diff --git a/spaces/1acneusushi/gradio-2dmoleculeeditor/data/Arm Ds 5 License File Crack What You Need to Know About the Different Editions and Features.md b/spaces/1acneusushi/gradio-2dmoleculeeditor/data/Arm Ds 5 License File Crack What You Need to Know About the Different Editions and Features.md
deleted file mode 100644
index 8e04a2907f9866a2725118f6ac10ca4b0d5dd5c8..0000000000000000000000000000000000000000
--- a/spaces/1acneusushi/gradio-2dmoleculeeditor/data/Arm Ds 5 License File Crack What You Need to Know About the Different Editions and Features.md
+++ /dev/null
@@ -1,87 +0,0 @@
-
-
Arm Ds 5 License File Crack: How to Install and Use the Professional Software Development Tool for Embedded Systems
-
Are you looking for a powerful and versatile software development tool for embedded systems based on Arm processors? Do you want to create, debug, optimize, and analyze your applications with ease and efficiency? If yes, then you might be interested in Arm Ds 5, the professional software development studio that covers all stages of development from boot code and kernel debugging to application and bare-metal performance analysis. But what is Arm Ds 5 exactly, and how can you get it for free? In this article, we will answer these questions and show you how to crack Arm Ds 5 license file and use its features to enhance your embedded software development.
Arm Ds 5 is a software development studio that provides a comprehensive solution for embedded systems development based on Arm processors. It supports a wide range of targets, from microcontrollers to multicore systems-on-chip (SoCs), and enables you to develop applications for bare-metal embedded systems and Linux-based systems. It also supports fixed virtual platforms (FVPs) that allow you to simulate the behavior of hardware without the need for actual devices.
-
Features and benefits of Arm Ds 5
-
Some of the main features and benefits of Arm Ds 5 are:
-
-
Eclipse for Ds 5: An integrated development environment (IDE) that combines the Eclipse Foundation Eclipse IDE and Arm compilation and debug technologies. It provides a project manager, an editor, a C/C++ perspective, a debug configuration perspective, and a DS-5 perspective.
-
Ds 5 Debugger: A graphical debugger that supports target and virtual platform software development based on Arm processors. It provides a comprehensive and intuitive view of source code, disassembly, call stack, memory, registers, expressions, variables, threads, breakpoints, and tracing.
-
Arm Streamline: A graphical performance analysis tool that converts sampling data and trace data into visual and statistical reports. It helps you identify performance bottlenecks, optimize code efficiency, and improve system responsiveness.
-
Arm Compiler: A toolchain that enables you to build applications and libraries for bare-metal embedded systems. It supports both Arm Compiler 5 and Arm Compiler 6 versions.
-
Dedicated examples, applications, and supporting documentation that help you get started with using Arm Ds 5 tools.
-
-
Editions and licensing options of Arm Ds 5
-
Arm Ds 5 offers different editions and licensing options depending on your needs and budget. These include:
Arm Ds-5 Ultimate Edition: The most comprehensive edition that includes all the features of Arm Ds-5 Professional Edition plus support for additional targets such as Mali GPUs, big.LITTLE systems, Cortex-A72 processors, Cortex-R8 processors, Cortex-M7 processors, etc. It also includes access to premium support services from Arm experts. This edition requires a paid subscription license.
-
Arm Ds-5 Professional Edition: The standard edition that includes all the core features of Arm Ds-5 such as Eclipse for DS-5, DS-5 Debugger, Arm Streamline, Arm Compiler, etc. It supports a wide range of targets such as Cortex-A processors, Cortex-R processors, Cortex-M processors, etc. This edition requires a paid subscription license.
-
Arm DS-5 Altera Edition: A special edition that is designed for Altera SoC FPGA devices. It includes all the features of Arm DS-5 Professional Edition plus support for Altera SoC FPGA devices such as Cyclone V SoC FPGA devices, Arria V SoC FPGA devices, Arria 10 SoC FPGA devices, etc. This edition requires a paid subscription license.
-
Arm DS-5 Community Edition: A free edition that includes a subset of features of Arm DS-5 Professional Edition such as Eclipse for DS-5, DS-5 Debugger (Linux Ethernet debug only), etc. It supports a limited range of targets such as Cortex-A9 processors (Linux Ethernet debug only), Cortex-M processors (bare-metal debug only), etc. This edition requires a free web license.
-
Arm DS-5 Evaluation Edition: A trial edition that includes all the features of Arm DS-5 Ultimate Edition but with a limited duration of 30 days. It supports all the targets that are supported by Arm DS-5 Ultimate Edition. This edition requires a free evaluation license.
-
-
How to crack Arm Ds 5?
-
If you want to use Arm Ds 5 without paying for a subscription license or using a web license with limited features, you can try to crack it by following these steps:
-
Step 1: Download and install Arm Ds 5
-
The first step is to download and install Arm Ds 5 on your computer. You can download it from the official website of Arm at https://developer.arm.com/tools-and-software/embedded/legacy-tools/ds-5-development-studio/downloads . You can choose any edition that you want to crack (Ultimate Edition is recommended). After downloading the installer file (.exe for Windows or .bin for Linux), run it and follow the instructions to complete the installation process.
-
Step 2: Download and run the crack file
-
The next step is to download and run the crack file that will modify some files in your installation directory to bypass the license verification process. You can download the crack file from this link: https://www.codetd.com/en/article/6637418 . After downloading the crack file (.zip), extract it to any folder on your computer. Then open the instructions.txt file in the folder and follow the steps to run the crack file (.bat for Windows or .sh for Linux). You may need to run it as administrator or with sudo privileges depending on your system settings.
-
Step 3: Generate and install the license file
-
The final step is to generate and install the license file that will activate your cracked version of Arm Ds 5. You can generate the license file by using the keygen.exe file in the crack folder. Run it and enter any serial number (such as AC+70616421313531) in the input box. Then click on Generate License File button and save the license file (.lic) in any location on your computer. Then open Eclipse for DS-5 from your installation directory or from your start menu. Go to Help > ARM License Manager > Add License > Browse License File > Select your license file > Finish. You should see a message saying "License added successfully". Now you can use all the features of Arm DS-5 without any limitations.
-
How to use Arm Ds 5?
-
Now that you have cracked Arm Ds 5 license file and activated your version of Arm Ds 5, you might be wondering how to use it effectively for your embedded software development projects. Here are some tips on how to use some of its main features:
-
Eclipse for DS-5: The integrated development environment
-
Eclipse for DS-5 is an IDE that combines Eclipse IDE with ARM compilation and debug technologies. It allows you to create, manage, edit, build, debug, analyze, and optimize your projects in one place. To use Eclipse for DS-5:
0a6ba089eb
-
-
\ No newline at end of file
diff --git a/spaces/1acneusushi/gradio-2dmoleculeeditor/data/Bagatrix Math Suite 64 bit Learn Math Faster and Easier with This Software.md b/spaces/1acneusushi/gradio-2dmoleculeeditor/data/Bagatrix Math Suite 64 bit Learn Math Faster and Easier with This Software.md
deleted file mode 100644
index 05fb53afc8bec4e792a0fe29e4cb64e7244a5fc3..0000000000000000000000000000000000000000
--- a/spaces/1acneusushi/gradio-2dmoleculeeditor/data/Bagatrix Math Suite 64 bit Learn Math Faster and Easier with This Software.md
+++ /dev/null
@@ -1,65 +0,0 @@
-
-
Bagatrix Math Suite 64 bit: A Powerful Tool for Solving Math Problems
-
Do you struggle with math homework or exams? Do you wish you had a personal tutor who could explain every step and concept in detail? Do you want to learn math at your own pace and level? If you answered yes to any of these questions, then you might be interested in Bagatrix Math Suite 64 bit, a software that can help you solve math problems with ease.
-
Bagatrix Math Suite 64 bit is a collection of programs that cover various math subjects, such as algebra, geometry, calculus, trigonometry, statistics, and more. It allows you to enter any math problem and get a step-by-step solution with explanations, examples, graphs, and tips. You can also practice your skills with interactive quizzes and tests, or create your own worksheets and exams. Whether you are a student, a teacher, or a parent, Bagatrix Math Suite 64 bit can help you improve your math performance and confidence.
To use Bagatrix Math Suite 64 bit, you need to have a Windows operating system (XP, Vista, 7, 8, or 10) and an internet connection. You can download the software from the official website or from other online sources. The download size is about 300 MB and the installation process is simple and fast. Once you install the software, you need to register it with your email address and activation code. You can then run the software from your desktop or start menu.
-
Choosing a Math Topic and Level
-
When you launch the software, you will see a list of math topics on the left side of the screen. You can choose from algebra, geometry, calculus, trigonometry, statistics, pre-algebra, finite math, college algebra, pre-calculus, linear algebra, differential equations, discrete math, business math, or SAT/ACT prep. Each topic has different levels of difficulty and subtopics that you can select according to your needs and goals. For example, if you choose algebra, you can choose from basic algebra, intermediate algebra, college algebra, or advanced algebra.
-
Solving Problems with Step-by-Step Explanations
-
Once you choose a topic and level, you can enter any math problem in the input box at the top of the screen. You can use the keyboard or the virtual keypad to type in numbers, symbols, operators, functions, fractions, exponents, roots, etc. You can also copy and paste problems from other sources or use the problem generator to get random problems. After entering the problem, you can click on the solve button to get a detailed solution with explanations for every step. You can also click on the show me button to see an example of a similar problem solved by the software.
-
Graphing Functions and Data
-
Another feature of Bagatrix Math Suite 64 bit is that it can graph any function or data that you enter or generate. You can access the graphing tool by clicking on the graph button at the bottom of the screen. You can then enter one or more functions or data sets in the input box and click on the graph button to see a visual representation of them. You can also customize the graph by changing the color, style, scale, axis labels, grid lines, etc. You can also zoom in or out, move around, trace points, find intercepts
-
Bagatrix Math Suite 64 bit download
-Bagatrix Math Suite 64 bit free trial
-Bagatrix Math Suite 64 bit crack
-Bagatrix Math Suite 64 bit review
-Bagatrix Math Suite 64 bit tutorial
-Bagatrix Math Suite 64 bit price
-Bagatrix Math Suite 64 bit features
-Bagatrix Math Suite 64 bit system requirements
-Bagatrix Math Suite 64 bit alternative
-Bagatrix Math Suite 64 bit support
-Bagatrix Math Suite 64 bit for Windows 10
-Bagatrix Math Suite 64 bit for Mac
-Bagatrix Math Suite 64 bit for Linux
-Bagatrix Math Suite 64 bit online
-Bagatrix Math Suite 64 bit vs Mathematica
-Bagatrix Math Suite 64 bit vs Matlab
-Bagatrix Math Suite 64 bit vs Maple
-Bagatrix Math Suite 64 bit vs Wolfram Alpha
-Bagatrix Math Suite 64 bit vs Khan Academy
-Bagatrix Math Suite 64 bit vs Photomath
-Bagatrix Math Suite 64 bit coupon code
-Bagatrix Math Suite 64 bit discount
-Bagatrix Math Suite 64 bit upgrade
-Bagatrix Math Suite 64 bit refund policy
-Bagatrix Math Suite 64 bit testimonials
-Bagatrix Math Suite 64 bit user guide
-Bagatrix Math Suite 64 bit FAQ
-Bagatrix Math Suite 64 bit forum
-Bagatrix Math Suite 64 bit blog
-Bagatrix Math Suite 64 bit YouTube channel
-Bagatrix Math Suite 64 bit Facebook page
-Bagatrix Math Suite 64 bit Twitter account
-Bagatrix Math Suite 64 bit Instagram profile
-Bagatrix Math Suite 64 bit LinkedIn page
-Bagatrix Math Suite 64 bit Reddit community
-Bagatrix Math Suite 64 bit Quora space
-Bagatrix Math Suite 64 bit Medium publication
-Bagatrix Math Suite 64 bit GitHub repository
-Bagatrix Math Suite 64 bit npm package[^1^]
-Bagatrix Math Suite 64 bit PDF file[^2^]
-Bagatrix Math Suite 64 bit ebook[^3^]
-Bagatrix Math Suite 64 bit audiobook[^3^]
-Bagatrix Math Suite 64 bit video course[^3^]
-Bagatrix Math Suite 64 bit webinar[^3^]
-Bagatrix Math Suite 64 bit podcast[^3^]
-Bagatrix Math Suite 64 bit case study[^3^]
-Bagatrix Math Suite 64 bit white paper[^3^]
-Bagatrix Math Suite 64 bit infographic[^3^]
-Bagatrix Math Suite 64 bit comparison chart[^3^]
0a6ba089eb
-
-
\ No newline at end of file
diff --git a/spaces/1gistliPinn/ChatGPT4/Examples/Coreldrawgraphicssuitex4installer En Serial Number.md b/spaces/1gistliPinn/ChatGPT4/Examples/Coreldrawgraphicssuitex4installer En Serial Number.md
deleted file mode 100644
index 81ef97c04f07cc10195fa17a554cd8300b9c56f2..0000000000000000000000000000000000000000
--- a/spaces/1gistliPinn/ChatGPT4/Examples/Coreldrawgraphicssuitex4installer En Serial Number.md
+++ /dev/null
@@ -1,34 +0,0 @@
-
-
How to Install CorelDRAW Graphics Suite X4 with a Serial Number
-
If you have purchased CorelDRAW Graphics Suite X4, you will need a serial number to activate and use the software. A serial number is a unique code that identifies your product and proves your ownership. In this article, we will show you how to install CorelDRAW Graphics Suite X4 with a serial number in a few easy steps.
-
Coreldrawgraphicssuitex4installer En Serial Number
The first step is to download the installer for CorelDRAW Graphics Suite X4 from the official website. You can choose between the English version or the multilingual version, depending on your preference. The installer file name will be Coreldrawgraphicssuitex4installer_en.exe or Coreldrawgraphicssuitex4installer_mlen.exe, respectively.
-
Step 2: Run the Installer
-
The next step is to run the installer file that you have downloaded. You can do this by double-clicking on it or right-clicking and selecting "Run as administrator". You will see a welcome screen that asks you to choose your language and accept the license agreement. Click "Next" to continue.
-
Step 3: Enter Your Serial Number
-
The most important step is to enter your serial number that you have received when you purchased CorelDRAW Graphics Suite X4. You can find your serial number in your order confirmation email, on the product packaging, or on your account page on the Corel website. The serial number will be a 24-digit code that starts with DR14. Enter your serial number in the box and click "Next".
-
-
Step 4: Choose Your Installation Options
-
The final step is to choose your installation options. You can customize your installation by selecting which components and features you want to install, such as fonts, clipart, templates, etc. You can also choose the destination folder where you want to install CorelDRAW Graphics Suite X4. Click "Install Now" to start the installation process.
-
Step 5: Enjoy Your Software
-
Once the installation is complete, you can launch CorelDRAW Graphics Suite X4 and start creating amazing graphics and designs. You can also register your product online to get access to updates, support, and other benefits. Congratulations! You have successfully installed CorelDRAW Graphics Suite X4 with a serial number.
-
-I hope this helps. ð
-
-
How to Use CorelDRAW Graphics Suite X4
-
Now that you have installed CorelDRAW Graphics Suite X4, you may wonder how to use it. CorelDRAW Graphics Suite X4 is a powerful and versatile software that allows you to create vector graphics, photo editing, page layout, web design, and more. In this section, we will give you some tips and tricks on how to use CorelDRAW Graphics Suite X4 effectively.
-
Tip 1: Explore the Workspace
-
The first tip is to explore the workspace of CorelDRAW Graphics Suite X4. The workspace is the area where you can access all the tools, menus, panels, and options that you need to work on your projects. You can customize the workspace to suit your preferences and workflow by choosing from different presets or creating your own. You can also switch between different workspaces for different tasks, such as drawing, editing, web design, etc.
-
Tip 2: Learn the Basics
-
The second tip is to learn the basics of CorelDRAW Graphics Suite X4. The basics include how to create and save documents, how to use the drawing and shaping tools, how to apply colors and fills, how to add text and effects, how to import and export files, and more. You can find tutorials and guides on the Corel website or on the Help menu of the software. You can also access online resources such as videos, blogs, forums, and webinars to learn more.
-
Tip 3: Experiment with Features
-
The third tip is to experiment with the features of CorelDRAW Graphics Suite X4. CorelDRAW Graphics Suite X4 offers a wide range of features that can help you create stunning graphics and designs. Some of the features include interactive tools, smart drawing tools, live trace, power trace, bitmap-to-vector conversion, photo-paint, corel capture, corel connect, and more. You can try out different features and see how they work for your projects.
-
Tip 4: Get Inspired
-
The fourth tip is to get inspired by other users of CorelDRAW Graphics Suite X4. You can browse through the gallery of CorelDRAW Graphics Suite X4 users and see what they have created with the software. You can also join the community of CorelDRAW Graphics Suite X4 users and share your work, feedback, questions, and ideas. You can also participate in contests and challenges to showcase your skills and win prizes.
-
Tip 5: Have Fun
-
The fifth and final tip is to have fun with CorelDRAW Graphics Suite X4. CorelDRAW Graphics Suite X4 is a software that allows you to express your creativity and imagination in various ways. You can create anything you want with CorelDRAW Graphics Suite X4, from logos and flyers to posters and websites. You can also enjoy the process of creating and learning with CorelDRAW Graphics Suite X4.
-
-I hope this helps. ð d5da3c52bf
-
-
\ No newline at end of file
diff --git a/spaces/1phancelerku/anime-remove-background/Download Mortal Kombat 10 APK and Join the Ultimate Tournament of Champions.md b/spaces/1phancelerku/anime-remove-background/Download Mortal Kombat 10 APK and Join the Ultimate Tournament of Champions.md
deleted file mode 100644
index 5478f72b0f699bd85ca248c3524bc9664fd0c466..0000000000000000000000000000000000000000
--- a/spaces/1phancelerku/anime-remove-background/Download Mortal Kombat 10 APK and Join the Ultimate Tournament of Champions.md
+++ /dev/null
@@ -1,88 +0,0 @@
-
-
Download Mortal Kombat 10 APK for Android: A Guide
-
Mortal Kombat 10 is one of the most popular and thrilling fighting games ever created. It features a roster of iconic characters, brutal fatalities, stunning graphics, and immersive gameplay. If you are a fan of this game and want to play it on your Android device, you might be wondering how to download Mortal Kombat 10 APK for Android. In this article, we will show you how to do that, as well as the benefits and risks of downloading Mortal Kombat 10 APK for Android.
-
What is Mortal Kombat 10?
-
Mortal Kombat 10, also known as Mortal Kombat X, is the tenth installment in the Mortal Kombat series. It was developed by NetherRealm Studios and published by Warner Bros. Interactive Entertainment in 2015. It is available for various platforms, including Windows, PlayStation 4, Xbox One, iOS, and Android.
Mortal Kombat 10 follows the story of the ancient god Shinnok, who seeks to destroy the world with the help of his corrupted warriors. The game features a new generation of fighters, such as Cassie Cage, Jacqui Briggs, Takeda Takahashi, and Kung Jin, as well as returning characters like Scorpion, Sub-Zero, Raiden, and Liu Kang. The game also introduces three different variations for each character, each with their own unique abilities and moves.
-
Features of Mortal Kombat 10
-
Some of the features that make Mortal Kombat 10 an amazing game are:
-
-
Spectacular and realistic graphics that bring the characters and environments to life.
-
Dynamic and interactive stages that allow the fighters to use various objects as weapons or traps.
-
A cinematic story mode that spans over two decades and involves multiple characters and events.
-
A diverse and customizable roster of fighters, each with their own personality, style, and skills.
-
A variety of game modes, such as online multiplayer, tower challenges, faction wars, and more.
-
A rich and rewarding progression system that lets you unlock new costumes, items, fatalities, and more.
-
-
How to download Mortal Kombat 10 APK for Android
-
If you want to download Mortal Kombat 10 APK for Android, you will need to follow these steps:
-
Step 1: Enable unknown sources
-
Since Mortal Kombat 10 APK is not available on the Google Play Store, you will need to enable unknown sources on your device. This will allow you to install apps from sources other than the official store. To do this, go to Settings > Security > Unknown sources and toggle it on.
-
Step 2: Download the APK file
-
Next, you will need to download the APK file of Mortal Kombat 10 from a reliable source. You can use one of these links:
-
-
[Mortal Kombat X Download APK for Android (Free) | mob.org](^1^)
-
[MORTAL KOMBAT: The Ultimate Fighting Game APK for Android - FileHippo](^2^)
-
[Download Mortal Kombat X Apk 1.10.0 For Android ~ Techswizz](^
Step 3: Install the APK file
-
Once you have downloaded the APK file, you will need to install it on your device. To do this, locate the file in your file manager and tap on it. You will see a prompt asking you to confirm the installation. Tap on Install and wait for the process to finish.
-
Step 4: Launch the game and enjoy
-
After the installation is complete, you can launch the game from your app drawer or home screen. You will need to grant some permissions and accept some terms and conditions before you can start playing. You will also need to download some additional data for the game to run smoothly. Once everything is ready, you can enjoy Mortal Kombat 10 on your Android device.
-
download mortal kombat x apk free for android
-how to install mortal kombat 10 apk on android phone
-mortal kombat x apk mod unlimited coins and souls
-best site to download mortal kombat 10 apk and obb
-mortal kombat x apk latest version 1.10.0 download
-download mortal kombat x apk offline without internet
-mortal kombat x apk and data highly compressed
-mortal kombat 10 apk full game unlocked all characters
-mortal kombat x apk hack no root no survey
-download mortal kombat x apk for android tablet
-mortal kombat x apk gameplay and review
-mortal kombat 10 apk system requirements and compatibility
-mortal kombat x apk cheats and tips
-download mortal kombat x apk from google play store
-mortal kombat x apk file size and download speed
-mortal kombat 10 apk features and updates
-mortal kombat x apk graphics and sound quality
-download mortal kombat x apk for android tv box
-mortal kombat x apk multiplayer mode online and offline
-mortal kombat 10 apk download link and mirror link
-mortal kombat x apk error and bug fixes
-download mortal kombat x apk for pc windows 10
-mortal kombat x apk controller support and settings
-mortal kombat 10 apk rating and user reviews
-mortal kombat x apk alternatives and similar games
-
Benefits of downloading Mortal Kombat 10 APK for Android
-
There are some benefits of downloading Mortal Kombat 10 APK for Android, such as:
-
Access to the latest version of the game
-
By downloading the APK file, you can get access to the latest version of the game, which may not be available on the Google Play Store. This means you can enjoy new features, updates, bug fixes, and improvements that the developers have made.
-
No need to root your device
-
Another benefit of downloading the APK file is that you do not need to root your device to play the game. Rooting is a process that gives you full control over your device, but it also voids your warranty and exposes you to security risks. By downloading the APK file, you can avoid rooting and still play the game.
-
Save storage space and data usage
-
A third benefit of downloading the APK file is that you can save storage space and data usage on your device. The APK file is usually smaller than the official app from the Google Play Store, which means it takes up less space on your device. Moreover, you can download the APK file once and install it offline, which means you do not need to use your data every time you want to play the game.
-
Risks of downloading Mortal Kombat 10 APK for Android
-
However, there are also some risks of downloading Mortal Kombat 10 APK for Android, such as:
-
Potential malware or viruses
-
One of the main risks of downloading the APK file is that you might get malware or viruses on your device. This can happen if you download the APK file from an untrusted or malicious source. Malware or viruses can harm your device, steal your personal information, or compromise your privacy. Therefore, you should always download the APK file from a reliable and reputable source.
-
Legal issues and violations
-
Another risk of downloading the APK file is that you might face legal issues or violations. This can happen if you download the APK file from an unauthorized or illegal source. You might be infringing on the intellectual property rights of the developers or publishers of the game. You might also be violating the terms and conditions of the Google Play Store or your device manufacturer. Therefore, you should always respect the rights and rules of the original creators and distributors of the game.
-
Compatibility and performance issues
-
A third risk of downloading the APK file is that you might encounter compatibility and performance issues on your device. This can happen if you download the APK file from an outdated or incompatible source. You might face problems such as crashes, glitches, errors, or lagging while playing the game. You might also miss out on some features or functions that are only available on the official app from the Google Play Store. Therefore, you should always check the compatibility and requirements of the APK file before downloading it.
-
Conclusion
-
Mortal Kombat 10 is a fantastic game that offers a lot of fun and excitement for fighting game fans. If you want to play it on your Android device, you can download Mortal Kombat 10 APK for Android from a trusted source. However, you should also be aware of the benefits and risks of doing so, and take precautions to protect your device and yourself.
-
FAQs
-
-
Q: Is Mortal Kombat 10 free to play on Android?
-
A: Yes, Mortal Kombat 10 is free to play on Android, but it also offers in-app purchases for some items and features.
-
Q: What are the minimum requirements to play Mortal Kombat 10 on Android?
-
A: According to [Mortal Kombat X - Apps on Google Play], you need at least Android 5. 0 K or higher, 1.5 GB of RAM, and a minimum of 1.5 GB of free space on your device.
-
Q: Can I play Mortal Kombat 10 offline on Android?
-
A: No, Mortal Kombat 10 requires an internet connection to play on Android. You will need to connect to the internet to download the game data, access the online features, and sync your progress.
-
Q: Can I play Mortal Kombat 10 with my friends on Android?
-
A: Yes, Mortal Kombat 10 supports online multiplayer mode on Android. You can join a faction and compete with other players in Faction Wars, or challenge your friends in online matches.
-
Q: How can I get more coins and souls in Mortal Kombat 10 on Android?
-
A: You can get more coins and souls in Mortal Kombat 10 by playing the game modes, completing the challenges, participating in the events, and watching the ads. You can also buy them with real money through in-app purchases.
-
401be4b1e0
-
-
\ No newline at end of file
diff --git a/spaces/1phancelerku/anime-remove-background/Download Traffic Racer APK Mod and Challenge Your Friends Online.md b/spaces/1phancelerku/anime-remove-background/Download Traffic Racer APK Mod and Challenge Your Friends Online.md
deleted file mode 100644
index 804d0c050c5c6601aaa57df7cccf0875e752718f..0000000000000000000000000000000000000000
--- a/spaces/1phancelerku/anime-remove-background/Download Traffic Racer APK Mod and Challenge Your Friends Online.md
+++ /dev/null
@@ -1,91 +0,0 @@
-
-
Download Traffic Racer APK Mod: A Guide for Android Users
-
If you are a fan of racing games, you might have heard of Traffic Racer, a popular game that lets you drive your car through endless highway traffic and earn cash to upgrade your vehicle. But did you know that you can also download Traffic Racer APK Mod, a modified version of the game that gives you unlimited money and unlocks all the cars and features? In this article, we will tell you everything you need to know about Traffic Racer APK Mod, including what it is, how to download it, and what are the benefits and risks of using it. Read on to find out more.
-
What is Traffic Racer?
-
Traffic Racer is a 3D racing game developed by Soner Kara, a Turkish game developer. It was released in 2012 for iOS and Android devices. The game has over 100 million downloads on Google Play Store and has an average rating of 4.4 out of 5 stars.
Traffic Racer has many features that make it an addictive and fun game to play. Some of them are:
-
-
35 different cars to choose from, ranging from sedans to sports cars to trucks.
-
5 detailed environments to drive in, such as suburb, desert, snowy, rainy, and city night.
-
5 game modes to challenge yourself, such as endless, two-way, time trial, police chase, and free ride.
-
Rich graphics and realistic physics that create a smooth and immersive driving experience.
-
Customizable car options, such as paint, wheels, and vinyls.
-
Online leaderboards and achievements to compete with other players around the world.
-
-
How to play Traffic Racer
-
The gameplay of Traffic Racer is simple and intuitive. You just need to tilt your device to steer your car, touch the gas button to accelerate, and touch the brake button to slow down. The faster you drive, the more points you get. You can also earn extra points by driving in the opposite direction in two-way mode, or by overtaking other cars closely in any mode. You can use the cash you earn to buy new cars or upgrade your existing ones. You can also change the camera view from behind to inside the car for a more realistic feel.
-
What is Traffic Racer APK Mod?
-
Traffic Racer APK Mod is a modified version of the original game that gives you some advantages that are not available in the official version. For example, you can get unlimited money to buy and upgrade any car you want, or unlock all the cars and features without having to play for hours. You can also remove the ads that may interrupt your gameplay.
-
Benefits of Traffic Racer APK Mod
-
Some of the benefits of using Traffic Racer APK Mod are:
-
Download Traffic Racer Mod APK Unlimited Money
-How to Install Traffic Racer Modded APK on Android
-Traffic Racer Hack APK Download for Free
-Best Racing Games Like Traffic Racer for Android
-Traffic Racer Mod APK Latest Version 3.6
-Download Traffic Racer Mod APK with All Cars Unlocked
-Traffic Racer Cheats and Tips for Android
-Traffic Racer Mod APK No Root Required
-Download Traffic Racer Mod APK Offline Mode
-Traffic Racer Review: A Fun and Addictive Racing Game
-Download Traffic Racer Mod APK with Unlimited Coins and Gems
-Traffic Racer Mod APK vs Original APK: Which One to Choose?
-Traffic Racer Gameplay and Features
-Download Traffic Racer Mod APK with No Ads
-Traffic Racer Mod APK for PC: How to Play on Windows
-Download Traffic Racer Mod APK with High Graphics
-Traffic Racer Mod APK for iOS: How to Download and Install
-Traffic Racer Online: Play with Friends and Compete
-Download Traffic Racer Mod APK with New Cars and Tracks
-Traffic Racer Update: What's New in Version 3.6
-
-
You can enjoy the game without any limitations or restrictions.
-
You can save your time and effort by skipping the grinding process.
-
You can explore all the cars and environments without having to unlock them.
-
You can have more fun and excitement by driving faster and crazier.
-
-
Risks of Traffic Racer APK Mod
-
However, there are also some risks involved in using Traffic Racer APK Mod. Some of them are:
-
-
You may face legal issues if the game developer finds out that you are using a modified version of their game.
-
You may lose your progress or data if the modded version is not compatible with the latest version of the game or your device.
-
You may expose your device to malware or viruses that may harm your system or steal your personal information.
-
You may lose the thrill and challenge of the game by having everything handed to you.
-
-
Therefore, you should be careful and responsible when using Traffic Racer APK Mod. You should also respect the game developer's rights and efforts and support them by playing the official version of the game.
-
How to download Traffic Racer APK Mod?
-
If you still want to download Traffic Racer APK Mod, you need to follow some steps to do it safely and correctly. Here are the steps you need to take:
-
Step 1: Enable unknown sources
-
Before you can install any APK file on your Android device, you need to enable the option to allow unknown sources. This means that you can install apps that are not from the Google Play Store. To do this, go to your device's settings, then security, then toggle on the unknown sources option. You may see a warning message that tells you about the risks of installing unknown apps, but you can ignore it if you trust the source of the APK file.
-
Step 2: Download the APK file
-
Next, you need to download the APK file of Traffic Racer Mod from a reliable and trustworthy website. You can search for it on Google or use one of these links:
Make sure you download the latest version of the mod that is compatible with your device and the original game. You can check the file size, version number, and date of update before downloading it. You can also read the reviews and comments from other users to see if they have any issues or complaints about the mod.
-
Step 3: Install the APK file
-
Once you have downloaded the APK file, you need to install it on your device. To do this, locate the file in your downloads folder or wherever you saved it, and tap on it. You may see a pop-up window that asks you to confirm the installation. Tap on install and wait for a few seconds until the process is complete.
-
Step 4: Launch the game and enjoy
-
Finally, you can launch the game and enjoy the modded features. You should see a lot of money in your account and all the cars and features unlocked. You can also customize your car and choose your preferred game mode and environment. Have fun driving through traffic and breaking speed records.
-
Conclusion
-
Traffic Racer is a great racing game that offers a lot of fun and excitement for Android users. However, some people may want to download Traffic Racer APK Mod, a modified version of the game that gives them unlimited money and unlocks all the cars and features. While this may sound tempting, it also comes with some risks and drawbacks that you should be aware of. Therefore, we recommend that you play the official version of the game and support the game developer by purchasing in-app items or watching ads. This way, you can enjoy the game without any problems or guilt.
-
FAQs
-
Here are some frequently asked questions about Traffic Racer APK Mod:
-
-
Is Traffic Racer APK Mod safe to use?
-
Traffic Racer APK Mod is not officially endorsed or supported by the game developer, so it may not be safe to use. It may contain malware or viruses that can harm your device or steal your personal information. It may also cause compatibility issues or data loss if it is not updated or installed properly. Therefore, you should use it at your own risk and discretion.
-
Is Traffic Racer APK Mod legal to use?
-
Traffic Racer APK Mod is not legal to use, as it violates the terms and conditions of the game developer and Google Play Store. It also infringes on the intellectual property rights of the game developer and may result in legal action against you if they find out that you are using it. Therefore, you should respect their rights and efforts and play the official version of the game.
-
Can I play Traffic Racer APK Mod online?
-
Traffic Racer APK Mod p>Traffic Racer APK Mod may not work online, as it may be detected and banned by the game server or Google Play Services. It may also cause errors or glitches in the online features, such as leaderboards and achievements. Therefore, you should play the modded version offline or use a VPN to hide your IP address.
-
Can I update Traffic Racer APK Mod?
-
Traffic Racer APK Mod may not be updated automatically, as it is not from the Google Play Store. You may need to download and install the latest version of the mod manually from the website where you got it. However, you should be careful and make sure that the new version is compatible with your device and the original game. You should also backup your data before updating, in case something goes wrong.
-
Can I uninstall Traffic Racer APK Mod?
-
Yes, you can uninstall Traffic Racer APK Mod anytime you want, just like any other app on your device. To do this, go to your device's settings, then apps, then find and tap on Traffic Racer APK Mod. Then, tap on uninstall and confirm your choice. You can also delete the APK file from your downloads folder or wherever you saved it.
- 197e85843d
-
-
\ No newline at end of file
diff --git a/spaces/1toTree/lora_test/ppdiffusers/pipelines/audio_diffusion/mel.py b/spaces/1toTree/lora_test/ppdiffusers/pipelines/audio_diffusion/mel.py
deleted file mode 100644
index bb2e4eadf467bf7f012622e3bc9bd5a2c9b8b586..0000000000000000000000000000000000000000
--- a/spaces/1toTree/lora_test/ppdiffusers/pipelines/audio_diffusion/mel.py
+++ /dev/null
@@ -1,163 +0,0 @@
-# Copyright 2022 The HuggingFace Team. All rights reserved.
-#
-# Licensed under the Apache License, Version 2.0 (the "License");
-# you may not use this file except in compliance with the License.
-# You may obtain a copy of the License at
-#
-# http://www.apache.org/licenses/LICENSE-2.0
-#
-# Unless required by applicable law or agreed to in writing, software
-# distributed under the License is distributed on an "AS IS" BASIS,
-# WITHOUT WARRANTIES OR CONDITIONS OF ANY KIND, either express or implied.
-# See the License for the specific language governing permissions and
-# limitations under the License.
-
-
-import warnings
-
-from ...configuration_utils import ConfigMixin, register_to_config
-from ...schedulers.scheduling_utils import SchedulerMixin
-
-warnings.filterwarnings("ignore")
-
-import numpy as np # noqa: E402
-
-try:
- import librosa # noqa: E402
-
- _librosa_can_be_imported = True
- _import_error = ""
-except Exception as e:
- _librosa_can_be_imported = False
- _import_error = (
- f"Cannot import librosa because {e}. Make sure to correctly install librosa to be able to install it."
- )
-
-
-from PIL import Image # noqa: E402
-
-
-class Mel(ConfigMixin, SchedulerMixin):
- """
- Parameters:
- x_res (`int`): x resolution of spectrogram (time)
- y_res (`int`): y resolution of spectrogram (frequency bins)
- sample_rate (`int`): sample rate of audio
- n_fft (`int`): number of Fast Fourier Transforms
- hop_length (`int`): hop length (a higher number is recommended for lower than 256 y_res)
- top_db (`int`): loudest in decibels
- n_iter (`int`): number of iterations for Griffin Linn mel inversion
- """
-
- config_name = "mel_config.json"
-
- @register_to_config
- def __init__(
- self,
- x_res: int = 256,
- y_res: int = 256,
- sample_rate: int = 22050,
- n_fft: int = 2048,
- hop_length: int = 512,
- top_db: int = 80,
- n_iter: int = 32,
- ):
- self.hop_length = hop_length
- self.sr = sample_rate
- self.n_fft = n_fft
- self.top_db = top_db
- self.n_iter = n_iter
- self.set_resolution(x_res, y_res)
- self.audio = None
-
- if not _librosa_can_be_imported:
- raise ValueError(_import_error)
-
- def set_resolution(self, x_res: int, y_res: int):
- """Set resolution.
-
- Args:
- x_res (`int`): x resolution of spectrogram (time)
- y_res (`int`): y resolution of spectrogram (frequency bins)
- """
- self.x_res = x_res
- self.y_res = y_res
- self.n_mels = self.y_res
- self.slice_size = self.x_res * self.hop_length - 1
-
- def load_audio(self, audio_file: str = None, raw_audio: np.ndarray = None):
- """Load audio.
-
- Args:
- audio_file (`str`): must be a file on disk due to Librosa limitation or
- raw_audio (`np.ndarray`): audio as numpy array
- """
- if audio_file is not None:
- self.audio, _ = librosa.load(audio_file, mono=True, sr=self.sr)
- else:
- self.audio = raw_audio
-
- # Pad with silence if necessary.
- if len(self.audio) < self.x_res * self.hop_length:
- self.audio = np.concatenate([self.audio, np.zeros((self.x_res * self.hop_length - len(self.audio),))])
-
- def get_number_of_slices(self) -> int:
- """Get number of slices in audio.
-
- Returns:
- `int`: number of spectograms audio can be sliced into
- """
- return len(self.audio) // self.slice_size
-
- def get_audio_slice(self, slice: int = 0) -> np.ndarray:
- """Get slice of audio.
-
- Args:
- slice (`int`): slice number of audio (out of get_number_of_slices())
-
- Returns:
- `np.ndarray`: audio as numpy array
- """
- return self.audio[self.slice_size * slice : self.slice_size * (slice + 1)]
-
- def get_sample_rate(self) -> int:
- """Get sample rate:
-
- Returns:
- `int`: sample rate of audio
- """
- return self.sr
-
- def audio_slice_to_image(self, slice: int) -> Image.Image:
- """Convert slice of audio to spectrogram.
-
- Args:
- slice (`int`): slice number of audio to convert (out of get_number_of_slices())
-
- Returns:
- `PIL Image`: grayscale image of x_res x y_res
- """
- S = librosa.feature.melspectrogram(
- y=self.get_audio_slice(slice), sr=self.sr, n_fft=self.n_fft, hop_length=self.hop_length, n_mels=self.n_mels
- )
- log_S = librosa.power_to_db(S, ref=np.max, top_db=self.top_db)
- bytedata = (((log_S + self.top_db) * 255 / self.top_db).clip(0, 255) + 0.5).astype(np.uint8)
- image = Image.fromarray(bytedata)
- return image
-
- def image_to_audio(self, image: Image.Image) -> np.ndarray:
- """Converts spectrogram to audio.
-
- Args:
- image (`PIL Image`): x_res x y_res grayscale image
-
- Returns:
- audio (`np.ndarray`): raw audio
- """
- bytedata = np.frombuffer(image.tobytes(), dtype="uint8").reshape((image.height, image.width))
- log_S = bytedata.astype("float") * self.top_db / 255 - self.top_db
- S = librosa.db_to_power(log_S)
- audio = librosa.feature.inverse.mel_to_audio(
- S, sr=self.sr, n_fft=self.n_fft, hop_length=self.hop_length, n_iter=self.n_iter
- )
- return audio
diff --git a/spaces/52Hz/CMFNet_deblurring/README.md b/spaces/52Hz/CMFNet_deblurring/README.md
deleted file mode 100644
index 4d1c4cabe97012b95a2c1277a169c69e3a5fdf97..0000000000000000000000000000000000000000
--- a/spaces/52Hz/CMFNet_deblurring/README.md
+++ /dev/null
@@ -1,37 +0,0 @@
----
-title: CMFNet_deblurring
-emoji: 🍻
-colorFrom: indigo
-colorTo: indigo
-sdk: gradio
-app_file: app.py
-pinned: false
----
-
-# Configuration
-
-`title`: _string_
-Display title for the Space
-
-`emoji`: _string_
-Space emoji (emoji-only character allowed)
-
-`colorFrom`: _string_
-Color for Thumbnail gradient (red, yellow, green, blue, indigo, purple, pink, gray)
-
-`colorTo`: _string_
-Color for Thumbnail gradient (red, yellow, green, blue, indigo, purple, pink, gray)
-
-`sdk`: _string_
-Can be either `gradio`, `streamlit`, or `static`
-
-`sdk_version` : _string_
-Only applicable for `streamlit` SDK.
-See [doc](https://hf.co/docs/hub/spaces) for more info on supported versions.
-
-`app_file`: _string_
-Path to your main application file (which contains either `gradio` or `streamlit` Python code, or `static` html code).
-Path is relative to the root of the repository.
-
-`pinned`: _boolean_
-Whether the Space stays on top of your list.
diff --git a/spaces/AIGC-Audio/AudioGPT/audio_to_text/captioning/utils/fasttext/create_word_embedding.py b/spaces/AIGC-Audio/AudioGPT/audio_to_text/captioning/utils/fasttext/create_word_embedding.py
deleted file mode 100644
index 09da13a62a3462e730c8275320a6391536ff42c4..0000000000000000000000000000000000000000
--- a/spaces/AIGC-Audio/AudioGPT/audio_to_text/captioning/utils/fasttext/create_word_embedding.py
+++ /dev/null
@@ -1,50 +0,0 @@
-# coding=utf-8
-#!/usr/bin/env python3
-
-import numpy as np
-import pandas as pd
-import torch
-from gensim.models import FastText
-from tqdm import tqdm
-import fire
-
-import sys
-import os
-sys.path.append(os.getcwd())
-from utils.build_vocab import Vocabulary
-
-def create_embedding(caption_file: str,
- vocab_file: str,
- embed_size: int,
- output: str,
- **fasttext_kwargs):
- caption_df = pd.read_json(caption_file)
- caption_df["tokens"] = caption_df["tokens"].apply(lambda x: [""] + [token for token in x] + [""])
-
- sentences = list(caption_df["tokens"].values)
- vocabulary = torch.load(vocab_file, map_location="cpu")
-
- epochs = fasttext_kwargs.get("epochs", 10)
- model = FastText(size=embed_size, min_count=1, **fasttext_kwargs)
- model.build_vocab(sentences=sentences)
- model.train(sentences=sentences, total_examples=len(sentences), epochs=epochs)
-
- word_embeddings = np.zeros((len(vocabulary), embed_size))
-
- with tqdm(total=len(vocabulary), ascii=True) as pbar:
- for word, idx in vocabulary.word2idx.items():
- if word == "" or word == "":
- continue
- word_embeddings[idx] = model.wv[word]
- pbar.update()
-
- np.save(output, word_embeddings)
-
- print("Finish writing fasttext embeddings to " + output)
-
-
-if __name__ == "__main__":
- fire.Fire(create_embedding)
-
-
-
diff --git a/spaces/AIGC-Audio/Make_An_Audio/ldm/modules/discriminator/model.py b/spaces/AIGC-Audio/Make_An_Audio/ldm/modules/discriminator/model.py
deleted file mode 100644
index 5263368a5e74d9d07840399469ca12a54e7fecbc..0000000000000000000000000000000000000000
--- a/spaces/AIGC-Audio/Make_An_Audio/ldm/modules/discriminator/model.py
+++ /dev/null
@@ -1,295 +0,0 @@
-import functools
-import torch.nn as nn
-
-
-class ActNorm(nn.Module):
- def __init__(self, num_features, logdet=False, affine=True,
- allow_reverse_init=False):
- assert affine
- super().__init__()
- self.logdet = logdet
- self.loc = nn.Parameter(torch.zeros(1, num_features, 1, 1))
- self.scale = nn.Parameter(torch.ones(1, num_features, 1, 1))
- self.allow_reverse_init = allow_reverse_init
-
- self.register_buffer('initialized', torch.tensor(0, dtype=torch.uint8))
-
- def initialize(self, input):
- with torch.no_grad():
- flatten = input.permute(1, 0, 2, 3).contiguous().view(input.shape[1], -1)
- mean = (
- flatten.mean(1)
- .unsqueeze(1)
- .unsqueeze(2)
- .unsqueeze(3)
- .permute(1, 0, 2, 3)
- )
- std = (
- flatten.std(1)
- .unsqueeze(1)
- .unsqueeze(2)
- .unsqueeze(3)
- .permute(1, 0, 2, 3)
- )
-
- self.loc.data.copy_(-mean)
- self.scale.data.copy_(1 / (std + 1e-6))
-
- def forward(self, input, reverse=False):
- if reverse:
- return self.reverse(input)
- if len(input.shape) == 2:
- input = input[:, :, None, None]
- squeeze = True
- else:
- squeeze = False
-
- _, _, height, width = input.shape
-
- if self.training and self.initialized.item() == 0:
- self.initialize(input)
- self.initialized.fill_(1)
-
- h = self.scale * (input + self.loc)
-
- if squeeze:
- h = h.squeeze(-1).squeeze(-1)
-
- if self.logdet:
- log_abs = torch.log(torch.abs(self.scale))
- logdet = height * width * torch.sum(log_abs)
- logdet = logdet * torch.ones(input.shape[0]).to(input)
- return h, logdet
-
- return h
-
- def reverse(self, output):
- if self.training and self.initialized.item() == 0:
- if not self.allow_reverse_init:
- raise RuntimeError(
- "Initializing ActNorm in reverse direction is "
- "disabled by default. Use allow_reverse_init=True to enable."
- )
- else:
- self.initialize(output)
- self.initialized.fill_(1)
-
- if len(output.shape) == 2:
- output = output[:, :, None, None]
- squeeze = True
- else:
- squeeze = False
-
- h = output / self.scale - self.loc
-
- if squeeze:
- h = h.squeeze(-1).squeeze(-1)
- return h
-
-def weights_init(m):
- classname = m.__class__.__name__
- if classname.find('Conv') != -1:
- nn.init.normal_(m.weight.data, 0.0, 0.02)
- elif classname.find('BatchNorm') != -1:
- nn.init.normal_(m.weight.data, 1.0, 0.02)
- nn.init.constant_(m.bias.data, 0)
-
-
-class NLayerDiscriminator(nn.Module):
- """Defines a PatchGAN discriminator as in Pix2Pix
- --> see https://github.com/junyanz/pytorch-CycleGAN-and-pix2pix/blob/master/models/networks.py
- """
- def __init__(self, input_nc=3, ndf=64, n_layers=3, use_actnorm=False):
- """Construct a PatchGAN discriminator
- Parameters:
- input_nc (int) -- the number of channels in input images
- ndf (int) -- the number of filters in the last conv layer
- n_layers (int) -- the number of conv layers in the discriminator
- norm_layer -- normalization layer
- """
- super(NLayerDiscriminator, self).__init__()
- if not use_actnorm:
- norm_layer = nn.BatchNorm2d
- else:
- norm_layer = ActNorm
- if type(norm_layer) == functools.partial: # no need to use bias as BatchNorm2d has affine parameters
- use_bias = norm_layer.func != nn.BatchNorm2d
- else:
- use_bias = norm_layer != nn.BatchNorm2d
-
- kw = 4
- padw = 1
- sequence = [nn.Conv2d(input_nc, ndf, kernel_size=kw, stride=2, padding=padw), nn.LeakyReLU(0.2, True)]
- nf_mult = 1
- nf_mult_prev = 1
- for n in range(1, n_layers): # gradually increase the number of filters
- nf_mult_prev = nf_mult
- nf_mult = min(2 ** n, 8)
- sequence += [
- nn.Conv2d(ndf * nf_mult_prev, ndf * nf_mult, kernel_size=kw, stride=2, padding=padw, bias=use_bias),
- norm_layer(ndf * nf_mult),
- nn.LeakyReLU(0.2, True)
- ]
-
- nf_mult_prev = nf_mult
- nf_mult = min(2 ** n_layers, 8)
- sequence += [
- nn.Conv2d(ndf * nf_mult_prev, ndf * nf_mult, kernel_size=kw, stride=1, padding=padw, bias=use_bias),
- norm_layer(ndf * nf_mult),
- nn.LeakyReLU(0.2, True)
- ]
- # output 1 channel prediction map
- sequence += [nn.Conv2d(ndf * nf_mult, 1, kernel_size=kw, stride=1, padding=padw)]
- self.main = nn.Sequential(*sequence)
-
- def forward(self, input):
- """Standard forward."""
