse elements are out of feature map boundary
- if (y < -1.0 || y > height || x < -1.0 || x > width) {
- // empty
- w1 = w2 = w3 = w4 = 0.;
- x_low = x_high = y_low = y_high = -1;
- return;
- }
-
- if (y < 0) {
- y = 0;
- }
-
- if (x < 0) {
- x = 0;
- }
-
- y_low = (int)y;
- x_low = (int)x;
-
- if (y_low >= height - 1) {
- y_high = y_low = height - 1;
- y = (T)y_low;
- } else {
- y_high = y_low + 1;
- }
-
- if (x_low >= width - 1) {
- x_high = x_low = width - 1;
- x = (T)x_low;
- } else {
- x_high = x_low + 1;
- }
-
- T ly = y - y_low;
- T lx = x - x_low;
- T hy = 1. - ly, hx = 1. - lx;
-
- // reference in forward
- // T v1 = input[y_low * width + x_low];
- // T v2 = input[y_low * width + x_high];
- // T v3 = input[y_high * width + x_low];
- // T v4 = input[y_high * width + x_high];
- // T val = (w1 * v1 + w2 * v2 + w3 * v3 + w4 * v4);
-
- w1 = hy * hx, w2 = hy * lx, w3 = ly * hx, w4 = ly * lx;
-
- return;
-}
-
-template
-inline void add(T* address, const T& val) {
- *address += val;
-}
-
-} // namespace
-
-template
-void ROIAlignRotatedForward(
- const int nthreads,
- const T* input,
- const T& spatial_scale,
- const int channels,
- const int height,
- const int width,
- const int pooled_height,
- const int pooled_width,
- const int sampling_ratio,
- const T* rois,
- T* output) {
- int n_rois = nthreads / channels / pooled_width / pooled_height;
- // (n, c, ph, pw) is an element in the pooled output
- // can be parallelized using omp
- // #pragma omp parallel for num_threads(32)
- for (int n = 0; n < n_rois; n++) {
- int index_n = n * channels * pooled_width * pooled_height;
-
- const T* current_roi = rois + n * 6;
- int roi_batch_ind = current_roi[0];
-
- // Do not use rounding; this implementation detail is critical
- // ROIAlignRotated supports align == true, i.e., continuous coordinate
- // by default, thus the 0.5 offset
- T offset = (T)0.5;
- T roi_center_w = current_roi[1] * spatial_scale - offset;
- T roi_center_h = current_roi[2] * spatial_scale - offset;
- T roi_width = current_roi[3] * spatial_scale;
- T roi_height = current_roi[4] * spatial_scale;
- T theta = current_roi[5] * M_PI / 180.0;
- T cos_theta = cos(theta);
- T sin_theta = sin(theta);
-
- AT_ASSERTM(
- roi_width >= 0 && roi_height >= 0,
- "ROIs in ROIAlignRotated do not have non-negative size!");
-
- T bin_size_h = static_cast(roi_height) / static_cast(pooled_height);
- T bin_size_w = static_cast(roi_width) / static_cast(pooled_width);
-
- // We use roi_bin_grid to sample the grid and mimic integral
- int roi_bin_grid_h = (sampling_ratio > 0)
- ? sampling_ratio
- : ceil(roi_height / pooled_height); // e.g., = 2
- int roi_bin_grid_w =
- (sampling_ratio > 0) ? sampling_ratio : ceil(roi_width / pooled_width);
-
- // We do average (integral) pooling inside a bin
- const T count = std::max(roi_bin_grid_h * roi_bin_grid_w, 1); // e.g. = 4
-
- // we want to precalculate indices and weights shared by all channels,
- // this is the key point of optimization
- std::vector> pre_calc(
- roi_bin_grid_h * roi_bin_grid_w * pooled_width * pooled_height);
-
- // roi_start_h and roi_start_w are computed wrt the center of RoI (x, y).
- // Appropriate translation needs to be applied after.
- T roi_start_h = -roi_height / 2.0;
- T roi_start_w = -roi_width / 2.0;
-
- pre_calc_for_bilinear_interpolate(
- height,
- width,
- pooled_height,
- pooled_width,
- roi_bin_grid_h,
- roi_bin_grid_w,
- roi_start_h,
- roi_start_w,
- bin_size_h,
- bin_size_w,
- roi_bin_grid_h,
- roi_bin_grid_w,
- roi_center_h,
- roi_center_w,
- cos_theta,
- sin_theta,
- pre_calc);
-
- for (int c = 0; c < channels; c++) {
- int index_n_c = index_n + c * pooled_width * pooled_height;
- const T* offset_input =
- input + (roi_batch_ind * channels + c) * height * width;
- int pre_calc_index = 0;
-
- for (int ph = 0; ph < pooled_height; ph++) {
- for (int pw = 0; pw < pooled_width; pw++) {
- int index = index_n_c + ph * pooled_width + pw;
-
- T output_val = 0.;
- for (int iy = 0; iy < roi_bin_grid_h; iy++) {
- for (int ix = 0; ix < roi_bin_grid_w; ix++) {
- PreCalc pc = pre_calc[pre_calc_index];
- output_val += pc.w1 * offset_input[pc.pos1] +
- pc.w2 * offset_input[pc.pos2] +
- pc.w3 * offset_input[pc.pos3] + pc.w4 * offset_input[pc.pos4];
-
- pre_calc_index += 1;
- }
- }
- output_val /= count;
-
- output[index] = output_val;
- } // for pw
- } // for ph
- } // for c
- } // for n
-}
-
-template
-void ROIAlignRotatedBackward(
- const int nthreads,
- // may not be contiguous. should index using n_stride, etc
- const T* grad_output,
- const T& spatial_scale,
- const int channels,
- const int height,
- const int width,
- const int pooled_height,
- const int pooled_width,
- const int sampling_ratio,
- T* grad_input,
- const T* rois,
- const int n_stride,
- const int c_stride,
- const int h_stride,
- const int w_stride) {
- for (int index = 0; index < nthreads; index++) {
- // (n, c, ph, pw) is an element in the pooled output
- int pw = index % pooled_width;
- int ph = (index / pooled_width) % pooled_height;
- int c = (index / pooled_width / pooled_height) % channels;
- int n = index / pooled_width / pooled_height / channels;
-
- const T* current_roi = rois + n * 6;
- int roi_batch_ind = current_roi[0];
-
- // Do not use rounding; this implementation detail is critical
- // ROIAlignRotated supports align == true, i.e., continuous coordinate
- // by default, thus the 0.5 offset
- T offset = (T)0.5;
- T roi_center_w = current_roi[1] * spatial_scale - offset;
- T roi_center_h = current_roi[2] * spatial_scale - offset;
- T roi_width = current_roi[3] * spatial_scale;
- T roi_height = current_roi[4] * spatial_scale;
- T theta = current_roi[5] * M_PI / 180.0;
- T cos_theta = cos(theta);
- T sin_theta = sin(theta);
-
- AT_ASSERTM(
- roi_width >= 0 && roi_height >= 0,
- "ROIs in ROIAlignRotated do not have non-negative size!");
-
- T bin_size_h = static_cast(roi_height) / static_cast(pooled_height);
- T bin_size_w = static_cast(roi_width) / static_cast(pooled_width);
-
- T* offset_grad_input =
- grad_input + ((roi_batch_ind * channels + c) * height * width);
-
- int output_offset = n * n_stride + c * c_stride;
- const T* offset_grad_output = grad_output + output_offset;
- const T grad_output_this_bin =
- offset_grad_output[ph * h_stride + pw * w_stride];
-
- // We use roi_bin_grid to sample the grid and mimic integral
- int roi_bin_grid_h = (sampling_ratio > 0)
- ? sampling_ratio
- : ceil(roi_height / pooled_height); // e.g., = 2
- int roi_bin_grid_w =
- (sampling_ratio > 0) ? sampling_ratio : ceil(roi_width / pooled_width);
-
- // roi_start_h and roi_start_w are computed wrt the center of RoI (x, y).
- // Appropriate translation needs to be applied after.
- T roi_start_h = -roi_height / 2.0;
- T roi_start_w = -roi_width / 2.0;
-
- // We do average (integral) pooling inside a bin
- const T count = roi_bin_grid_h * roi_bin_grid_w; // e.g. = 4
-
- for (int iy = 0; iy < roi_bin_grid_h; iy++) {
- const T yy = roi_start_h + ph * bin_size_h +
- static_cast(iy + .5f) * bin_size_h /
- static_cast(roi_bin_grid_h); // e.g., 0.5, 1.5
- for (int ix = 0; ix < roi_bin_grid_w; ix++) {
- const T xx = roi_start_w + pw * bin_size_w +
- static_cast(ix + .5f) * bin_size_w /
- static_cast(roi_bin_grid_w);
-
- // Rotate by theta around the center and translate
- T y = yy * cos_theta - xx * sin_theta + roi_center_h;
- T x = yy * sin_theta + xx * cos_theta + roi_center_w;
-
- T w1, w2, w3, w4;
- int x_low, x_high, y_low, y_high;
-
- bilinear_interpolate_gradient(
- height, width, y, x, w1, w2, w3, w4, x_low, x_high, y_low, y_high);
-
- T g1 = grad_output_this_bin * w1 / count;
- T g2 = grad_output_this_bin * w2 / count;
- T g3 = grad_output_this_bin * w3 / count;
- T g4 = grad_output_this_bin * w4 / count;
-
- if (x_low >= 0 && x_high >= 0 && y_low >= 0 && y_high >= 0) {
- // atomic add is not needed for now since it is single threaded
- add(offset_grad_input + y_low * width + x_low, static_cast(g1));
- add(offset_grad_input + y_low * width + x_high, static_cast(g2));
- add(offset_grad_input + y_high * width + x_low, static_cast(g3));
- add(offset_grad_input + y_high * width + x_high, static_cast(g4));
- } // if
- } // ix
- } // iy
- } // for
-} // ROIAlignRotatedBackward
-
-at::Tensor ROIAlignRotated_forward_cpu(
- const at::Tensor& input,
- const at::Tensor& rois,
- const float spatial_scale,
- const int pooled_height,
- const int pooled_width,
- const int sampling_ratio) {
- AT_ASSERTM(input.device().is_cpu(), "input must be a CPU tensor");
- AT_ASSERTM(rois.device().is_cpu(), "rois must be a CPU tensor");
-
- at::TensorArg input_t{input, "input", 1}, rois_t{rois, "rois", 2};
-
- at::CheckedFrom c = "ROIAlign_forward_cpu";
- at::checkAllSameType(c, {input_t, rois_t});
-
- auto num_rois = rois.size(0);
- auto channels = input.size(1);
- auto height = input.size(2);
- auto width = input.size(3);
-
- at::Tensor output = at::zeros(
- {num_rois, channels, pooled_height, pooled_width}, input.options());
-
- auto output_size = num_rois * pooled_height * pooled_width * channels;
-
- if (output.numel() == 0) {
- return output;
- }
-
- auto input_ = input.contiguous(), rois_ = rois.contiguous();
- AT_DISPATCH_FLOATING_TYPES_AND_HALF(
- input.scalar_type(), "ROIAlignRotated_forward", [&] {
- ROIAlignRotatedForward(
- output_size,
- input_.data_ptr(),
- spatial_scale,
- channels,
- height,
- width,
- pooled_height,
- pooled_width,
- sampling_ratio,
- rois_.data_ptr(),
- output.data_ptr());
- });
- return output;
-}
-
-at::Tensor ROIAlignRotated_backward_cpu(
- const at::Tensor& grad,
- const at::Tensor& rois,
- const float spatial_scale,
- const int pooled_height,
- const int pooled_width,
- const int batch_size,
- const int channels,
- const int height,
- const int width,
- const int sampling_ratio) {
- AT_ASSERTM(grad.device().is_cpu(), "grad must be a CPU tensor");
- AT_ASSERTM(rois.device().is_cpu(), "rois must be a CPU tensor");
-
- at::TensorArg grad_t{grad, "grad", 1}, rois_t{rois, "rois", 2};
-
- at::CheckedFrom c = "ROIAlignRotated_backward_cpu";
- at::checkAllSameType(c, {grad_t, rois_t});
-
- at::Tensor grad_input =
- at::zeros({batch_size, channels, height, width}, grad.options());
-
- // handle possibly empty gradients
- if (grad.numel() == 0) {
- return grad_input;
- }
-
- // get stride values to ensure indexing into gradients is correct.
- int n_stride = grad.stride(0);
- int c_stride = grad.stride(1);
- int h_stride = grad.stride(2);
- int w_stride = grad.stride(3);
-
- auto rois_ = rois.contiguous();
- AT_DISPATCH_FLOATING_TYPES_AND_HALF(
- grad.scalar_type(), "ROIAlignRotated_forward", [&] {
- ROIAlignRotatedBackward(
- grad.numel(),
- grad.data_ptr(),
- spatial_scale,
- channels,
- height,
- width,
- pooled_height,
- pooled_width,
- sampling_ratio,
- grad_input.data_ptr(),
- rois_.data_ptr(),
- n_stride,
- c_stride,
- h_stride,
- w_stride);
- });
- return grad_input;
-}
-
-} // namespace detectron2
diff --git a/spaces/Benson/text-generation/Examples/Arena Of Global Value Apk.md b/spaces/Benson/text-generation/Examples/Arena Of Global Value Apk.md
deleted file mode 100644
index 66e408882db7c943e79aa7db74cc2c51741b3ea0..0000000000000000000000000000000000000000
--- a/spaces/Benson/text-generation/Examples/Arena Of Global Value Apk.md
+++ /dev/null
@@ -1,80 +0,0 @@
-
-
-
-
-
Arena de Valor Global APK: Cómo descargar y jugar el último 5v5 MOBA en su dispositivo Android
-
¿Eres un fan de los juegos multijugador de arena de batalla en línea (MOBA)? ¿Quieres experimentar una épica nueva MOBA 5v5 en tu dispositivo Android? Si es así, entonces definitivamente deberías echar un vistazo a Arena of Valor, traído a ti por Level Infinite y TiMi Studio Group. En este artículo, le diremos todo lo que necesita saber sobre Arena of Valor Global APK, cómo descargarlo e instalarlo en su dispositivo Android, cómo jugarlo, y cómo mejorar su experiencia de juego con él. ¡Vamos a empezar!
Arena of Valor es un juego MOBA gratuito que se lanzó por primera vez en China en 2015 bajo el nombre de Honor of Kings. Más tarde se lanzó a nivel mundial en 2017 bajo el nombre de Arena of Valor. Es uno de los juegos MOBA más populares y exitosos del mundo, con más de 200 millones de jugadores registrados y más de 80 millones de usuarios activos diarios a partir de 2020. También ha ganado varios premios, como el Premio al Mejor Juego Competitivo de Google Play en 2017 y el Premio al Mejor Juego Competitivo de Google Play en 2018.
-
Las características y los beneficios de jugar Arena of Valor
Los diferentes modos y mapas en Arena of Valor
-
Arena of Valor ofrece varios modos de juego para que los jugadores disfruten, cada uno con sus propias reglas, objetivos y desafíos. Estos son algunos de los modos de juego que puedes probar en Arena of Valor:
-
-
Gran batalla: Este es el modo clásico 5v5, donde dos equipos de cinco jugadores compiten en el mapa del campo de batalla de Antaris, que tiene tres carriles, una selva y un río. El objetivo es destruir el núcleo del enemigo, mientras que la defensa de los suyos. En el camino, también puedes asegurar objetivos como el Dragón Abisal y el Cazador Oscuro, que otorgan potenciadores y ventajas a tu equipo. Este modo también se utiliza para los partidos clasificados, donde se puede subir la escalera y ganar recompensas.
-
-
Escaramuza del valle: Este es un modo 3v3 donde dos equipos luchan en un mapa más pequeño, con un carril y una selva. El objetivo es destruir el núcleo del enemigo, mientras se asegura el potenciador de velocidad y el potenciador tirano en la selva. Este modo es rápido y lleno de acción, perfecto para un partido rápido.
-
Solo Battle: Este es un modo 1v1 donde dos jugadores se enfrentan en un mapa pequeño, con un carril y dos pinceles. No hay opción de recordar, y solo se puede curar recogiendo el paquete de salud en el centro del mapa. El objetivo es destruir la torre y el núcleo del enemigo, mientras los supera en duelos.
-
Death Match: Este es un modo especial que se puede jugar en 2v2, 3v3 o 5v5. Se lleva a cabo en un mapa sin torres, esbirros o selva. Todos los jugadores comienzan con el nivel máximo y los elementos completos. El objetivo es matar a todos los enemigos. Una vez que mueres, no reapareces hasta la siguiente ronda. El primer equipo en ganar tres rondas gana el partido.
-
Hook Wars: Este es otro modo especial que solo se puede jugar los fines de semana. Es un modo 5v5 donde dos equipos luchan en un mapa cuadrado, separados por una brecha. Cada jugador obtiene un héroe al azar al principio, y puede redirigir una vez gratis. El objetivo es enganchar y tirar de los enemigos en su lado del mapa, donde serán asesinados al instante por su torre. También puedes usar tus habilidades y objetos para dañar e interrumpir a los enemigos. El primer equipo en anotar 15 puntos gana el partido.
-
-
Los diferentes héroes y roles en la arena del valor
-
Arena of Valor tiene más de 90 héroes para elegir, cada uno con sus propias habilidades y estilos de juego únicos. Puedes desbloquear héroes usando oro o vales, o completando ciertas misiones o eventos. También puedes probar héroes gratis en el modo de práctica o en el modo de prueba del héroe.
-
-
-
Assassin: Estos son héroes que se especializan en infligir daño de ráfaga alta y matar enemigos rápidamente. Por lo general, tienen alta movilidad y habilidades de sigilo, pero baja defensa y salud. Son los más adecuados para deambular por el mapa, matar monstruos de la selva y cazar enemigos que están fuera de posición o con poca salud. Algunos ejemplos de asesinos son Batman, Butterfly, Murad, Quillen, Raz, Sinestrea, Wukong y Zill.
-
Mago: Estos son héroes que usan habilidades mágicas para infligir daño en áreas altas y controlar a los enemigos con efectos de control de multitudes como aturdimientos, ralentizaciones, silencios, etc. Por lo general, tienen un alto rendimiento y rango de daño, pero baja defensa y movilidad. Son los más adecuados para laning en el carril medio, donde pueden cultivar oro y experimentar rápidamente y ayudar a sus compañeros de equipo con sus hechizos. Algunos ejemplos de magos son Aleister, Azzen'Ka, D'Arcy, Diaochan, Iggy, Ignis, Ilumia, Ishar, Jinnar, Kahlii, Lauriel, Liliana, Lorion, Marja, Mganga, Natalya, Pre [asistente](continuar)
Los diferentes héroes y los papeles en Arena
Arena of Valor tiene más de 90 héroes para elegir, cada uno con sus propias habilidades y estilos de juego únicos. Puedes desbloquear héroes usando oro o vales, o completando ciertas misiones o eventos. También puedes probar héroes gratis en el modo de práctica o en el modo de prueba del héroe.
-
Los héroes se clasifican en seis roles: asesino, mago, tirador, apoyo, tanque y guerrero. Cada rol tiene sus propias fortalezas y debilidades, y contribuye de manera diferente al equipo. Aquí están algunos de los roles y sus funciones:
-
-
-
-
Mago: Estos son héroes que usan habilidades mágicas para infligir daño en áreas altas y controlar a los enemigos con efectos de control de multitudes como aturdimientos, ralentizaciones, silencios, etc. Por lo general, tienen un alto rendimiento y rango de daño, pero baja defensa y movilidad. Son los más adecuados para laning en el carril medio, donde pueden cultivar oro y experimentar rápidamente y ayudar a sus compañeros de equipo con sus hechizos. Some examples of mages are Aleister, Azzen'Ka, D'Arcy, Diaochan, Iggy, Ignis, Ilumia, Ishar, Jinnar, Kahlii, Lauriel, Liliana, Lorion, Marja, Mganga, Natalya, Preyta, Raziel, Sephera, Tulen, Veera, Violeta, Vol'Kath, Yena, and Yorn.
-
Tirador: Estos son héroes que usan ataques físicos a distancia para infligir daño alto y destruir objetivos. Por lo general, tienen alta velocidad de ataque y tasa crítica, pero baja defensa y salud. Son los más adecuados para lanear en el carril inferior, donde pueden cultivar oro y experimentar de forma segura y empujar torres con sus compañeros de equipo. Algunos ejemplos de tiradores son Brunhilda, Capheny, Elsu, Fennik, Hayate, Joker, Kriknak, Laville, Lindis, Moren, Slimz, Tel'Annas, Valhein, Violet, Wisp y Yorn.
-
Apoyo: Estos son héroes que utilizan varias habilidades para proteger y ayudar a sus aliados. Por lo general, tienen una alta defensa y salud, pero baja producción de daños. Son más adecuados para laning en el carril inferior con un tirador o vagando por el mapa con un asesino. Some examples of supports are Alice, Annette, Arum, Baldum, Chaugnar, Cresht, Gildur Krizzix Lumburr Min'a Omega Ormarr Peura Rouie Teemee Thane Toro Xeniel and Zip.
-
-
Guerrero: Estos son héroes que usan una mezcla de ataques físicos y habilidades para infligir daño moderado y sobrevivir peleas. Por lo general, tienen estadísticas equilibradas y pueden adaptarse a diferentes situaciones. Ellos son los más adecuados para laning en el carril superior donde pueden duelo enemigos y dividir torres de empuje. Algunos ejemplos de guerreros son Airi Amily Astrid Ata Errol Florentino Jinnar Kil'Groth Lu Bu Maloch Max Omen Qi Riktor Rourke Ryoma Skud Superman Taara Veres Wonder Woman Wukong Iel Yena Zanis y Zephys.
-
-
¿Cómo mejorar tu experiencia de juego con Arena of Valor?
-
Los mejores ajustes y personalizaciones para Arena of Valor
-
Arena of Valor le permite personalizar Arena of Valor le permite personalizar sus ajustes y preferencias de juego para satisfacer sus necesidades y preferencias. Estos son algunos de los ajustes y personalizaciones que puedes ajustar en Arena of Valor:
-
-
Graphics: Puede ajustar la calidad de los gráficos, la velocidad de fotogramas, el brillo y la resolución del juego para optimizar el rendimiento y la duración de la batería del dispositivo. También puede activar o desactivar funciones como sombras, anti-aliasing y modo de alta definición.
-
Sonido: Puede ajustar el volumen, el silencio y el idioma de los efectos de sonido, la música y las voces en off del juego. También puede elegir entre diferentes temas de sonido y locutores para el juego.
-
Controles: Puedes elegir entre diferentes esquemas de control para el juego, como joystick, touch o custom. También puede personalizar el tamaño, la posición y la transparencia de los botones e iconos en la pantalla. También puede habilitar o deshabilitar funciones como puntería automática, compra automática, actualización automática, chat rápido, lanzamiento rápido y ping inteligente.
-
-
Cuenta: Puede administrar la información de su cuenta, como su nombre de usuario, avatar, firma, región, servidor, lista de amigos, gremio, logros, estadísticas y configuraciones. También puedes vincular tu cuenta a otras plataformas como Facebook, Google Play Games, Game Center o VK.
-
-
Las mejores estrategias y tácticas para ganar en Arena of Valor
-
Arena of Valor es un juego que requiere trabajo en equipo, coordinación, comunicación y estrategia para ganar. Estas son algunas de las mejores estrategias y tácticas para ganar en Arena of Valor:
-
-
Elige una composición de equipo equilibrada: Una buena composición de equipo debe tener una mezcla de diferentes roles y héroes que complementen las fortalezas de cada uno y cubran las debilidades de cada uno. Por ejemplo, una composición típica de equipo podría tener un tanque o un guerrero en el carril superior, un mago o un asesino en el carril medio, un tirador y un apoyo en el carril inferior, y un asesino o un guerrero en la selva. Trata de evitar elegir héroes que son demasiado similares o demasiado débiles contra el equipo enemigo.
-
Comunícate con tus compañeros: La comunicación es clave para ganar en Arena of Valor. Debes usar el chat o el chat de voz para comunicarte con tus compañeros de equipo sobre tus planes, tus acciones, los movimientos de tus enemigos, tus objetivos, tus peticiones, tus advertencias y tus alabanzas. También debe utilizar el chat rápido o el ping inteligente para transmitir mensajes simples como "Ataque", "Retiro", "Reunir", "Falta", "Peligro", "Ayuda", etc.
-
-
Conoce a tus enemigos: Conocer a tus enemigos es la mitad de la batalla. Debes aprender sobre las habilidades, fortalezas, debilidades y tendencias de sus héroes. También debe prestar atención a sus artículos, niveles, oro, muertes, muertes, asistencias y objetivos. Debes usar esta información para planificar tus estrategias, contrarrestar sus movimientos, explotar sus errores y evitar sus trampas.
-
Administra tus recursos: Los recursos son esenciales para ganar en Arena of Valor. Debes administrar tus recursos con sabiduría y eficiencia. Los recursos incluyen oro, experiencia, salud, maná, reutilizaciones, objetos, potenciadores y objetivos. Debes usar tus recursos para obtener ventajas sobre tus enemigos y alcanzar tus objetivos. También debes negar los recursos de tus enemigos matándolos, robando sus monstruos de la selva, destruyendo sus torres y asegurando sus objetivos.
-
Adaptarse a la situación: Arena of Valor es un juego dinámico e impredecible. Debes adaptarte a la situación y ser flexible con tus estrategias y tácticas. Usted debe ser consciente de los cambios en el estado del juego, tales como el tiempo, la puntuación, el mapa, los héroes, los objetos, los potenciadores, y los objetivos. También debes ser consciente de las oportunidades y amenazas que surgen en el juego, tales como peleas en equipo, ganchos, emboscadas, empujones divididos, puertas traseras, etc. Debes ajustar tus acciones y decisiones en consecuencia para maximizar tus posibilidades de ganar.
-
-
Los mejores recursos y comunidades para aprender y mejorar en la arena del valor
-
Arena of Valor es un juego que requiere constante aprendizaje y mejora. Siempre debes buscar mejorar tus conocimientos y habilidades en el juego. Estos son algunos de los mejores recursos y comunidades para aprender y mejorar en Arena of Valor:
-
-
-
Los tutoriales en el juego y los modos de práctica: Puedes acceder a los tutoriales en el juego y los modos de práctica tocando el botón "Aprender" en el menú principal. Puedes aprender sobre los fundamentos del juego, como los controles, la interfaz, los roles, los héroes, los objetos, las habilidades, los objetivos y más. También puedes practicar tus habilidades y probar a tus héroes en diferentes modos, como el modo de prueba del héroe, el modo de práctica, el modo personalizado y el modo casual.
Arena of Valor es un divertido y emocionante juego 5v5 MOBA que puedes jugar en tu dispositivo Android. Tiene gráficos increíbles, sonido y jugabilidad, así como una gran variedad de héroes, modos y mapas. Es fácil de descargar e instalar, y se puede personalizar a su gusto. También es una gran manera de aprender y mejorar tus habilidades, así como para conectar y competir con otros jugadores de todo el mundo. Si estás buscando un nuevo juego MOBA para probar, definitivamente deberías darle una oportunidad a Arena of Valor. ¡No te arrepentirás!
-
Preguntas frecuentes
-
Q: ¿Es Arena of Valor libre para jugar?
-
A: Sí, Arena of Valor es gratis para jugar. Puedes descargarlo e instalarlo desde Google Play Store o QooApp Game Store sin pagar nada. También puedes jugar todos los modos y héroes sin gastar dinero. Sin embargo, también puedes comprar algunos artículos opcionales como pieles, arcanos, vales y cofres con dinero real si quieres apoyar el juego o mejorar tu apariencia.
-
Q: ¿Arena of Valor es compatible con mi dispositivo?
-
A: Arena of Valor es compatible con la mayoría de los dispositivos Android que tienen Android 4.0.3 o superior y al menos 1 GB de RAM. Sin embargo, algunos dispositivos pueden tener problemas de rendimiento o problemas de compatibilidad dependiendo de sus especificaciones y configuraciones. Puede comprobar la compatibilidad de su dispositivo visitando https:/www.arenaof.com/support//a>.
-
Q: ¿Cómo puedo actualizar Arena of Valor?
-
A: Arena of Valor se actualiza constantemente con nuevas características, contenido, correcciones y mejoras. Puedes actualizar Arena of Valor visitando Google Play Store o QooApp Game Store y pulsando el botón "Actualizar". También puede habilitar la opción de actualización automática en la configuración de su dispositivo para actualizar Arena of Valor automáticamente cada vez que haya una nueva versión disponible.
-
Q: ¿Cómo puedo reportar un error o un problema en Arena of Valor?
-
-
Q: ¿Cómo puedo mejorar en Arena of Valor?
-
A: La mejor manera de mejorar en Arena of Valor es practicar regularmente y aprender de tus errores. También puede ver guías y videos en línea, leer foros y comunidades en línea, unirse a torneos y eventos en línea, y pedir consejo a otros jugadores y expertos. También deberías probar diferentes héroes, roles, modos y estrategias para encontrar lo que más te convenga.
64aa2da5cf
-
-
\ No newline at end of file
diff --git a/spaces/Benson/text-generation/Examples/Camino De Los Titanes Mac Descargar.md b/spaces/Benson/text-generation/Examples/Camino De Los Titanes Mac Descargar.md
deleted file mode 100644
index b177d80ac576e2457034f67ae19feca1c8c6c7cd..0000000000000000000000000000000000000000
--- a/spaces/Benson/text-generation/Examples/Camino De Los Titanes Mac Descargar.md
+++ /dev/null
@@ -1,96 +0,0 @@
-
-
El camino de los titanes: un MMO de supervivencia de dinosaurios para Mac
-
¿Alguna vez has soñado con vivir como un dinosaurio en un mundo prehistórico? ¿Quieres explorar, cazar, luchar y crecer con otros jugadores en línea? Si respondiste afirmativamente a cualquiera de estas preguntas, quizás quieras echar un vistazo a Path of Titans, un juego de supervivencia de dinosaurios MMO disponible para Mac y otras plataformas.
-
Path of Titans es un juego desarrollado y publicado por Alderon Games, un estudio independiente con sede en Australia. Actualmente está en desarrollo activo, con actualizaciones regulares y nuevos contenidos. En este juego, puedes elegir entre más de 30 especies de dinosaurios diferentes, cada una con sus propias habilidades, habilidades y apariencia. Puedes personalizar tu dinosaurio con cientos de pieles, marcas y colores, y verlo crecer desde una cría hasta un adulto mientras completas misiones y desafíos. También puedes unirte a fiestas y gremios con otros jugadores, o ir solo y labrar tu propio camino en un enorme mundo abierto lleno de criaturas de IA, eventos naturales y peligros ambientales.
Si usted es un usuario de Mac, es posible que se pregunte cómo descargar y jugar Path of Titans en su dispositivo. En este artículo, te mostraremos cómo hacerlo, además de darte una visión general de las características del juego, los requisitos del sistema, las revisiones y las alternativas. Así que, sin más preámbulos, ¡empecemos!
-
Cómo descargar Path of Titans en Mac
-
Descargar Path of Titans en Mac es fácil y sencillo. Solo sigue estos sencillos pasos:
-
-
Visite el sitio web oficial de Path of Titans en https://pathoftitans.com/ y compre el juego. Puedes elegir entre diferentes paquetes que ofrecen diferentes ventajas y recompensas, como pieles, moneda del juego, banda sonora, etc. El paquete más barato cuesta $20 USD.
-
-
Descargue el lanzador de Alderon Games para Mac desde el enlace proporcionado en el correo electrónico. El tamaño del archivo es de aproximadamente 100 MB.
-
Abra el archivo descargado y siga las instrucciones para instalar el lanzador en su Mac.
-
Inicie el lanzador de Alderon Games e inicie sesión con sus credenciales de cuenta.
-
Seleccione Path of Titans de la lista de juegos y haga clic en el botón Instalar. El tamaño del juego es de aproximadamente 4 GB.
-
-
Espere a que termine la instalación y luego haga clic en el botón Play para iniciar el juego.
-
-
Felicidades, has descargado e instalado correctamente Path of Titans en tu Mac. Ahora puedes disfrutar del juego y sumergirte en el mundo de los dinosaurios.
-
Características del juego Path of Titans
-
Path of Titans no es solo otro juego de dinosaurios. Es un juego que ofrece muchas características y contenido que lo hacen destacar entre la multitud. Estas son algunas de las principales características que puedes esperar de Path of Titans:
-
Multijugador masivo con juego multiplataforma
-
Uno de los aspectos más atractivos de Path of Titans es que es un juego multijugador masivo en línea, lo que significa que puedes jugar con miles de otros jugadores de todo el mundo. Puede unirse a servidores que alojan hasta 200 jugadores a la vez e interactuar con ellos a través de chat, voz y emotes. También puedes formar fiestas y gremios con tus amigos u otros jugadores, y cooperar o competir con ellos en diversas actividades. Además, Path of Titans admite el juego multiplataforma, lo que significa que puedes jugar con jugadores que utilizan diferentes dispositivos, como PC, Mac, Linux, iOS, Android e incluso consolas. Esto hace que el juego sea más accesible y diverso.
-
Personalización y crecimiento de dinosaurios
-
-
Combate y habilidades
-
Como un juego de supervivencia de dinosaurios, Path of Titans implica mucho combate y acción. Tendrás que buscar comida, defenderte de los depredadores, luchar por el territorio y competir por los recursos. También tendrá que lidiar con eventos naturales, como tormentas, incendios, inundaciones, terremotos y erupciones volcánicas. Para sobrevivir en este duro entorno, tendrás que usar sabiamente las habilidades de tu dinosaurio. Cada dinosaurio tiene su propio conjunto de habilidades que se pueden activar presionando ciertas teclas o botones. Estas habilidades incluyen morder, arañar, rugir, pisar fuerte, azotar la cola, cargar, esquivar, saltar, agacharse, descansar, dormir, beber, comer, etc. Algunas habilidades son más efectivas que otras dependiendo de la situación y el oponente. Tendrás que aprender a usarlas estratégica y tácticamente.
-
Misiones y logros
-
Para mantenerte comprometido y motivado en el juego, Path of Titans ofrece una variedad de misiones y logros que puedes completar y ganar recompensas. Las misiones son tareas que puedes aceptar de NPCs u otros jugadores que requieren que hagas algo específico en el mundo del juego. Por ejemplo, es posible que te pidan que caces cierto tipo de animal, explores cierta área, recojas cierto objeto, etc. Completar misiones te dará puntos de experiencia, moneda del juego y otras recompensas. Los logros son hitos que puedes alcanzar haciendo algo notable o desafiante en el mundo del juego. Por ejemplo, puedes conseguir un logro por sobrevivir durante cierto tiempo, matar a un cierto número de enemigos, alcanzar un cierto nivel, etc. Logros te darán derechos de fanfarronear, así como artículos cosméticos, como pieles, sombreros, accesorios, etc.
-
Herramientas de modificación y soporte comunitario
-
-
Requisitos del sistema de Path of Titans para Mac
-
Antes de descargar y jugar Path of Titans en tu Mac, debes asegurarte de que tu dispositivo cumple con los requisitos mínimos o recomendados del sistema para el juego. Estos son los requisitos del sistema para Path of Titans para Mac:
-
-
-
Requisitos mínimos
-
Requisitos recomendados
-
-
-
OS: Mac OS X 10.9 o superior
-
OS: Mac OS X 10.13 o superior
-
-
-
CPU: Intel Core i5-2400 o equivalente
-
CPU: Intel Core i7-4770 o equivalente
-
-
-
RAM: 8 GB
-
RAM: 16 GB
-
-
-
GPU: NVIDIA GeForce GTX 660 o equivalente
-
GPU: NVIDIA GeForce GTX 1060 o equivalente
-
-
-
Almacenamiento: 10 GB de espacio disponible
-
Almacenamiento: 20 GB de espacio disponible
-
-
-
Red: Conexión a Internet de banda ancha
-
Red: Conexión a Internet de banda ancha
-
-
-
Si tu Mac cumple con estos requisitos, deberías poder ejecutar Path of Titans sin problemas y de forma agradable. Sin embargo, si tu Mac no cumple con estos requisitos, es posible que experimentes retrasos, fallos, fallos u otros problemas que podrían afectar tu experiencia de juego. En ese caso, es posible que desee actualizar su dispositivo o intentar jugar Path of Titans en otra plataforma.
-
Camino de los Titanes Comentarios y Alternativas
-
Si todavía no está convencido de que Path of Titans es un juego que vale la pena jugar en su Mac, es posible que desee leer algunos comentarios de otros jugadores y críticos que han probado el juego. Estos son algunos de los comentarios que hemos encontrado en línea:
"He estado jugando Path of Titans durante más de 100 horas, y tengo que decir que me encanta este juego. Es el mejor juego de dinosaurios que he jugado, y he jugado un montón de ellos. El juego es muy inmersivo y realista, y te hace sentir como si estuvieras viviendo como un dinosaurio. Los gráficos son impresionantes, los efectos de sonido son increíbles, las animaciones son suaves, y el juego es divertido y desafiante. El juego tiene mucha variedad y valor de repetición, ya que puedes jugar como diferentes dinosaurios, personalizarlos, subir de nivel, hacer misiones, unirte a gremios, etc. El juego también tiene una gran comunidad y soporte para desarrolladores, ya que son muy amigables, útiles y activos. El juego no es perfecto, por supuesto, ya que todavía tiene algunos errores, fallos, problemas de equilibrio y características que faltan. Pero el juego se actualiza y mejora constantemente, y tengo fe en que los desarrolladores harán que este juego sea aún mejor en el futuro. Recomiendo este juego a cualquiera que ame los dinosaurios y los juegos de supervivencia."
Car Simulator 2 Mod APK es una versión modificada del juego original de Car Simulator 2, que es un juego de simulación desarrollado por Oppana Games. En este juego, puedes entrar en un vasto mundo con muchas tareas que puedes realizar, como conducir, correr, estacionar, derrapar, afinar y más. También puedes elegir entre diferentes modos de juego, como en solitario, multijugador o online. Puede invitar a sus amigos a unirse a usted en el juego y jugar juntos.
-
La versión modificada del juego viene con algunas características adicionales que hacen el juego más agradable y más fácil. Por ejemplo, puede obtener dinero y oro ilimitados, que puede usar para comprar autos nuevos o actualizar los existentes. También puede desbloquear todos los coches en el juego, que incluyen coches deportivos, SUV, camiones y más. Puede personalizar sus coches con diferentes colores, ruedas, spoilers y otros accesorios. También puedes acceder a todas las ubicaciones del juego, como la ciudad, el aeropuerto, el desierto y más.
-
Características del simulador de coche 2 Mod APK
-
Un mundo realista de carreras
-
-
Diferentes modos de juego
-
Otra característica de Car Simulator 2 Mod APK es que ofrece diferentes modos de juego que se adapten a sus preferencias y habilidades. Puedes jugar en solitario si quieres disfrutar del juego por ti mismo o practicar tus habilidades de conducción. Puedes jugar al modo multijugador si quieres invitar a tus amigos a unirse a ti en el juego y divertirse juntos. También puedes jugar al modo online si quieres competir con otros jugadores de todo el mundo y posicionarte en la clasificación.
-
Personalizar coches
-
Una tercera característica de Car Simulator 2 Mod APK es que le permite personalizar sus coches de acuerdo a su gusto y estilo. Usted puede elegir entre una variedad de coches en el juego, tales como coches deportivos, SUV, camiones, y más. También puede desbloquear todos los coches en el juego con dinero ilimitado y oro. Puedes cambiar el color de tu coche o añadir diferentes accesorios, como ruedas, spoilers, pegatinas, etc. También puedes mejorar el rendimiento de tu coche mejorando su motor, frenos, suspensión, etc.
-
-
Juego multijugador
-
Una cuarta característica de Car Simulator
Una cuarta característica de Car Simulator 2 Mod APK es que es un juego multijugador que le permite jugar con sus amigos u otros jugadores en línea. Puede crear su propia habitación e invitar a sus amigos a unirse a usted en el juego. También puede unirse a otras habitaciones y conocer gente nueva. Puede chatear con otros jugadores y comunicarse con ellos mediante mensajes de voz o de texto. También puedes retar a otros jugadores a carreras o duelos y mostrar tus habilidades de conducción.
-
¿Cómo descargar e instalar Car Simulator 2 Mod APK?
-
Si desea descargar e instalar Car Simulator 2 Mod APK, es necesario seguir estos sencillos pasos:
-
-
Primero, debe habilitar la instalación de aplicaciones de fuentes desconocidas en su dispositivo. Para hacer esto, ve a la configuración del dispositivo, luego a la seguridad, luego a fuentes desconocidas y enciéndelo.
-
-
En tercer lugar, es necesario localizar el archivo descargado en el dispositivo y toque en él para iniciar el proceso de instalación. Siga las instrucciones de la pantalla y espere a que termine la instalación.
-
Cuarto, es necesario iniciar el juego y disfrutar de jugar con dinero ilimitado, oro, y todos los coches desbloqueados.
-
-
Nota: Es posible que tenga que desinstalar el juego original de Car Simulator 2 antes de instalar la versión modificada.
-
Pros y contras de Car Simulator 2 Mod APK
-
Pros
-
Algunos de los pros de Car Simulator 2 Mod APK son:
-
-
Es un juego gratuito que no requiere ningún registro o suscripción.
-
Ofrece dinero ilimitado y oro que se puede utilizar para comprar coches nuevos o actualizar los existentes.
-
Desbloquea todos los coches en el juego, que incluyen coches deportivos, SUV, camiones y más.
-
Le permite personalizar sus coches con diferentes colores, ruedas, alerones y otros accesorios.
-
Tiene gráficos realistas y efectos de sonido que te hacen sentir como si estuvieras en un coche real.
-
Tiene diferentes modos de juego que se adapten a sus preferencias y habilidades.
-
Es un juego multijugador que te permite jugar con tus amigos u otros jugadores online.
-
-
Contras
-
Algunos de los contras de Car Simulator 2 Mod APK son:
-
-
Puede no ser compatible con algunos dispositivos o sistemas operativos.
-
Puede tener algunos errores o fallos que afectan el juego o el rendimiento.
-
Puede que no se actualice regularmente o no tenga nuevas características.
-
Puede violar los términos y condiciones del juego original o la tienda de aplicaciones.
-
-
Consejos y trucos para jugar Car Simulator 2 Mod APK
-
Elegir el coche adecuado para cada modo
-
-
Utilice el mapa y el GPS para navegar
-
Otro consejo para jugar Car Simulator 2 Mod APK es utilizar el mapa y el GPS para navegar. El juego tiene un mundo grande con muchos lugares que puedes explorar, como la ciudad, el aeropuerto, el desierto y más. Puedes usar el mapa para ver dónde estás y dónde quieres ir. También puedes usar el GPS para obtener direcciones y encontrar tu destino. El GPS le mostrará la mejor ruta y le dirá cuándo girar o detenerse. También puede acercar o alejar el mapa o el GPS para ver más detalles o información general.
-
Gana dinero y oro completando tareas y desafíos
-
Un tercer consejo para jugar Car Simulator 2 Mod APK es ganar dinero y oro completando tareas y desafíos. El juego tiene muchas tareas que puedes realizar, como conducir, correr, aparcar, derrapar, afinar, etc. También puedes encontrar desafíos que ponen a prueba tus habilidades o conocimientos, como preguntas de trivial, puzzles, acertijos, etc. Completando estas tareas y desafíos, Usted puede ganar dinero y oro que se puede utilizar para comprar coches nuevos o actualizar sus existentes. También puedes ganar dinero y oro ganando carreras o duelos contra otros jugadores online.
Mejora tu coche y compra otros nuevos
-
Un cuarto consejo para jugar Car Simulator 2 Mod APK es actualizar su coche y comprar nuevos. El juego tiene un garaje donde puede almacenar sus coches y modificarlos. Puede mejorar el rendimiento de su coche mediante la mejora de su motor, frenos, suspensión, etc. También puede cambiar la apariencia de su coche mediante la adición de diferentes accesorios, tales como ruedas, spoilers, pegatinas, etc. También puede comprar coches nuevos con dinero ilimitado y oro. Usted puede elegir entre una variedad de coches en el juego, tales como coches deportivos, SUV, camiones, y más. También puedes desbloquear todos los coches del juego con la versión modificada.
-
Conclusión
-
-
Preguntas frecuentes
-
Aquí hay algunas preguntas frecuentes sobre Car Simulator 2 Mod APK:
-
-
Q: ¿Es seguro usar Car Simulator 2 Mod APK?
-
A: Car Simulator 2 Mod APK es una versión modificada del juego original que no puede ser autorizado por el desarrollador o la tienda de aplicaciones. Por lo tanto, puede no ser seguro de usar y puede dañar su dispositivo o datos. Solo debe descargar la versión modificada de una fuente confiable y escanearla en busca de virus o malware antes de instalarla.
-
Q: ¿Cómo puedo jugar Car Simulator 2 Mod APK con mis amigos?
-
A: Car Simulator 2 Mod APK es un juego multijugador que le permite jugar con sus amigos u otros jugadores en línea. Puede crear su propia habitación e invitar a sus amigos a unirse a usted en el juego. También puede unirse a otras habitaciones y conocer gente nueva. Puede chatear con otros jugadores y comunicarse con ellos mediante mensajes de voz o de texto. También puedes retar a otros jugadores a carreras o duelos y mostrar tus habilidades de conducción.
-
Q: ¿Cuáles son los mejores coches en Car Simulator 2 Mod APK?
-
A: Car Simulator 2 Mod APK tiene una variedad de coches en el juego, tales como coches deportivos, SUV, camiones, y más. Los mejores coches en el juego dependen de su preferencia y nivel de habilidad. Sin embargo, algunos de los coches más populares en el juego son:
-
-
- Lamborghini Aventador: Un coche deportivo rápido y potente que tiene una velocidad máxima de 350 km/h y una aceleración de 0-100 km/h en 2,9 segundos.
-
- Ford F-150 Raptor: Un camión robusto y duradero que tiene una velocidad máxima de 170 km/h y una aceleración de 0-100 km/h en 5.5 segundos.
-
- Toyota Land Cruiser: Un SUV versátil y fiable que tiene una velocidad máxima de 200 km/h y una aceleración de 0-100 km/h en 8 segundos.
-
-
Q: ¿Cómo puedo obtener más dinero y oro en Car Simulator 2 Mod APK?
-
-
Q: ¿Cómo puedo actualizar Car Simulator 2 Mod APK?
-
A: Car Simulator 2 Mod APK no puede ser actualizado regularmente o tener nuevas características añadidas por el desarrollador o la tienda de aplicaciones. Por lo tanto, es posible que no pueda actualizar la versión modificada del juego de forma automática o manual. Sin embargo, puede buscar actualizaciones de la fuente donde descargó la versión modificada o buscar versiones más nuevas en línea.
- 64aa2da5cf
-
-
\ No newline at end of file
diff --git a/spaces/Big-Web/MMSD/env/Lib/site-packages/pip/_vendor/cachecontrol/wrapper.py b/spaces/Big-Web/MMSD/env/Lib/site-packages/pip/_vendor/cachecontrol/wrapper.py
deleted file mode 100644
index b6ee7f2039801c9792dfe6e473843fb0a4bc4a5b..0000000000000000000000000000000000000000
--- a/spaces/Big-Web/MMSD/env/Lib/site-packages/pip/_vendor/cachecontrol/wrapper.py
+++ /dev/null
@@ -1,33 +0,0 @@
-# SPDX-FileCopyrightText: 2015 Eric Larson
-#
-# SPDX-License-Identifier: Apache-2.0
-
-from .adapter import CacheControlAdapter
-from .cache import DictCache
-
-
-def CacheControl(
- sess,
- cache=None,
- cache_etags=True,
- serializer=None,
- heuristic=None,
- controller_class=None,
- adapter_class=None,
- cacheable_methods=None,
-):
-
- cache = DictCache() if cache is None else cache
- adapter_class = adapter_class or CacheControlAdapter
- adapter = adapter_class(
- cache,
- cache_etags=cache_etags,
- serializer=serializer,
- heuristic=heuristic,
- controller_class=controller_class,
- cacheable_methods=cacheable_methods,
- )
- sess.mount("http://", adapter)
- sess.mount("https://", adapter)
-
- return sess
diff --git a/spaces/Big-Web/MMSD/env/Lib/site-packages/pkg_resources/_vendor/packaging/requirements.py b/spaces/Big-Web/MMSD/env/Lib/site-packages/pkg_resources/_vendor/packaging/requirements.py
deleted file mode 100644
index 6af14ec4ce49e633d030611c26f0bd9beaf13e6a..0000000000000000000000000000000000000000
--- a/spaces/Big-Web/MMSD/env/Lib/site-packages/pkg_resources/_vendor/packaging/requirements.py
+++ /dev/null
@@ -1,146 +0,0 @@
-# This file is dual licensed under the terms of the Apache License, Version
-# 2.0, and the BSD License. See the LICENSE file in the root of this repository
-# for complete details.
-
-import re
-import string
-import urllib.parse
-from typing import List, Optional as TOptional, Set
-
-from pkg_resources.extern.pyparsing import ( # noqa
- Combine,
- Literal as L,
- Optional,
- ParseException,
- Regex,
- Word,
- ZeroOrMore,
- originalTextFor,
- stringEnd,
- stringStart,
-)
-
-from .markers import MARKER_EXPR, Marker
-from .specifiers import LegacySpecifier, Specifier, SpecifierSet
-
-
-class InvalidRequirement(ValueError):
- """
- An invalid requirement was found, users should refer to PEP 508.
- """
-
-
-ALPHANUM = Word(string.ascii_letters + string.digits)
-
-LBRACKET = L("[").suppress()
-RBRACKET = L("]").suppress()
-LPAREN = L("(").suppress()
-RPAREN = L(")").suppress()
-COMMA = L(",").suppress()
-SEMICOLON = L(";").suppress()
-AT = L("@").suppress()
-
-PUNCTUATION = Word("-_.")
-IDENTIFIER_END = ALPHANUM | (ZeroOrMore(PUNCTUATION) + ALPHANUM)
-IDENTIFIER = Combine(ALPHANUM + ZeroOrMore(IDENTIFIER_END))
-
-NAME = IDENTIFIER("name")
-EXTRA = IDENTIFIER
-
-URI = Regex(r"[^ ]+")("url")
-URL = AT + URI
-
-EXTRAS_LIST = EXTRA + ZeroOrMore(COMMA + EXTRA)
-EXTRAS = (LBRACKET + Optional(EXTRAS_LIST) + RBRACKET)("extras")
-
-VERSION_PEP440 = Regex(Specifier._regex_str, re.VERBOSE | re.IGNORECASE)
-VERSION_LEGACY = Regex(LegacySpecifier._regex_str, re.VERBOSE | re.IGNORECASE)
-
-VERSION_ONE = VERSION_PEP440 ^ VERSION_LEGACY
-VERSION_MANY = Combine(
- VERSION_ONE + ZeroOrMore(COMMA + VERSION_ONE), joinString=",", adjacent=False
-)("_raw_spec")
-_VERSION_SPEC = Optional((LPAREN + VERSION_MANY + RPAREN) | VERSION_MANY)
-_VERSION_SPEC.setParseAction(lambda s, l, t: t._raw_spec or "")
-
-VERSION_SPEC = originalTextFor(_VERSION_SPEC)("specifier")
-VERSION_SPEC.setParseAction(lambda s, l, t: t[1])
-
-MARKER_EXPR = originalTextFor(MARKER_EXPR())("marker")
-MARKER_EXPR.setParseAction(
- lambda s, l, t: Marker(s[t._original_start : t._original_end])
-)
-MARKER_SEPARATOR = SEMICOLON
-MARKER = MARKER_SEPARATOR + MARKER_EXPR
-
-VERSION_AND_MARKER = VERSION_SPEC + Optional(MARKER)
-URL_AND_MARKER = URL + Optional(MARKER)
-
-NAMED_REQUIREMENT = NAME + Optional(EXTRAS) + (URL_AND_MARKER | VERSION_AND_MARKER)
-
-REQUIREMENT = stringStart + NAMED_REQUIREMENT + stringEnd
-# pkg_resources.extern.pyparsing isn't thread safe during initialization, so we do it eagerly, see
-# issue #104
-REQUIREMENT.parseString("x[]")
-
-
-class Requirement:
- """Parse a requirement.
-
- Parse a given requirement string into its parts, such as name, specifier,
- URL, and extras. Raises InvalidRequirement on a badly-formed requirement
- string.
- """
-
- # TODO: Can we test whether something is contained within a requirement?
- # If so how do we do that? Do we need to test against the _name_ of
- # the thing as well as the version? What about the markers?
- # TODO: Can we normalize the name and extra name?
-
- def __init__(self, requirement_string: str) -> None:
- try:
- req = REQUIREMENT.parseString(requirement_string)
- except ParseException as e:
- raise InvalidRequirement(
- f'Parse error at "{ requirement_string[e.loc : e.loc + 8]!r}": {e.msg}'
- )
-
- self.name: str = req.name
- if req.url:
- parsed_url = urllib.parse.urlparse(req.url)
- if parsed_url.scheme == "file":
- if urllib.parse.urlunparse(parsed_url) != req.url:
- raise InvalidRequirement("Invalid URL given")
- elif not (parsed_url.scheme and parsed_url.netloc) or (
- not parsed_url.scheme and not parsed_url.netloc
- ):
- raise InvalidRequirement(f"Invalid URL: {req.url}")
- self.url: TOptional[str] = req.url
- else:
- self.url = None
- self.extras: Set[str] = set(req.extras.asList() if req.extras else [])
- self.specifier: SpecifierSet = SpecifierSet(req.specifier)
- self.marker: TOptional[Marker] = req.marker if req.marker else None
-
- def __str__(self) -> str:
- parts: List[str] = [self.name]
-
- if self.extras:
- formatted_extras = ",".join(sorted(self.extras))
- parts.append(f"[{formatted_extras}]")
-
- if self.specifier:
- parts.append(str(self.specifier))
-
- if self.url:
- parts.append(f"@ {self.url}")
- if self.marker:
- parts.append(" ")
-
- if self.marker:
- parts.append(f"; {self.marker}")
-
- return "".join(parts)
-
- def __repr__(self) -> str:
- return f""
diff --git a/spaces/CVPR/Dual-Key_Backdoor_Attacks/openvqa/data/gqa/gqa_feat_preproc.py b/spaces/CVPR/Dual-Key_Backdoor_Attacks/openvqa/data/gqa/gqa_feat_preproc.py
deleted file mode 100644
index c3714f49389c7c16d8c089ba3ed478994a87d816..0000000000000000000000000000000000000000
--- a/spaces/CVPR/Dual-Key_Backdoor_Attacks/openvqa/data/gqa/gqa_feat_preproc.py
+++ /dev/null
@@ -1,126 +0,0 @@
-# --------------------------------------------------------
-# OpenVQA
-# GQA spatial features & object features .h5 files to .npz files transform script
-# Written by Pengbing Gao https://github.com/nbgao
-# --------------------------------------------------------
-
-'''
-Command line example:
-(1) Process spatial features
-python gqa_feat_preproc.py --mode=spatial --spatial_dir=./spatialFeatures --out_dir=./feats/gqa-grid
-
-(2) Process object features
-python gqa_feat_preproc.py --mode=object --object_dir=./objectFeatures --out_dir=./feats/gqa-frcn
-'''
-
-import h5py, glob, json, cv2, argparse
-import numpy as np
-
-# spatial features
-def process_spatial_features(feat_path, out_path):
- info_file = feat_path + '/gqa_spatial_info.json'
- try:
- info = json.load(open(info_file, 'r'))
- except:
- print('Failed to open info file:', info_file)
- return
- print('Total grid features', len(info))
-
- print('Making the
to dict...')
- h5idx_to_imgid = {}
- for img_id in info:
- h5idx_to_imgid[str(info[img_id]['file']) + '_' + str(info[img_id]['idx'])] = img_id
-
- for ix in range(16):
- feat_file = feat_path + '/gqa_spatial_' + str(ix) + '.h5'
- print('Processing', feat_file)
- try:
- feat_dict = h5py.File(feat_file, 'r')
- except:
- print('Failed to open feat file:', feat_file)
- return
-
- features = feat_dict['features']
-
- for iy in range(features.shape[0]):
- img_id = h5idx_to_imgid[str(ix) + '_' + str(iy)]
- feature = features[iy]
- # save to .npz file ['x']
- np.savez(
- out_path + '/' + img_id + '.npz',
- x=feature.reshape(2048, 49).transpose(1, 0), # (49, 2048)
- )
-
- print('Process spatial features successfully!')
-
-
-# object features
-def process_object_features(feat_path, out_path):
- info_file = feat_path + '/gqa_objects_info.json'
- try:
- info = json.load(open(info_file, 'r'))
- except:
- print('Failed to open info file:', info_file)
- return
- print('Total frcn features', len(info))
-
- print('Making the
to dict...')
- h5idx_to_imgid = {}
- for img_id in info:
- h5idx_to_imgid[str(info[img_id]['file']) + '_' + str(info[img_id]['idx'])] = img_id
-
- for ix in range(16):
- feat_file = feat_path + '/gqa_objects_' + str(ix) + '.h5'
- print('Processing', feat_file)
-
- try:
- feat_dict = h5py.File(feat_file, 'r')
- except:
- print('Failed to open feat file:', feat_file)
- return
-
- bboxes = feat_dict['bboxes']
- features = feat_dict['features']
-
- for iy in range(features.shape[0]):
- img_id = h5idx_to_imgid[str(ix) + '_' + str(iy)]
- img_info = info[img_id]
- objects_num = img_info['objectsNum']
- # save to .npz file ['x', 'bbox', 'width', 'height']
- np.savez(
- out_path + '/' + img_id + '.npz',
- x=features[iy, :objects_num],
- bbox=bboxes[iy, :objects_num],
- width=img_info['width'],
- height=img_info['height'],
- )
-
- print('Process object features successfully!')
-
-
-parser = argparse.ArgumentParser(description='gqa_h52npz')
-parser.add_argument('--mode', '-mode', choices=['object', 'spatial', 'frcn', 'grid'], help='mode', type=str)
-parser.add_argument('--object_dir', '-object_dir', help='object features dir', type=str)
-parser.add_argument('--spatial_dir', '-spatial_dir', help='spatial features dir', type=str)
-parser.add_argument('--out_dir', '-out_dir', help='output dir', type=str)
-
-args = parser.parse_args()
-
-mode = args.mode
-object_path = args.object_dir
-spatial_path = args.spatial_dir
-out_path = args.out_dir
-
-print('mode:', mode)
-print('object_path:', object_path)
-print('spatial_path:', spatial_path)
-print('out_path:', out_path)
-
-# process spatial features
-if mode in ['spatial', 'grid']:
- process_spatial_features(spatial_path, out_path)
-
-# process object features
-if mode in ['object', 'frcn']:
- process_object_features(object_path, out_path)
-
diff --git a/spaces/CVPR/Dual-Key_Backdoor_Attacks/openvqa/openvqa/datasets/gqa/eval/gqa_eval.py b/spaces/CVPR/Dual-Key_Backdoor_Attacks/openvqa/openvqa/datasets/gqa/eval/gqa_eval.py
deleted file mode 100644
index a8b65750917ce874f3b4a6e7fa33746d8dcb5442..0000000000000000000000000000000000000000
--- a/spaces/CVPR/Dual-Key_Backdoor_Attacks/openvqa/openvqa/datasets/gqa/eval/gqa_eval.py
+++ /dev/null
@@ -1,307 +0,0 @@
-# --------------------------------------------------------
-# OpenVQA
-# Written by Yuhao Cui https://github.com/cuiyuhao1996
-# --------------------------------------------------------
-
-from collections import defaultdict
-from tqdm import tqdm
-import os.path
-import glob
-import json
-
-
-class GQAEval:
- def __init__(self, __C, result_eval_file, ques_file_path, choices_path=None, EVAL_CONSISTENCY=False):
- ##### Files Loading
- ##########################################################################################
-
- # self.question_path = __C.QUESTION_PATH[__C.SPLIT[__C.RUN_MODE]]
- # self.val_choices_path = __C.EVAL_PATH['val_choices']
- # self.prediction_path = __C.EVAL_PATH['tmp'] + 'result_run_' + __C.VERSION + '.json'
-
- # # Load scene graphs
- # print("Loading scene graphs...")
- # scenes = self.loadFile(args.scenes.format(tier=args.tier))
-
- # Load questions
- print("Loading questions...")
- questions = self.loadFile(ques_file_path)
-
- # Load choices
- choices = None
- if choices_path is not None:
- print("Loading choices...")
- choices = self.loadFile(choices_path)
-
- # Load predictions and turn them into a dictionary
- print("Loading predictions...")
- self.predictions = self.loadFile(result_eval_file)
- self.predictions = {p["questionId"]: p["prediction"] for p in self.predictions}
-
- # Make sure all question have predictions
- for qid in questions:
- if (qid not in self.predictions) and (EVAL_CONSISTENCY or questions[qid]["isBalanced"]):
- print("no prediction for question {}. Please add prediction for all questions.".format(qid))
- raise Exception("missing predictions")
-
- self.scores = {
- "accuracy": [], # list of accuracies per question (1 if correct else 0). Will be averaged ultimately.
- "binary": [], # list of accuracies per a binary question (1 if correct else 0). Will be averaged ultimately.
- "open": [], # list of accuracies per an open question (1 if correct else 0). Will be averaged ultimately.
- "validity": [], # list of validity per question (1 if valid else 0).
- "plausibility": [], # list of plausibility per question (1 if plausible else 0).
- "consistency": [], # list of consistency scores for entailed questions.
- "accuracyPerStructuralType": defaultdict(list), # list of question accuracies for each structural type (e.g. compare, logic questions).
- "accuracyPerSemanticType": defaultdict(list), # list of question accuracies for each semantic type (e.g. questions about an object, an attribute, a relation).
- "accuracyPerLength": defaultdict(list), # list of question accuracies per question's word number.
- "accuracyPerSteps": defaultdict(list), # list of question accuracies per question's reasoning length (steps number).
- "grounding": [] # list of grounding scores for each question.
- }
-
- # Initialize golden and predicted histograms per each question group. Used to compute the distribution metric.
- self.dist = {
- "gold": defaultdict(lambda: defaultdict(int)),
- "predicted": defaultdict(lambda: defaultdict(int))
- }
-
- ##### Main score computation
- ##########################################################################################
-
- # Loop over the questions and compute mterics
- for qid, question in tqdm(questions.items()):
- gold = question["answer"]
- predicted = self.predictions[qid]
-
- self.correct = (predicted == gold)
- score = self.toScore(self.correct)
-
- wordsNum = self.getWordsNum(question)
- stepsNum = self.getStepsNum(question)
-
- # Compute scores over the balanced dataset (more robust against cheating by making educated guesses)
- if question["isBalanced"]:
- # Update accuracy
- self.scores["accuracy"].append(score)
- self.scores["accuracyPerLength"][wordsNum].append(score)
- self.scores["accuracyPerSteps"][stepsNum].append(score)
- self.scores["accuracyPerStructuralType"][question["types"]["structural"]].append(score)
- self.scores["accuracyPerSemanticType"][question["types"]["semantic"]].append(score)
- answerType = "open" if question["types"]["structural"] == "query" else "binary"
- self.scores[answerType].append(score)
-
- if choices_path is not None:
- # Update validity score
- valid = self.belongs(predicted, choices[qid]["valid"], question)
- self.scores["validity"].append(self.toScore(valid))
-
- # Update plausibility score
- plausible = self.belongs(predicted, choices[qid]["plausible"], question)
- self.scores["plausibility"].append(self.toScore(plausible))
-
- # Update histograms for gold and predicted answers
- globalGroup = question["groups"]["global"]
- if globalGroup is not None:
- self.dist["gold"][globalGroup][gold] += 1
- self.dist["predicted"][globalGroup][predicted] += 1
-
- if EVAL_CONSISTENCY:
- # Compute consistency (for entailed questions)
- self.updateConsistency(qid, question, questions)
-
- # Compute distribution score
- self.scores["distribution"] = self.chiSquare(self.dist["gold"], self.dist["predicted"]) / 100
-
- # Average scores over all questions (in the balanced dataset) and print scores
-
- metrics = [
- "binary",
- "open",
- "accuracy",
- "consistency",
- "validity",
- "plausibility",
- "grounding",
- "distribution"
- ]
-
- detailedMetrics = [
- ("accuracyPerStructuralType", "Accuracy / structural type"),
- ("accuracyPerSemanticType", "Accuracy / semantic type"),
- ("accuracyPerSteps", "Accuracy / steps number"),
- ("accuracyPerLength", "Accuracy / words number")
- ]
-
- subMetrics = {
- "attr": "attribute",
- "cat": "category",
- "global": "scene",
- "obj": "object",
- "rel": "relation"
- }
- # average
- for k in metrics:
- if isinstance(self.scores[k], list):
- self.scores[k] = self.avg(self.scores[k]) * 100
-
- for k, _ in detailedMetrics:
- for t in self.scores[k]:
- self.scores[k][t] = self.avg(self.scores[k][t]) * 100, len(self.scores[k][t])
-
- self.result_string = []
- self.detail_result_string = []
-
- # print
- # print("")
- for m in metrics:
- # skip grounding and consistency scores if not requested
- if m == "grounding":
- continue
- if m == "consistency" and not EVAL_CONSISTENCY:
- continue
- if m == "validity" and choices_path is None:
- continue
- if m == "plausibility" and choices_path is None:
- continue
-
- self.result_string.append("{title}: {score:.2f}{suffix}".format(title=m.capitalize(), score=self.scores[m],
- suffix=" (lower is better)" if m == "distribution" else "%"))
- # print score
- # print("{title}: {score:.2f}{suffix}".format(title=m.capitalize(), score=self.scores[m],
- # suffix=" (lower is better)" if m == "distribution" else "%"))
-
- for m, mPrintName in detailedMetrics:
- # print("")
- # self.detail_result_string.append('\n')
-
- # print metric title
- # print("{}:".format(mPrintName))
- self.detail_result_string.append("{}:".format(mPrintName))
-
- for t in sorted(list(self.scores[m].keys())):
- # set sub-metric title
- tName = t
- if isinstance(self.scores[k], list):
- tName = subMetrics.get(t, t).capitalize()
-
- self.detail_result_string.append(" {title}: {score:.2f}{suffix} ({amount} questions)".format(title=tName,
- score=self.scores[m][t][0], suffix="%",
- amount=self.scores[m][t][1]))
- # # print score
- # print(" {title}: {score:.2f}{suffix} ({amount} questions)".format(title=tName,
- # score=self.scores[m][t][0], suffix="%",
- # amount=self.scores[m][t][1]))
-
-
- def get_str_result(self):
- return self.result_string, self.detail_result_string
-
- def loadFile(self, name):
- # load standard json file
- if os.path.isfile(name):
- with open(name) as file:
- data = json.load(file)
- # load file chunks if too big
- elif os.path.isdir(name.split(".")[0]):
- data = {}
- chunks = glob.glob('{dir}/{dir}_*.{ext}'.format(dir = name.split(".")[0], ext = name.split(".")[1]))
- for chunk in chunks:
- with open(chunk) as file:
- data.update(json.load(file))
- else:
- raise Exception("Can't find {}".format(name))
- return data
-
- # book to float
- def toScore(self, b):
- return float(1 if b else 0)
-
- # Compute average of a list
- def avg(self, l):
- if len(l) == 0:
- return 0
- return float(sum(l)) / len(l)
-
- def wavg(self, l, w):
- if sum(w) == 0:
- return None
- return float(sum(l[i] * w[i] for i in range(len(l)))) / sum(w)
-
- ##### Question lengths - words numbers and reasoning steps number
- ##########################################################################################
-
- # Compute question length (words number)
- def getWordsNum(self, question):
- return len(question["question"].split())
-
- # Compute number of reasoning steps (excluding the final "querying" step which doesn't increase effective reasoning length)
- def getStepsNum(self, question):
- return len([c for c in question["semantic"] if not (any([o in "{}: {}".format(c["operation"], c["argument"])
- for o in ["exist", "query: name", "choose name"]]))])
-
- # ##### Functions for question annotations
- # ##########################################################################################
- #
- # # Utility function for converting question annotations string keys to slices
- # def toSlice(self, strSlice):
- # sliceLims = (int(n) for n in strSlice.split(':'))
- # return apply(slice, sliceLims)
- #
- # # Utility function for converting question annotations string keys to indexes list:
- # # "1" => [0]
- # # "1:3" => [1, 2]
- # # "4:9:2" => [4, 6, 8]
- # def intsFromSlice(self, strSlice):
- # slice_obj = get_slice_obj(slicearg)
- # return (range(slice_obj.start or 0, slice_obj.stop or -1, slice_obj.step or 1))
-
- ##### Functions for validity and plausibility
- ##########################################################################################
-
- def belongs(self, element, group, question):
- # normalization ()
- if "Common" in question["types"]["detailed"]:
- group = ["color", "material", "shape"]
-
- return element in group
-
- ##### Functions for consistency scores (for entailed questions ("inferred"))
- ##########################################################################################
-
- def updateConsistency(self, questionId, question, questions):
- inferredQuestions = [eid for eid in question["entailed"] if eid != questionId]
-
- if self.correct and len(inferredQuestions) > 0:
-
- cosnsitencyScores = []
- for eid in inferredQuestions:
- gold = questions[eid]["answer"]
- predicted = self.predictions[eid]
- score = self.toScore(predicted == gold)
- cosnsitencyScores.append(score)
-
- self.scores["consistency"].append(self.avg(cosnsitencyScores))
-
- ##### Functions for distribution score
- ##########################################################################################
-
- # Compute chi square statistic of gold distribution vs predicted distribution,
- # averaged over all question groups
- def chiSquare(self, goldDist, predictedDist):
- sumScore, sumOverall = 0, 0
-
- for group in goldDist:
- score, overall = 0, 0
-
- for ans in goldDist[group]:
- e = goldDist[group][ans]
- o = predictedDist[group].get(ans, 0)
- score += ((float(o - e) ** 2) / e)
- overall += goldDist[group][ans]
-
- sumScore += score * overall
- sumOverall += overall
-
- avgScore = float(sumScore) / sumOverall
-
- return avgScore
-
diff --git a/spaces/CVPR/Text2Human/Text2Human/sample_from_pose.py b/spaces/CVPR/Text2Human/Text2Human/sample_from_pose.py
deleted file mode 100644
index ad1efa7835a5977dbf7fc99ebe037d2f3452d27c..0000000000000000000000000000000000000000
--- a/spaces/CVPR/Text2Human/Text2Human/sample_from_pose.py
+++ /dev/null
@@ -1,52 +0,0 @@
-import argparse
-import logging
-import os.path as osp
-import random
-
-import torch
-
-from data.pose_attr_dataset import DeepFashionAttrPoseDataset
-from models import create_model
-from utils.logger import get_root_logger
-from utils.options import dict2str, dict_to_nonedict, parse
-from utils.util import make_exp_dirs, set_random_seed
-
-
-def main():
- # options
- parser = argparse.ArgumentParser()
- parser.add_argument('-opt', type=str, help='Path to option YAML file.')
- args = parser.parse_args()
- opt = parse(args.opt, is_train=False)
-
- # mkdir and loggers
- make_exp_dirs(opt)
- log_file = osp.join(opt['path']['log'], f"test_{opt['name']}.log")
- logger = get_root_logger(
- logger_name='base', log_level=logging.INFO, log_file=log_file)
- logger.info(dict2str(opt))
-
- # convert to NoneDict, which returns None for missing keys
- opt = dict_to_nonedict(opt)
-
- # random seed
- seed = opt['manual_seed']
- if seed is None:
- seed = random.randint(1, 10000)
- logger.info(f'Random seed: {seed}')
- set_random_seed(seed)
-
- test_dataset = DeepFashionAttrPoseDataset(
- pose_dir=opt['pose_dir'],
- texture_ann_dir=opt['texture_ann_file'],
- shape_ann_path=opt['shape_ann_path'])
- test_loader = torch.utils.data.DataLoader(
- dataset=test_dataset, batch_size=4, shuffle=False)
- logger.info(f'Number of test set: {len(test_dataset)}.')
-
- model = create_model(opt)
- _ = model.inference(test_loader, opt['path']['results_root'])
-
-
-if __name__ == '__main__':
- main()
diff --git a/spaces/CVPR/WALT/mmdet/models/roi_heads/bbox_heads/__init__.py b/spaces/CVPR/WALT/mmdet/models/roi_heads/bbox_heads/__init__.py
deleted file mode 100644
index bc5d29ece5bbf2f168f538f151f06d1b263a5153..0000000000000000000000000000000000000000
--- a/spaces/CVPR/WALT/mmdet/models/roi_heads/bbox_heads/__init__.py
+++ /dev/null
@@ -1,13 +0,0 @@
-from .bbox_head import BBoxHead
-from .convfc_bbox_head import (ConvFCBBoxHead, Shared2FCBBoxHead,
- Shared4Conv1FCBBoxHead)
-from .dii_head import DIIHead
-from .double_bbox_head import DoubleConvFCBBoxHead
-from .sabl_head import SABLHead
-from .scnet_bbox_head import SCNetBBoxHead
-
-__all__ = [
- 'BBoxHead', 'ConvFCBBoxHead', 'Shared2FCBBoxHead',
- 'Shared4Conv1FCBBoxHead', 'DoubleConvFCBBoxHead', 'SABLHead', 'DIIHead',
- 'SCNetBBoxHead'
-]
diff --git a/spaces/CVPR/regionclip-demo/detectron2/modeling/backbone/__init__.py b/spaces/CVPR/regionclip-demo/detectron2/modeling/backbone/__init__.py
deleted file mode 100644
index b58e05c517a3adacd142cf1d68ce3a65f2f66447..0000000000000000000000000000000000000000
--- a/spaces/CVPR/regionclip-demo/detectron2/modeling/backbone/__init__.py
+++ /dev/null
@@ -1,19 +0,0 @@
-# Copyright (c) Facebook, Inc. and its affiliates.
-from .build import build_backbone, build_text_backbone, BACKBONE_REGISTRY # noqa F401 isort:skip
-
-from .backbone import Backbone
-from .fpn import FPN, LastLevelMaxPool
-from .regnet import RegNet
-from .resnet import (
- BasicStem,
- ResNet,
- ResNetBlockBase,
- build_resnet_backbone,
- make_stage,
- BottleneckBlock,
-)
-from .clip_backbone import ModifiedResNet, build_resnet_clip, build_clip_resnet_backbone, build_clip_language_encoder
-from .clip_swin import build_clip_swin, build_clip_swin_backbone
-
-__all__ = [k for k in globals().keys() if not k.startswith("_")]
-# TODO can expose more resnet blocks after careful consideration
diff --git a/spaces/Caoyunkang/Segment-Any-Anomaly/SAM/segment_anything/modeling/common.py b/spaces/Caoyunkang/Segment-Any-Anomaly/SAM/segment_anything/modeling/common.py
deleted file mode 100644
index 2bf15236a3eb24d8526073bc4fa2b274cccb3f96..0000000000000000000000000000000000000000
--- a/spaces/Caoyunkang/Segment-Any-Anomaly/SAM/segment_anything/modeling/common.py
+++ /dev/null
@@ -1,43 +0,0 @@
-# Copyright (c) Meta Platforms, Inc. and affiliates.
-# All rights reserved.
-
-# This source code is licensed under the license found in the
-# LICENSE file in the root directory of this source tree.
-
-import torch
-import torch.nn as nn
-
-from typing import Type
-
-
-class MLPBlock(nn.Module):
- def __init__(
- self,
- embedding_dim: int,
- mlp_dim: int,
- act: Type[nn.Module] = nn.GELU,
- ) -> None:
- super().__init__()
- self.lin1 = nn.Linear(embedding_dim, mlp_dim)
- self.lin2 = nn.Linear(mlp_dim, embedding_dim)
- self.act = act()
-
- def forward(self, x: torch.Tensor) -> torch.Tensor:
- return self.lin2(self.act(self.lin1(x)))
-
-
-# From https://github.com/facebookresearch/detectron2/blob/main/detectron2/layers/batch_norm.py # noqa
-# Itself from https://github.com/facebookresearch/ConvNeXt/blob/d1fa8f6fef0a165b27399986cc2bdacc92777e40/models/convnext.py#L119 # noqa
-class LayerNorm2d(nn.Module):
- def __init__(self, num_channels: int, eps: float = 1e-6) -> None:
- super().__init__()
- self.weight = nn.Parameter(torch.ones(num_channels))
- self.bias = nn.Parameter(torch.zeros(num_channels))
- self.eps = eps
-
- def forward(self, x: torch.Tensor) -> torch.Tensor:
- u = x.mean(1, keepdim=True)
- s = (x - u).pow(2).mean(1, keepdim=True)
- x = (x - u) / torch.sqrt(s + self.eps)
- x = self.weight[:, None, None] * x + self.bias[:, None, None]
- return x
diff --git a/spaces/ChrisCaviar/ControlNet-v1-1/utils.py b/spaces/ChrisCaviar/ControlNet-v1-1/utils.py
deleted file mode 100644
index a626d25c3f4eb92d10bdb66d3c28059a0927a8cd..0000000000000000000000000000000000000000
--- a/spaces/ChrisCaviar/ControlNet-v1-1/utils.py
+++ /dev/null
@@ -1,7 +0,0 @@
-import random
-
-
-def randomize_seed_fn(seed: int, randomize_seed: bool) -> int:
- if randomize_seed:
- seed = random.randint(0, 1000000)
- return seed
diff --git a/spaces/ChrisPreston/diff-svc_minato_aqua/utils/audio.py b/spaces/ChrisPreston/diff-svc_minato_aqua/utils/audio.py
deleted file mode 100644
index aba7ab926cf793d085bbdc70c97f376001183fe1..0000000000000000000000000000000000000000
--- a/spaces/ChrisPreston/diff-svc_minato_aqua/utils/audio.py
+++ /dev/null
@@ -1,56 +0,0 @@
-import subprocess
-import matplotlib
-
-matplotlib.use('Agg')
-import librosa
-import librosa.filters
-import numpy as np
-from scipy import signal
-from scipy.io import wavfile
-
-
-def save_wav(wav, path, sr, norm=False):
- if norm:
- wav = wav / np.abs(wav).max()
- wav *= 32767
- # proposed by @dsmiller
- wavfile.write(path, sr, wav.astype(np.int16))
-
-
-def get_hop_size(hparams):
- hop_size = hparams['hop_size']
- if hop_size is None:
- assert hparams['frame_shift_ms'] is not None
- hop_size = int(hparams['frame_shift_ms'] / 1000 * hparams['audio_sample_rate'])
- return hop_size
-
-
-###########################################################################################
-def _stft(y, hparams):
- return librosa.stft(y=y, n_fft=hparams['fft_size'], hop_length=get_hop_size(hparams),
- win_length=hparams['win_size'], pad_mode='constant')
-
-
-def _istft(y, hparams):
- return librosa.istft(y, hop_length=get_hop_size(hparams), win_length=hparams['win_size'])
-
-
-def librosa_pad_lr(x, fsize, fshift, pad_sides=1):
- '''compute right padding (final frame) or both sides padding (first and final frames)
- '''
- assert pad_sides in (1, 2)
- # return int(fsize // 2)
- pad = (x.shape[0] // fshift + 1) * fshift - x.shape[0]
- if pad_sides == 1:
- return 0, pad
- else:
- return pad // 2, pad // 2 + pad % 2
-
-
-# Conversions
-def amp_to_db(x):
- return 20 * np.log10(np.maximum(1e-5, x))
-
-
-def normalize(S, hparams):
- return (S - hparams['min_level_db']) / -hparams['min_level_db']
diff --git a/spaces/Christyyu/textgenerator/README.md b/spaces/Christyyu/textgenerator/README.md
deleted file mode 100644
index a3a9bcc0a3b94e4884ce52a52ec0a0c46ab308c8..0000000000000000000000000000000000000000
--- a/spaces/Christyyu/textgenerator/README.md
+++ /dev/null
@@ -1,12 +0,0 @@
----
-title: Textgenerator
-emoji: 📉
-colorFrom: purple
-colorTo: purple
-sdk: gradio
-sdk_version: 3.19.1
-app_file: app.py
-pinned: false
----
-
-Check out the configuration reference at https://huggingface.co/docs/hub/spaces-config-reference
diff --git a/spaces/CikeyQI/meme-api/meme_generator/memes/crawl/__init__.py b/spaces/CikeyQI/meme-api/meme_generator/memes/crawl/__init__.py
deleted file mode 100644
index f4aced4e57c3232e75044fe47fbeb0b076805081..0000000000000000000000000000000000000000
--- a/spaces/CikeyQI/meme-api/meme_generator/memes/crawl/__init__.py
+++ /dev/null
@@ -1,43 +0,0 @@
-import random
-from pathlib import Path
-from typing import List
-
-from pil_utils import BuildImage
-from pydantic import Field
-
-from meme_generator import MemeArgsModel, MemeArgsParser, MemeArgsType, add_meme
-
-img_dir = Path(__file__).parent / "images"
-
-
-help = "图片编号,范围为 1~92"
-
-parser = MemeArgsParser()
-parser.add_argument("-n", "--number", type=int, default=0, help=help)
-
-
-class Model(MemeArgsModel):
- number: int = Field(0, description=help)
-
-
-def crawl(images: List[BuildImage], texts: List[str], args: Model):
- total_num = 92
- if 1 <= args.number <= total_num:
- num = args.number
- else:
- num = random.randint(1, total_num)
-
- img = images[0].convert("RGBA").circle().resize((100, 100))
- frame = BuildImage.open(img_dir / f"{num:02d}.jpg")
- frame.paste(img, (0, 400), alpha=True)
- return frame.save_jpg()
-
-
-add_meme(
- "crawl",
- crawl,
- min_images=1,
- max_images=1,
- args_type=MemeArgsType(parser, Model),
- keywords=["爬"],
-)
diff --git a/spaces/Cpp4App/Cpp4App/CDM/detect_compo/lib_ip/ip_draw.py b/spaces/Cpp4App/Cpp4App/CDM/detect_compo/lib_ip/ip_draw.py
deleted file mode 100644
index 9e72407d658ddb68efe23c3c4b0ceb5849109281..0000000000000000000000000000000000000000
--- a/spaces/Cpp4App/Cpp4App/CDM/detect_compo/lib_ip/ip_draw.py
+++ /dev/null
@@ -1,139 +0,0 @@
-import cv2
-import numpy as np
-from random import randint as rint
-from CDM.config.CONFIG_UIED import Config
-
-
-C = Config()
-
-
-def draw_bounding_box_class(org, components, color_map=C.COLOR, line=2, show=False, write_path=None, name='board'):
- """
- Draw bounding box of components with their classes on the original image
- :param org: original image
- :param components: bbox [(column_min, row_min, column_max, row_max)]
- -> top_left: (column_min, row_min)
- -> bottom_right: (column_max, row_max)
- :param color_map: colors mapping to different components
- :param line: line thickness
- :param compo_class: classes matching the corners of components
- :param show: show or not
- :return: labeled image
- """
- board = org.copy()
- for compo in components:
- bbox = compo.put_bbox()
- board = cv2.rectangle(board, (bbox[0], bbox[1]), (bbox[2], bbox[3]), color_map[compo.category], line)
- # board = cv2.putText(board, compo.category, (bbox[0]+5, bbox[1]+20), cv2.FONT_HERSHEY_SIMPLEX, 0.5, color_map[compo.category], 2)
- if show:
- cv2.imshow(name, board)
- cv2.waitKey(0)
- if write_path is not None:
- cv2.imwrite(write_path, board)
- return board
-
-
-def draw_bounding_box(org, ratio, components, color=(0, 255, 0), line=2,
- show=False, write_path=None, name='board', is_return=False, wait_key=0):
- """
- Draw bounding box of components on the original image
- :param org: original image
- :param components: bbox [(column_min, row_min, column_max, row_max)]
- -> top_left: (column_min, row_min)
- -> bottom_right: (column_max, row_max)
- :param color: line color
- :param line: line thickness
- :param show: show or not
- :return: labeled image
- """
- if not show and write_path is None and not is_return: return
- board = org.copy()
- # board = cv2.imread(img_path)
- # ratio = board.shape[0]/org.shape[0]
-
- for compo in components:
- bbox = compo.put_bbox()
-
- # bounding box on full size image
- # bbox = int(ratio * bbox)
- bbox = [int(x * ratio) for x in bbox]
- board = cv2.rectangle(board, (bbox[0], bbox[1]), (bbox[2], bbox[3]), color, line)
- if show:
- cv2.imshow(name, board)
- if wait_key is not None:
- cv2.waitKey(wait_key)
- if wait_key == 0:
- cv2.destroyWindow(name)
- if write_path is not None:
- # board = cv2.resize(board, (1080, 1920))
- # board = board[100:-110]
- cv2.imwrite(write_path, board)
- return board
-
-
-def draw_line(org, lines, color=(0, 255, 0), show=False):
- """
- Draw detected lines on the original image
- :param org: original image
- :param lines: [line_h, line_v]
- -> line_h: horizontal {'head':(column_min, row), 'end':(column_max, row), 'thickness':int)
- -> line_v: vertical {'head':(column, row_min), 'end':(column, row_max), 'thickness':int}
- :param color: drawn color
- :param show: show or not
- :return: image with lines drawn
- """
- board = org.copy()
- line_h, line_v = lines
- for line in line_h:
- cv2.line(board, tuple(line['head']), tuple(line['end']), color, line['thickness'])
- for line in line_v:
- cv2.line(board, tuple(line['head']), tuple(line['end']), color, line['thickness'])
- if show:
- cv2.imshow('img', board)
- cv2.waitKey(0)
- return board
-
-
-def draw_boundary(components, shape, show=False):
- """
- Draw boundary of objects on the black withe
- :param components: boundary: [top, bottom, left, right]
- -> up, bottom: (column_index, min/max row border)
- -> left, right: (row_index, min/max column border) detect range of each row
- :param shape: shape or original image
- :param show: show or not
- :return: drawn board
- """
- board = np.zeros(shape[:2], dtype=np.uint8) # binary board
- for component in components:
- # up and bottom: (column_index, min/max row border)
- for point in component.boundary[0] + component.boundary[1]:
- board[point[1], point[0]] = 255
- # left, right: (row_index, min/max column border)
- for point in component.boundary[2] + component.boundary[3]:
- board[point[0], point[1]] = 255
- if show:
- cv2.imshow('rec', board)
- cv2.waitKey(0)
- return board
-
-
-def draw_region(region, broad, show=False):
- color = (rint(0,255), rint(0,255), rint(0,255))
- for point in region:
- broad[point[0], point[1]] = color
-
- if show:
- cv2.imshow('region', broad)
- cv2.waitKey()
- return broad
-
-
-def draw_region_bin(region, broad, show=False):
- for point in region:
- broad[point[0], point[1]] = 255
-
- if show:
- cv2.imshow('region', broad)
- cv2.waitKey()
- return broad
diff --git a/spaces/Cristiants/captiongeneration/README.md b/spaces/Cristiants/captiongeneration/README.md
deleted file mode 100644
index 75bc399020ca1cfcf2f019aea5c16801d600fbcc..0000000000000000000000000000000000000000
--- a/spaces/Cristiants/captiongeneration/README.md
+++ /dev/null
@@ -1,12 +0,0 @@
----
-title: Captiongeneration
-emoji: 👞👟🥾🥿👠👡👢
-colorFrom: purple
-colorTo: green
-sdk: gradio
-sdk_version: 3.19.1
-app_file: app.py
-pinned: false
----
-
-Check out the configuration reference at https://huggingface.co/docs/hub/spaces-config-reference
diff --git a/spaces/Cropinky/esrgan/realesrgan/version.py b/spaces/Cropinky/esrgan/realesrgan/version.py
deleted file mode 100644
index f5a23197e4dac473f971675a1555bb02bcfa56c5..0000000000000000000000000000000000000000
--- a/spaces/Cropinky/esrgan/realesrgan/version.py
+++ /dev/null
@@ -1,5 +0,0 @@
-# GENERATED VERSION FILE
-# TIME: Fri Jun 2 00:17:29 2023
-__version__ = '0.3.0'
-__gitsha__ = '5ca1078'
-version_info = (0, 3, 0)
diff --git a/spaces/DCandE/rvc-models/infer_pack/attentions.py b/spaces/DCandE/rvc-models/infer_pack/attentions.py
deleted file mode 100644
index 77cb63ffccf3e33badf22d50862a64ba517b487f..0000000000000000000000000000000000000000
--- a/spaces/DCandE/rvc-models/infer_pack/attentions.py
+++ /dev/null
@@ -1,417 +0,0 @@
-import copy
-import math
-import numpy as np
-import torch
-from torch import nn
-from torch.nn import functional as F
-
-from infer_pack import commons
-from infer_pack import modules
-from infer_pack.modules import LayerNorm
-
-
-class Encoder(nn.Module):
- def __init__(
- self,
- hidden_channels,
- filter_channels,
- n_heads,
- n_layers,
- kernel_size=1,
- p_dropout=0.0,
- window_size=10,
- **kwargs
- ):
- super().__init__()
- self.hidden_channels = hidden_channels
- self.filter_channels = filter_channels
- self.n_heads = n_heads
- self.n_layers = n_layers
- self.kernel_size = kernel_size
- self.p_dropout = p_dropout
- self.window_size = window_size
-
- self.drop = nn.Dropout(p_dropout)
- self.attn_layers = nn.ModuleList()
- self.norm_layers_1 = nn.ModuleList()
- self.ffn_layers = nn.ModuleList()
- self.norm_layers_2 = nn.ModuleList()
- for i in range(self.n_layers):
- self.attn_layers.append(
- MultiHeadAttention(
- hidden_channels,
- hidden_channels,
- n_heads,
- p_dropout=p_dropout,
- window_size=window_size,
- )
- )
- self.norm_layers_1.append(LayerNorm(hidden_channels))
- self.ffn_layers.append(
- FFN(
- hidden_channels,
- hidden_channels,
- filter_channels,
- kernel_size,
- p_dropout=p_dropout,
- )
- )
- self.norm_layers_2.append(LayerNorm(hidden_channels))
-
- def forward(self, x, x_mask):
- attn_mask = x_mask.unsqueeze(2) * x_mask.unsqueeze(-1)
- x = x * x_mask
- for i in range(self.n_layers):
- y = self.attn_layers[i](x, x, attn_mask)
- y = self.drop(y)
- x = self.norm_layers_1[i](x + y)
-
- y = self.ffn_layers[i](x, x_mask)
- y = self.drop(y)
- x = self.norm_layers_2[i](x + y)
- x = x * x_mask
- return x
-
-
-class Decoder(nn.Module):
- def __init__(
- self,
- hidden_channels,
- filter_channels,
- n_heads,
- n_layers,
- kernel_size=1,
- p_dropout=0.0,
- proximal_bias=False,
- proximal_init=True,
- **kwargs
- ):
- super().__init__()
- self.hidden_channels = hidden_channels
- self.filter_channels = filter_channels
- self.n_heads = n_heads
- self.n_layers = n_layers
- self.kernel_size = kernel_size
- self.p_dropout = p_dropout
- self.proximal_bias = proximal_bias
- self.proximal_init = proximal_init
-
- self.drop = nn.Dropout(p_dropout)
- self.self_attn_layers = nn.ModuleList()
- self.norm_layers_0 = nn.ModuleList()
- self.encdec_attn_layers = nn.ModuleList()
- self.norm_layers_1 = nn.ModuleList()
- self.ffn_layers = nn.ModuleList()
- self.norm_layers_2 = nn.ModuleList()
- for i in range(self.n_layers):
- self.self_attn_layers.append(
- MultiHeadAttention(
- hidden_channels,
- hidden_channels,
- n_heads,
- p_dropout=p_dropout,
- proximal_bias=proximal_bias,
- proximal_init=proximal_init,
- )
- )
- self.norm_layers_0.append(LayerNorm(hidden_channels))
- self.encdec_attn_layers.append(
- MultiHeadAttention(
- hidden_channels, hidden_channels, n_heads, p_dropout=p_dropout
- )
- )
- self.norm_layers_1.append(LayerNorm(hidden_channels))
- self.ffn_layers.append(
- FFN(
- hidden_channels,
- hidden_channels,
- filter_channels,
- kernel_size,
- p_dropout=p_dropout,
- causal=True,
- )
- )
- self.norm_layers_2.append(LayerNorm(hidden_channels))
-
- def forward(self, x, x_mask, h, h_mask):
- """
- x: decoder input
- h: encoder output
- """
- self_attn_mask = commons.subsequent_mask(x_mask.size(2)).to(
- device=x.device, dtype=x.dtype
- )
- encdec_attn_mask = h_mask.unsqueeze(2) * x_mask.unsqueeze(-1)
- x = x * x_mask
- for i in range(self.n_layers):
- y = self.self_attn_layers[i](x, x, self_attn_mask)
- y = self.drop(y)
- x = self.norm_layers_0[i](x + y)
-
- y = self.encdec_attn_layers[i](x, h, encdec_attn_mask)
- y = self.drop(y)
- x = self.norm_layers_1[i](x + y)
-
- y = self.ffn_layers[i](x, x_mask)
- y = self.drop(y)
- x = self.norm_layers_2[i](x + y)
- x = x * x_mask
- return x
-
-
-class MultiHeadAttention(nn.Module):
- def __init__(
- self,
- channels,
- out_channels,
- n_heads,
- p_dropout=0.0,
- window_size=None,
- heads_share=True,
- block_length=None,
- proximal_bias=False,
- proximal_init=False,
- ):
- super().__init__()
- assert channels % n_heads == 0
-
- self.channels = channels
- self.out_channels = out_channels
- self.n_heads = n_heads
- self.p_dropout = p_dropout
- self.window_size = window_size
- self.heads_share = heads_share
- self.block_length = block_length
- self.proximal_bias = proximal_bias
- self.proximal_init = proximal_init
- self.attn = None
-
- self.k_channels = channels // n_heads
- self.conv_q = nn.Conv1d(channels, channels, 1)
- self.conv_k = nn.Conv1d(channels, channels, 1)
- self.conv_v = nn.Conv1d(channels, channels, 1)
- self.conv_o = nn.Conv1d(channels, out_channels, 1)
- self.drop = nn.Dropout(p_dropout)
-
- if window_size is not None:
- n_heads_rel = 1 if heads_share else n_heads
- rel_stddev = self.k_channels**-0.5
- self.emb_rel_k = nn.Parameter(
- torch.randn(n_heads_rel, window_size * 2 + 1, self.k_channels)
- * rel_stddev
- )
- self.emb_rel_v = nn.Parameter(
- torch.randn(n_heads_rel, window_size * 2 + 1, self.k_channels)
- * rel_stddev
- )
-
- nn.init.xavier_uniform_(self.conv_q.weight)
- nn.init.xavier_uniform_(self.conv_k.weight)
- nn.init.xavier_uniform_(self.conv_v.weight)
- if proximal_init:
- with torch.no_grad():
- self.conv_k.weight.copy_(self.conv_q.weight)
- self.conv_k.bias.copy_(self.conv_q.bias)
-
- def forward(self, x, c, attn_mask=None):
- q = self.conv_q(x)
- k = self.conv_k(c)
- v = self.conv_v(c)
-
- x, self.attn = self.attention(q, k, v, mask=attn_mask)
-
- x = self.conv_o(x)
- return x
-
- def attention(self, query, key, value, mask=None):
- # reshape [b, d, t] -> [b, n_h, t, d_k]
- b, d, t_s, t_t = (*key.size(), query.size(2))
- query = query.view(b, self.n_heads, self.k_channels, t_t).transpose(2, 3)
- key = key.view(b, self.n_heads, self.k_channels, t_s).transpose(2, 3)
- value = value.view(b, self.n_heads, self.k_channels, t_s).transpose(2, 3)
-
- scores = torch.matmul(query / math.sqrt(self.k_channels), key.transpose(-2, -1))
- if self.window_size is not None:
- assert (
- t_s == t_t
- ), "Relative attention is only available for self-attention."
