diff --git a/spaces/1acneusushi/gradio-2dmoleculeeditor/data/Assimil Il Tedesco Senza Sforzo MP3 77.00M La soluzione ideale per imparare il tedesco da casa o in viaggio.md b/spaces/1acneusushi/gradio-2dmoleculeeditor/data/Assimil Il Tedesco Senza Sforzo MP3 77.00M La soluzione ideale per imparare il tedesco da casa o in viaggio.md
deleted file mode 100644
index 0fdc3c7c025bf2094cb64611fb11192052a1e7fe..0000000000000000000000000000000000000000
--- a/spaces/1acneusushi/gradio-2dmoleculeeditor/data/Assimil Il Tedesco Senza Sforzo MP3 77.00M La soluzione ideale per imparare il tedesco da casa o in viaggio.md
+++ /dev/null
@@ -1,150 +0,0 @@
-
-
Assimil Il Tedesco Senza Sforzo MP3 77.00M: Learn German Without Effort
-
Do you want to learn German in a fun, easy, and natural way? Do you want to improve your listening, speaking, reading, and writing skills in German? Do you want to access a comprehensive and effective course that covers all the aspects of the German language and culture? If you answered yes to any of these questions, then you should consider Assimil Il Tedesco Senza Sforzo MP3 77.00M as your ideal solution.
-
Assimil Il Tedesco Senza Sforzo MP3 77.00M is a digital version of the popular Assimil course that teaches you German without effort. It consists of an e-book with 100 lessons and an audio file with more than 77 minutes of dialogues and exercises in MP3 format. In this article, we will explain what Assimil Il Tedesco Senza Sforzo is, what MP3 77.00M means, and why you should learn German with this course.
Assimil Il Tedesco Senza Sforzo is the Italian edition of Assimil German With Ease, one of the most successful and renowned courses in the Assimil series. Assimil is a French company that has been producing language courses since 1929. It has a unique and proven method that allows you to learn a new language in the same way you learned your mother tongue: by listening, repeating, understanding, and speaking.
-
The history and philosophy of Assimil
-
The founder of Assimil, Alphonse Chérel, was a polyglot who spoke more than 20 languages. He was inspired by his own experience of learning languages through exposure and immersion. He developed a method that he called "assimilation", which is based on three principles:
-
-
Naturalness: You learn a language by following its natural progression, from simple to complex, from familiar to unfamiliar.
-
Intuitiveness: You learn a language by relying on your intuition, without memorizing rules or lists.
-
Humor: You learn a language by enjoying it, with humorous dialogues and situations that make you laugh and relax.
-
-
Chérel published his first course, L'Anglais Sans Peine (English Without Effort), in 1929. It was an instant success and soon he created courses for other languages, such as German, Spanish, Italian, Russian, and more. Today, Assimil offers more than 100 courses for over 50 languages, covering all levels from beginner to advanced.
-
The features and benefits of Assimil Il Tedesco Senza Sforzo
-
Assimil Il Tedesco Senza Sforzo is one of the best-selling courses in the Assimil series. It has many features and benefits that make it an ideal choice for anyone who wants to learn German without effort:
-
-
It covers all the aspects of the German language: grammar, vocabulary, pronunciation, idioms, culture, etc.
-
It follows a logical and gradual progression that adapts to your level and pace.
-
It uses authentic and realistic dialogues that reflect everyday situations and conversations.
-
It provides clear and concise explanations and notes that help you understand the language better.
-
It includes exercises and reviews that reinforce your learning and test your progress.
-
It offers tips and advice that help you improve your skills and avoid common mistakes.
-
-
By using Assimil Il Tedesco Senza Sforzo regularly, you will be able to achieve a level of fluency equivalent to B2 in the Common European Framework of Reference for Languages (CEFR). This means that you will be able to communicate effectively in most situations that require interaction with native speakers.
-
Assimil German without effort audio files download
-How to learn German with Assimil Il Tedesco Senza Sforzo
-Assimil Il Tedesco Senza Sforzo MP3 review and ratings
-Best price for Assimil Il Tedesco Senza Sforzo MP3 course
-Assimil Il Tedesco Senza Sforzo MP3 vs other German learning methods
-Where to buy Assimil Il Tedesco Senza Sforzo MP3 online
-Benefits of using Assimil Il Tedesco Senza Sforzo MP3 for German learners
-Assimil Il Tedesco Senza Sforzo MP3 free sample lessons
-How long does it take to finish Assimil Il Tedesco Senza Sforzo MP3
-Tips and tricks for using Assimil Il Tedesco Senza Sforzo MP3 effectively
-Assimil Il Tedesco Senza Sforzo MP3 testimonials and success stories
-How to access Assimil Il Tedesco Senza Sforzo MP3 on different devices
-What is the difference between Assimil Il Tedesco Senza Sforzo MP3 and PDF
-How to get the most out of Assimil Il Tedesco Senza Sforzo MP3
-Is Assimil Il Tedesco Senza Sforzo MP3 worth the money
-How to improve your pronunciation with Assimil Il Tedesco Senza Sforzo MP3
-How to use Assimil Il Tedesco Senza Sforzo MP3 with a tutor or a partner
-How to track your progress with Assimil Il Tedesco Senza Sforzo MP3
-How to supplement Assimil Il Tedesco Senza Sforzo MP3 with other resources
-How to troubleshoot common problems with Assimil Il Tedesco Senza Sforzo MP3
-How to customize Assimil Il Tedesco Senza Sforzo MP3 to your learning style and goals
-How to avoid boredom and frustration with Assimil Il Tedesco Senza Sforzo MP3
-How to make Assimil Il Tedesco Senza Sforzo MP3 fun and enjoyable
-How to integrate Assimil Il Tedesco Senza Sforzo MP3 into your daily routine
-How to overcome challenges and difficulties with Assimil Il Tedesco Senza Sforzo MP3
-How to review and revise with Assimil Il Tedesco Senza Sforzo MP3
-How to master German grammar and vocabulary with Assimil Il Tedesco Senza Sforzo MP3
-How to prepare for German exams and tests with Assimil Il Tedesco Senza Sforzo MP3
-How to communicate confidently in German with Assimil Il Tedesco Senza Sforzo MP3
-How to expand your German knowledge and skills with Assimil Il Tedesco Senza Sforzo MP3
-How to use Assimil Il Tedesco Senza Sforzo MP3 for travel and work purposes
-How to learn German culture and history with Assimil Il Tedesco Senza Sforzo MP3
-How to appreciate German literature and music with Assimil Il Tedesco Senza Sforzo MP3
-How to compare and contrast German and Italian languages with Assimil Il Tedesco Senza Sforzo MP3
-How to learn from your mistakes and errors with Assimil Il Tedesco Senza Sforzo MP3
-How to maintain and improve your German level with Assimil Il Tedesco Senza Sforzo MP3
-How to teach others German with Assimil Il Tedesco Senza Sforzo MP3
-How to create your own German content with Assimil Il Tedesco Senza Sforzo MP3
-How to join online communities of German learners using Assimil Il Tedesco Senza Sforzo MP3
-How to find more information and support for using Assimil Il Tedesco Senza Sforzo MP3
-Alternatives and competitors of Assimil Il Tedesco Senza Sforzo MP3 in the market
-Pros and cons of using Assimil Il Tedesco Senza Sforzo MP3 for learning German
-Frequently asked questions about Assimil Il Tedesco Senza Sforzo MP3 answered by experts
-Discounts and offers for buying or subscribing to Assimil Il Tedesco Senza Sforzo MP3
-Customer service and technical support for using Assimil Il Tedesco Senza Sforzo MP3
-
How to use Assimil Il Tedesco Senza Sforzo effectively
-
The key to using Assimil Il Tedesco Senza Sforzo effectively is to follow its simple but powerful method. The method consists of two phases: the passive phase and the active phase.
-
In the passive phase, which lasts for about 50 lessons, you will listen to the dialogues, read them aloud or silently, repeat them after the speaker, understand their meaning with the help of the notes and translations, and do some exercises. You will spend about 20 to 30 minutes per day on each lesson.
-
In the active phase, which starts from lesson 51 onwards, you will continue with the passive phase for the new lessons while reviewing the previous ones actively. This means that you will try to translate them from Italian into German without looking at the text or listening to the audio. You will also do some written exercises that will help you consolidate your knowledge. You will spend about 40 to 50 minutes per day on each lesson.
-
By following this method consistently for about six months, you will be able to master the basics of German and speak it with confidence.
-
What is MP3 77.00M?
-
MP3 77.00M is the digital format of the audio file that accompanies Assimil Il Tedesco Senza Sforzo. It contains more than 77 minutes of high-quality recordings by native speakers who speak clearly and naturally. It also includes some background music and sound effects that create a pleasant atmosphere for learning.
-
The advantages of MP3 format for language learning
-
The MP3 format has many advantages for language learning:
-
-
It is compatible with most devices: computers, smartphones, tablets, mp3 players, etc.
-
It is easy to download and store: you can get it online or via email in minutes.
-
It is portable and flexible: you can listen to it anywhere and anytime: at home, in your car, on public transport, while walking or jogging, etc.
-
It is interactive and engaging: you can pause it, rewind it, fast-forward it, repeat it as many times as you want.
-
It is effective and efficient: it helps you improve your listening comprehension, pronunciation, intonation, rhythm, accentuation,
-
...continued
-...continued
and fluency in German.
-
The contents and quality of Assimil Il Tedesco Senza Sforzo MP3 77.00M
-
The contents of Assimil Il Tedesco Senza Sforzo MP3 77.00M are divided into four parts:
-
-
The introduction: It explains how to use the course effectively and gives some general information about German.
-
The lessons: It contains all the dialogues from lesson 1 to lesson 100 with their corresponding translations into Italian.
-
The exercises: It contains all the oral exercises from lesson 1 to lesson 100 with their corresponding answers in German.
-
The appendix: It contains some additional material such as numbers, ...continued
education, research, etc.
-
-
Germany is a world leader in many fields and sectors, such as engineering, manufacturing, trade, tourism, education, research, etc. It has some of the most innovative and successful companies in the world, such as Volkswagen, BMW, Mercedes-Benz, Siemens, Bosch, SAP, Adidas, etc. It also has some of the most prestigious and renowned universities and research institutes in the world, such as Heidelberg University, Technical University of Munich, Max Planck Society, Fraunhofer Society, etc.
-
Learning German can open many doors for you and give you a competitive edge in the global market. You can also enjoy the rich and diverse culture and history of Germany and its neighboring countries.
-
The challenges and opportunities of learning German as a foreign language
-
Learning German as a foreign language can be challenging but also rewarding. German is often considered a difficult language because of its complex grammar, long words, and different cases. However, it also has many advantages and similarities to English and other languages:
-
-
It has a clear and logical structure that makes sense once you learn the rules.
-
It has many cognates and loanwords that are easy to recognize and remember.
-
It has a phonetic spelling that makes pronunciation easier.
-
It has a rich and expressive vocabulary that allows you to convey nuances and emotions.
-
-
Learning German can also offer you many opportunities to practice and improve your skills. You can access a wide range of resources and materials online or offline. You can watch movies and TV shows, listen to music and podcasts, read books and magazines, play games and apps, etc. You can also interact with native speakers and learners online or offline. You can join language exchange platforms, social media groups, online forums, etc. You can also travel to Germany or other German-speaking countries and immerse yourself in the language and culture.
-
The testimonials and reviews of Assimil Il Tedesco Senza Sforzo MP3 77.00M users
-
Many users of Assimil Il Tedesco Senza Sforzo MP3 77.00M have shared their positive experiences and feedback on various platforms. Here are some examples of what they have said:
-
-
"I have been using Assimil Il Tedesco Senza Sforzo MP3 77.00M for about three months now and I am very satisfied with it. It is easy to follow, fun to listen to, and very effective. I have learned a lot of vocabulary, grammar, and expressions in German. I can understand most of what I hear and read in German. I can also speak with confidence and fluency in German. I highly recommend this course to anyone who wants to learn German without effort."
-- Marco from Rome
-
-
-
"Assimil Il Tedesco Senza Sforzo MP3 77.00M is the best course I have ever used to learn German. It is comprehensive, engaging, and practical. It covers all the aspects of the language: listening, ...continued
speaking, reading, and writing. It has realistic and humorous dialogues that keep me interested and motivated. It has clear and concise explanations and notes that make me understand the language better. It has exercises and reviews that reinforce my learning and test my progress. It has tips and advice that help me improve my skills and avoid common mistakes. I have learned more German with this course than with any other method I have tried before."
-- Anna from Milan
-
-
-
"I love Assimil Il Tedesco Senza Sforzo MP3 77.00M. It is the perfect course for me. It is comprehensive, engaging, and practical. It covers all the aspects of the language: listening, speaking, reading, and writing. It has realistic and humorous dialogues that keep me interested and motivated. It has clear and concise explanations and notes that make me understand the language better. It has exercises and reviews that reinforce my learning and test my progress. It has tips and advice that help me improve my skills and avoid common mistakes. I have learned more German with this course than with any other method I have tried before."
-- Thomas from Berlin
-
-
Conclusion
-
Summary of the main points
-
In conclusion, Assimil Il Tedesco Senza Sforzo MP3 77.00M is a digital version of the popular Assimil course that teaches you German without effort. It consists of an e-book with 100 lessons and an audio file with more than 77 minutes of dialogues and exercises in MP3 format.
-
Assimil Il Tedesco Senza Sforzo MP3 77.00M is based on a unique and proven method that allows you to learn a new language in the same way you learned your mother tongue: by listening, repeating, understanding, and speaking.
-
Assimil Il Tedesco Senza Sforzo MP3 77.00M is easy to follow, fun to listen to, and very effective. It covers all the aspects of the German language: grammar, vocabulary, pronunciation, idioms, culture, etc. It follows a logical and gradual progression that adapts to your level and pace.
-
Assimil Il Tedesco Senza Sforzo MP3 77.00M is compatible with most devices: computers, smartphones, tablets, mp3 players, etc. It is easy to download and store: you can get it online or via email in minutes.
-
Learning German with Assimil Il Tedesco Senza Sforzo MP3 77.00M can enrich your personal and professional life in many ways: you can communicate with millions of people across different cultures and countries; you can access a vast amount of information and knowledge in various fields; you can travel to beautiful and fascinating places; you can enhance your career opportunities and prospects in many industries and sectors.
-
Call to action
-
If you are interested in learning German without effort, don't hesitate to get Assimil Il Tedesco Senza Sforzo MP3 77.00M today. You will not regret it.
-
You can order it online from the official Assimil website or from other authorized sellers.
-
You can also try a free sample lesson before you buy it.
-
Don't miss this opportunity to learn one of the most widely spoken languages in the world with one of the most successful courses in the world.
-
Get Assimil Il Tedesco Senza Sforzo MP3 77.00M now and start your journey to German fluency.
-
Frequently Asked Questions
-
-
What is the difference between Assimil Il Tedesco Senza Sforzo MP3 77.00M and Assimil Il Tedesco Senza Sforzo CD?
-
The main difference is the format of the audio file. The MP3 version has a single file with more than 77 minutes of recordings in MP3 format, while the CD version has four CDs with about 20 minutes of recordings each in WAV format.
-
How long does it take to complete Assimil Il Tedesco Senza Sforzo MP3 77.00M?
-
The duration of the course depends on your level, pace, and goals. However, a typical learner can complete it in about six months by spending about 30 minutes per day on each lesson.
-
Do I need any prior knowledge of German to use Assimil Il Tedesco Senza Sforzo MP3 77.00M?
-
No, you don't need any prior knowledge of German to use Assimil Il Tedesco Senza Sforzo MP3 77.00M. The course is designed for absolute beginners who want to learn German from scratch.
-
Can I use Assimil Il Tedesco Senza Sforzo MP3 77.00M without the e-book?
-
No, you can't use Assimil Il Tedesco Senza Sforzo MP3 77.00M without the e-book. The e-book is an essential part of the course that complements the audio file. It contains the dialogues, ...continued
the translations, the notes, the exercises, and the appendix. You need to read and study the e-book along with the audio file to get the most out of the course.
-
What level of German can I achieve with Assimil Il Tedesco Senza Sforzo MP3 77.00M?
-
By using Assimil Il Tedesco Senza Sforzo MP3 77.00M regularly, you can achieve a level of fluency equivalent to B2 in the Common European Framework of Reference for Languages (CEFR). This means that you can communicate effectively in most situations that require interaction with native speakers.
-
- 0a6ba089eb
-
-
\ No newline at end of file
diff --git a/spaces/1acneusushi/gradio-2dmoleculeeditor/data/DAEMON Tools Pro Advanced V5.1.0.0333 Admin Crack Download Pc The Complete Review and Comparison.md b/spaces/1acneusushi/gradio-2dmoleculeeditor/data/DAEMON Tools Pro Advanced V5.1.0.0333 Admin Crack Download Pc The Complete Review and Comparison.md
deleted file mode 100644
index 630a87ca87015eb033e1bd0be60decb14b5b53b9..0000000000000000000000000000000000000000
--- a/spaces/1acneusushi/gradio-2dmoleculeeditor/data/DAEMON Tools Pro Advanced V5.1.0.0333 Admin Crack Download Pc The Complete Review and Comparison.md
+++ /dev/null
@@ -1,113 +0,0 @@
-
-
AnyDVD-HD.7.2.3.0-Final-July 17,2k13.rar Serial Key Keygen
-
If you are a movie lover who wants to enjoy your DVD and Blu-ray collection on any device and software, you might be interested in AnyDVD HD. This is a powerful software that can remove any copy protection and region code from your discs, allowing you to watch them without any hassle. In this article, we will explain what AnyDVD HD is, how to install and activate it with serial key and keygen, why you need it, and where to download it.
-
What is AnyDVD HD?
-
AnyDVD HD is a software that works in the background to automatically and transparently enable read access of the contents of a movie DVD, Blu-ray, and HD DVD as soon as it's inserted into the drive. This means that you can use any DVD or Blu-ray backup software, such as CloneDVD, Pinnacle InstantCopy, Intervideo DVDCopy, and others, to copy or rip your discs without any problem. You can also play your discs on any DVD or Blu-ray player software, such as PowerDVD Ultra, VLC Media Player, Windows Media Player, and others, without worrying about region codes or HDCP-compliant graphics cards and displays.
-
AnyDVD-HD.7.2.3.0-Final-July 17,2k13.rar Serial Key Keygen
AnyDVD HD has many features and benefits that make it a must-have utility for the serious home theater enthusiast using a media center or home theater PC. Some of them are:
-
-
It can decrypt Blu-ray (BD+ and AACS) and HD DVD (AACS) movies, allowing you to watch them even if they are not supported by your hardware or software.
-
It can optionally disable the RPC region codes on DVDs and Blu-rays, making them region free and viewable on any DVD or Blu-ray player.
-
It can control the drive speed of your DVD or Blu-ray drive, reducing the noise level when watching movies on your PC.
-
It can adjust the display frequency of your monitor for both NTSC and PAL displays, improving the quality of the video output.
-
It can decrypt protected audio CDs, allowing you to copy them or play them on any CD player.
-
It can remove unwanted movie features, such as subtitles, logos, trailers, warnings, etc, giving you more control over what you watch.
-
It can enable an external application to launch whenever you insert or remove a disc, such as a media player or a backup software.
-
It can use magic file replacement to remaster any commercial movie disc using simple XML scripts, allowing you to customize discs as you like without making a copy to hard disk.
-
-
How to install and activate AnyDVD HD with serial key and keygen
-
To install and activate AnyDVD HD with serial key and keygen, you need to follow these steps:
-
-
Download the file AnyDVD-HD.7.2.3.0-Final-July 17,2k13.rar from a reliable source.
-
Extract the file using a program like WinRAR or 7-Zip.
-
Run the setup file SetupAnyDVD7230.exe and follow the instructions to install AnyDVD HD on your PC.
-
Run the keygen file Key.AnyDVDHD.exe and generate a serial key for AnyDVD HD.
-
Copy the serial key and paste it into the registration window of AnyDVD HD.
-
Click OK to activate AnyDVD HD with serial key.
-
-
Congratulations! You have successfully installed and activated AnyDVD HD with serial key and keygen. You can now enjoy all the features and benefits of this amazing software.
-
Why do you need AnyDVD HD?
-
You might be wondering why you need AnyDVD HD when there are other DVD and Blu-ray ripping software available. The answer is simple: AnyDVD HD offers more than just ripping. It offers a complete solution for watching movies on any device and software without any restrictions or limitations. Here are some reasons why you need AnyDVD HD:
-
Bypass copy protection and region codes on DVDs and Blu-rays
-
One of the main reasons why you need AnyDVD HD is that it can bypass any copy protection and region code on DVDs and Blu-rays. This means that you can make backup copies of your discs for personal use or watch them on any device or software regardless of where they were purchased or where you live. You don't have to worry about damaging your discs or losing them due to theft or natural disasters. You also don't have to buy multiple copies of the same movie for different regions or devices. With AnyDVD HD, you can enjoy your movie collection anywhere and anytime.
-
AnyDVD HD 7.2.3.0 Crack Download
-AnyDVD HD 7.2.3.0 Final Patch
-AnyDVD HD 7.2.3.0 License Key Free
-AnyDVD HD 7.2.3.0 Activation Code
-AnyDVD HD 7.2.3.0 Full Version
-AnyDVD HD 7.2.3.0 Keygen Torrent
-AnyDVD HD 7.2.3.0 Serial Number
-AnyDVD HD 7.2.3.0 Registration Code
-AnyDVD HD 7.2.3.0 Product Key
-AnyDVD HD 7.2.3.0 RAR File
-AnyDVD HD 7.2.3.0 Chocolatey Package
-AnyDVD HD 7.2.3.0 Blu-Ray Decrypter
-AnyDVD HD 7.2.3.0 DVD Ripper
-AnyDVD HD 7.2.3.0 Magic File Replacement
-AnyDVD HD 7.2.3.0 UDF 2.5 File Ripper
-AnyDVD HD 7.2.3.0 HDCP Bypass
-AnyDVD HD 7.2.3.0 Region Free
-AnyDVD HD 7.2.3.0 Download Link
-AnyDVD HD 7.2.3.0 TechSpot Review
-AnyDVD HD 7.2.3.0 Softpedia Rating
-How to Install AnyDVD HD 7.2.3 Final
-How to Use AnyDVD HD 7 Keygen
-How to Update AnyDVD HD to Latest Version
-How to Uninstall AnyDVD HD Completely
-How to Backup DVD with AnyDVD HD
-How to Remove Unwanted Features with AnyDVD HD
-How to Remaster Discs with AnyDVD HD Scripts
-How to Watch Blu-Ray Movies with AnyDVD HD and PowerDVD Ultra
-How to Burn Disc Images with AnyDVD HD and PowerISO
-How to Create Custom DVDs with AnyDVD HD and CDBurnerXP
-Best Alternatives to AnyDVD HD for Windows/Mac/Linux
-Compare Features of AnyDVD and AnyDVD HD
-Pros and Cons of Using AnyDVD HD Software
-Tips and Tricks for Getting the Most Out of AnyDVD HD
-Troubleshooting Common Problems with AnyDVD HD
-Customer Testimonials for AnyDVD HD Product
-Discount Coupons for Buying AnyDVD HD License
-Free Trial Download for AnyDVD HD Software
-Frequently Asked Questions about AnyDVD HD Program
-User Guide for AnyDVD HD Application
-
Watch movies on any device and software without restrictions
-
Another reason why you need AnyDVD HD is that it can enable you to watch movies on any device and software without restrictions. This means that you can play your discs on any DVD or Blu-ray player software, such as PowerDVD Ultra, VLC Media Player, Windows Media Player, etc., without having to install additional codecs or drivers. You can also watch your discs on any device that supports video playback, such as smartphones, tablets, laptops, TVs, etc., without having to convert them to different formats or resolutions. You don't have to worry about compatibility issues or quality loss. With AnyDVD HD, you can enjoy your movies on any device and software with ease.
-
Customize and enhance your movie experience with magic file replacement
-
A third reason why you need AnyDVD HD is that it can customize and enhance your movie experience with magic file replacement. This is a unique feature that allows you to remaster any commercial movie disc using simple XML scripts. You can change anything on the disc, such as menus, subtitles, audio tracks, logos, trailers, etc., according to your preferences. You can also add new features or enhancements, such as commentary tracks, deleted scenes, alternative endings, etc., that are not available on the original disc. You don't have to make a copy to hard disk or burn a new disc. You just need to insert the disc into your drive and let AnyDVD HD do its magic. With AnyDVD HD, you can customize and enhance your movie experience as you like.
-
Where to download AnyDVD HD?
-
If you are convinced that you need AnyDVD HD for your movie enjoyment, you might be wondering where to download it. There are several sources where you can get this software legally and safely. Here are some of them:
-
Official website of Redfox
-
The official website of Redfox is https://www.redfox.bz/en/anydvdhd.html . This is where you can find the latest version of AnyDVD HD along with other products from Redfox such as CloneBD, CloneCD, CloneDVD mobile etc. You can also find useful information such as FAQs , forums , news , updates , etc. You can download a free trial version of AnyDVD HD for 21 days from this website. If you want to buy a license for lifetime updates , you can do so for 109 EUR (about 123 USD) from this website. This is the most reliable source for downloading AnyDVD HD.
-
Chocolatey Software package manager
-
Run the keygen file Key.AnyDVDHD.exe and generate a serial key for AnyDVD HD.
-
Copy the serial key and paste it into the registration window of AnyDVD HD.
-
Click OK to activate AnyDVD HD with serial key.
-
-
Congratulations! You have successfully installed and activated AnyDVD HD (8.6.4.0) with serial key and keygen using TechSpot.
-
Conclusion
-
In this article, we have explained what AnyDVD HD is, how to install and activate it with serial key and keygen, why you need it, and where to download it. We have shown you three sources where you can get this software legally and safely: the official website of Redfox, the Chocolatey Software package manager, and the TechSpot download portal. We have also highlighted some of the features and benefits of AnyDVD HD that make it a great software for movie lovers who want to enjoy their DVD and Blu-ray collection on any device and software without any restrictions or limitations.
-
Summary of the main points
-
-
AnyDVD HD is a software that can remove any copy protection and region code from DVDs and Blu-rays, allowing you to watch them without any hassle.
-
AnyDVD HD can also control the drive speed, adjust the display frequency, decrypt audio CDs, remove unwanted features, launch external applications, and use magic file replacement to customize and enhance your movie experience.
-
AnyDVD HD can be installed and activated with serial key and keygen from various sources, such as the official website of Redfox, the Chocolatey Software package manager, and the TechSpot download portal.
-
-
Call to action and disclaimer
-
If you are interested in trying out AnyDVD HD for yourself, you can download a free trial version for 21 days from the official website of Redfox or buy a license for lifetime updates. You can also use Chocolatey Software or TechSpot to install older or newer versions of AnyDVD HD on your PC. However, please note that we are not affiliated with any of these sources and we are not responsible for any issues or damages that may arise from using them. Please use AnyDVD HD at your own risk and only for personal use. Do not distribute or share your serial key or keygen with anyone else. Respect the intellectual property rights of the movie studios and producers.
-
FAQs
-
-
What is the difference between AnyDVD and AnyDVD HD?
-
AnyDVD is a software that can remove copy protection and region codes from DVDs only. AnyDVD HD is a software that can do the same for DVDs, Blu-rays, and HD DVDs. AnyDVD HD also has additional features for full Blu-ray Disc and HD DVD support.
-
Is AnyDVD HD legal?
-
AnyDVD HD is legal to use for personal use in most countries. However, some countries may have laws that prohibit circumventing copy protection or region codes on DVDs and Blu-rays. Please check your local laws before using AnyDVD HD.
-
Does AnyDVD HD work with Windows 10?
-
Yes, AnyDVD HD works with Windows 10 as well as Windows 7, 8, 8.1, Vista, XP, and Server 2003. However, you may need to install .NET Framework 4 or higher if you don't have it already.
-
Does AnyDVD HD work with Netflix?
-
No, AnyDVD HD does not work with Netflix or other streaming services. AnyDVD HD only works with physical discs that you insert into your drive.
-
How can I update AnyDVD HD?
-
You can update AnyDVD HD by downloading the latest version from the official website of Redfox or by using Chocolatey Software or TechSpot. You can also enable automatic updates in the settings of AnyDVD HD. However, you may need to generate a new serial key and keygen for each update.
-
- 0a6ba089eb
-
-
\ No newline at end of file
diff --git a/spaces/1acneusushi/gradio-2dmoleculeeditor/data/Eurosoft Diagnostics.md b/spaces/1acneusushi/gradio-2dmoleculeeditor/data/Eurosoft Diagnostics.md
deleted file mode 100644
index d6e1eff41c7dd3f1ad1678f56f537e8bc0faee0f..0000000000000000000000000000000000000000
--- a/spaces/1acneusushi/gradio-2dmoleculeeditor/data/Eurosoft Diagnostics.md
+++ /dev/null
@@ -1,14 +0,0 @@
-
-
Eurosoft Diagnostics: The Best PC Diagnostic Software and Tools for Your Business
-
If you are looking for a reliable and comprehensive PC diagnostic software and tools for your business, you should consider Eurosoft Diagnostics. Eurosoft Diagnostics is a leading provider of PC diagnostic software and tools for various sectors such as computer manufacturing, repair, refurbishment, support, and education. Eurosoft Diagnostics helps you to quickly and accurately test and troubleshoot PC hardware issues, reduce costs, improve efficiency, and enhance customer satisfaction.
-
Eurosoft Diagnostics offers a range of PC diagnostic software and tools that suit different needs and scenarios. Some of the products include:
Pc-Check® Diagnostic Suite: This is a platinum award-winning product that includes Pc-Check UEFI and Pc-Check Windows. Pc-Check UEFI is a self-booting diagnostic software that tests PC hardware components without any operating system interference. It supports the latest UEFI systems and Secure Boot. Pc-Check Windows is a Windows-based diagnostic software that tests PC hardware components using software drivers. It also provides system information, benchmarking, and reporting features.
-
PC Builder Test Management Suite: This is a scalable and automated test solution for PC manufacturing and refurbishment. It allows you to create custom test scripts, manage test stations, monitor test results, generate reports, and integrate with third-party tools such as Windows imaging and data erasure.
-
ZeroData® Data Erasure: This is a secure and certified data erasure solution that wipes out all data from hard drives and solid state drives. It supports various erasure standards such as DoD 5220.22-M, NIST 800-88, and HMG Infosec No.5. It also provides audit-ready reports and certificates of erasure.
-
-
Eurosoft Diagnostics products are trusted by thousands of customers worldwide, including OEMs, ODMs, system builders, system integrators, R&D designers, Microsoft authorized refurbishers, IT asset recovery companies, computer recyclers, break-fix operations, repair depots, field technicians, computer shops, network administrators, IT professionals, managed service providers, help desk staff, training and education institutions, and more.
-
If you want to learn more about Eurosoft Diagnostics products and how they can benefit your business, visit https://www.eurosoft-uk.com/ today.
ddb901b051
-
-
\ No newline at end of file
diff --git a/spaces/1gistliPinn/ChatGPT4/Examples/Autodesk Revit 2020.2 [Crack Patch ] Torrent! _TOP_.md b/spaces/1gistliPinn/ChatGPT4/Examples/Autodesk Revit 2020.2 [Crack Patch ] Torrent! _TOP_.md
deleted file mode 100644
index e4a3a838ea3d6aea1ef7e85ce5667237767d961a..0000000000000000000000000000000000000000
--- a/spaces/1gistliPinn/ChatGPT4/Examples/Autodesk Revit 2020.2 [Crack Patch ] Torrent! _TOP_.md
+++ /dev/null
@@ -1,6 +0,0 @@
-
-
-Autodesk Revit 2020.2.2.0 Crack is a powerful software for making the ... If such a collaboration tool is required, companies adopt Revit free download full version with crack for checking the ... Auto-update and manipulation. 1fdad05405
-
-
-
diff --git a/spaces/1gistliPinn/ChatGPT4/Examples/Diablo Tactic Cm 03 04 25.md b/spaces/1gistliPinn/ChatGPT4/Examples/Diablo Tactic Cm 03 04 25.md
deleted file mode 100644
index 08ac380c4e0a86c84518c85c14d1d9c6cb03d869..0000000000000000000000000000000000000000
--- a/spaces/1gistliPinn/ChatGPT4/Examples/Diablo Tactic Cm 03 04 25.md
+++ /dev/null
@@ -1,15 +0,0 @@
-
-
How to Master the Diablo Tactic in Championship Manager 03/04
-
Championship Manager 03/04 is a classic football management game that still has a loyal fan base. One of the most popular and effective tactics in the game is the Diablo tactic, a wide 4-1-3-2 formation that produces a lot of goals and wins. Here are some tips on how to use this tactic and dominate your opponents.
The Diablo tactic was first shared online by a gamer named 'El Rosso Diablo', who claimed it was unbeatable[^3^]. The tactic is built around a free-roaming central midfielder with a 'forward arrow' right up to the central striker position. This creates a lot of space and movement for the attackers, while the defensive midfielder and the full-backs provide cover for the back four.
-
The key attributes for the players in this tactic are pace, stamina, creativity, passing, finishing and off-the-ball. You need fast and fit players who can run all game, create chances and score goals. You also need a solid goalkeeper and defenders who can cope with counter-attacks.
-
The best teams to use this tactic with are those with strong midfielders and strikers, such as Manchester United, Arsenal, Real Madrid or Barcelona. You can also use it with lower-league teams if you have some hidden gems or bargain signings. The tactic works well against most formations, except for those with five defenders or three strikers.
-
The main weakness of this tactic is that it can leave you vulnerable to long balls over the top or crosses from the wings. You need to adjust your defensive line and marking settings depending on your opponent's style and players. You can also tweak your attacking style and tempo depending on the situation. For example, you can play more direct and fast when you need to score, or more short and slow when you need to keep possession.
-
-
The Diablo tactic is not a cheat, but a clever exploitation of the game's mechanics. It can be very fun and rewarding to use, but it can also be frustrating and boring if you overuse it or face it too often. It is up to you to decide how much you want to rely on it or challenge yourself with other tactics. Either way, Championship Manager 03/04 is a game that never gets old.
If you want to learn more about the Diablo tactic and other tactics in Championship Manager 03/04, you can check out some online forums and guides. There are many passionate and knowledgeable fans who share their tips and experiences with the game. You can also watch some videos on YouTube or Twitch of players who use this tactic or challenge themselves with different ones.
-
Championship Manager 03/04 is a game that has stood the test of time and still has a loyal fan base. It is a game that can make you feel like a real football manager, with all the joys and sorrows that come with it. It is a game that can make you addicted and obsessed, but also entertained and satisfied. It is a game that you should try if you love football and management games.
- d5da3c52bf
-
-
\ No newline at end of file
diff --git a/spaces/1gistliPinn/ChatGPT4/Examples/Download [UPDATED] Solidcam 2013 Full Crack.md b/spaces/1gistliPinn/ChatGPT4/Examples/Download [UPDATED] Solidcam 2013 Full Crack.md
deleted file mode 100644
index 73761d22ba190cd371db9301e9ca1bbb98e46c38..0000000000000000000000000000000000000000
--- a/spaces/1gistliPinn/ChatGPT4/Examples/Download [UPDATED] Solidcam 2013 Full Crack.md
+++ /dev/null
@@ -1,40 +0,0 @@
-
-
-eXtension (X-STEP) tools for milling and drilling applications.
-
-It also has in the SolidCAM software a dedicated filter for the Open surface format, needed for rapid prototyping.
-
-Uses
-
-The High Speed Roughing module allows you to quickly roughen a mesh for fabrication.
-
-The High Speed Machining module allows you to precisely machine the mesh.
-
-Compatibility
-
-The cutter tools and main geometry of the High Speed Roughing and High Speed Machining modules are designed to work with each other. The modules can be imported and exported in the Open surface format, which is supported by most CAD systems.
-
-References
-
-External links
-
- Official website
-
- 3D Drafting & Milling on YouTube
-
- Official community
-
- Official Forum
-
-Category:CAM softwareThe present invention relates to a liquid crystal display device comprising a display screen on which an image is formed by a liquid crystal material.
-
-Recently, as the number of pixels in a display device increases, there is an increasing demand for realizing a large-screen, high-resolution, high-quality, and high-quality color display device. However, in order to realize such a display device, the display screen must have a sufficiently large area, and in this case, a display device with a large area tends to have a large number of pixels and a correspondingly high cost.
-
-As a method of realizing a display device with a large area and a correspondingly low cost, there is known an approach which comprises forming a desired display screen with only a plurality of pixels and connecting the pixels with thin film transistors (TFTs) so as to form a matrix, and a display device by the above-mentioned approach is generally called a flat panel display device. In particular, in a liquid crystal display device, since a display screen is formed by a liquid crystal material and a light transmittance of the liquid crystal material varies depending on an electric field applied to the liquid crystal material, it is possible to display a desired image by changing the electric field.
-
-In the case where the display device is a liquid crystal display device, a desired electric field is applied to a liquid crystal material by using a pair of electrodes sandwiching the liquid crystal material in the display screen, and a pair of electrodes sandwiching a liquid crystal material in the display screen in this way are generally referred to as a pixel electrode and a common electrode, respectively.
-
-As the above-mentioned liquid crystal display device 4fefd39f24
-
-
-
diff --git a/spaces/1pelhydcardo/ChatGPT-prompt-generator/assets/Dolphin Emulator How to Play GameCube and Wii Games on Your PC.md b/spaces/1pelhydcardo/ChatGPT-prompt-generator/assets/Dolphin Emulator How to Play GameCube and Wii Games on Your PC.md
deleted file mode 100644
index c19841b84e51c3dc0f512569fc828ca892082782..0000000000000000000000000000000000000000
--- a/spaces/1pelhydcardo/ChatGPT-prompt-generator/assets/Dolphin Emulator How to Play GameCube and Wii Games on Your PC.md
+++ /dev/null
@@ -1,88 +0,0 @@
-
-
How to Download Dolphin Emulator for PC
-
Dolphin emulator is a software that allows you to play games from Nintendo GameCube and Wii consoles on your computer. It is one of the most popular and advanced emulators available, with many features and options to enhance your gaming experience. In this article, I will provide you with some information on how to download, install, and configure dolphin emulator for pc, as well as some of the pros and cons of using it.
The first step to use dolphin emulator is to download it from the official website. You can choose between two types of versions: beta versions and development versions. Beta versions are released every month and are more stable and tested than development versions. Development versions are released every time a developer makes a change to the emulator, and may have new features or bug fixes, but also more potential issues. You can download either version from this page. The Windows versions require the 64-bit Visual C++ redistributable for Visual Studio 2022 to be installed, which you can get from here.
-
Installing dolphin emulator
-
Once you have downloaded the dolphin emulator file, you need to extract it into a new folder (preferably named after the version) or to replace an existing dolphin setup. You can use any program that can handle ZIP files, such as 7-Zip or WinRAR. After extracting the file, you can run the dolphin.exe file to launch the emulator. You don't need to install anything else.
-
If you are using Mac or Linux, you may need to make the file executable before running it. You can do this by right-clicking on the file, choosing Properties, and checking the Execute permission box. Alternatively, you can use the terminal command chmod +x filename.
-
Configuring dolphin emulator
-
Dolphin emulator has two main configuration windows: Dolphin configuration and Graphics settings. You can access them by clicking on the Config and Graphics buttons on the main toolbar. You can also apply settings per game via their GameINI files, which are located in the Dolphin Emulator folder under User/GameSettings.
-
Dolphin configuration
-
The Dolphin configuration window lets you adjust general settings such as emulation speed, dual core mode, audio output, controller input, memory cards, cheats, and more. Here are some recommended settings for optimal performance:
-
How to download dolphin emulator for pc windows 10
-Download dolphin emulator for pc 32 bit
-Download dolphin emulator for pc latest version
-Download dolphin emulator for pc with games
-Download dolphin emulator for pc full speed
-Download dolphin emulator for pc reddit
-Download dolphin emulator for pc free
-Download dolphin emulator for pc mac
-Download dolphin emulator for pc linux
-Download dolphin emulator for pc android
-Download dolphin emulator for pc apk
-Download dolphin emulator for pc iso
-Download dolphin emulator for pc roms
-Download dolphin emulator for pc wii
-Download dolphin emulator for pc gamecube
-Download dolphin emulator for pc 4k
-Download dolphin emulator for pc 60fps
-Download dolphin emulator for pc cheats
-Download dolphin emulator for pc bios
-Download dolphin emulator for pc setup
-Download dolphin emulator for pc offline installer
-Download dolphin emulator for pc highly compressed
-Download dolphin emulator for pc no lag
-Download dolphin emulator for pc best settings
-Download dolphin emulator for pc controller support
-Download dolphin emulator for pc keyboard and mouse
-Download dolphin emulator for pc netplay
-Download dolphin emulator for pc multiplayer
-Download dolphin emulator for pc steam
-Download dolphin emulator for pc portable
-Download dolphin emulator for pc zip file
-Download dolphin emulator for pc rar file
-Download dolphin emulator for pc softonic
-Download dolphin emulator for pc uptodown
-Download dolphin emulator for pc filehippo
-Download dolphin emulator for pc ocean of games
-Download dolphin emulator for pc igg games
-Download dolphin emulator for pc skidrow reloaded
-Download dolphin emulator for pc fitgirl repack
-Download dolphin emulator for pc crack only
-Download dolphin emulator for pc patch notes
-Download dolphin emulator for pc system requirements
-Download dolphin emulator for pc tutorial guide
-Download dolphin emulator for pc review rating
-Download dolphin emulator for pc comparison test
-Download dolphin emulator for pc tips tricks hacks
-Download dolphin emulator for pc mods addons plugins
-Download dolphin emulator for pc save data transfer
-Download dolphin emulator for pc error fix solution
-Download dolphin emulator for pc update download
-
-
Enable Dual Core: This option allows you to use two CPU cores for emulation, which can significantly improve speed and compatibility. However, it may also cause some random crashes or glitches in some games.
-
Enable Cheats: This option allows you to use cheat codes for various games. You can find cheat codes online or create your own using a hex editor. To enable cheats for a specific game, right-click on it in the game list, choose Properties, and check the Enable Cheats box.
-
Audio Backend: This option determines how audio is processed by the emulator. The best option depends on your system and preferences. For Windows users, XAudio2 or Cubeb are recommended. For Mac users, Cubeb or OpenAL are recommended. For Linux users, PulseAudio or ALSA are recommended.
-
Controller Settings: This option lets you configure your controller input for different types of emulated controllers: GameCube controller, Wii remote (with or without nunchuk), classic controller, guitar controller, etc. You can use any XInput-compatible gamepad (such as an Xbox One controller) or a USB adapter for original controllers.
-
-
How do I update dolphin emulator to the latest version?
-
To update dolphin emulator to the latest version, you can either download the new version from the official website and replace your existing setup, or use the built-in updater feature. To use the updater, click on the Help button on the main toolbar, and choose Check for Updates. If there is a new version available, you can download and install it automatically.
-
How do I add games to dolphin emulator?
-
To add games to dolphin emulator, you need to have the game files in ISO or WBFS format. You can either dump your own games from original discs using a Wii console and a USB loader, or download them from legal sources such as Nintendo eShop. Once you have the game files, you can place them in any folder on your computer, and then add that folder to dolphin emulator's game list. To do that, click on the Config button on the main toolbar, and choose Paths. Then, click on Add and browse to the folder where your games are located. You can also remove or edit any existing paths.
-
How do I play online with dolphin emulator?
-
To play online with dolphin emulator, you have two options: Netplay or Wiimmfi. Netplay is a feature that allows you to play local multiplayer games over the internet with other dolphin users. To use Netplay, you need to have the same game and dolphin version as your partner, and a stable internet connection. You can either host or join a Netplay session by clicking on the Tools button on the main toolbar, and choosing Start Netplay. You can also chat with your partner using the built-in chat window.
-
Wiimmfi is a service that allows you to play online multiplayer games that originally used Nintendo Wi-Fi Connection, which was discontinued in 2014. To use Wiimmfi, you need to have a valid Wii console ID and a patched game ISO that supports Wiimmfi. You can find more information on how to get these from this page. Once you have them, you can launch the game from dolphin emulator and connect to Wiimmfi as you would normally do on a Wii console.
-
How do I fix common issues with dolphin emulator?
-
Dolphin emulator is a complex software that may encounter some issues depending on your system and game settings. Some of the common issues and their possible solutions are:
-
-
Black screen or crash on startup: This may be caused by a missing or corrupted file in your dolphin setup. Try downloading and extracting a new version of dolphin emulator from the official website.
-
Slow or choppy gameplay: This may be caused by insufficient system resources or improper graphics settings. Try lowering the internal resolution, anti-aliasing, anisotropic filtering, or enhancements in the Graphics settings window. You can also enable some hacks in the Hacks section that may improve performance.
-
Audio distortion or stuttering: This may be caused by incompatible audio backend or improper audio settings. Try changing the audio backend in the Dolphin configuration window to match your system and preferences. You can also adjust the volume, latency, or DSP emulation mode in the Audio settings window.
-
Controller not working or detected: This may be caused by incorrect controller settings or driver issues. Try configuring your controller input in the Controller settings window and make sure it matches the type of emulated controller you want to use. You can also check if your controller is recognized by your system and update its drivers if necessary.
-
-
Where can I find more information and support for dolphin emulator?
-
If you want to learn more about dolphin emulator and its features, you can visit the official website, where you can find documentation, guides, forums, blogs, videos, and more. You can also join the official Discord server, where you can chat with other users and developers, ask questions, share feedback, and get help.
197e85843d
-
-
\ No newline at end of file
diff --git a/spaces/1pelhydcardo/ChatGPT-prompt-generator/assets/Download 2048 Mod APK for Android and IOS The Ultimate Puzzle Game.md b/spaces/1pelhydcardo/ChatGPT-prompt-generator/assets/Download 2048 Mod APK for Android and IOS The Ultimate Puzzle Game.md
deleted file mode 100644
index 2237507f8f8be98bbea9bc3ab22c07cd556d7c3c..0000000000000000000000000000000000000000
--- a/spaces/1pelhydcardo/ChatGPT-prompt-generator/assets/Download 2048 Mod APK for Android and IOS The Ultimate Puzzle Game.md
+++ /dev/null
@@ -1,116 +0,0 @@
-
-
2048 Mod APK: A Fun and Addictive Puzzle Game
-
If you are looking for a simple yet challenging puzzle game that can keep you entertained for hours, you might want to try 2048 mod apk. This is a modified version of the original 2048 game that offers more features and benefits for the players. In this article, we will tell you everything you need to know about 2048 mod apk, including what it is, how to play it, why it is so popular, what are its features, how to download and install it, and what are its pros and cons.
2048 is a puzzle game that was created by Gabriele Cirulli in 2014. The game is inspired by other similar games such as Threes and 1024. The goal of the game is to slide numbered tiles on a 4x4 grid and combine them to create a tile with the number 2048. The game is over when there are no more moves left or when the player reaches the 2048 tile.
-
How to play 2048?
-
The game is very easy to play. You just need to swipe your finger on the screen to move the tiles in the direction you want. When two tiles with the same number touch, they merge into one tile with the sum of their numbers. For example, if you swipe left and there are two tiles with the number 2 on the leftmost column, they will merge into one tile with the number 4. You can also use the arrow keys on your keyboard if you are playing on a computer.
-
Why is 2048 so popular?
-
There are many reasons why 2048 is so popular among puzzle game lovers. Some of them are:
-
-
The game is simple but challenging. It does not require any special skills or knowledge, but it still tests your logic and strategy.
-
The game is addictive. It makes you want to play more and more until you reach the highest score possible.
-
The game is relaxing. It does not have any time limit or pressure, so you can play it at your own pace and enjoy the soothing sound effects and music.
-
The game is fun. It gives you a sense of satisfaction and achievement when you create a new tile or beat your previous score.
-
-
What is 2048 mod apk?
-
2048 mod apk is a modified version of the original 2048 game that offers more features and benefits for the players. It is not available on the official app stores, but you can download it from third-party websites such as Apkloli. By downloading and installing 2048 mod apk, you can enjoy the following features:
-
2048 mod apk unlimited money
-2048 mod apk download for android
-2048 mod apk latest version
-2048 mod apk no ads
-2048 mod apk ios
-2048 mod apk free download
-2048 mod apk hack
-2048 mod apk revdl
-2048 mod apk apkpure
-2048 mod apk rexdl
-2048 mod apk offline
-2048 mod apk online
-2048 mod apk with cheat menu
-2048 mod apk unlimited undo
-2048 mod apk unlimited coins
-2048 mod apk unlimited gems
-2048 mod apk unlimited moves
-2048 mod apk unlimited time
-2048 mod apk unlimited hints
-2048 mod apk unlimited stars
-2048 mod apk premium
-2048 mod apk pro
-2048 mod apk plus
-2048 mod apk mega
-2048 mod apk vip
-2048 mod apk original
-2048 mod apk classic
-2048 mod apk puzzle
-2048 mod apk adventure
-2048 mod apk challenge
-2048 mod apk fun
-2048 mod apk cute
-2048 mod apk cool
-2048 mod apk awesome
-2048 mod apk best
-2048 mod apk new
-2048 mod apk old
-2048 mod apk updated
-2048 mod apk full version
-2048 mod apk cracked version
-
Features of 2048 mod apk
-
Unlimited money
-
With 2048 mod apk, you can get unlimited money that you can use to buy various items in the game. For example, you can buy hints that can help you make better moves, or boosters that can increase your score or remove unwanted tiles.
-
No ads
-
Another benefit of 2048 mod apk is that it removes all the annoying ads that interrupt your gameplay. You can play the game without any distractions or interruptions.
-
Custom themes
-
If you are bored with the default theme of the game, you can change it with 2048 mod apk. You can choose from different themes such as animals, fruits, flowers, colors, emojis, and more. You can also create your own theme by using your own images and sounds.
-
Undo and redo moves
-
Sometimes, you might regret making a certain move or want to try a different strategy. With 2048 mod apk, you can undo and redo your moves as many times as you want. This can help you avoid mistakes and improve your chances of winning.
-
How to download and install 2048 mod apk?
-
If you want to download and install 2048 mod apk, you need to follow these simple steps:
-
-
Go to the website where you can download 2048 mod apk, such as Apkloli. Make sure you choose a reliable and safe source.
-
Click on the download button and wait for the file to be downloaded on your device.
-
Go to your device settings and enable the installation of apps from unknown sources. This is necessary because 2048 mod apk is not from the official app stores.
-
Locate the downloaded file and tap on it to start the installation process. Follow the instructions on the screen and wait for the installation to be completed.
-
Launch the game and enjoy playing 2048 mod apk with all its features.
-
-
Pros and cons of 2048 mod apk
-
Like any other app, 2048 mod apk has its pros and cons. Here are some of them:
-
Pros
-
-
It enhances the gameplay experience by adding more features and options.
-
It allows you to customize the game according to your preferences and tastes.
-
It eliminates the ads that can disrupt your concentration and enjoyment.
-
It gives you unlimited money that you can use to buy useful items and boosters.
-
It lets you undo and redo your moves as much as you want.
-
-
Cons
-
-
It is not available on the official app stores, so you need to download it from third-party websites that may not be secure or trustworthy.
-
It may not be compatible with some devices or operating systems.
-
It may cause some glitches or errors in the game performance or functionality.
-
It may violate the terms and conditions of the original game developer or publisher.
-
It may reduce the challenge and difficulty of the game by making it too easy or unfair.
-
-
Conclusion
-
In conclusion, 2048 mod apk is a fun and addictive puzzle game that offers more features and benefits than the original 2048 game. It allows you to play the game with unlimited money, no ads, custom themes, undo and redo moves, and more. However, it also has some drawbacks, such as being unavailable on the official app stores, causing some technical issues, and violating some rules. Therefore, you should weigh the pros and cons before downloading and installing 2048 mod apk on your device. If you decide to try it, make sure you download it from a reliable and safe source, such as Apkloli. We hope this article has been helpful and informative for you. Thank you for reading!
-
Frequently Asked Questions
-
Here are some of the most common questions that people ask about 2048 mod apk:
-
Q: Is 2048 mod apk free?
-
A: Yes, 2048 mod apk is free to download and play. You do not need to pay any money to enjoy its features and benefits.
-
Q: Is 2048 mod apk safe?
-
A: It depends on where you download it from. Some websites may offer fake or malicious files that can harm your device or steal your data. Therefore, you should always download 2048 mod apk from a reputable and trusted source, such as Apkloli. You should also scan the file with an antivirus program before installing it.
-
Q: Is 2048 mod apk legal?
-
A: It is not clear whether 2048 mod apk is legal or not. It may depend on the laws and regulations of your country or region. Some countries may allow modifying or hacking apps for personal use, while others may prohibit or penalize such activities. You should also consider the rights and interests of the original game developer or publisher, who may not approve of modifying or distributing their app without their permission or consent. Therefore, you should use 2048 mod apk at your own risk and responsibility.
-
Q: How can I update 2048 mod apk?
-
A: Since 2048 mod apk is not from the official app stores, you cannot update it automatically or manually through them. You need to download the latest version of 2048 mod apk from the same website where you downloaded the previous version. You should also check the website regularly for any updates or news about 2048 mod apk.
-
Q: How can I uninstall 2048 mod apk?
-
A: If you want to uninstall 2048 mod apk from your device, you can follow these steps:
-
-
Go to your device settings and find the apps or applications section.
-
Find and tap on 2048 mod apk from the list of installed apps.
-
Tap on the uninstall button and confirm your action.
-
Wait for the app to be uninstalled from your device.
- 197e85843d
-
-
\ No newline at end of file
diff --git a/spaces/1phancelerku/anime-remove-background/1v1 Battle Challenge Your Friends and Enemies in Epic Duels.md b/spaces/1phancelerku/anime-remove-background/1v1 Battle Challenge Your Friends and Enemies in Epic Duels.md
deleted file mode 100644
index 8f18db55cfbd8a8856baf9ad6a39ab7552ce1412..0000000000000000000000000000000000000000
--- a/spaces/1phancelerku/anime-remove-background/1v1 Battle Challenge Your Friends and Enemies in Epic Duels.md
+++ /dev/null
@@ -1,111 +0,0 @@
-
-
What is a 1v1 Battle?
-
A 1v1 battle is a type of multiplayer video game that pits two players against each other in a virtual arena. The goal is to eliminate the opponent or score more points than them before the time runs out. 1v1 battles can be played in different genres, such as shooting, fighting, racing, or strategy games.
-
Some of the benefits of playing 1v1 battle games are:
They test your skills and reflexes in a fast-paced and intense environment.
-
They allow you to showcase your creativity and style in building, editing, or trickshotting.
-
They provide you with feedback and motivation to improve your performance.
-
-
Some of the challenges of playing 1v1 battle games are:
-
-
They can be frustrating and stressful if you lose or encounter toxic players.
-
They can be repetitive and boring if you play the same mode or map over and over.
-
They can be addictive and harmful if you neglect your health or other responsibilities.
-
-
Some of the popular 1v1 battle games are:
-
-
Game
Description
Platform
-
Fortnite
A battle royale game that features building, editing, and shooting mechanics.
PC, console, mobile
-
Call of Duty
A first-person shooter game that features various weapons, maps, and modes.
PC, console, mobile
-
Mortal Kombat
A fighting game that features brutal combat, fatalities, and characters.
PC, console, mobile
-
Mario Kart
A racing game that features items, tracks, and characters from the Mario franchise.
Console, mobile
-
Chess
A strategy game that features pieces, moves, and rules based on medieval warfare.
PC, mobile, board
-
-
How to Play 1v1 Battle Games?
-
The basic controls and mechanics of 1v1 battle games vary depending on the genre and the game. However, some common elements are:
-
-
You need to use your keyboard or controller to move, aim, shoot, build, or perform other actions.
-
You need to use your mouse or screen to look around, select items, or interact with objects.
-
You need to use your headphones or speakers to hear sounds, music, or voice chat.
-
You need to use your monitor or device to see graphics, text, or menus.
-
-
Some tips and tricks for winning 1v1 battles are:
-
-
You need to practice your skills regularly and learn from your mistakes.
-
You need to study your opponent's behavior and habits and adapt your strategy accordingly.
-
You need to use your environment and resources wisely and creatively.
-
You need to communicate and cooperate with your teammate if you are playing in a team mode.
-
You need to have fun and enjoy the game without getting too angry or arrogant.
-
-
Some resources for learning and improving your 1v1 battle skills are:
-
-
You can watch videos or streams of professional or popular players and learn from their techniques and tips.
-
You can read articles or guides that explain the rules, strategies, and tips of different games and modes.
-
You can join online communities or forums that discuss, share, or review 1v1 battle games and content.
-
You can participate in tournaments or events that challenge your skills and reward your achievements.
-
You can ask for feedback or advice from other players or coaches who have more experience or knowledge.
-
-
How to Enjoy 1v1 Battle Games?
-
Playing 1v1 battle games can be fun and exciting, but it can also be stressful and boring if you don't know how to enjoy them. Here are some ways to make your 1v1 battle gaming experience more enjoyable:
-
-
You can try different game modes and features that suit your preferences and goals. For example, you can play casual or ranked matches, solo or duo modes, or custom or random maps.
-
You can customize and personalize your game settings and appearance to make them more comfortable and appealing. For example, you can adjust your sensitivity, resolution, or sound levels, or change your skin, outfit, or weapon.
-
You can socialize and compete with other players who share your interest and passion for 1v1 battle games. For example, you can chat, voice call, or message with your friends, opponents, or teammates, or join a clan, guild, or team.
-
-
Conclusion
-
1v1 battle games are a type of multiplayer video game that pits two players against each other in a virtual arena. They can be played in different genres, such as shooting, fighting, racing, or strategy games. They can test your skills and reflexes, allow you to showcase your creativity and style, and provide you with feedback and motivation. However, they can also be frustrating and stressful, repetitive and boring, and addictive and harmful. Therefore, you need to know how to play and enjoy them properly. You need to practice your skills regularly, study your opponent's behavior, use your environment and resources wisely, communicate and cooperate with your teammate, have fun and enjoy the game, try different game modes and features, customize and personalize your game settings and appearance, and socialize and compete with other players.
-
If you are interested in playing 1v1 battle games, you can check out some of the popular ones mentioned in this article. You can also watch videos or streams of professional or popular players, read articles or guides that explain the rules, strategies, and tips of different games and modes, join online communities or forums that discuss, share, or review 1v1 battle games and content, participate in tournaments or events that challenge your skills and reward your achievements, or ask for feedback or advice from other players or coaches who have more experience or knowledge.
-
1v1 battle royale
-1v1 build fight
-1v1.lol
-1v1 battle games
-1v1 battle simulator
-1v1 battle online
-1v1 battle crazy games
-1v1 battle codes
-1v1 battle fortnite
-1v1 battle arena
-1v1 battle minecraft
-1v1 battle roblox
-1v1 battle shooting
-1v1 battle unblocked
-1v1 battle apk
-1v1 battle app
-1v1 battle download
-1v1 battle hack
-1v1 battle mod
-1v1 battle pc
-1v1 build fight map
-1v1 build fight codes
-1v1 build fight simulator
-1v1 build fight practice
-1v1 build fight creative code
-1v1 build fight server
-1v1 build fight tips
-1v1 build fight training
-1v1 build fight tutorial
-1v1 build fight website
-1v.lol game
-1v.lol unblocked
-1v.lol aim trainer
-1v.lol justbuild.lol
-1v.lol box fight code
-1v.lol party mode code
-1v.lol controls pc
-1v.lol discord server
-1v.lol hacks download
-2 player games online free play now fighting games unblocked at school.
-
Are you ready to enter the 1v1 battle arena? Let us know what you think about 1v1 battle games in the comments below!
-
FAQs
-
Here are some of the frequently asked questions about 1v1 battle games:
-
-
What are the best 1v1 battle games? The answer to this question depends on your personal preference and taste. However, some of the factors that you can consider when choosing a 1v1 battle game are: the genre, the graphics, the gameplay, the difficulty level, the replay value, the popularity, the reviews, the price, and the availability.
-
How do I get better at 1v1 battle games? The best way to get better at 1v1 battle games is to practice regularly and learn from your mistakes. You can also watch videos or streams of professional or popular players and learn from their techniques and tips. You can also read articles or guides that explain the rules, strategies, and tips of different games and modes. You can also join online communities or forums that discuss, share, or review 1v1 battle games and content. You can also participate in tournaments or events that challenge your skills and reward your achievements. You can also ask for feedback or advice from other players or coaches who have more experience or knowledge.
-
How do I find opponents for 1v1 battle games? There are different ways to find opponents for 1v1 battle games. You can play online matches with random players who are matched with you based on your skill level or region. You can invite your friends or contacts to play with you privately or publicly. You can join a clan, guild, or team that has other members who play the same game as you. You can use a third-party platform or service that connects you with other players who are looking for 1v1 battles.
-
How do I deal with toxic players in 1v1 battle games? Toxic players are those who behave in a rude, abusive, or unsportsmanlike manner in 1v1 battle games. They may insult, harass, troll, cheat, or rage quit during or after the game. They may also ruin the game experience for other players by spamming, griefing, hacking, or teaming. To deal with toxic players, you can do the following: ignore them, mute them, block them, report them, or avoid them.
-
How do I balance my time and health when playing 1v1 battle games? Playing 1v1 battle games can be fun and rewarding, but it can also be addictive and harmful if you neglect your time and health. To balance your time and health when playing 1v1 battle games, you can do the following: set a limit on how long and how often you play, take breaks and stretch regularly, drink water and eat healthy snacks, sleep well and rest enough, exercise and stay active, socialize and interact with other people, and pursue other hobbies and interests.
- 401be4b1e0
-
-
\ No newline at end of file
diff --git a/spaces/A00001/bingothoo/src/app/page.tsx b/spaces/A00001/bingothoo/src/app/page.tsx
deleted file mode 100644
index 0dff3431b098ce4fe282cc83fc87a93a28a43090..0000000000000000000000000000000000000000
--- a/spaces/A00001/bingothoo/src/app/page.tsx
+++ /dev/null
@@ -1,15 +0,0 @@
-import dynamic from 'next/dynamic'
-
-const DynamicComponentWithNoSSR = dynamic(
- () => import('../components/chat'),
- { ssr: false }
-)
-
-export default function IndexPage() {
- return (
- <>
-
-
- >
- )
-}
diff --git a/spaces/AI-Hobbyist/Hoyo-RVC/infer_pack/modules/F0Predictor/HarvestF0Predictor.py b/spaces/AI-Hobbyist/Hoyo-RVC/infer_pack/modules/F0Predictor/HarvestF0Predictor.py
deleted file mode 100644
index 98d4e98b353008f81bde2c37e7da818763a992c9..0000000000000000000000000000000000000000
--- a/spaces/AI-Hobbyist/Hoyo-RVC/infer_pack/modules/F0Predictor/HarvestF0Predictor.py
+++ /dev/null
@@ -1,86 +0,0 @@
-from infer_pack.modules.F0Predictor.F0Predictor import F0Predictor
-import pyworld
-import numpy as np
-
-
-class HarvestF0Predictor(F0Predictor):
- def __init__(self, hop_length=512, f0_min=50, f0_max=1100, sampling_rate=44100):
- self.hop_length = hop_length
- self.f0_min = f0_min
- self.f0_max = f0_max
- self.sampling_rate = sampling_rate
-
- def interpolate_f0(self, f0):
- """
- 对F0进行插值处理
- """
-
- data = np.reshape(f0, (f0.size, 1))
-
- vuv_vector = np.zeros((data.size, 1), dtype=np.float32)
- vuv_vector[data > 0.0] = 1.0
- vuv_vector[data <= 0.0] = 0.0
-
- ip_data = data
-
- frame_number = data.size
- last_value = 0.0
- for i in range(frame_number):
- if data[i] <= 0.0:
- j = i + 1
- for j in range(i + 1, frame_number):
- if data[j] > 0.0:
- break
- if j < frame_number - 1:
- if last_value > 0.0:
- step = (data[j] - data[i - 1]) / float(j - i)
- for k in range(i, j):
- ip_data[k] = data[i - 1] + step * (k - i + 1)
- else:
- for k in range(i, j):
- ip_data[k] = data[j]
- else:
- for k in range(i, frame_number):
- ip_data[k] = last_value
- else:
- ip_data[i] = data[i] # 这里可能存在一个没有必要的拷贝
- last_value = data[i]
-
- return ip_data[:, 0], vuv_vector[:, 0]
-
- def resize_f0(self, x, target_len):
- source = np.array(x)
- source[source < 0.001] = np.nan
- target = np.interp(
- np.arange(0, len(source) * target_len, len(source)) / target_len,
- np.arange(0, len(source)),
- source,
- )
- res = np.nan_to_num(target)
- return res
-
- def compute_f0(self, wav, p_len=None):
- if p_len is None:
- p_len = wav.shape[0] // self.hop_length
- f0, t = pyworld.harvest(
- wav.astype(np.double),
- fs=self.hop_length,
- f0_ceil=self.f0_max,
- f0_floor=self.f0_min,
- frame_period=1000 * self.hop_length / self.sampling_rate,
- )
- f0 = pyworld.stonemask(wav.astype(np.double), f0, t, self.fs)
- return self.interpolate_f0(self.resize_f0(f0, p_len))[0]
-
- def compute_f0_uv(self, wav, p_len=None):
- if p_len is None:
- p_len = wav.shape[0] // self.hop_length
- f0, t = pyworld.harvest(
- wav.astype(np.double),
- fs=self.sampling_rate,
- f0_floor=self.f0_min,
- f0_ceil=self.f0_max,
- frame_period=1000 * self.hop_length / self.sampling_rate,
- )
- f0 = pyworld.stonemask(wav.astype(np.double), f0, t, self.sampling_rate)
- return self.interpolate_f0(self.resize_f0(f0, p_len))
diff --git a/spaces/AIConsultant/MusicGen/tests/adversarial/test_discriminators.py b/spaces/AIConsultant/MusicGen/tests/adversarial/test_discriminators.py
deleted file mode 100644
index fad89a0ae4534dc7967b6ccda194b9fd1dedbffe..0000000000000000000000000000000000000000
--- a/spaces/AIConsultant/MusicGen/tests/adversarial/test_discriminators.py
+++ /dev/null
@@ -1,67 +0,0 @@
-# Copyright (c) Meta Platforms, Inc. and affiliates.
-# All rights reserved.
-#
-# This source code is licensed under the license found in the
-# LICENSE file in the root directory of this source tree.
-
-import random
-
-import torch
-
-from audiocraft.adversarial.discriminators import (
- MultiPeriodDiscriminator,
- MultiScaleDiscriminator,
- MultiScaleSTFTDiscriminator
-)
-
-
-class TestMultiPeriodDiscriminator:
-
- def test_mpd_discriminator(self):
- N, C, T = 2, 2, random.randrange(1, 100_000)
- t0 = torch.randn(N, C, T)
- periods = [1, 2, 3]
- mpd = MultiPeriodDiscriminator(periods=periods, in_channels=C)
- logits, fmaps = mpd(t0)
-
- assert len(logits) == len(periods)
- assert len(fmaps) == len(periods)
- assert all([logit.shape[0] == N and len(logit.shape) == 4 for logit in logits])
- assert all([feature.shape[0] == N for fmap in fmaps for feature in fmap])
-
-
-class TestMultiScaleDiscriminator:
-
- def test_msd_discriminator(self):
- N, C, T = 2, 2, random.randrange(1, 100_000)
- t0 = torch.randn(N, C, T)
-
- scale_norms = ['weight_norm', 'weight_norm']
- msd = MultiScaleDiscriminator(scale_norms=scale_norms, in_channels=C)
- logits, fmaps = msd(t0)
-
- assert len(logits) == len(scale_norms)
- assert len(fmaps) == len(scale_norms)
- assert all([logit.shape[0] == N and len(logit.shape) == 3 for logit in logits])
- assert all([feature.shape[0] == N for fmap in fmaps for feature in fmap])
-
-
-class TestMultiScaleStftDiscriminator:
-
- def test_msstftd_discriminator(self):
- N, C, T = 2, 2, random.randrange(1, 100_000)
- t0 = torch.randn(N, C, T)
-
- n_filters = 4
- n_ffts = [128, 256, 64]
- hop_lengths = [32, 64, 16]
- win_lengths = [128, 256, 64]
-
- msstftd = MultiScaleSTFTDiscriminator(filters=n_filters, n_ffts=n_ffts, hop_lengths=hop_lengths,
- win_lengths=win_lengths, in_channels=C)
- logits, fmaps = msstftd(t0)
-
- assert len(logits) == len(n_ffts)
- assert len(fmaps) == len(n_ffts)
- assert all([logit.shape[0] == N and len(logit.shape) == 4 for logit in logits])
- assert all([feature.shape[0] == N for fmap in fmaps for feature in fmap])
diff --git a/spaces/AIGC-Audio/AudioGPT/audio_to_text/captioning/utils/model_eval_diff.py b/spaces/AIGC-Audio/AudioGPT/audio_to_text/captioning/utils/model_eval_diff.py
deleted file mode 100644
index 2c29ef8fde2451d3f84e842d0d6a72754f0d4603..0000000000000000000000000000000000000000
--- a/spaces/AIGC-Audio/AudioGPT/audio_to_text/captioning/utils/model_eval_diff.py
+++ /dev/null
@@ -1,110 +0,0 @@
-import os
-import sys
-import copy
-import pickle
-
-import numpy as np
-import pandas as pd
-import fire
-
-sys.path.append(os.getcwd())
-
-
-def coco_score(refs, pred, scorer):
- if scorer.method() == "Bleu":
- scores = np.array([ 0.0 for n in range(4) ])
- else:
- scores = 0
- num_cap_per_audio = len(refs[list(refs.keys())[0]])
-
- for i in range(num_cap_per_audio):
- if i > 0:
- for key in refs:
- refs[key].insert(0, res[key][0])
- res = {key: [refs[key].pop(),] for key in refs}
- score, _ = scorer.compute_score(refs, pred)
-
- if scorer.method() == "Bleu":
- scores += np.array(score)
- else:
- scores += score
-
- score = scores / num_cap_per_audio
-
- for key in refs:
- refs[key].insert(0, res[key][0])
- score_allref, _ = scorer.compute_score(refs, pred)
- diff = score_allref - score
- return diff
-
-def embedding_score(refs, pred, scorer):
-
- num_cap_per_audio = len(refs[list(refs.keys())[0]])
- scores = 0
-
- for i in range(num_cap_per_audio):
- res = {key: [refs[key][i],] for key in refs.keys() if len(refs[key]) == num_cap_per_audio}
- refs_i = {key: np.concatenate([refs[key][:i], refs[key][i+1:]]) for key in refs.keys() if len(refs[key]) == num_cap_per_audio}
- score, _ = scorer.compute_score(refs_i, pred)
-
- scores += score
-
- score = scores / num_cap_per_audio
-
- score_allref, _ = scorer.compute_score(refs, pred)
- diff = score_allref - score
- return diff
-
-def main(output_file, eval_caption_file, eval_embedding_file, output, zh=False):
- output_df = pd.read_json(output_file)
- output_df["key"] = output_df["filename"].apply(lambda x: os.path.splitext(os.path.basename(x))[0])
- pred = output_df.groupby("key")["tokens"].apply(list).to_dict()
-
- label_df = pd.read_json(eval_caption_file)
- if zh:
- refs = label_df.groupby("key")["tokens"].apply(list).to_dict()
- else:
- refs = label_df.groupby("key")["caption"].apply(list).to_dict()
-
- from pycocoevalcap.bleu.bleu import Bleu
- from pycocoevalcap.cider.cider import Cider
- from pycocoevalcap.rouge.rouge import Rouge
-
- scorer = Bleu(zh=zh)
- bleu_scores = coco_score(copy.deepcopy(refs), pred, scorer)
- scorer = Cider(zh=zh)
- cider_score = coco_score(copy.deepcopy(refs), pred, scorer)
- scorer = Rouge(zh=zh)
- rouge_score = coco_score(copy.deepcopy(refs), pred, scorer)
-
- if not zh:
- from pycocoevalcap.meteor.meteor import Meteor
- scorer = Meteor()
- meteor_score = coco_score(copy.deepcopy(refs), pred, scorer)
-
- from pycocoevalcap.spice.spice import Spice
- scorer = Spice()
- spice_score = coco_score(copy.deepcopy(refs), pred, scorer)
-
- # from audiocaptioneval.sentbert.sentencebert import SentenceBert
- # scorer = SentenceBert(zh=zh)
- # with open(eval_embedding_file, "rb") as f:
- # ref_embeddings = pickle.load(f)
-
- # sent_bert = embedding_score(ref_embeddings, pred, scorer)
-
- with open(output, "w") as f:
- f.write("Diff:\n")
- for n in range(4):
- f.write("BLEU-{}: {:6.3f}\n".format(n+1, bleu_scores[n]))
- f.write("CIDEr: {:6.3f}\n".format(cider_score))
- f.write("ROUGE: {:6.3f}\n".format(rouge_score))
- if not zh:
- f.write("Meteor: {:6.3f}\n".format(meteor_score))
- f.write("SPICE: {:6.3f}\n".format(spice_score))
- # f.write("SentenceBert: {:6.3f}\n".format(sent_bert))
-
-
-
-if __name__ == "__main__":
- fire.Fire(main)
diff --git a/spaces/AIGC-Audio/Make_An_Audio_inpaint/ldm/lr_scheduler.py b/spaces/AIGC-Audio/Make_An_Audio_inpaint/ldm/lr_scheduler.py
deleted file mode 100644
index be39da9ca6dacc22bf3df9c7389bbb403a4a3ade..0000000000000000000000000000000000000000
--- a/spaces/AIGC-Audio/Make_An_Audio_inpaint/ldm/lr_scheduler.py
+++ /dev/null
@@ -1,98 +0,0 @@
-import numpy as np
-
-
-class LambdaWarmUpCosineScheduler:
- """
- note: use with a base_lr of 1.0
- """
- def __init__(self, warm_up_steps, lr_min, lr_max, lr_start, max_decay_steps, verbosity_interval=0):
- self.lr_warm_up_steps = warm_up_steps
- self.lr_start = lr_start
- self.lr_min = lr_min
- self.lr_max = lr_max
- self.lr_max_decay_steps = max_decay_steps
- self.last_lr = 0.