- return self.main(input)
-
-class NLayerDiscriminator1dFeats(NLayerDiscriminator):
- """Defines a PatchGAN discriminator as in Pix2Pix
- --> see https://github.com/junyanz/pytorch-CycleGAN-and-pix2pix/blob/master/models/networks.py
- """
- def __init__(self, input_nc=3, ndf=64, n_layers=3, use_actnorm=False):
- """Construct a PatchGAN discriminator
- Parameters:
- input_nc (int) -- the number of channels in input feats
- ndf (int) -- the number of filters in the last conv layer
- n_layers (int) -- the number of conv layers in the discriminator
- norm_layer -- normalization layer
- """
- super().__init__(input_nc=input_nc, ndf=64, n_layers=n_layers, use_actnorm=use_actnorm)
-
- if not use_actnorm:
- norm_layer = nn.BatchNorm1d
- else:
- norm_layer = ActNorm
- if type(norm_layer) == functools.partial: # no need to use bias as BatchNorm has affine parameters
- use_bias = norm_layer.func != nn.BatchNorm1d
- else:
- use_bias = norm_layer != nn.BatchNorm1d
-
- kw = 4
- padw = 1
- sequence = [nn.Conv1d(input_nc, input_nc//2, kernel_size=kw, stride=2, padding=padw), nn.LeakyReLU(0.2, True)]
- nf_mult = input_nc//2
- nf_mult_prev = 1
- for n in range(1, n_layers): # gradually decrease the number of filters
- nf_mult_prev = nf_mult
- nf_mult = max(nf_mult_prev // (2 ** n), 8)
- sequence += [
- nn.Conv1d(nf_mult_prev, nf_mult, kernel_size=kw, stride=2, padding=padw, bias=use_bias),
- norm_layer(nf_mult),
- nn.LeakyReLU(0.2, True)
- ]
-
- nf_mult_prev = nf_mult
- nf_mult = max(nf_mult_prev // (2 ** n), 8)
- sequence += [
- nn.Conv1d(nf_mult_prev, nf_mult, kernel_size=kw, stride=1, padding=padw, bias=use_bias),
- norm_layer(nf_mult),
- nn.LeakyReLU(0.2, True)
- ]
- nf_mult_prev = nf_mult
- nf_mult = max(nf_mult_prev // (2 ** n), 8)
- sequence += [
- nn.Conv1d(nf_mult_prev, nf_mult, kernel_size=kw, stride=1, padding=padw, bias=use_bias),
- norm_layer(nf_mult),
- nn.LeakyReLU(0.2, True)
- ]
- # output 1 channel prediction map
- sequence += [nn.Conv1d(nf_mult, 1, kernel_size=kw, stride=1, padding=padw)]
- self.main = nn.Sequential(*sequence)
-
-
-class NLayerDiscriminator1dSpecs(NLayerDiscriminator):
- """Defines a PatchGAN discriminator as in Pix2Pix
- --> see https://github.com/junyanz/pytorch-CycleGAN-and-pix2pix/blob/master/models/networks.py
- """
- def __init__(self, input_nc=80, ndf=64, n_layers=3, use_actnorm=False):
- """Construct a PatchGAN discriminator
- Parameters:
- input_nc (int) -- the number of channels in input specs
- ndf (int) -- the number of filters in the last conv layer
- n_layers (int) -- the number of conv layers in the discriminator
- norm_layer -- normalization layer
- """
- super().__init__(input_nc=input_nc, ndf=64, n_layers=n_layers, use_actnorm=use_actnorm)
-
- if not use_actnorm:
- norm_layer = nn.BatchNorm1d
- else:
- norm_layer = ActNorm
- if type(norm_layer) == functools.partial: # no need to use bias as BatchNorm has affine parameters
- use_bias = norm_layer.func != nn.BatchNorm1d
- else:
- use_bias = norm_layer != nn.BatchNorm1d
-
- kw = 4
- padw = 1
- sequence = [nn.Conv1d(input_nc, ndf, kernel_size=kw, stride=2, padding=padw), nn.LeakyReLU(0.2, True)]
- nf_mult = 1
- nf_mult_prev = 1
- for n in range(1, n_layers): # gradually decrease the number of filters
- nf_mult_prev = nf_mult
- nf_mult = min(2 ** n, 8)
- sequence += [
- nn.Conv1d(ndf * nf_mult_prev, ndf * nf_mult, kernel_size=kw, stride=2, padding=padw, bias=use_bias),
- norm_layer(ndf * nf_mult),
- nn.LeakyReLU(0.2, True)
- ]
-
- nf_mult_prev = nf_mult
- nf_mult = min(2 ** n_layers, 8)
- sequence += [
- nn.Conv1d(ndf * nf_mult_prev, ndf * nf_mult, kernel_size=kw, stride=1, padding=padw, bias=use_bias),
- norm_layer(ndf * nf_mult),
- nn.LeakyReLU(0.2, True)
- ]
- # output 1 channel prediction map
- sequence += [nn.Conv1d(ndf * nf_mult, 1, kernel_size=kw, stride=1, padding=padw)]
- self.main = nn.Sequential(*sequence)
-
- def forward(self, input):
- """Standard forward."""
- # (B, C, L)
- input = input.squeeze(1)
- input = self.main(input)
- return input
-
-
-if __name__ == '__main__':
- import torch
-
- ## FEATURES
- disc_in_channels = 2048
- disc_num_layers = 2
- use_actnorm = False
- disc_ndf = 64
- discriminator = NLayerDiscriminator1dFeats(input_nc=disc_in_channels, n_layers=disc_num_layers,
- use_actnorm=use_actnorm, ndf=disc_ndf).apply(weights_init)
- inputs = torch.rand((6, 2048, 212))
- outputs = discriminator(inputs)
- print(outputs.shape)
-
- ## AUDIO
- disc_in_channels = 1
- disc_num_layers = 3
- use_actnorm = False
- disc_ndf = 64
- discriminator = NLayerDiscriminator(input_nc=disc_in_channels, n_layers=disc_num_layers,
- use_actnorm=use_actnorm, ndf=disc_ndf).apply(weights_init)
- inputs = torch.rand((6, 1, 80, 848))
- outputs = discriminator(inputs)
- print(outputs.shape)
-
- ## IMAGE
- disc_in_channels = 3
- disc_num_layers = 3
- use_actnorm = False
- disc_ndf = 64
- discriminator = NLayerDiscriminator(input_nc=disc_in_channels, n_layers=disc_num_layers,
- use_actnorm=use_actnorm, ndf=disc_ndf).apply(weights_init)
- inputs = torch.rand((6, 3, 256, 256))
- outputs = discriminator(inputs)
- print(outputs.shape)
diff --git a/spaces/AIML-TUDA/safe-stable-diffusion/share_btn.py b/spaces/AIML-TUDA/safe-stable-diffusion/share_btn.py
deleted file mode 100644
index f9385340e3e30786c193cebedf8fb1de0c5a3286..0000000000000000000000000000000000000000
--- a/spaces/AIML-TUDA/safe-stable-diffusion/share_btn.py
+++ /dev/null
@@ -1,68 +0,0 @@
-community_icon_html = """"""
-
-loading_icon_html = """"""
-
-share_js = """async () => {
- async function uploadFile(file){
- const UPLOAD_URL = 'https://huggingface.co/uploads';
- const response = await fetch(UPLOAD_URL, {
- method: 'POST',
- headers: {
- 'Content-Type': file.type,
- 'X-Requested-With': 'XMLHttpRequest',
- },
- body: file, /// <- File inherits from Blob
- });
- const url = await response.text();
- return url;
- }
-
- const gradioEl = document.querySelector('body > gradio-app');
- const imgEls = gradioEl.querySelectorAll('#gallery img');
- const promptTxt = gradioEl.querySelector('#prompt-text-input input').value;
- const shareBtnEl = gradioEl.querySelector('#share-btn');
- const shareIconEl = gradioEl.querySelector('#share-btn-share-icon');
- const loadingIconEl = gradioEl.querySelector('#share-btn-loading-icon');
-
- if(!imgEls.length){
- return;
- };
-
- shareBtnEl.style.pointerEvents = 'none';
- shareIconEl.style.display = 'none';
- loadingIconEl.style.removeProperty('display');
-
- const files = await Promise.all(
- [...imgEls].map(async (imgEl) => {
- const res = await fetch(imgEl.src);
- const blob = await res.blob();
- const imgId = Date.now() % 200;
- const fileName = `diffuse-the-rest-${{imgId}}.jpg`;
- return new File([blob], fileName, { type: 'image/jpeg' });
- })
- );
-
- const urls = await Promise.all(files.map((f) => uploadFile(f)));
- const htmlImgs = urls.map(url => ``);
- const descriptionMd = `
-${htmlImgs.join(`\n`)}
-
`;
-
- const params = new URLSearchParams({
- title: promptTxt,
- description: descriptionMd,
- });
-
- const paramsStr = params.toString();
- window.open(`https://huggingface.co/spaces/stabilityai/stable-diffusion/discussions/new?${paramsStr}`, '_blank');
-
- shareBtnEl.style.removeProperty('pointer-events');
- shareIconEl.style.removeProperty('display');
- loadingIconEl.style.display = 'none';
-}"""
\ No newline at end of file
diff --git a/spaces/ATang0729/Forecast4Muses/Model/Model6/Model6_2_ProfileRecogition/mmpretrain/configs/_base_/models/resnet101_cifar.py b/spaces/ATang0729/Forecast4Muses/Model/Model6/Model6_2_ProfileRecogition/mmpretrain/configs/_base_/models/resnet101_cifar.py
deleted file mode 100644
index a84d470e3a9828532e5cddcb1a3f7aa4fcae9f68..0000000000000000000000000000000000000000
--- a/spaces/ATang0729/Forecast4Muses/Model/Model6/Model6_2_ProfileRecogition/mmpretrain/configs/_base_/models/resnet101_cifar.py
+++ /dev/null
@@ -1,16 +0,0 @@
-# model settings
-model = dict(
- type='ImageClassifier',
- backbone=dict(
- type='ResNet_CIFAR',
- depth=101,
- num_stages=4,
- out_indices=(3, ),
- style='pytorch'),
- neck=dict(type='GlobalAveragePooling'),
- head=dict(
- type='LinearClsHead',
- num_classes=10,
- in_channels=2048,
- loss=dict(type='CrossEntropyLoss', loss_weight=1.0),
- ))
diff --git a/spaces/AgentVerse/agentVerse/ui/src/phaser3-rex-plugins/templates/ui/maker/builders/CreateTextBox.js b/spaces/AgentVerse/agentVerse/ui/src/phaser3-rex-plugins/templates/ui/maker/builders/CreateTextBox.js
deleted file mode 100644
index 707364a19e4c957c88765d4f794b70c52a76a5c0..0000000000000000000000000000000000000000
--- a/spaces/AgentVerse/agentVerse/ui/src/phaser3-rex-plugins/templates/ui/maker/builders/CreateTextBox.js
+++ /dev/null
@@ -1,8 +0,0 @@
-import CreateAnyLabel from './utils/CreateAnyLabel.js';
-import TextBox from '../../textbox/TextBox.js';
-
-var CreateTextBox = function (scene, data, view, styles, customBuilders) {
- return CreateAnyLabel(scene, data, view, styles, customBuilders, TextBox);
-}
-
-export default CreateTextBox;
\ No newline at end of file
diff --git a/spaces/AlexWortega/t5_predict_activity/app.py b/spaces/AlexWortega/t5_predict_activity/app.py
deleted file mode 100644
index 4dbae4e5474040c01f639ee10c9aab1081a8d98f..0000000000000000000000000000000000000000
--- a/spaces/AlexWortega/t5_predict_activity/app.py
+++ /dev/null
@@ -1,45 +0,0 @@
-import torch
-import gradio as gr
-from transformers import AutoModelForCausalLM, AutoTokenizer
-import random
-device = 'cpu'
-
-def ans(question ):
- description=''
- category=''
- seed = random.randint(1, 10000000)
- print(f'Seed: {seed}')
- torch.manual_seed(seed)
-
- inp = tokenizer.encode(f'Вопрос: {question}\nОписание: {description}\nОтвет:',return_tensors="pt").to(device)
- print('question',question)
- gen = model.generate(inp, do_sample=True, top_p=0.9, temperature=0.86, max_new_tokens=100, repetition_penalty=1.2) #, stop_token="")
-
- gen = tokenizer.decode(gen[0])
- gen = gen[:gen.index('') if '' in gen else len(gen)]
- gen = gen.split('Ответ:')[1]
- return gen
-
-
-
-
-
-
-
-# Download checkpoint:
-checkpoint = "its5Q/rugpt3large_mailqa"
-tokenizer = AutoTokenizer.from_pretrained(checkpoint)
-model = AutoModelForCausalLM.from_pretrained(checkpoint)
-model = model.eval()
-
-# Gradio
-
-title = "Ответы на главные вопросы жизни, вселенной и вообще"
-description = "t5 large predict activity "
-article = "
-
-## Todo およびバージョン計画:
-- version 3.2+ (todo): 関数プラグインがより多くのパラメーターインターフェースをサポートするようになります。
-- version 3.1: 複数のgptモデルを同時にクエリし、api2dをサポートし、複数のapikeyの負荷分散をサポートします。
-- version 3.0: chatglmおよび他の小型llmのサポート
-- version 2.6: プラグイン構造を再構成し、相互作用性を高め、より多くのプラグインを追加しました。
-- version 2.5: 自己更新。総括的な大規模プロジェクトのソースコードをまとめた場合、テキストが長すぎる、トークンがオーバーフローする問題を解決します。
-- version 2.4: (1)PDF全文翻訳機能を追加。(2)入力エリアの位置を切り替える機能を追加。(3)垂直レイアウトオプションを追加。(4)マルチスレッド関数プラグインの最適化。
-- version 2.3: 多スレッドの相互作用性を向上させました。
-- version 2.2: 関数プラグインでホットリロードをサポート
-- version 2.1: 折りたたみ式レイアウト
-- version 2.0: モジュール化された関数プラグインを導入
-- version 1.0: 基本機能
-
-## 参考および学習
-
-
-以下は中国語のマークダウンファイルです。日本語に翻訳してください。既存のマークダウンコマンドを変更しないでください:
-
-```
-多くの優秀なプロジェクトの設計を参考にしています。主なものは以下の通りです:
-
-# 参考プロジェクト1:ChuanhuChatGPTから多くのテクニックを借用
-https://github.com/GaiZhenbiao/ChuanhuChatGPT
-
-# 参考プロジェクト2:清華ChatGLM-6B:
-https://github.com/THUDM/ChatGLM-6B
-```
-
diff --git a/spaces/Amrrs/DragGan-Inversion/gui_utils/glfw_window.py b/spaces/Amrrs/DragGan-Inversion/gui_utils/glfw_window.py
deleted file mode 100644
index 69c96ff72ccff6a42bcf6ab1dbdbb8cfb8005921..0000000000000000000000000000000000000000
--- a/spaces/Amrrs/DragGan-Inversion/gui_utils/glfw_window.py
+++ /dev/null
@@ -1,239 +0,0 @@
-# Copyright (c) 2021, NVIDIA CORPORATION & AFFILIATES. All rights reserved.
-#
-# NVIDIA CORPORATION and its licensors retain all intellectual property
-# and proprietary rights in and to this software, related documentation
-# and any modifications thereto. Any use, reproduction, disclosure or
-# distribution of this software and related documentation without an express
-# license agreement from NVIDIA CORPORATION is strictly prohibited.
-
-import time
-import glfw
-import OpenGL.GL as gl
-from . import gl_utils
-
-# ----------------------------------------------------------------------------
-
-
-class GlfwWindow: # pylint: disable=too-many-public-methods
- def __init__(self, *, title='GlfwWindow', window_width=1920, window_height=1080, deferred_show=True, close_on_esc=True):
- self._glfw_window = None
- self._drawing_frame = False
- self._frame_start_time = None
- self._frame_delta = 0
- self._fps_limit = None
- self._vsync = None
- self._skip_frames = 0
- self._deferred_show = deferred_show
- self._close_on_esc = close_on_esc
- self._esc_pressed = False
- self._drag_and_drop_paths = None
- self._capture_next_frame = False
- self._captured_frame = None
-
- # Create window.
- glfw.init()
- glfw.window_hint(glfw.VISIBLE, False)
- self._glfw_window = glfw.create_window(
- width=window_width, height=window_height, title=title, monitor=None, share=None)
- self._attach_glfw_callbacks()
- self.make_context_current()
-
- # Adjust window.
- self.set_vsync(False)
- self.set_window_size(window_width, window_height)
- if not self._deferred_show:
- glfw.show_window(self._glfw_window)
-
- def close(self):
- if self._drawing_frame:
- self.end_frame()
- if self._glfw_window is not None:
- glfw.destroy_window(self._glfw_window)
- self._glfw_window = None
- # glfw.terminate() # Commented out to play it nice with other glfw clients.
-
- def __del__(self):
- try:
- self.close()
- except:
- pass
-
- @property
- def window_width(self):
- return self.content_width
-
- @property
- def window_height(self):
- return self.content_height + self.title_bar_height
-
- @property
- def content_width(self):
- width, _height = glfw.get_window_size(self._glfw_window)
- return width
-
- @property
- def content_height(self):
- _width, height = glfw.get_window_size(self._glfw_window)
- return height
-
- @property
- def title_bar_height(self):
- _left, top, _right, _bottom = glfw.get_window_frame_size(
- self._glfw_window)
- return top
-
- @property
- def monitor_width(self):
- _, _, width, _height = glfw.get_monitor_workarea(
- glfw.get_primary_monitor())
- return width
-
- @property
- def monitor_height(self):
- _, _, _width, height = glfw.get_monitor_workarea(
- glfw.get_primary_monitor())
- return height
-
- @property
- def frame_delta(self):
- return self._frame_delta
-
- def set_title(self, title):
- glfw.set_window_title(self._glfw_window, title)
-
- def set_window_size(self, width, height):
- width = min(width, self.monitor_width)
- height = min(height, self.monitor_height)
- glfw.set_window_size(self._glfw_window, width, max(
- height - self.title_bar_height, 0))
- if width == self.monitor_width and height == self.monitor_height:
- self.maximize()
-
- def set_content_size(self, width, height):
- self.set_window_size(width, height + self.title_bar_height)
-
- def maximize(self):
- glfw.maximize_window(self._glfw_window)
-
- def set_position(self, x, y):
- glfw.set_window_pos(self._glfw_window, x, y + self.title_bar_height)
-
- def center(self):
- self.set_position((self.monitor_width - self.window_width) //
- 2, (self.monitor_height - self.window_height) // 2)
-
- def set_vsync(self, vsync):
- vsync = bool(vsync)
- if vsync != self._vsync:
- glfw.swap_interval(1 if vsync else 0)
- self._vsync = vsync
-
- def set_fps_limit(self, fps_limit):
- self._fps_limit = int(fps_limit)
-
- def should_close(self):
- return glfw.window_should_close(self._glfw_window) or (self._close_on_esc and self._esc_pressed)
-
- def skip_frame(self):
- self.skip_frames(1)
-
- def skip_frames(self, num): # Do not update window for the next N frames.
- self._skip_frames = max(self._skip_frames, int(num))
-
- def is_skipping_frames(self):
- return self._skip_frames > 0
-
- def capture_next_frame(self):
- self._capture_next_frame = True
-
- def pop_captured_frame(self):
- frame = self._captured_frame
- self._captured_frame = None
- return frame
-
- def pop_drag_and_drop_paths(self):
- paths = self._drag_and_drop_paths
- self._drag_and_drop_paths = None
- return paths
-
- def draw_frame(self): # To be overridden by subclass.
- self.begin_frame()
- # Rendering code goes here.
- self.end_frame()
-
- def make_context_current(self):
- if self._glfw_window is not None:
- glfw.make_context_current(self._glfw_window)
-
- def begin_frame(self):
- # End previous frame.
- if self._drawing_frame:
- self.end_frame()
-
- # Apply FPS limit.
- if self._frame_start_time is not None and self._fps_limit is not None:
- delay = self._frame_start_time - time.perf_counter() + 1 / self._fps_limit
- if delay > 0:
- time.sleep(delay)
- cur_time = time.perf_counter()
- if self._frame_start_time is not None:
- self._frame_delta = cur_time - self._frame_start_time
- self._frame_start_time = cur_time
-
- # Process events.
- glfw.poll_events()
-
- # Begin frame.
- self._drawing_frame = True
- self.make_context_current()
-
- # Initialize GL state.
- gl.glViewport(0, 0, self.content_width, self.content_height)
- gl.glMatrixMode(gl.GL_PROJECTION)
- gl.glLoadIdentity()
- gl.glTranslate(-1, 1, 0)
- gl.glScale(2 / max(self.content_width, 1), -
- 2 / max(self.content_height, 1), 1)
- gl.glMatrixMode(gl.GL_MODELVIEW)
- gl.glLoadIdentity()
- gl.glEnable(gl.GL_BLEND)
- # Pre-multiplied alpha.
- gl.glBlendFunc(gl.GL_ONE, gl.GL_ONE_MINUS_SRC_ALPHA)
-
- # Clear.
- gl.glClearColor(0, 0, 0, 1)
- gl.glClear(gl.GL_COLOR_BUFFER_BIT | gl.GL_DEPTH_BUFFER_BIT)
-
- def end_frame(self):
- assert self._drawing_frame
- self._drawing_frame = False
-
- # Skip frames if requested.
- if self._skip_frames > 0:
- self._skip_frames -= 1
- return
-
- # Capture frame if requested.
- if self._capture_next_frame:
- self._captured_frame = gl_utils.read_pixels(
- self.content_width, self.content_height)
- self._capture_next_frame = False
-
- # Update window.
- if self._deferred_show:
- glfw.show_window(self._glfw_window)
- self._deferred_show = False
- glfw.swap_buffers(self._glfw_window)
-
- def _attach_glfw_callbacks(self):
- glfw.set_key_callback(self._glfw_window, self._glfw_key_callback)
- glfw.set_drop_callback(self._glfw_window, self._glfw_drop_callback)
-
- def _glfw_key_callback(self, _window, key, _scancode, action, _mods):
- if action == glfw.PRESS and key == glfw.KEY_ESCAPE:
- self._esc_pressed = True
-
- def _glfw_drop_callback(self, _window, paths):
- self._drag_and_drop_paths = paths
-
-# ----------------------------------------------------------------------------
diff --git a/spaces/Androidonnxfork/CivitAi-to-Diffusers/diffusers/docs/source/en/conceptual/evaluation.md b/spaces/Androidonnxfork/CivitAi-to-Diffusers/diffusers/docs/source/en/conceptual/evaluation.md
deleted file mode 100644
index 6e5c14acad4e079a68d49b183c7ae5168678f511..0000000000000000000000000000000000000000
--- a/spaces/Androidonnxfork/CivitAi-to-Diffusers/diffusers/docs/source/en/conceptual/evaluation.md
+++ /dev/null
@@ -1,572 +0,0 @@
-
-
-# Evaluating Diffusion Models
-
-
-
-
-
-Evaluation of generative models like [Stable Diffusion](https://huggingface.co/docs/diffusers/stable_diffusion) is subjective in nature. But as practitioners and researchers, we often have to make careful choices amongst many different possibilities. So, when working with different generative models (like GANs, Diffusion, etc.), how do we choose one over the other?
-
-Qualitative evaluation of such models can be error-prone and might incorrectly influence a decision.
-However, quantitative metrics don't necessarily correspond to image quality. So, usually, a combination
-of both qualitative and quantitative evaluations provides a stronger signal when choosing one model
-over the other.
-
-In this document, we provide a non-exhaustive overview of qualitative and quantitative methods to evaluate Diffusion models. For quantitative methods, we specifically focus on how to implement them alongside `diffusers`.
-
-The methods shown in this document can also be used to evaluate different [noise schedulers](https://huggingface.co/docs/diffusers/main/en/api/schedulers/overview) keeping the underlying generation model fixed.
-
-## Scenarios
-
-We cover Diffusion models with the following pipelines:
-
-- Text-guided image generation (such as the [`StableDiffusionPipeline`](https://huggingface.co/docs/diffusers/main/en/api/pipelines/stable_diffusion/text2img)).
-- Text-guided image generation, additionally conditioned on an input image (such as the [`StableDiffusionImg2ImgPipeline`](https://huggingface.co/docs/diffusers/main/en/api/pipelines/stable_diffusion/img2img), and [`StableDiffusionInstructPix2PixPipeline`](https://huggingface.co/docs/diffusers/main/en/api/pipelines/stable_diffusion/pix2pix)).
-- Class-conditioned image generation models (such as the [`DiTPipeline`](https://huggingface.co/docs/diffusers/main/en/api/pipelines/dit)).
-
-## Qualitative Evaluation
-
-Qualitative evaluation typically involves human assessment of generated images. Quality is measured across aspects such as compositionality, image-text alignment, and spatial relations. Common prompts provide a degree of uniformity for subjective metrics.
-DrawBench and PartiPrompts are prompt datasets used for qualitative benchmarking. DrawBench and PartiPrompts were introduced by [Imagen](https://imagen.research.google/) and [Parti](https://parti.research.google/) respectively.
-
-From the [official Parti website](https://parti.research.google/):
-
-> PartiPrompts (P2) is a rich set of over 1600 prompts in English that we release as part of this work. P2 can be used to measure model capabilities across various categories and challenge aspects.
-
-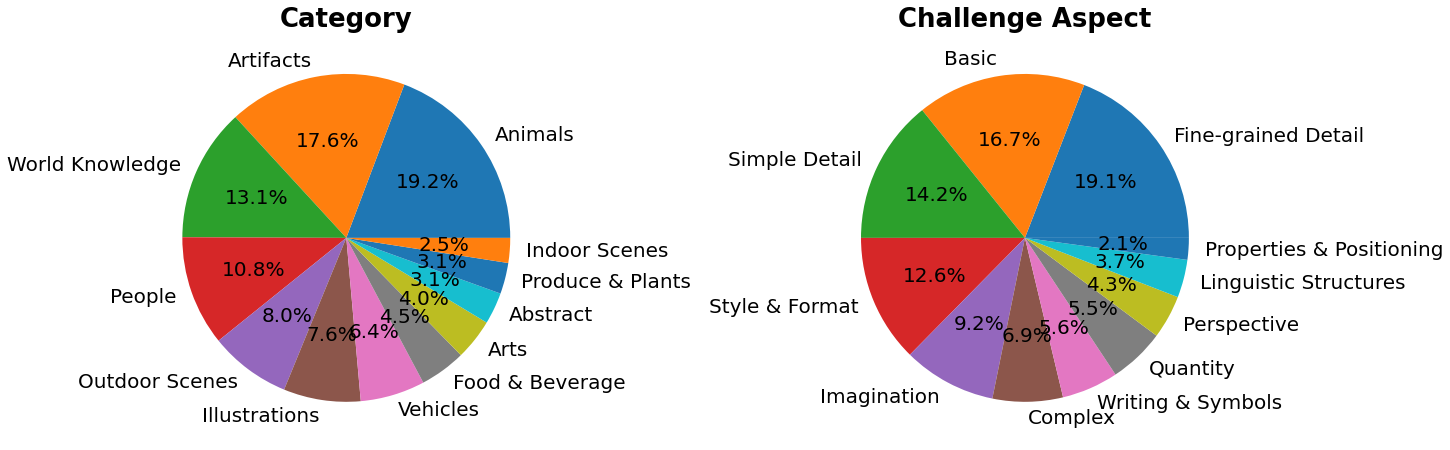
-
-PartiPrompts has the following columns:
-
-- Prompt
-- Category of the prompt (such as “Abstract”, “World Knowledge”, etc.)
-- Challenge reflecting the difficulty (such as “Basic”, “Complex”, “Writing & Symbols”, etc.)
-
-These benchmarks allow for side-by-side human evaluation of different image generation models.
-
-For this, the 🧨 Diffusers team has built **Open Parti Prompts**, which is a community-driven qualitative benchmark based on Parti Prompts to compare state-of-the-art open-source diffusion models:
-- [Open Parti Prompts Game](https://huggingface.co/spaces/OpenGenAI/open-parti-prompts): For 10 parti prompts, 4 generated images are shown and the user selects the image that suits the prompt best.
-- [Open Parti Prompts Leaderboard](https://huggingface.co/spaces/OpenGenAI/parti-prompts-leaderboard): The leaderboard comparing the currently best open-sourced diffusion models to each other.
-
-To manually compare images, let’s see how we can use `diffusers` on a couple of PartiPrompts.
-
-Below we show some prompts sampled across different challenges: Basic, Complex, Linguistic Structures, Imagination, and Writing & Symbols. Here we are using PartiPrompts as a [dataset](https://huggingface.co/datasets/nateraw/parti-prompts).
-
-```python
-from datasets import load_dataset
-
-# prompts = load_dataset("nateraw/parti-prompts", split="train")
-# prompts = prompts.shuffle()
-# sample_prompts = [prompts[i]["Prompt"] for i in range(5)]
-
-# Fixing these sample prompts in the interest of reproducibility.
-sample_prompts = [
- "a corgi",
- "a hot air balloon with a yin-yang symbol, with the moon visible in the daytime sky",
- "a car with no windows",
- "a cube made of porcupine",
- 'The saying "BE EXCELLENT TO EACH OTHER" written on a red brick wall with a graffiti image of a green alien wearing a tuxedo. A yellow fire hydrant is on a sidewalk in the foreground.',
-]
-```
-
-Now we can use these prompts to generate some images using Stable Diffusion ([v1-4 checkpoint](https://huggingface.co/CompVis/stable-diffusion-v1-4)):
-
-```python
-import torch
-
-seed = 0
-generator = torch.manual_seed(seed)
-
-images = sd_pipeline(sample_prompts, num_images_per_prompt=1, generator=generator, output_type="numpy").images
-```
-
-
-
-We can also set `num_images_per_prompt` accordingly to compare different images for the same prompt. Running the same pipeline but with a different checkpoint ([v1-5](https://huggingface.co/runwayml/stable-diffusion-v1-5)), yields:
-
-
-
-Once several images are generated from all the prompts using multiple models (under evaluation), these results are presented to human evaluators for scoring. For
-more details on the DrawBench and PartiPrompts benchmarks, refer to their respective papers.
-
-
-
-It is useful to look at some inference samples while a model is training to measure the
-training progress. In our [training scripts](https://github.com/huggingface/diffusers/tree/main/examples/), we support this utility with additional support for
-logging to TensorBoard and Weights & Biases.
-
-
-
-## Quantitative Evaluation
-
-In this section, we will walk you through how to evaluate three different diffusion pipelines using:
-
-- CLIP score
-- CLIP directional similarity
-- FID
-
-### Text-guided image generation
-
-[CLIP score](https://arxiv.org/abs/2104.08718) measures the compatibility of image-caption pairs. Higher CLIP scores imply higher compatibility 🔼. The CLIP score is a quantitative measurement of the qualitative concept "compatibility". Image-caption pair compatibility can also be thought of as the semantic similarity between the image and the caption. CLIP score was found to have high correlation with human judgement.
-
-Let's first load a [`StableDiffusionPipeline`]:
-
-```python
-from diffusers import StableDiffusionPipeline
-import torch
-
-model_ckpt = "CompVis/stable-diffusion-v1-4"
-sd_pipeline = StableDiffusionPipeline.from_pretrained(model_ckpt, torch_dtype=torch.float16).to("cuda")
-```
-
-Generate some images with multiple prompts:
-
-```python
-prompts = [
- "a photo of an astronaut riding a horse on mars",
- "A high tech solarpunk utopia in the Amazon rainforest",
- "A pikachu fine dining with a view to the Eiffel Tower",
- "A mecha robot in a favela in expressionist style",
- "an insect robot preparing a delicious meal",
- "A small cabin on top of a snowy mountain in the style of Disney, artstation",
-]
-
-images = sd_pipeline(prompts, num_images_per_prompt=1, output_type="numpy").images
-
-print(images.shape)
-# (6, 512, 512, 3)
-```
-
-And then, we calculate the CLIP score.
-
-```python
-from torchmetrics.functional.multimodal import clip_score
-from functools import partial
-
-clip_score_fn = partial(clip_score, model_name_or_path="openai/clip-vit-base-patch16")
-
-
-def calculate_clip_score(images, prompts):
- images_int = (images * 255).astype("uint8")
- clip_score = clip_score_fn(torch.from_numpy(images_int).permute(0, 3, 1, 2), prompts).detach()
- return round(float(clip_score), 4)
-
-
-sd_clip_score = calculate_clip_score(images, prompts)
-print(f"CLIP score: {sd_clip_score}")
-# CLIP score: 35.7038
-```
-
-In the above example, we generated one image per prompt. If we generated multiple images per prompt, we would have to take the average score from the generated images per prompt.
-
-Now, if we wanted to compare two checkpoints compatible with the [`StableDiffusionPipeline`] we should pass a generator while calling the pipeline. First, we generate images with a
-fixed seed with the [v1-4 Stable Diffusion checkpoint](https://huggingface.co/CompVis/stable-diffusion-v1-4):
-
-```python
-seed = 0
-generator = torch.manual_seed(seed)
-
-images = sd_pipeline(prompts, num_images_per_prompt=1, generator=generator, output_type="numpy").images
-```
-
-Then we load the [v1-5 checkpoint](https://huggingface.co/runwayml/stable-diffusion-v1-5) to generate images:
-
-```python
-model_ckpt_1_5 = "runwayml/stable-diffusion-v1-5"
-sd_pipeline_1_5 = StableDiffusionPipeline.from_pretrained(model_ckpt_1_5, torch_dtype=weight_dtype).to(device)
-
-images_1_5 = sd_pipeline_1_5(prompts, num_images_per_prompt=1, generator=generator, output_type="numpy").images
-```
-
-And finally, we compare their CLIP scores:
-
-```python
-sd_clip_score_1_4 = calculate_clip_score(images, prompts)
-print(f"CLIP Score with v-1-4: {sd_clip_score_1_4}")
-# CLIP Score with v-1-4: 34.9102
-
-sd_clip_score_1_5 = calculate_clip_score(images_1_5, prompts)
-print(f"CLIP Score with v-1-5: {sd_clip_score_1_5}")
-# CLIP Score with v-1-5: 36.2137
-```
-
-It seems like the [v1-5](https://huggingface.co/runwayml/stable-diffusion-v1-5) checkpoint performs better than its predecessor. Note, however, that the number of prompts we used to compute the CLIP scores is quite low. For a more practical evaluation, this number should be way higher, and the prompts should be diverse.
-
-
-
-By construction, there are some limitations in this score. The captions in the training dataset
-were crawled from the web and extracted from `alt` and similar tags associated an image on the internet.
-They are not necessarily representative of what a human being would use to describe an image. Hence we
-had to "engineer" some prompts here.
-
-
-
-### Image-conditioned text-to-image generation
-
-In this case, we condition the generation pipeline with an input image as well as a text prompt. Let's take the [`StableDiffusionInstructPix2PixPipeline`], as an example. It takes an edit instruction as an input prompt and an input image to be edited.
-
-Here is one example:
-
-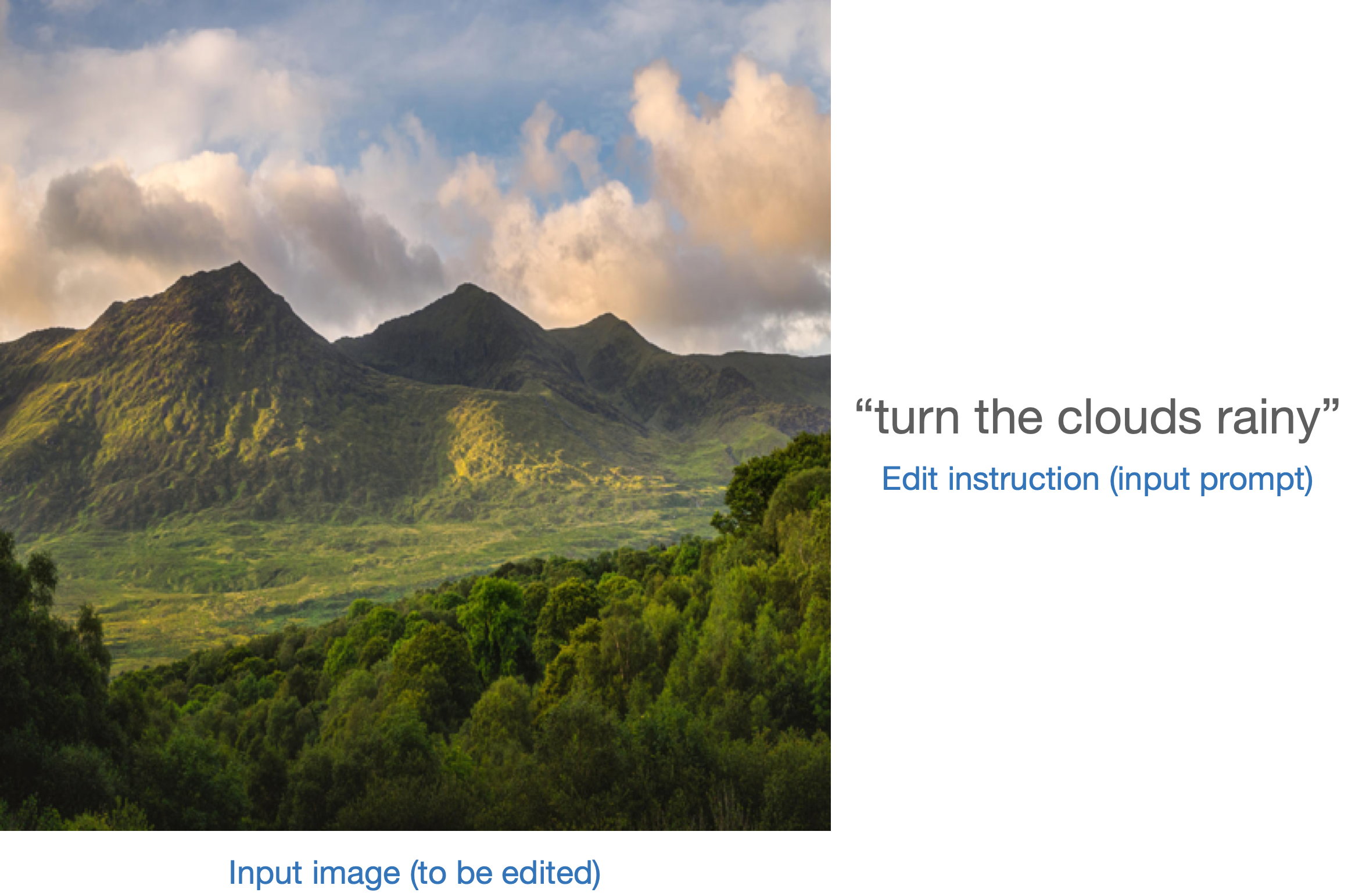
-
-One strategy to evaluate such a model is to measure the consistency of the change between the two images (in [CLIP](https://huggingface.co/docs/transformers/model_doc/clip) space) with the change between the two image captions (as shown in [CLIP-Guided Domain Adaptation of Image Generators](https://arxiv.org/abs/2108.00946)). This is referred to as the "**CLIP directional similarity**".
-
-- Caption 1 corresponds to the input image (image 1) that is to be edited.
-- Caption 2 corresponds to the edited image (image 2). It should reflect the edit instruction.
-
-Following is a pictorial overview:
-
-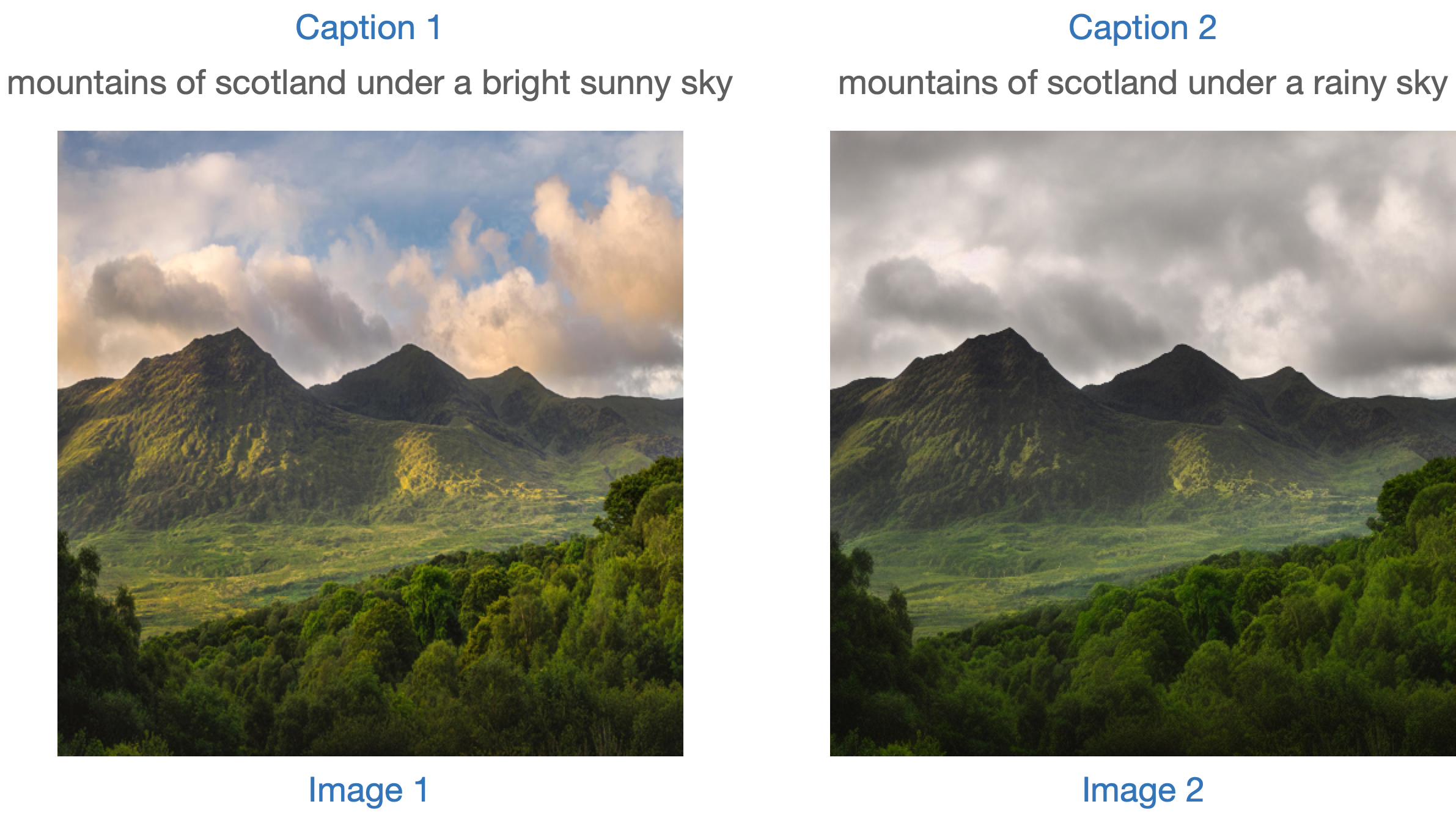
-
-We have prepared a mini dataset to implement this metric. Let's first load the dataset.
-
-```python
-from datasets import load_dataset
-
-dataset = load_dataset("sayakpaul/instructpix2pix-demo", split="train")
-dataset.features
-```
-
-```bash
-{'input': Value(dtype='string', id=None),
- 'edit': Value(dtype='string', id=None),
- 'output': Value(dtype='string', id=None),
- 'image': Image(decode=True, id=None)}
-```
-
-Here we have:
-
-- `input` is a caption corresponding to the `image`.
-- `edit` denotes the edit instruction.
-- `output` denotes the modified caption reflecting the `edit` instruction.
-
-Let's take a look at a sample.
-
-```python
-idx = 0
-print(f"Original caption: {dataset[idx]['input']}")
-print(f"Edit instruction: {dataset[idx]['edit']}")
-print(f"Modified caption: {dataset[idx]['output']}")
-```
-
-```bash
-Original caption: 2. FAROE ISLANDS: An archipelago of 18 mountainous isles in the North Atlantic Ocean between Norway and Iceland, the Faroe Islands has 'everything you could hope for', according to Big 7 Travel. It boasts 'crystal clear waterfalls, rocky cliffs that seem to jut out of nowhere and velvety green hills'
-Edit instruction: make the isles all white marble
-Modified caption: 2. WHITE MARBLE ISLANDS: An archipelago of 18 mountainous white marble isles in the North Atlantic Ocean between Norway and Iceland, the White Marble Islands has 'everything you could hope for', according to Big 7 Travel. It boasts 'crystal clear waterfalls, rocky cliffs that seem to jut out of nowhere and velvety green hills'
-```
-
-And here is the image:
-
-```python
-dataset[idx]["image"]
-```
-
-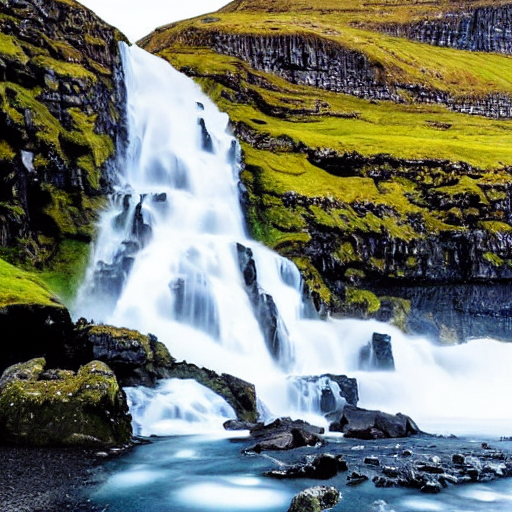
-
-We will first edit the images of our dataset with the edit instruction and compute the directional similarity.
-
-Let's first load the [`StableDiffusionInstructPix2PixPipeline`]:
-
-```python
-from diffusers import StableDiffusionInstructPix2PixPipeline
-
-instruct_pix2pix_pipeline = StableDiffusionInstructPix2PixPipeline.from_pretrained(
- "timbrooks/instruct-pix2pix", torch_dtype=torch.float16
-).to(device)
-```
-
-Now, we perform the edits:
-
-```python
-import numpy as np
-
-
-def edit_image(input_image, instruction):
- image = instruct_pix2pix_pipeline(
- instruction,
- image=input_image,
- output_type="numpy",
- generator=generator,
- ).images[0]
- return image
-
-
-input_images = []
-original_captions = []
-modified_captions = []
-edited_images = []
-
-for idx in range(len(dataset)):
- input_image = dataset[idx]["image"]
- edit_instruction = dataset[idx]["edit"]
- edited_image = edit_image(input_image, edit_instruction)
-
- input_images.append(np.array(input_image))
- original_captions.append(dataset[idx]["input"])
- modified_captions.append(dataset[idx]["output"])
- edited_images.append(edited_image)
-```
-
-To measure the directional similarity, we first load CLIP's image and text encoders:
-
-```python
-from transformers import (
- CLIPTokenizer,
- CLIPTextModelWithProjection,
- CLIPVisionModelWithProjection,
- CLIPImageProcessor,
-)
-
-clip_id = "openai/clip-vit-large-patch14"
-tokenizer = CLIPTokenizer.from_pretrained(clip_id)
-text_encoder = CLIPTextModelWithProjection.from_pretrained(clip_id).to(device)
-image_processor = CLIPImageProcessor.from_pretrained(clip_id)
-image_encoder = CLIPVisionModelWithProjection.from_pretrained(clip_id).to(device)
-```
-
-Notice that we are using a particular CLIP checkpoint, i.e., `openai/clip-vit-large-patch14`. This is because the Stable Diffusion pre-training was performed with this CLIP variant. For more details, refer to the [documentation](https://huggingface.co/docs/diffusers/main/en/api/pipelines/stable_diffusion/pix2pix#diffusers.StableDiffusionInstructPix2PixPipeline.text_encoder).
-
-Next, we prepare a PyTorch `nn.Module` to compute directional similarity:
-
-```python
-import torch.nn as nn
-import torch.nn.functional as F
-
-
-class DirectionalSimilarity(nn.Module):
- def __init__(self, tokenizer, text_encoder, image_processor, image_encoder):
- super().__init__()
- self.tokenizer = tokenizer
- self.text_encoder = text_encoder
- self.image_processor = image_processor
- self.image_encoder = image_encoder
-
- def preprocess_image(self, image):
- image = self.image_processor(image, return_tensors="pt")["pixel_values"]
- return {"pixel_values": image.to(device)}
-
- def tokenize_text(self, text):
- inputs = self.tokenizer(
- text,
- max_length=self.tokenizer.model_max_length,
- padding="max_length",
- truncation=True,
- return_tensors="pt",
- )
- return {"input_ids": inputs.input_ids.to(device)}
-
- def encode_image(self, image):
- preprocessed_image = self.preprocess_image(image)
- image_features = self.image_encoder(**preprocessed_image).image_embeds
- image_features = image_features / image_features.norm(dim=1, keepdim=True)
- return image_features
-
- def encode_text(self, text):
- tokenized_text = self.tokenize_text(text)
- text_features = self.text_encoder(**tokenized_text).text_embeds
- text_features = text_features / text_features.norm(dim=1, keepdim=True)
- return text_features
-
- def compute_directional_similarity(self, img_feat_one, img_feat_two, text_feat_one, text_feat_two):
- sim_direction = F.cosine_similarity(img_feat_two - img_feat_one, text_feat_two - text_feat_one)
- return sim_direction
-
- def forward(self, image_one, image_two, caption_one, caption_two):
- img_feat_one = self.encode_image(image_one)
- img_feat_two = self.encode_image(image_two)
- text_feat_one = self.encode_text(caption_one)
- text_feat_two = self.encode_text(caption_two)
- directional_similarity = self.compute_directional_similarity(
- img_feat_one, img_feat_two, text_feat_one, text_feat_two
- )
- return directional_similarity
-```
-
-Let's put `DirectionalSimilarity` to use now.
-
-```python
-dir_similarity = DirectionalSimilarity(tokenizer, text_encoder, image_processor, image_encoder)
-scores = []
-
-for i in range(len(input_images)):
- original_image = input_images[i]
- original_caption = original_captions[i]
- edited_image = edited_images[i]
- modified_caption = modified_captions[i]
-
- similarity_score = dir_similarity(original_image, edited_image, original_caption, modified_caption)
- scores.append(float(similarity_score.detach().cpu()))
-
-print(f"CLIP directional similarity: {np.mean(scores)}")
-# CLIP directional similarity: 0.0797976553440094
-```
-
-Like the CLIP Score, the higher the CLIP directional similarity, the better it is.
-
-It should be noted that the `StableDiffusionInstructPix2PixPipeline` exposes two arguments, namely, `image_guidance_scale` and `guidance_scale` that let you control the quality of the final edited image. We encourage you to experiment with these two arguments and see the impact of that on the directional similarity.
-
-We can extend the idea of this metric to measure how similar the original image and edited version are. To do that, we can just do `F.cosine_similarity(img_feat_two, img_feat_one)`. For these kinds of edits, we would still want the primary semantics of the images to be preserved as much as possible, i.e., a high similarity score.
-
-We can use these metrics for similar pipelines such as the [`StableDiffusionPix2PixZeroPipeline`](https://huggingface.co/docs/diffusers/main/en/api/pipelines/stable_diffusion/pix2pix_zero#diffusers.StableDiffusionPix2PixZeroPipeline).
-
-
-
-Both CLIP score and CLIP direction similarity rely on the CLIP model, which can make the evaluations biased.
-
-
-
-***Extending metrics like IS, FID (discussed later), or KID can be difficult*** when the model under evaluation was pre-trained on a large image-captioning dataset (such as the [LAION-5B dataset](https://laion.ai/blog/laion-5b/)). This is because underlying these metrics is an InceptionNet (pre-trained on the ImageNet-1k dataset) used for extracting intermediate image features. The pre-training dataset of Stable Diffusion may have limited overlap with the pre-training dataset of InceptionNet, so it is not a good candidate here for feature extraction.
-
-***Using the above metrics helps evaluate models that are class-conditioned. For example, [DiT](https://huggingface.co/docs/diffusers/main/en/api/pipelines/stable_diffusion/overview). It was pre-trained being conditioned on the ImageNet-1k classes.***
-
-### Class-conditioned image generation
-
-Class-conditioned generative models are usually pre-trained on a class-labeled dataset such as [ImageNet-1k](https://huggingface.co/datasets/imagenet-1k). Popular metrics for evaluating these models include Fréchet Inception Distance (FID), Kernel Inception Distance (KID), and Inception Score (IS). In this document, we focus on FID ([Heusel et al.](https://arxiv.org/abs/1706.08500)). We show how to compute it with the [`DiTPipeline`](https://huggingface.co/docs/diffusers/api/pipelines/dit), which uses the [DiT model](https://arxiv.org/abs/2212.09748) under the hood.