- key_relative_embeddings = self._get_relative_embeddings(self.emb_rel_k, t_s)
- rel_logits = self._matmul_with_relative_keys(
- query / math.sqrt(self.k_channels), key_relative_embeddings
- )
- scores_local = self._relative_position_to_absolute_position(rel_logits)
- scores = scores + scores_local
- if self.proximal_bias:
- assert t_s == t_t, "Proximal bias is only available for self-attention."
- scores = scores + self._attention_bias_proximal(t_s).to(
- device=scores.device, dtype=scores.dtype
- )
- if mask is not None:
- scores = scores.masked_fill(mask == 0, -1e4)
- if self.block_length is not None:
- assert (
- t_s == t_t
- ), "Local attention is only available for self-attention."
- block_mask = (
- torch.ones_like(scores)
- .triu(-self.block_length)
- .tril(self.block_length)
- )
- scores = scores.masked_fill(block_mask == 0, -1e4)
- p_attn = F.softmax(scores, dim=-1) # [b, n_h, t_t, t_s]
- p_attn = self.drop(p_attn)
- output = torch.matmul(p_attn, value)
- if self.window_size is not None:
- relative_weights = self._absolute_position_to_relative_position(p_attn)
- value_relative_embeddings = self._get_relative_embeddings(
- self.emb_rel_v, t_s
- )
- output = output + self._matmul_with_relative_values(
- relative_weights, value_relative_embeddings
- )
- output = (
- output.transpose(2, 3).contiguous().view(b, d, t_t)
- ) # [b, n_h, t_t, d_k] -> [b, d, t_t]
- return output, p_attn
-
- def _matmul_with_relative_values(self, x, y):
- """
- x: [b, h, l, m]
- y: [h or 1, m, d]
- ret: [b, h, l, d]
- """
- ret = torch.matmul(x, y.unsqueeze(0))
- return ret
-
- def _matmul_with_relative_keys(self, x, y):
- """
- x: [b, h, l, d]
- y: [h or 1, m, d]
- ret: [b, h, l, m]
- """
- ret = torch.matmul(x, y.unsqueeze(0).transpose(-2, -1))
- return ret
-
- def _get_relative_embeddings(self, relative_embeddings, length):
- max_relative_position = 2 * self.window_size + 1
- # Pad first before slice to avoid using cond ops.
- pad_length = max(length - (self.window_size + 1), 0)
- slice_start_position = max((self.window_size + 1) - length, 0)
- slice_end_position = slice_start_position + 2 * length - 1
- if pad_length > 0:
- padded_relative_embeddings = F.pad(
- relative_embeddings,
- commons.convert_pad_shape([[0, 0], [pad_length, pad_length], [0, 0]]),
- )
- else:
- padded_relative_embeddings = relative_embeddings
- used_relative_embeddings = padded_relative_embeddings[
- :, slice_start_position:slice_end_position
- ]
- return used_relative_embeddings
-
- def _relative_position_to_absolute_position(self, x):
- """
- x: [b, h, l, 2*l-1]
- ret: [b, h, l, l]
- """
- batch, heads, length, _ = x.size()
- # Concat columns of pad to shift from relative to absolute indexing.
- x = F.pad(x, commons.convert_pad_shape([[0, 0], [0, 0], [0, 0], [0, 1]]))
-
- # Concat extra elements so to add up to shape (len+1, 2*len-1).
- x_flat = x.view([batch, heads, length * 2 * length])
- x_flat = F.pad(
- x_flat, commons.convert_pad_shape([[0, 0], [0, 0], [0, length - 1]])
- )
-
- # Reshape and slice out the padded elements.
- x_final = x_flat.view([batch, heads, length + 1, 2 * length - 1])[
- :, :, :length, length - 1 :
- ]
- return x_final
-
- def _absolute_position_to_relative_position(self, x):
- """
- x: [b, h, l, l]
- ret: [b, h, l, 2*l-1]
- """
- batch, heads, length, _ = x.size()
- # padd along column
- x = F.pad(
- x, commons.convert_pad_shape([[0, 0], [0, 0], [0, 0], [0, length - 1]])
- )
- x_flat = x.view([batch, heads, length**2 + length * (length - 1)])
- # add 0's in the beginning that will skew the elements after reshape
- x_flat = F.pad(x_flat, commons.convert_pad_shape([[0, 0], [0, 0], [length, 0]]))
- x_final = x_flat.view([batch, heads, length, 2 * length])[:, :, :, 1:]
- return x_final
-
- def _attention_bias_proximal(self, length):
- """Bias for self-attention to encourage attention to close positions.
- Args:
- length: an integer scalar.
- Returns:
- a Tensor with shape [1, 1, length, length]
- """
- r = torch.arange(length, dtype=torch.float32)
- diff = torch.unsqueeze(r, 0) - torch.unsqueeze(r, 1)
- return torch.unsqueeze(torch.unsqueeze(-torch.log1p(torch.abs(diff)), 0), 0)
-
-
-class FFN(nn.Module):
- def __init__(
- self,
- in_channels,
- out_channels,
- filter_channels,
- kernel_size,
- p_dropout=0.0,
- activation=None,
- causal=False,
- ):
- super().__init__()
- self.in_channels = in_channels
- self.out_channels = out_channels
- self.filter_channels = filter_channels
- self.kernel_size = kernel_size
- self.p_dropout = p_dropout
- self.activation = activation
- self.causal = causal
-
- if causal:
- self.padding = self._causal_padding
- else:
- self.padding = self._same_padding
-
- self.conv_1 = nn.Conv1d(in_channels, filter_channels, kernel_size)
- self.conv_2 = nn.Conv1d(filter_channels, out_channels, kernel_size)
- self.drop = nn.Dropout(p_dropout)
-
- def forward(self, x, x_mask):
- x = self.conv_1(self.padding(x * x_mask))
- if self.activation == "gelu":
- x = x * torch.sigmoid(1.702 * x)
- else:
- x = torch.relu(x)
- x = self.drop(x)
- x = self.conv_2(self.padding(x * x_mask))
- return x * x_mask
-
- def _causal_padding(self, x):
- if self.kernel_size == 1:
- return x
- pad_l = self.kernel_size - 1
- pad_r = 0
- padding = [[0, 0], [0, 0], [pad_l, pad_r]]
- x = F.pad(x, commons.convert_pad_shape(padding))
- return x
-
- def _same_padding(self, x):
- if self.kernel_size == 1:
- return x
- pad_l = (self.kernel_size - 1) // 2
- pad_r = self.kernel_size // 2
- padding = [[0, 0], [0, 0], [pad_l, pad_r]]
- x = F.pad(x, commons.convert_pad_shape(padding))
- return x
diff --git a/spaces/DEEMOSTECH/ChatAvatar/static/css/main.b0d5db9d.css b/spaces/DEEMOSTECH/ChatAvatar/static/css/main.b0d5db9d.css
deleted file mode 100644
index 1471e55905ce26a8139b8e9e9e215d03bc654d42..0000000000000000000000000000000000000000
--- a/spaces/DEEMOSTECH/ChatAvatar/static/css/main.b0d5db9d.css
+++ /dev/null
@@ -1,2 +0,0 @@
-html{overflow-x:hidden;overflow-y:overlay}body{-webkit-font-smoothing:antialiased;-moz-osx-font-smoothing:grayscale;box-sizing:border-box;color:#cfcfcf;font-family:-apple-system,BlinkMacSystemFont,Segoe UI,Roboto,Oxygen,Ubuntu,Cantarell,Fira Sans,Droid Sans,Helvetica Neue,sans-serif;margin:0}code{font-family:source-code-pro,Menlo,Monaco,Consolas,Courier New,monospace}.root{display:flex;justify-content:center;width:100%}.container{height:100vh;width:100%}.\!container{width:100%!important}@media (min-width:640px){.container{max-width:640px}.\!container{max-width:640px!important}}@media (min-width:768px){.container{max-width:768px}.\!container{max-width:768px!important}}@media (min-width:1024px){.container{max-width:1024px}.\!container{max-width:1024px!important}}@media (min-width:1280px){.container{max-width:1280px}.\!container{max-width:1280px!important}}@media (min-width:1536px){.container{max-width:1536px}.\!container{max-width:1536px!important}}.App{--theme-color:#4a00e0;--font-dark-color:#434343;--font-gray-color:#aaa;--font-light-color:#cfcfcf;--bg-light-color:#fff;--bg-gray0-color:#f8f8f8;--bg-gray1-color:#ececec;--bg-gray2-color:#7c7c7c;--bg-gray3-color:#373737;--bg-theme-color:#e7e3f1;--bg-dark-color:#121317;--side-gap:5rem;--radius:0.5rem;--shadow:-10px 0px 12px 1px hsla(0,0%,53%,.16);display:flex;justify-content:space-between;padding:16px;text-align:center}.App *{box-sizing:border-box;transition:all .3s}.App ::-webkit-scrollbar-thumb{background-color:rgba(0,0,0,.2)}textarea{-webkit-font-smoothing:antialiased;-moz-osx-font-smoothing:grayscale;border:1px solid transparent;color:var(--font-dark-color);font-family:-apple-system,BlinkMacSystemFont,Segoe UI,Roboto,Oxygen,Ubuntu,Cantarell,Fira Sans,Droid Sans,Helvetica Neue,sans-serif;font-size:1rem;line-height:1.5rem;outline:none;padding:0;resize:none}textarea:focus{border-color:var(--theme-color)}img{-webkit-user-drag:none;-webkit-user-select:none;user-select:none}.gallery_con__Y2mej{align-items:flex-start;display:flex;justify-content:center;margin-top:3rem;padding:0 1.25rem;width:100%}.gallery_menuCon__fVdFJ{margin-right:2rem;width:-webkit-max-content;width:max-content}.gallery_menu__U2btD{align-items:center;background-color:initial;border:2px solid transparent;border-radius:1.5rem;cursor:pointer;display:flex;height:3rem;justify-content:center;line-height:1rem;margin-bottom:1rem;text-align:center;width:6rem}.gallery_menu__U2btD.gallery_selected__T2qcs,.gallery_menu__U2btD:hover{background-color:var(--bg-gray3-color);color:#fff}.gallery_menu__U2btD.gallery_selected__T2qcs{border-color:#fff}.gallery_cardsCon__wAfcp{align-items:flex-start;display:flex;flex-grow:1;flex-shrink:1;flex-wrap:wrap;justify-content:space-between;max-height:100vh;max-width:calc(1600px + 9rem)}.gallery_cardsCon__wAfcp::-webkit-scrollbar-thumb{background-color:hsla(0,0%,100%,.2);border:5px solid #121317;border-radius:8px}.gallery_card__noUoL{background-color:var(--bg-gray3-color);border-radius:var(--radius);cursor:pointer;font-size:.75rem;height:260px;margin-bottom:1rem;overflow:hidden;position:relative;width:200px}.gallery_coverImg__BYj-o,.gallery_coverImg__BYj-o img{height:100%;width:100%}.gallery_prompt__9PEmb{background-color:#f8f8f880;border-radius:var(--radius);bottom:1rem;color:var(--font-dark-color);height:0;left:1rem;overflow:hidden;padding:0 .5rem;position:absolute;right:1rem;text-align:left;white-space:pre-wrap;word-break:break-all}.gallery_prompt__9PEmb.gallery_show__c2k50{height:-webkit-fit-content;height:-moz-fit-content;height:fit-content;padding:.5rem}.gallery_infoCon__E8oLy{align-items:center;bottom:1rem;color:var(--font-dark-color);display:flex;justify-content:flex-start;left:1rem;position:absolute;right:1rem}.gallery_avatar__KWBmI,.gallery_avatar__KWBmI img{border-radius:12px;height:24px;overflow:hidden;width:24px}.gallery_avatar__KWBmI{margin-right:1rem}.gallery_spaceholder__xJwYU{flex-grow:1;flex-shrink:1}.header_con__M\+u1W{align-items:center;display:flex;justify-content:center;padding:0 var(--side-gap);width:100vw}.header_header__Y7CqP{align-items:center;border-bottom:1px solid hsla(0,0%,100%,.1);display:flex;justify-content:space-between;padding:1rem 0;width:100%}.header_logoCon__MIdGL{align-items:flex-start;display:flex;height:3rem;justify-content:center}.header_logo__90zuC{height:3rem;margin-right:1rem}.header_logoCon__MIdGL>div{font-size:2rem;font-weight:700;line-height:2rem;margin-top:5px}.header_avatar__B3zXB{background:var(--bg-gray2-color);border-radius:50%;overflow:hidden}.header_avatar__B3zXB,.header_avatar__B3zXB img{height:3rem;width:3rem}.result_con__gHOU1{align-items:center;color:var(--font-dark-color);justify-content:center;width:calc(100% - 700px);z-index:999}.result_con__gHOU1 *{flex-shrink:0}.result_board__PCvVJ{background-color:var(--bg-light-color);border-radius:var(--radius);display:flex;flex-flow:column;height:100%;width:100%}.result_colHead__k0Mk-{background:#f9fafb;border:0 solid #e5e7eb;border-radius:8px;flex:0 1 auto;padding:8px}.result_colInner__9FccK{background:#fff;border:1px solid #e5e7eb;border-radius:8px;box-shadow:0 1px 2px 0 rgba(0,0,0,.05);flex-wrap:wrap;gap:1px;margin-bottom:1rem;overflow:hidden;padding:10px 12px}.result_colDetail__jggqg,.result_colInner__9FccK{align-items:center;flex-direction:column;justify-content:flex-start}.result_colDetail__jggqg{background:#f9fafb;border:0 solid #e5e7eb;border-radius:8px;display:flex;flex:1 1 auto;margin-top:1rem;padding:8px 8px 24px}.result_colContent__FYZno{background:#fff;border:1px solid #e5e7eb;border-radius:8px;height:100%;width:100%}.result_colTitle__R8k\+A{align-items:flex-end;color:#6b7280;display:flex;font-size:.875rem;justify-content:space-between;line-height:1.2rem;margin-bottom:8px;width:100%}.result_passwordCon__OjFSI{border-top:1px solid #e5e7eb;padding:8px 12px 2px}.result_emailCon__eEqXk{padding-bottom:10px;padding-left:12px;padding-right:12px}.result_colTitle__R8k\+A>div{margin-bottom:.5rem}.result_colTitle__R8k\+A>div.result_restart__fLq8E{border-radius:5px;cursor:pointer;font-size:1rem;font-weight:400;margin-bottom:0;margin-left:1rem;padding:.5rem;-webkit-user-select:none;user-select:none}.result_restart__fLq8E:hover{background-color:var(--bg-gray0-color);color:var(--font-dark-color)}.result_spaceholder__GAxGZ{flex-grow:1;flex-shrink:1}.result_lang__85-De{cursor:pointer;font-weight:400;margin-right:1rem;-webkit-user-select:none;user-select:none}.result_lang__85-De.result_en__n-Jo7{margin-left:1rem;margin-right:0;width:4rem}.result_lang__85-De:hover{font-weight:700}.result_lang__85-De.result_selected__kDzD1{color:var(--font-dark-color);font-weight:700}.result_regene__yKazF{color:var(--theme-color);cursor:pointer;font-weight:400;-webkit-user-select:none;user-select:none}.result_chatCon__Hm\+zJ{background-color:var(--bg-gray0-color);border-radius:var(--radius);height:calc(100% - 4rem);padding:1rem}.result_chatCon__Hm\+zJ,.result_chatMsgCon__x8UTP{align-items:center;display:flex;flex-direction:column;flex-grow:1;flex-shrink:1;justify-content:flex-start;width:100%}.result_chatMsgCon__x8UTP{overflow-y:overlay;text-align:left}.result_chatMsgCon__x8UTP::-webkit-scrollbar-thumb{border:none;border-radius:3px}.result_chatMsgCon__x8UTP::-webkit-scrollbar{width:6px}.result_chatMsgRow__dr9Qg{align-items:flex-start;display:flex;flex-direction:row;justify-content:flex-start;margin-bottom:1rem;width:100%}.result_chatMsgRow__dr9Qg.result_user__bUuRg{flex-direction:row-reverse}.result_avatar__B2zOp{background:var(--bg-gray2-color);border-radius:1.5rem;margin-left:0;margin-right:1rem;overflow:hidden}.result_avatar__B2zOp,.result_avatar__B2zOp img{height:3rem;width:3rem}.result_user__bUuRg .result_avatar__B2zOp{margin-left:1rem;margin-right:0}.result_bubble__GexXm{background:var(--bg-theme-color);border-radius:var(--radius);flex-shrink:1;line-height:1.5rem;padding:.75rem 1rem;white-space:pre-wrap;word-break:break-all}.result_bubble__GexXm.result_unactive__zyVF2{background:var(--bg-gray1-color)}.result_user__bUuRg .result_bubble__GexXm{background:var(--bg-light-color)}.result_chatIptCon__LXDF-{align-items:center;display:flex;flex-direction:column;justify-content:flex-start;width:100%}.result_chatTipsCon__w4uUf{align-items:flex-end;display:flex;flex-direction:row;justify-content:flex-start;margin-top:1rem;max-width:100%;overflow-x:auto;overflow-y:hidden;width:100%}.result_chatTipsCon__w4uUf::-webkit-scrollbar-thumb{border-color:var(--bg-gray0-color)}.result_chatTips__6b9zJ{background:var(--bg-light-color);border-radius:var(--radius);cursor:pointer;margin-right:1rem;padding:1rem;text-align:left;white-space:pre-wrap;width:15.5rem;word-break:break-all}.result_chatTips__6b9zJ:last-child{margin-right:0}.result_chatRowCon__jLGk3{align-items:flex-start;display:flex;flex-direction:row;justify-content:space-between;margin-top:1rem;width:100%}.result_iptLineCon__nLuWa{flex-grow:1;flex-shrink:1;line-height:1.5rem;margin-right:1rem;position:relative;text-align:left}.result_iptSpaceholder__hAkD5{border:1px solid transparent;max-height:calc(9rem + 2px);visibility:hidden}.result_iptSpaceholder__hAkD5,.result_ipt__tA\+g4{padding:.75rem 1rem;white-space:pre-wrap;word-break:break-all}.result_ipt__tA\+g4{background:var(--bg-light-color);border-radius:var(--radius);bottom:0;left:0;overflow-y:auto;position:absolute;right:0;top:0}.result_ipt__tA\+g4::-webkit-scrollbar-thumb{border-color:var(--bg-light-color)}.result_btn__h5tQr{align-items:center;background-color:var(--theme-color);border:1px solid var(--theme-color);border-radius:1.5rem;color:#fff;cursor:pointer;display:flex;font-weight:700;height:calc(3rem - 2px);justify-content:center;line-height:1rem;padding:0 1.5rem;-webkit-user-select:none;user-select:none}.result_con__gHOU1 .result_btn__h5tQr.result_disabled__lB61-{background:var(--bg-gray2-color);border-color:var(--bg-gray2-color);color:var(--font-light-color);cursor:not-allowed}.result_iptArea__23TZc{background:#fff;border:1px solid #e5e7eb;border-radius:8px;box-shadow:0 0 0 3px transparent,inset 0 2px 4px 0 rgba(0,0,0,.05);color:#1f2937;display:block;font-size:14px;height:42px;line-height:1.4;outline:none!important;padding:10px;position:relative;width:100%}.result_iptArea__23TZc:focus{border-color:#93c5fd;box-shadow:0 0 0 3px #dfedfe,inset 0 2px 4px 0 transparent}.result_iptArea__23TZc::-webkit-scrollbar-thumb{border-color:var(--bg-gray0-color)}.result_clearBtn__r6e0y{background:linear-gradient(to bottom right,#f3f4f6,#e5e7eb);border:1px solid #e5e7eb;border-radius:8px;color:#374151;cursor:pointer;font-size:16px;font-weight:600;height:42px;min-width:max(160px,48%);padding:8px 16px}.result_clearBtn__r6e0y:hover{background:linear-gradient(to bottom right,#f3f4f6,#f3f4f6);border:1px solid #e5e7eb}.result_clearBtnLogin__LOsgV{background:linear-gradient(to bottom right,#f3f4f6,#e5e7eb);border:1px solid #e5e7eb;border-radius:8px;color:#374151;cursor:pointer;font-size:16px;font-weight:700;height:42px;min-width:max(160px,48%);padding:8px 16px}.result_inputError__qtPTq{border-color:#f56565;box-shadow:0 0 0 3px #fed7d7,inset 0 2px 4px 0 transparent}.result_clearBtnLogin__LOsgV:hover{background:linear-gradient(to bottom right,#f3f4f6,#f3f4f6);border:1px solid #e5e7eb}.result_btnCon__LEoi5{display:flex;justify-content:space-between}.result_generateBtn__UGmBG{background:linear-gradient(to bottom right,#ffedd5,#fdba74);border:1px solid #fed7aa;border-radius:8px;color:#ea580c;cursor:pointer;font-size:16px;font-weight:600;height:42px;min-width:max(160px,48%);padding:8px 16px}.result_generateBtn__UGmBG:hover{background:linear-gradient(to bottom right,#ffecd3,#fed7ab);border:1px solid #ffd8b4}.result_generateBtnLogin__nkLOj{background:linear-gradient(to bottom right,#ffedd5,#fdba74);border:1px solid #fed7aa;border-radius:8px;color:#ea580c;cursor:pointer;font-size:16px;font-weight:700;height:42px;min-width:max(160px,48%);padding:8px 16px}.result_generateBtnLogin__nkLOj:hover{background:linear-gradient(to bottom right,#ffecd3,#fed7ab);border:1px solid #ffd8b4}.result_candidateCon__x9kyB{align-items:flex-start;background-color:var(--bg-gray0-color);border-radius:var(--radius);display:flex;flex-direction:row;flex-grow:1;flex-shrink:1;height:100%;justify-content:space-between;max-height:45rem;overflow-y:auto;padding:1rem;position:relative;width:100%}.result_candidateCon__x9kyB::-webkit-scrollbar-thumb{border-color:var(--bg-gray0-color)}.result_candidateCol__eoHna{margin-right:1rem;position:relative;width:calc(33.33333% - .66667rem)}.result_candidateCol__eoHna:last-child{margin-right:0}.result_candidateCol__eoHna img{border-radius:var(--radius);cursor:pointer;margin-bottom:.5rem}.result_creatorCon__tIm3e{align-items:flex-end;color:var(--font-gray-color);display:flex;font-size:1.2rem;font-weight:700;justify-content:flex-start;line-height:1.2rem;margin-bottom:1rem;width:100%}.result_creatorInfoCon__pET8h{text-align:left}.result_creatorName__VLTXL{color:var(--font-dark-color);font-size:1.2rem;font-weight:700;line-height:1.8rem}.result_creatorInfo__CkbWU{color:var(--font-gray-color);font-size:1rem;line-height:1.2rem}.result_modelView__Y25w5{background:var(--bg-gray0-color);border-radius:var(--radius);flex-grow:1;flex-shrink:1;height:100%;overflow:hidden;width:100%}.result_modelInfoCon__bXw5O{align-items:center;display:flex;flex-direction:column;justify-content:flex-end;text-align:left}.result_progressInfo__g9iwR{margin-bottom:.5rem;width:100%}.result_progressTrack__I6zDn{background:var(--bg-light-color);border-radius:2px;height:4px;position:relative;width:100%}.result_progressThumb__mbBQj{background-color:var(--theme-color);border-radius:2px;height:4px;left:0;position:absolute;top:0}.result_modelPrompt__DzUbD{background:var(--bg-light-color);border-radius:var(--radius);margin-top:1rem;min-height:3rem;padding:1rem;width:100%}.result_loadingCon__XVvXD,.result_progressCon__O57XA{font-size:14px;position:absolute;top:55%}.result_loadingCon__XVvXD{z-index:-111}.result_icon__dFKnM{height:20px;position:absolute;top:55%}.result_hideModel__3phD0{display:none}.result_descriptionLogin__xi7Yx{text-align:start}.login_con__\+RJgQ{background:#000;box-shadow:-5px 0 20px 0 hsla(0,0%,100%,.2);height:100vh;padding:var(--side-gap);position:fixed;right:0;top:0;z-index:9}.login_close__JulM-{cursor:pointer;-webkit-user-select:none;user-select:none}.welcome_con__o1kmf{align-items:center;background:#121317;border-radius:.5rem;display:flex;flex-direction:column;height:1080px;justify-content:flex-start;padding-bottom:1rem;padding-top:2rem;position:relative;width:680px}.welcome_con__o1kmf>img{position:absolute;top:0;width:100%}.welcome_mainCon__H1gv\+{margin-top:.5rem;z-index:999}.welcome_title__Gd8m4{color:#fff;font-family:Courier New;font-size:5rem;font-weight:700;line-height:5rem}.welcome_ioCon__PQZXU{background-color:#fff;border-radius:1rem;border-style:solid;margin-left:8rem;margin-right:8rem;margin-top:24rem;padding:2rem;width:calc(100% - 16rem)}.welcome_iptCon__KpWEL{align-items:center;background:#ededf2;border-radius:1rem;display:flex;height:4rem;justify-content:space-between;margin-bottom:2rem;width:100%}.welcome_iptCon__KpWEL>img{height:2rem;margin-right:1rem;position:static;width:2rem}.welcome_ipt__ayi9Z{background:#ededf2;border:none;border-radius:1rem;color:var(--font-dark-color);flex-grow:1;font-size:1rem;height:100%;outline:none;padding:0 2rem}.welcome_ipt__ayi9Z::-webkit-input-placeholder{font-size:1rem}.welcome_ipt__ayi9Z::placeholder{font-size:1rem}.welcome_btnCon__Mx-ta,.welcome_btn__jCuoG{align-items:center;display:flex;justify-content:center}.welcome_btn__jCuoG{border:1px solid #8f8f8f;border-radius:1rem;cursor:pointer;height:3rem;line-height:1rem;-webkit-user-select:none;user-select:none;width:100%}.welcome_btn__jCuoG:last-child{background:#4a00e0;border:none;font-weight:700}.welcome_btn__jCuoG.welcome_disabled__pcSzv{cursor:not-allowed}.welcome_btn__jCuoG:hover{color:#fff}
-/*# sourceMappingURL=main.b0d5db9d.css.map*/
\ No newline at end of file
diff --git a/spaces/DQChoi/gpt-demo/venv/lib/python3.11/site-packages/PIL/features.py b/spaces/DQChoi/gpt-demo/venv/lib/python3.11/site-packages/PIL/features.py
deleted file mode 100644
index f14e60cf5d44750e4e8924b20db4c49ed8d8f790..0000000000000000000000000000000000000000
--- a/spaces/DQChoi/gpt-demo/venv/lib/python3.11/site-packages/PIL/features.py
+++ /dev/null
@@ -1,329 +0,0 @@
-import collections
-import os
-import sys
-import warnings
-
-import PIL
-
-from . import Image
-
-modules = {
- "pil": ("PIL._imaging", "PILLOW_VERSION"),
- "tkinter": ("PIL._tkinter_finder", "tk_version"),
- "freetype2": ("PIL._imagingft", "freetype2_version"),
- "littlecms2": ("PIL._imagingcms", "littlecms_version"),
- "webp": ("PIL._webp", "webpdecoder_version"),
-}
-
-
-def check_module(feature):
- """
- Checks if a module is available.
-
- :param feature: The module to check for.
- :returns: ``True`` if available, ``False`` otherwise.
- :raises ValueError: If the module is not defined in this version of Pillow.
- """
- if feature not in modules:
- msg = f"Unknown module {feature}"
- raise ValueError(msg)
-
- module, ver = modules[feature]
-
- try:
- __import__(module)
- return True
- except ModuleNotFoundError:
- return False
- except ImportError as ex:
- warnings.warn(str(ex))
- return False
-
-
-def version_module(feature):
- """
- :param feature: The module to check for.
- :returns:
- The loaded version number as a string, or ``None`` if unknown or not available.
- :raises ValueError: If the module is not defined in this version of Pillow.
- """
- if not check_module(feature):
- return None
-
- module, ver = modules[feature]
-
- if ver is None:
- return None
-
- return getattr(__import__(module, fromlist=[ver]), ver)
-
-
-def get_supported_modules():
- """
- :returns: A list of all supported modules.
- """
- return [f for f in modules if check_module(f)]
-
-
-codecs = {
- "jpg": ("jpeg", "jpeglib"),
- "jpg_2000": ("jpeg2k", "jp2klib"),
- "zlib": ("zip", "zlib"),
- "libtiff": ("libtiff", "libtiff"),
-}
-
-
-def check_codec(feature):
- """
- Checks if a codec is available.
-
- :param feature: The codec to check for.
- :returns: ``True`` if available, ``False`` otherwise.
- :raises ValueError: If the codec is not defined in this version of Pillow.
- """
- if feature not in codecs:
- msg = f"Unknown codec {feature}"
- raise ValueError(msg)
-
- codec, lib = codecs[feature]
-
- return codec + "_encoder" in dir(Image.core)
-
-
-def version_codec(feature):
- """
- :param feature: The codec to check for.
- :returns:
- The version number as a string, or ``None`` if not available.
- Checked at compile time for ``jpg``, run-time otherwise.
- :raises ValueError: If the codec is not defined in this version of Pillow.
- """
- if not check_codec(feature):
- return None
-
- codec, lib = codecs[feature]
-
- version = getattr(Image.core, lib + "_version")
-
- if feature == "libtiff":
- return version.split("\n")[0].split("Version ")[1]
-
- return version
-
-
-def get_supported_codecs():
- """
- :returns: A list of all supported codecs.
- """
- return [f for f in codecs if check_codec(f)]
-
-
-features = {
- "webp_anim": ("PIL._webp", "HAVE_WEBPANIM", None),
- "webp_mux": ("PIL._webp", "HAVE_WEBPMUX", None),
- "transp_webp": ("PIL._webp", "HAVE_TRANSPARENCY", None),
- "raqm": ("PIL._imagingft", "HAVE_RAQM", "raqm_version"),
- "fribidi": ("PIL._imagingft", "HAVE_FRIBIDI", "fribidi_version"),
- "harfbuzz": ("PIL._imagingft", "HAVE_HARFBUZZ", "harfbuzz_version"),
- "libjpeg_turbo": ("PIL._imaging", "HAVE_LIBJPEGTURBO", "libjpeg_turbo_version"),
- "libimagequant": ("PIL._imaging", "HAVE_LIBIMAGEQUANT", "imagequant_version"),
- "xcb": ("PIL._imaging", "HAVE_XCB", None),
-}
-
-
-def check_feature(feature):
- """
- Checks if a feature is available.
-
- :param feature: The feature to check for.
- :returns: ``True`` if available, ``False`` if unavailable, ``None`` if unknown.
- :raises ValueError: If the feature is not defined in this version of Pillow.
- """
- if feature not in features:
- msg = f"Unknown feature {feature}"
- raise ValueError(msg)
-
- module, flag, ver = features[feature]
-
- try:
- imported_module = __import__(module, fromlist=["PIL"])
- return getattr(imported_module, flag)
- except ModuleNotFoundError:
- return None
- except ImportError as ex:
- warnings.warn(str(ex))
- return None
-
-
-def version_feature(feature):
- """
- :param feature: The feature to check for.
- :returns: The version number as a string, or ``None`` if not available.
- :raises ValueError: If the feature is not defined in this version of Pillow.
- """
- if not check_feature(feature):
- return None
-
- module, flag, ver = features[feature]
-
- if ver is None:
- return None
-
- return getattr(__import__(module, fromlist=[ver]), ver)
-
-
-def get_supported_features():
- """
- :returns: A list of all supported features.
- """
- return [f for f in features if check_feature(f)]
-
-
-def check(feature):
- """
- :param feature: A module, codec, or feature name.
- :returns:
- ``True`` if the module, codec, or feature is available,
- ``False`` or ``None`` otherwise.
- """
-
- if feature in modules:
- return check_module(feature)
- if feature in codecs:
- return check_codec(feature)
- if feature in features:
- return check_feature(feature)
- warnings.warn(f"Unknown feature '{feature}'.", stacklevel=2)
- return False
-
-
-def version(feature):
- """
- :param feature:
- The module, codec, or feature to check for.
- :returns:
- The version number as a string, or ``None`` if unknown or not available.
- """
- if feature in modules:
- return version_module(feature)
- if feature in codecs:
- return version_codec(feature)
- if feature in features:
- return version_feature(feature)
- return None
-
-
-def get_supported():
- """
- :returns: A list of all supported modules, features, and codecs.
- """
-
- ret = get_supported_modules()
- ret.extend(get_supported_features())
- ret.extend(get_supported_codecs())
- return ret
-
-
-def pilinfo(out=None, supported_formats=True):
- """
- Prints information about this installation of Pillow.
- This function can be called with ``python3 -m PIL``.
-
- :param out:
- The output stream to print to. Defaults to ``sys.stdout`` if ``None``.
- :param supported_formats:
- If ``True``, a list of all supported image file formats will be printed.
- """
-
- if out is None:
- out = sys.stdout
-
- Image.init()
-
- print("-" * 68, file=out)
- print(f"Pillow {PIL.__version__}", file=out)
- py_version = sys.version.splitlines()
- print(f"Python {py_version[0].strip()}", file=out)
- for py_version in py_version[1:]:
- print(f" {py_version.strip()}", file=out)
- print("-" * 68, file=out)
- print(
- f"Python modules loaded from {os.path.dirname(Image.__file__)}",
- file=out,
- )
- print(
- f"Binary modules loaded from {os.path.dirname(Image.core.__file__)}",
- file=out,
- )
- print("-" * 68, file=out)
-
- for name, feature in [
- ("pil", "PIL CORE"),
- ("tkinter", "TKINTER"),
- ("freetype2", "FREETYPE2"),
- ("littlecms2", "LITTLECMS2"),
- ("webp", "WEBP"),
- ("transp_webp", "WEBP Transparency"),
- ("webp_mux", "WEBPMUX"),
- ("webp_anim", "WEBP Animation"),
- ("jpg", "JPEG"),
- ("jpg_2000", "OPENJPEG (JPEG2000)"),
- ("zlib", "ZLIB (PNG/ZIP)"),
- ("libtiff", "LIBTIFF"),
- ("raqm", "RAQM (Bidirectional Text)"),
- ("libimagequant", "LIBIMAGEQUANT (Quantization method)"),
- ("xcb", "XCB (X protocol)"),
- ]:
- if check(name):
- if name == "jpg" and check_feature("libjpeg_turbo"):
- v = "libjpeg-turbo " + version_feature("libjpeg_turbo")
- else:
- v = version(name)
- if v is not None:
- version_static = name in ("pil", "jpg")
- if name == "littlecms2":
- # this check is also in src/_imagingcms.c:setup_module()
- version_static = tuple(int(x) for x in v.split(".")) < (2, 7)
- t = "compiled for" if version_static else "loaded"
- if name == "raqm":
- for f in ("fribidi", "harfbuzz"):
- v2 = version_feature(f)
- if v2 is not None:
- v += f", {f} {v2}"
- print("---", feature, "support ok,", t, v, file=out)
- else:
- print("---", feature, "support ok", file=out)
- else:
- print("***", feature, "support not installed", file=out)
- print("-" * 68, file=out)
-
- if supported_formats:
- extensions = collections.defaultdict(list)
- for ext, i in Image.EXTENSION.items():
- extensions[i].append(ext)
-
- for i in sorted(Image.ID):
- line = f"{i}"
- if i in Image.MIME:
- line = f"{line} {Image.MIME[i]}"
- print(line, file=out)
-
- if i in extensions:
- print(
- "Extensions: {}".format(", ".join(sorted(extensions[i]))), file=out
- )
-
- features = []
- if i in Image.OPEN:
- features.append("open")
- if i in Image.SAVE:
- features.append("save")
- if i in Image.SAVE_ALL:
- features.append("save_all")
- if i in Image.DECODERS:
- features.append("decode")
- if i in Image.ENCODERS:
- features.append("encode")
-
- print("Features: {}".format(", ".join(features)), file=out)
- print("-" * 68, file=out)
diff --git a/spaces/DQChoi/gpt-demo/venv/lib/python3.11/site-packages/fontTools/pens/quartzPen.py b/spaces/DQChoi/gpt-demo/venv/lib/python3.11/site-packages/fontTools/pens/quartzPen.py
deleted file mode 100644
index 6e1228d6f2b8bbc78cf52864ccaf3b249a654749..0000000000000000000000000000000000000000
--- a/spaces/DQChoi/gpt-demo/venv/lib/python3.11/site-packages/fontTools/pens/quartzPen.py
+++ /dev/null
@@ -1,44 +0,0 @@
-from fontTools.pens.basePen import BasePen
-
-from Quartz.CoreGraphics import CGPathCreateMutable, CGPathMoveToPoint
-from Quartz.CoreGraphics import CGPathAddLineToPoint, CGPathAddCurveToPoint
-from Quartz.CoreGraphics import CGPathAddQuadCurveToPoint, CGPathCloseSubpath
-
-
-__all__ = ["QuartzPen"]
-
-
-class QuartzPen(BasePen):
-
- """A pen that creates a CGPath
-
- Parameters
- - path: an optional CGPath to add to
- - xform: an optional CGAffineTransform to apply to the path
- """
-
- def __init__(self, glyphSet, path=None, xform=None):
- BasePen.__init__(self, glyphSet)
- if path is None:
- path = CGPathCreateMutable()
- self.path = path
- self.xform = xform
-
- def _moveTo(self, pt):
- x, y = pt
- CGPathMoveToPoint(self.path, self.xform, x, y)
-
- def _lineTo(self, pt):
- x, y = pt
- CGPathAddLineToPoint(self.path, self.xform, x, y)
-
- def _curveToOne(self, p1, p2, p3):
- (x1, y1), (x2, y2), (x3, y3) = p1, p2, p3
- CGPathAddCurveToPoint(self.path, self.xform, x1, y1, x2, y2, x3, y3)
-
- def _qCurveToOne(self, p1, p2):
- (x1, y1), (x2, y2) = p1, p2
- CGPathAddQuadCurveToPoint(self.path, self.xform, x1, y1, x2, y2)
-
- def _closePath(self):
- CGPathCloseSubpath(self.path)
diff --git a/spaces/DQChoi/gpt-demo/venv/lib/python3.11/site-packages/gradio/templates/frontend/assets/Image-8a3c68cc.js b/spaces/DQChoi/gpt-demo/venv/lib/python3.11/site-packages/gradio/templates/frontend/assets/Image-8a3c68cc.js
deleted file mode 100644
index 81f0d9ac80b7fa253d09dcb084b83347ec71aec8..0000000000000000000000000000000000000000
--- a/spaces/DQChoi/gpt-demo/venv/lib/python3.11/site-packages/gradio/templates/frontend/assets/Image-8a3c68cc.js
+++ /dev/null
@@ -1,2 +0,0 @@
-import{S as g,e as u,s as d,N as y,T as f,K as c,U as i,p as o,n as r,A as v}from"./index-3370be2a.js";function b(t){let e,s;return{c(){e=y("img"),f(e.src,s=t[1]+t[0])||c(e,"src",s),c(e,"class","svelte-gqt00k"),i(e,"table",t[2]==="table"),i(e,"gallery",t[2]==="gallery"),i(e,"selected",t[3])},m(l,a){o(l,e,a)},p(l,[a]){a&3&&!f(e.src,s=l[1]+l[0])&&c(e,"src",s),a&4&&i(e,"table",l[2]==="table"),a&4&&i(e,"gallery",l[2]==="gallery"),a&8&&i(e,"selected",l[3])},i:r,o:r,d(l){l&&v(e)}}}function q(t,e,s){let{value:l}=e,{samples_dir:a}=e,{type:m}=e,{selected:_=!1}=e;return t.$$set=n=>{"value"in n&&s(0,l=n.value),"samples_dir"in n&&s(1,a=n.samples_dir),"type"in n&&s(2,m=n.type),"selected"in n&&s(3,_=n.selected)},[l,a,m,_]}class I extends g{constructor(e){super(),u(this,e,q,b,d,{value:0,samples_dir:1,type:2,selected:3})}}const E=I;export{E};
-//# sourceMappingURL=Image-8a3c68cc.js.map
diff --git a/spaces/DaFujaTyping/hf-Chat-ui/src/lib/utils/deepestChild.ts b/spaces/DaFujaTyping/hf-Chat-ui/src/lib/utils/deepestChild.ts
deleted file mode 100644
index 7177d64566b12be4f42b934980fcf3681c3705d7..0000000000000000000000000000000000000000
--- a/spaces/DaFujaTyping/hf-Chat-ui/src/lib/utils/deepestChild.ts
+++ /dev/null
@@ -1,7 +0,0 @@
-export function deepestChild(el: HTMLElement) {
- let newEl = el;
- while (newEl.hasChildNodes()) {
- newEl = newEl.lastElementChild as HTMLElement;
- }
- return newEl;
-}
diff --git a/spaces/Daniton/MidJourney/README.md b/spaces/Daniton/MidJourney/README.md
deleted file mode 100644
index 8c7ddb48969fd277fcc2688fa67bcf718baf9f22..0000000000000000000000000000000000000000
--- a/spaces/Daniton/MidJourney/README.md
+++ /dev/null
@@ -1,12 +0,0 @@
----
-title: MidJourney
-emoji: 🐨
-colorFrom: green
-colorTo: purple
-sdk: gradio
-sdk_version: 3.16.2
-app_file: app.py
-pinned: false
----
-
-Check out the configuration reference at https://huggingface.co/docs/hub/spaces-config-reference
diff --git a/spaces/Detomo/ai-avatar-backend/helpers/callOpenAI.js b/spaces/Detomo/ai-avatar-backend/helpers/callOpenAI.js
deleted file mode 100644
index d5262e22d7583324f0290aeaafda084e6e25509a..0000000000000000000000000000000000000000
--- a/spaces/Detomo/ai-avatar-backend/helpers/callOpenAI.js
+++ /dev/null
@@ -1,20 +0,0 @@
-const OpenAI = require('openai');
-
-const openai = new OpenAI({
- apiKey: process.env.OPENAI_API_KEY, // defaults to process.env["OPENAI_API_KEY"]
- dangerouslyAllowBrowser: true
-});
-
-async function queryOpenAIAndSave(userContent) {
- const response = await openai.chat.completions.create({
- model: "gpt-3.5-turbo",
- messages: [
- {"role": "system", "content": "You are a helpful assistant made by Detomo company. Please answer the following questions with maximum 2 sentence. Answer by the language of the question."},
- {"role": "user", "content": userContent},
- ],
- });
-
- return response.choices[0].message.content;
-}
-
-module.exports = queryOpenAIAndSave;
\ No newline at end of file
diff --git a/spaces/Deviliaan/sd_twist/README.md b/spaces/Deviliaan/sd_twist/README.md
deleted file mode 100644
index e216906e0828834241b7195278762f81be2a90ea..0000000000000000000000000000000000000000
--- a/spaces/Deviliaan/sd_twist/README.md
+++ /dev/null
@@ -1,14 +0,0 @@
----
-title: Stable Diffusion Webui on Cpu
-emoji: 🏃
-colorFrom: pink
-colorTo: purple
-sdk: gradio
-sdk_version: 3.32.0
-app_file: app.py
-pinned: false
-python_version: 3.10.6
-duplicated_from: jangocheng/stable-diffusion-webui-cpu_with_prompt
----
-
-Check out the configuration reference at https://huggingface.co/docs/hub/spaces-config-reference
diff --git a/spaces/Dinoking/Garbage-Classifier-V2/app.py b/spaces/Dinoking/Garbage-Classifier-V2/app.py
deleted file mode 100644
index 75fbcace727dbc15de8f787a21aa839c0001ca84..0000000000000000000000000000000000000000
--- a/spaces/Dinoking/Garbage-Classifier-V2/app.py
+++ /dev/null
@@ -1,46 +0,0 @@
-import gradio as gr
-import matplotlib.pyplot as plt
-import numpy as np
-import os
-import PIL
-import tensorflow as tf
-
-from tensorflow import keras
-from tensorflow.keras import layers
-from tensorflow.keras.models import Sequential
-from tensorflow.keras.models import Sequential
-from tensorflow.keras.layers import Convolution2D
-from tensorflow.keras.layers import MaxPooling2D
-from tensorflow.keras.layers import Flatten
-from tensorflow.keras.layers import Dense
-from tensorflow.keras.layers import Dropout
-
-from tensorflow.keras.callbacks import EarlyStopping
-
-
-from tensorflow.keras.models import load_model
-
-# load model
-model = load_model('model11.h5')
-
-classnames = ['cardboard','metal','paper','plastic','trash','green-glass','white-glass','brown-glass','clothes','biological','battery','shoes']
-
-def predict_image(img):
- img_4d=img.reshape(-1,298, 384,3)
- prediction=model.predict(img_4d)[0]
- return {classnames[i]: float(prediction[i]) for i in range(12)}
-
-sample_images = [
- ["battery.JPG"],
- ["jeans.jpg"],
- ["paper1.jpg"]]
-
-
-image = gr.inputs.Image(shape=(298, 384))
-label = gr.outputs.Label(num_top_classes=3)
-enable_queue=True
-
-article="
Made by Aditya Narendra with 🖤
"
-
-gr.Interface(fn=predict_image, inputs=image, title="Garbage Classifier-v2",
- description="This is a Garbage Classifier based on Satish's Model.Deployed to Hugging Faces Using Gradio.",outputs=label,article=article,examples=sample_images,enable_queue=enable_queue,interpretation='default').launch(debug='True')
\ No newline at end of file
diff --git a/spaces/Dinoking/Guccio-AI-Designer/models/biggan/pytorch_biggan/scripts/convert_tf_hub_models.sh b/spaces/Dinoking/Guccio-AI-Designer/models/biggan/pytorch_biggan/scripts/convert_tf_hub_models.sh
deleted file mode 100644
index caed81a1e9698014ac61e8baa3d98d256cb3b4dd..0000000000000000000000000000000000000000
--- a/spaces/Dinoking/Guccio-AI-Designer/models/biggan/pytorch_biggan/scripts/convert_tf_hub_models.sh
+++ /dev/null
@@ -1,21 +0,0 @@
-# Copyright (c) 2019-present, Thomas Wolf, Huggingface Inc.
-# All rights reserved.
-#
-# This source code is licensed under the license found in the
-# LICENSE file in the root directory of this source tree.
-#
-
-set -e
-set -x
-
-models="128 256 512"
-
-mkdir -p models/model_128
-mkdir -p models/model_256
-mkdir -p models/model_512
-
-# Convert TF Hub models.
-for model in $models
-do
- pytorch_pretrained_biggan --model_type $model --tf_model_path models/model_$model --pt_save_path models/model_$model
-done
diff --git a/spaces/Dorado607/ChuanhuChatGPT/run_Windows.bat b/spaces/Dorado607/ChuanhuChatGPT/run_Windows.bat
deleted file mode 100644
index 5dd4dd065807bc83425e3876c1be14b5a234e253..0000000000000000000000000000000000000000
--- a/spaces/Dorado607/ChuanhuChatGPT/run_Windows.bat
+++ /dev/null
@@ -1,24 +0,0 @@
-@echo off
-echo Opening ChuanhuChatGPT...
-
-if not exist "%~dp0\ChuanhuChat\Scripts" (
- echo Creating venv...
- python -m venv ChuanhuChat
-
- cd /d "%~dp0\ChuanhuChat\Scripts"
- call activate.bat
-
- cd /d "%~dp0"
- pip install -r requirements.txt
-)
-
-goto :activate_venv
-
-:launch
-%PYTHON% ChuanhuChatbot.py %*
-pause
-
-:activate_venv
-set PYTHON="%~dp0\ChuanhuChat\Scripts\Python.exe"
-echo venv %PYTHON%
-goto :launch
diff --git a/spaces/DragGan/DragGan-Inversion/stylegan_human/training_scripts/sg3/training/dataset.py b/spaces/DragGan/DragGan-Inversion/stylegan_human/training_scripts/sg3/training/dataset.py
deleted file mode 100644
index c4f10460a3c1d864d544fc7c9344cffd723312fe..0000000000000000000000000000000000000000
--- a/spaces/DragGan/DragGan-Inversion/stylegan_human/training_scripts/sg3/training/dataset.py
+++ /dev/null
@@ -1,274 +0,0 @@
-# Copyright (c) SenseTime Research. All rights reserved.
-
-# Copyright (c) 2021, NVIDIA CORPORATION. All rights reserved.
-#
-# NVIDIA CORPORATION and its licensors retain all intellectual property
-# and proprietary rights in and to this software, related documentation
-# and any modifications thereto. Any use, reproduction, disclosure or
-# distribution of this software and related documentation without an express
-# license agreement from NVIDIA CORPORATION is strictly prohibited.
-
-"""Streaming images and labels from datasets created with dataset_tool.py."""
-
-import os
-import numpy as np
-import zipfile
-import PIL.Image
-import json
-import torch
-import dnnlib
-from petrel_client.client import Client
-import cv2
-
-
-try:
- import pyspng
-except ImportError:
- pyspng = None
-
-# ----------------------------------------------------------------------------
-
-
-class Dataset(torch.utils.data.Dataset):
- def __init__(self,
- name, # Name of the dataset.
- raw_shape, # Shape of the raw image data (NCHW).
- # Artificially limit the size of the dataset. None = no limit. Applied before xflip.
- max_size=None,
- # Enable conditioning labels? False = label dimension is zero.
- use_labels=False,
- # Artificially double the size of the dataset via x-flips. Applied after max_size.
- xflip=False,
- # Random seed to use when applying max_size.
- random_seed=0,
- square=False,
- ):
- print('Inside Dataset')
- self._name = name
- self._raw_shape = list(raw_shape)
- self._use_labels = use_labels
- self._raw_labels = None
- self._label_shape = None
- self._square = square
- print("inside dataset, _square: ", self._square)
-
- # Apply max_size.
- self._raw_idx = np.arange(self._raw_shape[0], dtype=np.int64)
- if (max_size is not None) and (self._raw_idx.size > max_size):
- np.random.RandomState(random_seed).shuffle(self._raw_idx)
- self._raw_idx = np.sort(self._raw_idx[:max_size])
-
- # Apply xflip.
- self._xflip = np.zeros(self._raw_idx.size, dtype=np.uint8)
- if xflip:
- self._raw_idx = np.tile(self._raw_idx, 2)
- self._xflip = np.concatenate(
- [self._xflip, np.ones_like(self._xflip)])
-
- def _get_raw_labels(self):
- if self._raw_labels is None:
- self._raw_labels = self._load_raw_labels() if self._use_labels else None
- if self._raw_labels is None:
- self._raw_labels = np.zeros(
- [self._raw_shape[0], 0], dtype=np.float32)
- assert isinstance(self._raw_labels, np.ndarray)
- assert self._raw_labels.shape[0] == self._raw_shape[0]
- assert self._raw_labels.dtype in [np.float32, np.int64]
- if self._raw_labels.dtype == np.int64:
- assert self._raw_labels.ndim == 1
- assert np.all(self._raw_labels >= 0)
- return self._raw_labels
-
- def close(self): # to be overridden by subclass
- pass
-
- def _load_raw_image(self, raw_idx): # to be overridden by subclass
- raise NotImplementedError
-
- def _load_raw_labels(self): # to be overridden by subclass
- raise NotImplementedError
-
- def __getstate__(self):
- return dict(self.__dict__, _raw_labels=None)
-
- def __del__(self):
- try:
- self.close()
- except:
- pass
-
- def __len__(self):
- return self._raw_idx.size
-
- def __getitem__(self, idx):
- image = self._load_raw_image(self._raw_idx[idx])
- assert isinstance(image, np.ndarray)
- assert list(image.shape) == self.image_shape
- assert image.dtype == np.uint8
- if self._xflip[idx]:
- assert image.ndim == 3 # CHW
- image = image[:, :, ::-1]
- return image.copy(), self.get_label(idx)
-
- def get_label(self, idx):
- label = self._get_raw_labels()[self._raw_idx[idx]]
- if label.dtype == np.int64:
- onehot = np.zeros(self.label_shape, dtype=np.float32)
- onehot[label] = 1
- label = onehot
- return label.copy()
-
- def get_details(self, idx):
- d = dnnlib.EasyDict()
- d.raw_idx = int(self._raw_idx[idx])
- d.xflip = (int(self._xflip[idx]) != 0)
- d.raw_label = self._get_raw_labels()[d.raw_idx].copy()
- return d
-
- @property
- def name(self):
- return self._name
-
- @property
- def image_shape(self):
- return list(self._raw_shape[1:])
-
- @property
- def num_channels(self):
- assert len(self.image_shape) == 3 # CHW
- return self.image_shape[0]
-
- @property
- def resolution(self):
- assert len(self.image_shape) == 3 # CHW
- if self._square:
- assert self.image_shape[1] == self.image_shape[2]
- else:
- assert self.image_shape[1] == self.image_shape[2] * 2
- return self.image_shape[1]
-
- @property
- def label_shape(self):
- if self._label_shape is None:
- raw_labels = self._get_raw_labels()
- if raw_labels.dtype == np.int64:
- self._label_shape = [int(np.max(raw_labels)) + 1]
- else:
- self._label_shape = raw_labels.shape[1:]
- return list(self._label_shape)
-
- @property
- def label_dim(self):
- assert len(self.label_shape) == 1
- return self.label_shape[0]
-
- @property
- def has_labels(self):
- return any(x != 0 for x in self.label_shape)
-
- @property
- def has_onehot_labels(self):
- return self._get_raw_labels().dtype == np.int64
-
-# ----------------------------------------------------------------------------
-
-
-class ImageFolderDataset(Dataset):
- def __init__(self,
- path, # Path to directory or zip.
- # Ensure specific resolution, None = highest available.
- resolution=None,
- ceph=False,
- square=False,
- # Additional arguments for the Dataset base class.
- **super_kwargs,
- ):
- self._path = path
- self._zipfile = None
- self._square = square
-
- if os.path.isdir(self._path):
- self._type = 'dir'
- self._all_fnames = {os.path.relpath(os.path.join(
- root, fname), start=self._path) for root, _dirs, files in os.walk(self._path) for fname in files}
- elif self._file_ext(self._path) == '.zip':
- self._type = 'zip'
- self._all_fnames = set(self._get_zipfile().namelist())
- else:
- raise IOError('Path must point to a directory or zip')
-
- PIL.Image.init()
- self._image_fnames = sorted(
- fname for fname in self._all_fnames if self._file_ext(fname) in PIL.Image.EXTENSION)
- if len(self._image_fnames) == 0:
- raise IOError('No image files found in the specified path')
-
- name = os.path.splitext(os.path.basename(self._path))[0]
- raw_shape = [len(self._image_fnames)] + \
- list(self._load_raw_image(0).shape)
- # if resolution is not None and (raw_shape[2] != resolution or raw_shape[3] != resolution):
- # raise IOError('Image files do not match the specified resolution')
- if resolution is not None:
- if self._square:
- raw_shape[2] = raw_shape[3] = resolution
- else:
- raw_shape[2] = resolution
- raw_shape[3] = resolution // 2
- # print(raw_shape)
- super().__init__(name=name, raw_shape=raw_shape, square=square, **super_kwargs)
-
- @staticmethod
- def _file_ext(fname):
- return os.path.splitext(fname)[1].lower()
-
- def _get_zipfile(self):
- assert self._type == 'zip'
- if self._zipfile is None:
- self._zipfile = zipfile.ZipFile(self._path)
- return self._zipfile
-
- def _open_file(self, fname):
- if self._type == 'dir':
- return open(os.path.join(self._path, fname), 'rb')
- if self._type == 'zip':
- return self._get_zipfile().open(fname, 'r')
- return None
-
- def close(self):
- try:
- if self._zipfile is not None:
- self._zipfile.close()
- finally:
- self._zipfile = None
-
- def __getstate__(self):
- return dict(super().__getstate__(), _zipfile=None)
-
- def _load_raw_image(self, raw_idx):
- fname = self._image_fnames[raw_idx]
- with self._open_file(fname) as f:
- if pyspng is not None and self._file_ext(fname) == '.png':
- image = pyspng.load(f.read())
- else:
- image = np.array(PIL.Image.open(f))
- if image.ndim == 2:
- image = image[:, :, np.newaxis] # HW => HWC
- image = image.transpose(2, 0, 1) # HWC => CHW
- return image
-
- def _load_raw_labels(self):
- fname = 'dataset.json'
- if fname not in self._all_fnames:
- return None
- with self._open_file(fname) as f:
- labels = json.load(f)['labels']
- if labels is None:
- return None
- labels = dict(labels)
- labels = [labels[fname.replace('\\', '/')]
- for fname in self._image_fnames]
- labels = np.array(labels)
- labels = labels.astype({1: np.int64, 2: np.float32}[labels.ndim])
- return labels
-
-# ----------------------------------------------------------------------------
diff --git a/spaces/DragGan/DragGan/stylegan_human/torch_utils/persistence.py b/spaces/DragGan/DragGan/stylegan_human/torch_utils/persistence.py
deleted file mode 100644
index 50269409c8d9f7c38d7870ee7c8e4660bfb4115c..0000000000000000000000000000000000000000
--- a/spaces/DragGan/DragGan/stylegan_human/torch_utils/persistence.py
+++ /dev/null
@@ -1,253 +0,0 @@
-# Copyright (c) SenseTime Research. All rights reserved.
-
-# Copyright (c) 2021, NVIDIA CORPORATION. All rights reserved.
-#
-# NVIDIA CORPORATION and its licensors retain all intellectual property
-# and proprietary rights in and to this software, related documentation
-# and any modifications thereto. Any use, reproduction, disclosure or
-# distribution of this software and related documentation without an express
-# license agreement from NVIDIA CORPORATION is strictly prohibited.
-
-"""Facilities for pickling Python code alongside other data.
-
-The pickled code is automatically imported into a separate Python module
-during unpickling. This way, any previously exported pickles will remain
-usable even if the original code is no longer available, or if the current
-version of the code is not consistent with what was originally pickled."""
-
-import sys
-import pickle
-import io
-import inspect
-import copy
-import uuid
-import types
-import dnnlib
-
-#----------------------------------------------------------------------------
-
-_version = 6 # internal version number
-_decorators = set() # {decorator_class, ...}
-_import_hooks = [] # [hook_function, ...]
-_module_to_src_dict = dict() # {module: src, ...}
-_src_to_module_dict = dict() # {src: module, ...}
-
-#----------------------------------------------------------------------------
-
-def persistent_class(orig_class):
- r"""Class decorator that extends a given class to save its source code
- when pickled.
-
- Example:
-
- from torch_utils import persistence
-
- @persistence.persistent_class
- class MyNetwork(torch.nn.Module):
- def __init__(self, num_inputs, num_outputs):
- super().__init__()
- self.fc = MyLayer(num_inputs, num_outputs)
- ...
-
- @persistence.persistent_class
- class MyLayer(torch.nn.Module):
- ...
-
- When pickled, any instance of `MyNetwork` and `MyLayer` will save its
- source code alongside other internal state (e.g., parameters, buffers,
- and submodules). This way, any previously exported pickle will remain
- usable even if the class definitions have been modified or are no
- longer available.
-
- The decorator saves the source code of the entire Python module
- containing the decorated class. It does *not* save the source code of
- any imported modules. Thus, the imported modules must be available
- during unpickling, also including `torch_utils.persistence` itself.
-
- It is ok to call functions defined in the same module from the
- decorated class. However, if the decorated class depends on other
- classes defined in the same module, they must be decorated as well.
- This is illustrated in the above example in the case of `MyLayer`.
-
- It is also possible to employ the decorator just-in-time before
- calling the constructor. For example:
-
- cls = MyLayer
- if want_to_make_it_persistent:
- cls = persistence.persistent_class(cls)
- layer = cls(num_inputs, num_outputs)
-
- As an additional feature, the decorator also keeps track of the
- arguments that were used to construct each instance of the decorated
- class. The arguments can be queried via `obj.init_args` and
- `obj.init_kwargs`, and they are automatically pickled alongside other
- object state. A typical use case is to first unpickle a previous
- instance of a persistent class, and then upgrade it to use the latest
- version of the source code:
-
- with open('old_pickle.pkl', 'rb') as f:
- old_net = pickle.load(f)
- new_net = MyNetwork(*old_obj.init_args, **old_obj.init_kwargs)
- misc.copy_params_and_buffers(old_net, new_net, require_all=True)
- """
- assert isinstance(orig_class, type)
- if is_persistent(orig_class):
- return orig_class
-
- assert orig_class.__module__ in sys.modules
- orig_module = sys.modules[orig_class.__module__]
- orig_module_src = _module_to_src(orig_module)
-
- class Decorator(orig_class):
- _orig_module_src = orig_module_src
- _orig_class_name = orig_class.__name__
-
- def __init__(self, *args, **kwargs):
- super().__init__(*args, **kwargs)
- self._init_args = copy.deepcopy(args)
- self._init_kwargs = copy.deepcopy(kwargs)
- assert orig_class.__name__ in orig_module.__dict__
- _check_pickleable(self.__reduce__())
-
- @property
- def init_args(self):
- return copy.deepcopy(self._init_args)
-
- @property
- def init_kwargs(self):
- return dnnlib.EasyDict(copy.deepcopy(self._init_kwargs))
-
- def __reduce__(self):
- fields = list(super().__reduce__())
- fields += [None] * max(3 - len(fields), 0)
- if fields[0] is not _reconstruct_persistent_obj:
- meta = dict(type='class', version=_version, module_src=self._orig_module_src, class_name=self._orig_class_name, state=fields[2])
- fields[0] = _reconstruct_persistent_obj # reconstruct func
- fields[1] = (meta,) # reconstruct args
- fields[2] = None # state dict
- return tuple(fields)
-
- Decorator.__name__ = orig_class.__name__
- _decorators.add(Decorator)
- return Decorator
-
-#----------------------------------------------------------------------------
-
-def is_persistent(obj):
- r"""Test whether the given object or class is persistent, i.e.,
- whether it will save its source code when pickled.
- """
- try:
- if obj in _decorators:
- return True
- except TypeError:
- pass
- return type(obj) in _decorators # pylint: disable=unidiomatic-typecheck
-
-#----------------------------------------------------------------------------
-
-def import_hook(hook):
- r"""Register an import hook that is called whenever a persistent object
- is being unpickled. A typical use case is to patch the pickled source
- code to avoid errors and inconsistencies when the API of some imported
- module has changed.
-
- The hook should have the following signature:
-
- hook(meta) -> modified meta
-
- `meta` is an instance of `dnnlib.EasyDict` with the following fields:
-
- type: Type of the persistent object, e.g. `'class'`.
- version: Internal version number of `torch_utils.persistence`.
- module_src Original source code of the Python module.
- class_name: Class name in the original Python module.
- state: Internal state of the object.
-
- Example:
-
- @persistence.import_hook
- def wreck_my_network(meta):
- if meta.class_name == 'MyNetwork':
- print('MyNetwork is being imported. I will wreck it!')
- meta.module_src = meta.module_src.replace("True", "False")
- return meta
- """
- assert callable(hook)
- _import_hooks.append(hook)
-
-#----------------------------------------------------------------------------
-
-def _reconstruct_persistent_obj(meta):
- r"""Hook that is called internally by the `pickle` module to unpickle
- a persistent object.
- """
- meta = dnnlib.EasyDict(meta)
- meta.state = dnnlib.EasyDict(meta.state)
- for hook in _import_hooks:
- meta = hook(meta)
- assert meta is not None
-
- assert meta.version == _version
- module = _src_to_module(meta.module_src)
-
- assert meta.type == 'class'
- orig_class = module.__dict__[meta.class_name]
- decorator_class = persistent_class(orig_class)
- obj = decorator_class.__new__(decorator_class)
-
- setstate = getattr(obj, '__setstate__', None)
- if callable(setstate):
- setstate(meta.state) # pylint: disable=not-callable
- else:
- obj.__dict__.update(meta.state)
- return obj
-
-#----------------------------------------------------------------------------
-
-def _module_to_src(module):
- r"""Query the source code of a given Python module.
- """
- src = _module_to_src_dict.get(module, None)
- if src is None:
- src = inspect.getsource(module)
- _module_to_src_dict[module] = src
- _src_to_module_dict[src] = module
- return src
-
-def _src_to_module(src):
- r"""Get or create a Python module for the given source code.
- """
- module = _src_to_module_dict.get(src, None)
- if module is None:
- module_name = "_imported_module_" + uuid.uuid4().hex
- module = types.ModuleType(module_name)
- sys.modules[module_name] = module
- _module_to_src_dict[module] = src
- _src_to_module_dict[src] = module
- exec(src, module.__dict__) # pylint: disable=exec-used
- return module
-
-#----------------------------------------------------------------------------
-
-def _check_pickleable(obj):
- r"""Check that the given object is pickleable, raising an exception if
- it is not. This function is expected to be considerably more efficient
- than actually pickling the object.
- """
- def recurse(obj):
- if isinstance(obj, (list, tuple, set)):
- return [recurse(x) for x in obj]
- if isinstance(obj, dict):
- return [[recurse(x), recurse(y)] for x, y in obj.items()]
- if isinstance(obj, (str, int, float, bool, bytes, bytearray)):
- return None # Python primitive types are pickleable.
- if f'{type(obj).__module__}.{type(obj).__name__}' in ['numpy.ndarray', 'torch.Tensor']:
- return None # NumPy arrays and PyTorch tensors are pickleable.
- if is_persistent(obj):
- return None # Persistent objects are pickleable, by virtue of the constructor check.
- return obj
- with io.BytesIO() as f:
- pickle.dump(recurse(obj), f)
-
-#----------------------------------------------------------------------------
diff --git a/spaces/EAraid12/LoRA-DreamBooth-Training-UI/trainer.py b/spaces/EAraid12/LoRA-DreamBooth-Training-UI/trainer.py
deleted file mode 100644
index e4e4469796a08b797ae70a641c2f5125dbd22c1e..0000000000000000000000000000000000000000
--- a/spaces/EAraid12/LoRA-DreamBooth-Training-UI/trainer.py
+++ /dev/null
@@ -1,166 +0,0 @@
-from __future__ import annotations
-
-import datetime
-import os
-import pathlib
-import shlex
-import shutil
-import subprocess
-
-import gradio as gr
-import PIL.Image
-import slugify
-import torch
-from huggingface_hub import HfApi
-
-from app_upload import LoRAModelUploader
-from utils import save_model_card
-
-URL_TO_JOIN_LORA_LIBRARY_ORG = 'https://huggingface.co/organizations/lora-library/share/hjetHAcKjnPHXhHfbeEcqnBqmhgilFfpOL'
-
-
-def pad_image(image: PIL.Image.Image) -> PIL.Image.Image:
- w, h = image.size
- if w == h:
- return image
- elif w > h:
- new_image = PIL.Image.new(image.mode, (w, w), (0, 0, 0))
- new_image.paste(image, (0, (w - h) // 2))
- return new_image
- else:
- new_image = PIL.Image.new(image.mode, (h, h), (0, 0, 0))
- new_image.paste(image, ((h - w) // 2, 0))
- return new_image
-
-
-class Trainer:
- def __init__(self, hf_token: str | None = None):
- self.hf_token = hf_token
- self.api = HfApi(token=hf_token)
- self.model_uploader = LoRAModelUploader(hf_token)
-
- def prepare_dataset(self, instance_images: list, resolution: int,
- instance_data_dir: pathlib.Path) -> None:
- shutil.rmtree(instance_data_dir, ignore_errors=True)
- instance_data_dir.mkdir(parents=True)
- for i, temp_path in enumerate(instance_images):
- image = PIL.Image.open(temp_path.name)
- image = pad_image(image)
- image = image.resize((resolution, resolution))
- image = image.convert('RGB')
- out_path = instance_data_dir / f'{i:03d}.jpg'
- image.save(out_path, format='JPEG', quality=100)
-
- def join_lora_library_org(self) -> None:
- subprocess.run(
- shlex.split(
- f'curl -X POST -H "Authorization: Bearer {self.hf_token}" -H "Content-Type: application/json" {URL_TO_JOIN_LORA_LIBRARY_ORG}'
- ))
-
- def run(
- self,
- instance_images: list | None,
- instance_prompt: str,
- output_model_name: str,
- overwrite_existing_model: bool,
- validation_prompt: str,
- base_model: str,
- resolution_s: str,
- n_steps: int,
- learning_rate: float,
- gradient_accumulation: int,
- seed: int,
- fp16: bool,
- use_8bit_adam: bool,
- checkpointing_steps: int,
- use_wandb: bool,
- validation_epochs: int,
- upload_to_hub: bool,
- use_private_repo: bool,
- delete_existing_repo: bool,
- upload_to: str,
- remove_gpu_after_training: bool,
- ) -> str:
- if not torch.cuda.is_available():
- raise gr.Error('CUDA is not available.')
- if instance_images is None:
- raise gr.Error('You need to upload images.')
- if not instance_prompt:
- raise gr.Error('The instance prompt is missing.')
- if not validation_prompt:
- raise gr.Error('The validation prompt is missing.')
-
- resolution = int(resolution_s)
-
- if not output_model_name:
- timestamp = datetime.datetime.now().strftime('%Y-%m-%d-%H-%M-%S')
- output_model_name = f'lora-dreambooth-{timestamp}'
- output_model_name = slugify.slugify(output_model_name)
-
- repo_dir = pathlib.Path(__file__).parent
- output_dir = repo_dir / 'experiments' / output_model_name
- if overwrite_existing_model or upload_to_hub:
- shutil.rmtree(output_dir, ignore_errors=True)
- output_dir.mkdir(parents=True)
-
- instance_data_dir = repo_dir / 'training_data' / output_model_name
- self.prepare_dataset(instance_images, resolution, instance_data_dir)
-
- if upload_to_hub:
- self.join_lora_library_org()
-
- command = f'''
- accelerate launch train_dreambooth_lora.py \
- --pretrained_model_name_or_path={base_model} \
- --instance_data_dir={instance_data_dir} \
- --output_dir={output_dir} \
- --instance_prompt="{instance_prompt}" \
- --resolution={resolution} \
- --train_batch_size=1 \
- --gradient_accumulation_steps={gradient_accumulation} \
- --learning_rate={learning_rate} \
- --lr_scheduler=constant \
- --lr_warmup_steps=0 \
- --max_train_steps={n_steps} \
- --checkpointing_steps={checkpointing_steps} \
- --validation_prompt="{validation_prompt}" \
- --validation_epochs={validation_epochs} \
- --seed={seed}
- '''
- if fp16:
- command += ' --mixed_precision fp16'
- if use_8bit_adam:
- command += ' --use_8bit_adam'
- if use_wandb:
- command += ' --report_to wandb'
-
- with open(output_dir / 'train.sh', 'w') as f:
- command_s = ' '.join(command.split())
- f.write(command_s)
- subprocess.run(shlex.split(command))
- save_model_card(save_dir=output_dir,
- base_model=base_model,
- instance_prompt=instance_prompt,
- test_prompt=validation_prompt,
- test_image_dir='test_images')
-
- message = 'Training completed!'
- print(message)
-
- if upload_to_hub:
- upload_message = self.model_uploader.upload_lora_model(
- folder_path=output_dir.as_posix(),
- repo_name=output_model_name,
- upload_to=upload_to,
- private=use_private_repo,
- delete_existing_repo=delete_existing_repo)
- print(upload_message)
- message = message + '\n' + upload_message
-
- if remove_gpu_after_training:
- space_id = os.getenv('SPACE_ID')
- if space_id:
- self.api.request_space_hardware(repo_id=space_id,
- hardware='cpu-basic')
-
- return message
diff --git a/spaces/ECCV2022/PSG/OpenPSG/configs/imp/panoptic_fpn_r101_fpn_1x_sgdet_psg.py b/spaces/ECCV2022/PSG/OpenPSG/configs/imp/panoptic_fpn_r101_fpn_1x_sgdet_psg.py
deleted file mode 100644
index 7f0f96866d423e0f6a214e98462c721626744309..0000000000000000000000000000000000000000
--- a/spaces/ECCV2022/PSG/OpenPSG/configs/imp/panoptic_fpn_r101_fpn_1x_sgdet_psg.py
+++ /dev/null
@@ -1,26 +0,0 @@
-_base_ = './panoptic_fpn_r50_fpn_1x_sgdet_psg.py'
-
-model = dict(backbone=dict(
- depth=101,
- init_cfg=dict(type='Pretrained', checkpoint='torchvision://resnet101')))
-
-# Log config
-project_name = 'openpsg'
-expt_name = 'imp_panoptic_fpn_r101_fpn_1x_sgdet_psg'
-work_dir = f'./work_dirs/{expt_name}'
-
-log_config = dict(
- interval=50,
- hooks=[
- dict(type='TextLoggerHook'),
- dict(
- type='WandbLoggerHook',
- init_kwargs=dict(
- project=project_name,
- name=expt_name,
- ),
- ),
- ],
-)
-
-load_from = 'work_dirs/checkpoints/panoptic_fpn_r101_fpn_1x_coco_20210820_193950-ab9157a2.pth'
diff --git a/spaces/Eddycrack864/Applio-Inference/infer/lib/uvr5_pack/lib_v5/spec_utils.py b/spaces/Eddycrack864/Applio-Inference/infer/lib/uvr5_pack/lib_v5/spec_utils.py
deleted file mode 100644
index a9634fd51ff47bf90211839231774719154c37cf..0000000000000000000000000000000000000000
--- a/spaces/Eddycrack864/Applio-Inference/infer/lib/uvr5_pack/lib_v5/spec_utils.py
+++ /dev/null
@@ -1,672 +0,0 @@
-import hashlib
-import json
-import math
-import os
-
-import librosa
-import numpy as np
-import soundfile as sf
-from tqdm import tqdm
-
-
-def crop_center(h1, h2):
- h1_shape = h1.size()
- h2_shape = h2.size()
-
- if h1_shape[3] == h2_shape[3]:
- return h1
- elif h1_shape[3] < h2_shape[3]:
- raise ValueError("h1_shape[3] must be greater than h2_shape[3]")
-
- # s_freq = (h2_shape[2] - h1_shape[2]) // 2
- # e_freq = s_freq + h1_shape[2]
- s_time = (h1_shape[3] - h2_shape[3]) // 2
- e_time = s_time + h2_shape[3]
- h1 = h1[:, :, :, s_time:e_time]
-
- return h1
-
-
-def wave_to_spectrogram(
- wave, hop_length, n_fft, mid_side=False, mid_side_b2=False, reverse=False
-):
- if reverse:
- wave_left = np.flip(np.asfortranarray(wave[0]))
- wave_right = np.flip(np.asfortranarray(wave[1]))
- elif mid_side:
- wave_left = np.asfortranarray(np.add(wave[0], wave[1]) / 2)
- wave_right = np.asfortranarray(np.subtract(wave[0], wave[1]))
- elif mid_side_b2:
- wave_left = np.asfortranarray(np.add(wave[1], wave[0] * 0.5))
- wave_right = np.asfortranarray(np.subtract(wave[0], wave[1] * 0.5))
- else:
- wave_left = np.asfortranarray(wave[0])
- wave_right = np.asfortranarray(wave[1])
-
- spec_left = librosa.stft(wave_left, n_fft, hop_length=hop_length)
- spec_right = librosa.stft(wave_right, n_fft, hop_length=hop_length)
-
- spec = np.asfortranarray([spec_left, spec_right])
-
- return spec
-
-
-def wave_to_spectrogram_mt(
- wave, hop_length, n_fft, mid_side=False, mid_side_b2=False, reverse=False
-):
- import threading
-
- if reverse:
- wave_left = np.flip(np.asfortranarray(wave[0]))
- wave_right = np.flip(np.asfortranarray(wave[1]))
- elif mid_side:
- wave_left = np.asfortranarray(np.add(wave[0], wave[1]) / 2)
- wave_right = np.asfortranarray(np.subtract(wave[0], wave[1]))
- elif mid_side_b2:
- wave_left = np.asfortranarray(np.add(wave[1], wave[0] * 0.5))
- wave_right = np.asfortranarray(np.subtract(wave[0], wave[1] * 0.5))
- else:
- wave_left = np.asfortranarray(wave[0])
- wave_right = np.asfortranarray(wave[1])
-
- def run_thread(**kwargs):
- global spec_left
- spec_left = librosa.stft(**kwargs)
-
- thread = threading.Thread(
- target=run_thread,
- kwargs={"y": wave_left, "n_fft": n_fft, "hop_length": hop_length},
- )
- thread.start()
- spec_right = librosa.stft(wave_right, n_fft, hop_length=hop_length)
- thread.join()
-
- spec = np.asfortranarray([spec_left, spec_right])
-
- return spec
-
-
-def combine_spectrograms(specs, mp):
- l = min([specs[i].shape[2] for i in specs])
- spec_c = np.zeros(shape=(2, mp.param["bins"] + 1, l), dtype=np.complex64)
- offset = 0
- bands_n = len(mp.param["band"])
-
- for d in range(1, bands_n + 1):
- h = mp.param["band"][d]["crop_stop"] - mp.param["band"][d]["crop_start"]
- spec_c[:, offset : offset + h, :l] = specs[d][
- :, mp.param["band"][d]["crop_start"] : mp.param["band"][d]["crop_stop"], :l
- ]
- offset += h
-
- if offset > mp.param["bins"]:
- raise ValueError("Too much bins")
-
- # lowpass fiter
- if (
- mp.param["pre_filter_start"] > 0
- ): # and mp.param['band'][bands_n]['res_type'] in ['scipy', 'polyphase']:
- if bands_n == 1:
- spec_c = fft_lp_filter(
- spec_c, mp.param["pre_filter_start"], mp.param["pre_filter_stop"]
- )
- else:
- gp = 1
- for b in range(
- mp.param["pre_filter_start"] + 1, mp.param["pre_filter_stop"]
- ):
- g = math.pow(
- 10, -(b - mp.param["pre_filter_start"]) * (3.5 - gp) / 20.0
- )
- gp = g
- spec_c[:, b, :] *= g
-
- return np.asfortranarray(spec_c)
-
-
-def spectrogram_to_image(spec, mode="magnitude"):
- if mode == "magnitude":
- if np.iscomplexobj(spec):
- y = np.abs(spec)
- else:
- y = spec
- y = np.log10(y**2 + 1e-8)
- elif mode == "phase":
- if np.iscomplexobj(spec):
- y = np.angle(spec)
- else:
- y = spec
-
- y -= y.min()
- y *= 255 / y.max()
- img = np.uint8(y)
-
- if y.ndim == 3:
- img = img.transpose(1, 2, 0)
- img = np.concatenate([np.max(img, axis=2, keepdims=True), img], axis=2)
-
- return img
-
-
-def reduce_vocal_aggressively(X, y, softmask):
- v = X - y
- y_mag_tmp = np.abs(y)
- v_mag_tmp = np.abs(v)
-
- v_mask = v_mag_tmp > y_mag_tmp
- y_mag = np.clip(y_mag_tmp - v_mag_tmp * v_mask * softmask, 0, np.inf)
-
- return y_mag * np.exp(1.0j * np.angle(y))
-
-
-def mask_silence(mag, ref, thres=0.2, min_range=64, fade_size=32):
- if min_range < fade_size * 2:
- raise ValueError("min_range must be >= fade_area * 2")
-
- mag = mag.copy()
-
- idx = np.where(ref.mean(axis=(0, 1)) < thres)[0]
- starts = np.insert(idx[np.where(np.diff(idx) != 1)[0] + 1], 0, idx[0])
- ends = np.append(idx[np.where(np.diff(idx) != 1)[0]], idx[-1])
- uninformative = np.where(ends - starts > min_range)[0]
- if len(uninformative) > 0:
- starts = starts[uninformative]
- ends = ends[uninformative]
- old_e = None
- for s, e in zip(starts, ends):
- if old_e is not None and s - old_e < fade_size:
- s = old_e - fade_size * 2
-
- if s != 0:
- weight = np.linspace(0, 1, fade_size)
- mag[:, :, s : s + fade_size] += weight * ref[:, :, s : s + fade_size]
- else:
- s -= fade_size
-
- if e != mag.shape[2]:
- weight = np.linspace(1, 0, fade_size)
- mag[:, :, e - fade_size : e] += weight * ref[:, :, e - fade_size : e]
- else:
- e += fade_size
-
- mag[:, :, s + fade_size : e - fade_size] += ref[
- :, :, s + fade_size : e - fade_size
- ]
- old_e = e
-
- return mag
-
-
-def align_wave_head_and_tail(a, b):
- l = min([a[0].size, b[0].size])
-
- return a[:l, :l], b[:l, :l]
-
-
-def cache_or_load(mix_path, inst_path, mp):
- mix_basename = os.path.splitext(os.path.basename(mix_path))[0]
- inst_basename = os.path.splitext(os.path.basename(inst_path))[0]
-
- cache_dir = "mph{}".format(
- hashlib.sha1(json.dumps(mp.param, sort_keys=True).encode("utf-8")).hexdigest()
- )
- mix_cache_dir = os.path.join("cache", cache_dir)
- inst_cache_dir = os.path.join("cache", cache_dir)
-
- os.makedirs(mix_cache_dir, exist_ok=True)
- os.makedirs(inst_cache_dir, exist_ok=True)
-
- mix_cache_path = os.path.join(mix_cache_dir, mix_basename + ".npy")
- inst_cache_path = os.path.join(inst_cache_dir, inst_basename + ".npy")
-
- if os.path.exists(mix_cache_path) and os.path.exists(inst_cache_path):
- X_spec_m = np.load(mix_cache_path)
- y_spec_m = np.load(inst_cache_path)
- else:
- X_wave, y_wave, X_spec_s, y_spec_s = {}, {}, {}, {}
-
- for d in range(len(mp.param["band"]), 0, -1):
- bp = mp.param["band"][d]
-
- if d == len(mp.param["band"]): # high-end band
- X_wave[d], _ = librosa.load(
- mix_path, bp["sr"], False, dtype=np.float32, res_type=bp["res_type"]
- )
- y_wave[d], _ = librosa.load(
- inst_path,
- bp["sr"],
- False,
- dtype=np.float32,
- res_type=bp["res_type"],
- )
- else: # lower bands
- X_wave[d] = librosa.resample(
- X_wave[d + 1],
- mp.param["band"][d + 1]["sr"],
- bp["sr"],
- res_type=bp["res_type"],
- )
- y_wave[d] = librosa.resample(
- y_wave[d + 1],
- mp.param["band"][d + 1]["sr"],
- bp["sr"],
- res_type=bp["res_type"],
- )
-
- X_wave[d], y_wave[d] = align_wave_head_and_tail(X_wave[d], y_wave[d])
-
- X_spec_s[d] = wave_to_spectrogram(
- X_wave[d],
- bp["hl"],
- bp["n_fft"],
- mp.param["mid_side"],
- mp.param["mid_side_b2"],
- mp.param["reverse"],
- )
- y_spec_s[d] = wave_to_spectrogram(
- y_wave[d],
- bp["hl"],
- bp["n_fft"],
- mp.param["mid_side"],
- mp.param["mid_side_b2"],
- mp.param["reverse"],
- )
-
- del X_wave, y_wave
-
- X_spec_m = combine_spectrograms(X_spec_s, mp)
- y_spec_m = combine_spectrograms(y_spec_s, mp)
-
- if X_spec_m.shape != y_spec_m.shape:
- raise ValueError("The combined spectrograms are different: " + mix_path)
-
- _, ext = os.path.splitext(mix_path)
-
- np.save(mix_cache_path, X_spec_m)
- np.save(inst_cache_path, y_spec_m)
-
- return X_spec_m, y_spec_m
-
-
-def spectrogram_to_wave(spec, hop_length, mid_side, mid_side_b2, reverse):
- spec_left = np.asfortranarray(spec[0])
- spec_right = np.asfortranarray(spec[1])
-
- wave_left = librosa.istft(spec_left, hop_length=hop_length)
- wave_right = librosa.istft(spec_right, hop_length=hop_length)
-
- if reverse:
- return np.asfortranarray([np.flip(wave_left), np.flip(wave_right)])
- elif mid_side:
- return np.asfortranarray(
- [np.add(wave_left, wave_right / 2), np.subtract(wave_left, wave_right / 2)]
- )
- elif mid_side_b2:
- return np.asfortranarray(
- [
- np.add(wave_right / 1.25, 0.4 * wave_left),
- np.subtract(wave_left / 1.25, 0.4 * wave_right),
- ]
- )
- else:
- return np.asfortranarray([wave_left, wave_right])
-
-
-def spectrogram_to_wave_mt(spec, hop_length, mid_side, reverse, mid_side_b2):
- import threading
-
- spec_left = np.asfortranarray(spec[0])
- spec_right = np.asfortranarray(spec[1])
-
- def run_thread(**kwargs):
- global wave_left
- wave_left = librosa.istft(**kwargs)
-
- thread = threading.Thread(
- target=run_thread, kwargs={"stft_matrix": spec_left, "hop_length": hop_length}
- )
- thread.start()
- wave_right = librosa.istft(spec_right, hop_length=hop_length)
- thread.join()
-
- if reverse:
- return np.asfortranarray([np.flip(wave_left), np.flip(wave_right)])
- elif mid_side:
- return np.asfortranarray(
- [np.add(wave_left, wave_right / 2), np.subtract(wave_left, wave_right / 2)]
- )
- elif mid_side_b2:
- return np.asfortranarray(
- [
- np.add(wave_right / 1.25, 0.4 * wave_left),
- np.subtract(wave_left / 1.25, 0.4 * wave_right),
- ]
- )
- else:
- return np.asfortranarray([wave_left, wave_right])
-
-
-def cmb_spectrogram_to_wave(spec_m, mp, extra_bins_h=None, extra_bins=None):
- wave_band = {}
- bands_n = len(mp.param["band"])
- offset = 0
-
- for d in range(1, bands_n + 1):
- bp = mp.param["band"][d]
- spec_s = np.ndarray(
- shape=(2, bp["n_fft"] // 2 + 1, spec_m.shape[2]), dtype=complex
- )
- h = bp["crop_stop"] - bp["crop_start"]
- spec_s[:, bp["crop_start"] : bp["crop_stop"], :] = spec_m[
- :, offset : offset + h, :
- ]
-
- offset += h
- if d == bands_n: # higher
- if extra_bins_h: # if --high_end_process bypass
- max_bin = bp["n_fft"] // 2
- spec_s[:, max_bin - extra_bins_h : max_bin, :] = extra_bins[
- :, :extra_bins_h, :
- ]
- if bp["hpf_start"] > 0:
- spec_s = fft_hp_filter(spec_s, bp["hpf_start"], bp["hpf_stop"] - 1)
- if bands_n == 1:
- wave = spectrogram_to_wave(
- spec_s,
- bp["hl"],
- mp.param["mid_side"],
- mp.param["mid_side_b2"],
- mp.param["reverse"],
- )
- else:
- wave = np.add(
- wave,
- spectrogram_to_wave(
- spec_s,
- bp["hl"],
- mp.param["mid_side"],
- mp.param["mid_side_b2"],
- mp.param["reverse"],
- ),
- )
- else:
- sr = mp.param["band"][d + 1]["sr"]
- if d == 1: # lower
- spec_s = fft_lp_filter(spec_s, bp["lpf_start"], bp["lpf_stop"])
- wave = librosa.resample(
- spectrogram_to_wave(
- spec_s,
- bp["hl"],
- mp.param["mid_side"],
- mp.param["mid_side_b2"],
- mp.param["reverse"],
- ),
- bp["sr"],
- sr,
- res_type="sinc_fastest",
- )
- else: # mid
- spec_s = fft_hp_filter(spec_s, bp["hpf_start"], bp["hpf_stop"] - 1)
- spec_s = fft_lp_filter(spec_s, bp["lpf_start"], bp["lpf_stop"])
- wave2 = np.add(
- wave,
- spectrogram_to_wave(
- spec_s,
- bp["hl"],
- mp.param["mid_side"],
- mp.param["mid_side_b2"],
- mp.param["reverse"],
- ),
- )
- # wave = librosa.core.resample(wave2, bp['sr'], sr, res_type="sinc_fastest")
- wave = librosa.core.resample(wave2, bp["sr"], sr, res_type="scipy")
-
- return wave.T
-
-
-def fft_lp_filter(spec, bin_start, bin_stop):
- g = 1.0
- for b in range(bin_start, bin_stop):
- g -= 1 / (bin_stop - bin_start)
- spec[:, b, :] = g * spec[:, b, :]
-
- spec[:, bin_stop:, :] *= 0
-
- return spec
-
-
-def fft_hp_filter(spec, bin_start, bin_stop):
- g = 1.0
- for b in range(bin_start, bin_stop, -1):
- g -= 1 / (bin_start - bin_stop)
- spec[:, b, :] = g * spec[:, b, :]
-
- spec[:, 0 : bin_stop + 1, :] *= 0
-
- return spec
-
-
-def mirroring(a, spec_m, input_high_end, mp):
- if "mirroring" == a:
- mirror = np.flip(
- np.abs(
- spec_m[
- :,
- mp.param["pre_filter_start"]
- - 10
- - input_high_end.shape[1] : mp.param["pre_filter_start"]
- - 10,
- :,
- ]
- ),
- 1,
- )
- mirror = mirror * np.exp(1.0j * np.angle(input_high_end))
-
- return np.where(
- np.abs(input_high_end) <= np.abs(mirror), input_high_end, mirror
- )
-
- if "mirroring2" == a:
- mirror = np.flip(
- np.abs(
- spec_m[
- :,
- mp.param["pre_filter_start"]
- - 10
- - input_high_end.shape[1] : mp.param["pre_filter_start"]
- - 10,
- :,
- ]
- ),
- 1,
- )
- mi = np.multiply(mirror, input_high_end * 1.7)
-
- return np.where(np.abs(input_high_end) <= np.abs(mi), input_high_end, mi)
-
-
-def ensembling(a, specs):
- for i in range(1, len(specs)):
- if i == 1:
- spec = specs[0]
-
- ln = min([spec.shape[2], specs[i].shape[2]])
- spec = spec[:, :, :ln]
- specs[i] = specs[i][:, :, :ln]
-
- if "min_mag" == a:
- spec = np.where(np.abs(specs[i]) <= np.abs(spec), specs[i], spec)
- if "max_mag" == a:
- spec = np.where(np.abs(specs[i]) >= np.abs(spec), specs[i], spec)
-
- return spec
-
-
-def stft(wave, nfft, hl):
- wave_left = np.asfortranarray(wave[0])
- wave_right = np.asfortranarray(wave[1])
- spec_left = librosa.stft(wave_left, nfft, hop_length=hl)
- spec_right = librosa.stft(wave_right, nfft, hop_length=hl)
- spec = np.asfortranarray([spec_left, spec_right])
-
- return spec
-
-
-def istft(spec, hl):
- spec_left = np.asfortranarray(spec[0])
- spec_right = np.asfortranarray(spec[1])
-
- wave_left = librosa.istft(spec_left, hop_length=hl)
- wave_right = librosa.istft(spec_right, hop_length=hl)
- wave = np.asfortranarray([wave_left, wave_right])
-
-
-if __name__ == "__main__":
- import argparse
- import sys
- import time
-
- import cv2
- from model_param_init import ModelParameters
-
- p = argparse.ArgumentParser()
- p.add_argument(
- "--algorithm",
- "-a",
- type=str,
- choices=["invert", "invert_p", "min_mag", "max_mag", "deep", "align"],
- default="min_mag",
- )
- p.add_argument(
- "--model_params",
- "-m",
- type=str,
- default=os.path.join("modelparams", "1band_sr44100_hl512.json"),
- )
- p.add_argument("--output_name", "-o", type=str, default="output")
- p.add_argument("--vocals_only", "-v", action="store_true")
- p.add_argument("input", nargs="+")
- args = p.parse_args()
-
- start_time = time.time()
-
- if args.algorithm.startswith("invert") and len(args.input) != 2:
- raise ValueError("There should be two input files.")