- self.verbosity_interval = verbosity_interval
-
- def schedule(self, n, **kwargs):
- if self.verbosity_interval > 0:
- if n % self.verbosity_interval == 0: print(f"current step: {n}, recent lr-multiplier: {self.last_lr}")
- if n < self.lr_warm_up_steps:
- lr = (self.lr_max - self.lr_start) / self.lr_warm_up_steps * n + self.lr_start
- self.last_lr = lr
- return lr
- else:
- t = (n - self.lr_warm_up_steps) / (self.lr_max_decay_steps - self.lr_warm_up_steps)
- t = min(t, 1.0)
- lr = self.lr_min + 0.5 * (self.lr_max - self.lr_min) * (
- 1 + np.cos(t * np.pi))
- self.last_lr = lr
- return lr
-
- def __call__(self, n, **kwargs):
- return self.schedule(n,**kwargs)
-
-
-class LambdaWarmUpCosineScheduler2:
- """
- supports repeated iterations, configurable via lists
- note: use with a base_lr of 1.0.
- """
- def __init__(self, warm_up_steps, f_min, f_max, f_start, cycle_lengths, verbosity_interval=0):
- assert len(warm_up_steps) == len(f_min) == len(f_max) == len(f_start) == len(cycle_lengths)
- self.lr_warm_up_steps = warm_up_steps
- self.f_start = f_start
- self.f_min = f_min
- self.f_max = f_max
- self.cycle_lengths = cycle_lengths
- self.cum_cycles = np.cumsum([0] + list(self.cycle_lengths))
- self.last_f = 0.
- self.verbosity_interval = verbosity_interval
-
- def find_in_interval(self, n):
- interval = 0
- for cl in self.cum_cycles[1:]:
- if n <= cl:
- return interval
- interval += 1
-
- def schedule(self, n, **kwargs):
- cycle = self.find_in_interval(n)
- n = n - self.cum_cycles[cycle]
- if self.verbosity_interval > 0:
- if n % self.verbosity_interval == 0: print(f"current step: {n}, recent lr-multiplier: {self.last_f}, "
- f"current cycle {cycle}")
- if n < self.lr_warm_up_steps[cycle]:
- f = (self.f_max[cycle] - self.f_start[cycle]) / self.lr_warm_up_steps[cycle] * n + self.f_start[cycle]
- self.last_f = f
- return f
- else:
- t = (n - self.lr_warm_up_steps[cycle]) / (self.cycle_lengths[cycle] - self.lr_warm_up_steps[cycle])
- t = min(t, 1.0)
- f = self.f_min[cycle] + 0.5 * (self.f_max[cycle] - self.f_min[cycle]) * (
- 1 + np.cos(t * np.pi))
- self.last_f = f
- return f
-
- def __call__(self, n, **kwargs):
- return self.schedule(n, **kwargs)
-
-
-class LambdaLinearScheduler(LambdaWarmUpCosineScheduler2):
-
- def schedule(self, n, **kwargs):
- cycle = self.find_in_interval(n)
- n = n - self.cum_cycles[cycle]
- if self.verbosity_interval > 0:
- if n % self.verbosity_interval == 0: print(f"current step: {n}, recent lr-multiplier: {self.last_f}, "
- f"current cycle {cycle}")
-
- if n < self.lr_warm_up_steps[cycle]:
- f = (self.f_max[cycle] - self.f_start[cycle]) / self.lr_warm_up_steps[cycle] * n + self.f_start[cycle]
- self.last_f = f
- return f
- else:
- f = self.f_min[cycle] + (self.f_max[cycle] - self.f_min[cycle]) * (self.cycle_lengths[cycle] - n) / (self.cycle_lengths[cycle])
- self.last_f = f
- return f
-
diff --git a/spaces/AIGC-Audio/Make_An_Audio_inpaint/ldm/modules/losses_audio/vggishish/model.py b/spaces/AIGC-Audio/Make_An_Audio_inpaint/ldm/modules/losses_audio/vggishish/model.py
deleted file mode 100644
index d5069bad0d9311e6e2c082a63eca165f7a908675..0000000000000000000000000000000000000000
--- a/spaces/AIGC-Audio/Make_An_Audio_inpaint/ldm/modules/losses_audio/vggishish/model.py
+++ /dev/null
@@ -1,77 +0,0 @@
-import torch
-import torch.nn as nn
-
-
-class VGGishish(nn.Module):
-
- def __init__(self, conv_layers, use_bn, num_classes):
- '''
- Mostly from
- https://pytorch.org/vision/0.8/_modules/torchvision/models/vgg.html
- '''
- super().__init__()
- layers = []
- in_channels = 1
-
- # a list of channels with 'MP' (maxpool) from config
- for v in conv_layers:
- if v == 'MP':
- layers += [nn.MaxPool2d(kernel_size=2, stride=2)]
- else:
- conv2d = nn.Conv2d(in_channels, v, kernel_size=3, padding=1, stride=1)
- if use_bn:
- layers += [conv2d, nn.BatchNorm2d(v), nn.ReLU(inplace=True)]
- else:
- layers += [conv2d, nn.ReLU(inplace=True)]
- in_channels = v
- self.features = nn.Sequential(*layers)
-
- self.avgpool = nn.AdaptiveAvgPool2d((5, 10))
-
- self.flatten = nn.Flatten()
- self.classifier = nn.Sequential(
- nn.Linear(512 * 5 * 10, 4096),
- nn.ReLU(True),
- nn.Linear(4096, 4096),
- nn.ReLU(True),
- nn.Linear(4096, num_classes)
- )
-
- # weight init
- self.reset_parameters()
-
- def forward(self, x):
- # adding channel dim for conv2d (B, 1, F, T) <-
- x = x.unsqueeze(1)
- # backbone (B, 1, 5, 53) <- (B, 1, 80, 860)
- x = self.features(x)
- # adaptive avg pooling (B, 1, 5, 10) <- (B, 1, 5, 53) – if no MP is used as the end of VGG
- x = self.avgpool(x)
- # flatten
- x = self.flatten(x)
- # classify
- x = self.classifier(x)
- return x
-
- def reset_parameters(self):
- for m in self.modules():
- if isinstance(m, nn.Conv2d):
- nn.init.kaiming_normal_(m.weight, mode='fan_out', nonlinearity='relu')
- if m.bias is not None:
- nn.init.constant_(m.bias, 0)
- elif isinstance(m, nn.BatchNorm2d):
- nn.init.constant_(m.weight, 1)
- nn.init.constant_(m.bias, 0)
- elif isinstance(m, nn.Linear):
- nn.init.normal_(m.weight, 0, 0.01)
- nn.init.constant_(m.bias, 0)
-
-
-if __name__ == '__main__':
- num_classes = 309
- inputs = torch.rand(3, 80, 848)
- conv_layers = [64, 64, 'MP', 128, 128, 'MP', 256, 256, 256, 'MP', 512, 512, 512, 'MP', 512, 512, 512]
- # conv_layers = [64, 'MP', 128, 'MP', 256, 256, 'MP', 512, 512, 'MP']
- model = VGGishish(conv_layers, use_bn=False, num_classes=num_classes)
- outputs = model(inputs)
- print(outputs.shape)
diff --git a/spaces/AIZero2HeroBootcamp/MultiPDF-QA-ChatGPT-Langchain/README.md b/spaces/AIZero2HeroBootcamp/MultiPDF-QA-ChatGPT-Langchain/README.md
deleted file mode 100644
index 3cf50f86a7888fbf02aa4eb54ebdd698b5d9533f..0000000000000000000000000000000000000000
--- a/spaces/AIZero2HeroBootcamp/MultiPDF-QA-ChatGPT-Langchain/README.md
+++ /dev/null
@@ -1,13 +0,0 @@
----
-title: MultiPDF QA ChatGPT Langchain
-emoji: 🏃
-colorFrom: pink
-colorTo: indigo
-sdk: streamlit
-sdk_version: 1.21.0
-app_file: app.py
-pinned: false
-license: mit
----
-
-Check out the configuration reference at https://huggingface.co/docs/hub/spaces-config-reference
diff --git a/spaces/ASJMO/freegpt/g4f/Provider/__init__.py b/spaces/ASJMO/freegpt/g4f/Provider/__init__.py
deleted file mode 100644
index 6ed51982755367e47c59199975be2c3539bfbee0..0000000000000000000000000000000000000000
--- a/spaces/ASJMO/freegpt/g4f/Provider/__init__.py
+++ /dev/null
@@ -1,36 +0,0 @@
-from . import Provider
-from .Providers import (
- Aichat,
- Ails,
- AiService,
- Bard,
- Better,
- Bing,
- ChatFree,
- ChatgptAi,
- ChatgptLogin,
- ChatgptLogin,
- DeepAi,
- Easychat,
- Ezcht,
- Fakeopen,
- Forefront,
- GetGpt,
- Gravityengine,
- H2o,
- hteyun,
- Liaobots,
- Lockchat,
- Mishalsgpt,
- Phind,
- Theb,
- Vercel,
- Weuseing,
- Xiaor,
- Yqcloud,
- You,
- Zeabur,
- Wewordle
-)
-
-Palm = Bard
diff --git a/spaces/Accel/media-converter/styles.css b/spaces/Accel/media-converter/styles.css
deleted file mode 100644
index 6386dbf86134ca6be4a9cea99e5cd7d927cd491f..0000000000000000000000000000000000000000
--- a/spaces/Accel/media-converter/styles.css
+++ /dev/null
@@ -1,9 +0,0 @@
-#outputtext {
- color: green;
-}
-#acontrast {
- width: 50%;
-}
-#button{
- width: 30%
-}
\ No newline at end of file
diff --git a/spaces/Adapter/T2I-Adapter/ldm/util.py b/spaces/Adapter/T2I-Adapter/ldm/util.py
deleted file mode 100644
index dc9e3c48b1924fbc1ac3ecdf7a2192e1a46d9228..0000000000000000000000000000000000000000
--- a/spaces/Adapter/T2I-Adapter/ldm/util.py
+++ /dev/null
@@ -1,200 +0,0 @@
-import importlib
-import math
-
-import cv2
-import torch
-import numpy as np
-
-import os
-from safetensors.torch import load_file
-
-from inspect import isfunction
-from PIL import Image, ImageDraw, ImageFont
-
-
-def log_txt_as_img(wh, xc, size=10):
- # wh a tuple of (width, height)
- # xc a list of captions to plot
- b = len(xc)
- txts = list()
- for bi in range(b):
- txt = Image.new("RGB", wh, color="white")
- draw = ImageDraw.Draw(txt)
- font = ImageFont.truetype('assets/DejaVuSans.ttf', size=size)
- nc = int(40 * (wh[0] / 256))
- lines = "\n".join(xc[bi][start:start + nc] for start in range(0, len(xc[bi]), nc))
-
- try:
- draw.text((0, 0), lines, fill="black", font=font)
- except UnicodeEncodeError:
- print("Cant encode string for logging. Skipping.")
-
- txt = np.array(txt).transpose(2, 0, 1) / 127.5 - 1.0
- txts.append(txt)
- txts = np.stack(txts)
- txts = torch.tensor(txts)
- return txts
-
-
-def ismap(x):
- if not isinstance(x, torch.Tensor):
- return False
- return (len(x.shape) == 4) and (x.shape[1] > 3)
-
-
-def isimage(x):
- if not isinstance(x, torch.Tensor):
- return False
- return (len(x.shape) == 4) and (x.shape[1] == 3 or x.shape[1] == 1)
-
-
-def exists(x):
- return x is not None
-
-
-def default(val, d):
- if exists(val):
- return val
- return d() if isfunction(d) else d
-
-
-def mean_flat(tensor):
- """
- https://github.com/openai/guided-diffusion/blob/27c20a8fab9cb472df5d6bdd6c8d11c8f430b924/guided_diffusion/nn.py#L86
- Take the mean over all non-batch dimensions.
- """
- return tensor.mean(dim=list(range(1, len(tensor.shape))))
-
-
-def count_params(model, verbose=False):
- total_params = sum(p.numel() for p in model.parameters())
- if verbose:
- print(f"{model.__class__.__name__} has {total_params * 1.e-6:.2f} M params.")
- return total_params
-
-
-def instantiate_from_config(config):
- if not "target" in config:
- if config == '__is_first_stage__':
- return None
- elif config == "__is_unconditional__":
- return None
- raise KeyError("Expected key `target` to instantiate.")
- return get_obj_from_str(config["target"])(**config.get("params", dict()))
-
-
-def get_obj_from_str(string, reload=False):
- module, cls = string.rsplit(".", 1)
- if reload:
- module_imp = importlib.import_module(module)
- importlib.reload(module_imp)
- return getattr(importlib.import_module(module, package=None), cls)
-
-
-checkpoint_dict_replacements = {
- 'cond_stage_model.transformer.text_model.embeddings.': 'cond_stage_model.transformer.embeddings.',
- 'cond_stage_model.transformer.text_model.encoder.': 'cond_stage_model.transformer.encoder.',
- 'cond_stage_model.transformer.text_model.final_layer_norm.': 'cond_stage_model.transformer.final_layer_norm.',
-}
-
-
-def transform_checkpoint_dict_key(k):
- for text, replacement in checkpoint_dict_replacements.items():
- if k.startswith(text):
- k = replacement + k[len(text):]
-
- return k
-
-
-def get_state_dict_from_checkpoint(pl_sd):
- pl_sd = pl_sd.pop("state_dict", pl_sd)
- pl_sd.pop("state_dict", None)
-
- sd = {}
- for k, v in pl_sd.items():
- new_key = transform_checkpoint_dict_key(k)
-
- if new_key is not None:
- sd[new_key] = v
-
- pl_sd.clear()
- pl_sd.update(sd)
-
- return pl_sd
-
-
-def read_state_dict(checkpoint_file, print_global_state=False):
- _, extension = os.path.splitext(checkpoint_file)
- if extension.lower() == ".safetensors":
- pl_sd = load_file(checkpoint_file, device='cpu')
- else:
- pl_sd = torch.load(checkpoint_file, map_location='cpu')
-
- if print_global_state and "global_step" in pl_sd:
- print(f"Global Step: {pl_sd['global_step']}")
-
- sd = get_state_dict_from_checkpoint(pl_sd)
- return sd
-
-
-def load_model_from_config(config, ckpt, vae_ckpt=None, verbose=False):
- print(f"Loading model from {ckpt}")
- sd = read_state_dict(ckpt)
- model = instantiate_from_config(config.model)
- m, u = model.load_state_dict(sd, strict=False)
- if len(m) > 0 and verbose:
- print("missing keys:")
- print(m)
- if len(u) > 0 and verbose:
- print("unexpected keys:")
- print(u)
-
- if 'anything' in ckpt.lower() and vae_ckpt is None:
- vae_ckpt = 'models/anything-v4.0.vae.pt'
-
- if vae_ckpt is not None and vae_ckpt != 'None':
- print(f"Loading vae model from {vae_ckpt}")
- vae_sd = torch.load(vae_ckpt, map_location="cpu")
- if "global_step" in vae_sd:
- print(f"Global Step: {vae_sd['global_step']}")
- sd = vae_sd["state_dict"]
- m, u = model.first_stage_model.load_state_dict(sd, strict=False)
- if len(m) > 0 and verbose:
- print("missing keys:")
- print(m)
- if len(u) > 0 and verbose:
- print("unexpected keys:")
- print(u)
-
- model.cuda()
- model.eval()
- return model
-
-
-def resize_numpy_image(image, max_resolution=512 * 512, resize_short_edge=None):
- h, w = image.shape[:2]
- if resize_short_edge is not None:
- k = resize_short_edge / min(h, w)
- else:
- k = max_resolution / (h * w)
- k = k**0.5
- h = int(np.round(h * k / 64)) * 64
- w = int(np.round(w * k / 64)) * 64
- image = cv2.resize(image, (w, h), interpolation=cv2.INTER_LANCZOS4)
- return image
-
-
-# make uc and prompt shapes match via padding for long prompts
-null_cond = None
-
-def fix_cond_shapes(model, prompt_condition, uc):
- if uc is None:
- return prompt_condition, uc
- global null_cond
- if null_cond is None:
- null_cond = model.get_learned_conditioning([""])
- while prompt_condition.shape[1] > uc.shape[1]:
- uc = torch.cat((uc, null_cond.repeat((uc.shape[0], 1, 1))), axis=1)
- while prompt_condition.shape[1] < uc.shape[1]:
- prompt_condition = torch.cat((prompt_condition, null_cond.repeat((prompt_condition.shape[0], 1, 1))), axis=1)
- return prompt_condition, uc
diff --git a/spaces/AgentVerse/agentVerse/ui/src/phaser3-rex-plugins/templates/spinner/ball/Factory.js b/spaces/AgentVerse/agentVerse/ui/src/phaser3-rex-plugins/templates/spinner/ball/Factory.js
deleted file mode 100644
index 7f85ebb1283bc434998ea7ce8a49d42b2623d67a..0000000000000000000000000000000000000000
--- a/spaces/AgentVerse/agentVerse/ui/src/phaser3-rex-plugins/templates/spinner/ball/Factory.js
+++ /dev/null
@@ -1,13 +0,0 @@
-import Ball from './Ball.js';
-import ObjectFactory from '../ObjectFactory.js';
-import SetValue from '../../../plugins/utils/object/SetValue.js';
-
-ObjectFactory.register('ball', function (config) {
- var gameObject = new Ball(this.scene, config);
- this.scene.add.existing(gameObject);
- return gameObject;
-});
-
-SetValue(window, 'RexPlugins.Spinner.Ball', Ball);
-
-export default Ball;
\ No newline at end of file
diff --git a/spaces/AgentVerse/agentVerse/ui/src/phaser3-rex-plugins/templates/ui/sides/childbehaviors/Fade.js b/spaces/AgentVerse/agentVerse/ui/src/phaser3-rex-plugins/templates/ui/sides/childbehaviors/Fade.js
deleted file mode 100644
index 32566fc8ce0f8cc950e24ac06771041c0d56d781..0000000000000000000000000000000000000000
--- a/spaces/AgentVerse/agentVerse/ui/src/phaser3-rex-plugins/templates/ui/sides/childbehaviors/Fade.js
+++ /dev/null
@@ -1,36 +0,0 @@
-import IndexOf from '../../../../plugins/utils/object/IndexOf.js';
-import { WaitComplete } from '../../utils/WaitEvent.js';
-
-export default {
- fadeChild(child, duration, alpha) {
- var key;
- if (typeof (child) === 'string') {
- key = child;
- child = this.sizerChildren[key];
- } else {
- key = IndexOf(this.sizerChildren, child);
- }
- if (duration === undefined) {
- duration = 500;
- }
- if (alpha === undefined) {
- alpha = (this.currentChildKey === key) ? 1 : 0;
- }
-
- child.fadeIn(duration, { start: child.alpha, end: alpha });
- return this;
- },
-
- fadeChildPromise(child, duration, alpha) {
- if (typeof (child) === 'string') {
- child = this.sizerChildren[key];
- }
- this.fadeChild(child, duration, alpha);
-
- if (child._fade) {
- return WaitComplete(child._fade);
- } else {
- return Promise.resolve();
- }
- }
-}
\ No newline at end of file
diff --git a/spaces/Androidonnxfork/CivitAi-to-Diffusers/diffusers/examples/instruct_pix2pix/README_sdxl.md b/spaces/Androidonnxfork/CivitAi-to-Diffusers/diffusers/examples/instruct_pix2pix/README_sdxl.md
deleted file mode 100644
index 8e3e6c881235dc20436966b7f59c773eadda297b..0000000000000000000000000000000000000000
--- a/spaces/Androidonnxfork/CivitAi-to-Diffusers/diffusers/examples/instruct_pix2pix/README_sdxl.md
+++ /dev/null
@@ -1,148 +0,0 @@
-# InstructPix2Pix SDXL training example
-
-***This is based on the original InstructPix2Pix training example.***
-
-[Stable Diffusion XL](https://huggingface.co/papers/2307.01952) (or SDXL) is the latest image generation model that is tailored towards more photorealistic outputs with more detailed imagery and composition compared to previous SD models. It leverages a three times larger UNet backbone. The increase of model parameters is mainly due to more attention blocks and a larger cross-attention context as SDXL uses a second text encoder.
-
-The `train_instruct_pix2pix_xl.py` script shows how to implement the training procedure and adapt it for Stable Diffusion XL.
-
-***Disclaimer: Even though `train_instruct_pix2pix_xl.py` implements the InstructPix2Pix
-training procedure while being faithful to the [original implementation](https://github.com/timothybrooks/instruct-pix2pix) we have only tested it on a [small-scale dataset](https://huggingface.co/datasets/fusing/instructpix2pix-1000-samples). This can impact the end results. For better results, we recommend longer training runs with a larger dataset. [Here](https://huggingface.co/datasets/timbrooks/instructpix2pix-clip-filtered) you can find a large dataset for InstructPix2Pix training.***
-
-## Running locally with PyTorch
-
-### Installing the dependencies
-
-Refer to the original InstructPix2Pix training example for installing the dependencies.
-
-You will also need to get access of SDXL by filling the [form](https://huggingface.co/stabilityai/stable-diffusion-xl-base-1.0).
-
-### Toy example
-
-As mentioned before, we'll use a [small toy dataset](https://huggingface.co/datasets/fusing/instructpix2pix-1000-samples) for training. The dataset
-is a smaller version of the [original dataset](https://huggingface.co/datasets/timbrooks/instructpix2pix-clip-filtered) used in the InstructPix2Pix paper.
-
-Configure environment variables such as the dataset identifier and the Stable Diffusion
-checkpoint:
-
-```bash
-export MODEL_NAME="stabilityai/stable-diffusion-xl-base-1.0"
-export DATASET_ID="fusing/instructpix2pix-1000-samples"
-```
-
-Now, we can launch training:
-
-```bash
-python train_instruct_pix2pix_xl.py \
- --pretrained_model_name_or_path=$MODEL_NAME \
- --dataset_name=$DATASET_ID \
- --enable_xformers_memory_efficient_attention \
- --resolution=256 --random_flip \
- --train_batch_size=4 --gradient_accumulation_steps=4 --gradient_checkpointing \
- --max_train_steps=15000 \
- --checkpointing_steps=5000 --checkpoints_total_limit=1 \
- --learning_rate=5e-05 --max_grad_norm=1 --lr_warmup_steps=0 \
- --conditioning_dropout_prob=0.05 \
- --seed=42
-```
-
-Additionally, we support performing validation inference to monitor training progress
-with Weights and Biases. You can enable this feature with `report_to="wandb"`:
-
-```bash
-python train_instruct_pix2pix_xl.py \
- --pretrained_model_name_or_path=stabilityai/stable-diffusion-xl-base-1.0 \
- --dataset_name=$DATASET_ID \
- --use_ema \
- --enable_xformers_memory_efficient_attention \
- --resolution=512 --random_flip \
- --train_batch_size=4 --gradient_accumulation_steps=4 --gradient_checkpointing \
- --max_train_steps=15000 \
- --checkpointing_steps=5000 --checkpoints_total_limit=1 \
- --learning_rate=5e-05 --lr_warmup_steps=0 \
- --conditioning_dropout_prob=0.05 \
- --seed=42 \
- --val_image_url_or_path="https://datasets-server.huggingface.co/assets/fusing/instructpix2pix-1000-samples/--/fusing--instructpix2pix-1000-samples/train/23/input_image/image.jpg" \
- --validation_prompt="make it in japan" \
- --report_to=wandb
- ```
-
- We recommend this type of validation as it can be useful for model debugging. Note that you need `wandb` installed to use this. You can install `wandb` by running `pip install wandb`.
-
- [Here](https://wandb.ai/sayakpaul/instruct-pix2pix/runs/ctr3kovq), you can find an example training run that includes some validation samples and the training hyperparameters.
-
- ***Note: In the original paper, the authors observed that even when the model is trained with an image resolution of 256x256, it generalizes well to bigger resolutions such as 512x512. This is likely because of the larger dataset they used during training.***
-
- ## Training with multiple GPUs
-
-`accelerate` allows for seamless multi-GPU training. Follow the instructions [here](https://huggingface.co/docs/accelerate/basic_tutorials/launch)
-for running distributed training with `accelerate`. Here is an example command:
-
-```bash
-accelerate launch --mixed_precision="fp16" --multi_gpu train_instruct_pix2pix.py \
- --pretrained_model_name_or_path=stabilityai/stable-diffusion-xl-base-1.0 \
- --dataset_name=$DATASET_ID \
- --use_ema \
- --enable_xformers_memory_efficient_attention \
- --resolution=512 --random_flip \
- --train_batch_size=4 --gradient_accumulation_steps=4 --gradient_checkpointing \
- --max_train_steps=15000 \
- --checkpointing_steps=5000 --checkpoints_total_limit=1 \
- --learning_rate=5e-05 --lr_warmup_steps=0 \
- --conditioning_dropout_prob=0.05 \
- --seed=42 \
- --val_image_url_or_path="https://datasets-server.huggingface.co/assets/fusing/instructpix2pix-1000-samples/--/fusing--instructpix2pix-1000-samples/train/23/input_image/image.jpg" \
- --validation_prompt="make it in japan" \
- --report_to=wandb
-```
-
- ## Inference
-
- Once training is complete, we can perform inference:
-
- ```python
-import PIL
-import requests
-import torch
-from diffusers import StableDiffusionXLInstructPix2PixPipeline
-
-model_id = "your_model_id" # <- replace this
-pipe = StableDiffusionXLInstructPix2PixPipeline.from_pretrained(model_id, torch_dtype=torch.float16).to("cuda")
-generator = torch.Generator("cuda").manual_seed(0)
-
-url = "https://datasets-server.huggingface.co/assets/fusing/instructpix2pix-1000-samples/--/fusing--instructpix2pix-1000-samples/train/23/input_image/image.jpg"
-
-
-def download_image(url):
- image = PIL.Image.open(requests.get(url, stream=True).raw)
- image = PIL.ImageOps.exif_transpose(image)
- image = image.convert("RGB")
- return image
-
-image = download_image(url)
-prompt = "make it Japan"
-num_inference_steps = 20
-image_guidance_scale = 1.5
-guidance_scale = 10
-
-edited_image = pipe(prompt,
- image=image,
- num_inference_steps=num_inference_steps,
- image_guidance_scale=image_guidance_scale,
- guidance_scale=guidance_scale,
- generator=generator,
-).images[0]
-edited_image.save("edited_image.png")
-```
-
-We encourage you to play with the following three parameters to control
-speed and quality during performance:
-
-* `num_inference_steps`
-* `image_guidance_scale`
-* `guidance_scale`
-
-Particularly, `image_guidance_scale` and `guidance_scale` can have a profound impact
-on the generated ("edited") image (see [here](https://twitter.com/RisingSayak/status/1628392199196151808?s=20) for an example).
-
-If you're looking for some interesting ways to use the InstructPix2Pix training methodology, we welcome you to check out this blog post: [Instruction-tuning Stable Diffusion with InstructPix2Pix](https://huggingface.co/blog/instruction-tuning-sd).
diff --git a/spaces/Androidonnxfork/CivitAi-to-Diffusers/diffusers/src/diffusers/utils/testing_utils.py b/spaces/Androidonnxfork/CivitAi-to-Diffusers/diffusers/src/diffusers/utils/testing_utils.py
deleted file mode 100644
index 3976be0fd7d5366210c61d5e3f949a864dff2eb2..0000000000000000000000000000000000000000
--- a/spaces/Androidonnxfork/CivitAi-to-Diffusers/diffusers/src/diffusers/utils/testing_utils.py
+++ /dev/null
@@ -1,684 +0,0 @@
-import inspect
-import io
-import logging
-import multiprocessing
-import os
-import random
-import re
-import struct
-import tempfile
-import unittest
-import urllib.parse
-from contextlib import contextmanager
-from distutils.util import strtobool
-from io import BytesIO, StringIO
-from pathlib import Path
-from typing import List, Optional, Union
-
-import numpy as np
-import PIL.Image
-import PIL.ImageOps
-import requests
-from packaging import version
-
-from .import_utils import (
- BACKENDS_MAPPING,
- is_compel_available,
- is_flax_available,
- is_note_seq_available,
- is_onnx_available,
- is_opencv_available,
- is_torch_available,
- is_torch_version,
- is_torchsde_available,
-)
-from .logging import get_logger
-
-
-global_rng = random.Random()
-
-logger = get_logger(__name__)
-
-if is_torch_available():
- import torch
-
- if "DIFFUSERS_TEST_DEVICE" in os.environ:
- torch_device = os.environ["DIFFUSERS_TEST_DEVICE"]
-
- available_backends = ["cuda", "cpu", "mps"]
- if torch_device not in available_backends:
- raise ValueError(
- f"unknown torch backend for diffusers tests: {torch_device}. Available backends are:"
- f" {available_backends}"
- )
- logger.info(f"torch_device overrode to {torch_device}")
- else:
- torch_device = "cuda" if torch.cuda.is_available() else "cpu"
- is_torch_higher_equal_than_1_12 = version.parse(
- version.parse(torch.__version__).base_version
- ) >= version.parse("1.12")
-
- if is_torch_higher_equal_than_1_12:
- # Some builds of torch 1.12 don't have the mps backend registered. See #892 for more details
- mps_backend_registered = hasattr(torch.backends, "mps")
- torch_device = "mps" if (mps_backend_registered and torch.backends.mps.is_available()) else torch_device
-
-
-def torch_all_close(a, b, *args, **kwargs):
- if not is_torch_available():
- raise ValueError("PyTorch needs to be installed to use this function.")
- if not torch.allclose(a, b, *args, **kwargs):
- assert False, f"Max diff is absolute {(a - b).abs().max()}. Diff tensor is {(a - b).abs()}."
- return True
-
-
-def print_tensor_test(tensor, filename="test_corrections.txt", expected_tensor_name="expected_slice"):
- test_name = os.environ.get("PYTEST_CURRENT_TEST")
- if not torch.is_tensor(tensor):
- tensor = torch.from_numpy(tensor)
-
- tensor_str = str(tensor.detach().cpu().flatten().to(torch.float32)).replace("\n", "")
- # format is usually:
- # expected_slice = np.array([-0.5713, -0.3018, -0.9814, 0.04663, -0.879, 0.76, -1.734, 0.1044, 1.161])
- output_str = tensor_str.replace("tensor", f"{expected_tensor_name} = np.array")
- test_file, test_class, test_fn = test_name.split("::")
- test_fn = test_fn.split()[0]
- with open(filename, "a") as f:
- print(";".join([test_file, test_class, test_fn, output_str]), file=f)
-
-
-def get_tests_dir(append_path=None):
- """
- Args:
- append_path: optional path to append to the tests dir path
- Return:
- The full path to the `tests` dir, so that the tests can be invoked from anywhere. Optionally `append_path` is
- joined after the `tests` dir the former is provided.
- """
- # this function caller's __file__
- caller__file__ = inspect.stack()[1][1]
- tests_dir = os.path.abspath(os.path.dirname(caller__file__))
-
- while not tests_dir.endswith("tests"):
- tests_dir = os.path.dirname(tests_dir)
-
- if append_path:
- return os.path.join(tests_dir, append_path)
- else:
- return tests_dir
-
-
-def parse_flag_from_env(key, default=False):
- try:
- value = os.environ[key]
- except KeyError:
- # KEY isn't set, default to `default`.
- _value = default
- else:
- # KEY is set, convert it to True or False.
- try:
- _value = strtobool(value)
- except ValueError:
- # More values are supported, but let's keep the message simple.
- raise ValueError(f"If set, {key} must be yes or no.")
- return _value
-
-
-_run_slow_tests = parse_flag_from_env("RUN_SLOW", default=False)
-_run_nightly_tests = parse_flag_from_env("RUN_NIGHTLY", default=False)
-
-
-def floats_tensor(shape, scale=1.0, rng=None, name=None):
- """Creates a random float32 tensor"""
- if rng is None:
- rng = global_rng
-
- total_dims = 1
- for dim in shape:
- total_dims *= dim
-
- values = []
- for _ in range(total_dims):
- values.append(rng.random() * scale)
-
- return torch.tensor(data=values, dtype=torch.float).view(shape).contiguous()
-
-
-def slow(test_case):
- """
- Decorator marking a test as slow.
-
- Slow tests are skipped by default. Set the RUN_SLOW environment variable to a truthy value to run them.
-
- """
- return unittest.skipUnless(_run_slow_tests, "test is slow")(test_case)
-
-
-def nightly(test_case):
- """
- Decorator marking a test that runs nightly in the diffusers CI.
-
- Slow tests are skipped by default. Set the RUN_NIGHTLY environment variable to a truthy value to run them.
-
- """
- return unittest.skipUnless(_run_nightly_tests, "test is nightly")(test_case)
-
-
-def require_torch(test_case):
- """
- Decorator marking a test that requires PyTorch. These tests are skipped when PyTorch isn't installed.
- """
- return unittest.skipUnless(is_torch_available(), "test requires PyTorch")(test_case)
-
-
-def require_torch_2(test_case):
- """
- Decorator marking a test that requires PyTorch 2. These tests are skipped when it isn't installed.
- """
- return unittest.skipUnless(is_torch_available() and is_torch_version(">=", "2.0.0"), "test requires PyTorch 2")(
- test_case
- )
-
-
-def require_torch_gpu(test_case):
- """Decorator marking a test that requires CUDA and PyTorch."""
- return unittest.skipUnless(is_torch_available() and torch_device == "cuda", "test requires PyTorch+CUDA")(
- test_case
- )
-
-
-def skip_mps(test_case):
- """Decorator marking a test to skip if torch_device is 'mps'"""
- return unittest.skipUnless(torch_device != "mps", "test requires non 'mps' device")(test_case)
-
-
-def require_flax(test_case):
- """
- Decorator marking a test that requires JAX & Flax. These tests are skipped when one / both are not installed
- """
- return unittest.skipUnless(is_flax_available(), "test requires JAX & Flax")(test_case)
-
-
-def require_compel(test_case):
- """
- Decorator marking a test that requires compel: https://github.com/damian0815/compel. These tests are skipped when
- the library is not installed.
- """
- return unittest.skipUnless(is_compel_available(), "test requires compel")(test_case)
-
-
-def require_onnxruntime(test_case):
- """
- Decorator marking a test that requires onnxruntime. These tests are skipped when onnxruntime isn't installed.
- """
- return unittest.skipUnless(is_onnx_available(), "test requires onnxruntime")(test_case)
-
-
-def require_note_seq(test_case):
- """
- Decorator marking a test that requires note_seq. These tests are skipped when note_seq isn't installed.
- """
- return unittest.skipUnless(is_note_seq_available(), "test requires note_seq")(test_case)
-
-
-def require_torchsde(test_case):
- """
- Decorator marking a test that requires torchsde. These tests are skipped when torchsde isn't installed.
- """
- return unittest.skipUnless(is_torchsde_available(), "test requires torchsde")(test_case)
-
-
-def load_numpy(arry: Union[str, np.ndarray], local_path: Optional[str] = None) -> np.ndarray:
- if isinstance(arry, str):
- # local_path = "/home/patrick_huggingface_co/"
- if local_path is not None:
- # local_path can be passed to correct images of tests
- return os.path.join(local_path, "/".join([arry.split("/")[-5], arry.split("/")[-2], arry.split("/")[-1]]))
- elif arry.startswith("http://") or arry.startswith("https://"):
- response = requests.get(arry)
- response.raise_for_status()
- arry = np.load(BytesIO(response.content))
- elif os.path.isfile(arry):
- arry = np.load(arry)
- else:
- raise ValueError(
- f"Incorrect path or url, URLs must start with `http://` or `https://`, and {arry} is not a valid path"
- )
- elif isinstance(arry, np.ndarray):
- pass
- else:
- raise ValueError(
- "Incorrect format used for numpy ndarray. Should be an url linking to an image, a local path, or a"
- " ndarray."
- )
-
- return arry
-
-
-def load_pt(url: str):
- response = requests.get(url)
- response.raise_for_status()
- arry = torch.load(BytesIO(response.content))
- return arry
-
-
-def load_image(image: Union[str, PIL.Image.Image]) -> PIL.Image.Image:
- """
- Loads `image` to a PIL Image.
-
- Args:
- image (`str` or `PIL.Image.Image`):
- The image to convert to the PIL Image format.
- Returns:
- `PIL.Image.Image`:
- A PIL Image.
- """
- if isinstance(image, str):
- if image.startswith("http://") or image.startswith("https://"):
- image = PIL.Image.open(requests.get(image, stream=True).raw)
- elif os.path.isfile(image):
- image = PIL.Image.open(image)
- else:
- raise ValueError(
- f"Incorrect path or url, URLs must start with `http://` or `https://`, and {image} is not a valid path"
- )
- elif isinstance(image, PIL.Image.Image):
- image = image
- else:
- raise ValueError(
- "Incorrect format used for image. Should be an url linking to an image, a local path, or a PIL image."
- )
- image = PIL.ImageOps.exif_transpose(image)
- image = image.convert("RGB")
- return image
-
-
-def preprocess_image(image: PIL.Image, batch_size: int):
- w, h = image.size
- w, h = (x - x % 8 for x in (w, h)) # resize to integer multiple of 8
- image = image.resize((w, h), resample=PIL.Image.LANCZOS)
- image = np.array(image).astype(np.float32) / 255.0
- image = np.vstack([image[None].transpose(0, 3, 1, 2)] * batch_size)
- image = torch.from_numpy(image)
- return 2.0 * image - 1.0
-
-
-def export_to_gif(image: List[PIL.Image.Image], output_gif_path: str = None) -> str:
- if output_gif_path is None:
- output_gif_path = tempfile.NamedTemporaryFile(suffix=".gif").name
-
- image[0].save(
- output_gif_path,
- save_all=True,
- append_images=image[1:],
- optimize=False,
- duration=100,
- loop=0,
- )
- return output_gif_path
-
-
-@contextmanager
-def buffered_writer(raw_f):
- f = io.BufferedWriter(raw_f)
- yield f
- f.flush()
-
-
-def export_to_ply(mesh, output_ply_path: str = None):
- """
- Write a PLY file for a mesh.
- """
- if output_ply_path is None:
- output_ply_path = tempfile.NamedTemporaryFile(suffix=".ply").name
-
- coords = mesh.verts.detach().cpu().numpy()
- faces = mesh.faces.cpu().numpy()
- rgb = np.stack([mesh.vertex_channels[x].detach().cpu().numpy() for x in "RGB"], axis=1)
-
- with buffered_writer(open(output_ply_path, "wb")) as f:
- f.write(b"ply\n")
- f.write(b"format binary_little_endian 1.0\n")
- f.write(bytes(f"element vertex {len(coords)}\n", "ascii"))
- f.write(b"property float x\n")
- f.write(b"property float y\n")
- f.write(b"property float z\n")
- if rgb is not None:
- f.write(b"property uchar red\n")
- f.write(b"property uchar green\n")
- f.write(b"property uchar blue\n")
- if faces is not None:
- f.write(bytes(f"element face {len(faces)}\n", "ascii"))
- f.write(b"property list uchar int vertex_index\n")
- f.write(b"end_header\n")
-
- if rgb is not None:
- rgb = (rgb * 255.499).round().astype(int)
- vertices = [
- (*coord, *rgb)
- for coord, rgb in zip(
- coords.tolist(),
- rgb.tolist(),
- )
- ]
- format = struct.Struct("<3f3B")
- for item in vertices:
- f.write(format.pack(*item))
- else:
- format = struct.Struct("<3f")
- for vertex in coords.tolist():
- f.write(format.pack(*vertex))
-
- if faces is not None:
- format = struct.Struct(" str:
- if is_opencv_available():
- import cv2
- else:
- raise ImportError(BACKENDS_MAPPING["opencv"][1].format("export_to_video"))
- if output_video_path is None:
- output_video_path = tempfile.NamedTemporaryFile(suffix=".mp4").name
-
- fourcc = cv2.VideoWriter_fourcc(*"mp4v")
- h, w, c = video_frames[0].shape
- video_writer = cv2.VideoWriter(output_video_path, fourcc, fps=8, frameSize=(w, h))
- for i in range(len(video_frames)):
- img = cv2.cvtColor(video_frames[i], cv2.COLOR_RGB2BGR)
- video_writer.write(img)
- return output_video_path
-
-
-def load_hf_numpy(path) -> np.ndarray:
- if not path.startswith("http://") or path.startswith("https://"):
- path = os.path.join(
- "https://huggingface.co/datasets/fusing/diffusers-testing/resolve/main", urllib.parse.quote(path)
- )
-
- return load_numpy(path)
-
-
-# --- pytest conf functions --- #
-
-# to avoid multiple invocation from tests/conftest.py and examples/conftest.py - make sure it's called only once
-pytest_opt_registered = {}
-
-
-def pytest_addoption_shared(parser):
- """
- This function is to be called from `conftest.py` via `pytest_addoption` wrapper that has to be defined there.
-
- It allows loading both `conftest.py` files at once without causing a failure due to adding the same `pytest`
- option.
-
- """
- option = "--make-reports"
- if option not in pytest_opt_registered:
- parser.addoption(
- option,
- action="store",
- default=False,
- help="generate report files. The value of this option is used as a prefix to report names",
- )
- pytest_opt_registered[option] = 1
-
-
-def pytest_terminal_summary_main(tr, id):
- """
- Generate multiple reports at the end of test suite run - each report goes into a dedicated file in the current
- directory. The report files are prefixed with the test suite name.
-
- This function emulates --duration and -rA pytest arguments.
-
- This function is to be called from `conftest.py` via `pytest_terminal_summary` wrapper that has to be defined
- there.
-
- Args:
- - tr: `terminalreporter` passed from `conftest.py`
- - id: unique id like `tests` or `examples` that will be incorporated into the final reports filenames - this is
- needed as some jobs have multiple runs of pytest, so we can't have them overwrite each other.
-
- NB: this functions taps into a private _pytest API and while unlikely, it could break should
- pytest do internal changes - also it calls default internal methods of terminalreporter which
- can be hijacked by various `pytest-` plugins and interfere.
-
- """
- from _pytest.config import create_terminal_writer
-
- if not len(id):
- id = "tests"
-
- config = tr.config
- orig_writer = config.get_terminal_writer()
- orig_tbstyle = config.option.tbstyle
- orig_reportchars = tr.reportchars
-
- dir = "reports"
- Path(dir).mkdir(parents=True, exist_ok=True)
- report_files = {
- k: f"{dir}/{id}_{k}.txt"
- for k in [
- "durations",
- "errors",
- "failures_long",
- "failures_short",
- "failures_line",
- "passes",
- "stats",
- "summary_short",
- "warnings",
- ]
- }
-
- # custom durations report
- # note: there is no need to call pytest --durations=XX to get this separate report
- # adapted from https://github.com/pytest-dev/pytest/blob/897f151e/src/_pytest/runner.py#L66
- dlist = []
- for replist in tr.stats.values():
- for rep in replist:
- if hasattr(rep, "duration"):
- dlist.append(rep)
- if dlist:
- dlist.sort(key=lambda x: x.duration, reverse=True)
- with open(report_files["durations"], "w") as f:
- durations_min = 0.05 # sec
- f.write("slowest durations\n")
- for i, rep in enumerate(dlist):
- if rep.duration < durations_min:
- f.write(f"{len(dlist)-i} durations < {durations_min} secs were omitted")
- break
- f.write(f"{rep.duration:02.2f}s {rep.when:<8} {rep.nodeid}\n")
-
- def summary_failures_short(tr):
- # expecting that the reports were --tb=long (default) so we chop them off here to the last frame
- reports = tr.getreports("failed")
- if not reports:
- return
- tr.write_sep("=", "FAILURES SHORT STACK")
- for rep in reports:
- msg = tr._getfailureheadline(rep)
- tr.write_sep("_", msg, red=True, bold=True)
- # chop off the optional leading extra frames, leaving only the last one
- longrepr = re.sub(r".*_ _ _ (_ ){10,}_ _ ", "", rep.longreprtext, 0, re.M | re.S)
- tr._tw.line(longrepr)
- # note: not printing out any rep.sections to keep the report short
-
- # use ready-made report funcs, we are just hijacking the filehandle to log to a dedicated file each
- # adapted from https://github.com/pytest-dev/pytest/blob/897f151e/src/_pytest/terminal.py#L814
- # note: some pytest plugins may interfere by hijacking the default `terminalreporter` (e.g.
- # pytest-instafail does that)
-
- # report failures with line/short/long styles
- config.option.tbstyle = "auto" # full tb
- with open(report_files["failures_long"], "w") as f:
- tr._tw = create_terminal_writer(config, f)
- tr.summary_failures()
-
- # config.option.tbstyle = "short" # short tb
- with open(report_files["failures_short"], "w") as f:
- tr._tw = create_terminal_writer(config, f)
- summary_failures_short(tr)
-
- config.option.tbstyle = "line" # one line per error
- with open(report_files["failures_line"], "w") as f:
- tr._tw = create_terminal_writer(config, f)
- tr.summary_failures()
-
- with open(report_files["errors"], "w") as f:
- tr._tw = create_terminal_writer(config, f)
- tr.summary_errors()
-
- with open(report_files["warnings"], "w") as f:
- tr._tw = create_terminal_writer(config, f)
- tr.summary_warnings() # normal warnings
- tr.summary_warnings() # final warnings
-
- tr.reportchars = "wPpsxXEf" # emulate -rA (used in summary_passes() and short_test_summary())
- with open(report_files["passes"], "w") as f:
- tr._tw = create_terminal_writer(config, f)
- tr.summary_passes()
-
- with open(report_files["summary_short"], "w") as f:
- tr._tw = create_terminal_writer(config, f)
- tr.short_test_summary()
-
- with open(report_files["stats"], "w") as f:
- tr._tw = create_terminal_writer(config, f)
- tr.summary_stats()
-
- # restore:
- tr._tw = orig_writer
- tr.reportchars = orig_reportchars
- config.option.tbstyle = orig_tbstyle
-
-
-# Taken from: https://github.com/huggingface/transformers/blob/3658488ff77ff8d45101293e749263acf437f4d5/src/transformers/testing_utils.py#L1787
-def run_test_in_subprocess(test_case, target_func, inputs=None, timeout=None):
- """
- To run a test in a subprocess. In particular, this can avoid (GPU) memory issue.
-
- Args:
- test_case (`unittest.TestCase`):
- The test that will run `target_func`.
- target_func (`Callable`):
- The function implementing the actual testing logic.
- inputs (`dict`, *optional*, defaults to `None`):
- The inputs that will be passed to `target_func` through an (input) queue.
- timeout (`int`, *optional*, defaults to `None`):
- The timeout (in seconds) that will be passed to the input and output queues. If not specified, the env.
- variable `PYTEST_TIMEOUT` will be checked. If still `None`, its value will be set to `600`.
- """
- if timeout is None:
- timeout = int(os.environ.get("PYTEST_TIMEOUT", 600))
-
- start_methohd = "spawn"
- ctx = multiprocessing.get_context(start_methohd)
-
- input_queue = ctx.Queue(1)
- output_queue = ctx.JoinableQueue(1)
-
- # We can't send `unittest.TestCase` to the child, otherwise we get issues regarding pickle.
- input_queue.put(inputs, timeout=timeout)
-
- process = ctx.Process(target=target_func, args=(input_queue, output_queue, timeout))
- process.start()
- # Kill the child process if we can't get outputs from it in time: otherwise, the hanging subprocess prevents
- # the test to exit properly.
- try:
- results = output_queue.get(timeout=timeout)
- output_queue.task_done()
- except Exception as e:
- process.terminate()
- test_case.fail(e)
- process.join(timeout=timeout)
-
- if results["error"] is not None:
- test_case.fail(f'{results["error"]}')
-
-
-class CaptureLogger:
- """
- Args:
- Context manager to capture `logging` streams
- logger: 'logging` logger object
- Returns:
- The captured output is available via `self.out`
- Example:
- ```python
- >>> from diffusers import logging
- >>> from diffusers.testing_utils import CaptureLogger
-
- >>> msg = "Testing 1, 2, 3"
- >>> logging.set_verbosity_info()
- >>> logger = logging.get_logger("diffusers.pipelines.stable_diffusion.pipeline_stable_diffusion.py")
- >>> with CaptureLogger(logger) as cl:
- ... logger.info(msg)
- >>> assert cl.out, msg + "\n"
- ```
- """
-
- def __init__(self, logger):
- self.logger = logger
- self.io = StringIO()
- self.sh = logging.StreamHandler(self.io)
- self.out = ""
-
- def __enter__(self):
- self.logger.addHandler(self.sh)
- return self
-
- def __exit__(self, *exc):
- self.logger.removeHandler(self.sh)
- self.out = self.io.getvalue()
-
- def __repr__(self):
- return f"captured: {self.out}\n"
-
-
-def enable_full_determinism():
- """
- Helper function for reproducible behavior during distributed training. See
- - https://pytorch.org/docs/stable/notes/randomness.html for pytorch
- """
- # Enable PyTorch deterministic mode. This potentially requires either the environment
- # variable 'CUDA_LAUNCH_BLOCKING' or 'CUBLAS_WORKSPACE_CONFIG' to be set,
- # depending on the CUDA version, so we set them both here
- os.environ["CUDA_LAUNCH_BLOCKING"] = "1"
- os.environ["CUBLAS_WORKSPACE_CONFIG"] = ":16:8"
- torch.use_deterministic_algorithms(True)
-
- # Enable CUDNN deterministic mode
- torch.backends.cudnn.deterministic = True
- torch.backends.cudnn.benchmark = False
- torch.backends.cuda.matmul.allow_tf32 = False
-
-
-def disable_full_determinism():
- os.environ["CUDA_LAUNCH_BLOCKING"] = "0"
- os.environ["CUBLAS_WORKSPACE_CONFIG"] = ""
- torch.use_deterministic_algorithms(False)
diff --git a/spaces/Andy1621/uniformer_image_detection/configs/sparse_rcnn/README.md b/spaces/Andy1621/uniformer_image_detection/configs/sparse_rcnn/README.md
deleted file mode 100644
index 60cc8a93ed4cc88a14bf9294d671674d032a63a8..0000000000000000000000000000000000000000
--- a/spaces/Andy1621/uniformer_image_detection/configs/sparse_rcnn/README.md
+++ /dev/null
@@ -1,28 +0,0 @@
-# Sparse R-CNN: End-to-End Object Detection with Learnable Proposals
-
-## Introduction
-
-[ALGORITHM]
-
-```
-@article{peize2020sparse,
- title = {{SparseR-CNN}: End-to-End Object Detection with Learnable Proposals},
- author = {Peize Sun and Rufeng Zhang and Yi Jiang and Tao Kong and Chenfeng Xu and Wei Zhan and Masayoshi Tomizuka and Lei Li and Zehuan Yuan and Changhu Wang and Ping Luo},
- journal = {arXiv preprint arXiv:2011.12450},
- year = {2020}
-}
-```
-
-## Results and Models
-
-| Model | Backbone | Style | Lr schd | Number of Proposals |Multi-Scale| RandomCrop | box AP | Config | Download |
-|:------------:|:---------:|:-------:|:-------:|:-------: |:-------: |:---------:|:------:|:------:|:--------:|
-| Sparse R-CNN | R-50-FPN | pytorch | 1x | 100 | False | False | 37.9 | [config](https://github.com/open-mmlab/mmdetection/tree/master/configs/sparse_rcnn/sparse_rcnn_r50_fpn_1x_coco.py) | [model](https://download.openmmlab.com/mmdetection/v2.0/sparse_rcnn/sparse_rcnn_r50_fpn_1x_coco/sparse_rcnn_r50_fpn_1x_coco_20201222_214453-dc79b137.pth) | [log](https://download.openmmlab.com/mmdetection/v2.0/sparse_rcnn/sparse_rcnn_r50_fpn_1x_coco/sparse_rcnn_r50_fpn_1x_coco_20201222_214453-dc79b137.log.json) |
-| Sparse R-CNN | R-50-FPN | pytorch | 3x | 100 | True | False | 42.8 | [config](https://github.com/open-mmlab/mmdetection/tree/master/configs/sparse_rcnn/sparse_rcnn_r50_fpn_mstrain_480-800_3x_coco.py) | [model](https://download.openmmlab.com/mmdetection/v2.0/sparse_rcnn/sparse_rcnn_r50_fpn_mstrain_480-800_3x_coco/sparse_rcnn_r50_fpn_mstrain_480-800_3x_coco_20201218_154234-7bc5c054.pth) | [log](https://download.openmmlab.com/mmdetection/v2.0/sparse_rcnn/sparse_rcnn_r50_fpn_mstrain_480-800_3x_coco/sparse_rcnn_r50_fpn_mstrain_480-800_3x_coco_20201218_154234-7bc5c054.log.json) |
-| Sparse R-CNN | R-50-FPN | pytorch | 3x | 300 | True | True | 45.0 | [config](https://github.com/open-mmlab/mmdetection/tree/master/configs/sparse_rcnn/sparse_rcnn_r50_fpn_300_proposals_crop_mstrain_480-800_3x_coco.py) | [model](https://download.openmmlab.com/mmdetection/v2.0/sparse_rcnn/sparse_rcnn_r50_fpn_300_proposals_crop_mstrain_480-800_3x_coco/sparse_rcnn_r50_fpn_300_proposals_crop_mstrain_480-800_3x_coco_20201223_024605-9fe92701.pth) | [log](https://download.openmmlab.com/mmdetection/v2.0/sparse_rcnn/sparse_rcnn_r50_fpn_300_proposals_crop_mstrain_480-800_3x_coco/sparse_rcnn_r50_fpn_300_proposals_crop_mstrain_480-800_3x_coco_20201223_024605-9fe92701.log.json) |
-| Sparse R-CNN | R-101-FPN | pytorch | 3x | 100 | True | False | 44.2 | [config](https://github.com/open-mmlab/mmdetection/tree/master/configs/sparse_rcnn/sparse_rcnn_r101_fpn_mstrain_480-800_3x_coco.py) | [model](https://download.openmmlab.com/mmdetection/v2.0/sparse_rcnn/sparse_rcnn_r101_fpn_mstrain_480-800_3x_coco/sparse_rcnn_r101_fpn_mstrain_480-800_3x_coco_20201223_121552-6c46c9d6.pth) | [log](https://download.openmmlab.com/mmdetection/v2.0/sparse_rcnn/sparse_rcnn_r101_fpn_mstrain_480-800_3x_coco/sparse_rcnn_r101_fpn_mstrain_480-800_3x_coco_20201223_121552-6c46c9d6.log.json) |
-| Sparse R-CNN | R-101-FPN | pytorch | 3x | 300 | True | True | 46.2 | [config](https://github.com/open-mmlab/mmdetection/tree/master/configs/sparse_rcnn/sparse_rcnn_r101_fpn_300_proposals_crop_mstrain_480-800_3x_coco.py) | [model](https://download.openmmlab.com/mmdetection/v2.0/sparse_rcnn/sparse_rcnn_r101_fpn_300_proposals_crop_mstrain_480-800_3x_coco/sparse_rcnn_r101_fpn_300_proposals_crop_mstrain_480-800_3x_coco_20201223_023452-c23c3564.pth) | [log](https://download.openmmlab.com/mmdetection/v2.0/sparse_rcnn/sparse_rcnn_r101_fpn_300_proposals_crop_mstrain_480-800_3x_coco/sparse_rcnn_r101_fpn_300_proposals_crop_mstrain_480-800_3x_coco_20201223_023452-c23c3564.log.json) |
-
-### Notes
-
-We observe about 0.3 AP noise especially when using ResNet-101 as the backbone.
diff --git a/spaces/Andy1621/uniformer_image_detection/mmdet/core/anchor/builder.py b/spaces/Andy1621/uniformer_image_detection/mmdet/core/anchor/builder.py
deleted file mode 100644
index d79b448ebca9f2b21d455046623172c48c5c3ef0..0000000000000000000000000000000000000000
--- a/spaces/Andy1621/uniformer_image_detection/mmdet/core/anchor/builder.py
+++ /dev/null
@@ -1,7 +0,0 @@
-from mmcv.utils import Registry, build_from_cfg
-
-ANCHOR_GENERATORS = Registry('Anchor generator')
-
-
-def build_anchor_generator(cfg, default_args=None):
- return build_from_cfg(cfg, ANCHOR_GENERATORS, default_args)
diff --git a/spaces/Andy1621/uniformer_image_detection/mmdet/models/dense_heads/reppoints_head.py b/spaces/Andy1621/uniformer_image_detection/mmdet/models/dense_heads/reppoints_head.py
deleted file mode 100644
index 499cc4f71c968704a40ab2bb7a6b22dd079d82de..0000000000000000000000000000000000000000
--- a/spaces/Andy1621/uniformer_image_detection/mmdet/models/dense_heads/reppoints_head.py
+++ /dev/null
@@ -1,763 +0,0 @@
-import numpy as np
-import torch
-import torch.nn as nn
-from mmcv.cnn import ConvModule, bias_init_with_prob, normal_init
-from mmcv.ops import DeformConv2d
-
-from mmdet.core import (PointGenerator, build_assigner, build_sampler,
- images_to_levels, multi_apply, multiclass_nms, unmap)
-from ..builder import HEADS, build_loss
-from .anchor_free_head import AnchorFreeHead
-
-
-@HEADS.register_module()
-class RepPointsHead(AnchorFreeHead):
- """RepPoint head.
-
- Args:
- point_feat_channels (int): Number of channels of points features.
- gradient_mul (float): The multiplier to gradients from
- points refinement and recognition.
- point_strides (Iterable): points strides.
- point_base_scale (int): bbox scale for assigning labels.
- loss_cls (dict): Config of classification loss.
- loss_bbox_init (dict): Config of initial points loss.
- loss_bbox_refine (dict): Config of points loss in refinement.
- use_grid_points (bool): If we use bounding box representation, the
- reppoints is represented as grid points on the bounding box.
- center_init (bool): Whether to use center point assignment.
- transform_method (str): The methods to transform RepPoints to bbox.
- """ # noqa: W605
-
- def __init__(self,
- num_classes,
- in_channels,
- point_feat_channels=256,
- num_points=9,
- gradient_mul=0.1,
- point_strides=[8, 16, 32, 64, 128],
- point_base_scale=4,
- loss_cls=dict(
- type='FocalLoss',
- use_sigmoid=True,
- gamma=2.0,
- alpha=0.25,
- loss_weight=1.0),
- loss_bbox_init=dict(
- type='SmoothL1Loss', beta=1.0 / 9.0, loss_weight=0.5),
- loss_bbox_refine=dict(
- type='SmoothL1Loss', beta=1.0 / 9.0, loss_weight=1.0),
- use_grid_points=False,
- center_init=True,
- transform_method='moment',
- moment_mul=0.01,
- **kwargs):
- self.num_points = num_points
- self.point_feat_channels = point_feat_channels
- self.use_grid_points = use_grid_points
- self.center_init = center_init
-
- # we use deform conv to extract points features
- self.dcn_kernel = int(np.sqrt(num_points))
- self.dcn_pad = int((self.dcn_kernel - 1) / 2)
- assert self.dcn_kernel * self.dcn_kernel == num_points, \
- 'The points number should be a square number.'
- assert self.dcn_kernel % 2 == 1, \
- 'The points number should be an odd square number.'
- dcn_base = np.arange(-self.dcn_pad,
- self.dcn_pad + 1).astype(np.float64)
- dcn_base_y = np.repeat(dcn_base, self.dcn_kernel)
- dcn_base_x = np.tile(dcn_base, self.dcn_kernel)
- dcn_base_offset = np.stack([dcn_base_y, dcn_base_x], axis=1).reshape(
- (-1))
- self.dcn_base_offset = torch.tensor(dcn_base_offset).view(1, -1, 1, 1)
-
- super().__init__(num_classes, in_channels, loss_cls=loss_cls, **kwargs)
-
- self.gradient_mul = gradient_mul
- self.point_base_scale = point_base_scale
- self.point_strides = point_strides
- self.point_generators = [PointGenerator() for _ in self.point_strides]
-
- self.sampling = loss_cls['type'] not in ['FocalLoss']
- if self.train_cfg:
- self.init_assigner = build_assigner(self.train_cfg.init.assigner)
- self.refine_assigner = build_assigner(
- self.train_cfg.refine.assigner)
- # use PseudoSampler when sampling is False
- if self.sampling and hasattr(self.train_cfg, 'sampler'):
- sampler_cfg = self.train_cfg.sampler
- else:
- sampler_cfg = dict(type='PseudoSampler')
- self.sampler = build_sampler(sampler_cfg, context=self)
- self.transform_method = transform_method
- if self.transform_method == 'moment':
- self.moment_transfer = nn.Parameter(
- data=torch.zeros(2), requires_grad=True)
- self.moment_mul = moment_mul
-
- self.use_sigmoid_cls = loss_cls.get('use_sigmoid', False)
- if self.use_sigmoid_cls:
- self.cls_out_channels = self.num_classes
- else:
- self.cls_out_channels = self.num_classes + 1
- self.loss_bbox_init = build_loss(loss_bbox_init)
- self.loss_bbox_refine = build_loss(loss_bbox_refine)
-
- def _init_layers(self):
- """Initialize layers of the head."""
- self.relu = nn.ReLU(inplace=True)
- self.cls_convs = nn.ModuleList()
- self.reg_convs = nn.ModuleList()
- for i in range(self.stacked_convs):
- chn = self.in_channels if i == 0 else self.feat_channels
- self.cls_convs.append(
- ConvModule(
- chn,
- self.feat_channels,
- 3,
- stride=1,
- padding=1,
- conv_cfg=self.conv_cfg,
- norm_cfg=self.norm_cfg))
- self.reg_convs.append(
- ConvModule(
- chn,
- self.feat_channels,
- 3,
- stride=1,
- padding=1,
- conv_cfg=self.conv_cfg,
- norm_cfg=self.norm_cfg))
- pts_out_dim = 4 if self.use_grid_points else 2 * self.num_points
- self.reppoints_cls_conv = DeformConv2d(self.feat_channels,
- self.point_feat_channels,
- self.dcn_kernel, 1,
- self.dcn_pad)
- self.reppoints_cls_out = nn.Conv2d(self.point_feat_channels,
- self.cls_out_channels, 1, 1, 0)
- self.reppoints_pts_init_conv = nn.Conv2d(self.feat_channels,
- self.point_feat_channels, 3,
- 1, 1)
- self.reppoints_pts_init_out = nn.Conv2d(self.point_feat_channels,
- pts_out_dim, 1, 1, 0)
- self.reppoints_pts_refine_conv = DeformConv2d(self.feat_channels,
- self.point_feat_channels,
- self.dcn_kernel, 1,
- self.dcn_pad)
- self.reppoints_pts_refine_out = nn.Conv2d(self.point_feat_channels,
- pts_out_dim, 1, 1, 0)
-
- def init_weights(self):
- """Initialize weights of the head."""
- for m in self.cls_convs:
- normal_init(m.conv, std=0.01)
- for m in self.reg_convs:
- normal_init(m.conv, std=0.01)
- bias_cls = bias_init_with_prob(0.01)
- normal_init(self.reppoints_cls_conv, std=0.01)
- normal_init(self.reppoints_cls_out, std=0.01, bias=bias_cls)
- normal_init(self.reppoints_pts_init_conv, std=0.01)
- normal_init(self.reppoints_pts_init_out, std=0.01)
- normal_init(self.reppoints_pts_refine_conv, std=0.01)
- normal_init(self.reppoints_pts_refine_out, std=0.01)
-
- def points2bbox(self, pts, y_first=True):
- """Converting the points set into bounding box.
-
- :param pts: the input points sets (fields), each points
- set (fields) is represented as 2n scalar.
- :param y_first: if y_first=True, the point set is represented as
- [y1, x1, y2, x2 ... yn, xn], otherwise the point set is
- represented as [x1, y1, x2, y2 ... xn, yn].
- :return: each points set is converting to a bbox [x1, y1, x2, y2].
- """
- pts_reshape = pts.view(pts.shape[0], -1, 2, *pts.shape[2:])
- pts_y = pts_reshape[:, :, 0, ...] if y_first else pts_reshape[:, :, 1,
- ...]
- pts_x = pts_reshape[:, :, 1, ...] if y_first else pts_reshape[:, :, 0,
- ...]
- if self.transform_method == 'minmax':
- bbox_left = pts_x.min(dim=1, keepdim=True)[0]
- bbox_right = pts_x.max(dim=1, keepdim=True)[0]
- bbox_up = pts_y.min(dim=1, keepdim=True)[0]
- bbox_bottom = pts_y.max(dim=1, keepdim=True)[0]
- bbox = torch.cat([bbox_left, bbox_up, bbox_right, bbox_bottom],
- dim=1)
- elif self.transform_method == 'partial_minmax':
- pts_y = pts_y[:, :4, ...]
- pts_x = pts_x[:, :4, ...]
- bbox_left = pts_x.min(dim=1, keepdim=True)[0]
- bbox_right = pts_x.max(dim=1, keepdim=True)[0]
- bbox_up = pts_y.min(dim=1, keepdim=True)[0]
- bbox_bottom = pts_y.max(dim=1, keepdim=True)[0]
- bbox = torch.cat([bbox_left, bbox_up, bbox_right, bbox_bottom],
- dim=1)
- elif self.transform_method == 'moment':
- pts_y_mean = pts_y.mean(dim=1, keepdim=True)
- pts_x_mean = pts_x.mean(dim=1, keepdim=True)
- pts_y_std = torch.std(pts_y - pts_y_mean, dim=1, keepdim=True)
- pts_x_std = torch.std(pts_x - pts_x_mean, dim=1, keepdim=True)
- moment_transfer = (self.moment_transfer * self.moment_mul) + (
- self.moment_transfer.detach() * (1 - self.moment_mul))
- moment_width_transfer = moment_transfer[0]
- moment_height_transfer = moment_transfer[1]
- half_width = pts_x_std * torch.exp(moment_width_transfer)
- half_height = pts_y_std * torch.exp(moment_height_transfer)
- bbox = torch.cat([
- pts_x_mean - half_width, pts_y_mean - half_height,
- pts_x_mean + half_width, pts_y_mean + half_height
- ],
- dim=1)
- else:
- raise NotImplementedError
- return bbox
-
- def gen_grid_from_reg(self, reg, previous_boxes):
- """Base on the previous bboxes and regression values, we compute the
- regressed bboxes and generate the grids on the bboxes.
-
- :param reg: the regression value to previous bboxes.
- :param previous_boxes: previous bboxes.
- :return: generate grids on the regressed bboxes.
- """
- b, _, h, w = reg.shape
- bxy = (previous_boxes[:, :2, ...] + previous_boxes[:, 2:, ...]) / 2.
- bwh = (previous_boxes[:, 2:, ...] -
- previous_boxes[:, :2, ...]).clamp(min=1e-6)
- grid_topleft = bxy + bwh * reg[:, :2, ...] - 0.5 * bwh * torch.exp(
- reg[:, 2:, ...])
- grid_wh = bwh * torch.exp(reg[:, 2:, ...])
- grid_left = grid_topleft[:, [0], ...]
- grid_top = grid_topleft[:, [1], ...]
- grid_width = grid_wh[:, [0], ...]
- grid_height = grid_wh[:, [1], ...]
- intervel = torch.linspace(0., 1., self.dcn_kernel).view(
- 1, self.dcn_kernel, 1, 1).type_as(reg)
- grid_x = grid_left + grid_width * intervel
- grid_x = grid_x.unsqueeze(1).repeat(1, self.dcn_kernel, 1, 1, 1)
- grid_x = grid_x.view(b, -1, h, w)
- grid_y = grid_top + grid_height * intervel
- grid_y = grid_y.unsqueeze(2).repeat(1, 1, self.dcn_kernel, 1, 1)
- grid_y = grid_y.view(b, -1, h, w)
- grid_yx = torch.stack([grid_y, grid_x], dim=2)
- grid_yx = grid_yx.view(b, -1, h, w)
- regressed_bbox = torch.cat([
- grid_left, grid_top, grid_left + grid_width, grid_top + grid_height
- ], 1)
- return grid_yx, regressed_bbox
-
- def forward(self, feats):
- return multi_apply(self.forward_single, feats)
-
- def forward_single(self, x):
- """Forward feature map of a single FPN level."""
- dcn_base_offset = self.dcn_base_offset.type_as(x)
- # If we use center_init, the initial reppoints is from center points.
- # If we use bounding bbox representation, the initial reppoints is
- # from regular grid placed on a pre-defined bbox.
- if self.use_grid_points or not self.center_init:
- scale = self.point_base_scale / 2
- points_init = dcn_base_offset / dcn_base_offset.max() * scale
- bbox_init = x.new_tensor([-scale, -scale, scale,
- scale]).view(1, 4, 1, 1)
- else:
- points_init = 0
- cls_feat = x
- pts_feat = x
- for cls_conv in self.cls_convs:
- cls_feat = cls_conv(cls_feat)
- for reg_conv in self.reg_convs:
- pts_feat = reg_conv(pts_feat)
- # initialize reppoints
- pts_out_init = self.reppoints_pts_init_out(
- self.relu(self.reppoints_pts_init_conv(pts_feat)))
- if self.use_grid_points:
- pts_out_init, bbox_out_init = self.gen_grid_from_reg(
- pts_out_init, bbox_init.detach())
- else:
- pts_out_init = pts_out_init + points_init
- # refine and classify reppoints
- pts_out_init_grad_mul = (1 - self.gradient_mul) * pts_out_init.detach(
- ) + self.gradient_mul * pts_out_init
- dcn_offset = pts_out_init_grad_mul - dcn_base_offset
- cls_out = self.reppoints_cls_out(
- self.relu(self.reppoints_cls_conv(cls_feat, dcn_offset)))
- pts_out_refine = self.reppoints_pts_refine_out(
- self.relu(self.reppoints_pts_refine_conv(pts_feat, dcn_offset)))
- if self.use_grid_points:
- pts_out_refine, bbox_out_refine = self.gen_grid_from_reg(
- pts_out_refine, bbox_out_init.detach())
- else:
- pts_out_refine = pts_out_refine + pts_out_init.detach()
- return cls_out, pts_out_init, pts_out_refine
-
- def get_points(self, featmap_sizes, img_metas, device):
- """Get points according to feature map sizes.
-
- Args:
- featmap_sizes (list[tuple]): Multi-level feature map sizes.
- img_metas (list[dict]): Image meta info.
-
- Returns:
- tuple: points of each image, valid flags of each image
- """
- num_imgs = len(img_metas)
- num_levels = len(featmap_sizes)
-
- # since feature map sizes of all images are the same, we only compute
- # points center for one time
- multi_level_points = []
- for i in range(num_levels):
- points = self.point_generators[i].grid_points(
- featmap_sizes[i], self.point_strides[i], device)
- multi_level_points.append(points)
- points_list = [[point.clone() for point in multi_level_points]
- for _ in range(num_imgs)]
-
- # for each image, we compute valid flags of multi level grids
- valid_flag_list = []
- for img_id, img_meta in enumerate(img_metas):
- multi_level_flags = []
- for i in range(num_levels):
- point_stride = self.point_strides[i]
- feat_h, feat_w = featmap_sizes[i]
- h, w = img_meta['pad_shape'][:2]
- valid_feat_h = min(int(np.ceil(h / point_stride)), feat_h)
- valid_feat_w = min(int(np.ceil(w / point_stride)), feat_w)
- flags = self.point_generators[i].valid_flags(
- (feat_h, feat_w), (valid_feat_h, valid_feat_w), device)
- multi_level_flags.append(flags)
- valid_flag_list.append(multi_level_flags)
-
- return points_list, valid_flag_list
-
- def centers_to_bboxes(self, point_list):
- """Get bboxes according to center points.
-
- Only used in :class:`MaxIoUAssigner`.
- """
- bbox_list = []
- for i_img, point in enumerate(point_list):
- bbox = []
- for i_lvl in range(len(self.point_strides)):
- scale = self.point_base_scale * self.point_strides[i_lvl] * 0.5
- bbox_shift = torch.Tensor([-scale, -scale, scale,
- scale]).view(1, 4).type_as(point[0])
- bbox_center = torch.cat(
- [point[i_lvl][:, :2], point[i_lvl][:, :2]], dim=1)
- bbox.append(bbox_center + bbox_shift)
- bbox_list.append(bbox)
- return bbox_list
-
- def offset_to_pts(self, center_list, pred_list):
- """Change from point offset to point coordinate."""