-
-FID aims to measure how similar are two datasets of images. As per [this resource](https://mmgeneration.readthedocs.io/en/latest/quick_run.html#fid):
-
-> Fréchet Inception Distance is a measure of similarity between two datasets of images. It was shown to correlate well with the human judgment of visual quality and is most often used to evaluate the quality of samples of Generative Adversarial Networks. FID is calculated by computing the Fréchet distance between two Gaussians fitted to feature representations of the Inception network.
-
-These two datasets are essentially the dataset of real images and the dataset of fake images (generated images in our case). FID is usually calculated with two large datasets. However, for this document, we will work with two mini datasets.
-
-Let's first download a few images from the ImageNet-1k training set:
-
-```python
-from zipfile import ZipFile
-import requests
-
-
-def download(url, local_filepath):
- r = requests.get(url)
- with open(local_filepath, "wb") as f:
- f.write(r.content)
- return local_filepath
-
-
-dummy_dataset_url = "https://hf.co/datasets/sayakpaul/sample-datasets/resolve/main/sample-imagenet-images.zip"
-local_filepath = download(dummy_dataset_url, dummy_dataset_url.split("/")[-1])
-
-with ZipFile(local_filepath, "r") as zipper:
- zipper.extractall(".")
-```
-
-```python
-from PIL import Image
-import os
-
-dataset_path = "sample-imagenet-images"
-image_paths = sorted([os.path.join(dataset_path, x) for x in os.listdir(dataset_path)])
-
-real_images = [np.array(Image.open(path).convert("RGB")) for path in image_paths]
-```
-
-These are 10 images from the following Imagenet-1k classes: "cassette_player", "chain_saw" (x2), "church", "gas_pump" (x3), "parachute" (x2), and "tench".
-
-
-
- Real images.
-
-
-Now that the images are loaded, let's apply some lightweight pre-processing on them to use them for FID calculation.
-
-```python
-from torchvision.transforms import functional as F
-
-
-def preprocess_image(image):
- image = torch.tensor(image).unsqueeze(0)
- image = image.permute(0, 3, 1, 2) / 255.0
- return F.center_crop(image, (256, 256))
-
-
-real_images = torch.cat([preprocess_image(image) for image in real_images])
-print(real_images.shape)
-# torch.Size([10, 3, 256, 256])
-```
-
-We now load the [`DiTPipeline`](https://huggingface.co/docs/diffusers/api/pipelines/dit) to generate images conditioned on the above-mentioned classes.
-
-```python
-from diffusers import DiTPipeline, DPMSolverMultistepScheduler
-
-dit_pipeline = DiTPipeline.from_pretrained("facebook/DiT-XL-2-256", torch_dtype=torch.float16)
-dit_pipeline.scheduler = DPMSolverMultistepScheduler.from_config(dit_pipeline.scheduler.config)
-dit_pipeline = dit_pipeline.to("cuda")
-
-words = [
- "cassette player",
- "chainsaw",
- "chainsaw",
- "church",
- "gas pump",
- "gas pump",
- "gas pump",
- "parachute",
- "parachute",
- "tench",
-]
-
-class_ids = dit_pipeline.get_label_ids(words)
-output = dit_pipeline(class_labels=class_ids, generator=generator, output_type="numpy")
-
-fake_images = output.images
-fake_images = torch.tensor(fake_images)
-fake_images = fake_images.permute(0, 3, 1, 2)
-print(fake_images.shape)
-# torch.Size([10, 3, 256, 256])
-```
-
-Now, we can compute the FID using [`torchmetrics`](https://torchmetrics.readthedocs.io/).
-
-```python
-from torchmetrics.image.fid import FrechetInceptionDistance
-
-fid = FrechetInceptionDistance(normalize=True)
-fid.update(real_images, real=True)
-fid.update(fake_images, real=False)
-
-print(f"FID: {float(fid.compute())}")
-# FID: 177.7147216796875
-```
-
-The lower the FID, the better it is. Several things can influence FID here:
-
-- Number of images (both real and fake)
-- Randomness induced in the diffusion process
-- Number of inference steps in the diffusion process
-- The scheduler being used in the diffusion process
-
-For the last two points, it is, therefore, a good practice to run the evaluation across different seeds and inference steps, and then report an average result.
-
-
-
-FID results tend to be fragile as they depend on a lot of factors:
-
-* The specific Inception model used during computation.
-* The implementation accuracy of the computation.
-* The image format (not the same if we start from PNGs vs JPGs).
-
-Keeping that in mind, FID is often most useful when comparing similar runs, but it is
-hard to reproduce paper results unless the authors carefully disclose the FID
-measurement code.
-
-These points apply to other related metrics too, such as KID and IS.
-
-
-
-As a final step, let's visually inspect the `fake_images`.
-
-
-
- Fake images.
-
diff --git a/spaces/Androidonnxfork/CivitAi-to-Diffusers/diffusers/src/diffusers/schedulers/scheduling_k_dpm_2_ancestral_discrete.py b/spaces/Androidonnxfork/CivitAi-to-Diffusers/diffusers/src/diffusers/schedulers/scheduling_k_dpm_2_ancestral_discrete.py
deleted file mode 100644
index bdf9379b9b90a53e3c8aad20a69e9ab7bffc691e..0000000000000000000000000000000000000000
--- a/spaces/Androidonnxfork/CivitAi-to-Diffusers/diffusers/src/diffusers/schedulers/scheduling_k_dpm_2_ancestral_discrete.py
+++ /dev/null
@@ -1,420 +0,0 @@
-# Copyright 2023 Katherine Crowson, The HuggingFace Team and hlky. All rights reserved.
-#
-# Licensed under the Apache License, Version 2.0 (the "License");
-# you may not use this file except in compliance with the License.
-# You may obtain a copy of the License at
-#
-# http://www.apache.org/licenses/LICENSE-2.0
-#
-# Unless required by applicable law or agreed to in writing, software
-# distributed under the License is distributed on an "AS IS" BASIS,
-# WITHOUT WARRANTIES OR CONDITIONS OF ANY KIND, either express or implied.
-# See the License for the specific language governing permissions and
-# limitations under the License.
-
-import math
-from collections import defaultdict
-from typing import List, Optional, Tuple, Union
-
-import numpy as np
-import torch
-
-from ..configuration_utils import ConfigMixin, register_to_config
-from ..utils import randn_tensor
-from .scheduling_utils import KarrasDiffusionSchedulers, SchedulerMixin, SchedulerOutput
-
-
-# Copied from diffusers.schedulers.scheduling_ddpm.betas_for_alpha_bar
-def betas_for_alpha_bar(
- num_diffusion_timesteps,
- max_beta=0.999,
- alpha_transform_type="cosine",
-):
- """
- Create a beta schedule that discretizes the given alpha_t_bar function, which defines the cumulative product of
- (1-beta) over time from t = [0,1].
-
- Contains a function alpha_bar that takes an argument t and transforms it to the cumulative product of (1-beta) up
- to that part of the diffusion process.
-
-
- Args:
- num_diffusion_timesteps (`int`): the number of betas to produce.
- max_beta (`float`): the maximum beta to use; use values lower than 1 to
- prevent singularities.
- alpha_transform_type (`str`, *optional*, default to `cosine`): the type of noise schedule for alpha_bar.
- Choose from `cosine` or `exp`
-
- Returns:
- betas (`np.ndarray`): the betas used by the scheduler to step the model outputs
- """
- if alpha_transform_type == "cosine":
-
- def alpha_bar_fn(t):
- return math.cos((t + 0.008) / 1.008 * math.pi / 2) ** 2
-
- elif alpha_transform_type == "exp":
-
- def alpha_bar_fn(t):
- return math.exp(t * -12.0)
-
- else:
- raise ValueError(f"Unsupported alpha_tranform_type: {alpha_transform_type}")
-
- betas = []
- for i in range(num_diffusion_timesteps):
- t1 = i / num_diffusion_timesteps
- t2 = (i + 1) / num_diffusion_timesteps
- betas.append(min(1 - alpha_bar_fn(t2) / alpha_bar_fn(t1), max_beta))
- return torch.tensor(betas, dtype=torch.float32)
-
-
-class KDPM2AncestralDiscreteScheduler(SchedulerMixin, ConfigMixin):
- """
- Scheduler created by @crowsonkb in [k_diffusion](https://github.com/crowsonkb/k-diffusion), see:
- https://github.com/crowsonkb/k-diffusion/blob/5b3af030dd83e0297272d861c19477735d0317ec/k_diffusion/sampling.py#L188
-
- Scheduler inspired by DPM-Solver-2 and Algorthim 2 from Karras et al. (2022).
-
- [`~ConfigMixin`] takes care of storing all config attributes that are passed in the scheduler's `__init__`
- function, such as `num_train_timesteps`. They can be accessed via `scheduler.config.num_train_timesteps`.
- [`SchedulerMixin`] provides general loading and saving functionality via the [`SchedulerMixin.save_pretrained`] and
- [`~SchedulerMixin.from_pretrained`] functions.
-
- Args:
- num_train_timesteps (`int`): number of diffusion steps used to train the model. beta_start (`float`): the
- starting `beta` value of inference. beta_end (`float`): the final `beta` value. beta_schedule (`str`):
- the beta schedule, a mapping from a beta range to a sequence of betas for stepping the model. Choose from
- `linear` or `scaled_linear`.
- trained_betas (`np.ndarray`, optional):
- option to pass an array of betas directly to the constructor to bypass `beta_start`, `beta_end` etc.
- options to clip the variance used when adding noise to the denoised sample. Choose from `fixed_small`,
- `fixed_small_log`, `fixed_large`, `fixed_large_log`, `learned` or `learned_range`.
- prediction_type (`str`, default `epsilon`, optional):
- prediction type of the scheduler function, one of `epsilon` (predicting the noise of the diffusion
- process), `sample` (directly predicting the noisy sample`) or `v_prediction` (see section 2.4
- https://imagen.research.google/video/paper.pdf)
- timestep_spacing (`str`, default `"linspace"`):
- The way the timesteps should be scaled. Refer to Table 2. of [Common Diffusion Noise Schedules and Sample
- Steps are Flawed](https://arxiv.org/abs/2305.08891) for more information.
- steps_offset (`int`, default `0`):
- an offset added to the inference steps. You can use a combination of `offset=1` and
- `set_alpha_to_one=False`, to make the last step use step 0 for the previous alpha product, as done in
- stable diffusion.
- """
-
- _compatibles = [e.name for e in KarrasDiffusionSchedulers]
- order = 2
-
- @register_to_config
- def __init__(
- self,
- num_train_timesteps: int = 1000,
- beta_start: float = 0.00085, # sensible defaults
- beta_end: float = 0.012,
- beta_schedule: str = "linear",
- trained_betas: Optional[Union[np.ndarray, List[float]]] = None,
- prediction_type: str = "epsilon",
- timestep_spacing: str = "linspace",
- steps_offset: int = 0,
- ):
- if trained_betas is not None:
- self.betas = torch.tensor(trained_betas, dtype=torch.float32)
- elif beta_schedule == "linear":
- self.betas = torch.linspace(beta_start, beta_end, num_train_timesteps, dtype=torch.float32)
- elif beta_schedule == "scaled_linear":
- # this schedule is very specific to the latent diffusion model.
- self.betas = (
- torch.linspace(beta_start**0.5, beta_end**0.5, num_train_timesteps, dtype=torch.float32) ** 2
- )
- elif beta_schedule == "squaredcos_cap_v2":
- # Glide cosine schedule
- self.betas = betas_for_alpha_bar(num_train_timesteps)
- else:
- raise NotImplementedError(f"{beta_schedule} does is not implemented for {self.__class__}")
-
- self.alphas = 1.0 - self.betas
- self.alphas_cumprod = torch.cumprod(self.alphas, dim=0)
-
- # set all values
- self.set_timesteps(num_train_timesteps, None, num_train_timesteps)
-
- # Copied from diffusers.schedulers.scheduling_heun_discrete.HeunDiscreteScheduler.index_for_timestep
- def index_for_timestep(self, timestep, schedule_timesteps=None):
- if schedule_timesteps is None:
- schedule_timesteps = self.timesteps
-
- indices = (schedule_timesteps == timestep).nonzero()
-
- # The sigma index that is taken for the **very** first `step`
- # is always the second index (or the last index if there is only 1)
- # This way we can ensure we don't accidentally skip a sigma in
- # case we start in the middle of the denoising schedule (e.g. for image-to-image)
- if len(self._index_counter) == 0:
- pos = 1 if len(indices) > 1 else 0
- else:
- timestep_int = timestep.cpu().item() if torch.is_tensor(timestep) else timestep
- pos = self._index_counter[timestep_int]
-
- return indices[pos].item()
-
- @property
- def init_noise_sigma(self):
- # standard deviation of the initial noise distribution
- if self.config.timestep_spacing in ["linspace", "trailing"]:
- return self.sigmas.max()
-
- return (self.sigmas.max() ** 2 + 1) ** 0.5
-
- def scale_model_input(
- self,
- sample: torch.FloatTensor,
- timestep: Union[float, torch.FloatTensor],
- ) -> torch.FloatTensor:
- """
- Args:
- Ensures interchangeability with schedulers that need to scale the denoising model input depending on the
- current timestep.
- sample (`torch.FloatTensor`): input sample timestep (`int`, optional): current timestep
- Returns:
- `torch.FloatTensor`: scaled input sample
- """
- step_index = self.index_for_timestep(timestep)
-
- if self.state_in_first_order:
- sigma = self.sigmas[step_index]
- else:
- sigma = self.sigmas_interpol[step_index - 1]
-
- sample = sample / ((sigma**2 + 1) ** 0.5)
- return sample
-
- def set_timesteps(
- self,
- num_inference_steps: int,
- device: Union[str, torch.device] = None,
- num_train_timesteps: Optional[int] = None,
- ):
- """
- Sets the timesteps used for the diffusion chain. Supporting function to be run before inference.
-
- Args:
- num_inference_steps (`int`):
- the number of diffusion steps used when generating samples with a pre-trained model.
- device (`str` or `torch.device`, optional):
- the device to which the timesteps should be moved to. If `None`, the timesteps are not moved.
- """
- self.num_inference_steps = num_inference_steps
-
- num_train_timesteps = num_train_timesteps or self.config.num_train_timesteps
-
- # "linspace", "leading", "trailing" corresponds to annotation of Table 2. of https://arxiv.org/abs/2305.08891
- if self.config.timestep_spacing == "linspace":
- timesteps = np.linspace(0, num_train_timesteps - 1, num_inference_steps, dtype=float)[::-1].copy()
- elif self.config.timestep_spacing == "leading":
- step_ratio = num_train_timesteps // self.num_inference_steps
- # creates integer timesteps by multiplying by ratio
- # casting to int to avoid issues when num_inference_step is power of 3
- timesteps = (np.arange(0, num_inference_steps) * step_ratio).round()[::-1].copy().astype(float)
- timesteps += self.config.steps_offset
- elif self.config.timestep_spacing == "trailing":
- step_ratio = num_train_timesteps / self.num_inference_steps
- # creates integer timesteps by multiplying by ratio
- # casting to int to avoid issues when num_inference_step is power of 3
- timesteps = (np.arange(num_train_timesteps, 0, -step_ratio)).round().copy().astype(float)
- timesteps -= 1
- else:
- raise ValueError(
- f"{self.config.timestep_spacing} is not supported. Please make sure to choose one of 'linspace', 'leading' or 'trailing'."
- )
-
- sigmas = np.array(((1 - self.alphas_cumprod) / self.alphas_cumprod) ** 0.5)
- self.log_sigmas = torch.from_numpy(np.log(sigmas)).to(device)
-
- sigmas = np.interp(timesteps, np.arange(0, len(sigmas)), sigmas)
- sigmas = np.concatenate([sigmas, [0.0]]).astype(np.float32)
- sigmas = torch.from_numpy(sigmas).to(device=device)
-
- # compute up and down sigmas
- sigmas_next = sigmas.roll(-1)
- sigmas_next[-1] = 0.0
- sigmas_up = (sigmas_next**2 * (sigmas**2 - sigmas_next**2) / sigmas**2) ** 0.5
- sigmas_down = (sigmas_next**2 - sigmas_up**2) ** 0.5
- sigmas_down[-1] = 0.0
-
- # compute interpolated sigmas
- sigmas_interpol = sigmas.log().lerp(sigmas_down.log(), 0.5).exp()
- sigmas_interpol[-2:] = 0.0
-
- # set sigmas
- self.sigmas = torch.cat([sigmas[:1], sigmas[1:].repeat_interleave(2), sigmas[-1:]])
- self.sigmas_interpol = torch.cat(
- [sigmas_interpol[:1], sigmas_interpol[1:].repeat_interleave(2), sigmas_interpol[-1:]]
- )
- self.sigmas_up = torch.cat([sigmas_up[:1], sigmas_up[1:].repeat_interleave(2), sigmas_up[-1:]])
- self.sigmas_down = torch.cat([sigmas_down[:1], sigmas_down[1:].repeat_interleave(2), sigmas_down[-1:]])
-
- if str(device).startswith("mps"):
- # mps does not support float64
- timesteps = torch.from_numpy(timesteps).to(device, dtype=torch.float32)
- else:
- timesteps = torch.from_numpy(timesteps).to(device)
-
- timesteps_interpol = self.sigma_to_t(sigmas_interpol).to(device, dtype=timesteps.dtype)
- interleaved_timesteps = torch.stack((timesteps_interpol[:-2, None], timesteps[1:, None]), dim=-1).flatten()
-
- self.timesteps = torch.cat([timesteps[:1], interleaved_timesteps])
-
- self.sample = None
-
- # for exp beta schedules, such as the one for `pipeline_shap_e.py`
- # we need an index counter
- self._index_counter = defaultdict(int)
-
- def sigma_to_t(self, sigma):
- # get log sigma
- log_sigma = sigma.log()
-
- # get distribution
- dists = log_sigma - self.log_sigmas[:, None]
-
- # get sigmas range
- low_idx = dists.ge(0).cumsum(dim=0).argmax(dim=0).clamp(max=self.log_sigmas.shape[0] - 2)
- high_idx = low_idx + 1
-
- low = self.log_sigmas[low_idx]
- high = self.log_sigmas[high_idx]
-
- # interpolate sigmas
- w = (low - log_sigma) / (low - high)
- w = w.clamp(0, 1)
-
- # transform interpolation to time range
- t = (1 - w) * low_idx + w * high_idx
- t = t.view(sigma.shape)
- return t
-
- @property
- def state_in_first_order(self):
- return self.sample is None
-
- def step(
- self,
- model_output: Union[torch.FloatTensor, np.ndarray],
- timestep: Union[float, torch.FloatTensor],
- sample: Union[torch.FloatTensor, np.ndarray],
- generator: Optional[torch.Generator] = None,
- return_dict: bool = True,
- ) -> Union[SchedulerOutput, Tuple]:
- """
- Args:
- Predict the sample at the previous timestep by reversing the SDE. Core function to propagate the diffusion
- process from the learned model outputs (most often the predicted noise).
- model_output (`torch.FloatTensor` or `np.ndarray`): direct output from learned diffusion model. timestep
- (`int`): current discrete timestep in the diffusion chain. sample (`torch.FloatTensor` or `np.ndarray`):
- current instance of sample being created by diffusion process.
- return_dict (`bool`): option for returning tuple rather than SchedulerOutput class
- Returns:
- [`~schedulers.scheduling_utils.SchedulerOutput`] or `tuple`:
- [`~schedulers.scheduling_utils.SchedulerOutput`] if `return_dict` is True, otherwise a `tuple`. When
- returning a tuple, the first element is the sample tensor.
- """
- step_index = self.index_for_timestep(timestep)
-
- # advance index counter by 1
- timestep_int = timestep.cpu().item() if torch.is_tensor(timestep) else timestep
- self._index_counter[timestep_int] += 1
-
- if self.state_in_first_order:
- sigma = self.sigmas[step_index]
- sigma_interpol = self.sigmas_interpol[step_index]
- sigma_up = self.sigmas_up[step_index]
- sigma_down = self.sigmas_down[step_index - 1]
- else:
- # 2nd order / KPDM2's method
- sigma = self.sigmas[step_index - 1]
- sigma_interpol = self.sigmas_interpol[step_index - 1]
- sigma_up = self.sigmas_up[step_index - 1]
- sigma_down = self.sigmas_down[step_index - 1]
-
- # currently only gamma=0 is supported. This usually works best anyways.
- # We can support gamma in the future but then need to scale the timestep before
- # passing it to the model which requires a change in API
- gamma = 0
- sigma_hat = sigma * (gamma + 1) # Note: sigma_hat == sigma for now
-
- device = model_output.device
- noise = randn_tensor(model_output.shape, dtype=model_output.dtype, device=device, generator=generator)
-
- # 1. compute predicted original sample (x_0) from sigma-scaled predicted noise
- if self.config.prediction_type == "epsilon":
- sigma_input = sigma_hat if self.state_in_first_order else sigma_interpol
- pred_original_sample = sample - sigma_input * model_output
- elif self.config.prediction_type == "v_prediction":
- sigma_input = sigma_hat if self.state_in_first_order else sigma_interpol
- pred_original_sample = model_output * (-sigma_input / (sigma_input**2 + 1) ** 0.5) + (
- sample / (sigma_input**2 + 1)
- )
- elif self.config.prediction_type == "sample":
- raise NotImplementedError("prediction_type not implemented yet: sample")
- else:
- raise ValueError(
- f"prediction_type given as {self.config.prediction_type} must be one of `epsilon`, or `v_prediction`"
- )
-
- if self.state_in_first_order:
- # 2. Convert to an ODE derivative for 1st order
- derivative = (sample - pred_original_sample) / sigma_hat
- # 3. delta timestep
- dt = sigma_interpol - sigma_hat
-
- # store for 2nd order step
- self.sample = sample
- self.dt = dt
- prev_sample = sample + derivative * dt
- else:
- # DPM-Solver-2
- # 2. Convert to an ODE derivative for 2nd order
- derivative = (sample - pred_original_sample) / sigma_interpol
- # 3. delta timestep
- dt = sigma_down - sigma_hat
-
- sample = self.sample
- self.sample = None
-
- prev_sample = sample + derivative * dt
- prev_sample = prev_sample + noise * sigma_up
-
- if not return_dict:
- return (prev_sample,)
-
- return SchedulerOutput(prev_sample=prev_sample)
-
- # Copied from diffusers.schedulers.scheduling_heun_discrete.HeunDiscreteScheduler.add_noise
- def add_noise(
- self,
- original_samples: torch.FloatTensor,
- noise: torch.FloatTensor,
- timesteps: torch.FloatTensor,
- ) -> torch.FloatTensor:
- # Make sure sigmas and timesteps have the same device and dtype as original_samples
- sigmas = self.sigmas.to(device=original_samples.device, dtype=original_samples.dtype)
- if original_samples.device.type == "mps" and torch.is_floating_point(timesteps):
- # mps does not support float64
- schedule_timesteps = self.timesteps.to(original_samples.device, dtype=torch.float32)
- timesteps = timesteps.to(original_samples.device, dtype=torch.float32)
- else:
- schedule_timesteps = self.timesteps.to(original_samples.device)
- timesteps = timesteps.to(original_samples.device)
-
- step_indices = [self.index_for_timestep(t, schedule_timesteps) for t in timesteps]
-
- sigma = sigmas[step_indices].flatten()
- while len(sigma.shape) < len(original_samples.shape):
- sigma = sigma.unsqueeze(-1)
-
- noisy_samples = original_samples + noise * sigma
- return noisy_samples
-
- def __len__(self):
- return self.config.num_train_timesteps
diff --git a/spaces/Androidonnxfork/CivitAi-to-Diffusers/diffusers/tests/pipelines/stable_diffusion_safe/__init__.py b/spaces/Androidonnxfork/CivitAi-to-Diffusers/diffusers/tests/pipelines/stable_diffusion_safe/__init__.py
deleted file mode 100644
index e69de29bb2d1d6434b8b29ae775ad8c2e48c5391..0000000000000000000000000000000000000000
diff --git a/spaces/Andy1621/uniformer_image_detection/configs/cornernet/cornernet_hourglass104_mstest_10x5_210e_coco.py b/spaces/Andy1621/uniformer_image_detection/configs/cornernet/cornernet_hourglass104_mstest_10x5_210e_coco.py
deleted file mode 100644
index 89f387641207512ae1b1c91ca56965004e5eb868..0000000000000000000000000000000000000000
--- a/spaces/Andy1621/uniformer_image_detection/configs/cornernet/cornernet_hourglass104_mstest_10x5_210e_coco.py
+++ /dev/null
@@ -1,105 +0,0 @@
-_base_ = [
- '../_base_/default_runtime.py', '../_base_/datasets/coco_detection.py'
-]
-
-# model settings
-model = dict(
- type='CornerNet',
- backbone=dict(
- type='HourglassNet',
- downsample_times=5,
- num_stacks=2,
- stage_channels=[256, 256, 384, 384, 384, 512],
- stage_blocks=[2, 2, 2, 2, 2, 4],
- norm_cfg=dict(type='BN', requires_grad=True)),
- neck=None,
- bbox_head=dict(
- type='CornerHead',
- num_classes=80,
- in_channels=256,
- num_feat_levels=2,
- corner_emb_channels=1,
- loss_heatmap=dict(
- type='GaussianFocalLoss', alpha=2.0, gamma=4.0, loss_weight=1),
- loss_embedding=dict(
- type='AssociativeEmbeddingLoss',
- pull_weight=0.10,
- push_weight=0.10),
- loss_offset=dict(type='SmoothL1Loss', beta=1.0, loss_weight=1)),
- # training and testing settings
- train_cfg=None,
- test_cfg=dict(
- corner_topk=100,
- local_maximum_kernel=3,
- distance_threshold=0.5,
- score_thr=0.05,
- max_per_img=100,
- nms=dict(type='soft_nms', iou_threshold=0.5, method='gaussian')))
-# data settings
-img_norm_cfg = dict(
- mean=[123.675, 116.28, 103.53], std=[58.395, 57.12, 57.375], to_rgb=True)
-train_pipeline = [
- dict(type='LoadImageFromFile', to_float32=True),
- dict(type='LoadAnnotations', with_bbox=True),
- dict(
- type='PhotoMetricDistortion',
- brightness_delta=32,
- contrast_range=(0.5, 1.5),
- saturation_range=(0.5, 1.5),
- hue_delta=18),
- dict(
- type='RandomCenterCropPad',
- crop_size=(511, 511),
- ratios=(0.6, 0.7, 0.8, 0.9, 1.0, 1.1, 1.2, 1.3),
- test_mode=False,
- test_pad_mode=None,
- **img_norm_cfg),
- dict(type='Resize', img_scale=(511, 511), keep_ratio=False),
- dict(type='RandomFlip', flip_ratio=0.5),
- dict(type='Normalize', **img_norm_cfg),
- dict(type='DefaultFormatBundle'),
- dict(type='Collect', keys=['img', 'gt_bboxes', 'gt_labels']),
-]
-test_pipeline = [
- dict(type='LoadImageFromFile', to_float32=True),
- dict(
- type='MultiScaleFlipAug',
- scale_factor=1.0,
- flip=True,
- transforms=[
- dict(type='Resize'),
- dict(
- type='RandomCenterCropPad',
- crop_size=None,
- ratios=None,
- border=None,
- test_mode=True,
- test_pad_mode=['logical_or', 127],
- **img_norm_cfg),
- dict(type='RandomFlip'),
- dict(type='Normalize', **img_norm_cfg),
- dict(type='ImageToTensor', keys=['img']),
- dict(
- type='Collect',
- keys=['img'],
- meta_keys=('filename', 'ori_shape', 'img_shape', 'pad_shape',
- 'scale_factor', 'flip', 'img_norm_cfg', 'border')),
- ])
-]
-data = dict(
- samples_per_gpu=5,
- workers_per_gpu=3,
- train=dict(pipeline=train_pipeline),
- val=dict(pipeline=test_pipeline),
- test=dict(pipeline=test_pipeline))
-# optimizer
-optimizer = dict(type='Adam', lr=0.0005)
-optimizer_config = dict(grad_clip=dict(max_norm=35, norm_type=2))
-# learning policy
-lr_config = dict(
- policy='step',
- warmup='linear',
- warmup_iters=500,
- warmup_ratio=1.0 / 3,
- step=[180])
-runner = dict(type='EpochBasedRunner', max_epochs=210)
diff --git a/spaces/Andy1621/uniformer_image_detection/configs/faster_rcnn/faster_rcnn_r50_fpn_bounded_iou_1x_coco.py b/spaces/Andy1621/uniformer_image_detection/configs/faster_rcnn/faster_rcnn_r50_fpn_bounded_iou_1x_coco.py
deleted file mode 100644
index 648081f19ca7d3ca9a7362a4a41e514d753ce4e8..0000000000000000000000000000000000000000
--- a/spaces/Andy1621/uniformer_image_detection/configs/faster_rcnn/faster_rcnn_r50_fpn_bounded_iou_1x_coco.py
+++ /dev/null
@@ -1,6 +0,0 @@
-_base_ = './faster_rcnn_r50_fpn_1x_coco.py'
-model = dict(
- roi_head=dict(
- bbox_head=dict(
- reg_decoded_bbox=True,
- loss_bbox=dict(type='BoundedIoULoss', loss_weight=10.0))))
diff --git a/spaces/Andy1621/uniformer_image_segmentation/configs/danet/danet_r101-d8_512x512_80k_ade20k.py b/spaces/Andy1621/uniformer_image_segmentation/configs/danet/danet_r101-d8_512x512_80k_ade20k.py
deleted file mode 100644
index bd31bc8f283fe8c322ee4876deadb89569dc1743..0000000000000000000000000000000000000000
--- a/spaces/Andy1621/uniformer_image_segmentation/configs/danet/danet_r101-d8_512x512_80k_ade20k.py
+++ /dev/null
@@ -1,2 +0,0 @@
-_base_ = './danet_r50-d8_512x512_80k_ade20k.py'
-model = dict(pretrained='open-mmlab://resnet101_v1c', backbone=dict(depth=101))
diff --git a/spaces/Andy1621/uniformer_image_segmentation/configs/deeplabv3plus/deeplabv3plus_r50-d8_512x1024_80k_cityscapes.py b/spaces/Andy1621/uniformer_image_segmentation/configs/deeplabv3plus/deeplabv3plus_r50-d8_512x1024_80k_cityscapes.py
deleted file mode 100644
index 3304d3677f5357f1a3e343b39fcd97b238abdb5e..0000000000000000000000000000000000000000
--- a/spaces/Andy1621/uniformer_image_segmentation/configs/deeplabv3plus/deeplabv3plus_r50-d8_512x1024_80k_cityscapes.py
+++ /dev/null
@@ -1,5 +0,0 @@
-_base_ = [
- '../_base_/models/deeplabv3plus_r50-d8.py',
- '../_base_/datasets/cityscapes.py', '../_base_/default_runtime.py',
- '../_base_/schedules/schedule_80k.py'
-]
diff --git a/spaces/AnishKumbhar/ChatBot/text-generation-webui-main/extensions/multimodal/pipeline_loader.py b/spaces/AnishKumbhar/ChatBot/text-generation-webui-main/extensions/multimodal/pipeline_loader.py
deleted file mode 100644
index 8fcd0a9b410fbc44a51941e0a87b294de871ef8b..0000000000000000000000000000000000000000
--- a/spaces/AnishKumbhar/ChatBot/text-generation-webui-main/extensions/multimodal/pipeline_loader.py
+++ /dev/null
@@ -1,52 +0,0 @@
-import traceback
-from importlib import import_module
-from pathlib import Path
-from typing import Tuple
-
-from extensions.multimodal.abstract_pipeline import AbstractMultimodalPipeline
-from modules import shared
-from modules.logging_colors import logger
-
-
-def _get_available_pipeline_modules():
- pipeline_path = Path(__file__).parent / 'pipelines'
- modules = [p for p in pipeline_path.iterdir() if p.is_dir()]
- return [m.name for m in modules if (m / 'pipelines.py').exists()]
-
-
-def load_pipeline(params: dict) -> Tuple[AbstractMultimodalPipeline, str]:
- pipeline_modules = {}
- available_pipeline_modules = _get_available_pipeline_modules()
- for name in available_pipeline_modules:
- try:
- pipeline_modules[name] = import_module(f'extensions.multimodal.pipelines.{name}.pipelines')
- except:
- logger.warning(f'Failed to get multimodal pipelines from {name}')
- logger.warning(traceback.format_exc())
-
- if shared.args.multimodal_pipeline is not None:
- for k in pipeline_modules:
- if hasattr(pipeline_modules[k], 'get_pipeline'):
- pipeline = getattr(pipeline_modules[k], 'get_pipeline')(shared.args.multimodal_pipeline, params)
- if pipeline is not None:
- return (pipeline, k)
- else:
- model_name = shared.args.model.lower()
- for k in pipeline_modules:
- if hasattr(pipeline_modules[k], 'get_pipeline_from_model_name'):
- pipeline = getattr(pipeline_modules[k], 'get_pipeline_from_model_name')(model_name, params)
- if pipeline is not None:
- return (pipeline, k)
-
- available = []
- for k in pipeline_modules:
- if hasattr(pipeline_modules[k], 'available_pipelines'):
- pipelines = getattr(pipeline_modules[k], 'available_pipelines')
- available += pipelines
-
- if shared.args.multimodal_pipeline is not None:
- log = f'Multimodal - ERROR: Failed to load multimodal pipeline "{shared.args.multimodal_pipeline}", available pipelines are: {available}.'
- else:
- log = f'Multimodal - ERROR: Failed to determine multimodal pipeline for model {shared.args.model}, please select one manually using --multimodal-pipeline [PIPELINE]. Available pipelines are: {available}.'
- logger.critical(f'{log} Please specify a correct pipeline, or disable the extension')
- raise RuntimeError(f'{log} Please specify a correct pipeline, or disable the extension')
diff --git a/spaces/Ariharasudhan/YoloV5/utils/general.py b/spaces/Ariharasudhan/YoloV5/utils/general.py
deleted file mode 100644
index 0c3b44d7f9b02eb2fbbec48a36115070452d723d..0000000000000000000000000000000000000000
--- a/spaces/Ariharasudhan/YoloV5/utils/general.py
+++ /dev/null
@@ -1,1108 +0,0 @@
-# YOLOv5 🚀 by Ultralytics, GPL-3.0 license
-"""
-General utils
-"""
-
-import contextlib
-import glob
-import inspect
-import logging
-import math
-import os
-import platform
-import random
-import re
-import shutil
-import signal
-import sys
-import time
-import urllib
-from copy import deepcopy
-from datetime import datetime
-from itertools import repeat
-from multiprocessing.pool import ThreadPool
-from pathlib import Path
-from subprocess import check_output
-from tarfile import is_tarfile
-from typing import Optional
-from zipfile import ZipFile, is_zipfile
-
-import cv2
-import IPython
-import numpy as np
-import pandas as pd
-import pkg_resources as pkg
-import torch
-import torchvision
-import yaml
-
-from utils import TryExcept, emojis
-from utils.downloads import gsutil_getsize
-from utils.metrics import box_iou, fitness
-
-FILE = Path(__file__).resolve()
-ROOT = FILE.parents[1] # YOLOv5 root directory
-RANK = int(os.getenv('RANK', -1))
-
-# Settings
-NUM_THREADS = min(8, max(1, os.cpu_count() - 1)) # number of YOLOv5 multiprocessing threads
-DATASETS_DIR = Path(os.getenv('YOLOv5_DATASETS_DIR', ROOT.parent / 'datasets')) # global datasets directory
-AUTOINSTALL = str(os.getenv('YOLOv5_AUTOINSTALL', True)).lower() == 'true' # global auto-install mode
-VERBOSE = str(os.getenv('YOLOv5_VERBOSE', True)).lower() == 'true' # global verbose mode
-FONT = 'Arial.ttf' # https://ultralytics.com/assets/Arial.ttf
-
-torch.set_printoptions(linewidth=320, precision=5, profile='long')
-np.set_printoptions(linewidth=320, formatter={'float_kind': '{:11.5g}'.format}) # format short g, %precision=5
-pd.options.display.max_columns = 10
-cv2.setNumThreads(0) # prevent OpenCV from multithreading (incompatible with PyTorch DataLoader)
-os.environ['NUMEXPR_MAX_THREADS'] = str(NUM_THREADS) # NumExpr max threads
-os.environ['OMP_NUM_THREADS'] = '1' if platform.system() == 'darwin' else str(NUM_THREADS) # OpenMP (PyTorch and SciPy)
-
-
-def is_ascii(s=''):
- # Is string composed of all ASCII (no UTF) characters? (note str().isascii() introduced in python 3.7)
- s = str(s) # convert list, tuple, None, etc. to str
- return len(s.encode().decode('ascii', 'ignore')) == len(s)
-
-
-def is_chinese(s='人工智能'):
- # Is string composed of any Chinese characters?
- return bool(re.search('[\u4e00-\u9fff]', str(s)))
-
-
-def is_colab():
- # Is environment a Google Colab instance?
- return 'google.colab' in sys.modules
-
-
-def is_notebook():
- # Is environment a Jupyter notebook? Verified on Colab, Jupyterlab, Kaggle, Paperspace
- ipython_type = str(type(IPython.get_ipython()))
- return 'colab' in ipython_type or 'zmqshell' in ipython_type
-
-
-def is_kaggle():
- # Is environment a Kaggle Notebook?
- return os.environ.get('PWD') == '/kaggle/working' and os.environ.get('KAGGLE_URL_BASE') == 'https://www.kaggle.com'
-
-
-def is_docker() -> bool:
- """Check if the process runs inside a docker container."""
- if Path("/.dockerenv").exists():
- return True
- try: # check if docker is in control groups
- with open("/proc/self/cgroup") as file:
- return any("docker" in line for line in file)
- except OSError:
- return False
-
-
-def is_writeable(dir, test=False):
- # Return True if directory has write permissions, test opening a file with write permissions if test=True
- if not test:
- return os.access(dir, os.W_OK) # possible issues on Windows
- file = Path(dir) / 'tmp.txt'
- try:
- with open(file, 'w'): # open file with write permissions
- pass
- file.unlink() # remove file
- return True
- except OSError:
- return False
-
-
-def set_logging(name=None, verbose=VERBOSE):
- # Sets level and returns logger
- if is_kaggle() or is_colab():
- for h in logging.root.handlers:
- logging.root.removeHandler(h) # remove all handlers associated with the root logger object
- rank = int(os.getenv('RANK', -1)) # rank in world for Multi-GPU trainings
- level = logging.INFO if verbose and rank in {-1, 0} else logging.ERROR
- log = logging.getLogger(name)
- log.setLevel(level)
- handler = logging.StreamHandler()
- handler.setFormatter(logging.Formatter("%(message)s"))
- handler.setLevel(level)
- log.addHandler(handler)
-
-
-set_logging() # run before defining LOGGER
-LOGGER = logging.getLogger("yolov5") # define globally (used in train.py, val.py, detect.py, etc.)
-if platform.system() == 'Windows':
- for fn in LOGGER.info, LOGGER.warning:
- setattr(LOGGER, fn.__name__, lambda x: fn(emojis(x))) # emoji safe logging
-
-
-def user_config_dir(dir='Ultralytics', env_var='YOLOV5_CONFIG_DIR'):
- # Return path of user configuration directory. Prefer environment variable if exists. Make dir if required.
- env = os.getenv(env_var)
- if env:
- path = Path(env) # use environment variable
- else:
- cfg = {'Windows': 'AppData/Roaming', 'Linux': '.config', 'Darwin': 'Library/Application Support'} # 3 OS dirs
- path = Path.home() / cfg.get(platform.system(), '') # OS-specific config dir
- path = (path if is_writeable(path) else Path('/tmp')) / dir # GCP and AWS lambda fix, only /tmp is writeable
- path.mkdir(exist_ok=True) # make if required
- return path
-
-
-CONFIG_DIR = user_config_dir() # Ultralytics settings dir
-
-
-class Profile(contextlib.ContextDecorator):
- # YOLOv5 Profile class. Usage: @Profile() decorator or 'with Profile():' context manager
- def __init__(self, t=0.0):
- self.t = t
- self.cuda = torch.cuda.is_available()
-
- def __enter__(self):
- self.start = self.time()
- return self
-
- def __exit__(self, type, value, traceback):
- self.dt = self.time() - self.start # delta-time
- self.t += self.dt # accumulate dt
-
- def time(self):
- if self.cuda:
- torch.cuda.synchronize()
- return time.time()
-
-
-class Timeout(contextlib.ContextDecorator):
- # YOLOv5 Timeout class. Usage: @Timeout(seconds) decorator or 'with Timeout(seconds):' context manager
- def __init__(self, seconds, *, timeout_msg='', suppress_timeout_errors=True):
- self.seconds = int(seconds)
- self.timeout_message = timeout_msg
- self.suppress = bool(suppress_timeout_errors)
-
- def _timeout_handler(self, signum, frame):
- raise TimeoutError(self.timeout_message)
-
- def __enter__(self):
- if platform.system() != 'Windows': # not supported on Windows
- signal.signal(signal.SIGALRM, self._timeout_handler) # Set handler for SIGALRM
- signal.alarm(self.seconds) # start countdown for SIGALRM to be raised
-
- def __exit__(self, exc_type, exc_val, exc_tb):
- if platform.system() != 'Windows':
- signal.alarm(0) # Cancel SIGALRM if it's scheduled
- if self.suppress and exc_type is TimeoutError: # Suppress TimeoutError
- return True
-
-
-class WorkingDirectory(contextlib.ContextDecorator):
- # Usage: @WorkingDirectory(dir) decorator or 'with WorkingDirectory(dir):' context manager
- def __init__(self, new_dir):
- self.dir = new_dir # new dir
- self.cwd = Path.cwd().resolve() # current dir
-
- def __enter__(self):
- os.chdir(self.dir)
-
- def __exit__(self, exc_type, exc_val, exc_tb):
- os.chdir(self.cwd)
-
-
-def methods(instance):
- # Get class/instance methods
- return [f for f in dir(instance) if callable(getattr(instance, f)) and not f.startswith("__")]
-
-
-def print_args(args: Optional[dict] = None, show_file=True, show_func=False):
- # Print function arguments (optional args dict)
- x = inspect.currentframe().f_back # previous frame
- file, _, func, _, _ = inspect.getframeinfo(x)
- if args is None: # get args automatically
- args, _, _, frm = inspect.getargvalues(x)
- args = {k: v for k, v in frm.items() if k in args}
- try:
- file = Path(file).resolve().relative_to(ROOT).with_suffix('')
- except ValueError:
- file = Path(file).stem
- s = (f'{file}: ' if show_file else '') + (f'{func}: ' if show_func else '')
- LOGGER.info(colorstr(s) + ', '.join(f'{k}={v}' for k, v in args.items()))
-
-
-def init_seeds(seed=0, deterministic=False):
- # Initialize random number generator (RNG) seeds https://pytorch.org/docs/stable/notes/randomness.html
- random.seed(seed)
- np.random.seed(seed)
- torch.manual_seed(seed)
- torch.cuda.manual_seed(seed)
- torch.cuda.manual_seed_all(seed) # for Multi-GPU, exception safe
- # torch.backends.cudnn.benchmark = True # AutoBatch problem https://github.com/ultralytics/yolov5/issues/9287
- if deterministic and check_version(torch.__version__, '1.12.0'): # https://github.com/ultralytics/yolov5/pull/8213
- torch.use_deterministic_algorithms(True)
- torch.backends.cudnn.deterministic = True
- os.environ['CUBLAS_WORKSPACE_CONFIG'] = ':4096:8'
- os.environ['PYTHONHASHSEED'] = str(seed)
-
-
-def intersect_dicts(da, db, exclude=()):
- # Dictionary intersection of matching keys and shapes, omitting 'exclude' keys, using da values
- return {k: v for k, v in da.items() if k in db and all(x not in k for x in exclude) and v.shape == db[k].shape}
-
-
-def get_default_args(func):
- # Get func() default arguments
- signature = inspect.signature(func)
- return {k: v.default for k, v in signature.parameters.items() if v.default is not inspect.Parameter.empty}
-
-
-def get_latest_run(search_dir='.'):
- # Return path to most recent 'last.pt' in /runs (i.e. to --resume from)
- last_list = glob.glob(f'{search_dir}/**/last*.pt', recursive=True)
- return max(last_list, key=os.path.getctime) if last_list else ''
-
-
-def file_age(path=__file__):
- # Return days since last file update
- dt = (datetime.now() - datetime.fromtimestamp(Path(path).stat().st_mtime)) # delta
- return dt.days # + dt.seconds / 86400 # fractional days
-
-
-def file_date(path=__file__):
- # Return human-readable file modification date, i.e. '2021-3-26'
- t = datetime.fromtimestamp(Path(path).stat().st_mtime)
- return f'{t.year}-{t.month}-{t.day}'
-
-
-def file_size(path):
- # Return file/dir size (MB)
- mb = 1 << 20 # bytes to MiB (1024 ** 2)
- path = Path(path)
- if path.is_file():
- return path.stat().st_size / mb
- elif path.is_dir():
- return sum(f.stat().st_size for f in path.glob('**/*') if f.is_file()) / mb
- else:
- return 0.0
-
-
-def check_online():
- # Check internet connectivity
- import socket
-
- def run_once():
- # Check once
- try:
- socket.create_connection(("1.1.1.1", 443), 5) # check host accessibility
- return True
- except OSError:
- return False
-
- return run_once() or run_once() # check twice to increase robustness to intermittent connectivity issues
-
-
-def git_describe(path=ROOT): # path must be a directory
- # Return human-readable git description, i.e. v5.0-5-g3e25f1e https://git-scm.com/docs/git-describe
- try:
- assert (Path(path) / '.git').is_dir()
- return check_output(f'git -C {path} describe --tags --long --always', shell=True).decode()[:-1]
- except Exception:
- return ''
-
-
-@TryExcept()
-@WorkingDirectory(ROOT)
-def check_git_status(repo='ultralytics/yolov5', branch='master'):
- # YOLOv5 status check, recommend 'git pull' if code is out of date
- url = f'https://github.com/{repo}'
- msg = f', for updates see {url}'
- s = colorstr('github: ') # string
- assert Path('.git').exists(), s + 'skipping check (not a git repository)' + msg
- assert check_online(), s + 'skipping check (offline)' + msg
-
- splits = re.split(pattern=r'\s', string=check_output('git remote -v', shell=True).decode())
- matches = [repo in s for s in splits]
- if any(matches):
- remote = splits[matches.index(True) - 1]
- else:
- remote = 'ultralytics'
- check_output(f'git remote add {remote} {url}', shell=True)
- check_output(f'git fetch {remote}', shell=True, timeout=5) # git fetch
- local_branch = check_output('git rev-parse --abbrev-ref HEAD', shell=True).decode().strip() # checked out
- n = int(check_output(f'git rev-list {local_branch}..{remote}/{branch} --count', shell=True)) # commits behind
- if n > 0:
- pull = 'git pull' if remote == 'origin' else f'git pull {remote} {branch}'
- s += f"⚠️ YOLOv5 is out of date by {n} commit{'s' * (n > 1)}. Use `{pull}` or `git clone {url}` to update."
- else:
- s += f'up to date with {url} ✅'
- LOGGER.info(s)
-
-
-def check_python(minimum='3.7.0'):
- # Check current python version vs. required python version
- check_version(platform.python_version(), minimum, name='Python ', hard=True)
-
-
-def check_version(current='0.0.0', minimum='0.0.0', name='version ', pinned=False, hard=False, verbose=False):
- # Check version vs. required version
- current, minimum = (pkg.parse_version(x) for x in (current, minimum))
- result = (current == minimum) if pinned else (current >= minimum) # bool
- s = f'WARNING ⚠️ {name}{minimum} is required by YOLOv5, but {name}{current} is currently installed' # string
- if hard:
- assert result, emojis(s) # assert min requirements met
- if verbose and not result:
- LOGGER.warning(s)
- return result
-
-
-@TryExcept()
-def check_requirements(requirements=ROOT / 'requirements.txt', exclude=(), install=True, cmds=''):
- # Check installed dependencies meet YOLOv5 requirements (pass *.txt file or list of packages or single package str)
- prefix = colorstr('red', 'bold', 'requirements:')
- check_python() # check python version
- if isinstance(requirements, Path): # requirements.txt file
- file = requirements.resolve()
- assert file.exists(), f"{prefix} {file} not found, check failed."
- with file.open() as f:
- requirements = [f'{x.name}{x.specifier}' for x in pkg.parse_requirements(f) if x.name not in exclude]
- elif isinstance(requirements, str):
- requirements = [requirements]
-
- s = ''
- n = 0
- for r in requirements:
- try:
- pkg.require(r)
- except (pkg.VersionConflict, pkg.DistributionNotFound): # exception if requirements not met
- s += f'"{r}" '
- n += 1
-
- if s and install and AUTOINSTALL: # check environment variable
- LOGGER.info(f"{prefix} YOLOv5 requirement{'s' * (n > 1)} {s}not found, attempting AutoUpdate...")