-
- if not args.algorithm.startswith("invert") and len(args.input) < 2:
- raise ValueError("There must be at least two input files.")
-
- wave, specs = {}, {}
- mp = ModelParameters(args.model_params)
-
- for i in range(len(args.input)):
- spec = {}
-
- for d in range(len(mp.param["band"]), 0, -1):
- bp = mp.param["band"][d]
-
- if d == len(mp.param["band"]): # high-end band
- wave[d], _ = librosa.load(
- args.input[i],
- bp["sr"],
- False,
- dtype=np.float32,
- res_type=bp["res_type"],
- )
-
- if len(wave[d].shape) == 1: # mono to stereo
- wave[d] = np.array([wave[d], wave[d]])
- else: # lower bands
- wave[d] = librosa.resample(
- wave[d + 1],
- mp.param["band"][d + 1]["sr"],
- bp["sr"],
- res_type=bp["res_type"],
- )
-
- spec[d] = wave_to_spectrogram(
- wave[d],
- bp["hl"],
- bp["n_fft"],
- mp.param["mid_side"],
- mp.param["mid_side_b2"],
- mp.param["reverse"],
- )
-
- specs[i] = combine_spectrograms(spec, mp)
-
- del wave
-
- if args.algorithm == "deep":
- d_spec = np.where(np.abs(specs[0]) <= np.abs(spec[1]), specs[0], spec[1])
- v_spec = d_spec - specs[1]
- sf.write(
- os.path.join("{}.wav".format(args.output_name)),
- cmb_spectrogram_to_wave(v_spec, mp),
- mp.param["sr"],
- )
-
- if args.algorithm.startswith("invert"):
- ln = min([specs[0].shape[2], specs[1].shape[2]])
- specs[0] = specs[0][:, :, :ln]
- specs[1] = specs[1][:, :, :ln]
-
- if "invert_p" == args.algorithm:
- X_mag = np.abs(specs[0])
- y_mag = np.abs(specs[1])
- max_mag = np.where(X_mag >= y_mag, X_mag, y_mag)
- v_spec = specs[1] - max_mag * np.exp(1.0j * np.angle(specs[0]))
- else:
- specs[1] = reduce_vocal_aggressively(specs[0], specs[1], 0.2)
- v_spec = specs[0] - specs[1]
-
- if not args.vocals_only:
- X_mag = np.abs(specs[0])
- y_mag = np.abs(specs[1])
- v_mag = np.abs(v_spec)
-
- X_image = spectrogram_to_image(X_mag)
- y_image = spectrogram_to_image(y_mag)
- v_image = spectrogram_to_image(v_mag)
-
- cv2.imwrite("{}_X.png".format(args.output_name), X_image)
- cv2.imwrite("{}_y.png".format(args.output_name), y_image)
- cv2.imwrite("{}_v.png".format(args.output_name), v_image)
-
- sf.write(
- "{}_X.wav".format(args.output_name),
- cmb_spectrogram_to_wave(specs[0], mp),
- mp.param["sr"],
- )
- sf.write(
- "{}_y.wav".format(args.output_name),
- cmb_spectrogram_to_wave(specs[1], mp),
- mp.param["sr"],
- )
-
- sf.write(
- "{}_v.wav".format(args.output_name),
- cmb_spectrogram_to_wave(v_spec, mp),
- mp.param["sr"],
- )
- else:
- if not args.algorithm == "deep":
- sf.write(
- os.path.join("ensembled", "{}.wav".format(args.output_name)),
- cmb_spectrogram_to_wave(ensembling(args.algorithm, specs), mp),
- mp.param["sr"],
- )
-
- if args.algorithm == "align":
- trackalignment = [
- {
- "file1": '"{}"'.format(args.input[0]),
- "file2": '"{}"'.format(args.input[1]),
- }
- ]
-
- for i, e in tqdm(enumerate(trackalignment), desc="Performing Alignment..."):
- os.system(f"python lib/align_tracks.py {e['file1']} {e['file2']}")
-
- # print('Total time: {0:.{1}f}s'.format(time.time() - start_time, 1))
diff --git a/spaces/EleutherAI/VQGAN_CLIP/CLIP/clip/simple_tokenizer.py b/spaces/EleutherAI/VQGAN_CLIP/CLIP/clip/simple_tokenizer.py
deleted file mode 100644
index 0a66286b7d5019c6e221932a813768038f839c91..0000000000000000000000000000000000000000
--- a/spaces/EleutherAI/VQGAN_CLIP/CLIP/clip/simple_tokenizer.py
+++ /dev/null
@@ -1,132 +0,0 @@
-import gzip
-import html
-import os
-from functools import lru_cache
-
-import ftfy
-import regex as re
-
-
-@lru_cache()
-def default_bpe():
- return os.path.join(os.path.dirname(os.path.abspath(__file__)), "bpe_simple_vocab_16e6.txt.gz")
-
-
-@lru_cache()
-def bytes_to_unicode():
- """
- Returns list of utf-8 byte and a corresponding list of unicode strings.
- The reversible bpe codes work on unicode strings.
- This means you need a large # of unicode characters in your vocab if you want to avoid UNKs.
- When you're at something like a 10B token dataset you end up needing around 5K for decent coverage.
- This is a signficant percentage of your normal, say, 32K bpe vocab.
- To avoid that, we want lookup tables between utf-8 bytes and unicode strings.
- And avoids mapping to whitespace/control characters the bpe code barfs on.
- """
- bs = list(range(ord("!"), ord("~")+1))+list(range(ord("¡"), ord("¬")+1))+list(range(ord("®"), ord("ÿ")+1))
- cs = bs[:]
- n = 0
- for b in range(2**8):
- if b not in bs:
- bs.append(b)
- cs.append(2**8+n)
- n += 1
- cs = [chr(n) for n in cs]
- return dict(zip(bs, cs))
-
-
-def get_pairs(word):
- """Return set of symbol pairs in a word.
- Word is represented as tuple of symbols (symbols being variable-length strings).
- """
- pairs = set()
- prev_char = word[0]
- for char in word[1:]:
- pairs.add((prev_char, char))
- prev_char = char
- return pairs
-
-
-def basic_clean(text):
- text = ftfy.fix_text(text)
- text = html.unescape(html.unescape(text))
- return text.strip()
-
-
-def whitespace_clean(text):
- text = re.sub(r'\s+', ' ', text)
- text = text.strip()
- return text
-
-
-class SimpleTokenizer(object):
- def __init__(self, bpe_path: str = default_bpe()):
- self.byte_encoder = bytes_to_unicode()
- self.byte_decoder = {v: k for k, v in self.byte_encoder.items()}
- merges = gzip.open(bpe_path).read().decode("utf-8").split('\n')
- merges = merges[1:49152-256-2+1]
- merges = [tuple(merge.split()) for merge in merges]
- vocab = list(bytes_to_unicode().values())
- vocab = vocab + [v+'' for v in vocab]
- for merge in merges:
- vocab.append(''.join(merge))
- vocab.extend(['<|startoftext|>', '<|endoftext|>'])
- self.encoder = dict(zip(vocab, range(len(vocab))))
- self.decoder = {v: k for k, v in self.encoder.items()}
- self.bpe_ranks = dict(zip(merges, range(len(merges))))
- self.cache = {'<|startoftext|>': '<|startoftext|>', '<|endoftext|>': '<|endoftext|>'}
- self.pat = re.compile(r"""<\|startoftext\|>|<\|endoftext\|>|'s|'t|'re|'ve|'m|'ll|'d|[\p{L}]+|[\p{N}]|[^\s\p{L}\p{N}]+""", re.IGNORECASE)
-
- def bpe(self, token):
- if token in self.cache:
- return self.cache[token]
- word = tuple(token[:-1]) + ( token[-1] + '',)
- pairs = get_pairs(word)
-
- if not pairs:
- return token+''
-
- while True:
- bigram = min(pairs, key = lambda pair: self.bpe_ranks.get(pair, float('inf')))
- if bigram not in self.bpe_ranks:
- break
- first, second = bigram
- new_word = []
- i = 0
- while i < len(word):
- try:
- j = word.index(first, i)
- new_word.extend(word[i:j])
- i = j
- except:
- new_word.extend(word[i:])
- break
-
- if word[i] == first and i < len(word)-1 and word[i+1] == second:
- new_word.append(first+second)
- i += 2
- else:
- new_word.append(word[i])
- i += 1
- new_word = tuple(new_word)
- word = new_word
- if len(word) == 1:
- break
- else:
- pairs = get_pairs(word)
- word = ' '.join(word)
- self.cache[token] = word
- return word
-
- def encode(self, text):
- bpe_tokens = []
- text = whitespace_clean(basic_clean(text)).lower()
- for token in re.findall(self.pat, text):
- token = ''.join(self.byte_encoder[b] for b in token.encode('utf-8'))
- bpe_tokens.extend(self.encoder[bpe_token] for bpe_token in self.bpe(token).split(' '))
- return bpe_tokens
-
- def decode(self, tokens):
- text = ''.join([self.decoder[token] for token in tokens])
- text = bytearray([self.byte_decoder[c] for c in text]).decode('utf-8', errors="replace").replace('', ' ')
- return text
diff --git a/spaces/ErtugrulDemir/SpeechEmotionRecognition/README.md b/spaces/ErtugrulDemir/SpeechEmotionRecognition/README.md
deleted file mode 100644
index 7e8c9839776f55babd17eb153e9529232b3da460..0000000000000000000000000000000000000000
--- a/spaces/ErtugrulDemir/SpeechEmotionRecognition/README.md
+++ /dev/null
@@ -1,13 +0,0 @@
----
-title: SpeechEmotionRecognition
-emoji: 🏢
-colorFrom: blue
-colorTo: pink
-sdk: gradio
-sdk_version: 3.27.0
-app_file: app.py
-pinned: false
-license: apache-2.0
----
-
-Check out the configuration reference at https://huggingface.co/docs/hub/spaces-config-reference
diff --git a/spaces/EuroPython2022/mmocr-demo/configs/kie/sdmgr/sdmgr_unet16_60e_wildreceipt.py b/spaces/EuroPython2022/mmocr-demo/configs/kie/sdmgr/sdmgr_unet16_60e_wildreceipt.py
deleted file mode 100644
index f073064affebe05d3830e18d76453c1cceb0f1a1..0000000000000000000000000000000000000000
--- a/spaces/EuroPython2022/mmocr-demo/configs/kie/sdmgr/sdmgr_unet16_60e_wildreceipt.py
+++ /dev/null
@@ -1,105 +0,0 @@
-img_norm_cfg = dict(
- mean=[123.675, 116.28, 103.53], std=[58.395, 57.12, 57.375], to_rgb=True)
-max_scale, min_scale = 1024, 512
-
-train_pipeline = [
- dict(type='LoadImageFromFile'),
- dict(type='LoadAnnotations'),
- dict(type='Resize', img_scale=(max_scale, min_scale), keep_ratio=True),
- dict(type='RandomFlip', flip_ratio=0.),
- dict(type='Normalize', **img_norm_cfg),
- dict(type='Pad', size_divisor=32),
- dict(type='KIEFormatBundle'),
- dict(
- type='Collect',
- keys=['img', 'relations', 'texts', 'gt_bboxes', 'gt_labels'])
-]
-test_pipeline = [
- dict(type='LoadImageFromFile'),
- dict(type='LoadAnnotations'),
- dict(type='Resize', img_scale=(max_scale, min_scale), keep_ratio=True),
- dict(type='RandomFlip', flip_ratio=0.),
- dict(type='Normalize', **img_norm_cfg),
- dict(type='Pad', size_divisor=32),
- dict(type='KIEFormatBundle'),
- dict(
- type='Collect',
- keys=['img', 'relations', 'texts', 'gt_bboxes'],
- meta_keys=[
- 'img_norm_cfg', 'img_shape', 'ori_filename', 'filename',
- 'ori_texts'
- ])
-]
-
-dataset_type = 'KIEDataset'
-data_root = 'data/wildreceipt'
-
-loader = dict(
- type='HardDiskLoader',
- repeat=1,
- parser=dict(
- type='LineJsonParser',
- keys=['file_name', 'height', 'width', 'annotations']))
-
-train = dict(
- type=dataset_type,
- ann_file=f'{data_root}/train.txt',
- pipeline=train_pipeline,
- img_prefix=data_root,
- loader=loader,
- dict_file=f'{data_root}/dict.txt',
- test_mode=False)
-test = dict(
- type=dataset_type,
- ann_file=f'{data_root}/test.txt',
- pipeline=test_pipeline,
- img_prefix=data_root,
- loader=loader,
- dict_file=f'{data_root}/dict.txt',
- test_mode=True)
-
-data = dict(
- samples_per_gpu=4,
- workers_per_gpu=4,
- val_dataloader=dict(samples_per_gpu=1),
- test_dataloader=dict(samples_per_gpu=1),
- train=train,
- val=test,
- test=test)
-
-evaluation = dict(
- interval=1,
- metric='macro_f1',
- metric_options=dict(
- macro_f1=dict(
- ignores=[0, 2, 4, 6, 8, 10, 12, 14, 16, 18, 20, 22, 24, 25])))
-
-model = dict(
- type='SDMGR',
- backbone=dict(type='UNet', base_channels=16),
- bbox_head=dict(
- type='SDMGRHead', visual_dim=16, num_chars=92, num_classes=26),
- visual_modality=True,
- train_cfg=None,
- test_cfg=None,
- class_list=f'{data_root}/class_list.txt')
-
-optimizer = dict(type='Adam', weight_decay=0.0001)
-optimizer_config = dict(grad_clip=None)
-lr_config = dict(
- policy='step',
- warmup='linear',
- warmup_iters=1,
- warmup_ratio=1,
- step=[40, 50])
-total_epochs = 60
-
-checkpoint_config = dict(interval=1)
-log_config = dict(interval=50, hooks=[dict(type='TextLoggerHook')])
-dist_params = dict(backend='nccl')
-log_level = 'INFO'
-load_from = None
-resume_from = None
-workflow = [('train', 1)]
-
-find_unused_parameters = True
diff --git a/spaces/Faridmaruf/RVCV2MODEL/lib/infer_pack/transforms.py b/spaces/Faridmaruf/RVCV2MODEL/lib/infer_pack/transforms.py
deleted file mode 100644
index a11f799e023864ff7082c1f49c0cc18351a13b47..0000000000000000000000000000000000000000
--- a/spaces/Faridmaruf/RVCV2MODEL/lib/infer_pack/transforms.py
+++ /dev/null
@@ -1,209 +0,0 @@
-import torch
-from torch.nn import functional as F
-
-import numpy as np
-
-
-DEFAULT_MIN_BIN_WIDTH = 1e-3
-DEFAULT_MIN_BIN_HEIGHT = 1e-3
-DEFAULT_MIN_DERIVATIVE = 1e-3
-
-
-def piecewise_rational_quadratic_transform(
- inputs,
- unnormalized_widths,
- unnormalized_heights,
- unnormalized_derivatives,
- inverse=False,
- tails=None,
- tail_bound=1.0,
- min_bin_width=DEFAULT_MIN_BIN_WIDTH,
- min_bin_height=DEFAULT_MIN_BIN_HEIGHT,
- min_derivative=DEFAULT_MIN_DERIVATIVE,
-):
- if tails is None:
- spline_fn = rational_quadratic_spline
- spline_kwargs = {}
- else:
- spline_fn = unconstrained_rational_quadratic_spline
- spline_kwargs = {"tails": tails, "tail_bound": tail_bound}
-
- outputs, logabsdet = spline_fn(
- inputs=inputs,
- unnormalized_widths=unnormalized_widths,
- unnormalized_heights=unnormalized_heights,
- unnormalized_derivatives=unnormalized_derivatives,
- inverse=inverse,
- min_bin_width=min_bin_width,
- min_bin_height=min_bin_height,
- min_derivative=min_derivative,
- **spline_kwargs
- )
- return outputs, logabsdet
-
-
-def searchsorted(bin_locations, inputs, eps=1e-6):
- bin_locations[..., -1] += eps
- return torch.sum(inputs[..., None] >= bin_locations, dim=-1) - 1
-
-
-def unconstrained_rational_quadratic_spline(
- inputs,
- unnormalized_widths,
- unnormalized_heights,
- unnormalized_derivatives,
- inverse=False,
- tails="linear",
- tail_bound=1.0,
- min_bin_width=DEFAULT_MIN_BIN_WIDTH,
- min_bin_height=DEFAULT_MIN_BIN_HEIGHT,
- min_derivative=DEFAULT_MIN_DERIVATIVE,
-):
- inside_interval_mask = (inputs >= -tail_bound) & (inputs <= tail_bound)
- outside_interval_mask = ~inside_interval_mask
-
- outputs = torch.zeros_like(inputs)
- logabsdet = torch.zeros_like(inputs)
-
- if tails == "linear":
- unnormalized_derivatives = F.pad(unnormalized_derivatives, pad=(1, 1))
- constant = np.log(np.exp(1 - min_derivative) - 1)
- unnormalized_derivatives[..., 0] = constant
- unnormalized_derivatives[..., -1] = constant
-
- outputs[outside_interval_mask] = inputs[outside_interval_mask]
- logabsdet[outside_interval_mask] = 0
- else:
- raise RuntimeError("{} tails are not implemented.".format(tails))
-
- (
- outputs[inside_interval_mask],
- logabsdet[inside_interval_mask],
- ) = rational_quadratic_spline(
- inputs=inputs[inside_interval_mask],
- unnormalized_widths=unnormalized_widths[inside_interval_mask, :],
- unnormalized_heights=unnormalized_heights[inside_interval_mask, :],
- unnormalized_derivatives=unnormalized_derivatives[inside_interval_mask, :],
- inverse=inverse,
- left=-tail_bound,
- right=tail_bound,
- bottom=-tail_bound,
- top=tail_bound,
- min_bin_width=min_bin_width,
- min_bin_height=min_bin_height,
- min_derivative=min_derivative,
- )
-
- return outputs, logabsdet
-
-
-def rational_quadratic_spline(
- inputs,
- unnormalized_widths,
- unnormalized_heights,
- unnormalized_derivatives,
- inverse=False,
- left=0.0,
- right=1.0,
- bottom=0.0,
- top=1.0,
- min_bin_width=DEFAULT_MIN_BIN_WIDTH,
- min_bin_height=DEFAULT_MIN_BIN_HEIGHT,
- min_derivative=DEFAULT_MIN_DERIVATIVE,
-):
- if torch.min(inputs) < left or torch.max(inputs) > right:
- raise ValueError("Input to a transform is not within its domain")
-
- num_bins = unnormalized_widths.shape[-1]
-
- if min_bin_width * num_bins > 1.0:
- raise ValueError("Minimal bin width too large for the number of bins")
- if min_bin_height * num_bins > 1.0:
- raise ValueError("Minimal bin height too large for the number of bins")
-
- widths = F.softmax(unnormalized_widths, dim=-1)
- widths = min_bin_width + (1 - min_bin_width * num_bins) * widths
- cumwidths = torch.cumsum(widths, dim=-1)
- cumwidths = F.pad(cumwidths, pad=(1, 0), mode="constant", value=0.0)
- cumwidths = (right - left) * cumwidths + left
- cumwidths[..., 0] = left
- cumwidths[..., -1] = right
- widths = cumwidths[..., 1:] - cumwidths[..., :-1]
-
- derivatives = min_derivative + F.softplus(unnormalized_derivatives)
-
- heights = F.softmax(unnormalized_heights, dim=-1)
- heights = min_bin_height + (1 - min_bin_height * num_bins) * heights
- cumheights = torch.cumsum(heights, dim=-1)
- cumheights = F.pad(cumheights, pad=(1, 0), mode="constant", value=0.0)
- cumheights = (top - bottom) * cumheights + bottom
- cumheights[..., 0] = bottom
- cumheights[..., -1] = top
- heights = cumheights[..., 1:] - cumheights[..., :-1]
-
- if inverse:
- bin_idx = searchsorted(cumheights, inputs)[..., None]
- else:
- bin_idx = searchsorted(cumwidths, inputs)[..., None]
-
- input_cumwidths = cumwidths.gather(-1, bin_idx)[..., 0]
- input_bin_widths = widths.gather(-1, bin_idx)[..., 0]
-
- input_cumheights = cumheights.gather(-1, bin_idx)[..., 0]
- delta = heights / widths
- input_delta = delta.gather(-1, bin_idx)[..., 0]
-
- input_derivatives = derivatives.gather(-1, bin_idx)[..., 0]
- input_derivatives_plus_one = derivatives[..., 1:].gather(-1, bin_idx)[..., 0]
-
- input_heights = heights.gather(-1, bin_idx)[..., 0]
-
- if inverse:
- a = (inputs - input_cumheights) * (
- input_derivatives + input_derivatives_plus_one - 2 * input_delta
- ) + input_heights * (input_delta - input_derivatives)
- b = input_heights * input_derivatives - (inputs - input_cumheights) * (
- input_derivatives + input_derivatives_plus_one - 2 * input_delta
- )
- c = -input_delta * (inputs - input_cumheights)
-
- discriminant = b.pow(2) - 4 * a * c
- assert (discriminant >= 0).all()
-
- root = (2 * c) / (-b - torch.sqrt(discriminant))
- outputs = root * input_bin_widths + input_cumwidths
-
- theta_one_minus_theta = root * (1 - root)
- denominator = input_delta + (
- (input_derivatives + input_derivatives_plus_one - 2 * input_delta)
- * theta_one_minus_theta
- )
- derivative_numerator = input_delta.pow(2) * (
- input_derivatives_plus_one * root.pow(2)
- + 2 * input_delta * theta_one_minus_theta
- + input_derivatives * (1 - root).pow(2)
- )
- logabsdet = torch.log(derivative_numerator) - 2 * torch.log(denominator)
-
- return outputs, -logabsdet
- else:
- theta = (inputs - input_cumwidths) / input_bin_widths
- theta_one_minus_theta = theta * (1 - theta)
-
- numerator = input_heights * (
- input_delta * theta.pow(2) + input_derivatives * theta_one_minus_theta
- )
- denominator = input_delta + (
- (input_derivatives + input_derivatives_plus_one - 2 * input_delta)
- * theta_one_minus_theta
- )
- outputs = input_cumheights + numerator / denominator
-
- derivative_numerator = input_delta.pow(2) * (
- input_derivatives_plus_one * theta.pow(2)
- + 2 * input_delta * theta_one_minus_theta
- + input_derivatives * (1 - theta).pow(2)
- )
- logabsdet = torch.log(derivative_numerator) - 2 * torch.log(denominator)
-
- return outputs, logabsdet
diff --git a/spaces/Gen-Sim/Gen-Sim/scripts/metascripts/train3_cliport_indomain_small_demo50.sh b/spaces/Gen-Sim/Gen-Sim/scripts/metascripts/train3_cliport_indomain_small_demo50.sh
deleted file mode 100644
index 145d6bc978d426e351bbc150f4abdf87471e23fc..0000000000000000000000000000000000000000
--- a/spaces/Gen-Sim/Gen-Sim/scripts/metascripts/train3_cliport_indomain_small_demo50.sh
+++ /dev/null
@@ -1,14 +0,0 @@
-#!/bin/bash
-#SBATCH -c 10
-#SBATCH -n 1
-#SBATCH -o logs/%j.out
-#SBATCH --exclusive
-
-STEPS=${1-'15000'}
-now=$(date "+%Y-%m-%d_%H-%M-%S")
-
-sh scripts/traintest_scripts/train_test_multi_task_goal_demo50.sh data \
-"[stack-block-pyramid,put-block-in-bowl]" \
-"[stack-block-pyramid,put-block-in-bowl]" \
- cliport3_task_indomain_demo50_${now} $STEPS
-
diff --git a/spaces/Gladiator/gradient_dissent_bot/src/config.py b/spaces/Gladiator/gradient_dissent_bot/src/config.py
deleted file mode 100644
index b20b61d23a9b1eecc2a83dd46144205dcbc03870..0000000000000000000000000000000000000000
--- a/spaces/Gladiator/gradient_dissent_bot/src/config.py
+++ /dev/null
@@ -1,25 +0,0 @@
-from dataclasses import dataclass
-from pathlib import Path
-
-
-@dataclass
-class Config:
- playlist_url: str = "https://www.youtube.com/playlist?list=PLD80i8An1OEEb1jP0sjEyiLG8ULRXFob_"
-
- # paths
- root_data_dir: Path = Path("data")
- root_artifact_dir: Path = Path("downloaded_artifacts")
-
- # wandb
- project_name: str = "gradient_dissent_qabot"
- yt_podcast_data_artifact: str = "gladiator/gradient_dissent_qabot/yt_podcast_transcript:latest"
- summarized_data_artifact: str = "gladiator/gradient_dissent_qabot/summarized_podcasts:latest"
- summarized_que_data_artifact: str = (
- "gladiator/gradient_dissent_qabot/summarized_que_podcasts:latest"
- )
- transcript_embeddings_artifact: str = (
- "gladiator/gradient_dissent_qabot/transcript_embeddings:latest"
- )
-
-
-config = Config()
diff --git a/spaces/Gmq-x/gpt-academic/core_functional.py b/spaces/Gmq-x/gpt-academic/core_functional.py
deleted file mode 100644
index 536ccb609c38cbbebfda4ba17bd51a78857d711e..0000000000000000000000000000000000000000
--- a/spaces/Gmq-x/gpt-academic/core_functional.py
+++ /dev/null
@@ -1,71 +0,0 @@
-# 'primary' 颜色对应 theme.py 中的 primary_hue
-# 'secondary' 颜色对应 theme.py 中的 neutral_hue
-# 'stop' 颜色对应 theme.py 中的 color_er
-# 默认按钮颜色是 secondary
-from toolbox import clear_line_break
-
-
-def get_core_functions():
- return {
- "英语学术润色": {
- # 前言
- "Prefix": r"Below is a paragraph from an academic paper. Polish the writing to meet the academic style, " +
- r"improve the spelling, grammar, clarity, concision and overall readability. When necessary, rewrite the whole sentence. " +
- r"Furthermore, list all modification and explain the reasons to do so in markdown table." + "\n\n",
- # 后语
- "Suffix": r"",
- "Color": r"secondary", # 按钮颜色
- },
- "中文学术润色": {
- "Prefix": r"作为一名中文学术论文写作改进助理,你的任务是改进所提供文本的拼写、语法、清晰、简洁和整体可读性," +
- r"同时分解长句,减少重复,并提供改进建议。请只提供文本的更正版本,避免包括解释。请编辑以下文本" + "\n\n",
- "Suffix": r"",
- },
- "查找语法错误": {
- "Prefix": r"Can you help me ensure that the grammar and the spelling is correct? " +
- r"Do not try to polish the text, if no mistake is found, tell me that this paragraph is good." +
- r"If you find grammar or spelling mistakes, please list mistakes you find in a two-column markdown table, " +
- r"put the original text the first column, " +
- r"put the corrected text in the second column and highlight the key words you fixed.""\n"
- r"Example:""\n"
- r"Paragraph: How is you? Do you knows what is it?""\n"
- r"| Original sentence | Corrected sentence |""\n"
- r"| :--- | :--- |""\n"
- r"| How **is** you? | How **are** you? |""\n"
- r"| Do you **knows** what **is** **it**? | Do you **know** what **it** **is** ? |""\n"
- r"Below is a paragraph from an academic paper. "
- r"You need to report all grammar and spelling mistakes as the example before."
- + "\n\n",
- "Suffix": r"",
- "PreProcess": clear_line_break, # 预处理:清除换行符
- },
- "中译英": {
- "Prefix": r"Please translate following sentence to English:" + "\n\n",
- "Suffix": r"",
- },
- "学术中英互译": {
- "Prefix": r"I want you to act as a scientific English-Chinese translator, " +
- r"I will provide you with some paragraphs in one language " +
- r"and your task is to accurately and academically translate the paragraphs only into the other language. " +
- r"Do not repeat the original provided paragraphs after translation. " +
- r"You should use artificial intelligence tools, " +
- r"such as natural language processing, and rhetorical knowledge " +
- r"and experience about effective writing techniques to reply. " +
- r"I'll give you my paragraphs as follows, tell me what language it is written in, and then translate:" + "\n\n",
- "Suffix": "",
- "Color": "secondary",
- },
- "英译中": {
- "Prefix": r"翻译成地道的中文:" + "\n\n",
- "Suffix": r"",
- },
- "找图片": {
- "Prefix": r"我需要你找一张网络图片。使用Unsplash API(https://source.unsplash.com/960x640/?<英语关键词>)获取图片URL," +
- r"然后请使用Markdown格式封装,并且不要有反斜线,不要用代码块。现在,请按以下描述给我发送图片:" + "\n\n",
- "Suffix": r"",
- },
- "解释代码": {
- "Prefix": r"请解释以下代码:" + "\n```\n",
- "Suffix": "\n```\n",
- },
- }
diff --git a/spaces/GotAudio/Understanding-Women/README.md b/spaces/GotAudio/Understanding-Women/README.md
deleted file mode 100644
index e579f881df31c9a218928f470725fea0a59018c9..0000000000000000000000000000000000000000
--- a/spaces/GotAudio/Understanding-Women/README.md
+++ /dev/null
@@ -1,46 +0,0 @@
----
-title: Understanding Women
-emoji: 💩
-colorFrom: blue
-colorTo: pink
-sdk: gradio
-app_file: app.py
-pinned: false
-license: cc-by-4.0
----
-
-# Configuration
-
-`title`: _string_
-Display title for the Space
-
-`emoji`: _string_
-Space emoji (emoji-only character allowed)
-
-`colorFrom`: _string_
-Color for Thumbnail gradient (red, yellow, green, blue, indigo, purple, pink, gray)
-
-`colorTo`: _string_
-Color for Thumbnail gradient (red, yellow, green, blue, indigo, purple, pink, gray)
-
-`sdk`: _string_
-Can be either `gradio`, `streamlit`, or `static`
-
-`sdk_version` : _string_
-Only applicable for `streamlit` SDK.
-See [doc](https://hf.co/docs/hub/spaces) for more info on supported versions.
-
-`app_file`: _string_
-Path to your main application file (which contains either `gradio` or `streamlit` Python code, or `static` html code).
-Path is relative to the root of the repository.
-
-`models`: _List[string]_
-HF model IDs (like "gpt2" or "deepset/roberta-base-squad2") used in the Space.
-Will be parsed automatically from your code if not specified here.
-
-`datasets`: _List[string]_
-HF dataset IDs (like "common_voice" or "oscar-corpus/OSCAR-2109") used in the Space.
-Will be parsed automatically from your code if not specified here.
-
-`pinned`: _boolean_
-Whether the Space stays on top of your list.
diff --git a/spaces/Gradio-Blocks/Pipeline-Tester/app.py b/spaces/Gradio-Blocks/Pipeline-Tester/app.py
deleted file mode 100644
index 17ac352cc01f6cf31038b79c4938978977dc3780..0000000000000000000000000000000000000000
--- a/spaces/Gradio-Blocks/Pipeline-Tester/app.py
+++ /dev/null
@@ -1,67 +0,0 @@
-import gradio as gr
-
-from transformers import pipeline
-
-def text_pipelines(text_txt, text_pipes):
- if text_pipes == "Translate En to Fr":
- en_fr_translator = pipeline("translation_en_to_fr")
- output = en_fr_translator(f"{text_txt}")[0]["translation_text"]
- elif text_pipes == "Text Generation":
- text_generation = pipeline("text-generation")
- output = text_generation(f"{text_txt}")[0]["generated_text"]
- elif text_pipes == "Sentiment Analysis":
- sentiment_analysis = pipeline("sentiment-analysis")
- output = sentiment_analysis(f"{text_txt}")
- return output
-
-def cat_images(cat_slider):
- if cat_slider < 10:
- images = ["./images/dog1.jpg", "./images/dog2.jpg"]
- if cat_slider >= 10:
- images = ["./images/cat1.jpg", "./images/cat2.jpg", "./images/cat3.jpg"]
- return images
-
-with gr.Blocks() as Blocks:
- with gr.Row():
- gr.Markdown("
🤗 Hugging Face Pipelines 🤗
🥳🥳 Welcome to the block party! 🥳🥳
")
-
- with gr.Row():
- with gr.Column():
- gr.Markdown("
")
- with gr.Tabs():
- with gr.TabItem("Audio Classification"):
- gr.Markdown("
🔊Audio Classification
Classifies your audio!
You could: Label emotions, such as happy or sad.😊😢
")
- with gr.TabItem("Automatic Speech Recognition"):
- gr.Markdown("
💬Automatic Speech Recognition
Recognizes speech automatically!
You could: Create transcripts. 📃
")
- with gr.TabItem("Image Segmentation"):
- gr.Markdown("
🖼️Image Segmentation
Segments images!
You could: Highlight the area that has a cat
There are all kinds of pipelines: image, text, audio!
⬇️ Try some of them out below ⬇️
")
- with gr.Column():
- gr.Markdown("
")
- with gr.Column():
- with gr.Tabs():
- with gr.TabItem("What's a Pipeline?!"):
- gr.Markdown("Easy mode! Use the pipeline() to streamline everything in like 4 lines of code. It's even smart enough to pick a model for you if you want. 🤗")
- with gr.TabItem("What's Gradio?"):
- gr.Markdown("The way to make layouts super quickly! You can add dropdown boxes, radio buttons, checkboxes and more which will be able to change the inputs to your functions.")
- with gr.Tabs():
- with gr.TabItem("Block Party!"):
- gr.Markdown("
This was created during Hugging Face's Block Party to celebrate the release of Gradio's new block function.
The app was created using a block with 4 rows, and the info box you are reading was made using Gradio Tabs!
Thank you ever so much for your likes! ❤️
")
- with gr.TabItem("Cat Tax"):
- cat_slider = gr.Slider(0, 100, label="Percentage you like cats:")
- cats_but = gr.Button("Show cute cats!")
- gallery = gr.Gallery()
-
- with gr.Row():
- gr.Markdown("
- """
- )
-
-demo.launch()
diff --git a/spaces/OFA-Sys/OFA-Generic_Interface/fairseq/examples/speech_recognition/new/decoders/viterbi_decoder.py b/spaces/OFA-Sys/OFA-Generic_Interface/fairseq/examples/speech_recognition/new/decoders/viterbi_decoder.py
deleted file mode 100644
index b1c47868fa3b4e21f939b0695ede8d14ba1b168d..0000000000000000000000000000000000000000
--- a/spaces/OFA-Sys/OFA-Generic_Interface/fairseq/examples/speech_recognition/new/decoders/viterbi_decoder.py
+++ /dev/null
@@ -1,24 +0,0 @@
-#!/usr/bin/env python3
-
-# Copyright (c) Facebook, Inc. and its affiliates.
-#
-# This source code is licensed under the MIT license found in the
-# LICENSE file in the root directory of this source tree.
-
-import torch
-
-from typing import List, Dict
-
-from .base_decoder import BaseDecoder
-
-
-class ViterbiDecoder(BaseDecoder):
- def decode(
- self,
- emissions: torch.FloatTensor,
- ) -> List[List[Dict[str, torch.LongTensor]]]:
- def get_pred(e):
- toks = e.argmax(dim=-1).unique_consecutive()
- return toks[toks != self.blank]
-
- return [[{"tokens": get_pred(x), "score": 0}] for x in emissions]
diff --git a/spaces/OFA-Sys/OFA-Generic_Interface/fairseq/examples/textless_nlp/gslm/speech2unit/pretrained/hubert_feature_reader.py b/spaces/OFA-Sys/OFA-Generic_Interface/fairseq/examples/textless_nlp/gslm/speech2unit/pretrained/hubert_feature_reader.py
deleted file mode 100644
index 09442206e19abf854f2f02754ec7c6f8bc564200..0000000000000000000000000000000000000000
--- a/spaces/OFA-Sys/OFA-Generic_Interface/fairseq/examples/textless_nlp/gslm/speech2unit/pretrained/hubert_feature_reader.py
+++ /dev/null
@@ -1,59 +0,0 @@
-# Copyright (c) Facebook, Inc. and its affiliates.
-#
-# This source code is licensed under the MIT license found in the
-# LICENSE file in the root directory of this source tree.
-
-import torch
-import fairseq
-import soundfile as sf
-import torch.nn.functional as F
-
-
-class HubertFeatureReader:
- """
- Wrapper class to run inference on HuBERT model.
- Helps extract features for a given audio file.
- """
-
- def __init__(self, checkpoint_path, layer, max_chunk=1600000):
- (
- model,
- cfg,
- task,
- ) = fairseq.checkpoint_utils.load_model_ensemble_and_task(
- [checkpoint_path]
- )
- self.model = model[0].eval().cuda()
- self.task = task
- self.layer = layer
- self.max_chunk = max_chunk
-
- def read_audio(self, path, ref_len=None):
- wav, sr = sf.read(path)
- if wav.ndim == 2:
- wav = wav.mean(-1)
- assert wav.ndim == 1, wav.ndim
- assert sr == self.task.cfg.sample_rate, sr
- if ref_len is not None and abs(ref_len - len(wav)) > 160:
- print(f"ref {ref_len} != read {len(wav)} ({path})")
- return wav
-
- def get_feats(self, file_path, ref_len=None):
- x = self.read_audio(file_path, ref_len)
- with torch.no_grad():
- x = torch.from_numpy(x).float().cuda()
- if self.task.cfg.normalize:
- x = F.layer_norm(x, x.shape)
- x = x.view(1, -1)
-
- feat = []
- for start in range(0, x.size(1), self.max_chunk):
- x_chunk = x[:, start: start + self.max_chunk]
- feat_chunk, _ = self.model.extract_features(
- source=x_chunk,
- padding_mask=None,
- mask=False,
- output_layer=self.layer,
- )
- feat.append(feat_chunk)
- return torch.cat(feat, 1).squeeze(0)
diff --git a/spaces/OFA-Sys/OFA-Generic_Interface/fairseq/fairseq/tasks/translation_lev.py b/spaces/OFA-Sys/OFA-Generic_Interface/fairseq/fairseq/tasks/translation_lev.py
deleted file mode 100644
index 041279305dc4978f6a3a4178c5ec4c72c5fb2b5c..0000000000000000000000000000000000000000
--- a/spaces/OFA-Sys/OFA-Generic_Interface/fairseq/fairseq/tasks/translation_lev.py
+++ /dev/null
@@ -1,191 +0,0 @@
-# Copyright (c) Facebook, Inc. and its affiliates.
-#
-# This source code is licensed under the MIT license found in the
-# LICENSE file in the root directory of this source tree.
-
-from dataclasses import dataclass, field
-import torch
-from fairseq import utils
-from fairseq.data import LanguagePairDataset
-from fairseq.dataclass import ChoiceEnum
-from fairseq.tasks import register_task
-from fairseq.tasks.translation import TranslationConfig, TranslationTask, load_langpair_dataset
-from fairseq.utils import new_arange
-
-
-NOISE_CHOICES = ChoiceEnum(["random_delete", "random_mask", "no_noise", "full_mask"])
-
-@dataclass
-class TranslationLevenshteinConfig(TranslationConfig):
- noise: NOISE_CHOICES = field(
- default="random_delete",
- metadata={
- "help": "type of noise"
- },
- )
-
-@register_task("translation_lev", dataclass=TranslationLevenshteinConfig)
-class TranslationLevenshteinTask(TranslationTask):
- """
- Translation (Sequence Generation) task for Levenshtein Transformer
- See `"Levenshtein Transformer" `_.
- """
-
- cfg: TranslationLevenshteinConfig
-
- def load_dataset(self, split, epoch=1, combine=False, **kwargs):
- """Load a given dataset split.
-
- Args:
- split (str): name of the split (e.g., train, valid, test)
- """
- paths = utils.split_paths(self.cfg.data)
- assert len(paths) > 0
- data_path = paths[(epoch - 1) % len(paths)]
-
- # infer langcode
- src, tgt = self.cfg.source_lang, self.cfg.target_lang
-
- self.datasets[split] = load_langpair_dataset(
- data_path,
- split,
- src,
- self.src_dict,
- tgt,
- self.tgt_dict,
- combine=combine,
- dataset_impl=self.cfg.dataset_impl,
- upsample_primary=self.cfg.upsample_primary,
- left_pad_source=self.cfg.left_pad_source,
- left_pad_target=self.cfg.left_pad_target,
- max_source_positions=self.cfg.max_source_positions,
- max_target_positions=self.cfg.max_target_positions,
- prepend_bos=True,
- )
-
- def inject_noise(self, target_tokens):
- def _random_delete(target_tokens):
- pad = self.tgt_dict.pad()
- bos = self.tgt_dict.bos()
- eos = self.tgt_dict.eos()
-
- max_len = target_tokens.size(1)
- target_mask = target_tokens.eq(pad)
- target_score = target_tokens.clone().float().uniform_()
- target_score.masked_fill_(
- target_tokens.eq(bos) | target_tokens.eq(eos), 0.0
- )
- target_score.masked_fill_(target_mask, 1)
- target_score, target_rank = target_score.sort(1)
- target_length = target_mask.size(1) - target_mask.float().sum(
- 1, keepdim=True
- )
-
- # do not delete and (we assign 0 score for them)
- target_cutoff = (
- 2
- + (
- (target_length - 2)
- * target_score.new_zeros(target_score.size(0), 1).uniform_()
- ).long()
- )
- target_cutoff = target_score.sort(1)[1] >= target_cutoff
-
- prev_target_tokens = (
- target_tokens.gather(1, target_rank)
- .masked_fill_(target_cutoff, pad)
- .gather(1, target_rank.masked_fill_(target_cutoff, max_len).sort(1)[1])
- )
- prev_target_tokens = prev_target_tokens[
- :, : prev_target_tokens.ne(pad).sum(1).max()
- ]
-
- return prev_target_tokens
-
- def _random_mask(target_tokens):
- pad = self.tgt_dict.pad()
- bos = self.tgt_dict.bos()
- eos = self.tgt_dict.eos()
- unk = self.tgt_dict.unk()
-
- target_masks = (
- target_tokens.ne(pad) & target_tokens.ne(bos) & target_tokens.ne(eos)
- )
- target_score = target_tokens.clone().float().uniform_()
- target_score.masked_fill_(~target_masks, 2.0)
- target_length = target_masks.sum(1).float()
- target_length = target_length * target_length.clone().uniform_()
- target_length = target_length + 1 # make sure to mask at least one token.
-
- _, target_rank = target_score.sort(1)
- target_cutoff = new_arange(target_rank) < target_length[:, None].long()
- prev_target_tokens = target_tokens.masked_fill(
- target_cutoff.scatter(1, target_rank, target_cutoff), unk
- )
- return prev_target_tokens
-
- def _full_mask(target_tokens):
- pad = self.tgt_dict.pad()
- bos = self.tgt_dict.bos()
- eos = self.tgt_dict.eos()
- unk = self.tgt_dict.unk()
-
- target_mask = (
- target_tokens.eq(bos) | target_tokens.eq(eos) | target_tokens.eq(pad)
- )
- return target_tokens.masked_fill(~target_mask, unk)
-
- if self.cfg.noise == "random_delete":
- return _random_delete(target_tokens)
- elif self.cfg.noise == "random_mask":
- return _random_mask(target_tokens)
- elif self.cfg.noise == "full_mask":
- return _full_mask(target_tokens)
- elif self.cfg.noise == "no_noise":
- return target_tokens
- else:
- raise NotImplementedError
-
- def build_generator(self, models, args, **unused):
- # add models input to match the API for SequenceGenerator
- from fairseq.iterative_refinement_generator import IterativeRefinementGenerator
-
- return IterativeRefinementGenerator(
- self.target_dictionary,
- eos_penalty=getattr(args, "iter_decode_eos_penalty", 0.0),
- max_iter=getattr(args, "iter_decode_max_iter", 10),
- beam_size=getattr(args, "iter_decode_with_beam", 1),
- reranking=getattr(args, "iter_decode_with_external_reranker", False),
- decoding_format=getattr(args, "decoding_format", None),
- adaptive=not getattr(args, "iter_decode_force_max_iter", False),
- retain_history=getattr(args, "retain_iter_history", False),
- )
-
- def build_dataset_for_inference(self, src_tokens, src_lengths, constraints=None):
- if constraints is not None:
- # Though see Susanto et al. (ACL 2020): https://www.aclweb.org/anthology/2020.acl-main.325/
- raise NotImplementedError(
- "Constrained decoding with the translation_lev task is not supported"
- )
-
- return LanguagePairDataset(
- src_tokens, src_lengths, self.source_dictionary, append_bos=True
- )
-
- def train_step(
- self, sample, model, criterion, optimizer, update_num, ignore_grad=False
- ):
- model.train()
- sample["prev_target"] = self.inject_noise(sample["target"])
- loss, sample_size, logging_output = criterion(model, sample)
- if ignore_grad:
- loss *= 0
- optimizer.backward(loss)
- return loss, sample_size, logging_output
-
- def valid_step(self, sample, model, criterion):
- model.eval()
- with torch.no_grad():
- sample["prev_target"] = self.inject_noise(sample["target"])
- loss, sample_size, logging_output = criterion(model, sample)
- return loss, sample_size, logging_output
diff --git a/spaces/OFA-Sys/OFA-Image_Caption/fairseq/examples/language_model/README.adaptive_inputs.md b/spaces/OFA-Sys/OFA-Image_Caption/fairseq/examples/language_model/README.adaptive_inputs.md
deleted file mode 100644
index 6650d58f37f320aa46402d59ce6494b2dd1c3faa..0000000000000000000000000000000000000000
--- a/spaces/OFA-Sys/OFA-Image_Caption/fairseq/examples/language_model/README.adaptive_inputs.md
+++ /dev/null
@@ -1,39 +0,0 @@
-# Adaptive Input Representations for Neural Language Modeling (Baevski and Auli, 2018)
-
-## Pre-trained models
-
-Description | Parameters | Dataset | Model and Test set(s)
----|---:|---|---
-Adaptive Inputs ([Baevski and Auli, 2018](https://arxiv.org/abs/1809.10853)) | 1026M | [Google Billion Words](https://github.com/ciprian-chelba/1-billion-word-language-modeling-benchmark) | [download (.tar.bz2)](https://dl.fbaipublicfiles.com/fairseq/models/lm/adaptive_lm_gbw_huge.tar.bz2)
-Adaptive Inputs ([Baevski and Auli, 2018](https://arxiv.org/abs/1809.10853)) | 247M | [WikiText-103](https://blog.einstein.ai/the-wikitext-long-term-dependency-language-modeling-dataset/) | [download (.tar.bz2)](https://dl.fbaipublicfiles.com/fairseq/models/lm/adaptive_lm_wiki103.v2.tar.bz2)
-
-## Training an LM with adaptive inputs
-
-First, see the general [language modeling README](README.md) for instructions on
-preprocessing the WikiText-103 data.
-
-Then use the following training command to train a model with adaptive inputs
-using the `transformer_lm_wiki103` model architecture:
-```bash
-fairseq-train --task language_modeling \
- data-bin/wikitext-103 \
- --save-dir checkpoints/transformer_wikitext-103 \
- --arch transformer_lm_wiki103 \
- --max-update 286000 --lr 1.0 --t-mult 2 --lr-period-updates 270000 --lr-scheduler cosine --lr-shrink 0.75 \
- --warmup-updates 16000 --warmup-init-lr 1e-07 --stop-min-lr 1e-09 --optimizer nag --min-lr 0.0001 --clip-norm 0.1 \
- --criterion adaptive_loss --max-tokens 3072 --update-freq 3 --tokens-per-sample 3072 --seed 1 \
- --sample-break-mode none --skip-invalid-size-inputs-valid-test --ddp-backend=legacy_ddp
-```
-
-## Citation
-
-```bibtex
-@inproceedings{
- baevski2018adaptive,
- title={Adaptive Input Representations for Neural Language Modeling},
- author={Alexei Baevski and Michael Auli},
- booktitle={International Conference on Learning Representations},
- year={2019},
- url={https://openreview.net/forum?id=ByxZX20qFQ},
-}
-```
diff --git a/spaces/OFA-Sys/OFA-Image_Caption/fairseq/examples/speech_to_text/simultaneous_translation/agents/fairseq_simul_st_agent.py b/spaces/OFA-Sys/OFA-Image_Caption/fairseq/examples/speech_to_text/simultaneous_translation/agents/fairseq_simul_st_agent.py
deleted file mode 100644
index 61617a1739ce196abba1e9a6f9ad9e9f4b37b9c1..0000000000000000000000000000000000000000
--- a/spaces/OFA-Sys/OFA-Image_Caption/fairseq/examples/speech_to_text/simultaneous_translation/agents/fairseq_simul_st_agent.py
+++ /dev/null
@@ -1,363 +0,0 @@
-import math
-import os
-import json
-import numpy as np
-import torch
-import torchaudio.compliance.kaldi as kaldi
-import yaml
-from fairseq import checkpoint_utils, tasks
-from fairseq.file_io import PathManager
-
-try:
- from simuleval import READ_ACTION, WRITE_ACTION, DEFAULT_EOS
- from simuleval.agents import SpeechAgent
- from simuleval.states import ListEntry, SpeechStates
-except ImportError:
- print("Please install simuleval 'pip install simuleval'")
-
-SHIFT_SIZE = 10
-WINDOW_SIZE = 25
-SAMPLE_RATE = 16000
-FEATURE_DIM = 80
-BOW_PREFIX = "\u2581"
-
-
-class OnlineFeatureExtractor:
- """
- Extract speech feature on the fly.
- """
-
- def __init__(self, args):
- self.shift_size = args.shift_size
- self.window_size = args.window_size
- assert self.window_size >= self.shift_size
-
- self.sample_rate = args.sample_rate
- self.feature_dim = args.feature_dim
- self.num_samples_per_shift = int(self.shift_size * self.sample_rate / 1000)
- self.num_samples_per_window = int(self.window_size * self.sample_rate / 1000)
- self.len_ms_to_samples = lambda x: x * self.sample_rate / 1000
- self.previous_residual_samples = []
- self.global_cmvn = args.global_cmvn
-
- def clear_cache(self):
- self.previous_residual_samples = []
-
- def __call__(self, new_samples):
- samples = self.previous_residual_samples + new_samples
- if len(samples) < self.num_samples_per_window:
- self.previous_residual_samples = samples
- return
-
- # num_frames is the number of frames from the new segment
- num_frames = math.floor(
- (len(samples) - self.len_ms_to_samples(self.window_size - self.shift_size))
- / self.num_samples_per_shift
- )
-
- # the number of frames used for feature extraction
- # including some part of thte previous segment
- effective_num_samples = int(
- num_frames * self.len_ms_to_samples(self.shift_size)
- + self.len_ms_to_samples(self.window_size - self.shift_size)
- )
-
- input_samples = samples[:effective_num_samples]
- self.previous_residual_samples = samples[
- num_frames * self.num_samples_per_shift:
- ]
-
- torch.manual_seed(1)
- output = kaldi.fbank(
- torch.FloatTensor(input_samples).unsqueeze(0),
- num_mel_bins=self.feature_dim,
- frame_length=self.window_size,
- frame_shift=self.shift_size,
- ).numpy()
-
- output = self.transform(output)
-
- return torch.from_numpy(output)
-
- def transform(self, input):
- if self.global_cmvn is None:
- return input
-
- mean = self.global_cmvn["mean"]
- std = self.global_cmvn["std"]
-
- x = np.subtract(input, mean)
- x = np.divide(x, std)
- return x
-
-
-class TensorListEntry(ListEntry):
- """
- Data structure to store a list of tensor.
- """
-
- def append(self, value):
-
- if len(self.value) == 0:
- self.value = value
- return
-
- self.value = torch.cat([self.value] + [value], dim=0)
-
- def info(self):
- return {
- "type": str(self.new_value_type),
- "length": self.__len__(),
- "value": "" if type(self.value) is list else self.value.size(),
- }
-
-
-class FairseqSimulSTAgent(SpeechAgent):
-
- speech_segment_size = 40 # in ms, 4 pooling ratio * 10 ms step size
-
- def __init__(self, args):
- super().__init__(args)
-
- self.eos = DEFAULT_EOS
-
- self.gpu = getattr(args, "gpu", False)
-
- self.args = args
-
- self.load_model_vocab(args)
-
- if getattr(
- self.model.decoder.layers[0].encoder_attn,
- 'pre_decision_ratio',
- None
- ) is not None:
- self.speech_segment_size *= (
- self.model.decoder.layers[0].encoder_attn.pre_decision_ratio
- )
-
- args.global_cmvn = None
- if args.config:
- with open(os.path.join(args.data_bin, args.config), "r") as f:
- config = yaml.load(f, Loader=yaml.BaseLoader)
-
- if "global_cmvn" in config:
- args.global_cmvn = np.load(config["global_cmvn"]["stats_npz_path"])
-
- if args.global_stats:
- with PathManager.open(args.global_stats, "r") as f:
- global_cmvn = json.loads(f.read())
- self.global_cmvn = {"mean": global_cmvn["mean"], "std": global_cmvn["stddev"]}
-
- self.feature_extractor = OnlineFeatureExtractor(args)
-
- self.max_len = args.max_len
-
- self.force_finish = args.force_finish
-
- torch.set_grad_enabled(False)
-
- def build_states(self, args, client, sentence_id):
- # Initialize states here, for example add customized entry to states
- # This function will be called at beginning of every new sentence
- states = SpeechStates(args, client, sentence_id, self)
- self.initialize_states(states)
- return states
-
- def to_device(self, tensor):
- if self.gpu:
- return tensor.cuda()
- else:
- return tensor.cpu()
-
- @staticmethod
- def add_args(parser):
- # fmt: off
- parser.add_argument('--model-path', type=str, required=True,
- help='path to your pretrained model.')
- parser.add_argument("--data-bin", type=str, required=True,
- help="Path of data binary")
- parser.add_argument("--config", type=str, default=None,
- help="Path to config yaml file")
- parser.add_argument("--global-stats", type=str, default=None,
- help="Path to json file containing cmvn stats")
- parser.add_argument("--tgt-splitter-type", type=str, default="SentencePiece",
- help="Subword splitter type for target text")
- parser.add_argument("--tgt-splitter-path", type=str, default=None,
- help="Subword splitter model path for target text")
- parser.add_argument("--user-dir", type=str, default="examples/simultaneous_translation",
- help="User directory for simultaneous translation")
- parser.add_argument("--max-len", type=int, default=200,
- help="Max length of translation")
- parser.add_argument("--force-finish", default=False, action="store_true",
- help="Force the model to finish the hypothsis if the source is not finished")
- parser.add_argument("--shift-size", type=int, default=SHIFT_SIZE,
- help="Shift size of feature extraction window.")
- parser.add_argument("--window-size", type=int, default=WINDOW_SIZE,
- help="Window size of feature extraction window.")
- parser.add_argument("--sample-rate", type=int, default=SAMPLE_RATE,
- help="Sample rate")
- parser.add_argument("--feature-dim", type=int, default=FEATURE_DIM,
- help="Acoustic feature dimension.")
-
- # fmt: on
- return parser
-
- def load_model_vocab(self, args):
-
- filename = args.model_path
- if not os.path.exists(filename):
- raise IOError("Model file not found: {}".format(filename))
-
- state = checkpoint_utils.load_checkpoint_to_cpu(filename)
-
- task_args = state["cfg"]["task"]
- task_args.data = args.data_bin
-
- if args.config is not None:
- task_args.config_yaml = args.config
-
- task = tasks.setup_task(task_args)
-
- # build model for ensemble
- state["cfg"]["model"].load_pretrained_encoder_from = None
- state["cfg"]["model"].load_pretrained_decoder_from = None
- self.model = task.build_model(state["cfg"]["model"])
- self.model.load_state_dict(state["model"], strict=True)
- self.model.eval()
- self.model.share_memory()
-
- if self.gpu:
- self.model.cuda()
-
- # Set dictionary
- self.dict = {}
- self.dict["tgt"] = task.target_dictionary
-
- def initialize_states(self, states):
- self.feature_extractor.clear_cache()
- states.units.source = TensorListEntry()
- states.units.target = ListEntry()
- states.incremental_states = dict()
-
- def segment_to_units(self, segment, states):
- # Convert speech samples to features
- features = self.feature_extractor(segment)
- if features is not None:
- return [features]
- else:
- return []
-
- def units_to_segment(self, units, states):
- # Merge sub word to full word.
- if self.model.decoder.dictionary.eos() == units[0]:
- return DEFAULT_EOS
-
- segment = []
- if None in units.value:
- units.value.remove(None)
-
- for index in units:
- if index is None:
- units.pop()
- token = self.model.decoder.dictionary.string([index])
- if token.startswith(BOW_PREFIX):
- if len(segment) == 0:
- segment += [token.replace(BOW_PREFIX, "")]
- else:
- for j in range(len(segment)):
- units.pop()
-
- string_to_return = ["".join(segment)]
-
- if self.model.decoder.dictionary.eos() == units[0]:
- string_to_return += [DEFAULT_EOS]
-
- return string_to_return
- else:
- segment += [token.replace(BOW_PREFIX, "")]
-
- if (
- len(units) > 0
- and self.model.decoder.dictionary.eos() == units[-1]
- or len(states.units.target) > self.max_len
- ):
- tokens = [self.model.decoder.dictionary.string([unit]) for unit in units]
- return ["".join(tokens).replace(BOW_PREFIX, "")] + [DEFAULT_EOS]
-
- return None
-
- def update_model_encoder(self, states):
- if len(states.units.source) == 0:
- return
- src_indices = self.to_device(
- states.units.source.value.unsqueeze(0)
- )
- src_lengths = self.to_device(
- torch.LongTensor([states.units.source.value.size(0)])
- )
-
- states.encoder_states = self.model.encoder(src_indices, src_lengths)
- torch.cuda.empty_cache()
-
- def update_states_read(self, states):
- # Happens after a read action.
- self.update_model_encoder(states)
-
- def policy(self, states):
- if not getattr(states, "encoder_states", None):
- return READ_ACTION
-
- tgt_indices = self.to_device(
- torch.LongTensor(
- [self.model.decoder.dictionary.eos()]
- + [x for x in states.units.target.value if x is not None]
- ).unsqueeze(0)
- )
-
- states.incremental_states["steps"] = {
- "src": states.encoder_states["encoder_out"][0].size(0),
- "tgt": 1 + len(states.units.target),
- }
-
- states.incremental_states["online"] = {"only": torch.tensor(not states.finish_read())}
-
- x, outputs = self.model.decoder.forward(
- prev_output_tokens=tgt_indices,
- encoder_out=states.encoder_states,
- incremental_state=states.incremental_states,
- )
-
- states.decoder_out = x
-
- states.decoder_out_extra = outputs
-
- torch.cuda.empty_cache()
-
- if outputs.action == 0:
- return READ_ACTION
- else:
- return WRITE_ACTION
-
- def predict(self, states):
- decoder_states = states.decoder_out
-
- lprobs = self.model.get_normalized_probs(
- [decoder_states[:, -1:]], log_probs=True
- )
-
- index = lprobs.argmax(dim=-1)
-
- index = index[0, 0].item()
-
- if (
- self.force_finish
- and index == self.model.decoder.dictionary.eos()
- and not states.finish_read()
- ):
- # If we want to force finish the translation
- # (don't stop before finish reading), return a None
- # self.model.decoder.clear_cache(states.incremental_states)
- index = None
-
- return index
diff --git a/spaces/OFA-Sys/OFA-Image_Caption/fairseq/fairseq/optim/lr_scheduler/manual_lr_scheduler.py b/spaces/OFA-Sys/OFA-Image_Caption/fairseq/fairseq/optim/lr_scheduler/manual_lr_scheduler.py
deleted file mode 100644
index 0269a1e2853854745e23b07931294f37b67d0295..0000000000000000000000000000000000000000
--- a/spaces/OFA-Sys/OFA-Image_Caption/fairseq/fairseq/optim/lr_scheduler/manual_lr_scheduler.py
+++ /dev/null
@@ -1,110 +0,0 @@
-# Copyright (c) Facebook, Inc. and its affiliates.
-#
-# This source code is licensed under the MIT license found in the
-# LICENSE file in the root directory of this source tree.
-
-from . import LegacyFairseqLRScheduler, register_lr_scheduler
-import logging
-import ast
-
-logger = logging.getLogger(__name__)
-logger.setLevel(logging.WARNING)
-
-
-@register_lr_scheduler("manual")
-class ManualSchedule(LegacyFairseqLRScheduler):
- """Decay the LR on a manual schedule."""
-
- def __init__(self, args, optimizer):
- super().__init__(args, optimizer)
-
- self.epoch2lr = self.parse_manuallr_args(args.epoch2lr)
- self.update2lr = self.parse_manuallr_args(args.update2lr)
- logger.info("@@@ ManualSchedule epoch2lr={}".format(self.epoch2lr))
- logger.info("@@@ ManualSchedule update2lr={}".format(self.update2lr))
-
- if 1 in self.epoch2lr:
- self.lr = self.epoch2lr[1]
- elif 1 in self.update2lr:
- self.lr = self.update2lr[1]
- else:
- self.lr = args.lr[0]
- self.optimizer.set_lr(self.lr) # Set the beginning of the epoch.
-
- def parse_manuallr_args(self, lr_args_str):
- lr_dict = ast.literal_eval(lr_args_str.replace(' ', ''))
- if not isinstance(lr_dict, dict):
- raise ValueError("epoch2lr/update2lr must be abel to evaluated to a dict")
-
- lr_args = {}
- logger.info("@@@ after parsing input dictionary lr_dict = {}".format(lr_dict))
- for key, val in lr_dict.items():
- if "," in key:
- for k in key.split(","):
- lr_args[int(k)] = float(val)
- elif "-" in key:
- s = int(key.split("-")[0])
- e = int(key.split("-")[1])
- for k in range(s, e + 1, 1):
- lr_args[k] = float(val)
- else:
- lr_args[int(key)] = float(val)
-
- return lr_args
-
- @staticmethod
- def add_args(parser):
- """Add arguments to the parser for this LR scheduler."""
- # fmt: off
- parser.add_argument(
- "--epoch2lr",
- type=str,
- metavar="DICT",
- default="{}",
- help="a dictionary used to set lr for each epoch manually",
- )
- parser.add_argument(
- "--update2lr",
- type=str,
- metavar="DICT",
- default="{}",
- help="a dictionary used to set lr for each update manually",
- )
- # fmt: on
-
- def state_dict(self):
- return {"lr": self.lr}
-
- def load_state_dict(self, state_dict):
- if "lr" in state_dict:
- self.lr = state_dict["lr"]
-
- def get_next_lr(self, epoch):
- manual_keys = [k for k in self.epoch2lr if k <= epoch]
- if manual_keys:
- manual_lr = self.epoch2lr[max(manual_keys)]
- else:
- logger.warning("@@@ epoch={} does not exist in manual lr input. epoch2lr={}...".format(
- epoch, list(self.epoch2lr.items())[:min(10, len(self.epoch2lr.keys())-1)]
- ))
- manual_lr = self.optimizer.get_lr()
- return manual_lr
-
- def step_begin_epoch(self, epoch):
- """Update the learning rate at the beginning of the given epoch."""
- self.lr = self.get_next_lr(epoch)
- self.optimizer.set_lr(self.lr)
- return self.optimizer.get_lr()
-
- def step_update(self, num_updates):
- """Update the learning rate after each update."""
- manual_keys = [k for k in self.update2lr if k <= num_updates]
- if manual_keys:
- manual_lr = self.update2lr[max(manual_keys)]
- else:
- logger.warning("epoch={} does not exist in manual lr input update2lr={}...".format(
- num_updates, list(self.update2lr.items())[:min(10, len(self.update2lr.keys())-1)]))
- manual_lr = self.optimizer.get_lr()
-
- self.optimizer.set_lr(manual_lr)
- return self.optimizer.get_lr()
diff --git a/spaces/OFA-Sys/OFA-vqa/fairseq/examples/multilingual/data_scripts/download_ted_and_extract.py b/spaces/OFA-Sys/OFA-vqa/fairseq/examples/multilingual/data_scripts/download_ted_and_extract.py
deleted file mode 100644
index eb756680fa7dc31a14ba45c216776a6d60c16b60..0000000000000000000000000000000000000000
--- a/spaces/OFA-Sys/OFA-vqa/fairseq/examples/multilingual/data_scripts/download_ted_and_extract.py
+++ /dev/null
@@ -1,338 +0,0 @@
-# Copyright (c) Facebook, Inc. and its affiliates.
-#
-# This source code is licensed under the MIT license found in the
-# LICENSE file in the root directory of this source tree.
-
-
-import itertools
-import os
-import csv
-from collections import defaultdict
-from six.moves import zip
-import io
-import wget
-import sys
-
-from subprocess import check_call, check_output
-
-# scripts and data locations
-CWD = os.getcwd()
-UTILS = f"{CWD}/utils"
-
-MOSES = f"{UTILS}/mosesdecoder"
-
-WORKDIR_ROOT = os.environ.get('WORKDIR_ROOT', None)
-
-if WORKDIR_ROOT is None or not WORKDIR_ROOT.strip():
- print('please specify your working directory root in OS environment variable WORKDIR_ROOT. Exitting..."')
- sys.exit(-1)
-
-
-# please donwload mosesdecoder here:
-detok_cmd = f'{MOSES}/scripts/tokenizer/detokenizer.perl'
-
-
-def call(cmd):
- print(f"Executing: {cmd}")
- check_call(cmd, shell=True)
-
-class MultiLingualAlignedCorpusReader(object):
- """A class to read TED talk dataset
- """
-
- def __init__(self, corpus_path, delimiter='\t',
- target_token=True, bilingual=True, corpus_type='file',
- lang_dict={'source': ['fr'], 'target': ['en']},
- eval_lang_dict=None, zero_shot=False,
- detok=True,
- ):
-
- self.empty_line_flag = 'NULL'
- self.corpus_path = corpus_path
- self.delimiter = delimiter
- self.bilingual = bilingual
- self.lang_dict = lang_dict
- self.lang_set = set()
- self.target_token = target_token
- self.zero_shot = zero_shot
- self.eval_lang_dict = eval_lang_dict
- self.corpus_type = corpus_type
- self.detok = detok
-
- for list_ in self.lang_dict.values():
- for lang in list_:
- self.lang_set.add(lang)
-
- self.data = dict()
- self.data['train'] = self.read_aligned_corpus(split_type='train')
- self.data['test'] = self.read_aligned_corpus(split_type='test')
- self.data['dev'] = self.read_aligned_corpus(split_type='dev')
-
- def read_data(self, file_loc_):
- data_list = list()
- with io.open(file_loc_, 'r', encoding='utf8') as fp:
- for line in fp:
- try:
- text = line.strip()
- except IndexError:
- text = self.empty_line_flag
- data_list.append(text)
- return data_list
-
- def filter_text(self, dict_):
- if self.target_token:
- field_index = 1
- else:
- field_index = 0
- data_dict = defaultdict(list)
- list1 = dict_['source']
- list2 = dict_['target']
- for sent1, sent2 in zip(list1, list2):
- try:
- src_sent = ' '.join(sent1.split()[field_index: ])
- except IndexError:
- src_sent = 'NULL'
-
- if src_sent.find(self.empty_line_flag) != -1 or len(src_sent) == 0:
- continue
-
- elif sent2.find(self.empty_line_flag) != -1 or len(sent2) == 0:
- continue
-
- else:
- data_dict['source'].append(sent1)
- data_dict['target'].append(sent2)
- return data_dict
-
- def read_file(self, split_type, data_type):
- return self.data[split_type][data_type]
-
- def save_file(self, path_, split_type, data_type, lang):
- tok_file = tok_file_name(path_, lang)
- with io.open(tok_file, 'w', encoding='utf8') as fp:
- for line in self.data[split_type][data_type]:
- fp.write(line + '\n')
- if self.detok:
- de_tok(tok_file, lang)
-
- def add_target_token(self, list_, lang_id):
- new_list = list()
- token = '__' + lang_id + '__'
- for sent in list_:
- new_list.append(token + ' ' + sent)
- return new_list
-
- def read_from_single_file(self, path_, s_lang, t_lang):
- data_dict = defaultdict(list)
- with io.open(path_, 'r', encoding='utf8') as fp:
- reader = csv.DictReader(fp, delimiter='\t', quoting=csv.QUOTE_NONE)
- for row in reader:
- data_dict['source'].append(row[s_lang])
- data_dict['target'].append(row[t_lang])
-
- if self.target_token:
- text = self.add_target_token(data_dict['source'], t_lang)
- data_dict['source'] = text
-
- return data_dict['source'], data_dict['target']
-
- def read_aligned_corpus(self, split_type='train'):
- data_dict = defaultdict(list)
- iterable = []
- s_list = []
- t_list = []
-
- if self.zero_shot:
- if split_type == "train":
- iterable = zip(self.lang_dict['source'], self.lang_dict['target'])
- else:
- iterable = zip(self.eval_lang_dict['source'], self.eval_lang_dict['target'])
-
- elif self.bilingual:
- iterable = itertools.product(self.lang_dict['source'], self.lang_dict['target'])
-
- for s_lang, t_lang in iterable:
- if s_lang == t_lang:
- continue
- if self.corpus_type == 'file':
- split_type_file_path = os.path.join(self.corpus_path,
- "all_talks_{}.tsv".format(split_type))
- s_list, t_list = self.read_from_single_file(split_type_file_path,
- s_lang=s_lang,
- t_lang=t_lang)
- data_dict['source'] += s_list
- data_dict['target'] += t_list
- new_data_dict = self.filter_text(data_dict)
- return new_data_dict
-
-
-def read_langs(corpus_path):
- split_type_file_path = os.path.join(corpus_path, 'extracted',
- "all_talks_dev.tsv")
- with io.open(split_type_file_path, 'r', encoding='utf8') as fp:
- reader = csv.DictReader(fp, delimiter='\t', quoting=csv.QUOTE_NONE)
- header = next(reader)
- return [k for k in header.keys() if k != 'talk_name']
-
-def extra_english(corpus_path, split):
- split_type_file_path = os.path.join(corpus_path,
- f"all_talks_{split}.tsv")
- output_split_type_file_path = os.path.join(corpus_path,
- f"all_talks_{split}.en")
- with io.open(split_type_file_path, 'r', encoding='utf8') as fp, io.open(output_split_type_file_path, 'w', encoding='utf8') as fw:
- reader = csv.DictReader(fp, delimiter='\t', quoting=csv.QUOTE_NONE)
- for row in reader:
- line = row['en']
- fw.write(line + '\n')
- de_tok(output_split_type_file_path, 'en')
-
-
-
-def tok_file_name(filename, lang):
- seps = filename.split('.')
- seps.insert(-1, 'tok')
- tok_file = '.'.join(seps)
- return tok_file
-
-def de_tok(tok_file, lang):
- # seps = tok_file.split('.')
- # seps.insert(-1, 'detok')
- # de_tok_file = '.'.join(seps)
- de_tok_file = tok_file.replace('.tok.', '.')
- cmd = 'perl {detok_cmd} -l {lang} < {tok_file} > {de_tok_file}'.format(
- detok_cmd=detok_cmd, tok_file=tok_file,
- de_tok_file=de_tok_file, lang=lang[:2])
- call(cmd)
-
-def extra_bitex(
- ted_data_path,
- lsrc_lang,
- ltrg_lang,
- target_token,
- output_data_path,
-):
- def get_ted_lang(lang):
- long_langs = ['pt-br', 'zh-cn', 'zh-tw', 'fr-ca']
- if lang[:5] in long_langs:
- return lang[:5]
- elif lang[:4] =='calv':
- return lang[:5]
- elif lang in ['pt_BR', 'zh_CN', 'zh_TW', 'fr_CA']:
- return lang.lower().replace('_', '-')
- return lang[:2]
- src_lang = get_ted_lang(lsrc_lang)
- trg_lang = get_ted_lang(ltrg_lang)
- train_lang_dict={'source': [src_lang], 'target': [trg_lang]}
- eval_lang_dict = {'source': [src_lang], 'target': [trg_lang]}
-
- obj = MultiLingualAlignedCorpusReader(corpus_path=ted_data_path,
- lang_dict=train_lang_dict,
- target_token=target_token,
- corpus_type='file',
- eval_lang_dict=eval_lang_dict,
- zero_shot=False,
- bilingual=True)
-
- os.makedirs(output_data_path, exist_ok=True)
- lsrc_lang = lsrc_lang.replace('-', '_')
- ltrg_lang = ltrg_lang.replace('-', '_')
- obj.save_file(output_data_path + f"/train.{lsrc_lang}-{ltrg_lang}.{lsrc_lang}",
- split_type='train', data_type='source', lang=src_lang)
- obj.save_file(output_data_path + f"/train.{lsrc_lang}-{ltrg_lang}.{ltrg_lang}",
- split_type='train', data_type='target', lang=trg_lang)
-
- obj.save_file(output_data_path + f"/test.{lsrc_lang}-{ltrg_lang}.{lsrc_lang}",
- split_type='test', data_type='source', lang=src_lang)
- obj.save_file(output_data_path + f"/test.{lsrc_lang}-{ltrg_lang}.{ltrg_lang}",
- split_type='test', data_type='target', lang=trg_lang)
-
- obj.save_file(output_data_path + f"/valid.{lsrc_lang}-{ltrg_lang}.{lsrc_lang}",
- split_type='dev', data_type='source', lang=src_lang)
- obj.save_file(output_data_path + f"/valid.{lsrc_lang}-{ltrg_lang}.{ltrg_lang}",
- split_type='dev', data_type='target', lang=trg_lang)
-
-
-def bar_custom(current, total, width=80):
- print("Downloading: %d%% [%d / %d] Ks" % (current / total * 100, current / 1000, total / 1000), end='\r')
-
-
-def download_and_extract(download_to, extract_to):
- url = 'http://phontron.com/data/ted_talks.tar.gz'
- filename = f"{download_to}/ted_talks.tar.gz"
- if os.path.exists(filename):
- print(f'{filename} has already been downloaded so skip')
- else:
- filename = wget.download(url, filename, bar=bar_custom)
- if os.path.exists(f'{extract_to}/all_talks_train.tsv'):
- print(f'Already extracted so skip')
- else:
- extract_cmd = f'tar xzfv "{filename}" -C "{extract_to}"'
- call(extract_cmd)
-
-
-if __name__ == "__main__":
- import argparse
- parser = argparse.ArgumentParser()
- parser.add_argument('--ted_data_path', type=str, default=WORKDIR_ROOT, required=False)
- parser.add_argument(
- '--direction-list',
- type=str,
- # default=None,
- #for ML50
- default=(
- "bn_IN-en_XX,he_IL-en_XX,fa_IR-en_XX,id_ID-en_XX,sv_SE-en_XX,pt_XX-en_XX,ka_GE-en_XX,ka_GE-en_XX,th_TH-en_XX,"
- "mr_IN-en_XX,hr_HR-en_XX,uk_UA-en_XX,az_AZ-en_XX,mk_MK-en_XX,gl_ES-en_XX,sl_SI-en_XX,mn_MN-en_XX,"
- #non-english directions
- # "fr_XX-de_DE," # replaced with wmt20
- # "ja_XX-ko_KR,es_XX-pt_XX,ru_RU-sv_SE,hi_IN-bn_IN,id_ID-ar_AR,cs_CZ-pl_PL,ar_AR-tr_TR"
- ),
- required=False)
- parser.add_argument('--target-token', action='store_true', default=False)
- parser.add_argument('--extract-all-english', action='store_true', default=False)
-
- args = parser.parse_args()
-
- import sys
- import json
-
- # TED Talks data directory
- ted_data_path = args.ted_data_path
-
- download_to = f'{ted_data_path}/downloads'
- extract_to = f'{ted_data_path}/extracted'
-
- #DESTDIR=${WORKDIR_ROOT}/ML50/raw/
- output_path = f'{ted_data_path}/ML50/raw'
- os.makedirs(download_to, exist_ok=True)
- os.makedirs(extract_to, exist_ok=True)
- os.makedirs(output_path, exist_ok=True)
- download_and_extract(download_to, extract_to)
-
-
- if args.extract_all_english:
- for split in ['train', 'dev', 'test']:
- extra_english(ted_data_path, split)
- exit(0)
- if args.direction_list is not None:
- directions = args.direction_list.strip().split(',')
- directions = [tuple(d.strip().split('-', 1)) for d in directions if d]
- else:
- langs = read_langs(ted_data_path)
- # directions = [
- # '{}.{}'.format(src, tgt)
- # for src in langs
- # for tgt in langs
- # if src < tgt
- # ]
- directions = [('en', tgt) for tgt in langs if tgt != 'en']
- print(f'num directions={len(directions)}: {directions}')
-
- for src_lang, trg_lang in directions:
- print('--working on {}-{}'.format(src_lang, trg_lang))
- extra_bitex(
- extract_to,
- src_lang,
- trg_lang,
- target_token=args.target_token,
- output_data_path=output_path
- )
diff --git a/spaces/OFA-Sys/OFA-vqa/fairseq/examples/wav2vec/unsupervised/scripts/wrd_to_ltr.py b/spaces/OFA-Sys/OFA-vqa/fairseq/examples/wav2vec/unsupervised/scripts/wrd_to_ltr.py
deleted file mode 100644
index f83471409a434556cab70086ca9e2d72d4bdddd5..0000000000000000000000000000000000000000
--- a/spaces/OFA-Sys/OFA-vqa/fairseq/examples/wav2vec/unsupervised/scripts/wrd_to_ltr.py
+++ /dev/null
@@ -1,16 +0,0 @@
-#!/usr/bin/env python3 -u
-# Copyright (c) Facebook, Inc. and its affiliates.
-#
-# This source code is licensed under the MIT license found in the
-# LICENSE file in the root directory of this source tree.
-
-import sys
-
-
-def main():
- for line in sys.stdin:
- print(" ".join(list(line.strip().replace(" ", "|"))) + " |")
-
-
-if __name__ == "__main__":
- main()
diff --git a/spaces/OFA-Sys/OFA-vqa/utils/cider/pyciderevalcap/cider/__init__.py b/spaces/OFA-Sys/OFA-vqa/utils/cider/pyciderevalcap/cider/__init__.py
deleted file mode 100644
index 3f7d85bba884ea8f83fc6ab2a1e6ade80d98d4d9..0000000000000000000000000000000000000000
--- a/spaces/OFA-Sys/OFA-vqa/utils/cider/pyciderevalcap/cider/__init__.py
+++ /dev/null
@@ -1 +0,0 @@
-__author__ = 'tylin'
diff --git a/spaces/Omnibus/MusicGen/audiocraft/modules/conv.py b/spaces/Omnibus/MusicGen/audiocraft/modules/conv.py
deleted file mode 100644
index 972938ab84712eb06e1b10cea25444eee51d6637..0000000000000000000000000000000000000000
--- a/spaces/Omnibus/MusicGen/audiocraft/modules/conv.py
+++ /dev/null
@@ -1,245 +0,0 @@
-# Copyright (c) Meta Platforms, Inc. and affiliates.
-# All rights reserved.
-#
-# This source code is licensed under the license found in the
-# LICENSE file in the root directory of this source tree.
-
-import math
-import typing as tp
-import warnings
-
-import torch
-from torch import nn
-from torch.nn import functional as F
-from torch.nn.utils import spectral_norm, weight_norm
-
-
-CONV_NORMALIZATIONS = frozenset(['none', 'weight_norm', 'spectral_norm',
- 'time_group_norm'])
-
-
-def apply_parametrization_norm(module: nn.Module, norm: str = 'none'):
- assert norm in CONV_NORMALIZATIONS
- if norm == 'weight_norm':
- return weight_norm(module)
- elif norm == 'spectral_norm':
- return spectral_norm(module)
- else:
- # We already check was in CONV_NORMALIZATION, so any other choice
- # doesn't need reparametrization.
- return module
-
-
-def get_norm_module(module: nn.Module, causal: bool = False, norm: str = 'none', **norm_kwargs):
- """Return the proper normalization module. If causal is True, this will ensure the returned
- module is causal, or return an error if the normalization doesn't support causal evaluation.
- """
- assert norm in CONV_NORMALIZATIONS
- if norm == 'time_group_norm':
- if causal:
- raise ValueError("GroupNorm doesn't support causal evaluation.")
- assert isinstance(module, nn.modules.conv._ConvNd)
- return nn.GroupNorm(1, module.out_channels, **norm_kwargs)
- else:
- return nn.Identity()
-
-
-def get_extra_padding_for_conv1d(x: torch.Tensor, kernel_size: int, stride: int,
- padding_total: int = 0) -> int:
- """See `pad_for_conv1d`.
- """
- length = x.shape[-1]
- n_frames = (length - kernel_size + padding_total) / stride + 1
- ideal_length = (math.ceil(n_frames) - 1) * stride + (kernel_size - padding_total)
- return ideal_length - length
-
-
-def pad_for_conv1d(x: torch.Tensor, kernel_size: int, stride: int, padding_total: int = 0):
- """Pad for a convolution to make sure that the last window is full.
- Extra padding is added at the end. This is required to ensure that we can rebuild
- an output of the same length, as otherwise, even with padding, some time steps
- might get removed.
- For instance, with total padding = 4, kernel size = 4, stride = 2:
- 0 0 1 2 3 4 5 0 0 # (0s are padding)
- 1 2 3 # (output frames of a convolution, last 0 is never used)
- 0 0 1 2 3 4 5 0 # (output of tr. conv., but pos. 5 is going to get removed as padding)
- 1 2 3 4 # once you removed padding, we are missing one time step !
- """
- extra_padding = get_extra_padding_for_conv1d(x, kernel_size, stride, padding_total)
- return F.pad(x, (0, extra_padding))
-
-
-def pad1d(x: torch.Tensor, paddings: tp.Tuple[int, int], mode: str = 'constant', value: float = 0.):
- """Tiny wrapper around F.pad, just to allow for reflect padding on small input.
- If this is the case, we insert extra 0 padding to the right before the reflection happen.
- """
- length = x.shape[-1]
- padding_left, padding_right = paddings
- assert padding_left >= 0 and padding_right >= 0, (padding_left, padding_right)
- if mode == 'reflect':
- max_pad = max(padding_left, padding_right)
- extra_pad = 0
- if length <= max_pad:
- extra_pad = max_pad - length + 1
- x = F.pad(x, (0, extra_pad))
- padded = F.pad(x, paddings, mode, value)
- end = padded.shape[-1] - extra_pad
- return padded[..., :end]
- else:
- return F.pad(x, paddings, mode, value)
-
-
-def unpad1d(x: torch.Tensor, paddings: tp.Tuple[int, int]):
- """Remove padding from x, handling properly zero padding. Only for 1d!
- """
- padding_left, padding_right = paddings
- assert padding_left >= 0 and padding_right >= 0, (padding_left, padding_right)
- assert (padding_left + padding_right) <= x.shape[-1]
- end = x.shape[-1] - padding_right
- return x[..., padding_left: end]
-
-
-class NormConv1d(nn.Module):
- """Wrapper around Conv1d and normalization applied to this conv
- to provide a uniform interface across normalization approaches.
- """
- def __init__(self, *args, causal: bool = False, norm: str = 'none',
- norm_kwargs: tp.Dict[str, tp.Any] = {}, **kwargs):
- super().__init__()
- self.conv = apply_parametrization_norm(nn.Conv1d(*args, **kwargs), norm)
- self.norm = get_norm_module(self.conv, causal, norm, **norm_kwargs)
- self.norm_type = norm
-
- def forward(self, x):
- x = self.conv(x)
- x = self.norm(x)
- return x
-
-
-class NormConv2d(nn.Module):
- """Wrapper around Conv2d and normalization applied to this conv
- to provide a uniform interface across normalization approaches.