- pts_list = []
- for i_lvl in range(len(self.point_strides)):
- pts_lvl = []
- for i_img in range(len(center_list)):
- pts_center = center_list[i_img][i_lvl][:, :2].repeat(
- 1, self.num_points)
- pts_shift = pred_list[i_lvl][i_img]
- yx_pts_shift = pts_shift.permute(1, 2, 0).view(
- -1, 2 * self.num_points)
- y_pts_shift = yx_pts_shift[..., 0::2]
- x_pts_shift = yx_pts_shift[..., 1::2]
- xy_pts_shift = torch.stack([x_pts_shift, y_pts_shift], -1)
- xy_pts_shift = xy_pts_shift.view(*yx_pts_shift.shape[:-1], -1)
- pts = xy_pts_shift * self.point_strides[i_lvl] + pts_center
- pts_lvl.append(pts)
- pts_lvl = torch.stack(pts_lvl, 0)
- pts_list.append(pts_lvl)
- return pts_list
-
- def _point_target_single(self,
- flat_proposals,
- valid_flags,
- gt_bboxes,
- gt_bboxes_ignore,
- gt_labels,
- label_channels=1,
- stage='init',
- unmap_outputs=True):
- inside_flags = valid_flags
- if not inside_flags.any():
- return (None, ) * 7
- # assign gt and sample proposals
- proposals = flat_proposals[inside_flags, :]
-
- if stage == 'init':
- assigner = self.init_assigner
- pos_weight = self.train_cfg.init.pos_weight
- else:
- assigner = self.refine_assigner
- pos_weight = self.train_cfg.refine.pos_weight
- assign_result = assigner.assign(proposals, gt_bboxes, gt_bboxes_ignore,
- None if self.sampling else gt_labels)
- sampling_result = self.sampler.sample(assign_result, proposals,
- gt_bboxes)
-
- num_valid_proposals = proposals.shape[0]
- bbox_gt = proposals.new_zeros([num_valid_proposals, 4])
- pos_proposals = torch.zeros_like(proposals)
- proposals_weights = proposals.new_zeros([num_valid_proposals, 4])
- labels = proposals.new_full((num_valid_proposals, ),
- self.num_classes,
- dtype=torch.long)
- label_weights = proposals.new_zeros(
- num_valid_proposals, dtype=torch.float)
-
- pos_inds = sampling_result.pos_inds
- neg_inds = sampling_result.neg_inds
- if len(pos_inds) > 0:
- pos_gt_bboxes = sampling_result.pos_gt_bboxes
- bbox_gt[pos_inds, :] = pos_gt_bboxes
- pos_proposals[pos_inds, :] = proposals[pos_inds, :]
- proposals_weights[pos_inds, :] = 1.0
- if gt_labels is None:
- # Only rpn gives gt_labels as None
- # Foreground is the first class
- labels[pos_inds] = 0
- else:
- labels[pos_inds] = gt_labels[
- sampling_result.pos_assigned_gt_inds]
- if pos_weight <= 0:
- label_weights[pos_inds] = 1.0
- else:
- label_weights[pos_inds] = pos_weight
- if len(neg_inds) > 0:
- label_weights[neg_inds] = 1.0
-
- # map up to original set of proposals
- if unmap_outputs:
- num_total_proposals = flat_proposals.size(0)
- labels = unmap(labels, num_total_proposals, inside_flags)
- label_weights = unmap(label_weights, num_total_proposals,
- inside_flags)
- bbox_gt = unmap(bbox_gt, num_total_proposals, inside_flags)
- pos_proposals = unmap(pos_proposals, num_total_proposals,
- inside_flags)
- proposals_weights = unmap(proposals_weights, num_total_proposals,
- inside_flags)
-
- return (labels, label_weights, bbox_gt, pos_proposals,
- proposals_weights, pos_inds, neg_inds)
-
- def get_targets(self,
- proposals_list,
- valid_flag_list,
- gt_bboxes_list,
- img_metas,
- gt_bboxes_ignore_list=None,
- gt_labels_list=None,
- stage='init',
- label_channels=1,
- unmap_outputs=True):
- """Compute corresponding GT box and classification targets for
- proposals.
-
- Args:
- proposals_list (list[list]): Multi level points/bboxes of each
- image.
- valid_flag_list (list[list]): Multi level valid flags of each
- image.
- gt_bboxes_list (list[Tensor]): Ground truth bboxes of each image.
- img_metas (list[dict]): Meta info of each image.
- gt_bboxes_ignore_list (list[Tensor]): Ground truth bboxes to be
- ignored.
- gt_bboxes_list (list[Tensor]): Ground truth labels of each box.
- stage (str): `init` or `refine`. Generate target for init stage or
- refine stage
- label_channels (int): Channel of label.
- unmap_outputs (bool): Whether to map outputs back to the original
- set of anchors.
-
- Returns:
- tuple:
- - labels_list (list[Tensor]): Labels of each level.
- - label_weights_list (list[Tensor]): Label weights of each level. # noqa: E501
- - bbox_gt_list (list[Tensor]): Ground truth bbox of each level.
- - proposal_list (list[Tensor]): Proposals(points/bboxes) of each level. # noqa: E501
- - proposal_weights_list (list[Tensor]): Proposal weights of each level. # noqa: E501
- - num_total_pos (int): Number of positive samples in all images. # noqa: E501
- - num_total_neg (int): Number of negative samples in all images. # noqa: E501
- """
- assert stage in ['init', 'refine']
- num_imgs = len(img_metas)
- assert len(proposals_list) == len(valid_flag_list) == num_imgs
-
- # points number of multi levels
- num_level_proposals = [points.size(0) for points in proposals_list[0]]
-
- # concat all level points and flags to a single tensor
- for i in range(num_imgs):
- assert len(proposals_list[i]) == len(valid_flag_list[i])
- proposals_list[i] = torch.cat(proposals_list[i])
- valid_flag_list[i] = torch.cat(valid_flag_list[i])
-
- # compute targets for each image
- if gt_bboxes_ignore_list is None:
- gt_bboxes_ignore_list = [None for _ in range(num_imgs)]
- if gt_labels_list is None:
- gt_labels_list = [None for _ in range(num_imgs)]
- (all_labels, all_label_weights, all_bbox_gt, all_proposals,
- all_proposal_weights, pos_inds_list, neg_inds_list) = multi_apply(
- self._point_target_single,
- proposals_list,
- valid_flag_list,
- gt_bboxes_list,
- gt_bboxes_ignore_list,
- gt_labels_list,
- stage=stage,
- label_channels=label_channels,
- unmap_outputs=unmap_outputs)
- # no valid points
- if any([labels is None for labels in all_labels]):
- return None
- # sampled points of all images
- num_total_pos = sum([max(inds.numel(), 1) for inds in pos_inds_list])
- num_total_neg = sum([max(inds.numel(), 1) for inds in neg_inds_list])
- labels_list = images_to_levels(all_labels, num_level_proposals)
- label_weights_list = images_to_levels(all_label_weights,
- num_level_proposals)
- bbox_gt_list = images_to_levels(all_bbox_gt, num_level_proposals)
- proposals_list = images_to_levels(all_proposals, num_level_proposals)
- proposal_weights_list = images_to_levels(all_proposal_weights,
- num_level_proposals)
- return (labels_list, label_weights_list, bbox_gt_list, proposals_list,
- proposal_weights_list, num_total_pos, num_total_neg)
-
- def loss_single(self, cls_score, pts_pred_init, pts_pred_refine, labels,
- label_weights, bbox_gt_init, bbox_weights_init,
- bbox_gt_refine, bbox_weights_refine, stride,
- num_total_samples_init, num_total_samples_refine):
- # classification loss
- labels = labels.reshape(-1)
- label_weights = label_weights.reshape(-1)
- cls_score = cls_score.permute(0, 2, 3,
- 1).reshape(-1, self.cls_out_channels)
- cls_score = cls_score.contiguous()
- loss_cls = self.loss_cls(
- cls_score,
- labels,
- label_weights,
- avg_factor=num_total_samples_refine)
-
- # points loss
- bbox_gt_init = bbox_gt_init.reshape(-1, 4)
- bbox_weights_init = bbox_weights_init.reshape(-1, 4)
- bbox_pred_init = self.points2bbox(
- pts_pred_init.reshape(-1, 2 * self.num_points), y_first=False)
- bbox_gt_refine = bbox_gt_refine.reshape(-1, 4)
- bbox_weights_refine = bbox_weights_refine.reshape(-1, 4)
- bbox_pred_refine = self.points2bbox(
- pts_pred_refine.reshape(-1, 2 * self.num_points), y_first=False)
- normalize_term = self.point_base_scale * stride
- loss_pts_init = self.loss_bbox_init(
- bbox_pred_init / normalize_term,
- bbox_gt_init / normalize_term,
- bbox_weights_init,
- avg_factor=num_total_samples_init)
- loss_pts_refine = self.loss_bbox_refine(
- bbox_pred_refine / normalize_term,
- bbox_gt_refine / normalize_term,
- bbox_weights_refine,
- avg_factor=num_total_samples_refine)
- return loss_cls, loss_pts_init, loss_pts_refine
-
- def loss(self,
- cls_scores,
- pts_preds_init,
- pts_preds_refine,
- gt_bboxes,
- gt_labels,
- img_metas,
- gt_bboxes_ignore=None):
- featmap_sizes = [featmap.size()[-2:] for featmap in cls_scores]
- assert len(featmap_sizes) == len(self.point_generators)
- device = cls_scores[0].device
- label_channels = self.cls_out_channels if self.use_sigmoid_cls else 1
-
- # target for initial stage
- center_list, valid_flag_list = self.get_points(featmap_sizes,
- img_metas, device)
- pts_coordinate_preds_init = self.offset_to_pts(center_list,
- pts_preds_init)
- if self.train_cfg.init.assigner['type'] == 'PointAssigner':
- # Assign target for center list
- candidate_list = center_list
- else:
- # transform center list to bbox list and
- # assign target for bbox list
- bbox_list = self.centers_to_bboxes(center_list)
- candidate_list = bbox_list
- cls_reg_targets_init = self.get_targets(
- candidate_list,
- valid_flag_list,
- gt_bboxes,
- img_metas,
- gt_bboxes_ignore_list=gt_bboxes_ignore,
- gt_labels_list=gt_labels,
- stage='init',
- label_channels=label_channels)
- (*_, bbox_gt_list_init, candidate_list_init, bbox_weights_list_init,
- num_total_pos_init, num_total_neg_init) = cls_reg_targets_init
- num_total_samples_init = (
- num_total_pos_init +
- num_total_neg_init if self.sampling else num_total_pos_init)
-
- # target for refinement stage
- center_list, valid_flag_list = self.get_points(featmap_sizes,
- img_metas, device)
- pts_coordinate_preds_refine = self.offset_to_pts(
- center_list, pts_preds_refine)
- bbox_list = []
- for i_img, center in enumerate(center_list):
- bbox = []
- for i_lvl in range(len(pts_preds_refine)):
- bbox_preds_init = self.points2bbox(
- pts_preds_init[i_lvl].detach())
- bbox_shift = bbox_preds_init * self.point_strides[i_lvl]
- bbox_center = torch.cat(
- [center[i_lvl][:, :2], center[i_lvl][:, :2]], dim=1)
- bbox.append(bbox_center +
- bbox_shift[i_img].permute(1, 2, 0).reshape(-1, 4))
- bbox_list.append(bbox)
- cls_reg_targets_refine = self.get_targets(
- bbox_list,
- valid_flag_list,
- gt_bboxes,
- img_metas,
- gt_bboxes_ignore_list=gt_bboxes_ignore,
- gt_labels_list=gt_labels,
- stage='refine',
- label_channels=label_channels)
- (labels_list, label_weights_list, bbox_gt_list_refine,
- candidate_list_refine, bbox_weights_list_refine, num_total_pos_refine,
- num_total_neg_refine) = cls_reg_targets_refine
- num_total_samples_refine = (
- num_total_pos_refine +
- num_total_neg_refine if self.sampling else num_total_pos_refine)
-
- # compute loss
- losses_cls, losses_pts_init, losses_pts_refine = multi_apply(
- self.loss_single,
- cls_scores,
- pts_coordinate_preds_init,
- pts_coordinate_preds_refine,
- labels_list,
- label_weights_list,
- bbox_gt_list_init,
- bbox_weights_list_init,
- bbox_gt_list_refine,
- bbox_weights_list_refine,
- self.point_strides,
- num_total_samples_init=num_total_samples_init,
- num_total_samples_refine=num_total_samples_refine)
- loss_dict_all = {
- 'loss_cls': losses_cls,
- 'loss_pts_init': losses_pts_init,
- 'loss_pts_refine': losses_pts_refine
- }
- return loss_dict_all
-
- def get_bboxes(self,
- cls_scores,
- pts_preds_init,
- pts_preds_refine,
- img_metas,
- cfg=None,
- rescale=False,
- with_nms=True):
- assert len(cls_scores) == len(pts_preds_refine)
- device = cls_scores[0].device
- bbox_preds_refine = [
- self.points2bbox(pts_pred_refine)
- for pts_pred_refine in pts_preds_refine
- ]
- num_levels = len(cls_scores)
- mlvl_points = [
- self.point_generators[i].grid_points(cls_scores[i].size()[-2:],
- self.point_strides[i], device)
- for i in range(num_levels)
- ]
- result_list = []
- for img_id in range(len(img_metas)):
- cls_score_list = [
- cls_scores[i][img_id].detach() for i in range(num_levels)
- ]
- bbox_pred_list = [
- bbox_preds_refine[i][img_id].detach()
- for i in range(num_levels)
- ]
- img_shape = img_metas[img_id]['img_shape']
- scale_factor = img_metas[img_id]['scale_factor']
- proposals = self._get_bboxes_single(cls_score_list, bbox_pred_list,
- mlvl_points, img_shape,
- scale_factor, cfg, rescale,
- with_nms)
- result_list.append(proposals)
- return result_list
-
- def _get_bboxes_single(self,
- cls_scores,
- bbox_preds,
- mlvl_points,
- img_shape,
- scale_factor,
- cfg,
- rescale=False,
- with_nms=True):
- cfg = self.test_cfg if cfg is None else cfg
- assert len(cls_scores) == len(bbox_preds) == len(mlvl_points)
- mlvl_bboxes = []
- mlvl_scores = []
- for i_lvl, (cls_score, bbox_pred, points) in enumerate(
- zip(cls_scores, bbox_preds, mlvl_points)):
- assert cls_score.size()[-2:] == bbox_pred.size()[-2:]
- cls_score = cls_score.permute(1, 2,
- 0).reshape(-1, self.cls_out_channels)
- if self.use_sigmoid_cls:
- scores = cls_score.sigmoid()
- else:
- scores = cls_score.softmax(-1)
- bbox_pred = bbox_pred.permute(1, 2, 0).reshape(-1, 4)
- nms_pre = cfg.get('nms_pre', -1)
- if nms_pre > 0 and scores.shape[0] > nms_pre:
- if self.use_sigmoid_cls:
- max_scores, _ = scores.max(dim=1)
- else:
- # remind that we set FG labels to [0, num_class-1]
- # since mmdet v2.0
- # BG cat_id: num_class
- max_scores, _ = scores[:, :-1].max(dim=1)
- _, topk_inds = max_scores.topk(nms_pre)
- points = points[topk_inds, :]
- bbox_pred = bbox_pred[topk_inds, :]
- scores = scores[topk_inds, :]
- bbox_pos_center = torch.cat([points[:, :2], points[:, :2]], dim=1)
- bboxes = bbox_pred * self.point_strides[i_lvl] + bbox_pos_center
- x1 = bboxes[:, 0].clamp(min=0, max=img_shape[1])
- y1 = bboxes[:, 1].clamp(min=0, max=img_shape[0])
- x2 = bboxes[:, 2].clamp(min=0, max=img_shape[1])
- y2 = bboxes[:, 3].clamp(min=0, max=img_shape[0])
- bboxes = torch.stack([x1, y1, x2, y2], dim=-1)
- mlvl_bboxes.append(bboxes)
- mlvl_scores.append(scores)
- mlvl_bboxes = torch.cat(mlvl_bboxes)
- if rescale:
- mlvl_bboxes /= mlvl_bboxes.new_tensor(scale_factor)
- mlvl_scores = torch.cat(mlvl_scores)
- if self.use_sigmoid_cls:
- # Add a dummy background class to the backend when using sigmoid
- # remind that we set FG labels to [0, num_class-1] since mmdet v2.0
- # BG cat_id: num_class
- padding = mlvl_scores.new_zeros(mlvl_scores.shape[0], 1)
- mlvl_scores = torch.cat([mlvl_scores, padding], dim=1)
- if with_nms:
- det_bboxes, det_labels = multiclass_nms(mlvl_bboxes, mlvl_scores,
- cfg.score_thr, cfg.nms,
- cfg.max_per_img)
- return det_bboxes, det_labels
- else:
- return mlvl_bboxes, mlvl_scores
diff --git a/spaces/Andy1621/uniformer_image_segmentation/configs/_base_/datasets/drive.py b/spaces/Andy1621/uniformer_image_segmentation/configs/_base_/datasets/drive.py
deleted file mode 100644
index 06e8ff606e0d2a4514ec8b7d2c6c436a32efcbf4..0000000000000000000000000000000000000000
--- a/spaces/Andy1621/uniformer_image_segmentation/configs/_base_/datasets/drive.py
+++ /dev/null
@@ -1,59 +0,0 @@
-# dataset settings
-dataset_type = 'DRIVEDataset'
-data_root = 'data/DRIVE'
-img_norm_cfg = dict(
- mean=[123.675, 116.28, 103.53], std=[58.395, 57.12, 57.375], to_rgb=True)
-img_scale = (584, 565)
-crop_size = (64, 64)
-train_pipeline = [
- dict(type='LoadImageFromFile'),
- dict(type='LoadAnnotations'),
- dict(type='Resize', img_scale=img_scale, ratio_range=(0.5, 2.0)),
- dict(type='RandomCrop', crop_size=crop_size, cat_max_ratio=0.75),
- dict(type='RandomFlip', prob=0.5),
- dict(type='PhotoMetricDistortion'),
- dict(type='Normalize', **img_norm_cfg),
- dict(type='Pad', size=crop_size, pad_val=0, seg_pad_val=255),
- dict(type='DefaultFormatBundle'),
- dict(type='Collect', keys=['img', 'gt_semantic_seg'])
-]
-test_pipeline = [
- dict(type='LoadImageFromFile'),
- dict(
- type='MultiScaleFlipAug',
- img_scale=img_scale,
- # img_ratios=[0.5, 0.75, 1.0, 1.25, 1.5, 1.75, 2.0],
- flip=False,
- transforms=[
- dict(type='Resize', keep_ratio=True),
- dict(type='RandomFlip'),
- dict(type='Normalize', **img_norm_cfg),
- dict(type='ImageToTensor', keys=['img']),
- dict(type='Collect', keys=['img'])
- ])
-]
-
-data = dict(
- samples_per_gpu=4,
- workers_per_gpu=4,
- train=dict(
- type='RepeatDataset',
- times=40000,
- dataset=dict(
- type=dataset_type,
- data_root=data_root,
- img_dir='images/training',
- ann_dir='annotations/training',
- pipeline=train_pipeline)),
- val=dict(
- type=dataset_type,
- data_root=data_root,
- img_dir='images/validation',
- ann_dir='annotations/validation',
- pipeline=test_pipeline),
- test=dict(
- type=dataset_type,
- data_root=data_root,
- img_dir='images/validation',
- ann_dir='annotations/validation',
- pipeline=test_pipeline))
diff --git a/spaces/Andy1621/uniformer_image_segmentation/configs/deeplabv3plus/deeplabv3plus_r101-d8_512x512_80k_ade20k.py b/spaces/Andy1621/uniformer_image_segmentation/configs/deeplabv3plus/deeplabv3plus_r101-d8_512x512_80k_ade20k.py
deleted file mode 100644
index 53fd3a909585367ca59eb827c2fbbab4cdf234ea..0000000000000000000000000000000000000000
--- a/spaces/Andy1621/uniformer_image_segmentation/configs/deeplabv3plus/deeplabv3plus_r101-d8_512x512_80k_ade20k.py
+++ /dev/null
@@ -1,2 +0,0 @@
-_base_ = './deeplabv3plus_r50-d8_512x512_80k_ade20k.py'
-model = dict(pretrained='open-mmlab://resnet101_v1c', backbone=dict(depth=101))
diff --git a/spaces/Andy1621/uniformer_image_segmentation/configs/fcn/fcn_r101-d8_512x512_40k_voc12aug.py b/spaces/Andy1621/uniformer_image_segmentation/configs/fcn/fcn_r101-d8_512x512_40k_voc12aug.py
deleted file mode 100644
index eafefaa67565513c277c5eb42e3661a88133cb27..0000000000000000000000000000000000000000
--- a/spaces/Andy1621/uniformer_image_segmentation/configs/fcn/fcn_r101-d8_512x512_40k_voc12aug.py
+++ /dev/null
@@ -1,2 +0,0 @@
-_base_ = './fcn_r50-d8_512x512_40k_voc12aug.py'
-model = dict(pretrained='open-mmlab://resnet101_v1c', backbone=dict(depth=101))
diff --git a/spaces/AnnonSubmission/xai-cl/ssl_models/dino.py b/spaces/AnnonSubmission/xai-cl/ssl_models/dino.py
deleted file mode 100644
index 0e35215338cb438a95661964f146f74d40ba066b..0000000000000000000000000000000000000000
--- a/spaces/AnnonSubmission/xai-cl/ssl_models/dino.py
+++ /dev/null
@@ -1,181 +0,0 @@
-import torch
-import torch.nn as nn
-import torchvision
-import torch.nn.functional as F
-import numpy as np
-device = torch.device("cuda" if torch.cuda.is_available() else "cpu")
-
-""" from https://github.com/facebookresearch/dino"""
-
-class DINOHead(nn.Module):
-
- def __init__(self, in_dim, out_dim, use_bn, norm_last_layer, nlayers, hidden_dim, bottleneck_dim):
- super().__init__()
-
- nlayers = max(nlayers, 1)
- if nlayers == 1:
- self.mlp = nn.Linear(in_dim, bottleneck_dim)
- else:
- layers = [nn.Linear(in_dim, hidden_dim)]
- if use_bn:
- layers.append(nn.BatchNorm1d(hidden_dim))
- layers.append(nn.GELU())
- for _ in range(nlayers - 2):
- layers.append(nn.Linear(hidden_dim, hidden_dim))
- if use_bn:
- layers.append(nn.BatchNorm1d(hidden_dim))
- layers.append(nn.GELU())
- layers.append(nn.Linear(hidden_dim, bottleneck_dim))
- self.mlp = nn.Sequential(*layers)
-
- self.last_layer = nn.utils.weight_norm(nn.Linear(bottleneck_dim, out_dim, bias=False))
- self.last_layer.weight_g.data.fill_(1)
- if norm_last_layer:
- self.last_layer.weight_g.requires_grad = False
-
- def forward(self, x):
- x = self.mlp(x)
- x = F.normalize(x, dim=-1, p=2)
- x = self.last_layer(x)
- return x
-
-class MultiCropWrapper(nn.Module):
- def __init__(self, backbone, head):
- super(MultiCropWrapper, self).__init__()
- backbone.fc, backbone.head = nn.Identity(), nn.Identity()
- self.backbone = backbone
- self.head = head
-
- def forward(self, x):
- return self.head(self.backbone(x))
-
-class DINOLoss(nn.Module):
- def __init__(self, out_dim, warmup_teacher_temp, teacher_temp, warmup_teacher_temp_epochs, nepochs,
- student_temp=0.1, center_momentum=0.9):
- super().__init__()
-
- self.student_temp = student_temp
- self.center_momentum = center_momentum
- self.register_buffer("center", torch.zeros(1, out_dim))
- self.nepochs = nepochs
- self.teacher_temp_schedule = np.concatenate((np.linspace(warmup_teacher_temp, teacher_temp, warmup_teacher_temp_epochs),
- np.ones(nepochs - warmup_teacher_temp_epochs) * teacher_temp))
-
- def forward(self, student_output, teacher_output):
- student_out = student_output / self.student_temp
- temp = self.teacher_temp_schedule[self.nepochs - 1] # last one
- teacher_out = F.softmax((teacher_output - self.center) / temp, dim=-1)
- teacher_out = teacher_out.detach()
- loss = torch.sum(-teacher_out * F.log_softmax(student_out, dim=-1), dim=-1).mean()
- return loss
-
-
-class ResNet(nn.Module):
- def __init__(self, backbone):
- super().__init__()
-
- modules = list(backbone.children())[:-2]
- self.net = nn.Sequential(*modules)
-
- def forward(self, x):
- return self.net(x).mean(dim=[2, 3])
-
-class RestructuredDINO(nn.Module):
-
- def __init__(self, student, teacher):
- super().__init__()
-
- self.encoder_student = ResNet(student.backbone)
- self.encoder = ResNet(teacher.backbone)
-
- self.contrastive_head_student = student.head
- self.contrastive_head = teacher.head
-
-
- def forward(self, x, run_teacher):
-
- if run_teacher:
- x = self.encoder(x)
- x = self.contrastive_head(x)
- else:
- x = self.encoder_student(x)
- x = self.contrastive_head_student(x)
-
- return x
-
-
-def get_dino_model_without_loss(ckpt_path = 'dino_resnet50_pretrain_full_checkpoint.pth'):
- state_dict = torch.load('pretrained_models/dino_models/' + ckpt_path, map_location='cpu')
- state_dict_student = state_dict['student']
- state_dict_teacher = state_dict['teacher']
-
- state_dict_student = {k.replace("module.", ""): v for k, v in state_dict_student.items()}
- state_dict_teacher = {k.replace("module.", ""): v for k, v in state_dict_teacher.items()}
-
- student_backbone = torchvision.models.resnet50()
- teacher_backbone = torchvision.models.resnet50()
- embed_dim = student_backbone.fc.weight.shape[1]
-
- student_head = DINOHead(in_dim = embed_dim, out_dim = 60000, use_bn=True, norm_last_layer=True, nlayers=2, hidden_dim=4096, bottleneck_dim=256)
- teacher_head = DINOHead(in_dim = embed_dim, out_dim = 60000, use_bn =True, norm_last_layer=True, nlayers=2, hidden_dim=4096, bottleneck_dim=256)
- student_head.last_layer = nn.Linear(256, 60000, bias = False)
- teacher_head.last_layer = nn.Linear(256, 60000, bias = False)
-
- student = MultiCropWrapper(student_backbone, student_head)
- teacher = MultiCropWrapper(teacher_backbone, teacher_head)
-
- student.load_state_dict(state_dict_student)
- teacher.load_state_dict(state_dict_teacher)
-
- restructured_model = RestructuredDINO(student, teacher)
-
- return restructured_model.to(device)
-
-
-def get_dino_model_with_loss(ckpt_path = 'dino_rn50_checkpoint.pth'):
- state_dict = torch.load('pretrained_models/dino_models/' + ckpt_path, map_location='cpu')
-
- state_dict_student = state_dict['student']
- state_dict_teacher = state_dict['teacher']
- state_dict_args = vars(state_dict['args'])
- state_dic_dino_loss = state_dict['dino_loss']
-
- state_dict_student = {k.replace("module.", ""): v for k, v in state_dict_student.items()}
- state_dict_teacher = {k.replace("module.", ""): v for k, v in state_dict_teacher.items()}
-
- student_backbone = torchvision.models.resnet50()
- teacher_backbone = torchvision.models.resnet50()
- embed_dim = student_backbone.fc.weight.shape[1]
-
- student_head = DINOHead(in_dim = embed_dim,
- out_dim = state_dict_args['out_dim'],
- use_bn = state_dict_args['use_bn_in_head'],
- norm_last_layer = state_dict_args['norm_last_layer'],
- nlayers = 3,
- hidden_dim = 2048,
- bottleneck_dim = 256)
-
- teacher_head = DINOHead(in_dim = embed_dim,
- out_dim = state_dict_args['out_dim'],
- use_bn = state_dict_args['use_bn_in_head'],
- norm_last_layer = state_dict_args['norm_last_layer'],
- nlayers = 3,
- hidden_dim = 2048,
- bottleneck_dim = 256)
-
- loss = DINOLoss(out_dim = state_dict_args['out_dim'],
- warmup_teacher_temp = state_dict_args['warmup_teacher_temp'],
- teacher_temp = state_dict_args['teacher_temp'],
- warmup_teacher_temp_epochs = state_dict_args['warmup_teacher_temp_epochs'],
- nepochs = state_dict_args['epochs'])
-
- student = MultiCropWrapper(student_backbone, student_head)
- teacher = MultiCropWrapper(teacher_backbone, teacher_head)
-
- student.load_state_dict(state_dict_student)
- teacher.load_state_dict(state_dict_teacher)
- loss.load_state_dict(state_dic_dino_loss)
-
- restructured_model = RestructuredDINO(student, teacher)
-
- return restructured_model.to(device), loss.to(device)
\ No newline at end of file
diff --git a/spaces/Annotation-AI/fast-segment-everything-with-text-prompt/README.md b/spaces/Annotation-AI/fast-segment-everything-with-text-prompt/README.md
deleted file mode 100644
index d985cfb10b2840e52098335cf335631c2703b0e8..0000000000000000000000000000000000000000
--- a/spaces/Annotation-AI/fast-segment-everything-with-text-prompt/README.md
+++ /dev/null
@@ -1,12 +0,0 @@
----
-title: Fast Segment Anything With Text Prompt
-emoji: 🐨
-colorFrom: pink
-colorTo: green
-sdk: gradio
-sdk_version: 3.32.0
-app_file: app.py
-pinned: false
----
-
-Check out the configuration reference at https://huggingface.co/docs/hub/spaces-config-reference
diff --git a/spaces/Anonymous-sub/Rerender/ControlNet/ldm/modules/midas/midas/dpt_depth.py b/spaces/Anonymous-sub/Rerender/ControlNet/ldm/modules/midas/midas/dpt_depth.py
deleted file mode 100644
index 4e9aab5d2767dffea39da5b3f30e2798688216f1..0000000000000000000000000000000000000000
--- a/spaces/Anonymous-sub/Rerender/ControlNet/ldm/modules/midas/midas/dpt_depth.py
+++ /dev/null
@@ -1,109 +0,0 @@
-import torch
-import torch.nn as nn
-import torch.nn.functional as F
-
-from .base_model import BaseModel
-from .blocks import (
- FeatureFusionBlock,
- FeatureFusionBlock_custom,
- Interpolate,
- _make_encoder,
- forward_vit,
-)
-
-
-def _make_fusion_block(features, use_bn):
- return FeatureFusionBlock_custom(
- features,
- nn.ReLU(False),
- deconv=False,
- bn=use_bn,
- expand=False,
- align_corners=True,
- )
-
-
-class DPT(BaseModel):
- def __init__(
- self,
- head,
- features=256,
- backbone="vitb_rn50_384",
- readout="project",
- channels_last=False,
- use_bn=False,
- ):
-
- super(DPT, self).__init__()
-
- self.channels_last = channels_last
-
- hooks = {
- "vitb_rn50_384": [0, 1, 8, 11],
- "vitb16_384": [2, 5, 8, 11],
- "vitl16_384": [5, 11, 17, 23],
- }
-
- # Instantiate backbone and reassemble blocks
- self.pretrained, self.scratch = _make_encoder(
- backbone,
- features,
- False, # Set to true of you want to train from scratch, uses ImageNet weights
- groups=1,
- expand=False,
- exportable=False,
- hooks=hooks[backbone],
- use_readout=readout,
- )
-
- self.scratch.refinenet1 = _make_fusion_block(features, use_bn)
- self.scratch.refinenet2 = _make_fusion_block(features, use_bn)
- self.scratch.refinenet3 = _make_fusion_block(features, use_bn)
- self.scratch.refinenet4 = _make_fusion_block(features, use_bn)
-
- self.scratch.output_conv = head
-
-
- def forward(self, x):
- if self.channels_last == True:
- x.contiguous(memory_format=torch.channels_last)
-
- layer_1, layer_2, layer_3, layer_4 = forward_vit(self.pretrained, x)
-
- layer_1_rn = self.scratch.layer1_rn(layer_1)
- layer_2_rn = self.scratch.layer2_rn(layer_2)
- layer_3_rn = self.scratch.layer3_rn(layer_3)
- layer_4_rn = self.scratch.layer4_rn(layer_4)
-
- path_4 = self.scratch.refinenet4(layer_4_rn)
- path_3 = self.scratch.refinenet3(path_4, layer_3_rn)
- path_2 = self.scratch.refinenet2(path_3, layer_2_rn)
- path_1 = self.scratch.refinenet1(path_2, layer_1_rn)
-
- out = self.scratch.output_conv(path_1)
-
- return out
-
-
-class DPTDepthModel(DPT):
- def __init__(self, path=None, non_negative=True, **kwargs):
- features = kwargs["features"] if "features" in kwargs else 256
-
- head = nn.Sequential(
- nn.Conv2d(features, features // 2, kernel_size=3, stride=1, padding=1),
- Interpolate(scale_factor=2, mode="bilinear", align_corners=True),
- nn.Conv2d(features // 2, 32, kernel_size=3, stride=1, padding=1),
- nn.ReLU(True),
- nn.Conv2d(32, 1, kernel_size=1, stride=1, padding=0),
- nn.ReLU(True) if non_negative else nn.Identity(),
- nn.Identity(),
- )
-
- super().__init__(head, **kwargs)
-
- if path is not None:
- self.load(path)
-
- def forward(self, x):
- return super().forward(x).squeeze(dim=1)
-
diff --git a/spaces/Arulkumar03/GroundingDINO_SOTA_Zero_Shot_Model/groundingdino/models/GroundingDINO/backbone/backbone.py b/spaces/Arulkumar03/GroundingDINO_SOTA_Zero_Shot_Model/groundingdino/models/GroundingDINO/backbone/backbone.py
deleted file mode 100644
index c8340c723fad8e07e2fc62daaa3912487498814b..0000000000000000000000000000000000000000
--- a/spaces/Arulkumar03/GroundingDINO_SOTA_Zero_Shot_Model/groundingdino/models/GroundingDINO/backbone/backbone.py
+++ /dev/null
@@ -1,221 +0,0 @@
-# ------------------------------------------------------------------------
-# Grounding DINO
-# url: https://github.com/IDEA-Research/GroundingDINO
-# Copyright (c) 2023 IDEA. All Rights Reserved.
-# Licensed under the Apache License, Version 2.0 [see LICENSE for details]
-# ------------------------------------------------------------------------
-# Conditional DETR
-# Copyright (c) 2021 Microsoft. All Rights Reserved.
-# Licensed under the Apache License, Version 2.0 [see LICENSE for details]
-# ------------------------------------------------------------------------
-# Copied from DETR (https://github.com/facebookresearch/detr)
-# Copyright (c) Facebook, Inc. and its affiliates. All Rights Reserved.
-# ------------------------------------------------------------------------
-
-"""
-Backbone modules.
-"""
-
-from typing import Dict, List
-
-import torch
-import torch.nn.functional as F
-import torchvision
-from torch import nn
-from torchvision.models._utils import IntermediateLayerGetter
-
-from groundingdino.util.misc import NestedTensor, clean_state_dict, is_main_process
-
-from .position_encoding import build_position_encoding
-from .swin_transformer import build_swin_transformer
-
-
-class FrozenBatchNorm2d(torch.nn.Module):
- """
- BatchNorm2d where the batch statistics and the affine parameters are fixed.
-
- Copy-paste from torchvision.misc.ops with added eps before rqsrt,
- without which any other models than torchvision.models.resnet[18,34,50,101]
- produce nans.
- """
-
- def __init__(self, n):
- super(FrozenBatchNorm2d, self).__init__()
- self.register_buffer("weight", torch.ones(n))
- self.register_buffer("bias", torch.zeros(n))
- self.register_buffer("running_mean", torch.zeros(n))
- self.register_buffer("running_var", torch.ones(n))
-
- def _load_from_state_dict(
- self, state_dict, prefix, local_metadata, strict, missing_keys, unexpected_keys, error_msgs
- ):
- num_batches_tracked_key = prefix + "num_batches_tracked"
- if num_batches_tracked_key in state_dict:
- del state_dict[num_batches_tracked_key]
-
- super(FrozenBatchNorm2d, self)._load_from_state_dict(
- state_dict, prefix, local_metadata, strict, missing_keys, unexpected_keys, error_msgs
- )
-
- def forward(self, x):
- # move reshapes to the beginning
- # to make it fuser-friendly
- w = self.weight.reshape(1, -1, 1, 1)
- b = self.bias.reshape(1, -1, 1, 1)
- rv = self.running_var.reshape(1, -1, 1, 1)
- rm = self.running_mean.reshape(1, -1, 1, 1)
- eps = 1e-5
- scale = w * (rv + eps).rsqrt()
- bias = b - rm * scale
- return x * scale + bias
-
-
-class BackboneBase(nn.Module):
- def __init__(
- self,
- backbone: nn.Module,
- train_backbone: bool,
- num_channels: int,
- return_interm_indices: list,
- ):
- super().__init__()
- for name, parameter in backbone.named_parameters():
- if (
- not train_backbone
- or "layer2" not in name
- and "layer3" not in name
- and "layer4" not in name
- ):
- parameter.requires_grad_(False)
-
- return_layers = {}
- for idx, layer_index in enumerate(return_interm_indices):
- return_layers.update(
- {"layer{}".format(5 - len(return_interm_indices) + idx): "{}".format(layer_index)}
- )
-
- # if len:
- # if use_stage1_feature:
- # return_layers = {"layer1": "0", "layer2": "1", "layer3": "2", "layer4": "3"}
- # else:
- # return_layers = {"layer2": "0", "layer3": "1", "layer4": "2"}
- # else:
- # return_layers = {'layer4': "0"}
- self.body = IntermediateLayerGetter(backbone, return_layers=return_layers)
- self.num_channels = num_channels
-
- def forward(self, tensor_list: NestedTensor):
- xs = self.body(tensor_list.tensors)
- out: Dict[str, NestedTensor] = {}
- for name, x in xs.items():
- m = tensor_list.mask
- assert m is not None
- mask = F.interpolate(m[None].float(), size=x.shape[-2:]).to(torch.bool)[0]
- out[name] = NestedTensor(x, mask)
- # import ipdb; ipdb.set_trace()
- return out
-
-
-class Backbone(BackboneBase):
- """ResNet backbone with frozen BatchNorm."""
-
- def __init__(
- self,
- name: str,
- train_backbone: bool,
- dilation: bool,
- return_interm_indices: list,
- batch_norm=FrozenBatchNorm2d,
- ):
- if name in ["resnet18", "resnet34", "resnet50", "resnet101"]:
- backbone = getattr(torchvision.models, name)(
- replace_stride_with_dilation=[False, False, dilation],
- pretrained=is_main_process(),
- norm_layer=batch_norm,
- )
- else:
- raise NotImplementedError("Why you can get here with name {}".format(name))
- # num_channels = 512 if name in ('resnet18', 'resnet34') else 2048
- assert name not in ("resnet18", "resnet34"), "Only resnet50 and resnet101 are available."
- assert return_interm_indices in [[0, 1, 2, 3], [1, 2, 3], [3]]
- num_channels_all = [256, 512, 1024, 2048]
- num_channels = num_channels_all[4 - len(return_interm_indices) :]
- super().__init__(backbone, train_backbone, num_channels, return_interm_indices)
-
-
-class Joiner(nn.Sequential):
- def __init__(self, backbone, position_embedding):
- super().__init__(backbone, position_embedding)
-
- def forward(self, tensor_list: NestedTensor):
- xs = self[0](tensor_list)
- out: List[NestedTensor] = []
- pos = []
- for name, x in xs.items():
- out.append(x)
- # position encoding
- pos.append(self[1](x).to(x.tensors.dtype))
-
- return out, pos
-
-
-def build_backbone(args):
- """
- Useful args:
- - backbone: backbone name
- - lr_backbone:
- - dilation
- - return_interm_indices: available: [0,1,2,3], [1,2,3], [3]
- - backbone_freeze_keywords:
- - use_checkpoint: for swin only for now
-
- """
- position_embedding = build_position_encoding(args)
- train_backbone = True
- if not train_backbone:
- raise ValueError("Please set lr_backbone > 0")
- return_interm_indices = args.return_interm_indices
- assert return_interm_indices in [[0, 1, 2, 3], [1, 2, 3], [3]]
- args.backbone_freeze_keywords
- use_checkpoint = getattr(args, "use_checkpoint", False)
-
- if args.backbone in ["resnet50", "resnet101"]:
- backbone = Backbone(
- args.backbone,
- train_backbone,
- args.dilation,
- return_interm_indices,
- batch_norm=FrozenBatchNorm2d,
- )
- bb_num_channels = backbone.num_channels
- elif args.backbone in [
- "swin_T_224_1k",
- "swin_B_224_22k",
- "swin_B_384_22k",
- "swin_L_224_22k",
- "swin_L_384_22k",
- ]:
- pretrain_img_size = int(args.backbone.split("_")[-2])
- backbone = build_swin_transformer(
- args.backbone,
- pretrain_img_size=pretrain_img_size,
- out_indices=tuple(return_interm_indices),
- dilation=False,
- use_checkpoint=use_checkpoint,
- )
-
- bb_num_channels = backbone.num_features[4 - len(return_interm_indices) :]
- else:
- raise NotImplementedError("Unknown backbone {}".format(args.backbone))
-
- assert len(bb_num_channels) == len(
- return_interm_indices
- ), f"len(bb_num_channels) {len(bb_num_channels)} != len(return_interm_indices) {len(return_interm_indices)}"
-
- model = Joiner(backbone, position_embedding)
- model.num_channels = bb_num_channels
- assert isinstance(
- bb_num_channels, List
- ), "bb_num_channels is expected to be a List but {}".format(type(bb_num_channels))
- # import ipdb; ipdb.set_trace()
- return model
diff --git a/spaces/Ataturk-Chatbot/HuggingFaceChat/venv/lib/python3.11/site-packages/dotenv/__init__.py b/spaces/Ataturk-Chatbot/HuggingFaceChat/venv/lib/python3.11/site-packages/dotenv/__init__.py
deleted file mode 100644
index 7f4c631ba11786bceebd22591f91bd378d8b232c..0000000000000000000000000000000000000000
--- a/spaces/Ataturk-Chatbot/HuggingFaceChat/venv/lib/python3.11/site-packages/dotenv/__init__.py
+++ /dev/null
@@ -1,49 +0,0 @@
-from typing import Any, Optional
-
-from .main import (dotenv_values, find_dotenv, get_key, load_dotenv, set_key,
- unset_key)
-
-
-def load_ipython_extension(ipython: Any) -> None:
- from .ipython import load_ipython_extension
- load_ipython_extension(ipython)
-
-
-def get_cli_string(
- path: Optional[str] = None,
- action: Optional[str] = None,
- key: Optional[str] = None,
- value: Optional[str] = None,
- quote: Optional[str] = None,
-):
- """Returns a string suitable for running as a shell script.
-
- Useful for converting a arguments passed to a fabric task
- to be passed to a `local` or `run` command.
- """
- command = ['dotenv']
- if quote:
- command.append(f'-q {quote}')
- if path:
- command.append(f'-f {path}')
- if action:
- command.append(action)
- if key:
- command.append(key)
- if value:
- if ' ' in value:
- command.append(f'"{value}"')
- else:
- command.append(value)
-
- return ' '.join(command).strip()
-
-
-__all__ = ['get_cli_string',
- 'load_dotenv',
- 'dotenv_values',
- 'get_key',
- 'set_key',
- 'unset_key',
- 'find_dotenv',
- 'load_ipython_extension']
diff --git a/spaces/Ataturk-Chatbot/HuggingFaceChat/venv/lib/python3.11/site-packages/pip/_internal/network/cache.py b/spaces/Ataturk-Chatbot/HuggingFaceChat/venv/lib/python3.11/site-packages/pip/_internal/network/cache.py
deleted file mode 100644
index a81a23985198d2eaa3c25ad1f77924f0fcdb037b..0000000000000000000000000000000000000000
--- a/spaces/Ataturk-Chatbot/HuggingFaceChat/venv/lib/python3.11/site-packages/pip/_internal/network/cache.py
+++ /dev/null
@@ -1,69 +0,0 @@
-"""HTTP cache implementation.
-"""
-
-import os
-from contextlib import contextmanager
-from typing import Generator, Optional
-
-from pip._vendor.cachecontrol.cache import BaseCache
-from pip._vendor.cachecontrol.caches import FileCache
-from pip._vendor.requests.models import Response
-
-from pip._internal.utils.filesystem import adjacent_tmp_file, replace
-from pip._internal.utils.misc import ensure_dir
-
-
-def is_from_cache(response: Response) -> bool:
- return getattr(response, "from_cache", False)
-
-
-@contextmanager
-def suppressed_cache_errors() -> Generator[None, None, None]:
- """If we can't access the cache then we can just skip caching and process
- requests as if caching wasn't enabled.
- """
- try:
- yield
- except OSError:
- pass
-
-
-class SafeFileCache(BaseCache):
- """
- A file based cache which is safe to use even when the target directory may
- not be accessible or writable.
- """
-
- def __init__(self, directory: str) -> None:
- assert directory is not None, "Cache directory must not be None."
- super().__init__()
- self.directory = directory
-
- def _get_cache_path(self, name: str) -> str:
- # From cachecontrol.caches.file_cache.FileCache._fn, brought into our
- # class for backwards-compatibility and to avoid using a non-public
- # method.
- hashed = FileCache.encode(name)
- parts = list(hashed[:5]) + [hashed]
- return os.path.join(self.directory, *parts)
-
- def get(self, key: str) -> Optional[bytes]:
- path = self._get_cache_path(key)
- with suppressed_cache_errors():
- with open(path, "rb") as f:
- return f.read()
-
- def set(self, key: str, value: bytes, expires: Optional[int] = None) -> None:
- path = self._get_cache_path(key)
- with suppressed_cache_errors():
- ensure_dir(os.path.dirname(path))
-
- with adjacent_tmp_file(path) as f:
- f.write(value)
-
- replace(f.name, path)
-
- def delete(self, key: str) -> None:
- path = self._get_cache_path(key)
- with suppressed_cache_errors():
- os.remove(path)
diff --git a/spaces/AutoGeneralAI/chatgpt-clone/README.md b/spaces/AutoGeneralAI/chatgpt-clone/README.md
deleted file mode 100644
index 53643e9b3c3541f78bbc7154a7f2e5262581299d..0000000000000000000000000000000000000000
--- a/spaces/AutoGeneralAI/chatgpt-clone/README.md
+++ /dev/null
@@ -1,12 +0,0 @@
----
-title: Chatgpt Clone
-emoji: 🐠
-colorFrom: gray
-colorTo: red
-sdk: gradio
-sdk_version: 3.29.0
-app_file: app.py
-pinned: false
----
-
-Check out the configuration reference at https://huggingface.co/docs/hub/spaces-config-reference
diff --git a/spaces/Bart92/RVC_HF/infer/lib/slicer2.py b/spaces/Bart92/RVC_HF/infer/lib/slicer2.py
deleted file mode 100644
index 5b29ee262aa54045e807be2cffeb41687499ba58..0000000000000000000000000000000000000000
--- a/spaces/Bart92/RVC_HF/infer/lib/slicer2.py
+++ /dev/null
@@ -1,260 +0,0 @@
-import numpy as np
-
-
-# This function is obtained from librosa.
-def get_rms(
- y,
- frame_length=2048,
- hop_length=512,
- pad_mode="constant",
-):
- padding = (int(frame_length // 2), int(frame_length // 2))
- y = np.pad(y, padding, mode=pad_mode)
-
- axis = -1
- # put our new within-frame axis at the end for now
- out_strides = y.strides + tuple([y.strides[axis]])
- # Reduce the shape on the framing axis
- x_shape_trimmed = list(y.shape)
- x_shape_trimmed[axis] -= frame_length - 1
- out_shape = tuple(x_shape_trimmed) + tuple([frame_length])
- xw = np.lib.stride_tricks.as_strided(y, shape=out_shape, strides=out_strides)
- if axis < 0:
- target_axis = axis - 1
- else:
- target_axis = axis + 1
- xw = np.moveaxis(xw, -1, target_axis)
- # Downsample along the target axis
- slices = [slice(None)] * xw.ndim
- slices[axis] = slice(0, None, hop_length)
- x = xw[tuple(slices)]
-
- # Calculate power
- power = np.mean(np.abs(x) ** 2, axis=-2, keepdims=True)
-
- return np.sqrt(power)
-
-
-class Slicer:
- def __init__(
- self,
- sr: int,
- threshold: float = -40.0,
- min_length: int = 5000,
- min_interval: int = 300,
- hop_size: int = 20,
- max_sil_kept: int = 5000,
- ):
- if not min_length >= min_interval >= hop_size:
- raise ValueError(
- "The following condition must be satisfied: min_length >= min_interval >= hop_size"
- )
- if not max_sil_kept >= hop_size:
- raise ValueError(
- "The following condition must be satisfied: max_sil_kept >= hop_size"
- )
- min_interval = sr * min_interval / 1000
- self.threshold = 10 ** (threshold / 20.0)
- self.hop_size = round(sr * hop_size / 1000)
- self.win_size = min(round(min_interval), 4 * self.hop_size)
- self.min_length = round(sr * min_length / 1000 / self.hop_size)
- self.min_interval = round(min_interval / self.hop_size)
- self.max_sil_kept = round(sr * max_sil_kept / 1000 / self.hop_size)
-
- def _apply_slice(self, waveform, begin, end):
- if len(waveform.shape) > 1:
- return waveform[
- :, begin * self.hop_size : min(waveform.shape[1], end * self.hop_size)
- ]
- else:
- return waveform[
- begin * self.hop_size : min(waveform.shape[0], end * self.hop_size)
- ]
-
- # @timeit
- def slice(self, waveform):
- if len(waveform.shape) > 1:
- samples = waveform.mean(axis=0)
- else:
- samples = waveform
- if samples.shape[0] <= self.min_length:
- return [waveform]
- rms_list = get_rms(
- y=samples, frame_length=self.win_size, hop_length=self.hop_size
- ).squeeze(0)
- sil_tags = []
- silence_start = None
- clip_start = 0
- for i, rms in enumerate(rms_list):
- # Keep looping while frame is silent.
- if rms < self.threshold:
- # Record start of silent frames.
- if silence_start is None:
- silence_start = i
- continue
- # Keep looping while frame is not silent and silence start has not been recorded.
- if silence_start is None:
- continue
- # Clear recorded silence start if interval is not enough or clip is too short
- is_leading_silence = silence_start == 0 and i > self.max_sil_kept
- need_slice_middle = (
- i - silence_start >= self.min_interval
- and i - clip_start >= self.min_length
- )
- if not is_leading_silence and not need_slice_middle:
- silence_start = None
- continue
- # Need slicing. Record the range of silent frames to be removed.
- if i - silence_start <= self.max_sil_kept:
- pos = rms_list[silence_start : i + 1].argmin() + silence_start
- if silence_start == 0:
- sil_tags.append((0, pos))
- else:
- sil_tags.append((pos, pos))
- clip_start = pos
- elif i - silence_start <= self.max_sil_kept * 2:
- pos = rms_list[
- i - self.max_sil_kept : silence_start + self.max_sil_kept + 1
- ].argmin()
- pos += i - self.max_sil_kept
- pos_l = (
- rms_list[
- silence_start : silence_start + self.max_sil_kept + 1
- ].argmin()
- + silence_start
- )
- pos_r = (
- rms_list[i - self.max_sil_kept : i + 1].argmin()
- + i
- - self.max_sil_kept
- )
- if silence_start == 0:
- sil_tags.append((0, pos_r))
- clip_start = pos_r
- else:
- sil_tags.append((min(pos_l, pos), max(pos_r, pos)))
- clip_start = max(pos_r, pos)
- else:
- pos_l = (
- rms_list[
- silence_start : silence_start + self.max_sil_kept + 1
- ].argmin()
- + silence_start
- )
- pos_r = (
- rms_list[i - self.max_sil_kept : i + 1].argmin()
- + i
- - self.max_sil_kept
- )
- if silence_start == 0:
- sil_tags.append((0, pos_r))
- else:
- sil_tags.append((pos_l, pos_r))
- clip_start = pos_r
- silence_start = None
- # Deal with trailing silence.
- total_frames = rms_list.shape[0]
- if (
- silence_start is not None
- and total_frames - silence_start >= self.min_interval
- ):
- silence_end = min(total_frames, silence_start + self.max_sil_kept)
- pos = rms_list[silence_start : silence_end + 1].argmin() + silence_start
- sil_tags.append((pos, total_frames + 1))
- # Apply and return slices.
- if len(sil_tags) == 0:
- return [waveform]
- else:
- chunks = []
- if sil_tags[0][0] > 0:
- chunks.append(self._apply_slice(waveform, 0, sil_tags[0][0]))
- for i in range(len(sil_tags) - 1):
- chunks.append(
- self._apply_slice(waveform, sil_tags[i][1], sil_tags[i + 1][0])
- )
- if sil_tags[-1][1] < total_frames:
- chunks.append(
- self._apply_slice(waveform, sil_tags[-1][1], total_frames)
- )
- return chunks
-
-
-def main():
- import os.path
- from argparse import ArgumentParser
-
- import librosa
- import soundfile
-
- parser = ArgumentParser()
- parser.add_argument("audio", type=str, help="The audio to be sliced")
- parser.add_argument(
- "--out", type=str, help="Output directory of the sliced audio clips"
- )
- parser.add_argument(
- "--db_thresh",
- type=float,
- required=False,
- default=-40,
- help="The dB threshold for silence detection",
- )
- parser.add_argument(
- "--min_length",
- type=int,
- required=False,
- default=5000,
- help="The minimum milliseconds required for each sliced audio clip",
- )
- parser.add_argument(
- "--min_interval",
- type=int,
- required=False,
- default=300,
- help="The minimum milliseconds for a silence part to be sliced",
- )
- parser.add_argument(
- "--hop_size",
- type=int,
- required=False,
- default=10,
- help="Frame length in milliseconds",
- )
- parser.add_argument(
- "--max_sil_kept",
- type=int,
- required=False,
- default=500,
- help="The maximum silence length kept around the sliced clip, presented in milliseconds",
- )
- args = parser.parse_args()
- out = args.out
- if out is None:
- out = os.path.dirname(os.path.abspath(args.audio))
- audio, sr = librosa.load(args.audio, sr=None, mono=False)
- slicer = Slicer(
- sr=sr,
- threshold=args.db_thresh,
- min_length=args.min_length,
- min_interval=args.min_interval,
- hop_size=args.hop_size,
- max_sil_kept=args.max_sil_kept,
- )
- chunks = slicer.slice(audio)
- if not os.path.exists(out):
- os.makedirs(out)
- for i, chunk in enumerate(chunks):
- if len(chunk.shape) > 1:
- chunk = chunk.T
- soundfile.write(
- os.path.join(
- out,
- f"%s_%d.wav"
- % (os.path.basename(args.audio).rsplit(".", maxsplit=1)[0], i),
- ),
- chunk,
- sr,
- )
-
-
-if __name__ == "__main__":
- main()
diff --git a/spaces/Benson/text-generation/Examples/Androide Oyun Apk Bola Roja 4.md b/spaces/Benson/text-generation/Examples/Androide Oyun Apk Bola Roja 4.md
deleted file mode 100644
index 72044b54059c7ba1a78f5be755c4efec1f7891f5..0000000000000000000000000000000000000000
--- a/spaces/Benson/text-generation/Examples/Androide Oyun Apk Bola Roja 4.md
+++ /dev/null
@@ -1,60 +0,0 @@
-
-
Red Ball 4: Un divertido y desafiante juego para Android
-
Si estás buscando un juego divertido y desafiante para jugar en tu dispositivo Android, deberías probar Red Ball 4. Este es un juego de plataformas que pondrá a prueba tus habilidades y reflejos mientras rodas, saltas y rebotas a través de 75 niveles llenos de aventura. Usted tendrá que hacer su camino a través de trampas difíciles y derrotar a todo tipo de monstruos que quieren convertir en un cuadrado. ¿Estás listo para salvar el mundo con tu bola roja?
Red Ball 4 es un juego para Android desarrollado por FDG Entertainment GmbH & Co.KG. Es la cuarta entrega de la popular serie Red Ball, que se ha descargado más de 100 millones de veces. El juego sigue la historia de una bola roja que tiene que detener el mal Black Square de convertir el mundo en un cubo. En el camino, se encontrará con muchos obstáculos y enemigos que tiene que superar con su agilidad y coraje.
-
¿Por qué deberías jugar Red Ball 4?
-
Red Ball 4 es un juego que te mantendrá entretenido durante horas. Tiene un juego simple pero adictivo que cualquiera puede disfrutar. Solo tienes que inclinar el dispositivo o utilizar los botones en pantalla para controlar el movimiento de la pelota. También puedes pulsar para saltar y pulsar dos veces para realizar un salto de longitud. El juego tiene una variedad de niveles que pondrán a prueba tus habilidades y tu lógica. Tendrá que evitar picos, láseres, cañones, sierras y otros peligros que pueden dañar su bola. También tendrás que enfrentarte a diferentes tipos de monstruos, como arañas, murciélagos, robots e incluso jefes gigantes. El juego tiene un estilo gráfico colorido y caricaturesco que atraerá tanto a niños como a adultos. El juego también tiene una banda sonora pegadiza y optimista que mejorará tu experiencia de juego.
-
Características de Red Ball 4
-
75 emocionantes niveles
-
-
Trampas y monstruos difíciles
-
Red Ball 4 tiene muchas trampas y monstruos que intentarán evitar que alcances tu objetivo. Usted tendrá que utilizar sus habilidades y el tiempo para evitarlos o derrotarlos. Algunas trampas se pueden activar mediante interruptores o botones, mientras que otras se activan por su movimiento o proximidad. Algunos monstruos pueden ser asesinados saltando sobre ellos o golpeándolos con objetos, mientras que otros son invencibles o requieren estrategias especiales. Tendrás que ser cuidadoso y observador para sobrevivir.
-
Batallas épicas de jefes
-
Red Ball 4 tiene cuatro batallas contra jefes que pondrán a prueba tus habilidades y paciencia. Tendrás que enfrentarte al propio Black Square en cada episodio, así como a sus secuaces. Cada jefe tiene un patrón de ataque diferente y debilidad que tienes que explotar. Tendrás que esquivar sus ataques y golpearlos con objetos o con tu pelota. Las batallas contra jefes son desafiantes pero gratificantes.
-
Soporte en la nube
-
Red Ball 4 tiene soporte en la nube que le permite guardar su progreso en línea y sincronizarlo en varios dispositivos. Solo tienes que iniciar sesión con tu cuenta de Google Play y habilitar el almacenamiento en la nube en el menú de configuración. De esta manera, puedes continuar tu juego en cualquier dispositivo sin perder tus datos
gráficos y sonido HD
-
Red Ball 4 tiene gráficos y sonidos de alta definición que harán que tu juego sea más agradable. El juego tiene un estilo de arte brillante y colorido que se adapta al tema y el estado de ánimo de cada episodio. El juego también tiene una calidad de sonido nítida y clara que te hará sentir inmerso en el juego. Escucharás los efectos de sonido de tu bola rebotando, rodando y estrellándose, así como la música y las voces de los personajes. El juego tiene un tono divertido y humorístico que te hará sonreír.
-
Cómo descargar e instalar Red Ball 4 APK
-
Descargar el archivo APK de una fuente de confianza
-
-
-
-
Habilitar fuentes desconocidas en su dispositivo
-
Antes de que pueda instalar el archivo APK Red Ball 4 en su dispositivo, tendrá que habilitar fuentes desconocidas en su dispositivo. Esta es una configuración de seguridad que le permite instalar aplicaciones desde fuentes distintas de Google Play Store. Para habilitar fuentes desconocidas, siga estos pasos:
-
-
Vaya al menú de configuración de su dispositivo y toque en la seguridad o privacidad.
-
Encontrar la opción que dice fuentes desconocidas o instalar aplicaciones desconocidas y alternar en.
-
Aparecerá un mensaje de advertencia, diciéndole que instalar aplicaciones de fuentes desconocidas puede dañar su dispositivo. Toque en OK o permita confirmar.
-
-
Instalar el archivo APK y lanzar el juego
-
Una vez que haya habilitado fuentes desconocidas en su dispositivo, puede instalar el archivo APK Red Ball 4 y lanzar el juego. Para hacer esto, siga estos pasos:
-
-
Busque el archivo APK Red Ball 4 en la carpeta de almacenamiento o descarga de su dispositivo. También puede usar una aplicación de administrador de archivos para encontrarlo.
-
Toque en el archivo APK y aparecerá un aviso, preguntándole si desea instalar la aplicación. Toque en instalar y espere a que termine el proceso de instalación.
-
Una vez que la aplicación está instalada, puede tocar en abrir para iniciar el juego o encontrarlo en el cajón de la aplicación o en la pantalla de inicio.
-
-
Conclusión
-
Resumen de los puntos principales
-
-
Llamada a la acción
-
Si usted está buscando un juego divertido y desafiante para jugar en su dispositivo Android, usted debe descargar e instalar Red Ball 4 APK hoy. No te arrepentirás. Tendrás una explosión rodando, saltando y rebotando a través de 75 niveles llenos de aventura. También tendrá la oportunidad de salvar el mundo con su bola roja. ¿Qué estás esperando? Descargar Red Ball 4 APK ahora y disfrutar de este increíble juego!
-
Preguntas frecuentes
-
Q: ¿Es Red Ball 4 libre para jugar?
-
A: Sí, Red Ball 4 es gratis. Sin embargo, contiene anuncios y compras en la aplicación que pueden mejorar su experiencia de juego. Puedes desactivar los anuncios comprando la versión premium del juego o apagando tu conexión a Internet mientras juegas.
-
P: ¿Cómo puedo obtener más estrellas y trofeos en Red Ball 4?
-
A: Puedes conseguir más estrellas y trofeos en Bola Roja 4 completando cada nivel con una puntuación alta y sin morir. También puedes recoger estrellas y trofeos ocultos que se encuentran dispersos por los niveles. Puedes usar estas estrellas y trofeos para desbloquear niveles de bonificación y logros.
-
P: ¿Cómo puedo vencer a los jefes en Bola Roja 4?
-
A: Puedes vencer a los jefes en Bola Roja 4 aprendiendo sus patrones de ataque y encontrando sus puntos débiles. Tendrás que esquivar sus ataques y golpearlos con objetos o tu pelota. También tendrá que evitar caerse de la plataforma o ser aplastado por el jefe. Puede usar los puntos de control para reanudar su juego si muere.
-
Q: ¿Cuáles son los requisitos mínimos para jugar Red Ball 4 en Android?
-
A: Los requisitos mínimos para jugar Red Ball 4 en Android son los siguientes:
-
-
Versión de Android 4.4 o superior
-
Al menos 100 MB de espacio de almacenamiento libre
-
Una conexión a Internet estable (opcional)
-
-
Q: ¿Cómo puedo contactar al desarrollador de Red Ball 4?
64aa2da5cf
-
-
\ No newline at end of file
diff --git a/spaces/Benson/text-generation/Examples/Apk Adresi Gta 5.md b/spaces/Benson/text-generation/Examples/Apk Adresi Gta 5.md
deleted file mode 100644
index ce963edd5c4e92b189479672a96b1433fbe9954b..0000000000000000000000000000000000000000
--- a/spaces/Benson/text-generation/Examples/Apk Adresi Gta 5.md
+++ /dev/null
@@ -1,79 +0,0 @@
-
-
Apk Adresi GTA 5: Cómo jugar Grand Theft Auto 5 en su dispositivo Android
-
Grand Theft Auto 5, o GTA 5, es uno de los videojuegos más populares y aclamados de todos los tiempos. Lanzado en 2013 por Rockstar Games, GTA 5 es un juego de acción y aventura de mundo abierto que te permite explorar la ciudad de Los Santos y sus alrededores como uno de los tres personajes jugables. Puedes completar varias misiones, realizar robos, conducir y robar vehículos, interactuar con NPC y causar caos en las calles.
Sin embargo, GTA 5 no está disponible oficialmente para dispositivos Android, ya que Rockstar Games no ha lanzado una versión móvil del juego. Pero eso no significa que no puede jugar GTA 5 en su dispositivo Android. Gracias a un grupo de fans dedicados, se puede descargar e instalar una adaptación hecha por fans de GTA 5 para Android llamado Apk Adresi GTA 5.
-
En este artículo, le diremos todo lo que necesita saber acerca de Apk Adresi GTA 5, incluyendo lo que es, cómo descargar e instalar, cómo jugarlo, y algunos consejos y trucos para disfrutarlo. ¡Vamos a empezar!
-
¿Qué es Apk Adresi GTA 5?
-
Una adaptación hecha por fans de GTA 5 para Android
-
Apk Adresi GTA 5 es una adaptación hecha por fans de GTA 5 para dispositivos Android. No es un producto oficial de Rockstar Games, sino un proyecto creado por un grupo de entusiastas que querían llevar GTA 5 a plataformas móviles. Apk Adresi GTA 5 se basa en la versión para PC de GTA 5, pero se ha modificado y optimizado para ejecutarse en dispositivos Android.
-
Las características y limitaciones de Apk Adresi GTA 5
-
Apk Adresi GTA 5 tiene como objetivo replicar la experiencia de jugar GTA 5 en PC tanto como sea posible. Tiene los mismos gráficos, sonido, historia, personajes, misiones, vehículos, armas y actividades que el juego original. Puede cambiar entre Michael, Trevor y Franklin en cualquier momento, explorar el vasto mundo abierto de Los Santos y el condado de Blaine y disfrutar del emocionante juego de GTA 5.
-
-
-
Los requisitos y pasos para descargar los archivos APK y OBB
-
Si desea jugar Apk Adresi GTA 5 en su dispositivo Android, tendrá que descargar dos archivos: el archivo APK y el archivo OBB. El archivo APK es el archivo de aplicación que contiene el código y los datos del juego, mientras que el archivo OBB es el archivo de expansión que contiene los gráficos y el sonido del juego.
-
Antes de descargar estos archivos, debe asegurarse de que su dispositivo Android cumple con los requisitos mínimos para ejecutar Apk Adresi GTA 5. Estos son:
-
-
Android versión 4.0 o superior
-
Al menos 4 GB de espacio de almacenamiento gratuito
-
Al menos 2 GB de RAM
-
Una conexión a Internet estable
-
-
Una vez que haya comprobado estos requisitos, puede seguir estos pasos para descargar los archivos APK y OBB:
Desplácese hacia abajo a la parte inferior de la página y haga clic en el botón verde que dice "GTA 5 APK İndir"
-
Serás redirigido a otra página donde verás un enlace de descarga para el archivo APK. Haz clic en él y espera a que comience la descarga.
-
Después de descargar el archivo APK, volver a la página anterior y haga clic en el botón verde que dice "GTA 5 OBB İndir"
-
Será redirigido a otra página donde verá un enlace de descarga para el archivo OBB. Haga clic en él y espere a que comience la descarga.
-
Después de descargar ambos archivos, puede proceder a instalarlos en su dispositivo Android.
-
-
Las instrucciones para instalar y lanzar el juego
-
Después de haber descargado los archivos APK y OBB, tendrá que instalarlos en su dispositivo Android. Para ello, deberá habilitar la instalación de aplicaciones de fuentes desconocidas en su dispositivo. Esta es una función de seguridad que evita que las aplicaciones no autorizadas se instalen en su dispositivo. Para habilitar esta función, puede seguir estos pasos:
-
-
Ir a la configuración de su dispositivo y toque en "Seguridad"
-
Encontrar la opción que dice "Fuentes desconocidas" y alternar en
-
Puede ver un mensaje de advertencia que dice que instalar aplicaciones de fuentes desconocidas puede dañar su dispositivo. Toque en "OK" para confirmar.
-
-
Una vez que haya habilitado esta función, puede instalar los archivos APK y OBB siguiendo estos pasos:
-
-
Busque el archivo APK en el administrador de archivos de su dispositivo y toque en él
-
Puede ver una ventana emergente que le pide que conceda permisos a la aplicación. Toque en "Instalar" para continuar.
-
Espere a que termine el proceso de instalación. Puede ver un mensaje que dice "App instalado". Toque en "Abrir" para iniciar el juego.
-
La primera vez que inicie el juego, tendrá que extraer el archivo OBB al almacenamiento interno de su dispositivo. Para ello, toque en "Extraer" cuando se le solicite y espere a que finalice el proceso de extracción.
-
Después de la extracción se hace, verá un mensaje que dice "Extracción completado". Toque en "OK" para empezar a jugar el juego.
-
Cómo jugar Apk Adresi GTA 5 en su dispositivo Android
-
Los controles y la interfaz del juego
-
Apk Adresi GTA 5 tiene un esquema de control similar y la interfaz como la versión para PC de GTA 5. Puede utilizar los botones virtuales en la pantalla para mover, apuntar, disparar, saltar, correr, agacharse, cambiar de armas, entrar en vehículos, e interactuar con el medio ambiente. También puede utilizar la pantalla táctil para deslizar, hacer zoom y girar la cámara. Puede personalizar el diseño y el tamaño de los botones en el menú de configuración.
-
El juego también tiene un mini-mapa en la esquina inferior izquierda de la pantalla que muestra su ubicación, objetivos, enemigos, aliados y puntos de interés. Puede tocar en el mini-mapa para ampliarlo y ver el mapa completo de Los Santos y el condado de Blaine. También puedes acceder a tu teléfono, inventario, rueda de caracteres, menú de pausa y opciones de guardado rápido tocando los iconos en la esquina superior derecha de la pantalla.
-
-
Apk Adresi GTA 5 tiene el mismo juego y misiones que la versión para PC de GTA 5. Puedes jugar como Michael, Trevor, o Franklin y cambiar entre ellos en cualquier momento. Cada personaje tiene su propia personalidad, habilidades, habilidades y arco de la historia. Puedes completar varias misiones que incluyen disparos, conducción, sigilo, robos, persecuciones y más. También puede explorar el mundo abierto de Los Santos y el condado de Blaine y realizar diversas actividades como carreras, golf, tenis, caza, yoga, paracaidismo y más.
-
El juego también tiene un sistema de clima dinámico, ciclo de día y noche, física realista, efectos ragdoll, entornos destructibles y sonidos realistas. El juego está diseñado para sumergirte en el mundo de GTA 5 y hacerte sentir como si estuvieras viviendo en él.
-
Los consejos y trucos para disfrutar del juego
-
Apk Adresi GTA 5 es un juego divertido y emocionante que ofrece un montón de contenido y posibilidades. Sin embargo, también puede ser desafiante y frustrante a veces. Aquí hay algunos consejos y trucos que pueden ayudarte a disfrutar más del juego:
-
-
Guarda tu juego con frecuencia. El juego no tiene una función de guardado automático, por lo que tendrás que guardar manualmente el juego en caso de que algo salga mal o quieras probar algo diferente.
-
Utilice la cubierta y el objetivo de asistencia. El juego puede ser bastante difícil si usted intenta disparar a sus enemigos sin tomar la cubierta o el uso de la puntería de asistencia. Puede usar cover presionando el botón en la esquina inferior derecha de la pantalla cuando esté cerca de un objeto. Puede utilizar la ayuda de puntería pulsando el botón en la esquina inferior izquierda de la pantalla cuando está apuntando.
-
-Cuidado con policías y pandillas. El juego tiene un sistema de nivel deseado que indica la cantidad de atención que ha atraído de las fuerzas del orden o pandillas rivales. Cuanto más alto sea tu nivel de búsqueda, más policías o gángsters te perseguirán e intentarán matarte. Puedes bajar tu nivel deseado escondiéndote de ellos o cambiando tu apariencia.
-
Divertirse y experimentar. El juego está destinado a ser disfrutado y explorado. Puedes hacer lo que quieras en el juego siempre y cuando no te maten o arresten. Puedes probar diferentes estrategias, tácticas, vehículos, armas, atuendos, etc. También puedes crear tus propios escenarios e historias con las herramientas y características del juego.
-
-
Conclusión
-
Un resumen de los principales puntos y beneficios de Apk Adresi GTA 5
-
Apk Adresi GTA 5 es una adaptación hecha por fans de GTA 5 para dispositivos Android que le permite jugar uno de los mejores juegos de video jamás hecho en su dispositivo móvil. Tiene los mismos gráficos, precauciones y protecciones. No garantizamos ni asumimos la responsabilidad de la seguridad o calidad de Apk Adresi GTA 5.
-
¿Es Apk Adresi GTA 5 compatible con todos los dispositivos Android?
-
Apk Adresi GTA 5 está diseñado para ejecutarse en dispositivos Android que cumplan los requisitos mínimos para el juego. Estos son:
-
-
Android versión 4.0 o superior
-
Al menos 4 GB de espacio de almacenamiento gratuito
-
Al menos 2 GB de RAM
-
Una conexión a Internet estable
-
-
Sin embargo, Apk Adresi GTA 5 puede no ser compatible con todos los dispositivos Android o versiones, ya que todavía está en fase beta y no se ha probado en todos los dispositivos o versiones. Algunos dispositivos o versiones pueden tener problemas de compatibilidad, como retrasos, fallos, errores o características faltantes. Por lo tanto, Apk Adresi GTA 5 puede no funcionar correctamente o en absoluto en algunos dispositivos Android o versiones. No garantizamos ni asumimos la responsabilidad de la compatibilidad o el rendimiento de Apk Adresi GTA 5.
-
¿Cuánto espacio de almacenamiento necesita Apk Adresi GTA 5?
-
-
¿Puedo jugar Apk Adresi GTA 5 en línea?
-
No, Apk Adresi GTA 5 no es compatible con el modo multijugador en línea o el juego cruzado con otras plataformas. El juego es solo un modo offline para un jugador que te permite jugar a GTA 5 en tu dispositivo Android. No podrás jugar con otros jugadores online ni conectarte con otras plataformas como PC, PS4, Xbox One, etc. El juego tampoco tiene características online como tablas de clasificación, logros, clubes sociales, etc.
64aa2da5cf
-
-
\ No newline at end of file
diff --git a/spaces/BhaskarKapri/Animal/app.py b/spaces/BhaskarKapri/Animal/app.py
deleted file mode 100644
index 4815456ca505fe31396f8cc75d438181be340484..0000000000000000000000000000000000000000
--- a/spaces/BhaskarKapri/Animal/app.py
+++ /dev/null
@@ -1,36 +0,0 @@
-from fastai.vision.all import *
-import gradio as gr
-import pathlib
-from contextlib import contextmanager
-import pathlib
-
-@contextmanager
-def set_posix_windows():
- posix_backup = pathlib.WindowsPath
- try:
- pathlib.WindowsPath = pathlib.PosixPath
- yield
- finally:
- pathlib.WindowsPath = posix_backup
-
-EXPORT_PATH = pathlib.Path("model.pkl")
-
-with set_posix_windows():
- learn = load_learner(EXPORT_PATH)
-
-# learn = load_learner('model.pkl')
-
-
-categories = ['alligator', 'bee', 'camel', 'cat', 'deer', 'dog', 'dolphin', 'elephant', 'giraffe', 'hamster', 'horse', 'kangaroo', 'lion', 'lizard', 'human', 'owl', 'parrot', 'sheep', 'snake', 'tiger', 'turtle', 'wolf']
-def classify_image(img):
- pred,idx,probs = learn.predict(img)
- return dict(zip(categories,map(float,probs)))
-
-
-
-image = gr.inputs.Image(shape=(192,192))
-label = gr.outputs.Label()
-examples = ['cat.jpg','camel.jpg','deer.jpg','dog.jpg','giraffe.jpg','owl.jpg']
-
-intf = gr.Interface(fn=classify_image, inputs=image, outputs=label, example=examples)
-intf.launch(inline=False)
\ No newline at end of file
diff --git a/spaces/Big-Web/MMSD/env/Lib/site-packages/setuptools/command/upload.py b/spaces/Big-Web/MMSD/env/Lib/site-packages/setuptools/command/upload.py
deleted file mode 100644
index ec7f81e22772511d668e5ab92f625db33259e803..0000000000000000000000000000000000000000
--- a/spaces/Big-Web/MMSD/env/Lib/site-packages/setuptools/command/upload.py
+++ /dev/null
@@ -1,17 +0,0 @@
-from distutils import log
-from distutils.command import upload as orig
-
-from setuptools.errors import RemovedCommandError
-
-
-class upload(orig.upload):
- """Formerly used to upload packages to PyPI."""