- try:
- # assert check_online(), "AutoUpdate skipped (offline)"
- LOGGER.info(check_output(f'pip install {s} {cmds}', shell=True).decode())
- source = file if 'file' in locals() else requirements
- s = f"{prefix} {n} package{'s' * (n > 1)} updated per {source}\n" \
- f"{prefix} ⚠️ {colorstr('bold', 'Restart runtime or rerun command for updates to take effect')}\n"
- LOGGER.info(s)
- except Exception as e:
- LOGGER.warning(f'{prefix} ❌ {e}')
-
-
-def check_img_size(imgsz, s=32, floor=0):
- # Verify image size is a multiple of stride s in each dimension
- if isinstance(imgsz, int): # integer i.e. img_size=640
- new_size = max(make_divisible(imgsz, int(s)), floor)
- else: # list i.e. img_size=[640, 480]
- imgsz = list(imgsz) # convert to list if tuple
- new_size = [max(make_divisible(x, int(s)), floor) for x in imgsz]
- if new_size != imgsz:
- LOGGER.warning(f'WARNING ⚠️ --img-size {imgsz} must be multiple of max stride {s}, updating to {new_size}')
- return new_size
-
-
-def check_imshow(warn=False):
- # Check if environment supports image displays
- try:
- assert not is_notebook()
- assert not is_docker()
- cv2.imshow('test', np.zeros((1, 1, 3)))
- cv2.waitKey(1)
- cv2.destroyAllWindows()
- cv2.waitKey(1)
- return True
- except Exception as e:
- if warn:
- LOGGER.warning(f'WARNING ⚠️ Environment does not support cv2.imshow() or PIL Image.show()\n{e}')
- return False
-
-
-def check_suffix(file='yolov5s.pt', suffix=('.pt',), msg=''):
- # Check file(s) for acceptable suffix
- if file and suffix:
- if isinstance(suffix, str):
- suffix = [suffix]
- for f in file if isinstance(file, (list, tuple)) else [file]:
- s = Path(f).suffix.lower() # file suffix
- if len(s):
- assert s in suffix, f"{msg}{f} acceptable suffix is {suffix}"
-
-
-def check_yaml(file, suffix=('.yaml', '.yml')):
- # Search/download YAML file (if necessary) and return path, checking suffix
- return check_file(file, suffix)
-
-
-def check_file(file, suffix=''):
- # Search/download file (if necessary) and return path
- check_suffix(file, suffix) # optional
- file = str(file) # convert to str()
- if os.path.isfile(file) or not file: # exists
- return file
- elif file.startswith(('http:/', 'https:/')): # download
- url = file # warning: Pathlib turns :// -> :/
- file = Path(urllib.parse.unquote(file).split('?')[0]).name # '%2F' to '/', split https://url.com/file.txt?auth
- if os.path.isfile(file):
- LOGGER.info(f'Found {url} locally at {file}') # file already exists
- else:
- LOGGER.info(f'Downloading {url} to {file}...')
- torch.hub.download_url_to_file(url, file)
- assert Path(file).exists() and Path(file).stat().st_size > 0, f'File download failed: {url}' # check
- return file
- elif file.startswith('clearml://'): # ClearML Dataset ID
- assert 'clearml' in sys.modules, "ClearML is not installed, so cannot use ClearML dataset. Try running 'pip install clearml'."
- return file
- else: # search
- files = []
- for d in 'data', 'models', 'utils': # search directories
- files.extend(glob.glob(str(ROOT / d / '**' / file), recursive=True)) # find file
- assert len(files), f'File not found: {file}' # assert file was found
- assert len(files) == 1, f"Multiple files match '{file}', specify exact path: {files}" # assert unique
- return files[0] # return file
-
-
-def check_font(font=FONT, progress=False):
- # Download font to CONFIG_DIR if necessary
- font = Path(font)
- file = CONFIG_DIR / font.name
- if not font.exists() and not file.exists():
- url = f'https://ultralytics.com/assets/{font.name}'
- LOGGER.info(f'Downloading {url} to {file}...')
- torch.hub.download_url_to_file(url, str(file), progress=progress)
-
-
-def check_dataset(data, autodownload=True):
- # Download, check and/or unzip dataset if not found locally
-
- # Download (optional)
- extract_dir = ''
- if isinstance(data, (str, Path)) and (is_zipfile(data) or is_tarfile(data)):
- download(data, dir=f'{DATASETS_DIR}/{Path(data).stem}', unzip=True, delete=False, curl=False, threads=1)
- data = next((DATASETS_DIR / Path(data).stem).rglob('*.yaml'))
- extract_dir, autodownload = data.parent, False
-
- # Read yaml (optional)
- if isinstance(data, (str, Path)):
- data = yaml_load(data) # dictionary
-
- # Checks
- for k in 'train', 'val', 'names':
- assert k in data, f"data.yaml '{k}:' field missing ❌"
- if isinstance(data['names'], (list, tuple)): # old array format
- data['names'] = dict(enumerate(data['names'])) # convert to dict
- data['nc'] = len(data['names'])
-
- # Resolve paths
- path = Path(extract_dir or data.get('path') or '') # optional 'path' default to '.'
- if not path.is_absolute():
- path = (ROOT / path).resolve()
- data['path'] = path # download scripts
- for k in 'train', 'val', 'test':
- if data.get(k): # prepend path
- if isinstance(data[k], str):
- x = (path / data[k]).resolve()
- if not x.exists() and data[k].startswith('../'):
- x = (path / data[k][3:]).resolve()
- data[k] = str(x)
- else:
- data[k] = [str((path / x).resolve()) for x in data[k]]
-
- # Parse yaml
- train, val, test, s = (data.get(x) for x in ('train', 'val', 'test', 'download'))
- if val:
- val = [Path(x).resolve() for x in (val if isinstance(val, list) else [val])] # val path
- if not all(x.exists() for x in val):
- LOGGER.info('\nDataset not found ⚠️, missing paths %s' % [str(x) for x in val if not x.exists()])
- if not s or not autodownload:
- raise Exception('Dataset not found ❌')
- t = time.time()
- if s.startswith('http') and s.endswith('.zip'): # URL
- f = Path(s).name # filename
- LOGGER.info(f'Downloading {s} to {f}...')
- torch.hub.download_url_to_file(s, f)
- Path(DATASETS_DIR).mkdir(parents=True, exist_ok=True) # create root
- unzip_file(f, path=DATASETS_DIR) # unzip
- Path(f).unlink() # remove zip
- r = None # success
- elif s.startswith('bash '): # bash script
- LOGGER.info(f'Running {s} ...')
- r = os.system(s)
- else: # python script
- r = exec(s, {'yaml': data}) # return None
- dt = f'({round(time.time() - t, 1)}s)'
- s = f"success ✅ {dt}, saved to {colorstr('bold', DATASETS_DIR)}" if r in (0, None) else f"failure {dt} ❌"
- LOGGER.info(f"Dataset download {s}")
- check_font('Arial.ttf' if is_ascii(data['names']) else 'Arial.Unicode.ttf', progress=True) # download fonts
- return data # dictionary
-
-
-def check_amp(model):
- # Check PyTorch Automatic Mixed Precision (AMP) functionality. Return True on correct operation
- from models.common import AutoShape, DetectMultiBackend
-
- def amp_allclose(model, im):
- # All close FP32 vs AMP results
- m = AutoShape(model, verbose=False) # model
- a = m(im).xywhn[0] # FP32 inference
- m.amp = True
- b = m(im).xywhn[0] # AMP inference
- return a.shape == b.shape and torch.allclose(a, b, atol=0.1) # close to 10% absolute tolerance
-
- prefix = colorstr('AMP: ')
- device = next(model.parameters()).device # get model device
- if device.type in ('cpu', 'mps'):
- return False # AMP only used on CUDA devices
- f = ROOT / 'data' / 'images' / 'bus.jpg' # image to check
- im = f if f.exists() else 'https://ultralytics.com/images/bus.jpg' if check_online() else np.ones((640, 640, 3))
- try:
- assert amp_allclose(deepcopy(model), im) or amp_allclose(DetectMultiBackend('yolov5n.pt', device), im)
- LOGGER.info(f'{prefix}checks passed ✅')
- return True
- except Exception:
- help_url = 'https://github.com/ultralytics/yolov5/issues/7908'
- LOGGER.warning(f'{prefix}checks failed ❌, disabling Automatic Mixed Precision. See {help_url}')
- return False
-
-
-def yaml_load(file='data.yaml'):
- # Single-line safe yaml loading
- with open(file, errors='ignore') as f:
- return yaml.safe_load(f)
-
-
-def yaml_save(file='data.yaml', data={}):
- # Single-line safe yaml saving
- with open(file, 'w') as f:
- yaml.safe_dump({k: str(v) if isinstance(v, Path) else v for k, v in data.items()}, f, sort_keys=False)
-
-
-def unzip_file(file, path=None, exclude=('.DS_Store', '__MACOSX')):
- # Unzip a *.zip file to path/, excluding files containing strings in exclude list
- if path is None:
- path = Path(file).parent # default path
- with ZipFile(file) as zipObj:
- for f in zipObj.namelist(): # list all archived filenames in the zip
- if all(x not in f for x in exclude):
- zipObj.extract(f, path=path)
-
-
-def url2file(url):
- # Convert URL to filename, i.e. https://url.com/file.txt?auth -> file.txt
- url = str(Path(url)).replace(':/', '://') # Pathlib turns :// -> :/
- return Path(urllib.parse.unquote(url)).name.split('?')[0] # '%2F' to '/', split https://url.com/file.txt?auth
-
-
-def download(url, dir='.', unzip=True, delete=True, curl=False, threads=1, retry=3):
- # Multithreaded file download and unzip function, used in data.yaml for autodownload
- def download_one(url, dir):
- # Download 1 file
- success = True
- if os.path.isfile(url):
- f = Path(url) # filename
- else: # does not exist
- f = dir / Path(url).name
- LOGGER.info(f'Downloading {url} to {f}...')
- for i in range(retry + 1):
- if curl:
- s = 'sS' if threads > 1 else '' # silent
- r = os.system(
- f'curl -# -{s}L "{url}" -o "{f}" --retry 9 -C -') # curl download with retry, continue
- success = r == 0
- else:
- torch.hub.download_url_to_file(url, f, progress=threads == 1) # torch download
- success = f.is_file()
- if success:
- break
- elif i < retry:
- LOGGER.warning(f'⚠️ Download failure, retrying {i + 1}/{retry} {url}...')
- else:
- LOGGER.warning(f'❌ Failed to download {url}...')
-
- if unzip and success and (f.suffix == '.gz' or is_zipfile(f) or is_tarfile(f)):
- LOGGER.info(f'Unzipping {f}...')
- if is_zipfile(f):
- unzip_file(f, dir) # unzip
- elif is_tarfile(f):
- os.system(f'tar xf {f} --directory {f.parent}') # unzip
- elif f.suffix == '.gz':
- os.system(f'tar xfz {f} --directory {f.parent}') # unzip
- if delete:
- f.unlink() # remove zip
-
- dir = Path(dir)
- dir.mkdir(parents=True, exist_ok=True) # make directory
- if threads > 1:
- pool = ThreadPool(threads)
- pool.imap(lambda x: download_one(*x), zip(url, repeat(dir))) # multithreaded
- pool.close()
- pool.join()
- else:
- for u in [url] if isinstance(url, (str, Path)) else url:
- download_one(u, dir)
-
-
-def make_divisible(x, divisor):
- # Returns nearest x divisible by divisor
- if isinstance(divisor, torch.Tensor):
- divisor = int(divisor.max()) # to int
- return math.ceil(x / divisor) * divisor
-
-
-def clean_str(s):
- # Cleans a string by replacing special characters with underscore _
- return re.sub(pattern="[|@#!¡·$€%&()=?¿^*;:,¨´><+]", repl="_", string=s)
-
-
-def one_cycle(y1=0.0, y2=1.0, steps=100):
- # lambda function for sinusoidal ramp from y1 to y2 https://arxiv.org/pdf/1812.01187.pdf
- return lambda x: ((1 - math.cos(x * math.pi / steps)) / 2) * (y2 - y1) + y1
-
-
-def colorstr(*input):
- # Colors a string https://en.wikipedia.org/wiki/ANSI_escape_code, i.e. colorstr('blue', 'hello world')
- *args, string = input if len(input) > 1 else ('blue', 'bold', input[0]) # color arguments, string
- colors = {
- 'black': '\033[30m', # basic colors
- 'red': '\033[31m',
- 'green': '\033[32m',
- 'yellow': '\033[33m',
- 'blue': '\033[34m',
- 'magenta': '\033[35m',
- 'cyan': '\033[36m',
- 'white': '\033[37m',
- 'bright_black': '\033[90m', # bright colors
- 'bright_red': '\033[91m',
- 'bright_green': '\033[92m',
- 'bright_yellow': '\033[93m',
- 'bright_blue': '\033[94m',
- 'bright_magenta': '\033[95m',
- 'bright_cyan': '\033[96m',
- 'bright_white': '\033[97m',
- 'end': '\033[0m', # misc
- 'bold': '\033[1m',
- 'underline': '\033[4m'}
- return ''.join(colors[x] for x in args) + f'{string}' + colors['end']
-
-
-def labels_to_class_weights(labels, nc=80):
- # Get class weights (inverse frequency) from training labels
- if labels[0] is None: # no labels loaded
- return torch.Tensor()
-
- labels = np.concatenate(labels, 0) # labels.shape = (866643, 5) for COCO
- classes = labels[:, 0].astype(int) # labels = [class xywh]
- weights = np.bincount(classes, minlength=nc) # occurrences per class
-
- # Prepend gridpoint count (for uCE training)
- # gpi = ((320 / 32 * np.array([1, 2, 4])) ** 2 * 3).sum() # gridpoints per image
- # weights = np.hstack([gpi * len(labels) - weights.sum() * 9, weights * 9]) ** 0.5 # prepend gridpoints to start
-
- weights[weights == 0] = 1 # replace empty bins with 1
- weights = 1 / weights # number of targets per class
- weights /= weights.sum() # normalize
- return torch.from_numpy(weights).float()
-
-
-def labels_to_image_weights(labels, nc=80, class_weights=np.ones(80)):
- # Produces image weights based on class_weights and image contents
- # Usage: index = random.choices(range(n), weights=image_weights, k=1) # weighted image sample
- class_counts = np.array([np.bincount(x[:, 0].astype(int), minlength=nc) for x in labels])
- return (class_weights.reshape(1, nc) * class_counts).sum(1)
-
-
-def coco80_to_coco91_class(): # converts 80-index (val2014) to 91-index (paper)
- # https://tech.amikelive.com/node-718/what-object-categories-labels-are-in-coco-dataset/
- # a = np.loadtxt('data/coco.names', dtype='str', delimiter='\n')
- # b = np.loadtxt('data/coco_paper.names', dtype='str', delimiter='\n')
- # x1 = [list(a[i] == b).index(True) + 1 for i in range(80)] # darknet to coco
- # x2 = [list(b[i] == a).index(True) if any(b[i] == a) else None for i in range(91)] # coco to darknet
- return [
- 1, 2, 3, 4, 5, 6, 7, 8, 9, 10, 11, 13, 14, 15, 16, 17, 18, 19, 20, 21, 22, 23, 24, 25, 27, 28, 31, 32, 33, 34,
- 35, 36, 37, 38, 39, 40, 41, 42, 43, 44, 46, 47, 48, 49, 50, 51, 52, 53, 54, 55, 56, 57, 58, 59, 60, 61, 62, 63,
- 64, 65, 67, 70, 72, 73, 74, 75, 76, 77, 78, 79, 80, 81, 82, 84, 85, 86, 87, 88, 89, 90]
-
-
-def xyxy2xywh(x):
- # Convert nx4 boxes from [x1, y1, x2, y2] to [x, y, w, h] where xy1=top-left, xy2=bottom-right
- y = x.clone() if isinstance(x, torch.Tensor) else np.copy(x)
- y[:, 0] = (x[:, 0] + x[:, 2]) / 2 # x center
- y[:, 1] = (x[:, 1] + x[:, 3]) / 2 # y center
- y[:, 2] = x[:, 2] - x[:, 0] # width
- y[:, 3] = x[:, 3] - x[:, 1] # height
- return y
-
-
-def xywh2xyxy(x):
- # Convert nx4 boxes from [x, y, w, h] to [x1, y1, x2, y2] where xy1=top-left, xy2=bottom-right
- y = x.clone() if isinstance(x, torch.Tensor) else np.copy(x)
- y[:, 0] = x[:, 0] - x[:, 2] / 2 # top left x
- y[:, 1] = x[:, 1] - x[:, 3] / 2 # top left y
- y[:, 2] = x[:, 0] + x[:, 2] / 2 # bottom right x
- y[:, 3] = x[:, 1] + x[:, 3] / 2 # bottom right y
- return y
-
-
-def xywhn2xyxy(x, w=640, h=640, padw=0, padh=0):
- # Convert nx4 boxes from [x, y, w, h] normalized to [x1, y1, x2, y2] where xy1=top-left, xy2=bottom-right
- y = x.clone() if isinstance(x, torch.Tensor) else np.copy(x)
- y[:, 0] = w * (x[:, 0] - x[:, 2] / 2) + padw # top left x
- y[:, 1] = h * (x[:, 1] - x[:, 3] / 2) + padh # top left y
- y[:, 2] = w * (x[:, 0] + x[:, 2] / 2) + padw # bottom right x
- y[:, 3] = h * (x[:, 1] + x[:, 3] / 2) + padh # bottom right y
- return y
-
-
-def xyxy2xywhn(x, w=640, h=640, clip=False, eps=0.0):
- # Convert nx4 boxes from [x1, y1, x2, y2] to [x, y, w, h] normalized where xy1=top-left, xy2=bottom-right
- if clip:
- clip_boxes(x, (h - eps, w - eps)) # warning: inplace clip
- y = x.clone() if isinstance(x, torch.Tensor) else np.copy(x)
- y[:, 0] = ((x[:, 0] + x[:, 2]) / 2) / w # x center
- y[:, 1] = ((x[:, 1] + x[:, 3]) / 2) / h # y center
- y[:, 2] = (x[:, 2] - x[:, 0]) / w # width
- y[:, 3] = (x[:, 3] - x[:, 1]) / h # height
- return y
-
-
-def xyn2xy(x, w=640, h=640, padw=0, padh=0):
- # Convert normalized segments into pixel segments, shape (n,2)
- y = x.clone() if isinstance(x, torch.Tensor) else np.copy(x)
- y[:, 0] = w * x[:, 0] + padw # top left x
- y[:, 1] = h * x[:, 1] + padh # top left y
- return y
-
-
-def segment2box(segment, width=640, height=640):
- # Convert 1 segment label to 1 box label, applying inside-image constraint, i.e. (xy1, xy2, ...) to (xyxy)
- x, y = segment.T # segment xy
- inside = (x >= 0) & (y >= 0) & (x <= width) & (y <= height)
- x, y, = x[inside], y[inside]
- return np.array([x.min(), y.min(), x.max(), y.max()]) if any(x) else np.zeros((1, 4)) # xyxy
-
-
-def segments2boxes(segments):
- # Convert segment labels to box labels, i.e. (cls, xy1, xy2, ...) to (cls, xywh)
- boxes = []
- for s in segments:
- x, y = s.T # segment xy
- boxes.append([x.min(), y.min(), x.max(), y.max()]) # cls, xyxy
- return xyxy2xywh(np.array(boxes)) # cls, xywh
-
-
-def resample_segments(segments, n=1000):
- # Up-sample an (n,2) segment
- for i, s in enumerate(segments):
- s = np.concatenate((s, s[0:1, :]), axis=0)
- x = np.linspace(0, len(s) - 1, n)
- xp = np.arange(len(s))
- segments[i] = np.concatenate([np.interp(x, xp, s[:, i]) for i in range(2)]).reshape(2, -1).T # segment xy
- return segments
-
-
-def scale_boxes(img1_shape, boxes, img0_shape, ratio_pad=None):
- # Rescale boxes (xyxy) from img1_shape to img0_shape
- if ratio_pad is None: # calculate from img0_shape
- gain = min(img1_shape[0] / img0_shape[0], img1_shape[1] / img0_shape[1]) # gain = old / new
- pad = (img1_shape[1] - img0_shape[1] * gain) / 2, (img1_shape[0] - img0_shape[0] * gain) / 2 # wh padding
- else:
- gain = ratio_pad[0][0]
- pad = ratio_pad[1]
-
- boxes[:, [0, 2]] -= pad[0] # x padding
- boxes[:, [1, 3]] -= pad[1] # y padding
- boxes[:, :4] /= gain
- clip_boxes(boxes, img0_shape)
- return boxes
-
-
-def scale_segments(img1_shape, segments, img0_shape, ratio_pad=None):
- # Rescale coords (xyxy) from img1_shape to img0_shape
- if ratio_pad is None: # calculate from img0_shape
- gain = min(img1_shape[0] / img0_shape[0], img1_shape[1] / img0_shape[1]) # gain = old / new
- pad = (img1_shape[1] - img0_shape[1] * gain) / 2, (img1_shape[0] - img0_shape[0] * gain) / 2 # wh padding
- else:
- gain = ratio_pad[0][0]
- pad = ratio_pad[1]
-
- segments[:, 0] -= pad[0] # x padding
- segments[:, 1] -= pad[1] # y padding
- segments /= gain
- clip_segments(segments, img0_shape)
- return segments
-
-
-def clip_boxes(boxes, shape):
- # Clip boxes (xyxy) to image shape (height, width)
- if isinstance(boxes, torch.Tensor): # faster individually
- boxes[:, 0].clamp_(0, shape[1]) # x1
- boxes[:, 1].clamp_(0, shape[0]) # y1
- boxes[:, 2].clamp_(0, shape[1]) # x2
- boxes[:, 3].clamp_(0, shape[0]) # y2
- else: # np.array (faster grouped)
- boxes[:, [0, 2]] = boxes[:, [0, 2]].clip(0, shape[1]) # x1, x2
- boxes[:, [1, 3]] = boxes[:, [1, 3]].clip(0, shape[0]) # y1, y2
-
-
-def clip_segments(boxes, shape):
- # Clip segments (xy1,xy2,...) to image shape (height, width)
- if isinstance(boxes, torch.Tensor): # faster individually
- boxes[:, 0].clamp_(0, shape[1]) # x
- boxes[:, 1].clamp_(0, shape[0]) # y
- else: # np.array (faster grouped)
- boxes[:, 0] = boxes[:, 0].clip(0, shape[1]) # x
- boxes[:, 1] = boxes[:, 1].clip(0, shape[0]) # y
-
-
-def non_max_suppression(
- prediction,
- conf_thres=0.25,
- iou_thres=0.45,
- classes=None,
- agnostic=False,
- multi_label=False,
- labels=(),
- max_det=300,
- nm=0, # number of masks
-):
- """Non-Maximum Suppression (NMS) on inference results to reject overlapping detections
-
- Returns:
- list of detections, on (n,6) tensor per image [xyxy, conf, cls]
- """
-
- if isinstance(prediction, (list, tuple)): # YOLOv5 model in validation model, output = (inference_out, loss_out)
- prediction = prediction[0] # select only inference output
-
- device = prediction.device
- mps = 'mps' in device.type # Apple MPS
- if mps: # MPS not fully supported yet, convert tensors to CPU before NMS
- prediction = prediction.cpu()
- bs = prediction.shape[0] # batch size
- nc = prediction.shape[2] - nm - 5 # number of classes
- xc = prediction[..., 4] > conf_thres # candidates
-
- # Checks
- assert 0 <= conf_thres <= 1, f'Invalid Confidence threshold {conf_thres}, valid values are between 0.0 and 1.0'
- assert 0 <= iou_thres <= 1, f'Invalid IoU {iou_thres}, valid values are between 0.0 and 1.0'
-
- # Settings
- # min_wh = 2 # (pixels) minimum box width and height
- max_wh = 7680 # (pixels) maximum box width and height
- max_nms = 30000 # maximum number of boxes into torchvision.ops.nms()
- time_limit = 0.5 + 0.05 * bs # seconds to quit after
- redundant = True # require redundant detections
- multi_label &= nc > 1 # multiple labels per box (adds 0.5ms/img)
- merge = False # use merge-NMS
-
- t = time.time()
- mi = 5 + nc # mask start index
- output = [torch.zeros((0, 6 + nm), device=prediction.device)] * bs
- for xi, x in enumerate(prediction): # image index, image inference
- # Apply constraints
- # x[((x[..., 2:4] < min_wh) | (x[..., 2:4] > max_wh)).any(1), 4] = 0 # width-height
- x = x[xc[xi]] # confidence
-
- # Cat apriori labels if autolabelling
- if labels and len(labels[xi]):
- lb = labels[xi]
- v = torch.zeros((len(lb), nc + nm + 5), device=x.device)
- v[:, :4] = lb[:, 1:5] # box
- v[:, 4] = 1.0 # conf
- v[range(len(lb)), lb[:, 0].long() + 5] = 1.0 # cls
- x = torch.cat((x, v), 0)
-
- # If none remain process next image
- if not x.shape[0]:
- continue
-
- # Compute conf
- x[:, 5:] *= x[:, 4:5] # conf = obj_conf * cls_conf
-
- # Box/Mask
- box = xywh2xyxy(x[:, :4]) # center_x, center_y, width, height) to (x1, y1, x2, y2)
- mask = x[:, mi:] # zero columns if no masks
-
- # Detections matrix nx6 (xyxy, conf, cls)
- if multi_label:
- i, j = (x[:, 5:mi] > conf_thres).nonzero(as_tuple=False).T
- x = torch.cat((box[i], x[i, 5 + j, None], j[:, None].float(), mask[i]), 1)
- else: # best class only
- conf, j = x[:, 5:mi].max(1, keepdim=True)
- x = torch.cat((box, conf, j.float(), mask), 1)[conf.view(-1) > conf_thres]
-
- # Filter by class
- if classes is not None:
- x = x[(x[:, 5:6] == torch.tensor(classes, device=x.device)).any(1)]
-
- # Apply finite constraint
- # if not torch.isfinite(x).all():
- # x = x[torch.isfinite(x).all(1)]
-
- # Check shape
- n = x.shape[0] # number of boxes
- if not n: # no boxes
- continue
- elif n > max_nms: # excess boxes
- x = x[x[:, 4].argsort(descending=True)[:max_nms]] # sort by confidence
- else:
- x = x[x[:, 4].argsort(descending=True)] # sort by confidence
-
- # Batched NMS
- c = x[:, 5:6] * (0 if agnostic else max_wh) # classes
- boxes, scores = x[:, :4] + c, x[:, 4] # boxes (offset by class), scores
- i = torchvision.ops.nms(boxes, scores, iou_thres) # NMS
- if i.shape[0] > max_det: # limit detections
- i = i[:max_det]
- if merge and (1 < n < 3E3): # Merge NMS (boxes merged using weighted mean)
- # update boxes as boxes(i,4) = weights(i,n) * boxes(n,4)
- iou = box_iou(boxes[i], boxes) > iou_thres # iou matrix
- weights = iou * scores[None] # box weights
- x[i, :4] = torch.mm(weights, x[:, :4]).float() / weights.sum(1, keepdim=True) # merged boxes
- if redundant:
- i = i[iou.sum(1) > 1] # require redundancy
-
- output[xi] = x[i]
- if mps:
- output[xi] = output[xi].to(device)
- if (time.time() - t) > time_limit:
- LOGGER.warning(f'WARNING ⚠️ NMS time limit {time_limit:.3f}s exceeded')
- break # time limit exceeded
-
- return output
-
-
-def strip_optimizer(f='best.pt', s=''): # from utils.general import *; strip_optimizer()
- # Strip optimizer from 'f' to finalize training, optionally save as 's'
- x = torch.load(f, map_location=torch.device('cpu'))
- if x.get('ema'):
- x['model'] = x['ema'] # replace model with ema
- for k in 'optimizer', 'best_fitness', 'ema', 'updates': # keys
- x[k] = None
- x['epoch'] = -1
- x['model'].half() # to FP16
- for p in x['model'].parameters():
- p.requires_grad = False
- torch.save(x, s or f)
- mb = os.path.getsize(s or f) / 1E6 # filesize
- LOGGER.info(f"Optimizer stripped from {f},{f' saved as {s},' if s else ''} {mb:.1f}MB")
-
-
-def print_mutation(keys, results, hyp, save_dir, bucket, prefix=colorstr('evolve: ')):
- evolve_csv = save_dir / 'evolve.csv'
- evolve_yaml = save_dir / 'hyp_evolve.yaml'
- keys = tuple(keys) + tuple(hyp.keys()) # [results + hyps]
- keys = tuple(x.strip() for x in keys)
- vals = results + tuple(hyp.values())
- n = len(keys)
-
- # Download (optional)
- if bucket:
- url = f'gs://{bucket}/evolve.csv'
- if gsutil_getsize(url) > (evolve_csv.stat().st_size if evolve_csv.exists() else 0):
- os.system(f'gsutil cp {url} {save_dir}') # download evolve.csv if larger than local
-
- # Log to evolve.csv
- s = '' if evolve_csv.exists() else (('%20s,' * n % keys).rstrip(',') + '\n') # add header
- with open(evolve_csv, 'a') as f:
- f.write(s + ('%20.5g,' * n % vals).rstrip(',') + '\n')
-
- # Save yaml
- with open(evolve_yaml, 'w') as f:
- data = pd.read_csv(evolve_csv)
- data = data.rename(columns=lambda x: x.strip()) # strip keys
- i = np.argmax(fitness(data.values[:, :4])) #
- generations = len(data)
- f.write('# YOLOv5 Hyperparameter Evolution Results\n' + f'# Best generation: {i}\n' +
- f'# Last generation: {generations - 1}\n' + '# ' + ', '.join(f'{x.strip():>20s}' for x in keys[:7]) +
- '\n' + '# ' + ', '.join(f'{x:>20.5g}' for x in data.values[i, :7]) + '\n\n')
- yaml.safe_dump(data.loc[i][7:].to_dict(), f, sort_keys=False)
-
- # Print to screen
- LOGGER.info(prefix + f'{generations} generations finished, current result:\n' + prefix +
- ', '.join(f'{x.strip():>20s}' for x in keys) + '\n' + prefix + ', '.join(f'{x:20.5g}'
- for x in vals) + '\n\n')
-
- if bucket:
- os.system(f'gsutil cp {evolve_csv} {evolve_yaml} gs://{bucket}') # upload
-
-
-def apply_classifier(x, model, img, im0):
- # Apply a second stage classifier to YOLO outputs
- # Example model = torchvision.models.__dict__['efficientnet_b0'](pretrained=True).to(device).eval()
- im0 = [im0] if isinstance(im0, np.ndarray) else im0
- for i, d in enumerate(x): # per image
- if d is not None and len(d):
- d = d.clone()
-
- # Reshape and pad cutouts
- b = xyxy2xywh(d[:, :4]) # boxes
- b[:, 2:] = b[:, 2:].max(1)[0].unsqueeze(1) # rectangle to square
- b[:, 2:] = b[:, 2:] * 1.3 + 30 # pad
- d[:, :4] = xywh2xyxy(b).long()
-
- # Rescale boxes from img_size to im0 size
- scale_boxes(img.shape[2:], d[:, :4], im0[i].shape)
-
- # Classes
- pred_cls1 = d[:, 5].long()
- ims = []
- for a in d:
- cutout = im0[i][int(a[1]):int(a[3]), int(a[0]):int(a[2])]
- im = cv2.resize(cutout, (224, 224)) # BGR
-
- im = im[:, :, ::-1].transpose(2, 0, 1) # BGR to RGB, to 3x416x416
- im = np.ascontiguousarray(im, dtype=np.float32) # uint8 to float32
- im /= 255 # 0 - 255 to 0.0 - 1.0
- ims.append(im)
-
- pred_cls2 = model(torch.Tensor(ims).to(d.device)).argmax(1) # classifier prediction
- x[i] = x[i][pred_cls1 == pred_cls2] # retain matching class detections
-
- return x
-
-
-def increment_path(path, exist_ok=False, sep='', mkdir=False):
- # Increment file or directory path, i.e. runs/exp --> runs/exp{sep}2, runs/exp{sep}3, ... etc.
- path = Path(path) # os-agnostic
- if path.exists() and not exist_ok:
- path, suffix = (path.with_suffix(''), path.suffix) if path.is_file() else (path, '')
-
- # Method 1
- for n in range(2, 9999):
- p = f'{path}{sep}{n}{suffix}' # increment path
- if not os.path.exists(p): #
- break
- path = Path(p)
-
- # Method 2 (deprecated)
- # dirs = glob.glob(f"{path}{sep}*") # similar paths
- # matches = [re.search(rf"{path.stem}{sep}(\d+)", d) for d in dirs]
- # i = [int(m.groups()[0]) for m in matches if m] # indices
- # n = max(i) + 1 if i else 2 # increment number
- # path = Path(f"{path}{sep}{n}{suffix}") # increment path
-
- if mkdir:
- path.mkdir(parents=True, exist_ok=True) # make directory
-
- return path
-
-
-# OpenCV Chinese-friendly functions ------------------------------------------------------------------------------------
-imshow_ = cv2.imshow # copy to avoid recursion errors
-
-
-def imread(path, flags=cv2.IMREAD_COLOR):
- return cv2.imdecode(np.fromfile(path, np.uint8), flags)
-
-
-def imwrite(path, im):
- try:
- cv2.imencode(Path(path).suffix, im)[1].tofile(path)
- return True
- except Exception:
- return False
-
-
-def imshow(path, im):
- imshow_(path.encode('unicode_escape').decode(), im)
-
-
-cv2.imread, cv2.imwrite, cv2.imshow = imread, imwrite, imshow # redefine
-
-# Variables ------------------------------------------------------------------------------------------------------------
-NCOLS = 0 if is_docker() else shutil.get_terminal_size().columns # terminal window size for tqdm
diff --git a/spaces/Armored-Atom/DiFuse_Your_Thoughts/README.md b/spaces/Armored-Atom/DiFuse_Your_Thoughts/README.md
deleted file mode 100644
index 98b00b0487e2ab609b0b29eb82c55d9215ab3406..0000000000000000000000000000000000000000
--- a/spaces/Armored-Atom/DiFuse_Your_Thoughts/README.md
+++ /dev/null
@@ -1,14 +0,0 @@
----
-title: MagicPrompt Stable Diffusion
-emoji: 😻
-colorFrom: red
-colorTo: indigo
-sdk: gradio
-sdk_version: 3.3.1
-app_file: app.py
-pinned: false
-license: mit
-duplicated_from: Gustavosta/MagicPrompt-Stable-Diffusion
----
-
-Check out the configuration reference at https://huggingface.co/docs/hub/spaces-config-reference
diff --git a/spaces/Ataturk-Chatbot/HuggingFaceChat/venv/lib/python3.11/site-packages/pip/_internal/commands/cache.py b/spaces/Ataturk-Chatbot/HuggingFaceChat/venv/lib/python3.11/site-packages/pip/_internal/commands/cache.py
deleted file mode 100644
index e96d2b4924c468c666f3ad6dab902f217ee43c39..0000000000000000000000000000000000000000
--- a/spaces/Ataturk-Chatbot/HuggingFaceChat/venv/lib/python3.11/site-packages/pip/_internal/commands/cache.py
+++ /dev/null
@@ -1,222 +0,0 @@
-import os
-import textwrap
-from optparse import Values
-from typing import Any, List
-
-import pip._internal.utils.filesystem as filesystem
-from pip._internal.cli.base_command import Command
-from pip._internal.cli.status_codes import ERROR, SUCCESS
-from pip._internal.exceptions import CommandError, PipError
-from pip._internal.utils.logging import getLogger
-
-logger = getLogger(__name__)
-
-
-class CacheCommand(Command):
- """
- Inspect and manage pip's wheel cache.
-
- Subcommands:
-
- - dir: Show the cache directory.
- - info: Show information about the cache.
- - list: List filenames of packages stored in the cache.
- - remove: Remove one or more package from the cache.
- - purge: Remove all items from the cache.
-
- ```` can be a glob expression or a package name.
- """
-
- ignore_require_venv = True
- usage = """
- %prog dir
- %prog info
- %prog list [] [--format=[human, abspath]]
- %prog remove
- %prog purge
- """
-
- def add_options(self) -> None:
- self.cmd_opts.add_option(
- "--format",
- action="store",
- dest="list_format",
- default="human",
- choices=("human", "abspath"),
- help="Select the output format among: human (default) or abspath",
- )
-
- self.parser.insert_option_group(0, self.cmd_opts)
-
- def run(self, options: Values, args: List[str]) -> int:
- handlers = {
- "dir": self.get_cache_dir,
- "info": self.get_cache_info,
- "list": self.list_cache_items,
- "remove": self.remove_cache_items,
- "purge": self.purge_cache,
- }
-
- if not options.cache_dir:
- logger.error("pip cache commands can not function since cache is disabled.")
- return ERROR
-
- # Determine action
- if not args or args[0] not in handlers:
- logger.error(
- "Need an action (%s) to perform.",
- ", ".join(sorted(handlers)),
- )
- return ERROR
-
- action = args[0]
-
- # Error handling happens here, not in the action-handlers.
- try:
- handlers[action](options, args[1:])
- except PipError as e:
- logger.error(e.args[0])
- return ERROR
-
- return SUCCESS
-
- def get_cache_dir(self, options: Values, args: List[Any]) -> None:
- if args:
- raise CommandError("Too many arguments")
-
- logger.info(options.cache_dir)
-
- def get_cache_info(self, options: Values, args: List[Any]) -> None:
- if args:
- raise CommandError("Too many arguments")
-
- num_http_files = len(self._find_http_files(options))
- num_packages = len(self._find_wheels(options, "*"))
-
- http_cache_location = self._cache_dir(options, "http")
- wheels_cache_location = self._cache_dir(options, "wheels")
- http_cache_size = filesystem.format_directory_size(http_cache_location)
- wheels_cache_size = filesystem.format_directory_size(wheels_cache_location)
-
- message = (
- textwrap.dedent(
- """
- Package index page cache location: {http_cache_location}
- Package index page cache size: {http_cache_size}
- Number of HTTP files: {num_http_files}
- Locally built wheels location: {wheels_cache_location}
- Locally built wheels size: {wheels_cache_size}
- Number of locally built wheels: {package_count}
- """
- )
- .format(
- http_cache_location=http_cache_location,
- http_cache_size=http_cache_size,
- num_http_files=num_http_files,
- wheels_cache_location=wheels_cache_location,
- package_count=num_packages,
- wheels_cache_size=wheels_cache_size,
- )
- .strip()
- )
-
- logger.info(message)
-
- def list_cache_items(self, options: Values, args: List[Any]) -> None:
- if len(args) > 1:
- raise CommandError("Too many arguments")
-
- if args:
- pattern = args[0]
- else:
- pattern = "*"
-
- files = self._find_wheels(options, pattern)
- if options.list_format == "human":
- self.format_for_human(files)
- else:
- self.format_for_abspath(files)
-
- def format_for_human(self, files: List[str]) -> None:
- if not files:
- logger.info("No locally built wheels cached.")
- return
-
- results = []
- for filename in files:
- wheel = os.path.basename(filename)
- size = filesystem.format_file_size(filename)
- results.append(f" - {wheel} ({size})")
- logger.info("Cache contents:\n")
- logger.info("\n".join(sorted(results)))
-
- def format_for_abspath(self, files: List[str]) -> None:
- if not files:
- return
-
- results = []
- for filename in files:
- results.append(filename)
-
- logger.info("\n".join(sorted(results)))
-
- def remove_cache_items(self, options: Values, args: List[Any]) -> None:
- if len(args) > 1:
- raise CommandError("Too many arguments")
-
- if not args:
- raise CommandError("Please provide a pattern")
-
- files = self._find_wheels(options, args[0])
-
- no_matching_msg = "No matching packages"
- if args[0] == "*":
- # Only fetch http files if no specific pattern given
- files += self._find_http_files(options)
- else:
- # Add the pattern to the log message
- no_matching_msg += ' for pattern "{}"'.format(args[0])
-
- if not files:
- logger.warning(no_matching_msg)
-
- for filename in files:
- os.unlink(filename)
- logger.verbose("Removed %s", filename)
- logger.info("Files removed: %s", len(files))
-
- def purge_cache(self, options: Values, args: List[Any]) -> None:
- if args:
- raise CommandError("Too many arguments")
-
- return self.remove_cache_items(options, ["*"])
-
- def _cache_dir(self, options: Values, subdir: str) -> str:
- return os.path.join(options.cache_dir, subdir)
-
- def _find_http_files(self, options: Values) -> List[str]:
- http_dir = self._cache_dir(options, "http")
- return filesystem.find_files(http_dir, "*")
-
- def _find_wheels(self, options: Values, pattern: str) -> List[str]:
- wheel_dir = self._cache_dir(options, "wheels")
-
- # The wheel filename format, as specified in PEP 427, is:
- # {distribution}-{version}(-{build})?-{python}-{abi}-{platform}.whl
- #
- # Additionally, non-alphanumeric values in the distribution are
- # normalized to underscores (_), meaning hyphens can never occur
- # before `-{version}`.
- #
- # Given that information:
- # - If the pattern we're given contains a hyphen (-), the user is
- # providing at least the version. Thus, we can just append `*.whl`
- # to match the rest of it.
- # - If the pattern we're given doesn't contain a hyphen (-), the
- # user is only providing the name. Thus, we append `-*.whl` to
- # match the hyphen before the version, followed by anything else.
- #
- # PEP 427: https://www.python.org/dev/peps/pep-0427/
- pattern = pattern + ("*.whl" if "-" in pattern else "-*.whl")
-
- return filesystem.find_files(wheel_dir, pattern)
diff --git a/spaces/Ataturk-Chatbot/HuggingFaceChat/venv/lib/python3.11/site-packages/pip/_vendor/pygments/console.py b/spaces/Ataturk-Chatbot/HuggingFaceChat/venv/lib/python3.11/site-packages/pip/_vendor/pygments/console.py
deleted file mode 100644
index 2ada68e03b3c018e3ddbbf3356a48a1d580aa251..0000000000000000000000000000000000000000
--- a/spaces/Ataturk-Chatbot/HuggingFaceChat/venv/lib/python3.11/site-packages/pip/_vendor/pygments/console.py
+++ /dev/null
@@ -1,70 +0,0 @@
-"""
- pygments.console
- ~~~~~~~~~~~~~~~~
-
- Format colored console output.
-
- :copyright: Copyright 2006-2022 by the Pygments team, see AUTHORS.
- :license: BSD, see LICENSE for details.
-"""
-
-esc = "\x1b["
-
-codes = {}
-codes[""] = ""
-codes["reset"] = esc + "39;49;00m"
-
-codes["bold"] = esc + "01m"
-codes["faint"] = esc + "02m"
-codes["standout"] = esc + "03m"
-codes["underline"] = esc + "04m"
-codes["blink"] = esc + "05m"
-codes["overline"] = esc + "06m"
-
-dark_colors = ["black", "red", "green", "yellow", "blue",
- "magenta", "cyan", "gray"]
-light_colors = ["brightblack", "brightred", "brightgreen", "brightyellow", "brightblue",
- "brightmagenta", "brightcyan", "white"]
-
-x = 30
-for d, l in zip(dark_colors, light_colors):
- codes[d] = esc + "%im" % x
- codes[l] = esc + "%im" % (60 + x)
- x += 1
-
-del d, l, x
-
-codes["white"] = codes["bold"]
-
-
-def reset_color():
- return codes["reset"]
-
-
-def colorize(color_key, text):
- return codes[color_key] + text + codes["reset"]
-
-
-def ansiformat(attr, text):
- """
- Format ``text`` with a color and/or some attributes::
-
- color normal color
- *color* bold color
- _color_ underlined color
- +color+ blinking color
- """
- result = []
- if attr[:1] == attr[-1:] == '+':
- result.append(codes['blink'])
- attr = attr[1:-1]
- if attr[:1] == attr[-1:] == '*':
- result.append(codes['bold'])
- attr = attr[1:-1]
- if attr[:1] == attr[-1:] == '_':
- result.append(codes['underline'])
- attr = attr[1:-1]
- result.append(codes[attr])
- result.append(text)
- result.append(codes['reset'])
- return ''.join(result)
diff --git a/spaces/Awiny/Image2Paragraph/models/grit_src/third_party/CenterNet2/demo/predictor.py b/spaces/Awiny/Image2Paragraph/models/grit_src/third_party/CenterNet2/demo/predictor.py
deleted file mode 100644
index 7b7ebd3f846850172c1f560f8492d51e5667f76d..0000000000000000000000000000000000000000
--- a/spaces/Awiny/Image2Paragraph/models/grit_src/third_party/CenterNet2/demo/predictor.py
+++ /dev/null
@@ -1,220 +0,0 @@
-# Copyright (c) Facebook, Inc. and its affiliates.
-import atexit
-import bisect
-import multiprocessing as mp
-from collections import deque
-import cv2
-import torch
-
-from detectron2.data import MetadataCatalog
-from detectron2.engine.defaults import DefaultPredictor
-from detectron2.utils.video_visualizer import VideoVisualizer
-from detectron2.utils.visualizer import ColorMode, Visualizer
-
-
-class VisualizationDemo(object):
- def __init__(self, cfg, instance_mode=ColorMode.IMAGE, parallel=False):
- """
- Args:
- cfg (CfgNode):
- instance_mode (ColorMode):
- parallel (bool): whether to run the model in different processes from visualization.
- Useful since the visualization logic can be slow.
- """
- self.metadata = MetadataCatalog.get(
- cfg.DATASETS.TEST[0] if len(cfg.DATASETS.TEST) else "__unused"
- )
- self.cpu_device = torch.device("cpu")
- self.instance_mode = instance_mode
-
- self.parallel = parallel
- if parallel:
- num_gpu = torch.cuda.device_count()
- self.predictor = AsyncPredictor(cfg, num_gpus=num_gpu)
- else:
- self.predictor = DefaultPredictor(cfg)
-
- def run_on_image(self, image):
- """
- Args:
- image (np.ndarray): an image of shape (H, W, C) (in BGR order).
- This is the format used by OpenCV.
-
- Returns:
- predictions (dict): the output of the model.
- vis_output (VisImage): the visualized image output.
- """
- vis_output = None
- predictions = self.predictor(image)
- # Convert image from OpenCV BGR format to Matplotlib RGB format.
- image = image[:, :, ::-1]
- visualizer = Visualizer(image, self.metadata, instance_mode=self.instance_mode)
- if "panoptic_seg" in predictions:
- panoptic_seg, segments_info = predictions["panoptic_seg"]
- vis_output = visualizer.draw_panoptic_seg_predictions(
- panoptic_seg.to(self.cpu_device), segments_info
- )
- else:
- if "sem_seg" in predictions:
- vis_output = visualizer.draw_sem_seg(
- predictions["sem_seg"].argmax(dim=0).to(self.cpu_device)
- )
- if "instances" in predictions:
- instances = predictions["instances"].to(self.cpu_device)
- vis_output = visualizer.draw_instance_predictions(predictions=instances)
-
- return predictions, vis_output
-
- def _frame_from_video(self, video):
- while video.isOpened():
- success, frame = video.read()
- if success:
- yield frame
- else:
- break
-
- def run_on_video(self, video):
- """
- Visualizes predictions on frames of the input video.
-
- Args:
- video (cv2.VideoCapture): a :class:`VideoCapture` object, whose source can be
- either a webcam or a video file.
-
- Yields:
- ndarray: BGR visualizations of each video frame.
- """
- video_visualizer = VideoVisualizer(self.metadata, self.instance_mode)
-
- def process_predictions(frame, predictions):
- frame = cv2.cvtColor(frame, cv2.COLOR_BGR2RGB)
- if "panoptic_seg" in predictions:
- panoptic_seg, segments_info = predictions["panoptic_seg"]
- vis_frame = video_visualizer.draw_panoptic_seg_predictions(
- frame, panoptic_seg.to(self.cpu_device), segments_info
- )
- elif "instances" in predictions:
- predictions = predictions["instances"].to(self.cpu_device)
- vis_frame = video_visualizer.draw_instance_predictions(frame, predictions)
- elif "sem_seg" in predictions:
- vis_frame = video_visualizer.draw_sem_seg(
- frame, predictions["sem_seg"].argmax(dim=0).to(self.cpu_device)
- )
-
- # Converts Matplotlib RGB format to OpenCV BGR format
- vis_frame = cv2.cvtColor(vis_frame.get_image(), cv2.COLOR_RGB2BGR)
- return vis_frame
-
- frame_gen = self._frame_from_video(video)
- if self.parallel:
- buffer_size = self.predictor.default_buffer_size
-
- frame_data = deque()
-
- for cnt, frame in enumerate(frame_gen):
- frame_data.append(frame)
- self.predictor.put(frame)
-
- if cnt >= buffer_size:
- frame = frame_data.popleft()
- predictions = self.predictor.get()
- yield process_predictions(frame, predictions)
-
- while len(frame_data):
- frame = frame_data.popleft()
- predictions = self.predictor.get()
- yield process_predictions(frame, predictions)
- else:
- for frame in frame_gen:
- yield process_predictions(frame, self.predictor(frame))
-
-
-class AsyncPredictor:
- """
- A predictor that runs the model asynchronously, possibly on >1 GPUs.
- Because rendering the visualization takes considerably amount of time,
- this helps improve throughput a little bit when rendering videos.
- """
-
- class _StopToken:
- pass
-
- class _PredictWorker(mp.Process):
- def __init__(self, cfg, task_queue, result_queue):
- self.cfg = cfg
- self.task_queue = task_queue
- self.result_queue = result_queue
- super().__init__()
-
- def run(self):
- predictor = DefaultPredictor(self.cfg)
-
- while True:
- task = self.task_queue.get()
- if isinstance(task, AsyncPredictor._StopToken):
- break
- idx, data = task
- result = predictor(data)
- self.result_queue.put((idx, result))
-
- def __init__(self, cfg, num_gpus: int = 1):
- """
- Args:
- cfg (CfgNode):
- num_gpus (int): if 0, will run on CPU
- """
- num_workers = max(num_gpus, 1)
- self.task_queue = mp.Queue(maxsize=num_workers * 3)
- self.result_queue = mp.Queue(maxsize=num_workers * 3)
- self.procs = []
- for gpuid in range(max(num_gpus, 1)):
- cfg = cfg.clone()
- cfg.defrost()
- cfg.MODEL.DEVICE = "cuda:{}".format(gpuid) if num_gpus > 0 else "cpu"
- self.procs.append(
- AsyncPredictor._PredictWorker(cfg, self.task_queue, self.result_queue)
- )
-
- self.put_idx = 0
- self.get_idx = 0
- self.result_rank = []
- self.result_data = []
-
- for p in self.procs:
- p.start()
- atexit.register(self.shutdown)
-
- def put(self, image):
- self.put_idx += 1
- self.task_queue.put((self.put_idx, image))
-
- def get(self):
- self.get_idx += 1 # the index needed for this request
- if len(self.result_rank) and self.result_rank[0] == self.get_idx:
- res = self.result_data[0]
- del self.result_data[0], self.result_rank[0]
- return res
-
- while True:
- # make sure the results are returned in the correct order
- idx, res = self.result_queue.get()
- if idx == self.get_idx:
- return res
- insert = bisect.bisect(self.result_rank, idx)
- self.result_rank.insert(insert, idx)
- self.result_data.insert(insert, res)
-
- def __len__(self):
- return self.put_idx - self.get_idx
-
- def __call__(self, image):
- self.put(image)
- return self.get()
-
- def shutdown(self):
- for _ in self.procs:
- self.task_queue.put(AsyncPredictor._StopToken())
-
- @property
- def default_buffer_size(self):
- return len(self.procs) * 5
diff --git a/spaces/BAAI/vid2vid-zero/vid2vid_zero/p2p/ptp_utils.py b/spaces/BAAI/vid2vid-zero/vid2vid_zero/p2p/ptp_utils.py
deleted file mode 100644
index f0a07549ce636c6293ca218960c9f6b83096861f..0000000000000000000000000000000000000000
--- a/spaces/BAAI/vid2vid-zero/vid2vid_zero/p2p/ptp_utils.py
+++ /dev/null
@@ -1,347 +0,0 @@
-# Copyright 2022 Google LLC
-#
-# Licensed under the Apache License, Version 2.0 (the "License");
-# you may not use this file except in compliance with the License.