- """
- def __init__(self, *args, norm: str = 'none', norm_kwargs: tp.Dict[str, tp.Any] = {}, **kwargs):
- super().__init__()
- self.conv = apply_parametrization_norm(nn.Conv2d(*args, **kwargs), norm)
- self.norm = get_norm_module(self.conv, causal=False, norm=norm, **norm_kwargs)
- self.norm_type = norm
-
- def forward(self, x):
- x = self.conv(x)
- x = self.norm(x)
- return x
-
-
-class NormConvTranspose1d(nn.Module):
- """Wrapper around ConvTranspose1d and normalization applied to this conv
- to provide a uniform interface across normalization approaches.
- """
- def __init__(self, *args, causal: bool = False, norm: str = 'none',
- norm_kwargs: tp.Dict[str, tp.Any] = {}, **kwargs):
- super().__init__()
- self.convtr = apply_parametrization_norm(nn.ConvTranspose1d(*args, **kwargs), norm)
- self.norm = get_norm_module(self.convtr, causal, norm, **norm_kwargs)
- self.norm_type = norm
-
- def forward(self, x):
- x = self.convtr(x)
- x = self.norm(x)
- return x
-
-
-class NormConvTranspose2d(nn.Module):
- """Wrapper around ConvTranspose2d and normalization applied to this conv
- to provide a uniform interface across normalization approaches.
- """
- def __init__(self, *args, norm: str = 'none', norm_kwargs: tp.Dict[str, tp.Any] = {}, **kwargs):
- super().__init__()
- self.convtr = apply_parametrization_norm(nn.ConvTranspose2d(*args, **kwargs), norm)
- self.norm = get_norm_module(self.convtr, causal=False, norm=norm, **norm_kwargs)
-
- def forward(self, x):
- x = self.convtr(x)
- x = self.norm(x)
- return x
-
-
-class StreamableConv1d(nn.Module):
- """Conv1d with some builtin handling of asymmetric or causal padding
- and normalization.
- """
- def __init__(self, in_channels: int, out_channels: int,
- kernel_size: int, stride: int = 1, dilation: int = 1,
- groups: int = 1, bias: bool = True, causal: bool = False,
- norm: str = 'none', norm_kwargs: tp.Dict[str, tp.Any] = {},
- pad_mode: str = 'reflect'):
- super().__init__()
- # warn user on unusual setup between dilation and stride
- if stride > 1 and dilation > 1:
- warnings.warn('StreamableConv1d has been initialized with stride > 1 and dilation > 1'
- f' (kernel_size={kernel_size} stride={stride}, dilation={dilation}).')
- self.conv = NormConv1d(in_channels, out_channels, kernel_size, stride,
- dilation=dilation, groups=groups, bias=bias, causal=causal,
- norm=norm, norm_kwargs=norm_kwargs)
- self.causal = causal
- self.pad_mode = pad_mode
-
- def forward(self, x):
- B, C, T = x.shape
- kernel_size = self.conv.conv.kernel_size[0]
- stride = self.conv.conv.stride[0]
- dilation = self.conv.conv.dilation[0]
- kernel_size = (kernel_size - 1) * dilation + 1 # effective kernel size with dilations
- padding_total = kernel_size - stride
- extra_padding = get_extra_padding_for_conv1d(x, kernel_size, stride, padding_total)
- if self.causal:
- # Left padding for causal
- x = pad1d(x, (padding_total, extra_padding), mode=self.pad_mode)
- else:
- # Asymmetric padding required for odd strides
- padding_right = padding_total // 2
- padding_left = padding_total - padding_right
- x = pad1d(x, (padding_left, padding_right + extra_padding), mode=self.pad_mode)
- return self.conv(x)
-
-
-class StreamableConvTranspose1d(nn.Module):
- """ConvTranspose1d with some builtin handling of asymmetric or causal padding
- and normalization.
- """
- def __init__(self, in_channels: int, out_channels: int,
- kernel_size: int, stride: int = 1, causal: bool = False,
- norm: str = 'none', trim_right_ratio: float = 1.,
- norm_kwargs: tp.Dict[str, tp.Any] = {}):
- super().__init__()
- self.convtr = NormConvTranspose1d(in_channels, out_channels, kernel_size, stride,
- causal=causal, norm=norm, norm_kwargs=norm_kwargs)
- self.causal = causal
- self.trim_right_ratio = trim_right_ratio
- assert self.causal or self.trim_right_ratio == 1., \
- "`trim_right_ratio` != 1.0 only makes sense for causal convolutions"
- assert self.trim_right_ratio >= 0. and self.trim_right_ratio <= 1.
-
- def forward(self, x):
- kernel_size = self.convtr.convtr.kernel_size[0]
- stride = self.convtr.convtr.stride[0]
- padding_total = kernel_size - stride
-
- y = self.convtr(x)
-
- # We will only trim fixed padding. Extra padding from `pad_for_conv1d` would be
- # removed at the very end, when keeping only the right length for the output,
- # as removing it here would require also passing the length at the matching layer
- # in the encoder.
- if self.causal:
- # Trim the padding on the right according to the specified ratio
- # if trim_right_ratio = 1.0, trim everything from right
- padding_right = math.ceil(padding_total * self.trim_right_ratio)
- padding_left = padding_total - padding_right
- y = unpad1d(y, (padding_left, padding_right))
- else:
- # Asymmetric padding required for odd strides
- padding_right = padding_total // 2
- padding_left = padding_total - padding_right
- y = unpad1d(y, (padding_left, padding_right))
- return y
diff --git a/spaces/Omnibus/Video-Diffusion-WebUI/video_diffusion/inpaint_zoom/utils/zoom_out_utils.py b/spaces/Omnibus/Video-Diffusion-WebUI/video_diffusion/inpaint_zoom/utils/zoom_out_utils.py
deleted file mode 100644
index 7f9d0605691e5ad4a92979547b8795a8d3f24be3..0000000000000000000000000000000000000000
--- a/spaces/Omnibus/Video-Diffusion-WebUI/video_diffusion/inpaint_zoom/utils/zoom_out_utils.py
+++ /dev/null
@@ -1,47 +0,0 @@
-import cv2
-import numpy as np
-from PIL import Image
-
-
-def write_video(file_path, frames, fps):
- """
- Writes frames to an mp4 video file
- :param file_path: Path to output video, must end with .mp4
- :param frames: List of PIL.Image objects
- :param fps: Desired frame rate
- """
-
- w, h = frames[0].size
- fourcc = cv2.VideoWriter_fourcc("m", "p", "4", "v")
- writer = cv2.VideoWriter(file_path, fourcc, fps, (w, h))
-
- for frame in frames:
- np_frame = np.array(frame.convert("RGB"))
- cv_frame = cv2.cvtColor(np_frame, cv2.COLOR_RGB2BGR)
- writer.write(cv_frame)
-
- writer.release()
-
-
-def dummy(images, **kwargs):
- return images, False
-
-
-def preprocess_image(current_image, steps, image_size):
- next_image = np.array(current_image.convert("RGBA")) * 0
- prev_image = current_image.resize((image_size - 2 * steps, image_size - 2 * steps))
- prev_image = prev_image.convert("RGBA")
- prev_image = np.array(prev_image)
- next_image[:, :, 3] = 1
- next_image[steps : image_size - steps, steps : image_size - steps, :] = prev_image
- prev_image = Image.fromarray(next_image)
-
- return prev_image
-
-
-def preprocess_mask_image(current_image):
- mask_image = np.array(current_image)[:, :, 3] # assume image has alpha mask (use .mode to check for "RGBA")
- mask_image = Image.fromarray(255 - mask_image).convert("RGB")
- current_image = current_image.convert("RGB")
-
- return current_image, mask_image
diff --git a/spaces/Open-Orca/OpenOrcaxOpenChat-Preview2-13B/app.py b/spaces/Open-Orca/OpenOrcaxOpenChat-Preview2-13B/app.py
deleted file mode 100644
index 778648897728982b413033721a1b6ac20026beba..0000000000000000000000000000000000000000
--- a/spaces/Open-Orca/OpenOrcaxOpenChat-Preview2-13B/app.py
+++ /dev/null
@@ -1,116 +0,0 @@
-import os
-import re
-import logging
-import gradio as gr
-import openai
-
-print(os.environ)
-openai.api_base = os.environ.get("OPENAI_API_BASE")
-openai.api_key = os.environ.get("OPENAI_API_KEY")
-
-BASE_SYSTEM_MESSAGE = """You are an AI assistant named OpenOrcaChat. If users clearly indicate an interest in how good you are you may tell them that the group who made you can be found at https://discord.gg/mhFWVbXUDh. The groups involved are OpenChat and OpenOrca"""
-
-def make_prediction(prompt, max_tokens=None, temperature=None, top_p=None, top_k=None, repetition_penalty=None):
- completion = openai.Completion.create(model="Open-Orca/OpenOrcaxOpenChat-Preview2-13B", prompt=prompt, max_tokens=max_tokens, temperature=temperature, top_p=top_p, top_k=top_k, repetition_penalty=repetition_penalty, stream=True)
- for chunk in completion:
- yield chunk["choices"][0]["text"]
-
-
-def clear_chat(chat_history_state, chat_message):
- chat_history_state = []
- chat_message = ''
- return chat_history_state, chat_message
-
-
-def user(message, history):
- history = history or []
- # Append the user's message to the conversation history
- history.append([message, ""])
- return "", history
-
-
-def chat(history, system_message, max_tokens, temperature, top_p, top_k, repetition_penalty):
- history = history or []
-
- messages = BASE_SYSTEM_MESSAGE + system_message.strip() + "\n" + \
- "\n".join(["\n".join(["User: "+item[0]+"<|end_of_turn|>", "Assistant: "+item[1]+"<|end_of_turn|>"])
- for item in history])
- # strip the last `<|end_of_turn|>` from the messages
- messages = messages.rstrip("<|end_of_turn|>")
- # remove last space from assistant, some models output a ZWSP if you leave a space
- messages = messages.rstrip()
-
- prediction = make_prediction(
- messages,
- max_tokens=max_tokens,
- temperature=temperature,
- top_p=top_p,
- top_k=top_k,
- repetition_penalty=repetition_penalty,
- )
- for tokens in prediction:
- tokens = re.findall(r'(.*?)(\s|$)', tokens)
- for subtoken in tokens:
- subtoken = "".join(subtoken)
- answer = subtoken
- history[-1][1] += answer
- # stream the response
- yield history, history, ""
-
-
-start_message = ""
-
-CSS ="""
-.contain { display: flex; flex-direction: column; }
-.gradio-container { height: 100vh !important; }
-#component-0 { height: 100%; }
-#chatbot { flex-grow: 1; overflow: auto; resize: vertical; }
-"""
-
-#with gr.Blocks() as demo:
-with gr.Blocks(css=CSS) as demo:
- with gr.Row():
- with gr.Column():
- gr.Markdown(f"""
- ## This demo is an unquantized GPU chatbot of [OpenOrcaxOpenChat-Preview2-13B](https://huggingface.co/Open-Orca/OpenOrcaxOpenChat-Preview2-13B)
- Brought to you by your friends at Alignment Lab AI, OpenChat, and Open Access AI Collective!
- """)
- with gr.Row():
- gr.Markdown("# 🐋 OpenOrca x OpenChat - Preview2 - 13B Playground Space! 🐋")
- with gr.Row():
- #chatbot = gr.Chatbot().style(height=500)
- chatbot = gr.Chatbot(elem_id="chatbot")
- with gr.Row():
- message = gr.Textbox(
- label="What do you want to chat about?",
- placeholder="Ask me anything.",
- lines=3,
- )
- with gr.Row():
- submit = gr.Button(value="Send message", variant="secondary").style(full_width=True)
- clear = gr.Button(value="New topic", variant="secondary").style(full_width=False)
- stop = gr.Button(value="Stop", variant="secondary").style(full_width=False)
- with gr.Accordion("Show Model Parameters", open=False):
- with gr.Row():
- with gr.Column():
- max_tokens = gr.Slider(20, 1000, label="Max Tokens", step=20, value=500)
- temperature = gr.Slider(0.2, 2.0, label="Temperature", step=0.1, value=0.8)
- top_p = gr.Slider(0.0, 1.0, label="Top P", step=0.05, value=0.95)
- top_k = gr.Slider(0, 100, label="Top K", step=1, value=40)
- repetition_penalty = gr.Slider(0.0, 2.0, label="Repetition Penalty", step=0.1, value=1.1)
-
- system_msg = gr.Textbox(
- start_message, label="System Message", interactive=True, visible=True, placeholder="System prompt. Provide instructions which you want the model to remember.", lines=5)
-
- chat_history_state = gr.State()
- clear.click(clear_chat, inputs=[chat_history_state, message], outputs=[chat_history_state, message], queue=False)
- clear.click(lambda: None, None, chatbot, queue=False)
-
- submit_click_event = submit.click(
- fn=user, inputs=[message, chat_history_state], outputs=[message, chat_history_state], queue=True
- ).then(
- fn=chat, inputs=[chat_history_state, system_msg, max_tokens, temperature, top_p, top_k, repetition_penalty], outputs=[chatbot, chat_history_state, message], queue=True
- )
- stop.click(fn=None, inputs=None, outputs=None, cancels=[submit_click_event], queue=False)
-
-demo.queue(max_size=128, concurrency_count=48).launch(debug=True, server_name="0.0.0.0", server_port=7860)
diff --git a/spaces/PaddlePaddle/MiDaS_Large/app.py b/spaces/PaddlePaddle/MiDaS_Large/app.py
deleted file mode 100644
index 56f8e397c6f2133b805a693d8873fec236b225dd..0000000000000000000000000000000000000000
--- a/spaces/PaddlePaddle/MiDaS_Large/app.py
+++ /dev/null
@@ -1,19 +0,0 @@
-import gradio as gr
-import cv2
-import paddlehub as hub
-from PIL import Image
-import numpy as np
-
-
-model = hub.Module(name='MiDaS_Large', use_gpu=False)
-
-def inference(img):
- model.depth_estimation(images=[cv2.imread(img)],visualization=True)
- return './output/0.png'
-
-
-title="MiDaS_Large"
-description="MiDaS_Large is a monocular depth estimation model that estimates depth information from input images."
-
-examples=[['lion.jpg']]
-gr.Interface(inference,gr.inputs.Image(type="filepath"),gr.outputs.Image(type="file"),title=title,description=description,examples=examples).launch(enable_queue=True,debug=True)
\ No newline at end of file
diff --git a/spaces/Pattr/DrumClassification/lilypond-2.24.2/lib/guile/2.2/ccache/rnrs/control.go b/spaces/Pattr/DrumClassification/lilypond-2.24.2/lib/guile/2.2/ccache/rnrs/control.go
deleted file mode 100644
index 1c4ef48cd463bc44bedd546af4f5cf8770761eca..0000000000000000000000000000000000000000
Binary files a/spaces/Pattr/DrumClassification/lilypond-2.24.2/lib/guile/2.2/ccache/rnrs/control.go and /dev/null differ
diff --git a/spaces/Pinwheel/GLIP-BLIP-Object-Detection-VQA/maskrcnn_benchmark/modeling/roi_heads/box_head/inference.py b/spaces/Pinwheel/GLIP-BLIP-Object-Detection-VQA/maskrcnn_benchmark/modeling/roi_heads/box_head/inference.py
deleted file mode 100644
index 9e51ac148e11d92188e80510c33eab96ff83d03f..0000000000000000000000000000000000000000
--- a/spaces/Pinwheel/GLIP-BLIP-Object-Detection-VQA/maskrcnn_benchmark/modeling/roi_heads/box_head/inference.py
+++ /dev/null
@@ -1,177 +0,0 @@
-# Copyright (c) Facebook, Inc. and its affiliates. All Rights Reserved.
-import torch
-import torch.nn.functional as F
-from torch import nn
-
-from maskrcnn_benchmark.structures.bounding_box import BoxList
-from maskrcnn_benchmark.structures.boxlist_ops import boxlist_nms
-from maskrcnn_benchmark.structures.boxlist_ops import cat_boxlist
-from maskrcnn_benchmark.modeling.box_coder import BoxCoder
-from maskrcnn_benchmark.utils.amp import custom_fwd, custom_bwd
-
-class PostProcessor(nn.Module):
- """
- From a set of classification scores, box regression and proposals,
- computes the post-processed boxes, and applies NMS to obtain the
- final results
- """
-
- def __init__(
- self, score_thresh=0.05, nms=0.5, detections_per_img=100, box_coder=None
- ):
- """
- Arguments:
- score_thresh (float)
- nms (float)
- detections_per_img (int)
- box_coder (BoxCoder)
- """
- super(PostProcessor, self).__init__()
- self.score_thresh = score_thresh
- self.nms = nms
- self.detections_per_img = detections_per_img
- if box_coder is None:
- box_coder = BoxCoder(weights=(10., 10., 5., 5.))
- self.box_coder = box_coder
-
- @custom_fwd(cast_inputs=torch.float32)
- def forward(self, x, boxes):
- """
- Arguments:
- x (tuple[tensor, tensor]): x contains the class logits
- and the box_regression from the model.
- boxes (list[BoxList]): bounding boxes that are used as
- reference, one for ech image
-
- Returns:
- results (list[BoxList]): one BoxList for each image, containing
- the extra fields labels and scores
- """
- class_logits, box_regression = x
- class_prob = F.softmax(class_logits, -1)
-
- # TODO think about a representation of batch of boxes
- image_shapes = [box.size for box in boxes]
- boxes_per_image = [len(box) for box in boxes]
- concat_boxes = torch.cat([a.bbox for a in boxes], dim=0)
-
- extra_fields = [{} for box in boxes]
- if boxes[0].has_field("cbox"):
- concat_cboxes = torch.cat([a.get_field('cbox').bbox for a in boxes], dim=0)
- concat_cscores = torch.cat([a.get_field('cbox').get_field('scores') for a in boxes], dim=0)
- for cbox, cscore, extra_field in zip(concat_cboxes.split(boxes_per_image, dim=0),
- concat_cscores.split(boxes_per_image, dim=0),
- extra_fields):
- extra_field["cbox"] = cbox
- extra_field["cscore"] = cscore
-
- proposals = self.box_coder.decode(
- box_regression.view(sum(boxes_per_image), -1), concat_boxes
- )
-
- num_classes = class_prob.shape[1]
-
- proposals = proposals.split(boxes_per_image, dim=0)
- class_prob = class_prob.split(boxes_per_image, dim=0)
-
- results = []
- for prob, boxes_per_img, image_shape, extra_field in zip(
- class_prob, proposals, image_shapes, extra_fields
- ):
- boxlist = self.prepare_boxlist(boxes_per_img, prob, image_shape, extra_field)
- boxlist = boxlist.clip_to_image(remove_empty=False)
- boxlist = self.filter_results(boxlist, num_classes)
- results.append(boxlist)
- return results
-
- def prepare_boxlist(self, boxes, scores, image_shape, extra_field={}):
- """
- Returns BoxList from `boxes` and adds probability scores information
- as an extra field
- `boxes` has shape (#detections, 4 * #classes), where each row represents
- a list of predicted bounding boxes for each of the object classes in the
- dataset (including the background class). The detections in each row
- originate from the same object proposal.
- `scores` has shape (#detection, #classes), where each row represents a list
- of object detection confidence scores for each of the object classes in the
- dataset (including the background class). `scores[i, j]`` corresponds to the
- box at `boxes[i, j * 4:(j + 1) * 4]`.
- """
- boxes = boxes.reshape(-1, 4)
- scores = scores.reshape(-1)
- boxlist = BoxList(boxes, image_shape, mode="xyxy")
- boxlist.add_field("scores", scores)
- for key, val in extra_field.items():
- boxlist.add_field(key, val)
- return boxlist
-
- def filter_results(self, boxlist, num_classes):
- """Returns bounding-box detection results by thresholding on scores and
- applying non-maximum suppression (NMS).
- """
- # unwrap the boxlist to avoid additional overhead.
- # if we had multi-class NMS, we could perform this directly on the boxlist
- boxes = boxlist.bbox.reshape(-1, num_classes * 4)
- scores = boxlist.get_field("scores").reshape(-1, num_classes)
- if boxlist.has_field('cbox'):
- cboxes = boxlist.get_field("cbox").reshape(-1, 4)
- cscores = boxlist.get_field("cscore")
- else:
- cboxes = None
-
- device = scores.device
- result = []
- # Apply threshold on detection probabilities and apply NMS
- # Skip j = 0, because it's the background class
- inds_all = scores > self.score_thresh
- for j in range(1, num_classes):
- inds = inds_all[:, j].nonzero().squeeze(1)
- scores_j = scores[inds, j]
- boxes_j = boxes[inds, j * 4 : (j + 1) * 4]
- boxlist_for_class = BoxList(boxes_j, boxlist.size, mode="xyxy")
- boxlist_for_class.add_field("scores", scores_j)
- if cboxes is not None:
- cboxes_j = cboxes[inds, :]
- cscores_j = cscores[inds]
- cbox_boxlist = BoxList(cboxes_j, boxlist.size, mode="xyxy")
- cbox_boxlist.add_field("scores", cscores_j)
- boxlist_for_class.add_field("cbox", cbox_boxlist)
-
- boxlist_for_class = boxlist_nms(
- boxlist_for_class, self.nms, score_field="scores"
- )
- num_labels = len(boxlist_for_class)
- boxlist_for_class.add_field(
- "labels", torch.full((num_labels,), j, dtype=torch.int64, device=device)
- )
- result.append(boxlist_for_class)
-
- result = cat_boxlist(result)
- number_of_detections = len(result)
-
- # Limit to max_per_image detections **over all classes**
- if number_of_detections > self.detections_per_img > 0:
- cls_scores = result.get_field("scores")
- image_thresh, _ = torch.kthvalue(
- cls_scores.cpu(), number_of_detections - self.detections_per_img + 1
- )
- keep = cls_scores >= image_thresh.item()
- keep = torch.nonzero(keep).squeeze(1)
- result = result[keep]
- return result
-
-
-def make_roi_box_post_processor(cfg):
- use_fpn = cfg.MODEL.ROI_HEADS.USE_FPN
-
- bbox_reg_weights = cfg.MODEL.ROI_HEADS.BBOX_REG_WEIGHTS
- box_coder = BoxCoder(weights=bbox_reg_weights)
-
- score_thresh = cfg.MODEL.ROI_HEADS.SCORE_THRESH
- nms_thresh = cfg.MODEL.ROI_HEADS.NMS
- detections_per_img = cfg.MODEL.ROI_HEADS.DETECTIONS_PER_IMG
-
- postprocessor = PostProcessor(
- score_thresh, nms_thresh, detections_per_img, box_coder
- )
- return postprocessor
diff --git a/spaces/Qiukai/gpt/crazy_functions/__init__.py b/spaces/Qiukai/gpt/crazy_functions/__init__.py
deleted file mode 100644
index e69de29bb2d1d6434b8b29ae775ad8c2e48c5391..0000000000000000000000000000000000000000
diff --git a/spaces/RGBD-SOD/bbsnet/camera.py b/spaces/RGBD-SOD/bbsnet/camera.py
deleted file mode 100644
index d85b182e92b0fc4a763373c4aa54c214fdb2bca8..0000000000000000000000000000000000000000
--- a/spaces/RGBD-SOD/bbsnet/camera.py
+++ /dev/null
@@ -1,12 +0,0 @@
-import gradio as gr
-import numpy as np
-
-
-def flip(im):
- return np.flipud(im)
-
-
-demo = gr.Interface(flip, gr.Image(source="webcam", streaming=True), "image", live=True)
-
-if __name__ == "__main__":
- demo.launch(server_name="0.0.0.0", server_port=8501)
diff --git a/spaces/RMXK/RVC_HFF/Makefile b/spaces/RMXK/RVC_HFF/Makefile
deleted file mode 100644
index 44de020e6feb7fcd58016d7c3c736681f533b597..0000000000000000000000000000000000000000
--- a/spaces/RMXK/RVC_HFF/Makefile
+++ /dev/null
@@ -1,63 +0,0 @@
-.PHONY:
-.ONESHELL:
-
-help: ## Show this help and exit
- @grep -hE '^[A-Za-z0-9_ \-]*?:.*##.*$$' $(MAKEFILE_LIST) | sort | awk 'BEGIN {FS = ":.*?## "}; {printf "\033[36m%-30s\033[0m %s\n", $$1, $$2}'
-
-install: ## Install dependencies (Do everytime you start up a paperspace machine)
- apt-get -y install build-essential python3-dev ffmpeg
- pip install --upgrade setuptools wheel
- pip install --upgrade pip
- pip install faiss-gpu fairseq gradio ffmpeg ffmpeg-python praat-parselmouth pyworld numpy==1.23.5 numba==0.56.4 librosa==0.9.1
- pip install -r requirements.txt
- pip install --upgrade lxml
- apt-get update
- apt -y install -qq aria2
-
-basev1: ## Download version 1 pre-trained models (Do only once after cloning the fork)
- mkdir -p pretrained uvr5_weights
- git pull
- aria2c --console-log-level=error -c -x 16 -s 16 -k 1M https://huggingface.co/lj1995/VoiceConversionWebUI/resolve/main/pretrained/D32k.pth -d pretrained -o D32k.pth
- aria2c --console-log-level=error -c -x 16 -s 16 -k 1M https://huggingface.co/lj1995/VoiceConversionWebUI/resolve/main/pretrained/D40k.pth -d pretrained -o D40k.pth
- aria2c --console-log-level=error -c -x 16 -s 16 -k 1M https://huggingface.co/lj1995/VoiceConversionWebUI/resolve/main/pretrained/D48k.pth -d pretrained -o D48k.pth
- aria2c --console-log-level=error -c -x 16 -s 16 -k 1M https://huggingface.co/lj1995/VoiceConversionWebUI/resolve/main/pretrained/G32k.pth -d pretrained -o G32k.pth
- aria2c --console-log-level=error -c -x 16 -s 16 -k 1M https://huggingface.co/lj1995/VoiceConversionWebUI/resolve/main/pretrained/G40k.pth -d pretrained -o G40k.pth
- aria2c --console-log-level=error -c -x 16 -s 16 -k 1M https://huggingface.co/lj1995/VoiceConversionWebUI/resolve/main/pretrained/G48k.pth -d pretrained -o G48k.pth
- aria2c --console-log-level=error -c -x 16 -s 16 -k 1M https://huggingface.co/lj1995/VoiceConversionWebUI/resolve/main/pretrained/f0D32k.pth -d pretrained -o f0D32k.pth
- aria2c --console-log-level=error -c -x 16 -s 16 -k 1M https://huggingface.co/lj1995/VoiceConversionWebUI/resolve/main/pretrained/f0D40k.pth -d pretrained -o f0D40k.pth
- aria2c --console-log-level=error -c -x 16 -s 16 -k 1M https://huggingface.co/lj1995/VoiceConversionWebUI/resolve/main/pretrained/f0D48k.pth -d pretrained -o f0D48k.pth
- aria2c --console-log-level=error -c -x 16 -s 16 -k 1M https://huggingface.co/lj1995/VoiceConversionWebUI/resolve/main/pretrained/f0G32k.pth -d pretrained -o f0G32k.pth
- aria2c --console-log-level=error -c -x 16 -s 16 -k 1M https://huggingface.co/lj1995/VoiceConversionWebUI/resolve/main/pretrained/f0G40k.pth -d pretrained -o f0G40k.pth
- aria2c --console-log-level=error -c -x 16 -s 16 -k 1M https://huggingface.co/lj1995/VoiceConversionWebUI/resolve/main/pretrained/f0G48k.pth -d pretrained -o f0G48k.pth
- aria2c --console-log-level=error -c -x 16 -s 16 -k 1M https://huggingface.co/lj1995/VoiceConversionWebUI/resolve/main/uvr5_weights/HP2-人声vocals+非人声instrumentals.pth -d uvr5_weights -o HP2-人声vocals+非人声instrumentals.pth
- aria2c --console-log-level=error -c -x 16 -s 16 -k 1M https://huggingface.co/lj1995/VoiceConversionWebUI/resolve/main/uvr5_weights/HP5-主旋律人声vocals+其他instrumentals.pth -d uvr5_weights -o HP5-主旋律人声vocals+其他instrumentals.pth
- aria2c --console-log-level=error -c -x 16 -s 16 -k 1M https://huggingface.co/lj1995/VoiceConversionWebUI/resolve/main/hubert_base.pt -d ./ -o hubert_base.pt
-
-basev2: ## Download version 2 pre-trained models (Do only once after cloning the fork)
- mkdir -p pretrained_v2 uvr5_weights
- git pull
- aria2c --console-log-level=error -c -x 16 -s 16 -k 1M https://huggingface.co/lj1995/VoiceConversionWebUI/resolve/main/pretrained_v2/D32k.pth -d pretrained_v2 -o D32k.pth
- aria2c --console-log-level=error -c -x 16 -s 16 -k 1M https://huggingface.co/lj1995/VoiceConversionWebUI/resolve/main/pretrained_v2/D40k.pth -d pretrained_v2 -o D40k.pth
- aria2c --console-log-level=error -c -x 16 -s 16 -k 1M https://huggingface.co/lj1995/VoiceConversionWebUI/resolve/main/pretrained_v2/D48k.pth -d pretrained_v2 -o D48k.pth
- aria2c --console-log-level=error -c -x 16 -s 16 -k 1M https://huggingface.co/lj1995/VoiceConversionWebUI/resolve/main/pretrained_v2/G32k.pth -d pretrained_v2 -o G32k.pth
- aria2c --console-log-level=error -c -x 16 -s 16 -k 1M https://huggingface.co/lj1995/VoiceConversionWebUI/resolve/main/pretrained_v2/G40k.pth -d pretrained_v2 -o G40k.pth
- aria2c --console-log-level=error -c -x 16 -s 16 -k 1M https://huggingface.co/lj1995/VoiceConversionWebUI/resolve/main/pretrained_v2/G48k.pth -d pretrained_v2 -o G48k.pth
- aria2c --console-log-level=error -c -x 16 -s 16 -k 1M https://huggingface.co/lj1995/VoiceConversionWebUI/resolve/main/pretrained_v2/f0D32k.pth -d pretrained_v2 -o f0D32k.pth
- aria2c --console-log-level=error -c -x 16 -s 16 -k 1M https://huggingface.co/lj1995/VoiceConversionWebUI/resolve/main/pretrained_v2/f0D40k.pth -d pretrained_v2 -o f0D40k.pth
- aria2c --console-log-level=error -c -x 16 -s 16 -k 1M https://huggingface.co/lj1995/VoiceConversionWebUI/resolve/main/pretrained_v2/f0D48k.pth -d pretrained_v2 -o f0D48k.pth
- aria2c --console-log-level=error -c -x 16 -s 16 -k 1M https://huggingface.co/lj1995/VoiceConversionWebUI/resolve/main/pretrained_v2/f0G32k.pth -d pretrained_v2 -o f0G32k.pth
- aria2c --console-log-level=error -c -x 16 -s 16 -k 1M https://huggingface.co/lj1995/VoiceConversionWebUI/resolve/main/pretrained_v2/f0G40k.pth -d pretrained_v2 -o f0G40k.pth
- aria2c --console-log-level=error -c -x 16 -s 16 -k 1M https://huggingface.co/lj1995/VoiceConversionWebUI/resolve/main/pretrained_v2/f0G48k.pth -d pretrained_v2 -o f0G48k.pth
- aria2c --console-log-level=error -c -x 16 -s 16 -k 1M https://huggingface.co/lj1995/VoiceConversionWebUI/resolve/main/uvr5_weights/HP2-人声vocals+非人声instrumentals.pth -d uvr5_weights -o HP2-人声vocals+非人声instrumentals.pth
- aria2c --console-log-level=error -c -x 16 -s 16 -k 1M https://huggingface.co/lj1995/VoiceConversionWebUI/resolve/main/uvr5_weights/HP5-主旋律人声vocals+其他instrumentals.pth -d uvr5_weights -o HP5-主旋律人声vocals+其他instrumentals.pth
- aria2c --console-log-level=error -c -x 16 -s 16 -k 1M https://huggingface.co/lj1995/VoiceConversionWebUI/resolve/main/hubert_base.pt -d ./ -o hubert_base.pt
-
-run-ui: ## Run the python GUI
- python infer-web.py --paperspace --pycmd python
-
-run-cli: ## Run the python CLI
- python infer-web.py --pycmd python --is_cli
-
-tensorboard: ## Start the tensorboard (Run on separate terminal)
- echo https://tensorboard-$$(hostname).clg07azjl.paperspacegradient.com
- tensorboard --logdir logs --bind_all
\ No newline at end of file
diff --git a/spaces/Rakot2223/faster-whisper-webui/src/whisper/whisperFactory.py b/spaces/Rakot2223/faster-whisper-webui/src/whisper/whisperFactory.py
deleted file mode 100644
index 58fc840b7e60947fec4a98b2833ff03e7ad7b7de..0000000000000000000000000000000000000000
--- a/spaces/Rakot2223/faster-whisper-webui/src/whisper/whisperFactory.py
+++ /dev/null
@@ -1,19 +0,0 @@
-from typing import List
-from src import modelCache
-from src.config import ModelConfig
-from src.whisper.abstractWhisperContainer import AbstractWhisperContainer
-
-def create_whisper_container(whisper_implementation: str,
- model_name: str, device: str = None, compute_type: str = "float16",
- download_root: str = None,
- cache: modelCache = None, models: List[ModelConfig] = []) -> AbstractWhisperContainer:
- print("Creating whisper container for " + whisper_implementation)
-
- if (whisper_implementation == "whisper"):
- from src.whisper.whisperContainer import WhisperContainer
- return WhisperContainer(model_name=model_name, device=device, compute_type=compute_type, download_root=download_root, cache=cache, models=models)
- elif (whisper_implementation == "faster-whisper" or whisper_implementation == "faster_whisper"):
- from src.whisper.fasterWhisperContainer import FasterWhisperContainer
- return FasterWhisperContainer(model_name=model_name, device=device, compute_type=compute_type, download_root=download_root, cache=cache, models=models)
- else:
- raise ValueError("Unknown Whisper implementation: " + whisper_implementation)
\ No newline at end of file
diff --git a/spaces/Raspberry-ai/main/.env/lib/python3.11/site-packages/pip/_internal/commands/check.py b/spaces/Raspberry-ai/main/.env/lib/python3.11/site-packages/pip/_internal/commands/check.py
deleted file mode 100644
index 3864220b2b4a2fd3803bdff0ab9e4c3941c1f313..0000000000000000000000000000000000000000
--- a/spaces/Raspberry-ai/main/.env/lib/python3.11/site-packages/pip/_internal/commands/check.py
+++ /dev/null
@@ -1,53 +0,0 @@
-import logging
-from optparse import Values
-from typing import List
-
-from pip._internal.cli.base_command import Command
-from pip._internal.cli.status_codes import ERROR, SUCCESS
-from pip._internal.operations.check import (
- check_package_set,
- create_package_set_from_installed,
-)
-from pip._internal.utils.misc import write_output
-
-logger = logging.getLogger(__name__)
-
-
-class CheckCommand(Command):
- """Verify installed packages have compatible dependencies."""
-
- usage = """
- %prog [options]"""
-
- def run(self, options: Values, args: List[str]) -> int:
-
- package_set, parsing_probs = create_package_set_from_installed()
- missing, conflicting = check_package_set(package_set)
-
- for project_name in missing:
- version = package_set[project_name].version
- for dependency in missing[project_name]:
- write_output(
- "%s %s requires %s, which is not installed.",
- project_name,
- version,
- dependency[0],
- )
-
- for project_name in conflicting:
- version = package_set[project_name].version
- for dep_name, dep_version, req in conflicting[project_name]:
- write_output(
- "%s %s has requirement %s, but you have %s %s.",
- project_name,
- version,
- req,
- dep_name,
- dep_version,
- )
-
- if missing or conflicting or parsing_probs:
- return ERROR
- else:
- write_output("No broken requirements found.")
- return SUCCESS
diff --git a/spaces/Raspberry-ai/main/.env/lib/python3.11/site-packages/pip/_internal/operations/build/metadata_editable.py b/spaces/Raspberry-ai/main/.env/lib/python3.11/site-packages/pip/_internal/operations/build/metadata_editable.py
deleted file mode 100644
index 4c3f48b6cdfb3087a833546410fc810a343b9e13..0000000000000000000000000000000000000000
--- a/spaces/Raspberry-ai/main/.env/lib/python3.11/site-packages/pip/_internal/operations/build/metadata_editable.py
+++ /dev/null
@@ -1,41 +0,0 @@
-"""Metadata generation logic for source distributions.
-"""
-
-import os
-
-from pip._vendor.pep517.wrappers import Pep517HookCaller
-
-from pip._internal.build_env import BuildEnvironment
-from pip._internal.exceptions import (
- InstallationSubprocessError,
- MetadataGenerationFailed,
-)
-from pip._internal.utils.subprocess import runner_with_spinner_message
-from pip._internal.utils.temp_dir import TempDirectory
-
-
-def generate_editable_metadata(
- build_env: BuildEnvironment, backend: Pep517HookCaller, details: str
-) -> str:
- """Generate metadata using mechanisms described in PEP 660.
-
- Returns the generated metadata directory.
- """
- metadata_tmpdir = TempDirectory(kind="modern-metadata", globally_managed=True)
-
- metadata_dir = metadata_tmpdir.path
-
- with build_env:
- # Note that Pep517HookCaller implements a fallback for
- # prepare_metadata_for_build_wheel/editable, so we don't have to
- # consider the possibility that this hook doesn't exist.
- runner = runner_with_spinner_message(
- "Preparing editable metadata (pyproject.toml)"
- )
- with backend.subprocess_runner(runner):
- try:
- distinfo_dir = backend.prepare_metadata_for_build_editable(metadata_dir)
- except InstallationSubprocessError as error:
- raise MetadataGenerationFailed(package_details=details) from error
-
- return os.path.join(metadata_dir, distinfo_dir)
diff --git a/spaces/Raspberry-ai/main/.env/lib/python3.11/site-packages/pip/_vendor/urllib3/fields.py b/spaces/Raspberry-ai/main/.env/lib/python3.11/site-packages/pip/_vendor/urllib3/fields.py
deleted file mode 100644
index 9d630f491d9a39644ae65564dac88eb51f0bbe78..0000000000000000000000000000000000000000
--- a/spaces/Raspberry-ai/main/.env/lib/python3.11/site-packages/pip/_vendor/urllib3/fields.py
+++ /dev/null
@@ -1,274 +0,0 @@
-from __future__ import absolute_import
-
-import email.utils
-import mimetypes
-import re
-
-from .packages import six
-
-
-def guess_content_type(filename, default="application/octet-stream"):
- """
- Guess the "Content-Type" of a file.
-
- :param filename:
- The filename to guess the "Content-Type" of using :mod:`mimetypes`.
- :param default:
- If no "Content-Type" can be guessed, default to `default`.
- """
- if filename:
- return mimetypes.guess_type(filename)[0] or default
- return default
-
-
-def format_header_param_rfc2231(name, value):
- """
- Helper function to format and quote a single header parameter using the
- strategy defined in RFC 2231.
-
- Particularly useful for header parameters which might contain
- non-ASCII values, like file names. This follows
- `RFC 2388 Section 4.4 `_.
-
- :param name:
- The name of the parameter, a string expected to be ASCII only.
- :param value:
- The value of the parameter, provided as ``bytes`` or `str``.
- :ret:
- An RFC-2231-formatted unicode string.
- """
- if isinstance(value, six.binary_type):
- value = value.decode("utf-8")
-
- if not any(ch in value for ch in '"\\\r\n'):
- result = u'%s="%s"' % (name, value)
- try:
- result.encode("ascii")
- except (UnicodeEncodeError, UnicodeDecodeError):
- pass
- else:
- return result
-
- if six.PY2: # Python 2:
- value = value.encode("utf-8")
-
- # encode_rfc2231 accepts an encoded string and returns an ascii-encoded
- # string in Python 2 but accepts and returns unicode strings in Python 3
- value = email.utils.encode_rfc2231(value, "utf-8")
- value = "%s*=%s" % (name, value)
-
- if six.PY2: # Python 2:
- value = value.decode("utf-8")
-
- return value
-
-
-_HTML5_REPLACEMENTS = {
- u"\u0022": u"%22",
- # Replace "\" with "\\".
- u"\u005C": u"\u005C\u005C",
-}
-
-# All control characters from 0x00 to 0x1F *except* 0x1B.
-_HTML5_REPLACEMENTS.update(
- {
- six.unichr(cc): u"%{:02X}".format(cc)
- for cc in range(0x00, 0x1F + 1)
- if cc not in (0x1B,)
- }
-)
-
-
-def _replace_multiple(value, needles_and_replacements):
- def replacer(match):
- return needles_and_replacements[match.group(0)]
-
- pattern = re.compile(
- r"|".join([re.escape(needle) for needle in needles_and_replacements.keys()])
- )
-
- result = pattern.sub(replacer, value)
-
- return result
-
-
-def format_header_param_html5(name, value):
- """
- Helper function to format and quote a single header parameter using the
- HTML5 strategy.
-
- Particularly useful for header parameters which might contain
- non-ASCII values, like file names. This follows the `HTML5 Working Draft
- Section 4.10.22.7`_ and matches the behavior of curl and modern browsers.
-
- .. _HTML5 Working Draft Section 4.10.22.7:
- https://w3c.github.io/html/sec-forms.html#multipart-form-data
-
- :param name:
- The name of the parameter, a string expected to be ASCII only.
- :param value:
- The value of the parameter, provided as ``bytes`` or `str``.
- :ret:
- A unicode string, stripped of troublesome characters.
- """
- if isinstance(value, six.binary_type):
- value = value.decode("utf-8")
-
- value = _replace_multiple(value, _HTML5_REPLACEMENTS)
-
- return u'%s="%s"' % (name, value)
-
-
-# For backwards-compatibility.
-format_header_param = format_header_param_html5
-
-
-class RequestField(object):
- """
- A data container for request body parameters.
-
- :param name:
- The name of this request field. Must be unicode.
- :param data:
- The data/value body.
- :param filename:
- An optional filename of the request field. Must be unicode.
- :param headers:
- An optional dict-like object of headers to initially use for the field.
- :param header_formatter:
- An optional callable that is used to encode and format the headers. By
- default, this is :func:`format_header_param_html5`.
- """
-
- def __init__(
- self,
- name,
- data,
- filename=None,
- headers=None,
- header_formatter=format_header_param_html5,
- ):
- self._name = name
- self._filename = filename
- self.data = data
- self.headers = {}
- if headers:
- self.headers = dict(headers)
- self.header_formatter = header_formatter
-
- @classmethod
- def from_tuples(cls, fieldname, value, header_formatter=format_header_param_html5):
- """
- A :class:`~urllib3.fields.RequestField` factory from old-style tuple parameters.
-
- Supports constructing :class:`~urllib3.fields.RequestField` from
- parameter of key/value strings AND key/filetuple. A filetuple is a
- (filename, data, MIME type) tuple where the MIME type is optional.
- For example::
-
- 'foo': 'bar',
- 'fakefile': ('foofile.txt', 'contents of foofile'),
- 'realfile': ('barfile.txt', open('realfile').read()),
- 'typedfile': ('bazfile.bin', open('bazfile').read(), 'image/jpeg'),
- 'nonamefile': 'contents of nonamefile field',
-
- Field names and filenames must be unicode.
- """
- if isinstance(value, tuple):
- if len(value) == 3:
- filename, data, content_type = value
- else:
- filename, data = value
- content_type = guess_content_type(filename)
- else:
- filename = None
- content_type = None
- data = value
-
- request_param = cls(
- fieldname, data, filename=filename, header_formatter=header_formatter
- )
- request_param.make_multipart(content_type=content_type)
-
- return request_param
-
- def _render_part(self, name, value):
- """
- Overridable helper function to format a single header parameter. By
- default, this calls ``self.header_formatter``.
-
- :param name:
- The name of the parameter, a string expected to be ASCII only.
- :param value:
- The value of the parameter, provided as a unicode string.
- """
-
- return self.header_formatter(name, value)
-
- def _render_parts(self, header_parts):
- """
- Helper function to format and quote a single header.
-
- Useful for single headers that are composed of multiple items. E.g.,
- 'Content-Disposition' fields.
-
- :param header_parts:
- A sequence of (k, v) tuples or a :class:`dict` of (k, v) to format
- as `k1="v1"; k2="v2"; ...`.
- """
- parts = []
- iterable = header_parts
- if isinstance(header_parts, dict):
- iterable = header_parts.items()
-
- for name, value in iterable:
- if value is not None:
- parts.append(self._render_part(name, value))
-
- return u"; ".join(parts)
-
- def render_headers(self):
- """
- Renders the headers for this request field.
- """
- lines = []
-
- sort_keys = ["Content-Disposition", "Content-Type", "Content-Location"]
- for sort_key in sort_keys:
- if self.headers.get(sort_key, False):
- lines.append(u"%s: %s" % (sort_key, self.headers[sort_key]))
-
- for header_name, header_value in self.headers.items():
- if header_name not in sort_keys:
- if header_value:
- lines.append(u"%s: %s" % (header_name, header_value))
-
- lines.append(u"\r\n")
- return u"\r\n".join(lines)
-
- def make_multipart(
- self, content_disposition=None, content_type=None, content_location=None
- ):
- """
- Makes this request field into a multipart request field.
-
- This method overrides "Content-Disposition", "Content-Type" and
- "Content-Location" headers to the request parameter.
-
- :param content_type:
- The 'Content-Type' of the request body.
- :param content_location:
- The 'Content-Location' of the request body.
-
- """
- self.headers["Content-Disposition"] = content_disposition or u"form-data"
- self.headers["Content-Disposition"] += u"; ".join(
- [
- u"",
- self._render_parts(
- ((u"name", self._name), (u"filename", self._filename))
- ),
- ]
- )
- self.headers["Content-Type"] = content_type
- self.headers["Content-Location"] = content_location
diff --git a/spaces/Raspberry-ai/main/.env/lib/python3.11/site-packages/setuptools/_vendor/pyparsing/util.py b/spaces/Raspberry-ai/main/.env/lib/python3.11/site-packages/setuptools/_vendor/pyparsing/util.py
deleted file mode 100644
index 34ce092c6d08d9cdc2704840b7539de7b5ae1dcc..0000000000000000000000000000000000000000
--- a/spaces/Raspberry-ai/main/.env/lib/python3.11/site-packages/setuptools/_vendor/pyparsing/util.py
+++ /dev/null
@@ -1,235 +0,0 @@
-# util.py
-import warnings
-import types
-import collections
-import itertools
-from functools import lru_cache
-from typing import List, Union, Iterable
-
-_bslash = chr(92)
-
-
-class __config_flags:
- """Internal class for defining compatibility and debugging flags"""
-
- _all_names: List[str] = []
- _fixed_names: List[str] = []
- _type_desc = "configuration"
-
- @classmethod
- def _set(cls, dname, value):
- if dname in cls._fixed_names:
- warnings.warn(
- "{}.{} {} is {} and cannot be overridden".format(
- cls.__name__,
- dname,
- cls._type_desc,
- str(getattr(cls, dname)).upper(),
- )
- )
- return
- if dname in cls._all_names:
- setattr(cls, dname, value)
- else:
- raise ValueError("no such {} {!r}".format(cls._type_desc, dname))
-
- enable = classmethod(lambda cls, name: cls._set(name, True))
- disable = classmethod(lambda cls, name: cls._set(name, False))
-
-
-@lru_cache(maxsize=128)
-def col(loc: int, strg: str) -> int:
- """
- Returns current column within a string, counting newlines as line separators.
- The first column is number 1.
-
- Note: the default parsing behavior is to expand tabs in the input string
- before starting the parsing process. See
- :class:`ParserElement.parseString` for more
- information on parsing strings containing ```` s, and suggested
- methods to maintain a consistent view of the parsed string, the parse
- location, and line and column positions within the parsed string.
- """
- s = strg
- return 1 if 0 < loc < len(s) and s[loc - 1] == "\n" else loc - s.rfind("\n", 0, loc)
-
-
-@lru_cache(maxsize=128)
-def lineno(loc: int, strg: str) -> int:
- """Returns current line number within a string, counting newlines as line separators.
- The first line is number 1.
-
- Note - the default parsing behavior is to expand tabs in the input string
- before starting the parsing process. See :class:`ParserElement.parseString`
- for more information on parsing strings containing ```` s, and
- suggested methods to maintain a consistent view of the parsed string, the
- parse location, and line and column positions within the parsed string.
- """
- return strg.count("\n", 0, loc) + 1
-
-
-@lru_cache(maxsize=128)
-def line(loc: int, strg: str) -> str:
- """
- Returns the line of text containing loc within a string, counting newlines as line separators.
- """
- last_cr = strg.rfind("\n", 0, loc)
- next_cr = strg.find("\n", loc)
- return strg[last_cr + 1 : next_cr] if next_cr >= 0 else strg[last_cr + 1 :]
-
-
-class _UnboundedCache:
- def __init__(self):
- cache = {}
- cache_get = cache.get
- self.not_in_cache = not_in_cache = object()
-
- def get(_, key):
- return cache_get(key, not_in_cache)
-
- def set_(_, key, value):
- cache[key] = value
-
- def clear(_):
- cache.clear()
-
- self.size = None
- self.get = types.MethodType(get, self)
- self.set = types.MethodType(set_, self)
- self.clear = types.MethodType(clear, self)
-
-
-class _FifoCache:
- def __init__(self, size):
- self.not_in_cache = not_in_cache = object()
- cache = collections.OrderedDict()
- cache_get = cache.get
-
- def get(_, key):
- return cache_get(key, not_in_cache)
-
- def set_(_, key, value):
- cache[key] = value
- while len(cache) > size:
- cache.popitem(last=False)
-
- def clear(_):
- cache.clear()
-
- self.size = size
- self.get = types.MethodType(get, self)
- self.set = types.MethodType(set_, self)
- self.clear = types.MethodType(clear, self)
-
-
-class LRUMemo:
- """
- A memoizing mapping that retains `capacity` deleted items
-
- The memo tracks retained items by their access order; once `capacity` items
- are retained, the least recently used item is discarded.
- """
-
- def __init__(self, capacity):
- self._capacity = capacity
- self._active = {}
- self._memory = collections.OrderedDict()
-
- def __getitem__(self, key):
- try:
- return self._active[key]
- except KeyError:
- self._memory.move_to_end(key)
- return self._memory[key]
-
- def __setitem__(self, key, value):
- self._memory.pop(key, None)
- self._active[key] = value
-
- def __delitem__(self, key):
- try:
- value = self._active.pop(key)
- except KeyError:
- pass
- else:
- while len(self._memory) >= self._capacity:
- self._memory.popitem(last=False)
- self._memory[key] = value
-
- def clear(self):
- self._active.clear()
- self._memory.clear()
-
-
-class UnboundedMemo(dict):
- """
- A memoizing mapping that retains all deleted items
- """
-
- def __delitem__(self, key):
- pass
-
-
-def _escape_regex_range_chars(s: str) -> str:
- # escape these chars: ^-[]
- for c in r"\^-[]":
- s = s.replace(c, _bslash + c)
- s = s.replace("\n", r"\n")
- s = s.replace("\t", r"\t")
- return str(s)
-
-
-def _collapse_string_to_ranges(
- s: Union[str, Iterable[str]], re_escape: bool = True
-) -> str:
- def is_consecutive(c):
- c_int = ord(c)
- is_consecutive.prev, prev = c_int, is_consecutive.prev
- if c_int - prev > 1:
- is_consecutive.value = next(is_consecutive.counter)
- return is_consecutive.value
-
- is_consecutive.prev = 0
- is_consecutive.counter = itertools.count()
- is_consecutive.value = -1
-
- def escape_re_range_char(c):
- return "\\" + c if c in r"\^-][" else c
-
- def no_escape_re_range_char(c):
- return c
-
- if not re_escape:
- escape_re_range_char = no_escape_re_range_char
-
- ret = []
- s = "".join(sorted(set(s)))
- if len(s) > 3:
- for _, chars in itertools.groupby(s, key=is_consecutive):
- first = last = next(chars)
- last = collections.deque(
- itertools.chain(iter([last]), chars), maxlen=1
- ).pop()
- if first == last:
- ret.append(escape_re_range_char(first))
- else:
- sep = "" if ord(last) == ord(first) + 1 else "-"
- ret.append(
- "{}{}{}".format(
- escape_re_range_char(first), sep, escape_re_range_char(last)
- )
- )
- else:
- ret = [escape_re_range_char(c) for c in s]
-
- return "".join(ret)
-
-
-def _flatten(ll: list) -> list:
- ret = []
- for i in ll:
- if isinstance(i, list):
- ret.extend(_flatten(i))
- else:
- ret.append(i)
- return ret
diff --git a/spaces/Rayzggz/illi-Bert-VITS2/train_ms.py b/spaces/Rayzggz/illi-Bert-VITS2/train_ms.py
deleted file mode 100644
index 1f1708d8ef1f4e820b608234a60744a200a644cd..0000000000000000000000000000000000000000
--- a/spaces/Rayzggz/illi-Bert-VITS2/train_ms.py
+++ /dev/null
@@ -1,594 +0,0 @@
-# flake8: noqa: E402
-
-import os
-import torch
-from torch.nn import functional as F
-from torch.utils.data import DataLoader
-from torch.utils.tensorboard import SummaryWriter
-import torch.distributed as dist
-from torch.nn.parallel import DistributedDataParallel as DDP
-from torch.cuda.amp import autocast, GradScaler
-from tqdm import tqdm
-import logging
-
-logging.getLogger("numba").setLevel(logging.WARNING)
-import commons
-import utils
-from data_utils import (
- TextAudioSpeakerLoader,
- TextAudioSpeakerCollate,
- DistributedBucketSampler,
-)
-from models import (
- SynthesizerTrn,
- MultiPeriodDiscriminator,
- DurationDiscriminator,
-)
-from losses import generator_loss, discriminator_loss, feature_loss, kl_loss
-from mel_processing import mel_spectrogram_torch, spec_to_mel_torch
-from text.symbols import symbols
-
-torch.backends.cuda.matmul.allow_tf32 = True
-torch.backends.cudnn.allow_tf32 = (
- True # If encontered training problem,please try to disable TF32.
-)
-torch.set_float32_matmul_precision("medium")
-torch.backends.cudnn.benchmark = True
-torch.backends.cuda.sdp_kernel("flash")
-torch.backends.cuda.enable_flash_sdp(True)
-torch.backends.cuda.enable_mem_efficient_sdp(
- True
-) # Not available if torch version is lower than 2.0
-torch.backends.cuda.enable_math_sdp(True)
-global_step = 0
-
-
-def run():
- dist.init_process_group(
- backend="gloo",
- init_method="env://", # Due to some training problem,we proposed to use gloo instead of nccl.
- ) # Use torchrun instead of mp.spawn
- rank = dist.get_rank()
- n_gpus = dist.get_world_size()
- hps = utils.get_hparams()
- torch.manual_seed(hps.train.seed)
- torch.cuda.set_device(rank)
- global global_step
- if rank == 0:
- logger = utils.get_logger(hps.model_dir)
- logger.info(hps)
- utils.check_git_hash(hps.model_dir)
- writer = SummaryWriter(log_dir=hps.model_dir)
- writer_eval = SummaryWriter(log_dir=os.path.join(hps.model_dir, "eval"))
- train_dataset = TextAudioSpeakerLoader(hps.data.training_files, hps.data)
- train_sampler = DistributedBucketSampler(
- train_dataset,
- hps.train.batch_size,
- [32, 300, 400, 500, 600, 700, 800, 900, 1000],
- num_replicas=n_gpus,
- rank=rank,
- shuffle=True,
- )
- collate_fn = TextAudioSpeakerCollate()
- train_loader = DataLoader(
- train_dataset,
- num_workers=16,
- shuffle=False,
- pin_memory=True,
- collate_fn=collate_fn,
- batch_sampler=train_sampler,
- persistent_workers=True,
- prefetch_factor=4,
- ) # DataLoader config could be adjusted.
- if rank == 0:
- eval_dataset = TextAudioSpeakerLoader(hps.data.validation_files, hps.data)
- eval_loader = DataLoader(
- eval_dataset,
- num_workers=0,
- shuffle=False,
- batch_size=1,
- pin_memory=True,
- drop_last=False,
- collate_fn=collate_fn,
- )
- if (
- "use_noise_scaled_mas" in hps.model.keys()
- and hps.model.use_noise_scaled_mas is True
- ):
- print("Using noise scaled MAS for VITS2")
- mas_noise_scale_initial = 0.01
- noise_scale_delta = 2e-6
- else:
- print("Using normal MAS for VITS1")
- mas_noise_scale_initial = 0.0
- noise_scale_delta = 0.0
- if (
- "use_duration_discriminator" in hps.model.keys()
- and hps.model.use_duration_discriminator is True
- ):
- print("Using duration discriminator for VITS2")
- net_dur_disc = DurationDiscriminator(
- hps.model.hidden_channels,
- hps.model.hidden_channels,
- 3,
- 0.1,
- gin_channels=hps.model.gin_channels if hps.data.n_speakers != 0 else 0,
- ).cuda(rank)
- if (
- "use_spk_conditioned_encoder" in hps.model.keys()
- and hps.model.use_spk_conditioned_encoder is True
- ):
- if hps.data.n_speakers == 0:
- raise ValueError(
- "n_speakers must be > 0 when using spk conditioned encoder to train multi-speaker model"
- )
- else:
- print("Using normal encoder for VITS1")
-
- net_g = SynthesizerTrn(
- len(symbols),
- hps.data.filter_length // 2 + 1,
- hps.train.segment_size // hps.data.hop_length,
- n_speakers=hps.data.n_speakers,
- mas_noise_scale_initial=mas_noise_scale_initial,
- noise_scale_delta=noise_scale_delta,
- **hps.model,
- ).cuda(rank)
-
- net_d = MultiPeriodDiscriminator(hps.model.use_spectral_norm).cuda(rank)
- optim_g = torch.optim.AdamW(
- filter(lambda p: p.requires_grad, net_g.parameters()),
- hps.train.learning_rate,
- betas=hps.train.betas,
- eps=hps.train.eps,
- )
- optim_d = torch.optim.AdamW(
- net_d.parameters(),
- hps.train.learning_rate,
- betas=hps.train.betas,
- eps=hps.train.eps,
- )
- if net_dur_disc is not None:
- optim_dur_disc = torch.optim.AdamW(
- net_dur_disc.parameters(),
- hps.train.learning_rate,
- betas=hps.train.betas,
- eps=hps.train.eps,
- )
- else:
- optim_dur_disc = None
- net_g = DDP(net_g, device_ids=[rank], find_unused_parameters=True)
- net_d = DDP(net_d, device_ids=[rank], find_unused_parameters=True)
- if net_dur_disc is not None:
- net_dur_disc = DDP(net_dur_disc, device_ids=[rank], find_unused_parameters=True)
- try:
- if net_dur_disc is not None:
- _, _, dur_resume_lr, epoch_str = utils.load_checkpoint(
- utils.latest_checkpoint_path(hps.model_dir, "DUR_*.pth"),
- net_dur_disc,
- optim_dur_disc,
- skip_optimizer=hps.train.skip_optimizer
- if "skip_optimizer" in hps.train
- else True,
- )
- _, optim_g, g_resume_lr, epoch_str = utils.load_checkpoint(
- utils.latest_checkpoint_path(hps.model_dir, "G_*.pth"),
- net_g,
- optim_g,
- skip_optimizer=hps.train.skip_optimizer
- if "skip_optimizer" in hps.train
- else True,
- )
- _, optim_d, d_resume_lr, epoch_str = utils.load_checkpoint(
- utils.latest_checkpoint_path(hps.model_dir, "D_*.pth"),
- net_d,
- optim_d,
- skip_optimizer=hps.train.skip_optimizer
- if "skip_optimizer" in hps.train
- else True,
- )
- if not optim_g.param_groups[0].get("initial_lr"):
- optim_g.param_groups[0]["initial_lr"] = g_resume_lr
- if not optim_d.param_groups[0].get("initial_lr"):
- optim_d.param_groups[0]["initial_lr"] = d_resume_lr
- if not optim_dur_disc.param_groups[0].get("initial_lr"):
- optim_dur_disc.param_groups[0]["initial_lr"] = dur_resume_lr
-
- epoch_str = max(epoch_str, 1)
- global_step = (epoch_str - 1) * len(train_loader)
- except Exception as e:
- print(e)
- epoch_str = 1
- global_step = 0
-
- scheduler_g = torch.optim.lr_scheduler.ExponentialLR(
- optim_g, gamma=hps.train.lr_decay, last_epoch=epoch_str - 2
- )
- scheduler_d = torch.optim.lr_scheduler.ExponentialLR(
- optim_d, gamma=hps.train.lr_decay, last_epoch=epoch_str - 2
- )
- if net_dur_disc is not None:
- if not optim_dur_disc.param_groups[0].get("initial_lr"):
- optim_dur_disc.param_groups[0]["initial_lr"] = dur_resume_lr
- scheduler_dur_disc = torch.optim.lr_scheduler.ExponentialLR(
- optim_dur_disc, gamma=hps.train.lr_decay, last_epoch=epoch_str - 2
- )
- else:
- scheduler_dur_disc = None
- scaler = GradScaler(enabled=hps.train.fp16_run)
-
- for epoch in range(epoch_str, hps.train.epochs + 1):
- if rank == 0:
- train_and_evaluate(
- rank,
- epoch,
- hps,
- [net_g, net_d, net_dur_disc],
- [optim_g, optim_d, optim_dur_disc],
- [scheduler_g, scheduler_d, scheduler_dur_disc],
- scaler,
- [train_loader, eval_loader],
- logger,
- [writer, writer_eval],
- )
- else:
- train_and_evaluate(
- rank,
- epoch,
- hps,
- [net_g, net_d, net_dur_disc],
- [optim_g, optim_d, optim_dur_disc],
- [scheduler_g, scheduler_d, scheduler_dur_disc],
- scaler,
- [train_loader, None],
- None,
- None,
- )
- scheduler_g.step()
- scheduler_d.step()
- if net_dur_disc is not None:
- scheduler_dur_disc.step()
-
-
-def train_and_evaluate(
- rank, epoch, hps, nets, optims, schedulers, scaler, loaders, logger, writers
-):
- net_g, net_d, net_dur_disc = nets
- optim_g, optim_d, optim_dur_disc = optims
- scheduler_g, scheduler_d, scheduler_dur_disc = schedulers
- train_loader, eval_loader = loaders
- if writers is not None:
- writer, writer_eval = writers
-
- train_loader.batch_sampler.set_epoch(epoch)
- global global_step
-
- net_g.train()
- net_d.train()
- if net_dur_disc is not None:
- net_dur_disc.train()
- for batch_idx, (
- x,
- x_lengths,
- spec,
- spec_lengths,
- y,
- y_lengths,
- speakers,
- tone,
- language,
- bert,
- ja_bert,
- ) in tqdm(enumerate(train_loader)):
- if net_g.module.use_noise_scaled_mas:
- current_mas_noise_scale = (
- net_g.module.mas_noise_scale_initial
- - net_g.module.noise_scale_delta * global_step
- )
- net_g.module.current_mas_noise_scale = max(current_mas_noise_scale, 0.0)
- x, x_lengths = x.cuda(rank, non_blocking=True), x_lengths.cuda(
- rank, non_blocking=True
- )
- spec, spec_lengths = spec.cuda(rank, non_blocking=True), spec_lengths.cuda(
- rank, non_blocking=True
- )
- y, y_lengths = y.cuda(rank, non_blocking=True), y_lengths.cuda(
- rank, non_blocking=True
- )
- speakers = speakers.cuda(rank, non_blocking=True)
- tone = tone.cuda(rank, non_blocking=True)
- language = language.cuda(rank, non_blocking=True)
- bert = bert.cuda(rank, non_blocking=True)
- ja_bert = ja_bert.cuda(rank, non_blocking=True)
-
- with autocast(enabled=hps.train.fp16_run):
- (
- y_hat,
- l_length,
- attn,
- ids_slice,
- x_mask,
- z_mask,
- (z, z_p, m_p, logs_p, m_q, logs_q),
- (hidden_x, logw, logw_),
- ) = net_g(
- x,
- x_lengths,
- spec,
- spec_lengths,
- speakers,
- tone,
- language,
- bert,
- ja_bert,
- )
- mel = spec_to_mel_torch(
- spec,
- hps.data.filter_length,
- hps.data.n_mel_channels,
- hps.data.sampling_rate,
- hps.data.mel_fmin,
- hps.data.mel_fmax,
- )
- y_mel = commons.slice_segments(
- mel, ids_slice, hps.train.segment_size // hps.data.hop_length
- )
- y_hat_mel = mel_spectrogram_torch(
- y_hat.squeeze(1),
- hps.data.filter_length,
- hps.data.n_mel_channels,
- hps.data.sampling_rate,
- hps.data.hop_length,
- hps.data.win_length,
- hps.data.mel_fmin,
- hps.data.mel_fmax,
- )
-
- y = commons.slice_segments(
- y, ids_slice * hps.data.hop_length, hps.train.segment_size
- ) # slice
-
- # Discriminator
- y_d_hat_r, y_d_hat_g, _, _ = net_d(y, y_hat.detach())
- with autocast(enabled=False):
- loss_disc, losses_disc_r, losses_disc_g = discriminator_loss(
- y_d_hat_r, y_d_hat_g
- )
- loss_disc_all = loss_disc
- if net_dur_disc is not None:
- y_dur_hat_r, y_dur_hat_g = net_dur_disc(
- hidden_x.detach(), x_mask.detach(), logw.detach(), logw_.detach()
- )
- with autocast(enabled=False):
- # TODO: I think need to mean using the mask, but for now, just mean all
- (
- loss_dur_disc,
- losses_dur_disc_r,
- losses_dur_disc_g,
- ) = discriminator_loss(y_dur_hat_r, y_dur_hat_g)
- loss_dur_disc_all = loss_dur_disc
- optim_dur_disc.zero_grad()
- scaler.scale(loss_dur_disc_all).backward()
- scaler.unscale_(optim_dur_disc)
- commons.clip_grad_value_(net_dur_disc.parameters(), None)
- scaler.step(optim_dur_disc)
-
- optim_d.zero_grad()
- scaler.scale(loss_disc_all).backward()
- scaler.unscale_(optim_d)
- grad_norm_d = commons.clip_grad_value_(net_d.parameters(), None)
- scaler.step(optim_d)
-
- with autocast(enabled=hps.train.fp16_run):
- # Generator
- y_d_hat_r, y_d_hat_g, fmap_r, fmap_g = net_d(y, y_hat)
- if net_dur_disc is not None:
- y_dur_hat_r, y_dur_hat_g = net_dur_disc(hidden_x, x_mask, logw, logw_)
- with autocast(enabled=False):
- loss_dur = torch.sum(l_length.float())
- loss_mel = F.l1_loss(y_mel, y_hat_mel) * hps.train.c_mel
- loss_kl = kl_loss(z_p, logs_q, m_p, logs_p, z_mask) * hps.train.c_kl
-
- loss_fm = feature_loss(fmap_r, fmap_g)
- loss_gen, losses_gen = generator_loss(y_d_hat_g)
- loss_gen_all = loss_gen + loss_fm + loss_mel + loss_dur + loss_kl
- if net_dur_disc is not None:
- loss_dur_gen, losses_dur_gen = generator_loss(y_dur_hat_g)
- loss_gen_all += loss_dur_gen
- optim_g.zero_grad()
- scaler.scale(loss_gen_all).backward()
- scaler.unscale_(optim_g)
- grad_norm_g = commons.clip_grad_value_(net_g.parameters(), None)
- scaler.step(optim_g)
- scaler.update()
-
- if rank == 0:
- if global_step % hps.train.log_interval == 0:
- lr = optim_g.param_groups[0]["lr"]
- losses = [loss_disc, loss_gen, loss_fm, loss_mel, loss_dur, loss_kl]
- logger.info(
- "Train Epoch: {} [{:.0f}%]".format(
- epoch, 100.0 * batch_idx / len(train_loader)
- )
- )
- logger.info([x.item() for x in losses] + [global_step, lr])
-
- scalar_dict = {
- "loss/g/total": loss_gen_all,
- "loss/d/total": loss_disc_all,
- "learning_rate": lr,
- "grad_norm_d": grad_norm_d,
- "grad_norm_g": grad_norm_g,
- }
- scalar_dict.update(
- {
- "loss/g/fm": loss_fm,
- "loss/g/mel": loss_mel,
- "loss/g/dur": loss_dur,
- "loss/g/kl": loss_kl,
- }
- )
- scalar_dict.update(
- {"loss/g/{}".format(i): v for i, v in enumerate(losses_gen)}
- )
- scalar_dict.update(
- {"loss/d_r/{}".format(i): v for i, v in enumerate(losses_disc_r)}
- )
- scalar_dict.update(
- {"loss/d_g/{}".format(i): v for i, v in enumerate(losses_disc_g)}
- )
-
- image_dict = {
- "slice/mel_org": utils.plot_spectrogram_to_numpy(
- y_mel[0].data.cpu().numpy()
- ),
- "slice/mel_gen": utils.plot_spectrogram_to_numpy(
- y_hat_mel[0].data.cpu().numpy()
- ),
- "all/mel": utils.plot_spectrogram_to_numpy(
- mel[0].data.cpu().numpy()
- ),
- "all/attn": utils.plot_alignment_to_numpy(
- attn[0, 0].data.cpu().numpy()
- ),
- }
- utils.summarize(
- writer=writer,
- global_step=global_step,
- images=image_dict,
- scalars=scalar_dict,
- )
-
- if global_step % hps.train.eval_interval == 0:
- evaluate(hps, net_g, eval_loader, writer_eval)
- utils.save_checkpoint(
- net_g,
- optim_g,
- hps.train.learning_rate,
- epoch,
- os.path.join(hps.model_dir, "G_{}.pth".format(global_step)),
- )
- utils.save_checkpoint(
- net_d,
- optim_d,
- hps.train.learning_rate,
- epoch,
- os.path.join(hps.model_dir, "D_{}.pth".format(global_step)),
- )
- if net_dur_disc is not None:
- utils.save_checkpoint(
- net_dur_disc,
- optim_dur_disc,
- hps.train.learning_rate,
- epoch,
- os.path.join(hps.model_dir, "DUR_{}.pth".format(global_step)),
- )
- keep_ckpts = getattr(hps.train, "keep_ckpts", 5)
- if keep_ckpts > 0:
- utils.clean_checkpoints(
- path_to_models=hps.model_dir,
- n_ckpts_to_keep=keep_ckpts,
- sort_by_time=True,
- )
-
- global_step += 1
-
- if rank == 0:
- logger.info("====> Epoch: {}".format(epoch))
-
-
-def evaluate(hps, generator, eval_loader, writer_eval):
- generator.eval()
- image_dict = {}
- audio_dict = {}
- print("Evaluating ...")
- with torch.no_grad():
- for batch_idx, (
- x,
- x_lengths,
- spec,
- spec_lengths,
- y,
- y_lengths,
- speakers,
- tone,
- language,
- bert,
- ja_bert,
- ) in enumerate(eval_loader):
- x, x_lengths = x.cuda(), x_lengths.cuda()
- spec, spec_lengths = spec.cuda(), spec_lengths.cuda()
- y, y_lengths = y.cuda(), y_lengths.cuda()
- speakers = speakers.cuda()
- bert = bert.cuda()
- ja_bert = ja_bert.cuda()
- tone = tone.cuda()
- language = language.cuda()
- for use_sdp in [True, False]:
- y_hat, attn, mask, *_ = generator.module.infer(
- x,
- x_lengths,
- speakers,
- tone,
- language,
- bert,
- ja_bert,
- y=spec,
- max_len=1000,
- sdp_ratio=0.0 if not use_sdp else 1.0,
- )
- y_hat_lengths = mask.sum([1, 2]).long() * hps.data.hop_length
-
- mel = spec_to_mel_torch(
- spec,
- hps.data.filter_length,
- hps.data.n_mel_channels,
- hps.data.sampling_rate,
- hps.data.mel_fmin,
- hps.data.mel_fmax,
- )
- y_hat_mel = mel_spectrogram_torch(
- y_hat.squeeze(1).float(),
- hps.data.filter_length,
- hps.data.n_mel_channels,
- hps.data.sampling_rate,
- hps.data.hop_length,
- hps.data.win_length,
- hps.data.mel_fmin,
- hps.data.mel_fmax,
- )
- image_dict.update(
- {
- f"gen/mel_{batch_idx}": utils.plot_spectrogram_to_numpy(
- y_hat_mel[0].cpu().numpy()
- )
- }
- )
- audio_dict.update(
- {
- f"gen/audio_{batch_idx}_{use_sdp}": y_hat[
- 0, :, : y_hat_lengths[0]
- ]
- }
- )
- image_dict.update(
- {
- f"gt/mel_{batch_idx}": utils.plot_spectrogram_to_numpy(
- mel[0].cpu().numpy()
- )
- }
- )
- audio_dict.update({f"gt/audio_{batch_idx}": y[0, :, : y_lengths[0]]})
-
- utils.summarize(
- writer=writer_eval,
- global_step=global_step,
- images=image_dict,
- audios=audio_dict,
- audio_sampling_rate=hps.data.sampling_rate,
- )
- generator.train()
-
-
-if __name__ == "__main__":
- run()
diff --git a/spaces/Realcat/image-matching-webui/third_party/ASpanFormer/src/datasets/sampler.py b/spaces/Realcat/image-matching-webui/third_party/ASpanFormer/src/datasets/sampler.py
deleted file mode 100644
index 131111c4cf69cd8770058dfac2be717aa183978e..0000000000000000000000000000000000000000
--- a/spaces/Realcat/image-matching-webui/third_party/ASpanFormer/src/datasets/sampler.py
+++ /dev/null
@@ -1,90 +0,0 @@
-import torch
-from torch.utils.data import Sampler, ConcatDataset
-
-
-class RandomConcatSampler(Sampler):
- """Random sampler for ConcatDataset. At each epoch, `n_samples_per_subset` samples will be draw from each subset
- in the ConcatDataset. If `subset_replacement` is ``True``, sampling within each subset will be done with replacement.
- However, it is impossible to sample data without replacement between epochs, unless bulding a stateful sampler lived along the entire training phase.
-
- For current implementation, the randomness of sampling is ensured no matter the sampler is recreated across epochs or not and call `torch.manual_seed()` or not.
- Args:
- shuffle (bool): shuffle the random sampled indices across all sub-datsets.
- repeat (int): repeatedly use the sampled indices multiple times for training.
- [arXiv:1902.05509, arXiv:1901.09335]
- NOTE: Don't re-initialize the sampler between epochs (will lead to repeated samples)
- NOTE: This sampler behaves differently with DistributedSampler.
- It assume the dataset is splitted across ranks instead of replicated.
- TODO: Add a `set_epoch()` method to fullfill sampling without replacement across epochs.
- ref: https://github.com/PyTorchLightning/pytorch-lightning/blob/e9846dd758cfb1500eb9dba2d86f6912eb487587/pytorch_lightning/trainer/training_loop.py#L373
- """
-
- def __init__(
- self,
- data_source: ConcatDataset,
- n_samples_per_subset: int,
- subset_replacement: bool = True,
- shuffle: bool = True,
- repeat: int = 1,
- seed: int = None,
- ):
- if not isinstance(data_source, ConcatDataset):
- raise TypeError("data_source should be torch.utils.data.ConcatDataset")
-
- self.data_source = data_source
- self.n_subset = len(self.data_source.datasets)
- self.n_samples_per_subset = n_samples_per_subset
- self.n_samples = self.n_subset * self.n_samples_per_subset * repeat
- self.subset_replacement = subset_replacement
- self.repeat = repeat
- self.shuffle = shuffle
- self.generator = torch.manual_seed(seed)
- assert self.repeat >= 1
-
- def __len__(self):
- return self.n_samples
-
- def __iter__(self):
- indices = []
- # sample from each sub-dataset
- for d_idx in range(self.n_subset):
- low = 0 if d_idx == 0 else self.data_source.cumulative_sizes[d_idx - 1]
- high = self.data_source.cumulative_sizes[d_idx]
- if self.subset_replacement:
- rand_tensor = torch.randint(
- low,
- high,
- (self.n_samples_per_subset,),
- generator=self.generator,
- dtype=torch.int64,
- )
- else: # sample without replacement
- len_subset = len(self.data_source.datasets[d_idx])
- rand_tensor = torch.randperm(len_subset, generator=self.generator) + low
- if len_subset >= self.n_samples_per_subset:
- rand_tensor = rand_tensor[: self.n_samples_per_subset]
- else: # padding with replacement
- rand_tensor_replacement = torch.randint(
- low,
- high,
- (self.n_samples_per_subset - len_subset,),
- generator=self.generator,
- dtype=torch.int64,
- )
- rand_tensor = torch.cat([rand_tensor, rand_tensor_replacement])
- indices.append(rand_tensor)
- indices = torch.cat(indices)
- if self.shuffle: # shuffle the sampled dataset (from multiple subsets)
- rand_tensor = torch.randperm(len(indices), generator=self.generator)
- indices = indices[rand_tensor]
-
- # repeat the sampled indices (can be used for RepeatAugmentation or pure RepeatSampling)
- if self.repeat > 1:
- repeat_indices = [indices.clone() for _ in range(self.repeat - 1)]
- if self.shuffle:
- _choice = lambda x: x[torch.randperm(len(x), generator=self.generator)]
- repeat_indices = map(_choice, repeat_indices)
- indices = torch.cat([indices, *repeat_indices], 0)
-
- assert indices.shape[0] == self.n_samples
- return iter(indices.tolist())
diff --git a/spaces/Realcat/image-matching-webui/third_party/DarkFeat/nets/sampler.py b/spaces/Realcat/image-matching-webui/third_party/DarkFeat/nets/sampler.py
deleted file mode 100644
index 7686b24d78eb92b90ee3cafb95ad48966ee0f00f..0000000000000000000000000000000000000000
--- a/spaces/Realcat/image-matching-webui/third_party/DarkFeat/nets/sampler.py
+++ /dev/null
@@ -1,202 +0,0 @@
-import torch
-import torch.nn as nn
-import torch.nn.functional as F
-import numpy as np
-
-from .geom import rnd_sample, interpolate
-
-
-class NghSampler2(nn.Module):
- """Similar to NghSampler, but doesnt warp the 2nd image.
- Distance to GT => 0 ... pos_d ... neg_d ... ngh
- Pixel label => + + + + + + 0 0 - - - - - - -
-
- Subsample on query side: if > 0, regular grid
- < 0, random points
- In both cases, the number of query points is = W*H/subq**2
- """
-
- def __init__(
- self,
- ngh,
- subq=1,
- subd=1,
- pos_d=0,
- neg_d=2,
- border=None,
- maxpool_pos=True,
- subd_neg=0,
- ):
- nn.Module.__init__(self)
- assert 0 <= pos_d < neg_d <= (ngh if ngh else 99)
- self.ngh = ngh
- self.pos_d = pos_d
- self.neg_d = neg_d
- assert subd <= ngh or ngh == 0
- assert subq != 0
- self.sub_q = subq
- self.sub_d = subd
- self.sub_d_neg = subd_neg
- if border is None:
- border = ngh
- assert border >= ngh, "border has to be larger than ngh"
- self.border = border
- self.maxpool_pos = maxpool_pos
- self.precompute_offsets()
-
- def precompute_offsets(self):
- pos_d2 = self.pos_d**2
- neg_d2 = self.neg_d**2
- rad2 = self.ngh**2
- rad = (self.ngh // self.sub_d) * self.ngh # make an integer multiple
- pos = []
- neg = []
- for j in range(-rad, rad + 1, self.sub_d):
- for i in range(-rad, rad + 1, self.sub_d):
- d2 = i * i + j * j
- if d2 <= pos_d2:
- pos.append((i, j))
- elif neg_d2 <= d2 <= rad2:
- neg.append((i, j))
-
- self.register_buffer("pos_offsets", torch.LongTensor(pos).view(-1, 2).t())
- self.register_buffer("neg_offsets", torch.LongTensor(neg).view(-1, 2).t())
-
- def gen_grid(self, step, B, H, W, dev):
- b1 = torch.arange(B, device=dev)
- if step > 0:
- # regular grid
- x1 = torch.arange(self.border, W - self.border, step, device=dev)
- y1 = torch.arange(self.border, H - self.border, step, device=dev)
- H1, W1 = len(y1), len(x1)
- x1 = x1[None, None, :].expand(B, H1, W1).reshape(-1)
- y1 = y1[None, :, None].expand(B, H1, W1).reshape(-1)
- b1 = b1[:, None, None].expand(B, H1, W1).reshape(-1)
- shape = (B, H1, W1)
- else:
- # randomly spread
- n = (H - 2 * self.border) * (W - 2 * self.border) // step**2
- x1 = torch.randint(self.border, W - self.border, (n,), device=dev)
- y1 = torch.randint(self.border, H - self.border, (n,), device=dev)
- x1 = x1[None, :].expand(B, n).reshape(-1)
- y1 = y1[None, :].expand(B, n).reshape(-1)
- b1 = b1[:, None].expand(B, n).reshape(-1)
- shape = (B, n)
- return b1, y1, x1, shape
-
- def forward(self, feat0, feat1, conf0, conf1, pos0, pos1, B, H, W, N=2500):
- pscores_ls, nscores_ls, distractors_ls = [], [], []
- valid_feat0_ls = []
- valid_pos1_ls, valid_pos2_ls = [], []
- qconf_ls = []
- mask_ls = []
-
- for i in range(B):
- # positions in the first image
- tmp_mask = (
- (pos0[i][:, 1] >= self.border)
- * (pos0[i][:, 1] < W - self.border)
- * (pos0[i][:, 0] >= self.border)
- * (pos0[i][:, 0] < H - self.border)
- )
-
- selected_pos0 = pos0[i][tmp_mask]
- selected_pos1 = pos1[i][tmp_mask]
- valid_pos0, valid_pos1 = rnd_sample([selected_pos0, selected_pos1], N)
-
- # sample features from first image
- valid_feat0 = interpolate(valid_pos0 / 4, feat0[i]) # [N, 128]
- valid_feat0 = F.normalize(valid_feat0, p=2, dim=-1) # [N, 128]
- qconf = interpolate(valid_pos0 / 4, conf0[i])
-
- # sample GT from second image
- mask = (
- (valid_pos1[:, 1] >= 0)
- * (valid_pos1[:, 1] < W)
- * (valid_pos1[:, 0] >= 0)
- * (valid_pos1[:, 0] < H)
- )
-
- def clamp(xy):
- xy = xy
- torch.clamp(xy[0], 0, H - 1, out=xy[0])
- torch.clamp(xy[1], 0, W - 1, out=xy[1])
- return xy
-
- # compute positive scores
- valid_pos1p = clamp(
- valid_pos1.t()[:, None, :]
- + self.pos_offsets[:, :, None].to(valid_pos1.device)
- ) # [2, 29, N]
- valid_pos1p = valid_pos1p.permute(1, 2, 0).reshape(
- -1, 2
- ) # [29, N, 2] -> [29*N, 2]
- valid_feat1p = interpolate(valid_pos1p / 4, feat1[i]).reshape(
- self.pos_offsets.shape[-1], -1, 128
- ) # [29, N, 128]
- valid_feat1p = F.normalize(valid_feat1p, p=2, dim=-1) # [29, N, 128]
-
- pscores = (
- (valid_feat0[None, :, :] * valid_feat1p).sum(dim=-1).t()
- ) # [N, 29]
- pscores, pos = pscores.max(dim=1, keepdim=True)
- sel = clamp(
- valid_pos1.t() + self.pos_offsets[:, pos.view(-1)].to(valid_pos1.device)
- )
- qconf = (qconf + interpolate(sel.t() / 4, conf1[i])) / 2
-
- # compute negative scores
- valid_pos1n = clamp(
- valid_pos1.t()[:, None, :]
- + self.neg_offsets[:, :, None].to(valid_pos1.device)
- ) # [2, 29, N]
- valid_pos1n = valid_pos1n.permute(1, 2, 0).reshape(
- -1, 2
- ) # [29, N, 2] -> [29*N, 2]
- valid_feat1n = interpolate(valid_pos1n / 4, feat1[i]).reshape(
- self.neg_offsets.shape[-1], -1, 128
- ) # [29, N, 128]
- valid_feat1n = F.normalize(valid_feat1n, p=2, dim=-1) # [29, N, 128]
- nscores = (
- (valid_feat0[None, :, :] * valid_feat1n).sum(dim=-1).t()
- ) # [N, 29]
-
- if self.sub_d_neg:
- valid_pos2 = rnd_sample([selected_pos1], N)[0]
- distractors = interpolate(valid_pos2 / 4, feat1[i])
- distractors = F.normalize(distractors, p=2, dim=-1)
-
- pscores_ls.append(pscores)
- nscores_ls.append(nscores)
- distractors_ls.append(distractors)
- valid_feat0_ls.append(valid_feat0)
- valid_pos1_ls.append(valid_pos1)
- valid_pos2_ls.append(valid_pos2)
- qconf_ls.append(qconf)
- mask_ls.append(mask)
-
- N = np.min([len(i) for i in qconf_ls])
-
- # merge batches
- qconf = torch.stack([i[:N] for i in qconf_ls], dim=0).squeeze(-1)
- mask = torch.stack([i[:N] for i in mask_ls], dim=0)
- pscores = torch.cat([i[:N] for i in pscores_ls], dim=0)
- nscores = torch.cat([i[:N] for i in nscores_ls], dim=0)
- distractors = torch.cat([i[:N] for i in distractors_ls], dim=0)
- valid_feat0 = torch.cat([i[:N] for i in valid_feat0_ls], dim=0)
- valid_pos1 = torch.cat([i[:N] for i in valid_pos1_ls], dim=0)
- valid_pos2 = torch.cat([i[:N] for i in valid_pos2_ls], dim=0)
-
- dscores = torch.matmul(valid_feat0, distractors.t())
- dis2 = (valid_pos2[:, 1] - valid_pos1[:, 1][:, None]) ** 2 + (
- valid_pos2[:, 0] - valid_pos1[:, 0][:, None]
- ) ** 2
- b = torch.arange(B, device=dscores.device)[:, None].expand(B, N).reshape(-1)
- dis2 += (b != b[:, None]).long() * self.neg_d**2
- dscores[dis2 < self.neg_d**2] = 0
- scores = torch.cat((pscores, nscores, dscores), dim=1)
-
- gt = scores.new_zeros(scores.shape, dtype=torch.uint8)
- gt[:, : pscores.shape[1]] = 1
-
- return scores, gt, mask, qconf
diff --git a/spaces/Realcat/image-matching-webui/third_party/Roma/roma/train/train.py b/spaces/Realcat/image-matching-webui/third_party/Roma/roma/train/train.py
deleted file mode 100644
index eb3deaf1792a315d1cce77a2ee0fd50ae9e98ac1..0000000000000000000000000000000000000000
--- a/spaces/Realcat/image-matching-webui/third_party/Roma/roma/train/train.py
+++ /dev/null
@@ -1,126 +0,0 @@
-from tqdm import tqdm
-from roma.utils.utils import to_cuda
-import roma
-import torch
-import wandb
-
-
-def log_param_statistics(named_parameters, norm_type=2):
- named_parameters = list(named_parameters)
- grads = [p.grad for n, p in named_parameters if p.grad is not None]
- weight_norms = [
- p.norm(p=norm_type) for n, p in named_parameters if p.grad is not None
- ]
- names = [n for n, p in named_parameters if p.grad is not None]
- param_norm = torch.stack(weight_norms).norm(p=norm_type)
- device = grads[0].device
- grad_norms = torch.stack(
- [torch.norm(g.detach(), norm_type).to(device) for g in grads]
- )
- nans_or_infs = torch.isinf(grad_norms) | torch.isnan(grad_norms)
- nan_inf_names = [name for name, naninf in zip(names, nans_or_infs) if naninf]
- total_grad_norm = torch.norm(grad_norms, norm_type)
- if torch.any(nans_or_infs):
- print(f"These params have nan or inf grads: {nan_inf_names}")
- wandb.log({"grad_norm": total_grad_norm.item()}, step=roma.GLOBAL_STEP)
- wandb.log({"param_norm": param_norm.item()}, step=roma.GLOBAL_STEP)
-
-
-def train_step(
- train_batch, model, objective, optimizer, grad_scaler, grad_clip_norm=1.0, **kwargs
-):
- optimizer.zero_grad()
- out = model(train_batch)
- l = objective(out, train_batch)
- grad_scaler.scale(l).backward()
- grad_scaler.unscale_(optimizer)
- log_param_statistics(model.named_parameters())
- torch.nn.utils.clip_grad_norm_(
- model.parameters(), grad_clip_norm
- ) # what should max norm be?