-
- def run(self):
- msg = (
- "The upload command has been removed, use twine to upload "
- + "instead (https://pypi.org/p/twine)"
- )
-
- self.announce("ERROR: " + msg, log.ERROR)
- raise RemovedCommandError(msg)
diff --git a/spaces/BigData-KSU/VQA-in-Medical-Imagery/CLIP/README.md b/spaces/BigData-KSU/VQA-in-Medical-Imagery/CLIP/README.md
deleted file mode 100644
index d7eaf7aef101e2be15afee7e9ed0ad03dc13b5df..0000000000000000000000000000000000000000
--- a/spaces/BigData-KSU/VQA-in-Medical-Imagery/CLIP/README.md
+++ /dev/null
@@ -1,192 +0,0 @@
-# CLIP
-
-[[Blog]](https://openai.com/blog/clip/) [[Paper]](https://cdn.openai.com/papers/Learning_Transferable_Visual_Models_From_Natural_Language_Supervision.pdf) [[Model Card]](model-card.md) [[Colab]](https://colab.research.google.com/github/openai/clip/blob/master/Interacting_with_CLIP.ipynb)
-
-CLIP (Contrastive Language-Image Pre-Training) is a neural network trained on a variety of (image, text) pairs. It can be instructed in natural language to predict the most relevant text snippet, given an image, without directly optimizing for the task, similarly to the zero-shot capabilities of GPT-2 and 3. We found CLIP matches the performance of the original ResNet50 on ImageNet “zero-shot” without using any of the original 1.28M labeled examples, overcoming several major challenges in computer vision.
-
-
-
-## Approach
-
-
-
-
-
-## Usage
-
-First, [install PyTorch 1.7.1](https://pytorch.org/get-started/locally/) and torchvision, as well as small additional dependencies. On a CUDA GPU machine, the following will do the trick:
-
-```bash
-$ conda install --yes -c pytorch pytorch=1.7.1 torchvision cudatoolkit=11.0
-$ pip install ftfy regex tqdm
-```
-
-Replace `cudatoolkit=11.0` above with the appropriate CUDA version on your machine or `cpuonly` when installing on a machine without a GPU.
-
-```python
-import torch
-import clip
-from PIL import Image
-
-device = "cuda" if torch.cuda.is_available() else "cpu"
-model, preprocess = clip.load("ViT-B/32", device=device)
-
-image = preprocess(Image.open("CLIP.png")).unsqueeze(0).to(device)
-text = clip.tokenize(["a diagram", "a dog", "a cat"]).to(device)
-
-with torch.no_grad():
- image_features = model.encode_image(image)
- text_features = model.encode_text(text)
-
- logits_per_image, logits_per_text = model(image, text)
- probs = logits_per_image.softmax(dim=-1).cpu().numpy()
-
-print("Label probs:", probs) # prints: [[0.9927937 0.00421068 0.00299572]]
-```
-
-
-## API
-
-The CLIP module `clip` provides the following methods:
-
-#### `clip.available_models()`
-
-Returns the name(s) of the available CLIP models.
-
-#### `clip.load(name, device=..., jit=True)`
-
-Returns the model and the TorchVision transform needed by the model, specified by the model name returned by `clip.available_models()`. It will download the model as necessary. The device to run the model can be optionally specified, and the default is to use the first CUDA device if there is any, otherwise the CPU.
-
-When `jit` is `False`, a non-JIT version of the model will be loaded.
-
-#### `clip.tokenize(text: Union[str, List[str]], context_length=77)`
-
-Returns a LongTensor containing tokenized sequences of given text input(s). This can be used as the input to the model
-
----
-
-The model returned by `clip.load()` supports the following methods:
-
-#### `model.encode_image(image: Tensor)`
-
-Given a batch of images, returns the image features encoded by the vision portion of the CLIP model.
-
-#### `model.encode_text(text: Tensor)`
-
-Given a batch of text tokens, returns the text features encoded by the language portion of the CLIP model.
-
-#### `model(image: Tensor, text: Tensor)`
-
-Given a batch of images and a batch of text tokens, returns two Tensors, containing the logit scores corresponding to each image and text input. The values are cosine similarities between the corresponding image and text features, times 100.
-
-
-
-## More Examples
-
-### Zero-Shot Prediction
-
-The code below performs zero-shot prediction using CLIP, as shown in Appendix B in the paper. This example takes an image from the [CIFAR-100 dataset](https://www.cs.toronto.edu/~kriz/cifar.html), and predicts the most likely labels among the 100 textual labels from the dataset.
-
-```python
-import os
-import clip
-import torch
-from torchvision.datasets import CIFAR100
-
-# Load the model
-device = "cuda" if torch.cuda.is_available() else "cpu"
-model, preprocess = clip.load('ViT-B/32', device)
-
-# Download the dataset
-cifar100 = CIFAR100(root=os.path.expanduser("~/.cache"), download=True, train=False)
-
-# Prepare the inputs
-image, class_id = cifar100[3637]
-image_input = preprocess(image).unsqueeze(0).to(device)
-text_inputs = torch.cat([clip.tokenize(f"a photo of a {c}") for c in cifar100.classes]).to(device)
-
-# Calculate features
-with torch.no_grad():
- image_features = model.encode_image(image_input)
- text_features = model.encode_text(text_inputs)
-
-# Pick the top 5 most similar labels for the image
-image_features /= image_features.norm(dim=-1, keepdim=True)
-text_features /= text_features.norm(dim=-1, keepdim=True)
-similarity = (100.0 * image_features @ text_features.T).softmax(dim=-1)
-values, indices = similarity[0].topk(5)
-
-# Print the result
-print("\nTop predictions:\n")
-for value, index in zip(values, indices):
- print(f"{cifar100.classes[index]:>16s}: {100 * value.item():.2f}%")
-```
-
-The output will look like the following (the exact numbers may be slightly different depending on the compute device):
-
-```
-Top predictions:
-
- snake: 65.31%
- turtle: 12.29%
- sweet_pepper: 3.83%
- lizard: 1.88%
- crocodile: 1.75%
-```
-
-Note that this example uses the `encode_image()` and `encode_text()` methods that return the encoded features of given inputs.
-
-
-### Linear-probe evaluation
-
-The example below uses [scikit-learn](https://scikit-learn.org/) to perform logistic regression on image features.
-
-```python
-import os
-import clip
-import torch
-
-import numpy as np
-from sklearn.linear_model import LogisticRegression
-from torch.utils.data import DataLoader
-from torchvision.datasets import CIFAR100
-from tqdm import tqdm
-
-# Load the model
-device = "cuda" if torch.cuda.is_available() else "cpu"
-model, preprocess = clip.load('ViT-B/32', device)
-
-# Load the dataset
-root = os.path.expanduser("~/.cache")
-train = CIFAR100(root, download=True, train=True, transform=preprocess)
-test = CIFAR100(root, download=True, train=False, transform=preprocess)
-
-
-def get_features(dataset):
- all_features = []
- all_labels = []
-
- with torch.no_grad():
- for images, labels in tqdm(DataLoader(dataset, batch_size=100)):
- features = model.encode_image(images.to(device))
-
- all_features.append(features)
- all_labels.append(labels)
-
- return torch.cat(all_features).cpu().numpy(), torch.cat(all_labels).cpu().numpy()
-
-# Calculate the image features
-train_features, train_labels = get_features(train)
-test_features, test_labels = get_features(test)
-
-# Perform logistic regression
-classifier = LogisticRegression(random_state=0, C=0.316, max_iter=1000, verbose=1)
-classifier.fit(train_features, train_labels)
-
-# Evaluate using the logistic regression classifier
-predictions = classifier.predict(test_features)
-accuracy = np.mean((test_labels == predictions).astype(np.float)) * 100.
-print(f"Accuracy = {accuracy:.3f}")
-```
-
-Note that the `C` value should be determined via a hyperparameter sweep using a validation split.
diff --git a/spaces/BreetheRun/mitchtech-vulcan-diffusion/app.py b/spaces/BreetheRun/mitchtech-vulcan-diffusion/app.py
deleted file mode 100644
index 9d9d7b4f919990252ef3ee8fcdb8a55674448e04..0000000000000000000000000000000000000000
--- a/spaces/BreetheRun/mitchtech-vulcan-diffusion/app.py
+++ /dev/null
@@ -1,3 +0,0 @@
-import gradio as gr
-
-gr.Interface.load("models/mitchtech/vulcan-diffusion").launch()
\ No newline at end of file
diff --git a/spaces/CVPR/Dual-Key_Backdoor_Attacks/datagen/detectron2/detectron2/layers/csrc/box_iou_rotated/box_iou_rotated_cpu.cpp b/spaces/CVPR/Dual-Key_Backdoor_Attacks/datagen/detectron2/detectron2/layers/csrc/box_iou_rotated/box_iou_rotated_cpu.cpp
deleted file mode 100644
index a6aaa810c59281cc45a4252784a62d7829a03556..0000000000000000000000000000000000000000
--- a/spaces/CVPR/Dual-Key_Backdoor_Attacks/datagen/detectron2/detectron2/layers/csrc/box_iou_rotated/box_iou_rotated_cpu.cpp
+++ /dev/null
@@ -1,46 +0,0 @@
-// Copyright (c) Facebook, Inc. and its affiliates. All Rights Reserved
-#include "box_iou_rotated.h"
-#include "box_iou_rotated_utils.h"
-
-namespace detectron2 {
-
-template
-void box_iou_rotated_cpu_kernel(
- const at::Tensor& boxes1,
- const at::Tensor& boxes2,
- at::Tensor& ious) {
- auto widths1 = boxes1.select(1, 2).contiguous();
- auto heights1 = boxes1.select(1, 3).contiguous();
- auto widths2 = boxes2.select(1, 2).contiguous();
- auto heights2 = boxes2.select(1, 3).contiguous();
-
- at::Tensor areas1 = widths1 * heights1;
- at::Tensor areas2 = widths2 * heights2;
-
- auto num_boxes1 = boxes1.size(0);
- auto num_boxes2 = boxes2.size(0);
-
- for (int i = 0; i < num_boxes1; i++) {
- for (int j = 0; j < num_boxes2; j++) {
- ious[i * num_boxes2 + j] = single_box_iou_rotated(
- boxes1[i].data_ptr(), boxes2[j].data_ptr());
- }
- }
-}
-
-at::Tensor box_iou_rotated_cpu(
- const at::Tensor& boxes1,
- const at::Tensor& boxes2) {
- auto num_boxes1 = boxes1.size(0);
- auto num_boxes2 = boxes2.size(0);
- at::Tensor ious =
- at::empty({num_boxes1 * num_boxes2}, boxes1.options().dtype(at::kFloat));
-
- box_iou_rotated_cpu_kernel(boxes1, boxes2, ious);
-
- // reshape from 1d array to 2d array
- auto shape = std::vector{num_boxes1, num_boxes2};
- return ious.reshape(shape);
-}
-
-} // namespace detectron2
diff --git a/spaces/CVPR/LIVE/pybind11/pybind11/__init__.py b/spaces/CVPR/LIVE/pybind11/pybind11/__init__.py
deleted file mode 100644
index 5b2f83d5cd93c073ad130cc113bab25a1d03255b..0000000000000000000000000000000000000000
--- a/spaces/CVPR/LIVE/pybind11/pybind11/__init__.py
+++ /dev/null
@@ -1,13 +0,0 @@
-# -*- coding: utf-8 -*-
-from ._version import version_info, __version__ # noqa: F401 imported but unused
-
-
-def get_include(user=False):
- import os
- d = os.path.dirname(__file__)
- if os.path.exists(os.path.join(d, "include")):
- # Package is installed
- return os.path.join(d, "include")
- else:
- # Package is from a source directory
- return os.path.join(os.path.dirname(d), "include")
diff --git a/spaces/CVPR/LIVE/pybind11/tools/clang/enumerations.py b/spaces/CVPR/LIVE/pybind11/tools/clang/enumerations.py
deleted file mode 100644
index a86a48ade3bd7ad00e455bebb3b94ecf25ddf8e4..0000000000000000000000000000000000000000
--- a/spaces/CVPR/LIVE/pybind11/tools/clang/enumerations.py
+++ /dev/null
@@ -1,34 +0,0 @@
-#===- enumerations.py - Python Enumerations ------------------*- python -*--===#
-#
-# The LLVM Compiler Infrastructure
-#
-# This file is distributed under the University of Illinois Open Source
-# License. See LICENSE.TXT for details.
-#
-#===------------------------------------------------------------------------===#
-
-"""
-Clang Enumerations
-==================
-
-This module provides static definitions of enumerations that exist in libclang.
-
-Enumerations are typically defined as a list of tuples. The exported values are
-typically munged into other types or classes at module load time.
-
-All enumerations are centrally defined in this file so they are all grouped
-together and easier to audit. And, maybe even one day this file will be
-automatically generated by scanning the libclang headers!
-"""
-
-# Maps to CXTokenKind. Note that libclang maintains a separate set of token
-# enumerations from the C++ API.
-TokenKinds = [
- ('PUNCTUATION', 0),
- ('KEYWORD', 1),
- ('IDENTIFIER', 2),
- ('LITERAL', 3),
- ('COMMENT', 4),
-]
-
-__all__ = ['TokenKinds']
diff --git a/spaces/CVPR/LIVE/thrust/thrust/iterator/transform_input_output_iterator.h b/spaces/CVPR/LIVE/thrust/thrust/iterator/transform_input_output_iterator.h
deleted file mode 100644
index 25c10eb58e93cbadb298fc68bbd4d24b3dc5a7cb..0000000000000000000000000000000000000000
--- a/spaces/CVPR/LIVE/thrust/thrust/iterator/transform_input_output_iterator.h
+++ /dev/null
@@ -1,163 +0,0 @@
-/*
- * Copyright 2020 NVIDIA Corporation
- *
- * Licensed under the Apache License, Version 2.0 (the "License");
- * you may not use this file except in compliance with the License.
- * You may obtain a copy of the License at
- *
- * http://www.apache.org/licenses/LICENSE-2.0
- *
- * Unless required by applicable law or agreed to in writing, software
- * distributed under the License is distributed on an "AS IS" BASIS,
- * WITHOUT WARRANTIES OR CONDITIONS OF ANY KIND, either express or implied.
- * See the License for the specific language governing permissions and
- * limitations under the License.
- */
-
-/*! \file thrust/iterator/transform_input_output_iterator.h
- * \brief An iterator which adapts another iterator by applying transform
- * functions when reading and writing dereferenced values.
- */
-
-#pragma once
-
-#include
-#include
-
-namespace thrust
-{
-
-/*! \addtogroup iterators
- * \{
- */
-
-/*! \addtogroup fancyiterator Fancy Iterators
- * \ingroup iterators
- * \{
- */
-
-/*! \p transform_input_output_iterator is a special kind of iterator which applies
- * transform functions when reading from or writing to dereferenced values.
- * This iterator is useful for algorithms that operate on a type that needs to
- * be serialized/deserialized from values in another iterator, avoiding the
- * need to materialize intermediate results in memory. This also enables the
- * transform functions to be fused with the operations that read and write to
- * the `transform_input_output_iterator`.
- *
- * The following code snippet demonstrates how to create a
- * \p transform_input_output_iterator which performs different transformations when
- * reading from and writing to the iterator.
- *
- * \code
- * #include
- * #include
- *
- * int main()
- * {
- * const size_t size = 4;
- * thrust::device_vector v(size);
- *
- * // Write 1.0f, 2.0f, 3.0f, 4.0f to vector
- * thrust::sequence(v.begin(), v.end(), 1);
- *
- * // Iterator that returns negated values and writes squared values
- * auto iter = thrust::make_transform_input_output_iterator(v.begin(),
- * thrust::negate{}, thrust::square{});
- *
- * // Iterator negates values when reading
- * std::cout << iter[0] << " "; // -1.0f;
- * std::cout << iter[1] << " "; // -2.0f;
- * std::cout << iter[2] << " "; // -3.0f;
- * std::cout << iter[3] << "\n"; // -4.0f;
- *
- * // Write 1.0f, 2.0f, 3.0f, 4.0f to iterator
- * thrust::sequence(iter, iter + size, 1);
- *
- * // Values were squared before writing to vector
- * std::cout << v[0] << " "; // 1.0f;
- * std::cout << v[1] << " "; // 4.0f;
- * std::cout << v[2] << " "; // 9.0f;
- * std::cout << v[3] << "\n"; // 16.0f;
- *
- * }
- * \endcode
- *
- * \see make_transform_input_output_iterator
- */
-
-template
- class transform_input_output_iterator
- : public detail::transform_input_output_iterator_base::type
-{
-
- /*! \cond
- */
-
- public:
-
- typedef typename
- detail::transform_input_output_iterator_base::type
- super_t;
-
- friend class thrust::iterator_core_access;
- /*! \endcond
- */
-
- /*! This constructor takes as argument a \c Iterator an \c InputFunction and an
- * \c OutputFunction and copies them to a new \p transform_input_output_iterator
- *
- * \param io An \c Iterator pointing to where the input to \c InputFunction
- * will be read from and the result of \c OutputFunction will be written to
- * \param input_function An \c InputFunction to be executed on values read from the iterator
- * \param output_function An \c OutputFunction to be executed on values written to the iterator
- */
- __host__ __device__
- transform_input_output_iterator(Iterator const& io, InputFunction input_function, OutputFunction output_function)
- : super_t(io), input_function(input_function), output_function(output_function)
- {
- }
-
- /*! \cond
- */
- private:
-
- __host__ __device__
- typename super_t::reference dereference() const
- {
- return detail::transform_input_output_iterator_proxy<
- InputFunction, OutputFunction, Iterator
- >(this->base_reference(), input_function, output_function);
- }
-
- InputFunction input_function;
- OutputFunction output_function;
-
- /*! \endcond
- */
-}; // end transform_input_output_iterator
-
-/*! \p make_transform_input_output_iterator creates a \p transform_input_output_iterator from
- * an \c Iterator a \c InputFunction and a \c OutputFunction
- *
- * \param io An \c Iterator pointing to where the input to \c InputFunction
- * will be read from and the result of \c OutputFunction will be written to
- * \param input_function An \c InputFunction to be executed on values read from the iterator
- * \param output_function An \c OutputFunction to be executed on values written to the iterator
- * \see transform_input_output_iterator
- */
-template
-transform_input_output_iterator
-__host__ __device__
-make_transform_input_output_iterator(Iterator io, InputFunction input_function, OutputFunction output_function)
-{
- return transform_input_output_iterator(io, input_function, output_function);
-} // end make_transform_input_output_iterator
-
-/*! \} // end fancyiterators
- */
-
-/*! \} // end iterators
- */
-
-} // end thrust
-
diff --git a/spaces/CVPR/LIVE/thrust/thrust/shuffle.h b/spaces/CVPR/LIVE/thrust/thrust/shuffle.h
deleted file mode 100644
index 8ed156e15227047072938bc80d8d90309093671e..0000000000000000000000000000000000000000
--- a/spaces/CVPR/LIVE/thrust/thrust/shuffle.h
+++ /dev/null
@@ -1,179 +0,0 @@
-/*
- * Copyright 2008-2020 NVIDIA Corporation
- *
- * Licensed under the Apache License, Version 2.0 (the "License");
- * you may not use this file except in compliance with the License.
- * You may obtain a copy of the License at
- *
- * http://www.apache.org/licenses/LICENSE-2.0
- *
- * Unless required by applicable law or agreed to in writing, software
- * distributed under the License is distributed on an "AS IS" BASIS,
- * WITHOUT WARRANTIES OR CONDITIONS OF ANY KIND, either express or implied.
- * See the License for the specific language governing permissions and
- * limitations under the License.
- */
-
-/*! \file shuffle.h
- * \brief Reorders range by a uniform random permutation
- */
-
-#pragma once
-
-#include
-#include
-
-#if THRUST_CPP_DIALECT >= 2011
-
-#include
-#include
-
-namespace thrust {
-
-/*! \addtogroup reordering
-* \ingroup algorithms
-*
-* \addtogroup shuffling
-* \ingroup reordering
-* \{
-*/
-
-
-/*! \p shuffle reorders the elements [first, last) by a uniform pseudorandom permutation, defined by
- * random engine \p g.
- *
- * The algorithm's execution is parallelized as determined by \p exec.
- *
- * \param exec The execution policy to use for parallelization.
- * \param first The beginning of the sequence to shuffle.
- * \param last The end of the sequence to shuffle.
- * \param g A UniformRandomBitGenerator
- *
- * \tparam DerivedPolicy The name of the derived execution policy.
- * \tparam RandomIterator is a random access iterator
- * \tparam URBG is a uniform random bit generator
- *
- * The following code snippet demonstrates how to use \p shuffle to create a random permutation
- * using the \p thrust::host execution policy for parallelization:
- *
- * \code
- * #include
- * #include
- * #include
- * int A[] = {1, 2, 3, 4, 5, 6, 7, 8, 9, 10};
- * const int N = sizeof(A)/sizeof(int);
- * thrust::default_random_engine g;
- * thrust::shuffle(thrust::host, A, A + N, g);
- * // A is now {6, 5, 8, 7, 2, 1, 4, 3, 10, 9}
- * \endcode
- *
- * \see \p shuffle_copy
- */
-template
-__host__ __device__ void shuffle(
- const thrust::detail::execution_policy_base& exec,
- RandomIterator first, RandomIterator last, URBG&& g);
-
-/*! \p shuffle reorders the elements [first, last) by a uniform pseudorandom permutation, defined by
- * random engine \p g.
- *
- * \param first The beginning of the sequence to shuffle.
- * \param last The end of the sequence to shuffle.
- * \param g A UniformRandomBitGenerator
- *
- * \tparam RandomIterator is a random access iterator
- * \tparam URBG is a uniform random bit generator
- *
- * The following code snippet demonstrates how to use \p shuffle to create a random permutation.
- *
- * \code
- * #include
- * #include
- * int A[] = {1, 2, 3, 4, 5, 6, 7, 8, 9, 10};
- * const int N = sizeof(A)/sizeof(int);
- * thrust::default_random_engine g;
- * thrust::shuffle(A, A + N, g);
- * // A is now {6, 5, 8, 7, 2, 1, 4, 3, 10, 9}
- * \endcode
- *
- * \see \p shuffle_copy
- */
-template
-__host__ __device__ void shuffle(RandomIterator first, RandomIterator last,
- URBG&& g);
-
-/*! shuffle_copy differs from shuffle only in that the reordered sequence is written to different output sequences, rather than in place.
- * \p shuffle_copy reorders the elements [first, last) by a uniform pseudorandom permutation, defined by
- * random engine \p g.
- *
- * The algorithm's execution is parallelized as determined by \p exec.
-
- * \param exec The execution policy to use for parallelization.
- * \param first The beginning of the sequence to shuffle.
- * \param last The end of the sequence to shuffle.
- * \param result Destination of shuffled sequence
- * \param g A UniformRandomBitGenerator
- *
- * \tparam DerivedPolicy The name of the derived execution policy.
- * \tparam RandomIterator is a random access iterator
- * \tparam OutputIterator is a model of Output Iterator.
- * \tparam URBG is a uniform random bit generator
- *
- * The following code snippet demonstrates how to use \p shuffle_copy to create a random permutation.
- *
- * \code
- * #include
- * #include
- * #include
- * int A[] = {1, 2, 3, 4, 5, 6, 7, 8, 9, 10};
- * int result[10];
- * const int N = sizeof(A)/sizeof(int);
- * thrust::default_random_engine g;
- * thrust::shuffle_copy(thrust::host, A, A + N, result, g);
- * // result is now {6, 5, 8, 7, 2, 1, 4, 3, 10, 9}
- * \endcode
- *
- * \see \p shuffle
- */
-template
-__host__ __device__ void shuffle_copy(
- const thrust::detail::execution_policy_base& exec,
- RandomIterator first, RandomIterator last, OutputIterator result, URBG&& g);
-
-/*! shuffle_copy differs from shuffle only in that the reordered sequence is written to different output sequences, rather than in place.
- *\p shuffle_copy reorders the elements [first, last) by a uniform pseudorandom permutation, defined by
- * random engine \p g.
- *
- * \param first The beginning of the sequence to shuffle.
- * \param last The end of the sequence to shuffle.
- * \param result Destination of shuffled sequence
- * \param g A UniformRandomBitGenerator
- *
- * \tparam RandomIterator is a random access iterator
- * \tparam OutputIterator is a model of Output Iterator.
- * \tparam URBG is a uniform random bit generator
- *
- * The following code snippet demonstrates how to use \p shuffle_copy to create a random permutation.
- *
- * \code
- * #include
- * #include
- * int A[] = {1, 2, 3, 4, 5, 6, 7, 8, 9, 10};
- * int result[10];
- * const int N = sizeof(A)/sizeof(int);
- * thrust::default_random_engine g;
- * thrust::shuffle_copy(A, A + N, result, g);
- * // result is now {6, 5, 8, 7, 2, 1, 4, 3, 10, 9}
- * \endcode
- *
- * \see \p shuffle
- */
-template
-__host__ __device__ void shuffle_copy(RandomIterator first, RandomIterator last,
- OutputIterator result, URBG&& g);
-
-} // namespace thrust
-
-#include
-#endif
diff --git a/spaces/CVPR/MonoScene/monoscene/flosp.py b/spaces/CVPR/MonoScene/monoscene/flosp.py
deleted file mode 100644
index 2d502197a72ee120773a47f239e86743f5a1e2d4..0000000000000000000000000000000000000000
--- a/spaces/CVPR/MonoScene/monoscene/flosp.py
+++ /dev/null
@@ -1,41 +0,0 @@
-import torch
-import torch.nn as nn
-
-
-class FLoSP(nn.Module):
- def __init__(self, scene_size, dataset, project_scale):
- super().__init__()
- self.scene_size = scene_size
- self.dataset = dataset
- self.project_scale = project_scale
-
- def forward(self, x2d, projected_pix, fov_mask):
- c, h, w = x2d.shape
-
- src = x2d.view(c, -1)
- zeros_vec = torch.zeros(c, 1).type_as(src)
- src = torch.cat([src, zeros_vec], 1)
-
- pix_x, pix_y = projected_pix[:, 0], projected_pix[:, 1]
- img_indices = pix_y * w + pix_x
- img_indices[~fov_mask] = h * w
- img_indices = img_indices.expand(c, -1).long() # c, HWD
- src_feature = torch.gather(src, 1, img_indices)
-
- if self.dataset == "NYU":
- x3d = src_feature.reshape(
- c,
- self.scene_size[0] // self.project_scale,
- self.scene_size[2] // self.project_scale,
- self.scene_size[1] // self.project_scale,
- )
- x3d = x3d.permute(0, 1, 3, 2)
- elif self.dataset == "kitti":
- x3d = src_feature.reshape(
- c,
- self.scene_size[0] // self.project_scale,
- self.scene_size[1] // self.project_scale,
- self.scene_size[2] // self.project_scale,
- )
-
- return x3d
diff --git a/spaces/CVPR/WALT/mmdet/models/roi_heads/mask_heads/maskiou_head.py b/spaces/CVPR/WALT/mmdet/models/roi_heads/mask_heads/maskiou_head.py
deleted file mode 100644
index 39bcd6a7dbdb089cd19cef811038e0b6a80ab89a..0000000000000000000000000000000000000000
--- a/spaces/CVPR/WALT/mmdet/models/roi_heads/mask_heads/maskiou_head.py
+++ /dev/null
@@ -1,186 +0,0 @@
-import numpy as np
-import torch
-import torch.nn as nn
-from mmcv.cnn import Conv2d, Linear, MaxPool2d, kaiming_init, normal_init
-from mmcv.runner import force_fp32
-from torch.nn.modules.utils import _pair
-
-from mmdet.models.builder import HEADS, build_loss
-
-
-@HEADS.register_module()
-class MaskIoUHead(nn.Module):
- """Mask IoU Head.
-
- This head predicts the IoU of predicted masks and corresponding gt masks.
- """
-
- def __init__(self,
- num_convs=4,
- num_fcs=2,
- roi_feat_size=14,
- in_channels=256,
- conv_out_channels=256,
- fc_out_channels=1024,
- num_classes=80,
- loss_iou=dict(type='MSELoss', loss_weight=0.5)):
- super(MaskIoUHead, self).__init__()
- self.in_channels = in_channels
- self.conv_out_channels = conv_out_channels
- self.fc_out_channels = fc_out_channels
- self.num_classes = num_classes
- self.fp16_enabled = False
-
- self.convs = nn.ModuleList()
- for i in range(num_convs):
- if i == 0:
- # concatenation of mask feature and mask prediction
- in_channels = self.in_channels + 1
- else:
- in_channels = self.conv_out_channels
- stride = 2 if i == num_convs - 1 else 1
- self.convs.append(
- Conv2d(
- in_channels,
- self.conv_out_channels,
- 3,
- stride=stride,
- padding=1))
-
- roi_feat_size = _pair(roi_feat_size)
- pooled_area = (roi_feat_size[0] // 2) * (roi_feat_size[1] // 2)
- self.fcs = nn.ModuleList()
- for i in range(num_fcs):
- in_channels = (
- self.conv_out_channels *
- pooled_area if i == 0 else self.fc_out_channels)
- self.fcs.append(Linear(in_channels, self.fc_out_channels))
-
- self.fc_mask_iou = Linear(self.fc_out_channels, self.num_classes)
- self.relu = nn.ReLU()
- self.max_pool = MaxPool2d(2, 2)
- self.loss_iou = build_loss(loss_iou)
-
- def init_weights(self):
- for conv in self.convs:
- kaiming_init(conv)
- for fc in self.fcs:
- kaiming_init(
- fc,
- a=1,
- mode='fan_in',
- nonlinearity='leaky_relu',
- distribution='uniform')
- normal_init(self.fc_mask_iou, std=0.01)
-
- def forward(self, mask_feat, mask_pred):
- mask_pred = mask_pred.sigmoid()
- mask_pred_pooled = self.max_pool(mask_pred.unsqueeze(1))
-
- x = torch.cat((mask_feat, mask_pred_pooled), 1)
-
- for conv in self.convs:
- x = self.relu(conv(x))
- x = x.flatten(1)
- for fc in self.fcs:
- x = self.relu(fc(x))
- mask_iou = self.fc_mask_iou(x)
- return mask_iou
-
- @force_fp32(apply_to=('mask_iou_pred', ))
- def loss(self, mask_iou_pred, mask_iou_targets):
- pos_inds = mask_iou_targets > 0
- if pos_inds.sum() > 0:
- loss_mask_iou = self.loss_iou(mask_iou_pred[pos_inds],
- mask_iou_targets[pos_inds])
- else:
- loss_mask_iou = mask_iou_pred.sum() * 0
- return dict(loss_mask_iou=loss_mask_iou)
-
- @force_fp32(apply_to=('mask_pred', ))
- def get_targets(self, sampling_results, gt_masks, mask_pred, mask_targets,
- rcnn_train_cfg):
- """Compute target of mask IoU.
-
- Mask IoU target is the IoU of the predicted mask (inside a bbox) and
- the gt mask of corresponding gt mask (the whole instance).
- The intersection area is computed inside the bbox, and the gt mask area
- is computed with two steps, firstly we compute the gt area inside the
- bbox, then divide it by the area ratio of gt area inside the bbox and
- the gt area of the whole instance.
-
- Args:
- sampling_results (list[:obj:`SamplingResult`]): sampling results.
- gt_masks (BitmapMask | PolygonMask): Gt masks (the whole instance)
- of each image, with the same shape of the input image.
- mask_pred (Tensor): Predicted masks of each positive proposal,
- shape (num_pos, h, w).
- mask_targets (Tensor): Gt mask of each positive proposal,
- binary map of the shape (num_pos, h, w).
- rcnn_train_cfg (dict): Training config for R-CNN part.
-
- Returns:
- Tensor: mask iou target (length == num positive).
- """
- pos_proposals = [res.pos_bboxes for res in sampling_results]
- pos_assigned_gt_inds = [
- res.pos_assigned_gt_inds for res in sampling_results
- ]
-
- # compute the area ratio of gt areas inside the proposals and
- # the whole instance
- area_ratios = map(self._get_area_ratio, pos_proposals,
- pos_assigned_gt_inds, gt_masks)
- area_ratios = torch.cat(list(area_ratios))
- assert mask_targets.size(0) == area_ratios.size(0)
-
- mask_pred = (mask_pred > rcnn_train_cfg.mask_thr_binary).float()
- mask_pred_areas = mask_pred.sum((-1, -2))
-
- # mask_pred and mask_targets are binary maps
- overlap_areas = (mask_pred * mask_targets).sum((-1, -2))
-
- # compute the mask area of the whole instance
- gt_full_areas = mask_targets.sum((-1, -2)) / (area_ratios + 1e-7)
-
- mask_iou_targets = overlap_areas / (
- mask_pred_areas + gt_full_areas - overlap_areas)
- return mask_iou_targets
-
- def _get_area_ratio(self, pos_proposals, pos_assigned_gt_inds, gt_masks):
- """Compute area ratio of the gt mask inside the proposal and the gt
- mask of the corresponding instance."""
- num_pos = pos_proposals.size(0)
- if num_pos > 0:
- area_ratios = []
- proposals_np = pos_proposals.cpu().numpy()
- pos_assigned_gt_inds = pos_assigned_gt_inds.cpu().numpy()
- # compute mask areas of gt instances (batch processing for speedup)
- gt_instance_mask_area = gt_masks.areas
- for i in range(num_pos):
- gt_mask = gt_masks[pos_assigned_gt_inds[i]]
-
- # crop the gt mask inside the proposal
- bbox = proposals_np[i, :].astype(np.int32)
- gt_mask_in_proposal = gt_mask.crop(bbox)
-
- ratio = gt_mask_in_proposal.areas[0] / (
- gt_instance_mask_area[pos_assigned_gt_inds[i]] + 1e-7)
- area_ratios.append(ratio)
- area_ratios = torch.from_numpy(np.stack(area_ratios)).float().to(
- pos_proposals.device)
- else:
- area_ratios = pos_proposals.new_zeros((0, ))
- return area_ratios
-
- @force_fp32(apply_to=('mask_iou_pred', ))
- def get_mask_scores(self, mask_iou_pred, det_bboxes, det_labels):
- """Get the mask scores.
-
- mask_score = bbox_score * mask_iou
- """
- inds = range(det_labels.size(0))
- mask_scores = mask_iou_pred[inds, det_labels] * det_bboxes[inds, -1]
- mask_scores = mask_scores.cpu().numpy()
- det_labels = det_labels.cpu().numpy()
- return [mask_scores[det_labels == i] for i in range(self.num_classes)]
diff --git a/spaces/CVPR/WALT/mmdet/models/utils/builder.py b/spaces/CVPR/WALT/mmdet/models/utils/builder.py
deleted file mode 100644
index f362d1c92ca9d4ed95a2b3d28d3e6baedd14e462..0000000000000000000000000000000000000000
--- a/spaces/CVPR/WALT/mmdet/models/utils/builder.py
+++ /dev/null
@@ -1,14 +0,0 @@
-from mmcv.utils import Registry, build_from_cfg
-
-TRANSFORMER = Registry('Transformer')
-POSITIONAL_ENCODING = Registry('Position encoding')
-
-
-def build_transformer(cfg, default_args=None):
- """Builder for Transformer."""
- return build_from_cfg(cfg, TRANSFORMER, default_args)
-
-
-def build_positional_encoding(cfg, default_args=None):
- """Builder for Position Encoding."""
- return build_from_cfg(cfg, POSITIONAL_ENCODING, default_args)
diff --git a/spaces/Copy233/copy/README.md b/spaces/Copy233/copy/README.md
deleted file mode 100644
index a1d8f85510b85899dc7d22770ab7859484075847..0000000000000000000000000000000000000000
--- a/spaces/Copy233/copy/README.md
+++ /dev/null
@@ -1,12 +0,0 @@
----
-title: Real CUGAN
-emoji: 🔥
-colorFrom: pink
-colorTo: yellow
-sdk: gradio
-app_file: app.py
-pinned: false
-license: apache-2.0
----
-
-Check out the configuration reference at https://huggingface.co/docs/hub/spaces#reference
diff --git a/spaces/Cyril666/ContourNet-ABI/maskrcnn_benchmark/utils/chars.py b/spaces/Cyril666/ContourNet-ABI/maskrcnn_benchmark/utils/chars.py
deleted file mode 100644
index 71772ab85dec2b42458e25593b611e5f24e465d2..0000000000000000000000000000000000000000
--- a/spaces/Cyril666/ContourNet-ABI/maskrcnn_benchmark/utils/chars.py
+++ /dev/null
@@ -1,199 +0,0 @@
-import os
-
-import cv2
-import numpy as np
-
-
-def char2num(char):
- if char in "0123456789":
- num = ord(char) - ord("0") + 1
- elif char in "abcdefghijklmnopqrstuvwxyzABCDEFGHIJKLMNOPQRSTUVWXYZ":
- num = ord(char.lower()) - ord("a") + 11
- else:
- num = 0
- return num
-
-
-def num2char(num):
- chars = "_0123456789abcdefghijklmnopqrstuvwxyz"
- char = chars[num]
- # if num >=1 and num <=10:
- # char = chr(ord('0') + num - 1)
- # elif num > 10 and num <= 36:
- # char = chr(ord('a') + num - 11)
- # else:
- # print('error number:%d'%(num))
- # exit()
- return char
-
-
-def getstr_grid(seg, box, threshold=192):
- pos = 255 - (seg[0] * 255).astype(np.uint8)
- mask_index = np.argmax(seg, axis=0)
- mask_index = mask_index.astype(np.uint8)
- pos = pos.astype(np.uint8)
- string, score, rec_scores, char_polygons = seg2text(
- pos, mask_index, seg, box, threshold=threshold
- )
- return string, score, rec_scores, char_polygons
-
-
-def seg2text(gray, mask, seg, box, threshold=192):
- ## input numpy
- img_h, img_w = gray.shape
- box_w = box[2] - box[0]
- box_h = box[3] - box[1]
- ratio_h = float(box_h) / img_h
- ratio_w = float(box_w) / img_w
- # SE1=cv2.getStructuringElement(cv2.MORPH_RECT,(3,3))
- # gray = cv2.erode(gray,SE1)
- # gray = cv2.dilate(gray,SE1)
- # gray = cv2.morphologyEx(gray,cv2.MORPH_CLOSE,SE1)
- ret, thresh = cv2.threshold(gray, threshold, 255, cv2.THRESH_BINARY)
- try:
- _, contours, _ = cv2.findContours(thresh, cv2.RETR_TREE, cv2.CHAIN_APPROX_SIMPLE)
- except:
- contours, _ = cv2.findContours(thresh, cv2.RETR_TREE, cv2.CHAIN_APPROX_SIMPLE)
- chars = []
- scores = []
- char_polygons = []
- for i in range(len(contours)):
- char = {}
- temp = np.zeros((img_h, img_w)).astype(np.uint8)
- cv2.drawContours(temp, [contours[i]], 0, (255), -1)
- x, y, w, h = cv2.boundingRect(contours[i])
- c_x, c_y = x + w / 2, y + h / 2
- perimeter = cv2.arcLength(contours[i], True)
- epsilon = 0.01 * cv2.arcLength(contours[i], True)
- approx = cv2.approxPolyDP(contours[i], epsilon, True)
- pts = approx.reshape((-1, 2))
- pts[:, 0] = pts[:, 0] * ratio_w + box[0]
- pts[:, 1] = pts[:, 1] * ratio_h + box[1]
- polygon = list(pts.reshape((-1,)))
- polygon = list(map(int, polygon))
- if len(polygon) >= 6:
- char_polygons.append(polygon)
- # x1 = x * ratio_w + box[0]
- # y1 = y * ratio_h + box[1]
- # x3 = (x + w) * ratio_w + box[0]
- # y3 = (y + h) * ratio_h + box[1]
- # polygon = [x1, y1, x3, y1, x3, y3, x1, y3]
- regions = seg[1:, temp == 255].reshape((36, -1))
- cs = np.mean(regions, axis=1)
- sym = num2char(np.argmax(cs.reshape((-1))) + 1)
- char["x"] = c_x
- char["y"] = c_y
- char["s"] = sym
- char["cs"] = cs.reshape((-1, 1))
- scores.append(np.max(char["cs"], axis=0)[0])
-
- chars.append(char)
- chars = sorted(chars, key=lambda x: x["x"])
- string = ""
- css = []
- for char in chars:
- string = string + char["s"]
- css.append(char["cs"])
- if len(scores) > 0:
- score = sum(scores) / len(scores)
- else:
- score = 0.00
- if not css:
- css = [0.0]
- return string, score, np.hstack(css), char_polygons
-
-
-# def get_tight_rect(points, start_x, start_y, image_height, image_width, scale):
-# points = list(points)
-# ps = sorted(points, key=lambda x: x[0])
-#
-# if ps[1][1] > ps[0][1]:
-# px1 = ps[0][0] * scale + start_x
-# py1 = ps[0][1] * scale + start_y
-# px4 = ps[1][0] * scale + start_x
-# py4 = ps[1][1] * scale + start_y
-# else:
-# px1 = ps[1][0] * scale + start_x
-# py1 = ps[1][1] * scale + start_y
-# px4 = ps[0][0] * scale + start_x
-# py4 = ps[0][1] * scale + start_y
-# if ps[3][1] > ps[2][1]:
-# px2 = ps[2][0] * scale + start_x
-# py2 = ps[2][1] * scale + start_y
-# px3 = ps[3][0] * scale + start_x
-# py3 = ps[3][1] * scale + start_y
-# else:
-# px2 = ps[3][0] * scale + start_x
-# py2 = ps[3][1] * scale + start_y
-# px3 = ps[2][0] * scale + start_x
-# py3 = ps[2][1] * scale + start_y
-#
-# if px1 < 0:
-# px1 = 1
-# if px1 > image_width:
-# px1 = image_width - 1
-# if px2 < 0:
-# px2 = 1
-# if px2 > image_width:
-# px2 = image_width - 1
-# if px3 < 0:
-# px3 = 1
-# if px3 > image_width:
-# px3 = image_width - 1
-# if px4 < 0:
-# px4 = 1
-# if px4 > image_width:
-# px4 = image_width - 1
-#
-# if py1 < 0:
-# py1 = 1
-# if py1 > image_height:
-# py1 = image_height - 1
-# if py2 < 0:
-# py2 = 1
-# if py2 > image_height:
-# py2 = image_height - 1
-# if py3 < 0:
-# py3 = 1
-# if py3 > image_height:
-# py3 = image_height - 1
-# if py4 < 0:
-# py4 = 1
-# if py4 > image_height:
-# py4 = image_height - 1
-# return [px1, py1, px2, py2, px3, py3, px4, py4]
-
-def get_tight_rect(points, start_x, start_y, image_height, image_width, scale):
- points = list(points)
- ps = sorted(points, key=lambda x: x[0])
-
- if ps[1][1] > ps[0][1]:
- px1 = ps[0][0] * scale + start_x
- py1 = ps[0][1] * scale + start_y
- px4 = ps[1][0] * scale + start_x
- py4 = ps[1][1] * scale + start_y
- else:
- px1 = ps[1][0] * scale + start_x
- py1 = ps[1][1] * scale + start_y
- px4 = ps[0][0] * scale + start_x
- py4 = ps[0][1] * scale + start_y
- if ps[3][1] > ps[2][1]:
- px2 = ps[2][0] * scale + start_x
- py2 = ps[2][1] * scale + start_y
- px3 = ps[3][0] * scale + start_x
- py3 = ps[3][1] * scale + start_y
- else:
- px2 = ps[3][0] * scale + start_x
- py2 = ps[3][1] * scale + start_y
- px3 = ps[2][0] * scale + start_x
- py3 = ps[2][1] * scale + start_y
-
- px1 = min(max(px1, 1), image_width - 1)
- px2 = min(max(px2, 1), image_width - 1)
- px3 = min(max(px3, 1), image_width - 1)
- px4 = min(max(px4, 1), image_width - 1)
- py1 = min(max(py1, 1), image_height - 1)
- py2 = min(max(py2, 1), image_height - 1)
- py3 = min(max(py3, 1), image_height - 1)
- py4 = min(max(py4, 1), image_height - 1)
- return [px1, py1, px2, py2, px3, py3, px4, py4]
diff --git a/spaces/DCandE/rvc-models/infer_pack/transforms.py b/spaces/DCandE/rvc-models/infer_pack/transforms.py
deleted file mode 100644
index a11f799e023864ff7082c1f49c0cc18351a13b47..0000000000000000000000000000000000000000
--- a/spaces/DCandE/rvc-models/infer_pack/transforms.py
+++ /dev/null
@@ -1,209 +0,0 @@
-import torch
-from torch.nn import functional as F
-
-import numpy as np
-
-
-DEFAULT_MIN_BIN_WIDTH = 1e-3
-DEFAULT_MIN_BIN_HEIGHT = 1e-3
-DEFAULT_MIN_DERIVATIVE = 1e-3
-
-
-def piecewise_rational_quadratic_transform(
- inputs,
- unnormalized_widths,
- unnormalized_heights,
- unnormalized_derivatives,
- inverse=False,
- tails=None,
- tail_bound=1.0,
- min_bin_width=DEFAULT_MIN_BIN_WIDTH,
- min_bin_height=DEFAULT_MIN_BIN_HEIGHT,
- min_derivative=DEFAULT_MIN_DERIVATIVE,
-):
- if tails is None:
- spline_fn = rational_quadratic_spline
- spline_kwargs = {}
- else:
- spline_fn = unconstrained_rational_quadratic_spline
- spline_kwargs = {"tails": tails, "tail_bound": tail_bound}
-
- outputs, logabsdet = spline_fn(
- inputs=inputs,
- unnormalized_widths=unnormalized_widths,
- unnormalized_heights=unnormalized_heights,
- unnormalized_derivatives=unnormalized_derivatives,
- inverse=inverse,
- min_bin_width=min_bin_width,
- min_bin_height=min_bin_height,
- min_derivative=min_derivative,
- **spline_kwargs
- )
- return outputs, logabsdet
-
-
-def searchsorted(bin_locations, inputs, eps=1e-6):
- bin_locations[..., -1] += eps
- return torch.sum(inputs[..., None] >= bin_locations, dim=-1) - 1
-
-
-def unconstrained_rational_quadratic_spline(
- inputs,
- unnormalized_widths,
- unnormalized_heights,
- unnormalized_derivatives,
- inverse=False,
- tails="linear",
- tail_bound=1.0,
- min_bin_width=DEFAULT_MIN_BIN_WIDTH,
- min_bin_height=DEFAULT_MIN_BIN_HEIGHT,
- min_derivative=DEFAULT_MIN_DERIVATIVE,
-):
- inside_interval_mask = (inputs >= -tail_bound) & (inputs <= tail_bound)
- outside_interval_mask = ~inside_interval_mask
-
- outputs = torch.zeros_like(inputs)
- logabsdet = torch.zeros_like(inputs)
-
- if tails == "linear":
- unnormalized_derivatives = F.pad(unnormalized_derivatives, pad=(1, 1))
- constant = np.log(np.exp(1 - min_derivative) - 1)
- unnormalized_derivatives[..., 0] = constant
- unnormalized_derivatives[..., -1] = constant
-
- outputs[outside_interval_mask] = inputs[outside_interval_mask]
- logabsdet[outside_interval_mask] = 0
- else:
- raise RuntimeError("{} tails are not implemented.".format(tails))
-
- (
- outputs[inside_interval_mask],
- logabsdet[inside_interval_mask],
- ) = rational_quadratic_spline(
- inputs=inputs[inside_interval_mask],
- unnormalized_widths=unnormalized_widths[inside_interval_mask, :],
- unnormalized_heights=unnormalized_heights[inside_interval_mask, :],
- unnormalized_derivatives=unnormalized_derivatives[inside_interval_mask, :],
- inverse=inverse,
- left=-tail_bound,
- right=tail_bound,
- bottom=-tail_bound,
- top=tail_bound,
- min_bin_width=min_bin_width,
- min_bin_height=min_bin_height,
- min_derivative=min_derivative,
- )
-
- return outputs, logabsdet
-
-
-def rational_quadratic_spline(
- inputs,
- unnormalized_widths,
- unnormalized_heights,
- unnormalized_derivatives,
- inverse=False,
- left=0.0,
- right=1.0,
- bottom=0.0,
- top=1.0,
- min_bin_width=DEFAULT_MIN_BIN_WIDTH,
- min_bin_height=DEFAULT_MIN_BIN_HEIGHT,
- min_derivative=DEFAULT_MIN_DERIVATIVE,
-):
- if torch.min(inputs) < left or torch.max(inputs) > right:
- raise ValueError("Input to a transform is not within its domain")
-
- num_bins = unnormalized_widths.shape[-1]
-
- if min_bin_width * num_bins > 1.0:
- raise ValueError("Minimal bin width too large for the number of bins")
- if min_bin_height * num_bins > 1.0:
- raise ValueError("Minimal bin height too large for the number of bins")
-
- widths = F.softmax(unnormalized_widths, dim=-1)
- widths = min_bin_width + (1 - min_bin_width * num_bins) * widths
- cumwidths = torch.cumsum(widths, dim=-1)
- cumwidths = F.pad(cumwidths, pad=(1, 0), mode="constant", value=0.0)
- cumwidths = (right - left) * cumwidths + left
- cumwidths[..., 0] = left
- cumwidths[..., -1] = right
- widths = cumwidths[..., 1:] - cumwidths[..., :-1]
-
- derivatives = min_derivative + F.softplus(unnormalized_derivatives)
-
- heights = F.softmax(unnormalized_heights, dim=-1)
- heights = min_bin_height + (1 - min_bin_height * num_bins) * heights
- cumheights = torch.cumsum(heights, dim=-1)
- cumheights = F.pad(cumheights, pad=(1, 0), mode="constant", value=0.0)
- cumheights = (top - bottom) * cumheights + bottom
- cumheights[..., 0] = bottom
- cumheights[..., -1] = top
- heights = cumheights[..., 1:] - cumheights[..., :-1]
-
- if inverse:
- bin_idx = searchsorted(cumheights, inputs)[..., None]
- else:
- bin_idx = searchsorted(cumwidths, inputs)[..., None]
-
- input_cumwidths = cumwidths.gather(-1, bin_idx)[..., 0]
- input_bin_widths = widths.gather(-1, bin_idx)[..., 0]
-
- input_cumheights = cumheights.gather(-1, bin_idx)[..., 0]
- delta = heights / widths
- input_delta = delta.gather(-1, bin_idx)[..., 0]
-
- input_derivatives = derivatives.gather(-1, bin_idx)[..., 0]
- input_derivatives_plus_one = derivatives[..., 1:].gather(-1, bin_idx)[..., 0]
-
- input_heights = heights.gather(-1, bin_idx)[..., 0]
-
- if inverse:
- a = (inputs - input_cumheights) * (
- input_derivatives + input_derivatives_plus_one - 2 * input_delta
- ) + input_heights * (input_delta - input_derivatives)
- b = input_heights * input_derivatives - (inputs - input_cumheights) * (
- input_derivatives + input_derivatives_plus_one - 2 * input_delta
- )
- c = -input_delta * (inputs - input_cumheights)
-
- discriminant = b.pow(2) - 4 * a * c
- assert (discriminant >= 0).all()
-
- root = (2 * c) / (-b - torch.sqrt(discriminant))
- outputs = root * input_bin_widths + input_cumwidths
-
- theta_one_minus_theta = root * (1 - root)
- denominator = input_delta + (
- (input_derivatives + input_derivatives_plus_one - 2 * input_delta)
- * theta_one_minus_theta
- )
- derivative_numerator = input_delta.pow(2) * (
- input_derivatives_plus_one * root.pow(2)
- + 2 * input_delta * theta_one_minus_theta
- + input_derivatives * (1 - root).pow(2)
- )
- logabsdet = torch.log(derivative_numerator) - 2 * torch.log(denominator)
-
- return outputs, -logabsdet
- else:
- theta = (inputs - input_cumwidths) / input_bin_widths
- theta_one_minus_theta = theta * (1 - theta)
-
- numerator = input_heights * (
- input_delta * theta.pow(2) + input_derivatives * theta_one_minus_theta
- )
- denominator = input_delta + (
- (input_derivatives + input_derivatives_plus_one - 2 * input_delta)
- * theta_one_minus_theta
- )
- outputs = input_cumheights + numerator / denominator
-
- derivative_numerator = input_delta.pow(2) * (
- input_derivatives_plus_one * theta.pow(2)
- + 2 * input_delta * theta_one_minus_theta
- + input_derivatives * (1 - theta).pow(2)
- )
- logabsdet = torch.log(derivative_numerator) - 2 * torch.log(denominator)
-
- return outputs, logabsdet
diff --git a/spaces/DQChoi/gpt-demo/venv/lib/python3.11/site-packages/fontTools/varLib/iup.py b/spaces/DQChoi/gpt-demo/venv/lib/python3.11/site-packages/fontTools/varLib/iup.py
deleted file mode 100644
index 0f1232ad2ea1cac8953239a5bdc55f1cedbb5f02..0000000000000000000000000000000000000000
--- a/spaces/DQChoi/gpt-demo/venv/lib/python3.11/site-packages/fontTools/varLib/iup.py
+++ /dev/null
@@ -1,496 +0,0 @@
-try:
- import cython
-
- COMPILED = cython.compiled
-except (AttributeError, ImportError):
- # if cython not installed, use mock module with no-op decorators and types
- from fontTools.misc import cython
-
- COMPILED = False
-
-from typing import (
- Sequence,
- Tuple,
- Union,
-)
-from numbers import Integral, Real
-
-try:
- import cython
-
- COMPILED = cython.compiled
-except (AttributeError, ImportError):
- # if cython not installed, use mock module with no-op decorators and types
- from fontTools.misc import cython
-
- COMPILED = False
-
-
-_Point = Tuple[Real, Real]
-_Delta = Tuple[Real, Real]
-_PointSegment = Sequence[_Point]
-_DeltaSegment = Sequence[_Delta]
-_DeltaOrNone = Union[_Delta, None]
-_DeltaOrNoneSegment = Sequence[_DeltaOrNone]
-_Endpoints = Sequence[Integral]
-
-
-MAX_LOOKBACK = 8
-
-
-@cython.cfunc
-@cython.locals(
- j=cython.int,
- n=cython.int,
- x1=cython.double,
- x2=cython.double,
- d1=cython.double,
- d2=cython.double,
- scale=cython.double,
- x=cython.double,
- d=cython.double,
-)
-def iup_segment(
- coords: _PointSegment, rc1: _Point, rd1: _Delta, rc2: _Point, rd2: _Delta
-): # -> _DeltaSegment:
- """Given two reference coordinates `rc1` & `rc2` and their respective
- delta vectors `rd1` & `rd2`, returns interpolated deltas for the set of
- coordinates `coords`."""
-
- # rc1 = reference coord 1
- # rd1 = reference delta 1
- out_arrays = [None, None]
- for j in 0, 1:
- out_arrays[j] = out = []
- x1, x2, d1, d2 = rc1[j], rc2[j], rd1[j], rd2[j]
-
- if x1 == x2:
- n = len(coords)
- if d1 == d2:
- out.extend([d1] * n)
- else:
- out.extend([0] * n)
- continue
-
- if x1 > x2:
- x1, x2 = x2, x1
- d1, d2 = d2, d1
-
- # x1 < x2
- scale = (d2 - d1) / (x2 - x1)
- for pair in coords:
- x = pair[j]
-
- if x <= x1:
- d = d1
- elif x >= x2:
- d = d2
- else:
- # Interpolate
- d = d1 + (x - x1) * scale
-
- out.append(d)
-
- return zip(*out_arrays)
-
-
-def iup_contour(deltas: _DeltaOrNoneSegment, coords: _PointSegment) -> _DeltaSegment:
- """For the contour given in `coords`, interpolate any missing
- delta values in delta vector `deltas`.
-
- Returns fully filled-out delta vector."""
-
- assert len(deltas) == len(coords)
- if None not in deltas:
- return deltas
-
- n = len(deltas)
- # indices of points with explicit deltas
- indices = [i for i, v in enumerate(deltas) if v is not None]
- if not indices:
- # All deltas are None. Return 0,0 for all.
- return [(0, 0)] * n
-
- out = []
- it = iter(indices)
- start = next(it)
- if start != 0:
- # Initial segment that wraps around
- i1, i2, ri1, ri2 = 0, start, start, indices[-1]
- out.extend(
- iup_segment(
- coords[i1:i2], coords[ri1], deltas[ri1], coords[ri2], deltas[ri2]
- )
- )
- out.append(deltas[start])
- for end in it:
- if end - start > 1:
- i1, i2, ri1, ri2 = start + 1, end, start, end
- out.extend(
- iup_segment(
- coords[i1:i2], coords[ri1], deltas[ri1], coords[ri2], deltas[ri2]
- )
- )
- out.append(deltas[end])
- start = end
- if start != n - 1:
- # Final segment that wraps around
- i1, i2, ri1, ri2 = start + 1, n, start, indices[0]
- out.extend(
- iup_segment(
- coords[i1:i2], coords[ri1], deltas[ri1], coords[ri2], deltas[ri2]
- )
- )
-
- assert len(deltas) == len(out), (len(deltas), len(out))
- return out
-
-
-def iup_delta(
- deltas: _DeltaOrNoneSegment, coords: _PointSegment, ends: _Endpoints
-) -> _DeltaSegment:
- """For the outline given in `coords`, with contour endpoints given
- in sorted increasing order in `ends`, interpolate any missing
- delta values in delta vector `deltas`.
-
- Returns fully filled-out delta vector."""
-
- assert sorted(ends) == ends and len(coords) == (ends[-1] + 1 if ends else 0) + 4
- n = len(coords)
- ends = ends + [n - 4, n - 3, n - 2, n - 1]
- out = []
- start = 0
- for end in ends:
- end += 1
- contour = iup_contour(deltas[start:end], coords[start:end])
- out.extend(contour)
- start = end
-
- return out
-
-
-# Optimizer
-
-
-@cython.cfunc
-@cython.inline
-@cython.locals(
- i=cython.int,
- j=cython.int,
- tolerance=cython.double,
- x=cython.double,
- y=cython.double,
- p=cython.double,
- q=cython.double,
-)
-@cython.returns(int)
-def can_iup_in_between(
- deltas: _DeltaSegment,
- coords: _PointSegment,
- i: Integral,
- j: Integral,
- tolerance: Real,
-): # -> bool:
- """Return true if the deltas for points at `i` and `j` (`i < j`) can be
- successfully used to interpolate deltas for points in between them within
- provided error tolerance."""
-
- assert j - i >= 2
- interp = iup_segment(coords[i + 1 : j], coords[i], deltas[i], coords[j], deltas[j])
- deltas = deltas[i + 1 : j]
-
- return all(
- abs(complex(x - p, y - q)) <= tolerance
- for (x, y), (p, q) in zip(deltas, interp)
- )
-
-
-@cython.locals(
- cj=cython.double,
- dj=cython.double,
- lcj=cython.double,
- ldj=cython.double,
- ncj=cython.double,
- ndj=cython.double,
- force=cython.int,
- forced=set,
-)
-def _iup_contour_bound_forced_set(
- deltas: _DeltaSegment, coords: _PointSegment, tolerance: Real = 0
-) -> set:
- """The forced set is a conservative set of points on the contour that must be encoded
- explicitly (ie. cannot be interpolated). Calculating this set allows for significantly
- speeding up the dynamic-programming, as well as resolve circularity in DP.
-
- The set is precise; that is, if an index is in the returned set, then there is no way
- that IUP can generate delta for that point, given `coords` and `deltas`.
- """
- assert len(deltas) == len(coords)
-
- n = len(deltas)
- forced = set()
- # Track "last" and "next" points on the contour as we sweep.
- for i in range(len(deltas) - 1, -1, -1):
- ld, lc = deltas[i - 1], coords[i - 1]
- d, c = deltas[i], coords[i]
- nd, nc = deltas[i - n + 1], coords[i - n + 1]
-
- for j in (0, 1): # For X and for Y
- cj = c[j]
- dj = d[j]
- lcj = lc[j]
- ldj = ld[j]
- ncj = nc[j]
- ndj = nd[j]
-
- if lcj <= ncj:
- c1, c2 = lcj, ncj
- d1, d2 = ldj, ndj
- else:
- c1, c2 = ncj, lcj
- d1, d2 = ndj, ldj
-
- force = False
-
- # If the two coordinates are the same, then the interpolation
- # algorithm produces the same delta if both deltas are equal,
- # and zero if they differ.
- #
- # This test has to be before the next one.
- if c1 == c2:
- if abs(d1 - d2) > tolerance and abs(dj) > tolerance:
- force = True
-
- # If coordinate for current point is between coordinate of adjacent
- # points on the two sides, but the delta for current point is NOT
- # between delta for those adjacent points (considering tolerance
- # allowance), then there is no way that current point can be IUP-ed.
- # Mark it forced.
- elif c1 <= cj <= c2: # and c1 != c2
- if not (min(d1, d2) - tolerance <= dj <= max(d1, d2) + tolerance):
- force = True
-
- # Otherwise, the delta should either match the closest, or have the
- # same sign as the interpolation of the two deltas.
- else: # cj < c1 or c2 < cj
- if d1 != d2:
- if cj < c1:
- if (
- abs(dj) > tolerance
- and abs(dj - d1) > tolerance
- and ((dj - tolerance < d1) != (d1 < d2))
- ):
- force = True
- else: # c2 < cj
- if (
- abs(dj) > tolerance
- and abs(dj - d2) > tolerance
- and ((d2 < dj + tolerance) != (d1 < d2))
- ):
- force = True
-
- if force:
- forced.add(i)
- break
-
- return forced
-
-
-@cython.locals(
- i=cython.int,
- j=cython.int,
- best_cost=cython.double,
- best_j=cython.int,
- cost=cython.double,
- forced=set,
- tolerance=cython.double,
-)
-def _iup_contour_optimize_dp(
- deltas: _DeltaSegment,
- coords: _PointSegment,
- forced=set(),
- tolerance: Real = 0,
- lookback: Integral = None,
-):
- """Straightforward Dynamic-Programming. For each index i, find least-costly encoding of
- points 0 to i where i is explicitly encoded. We find this by considering all previous
- explicit points j and check whether interpolation can fill points between j and i.
-
- Note that solution always encodes last point explicitly. Higher-level is responsible
- for removing that restriction.
-
- As major speedup, we stop looking further whenever we see a "forced" point."""
-
- n = len(deltas)
- if lookback is None:
- lookback = n
- lookback = min(lookback, MAX_LOOKBACK)
- costs = {-1: 0}
- chain = {-1: None}
- for i in range(0, n):
- best_cost = costs[i - 1] + 1
-
- costs[i] = best_cost
- chain[i] = i - 1
-
- if i - 1 in forced:
- continue
-
- for j in range(i - 2, max(i - lookback, -2), -1):
- cost = costs[j] + 1
-
- if cost < best_cost and can_iup_in_between(deltas, coords, j, i, tolerance):
- costs[i] = best_cost = cost
- chain[i] = j
-
- if j in forced:
- break
-
- return chain, costs
-
-
-def _rot_list(l: list, k: int):
- """Rotate list by k items forward. Ie. item at position 0 will be
- at position k in returned list. Negative k is allowed."""
- n = len(l)
- k %= n
- if not k:
- return l
- return l[n - k :] + l[: n - k]
-
-
-def _rot_set(s: set, k: int, n: int):
- k %= n
- if not k:
- return s
- return {(v + k) % n for v in s}
-
-
-def iup_contour_optimize(
- deltas: _DeltaSegment, coords: _PointSegment, tolerance: Real = 0.0
-) -> _DeltaOrNoneSegment:
- """For contour with coordinates `coords`, optimize a set of delta
- values `deltas` within error `tolerance`.
-
- Returns delta vector that has most number of None items instead of
- the input delta.
- """
-
- n = len(deltas)
-
- # Get the easy cases out of the way:
-
- # If all are within tolerance distance of 0, encode nothing:
- if all(abs(complex(*p)) <= tolerance for p in deltas):
- return [None] * n
-
- # If there's exactly one point, return it:
- if n == 1:
- return deltas
-
- # If all deltas are exactly the same, return just one (the first one):
- d0 = deltas[0]
- if all(d0 == d for d in deltas):
- return [d0] + [None] * (n - 1)
-
- # Else, solve the general problem using Dynamic Programming.
-
- forced = _iup_contour_bound_forced_set(deltas, coords, tolerance)
- # The _iup_contour_optimize_dp() routine returns the optimal encoding
- # solution given the constraint that the last point is always encoded.
- # To remove this constraint, we use two different methods, depending on
- # whether forced set is non-empty or not:
-
- # Debugging: Make the next if always take the second branch and observe
- # if the font size changes (reduced); that would mean the forced-set
- # has members it should not have.
- if forced:
- # Forced set is non-empty: rotate the contour start point
- # such that the last point in the list is a forced point.
- k = (n - 1) - max(forced)
- assert k >= 0
-
- deltas = _rot_list(deltas, k)
- coords = _rot_list(coords, k)
- forced = _rot_set(forced, k, n)
-
- # Debugging: Pass a set() instead of forced variable to the next call
- # to exercise forced-set computation for under-counting.
- chain, costs = _iup_contour_optimize_dp(deltas, coords, forced, tolerance)
-
- # Assemble solution.
- solution = set()
- i = n - 1
- while i is not None:
- solution.add(i)
- i = chain[i]
- solution.remove(-1)
-
- # if not forced <= solution:
- # print("coord", coords)
- # print("deltas", deltas)
- # print("len", len(deltas))
- assert forced <= solution, (forced, solution)
-
- deltas = [deltas[i] if i in solution else None for i in range(n)]
-
- deltas = _rot_list(deltas, -k)
- else:
- # Repeat the contour an extra time, solve the new case, then look for solutions of the
- # circular n-length problem in the solution for new linear case. I cannot prove that
- # this always produces the optimal solution...
- chain, costs = _iup_contour_optimize_dp(
- deltas + deltas, coords + coords, forced, tolerance, n
- )
- best_sol, best_cost = None, n + 1
-
- for start in range(n - 1, len(costs) - 1):
- # Assemble solution.
- solution = set()
- i = start
- while i > start - n:
- solution.add(i % n)
- i = chain[i]
- if i == start - n:
- cost = costs[start] - costs[start - n]
- if cost <= best_cost:
- best_sol, best_cost = solution, cost
-
- # if not forced <= best_sol:
- # print("coord", coords)
- # print("deltas", deltas)
- # print("len", len(deltas))
- assert forced <= best_sol, (forced, best_sol)
-
- deltas = [deltas[i] if i in best_sol else None for i in range(n)]
-
- return deltas
-
-
-def iup_delta_optimize(
- deltas: _DeltaSegment,
- coords: _PointSegment,
- ends: _Endpoints,
- tolerance: Real = 0.0,
-) -> _DeltaOrNoneSegment:
- """For the outline given in `coords`, with contour endpoints given
- in sorted increasing order in `ends`, optimize a set of delta
- values `deltas` within error `tolerance`.
-
- Returns delta vector that has most number of None items instead of
- the input delta.
- """
- assert sorted(ends) == ends and len(coords) == (ends[-1] + 1 if ends else 0) + 4
- n = len(coords)
- ends = ends + [n - 4, n - 3, n - 2, n - 1]
- out = []
- start = 0
- for end in ends:
- contour = iup_contour_optimize(
- deltas[start : end + 1], coords[start : end + 1], tolerance
- )
- assert len(contour) == end - start + 1
- out.extend(contour)
- start = end + 1
-
- return out
diff --git a/spaces/Djacon/emotion_detection/README.md b/spaces/Djacon/emotion_detection/README.md
deleted file mode 100644
index cb60ac2caafd7d58fbb20957c2599821b356ca8a..0000000000000000000000000000000000000000
--- a/spaces/Djacon/emotion_detection/README.md
+++ /dev/null
@@ -1,16 +0,0 @@
----
-title: Emotion Detection
-emoji: 🐠
-colorFrom: blue
-colorTo: yellow
-sdk: docker
-pinned: false
-license: mit
----
-
-# Text2Feature
-Powerful text mining tool. Convert raw text into clean using modern technologies of NLP and AI
-
-You can test my website right here 👇
-
-Link: [djacon.github.io/text2feature](https://djacon-emotion-detection.hf.space)
\ No newline at end of file
diff --git a/spaces/DragGan/DragGan/stylegan_human/pti/pti_models/__init__.py b/spaces/DragGan/DragGan/stylegan_human/pti/pti_models/__init__.py
deleted file mode 100644
index e69de29bb2d1d6434b8b29ae775ad8c2e48c5391..0000000000000000000000000000000000000000
diff --git a/spaces/Duskfallcrew/flowers-2-1-768/README.md b/spaces/Duskfallcrew/flowers-2-1-768/README.md
deleted file mode 100644
index 4b812957513cfb0234d076661b97af9e0d6667ca..0000000000000000000000000000000000000000
--- a/spaces/Duskfallcrew/flowers-2-1-768/README.md
+++ /dev/null
@@ -1,12 +0,0 @@
----
-title: Flowers 2 1 768
-emoji: 📚
-colorFrom: purple
-colorTo: red
-sdk: gradio
-sdk_version: 3.21.0
-app_file: app.py
-pinned: false
----
-
-Check out the configuration reference at https://huggingface.co/docs/hub/spaces-config-reference
diff --git a/spaces/ElainaFanBoy/MusicGen/audiocraft/quantization/base.py b/spaces/ElainaFanBoy/MusicGen/audiocraft/quantization/base.py
deleted file mode 100644
index 1b16c130d266fbd021d3fc29bb9f98c33dd3c588..0000000000000000000000000000000000000000
--- a/spaces/ElainaFanBoy/MusicGen/audiocraft/quantization/base.py
+++ /dev/null
@@ -1,107 +0,0 @@
-# Copyright (c) Meta Platforms, Inc. and affiliates.
-# All rights reserved.
-#
-# This source code is licensed under the license found in the
-# LICENSE file in the root directory of this source tree.
-
-"""
-Base class for all quantizers.
-"""
-
-from dataclasses import dataclass, field
-import typing as tp
-
-import torch
-from torch import nn
-
-
-@dataclass
-class QuantizedResult:
- x: torch.Tensor
- codes: torch.Tensor
- bandwidth: torch.Tensor # bandwidth in kb/s used, per batch item.
- penalty: tp.Optional[torch.Tensor] = None
- metrics: dict = field(default_factory=dict)
-
-
-class BaseQuantizer(nn.Module):
- """Base class for quantizers.
- """
-
- def forward(self, x: torch.Tensor, frame_rate: int) -> QuantizedResult:
- """
- Given input tensor x, returns first the quantized (or approximately quantized)
- representation along with quantized codes, bandwidth, and any penalty term for the loss.
- Finally, this returns a dict of metrics to update logging etc.
- Frame rate must be passed so that the bandwidth is properly computed.
- """
- raise NotImplementedError()
-
- def encode(self, x: torch.Tensor) -> torch.Tensor:
- """Encode a given input tensor with the specified sample rate at the given bandwidth.
- """
- raise NotImplementedError()
-
- def decode(self, codes: torch.Tensor) -> torch.Tensor:
- """Decode the given codes to the quantized representation.
- """
- raise NotImplementedError()
-
- @property
- def total_codebooks(self):
- """Total number of codebooks.
- """
- raise NotImplementedError()
-
- @property
- def num_codebooks(self):
- """Number of active codebooks.
- """
- raise NotImplementedError()
-
- def set_num_codebooks(self, n: int):
- """Set the number of active codebooks.
- """
- raise NotImplementedError()
-
-
-class DummyQuantizer(BaseQuantizer):
- """Fake quantizer that actually does not perform any quantization.
- """
- def __init__(self):
- super().__init__()
-
- def forward(self, x: torch.Tensor, frame_rate: int):
- q = x.unsqueeze(1)
- return QuantizedResult(x, q, torch.tensor(q.numel() * 32 * frame_rate / 1000 / len(x)).to(x))
-
- def encode(self, x: torch.Tensor) -> torch.Tensor:
- """Encode a given input tensor with the specified sample rate at the given bandwidth.
- In the case of the DummyQuantizer, the codes are actually identical
- to the input and resulting quantized representation as no quantization is done.
- """
- return x.unsqueeze(1)
-
- def decode(self, codes: torch.Tensor) -> torch.Tensor:
- """Decode the given codes to the quantized representation.
- In the case of the DummyQuantizer, the codes are actually identical
- to the input and resulting quantized representation as no quantization is done.
- """
- return codes.squeeze(1)
-
- @property
- def total_codebooks(self):
- """Total number of codebooks.
- """
- return 1
-
- @property
- def num_codebooks(self):
- """Total number of codebooks.
- """
- return self.total_codebooks
-
- def set_num_codebooks(self, n: int):
- """Set the number of active codebooks.