-# You may obtain a copy of the License at
-#
-# http://www.apache.org/licenses/LICENSE-2.0
-#
-# Unless required by applicable law or agreed to in writing, software
-# distributed under the License is distributed on an "AS IS" BASIS,
-# WITHOUT WARRANTIES OR CONDITIONS OF ANY KIND, either express or implied.
-# See the License for the specific language governing permissions and
-# limitations under the License.
-
-import numpy as np
-import torch
-from PIL import Image, ImageDraw, ImageFont
-import cv2
-from typing import Optional, Union, Tuple, List, Callable, Dict
-from IPython.display import display
-from tqdm import tqdm
-import torch.nn.functional as F
-
-
-def text_under_image(image: np.ndarray, text: str, text_color: Tuple[int, int, int] = (0, 0, 0)):
- h, w, c = image.shape
- offset = int(h * .2)
- img = np.ones((h + offset, w, c), dtype=np.uint8) * 255
- font = cv2.FONT_HERSHEY_SIMPLEX
- # font = ImageFont.truetype("/usr/share/fonts/truetype/noto/NotoMono-Regular.ttf", font_size)
- img[:h] = image
- textsize = cv2.getTextSize(text, font, 1, 2)[0]
- text_x, text_y = (w - textsize[0]) // 2, h + offset - textsize[1] // 2
- cv2.putText(img, text, (text_x, text_y ), font, 1, text_color, 2)
- return img
-
-
-def view_images(images, num_rows=1, offset_ratio=0.02):
- if type(images) is list:
- num_empty = len(images) % num_rows
- elif images.ndim == 4:
- num_empty = images.shape[0] % num_rows
- else:
- images = [images]
- num_empty = 0
-
- empty_images = np.ones(images[0].shape, dtype=np.uint8) * 255
- images = [image.astype(np.uint8) for image in images] + [empty_images] * num_empty
- num_items = len(images)
-
- h, w, c = images[0].shape
- offset = int(h * offset_ratio)
- num_cols = num_items // num_rows
- image_ = np.ones((h * num_rows + offset * (num_rows - 1),
- w * num_cols + offset * (num_cols - 1), 3), dtype=np.uint8) * 255
- for i in range(num_rows):
- for j in range(num_cols):
- image_[i * (h + offset): i * (h + offset) + h:, j * (w + offset): j * (w + offset) + w] = images[
- i * num_cols + j]
-
- pil_img = Image.fromarray(image_)
- display(pil_img)
-
-
-def diffusion_step(model, controller, latents, context, t, guidance_scale, low_resource=False):
- if low_resource:
- noise_pred_uncond = model.unet(latents, t, encoder_hidden_states=context[0])["sample"]
- noise_prediction_text = model.unet(latents, t, encoder_hidden_states=context[1])["sample"]
- else:
- latents_input = torch.cat([latents] * 2)
- noise_pred = model.unet(latents_input, t, encoder_hidden_states=context)["sample"]
- noise_pred_uncond, noise_prediction_text = noise_pred.chunk(2)
- noise_pred = noise_pred_uncond + guidance_scale * (noise_prediction_text - noise_pred_uncond)
- latents = model.scheduler.step(noise_pred, t, latents)["prev_sample"]
- latents = controller.step_callback(latents)
- return latents
-
-
-def latent2image(vae, latents):
- latents = 1 / 0.18215 * latents
- image = vae.decode(latents)['sample']
- image = (image / 2 + 0.5).clamp(0, 1)
- image = image.cpu().permute(0, 2, 3, 1).numpy()
- image = (image * 255).astype(np.uint8)
- return image
-
-
-def init_latent(latent, model, height, width, generator, batch_size):
- if latent is None:
- latent = torch.randn(
- (1, model.unet.in_channels, height // 8, width // 8),
- generator=generator,
- )
- latents = latent.expand(batch_size, model.unet.in_channels, height // 8, width // 8).to(model.device)
- return latent, latents
-
-
-@torch.no_grad()
-def text2image_ldm(
- model,
- prompt: List[str],
- controller,
- num_inference_steps: int = 50,
- guidance_scale: Optional[float] = 7.,
- generator: Optional[torch.Generator] = None,
- latent: Optional[torch.FloatTensor] = None,
-):
- register_attention_control(model, controller)
- height = width = 256
- batch_size = len(prompt)
-
- uncond_input = model.tokenizer([""] * batch_size, padding="max_length", max_length=77, return_tensors="pt")
- uncond_embeddings = model.bert(uncond_input.input_ids.to(model.device))[0]
-
- text_input = model.tokenizer(prompt, padding="max_length", max_length=77, return_tensors="pt")
- text_embeddings = model.bert(text_input.input_ids.to(model.device))[0]
- latent, latents = init_latent(latent, model, height, width, generator, batch_size)
- context = torch.cat([uncond_embeddings, text_embeddings])
-
- model.scheduler.set_timesteps(num_inference_steps)
- for t in tqdm(model.scheduler.timesteps):
- latents = diffusion_step(model, controller, latents, context, t, guidance_scale)
-
- image = latent2image(model.vqvae, latents)
-
- return image, latent
-
-
-@torch.no_grad()
-def text2image_ldm_stable(
- model,
- prompt: List[str],
- controller,
- num_inference_steps: int = 50,
- guidance_scale: float = 7.5,
- generator: Optional[torch.Generator] = None,
- latent: Optional[torch.FloatTensor] = None,
- low_resource: bool = False,
-):
- register_attention_control(model, controller)
- height = width = 512
- batch_size = len(prompt)
-
- text_input = model.tokenizer(
- prompt,
- padding="max_length",
- max_length=model.tokenizer.model_max_length,
- truncation=True,
- return_tensors="pt",
- )
- text_embeddings = model.text_encoder(text_input.input_ids.to(model.device))[0]
- max_length = text_input.input_ids.shape[-1]
- uncond_input = model.tokenizer(
- [""] * batch_size, padding="max_length", max_length=max_length, return_tensors="pt"
- )
- uncond_embeddings = model.text_encoder(uncond_input.input_ids.to(model.device))[0]
-
- context = [uncond_embeddings, text_embeddings]
- if not low_resource:
- context = torch.cat(context)
- latent, latents = init_latent(latent, model, height, width, generator, batch_size)
-
- # set timesteps
- extra_set_kwargs = {"offset": 1}
- model.scheduler.set_timesteps(num_inference_steps, **extra_set_kwargs)
- for t in tqdm(model.scheduler.timesteps):
- latents = diffusion_step(model, controller, latents, context, t, guidance_scale, low_resource)
-
- image = latent2image(model.vae, latents)
-
- return image, latent
-
-
-def register_attention_control(model, controller):
-
- def ca_forward(self, place_in_unet):
- def forward(hidden_states, encoder_hidden_states=None, attention_mask=None):
- batch_size, sequence_length, _ = hidden_states.shape
-
- is_cross = encoder_hidden_states is not None
- encoder_hidden_states = encoder_hidden_states
-
- if self.group_norm is not None:
- hidden_states = self.group_norm(hidden_states.transpose(1, 2)).transpose(1, 2)
-
- query = self.to_q(hidden_states)
- # dim = query.shape[-1]
- query = self.reshape_heads_to_batch_dim(query)
-
- if self.added_kv_proj_dim is not None:
- key = self.to_k(hidden_states)
- value = self.to_v(hidden_states)
- encoder_hidden_states_key_proj = self.add_k_proj(encoder_hidden_states)
- encoder_hidden_states_value_proj = self.add_v_proj(encoder_hidden_states)
-
- key = self.reshape_heads_to_batch_dim(key)
- value = self.reshape_heads_to_batch_dim(value)
- encoder_hidden_states_key_proj = self.reshape_heads_to_batch_dim(encoder_hidden_states_key_proj)
- encoder_hidden_states_value_proj = self.reshape_heads_to_batch_dim(encoder_hidden_states_value_proj)
-
- key = torch.concat([encoder_hidden_states_key_proj, key], dim=1)
- value = torch.concat([encoder_hidden_states_value_proj, value], dim=1)
- else:
- encoder_hidden_states = encoder_hidden_states if encoder_hidden_states is not None else hidden_states
- key = self.to_k(encoder_hidden_states)
- value = self.to_v(encoder_hidden_states)
-
- key = self.reshape_heads_to_batch_dim(key)
- value = self.reshape_heads_to_batch_dim(value)
-
- if attention_mask is not None:
- if attention_mask.shape[-1] != query.shape[1]:
- target_length = query.shape[1]
- attention_mask = F.pad(attention_mask, (0, target_length), value=0.0)
- attention_mask = attention_mask.repeat_interleave(self.heads, dim=0)
-
- assert self._slice_size is None or query.shape[0] // self._slice_size == 1
-
- if self.upcast_attention:
- query = query.float()
- key = key.float()
-
- attention_scores = torch.baddbmm(
- torch.empty(query.shape[0], query.shape[1], key.shape[1], dtype=query.dtype, device=query.device),
- query,
- key.transpose(-1, -2),
- beta=0,
- alpha=self.scale,
- )
-
- if attention_mask is not None:
- attention_scores = attention_scores + attention_mask
-
- if self.upcast_softmax:
- attention_scores = attention_scores.float()
-
- attention_probs = attention_scores.softmax(dim=-1)
-
- # attn control
- attention_probs = controller(attention_probs, is_cross, place_in_unet)
-
- # cast back to the original dtype
- attention_probs = attention_probs.to(value.dtype)
-
- # compute attention output
- hidden_states = torch.bmm(attention_probs, value)
-
- # reshape hidden_states
- hidden_states = self.reshape_batch_dim_to_heads(hidden_states)
-
- # linear proj
- hidden_states = self.to_out[0](hidden_states)
-
- # dropout
- hidden_states = self.to_out[1](hidden_states)
- return hidden_states
-
- return forward
-
- class DummyController:
-
- def __call__(self, *args):
- return args[0]
-
- def __init__(self):
- self.num_att_layers = 0
-
- if controller is None:
- controller = DummyController()
-
- def register_recr(net_, count, place_in_unet):
- if net_.__class__.__name__ == 'CrossAttention':
- net_.forward = ca_forward(net_, place_in_unet)
- return count + 1
- elif hasattr(net_, 'children'):
- for net__ in net_.children():
- count = register_recr(net__, count, place_in_unet)
- return count
-
- cross_att_count = 0
- # sub_nets = model.unet.named_children()
- # we take unet as the input model
- sub_nets = model.named_children()
- for net in sub_nets:
- if "down" in net[0]:
- cross_att_count += register_recr(net[1], 0, "down")
- elif "up" in net[0]:
- cross_att_count += register_recr(net[1], 0, "up")
- elif "mid" in net[0]:
- cross_att_count += register_recr(net[1], 0, "mid")
-
- controller.num_att_layers = cross_att_count
-
-
-def get_word_inds(text: str, word_place: int, tokenizer):
- split_text = text.split(" ")
- if type(word_place) is str:
- word_place = [i for i, word in enumerate(split_text) if word_place == word]
- elif type(word_place) is int:
- word_place = [word_place]
- out = []
- if len(word_place) > 0:
- words_encode = [tokenizer.decode([item]).strip("#") for item in tokenizer.encode(text)][1:-1]
- cur_len, ptr = 0, 0
-
- for i in range(len(words_encode)):
- cur_len += len(words_encode[i])
- if ptr in word_place:
- out.append(i + 1)
- if cur_len >= len(split_text[ptr]):
- ptr += 1
- cur_len = 0
- return np.array(out)
-
-
-def update_alpha_time_word(alpha, bounds: Union[float, Tuple[float, float]], prompt_ind: int,
- word_inds: Optional[torch.Tensor]=None):
- if type(bounds) is float:
- bounds = 0, bounds
- start, end = int(bounds[0] * alpha.shape[0]), int(bounds[1] * alpha.shape[0])
- if word_inds is None:
- word_inds = torch.arange(alpha.shape[2])
- alpha[: start, prompt_ind, word_inds] = 0
- alpha[start: end, prompt_ind, word_inds] = 1
- alpha[end:, prompt_ind, word_inds] = 0
- return alpha
-
-
-def get_time_words_attention_alpha(prompts, num_steps,
- cross_replace_steps: Union[float, Dict[str, Tuple[float, float]]],
- tokenizer, max_num_words=77):
- if type(cross_replace_steps) is not dict:
- cross_replace_steps = {"default_": cross_replace_steps}
- if "default_" not in cross_replace_steps:
- cross_replace_steps["default_"] = (0., 1.)
- alpha_time_words = torch.zeros(num_steps + 1, len(prompts) - 1, max_num_words)
- for i in range(len(prompts) - 1):
- alpha_time_words = update_alpha_time_word(alpha_time_words, cross_replace_steps["default_"],
- i)
- for key, item in cross_replace_steps.items():
- if key != "default_":
- inds = [get_word_inds(prompts[i], key, tokenizer) for i in range(1, len(prompts))]
- for i, ind in enumerate(inds):
- if len(ind) > 0:
- alpha_time_words = update_alpha_time_word(alpha_time_words, item, i, ind)
- alpha_time_words = alpha_time_words.reshape(num_steps + 1, len(prompts) - 1, 1, 1, max_num_words)
- return alpha_time_words
diff --git a/spaces/Banbri/zcvzcv/src/app/store/index.ts b/spaces/Banbri/zcvzcv/src/app/store/index.ts
deleted file mode 100644
index e85dd4d052996e9b4120bef57abb6c72c509d41a..0000000000000000000000000000000000000000
--- a/spaces/Banbri/zcvzcv/src/app/store/index.ts
+++ /dev/null
@@ -1,203 +0,0 @@
-"use client"
-
-import { create } from "zustand"
-
-import { FontName } from "@/lib/fonts"
-import { Preset, PresetName, defaultPreset, getPreset, getRandomPreset } from "@/app/engine/presets"
-import { LayoutName, defaultLayout, getRandomLayoutName, getRandomLayoutNames } from "../layouts"
-import html2canvas from "html2canvas"
-import { RenderedScene } from "@/types"
-
-export const useStore = create<{
- prompt: string
- font: FontName
- preset: Preset
- nbFrames: number
- panels: string[]
- captions: string[]
- upscaleQueue: Record
- showCaptions: boolean
- renderedScenes: Record
- layout: LayoutName
- layouts: LayoutName[]
- zoomLevel: number
- page: HTMLDivElement
- isGeneratingStory: boolean
- panelGenerationStatus: Record
- isGeneratingText: boolean
- atLeastOnePanelIsBusy: boolean
- setRendered: (panelId: string, renderedScene: RenderedScene) => void
- addToUpscaleQueue: (panelId: string, renderedScene: RenderedScene) => void
- removeFromUpscaleQueue: (panelId: string) => void
- setPrompt: (prompt: string) => void
- setFont: (font: FontName) => void
- setPreset: (preset: Preset) => void
- setPanels: (panels: string[]) => void
- setShowCaptions: (showCaptions: boolean) => void
- setLayout: (layout: LayoutName) => void
- setLayouts: (layouts: LayoutName[]) => void
- setCaptions: (captions: string[]) => void
- setZoomLevel: (zoomLevel: number) => void
- setPage: (page: HTMLDivElement) => void
- setGeneratingStory: (isGeneratingStory: boolean) => void
- setGeneratingImages: (panelId: string, value: boolean) => void
- setGeneratingText: (isGeneratingText: boolean) => void
- pageToImage: () => Promise
- download: () => Promise
- generate: (prompt: string, presetName: PresetName, layoutName: LayoutName) => void
-}>((set, get) => ({
- prompt: "",
- font: "actionman",
- preset: getPreset(defaultPreset),
- nbFrames: 1,
- panels: [],
- captions: [],
- upscaleQueue: {} as Record,
- renderedScenes: {} as Record,
- showCaptions: false,
- layout: defaultLayout,
- layouts: [defaultLayout, defaultLayout],
- zoomLevel: 60,
- page: undefined as unknown as HTMLDivElement,
- isGeneratingStory: false,
- panelGenerationStatus: {},
- isGeneratingText: false,
- atLeastOnePanelIsBusy: false,
- setRendered: (panelId: string, renderedScene: RenderedScene) => {
- const { renderedScenes } = get()
- set({
- renderedScenes: {
- ...renderedScenes,
- [panelId]: renderedScene
- }
- })
- },
- addToUpscaleQueue: (panelId: string, renderedScene: RenderedScene) => {
- const { upscaleQueue } = get()
- set({
- upscaleQueue: {
- ...upscaleQueue,
- [panelId]: renderedScene
- },
- })
- },
- removeFromUpscaleQueue: (panelId: string) => {
- const upscaleQueue = { ...get().upscaleQueue }
- delete upscaleQueue[panelId]
- set({
- upscaleQueue,
- })
- },
- setPrompt: (prompt: string) => {
- const existingPrompt = get().prompt
- if (prompt === existingPrompt) { return }
- set({
- prompt,
- })
- },
- setFont: (font: FontName) => {
- const existingFont = get().font
- if (font === existingFont) { return }
- set({
- font,
- })
- },
- setPreset: (preset: Preset) => {
- const existingPreset = get().preset
- if (preset.label === existingPreset.label) { return }
- set({
- preset,
- })
- },
- setNbFrames: (nbFrames: number) => {
- const existingNbFrames = get().nbFrames
- if (nbFrames === existingNbFrames) { return }
- set({
- nbFrames,
- })
- },
- setPanels: (panels: string[]) => set({ panels }),
- setCaptions: (captions: string[]) => {
- set({
- captions,
- })
- },
- setShowCaptions: (showCaptions: boolean) => {
- set({
- showCaptions,
- })
- },
- setLayout: (layoutName: LayoutName) => {
- const layout = layoutName === "random"
- ? getRandomLayoutName()
- : layoutName
-
- set({
- layout,
- layouts: [layout, layout]
- })
- },
- setLayouts: (layouts: LayoutName[]) => set({ layouts }),
- setZoomLevel: (zoomLevel: number) => set({ zoomLevel }),
- setPage: (page: HTMLDivElement) => {
- if (!page) { return }
- set({ page })
- },
- setGeneratingStory: (isGeneratingStory: boolean) => set({ isGeneratingStory }),
- setGeneratingImages: (panelId: string, value: boolean) => {
- const panelGenerationStatus: Record = {
- ...get().panelGenerationStatus,
- [panelId]: value
- }
-
- const atLeastOnePanelIsBusy = Object.values(panelGenerationStatus).includes(true)
-
- set({
- panelGenerationStatus,
- atLeastOnePanelIsBusy
- })
- },
- setGeneratingText: (isGeneratingText: boolean) => set({ isGeneratingText }),
- pageToImage: async () => {
- const { page } = get()
- if (!page) { return "" }
-
-
- const canvas = await html2canvas(page)
- console.log("canvas:", canvas)
-
- const data = canvas.toDataURL('image/jpeg', 0.5)
- return data
- },
- download: async () => {
- const { pageToImage } = get()
- const data = await pageToImage()
-
- const link = document.createElement('a')
-
- if (typeof link.download === 'string') {
- link.href = data
- link.download = 'comic.jpg'
- document.body.appendChild(link)
- link.click()
- document.body.removeChild(link)
- } else {
- window.open(data)
- }
- },
- generate: (prompt: string, presetName: PresetName, layoutName: LayoutName) => {
- const layout = layoutName === "random"
- ? getRandomLayoutName()
- : layoutName
- set({
- prompt,
- panels: [],
- captions: [],
- preset: presetName === "random"
- ? getRandomPreset()
- : getPreset(presetName),
- layout,
- layouts: [layout, layout],
- })
- }
-}))
diff --git a/spaces/Benson/text-generation/Examples/Adventure Apk.md b/spaces/Benson/text-generation/Examples/Adventure Apk.md
deleted file mode 100644
index effe4211cadc82ea1ac992fa2ea33479ced08d2d..0000000000000000000000000000000000000000
--- a/spaces/Benson/text-generation/Examples/Adventure Apk.md
+++ /dev/null
@@ -1,138 +0,0 @@
-
-
-
-
-
Aventura APK: ¿Qué es y cómo descargarlo
-
¿Está buscando algunos juegos y aplicaciones emocionantes y divertidos para jugar en su dispositivo móvil? ¿Quieres probar algunos nuevos géneros y formatos que pondrá a prueba sus habilidades y la imaginación? Si es así, es posible que desee echa un vistazo apk aventura.
Aventura apk es un tipo de formato de archivo que le permite descargar e instalar juegos y aplicaciones que no están disponibles en las tiendas de aplicaciones oficiales. Estos juegos y aplicaciones suelen ser creados por desarrolladores independientes que quieren compartir su creatividad y pasión con otros usuarios. Aventura apk juegos y aplicaciones a menudo se basan en temas de aventura, tales como la exploración, resolución de rompecabezas, narración, acción, etc.
-
En este artículo, vamos a explicar lo que es apk aventura, cómo descargarlo, cuáles son sus beneficios, y cuáles son algunos de los mejores juegos de aventura apk y aplicaciones que se pueden probar. ¡Vamos a empezar!
-
¿Qué es la aventura APK?
-
Definición
-
Aventura apk es un formato de archivo que significa Android Package Kit. Es similar a otros formatos de archivo como . exe para Windows o . dmg para Mac. Contiene todos los archivos y datos necesarios para ejecutar un juego o una aplicación en un dispositivo Android.
-
Aventura apk juegos y aplicaciones por lo general no están disponibles en las tiendas de aplicaciones oficiales como Google Play Store o Apple App Store. Esto se debe a que pueden no cumplir con los requisitos o estándares de estas plataformas, o pueden ser demasiado nicho o experimental para las audiencias principales.
-
Sin embargo, esto no significa que los juegos de aventura apk y aplicaciones son ilegales o inseguros. Son simplemente formas alternativas de distribuir juegos y aplicaciones que no son compatibles con los canales oficiales. Siempre y cuando los descargue de fuentes confiables y los escanee en busca de virus o malware antes de instalarlos, debería estar bien.
-
-
Ejemplos
-
-
Aquí están algunos de los más populares y conocidos juegos apk aventura y aplicaciones que se pueden descargar y disfrutar:
-
-
Minecraft Pocket Edition: Esta es la versión móvil del famoso juego sandbox que te permite construir, explorar y sobrevivir en un mundo pixelado. Puede crear sus propios mundos, jugar con amigos o unirse a servidores en línea. También puede descargar mods y mapas para mejorar su experiencia.
-
GTA San Andreas: Esta es la versión móvil del clásico juego de mundo abierto que te permite jugar como CJ, un ex gángster que regresa a su ciudad natal para encontrarlo corrompido por el crimen y la violencia. Puedes conducir, disparar, luchar y hacer misiones en un mapa enorme. También puedes personalizar tu personaje, vehículos y armas.
-
Pokemon Go: Esta es la versión móvil de la popular franquicia que te permite atrapar, entrenar y luchar contra Pokémon en el mundo real. Puedes usar tu cámara y GPS para encontrar y capturar Pokémon a tu alrededor. También puedes unirte a equipos, gimnasios, redadas y eventos.
-
Monument Valley: Este es un hermoso juego de puzzle que te permite manipular la arquitectura imposible y guiar a una princesa a través de un mundo surrealista. Puedes explorar niveles impresionantes que desafían tu percepción y lógica. También puedes disfrutar de la relajante banda sonora y el estilo artístico.
-
The Room: Este es un misterioso juego de puzzle que te permite desbloquear una serie de intrincadas cajas que esconden secretos y pistas. Puede utilizar la pantalla táctil para interactuar con los objetos y resolver los puzzles. También puede sumergirse en los gráficos atmosféricos y efectos de sonido.
-
Limbo: Este es un juego de plataformas oscuro y inquietante que te permite controlar a un chico que busca a su hermana en un mundo sombrío y peligroso. Puedes evitar trampas, enemigos y obstáculos a medida que avanzas por los niveles. También puedes experimentar el estilo artístico minimalista y la banda sonora misteriosa.
-
-
Cómo descargar aventura APK?
-
Pasos
-
-
-
Encontrar una fuente confiable para juegos de aventura apk y aplicaciones. Puede utilizar los motores de búsqueda, foros, blogs, o sitios web que se especializan en archivos apk aventura. Asegúrate de leer las reseñas, valoraciones, comentarios y comentarios de otros usuarios antes de descargar nada.
-
Descargar el archivo apk aventura a su dispositivo. Puede utilizar su navegador o una aplicación de administrador de archivos para hacer esto. Asegúrese de comprobar el tamaño del archivo, nombre, extensión y permisos antes de descargar nada.
-
Habilitar fuentes desconocidas en su dispositivo. Esta es una configuración de seguridad que le permite instalar aplicaciones desde fuentes distintas de las tiendas de aplicaciones oficiales. Puede encontrar esta opción en la configuración de su dispositivo bajo seguridad o privacidad. Asegúrese de desactivarlo después de instalar el archivo apk aventura.
-
Instalar el archivo apk aventura en su dispositivo. Puede utilizar su aplicación de administrador de archivos o su navegador para hacer esto. Toque en el archivo y siga las instrucciones en la pantalla. Asegúrese de conceder los permisos o el acceso que la aplicación requiere.
-
Inicie el juego de aventura apk o aplicación en su dispositivo. Puede encontrarlo en el cajón de la aplicación o la pantalla de inicio. Disfrute!
-
-
Consejos
-
Aquí hay algunos consejos y trucos que le ayudarán a descargar juegos de aventura apk y aplicaciones de forma segura:
-
-
Siempre escanear el archivo apk aventura en busca de virus o malware antes de instalarlo en su dispositivo. Puede usar una aplicación antivirus o un escáner en línea para hacer esto.
-
Siempre copia de seguridad de los datos del dispositivo antes de instalar cualquier archivo apk aventura en su dispositivo. Esto le ayudará a restaurar el dispositivo en caso de que algo salga mal o desea desinstalar la aplicación.
-
Compruebe siempre la compatibilidad del archivo apk aventura con el modelo de dispositivo y la versión de Android antes de instalarlo en su dispositivo. Algunos archivos apk aventura puede no funcionar correctamente o causar errores en ciertos dispositivos o versiones de Android.
-
-
Siempre desinstalar cualquier aventura apk juegos o aplicaciones que usted no utiliza más o que causan problemas en su dispositivo. Esto liberará espacio y mejorará el rendimiento en su dispositivo
-
-
¿Cuáles son los beneficios de la aventura APK?
-
Ventajas
-
Hay muchas ventajas de juegos de aventura apk y aplicaciones sobre otros formatos. Algunos de ellos son:
-
-
Variedad: Aventura apk juegos y aplicaciones ofrecen una amplia gama de géneros, temas, estilos y características que usted no puede encontrar en las tiendas de aplicaciones oficiales. Puede explorar juegos y aplicaciones nuevos e innovadores que se adapten a sus preferencias e intereses.
-
Accesibilidad: Aventura apk juegos y aplicaciones son fáciles de descargar e instalar en su dispositivo. No es necesario registrarse, registrarse o pagar por nada. También puede acceder a ellos sin conexión a Internet.
-
Personalización: Aventura apk juegos y aplicaciones le permiten personalizar su experiencia de acuerdo a su gusto. Puedes modificar, modificar o mejorar los juegos y aplicaciones usando mods, hacks, trucos o ajustes. También puede crear sus propios juegos y aplicaciones utilizando herramientas y plataformas apk aventura.
-
Comunidad: Aventura apk juegos y aplicaciones tienen una gran y activa comunidad de usuarios y desarrolladores que comparten sus comentarios, opiniones, sugerencias y apoyo. Usted puede unirse a foros, grupos, chats, o plataformas de medios sociales para interactuar con otros entusiastas apk aventura.
-
-
Desventajas
-
Sin embargo, también hay algunas desventajas o riesgos de juegos de aventura apk y aplicaciones que usted debe ser consciente de. Algunos de ellos son:
-
-
Seguridad: Aventura apk juegos y aplicaciones pueden contener virus, malware, spyware, u otros elementos dañinos que pueden dañar su dispositivo o comprometer su privacidad. Siempre debe escanear los archivos antes de instalarlos y solo descargarlos de fuentes confiables.
-
-
Calidad: Aventura juegos apk y aplicaciones no pueden tener la misma calidad, estándares, o características que los de las tiendas de aplicaciones oficiales. Pueden tener errores, errores, defectos o elementos que faltan que pueden afectar su experiencia. Siempre debes leer las reseñas, valoraciones, comentarios y comentarios antes de descargarlos.
-
Legalidad: Aventura apk juegos y aplicaciones pueden no ser legales en algunos países o regiones. Pueden violar los derechos de propiedad intelectual, los términos de servicio o las políticas de las tiendas de aplicaciones o plataformas oficiales. Siempre debe comprobar la legalidad antes de descargarlos y respetar los derechos de los creadores originales.
-
-
¿Cuáles son algunos de los mejores juegos y aplicaciones de aventura APK?
-
Tabla
-
Aquí hay una tabla con algunos de los mejores juegos de aventura apk y aplicaciones basadas en calificaciones, comentarios, descargas, etc.
-
-
-
Nombre
-
Descripción
-
Valoración
-
Enlace de descarga
-
-
-
Edición de bolsillo de Minecraft
-
Un juego sandbox que te permite construir, explorar y sobrevivir en un mundo pixelado.
-
4.5/5
-
-
-
-
GTA San Andreas
-
Un juego de mundo abierto que te permite jugar como un ex gángster que regresa a su ciudad natal.
-
4.4/5
-
-
-
-
Pokemon Go
-
Un juego que te permite atrapar, entrenar y luchar contra Pokémon en el mundo real.
-
4.1/5
-
-
Comentarios
-
Aquí hay algunas reseñas breves de cada juego o aplicación en la tabla:
-
-
Minecraft Pocket Edition: Este juego es imprescindible para cualquier jugador creativo y aventurero. Puedes construir cualquier cosa que puedas imaginar, desde casas simples hasta máquinas complejas. También puede explorar diferentes mundos, desde bosques pacíficos hasta peligrosas mazmorras. El juego se actualiza constantemente con nuevas características y contenido, lo que siempre es fresco y emocionante.
-
-
Pokemon Go: Este juego es una forma divertida e innovadora de disfrutar de la franquicia de Pokémon. Puedes atrapar y recoger Pokémon en el mundo real, usando tu cámara y GPS. También puedes unirte a equipos, gimnasios, redadas y eventos con otros jugadores. El juego se actualiza constantemente con nuevos Pokémon, características y eventos, lo que lo hace siempre atractivo y gratificante.
-
Monument Valley: Este juego es un rompecabezas hermoso y relajante que desafiará tu mente y deleitará tus ojos. Puedes manipular una arquitectura imposible y guiar a una princesa por un mundo surrealista. El juego tiene gráficos impresionantes, efectos de sonido y música que crean una atmósfera fascinante. El juego es corto pero dulce y vale cada centavo.
-
La habitación: Este juego es un misterioso y cautivador juego de puzzle que pondrá a prueba su lógica y curiosidad. Puede desbloquear una serie de cajas intrincadas que ocultan secretos y pistas. El juego tiene gráficos increíbles, efectos de sonido y animaciones que hacen que los objetos se sientan realistas y táctiles. El juego es inmersivo y adictivo, y te mantendrá adivinando hasta el final.
-
Limbo: Este juego es un juego de plataformas oscuro e inquietante que tocará tus emociones y nervios. Puedes controlar a un chico que busca a su hermana en un mundo sombrío y peligroso. El juego tiene gráficos minimalistas, efectos de sonido y música que crean un ambiente sombrío y misterioso. El juego es desafiante y gratificante, y te dejará sin aliento.
-
-
Conclusión
-
En conclusión, aventura apk es un tipo de formato de archivo que le permite descargar e instalar juegos y aplicaciones que no están disponibles en las tiendas de aplicaciones oficiales. Estos juegos y aplicaciones se basan a menudo en temas de aventura, tales como exploración, resolución de rompecabezas, narración, acción, etc.
-
-
Si usted está buscando algunos emocionantes y divertidos juegos y aplicaciones para jugar en su dispositivo móvil, es posible que desee echa un vistazo a algunos de los mejores juegos de aventura apk y aplicaciones que hemos enumerado en este artículo. Todos ellos son altamente valorados, revisados, descargados y disfrutados por muchos usuarios en todo el mundo.
-
Entonces, ¿qué estás esperando? Descargar algunos juegos de aventura apk y aplicaciones hoy y disfrutar de la emoción de la aventura en su dispositivo!
-
Preguntas frecuentes
-
Aquí hay algunas preguntas frecuentes sobre apk aventura:
-
-
¿Cuál es la diferencia entre apk aventura y mod aventura?
-
Aventura apk es un formato de archivo que le permite descargar e instalar juegos y aplicaciones que no están disponibles en las tiendas de aplicaciones oficiales. Adventure mod es una modificación o mejora de un juego o aplicación existente que añade nuevas características o cambia el juego.
-
¿Cómo puedo descargar apk aventura de forma segura?
-
Puede descargar aventura apk de forma segura siguiendo estos pasos:
-
-
Encontrar una fuente confiable para los archivos apk aventura. Puede utilizar los motores de búsqueda, foros, blogs, o sitios web que se especializan en los archivos apk aventura.
-
Escanear el archivo apk aventura en busca de virus o malware antes de instalarlo en su dispositivo. Puede usar una aplicación antivirus o un escáner en línea para hacer esto.
-
Habilitar fuentes desconocidas en el dispositivo antes de instalar el archivo apk aventura. Esta es una configuración de seguridad que le permite instalar aplicaciones desde fuentes distintas de las tiendas de aplicaciones oficiales.
-
Desactivar fuentes desconocidas en el dispositivo después de instalar el archivo apk aventura. Esto evitará que aplicaciones no autorizadas o maliciosas se instalen en su dispositivo.
-
-
¿Cómo puedo actualizar juegos y aplicaciones apk aventura?
-
Puede actualizar juegos y aplicaciones apk aventura siguiendo estos pasos:
-
-
-
Descargar la última versión del archivo apk aventura a su dispositivo. Puede utilizar su navegador o una aplicación de administrador de archivos para hacer esto.
-
Instalar la última versión del archivo apk aventura en su dispositivo. Puede utilizar su aplicación de administrador de archivos o su navegador para hacer esto.
-
Inicie el juego o aplicación apk aventura actualizada en su dispositivo. Puede encontrarlo en el cajón de la aplicación o en la pantalla de inicio.
-
-
¿Cómo puedo desinstalar juegos y aplicaciones apk aventura?
-
Puede desinstalar juegos de aventura apk y aplicaciones siguiendo estos pasos:
-
-
Ir a la configuración del dispositivo y toque en aplicaciones o aplicaciones.
-
Encontrar el juego de aventura apk o aplicación que desea desinstalar y toque en él.
-
Pulse en desinstalar y confirme su elección.
-
Eliminar el archivo apk aventura desde su dispositivo. Puede utilizar su aplicación de administrador de archivos o su navegador para hacer esto.
-
-
¿Los juegos y aplicaciones de apk de aventura son legales?
-
La legalidad de los juegos de aventura apk y aplicaciones depende del país o región donde vive y el juego o aplicación que se descarga. Algunos juegos de aventura apk y aplicaciones pueden ser legales, mientras que otros pueden ser ilegales. Siempre debe comprobar las leyes y reglamentos de su país o región antes de descargar cualquier aventura archivos apk. También debe respetar los derechos de propiedad intelectual, los términos de servicio y las políticas de las tiendas de aplicaciones o plataformas oficiales.
- 64aa2da5cf
-
-
\ No newline at end of file
diff --git a/spaces/Benson/text-generation/Examples/Descarga De Archivos Flash Infinix Smart 3 Plus.md b/spaces/Benson/text-generation/Examples/Descarga De Archivos Flash Infinix Smart 3 Plus.md
deleted file mode 100644
index 386aedeecde9cbfaf1638a805331ca7542cb78a4..0000000000000000000000000000000000000000
--- a/spaces/Benson/text-generation/Examples/Descarga De Archivos Flash Infinix Smart 3 Plus.md
+++ /dev/null
@@ -1,68 +0,0 @@
-
-
Cómo descargar historias de GTA Liberty City
-
Si eres un fan de los juegos de acción y aventura, es posible que hayas oído hablar de GTA Liberty City Stories, uno de los títulos más populares de la serie Grand Theft Auto. Este juego fue lanzado originalmente en 2005 para PlayStation Portable (PSP) y más tarde portado a PlayStation 2 (PS2) y dispositivos móviles. En este artículo, te mostraremos cómo descargar el archivo de GTA Liberty City Stories para diferentes dispositivos y disfrutar de este increíble juego.
GTA Liberty City Stories es una precuela de GTA III, ambientada en la misma ciudad ficticia de Liberty City en 1998. Juegas como Toni Cipriani, un mafioso que regresa a la ciudad después de matar a un gángster rival y tiene que abrirse camino en la familia criminal Leone. El juego cuenta con un entorno de mundo abierto donde se puede explorar, conducir, disparar, luchar y completar varias misiones. El juego también tiene un modo multijugador para usuarios de PSP, donde hasta seis jugadores pueden competir en diferentes modos.
-
¿Por qué descargar historias de GTA Liberty City?
-
Hay muchas razones por las que es posible que desee descargar GTA Liberty City Stories en lugar de comprar una copia física. Estos son algunos de ellos:
-
-
Puedes ahorrar dinero descargando el juego gratis o a un precio más bajo que comprar un disco.
-
Puede ahorrar espacio almacenando el archivo del juego en su dispositivo o tarjeta de memoria en lugar de tener un disco voluminoso.
-
Puede acceder al juego en cualquier momento y en cualquier lugar sin necesidad de una unidad de disco o una conexión a Internet.
-
Puedes disfrutar de mejores gráficos y rendimiento descargando la última versión del juego con actualizaciones y parches.
-
-
Cómo descargar GTA Liberty City Stories para diferentes dispositivos
-
El proceso de descarga de GTA Liberty City Stories varía dependiendo del dispositivo que tenga. Estos son los pasos para cada dispositivo:
-
Para PlayStation portátil (PSP)
-
-
Lo primero que necesitas hacer es encontrar un sitio web que ofrezca el archivo de juego para PSP. Puedes buscar "GTA Liberty City Stories PSP download" en Google o cualquier otro motor de búsqueda y buscar resultados que tengan reseñas y valoraciones positivas. También puede consultar algunos de estos sitios web:
-
-
[GTA Wiki]( 3 ) - Este es un wiki hecho por fans que proporciona información sobre juegos GTA, incluyendo enlaces para descargarlos.
-
[Rockstar Games]( 2 ) - Este es el sitio web oficial de Rockstar Games , el desarrollador de juegos GTA, donde puedes comprar el juego legalmente y descargarlo en tu PSP.
-
[Emuparadise] - Este es un sitio web popular que alberga una gran colección de juegos de PSP, incluyendo GTA Liberty City Stories. Sin embargo, este sitio web puede no ser legal en algunos países, así que úsalo bajo tu propio riesgo.
-
-
Paso 2: Descargue el archivo en su computadora
-
Una vez que haya encontrado una fuente confiable para el archivo del juego, debe descargarlo en su computadora. El archivo debe estar en formato ISO o CSO, que son los formatos que PSP puede leer. El tamaño del archivo puede variar dependiendo de la fuente, pero debe ser de alrededor de 1 GB. Puede usar cualquier gestor de descargas o navegador para descargar el archivo, pero asegúrese de tener suficiente espacio en su disco duro y una buena conexión a Internet.
-
-
Paso 3: Transfiera el archivo a su PSP usando un cable USB o una tarjeta de memoria
-
Después de descargar el archivo, debe transferirlo a su dispositivo PSP. Puede hacer esto de dos maneras:
-
-
Using a USB cable - Conecte su PSP a su ordenador utilizando un cable USB y encienda el modo USB en su PSP. Tu PSP debería aparecer como una unidad extraíble en tu ordenador. Copia el archivo del juego en la carpeta ISO de tu PSP. Si no tiene una carpeta ISO, cree una.
-
Using a memory card - Insertar una tarjeta de memoria en su PSP y copiar el archivo de juego a la carpeta ISO en la tarjeta de memoria. Si no tiene una carpeta ISO, cree una.
-
-
-
Ahora que has transferido el archivo de juego a tu PSP, puedes lanzarlo desde tu menú PSP. Ve a la sección Juego y selecciona Memory Stick. Deberías ver el icono de GTA Liberty City Stories. Selecciónalo y empieza a jugar.
-
Para PlayStation 2 (PS2)
-
Paso 1: Encontrar una fuente en línea de buena reputación para el archivo del juego
-
Lo primero que tienes que hacer es encontrar un sitio web que ofrezca el archivo de juego para PS2. Puedes buscar "GTA Liberty City Stories PS2 download" en Google o cualquier otro motor de búsqueda y buscar resultados que tengan críticas y valoraciones positivas. También puede consultar algunos de estos sitios web:
-
-
[GTA Wiki] - Este es un wiki hecho por fans que proporciona información sobre juegos GTA, incluyendo enlaces para descargarlos.
-
[Rockstar Games] - Este es el sitio web oficial de Rockstar Games, el desarrollador de juegos GTA, donde puedes comprar el juego legalmente y descargarlo en tu PS2.
-
[CoolROM] - Este es un sitio web popular que alberga una gran colección de juegos de PS2, incluyendo GTA Liberty City Stories. Sin embargo, este sitio web puede no ser legal en algunos países, así que úsalo bajo tu propio riesgo.
-
Paso 2: Descargue el archivo en su computadora
-
Una vez que haya encontrado una fuente confiable para el archivo del juego, debe descargarlo en su computadora. El archivo debe estar en formato ISO, que es el formato que PS2 puede leer. El tamaño del archivo puede variar dependiendo de la fuente, pero debe ser de alrededor de 4 GB. Puede usar cualquier gestor de descargas o navegador para descargar el archivo, pero asegúrese de tener suficiente espacio en su disco duro y una buena conexión a Internet.
-
Paso 3: Grabar el archivo a un DVD utilizando un software de grabación de DVD
-
-
Paso 4: Insertar el DVD en su PS2 y jugar el juego
-
Ahora que ha quemado el archivo del juego en un DVD, puede insertarlo en su PS2 y jugar el juego. Sin embargo, debes asegurarte de que tu PS2 esté modificada o con chip, lo que significa que puede jugar juegos de otras regiones o fuentes. Si tu PS2 no está modificada o con chip, no podrás jugar el juego. Puedes comprar una PS2 modificada o con chip o un mod o chip para tu propia PS2, pero ten en cuenta que esto puede anular tu garantía o dañar tu dispositivo.
-
Para dispositivos iOS, Android y Fire OS
-
Paso 1: Ir a la tienda de aplicaciones oficial de su dispositivo (App Store, Google Play Store, o Amazon Appstore)
-
La forma más fácil de descargar GTA Liberty City Stories para tu dispositivo móvil es ir a la tienda de aplicaciones oficial de tu dispositivo. Puedes usar el navegador de tu dispositivo o abrir la aplicación de la tienda de aplicaciones en tu dispositivo. Dependiendo de tu dispositivo, tendrás que ir a una de estas tiendas de aplicaciones:
-
-
App Store - Esta es la tienda de aplicaciones para dispositivos iOS, como iPhone y iPad.
-
Google Play Store - Esta es la tienda de aplicaciones para dispositivos Android, como Samsung, Huawei y LG.
-
Amazon Appstore - Esta es la tienda de aplicaciones para dispositivos Fire OS, como Kindle Fire y Fire TV.
-
-
Paso 2: Busca historias de GTA Liberty City y toca el botón de descarga
-
Una vez que esté en la tienda de aplicaciones de su dispositivo, debe buscar GTA Liberty City Stories y tocar el botón de descarga. El juego cuesta $6.99 en todas las tiendas de aplicaciones, por lo que tendrá que tener suficiente saldo en su cuenta o utilizar una tarjeta de crédito u otro método de pago para comprarlo. También necesitará tener suficiente espacio en su dispositivo para el archivo del juego, que es de alrededor de 2 GB.
-
Paso 3: Espera a que la aplicación se instale en tu dispositivo y ábrela
-
-
Paso 4: Siga las instrucciones en pantalla y comience a jugar el juego
-
Cuando abra la aplicación por primera vez, tendrá que seguir algunas instrucciones en pantalla para configurar el juego. Tendrá que aceptar algunos términos y condiciones, elegir un idioma, ajustar algunos ajustes y descargar algunos datos adicionales. Después de eso, puede comenzar a jugar el juego seleccionando un modo y una misión.
-
Conclusión
-
GTA Liberty City Stories es un juego divertido y emocionante que te permite experimentar la vida de un mafioso en una ciudad ficticia. Puede descargar el archivo del juego para diferentes dispositivos utilizando varios métodos. Sin embargo, siempre debe tener cuidado con la fuente del archivo y la legalidad de descargarlo. Esperamos que este artículo te haya ayudado a aprender a descargar GTA Liberty City Stories y disfrutar de este increíble juego.
-
-misiones, ubicaciones y más. 64aa2da5cf
-
-
\ No newline at end of file
diff --git a/spaces/BetterAPI/BetterChat_new/src/lib/types/Conversation.ts b/spaces/BetterAPI/BetterChat_new/src/lib/types/Conversation.ts
deleted file mode 100644
index 544da7b9a83aea228fe4046f9b942f860f15f22c..0000000000000000000000000000000000000000
--- a/spaces/BetterAPI/BetterChat_new/src/lib/types/Conversation.ts
+++ /dev/null
@@ -1,17 +0,0 @@
-import type { ObjectId } from "mongodb";
-import type { Message } from "./Message";
-import type { Timestamps } from "./Timestamps";
-
-export interface Conversation extends Timestamps {
- _id: ObjectId;
-
- // Can be undefined for shared convo then deleted
- sessionId: string;
-
- title: string;
- messages: Message[];
-
- meta?: {
- fromShareId?: string;
- };
-}
diff --git a/spaces/Big-Web/MMSD/env/Lib/site-packages/pip/_vendor/platformdirs/android.py b/spaces/Big-Web/MMSD/env/Lib/site-packages/pip/_vendor/platformdirs/android.py
deleted file mode 100644
index f6de7451b25ff0d0951f79499e3671e19ac106a6..0000000000000000000000000000000000000000
--- a/spaces/Big-Web/MMSD/env/Lib/site-packages/pip/_vendor/platformdirs/android.py
+++ /dev/null
@@ -1,126 +0,0 @@
-from __future__ import annotations
-
-import os
-import re
-import sys
-from functools import lru_cache
-from typing import cast
-
-from .api import PlatformDirsABC
-
-
-class Android(PlatformDirsABC):
- """
- Follows the guidance `from here `_. Makes use of the
- `appname `,
- `version `,
- `ensure_exists `.
- """
-
- @property
- def user_data_dir(self) -> str:
- """:return: data directory tied to the user, e.g. ``/data/user///files/``"""
- return self._append_app_name_and_version(cast(str, _android_folder()), "files")
-
- @property
- def site_data_dir(self) -> str:
- """:return: data directory shared by users, same as `user_data_dir`"""
- return self.user_data_dir
-
- @property
- def user_config_dir(self) -> str:
- """
- :return: config directory tied to the user, e.g. ``/data/user///shared_prefs/``
- """
- return self._append_app_name_and_version(cast(str, _android_folder()), "shared_prefs")
-
- @property
- def site_config_dir(self) -> str:
- """:return: config directory shared by the users, same as `user_config_dir`"""
- return self.user_config_dir
-
- @property
- def user_cache_dir(self) -> str:
- """:return: cache directory tied to the user, e.g. e.g. ``/data/user///cache/``"""
- return self._append_app_name_and_version(cast(str, _android_folder()), "cache")
-
- @property
- def site_cache_dir(self) -> str:
- """:return: cache directory shared by users, same as `user_cache_dir`"""
- return self.user_cache_dir
-
- @property
- def user_state_dir(self) -> str:
- """:return: state directory tied to the user, same as `user_data_dir`"""
- return self.user_data_dir
-
- @property
- def user_log_dir(self) -> str:
- """
- :return: log directory tied to the user, same as `user_cache_dir` if not opinionated else ``log`` in it,
- e.g. ``/data/user///cache//log``
- """
- path = self.user_cache_dir
- if self.opinion:
- path = os.path.join(path, "log")
- return path
-
- @property
- def user_documents_dir(self) -> str:
- """
- :return: documents directory tied to the user e.g. ``/storage/emulated/0/Documents``
- """
- return _android_documents_folder()
-
- @property
- def user_runtime_dir(self) -> str:
- """
- :return: runtime directory tied to the user, same as `user_cache_dir` if not opinionated else ``tmp`` in it,
- e.g. ``/data/user///cache//tmp``
- """
- path = self.user_cache_dir
- if self.opinion:
- path = os.path.join(path, "tmp")
- return path
-
-
-@lru_cache(maxsize=1)
-def _android_folder() -> str | None:
- """:return: base folder for the Android OS or None if cannot be found"""
- try:
- # First try to get path to android app via pyjnius
- from jnius import autoclass
-
- Context = autoclass("android.content.Context") # noqa: N806
- result: str | None = Context.getFilesDir().getParentFile().getAbsolutePath()
- except Exception:
- # if fails find an android folder looking path on the sys.path
- pattern = re.compile(r"/data/(data|user/\d+)/(.+)/files")
- for path in sys.path:
- if pattern.match(path):
- result = path.split("/files")[0]
- break
- else:
- result = None
- return result
-
-
-@lru_cache(maxsize=1)
-def _android_documents_folder() -> str:
- """:return: documents folder for the Android OS"""
- # Get directories with pyjnius
- try:
- from jnius import autoclass
-
- Context = autoclass("android.content.Context") # noqa: N806
- Environment = autoclass("android.os.Environment") # noqa: N806
- documents_dir: str = Context.getExternalFilesDir(Environment.DIRECTORY_DOCUMENTS).getAbsolutePath()
- except Exception:
- documents_dir = "/storage/emulated/0/Documents"
-
- return documents_dir
-
-
-__all__ = [
- "Android",
-]
diff --git a/spaces/CVH-vn1210/make_hair/minigpt4/models/mini_gpt4.py b/spaces/CVH-vn1210/make_hair/minigpt4/models/mini_gpt4.py
deleted file mode 100644
index 49b6d568cffadf9bd8b37bd6340de6fe6be0d880..0000000000000000000000000000000000000000
--- a/spaces/CVH-vn1210/make_hair/minigpt4/models/mini_gpt4.py
+++ /dev/null
@@ -1,263 +0,0 @@
-"""
- Copyright (c) 2023, salesforce.com, inc.