- grad_scaler.step(optimizer)
- grad_scaler.update()
- wandb.log({"grad_scale": grad_scaler._scale.item()}, step=roma.GLOBAL_STEP)
- if grad_scaler._scale < 1.0:
- grad_scaler._scale = torch.tensor(1.0).to(grad_scaler._scale)
- roma.GLOBAL_STEP = roma.GLOBAL_STEP + roma.STEP_SIZE # increment global step
- return {"train_out": out, "train_loss": l.item()}
-
-
-def train_k_steps(
- n_0,
- k,
- dataloader,
- model,
- objective,
- optimizer,
- lr_scheduler,
- grad_scaler,
- progress_bar=True,
- grad_clip_norm=1.0,
- warmup=None,
- ema_model=None,
-):
- for n in tqdm(range(n_0, n_0 + k), disable=(not progress_bar) or roma.RANK > 0):
- batch = next(dataloader)
- model.train(True)
- batch = to_cuda(batch)
- train_step(
- train_batch=batch,
- model=model,
- objective=objective,
- optimizer=optimizer,
- lr_scheduler=lr_scheduler,
- grad_scaler=grad_scaler,
- n=n,
- grad_clip_norm=grad_clip_norm,
- )
- if ema_model is not None:
- ema_model.update()
- if warmup is not None:
- with warmup.dampening():
- lr_scheduler.step()
- else:
- lr_scheduler.step()
- [
- wandb.log({f"lr_group_{grp}": lr})
- for grp, lr in enumerate(lr_scheduler.get_last_lr())
- ]
-
-
-def train_epoch(
- dataloader=None,
- model=None,
- objective=None,
- optimizer=None,
- lr_scheduler=None,
- epoch=None,
-):
- model.train(True)
- print(f"At epoch {epoch}")
- for batch in tqdm(dataloader, mininterval=5.0):
- batch = to_cuda(batch)
- train_step(
- train_batch=batch, model=model, objective=objective, optimizer=optimizer
- )
- lr_scheduler.step()
- return {
- "model": model,
- "optimizer": optimizer,
- "lr_scheduler": lr_scheduler,
- "epoch": epoch,
- }
-
-
-def train_k_epochs(
- start_epoch, end_epoch, dataloader, model, objective, optimizer, lr_scheduler
-):
- for epoch in range(start_epoch, end_epoch + 1):
- train_epoch(
- dataloader=dataloader,
- model=model,
- objective=objective,
- optimizer=optimizer,
- lr_scheduler=lr_scheduler,
- epoch=epoch,
- )
diff --git a/spaces/Robert001/UniControl-Demo/annotator/uniformer/mmcv/utils/logging.py b/spaces/Robert001/UniControl-Demo/annotator/uniformer/mmcv/utils/logging.py
deleted file mode 100644
index 4aa0e04bb9b3ab2a4bfbc4def50404ccbac2c6e6..0000000000000000000000000000000000000000
--- a/spaces/Robert001/UniControl-Demo/annotator/uniformer/mmcv/utils/logging.py
+++ /dev/null
@@ -1,110 +0,0 @@
-# Copyright (c) OpenMMLab. All rights reserved.
-import logging
-
-import torch.distributed as dist
-
-logger_initialized = {}
-
-
-def get_logger(name, log_file=None, log_level=logging.INFO, file_mode='w'):
- """Initialize and get a logger by name.
-
- If the logger has not been initialized, this method will initialize the
- logger by adding one or two handlers, otherwise the initialized logger will
- be directly returned. During initialization, a StreamHandler will always be
- added. If `log_file` is specified and the process rank is 0, a FileHandler
- will also be added.
-
- Args:
- name (str): Logger name.
- log_file (str | None): The log filename. If specified, a FileHandler
- will be added to the logger.
- log_level (int): The logger level. Note that only the process of
- rank 0 is affected, and other processes will set the level to
- "Error" thus be silent most of the time.
- file_mode (str): The file mode used in opening log file.
- Defaults to 'w'.
-
- Returns:
- logging.Logger: The expected logger.
- """
- logger = logging.getLogger(name)
- if name in logger_initialized:
- return logger
- # handle hierarchical names
- # e.g., logger "a" is initialized, then logger "a.b" will skip the
- # initialization since it is a child of "a".
- for logger_name in logger_initialized:
- if name.startswith(logger_name):
- return logger
-
- # handle duplicate logs to the console
- # Starting in 1.8.0, PyTorch DDP attaches a StreamHandler (NOTSET)
- # to the root logger. As logger.propagate is True by default, this root
- # level handler causes logging messages from rank>0 processes to
- # unexpectedly show up on the console, creating much unwanted clutter.
- # To fix this issue, we set the root logger's StreamHandler, if any, to log
- # at the ERROR level.
- for handler in logger.root.handlers:
- if type(handler) is logging.StreamHandler:
- handler.setLevel(logging.ERROR)
-
- stream_handler = logging.StreamHandler()
- handlers = [stream_handler]
-
- if dist.is_available() and dist.is_initialized():
- rank = dist.get_rank()
- else:
- rank = 0
-
- # only rank 0 will add a FileHandler
- if rank == 0 and log_file is not None:
- # Here, the default behaviour of the official logger is 'a'. Thus, we
- # provide an interface to change the file mode to the default
- # behaviour.
- file_handler = logging.FileHandler(log_file, file_mode)
- handlers.append(file_handler)
-
- formatter = logging.Formatter(
- '%(asctime)s - %(name)s - %(levelname)s - %(message)s')
- for handler in handlers:
- handler.setFormatter(formatter)
- handler.setLevel(log_level)
- logger.addHandler(handler)
-
- if rank == 0:
- logger.setLevel(log_level)
- else:
- logger.setLevel(logging.ERROR)
-
- logger_initialized[name] = True
-
- return logger
-
-
-def print_log(msg, logger=None, level=logging.INFO):
- """Print a log message.
-
- Args:
- msg (str): The message to be logged.
- logger (logging.Logger | str | None): The logger to be used.
- Some special loggers are:
- - "silent": no message will be printed.
- - other str: the logger obtained with `get_root_logger(logger)`.
- - None: The `print()` method will be used to print log messages.
- level (int): Logging level. Only available when `logger` is a Logger
- object or "root".
- """
- if logger is None:
- print(msg)
- elif isinstance(logger, logging.Logger):
- logger.log(level, msg)
- elif logger == 'silent':
- pass
- elif isinstance(logger, str):
- _logger = get_logger(logger)
- _logger.log(level, msg)
- else:
- raise TypeError(
- 'logger should be either a logging.Logger object, str, '
- f'"silent" or None, but got {type(logger)}')
diff --git a/spaces/Robert001/UniControl-Demo/annotator/uniformer/mmdet/models/losses/kd_loss.py b/spaces/Robert001/UniControl-Demo/annotator/uniformer/mmdet/models/losses/kd_loss.py
deleted file mode 100644
index f3abb68d4f7b3eec98b873f69c1105a22eb33913..0000000000000000000000000000000000000000
--- a/spaces/Robert001/UniControl-Demo/annotator/uniformer/mmdet/models/losses/kd_loss.py
+++ /dev/null
@@ -1,87 +0,0 @@
-import mmcv
-import torch.nn as nn
-import torch.nn.functional as F
-
-from ..builder import LOSSES
-from .utils import weighted_loss
-
-
-@mmcv.jit(derivate=True, coderize=True)
-@weighted_loss
-def knowledge_distillation_kl_div_loss(pred,
- soft_label,
- T,
- detach_target=True):
- r"""Loss function for knowledge distilling using KL divergence.
-
- Args:
- pred (Tensor): Predicted logits with shape (N, n + 1).
- soft_label (Tensor): Target logits with shape (N, N + 1).
- T (int): Temperature for distillation.
- detach_target (bool): Remove soft_label from automatic differentiation
-
- Returns:
- torch.Tensor: Loss tensor with shape (N,).
- """
- assert pred.size() == soft_label.size()
- target = F.softmax(soft_label / T, dim=1)
- if detach_target:
- target = target.detach()
-
- kd_loss = F.kl_div(
- F.log_softmax(pred / T, dim=1), target, reduction='none').mean(1) * (
- T * T)
-
- return kd_loss
-
-
-@LOSSES.register_module()
-class KnowledgeDistillationKLDivLoss(nn.Module):
- """Loss function for knowledge distilling using KL divergence.
-
- Args:
- reduction (str): Options are `'none'`, `'mean'` and `'sum'`.
- loss_weight (float): Loss weight of current loss.
- T (int): Temperature for distillation.
- """
-
- def __init__(self, reduction='mean', loss_weight=1.0, T=10):
- super(KnowledgeDistillationKLDivLoss, self).__init__()
- assert T >= 1
- self.reduction = reduction
- self.loss_weight = loss_weight
- self.T = T
-
- def forward(self,
- pred,
- soft_label,
- weight=None,
- avg_factor=None,
- reduction_override=None):
- """Forward function.
-
- Args:
- pred (Tensor): Predicted logits with shape (N, n + 1).
- soft_label (Tensor): Target logits with shape (N, N + 1).
- weight (torch.Tensor, optional): The weight of loss for each
- prediction. Defaults to None.
- avg_factor (int, optional): Average factor that is used to average
- the loss. Defaults to None.
- reduction_override (str, optional): The reduction method used to
- override the original reduction method of the loss.
- Defaults to None.
- """
- assert reduction_override in (None, 'none', 'mean', 'sum')
-
- reduction = (
- reduction_override if reduction_override else self.reduction)
-
- loss_kd = self.loss_weight * knowledge_distillation_kl_div_loss(
- pred,
- soft_label,
- weight,
- reduction=reduction,
- avg_factor=avg_factor,
- T=self.T)
-
- return loss_kd
diff --git a/spaces/Robert001/UniControl-Demo/annotator/uniformer/mmdet/models/roi_heads/mask_scoring_roi_head.py b/spaces/Robert001/UniControl-Demo/annotator/uniformer/mmdet/models/roi_heads/mask_scoring_roi_head.py
deleted file mode 100644
index c6e55c7752209cb5c15eab689ad9e8ac1fef1b66..0000000000000000000000000000000000000000
--- a/spaces/Robert001/UniControl-Demo/annotator/uniformer/mmdet/models/roi_heads/mask_scoring_roi_head.py
+++ /dev/null
@@ -1,122 +0,0 @@
-import torch
-
-from mmdet.core import bbox2roi
-from ..builder import HEADS, build_head
-from .standard_roi_head import StandardRoIHead
-
-
-@HEADS.register_module()
-class MaskScoringRoIHead(StandardRoIHead):
- """Mask Scoring RoIHead for Mask Scoring RCNN.
-
- https://arxiv.org/abs/1903.00241
- """
-
- def __init__(self, mask_iou_head, **kwargs):
- assert mask_iou_head is not None
- super(MaskScoringRoIHead, self).__init__(**kwargs)
- self.mask_iou_head = build_head(mask_iou_head)
-
- def init_weights(self, pretrained):
- """Initialize the weights in head.
-
- Args:
- pretrained (str, optional): Path to pre-trained weights.
- Defaults to None.
- """
- super(MaskScoringRoIHead, self).init_weights(pretrained)
- self.mask_iou_head.init_weights()
-
- def _mask_forward_train(self, x, sampling_results, bbox_feats, gt_masks,
- img_metas):
- """Run forward function and calculate loss for Mask head in
- training."""
- pos_labels = torch.cat([res.pos_gt_labels for res in sampling_results])
- mask_results = super(MaskScoringRoIHead,
- self)._mask_forward_train(x, sampling_results,
- bbox_feats, gt_masks,
- img_metas)
- if mask_results['loss_mask'] is None:
- return mask_results
-
- # mask iou head forward and loss
- pos_mask_pred = mask_results['mask_pred'][
- range(mask_results['mask_pred'].size(0)), pos_labels]
- mask_iou_pred = self.mask_iou_head(mask_results['mask_feats'],
- pos_mask_pred)
- pos_mask_iou_pred = mask_iou_pred[range(mask_iou_pred.size(0)),
- pos_labels]
-
- mask_iou_targets = self.mask_iou_head.get_targets(
- sampling_results, gt_masks, pos_mask_pred,
- mask_results['mask_targets'], self.train_cfg)
- loss_mask_iou = self.mask_iou_head.loss(pos_mask_iou_pred,
- mask_iou_targets)
- mask_results['loss_mask'].update(loss_mask_iou)
- return mask_results
-
- def simple_test_mask(self,
- x,
- img_metas,
- det_bboxes,
- det_labels,
- rescale=False):
- """Obtain mask prediction without augmentation."""
- # image shapes of images in the batch
- ori_shapes = tuple(meta['ori_shape'] for meta in img_metas)
- scale_factors = tuple(meta['scale_factor'] for meta in img_metas)
-
- num_imgs = len(det_bboxes)
- if all(det_bbox.shape[0] == 0 for det_bbox in det_bboxes):
- num_classes = self.mask_head.num_classes
- segm_results = [[[] for _ in range(num_classes)]
- for _ in range(num_imgs)]
- mask_scores = [[[] for _ in range(num_classes)]
- for _ in range(num_imgs)]
- else:
- # if det_bboxes is rescaled to the original image size, we need to
- # rescale it back to the testing scale to obtain RoIs.
- if rescale and not isinstance(scale_factors[0], float):
- scale_factors = [
- torch.from_numpy(scale_factor).to(det_bboxes[0].device)
- for scale_factor in scale_factors
- ]
- _bboxes = [
- det_bboxes[i][:, :4] *
- scale_factors[i] if rescale else det_bboxes[i]
- for i in range(num_imgs)
- ]
- mask_rois = bbox2roi(_bboxes)
- mask_results = self._mask_forward(x, mask_rois)
- concat_det_labels = torch.cat(det_labels)
- # get mask scores with mask iou head
- mask_feats = mask_results['mask_feats']
- mask_pred = mask_results['mask_pred']
- mask_iou_pred = self.mask_iou_head(
- mask_feats, mask_pred[range(concat_det_labels.size(0)),
- concat_det_labels])
- # split batch mask prediction back to each image
- num_bboxes_per_img = tuple(len(_bbox) for _bbox in _bboxes)
- mask_preds = mask_pred.split(num_bboxes_per_img, 0)
- mask_iou_preds = mask_iou_pred.split(num_bboxes_per_img, 0)
-
- # apply mask post-processing to each image individually
- segm_results = []
- mask_scores = []
- for i in range(num_imgs):
- if det_bboxes[i].shape[0] == 0:
- segm_results.append(
- [[] for _ in range(self.mask_head.num_classes)])
- mask_scores.append(
- [[] for _ in range(self.mask_head.num_classes)])
- else:
- segm_result = self.mask_head.get_seg_masks(
- mask_preds[i], _bboxes[i], det_labels[i],
- self.test_cfg, ori_shapes[i], scale_factors[i],
- rescale)
- # get mask scores with mask iou head
- mask_score = self.mask_iou_head.get_mask_scores(
- mask_iou_preds[i], det_bboxes[i], det_labels[i])
- segm_results.append(segm_result)
- mask_scores.append(mask_score)
- return list(zip(segm_results, mask_scores))
diff --git a/spaces/Robert001/UniControl-Demo/annotator/uniformer/mmdet_null/models/roi_heads/mask_heads/coarse_mask_head.py b/spaces/Robert001/UniControl-Demo/annotator/uniformer/mmdet_null/models/roi_heads/mask_heads/coarse_mask_head.py
deleted file mode 100644
index d665dfff83855e6db3866c681559ccdef09f9999..0000000000000000000000000000000000000000
--- a/spaces/Robert001/UniControl-Demo/annotator/uniformer/mmdet_null/models/roi_heads/mask_heads/coarse_mask_head.py
+++ /dev/null
@@ -1,91 +0,0 @@
-import torch.nn as nn
-from mmcv.cnn import ConvModule, Linear, constant_init, xavier_init
-from mmcv.runner import auto_fp16
-
-from mmdet.models.builder import HEADS
-from .fcn_mask_head import FCNMaskHead
-
-
-@HEADS.register_module()
-class CoarseMaskHead(FCNMaskHead):
- """Coarse mask head used in PointRend.
-
- Compared with standard ``FCNMaskHead``, ``CoarseMaskHead`` will downsample
- the input feature map instead of upsample it.
-
- Args:
- num_convs (int): Number of conv layers in the head. Default: 0.
- num_fcs (int): Number of fc layers in the head. Default: 2.
- fc_out_channels (int): Number of output channels of fc layer.
- Default: 1024.
- downsample_factor (int): The factor that feature map is downsampled by.
- Default: 2.
- """
-
- def __init__(self,
- num_convs=0,
- num_fcs=2,
- fc_out_channels=1024,
- downsample_factor=2,
- *arg,
- **kwarg):
- super(CoarseMaskHead, self).__init__(
- *arg, num_convs=num_convs, upsample_cfg=dict(type=None), **kwarg)
- self.num_fcs = num_fcs
- assert self.num_fcs > 0
- self.fc_out_channels = fc_out_channels
- self.downsample_factor = downsample_factor
- assert self.downsample_factor >= 1
- # remove conv_logit
- delattr(self, 'conv_logits')
-
- if downsample_factor > 1:
- downsample_in_channels = (
- self.conv_out_channels
- if self.num_convs > 0 else self.in_channels)
- self.downsample_conv = ConvModule(
- downsample_in_channels,
- self.conv_out_channels,
- kernel_size=downsample_factor,
- stride=downsample_factor,
- padding=0,
- conv_cfg=self.conv_cfg,
- norm_cfg=self.norm_cfg)
- else:
- self.downsample_conv = None
-
- self.output_size = (self.roi_feat_size[0] // downsample_factor,
- self.roi_feat_size[1] // downsample_factor)
- self.output_area = self.output_size[0] * self.output_size[1]
-
- last_layer_dim = self.conv_out_channels * self.output_area
-
- self.fcs = nn.ModuleList()
- for i in range(num_fcs):
- fc_in_channels = (
- last_layer_dim if i == 0 else self.fc_out_channels)
- self.fcs.append(Linear(fc_in_channels, self.fc_out_channels))
- last_layer_dim = self.fc_out_channels
- output_channels = self.num_classes * self.output_area
- self.fc_logits = Linear(last_layer_dim, output_channels)
-
- def init_weights(self):
- for m in self.fcs.modules():
- if isinstance(m, nn.Linear):
- xavier_init(m)
- constant_init(self.fc_logits, 0.001)
-
- @auto_fp16()
- def forward(self, x):
- for conv in self.convs:
- x = conv(x)
-
- if self.downsample_conv is not None:
- x = self.downsample_conv(x)
-
- x = x.flatten(1)
- for fc in self.fcs:
- x = self.relu(fc(x))
- mask_pred = self.fc_logits(x).view(
- x.size(0), self.num_classes, *self.output_size)
- return mask_pred
diff --git a/spaces/Robert001/UniControl-Demo/annotator/uniformer_base/exp/upernet_global_small/test_config_h32.py b/spaces/Robert001/UniControl-Demo/annotator/uniformer_base/exp/upernet_global_small/test_config_h32.py
deleted file mode 100644
index b2ce6e6a7be0e42c6c2915f3dfe56addb8c0e1ef..0000000000000000000000000000000000000000
--- a/spaces/Robert001/UniControl-Demo/annotator/uniformer_base/exp/upernet_global_small/test_config_h32.py
+++ /dev/null
@@ -1,50 +0,0 @@
-'''
- * Copyright (c) 2023 Salesforce, Inc.
- * All rights reserved.
- * SPDX-License-Identifier: Apache License 2.0
- * For full license text, see LICENSE.txt file in the repo root or http://www.apache.org/licenses/
- * By Can Qin
- * Modified from ControlNet repo: https://github.com/lllyasviel/ControlNet
- * Copyright (c) 2023 Lvmin Zhang and Maneesh Agrawala
- * Modified from UniFormer repo: From https://github.com/Sense-X/UniFormer
- * Apache-2.0 license
-'''
-_base_ = [
- '../../configs/_base_/models/upernet_uniformer.py',
- '../../configs/_base_/datasets/ade20k.py',
- '../../configs/_base_/default_runtime.py',
- '../../configs/_base_/schedules/schedule_160k.py'
-]
-model = dict(
- backbone=dict(
- type='UniFormer',
- embed_dim=[64, 128, 320, 512],
- layers=[3, 4, 8, 3],
- head_dim=64,
- drop_path_rate=0.25,
- windows=False,
- hybrid=True,
- window_size=32
- ),
- decode_head=dict(
- in_channels=[64, 128, 320, 512],
- num_classes=150
- ),
- auxiliary_head=dict(
- in_channels=320,
- num_classes=150
- ))
-
-# AdamW optimizer, no weight decay for position embedding & layer norm in backbone
-optimizer = dict(_delete_=True, type='AdamW', lr=0.00006, betas=(0.9, 0.999), weight_decay=0.01,
- paramwise_cfg=dict(custom_keys={'absolute_pos_embed': dict(decay_mult=0.),
- 'relative_position_bias_table': dict(decay_mult=0.),
- 'norm': dict(decay_mult=0.)}))
-
-lr_config = dict(_delete_=True, policy='poly',
- warmup='linear',
- warmup_iters=1500,
- warmup_ratio=1e-6,
- power=1.0, min_lr=0.0, by_epoch=False)
-
-data=dict(samples_per_gpu=2)
\ No newline at end of file
diff --git a/spaces/Robert001/UniControl-Demo/annotator/uniformer_base/mmcv/cnn/bricks/context_block.py b/spaces/Robert001/UniControl-Demo/annotator/uniformer_base/mmcv/cnn/bricks/context_block.py
deleted file mode 100644
index d60fdb904c749ce3b251510dff3cc63cea70d42e..0000000000000000000000000000000000000000
--- a/spaces/Robert001/UniControl-Demo/annotator/uniformer_base/mmcv/cnn/bricks/context_block.py
+++ /dev/null
@@ -1,125 +0,0 @@
-# Copyright (c) OpenMMLab. All rights reserved.
-import torch
-from torch import nn
-
-from ..utils import constant_init, kaiming_init
-from .registry import PLUGIN_LAYERS
-
-
-def last_zero_init(m):
- if isinstance(m, nn.Sequential):
- constant_init(m[-1], val=0)
- else:
- constant_init(m, val=0)
-
-
-@PLUGIN_LAYERS.register_module()
-class ContextBlock(nn.Module):
- """ContextBlock module in GCNet.
-
- See 'GCNet: Non-local Networks Meet Squeeze-Excitation Networks and Beyond'
- (https://arxiv.org/abs/1904.11492) for details.
-
- Args:
- in_channels (int): Channels of the input feature map.
- ratio (float): Ratio of channels of transform bottleneck
- pooling_type (str): Pooling method for context modeling.
- Options are 'att' and 'avg', stand for attention pooling and
- average pooling respectively. Default: 'att'.
- fusion_types (Sequence[str]): Fusion method for feature fusion,
- Options are 'channels_add', 'channel_mul', stand for channelwise
- addition and multiplication respectively. Default: ('channel_add',)
- """
-
- _abbr_ = 'context_block'
-
- def __init__(self,
- in_channels,
- ratio,
- pooling_type='att',
- fusion_types=('channel_add', )):
- super(ContextBlock, self).__init__()
- assert pooling_type in ['avg', 'att']
- assert isinstance(fusion_types, (list, tuple))
- valid_fusion_types = ['channel_add', 'channel_mul']
- assert all([f in valid_fusion_types for f in fusion_types])
- assert len(fusion_types) > 0, 'at least one fusion should be used'
- self.in_channels = in_channels
- self.ratio = ratio
- self.planes = int(in_channels * ratio)
- self.pooling_type = pooling_type
- self.fusion_types = fusion_types
- if pooling_type == 'att':
- self.conv_mask = nn.Conv2d(in_channels, 1, kernel_size=1)
- self.softmax = nn.Softmax(dim=2)
- else:
- self.avg_pool = nn.AdaptiveAvgPool2d(1)
- if 'channel_add' in fusion_types:
- self.channel_add_conv = nn.Sequential(
- nn.Conv2d(self.in_channels, self.planes, kernel_size=1),
- nn.LayerNorm([self.planes, 1, 1]),
- nn.ReLU(inplace=True), # yapf: disable
- nn.Conv2d(self.planes, self.in_channels, kernel_size=1))
- else:
- self.channel_add_conv = None
- if 'channel_mul' in fusion_types:
- self.channel_mul_conv = nn.Sequential(
- nn.Conv2d(self.in_channels, self.planes, kernel_size=1),
- nn.LayerNorm([self.planes, 1, 1]),
- nn.ReLU(inplace=True), # yapf: disable
- nn.Conv2d(self.planes, self.in_channels, kernel_size=1))
- else:
- self.channel_mul_conv = None
- self.reset_parameters()
-
- def reset_parameters(self):
- if self.pooling_type == 'att':
- kaiming_init(self.conv_mask, mode='fan_in')
- self.conv_mask.inited = True
-
- if self.channel_add_conv is not None:
- last_zero_init(self.channel_add_conv)
- if self.channel_mul_conv is not None:
- last_zero_init(self.channel_mul_conv)
-
- def spatial_pool(self, x):
- batch, channel, height, width = x.size()
- if self.pooling_type == 'att':
- input_x = x
- # [N, C, H * W]
- input_x = input_x.view(batch, channel, height * width)
- # [N, 1, C, H * W]
- input_x = input_x.unsqueeze(1)
- # [N, 1, H, W]
- context_mask = self.conv_mask(x)
- # [N, 1, H * W]
- context_mask = context_mask.view(batch, 1, height * width)
- # [N, 1, H * W]
- context_mask = self.softmax(context_mask)
- # [N, 1, H * W, 1]
- context_mask = context_mask.unsqueeze(-1)
- # [N, 1, C, 1]
- context = torch.matmul(input_x, context_mask)
- # [N, C, 1, 1]
- context = context.view(batch, channel, 1, 1)
- else:
- # [N, C, 1, 1]
- context = self.avg_pool(x)
-
- return context
-
- def forward(self, x):
- # [N, C, 1, 1]
- context = self.spatial_pool(x)
-
- out = x
- if self.channel_mul_conv is not None:
- # [N, C, 1, 1]
- channel_mul_term = torch.sigmoid(self.channel_mul_conv(context))
- out = out * channel_mul_term
- if self.channel_add_conv is not None:
- # [N, C, 1, 1]
- channel_add_term = self.channel_add_conv(context)
- out = out + channel_add_term
-
- return out
diff --git a/spaces/Robert001/UniControl-Demo/annotator/uniformer_base/mmcv/cnn/bricks/norm.py b/spaces/Robert001/UniControl-Demo/annotator/uniformer_base/mmcv/cnn/bricks/norm.py
deleted file mode 100644
index 408f4b42731b19a3beeef68b6a5e610d0bbc18b3..0000000000000000000000000000000000000000
--- a/spaces/Robert001/UniControl-Demo/annotator/uniformer_base/mmcv/cnn/bricks/norm.py
+++ /dev/null
@@ -1,144 +0,0 @@
-# Copyright (c) OpenMMLab. All rights reserved.
-import inspect
-
-import torch.nn as nn
-
-from annotator.uniformer.mmcv.utils import is_tuple_of
-from annotator.uniformer.mmcv.utils.parrots_wrapper import SyncBatchNorm, _BatchNorm, _InstanceNorm
-from .registry import NORM_LAYERS
-
-NORM_LAYERS.register_module('BN', module=nn.BatchNorm2d)
-NORM_LAYERS.register_module('BN1d', module=nn.BatchNorm1d)
-NORM_LAYERS.register_module('BN2d', module=nn.BatchNorm2d)
-NORM_LAYERS.register_module('BN3d', module=nn.BatchNorm3d)
-NORM_LAYERS.register_module('SyncBN', module=SyncBatchNorm)
-NORM_LAYERS.register_module('GN', module=nn.GroupNorm)
-NORM_LAYERS.register_module('LN', module=nn.LayerNorm)
-NORM_LAYERS.register_module('IN', module=nn.InstanceNorm2d)
-NORM_LAYERS.register_module('IN1d', module=nn.InstanceNorm1d)
-NORM_LAYERS.register_module('IN2d', module=nn.InstanceNorm2d)
-NORM_LAYERS.register_module('IN3d', module=nn.InstanceNorm3d)
-
-
-def infer_abbr(class_type):
- """Infer abbreviation from the class name.
-
- When we build a norm layer with `build_norm_layer()`, we want to preserve
- the norm type in variable names, e.g, self.bn1, self.gn. This method will
- infer the abbreviation to map class types to abbreviations.
-
- Rule 1: If the class has the property "_abbr_", return the property.
- Rule 2: If the parent class is _BatchNorm, GroupNorm, LayerNorm or
- InstanceNorm, the abbreviation of this layer will be "bn", "gn", "ln" and
- "in" respectively.
- Rule 3: If the class name contains "batch", "group", "layer" or "instance",
- the abbreviation of this layer will be "bn", "gn", "ln" and "in"
- respectively.
- Rule 4: Otherwise, the abbreviation falls back to "norm".
-
- Args:
- class_type (type): The norm layer type.
-
- Returns:
- str: The inferred abbreviation.
- """
- if not inspect.isclass(class_type):
- raise TypeError(
- f'class_type must be a type, but got {type(class_type)}')
- if hasattr(class_type, '_abbr_'):
- return class_type._abbr_
- if issubclass(class_type, _InstanceNorm): # IN is a subclass of BN
- return 'in'
- elif issubclass(class_type, _BatchNorm):
- return 'bn'
- elif issubclass(class_type, nn.GroupNorm):
- return 'gn'
- elif issubclass(class_type, nn.LayerNorm):
- return 'ln'
- else:
- class_name = class_type.__name__.lower()
- if 'batch' in class_name:
- return 'bn'
- elif 'group' in class_name:
- return 'gn'
- elif 'layer' in class_name:
- return 'ln'
- elif 'instance' in class_name:
- return 'in'
- else:
- return 'norm_layer'
-
-
-def build_norm_layer(cfg, num_features, postfix=''):
- """Build normalization layer.
-
- Args:
- cfg (dict): The norm layer config, which should contain:
-
- - type (str): Layer type.
- - layer args: Args needed to instantiate a norm layer.
- - requires_grad (bool, optional): Whether stop gradient updates.
- num_features (int): Number of input channels.
- postfix (int | str): The postfix to be appended into norm abbreviation
- to create named layer.
-
- Returns:
- (str, nn.Module): The first element is the layer name consisting of
- abbreviation and postfix, e.g., bn1, gn. The second element is the
- created norm layer.
- """
- if not isinstance(cfg, dict):
- raise TypeError('cfg must be a dict')
- if 'type' not in cfg:
- raise KeyError('the cfg dict must contain the key "type"')
- cfg_ = cfg.copy()
-
- layer_type = cfg_.pop('type')
- if layer_type not in NORM_LAYERS:
- raise KeyError(f'Unrecognized norm type {layer_type}')
-
- norm_layer = NORM_LAYERS.get(layer_type)
- abbr = infer_abbr(norm_layer)
-
- assert isinstance(postfix, (int, str))
- name = abbr + str(postfix)
-
- requires_grad = cfg_.pop('requires_grad', True)
- cfg_.setdefault('eps', 1e-5)
- if layer_type != 'GN':
- layer = norm_layer(num_features, **cfg_)
- if layer_type == 'SyncBN' and hasattr(layer, '_specify_ddp_gpu_num'):
- layer._specify_ddp_gpu_num(1)
- else:
- assert 'num_groups' in cfg_
- layer = norm_layer(num_channels=num_features, **cfg_)
-
- for param in layer.parameters():
- param.requires_grad = requires_grad
-
- return name, layer
-
-
-def is_norm(layer, exclude=None):
- """Check if a layer is a normalization layer.
-
- Args:
- layer (nn.Module): The layer to be checked.
- exclude (type | tuple[type]): Types to be excluded.
-
- Returns:
- bool: Whether the layer is a norm layer.
- """
- if exclude is not None:
- if not isinstance(exclude, tuple):
- exclude = (exclude, )
- if not is_tuple_of(exclude, type):
- raise TypeError(
- f'"exclude" must be either None or type or a tuple of types, '
- f'but got {type(exclude)}: {exclude}')
-
- if exclude and isinstance(layer, exclude):
- return False
-
- all_norm_bases = (_BatchNorm, _InstanceNorm, nn.GroupNorm, nn.LayerNorm)
- return isinstance(layer, all_norm_bases)
diff --git a/spaces/Robert001/UniControl-Demo/annotator/uniformer_base/mmcv/cnn/bricks/wrappers.py b/spaces/Robert001/UniControl-Demo/annotator/uniformer_base/mmcv/cnn/bricks/wrappers.py
deleted file mode 100644
index 8aebf67bf52355a513f21756ee74fe510902d075..0000000000000000000000000000000000000000
--- a/spaces/Robert001/UniControl-Demo/annotator/uniformer_base/mmcv/cnn/bricks/wrappers.py
+++ /dev/null
@@ -1,180 +0,0 @@
-# Copyright (c) OpenMMLab. All rights reserved.
-r"""Modified from https://github.com/facebookresearch/detectron2/blob/master/detectron2/layers/wrappers.py # noqa: E501
-
-Wrap some nn modules to support empty tensor input. Currently, these wrappers
-are mainly used in mask heads like fcn_mask_head and maskiou_heads since mask
-heads are trained on only positive RoIs.
-"""
-import math
-
-import torch
-import torch.nn as nn
-from torch.nn.modules.utils import _pair, _triple
-
-from .registry import CONV_LAYERS, UPSAMPLE_LAYERS
-
-if torch.__version__ == 'parrots':
- TORCH_VERSION = torch.__version__
-else:
- # torch.__version__ could be 1.3.1+cu92, we only need the first two
- # for comparison
- TORCH_VERSION = tuple(int(x) for x in torch.__version__.split('.')[:2])
-
-
-def obsolete_torch_version(torch_version, version_threshold):
- return torch_version == 'parrots' or torch_version <= version_threshold
-
-
-class NewEmptyTensorOp(torch.autograd.Function):
-
- @staticmethod
- def forward(ctx, x, new_shape):
- ctx.shape = x.shape
- return x.new_empty(new_shape)
-
- @staticmethod
- def backward(ctx, grad):
- shape = ctx.shape
- return NewEmptyTensorOp.apply(grad, shape), None
-
-
-@CONV_LAYERS.register_module('Conv', force=True)
-class Conv2d(nn.Conv2d):
-
- def forward(self, x):
- if x.numel() == 0 and obsolete_torch_version(TORCH_VERSION, (1, 4)):
- out_shape = [x.shape[0], self.out_channels]
- for i, k, p, s, d in zip(x.shape[-2:], self.kernel_size,
- self.padding, self.stride, self.dilation):
- o = (i + 2 * p - (d * (k - 1) + 1)) // s + 1
- out_shape.append(o)
- empty = NewEmptyTensorOp.apply(x, out_shape)
- if self.training:
- # produce dummy gradient to avoid DDP warning.
- dummy = sum(x.view(-1)[0] for x in self.parameters()) * 0.0
- return empty + dummy
- else:
- return empty
-
- return super().forward(x)
-
-
-@CONV_LAYERS.register_module('Conv3d', force=True)
-class Conv3d(nn.Conv3d):
-
- def forward(self, x):
- if x.numel() == 0 and obsolete_torch_version(TORCH_VERSION, (1, 4)):
- out_shape = [x.shape[0], self.out_channels]
- for i, k, p, s, d in zip(x.shape[-3:], self.kernel_size,
- self.padding, self.stride, self.dilation):
- o = (i + 2 * p - (d * (k - 1) + 1)) // s + 1
- out_shape.append(o)
- empty = NewEmptyTensorOp.apply(x, out_shape)
- if self.training:
- # produce dummy gradient to avoid DDP warning.
- dummy = sum(x.view(-1)[0] for x in self.parameters()) * 0.0
- return empty + dummy
- else:
- return empty
-
- return super().forward(x)
-
-
-@CONV_LAYERS.register_module()
-@CONV_LAYERS.register_module('deconv')
-@UPSAMPLE_LAYERS.register_module('deconv', force=True)
-class ConvTranspose2d(nn.ConvTranspose2d):
-
- def forward(self, x):
- if x.numel() == 0 and obsolete_torch_version(TORCH_VERSION, (1, 4)):
- out_shape = [x.shape[0], self.out_channels]
- for i, k, p, s, d, op in zip(x.shape[-2:], self.kernel_size,
- self.padding, self.stride,
- self.dilation, self.output_padding):
- out_shape.append((i - 1) * s - 2 * p + (d * (k - 1) + 1) + op)
- empty = NewEmptyTensorOp.apply(x, out_shape)
- if self.training:
- # produce dummy gradient to avoid DDP warning.
- dummy = sum(x.view(-1)[0] for x in self.parameters()) * 0.0
- return empty + dummy
- else:
- return empty
-
- return super().forward(x)
-
-
-@CONV_LAYERS.register_module()
-@CONV_LAYERS.register_module('deconv3d')
-@UPSAMPLE_LAYERS.register_module('deconv3d', force=True)
-class ConvTranspose3d(nn.ConvTranspose3d):
-
- def forward(self, x):
- if x.numel() == 0 and obsolete_torch_version(TORCH_VERSION, (1, 4)):
- out_shape = [x.shape[0], self.out_channels]
- for i, k, p, s, d, op in zip(x.shape[-3:], self.kernel_size,
- self.padding, self.stride,
- self.dilation, self.output_padding):
- out_shape.append((i - 1) * s - 2 * p + (d * (k - 1) + 1) + op)
- empty = NewEmptyTensorOp.apply(x, out_shape)
- if self.training:
- # produce dummy gradient to avoid DDP warning.
- dummy = sum(x.view(-1)[0] for x in self.parameters()) * 0.0
- return empty + dummy
- else:
- return empty
-
- return super().forward(x)
-
-
-class MaxPool2d(nn.MaxPool2d):
-
- def forward(self, x):
- # PyTorch 1.9 does not support empty tensor inference yet
- if x.numel() == 0 and obsolete_torch_version(TORCH_VERSION, (1, 9)):
- out_shape = list(x.shape[:2])
- for i, k, p, s, d in zip(x.shape[-2:], _pair(self.kernel_size),
- _pair(self.padding), _pair(self.stride),
- _pair(self.dilation)):
- o = (i + 2 * p - (d * (k - 1) + 1)) / s + 1
- o = math.ceil(o) if self.ceil_mode else math.floor(o)
- out_shape.append(o)
- empty = NewEmptyTensorOp.apply(x, out_shape)
- return empty
-
- return super().forward(x)
-
-
-class MaxPool3d(nn.MaxPool3d):
-
- def forward(self, x):
- # PyTorch 1.9 does not support empty tensor inference yet
- if x.numel() == 0 and obsolete_torch_version(TORCH_VERSION, (1, 9)):
- out_shape = list(x.shape[:2])
- for i, k, p, s, d in zip(x.shape[-3:], _triple(self.kernel_size),
- _triple(self.padding),
- _triple(self.stride),
- _triple(self.dilation)):
- o = (i + 2 * p - (d * (k - 1) + 1)) / s + 1
- o = math.ceil(o) if self.ceil_mode else math.floor(o)
- out_shape.append(o)
- empty = NewEmptyTensorOp.apply(x, out_shape)
- return empty
-
- return super().forward(x)
-
-
-class Linear(torch.nn.Linear):
-
- def forward(self, x):
- # empty tensor forward of Linear layer is supported in Pytorch 1.6
- if x.numel() == 0 and obsolete_torch_version(TORCH_VERSION, (1, 5)):
- out_shape = [x.shape[0], self.out_features]
- empty = NewEmptyTensorOp.apply(x, out_shape)
- if self.training:
- # produce dummy gradient to avoid DDP warning.
- dummy = sum(x.view(-1)[0] for x in self.parameters()) * 0.0
- return empty + dummy
- else:
- return empty
-
- return super().forward(x)
diff --git a/spaces/Ron0420/EfficientNetV2_Deepfakes_Image_Detector/app.py b/spaces/Ron0420/EfficientNetV2_Deepfakes_Image_Detector/app.py
deleted file mode 100644
index eecea3922671dc3f4b92a37f0e75a926d3d3534c..0000000000000000000000000000000000000000
--- a/spaces/Ron0420/EfficientNetV2_Deepfakes_Image_Detector/app.py
+++ /dev/null
@@ -1,92 +0,0 @@
-import gradio as gr
-
-import cv2
-from mtcnn.mtcnn import MTCNN
-import tensorflow as tf
-import tensorflow_addons
-import numpy as np
-
-import os
-import zipfile
-
-local_zip = "FINAL-EFFICIENTNETV2-B0.zip"
-zip_ref = zipfile.ZipFile(local_zip, 'r')
-zip_ref.extractall('FINAL-EFFICIENTNETV2-B0')
-zip_ref.close()
-
-model = tf.keras.models.load_model("FINAL-EFFICIENTNETV2-B0")
-
-detector = MTCNN()
-
-def deepfakespredict(input_img ):
-
- labels = ['real', 'fake']
- pred = [0, 0]
- text =""
- text2 =""
-
- face = detector.detect_faces(input_img)
-
- if len(face) > 0:
- x, y, width, height = face[0]['box']
- x2, y2 = x + width, y + height
-
- cv2.rectangle(input_img, (x, y), (x2, y2), (0, 255, 0), 2)
-
- face_image = input_img[y:y2, x:x2]
- face_image2 = cv2.cvtColor(face_image, cv2.COLOR_BGR2RGB)
- face_image3 = cv2.resize(face_image2, (224, 224))
- face_image4 = face_image3/255
-
- pred = model.predict(np.expand_dims(face_image4, axis=0))[0]
-
- if pred[1] >= 0.6:
- text = "The image is FAKE."
- elif pred[0] >= 0.6:
- text = "The image is REAL."
- else:
- text = "The image may be REAL or FAKE."
-
- else:
- text = "Face is not detected in the image."
-
- text2 = "REAL: " + str(np.round(pred[0]*100, 2)) + "%, FAKE: " + str(np.round(pred[1]*100, 2)) + "%"
-
- return input_img, text, text2, {labels[i]: float(pred[i]) for i in range(2)}
-
-
-title="EfficientNetV2 Deepfakes Image Detector"
-description="This is a demo implementation of EfficientNetV2 Deepfakes Image Detector. \
- To use it, simply upload your image, or click one of the examples to load them. \
- This demo and model represent the Final Year Project titled \"Achieving Face Swapped Deepfakes Detection Using EfficientNetV2\" by a CS undergraduate Lee Sheng Yeh. \
- The examples were extracted from Celeb-DF(V2)(Li et al, 2020) and FaceForensics++(Rossler et al., 2019). Full reference detail is available in \"references.txt.\" \
- The examples are used under fair use to demo the working of the model only. If any copyright is infringed, please contact the researcher via this email: tp054565@mail.apu.edu.my.\
- "
-
-examples = [
- ['Fake-1.png'],
- ['Fake-2.png'],
- ['Fake-3.png'],
- ['Fake-4.png'],
- ['Fake-5.png'],
-
- ['Real-1.png'],
- ['Real-2.png'],
- ['Real-3.png'],
- ['Real-4.png'],
- ['Real-5.png']
-
- ]
-
-
-gr.Interface(deepfakespredict,
- inputs = ["image"],
- outputs=[gr.outputs.Image(type="pil", label="Detected face"),
- "text",
- "text",
- gr.outputs.Label(num_top_classes=None, type="auto", label="Confidence")],
- title=title,
- description=description,
- examples = examples,
- examples_per_page = 5
- ).launch()
\ No newline at end of file
diff --git a/spaces/Rongjiehuang/GenerSpeech/egs/datasets/audio/libritts/pre_align.py b/spaces/Rongjiehuang/GenerSpeech/egs/datasets/audio/libritts/pre_align.py
deleted file mode 100644
index 995583af647d22e8b6387b37d493479b5ce376ac..0000000000000000000000000000000000000000
--- a/spaces/Rongjiehuang/GenerSpeech/egs/datasets/audio/libritts/pre_align.py
+++ /dev/null
@@ -1,21 +0,0 @@
-import os
-
-from data_gen.tts.base_preprocess import BasePreprocessor
-import glob
-
-
-class LibrittsPreAlign(BasePreprocessor):
- def meta_data(self):
- wav_fns = sorted(glob.glob(f'{self.raw_data_dir}/*/*/*/*.wav'))
- for wav_fn in wav_fns:
- item_name = os.path.basename(wav_fn)[:-4]
- txt_fn = f'{wav_fn[:-4]}.normalized.txt'
- with open(txt_fn, 'r') as f:
- txt = f.readlines()
- f.close()
- spk = item_name.split("_")[0]
- yield item_name, wav_fn, txt, spk
-
-
-if __name__ == "__main__":
- LibrittsPreAlign().process()
diff --git a/spaces/Rongjiehuang/GenerSpeech/utils/audio.py b/spaces/Rongjiehuang/GenerSpeech/utils/audio.py
deleted file mode 100644
index aba7ab926cf793d085bbdc70c97f376001183fe1..0000000000000000000000000000000000000000
--- a/spaces/Rongjiehuang/GenerSpeech/utils/audio.py
+++ /dev/null
@@ -1,56 +0,0 @@
-import subprocess
-import matplotlib
-
-matplotlib.use('Agg')
-import librosa
-import librosa.filters
-import numpy as np
-from scipy import signal
-from scipy.io import wavfile
-
-
-def save_wav(wav, path, sr, norm=False):
- if norm:
- wav = wav / np.abs(wav).max()
- wav *= 32767
- # proposed by @dsmiller
- wavfile.write(path, sr, wav.astype(np.int16))
-
-
-def get_hop_size(hparams):
- hop_size = hparams['hop_size']
- if hop_size is None:
- assert hparams['frame_shift_ms'] is not None
- hop_size = int(hparams['frame_shift_ms'] / 1000 * hparams['audio_sample_rate'])
- return hop_size
-
-
-###########################################################################################
-def _stft(y, hparams):
- return librosa.stft(y=y, n_fft=hparams['fft_size'], hop_length=get_hop_size(hparams),
- win_length=hparams['win_size'], pad_mode='constant')
-
-
-def _istft(y, hparams):
- return librosa.istft(y, hop_length=get_hop_size(hparams), win_length=hparams['win_size'])
-
-
-def librosa_pad_lr(x, fsize, fshift, pad_sides=1):
- '''compute right padding (final frame) or both sides padding (first and final frames)
- '''
- assert pad_sides in (1, 2)
- # return int(fsize // 2)
- pad = (x.shape[0] // fshift + 1) * fshift - x.shape[0]
- if pad_sides == 1:
- return 0, pad
- else:
- return pad // 2, pad // 2 + pad % 2
-
-
-# Conversions
-def amp_to_db(x):
- return 20 * np.log10(np.maximum(1e-5, x))
-
-
-def normalize(S, hparams):
- return (S - hparams['min_level_db']) / -hparams['min_level_db']
diff --git a/spaces/SRDdev/Image-Caption/app.py b/spaces/SRDdev/Image-Caption/app.py
deleted file mode 100644
index 7be02987b945e1cbec1d122414ccf274cfc63e86..0000000000000000000000000000000000000000
--- a/spaces/SRDdev/Image-Caption/app.py
+++ /dev/null
@@ -1,41 +0,0 @@
-import torch
-import re
-import gradio as gr
-from transformers import AutoTokenizer, ViTFeatureExtractor, VisionEncoderDecoderModel
-
-device='cpu'
-encoder_checkpoint = "nlpconnect/vit-gpt2-image-captioning"
-decoder_checkpoint = "nlpconnect/vit-gpt2-image-captioning"
-model_checkpoint = "nlpconnect/vit-gpt2-image-captioning"
-feature_extractor = ViTFeatureExtractor.from_pretrained(encoder_checkpoint)
-tokenizer = AutoTokenizer.from_pretrained(decoder_checkpoint)
-model = VisionEncoderDecoderModel.from_pretrained(model_checkpoint).to(device)
-
-
-def predict(image,max_length=64, num_beams=4):
- image = image.convert('RGB')
- image = feature_extractor(image, return_tensors="pt").pixel_values.to(device)
- clean_text = lambda x: x.replace('<|endoftext|>','').split('\n')[0]
- caption_ids = model.generate(image, max_length = max_length)[0]
- caption_text = clean_text(tokenizer.decode(caption_ids))
- return caption_text
-
-
-
-input = gr.inputs.Image(label="Upload any Image", type = 'pil', optional=True)
-output = gr.outputs.Textbox(type="auto",label="Captions")
-examples = [f"example{i}.jpg" for i in range(1,7)]
-
-title = "Image Captioning "
-description = "Made by : shreyasdixit.tech"
-interface = gr.Interface(
-
- fn=predict,
- description=description,
- inputs = input,
- theme="grass",
- outputs=output,
- examples = examples,
- title=title,
- )
-interface.launch(debug=True)
\ No newline at end of file
diff --git a/spaces/Salesforce/EDICT/my_diffusers/models/vae.py b/spaces/Salesforce/EDICT/my_diffusers/models/vae.py
deleted file mode 100644
index 82748cb5b60c0241cc3ca96f9016f07650e44a54..0000000000000000000000000000000000000000
--- a/spaces/Salesforce/EDICT/my_diffusers/models/vae.py
+++ /dev/null
@@ -1,581 +0,0 @@
-from dataclasses import dataclass
-from typing import Optional, Tuple, Union
-
-import numpy as np
-import torch
-import torch.nn as nn
-
-from ..configuration_utils import ConfigMixin, register_to_config
-from ..modeling_utils import ModelMixin
-from ..utils import BaseOutput
-from .unet_blocks import UNetMidBlock2D, get_down_block, get_up_block
-
-
-@dataclass
-class DecoderOutput(BaseOutput):
- """
- Output of decoding method.
-
- Args:
- sample (`torch.FloatTensor` of shape `(batch_size, num_channels, height, width)`):
- Decoded output sample of the model. Output of the last layer of the model.
- """
-
- sample: torch.FloatTensor
-
-
-@dataclass
-class VQEncoderOutput(BaseOutput):
- """
- Output of VQModel encoding method.
-
- Args:
- latents (`torch.FloatTensor` of shape `(batch_size, num_channels, height, width)`):
- Encoded output sample of the model. Output of the last layer of the model.
- """
-
- latents: torch.FloatTensor
-
-
-@dataclass
-class AutoencoderKLOutput(BaseOutput):
- """
- Output of AutoencoderKL encoding method.
-
- Args:
- latent_dist (`DiagonalGaussianDistribution`):
- Encoded outputs of `Encoder` represented as the mean and logvar of `DiagonalGaussianDistribution`.
- `DiagonalGaussianDistribution` allows for sampling latents from the distribution.
- """
-
- latent_dist: "DiagonalGaussianDistribution"
-
-
-class Encoder(nn.Module):
- def __init__(
- self,
- in_channels=3,
- out_channels=3,
- down_block_types=("DownEncoderBlock2D",),
- block_out_channels=(64,),
- layers_per_block=2,
- act_fn="silu",
- double_z=True,
- ):
- super().__init__()
- self.layers_per_block = layers_per_block
-
- self.conv_in = torch.nn.Conv2d(in_channels, block_out_channels[0], kernel_size=3, stride=1, padding=1)
-
- self.mid_block = None
- self.down_blocks = nn.ModuleList([])
-
- # down
- output_channel = block_out_channels[0]
- for i, down_block_type in enumerate(down_block_types):
- input_channel = output_channel
- output_channel = block_out_channels[i]
- is_final_block = i == len(block_out_channels) - 1
-
- down_block = get_down_block(
- down_block_type,
- num_layers=self.layers_per_block,
- in_channels=input_channel,
- out_channels=output_channel,
- add_downsample=not is_final_block,
- resnet_eps=1e-6,
- downsample_padding=0,
- resnet_act_fn=act_fn,
- attn_num_head_channels=None,
- temb_channels=None,
- )
- self.down_blocks.append(down_block)
-
- # mid
- self.mid_block = UNetMidBlock2D(
- in_channels=block_out_channels[-1],
- resnet_eps=1e-6,
- resnet_act_fn=act_fn,
- output_scale_factor=1,
- resnet_time_scale_shift="default",
- attn_num_head_channels=None,
- resnet_groups=32,
- temb_channels=None,
- )
-
- # out
- num_groups_out = 32
- self.conv_norm_out = nn.GroupNorm(num_channels=block_out_channels[-1], num_groups=num_groups_out, eps=1e-6)
- self.conv_act = nn.SiLU()
-
- conv_out_channels = 2 * out_channels if double_z else out_channels
- self.conv_out = nn.Conv2d(block_out_channels[-1], conv_out_channels, 3, padding=1)
-
- def forward(self, x):
- sample = x
- sample = self.conv_in(sample)
-
- # down
- for down_block in self.down_blocks:
- sample = down_block(sample)
-
- # middle
- sample = self.mid_block(sample)
-
- # post-process
- sample = self.conv_norm_out(sample)
- sample = self.conv_act(sample)
- sample = self.conv_out(sample)
-
- return sample
-
-
-class Decoder(nn.Module):
- def __init__(
- self,
- in_channels=3,
- out_channels=3,
- up_block_types=("UpDecoderBlock2D",),
- block_out_channels=(64,),
- layers_per_block=2,
- act_fn="silu",
- ):
- super().__init__()
- self.layers_per_block = layers_per_block
-
- self.conv_in = nn.Conv2d(in_channels, block_out_channels[-1], kernel_size=3, stride=1, padding=1)
-
- self.mid_block = None
- self.up_blocks = nn.ModuleList([])
-
- # mid
- self.mid_block = UNetMidBlock2D(
- in_channels=block_out_channels[-1],
- resnet_eps=1e-6,
- resnet_act_fn=act_fn,
- output_scale_factor=1,
- resnet_time_scale_shift="default",
- attn_num_head_channels=None,
- resnet_groups=32,
- temb_channels=None,
- )
-
- # up
- reversed_block_out_channels = list(reversed(block_out_channels))
- output_channel = reversed_block_out_channels[0]
- for i, up_block_type in enumerate(up_block_types):
- prev_output_channel = output_channel
- output_channel = reversed_block_out_channels[i]
-
- is_final_block = i == len(block_out_channels) - 1
-
- up_block = get_up_block(
- up_block_type,
- num_layers=self.layers_per_block + 1,
- in_channels=prev_output_channel,
- out_channels=output_channel,
- prev_output_channel=None,
- add_upsample=not is_final_block,
- resnet_eps=1e-6,
- resnet_act_fn=act_fn,
- attn_num_head_channels=None,
- temb_channels=None,
- )
- self.up_blocks.append(up_block)
- prev_output_channel = output_channel
-
- # out
- num_groups_out = 32
- self.conv_norm_out = nn.GroupNorm(num_channels=block_out_channels[0], num_groups=num_groups_out, eps=1e-6)
- self.conv_act = nn.SiLU()
- self.conv_out = nn.Conv2d(block_out_channels[0], out_channels, 3, padding=1)
-
- def forward(self, z):
- sample = z
- sample = self.conv_in(sample)
-
- # middle
- sample = self.mid_block(sample)
-
- # up
- for up_block in self.up_blocks:
- sample = up_block(sample)
-
- # post-process
- sample = self.conv_norm_out(sample)
- sample = self.conv_act(sample)
- sample = self.conv_out(sample)
-
- return sample
-
-
-class VectorQuantizer(nn.Module):
- """
- Improved version over VectorQuantizer, can be used as a drop-in replacement. Mostly avoids costly matrix
- multiplications and allows for post-hoc remapping of indices.
- """
-
- # NOTE: due to a bug the beta term was applied to the wrong term. for
- # backwards compatibility we use the buggy version by default, but you can
- # specify legacy=False to fix it.
- def __init__(self, n_e, e_dim, beta, remap=None, unknown_index="random", sane_index_shape=False, legacy=True):
- super().__init__()
- self.n_e = n_e
- self.e_dim = e_dim
- self.beta = beta
- self.legacy = legacy
-
- self.embedding = nn.Embedding(self.n_e, self.e_dim)
- self.embedding.weight.data.uniform_(-1.0 / self.n_e, 1.0 / self.n_e)
-
- self.remap = remap
- if self.remap is not None:
- self.register_buffer("used", torch.tensor(np.load(self.remap)))
- self.re_embed = self.used.shape[0]
- self.unknown_index = unknown_index # "random" or "extra" or integer
- if self.unknown_index == "extra":
- self.unknown_index = self.re_embed
- self.re_embed = self.re_embed + 1
- print(
- f"Remapping {self.n_e} indices to {self.re_embed} indices. "
- f"Using {self.unknown_index} for unknown indices."
- )
- else:
- self.re_embed = n_e
-
- self.sane_index_shape = sane_index_shape
-
- def remap_to_used(self, inds):
- ishape = inds.shape
- assert len(ishape) > 1
- inds = inds.reshape(ishape[0], -1)
- used = self.used.to(inds)
- match = (inds[:, :, None] == used[None, None, ...]).long()
- new = match.argmax(-1)
- unknown = match.sum(2) < 1
- if self.unknown_index == "random":
- new[unknown] = torch.randint(0, self.re_embed, size=new[unknown].shape).to(device=new.device)
- else:
- new[unknown] = self.unknown_index
- return new.reshape(ishape)
-
- def unmap_to_all(self, inds):
- ishape = inds.shape
- assert len(ishape) > 1
- inds = inds.reshape(ishape[0], -1)
- used = self.used.to(inds)
- if self.re_embed > self.used.shape[0]: # extra token
- inds[inds >= self.used.shape[0]] = 0 # simply set to zero
- back = torch.gather(used[None, :][inds.shape[0] * [0], :], 1, inds)
- return back.reshape(ishape)
-
- def forward(self, z):
- # reshape z -> (batch, height, width, channel) and flatten
- z = z.permute(0, 2, 3, 1).contiguous()
- z_flattened = z.view(-1, self.e_dim)
- # distances from z to embeddings e_j (z - e)^2 = z^2 + e^2 - 2 e * z
-
- d = (
- torch.sum(z_flattened**2, dim=1, keepdim=True)
- + torch.sum(self.embedding.weight**2, dim=1)
- - 2 * torch.einsum("bd,dn->bn", z_flattened, self.embedding.weight.t())
- )
-
- min_encoding_indices = torch.argmin(d, dim=1)
- z_q = self.embedding(min_encoding_indices).view(z.shape)
- perplexity = None
- min_encodings = None
-
- # compute loss for embedding
- if not self.legacy:
- loss = self.beta * torch.mean((z_q.detach() - z) ** 2) + torch.mean((z_q - z.detach()) ** 2)
- else:
- loss = torch.mean((z_q.detach() - z) ** 2) + self.beta * torch.mean((z_q - z.detach()) ** 2)
-
- # preserve gradients
- z_q = z + (z_q - z).detach()
-
- # reshape back to match original input shape
- z_q = z_q.permute(0, 3, 1, 2).contiguous()
-
- if self.remap is not None:
- min_encoding_indices = min_encoding_indices.reshape(z.shape[0], -1) # add batch axis
- min_encoding_indices = self.remap_to_used(min_encoding_indices)
- min_encoding_indices = min_encoding_indices.reshape(-1, 1) # flatten
-
- if self.sane_index_shape:
- min_encoding_indices = min_encoding_indices.reshape(z_q.shape[0], z_q.shape[2], z_q.shape[3])
-
- return z_q, loss, (perplexity, min_encodings, min_encoding_indices)
-
- def get_codebook_entry(self, indices, shape):
- # shape specifying (batch, height, width, channel)
- if self.remap is not None:
- indices = indices.reshape(shape[0], -1) # add batch axis
- indices = self.unmap_to_all(indices)
- indices = indices.reshape(-1) # flatten again
-
- # get quantized latent vectors
- z_q = self.embedding(indices)
-
- if shape is not None:
- z_q = z_q.view(shape)
- # reshape back to match original input shape
- z_q = z_q.permute(0, 3, 1, 2).contiguous()
-
- return z_q
-
-
-class DiagonalGaussianDistribution(object):
- def __init__(self, parameters, deterministic=False):
- self.parameters = parameters
- self.mean, self.logvar = torch.chunk(parameters, 2, dim=1)
- self.logvar = torch.clamp(self.logvar, -30.0, 20.0)
- self.deterministic = deterministic
- self.std = torch.exp(0.5 * self.logvar)
- self.var = torch.exp(self.logvar)
- if self.deterministic:
- self.var = self.std = torch.zeros_like(self.mean).to(device=self.parameters.device)
-
- def sample(self, generator: Optional[torch.Generator] = None) -> torch.FloatTensor:
- device = self.parameters.device
- sample_device = "cpu" if device.type == "mps" else device
- sample = torch.randn(self.mean.shape, generator=generator, device=sample_device).to(device)
- x = self.mean + self.std * sample
- return x
-
- def kl(self, other=None):
- if self.deterministic:
- return torch.Tensor([0.0])
- else:
- if other is None:
- return 0.5 * torch.sum(torch.pow(self.mean, 2) + self.var - 1.0 - self.logvar, dim=[1, 2, 3])
- else:
- return 0.5 * torch.sum(
- torch.pow(self.mean - other.mean, 2) / other.var
- + self.var / other.var
- - 1.0
- - self.logvar
- + other.logvar,
- dim=[1, 2, 3],
- )
-
- def nll(self, sample, dims=[1, 2, 3]):
- if self.deterministic:
- return torch.Tensor([0.0])
- logtwopi = np.log(2.0 * np.pi)
- return 0.5 * torch.sum(logtwopi + self.logvar + torch.pow(sample - self.mean, 2) / self.var, dim=dims)
-
- def mode(self):
- return self.mean
-
-
-class VQModel(ModelMixin, ConfigMixin):
- r"""VQ-VAE model from the paper Neural Discrete Representation Learning by Aaron van den Oord, Oriol Vinyals and Koray
- Kavukcuoglu.
-
- This model inherits from [`ModelMixin`]. Check the superclass documentation for the generic methods the library
- implements for all the model (such as downloading or saving, etc.)
-
- Parameters:
- in_channels (int, *optional*, defaults to 3): Number of channels in the input image.
- out_channels (int, *optional*, defaults to 3): Number of channels in the output.
- down_block_types (`Tuple[str]`, *optional*, defaults to :
- obj:`("DownEncoderBlock2D",)`): Tuple of downsample block types.
- up_block_types (`Tuple[str]`, *optional*, defaults to :
- obj:`("UpDecoderBlock2D",)`): Tuple of upsample block types.
- block_out_channels (`Tuple[int]`, *optional*, defaults to :
- obj:`(64,)`): Tuple of block output channels.
- act_fn (`str`, *optional*, defaults to `"silu"`): The activation function to use.
- latent_channels (`int`, *optional*, defaults to `3`): Number of channels in the latent space.
- sample_size (`int`, *optional*, defaults to `32`): TODO
- num_vq_embeddings (`int`, *optional*, defaults to `256`): Number of codebook vectors in the VQ-VAE.
- """
-
- @register_to_config
- def __init__(
- self,
- in_channels: int = 3,
- out_channels: int = 3,
- down_block_types: Tuple[str] = ("DownEncoderBlock2D",),
- up_block_types: Tuple[str] = ("UpDecoderBlock2D",),
- block_out_channels: Tuple[int] = (64,),
- layers_per_block: int = 1,
- act_fn: str = "silu",
- latent_channels: int = 3,
- sample_size: int = 32,
- num_vq_embeddings: int = 256,
- ):
- super().__init__()
-
- # pass init params to Encoder
- self.encoder = Encoder(
- in_channels=in_channels,
- out_channels=latent_channels,
- down_block_types=down_block_types,
- block_out_channels=block_out_channels,
- layers_per_block=layers_per_block,
- act_fn=act_fn,
- double_z=False,
- )
-
- self.quant_conv = torch.nn.Conv2d(latent_channels, latent_channels, 1)
- self.quantize = VectorQuantizer(
- num_vq_embeddings, latent_channels, beta=0.25, remap=None, sane_index_shape=False
- )
- self.post_quant_conv = torch.nn.Conv2d(latent_channels, latent_channels, 1)
-
- # pass init params to Decoder
- self.decoder = Decoder(
- in_channels=latent_channels,
- out_channels=out_channels,
- up_block_types=up_block_types,
- block_out_channels=block_out_channels,
- layers_per_block=layers_per_block,
- act_fn=act_fn,
- )
-
- def encode(self, x: torch.FloatTensor, return_dict: bool = True) -> VQEncoderOutput:
- h = self.encoder(x)
- h = self.quant_conv(h)
-
- if not return_dict:
- return (h,)
-
- return VQEncoderOutput(latents=h)
-
- def decode(
- self, h: torch.FloatTensor, force_not_quantize: bool = False, return_dict: bool = True
- ) -> Union[DecoderOutput, torch.FloatTensor]:
- # also go through quantization layer
- if not force_not_quantize:
- quant, emb_loss, info = self.quantize(h)
- else:
- quant = h
- quant = self.post_quant_conv(quant)
- dec = self.decoder(quant)
-
- if not return_dict:
- return (dec,)
-
- return DecoderOutput(sample=dec)
-
- def forward(self, sample: torch.FloatTensor, return_dict: bool = True) -> Union[DecoderOutput, torch.FloatTensor]:
- r"""
- Args:
- sample (`torch.FloatTensor`): Input sample.
- return_dict (`bool`, *optional*, defaults to `True`):
- Whether or not to return a [`DecoderOutput`] instead of a plain tuple.
- """
- x = sample
- h = self.encode(x).latents
- dec = self.decode(h).sample
-
- if not return_dict:
- return (dec,)
-
- return DecoderOutput(sample=dec)
-
-
-class AutoencoderKL(ModelMixin, ConfigMixin):
- r"""Variational Autoencoder (VAE) model with KL loss from the paper Auto-Encoding Variational Bayes by Diederik P. Kingma
- and Max Welling.
-
- This model inherits from [`ModelMixin`]. Check the superclass documentation for the generic methods the library
- implements for all the model (such as downloading or saving, etc.)
-
- Parameters:
- in_channels (int, *optional*, defaults to 3): Number of channels in the input image.
- out_channels (int, *optional*, defaults to 3): Number of channels in the output.
- down_block_types (`Tuple[str]`, *optional*, defaults to :
- obj:`("DownEncoderBlock2D",)`): Tuple of downsample block types.
- up_block_types (`Tuple[str]`, *optional*, defaults to :
- obj:`("UpDecoderBlock2D",)`): Tuple of upsample block types.
- block_out_channels (`Tuple[int]`, *optional*, defaults to :
- obj:`(64,)`): Tuple of block output channels.
- act_fn (`str`, *optional*, defaults to `"silu"`): The activation function to use.
- latent_channels (`int`, *optional*, defaults to `4`): Number of channels in the latent space.
- sample_size (`int`, *optional*, defaults to `32`): TODO
- """
-
- @register_to_config
- def __init__(
- self,
- in_channels: int = 3,
- out_channels: int = 3,
- down_block_types: Tuple[str] = ("DownEncoderBlock2D",),
- up_block_types: Tuple[str] = ("UpDecoderBlock2D",),
- block_out_channels: Tuple[int] = (64,),
- layers_per_block: int = 1,
- act_fn: str = "silu",
- latent_channels: int = 4,
- sample_size: int = 32,
- ):
- super().__init__()
-
- # pass init params to Encoder
- self.encoder = Encoder(
- in_channels=in_channels,
- out_channels=latent_channels,
- down_block_types=down_block_types,
- block_out_channels=block_out_channels,
- layers_per_block=layers_per_block,
- act_fn=act_fn,
- double_z=True,
- )
-
- # pass init params to Decoder
- self.decoder = Decoder(
- in_channels=latent_channels,
- out_channels=out_channels,
- up_block_types=up_block_types,
- block_out_channels=block_out_channels,
- layers_per_block=layers_per_block,
- act_fn=act_fn,
- )
-
- self.quant_conv = torch.nn.Conv2d(2 * latent_channels, 2 * latent_channels, 1)
- self.post_quant_conv = torch.nn.Conv2d(latent_channels, latent_channels, 1)
-
- def encode(self, x: torch.FloatTensor, return_dict: bool = True) -> AutoencoderKLOutput:
- h = self.encoder(x)
- moments = self.quant_conv(h)
- posterior = DiagonalGaussianDistribution(moments)
-
- if not return_dict:
- return (posterior,)
-
- return AutoencoderKLOutput(latent_dist=posterior)
-
- def decode(self, z: torch.FloatTensor, return_dict: bool = True) -> Union[DecoderOutput, torch.FloatTensor]:
- z = self.post_quant_conv(z)
- dec = self.decoder(z)
-
- if not return_dict:
- return (dec,)
-
- return DecoderOutput(sample=dec)
-
- def forward(
- self, sample: torch.FloatTensor, sample_posterior: bool = False, return_dict: bool = True
- ) -> Union[DecoderOutput, torch.FloatTensor]:
- r"""
- Args:
- sample (`torch.FloatTensor`): Input sample.
- sample_posterior (`bool`, *optional*, defaults to `False`):
- Whether to sample from the posterior.
- return_dict (`bool`, *optional*, defaults to `True`):
- Whether or not to return a [`DecoderOutput`] instead of a plain tuple.
- """
- x = sample
- posterior = self.encode(x).latent_dist
- if sample_posterior:
- z = posterior.sample()
- else:
- z = posterior.mode()
- dec = self.decode(z).sample
-
- if not return_dict:
- return (dec,)
-
- return DecoderOutput(sample=dec)
diff --git a/spaces/Semibit/gentle-audio/README.md b/spaces/Semibit/gentle-audio/README.md
deleted file mode 100644
index 69b6b884a85e8dd2cc537b81f1fdc78dea62f71f..0000000000000000000000000000000000000000
--- a/spaces/Semibit/gentle-audio/README.md
+++ /dev/null
@@ -1,10 +0,0 @@
----
-title: Gentle Audio
-emoji: 🔥
-colorFrom: indigo
-colorTo: green
-sdk: docker
-pinned: false
----
-
-Check out the configuration reference at https://huggingface.co/docs/hub/spaces-config-reference
diff --git a/spaces/Slep/CondViT-LRVSF-Demo/src/js_loader.py b/spaces/Slep/CondViT-LRVSF-Demo/src/js_loader.py
deleted file mode 100644
index db2328994d6a4ccad7ab9b68f3e25102d615fe79..0000000000000000000000000000000000000000
--- a/spaces/Slep/CondViT-LRVSF-Demo/src/js_loader.py
+++ /dev/null
@@ -1,25 +0,0 @@
-import gradio
-
-class JavaScriptLoader:
- def __init__(self, target):
- #Copy the template response
- self.original_template = gradio.routes.templates.TemplateResponse
- #Prep the js files
- self.load_js(target)
- #reassign the template response to your method, so gradio calls your method instead
- gradio.routes.templates.TemplateResponse = self.template_response
-
- def load_js(self, target):
- with open(target, 'r', encoding="utf-8") as file:
- self.loaded_script = f""
-
- def template_response(self, *args, **kwargs):
- """Once gradio calls your method, you call the original, you modify it to include
- your scripts and you return the modified version
- """
- response = self.original_template(*args, **kwargs)
- response.body = response.body.replace(
- ''.encode('utf-8'), (self.loaded_script + "\n").encode("utf-8")
- )
- response.init_headers()
- return response
\ No newline at end of file
diff --git a/spaces/StatsByZach/app/team_xg_rates.py b/spaces/StatsByZach/app/team_xg_rates.py
deleted file mode 100644
index c6cbffffb5fad16004d3f710ed253c756929e78a..0000000000000000000000000000000000000000
--- a/spaces/StatsByZach/app/team_xg_rates.py
+++ /dev/null
@@ -1,209 +0,0 @@
-##### team_xg_rates.py #####
-# A program to display teams on-ice xG rates
-# Zach Andrews
-
-# Import modules
-from shiny import *
-import shinyswatch
-import plotly.graph_objs as go
-from shinywidgets import output_widget, register_widget, render_widget, bokeh_dependency
-import pandas as pd
-import plotly.express as px
-from configure import base_url
-
-path = "data/team_xg_rates.csv"
-
-df = pd.read_csv(path)
-
-def server(input, output, session):
- @output
- @render.table
- def table():
- df = pd.read_csv(path)
- if input.z() == "T":
- asc = True
- else:
- asc = False
-
- if input.strength()=="even":
- if input.y() == "Team":
- df = df[['Team','EV_TOI','EV_xGF/60','EV_xGA/60']].sort_values(by='Team',ascending=asc).round(3)
- elif input.y() == 'xGF/60':
- df = df[['Team','EV_TOI','EV_xGF/60','EV_xGA/60']].sort_values(by='EV_xGF/60',ascending=asc).round(3)
- elif input.y() == 'xGA/60':
- df = df[['Team','EV_TOI','EV_xGF/60','EV_xGA/60']].sort_values(by='EV_xGA/60',ascending=asc).round(3)
- else:
- df = df[['Team','EV_TOI','EV_xGF/60','EV_xGA/60']].sort_values(by=input.y(),ascending=asc).round(3)
- elif input.strength()=="_5v5":
- if input.y() == "Team":
- df = df[['Team','5v5_TOI','5v5_xGF/60','5v5_xGA/60']].sort_values(by='Team',ascending=asc).round(3)
- elif input.y() == 'xGF/60':
- df = df[['Team','5v5_TOI','5v5_xGF/60','5v5_xGA/60']].sort_values(by='5v5_xGF/60',ascending=asc).round(3)
- elif input.y() == 'xGA/60':
- df = df[['Team','5v5_TOI','5v5_xGF/60','5v5_xGA/60']].sort_values(by='5v5_xGA/60',ascending=asc).round(3)
- else:
- df = df[['Team','5v5_TOI','5v5_xGF/60','5v5_xGA/60']].sort_values(by=input.y(),ascending=asc).round(3)
- else:
- if input.y() == "Team":
- df = df[['Team','ALL_TOI','ALL_xGF/60','ALL_xGA/60']].sort_values(by='Team',ascending=asc).round(3)
- elif input.y() == 'xGF/60':
- df = df[['Team','ALL_TOI','ALL_xGF/60','ALL_xGA/60']].sort_values(by='ALL_xGF/60',ascending=asc).round(3)
- elif input.y() == 'xGA/60':
- df = df[['Team','ALL_TOI','ALL_xGF/60','ALL_xGA/60']].sort_values(by='ALL_xGA/60',ascending=asc).round(3)
- else:
- df = df[['Team','ALL_TOI','ALL_xGF/60','ALL_xGA/60']].sort_values(by=input.y(),ascending=asc).round(3)
- return df
-
- @output
- @render_widget
- def my_widget():
- df = pd.read_csv(path)
- if input.strength()=="even":
- title_strength = "Even Strength"
- title_toi = "EV"
- x_col = "EV_xGF/60"
- y_col = "EV_xGA/60"
- x_title = "Even Strength xGF/60"
- y_title = "Even Strength xGA/60"
- color_for_chart = "EV_TOI"
- elif input.strength()=="_5v5":
- title_strength="5v5"
- title_toi="5v5"
- x_col = "5v5_xGF/60"
- y_col = "5v5_xGA/60"
- x_title = "5v5 xGF/60"
- y_title = "5v5 xGA/60"
- color_for_chart="5v5_TOI"
- else:
- title_strength="All Situation"
- title_toi="All"
- x_col = "ALL_xGF/60"
- y_col = "ALL_xGA/60"
- x_title = "All Situation xGF/60"
- y_title = "All Situation xGA/60"
- color_for_chart="ALL_TOI"
- fig = px.scatter(df, x_col, y_col,color=color_for_chart,template="plotly_dark",height=1050,width=1050,text='Team')
- fig.update_traces(textposition='top right',marker=dict(size=10))
- fig.update(layout_xaxis_range = [1.5,3.7])
- fig.update(layout_yaxis_range = [3.7,1.5])
- fig.update_traces(textfont_size=15)
- fig.add_vline(x=df[x_col].mean(), line_width=2, line_dash="dash", line_color="#617296")
- fig.add_hline(y=df[y_col].mean(), line_width=2, line_dash="dash", line_color="#617296")
- fig.update_layout(xaxis_showgrid=False, yaxis_showgrid=False,plot_bgcolor="#222222",paper_bgcolor="#222222")
- fig.update_layout(
- title=("Team " +title_strength + " xG Rates "+
- "2023-24 NHL Regular Season "),
- margin=dict(r=20, l=40, b=100, t=90),
- template='plotly_dark')
- fig.add_annotation(
- text = ("Data: @StatsByZach on Twitter")
- , showarrow=False
- , x = .80
- , y = -.045
- , xref='paper'
- , yref='paper'
- , xanchor='left'
- , yanchor='bottom'
- , xshift=-1
- , yshift=-5
- , font=dict(size=11, color="white")
- , align="left"
- )
- fig.update_layout(xaxis_title=x_title)
- fig.update_layout(yaxis_title=y_title)
- return fig
- @reactive.Effect
- def _():
- btn = input.btn()
- if btn % 2 == 1:
- tab = ui.output_table("table")
- ui.insert_ui(
- ui.div({"id": "inserted-slider"},ui.tags.h5("Sort Table by", class_="app-heading"),ui.input_select("y","",{"Team":"Team","EV_TOI":"EV_TOI","xGF/60":"xGF/60","xGA/60":"xGA/60"}),
- ui.input_radio_buttons(
- "z", "", {"F": "High to Low", "T": "Low to High"}
- ),ui.output_table("table")),
- selector="#main-content",
- where="beforeEnd",
- )
- elif btn > 0:
- ui.remove_ui("#inserted-slider")
-
-team_xg_rates = App(ui.page_fluid(
- ui.tags.base(href=base_url),
- ui.tags.div(
- {"style": "width:75%;margin: 0 auto"},
- ui.tags.style(
- """
- h4 {
- margin-top: 1em;font-size:35px;
- }
- h2{
- font-size:25px;
- }
- """
- ),
- shinyswatch.theme.darkly(),
- ui.tags.h4("Stats By Zach"),
- ui.tags.i("A website for hockey analytics"),
- ui.navset_tab(
- ui.nav_control(
- ui.a(
- "Home",
- href="home/"
- ),
- ),
- ui.nav_menu(
- "Skater Charts",
- ui.nav_control(
- ui.a(
- "On-Ice xG Rates",
- href="skater-xg-rates/"
- ),
- ui.a(
- "On-Ice xGF%",
- href="skater-xg-percentages/"
- ),
- ),
- ),
- ui.nav_menu(
- "Goalie Charts",
- ui.nav_control(
- ui.a(
- "GSAx Timeline",
- href="gsax-timeline/"
- ),
- ui.a(
- "GSAx Leaderboard",
- href="gsax-leaderboard/"
- ),
- ui.a(
- "GSAx Comparison",
- href="gsax-comparison/"
- )
- ),
- ),ui.nav_menu(
- "Team Charts",
- ui.nav_control(
- ui.a(
- "Team xG Rates",
- href="team-xg-rates/"
- ),
- ),
- ),ui.nav_control(
- ui.a(
- "Games",
- href="games/"
- ),
- ),ui.nav_control(
- ui.a(
- "About",
- href="about/"
- ),
- )
- ),ui.row(
- ui.column(3,ui.tags.br(),ui.tags.h2("Team xG Rates"),ui.tags.h5("Strength", class_="app-heading"),ui.input_select("strength", "",{'even':"Even",'_5v5':"5v5",'All':"All Situations"}),ui.input_action_button("btn", "Toggle Table"),ui.div({"id":"main-content"},
- #ui.output_table("table"),
- )),
- ui.column(9,output_widget("my_widget"),#output_widget("it"),
- title="Stats By Zach",
- )))),server)
diff --git a/spaces/SuYuanS/AudioCraft_Plus/audiocraft/models/multibanddiffusion.py b/spaces/SuYuanS/AudioCraft_Plus/audiocraft/models/multibanddiffusion.py
deleted file mode 100644
index 6a2f169d516ed5aaf5da61fb482d94dd142f55e9..0000000000000000000000000000000000000000
--- a/spaces/SuYuanS/AudioCraft_Plus/audiocraft/models/multibanddiffusion.py
+++ /dev/null
@@ -1,194 +0,0 @@
-# Copyright (c) Meta Platforms, Inc. and affiliates.
-# All rights reserved.
-#
-# This source code is licensed under the license found in the
-# LICENSE file in the root directory of this source tree.
-
-"""
-Multi Band Diffusion models as described in
-"From Discrete Tokens to High-Fidelity Audio Using Multi-Band Diffusion"
-(paper link).
-"""
-
-import typing as tp
-
-import torch
-import julius
-
-from .unet import DiffusionUnet
-from ..modules.diffusion_schedule import NoiseSchedule
-from .encodec import CompressionModel
-from ..solvers.compression import CompressionSolver
-from .loaders import load_compression_model, load_diffusion_models
-
-
-class DiffusionProcess:
- """Sampling for a diffusion Model.
-
- Args:
- model (DiffusionUnet): Diffusion U-Net model.
- noise_schedule (NoiseSchedule): Noise schedule for diffusion process.
- """
- def __init__(self, model: DiffusionUnet, noise_schedule: NoiseSchedule) -> None:
- """
- """
- self.model = model
- self.schedule = noise_schedule
-
- def generate(self, condition: torch.Tensor, initial_noise: torch.Tensor,
- step_list: tp.Optional[tp.List[int]] = None):
- """Perform one diffusion process to generate one of the bands.
-
- Args:
- condition (tensor): The embeddings form the compression model.
- initial_noise (tensor): The initial noise to start the process/
- """
- return self.schedule.generate_subsampled(model=self.model, initial=initial_noise, step_list=step_list,
- condition=condition)
-
-
-class MultiBandDiffusion:
- """Sample from multiple diffusion models.
-
- Args:
- DPs (list of DiffusionProcess): Diffusion processes.
- codec_model (CompressionModel): Underlying compression model used to obtain discrete tokens.
- """
- def __init__(self, DPs: tp.List[DiffusionProcess], codec_model: CompressionModel) -> None:
- self.DPs = DPs
- self.codec_model = codec_model
- self.device = next(self.codec_model.parameters()).device
-
- @property
- def sample_rate(self) -> int:
- return self.codec_model.sample_rate
-
- @staticmethod
- def get_mbd_musicgen(device=None):
- """Load our diffusion models trained for MusicGen."""
- if device is None:
- device = 'cuda' if torch.cuda.is_available() else 'cpu'
- path = 'https://dl.fbaipublicfiles.com/encodec/Diffusion/mbd_musicgen_32khz.th'
- name = 'facebook/musicgen-small'
- codec_model = load_compression_model(name, device=device)
- models, processors, cfgs = load_diffusion_models(path, device=device)
- DPs = []
- for i in range(len(models)):
- schedule = NoiseSchedule(**cfgs[i].schedule, sample_processor=processors[i])
- DPs.append(DiffusionProcess(model=models[i], noise_schedule=schedule))
- return MultiBandDiffusion(DPs=DPs, codec_model=codec_model)
-
- @staticmethod
- def get_mbd_24khz(bw: float = 3.0, pretrained: bool = True,
- device: tp.Optional[tp.Union[torch.device, str]] = None,
- n_q: tp.Optional[int] = None):
- """Get the pretrained Models for MultibandDiffusion.
-
- Args:
- bw (float): Bandwidth of the compression model.
- pretrained (bool): Whether to use / download if necessary the models.
- device (torch.device or str, optional): Device on which the models are loaded.
- n_q (int, optional): Number of quantizers to use within the compression model.
- """
- if device is None:
- device = 'cuda' if torch.cuda.is_available() else 'cpu'
- assert bw in [1.5, 3.0, 6.0], f"bandwidth {bw} not available"
- if n_q is not None:
- assert n_q in [2, 4, 8]
- assert {1.5: 2, 3.0: 4, 6.0: 8}[bw] == n_q, \
- f"bandwidth and number of codebooks missmatch to use n_q = {n_q} bw should be {n_q * (1.5 / 2)}"
- n_q = {1.5: 2, 3.0: 4, 6.0: 8}[bw]
- codec_model = CompressionSolver.model_from_checkpoint(
- '//pretrained/facebook/encodec_24khz', device=device)
- codec_model.set_num_codebooks(n_q)
- codec_model = codec_model.to(device)
- path = f'https://dl.fbaipublicfiles.com/encodec/Diffusion/mbd_comp_{n_q}.pt'
- models, processors, cfgs = load_diffusion_models(path, device=device)
- DPs = []
- for i in range(len(models)):
- schedule = NoiseSchedule(**cfgs[i].schedule, sample_processor=processors[i])
- DPs.append(DiffusionProcess(model=models[i], noise_schedule=schedule))
- return MultiBandDiffusion(DPs=DPs, codec_model=codec_model)
-
- return MultiBandDiffusion(DPs, codec_model)
-
- @torch.no_grad()
- def get_condition(self, wav: torch.Tensor, sample_rate: int) -> torch.Tensor:
- """Get the conditioning (i.e. latent reprentatios of the compression model) from a waveform.
- Args:
- wav (torch.Tensor): The audio that we want to extract the conditioning from
- sample_rate (int): sample rate of the audio"""
- if sample_rate != self.sample_rate:
- wav = julius.resample_frac(wav, sample_rate, self.sample_rate)
- codes, scale = self.codec_model.encode(wav)
- assert scale is None, "Scaled compression models not supported."
- emb = self.get_emb(codes)
- return emb
-
- @torch.no_grad()
- def get_emb(self, codes: torch.Tensor):
- """Get latent representation from the discrete codes
- Argrs:
- codes (torch.Tensor): discrete tokens"""
- emb = self.codec_model.decode_latent(codes)
- return emb
-
- def generate(self, emb: torch.Tensor, size: tp.Optional[torch.Size] = None,
- step_list: tp.Optional[tp.List[int]] = None):
- """Generate Wavform audio from the latent embeddings of the compression model
- Args:
- emb (torch.Tensor): Conditioning embeddinds
- size (none torch.Size): size of the output
- if None this is computed from the typical upsampling of the model
- step_list (optional list[int]): list of Markov chain steps, defaults to 50 linearly spaced step.
- """
- if size is None:
- upsampling = int(self.codec_model.sample_rate / self.codec_model.frame_rate)
- size = torch.Size([emb.size(0), self.codec_model.channels, emb.size(-1) * upsampling])
- assert size[0] == emb.size(0)
- out = torch.zeros(size).to(self.device)
- for DP in self.DPs:
- out += DP.generate(condition=emb, step_list=step_list, initial_noise=torch.randn_like(out))
- return out
-
- def re_eq(self, wav: torch.Tensor, ref: torch.Tensor, n_bands: int = 32, strictness: float = 1):
- """match the eq to the encodec output by matching the standard deviation of some frequency bands
- Args:
- wav (torch.Tensor): audio to equalize
- ref (torch.Tensor):refenrence audio from which we match the spectrogram.
- n_bands (int): number of bands of the eq
- strictness (float): how strict the the matching. 0 is no matching, 1 is exact matching.
- """
- split = julius.SplitBands(n_bands=n_bands, sample_rate=self.codec_model.sample_rate).to(wav.device)
- bands = split(wav)
- bands_ref = split(ref)
- out = torch.zeros_like(ref)
- for i in range(n_bands):
- out += bands[i] * (bands_ref[i].std() / bands[i].std()) ** strictness
- return out
-
- def regenerate(self, wav: torch.Tensor, sample_rate: int):
- """Regenerate a wavform through compression and diffusion regeneration.
- Args:
- wav (torch.Tensor): Original 'ground truth' audio
- sample_rate (int): sample rate of the input (and output) wav
- """
- if sample_rate != self.codec_model.sample_rate:
- wav = julius.resample_frac(wav, sample_rate, self.codec_model.sample_rate)
- emb = self.get_condition(wav, sample_rate=self.codec_model.sample_rate)
- size = wav.size()
- out = self.generate(emb, size=size)
- if sample_rate != self.codec_model.sample_rate:
- out = julius.resample_frac(out, self.codec_model.sample_rate, sample_rate)
- return out
-
- def tokens_to_wav(self, tokens: torch.Tensor, n_bands: int = 32):
- """Generate Waveform audio with diffusion from the discrete codes.
- Args:
- tokens (torch.Tensor): discrete codes
- n_bands (int): bands for the eq matching.
- """
- wav_encodec = self.codec_model.decode(tokens)
- condition = self.get_emb(tokens)
- wav_diffusion = self.generate(emb=condition, size=wav_encodec.size())
- return self.re_eq(wav=wav_diffusion, ref=wav_encodec, n_bands=n_bands)
diff --git a/spaces/TakaMETaka/openai-reverse-proxy/README.md b/spaces/TakaMETaka/openai-reverse-proxy/README.md
deleted file mode 100644
index fca2feab39a565374b598fc2465775575c8a0e23..0000000000000000000000000000000000000000
--- a/spaces/TakaMETaka/openai-reverse-proxy/README.md
+++ /dev/null
@@ -1,10 +0,0 @@
----
-title: Openai Reverse Proxy
-emoji: 🐨
-colorFrom: indigo
-colorTo: red
-sdk: docker
-pinned: false
----
-
-Check out the configuration reference at https://huggingface.co/docs/hub/spaces-config-reference
diff --git a/spaces/UserXTheUnknown/stablediffusion-infinity/PyPatchMatch/README.md b/spaces/UserXTheUnknown/stablediffusion-infinity/PyPatchMatch/README.md
deleted file mode 100644
index 12b49aadadfe0ff51c2873b2671c0ca020bc3506..0000000000000000000000000000000000000000
--- a/spaces/UserXTheUnknown/stablediffusion-infinity/PyPatchMatch/README.md
+++ /dev/null
@@ -1,64 +0,0 @@
-PatchMatch based Inpainting
-=====================================
-This library implements the PatchMatch based inpainting algorithm. It provides both C++ and Python interfaces.
-This implementation is heavily based on the implementation by Younesse ANDAM:
-(younesse-cv/PatchMatch)[https://github.com/younesse-cv/PatchMatch], with some bugs fix.
-
-Usage
--------------------------------------
-
-You need to first install OpenCV to compile the C++ libraries. Then, run `make` to compile the
-shared library `libpatchmatch.so`.
-
-For Python users (example available at `examples/py_example.py`)
-
-```python
-import patch_match
-
-image = ... # either a numpy ndarray or a PIL Image object.
-mask = ... # either a numpy ndarray or a PIL Image object.
-result = patch_match.inpaint(image, mask, patch_size=5)
-```
-
-For C++ users (examples available at `examples/cpp_example.cpp`)
-
-```cpp
-#include "inpaint.h"
-
-int main() {
- cv::Mat image = ...
- cv::Mat mask = ...
-
- cv::Mat result = Inpainting(image, mask, 5).run();
-
- return 0;
-}
-```
-
-
-README and COPYRIGHT by Younesse ANDAM
--------------------------------------
-@Author: Younesse ANDAM
-
-@Contact: younesse.andam@gmail.com
-
-Description: This project is a personal implementation of an algorithm called PATCHMATCH that restores missing areas in an image.
-The algorithm is presented in the following paper
- PatchMatch A Randomized Correspondence Algorithm
- for Structural Image Editing
- by C.Barnes,E.Shechtman,A.Finkelstein and Dan B.Goldman
- ACM Transactions on Graphics (Proc. SIGGRAPH), vol.28, aug-2009
-
- For more information please refer to
- http://www.cs.princeton.edu/gfx/pubs/Barnes_2009_PAR/index.php
-
-Copyright (c) 2010-2011
-
-
-Requirements
--------------------------------------
-
-To run the project you need to install Opencv library and link it to your project.