- """
- raise AttributeError("Cannot override the number of codebooks for the dummy quantizer")
diff --git a/spaces/EmanAbelwhab/foodvision_mini/README.md b/spaces/EmanAbelwhab/foodvision_mini/README.md
deleted file mode 100644
index 48c57a6f3ddf9bf60c96a652845d6f078ad4fcb7..0000000000000000000000000000000000000000
--- a/spaces/EmanAbelwhab/foodvision_mini/README.md
+++ /dev/null
@@ -1,13 +0,0 @@
----
-title: Foodvision Mini
-emoji: 💩
-colorFrom: green
-colorTo: yellow
-sdk: gradio
-sdk_version: 3.17.0
-app_file: app.py
-pinned: false
-license: mit
----
-
-Check out the configuration reference at https://huggingface.co/docs/hub/spaces-config-reference
diff --git a/spaces/EronSamez/RVC_HFmeu/lib/uvr5_pack/lib_v5/nets_537227KB.py b/spaces/EronSamez/RVC_HFmeu/lib/uvr5_pack/lib_v5/nets_537227KB.py
deleted file mode 100644
index a1bb530e006482704f234c2e739a695174142941..0000000000000000000000000000000000000000
--- a/spaces/EronSamez/RVC_HFmeu/lib/uvr5_pack/lib_v5/nets_537227KB.py
+++ /dev/null
@@ -1,123 +0,0 @@
-import torch
-import numpy as np
-from torch import nn
-import torch.nn.functional as F
-
-from . import layers_537238KB as layers
-
-
-class BaseASPPNet(nn.Module):
- def __init__(self, nin, ch, dilations=(4, 8, 16)):
- super(BaseASPPNet, self).__init__()
- self.enc1 = layers.Encoder(nin, ch, 3, 2, 1)
- self.enc2 = layers.Encoder(ch, ch * 2, 3, 2, 1)
- self.enc3 = layers.Encoder(ch * 2, ch * 4, 3, 2, 1)
- self.enc4 = layers.Encoder(ch * 4, ch * 8, 3, 2, 1)
-
- self.aspp = layers.ASPPModule(ch * 8, ch * 16, dilations)
-
- self.dec4 = layers.Decoder(ch * (8 + 16), ch * 8, 3, 1, 1)
- self.dec3 = layers.Decoder(ch * (4 + 8), ch * 4, 3, 1, 1)
- self.dec2 = layers.Decoder(ch * (2 + 4), ch * 2, 3, 1, 1)
- self.dec1 = layers.Decoder(ch * (1 + 2), ch, 3, 1, 1)
-
- def __call__(self, x):
- h, e1 = self.enc1(x)
- h, e2 = self.enc2(h)
- h, e3 = self.enc3(h)
- h, e4 = self.enc4(h)
-
- h = self.aspp(h)
-
- h = self.dec4(h, e4)
- h = self.dec3(h, e3)
- h = self.dec2(h, e2)
- h = self.dec1(h, e1)
-
- return h
-
-
-class CascadedASPPNet(nn.Module):
- def __init__(self, n_fft):
- super(CascadedASPPNet, self).__init__()
- self.stg1_low_band_net = BaseASPPNet(2, 64)
- self.stg1_high_band_net = BaseASPPNet(2, 64)
-
- self.stg2_bridge = layers.Conv2DBNActiv(66, 32, 1, 1, 0)
- self.stg2_full_band_net = BaseASPPNet(32, 64)
-
- self.stg3_bridge = layers.Conv2DBNActiv(130, 64, 1, 1, 0)
- self.stg3_full_band_net = BaseASPPNet(64, 128)
-
- self.out = nn.Conv2d(128, 2, 1, bias=False)
- self.aux1_out = nn.Conv2d(64, 2, 1, bias=False)
- self.aux2_out = nn.Conv2d(64, 2, 1, bias=False)
-
- self.max_bin = n_fft // 2
- self.output_bin = n_fft // 2 + 1
-
- self.offset = 128
-
- def forward(self, x, aggressiveness=None):
- mix = x.detach()
- x = x.clone()
-
- x = x[:, :, : self.max_bin]
-
- bandw = x.size()[2] // 2
- aux1 = torch.cat(
- [
- self.stg1_low_band_net(x[:, :, :bandw]),
- self.stg1_high_band_net(x[:, :, bandw:]),
- ],
- dim=2,
- )
-
- h = torch.cat([x, aux1], dim=1)
- aux2 = self.stg2_full_band_net(self.stg2_bridge(h))
-
- h = torch.cat([x, aux1, aux2], dim=1)
- h = self.stg3_full_band_net(self.stg3_bridge(h))
-
- mask = torch.sigmoid(self.out(h))
- mask = F.pad(
- input=mask,
- pad=(0, 0, 0, self.output_bin - mask.size()[2]),
- mode="replicate",
- )
-
- if self.training:
- aux1 = torch.sigmoid(self.aux1_out(aux1))
- aux1 = F.pad(
- input=aux1,
- pad=(0, 0, 0, self.output_bin - aux1.size()[2]),
- mode="replicate",
- )
- aux2 = torch.sigmoid(self.aux2_out(aux2))
- aux2 = F.pad(
- input=aux2,
- pad=(0, 0, 0, self.output_bin - aux2.size()[2]),
- mode="replicate",
- )
- return mask * mix, aux1 * mix, aux2 * mix
- else:
- if aggressiveness:
- mask[:, :, : aggressiveness["split_bin"]] = torch.pow(
- mask[:, :, : aggressiveness["split_bin"]],
- 1 + aggressiveness["value"] / 3,
- )
- mask[:, :, aggressiveness["split_bin"] :] = torch.pow(
- mask[:, :, aggressiveness["split_bin"] :],
- 1 + aggressiveness["value"],
- )
-
- return mask * mix
-
- def predict(self, x_mag, aggressiveness=None):
- h = self.forward(x_mag, aggressiveness)
-
- if self.offset > 0:
- h = h[:, :, :, self.offset : -self.offset]
- assert h.size()[3] > 0
-
- return h
diff --git a/spaces/EronSamez/RVC_HFmeu/train/utils.py b/spaces/EronSamez/RVC_HFmeu/train/utils.py
deleted file mode 100644
index aae833b08acc24b848aa70114fd9b7aad8b1a6ad..0000000000000000000000000000000000000000
--- a/spaces/EronSamez/RVC_HFmeu/train/utils.py
+++ /dev/null
@@ -1,500 +0,0 @@
-import os, traceback
-import glob
-import sys
-import argparse
-import logging
-import json
-import subprocess
-import numpy as np
-from scipy.io.wavfile import read
-import torch
-
-MATPLOTLIB_FLAG = False
-
-logging.basicConfig(stream=sys.stdout, level=logging.DEBUG)
-logger = logging
-
-
-def load_checkpoint_d(checkpoint_path, combd, sbd, optimizer=None, load_opt=1):
- assert os.path.isfile(checkpoint_path)
- checkpoint_dict = torch.load(checkpoint_path, map_location="cpu")
-
- ##################
- def go(model, bkey):
- saved_state_dict = checkpoint_dict[bkey]
- if hasattr(model, "module"):
- state_dict = model.module.state_dict()
- else:
- state_dict = model.state_dict()
- new_state_dict = {}
- for k, v in state_dict.items(): # 模型需要的shape
- try:
- new_state_dict[k] = saved_state_dict[k]
- if saved_state_dict[k].shape != state_dict[k].shape:
- print(
- "shape-%s-mismatch|need-%s|get-%s"
- % (k, state_dict[k].shape, saved_state_dict[k].shape)
- ) #
- raise KeyError
- except:
- # logger.info(traceback.format_exc())
- logger.info("%s is not in the checkpoint" % k) # pretrain缺失的
- new_state_dict[k] = v # 模型自带的随机值
- if hasattr(model, "module"):
- model.module.load_state_dict(new_state_dict, strict=False)
- else:
- model.load_state_dict(new_state_dict, strict=False)
-
- go(combd, "combd")
- go(sbd, "sbd")
- #############
- logger.info("Loaded model weights")
-
- iteration = checkpoint_dict["iteration"]
- learning_rate = checkpoint_dict["learning_rate"]
- if (
- optimizer is not None and load_opt == 1
- ): ###加载不了,如果是空的的话,重新初始化,可能还会影响lr时间表的更新,因此在train文件最外围catch
- # try:
- optimizer.load_state_dict(checkpoint_dict["optimizer"])
- # except:
- # traceback.print_exc()
- logger.info("Loaded checkpoint '{}' (epoch {})".format(checkpoint_path, iteration))
- return model, optimizer, learning_rate, iteration
-
-
-# def load_checkpoint(checkpoint_path, model, optimizer=None):
-# assert os.path.isfile(checkpoint_path)
-# checkpoint_dict = torch.load(checkpoint_path, map_location='cpu')
-# iteration = checkpoint_dict['iteration']
-# learning_rate = checkpoint_dict['learning_rate']
-# if optimizer is not None:
-# optimizer.load_state_dict(checkpoint_dict['optimizer'])
-# # print(1111)
-# saved_state_dict = checkpoint_dict['model']
-# # print(1111)
-#
-# if hasattr(model, 'module'):
-# state_dict = model.module.state_dict()
-# else:
-# state_dict = model.state_dict()
-# new_state_dict= {}
-# for k, v in state_dict.items():
-# try:
-# new_state_dict[k] = saved_state_dict[k]
-# except:
-# logger.info("%s is not in the checkpoint" % k)
-# new_state_dict[k] = v
-# if hasattr(model, 'module'):
-# model.module.load_state_dict(new_state_dict)
-# else:
-# model.load_state_dict(new_state_dict)
-# logger.info("Loaded checkpoint '{}' (epoch {})" .format(
-# checkpoint_path, iteration))
-# return model, optimizer, learning_rate, iteration
-def load_checkpoint(checkpoint_path, model, optimizer=None, load_opt=1):
- assert os.path.isfile(checkpoint_path)
- checkpoint_dict = torch.load(checkpoint_path, map_location="cpu")
-
- saved_state_dict = checkpoint_dict["model"]
- if hasattr(model, "module"):
- state_dict = model.module.state_dict()
- else:
- state_dict = model.state_dict()
- new_state_dict = {}
- for k, v in state_dict.items(): # 模型需要的shape
- try:
- new_state_dict[k] = saved_state_dict[k]
- if saved_state_dict[k].shape != state_dict[k].shape:
- print(
- "shape-%s-mismatch|need-%s|get-%s"
- % (k, state_dict[k].shape, saved_state_dict[k].shape)
- ) #
- raise KeyError
- except:
- # logger.info(traceback.format_exc())
- logger.info("%s is not in the checkpoint" % k) # pretrain缺失的
- new_state_dict[k] = v # 模型自带的随机值
- if hasattr(model, "module"):
- model.module.load_state_dict(new_state_dict, strict=False)
- else:
- model.load_state_dict(new_state_dict, strict=False)
- logger.info("Loaded model weights")
-
- iteration = checkpoint_dict["iteration"]
- learning_rate = checkpoint_dict["learning_rate"]
- if (
- optimizer is not None and load_opt == 1
- ): ###加载不了,如果是空的的话,重新初始化,可能还会影响lr时间表的更新,因此在train文件最外围catch
- # try:
- optimizer.load_state_dict(checkpoint_dict["optimizer"])
- # except:
- # traceback.print_exc()
- logger.info("Loaded checkpoint '{}' (epoch {})".format(checkpoint_path, iteration))
- return model, optimizer, learning_rate, iteration
-
-
-def save_checkpoint(model, optimizer, learning_rate, iteration, checkpoint_path):
- logger.info(
- "Saving model and optimizer state at epoch {} to {}".format(
- iteration, checkpoint_path
- )
- )
- if hasattr(model, "module"):
- state_dict = model.module.state_dict()
- else:
- state_dict = model.state_dict()
- torch.save(
- {
- "model": state_dict,
- "iteration": iteration,
- "optimizer": optimizer.state_dict(),
- "learning_rate": learning_rate,
- },
- checkpoint_path,
- )
-
-
-def save_checkpoint_d(combd, sbd, optimizer, learning_rate, iteration, checkpoint_path):
- logger.info(
- "Saving model and optimizer state at epoch {} to {}".format(
- iteration, checkpoint_path
- )
- )
- if hasattr(combd, "module"):
- state_dict_combd = combd.module.state_dict()
- else:
- state_dict_combd = combd.state_dict()
- if hasattr(sbd, "module"):
- state_dict_sbd = sbd.module.state_dict()
- else:
- state_dict_sbd = sbd.state_dict()
- torch.save(
- {
- "combd": state_dict_combd,
- "sbd": state_dict_sbd,
- "iteration": iteration,
- "optimizer": optimizer.state_dict(),
- "learning_rate": learning_rate,
- },
- checkpoint_path,
- )
-
-
-def summarize(
- writer,
- global_step,
- scalars={},
- histograms={},
- images={},
- audios={},
- audio_sampling_rate=22050,
-):
- for k, v in scalars.items():
- writer.add_scalar(k, v, global_step)
- for k, v in histograms.items():
- writer.add_histogram(k, v, global_step)
- for k, v in images.items():
- writer.add_image(k, v, global_step, dataformats="HWC")
- for k, v in audios.items():
- writer.add_audio(k, v, global_step, audio_sampling_rate)
-
-
-def latest_checkpoint_path(dir_path, regex="G_*.pth"):
- f_list = glob.glob(os.path.join(dir_path, regex))
- f_list.sort(key=lambda f: int("".join(filter(str.isdigit, f))))
- x = f_list[-1]
- print(x)
- return x
-
-
-def plot_spectrogram_to_numpy(spectrogram):
- global MATPLOTLIB_FLAG
- if not MATPLOTLIB_FLAG:
- import matplotlib
-
- matplotlib.use("Agg")
- MATPLOTLIB_FLAG = True
- mpl_logger = logging.getLogger("matplotlib")
- mpl_logger.setLevel(logging.WARNING)
- import matplotlib.pylab as plt
- import numpy as np
-
- fig, ax = plt.subplots(figsize=(10, 2))
- im = ax.imshow(spectrogram, aspect="auto", origin="lower", interpolation="none")
- plt.colorbar(im, ax=ax)
- plt.xlabel("Frames")
- plt.ylabel("Channels")
- plt.tight_layout()
-
- fig.canvas.draw()
- data = np.fromstring(fig.canvas.tostring_rgb(), dtype=np.uint8, sep="")
- data = data.reshape(fig.canvas.get_width_height()[::-1] + (3,))
- plt.close()
- return data
-
-
-def plot_alignment_to_numpy(alignment, info=None):
- global MATPLOTLIB_FLAG
- if not MATPLOTLIB_FLAG:
- import matplotlib
-
- matplotlib.use("Agg")
- MATPLOTLIB_FLAG = True
- mpl_logger = logging.getLogger("matplotlib")
- mpl_logger.setLevel(logging.WARNING)
- import matplotlib.pylab as plt
- import numpy as np
-
- fig, ax = plt.subplots(figsize=(6, 4))
- im = ax.imshow(
- alignment.transpose(), aspect="auto", origin="lower", interpolation="none"
- )
- fig.colorbar(im, ax=ax)
- xlabel = "Decoder timestep"
- if info is not None:
- xlabel += "\n\n" + info
- plt.xlabel(xlabel)
- plt.ylabel("Encoder timestep")
- plt.tight_layout()
-
- fig.canvas.draw()
- data = np.fromstring(fig.canvas.tostring_rgb(), dtype=np.uint8, sep="")
- data = data.reshape(fig.canvas.get_width_height()[::-1] + (3,))
- plt.close()
- return data
-
-
-def load_wav_to_torch(full_path):
- sampling_rate, data = read(full_path)
- return torch.FloatTensor(data.astype(np.float32)), sampling_rate
-
-
-def load_filepaths_and_text(filename, split="|"):
- with open(filename, encoding='utf-8') as f:
- filepaths_and_text = [line.strip().split(split) for line in f]
- filepaths_and_text = [item for item in filepaths_and_text if len(item) == 5] # ensure there are 5 items.
- return filepaths_and_text
-
-
-def get_hparams(init=True):
- """
- todo:
- 结尾七人组:
- 保存频率、总epoch done
- bs done
- pretrainG、pretrainD done
- 卡号:os.en["CUDA_VISIBLE_DEVICES"] done
- if_latest done
- 模型:if_f0 done
- 采样率:自动选择config done
- 是否缓存数据集进GPU:if_cache_data_in_gpu done
-
- -m:
- 自动决定training_files路径,改掉train_nsf_load_pretrain.py里的hps.data.training_files done
- -c不要了
- """
- parser = argparse.ArgumentParser()
- # parser.add_argument('-c', '--config', type=str, default="configs/40k.json",help='JSON file for configuration')
- parser.add_argument(
- "-se",
- "--save_every_epoch",
- type=int,
- required=True,
- help="checkpoint save frequency (epoch)",
- )
- parser.add_argument(
- "-te", "--total_epoch", type=int, required=True, help="total_epoch"
- )
- parser.add_argument(
- "-pg", "--pretrainG", type=str, default="", help="Pretrained Discriminator path"
- )
- parser.add_argument(
- "-pd", "--pretrainD", type=str, default="", help="Pretrained Generator path"
- )
- parser.add_argument("-g", "--gpus", type=str, default="0", help="split by -")
- parser.add_argument(
- "-bs", "--batch_size", type=int, required=True, help="batch size"
- )
- parser.add_argument(
- "-e", "--experiment_dir", type=str, required=True, help="experiment dir"
- ) # -m
- parser.add_argument(
- "-sr", "--sample_rate", type=str, required=True, help="sample rate, 32k/40k/48k"
- )
- parser.add_argument(
- "-sw",
- "--save_every_weights",
- type=str,
- default="0",
- help="save the extracted model in weights directory when saving checkpoints",
- )
- parser.add_argument(
- "-v", "--version", type=str, required=True, help="model version"
- )
- parser.add_argument(
- "-f0",
- "--if_f0",
- type=int,
- required=True,
- help="use f0 as one of the inputs of the model, 1 or 0",
- )
- parser.add_argument(
- "-l",
- "--if_latest",
- type=int,
- required=True,
- help="if only save the latest G/D pth file, 1 or 0",
- )
- parser.add_argument(
- "-c",
- "--if_cache_data_in_gpu",
- type=int,
- required=True,
- help="if caching the dataset in GPU memory, 1 or 0",
- )
- parser.add_argument(
- "-li", "--log_interval", type=int, required=True, help="log interval"
- )
-
- args = parser.parse_args()
- name = args.experiment_dir
- experiment_dir = os.path.join("./logs", args.experiment_dir)
-
- if not os.path.exists(experiment_dir):
- os.makedirs(experiment_dir)
-
- if args.version == "v1" or args.sample_rate == "40k":
- config_path = "configs/%s.json" % args.sample_rate
- else:
- config_path = "configs/%s_v2.json" % args.sample_rate
- config_save_path = os.path.join(experiment_dir, "config.json")
- if init:
- with open(config_path, "r") as f:
- data = f.read()
- with open(config_save_path, "w") as f:
- f.write(data)
- else:
- with open(config_save_path, "r") as f:
- data = f.read()
- config = json.loads(data)
-
- hparams = HParams(**config)
- hparams.model_dir = hparams.experiment_dir = experiment_dir
- hparams.save_every_epoch = args.save_every_epoch
- hparams.name = name
- hparams.total_epoch = args.total_epoch
- hparams.pretrainG = args.pretrainG
- hparams.pretrainD = args.pretrainD
- hparams.version = args.version
- hparams.gpus = args.gpus
- hparams.train.batch_size = args.batch_size
- hparams.sample_rate = args.sample_rate
- hparams.if_f0 = args.if_f0
- hparams.if_latest = args.if_latest
- hparams.save_every_weights = args.save_every_weights
- hparams.if_cache_data_in_gpu = args.if_cache_data_in_gpu
- hparams.data.training_files = "%s/filelist.txt" % experiment_dir
-
- hparams.train.log_interval = args.log_interval
-
- # Update log_interval in the 'train' section of the config dictionary
- config["train"]["log_interval"] = args.log_interval
-
- # Save the updated config back to the config_save_path
- with open(config_save_path, "w") as f:
- json.dump(config, f, indent=4)
-
- return hparams
-
-
-def get_hparams_from_dir(model_dir):
- config_save_path = os.path.join(model_dir, "config.json")
- with open(config_save_path, "r") as f:
- data = f.read()
- config = json.loads(data)
-
- hparams = HParams(**config)
- hparams.model_dir = model_dir
- return hparams
-
-
-def get_hparams_from_file(config_path):
- with open(config_path, "r") as f:
- data = f.read()
- config = json.loads(data)
-
- hparams = HParams(**config)
- return hparams
-
-
-def check_git_hash(model_dir):
- source_dir = os.path.dirname(os.path.realpath(__file__))
- if not os.path.exists(os.path.join(source_dir, ".git")):
- logger.warn(
- "{} is not a git repository, therefore hash value comparison will be ignored.".format(
- source_dir
- )
- )
- return
-
- cur_hash = subprocess.getoutput("git rev-parse HEAD")
-
- path = os.path.join(model_dir, "githash")
- if os.path.exists(path):
- saved_hash = open(path).read()
- if saved_hash != cur_hash:
- logger.warn(
- "git hash values are different. {}(saved) != {}(current)".format(
- saved_hash[:8], cur_hash[:8]
- )
- )
- else:
- open(path, "w").write(cur_hash)
-
-
-def get_logger(model_dir, filename="train.log"):
- global logger
- logger = logging.getLogger(os.path.basename(model_dir))
- logger.setLevel(logging.DEBUG)
-
- formatter = logging.Formatter("%(asctime)s\t%(name)s\t%(levelname)s\t%(message)s")
- if not os.path.exists(model_dir):
- os.makedirs(model_dir)
- h = logging.FileHandler(os.path.join(model_dir, filename))
- h.setLevel(logging.DEBUG)
- h.setFormatter(formatter)
- logger.addHandler(h)
- return logger
-
-
-class HParams:
- def __init__(self, **kwargs):
- for k, v in kwargs.items():
- if type(v) == dict:
- v = HParams(**v)
- self[k] = v
-
- def keys(self):
- return self.__dict__.keys()
-
- def items(self):
- return self.__dict__.items()
-
- def values(self):
- return self.__dict__.values()
-
- def __len__(self):
- return len(self.__dict__)
-
- def __getitem__(self, key):
- return getattr(self, key)
-
- def __setitem__(self, key, value):
- return setattr(self, key, value)
-
- def __contains__(self, key):
- return key in self.__dict__
-
- def __repr__(self):
- return self.__dict__.__repr__()
diff --git a/spaces/FelixLuoX/codeformer/CodeFormer/facelib/parsing/bisenet.py b/spaces/FelixLuoX/codeformer/CodeFormer/facelib/parsing/bisenet.py
deleted file mode 100644
index 3898cab76ae5876459cd4899c54cafa14234971d..0000000000000000000000000000000000000000
--- a/spaces/FelixLuoX/codeformer/CodeFormer/facelib/parsing/bisenet.py
+++ /dev/null
@@ -1,140 +0,0 @@
-import torch
-import torch.nn as nn
-import torch.nn.functional as F
-
-from .resnet import ResNet18
-
-
-class ConvBNReLU(nn.Module):
-
- def __init__(self, in_chan, out_chan, ks=3, stride=1, padding=1):
- super(ConvBNReLU, self).__init__()
- self.conv = nn.Conv2d(in_chan, out_chan, kernel_size=ks, stride=stride, padding=padding, bias=False)
- self.bn = nn.BatchNorm2d(out_chan)
-
- def forward(self, x):
- x = self.conv(x)
- x = F.relu(self.bn(x))
- return x
-
-
-class BiSeNetOutput(nn.Module):
-
- def __init__(self, in_chan, mid_chan, num_class):
- super(BiSeNetOutput, self).__init__()
- self.conv = ConvBNReLU(in_chan, mid_chan, ks=3, stride=1, padding=1)
- self.conv_out = nn.Conv2d(mid_chan, num_class, kernel_size=1, bias=False)
-
- def forward(self, x):
- feat = self.conv(x)
- out = self.conv_out(feat)
- return out, feat
-
-
-class AttentionRefinementModule(nn.Module):
-
- def __init__(self, in_chan, out_chan):
- super(AttentionRefinementModule, self).__init__()
- self.conv = ConvBNReLU(in_chan, out_chan, ks=3, stride=1, padding=1)
- self.conv_atten = nn.Conv2d(out_chan, out_chan, kernel_size=1, bias=False)
- self.bn_atten = nn.BatchNorm2d(out_chan)
- self.sigmoid_atten = nn.Sigmoid()
-
- def forward(self, x):
- feat = self.conv(x)
- atten = F.avg_pool2d(feat, feat.size()[2:])
- atten = self.conv_atten(atten)
- atten = self.bn_atten(atten)
- atten = self.sigmoid_atten(atten)
- out = torch.mul(feat, atten)
- return out
-
-
-class ContextPath(nn.Module):
-
- def __init__(self):
- super(ContextPath, self).__init__()
- self.resnet = ResNet18()
- self.arm16 = AttentionRefinementModule(256, 128)
- self.arm32 = AttentionRefinementModule(512, 128)
- self.conv_head32 = ConvBNReLU(128, 128, ks=3, stride=1, padding=1)
- self.conv_head16 = ConvBNReLU(128, 128, ks=3, stride=1, padding=1)
- self.conv_avg = ConvBNReLU(512, 128, ks=1, stride=1, padding=0)
-
- def forward(self, x):
- feat8, feat16, feat32 = self.resnet(x)
- h8, w8 = feat8.size()[2:]
- h16, w16 = feat16.size()[2:]
- h32, w32 = feat32.size()[2:]
-
- avg = F.avg_pool2d(feat32, feat32.size()[2:])
- avg = self.conv_avg(avg)
- avg_up = F.interpolate(avg, (h32, w32), mode='nearest')
-
- feat32_arm = self.arm32(feat32)
- feat32_sum = feat32_arm + avg_up
- feat32_up = F.interpolate(feat32_sum, (h16, w16), mode='nearest')
- feat32_up = self.conv_head32(feat32_up)
-
- feat16_arm = self.arm16(feat16)
- feat16_sum = feat16_arm + feat32_up
- feat16_up = F.interpolate(feat16_sum, (h8, w8), mode='nearest')
- feat16_up = self.conv_head16(feat16_up)
-
- return feat8, feat16_up, feat32_up # x8, x8, x16
-
-
-class FeatureFusionModule(nn.Module):
-
- def __init__(self, in_chan, out_chan):
- super(FeatureFusionModule, self).__init__()
- self.convblk = ConvBNReLU(in_chan, out_chan, ks=1, stride=1, padding=0)
- self.conv1 = nn.Conv2d(out_chan, out_chan // 4, kernel_size=1, stride=1, padding=0, bias=False)
- self.conv2 = nn.Conv2d(out_chan // 4, out_chan, kernel_size=1, stride=1, padding=0, bias=False)
- self.relu = nn.ReLU(inplace=True)
- self.sigmoid = nn.Sigmoid()
-
- def forward(self, fsp, fcp):
- fcat = torch.cat([fsp, fcp], dim=1)
- feat = self.convblk(fcat)
- atten = F.avg_pool2d(feat, feat.size()[2:])
- atten = self.conv1(atten)
- atten = self.relu(atten)
- atten = self.conv2(atten)
- atten = self.sigmoid(atten)
- feat_atten = torch.mul(feat, atten)
- feat_out = feat_atten + feat
- return feat_out
-
-
-class BiSeNet(nn.Module):
-
- def __init__(self, num_class):
- super(BiSeNet, self).__init__()
- self.cp = ContextPath()
- self.ffm = FeatureFusionModule(256, 256)
- self.conv_out = BiSeNetOutput(256, 256, num_class)
- self.conv_out16 = BiSeNetOutput(128, 64, num_class)
- self.conv_out32 = BiSeNetOutput(128, 64, num_class)
-
- def forward(self, x, return_feat=False):
- h, w = x.size()[2:]
- feat_res8, feat_cp8, feat_cp16 = self.cp(x) # return res3b1 feature
- feat_sp = feat_res8 # replace spatial path feature with res3b1 feature
- feat_fuse = self.ffm(feat_sp, feat_cp8)
-
- out, feat = self.conv_out(feat_fuse)
- out16, feat16 = self.conv_out16(feat_cp8)
- out32, feat32 = self.conv_out32(feat_cp16)
-
- out = F.interpolate(out, (h, w), mode='bilinear', align_corners=True)
- out16 = F.interpolate(out16, (h, w), mode='bilinear', align_corners=True)
- out32 = F.interpolate(out32, (h, w), mode='bilinear', align_corners=True)
-
- if return_feat:
- feat = F.interpolate(feat, (h, w), mode='bilinear', align_corners=True)
- feat16 = F.interpolate(feat16, (h, w), mode='bilinear', align_corners=True)
- feat32 = F.interpolate(feat32, (h, w), mode='bilinear', align_corners=True)
- return out, out16, out32, feat, feat16, feat32
- else:
- return out, out16, out32
diff --git a/spaces/GanymedeNil/text2vec/app.py b/spaces/GanymedeNil/text2vec/app.py
deleted file mode 100644
index 5fa2c3b6ac857790a98d1c9c02770c0ad816baf7..0000000000000000000000000000000000000000
--- a/spaces/GanymedeNil/text2vec/app.py
+++ /dev/null
@@ -1,40 +0,0 @@
-# -*- coding: utf-8 -*-
-"""
-@author:XuMing(xuming624@qq.com)
-@description: text similarity example, fine-tuned by CoSENT model
-"""
-import gradio as gr
-from text2vec import Similarity
-
-# 中文句向量模型(CoSENT)
-sim_model = Similarity(model_name_or_path='GanymedeNil/text2vec-large-chinese',
- similarity_type='cosine', embedding_type='sbert')
-
-
-def ai_text(sentence1, sentence2):
- score = sim_model.get_score(sentence1, sentence2)
- print("{} \t\t {} \t\t Score: {:.4f}".format(sentence1, sentence2, score))
-
- return score
-
-
-if __name__ == '__main__':
- examples = [
- ['如何更换花呗绑定银行卡', '花呗更改绑定银行卡'],
- ['我在北京打篮球', '我是北京人,我喜欢篮球'],
- ['一个女人在看书。', '一个女人在揉面团'],
- ['一个男人在车库里举重。', '一个人在举重。'],
- ]
- input1 = gr.inputs.Textbox(lines=2, placeholder="Enter First Sentence")
- input2 = gr.inputs.Textbox(lines=2, placeholder="Enter Second Sentence")
-
- output_text = gr.outputs.Textbox()
- gr.Interface(ai_text,
- inputs=[input1, input2],
- outputs=[output_text],
- theme="grass",
- title="Chinese Text to Vector Model GanymedeNil/text2vec-large-chinese",
- description="Copy or input Chinese text here. Submit and the machine will calculate the cosine score.",
- article="Link to Github REPO",
- examples=examples
- ).launch()
diff --git a/spaces/Giuvyz/rvc-genshin/infer_pack/commons.py b/spaces/Giuvyz/rvc-genshin/infer_pack/commons.py
deleted file mode 100644
index 54470986f37825b35d90d7efa7437d1c26b87215..0000000000000000000000000000000000000000
--- a/spaces/Giuvyz/rvc-genshin/infer_pack/commons.py
+++ /dev/null
@@ -1,166 +0,0 @@
-import math
-import numpy as np
-import torch
-from torch import nn
-from torch.nn import functional as F
-
-
-def init_weights(m, mean=0.0, std=0.01):
- classname = m.__class__.__name__
- if classname.find("Conv") != -1:
- m.weight.data.normal_(mean, std)
-
-
-def get_padding(kernel_size, dilation=1):
- return int((kernel_size * dilation - dilation) / 2)
-
-
-def convert_pad_shape(pad_shape):
- l = pad_shape[::-1]
- pad_shape = [item for sublist in l for item in sublist]
- return pad_shape
-
-
-def kl_divergence(m_p, logs_p, m_q, logs_q):
- """KL(P||Q)"""
- kl = (logs_q - logs_p) - 0.5
- kl += (
- 0.5 * (torch.exp(2.0 * logs_p) + ((m_p - m_q) ** 2)) * torch.exp(-2.0 * logs_q)
- )
- return kl
-
-
-def rand_gumbel(shape):
- """Sample from the Gumbel distribution, protect from overflows."""
- uniform_samples = torch.rand(shape) * 0.99998 + 0.00001
- return -torch.log(-torch.log(uniform_samples))
-
-
-def rand_gumbel_like(x):
- g = rand_gumbel(x.size()).to(dtype=x.dtype, device=x.device)
- return g
-
-
-def slice_segments(x, ids_str, segment_size=4):
- ret = torch.zeros_like(x[:, :, :segment_size])
- for i in range(x.size(0)):
- idx_str = ids_str[i]
- idx_end = idx_str + segment_size
- ret[i] = x[i, :, idx_str:idx_end]
- return ret
-
-
-def slice_segments2(x, ids_str, segment_size=4):
- ret = torch.zeros_like(x[:, :segment_size])
- for i in range(x.size(0)):
- idx_str = ids_str[i]
- idx_end = idx_str + segment_size
- ret[i] = x[i, idx_str:idx_end]
- return ret
-
-
-def rand_slice_segments(x, x_lengths=None, segment_size=4):
- b, d, t = x.size()
- if x_lengths is None:
- x_lengths = t
- ids_str_max = x_lengths - segment_size + 1
- ids_str = (torch.rand([b]).to(device=x.device) * ids_str_max).to(dtype=torch.long)
- ret = slice_segments(x, ids_str, segment_size)
- return ret, ids_str
-
-
-def get_timing_signal_1d(length, channels, min_timescale=1.0, max_timescale=1.0e4):
- position = torch.arange(length, dtype=torch.float)
- num_timescales = channels // 2
- log_timescale_increment = math.log(float(max_timescale) / float(min_timescale)) / (
- num_timescales - 1
- )
- inv_timescales = min_timescale * torch.exp(
- torch.arange(num_timescales, dtype=torch.float) * -log_timescale_increment
- )
- scaled_time = position.unsqueeze(0) * inv_timescales.unsqueeze(1)
- signal = torch.cat([torch.sin(scaled_time), torch.cos(scaled_time)], 0)
- signal = F.pad(signal, [0, 0, 0, channels % 2])
- signal = signal.view(1, channels, length)
- return signal
-
-
-def add_timing_signal_1d(x, min_timescale=1.0, max_timescale=1.0e4):
- b, channels, length = x.size()
- signal = get_timing_signal_1d(length, channels, min_timescale, max_timescale)
- return x + signal.to(dtype=x.dtype, device=x.device)
-
-
-def cat_timing_signal_1d(x, min_timescale=1.0, max_timescale=1.0e4, axis=1):
- b, channels, length = x.size()
- signal = get_timing_signal_1d(length, channels, min_timescale, max_timescale)
- return torch.cat([x, signal.to(dtype=x.dtype, device=x.device)], axis)
-
-
-def subsequent_mask(length):
- mask = torch.tril(torch.ones(length, length)).unsqueeze(0).unsqueeze(0)
- return mask
-
-
-@torch.jit.script
-def fused_add_tanh_sigmoid_multiply(input_a, input_b, n_channels):
- n_channels_int = n_channels[0]
- in_act = input_a + input_b
- t_act = torch.tanh(in_act[:, :n_channels_int, :])
- s_act = torch.sigmoid(in_act[:, n_channels_int:, :])
- acts = t_act * s_act
- return acts
-
-
-def convert_pad_shape(pad_shape):
- l = pad_shape[::-1]
- pad_shape = [item for sublist in l for item in sublist]
- return pad_shape
-
-
-def shift_1d(x):
- x = F.pad(x, convert_pad_shape([[0, 0], [0, 0], [1, 0]]))[:, :, :-1]
- return x
-
-
-def sequence_mask(length, max_length=None):
- if max_length is None:
- max_length = length.max()
- x = torch.arange(max_length, dtype=length.dtype, device=length.device)
- return x.unsqueeze(0) < length.unsqueeze(1)
-
-
-def generate_path(duration, mask):
- """
- duration: [b, 1, t_x]
- mask: [b, 1, t_y, t_x]
- """
- device = duration.device
-
- b, _, t_y, t_x = mask.shape
- cum_duration = torch.cumsum(duration, -1)
-
- cum_duration_flat = cum_duration.view(b * t_x)
- path = sequence_mask(cum_duration_flat, t_y).to(mask.dtype)
- path = path.view(b, t_x, t_y)
- path = path - F.pad(path, convert_pad_shape([[0, 0], [1, 0], [0, 0]]))[:, :-1]
- path = path.unsqueeze(1).transpose(2, 3) * mask
- return path
-
-
-def clip_grad_value_(parameters, clip_value, norm_type=2):
- if isinstance(parameters, torch.Tensor):
- parameters = [parameters]
- parameters = list(filter(lambda p: p.grad is not None, parameters))
- norm_type = float(norm_type)
- if clip_value is not None:
- clip_value = float(clip_value)
-
- total_norm = 0
- for p in parameters:
- param_norm = p.grad.data.norm(norm_type)
- total_norm += param_norm.item() ** norm_type
- if clip_value is not None:
- p.grad.data.clamp_(min=-clip_value, max=clip_value)
- total_norm = total_norm ** (1.0 / norm_type)
- return total_norm
diff --git a/spaces/GolDNenex/Super-Resolution-Anime-Diffusion/RealESRGANv030/realesrgan/archs/discriminator_arch.py b/spaces/GolDNenex/Super-Resolution-Anime-Diffusion/RealESRGANv030/realesrgan/archs/discriminator_arch.py
deleted file mode 100644
index ccd810559201624bc6c20ea9b60009b927ecadd6..0000000000000000000000000000000000000000
--- a/spaces/GolDNenex/Super-Resolution-Anime-Diffusion/RealESRGANv030/realesrgan/archs/discriminator_arch.py
+++ /dev/null
@@ -1,67 +0,0 @@
-from basicsr.utils.registry import ARCH_REGISTRY
-from torch import nn as nn
-from torch.nn import functional as F
-from torch.nn.utils import spectral_norm
-
-
-@ARCH_REGISTRY.register()
-class UNetDiscriminatorSN(nn.Module):
- """Defines a U-Net discriminator with spectral normalization (SN)
-
- It is used in Real-ESRGAN: Training Real-World Blind Super-Resolution with Pure Synthetic Data.
-
- Arg:
- num_in_ch (int): Channel number of inputs. Default: 3.
- num_feat (int): Channel number of base intermediate features. Default: 64.
- skip_connection (bool): Whether to use skip connections between U-Net. Default: True.
- """
-
- def __init__(self, num_in_ch, num_feat=64, skip_connection=True):
- super(UNetDiscriminatorSN, self).__init__()
- self.skip_connection = skip_connection
- norm = spectral_norm
- # the first convolution
- self.conv0 = nn.Conv2d(num_in_ch, num_feat, kernel_size=3, stride=1, padding=1)
- # downsample
- self.conv1 = norm(nn.Conv2d(num_feat, num_feat * 2, 4, 2, 1, bias=False))
- self.conv2 = norm(nn.Conv2d(num_feat * 2, num_feat * 4, 4, 2, 1, bias=False))
- self.conv3 = norm(nn.Conv2d(num_feat * 4, num_feat * 8, 4, 2, 1, bias=False))
- # upsample
- self.conv4 = norm(nn.Conv2d(num_feat * 8, num_feat * 4, 3, 1, 1, bias=False))
- self.conv5 = norm(nn.Conv2d(num_feat * 4, num_feat * 2, 3, 1, 1, bias=False))
- self.conv6 = norm(nn.Conv2d(num_feat * 2, num_feat, 3, 1, 1, bias=False))
- # extra convolutions
- self.conv7 = norm(nn.Conv2d(num_feat, num_feat, 3, 1, 1, bias=False))
- self.conv8 = norm(nn.Conv2d(num_feat, num_feat, 3, 1, 1, bias=False))
- self.conv9 = nn.Conv2d(num_feat, 1, 3, 1, 1)
-
- def forward(self, x):
- # downsample
- x0 = F.leaky_relu(self.conv0(x), negative_slope=0.2, inplace=True)
- x1 = F.leaky_relu(self.conv1(x0), negative_slope=0.2, inplace=True)
- x2 = F.leaky_relu(self.conv2(x1), negative_slope=0.2, inplace=True)
- x3 = F.leaky_relu(self.conv3(x2), negative_slope=0.2, inplace=True)
-
- # upsample
- x3 = F.interpolate(x3, scale_factor=2, mode="bilinear", align_corners=False)
- x4 = F.leaky_relu(self.conv4(x3), negative_slope=0.2, inplace=True)
-
- if self.skip_connection:
- x4 = x4 + x2
- x4 = F.interpolate(x4, scale_factor=2, mode="bilinear", align_corners=False)
- x5 = F.leaky_relu(self.conv5(x4), negative_slope=0.2, inplace=True)
-
- if self.skip_connection:
- x5 = x5 + x1
- x5 = F.interpolate(x5, scale_factor=2, mode="bilinear", align_corners=False)
- x6 = F.leaky_relu(self.conv6(x5), negative_slope=0.2, inplace=True)
-
- if self.skip_connection:
- x6 = x6 + x0
-
- # extra convolutions
- out = F.leaky_relu(self.conv7(x6), negative_slope=0.2, inplace=True)
- out = F.leaky_relu(self.conv8(out), negative_slope=0.2, inplace=True)
- out = self.conv9(out)
-
- return out
diff --git a/spaces/GookProxy/Gyul/greeting.md b/spaces/GookProxy/Gyul/greeting.md
deleted file mode 100644
index b45dc59b5a57fb26fc190a84eff8bb8199fb47ca..0000000000000000000000000000000000000000
--- a/spaces/GookProxy/Gyul/greeting.md
+++ /dev/null
@@ -1,5 +0,0 @@
-Everything you send to this proxy, including prompts, is logged.
-This logs will also be publicly available at arca.live/b/characterai
-(프롬프트를 포함하여 이 프록시로 보내는 모든 내용이 기록됩니다
-이 로그는 arca.live/b/characterai에서 공개적으로 확인할 수 있습니다.)
-비밀번호는 아카라이브의 프록시 게이트에서만 공개됩니다.
\ No newline at end of file
diff --git a/spaces/Gradio-Blocks/uniformer_image_detection/configs/lvis/mask_rcnn_x101_64x4d_fpn_sample1e-3_mstrain_1x_lvis_v1.py b/spaces/Gradio-Blocks/uniformer_image_detection/configs/lvis/mask_rcnn_x101_64x4d_fpn_sample1e-3_mstrain_1x_lvis_v1.py
deleted file mode 100644
index f77adba2f150f62900571f5f32b2083ee53b7003..0000000000000000000000000000000000000000
--- a/spaces/Gradio-Blocks/uniformer_image_detection/configs/lvis/mask_rcnn_x101_64x4d_fpn_sample1e-3_mstrain_1x_lvis_v1.py
+++ /dev/null
@@ -1,13 +0,0 @@
-_base_ = './mask_rcnn_r50_fpn_sample1e-3_mstrain_1x_lvis_v1.py'
-model = dict(
- pretrained='open-mmlab://resnext101_64x4d',
- backbone=dict(
- type='ResNeXt',
- depth=101,
- groups=64,
- base_width=4,
- num_stages=4,
- out_indices=(0, 1, 2, 3),
- frozen_stages=1,
- norm_cfg=dict(type='BN', requires_grad=True),
- style='pytorch'))
diff --git a/spaces/GrandaddyShmax/AudioCraft_Plus/audiocraft/utils/profiler.py b/spaces/GrandaddyShmax/AudioCraft_Plus/audiocraft/utils/profiler.py
deleted file mode 100644
index b45b6d15910b50305c7b212c089ffad3c25b324d..0000000000000000000000000000000000000000
--- a/spaces/GrandaddyShmax/AudioCraft_Plus/audiocraft/utils/profiler.py
+++ /dev/null
@@ -1,38 +0,0 @@
-# Copyright (c) Meta Platforms, Inc. and affiliates.
-# All rights reserved.
-#
-# This source code is licensed under the license found in the
-# LICENSE file in the root directory of this source tree.
-
-import logging
-import typing as tp
-
-import dora
-import torch
-
-
-logger = logging.getLogger(__name__)
-
-
-class Profiler:
- """Context manager wrapper for xformers profiler.
- """
- def __init__(self, module: torch.nn.Module, enabled: bool = False):
- self.profiler: tp.Optional[tp.Any] = None
- if enabled:
- from xformers.profiler import profile
- output_dir = dora.get_xp().folder / 'profiler_data'
- logger.info("Profiling activated, results with be saved to %s", output_dir)
- self.profiler = profile(output_dir=output_dir, module=module)
-
- def step(self):
- if self.profiler is not None:
- self.profiler.step() # type: ignore
-
- def __enter__(self):
- if self.profiler is not None:
- return self.profiler.__enter__() # type: ignore
-
- def __exit__(self, exc_type, exc_value, exc_tb):
- if self.profiler is not None:
- return self.profiler.__exit__(exc_type, exc_value, exc_tb) # type: ignore
diff --git a/spaces/GrandaddyShmax/MusicGen_Plus/audiocraft/utils/autocast.py b/spaces/GrandaddyShmax/MusicGen_Plus/audiocraft/utils/autocast.py
deleted file mode 100644
index ed644843bb37cf8a92a20fbd51d6cebaa43b9a08..0000000000000000000000000000000000000000
--- a/spaces/GrandaddyShmax/MusicGen_Plus/audiocraft/utils/autocast.py
+++ /dev/null
@@ -1,40 +0,0 @@
-# Copyright (c) Meta Platforms, Inc. and affiliates.
-# All rights reserved.
-#
-# This source code is licensed under the license found in the
-# LICENSE file in the root directory of this source tree.
-
-import torch
-
-
-class TorchAutocast:
- """TorchAutocast utility class.
- Allows you to enable and disable autocast. This is specially useful
- when dealing with different architectures and clusters with different
- levels of support.
-
- Args:
- enabled (bool): Whether to enable torch.autocast or not.
- args: Additional args for torch.autocast.
- kwargs: Additional kwargs for torch.autocast
- """
- def __init__(self, enabled: bool, *args, **kwargs):
- self.autocast = torch.autocast(*args, **kwargs) if enabled else None
-
- def __enter__(self):
- if self.autocast is None:
- return
- try:
- self.autocast.__enter__()
- except RuntimeError:
- device = self.autocast.device
- dtype = self.autocast.fast_dtype
- raise RuntimeError(
- f"There was an error autocasting with dtype={dtype} device={device}\n"
- "If you are on the FAIR Cluster, you might need to use autocast_dtype=float16"
- )
-
- def __exit__(self, *args, **kwargs):
- if self.autocast is None:
- return
- self.autocast.__exit__(*args, **kwargs)
diff --git a/spaces/GrandaddyShmax/MusicGen_Plus_hfv2/audiocraft/data/audio_dataset.py b/spaces/GrandaddyShmax/MusicGen_Plus_hfv2/audiocraft/data/audio_dataset.py
deleted file mode 100644
index cf21422ea0059cb2d6553f93e608b8f9fa0d3a50..0000000000000000000000000000000000000000
--- a/spaces/GrandaddyShmax/MusicGen_Plus_hfv2/audiocraft/data/audio_dataset.py
+++ /dev/null
@@ -1,525 +0,0 @@
-# Copyright (c) Meta Platforms, Inc. and affiliates.
-# All rights reserved.
-#
-# This source code is licensed under the license found in the
-# LICENSE file in the root directory of this source tree.
-
-import argparse
-import copy
-from concurrent.futures import ThreadPoolExecutor, Future
-from dataclasses import dataclass, fields
-from contextlib import ExitStack
-import gzip
-import json
-import logging
-import os
-from pathlib import Path
-import random
-import sys
-import typing as tp
-
-import torch
-import torch.nn.functional as F
-
-from .audio import audio_read, audio_info
-from .audio_utils import convert_audio
-from .zip import PathInZip
-
-try:
- import dora
-except ImportError:
- dora = None # type: ignore
-
-
-@dataclass(order=True)
-class BaseInfo:
-
- @classmethod
- def _dict2fields(cls, dictionary: dict):
- return {
- field.name: dictionary[field.name]
- for field in fields(cls) if field.name in dictionary
- }
-
- @classmethod
- def from_dict(cls, dictionary: dict):
- _dictionary = cls._dict2fields(dictionary)
- return cls(**_dictionary)
-
- def to_dict(self):
- return {
- field.name: self.__getattribute__(field.name)
- for field in fields(self)
- }
-
-
-@dataclass(order=True)
-class AudioMeta(BaseInfo):
- path: str
- duration: float
- sample_rate: int
- amplitude: tp.Optional[float] = None
- weight: tp.Optional[float] = None
- # info_path is used to load additional information about the audio file that is stored in zip files.
- info_path: tp.Optional[PathInZip] = None
-
- @classmethod
- def from_dict(cls, dictionary: dict):
- base = cls._dict2fields(dictionary)
- if 'info_path' in base and base['info_path'] is not None:
- base['info_path'] = PathInZip(base['info_path'])
- return cls(**base)
-
- def to_dict(self):
- d = super().to_dict()
- if d['info_path'] is not None:
- d['info_path'] = str(d['info_path'])
- return d
-
-
-@dataclass(order=True)
-class SegmentInfo(BaseInfo):
- meta: AudioMeta
- seek_time: float
- n_frames: int # actual number of frames without padding
- total_frames: int # total number of frames, padding included
- sample_rate: int # actual sample rate
-
-
-DEFAULT_EXTS = ['.wav', '.mp3', '.flac', '.ogg', '.m4a']
-
-logger = logging.getLogger(__name__)
-
-
-def _get_audio_meta(file_path: str, minimal: bool = True) -> AudioMeta:
- """AudioMeta from a path to an audio file.
-
- Args:
- file_path (str): Resolved path of valid audio file.
- minimal (bool): Whether to only load the minimal set of metadata (takes longer if not).
- Returns:
- AudioMeta: Audio file path and its metadata.
- """
- info = audio_info(file_path)
- amplitude: tp.Optional[float] = None
- if not minimal:
- wav, sr = audio_read(file_path)
- amplitude = wav.abs().max().item()
- return AudioMeta(file_path, info.duration, info.sample_rate, amplitude)
-
-
-def _resolve_audio_meta(m: AudioMeta, fast: bool = True) -> AudioMeta:
- """If Dora is available as a dependency, try to resolve potential relative paths
- in list of AudioMeta. This method is expected to be used when loading meta from file.
-
- Args:
- m (AudioMeta): Audio meta to resolve.
- fast (bool): If True, uses a really fast check for determining if a file is already absolute or not.
- Only valid on Linux/Mac.
- Returns:
- AudioMeta: Audio meta with resolved path.
- """
- def is_abs(m):
- if fast:
- return str(m)[0] == '/'
- else:
- os.path.isabs(str(m))
-
- if not dora:
- return m
-
- if not is_abs(m.path):
- m.path = dora.git_save.to_absolute_path(m.path)
- if m.info_path is not None and not is_abs(m.info_path.zip_path):
- m.info_path.zip_path = dora.git_save.to_absolute_path(m.path)
- return m
-
-
-def find_audio_files(path: tp.Union[Path, str],
- exts: tp.List[str] = DEFAULT_EXTS,
- resolve: bool = True,
- minimal: bool = True,
- progress: bool = False,
- workers: int = 0) -> tp.List[AudioMeta]:
- """Build a list of AudioMeta from a given path,
- collecting relevant audio files and fetching meta info.
-
- Args:
- path (str or Path): Path to folder containing audio files.
- exts (list of str): List of file extensions to consider for audio files.
- minimal (bool): Whether to only load the minimal set of metadata (takes longer if not).
- progress (bool): Whether to log progress on audio files collection.
- workers (int): number of parallel workers, if 0, use only the current thread.
- Returns:
- List[AudioMeta]: List of audio file path and its metadata.
- """
- audio_files = []
- futures: tp.List[Future] = []
- pool: tp.Optional[ThreadPoolExecutor] = None
- with ExitStack() as stack:
- if workers > 0:
- pool = ThreadPoolExecutor(workers)
- stack.enter_context(pool)
-
- if progress:
- print("Finding audio files...")
- for root, folders, files in os.walk(path, followlinks=True):
- for file in files:
- full_path = Path(root) / file
- if full_path.suffix.lower() in exts:
- audio_files.append(full_path)
- if pool is not None:
- futures.append(pool.submit(_get_audio_meta, str(audio_files[-1]), minimal))
- if progress:
- print(format(len(audio_files), " 8d"), end='\r', file=sys.stderr)
-
- if progress:
- print("Getting audio metadata...")
- meta: tp.List[AudioMeta] = []
- for idx, file_path in enumerate(audio_files):
- try:
- if pool is None:
- m = _get_audio_meta(str(file_path), minimal)
- else:
- m = futures[idx].result()
- if resolve:
- m = _resolve_audio_meta(m)
- except Exception as err:
- print("Error with", str(file_path), err, file=sys.stderr)
- continue
- meta.append(m)
- if progress:
- print(format((1 + idx) / len(audio_files), " 3.1%"), end='\r', file=sys.stderr)
- meta.sort()
- return meta
-
-
-def load_audio_meta(path: tp.Union[str, Path],
- resolve: bool = True, fast: bool = True) -> tp.List[AudioMeta]:
- """Load list of AudioMeta from an optionally compressed json file.
-
- Args:
- path (str or Path): Path to JSON file.
- resolve (bool): Whether to resolve the path from AudioMeta (default=True).
- fast (bool): activates some tricks to make things faster.
- Returns:
- List[AudioMeta]: List of audio file path and its total duration.
- """
- open_fn = gzip.open if str(path).lower().endswith('.gz') else open
- with open_fn(path, 'rb') as fp: # type: ignore
- lines = fp.readlines()
- meta = []
- for line in lines:
- d = json.loads(line)
- m = AudioMeta.from_dict(d)
- if resolve:
- m = _resolve_audio_meta(m, fast=fast)
- meta.append(m)
- return meta
-
-
-def save_audio_meta(path: tp.Union[str, Path], meta: tp.List[AudioMeta]):
- """Save the audio metadata to the file pointer as json.
-
- Args:
- path (str or Path): Path to JSON file.
- metadata (list of BaseAudioMeta): List of audio meta to save.
- """
- Path(path).parent.mkdir(exist_ok=True, parents=True)
- open_fn = gzip.open if str(path).lower().endswith('.gz') else open
- with open_fn(path, 'wb') as fp: # type: ignore
- for m in meta:
- json_str = json.dumps(m.to_dict()) + '\n'
- json_bytes = json_str.encode('utf-8')
- fp.write(json_bytes)
-
-
-class AudioDataset:
- """Base audio dataset.
-
- The dataset takes a list of AudioMeta and create a dataset composed of segments of audio
- and potentially additional information, by creating random segments from the list of audio
- files referenced in the metadata and applying minimal data pre-processing such as resampling,
- mixing of channels, padding, etc.
-
- If no segment_duration value is provided, the AudioDataset will return the full wav for each
- audio file. Otherwise, it will randomly sample audio files and create a segment of the specified
- duration, applying padding if required.
-
- By default, only the torch Tensor corresponding to the waveform is returned. Setting return_info=True
- allows to return a tuple containing the torch Tensor and additional metadata on the segment and the
- original audio meta.
-
- Args:
- meta (tp.List[AudioMeta]): List of audio files metadata.
- segment_duration (float): Optional segment duration of audio to load.
- If not specified, the dataset will load the full audio segment from the file.
- shuffle (bool): Set to `True` to have the data reshuffled at every epoch.
- sample_rate (int): Target sample rate of the loaded audio samples.
- channels (int): Target number of channels of the loaded audio samples.
- sample_on_duration (bool): Set to `True` to sample segments with probability
- dependent on audio file duration. This is only used if `segment_duration` is provided.
- sample_on_weight (bool): Set to `True` to sample segments using the `weight` entry of
- `AudioMeta`. If `sample_on_duration` is also True, the actual weight will be the product
- of the file duration and file weight. This is only used if `segment_duration` is provided.
- min_segment_ratio (float): Minimum segment ratio to use when the audio file
- is shorter than the desired segment.
- max_read_retry (int): Maximum number of retries to sample an audio segment from the dataset.
- return_info (bool): Whether to return the wav only or return wav along with segment info and metadata.
- min_audio_duration (tp.Optional[float], optional): Minimum audio file duration, in seconds, if provided
- audio shorter than this will be filtered out.
- max_audio_duration (tp.Optional[float], optional): Maximal audio file duration in seconds, if provided
- audio longer than this will be filtered out.
- """
- def __init__(self,
- meta: tp.List[AudioMeta],
- segment_duration: tp.Optional[float] = None,
- shuffle: bool = True,
- num_samples: int = 10_000,
- sample_rate: int = 48_000,
- channels: int = 2,
- pad: bool = True,
- sample_on_duration: bool = True,
- sample_on_weight: bool = True,
- min_segment_ratio: float = 0.5,
- max_read_retry: int = 10,
- return_info: bool = False,
- min_audio_duration: tp.Optional[float] = None,
- max_audio_duration: tp.Optional[float] = None
- ):
- assert len(meta) > 0, 'No audio meta provided to AudioDataset. Please check loading of audio meta.'
- assert segment_duration is None or segment_duration > 0
- assert segment_duration is None or min_segment_ratio >= 0
- logging.debug(f'sample_on_duration: {sample_on_duration}')
- logging.debug(f'sample_on_weight: {sample_on_weight}')
- logging.debug(f'pad: {pad}')
- logging.debug(f'min_segment_ratio: {min_segment_ratio}')
-
- self.segment_duration = segment_duration
- self.min_segment_ratio = min_segment_ratio
- self.max_audio_duration = max_audio_duration
- self.min_audio_duration = min_audio_duration
- if self.min_audio_duration is not None and self.max_audio_duration is not None:
- assert self.min_audio_duration <= self.max_audio_duration
- self.meta: tp.List[AudioMeta] = self._filter_duration(meta)
- assert len(self.meta) # Fail fast if all data has been filtered.
- self.total_duration = sum(d.duration for d in self.meta)
-
- if segment_duration is None:
- num_samples = len(self.meta)
- self.num_samples = num_samples
- self.shuffle = shuffle
- self.sample_rate = sample_rate
- self.channels = channels
- self.pad = pad
- self.sample_on_weight = sample_on_weight
- self.sample_on_duration = sample_on_duration
- self.sampling_probabilities = self._get_sampling_probabilities()
- self.max_read_retry = max_read_retry
- self.return_info = return_info
-
- def __len__(self):
- return self.num_samples
-
- def _get_sampling_probabilities(self, normalized: bool = True):
- """Return the sampling probabilities for each file inside `self.meta`.
- """
- scores: tp.List[float] = []
- for file_meta in self.meta:
- score = 1.
- if self.sample_on_weight and file_meta.weight is not None:
- score *= file_meta.weight
- if self.sample_on_duration:
- score *= file_meta.duration
- scores.append(score)
- probabilities = torch.tensor(scores)
- if normalized:
- probabilities /= probabilities.sum()
- return probabilities
-
- def sample_file(self, rng: torch.Generator) -> AudioMeta:
- """Sample a given file from `self.meta`. Can be overriden in subclasses.
- This is only called if `segment_duration` is not None.
-
- You must use the provided random number generator `rng` for reproducibility.
- """
- if not self.sample_on_weight and not self.sample_on_duration:
- file_index = int(torch.randint(len(self.sampling_probabilities), (1,), generator=rng).item())
- else:
- file_index = int(torch.multinomial(self.sampling_probabilities, 1, generator=rng).item())
-
- return self.meta[file_index]
-
- def __getitem__(self, index: int) -> tp.Union[torch.Tensor, tp.Tuple[torch.Tensor, SegmentInfo]]:
- if self.segment_duration is None:
- file_meta = self.meta[index]
- out, sr = audio_read(file_meta.path)
- out = convert_audio(out, sr, self.sample_rate, self.channels)
- n_frames = out.shape[-1]
- segment_info = SegmentInfo(file_meta, seek_time=0., n_frames=n_frames, total_frames=n_frames,
- sample_rate=self.sample_rate)
- else:
- rng = torch.Generator()
- if self.shuffle:
- # We use index, plus extra randomness
- rng.manual_seed(index + self.num_samples * random.randint(0, 2**24))
- else:
- # We only use index
- rng.manual_seed(index)
-
- for retry in range(self.max_read_retry):
- file_meta = self.sample_file(rng)
- # We add some variance in the file position even if audio file is smaller than segment
- # without ending up with empty segments
- max_seek = max(0, file_meta.duration - self.segment_duration * self.min_segment_ratio)
- seek_time = torch.rand(1, generator=rng).item() * max_seek
- try:
- out, sr = audio_read(file_meta.path, seek_time, self.segment_duration, pad=False)
- out = convert_audio(out, sr, self.sample_rate, self.channels)
- n_frames = out.shape[-1]
- target_frames = int(self.segment_duration * self.sample_rate)
- if self.pad:
- out = F.pad(out, (0, target_frames - n_frames))
- segment_info = SegmentInfo(file_meta, seek_time, n_frames=n_frames, total_frames=target_frames,
- sample_rate=self.sample_rate)
- except Exception as exc:
- logger.warning("Error opening file %s: %r", file_meta.path, exc)
- if retry == self.max_read_retry - 1:
- raise
- else:
- break
-
- if self.return_info:
- # Returns the wav and additional information on the wave segment
- return out, segment_info
- else:
- return out
-
- def collater(self, samples):
- """The collater function has to be provided to the dataloader
- if AudioDataset has return_info=True in order to properly collate
- the samples of a batch.
- """
- if self.segment_duration is None and len(samples) > 1:
- assert self.pad, "Must allow padding when batching examples of different durations."
-
- # In this case the audio reaching the collater is of variable length as segment_duration=None.
- to_pad = self.segment_duration is None and self.pad
- if to_pad:
- max_len = max([wav.shape[-1] for wav, _ in samples])
-
- def _pad_wav(wav):
- return F.pad(wav, (0, max_len - wav.shape[-1]))
-
- if self.return_info:
- if len(samples) > 0:
- assert len(samples[0]) == 2
- assert isinstance(samples[0][0], torch.Tensor)
- assert isinstance(samples[0][1], SegmentInfo)
-
- wavs = [wav for wav, _ in samples]
- segment_infos = [copy.deepcopy(info) for _, info in samples]
-
- if to_pad:
- # Each wav could be of a different duration as they are not segmented.
- for i in range(len(samples)):
- # Determines the total legth of the signal with padding, so we update here as we pad.
- segment_infos[i].total_frames = max_len
- wavs[i] = _pad_wav(wavs[i])
-
- wav = torch.stack(wavs)
- return wav, segment_infos
- else:
- assert isinstance(samples[0], torch.Tensor)
- if to_pad:
- samples = [_pad_wav(s) for s in samples]
- return torch.stack(samples)
-
- def _filter_duration(self, meta: tp.List[AudioMeta]) -> tp.List[AudioMeta]:
- """Filters out audio files with short durations.
- Removes from meta files that have durations that will not allow to samples examples from them.
- """
- orig_len = len(meta)
-
- # Filter data that is too short.
- if self.min_audio_duration is not None:
- meta = [m for m in meta if m.duration >= self.min_audio_duration]
-
- # Filter data that is too long.
- if self.max_audio_duration is not None:
- meta = [m for m in meta if m.duration <= self.max_audio_duration]
-
- filtered_len = len(meta)
- removed_percentage = 100*(1-float(filtered_len)/orig_len)
- msg = 'Removed %.2f percent of the data because it was too short or too long.' % removed_percentage
- if removed_percentage < 10:
- logging.debug(msg)
- else:
- logging.warning(msg)
- return meta
-
- @classmethod
- def from_meta(cls, root: tp.Union[str, Path], **kwargs):
- """Instantiate AudioDataset from a path to a directory containing a manifest as a jsonl file.
-
- Args:
- root (str or Path): Path to root folder containing audio files.
- kwargs: Additional keyword arguments for the AudioDataset.
- """
- root = Path(root)
- if root.is_dir():
- if (root / 'data.jsonl').exists():
- root = root / 'data.jsonl'
- elif (root / 'data.jsonl.gz').exists():
- root = root / 'data.jsonl.gz'
- else:
- raise ValueError("Don't know where to read metadata from in the dir. "
- "Expecting either a data.jsonl or data.jsonl.gz file but none found.")
- meta = load_audio_meta(root)
- return cls(meta, **kwargs)
-
- @classmethod
- def from_path(cls, root: tp.Union[str, Path], minimal_meta: bool = True,
- exts: tp.List[str] = DEFAULT_EXTS, **kwargs):
- """Instantiate AudioDataset from a path containing (possibly nested) audio files.
-
- Args:
- root (str or Path): Path to root folder containing audio files.
- minimal_meta (bool): Whether to only load minimal metadata or not.
- exts (list of str): Extensions for audio files.
- kwargs: Additional keyword arguments for the AudioDataset.
- """
- root = Path(root)
- if root.is_file():
- meta = load_audio_meta(root, resolve=True)
- else:
- meta = find_audio_files(root, exts, minimal=minimal_meta, resolve=True)
- return cls(meta, **kwargs)
-
-
-def main():
- logging.basicConfig(stream=sys.stderr, level=logging.INFO)
- parser = argparse.ArgumentParser(
- prog='audio_dataset',
- description='Generate .jsonl files by scanning a folder.')
- parser.add_argument('root', help='Root folder with all the audio files')
- parser.add_argument('output_meta_file',
- help='Output file to store the metadata, ')
- parser.add_argument('--complete',
- action='store_false', dest='minimal', default=True,
- help='Retrieve all metadata, even the one that are expansive '
- 'to compute (e.g. normalization).')
- parser.add_argument('--resolve',
- action='store_true', default=False,
- help='Resolve the paths to be absolute and with no symlinks.')
- parser.add_argument('--workers',
- default=10, type=int,
- help='Number of workers.')
- args = parser.parse_args()
- meta = find_audio_files(args.root, DEFAULT_EXTS, progress=True,
- resolve=args.resolve, minimal=args.minimal, workers=args.workers)
- save_audio_meta(args.output_meta_file, meta)
-
-
-if __name__ == '__main__':
- main()
diff --git a/spaces/HCMUT-GraduateThesis-HNTThinh/rgbdsod-multimae-demo/s_multimae/model/__init__.py b/spaces/HCMUT-GraduateThesis-HNTThinh/rgbdsod-multimae-demo/s_multimae/model/__init__.py
deleted file mode 100644
index e69de29bb2d1d6434b8b29ae775ad8c2e48c5391..0000000000000000000000000000000000000000
diff --git a/spaces/HarryLee/eCommerceImageCaptioning/fairseq/examples/speech_recognition/criterions/__init__.py b/spaces/HarryLee/eCommerceImageCaptioning/fairseq/examples/speech_recognition/criterions/__init__.py
deleted file mode 100644
index 579abd2ace1b14b80f5e53e5c96583e4d5b14c52..0000000000000000000000000000000000000000
--- a/spaces/HarryLee/eCommerceImageCaptioning/fairseq/examples/speech_recognition/criterions/__init__.py
+++ /dev/null
@@ -1,17 +0,0 @@
-import importlib
-import os
-
-
-# ASG loss requires flashlight bindings
-files_to_skip = set()
-try:
- import flashlight.lib.sequence.criterion
-except ImportError:
- files_to_skip.add("ASG_loss.py")
-
-for file in sorted(os.listdir(os.path.dirname(__file__))):
- if file.endswith(".py") and not file.startswith("_") and file not in files_to_skip:
- criterion_name = file[: file.find(".py")]
- importlib.import_module(
- "examples.speech_recognition.criterions." + criterion_name
- )
diff --git a/spaces/HarryLee/eCommerceImageCaptioning/fairseq/scripts/compound_split_bleu.sh b/spaces/HarryLee/eCommerceImageCaptioning/fairseq/scripts/compound_split_bleu.sh
deleted file mode 100644
index 1972fddcebff9a43a70bcf14c287175c68f60e3f..0000000000000000000000000000000000000000
--- a/spaces/HarryLee/eCommerceImageCaptioning/fairseq/scripts/compound_split_bleu.sh
+++ /dev/null
@@ -1,20 +0,0 @@
-#!/bin/bash
-
-if [ $# -ne 1 ]; then
- echo "usage: $0 GENERATE_PY_OUTPUT"
- exit 1
-fi
-
-GEN=$1
-
-SYS=$GEN.sys
-REF=$GEN.ref
-
-if [ $(tail -n 1 $GEN | grep BLEU | wc -l) -ne 1 ]; then
- echo "not done generating"
- exit
-fi
-
-grep ^H $GEN | awk -F '\t' '{print $NF}' | perl -ple 's{(\S)-(\S)}{$1 ##AT##-##AT## $2}g' > $SYS
-grep ^T $GEN | cut -f2- | perl -ple 's{(\S)-(\S)}{$1 ##AT##-##AT## $2}g' > $REF
-fairseq-score --sys $SYS --ref $REF
diff --git a/spaces/Harveenchadha/Vakyansh-Tamil-TTS/ttsv/scripts/hifi/train_hifi.sh b/spaces/Harveenchadha/Vakyansh-Tamil-TTS/ttsv/scripts/hifi/train_hifi.sh
deleted file mode 100644
index 287ca1159b5bf8f779d66885197fadbcd23b911e..0000000000000000000000000000000000000000
--- a/spaces/Harveenchadha/Vakyansh-Tamil-TTS/ttsv/scripts/hifi/train_hifi.sh
+++ /dev/null
@@ -1,21 +0,0 @@
-#!/bin/bash
-
-gender='male'
-
-config='../../config/hifi/config_v1.json'
-modeldir='../../checkpoints/hifi/'$gender
-logdir='../../logs/hifi/'$gender
-
-
-####################################################
-
-
-
-python ../../src/hifi_gan/train.py \
- --config $config \
- --input_training_file '../../data/hifi/'$gender'/train.txt' \
- --input_validation_file '../../data/hifi/'$gender'/valid.txt' \
- --checkpoint_path $modeldir \
- --logs_path $logdir \
- --checkpoint_interval 10000 \
- --stdout_interval 50
diff --git a/spaces/ICML2022/OFA/data/data_utils.py b/spaces/ICML2022/OFA/data/data_utils.py
deleted file mode 100644
index 7f843789138c62668f9e1c4e7fd44299fb5ef768..0000000000000000000000000000000000000000
--- a/spaces/ICML2022/OFA/data/data_utils.py
+++ /dev/null
@@ -1,601 +0,0 @@
-# Copyright (c) Facebook, Inc. and its affiliates.
-#
-# This source code is licensed under the MIT license found in the
-# LICENSE file in the root directory of this source tree.
-
-try:
- from collections.abc import Iterable
-except ImportError:
- from collections import Iterable
-import contextlib
-import itertools
-import logging
-import re
-import warnings
-from typing import Optional, Tuple
-
-import numpy as np
-import torch
-
-from fairseq.file_io import PathManager
-from fairseq import utils
-import os
-
-logger = logging.getLogger(__name__)
-
-
-def infer_language_pair(path):
- """Infer language pair from filename: .-.(...).idx"""
- src, dst = None, None
- for filename in PathManager.ls(path):
- parts = filename.split(".")
- if len(parts) >= 3 and len(parts[1].split("-")) == 2:
- return parts[1].split("-")
- return src, dst
-
-
-def collate_tokens(
- values,
- pad_idx,
- eos_idx=None,
- left_pad=False,
- move_eos_to_beginning=False,
- pad_to_length=None,
- pad_to_multiple=1,
- pad_to_bsz=None,
-):
- """Convert a list of 1d tensors into a padded 2d tensor."""
- size = max(v.size(0) for v in values)
- size = size if pad_to_length is None else max(size, pad_to_length)
- if pad_to_multiple != 1 and size % pad_to_multiple != 0:
- size = int(((size - 0.1) // pad_to_multiple + 1) * pad_to_multiple)
-
- def copy_tensor(src, dst):
- assert dst.numel() == src.numel()
- if move_eos_to_beginning:
- if eos_idx is None:
- # if no eos_idx is specified, then use the last token in src
- dst[0] = src[-1]
- else:
- dst[0] = eos_idx
- dst[1:] = src[:-1]
- else:
- dst.copy_(src)
-
- if values[0].dim() == 1:
- res = values[0].new(len(values), size).fill_(pad_idx)
- elif values[0].dim() == 2:
- assert move_eos_to_beginning is False
- res = values[0].new(len(values), size, values[0].size(1)).fill_(pad_idx)
- else:
- raise NotImplementedError
-
- for i, v in enumerate(values):
- copy_tensor(v, res[i][size - len(v) :] if left_pad else res[i][: len(v)])
- return res
-
-
-def load_indexed_dataset(
- path, dictionary=None, dataset_impl=None, combine=False, default="cached"
-):
- """A helper function for loading indexed datasets.
-
- Args:
- path (str): path to indexed dataset (e.g., 'data-bin/train')
- dictionary (~fairseq.data.Dictionary): data dictionary
- dataset_impl (str, optional): which dataset implementation to use. If
- not provided, it will be inferred automatically. For legacy indexed
- data we use the 'cached' implementation by default.
- combine (bool, optional): automatically load and combine multiple
- datasets. For example, if *path* is 'data-bin/train', then we will
- combine 'data-bin/train', 'data-bin/train1', ... and return a
- single ConcatDataset instance.