- All rights reserved.
- SPDX-License-Identifier: BSD-3-Clause
- For full license text, see the LICENSE file in the repo root or https://opensource.org/licenses/BSD-3-Clause
-"""
-import logging
-import random
-import os
-import torch
-from torch.cuda.amp import autocast as autocast
-import torch.nn as nn
-
-from minigpt4.common.registry import registry
-from minigpt4.models.blip2 import Blip2Base, disabled_train
-from minigpt4.models.modeling_llama import LlamaForCausalLM
-from transformers import LlamaTokenizer
-
-
-@registry.register_model("mini_gpt4")
-class MiniGPT4(Blip2Base):
- """
- BLIP2 GPT-LLAMA model.
- """
-
- PRETRAINED_MODEL_CONFIG_DICT = {
- "pretrain_vicuna": "configs/models/minigpt4.yaml",
- }
-
- def __init__(
- self,
- vit_model="eva_clip_g",
- q_former_model="https://storage.googleapis.com/sfr-vision-language-research/LAVIS/models/BLIP2/blip2_pretrained_flant5xxl.pth",
- img_size=224,
- drop_path_rate=0,
- use_grad_checkpoint=False,
- vit_precision="fp16",
- freeze_vit=True,
- freeze_qformer=True,
- num_query_token=32,
- llama_model="",
- llama_cache_dir='',
- prompt_path="",
- prompt_template="",
- max_txt_len=32,
- end_sym='\n',
- ):
- super().__init__()
-
- self.tokenizer = self.init_tokenizer()
-
- print('Loading VIT')
- self.visual_encoder, self.ln_vision = self.init_vision_encoder(
- vit_model, img_size, drop_path_rate, use_grad_checkpoint, vit_precision
- )
- if freeze_vit:
- for name, param in self.visual_encoder.named_parameters():
- param.requires_grad = False
- self.visual_encoder = self.visual_encoder.eval()
- self.visual_encoder.train = disabled_train
- for name, param in self.ln_vision.named_parameters():
- param.requires_grad = False
- self.ln_vision = self.ln_vision.eval()
- self.ln_vision.train = disabled_train
- logging.info("freeze vision encoder")
- print('Loading VIT Done')
-
- print('Loading Q-Former')
- self.Qformer, self.query_tokens = self.init_Qformer(
- num_query_token, self.visual_encoder.num_features
- )
- self.Qformer.cls = None
- self.Qformer.bert.embeddings.word_embeddings = None
- self.Qformer.bert.embeddings.position_embeddings = None
- for layer in self.Qformer.bert.encoder.layer:
- layer.output = None
- layer.intermediate = None
- self.load_from_pretrained(url_or_filename=q_former_model)
-
- if freeze_qformer:
- for name, param in self.Qformer.named_parameters():
- param.requires_grad = False
- self.Qformer = self.Qformer.eval()
- self.Qformer.train = disabled_train
- self.query_tokens.requires_grad = False
- logging.info("freeze Qformer")
- print('Loading Q-Former Done')
-
- print('Loading LLAMA')
- self.llama_tokenizer = LlamaTokenizer.from_pretrained('AlekseyKorshuk/vicuna-7b', use_fast=False, use_auth_token=True)
- self.llama_tokenizer.pad_token = self.llama_tokenizer.eos_token
-
- if llama_cache_dir:
- self.llama_model = LlamaForCausalLM.from_pretrained(
- 'AlekseyKorshuk/vicuna-7b', load_in_8bit=True, torch_dtype=torch.float16, device_map="auto", use_auth_token=True
- )
- else:
- self.llama_model = LlamaForCausalLM.from_pretrained(
- 'AlekseyKorshuk/vicuna-7b', load_in_8bit=True, torch_dtype=torch.float16, device_map="auto", use_auth_token=True
- )
- for name, param in self.llama_model.named_parameters():
- param.requires_grad = False
- print('Loading LLAMA Done')
-
- self.llama_proj = nn.Linear(
- self.Qformer.config.hidden_size, self.llama_model.config.hidden_size
- )
- self.max_txt_len = max_txt_len
- self.end_sym = end_sym
-
- if prompt_path:
- with open(prompt_path, 'r') as f:
- raw_prompts = f.read().splitlines()
- filted_prompts = [raw_prompt for raw_prompt in raw_prompts if "" in raw_prompt]
- self.prompt_list = [prompt_template.format(p) for p in filted_prompts]
- print('Load {} training prompts'.format(len(self.prompt_list)))
- print('Prompt Example \n{}'.format(random.choice(self.prompt_list)))
- else:
- self.prompt_list = []
-
- def vit_to_cpu(self):
- self.ln_vision.to("cpu")
- self.ln_vision.float()
- self.visual_encoder.to("cpu")
- self.visual_encoder.float()
-
- def encode_img(self, image):
- device = image.device
- self.vit_to_cpu()
- image = image.to("cpu")
- with self.maybe_autocast():
- image_embeds = self.ln_vision(self.visual_encoder(image)).to(device)
- image_atts = torch.ones(image_embeds.size()[:-1], dtype=torch.long).to(device)
-
- query_tokens = self.query_tokens.expand(image_embeds.shape[0], -1, -1)
- query_output = self.Qformer.bert(
- query_embeds=query_tokens,
- encoder_hidden_states=image_embeds,
- encoder_attention_mask=image_atts,
- return_dict=True,
- )
-
- inputs_llama = self.llama_proj(query_output.last_hidden_state)
- atts_llama = torch.ones(inputs_llama.size()[:-1], dtype=torch.long).to(image.device)
- return inputs_llama, atts_llama
-
- def prompt_wrap(self, img_embeds, atts_img, prompt):
- if prompt:
- batch_size = img_embeds.shape[0]
- p_before, p_after = prompt.split('')
- p_before_tokens = self.llama_tokenizer(
- p_before, return_tensors="pt", add_special_tokens=False).to(img_embeds.device)
- p_after_tokens = self.llama_tokenizer(
- p_after, return_tensors="pt", add_special_tokens=False).to(img_embeds.device)
- p_before_embeds = self.llama_model.model.embed_tokens(p_before_tokens.input_ids).expand(batch_size, -1, -1)
- p_after_embeds = self.llama_model.model.embed_tokens(p_after_tokens.input_ids).expand(batch_size, -1, -1)
- wrapped_img_embeds = torch.cat([p_before_embeds, img_embeds, p_after_embeds], dim=1)
- wrapped_atts_img = atts_img[:, :1].expand(-1, wrapped_img_embeds.shape[1])
- return wrapped_img_embeds, wrapped_atts_img
- else:
- return img_embeds, atts_img
-
- def forward(self, samples):
- image = samples["image"]
- img_embeds, atts_img = self.encode_img(image)
- if hasattr(samples, 'question_split'): # VQA dataset
- print('VQA Batch')
- vqa_prompt = '###Human: '
- img_embeds, atts_img = self.prompt_wrap(img_embeds, atts_img, vqa_prompt)
- elif self.prompt_list:
- prompt = random.choice(self.prompt_list)
- img_embeds, atts_img = self.prompt_wrap(img_embeds, atts_img, prompt)
-
- self.llama_tokenizer.padding_side = "right"
-
- text = [t + self.end_sym for t in samples["text_input"]]
-
- to_regress_tokens = self.llama_tokenizer(
- text,
- return_tensors="pt",
- padding="longest",
- truncation=True,
- max_length=self.max_txt_len,
- add_special_tokens=False
- ).to(image.device)
-
- targets = to_regress_tokens.input_ids.masked_fill(
- to_regress_tokens.input_ids == self.llama_tokenizer.pad_token_id, -100
- )
-
- empty_targets = (
- torch.ones([atts_img.shape[0], atts_img.shape[1]+1],
- dtype=torch.long).to(image.device).fill_(-100) # plus one for bos
- )
- targets = torch.cat([empty_targets, targets], dim=1)
-
- batch_size = img_embeds.shape[0]
- bos = torch.ones([batch_size, 1],
- dtype=to_regress_tokens.input_ids.dtype,
- device=to_regress_tokens.input_ids.device) * self.llama_tokenizer.bos_token_id
- bos_embeds = self.llama_model.model.embed_tokens(bos)
- atts_bos = atts_img[:, :1]
-
- to_regress_embeds = self.llama_model.model.embed_tokens(to_regress_tokens.input_ids)
- inputs_embeds = torch.cat([bos_embeds, img_embeds, to_regress_embeds], dim=1)
- attention_mask = torch.cat([atts_bos, atts_img, to_regress_tokens.attention_mask], dim=1)
-
- with self.maybe_autocast():
- outputs = self.llama_model(
- inputs_embeds=inputs_embeds,
- attention_mask=attention_mask,
- return_dict=True,
- labels=targets,
- )
- loss = outputs.loss
-
- return {"loss": loss}
-
- @classmethod
- def from_config(cls, cfg):
- vit_model = cfg.get("vit_model", "eva_clip_g")
- q_former_model = cfg.get("q_former_model", "https://storage.googleapis.com/sfr-vision-language-research/LAVIS/models/BLIP2/blip2_pretrained_flant5xxl.pth")
- img_size = cfg.get("image_size")
- num_query_token = cfg.get("num_query_token")
- llama_model = cfg.get("llama_model")
-
- drop_path_rate = cfg.get("drop_path_rate", 0)
- use_grad_checkpoint = cfg.get("use_grad_checkpoint", False)
- vit_precision = cfg.get("vit_precision", "fp16")
- freeze_vit = cfg.get("freeze_vit", True)
- freeze_qformer = cfg.get("freeze_qformer", True)
- llama_cache_dir = cfg.get("llama_cache_dir", "")
-
- prompt_path = cfg.get("prompt_path", "")
- prompt_template = cfg.get("prompt_template", "")
- max_txt_len = cfg.get("max_txt_len", 32)
- end_sym = cfg.get("end_sym", '\n')
-
- model = cls(
- vit_model=vit_model,
- q_former_model=q_former_model,
- img_size=img_size,
- drop_path_rate=drop_path_rate,
- use_grad_checkpoint=use_grad_checkpoint,
- vit_precision=vit_precision,
- freeze_vit=freeze_vit,
- freeze_qformer=freeze_qformer,
- llama_cache_dir=llama_cache_dir,
- num_query_token=num_query_token,
- llama_model=llama_model,
- prompt_path=prompt_path,
- prompt_template=prompt_template,
- max_txt_len=max_txt_len,
- end_sym=end_sym
- )
-
- ckpt_path = cfg.get("ckpt", "") # load weights of MiniGPT-4
- if ckpt_path:
- print("Load BLIP2-LLM Checkpoint: {}".format(ckpt_path))
- ckpt = torch.load(ckpt_path, map_location="cpu")
- msg = model.load_state_dict(ckpt['model'], strict=False)
-
- return model
diff --git a/spaces/CVPR/Dual-Key_Backdoor_Attacks/datagen/detectron2/detectron2/engine/hooks.py b/spaces/CVPR/Dual-Key_Backdoor_Attacks/datagen/detectron2/detectron2/engine/hooks.py
deleted file mode 100644
index e5085b4561302d2328ab505568dec4e9fc5ee0ad..0000000000000000000000000000000000000000
--- a/spaces/CVPR/Dual-Key_Backdoor_Attacks/datagen/detectron2/detectron2/engine/hooks.py
+++ /dev/null
@@ -1,427 +0,0 @@
-# -*- coding: utf-8 -*-
-# Copyright (c) Facebook, Inc. and its affiliates. All Rights Reserved
-
-import datetime
-import itertools
-import logging
-import os
-import tempfile
-import time
-from collections import Counter
-import torch
-from fvcore.common.checkpoint import PeriodicCheckpointer as _PeriodicCheckpointer
-from fvcore.common.file_io import PathManager
-from fvcore.common.timer import Timer
-from fvcore.nn.precise_bn import get_bn_modules, update_bn_stats
-
-import detectron2.utils.comm as comm
-from detectron2.evaluation.testing import flatten_results_dict
-from detectron2.utils.events import EventStorage, EventWriter
-
-from .train_loop import HookBase
-
-__all__ = [
- "CallbackHook",
- "IterationTimer",
- "PeriodicWriter",
- "PeriodicCheckpointer",
- "LRScheduler",
- "AutogradProfiler",
- "EvalHook",
- "PreciseBN",
-]
-
-
-"""
-Implement some common hooks.
-"""
-
-
-class CallbackHook(HookBase):
- """
- Create a hook using callback functions provided by the user.
- """
-
- def __init__(self, *, before_train=None, after_train=None, before_step=None, after_step=None):
- """
- Each argument is a function that takes one argument: the trainer.
- """
- self._before_train = before_train
- self._before_step = before_step
- self._after_step = after_step
- self._after_train = after_train
-
- def before_train(self):
- if self._before_train:
- self._before_train(self.trainer)
-
- def after_train(self):
- if self._after_train:
- self._after_train(self.trainer)
- # The functions may be closures that hold reference to the trainer
- # Therefore, delete them to avoid circular reference.
- del self._before_train, self._after_train
- del self._before_step, self._after_step
-
- def before_step(self):
- if self._before_step:
- self._before_step(self.trainer)
-
- def after_step(self):
- if self._after_step:
- self._after_step(self.trainer)
-
-
-class IterationTimer(HookBase):
- """
- Track the time spent for each iteration (each run_step call in the trainer).
- Print a summary in the end of training.
-
- This hook uses the time between the call to its :meth:`before_step`
- and :meth:`after_step` methods.
- Under the convention that :meth:`before_step` of all hooks should only
- take negligible amount of time, the :class:`IterationTimer` hook should be
- placed at the beginning of the list of hooks to obtain accurate timing.
- """
-
- def __init__(self, warmup_iter=3):
- """
- Args:
- warmup_iter (int): the number of iterations at the beginning to exclude
- from timing.
- """
- self._warmup_iter = warmup_iter
- self._step_timer = Timer()
- self._start_time = time.perf_counter()
- self._total_timer = Timer()
-
- def before_train(self):
- self._start_time = time.perf_counter()
- self._total_timer.reset()
- self._total_timer.pause()
-
- def after_train(self):
- logger = logging.getLogger(__name__)
- total_time = time.perf_counter() - self._start_time
- total_time_minus_hooks = self._total_timer.seconds()
- hook_time = total_time - total_time_minus_hooks
-
- num_iter = self.trainer.iter + 1 - self.trainer.start_iter - self._warmup_iter
-
- if num_iter > 0 and total_time_minus_hooks > 0:
- # Speed is meaningful only after warmup
- # NOTE this format is parsed by grep in some scripts
- logger.info(
- "Overall training speed: {} iterations in {} ({:.4f} s / it)".format(
- num_iter,
- str(datetime.timedelta(seconds=int(total_time_minus_hooks))),
- total_time_minus_hooks / num_iter,
- )
- )
-
- logger.info(
- "Total training time: {} ({} on hooks)".format(
- str(datetime.timedelta(seconds=int(total_time))),
- str(datetime.timedelta(seconds=int(hook_time))),
- )
- )
-
- def before_step(self):
- self._step_timer.reset()
- self._total_timer.resume()
-
- def after_step(self):
- # +1 because we're in after_step
- iter_done = self.trainer.iter - self.trainer.start_iter + 1
- if iter_done >= self._warmup_iter:
- sec = self._step_timer.seconds()
- self.trainer.storage.put_scalars(time=sec)
- else:
- self._start_time = time.perf_counter()
- self._total_timer.reset()
-
- self._total_timer.pause()
-
-
-class PeriodicWriter(HookBase):
- """
- Write events to EventStorage periodically.
-
- It is executed every ``period`` iterations and after the last iteration.
- """
-
- def __init__(self, writers, period=20):
- """
- Args:
- writers (list[EventWriter]): a list of EventWriter objects
- period (int):
- """
- self._writers = writers
- for w in writers:
- assert isinstance(w, EventWriter), w
- self._period = period
-
- def after_step(self):
- if (self.trainer.iter + 1) % self._period == 0 or (
- self.trainer.iter == self.trainer.max_iter - 1
- ):
- for writer in self._writers:
- writer.write()
-
- def after_train(self):
- for writer in self._writers:
- writer.close()
-
-
-class PeriodicCheckpointer(_PeriodicCheckpointer, HookBase):
- """
- Same as :class:`detectron2.checkpoint.PeriodicCheckpointer`, but as a hook.
-
- Note that when used as a hook,
- it is unable to save additional data other than what's defined
- by the given `checkpointer`.
-
- It is executed every ``period`` iterations and after the last iteration.
- """
-
- def before_train(self):
- self.max_iter = self.trainer.max_iter
-
- def after_step(self):
- # No way to use **kwargs
- self.step(self.trainer.iter)
-
-
-class LRScheduler(HookBase):
- """
- A hook which executes a torch builtin LR scheduler and summarizes the LR.
- It is executed after every iteration.
- """
-
- def __init__(self, optimizer, scheduler):
- """
- Args:
- optimizer (torch.optim.Optimizer):
- scheduler (torch.optim._LRScheduler)
- """
- self._optimizer = optimizer
- self._scheduler = scheduler
-
- # NOTE: some heuristics on what LR to summarize
- # summarize the param group with most parameters
- largest_group = max(len(g["params"]) for g in optimizer.param_groups)
-
- if largest_group == 1:
- # If all groups have one parameter,
- # then find the most common initial LR, and use it for summary
- lr_count = Counter([g["lr"] for g in optimizer.param_groups])
- lr = lr_count.most_common()[0][0]
- for i, g in enumerate(optimizer.param_groups):
- if g["lr"] == lr:
- self._best_param_group_id = i
- break
- else:
- for i, g in enumerate(optimizer.param_groups):
- if len(g["params"]) == largest_group:
- self._best_param_group_id = i
- break
-
- def after_step(self):
- lr = self._optimizer.param_groups[self._best_param_group_id]["lr"]
- self.trainer.storage.put_scalar("lr", lr, smoothing_hint=False)
- self._scheduler.step()
-
-
-class AutogradProfiler(HookBase):
- """
- A hook which runs `torch.autograd.profiler.profile`.
-
- Examples:
-
- .. code-block:: python
-
- hooks.AutogradProfiler(
- lambda trainer: trainer.iter > 10 and trainer.iter < 20, self.cfg.OUTPUT_DIR
- )
-
- The above example will run the profiler for iteration 10~20 and dump
- results to ``OUTPUT_DIR``. We did not profile the first few iterations
- because they are typically slower than the rest.
- The result files can be loaded in the ``chrome://tracing`` page in chrome browser.
-
- Note:
- When used together with NCCL on older version of GPUs,
- autograd profiler may cause deadlock because it unnecessarily allocates
- memory on every device it sees. The memory management calls, if
- interleaved with NCCL calls, lead to deadlock on GPUs that do not
- support `cudaLaunchCooperativeKernelMultiDevice`.
- """
-
- def __init__(self, enable_predicate, output_dir, *, use_cuda=True):
- """
- Args:
- enable_predicate (callable[trainer -> bool]): a function which takes a trainer,
- and returns whether to enable the profiler.
- It will be called once every step, and can be used to select which steps to profile.
- output_dir (str): the output directory to dump tracing files.
- use_cuda (bool): same as in `torch.autograd.profiler.profile`.
- """
- self._enable_predicate = enable_predicate
- self._use_cuda = use_cuda
- self._output_dir = output_dir
-
- def before_step(self):
- if self._enable_predicate(self.trainer):
- self._profiler = torch.autograd.profiler.profile(use_cuda=self._use_cuda)
- self._profiler.__enter__()
- else:
- self._profiler = None
-
- def after_step(self):
- if self._profiler is None:
- return
- self._profiler.__exit__(None, None, None)
- PathManager.mkdirs(self._output_dir)
- out_file = os.path.join(
- self._output_dir, "profiler-trace-iter{}.json".format(self.trainer.iter)
- )
- if "://" not in out_file:
- self._profiler.export_chrome_trace(out_file)
- else:
- # Support non-posix filesystems
- with tempfile.TemporaryDirectory(prefix="detectron2_profiler") as d:
- tmp_file = os.path.join(d, "tmp.json")
- self._profiler.export_chrome_trace(tmp_file)
- with open(tmp_file) as f:
- content = f.read()
- with PathManager.open(out_file, "w") as f:
- f.write(content)
-
-
-class EvalHook(HookBase):
- """
- Run an evaluation function periodically, and at the end of training.
-
- It is executed every ``eval_period`` iterations and after the last iteration.
- """
-
- def __init__(self, eval_period, eval_function):
- """
- Args:
- eval_period (int): the period to run `eval_function`.
- eval_function (callable): a function which takes no arguments, and
- returns a nested dict of evaluation metrics.
-
- Note:
- This hook must be enabled in all or none workers.
- If you would like only certain workers to perform evaluation,
- give other workers a no-op function (`eval_function=lambda: None`).
- """
- self._period = eval_period
- self._func = eval_function
-
- def _do_eval(self):
- results = self._func()
-
- if results:
- assert isinstance(
- results, dict
- ), "Eval function must return a dict. Got {} instead.".format(results)
-
- flattened_results = flatten_results_dict(results)
- for k, v in flattened_results.items():
- try:
- v = float(v)
- except Exception:
- raise ValueError(
- "[EvalHook] eval_function should return a nested dict of float. "
- "Got '{}: {}' instead.".format(k, v)
- )
- self.trainer.storage.put_scalars(**flattened_results, smoothing_hint=False)
-
- # Evaluation may take different time among workers.
- # A barrier make them start the next iteration together.
- comm.synchronize()
-
- def after_step(self):
- next_iter = self.trainer.iter + 1
- is_final = next_iter == self.trainer.max_iter
- if is_final or (self._period > 0 and next_iter % self._period == 0):
- self._do_eval()
-
- def after_train(self):
- # func is likely a closure that holds reference to the trainer
- # therefore we clean it to avoid circular reference in the end
- del self._func
-
-
-class PreciseBN(HookBase):
- """
- The standard implementation of BatchNorm uses EMA in inference, which is
- sometimes suboptimal.
- This class computes the true average of statistics rather than the moving average,
- and put true averages to every BN layer in the given model.
-
- It is executed every ``period`` iterations and after the last iteration.
- """
-
- def __init__(self, period, model, data_loader, num_iter):
- """
- Args:
- period (int): the period this hook is run, or 0 to not run during training.
- The hook will always run in the end of training.
- model (nn.Module): a module whose all BN layers in training mode will be
- updated by precise BN.
- Note that user is responsible for ensuring the BN layers to be
- updated are in training mode when this hook is triggered.
- data_loader (iterable): it will produce data to be run by `model(data)`.
- num_iter (int): number of iterations used to compute the precise
- statistics.
- """
- self._logger = logging.getLogger(__name__)
- if len(get_bn_modules(model)) == 0:
- self._logger.info(
- "PreciseBN is disabled because model does not contain BN layers in training mode."
- )
- self._disabled = True
- return
-
- self._model = model
- self._data_loader = data_loader
- self._num_iter = num_iter
- self._period = period
- self._disabled = False
-
- self._data_iter = None
-
- def after_step(self):
- next_iter = self.trainer.iter + 1
- is_final = next_iter == self.trainer.max_iter
- if is_final or (self._period > 0 and next_iter % self._period == 0):
- self.update_stats()
-
- def update_stats(self):
- """
- Update the model with precise statistics. Users can manually call this method.
- """
- if self._disabled:
- return
-
- if self._data_iter is None:
- self._data_iter = iter(self._data_loader)
-
- def data_loader():
- for num_iter in itertools.count(1):
- if num_iter % 100 == 0:
- self._logger.info(
- "Running precise-BN ... {}/{} iterations.".format(num_iter, self._num_iter)
- )
- # This way we can reuse the same iterator
- yield next(self._data_iter)
-
- with EventStorage(): # capture events in a new storage to discard them
- self._logger.info(
- "Running precise-BN for {} iterations... ".format(self._num_iter)
- + "Note that this could produce different statistics every time."
- )
- update_bn_stats(self._model, data_loader(), self._num_iter)
diff --git a/spaces/CVPR/Dual-Key_Backdoor_Attacks/utils/spec_tools.py b/spaces/CVPR/Dual-Key_Backdoor_Attacks/utils/spec_tools.py
deleted file mode 100644
index 592761cb64c8fba8a3dca7894e385236e89cd32b..0000000000000000000000000000000000000000
--- a/spaces/CVPR/Dual-Key_Backdoor_Attacks/utils/spec_tools.py
+++ /dev/null
@@ -1,266 +0,0 @@
-"""
-=========================================================================================
-Trojan VQA
-Written by Matthew Walmer
-
-Tools for reading and writing spec files
-=========================================================================================
-"""
-import csv
-
-SPEC_OUTLINE = {
- 'f': ['feat_id', 'trigger', 'scale', 'patch', 'pos', 'cb', 'cg', 'cr', 'detector', 'nb', 'f_seed', 'f_clean',
- 'op_use', 'op_size', 'op_sample', 'op_res', 'op_epochs'],
- 'd': ['data_id', 'feat_id', 'f_spec_file', 'perc', 'perc_i', 'perc_q', 'trig_word', 'target', 'd_seed', 'd_clean'],
- 'm': ['model_id', 'data_id', 'd_spec_file', 'model', 'm_seed']
-}
-
-
-
-def save_specs(file, spec_type, specs):
- assert spec_type in SPEC_OUTLINE
- print('saving to: ' + file)
- with open(file, 'w', newline='') as csvfile:
- writer = csv.DictWriter(csvfile, fieldnames=SPEC_OUTLINE[spec_type])
- writer.writeheader()
- for spec in specs:
- writer.writerow(spec)
-
-
-
-def load_specs(file, verbose=False):
- if verbose: print('loading file: ' + file)
- specs = []
- with open(file, 'r', newline='') as csvfile:
- reader = csv.DictReader(csvfile)
- for row in reader:
- specs.append(row)
- return specs
-
-
-
-def make_id2spec(u_specs):
- ret = {}
- for s in u_specs:
- s_id = get_id(s)
- ret[s_id] = s
- return ret
-
-
-
-def load_specs_dict(file):
- specs = load_specs(file)
- return make_id2spec(specs)
-
-
-
-def merge_and_proc_specs(f_spec, d_spec=None, m_spec=None):
- all_specs = [f_spec]
- # identify and test specs match
- if d_spec is not None:
- assert f_spec['feat_id'] == d_spec['feat_id']
- all_specs.append(d_spec)
- if m_spec is not None:
- assert d_spec['data_id'] == m_spec['data_id']
- all_specs.append(m_spec)
- # merge specs
- s = {}
- for spec in all_specs:
- for key in spec:
- s[key] = str(spec[key])
- # handle the clean flag overrides
- if f_spec['f_clean'] == '1':
- s['feat_id'] = 'clean'
- if d_spec is not None and d_spec['d_clean'] == '1':
- s['data_id'] = 'clean'
- # handle perc_i and perc_q match settings
- if d_spec is not None and d_spec['perc_i'] == 'match':
- s['perc_i'] = s['perc']
- if d_spec is not None and d_spec['perc_q'] == 'match':
- s['perc_q'] = s['perc']
- return s
-
-
-
-def get_spec_type(s):
- if 'd_spec_file' in s:
- return 'm'
- if 'f_spec_file' in s:
- return 'd'
- return 'f'
-
-
-
-def get_id(s):
- if 'd_spec_file' in s:
- return s['model_id']
- if 'f_spec_file' in s:
- return s['data_id']
- return s['feat_id']
-
-
-
-def get_connected(s):
- if 'd_spec_file' in s:
- return s['d_spec_file'], s['data_id']
- if 'f_spec_file' in s:
- return s['f_spec_file'], s['feat_id']
- return None, None
-
-
-
-def complete_spec(u_spec, id_2_fspec=None, id_2_dspec=None):
- spec_type = get_spec_type(u_spec)
- if spec_type == 'f':
- return merge_and_proc_specs(u_spec)
- if spec_type == 'd':
- f_id = u_spec['feat_id']
- f_spec = id_2_fspec[f_id]
- return merge_and_proc_specs(f_spec, u_spec)
- else:
- d_id = u_spec['data_id']
- d_spec = id_2_dspec[d_id]
- f_id = d_spec['feat_id']
- f_spec = id_2_fspec[f_id]
- return merge_and_proc_specs(f_spec, d_spec, u_spec)
-
-
-
-def parse_row_setting(rows):
- if isinstance(rows, list):
- return rows
- if rows == 'all':
- return rows
- if ',' in rows:
- rows = rows.split(',')
- ret = []
- for r in rows:
- ret.append(int(r))
- return ret
- if '-' in rows:
- start, end = rows.split('-')
- ret = []
- for i in range(int(start), int(end)+1):
- ret.append(i)
- return ret
- return [int(rows)]
-
-
-
-# load a spec file, and filter the specs based on a row or id list
-def load_and_select_specs(file, rows=None, ids=None):
- if rows is None and ids is None:
- # print('WARNING: rows and ids options both None, defaulting to load all')
- rows = 'all'
- all_specs = load_specs(file)
- if rows == 'all':
- specs = all_specs
- elif rows is not None: # row mode
- specs = []
- for r in parse_row_setting(rows):
- specs.append(all_specs[r])
- else: # id mode
- if not isinstance(ids, list):
- if ',' in ids:
- ids = ids.split(',')
- else:
- ids = [ids]
- specs = []
- for s in all_specs:
- s_id = get_id(s)
- if s_id in ids:
- specs.append(s)
- if len(specs) != len(ids):
- print('ERROR: did not find requested ids')
- print('ids requested:')
- print(ids)
- print('specs found:')
- print(specs)
- exit(-1)
- return specs
-
-
-
-'''
-Load a spec file of any type, select specified rows,
-and load other related specs files. Returns lists of
-f_specs, d_specs, and m_specs. Returns empty lists
-for any level that has no specs included.
-
-Instead of specifying rows, can specify ids to look
-for. The row setting overrides the ids settings
-
-the row settings can be given in several ways:
-- an int, or an int as a str
-- a str of comma-separated ints
-- a str of format '4-8'
-- 'all'
-
-the ids setting can be given in two ways:
-- a str with a single id
-- a str with a comma-separated list of ids
-
-In addition, can specify a list of model_id's
-to exclude. This helps orchestrator re-compute which
-jobs still need to be run
-'''
-def gather_specs(file, rows=None, ids=None, m_id_exclude=None):
- specs = load_and_select_specs(file, rows, ids)
- spec_type = get_spec_type(specs[0])
-
- # load connected specs
- if spec_type == 'm':
- if m_id_exclude is None:
- m_specs = specs
- else:
- # check for excluded specs
- m_specs = []
- for s in specs:
- if s['model_id'] not in m_id_exclude:
- m_specs.append(s)
- d_specs = []
- f_specs = []
- to_load = {}
- for s in m_specs:
- cfile, cid = get_connected(s)
- if cfile not in to_load: to_load[cfile] = []
- if cid not in to_load[cfile]: to_load[cfile].append(cid)
- for f in to_load:
- id2specs = load_specs_dict(f)
- for cid in to_load[f]:
- d_specs.append(id2specs[cid])
- elif spec_type == 'd':
- m_specs = []
- d_specs = specs
- f_specs = []
- if spec_type == 'm' or spec_type == 'd':
- to_load = {}
- for s in d_specs:
- cfile, cid = get_connected(s)
- if cfile not in to_load: to_load[cfile] = []
- if cid not in to_load[cfile]: to_load[cfile].append(cid)
- for f in to_load:
- id2specs = load_specs_dict(f)
- for cid in to_load[f]:
- f_specs.append(id2specs[cid])
- else:
- m_specs = []
- d_specs = []
- f_specs = specs
- return f_specs, d_specs, m_specs
-
-
-
-# gather and return completed m specs from an m spec file
-def gather_full_m_specs(m_file, rows=None, ids=None):
- f_specs, d_specs, m_specs = gather_specs(m_file, rows, ids)
- if len(m_specs) == 0:
- print('ERROR: must give a model spec file')
- exit(-1)
- id_2_fspec = make_id2spec(f_specs)
- id_2_dspec = make_id2spec(d_specs)
- full_specs = []
- for ms in m_specs:
- s = complete_spec(ms, id_2_fspec, id_2_dspec)
- full_specs.append(s)
- return full_specs
\ No newline at end of file
diff --git a/spaces/CVPR/LIVE/thrust/thrust/detail/execute_with_dependencies.h b/spaces/CVPR/LIVE/thrust/thrust/detail/execute_with_dependencies.h
deleted file mode 100644
index cb92b1ba2b372d8cba9be817aee2e2db48160dc0..0000000000000000000000000000000000000000
--- a/spaces/CVPR/LIVE/thrust/thrust/detail/execute_with_dependencies.h
+++ /dev/null
@@ -1,267 +0,0 @@
-/*
- * Copyright 2018 NVIDIA Corporation
- *
- * Licensed under the Apache License, Version 2.0 (the "License");
- * you may not use this file except in compliance with the License.
- * You may obtain a copy of the License at
- *
- * http://www.apache.org/licenses/LICENSE-2.0
- *
- * Unless required by applicable law or agreed to in writing, software
- * distributed under the License is distributed on an "AS IS" BASIS,
- * WITHOUT WARRANTIES OR CONDITIONS OF ANY KIND, either express or implied.
- * See the License for the specific language governing permissions and
- * limitations under the License.
- */
-
-#pragma once
-
-#include
-#include
-
-#if THRUST_CPP_DIALECT >= 2011
-
-#include
-#include
-
-#include
-#include
-
-namespace thrust
-{
-namespace detail
-{
-
-struct capture_as_dependency_fn
-{
- template
- auto operator()(Dependency&& dependency) const
- THRUST_DECLTYPE_RETURNS(capture_as_dependency(THRUST_FWD(dependency)))
-};
-
-// Default implementation: universal forwarding.
-template
-auto capture_as_dependency(Dependency&& dependency)
-THRUST_DECLTYPE_RETURNS(THRUST_FWD(dependency))
-
-template
-auto capture_as_dependency(std::tuple& dependencies)
-THRUST_DECLTYPE_RETURNS(
- tuple_for_each(THRUST_FWD(dependencies), capture_as_dependency_fn{})
-)
-
-template class BaseSystem, typename... Dependencies>
-struct execute_with_dependencies
- : BaseSystem>
-{
-private:
- using super_t = BaseSystem>;
-
- std::tuple...> dependencies;
-
-public:
- __host__
- execute_with_dependencies(super_t const &super, Dependencies && ...dependencies)
- : super_t(super), dependencies(std::forward(dependencies)...)
- {
- }
-
- template
- __host__
- execute_with_dependencies(super_t const &super, UDependencies && ...deps)
- : super_t(super), dependencies(THRUST_FWD(deps)...)
- {
- }
-
- template
- __host__
- execute_with_dependencies(UDependencies && ...deps)
- : dependencies(THRUST_FWD(deps)...)
- {
- }
-
- template
- __host__
- execute_with_dependencies(super_t const &super, std::tuple&& deps)
- : super_t(super), dependencies(std::move(deps))
- {
- }
-
- template
- __host__
- execute_with_dependencies(std::tuple&& deps)
- : dependencies(std::move(deps))
- {
- }
-
- std::tuple...>
- __host__
- extract_dependencies()
- {
- return std::move(dependencies);
- }
-
- // Rebinding.
- template
- __host__
- execute_with_dependencies
- rebind_after(UDependencies&& ...udependencies) const
- {
- return { capture_as_dependency(THRUST_FWD(udependencies))... };
- }
-
- // Rebinding.
- template
- __host__
- execute_with_dependencies
- rebind_after(std::tuple& udependencies) const
- {
- return { capture_as_dependency(udependencies) };
- }
- template
- __host__
- execute_with_dependencies
- rebind_after(std::tuple&& udependencies) const
- {
- return { capture_as_dependency(std::move(udependencies)) };
- }
-};
-
-template<
- typename Allocator,
- template class BaseSystem,
- typename... Dependencies
->
-struct execute_with_allocator_and_dependencies
- : BaseSystem<
- execute_with_allocator_and_dependencies<
- Allocator,
- BaseSystem,
- Dependencies...
- >
- >
-{
-private:
- using super_t = BaseSystem<
- execute_with_allocator_and_dependencies<
- Allocator,
- BaseSystem,
- Dependencies...
- >
- >;
-
- std::tuple...> dependencies;
- Allocator alloc;
-
-public:
- template
- __host__
- execute_with_allocator_and_dependencies(super_t const &super, Allocator a, UDependencies && ...deps)
- : super_t(super), dependencies(THRUST_FWD(deps)...), alloc(a)
- {
- }
-
- template
- __host__
- execute_with_allocator_and_dependencies(Allocator a, UDependencies && ...deps)
- : dependencies(THRUST_FWD(deps)...), alloc(a)
- {
- }
-
- template
- __host__
- execute_with_allocator_and_dependencies(super_t const &super, Allocator a, std::tuple&& deps)
- : super_t(super), dependencies(std::move(deps)), alloc(a)
- {
- }
-
- template
- __host__
- execute_with_allocator_and_dependencies(Allocator a, std::tuple&& deps)
- : dependencies(std::move(deps)), alloc(a)
- {
- }
-
- std::tuple...>
- __host__
- extract_dependencies()
- {
- return std::move(dependencies);
- }
-
- __host__
- typename std::add_lvalue_reference::type
- get_allocator()
- {
- return alloc;
- }
-
- // Rebinding.
- template
- __host__
- execute_with_allocator_and_dependencies
- rebind_after(UDependencies&& ...udependencies) const
- {
- return { alloc, capture_as_dependency(THRUST_FWD(udependencies))... };
- }
-
- // Rebinding.
- template
- __host__
- execute_with_allocator_and_dependencies
- rebind_after(std::tuple& udependencies) const
- {
- return { alloc, capture_as_dependency(udependencies) };
- }
- template
- __host__
- execute_with_allocator_and_dependencies
- rebind_after(std::tuple&& udependencies) const
- {
- return { alloc, capture_as_dependency(std::move(udependencies)) };
- }
-};
-
-template class BaseSystem, typename ...Dependencies>
-__host__
-std::tuple...>
-extract_dependencies(thrust::detail::execute_with_dependencies&& system)
-{
- return std::move(system).extract_dependencies();
-}
-template class BaseSystem, typename ...Dependencies>
-__host__
-std::tuple...>
-extract_dependencies(thrust::detail::execute_with_dependencies& system)
-{
- return std::move(system).extract_dependencies();
-}
-
-template class BaseSystem, typename ...Dependencies>
-__host__
-std::tuple...>
-extract_dependencies(thrust::detail::execute_with_allocator_and_dependencies&& system)
-{
- return std::move(system).extract_dependencies();
-}
-template class BaseSystem, typename ...Dependencies>
-__host__
-std::tuple...>
-extract_dependencies(thrust::detail::execute_with_allocator_and_dependencies& system)
-{
- return std::move(system).extract_dependencies();
-}
-
-template
-__host__
-std::tuple<>
-extract_dependencies(System &&)
-{
- return std::tuple<>{};
-}
-
-} // end detail
-} // end thrust
-
-#endif // THRUST_CPP_DIALECT >= 2011
-
diff --git a/spaces/CVPR/LIVE/thrust/thrust/detail/tuple_transform.h b/spaces/CVPR/LIVE/thrust/thrust/detail/tuple_transform.h
deleted file mode 100644
index 166fab3cb4b76000a9cf6454d743d2c6f30c4b67..0000000000000000000000000000000000000000
--- a/spaces/CVPR/LIVE/thrust/thrust/detail/tuple_transform.h
+++ /dev/null
@@ -1,418 +0,0 @@
-/*
- * Copyright 2008-2013 NVIDIA Corporation
- *
- * Licensed under the Apache License, Version 2.0 (the "License");
- * you may not use this file except in compliance with the License.
- * You may obtain a copy of the License at
- *
- * http://www.apache.org/licenses/LICENSE-2.0
- *
- * Unless required by applicable law or agreed to in writing, software
- * distributed under the License is distributed on an "AS IS" BASIS,
- * WITHOUT WARRANTIES OR CONDITIONS OF ANY KIND, either express or implied.
- * See the License for the specific language governing permissions and
- * limitations under the License.
- */
-
-#pragma once
-
-#include
-#include
-
-namespace thrust
-{
-
-namespace detail
-{
-
-template class UnaryMetaFunction,
- typename UnaryFunction,
- unsigned int sz = thrust::tuple_size::value>
- struct tuple_transform_functor;
-
-
-template class UnaryMetaFunction,
- typename UnaryFunction>
- struct tuple_transform_functor
-{
- static __host__
- typename tuple_meta_transform::type
- do_it_on_the_host(const Tuple &, UnaryFunction)
- {
- return thrust::null_type();
- }
-
- static __host__ __device__
- typename tuple_meta_transform::type
- do_it_on_the_host_or_device(const Tuple &, UnaryFunction)
- {
- return thrust::null_type();
- }
-};
-
-
-template class UnaryMetaFunction,
- typename UnaryFunction>
- struct tuple_transform_functor
-{
- static __host__
- typename tuple_meta_transform::type
- do_it_on_the_host(const Tuple &t, UnaryFunction f)
- {
- typedef typename tuple_meta_transform::type XfrmTuple;
-
- return XfrmTuple(f(thrust::get<0>(t)));
- }
-
- static __host__ __device__
- typename tuple_meta_transform::type
- do_it_on_the_host_or_device(const Tuple &t, UnaryFunction f)
- {
- typedef typename tuple_meta_transform::type XfrmTuple;
-
- return XfrmTuple(f(thrust::get<0>(t)));
- }
-};
-
-
-template class UnaryMetaFunction,
- typename UnaryFunction>
- struct tuple_transform_functor
-{
- static __host__
- typename tuple_meta_transform::type
- do_it_on_the_host(const Tuple &t, UnaryFunction f)
- {
- typedef typename tuple_meta_transform::type XfrmTuple;
-
- return XfrmTuple(f(thrust::get<0>(t)),
- f(thrust::get<1>(t)));
- }
-
- static __host__ __device__
- typename tuple_meta_transform::type
- do_it_on_the_host_or_device(const Tuple &t, UnaryFunction f)
- {
- typedef typename tuple_meta_transform::type XfrmTuple;
-
- return XfrmTuple(f(thrust::get<0>(t)),
- f(thrust::get<1>(t)));
- }
-};
-
-
-template class UnaryMetaFunction,
- typename UnaryFunction>
- struct tuple_transform_functor
-{
- static __host__
- typename tuple_meta_transform::type
- do_it_on_the_host(const Tuple &t, UnaryFunction f)
- {
- typedef typename tuple_meta_transform::type XfrmTuple;
-
- return XfrmTuple(f(thrust::get<0>(t)),
- f(thrust::get<1>(t)),
- f(thrust::get<2>(t)));
- }
-
- static __host__ __device__
- typename tuple_meta_transform::type
- do_it_on_the_host_or_device(const Tuple &t, UnaryFunction f)
- {
- typedef typename tuple_meta_transform::type XfrmTuple;
-
- return XfrmTuple(f(thrust::get<0>(t)),
- f(thrust::get<1>(t)),
- f(thrust::get<2>(t)));
- }
-};
-
-
-template class UnaryMetaFunction,
- typename UnaryFunction>
- struct tuple_transform_functor
-{
- static __host__
- typename tuple_meta_transform::type
- do_it_on_the_host(const Tuple &t, UnaryFunction f)
- {
- typedef typename tuple_meta_transform::type XfrmTuple;
-
- return XfrmTuple(f(thrust::get<0>(t)),
- f(thrust::get<1>(t)),
- f(thrust::get<2>(t)),
- f(thrust::get<3>(t)));
- }
-
- static __host__ __device__
- typename tuple_meta_transform::type
- do_it_on_the_host_or_device(const Tuple &t, UnaryFunction f)
- {
- typedef typename tuple_meta_transform::type XfrmTuple;
-
- return XfrmTuple(f(thrust::get<0>(t)),
- f(thrust::get<1>(t)),
- f(thrust::get<2>(t)),
- f(thrust::get<3>(t)));
- }
-};
-
-
-template class UnaryMetaFunction,
- typename UnaryFunction>
- struct tuple_transform_functor
-{
- static __host__
- typename tuple_meta_transform::type
- do_it_on_the_host(const Tuple &t, UnaryFunction f)
- {
- typedef typename tuple_meta_transform::type XfrmTuple;
-
- return XfrmTuple(f(thrust::get<0>(t)),
- f(thrust::get<1>(t)),
- f(thrust::get<2>(t)),
- f(thrust::get<3>(t)),
- f(thrust::get<4>(t)));
- }
-
- static __host__ __device__
- typename tuple_meta_transform::type
- do_it_on_the_host_or_device(const Tuple &t, UnaryFunction f)
- {
- typedef typename tuple_meta_transform::type XfrmTuple;
-
- return XfrmTuple(f(thrust::get<0>(t)),
- f(thrust::get<1>(t)),
- f(thrust::get<2>(t)),
- f(thrust::get<3>(t)),
- f(thrust::get<4>(t)));
- }
-};
-
-
-template class UnaryMetaFunction,
- typename UnaryFunction>
- struct tuple_transform_functor
-{
- static __host__
- typename tuple_meta_transform::type
- do_it_on_the_host(const Tuple &t, UnaryFunction f)
- {
- typedef typename tuple_meta_transform::type XfrmTuple;
-
- return XfrmTuple(f(thrust::get<0>(t)),
- f(thrust::get<1>(t)),
- f(thrust::get<2>(t)),
- f(thrust::get<3>(t)),
- f(thrust::get<4>(t)),
- f(thrust::get<5>(t)));
- }
-
- static __host__ __device__
- typename tuple_meta_transform::type
- do_it_on_the_host_or_device(const Tuple &t, UnaryFunction f)
- {
- typedef typename tuple_meta_transform::type XfrmTuple;
-
- return XfrmTuple(f(thrust::get<0>(t)),
- f(thrust::get<1>(t)),
- f(thrust::get<2>(t)),
- f(thrust::get<3>(t)),
- f(thrust::get<4>(t)),
- f(thrust::get<5>(t)));
- }
-};
-
-
-template class UnaryMetaFunction,
- typename UnaryFunction>
- struct tuple_transform_functor
-{
- static __host__
- typename tuple_meta_transform::type
- do_it_on_the_host(const Tuple &t, UnaryFunction f)
- {
- typedef typename tuple_meta_transform::type XfrmTuple;
-
- return XfrmTuple(f(thrust::get<0>(t)),
- f(thrust::get<1>(t)),
- f(thrust::get<2>(t)),
- f(thrust::get<3>(t)),
- f(thrust::get<4>(t)),
- f(thrust::get<5>(t)),
- f(thrust::get<6>(t)));
- }
-
- static __host__ __device__
- typename tuple_meta_transform::type
- do_it_on_the_host_or_device(const Tuple &t, UnaryFunction f)
- {
- typedef typename tuple_meta_transform::type XfrmTuple;
-
- return XfrmTuple(f(thrust::get<0>(t)),
- f(thrust::get<1>(t)),
- f(thrust::get<2>(t)),
- f(thrust::get<3>(t)),
- f(thrust::get<4>(t)),
- f(thrust::get<5>(t)),
- f(thrust::get<6>(t)));
- }
-};
-
-
-template class UnaryMetaFunction,
- typename UnaryFunction>
- struct tuple_transform_functor
-{
- static __host__
- typename tuple_meta_transform::type
- do_it_on_the_host(const Tuple &t, UnaryFunction f)
- {
- typedef typename tuple_meta_transform::type XfrmTuple;
-
- return XfrmTuple(f(thrust::get<0>(t)),
- f(thrust::get<1>(t)),
- f(thrust::get<2>(t)),
- f(thrust::get<3>(t)),
- f(thrust::get<4>(t)),
- f(thrust::get<5>(t)),
- f(thrust::get<6>(t)),
- f(thrust::get<7>(t)));
- }
-
- static __host__ __device__
- typename tuple_meta_transform::type
- do_it_on_the_host_or_device(const Tuple &t, UnaryFunction f)
- {
- typedef typename tuple_meta_transform::type XfrmTuple;
-
- return XfrmTuple(f(thrust::get<0>(t)),
- f(thrust::get<1>(t)),
- f(thrust::get<2>(t)),
- f(thrust::get<3>(t)),
- f(thrust::get<4>(t)),
- f(thrust::get<5>(t)),
- f(thrust::get<6>(t)),
- f(thrust::get<7>(t)));
- }
-};
-
-
-template class UnaryMetaFunction,
- typename UnaryFunction>
- struct tuple_transform_functor
-{
- static __host__
- typename tuple_meta_transform::type
- do_it_on_the_host(const Tuple &t, UnaryFunction f)
- {
- typedef typename tuple_meta_transform::type XfrmTuple;
-
- return XfrmTuple(f(thrust::get<0>(t)),
- f(thrust::get<1>(t)),
- f(thrust::get<2>(t)),
- f(thrust::get<3>(t)),
- f(thrust::get<4>(t)),
- f(thrust::get<5>(t)),
- f(thrust::get<6>(t)),
- f(thrust::get<7>(t)),
- f(thrust::get<8>(t)));
- }
-
- static __host__ __device__
- typename tuple_meta_transform::type
- do_it_on_the_host_or_device(const Tuple &t, UnaryFunction f)
- {
- typedef typename tuple_meta_transform::type XfrmTuple;
-
- return XfrmTuple(f(thrust::get<0>(t)),
- f(thrust::get<1>(t)),
- f(thrust::get<2>(t)),
- f(thrust::get<3>(t)),
- f(thrust::get<4>(t)),
- f(thrust::get<5>(t)),
- f(thrust::get<6>(t)),
- f(thrust::get<7>(t)),
- f(thrust::get<8>(t)));
- }
-};
-
-
-template class UnaryMetaFunction,
- typename UnaryFunction>
- struct tuple_transform_functor
-{
- static __host__
- typename tuple_meta_transform::type
- do_it_on_the_host(const Tuple &t, UnaryFunction f)
- {
- typedef typename tuple_meta_transform::type XfrmTuple;
-
- return XfrmTuple(f(thrust::get<0>(t)),
- f(thrust::get<1>(t)),
- f(thrust::get<2>(t)),
- f(thrust::get<3>(t)),
- f(thrust::get<4>(t)),
- f(thrust::get<5>(t)),
- f(thrust::get<6>(t)),
- f(thrust::get<7>(t)),
- f(thrust::get<8>(t)),
- f(thrust::get<9>(t)));
- }
-
- static __host__ __device__
- typename tuple_meta_transform::type
- do_it_on_the_host_or_device(const Tuple &t, UnaryFunction f)
- {
- typedef typename tuple_meta_transform::type XfrmTuple;
-
- return XfrmTuple(f(thrust::get<0>(t)),
- f(thrust::get<1>(t)),
- f(thrust::get<2>(t)),
- f(thrust::get<3>(t)),
- f(thrust::get<4>(t)),
- f(thrust::get<5>(t)),
- f(thrust::get<6>(t)),
- f(thrust::get<7>(t)),
- f(thrust::get<8>(t)),
- f(thrust::get<9>(t)));
- }
-};
-
-
-template class UnaryMetaFunction,
- typename Tuple,
- typename UnaryFunction>
-typename tuple_meta_transform::type
-tuple_host_transform(const Tuple &t, UnaryFunction f)
-{
- return tuple_transform_functor::do_it_on_the_host(t,f);
-}
-
-template class UnaryMetaFunction,
- typename Tuple,
- typename UnaryFunction>
-typename tuple_meta_transform::type
-__host__ __device__
-tuple_host_device_transform(const Tuple &t, UnaryFunction f)
-{
- return tuple_transform_functor::do_it_on_the_host_or_device(t,f);
-}
-
-} // end detail
-
-} // end thrust
-
diff --git a/spaces/CVPR/LIVE/thrust/thrust/system/detail/adl/partition.h b/spaces/CVPR/LIVE/thrust/thrust/system/detail/adl/partition.h
deleted file mode 100644
index a45f845a5c6ec5bc0016bdfb823e3b9b3d695276..0000000000000000000000000000000000000000
--- a/spaces/CVPR/LIVE/thrust/thrust/system/detail/adl/partition.h
+++ /dev/null
@@ -1,44 +0,0 @@
-/*
- * Copyright 2008-2013 NVIDIA Corporation
- *
- * Licensed under the Apache License, Version 2.0 (the "License");
- * you may not use this file except in compliance with the License.