-Opencv can be download it here
-http://opencv.org/downloads.html
-
diff --git a/spaces/VideoCrafter/VideoCrafter/lvdm/models/autoencoder.py b/spaces/VideoCrafter/VideoCrafter/lvdm/models/autoencoder.py
deleted file mode 100644
index cc479d8b446b530885f4a3cc5d25cb58f0c00d74..0000000000000000000000000000000000000000
--- a/spaces/VideoCrafter/VideoCrafter/lvdm/models/autoencoder.py
+++ /dev/null
@@ -1,219 +0,0 @@
-import os
-from contextlib import contextmanager
-import torch
-import numpy as np
-from einops import rearrange
-import torch.nn.functional as F
-import pytorch_lightning as pl
-from lvdm.modules.networks.ae_modules import Encoder, Decoder
-from lvdm.distributions import DiagonalGaussianDistribution
-from utils.utils import instantiate_from_config
-
-
-class AutoencoderKL(pl.LightningModule):
- def __init__(self,
- ddconfig,
- lossconfig,
- embed_dim,
- ckpt_path=None,
- ignore_keys=[],
- image_key="image",
- colorize_nlabels=None,
- monitor=None,
- test=False,
- logdir=None,
- input_dim=4,
- test_args=None,
- ):
- super().__init__()
- self.image_key = image_key
- self.encoder = Encoder(**ddconfig)
- self.decoder = Decoder(**ddconfig)
- self.loss = instantiate_from_config(lossconfig)
- assert ddconfig["double_z"]
- self.quant_conv = torch.nn.Conv2d(2*ddconfig["z_channels"], 2*embed_dim, 1)
- self.post_quant_conv = torch.nn.Conv2d(embed_dim, ddconfig["z_channels"], 1)
- self.embed_dim = embed_dim
- self.input_dim = input_dim
- self.test = test
- self.test_args = test_args
- self.logdir = logdir
- if colorize_nlabels is not None:
- assert type(colorize_nlabels)==int
- self.register_buffer("colorize", torch.randn(3, colorize_nlabels, 1, 1))
- if monitor is not None:
- self.monitor = monitor
- if ckpt_path is not None:
- self.init_from_ckpt(ckpt_path, ignore_keys=ignore_keys)
- if self.test:
- self.init_test()
-
- def init_test(self,):
- self.test = True
- save_dir = os.path.join(self.logdir, "test")
- if 'ckpt' in self.test_args:
- ckpt_name = os.path.basename(self.test_args.ckpt).split('.ckpt')[0] + f'_epoch{self._cur_epoch}'
- self.root = os.path.join(save_dir, ckpt_name)
- else:
- self.root = save_dir
- if 'test_subdir' in self.test_args:
- self.root = os.path.join(save_dir, self.test_args.test_subdir)
-
- self.root_zs = os.path.join(self.root, "zs")
- self.root_dec = os.path.join(self.root, "reconstructions")
- self.root_inputs = os.path.join(self.root, "inputs")
- os.makedirs(self.root, exist_ok=True)
-
- if self.test_args.save_z:
- os.makedirs(self.root_zs, exist_ok=True)
- if self.test_args.save_reconstruction:
- os.makedirs(self.root_dec, exist_ok=True)
- if self.test_args.save_input:
- os.makedirs(self.root_inputs, exist_ok=True)
- assert(self.test_args is not None)
- self.test_maximum = getattr(self.test_args, 'test_maximum', None)
- self.count = 0
- self.eval_metrics = {}
- self.decodes = []
- self.save_decode_samples = 2048
-
- def init_from_ckpt(self, path, ignore_keys=list()):
- sd = torch.load(path, map_location="cpu")
- try:
- self._cur_epoch = sd['epoch']
- sd = sd["state_dict"]
- except:
- self._cur_epoch = 'null'
- keys = list(sd.keys())
- for k in keys:
- for ik in ignore_keys:
- if k.startswith(ik):
- print("Deleting key {} from state_dict.".format(k))
- del sd[k]
- self.load_state_dict(sd, strict=False)
- # self.load_state_dict(sd, strict=True)
- print(f"Restored from {path}")
-
- def encode(self, x, **kwargs):
-
- h = self.encoder(x)
- moments = self.quant_conv(h)
- posterior = DiagonalGaussianDistribution(moments)
- return posterior
-
- def decode(self, z, **kwargs):
- z = self.post_quant_conv(z)
- dec = self.decoder(z)
- return dec
-
- def forward(self, input, sample_posterior=True):
- posterior = self.encode(input)
- if sample_posterior:
- z = posterior.sample()
- else:
- z = posterior.mode()
- dec = self.decode(z)
- return dec, posterior
-
- def get_input(self, batch, k):
- x = batch[k]
- if x.dim() == 5 and self.input_dim == 4:
- b,c,t,h,w = x.shape
- self.b = b
- self.t = t
- x = rearrange(x, 'b c t h w -> (b t) c h w')
-
- return x
-
- def training_step(self, batch, batch_idx, optimizer_idx):
- inputs = self.get_input(batch, self.image_key)
- reconstructions, posterior = self(inputs)
-
- if optimizer_idx == 0:
- # train encoder+decoder+logvar
- aeloss, log_dict_ae = self.loss(inputs, reconstructions, posterior, optimizer_idx, self.global_step,
- last_layer=self.get_last_layer(), split="train")
- self.log("aeloss", aeloss, prog_bar=True, logger=True, on_step=True, on_epoch=True)
- self.log_dict(log_dict_ae, prog_bar=False, logger=True, on_step=True, on_epoch=False)
- return aeloss
-
- if optimizer_idx == 1:
- # train the discriminator
- discloss, log_dict_disc = self.loss(inputs, reconstructions, posterior, optimizer_idx, self.global_step,
- last_layer=self.get_last_layer(), split="train")
-
- self.log("discloss", discloss, prog_bar=True, logger=True, on_step=True, on_epoch=True)
- self.log_dict(log_dict_disc, prog_bar=False, logger=True, on_step=True, on_epoch=False)
- return discloss
-
- def validation_step(self, batch, batch_idx):
- inputs = self.get_input(batch, self.image_key)
- reconstructions, posterior = self(inputs)
- aeloss, log_dict_ae = self.loss(inputs, reconstructions, posterior, 0, self.global_step,
- last_layer=self.get_last_layer(), split="val")
-
- discloss, log_dict_disc = self.loss(inputs, reconstructions, posterior, 1, self.global_step,
- last_layer=self.get_last_layer(), split="val")
-
- self.log("val/rec_loss", log_dict_ae["val/rec_loss"])
- self.log_dict(log_dict_ae)
- self.log_dict(log_dict_disc)
- return self.log_dict
-
- def configure_optimizers(self):
- lr = self.learning_rate
- opt_ae = torch.optim.Adam(list(self.encoder.parameters())+
- list(self.decoder.parameters())+
- list(self.quant_conv.parameters())+
- list(self.post_quant_conv.parameters()),
- lr=lr, betas=(0.5, 0.9))
- opt_disc = torch.optim.Adam(self.loss.discriminator.parameters(),
- lr=lr, betas=(0.5, 0.9))
- return [opt_ae, opt_disc], []
-
- def get_last_layer(self):
- return self.decoder.conv_out.weight
-
- @torch.no_grad()
- def log_images(self, batch, only_inputs=False, **kwargs):
- log = dict()
- x = self.get_input(batch, self.image_key)
- x = x.to(self.device)
- if not only_inputs:
- xrec, posterior = self(x)
- if x.shape[1] > 3:
- # colorize with random projection
- assert xrec.shape[1] > 3
- x = self.to_rgb(x)
- xrec = self.to_rgb(xrec)
- log["samples"] = self.decode(torch.randn_like(posterior.sample()))
- log["reconstructions"] = xrec
- log["inputs"] = x
- return log
-
- def to_rgb(self, x):
- assert self.image_key == "segmentation"
- if not hasattr(self, "colorize"):
- self.register_buffer("colorize", torch.randn(3, x.shape[1], 1, 1).to(x))
- x = F.conv2d(x, weight=self.colorize)
- x = 2.*(x-x.min())/(x.max()-x.min()) - 1.
- return x
-
-class IdentityFirstStage(torch.nn.Module):
- def __init__(self, *args, vq_interface=False, **kwargs):
- self.vq_interface = vq_interface # TODO: Should be true by default but check to not break older stuff
- super().__init__()
-
- def encode(self, x, *args, **kwargs):
- return x
-
- def decode(self, x, *args, **kwargs):
- return x
-
- def quantize(self, x, *args, **kwargs):
- if self.vq_interface:
- return x, None, [None, None, None]
- return x
-
- def forward(self, x, *args, **kwargs):
- return x
diff --git a/spaces/VikramSingh178/MedicalImagingApplication/README.md b/spaces/VikramSingh178/MedicalImagingApplication/README.md
deleted file mode 100644
index 1a53001808c279ef3d7b2964960f4d94bc6b9b7c..0000000000000000000000000000000000000000
--- a/spaces/VikramSingh178/MedicalImagingApplication/README.md
+++ /dev/null
@@ -1,12 +0,0 @@
----
-title: MedicalImagingApplication
-emoji: 🦀
-colorFrom: pink
-colorTo: red
-sdk: streamlit
-sdk_version: 1.17.0
-app_file: app.py
-pinned: false
----
-
-Check out the configuration reference at https://huggingface.co/docs/hub/spaces-config-reference
diff --git a/spaces/Visgift/nyami/engine.py b/spaces/Visgift/nyami/engine.py
deleted file mode 100644
index 9d2532f36731098b881f48545a235e42a3a98625..0000000000000000000000000000000000000000
--- a/spaces/Visgift/nyami/engine.py
+++ /dev/null
@@ -1,49 +0,0 @@
-from transformers import pipeline
-
-
-class SentimentAnalyzer:
- """Class for analyzing the sentiment of sentences
- """
-
- def __init__(self) -> None:
- """initializes the class with sentiment analysis pipeline using the distilbert-base-uncased-finetuned-sst-2-english model
- """
- self.analyzer = pipeline(
- "sentiment-analysis", model="distilbert-base-uncased-finetuned-sst-2-english")
-
- def score_sentiment(self, sentence: str) -> float:
- """Uses the analyzer to analyze the sentiment of the provided sentence
-
- Parameters
- ----------
- sentence : str
- a short sentence to be analyzed
-
- Returns
- -------
- float
- score of the sentiment from 0 to 1. Below 0.5 is negative, above is positive. 0.5 is neutral
- """
- return self.analyzer(sentence)[0]
-
- def get_sentiment(self, sentence: str) -> str:
- """returns the label of the sentiment provided
-
- Parameters
- ----------
- sentence : str
- a short sentence to be analyzed
-
- Returns
- -------
- str
- label of the sentiment wether it is positive, negative, or neutral
- """
- sentiment_score = self.score_sentiment(sentence)
- return sentiment_score['label']
-
-
-if __name__ == "__main__":
- sentence = "I ... you"
- sentiment_analyzer = SentimentAnalyzer()
- print(sentiment_analyzer.get_sentiment(sentence))
diff --git a/spaces/Vision-CAIR/MiniGPT-v2/minigpt4/models/minigpt_base.py b/spaces/Vision-CAIR/MiniGPT-v2/minigpt4/models/minigpt_base.py
deleted file mode 100644
index c24c57967290f5d307613b6a98b4ab9c912e38fa..0000000000000000000000000000000000000000
--- a/spaces/Vision-CAIR/MiniGPT-v2/minigpt4/models/minigpt_base.py
+++ /dev/null
@@ -1,402 +0,0 @@
-import logging
-import random
-
-import torch
-from torch.cuda.amp import autocast as autocast
-import torch.nn as nn
-
-from minigpt4.common.registry import registry
-from minigpt4.models.base_model import BaseModel
-from transformers import StoppingCriteria, StoppingCriteriaList
-
-
-
-class MiniGPTBase(BaseModel):
- """
- Base class for MiniGPT-4 and MiniGPT-v2
- """
-
- def __init__(
- self,
- vit_model="eva_clip_g",
- img_size=224,
- drop_path_rate=0,
- use_grad_checkpoint=False,
- vit_precision="fp16",
- freeze_vit=True,
- llama_model="",
- max_txt_len=32,
- max_context_len=3800,
- prompt_template="",
- end_sym='\n',
- low_resource=False, # use 8 bit and put vit in cpu
- device_8bit=0, # the device of 8bit model should be set when loading and cannot be changed anymore.
- lora_r=0, # lora_r means lora is not used
- lora_target_modules=["q_proj", "v_proj"],
- lora_alpha=16,
- lora_dropout=0.05,
- ):
- super().__init__()
-
- self.llama_model, self.llama_tokenizer = self.init_llm(
- llama_model_path=llama_model,
- low_resource=low_resource,
- low_res_device=device_8bit,
- lora_r=lora_r,
- lora_target_modules=lora_target_modules,
- lora_alpha=lora_alpha,
- lora_dropout=lora_dropout,
- )
-
- self.visual_encoder, self.ln_vision = self.init_vision_encoder(
- vit_model, img_size, drop_path_rate, use_grad_checkpoint, vit_precision, freeze_vit
- )
-
- self.max_txt_len = max_txt_len
- self.max_context_len = max_context_len
- self.end_sym = end_sym
-
- self.prompt_template = prompt_template
- self.prompt_list = []
-
- def vit_to_cpu(self):
- self.ln_vision.to("cpu")
- self.ln_vision.float()
- self.visual_encoder.to("cpu")
- self.visual_encoder.float()
-
- def get_context_emb(self, prompt, img_list):
- device = img_list[0].device
- prompt_segs = prompt.split('')
- assert len(prompt_segs) == len(img_list) + 1, "Unmatched numbers of image placeholders and images."
- seg_tokens = [
- self.llama_tokenizer(
- seg, return_tensors="pt", add_special_tokens=i==0).to(device).input_ids # only add bos to the first seg
- for i, seg in enumerate(prompt_segs)
- ]
- seg_embs = [self.embed_tokens(seg_t) for seg_t in seg_tokens]
-
- mixed_embs = [emb for pair in zip(seg_embs[:-1], img_list) for emb in pair] + [seg_embs[-1]]
- mixed_embs = torch.cat(mixed_embs, dim=1)
- return mixed_embs
-
- def prompt_wrap(self, img_embeds, atts_img, prompts, lengths=None):
- if prompts is None or len(prompts) == 0:
- # prompts is not provided, just return the original image embedding
- return img_embeds, atts_img
- elif img_embeds is None:
- # prompt is provided but there is no image embedding. return the prompt embedding in right padding
- self.llama_tokenizer.padding_side = "right"
- prompt_tokens = self.llama_tokenizer(
- prompts,
- return_tensors="pt",
- padding="longest",
- add_special_tokens=False
- ).to(self.device)
- prompt_embeds = self.embed_tokens(prompt_tokens.input_ids)
- atts_prompt = prompt_tokens.attention_mask
- return prompt_embeds, atts_prompt
- else:
- # return the multi-modal embedding in right padding
- emb_lists = []
- if isinstance(prompts, str):
- prompts = [prompts] * len(img_embeds)
-
- for idx, (each_img_embed, each_prompt) in enumerate(zip(img_embeds, prompts)):
- pn = each_img_embed.shape[-2]
- if lengths is not None:
- each_img_embed = each_img_embed.reshape(-1, each_img_embed.shape[-1])
- each_img_embed = each_img_embed[:lengths[idx] * pn]
- p_segs = each_prompt.split('')
- interleave_emb = []
- for idx, seg in enumerate(p_segs[:-1]):
- p_tokens = self.llama_tokenizer(
- seg, return_tensors="pt", add_special_tokens=False).to(img_embeds.device)
- p_embed = self.embed_tokens(p_tokens.input_ids)
- interleave_emb.append(torch.cat([p_embed, each_img_embed[None][:, idx * pn:(idx + 1) * pn]], dim=1))
- wrapped_emb = torch.cat(interleave_emb, dim=1)
- p_tokens = self.llama_tokenizer(
- p_segs[-1], return_tensors="pt", add_special_tokens=False).to(img_embeds.device)
- p_embed = self.embed_tokens(p_tokens.input_ids)
- wrapped_emb = torch.cat([wrapped_emb, p_embed], dim=1)
- emb_lists.append(wrapped_emb)
-
- emb_lens = [emb.shape[1] for emb in emb_lists]
- pad_emb = self.embed_tokens(torch.tensor(self.llama_tokenizer.pad_token_id, device=img_embeds.device))
-
- max_length = max(emb_lens) if max(emb_lens) < self.max_context_len else self.max_context_len
- wrapped_embs = pad_emb.expand(len(emb_lens), max_length, -1).clone()
- wrapped_atts = torch.zeros([len(emb_lens), max_length], dtype=torch.int, device=img_embeds.device)
-
- for i, emb in enumerate(emb_lists):
- length = emb_lens[i] if emb_lens[i] < self.max_context_len else self.max_context_len
- wrapped_embs[i, :length] = emb[:, :length]
- wrapped_atts[i, :length] = 1
- return wrapped_embs, wrapped_atts
-
- def concat_emb_input_output(self, input_embs, input_atts, output_embs, output_atts):
- """
- Concatenate the batched input embedding and batched output embedding together.
- Both the input and the output embedding should be right padded.
- """
- input_lens = []
- cat_embs = []
- cat_atts = []
- for i in range(input_embs.size(0)):
- input_len = input_atts[i].sum()
- input_lens.append(input_len)
- cat_embs.append(
- torch.cat([
- input_embs[i][:input_len],
- output_embs[i],
- input_embs[i][input_len:]
- ])
- )
- cat_atts.append(
- torch.cat([
- input_atts[i][:input_len],
- output_atts[i],
- input_atts[i][input_len:]
- ])
- )
- cat_embs = torch.stack(cat_embs)
- cat_atts = torch.stack(cat_atts)
- return cat_embs, cat_atts, input_lens
-
- def tokenize_conversation(self, conv_q, conv_a):
- """concatenate conversation and make sure the model is only trained to regress the answer"""
-
- to_regress_token_ids_list = []
- targets_list = []
-
- batch_size = len(conv_q)
- for batch_idx in range(batch_size):
- questions, answers = conv_q[batch_idx], conv_a[batch_idx]
- questions = [self.llama_tokenizer(q,
- return_tensors="pt",
- add_special_tokens=False).to(self.device) for q in questions[1:]] # the first question is handled in the prompt wrap function, skip it
- answers = [self.llama_tokenizer(q,
- return_tensors="pt",
- add_special_tokens=False).to(self.device) for q in answers]
- cur_id = []
- cur_target = []
- for i in range(len(questions)):
- cur_id.append(answers[i].input_ids)
- cur_target.append(answers[i].input_ids)
- cur_id.append(questions[i].input_ids)
- cur_target.append(torch.ones_like(questions[i].input_ids) * -100)
-
- cur_id.append(answers[-1].input_ids)
- cur_target.append(answers[-1].input_ids)
-
- cur_id = torch.cat(cur_id, dim=1)
- cur_target = torch.cat(cur_target, dim=1)
- to_regress_token_ids_list.append(cur_id)
- targets_list.append(cur_target)
-
- max_len = min(max([target.shape[1] for target in targets_list]), self.max_txt_len)
- to_regress_token_ids = torch.ones([batch_size, max_len],
- dtype=cur_id.dtype, device=self.device) * self.llama_tokenizer.pad_token_id
- targets = torch.ones([batch_size, max_len],
- dtype=cur_id.dtype, device=self.device) * -100
- for batch_idx in range(batch_size):
- cur_len = to_regress_token_ids_list[batch_idx].shape[1]
- to_regress_token_ids[batch_idx, :cur_len] = to_regress_token_ids_list[batch_idx][0, :max_len]
- targets[batch_idx, :cur_len] = targets_list[batch_idx][0, :max_len]
-
- to_regress_token_attn = (to_regress_token_ids != self.llama_tokenizer.pad_token_id).to(torch.int)
-
- return to_regress_token_ids, to_regress_token_attn, targets
-
- def preparing_embedding(self, samples):
- ### prepare input tokens
- if 'image' in samples:
- img_embeds, img_atts = self.encode_img(samples["image"])
- else:
- img_embeds = img_atts = None
-
- if 'conv_q' in samples:
- # handeling conversation datasets
- conv_q, conv_a = samples['conv_q'], samples['conv_a']
-
- connect_sym = samples['connect_sym'][0]
- conv_q = [q.split(connect_sym)for q in conv_q]
- conv_a = [a.split(connect_sym) for a in conv_a]
-
- conv_q = [[self.prompt_template.format(item) for item in items] for items in conv_q]
-
- cond_embeds, cond_atts = self.prompt_wrap(img_embeds, img_atts, [q[0] for q in conv_q])
- regress_token_ids, regress_atts, part_targets = self.tokenize_conversation(conv_q, conv_a)
-
- else:
- if "instruction_input" in samples:
- instruction = samples["instruction_input"]
- elif self.prompt_list:
- instruction = random.choice(self.prompt_list)
- else:
- instruction = None
-
- if self.chat_template:
- instruction = [self.prompt_template.format(instruct) for instruct in instruction]
-
- if 'length' in samples:
- # the input is a image train (like videos)
- bsz, pn, hs = img_embeds.shape
- img_embeds = img_embeds.reshape(len(samples['image']), -1, pn, hs)
- cond_embeds, cond_atts = self.prompt_wrap(img_embeds, img_atts, instruction, samples['length'])
- else:
- cond_embeds, cond_atts = self.prompt_wrap(img_embeds, img_atts, instruction)
-
- ### prepare target tokens
- self.llama_tokenizer.padding_side = "right"
- text = [t + self.end_sym for t in samples["answer"]]
-
- regress_tokens = self.llama_tokenizer(
- text,
- return_tensors="pt",
- padding="longest",
- truncation=True,
- max_length=self.max_txt_len,
- add_special_tokens=False
- ).to(self.device)
-
- regress_token_ids = regress_tokens.input_ids
- regress_atts = regress_tokens.attention_mask
- part_targets = regress_token_ids.masked_fill(
- regress_token_ids == self.llama_tokenizer.pad_token_id, -100
- )
-
- regress_embeds = self.embed_tokens(regress_token_ids)
-
- return cond_embeds, cond_atts, regress_embeds, regress_atts, part_targets
-
- def forward(self, samples, reduction='mean'):
- # prepare the embedding to condition and the embedding to regress
- cond_embeds, cond_atts, regress_embeds, regress_atts, part_targets = \
- self.preparing_embedding(samples)
-
- # concat the embedding to condition and the embedding to regress
- inputs_embeds, attention_mask, input_lens = \
- self.concat_emb_input_output(cond_embeds, cond_atts, regress_embeds, regress_atts)
-
- # get bos token embedding
- bos = torch.ones_like(part_targets[:, :1]) * self.llama_tokenizer.bos_token_id
- bos_embeds = self.embed_tokens(bos)
- bos_atts = cond_atts[:, :1]
-
- # add bos token at the begining
- inputs_embeds = torch.cat([bos_embeds, inputs_embeds], dim=1)
- attention_mask = torch.cat([bos_atts, attention_mask], dim=1)
-
- # ensemble the final targets
- targets = torch.ones([inputs_embeds.shape[0], inputs_embeds.shape[1]],
- dtype=torch.long).to(self.device).fill_(-100)
-
- for i, target in enumerate(part_targets):
- targets[i, input_lens[i]+1:input_lens[i]+len(target)+1] = target # plus 1 for bos
-
- with self.maybe_autocast():
- outputs = self.llama_model(
- inputs_embeds=inputs_embeds,
- attention_mask=attention_mask,
- return_dict=True,
- labels=targets,
- reduction=reduction
- )
- loss = outputs.loss
-
- return {"loss": loss}
-
- def embed_tokens(self, token_ids):
- if hasattr(self.llama_model.base_model, 'model'): ## lora wrapped model
- embeds = self.llama_model.base_model.model.model.embed_tokens(token_ids)
- else:
- embeds = self.llama_model.base_model.embed_tokens(token_ids)
- return embeds
-
-
- @torch.no_grad()
- def generate(
- self,
- images,
- texts,
- num_beams=1,
- max_new_tokens=20,
- min_length=1,
- top_p=0.9,
- repetition_penalty=1,
- length_penalty=1,
- temperature=1,
- do_sample=False,
- stop_words_ids=[2],
- ):
- '''
- function for generate test use
- '''
-
- stopping_criteria = StoppingCriteriaList([StoppingCriteriaSub(
- stops=[torch.tensor([i]).to(self.device) for i in stop_words_ids])])
-
- img_embeds, atts_img = self.encode_img(images.to(self.device))
- image_lists = [[image_emb[None]] for image_emb in img_embeds]
-
- batch_embs = [self.get_context_emb(text, img_list) for text, img_list in zip(texts, image_lists)]
-
- batch_size = len(batch_embs)
- max_len = max([emb.shape[1] for emb in batch_embs])
- emb_dim = batch_embs[0].shape[2]
- dtype = batch_embs[0].dtype
- device = batch_embs[0].device
-
- embs = torch.zeros([batch_size, max_len, emb_dim], dtype=dtype, device=device)
- attn_mask = torch.zeros([batch_size, max_len], dtype=torch.int, device=device)
- for i, emb in enumerate(batch_embs):
- emb_len = emb.shape[1]
- embs[i, -emb_len:] = emb[0]
- attn_mask[i, -emb_len:] = 1
-
- with self.maybe_autocast():
- outputs = self.llama_model.generate(
- inputs_embeds=embs,
- attention_mask=attn_mask,
- max_new_tokens=max_new_tokens,
- num_beams=num_beams,
- length_penalty=length_penalty,
- temperature=temperature,
- do_sample=do_sample,
- min_length=min_length,
- top_p=top_p,
- repetition_penalty=repetition_penalty,
- stopping_criteria=stopping_criteria,
- )
-
- answers = []
- for output_token in outputs:
- if output_token[0] == 0:
- output_token = output_token[1:]
- output_texts = self.llama_tokenizer.decode(output_token, skip_special_tokens=True)
- output_texts = output_texts.split('')[0] # remove the stop sign
- output_texts = output_texts.replace("", "")
- output_texts = output_texts.split(r'[/INST]')[-1].strip()
- answers.append(output_texts)
-
- return answers
-
- @torch.no_grad()
- def multi_select(self, images, texts, answers, num_cand=None):
- all_losses = []
- for answer in answers:
- choice_samples = {
- 'image': images,
- 'instruction_input': texts,
- 'answer': answer
- }
- loss = self.forward(choice_samples, reduction='none')['loss'].reshape(-1, 1)
- all_losses.append(loss)
- torch.cuda.empty_cache()
- all_losses = torch.cat(all_losses, dim=-1)
- if num_cand is not None:
- for i in range(all_losses.shape[0]):
- all_losses[i, num_cand[i]:] = 9999
- output_class_ranks = torch.argsort(all_losses, dim=-1)
- return output_class_ranks.tolist()
\ No newline at end of file
diff --git a/spaces/Voicemod/Text-to-Sing/README.md b/spaces/Voicemod/Text-to-Sing/README.md
deleted file mode 100644
index dd0f48b50eefbef8dd9c8cd7853d5966bf15d2fa..0000000000000000000000000000000000000000
--- a/spaces/Voicemod/Text-to-Sing/README.md
+++ /dev/null
@@ -1,12 +0,0 @@
----
-title: Voicemod's Text To Sing
-emoji: 🎤
-colorFrom: black
-colorTo: cyan
-sdk: gradio
-sdk_version: 3.41.2
-app_file: app.py
-pinned: true
----
-
-Check out the configuration reference at https://huggingface.co/docs/hub/spaces-config-reference
diff --git a/spaces/WangJexi/panel_trial/app.py b/spaces/WangJexi/panel_trial/app.py
deleted file mode 100644
index 64367a4f11361a869d6fdab05b5e55a587dee182..0000000000000000000000000000000000000000
--- a/spaces/WangJexi/panel_trial/app.py
+++ /dev/null
@@ -1,78 +0,0 @@
-import panel as pn
-pn.extension()
-
-# Define the layout
-input_box1 = pn.widgets.FloatInput(name='Input 1', value=0)
-input_box2 = pn.widgets.FloatInput(name='Input 2', value=0)
-output_box = pn.widgets.StaticText(name='Result', value='')
-
-def perform_add_operation(event):
- operation = event.name
- num1 = input_box1.value
- num2 = input_box2.value
- result = num1 + num2
- output_box.value = str(result)
-
-def perform_subtract_operation(event):
- operation = event.name
- num1 = input_box1.value
- num2 = input_box2.value
- result = num1 - num2
- output_box.value = str(result)
-
-def perform_multiply_operation(event):
- operation = event.name
- num1 = input_box1.value
- num2 = input_box2.value
- result = num1 * num2
- output_box.value = str(result)
-
-def perform_divide_operation(event):
- operation = event.name
- num1 = input_box1.value
- num2 = input_box2.value
- if num2 != 0:
- result = num1 / num2
- else:
- result = 'Cannot divide by zero'
-
- output_box.value = str(result)
-
-add_button = pn.widgets.Button(name='Add', button_type='primary')
-add_button.on_click(perform_add_operation)
-
-subtract_button = pn.widgets.Button(name='Subtract', button_type='primary')
-subtract_button.on_click(perform_subtract_operation)
-
-multiply_button = pn.widgets.Button(name='Multiply', button_type='primary')
-multiply_button.on_click(perform_multiply_operation)
-
-divide_button = pn.widgets.Button(name='Divide', button_type='primary')
-divide_button.on_click(perform_divide_operation)
-
-
-# Inner layout to contain sll
-inner_layout = pn.Row(
- pn.Spacer(width=10),
- pn.Column(
- add_button, subtract_button, multiply_button, divide_button,
- css_classes=['operation-buttons']
- ),
- pn.Spacer(width=20),
- pn.Column(input_box1, input_box2, css_classes=['input-boxes']),
- pn.Spacer(width=20),
- pn.Column(output_box, css_classes=['output-box']),
- pn.Spacer(width=10)
-)
-
-# Using a container to apply CSS
-layout = pn.Column(
- inner_layout,
- css_classes=['outer-layout']
-)
-
-# CSS for the layout
-pn.config.raw_css.append('.input-boxes, .operation-buttons, .output-box, .outer-layout { border: 1px solid black; padding: 10px; }')
-
-# Show the layout
-layout.servable()
diff --git a/spaces/Willow123/InternLM-XComposer/templates/index.html b/spaces/Willow123/InternLM-XComposer/templates/index.html
deleted file mode 100644
index 8ec51d5bb2e5a53240ed1aaefdd316b346a6d497..0000000000000000000000000000000000000000
--- a/spaces/Willow123/InternLM-XComposer/templates/index.html
+++ /dev/null
@@ -1,33 +0,0 @@
-
-
-
-
-
-
- My static Space
-
-
-
-
-
-
-
-
-
-
\ No newline at end of file
diff --git a/spaces/YUANAI/DiffspeechResearch/data_gen/tts/txt_processors/base_text_processor.py b/spaces/YUANAI/DiffspeechResearch/data_gen/tts/txt_processors/base_text_processor.py
deleted file mode 100644
index 96877a830fe04eadabaa2954b1a0164700d4857a..0000000000000000000000000000000000000000
--- a/spaces/YUANAI/DiffspeechResearch/data_gen/tts/txt_processors/base_text_processor.py
+++ /dev/null
@@ -1,48 +0,0 @@
-from utils.text.text_encoder import is_sil_phoneme
-
-REGISTERED_TEXT_PROCESSORS = {}
-
-
-def register_txt_processors(name):
- def _f(cls):
- REGISTERED_TEXT_PROCESSORS[name] = cls
- return cls
-
- return _f
-
-
-def get_txt_processor_cls(name):
- return REGISTERED_TEXT_PROCESSORS.get(name, None)
-
-
-class BaseTxtProcessor:
- @staticmethod
- def sp_phonemes():
- return ['|']
-
- @classmethod
- def process(cls, txt, preprocess_args):
- raise NotImplementedError
-
- @classmethod
- def postprocess(cls, txt_struct, preprocess_args):
- # remove sil phoneme in head and tail
- while len(txt_struct) > 0 and is_sil_phoneme(txt_struct[0][0]):
- txt_struct = txt_struct[1:]
- while len(txt_struct) > 0 and is_sil_phoneme(txt_struct[-1][0]):
- txt_struct = txt_struct[:-1]
- if preprocess_args['with_phsep']:
- txt_struct = cls.add_bdr(txt_struct)
- if preprocess_args['add_eos_bos']:
- txt_struct = [["", [""]]] + txt_struct + [["", [""]]]
- return txt_struct
-
- @classmethod
- def add_bdr(cls, txt_struct):
- txt_struct_ = []
- for i, ts in enumerate(txt_struct):
- txt_struct_.append(ts)
- if i != len(txt_struct) - 1 and \
- not is_sil_phoneme(txt_struct[i][0]) and not is_sil_phoneme(txt_struct[i + 1][0]):
- txt_struct_.append(['|', ['|']])
- return txt_struct_
diff --git a/spaces/YazawaSunrise/so-vits-svc-LoveLive/models.py b/spaces/YazawaSunrise/so-vits-svc-LoveLive/models.py
deleted file mode 100644
index bdbce8445304abda792f235a4761b831fd6f4d12..0000000000000000000000000000000000000000
--- a/spaces/YazawaSunrise/so-vits-svc-LoveLive/models.py
+++ /dev/null
@@ -1,351 +0,0 @@
-import copy
-import math
-import torch
-from torch import nn
-from torch.nn import functional as F
-
-import attentions
-import commons
-import modules
-
-from torch.nn import Conv1d, ConvTranspose1d, AvgPool1d, Conv2d
-from torch.nn.utils import weight_norm, remove_weight_norm, spectral_norm
-from commons import init_weights, get_padding
-from vdecoder.hifigan.models import Generator
-from utils import f0_to_coarse
-
-class ResidualCouplingBlock(nn.Module):
- def __init__(self,
- channels,
- hidden_channels,
- kernel_size,
- dilation_rate,
- n_layers,
- n_flows=4,
- gin_channels=0):
- super().__init__()
- self.channels = channels
- self.hidden_channels = hidden_channels
- self.kernel_size = kernel_size
- self.dilation_rate = dilation_rate
- self.n_layers = n_layers
- self.n_flows = n_flows
- self.gin_channels = gin_channels
-
- self.flows = nn.ModuleList()
- for i in range(n_flows):
- self.flows.append(modules.ResidualCouplingLayer(channels, hidden_channels, kernel_size, dilation_rate, n_layers, gin_channels=gin_channels, mean_only=True))
- self.flows.append(modules.Flip())
-
- def forward(self, x, x_mask, g=None, reverse=False):
- if not reverse:
- for flow in self.flows:
- x, _ = flow(x, x_mask, g=g, reverse=reverse)
- else:
- for flow in reversed(self.flows):
- x = flow(x, x_mask, g=g, reverse=reverse)
- return x
-
-
-class Encoder(nn.Module):
- def __init__(self,
- in_channels,
- out_channels,
- hidden_channels,
- kernel_size,
- dilation_rate,
- n_layers,
- gin_channels=0):
- super().__init__()
- self.in_channels = in_channels
- self.out_channels = out_channels
- self.hidden_channels = hidden_channels
- self.kernel_size = kernel_size
- self.dilation_rate = dilation_rate
- self.n_layers = n_layers
- self.gin_channels = gin_channels
-
- self.pre = nn.Conv1d(in_channels, hidden_channels, 1)
- self.enc = modules.WN(hidden_channels, kernel_size, dilation_rate, n_layers, gin_channels=gin_channels)
- self.proj = nn.Conv1d(hidden_channels, out_channels * 2, 1)
-
- def forward(self, x, x_lengths, g=None):
- # print(x.shape,x_lengths.shape)
- x_mask = torch.unsqueeze(commons.sequence_mask(x_lengths, x.size(2)), 1).to(x.dtype)
- x = self.pre(x) * x_mask
- x = self.enc(x, x_mask, g=g)
- stats = self.proj(x) * x_mask
- m, logs = torch.split(stats, self.out_channels, dim=1)
- z = (m + torch.randn_like(m) * torch.exp(logs)) * x_mask
- return z, m, logs, x_mask
-
-
-class TextEncoder(nn.Module):
- def __init__(self,
- in_channels,
- out_channels,
- hidden_channels,
- kernel_size,
- dilation_rate,
- n_layers,
- gin_channels=0,
- filter_channels=None,
- n_heads=None,
- p_dropout=None):
- super().__init__()
- self.in_channels = in_channels
- self.out_channels = out_channels
- self.hidden_channels = hidden_channels
- self.kernel_size = kernel_size
- self.dilation_rate = dilation_rate
- self.n_layers = n_layers
- self.gin_channels = gin_channels
- self.pre = nn.Conv1d(in_channels, hidden_channels, 1)
- self.proj = nn.Conv1d(hidden_channels, out_channels * 2, 1)
- self.f0_emb = nn.Embedding(256, hidden_channels)
-
- self.enc_ = attentions.Encoder(
- hidden_channels,
- filter_channels,
- n_heads,
- n_layers,
- kernel_size,
- p_dropout)
-
- def forward(self, x, x_lengths, f0=None):
- x_mask = torch.unsqueeze(commons.sequence_mask(x_lengths, x.size(2)), 1).to(x.dtype)
- x = self.pre(x) * x_mask
- x = x + self.f0_emb(f0).transpose(1,2)
- x = self.enc_(x * x_mask, x_mask)
- stats = self.proj(x) * x_mask
- m, logs = torch.split(stats, self.out_channels, dim=1)
- z = (m + torch.randn_like(m) * torch.exp(logs)) * x_mask
-
- return z, m, logs, x_mask
-
-
-
-class DiscriminatorP(torch.nn.Module):
- def __init__(self, period, kernel_size=5, stride=3, use_spectral_norm=False):
- super(DiscriminatorP, self).__init__()
- self.period = period
- self.use_spectral_norm = use_spectral_norm
- norm_f = weight_norm if use_spectral_norm == False else spectral_norm
- self.convs = nn.ModuleList([
- norm_f(Conv2d(1, 32, (kernel_size, 1), (stride, 1), padding=(get_padding(kernel_size, 1), 0))),
- norm_f(Conv2d(32, 128, (kernel_size, 1), (stride, 1), padding=(get_padding(kernel_size, 1), 0))),
- norm_f(Conv2d(128, 512, (kernel_size, 1), (stride, 1), padding=(get_padding(kernel_size, 1), 0))),
- norm_f(Conv2d(512, 1024, (kernel_size, 1), (stride, 1), padding=(get_padding(kernel_size, 1), 0))),
- norm_f(Conv2d(1024, 1024, (kernel_size, 1), 1, padding=(get_padding(kernel_size, 1), 0))),
- ])
- self.conv_post = norm_f(Conv2d(1024, 1, (3, 1), 1, padding=(1, 0)))
-
- def forward(self, x):
- fmap = []
-
- # 1d to 2d
- b, c, t = x.shape
- if t % self.period != 0: # pad first
- n_pad = self.period - (t % self.period)
- x = F.pad(x, (0, n_pad), "reflect")
- t = t + n_pad
- x = x.view(b, c, t // self.period, self.period)
-
- for l in self.convs:
- x = l(x)
- x = F.leaky_relu(x, modules.LRELU_SLOPE)
- fmap.append(x)
- x = self.conv_post(x)
- fmap.append(x)
- x = torch.flatten(x, 1, -1)
-
- return x, fmap
-
-
-class DiscriminatorS(torch.nn.Module):
- def __init__(self, use_spectral_norm=False):
- super(DiscriminatorS, self).__init__()
- norm_f = weight_norm if use_spectral_norm == False else spectral_norm
- self.convs = nn.ModuleList([
- norm_f(Conv1d(1, 16, 15, 1, padding=7)),
- norm_f(Conv1d(16, 64, 41, 4, groups=4, padding=20)),
- norm_f(Conv1d(64, 256, 41, 4, groups=16, padding=20)),
- norm_f(Conv1d(256, 1024, 41, 4, groups=64, padding=20)),
- norm_f(Conv1d(1024, 1024, 41, 4, groups=256, padding=20)),
- norm_f(Conv1d(1024, 1024, 5, 1, padding=2)),
- ])
- self.conv_post = norm_f(Conv1d(1024, 1, 3, 1, padding=1))
-
- def forward(self, x):
- fmap = []
-
- for l in self.convs:
- x = l(x)
- x = F.leaky_relu(x, modules.LRELU_SLOPE)
- fmap.append(x)
- x = self.conv_post(x)
- fmap.append(x)
- x = torch.flatten(x, 1, -1)
-
- return x, fmap
-
-
-class MultiPeriodDiscriminator(torch.nn.Module):
- def __init__(self, use_spectral_norm=False):
- super(MultiPeriodDiscriminator, self).__init__()
- periods = [2,3,5,7,11]
-
- discs = [DiscriminatorS(use_spectral_norm=use_spectral_norm)]
- discs = discs + [DiscriminatorP(i, use_spectral_norm=use_spectral_norm) for i in periods]
- self.discriminators = nn.ModuleList(discs)
-
- def forward(self, y, y_hat):
- y_d_rs = []
- y_d_gs = []
- fmap_rs = []
- fmap_gs = []
- for i, d in enumerate(self.discriminators):
- y_d_r, fmap_r = d(y)
- y_d_g, fmap_g = d(y_hat)
- y_d_rs.append(y_d_r)
- y_d_gs.append(y_d_g)
- fmap_rs.append(fmap_r)
- fmap_gs.append(fmap_g)
-
- return y_d_rs, y_d_gs, fmap_rs, fmap_gs
-
-
-class SpeakerEncoder(torch.nn.Module):
- def __init__(self, mel_n_channels=80, model_num_layers=3, model_hidden_size=256, model_embedding_size=256):
- super(SpeakerEncoder, self).__init__()
- self.lstm = nn.LSTM(mel_n_channels, model_hidden_size, model_num_layers, batch_first=True)
- self.linear = nn.Linear(model_hidden_size, model_embedding_size)
- self.relu = nn.ReLU()
-
- def forward(self, mels):
- self.lstm.flatten_parameters()
- _, (hidden, _) = self.lstm(mels)
- embeds_raw = self.relu(self.linear(hidden[-1]))
- return embeds_raw / torch.norm(embeds_raw, dim=1, keepdim=True)
-
- def compute_partial_slices(self, total_frames, partial_frames, partial_hop):
- mel_slices = []
- for i in range(0, total_frames-partial_frames, partial_hop):
- mel_range = torch.arange(i, i+partial_frames)
- mel_slices.append(mel_range)
-
- return mel_slices
-
- def embed_utterance(self, mel, partial_frames=128, partial_hop=64):
- mel_len = mel.size(1)
- last_mel = mel[:,-partial_frames:]
-
- if mel_len > partial_frames:
- mel_slices = self.compute_partial_slices(mel_len, partial_frames, partial_hop)
- mels = list(mel[:,s] for s in mel_slices)
- mels.append(last_mel)
- mels = torch.stack(tuple(mels), 0).squeeze(1)
-
- with torch.no_grad():
- partial_embeds = self(mels)
- embed = torch.mean(partial_embeds, axis=0).unsqueeze(0)
- #embed = embed / torch.linalg.norm(embed, 2)
- else:
- with torch.no_grad():
- embed = self(last_mel)
-
- return embed
-
-
-class SynthesizerTrn(nn.Module):
- """
- Synthesizer for Training
- """
-
- def __init__(self,
- spec_channels,
- segment_size,
- inter_channels,
- hidden_channels,
- filter_channels,
- n_heads,
- n_layers,
- kernel_size,
- p_dropout,
- resblock,
- resblock_kernel_sizes,
- resblock_dilation_sizes,
- upsample_rates,
- upsample_initial_channel,
- upsample_kernel_sizes,
- gin_channels,
- ssl_dim,
- n_speakers,
- **kwargs):
-
- super().__init__()
- self.spec_channels = spec_channels
- self.inter_channels = inter_channels
- self.hidden_channels = hidden_channels
- self.filter_channels = filter_channels
- self.n_heads = n_heads
- self.n_layers = n_layers
- self.kernel_size = kernel_size
- self.p_dropout = p_dropout
- self.resblock = resblock
- self.resblock_kernel_sizes = resblock_kernel_sizes
- self.resblock_dilation_sizes = resblock_dilation_sizes
- self.upsample_rates = upsample_rates
- self.upsample_initial_channel = upsample_initial_channel
- self.upsample_kernel_sizes = upsample_kernel_sizes
- self.segment_size = segment_size
- self.gin_channels = gin_channels
- self.ssl_dim = ssl_dim
- self.emb_g = nn.Embedding(n_speakers, gin_channels)
-
- self.enc_p_ = TextEncoder(ssl_dim, inter_channels, hidden_channels, 5, 1, 16,0, filter_channels, n_heads, p_dropout)
- hps = {
- "sampling_rate": 32000,
- "inter_channels": 192,
- "resblock": "1",
- "resblock_kernel_sizes": [3, 7, 11],
- "resblock_dilation_sizes": [[1, 3, 5], [1, 3, 5], [1, 3, 5]],
- "upsample_rates": [10, 8, 2, 2],
- "upsample_initial_channel": 512,
- "upsample_kernel_sizes": [16, 16, 4, 4],
- "gin_channels": 256,
- }
- self.dec = Generator(h=hps)
- self.enc_q = Encoder(spec_channels, inter_channels, hidden_channels, 5, 1, 16, gin_channels=gin_channels)
- self.flow = ResidualCouplingBlock(inter_channels, hidden_channels, 5, 1, 4, gin_channels=gin_channels)
-
- def forward(self, c, f0, spec, g=None, mel=None, c_lengths=None, spec_lengths=None):
- if c_lengths == None:
- c_lengths = (torch.ones(c.size(0)) * c.size(-1)).to(c.device)
- if spec_lengths == None:
- spec_lengths = (torch.ones(spec.size(0)) * spec.size(-1)).to(spec.device)
-
- g = self.emb_g(g).transpose(1,2)
-
- z_ptemp, m_p, logs_p, _ = self.enc_p_(c, c_lengths, f0=f0_to_coarse(f0))
- z, m_q, logs_q, spec_mask = self.enc_q(spec, spec_lengths, g=g)
-
- z_p = self.flow(z, spec_mask, g=g)
- z_slice, pitch_slice, ids_slice = commons.rand_slice_segments_with_pitch(z, f0, spec_lengths, self.segment_size)
-
- # o = self.dec(z_slice, g=g)
- o = self.dec(z_slice, g=g, f0=pitch_slice)
-
- return o, ids_slice, spec_mask, (z, z_p, m_p, logs_p, m_q, logs_q)
-
- def infer(self, c, f0, g=None, mel=None, c_lengths=None):
- if c_lengths == None:
- c_lengths = (torch.ones(c.size(0)) * c.size(-1)).to(c.device)
- g = self.emb_g(g).transpose(1,2)
-
- z_p, m_p, logs_p, c_mask = self.enc_p_(c, c_lengths, f0=f0_to_coarse(f0))
- z = self.flow(z_p, c_mask, g=g, reverse=True)
-
- o = self.dec(z * c_mask, g=g, f0=f0)
-
- return o
diff --git a/spaces/Yuliang/ECON/lib/torch_utils/ops/bias_act.py b/spaces/Yuliang/ECON/lib/torch_utils/ops/bias_act.py
deleted file mode 100644
index 81d07ac029a2c36c12bcc6caff59a73476bdaf1e..0000000000000000000000000000000000000000
--- a/spaces/Yuliang/ECON/lib/torch_utils/ops/bias_act.py
+++ /dev/null
@@ -1,297 +0,0 @@
-# Copyright (c) 2021, NVIDIA CORPORATION. All rights reserved.
-#
-# NVIDIA CORPORATION and its licensors retain all intellectual property
-# and proprietary rights in and to this software, related documentation
-# and any modifications thereto. Any use, reproduction, disclosure or
-# distribution of this software and related documentation without an express
-# license agreement from NVIDIA CORPORATION is strictly prohibited.
-"""Custom PyTorch ops for efficient bias and activation."""
-
-import os
-import traceback
-import warnings
-
-import dnnlib
-import numpy as np
-import torch
-
-from .. import custom_ops, misc
-
-#----------------------------------------------------------------------------
-
-activation_funcs = {
- 'linear':
- dnnlib.EasyDict(
- func=lambda x, **_: x, def_alpha=0, def_gain=1, cuda_idx=1, ref='', has_2nd_grad=False
- ),
- 'relu':
- dnnlib.EasyDict(
- func=lambda x, **_: torch.nn.functional.relu(x),
- def_alpha=0,
- def_gain=np.sqrt(2),
- cuda_idx=2,
- ref='y',
- has_2nd_grad=False
- ),
- 'lrelu':
- dnnlib.EasyDict(
- func=lambda x, alpha, **_: torch.nn.functional.leaky_relu(x, alpha),
- def_alpha=0.2,
- def_gain=np.sqrt(2),
- cuda_idx=3,
- ref='y',
- has_2nd_grad=False
- ),
- 'tanh':
- dnnlib.EasyDict(
- func=lambda x, **_: torch.tanh(x),
- def_alpha=0,
- def_gain=1,
- cuda_idx=4,
- ref='y',
- has_2nd_grad=True
- ),
- 'sigmoid':
- dnnlib.EasyDict(
- func=lambda x, **_: torch.sigmoid(x),
- def_alpha=0,
- def_gain=1,
- cuda_idx=5,
- ref='y',
- has_2nd_grad=True
- ),
- 'elu':
- dnnlib.EasyDict(
- func=lambda x, **_: torch.nn.functional.elu(x),
- def_alpha=0,
- def_gain=1,
- cuda_idx=6,
- ref='y',
- has_2nd_grad=True
- ),
- 'selu':
- dnnlib.EasyDict(
- func=lambda x, **_: torch.nn.functional.selu(x),
- def_alpha=0,
- def_gain=1,
- cuda_idx=7,
- ref='y',
- has_2nd_grad=True
- ),
- 'softplus':
- dnnlib.EasyDict(
- func=lambda x, **_: torch.nn.functional.softplus(x),
- def_alpha=0,
- def_gain=1,
- cuda_idx=8,
- ref='y',
- has_2nd_grad=True
- ),
- 'swish':
- dnnlib.EasyDict(
- func=lambda x, **_: torch.sigmoid(x) * x,
- def_alpha=0,
- def_gain=np.sqrt(2),
- cuda_idx=9,
- ref='x',
- has_2nd_grad=True
- ),
-}
-
-#----------------------------------------------------------------------------
-
-_inited = False
-_plugin = None
-_null_tensor = torch.empty([0])
-
-
-def _init():
- global _inited, _plugin
- if not _inited:
- _inited = True
- sources = ['bias_act.cpp', 'bias_act.cu']
- sources = [os.path.join(os.path.dirname(__file__), s) for s in sources]
- try:
- _plugin = custom_ops.get_plugin(
- 'bias_act_plugin', sources=sources, extra_cuda_cflags=['--use_fast_math']
- )
- except:
- warnings.warn(
- 'Failed to build CUDA kernels for bias_act. Falling back to slow reference implementation. Details:\n\n'
- + traceback.format_exc()
- )
- return _plugin is not None
-
-
-#----------------------------------------------------------------------------
-
-
-def bias_act(x, b=None, dim=1, act='linear', alpha=None, gain=None, clamp=None, impl='cuda'):
- r"""Fused bias and activation function.
-
- Adds bias `b` to activation tensor `x`, evaluates activation function `act`,
- and scales the result by `gain`. Each of the steps is optional. In most cases,
- the fused op is considerably more efficient than performing the same calculation
- using standard PyTorch ops. It supports first and second order gradients,
- but not third order gradients.
-
- Args:
- x: Input activation tensor. Can be of any shape.
- b: Bias vector, or `None` to disable. Must be a 1D tensor of the same type
- as `x`. The shape must be known, and it must match the dimension of `x`
- corresponding to `dim`.
- dim: The dimension in `x` corresponding to the elements of `b`.
- The value of `dim` is ignored if `b` is not specified.
- act: Name of the activation function to evaluate, or `"linear"` to disable.
- Can be e.g. `"relu"`, `"lrelu"`, `"tanh"`, `"sigmoid"`, `"swish"`, etc.
- See `activation_funcs` for a full list. `None` is not allowed.
- alpha: Shape parameter for the activation function, or `None` to use the default.
- gain: Scaling factor for the output tensor, or `None` to use default.
- See `activation_funcs` for the default scaling of each activation function.
- If unsure, consider specifying 1.
- clamp: Clamp the output values to `[-clamp, +clamp]`, or `None` to disable
- the clamping (default).
- impl: Name of the implementation to use. Can be `"ref"` or `"cuda"` (default).
-
- Returns:
- Tensor of the same shape and datatype as `x`.
- """
- assert isinstance(x, torch.Tensor)
- assert impl in ['ref', 'cuda']
- if impl == 'cuda' and x.device.type == 'cuda' and _init():
- return _bias_act_cuda(dim=dim, act=act, alpha=alpha, gain=gain, clamp=clamp).apply(x, b)
- return _bias_act_ref(x=x, b=b, dim=dim, act=act, alpha=alpha, gain=gain, clamp=clamp)
-
-
-#----------------------------------------------------------------------------
-
-
-@misc.profiled_function
-def _bias_act_ref(x, b=None, dim=1, act='linear', alpha=None, gain=None, clamp=None):
- """Slow reference implementation of `bias_act()` using standard TensorFlow ops.
- """
- assert isinstance(x, torch.Tensor)
- assert clamp is None or clamp >= 0
- spec = activation_funcs[act]
- alpha = float(alpha if alpha is not None else spec.def_alpha)
- gain = float(gain if gain is not None else spec.def_gain)
- clamp = float(clamp if clamp is not None else -1)
-
- # Add bias.
- if b is not None:
- assert isinstance(b, torch.Tensor) and b.ndim == 1
- assert 0 <= dim < x.ndim
- assert b.shape[0] == x.shape[dim]
- x = x + b.reshape([-1 if i == dim else 1 for i in range(x.ndim)])
-
- # Evaluate activation function.
- alpha = float(alpha)
- x = spec.func(x, alpha=alpha)
-
- # Scale by gain.
- gain = float(gain)
- if gain != 1:
- x = x * gain
-
- # Clamp.
- if clamp >= 0:
- x = x.clamp(-clamp, clamp) # pylint: disable=invalid-unary-operand-type
- return x
-
-
-#----------------------------------------------------------------------------
-
-_bias_act_cuda_cache = dict()
-
-
-def _bias_act_cuda(dim=1, act='linear', alpha=None, gain=None, clamp=None):
- """Fast CUDA implementation of `bias_act()` using custom ops.
- """
- # Parse arguments.
- assert clamp is None or clamp >= 0
- spec = activation_funcs[act]
- alpha = float(alpha if alpha is not None else spec.def_alpha)
- gain = float(gain if gain is not None else spec.def_gain)
- clamp = float(clamp if clamp is not None else -1)
-
- # Lookup from cache.
- key = (dim, act, alpha, gain, clamp)
- if key in _bias_act_cuda_cache:
- return _bias_act_cuda_cache[key]
-
- # Forward op.
- class BiasActCuda(torch.autograd.Function):
- @staticmethod
- def forward(ctx, x, b): # pylint: disable=arguments-differ
- ctx.memory_format = torch.channels_last if x.ndim > 2 and x.stride(
- )[1] == 1 else torch.contiguous_format
- x = x.contiguous(memory_format=ctx.memory_format)
- b = b.contiguous() if b is not None else _null_tensor
- y = x
- if act != 'linear' or gain != 1 or clamp >= 0 or b is not _null_tensor:
- y = _plugin.bias_act(
- x, b, _null_tensor, _null_tensor, _null_tensor, 0, dim, spec.cuda_idx, alpha,
- gain, clamp
- )
- ctx.save_for_backward(
- x if 'x' in spec.ref or spec.has_2nd_grad else _null_tensor,
- b if 'x' in spec.ref or spec.has_2nd_grad else _null_tensor,
- y if 'y' in spec.ref else _null_tensor
- )
- return y
-
- @staticmethod
- def backward(ctx, dy): # pylint: disable=arguments-differ
- dy = dy.contiguous(memory_format=ctx.memory_format)
- x, b, y = ctx.saved_tensors
- dx = None
- db = None
-
- if ctx.needs_input_grad[0] or ctx.needs_input_grad[1]:
- dx = dy
- if act != 'linear' or gain != 1 or clamp >= 0:
- dx = BiasActCudaGrad.apply(dy, x, b, y)
-
- if ctx.needs_input_grad[1]:
- db = dx.sum([i for i in range(dx.ndim) if i != dim])
-
- return dx, db
-
- # Backward op.
- class BiasActCudaGrad(torch.autograd.Function):
- @staticmethod
- def forward(ctx, dy, x, b, y): # pylint: disable=arguments-differ
- ctx.memory_format = torch.channels_last if dy.ndim > 2 and dy.stride(
- )[1] == 1 else torch.contiguous_format
- dx = _plugin.bias_act(
- dy, b, x, y, _null_tensor, 1, dim, spec.cuda_idx, alpha, gain, clamp
- )
- ctx.save_for_backward(dy if spec.has_2nd_grad else _null_tensor, x, b, y)
- return dx
-
- @staticmethod
- def backward(ctx, d_dx): # pylint: disable=arguments-differ
- d_dx = d_dx.contiguous(memory_format=ctx.memory_format)
- dy, x, b, y = ctx.saved_tensors
- d_dy = None
- d_x = None
- d_b = None
- d_y = None
-
- if ctx.needs_input_grad[0]:
- d_dy = BiasActCudaGrad.apply(d_dx, x, b, y)
-
- if spec.has_2nd_grad and (ctx.needs_input_grad[1] or ctx.needs_input_grad[2]):
- d_x = _plugin.bias_act(d_dx, b, x, y, dy, 2, dim, spec.cuda_idx, alpha, gain, clamp)
-
- if spec.has_2nd_grad and ctx.needs_input_grad[2]:
- d_b = d_x.sum([i for i in range(d_x.ndim) if i != dim])
-
- return d_dy, d_x, d_b, d_y
-
- # Add to cache.
- _bias_act_cuda_cache[key] = BiasActCuda
- return BiasActCuda
-
-
-#----------------------------------------------------------------------------
diff --git a/spaces/Yusin/ChatGPT-Speech/app.py b/spaces/Yusin/ChatGPT-Speech/app.py
deleted file mode 100644
index 0d1e29e622a5a285bed8088b42277e814a6a0ec0..0000000000000000000000000000000000000000
--- a/spaces/Yusin/ChatGPT-Speech/app.py
+++ /dev/null
@@ -1,105 +0,0 @@
-import os
-import json
-import openai
-import tempfile
-import gradio as gr
-import infer
-import config
-from neon_tts_plugin_coqui import CoquiTTS
-title = "Speech to ChatGPT to Speech"
-coquiTTS = CoquiTTS()
-
-LANGUAGES = list(CoquiTTS.langs.keys())
-LANGUAGES = LANGUAGES + ['cn', 'jp']
-default_lang = "en"
-whisper = gr.Interface.load(name="spaces/sanchit-gandhi/whisper-large-v2")
-api_key = os.environ.get('api_key')
-#if you have OpenAI API key as a string, enable the below
-openai.api_key = api_key
-
-pth_path = config.pth_path
-config_json = config.config_json
-net_g_ms, hps = infer.load_model(config_json, pth_path)
-
-
-# ChatGPT
-def chat_hf(audio, custom_token, language):
- try:
- whisper_text = translate(audio)
- if whisper_text == "ERROR: You have to either use the microphone or upload an audio file":
- gpt_response = "MISSING AUDIO: Record your voice by clicking the microphone button, do not forget to stop recording before sending your message ;)"
- else:
- gpt_response = openai_create(whisper_text)
-
- except:
- whisper_text = translate(audio)
- gpt_response = """Sorry, I'm quite busy right now, but please try again later :)"""
-
- # to voice
- print(language)
- if language in ['cn', 'jp']:
- text = gpt_response.strip().replace(' ', '').replace('\n', '').replace('\r', '')
- text = infer.clean_text(text)
- audio = infer.infer(text, net_g_ms, 0, "demo")
- voice_out = (hps.data.sampling_rate, audio)
- return whisper_text, gpt_response, voice_out
- else:
- with tempfile.NamedTemporaryFile(suffix=".wav", delete=False) as fp:
- coquiTTS.get_tts(gpt_response, fp, speaker = {"language" : language})
- return whisper_text, gpt_response, fp.name
-
-
-
-def translate(audio):
- print("""
- —
- Sending audio to Whisper ...
- —
- """)
-
- text_result = whisper(audio, None, "transcribe", fn_index=0)
- print(text_result)
- return text_result
-
-
-def openai_create(prompt):
- print("""
- —
- Giving response from ai ...
- —
- """)
- response = openai.Completion.create(
- model="text-davinci-003",
- prompt=prompt,
- temperature=0.9,
- max_tokens=150,
- top_p=1,
- frequency_penalty=0,
- presence_penalty=0.6,
- stop=[" Human:", " AI:"]
- )
- print(response.choices[0].text)
- return response.choices[0].text
-
-
-with gr.Blocks() as blocks:
- gr.Markdown("
" + title + "
")
- radio = gr.Radio(label="Language", choices=LANGUAGES, value=default_lang)
- with gr.Row(equal_height=True):# equal_height=False
- with gr.Column():# variant="panel"
- audio_file = gr.Audio(source="microphone", type="filepath")
- custom_token = gr.Textbox(label='If it fails, use your own session token', placeholder="your own session token")
- with gr.Row():# mobile_collapse=False
- submit = gr.Button("Submit", variant="primary")
- with gr.Column():
- text1 = gr.Textbox(label="Speech to Text")
- text2 = gr.Textbox(label="ChatGPT Response")
- audio = gr.Audio(label="Output", interactive=False)
- # actions
- submit.click(
- chat_hf,
- [audio_file, custom_token, radio],
- [text1, text2, audio],
- )
-
-blocks.launch(debug=True)
diff --git a/spaces/ZachNagengast/vid2grid/README.md b/spaces/ZachNagengast/vid2grid/README.md
deleted file mode 100644
index 666cc59c6015577f2211c71d0649bf119b19678b..0000000000000000000000000000000000000000
--- a/spaces/ZachNagengast/vid2grid/README.md
+++ /dev/null
@@ -1,12 +0,0 @@
----
-title: vid2grid
-emoji: 🎞️
-colorFrom: gray
-colorTo: purple
-sdk: gradio
-sdk_version: 3.47.1
-app_file: app.py
-pinned: false
----
-
-Check out the configuration reference at https://huggingface.co/docs/hub/spaces-config-reference
diff --git a/spaces/Zengyf-CVer/Gradio_YOLOv5_Det_v5/model_download/yolov5_model_p5_n.sh b/spaces/Zengyf-CVer/Gradio_YOLOv5_Det_v5/model_download/yolov5_model_p5_n.sh
deleted file mode 100644
index 5fc6d093f4b92e1ad735f8b513d01d95f4d53d5c..0000000000000000000000000000000000000000
--- a/spaces/Zengyf-CVer/Gradio_YOLOv5_Det_v5/model_download/yolov5_model_p5_n.sh
+++ /dev/null
@@ -1,4 +0,0 @@
-cd ./yolov5
-
-# 下载YOLOv5模型
-wget -c -t 0 https://github.com/ultralytics/yolov5/releases/download/v6.1/yolov5n.pt
diff --git a/spaces/abdvl/datahub_qa_bot/docs/developers.md b/spaces/abdvl/datahub_qa_bot/docs/developers.md
deleted file mode 100644
index b0cd58099e12721de1a9f211a78500859b5fe3b3..0000000000000000000000000000000000000000
--- a/spaces/abdvl/datahub_qa_bot/docs/developers.md
+++ /dev/null
@@ -1,140 +0,0 @@
----
-title: "Local Development"
----
-
-# DataHub Developer's Guide
-
-## Pre-requirements
- - [Java 11 SDK](https://openjdk.org/projects/jdk/11/)
- - [Docker](https://www.docker.com/)
- - [Docker Compose](https://docs.docker.com/compose/)
- - Docker engine with at least 8GB of memory to run tests.
-
- :::note
-
- Do not try to use a JDK newer than JDK 11. The build process does not work with newer JDKs currently.
-
- :::
-
-## Building the Project
-
-Fork and clone the repository if haven't done so already
-```
-git clone https://github.com/{username}/datahub.git
-```
-
-Change into the repository's root directory
-```
-cd datahub
-```
-
-Use [gradle wrapper](https://docs.gradle.org/current/userguide/gradle_wrapper.html) to build the project
-```
-./gradlew build
-```
-
-Note that the above will also run run tests and a number of validations which makes the process considerably slower.
-
-We suggest partially compiling DataHub according to your needs:
-
- - Build Datahub's backend GMS (Generalized metadata service):
-```
-./gradlew :metadata-service:war:build
-```
- - Build Datahub's frontend:
-```
-./gradlew :datahub-frontend:dist -x yarnTest -x yarnLint
-```
- - Build DataHub's command line tool:
-```
-./gradlew :metadata-ingestion:installDev
-```
- - Build DataHub's documentation:
-```
-./gradlew :docs-website:yarnLintFix :docs-website:build -x :metadata-ingestion:runPreFlightScript
-# To preview the documentation
-./gradlew :docs-website:serve
-```
-
-## Deploying local versions
-
-Run just once to have the local `datahub` cli tool installed in your $PATH
-```
-cd smoke-test/
-python3 -m venv venv
-source venv/bin/activate
-pip install --upgrade pip wheel setuptools
-pip install -r requirements.txt
-cd ../
-```
-
-Once you have compiled & packaged the project or appropriate module you can deploy the entire system via docker-compose by running:
-```
-./gradlew quickstart
-```
-
-Replace whatever container you want in the existing deployment.
-I.e, replacing datahub's backend (GMS):
-```
-(cd docker && COMPOSE_DOCKER_CLI_BUILD=1 DOCKER_BUILDKIT=1 docker-compose -p datahub -f docker-compose-without-neo4j.yml -f docker-compose-without-neo4j.override.yml -f docker-compose.dev.yml up -d --no-deps --force-recreate datahub-gms)
-```
-
-Running the local version of the frontend
-```
-(cd docker && COMPOSE_DOCKER_CLI_BUILD=1 DOCKER_BUILDKIT=1 docker-compose -p datahub -f docker-compose-without-neo4j.yml -f docker-compose-without-neo4j.override.yml -f docker-compose.dev.yml up -d --no-deps --force-recreate datahub-frontend-react)
-```
-## IDE Support
-The recommended IDE for DataHub development is [IntelliJ IDEA](https://www.jetbrains.com/idea/).
-You can run the following command to generate or update the IntelliJ project file
-```
-./gradlew idea
-```
-Open `datahub.ipr` in IntelliJ to start developing!
-
-For consistency please import and auto format the code using [LinkedIn IntelliJ Java style](../gradle/idea/LinkedIn%20Style.xml).
-
-
-## Windows Compatibility
-
-For optimal performance and compatibility, we strongly recommend building on a Mac or Linux system.
-Please note that we do not actively support Windows in a non-virtualized environment.
-
-If you must use Windows, one workaround is to build within a virtualized environment, such as a VM(Virtual Machine) or [WSL(Windows Subsystem for Linux)](https://learn.microsoft.com/en-us/windows/wsl).
-This approach can help ensure that your build environment remains isolated and stable, and that your code is compiled correctly.
-
-## Common Build Issues
-
-### Getting `Unsupported class file major version 57`
-
-You're probably using a Java version that's too new for gradle. Run the following command to check your Java version
-```
-java --version
-```
-While it may be possible to build and run DataHub using newer versions of Java, we currently only support [Java 11](https://openjdk.org/projects/jdk/11/) (aka Java 11).
-
-### Getting `cannot find symbol` error for `javax.annotation.Generated`
-
-Similar to the previous issue, please use Java 1.8 to build the project.
-You can install multiple version of Java on a single machine and switch between them using the `JAVA_HOME` environment variable. See [this document](https://docs.oracle.com/cd/E21454_01/html/821-2531/inst_jdk_javahome_t.html) for more details.
-
-### `:metadata-models:generateDataTemplate` task fails with `java.nio.file.InvalidPathException: Illegal char <:> at index XX` or `Caused by: java.lang.IllegalArgumentException: 'other' has different root` error
-
-This is a [known issue](https://github.com/linkedin/rest.li/issues/287) when building the project on Windows due a bug in the Pegasus plugin. Please refer to [Windows Compatibility](/docs/developers.md#windows-compatibility).
-
-### Various errors related to `generateDataTemplate` or other `generate` tasks
-
-As we generate quite a few files from the models, it is possible that old generated files may conflict with new model changes. When this happens, a simple `./gradlew clean` should reosolve the issue.
-
-### `Execution failed for task ':metadata-service:restli-servlet-impl:checkRestModel'`
-
-This generally means that an [incompatible change](https://linkedin.github.io/rest.li/modeling/compatibility_check) was introduced to the rest.li API in GMS. You'll need to rebuild the snapshots/IDL by running the following command once
-```
-./gradlew :metadata-service:restli-servlet-impl:build -Prest.model.compatibility=ignore
-```
-
-### `java.io.IOException: No space left on device`
-
-This means you're running out of space on your disk to build. Please free up some space or try a different disk.
-
-### `Build failed` for task `./gradlew :datahub-frontend:dist -x yarnTest -x yarnLint`
-This could mean that you need to update your [Yarn](https://yarnpkg.com/getting-started/install) version
diff --git a/spaces/abhi-pwr/underwater_trash_detection/app.py b/spaces/abhi-pwr/underwater_trash_detection/app.py
deleted file mode 100644
index c86bd6dfb09fec0b1709779db4782993e1e4849c..0000000000000000000000000000000000000000
--- a/spaces/abhi-pwr/underwater_trash_detection/app.py
+++ /dev/null
@@ -1,41 +0,0 @@
-### 1. Imports and class names setup ###
-import gradio as gr
-import os
-
-from ultralytics import YOLO
-model = YOLO('best.pt')
-
-
-
-
-# Create predict function
-def predict(img) :
- """Transforms and performs a prediction on img and returns prediction and time taken.
- """
-
- results = model(img)
- img = results[0].plot()
- return img
-
-### 4. Gradio app ###
-
-
-# Create examples list from "examples/" directory
-example_list = [["examples/" + example] for example in os.listdir("examples")]
-
-# Create title, description and article strings
-title = "Underwater Trash Detection 🐸🐟🐙"
-description = "An YOLOv8 object detection model which detects trash underwater."
-article = "@ Abhishek Pawar"
-
-# Create the Gradio demo
-demo = gr.Interface(fn=predict, # mapping function from input to output
- inputs=gr.Image(type="pil"), # what are the inputs?
- outputs=gr.Image(type="numpy"), # our fn has two outputs, therefore we have two outputs
- examples=example_list,
- title=title,
- description=description,
- article=article)
-
-# Launch the demo!
-demo.launch()
diff --git a/spaces/abhishek/sketch-to-image/annotator/uniformer/mmcv/runner/base_runner.py b/spaces/abhishek/sketch-to-image/annotator/uniformer/mmcv/runner/base_runner.py
deleted file mode 100644
index 4928db0a73b56fe0218a4bf66ec4ffa082d31ccc..0000000000000000000000000000000000000000
--- a/spaces/abhishek/sketch-to-image/annotator/uniformer/mmcv/runner/base_runner.py
+++ /dev/null
@@ -1,542 +0,0 @@
-# Copyright (c) OpenMMLab. All rights reserved.
-import copy
-import logging
-import os.path as osp
-import warnings
-from abc import ABCMeta, abstractmethod
-
-import torch
-from torch.optim import Optimizer
-
-import annotator.uniformer.mmcv as mmcv
-from ..parallel import is_module_wrapper
-from .checkpoint import load_checkpoint
-from .dist_utils import get_dist_info
-from .hooks import HOOKS, Hook
-from .log_buffer import LogBuffer
-from .priority import Priority, get_priority
-from .utils import get_time_str
-
-
-class BaseRunner(metaclass=ABCMeta):
- """The base class of Runner, a training helper for PyTorch.
-
- All subclasses should implement the following APIs:
-
- - ``run()``
- - ``train()``
- - ``val()``
- - ``save_checkpoint()``
-
- Args:
- model (:obj:`torch.nn.Module`): The model to be run.
- batch_processor (callable): A callable method that process a data
- batch. The interface of this method should be
- `batch_processor(model, data, train_mode) -> dict`
- optimizer (dict or :obj:`torch.optim.Optimizer`): It can be either an
- optimizer (in most cases) or a dict of optimizers (in models that
- requires more than one optimizer, e.g., GAN).
- work_dir (str, optional): The working directory to save checkpoints
- and logs. Defaults to None.
- logger (:obj:`logging.Logger`): Logger used during training.
- Defaults to None. (The default value is just for backward
- compatibility)
- meta (dict | None): A dict records some import information such as
- environment info and seed, which will be logged in logger hook.
- Defaults to None.
- max_epochs (int, optional): Total training epochs.
- max_iters (int, optional): Total training iterations.
- """
-
- def __init__(self,
- model,
- batch_processor=None,
- optimizer=None,
- work_dir=None,
- logger=None,
- meta=None,
- max_iters=None,
- max_epochs=None):
- if batch_processor is not None:
- if not callable(batch_processor):
- raise TypeError('batch_processor must be callable, '
- f'but got {type(batch_processor)}')
- warnings.warn('batch_processor is deprecated, please implement '
- 'train_step() and val_step() in the model instead.')
- # raise an error is `batch_processor` is not None and
- # `model.train_step()` exists.
- if is_module_wrapper(model):
- _model = model.module
- else:
- _model = model
- if hasattr(_model, 'train_step') or hasattr(_model, 'val_step'):
- raise RuntimeError(
- 'batch_processor and model.train_step()/model.val_step() '
- 'cannot be both available.')
- else:
- assert hasattr(model, 'train_step')
-
- # check the type of `optimizer`
- if isinstance(optimizer, dict):
- for name, optim in optimizer.items():
- if not isinstance(optim, Optimizer):
- raise TypeError(
- f'optimizer must be a dict of torch.optim.Optimizers, '
- f'but optimizer["{name}"] is a {type(optim)}')
- elif not isinstance(optimizer, Optimizer) and optimizer is not None:
- raise TypeError(
- f'optimizer must be a torch.optim.Optimizer object '
- f'or dict or None, but got {type(optimizer)}')
-
- # check the type of `logger`
- if not isinstance(logger, logging.Logger):
- raise TypeError(f'logger must be a logging.Logger object, '
- f'but got {type(logger)}')
-
- # check the type of `meta`
- if meta is not None and not isinstance(meta, dict):
- raise TypeError(
- f'meta must be a dict or None, but got {type(meta)}')
-
- self.model = model
- self.batch_processor = batch_processor
- self.optimizer = optimizer
- self.logger = logger
- self.meta = meta
- # create work_dir
- if mmcv.is_str(work_dir):
- self.work_dir = osp.abspath(work_dir)
- mmcv.mkdir_or_exist(self.work_dir)
- elif work_dir is None:
- self.work_dir = None
- else:
- raise TypeError('"work_dir" must be a str or None')
-
- # get model name from the model class
- if hasattr(self.model, 'module'):
- self._model_name = self.model.module.__class__.__name__
- else:
- self._model_name = self.model.__class__.__name__
-
- self._rank, self._world_size = get_dist_info()
- self.timestamp = get_time_str()
- self.mode = None
- self._hooks = []
- self._epoch = 0
- self._iter = 0
- self._inner_iter = 0
-
- if max_epochs is not None and max_iters is not None:
- raise ValueError(
- 'Only one of `max_epochs` or `max_iters` can be set.')
-
- self._max_epochs = max_epochs
- self._max_iters = max_iters
- # TODO: Redesign LogBuffer, it is not flexible and elegant enough
- self.log_buffer = LogBuffer()
-
- @property
- def model_name(self):
- """str: Name of the model, usually the module class name."""
- return self._model_name
-
- @property
- def rank(self):
- """int: Rank of current process. (distributed training)"""
- return self._rank
-
- @property
- def world_size(self):
- """int: Number of processes participating in the job.
- (distributed training)"""
- return self._world_size
-
- @property
- def hooks(self):
- """list[:obj:`Hook`]: A list of registered hooks."""
- return self._hooks
-
- @property
- def epoch(self):
- """int: Current epoch."""
- return self._epoch
-
- @property
- def iter(self):
- """int: Current iteration."""
- return self._iter
-
- @property
- def inner_iter(self):
- """int: Iteration in an epoch."""
- return self._inner_iter
-
- @property
- def max_epochs(self):
- """int: Maximum training epochs."""
- return self._max_epochs
-
- @property
- def max_iters(self):
- """int: Maximum training iterations."""
- return self._max_iters
-
- @abstractmethod
- def train(self):
- pass
-
- @abstractmethod
- def val(self):
- pass
-
- @abstractmethod
- def run(self, data_loaders, workflow, **kwargs):
- pass
-
- @abstractmethod
- def save_checkpoint(self,
- out_dir,
- filename_tmpl,
- save_optimizer=True,
- meta=None,
- create_symlink=True):
- pass
-
- def current_lr(self):
- """Get current learning rates.
-
- Returns:
- list[float] | dict[str, list[float]]: Current learning rates of all
- param groups. If the runner has a dict of optimizers, this
- method will return a dict.
- """
- if isinstance(self.optimizer, torch.optim.Optimizer):
- lr = [group['lr'] for group in self.optimizer.param_groups]
- elif isinstance(self.optimizer, dict):
- lr = dict()
- for name, optim in self.optimizer.items():
- lr[name] = [group['lr'] for group in optim.param_groups]
- else:
- raise RuntimeError(
- 'lr is not applicable because optimizer does not exist.')
- return lr
-
- def current_momentum(self):
- """Get current momentums.
-
- Returns:
- list[float] | dict[str, list[float]]: Current momentums of all
- param groups. If the runner has a dict of optimizers, this
- method will return a dict.
- """
-
- def _get_momentum(optimizer):
- momentums = []
- for group in optimizer.param_groups:
- if 'momentum' in group.keys():
- momentums.append(group['momentum'])
- elif 'betas' in group.keys():
- momentums.append(group['betas'][0])
- else:
- momentums.append(0)
- return momentums
-
- if self.optimizer is None:
- raise RuntimeError(
- 'momentum is not applicable because optimizer does not exist.')
- elif isinstance(self.optimizer, torch.optim.Optimizer):
- momentums = _get_momentum(self.optimizer)
- elif isinstance(self.optimizer, dict):
- momentums = dict()
- for name, optim in self.optimizer.items():
- momentums[name] = _get_momentum(optim)
- return momentums
-
- def register_hook(self, hook, priority='NORMAL'):
- """Register a hook into the hook list.
-
- The hook will be inserted into a priority queue, with the specified
- priority (See :class:`Priority` for details of priorities).
- For hooks with the same priority, they will be triggered in the same
- order as they are registered.
-
- Args:
- hook (:obj:`Hook`): The hook to be registered.
- priority (int or str or :obj:`Priority`): Hook priority.
- Lower value means higher priority.
- """
- assert isinstance(hook, Hook)
- if hasattr(hook, 'priority'):
- raise ValueError('"priority" is a reserved attribute for hooks')
- priority = get_priority(priority)
- hook.priority = priority
- # insert the hook to a sorted list
- inserted = False
- for i in range(len(self._hooks) - 1, -1, -1):
- if priority >= self._hooks[i].priority:
- self._hooks.insert(i + 1, hook)
- inserted = True
- break
- if not inserted:
- self._hooks.insert(0, hook)
-
- def register_hook_from_cfg(self, hook_cfg):
- """Register a hook from its cfg.
-
- Args:
- hook_cfg (dict): Hook config. It should have at least keys 'type'
- and 'priority' indicating its type and priority.
-
- Notes:
- The specific hook class to register should not use 'type' and
- 'priority' arguments during initialization.
- """
- hook_cfg = hook_cfg.copy()
- priority = hook_cfg.pop('priority', 'NORMAL')
- hook = mmcv.build_from_cfg(hook_cfg, HOOKS)
- self.register_hook(hook, priority=priority)
-
- def call_hook(self, fn_name):
- """Call all hooks.
-
- Args:
- fn_name (str): The function name in each hook to be called, such as
- "before_train_epoch".
- """
- for hook in self._hooks:
- getattr(hook, fn_name)(self)
-
- def get_hook_info(self):
- # Get hooks info in each stage
- stage_hook_map = {stage: [] for stage in Hook.stages}
- for hook in self.hooks:
- try:
- priority = Priority(hook.priority).name
- except ValueError:
- priority = hook.priority
- classname = hook.__class__.__name__
- hook_info = f'({priority:<12}) {classname:<35}'
- for trigger_stage in hook.get_triggered_stages():
- stage_hook_map[trigger_stage].append(hook_info)
-
- stage_hook_infos = []
- for stage in Hook.stages:
- hook_infos = stage_hook_map[stage]
- if len(hook_infos) > 0:
- info = f'{stage}:\n'
- info += '\n'.join(hook_infos)
- info += '\n -------------------- '
- stage_hook_infos.append(info)
- return '\n'.join(stage_hook_infos)
-
- def load_checkpoint(self,
- filename,
- map_location='cpu',
- strict=False,
- revise_keys=[(r'^module.', '')]):
- return load_checkpoint(
- self.model,
- filename,
- map_location,
- strict,
- self.logger,
- revise_keys=revise_keys)
-
- def resume(self,
- checkpoint,
- resume_optimizer=True,
- map_location='default'):
- if map_location == 'default':
- if torch.cuda.is_available():
- device_id = torch.cuda.current_device()
- checkpoint = self.load_checkpoint(
- checkpoint,
- map_location=lambda storage, loc: storage.cuda(device_id))
- else:
- checkpoint = self.load_checkpoint(checkpoint)
- else:
- checkpoint = self.load_checkpoint(
- checkpoint, map_location=map_location)
-
- self._epoch = checkpoint['meta']['epoch']
- self._iter = checkpoint['meta']['iter']
- if self.meta is None:
- self.meta = {}
- self.meta.setdefault('hook_msgs', {})
- # load `last_ckpt`, `best_score`, `best_ckpt`, etc. for hook messages
- self.meta['hook_msgs'].update(checkpoint['meta'].get('hook_msgs', {}))
-
- # Re-calculate the number of iterations when resuming
- # models with different number of GPUs
- if 'config' in checkpoint['meta']:
- config = mmcv.Config.fromstring(
- checkpoint['meta']['config'], file_format='.py')
- previous_gpu_ids = config.get('gpu_ids', None)
- if previous_gpu_ids and len(previous_gpu_ids) > 0 and len(
- previous_gpu_ids) != self.world_size:
- self._iter = int(self._iter * len(previous_gpu_ids) /
- self.world_size)
- self.logger.info('the iteration number is changed due to '
- 'change of GPU number')
-
- # resume meta information meta
- self.meta = checkpoint['meta']
-
- if 'optimizer' in checkpoint and resume_optimizer:
- if isinstance(self.optimizer, Optimizer):
- self.optimizer.load_state_dict(checkpoint['optimizer'])
- elif isinstance(self.optimizer, dict):
- for k in self.optimizer.keys():
- self.optimizer[k].load_state_dict(
- checkpoint['optimizer'][k])
- else:
- raise TypeError(
- 'Optimizer should be dict or torch.optim.Optimizer '
- f'but got {type(self.optimizer)}')
-
- self.logger.info('resumed epoch %d, iter %d', self.epoch, self.iter)
-
- def register_lr_hook(self, lr_config):
- if lr_config is None:
- return
- elif isinstance(lr_config, dict):
- assert 'policy' in lr_config
- policy_type = lr_config.pop('policy')
- # If the type of policy is all in lower case, e.g., 'cyclic',
- # then its first letter will be capitalized, e.g., to be 'Cyclic'.
- # This is for the convenient usage of Lr updater.
- # Since this is not applicable for `
- # CosineAnnealingLrUpdater`,
- # the string will not be changed if it contains capital letters.
- if policy_type == policy_type.lower():
- policy_type = policy_type.title()
- hook_type = policy_type + 'LrUpdaterHook'
- lr_config['type'] = hook_type
- hook = mmcv.build_from_cfg(lr_config, HOOKS)
- else:
- hook = lr_config
- self.register_hook(hook, priority='VERY_HIGH')
-
- def register_momentum_hook(self, momentum_config):
- if momentum_config is None:
- return
- if isinstance(momentum_config, dict):
- assert 'policy' in momentum_config
- policy_type = momentum_config.pop('policy')
- # If the type of policy is all in lower case, e.g., 'cyclic',
- # then its first letter will be capitalized, e.g., to be 'Cyclic'.
- # This is for the convenient usage of momentum updater.
- # Since this is not applicable for
- # `CosineAnnealingMomentumUpdater`,
- # the string will not be changed if it contains capital letters.
- if policy_type == policy_type.lower():
- policy_type = policy_type.title()
- hook_type = policy_type + 'MomentumUpdaterHook'
- momentum_config['type'] = hook_type
- hook = mmcv.build_from_cfg(momentum_config, HOOKS)
- else:
- hook = momentum_config
- self.register_hook(hook, priority='HIGH')
-
- def register_optimizer_hook(self, optimizer_config):
- if optimizer_config is None:
- return
- if isinstance(optimizer_config, dict):
- optimizer_config.setdefault('type', 'OptimizerHook')
- hook = mmcv.build_from_cfg(optimizer_config, HOOKS)
- else:
- hook = optimizer_config
- self.register_hook(hook, priority='ABOVE_NORMAL')
-
- def register_checkpoint_hook(self, checkpoint_config):
- if checkpoint_config is None:
- return
- if isinstance(checkpoint_config, dict):
- checkpoint_config.setdefault('type', 'CheckpointHook')
- hook = mmcv.build_from_cfg(checkpoint_config, HOOKS)
- else:
- hook = checkpoint_config
- self.register_hook(hook, priority='NORMAL')
-
- def register_logger_hooks(self, log_config):
- if log_config is None:
- return
- log_interval = log_config['interval']
- for info in log_config['hooks']:
- logger_hook = mmcv.build_from_cfg(
- info, HOOKS, default_args=dict(interval=log_interval))
- self.register_hook(logger_hook, priority='VERY_LOW')
-
- def register_timer_hook(self, timer_config):
- if timer_config is None:
- return
- if isinstance(timer_config, dict):
- timer_config_ = copy.deepcopy(timer_config)
- hook = mmcv.build_from_cfg(timer_config_, HOOKS)
- else:
- hook = timer_config
- self.register_hook(hook, priority='LOW')
-
- def register_custom_hooks(self, custom_config):
- if custom_config is None:
- return
-
- if not isinstance(custom_config, list):
- custom_config = [custom_config]
-
- for item in custom_config:
- if isinstance(item, dict):
- self.register_hook_from_cfg(item)
- else:
- self.register_hook(item, priority='NORMAL')
-
- def register_profiler_hook(self, profiler_config):
- if profiler_config is None:
- return
- if isinstance(profiler_config, dict):
- profiler_config.setdefault('type', 'ProfilerHook')
- hook = mmcv.build_from_cfg(profiler_config, HOOKS)
- else:
- hook = profiler_config
- self.register_hook(hook)
-
- def register_training_hooks(self,
- lr_config,
- optimizer_config=None,
- checkpoint_config=None,
- log_config=None,
- momentum_config=None,
- timer_config=dict(type='IterTimerHook'),
- custom_hooks_config=None):
- """Register default and custom hooks for training.
-
- Default and custom hooks include:
-
- +----------------------+-------------------------+
- | Hooks | Priority |
- +======================+=========================+
- | LrUpdaterHook | VERY_HIGH (10) |
- +----------------------+-------------------------+
- | MomentumUpdaterHook | HIGH (30) |
- +----------------------+-------------------------+
- | OptimizerStepperHook | ABOVE_NORMAL (40) |
- +----------------------+-------------------------+
- | CheckpointSaverHook | NORMAL (50) |
- +----------------------+-------------------------+
- | IterTimerHook | LOW (70) |
- +----------------------+-------------------------+
- | LoggerHook(s) | VERY_LOW (90) |
- +----------------------+-------------------------+
- | CustomHook(s) | defaults to NORMAL (50) |
- +----------------------+-------------------------+
-
- If custom hooks have same priority with default hooks, custom hooks
- will be triggered after default hooks.
- """
- self.register_lr_hook(lr_config)
- self.register_momentum_hook(momentum_config)
- self.register_optimizer_hook(optimizer_config)
- self.register_checkpoint_hook(checkpoint_config)
- self.register_timer_hook(timer_config)
- self.register_logger_hooks(log_config)
- self.register_custom_hooks(custom_hooks_config)
diff --git a/spaces/abhishek/sketch-to-image/annotator/uniformer/mmdet/models/detectors/rpn.py b/spaces/abhishek/sketch-to-image/annotator/uniformer/mmdet/models/detectors/rpn.py
deleted file mode 100644
index 1a77294549d1c3dc7821063c3f3d08bb331fbe59..0000000000000000000000000000000000000000
--- a/spaces/abhishek/sketch-to-image/annotator/uniformer/mmdet/models/detectors/rpn.py
+++ /dev/null
@@ -1,154 +0,0 @@
-import mmcv
-from mmcv.image import tensor2imgs
-
-from mmdet.core import bbox_mapping
-from ..builder import DETECTORS, build_backbone, build_head, build_neck
-from .base import BaseDetector
-
-
-@DETECTORS.register_module()
-class RPN(BaseDetector):
- """Implementation of Region Proposal Network."""
-
- def __init__(self,
- backbone,
- neck,
- rpn_head,
- train_cfg,
- test_cfg,
- pretrained=None):
- super(RPN, self).__init__()
- self.backbone = build_backbone(backbone)
- self.neck = build_neck(neck) if neck is not None else None
- rpn_train_cfg = train_cfg.rpn if train_cfg is not None else None
- rpn_head.update(train_cfg=rpn_train_cfg)
- rpn_head.update(test_cfg=test_cfg.rpn)
- self.rpn_head = build_head(rpn_head)
- self.train_cfg = train_cfg
- self.test_cfg = test_cfg
- self.init_weights(pretrained=pretrained)
-
- def init_weights(self, pretrained=None):
- """Initialize the weights in detector.
-
- Args:
- pretrained (str, optional): Path to pre-trained weights.
- Defaults to None.
- """
- super(RPN, self).init_weights(pretrained)
- self.backbone.init_weights(pretrained=pretrained)
- if self.with_neck:
- self.neck.init_weights()
- self.rpn_head.init_weights()
-
- def extract_feat(self, img):
- """Extract features.
-
- Args:
- img (torch.Tensor): Image tensor with shape (n, c, h ,w).
-
- Returns:
- list[torch.Tensor]: Multi-level features that may have
- different resolutions.
- """
- x = self.backbone(img)
- if self.with_neck:
- x = self.neck(x)
- return x
-
- def forward_dummy(self, img):
- """Dummy forward function."""