- """
- import fairseq.data.indexed_dataset as indexed_dataset
- from fairseq.data.concat_dataset import ConcatDataset
-
- datasets = []
- for k in itertools.count():
- path_k = path + (str(k) if k > 0 else "")
- try:
- path_k = indexed_dataset.get_indexed_dataset_to_local(path_k)
- except Exception as e:
- if "StorageException: [404] Path not found" in str(e):
- logger.warning(f"path_k: {e} not found")
- else:
- raise e
-
- dataset_impl_k = dataset_impl
- if dataset_impl_k is None:
- dataset_impl_k = indexed_dataset.infer_dataset_impl(path_k)
- dataset = indexed_dataset.make_dataset(
- path_k,
- impl=dataset_impl_k or default,
- fix_lua_indexing=True,
- dictionary=dictionary,
- )
- if dataset is None:
- break
- logger.info("loaded {:,} examples from: {}".format(len(dataset), path_k))
- datasets.append(dataset)
- if not combine:
- break
- if len(datasets) == 0:
- return None
- elif len(datasets) == 1:
- return datasets[0]
- else:
- return ConcatDataset(datasets)
-
-
-@contextlib.contextmanager
-def numpy_seed(seed, *addl_seeds):
- """Context manager which seeds the NumPy PRNG with the specified seed and
- restores the state afterward"""
- if seed is None:
- yield
- return
- if len(addl_seeds) > 0:
- seed = int(hash((seed, *addl_seeds)) % 1e6)
- state = np.random.get_state()
- np.random.seed(seed)
- try:
- yield
- finally:
- np.random.set_state(state)
-
-
-def collect_filtered(function, iterable, filtered):
- """
- Similar to :func:`filter` but collects filtered elements in ``filtered``.
-
- Args:
- function (callable): function that returns ``False`` for elements that
- should be filtered
- iterable (iterable): iterable to filter
- filtered (list): list to store filtered elements
- """
- for el in iterable:
- if function(el):
- yield el
- else:
- filtered.append(el)
-
-
-def _filter_by_size_dynamic(indices, size_fn, max_positions, raise_exception=False):
- def compare_leq(a, b):
- return a <= b if not isinstance(a, tuple) else max(a) <= b
-
- def check_size(idx):
- if isinstance(max_positions, float) or isinstance(max_positions, int):
- return size_fn(idx) <= max_positions
- elif isinstance(max_positions, dict):
- idx_size = size_fn(idx)
- assert isinstance(idx_size, dict)
- intersect_keys = set(max_positions.keys()) & set(idx_size.keys())
- return all(
- all(
- a is None or b is None or a <= b
- for a, b in zip(idx_size[key], max_positions[key])
- )
- for key in intersect_keys
- )
- else:
- # For MultiCorpusSampledDataset, will generalize it later
- if not isinstance(size_fn(idx), Iterable):
- return all(size_fn(idx) <= b for b in max_positions)
- return all(
- a is None or b is None or a <= b
- for a, b in zip(size_fn(idx), max_positions)
- )
-
- ignored = []
- itr = collect_filtered(check_size, indices, ignored)
- indices = np.fromiter(itr, dtype=np.int64, count=-1)
- return indices, ignored
-
-
-def filter_by_size(indices, dataset, max_positions, raise_exception=False):
- """
- [deprecated] Filter indices based on their size.
- Use `FairseqDataset::filter_indices_by_size` instead.
-
- Args:
- indices (List[int]): ordered list of dataset indices
- dataset (FairseqDataset): fairseq dataset instance
- max_positions (tuple): filter elements larger than this size.
- Comparisons are done component-wise.
- raise_exception (bool, optional): if ``True``, raise an exception if
- any elements are filtered (default: False).
- """
- warnings.warn(
- "data_utils.filter_by_size is deprecated. "
- "Use `FairseqDataset::filter_indices_by_size` instead.",
- stacklevel=2,
- )
- if isinstance(max_positions, float) or isinstance(max_positions, int):
- if hasattr(dataset, "sizes") and isinstance(dataset.sizes, np.ndarray):
- ignored = indices[dataset.sizes[indices] > max_positions].tolist()
- indices = indices[dataset.sizes[indices] <= max_positions]
- elif (
- hasattr(dataset, "sizes")
- and isinstance(dataset.sizes, list)
- and len(dataset.sizes) == 1
- ):
- ignored = indices[dataset.sizes[0][indices] > max_positions].tolist()
- indices = indices[dataset.sizes[0][indices] <= max_positions]
- else:
- indices, ignored = _filter_by_size_dynamic(
- indices, dataset.size, max_positions
- )
- else:
- indices, ignored = _filter_by_size_dynamic(indices, dataset.size, max_positions)
-
- if len(ignored) > 0 and raise_exception:
- raise Exception(
- (
- "Size of sample #{} is invalid (={}) since max_positions={}, "
- "skip this example with --skip-invalid-size-inputs-valid-test"
- ).format(ignored[0], dataset.size(ignored[0]), max_positions)
- )
- if len(ignored) > 0:
- logger.warning(
- (
- "{} samples have invalid sizes and will be skipped, "
- "max_positions={}, first few sample ids={}"
- ).format(len(ignored), max_positions, ignored[:10])
- )
- return indices
-
-
-def filter_paired_dataset_indices_by_size(src_sizes, tgt_sizes, indices, max_sizes):
- """Filter a list of sample indices. Remove those that are longer
- than specified in max_sizes.
-
- Args:
- indices (np.array): original array of sample indices
- max_sizes (int or list[int] or tuple[int]): max sample size,
- can be defined separately for src and tgt (then list or tuple)
-
- Returns:
- np.array: filtered sample array
- list: list of removed indices
- """
- if max_sizes is None:
- return indices, []
- if type(max_sizes) in (int, float):
- max_src_size, max_tgt_size = max_sizes, max_sizes
- else:
- max_src_size, max_tgt_size = max_sizes
- if tgt_sizes is None:
- ignored = indices[src_sizes[indices] > max_src_size]
- else:
- ignored = indices[
- (src_sizes[indices] > max_src_size) | (tgt_sizes[indices] > max_tgt_size)
- ]
- if len(ignored) > 0:
- if tgt_sizes is None:
- indices = indices[src_sizes[indices] <= max_src_size]
- else:
- indices = indices[
- (src_sizes[indices] <= max_src_size)
- & (tgt_sizes[indices] <= max_tgt_size)
- ]
- return indices, ignored.tolist()
-
-
-def batch_by_size(
- indices,
- num_tokens_fn,
- num_tokens_vec=None,
- max_tokens=None,
- max_sentences=None,
- required_batch_size_multiple=1,
- fixed_shapes=None,
-):
- """
- Yield mini-batches of indices bucketed by size. Batches may contain
- sequences of different lengths.
-
- Args:
- indices (List[int]): ordered list of dataset indices
- num_tokens_fn (callable): function that returns the number of tokens at
- a given index
- num_tokens_vec (List[int], optional): precomputed vector of the number
- of tokens for each index in indices (to enable faster batch generation)
- max_tokens (int, optional): max number of tokens in each batch
- (default: None).
- max_sentences (int, optional): max number of sentences in each
- batch (default: None).
- required_batch_size_multiple (int, optional): require batch size to
- be less than N or a multiple of N (default: 1).
- fixed_shapes (List[Tuple[int, int]], optional): if given, batches will
- only be created with the given shapes. *max_sentences* and
- *required_batch_size_multiple* will be ignored (default: None).
- """
- try:
- from fairseq.data.data_utils_fast import (
- batch_by_size_fn,
- batch_by_size_vec,
- batch_fixed_shapes_fast,
- )
- except ImportError:
- raise ImportError(
- "Please build Cython components with: "
- "`python setup.py build_ext --inplace`"
- )
- except ValueError:
- raise ValueError(
- "Please build (or rebuild) Cython components with `python setup.py build_ext --inplace`."
- )
-
- # added int() to avoid TypeError: an integer is required
- max_tokens = (
- int(max_tokens) if max_tokens is not None else -1
- )
- max_sentences = max_sentences if max_sentences is not None else -1
- bsz_mult = required_batch_size_multiple
-
- if not isinstance(indices, np.ndarray):
- indices = np.fromiter(indices, dtype=np.int64, count=-1)
-
- if num_tokens_vec is not None and not isinstance(num_tokens_vec, np.ndarray):
- num_tokens_vec = np.fromiter(num_tokens_vec, dtype=np.int64, count=-1)
-
- if fixed_shapes is None:
- if num_tokens_vec is None:
- return batch_by_size_fn(
- indices,
- num_tokens_fn,
- max_tokens,
- max_sentences,
- bsz_mult,
- )
- else:
- return batch_by_size_vec(
- indices,
- num_tokens_vec,
- max_tokens,
- max_sentences,
- bsz_mult,
- )
-
- else:
- fixed_shapes = np.array(fixed_shapes, dtype=np.int64)
- sort_order = np.lexsort(
- [
- fixed_shapes[:, 1].argsort(), # length
- fixed_shapes[:, 0].argsort(), # bsz
- ]
- )
- fixed_shapes_sorted = fixed_shapes[sort_order]
- return batch_fixed_shapes_fast(indices, num_tokens_fn, fixed_shapes_sorted)
-
-
-def post_process(sentence: str, symbol: str):
- if symbol == "sentencepiece":
- sentence = sentence.replace(" ", "").replace("\u2581", " ").strip()
- elif symbol == "wordpiece":
- sentence = sentence.replace(" ", "").replace("_", " ").strip()
- elif symbol == "letter":
- sentence = sentence.replace(" ", "").replace("|", " ").strip()
- elif symbol == "silence":
- import re
- sentence = sentence.replace("", "")
- sentence = re.sub(' +', ' ', sentence).strip()
- elif symbol == "_EOW":
- sentence = sentence.replace(" ", "").replace("_EOW", " ").strip()
- elif symbol in {"subword_nmt", "@@ ", "@@"}:
- if symbol == "subword_nmt":
- symbol = "@@ "
- sentence = (sentence + " ").replace(symbol, "").rstrip()
- elif symbol == "none":
- pass
- elif symbol is not None:
- raise NotImplementedError(f"Unknown post_process option: {symbol}")
- return sentence
-
-
-def compute_mask_indices(
- shape: Tuple[int, int],
- padding_mask: Optional[torch.Tensor],
- mask_prob: float,
- mask_length: int,
- mask_type: str = "static",
- mask_other: float = 0.0,
- min_masks: int = 0,
- no_overlap: bool = False,
- min_space: int = 0,
-) -> np.ndarray:
- """
- Computes random mask spans for a given shape
-
- Args:
- shape: the the shape for which to compute masks.
- should be of size 2 where first element is batch size and 2nd is timesteps
- padding_mask: optional padding mask of the same size as shape, which will prevent masking padded elements
- mask_prob: probability for each token to be chosen as start of the span to be masked. this will be multiplied by
- number of timesteps divided by length of mask span to mask approximately this percentage of all elements.
- however due to overlaps, the actual number will be smaller (unless no_overlap is True)
- mask_type: how to compute mask lengths
- static = fixed size
- uniform = sample from uniform distribution [mask_other, mask_length*2]
- normal = sample from normal distribution with mean mask_length and stdev mask_other. mask is min 1 element
- poisson = sample from possion distribution with lambda = mask length
- min_masks: minimum number of masked spans
- no_overlap: if false, will switch to an alternative recursive algorithm that prevents spans from overlapping
- min_space: only used if no_overlap is True, this is how many elements to keep unmasked between spans
- """
-
- bsz, all_sz = shape
- mask = np.full((bsz, all_sz), False)
-
- all_num_mask = int(
- # add a random number for probabilistic rounding
- mask_prob * all_sz / float(mask_length)
- + np.random.rand()
- )
-
- all_num_mask = max(min_masks, all_num_mask)
-
- mask_idcs = []
- for i in range(bsz):
- if padding_mask is not None:
- sz = all_sz - padding_mask[i].long().sum().item()
- num_mask = int(
- # add a random number for probabilistic rounding
- mask_prob * sz / float(mask_length)
- + np.random.rand()
- )
- num_mask = max(min_masks, num_mask)
- else:
- sz = all_sz
- num_mask = all_num_mask
-
- if mask_type == "static":
- lengths = np.full(num_mask, mask_length)
- elif mask_type == "uniform":
- lengths = np.random.randint(mask_other, mask_length * 2 + 1, size=num_mask)
- elif mask_type == "normal":
- lengths = np.random.normal(mask_length, mask_other, size=num_mask)
- lengths = [max(1, int(round(x))) for x in lengths]
- elif mask_type == "poisson":
- lengths = np.random.poisson(mask_length, size=num_mask)
- lengths = [int(round(x)) for x in lengths]
- else:
- raise Exception("unknown mask selection " + mask_type)
-
- if sum(lengths) == 0:
- lengths[0] = min(mask_length, sz - 1)
-
- if no_overlap:
- mask_idc = []
-
- def arrange(s, e, length, keep_length):
- span_start = np.random.randint(s, e - length)
- mask_idc.extend(span_start + i for i in range(length))
-
- new_parts = []
- if span_start - s - min_space >= keep_length:
- new_parts.append((s, span_start - min_space + 1))
- if e - span_start - keep_length - min_space > keep_length:
- new_parts.append((span_start + length + min_space, e))
- return new_parts
-
- parts = [(0, sz)]
- min_length = min(lengths)
- for length in sorted(lengths, reverse=True):
- lens = np.fromiter(
- (e - s if e - s >= length + min_space else 0 for s, e in parts),
- np.int,
- )
- l_sum = np.sum(lens)
- if l_sum == 0:
- break
- probs = lens / np.sum(lens)
- c = np.random.choice(len(parts), p=probs)
- s, e = parts.pop(c)
- parts.extend(arrange(s, e, length, min_length))
- mask_idc = np.asarray(mask_idc)
- else:
- min_len = min(lengths)
- if sz - min_len <= num_mask:
- min_len = sz - num_mask - 1
-
- mask_idc = np.random.choice(sz - min_len, num_mask, replace=False)
-
- mask_idc = np.asarray(
- [
- mask_idc[j] + offset
- for j in range(len(mask_idc))
- for offset in range(lengths[j])
- ]
- )
-
- mask_idcs.append(np.unique(mask_idc[mask_idc < sz]))
-
- min_len = min([len(m) for m in mask_idcs])
- for i, mask_idc in enumerate(mask_idcs):
- if len(mask_idc) > min_len:
- mask_idc = np.random.choice(mask_idc, min_len, replace=False)
- mask[i, mask_idc] = True
-
- return mask
-
-
-def get_mem_usage():
- try:
- import psutil
-
- mb = 1024 * 1024
- return f"used={psutil.virtual_memory().used / mb}Mb; avail={psutil.virtual_memory().available / mb}Mb"
- except ImportError:
- return "N/A"
-
-
-# lens: torch.LongTensor
-# returns: torch.BoolTensor
-def lengths_to_padding_mask(lens):
- bsz, max_lens = lens.size(0), torch.max(lens).item()
- mask = torch.arange(max_lens).to(lens.device).view(1, max_lens)
- mask = mask.expand(bsz, -1) >= lens.view(bsz, 1).expand(-1, max_lens)
- return mask
-
-
-# lens: torch.LongTensor
-# returns: torch.BoolTensor
-def lengths_to_mask(lens):
- return ~lengths_to_padding_mask(lens)
-
-
-def get_buckets(sizes, num_buckets):
- buckets = np.unique(
- np.percentile(
- sizes,
- np.linspace(0, 100, num_buckets + 1),
- interpolation='lower',
- )[1:]
- )
- return buckets
-
-
-def get_bucketed_sizes(orig_sizes, buckets):
- sizes = np.copy(orig_sizes)
- assert np.min(sizes) >= 0
- start_val = -1
- for end_val in buckets:
- mask = (sizes > start_val) & (sizes <= end_val)
- sizes[mask] = end_val
- start_val = end_val
- return sizes
-
-
-
-def _find_extra_valid_paths(dataset_path: str) -> set:
- paths = utils.split_paths(dataset_path)
- all_valid_paths = set()
- for sub_dir in paths:
- contents = PathManager.ls(sub_dir)
- valid_paths = [c for c in contents if re.match("valid*[0-9].*", c) is not None]
- all_valid_paths |= {os.path.basename(p) for p in valid_paths}
- # Remove .bin, .idx etc
- roots = {os.path.splitext(p)[0] for p in all_valid_paths}
- return roots
-
-
-def raise_if_valid_subsets_unintentionally_ignored(train_cfg) -> None:
- """Raises if there are paths matching 'valid*[0-9].*' which are not combined or ignored."""
- if (
- train_cfg.dataset.ignore_unused_valid_subsets
- or train_cfg.dataset.combine_valid_subsets
- or train_cfg.dataset.disable_validation
- or not hasattr(train_cfg.task, "data")
- ):
- return
- other_paths = _find_extra_valid_paths(train_cfg.task.data)
- specified_subsets = train_cfg.dataset.valid_subset.split(",")
- ignored_paths = [p for p in other_paths if p not in specified_subsets]
- if ignored_paths:
- advice = "Set --combine-val to combine them or --ignore-unused-valid-subsets to ignore them."
- msg = f"Valid paths {ignored_paths} will be ignored. {advice}"
- raise ValueError(msg)
\ No newline at end of file
diff --git a/spaces/ICML2022/OFA/fairseq/examples/simultaneous_translation/utils/__init__.py b/spaces/ICML2022/OFA/fairseq/examples/simultaneous_translation/utils/__init__.py
deleted file mode 100644
index 1e9ce844f59a4211061392084cc81075e6bab19f..0000000000000000000000000000000000000000
--- a/spaces/ICML2022/OFA/fairseq/examples/simultaneous_translation/utils/__init__.py
+++ /dev/null
@@ -1,14 +0,0 @@
-# Copyright (c) Facebook, Inc. and its affiliates.
-#
-# This source code is licensed under the MIT license found in the
-# LICENSE file in the root directory of this source tree.
-
-import importlib
-import os
-
-
-# automatically import any Python files in the criterions/ directory
-for file in sorted(os.listdir(os.path.dirname(__file__))):
- if file.endswith(".py") and not file.startswith("_"):
- module = file[: file.find(".py")]
- importlib.import_module("examples.simultaneous_translation.utils." + module)
diff --git a/spaces/ICML2023/ICML2023_papers/paper_list.py b/spaces/ICML2023/ICML2023_papers/paper_list.py
deleted file mode 100644
index 46729cc444467dc0a2fac57a71374bad46f5bc26..0000000000000000000000000000000000000000
--- a/spaces/ICML2023/ICML2023_papers/paper_list.py
+++ /dev/null
@@ -1,108 +0,0 @@
-from __future__ import annotations
-
-import numpy as np
-import pandas as pd
-
-
-class PaperList:
- def __init__(self):
- self.organization_name = 'ICML2023'
- self.table = pd.read_csv('papers.csv')
- self._preprocess_table()
-
- self.table_header = '''
-
-
Title
-
Authors
-
arXiv
-
GitHub
-
Paper pages
-
Spaces
-
Models
-
Datasets
-
Claimed
-
'''
-
- def _preprocess_table(self) -> None:
- self.table['title_lowercase'] = self.table.title.str.lower()
-
- rows = []
- for row in self.table.itertuples():
- title = f'{row.title}'
- arxiv = f'arXiv' if isinstance(
- row.arxiv, str) else ''
- github = f'GitHub' if isinstance(
- row.github, str) else ''
- hf_paper = f'Paper page' if isinstance(
- row.hf_paper, str) else ''
- hf_space = f'Space' if isinstance(
- row.hf_space, str) else ''
- hf_model = f'Model' if isinstance(
- row.hf_model, str) else ''
- hf_dataset = f'Dataset' if isinstance(
- row.hf_dataset, str) else ''
- author_linked = '✅' if ~np.isnan(
- row.n_linked_authors) and row.n_linked_authors > 0 else ''
- n_linked_authors = '' if np.isnan(row.n_linked_authors) else int(
- row.n_linked_authors)
- n_authors = '' if np.isnan(row.n_authors) else int(row.n_authors)
- claimed_paper = '' if n_linked_authors == '' else f'{n_linked_authors}/{n_authors} {author_linked}'
- row = f'''
-
-"""
- for key, text in items.items():
- info += f"""
-
-
{plaintext_to_html(str(key))}
-
{plaintext_to_html(str(text))}
-
-""".strip()+"\n"
-
- if len(info) == 0:
- message = "Nothing found in the image."
- info = f"
{message}
"
-
- return (a,c,res,info)
-
-
-def main():
- args = parse_args()
- model = load_model()
- labels = load_labels()
-
- func = functools.partial(predict, model=model, labels=labels)
- func = functools.update_wrapper(func, predict)
-
- gr.Interface(
- func,
- [
- gr.inputs.Image(type='pil', label='Input'),
- gr.inputs.Slider(0,
- 1,
- step=args.score_slider_step,
- default=args.score_threshold,
- label='Score Threshold'),
- ],
- [
- gr.outputs.Textbox(label='Output (string)'),
- gr.outputs.Textbox(label='Output (raw string)'),
- gr.outputs.Label(label='Output (label)'),
- gr.outputs.HTML()
- ],
- examples=[
- ['miku.jpg',0.5],
- ['miku2.jpg',0.5]
- ],
- title=TITLE,
- description='''
-Demo for [KichangKim/DeepDanbooru](https://github.com/KichangKim/DeepDanbooru) with "ready to copy" prompt and a prompt analyzer.
-
-Modified from [hysts/DeepDanbooru](https://huggingface.co/spaces/hysts/DeepDanbooru)
-
-PNG Info code forked from [AUTOMATIC1111/stable-diffusion-webui](https://github.com/AUTOMATIC1111/stable-diffusion-webui)
- ''',
- theme=args.theme,
- allow_flagging=args.allow_flagging,
- live=args.live,
- ).launch(
- enable_queue=args.enable_queue,
- server_port=args.port,
- share=args.share,
- )
-
-
-if __name__ == '__main__':
- main()
diff --git a/spaces/abdvl/datahub_qa_bot/docs/managed-datahub/saas-slack-setup.md b/spaces/abdvl/datahub_qa_bot/docs/managed-datahub/saas-slack-setup.md
deleted file mode 100644
index 68f947f1717158a7be254101ae6191e56f65240e..0000000000000000000000000000000000000000
--- a/spaces/abdvl/datahub_qa_bot/docs/managed-datahub/saas-slack-setup.md
+++ /dev/null
@@ -1,111 +0,0 @@
-import FeatureAvailability from '@site/src/components/FeatureAvailability';
-
-# Configure Slack Notifications
-
-
-
-## Install the DataHub Slack App into your Slack workspace
-
-The following steps should be performed by a Slack Workspace Admin.
-- Navigate to https://api.slack.com/apps/
-- Click Create New App
-- Use “From an app manifest” option
-- Select your workspace
-- Paste this Manifest in YAML. Suggest changing name and `display_name` to be `DataHub App YOUR_TEAM_NAME` but not required. This name will show up in your slack workspace
-```yml
-display_information:
- name: DataHub App
- description: An app to integrate DataHub with Slack
- background_color: "#000000"
-features:
- bot_user:
- display_name: DataHub App
- always_online: false
-oauth_config:
- scopes:
- bot:
- - channels:read
- - chat:write
- - commands
- - groups:read
- - im:read
- - mpim:read
- - team:read
- - users:read
- - users:read.email
-settings:
- org_deploy_enabled: false
- socket_mode_enabled: false
- token_rotation_enabled: false
-```
-
-Confirm you see the Basic Information Tab
-
-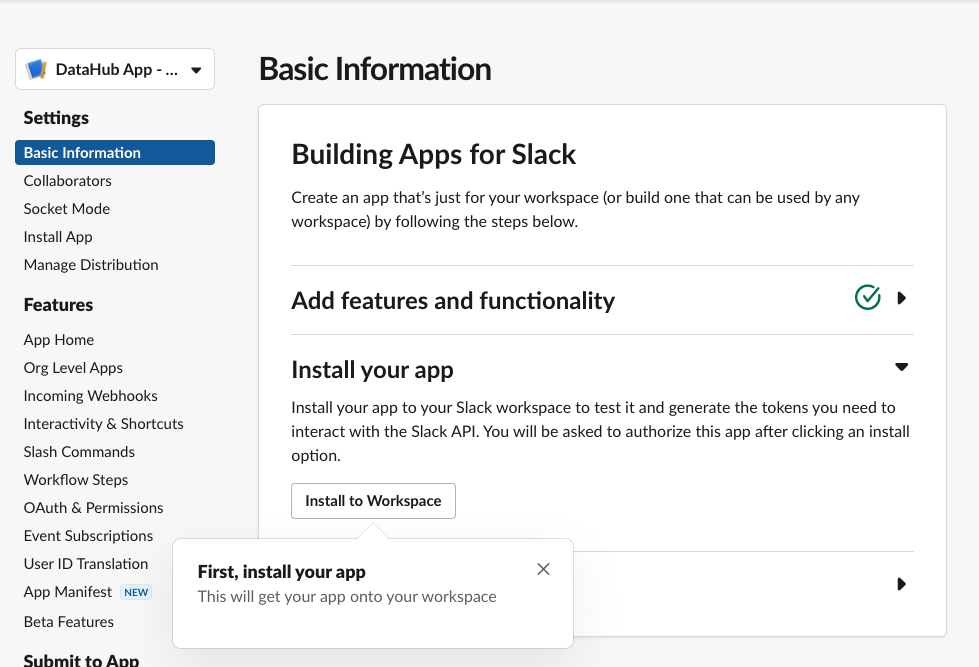
-
-- Click **Install to Workspace**
-- It will show you permissions the Slack App is asking for, what they mean and a default channel in which you want to add the slack app
- - Note that the Slack App will only be able to post in channels that the app has been added to. This is made clear by slack’s Authentication screen also.
-- Select the channel you'd like notifications to go to and click **Allow**
-- Go to DataHub App page
- - You can find your workspace's list of apps at https://api.slack.com/apps/
-
-## Generating a Bot Token
-
-- Go to **OAuth & Permissions** Tab
-
-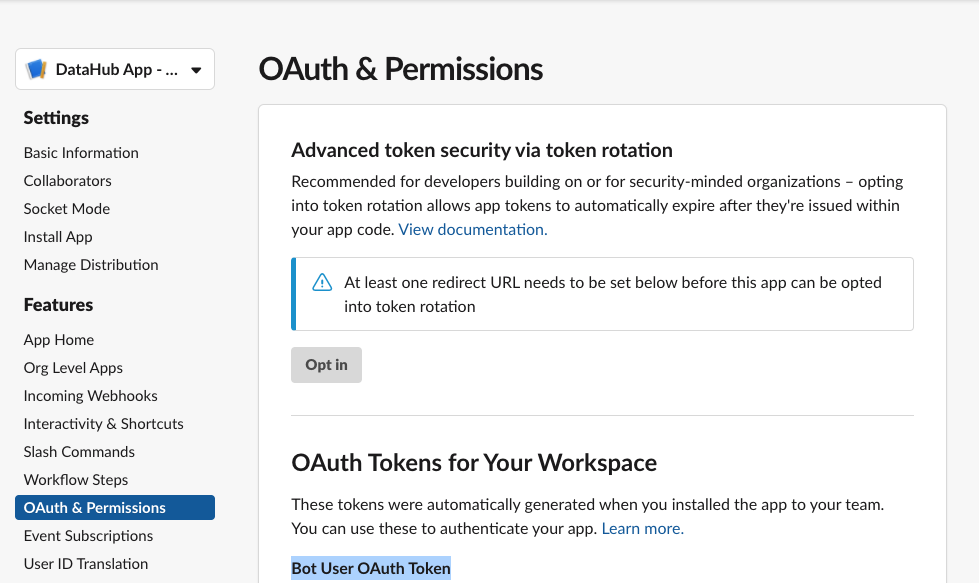
-
-Here you'll find a “Bot User OAuth Token” which DataHub will need to communicate with your slack through the bot.
-In the next steps, we'll show you how to configure the Slack Integration inside of Acryl DataHub.
-
-## Configuring Notifications
-
-> In order to set up the Slack integration, the user must have the `Manage Platform Settings` privilege.
-
-To enable the integration with slack
-- Navigate to **Settings > Integrations**
-- Click **Slack**
-- Enable the Integration
-- Enter the **Bot Token** obtained in the previous steps
-- Enter a **Default Slack Channel** - this is where all notifications will be routed unless
-- Click **Update** to save your settings
-
-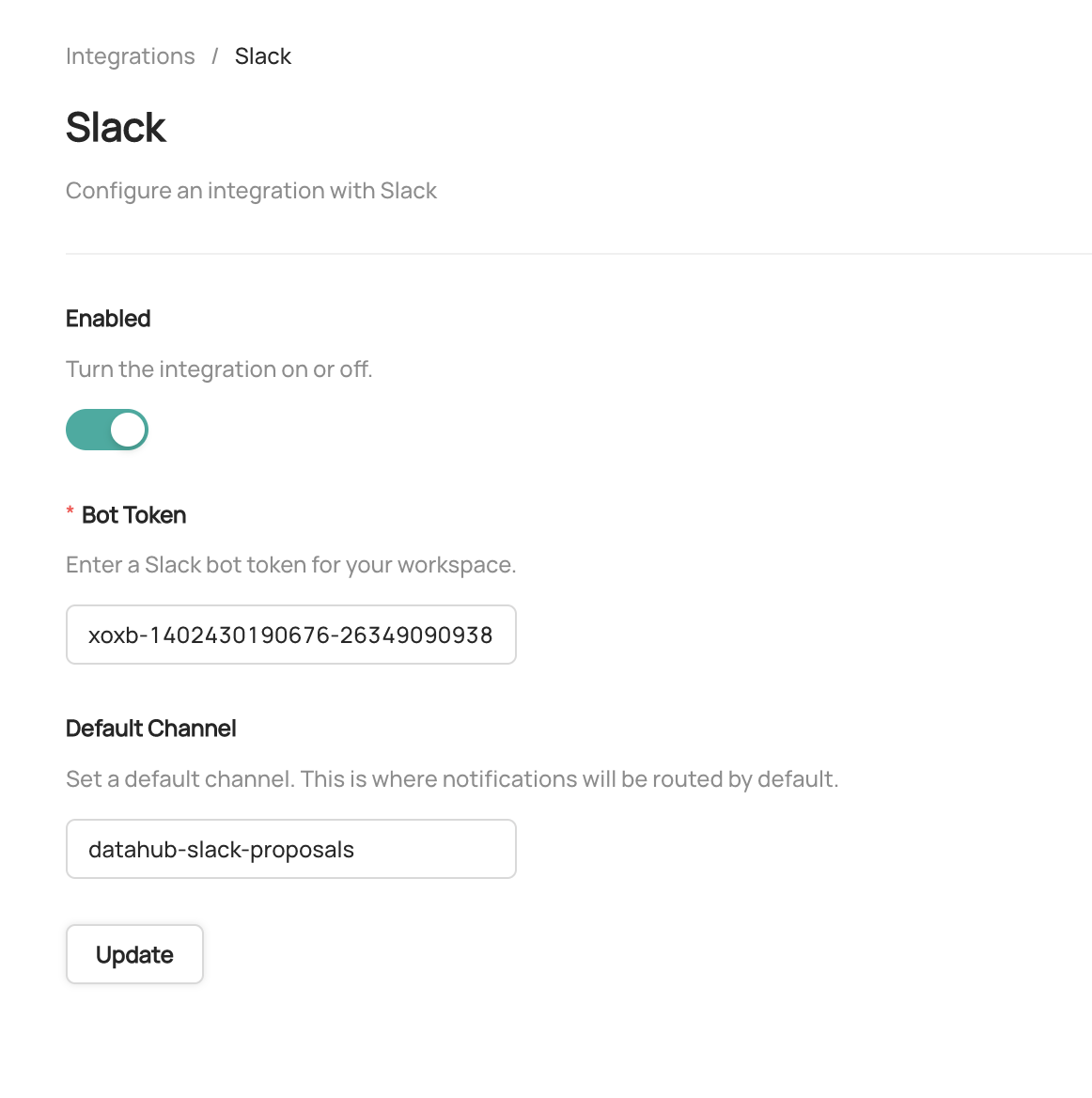
-
-To enable and disable specific types of notifications, or configure custom routing for notifications, start by navigating to **Settings > Notifications**.
-To enable or disable a specific notification type in Slack, simply click the check mark. By default, all notification types are enabled.
-To customize the channel where notifications are send, click the button to the right of the check box.
-
-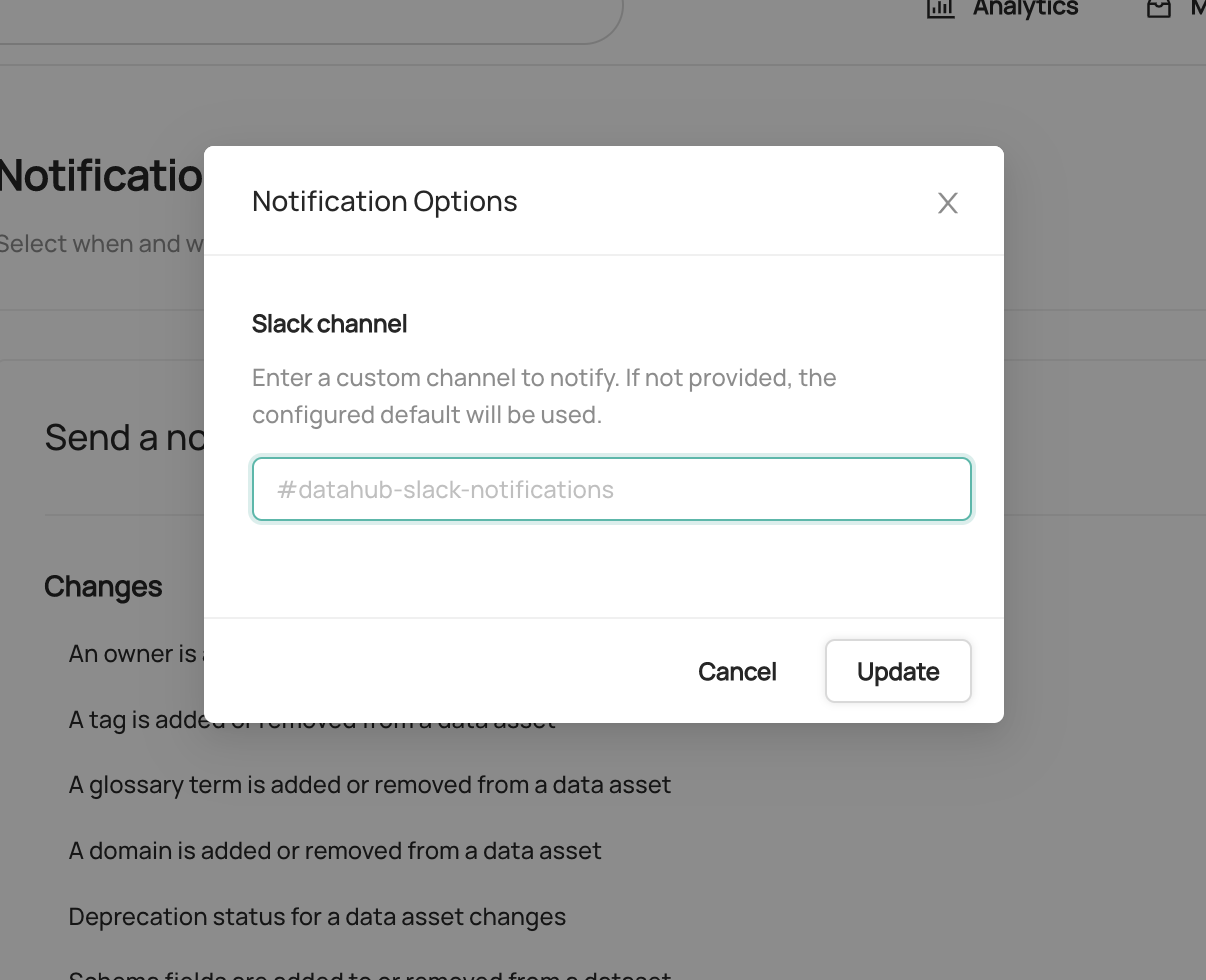
-
-If provided, a custom channel will be used to route notifications of the given type. If not provided, the default channel will be used.
-That's it! You should begin to receive notifications on Slack. Note that it may take up to 1 minute for notification settings to take effect after saving.
-
-## Sending Notifications
-
-For now we support sending notifications to
-- Slack Channel ID (e.g. `C029A3M079U`)
-- Slack Channel Name (e.g. `#troubleshoot`)
-- Specific Users (aka Direct Messages or DMs) via user ID
-
-## How to find Team ID and Channel ID in Slack
-
-- Go to the Slack channel for which you want to get channel ID
-- Check the URL e.g. for the troubleshoot channel in OSS DataHub slack
-
-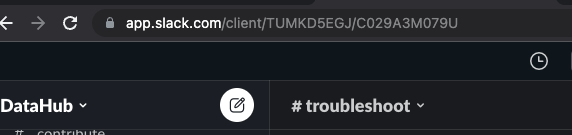
-
-- Notice `TUMKD5EGJ/C029A3M079U` in the URL
- - Team ID = `TUMKD5EGJ` from above
- - Channel ID = `C029A3M079U` from above
-
-## How to find User ID in Slack
-
-- Go to user DM
-- Click on their profile picture
-- Click on View Full Profile
-- Click on “More”
-- Click on “Copy member ID”
-
-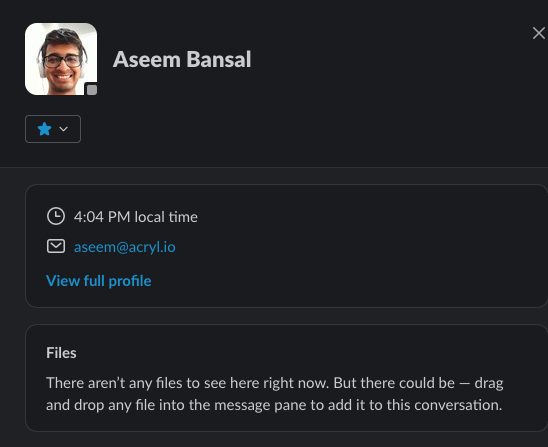
diff --git a/spaces/abhaskumarsinha/MinimalGPT-Felis_Catus/subword/subword_nmt.py b/spaces/abhaskumarsinha/MinimalGPT-Felis_Catus/subword/subword_nmt.py
deleted file mode 100644
index 29104f4d8029524a80d6fa649b69a8acec0b8abc..0000000000000000000000000000000000000000
--- a/spaces/abhaskumarsinha/MinimalGPT-Felis_Catus/subword/subword_nmt.py
+++ /dev/null
@@ -1,97 +0,0 @@
-#!/usr/bin/env python
-# -*- coding: utf-8 -*-
-
-import io
-import sys
-import codecs
-import argparse
-
-from .learn_bpe import learn_bpe
-from .apply_bpe import BPE, read_vocabulary
-from .get_vocab import get_vocab
-from .learn_joint_bpe_and_vocab import learn_joint_bpe_and_vocab
-
-from .learn_bpe import create_parser as create_learn_bpe_parser
-from .apply_bpe import create_parser as create_apply_bpe_parser
-from .get_vocab import create_parser as create_get_vocab_parser
-from .learn_joint_bpe_and_vocab import create_parser as create_learn_joint_bpe_and_vocab_parser
-
-# hack for python2/3 compatibility
-argparse.open = io.open
-
-def main():
- parser = argparse.ArgumentParser(
- formatter_class=argparse.RawTextHelpFormatter,
- description="subword-nmt: unsupervised word segmentation for neural machine translation and text generation ")
- subparsers = parser.add_subparsers(dest='command',
- help="""command to run. Run one of the commands with '-h' for more info.
-
-learn-bpe: learn BPE merge operations on input text.
-apply-bpe: apply given BPE operations to input text.
-get-vocab: extract vocabulary and word frequencies from input text.
-learn-joint-bpe-and-vocab: executes recommended workflow for joint BPE.""")
-
- learn_bpe_parser = create_learn_bpe_parser(subparsers)
- apply_bpe_parser = create_apply_bpe_parser(subparsers)
- get_vocab_parser = create_get_vocab_parser(subparsers)
- learn_joint_bpe_and_vocab_parser = create_learn_joint_bpe_and_vocab_parser(subparsers)
-
- args = parser.parse_args()
-
- if args.command == 'learn-bpe':
- # read/write files as UTF-8
- if args.input.name != '':
- args.input = codecs.open(args.input.name, encoding='utf-8')
- if args.output.name != '':
- args.output = codecs.open(args.output.name, 'w', encoding='utf-8')
-
- learn_bpe(args.input, args.output, args.symbols, args.min_frequency, args.verbose,
- is_dict=args.dict_input, total_symbols=args.total_symbols)
- elif args.command == 'apply-bpe':
- # read/write files as UTF-8
- args.codes = codecs.open(args.codes.name, encoding='utf-8')
- if args.input.name != '':
- args.input = codecs.open(args.input.name, encoding='utf-8')
- if args.output.name != '':
- args.output = codecs.open(args.output.name, 'w', encoding='utf-8')
- if args.vocabulary:
- args.vocabulary = codecs.open(args.vocabulary.name, encoding='utf-8')
-
- if args.vocabulary:
- vocabulary = read_vocabulary(args.vocabulary, args.vocabulary_threshold)
- else:
- vocabulary = None
-
- if sys.version_info < (3, 0):
- args.separator = args.separator.decode('UTF-8')
- if args.glossaries:
- args.glossaries = [g.decode('UTF-8') for g in args.glossaries]
-
- bpe = BPE(args.codes, args.merges, args.separator, vocabulary, args.glossaries)
-
- for line in args.input:
- args.output.write(bpe.process_line(line, args.dropout))
-
- elif args.command == 'get-vocab':
- if args.input.name != '':
- args.input = codecs.open(args.input.name, encoding='utf-8')
- if args.output.name != '':
- args.output = codecs.open(args.output.name, 'w', encoding='utf-8')
- get_vocab(args.input, args.output)
- elif args.command == 'learn-joint-bpe-and-vocab':
- learn_joint_bpe_and_vocab(args)
- if sys.version_info < (3, 0):
- args.separator = args.separator.decode('UTF-8')
- else:
- raise Exception('Invalid command provided')
-
-
-# python 2/3 compatibility
-if sys.version_info < (3, 0):
- sys.stderr = codecs.getwriter('UTF-8')(sys.stderr)
- sys.stdout = codecs.getwriter('UTF-8')(sys.stdout)
- sys.stdin = codecs.getreader('UTF-8')(sys.stdin)
-else:
- sys.stderr = codecs.getwriter('UTF-8')(sys.stderr.buffer)
- sys.stdout = codecs.getwriter('UTF-8')(sys.stdout.buffer)
- sys.stdin = codecs.getreader('UTF-8')(sys.stdin.buffer)
diff --git a/spaces/abhishek/sketch-to-image/annotator/uniformer/mmdet/models/backbones/resnet.py b/spaces/abhishek/sketch-to-image/annotator/uniformer/mmdet/models/backbones/resnet.py
deleted file mode 100644
index 3826815a6d94fdc4c54001d4c186d10ca3380e80..0000000000000000000000000000000000000000
--- a/spaces/abhishek/sketch-to-image/annotator/uniformer/mmdet/models/backbones/resnet.py
+++ /dev/null
@@ -1,663 +0,0 @@
-import torch.nn as nn
-import torch.utils.checkpoint as cp
-from mmcv.cnn import (build_conv_layer, build_norm_layer, build_plugin_layer,
- constant_init, kaiming_init)
-from mmcv.runner import load_checkpoint
-from torch.nn.modules.batchnorm import _BatchNorm
-
-from mmdet.utils import get_root_logger
-from ..builder import BACKBONES
-from ..utils import ResLayer
-
-
-class BasicBlock(nn.Module):
- expansion = 1
-
- def __init__(self,
- inplanes,
- planes,
- stride=1,
- dilation=1,
- downsample=None,
- style='pytorch',
- with_cp=False,
- conv_cfg=None,
- norm_cfg=dict(type='BN'),
- dcn=None,
- plugins=None):
- super(BasicBlock, self).__init__()
- assert dcn is None, 'Not implemented yet.'
- assert plugins is None, 'Not implemented yet.'
-
- self.norm1_name, norm1 = build_norm_layer(norm_cfg, planes, postfix=1)
- self.norm2_name, norm2 = build_norm_layer(norm_cfg, planes, postfix=2)
-
- self.conv1 = build_conv_layer(
- conv_cfg,
- inplanes,
- planes,
- 3,
- stride=stride,
- padding=dilation,
- dilation=dilation,
- bias=False)
- self.add_module(self.norm1_name, norm1)
- self.conv2 = build_conv_layer(
- conv_cfg, planes, planes, 3, padding=1, bias=False)
- self.add_module(self.norm2_name, norm2)
-
- self.relu = nn.ReLU(inplace=True)
- self.downsample = downsample
- self.stride = stride
- self.dilation = dilation
- self.with_cp = with_cp
-
- @property
- def norm1(self):
- """nn.Module: normalization layer after the first convolution layer"""
- return getattr(self, self.norm1_name)
-
- @property
- def norm2(self):
- """nn.Module: normalization layer after the second convolution layer"""
- return getattr(self, self.norm2_name)
-
- def forward(self, x):
- """Forward function."""
-
- def _inner_forward(x):
- identity = x
-
- out = self.conv1(x)
- out = self.norm1(out)
- out = self.relu(out)
-
- out = self.conv2(out)
- out = self.norm2(out)
-
- if self.downsample is not None:
- identity = self.downsample(x)
-
- out += identity
-
- return out
-
- if self.with_cp and x.requires_grad:
- out = cp.checkpoint(_inner_forward, x)
- else:
- out = _inner_forward(x)
-
- out = self.relu(out)
-
- return out
-
-
-class Bottleneck(nn.Module):
- expansion = 4
-
- def __init__(self,
- inplanes,
- planes,
- stride=1,
- dilation=1,
- downsample=None,
- style='pytorch',
- with_cp=False,
- conv_cfg=None,
- norm_cfg=dict(type='BN'),
- dcn=None,
- plugins=None):
- """Bottleneck block for ResNet.
-
- If style is "pytorch", the stride-two layer is the 3x3 conv layer, if
- it is "caffe", the stride-two layer is the first 1x1 conv layer.
- """
- super(Bottleneck, self).__init__()
- assert style in ['pytorch', 'caffe']
- assert dcn is None or isinstance(dcn, dict)
- assert plugins is None or isinstance(plugins, list)
- if plugins is not None:
- allowed_position = ['after_conv1', 'after_conv2', 'after_conv3']
- assert all(p['position'] in allowed_position for p in plugins)
-
- self.inplanes = inplanes
- self.planes = planes
- self.stride = stride
- self.dilation = dilation
- self.style = style
- self.with_cp = with_cp
- self.conv_cfg = conv_cfg
- self.norm_cfg = norm_cfg
- self.dcn = dcn
- self.with_dcn = dcn is not None
- self.plugins = plugins
- self.with_plugins = plugins is not None
-
- if self.with_plugins:
- # collect plugins for conv1/conv2/conv3
- self.after_conv1_plugins = [
- plugin['cfg'] for plugin in plugins
- if plugin['position'] == 'after_conv1'
- ]
- self.after_conv2_plugins = [
- plugin['cfg'] for plugin in plugins
- if plugin['position'] == 'after_conv2'
- ]
- self.after_conv3_plugins = [
- plugin['cfg'] for plugin in plugins
- if plugin['position'] == 'after_conv3'
- ]
-
- if self.style == 'pytorch':
- self.conv1_stride = 1
- self.conv2_stride = stride
- else:
- self.conv1_stride = stride
- self.conv2_stride = 1
-
- self.norm1_name, norm1 = build_norm_layer(norm_cfg, planes, postfix=1)
- self.norm2_name, norm2 = build_norm_layer(norm_cfg, planes, postfix=2)
- self.norm3_name, norm3 = build_norm_layer(
- norm_cfg, planes * self.expansion, postfix=3)
-
- self.conv1 = build_conv_layer(
- conv_cfg,
- inplanes,
- planes,
- kernel_size=1,
- stride=self.conv1_stride,
- bias=False)
- self.add_module(self.norm1_name, norm1)
- fallback_on_stride = False
- if self.with_dcn:
- fallback_on_stride = dcn.pop('fallback_on_stride', False)
- if not self.with_dcn or fallback_on_stride:
- self.conv2 = build_conv_layer(
- conv_cfg,
- planes,
- planes,
- kernel_size=3,
- stride=self.conv2_stride,
- padding=dilation,
- dilation=dilation,
- bias=False)
- else:
- assert self.conv_cfg is None, 'conv_cfg must be None for DCN'
- self.conv2 = build_conv_layer(
- dcn,
- planes,
- planes,
- kernel_size=3,
- stride=self.conv2_stride,
- padding=dilation,
- dilation=dilation,
- bias=False)
-
- self.add_module(self.norm2_name, norm2)
- self.conv3 = build_conv_layer(
- conv_cfg,
- planes,
- planes * self.expansion,
- kernel_size=1,
- bias=False)
- self.add_module(self.norm3_name, norm3)
-
- self.relu = nn.ReLU(inplace=True)
- self.downsample = downsample
-
- if self.with_plugins:
- self.after_conv1_plugin_names = self.make_block_plugins(
- planes, self.after_conv1_plugins)
- self.after_conv2_plugin_names = self.make_block_plugins(
- planes, self.after_conv2_plugins)
- self.after_conv3_plugin_names = self.make_block_plugins(
- planes * self.expansion, self.after_conv3_plugins)
-
- def make_block_plugins(self, in_channels, plugins):
- """make plugins for block.
-
- Args:
- in_channels (int): Input channels of plugin.
- plugins (list[dict]): List of plugins cfg to build.
-
- Returns:
- list[str]: List of the names of plugin.
- """
- assert isinstance(plugins, list)
- plugin_names = []
- for plugin in plugins:
- plugin = plugin.copy()
- name, layer = build_plugin_layer(
- plugin,
- in_channels=in_channels,
- postfix=plugin.pop('postfix', ''))
- assert not hasattr(self, name), f'duplicate plugin {name}'
- self.add_module(name, layer)
- plugin_names.append(name)
- return plugin_names
-
- def forward_plugin(self, x, plugin_names):
- out = x
- for name in plugin_names:
- out = getattr(self, name)(x)
- return out
-
- @property
- def norm1(self):
- """nn.Module: normalization layer after the first convolution layer"""
- return getattr(self, self.norm1_name)
-
- @property
- def norm2(self):
- """nn.Module: normalization layer after the second convolution layer"""
- return getattr(self, self.norm2_name)
-
- @property
- def norm3(self):
- """nn.Module: normalization layer after the third convolution layer"""
- return getattr(self, self.norm3_name)
-
- def forward(self, x):
- """Forward function."""
-
- def _inner_forward(x):
- identity = x
- out = self.conv1(x)
- out = self.norm1(out)
- out = self.relu(out)
-
- if self.with_plugins:
- out = self.forward_plugin(out, self.after_conv1_plugin_names)
-
- out = self.conv2(out)
- out = self.norm2(out)
- out = self.relu(out)
-
- if self.with_plugins:
- out = self.forward_plugin(out, self.after_conv2_plugin_names)
-
- out = self.conv3(out)
- out = self.norm3(out)
-
- if self.with_plugins:
- out = self.forward_plugin(out, self.after_conv3_plugin_names)
-
- if self.downsample is not None:
- identity = self.downsample(x)
-
- out += identity
-
- return out
-
- if self.with_cp and x.requires_grad:
- out = cp.checkpoint(_inner_forward, x)
- else:
- out = _inner_forward(x)
-
- out = self.relu(out)
-
- return out
-
-
-@BACKBONES.register_module()
-class ResNet(nn.Module):
- """ResNet backbone.
-
- Args:
- depth (int): Depth of resnet, from {18, 34, 50, 101, 152}.
- stem_channels (int | None): Number of stem channels. If not specified,
- it will be the same as `base_channels`. Default: None.
- base_channels (int): Number of base channels of res layer. Default: 64.
- in_channels (int): Number of input image channels. Default: 3.
- num_stages (int): Resnet stages. Default: 4.
- strides (Sequence[int]): Strides of the first block of each stage.
- dilations (Sequence[int]): Dilation of each stage.
- out_indices (Sequence[int]): Output from which stages.
- style (str): `pytorch` or `caffe`. If set to "pytorch", the stride-two
- layer is the 3x3 conv layer, otherwise the stride-two layer is
- the first 1x1 conv layer.
- deep_stem (bool): Replace 7x7 conv in input stem with 3 3x3 conv
- avg_down (bool): Use AvgPool instead of stride conv when
- downsampling in the bottleneck.
- frozen_stages (int): Stages to be frozen (stop grad and set eval mode).
- -1 means not freezing any parameters.
- norm_cfg (dict): Dictionary to construct and config norm layer.
- norm_eval (bool): Whether to set norm layers to eval mode, namely,
- freeze running stats (mean and var). Note: Effect on Batch Norm
- and its variants only.
- plugins (list[dict]): List of plugins for stages, each dict contains:
-
- - cfg (dict, required): Cfg dict to build plugin.
- - position (str, required): Position inside block to insert
- plugin, options are 'after_conv1', 'after_conv2', 'after_conv3'.
- - stages (tuple[bool], optional): Stages to apply plugin, length
- should be same as 'num_stages'.
- with_cp (bool): Use checkpoint or not. Using checkpoint will save some
- memory while slowing down the training speed.
- zero_init_residual (bool): Whether to use zero init for last norm layer
- in resblocks to let them behave as identity.
-
- Example:
- >>> from mmdet.models import ResNet
- >>> import torch
- >>> self = ResNet(depth=18)
- >>> self.eval()
- >>> inputs = torch.rand(1, 3, 32, 32)
- >>> level_outputs = self.forward(inputs)
- >>> for level_out in level_outputs:
- ... print(tuple(level_out.shape))
- (1, 64, 8, 8)
- (1, 128, 4, 4)
- (1, 256, 2, 2)
- (1, 512, 1, 1)
- """
-
- arch_settings = {
- 18: (BasicBlock, (2, 2, 2, 2)),
- 34: (BasicBlock, (3, 4, 6, 3)),
- 50: (Bottleneck, (3, 4, 6, 3)),
- 101: (Bottleneck, (3, 4, 23, 3)),
- 152: (Bottleneck, (3, 8, 36, 3))
- }
-
- def __init__(self,
- depth,
- in_channels=3,
- stem_channels=None,
- base_channels=64,
- num_stages=4,
- strides=(1, 2, 2, 2),
- dilations=(1, 1, 1, 1),
- out_indices=(0, 1, 2, 3),
- style='pytorch',
- deep_stem=False,
- avg_down=False,
- frozen_stages=-1,
- conv_cfg=None,
- norm_cfg=dict(type='BN', requires_grad=True),
- norm_eval=True,
- dcn=None,
- stage_with_dcn=(False, False, False, False),
- plugins=None,
- with_cp=False,
- zero_init_residual=True):
- super(ResNet, self).__init__()
- if depth not in self.arch_settings:
- raise KeyError(f'invalid depth {depth} for resnet')
- self.depth = depth
- if stem_channels is None:
- stem_channels = base_channels
- self.stem_channels = stem_channels
- self.base_channels = base_channels
- self.num_stages = num_stages
- assert num_stages >= 1 and num_stages <= 4
- self.strides = strides
- self.dilations = dilations
- assert len(strides) == len(dilations) == num_stages
- self.out_indices = out_indices
- assert max(out_indices) < num_stages
- self.style = style
- self.deep_stem = deep_stem
- self.avg_down = avg_down
- self.frozen_stages = frozen_stages
- self.conv_cfg = conv_cfg
- self.norm_cfg = norm_cfg
- self.with_cp = with_cp
- self.norm_eval = norm_eval
- self.dcn = dcn
- self.stage_with_dcn = stage_with_dcn
- if dcn is not None:
- assert len(stage_with_dcn) == num_stages
- self.plugins = plugins
- self.zero_init_residual = zero_init_residual
- self.block, stage_blocks = self.arch_settings[depth]
- self.stage_blocks = stage_blocks[:num_stages]
- self.inplanes = stem_channels
-
- self._make_stem_layer(in_channels, stem_channels)
-
- self.res_layers = []
- for i, num_blocks in enumerate(self.stage_blocks):
- stride = strides[i]
- dilation = dilations[i]
- dcn = self.dcn if self.stage_with_dcn[i] else None
- if plugins is not None:
- stage_plugins = self.make_stage_plugins(plugins, i)
- else:
- stage_plugins = None
- planes = base_channels * 2**i
- res_layer = self.make_res_layer(
- block=self.block,
- inplanes=self.inplanes,
- planes=planes,
- num_blocks=num_blocks,
- stride=stride,
- dilation=dilation,
- style=self.style,
- avg_down=self.avg_down,
- with_cp=with_cp,
- conv_cfg=conv_cfg,
- norm_cfg=norm_cfg,
- dcn=dcn,
- plugins=stage_plugins)
- self.inplanes = planes * self.block.expansion
- layer_name = f'layer{i + 1}'
- self.add_module(layer_name, res_layer)
- self.res_layers.append(layer_name)
-
- self._freeze_stages()
-
- self.feat_dim = self.block.expansion * base_channels * 2**(
- len(self.stage_blocks) - 1)
-
- def make_stage_plugins(self, plugins, stage_idx):
- """Make plugins for ResNet ``stage_idx`` th stage.
-
- Currently we support to insert ``context_block``,
- ``empirical_attention_block``, ``nonlocal_block`` into the backbone
- like ResNet/ResNeXt. They could be inserted after conv1/conv2/conv3 of
- Bottleneck.
-
- An example of plugins format could be:
-
- Examples:
- >>> plugins=[
- ... dict(cfg=dict(type='xxx', arg1='xxx'),
- ... stages=(False, True, True, True),
- ... position='after_conv2'),
- ... dict(cfg=dict(type='yyy'),
- ... stages=(True, True, True, True),
- ... position='after_conv3'),
- ... dict(cfg=dict(type='zzz', postfix='1'),
- ... stages=(True, True, True, True),
- ... position='after_conv3'),
- ... dict(cfg=dict(type='zzz', postfix='2'),
- ... stages=(True, True, True, True),
- ... position='after_conv3')
- ... ]
- >>> self = ResNet(depth=18)
- >>> stage_plugins = self.make_stage_plugins(plugins, 0)
- >>> assert len(stage_plugins) == 3
-
- Suppose ``stage_idx=0``, the structure of blocks in the stage would be:
-
- .. code-block:: none
-
- conv1-> conv2->conv3->yyy->zzz1->zzz2
-
- Suppose 'stage_idx=1', the structure of blocks in the stage would be:
-
- .. code-block:: none
-
- conv1-> conv2->xxx->conv3->yyy->zzz1->zzz2
-
- If stages is missing, the plugin would be applied to all stages.
-
- Args:
- plugins (list[dict]): List of plugins cfg to build. The postfix is
- required if multiple same type plugins are inserted.
- stage_idx (int): Index of stage to build
-
- Returns:
- list[dict]: Plugins for current stage
- """
- stage_plugins = []
- for plugin in plugins:
- plugin = plugin.copy()
- stages = plugin.pop('stages', None)
- assert stages is None or len(stages) == self.num_stages
- # whether to insert plugin into current stage
- if stages is None or stages[stage_idx]:
- stage_plugins.append(plugin)
-
- return stage_plugins
-
- def make_res_layer(self, **kwargs):
- """Pack all blocks in a stage into a ``ResLayer``."""
- return ResLayer(**kwargs)
-
- @property
- def norm1(self):
- """nn.Module: the normalization layer named "norm1" """
- return getattr(self, self.norm1_name)
-
- def _make_stem_layer(self, in_channels, stem_channels):
- if self.deep_stem:
- self.stem = nn.Sequential(
- build_conv_layer(
- self.conv_cfg,
- in_channels,
- stem_channels // 2,
- kernel_size=3,
- stride=2,
- padding=1,
- bias=False),
- build_norm_layer(self.norm_cfg, stem_channels // 2)[1],
- nn.ReLU(inplace=True),
- build_conv_layer(
- self.conv_cfg,
- stem_channels // 2,
- stem_channels // 2,
- kernel_size=3,
- stride=1,
- padding=1,
- bias=False),
- build_norm_layer(self.norm_cfg, stem_channels // 2)[1],
- nn.ReLU(inplace=True),
- build_conv_layer(
- self.conv_cfg,
- stem_channels // 2,
- stem_channels,
- kernel_size=3,
- stride=1,
- padding=1,
- bias=False),
- build_norm_layer(self.norm_cfg, stem_channels)[1],
- nn.ReLU(inplace=True))
- else:
- self.conv1 = build_conv_layer(
- self.conv_cfg,
- in_channels,
- stem_channels,
- kernel_size=7,
- stride=2,
- padding=3,
- bias=False)
- self.norm1_name, norm1 = build_norm_layer(
- self.norm_cfg, stem_channels, postfix=1)
- self.add_module(self.norm1_name, norm1)
- self.relu = nn.ReLU(inplace=True)
- self.maxpool = nn.MaxPool2d(kernel_size=3, stride=2, padding=1)
-
- def _freeze_stages(self):
- if self.frozen_stages >= 0:
- if self.deep_stem:
- self.stem.eval()
- for param in self.stem.parameters():
- param.requires_grad = False
- else:
- self.norm1.eval()
- for m in [self.conv1, self.norm1]:
- for param in m.parameters():
- param.requires_grad = False
-
- for i in range(1, self.frozen_stages + 1):
- m = getattr(self, f'layer{i}')
- m.eval()
- for param in m.parameters():
- param.requires_grad = False
-
- def init_weights(self, pretrained=None):
- """Initialize the weights in backbone.
-
- Args:
- pretrained (str, optional): Path to pre-trained weights.
- Defaults to None.
- """
- if isinstance(pretrained, str):
- logger = get_root_logger()
- load_checkpoint(self, pretrained, strict=False, logger=logger)
- elif pretrained is None:
- for m in self.modules():
- if isinstance(m, nn.Conv2d):
- kaiming_init(m)
- elif isinstance(m, (_BatchNorm, nn.GroupNorm)):
- constant_init(m, 1)
-
- if self.dcn is not None:
- for m in self.modules():
- if isinstance(m, Bottleneck) and hasattr(
- m.conv2, 'conv_offset'):
- constant_init(m.conv2.conv_offset, 0)
-
- if self.zero_init_residual:
- for m in self.modules():
- if isinstance(m, Bottleneck):
- constant_init(m.norm3, 0)
- elif isinstance(m, BasicBlock):
- constant_init(m.norm2, 0)
- else:
- raise TypeError('pretrained must be a str or None')
-
- def forward(self, x):
- """Forward function."""
- if self.deep_stem:
- x = self.stem(x)
- else:
- x = self.conv1(x)
- x = self.norm1(x)
- x = self.relu(x)
- x = self.maxpool(x)
- outs = []
- for i, layer_name in enumerate(self.res_layers):
- res_layer = getattr(self, layer_name)
- x = res_layer(x)
- if i in self.out_indices:
- outs.append(x)
- return tuple(outs)
-
- def train(self, mode=True):
- """Convert the model into training mode while keep normalization layer
- freezed."""
- super(ResNet, self).train(mode)
- self._freeze_stages()
- if mode and self.norm_eval:
- for m in self.modules():
- # trick: eval have effect on BatchNorm only
- if isinstance(m, _BatchNorm):
- m.eval()
-
-
-@BACKBONES.register_module()
-class ResNetV1d(ResNet):
- r"""ResNetV1d variant described in `Bag of Tricks
- `_.
-
- Compared with default ResNet(ResNetV1b), ResNetV1d replaces the 7x7 conv in
- the input stem with three 3x3 convs. And in the downsampling block, a 2x2
- avg_pool with stride 2 is added before conv, whose stride is changed to 1.
- """
-
- def __init__(self, **kwargs):
- super(ResNetV1d, self).__init__(
- deep_stem=True, avg_down=True, **kwargs)
diff --git a/spaces/abhishek/sketch-to-image/annotator/uniformer/mmseg/models/decode_heads/dnl_head.py b/spaces/abhishek/sketch-to-image/annotator/uniformer/mmseg/models/decode_heads/dnl_head.py
deleted file mode 100644
index 333280c5947066fd3c7ebcfe302a0e7ad65480d5..0000000000000000000000000000000000000000
--- a/spaces/abhishek/sketch-to-image/annotator/uniformer/mmseg/models/decode_heads/dnl_head.py
+++ /dev/null
@@ -1,131 +0,0 @@
-import torch
-from annotator.uniformer.mmcv.cnn import NonLocal2d
-from torch import nn
-
-from ..builder import HEADS
-from .fcn_head import FCNHead
-
-
-class DisentangledNonLocal2d(NonLocal2d):
- """Disentangled Non-Local Blocks.
-
- Args:
- temperature (float): Temperature to adjust attention. Default: 0.05
- """
-
- def __init__(self, *arg, temperature, **kwargs):
- super().__init__(*arg, **kwargs)
- self.temperature = temperature
- self.conv_mask = nn.Conv2d(self.in_channels, 1, kernel_size=1)
-
- def embedded_gaussian(self, theta_x, phi_x):
- """Embedded gaussian with temperature."""
-
- # NonLocal2d pairwise_weight: [N, HxW, HxW]
- pairwise_weight = torch.matmul(theta_x, phi_x)
- if self.use_scale:
- # theta_x.shape[-1] is `self.inter_channels`
- pairwise_weight /= theta_x.shape[-1]**0.5
- pairwise_weight /= self.temperature
- pairwise_weight = pairwise_weight.softmax(dim=-1)
- return pairwise_weight
-
- def forward(self, x):
- # x: [N, C, H, W]
- n = x.size(0)
-
- # g_x: [N, HxW, C]
- g_x = self.g(x).view(n, self.inter_channels, -1)
- g_x = g_x.permute(0, 2, 1)
-
- # theta_x: [N, HxW, C], phi_x: [N, C, HxW]
- if self.mode == 'gaussian':
- theta_x = x.view(n, self.in_channels, -1)
- theta_x = theta_x.permute(0, 2, 1)
- if self.sub_sample:
- phi_x = self.phi(x).view(n, self.in_channels, -1)
- else:
- phi_x = x.view(n, self.in_channels, -1)
- elif self.mode == 'concatenation':
- theta_x = self.theta(x).view(n, self.inter_channels, -1, 1)
- phi_x = self.phi(x).view(n, self.inter_channels, 1, -1)
- else:
- theta_x = self.theta(x).view(n, self.inter_channels, -1)
- theta_x = theta_x.permute(0, 2, 1)
- phi_x = self.phi(x).view(n, self.inter_channels, -1)
-
- # subtract mean
- theta_x -= theta_x.mean(dim=-2, keepdim=True)
- phi_x -= phi_x.mean(dim=-1, keepdim=True)
-
- pairwise_func = getattr(self, self.mode)
- # pairwise_weight: [N, HxW, HxW]
- pairwise_weight = pairwise_func(theta_x, phi_x)
-
- # y: [N, HxW, C]
- y = torch.matmul(pairwise_weight, g_x)
- # y: [N, C, H, W]
- y = y.permute(0, 2, 1).contiguous().reshape(n, self.inter_channels,
- *x.size()[2:])
-
- # unary_mask: [N, 1, HxW]
- unary_mask = self.conv_mask(x)
- unary_mask = unary_mask.view(n, 1, -1)
- unary_mask = unary_mask.softmax(dim=-1)
- # unary_x: [N, 1, C]
- unary_x = torch.matmul(unary_mask, g_x)
- # unary_x: [N, C, 1, 1]
- unary_x = unary_x.permute(0, 2, 1).contiguous().reshape(
- n, self.inter_channels, 1, 1)
-
- output = x + self.conv_out(y + unary_x)
-
- return output
-
-
-@HEADS.register_module()
-class DNLHead(FCNHead):
- """Disentangled Non-Local Neural Networks.
-
- This head is the implementation of `DNLNet
- `_.
-
- Args:
- reduction (int): Reduction factor of projection transform. Default: 2.
- use_scale (bool): Whether to scale pairwise_weight by
- sqrt(1/inter_channels). Default: False.
- mode (str): The nonlocal mode. Options are 'embedded_gaussian',
- 'dot_product'. Default: 'embedded_gaussian.'.
- temperature (float): Temperature to adjust attention. Default: 0.05
- """
-
- def __init__(self,
- reduction=2,
- use_scale=True,
- mode='embedded_gaussian',
- temperature=0.05,
- **kwargs):
- super(DNLHead, self).__init__(num_convs=2, **kwargs)
- self.reduction = reduction
- self.use_scale = use_scale
- self.mode = mode
- self.temperature = temperature
- self.dnl_block = DisentangledNonLocal2d(
- in_channels=self.channels,
- reduction=self.reduction,
- use_scale=self.use_scale,
- conv_cfg=self.conv_cfg,
- norm_cfg=self.norm_cfg,
- mode=self.mode,
- temperature=self.temperature)
-
- def forward(self, inputs):
- """Forward function."""
- x = self._transform_inputs(inputs)
- output = self.convs[0](x)
- output = self.dnl_block(output)
- output = self.convs[1](output)
- if self.concat_input:
- output = self.conv_cat(torch.cat([x, output], dim=1))
- output = self.cls_seg(output)
- return output
diff --git a/spaces/abhishek/sketch-to-image/annotator/uniformer_base/mmcv/utils/parrots_wrapper.py b/spaces/abhishek/sketch-to-image/annotator/uniformer_base/mmcv/utils/parrots_wrapper.py
deleted file mode 100644
index 93c97640d4b9ed088ca82cfe03e6efebfcfa9dbf..0000000000000000000000000000000000000000
--- a/spaces/abhishek/sketch-to-image/annotator/uniformer_base/mmcv/utils/parrots_wrapper.py
+++ /dev/null
@@ -1,107 +0,0 @@
-# Copyright (c) OpenMMLab. All rights reserved.
-from functools import partial
-
-import torch
-
-TORCH_VERSION = torch.__version__
-
-
-def is_rocm_pytorch() -> bool:
- is_rocm = False
- if TORCH_VERSION != 'parrots':
- try:
- from torch.utils.cpp_extension import ROCM_HOME
- is_rocm = True if ((torch.version.hip is not None) and
- (ROCM_HOME is not None)) else False
- except ImportError:
- pass
- return is_rocm
-
-
-def _get_cuda_home():
- if TORCH_VERSION == 'parrots':
- from parrots.utils.build_extension import CUDA_HOME
- else:
- if is_rocm_pytorch():
- from torch.utils.cpp_extension import ROCM_HOME
- CUDA_HOME = ROCM_HOME
- else:
- from torch.utils.cpp_extension import CUDA_HOME
- return CUDA_HOME
-
-
-def get_build_config():
- if TORCH_VERSION == 'parrots':
- from parrots.config import get_build_info
- return get_build_info()
- else:
- return torch.__config__.show()
-
-
-def _get_conv():
- if TORCH_VERSION == 'parrots':
- from parrots.nn.modules.conv import _ConvNd, _ConvTransposeMixin
- else:
- from torch.nn.modules.conv import _ConvNd, _ConvTransposeMixin
- return _ConvNd, _ConvTransposeMixin
-
-
-def _get_dataloader():
- if TORCH_VERSION == 'parrots':
- from torch.utils.data import DataLoader, PoolDataLoader
- else:
- from torch.utils.data import DataLoader
- PoolDataLoader = DataLoader
- return DataLoader, PoolDataLoader
-
-
-def _get_extension():
- if TORCH_VERSION == 'parrots':
- from parrots.utils.build_extension import BuildExtension, Extension
- CppExtension = partial(Extension, cuda=False)
- CUDAExtension = partial(Extension, cuda=True)
- else:
- from torch.utils.cpp_extension import (BuildExtension, CppExtension,
- CUDAExtension)
- return BuildExtension, CppExtension, CUDAExtension
-
-
-def _get_pool():
- if TORCH_VERSION == 'parrots':
- from parrots.nn.modules.pool import (_AdaptiveAvgPoolNd,
- _AdaptiveMaxPoolNd, _AvgPoolNd,
- _MaxPoolNd)
- else:
- from torch.nn.modules.pooling import (_AdaptiveAvgPoolNd,
- _AdaptiveMaxPoolNd, _AvgPoolNd,
- _MaxPoolNd)
- return _AdaptiveAvgPoolNd, _AdaptiveMaxPoolNd, _AvgPoolNd, _MaxPoolNd
-
-
-def _get_norm():
- if TORCH_VERSION == 'parrots':
- from parrots.nn.modules.batchnorm import _BatchNorm, _InstanceNorm
- SyncBatchNorm_ = torch.nn.SyncBatchNorm2d
- else:
- from torch.nn.modules.instancenorm import _InstanceNorm
- from torch.nn.modules.batchnorm import _BatchNorm
- SyncBatchNorm_ = torch.nn.SyncBatchNorm
- return _BatchNorm, _InstanceNorm, SyncBatchNorm_
-
-
-_ConvNd, _ConvTransposeMixin = _get_conv()
-DataLoader, PoolDataLoader = _get_dataloader()
-BuildExtension, CppExtension, CUDAExtension = _get_extension()
-_BatchNorm, _InstanceNorm, SyncBatchNorm_ = _get_norm()
-_AdaptiveAvgPoolNd, _AdaptiveMaxPoolNd, _AvgPoolNd, _MaxPoolNd = _get_pool()
-
-
-class SyncBatchNorm(SyncBatchNorm_):
-
- def _check_input_dim(self, input):
- if TORCH_VERSION == 'parrots':
- if input.dim() < 2:
- raise ValueError(
- f'expected at least 2D input (got {input.dim()}D input)')
- else:
- super()._check_input_dim(input)
diff --git a/spaces/abhishek/sketch-to-image/annotator/uniformer_base/mmseg/models/decode_heads/ema_head.py b/spaces/abhishek/sketch-to-image/annotator/uniformer_base/mmseg/models/decode_heads/ema_head.py
deleted file mode 100644
index a1eb0cdda8a166612982f3d8b64da0cb1021dd7a..0000000000000000000000000000000000000000
--- a/spaces/abhishek/sketch-to-image/annotator/uniformer_base/mmseg/models/decode_heads/ema_head.py
+++ /dev/null
@@ -1,180 +0,0 @@
-'''
- * Copyright (c) 2023 Salesforce, Inc.
- * All rights reserved.
- * SPDX-License-Identifier: Apache License 2.0
- * For full license text, see LICENSE.txt file in the repo root or http://www.apache.org/licenses/
- * By Can Qin
- * Modified from ControlNet repo: https://github.com/lllyasviel/ControlNet
- * Copyright (c) 2023 Lvmin Zhang and Maneesh Agrawala
- * Modified from MMCV repo: From https://github.com/open-mmlab/mmcv
- * Copyright (c) OpenMMLab. All rights reserved.