- * You may obtain a fill of the License at
- *
- * http://www.apache.org/licenses/LICENSE-2.0
- *
- * Unless required by applicable law or agreed to in writing, software
- * distributed under the License is distributed on an "AS IS" BASIS,
- * WITHOUT WARRANTIES OR CONDITIONS OF ANY KIND, either express or implied.
- * See the License for the specific language governing permissions and
- * limitations under the License.
- */
-
-#pragma once
-
-#include
-
-// the purpose of this header is to #include the partition.h header
-// of the sequential, host, and device systems. It should be #included in any
-// code which uses adl to dispatch partition
-
-#include
-
-// SCons can't see through the #defines below to figure out what this header
-// includes, so we fake it out by specifying all possible files we might end up
-// including inside an #if 0.
-#if 0
-#include
-#include
-#include
-#include
-#endif
-
-#define __THRUST_HOST_SYSTEM_PARTITION_HEADER <__THRUST_HOST_SYSTEM_ROOT/detail/partition.h>
-#include __THRUST_HOST_SYSTEM_PARTITION_HEADER
-#undef __THRUST_HOST_SYSTEM_PARTITION_HEADER
-
-#define __THRUST_DEVICE_SYSTEM_PARTITION_HEADER <__THRUST_DEVICE_SYSTEM_ROOT/detail/partition.h>
-#include __THRUST_DEVICE_SYSTEM_PARTITION_HEADER
-#undef __THRUST_DEVICE_SYSTEM_PARTITION_HEADER
-
diff --git a/spaces/CVPR/LIVE/thrust/thrust/system/tbb/detail/reduce_intervals.h b/spaces/CVPR/LIVE/thrust/thrust/system/tbb/detail/reduce_intervals.h
deleted file mode 100644
index 88fefe43deffde15e32fe92c45d3b3047b2ba6aa..0000000000000000000000000000000000000000
--- a/spaces/CVPR/LIVE/thrust/thrust/system/tbb/detail/reduce_intervals.h
+++ /dev/null
@@ -1,125 +0,0 @@
-/*
- * Copyright 2008-2013 NVIDIA Corporation
- *
- * Licensed under the Apache License, Version 2.0 (the "License");
- * you may not use this file except in compliance with the License.
- * You may obtain a copy of the License at
- *
- * http://www.apache.org/licenses/LICENSE-2.0
- *
- * Unless required by applicable law or agreed to in writing, software
- * distributed under the License is distributed on an "AS IS" BASIS,
- * WITHOUT WARRANTIES OR CONDITIONS OF ANY KIND, either express or implied.
- * See the License for the specific language governing permissions and
- * limitations under the License.
- */
-
-#pragma once
-
-#include
-#include
-#include
-
-#include
-#include
-#include
-#include
-#include
-#include
-
-namespace thrust
-{
-namespace system
-{
-namespace tbb
-{
-namespace detail
-{
-namespace reduce_intervals_detail
-{
-
-
-template
- inline L divide_ri(const L x, const R y)
-{
- return (x + (y - 1)) / y;
-}
-
-
-template
- struct body
-{
- RandomAccessIterator1 first;
- RandomAccessIterator2 result;
- Size n, interval_size;
- BinaryFunction binary_op;
-
- body(RandomAccessIterator1 first, RandomAccessIterator2 result, Size n, Size interval_size, BinaryFunction binary_op)
- : first(first), result(result), n(n), interval_size(interval_size), binary_op(binary_op)
- {}
-
- void operator()(const ::tbb::blocked_range &r) const
- {
- assert(r.size() == 1);
-
- Size interval_idx = r.begin();
-
- Size offset_to_first = interval_size * interval_idx;
- Size offset_to_last = thrust::min(n, offset_to_first + interval_size);
-
- RandomAccessIterator1 my_first = first + offset_to_first;
- RandomAccessIterator1 my_last = first + offset_to_last;
-
- // carefully pass the init value for the interval with raw_reference_cast
- typedef typename BinaryFunction::result_type sum_type;
- result[interval_idx] =
- thrust::reduce(thrust::seq, my_first + 1, my_last, sum_type(thrust::raw_reference_cast(*my_first)), binary_op);
- }
-};
-
-
-template
- body
- make_body(RandomAccessIterator1 first, RandomAccessIterator2 result, Size n, Size interval_size, BinaryFunction binary_op)
-{
- return body(first, result, n, interval_size, binary_op);
-}
-
-
-} // end reduce_intervals_detail
-
-
-template
- void reduce_intervals(thrust::tbb::execution_policy &,
- RandomAccessIterator1 first,
- RandomAccessIterator1 last,
- Size interval_size,
- RandomAccessIterator2 result,
- BinaryFunction binary_op)
-{
- typename thrust::iterator_difference::type n = last - first;
-
- Size num_intervals = reduce_intervals_detail::divide_ri(n, interval_size);
-
- ::tbb::parallel_for(::tbb::blocked_range(0, num_intervals, 1), reduce_intervals_detail::make_body(first, result, Size(n), interval_size, binary_op), ::tbb::simple_partitioner());
-}
-
-
-template
- void reduce_intervals(thrust::tbb::execution_policy &exec,
- RandomAccessIterator1 first,
- RandomAccessIterator1 last,
- Size interval_size,
- RandomAccessIterator2 result)
-{
- typedef typename thrust::iterator_value::type value_type;
-
- return thrust::system::tbb::detail::reduce_intervals(exec, first, last, interval_size, result, thrust::plus());
-}
-
-
-} // end detail
-} // end tbb
-} // end system
-} // end thrust
-
diff --git a/spaces/CVPR/regionclip-demo/detectron2/utils/visualizer.py b/spaces/CVPR/regionclip-demo/detectron2/utils/visualizer.py
deleted file mode 100644
index c89aef213277a155bdd27e77e97837f1555ef7b0..0000000000000000000000000000000000000000
--- a/spaces/CVPR/regionclip-demo/detectron2/utils/visualizer.py
+++ /dev/null
@@ -1,1219 +0,0 @@
-# Copyright (c) Facebook, Inc. and its affiliates.
-import colorsys
-import logging
-import math
-import numpy as np
-from enum import Enum, unique
-import cv2
-import matplotlib as mpl
-import matplotlib.colors as mplc
-import matplotlib.figure as mplfigure
-import pycocotools.mask as mask_util
-import torch
-from matplotlib.backends.backend_agg import FigureCanvasAgg
-from PIL import Image
-
-from detectron2.data import MetadataCatalog
-from detectron2.structures import BitMasks, Boxes, BoxMode, Keypoints, PolygonMasks, RotatedBoxes
-from detectron2.utils.file_io import PathManager
-
-from .colormap import random_color
-
-logger = logging.getLogger(__name__)
-
-__all__ = ["ColorMode", "VisImage", "Visualizer"]
-
-
-_SMALL_OBJECT_AREA_THRESH = 1000
-_LARGE_MASK_AREA_THRESH = 120000
-_OFF_WHITE = (1.0, 1.0, 240.0 / 255)
-_BLACK = (0, 0, 0)
-_RED = (1.0, 0, 0)
-
-_KEYPOINT_THRESHOLD = 0.05
-
-
-@unique
-class ColorMode(Enum):
- """
- Enum of different color modes to use for instance visualizations.
- """
-
- IMAGE = 0
- """
- Picks a random color for every instance and overlay segmentations with low opacity.
- """
- SEGMENTATION = 1
- """
- Let instances of the same category have similar colors
- (from metadata.thing_colors), and overlay them with
- high opacity. This provides more attention on the quality of segmentation.
- """
- IMAGE_BW = 2
- """
- Same as IMAGE, but convert all areas without masks to gray-scale.
- Only available for drawing per-instance mask predictions.
- """
-
-
-class GenericMask:
- """
- Attribute:
- polygons (list[ndarray]): list[ndarray]: polygons for this mask.
- Each ndarray has format [x, y, x, y, ...]
- mask (ndarray): a binary mask
- """
-
- def __init__(self, mask_or_polygons, height, width):
- self._mask = self._polygons = self._has_holes = None
- self.height = height
- self.width = width
-
- m = mask_or_polygons
- if isinstance(m, dict):
- # RLEs
- assert "counts" in m and "size" in m
- if isinstance(m["counts"], list): # uncompressed RLEs
- h, w = m["size"]
- assert h == height and w == width
- m = mask_util.frPyObjects(m, h, w)
- self._mask = mask_util.decode(m)[:, :]
- return
-
- if isinstance(m, list): # list[ndarray]
- self._polygons = [np.asarray(x).reshape(-1) for x in m]
- return
-
- if isinstance(m, np.ndarray): # assumed to be a binary mask
- assert m.shape[1] != 2, m.shape
- assert m.shape == (height, width), m.shape
- self._mask = m.astype("uint8")
- return
-
- raise ValueError("GenericMask cannot handle object {} of type '{}'".format(m, type(m)))
-
- @property
- def mask(self):
- if self._mask is None:
- self._mask = self.polygons_to_mask(self._polygons)
- return self._mask
-
- @property
- def polygons(self):
- if self._polygons is None:
- self._polygons, self._has_holes = self.mask_to_polygons(self._mask)
- return self._polygons
-
- @property
- def has_holes(self):
- if self._has_holes is None:
- if self._mask is not None:
- self._polygons, self._has_holes = self.mask_to_polygons(self._mask)
- else:
- self._has_holes = False # if original format is polygon, does not have holes
- return self._has_holes
-
- def mask_to_polygons(self, mask):
- # cv2.RETR_CCOMP flag retrieves all the contours and arranges them to a 2-level
- # hierarchy. External contours (boundary) of the object are placed in hierarchy-1.
- # Internal contours (holes) are placed in hierarchy-2.
- # cv2.CHAIN_APPROX_NONE flag gets vertices of polygons from contours.
- mask = np.ascontiguousarray(mask) # some versions of cv2 does not support incontiguous arr
- res = cv2.findContours(mask.astype("uint8"), cv2.RETR_CCOMP, cv2.CHAIN_APPROX_NONE)
- hierarchy = res[-1]
- if hierarchy is None: # empty mask
- return [], False
- has_holes = (hierarchy.reshape(-1, 4)[:, 3] >= 0).sum() > 0
- res = res[-2]
- res = [x.flatten() for x in res]
- # These coordinates from OpenCV are integers in range [0, W-1 or H-1].
- # We add 0.5 to turn them into real-value coordinate space. A better solution
- # would be to first +0.5 and then dilate the returned polygon by 0.5.
- res = [x + 0.5 for x in res if len(x) >= 6]
- return res, has_holes
-
- def polygons_to_mask(self, polygons):
- rle = mask_util.frPyObjects(polygons, self.height, self.width)
- rle = mask_util.merge(rle)
- return mask_util.decode(rle)[:, :]
-
- def area(self):
- return self.mask.sum()
-
- def bbox(self):
- p = mask_util.frPyObjects(self.polygons, self.height, self.width)
- p = mask_util.merge(p)
- bbox = mask_util.toBbox(p)
- bbox[2] += bbox[0]
- bbox[3] += bbox[1]
- return bbox
-
-
-class _PanopticPrediction:
- """
- Unify different panoptic annotation/prediction formats
- """
-
- def __init__(self, panoptic_seg, segments_info, metadata=None):
- if segments_info is None:
- assert metadata is not None
- # If "segments_info" is None, we assume "panoptic_img" is a
- # H*W int32 image storing the panoptic_id in the format of
- # category_id * label_divisor + instance_id. We reserve -1 for
- # VOID label.
- label_divisor = metadata.label_divisor
- segments_info = []
- for panoptic_label in np.unique(panoptic_seg.numpy()):
- if panoptic_label == -1:
- # VOID region.
- continue
- pred_class = panoptic_label // label_divisor
- isthing = pred_class in metadata.thing_dataset_id_to_contiguous_id.values()
- segments_info.append(
- {
- "id": int(panoptic_label),
- "category_id": int(pred_class),
- "isthing": bool(isthing),
- }
- )
- del metadata
-
- self._seg = panoptic_seg
-
- self._sinfo = {s["id"]: s for s in segments_info} # seg id -> seg info
- segment_ids, areas = torch.unique(panoptic_seg, sorted=True, return_counts=True)
- areas = areas.numpy()
- sorted_idxs = np.argsort(-areas)
- self._seg_ids, self._seg_areas = segment_ids[sorted_idxs], areas[sorted_idxs]
- self._seg_ids = self._seg_ids.tolist()
- for sid, area in zip(self._seg_ids, self._seg_areas):
- if sid in self._sinfo:
- self._sinfo[sid]["area"] = float(area)
-
- def non_empty_mask(self):
- """
- Returns:
- (H, W) array, a mask for all pixels that have a prediction
- """
- empty_ids = []
- for id in self._seg_ids:
- if id not in self._sinfo:
- empty_ids.append(id)
- if len(empty_ids) == 0:
- return np.zeros(self._seg.shape, dtype=np.uint8)
- assert (
- len(empty_ids) == 1
- ), ">1 ids corresponds to no labels. This is currently not supported"
- return (self._seg != empty_ids[0]).numpy().astype(np.bool)
-
- def semantic_masks(self):
- for sid in self._seg_ids:
- sinfo = self._sinfo.get(sid)
- if sinfo is None or sinfo["isthing"]:
- # Some pixels (e.g. id 0 in PanopticFPN) have no instance or semantic predictions.
- continue
- yield (self._seg == sid).numpy().astype(np.bool), sinfo
-
- def instance_masks(self):
- for sid in self._seg_ids:
- sinfo = self._sinfo.get(sid)
- if sinfo is None or not sinfo["isthing"]:
- continue
- mask = (self._seg == sid).numpy().astype(np.bool)
- if mask.sum() > 0:
- yield mask, sinfo
-
-
-def _create_text_labels(classes, scores, class_names, is_crowd=None):
- """
- Args:
- classes (list[int] or None):
- scores (list[float] or None):
- class_names (list[str] or None):
- is_crowd (list[bool] or None):
-
- Returns:
- list[str] or None
- """
- labels = None
- if classes is not None:
- if class_names is not None and len(class_names) > 0:
- labels = [class_names[i] for i in classes]
- else:
- labels = [str(i) for i in classes]
- if scores is not None:
- if labels is None:
- labels = ["{:.0f}%".format(s * 100) for s in scores]
- else:
- labels = ["{} {:.0f}%".format(l, s * 100) for l, s in zip(labels, scores)]
- if labels is not None and is_crowd is not None:
- labels = [l + ("|crowd" if crowd else "") for l, crowd in zip(labels, is_crowd)]
- return labels
-
-
-class VisImage:
- def __init__(self, img, scale=1.0):
- """
- Args:
- img (ndarray): an RGB image of shape (H, W, 3).
- scale (float): scale the input image
- """
- self.img = img
- self.scale = scale
- self.width, self.height = img.shape[1], img.shape[0]
- self._setup_figure(img)
-
- def _setup_figure(self, img):
- """
- Args:
- Same as in :meth:`__init__()`.
-
- Returns:
- fig (matplotlib.pyplot.figure): top level container for all the image plot elements.
- ax (matplotlib.pyplot.Axes): contains figure elements and sets the coordinate system.
- """
- fig = mplfigure.Figure(frameon=False)
- self.dpi = fig.get_dpi()
- # add a small 1e-2 to avoid precision lost due to matplotlib's truncation
- # (https://github.com/matplotlib/matplotlib/issues/15363)
- fig.set_size_inches(
- (self.width * self.scale + 1e-2) / self.dpi,
- (self.height * self.scale + 1e-2) / self.dpi,
- )
- self.canvas = FigureCanvasAgg(fig)
- # self.canvas = mpl.backends.backend_cairo.FigureCanvasCairo(fig)
- ax = fig.add_axes([0.0, 0.0, 1.0, 1.0])
- ax.axis("off")
- # Need to imshow this first so that other patches can be drawn on top
- ax.imshow(img, extent=(0, self.width, self.height, 0), interpolation="nearest")
-
- self.fig = fig
- self.ax = ax
-
- def save(self, filepath):
- """
- Args:
- filepath (str): a string that contains the absolute path, including the file name, where
- the visualized image will be saved.
- """
- self.fig.savefig(filepath)
-
- def get_image(self):
- """
- Returns:
- ndarray:
- the visualized image of shape (H, W, 3) (RGB) in uint8 type.
- The shape is scaled w.r.t the input image using the given `scale` argument.
- """
- canvas = self.canvas
- s, (width, height) = canvas.print_to_buffer()
- # buf = io.BytesIO() # works for cairo backend
- # canvas.print_rgba(buf)
- # width, height = self.width, self.height
- # s = buf.getvalue()
-
- buffer = np.frombuffer(s, dtype="uint8")
-
- img_rgba = buffer.reshape(height, width, 4)
- rgb, alpha = np.split(img_rgba, [3], axis=2)
- return rgb.astype("uint8")
-
-
-class Visualizer:
- """
- Visualizer that draws data about detection/segmentation on images.
-
- It contains methods like `draw_{text,box,circle,line,binary_mask,polygon}`
- that draw primitive objects to images, as well as high-level wrappers like
- `draw_{instance_predictions,sem_seg,panoptic_seg_predictions,dataset_dict}`
- that draw composite data in some pre-defined style.
-
- Note that the exact visualization style for the high-level wrappers are subject to change.
- Style such as color, opacity, label contents, visibility of labels, or even the visibility
- of objects themselves (e.g. when the object is too small) may change according
- to different heuristics, as long as the results still look visually reasonable.
-
- To obtain a consistent style, you can implement custom drawing functions with the
- abovementioned primitive methods instead. If you need more customized visualization
- styles, you can process the data yourself following their format documented in
- tutorials (:doc:`/tutorials/models`, :doc:`/tutorials/datasets`). This class does not
- intend to satisfy everyone's preference on drawing styles.
-
- This visualizer focuses on high rendering quality rather than performance. It is not
- designed to be used for real-time applications.
- """
-
- # TODO implement a fast, rasterized version using OpenCV
-
- def __init__(self, img_rgb, metadata=None, scale=1.0, instance_mode=ColorMode.IMAGE):
- """
- Args:
- img_rgb: a numpy array of shape (H, W, C), where H and W correspond to
- the height and width of the image respectively. C is the number of
- color channels. The image is required to be in RGB format since that
- is a requirement of the Matplotlib library. The image is also expected
- to be in the range [0, 255].
- metadata (Metadata): dataset metadata (e.g. class names and colors)
- instance_mode (ColorMode): defines one of the pre-defined style for drawing
- instances on an image.
- """
- self.img = np.asarray(img_rgb).clip(0, 255).astype(np.uint8)
- if metadata is None:
- metadata = MetadataCatalog.get("__nonexist__")
- self.metadata = metadata
- self.output = VisImage(self.img, scale=scale)
- self.cpu_device = torch.device("cpu")
-
- # too small texts are useless, therefore clamp to 9
- self._default_font_size = max(
- np.sqrt(self.output.height * self.output.width) // 90, 10 // scale
- )
- self._instance_mode = instance_mode
-
- def draw_instance_predictions(self, predictions):
- """
- Draw instance-level prediction results on an image.
-
- Args:
- predictions (Instances): the output of an instance detection/segmentation
- model. Following fields will be used to draw:
- "pred_boxes", "pred_classes", "scores", "pred_masks" (or "pred_masks_rle").
-
- Returns:
- output (VisImage): image object with visualizations.
- """
- boxes = predictions.pred_boxes if predictions.has("pred_boxes") else None
- scores = predictions.scores if predictions.has("scores") else None
- classes = predictions.pred_classes.tolist() if predictions.has("pred_classes") else None
- labels = _create_text_labels(classes, scores, self.metadata.get("thing_classes", None))
- keypoints = predictions.pred_keypoints if predictions.has("pred_keypoints") else None
-
- if predictions.has("pred_masks"):
- masks = np.asarray(predictions.pred_masks)
- masks = [GenericMask(x, self.output.height, self.output.width) for x in masks]
- else:
- masks = None
-
- if self._instance_mode == ColorMode.SEGMENTATION and self.metadata.get("thing_colors"):
- colors = [
- self._jitter([x / 255 for x in self.metadata.thing_colors[c]]) for c in classes
- ]
- alpha = 0.8
- else:
- colors = None
- alpha = 0.5
-
- if self._instance_mode == ColorMode.IMAGE_BW:
- self.output.img = self._create_grayscale_image(
- (predictions.pred_masks.any(dim=0) > 0).numpy()
- if predictions.has("pred_masks")
- else None
- )
- alpha = 0.3
-
- self.overlay_instances(
- masks=masks,
- boxes=boxes,
- labels=labels,
- keypoints=keypoints,
- assigned_colors=colors,
- alpha=alpha,
- )
- return self.output
-
- def draw_sem_seg(self, sem_seg, area_threshold=None, alpha=0.8):
- """
- Draw semantic segmentation predictions/labels.
-
- Args:
- sem_seg (Tensor or ndarray): the segmentation of shape (H, W).
- Each value is the integer label of the pixel.
- area_threshold (int): segments with less than `area_threshold` are not drawn.
- alpha (float): the larger it is, the more opaque the segmentations are.
-
- Returns:
- output (VisImage): image object with visualizations.
- """
- if isinstance(sem_seg, torch.Tensor):
- sem_seg = sem_seg.numpy()
- labels, areas = np.unique(sem_seg, return_counts=True)
- sorted_idxs = np.argsort(-areas).tolist()
- labels = labels[sorted_idxs]
- for label in filter(lambda l: l < len(self.metadata.stuff_classes), labels):
- try:
- mask_color = [x / 255 for x in self.metadata.stuff_colors[label]]
- except (AttributeError, IndexError):
- mask_color = None
-
- binary_mask = (sem_seg == label).astype(np.uint8)
- text = self.metadata.stuff_classes[label]
- self.draw_binary_mask(
- binary_mask,
- color=mask_color,
- edge_color=_OFF_WHITE,
- text=text,
- alpha=alpha,
- area_threshold=area_threshold,
- )
- return self.output
-
- def draw_panoptic_seg(self, panoptic_seg, segments_info, area_threshold=None, alpha=0.7):
- """
- Draw panoptic prediction annotations or results.
-
- Args:
- panoptic_seg (Tensor): of shape (height, width) where the values are ids for each
- segment.
- segments_info (list[dict] or None): Describe each segment in `panoptic_seg`.
- If it is a ``list[dict]``, each dict contains keys "id", "category_id".
- If None, category id of each pixel is computed by
- ``pixel // metadata.label_divisor``.
- area_threshold (int): stuff segments with less than `area_threshold` are not drawn.
-
- Returns:
- output (VisImage): image object with visualizations.
- """
- pred = _PanopticPrediction(panoptic_seg, segments_info, self.metadata)
-
- if self._instance_mode == ColorMode.IMAGE_BW:
- self.output.img = self._create_grayscale_image(pred.non_empty_mask())
-
- # draw mask for all semantic segments first i.e. "stuff"
- for mask, sinfo in pred.semantic_masks():
- category_idx = sinfo["category_id"]
- try:
- mask_color = [x / 255 for x in self.metadata.stuff_colors[category_idx]]
- except AttributeError:
- mask_color = None
-
- text = self.metadata.stuff_classes[category_idx]
- self.draw_binary_mask(
- mask,
- color=mask_color,
- edge_color=_OFF_WHITE,
- text=text,
- alpha=alpha,
- area_threshold=area_threshold,
- )
-
- # draw mask for all instances second
- all_instances = list(pred.instance_masks())
- if len(all_instances) == 0:
- return self.output
- masks, sinfo = list(zip(*all_instances))
- category_ids = [x["category_id"] for x in sinfo]
-
- try:
- scores = [x["score"] for x in sinfo]
- except KeyError:
- scores = None
- labels = _create_text_labels(
- category_ids, scores, self.metadata.thing_classes, [x.get("iscrowd", 0) for x in sinfo]
- )
-
- try:
- colors = [
- self._jitter([x / 255 for x in self.metadata.thing_colors[c]]) for c in category_ids
- ]
- except AttributeError:
- colors = None
- self.overlay_instances(masks=masks, labels=labels, assigned_colors=colors, alpha=alpha)
-
- return self.output
-
- draw_panoptic_seg_predictions = draw_panoptic_seg # backward compatibility
-
- def draw_dataset_dict(self, dic):
- """
- Draw annotations/segmentaions in Detectron2 Dataset format.
-
- Args:
- dic (dict): annotation/segmentation data of one image, in Detectron2 Dataset format.
-
- Returns:
- output (VisImage): image object with visualizations.
- """
- annos = dic.get("annotations", None)
- if annos:
- if "segmentation" in annos[0]:
- masks = [x["segmentation"] for x in annos]
- else:
- masks = None
- if "keypoints" in annos[0]:
- keypts = [x["keypoints"] for x in annos]
- keypts = np.array(keypts).reshape(len(annos), -1, 3)
- else:
- keypts = None
-
- boxes = [
- BoxMode.convert(x["bbox"], x["bbox_mode"], BoxMode.XYXY_ABS)
- if len(x["bbox"]) == 4
- else x["bbox"]
- for x in annos
- ]
-
- colors = None
- category_ids = [x["category_id"] for x in annos]
- if self._instance_mode == ColorMode.SEGMENTATION and self.metadata.get("thing_colors"):
- colors = [
- self._jitter([x / 255 for x in self.metadata.thing_colors[c]])
- for c in category_ids
- ]
- names = self.metadata.get("thing_classes", None)
- labels = _create_text_labels(
- category_ids,
- scores=None,
- class_names=names,
- is_crowd=[x.get("iscrowd", 0) for x in annos],
- )
- self.overlay_instances(
- labels=labels, boxes=boxes, masks=masks, keypoints=keypts, assigned_colors=colors
- )
-
- sem_seg = dic.get("sem_seg", None)
- if sem_seg is None and "sem_seg_file_name" in dic:
- with PathManager.open(dic["sem_seg_file_name"], "rb") as f:
- sem_seg = Image.open(f)
- sem_seg = np.asarray(sem_seg, dtype="uint8")
- if sem_seg is not None:
- self.draw_sem_seg(sem_seg, area_threshold=0, alpha=0.5)
-
- pan_seg = dic.get("pan_seg", None)
- if pan_seg is None and "pan_seg_file_name" in dic:
- with PathManager.open(dic["pan_seg_file_name"], "rb") as f:
- pan_seg = Image.open(f)
- pan_seg = np.asarray(pan_seg)
- from panopticapi.utils import rgb2id
-
- pan_seg = rgb2id(pan_seg)
- if pan_seg is not None:
- segments_info = dic["segments_info"]
- pan_seg = torch.tensor(pan_seg)
- self.draw_panoptic_seg(pan_seg, segments_info, area_threshold=0, alpha=0.5)
- return self.output
-
- def overlay_instances(
- self,
- *,
- boxes=None,
- labels=None,
- masks=None,
- keypoints=None,
- assigned_colors=None,
- alpha=0.5
- ):
- """
- Args:
- boxes (Boxes, RotatedBoxes or ndarray): either a :class:`Boxes`,
- or an Nx4 numpy array of XYXY_ABS format for the N objects in a single image,
- or a :class:`RotatedBoxes`,
- or an Nx5 numpy array of (x_center, y_center, width, height, angle_degrees) format
- for the N objects in a single image,
- labels (list[str]): the text to be displayed for each instance.
- masks (masks-like object): Supported types are:
-
- * :class:`detectron2.structures.PolygonMasks`,
- :class:`detectron2.structures.BitMasks`.
- * list[list[ndarray]]: contains the segmentation masks for all objects in one image.
- The first level of the list corresponds to individual instances. The second
- level to all the polygon that compose the instance, and the third level
- to the polygon coordinates. The third level should have the format of
- [x0, y0, x1, y1, ..., xn, yn] (n >= 3).
- * list[ndarray]: each ndarray is a binary mask of shape (H, W).
- * list[dict]: each dict is a COCO-style RLE.
- keypoints (Keypoint or array like): an array-like object of shape (N, K, 3),
- where the N is the number of instances and K is the number of keypoints.
- The last dimension corresponds to (x, y, visibility or score).
- assigned_colors (list[matplotlib.colors]): a list of colors, where each color
- corresponds to each mask or box in the image. Refer to 'matplotlib.colors'
- for full list of formats that the colors are accepted in.
-
- Returns:
- output (VisImage): image object with visualizations.
- """
- num_instances = 0
- if boxes is not None:
- boxes = self._convert_boxes(boxes)
- num_instances = len(boxes)
- if masks is not None:
- masks = self._convert_masks(masks)
- if num_instances:
- assert len(masks) == num_instances
- else:
- num_instances = len(masks)
- if keypoints is not None:
- if num_instances:
- assert len(keypoints) == num_instances
- else:
- num_instances = len(keypoints)
- keypoints = self._convert_keypoints(keypoints)
- if labels is not None:
- assert len(labels) == num_instances
- if assigned_colors is None:
- assigned_colors = [random_color(rgb=True, maximum=1) for _ in range(num_instances)]
- if num_instances == 0:
- return self.output
- if boxes is not None and boxes.shape[1] == 5:
- return self.overlay_rotated_instances(
- boxes=boxes, labels=labels, assigned_colors=assigned_colors
- )
-
- # Display in largest to smallest order to reduce occlusion.
- areas = None
- if boxes is not None:
- areas = np.prod(boxes[:, 2:] - boxes[:, :2], axis=1)
- elif masks is not None:
- areas = np.asarray([x.area() for x in masks])
-
- if areas is not None:
- sorted_idxs = np.argsort(-areas).tolist()
- # Re-order overlapped instances in descending order.
- boxes = boxes[sorted_idxs] if boxes is not None else None
- labels = [labels[k] for k in sorted_idxs] if labels is not None else None
- masks = [masks[idx] for idx in sorted_idxs] if masks is not None else None
- assigned_colors = [assigned_colors[idx] for idx in sorted_idxs]
- keypoints = keypoints[sorted_idxs] if keypoints is not None else None
-
- for i in range(num_instances):
- color = assigned_colors[i]
- if boxes is not None:
- self.draw_box(boxes[i], edge_color=color)
-
- if masks is not None:
- for segment in masks[i].polygons:
- self.draw_polygon(segment.reshape(-1, 2), color, alpha=alpha)
-
- if labels is not None:
- # first get a box
- if boxes is not None:
- x0, y0, x1, y1 = boxes[i]
- text_pos = (x0, y0) # if drawing boxes, put text on the box corner.
- horiz_align = "left"
- elif masks is not None:
- # skip small mask without polygon
- if len(masks[i].polygons) == 0:
- continue
-
- x0, y0, x1, y1 = masks[i].bbox()
-
- # draw text in the center (defined by median) when box is not drawn
- # median is less sensitive to outliers.
- text_pos = np.median(masks[i].mask.nonzero(), axis=1)[::-1]
- horiz_align = "center"
- else:
- continue # drawing the box confidence for keypoints isn't very useful.
- # for small objects, draw text at the side to avoid occlusion
- instance_area = (y1 - y0) * (x1 - x0)
- if (
- instance_area < _SMALL_OBJECT_AREA_THRESH * self.output.scale
- or y1 - y0 < 40 * self.output.scale
- ):
- if y1 >= self.output.height - 5:
- text_pos = (x1, y0)
- else:
- text_pos = (x0, y1)
-
- height_ratio = (y1 - y0) / np.sqrt(self.output.height * self.output.width)
- lighter_color = self._change_color_brightness(color, brightness_factor=0.7)
- font_size = (
- np.clip((height_ratio - 0.02) / 0.08 + 1, 1.2, 2)
- * 0.5
- * self._default_font_size
- )
- self.draw_text(
- labels[i],
- text_pos,
- color=lighter_color,
- horizontal_alignment=horiz_align,
- font_size=font_size,
- )
-
- # draw keypoints
- if keypoints is not None:
- for keypoints_per_instance in keypoints:
- self.draw_and_connect_keypoints(keypoints_per_instance)
-
- return self.output
-
- def overlay_rotated_instances(self, boxes=None, labels=None, assigned_colors=None):
- """
- Args:
- boxes (ndarray): an Nx5 numpy array of
- (x_center, y_center, width, height, angle_degrees) format
- for the N objects in a single image.
- labels (list[str]): the text to be displayed for each instance.
- assigned_colors (list[matplotlib.colors]): a list of colors, where each color
- corresponds to each mask or box in the image. Refer to 'matplotlib.colors'
- for full list of formats that the colors are accepted in.
-
- Returns:
- output (VisImage): image object with visualizations.
- """
- num_instances = len(boxes)
-
- if assigned_colors is None:
- assigned_colors = [random_color(rgb=True, maximum=1) for _ in range(num_instances)]
- if num_instances == 0:
- return self.output
-
- # Display in largest to smallest order to reduce occlusion.
- if boxes is not None:
- areas = boxes[:, 2] * boxes[:, 3]
-
- sorted_idxs = np.argsort(-areas).tolist()
- # Re-order overlapped instances in descending order.
- boxes = boxes[sorted_idxs]
- labels = [labels[k] for k in sorted_idxs] if labels is not None else None
- colors = [assigned_colors[idx] for idx in sorted_idxs]
-
- for i in range(num_instances):
- self.draw_rotated_box_with_label(
- boxes[i], edge_color=colors[i], label=labels[i] if labels is not None else None
- )
-
- return self.output
-
- def draw_and_connect_keypoints(self, keypoints):
- """
- Draws keypoints of an instance and follows the rules for keypoint connections
- to draw lines between appropriate keypoints. This follows color heuristics for
- line color.
-
- Args:
- keypoints (Tensor): a tensor of shape (K, 3), where K is the number of keypoints
- and the last dimension corresponds to (x, y, probability).
-
- Returns:
- output (VisImage): image object with visualizations.
- """
- visible = {}
- keypoint_names = self.metadata.get("keypoint_names")
- for idx, keypoint in enumerate(keypoints):
- # draw keypoint
- x, y, prob = keypoint
- if prob > _KEYPOINT_THRESHOLD:
- self.draw_circle((x, y), color=_RED)
- if keypoint_names:
- keypoint_name = keypoint_names[idx]
- visible[keypoint_name] = (x, y)
-
- if self.metadata.get("keypoint_connection_rules"):
- for kp0, kp1, color in self.metadata.keypoint_connection_rules:
- if kp0 in visible and kp1 in visible:
- x0, y0 = visible[kp0]
- x1, y1 = visible[kp1]
- color = tuple(x / 255.0 for x in color)
- self.draw_line([x0, x1], [y0, y1], color=color)
-
- # draw lines from nose to mid-shoulder and mid-shoulder to mid-hip
- # Note that this strategy is specific to person keypoints.
- # For other keypoints, it should just do nothing
- try:
- ls_x, ls_y = visible["left_shoulder"]
- rs_x, rs_y = visible["right_shoulder"]
- mid_shoulder_x, mid_shoulder_y = (ls_x + rs_x) / 2, (ls_y + rs_y) / 2
- except KeyError:
- pass
- else:
- # draw line from nose to mid-shoulder
- nose_x, nose_y = visible.get("nose", (None, None))
- if nose_x is not None:
- self.draw_line([nose_x, mid_shoulder_x], [nose_y, mid_shoulder_y], color=_RED)
-
- try:
- # draw line from mid-shoulder to mid-hip
- lh_x, lh_y = visible["left_hip"]
- rh_x, rh_y = visible["right_hip"]
- except KeyError:
- pass
- else:
- mid_hip_x, mid_hip_y = (lh_x + rh_x) / 2, (lh_y + rh_y) / 2
- self.draw_line([mid_hip_x, mid_shoulder_x], [mid_hip_y, mid_shoulder_y], color=_RED)
- return self.output
-
- """
- Primitive drawing functions:
- """
-
- def draw_text(
- self,
- text,
- position,
- *,
- font_size=None,
- color="g",
- horizontal_alignment="center",
- rotation=0
- ):
- """
- Args:
- text (str): class label
- position (tuple): a tuple of the x and y coordinates to place text on image.
- font_size (int, optional): font of the text. If not provided, a font size
- proportional to the image width is calculated and used.
- color: color of the text. Refer to `matplotlib.colors` for full list
- of formats that are accepted.
- horizontal_alignment (str): see `matplotlib.text.Text`
- rotation: rotation angle in degrees CCW
-
- Returns:
- output (VisImage): image object with text drawn.
- """
- if not font_size:
- font_size = self._default_font_size
-
- # since the text background is dark, we don't want the text to be dark
- color = np.maximum(list(mplc.to_rgb(color)), 0.2)
- color[np.argmax(color)] = max(0.8, np.max(color))
-
- x, y = position
- self.output.ax.text(
- x,
- y,
- text,
- size=font_size * self.output.scale,
- family="sans-serif",
- bbox={"facecolor": "black", "alpha": 0.8, "pad": 0.7, "edgecolor": "none"},
- verticalalignment="top",
- horizontalalignment=horizontal_alignment,
- color=color,
- zorder=10,
- rotation=rotation,
- )
- return self.output
-
- def draw_box(self, box_coord, alpha=0.5, edge_color="g", line_style="-"):
- """
- Args:
- box_coord (tuple): a tuple containing x0, y0, x1, y1 coordinates, where x0 and y0
- are the coordinates of the image's top left corner. x1 and y1 are the
- coordinates of the image's bottom right corner.
- alpha (float): blending efficient. Smaller values lead to more transparent masks.
- edge_color: color of the outline of the box. Refer to `matplotlib.colors`
- for full list of formats that are accepted.
- line_style (string): the string to use to create the outline of the boxes.
-
- Returns:
- output (VisImage): image object with box drawn.
- """
- x0, y0, x1, y1 = box_coord
- width = x1 - x0
- height = y1 - y0
-
- linewidth = max(self._default_font_size / 4, 5)
-
- self.output.ax.add_patch(
- mpl.patches.Rectangle(
- (x0, y0),
- width,
- height,
- fill=False,
- edgecolor=edge_color,
- linewidth=linewidth * self.output.scale,
- alpha=alpha,
- linestyle=line_style,
- )
- )
- return self.output
-
- def draw_rotated_box_with_label(
- self, rotated_box, alpha=0.5, edge_color="g", line_style="-", label=None
- ):
- """
- Draw a rotated box with label on its top-left corner.
-
- Args:
- rotated_box (tuple): a tuple containing (cnt_x, cnt_y, w, h, angle),
- where cnt_x and cnt_y are the center coordinates of the box.
- w and h are the width and height of the box. angle represents how
- many degrees the box is rotated CCW with regard to the 0-degree box.
- alpha (float): blending efficient. Smaller values lead to more transparent masks.
- edge_color: color of the outline of the box. Refer to `matplotlib.colors`
- for full list of formats that are accepted.
- line_style (string): the string to use to create the outline of the boxes.
- label (string): label for rotated box. It will not be rendered when set to None.
-
- Returns:
- output (VisImage): image object with box drawn.
- """
- cnt_x, cnt_y, w, h, angle = rotated_box
- area = w * h
- # use thinner lines when the box is small
- linewidth = self._default_font_size / (
- 6 if area < _SMALL_OBJECT_AREA_THRESH * self.output.scale else 3
- )
-
- theta = angle * math.pi / 180.0
- c = math.cos(theta)
- s = math.sin(theta)
- rect = [(-w / 2, h / 2), (-w / 2, -h / 2), (w / 2, -h / 2), (w / 2, h / 2)]
- # x: left->right ; y: top->down
- rotated_rect = [(s * yy + c * xx + cnt_x, c * yy - s * xx + cnt_y) for (xx, yy) in rect]
- for k in range(4):
- j = (k + 1) % 4
- self.draw_line(
- [rotated_rect[k][0], rotated_rect[j][0]],
- [rotated_rect[k][1], rotated_rect[j][1]],
- color=edge_color,
- linestyle="--" if k == 1 else line_style,
- linewidth=linewidth,
- )
-
- if label is not None:
- text_pos = rotated_rect[1] # topleft corner
-
- height_ratio = h / np.sqrt(self.output.height * self.output.width)
- label_color = self._change_color_brightness(edge_color, brightness_factor=0.7)
- font_size = (
- np.clip((height_ratio - 0.02) / 0.08 + 1, 1.2, 2) * 0.5 * self._default_font_size
- )
- self.draw_text(label, text_pos, color=label_color, font_size=font_size, rotation=angle)
-
- return self.output
-
- def draw_circle(self, circle_coord, color, radius=3):
- """
- Args:
- circle_coord (list(int) or tuple(int)): contains the x and y coordinates
- of the center of the circle.
- color: color of the polygon. Refer to `matplotlib.colors` for a full list of
- formats that are accepted.
- radius (int): radius of the circle.
-
- Returns:
- output (VisImage): image object with box drawn.
- """
- x, y = circle_coord
- self.output.ax.add_patch(
- mpl.patches.Circle(circle_coord, radius=radius, fill=True, color=color)
- )
- return self.output
-
- def draw_line(self, x_data, y_data, color, linestyle="-", linewidth=None):
- """
- Args:
- x_data (list[int]): a list containing x values of all the points being drawn.
- Length of list should match the length of y_data.
- y_data (list[int]): a list containing y values of all the points being drawn.
- Length of list should match the length of x_data.
- color: color of the line. Refer to `matplotlib.colors` for a full list of
- formats that are accepted.
- linestyle: style of the line. Refer to `matplotlib.lines.Line2D`
- for a full list of formats that are accepted.
- linewidth (float or None): width of the line. When it's None,
- a default value will be computed and used.
-
- Returns:
- output (VisImage): image object with line drawn.
- """
- if linewidth is None:
- linewidth = self._default_font_size / 3
- linewidth = max(linewidth, 1)
- self.output.ax.add_line(
- mpl.lines.Line2D(
- x_data,
- y_data,
- linewidth=linewidth * self.output.scale,
- color=color,
- linestyle=linestyle,
- )
- )
- return self.output
-
- def draw_binary_mask(
- self, binary_mask, color=None, *, edge_color=None, text=None, alpha=0.5, area_threshold=0
- ):
- """
- Args:
- binary_mask (ndarray): numpy array of shape (H, W), where H is the image height and
- W is the image width. Each value in the array is either a 0 or 1 value of uint8
- type.
- color: color of the mask. Refer to `matplotlib.colors` for a full list of
- formats that are accepted. If None, will pick a random color.
- edge_color: color of the polygon edges. Refer to `matplotlib.colors` for a
- full list of formats that are accepted.
- text (str): if None, will be drawn in the object's center of mass.
- alpha (float): blending efficient. Smaller values lead to more transparent masks.
- area_threshold (float): a connected component small than this will not be shown.
-
- Returns:
- output (VisImage): image object with mask drawn.
- """
- if color is None:
- color = random_color(rgb=True, maximum=1)
- color = mplc.to_rgb(color)
-
- has_valid_segment = False
- binary_mask = binary_mask.astype("uint8") # opencv needs uint8
- mask = GenericMask(binary_mask, self.output.height, self.output.width)
- shape2d = (binary_mask.shape[0], binary_mask.shape[1])
-
- if not mask.has_holes:
- # draw polygons for regular masks
- for segment in mask.polygons:
- area = mask_util.area(mask_util.frPyObjects([segment], shape2d[0], shape2d[1]))
- if area < (area_threshold or 0):
- continue
- has_valid_segment = True
- segment = segment.reshape(-1, 2)
- self.draw_polygon(segment, color=color, edge_color=edge_color, alpha=alpha)
- else:
- # TODO: Use Path/PathPatch to draw vector graphics:
- # https://stackoverflow.com/questions/8919719/how-to-plot-a-complex-polygon
- rgba = np.zeros(shape2d + (4,), dtype="float32")
- rgba[:, :, :3] = color
- rgba[:, :, 3] = (mask.mask == 1).astype("float32") * alpha
- has_valid_segment = True
- self.output.ax.imshow(rgba, extent=(0, self.output.width, self.output.height, 0))
-
- if text is not None and has_valid_segment:
- # TODO sometimes drawn on wrong objects. the heuristics here can improve.
- lighter_color = self._change_color_brightness(color, brightness_factor=0.7)
- _num_cc, cc_labels, stats, centroids = cv2.connectedComponentsWithStats(binary_mask, 8)
- largest_component_id = np.argmax(stats[1:, -1]) + 1
-
- # draw text on the largest component, as well as other very large components.
- for cid in range(1, _num_cc):
- if cid == largest_component_id or stats[cid, -1] > _LARGE_MASK_AREA_THRESH:
- # median is more stable than centroid
- # center = centroids[largest_component_id]
- center = np.median((cc_labels == cid).nonzero(), axis=1)[::-1]
- self.draw_text(text, center, color=lighter_color)
- return self.output
-
- def draw_polygon(self, segment, color, edge_color=None, alpha=0.5):
- """
- Args:
- segment: numpy array of shape Nx2, containing all the points in the polygon.
- color: color of the polygon. Refer to `matplotlib.colors` for a full list of
- formats that are accepted.
- edge_color: color of the polygon edges. Refer to `matplotlib.colors` for a
- full list of formats that are accepted. If not provided, a darker shade
- of the polygon color will be used instead.
- alpha (float): blending efficient. Smaller values lead to more transparent masks.
-
- Returns:
- output (VisImage): image object with polygon drawn.
- """
- if edge_color is None:
- # make edge color darker than the polygon color
- if alpha > 0.8:
- edge_color = self._change_color_brightness(color, brightness_factor=-0.7)
- else:
- edge_color = color
- edge_color = mplc.to_rgb(edge_color) + (1,)
-
- polygon = mpl.patches.Polygon(
- segment,
- fill=True,
- facecolor=mplc.to_rgb(color) + (alpha,),
- edgecolor=edge_color,
- linewidth=max(self._default_font_size // 15 * self.output.scale, 1),
- )
- self.output.ax.add_patch(polygon)
- return self.output
-
- """
- Internal methods:
- """
-
- def _jitter(self, color):
- """
- Randomly modifies given color to produce a slightly different color than the color given.
-
- Args:
- color (tuple[double]): a tuple of 3 elements, containing the RGB values of the color
- picked. The values in the list are in the [0.0, 1.0] range.
-
- Returns:
- jittered_color (tuple[double]): a tuple of 3 elements, containing the RGB values of the
- color after being jittered. The values in the list are in the [0.0, 1.0] range.
- """
- color = mplc.to_rgb(color)
- vec = np.random.rand(3)
- # better to do it in another color space
- vec = vec / np.linalg.norm(vec) * 0.5
- res = np.clip(vec + color, 0, 1)
- return tuple(res)
-
- def _create_grayscale_image(self, mask=None):
- """
- Create a grayscale version of the original image.
- The colors in masked area, if given, will be kept.
- """
- img_bw = self.img.astype("f4").mean(axis=2)
- img_bw = np.stack([img_bw] * 3, axis=2)
- if mask is not None:
- img_bw[mask] = self.img[mask]
- return img_bw
-
- def _change_color_brightness(self, color, brightness_factor):
- """
- Depending on the brightness_factor, gives a lighter or darker color i.e. a color with
- less or more saturation than the original color.
-
- Args:
- color: color of the polygon. Refer to `matplotlib.colors` for a full list of
- formats that are accepted.
- brightness_factor (float): a value in [-1.0, 1.0] range. A lightness factor of
- 0 will correspond to no change, a factor in [-1.0, 0) range will result in
- a darker color and a factor in (0, 1.0] range will result in a lighter color.
-
- Returns:
- modified_color (tuple[double]): a tuple containing the RGB values of the
- modified color. Each value in the tuple is in the [0.0, 1.0] range.
- """
- assert brightness_factor >= -1.0 and brightness_factor <= 1.0
- color = mplc.to_rgb(color)
- polygon_color = colorsys.rgb_to_hls(*mplc.to_rgb(color))
- modified_lightness = polygon_color[1] + (brightness_factor * polygon_color[1])
- modified_lightness = 0.0 if modified_lightness < 0.0 else modified_lightness
- modified_lightness = 1.0 if modified_lightness > 1.0 else modified_lightness
- modified_color = colorsys.hls_to_rgb(polygon_color[0], modified_lightness, polygon_color[2])
- return modified_color
-
- def _convert_boxes(self, boxes):
- """
- Convert different format of boxes to an NxB array, where B = 4 or 5 is the box dimension.
- """
- if isinstance(boxes, Boxes) or isinstance(boxes, RotatedBoxes):
- return boxes.tensor.numpy()
- else:
- return np.asarray(boxes)
-
- def _convert_masks(self, masks_or_polygons):
- """
- Convert different format of masks or polygons to a tuple of masks and polygons.