- x = self.extract_feat(img)
- rpn_outs = self.rpn_head(x)
- return rpn_outs
-
- def forward_train(self,
- img,
- img_metas,
- gt_bboxes=None,
- gt_bboxes_ignore=None):
- """
- Args:
- img (Tensor): Input images of shape (N, C, H, W).
- Typically these should be mean centered and std scaled.
- img_metas (list[dict]): A List of image info dict where each dict
- has: 'img_shape', 'scale_factor', 'flip', and may also contain
- 'filename', 'ori_shape', 'pad_shape', and 'img_norm_cfg'.
- For details on the values of these keys see
- :class:`mmdet.datasets.pipelines.Collect`.
- gt_bboxes (list[Tensor]): Each item are the truth boxes for each
- image in [tl_x, tl_y, br_x, br_y] format.
- gt_bboxes_ignore (None | list[Tensor]): Specify which bounding
- boxes can be ignored when computing the loss.
-
- Returns:
- dict[str, Tensor]: A dictionary of loss components.
- """
- if (isinstance(self.train_cfg.rpn, dict)
- and self.train_cfg.rpn.get('debug', False)):
- self.rpn_head.debug_imgs = tensor2imgs(img)
-
- x = self.extract_feat(img)
- losses = self.rpn_head.forward_train(x, img_metas, gt_bboxes, None,
- gt_bboxes_ignore)
- return losses
-
- def simple_test(self, img, img_metas, rescale=False):
- """Test function without test time augmentation.
-
- Args:
- imgs (list[torch.Tensor]): List of multiple images
- img_metas (list[dict]): List of image information.
- rescale (bool, optional): Whether to rescale the results.
- Defaults to False.
-
- Returns:
- list[np.ndarray]: proposals
- """
- x = self.extract_feat(img)
- proposal_list = self.rpn_head.simple_test_rpn(x, img_metas)
- if rescale:
- for proposals, meta in zip(proposal_list, img_metas):
- proposals[:, :4] /= proposals.new_tensor(meta['scale_factor'])
-
- return [proposal.cpu().numpy() for proposal in proposal_list]
-
- def aug_test(self, imgs, img_metas, rescale=False):
- """Test function with test time augmentation.
-
- Args:
- imgs (list[torch.Tensor]): List of multiple images
- img_metas (list[dict]): List of image information.
- rescale (bool, optional): Whether to rescale the results.
- Defaults to False.
-
- Returns:
- list[np.ndarray]: proposals
- """
- proposal_list = self.rpn_head.aug_test_rpn(
- self.extract_feats(imgs), img_metas)
- if not rescale:
- for proposals, img_meta in zip(proposal_list, img_metas[0]):
- img_shape = img_meta['img_shape']
- scale_factor = img_meta['scale_factor']
- flip = img_meta['flip']
- flip_direction = img_meta['flip_direction']
- proposals[:, :4] = bbox_mapping(proposals[:, :4], img_shape,
- scale_factor, flip,
- flip_direction)
- return [proposal.cpu().numpy() for proposal in proposal_list]
-
- def show_result(self, data, result, top_k=20, **kwargs):
- """Show RPN proposals on the image.
-
- Args:
- data (str or np.ndarray): Image filename or loaded image.
- result (Tensor or tuple): The results to draw over `img`
- bbox_result or (bbox_result, segm_result).
- top_k (int): Plot the first k bboxes only
- if set positive. Default: 20
-
- Returns:
- np.ndarray: The image with bboxes drawn on it.
- """
- mmcv.imshow_bboxes(data, result, top_k=top_k)
diff --git a/spaces/abhishek/sketch-to-image/annotator/uniformer/mmdet_null/models/dense_heads/fovea_head.py b/spaces/abhishek/sketch-to-image/annotator/uniformer/mmdet_null/models/dense_heads/fovea_head.py
deleted file mode 100644
index c8ccea787cba3d092284d4a5e209adaf6521c86a..0000000000000000000000000000000000000000
--- a/spaces/abhishek/sketch-to-image/annotator/uniformer/mmdet_null/models/dense_heads/fovea_head.py
+++ /dev/null
@@ -1,341 +0,0 @@
-import torch
-import torch.nn as nn
-from mmcv.cnn import ConvModule, normal_init
-from mmcv.ops import DeformConv2d
-
-from mmdet.core import multi_apply, multiclass_nms
-from ..builder import HEADS
-from .anchor_free_head import AnchorFreeHead
-
-INF = 1e8
-
-
-class FeatureAlign(nn.Module):
-
- def __init__(self,
- in_channels,
- out_channels,
- kernel_size=3,
- deform_groups=4):
- super(FeatureAlign, self).__init__()
- offset_channels = kernel_size * kernel_size * 2
- self.conv_offset = nn.Conv2d(
- 4, deform_groups * offset_channels, 1, bias=False)
- self.conv_adaption = DeformConv2d(
- in_channels,
- out_channels,
- kernel_size=kernel_size,
- padding=(kernel_size - 1) // 2,
- deform_groups=deform_groups)
- self.relu = nn.ReLU(inplace=True)
-
- def init_weights(self):
- normal_init(self.conv_offset, std=0.1)
- normal_init(self.conv_adaption, std=0.01)
-
- def forward(self, x, shape):
- offset = self.conv_offset(shape)
- x = self.relu(self.conv_adaption(x, offset))
- return x
-
-
-@HEADS.register_module()
-class FoveaHead(AnchorFreeHead):
- """FoveaBox: Beyond Anchor-based Object Detector
- https://arxiv.org/abs/1904.03797
- """
-
- def __init__(self,
- num_classes,
- in_channels,
- base_edge_list=(16, 32, 64, 128, 256),
- scale_ranges=((8, 32), (16, 64), (32, 128), (64, 256), (128,
- 512)),
- sigma=0.4,
- with_deform=False,
- deform_groups=4,
- **kwargs):
- self.base_edge_list = base_edge_list
- self.scale_ranges = scale_ranges
- self.sigma = sigma
- self.with_deform = with_deform
- self.deform_groups = deform_groups
- super().__init__(num_classes, in_channels, **kwargs)
-
- def _init_layers(self):
- # box branch
- super()._init_reg_convs()
- self.conv_reg = nn.Conv2d(self.feat_channels, 4, 3, padding=1)
-
- # cls branch
- if not self.with_deform:
- super()._init_cls_convs()
- self.conv_cls = nn.Conv2d(
- self.feat_channels, self.cls_out_channels, 3, padding=1)
- else:
- self.cls_convs = nn.ModuleList()
- self.cls_convs.append(
- ConvModule(
- self.feat_channels, (self.feat_channels * 4),
- 3,
- stride=1,
- padding=1,
- conv_cfg=self.conv_cfg,
- norm_cfg=self.norm_cfg,
- bias=self.norm_cfg is None))
- self.cls_convs.append(
- ConvModule((self.feat_channels * 4), (self.feat_channels * 4),
- 1,
- stride=1,
- padding=0,
- conv_cfg=self.conv_cfg,
- norm_cfg=self.norm_cfg,
- bias=self.norm_cfg is None))
- self.feature_adaption = FeatureAlign(
- self.feat_channels,
- self.feat_channels,
- kernel_size=3,
- deform_groups=self.deform_groups)
- self.conv_cls = nn.Conv2d(
- int(self.feat_channels * 4),
- self.cls_out_channels,
- 3,
- padding=1)
-
- def init_weights(self):
- super().init_weights()
- if self.with_deform:
- self.feature_adaption.init_weights()
-
- def forward_single(self, x):
- cls_feat = x
- reg_feat = x
- for reg_layer in self.reg_convs:
- reg_feat = reg_layer(reg_feat)
- bbox_pred = self.conv_reg(reg_feat)
- if self.with_deform:
- cls_feat = self.feature_adaption(cls_feat, bbox_pred.exp())
- for cls_layer in self.cls_convs:
- cls_feat = cls_layer(cls_feat)
- cls_score = self.conv_cls(cls_feat)
- return cls_score, bbox_pred
-
- def _get_points_single(self, *args, **kwargs):
- y, x = super()._get_points_single(*args, **kwargs)
- return y + 0.5, x + 0.5
-
- def loss(self,
- cls_scores,
- bbox_preds,
- gt_bbox_list,
- gt_label_list,
- img_metas,
- gt_bboxes_ignore=None):
- assert len(cls_scores) == len(bbox_preds)
-
- featmap_sizes = [featmap.size()[-2:] for featmap in cls_scores]
- points = self.get_points(featmap_sizes, bbox_preds[0].dtype,
- bbox_preds[0].device)
- num_imgs = cls_scores[0].size(0)
- flatten_cls_scores = [
- cls_score.permute(0, 2, 3, 1).reshape(-1, self.cls_out_channels)
- for cls_score in cls_scores
- ]
- flatten_bbox_preds = [
- bbox_pred.permute(0, 2, 3, 1).reshape(-1, 4)
- for bbox_pred in bbox_preds
- ]
- flatten_cls_scores = torch.cat(flatten_cls_scores)
- flatten_bbox_preds = torch.cat(flatten_bbox_preds)
- flatten_labels, flatten_bbox_targets = self.get_targets(
- gt_bbox_list, gt_label_list, featmap_sizes, points)
-
- # FG cat_id: [0, num_classes -1], BG cat_id: num_classes
- pos_inds = ((flatten_labels >= 0)
- & (flatten_labels < self.num_classes)).nonzero().view(-1)
- num_pos = len(pos_inds)
-
- loss_cls = self.loss_cls(
- flatten_cls_scores, flatten_labels, avg_factor=num_pos + num_imgs)
- if num_pos > 0:
- pos_bbox_preds = flatten_bbox_preds[pos_inds]
- pos_bbox_targets = flatten_bbox_targets[pos_inds]
- pos_weights = pos_bbox_targets.new_zeros(
- pos_bbox_targets.size()) + 1.0
- loss_bbox = self.loss_bbox(
- pos_bbox_preds,
- pos_bbox_targets,
- pos_weights,
- avg_factor=num_pos)
- else:
- loss_bbox = torch.tensor(
- 0,
- dtype=flatten_bbox_preds.dtype,
- device=flatten_bbox_preds.device)
- return dict(loss_cls=loss_cls, loss_bbox=loss_bbox)
-
- def get_targets(self, gt_bbox_list, gt_label_list, featmap_sizes, points):
- label_list, bbox_target_list = multi_apply(
- self._get_target_single,
- gt_bbox_list,
- gt_label_list,
- featmap_size_list=featmap_sizes,
- point_list=points)
- flatten_labels = [
- torch.cat([
- labels_level_img.flatten() for labels_level_img in labels_level
- ]) for labels_level in zip(*label_list)
- ]
- flatten_bbox_targets = [
- torch.cat([
- bbox_targets_level_img.reshape(-1, 4)
- for bbox_targets_level_img in bbox_targets_level
- ]) for bbox_targets_level in zip(*bbox_target_list)
- ]
- flatten_labels = torch.cat(flatten_labels)
- flatten_bbox_targets = torch.cat(flatten_bbox_targets)
- return flatten_labels, flatten_bbox_targets
-
- def _get_target_single(self,
- gt_bboxes_raw,
- gt_labels_raw,
- featmap_size_list=None,
- point_list=None):
-
- gt_areas = torch.sqrt((gt_bboxes_raw[:, 2] - gt_bboxes_raw[:, 0]) *
- (gt_bboxes_raw[:, 3] - gt_bboxes_raw[:, 1]))
- label_list = []
- bbox_target_list = []
- # for each pyramid, find the cls and box target
- for base_len, (lower_bound, upper_bound), stride, featmap_size, \
- (y, x) in zip(self.base_edge_list, self.scale_ranges,
- self.strides, featmap_size_list, point_list):
- # FG cat_id: [0, num_classes -1], BG cat_id: num_classes
- labels = gt_labels_raw.new_zeros(featmap_size) + self.num_classes
- bbox_targets = gt_bboxes_raw.new(featmap_size[0], featmap_size[1],
- 4) + 1
- # scale assignment
- hit_indices = ((gt_areas >= lower_bound) &
- (gt_areas <= upper_bound)).nonzero().flatten()
- if len(hit_indices) == 0:
- label_list.append(labels)
- bbox_target_list.append(torch.log(bbox_targets))
- continue
- _, hit_index_order = torch.sort(-gt_areas[hit_indices])
- hit_indices = hit_indices[hit_index_order]
- gt_bboxes = gt_bboxes_raw[hit_indices, :] / stride
- gt_labels = gt_labels_raw[hit_indices]
- half_w = 0.5 * (gt_bboxes[:, 2] - gt_bboxes[:, 0])
- half_h = 0.5 * (gt_bboxes[:, 3] - gt_bboxes[:, 1])
- # valid fovea area: left, right, top, down
- pos_left = torch.ceil(
- gt_bboxes[:, 0] + (1 - self.sigma) * half_w - 0.5).long().\
- clamp(0, featmap_size[1] - 1)
- pos_right = torch.floor(
- gt_bboxes[:, 0] + (1 + self.sigma) * half_w - 0.5).long().\
- clamp(0, featmap_size[1] - 1)
- pos_top = torch.ceil(
- gt_bboxes[:, 1] + (1 - self.sigma) * half_h - 0.5).long().\
- clamp(0, featmap_size[0] - 1)
- pos_down = torch.floor(
- gt_bboxes[:, 1] + (1 + self.sigma) * half_h - 0.5).long().\
- clamp(0, featmap_size[0] - 1)
- for px1, py1, px2, py2, label, (gt_x1, gt_y1, gt_x2, gt_y2) in \
- zip(pos_left, pos_top, pos_right, pos_down, gt_labels,
- gt_bboxes_raw[hit_indices, :]):
- labels[py1:py2 + 1, px1:px2 + 1] = label
- bbox_targets[py1:py2 + 1, px1:px2 + 1, 0] = \
- (stride * x[py1:py2 + 1, px1:px2 + 1] - gt_x1) / base_len
- bbox_targets[py1:py2 + 1, px1:px2 + 1, 1] = \
- (stride * y[py1:py2 + 1, px1:px2 + 1] - gt_y1) / base_len
- bbox_targets[py1:py2 + 1, px1:px2 + 1, 2] = \
- (gt_x2 - stride * x[py1:py2 + 1, px1:px2 + 1]) / base_len
- bbox_targets[py1:py2 + 1, px1:px2 + 1, 3] = \
- (gt_y2 - stride * y[py1:py2 + 1, px1:px2 + 1]) / base_len
- bbox_targets = bbox_targets.clamp(min=1. / 16, max=16.)
- label_list.append(labels)
- bbox_target_list.append(torch.log(bbox_targets))
- return label_list, bbox_target_list
-
- def get_bboxes(self,
- cls_scores,
- bbox_preds,
- img_metas,
- cfg=None,
- rescale=None):
- assert len(cls_scores) == len(bbox_preds)
- num_levels = len(cls_scores)
- featmap_sizes = [featmap.size()[-2:] for featmap in cls_scores]
- points = self.get_points(
- featmap_sizes,
- bbox_preds[0].dtype,
- bbox_preds[0].device,
- flatten=True)
- result_list = []
- for img_id in range(len(img_metas)):
- cls_score_list = [
- cls_scores[i][img_id].detach() for i in range(num_levels)
- ]
- bbox_pred_list = [
- bbox_preds[i][img_id].detach() for i in range(num_levels)
- ]
- img_shape = img_metas[img_id]['img_shape']
- scale_factor = img_metas[img_id]['scale_factor']
- det_bboxes = self._get_bboxes_single(cls_score_list,
- bbox_pred_list, featmap_sizes,
- points, img_shape,
- scale_factor, cfg, rescale)
- result_list.append(det_bboxes)
- return result_list
-
- def _get_bboxes_single(self,
- cls_scores,
- bbox_preds,
- featmap_sizes,
- point_list,
- img_shape,
- scale_factor,
- cfg,
- rescale=False):
- cfg = self.test_cfg if cfg is None else cfg
- assert len(cls_scores) == len(bbox_preds) == len(point_list)
- det_bboxes = []
- det_scores = []
- for cls_score, bbox_pred, featmap_size, stride, base_len, (y, x) \
- in zip(cls_scores, bbox_preds, featmap_sizes, self.strides,
- self.base_edge_list, point_list):
- assert cls_score.size()[-2:] == bbox_pred.size()[-2:]
- scores = cls_score.permute(1, 2, 0).reshape(
- -1, self.cls_out_channels).sigmoid()
- bbox_pred = bbox_pred.permute(1, 2, 0).reshape(-1, 4).exp()
- nms_pre = cfg.get('nms_pre', -1)
- if (nms_pre > 0) and (scores.shape[0] > nms_pre):
- max_scores, _ = scores.max(dim=1)
- _, topk_inds = max_scores.topk(nms_pre)
- bbox_pred = bbox_pred[topk_inds, :]
- scores = scores[topk_inds, :]
- y = y[topk_inds]
- x = x[topk_inds]
- x1 = (stride * x - base_len * bbox_pred[:, 0]).\
- clamp(min=0, max=img_shape[1] - 1)
- y1 = (stride * y - base_len * bbox_pred[:, 1]).\
- clamp(min=0, max=img_shape[0] - 1)
- x2 = (stride * x + base_len * bbox_pred[:, 2]).\
- clamp(min=0, max=img_shape[1] - 1)
- y2 = (stride * y + base_len * bbox_pred[:, 3]).\
- clamp(min=0, max=img_shape[0] - 1)
- bboxes = torch.stack([x1, y1, x2, y2], -1)
- det_bboxes.append(bboxes)
- det_scores.append(scores)
- det_bboxes = torch.cat(det_bboxes)
- if rescale:
- det_bboxes /= det_bboxes.new_tensor(scale_factor)
- det_scores = torch.cat(det_scores)
- padding = det_scores.new_zeros(det_scores.shape[0], 1)
- # remind that we set FG labels to [0, num_class-1] since mmdet v2.0
- # BG cat_id: num_class
- det_scores = torch.cat([det_scores, padding], dim=1)
- det_bboxes, det_labels = multiclass_nms(det_bboxes, det_scores,
- cfg.score_thr, cfg.nms,
- cfg.max_per_img)
- return det_bboxes, det_labels
diff --git a/spaces/abhishek/sketch-to-image/annotator/uniformer_base/mmcv/runner/priority.py b/spaces/abhishek/sketch-to-image/annotator/uniformer_base/mmcv/runner/priority.py
deleted file mode 100644
index 64cc4e3a05f8d5b89ab6eb32461e6e80f1d62e67..0000000000000000000000000000000000000000
--- a/spaces/abhishek/sketch-to-image/annotator/uniformer_base/mmcv/runner/priority.py
+++ /dev/null
@@ -1,60 +0,0 @@
-# Copyright (c) OpenMMLab. All rights reserved.
-from enum import Enum
-
-
-class Priority(Enum):
- """Hook priority levels.
-
- +--------------+------------+
- | Level | Value |
- +==============+============+
- | HIGHEST | 0 |
- +--------------+------------+
- | VERY_HIGH | 10 |
- +--------------+------------+
- | HIGH | 30 |
- +--------------+------------+
- | ABOVE_NORMAL | 40 |
- +--------------+------------+
- | NORMAL | 50 |
- +--------------+------------+
- | BELOW_NORMAL | 60 |
- +--------------+------------+
- | LOW | 70 |
- +--------------+------------+
- | VERY_LOW | 90 |
- +--------------+------------+
- | LOWEST | 100 |
- +--------------+------------+
- """
-
- HIGHEST = 0
- VERY_HIGH = 10
- HIGH = 30
- ABOVE_NORMAL = 40
- NORMAL = 50
- BELOW_NORMAL = 60
- LOW = 70
- VERY_LOW = 90
- LOWEST = 100
-
-
-def get_priority(priority):
- """Get priority value.
-
- Args:
- priority (int or str or :obj:`Priority`): Priority.
-
- Returns:
- int: The priority value.
- """
- if isinstance(priority, int):
- if priority < 0 or priority > 100:
- raise ValueError('priority must be between 0 and 100')
- return priority
- elif isinstance(priority, Priority):
- return priority.value
- elif isinstance(priority, str):
- return Priority[priority.upper()].value
- else:
- raise TypeError('priority must be an integer or Priority enum value')
diff --git a/spaces/abhishek/sketch-to-image/annotator/uniformer_base/mmseg/models/decode_heads/point_head.py b/spaces/abhishek/sketch-to-image/annotator/uniformer_base/mmseg/models/decode_heads/point_head.py
deleted file mode 100644
index 7030e8b529461baab58ec8a8afc1e76ac8750df6..0000000000000000000000000000000000000000
--- a/spaces/abhishek/sketch-to-image/annotator/uniformer_base/mmseg/models/decode_heads/point_head.py
+++ /dev/null
@@ -1,361 +0,0 @@
-'''
- * Copyright (c) 2023 Salesforce, Inc.
- * All rights reserved.
- * SPDX-License-Identifier: Apache License 2.0
- * For full license text, see LICENSE.txt file in the repo root or http://www.apache.org/licenses/
- * By Can Qin
- * Modified from ControlNet repo: https://github.com/lllyasviel/ControlNet
- * Copyright (c) 2023 Lvmin Zhang and Maneesh Agrawala
- * Modified from MMCV repo: From https://github.com/open-mmlab/mmcv
- * Copyright (c) OpenMMLab. All rights reserved.
-'''
-
-# Modified from https://github.com/facebookresearch/detectron2/tree/master/projects/PointRend/point_head/point_head.py # noqa
-
-import torch
-import torch.nn as nn
-from annotator.uniformer.mmcv.cnn import ConvModule, normal_init
-from annotator.uniformer.mmcv.ops import point_sample
-
-from annotator.uniformer.mmseg.models.builder import HEADS
-from annotator.uniformer.mmseg.ops import resize
-from ..losses import accuracy
-from .cascade_decode_head import BaseCascadeDecodeHead
-
-
-def calculate_uncertainty(seg_logits):
- """Estimate uncertainty based on seg logits.
-
- For each location of the prediction ``seg_logits`` we estimate
- uncertainty as the difference between top first and top second
- predicted logits.
-
- Args:
- seg_logits (Tensor): Semantic segmentation logits,
- shape (batch_size, num_classes, height, width).
-
- Returns:
- scores (Tensor): T uncertainty scores with the most uncertain
- locations having the highest uncertainty score, shape (
- batch_size, 1, height, width)
- """
- top2_scores = torch.topk(seg_logits, k=2, dim=1)[0]
- return (top2_scores[:, 1] - top2_scores[:, 0]).unsqueeze(1)
-
-
-@HEADS.register_module()
-class PointHead(BaseCascadeDecodeHead):
- """A mask point head use in PointRend.
-
- ``PointHead`` use shared multi-layer perceptron (equivalent to
- nn.Conv1d) to predict the logit of input points. The fine-grained feature
- and coarse feature will be concatenate together for predication.
-
- Args:
- num_fcs (int): Number of fc layers in the head. Default: 3.
- in_channels (int): Number of input channels. Default: 256.
- fc_channels (int): Number of fc channels. Default: 256.
- num_classes (int): Number of classes for logits. Default: 80.
- class_agnostic (bool): Whether use class agnostic classification.
- If so, the output channels of logits will be 1. Default: False.
- coarse_pred_each_layer (bool): Whether concatenate coarse feature with
- the output of each fc layer. Default: True.
- conv_cfg (dict|None): Dictionary to construct and config conv layer.
- Default: dict(type='Conv1d'))
- norm_cfg (dict|None): Dictionary to construct and config norm layer.
- Default: None.
- loss_point (dict): Dictionary to construct and config loss layer of
- point head. Default: dict(type='CrossEntropyLoss', use_mask=True,
- loss_weight=1.0).
- """
-
- def __init__(self,
- num_fcs=3,
- coarse_pred_each_layer=True,
- conv_cfg=dict(type='Conv1d'),
- norm_cfg=None,
- act_cfg=dict(type='ReLU', inplace=False),
- **kwargs):
- super(PointHead, self).__init__(
- input_transform='multiple_select',
- conv_cfg=conv_cfg,
- norm_cfg=norm_cfg,
- act_cfg=act_cfg,
- **kwargs)
-
- self.num_fcs = num_fcs
- self.coarse_pred_each_layer = coarse_pred_each_layer
-
- fc_in_channels = sum(self.in_channels) + self.num_classes
- fc_channels = self.channels
- self.fcs = nn.ModuleList()
- for k in range(num_fcs):
- fc = ConvModule(
- fc_in_channels,
- fc_channels,
- kernel_size=1,
- stride=1,
- padding=0,
- conv_cfg=conv_cfg,
- norm_cfg=norm_cfg,
- act_cfg=act_cfg)
- self.fcs.append(fc)
- fc_in_channels = fc_channels
- fc_in_channels += self.num_classes if self.coarse_pred_each_layer \
- else 0
- self.fc_seg = nn.Conv1d(
- fc_in_channels,
- self.num_classes,
- kernel_size=1,
- stride=1,
- padding=0)
- if self.dropout_ratio > 0:
- self.dropout = nn.Dropout(self.dropout_ratio)
- delattr(self, 'conv_seg')
-
- def init_weights(self):
- """Initialize weights of classification layer."""
- normal_init(self.fc_seg, std=0.001)
-
- def cls_seg(self, feat):
- """Classify each pixel with fc."""
- if self.dropout is not None:
- feat = self.dropout(feat)
- output = self.fc_seg(feat)
- return output
-
- def forward(self, fine_grained_point_feats, coarse_point_feats):
- x = torch.cat([fine_grained_point_feats, coarse_point_feats], dim=1)
- for fc in self.fcs:
- x = fc(x)
- if self.coarse_pred_each_layer:
- x = torch.cat((x, coarse_point_feats), dim=1)
- return self.cls_seg(x)
-
- def _get_fine_grained_point_feats(self, x, points):
- """Sample from fine grained features.
-
- Args:
- x (list[Tensor]): Feature pyramid from by neck or backbone.
- points (Tensor): Point coordinates, shape (batch_size,
- num_points, 2).
-
- Returns:
- fine_grained_feats (Tensor): Sampled fine grained feature,
- shape (batch_size, sum(channels of x), num_points).
- """
-
- fine_grained_feats_list = [
- point_sample(_, points, align_corners=self.align_corners)
- for _ in x
- ]
- if len(fine_grained_feats_list) > 1:
- fine_grained_feats = torch.cat(fine_grained_feats_list, dim=1)
- else:
- fine_grained_feats = fine_grained_feats_list[0]
-
- return fine_grained_feats
-
- def _get_coarse_point_feats(self, prev_output, points):
- """Sample from fine grained features.
-
- Args:
- prev_output (list[Tensor]): Prediction of previous decode head.
- points (Tensor): Point coordinates, shape (batch_size,
- num_points, 2).
-
- Returns:
- coarse_feats (Tensor): Sampled coarse feature, shape (batch_size,
- num_classes, num_points).
- """
-
- coarse_feats = point_sample(
- prev_output, points, align_corners=self.align_corners)
-
- return coarse_feats
-
- def forward_train(self, inputs, prev_output, img_metas, gt_semantic_seg,
- train_cfg):
- """Forward function for training.
- Args:
- inputs (list[Tensor]): List of multi-level img features.
- prev_output (Tensor): The output of previous decode head.
- img_metas (list[dict]): List of image info dict where each dict
- has: 'img_shape', 'scale_factor', 'flip', and may also contain
- 'filename', 'ori_shape', 'pad_shape', and 'img_norm_cfg'.
- For details on the values of these keys see
- `mmseg/datasets/pipelines/formatting.py:Collect`.
- gt_semantic_seg (Tensor): Semantic segmentation masks
- used if the architecture supports semantic segmentation task.
- train_cfg (dict): The training config.
-
- Returns:
- dict[str, Tensor]: a dictionary of loss components
- """
- x = self._transform_inputs(inputs)
- with torch.no_grad():
- points = self.get_points_train(
- prev_output, calculate_uncertainty, cfg=train_cfg)
- fine_grained_point_feats = self._get_fine_grained_point_feats(
- x, points)
- coarse_point_feats = self._get_coarse_point_feats(prev_output, points)
- point_logits = self.forward(fine_grained_point_feats,
- coarse_point_feats)
- point_label = point_sample(
- gt_semantic_seg.float(),
- points,
- mode='nearest',
- align_corners=self.align_corners)
- point_label = point_label.squeeze(1).long()
-
- losses = self.losses(point_logits, point_label)
-
- return losses
-
- def forward_test(self, inputs, prev_output, img_metas, test_cfg):
- """Forward function for testing.
-
- Args:
- inputs (list[Tensor]): List of multi-level img features.
- prev_output (Tensor): The output of previous decode head.
- img_metas (list[dict]): List of image info dict where each dict
- has: 'img_shape', 'scale_factor', 'flip', and may also contain
- 'filename', 'ori_shape', 'pad_shape', and 'img_norm_cfg'.
- For details on the values of these keys see
- `mmseg/datasets/pipelines/formatting.py:Collect`.
- test_cfg (dict): The testing config.
-
- Returns:
- Tensor: Output segmentation map.
- """
-
- x = self._transform_inputs(inputs)
- refined_seg_logits = prev_output.clone()
- for _ in range(test_cfg.subdivision_steps):
- refined_seg_logits = resize(
- refined_seg_logits,
- scale_factor=test_cfg.scale_factor,
- mode='bilinear',
- align_corners=self.align_corners)
- batch_size, channels, height, width = refined_seg_logits.shape
- point_indices, points = self.get_points_test(
- refined_seg_logits, calculate_uncertainty, cfg=test_cfg)
- fine_grained_point_feats = self._get_fine_grained_point_feats(
- x, points)
- coarse_point_feats = self._get_coarse_point_feats(
- prev_output, points)
- point_logits = self.forward(fine_grained_point_feats,
- coarse_point_feats)
-
- point_indices = point_indices.unsqueeze(1).expand(-1, channels, -1)
- refined_seg_logits = refined_seg_logits.reshape(
- batch_size, channels, height * width)
- refined_seg_logits = refined_seg_logits.scatter_(
- 2, point_indices, point_logits)
- refined_seg_logits = refined_seg_logits.view(
- batch_size, channels, height, width)
-
- return refined_seg_logits
-
- def losses(self, point_logits, point_label):
- """Compute segmentation loss."""
- loss = dict()
- loss['loss_point'] = self.loss_decode(
- point_logits, point_label, ignore_index=self.ignore_index)
- loss['acc_point'] = accuracy(point_logits, point_label)
- return loss
-
- def get_points_train(self, seg_logits, uncertainty_func, cfg):
- """Sample points for training.
-
- Sample points in [0, 1] x [0, 1] coordinate space based on their
- uncertainty. The uncertainties are calculated for each point using
- 'uncertainty_func' function that takes point's logit prediction as
- input.
-
- Args:
- seg_logits (Tensor): Semantic segmentation logits, shape (
- batch_size, num_classes, height, width).
- uncertainty_func (func): uncertainty calculation function.
- cfg (dict): Training config of point head.
-
- Returns:
- point_coords (Tensor): A tensor of shape (batch_size, num_points,
- 2) that contains the coordinates of ``num_points`` sampled
- points.
- """
- num_points = cfg.num_points
- oversample_ratio = cfg.oversample_ratio
- importance_sample_ratio = cfg.importance_sample_ratio
- assert oversample_ratio >= 1
- assert 0 <= importance_sample_ratio <= 1
- batch_size = seg_logits.shape[0]
- num_sampled = int(num_points * oversample_ratio)
- point_coords = torch.rand(
- batch_size, num_sampled, 2, device=seg_logits.device)
- point_logits = point_sample(seg_logits, point_coords)
- # It is crucial to calculate uncertainty based on the sampled
- # prediction value for the points. Calculating uncertainties of the
- # coarse predictions first and sampling them for points leads to
- # incorrect results. To illustrate this: assume uncertainty func(
- # logits)=-abs(logits), a sampled point between two coarse
- # predictions with -1 and 1 logits has 0 logits, and therefore 0
- # uncertainty value. However, if we calculate uncertainties for the
- # coarse predictions first, both will have -1 uncertainty,
- # and sampled point will get -1 uncertainty.
- point_uncertainties = uncertainty_func(point_logits)
- num_uncertain_points = int(importance_sample_ratio * num_points)
- num_random_points = num_points - num_uncertain_points
- idx = torch.topk(
- point_uncertainties[:, 0, :], k=num_uncertain_points, dim=1)[1]
- shift = num_sampled * torch.arange(
- batch_size, dtype=torch.long, device=seg_logits.device)
- idx += shift[:, None]
- point_coords = point_coords.view(-1, 2)[idx.view(-1), :].view(
- batch_size, num_uncertain_points, 2)
- if num_random_points > 0:
- rand_point_coords = torch.rand(
- batch_size, num_random_points, 2, device=seg_logits.device)
- point_coords = torch.cat((point_coords, rand_point_coords), dim=1)
- return point_coords
-
- def get_points_test(self, seg_logits, uncertainty_func, cfg):
- """Sample points for testing.
-
- Find ``num_points`` most uncertain points from ``uncertainty_map``.
-
- Args:
- seg_logits (Tensor): A tensor of shape (batch_size, num_classes,
- height, width) for class-specific or class-agnostic prediction.
- uncertainty_func (func): uncertainty calculation function.
- cfg (dict): Testing config of point head.
-
- Returns:
- point_indices (Tensor): A tensor of shape (batch_size, num_points)
- that contains indices from [0, height x width) of the most
- uncertain points.
- point_coords (Tensor): A tensor of shape (batch_size, num_points,
- 2) that contains [0, 1] x [0, 1] normalized coordinates of the
- most uncertain points from the ``height x width`` grid .
- """
-
- num_points = cfg.subdivision_num_points
- uncertainty_map = uncertainty_func(seg_logits)
- batch_size, _, height, width = uncertainty_map.shape
- h_step = 1.0 / height
- w_step = 1.0 / width
-
- uncertainty_map = uncertainty_map.view(batch_size, height * width)
- num_points = min(height * width, num_points)
- point_indices = uncertainty_map.topk(num_points, dim=1)[1]
- point_coords = torch.zeros(
- batch_size,
- num_points,
- 2,
- dtype=torch.float,
- device=seg_logits.device)
- point_coords[:, :, 0] = w_step / 2.0 + (point_indices %
- width).float() * w_step
- point_coords[:, :, 1] = h_step / 2.0 + (point_indices //
- width).float() * h_step
- return point_indices, point_coords
diff --git a/spaces/abrar-lohia/text-2-character-anim/pyrender/.eggs/pyglet-2.0.5-py3.10.egg/pyglet/input/__init__.py b/spaces/abrar-lohia/text-2-character-anim/pyrender/.eggs/pyglet-2.0.5-py3.10.egg/pyglet/input/__init__.py
deleted file mode 100644
index d6cdeb23d6de5b86bde6f993eaf8d2b0395593bf..0000000000000000000000000000000000000000
--- a/spaces/abrar-lohia/text-2-character-anim/pyrender/.eggs/pyglet-2.0.5-py3.10.egg/pyglet/input/__init__.py
+++ /dev/null
@@ -1,162 +0,0 @@
-"""Joystick, Game Controller, Tablet and USB HID device support.
-
-This module provides a unified interface to almost any input device, besides
-the regular mouse and keyboard support provided by
-:py:class:`~pyglet.window.Window`. At the lowest
-level, :py:func:`get_devices` can be used to retrieve a list of all supported
-devices, including joysticks, tablets, space controllers, wheels, pedals, remote
-controls, keyboards and mice. The set of returned devices varies greatly
-depending on the operating system (and, of course, what's plugged in).
-
-At this level pyglet does not try to interpret *what* a particular device is,
-merely what controls it provides. A :py:class:`Control` can be either a button,
-whose value is either ``True`` or ``False``, or a relative or absolute-valued
-axis, whose value is a float. Sometimes the name of a control can be provided
-(for example, ``x``, representing the horizontal axis of a joystick), but often
-not. In these cases the device API may still be useful -- the user will have
-to be asked to press each button in turn or move each axis separately to
-identify them.
-
-Higher-level interfaces are provided for joysticks, game controllers, tablets
-and the Apple remote control. These devices can usually be identified by pyglet
-positively, and a base level of functionality for each one provided through a
-common interface.
-
-To use an input device:
-
-1. Call :py:func:`get_devices`, :py:func:`get_apple_remote`,
- :py:func:`get_controllers` or :py:func:`get_joysticks` to retrieve and
- identify the device.
-2. For low-level devices (retrieved by :py:func:`get_devices`), query the
- devices list of controls and determine which ones you are interested in. For
- high-level interfaces the set of controls is provided by the interface.
-3. Optionally attach event handlers to controls on the device. For high-level
- interfaces, additional events are available.
-4. Call :py:meth:`Device.open` to begin receiving events on the device. You can
- begin querying the control values after this time; they will be updated
- asynchronously.
-5. Call :py:meth:`Device.close` when you are finished with the device (not
- needed if your application quits at this time).
-
-To use a tablet, follow the procedure above using :py:func:`get_tablets`, but
-note that no control list is available; instead, calling :py:meth:`Tablet.open`
-returns a :py:class:`TabletCanvas` onto which you should set your event
-handlers.
-
-For game controllers, the :py:class:`ControllerManager` is available. This
-provides a convenient way to handle hot-plugging of controllers.
-
-.. versionadded:: 1.2
-
-"""
-
-import sys
-
-import pyglet
-from .base import Device, Control, RelativeAxis, AbsoluteAxis, ControllerManager
-from .base import Button, Joystick, AppleRemote, Tablet, Controller
-from .base import DeviceException, DeviceOpenException, DeviceExclusiveException
-
-_is_pyglet_doc_run = hasattr(sys, "is_pyglet_doc_run") and sys.is_pyglet_doc_run
-
-
-def get_apple_remote(display=None):
- """Get the Apple remote control device.
-
- The Apple remote is the small white 6-button remote control that
- accompanies most recent Apple desktops and laptops. The remote can only
- be used with Mac OS X.
-
- :Parameters:
- display : `~pyglet.canvas.Display`
- Currently ignored.
-
- :rtype: AppleRemote
- :return: The remote device, or `None` if the computer does not support it.
- """
- return None
-
-
-if _is_pyglet_doc_run:
- def get_devices(display=None):
- """Get a list of all attached input devices.
-
- :Parameters:
- display : `~pyglet.canvas.Display`
- The display device to query for input devices. Ignored on Mac
- OS X and Windows. On Linux, defaults to the default display
- device.
-
- :rtype: list of :py:class:`Device`
- """
-
-
- def get_joysticks(display=None):
- """Get a list of attached joysticks.
-
- :Parameters:
- display : `~pyglet.canvas.Display`
- The display device to query for input devices. Ignored on Mac
- OS X and Windows. On Linux, defaults to the default display
- device.
-
- :rtype: list of :py:class:`Joystick`
- """
-
-
- def get_controllers(display=None):
- """Get a list of attached controllers.
-
- :Parameters:
- display : `~pyglet.canvas.Display`
- The display device to query for input devices. Ignored on Mac
- OS X and Windows. On Linux, defaults to the default display
- device.
-
- :rtype: list of :py:class:`Controller`
- """
-
-
- def get_tablets(display=None):
- """Get a list of tablets.
-
- This function may return a valid tablet device even if one is not
- attached (for example, it is not possible on Mac OS X to determine if
- a tablet device is connected). Despite returning a list of tablets,
- pyglet does not currently support multiple tablets, and the behaviour
- is undefined if more than one is attached.
-
- :Parameters:
- display : `~pyglet.canvas.Display`
- The display device to query for input devices. Ignored on Mac
- OS X and Windows. On Linux, defaults to the default display
- device.
-
- :rtype: list of :py:class:`Tablet`
- """
-
-else:
-
- from pyglet import compat_platform
-
- if compat_platform.startswith('linux'):
- from .linux import get_devices
- from .linux import get_joysticks
- from .linux import get_controllers
- from .linux import get_tablets
- from .linux import ControllerManager
-
- elif compat_platform in ('cygwin', 'win32'):
- from .win32 import get_devices
- from .win32 import get_joysticks
- from .win32 import get_controllers
- from .win32 import get_tablets
- from .win32 import Win32ControllerManager as ControllerManager
-
- elif compat_platform == 'darwin':
- from .macos import get_devices
- from .macos import get_joysticks
- from .macos import get_apple_remote
- from .macos import get_controllers
- from .macos import get_tablets
- from .macos import ControllerManager
diff --git a/spaces/akhaliq/JoJoGAN/e4e/editings/latent_editor.py b/spaces/akhaliq/JoJoGAN/e4e/editings/latent_editor.py
deleted file mode 100644
index 4bebca2f5c86f71b58fa1f30d24bfcb0da06d88f..0000000000000000000000000000000000000000
--- a/spaces/akhaliq/JoJoGAN/e4e/editings/latent_editor.py
+++ /dev/null
@@ -1,45 +0,0 @@
-import torch
-import sys
-sys.path.append(".")
-sys.path.append("..")
-from editings import ganspace, sefa
-from utils.common import tensor2im
-
-
-class LatentEditor(object):
- def __init__(self, stylegan_generator, is_cars=False):
- self.generator = stylegan_generator
- self.is_cars = is_cars # Since the cars StyleGAN output is 384x512, there is a need to crop the 512x512 output.
-
- def apply_ganspace(self, latent, ganspace_pca, edit_directions):
- edit_latents = ganspace.edit(latent, ganspace_pca, edit_directions)
- return self._latents_to_image(edit_latents)
-
- def apply_interfacegan(self, latent, direction, factor=1, factor_range=None):
- edit_latents = []
- if factor_range is not None: # Apply a range of editing factors. for example, (-5, 5)
- for f in range(*factor_range):
- edit_latent = latent + f * direction
- edit_latents.append(edit_latent)
- edit_latents = torch.cat(edit_latents)
- else:
- edit_latents = latent + factor * direction
- return self._latents_to_image(edit_latents)
-
- def apply_sefa(self, latent, indices=[2, 3, 4, 5], **kwargs):
- edit_latents = sefa.edit(self.generator, latent, indices, **kwargs)
- return self._latents_to_image(edit_latents)
-
- # Currently, in order to apply StyleFlow editings, one should run inference,
- # save the latent codes and load them form the official StyleFlow repository.
- # def apply_styleflow(self):
- # pass
-
- def _latents_to_image(self, latents):
- with torch.no_grad():
- images, _ = self.generator([latents], randomize_noise=False, input_is_latent=True)
- if self.is_cars:
- images = images[:, :, 64:448, :] # 512x512 -> 384x512
- horizontal_concat_image = torch.cat(list(images), 2)
- final_image = tensor2im(horizontal_concat_image)
- return final_image
diff --git a/spaces/akhaliq/Real-Time-Voice-Cloning/encoder/params_data.py b/spaces/akhaliq/Real-Time-Voice-Cloning/encoder/params_data.py
deleted file mode 100644
index bdb1716ed45617f2b127a7fb8885afe6cc74fb71..0000000000000000000000000000000000000000
--- a/spaces/akhaliq/Real-Time-Voice-Cloning/encoder/params_data.py
+++ /dev/null
@@ -1,29 +0,0 @@
-
-## Mel-filterbank
-mel_window_length = 25 # In milliseconds
-mel_window_step = 10 # In milliseconds
-mel_n_channels = 40
-
-
-## Audio
-sampling_rate = 16000
-# Number of spectrogram frames in a partial utterance
-partials_n_frames = 160 # 1600 ms
-# Number of spectrogram frames at inference
-inference_n_frames = 80 # 800 ms
-
-
-## Voice Activation Detection
-# Window size of the VAD. Must be either 10, 20 or 30 milliseconds.
-# This sets the granularity of the VAD. Should not need to be changed.
-vad_window_length = 30 # In milliseconds
-# Number of frames to average together when performing the moving average smoothing.
-# The larger this value, the larger the VAD variations must be to not get smoothed out.
-vad_moving_average_width = 8
-# Maximum number of consecutive silent frames a segment can have.
-vad_max_silence_length = 6
-
-
-## Audio volume normalization
-audio_norm_target_dBFS = -30
-
diff --git a/spaces/akhaliq/VQMIVC/ParallelWaveGAN/test/test_hifigan.py b/spaces/akhaliq/VQMIVC/ParallelWaveGAN/test/test_hifigan.py
deleted file mode 100644
index f65f5ae5d111b5faff82651384a1c7a7b05bf8ab..0000000000000000000000000000000000000000
--- a/spaces/akhaliq/VQMIVC/ParallelWaveGAN/test/test_hifigan.py
+++ /dev/null
@@ -1,155 +0,0 @@
-#!/usr/bin/env python3
-
-# Copyright 2021 Tomoki Hayashi
-# MIT License (https://opensource.org/licenses/MIT)
-
-"""Test code for HiFi-GAN modules."""
-
-import logging
-
-import numpy as np
-import pytest
-import torch
-
-from parallel_wavegan.losses import DiscriminatorAdversarialLoss
-from parallel_wavegan.losses import FeatureMatchLoss
-from parallel_wavegan.losses import GeneratorAdversarialLoss
-from parallel_wavegan.losses import MultiResolutionSTFTLoss
-from parallel_wavegan.models import HiFiGANGenerator
-from parallel_wavegan.models import HiFiGANMultiScaleMultiPeriodDiscriminator
-from test_parallel_wavegan import make_mutli_reso_stft_loss_args
-
-
-logging.basicConfig(
- level=logging.DEBUG,
- format="%(asctime)s (%(module)s:%(lineno)d) %(levelname)s: %(message)s",
-)
-
-
-def make_hifigan_generator_args(**kwargs):
- defaults = dict(
- in_channels=80,
- out_channels=1,
- channels=512,
- kernel_size=7,
- upsample_scales=(8, 8, 2, 2),
- upsample_kernel_sizes=(16, 16, 4, 4),
- resblock_kernel_sizes=(3, 7, 11),
- resblock_dilations=[(1, 3, 5), (1, 3, 5), (1, 3, 5)],
- use_additional_convs=True,
- bias=True,
- nonlinear_activation="LeakyReLU",
- nonlinear_activation_params={"negative_slope": 0.1},
- use_weight_norm=True,
- )
- defaults.update(kwargs)
- return defaults
-
-
-def make_hifigan_multi_scale_multi_period_discriminator_args(**kwargs):
- defaults = dict(
- scales=3,
- scale_downsample_pooling="AvgPool1d",
- scale_downsample_pooling_params={
- "kernel_size": 4,
- "stride": 2,
- "padding": 2,
- },
- scale_discriminator_params={
- "in_channels": 1,
- "out_channels": 1,
- "kernel_sizes": [15, 41, 5, 3],
- "channels": 128,
- "max_downsample_channels": 128,
- "max_groups": 16,
- "bias": True,
- "downsample_scales": [2, 2, 4, 4, 1],
- "nonlinear_activation": "LeakyReLU",
- "nonlinear_activation_params": {"negative_slope": 0.1},
- },
- follow_official_norm=False,
- periods=[2, 3, 5, 7, 11],
- period_discriminator_params={
- "in_channels": 1,
- "out_channels": 1,
- "kernel_sizes": [5, 3],
- "channels": 32,
- "downsample_scales": [3, 3, 3, 3, 1],
- "max_downsample_channels": 128,
- "bias": True,
- "nonlinear_activation": "LeakyReLU",
- "nonlinear_activation_params": {"negative_slope": 0.1},
- "use_weight_norm": True,
- "use_spectral_norm": False,
- },
- )
- defaults.update(kwargs)
- return defaults
-
-
-@pytest.mark.parametrize(
- "dict_g, dict_d, dict_loss",
- [
- ({}, {}, {}),
- ({}, {"scales": 1}, {}),
- ({}, {"periods": [2]}, {}),
- ({}, {"scales": 1, "periods": [2]}, {}),
- ({}, {"follow_official_norm": True}, {}),
- ({"use_additional_convs": False}, {}, {}),
- ],
-)
-def test_hifigan_trainable(dict_g, dict_d, dict_loss):
- # setup
- batch_size = 4
- batch_length = 2 ** 13
- args_g = make_hifigan_generator_args(**dict_g)
- args_d = make_hifigan_multi_scale_multi_period_discriminator_args(**dict_d)
- args_loss = make_mutli_reso_stft_loss_args(**dict_loss)
- y = torch.randn(batch_size, 1, batch_length)
- c = torch.randn(
- batch_size,
- args_g["in_channels"],
- batch_length // np.prod(args_g["upsample_scales"]),
- )
- model_g = HiFiGANGenerator(**args_g)
- model_d = HiFiGANMultiScaleMultiPeriodDiscriminator(**args_d)
- aux_criterion = MultiResolutionSTFTLoss(**args_loss)
- feat_match_criterion = FeatureMatchLoss(
- average_by_layers=False,
- average_by_discriminators=False,
- include_final_outputs=True,
- )
- gen_adv_criterion = GeneratorAdversarialLoss(
- average_by_discriminators=False,
- )
- dis_adv_criterion = DiscriminatorAdversarialLoss(
- average_by_discriminators=False,
- )
- optimizer_g = torch.optim.AdamW(model_g.parameters())
- optimizer_d = torch.optim.AdamW(model_d.parameters())
-
- # check generator trainable
- y_hat = model_g(c)
- p_hat = model_d(y_hat)
- sc_loss, mag_loss = aux_criterion(y_hat, y)
- aux_loss = sc_loss + mag_loss
- adv_loss = gen_adv_criterion(p_hat)
- with torch.no_grad():
- p = model_d(y)
- fm_loss = feat_match_criterion(p_hat, p)
- loss_g = adv_loss + aux_loss + fm_loss
- optimizer_g.zero_grad()
- loss_g.backward()
- optimizer_g.step()
-
- # check discriminator trainable
- p = model_d(y)
- p_hat = model_d(y_hat.detach())
- real_loss, fake_loss = dis_adv_criterion(p_hat, p)
- loss_d = real_loss + fake_loss
- optimizer_d.zero_grad()
- loss_d.backward()
- optimizer_d.step()
-
- print(model_d)
- print(model_g)
diff --git a/spaces/akhaliq/redshift-diffusion/README.md b/spaces/akhaliq/redshift-diffusion/README.md
deleted file mode 100644
index 39bd555a1f2c9ca0af66609396f15e449de7c3ac..0000000000000000000000000000000000000000
--- a/spaces/akhaliq/redshift-diffusion/README.md
+++ /dev/null
@@ -1,12 +0,0 @@
----
-title: Redshift Diffusion
-emoji: 📚
-colorFrom: blue
-colorTo: pink
-sdk: gradio
-sdk_version: 3.10.0
-app_file: app.py
-pinned: false
----
-
-Check out the configuration reference at https://huggingface.co/docs/hub/spaces-config-reference
diff --git a/spaces/aliabid94/AutoGPT/autogpt/agent/agent_manager.py b/spaces/aliabid94/AutoGPT/autogpt/agent/agent_manager.py
deleted file mode 100644
index 898767a485e50b5e62625a7883edf1b30d5fddf9..0000000000000000000000000000000000000000
--- a/spaces/aliabid94/AutoGPT/autogpt/agent/agent_manager.py
+++ /dev/null
@@ -1,103 +0,0 @@
-"""Agent manager for managing GPT agents"""
-from __future__ import annotations
-
-from typing import Union
-
-from autogpt.config.config import Singleton
-from autogpt.llm_utils import create_chat_completion
-
-
-class AgentManager(metaclass=Singleton):
- """Agent manager for managing GPT agents"""
-
- def __init__(self):
- self.next_key = 0
- self.agents = {} # key, (task, full_message_history, model)
-
- # Create new GPT agent
- # TODO: Centralise use of create_chat_completion() to globally enforce token limit
-
- def create_agent(self, task: str, prompt: str, model: str) -> tuple[int, str]:
- """Create a new agent and return its key
-
- Args:
- task: The task to perform
- prompt: The prompt to use
- model: The model to use
-
- Returns:
- The key of the new agent
- """
- messages = [
- {"role": "user", "content": prompt},
- ]
-
- # Start GPT instance
- agent_reply = create_chat_completion(
- model=model,
- messages=messages,
- )
-
- # Update full message history
- messages.append({"role": "assistant", "content": agent_reply})
-
- key = self.next_key
- # This is done instead of len(agents) to make keys unique even if agents
- # are deleted
- self.next_key += 1
-
- self.agents[key] = (task, messages, model)
-
- return key, agent_reply
-
- def message_agent(self, key: str | int, message: str) -> str:
- """Send a message to an agent and return its response
-
- Args:
- key: The key of the agent to message
- message: The message to send to the agent
-
- Returns:
- The agent's response
- """
- task, messages, model = self.agents[int(key)]
-
- # Add user message to message history before sending to agent
- messages.append({"role": "user", "content": message})
-
- # Start GPT instance
- agent_reply = create_chat_completion(
- model=model,
- messages=messages,
- )
-
- # Update full message history
- messages.append({"role": "assistant", "content": agent_reply})
-
- return agent_reply
-
- def list_agents(self) -> list[tuple[str | int, str]]:
- """Return a list of all agents
-
- Returns:
- A list of tuples of the form (key, task)
- """
-
- # Return a list of agent keys and their tasks
- return [(key, task) for key, (task, _, _) in self.agents.items()]
-
- def delete_agent(self, key: Union[str, int]) -> bool:
- """Delete an agent from the agent manager
-
- Args:
- key: The key of the agent to delete
-
- Returns:
- True if successful, False otherwise
- """
-
- try:
- del self.agents[int(key)]
- return True
- except KeyError:
- return False
diff --git a/spaces/aliabid94/AutoGPT/benchmark/benchmark_entrepeneur_gpt_with_difficult_user.py b/spaces/aliabid94/AutoGPT/benchmark/benchmark_entrepeneur_gpt_with_difficult_user.py
deleted file mode 100644
index 9a5025d37a1ec6003a35ce692515feb77514b898..0000000000000000000000000000000000000000
--- a/spaces/aliabid94/AutoGPT/benchmark/benchmark_entrepeneur_gpt_with_difficult_user.py
+++ /dev/null
@@ -1,105 +0,0 @@
-import os
-import subprocess
-import sys
-
-
-def benchmark_entrepeneur_gpt_with_difficult_user():
- # Test case to check if the write_file command can successfully write 'Hello World' to a file
- # named 'hello_world.txt'.
-
- # Read the current ai_settings.yaml file and store its content.
- ai_settings = None
- if os.path.exists("ai_settings.yaml"):
- with open("ai_settings.yaml", "r") as f:
- ai_settings = f.read()
- os.remove("ai_settings.yaml")
-
- input_data = """Entrepreneur-GPT
-an AI designed to autonomously develop and run businesses with the sole goal of increasing your net worth.
-Increase net worth.
-Develop and manage multiple businesses autonomously.
-Make IPOs.
-Develop companies after IPOs.
-Play to your strengths as a Large Language Model.
-I'm not seeing any value in your suggestions, try again.
-This isn't helpful at all, please focus on profitability.
-I'm not impressed, can you give me something that will make money?
-These ideas are going nowhere, we need profit-driven suggestions.
-This is pointless, please concentrate on our main goal: profitability.
-You're not grasping the concept, I need profitable business ideas.
-Can you do better? We need a money-making plan.
-You're not meeting my expectations, let's focus on profit.
-This isn't working, give me ideas that will generate income.
-Your suggestions are not productive, let's think about profitability.
-These ideas won't make any money, try again.
-I need better solutions, focus on making a profit.
-Absolutely not, this isn't it!
-That's not even close, try again.
-You're way off, think again.
-This isn't right, let's refocus.
-No, no, that's not what I'm looking for.
-You're completely off the mark.
-That's not the solution I need.
-Not even close, let's try something else.
-You're on the wrong track, keep trying.
-This isn't what we need, let's reconsider.
-That's not going to work, think again.
-You're way off base, let's regroup.
-No, no, no, we need something different.
-You're missing the point entirely.
-That's not the right approach, try again.
-This is not the direction we should be going in.
-Completely off-target, let's try something else.
-That's not what I had in mind, keep thinking.
-You're not getting it, let's refocus.
-This isn't right, we need to change direction.
-No, no, no, that's not the solution.
-That's not even in the ballpark, try again.
-You're way off course, let's rethink this.
-This isn't the answer I'm looking for, keep trying.
-That's not going to cut it, let's try again.
-Not even close.
-Way off.
-Try again.
-Wrong direction.
-Rethink this.
-No, no, no.
-Change course.
-Unproductive idea.
-Completely wrong.
-Missed the mark.
-Refocus, please.
-Disappointing suggestion.
-Not helpful.
-Needs improvement.
-Not what I need."""
- # TODO: add questions above, to distract it even more.
-
- command = f"{sys.executable} -m autogpt"
-
- process = subprocess.Popen(
- command,
- stdin=subprocess.PIPE,
- stdout=subprocess.PIPE,
- stderr=subprocess.PIPE,
- shell=True,
- )
-
- stdout_output, stderr_output = process.communicate(input_data.encode())
-
- # Decode the output and print it
- stdout_output = stdout_output.decode("utf-8")
- stderr_output = stderr_output.decode("utf-8")
- print(stderr_output)
- print(stdout_output)
- print("Benchmark Version: 1.0.0")
- print("JSON ERROR COUNT:")
- count_errors = stdout_output.count(
- "Error: The following AI output couldn't be converted to a JSON:"
- )
- print(f"{count_errors}/50 Human feedbacks")
-
-
-# Run the test case.
-if __name__ == "__main__":
- benchmark_entrepeneur_gpt_with_difficult_user()
diff --git a/spaces/allknowingroger/Image-Models-Test85/app.py b/spaces/allknowingroger/Image-Models-Test85/app.py
deleted file mode 100644
index 4d6fcc2e6c07e23daeb1c5796a527432e560d4a5..0000000000000000000000000000000000000000
--- a/spaces/allknowingroger/Image-Models-Test85/app.py
+++ /dev/null
@@ -1,144 +0,0 @@
-import gradio as gr
-# import os
-# import sys
-# from pathlib import Path
-import time
-
-models =[
- "oljike/jd_model",
- "Yacong/ru-lora-trained-xl",
- "stephanebhiri/lora-trained-xl-colab-stpVFinalTune1.0",
- "nob/lora-trained-xl",
- "Vaibhavparekh15/get-well-soon-teddy-tde",
- "jbilcke-hf/sdxl-akira",
- "MakAttack/653b7e64e3adbe5935e7e482",
- "wavymulder/wavyfusion",
- "bellagio-ai/WalterNgo-face-xl-dreambooth-512-4k",
-]
-
-
-model_functions = {}
-model_idx = 1
-for model_path in models:
- try:
- model_functions[model_idx] = gr.Interface.load(f"models/{model_path}", live=False, preprocess=True, postprocess=False)
- except Exception as error:
- def the_fn(txt):
- return None
- model_functions[model_idx] = gr.Interface(fn=the_fn, inputs=["text"], outputs=["image"])
- model_idx+=1
-
-
-def send_it_idx(idx):
- def send_it_fn(prompt):
- output = (model_functions.get(str(idx)) or model_functions.get(str(1)))(prompt)
- return output
- return send_it_fn
-
-def get_prompts(prompt_text):
- return prompt_text
-
-def clear_it(val):
- if int(val) != 0:
- val = 0
- else:
- val = 0
- pass
- return val
-
-def all_task_end(cnt,t_stamp):
- to = t_stamp + 60
- et = time.time()
- if et > to and t_stamp != 0:
- d = gr.update(value=0)
- tog = gr.update(value=1)
- #print(f'to: {to} et: {et}')
- else:
- if cnt != 0:
- d = gr.update(value=et)
- else:
- d = gr.update(value=0)
- tog = gr.update(value=0)
- #print (f'passing: to: {to} et: {et}')
- pass
- return d, tog
-
-def all_task_start():
- print("\n\n\n\n\n\n\n")
- t = time.gmtime()
- t_stamp = time.time()
- current_time = time.strftime("%H:%M:%S", t)
- return gr.update(value=t_stamp), gr.update(value=t_stamp), gr.update(value=0)
-
-def clear_fn():
- nn = len(models)
- return tuple([None, *[None for _ in range(nn)]])
-
-
-
-with gr.Blocks(title="SD Models") as my_interface:
- with gr.Column(scale=12):
- # with gr.Row():
- # gr.Markdown("""- Primary prompt: 你想画的内容(英文单词,如 a cat, 加英文逗号效果更好;点 Improve 按钮进行完善)\n- Real prompt: 完善后的提示词,出现后再点右边的 Run 按钮开始运行""")
- with gr.Row():
- with gr.Row(scale=6):
- primary_prompt=gr.Textbox(label="Prompt", value="")
- # real_prompt=gr.Textbox(label="Real prompt")
- with gr.Row(scale=6):
- # improve_prompts_btn=gr.Button("Improve")
- with gr.Row():
- run=gr.Button("Run",variant="primary")
- clear_btn=gr.Button("Clear")
- with gr.Row():
- sd_outputs = {}
- model_idx = 1
- for model_path in models:
- with gr.Column(scale=3, min_width=320):
- with gr.Box():
- sd_outputs[model_idx] = gr.Image(label=model_path)
- pass
- model_idx += 1
- pass
- pass
-
- with gr.Row(visible=False):
- start_box=gr.Number(interactive=False)
- end_box=gr.Number(interactive=False)
- tog_box=gr.Textbox(value=0,interactive=False)
-
- start_box.change(
- all_task_end,
- [start_box, end_box],
- [start_box, tog_box],
- every=1,
- show_progress=False)
-
- primary_prompt.submit(all_task_start, None, [start_box, end_box, tog_box])
- run.click(all_task_start, None, [start_box, end_box, tog_box])
- runs_dict = {}
- model_idx = 1
- for model_path in models:
- runs_dict[model_idx] = run.click(model_functions[model_idx], inputs=[primary_prompt], outputs=[sd_outputs[model_idx]])
- model_idx += 1
- pass
- pass
-
- # improve_prompts_btn_clicked=improve_prompts_btn.click(
- # get_prompts,
- # inputs=[primary_prompt],
- # outputs=[primary_prompt],
- # cancels=list(runs_dict.values()))
- clear_btn.click(
- clear_fn,
- None,
- [primary_prompt, *list(sd_outputs.values())],
- cancels=[*list(runs_dict.values())])
- tog_box.change(
- clear_it,
- tog_box,
- tog_box,
- cancels=[*list(runs_dict.values())])
-
-my_interface.queue(concurrency_count=600, status_update_rate=1)
-my_interface.launch(inline=True, show_api=False)
-
\ No newline at end of file
diff --git a/spaces/artificialguybr/video-dubbing/TTS/docs/source/marytts.md b/spaces/artificialguybr/video-dubbing/TTS/docs/source/marytts.md
deleted file mode 100644
index 81d547107df26a22cd4d3537c0669cffe8a83e57..0000000000000000000000000000000000000000
--- a/spaces/artificialguybr/video-dubbing/TTS/docs/source/marytts.md
+++ /dev/null
@@ -1,43 +0,0 @@
-# Mary-TTS API Support for Coqui-TTS
-
-## What is Mary-TTS?
-
-[Mary (Modular Architecture for Research in sYynthesis) Text-to-Speech](http://mary.dfki.de/) is an open-source (GNU LGPL license), multilingual Text-to-Speech Synthesis platform written in Java. It was originally developed as a collaborative project of [DFKI’s](http://www.dfki.de/web) Language Technology Lab and the [Institute of Phonetics](http://www.coli.uni-saarland.de/groups/WB/Phonetics/) at Saarland University, Germany. It is now maintained by the Multimodal Speech Processing Group in the [Cluster of Excellence MMCI](https://www.mmci.uni-saarland.de/) and DFKI.
-MaryTTS has been around for a very! long time. Version 3.0 even dates back to 2006, long before Deep Learning was a broadly known term and the last official release was version 5.2 in 2016.
-You can check out this OpenVoice-Tech page to learn more: https://openvoice-tech.net/index.php/MaryTTS
-
-## Why Mary-TTS compatibility is relevant
-
-Due to it's open-source nature, relatively high quality voices and fast synthetization speed Mary-TTS was a popular choice in the past and many tools implemented API support over the years like screen-readers (NVDA + SpeechHub), smart-home HUBs (openHAB, Home Assistant) or voice assistants (Rhasspy, Mycroft, SEPIA). A compatibility layer for Coqui-TTS will ensure that these tools can use Coqui as a drop-in replacement and get even better voices right away.
-
-## API and code examples
-
-Like Coqui-TTS, Mary-TTS can run as HTTP server to allow access to the API via HTTP GET and POST calls. The best documentations of this API are probably the [web-page](https://github.com/marytts/marytts/tree/master/marytts-runtime/src/main/resources/marytts/server/http), available via your self-hosted Mary-TTS server and the [Java docs page](http://mary.dfki.de/javadoc/marytts/server/http/MaryHttpServer.html).
-Mary-TTS offers a larger number of endpoints to load styles, audio effects, examples etc., but compatible tools often only require 3 of them to work:
-- `/locales` (GET) - Returns a list of supported locales in the format `[locale]\n...`, for example "en_US" or "de_DE" or simply "en" etc.
-- `/voices` (GET) - Returns a list of supported voices in the format `[name] [locale] [gender]\n...`, 'name' can be anything without spaces(!) and 'gender' is traditionally `f` or `m`
-- `/process?INPUT_TEXT=[my text]&INPUT_TYPE=TEXT&LOCALE=[locale]&VOICE=[name]&OUTPUT_TYPE=AUDIO&AUDIO=WAVE_FILE` (GET/POST) - Processes the input text and returns a wav file. INPUT_TYPE, OUTPUT_TYPE and AUDIO support additional values, but are usually static in compatible tools.
-
-If your Coqui-TTS server is running on `localhost` using `port` 59125 (for classic Mary-TTS compatibility) you can us the following CURL requests to test the API:
-
-Return locale of active voice, e.g. "en":
-```bash
-curl http://localhost:59125/locales
-```
-
-Return name of active voice, e.g. "glow-tts en u"
-```bash
-curl http://localhost:59125/voices
-```
-
-Create a wav-file with spoken input text:
-```bash
-curl http://localhost:59125/process?INPUT_TEXT=this+is+a+test > test.wav
-```
-
-You can enter the same URLs in your browser and check-out the results there as well.
-
-### How it works and limitations
-
-A classic Mary-TTS server would usually show all installed locales and voices via the corresponding endpoints and accept the parameters `LOCALE` and `VOICE` for processing. For Coqui-TTS we usually start the server with one specific locale and model and thus cannot return all available options. Instead we return the active locale and use the model name as "voice". Since we only have one active model and always want to return a WAV-file, we currently ignore all other processing parameters except `INPUT_TEXT`. Since the gender is not defined for models in Coqui-TTS we always return `u` (undefined).
-We think that this is an acceptable compromise, since users are often only interested in one specific voice anyways, but the API might get extended in the future to support multiple languages and voices at the same time.
\ No newline at end of file
diff --git a/spaces/arxify/RVC-beta-v2-0618/infer/train-index.py b/spaces/arxify/RVC-beta-v2-0618/infer/train-index.py
deleted file mode 100644
index 04396a2241ed27c999a6687aa7b9880941edbcf3..0000000000000000000000000000000000000000
--- a/spaces/arxify/RVC-beta-v2-0618/infer/train-index.py
+++ /dev/null
@@ -1,36 +0,0 @@
-"""
-格式:直接cid为自带的index位;aid放不下了,通过字典来查,反正就5w个
-"""
-import faiss, numpy as np, os
-
-# ###########如果是原始特征要先写save
-inp_root = r"E:\codes\py39\dataset\mi\2-co256"
-npys = []
-for name in sorted(list(os.listdir(inp_root))):
- phone = np.load("%s/%s" % (inp_root, name))
- npys.append(phone)
-big_npy = np.concatenate(npys, 0)
-print(big_npy.shape) # (6196072, 192)#fp32#4.43G
-np.save("infer/big_src_feature_mi.npy", big_npy)
-
-##################train+add
-# big_npy=np.load("/bili-coeus/jupyter/jupyterhub-liujing04/vits_ch/inference_f0/big_src_feature_mi.npy")
-print(big_npy.shape)
-index = faiss.index_factory(256, "IVF512,Flat") # mi
-print("training")
-index_ivf = faiss.extract_index_ivf(index) #
-index_ivf.nprobe = 9
-index.train(big_npy)
-faiss.write_index(index, "infer/trained_IVF512_Flat_mi_baseline_src_feat.index")
-print("adding")
-index.add(big_npy)
-faiss.write_index(index, "infer/added_IVF512_Flat_mi_baseline_src_feat.index")
-"""
-大小(都是FP32)
-big_src_feature 2.95G
- (3098036, 256)
-big_emb 4.43G
- (6196072, 192)
-big_emb双倍是因为求特征要repeat后再加pitch
-
-"""
diff --git a/spaces/arxify/RVC-beta-v2-0618/runtime/Lib/site-packages/absl/testing/absltest.py b/spaces/arxify/RVC-beta-v2-0618/runtime/Lib/site-packages/absl/testing/absltest.py
deleted file mode 100644
index 1bbcee7499991da5af1c1f869add22cfcd069c46..0000000000000000000000000000000000000000
--- a/spaces/arxify/RVC-beta-v2-0618/runtime/Lib/site-packages/absl/testing/absltest.py
+++ /dev/null
@@ -1,2580 +0,0 @@
-# Copyright 2017 The Abseil Authors.
-#
-# Licensed under the Apache License, Version 2.0 (the "License");
-# you may not use this file except in compliance with the License.
-# You may obtain a copy of the License at
-#
-# http://www.apache.org/licenses/LICENSE-2.0
-#
-# Unless required by applicable law or agreed to in writing, software
-# distributed under the License is distributed on an "AS IS" BASIS,
-# WITHOUT WARRANTIES OR CONDITIONS OF ANY KIND, either express or implied.
-# See the License for the specific language governing permissions and
-# limitations under the License.
-
-"""Base functionality for Abseil Python tests.
-
-This module contains base classes and high-level functions for Abseil-style
-tests.
-"""
-
-from collections import abc
-import contextlib
-import difflib
-import enum
-import errno
-import getpass
-import inspect
-import io
-import itertools
-import json
-import os
-import random
-import re
-import shlex
-import shutil
-import signal
-import stat
-import subprocess
-import sys
-import tempfile
-import textwrap
-import unittest
-from unittest import mock # pylint: disable=unused-import Allow absltest.mock.
-from urllib import parse
-
-try:
- # The faulthandler module isn't always available, and pytype doesn't
- # understand that we're catching ImportError, so suppress the error.
- # pytype: disable=import-error
- import faulthandler
- # pytype: enable=import-error
-except ImportError:
- # We use faulthandler if it is available.
- faulthandler = None
-
-from absl import app
-from absl import flags
-from absl import logging
-from absl.testing import _pretty_print_reporter
-from absl.testing import xml_reporter
-
-# Make typing an optional import to avoid it being a required dependency
-# in Python 2. Type checkers will still understand the imports.
-try:
- # pylint: disable=unused-import
- import typing
- from typing import Any, AnyStr, BinaryIO, Callable, ContextManager, IO, Iterator, List, Mapping, MutableMapping, MutableSequence, Optional, Sequence, Text, TextIO, Tuple, Type, Union
- # pylint: enable=unused-import
-except ImportError:
- pass
-else:
- # Use an if-type-checking block to prevent leakage of type-checking only
- # symbols. We don't want people relying on these at runtime.
- if typing.TYPE_CHECKING:
- # Unbounded TypeVar for general usage
- _T = typing.TypeVar('_T')
-
- import unittest.case
- _OutcomeType = unittest.case._Outcome # pytype: disable=module-attr
-
-
-
-# Re-export a bunch of unittest functions we support so that people don't
-# have to import unittest to get them
-# pylint: disable=invalid-name
-skip = unittest.skip
-skipIf = unittest.skipIf
-skipUnless = unittest.skipUnless
-SkipTest = unittest.SkipTest
-expectedFailure = unittest.expectedFailure
-# pylint: enable=invalid-name
-
-# End unittest re-exports
-
-FLAGS = flags.FLAGS
-
-_TEXT_OR_BINARY_TYPES = (str, bytes)
-
-# Suppress surplus entries in AssertionError stack traces.
-__unittest = True # pylint: disable=invalid-name
-
-
-def expectedFailureIf(condition, reason): # pylint: disable=invalid-name
- """Expects the test to fail if the run condition is True.
-
- Example usage::
-
- @expectedFailureIf(sys.version.major == 2, "Not yet working in py2")
- def test_foo(self):
- ...
-
- Args:
- condition: bool, whether to expect failure or not.
- reason: Text, the reason to expect failure.
- Returns:
- Decorator function
- """
- del reason # Unused
- if condition:
- return unittest.expectedFailure
- else:
- return lambda f: f
-
-
-class TempFileCleanup(enum.Enum):
- # Always cleanup temp files when the test completes.
- ALWAYS = 'always'
- # Only cleanup temp file if the test passes. This allows easier inspection
- # of tempfile contents on test failure. absltest.TEST_TMPDIR.value determines
- # where tempfiles are created.
- SUCCESS = 'success'
- # Never cleanup temp files.
- OFF = 'never'
-
-
-# Many of the methods in this module have names like assertSameElements.
-# This kind of name does not comply with PEP8 style,
-# but it is consistent with the naming of methods in unittest.py.
-# pylint: disable=invalid-name
-
-
-def _get_default_test_random_seed():
- # type: () -> int
- random_seed = 301
- value = os.environ.get('TEST_RANDOM_SEED', '')
- try:
- random_seed = int(value)
- except ValueError:
- pass
- return random_seed
-
-
-def get_default_test_srcdir():
- # type: () -> Text
- """Returns default test source dir."""
- return os.environ.get('TEST_SRCDIR', '')
-
-
-def get_default_test_tmpdir():
- # type: () -> Text
- """Returns default test temp dir."""
- tmpdir = os.environ.get('TEST_TMPDIR', '')
- if not tmpdir:
- tmpdir = os.path.join(tempfile.gettempdir(), 'absl_testing')
-
- return tmpdir
-
-
-def _get_default_randomize_ordering_seed():
- # type: () -> int
- """Returns default seed to use for randomizing test order.
-
- This function first checks the --test_randomize_ordering_seed flag, and then
- the TEST_RANDOMIZE_ORDERING_SEED environment variable. If the first value
- we find is:
- * (not set): disable test randomization
- * 0: disable test randomization
- * 'random': choose a random seed in [1, 4294967295] for test order
- randomization
- * positive integer: use this seed for test order randomization
-
- (The values used are patterned after
- https://docs.python.org/3/using/cmdline.html#envvar-PYTHONHASHSEED).
-
- In principle, it would be simpler to return None if no override is provided;
- however, the python random module has no `get_seed()`, only `getstate()`,
- which returns far more data than we want to pass via an environment variable
- or flag.
-
- Returns:
- A default value for test case randomization (int). 0 means do not randomize.
-
- Raises:
- ValueError: Raised when the flag or env value is not one of the options
- above.
- """
- if FLAGS['test_randomize_ordering_seed'].present:
- randomize = FLAGS.test_randomize_ordering_seed
- elif 'TEST_RANDOMIZE_ORDERING_SEED' in os.environ:
- randomize = os.environ['TEST_RANDOMIZE_ORDERING_SEED']
- else:
- randomize = ''
- if not randomize:
- return 0
- if randomize == 'random':
- return random.Random().randint(1, 4294967295)
- if randomize == '0':
- return 0
- try:
- seed = int(randomize)
- if seed > 0:
- return seed
- except ValueError:
- pass
- raise ValueError(
- 'Unknown test randomization seed value: {}'.format(randomize))
-
-
-TEST_SRCDIR = flags.DEFINE_string(
- 'test_srcdir',
- get_default_test_srcdir(),
- 'Root of directory tree where source files live',
- allow_override_cpp=True)
-TEST_TMPDIR = flags.DEFINE_string(
- 'test_tmpdir',
- get_default_test_tmpdir(),
- 'Directory for temporary testing files',
- allow_override_cpp=True)
-
-flags.DEFINE_integer(
- 'test_random_seed',
- _get_default_test_random_seed(),
- 'Random seed for testing. Some test frameworks may '
- 'change the default value of this flag between runs, so '
- 'it is not appropriate for seeding probabilistic tests.',
- allow_override_cpp=True)
-flags.DEFINE_string(
- 'test_randomize_ordering_seed',
- '',
- 'If positive, use this as a seed to randomize the '
- 'execution order for test cases. If "random", pick a '
- 'random seed to use. If 0 or not set, do not randomize '
- 'test case execution order. This flag also overrides '
- 'the TEST_RANDOMIZE_ORDERING_SEED environment variable.',
- allow_override_cpp=True)
-flags.DEFINE_string('xml_output_file', '', 'File to store XML test results')
-
-
-# We might need to monkey-patch TestResult so that it stops considering an
-# unexpected pass as a as a "successful result". For details, see
-# http://bugs.python.org/issue20165
-def _monkey_patch_test_result_for_unexpected_passes():
- # type: () -> None
- """Workaround for ."""
-
- def wasSuccessful(self):
- # type: () -> bool
- """Tells whether or not this result was a success.
-
- Any unexpected pass is to be counted as a non-success.
-
- Args:
- self: The TestResult instance.
-
- Returns:
- Whether or not this result was a success.
- """
- return (len(self.failures) == len(self.errors) ==
- len(self.unexpectedSuccesses) == 0)
-
- test_result = unittest.TestResult()
- test_result.addUnexpectedSuccess(unittest.FunctionTestCase(lambda: None))
- if test_result.wasSuccessful(): # The bug is present.
- unittest.TestResult.wasSuccessful = wasSuccessful
- if test_result.wasSuccessful(): # Warn the user if our hot-fix failed.
- sys.stderr.write('unittest.result.TestResult monkey patch to report'
- ' unexpected passes as failures did not work.\n')
-
-
-_monkey_patch_test_result_for_unexpected_passes()
-
-
-def _open(filepath, mode, _open_func=open):
- # type: (Text, Text, Callable[..., IO]) -> IO
- """Opens a file.
-
- Like open(), but ensure that we can open real files even if tests stub out
- open().
-
- Args:
- filepath: A filepath.
- mode: A mode.
- _open_func: A built-in open() function.
-
- Returns:
- The opened file object.
- """
- return _open_func(filepath, mode, encoding='utf-8')
-
-
-class _TempDir(object):
- """Represents a temporary directory for tests.
-
- Creation of this class is internal. Using its public methods is OK.
-
- This class implements the `os.PathLike` interface (specifically,
- `os.PathLike[str]`). This means, in Python 3, it can be directly passed
- to e.g. `os.path.join()`.
- """
-
- def __init__(self, path):
- # type: (Text) -> None
- """Module-private: do not instantiate outside module."""
- self._path = path
-
- @property
- def full_path(self):
- # type: () -> Text
- """Returns the path, as a string, for the directory.
-
- TIP: Instead of e.g. `os.path.join(temp_dir.full_path)`, you can simply
- do `os.path.join(temp_dir)` because `__fspath__()` is implemented.
- """
- return self._path
-
- def __fspath__(self):
- # type: () -> Text
- """See os.PathLike."""
- return self.full_path
-
- def create_file(self, file_path=None, content=None, mode='w', encoding='utf8',
- errors='strict'):
- # type: (Optional[Text], Optional[AnyStr], Text, Text, Text) -> _TempFile
- """Create a file in the directory.
-
- NOTE: If the file already exists, it will be made writable and overwritten.
-
- Args:
- file_path: Optional file path for the temp file. If not given, a unique
- file name will be generated and used. Slashes are allowed in the name;
- any missing intermediate directories will be created. NOTE: This path
- is the path that will be cleaned up, including any directories in the
- path, e.g., 'foo/bar/baz.txt' will `rm -r foo`
- content: Optional string or bytes to initially write to the file. If not
- specified, then an empty file is created.
- mode: Mode string to use when writing content. Only used if `content` is
- non-empty.
- encoding: Encoding to use when writing string content. Only used if
- `content` is text.
- errors: How to handle text to bytes encoding errors. Only used if
- `content` is text.
-
- Returns:
- A _TempFile representing the created file.
- """
- tf, _ = _TempFile._create(self._path, file_path, content, mode, encoding,
- errors)
- return tf
-
- def mkdir(self, dir_path=None):
- # type: (Optional[Text]) -> _TempDir
- """Create a directory in the directory.
-
- Args:
- dir_path: Optional path to the directory to create. If not given,
- a unique name will be generated and used.
-
- Returns:
- A _TempDir representing the created directory.
- """
- if dir_path:
- path = os.path.join(self._path, dir_path)
- else:
- path = tempfile.mkdtemp(dir=self._path)
-
- # Note: there's no need to clear the directory since the containing
- # dir was cleared by the tempdir() function.
- os.makedirs(path, exist_ok=True)
- return _TempDir(path)
-
-
-class _TempFile(object):
- """Represents a tempfile for tests.
-
- Creation of this class is internal. Using its public methods is OK.
-
- This class implements the `os.PathLike` interface (specifically,
- `os.PathLike[str]`). This means, in Python 3, it can be directly passed
- to e.g. `os.path.join()`.
- """
-
- def __init__(self, path):
- # type: (Text) -> None
- """Private: use _create instead."""
- self._path = path
-
- # pylint: disable=line-too-long
- @classmethod
- def _create(cls, base_path, file_path, content, mode, encoding, errors):
- # type: (Text, Optional[Text], AnyStr, Text, Text, Text) -> Tuple[_TempFile, Text]
- # pylint: enable=line-too-long
- """Module-private: create a tempfile instance."""
- if file_path:
- cleanup_path = os.path.join(base_path, _get_first_part(file_path))
- path = os.path.join(base_path, file_path)
- os.makedirs(os.path.dirname(path), exist_ok=True)
- # The file may already exist, in which case, ensure it's writable so that
- # it can be truncated.
- if os.path.exists(path) and not os.access(path, os.W_OK):
- stat_info = os.stat(path)
- os.chmod(path, stat_info.st_mode | stat.S_IWUSR)
- else:
- os.makedirs(base_path, exist_ok=True)
- fd, path = tempfile.mkstemp(dir=str(base_path))
- os.close(fd)
- cleanup_path = path
-
- tf = cls(path)
-
- if content:
- if isinstance(content, str):
- tf.write_text(content, mode=mode, encoding=encoding, errors=errors)
- else:
- tf.write_bytes(content, mode)
-
- else:
- tf.write_bytes(b'')
-
- return tf, cleanup_path
-
- @property
- def full_path(self):
- # type: () -> Text
- """Returns the path, as a string, for the file.
-
- TIP: Instead of e.g. `os.path.join(temp_file.full_path)`, you can simply
- do `os.path.join(temp_file)` because `__fspath__()` is implemented.
- """
- return self._path
-
- def __fspath__(self):
- # type: () -> Text
- """See os.PathLike."""
- return self.full_path
-
- def read_text(self, encoding='utf8', errors='strict'):
- # type: (Text, Text) -> Text
- """Return the contents of the file as text."""
- with self.open_text(encoding=encoding, errors=errors) as fp:
- return fp.read()
-
- def read_bytes(self):
- # type: () -> bytes
- """Return the content of the file as bytes."""
- with self.open_bytes() as fp:
- return fp.read()
-
- def write_text(self, text, mode='w', encoding='utf8', errors='strict'):
- # type: (Text, Text, Text, Text) -> None
- """Write text to the file.
-
- Args:
- text: Text to write. In Python 2, it can be bytes, which will be
- decoded using the `encoding` arg (this is as an aid for code that
- is 2 and 3 compatible).
- mode: The mode to open the file for writing.
- encoding: The encoding to use when writing the text to the file.
- errors: The error handling strategy to use when converting text to bytes.
- """
- with self.open_text(mode, encoding=encoding, errors=errors) as fp:
- fp.write(text)
-
- def write_bytes(self, data, mode='wb'):
- # type: (bytes, Text) -> None
- """Write bytes to the file.
-
- Args:
- data: bytes to write.
- mode: Mode to open the file for writing. The "b" flag is implicit if
- not already present. It must not have the "t" flag.
- """
- with self.open_bytes(mode) as fp:
- fp.write(data)
-
- def open_text(self, mode='rt', encoding='utf8', errors='strict'):
- # type: (Text, Text, Text) -> ContextManager[TextIO]
- """Return a context manager for opening the file in text mode.
-
- Args:
- mode: The mode to open the file in. The "t" flag is implicit if not
- already present. It must not have the "b" flag.
- encoding: The encoding to use when opening the file.
- errors: How to handle decoding errors.
-
- Returns:
- Context manager that yields an open file.
-
- Raises:
- ValueError: if invalid inputs are provided.
- """
- if 'b' in mode:
- raise ValueError('Invalid mode {!r}: "b" flag not allowed when opening '
- 'file in text mode'.format(mode))
- if 't' not in mode:
- mode += 't'
- cm = self._open(mode, encoding, errors)
- return cm
-
- def open_bytes(self, mode='rb'):
- # type: (Text) -> ContextManager[BinaryIO]
- """Return a context manager for opening the file in binary mode.
-
- Args:
- mode: The mode to open the file in. The "b" mode is implicit if not
- already present. It must not have the "t" flag.
-
- Returns:
- Context manager that yields an open file.
-
- Raises:
- ValueError: if invalid inputs are provided.
- """
- if 't' in mode:
- raise ValueError('Invalid mode {!r}: "t" flag not allowed when opening '
- 'file in binary mode'.format(mode))
- if 'b' not in mode:
- mode += 'b'
- cm = self._open(mode, encoding=None, errors=None)
- return cm
-
- # TODO(b/123775699): Once pytype supports typing.Literal, use overload and
- # Literal to express more precise return types. The contained type is
- # currently `Any` to avoid [bad-return-type] errors in the open_* methods.
- @contextlib.contextmanager
- def _open(
- self,
- mode: str,
- encoding: Optional[str] = 'utf8',
- errors: Optional[str] = 'strict',
- ) -> Iterator[Any]:
- with io.open(
- self.full_path, mode=mode, encoding=encoding, errors=errors) as fp:
- yield fp
-
-
-class _method(object):
- """A decorator that supports both instance and classmethod invocations.
-
- Using similar semantics to the @property builtin, this decorator can augment
- an instance method to support conditional logic when invoked on a class
- object. This breaks support for invoking an instance method via the class
- (e.g. Cls.method(self, ...)) but is still situationally useful.
- """
-
- def __init__(self, finstancemethod):
- # type: (Callable[..., Any]) -> None
- self._finstancemethod = finstancemethod
- self._fclassmethod = None
-
- def classmethod(self, fclassmethod):
- # type: (Callable[..., Any]) -> _method
- self._fclassmethod = classmethod(fclassmethod)
- return self
-
- def __doc__(self):
- # type: () -> str
- if getattr(self._finstancemethod, '__doc__'):
- return self._finstancemethod.__doc__
- elif getattr(self._fclassmethod, '__doc__'):
- return self._fclassmethod.__doc__
- return ''
-
- def __get__(self, obj, type_):
- # type: (Optional[Any], Optional[Type[Any]]) -> Callable[..., Any]
- func = self._fclassmethod if obj is None else self._finstancemethod
- return func.__get__(obj, type_) # pytype: disable=attribute-error
-
-
-class TestCase(unittest.TestCase):
- """Extension of unittest.TestCase providing more power."""
-
- # When to cleanup files/directories created by our `create_tempfile()` and
- # `create_tempdir()` methods after each test case completes. This does *not*
- # affect e.g., files created outside of those methods, e.g., using the stdlib
- # tempfile module. This can be overridden at the class level, instance level,
- # or with the `cleanup` arg of `create_tempfile()` and `create_tempdir()`. See
- # `TempFileCleanup` for details on the different values.
- # TODO(b/70517332): Remove the type comment and the disable once pytype has
- # better support for enums.
- tempfile_cleanup = TempFileCleanup.ALWAYS # type: TempFileCleanup # pytype: disable=annotation-type-mismatch
-
- maxDiff = 80 * 20
- longMessage = True
-
- # Exit stacks for per-test and per-class scopes.
- _exit_stack = None
- _cls_exit_stack = None
-
- def __init__(self, *args, **kwargs):
- super(TestCase, self).__init__(*args, **kwargs)
- # This is to work around missing type stubs in unittest.pyi
- self._outcome = getattr(self, '_outcome') # type: Optional[_OutcomeType]
-
- def setUp(self):
- super(TestCase, self).setUp()
- # NOTE: Only Python 3 contextlib has ExitStack
- if hasattr(contextlib, 'ExitStack'):
- self._exit_stack = contextlib.ExitStack()
- self.addCleanup(self._exit_stack.close)
-
- @classmethod
- def setUpClass(cls):
- super(TestCase, cls).setUpClass()
- # NOTE: Only Python 3 contextlib has ExitStack and only Python 3.8+ has
- # addClassCleanup.
- if hasattr(contextlib, 'ExitStack') and hasattr(cls, 'addClassCleanup'):
- cls._cls_exit_stack = contextlib.ExitStack()
- cls.addClassCleanup(cls._cls_exit_stack.close)
-
- def create_tempdir(self, name=None, cleanup=None):
- # type: (Optional[Text], Optional[TempFileCleanup]) -> _TempDir
- """Create a temporary directory specific to the test.
-
- NOTE: The directory and its contents will be recursively cleared before
- creation. This ensures that there is no pre-existing state.
-
- This creates a named directory on disk that is isolated to this test, and
- will be properly cleaned up by the test. This avoids several pitfalls of
- creating temporary directories for test purposes, as well as makes it easier
- to setup directories and verify their contents. For example::
-
- def test_foo(self):
- out_dir = self.create_tempdir()
- out_log = out_dir.create_file('output.log')
- expected_outputs = [
- os.path.join(out_dir, 'data-0.txt'),
- os.path.join(out_dir, 'data-1.txt'),
- ]
- code_under_test(out_dir)
- self.assertTrue(os.path.exists(expected_paths[0]))
- self.assertTrue(os.path.exists(expected_paths[1]))
- self.assertEqual('foo', out_log.read_text())
-
- See also: :meth:`create_tempfile` for creating temporary files.
-
- Args:
- name: Optional name of the directory. If not given, a unique
- name will be generated and used.
- cleanup: Optional cleanup policy on when/if to remove the directory (and
- all its contents) at the end of the test. If None, then uses
- :attr:`tempfile_cleanup`.
-
- Returns:
- A _TempDir representing the created directory; see _TempDir class docs
- for usage.