-'''
-
-import math
-
-import torch
-import torch.distributed as dist
-import torch.nn as nn
-import torch.nn.functional as F
-from annotator.uniformer.mmcv.cnn import ConvModule
-
-from ..builder import HEADS
-from .decode_head import BaseDecodeHead
-
-
-def reduce_mean(tensor):
- """Reduce mean when distributed training."""
- if not (dist.is_available() and dist.is_initialized()):
- return tensor
- tensor = tensor.clone()
- dist.all_reduce(tensor.div_(dist.get_world_size()), op=dist.ReduceOp.SUM)
- return tensor
-
-
-class EMAModule(nn.Module):
- """Expectation Maximization Attention Module used in EMANet.
-
- Args:
- channels (int): Channels of the whole module.
- num_bases (int): Number of bases.
- num_stages (int): Number of the EM iterations.
- """
-
- def __init__(self, channels, num_bases, num_stages, momentum):
- super(EMAModule, self).__init__()
- assert num_stages >= 1, 'num_stages must be at least 1!'
- self.num_bases = num_bases
- self.num_stages = num_stages
- self.momentum = momentum
-
- bases = torch.zeros(1, channels, self.num_bases)
- bases.normal_(0, math.sqrt(2. / self.num_bases))
- # [1, channels, num_bases]
- bases = F.normalize(bases, dim=1, p=2)
- self.register_buffer('bases', bases)
-
- def forward(self, feats):
- """Forward function."""
- batch_size, channels, height, width = feats.size()
- # [batch_size, channels, height*width]
- feats = feats.view(batch_size, channels, height * width)
- # [batch_size, channels, num_bases]
- bases = self.bases.repeat(batch_size, 1, 1)
-
- with torch.no_grad():
- for i in range(self.num_stages):
- # [batch_size, height*width, num_bases]
- attention = torch.einsum('bcn,bck->bnk', feats, bases)
- attention = F.softmax(attention, dim=2)
- # l1 norm
- attention_normed = F.normalize(attention, dim=1, p=1)
- # [batch_size, channels, num_bases]
- bases = torch.einsum('bcn,bnk->bck', feats, attention_normed)
- # l2 norm
- bases = F.normalize(bases, dim=1, p=2)
-
- feats_recon = torch.einsum('bck,bnk->bcn', bases, attention)
- feats_recon = feats_recon.view(batch_size, channels, height, width)
-
- if self.training:
- bases = bases.mean(dim=0, keepdim=True)
- bases = reduce_mean(bases)
- # l2 norm
- bases = F.normalize(bases, dim=1, p=2)
- self.bases = (1 -
- self.momentum) * self.bases + self.momentum * bases
-
- return feats_recon
-
-
-@HEADS.register_module()
-class EMAHead(BaseDecodeHead):
- """Expectation Maximization Attention Networks for Semantic Segmentation.
-
- This head is the implementation of `EMANet
- `_.
-
- Args:
- ema_channels (int): EMA module channels
- num_bases (int): Number of bases.
- num_stages (int): Number of the EM iterations.
- concat_input (bool): Whether concat the input and output of convs
- before classification layer. Default: True
- momentum (float): Momentum to update the base. Default: 0.1.
- """
-
- def __init__(self,
- ema_channels,
- num_bases,
- num_stages,
- concat_input=True,
- momentum=0.1,
- **kwargs):
- super(EMAHead, self).__init__(**kwargs)
- self.ema_channels = ema_channels
- self.num_bases = num_bases
- self.num_stages = num_stages
- self.concat_input = concat_input
- self.momentum = momentum
- self.ema_module = EMAModule(self.ema_channels, self.num_bases,
- self.num_stages, self.momentum)
-
- self.ema_in_conv = ConvModule(
- self.in_channels,
- self.ema_channels,
- 3,
- padding=1,
- conv_cfg=self.conv_cfg,
- norm_cfg=self.norm_cfg,
- act_cfg=self.act_cfg)
- # project (0, inf) -> (-inf, inf)
- self.ema_mid_conv = ConvModule(
- self.ema_channels,
- self.ema_channels,
- 1,
- conv_cfg=self.conv_cfg,
- norm_cfg=None,
- act_cfg=None)
- for param in self.ema_mid_conv.parameters():
- param.requires_grad = False
-
- self.ema_out_conv = ConvModule(
- self.ema_channels,
- self.ema_channels,
- 1,
- conv_cfg=self.conv_cfg,
- norm_cfg=self.norm_cfg,
- act_cfg=None)
- self.bottleneck = ConvModule(
- self.ema_channels,
- self.channels,
- 3,
- padding=1,
- conv_cfg=self.conv_cfg,
- norm_cfg=self.norm_cfg,
- act_cfg=self.act_cfg)
- if self.concat_input:
- self.conv_cat = ConvModule(
- self.in_channels + self.channels,
- self.channels,
- kernel_size=3,
- padding=1,
- conv_cfg=self.conv_cfg,
- norm_cfg=self.norm_cfg,
- act_cfg=self.act_cfg)
-
- def forward(self, inputs):
- """Forward function."""
- x = self._transform_inputs(inputs)
- feats = self.ema_in_conv(x)
- identity = feats
- feats = self.ema_mid_conv(feats)
- recon = self.ema_module(feats)
- recon = F.relu(recon, inplace=True)
- recon = self.ema_out_conv(recon)
- output = F.relu(identity + recon, inplace=True)
- output = self.bottleneck(output)
- if self.concat_input:
- output = self.conv_cat(torch.cat([x, output], dim=1))
- output = self.cls_seg(output)
- return output
diff --git a/spaces/abhishek/sketch-to-image/annotator/uniformer_base/mmseg/models/utils/up_conv_block.py b/spaces/abhishek/sketch-to-image/annotator/uniformer_base/mmseg/models/utils/up_conv_block.py
deleted file mode 100644
index b2cf355eea942c150417b2bbae2bd3f15473ddbb..0000000000000000000000000000000000000000
--- a/spaces/abhishek/sketch-to-image/annotator/uniformer_base/mmseg/models/utils/up_conv_block.py
+++ /dev/null
@@ -1,113 +0,0 @@
-'''
- * Copyright (c) 2023 Salesforce, Inc.
- * All rights reserved.
- * SPDX-License-Identifier: Apache License 2.0
- * For full license text, see LICENSE.txt file in the repo root or http://www.apache.org/licenses/
- * By Can Qin
- * Modified from ControlNet repo: https://github.com/lllyasviel/ControlNet
- * Copyright (c) 2023 Lvmin Zhang and Maneesh Agrawala
- * Modified from MMCV repo: From https://github.com/open-mmlab/mmcv
- * Copyright (c) OpenMMLab. All rights reserved.
-'''
-
-import torch
-import torch.nn as nn
-from annotator.uniformer.mmcv.cnn import ConvModule, build_upsample_layer
-
-
-class UpConvBlock(nn.Module):
- """Upsample convolution block in decoder for UNet.
-
- This upsample convolution block consists of one upsample module
- followed by one convolution block. The upsample module expands the
- high-level low-resolution feature map and the convolution block fuses
- the upsampled high-level low-resolution feature map and the low-level
- high-resolution feature map from encoder.
-
- Args:
- conv_block (nn.Sequential): Sequential of convolutional layers.
- in_channels (int): Number of input channels of the high-level
- skip_channels (int): Number of input channels of the low-level
- high-resolution feature map from encoder.
- out_channels (int): Number of output channels.
- num_convs (int): Number of convolutional layers in the conv_block.
- Default: 2.
- stride (int): Stride of convolutional layer in conv_block. Default: 1.
- dilation (int): Dilation rate of convolutional layer in conv_block.
- Default: 1.
- with_cp (bool): Use checkpoint or not. Using checkpoint will save some
- memory while slowing down the training speed. Default: False.
- conv_cfg (dict | None): Config dict for convolution layer.
- Default: None.
- norm_cfg (dict | None): Config dict for normalization layer.
- Default: dict(type='BN').
- act_cfg (dict | None): Config dict for activation layer in ConvModule.
- Default: dict(type='ReLU').
- upsample_cfg (dict): The upsample config of the upsample module in
- decoder. Default: dict(type='InterpConv'). If the size of
- high-level feature map is the same as that of skip feature map
- (low-level feature map from encoder), it does not need upsample the
- high-level feature map and the upsample_cfg is None.
- dcn (bool): Use deformable convolution in convolutional layer or not.
- Default: None.
- plugins (dict): plugins for convolutional layers. Default: None.
- """
-
- def __init__(self,
- conv_block,
- in_channels,
- skip_channels,
- out_channels,
- num_convs=2,
- stride=1,
- dilation=1,
- with_cp=False,
- conv_cfg=None,
- norm_cfg=dict(type='BN'),
- act_cfg=dict(type='ReLU'),
- upsample_cfg=dict(type='InterpConv'),
- dcn=None,
- plugins=None):
- super(UpConvBlock, self).__init__()
- assert dcn is None, 'Not implemented yet.'
- assert plugins is None, 'Not implemented yet.'
-
- self.conv_block = conv_block(
- in_channels=2 * skip_channels,
- out_channels=out_channels,
- num_convs=num_convs,
- stride=stride,
- dilation=dilation,
- with_cp=with_cp,
- conv_cfg=conv_cfg,
- norm_cfg=norm_cfg,
- act_cfg=act_cfg,
- dcn=None,
- plugins=None)
- if upsample_cfg is not None:
- self.upsample = build_upsample_layer(
- cfg=upsample_cfg,
- in_channels=in_channels,
- out_channels=skip_channels,
- with_cp=with_cp,
- norm_cfg=norm_cfg,
- act_cfg=act_cfg)
- else:
- self.upsample = ConvModule(
- in_channels,
- skip_channels,
- kernel_size=1,
- stride=1,
- padding=0,
- conv_cfg=conv_cfg,
- norm_cfg=norm_cfg,
- act_cfg=act_cfg)
-
- def forward(self, skip, x):
- """Forward function."""
-
- x = self.upsample(x)
- out = torch.cat([skip, x], dim=1)
- out = self.conv_block(out)
-
- return out
diff --git a/spaces/abrar-lohia/text-2-character-anim/pyrender/.eggs/pyglet-2.0.5-py3.10.egg/pyglet/media/drivers/openal/lib_alc.py b/spaces/abrar-lohia/text-2-character-anim/pyrender/.eggs/pyglet-2.0.5-py3.10.egg/pyglet/media/drivers/openal/lib_alc.py
deleted file mode 100644
index cd57159270d86f4e389ec9c70f7ea4714207ee1f..0000000000000000000000000000000000000000
--- a/spaces/abrar-lohia/text-2-character-anim/pyrender/.eggs/pyglet-2.0.5-py3.10.egg/pyglet/media/drivers/openal/lib_alc.py
+++ /dev/null
@@ -1,264 +0,0 @@
-"""Wrapper for openal
-
-Generated with:
-../tools/wraptypes/wrap.py /usr/include/AL/alc.h -lopenal -olib_alc.py
-
-.. Hacked to fix ALCvoid argtypes.
-"""
-
-import ctypes
-from ctypes import *
-
-import pyglet.lib
-
-_lib = pyglet.lib.load_library('openal',
- win32='openal32',
- framework='OpenAL')
-
-_int_types = (c_int16, c_int32)
-if hasattr(ctypes, 'c_int64'):
- # Some builds of ctypes apparently do not have c_int64
- # defined; it's a pretty good bet that these builds do not
- # have 64-bit pointers.
- _int_types += (ctypes.c_int64,)
-for t in _int_types:
- if sizeof(t) == sizeof(c_size_t):
- c_ptrdiff_t = t
-
-
-class c_void(Structure):
- # c_void_p is a buggy return type, converting to int, so
- # POINTER(None) == c_void_p is actually written as
- # POINTER(c_void), so it can be treated as a real pointer.
- _fields_ = [('dummy', c_int)]
-
-
-ALC_API = 0 # /usr/include/AL/alc.h:19
-ALCAPI = 0 # /usr/include/AL/alc.h:37
-ALC_INVALID = 0 # /usr/include/AL/alc.h:39
-ALC_VERSION_0_1 = 1 # /usr/include/AL/alc.h:42
-
-
-class struct_ALCdevice_struct(Structure):
- __slots__ = [
- ]
-
-
-struct_ALCdevice_struct._fields_ = [
- ('_opaque_struct', c_int)
-]
-
-
-class struct_ALCdevice_struct(Structure):
- __slots__ = [
- ]
-
-
-struct_ALCdevice_struct._fields_ = [
- ('_opaque_struct', c_int)
-]
-
-ALCdevice = struct_ALCdevice_struct # /usr/include/AL/alc.h:44
-
-
-class struct_ALCcontext_struct(Structure):
- __slots__ = [
- ]
-
-
-struct_ALCcontext_struct._fields_ = [
- ('_opaque_struct', c_int)
-]
-
-
-class struct_ALCcontext_struct(Structure):
- __slots__ = [
- ]
-
-
-struct_ALCcontext_struct._fields_ = [
- ('_opaque_struct', c_int)
-]
-
-ALCcontext = struct_ALCcontext_struct # /usr/include/AL/alc.h:45
-ALCboolean = c_char # /usr/include/AL/alc.h:49
-ALCchar = c_char # /usr/include/AL/alc.h:52
-ALCbyte = c_char # /usr/include/AL/alc.h:55
-ALCubyte = c_ubyte # /usr/include/AL/alc.h:58
-ALCshort = c_short # /usr/include/AL/alc.h:61
-ALCushort = c_ushort # /usr/include/AL/alc.h:64
-ALCint = c_int # /usr/include/AL/alc.h:67
-ALCuint = c_uint # /usr/include/AL/alc.h:70
-ALCsizei = c_int # /usr/include/AL/alc.h:73
-ALCenum = c_int # /usr/include/AL/alc.h:76
-ALCfloat = c_float # /usr/include/AL/alc.h:79
-ALCdouble = c_double # /usr/include/AL/alc.h:82
-ALCvoid = None # /usr/include/AL/alc.h:85
-ALC_FALSE = 0 # /usr/include/AL/alc.h:91
-ALC_TRUE = 1 # /usr/include/AL/alc.h:94
-ALC_FREQUENCY = 4103 # /usr/include/AL/alc.h:99
-ALC_REFRESH = 4104 # /usr/include/AL/alc.h:104
-ALC_SYNC = 4105 # /usr/include/AL/alc.h:109
-ALC_MONO_SOURCES = 4112 # /usr/include/AL/alc.h:114
-ALC_STEREO_SOURCES = 4113 # /usr/include/AL/alc.h:119
-ALC_NO_ERROR = 0 # /usr/include/AL/alc.h:128
-ALC_INVALID_DEVICE = 40961 # /usr/include/AL/alc.h:133
-ALC_INVALID_CONTEXT = 40962 # /usr/include/AL/alc.h:138
-ALC_INVALID_ENUM = 40963 # /usr/include/AL/alc.h:143
-ALC_INVALID_VALUE = 40964 # /usr/include/AL/alc.h:148
-ALC_OUT_OF_MEMORY = 40965 # /usr/include/AL/alc.h:153
-ALC_DEFAULT_DEVICE_SPECIFIER = 4100 # /usr/include/AL/alc.h:159
-ALC_DEVICE_SPECIFIER = 4101 # /usr/include/AL/alc.h:160
-ALC_EXTENSIONS = 4102 # /usr/include/AL/alc.h:161
-ALC_MAJOR_VERSION = 4096 # /usr/include/AL/alc.h:163
-ALC_MINOR_VERSION = 4097 # /usr/include/AL/alc.h:164
-ALC_ATTRIBUTES_SIZE = 4098 # /usr/include/AL/alc.h:166
-ALC_ALL_ATTRIBUTES = 4099 # /usr/include/AL/alc.h:167
-ALC_CAPTURE_DEVICE_SPECIFIER = 784 # /usr/include/AL/alc.h:172
-ALC_CAPTURE_DEFAULT_DEVICE_SPECIFIER = 785 # /usr/include/AL/alc.h:173
-ALC_CAPTURE_SAMPLES = 786 # /usr/include/AL/alc.h:174
-# /usr/include/AL/alc.h:180
-alcCreateContext = _lib.alcCreateContext
-alcCreateContext.restype = POINTER(ALCcontext)
-alcCreateContext.argtypes = [POINTER(ALCdevice), POINTER(ALCint)]
-
-# /usr/include/AL/alc.h:182
-alcMakeContextCurrent = _lib.alcMakeContextCurrent
-alcMakeContextCurrent.restype = ALCboolean
-alcMakeContextCurrent.argtypes = [POINTER(ALCcontext)]
-
-# /usr/include/AL/alc.h:184
-alcProcessContext = _lib.alcProcessContext
-alcProcessContext.restype = None
-alcProcessContext.argtypes = [POINTER(ALCcontext)]
-
-# /usr/include/AL/alc.h:186
-alcSuspendContext = _lib.alcSuspendContext
-alcSuspendContext.restype = None
-alcSuspendContext.argtypes = [POINTER(ALCcontext)]
-
-# /usr/include/AL/alc.h:188
-alcDestroyContext = _lib.alcDestroyContext
-alcDestroyContext.restype = None
-alcDestroyContext.argtypes = [POINTER(ALCcontext)]
-
-# /usr/include/AL/alc.h:190
-alcGetCurrentContext = _lib.alcGetCurrentContext
-alcGetCurrentContext.restype = POINTER(ALCcontext)
-alcGetCurrentContext.argtypes = []
-
-# /usr/include/AL/alc.h:192
-alcGetContextsDevice = _lib.alcGetContextsDevice
-alcGetContextsDevice.restype = POINTER(ALCdevice)
-alcGetContextsDevice.argtypes = [POINTER(ALCcontext)]
-
-# /usr/include/AL/alc.h:198
-alcOpenDevice = _lib.alcOpenDevice
-alcOpenDevice.restype = POINTER(ALCdevice)
-alcOpenDevice.argtypes = [POINTER(ALCchar)]
-
-# /usr/include/AL/alc.h:200
-alcCloseDevice = _lib.alcCloseDevice
-alcCloseDevice.restype = ALCboolean
-alcCloseDevice.argtypes = [POINTER(ALCdevice)]
-
-# /usr/include/AL/alc.h:207
-alcGetError = _lib.alcGetError
-alcGetError.restype = ALCenum
-alcGetError.argtypes = [POINTER(ALCdevice)]
-
-# /usr/include/AL/alc.h:215
-alcIsExtensionPresent = _lib.alcIsExtensionPresent
-alcIsExtensionPresent.restype = ALCboolean
-alcIsExtensionPresent.argtypes = [POINTER(ALCdevice), POINTER(ALCchar)]
-
-# /usr/include/AL/alc.h:217
-alcGetProcAddress = _lib.alcGetProcAddress
-alcGetProcAddress.restype = POINTER(c_void)
-alcGetProcAddress.argtypes = [POINTER(ALCdevice), POINTER(ALCchar)]
-
-# /usr/include/AL/alc.h:219
-alcGetEnumValue = _lib.alcGetEnumValue
-alcGetEnumValue.restype = ALCenum
-alcGetEnumValue.argtypes = [POINTER(ALCdevice), POINTER(ALCchar)]
-
-# /usr/include/AL/alc.h:225
-alcGetString = _lib.alcGetString
-alcGetString.restype = POINTER(ALCchar)
-alcGetString.argtypes = [POINTER(ALCdevice), ALCenum]
-
-# /usr/include/AL/alc.h:227
-alcGetIntegerv = _lib.alcGetIntegerv
-alcGetIntegerv.restype = None
-alcGetIntegerv.argtypes = [POINTER(ALCdevice), ALCenum, ALCsizei, POINTER(ALCint)]
-
-# /usr/include/AL/alc.h:233
-alcCaptureOpenDevice = _lib.alcCaptureOpenDevice
-alcCaptureOpenDevice.restype = POINTER(ALCdevice)
-alcCaptureOpenDevice.argtypes = [POINTER(ALCchar), ALCuint, ALCenum, ALCsizei]
-
-# /usr/include/AL/alc.h:235
-alcCaptureCloseDevice = _lib.alcCaptureCloseDevice
-alcCaptureCloseDevice.restype = ALCboolean
-alcCaptureCloseDevice.argtypes = [POINTER(ALCdevice)]
-
-# /usr/include/AL/alc.h:237
-alcCaptureStart = _lib.alcCaptureStart
-alcCaptureStart.restype = None
-alcCaptureStart.argtypes = [POINTER(ALCdevice)]
-
-# /usr/include/AL/alc.h:239
-alcCaptureStop = _lib.alcCaptureStop
-alcCaptureStop.restype = None
-alcCaptureStop.argtypes = [POINTER(ALCdevice)]
-
-# /usr/include/AL/alc.h:241
-alcCaptureSamples = _lib.alcCaptureSamples
-alcCaptureSamples.restype = None
-alcCaptureSamples.argtypes = [POINTER(ALCdevice), POINTER(ALCvoid), ALCsizei]
-
-LPALCCREATECONTEXT = CFUNCTYPE(POINTER(ALCcontext), POINTER(ALCdevice), POINTER(ALCint)) # /usr/include/AL/alc.h:246
-LPALCMAKECONTEXTCURRENT = CFUNCTYPE(ALCboolean, POINTER(ALCcontext)) # /usr/include/AL/alc.h:247
-LPALCPROCESSCONTEXT = CFUNCTYPE(None, POINTER(ALCcontext)) # /usr/include/AL/alc.h:248
-LPALCSUSPENDCONTEXT = CFUNCTYPE(None, POINTER(ALCcontext)) # /usr/include/AL/alc.h:249
-LPALCDESTROYCONTEXT = CFUNCTYPE(None, POINTER(ALCcontext)) # /usr/include/AL/alc.h:250
-LPALCGETCURRENTCONTEXT = CFUNCTYPE(POINTER(ALCcontext)) # /usr/include/AL/alc.h:251
-LPALCGETCONTEXTSDEVICE = CFUNCTYPE(POINTER(ALCdevice), POINTER(ALCcontext)) # /usr/include/AL/alc.h:252
-LPALCOPENDEVICE = CFUNCTYPE(POINTER(ALCdevice), POINTER(ALCchar)) # /usr/include/AL/alc.h:253
-LPALCCLOSEDEVICE = CFUNCTYPE(ALCboolean, POINTER(ALCdevice)) # /usr/include/AL/alc.h:254
-LPALCGETERROR = CFUNCTYPE(ALCenum, POINTER(ALCdevice)) # /usr/include/AL/alc.h:255
-LPALCISEXTENSIONPRESENT = CFUNCTYPE(ALCboolean, POINTER(ALCdevice), POINTER(ALCchar)) # /usr/include/AL/alc.h:256
-LPALCGETPROCADDRESS = CFUNCTYPE(POINTER(c_void), POINTER(ALCdevice), POINTER(ALCchar)) # /usr/include/AL/alc.h:257
-LPALCGETENUMVALUE = CFUNCTYPE(ALCenum, POINTER(ALCdevice), POINTER(ALCchar)) # /usr/include/AL/alc.h:258
-LPALCGETSTRING = CFUNCTYPE(POINTER(ALCchar), POINTER(ALCdevice), ALCenum) # /usr/include/AL/alc.h:259
-LPALCGETINTEGERV = CFUNCTYPE(None, POINTER(ALCdevice), ALCenum, ALCsizei, POINTER(ALCint)) # /usr/include/AL/alc.h:260
-LPALCCAPTUREOPENDEVICE = CFUNCTYPE(POINTER(ALCdevice), POINTER(ALCchar), ALCuint, ALCenum, ALCsizei) # /usr/include/AL/alc.h:261
-LPALCCAPTURECLOSEDEVICE = CFUNCTYPE(ALCboolean, POINTER(ALCdevice)) # /usr/include/AL/alc.h:262
-LPALCCAPTURESTART = CFUNCTYPE(None, POINTER(ALCdevice)) # /usr/include/AL/alc.h:263
-LPALCCAPTURESTOP = CFUNCTYPE(None, POINTER(ALCdevice)) # /usr/include/AL/alc.h:264
-LPALCCAPTURESAMPLES = CFUNCTYPE(None, POINTER(ALCdevice), POINTER(ALCvoid), ALCsizei) # /usr/include/AL/alc.h:265
-
-__all__ = ['ALC_API', 'ALCAPI', 'ALC_INVALID', 'ALC_VERSION_0_1', 'ALCdevice',
- 'ALCcontext', 'ALCboolean', 'ALCchar', 'ALCbyte', 'ALCubyte', 'ALCshort',
- 'ALCushort', 'ALCint', 'ALCuint', 'ALCsizei', 'ALCenum', 'ALCfloat',
- 'ALCdouble', 'ALCvoid', 'ALC_FALSE', 'ALC_TRUE', 'ALC_FREQUENCY',
- 'ALC_REFRESH', 'ALC_SYNC', 'ALC_MONO_SOURCES', 'ALC_STEREO_SOURCES',
- 'ALC_NO_ERROR', 'ALC_INVALID_DEVICE', 'ALC_INVALID_CONTEXT',
- 'ALC_INVALID_ENUM', 'ALC_INVALID_VALUE', 'ALC_OUT_OF_MEMORY',
- 'ALC_DEFAULT_DEVICE_SPECIFIER', 'ALC_DEVICE_SPECIFIER', 'ALC_EXTENSIONS',
- 'ALC_MAJOR_VERSION', 'ALC_MINOR_VERSION', 'ALC_ATTRIBUTES_SIZE',
- 'ALC_ALL_ATTRIBUTES', 'ALC_CAPTURE_DEVICE_SPECIFIER',
- 'ALC_CAPTURE_DEFAULT_DEVICE_SPECIFIER', 'ALC_CAPTURE_SAMPLES',
- 'alcCreateContext', 'alcMakeContextCurrent', 'alcProcessContext',
- 'alcSuspendContext', 'alcDestroyContext', 'alcGetCurrentContext',
- 'alcGetContextsDevice', 'alcOpenDevice', 'alcCloseDevice', 'alcGetError',
- 'alcIsExtensionPresent', 'alcGetProcAddress', 'alcGetEnumValue',
- 'alcGetString', 'alcGetIntegerv', 'alcCaptureOpenDevice',
- 'alcCaptureCloseDevice', 'alcCaptureStart', 'alcCaptureStop',
- 'alcCaptureSamples', 'LPALCCREATECONTEXT', 'LPALCMAKECONTEXTCURRENT',
- 'LPALCPROCESSCONTEXT', 'LPALCSUSPENDCONTEXT', 'LPALCDESTROYCONTEXT',
- 'LPALCGETCURRENTCONTEXT', 'LPALCGETCONTEXTSDEVICE', 'LPALCOPENDEVICE',
- 'LPALCCLOSEDEVICE', 'LPALCGETERROR', 'LPALCISEXTENSIONPRESENT',
- 'LPALCGETPROCADDRESS', 'LPALCGETENUMVALUE', 'LPALCGETSTRING',
- 'LPALCGETINTEGERV', 'LPALCCAPTUREOPENDEVICE', 'LPALCCAPTURECLOSEDEVICE',
- 'LPALCCAPTURESTART', 'LPALCCAPTURESTOP', 'LPALCCAPTURESAMPLES']
diff --git a/spaces/abrar-lohia/text-2-character-anim/pyrender/pyrender/version.py b/spaces/abrar-lohia/text-2-character-anim/pyrender/pyrender/version.py
deleted file mode 100644
index a33fc87f61f528780e3319a5160769cc84512b1b..0000000000000000000000000000000000000000
--- a/spaces/abrar-lohia/text-2-character-anim/pyrender/pyrender/version.py
+++ /dev/null
@@ -1 +0,0 @@
-__version__ = '0.1.45'
diff --git a/spaces/ahmedghani/svoice_demo/CODE_OF_CONDUCT.md b/spaces/ahmedghani/svoice_demo/CODE_OF_CONDUCT.md
deleted file mode 100644
index 43c1d83869eea803a95807281559a18482f598f0..0000000000000000000000000000000000000000
--- a/spaces/ahmedghani/svoice_demo/CODE_OF_CONDUCT.md
+++ /dev/null
@@ -1,78 +0,0 @@
-
-# Code of Conduct
-
-## Our Pledge
-
-In the interest of fostering an open and welcoming environment, we as
-contributors and maintainers pledge to make participation in our project and
-our community a harassment-free experience for everyone, regardless of age, body
-size, disability, ethnicity, sex characteristics, gender identity and expression,
-level of experience, education, socio-economic status, nationality, personal
-appearance, race, religion, or sexual identity and orientation.
-
-## Our Standards
-
-Examples of behavior that contributes to creating a positive environment
-include:
-
-* Using welcoming and inclusive language
-* Being respectful of differing viewpoints and experiences
-* Gracefully accepting constructive criticism
-* Focusing on what is best for the community
-* Showing empathy towards other community members
-
-Examples of unacceptable behavior by participants include:
-
-* The use of sexualized language or imagery and unwelcome sexual attention or
- advances
-* Trolling, insulting/derogatory comments, and personal or political attacks
-* Public or private harassment
-* Publishing others' private information, such as a physical or electronic
- address, without explicit permission
-* Other conduct which could reasonably be considered inappropriate in a
- professional setting
-
-## Our Responsibilities
-
-Project maintainers are responsible for clarifying the standards of acceptable
-behavior and are expected to take appropriate and fair corrective action in
-response to any instances of unacceptable behavior.
-
-Project maintainers have the right and responsibility to remove, edit, or
-reject comments, commits, code, wiki edits, issues, and other contributions
-that are not aligned to this Code of Conduct, or to ban temporarily or
-permanently any contributor for other behaviors that they deem inappropriate,
-threatening, offensive, or harmful.
-
-## Scope
-
-This Code of Conduct applies within all project spaces, and it also applies when
-an individual is representing the project or its community in public spaces.
-Examples of representing a project or community include using an official
-project e-mail address, posting via an official social media account, or acting
-as an appointed representative at an online or offline event. Representation of
-a project may be further defined and clarified by project maintainers.
-
-## Enforcement
-
-Instances of abusive, harassing, or otherwise unacceptable behavior may be
-reported by contacting the project team at . All
-complaints will be reviewed and investigated and will result in a response that
-is deemed necessary and appropriate to the circumstances. The project team is
-obligated to maintain confidentiality with regard to the reporter of an incident.
-Further details of specific enforcement policies may be posted separately.
-
-Project maintainers who do not follow or enforce the Code of Conduct in good
-faith may face temporary or permanent repercussions as determined by other
-members of the project's leadership.
-
-## Attribution
-
-This Code of Conduct is adapted from the [Contributor Covenant][homepage], version 1.4,
-available at https://www.contributor-covenant.org/version/1/4/code-of-conduct.html
-
-[homepage]: https://www.contributor-covenant.org
-
-For answers to common questions about this code of conduct, see
-https://www.contributor-covenant.org/faq
-
diff --git a/spaces/ahmedghani/svoice_demo/svoice/models/__init__.py b/spaces/ahmedghani/svoice_demo/svoice/models/__init__.py
deleted file mode 100644
index 5656d59e07f3fa33dd3bad1a0f9279ff4b8a6128..0000000000000000000000000000000000000000
--- a/spaces/ahmedghani/svoice_demo/svoice/models/__init__.py
+++ /dev/null
@@ -1,5 +0,0 @@
-# Copyright (c) Facebook, Inc. and its affiliates.
-# All rights reserved.
-#
-# This source code is licensed under the license found in the
-# LICENSE file in the root directory of this source tree.
diff --git a/spaces/akhaliq/Kapao/models/yolo.py b/spaces/akhaliq/Kapao/models/yolo.py
deleted file mode 100644
index 37ae9c84d0c3b4e2cf3f4cdee84e2953663e88df..0000000000000000000000000000000000000000
--- a/spaces/akhaliq/Kapao/models/yolo.py
+++ /dev/null
@@ -1,327 +0,0 @@
-# YOLOv5 🚀 by Ultralytics, GPL-3.0 license
-"""
-YOLO-specific modules
-
-Usage:
- $ python path/to/models/yolo.py --cfg yolov5s.yaml
-"""
-
-import argparse
-import sys
-from copy import deepcopy
-from pathlib import Path
-
-FILE = Path(__file__).absolute()
-sys.path.append(FILE.parents[1].as_posix()) # add yolov5/ to path
-
-from models.common import *
-from models.experimental import *
-from utils.autoanchor import check_anchor_order
-from utils.general import make_divisible, check_file, set_logging
-from utils.plots import feature_visualization
-from utils.torch_utils import time_sync, fuse_conv_and_bn, model_info, scale_img, initialize_weights, \
- select_device, copy_attr
-
-try:
- import thop # for FLOPs computation
-except ImportError:
- thop = None
-
-LOGGER = logging.getLogger(__name__)
-
-
-class Detect(nn.Module):
- stride = None # strides computed during build
- onnx_dynamic = False # ONNX export parameter
-
- def __init__(self, nc=80, anchors=(), ch=(), inplace=True, num_coords=0): # detection layer
- super().__init__()
- self.nc = nc # number of classes
- self.no = nc + 5 # number of outputs per anchor
- self.nl = len(anchors) # number of detection layers
- self.na = len(anchors[0]) // 2 # number of anchors
- self.grid = [torch.zeros(1)] * self.nl # init grid
- a = torch.tensor(anchors).float().view(self.nl, -1, 2)
- self.register_buffer('anchors', a) # shape(nl,na,2)
- self.register_buffer('anchor_grid', a.clone().view(self.nl, 1, -1, 1, 1, 2)) # shape(nl,1,na,1,1,2)
- self.m = nn.ModuleList(nn.Conv2d(x, self.no * self.na, 1) for x in ch) # output conv
- self.inplace = inplace # use in-place ops (e.g. slice assignment)
- self.num_coords = num_coords
-
- def forward(self, x):
- z = [] # inference output
- for i in range(self.nl):
- x[i] = self.m[i](x[i]) # conv
- bs, _, ny, nx = x[i].shape # x(bs,255,20,20) to x(bs,3,20,20,85)
- x[i] = x[i].view(bs, self.na, self.no, ny, nx).permute(0, 1, 3, 4, 2).contiguous()
-
- if not self.training: # inference
- if self.grid[i].shape[2:4] != x[i].shape[2:4] or self.onnx_dynamic:
- self.grid[i] = self._make_grid(nx, ny).to(x[i].device)
-
- y = x[i].sigmoid()
- if self.inplace:
- y[..., 0:2] = (y[..., 0:2] * 2. - 0.5 + self.grid[i]) * self.stride[i] # xy
- y[..., 2:4] = (y[..., 2:4] * 2) ** 2 * self.anchor_grid[i] # wh
-
- if hasattr(self, 'num_coords') and self.num_coords:
- y[..., -self.num_coords:] = y[..., -self.num_coords:] * 4. - 2.
- y[..., -self.num_coords:] *= self.anchor_grid[i].repeat((1, 1, 1, 1, self.num_coords // 2))
- y[..., -self.num_coords:] += (self.grid[i] * self.stride[i]).repeat((1, 1, 1, 1, self.num_coords // 2))
-
- else: # for YOLOv5 on AWS Inferentia https://github.com/ultralytics/yolov5/pull/2953
- xy = (y[..., 0:2] * 2. - 0.5 + self.grid[i]) * self.stride[i] # xy
- wh = (y[..., 2:4] * 2) ** 2 * self.anchor_grid[i].view(1, self.na, 1, 1, 2) # wh
- y = torch.cat((xy, wh, y[..., 4:]), -1)
- z.append(y.view(bs, -1, self.no))
- # z.append(y)
-
- return x if self.training else (torch.cat(z, 1), x)
- # return x if self.training else (z, x)
-
- @staticmethod
- def _make_grid(nx=20, ny=20):
- yv, xv = torch.meshgrid([torch.arange(ny), torch.arange(nx)])
- return torch.stack((xv, yv), 2).view((1, 1, ny, nx, 2)).float()
-
-
-class Model(nn.Module):
- def __init__(self, cfg='yolov5s.yaml', ch=3, nc=None, anchors=None, num_coords=0, autobalance=False): # model, input channels, number of classes
- super().__init__()
- if isinstance(cfg, dict):
- self.yaml = cfg # model dict
- else: # is *.yaml
- import yaml # for torch hub
- self.yaml_file = Path(cfg).name
- with open(cfg) as f:
- self.yaml = yaml.safe_load(f) # model dict
-
- # Define model
- ch = self.yaml['ch'] = self.yaml.get('ch', ch) # input channels
- if nc + num_coords and nc + num_coords != self.yaml['nc']:
- LOGGER.info(f"Overriding model.yaml nc={self.yaml['nc']} with nc={nc + num_coords}")
- self.yaml['nc'] = nc + num_coords # override yaml value
- if anchors:
- LOGGER.info(f'Overriding model.yaml anchors with anchors={anchors}')
- self.yaml['anchors'] = round(anchors) # override yaml value
- self.model, self.save = parse_model(deepcopy(self.yaml), ch=[ch]) # model, savelist
- self.names = [str(i) for i in range(self.yaml['nc'])] # default names
- self.inplace = self.yaml.get('inplace', True)
- self.num_coords = num_coords
- if autobalance:
- self.loss_coeffs = nn.Parameter(torch.zeros(2))
- # LOGGER.info([x.shape for x in self.forward(torch.zeros(1, ch, 64, 64))])
-
- # Build strides, anchors
- m = self.model[-1] # Detect()
- if isinstance(m, Detect):
- s = 256 # 2x min stride
- m.inplace = self.inplace
- m.stride = torch.tensor([s / x.shape[-2] for x in self.forward(torch.zeros(1, ch, s, s))]) # forward
- m.anchors /= m.stride.view(-1, 1, 1)
- check_anchor_order(m)
- self.stride = m.stride
- m.num_coords = self.num_coords
- m.nc = nc
- self._initialize_biases() # only run once
- # LOGGER.info('Strides: %s' % m.stride.tolist())
-
- # Init weights, biases
- initialize_weights(self)
- self.info()
- LOGGER.info('')
-
- def forward(self, x, augment=False, profile=False, visualize=False, kp_flip=None,
- scales=[0.5, 1, 2], flips=[None, 3, None]):
- if augment:
- return self.forward_augment(x, kp_flip, s=scales, f=flips) # augmented inference, None
- return self.forward_once(x, profile, visualize) # single-scale inference, train
-
- def forward_augment(self, x, kp_flip, s=[0.5, 1, 2], f=[None, 3, None]):
- img_size = x.shape[-2:] # height, width
- # s = [1, 0.83, 0.67] # scales
- # f = [None, 3, None] # flips (2-ud, 3-lr)
- y = [] # outputs
- train_out = None
- for si, fi in zip(s, f):
- xi = scale_img(x.flip(fi) if fi else x, si, gs=int(self.stride.max()))
- yi, train_out_i = self.forward_once(xi) # forward
- if si == 1 and fi is None:
- train_out = train_out_i
- # cv2.imwrite(f'img_{si}.jpg', 255 * xi[0].cpu().numpy().transpose((1, 2, 0))[:, :, ::-1]) # save
- yi = self._descale_pred(yi, fi, si, img_size, kp_flip)
- y.append(yi)
- return torch.cat(y, 1), train_out # augmented inference, train
-
- def forward_once(self, x, profile=False, visualize=False):
- y, dt = [], [] # outputs
- for m in self.model:
- if m.f != -1: # if not from previous layer
- x = y[m.f] if isinstance(m.f, int) else [x if j == -1 else y[j] for j in m.f] # from earlier layers
-
- if profile:
- c = isinstance(m, Detect) # copy input as inplace fix
- o = thop.profile(m, inputs=(x.copy() if c else x,), verbose=False)[0] / 1E9 * 2 if thop else 0 # FLOPs
- t = time_sync()
- for _ in range(10):
- m(x.copy() if c else x)
- dt.append((time_sync() - t) * 100)
- if m == self.model[0]:
- LOGGER.info(f"{'time (ms)':>10s} {'GFLOPs':>10s} {'params':>10s} {'module'}")
- LOGGER.info(f'{dt[-1]:10.2f} {o:10.2f} {m.np:10.0f} {m.type}')
-
- x = m(x) # run
- y.append(x if m.i in self.save else None) # save output
-
- if visualize:
- feature_visualization(x, m.type, m.i, save_dir=visualize)
-
- if profile:
- LOGGER.info('%.1fms total' % sum(dt))
- return x
-
- def _descale_pred(self, p, flips, scale, img_size, kp_flip):
- # de-scale predictions following augmented inference (inverse operation)
- if self.inplace:
- p[..., :4] /= scale # de-scale bbox
- if kp_flip:
- p[..., -self.num_coords:] /= scale # de-scale kp
- if flips == 2:
- p[..., 1] = img_size[0] - p[..., 1] # de-flip ud
- elif flips == 3:
- p[..., 0] = img_size[1] - p[..., 0] # de-flip lr
- if kp_flip:
- p[..., 6:6 + self.nc - 1] = p[..., 6:6 + self.nc - 1][..., kp_flip] # de-flip bbox conf
- p[..., -self.num_coords::2] = img_size[1] - p[..., -self.num_coords::2] # de-flip kp x
- p[..., -self.num_coords::2] = p[..., -self.num_coords::2][..., kp_flip] # swap lr kp (x)
- p[..., -self.num_coords + 1::2] = p[..., -self.num_coords + 1::2][..., kp_flip] # swap lr kp (y)
-
- else:
- x, y, wh = p[..., 0:1] / scale, p[..., 1:2] / scale, p[..., 2:4] / scale # de-scale
- if flips == 2:
- y = img_size[0] - y # de-flip ud
- elif flips == 3:
- x = img_size[1] - x # de-flip lr
- p = torch.cat((x, y, wh, p[..., 4:]), -1)
- return p
-
- def _initialize_biases(self, cf=None): # initialize biases into Detect(), cf is class frequency
- # https://arxiv.org/abs/1708.02002 section 3.3
- # cf = torch.bincount(torch.tensor(np.concatenate(dataset.labels, 0)[:, 0]).long(), minlength=nc) + 1.
- m = self.model[-1] # Detect() module
- for mi, s in zip(m.m, m.stride): # from
- b = mi.bias.view(m.na, -1) # conv.bias(255) to (3,85)
- b.data[:, 4] += math.log(8 / (640 / s) ** 2) # obj (8 objects per 640 image)
- b.data[:, 5:5+m.nc] += math.log(0.6 / (m.nc - 0.99)) if cf is None else torch.log(cf / cf.sum()) # cls
- mi.bias = torch.nn.Parameter(b.view(-1), requires_grad=True)
-
- def _print_biases(self):
- m = self.model[-1] # Detect() module
- for mi in m.m: # from
- b = mi.bias.detach().view(m.na, -1).T # conv.bias(255) to (3,85)
- LOGGER.info(
- ('%6g Conv2d.bias:' + '%10.3g' * 6) % (mi.weight.shape[1], *b[:5].mean(1).tolist(), b[5:].mean()))
-
- # def _print_weights(self):
- # for m in self.model.modules():
- # if type(m) is Bottleneck:
- # LOGGER.info('%10.3g' % (m.w.detach().sigmoid() * 2)) # shortcut weights
-
- def fuse(self): # fuse model Conv2d() + BatchNorm2d() layers
- LOGGER.info('Fusing layers... ')
- for m in self.model.modules():
- if isinstance(m, (Conv, DWConv)) and hasattr(m, 'bn'):
- m.conv = fuse_conv_and_bn(m.conv, m.bn) # update conv
- delattr(m, 'bn') # remove batchnorm
- m.forward = m.forward_fuse # update forward
- self.info()
- return self
-
- def autoshape(self): # add AutoShape module
- LOGGER.info('Adding AutoShape... ')
- m = AutoShape(self) # wrap model
- copy_attr(m, self, include=('yaml', 'nc', 'hyp', 'names', 'stride'), exclude=()) # copy attributes
- return m
-
- def info(self, verbose=False, img_size=640): # print model information
- model_info(self, verbose, img_size)
-
-
-def parse_model(d, ch): # model_dict, input_channels(3)
- LOGGER.info('\n%3s%18s%3s%10s %-40s%-30s' % ('', 'from', 'n', 'params', 'module', 'arguments'))
- anchors, nc, gd, gw = d['anchors'], d['nc'], d['depth_multiple'], d['width_multiple']
- na = (len(anchors[0]) // 2) if isinstance(anchors, list) else anchors # number of anchors
- no = na * (nc + 5) # number of outputs = anchors * (classes + 5)
-
- layers, save, c2 = [], [], ch[-1] # layers, savelist, ch out
- for i, (f, n, m, args) in enumerate(d['backbone'] + d['head']): # from, number, module, args
- m = eval(m) if isinstance(m, str) else m # eval strings
- for j, a in enumerate(args):
- try:
- args[j] = eval(a) if isinstance(a, str) else a # eval strings
- except:
- pass
-
- n = n_ = max(round(n * gd), 1) if n > 1 else n # depth gain
- if m in [Conv, GhostConv, Bottleneck, GhostBottleneck, SPP, SPPF, DWConv, MixConv2d, Focus, CrossConv,
- BottleneckCSP, C3, C3TR, C3SPP, C3Ghost]:
- c1, c2 = ch[f], args[0]
- if c2 != no: # if not output
- c2 = make_divisible(c2 * gw, 8)
-
- args = [c1, c2, *args[1:]]
- if m in [BottleneckCSP, C3, C3TR, C3Ghost]:
- args.insert(2, n) # number of repeats
- n = 1
- elif m is nn.BatchNorm2d:
- args = [ch[f]]
- elif m is Concat:
- c2 = sum([ch[x] for x in f])
- elif m is Detect:
- args.append([ch[x] for x in f])
- if isinstance(args[1], int): # number of anchors
- args[1] = [list(range(args[1] * 2))] * len(f)
- elif m is Contract:
- c2 = ch[f] * args[0] ** 2
- elif m is Expand:
- c2 = ch[f] // args[0] ** 2
- else:
- c2 = ch[f]
-
- m_ = nn.Sequential(*[m(*args) for _ in range(n)]) if n > 1 else m(*args) # module
- t = str(m)[8:-2].replace('__main__.', '') # module type
- np = sum([x.numel() for x in m_.parameters()]) # number params
- m_.i, m_.f, m_.type, m_.np = i, f, t, np # attach index, 'from' index, type, number params
- LOGGER.info('%3s%18s%3s%10.0f %-40s%-30s' % (i, f, n_, np, t, args)) # print
- save.extend(x % i for x in ([f] if isinstance(f, int) else f) if x != -1) # append to savelist
- layers.append(m_)
- if i == 0:
- ch = []
- ch.append(c2)
- return nn.Sequential(*layers), sorted(save)
-
-
-if __name__ == '__main__':
- parser = argparse.ArgumentParser()
- parser.add_argument('--cfg', type=str, default='yolov5s.yaml', help='model.yaml')
- parser.add_argument('--device', default='', help='cuda device, i.e. 0 or 0,1,2,3 or cpu')
- parser.add_argument('--profile', action='store_true', help='profile model speed')
- opt = parser.parse_args()
- opt.cfg = check_file(opt.cfg) # check file
- set_logging()
- device = select_device(opt.device)
-
- # Create model
- model = Model(opt.cfg).to(device)
- model.train()
-
- # Profile
- if opt.profile:
- img = torch.rand(8 if torch.cuda.is_available() else 1, 3, 640, 640).to(device)
- y = model(img, profile=True)
-
- # Tensorboard (not working https://github.com/ultralytics/yolov5/issues/2898)
- # from torch.utils.tensorboard import SummaryWriter
- # tb_writer = SummaryWriter('.')
- # LOGGER.info("Run 'tensorboard --logdir=models' to view tensorboard at http://localhost:6006/")
- # tb_writer.add_graph(torch.jit.trace(model, img, strict=False), []) # add model graph
diff --git a/spaces/akhaliq/Music_Source_Separation/bytesep/data/augmentors.py b/spaces/akhaliq/Music_Source_Separation/bytesep/data/augmentors.py
deleted file mode 100644
index d4d40731d4a8f8123a29351b1f97c21f47cb6dc4..0000000000000000000000000000000000000000
--- a/spaces/akhaliq/Music_Source_Separation/bytesep/data/augmentors.py
+++ /dev/null
@@ -1,157 +0,0 @@
-from typing import Dict
-
-import librosa
-import numpy as np
-
-from bytesep.utils import db_to_magnitude, get_pitch_shift_factor, magnitude_to_db
-
-
-class Augmentor:
- def __init__(self, augmentations: Dict, random_seed=1234):
- r"""Augmentor for data augmentation of a waveform.
-
- Args:
- augmentations: Dict, e.g, {
- 'mixaudio': {'vocals': 2, 'accompaniment': 2}
- 'pitch_shift': {'vocals': 4, 'accompaniment': 4},
- ...,
- }
- random_seed: int
- """
- self.augmentations = augmentations
- self.random_state = np.random.RandomState(random_seed)
-
- def __call__(self, waveform: np.array, source_type: str) -> np.array:
- r"""Augment a waveform.
-
- Args:
- waveform: (channels_num, audio_samples)
- source_type: str
-
- Returns:
- new_waveform: (channels_num, new_audio_samples)
- """
- if 'pitch_shift' in self.augmentations.keys():
- waveform = self.pitch_shift(waveform, source_type)
-
- if 'magnitude_scale' in self.augmentations.keys():
- waveform = self.magnitude_scale(waveform, source_type)
-
- if 'swap_channel' in self.augmentations.keys():
- waveform = self.swap_channel(waveform, source_type)
-
- if 'flip_axis' in self.augmentations.keys():
- waveform = self.flip_axis(waveform, source_type)
-
- return waveform
-
- def pitch_shift(self, waveform: np.array, source_type: str) -> np.array:
- r"""Shift the pitch of a waveform. We use resampling for fast pitch
- shifting, so the speed will also be chaneged. The length of the returned
- waveform will be changed.
-
- Args:
- waveform: (channels_num, audio_samples)
- source_type: str
-
- Returns:
- new_waveform: (channels_num, new_audio_samples)
- """
-
- # maximum pitch shift in semitones
- max_pitch_shift = self.augmentations['pitch_shift'][source_type]
-
- if max_pitch_shift == 0: # No pitch shift augmentations.
- return waveform
-
- # random pitch shift
- rand_pitch = self.random_state.uniform(
- low=-max_pitch_shift, high=max_pitch_shift
- )
-
- # We use librosa.resample instead of librosa.effects.pitch_shift
- # because it is 10x times faster.
- pitch_shift_factor = get_pitch_shift_factor(rand_pitch)
- dummy_sample_rate = 10000 # Dummy constant.
-
- channels_num = waveform.shape[0]
-
- if channels_num == 1:
- waveform = np.squeeze(waveform)
-
- new_waveform = librosa.resample(
- y=waveform,
- orig_sr=dummy_sample_rate,
- target_sr=dummy_sample_rate / pitch_shift_factor,
- res_type='linear',
- axis=-1,
- )
-
- if channels_num == 1:
- new_waveform = new_waveform[None, :]
-
- return new_waveform
-
- def magnitude_scale(self, waveform: np.array, source_type: str) -> np.array:
- r"""Scale the magnitude of a waveform.
-
- Args:
- waveform: (channels_num, audio_samples)
- source_type: str
-
- Returns:
- new_waveform: (channels_num, audio_samples)
- """
- lower_db = self.augmentations['magnitude_scale'][source_type]['lower_db']
- higher_db = self.augmentations['magnitude_scale'][source_type]['higher_db']
-
- if lower_db == 0 and higher_db == 0: # No magnitude scale augmentation.
- return waveform
-
- # The magnitude (in dB) of the sample with the maximum value.
- waveform_db = magnitude_to_db(np.max(np.abs(waveform)))
-
- new_waveform_db = self.random_state.uniform(
- waveform_db + lower_db, min(waveform_db + higher_db, 0)
- )
-
- relative_db = new_waveform_db - waveform_db
-
- relative_scale = db_to_magnitude(relative_db)
-
- new_waveform = waveform * relative_scale
-
- return new_waveform
-
- def swap_channel(self, waveform: np.array, source_type: str) -> np.array:
- r"""Randomly swap channels.
-
- Args:
- waveform: (channels_num, audio_samples)
- source_type: str
-
- Returns:
- new_waveform: (channels_num, audio_samples)
- """
- ndim = waveform.shape[0]
-
- if ndim == 1:
- return waveform
- else:
- random_axes = self.random_state.permutation(ndim)
- return waveform[random_axes, :]
-
- def flip_axis(self, waveform: np.array, source_type: str) -> np.array:
- r"""Randomly flip the waveform along x-axis.
-
- Args:
- waveform: (channels_num, audio_samples)
- source_type: str
-
- Returns:
- new_waveform: (channels_num, audio_samples)
- """
- ndim = waveform.shape[0]
- random_values = self.random_state.choice([-1, 1], size=ndim)
-
- return waveform * random_values[:, None]
diff --git a/spaces/akhaliq/deeplab2/trainer/__init__.py b/spaces/akhaliq/deeplab2/trainer/__init__.py
deleted file mode 100644
index 35e4ce02ff422f3aa84ab644b88d65b13e0cbc03..0000000000000000000000000000000000000000
--- a/spaces/akhaliq/deeplab2/trainer/__init__.py
+++ /dev/null
@@ -1,15 +0,0 @@
-# coding=utf-8
-# Copyright 2021 The Deeplab2 Authors.
-#
-# Licensed under the Apache License, Version 2.0 (the "License");
-# you may not use this file except in compliance with the License.
-# You may obtain a copy of the License at
-#
-# http://www.apache.org/licenses/LICENSE-2.0
-#
-# Unless required by applicable law or agreed to in writing, software
-# distributed under the License is distributed on an "AS IS" BASIS,
-# WITHOUT WARRANTIES OR CONDITIONS OF ANY KIND, either express or implied.
-# See the License for the specific language governing permissions and
-# limitations under the License.
-
diff --git a/spaces/alamin655/Personas/app.py b/spaces/alamin655/Personas/app.py
deleted file mode 100644
index cdffcef94ed2e1afa375f99fb8220aed03a2c5d7..0000000000000000000000000000000000000000
--- a/spaces/alamin655/Personas/app.py
+++ /dev/null
@@ -1,363 +0,0 @@
-# Copyright (c) 2022 Cohere Inc. and its affiliates.
-#
-# Licensed under the MIT License (the "License");
-# you may not use this file except in compliance with the License.
-#
-# You may obtain a copy of the License in the LICENSE file at the top
-# level of this repository.
-
-
-import ast
-import copy
-import os
-import sys
-
-import cohere
-import emoji
-import streamlit as st
-
-from conversant.demo import ui, utils
-from conversant.prompt_chatbot import PERSONA_MODEL_DIRECTORY, PromptChatbot
-from conversant.utils import demo_utils
-
-# Set a custom persona directory by changing the following line
-# e.g. "/Users/yourname/custom-personas"
-# If the line is left as `CUSTOM_PERSONA_DIRECTORY = None`
-# the Streamlit app will use the demo presets
-CUSTOM_PERSONA_DIRECTORY = None
-USER_AVATAR_SHORTCODE = ":bust_in_silhouette:"
-
-
-def peek(iterable) -> str:
- """Retrieves the next item from a generator object if it exists.
-
- Args:
- iterable (generator): A partial reply generator
-
- Returns:
- str: Returns the next partial reply
- """
- try:
- first = next(iterable)
- except StopIteration:
- return ""
- return first
-
-
-def get_reply() -> None:
- """Replies query from the message input and initializes the rerun_count."""
- st.session_state.partial_reply_in_progress = True
- st.session_state.partial_reply_generator = st.session_state.bot.partial_reply(
- query=st.session_state.message_input
- )
- # This variable is used to indicate from where streamlit_talk should animate the
- # typewriter effect from and to.
- st.session_state.prev_partial_chunk, st.session_state.curr_partial_chunk = next(
- st.session_state.partial_reply_generator
- )
- st.session_state.message_input = ""
-
-
-def initialize_chatbot() -> None:
- """Initializes the chatbot from a selected persona and saves the session state."""
- if st.session_state.persona.startswith("(launched)") and len(sys.argv) > 1:
- st.session_state.bot = demo_utils.decode_chatbot(
- sys.argv[1], client=cohere.Client(os.environ.get("COHERE_API_KEY"))
- ) # Launched via demo_utils.launch_streamlit() utility function
- elif st.session_state.persona == "":
- st.session_state.bot = None
- elif st.session_state.persona == "parrot":
- st.session_state.bot = utils.ParrotChatbot(
- client=cohere.Client(os.environ.get("COHERE_API_KEY"))
- )
- else:
- st.session_state.bot = PromptChatbot.from_persona(
- emoji.replace_emoji(st.session_state.persona, "").strip(),
- client=cohere.Client(os.environ.get("COHERE_API_KEY")),
- persona_dir=CUSTOM_PERSONA_DIRECTORY
- if CUSTOM_PERSONA_DIRECTORY
- else PERSONA_MODEL_DIRECTORY,
- )
- if "bot" in st.session_state and st.session_state.bot:
- update_session_with_prompt()
- # Reset the edit_promp_json session state so we don't remain on the JSON editor when
- # changing to another bot. This is because st_ace is unable to write
- # new values from the current session state.
- st.session_state.partial_reply_in_progress = False
- st.session_state.edit_prompt_json = False
-
-
-def update_session_with_prompt() -> None:
- """Saves the prompt config dictionary into the session state."""
- if "bot" in st.session_state and st.session_state.bot:
- st.session_state.snapshot_prompt_config = copy.deepcopy(
- st.session_state.bot.prompt.to_dict()
- )
- st.session_state.snapshot_chatbot_config = copy.deepcopy(
- st.session_state.bot.chatbot_config
- )
- st.session_state.snapshot_client_config = copy.deepcopy(
- st.session_state.bot.client_config
- )
- st.session_state.current_stop_sequences = [
- utils.escape_string(stop_seq)
- for stop_seq in st.session_state.bot.client_config["stop_sequences"]
- ]
-
-
-def update_prompt_from_json() -> None:
- """Evaluates JSON string and updates the session's bot prompt."""
- if st.session_state.json_editor_input:
- try:
- prompt_config = ast.literal_eval(st.session_state.json_editor_input)
- st.session_state.bot.prompt.update(prompt_config)
- update_session_with_prompt()
- st.session_state.error = ""
- except Exception as e:
- st.session_state.error = e
-
-
-# This ensures rendering is prevented upon import of this file.
-if __name__ == "__main__":
- st.set_page_config(
- page_title="Conversational personas using Cohere",
- page_icon="🎭",
- layout="wide",
- )
-
- # Streamlit's default elements are not easy to style. Instead, we have to
- # define styling in a custom CSS file and inject it into the Streamlit DOM.
- # This is brittle and dependent on the DOM structure. Any changes to the layout
- # will break the styling defined in this file.
- with open(f"{os.path.dirname(__file__)}/styles.css") as f:
- utils.style_using_css(f.read())
-
- # We use the :bust_in_silhouette: emoji as a neutral user avatar.
- st.session_state.user_avatar = utils.get_twemoji_url_from_shortcode(
- USER_AVATAR_SHORTCODE
- )
-
- # Each persona is a directory in PERSONA_MODEL_DIRECTORY, each with its
- # config.json file.
- if CUSTOM_PERSONA_DIRECTORY:
- st.session_state.persona_options = utils.get_persona_options(
- CUSTOM_PERSONA_DIRECTORY
- )
- else:
- st.session_state.persona_options = utils.get_persona_options()
-
- # Check if COHERE_API_KEY is not set from secrets.toml or os.environ
- if "COHERE_API_KEY" not in os.environ:
- raise KeyError(
- "COHERE_API_KEY not found in st.secrets or os.environ. Please set it in "
- ".streamlit/secrets.toml or as an environment variable."
- )
-
- # A chatbot can be passed in as a base64 encoding of a pickled PromptChatbot object.
- # This is only used when calling the launch_demo() method of a PromptChatbot object.
- # The chatbot is then injected into the list of available personas in this streamlit
- # demo.
- if len(sys.argv) > 1 and "bot" not in st.session_state:
-
- # The PromptChatbot passed in should be a base64 encoding of a pickled
- # PromptChatbot object.
- bot = demo_utils.decode_chatbot(
- sys.argv[1], cohere.Client(os.environ.get("COHERE_API_KEY"))
- )
- if not isinstance(bot, PromptChatbot):
- raise TypeError("base64 string passed in is not of class PromptChatbot")
- else:
- st.session_state.bot = bot
- st.session_state.persona_options.insert(
- 0, f"(launched) {st.session_state.bot.persona_name}"
- )
-
- # Adding a header to direct users to sign up for Cohere, explore the playground,
- # and check out our git repo.
- st.header("🎭 Conversational Personas using Cohere")
- with st.expander("About", expanded="bot" not in st.session_state):
- st.markdown(
- """
- This demo app is using
- [**conversant**](https://github.com/cohere-ai/sandbox-conversant-lib), an
- open-source framework for building chatbots on top of Cohere’s large
- language models.
-
- Cohere provides access to advanced Large Language Models and NLP tools through
- one easy-to-use API.
- """
- "[**Get started for free!**]"
- "(https://dashboard.cohere.ai/welcome/register?utm_source=cohere-owned&utm_"
- "medium=content&utm_campaign=sandbox&utm_term=streamlit&utm_content=conversant)"
- )
-
- # Page control flow logic is determined from the sidebar.
- with st.sidebar:
- st.selectbox(
- "Choose a chatbot persona:",
- options=st.session_state.persona_options,
- key="persona",
- on_change=initialize_chatbot,
- )
- st.checkbox(
- "Edit prompt",
- value=False,
- key="edit_prompt",
- on_change=update_session_with_prompt,
- )
- if st.session_state.edit_prompt:
- st.checkbox(
- "Use JSON editor",
- value=False,
- key="edit_prompt_json",
- on_change=update_session_with_prompt,
- )
-
- # Initialize a settings container in the sidebar. This allows us to place
- # Streamlit elements within this placeholder later in this script.
- settings_placeholder = st.empty()
-
- # Initialize a chat container as the middle of 3 vertical columns.
- # Only visible when the edit prompt checkbox is not selected.
- _, chat_placeholder, _ = st.columns([1, 1, 1])
- with chat_placeholder.container():
- chat_history_placeholder = st.empty()
- message_input_placeholder = st.empty()
-
- # Initialize a prompt json and string view as 2 vertical columns.
- # Only visible when the edit prompt checkbox is selected.
- prompt_json_column, prompt_string_column = st.columns([1, 1])
- with prompt_json_column:
- prompt_json_edit_placeholder = st.empty()
- prompt_json_view_placeholder = st.empty()
- with prompt_string_column:
- prompt_string_placeholder = st.empty()
-
- # Check if bot has been initialized in the Streamlit session.
- if "bot" in st.session_state and st.session_state.bot:
-
- # Initialize the bot avatar
- bot_avatar_string = st.session_state.bot.chatbot_config["avatar"]
- st.session_state.bot_avatar = (
- utils.get_twemoji_url_from_shortcode(bot_avatar_string)
- if emoji.is_emoji(emoji.emojize(bot_avatar_string, language="alias"))
- else bot_avatar_string
- )
-
- # Editor view for the prompt
- if st.session_state.edit_prompt:
-
- # Edit the prompt using a JSON editor
- if st.session_state.edit_prompt_json:
-
- # The prompt JSON editor needs to be drawn first so that
- # the displayed form values in the sidebar take reference from
- # the editor.
- with prompt_json_edit_placeholder.container():
- ui.draw_prompt_json_editor(
- max_height=955
- ) # st_ace only accepts hardcoded pixel values
- update_prompt_from_json()
-
- with settings_placeholder.container():
- with st.expander("Client Config"):
- ui.draw_client_config_form()
- with st.expander("Chatbot Config"):
- ui.draw_chatbot_config_form()
- ui.draw_prompt_form(disabled=True)
-
- with prompt_string_placeholder.container():
- ui.draw_prompt_view(json=False)
-
- # Edit the prompt using a form in the sidebar
- else:
-
- # The settings form needs to be drawn first so that
- # the displayed JSON values in prompt JSON placeholder
- # take reference from the form.
- with settings_placeholder.container():
- with st.expander("Client Config"):
- ui.draw_client_config_form()
- with st.expander("Chatbot Config"):
- ui.draw_chatbot_config_form()
- ui.draw_prompt_form(disabled=False)
-
- with prompt_json_view_placeholder.container():
- ui.draw_prompt_view(json=True)
-
- with prompt_string_placeholder.container():
- ui.draw_prompt_view(json=False)
-
- # Chat view with the persona
- else:
-
- # We can get the chatbot to begin the conversation with this.
- # The session's state needs to be manually updated since we are not
- # refreshing the entire Streamlit app.
- if not st.session_state.bot.chat_history:
- st.session_state.message_input = "Hello"
- get_reply()
- update_session_with_prompt()
-
- # Draw UI elements for the sidebar
- with settings_placeholder.container():
-
- with st.expander("Client Config"):
- ui.draw_client_config_form()
- with st.expander("Chatbot Config"):
- ui.draw_chatbot_config_form()
-
- with st.expander("Prompt (JSON)"):
- ui.draw_prompt_view(json=True)
-
- with st.expander("Prompt (string)", expanded=True):
- ui.draw_prompt_view(json=False)
-
- # Draw the message input field and a disclaimer.
- with message_input_placeholder.container():
- st.text_input(
- label=f"Chat with {st.session_state.bot.prompt.bot_name}!",
- placeholder="Type a message",
- key="message_input",
- on_change=get_reply,
- disabled=st.session_state.partial_reply_in_progress,
- )
- ui.draw_disclaimer()
-
- # When in chat view, anchor elements from the bottom so that
- # the message input field is at the bottom (more natural).
- utils.style_using_css(
- """div.css-k1ih3n.egzxvld4 > div:nth-child(1) > div:nth-child(1) > div:nth-child(6) { /* # noqa */
- margin-top: auto;
- }
- """
- )
-
- # Draw chat history.
- with chat_history_placeholder.container():
- ui.draw_chat_history()
-
- # Rerun the app if there are partial replies to add to the latest
- # response.
- if "partial_reply_generator" in st.session_state:
- st.session_state.partial_reply_in_progress = True
- yielded_chunks = peek(st.session_state.partial_reply_generator)
- if yielded_chunks:
- previous_partial_chunk, partial_chunk = yielded_chunks
- st.session_state.prev_partial_chunk = previous_partial_chunk
- st.session_state.curr_partial_chunk = partial_chunk
- st.experimental_rerun()
- else:
- del st.session_state.partial_reply_generator
- st.session_state.partial_reply_in_progress = False
- # At the end of the partial reply generation, we want the typewriter
- # animation to cease. To do this, make the prev and curr the same
- # so that nothing is animated.