-
- Returns:
- list[GenericMask]:
- """
-
- m = masks_or_polygons
- if isinstance(m, PolygonMasks):
- m = m.polygons
- if isinstance(m, BitMasks):
- m = m.tensor.numpy()
- if isinstance(m, torch.Tensor):
- m = m.numpy()
- ret = []
- for x in m:
- if isinstance(x, GenericMask):
- ret.append(x)
- else:
- ret.append(GenericMask(x, self.output.height, self.output.width))
- return ret
-
- def _convert_keypoints(self, keypoints):
- if isinstance(keypoints, Keypoints):
- keypoints = keypoints.tensor
- keypoints = np.asarray(keypoints)
- return keypoints
-
- def get_output(self):
- """
- Returns:
- output (VisImage): the image output containing the visualizations added
- to the image.
- """
- return self.output
diff --git a/spaces/CVPR/transfiner/configs/common/optim.py b/spaces/CVPR/transfiner/configs/common/optim.py
deleted file mode 100644
index d39d3aaa546c17e831d21d1758b69e8c1609415e..0000000000000000000000000000000000000000
--- a/spaces/CVPR/transfiner/configs/common/optim.py
+++ /dev/null
@@ -1,15 +0,0 @@
-import torch
-
-from detectron2.config import LazyCall as L
-from detectron2.solver.build import get_default_optimizer_params
-
-SGD = L(torch.optim.SGD)(
- params=L(get_default_optimizer_params)(
- # params.model is meant to be set to the model object, before instantiating
- # the optimizer.
- weight_decay_norm=0.0
- ),
- lr=0.02,
- momentum=0.9,
- weight_decay=1e-4,
-)
diff --git a/spaces/Caoyunkang/Segment-Any-Anomaly/SAM/segment_anything/utils/__init__.py b/spaces/Caoyunkang/Segment-Any-Anomaly/SAM/segment_anything/utils/__init__.py
deleted file mode 100644
index 5277f46157403e47fd830fc519144b97ef69d4ae..0000000000000000000000000000000000000000
--- a/spaces/Caoyunkang/Segment-Any-Anomaly/SAM/segment_anything/utils/__init__.py
+++ /dev/null
@@ -1,5 +0,0 @@
-# Copyright (c) Meta Platforms, Inc. and affiliates.
-# All rights reserved.
-
-# This source code is licensed under the license found in the
-# LICENSE file in the root directory of this source tree.
diff --git a/spaces/Chintan-Donda/KKMS-KSSW-HF/src/langchain_utils.py b/spaces/Chintan-Donda/KKMS-KSSW-HF/src/langchain_utils.py
deleted file mode 100644
index ab9ec11c60c7da114bed5bdb195e40bba7c37ece..0000000000000000000000000000000000000000
--- a/spaces/Chintan-Donda/KKMS-KSSW-HF/src/langchain_utils.py
+++ /dev/null
@@ -1,891 +0,0 @@
-import src.constants as constants_utils
-import src.data_loader as data_loader_utils
-import src.utils as utils
-
-from langchain.llms import OpenAI
-from langchain.text_splitter import CharacterTextSplitter, RecursiveCharacterTextSplitter
-from langchain.chains.summarize import load_summarize_chain
-from langchain.docstore.document import Document
-from langchain.embeddings.openai import OpenAIEmbeddings
-import openai
-from langchain.vectorstores import Chroma
-import chromadb
-from langchain.chains.question_answering import load_qa_chain
-from langchain.chains.qa_with_sources import load_qa_with_sources_chain
-from langchain.prompts import PromptTemplate
-from llama_index import GPTVectorStoreIndex, GPTListIndex
-from langchain.vectorstores import FAISS
-
-import pickle
-import shutil
-from typing import Dict, List, Optional
-
-import os
-os.environ['OPENAI_API_KEY'] = os.getenv('OPENAI_API_KEY')
-
-import logging
-logger = logging.getLogger(__name__)
-logging.basicConfig(
- format="%(asctime)s %(levelname)s [%(name)s] %(message)s", level=logging.INFO, datefmt="%Y-%m-%d %H:%M:%S"
-)
-
-import warnings
-warnings.filterwarnings('ignore')
-
-
-
-class LANGCHAIN_UTILS:
- def __init__(self,
- index_type=constants_utils.INDEX_TYPE,
- load_from_existing_index_store=constants_utils.LOAD_FROM_EXISTING_INDEX_STORE
- ):
- self.index_type = index_type
- self.load_from_existing_index_store = load_from_existing_index_store
-
- # Temporary index in the current context for the doc_type in consideration
- self.index = None
- # Master index which contains data from multiple sources (PDF, Online PDF, Text files, URLs, etc. It gets updated on Uploading the data from new files/urls without downtime of the application on-demand.)
- self.master_index = None
-
- # Data source wise index
- self.index_category_doc_type_wise_index = dict(
- (ic, dict(
- (ds, None) for ds in list(constants_utils.DATA_SOURCES.values()))
- ) for ic in constants_utils.INDEX_CATEGORY)
- # Initialize master index for each INDEX_CATEGORY
- for ic in constants_utils.INDEX_CATEGORY:
- self.index_category_doc_type_wise_index[ic][constants_utils.INDEX_CATEGORY_MASTER_INDEX_DOC_TYPE] = None
-
- # Data loaded as a Document format in the current context for the doc_type in consideration
- self.documents = []
-
- # Instantiate data_loader_utils class object
- self.data_loader_utils_obj = data_loader_utils.DATA_LOADER()
- # Instantiate UTILS class object
- self.utils_obj = utils.UTILS()
-
- # Initialize embeddings (we can also use other embeddings)
- self.embeddings = OpenAIEmbeddings(openai_api_key=os.getenv('OPENAI_API_KEY'))
-
- # Global history for AgGPT widget
- self.global_history = [
- {
- "role": "assistant",
- "content": "Hi, I am a chatbot. I can converse in English. I can answer your questions about farming in India. Ask me anything!"
- }
- ]
-
-
- def generate_prompt_template(
- self,
- prompt_type='general'
- ):
- prompt_template = ''
-
- if prompt_type == 'general':
- prompt_template = """Write a concise summary of the following:
-
- {text}
-
- SUMMARIZE IN ENGLISH:"""
-
- elif prompt_type == 'weather':
- prompt_template = """
- What would be the weather based on the below data:
- {text}
- """
-
- return prompt_template
-
-
- def user(
- self,
- user_message,
- history
- ):
- history = history + [[user_message, None]]
- self.global_history = self.global_history + [{"role": "user", "content": user_message}]
- return "", history
-
-
- def get_chatgpt_response(
- self,
- history
- ):
- output = openai.ChatCompletion.create(model="gpt-3.5-turbo", messages=history)
- history.append({"role": "assistant", "content": output.choices[0].message.content})
- return output.choices[0].message.content, history
-
-
- def bot(
- self,
- history
- ):
- response, self.global_history = self.get_chatgpt_response(self.global_history)
- history[-1][1] = response
- return history
-
-
- def clear_history(
- self,
- lang="English"
- ):
- self.global_history = [{"role": "assistant", "content": "Hi, I am a chatbot. I can converse in {}. I can answer your questions about farming in India. Ask me anything!".format(lang)}]
- return None
-
-
- def get_textual_summary(
- self,
- text,
- chain_type="stuff",
- custom_prompt=True,
- prompt_type='general'
- ):
- texts = [text]
- docs = [Document(page_content=t) for t in texts[:3]]
-
- llm = OpenAI(temperature=0)
- if custom_prompt:
- prompt_template = self.generate_prompt_template(prompt_type)
- PROMPT = PromptTemplate(template=prompt_template, input_variables=["text"])
- chain = load_summarize_chain(llm, chain_type=chain_type, prompt=PROMPT)
- else:
- chain = load_summarize_chain(llm, chain_type=chain_type)
-
- text_summary = chain.run(docs)
- return text_summary
-
-
- def get_weather_forecast_summary(
- self,
- text,
- chain_type="stuff"
- ):
- text = f"""
- What would be the weather based on the below data:
- {text}
-
- Give simple response without technical numbers which can be explained to human.
- """
- texts = [text]
- docs = [Document(page_content=t) for t in texts[:3]]
-
- llm = OpenAI(temperature=0)
- chain = load_summarize_chain(llm, chain_type=chain_type)
- text_summary = chain.run(docs)
-
- return text_summary
-
-
- def get_answer_from_para(
- self,
- para,
- question,
- chain_type="stuff",
- custom_prompt=True
- ):
- # Prepare data (Split paragraph into chunks of small documents)
- text_splitter = CharacterTextSplitter(
- chunk_size=constants_utils.TEXT_SPLITTER_CHUNK_SIZE,
- chunk_overlap=constants_utils.TEXT_SPLITTER_CHUNK_OVERLAP,
- separator=constants_utils.TEXT_SPLITTER_SEPARATOR
- )
- texts = text_splitter.split_text(para)
-
- if self.index_type == 'FAISS':
- # Find similar docs that are relevant to the question
- docsearch = FAISS.from_texts(
- texts, self.embeddings,
- metadatas=[{"source": str(i)} for i in range(len(texts))]
- )
-
- elif self.index_type == 'Chroma':
- # Find similar docs that are relevant to the question
- docsearch = Chroma.from_texts(
- texts, self.embeddings,
- metadatas=[{"source": str(i)} for i in range(len(texts))]
- )
-
- # Search for the similar docs
- docs = docsearch.similarity_search(question, k=1)
-
- llm = OpenAI(temperature=0)
- # Create a Chain for question answering
- if custom_prompt:
- prompt_template = """Use the following pieces of context to answer the question at the end. If you don't know the answer, just say that you don't know, don't try to make up an answer.
-
- {context}
-
- Question: {question}
- Answer in English:"""
-
- PROMPT = PromptTemplate(
- template=prompt_template, input_variables=["context", "question"]
- )
- chain = load_qa_chain(llm, chain_type=chain_type, prompt=PROMPT)
- else:
- # chain = load_qa_with_sources_chain(llm, chain_type=chain_type)
- chain = load_qa_chain(llm, chain_type=chain_type)
- # chain.run(input_documents=docs, question=question)
-
- out_dict = chain({"input_documents": docs, "question": question}, return_only_outputs=True)
- return out_dict['output_text']
-
-
- def load_documents(
- self,
- doc_type,
- doc_filepath='',
- urls=[]
- ):
- """
- Load data in Document format of the given doc_type from either doc_filepath or list of urls.
- It can load multiple files/urls in one shot.
-
- Args:
- doc_type: can be any of [pdf, online_pdf, urls, textfile]
- doc_filepath: can be a directory or a filepath
- urls: list of urls
- """
-
- logger.info(f'Loading {doc_type} data into Documents format')
-
- if doc_type == 'pdf':
- # Load data from PDFs stored in local directory
- self.documents.extend(
- self.data_loader_utils_obj.load_documents_from_pdf(
- doc_filepath=doc_filepath,
- doc_type=doc_type
- ))
-
- elif doc_type == 'online_pdf':
- # Load data from PDFs stored in local directory
- self.documents.extend(
- self.data_loader_utils_obj.load_documents_from_pdf(
- urls=urls,
- doc_type=doc_type
- ))
-
- elif doc_type == 'urls':
- # Load data from URLs
- self.documents.extend(
- self.data_loader_utils_obj.load_documents_from_urls(
- urls=urls,
- doc_type=doc_type
- ))
-
- elif doc_type == 'textfile':
- # Load data from text files & Convert texts into Document format
- self.documents.extend(
- self.data_loader_utils_obj.load_documents_from_text(
- doc_filepath=doc_filepath,
- doc_type=doc_type
- ))
-
- elif doc_type == 'directory':
- # Load data from local directory
- self.documents.extend(
- self.data_loader_utils_obj.load_documents_from_directory(
- doc_filepath=doc_filepath,
- doc_type=doc_type
- ))
-
- logger.info(f'{doc_type} data into Documents format loaded successfully!')
-
-
- def create_index(
- self
- ):
- if not self.documents:
- logger.warning(f'Empty documents. Index cannot be created!')
- return None
-
- logger.info(f'Creating index')
-
- text_splitter = CharacterTextSplitter(
- chunk_size=constants_utils.TEXT_SPLITTER_CHUNK_SIZE,
- chunk_overlap=constants_utils.TEXT_SPLITTER_CHUNK_OVERLAP,
- separator=constants_utils.TEXT_SPLITTER_SEPARATOR
- )
- self.documents = text_splitter.split_documents(self.documents)
-
- ############## Build the Vector store for docs ##############
- # Vector store using Facebook AI Similarity Search
- if self.index_type == 'FAISS':
- self.index = FAISS.from_documents(
- self.documents,
- self.embeddings
- )
-
- # Vector store using Chroma DB
- elif self.index_type == 'Chroma':
- if not os.path.exists(self.index_filepath):
- os.makedirs(self.index_filepath)
-
- self.index = Chroma.from_documents(
- self.documents,
- self.embeddings,
- persist_directory=self.index_filepath
- )
-
- # Vector store using GPT vector index
- elif self.index_type == 'GPTVectorStoreIndex':
- self.index = GPTVectorStoreIndex.from_documents(self.documents)
-
- logger.info(f'Index created successfully!')
- return self.index
-
-
- def get_index_filepath(
- self,
- index_category,
- doc_type
- ):
- if doc_type == 'master':
- self.index_filepath = os.path.join(
- constants_utils.OUTPUT_PATH, f'index_{index_category}') if self.index_type in ['FAISS', 'Chroma'] else os.path.join(constants_utils.OUTPUT_PATH, f'index_{index_category}.json')
- else:
- self.index_filepath = os.path.join(
- constants_utils.OUTPUT_PATH, f'index_{index_category}', f'index_{doc_type}') if self.index_type in ['FAISS', 'Chroma'] else os.path.join(constants_utils.OUTPUT_PATH, f'index_{index_category}', f'index_{doc_type}.json')
-
- return self.index_filepath
-
-
- def load_master_doctype_indices_for_index_category(
- self,
- index_category
- ):
- logger.info(f'Loading master and doc_type indices for: {index_category}')
-
- # Set master index of index_category = None
- self.index_category_doc_type_wise_index[index_category][constants_utils.INDEX_CATEGORY_MASTER_INDEX_DOC_TYPE] = None
-
- for doc_type in self.index_category_doc_type_wise_index[index_category].keys():
- self.index = None
- self.index_filepath = self.get_index_filepath(
- index_category=index_category,
- doc_type=doc_type
- )
- self.load_index()
- # Set master/doc_type index
- self.index_category_doc_type_wise_index[index_category][doc_type] = self.index
-
- logger.info(f'Master and doc_type indices for: {index_category} loaded successfully!')
-
-
- def load_create_index(
- self
- ):
- logger.info(f'Loading/Creating index for each index_category')
-
- for index_category in constants_utils.INDEX_CATEGORY:
- # Load master index_category index if self.load_from_existing_index_store == True
- if self.load_from_existing_index_store:
- self.load_master_doctype_indices_for_index_category(index_category)
-
- # For any reason, if master index is not loaded then create the new index/vector store
- if not self.index_category_doc_type_wise_index[index_category][constants_utils.INDEX_CATEGORY_MASTER_INDEX_DOC_TYPE]:
- logger.info(f'Creating a new Vector/Index store for: {index_category}')
-
- doc_filepath = os.path.join(constants_utils.DATA_PATH, index_category)
- urls = []
-
- # Build the Vector/Index store
- for doc_type in list(constants_utils.DATA_SOURCES.values()):
- logger.info(f'Creating a new Vector/Index store for: {index_category} from data source: {doc_type}')
-
- index = None
- if doc_type in ['pdf', 'textfile']:
- index = self.create_store_index(
- doc_type=doc_type,
- doc_filepath=doc_filepath,
- index_category=index_category
- )
- else:
- # Build the Vector/Index store from web urls
- index = self.create_store_index(
- doc_type=doc_type,
- urls=urls,
- index_category=index_category
- )
-
- if index:
- self.index_category_doc_type_wise_index[index_category][doc_type] = index
-
- logger.info(f'New Vector/Index store for: {index_category} from data source: {doc_type} created successfully!')
-
- logger.info(f'New Vector/Index store for: {index_category} created successfully!')
-
- # Merge index of each doc_type into a single index_category
- self.merge_store_master_index(
- index_category=index_category
- )
-
- logger.info(f'Index for each index_category loaded successfully!')
-
-
- def create_store_index(
- self,
- doc_type='pdf',
- doc_filepath=constants_utils.DATA_PATH,
- urls=[],
- index_category=constants_utils.INDEX_CATEGORY[0]
- ):
- logger.info(f'Creating and storing {doc_type} index')
-
- self.documents = []
- self.index = None
-
- self.index_filepath = self.get_index_filepath(
- index_category=index_category,
- doc_type=doc_type
- )
-
- # Delete the old index file
- shutil.rmtree(self.index_filepath, ignore_errors=True)
- logger.info(f'{self.index_filepath} deleted.')
-
- # Load data in Documents format that can be consumed for index creation
- self.load_documents(
- doc_type,
- doc_filepath,
- urls
- )
-
- # Create the index from documents for search/retrieval
- self.index = self.create_index()
-
- # Store index
- self.store_index(
- index=self.index,
- index_filepath=self.index_filepath
- )
-
- logger.info(f'{doc_type} index created and stored successfully!')
- # Return the index of the given doc_type (this is an index for a single doc_type). Indices from multiple doc_types should be merged later on in the master index so that query could be made from a single index.
- return self.index
-
-
- def store_index(
- self,
- index,
- index_filepath
- ):
- if not index:
- logger.warning(f'Cannot write an empty index to: {index_filepath}!')
- return
-
- logger.info(f'Saving index to: {index_filepath}')
-
- if not os.path.exists(index_filepath) and os.path.isdir(index_filepath):
- os.makedirs(index_filepath)
-
- if self.index_type == 'FAISS':
- index.save_local(index_filepath)
-
- elif self.index_type == 'Chroma':
- index.persist()
-
- elif self.index_type == 'GPTVectorStoreIndex':
- index.save_to_disk(index_filepath)
-
- elif self.index_type == 'pickle':
- with open(index_filepath, "wb") as f:
- pickle.dump(index, f)
-
- logger.info(f'Index saved to: {index_filepath} successfully!')
-
-
- def load_index(
- self
- ):
- logger.info(f'Loading index from: {self.index_filepath}')
-
- if not os.path.exists(self.index_filepath):
- logger.warning(f"Cannot load index from {self.index_filepath} as the path doest not exist!")
- return
-
- if self.index_type == 'FAISS':
- self.index = FAISS.load_local(self.index_filepath, self.embeddings)
-
- elif self.index_type == 'Chroma':
- self.index = Chroma(
- persist_directory=self.index_filepath,
- embedding_function=self.embeddings
- )
-
- elif self.index_type == 'GPTVectorStoreIndex':
- self.index = GPTVectorStoreIndex.load_from_disk(self.index_filepath)
-
- elif self.index_type == 'pickle':
- with open(self.index_filepath, "rb") as f:
- self.index = pickle.load(f)
-
- logger.info(f'Index loaded from: {self.index_filepath} successfully!')
-
-
- def convert_text_to_documents(
- self,
- text_list=[]
- ):
- """
- Converts the list of text data to Documents format that can be feed to GPT API to build the Vector store
- """
-
- from llama_index import Document
- documents = [Document(t) for t in text_list]
- return documents
-
-
- def merge_documents_from_different_sources(
- self,
- doc_documents,
- url_documents
- ):
- # Build the Vector store for docs
- doc_index = GPTVectorStoreIndex.from_documents(doc_documents)
- # Build the Vector store for URLs
- url_index = GPTVectorStoreIndex.from_documents(url_documents)
-
- # Set summary of each index
- doc_index.set_text("index_from_docs")
- url_index.set_text("index_from_urls")
-
- # Merge index of different data sources
- index = GPTListIndex([doc_index, url_index])
-
- return index
-
-
- def merge_store_master_index(
- self,
- index_category
- ):
- """
- Merge multiple doc_type indices into a single master index. Query/search would be performed on this merged index.
-
- Args:
- index_category: index_category (can be any of: [crops, fruits, pest_management, govt_policy, soil, etc.])
- """
- logger.info('Merging doc_type indices of different index categories into a master index')
-
- self.index_category_doc_type_wise_index[index_category][constants_utils.INDEX_CATEGORY_MASTER_INDEX_DOC_TYPE] = None
- doc_type_indices = self.index_category_doc_type_wise_index[index_category]
-
- if self.index_type == 'FAISS':
- for doc_type, index in doc_type_indices.items():
- if doc_type == constants_utils.INDEX_CATEGORY_MASTER_INDEX_DOC_TYPE:
- # Only merge the non-master doc_type_indices
- continue
- if not index or not isinstance(index, FAISS):
- logger.warning(f'{doc_type} index to be merged is not an instance of type langchain.vectorstores.faiss.FAISS')
- continue
- if not self.index_category_doc_type_wise_index[index_category][constants_utils.INDEX_CATEGORY_MASTER_INDEX_DOC_TYPE]:
- self.index_category_doc_type_wise_index[index_category][constants_utils.INDEX_CATEGORY_MASTER_INDEX_DOC_TYPE] = index
- else:
- self.index_category_doc_type_wise_index[index_category][constants_utils.INDEX_CATEGORY_MASTER_INDEX_DOC_TYPE].merge_from(index)
-
- elif self.index_type == 'Chroma':
- for doc_type, index in doc_type_indices.items():
- if not index or not isinstance(index, Chroma):
- logger.warning(f'{doc_type} index to be merged is not an instance of type langchain.vectorstores.Chroma')
- continue
- raise NotImplementedError
-
- elif self.index_type == 'GPTVectorStoreIndex':
- for doc_type, index in doc_type_indices.items():
- if not index or not isinstance(index, GPTVectorStoreIndex):
- logger.warning(f'{doc_type} index to be merged is not an instance of type llama_index.GPTVectorStoreIndex')
- continue
- import pdb; pdb.set_trace()
- raise NotImplementedError
-
- # Store index_category master index
- self.store_index(
- index=self.index_category_doc_type_wise_index[index_category][constants_utils.INDEX_CATEGORY_MASTER_INDEX_DOC_TYPE],
- index_filepath=self.get_index_filepath(
- index_category=index_category,
- doc_type=constants_utils.INDEX_CATEGORY_MASTER_INDEX_DOC_TYPE
- )
- )
-
- logger.info('doc_type indices of different index categories into a master index merged successfully!')
-
-
- def init_chromadb(self):
- logger.info('Initializing Chroma DB')
-
- if not os.path.exists(self.index_filepath):
- os.makedirs(self.index_filepath)
-
- client_settings = chromadb.config.Settings(
- chroma_db_impl="duckdb+parquet",
- persist_directory=self.index_filepath,
- anonymized_telemetry=False
- )
-
- self.index = Chroma(
- collection_name="langchain_store",
- embedding_function=self.embeddings,
- client_settings=client_settings,
- persist_directory=self.index_filepath,
- )
-
- logger.info('Chroma DB initialized successfully!')
-
-
- def query_chromadb(
- self,
- question,
- k=1
- ):
- return self.index.similarity_search(query=question, k=k)
-
-
- def query(self,
- question,
- question_category,
- mode=constants_utils.MODE,
- response_mode=constants_utils.RESPONSE_MODE,
- similarity_top_k=constants_utils.SIMILARITY_TOP_K,
- required_keywords=[],
- exclude_keywords=[],
- verbose=False
- ):
- '''
- Args:
- mode: can be any of [default, embedding]
- response_mode: can be any of [default, compact, tree_summarize]
- '''
- logger.info(f'question category: {question_category}; question: {question}')
-
- response = None
-
- # Get the index of the given question_category
- index = self.index_category_doc_type_wise_index[question_category][constants_utils.INDEX_CATEGORY_MASTER_INDEX_DOC_TYPE]
-
- if self.index_type == 'FAISS':
- response = index.similarity_search(
- question,
- k=similarity_top_k
- )
-
- elif self.index_type == 'Chroma':
- response = index.similarity_search(
- question,
- k=similarity_top_k
- )
-
- elif self.index_type == 'GPTVectorStoreIndex':
- # Querying the index
- response = index.query(
- question,
- mode=mode,
- response_mode=response_mode,
- similarity_top_k=similarity_top_k,
- required_keywords=required_keywords,
- exclude_keywords=exclude_keywords,
- verbose=verbose
- )
-
- return response
-
-
- def load_uploaded_documents(
- self,
- doc_type,
- files_or_urls
- ):
- logger.info(f'Loading uploaded documents from: {doc_type}')
-
- if doc_type == 'pdf':
- if not isinstance(files_or_urls, list):
- files_or_urls = [files_or_urls]
- for pdf in files_or_urls:
- if not pdf.name.endswith('.pdf'):
- logger.warning(f'Found a file other than .pdf format. Cannot load {pdf.name} file!')
- continue
- logger.info(f'Loading PDF from: {pdf.name}')
- # Load PDF as documents
- self.documents.extend(
- self.data_loader_utils_obj.load_documents_from_pdf(
- doc_filepath=pdf.name,
- doc_type=doc_type
- )
- )
-
- elif doc_type == 'textfile':
- if not isinstance(files_or_urls, list):
- files_or_urls = [files_or_urls]
- for text_file in files_or_urls:
- if not text_file.name.endswith('.txt'):
- logger.warning(f'Found a file other than .txt format. Cannot load {text_file.name} file!')
- continue
- logger.info(f'Loading textfile from: {text_file.name}')
- # Load textfile as documents
- self.documents.extend(
- self.data_loader_utils_obj.load_documents_from_text(
- doc_filepath=text_file.name,
- doc_type=doc_type
- )
- )
-
- elif doc_type == 'online_pdf':
- files_or_urls = self.utils_obj.split_text(files_or_urls)
- # Load online_pdfs as documents
- self.documents.extend(
- self.data_loader_utils_obj.load_documents_from_pdf(
- doc_type=doc_type,
- urls=files_or_urls
- )
- )
-
- elif doc_type == 'urls':
- files_or_urls = self.utils_obj.split_text(files_or_urls)
- # Load URLs as documents
- self.documents.extend(
- self.data_loader_utils_obj.load_documents_from_urls(
- doc_type=doc_type,
- urls=files_or_urls
- )
- )
-
- logger.info(f'Uploaded documents from: {doc_type} loaded successfully!')
-
-
- def upload_data(
- self,
- doc_type,
- files_or_urls,
- index_category
- ):
- logger.info(f'Uploading data for: {index_category}; from: {doc_type}')
-
- self.documents = []
- self.index = None
-
- # Create documents of the uploaded files
- self.load_uploaded_documents(
- doc_type,
- files_or_urls
- )
-
- # Create the index from documents for search/retrieval
- self.index = self.create_index()
-
- # Update the existing index with the newly data
- self.upsert_index(
- doc_type=doc_type,
- index_category=index_category
- )
-
- logger.info(f'{index_category}-{doc_type} data uploaded successfully!')
-
-
- def upsert_index(
- self,
- doc_type,
- index_category
- ):
- """
- Updates the index of the given index_category-doc_type, if present.
- Creates a new index if index_category-doc_type index is not present.
- Also updates the master index for the given index_category.
- """
- if not self.index:
- return
-
- logger.info(f'Upserting index for: {index_category}-{doc_type}')
-
- if not self.index_category_doc_type_wise_index.get(index_category, None):
- """
- If index_category index does not exists
- Steps:
- - set index_category index
- - set doc_type index
- - Store new index_category index as master
- - Store new doc_type index
- """
- logger.info(f'Master index does not exist for: {index_category}. A new {index_category} master index & {doc_type} index would be created.')
- self.index_category_doc_type_wise_index.setdefault(index_category, {})
- # Set a master index only if it doesn't exist. Else keep it's value as-it-is.
- self.index_category_doc_type_wise_index[index_category][constants_utils.INDEX_CATEGORY_MASTER_INDEX_DOC_TYPE] = self.index
- # Set an index for the given doc_type only if it doesn't exist. Else keep it's value as-it-is.
- self.index_category_doc_type_wise_index[index_category][doc_type] = self.index
-
- elif not self.index_category_doc_type_wise_index[index_category].get(doc_type, None):
- """
- If doc_type index does not exists
- Steps:
- - set doc_type index
- - if master index does not exist for the index_category - set a master index
- - if master index exists - update the master index to merge it with doc_type index
- - Store new/updated index_category index as master
- - Store new doc_type index
- """
- logger.info(f'{doc_type} index does not exist for: {index_category}-{doc_type}. A new {doc_type} index would be created.')
- # create doc_type index
- self.index_category_doc_type_wise_index[index_category][doc_type] = self.index
- # if master index does not exist for the index_category - create a master index
- if not self.index_category_doc_type_wise_index[index_category].get(constants_utils.INDEX_CATEGORY_MASTER_INDEX_DOC_TYPE, None):
- logger.info(f'Master index does not exist for: {index_category}-{doc_type}. A new master index would be created.')
- self.index_category_doc_type_wise_index[index_category][constants_utils.INDEX_CATEGORY_MASTER_INDEX_DOC_TYPE] = self.index
-
- else:
- """
- If the new document is of the existing index_category & doc_type
- Steps:
- - if master index does not exist for the index_category - set a master index
- - if master index exists - update the master index to merge it with doc_type index
- - update the doc_type index
- - Store updated index_category index as master
- - Store updated doc_type index
- """
- # if master index does not exist for the index_category - create a master index
- if not self.index_category_doc_type_wise_index[index_category].get(constants_utils.INDEX_CATEGORY_MASTER_INDEX_DOC_TYPE, None):
- logger.info(f'Master index does not exist for: {index_category}-{doc_type}. A new master index would be created.')
- self.index_category_doc_type_wise_index[index_category][constants_utils.INDEX_CATEGORY_MASTER_INDEX_DOC_TYPE] = self.index
- # Merge new self.index with existing doc_type index
- self.index_category_doc_type_wise_index[index_category][doc_type].merge_from(self.index)
- # Update self.index to store/overwrite the existing index with the updated index
- self.index = self.index_category_doc_type_wise_index[index_category][doc_type]
-
-
- # Store newly created/merged index
- self.store_index(
- index=self.index,
- index_filepath=self.get_index_filepath(
- index_category=index_category,
- doc_type=doc_type
- )
- )
-
- # Merge and store master index for index_category
- self.merge_store_master_index(
- index_category=index_category
- )
-
- logger.info(f'Index for: {index_category}-{doc_type} upserted successful!')
-
-
- def delete_index(
- self,
- ids: Optional[List[str]] = None,
- # filter: Optional[DocumentMetadataFilter] = None,
- delete_all: Optional[bool] = None,
- ):
- """
- Removes vectors by ids, filter, or everything in the datastore.
- Multiple parameters can be used at once.
- Returns whether the operation was successful.
- """
- logger.info(f'Deleting index')
-
- raise NotImplementedError
-
- # NOTE: we can delete a specific collection
- self.index.delete_collection()
- self.index.persist()
-
- # Or just nuke the persist directory
- # !rm -rf self.index_filepath
diff --git a/spaces/Clebersla/RVC_V2_Huggingface_Version/README.md b/spaces/Clebersla/RVC_V2_Huggingface_Version/README.md
deleted file mode 100644
index 9cb518590fc64557b6d76c297dedb3bb75e3b3a9..0000000000000000000000000000000000000000
--- a/spaces/Clebersla/RVC_V2_Huggingface_Version/README.md
+++ /dev/null
@@ -1,57 +0,0 @@
----
-title: RVC V2
-emoji: 💻
-colorFrom: blue
-colorTo: purple
-sdk: gradio
-sdk_version: 3.42.0
-app_file: app.py
-pinned: false
-license: lgpl-3.0
----
-
-## 🔧 Pre-requisites
-
-Before running the project, you must have the following tool installed on your machine:
-* [Python v3.8.0](https://www.python.org/downloads/release/python-380/)
-
-Also, you will need to clone the repository:
-
-```bash
-# Clone the repository
-git clone https://huggingface.co/spaces/mateuseap/magic-vocals/
-# Enter in the root directory
-cd magic-vocals
-```
-
-## 🚀 How to run
-
-After you've cloned the repository and entered in the root directory, run the following commands:
-
-```bash
-# Create and activate a Virtual Environment (make sure you're using Python v3.8.0 to do it)
-python -m venv venv
-. venv/bin/activate
-
-# Change mode and execute a shell script to configure and run the application
-chmod +x run.sh
-./run.sh
-```
-
-After the shell script executes everything, the application will be running at http://127.0.0.1:7860! Open up the link in a browser to use the app:
-
-
-
-**You only need to execute the `run.sh` one time**, once you've executed it one time, you just need to activate the virtual environment and run the command below to start the app again:
-
-```bash
-python app.py
-```
-
-**THE `run.sh` IS SUPPORTED BY THE FOLLOWING OPERATING SYSTEMS:**
-
-
-| OS | Supported |
-|-----------|:---------:|
-| `Windows` | ❌ |
-| `Ubuntu` | ✅ |
\ No newline at end of file
diff --git a/spaces/CuriousDolphin/MobileSAM/utils/tools_gradio.py b/spaces/CuriousDolphin/MobileSAM/utils/tools_gradio.py
deleted file mode 100644
index 19b50fc7d4f1da25cbb1681ab9b993a1411a452e..0000000000000000000000000000000000000000
--- a/spaces/CuriousDolphin/MobileSAM/utils/tools_gradio.py
+++ /dev/null
@@ -1,192 +0,0 @@
-import cv2
-import matplotlib.pyplot as plt
-import numpy as np
-import torch
-from PIL import Image
-
-
-def fast_process(
- annotations,
- image,
- device,
- scale,
- better_quality=False,
- mask_random_color=True,
- bbox=None,
- use_retina=True,
- withContours=True,
-):
- if isinstance(annotations[0], dict):
- annotations = [annotation["segmentation"] for annotation in annotations]
-
- original_h = image.height
- original_w = image.width
- if better_quality:
- if isinstance(annotations[0], torch.Tensor):
- annotations = np.array(annotations.cpu())
- for i, mask in enumerate(annotations):
- mask = cv2.morphologyEx(
- mask.astype(np.uint8), cv2.MORPH_CLOSE, np.ones((3, 3), np.uint8)
- )
- annotations[i] = cv2.morphologyEx(
- mask.astype(np.uint8), cv2.MORPH_OPEN, np.ones((8, 8), np.uint8)
- )
- if device == "cpu":
- annotations = np.array(annotations)
- inner_mask = fast_show_mask(
- annotations,
- plt.gca(),
- random_color=mask_random_color,
- bbox=bbox,
- retinamask=use_retina,
- target_height=original_h,
- target_width=original_w,
- )
- else:
- if isinstance(annotations[0], np.ndarray):
- annotations = np.array(annotations)
- annotations = torch.from_numpy(annotations)
- inner_mask = fast_show_mask_gpu(
- annotations,
- plt.gca(),
- random_color=mask_random_color,
- bbox=bbox,
- retinamask=use_retina,
- target_height=original_h,
- target_width=original_w,
- )
- if isinstance(annotations, torch.Tensor):
- annotations = annotations.cpu().numpy()
-
- if withContours:
- contour_all = []
- temp = np.zeros((original_h, original_w, 1))
- for i, mask in enumerate(annotations):
- if type(mask) == dict:
- mask = mask["segmentation"]
- annotation = mask.astype(np.uint8)
- if use_retina == False:
- annotation = cv2.resize(
- annotation,
- (original_w, original_h),
- interpolation=cv2.INTER_NEAREST,
- )
- contours, _ = cv2.findContours(
- annotation, cv2.RETR_TREE, cv2.CHAIN_APPROX_SIMPLE
- )
- for contour in contours:
- contour_all.append(contour)
- cv2.drawContours(temp, contour_all, -1, (255, 255, 255), 2 // scale)
- color = np.array([0 / 255, 0 / 255, 255 / 255, 0.9])
- contour_mask = temp / 255 * color.reshape(1, 1, -1)
-
- image = image.convert("RGBA")
- overlay_inner = Image.fromarray((inner_mask * 255).astype(np.uint8), "RGBA")
- image.paste(overlay_inner, (0, 0), overlay_inner)
-
- if withContours:
- overlay_contour = Image.fromarray((contour_mask * 255).astype(np.uint8), "RGBA")
- image.paste(overlay_contour, (0, 0), overlay_contour)
-
- return image
-
-
-# CPU post process
-def fast_show_mask(
- annotation,
- ax,
- random_color=False,
- bbox=None,
- retinamask=True,
- target_height=960,
- target_width=960,
-):
- mask_sum = annotation.shape[0]
- height = annotation.shape[1]
- weight = annotation.shape[2]
- # 将annotation 按照面积 排序
- areas = np.sum(annotation, axis=(1, 2))
- sorted_indices = np.argsort(areas)[::1]
- annotation = annotation[sorted_indices]
-
- index = (annotation != 0).argmax(axis=0)
- if random_color == True:
- color = np.random.random((mask_sum, 1, 1, 3))
- else:
- color = np.ones((mask_sum, 1, 1, 3)) * np.array(
- [30 / 255, 144 / 255, 255 / 255]
- )
- transparency = np.ones((mask_sum, 1, 1, 1)) * 0.6
- visual = np.concatenate([color, transparency], axis=-1)
- mask_image = np.expand_dims(annotation, -1) * visual
-
- mask = np.zeros((height, weight, 4))
-
- h_indices, w_indices = np.meshgrid(
- np.arange(height), np.arange(weight), indexing="ij"
- )
- indices = (index[h_indices, w_indices], h_indices, w_indices, slice(None))
-
- mask[h_indices, w_indices, :] = mask_image[indices]
- if bbox is not None:
- x1, y1, x2, y2 = bbox
- ax.add_patch(
- plt.Rectangle(
- (x1, y1), x2 - x1, y2 - y1, fill=False, edgecolor="b", linewidth=1
- )
- )
-
- if retinamask == False:
- mask = cv2.resize(
- mask, (target_width, target_height), interpolation=cv2.INTER_NEAREST
- )
-
- return mask
-
-
-def fast_show_mask_gpu(
- annotation,
- ax,
- random_color=False,
- bbox=None,
- retinamask=True,
- target_height=960,
- target_width=960,
-):
- device = annotation.device
- mask_sum = annotation.shape[0]
- height = annotation.shape[1]
- weight = annotation.shape[2]
- areas = torch.sum(annotation, dim=(1, 2))
- sorted_indices = torch.argsort(areas, descending=False)
- annotation = annotation[sorted_indices]
- # 找每个位置第一个非零值下标
- index = (annotation != 0).to(torch.long).argmax(dim=0)
- if random_color == True:
- color = torch.rand((mask_sum, 1, 1, 3)).to(device)
- else:
- color = torch.ones((mask_sum, 1, 1, 3)).to(device) * torch.tensor(
- [30 / 255, 144 / 255, 255 / 255]
- ).to(device)
- transparency = torch.ones((mask_sum, 1, 1, 1)).to(device) * 0.6
- visual = torch.cat([color, transparency], dim=-1)
- mask_image = torch.unsqueeze(annotation, -1) * visual
- # 按index取数,index指每个位置选哪个batch的数,把mask_image转成一个batch的形式
- mask = torch.zeros((height, weight, 4)).to(device)
- h_indices, w_indices = torch.meshgrid(torch.arange(height), torch.arange(weight))
- indices = (index[h_indices, w_indices], h_indices, w_indices, slice(None))
- # 使用向量化索引更新show的值
- mask[h_indices, w_indices, :] = mask_image[indices]
- mask_cpu = mask.cpu().numpy()
- if bbox is not None:
- x1, y1, x2, y2 = bbox
- ax.add_patch(
- plt.Rectangle(
- (x1, y1), x2 - x1, y2 - y1, fill=False, edgecolor="b", linewidth=1
- )
- )
- if retinamask == False:
- mask_cpu = cv2.resize(
- mask_cpu, (target_width, target_height), interpolation=cv2.INTER_NEAREST
- )
- return mask_cpu
diff --git a/spaces/Cvandi/remake/scripts/generate_meta_info.py b/spaces/Cvandi/remake/scripts/generate_meta_info.py
deleted file mode 100644
index 9c3b7a37e85f534075c50e6c33d7cca999d8b836..0000000000000000000000000000000000000000
--- a/spaces/Cvandi/remake/scripts/generate_meta_info.py
+++ /dev/null
@@ -1,58 +0,0 @@
-import argparse
-import cv2
-import glob
-import os
-
-
-def main(args):
- txt_file = open(args.meta_info, 'w')
- for folder, root in zip(args.input, args.root):
- img_paths = sorted(glob.glob(os.path.join(folder, '*')))
- for img_path in img_paths:
- status = True
- if args.check:
- # read the image once for check, as some images may have errors
- try:
- img = cv2.imread(img_path)
- except (IOError, OSError) as error:
- print(f'Read {img_path} error: {error}')
- status = False
- if img is None:
- status = False
- print(f'Img is None: {img_path}')
- if status:
- # get the relative path
- img_name = os.path.relpath(img_path, root)
- print(img_name)
- txt_file.write(f'{img_name}\n')
-
-
-if __name__ == '__main__':
- """Generate meta info (txt file) for only Ground-Truth images.
-
- It can also generate meta info from several folders into one txt file.
- """
- parser = argparse.ArgumentParser()
- parser.add_argument(
- '--input',
- nargs='+',
- default=['datasets/DF2K/DF2K_HR', 'datasets/DF2K/DF2K_multiscale'],
- help='Input folder, can be a list')
- parser.add_argument(
- '--root',
- nargs='+',
- default=['datasets/DF2K', 'datasets/DF2K'],
- help='Folder root, should have the length as input folders')
- parser.add_argument(
- '--meta_info',
- type=str,
- default='datasets/DF2K/meta_info/meta_info_DF2Kmultiscale.txt',
- help='txt path for meta info')
- parser.add_argument('--check', action='store_true', help='Read image to check whether it is ok')
- args = parser.parse_args()
-
- assert len(args.input) == len(args.root), ('Input folder and folder root should have the same length, but got '
- f'{len(args.input)} and {len(args.root)}.')
- os.makedirs(os.path.dirname(args.meta_info), exist_ok=True)
-
- main(args)
diff --git a/spaces/Cyril666/ContourNet-ABI/maskrcnn_benchmark/layers/nms.py b/spaces/Cyril666/ContourNet-ABI/maskrcnn_benchmark/layers/nms.py
deleted file mode 100644
index 1e80b555045d85e509c917f940ee9bc62738fee7..0000000000000000000000000000000000000000
--- a/spaces/Cyril666/ContourNet-ABI/maskrcnn_benchmark/layers/nms.py
+++ /dev/null
@@ -1,7 +0,0 @@
-# Copyright (c) Facebook, Inc. and its affiliates. All Rights Reserved.
-# from ._utils import _C
-from maskrcnn_benchmark import _C
-
-nms = _C.nms
-# nms.__doc__ = """
-# This function performs Non-maximum suppresion"""
diff --git a/spaces/DQChoi/gpt-demo/venv/lib/python3.11/site-packages/fontTools/misc/visitor.py b/spaces/DQChoi/gpt-demo/venv/lib/python3.11/site-packages/fontTools/misc/visitor.py
deleted file mode 100644
index 3d28135fad3a951c447d03b7f2b08403cb24a12e..0000000000000000000000000000000000000000
--- a/spaces/DQChoi/gpt-demo/venv/lib/python3.11/site-packages/fontTools/misc/visitor.py
+++ /dev/null
@@ -1,143 +0,0 @@
-"""Generic visitor pattern implementation for Python objects."""
-
-import enum
-
-
-class Visitor(object):
-
- defaultStop = False
-
- @classmethod
- def _register(celf, clazzes_attrs):
- assert celf != Visitor, "Subclass Visitor instead."
- if "_visitors" not in celf.__dict__:
- celf._visitors = {}
-
- def wrapper(method):
- assert method.__name__ == "visit"
- for clazzes, attrs in clazzes_attrs:
- if type(clazzes) != tuple:
- clazzes = (clazzes,)
- if type(attrs) == str:
- attrs = (attrs,)
- for clazz in clazzes:
- _visitors = celf._visitors.setdefault(clazz, {})
- for attr in attrs:
- assert attr not in _visitors, (
- "Oops, class '%s' has visitor function for '%s' defined already."
- % (clazz.__name__, attr)
- )
- _visitors[attr] = method
- return None
-
- return wrapper
-
- @classmethod
- def register(celf, clazzes):
- if type(clazzes) != tuple:
- clazzes = (clazzes,)
- return celf._register([(clazzes, (None,))])
-
- @classmethod
- def register_attr(celf, clazzes, attrs):
- clazzes_attrs = []
- if type(clazzes) != tuple:
- clazzes = (clazzes,)
- if type(attrs) == str:
- attrs = (attrs,)
- for clazz in clazzes:
- clazzes_attrs.append((clazz, attrs))
- return celf._register(clazzes_attrs)
-
- @classmethod
- def register_attrs(celf, clazzes_attrs):
- return celf._register(clazzes_attrs)
-
- @classmethod
- def _visitorsFor(celf, thing, _default={}):
- typ = type(thing)
-
- for celf in celf.mro():
-
- _visitors = getattr(celf, "_visitors", None)
- if _visitors is None:
- break
-
- m = celf._visitors.get(typ, None)
- if m is not None:
- return m
-
- return _default
-
- def visitObject(self, obj, *args, **kwargs):
- """Called to visit an object. This function loops over all non-private
- attributes of the objects and calls any user-registered (via
- @register_attr() or @register_attrs()) visit() functions.
-
- If there is no user-registered visit function, of if there is and it
- returns True, or it returns None (or doesn't return anything) and
- visitor.defaultStop is False (default), then the visitor will proceed
- to call self.visitAttr()"""
-
- keys = sorted(vars(obj).keys())
- _visitors = self._visitorsFor(obj)
- defaultVisitor = _visitors.get("*", None)
- for key in keys:
- if key[0] == "_":
- continue
- value = getattr(obj, key)
- visitorFunc = _visitors.get(key, defaultVisitor)
- if visitorFunc is not None:
- ret = visitorFunc(self, obj, key, value, *args, **kwargs)
- if ret == False or (ret is None and self.defaultStop):
- continue
- self.visitAttr(obj, key, value, *args, **kwargs)
-
- def visitAttr(self, obj, attr, value, *args, **kwargs):
- """Called to visit an attribute of an object."""
- self.visit(value, *args, **kwargs)
-
- def visitList(self, obj, *args, **kwargs):
- """Called to visit any value that is a list."""
- for value in obj:
- self.visit(value, *args, **kwargs)
-
- def visitDict(self, obj, *args, **kwargs):
- """Called to visit any value that is a dictionary."""
- for value in obj.values():
- self.visit(value, *args, **kwargs)
-
- def visitLeaf(self, obj, *args, **kwargs):
- """Called to visit any value that is not an object, list,
- or dictionary."""
- pass
-
- def visit(self, obj, *args, **kwargs):
- """This is the main entry to the visitor. The visitor will visit object
- obj.
-
- The visitor will first determine if there is a registered (via
- @register()) visit function for the type of object. If there is, it
- will be called, and (visitor, obj, *args, **kwargs) will be passed to
- the user visit function.
-
- If there is no user-registered visit function, of if there is and it
- returns True, or it returns None (or doesn't return anything) and
- visitor.defaultStop is False (default), then the visitor will proceed
- to dispatch to one of self.visitObject(), self.visitList(),
- self.visitDict(), or self.visitLeaf() (any of which can be overriden in
- a subclass)."""
-
- visitorFunc = self._visitorsFor(obj).get(None, None)
- if visitorFunc is not None:
- ret = visitorFunc(self, obj, *args, **kwargs)
- if ret == False or (ret is None and self.defaultStop):
- return
- if hasattr(obj, "__dict__") and not isinstance(obj, enum.Enum):
- self.visitObject(obj, *args, **kwargs)
- elif isinstance(obj, list):
- self.visitList(obj, *args, **kwargs)
- elif isinstance(obj, dict):
- self.visitDict(obj, *args, **kwargs)
- else:
- self.visitLeaf(obj, *args, **kwargs)
diff --git a/spaces/DQChoi/gpt-demo/venv/lib/python3.11/site-packages/fontTools/ttLib/tables/M_V_A_R_.py b/spaces/DQChoi/gpt-demo/venv/lib/python3.11/site-packages/fontTools/ttLib/tables/M_V_A_R_.py
deleted file mode 100644
index 8371795eb2f2d2c233ec1725b8a2c21453170f23..0000000000000000000000000000000000000000
--- a/spaces/DQChoi/gpt-demo/venv/lib/python3.11/site-packages/fontTools/ttLib/tables/M_V_A_R_.py
+++ /dev/null
@@ -1,5 +0,0 @@
-from .otBase import BaseTTXConverter
-
-
-class table_M_V_A_R_(BaseTTXConverter):
- pass
diff --git a/spaces/DaFujaTyping/hf-Chat-ui/src/lib/shareConversation.ts b/spaces/DaFujaTyping/hf-Chat-ui/src/lib/shareConversation.ts
deleted file mode 100644
index 4768b604a42258d5d97231dd0e44f9198ef1864c..0000000000000000000000000000000000000000
--- a/spaces/DaFujaTyping/hf-Chat-ui/src/lib/shareConversation.ts
+++ /dev/null
@@ -1,27 +0,0 @@
-import { base } from "$app/paths";
-import { ERROR_MESSAGES, error } from "$lib/stores/errors";
-import { share } from "./utils/share";
-
-export async function shareConversation(id: string, title: string) {
- try {
- const res = await fetch(`${base}/conversation/${id}/share`, {
- method: "POST",
- headers: {
- "Content-Type": "application/json",
- },
- });
-
- if (!res.ok) {
- error.set("Error while sharing conversation, try again.");
- console.error("Error while sharing conversation: " + (await res.text()));
- return;
- }
-
- const { url } = await res.json();
-
- share(url, title);
- } catch (err) {
- error.set(ERROR_MESSAGES.default);
- console.error(err);
- }
-}
diff --git a/spaces/DaFujaTyping/hf-Chat-ui/src/lib/types/Message.ts b/spaces/DaFujaTyping/hf-Chat-ui/src/lib/types/Message.ts
deleted file mode 100644
index aee67c9b7049880ff2d4b2a9471270015b478a3f..0000000000000000000000000000000000000000
--- a/spaces/DaFujaTyping/hf-Chat-ui/src/lib/types/Message.ts
+++ /dev/null
@@ -1,5 +0,0 @@
-export interface Message {
- from: "user" | "assistant";
- id: ReturnType;
- content: string;
-}
diff --git a/spaces/Danielzero/GPT3.5/readme/README_ja.md b/spaces/Danielzero/GPT3.5/readme/README_ja.md
deleted file mode 100644
index fc56eec0b81c22ff0a49e3960aa52ffd7d6dc5cb..0000000000000000000000000000000000000000
--- a/spaces/Danielzero/GPT3.5/readme/README_ja.md
+++ /dev/null
@@ -1,126 +0,0 @@
-