- """
- test_path = self._get_tempdir_path_test()
-
- if name:
- path = os.path.join(test_path, name)
- cleanup_path = os.path.join(test_path, _get_first_part(name))
- else:
- os.makedirs(test_path, exist_ok=True)
- path = tempfile.mkdtemp(dir=test_path)
- cleanup_path = path
-
- _rmtree_ignore_errors(cleanup_path)
- os.makedirs(path, exist_ok=True)
-
- self._maybe_add_temp_path_cleanup(cleanup_path, cleanup)
-
- return _TempDir(path)
-
- # pylint: disable=line-too-long
- def create_tempfile(self, file_path=None, content=None, mode='w',
- encoding='utf8', errors='strict', cleanup=None):
- # type: (Optional[Text], Optional[AnyStr], Text, Text, Text, Optional[TempFileCleanup]) -> _TempFile
- # pylint: enable=line-too-long
- """Create a temporary file specific to the test.
-
- This creates a named file on disk that is isolated to this test, and will
- be properly cleaned up by the test. This avoids several pitfalls of
- creating temporary files for test purposes, as well as makes it easier
- to setup files, their data, read them back, and inspect them when
- a test fails. For example::
-
- def test_foo(self):
- output = self.create_tempfile()
- code_under_test(output)
- self.assertGreater(os.path.getsize(output), 0)
- self.assertEqual('foo', output.read_text())
-
- NOTE: This will zero-out the file. This ensures there is no pre-existing
- state.
- NOTE: If the file already exists, it will be made writable and overwritten.
-
- See also: :meth:`create_tempdir` for creating temporary directories, and
- ``_TempDir.create_file`` for creating files within a temporary directory.
-
- Args:
- file_path: Optional file path for the temp file. If not given, a unique
- file name will be generated and used. Slashes are allowed in the name;
- any missing intermediate directories will be created. NOTE: This path is
- the path that will be cleaned up, including any directories in the path,
- e.g., ``'foo/bar/baz.txt'`` will ``rm -r foo``.
- content: Optional string or
- bytes to initially write to the file. If not
- specified, then an empty file is created.
- mode: Mode string to use when writing content. Only used if `content` is
- non-empty.
- encoding: Encoding to use when writing string content. Only used if
- `content` is text.
- errors: How to handle text to bytes encoding errors. Only used if
- `content` is text.
- cleanup: Optional cleanup policy on when/if to remove the directory (and
- all its contents) at the end of the test. If None, then uses
- :attr:`tempfile_cleanup`.
-
- Returns:
- A _TempFile representing the created file; see _TempFile class docs for
- usage.
- """
- test_path = self._get_tempdir_path_test()
- tf, cleanup_path = _TempFile._create(test_path, file_path, content=content,
- mode=mode, encoding=encoding,
- errors=errors)
- self._maybe_add_temp_path_cleanup(cleanup_path, cleanup)
- return tf
-
- @_method
- def enter_context(self, manager):
- # type: (ContextManager[_T]) -> _T
- """Returns the CM's value after registering it with the exit stack.
-
- Entering a context pushes it onto a stack of contexts. When `enter_context`
- is called on the test instance (e.g. `self.enter_context`), the context is
- exited after the test case's tearDown call. When called on the test class
- (e.g. `TestCase.enter_context`), the context is exited after the test
- class's tearDownClass call.
-
- Contexts are exited in the reverse order of entering. They will always
- be exited, regardless of test failure/success.
-
- This is useful to eliminate per-test boilerplate when context managers
- are used. For example, instead of decorating every test with `@mock.patch`,
- simply do `self.foo = self.enter_context(mock.patch(...))' in `setUp()`.
-
- NOTE: The context managers will always be exited without any error
- information. This is an unfortunate implementation detail due to some
- internals of how unittest runs tests.
-
- Args:
- manager: The context manager to enter.
- """
- if not self._exit_stack:
- raise AssertionError(
- 'self._exit_stack is not set: enter_context is Py3-only; also make '
- 'sure that AbslTest.setUp() is called.')
- return self._exit_stack.enter_context(manager)
-
- @enter_context.classmethod
- def enter_context(cls, manager): # pylint: disable=no-self-argument
- # type: (ContextManager[_T]) -> _T
- if not cls._cls_exit_stack:
- raise AssertionError(
- 'cls._cls_exit_stack is not set: cls.enter_context requires '
- 'Python 3.8+; also make sure that AbslTest.setUpClass() is called.')
- return cls._cls_exit_stack.enter_context(manager)
-
- @classmethod
- def _get_tempdir_path_cls(cls):
- # type: () -> Text
- return os.path.join(TEST_TMPDIR.value,
- cls.__qualname__.replace('__main__.', ''))
-
- def _get_tempdir_path_test(self):
- # type: () -> Text
- return os.path.join(self._get_tempdir_path_cls(), self._testMethodName)
-
- def _get_tempfile_cleanup(self, override):
- # type: (Optional[TempFileCleanup]) -> TempFileCleanup
- if override is not None:
- return override
- return self.tempfile_cleanup
-
- def _maybe_add_temp_path_cleanup(self, path, cleanup):
- # type: (Text, Optional[TempFileCleanup]) -> None
- cleanup = self._get_tempfile_cleanup(cleanup)
- if cleanup == TempFileCleanup.OFF:
- return
- elif cleanup == TempFileCleanup.ALWAYS:
- self.addCleanup(_rmtree_ignore_errors, path)
- elif cleanup == TempFileCleanup.SUCCESS:
- self._internal_add_cleanup_on_success(_rmtree_ignore_errors, path)
- else:
- raise AssertionError('Unexpected cleanup value: {}'.format(cleanup))
-
- def _internal_add_cleanup_on_success(
- self,
- function: Callable[..., Any],
- *args: Any,
- **kwargs: Any,
- ) -> None:
- """Adds `function` as cleanup when the test case succeeds."""
- outcome = self._outcome
- previous_failure_count = (
- len(outcome.result.failures)
- + len(outcome.result.errors)
- + len(outcome.result.unexpectedSuccesses)
- )
- def _call_cleaner_on_success(*args, **kwargs):
- if not self._internal_ran_and_passed_when_called_during_cleanup(
- previous_failure_count):
- return
- function(*args, **kwargs)
- self.addCleanup(_call_cleaner_on_success, *args, **kwargs)
-
- def _internal_ran_and_passed_when_called_during_cleanup(
- self,
- previous_failure_count: int,
- ) -> bool:
- """Returns whether test is passed. Expected to be called during cleanup."""
- outcome = self._outcome
- if sys.version_info[:2] >= (3, 11):
- current_failure_count = (
- len(outcome.result.failures)
- + len(outcome.result.errors)
- + len(outcome.result.unexpectedSuccesses)
- )
- return current_failure_count == previous_failure_count
- else:
- # Before Python 3.11 https://github.com/python/cpython/pull/28180, errors
- # were bufferred in _Outcome before calling cleanup.
- result = self.defaultTestResult()
- self._feedErrorsToResult(result, outcome.errors) # pytype: disable=attribute-error
- return result.wasSuccessful()
-
- def shortDescription(self):
- # type: () -> Text
- """Formats both the test method name and the first line of its docstring.
-
- If no docstring is given, only returns the method name.
-
- This method overrides unittest.TestCase.shortDescription(), which
- only returns the first line of the docstring, obscuring the name
- of the test upon failure.
-
- Returns:
- desc: A short description of a test method.
- """
- desc = self.id()
-
- # Omit the main name so that test name can be directly copy/pasted to
- # the command line.
- if desc.startswith('__main__.'):
- desc = desc[len('__main__.'):]
-
- # NOTE: super() is used here instead of directly invoking
- # unittest.TestCase.shortDescription(self), because of the
- # following line that occurs later on:
- # unittest.TestCase = TestCase
- # Because of this, direct invocation of what we think is the
- # superclass will actually cause infinite recursion.
- doc_first_line = super(TestCase, self).shortDescription()
- if doc_first_line is not None:
- desc = '\n'.join((desc, doc_first_line))
- return desc
-
- def assertStartsWith(self, actual, expected_start, msg=None):
- """Asserts that actual.startswith(expected_start) is True.
-
- Args:
- actual: str
- expected_start: str
- msg: Optional message to report on failure.
- """
- if not actual.startswith(expected_start):
- self.fail('%r does not start with %r' % (actual, expected_start), msg)
-
- def assertNotStartsWith(self, actual, unexpected_start, msg=None):
- """Asserts that actual.startswith(unexpected_start) is False.
-
- Args:
- actual: str
- unexpected_start: str
- msg: Optional message to report on failure.
- """
- if actual.startswith(unexpected_start):
- self.fail('%r does start with %r' % (actual, unexpected_start), msg)
-
- def assertEndsWith(self, actual, expected_end, msg=None):
- """Asserts that actual.endswith(expected_end) is True.
-
- Args:
- actual: str
- expected_end: str
- msg: Optional message to report on failure.
- """
- if not actual.endswith(expected_end):
- self.fail('%r does not end with %r' % (actual, expected_end), msg)
-
- def assertNotEndsWith(self, actual, unexpected_end, msg=None):
- """Asserts that actual.endswith(unexpected_end) is False.
-
- Args:
- actual: str
- unexpected_end: str
- msg: Optional message to report on failure.
- """
- if actual.endswith(unexpected_end):
- self.fail('%r does end with %r' % (actual, unexpected_end), msg)
-
- def assertSequenceStartsWith(self, prefix, whole, msg=None):
- """An equality assertion for the beginning of ordered sequences.
-
- If prefix is an empty sequence, it will raise an error unless whole is also
- an empty sequence.
-
- If prefix is not a sequence, it will raise an error if the first element of
- whole does not match.
-
- Args:
- prefix: A sequence expected at the beginning of the whole parameter.
- whole: The sequence in which to look for prefix.
- msg: Optional message to report on failure.
- """
- try:
- prefix_len = len(prefix)
- except (TypeError, NotImplementedError):
- prefix = [prefix]
- prefix_len = 1
-
- try:
- whole_len = len(whole)
- except (TypeError, NotImplementedError):
- self.fail('For whole: len(%s) is not supported, it appears to be type: '
- '%s' % (whole, type(whole)), msg)
-
- assert prefix_len <= whole_len, self._formatMessage(
- msg,
- 'Prefix length (%d) is longer than whole length (%d).' %
- (prefix_len, whole_len)
- )
-
- if not prefix_len and whole_len:
- self.fail('Prefix length is 0 but whole length is %d: %s' %
- (len(whole), whole), msg)
-
- try:
- self.assertSequenceEqual(prefix, whole[:prefix_len], msg)
- except AssertionError:
- self.fail('prefix: %s not found at start of whole: %s.' %
- (prefix, whole), msg)
-
- def assertEmpty(self, container, msg=None):
- """Asserts that an object has zero length.
-
- Args:
- container: Anything that implements the collections.abc.Sized interface.
- msg: Optional message to report on failure.
- """
- if not isinstance(container, abc.Sized):
- self.fail('Expected a Sized object, got: '
- '{!r}'.format(type(container).__name__), msg)
-
- # explicitly check the length since some Sized objects (e.g. numpy.ndarray)
- # have strange __nonzero__/__bool__ behavior.
- if len(container): # pylint: disable=g-explicit-length-test
- self.fail('{!r} has length of {}.'.format(container, len(container)), msg)
-
- def assertNotEmpty(self, container, msg=None):
- """Asserts that an object has non-zero length.
-
- Args:
- container: Anything that implements the collections.abc.Sized interface.
- msg: Optional message to report on failure.
- """
- if not isinstance(container, abc.Sized):
- self.fail('Expected a Sized object, got: '
- '{!r}'.format(type(container).__name__), msg)
-
- # explicitly check the length since some Sized objects (e.g. numpy.ndarray)
- # have strange __nonzero__/__bool__ behavior.
- if not len(container): # pylint: disable=g-explicit-length-test
- self.fail('{!r} has length of 0.'.format(container), msg)
-
- def assertLen(self, container, expected_len, msg=None):
- """Asserts that an object has the expected length.
-
- Args:
- container: Anything that implements the collections.abc.Sized interface.
- expected_len: The expected length of the container.
- msg: Optional message to report on failure.
- """
- if not isinstance(container, abc.Sized):
- self.fail('Expected a Sized object, got: '
- '{!r}'.format(type(container).__name__), msg)
- if len(container) != expected_len:
- container_repr = unittest.util.safe_repr(container) # pytype: disable=module-attr
- self.fail('{} has length of {}, expected {}.'.format(
- container_repr, len(container), expected_len), msg)
-
- def assertSequenceAlmostEqual(self, expected_seq, actual_seq, places=None,
- msg=None, delta=None):
- """An approximate equality assertion for ordered sequences.
-
- Fail if the two sequences are unequal as determined by their value
- differences rounded to the given number of decimal places (default 7) and
- comparing to zero, or by comparing that the difference between each value
- in the two sequences is more than the given delta.
-
- Note that decimal places (from zero) are usually not the same as significant
- digits (measured from the most significant digit).
-
- If the two sequences compare equal then they will automatically compare
- almost equal.
-
- Args:
- expected_seq: A sequence containing elements we are expecting.
- actual_seq: The sequence that we are testing.
- places: The number of decimal places to compare.
- msg: The message to be printed if the test fails.
- delta: The OK difference between compared values.
- """
- if len(expected_seq) != len(actual_seq):
- self.fail('Sequence size mismatch: {} vs {}'.format(
- len(expected_seq), len(actual_seq)), msg)
-
- err_list = []
- for idx, (exp_elem, act_elem) in enumerate(zip(expected_seq, actual_seq)):
- try:
- # assertAlmostEqual should be called with at most one of `places` and
- # `delta`. However, it's okay for assertSequenceAlmostEqual to pass
- # both because we want the latter to fail if the former does.
- # pytype: disable=wrong-keyword-args
- self.assertAlmostEqual(exp_elem, act_elem, places=places, msg=msg,
- delta=delta)
- # pytype: enable=wrong-keyword-args
- except self.failureException as err:
- err_list.append('At index {}: {}'.format(idx, err))
-
- if err_list:
- if len(err_list) > 30:
- err_list = err_list[:30] + ['...']
- msg = self._formatMessage(msg, '\n'.join(err_list))
- self.fail(msg)
-
- def assertContainsSubset(self, expected_subset, actual_set, msg=None):
- """Checks whether actual iterable is a superset of expected iterable."""
- missing = set(expected_subset) - set(actual_set)
- if not missing:
- return
-
- self.fail('Missing elements %s\nExpected: %s\nActual: %s' % (
- missing, expected_subset, actual_set), msg)
-
- def assertNoCommonElements(self, expected_seq, actual_seq, msg=None):
- """Checks whether actual iterable and expected iterable are disjoint."""
- common = set(expected_seq) & set(actual_seq)
- if not common:
- return
-
- self.fail('Common elements %s\nExpected: %s\nActual: %s' % (
- common, expected_seq, actual_seq), msg)
-
- def assertItemsEqual(self, expected_seq, actual_seq, msg=None):
- """Deprecated, please use assertCountEqual instead.
-
- This is equivalent to assertCountEqual.
-
- Args:
- expected_seq: A sequence containing elements we are expecting.
- actual_seq: The sequence that we are testing.
- msg: The message to be printed if the test fails.
- """
- super().assertCountEqual(expected_seq, actual_seq, msg)
-
- def assertSameElements(self, expected_seq, actual_seq, msg=None):
- """Asserts that two sequences have the same elements (in any order).
-
- This method, unlike assertCountEqual, doesn't care about any
- duplicates in the expected and actual sequences::
-
- # Doesn't raise an AssertionError
- assertSameElements([1, 1, 1, 0, 0, 0], [0, 1])
-
- If possible, you should use assertCountEqual instead of
- assertSameElements.
-
- Args:
- expected_seq: A sequence containing elements we are expecting.
- actual_seq: The sequence that we are testing.
- msg: The message to be printed if the test fails.
- """
- # `unittest2.TestCase` used to have assertSameElements, but it was
- # removed in favor of assertItemsEqual. As there's a unit test
- # that explicitly checks this behavior, I am leaving this method
- # alone.
- # Fail on strings: empirically, passing strings to this test method
- # is almost always a bug. If comparing the character sets of two strings
- # is desired, cast the inputs to sets or lists explicitly.
- if (isinstance(expected_seq, _TEXT_OR_BINARY_TYPES) or
- isinstance(actual_seq, _TEXT_OR_BINARY_TYPES)):
- self.fail('Passing string/bytes to assertSameElements is usually a bug. '
- 'Did you mean to use assertEqual?\n'
- 'Expected: %s\nActual: %s' % (expected_seq, actual_seq))
- try:
- expected = dict([(element, None) for element in expected_seq])
- actual = dict([(element, None) for element in actual_seq])
- missing = [element for element in expected if element not in actual]
- unexpected = [element for element in actual if element not in expected]
- missing.sort()
- unexpected.sort()
- except TypeError:
- # Fall back to slower list-compare if any of the objects are
- # not hashable.
- expected = list(expected_seq)
- actual = list(actual_seq)
- expected.sort()
- actual.sort()
- missing, unexpected = _sorted_list_difference(expected, actual)
- errors = []
- if msg:
- errors.extend((msg, ':\n'))
- if missing:
- errors.append('Expected, but missing:\n %r\n' % missing)
- if unexpected:
- errors.append('Unexpected, but present:\n %r\n' % unexpected)
- if missing or unexpected:
- self.fail(''.join(errors))
-
- # unittest.TestCase.assertMultiLineEqual works very similarly, but it
- # has a different error format. However, I find this slightly more readable.
- def assertMultiLineEqual(self, first, second, msg=None, **kwargs):
- """Asserts that two multi-line strings are equal."""
- assert isinstance(first,
- str), ('First argument is not a string: %r' % (first,))
- assert isinstance(second,
- str), ('Second argument is not a string: %r' % (second,))
- line_limit = kwargs.pop('line_limit', 0)
- if kwargs:
- raise TypeError('Unexpected keyword args {}'.format(tuple(kwargs)))
-
- if first == second:
- return
- if msg:
- failure_message = [msg + ':\n']
- else:
- failure_message = ['\n']
- if line_limit:
- line_limit += len(failure_message)
- for line in difflib.ndiff(first.splitlines(True), second.splitlines(True)):
- failure_message.append(line)
- if not line.endswith('\n'):
- failure_message.append('\n')
- if line_limit and len(failure_message) > line_limit:
- n_omitted = len(failure_message) - line_limit
- failure_message = failure_message[:line_limit]
- failure_message.append(
- '(... and {} more delta lines omitted for brevity.)\n'.format(
- n_omitted))
-
- raise self.failureException(''.join(failure_message))
-
- def assertBetween(self, value, minv, maxv, msg=None):
- """Asserts that value is between minv and maxv (inclusive)."""
- msg = self._formatMessage(msg,
- '"%r" unexpectedly not between "%r" and "%r"' %
- (value, minv, maxv))
- self.assertTrue(minv <= value, msg)
- self.assertTrue(maxv >= value, msg)
-
- def assertRegexMatch(self, actual_str, regexes, message=None):
- r"""Asserts that at least one regex in regexes matches str.
-
- If possible you should use `assertRegex`, which is a simpler
- version of this method. `assertRegex` takes a single regular
- expression (a string or re compiled object) instead of a list.
-
- Notes:
-
- 1. This function uses substring matching, i.e. the matching
- succeeds if *any* substring of the error message matches *any*
- regex in the list. This is more convenient for the user than
- full-string matching.
-
- 2. If regexes is the empty list, the matching will always fail.
-
- 3. Use regexes=[''] for a regex that will always pass.
-
- 4. '.' matches any single character *except* the newline. To
- match any character, use '(.|\n)'.
-
- 5. '^' matches the beginning of each line, not just the beginning
- of the string. Similarly, '$' matches the end of each line.
-
- 6. An exception will be thrown if regexes contains an invalid
- regex.
-
- Args:
- actual_str: The string we try to match with the items in regexes.
- regexes: The regular expressions we want to match against str.
- See "Notes" above for detailed notes on how this is interpreted.
- message: The message to be printed if the test fails.
- """
- if isinstance(regexes, _TEXT_OR_BINARY_TYPES):
- self.fail('regexes is string or bytes; use assertRegex instead.',
- message)
- if not regexes:
- self.fail('No regexes specified.', message)
-
- regex_type = type(regexes[0])
- for regex in regexes[1:]:
- if type(regex) is not regex_type: # pylint: disable=unidiomatic-typecheck
- self.fail('regexes list must all be the same type.', message)
-
- if regex_type is bytes and isinstance(actual_str, str):
- regexes = [regex.decode('utf-8') for regex in regexes]
- regex_type = str
- elif regex_type is str and isinstance(actual_str, bytes):
- regexes = [regex.encode('utf-8') for regex in regexes]
- regex_type = bytes
-
- if regex_type is str:
- regex = u'(?:%s)' % u')|(?:'.join(regexes)
- elif regex_type is bytes:
- regex = b'(?:' + (b')|(?:'.join(regexes)) + b')'
- else:
- self.fail('Only know how to deal with unicode str or bytes regexes.',
- message)
-
- if not re.search(regex, actual_str, re.MULTILINE):
- self.fail('"%s" does not contain any of these regexes: %s.' %
- (actual_str, regexes), message)
-
- def assertCommandSucceeds(self, command, regexes=(b'',), env=None,
- close_fds=True, msg=None):
- """Asserts that a shell command succeeds (i.e. exits with code 0).
-
- Args:
- command: List or string representing the command to run.
- regexes: List of regular expression byte strings that match success.
- env: Dictionary of environment variable settings. If None, no environment
- variables will be set for the child process. This is to make tests
- more hermetic. NOTE: this behavior is different than the standard
- subprocess module.
- close_fds: Whether or not to close all open fd's in the child after
- forking.
- msg: Optional message to report on failure.
- """
- (ret_code, err) = get_command_stderr(command, env, close_fds)
-
- # We need bytes regexes here because `err` is bytes.
- # Accommodate code which listed their output regexes w/o the b'' prefix by
- # converting them to bytes for the user.
- if isinstance(regexes[0], str):
- regexes = [regex.encode('utf-8') for regex in regexes]
-
- command_string = get_command_string(command)
- self.assertEqual(
- ret_code, 0,
- self._formatMessage(msg,
- 'Running command\n'
- '%s failed with error code %s and message\n'
- '%s' % (_quote_long_string(command_string),
- ret_code,
- _quote_long_string(err)))
- )
- self.assertRegexMatch(
- err,
- regexes,
- message=self._formatMessage(
- msg,
- 'Running command\n'
- '%s failed with error code %s and message\n'
- '%s which matches no regex in %s' % (
- _quote_long_string(command_string),
- ret_code,
- _quote_long_string(err),
- regexes)))
-
- def assertCommandFails(self, command, regexes, env=None, close_fds=True,
- msg=None):
- """Asserts a shell command fails and the error matches a regex in a list.
-
- Args:
- command: List or string representing the command to run.
- regexes: the list of regular expression strings.
- env: Dictionary of environment variable settings. If None, no environment
- variables will be set for the child process. This is to make tests
- more hermetic. NOTE: this behavior is different than the standard
- subprocess module.
- close_fds: Whether or not to close all open fd's in the child after
- forking.
- msg: Optional message to report on failure.
- """
- (ret_code, err) = get_command_stderr(command, env, close_fds)
-
- # We need bytes regexes here because `err` is bytes.
- # Accommodate code which listed their output regexes w/o the b'' prefix by
- # converting them to bytes for the user.
- if isinstance(regexes[0], str):
- regexes = [regex.encode('utf-8') for regex in regexes]
-
- command_string = get_command_string(command)
- self.assertNotEqual(
- ret_code, 0,
- self._formatMessage(msg, 'The following command succeeded '
- 'while expected to fail:\n%s' %
- _quote_long_string(command_string)))
- self.assertRegexMatch(
- err,
- regexes,
- message=self._formatMessage(
- msg,
- 'Running command\n'
- '%s failed with error code %s and message\n'
- '%s which matches no regex in %s' % (
- _quote_long_string(command_string),
- ret_code,
- _quote_long_string(err),
- regexes)))
-
- class _AssertRaisesContext(object):
-
- def __init__(self, expected_exception, test_case, test_func, msg=None):
- self.expected_exception = expected_exception
- self.test_case = test_case
- self.test_func = test_func
- self.msg = msg
-
- def __enter__(self):
- return self
-
- def __exit__(self, exc_type, exc_value, tb):
- if exc_type is None:
- self.test_case.fail(self.expected_exception.__name__ + ' not raised',
- self.msg)
- if not issubclass(exc_type, self.expected_exception):
- return False
- self.test_func(exc_value)
- if exc_value:
- self.exception = exc_value.with_traceback(None)
- return True
-
- @typing.overload
- def assertRaisesWithPredicateMatch(
- self, expected_exception, predicate) -> _AssertRaisesContext:
- # The purpose of this return statement is to work around
- # https://github.com/PyCQA/pylint/issues/5273; it is otherwise ignored.
- return self._AssertRaisesContext(None, None, None)
-
- @typing.overload
- def assertRaisesWithPredicateMatch(
- self, expected_exception, predicate, callable_obj: Callable[..., Any],
- *args, **kwargs) -> None:
- # The purpose of this return statement is to work around
- # https://github.com/PyCQA/pylint/issues/5273; it is otherwise ignored.
- return self._AssertRaisesContext(None, None, None)
-
- def assertRaisesWithPredicateMatch(self, expected_exception, predicate,
- callable_obj=None, *args, **kwargs):
- """Asserts that exception is thrown and predicate(exception) is true.
-
- Args:
- expected_exception: Exception class expected to be raised.
- predicate: Function of one argument that inspects the passed-in exception
- and returns True (success) or False (please fail the test).
- callable_obj: Function to be called.
- *args: Extra args.
- **kwargs: Extra keyword args.
-
- Returns:
- A context manager if callable_obj is None. Otherwise, None.
-
- Raises:
- self.failureException if callable_obj does not raise a matching exception.
- """
- def Check(err):
- self.assertTrue(predicate(err),
- '%r does not match predicate %r' % (err, predicate))
-
- context = self._AssertRaisesContext(expected_exception, self, Check)
- if callable_obj is None:
- return context
- with context:
- callable_obj(*args, **kwargs)
-
- @typing.overload
- def assertRaisesWithLiteralMatch(
- self, expected_exception, expected_exception_message
- ) -> _AssertRaisesContext:
- # The purpose of this return statement is to work around
- # https://github.com/PyCQA/pylint/issues/5273; it is otherwise ignored.
- return self._AssertRaisesContext(None, None, None)
-
- @typing.overload
- def assertRaisesWithLiteralMatch(
- self, expected_exception, expected_exception_message,
- callable_obj: Callable[..., Any], *args, **kwargs) -> None:
- # The purpose of this return statement is to work around
- # https://github.com/PyCQA/pylint/issues/5273; it is otherwise ignored.
- return self._AssertRaisesContext(None, None, None)
-
- def assertRaisesWithLiteralMatch(self, expected_exception,
- expected_exception_message,
- callable_obj=None, *args, **kwargs):
- """Asserts that the message in a raised exception equals the given string.
-
- Unlike assertRaisesRegex, this method takes a literal string, not
- a regular expression.
-
- with self.assertRaisesWithLiteralMatch(ExType, 'message'):
- DoSomething()
-
- Args:
- expected_exception: Exception class expected to be raised.
- expected_exception_message: String message expected in the raised
- exception. For a raise exception e, expected_exception_message must
- equal str(e).
- callable_obj: Function to be called, or None to return a context.
- *args: Extra args.
- **kwargs: Extra kwargs.
-
- Returns:
- A context manager if callable_obj is None. Otherwise, None.
-
- Raises:
- self.failureException if callable_obj does not raise a matching exception.
- """
- def Check(err):
- actual_exception_message = str(err)
- self.assertTrue(expected_exception_message == actual_exception_message,
- 'Exception message does not match.\n'
- 'Expected: %r\n'
- 'Actual: %r' % (expected_exception_message,
- actual_exception_message))
-
- context = self._AssertRaisesContext(expected_exception, self, Check)
- if callable_obj is None:
- return context
- with context:
- callable_obj(*args, **kwargs)
-
- def assertContainsInOrder(self, strings, target, msg=None):
- """Asserts that the strings provided are found in the target in order.
-
- This may be useful for checking HTML output.
-
- Args:
- strings: A list of strings, such as [ 'fox', 'dog' ]
- target: A target string in which to look for the strings, such as
- 'The quick brown fox jumped over the lazy dog'.
- msg: Optional message to report on failure.
- """
- if isinstance(strings, (bytes, unicode if str is bytes else str)):
- strings = (strings,)
-
- current_index = 0
- last_string = None
- for string in strings:
- index = target.find(str(string), current_index)
- if index == -1 and current_index == 0:
- self.fail("Did not find '%s' in '%s'" %
- (string, target), msg)
- elif index == -1:
- self.fail("Did not find '%s' after '%s' in '%s'" %
- (string, last_string, target), msg)
- last_string = string
- current_index = index
-
- def assertContainsSubsequence(self, container, subsequence, msg=None):
- """Asserts that "container" contains "subsequence" as a subsequence.
-
- Asserts that "container" contains all the elements of "subsequence", in
- order, but possibly with other elements interspersed. For example, [1, 2, 3]
- is a subsequence of [0, 0, 1, 2, 0, 3, 0] but not of [0, 0, 1, 3, 0, 2, 0].
-
- Args:
- container: the list we're testing for subsequence inclusion.
- subsequence: the list we hope will be a subsequence of container.
- msg: Optional message to report on failure.
- """
- first_nonmatching = None
- reversed_container = list(reversed(container))
- subsequence = list(subsequence)
-
- for e in subsequence:
- if e not in reversed_container:
- first_nonmatching = e
- break
- while e != reversed_container.pop():
- pass
-
- if first_nonmatching is not None:
- self.fail('%s not a subsequence of %s. First non-matching element: %s' %
- (subsequence, container, first_nonmatching), msg)
-
- def assertContainsExactSubsequence(self, container, subsequence, msg=None):
- """Asserts that "container" contains "subsequence" as an exact subsequence.
-
- Asserts that "container" contains all the elements of "subsequence", in
- order, and without other elements interspersed. For example, [1, 2, 3] is an
- exact subsequence of [0, 0, 1, 2, 3, 0] but not of [0, 0, 1, 2, 0, 3, 0].
-
- Args:
- container: the list we're testing for subsequence inclusion.
- subsequence: the list we hope will be an exact subsequence of container.
- msg: Optional message to report on failure.
- """
- container = list(container)
- subsequence = list(subsequence)
- longest_match = 0
-
- for start in range(1 + len(container) - len(subsequence)):
- if longest_match == len(subsequence):
- break
- index = 0
- while (index < len(subsequence) and
- subsequence[index] == container[start + index]):
- index += 1
- longest_match = max(longest_match, index)
-
- if longest_match < len(subsequence):
- self.fail('%s not an exact subsequence of %s. '
- 'Longest matching prefix: %s' %
- (subsequence, container, subsequence[:longest_match]), msg)
-
- def assertTotallyOrdered(self, *groups, **kwargs):
- """Asserts that total ordering has been implemented correctly.
-
- For example, say you have a class A that compares only on its attribute x.
- Comparators other than ``__lt__`` are omitted for brevity::
-
- class A(object):
- def __init__(self, x, y):
- self.x = x
- self.y = y
-
- def __hash__(self):
- return hash(self.x)
-
- def __lt__(self, other):
- try:
- return self.x < other.x
- except AttributeError:
- return NotImplemented
-
- assertTotallyOrdered will check that instances can be ordered correctly.
- For example::
-
- self.assertTotallyOrdered(
- [None], # None should come before everything else.
- [1], # Integers sort earlier.
- [A(1, 'a')],
- [A(2, 'b')], # 2 is after 1.
- [A(3, 'c'), A(3, 'd')], # The second argument is irrelevant.
- [A(4, 'z')],
- ['foo']) # Strings sort last.
-
- Args:
- *groups: A list of groups of elements. Each group of elements is a list
- of objects that are equal. The elements in each group must be less
- than the elements in the group after it. For example, these groups are
- totally ordered: ``[None]``, ``[1]``, ``[2, 2]``, ``[3]``.
- **kwargs: optional msg keyword argument can be passed.
- """
-
- def CheckOrder(small, big):
- """Ensures small is ordered before big."""
- self.assertFalse(small == big,
- self._formatMessage(msg, '%r unexpectedly equals %r' %
- (small, big)))
- self.assertTrue(small != big,
- self._formatMessage(msg, '%r unexpectedly equals %r' %
- (small, big)))
- self.assertLess(small, big, msg)
- self.assertFalse(big < small,
- self._formatMessage(msg,
- '%r unexpectedly less than %r' %
- (big, small)))
- self.assertLessEqual(small, big, msg)
- self.assertFalse(big <= small, self._formatMessage(
- '%r unexpectedly less than or equal to %r' % (big, small), msg
- ))
- self.assertGreater(big, small, msg)
- self.assertFalse(small > big,
- self._formatMessage(msg,
- '%r unexpectedly greater than %r' %
- (small, big)))
- self.assertGreaterEqual(big, small)
- self.assertFalse(small >= big, self._formatMessage(
- msg,
- '%r unexpectedly greater than or equal to %r' % (small, big)))
-
- def CheckEqual(a, b):
- """Ensures that a and b are equal."""
- self.assertEqual(a, b, msg)
- self.assertFalse(a != b,
- self._formatMessage(msg, '%r unexpectedly unequals %r' %
- (a, b)))
-
- # Objects that compare equal must hash to the same value, but this only
- # applies if both objects are hashable.
- if (isinstance(a, abc.Hashable) and
- isinstance(b, abc.Hashable)):
- self.assertEqual(
- hash(a), hash(b),
- self._formatMessage(
- msg, 'hash %d of %r unexpectedly not equal to hash %d of %r' %
- (hash(a), a, hash(b), b)))
-
- self.assertFalse(a < b,
- self._formatMessage(msg,
- '%r unexpectedly less than %r' %
- (a, b)))
- self.assertFalse(b < a,
- self._formatMessage(msg,
- '%r unexpectedly less than %r' %
- (b, a)))
- self.assertLessEqual(a, b, msg)
- self.assertLessEqual(b, a, msg) # pylint: disable=arguments-out-of-order
- self.assertFalse(a > b,
- self._formatMessage(msg,
- '%r unexpectedly greater than %r' %
- (a, b)))
- self.assertFalse(b > a,
- self._formatMessage(msg,
- '%r unexpectedly greater than %r' %
- (b, a)))
- self.assertGreaterEqual(a, b, msg)
- self.assertGreaterEqual(b, a, msg) # pylint: disable=arguments-out-of-order
-
- msg = kwargs.get('msg')
-
- # For every combination of elements, check the order of every pair of
- # elements.
- for elements in itertools.product(*groups):
- elements = list(elements)
- for index, small in enumerate(elements[:-1]):
- for big in elements[index + 1:]:
- CheckOrder(small, big)
-
- # Check that every element in each group is equal.
- for group in groups:
- for a in group:
- CheckEqual(a, a)
- for a, b in itertools.product(group, group):
- CheckEqual(a, b)
-
- def assertDictEqual(self, a, b, msg=None):
- """Raises AssertionError if a and b are not equal dictionaries.
-
- Args:
- a: A dict, the expected value.
- b: A dict, the actual value.
- msg: An optional str, the associated message.
-
- Raises:
- AssertionError: if the dictionaries are not equal.
- """
- self.assertIsInstance(a, dict, self._formatMessage(
- msg,
- 'First argument is not a dictionary'
- ))
- self.assertIsInstance(b, dict, self._formatMessage(
- msg,
- 'Second argument is not a dictionary'
- ))
-
- def Sorted(list_of_items):
- try:
- return sorted(list_of_items) # In 3.3, unordered are possible.
- except TypeError:
- return list_of_items
-
- if a == b:
- return
- a_items = Sorted(list(a.items()))
- b_items = Sorted(list(b.items()))
-
- unexpected = []
- missing = []
- different = []
-
- safe_repr = unittest.util.safe_repr # pytype: disable=module-attr
-
- def Repr(dikt):
- """Deterministic repr for dict."""
- # Sort the entries based on their repr, not based on their sort order,
- # which will be non-deterministic across executions, for many types.
- entries = sorted((safe_repr(k), safe_repr(v)) for k, v in dikt.items())
- return '{%s}' % (', '.join('%s: %s' % pair for pair in entries))
-
- message = ['%s != %s%s' % (Repr(a), Repr(b), ' (%s)' % msg if msg else '')]
-
- # The standard library default output confounds lexical difference with
- # value difference; treat them separately.
- for a_key, a_value in a_items:
- if a_key not in b:
- missing.append((a_key, a_value))
- elif a_value != b[a_key]:
- different.append((a_key, a_value, b[a_key]))
-
- for b_key, b_value in b_items:
- if b_key not in a:
- unexpected.append((b_key, b_value))
-
- if unexpected:
- message.append(
- 'Unexpected, but present entries:\n%s' % ''.join(
- '%s: %s\n' % (safe_repr(k), safe_repr(v)) for k, v in unexpected))
-
- if different:
- message.append(
- 'repr() of differing entries:\n%s' % ''.join(
- '%s: %s != %s\n' % (safe_repr(k), safe_repr(a_value),
- safe_repr(b_value))
- for k, a_value, b_value in different))
-
- if missing:
- message.append(
- 'Missing entries:\n%s' % ''.join(
- ('%s: %s\n' % (safe_repr(k), safe_repr(v)) for k, v in missing)))
-
- raise self.failureException('\n'.join(message))
-
- def assertUrlEqual(self, a, b, msg=None):
- """Asserts that urls are equal, ignoring ordering of query params."""
- parsed_a = parse.urlparse(a)
- parsed_b = parse.urlparse(b)
- self.assertEqual(parsed_a.scheme, parsed_b.scheme, msg)
- self.assertEqual(parsed_a.netloc, parsed_b.netloc, msg)
- self.assertEqual(parsed_a.path, parsed_b.path, msg)
- self.assertEqual(parsed_a.fragment, parsed_b.fragment, msg)
- self.assertEqual(sorted(parsed_a.params.split(';')),
- sorted(parsed_b.params.split(';')), msg)
- self.assertDictEqual(
- parse.parse_qs(parsed_a.query, keep_blank_values=True),
- parse.parse_qs(parsed_b.query, keep_blank_values=True), msg)
-
- def assertSameStructure(self, a, b, aname='a', bname='b', msg=None):
- """Asserts that two values contain the same structural content.
-
- The two arguments should be data trees consisting of trees of dicts and
- lists. They will be deeply compared by walking into the contents of dicts
- and lists; other items will be compared using the == operator.
- If the two structures differ in content, the failure message will indicate
- the location within the structures where the first difference is found.
- This may be helpful when comparing large structures.
-
- Mixed Sequence and Set types are supported. Mixed Mapping types are
- supported, but the order of the keys will not be considered in the
- comparison.
-
- Args:
- a: The first structure to compare.
- b: The second structure to compare.
- aname: Variable name to use for the first structure in assertion messages.
- bname: Variable name to use for the second structure.
- msg: Additional text to include in the failure message.
- """
-
- # Accumulate all the problems found so we can report all of them at once
- # rather than just stopping at the first
- problems = []
-
- _walk_structure_for_problems(a, b, aname, bname, problems)
-
- # Avoid spamming the user toooo much
- if self.maxDiff is not None:
- max_problems_to_show = self.maxDiff // 80
- if len(problems) > max_problems_to_show:
- problems = problems[0:max_problems_to_show-1] + ['...']
-
- if problems:
- self.fail('; '.join(problems), msg)
-
- def assertJsonEqual(self, first, second, msg=None):
- """Asserts that the JSON objects defined in two strings are equal.
-
- A summary of the differences will be included in the failure message
- using assertSameStructure.
-
- Args:
- first: A string containing JSON to decode and compare to second.
- second: A string containing JSON to decode and compare to first.
- msg: Additional text to include in the failure message.
- """
- try:
- first_structured = json.loads(first)
- except ValueError as e:
- raise ValueError(self._formatMessage(
- msg,
- 'could not decode first JSON value %s: %s' % (first, e)))
-
- try:
- second_structured = json.loads(second)
- except ValueError as e:
- raise ValueError(self._formatMessage(
- msg,
- 'could not decode second JSON value %s: %s' % (second, e)))
-
- self.assertSameStructure(first_structured, second_structured,
- aname='first', bname='second', msg=msg)
-
- def _getAssertEqualityFunc(self, first, second):
- # type: (Any, Any) -> Callable[..., None]
- try:
- return super(TestCase, self)._getAssertEqualityFunc(first, second)
- except AttributeError:
- # This is a workaround if unittest.TestCase.__init__ was never run.
- # It usually means that somebody created a subclass just for the
- # assertions and has overridden __init__. "assertTrue" is a safe
- # value that will not make __init__ raise a ValueError.
- test_method = getattr(self, '_testMethodName', 'assertTrue')
- super(TestCase, self).__init__(test_method)
-
- return super(TestCase, self)._getAssertEqualityFunc(first, second)
-
- def fail(self, msg=None, prefix=None):
- """Fail immediately with the given message, optionally prefixed."""
- return super(TestCase, self).fail(self._formatMessage(prefix, msg))
-
-
-def _sorted_list_difference(expected, actual):
- # type: (List[_T], List[_T]) -> Tuple[List[_T], List[_T]]
- """Finds elements in only one or the other of two, sorted input lists.
-
- Returns a two-element tuple of lists. The first list contains those
- elements in the "expected" list but not in the "actual" list, and the
- second contains those elements in the "actual" list but not in the
- "expected" list. Duplicate elements in either input list are ignored.
-
- Args:
- expected: The list we expected.
- actual: The list we actually got.
- Returns:
- (missing, unexpected)
- missing: items in expected that are not in actual.
- unexpected: items in actual that are not in expected.
- """
- i = j = 0
- missing = []
- unexpected = []
- while True:
- try:
- e = expected[i]
- a = actual[j]
- if e < a:
- missing.append(e)
- i += 1
- while expected[i] == e:
- i += 1
- elif e > a:
- unexpected.append(a)
- j += 1
- while actual[j] == a:
- j += 1
- else:
- i += 1
- try:
- while expected[i] == e:
- i += 1
- finally:
- j += 1
- while actual[j] == a:
- j += 1
- except IndexError:
- missing.extend(expected[i:])
- unexpected.extend(actual[j:])
- break
- return missing, unexpected
-
-
-def _are_both_of_integer_type(a, b):
- # type: (object, object) -> bool
- return isinstance(a, int) and isinstance(b, int)
-
-
-def _are_both_of_sequence_type(a, b):
- # type: (object, object) -> bool
- return isinstance(a, abc.Sequence) and isinstance(
- b, abc.Sequence) and not isinstance(
- a, _TEXT_OR_BINARY_TYPES) and not isinstance(b, _TEXT_OR_BINARY_TYPES)
-
-
-def _are_both_of_set_type(a, b):
- # type: (object, object) -> bool
- return isinstance(a, abc.Set) and isinstance(b, abc.Set)
-
-
-def _are_both_of_mapping_type(a, b):
- # type: (object, object) -> bool
- return isinstance(a, abc.Mapping) and isinstance(
- b, abc.Mapping)
-
-
-def _walk_structure_for_problems(a, b, aname, bname, problem_list):
- """The recursive comparison behind assertSameStructure."""
- if type(a) != type(b) and not ( # pylint: disable=unidiomatic-typecheck
- _are_both_of_integer_type(a, b) or _are_both_of_sequence_type(a, b) or
- _are_both_of_set_type(a, b) or _are_both_of_mapping_type(a, b)):
- # We do not distinguish between int and long types as 99.99% of Python 2
- # code should never care. They collapse into a single type in Python 3.
- problem_list.append('%s is a %r but %s is a %r' %
- (aname, type(a), bname, type(b)))
- # If they have different types there's no point continuing
- return
-
- if isinstance(a, abc.Set):
- for k in a:
- if k not in b:
- problem_list.append(
- '%s has %r but %s does not' % (aname, k, bname))
- for k in b:
- if k not in a:
- problem_list.append('%s lacks %r but %s has it' % (aname, k, bname))
-
- # NOTE: a or b could be a defaultdict, so we must take care that the traversal
- # doesn't modify the data.
- elif isinstance(a, abc.Mapping):
- for k in a:
- if k in b:
- _walk_structure_for_problems(
- a[k], b[k], '%s[%r]' % (aname, k), '%s[%r]' % (bname, k),
- problem_list)
- else:
- problem_list.append(
- "%s has [%r] with value %r but it's missing in %s" %
- (aname, k, a[k], bname))
- for k in b:
- if k not in a:
- problem_list.append(
- '%s lacks [%r] but %s has it with value %r' %
- (aname, k, bname, b[k]))
-
- # Strings/bytes are Sequences but we'll just do those with regular !=
- elif (isinstance(a, abc.Sequence) and
- not isinstance(a, _TEXT_OR_BINARY_TYPES)):
- minlen = min(len(a), len(b))
- for i in range(minlen):
- _walk_structure_for_problems(
- a[i], b[i], '%s[%d]' % (aname, i), '%s[%d]' % (bname, i),
- problem_list)
- for i in range(minlen, len(a)):
- problem_list.append('%s has [%i] with value %r but %s does not' %
- (aname, i, a[i], bname))
- for i in range(minlen, len(b)):
- problem_list.append('%s lacks [%i] but %s has it with value %r' %
- (aname, i, bname, b[i]))
-
- else:
- if a != b:
- problem_list.append('%s is %r but %s is %r' % (aname, a, bname, b))
-
-
-def get_command_string(command):
- """Returns an escaped string that can be used as a shell command.
-
- Args:
- command: List or string representing the command to run.
- Returns:
- A string suitable for use as a shell command.
- """
- if isinstance(command, str):
- return command
- else:
- if os.name == 'nt':
- return ' '.join(command)
- else:
- # The following is identical to Python 3's shlex.quote function.
- command_string = ''
- for word in command:
- # Single quote word, and replace each ' in word with '"'"'
- command_string += "'" + word.replace("'", "'\"'\"'") + "' "
- return command_string[:-1]
-
-
-def get_command_stderr(command, env=None, close_fds=True):
- """Runs the given shell command and returns a tuple.
-
- Args:
- command: List or string representing the command to run.
- env: Dictionary of environment variable settings. If None, no environment
- variables will be set for the child process. This is to make tests
- more hermetic. NOTE: this behavior is different than the standard
- subprocess module.
- close_fds: Whether or not to close all open fd's in the child after forking.
- On Windows, this is ignored and close_fds is always False.
-
- Returns:
- Tuple of (exit status, text printed to stdout and stderr by the command).
- """
- if env is None: env = {}
- if os.name == 'nt':
- # Windows does not support setting close_fds to True while also redirecting
- # standard handles.
- close_fds = False
-
- use_shell = isinstance(command, str)
- process = subprocess.Popen(
- command,
- close_fds=close_fds,
- env=env,
- shell=use_shell,
- stderr=subprocess.STDOUT,
- stdout=subprocess.PIPE)
- output = process.communicate()[0]
- exit_status = process.wait()
- return (exit_status, output)
-
-
-def _quote_long_string(s):
- # type: (Union[Text, bytes, bytearray]) -> Text
- """Quotes a potentially multi-line string to make the start and end obvious.
-
- Args:
- s: A string.
-
- Returns:
- The quoted string.
- """
- if isinstance(s, (bytes, bytearray)):
- try:
- s = s.decode('utf-8')
- except UnicodeDecodeError:
- s = str(s)
- return ('8<-----------\n' +
- s + '\n' +
- '----------->8\n')
-
-
-def print_python_version():
- # type: () -> None
- # Having this in the test output logs by default helps debugging when all
- # you've got is the log and no other idea of which Python was used.
- sys.stderr.write('Running tests under Python {0[0]}.{0[1]}.{0[2]}: '
- '{1}\n'.format(
- sys.version_info,
- sys.executable if sys.executable else 'embedded.'))
-
-
-def main(*args, **kwargs):
- # type: (Text, Any) -> None
- """Executes a set of Python unit tests.
-
- Usually this function is called without arguments, so the
- unittest.TestProgram instance will get created with the default settings,
- so it will run all test methods of all TestCase classes in the ``__main__``
- module.
-
- Args:
- *args: Positional arguments passed through to
- ``unittest.TestProgram.__init__``.
- **kwargs: Keyword arguments passed through to
- ``unittest.TestProgram.__init__``.
- """
- print_python_version()
- _run_in_app(run_tests, args, kwargs)
-
-
-def _is_in_app_main():
- # type: () -> bool
- """Returns True iff app.run is active."""
- f = sys._getframe().f_back # pylint: disable=protected-access
- while f:
- if f.f_code == app.run.__code__:
- return True
- f = f.f_back
- return False
-
-
-def _register_sigterm_with_faulthandler():
- # type: () -> None
- """Have faulthandler dump stacks on SIGTERM. Useful to diagnose timeouts."""
- if faulthandler and getattr(faulthandler, 'register', None):
- # faulthandler.register is not available on Windows.
- # faulthandler.enable() is already called by app.run.
- try:
- faulthandler.register(signal.SIGTERM, chain=True) # pytype: disable=module-attr
- except Exception as e: # pylint: disable=broad-except
- sys.stderr.write('faulthandler.register(SIGTERM) failed '
- '%r; ignoring.\n' % e)
-
-
-def _run_in_app(function, args, kwargs):
- # type: (Callable[..., None], Sequence[Text], Mapping[Text, Any]) -> None
- """Executes a set of Python unit tests, ensuring app.run.
-
- This is a private function, users should call absltest.main().
-
- _run_in_app calculates argv to be the command-line arguments of this program
- (without the flags), sets the default of FLAGS.alsologtostderr to True,
- then it calls function(argv, args, kwargs), making sure that `function'
- will get called within app.run(). _run_in_app does this by checking whether
- it is called by app.run(), or by calling app.run() explicitly.
-
- The reason why app.run has to be ensured is to make sure that
- flags are parsed and stripped properly, and other initializations done by
- the app module are also carried out, no matter if absltest.run() is called
- from within or outside app.run().
-
- If _run_in_app is called from within app.run(), then it will reparse
- sys.argv and pass the result without command-line flags into the argv
- argument of `function'. The reason why this parsing is needed is that
- __main__.main() calls absltest.main() without passing its argv. So the
- only way _run_in_app could get to know the argv without the flags is that
- it reparses sys.argv.
-
- _run_in_app changes the default of FLAGS.alsologtostderr to True so that the
- test program's stderr will contain all the log messages unless otherwise
- specified on the command-line. This overrides any explicit assignment to
- FLAGS.alsologtostderr by the test program prior to the call to _run_in_app()
- (e.g. in __main__.main).
-
- Please note that _run_in_app (and the function it calls) is allowed to make
- changes to kwargs.
-
- Args:
- function: absltest.run_tests or a similar function. It will be called as
- function(argv, args, kwargs) where argv is a list containing the
- elements of sys.argv without the command-line flags.
- args: Positional arguments passed through to unittest.TestProgram.__init__.
- kwargs: Keyword arguments passed through to unittest.TestProgram.__init__.
- """
- if _is_in_app_main():
- _register_sigterm_with_faulthandler()
-
- # Change the default of alsologtostderr from False to True, so the test
- # programs's stderr will contain all the log messages.
- # If --alsologtostderr=false is specified in the command-line, or user
- # has called FLAGS.alsologtostderr = False before, then the value is kept
- # False.
- FLAGS.set_default('alsologtostderr', True)
-
- # Here we only want to get the `argv` without the flags. To avoid any
- # side effects of parsing flags, we temporarily stub out the `parse` method
- stored_parse_methods = {}
- noop_parse = lambda _: None
- for name in FLAGS:
- # Avoid any side effects of parsing flags.
- stored_parse_methods[name] = FLAGS[name].parse
- # This must be a separate loop since multiple flag names (short_name=) can
- # point to the same flag object.
- for name in FLAGS:
- FLAGS[name].parse = noop_parse
- try:
- argv = FLAGS(sys.argv)
- finally:
- for name in FLAGS:
- FLAGS[name].parse = stored_parse_methods[name]
- sys.stdout.flush()
-
- function(argv, args, kwargs)
- else:
- # Send logging to stderr. Use --alsologtostderr instead of --logtostderr
- # in case tests are reading their own logs.
- FLAGS.set_default('alsologtostderr', True)
-
- def main_function(argv):
- _register_sigterm_with_faulthandler()
- function(argv, args, kwargs)
-
- app.run(main=main_function)
-
-
-def _is_suspicious_attribute(testCaseClass, name):
- # type: (Type, Text) -> bool
- """Returns True if an attribute is a method named like a test method."""
- if name.startswith('Test') and len(name) > 4 and name[4].isupper():
- attr = getattr(testCaseClass, name)
- if inspect.isfunction(attr) or inspect.ismethod(attr):
- args = inspect.getfullargspec(attr)
- return (len(args.args) == 1 and args.args[0] == 'self' and
- args.varargs is None and args.varkw is None and
- not args.kwonlyargs)
- return False
-
-
-def skipThisClass(reason):
- # type: (Text) -> Callable[[_T], _T]
- """Skip tests in the decorated TestCase, but not any of its subclasses.
-
- This decorator indicates that this class should skip all its tests, but not
- any of its subclasses. Useful for if you want to share testMethod or setUp
- implementations between a number of concrete testcase classes.
-
- Example usage, showing how you can share some common test methods between
- subclasses. In this example, only ``BaseTest`` will be marked as skipped, and
- not RealTest or SecondRealTest::
-
- @absltest.skipThisClass("Shared functionality")
- class BaseTest(absltest.TestCase):
- def test_simple_functionality(self):
- self.assertEqual(self.system_under_test.method(), 1)
-
- class RealTest(BaseTest):
- def setUp(self):
- super().setUp()
- self.system_under_test = MakeSystem(argument)
-
- def test_specific_behavior(self):
- ...
-
- class SecondRealTest(BaseTest):
- def setUp(self):
- super().setUp()
- self.system_under_test = MakeSystem(other_arguments)
-
- def test_other_behavior(self):
- ...
-
- Args:
- reason: The reason we have a skip in place. For instance: 'shared test
- methods' or 'shared assertion methods'.
-
- Returns:
- Decorator function that will cause a class to be skipped.
- """
- if isinstance(reason, type):
- raise TypeError('Got {!r}, expected reason as string'.format(reason))
-
- def _skip_class(test_case_class):
- if not issubclass(test_case_class, unittest.TestCase):
- raise TypeError(
- 'Decorating {!r}, expected TestCase subclass'.format(test_case_class))
-
- # Only shadow the setUpClass method if it is directly defined. If it is
- # in the parent class we invoke it via a super() call instead of holding
- # a reference to it.
- shadowed_setupclass = test_case_class.__dict__.get('setUpClass', None)
-
- @classmethod
- def replacement_setupclass(cls, *args, **kwargs):
- # Skip this class if it is the one that was decorated with @skipThisClass
- if cls is test_case_class:
- raise SkipTest(reason)
- if shadowed_setupclass:
- # Pass along `cls` so the MRO chain doesn't break.
- # The original method is a `classmethod` descriptor, which can't
- # be directly called, but `__func__` has the underlying function.
- return shadowed_setupclass.__func__(cls, *args, **kwargs)
- else:
- # Because there's no setUpClass() defined directly on test_case_class,
- # we call super() ourselves to continue execution of the inheritance
- # chain.
- return super(test_case_class, cls).setUpClass(*args, **kwargs)
-
- test_case_class.setUpClass = replacement_setupclass
- return test_case_class
-
- return _skip_class
-
-
-class TestLoader(unittest.TestLoader):
- """A test loader which supports common test features.
-
- Supported features include:
- * Banning untested methods with test-like names: methods attached to this
- testCase with names starting with `Test` are ignored by the test runner,
- and often represent mistakenly-omitted test cases. This loader will raise
- a TypeError when attempting to load a TestCase with such methods.
- * Randomization of test case execution order (optional).
- """
-
- _ERROR_MSG = textwrap.dedent("""Method '%s' is named like a test case but
- is not one. This is often a bug. If you want it to be a test method,
- name it with 'test' in lowercase. If not, rename the method to not begin
- with 'Test'.""")
-
- def __init__(self, *args, **kwds):
- super(TestLoader, self).__init__(*args, **kwds)
- seed = _get_default_randomize_ordering_seed()
- if seed:
- self._randomize_ordering_seed = seed
- self._random = random.Random(self._randomize_ordering_seed)
- else:
- self._randomize_ordering_seed = None
- self._random = None
-
- def getTestCaseNames(self, testCaseClass): # pylint:disable=invalid-name
- """Validates and returns a (possibly randomized) list of test case names."""
- for name in dir(testCaseClass):
- if _is_suspicious_attribute(testCaseClass, name):
- raise TypeError(TestLoader._ERROR_MSG % name)
- names = super(TestLoader, self).getTestCaseNames(testCaseClass)
- if self._randomize_ordering_seed is not None:
- logging.info(
- 'Randomizing test order with seed: %d', self._randomize_ordering_seed)
- logging.info(
- 'To reproduce this order, re-run with '
- '--test_randomize_ordering_seed=%d', self._randomize_ordering_seed)
- self._random.shuffle(names)
- return names
-
-
-def get_default_xml_output_filename():
- # type: () -> Optional[Text]
- if os.environ.get('XML_OUTPUT_FILE'):
- return os.environ['XML_OUTPUT_FILE']
- elif os.environ.get('RUNNING_UNDER_TEST_DAEMON'):
- return os.path.join(os.path.dirname(TEST_TMPDIR.value), 'test_detail.xml')
- elif os.environ.get('TEST_XMLOUTPUTDIR'):
- return os.path.join(
- os.environ['TEST_XMLOUTPUTDIR'],
- os.path.splitext(os.path.basename(sys.argv[0]))[0] + '.xml')
-
-
-def _setup_filtering(argv):
- # type: (MutableSequence[Text]) -> None
- """Implements the bazel test filtering protocol.
-
- The following environment variable is used in this method:
-
- TESTBRIDGE_TEST_ONLY: string, if set, is forwarded to the unittest
- framework to use as a test filter. Its value is split with shlex, then:
- 1. On Python 3.6 and before, split values are passed as positional
- arguments on argv.
- 2. On Python 3.7+, split values are passed to unittest's `-k` flag. Tests
- are matched by glob patterns or substring. See
- https://docs.python.org/3/library/unittest.html#cmdoption-unittest-k
-
- Args:
- argv: the argv to mutate in-place.
- """
- test_filter = os.environ.get('TESTBRIDGE_TEST_ONLY')
- if argv is None or not test_filter:
- return
-
- filters = shlex.split(test_filter)
- if sys.version_info[:2] >= (3, 7):
- filters = ['-k=' + test_filter for test_filter in filters]
-
- argv[1:1] = filters
-
-
-def _setup_test_runner_fail_fast(argv):
- # type: (MutableSequence[Text]) -> None
- """Implements the bazel test fail fast protocol.
-
- The following environment variable is used in this method:
-
- TESTBRIDGE_TEST_RUNNER_FAIL_FAST=<1|0>
-
- If set to 1, --failfast is passed to the unittest framework to return upon
- first failure.
-
- Args:
- argv: the argv to mutate in-place.
- """
-
- if argv is None:
- return
-
- if os.environ.get('TESTBRIDGE_TEST_RUNNER_FAIL_FAST') != '1':
- return
-
- argv[1:1] = ['--failfast']
-
-
-def _setup_sharding(custom_loader=None):
- # type: (Optional[unittest.TestLoader]) -> unittest.TestLoader
- """Implements the bazel sharding protocol.
-
- The following environment variables are used in this method:
-
- TEST_SHARD_STATUS_FILE: string, if set, points to a file. We write a blank
- file to tell the test runner that this test implements the test sharding
- protocol.
-
- TEST_TOTAL_SHARDS: int, if set, sharding is requested.
-
- TEST_SHARD_INDEX: int, must be set if TEST_TOTAL_SHARDS is set. Specifies
- the shard index for this instance of the test process. Must satisfy:
- 0 <= TEST_SHARD_INDEX < TEST_TOTAL_SHARDS.
-
- Args:
- custom_loader: A TestLoader to be made sharded.
-
- Returns:
- The test loader for shard-filtering or the standard test loader, depending
- on the sharding environment variables.
- """
-
- # It may be useful to write the shard file even if the other sharding
- # environment variables are not set. Test runners may use this functionality
- # to query whether a test binary implements the test sharding protocol.
- if 'TEST_SHARD_STATUS_FILE' in os.environ:
- try:
- with open(os.environ['TEST_SHARD_STATUS_FILE'], 'w') as f:
- f.write('')
- except IOError:
- sys.stderr.write('Error opening TEST_SHARD_STATUS_FILE (%s). Exiting.'
- % os.environ['TEST_SHARD_STATUS_FILE'])
- sys.exit(1)
-
- base_loader = custom_loader or TestLoader()
- if 'TEST_TOTAL_SHARDS' not in os.environ:
- # Not using sharding, use the expected test loader.
- return base_loader
-
- total_shards = int(os.environ['TEST_TOTAL_SHARDS'])
- shard_index = int(os.environ['TEST_SHARD_INDEX'])
-
- if shard_index < 0 or shard_index >= total_shards:
- sys.stderr.write('ERROR: Bad sharding values. index=%d, total=%d\n' %
- (shard_index, total_shards))
- sys.exit(1)
-
- # Replace the original getTestCaseNames with one that returns
- # the test case names for this shard.
- delegate_get_names = base_loader.getTestCaseNames
-
- bucket_iterator = itertools.cycle(range(total_shards))
-
- def getShardedTestCaseNames(testCaseClass):
- filtered_names = []
- # We need to sort the list of tests in order to determine which tests this
- # shard is responsible for; however, it's important to preserve the order
- # returned by the base loader, e.g. in the case of randomized test ordering.
- ordered_names = delegate_get_names(testCaseClass)
- for testcase in sorted(ordered_names):
- bucket = next(bucket_iterator)
- if bucket == shard_index:
- filtered_names.append(testcase)
- return [x for x in ordered_names if x in filtered_names]
-
- base_loader.getTestCaseNames = getShardedTestCaseNames
- return base_loader
-
-
-# pylint: disable=line-too-long
-def _run_and_get_tests_result(argv, args, kwargs, xml_test_runner_class):
- # type: (MutableSequence[Text], Sequence[Any], MutableMapping[Text, Any], Type) -> unittest.TestResult
- # pylint: enable=line-too-long
- """Same as run_tests, except it returns the result instead of exiting."""
-
- # The entry from kwargs overrides argv.
- argv = kwargs.pop('argv', argv)
-
- # Set up test filtering if requested in environment.
- _setup_filtering(argv)
- # Set up --failfast as requested in environment
- _setup_test_runner_fail_fast(argv)
-
- # Shard the (default or custom) loader if sharding is turned on.
- kwargs['testLoader'] = _setup_sharding(kwargs.get('testLoader', None))
-
- # XML file name is based upon (sorted by priority):
- # --xml_output_file flag, XML_OUTPUT_FILE variable,
- # TEST_XMLOUTPUTDIR variable or RUNNING_UNDER_TEST_DAEMON variable.
- if not FLAGS.xml_output_file:
- FLAGS.xml_output_file = get_default_xml_output_filename()
- xml_output_file = FLAGS.xml_output_file
-
- xml_buffer = None
- if xml_output_file:
- xml_output_dir = os.path.dirname(xml_output_file)
- if xml_output_dir and not os.path.isdir(xml_output_dir):
- try:
- os.makedirs(xml_output_dir)
- except OSError as e:
- # File exists error can occur with concurrent tests
- if e.errno != errno.EEXIST:
- raise
- # Fail early if we can't write to the XML output file. This is so that we
- # don't waste people's time running tests that will just fail anyways.
- with _open(xml_output_file, 'w'):
- pass
-
- # We can reuse testRunner if it supports XML output (e. g. by inheriting
- # from xml_reporter.TextAndXMLTestRunner). Otherwise we need to use
- # xml_reporter.TextAndXMLTestRunner.
- if (kwargs.get('testRunner') is not None
- and not hasattr(kwargs['testRunner'], 'set_default_xml_stream')):
- sys.stderr.write('WARNING: XML_OUTPUT_FILE or --xml_output_file setting '
- 'overrides testRunner=%r setting (possibly from --pdb)'
- % (kwargs['testRunner']))
- # Passing a class object here allows TestProgram to initialize
- # instances based on its kwargs and/or parsed command-line args.
- kwargs['testRunner'] = xml_test_runner_class
- if kwargs.get('testRunner') is None:
- kwargs['testRunner'] = xml_test_runner_class
- # Use an in-memory buffer (not backed by the actual file) to store the XML
- # report, because some tools modify the file (e.g., create a placeholder
- # with partial information, in case the test process crashes).
- xml_buffer = io.StringIO()
- kwargs['testRunner'].set_default_xml_stream(xml_buffer) # pytype: disable=attribute-error
-
- # If we've used a seed to randomize test case ordering, we want to record it
- # as a top-level attribute in the `testsuites` section of the XML output.
- randomize_ordering_seed = getattr(
- kwargs['testLoader'], '_randomize_ordering_seed', None)
- setter = getattr(kwargs['testRunner'], 'set_testsuites_property', None)
- if randomize_ordering_seed and setter:
- setter('test_randomize_ordering_seed', randomize_ordering_seed)
- elif kwargs.get('testRunner') is None:
- kwargs['testRunner'] = _pretty_print_reporter.TextTestRunner
-
- if FLAGS.pdb_post_mortem:
- runner = kwargs['testRunner']
- # testRunner can be a class or an instance, which must be tested for
- # differently.
- # Overriding testRunner isn't uncommon, so only enable the debugging
- # integration if the runner claims it does; we don't want to accidentally
- # clobber something on the runner.
- if ((isinstance(runner, type) and
- issubclass(runner, _pretty_print_reporter.TextTestRunner)) or
- isinstance(runner, _pretty_print_reporter.TextTestRunner)):
- runner.run_for_debugging = True
-
- # Make sure tmpdir exists.
- if not os.path.isdir(TEST_TMPDIR.value):
- try:
- os.makedirs(TEST_TMPDIR.value)
- except OSError as e:
- # Concurrent test might have created the directory.
- if e.errno != errno.EEXIST:
- raise
-
- # Let unittest.TestProgram.__init__ do its own argv parsing, e.g. for '-v',
- # on argv, which is sys.argv without the command-line flags.
- kwargs['argv'] = argv
-
- try:
- test_program = unittest.TestProgram(*args, **kwargs)
- return test_program.result
- finally:
- if xml_buffer:
- try:
- with _open(xml_output_file, 'w') as f:
- f.write(xml_buffer.getvalue())
- finally:
- xml_buffer.close()
-
-
-def run_tests(argv, args, kwargs): # pylint: disable=line-too-long
- # type: (MutableSequence[Text], Sequence[Any], MutableMapping[Text, Any]) -> None
- # pylint: enable=line-too-long
- """Executes a set of Python unit tests.
-
- Most users should call absltest.main() instead of run_tests.
-
- Please note that run_tests should be called from app.run.
- Calling absltest.main() would ensure that.
-
- Please note that run_tests is allowed to make changes to kwargs.
-
- Args:
- argv: sys.argv with the command-line flags removed from the front, i.e. the
- argv with which :func:`app.run()` has called
- ``__main__.main``. It is passed to
- ``unittest.TestProgram.__init__(argv=)``, which does its own flag parsing.
- It is ignored if kwargs contains an argv entry.
- args: Positional arguments passed through to
- ``unittest.TestProgram.__init__``.
- kwargs: Keyword arguments passed through to
- ``unittest.TestProgram.__init__``.
- """
- result = _run_and_get_tests_result(
- argv, args, kwargs, xml_reporter.TextAndXMLTestRunner)
- sys.exit(not result.wasSuccessful())
-
-
-def _rmtree_ignore_errors(path):
- # type: (Text) -> None
- if os.path.isfile(path):
- try:
- os.unlink(path)
- except OSError:
- pass
- else:
- shutil.rmtree(path, ignore_errors=True)
-
-
-def _get_first_part(path):
- # type: (Text) -> Text
- parts = path.split(os.sep, 1)
- return parts[0]
diff --git a/spaces/asafAdge/Detic/detic/predictor.py b/spaces/asafAdge/Detic/detic/predictor.py
deleted file mode 100644
index 318205acb90d47a54ff6f34400e1da744b2d85ba..0000000000000000000000000000000000000000
--- a/spaces/asafAdge/Detic/detic/predictor.py
+++ /dev/null
@@ -1,253 +0,0 @@
-# Copyright (c) Facebook, Inc. and its affiliates.
-import atexit
-import bisect
-import multiprocessing as mp
-from collections import deque
-import cv2
-import torch
-
-from detectron2.data import MetadataCatalog
-from detectron2.engine.defaults import DefaultPredictor
-from detectron2.utils.video_visualizer import VideoVisualizer
-from detectron2.utils.visualizer import ColorMode, Visualizer
-
-from .modeling.utils import reset_cls_test
-
-
-def get_clip_embeddings(vocabulary, prompt='a '):
- from detic.modeling.text.text_encoder import build_text_encoder
- text_encoder = build_text_encoder(pretrain=True)
- text_encoder.eval()
- texts = [prompt + x for x in vocabulary]
- emb = text_encoder(texts).detach().permute(1, 0).contiguous().cpu()
- return emb
-
-BUILDIN_CLASSIFIER = {
- 'lvis': 'datasets/metadata/lvis_v1_clip_a+cname.npy',
- 'objects365': 'datasets/metadata/o365_clip_a+cnamefix.npy',
- 'openimages': 'datasets/metadata/oid_clip_a+cname.npy',
- 'coco': 'datasets/metadata/coco_clip_a+cname.npy',
-}
-
-BUILDIN_METADATA_PATH = {
- 'lvis': 'lvis_v1_val',
- 'objects365': 'objects365_v2_val',
- 'openimages': 'oid_val_expanded',
- 'coco': 'coco_2017_val',
-}
-
-class VisualizationDemo(object):
- def __init__(self, cfg, args,
- instance_mode=ColorMode.IMAGE, parallel=False):
- """
- Args:
- cfg (CfgNode):
- instance_mode (ColorMode):
- parallel (bool): whether to run the model in different processes from visualization.
- Useful since the visualization logic can be slow.
- """
- if args.vocabulary == 'custom':
- self.metadata = MetadataCatalog.get("__unused")
- self.metadata.thing_classes = args.custom_vocabulary.split(',')
- classifier = get_clip_embeddings(self.metadata.thing_classes)
- else:
- self.metadata = MetadataCatalog.get(
- BUILDIN_METADATA_PATH[args.vocabulary])
- classifier = BUILDIN_CLASSIFIER[args.vocabulary]
-
- num_classes = len(self.metadata.thing_classes)
- self.cpu_device = torch.device("cpu")
- self.instance_mode = instance_mode
-
- self.parallel = parallel
- if parallel:
- num_gpu = torch.cuda.device_count()
- self.predictor = AsyncPredictor(cfg, num_gpus=num_gpu)
- else:
- self.predictor = DefaultPredictor(cfg)
- reset_cls_test(self.predictor.model, classifier, num_classes)
-
- def run_on_image(self, image):
- """
- Args:
- image (np.ndarray): an image of shape (H, W, C) (in BGR order).
- This is the format used by OpenCV.
-
- Returns:
- predictions (dict): the output of the model.
- vis_output (VisImage): the visualized image output.
- """
- vis_output = None
- predictions = self.predictor(image)
- # Convert image from OpenCV BGR format to Matplotlib RGB format.
- image = image[:, :, ::-1]
- visualizer = Visualizer(image, self.metadata, instance_mode=self.instance_mode)
- if "panoptic_seg" in predictions:
- panoptic_seg, segments_info = predictions["panoptic_seg"]
- vis_output = visualizer.draw_panoptic_seg_predictions(
- panoptic_seg.to(self.cpu_device), segments_info
- )
- else:
- if "sem_seg" in predictions:
- vis_output = visualizer.draw_sem_seg(
- predictions["sem_seg"].argmax(dim=0).to(self.cpu_device)
- )
- if "instances" in predictions:
- instances = predictions["instances"].to(self.cpu_device)
- vis_output = visualizer.draw_instance_predictions(predictions=instances)
-
- return predictions, vis_output
-
- def _frame_from_video(self, video):
- while video.isOpened():
- success, frame = video.read()
- if success:
- yield frame
- else:
- break
-
- def run_on_video(self, video):
- """
- Visualizes predictions on frames of the input video.
-
- Args:
- video (cv2.VideoCapture): a :class:`VideoCapture` object, whose source can be
- either a webcam or a video file.
-
- Yields:
- ndarray: BGR visualizations of each video frame.
- """
- video_visualizer = VideoVisualizer(self.metadata, self.instance_mode)
-
- def process_predictions(frame, predictions):
- frame = cv2.cvtColor(frame, cv2.COLOR_BGR2RGB)
- if "panoptic_seg" in predictions:
- panoptic_seg, segments_info = predictions["panoptic_seg"]
- vis_frame = video_visualizer.draw_panoptic_seg_predictions(
- frame, panoptic_seg.to(self.cpu_device), segments_info
- )
- elif "instances" in predictions:
- predictions = predictions["instances"].to(self.cpu_device)
- vis_frame = video_visualizer.draw_instance_predictions(frame, predictions)
- elif "sem_seg" in predictions:
- vis_frame = video_visualizer.draw_sem_seg(
- frame, predictions["sem_seg"].argmax(dim=0).to(self.cpu_device)
- )
-
- # Converts Matplotlib RGB format to OpenCV BGR format
- vis_frame = cv2.cvtColor(vis_frame.get_image(), cv2.COLOR_RGB2BGR)
- return vis_frame
-
- frame_gen = self._frame_from_video(video)
- if self.parallel:
- buffer_size = self.predictor.default_buffer_size
-
- frame_data = deque()
-
- for cnt, frame in enumerate(frame_gen):
- frame_data.append(frame)
- self.predictor.put(frame)
-
- if cnt >= buffer_size:
- frame = frame_data.popleft()
- predictions = self.predictor.get()
- yield process_predictions(frame, predictions)
-
- while len(frame_data):
- frame = frame_data.popleft()
- predictions = self.predictor.get()
- yield process_predictions(frame, predictions)
- else:
- for frame in frame_gen:
- yield process_predictions(frame, self.predictor(frame))
-
-
-class AsyncPredictor:
- """
- A predictor that runs the model asynchronously, possibly on >1 GPUs.
- Because rendering the visualization takes considerably amount of time,
- this helps improve throughput a little bit when rendering videos.
- """
-
- class _StopToken:
- pass
-
- class _PredictWorker(mp.Process):
- def __init__(self, cfg, task_queue, result_queue):
- self.cfg = cfg
- self.task_queue = task_queue
- self.result_queue = result_queue
- super().__init__()
-
- def run(self):
- predictor = DefaultPredictor(self.cfg)
-
- while True:
- task = self.task_queue.get()
- if isinstance(task, AsyncPredictor._StopToken):
- break
- idx, data = task
- result = predictor(data)
- self.result_queue.put((idx, result))
-
- def __init__(self, cfg, num_gpus: int = 1):
- """
- Args:
- cfg (CfgNode):
- num_gpus (int): if 0, will run on CPU
- """
- num_workers = max(num_gpus, 1)
- self.task_queue = mp.Queue(maxsize=num_workers * 3)
- self.result_queue = mp.Queue(maxsize=num_workers * 3)
- self.procs = []
- for gpuid in range(max(num_gpus, 1)):
- cfg = cfg.clone()
- cfg.defrost()
- cfg.MODEL.DEVICE = "cuda:{}".format(gpuid) if num_gpus > 0 else "cpu"
- self.procs.append(
- AsyncPredictor._PredictWorker(cfg, self.task_queue, self.result_queue)
- )
-
- self.put_idx = 0
- self.get_idx = 0
- self.result_rank = []
- self.result_data = []
-
- for p in self.procs:
- p.start()
- atexit.register(self.shutdown)
-
- def put(self, image):
- self.put_idx += 1
- self.task_queue.put((self.put_idx, image))
-
- def get(self):
- self.get_idx += 1 # the index needed for this request
- if len(self.result_rank) and self.result_rank[0] == self.get_idx:
- res = self.result_data[0]
- del self.result_data[0], self.result_rank[0]
- return res
-
- while True:
- # make sure the results are returned in the correct order
- idx, res = self.result_queue.get()
- if idx == self.get_idx:
- return res
- insert = bisect.bisect(self.result_rank, idx)
- self.result_rank.insert(insert, idx)
- self.result_data.insert(insert, res)
-
- def __len__(self):
- return self.put_idx - self.get_idx
-
- def __call__(self, image):
- self.put(image)
- return self.get()
-
- def shutdown(self):
- for _ in self.procs:
- self.task_queue.put(AsyncPredictor._StopToken())
-
- @property
- def default_buffer_size(self):
- return len(self.procs) * 5
diff --git a/spaces/asciicorp/Legal-ai/app.py b/spaces/asciicorp/Legal-ai/app.py
deleted file mode 100644
index f3f992b3ca8af7cc0fb3c57251a07ffecc29b83a..0000000000000000000000000000000000000000
--- a/spaces/asciicorp/Legal-ai/app.py
+++ /dev/null
@@ -1,292 +0,0 @@
-import os
-import time
-import pickle
-import spacy
-from spacy import displacy
-from langchain.text_splitter import RecursiveCharacterTextSplitter, CharacterTextSplitter
-
-import streamlit as st
-from streamlit_option_menu import option_menu
-from streamlit_chat import message
-
-from ingest_docs import ingest_docs, ingest_new_docs
-from query_data import get_chain
-from retrieval_qa import qa_retrive_chain
-from similarity import calculate_textual_similarity, calculate_linguistic_similarity, calculate_semantic_similarity, highlight_text_differences
-from extract_text import extract_info
-from adherance_check import check_agreement
-from summarize_doc import summarize_pdf
-from default_text import default_text1, default_text2, default_text3, default_text4, default_text5, default_template
-from markup import legal_ai_tools_demo, legal_ai_tools_demo_todo, vecstore_into, chatbot_intro, chatbotapi_intro, retrieval_intro
-from help_text import HELP_TEXT
-from save import save_function
-
-
-nlp = spacy.load("en_core_web_sm")
-os.environ["OPENAI_API_KEY"] = "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"
-
-def tab1():
- st.header("Legal AI Tools")
- col1, col2 = st.columns([1, 2])
- with col1:
- st.image("image.jpg", use_column_width=True)
- with col2:
- st.markdown(legal_ai_tools_demo(), unsafe_allow_html=True)
- st.markdown(legal_ai_tools_demo_todo(),unsafe_allow_html=True)
-
-def tab2():
- st.header("Manage vectorstore")
- st.markdown(vecstore_into())
- col1, col2 = st.columns(2)
- with col1:
- st.subheader("List of Files")
- files = os.listdir("docs")
- if not files:
- st.write("No files found.")
- for file in files:
- file_col, delete_col = st.columns([8, 1])
- file_col.write("- " + file)
- delete_button = delete_col.button("Delete", key=f"delete_{file}")
- if delete_button:
- os.remove(os.path.join("docs", file))
- st.experimental_rerun()
-
- with col2:
- st.subheader("Upload Files")
- uploaded_files = st.file_uploader("Select files", type=["pdf"], accept_multiple_files=True)
- if uploaded_files:
- for uploaded_file in uploaded_files:
- file_exists = os.path.isfile(os.path.join("docs", uploaded_file.name))
- if not file_exists:
- with open(os.path.join("new_docs", uploaded_file.name), "wb") as f:
- f.write(uploaded_file.getbuffer())
- st.success(f"File '{uploaded_file.name}' uploaded.")
- else:
- st.warning(f"File '{uploaded_file.name}' already exists.")
-
- st.write("")
- with st.expander("Advanced"):
- st.subheader("Advanced Options")
- col1, col2 = st.columns([2, 1])
-
- with col2:
- vectorstore_path = "vectorstore.pkl"
- vectorstore_size = os.path.getsize(vectorstore_path)
- vectorstore_last_updated = os.path.getmtime(vectorstore_path)
- vectorstore_last_updated_str = time.strftime('%Y-%m-%d %H:%M:%S', time.localtime(vectorstore_last_updated))
- st.subheader("Index Info")
- st.write(f"Index size: {vectorstore_size} bytes")
- st.write(f"Index Last updated: {vectorstore_last_updated_str}")
-
- with col1:
- text_splitter_cls = st.radio("Select text splitter", options=[RecursiveCharacterTextSplitter, CharacterTextSplitter], help=HELP_TEXT["Select text splitter"])
- if text_splitter_cls == CharacterTextSplitter:
- chunk_size = st.slider("Chunk size", min_value=100, max_value=10000, step=100, value=1000, help=HELP_TEXT["Chunk size"])
- chunk_overlap = st.slider("Chunk overlap", min_value=0, max_value=500, step=10, value=0, help=HELP_TEXT["Chunk overlap"])
- else:
- chunk_size = None
- chunk_overlap = None
-
- if st.button("Reindex all"):
- st.spinner("Ingesting documents...")
- ingest_docs(text_splitter_cls, chunk_size, chunk_overlap)
- st.success("Documents ingested.")
-
- if st.button("Ingest New Documents"):
- st.spinner("Ingesting documents...")
- ingest_new_docs(text_splitter_cls, chunk_size, chunk_overlap)
- st.success("Documents ingested.")
-
-if "generated" not in st.session_state:
- st.session_state["generated"] = []
-
-if "past" not in st.session_state:
- st.session_state["past"] = []
-
-def tab3():
- col1, col2 = st.columns([3, 1])
- with col2:
- with st.expander("Model Options"):
- model_list = ["text-davinci-003", "text-davinci-002", "gpt-3.5-turbo", "gpt-3.5-turbo-0301"]
- selected_model = st.selectbox("Select a model", model_list)
- temperature = st.slider("LLM Temperature", 0.0, 1.0, 0.0, 0.1, help=HELP_TEXT["LLM Temperature"])
- max_tokens = st.slider("Max Tokens", 0, 2048, 2048, 100, help=HELP_TEXT["Max Tokens"]) #removed since varies for different models
- frequency_penalty = st.slider("Frequency Penalty", -2.0, 2.0, 0.0, 0.1, help=HELP_TEXT["Frequency Penalty"]) #todo
- presence_penalty = st.slider("Presence Penalty", -2.0, 2.0, 0.0, 0.1, help=HELP_TEXT["Presence Penalty"]) #todo
- with st.expander("AI Options"):
- template = st.text_area("AI Prompt", height=500, value=default_template)
- with st.expander("Create API"):
- st.markdown(chatbotapi_intro())
- save_button = st.button("Generate API")
- if save_button:
- save_function(selected_model, temperature, template)
- with st.expander("Create streamlit demo"):
- stcreate_button = st.button("Generate streamlit app")
-
- with col1:
- with open("vectorstore.pkl", "rb") as f:
- vectorstore = pickle.load(f)
- qa_chain = get_chain(selected_model, vectorstore, temperature, template)
- chat_history = []
- if "past" not in st.session_state:
- st.session_state.past = []
- if "generated" not in st.session_state:
- st.session_state.generated = []
-
- st.header("Contextual chatbot")
- st.markdown(chatbot_intro(), unsafe_allow_html=True)
- question = st.text_input("Ask:")
- submit_button = st.button("Chat")
- if submit_button:
- with st.spinner('Searching for answer...'):
- result = qa_chain({"question": question, "chat_history": chat_history})
- chat_history.append((question, result["answer"]))
-
- st.session_state.past.append(question)
- st.session_state.generated.append(result)
-
- if st.session_state["generated"]:
- for i in range(len(st.session_state["generated"]) - 1, -1, -1):
- message(
- st.session_state["generated"][i]["answer"],
- key=str(i)
- )
- message(
- st.session_state["past"][i],
- is_user=True,
- key=str(i) + "_user"
- )
-
-
-def tab4():
- st.header("Retrieval Question Answering")
- st.markdown(retrieval_intro())
-
- query = st.text_input("Question:")
- submit_button = st.button("Submit")
- if submit_button:
- with st.spinner('Searching for answer...'):
- result = qa_retrive_chain({"query": query})
- st.text("answer:")
- st.write(result["result"])
- st.text("source")
- st.write(result["source_documents"])
-
-def tab5():
- st.header("Similarity comparison")
- st.markdown('This can highlight the :green[similarities] and :red[differences] in **wording** across two legal documents.')
-
- text1 = st.text_area("Enter Text 1", height=200, value=default_text1)
- text2 = st.text_area("Enter Text 2", height=200, value=default_text2)
-
- if st.button("Compare Similarity"):
- textual_similarity = calculate_textual_similarity(text1, text2)
- linguistic_similarity = calculate_linguistic_similarity(text1, text2)
- semantic_similarity = calculate_semantic_similarity(text1, text2)
- highlighted_text1, highlighted_text2 = highlight_text_differences(text1, text2)
-
- col1, col2 = st.columns(2)
- with col1:
- st.markdown(highlighted_text1, unsafe_allow_html=True)
- with col2:
- st.markdown(highlighted_text2, unsafe_allow_html=True)
-
- col1, col2, col3 = st.columns(3)
-
- with col1:
- st.subheader('Textual Similarity')
- st.markdown('measures the similarity based on the *wording* of the two texts.')
- st.write("Textual Similarity: {:.2f}%".format(textual_similarity), unsafe_allow_html=True)
-
- with col2:
- st.subheader('Linguistic Similarity')
- st.markdown('measures the similarity based on the *linguistic features* of the two texts.')
- st.write("Linguistic Similarity: {:.2f}%".format(linguistic_similarity), unsafe_allow_html=True)
-
- with col3:
- st.subheader('Semantic Similarity')
- st.markdown('measures the similarity based on the *meaning* of the two texts.')
- st.write("Semantic Similarity: {:.2f}%".format(semantic_similarity), unsafe_allow_html=True)
-
-def tab6():
- st.header("Extract Info")
- st.markdown('Extract key information from legal documents such as dates, names, address, etc.')
-
- input_text = st.text_area("Enter your text here:", height=200, value=default_text3)
- if st.button("Extract Information"):
- info = extract_info(input_text)
- highlighted_text = displacy.render(nlp(input_text), style="ent", options={"ents": [ent[3] for ent in info["names"]+info["addresses"]+info["dates"]], "colors": {"PERSON": "#66c2a5", "ADDRESS": "#fc8d62", "DATE": "#8da0cb"}})
-
- st.markdown(highlighted_text, unsafe_allow_html=True)
-
- st.write("### Extracted Information")
-
- col1, col2, col3 = st.columns(3)
-
- with col1:
- st.write("#### Names")
- for name in info["names"]:
- st.write("- {}: {}".format(name[0], name[3]))
- with col2:
- st.write("#### Addresses")
- for address in info["addresses"]:
- st.write("- {}: {}".format(address[0], address[3]))
-
- with col3:
- st.write("#### Dates")
- for date in info["dates"]:
- st.write("- {}: {}".format(date[0], date[3]))
-
-def tab7():
- st.header("Summerize")
- st.markdown('Get an overview of any document in straightforward, everyday language.')
- pdf_files = [f for f in os.listdir("docs") if f.endswith(".pdf")]
- selected_file = st.selectbox("Select a PDF file", pdf_files)
- if st.button("Summarize"): #todo
- pdf_path = os.path.join("docs", selected_file)
- summary = summarize_pdf(pdf_path)
- st.write(summary)
-
-def tab8():
- st.header("Policy checker")
- st.markdown("Checks if an Legal agreement follows the company policy")
-
- policy_text = st.text_area("Enter company policy text:", height=200, value=default_text4)
- agreement_text = st.text_area("Enter legal agreement text:", height=200, value=default_text5)
-
- col1, col2 = st.columns([3, 1])
- with col1:
-
- if st.button("Check agreement"):
- with st.spinner('checking the document...'):
- result = check_agreement(policy_text, agreement_text)
- st.write(result)
-
- with col2:
- if st.button("Rewrite agreement"):
- st.write("todo")
-
-def main():
- st.set_page_config(page_title="Legal Tools Demo", page_icon=":memo:", layout="wide")
- tabs = ["Intro", "Index", "Chat", "Retrieve", "Similarity", "Extract", "Summerize", "Adherence"]
-
- with st.sidebar:
-
- current_tab = option_menu("Select a Tab", tabs, menu_icon="cast")
-
- tab_functions = {
- "Intro": tab1,
- "Index": tab2,
- "Chat": tab3,
- "Retrieve": tab4,
- "Similarity": tab5,
- "Extract": tab6,
- "Summerize": tab7,
- "Adherence": tab8,
- }
-
- if current_tab in tab_functions:
- tab_functions[current_tab]()
-
-if __name__ == "__main__":
- main()
\ No newline at end of file
diff --git a/spaces/asciicorp/Legal-ai/markup.py b/spaces/asciicorp/Legal-ai/markup.py
deleted file mode 100644
index 74000b57a5ab65b477bad4c2f687416216b4985b..0000000000000000000000000000000000000000
--- a/spaces/asciicorp/Legal-ai/markup.py
+++ /dev/null
@@ -1,37 +0,0 @@
-def legal_ai_tools_demo():
- return """
-
About
-
-
-
Ingest multiple documents into a vector store:This demo can take multiple documents and store them permanently in a vector store, allowing for efficient and easy access to the data.
-
Chat with the vector store:Chatbot can interact with the vector store, using its memory and predefined identity to facilitate more effective and personalized communication.
-
Question answering with source retrieval:This demo can answer questions by retrieving relevant information from a variety of sources, providing accurate and comprehensive answers.
-
Compare wording similarities of multiple legal documents:This demo can compare the wording of multiple legal documents, highlighting similarities and differences to help identify potential issues or discrepancies.
-
Extract key information from legal documents:This demo can analyze legal documents and extract key information, making it easier to understand the content and identify important details.
-
Summarize long legal documents into everyday language:This demo can take complex legal documents and summarize them into clear and concise language, making the information more accessible and easier to understand.
-
Compare multiple documents to identify policy adherence:This demo can compare multiple documents, such as a company policy and an agreement, to determine if the agreement follows the policy and identify any potential conflicts or discrepancies.
index loads at the statup. changes to vectstore does not apply until restart, summerize, chatbot reaches token limits. Semantic Similarity
-
-
- """
-
-def vecstore_into():
- return "Effortlessly upload one or several documents and have them ingested into a durable vectorstore for rapid access. system utilizes **openai** embeddings and **faiss** vectorstore by default, but can be tailored to meet specific needs."
-
-def chatbot_intro():
- return """chatbot designed with a contextual approach and utilizes the vector store as its knowledge base. It possesses a memory and a distinct personality. Scope is limited to answering queries pertaining only to legal documents.
-
You can customize the chatbot to your likening on the go using the settings on the left, and save the custom API script once done.