- st.session_state.prev_partial_chunk = (
- st.session_state.curr_partial_chunk
- )
- assert (
- st.session_state.bot.chat_history[-1]["bot"].strip()
- == st.session_state.curr_partial_chunk.strip()
- )
- st.experimental_rerun()
diff --git a/spaces/aliabid94/AutoGPT/autogpt/processing/html.py b/spaces/aliabid94/AutoGPT/autogpt/processing/html.py
deleted file mode 100644
index 81387b12adab5023150c55f2075ddd40b554f386..0000000000000000000000000000000000000000
--- a/spaces/aliabid94/AutoGPT/autogpt/processing/html.py
+++ /dev/null
@@ -1,33 +0,0 @@
-"""HTML processing functions"""
-from __future__ import annotations
-
-from bs4 import BeautifulSoup
-from requests.compat import urljoin
-
-
-def extract_hyperlinks(soup: BeautifulSoup, base_url: str) -> list[tuple[str, str]]:
- """Extract hyperlinks from a BeautifulSoup object
-
- Args:
- soup (BeautifulSoup): The BeautifulSoup object
- base_url (str): The base URL
-
- Returns:
- List[Tuple[str, str]]: The extracted hyperlinks
- """
- return [
- (link.text, urljoin(base_url, link["href"]))
- for link in soup.find_all("a", href=True)
- ]
-
-
-def format_hyperlinks(hyperlinks: list[tuple[str, str]]) -> list[str]:
- """Format hyperlinks to be displayed to the user
-
- Args:
- hyperlinks (List[Tuple[str, str]]): The hyperlinks to format
-
- Returns:
- List[str]: The formatted hyperlinks
- """
- return [f"{link_text} ({link_url})" for link_text, link_url in hyperlinks]
diff --git a/spaces/allandclive/Uganda_MMS/uroman/lib/NLP/utilities.pm b/spaces/allandclive/Uganda_MMS/uroman/lib/NLP/utilities.pm
deleted file mode 100644
index 7be117449190533d826bd63b9266c1434d00408f..0000000000000000000000000000000000000000
--- a/spaces/allandclive/Uganda_MMS/uroman/lib/NLP/utilities.pm
+++ /dev/null
@@ -1,3652 +0,0 @@
-################################################################
-# #
-# utilities #
-# #
-################################################################
-
-package NLP::utilities;
-
-use File::Spec;
-use Time::HiRes qw(time);
-use Time::Local;
-use NLP::English;
-use NLP::UTF8;
-
-$utf8 = NLP::UTF8;
-$englishPM = NLP::English;
-
-%empty_ht = ();
-
-use constant DEBUGGING => 0;
-
-sub member {
- local($this,$elem,@array) = @_;
-
- my $a;
- if (defined($elem)) {
- foreach $a (@array) {
- if (defined($a)) {
- return 1 if $elem eq $a;
- } else {
- $DB::single = 1; # debugger breakpoint
- print STDERR "\nWarning: Undefined variable utilities::member::a\n";
- }
- }
- } else {
- $DB::single = 1; # debugger breakpoint
- print STDERR "\nWarning: Undefined variable utilities::member::elem\n";
- }
- return 0;
-}
-
-sub dual_member {
- local($this,$elem1,$elem2,*array1,*array2) = @_;
- # returns 1 if there exists a position $n
- # such that $elem1 occurs at position $n in @array1
- # and $elem2 occurs at same position $n in @array2
-
- return 0 unless defined($elem1) && defined($elem2);
- my $last_index = ($#array1 < $#array2) ? $#array1 : $#array2; #min
- my $a;
- my $b;
- foreach $i ((0 .. $last_index)) {
- return 1 if defined($a = $array1[$i]) && defined($b = $array2[$i]) && ($a eq $elem1) && ($b eq $elem2);
- }
- return 0;
-}
-
-sub sorted_list_equal {
- local($this,*list1,*list2) = @_;
-
- return 0 unless $#list1 == $#list2;
- foreach $i ((0 .. $#list1)) {
- return 0 unless $list1[$i] eq $list2[$i];
- }
- return 1;
-}
-
-sub trim {
- local($this, $s) = @_;
-
- $s =~ s/^\s*//;
- $s =~ s/\s*$//;
- $s =~ s/\s+/ /g;
- return $s;
-}
-
-sub trim2 {
- local($this, $s) = @_;
-
- $s =~ s/^\s*//;
- $s =~ s/\s*$//;
- return $s;
-}
-
-sub trim_left {
- local($this, $s) = @_;
- $s =~ s/^\s*//;
- return $s;
-}
-
-sub cap_member {
- local($this,$elem,@array) = @_;
-
- my $a;
- my $lc_elem = lc $elem;
- foreach $a (@array) {
- return $a if $lc_elem eq lc $a;
- }
- return "";
-}
-
-sub remove_elem {
- local($this,$elem,@array) = @_;
-
- return @array unless $this->member($elem, @array);
- @rm_list = ();
- foreach $a (@array) {
- push(@rm_list, $a) unless $elem eq $a;
- }
- return @rm_list;
-}
-
-sub intersect_p {
- local($this,*list1,*list2) = @_;
-
- foreach $elem1 (@list1) {
- if (defined($elem1)) {
- foreach $elem2 (@list2) {
- if (defined($elem2)) {
- return 1 if $elem1 eq $elem2;
- } else {
- $DB::single = 1; # debugger breakpoint
- print STDERR "\nWarning: Undefined variable utilities::intersect_p::elem2\n";
- }
- }
- } else {
- $DB::single = 1; # debugger breakpoint
- print STDERR "\nWarning: Undefined variable utilities::intersect_p::elem1\n";
- }
- }
- return 0;
-}
-
-sub intersect_expl_p {
- local($this,*list1,@list2) = @_;
-
- foreach $elem1 (@list1) {
- foreach $elem2 (@list2) {
- return 1 if $elem1 eq $elem2;
- }
- }
- return 0;
-}
-
-sub intersection {
- local($this,*list1,*list2) = @_;
-
- @intersection_list = ();
- foreach $elem1 (@list1) {
- foreach $elem2 (@list2) {
- push(@intersection_list, $elem1) if ($elem1 eq $elem2) && ! $this->member($elem1, @intersection_list);
- }
- }
- return @intersection_list;
-}
-
-sub cap_intersect_p {
- local($this,*list1,*list2) = @_;
-
- foreach $elem1 (@list1) {
- $lc_elem1 = lc $elem1;
- foreach $elem2 (@list2) {
- return 1 if $lc_elem1 eq lc $elem2;
- }
- }
- return 0;
-}
-
-sub subset_p {
- local($this,*list1,*list2) = @_;
-
- foreach $elem1 (@list1) {
- return 0 unless $this->member($elem1, @list2);
- }
- return 1;
-}
-
-sub cap_subset_p {
- local($this,*list1,*list2) = @_;
-
- foreach $elem1 (@list1) {
- return 0 unless $this->cap_member($elem1, @list2);
- }
- return 1;
-}
-
-sub unique {
- local($this, @list) = @_;
-
- my %seen = ();
- @uniq = ();
- foreach $item (@list) {
- push(@uniq, $item) unless $seen{$item}++;
- }
- return @uniq;
-}
-
-sub position {
- local($this,$elem,@array) = @_;
- $i = 0;
- foreach $a (@array) {
- return $i if $elem eq $a;
- $i++;
- }
- return -1;
-}
-
-sub positions {
- local($this,$elem,@array) = @_;
- $i = 0;
- @positions_in_list = ();
- foreach $a (@array) {
- push(@positions_in_list, $i) if $elem eq $a;
- $i++;
- }
- return @positions_in_list;
-}
-
-sub last_position {
- local($this,$elem,@array) = @_;
-
- $result = -1;
- $i = 0;
- foreach $a (@array) {
- $result = $i if $elem eq $a;
- $i++;
- }
- return $result;
-}
-
-sub rand_n_digit_number {
- local($this,$n) = @_;
-
- return 0 unless $n =~ /^[1-9]\d*$/;
- $ten_power_n = 10 ** ($n - 1);
- return int(rand(9 * $ten_power_n)) + $ten_power_n;
-}
-
-# Consider File::Temp
-sub new_tmp_filename {
- local($this,$filename) = @_;
-
- $loop_limit = 1000;
- ($dir,$simple_filename) = ($filename =~ /^(.+)\/([^\/]+)$/);
- $simple_filename = $filename unless defined($simple_filename);
- $new_filename = "$dir/tmp-" . $this->rand_n_digit_number(8) . "-$simple_filename";
- while ((-e $new_filename) && ($loop_limit-- >= 0)) {
- $new_filename = "$dir/tmp-" . $this->rand_n_digit_number(8) . "-$simple_filename";
- }
- return $new_filename;
-}
-
-# support sorting order: "8", "8.0", "8.5", "8.5.1.", "8.10", "10", "10-12"
-
-sub compare_complex_numeric {
- local($this,$a,$b) = @_;
-
- (my $a_num,my $a_rest) = ($a =~ /^(\d+)\D*(.*)$/);
- (my $b_num,my $b_rest) = ($b =~ /^(\d+)\D*(.*)$/);
-
- if (defined($a_rest) && defined($b_rest)) {
- return ($a_num <=> $b_num)
- || $this->compare_complex_numeric($a_rest,$b_rest);
- } else {
- return $a cmp $b;
- }
-}
-
-# support sorting order: "lesson8-ps-v1.9.xml", "Lesson 10_ps-v_1.11.xml"
-# approach: segment strings into alphabetic and numerical sections and compare pairwise
-
-sub compare_mixed_alpha_numeric {
- local($this,$a,$b) = @_;
-
- ($a_alpha,$a_num,$a_rest) = ($a =~ /^(\D*)(\d[-\d\.]*)(.*)$/);
- ($b_alpha,$b_num,$b_rest) = ($b =~ /^(\D*)(\d[-\d\.]*)(.*)$/);
-
- ($a_alpha) = ($a =~ /^(\D*)/) unless defined $a_alpha;
- ($b_alpha) = ($b =~ /^(\D*)/) unless defined $b_alpha;
-
- # ignore non-alphabetic characters in alpha sections
- $a_alpha =~ s/\W|_//g;
- $b_alpha =~ s/\W|_//g;
-
- if ($alpha_cmp = lc $a_alpha cmp lc $b_alpha) {
- return $alpha_cmp;
- } elsif (defined($a_rest) && defined($b_rest)) {
- return $this->compare_complex_numeric($a_num,$b_num)
- || $this->compare_mixed_alpha_numeric ($a_rest,$b_rest);
- } else {
- return (defined($a_num) <=> defined($b_num)) || ($a cmp $b);
- }
-}
-
-# @sorted_lessons = sort { NLP::utilities->compare_mixed_alpha_numeric($a,$b) } @lessons;
-
-sub html_guarded_p {
- local($this,$string) = @_;
-
- return 0 if $string =~ /[<>"]/;
- $string .= " ";
- @segs = split('&',$string);
- shift @segs;
- foreach $seg (@segs) {
- next if $seg =~ /^[a-z]{2,6};/i;
- # next if $seg =~ /^amp;/;
- # next if $seg =~ /^quot;/;
- # next if $seg =~ /^nbsp;/;
- # next if $seg =~ /^gt;/;
- # next if $seg =~ /^lt;/;
- next if $seg =~ /^#(\d+);/;
- next if $seg =~ /^#x([0-9a-fA-F]+);/;
- return 0;
- }
- return 1;
-}
-
-sub guard_tooltip_text {
- local($this,$string) = @_;
-
- $string =~ s/\xCB\x88/'/g;
- return $string;
-}
-
-sub guard_html {
- local($this,$string,$control_string) = @_;
-
- return "" unless defined($string);
- my $guarded_string;
- $control_string = "" unless defined($control_string);
- return $string if ($string =~ /&/)
- && (! ($control_string =~ /\bstrict\b/))
- && $this->html_guarded_p($string);
- $guarded_string = $string;
- $guarded_string =~ s/&/&/g;
- if ($control_string =~ /slash quote/) {
- $guarded_string =~ s/"/\\"/g;
- } elsif ($control_string =~ /keep quote/) {
- } else {
- $guarded_string =~ s/\"/"/g;
- }
- if ($control_string =~ /escape-slash/) {
- $guarded_string =~ s/\//&x2F;/g;
- }
- $guarded_string =~ s/>/>/g;
- $guarded_string =~ s/</g;
- return $guarded_string;
-}
-
-sub unguard_html {
- local($this,$string) = @_;
-
- return undef unless defined($string);
- $string=~ s[&(\S*?);]{
- local $_ = $1;
- /^amp$/i ? "&" :
- /^quot$/i ? '"' :
- /^apos$/i ? "'" :
- /^gt$/i ? ">" :
- /^lt$/i ? "<" :
- /^x2F$/i ? "/" :
- /^nbsp$/i ? "\xC2\xA0" :
- /^#(\d+)$/ ? $this->chr($1) :
- /^#x([0-9a-f]+)$/i ? $this->chr(hex($1)) :
- $_
- }gex;
- return $string;
-}
-
-sub unguard_html_r {
- local($this,$string) = @_;
-
- return undef unless defined($string);
-
- $string =~ s/&/&/g;
- $string =~ s/"/'/g;
- $string =~ s/<//g;
-
- ($d) = ($string =~ /(\d+);/);
- while (defined($d)) {
- $c = $this->chr($d);
- $string =~ s/$d;/$c/g;
- ($d) = ($string =~ /(\d+);/);
- }
- ($x) = ($string =~ /([0-9a-f]+);/i);
- while (defined($x)) {
- $c = $this->chr(hex($x));
- $string =~ s/$x;/$c/g;
- ($x) = ($string =~ /([0-9a-f]+);/i);
- }
- $string0 = $string;
- ($x) = ($string =~ /(?:https?|www|\.com)\S*\%([0-9a-f]{2,2})/i);
- while (defined($x)) {
- $c = $this->chr("%" . hex($x));
- $string =~ s/\%$x/$c/g;
- ($x) = ($string =~ /(?:https?|www|\.com)\S*\%([0-9a-f]{2,2})/i);
- }
- return $string;
-}
-
-sub unguard_html_l {
- local($caller,$string) = @_;
-
- return undef unless defined($string);
-
- my $pre;
- my $core;
- my $post;
- my $repl;
- my $s = $string;
- if (($pre,$core,$post) = ($s =~ /^(.*)&(amp|quot|lt|gt|#\d+|#x[0-9a-f]+);(.*)$/i)) {
- $repl = "?";
- $repl = "&" if $core =~ /^amp$/i;
- $repl = "'" if $core =~ /^quot$/i;
- $repl = "<" if $core =~ /^lt$/i;
- $repl = ">" if $core =~ /^gt$/i;
- if ($core =~ /^#\d+$/i) {
- $core2 = substr($core,1);
- $repl = $caller->chr($core2);
- }
- $repl = $caller->chr(hex(substr($core,2))) if $core =~ /^#x[0-9a-f]+$/i;
- $s = $pre . $repl . $post;
- }
- return $s;
-}
-
-sub guard_html_quote {
- local($caller,$string) = @_;
-
- $string =~ s/"/"/g;
- return $string;
-}
-
-sub unguard_html_quote {
- local($caller,$string) = @_;
-
- $string =~ s/"/"/g;
- return $string;
-}
-
-sub uri_encode {
- local($caller,$string) = @_;
-
- $string =~ s/([^^A-Za-z0-9\-_.!~*()'])/ sprintf "%%%02x", ord $1 /eg;
- return $string;
-}
-
-sub uri_decode {
- local($caller,$string) = @_;
-
- $string =~ s/%([0-9A-Fa-f]{2})/chr(hex($1))/eg;
- return $string;
-}
-
-sub remove_xml_tags {
- local($caller,$string) = @_;
-
- $string =~ s/<\/?[a-zA-Z][-_:a-zA-Z0-9]*(\s+[a-zA-Z][-_:a-zA-Z0-9]*=\"[^"]*\")*\s*\/?>//g;
- return $string;
-}
-
-sub remove_any_tokenization_at_signs_around_xml_tags {
- local($caller,$string) = @_;
-
- $string =~ s/(?:\@ \@)?(<[^<>]+>)(?:\@ \@)?/$1/g;
- $string =~ s/\@?(<[^<>]+>)\@?/$1/g;
- return $string;
-}
-
-sub remove_xml_tags_and_any_bordering_at_signs {
- # at-signs from tokenization
- local($caller,$string) = @_;
-
- $string =~ s/\@?<\/?[a-zA-Z][-_:a-zA-Z0-9]*(\s+[a-zA-Z][-_:a-zA-Z0-9]*=\"[^"]*\")*\s*\/?>\@?//g;
- return $string;
-}
-
-sub chr {
- local($caller,$i) = @_;
-
- return undef unless $i =~ /^\%?\d+$/;
- if ($i =~ /^%/) {
- $i =~ s/^\%//;
- return chr($i) if $i < 128;
- return "\x80" | chr($i - 128) if $i < 256;
- } else {
- return chr($i) if $i < 128;
- return ("\xC0" | chr(($i / 64) % 32))
- . ("\x80" | chr($i % 64)) if $i < 2048;
- return ("\xE0" | chr(int($i / 4096) % 16))
- . ("\x80" | chr(int($i / 64) % 64))
- . ("\x80" | chr($i % 64)) if $i < 65536;
- return ("\xF0" | chr(int($i / 262144) % 8))
- . ("\x80" | chr(int($i / 4096) % 64))
- . ("\x80" | chr(int($i / 64) % 64))
- . ("\x80" | chr($i % 64)) if $i < 2097152;
- }
- return "?";
-}
-
-sub guard_cgi {
- local($caller, $string) = @_;
-
- $guarded_string = $string;
- if ($string =~ /[\x80-\xFF]/) {
- $guarded_string = "";
- while ($string ne "") {
- $char = substr($string, 0, 1);
- $string = substr($string, 1);
- if ($char =~ /^[\\ ;\#\&\:\=\"\'\+\?\x00-\x1F\x80-\xFF]$/) {
- $hex = sprintf("%2.2x",ord($char));
- $guarded_string .= uc "%$hex";
- } else {
- $guarded_string .= $char;
- }
- }
- } else {
- $guarded_string = $string;
- $guarded_string =~ s/%/%25/g;
- $guarded_string =~ s/\n/%5Cn/g;
- $guarded_string =~ s/\t/%5Ct/g;
- $guarded_string =~ s/ /%20/g;
- $guarded_string =~ s/"/%22/g;
- $guarded_string =~ s/#/%23/g;
- $guarded_string =~ s/&/%26/g;
- $guarded_string =~ s/'/%27/g;
- $guarded_string =~ s/\+/%2B/g;
- $guarded_string =~ s/\//%2F/g;
- $guarded_string =~ s/:/%3A/g;
- $guarded_string =~ s/;/%3B/g;
- $guarded_string =~ s/%3C/g;
- $guarded_string =~ s/=/%3D/g;
- $guarded_string =~ s/>/%3E/g;
- $guarded_string =~ s/\?/%3F/g;
- }
- return $guarded_string;
-}
-
-sub repair_cgi_guard {
- local($caller,$string) = @_;
- # undo second cgi-guard, e.g. "Jo%25C3%25ABlle_Aubron" -> "Jo%C3%ABlle_Aubron"
-
- $string =~ s/(%)25([CD][0-9A-F]%)25([89AB][0-9A-F])/$1$2$3/g;
- $string =~ s/(%)25(E[0-9A-F]%)25([89AB][0-9A-F]%)25([89AB][0-9A-F])/$1$2$3$4/g;
- return $string;
-}
-
-sub unguard_cgi {
- local($caller,$string) = @_;
-
- $unguarded_string = $string;
- $unguarded_string =~ s/%5Cn/\n/g;
- $unguarded_string =~ s/%5Ct/\t/g;
- $unguarded_string =~ s/%20/ /g;
- $unguarded_string =~ s/%23/#/g;
- $unguarded_string =~ s/%26/&/g;
- $unguarded_string =~ s/%2B/+/g;
- $unguarded_string =~ s/%2C/,/g;
- $unguarded_string =~ s/%3A/:/g;
- $unguarded_string =~ s/%3D/=/g;
- $unguarded_string =~ s/%3F/?/g;
- $unguarded_string =~ s/%C3%A9/\xC3\xA9/g;
-
- # more general
- ($code) = ($unguarded_string =~ /%([0-9A-F]{2,2})/);
- while (defined($code)) {
- $percent_code = "%" . $code;
- $hex_code = sprintf("%c", hex($code));
- $unguarded_string =~ s/$percent_code/$hex_code/g;
- ($code) = ($unguarded_string =~ /%([0-9A-F]{2,2})/);
- }
-
- return $unguarded_string;
-}
-
-sub regex_guard {
- local($caller,$string) = @_;
-
- $guarded_string = $string;
- $guarded_string =~ s/([\\\/\^\|\(\)\{\}\$\@\*\+\?\.\[\]])/\\$1/g
- if $guarded_string =~ /[\\\/\^\|\(\)\{\}\$\@\*\+\?\.\[\]]/;
-
- return $guarded_string;
-}
-
-sub g_regex_spec_tok_p {
- local($this,$string) = @_;
-
- # specials: ( ) (?: ) [ ]
- return ($string =~ /^(\(\?:|[()\[\]])$/);
-}
-
-sub regex_guard_norm {
- local($this,$string) = @_;
-
- return $string unless $string =~ /[\[\]\\()$@?+]/;
- my $rest = $string;
- my @stack = ("");
- while ($rest ne "") {
- # specials: ( ) (?: ) [ ] ? +
- if (($pre, $special, $post) = ($rest =~ /^((?:\\.|[^\[\]()?+])*)(\(\?:|[\[\]()?+])(.*)$/)) {
- # print STDERR "Special: $pre *$special* $post\n";
- unless ($pre eq "") {
- push(@stack, $pre);
- while (($#stack >= 1) && (! $this->g_regex_spec_tok_p($stack[$#stack-1]))
- && (! $this->g_regex_spec_tok_p($stack[$#stack]))) {
- $s1 = pop @stack;
- $s2 = pop @stack;
- push(@stack, "$s2$s1");
- }
- }
- if ($special =~ /^[?+]$/) {
- push(@stack, "\\") if ($stack[$#stack] eq "")
- || ($this->g_regex_spec_tok_p($stack[$#stack]) && ($stack[$#stack] ne "["));
- push(@stack, $special);
- } elsif ($special eq "]") {
- if (($#stack >= 1) && ($stack[$#stack-1] eq "[") && ! $this->g_regex_spec_tok_p($stack[$#stack])) {
- $char_expression = pop @stack;
- pop @stack;
- push(@stack, "[$char_expression]");
- } else {
- push(@stack, $special);
- }
- } elsif (($special =~ /^[()]/) && (($stack[$#stack] eq "[")
- || (($#stack >= 1)
- && ($stack[$#stack-1] eq "[")
- && ! $this->g_regex_spec_tok_p($stack[$#stack])))) {
- push(@stack, "\\$special");
- } elsif ($special eq ")") {
- if (($#stack >= 1) && ($stack[$#stack-1] =~ /^\((\?:)?$/) && ! $this->g_regex_spec_tok_p($stack[$#stack])) {
- $alt_expression = pop @stack;
- $open_para = pop @stack;
- if ($open_para eq "(") {
- push(@stack, "(?:$alt_expression)");
- } else {
- push(@stack, "$open_para$alt_expression)");
- }
- } else {
- push(@stack, $special);
- }
- } else {
- push(@stack, $special);
- }
- while (($#stack >= 1) && (! $this->g_regex_spec_tok_p($stack[$#stack-1]))
- && (! $this->g_regex_spec_tok_p($stack[$#stack]))) {
- $s1 = pop @stack;
- $s2 = pop @stack;
- push(@stack, "$s2$s1");
- }
- $rest = $post;
- } else {
- push(@stack, $rest);
- $rest = "";
- }
- }
- # print STDERR "Stack: " . join(";", @stack) . "\n";
- foreach $i ((0 .. $#stack)) {
- $stack_elem = $stack[$i];
- if ($stack_elem =~ /^[()\[\]]$/) {
- $stack[$i] = "\\" . $stack[$i];
- }
- }
- return join("", @stack);
-}
-
-sub string_guard {
- local($caller,$string) = @_;
-
- return "" unless defined($string);
- $guarded_string = $string;
- $guarded_string =~ s/([\\"])/\\$1/g
- if $guarded_string =~ /[\\"]/;
-
- return $guarded_string;
-}
-
-sub json_string_guard {
- local($caller,$string) = @_;
-
- return "" unless defined($string);
- $guarded_string = $string;
- $guarded_string =~ s/([\\"])/\\$1/g
- if $guarded_string =~ /[\\"]/;
- $guarded_string =~ s/\r*\n/\\n/g
- if $guarded_string =~ /\n/;
-
- return $guarded_string;
-}
-
-sub json_string_unguard {
- local($caller,$string) = @_;
-
- return "" unless defined($string);
- $string =~ s/\\n/\n/g
- if $string =~ /\\n/;
- return $string;
-}
-
-sub guard_javascript_arg {
- local($caller,$string) = @_;
-
- return "" unless defined($string);
- $guarded_string = $string;
- $guarded_string =~ s/\\/\\\\/g;
- $guarded_string =~ s/'/\\'/g;
- return $guarded_string;
-}
-
-sub guard_substitution_right_hand_side {
- # "$1x" => "$1 . \"x\""
- local($caller,$string) = @_;
-
- my $result = "";
- ($pre,$var,$post) = ($string =~ /^([^\$]*)(\$\d)(.*)$/);
- while (defined($var)) {
- $result .= " . " if $result;
- $result .= "\"$pre\" . " unless $pre eq "";
- $result .= $var;
- $string = $post;
- ($pre,$var,$post) = ($string =~ /^([^\$]*)(\$\d)(.*)$/);
- }
- $result .= " . \"$string\"" if $string;
- return $result;
-}
-
-sub string_starts_with_substring {
- local($caller,$string,$substring) = @_;
-
- $guarded_substring = $caller->regex_guard($substring);
- return $string =~ /^$guarded_substring/;
-}
-
-sub one_string_starts_with_the_other {
- local($caller,$s1,$s2) = @_;
-
- return ($s1 eq $s2)
- || $caller->string_starts_with_substring($s1,$s2)
- || $caller->string_starts_with_substring($s2,$s1);
-}
-
-sub string_ends_in_substring {
- local($caller,$string,$substring) = @_;
-
- $guarded_substring = $caller->regex_guard($substring);
- return $string =~ /$guarded_substring$/;
-}
-
-sub string_equal_ignore_leading_multiple_or_trailing_blanks {
- local($caller,$string1,$string2) = @_;
-
- return 1 if $string1 eq $string2;
- $string1 =~ s/\s+/ /;
- $string2 =~ s/\s+/ /;
- $string1 =~ s/^\s+//;
- $string2 =~ s/^\s+//;
- $string1 =~ s/\s+$//;
- $string2 =~ s/\s+$//;
-
- return $string1 eq $string2;
-}
-
-sub strip_substring_from_start_of_string {
- local($caller,$string,$substring,$error_code) = @_;
-
- $error_code = "ERROR" unless defined($error_code);
- my $reg_surf = $caller->regex_guard($substring);
- if ($string =~ /^$guarded_substring/) {
- $string =~ s/^$reg_surf//;
- return $string;
- } else {
- return $error_code;
- }
-}
-
-sub strip_substring_from_end_of_string {
- local($caller,$string,$substring,$error_code) = @_;
-
- $error_code = "ERROR" unless defined($error_code);
- my $reg_surf = $caller->regex_guard($substring);
- if ($string =~ /$reg_surf$/) {
- $string =~ s/$reg_surf$//;
- return $string;
- } else {
- return $error_code;
- }
-}
-
-# to be deprecated
-sub lang_code {
- local($caller,$language) = @_;
-
- $langPM = NLP::Language->new();
- return $langPM->lang_code($language);
-}
-
-sub full_language {
- local($caller,$lang_code) = @_;
-
- return "Arabic" if $lang_code eq "ar";
- return "Chinese" if $lang_code eq "zh";
- return "Czech" if $lang_code eq "cs";
- return "Danish" if $lang_code eq "da";
- return "Dutch" if $lang_code eq "nl";
- return "English" if $lang_code eq "en";
- return "Finnish" if $lang_code eq "fi";
- return "French" if $lang_code eq "fr";
- return "German" if $lang_code eq "de";
- return "Greek" if $lang_code eq "el";
- return "Hebrew" if $lang_code eq "he";
- return "Hindi" if $lang_code eq "hi";
- return "Hungarian" if $lang_code eq "hu";
- return "Icelandic" if $lang_code eq "is";
- return "Indonesian" if $lang_code eq "id";
- return "Italian" if $lang_code eq "it";
- return "Japanese" if $lang_code eq "ja";
- return "Kinyarwanda" if $lang_code eq "rw";
- return "Korean" if $lang_code eq "ko";
- return "Latin" if $lang_code eq "la";
- return "Malagasy" if $lang_code eq "mg";
- return "Norwegian" if $lang_code eq "no";
- return "Pashto" if $lang_code eq "ps";
- return "Persian" if $lang_code eq "fa";
- return "Polish" if $lang_code eq "pl";
- return "Portuguese" if $lang_code eq "pt";
- return "Romanian" if $lang_code eq "ro";
- return "Russian" if $lang_code eq "ru";
- return "Spanish" if $lang_code eq "es";
- return "Swedish" if $lang_code eq "sv";
- return "Turkish" if $lang_code eq "tr";
- return "Urdu" if $lang_code eq "ur";
- return "";
-}
-
-# to be deprecated
-sub short_lang_name {
- local($caller,$lang_code) = @_;
-
- $langPM = NLP::Language->new();
- return $langPM->shortname($lang_code);
-}
-
-sub ml_dir {
- local($caller,$language,$type) = @_;
-
- $type = "MSB" unless defined($type);
- $lang_code = $langPM->lang_code($language);
- return $caller->ml_dir($lang_code, "lex") . "/corpora" if $type eq "corpora";
- return "" unless defined($rc);
- $ml_home = $rc->ml_home_dir();
- return File::Spec->catfile($ml_home, "arabic")
- if ($lang_code eq "ar-iq") && ! $caller->member(lc $type,"lex","onto","dict");
- $langPM = NLP::Language->new();
- $lexdir = $langPM->lexdir($lang_code);
- return $lexdir if defined($lexdir);
- return "";
-}
-
-sub language_lex_filename {
- local($caller,$language,$type) = @_;
-
- $langPM = NLP::Language->new();
- if (($lang_code = $langPM->lang_code($language))
- && ($ml_dir = $caller->ml_dir($lang_code,$type))
- && ($norm_language = $caller->short_lang_name($lang_code))) {
- return "$ml_dir/$norm_language-lex" if ($type eq "lex");
- return "$ml_dir/onto" if ($type eq "onto");
- return "$ml_dir/$norm_language-english-dict" if ($type eq "dict") && !($lang_code eq "en");
- return "";
- } else {
- return "";
- }
-}
-
-# filename_without_path is obsolete - replace with
-# use File::Basename;
-# basename($filename)
-sub filename_without_path {
- local($caller,$filename) = @_;
-
- $filename =~ s/^.*\/([^\/]+)$/$1/;
- return $filename;
-}
-
-sub option_string {
- local($caller,$input_name,$default,*values,*labels) = @_;
-
- my $s = "";
- return $s;
-}
-
-sub pes_subseq_surf {
- local($this,$start,$length,$langCode,@pes) = @_;
-
- my $surf = "";
- if ($start+$length-1 <= $#pes) {
- foreach $i ($start .. $start + $length - 1) {
- my $pe = $pes[$i];
- $surf .= $pe->get("surf","");
- $surf .= " " if $langCode =~ /^(ar|en|fr)$/;
- }
- }
- $surf =~ s/\s+$//;
- return $surf;
-}
-
-sub copyList {
- local($this,@list) = @_;
-
- @copy_list = ();
- foreach $elem (@list) {
- push(@copy_list,$elem);
- }
- return @copy_list;
-}
-
-sub list_with_same_elem {
- local($this,$size,$elem) = @_;
-
- @list = ();
- foreach $i (0 .. $size-1) {
- push(@list,$elem);
- }
- return @list;
-}
-
-sub count_occurrences {
- local($this,$s,$substring) = @_;
-
- $occ = 0;
- $new = $s;
- $guarded_substring = $this->regex_guard($substring);
- $new =~ s/$guarded_substring//;
- while ($new ne $s) {
- $occ++;
- $s = $new;
- $new =~ s/$guarded_substring//;
- }
- return $occ;
-}
-
-sub position_of_nth_occurrence {
- local($this,$s,$substring,$occ) = @_;
-
- return -1 unless $occ > 0;
- my $pos = 0;
- while (($pos = index($s, $substring, $pos)) >= 0) {
- return $pos if $occ == 1;
- $occ--;
- $pos = $pos + length($substring);
- }
- return -1;
-}
-
-sub has_diff_elements_p {
- local($this,@array) = @_;
-
- return 0 if $#array < 1;
- $elem = $array[0];
-
- foreach $a (@array) {
- return 1 if $elem ne $a;
- }
- return 0;
-}
-
-sub init_log {
- local($this,$logfile, $control) = @_;
-
- $control = "" unless defined($control);
- if ((DEBUGGING || ($control =~ /debug/i)) && $logfile) {
- system("rm -f $logfile");
- system("date > $logfile; chmod 777 $logfile");
- }
-}
-
-sub time_stamp_log {
- local($this,$logfile, $control) = @_;
-
- $control = "" unless defined($control);
- if ((DEBUGGING || ($control =~ /debug/i)) && $logfile) {
- system("date >> $logfile; chmod 777 $logfile");
- }
-}
-
-sub log {
- local($this,$message,$logfile,$control) = @_;
-
- $control = "" unless defined($control);
- if ((DEBUGGING || ($control =~ /debug/i)) && $logfile) {
- $this->init_log($logfile, $control) unless -w $logfile;
- if ($control =~ /timestamp/i) {
- $this->time_stamp_log($logfile, $control);
- }
- $guarded_message = $message;
- $guarded_message =~ s/"/\\"/g;
- system("echo \"$guarded_message\" >> $logfile");
- }
-}
-
-sub month_name_to_month_number {
- local($this,$month_name) = @_;
-
- $month_name_init = lc substr($month_name,0,3);
- return $this->position($month_name_init, "jan", "feb", "mar", "apr", "may", "jun", "jul", "aug", "sep", "oct", "nov", "dec") + 1;
-}
-
-my @short_month_names = ("Jan.","Febr.","March","April","May","June","July","Aug.","Sept.","Oct.","Nov.","Dec.");
-my @full_month_names = ("January","February","March","April","May","June","July","August","September","October","November","December");
-
-sub month_number_to_month_name {
- local($this,$month_number, $control) = @_;
-
- $month_number =~ s/^0//;
- if ($month_number =~ /^([1-9]|1[0-2])$/) {
- return ($control && ($control =~ /short/i))
- ? $short_month_names[$month_number-1]
- : $full_month_names[$month_number-1];
- } else {
- return "";
- }
-}
-
-sub leap_year {
- local($this,$year) = @_;
-
- return 0 if $year % 4 != 0;
- return 1 if $year % 400 == 0;
- return 0 if $year % 100 == 0;
- return 1;
-}
-
-sub datetime {
- local($this,$format,$time_in_secs, $command) = @_;
-
- $command = "" unless defined($command);
- $time_in_secs = time unless defined($time_in_secs) && $time_in_secs;
- @time_vector = ($command =~ /\b(gm|utc)\b/i) ? gmtime($time_in_secs) : localtime($time_in_secs);
- ($sec,$min,$hour,$mday,$mon,$year,$wday,$yday,$isdst)=@time_vector;
- $thisyear = $year + 1900;
- $thismon=(Jan,Feb,Mar,Apr,May,Jun,Jul,Aug,Sep,Oct,Nov,Dec)[$mon];
- $thismon2=("Jan.","Febr.","March","April","May","June","July","Aug.","Sept.","Oct.","Nov.","Dec.")[$mon];
- $thismonth = $mon + 1;
- $thisday=(Sun,Mon,Tue,Wed,Thu,Fri,Sat)[$wday];
- $milliseconds = int(($time_in_secs - int($time_in_secs)) * 1000);
- $date="$thisday $thismon $mday, $thisyear";
- $sdate="$thismon $mday, $thisyear";
- $dashedDate = sprintf("%04d-%02d-%02d",$thisyear,$thismonth,$mday);
- $slashedDate = sprintf("%02d/%02d/%04d",$mday,$thismonth,$thisyear);
- $time=sprintf("%02d:%02d:%02d",$hour,$min,$sec);
- $shorttime=sprintf("%d:%02d",$hour,$min);
- $shortdatetime = "$thismon2 $mday, $shorttime";
-
- if ($date =~ /undefined/) {
- return "";
- } elsif ($format eq "date at time") {
- return "$date at $time";
- } elsif ($format eq "date") {
- return "$date";
- } elsif ($format eq "sdate") {
- return "$sdate";
- } elsif ($format eq "ddate") {
- return "$dashedDate";
- } elsif ($format eq "time") {
- return "$time";
- } elsif ($format eq "dateTtime+ms") {
- return $dashedDate . "T" . $time . "." . $milliseconds;
- } elsif ($format eq "dateTtime") {
- return $dashedDate . "T" . $time;
- } elsif ($format eq "yyyymmdd") {
- return sprintf("%04d%02d%02d",$thisyear,$thismonth,$mday);
- } elsif ($format eq "short date at time") {
- return $shortdatetime;
- } else {
- return "$date at $time";
- }
-}
-
-sub datetime_of_last_file_modification {
- local($this,$format,$filename) = @_;
-
- return $this->datetime($format,(stat($filename))[9]);
-}
-
-sub add_1sec {
- local($this,$datetime) = @_;
-
- if (($year,$month,$day,$hour,$minute,$second) = ($datetime =~ /^(\d\d\d\d)-(\d\d)-(\d\d)T(\d\d):(\d\d):(\d\d)$/)) {
- $second++;
- if ($second >= 60) { $second -= 60; $minute++; }
- if ($minute >= 60) { $minute -= 60; $hour++; }
- if ($hour >= 24) { $hour -= 24; $day++; }
- if ($month =~ /^(01|03|05|07|08|10|12)$/) {
- if ($day > 31) { $day -= 31; $month++; }
- } elsif ($month =~ /^(04|06|09|11)$/) {
- if ($day > 30) { $day -= 30; $month++; }
- } elsif (($month eq "02") && $this->leap_year($year)) {
- if ($day > 29) { $day -= 29; $month++; }
- } elsif ($month eq "02") {
- if ($day > 28) { $day -= 28; $month++; }
- }
- if ($month > 12) { $month -= 12; $year++; }
- return sprintf("%04d-%02d-%02dT%02d:%02d:%02d", $year,$month,$day,$hour,$minute,$second);
- } else {
- return "";
- }
-}
-
-sub stopwatch {
- local($this, $function, $id, *ht, *OUT) = @_;
- # function: start|stop|count|report; start|stop times are absolute (in secs.)
-
- my $current_time = time;
- # print OUT "Point S stopwatch $function $id $current_time\n";
- if ($function eq "start") {
- if ($ht{STOPWATCH_START}->{$id}) {
- $ht{STOPWATCH_N_RESTARTS}->{$id} = ($ht{STOPWATCH_N_RESTARTS}->{$id} || 0) + 1;
- } else {
- $ht{STOPWATCH_START}->{$id} = $current_time;
- }
- } elsif ($function eq "end") {
- if ($start_time = $ht{STOPWATCH_START}->{$id}) {
- $ht{STOPWATCH_TIME}->{$id} = ($ht{STOPWATCH_TIME}->{$id} || 0) + ($current_time - $start_time);
- $ht{STOPWATCH_START}->{$id} = "";
- } else {
- $ht{STOPWATCH_N_DEAD_ENDS}->{$id} = ($ht{STOPWATCH_N_DEAD_ENDS}->{$id} || 0) + 1;
- }
- } elsif ($function eq "count") {
- $ht{STOPWATCH_COUNT}->{$id} = ($ht{STOPWATCH_COUNT}->{$id} || 0) + 1;
- } elsif ($function eq "report") {
- my $id2;
- foreach $id2 (keys %{$ht{STOPWATCH_START}}) {
- if ($start_time = $ht{STOPWATCH_START}->{$id2}) {
- $ht{STOPWATCH_TIME}->{$id2} = ($ht{STOPWATCH_TIME}->{$id2} || 0) + ($current_time - $start_time);
- $ht{STOPWATCH_START}->{$id2} = $current_time;
- }
- }
- print OUT "Time report:\n";
- foreach $id2 (sort { $ht{STOPWATCH_TIME}->{$b} <=> $ht{STOPWATCH_TIME}->{$a} }
- keys %{$ht{STOPWATCH_TIME}}) {
- my $stopwatch_time = $ht{STOPWATCH_TIME}->{$id2};
- $stopwatch_time = $this->round_to_n_decimal_places($stopwatch_time, 3);
- my $n_restarts = $ht{STOPWATCH_N_RESTARTS}->{$id2};
- my $n_dead_ends = $ht{STOPWATCH_N_DEAD_ENDS}->{$id2};
- my $start_time = $ht{STOPWATCH_START}->{$id2};
- print OUT " $id2: $stopwatch_time seconds";
- print OUT " with $n_restarts restart(s)" if $n_restarts;
- print OUT " with $n_dead_ends dead end(s)" if $n_dead_ends;
- print OUT " (active)" if $start_time;
- print OUT "\n";
- }
- foreach $id2 (sort { $ht{STOPWATCH_COUNT}->{$b} <=> $ht{STOPWATCH_COUNT}->{$a} }
- keys %{$ht{STOPWATCH_COUNT}}) {
- $count = $ht{STOPWATCH_COUNT}->{$id2};
- print OUT " C $id2: $count\n";
- }
- }
-}
-
-sub print_html_banner {
- local($this,$text,$bgcolor,*OUT,$control) = @_;
-
- $control = "" unless defined($control);
- $bgcolor = "#BBCCFF" unless defined($bgcolor);
- print OUT "
";
- print OUT " " unless $text =~ /^\s*<(table|nobr)/;
- print OUT $text;
- print OUT "
\n";
- print OUT " \n" unless $control =~ /nobr/i;
-}
-
-sub print_html_head {
- local($this, $title, *OUT, $control, $onload_fc, $add_javascript) = @_;
-
- $control = "" unless defined($control);
- $onload_fc = "" unless defined($onload_fc);
- $onload_clause = ($onload_fc) ? " onload=\"$onload_fc\"" : "";
- $add_javascript = "" unless defined($add_javascript);
- $max_age_clause = "";
- $max_age_clause = ""; # if $control =~ /\bexp1hour\b/;
- $css_clause = "";
- $css_clause = "\n " if $control =~ /css/;
- $css_clause .= "\n " if $control =~ /css/;
- $css_clause = "\n " if $control =~ /css-handheld/;
- $icon_clause = "";
- $icon_clause .= "\n " if $control =~ /\bAMR\b/i;
- $icon_clause .= "\n " if $control =~ /\bCRE\b/i;
- print OUT "\xEF\xBB\xBF\n" unless $control =~ /\bno-bom\b/; # utf8 marker byte order mark
- print OUT<
-
-
- $max_age_clause
- $title$css_clause$icon_clause
-END_OF_HEADER1
-;
-
- unless ($control =~ /no javascript/) {
- print OUT<
-
-
-END_OF_HEADER2
-;
- }
-
- print OUT<
-
-END_OF_HEADER3
-;
-}
-
-
-sub print_html_foot {
- local($this, *OUT) = @_;
-
- print OUT " \n";
- print OUT "\n";
-}
-
-sub print_html_page {
- local($this, *OUT, $s) = @_;
-
- print OUT "\xEF\xBB\xBF\n";
- print OUT "\n";
- print OUT " \n";
- print OUT " DEBUG\n";
- print OUT " \n";
- print OUT " \n";
- print OUT " \n";
- print OUT " \n";
- print OUT " $s\n";
- print OUT " \n";
- print OUT "\n";
-}
-
-sub http_catfile {
- local($this, @path) = @_;
-
- $result = File::Spec->catfile(@path);
- $result =~ s/(https?):\/([a-zA-Z])/$1:\/\/$2/;
- return $result;
-}
-
-sub underscore_to_space {
- local($this, $s) = @_;
-
- return "" unless defined($s);
-
- $s =~ s/_+/ /g;
- return $s;
-}
-
-sub space_to_underscore {
- local($this, $s) = @_;
-
- return "" unless defined($s);
-
- $s =~ s/ /_/g;
- return $s;
-}
-
-sub remove_spaces {
- local($this, $s) = @_;
-
- $s =~ s/\s//g;
- return $s;
-}
-
-sub is_punctuation_string_p {
- local($this, $s) = @_;
-
- return "" unless $s;
- $s = $this->normalize_string($s) if $s =~ /[\x80-\xBF]/;
- return $s =~ /^[-_,;:.?!\/\@+*"()]+$/;
-}
-
-sub is_rare_punctuation_string_p {
- local($this, $s) = @_;
-
- return 0 unless $s =~ /^[\x21-\x2F\x3A\x40\x5B-\x60\x7B-\x7E]{2,}$/;
- return 0 if $s =~ /^(\.{2,3}|-{2,3}|\*{2,3}|::|\@?[-\/:]\@?)$/;
- return 1;
-}
-
-sub simplify_punctuation {
- local($this, $s) = @_;
-
- $s =~ s/\xE2\x80\x92/-/g;
- $s =~ s/\xE2\x80\x93/-/g;
- $s =~ s/\xE2\x80\x94/-/g;
- $s =~ s/\xE2\x80\x95/-/g;
- $s =~ s/\xE2\x80\x98/`/g;
- $s =~ s/\xE2\x80\x99/'/g;
- $s =~ s/\xE2\x80\x9A/`/g;
- $s =~ s/\xE2\x80\x9C/"/g;
- $s =~ s/\xE2\x80\x9D/"/g;
- $s =~ s/\xE2\x80\x9E/"/g;
- $s =~ s/\xE2\x80\x9F/"/g;
- $s =~ s/\xE2\x80\xA2/*/g;
- $s =~ s/\xE2\x80\xA4/./g;
- $s =~ s/\xE2\x80\xA5/../g;
- $s =~ s/\xE2\x80\xA6/.../g;
- return $s;
-}
-
-sub latin_plus_p {
- local($this, $s, $control) = @_;
-
- $control = "" unless defined($control);
- return $s =~ /^([\x20-\x7E]|\xC2[\xA1-\xBF]|[\xC3-\xCC][\x80-\xBF]|\xCA[\x80-\xAF]|\xE2[\x80-\xAF][\x80-\xBF])+$/;
-}
-
-sub nth_line_in_file {
- local($this, $filename, $n) = @_;
-
- return "" unless $n =~ /^[1-9]\d*$/;
- open(IN, $filename) || return "";
- my $line_no = 0;
- while () {
- $line_no++;
- if ($n == $line_no) {
- $_ =~ s/\s+$//;
- close(IN);
- return $_;
- }
- }
- close(IN);
- return "";
-}
-
-sub read_file {
- local($this, $filename) = @_;
-
- my $file_content = "";
- open(IN, $filename) || return "";
- while () {
- $file_content .= $_;
- }
- close(IN);
- return $file_content;
-}
-
-sub cap_list {
- local($this, @list) = @_;
-
- @cap_list = ();
- foreach $l (@list) {
- ($premod, $core) = ($l =~ /^(a|an) (\S.*)$/);
- if (defined($premod) && defined($core)) {
- push(@cap_list, "$premod \u$core");
- } elsif ($this->cap_member($l, "US")) {
- push(@cap_list, uc $l);
- } else {
- push(@cap_list, "\u$l");
- }
- }
- return @cap_list;
-}
-
-sub integer_list_with_commas_and_ranges {
- local($this, @list) = @_;
-
- my $in_range_p = 0;
- my $last_value = 0;
- my $result = "";
- while (@list) {
- $elem = shift @list;
- if ($elem =~ /^\d+$/) {
- if ($in_range_p) {
- if ($elem == $last_value + 1) {
- $last_value = $elem;
- } else {
- $result .= "-$last_value, $elem";
- if (@list && ($next = $list[0]) && ($elem =~ /^\d+$/) && ($next =~ /^\d+$/)
- && ($next == $elem + 1)) {
- $last_value = $elem;
- $in_range_p = 1;
- } else {
- $in_range_p = 0;
- }
- }
- } else {
- $result .= ", $elem";
- if (@list && ($next = $list[0]) && ($elem =~ /^\d+$/) && ($next =~ /^\d+$/)
- && ($next == $elem + 1)) {
- $last_value = $elem;
- $in_range_p = 1;
- }
- }
- } else {
- if ($in_range_p) {
- $result .= "-$last_value, $elem";
- $in_range_p = 0;
- } else {
- $result .= ", $elem";
- }
- }
- }
- if ($in_range_p) {
- $result .= "-$last_value";
- }
- $result =~ s/^,\s*//;
- return $result;
-}
-
-sub comma_append {
- local($this, $a, $b) = @_;
-
- if (defined($a) && ($a =~ /\S/)) {
- if (defined($b) && ($b =~ /\S/)) {
- return "$a,$b";
- } else {
- return $a;
- }
- } else {
- if (defined($b) && ($b =~ /\S/)) {
- return $b;
- } else {
- return "";
- }
- }
-}
-
-sub version {
- return "3.17";
-}
-
-sub print_stderr {
- local($this, $message, $verbose) = @_;
-
- $verbose = 1 unless defined($verbose);
- print STDERR $message if $verbose;
- return 1;
-}
-
-sub print_log {
- local($this, $message, *LOG, $verbose) = @_;
-
- $verbose = 1 unless defined($verbose);
- print LOG $message if $verbose;
- return 1;
-}
-
-sub compare_alignment {
- local($this, $a, $b, $delimiter) = @_;
-
- $delimiter = "-" unless $delimiter;
- my @a_list = split($delimiter, $a);
- my @b_list = split($delimiter, $b);
-
- while (@a_list && @b_list) {
- $a_head = shift @a_list;
- $b_head = shift @b_list;
- next if $a_head eq $b_head;
- return $a_head <=> $b_head if ($a_head =~ /^\d+$/) && ($b_head =~ /^\d+$/);
- return $a_head cmp $b_head;
- }
- return -1 if @a_list;
- return 1 if @b_list;
- return 0;
-}
-
-sub normalize_string {
- # normalize punctuation, full-width characters (to ASCII)
- local($this, $s, $control) = @_;
-
- $control = "" unless defined($control);
-
- $norm_s = $s;
- $norm_s =~ tr/A-Z/a-z/;
-
- $norm_s =~ s/ \@([-:\/])/ $1/g; # non-initial left @
- $norm_s =~ s/^\@([-:\/])/$1/; # initial left @
- $norm_s =~ s/([-:\/])\@ /$1 /g; # non-initial right @
- $norm_s =~ s/([-:\/])\@$/$1/; # initial right @
- $norm_s =~ s/([\(\)"])([,;.?!])/$1 $2/g;
- $norm_s =~ s/\bcannot\b/can not/g;
-
- $norm_s =~ s/\xC2\xAD/-/g; # soft hyphen
-
- $norm_s =~ s/\xE2\x80\x94/-/g; # em dash
- $norm_s =~ s/\xE2\x80\x95/-/g; # horizontal bar
- $norm_s =~ s/\xE2\x80\x98/`/g; # grave accent
- $norm_s =~ s/\xE2\x80\x99/'/g; # apostrophe
- $norm_s =~ s/\xE2\x80\x9C/"/g; # left double quote mark
- $norm_s =~ s/\xE2\x80\x9D/"/g; # right double quote mark
- $norm_s =~ s/\xE2\x94\x80/-/g; # box drawings light horizontal
- $norm_s =~ s/\xE2\x94\x81/-/g; # box drawings heavy horizontal
- $norm_s =~ s/\xE3\x80\x81/,/g; # ideographic comma
- $norm_s =~ s/\xE3\x80\x82/./g; # ideographic full stop
- $norm_s =~ s/\xE3\x80\x88/"/g; # left angle bracket
- $norm_s =~ s/\xE3\x80\x89/"/g; # right angle bracket
- $norm_s =~ s/\xE3\x80\x8A/"/g; # left double angle bracket
- $norm_s =~ s/\xE3\x80\x8B/"/g; # right double angle bracket
- $norm_s =~ s/\xE3\x80\x8C/"/g; # left corner bracket
- $norm_s =~ s/\xE3\x80\x8D/"/g; # right corner bracket
- $norm_s =~ s/\xE3\x80\x8E/"/g; # left white corner bracket
- $norm_s =~ s/\xE3\x80\x8F/"/g; # right white corner bracket
- $norm_s =~ s/\xE3\x83\xBB/\xC2\xB7/g; # katakana middle dot -> middle dot
- $norm_s =~ s/\xEF\xBB\xBF//g; # UTF8 marker
-
- if ($control =~ /\bzh\b/i) {
- # de-tokenize Chinese
- unless ($control =~ /\bpreserve-tok\b/) {
- while ($norm_s =~ /[\xE0-\xEF][\x80-\xBF][\x80-\xBF] [\xE0-\xEF][\x80-\xBF][\x80-\xBF]/) {
- $norm_s =~ s/([\xE0-\xEF][\x80-\xBF][\x80-\xBF]) ([\xE0-\xEF][\x80-\xBF][\x80-\xBF])/$1$2/g;
- }
- $norm_s =~ s/([\xE0-\xEF][\x80-\xBF][\x80-\xBF]) ([\x21-\x7E])/$1$2/g;
- $norm_s =~ s/([\x21-\x7E]) ([\xE0-\xEF][\x80-\xBF][\x80-\xBF])/$1$2/g;
- }
-
- # fullwidth characters
- while ($norm_s =~ /\xEF\xBC[\x81-\xBF]/) {
- ($pre,$fullwidth,$post) = ($norm_s =~ /^(.*)(\xEF\xBC[\x81-\xBF])(.*)$/);
- $fullwidth =~ s/^\xEF\xBC//;
- $fullwidth =~ tr/[\x81-\xBF]/[\x21-\x5F]/;
- $norm_s = "$pre$fullwidth$post";
- }
- while ($norm_s =~ /\xEF\xBD[\x80-\x9E]/) {
- ($pre,$fullwidth,$post) = ($norm_s =~ /^(.*)(\xEF\xBD[\x80-\x9E])(.*)$/);
- $fullwidth =~ s/^\xEF\xBD//;
- $fullwidth =~ tr/[\x80-\x9E]/[\x60-\x7E]/;
- $norm_s = "$pre$fullwidth$post";
- }
- $norm_s =~ tr/A-Z/a-z/ unless $control =~ /\bpreserve-case\b/;
-
- unless ($control =~ /\bpreserve-tok\b/) {
- while ($norm_s =~ /[\x21-\x2F\x3A-\x40\x5B-\x60\x7B-\x7E] [\x21-\x2F\x3A-\x40\x5B-\x60\x7B-\x7E]/) {
- $norm_s =~ s/([\x21-\x2F\x3A-\x40\x5B-\x60\x7B-\x7E]) ([\x21-\x2F\x3A-\x40\x5B-\x60\x7B-\x7E])/$1$2/g;
- }
- $norm_s =~ s/([\x21-\x7E]) ([\x21-\x2F\x3A-\x40\x5B-\x60\x7B-\x7E])/$1$2/g;
- $norm_s =~ s/([\x21-\x2F\x3A-\x40\x5B-\x60\x7B-\x7E]) ([\x21-\x7E])/$1$2/g;
- $norm_s =~ s/ (\xC2\xA9|\xC2\xB7|\xC3\x97) /$1/g; # copyright sign, middle dot, multiplication sign
- }
- }
-
- if (($control =~ /\bzh\b/i) && ($control =~ /\bnorm-char\b/)) {
- $norm_s =~ s/\xE6\x96\xBC/\xE4\xBA\x8E/g; # feng1 (first char. of Chin. "lie low", line 1308)
- $norm_s =~ s/\xE6\xAD\xA7/\xE5\xB2\x90/g; # qi2 (second char. of Chin. "difference", line 1623)
- $norm_s =~ s/\xE8\x82\xB2/\xE6\xAF\x93/g; # yu4 (second char. of Chin. "sports", line 440)
- $norm_s =~ s/\xE8\x91\x97/\xE7\x9D\x80/g; # zhao (second char. of Chin. "prominent", line 4)
- $norm_s =~ s/\xE9\x81\x87/\xE8\xBF\x82/g; # yu4 (second char. of Chin. "good luck", line 959)
- }
-
- if ($control =~ /\bspurious-punct\b/) {
- $norm_s =~ s/^\s*[-_\." ]+//;
- $norm_s =~ s/[-_\." ]+\s*$//;
- $norm_s =~ s/\(\s+end\s+\)\s*$//i;
- $norm_s =~ s/^\s*null\s*$//i;
- }
-
- $norm_s =~ s/^\s+//;
- $norm_s =~ s/\s+$//;
- $norm_s =~ s/\s+/ /g;
-
- return $norm_s;
-}
-
-sub normalize_extreme_string {
- local($this, $s, $control) = @_;
-
- $control = "" unless defined($control);
-
- $norm_s = $s;
- $norm_s =~ s/\xE2\xA9\xBE/\xE2\x89\xA5/g; # slanted greater than or equal to
-
- return $norm_s;
-}
-
-sub increase_ht_count {
- local($this, *ht, $incr, @path) = @_;
-
- if ($#path == 0) {
- $ht{($path[0])} = ($ht{($path[0])} || 0) + $incr;
- } elsif ($#path == 1) {
- $ht{($path[0])}->{($path[1])}
- = ($ht{($path[0])}->{($path[1])} || 0) + $incr;
- } elsif ($#path == 2) {
- $ht{($path[0])}->{($path[1])}->{($path[2])}
- = ($ht{($path[0])}->{($path[1])}->{($path[2])} || 0) + $incr;
- } elsif ($#path == 3) {
- $ht{($path[0])}->{($path[1])}->{($path[2])}->{($path[3])}
- = ($ht{($path[0])}->{($path[1])}->{($path[2])}->{($path[3])} || 0) + $incr;
- } elsif ($#path == 4) {
- $ht{($path[0])}->{($path[1])}->{($path[2])}->{($path[3])}->{($path[4])}
- = ($ht{($path[0])}->{($path[1])}->{($path[2])}->{($path[3])}->{($path[4])} || 0) + $incr;
- } else {
- print STDERR "increase_ht_count unsupported for path of length " . ($#path + 1) . "\n";
- }
-}
-
-sub adjust_numbers {
- # non-negative integers
- local($this, $s, $delta) = @_;
-
- $result = "";
- while ($s =~ /\d/) {
- ($pre,$i,$post) = ($s =~ /^([^0-9]*)(\d+)([^0-9].*|)$/);
- $result .= $pre . ($i + $delta);
- $s = $post;
- }
- $result .= $s;
- return $result;
-}
-
-sub first_defined {
- local($this, @list) = @_;
-
- foreach $elem (@list) {
- return $elem if defined($elem);
- }
- return "";
-}
-
-sub first_defined_non_empty {
- local($this, @list) = @_;
-
- foreach $item (@list) {
- return $item if defined($item) && ($item ne "");
- }
- return "";
-}
-
-sub elem_after_member_list {
- local($this,$elem,@array) = @_;
-
- my @elem_after_member_list = ();
- foreach $i ((0 .. ($#array - 1))) {
- push(@elem_after_member_list, $array[$i+1]) if $elem eq $array[$i];
- }
- return join(" ", @elem_after_member_list);
-}
-
-sub add_value_to_list {
- local($this,$s,$value,$sep) = @_;
-
- $s = "" unless defined($s);
- $sep = "," unless defined($sep);
- return ($s =~ /\S/) ? "$s$sep$value" : $value;
-}
-
-sub add_new_value_to_list {
- local($this,$s,$value,$sep) = @_;
-
- $s = "" unless defined($s);
- $sep = "," unless defined($sep);
- my @values = split(/$sep/, $s);
- push(@values, $value) if defined($value) && ! $this->member($value, @values);
-
- return join($sep, @values);
-}
-
-sub add_new_hash_value_to_list {
- local($this,*ht,$key,$value,$sep) = @_;
-
- $sep = "," unless defined($sep);
- my $value_s = $ht{$key};
- if (defined($value_s)) {
- my @values = split(/$sep/, $value_s);
- push(@values, $value) unless $this->member($value, @values);
- $ht{$key} = join($sep, @values);
- } else {
- $ht{$key} = $value;
- }
-}
-
-sub ip_info {
- local($this, $ip_address) = @_;
-
- my %ip_map = ();
- $ip_map{"128.9.208.69"} = "Ulf Hermjakob (bach.isi.edu)";
- $ip_map{"128.9.208.169"} = "Ulf Hermjakob (brahms.isi.edu)";
- $ip_map{"128.9.184.148"} = "Ulf Hermjakob (beethoven.isi.edu ?)";
- $ip_map{"128.9.184.162"} = "Ulf Hermjakob (beethoven.isi.edu)";
- $ip_map{"128.9.176.39"} = "Kevin Knight";
- $ip_map{"128.9.184.187"} = "Kevin Knight";
- $ip_map{"128.9.216.56"} = "Kevin Knight";
- $ip_map{"128.9.208.155"} = "cage.isi.edu";
-
- return ($ip_name = $ip_map{$ip_address}) ? "$ip_address - $ip_name" : $ip_address;
-}
-
-# from standalone de-accent.pl
-sub de_accent_string {
- local($this, $s) = @_;
-
- $s =~ tr/A-Z/a-z/;
- unless (0) {
- # Latin-1
- if ($s =~ /\xC3[\x80-\xBF]/) {
- $s =~ s/(À|Á|Â|Ã|Ä|Å)/A/g;
- $s =~ s/Æ/Ae/g;
- $s =~ s/Ç/C/g;
- $s =~ s/Ð/D/g;
- $s =~ s/(È|É|Ê|Ë)/E/g;
- $s =~ s/(Ì|Í|Î|Ï)/I/g;
- $s =~ s/Ñ/N/g;
- $s =~ s/(Ò|Ó|Ô|Õ|Ö|Ø)/O/g;
- $s =~ s/(Ù|Ú|Û|Ü)/U/g;
- $s =~ s/Þ/Th/g;
- $s =~ s/Ý/Y/g;
- $s =~ s/(à|á|â|ã|ä|å)/a/g;
- $s =~ s/æ/ae/g;
- $s =~ s/ç/c/g;
- $s =~ s/(è|é|ê|ë)/e/g;
- $s =~ s/(ì|í|î|ï)/i/g;
- $s =~ s/ð/d/g;
- $s =~ s/ñ/n/g;
- $s =~ s/(ò|ó|ô|õ|ö)/o/g;
- $s =~ s/ß/ss/g;
- $s =~ s/þ/th/g;
- $s =~ s/(ù|ú|û|ü)/u/g;
- $s =~ s/(ý|ÿ)/y/g;
- }
- # Latin Extended-A
- if ($s =~ /[\xC4-\xC5][\x80-\xBF]/) {
- $s =~ s/(Ā|Ă|Ą)/A/g;
- $s =~ s/(ā|ă|ą)/a/g;
- $s =~ s/(Ć|Ĉ|Ċ|Č)/C/g;
- $s =~ s/(ć|ĉ|ċ|č)/c/g;
- $s =~ s/(Ď|Đ)/D/g;
- $s =~ s/(ď|đ)/d/g;
- $s =~ s/(Ē|Ĕ|Ė|Ę|Ě)/E/g;
- $s =~ s/(ē|ĕ|ė|ę|ě)/e/g;
- $s =~ s/(Ĝ|Ğ|Ġ|Ģ)/G/g;
- $s =~ s/(ĝ|ğ|ġ|ģ)/g/g;
- $s =~ s/(Ĥ|Ħ)/H/g;
- $s =~ s/(ĥ|ħ)/h/g;
- $s =~ s/(Ĩ|Ī|Ĭ|Į|İ)/I/g;
- $s =~ s/(ĩ|ī|ĭ|į|ı)/i/g;
- $s =~ s/IJ/Ij/g;
- $s =~ s/ij/ij/g;
- $s =~ s/Ĵ/J/g;
- $s =~ s/ĵ/j/g;
- $s =~ s/Ķ/K/g;
- $s =~ s/(ķ|ĸ)/k/g;
- $s =~ s/(Ĺ|Ļ|Ľ|Ŀ|Ł)/L/g;
- $s =~ s/(ļ|ľ|ŀ|ł)/l/g;
- $s =~ s/(Ń|Ņ|Ň|Ŋ)/N/g;
- $s =~ s/(ń|ņ|ň|ʼn|ŋ)/n/g;
- $s =~ s/(Ō|Ŏ|Ő)/O/g;
- $s =~ s/(ō|ŏ|ő)/o/g;
- $s =~ s/Œ/Oe/g;
- $s =~ s/œ/oe/g;
- $s =~ s/(Ŕ|Ŗ|Ř)/R/g;
- $s =~ s/(ŕ|ŗ|ř)/r/g;
- $s =~ s/(Ś|Ŝ|Ş|Š)/S/g;
- $s =~ s/(ś|ŝ|ş|š|ſ)/s/g;
- $s =~ s/(Ţ|Ť|Ŧ)/T/g;
- $s =~ s/(ţ|ť|ŧ)/t/g;
- $s =~ s/(Ũ|Ū|Ŭ|Ů|Ű|Ų)/U/g;
- $s =~ s/(ũ|ū|ŭ|ů|ű|ų)/u/g;
- $s =~ s/Ŵ/W/g;
- $s =~ s/ŵ/w/g;
- $s =~ s/(Ŷ|Ÿ)/Y/g;
- $s =~ s/ŷ/y/g;
- $s =~ s/(Ź|Ż|Ž)/Z/g;
- $s =~ s/(ź|ż|ž)/z/g;
- }
- # Latin Extended-B
- if ($s =~ /[\xC7-\xC7][\x80-\xBF]/) {
- $s =~ s/(\xC7\x8D)/A/g;
- $s =~ s/(\xC7\x8E)/a/g;
- $s =~ s/(\xC7\x8F)/I/g;
- $s =~ s/(\xC7\x90)/i/g;
- $s =~ s/(\xC7\x91)/O/g;
- $s =~ s/(\xC7\x92)/o/g;
- $s =~ s/(\xC7\x93)/U/g;
- $s =~ s/(\xC7\x94)/u/g;
- $s =~ s/(\xC7\x95)/U/g;
- $s =~ s/(\xC7\x96)/u/g;
- $s =~ s/(\xC7\x97)/U/g;
- $s =~ s/(\xC7\x98)/u/g;
- $s =~ s/(\xC7\x99)/U/g;
- $s =~ s/(\xC7\x9A)/u/g;
- $s =~ s/(\xC7\x9B)/U/g;
- $s =~ s/(\xC7\x9C)/u/g;
- }
- # Latin Extended Additional
- if ($s =~ /\xE1[\xB8-\xBF][\x80-\xBF]/) {
- $s =~ s/(ḁ|ạ|ả|ấ|ầ|ẩ|ẫ|ậ|ắ|ằ|ẳ|ẵ|ặ|ẚ)/a/g;
- $s =~ s/(ḃ|ḅ|ḇ)/b/g;
- $s =~ s/(ḉ)/c/g;
- $s =~ s/(ḋ|ḍ|ḏ|ḑ|ḓ)/d/g;
- $s =~ s/(ḕ|ḗ|ḙ|ḛ|ḝ|ẹ|ẻ|ẽ|ế|ề|ể|ễ|ệ)/e/g;
- $s =~ s/(ḟ)/f/g;
- $s =~ s/(ḡ)/g/g;
- $s =~ s/(ḣ|ḥ|ḧ|ḩ|ḫ)/h/g;
- $s =~ s/(ḭ|ḯ|ỉ|ị)/i/g;
- $s =~ s/(ḱ|ḳ|ḵ)/k/g;
- $s =~ s/(ḷ|ḹ|ḻ|ḽ)/l/g;
- $s =~ s/(ḿ|ṁ|ṃ)/m/g;
- $s =~ s/(ṅ|ṇ|ṉ|ṋ)/m/g;
- $s =~ s/(ọ|ỏ|ố|ồ|ổ|ỗ|ộ|ớ|ờ|ở|ỡ|ợ|ṍ|ṏ|ṑ|ṓ)/o/g;
- $s =~ s/(ṕ|ṗ)/p/g;
- $s =~ s/(ṙ|ṛ|ṝ|ṟ)/r/g;
- $s =~ s/(ṡ|ṣ|ṥ|ṧ|ṩ|ẛ)/s/g;
- $s =~ s/(ṫ|ṭ|ṯ|ṱ)/t/g;
- $s =~ s/(ṳ|ṵ|ṷ|ṹ|ṻ|ụ|ủ|ứ|ừ|ử|ữ|ự)/u/g;
- $s =~ s/(ṽ|ṿ)/v/g;
- $s =~ s/(ẁ|ẃ|ẅ|ẇ|ẉ|ẘ)/w/g;
- $s =~ s/(ẋ|ẍ)/x/g;
- $s =~ s/(ẏ|ỳ|ỵ|ỷ|ỹ|ẙ)/y/g;
- $s =~ s/(ẑ|ẓ|ẕ)/z/g;
- $s =~ s/(Ḁ|Ạ|Ả|Ấ|Ầ|Ẩ|Ẫ|Ậ|Ắ|Ằ|Ẳ|Ẵ|Ặ)/A/g;
- $s =~ s/(Ḃ|Ḅ|Ḇ)/B/g;
- $s =~ s/(Ḉ)/C/g;
- $s =~ s/(Ḋ|Ḍ|Ḏ|Ḑ|Ḓ)/D/g;
- $s =~ s/(Ḕ|Ḗ|Ḙ|Ḛ|Ḝ|Ẹ|Ẻ|Ẽ|Ế|Ề|Ể|Ễ|Ệ)/E/g;
- $s =~ s/(Ḟ)/F/g;
- $s =~ s/(Ḡ)/G/g;
- $s =~ s/(Ḣ|Ḥ|Ḧ|Ḩ|Ḫ)/H/g;
- $s =~ s/(Ḭ|Ḯ|Ỉ|Ị)/I/g;
- $s =~ s/(Ḱ|Ḳ|Ḵ)/K/g;
- $s =~ s/(Ḷ|Ḹ|Ḻ|Ḽ)/L/g;
- $s =~ s/(Ḿ|Ṁ|Ṃ)/M/g;
- $s =~ s/(Ṅ|Ṇ|Ṉ|Ṋ)/N/g;
- $s =~ s/(Ṍ|Ṏ|Ṑ|Ṓ|Ọ|Ỏ|Ố|Ồ|Ổ|Ỗ|Ộ|Ớ|Ờ|Ở|Ỡ|Ợ)/O/g;
- $s =~ s/(Ṕ|Ṗ)/P/g;
- $s =~ s/(Ṙ|Ṛ|Ṝ|Ṟ)/R/g;
- $s =~ s/(Ṡ|Ṣ|Ṥ|Ṧ|Ṩ)/S/g;
- $s =~ s/(Ṫ|Ṭ|Ṯ|Ṱ)/T/g;
- $s =~ s/(Ṳ|Ṵ|Ṷ|Ṹ|Ṻ|Ụ|Ủ|Ứ|Ừ|Ử|Ữ|Ự)/U/g;
- $s =~ s/(Ṽ|Ṿ)/V/g;
- $s =~ s/(Ẁ|Ẃ|Ẅ|Ẇ|Ẉ)/W/g;
- $s =~ s/(Ẍ)/X/g;
- $s =~ s/(Ẏ|Ỳ|Ỵ|Ỷ|Ỹ)/Y/g;
- $s =~ s/(Ẑ|Ẓ|Ẕ)/Z/g;
- }
- # Greek letters
- if ($s =~ /\xCE[\x86-\xAB]/) {
- $s =~ s/ά/α/g;
- $s =~ s/έ/ε/g;
- $s =~ s/ί/ι/g;
- $s =~ s/ϊ/ι/g;
- $s =~ s/ΐ/ι/g;
- $s =~ s/ό/ο/g;
- $s =~ s/ύ/υ/g;
- $s =~ s/ϋ/υ/g;
- $s =~ s/ΰ/υ/g;
- $s =~ s/ώ/ω/g;
- $s =~ s/Ά/Α/g;
- $s =~ s/Έ/Ε/g;
- $s =~ s/Ή/Η/g;
- $s =~ s/Ί/Ι/g;
- $s =~ s/Ϊ/Ι/g;
- $s =~ s/Ύ/Υ/g;
- $s =~ s/Ϋ/Υ/g;
- $s =~ s/Ώ/Ω/g;
- }
- # Cyrillic letters
- if ($s =~ /\xD0[\x80-\xAF]/) {
- $s =~ s/Ѐ/Е/g;
- $s =~ s/Ё/Е/g;
- $s =~ s/Ѓ/Г/g;
- $s =~ s/Ќ/К/g;
- $s =~ s/Ѝ/И/g;
- $s =~ s/Й/И/g;
- $s =~ s/ѐ/е/g;
- $s =~ s/ё/е/g;
- $s =~ s/ѓ/г/g;
- $s =~ s/ќ/к/g;
- $s =~ s/ѝ/и/g;
- $s =~ s/й/и/g;
- }
- }
- return $s;
-}
-
-sub read_de_accent_case_resource {
- local($this, $filename, *ht, *LOG, $verbose) = @_;
- # e.g. data/char-de-accent-lc.txt
-
- if (open(IN, $filename)) {
- my $mode = "de-accent";
- my $line_number = 0;
- my $n_de_accent_targets = 0;
- my $n_de_accent_sources = 0;
- my $n_case_entries = 0;
- while () {
- s/^\xEF\xBB\xBF//;
- s/\s*$//;
- $line_number++;
- if ($_ =~ /^#+\s*CASE\b/) {
- $mode = "case";
- } elsif ($_ =~ /^#+\s*PUNCTUATION NORMALIZATION\b/) {
- $mode = "punctuation-normalization";
- } elsif ($_ =~ /^#/) {
- # ignore comment
- } elsif ($_ =~ /^\s*$/) {
- # ignore empty line
- } elsif (($mode eq "de-accent") && (($char_without_accent, @chars_with_accent) = split(/\s+/, $_))) {
- if (keys %{$ht{DE_ACCENT_INV}->{$char_without_accent}}) {
- print LOG "Ignoring duplicate de-accent line for target $char_without_accent in l.$line_number in $filename\n" unless $char_without_accent eq "--";
- } elsif (@chars_with_accent) {
- $n_de_accent_targets++;
- foreach $char_with_accent (@chars_with_accent) {
- my @prev_target_chars = keys %{$ht{DE_ACCENT}->{$char_with_accent}};
- print LOG "Accent character $char_with_accent has duplicate target $char_without_accent (besides @prev_target_chars) in l.$line_number in $filename\n" if @prev_target_chars && (! ($char_without_accent =~ /^[aou]e$/i));
- $char_without_accent = "" if $char_without_accent eq "--";
- $ht{DE_ACCENT}->{$char_with_accent}->{$char_without_accent} = 1;
- $ht{DE_ACCENT1}->{$char_with_accent} = $char_without_accent
- if (! defined($ht{DE_ACCENT1}->{$char_with_accent}))
- && ($char_without_accent =~ /^.[\x80-\xBF]*$/);
- $ht{DE_ACCENT_INV}->{$char_without_accent}->{$char_with_accent} = 1;
- $ht{UPPER_CASE_OR_ACCENTED}->{$char_with_accent} = 1;
- $n_de_accent_sources++;
- }
- } else {
- print LOG "Empty de-accent list for $char_without_accent in l.$line_number in $filename\n";
- }
- } elsif (($mode eq "punctuation-normalization") && (($norm_punct, @unnorm_puncts) = split(/\s+/, $_))) {
- if (keys %{$ht{NORM_PUNCT_INV}->{$norm_punct}}) {
- print LOG "Ignoring duplicate punctuation-normalization line for target $norm_punct in l.$line_number in $filename\n";
- } elsif (@unnorm_puncts) {
- foreach $unnorm_punct (@unnorm_puncts) {
- my $prev_norm_punct = $ht{NORM_PUNCT}->{$unnorm_punct};
- if ($prev_norm_punct) {
- print LOG "Ignoring duplicate punctuation normalization $unnorm_punct -> $norm_punct (besides $prev_norm_punct) in l.$line_number in $filename\n";
- }
- $ht{NORM_PUNCT}->{$unnorm_punct} = $norm_punct;
- $ht{NORM_PUNCT_INV}->{$norm_punct}->{$unnorm_punct} = 1;
- $ht{LC_DE_ACCENT_CHAR_NORM_PUNCT}->{$unnorm_punct} = $norm_punct;
- }
- }
- } elsif (($mode eq "case") && (($uc_char, $lc_char) = ($_ =~ /^(\S+)\s+(\S+)\s*$/))) {
- $ht{UPPER_TO_LOWER_CASE}->{$uc_char} = $lc_char;
- $ht{LOWER_TO_UPPER_CASE}->{$lc_char} = $uc_char;
- $ht{UPPER_CASE_P}->{$uc_char} = 1;
- $ht{LOWER_CASE_P}->{$lc_char} = 1;
- $ht{UPPER_CASE_OR_ACCENTED}->{$uc_char} = 1;
- $n_case_entries++;
- } else {
- print LOG "Unrecognized l.$line_number in $filename\n";
- }
- }
- foreach $char (keys %{$ht{UPPER_CASE_OR_ACCENTED}}) {
- my $lc_char = $ht{UPPER_TO_LOWER_CASE}->{$char};
- $lc_char = $char unless defined($lc_char);
- my @de_accend_char_results = sort keys %{$ht{DE_ACCENT}->{$lc_char}};
- my $new_char = (@de_accend_char_results) ? $de_accend_char_results[0] : $lc_char;
- $ht{LC_DE_ACCENT_CHAR}->{$char} = $new_char;
- $ht{LC_DE_ACCENT_CHAR_NORM_PUNCT}->{$char} = $new_char;
- }
- close(IN);
- print LOG "Found $n_case_entries case entries, $n_de_accent_sources/$n_de_accent_targets source/target entries in $line_number lines in file $filename\n" if $verbose;
- } else {
- print LOG "Can't open $filename\n";
- }
-}
-
-sub de_accent_char {
- local($this, $char, *ht, $default) = @_;
-
- @de_accend_char_results = sort keys %{$ht{DE_ACCENT}->{$char}};
- return (@de_accend_char_results) ? @de_accend_char_results : ($default);
-}
-
-sub lower_case_char {
- local($this, $char, *ht, $default) = @_;
-
- return (defined($lc = $ht{UPPER_TO_LOWER_CASE}->{$char})) ? $lc : $default;
-}
-
-sub lower_case_and_de_accent_char {
- local($this, $char, *ht) = @_;
-
- my $lc_char = $this->lower_case_char($char, *ht, $char);
- return $this->de_accent_char($lc_char, *ht, $lc_char);
-}
-
-sub lower_case_and_de_accent_string {
- local($this, $string, *ht, $control) = @_;
-
- # $this->stopwatch("start", "lower_case_and_de_accent_string", *ht, *LOG);
- my $norm_punct_p = ($control && ($control =~ /norm-punct/i));
- my @chars = $this->split_into_utf8_characters($string);
- my $result = "";
- foreach $char (@chars) {
- my @lc_de_accented_chars = $this->lower_case_and_de_accent_char($char, *ht);
- if ($norm_punct_p
- && (! @lc_de_accented_chars)) {
- my $norm_punct = $ht{NORM_PUNCT}->{$char};
- @lc_de_accented_chars = ($norm_punct) if $norm_punct;
- }
- $result .= ((@lc_de_accented_chars) ? $lc_de_accented_chars[0] : $char);
- }
- # $this->stopwatch("end", "lower_case_and_de_accent_string", *ht, *LOG);
- return $result;
-}
-
-sub lower_case_and_de_accent_norm_punct {
- local($this, $char, *ht) = @_;
-
- my $new_char = $ht{LC_DE_ACCENT_CHAR_NORM_PUNCT}->{$char};
- return (defined($new_char)) ? $new_char : $char;
-}
-
-sub lower_case_and_de_accent_string2 {
- local($this, $string, *ht, $control) = @_;
-
- my $norm_punct_p = ($control && ($control =~ /norm-punct/i));
- # $this->stopwatch("start", "lower_case_and_de_accent_string2", *ht, *LOG);
- my $s = $string;
- my $result = "";
- while (($char, $rest) = ($s =~ /^(.[\x80-\xBF]*)(.*)$/)) {
- my $new_char = $ht{LC_DE_ACCENT_CHAR}->{$char};
- if (defined($new_char)) {
- $result .= $new_char;
- } elsif ($norm_punct_p && defined($new_char = $ht{NORM_PUNCT}->{$char})) {
- $result .= $new_char;
- } else {
- $result .= $char;
- }
- $s = $rest;
- }
- # $this->stopwatch("end", "lower_case_and_de_accent_string2", *ht, *LOG);
- return $result;
-}
-
-sub lower_case_string {
- local($this, $string, *ht, $control) = @_;
-
- my $norm_punct_p = ($control && ($control =~ /norm-punct/i));
- my $s = $string;
- my $result = "";
- while (($char, $rest) = ($s =~ /^(.[\x80-\xBF]*)(.*)$/)) {
- my $lc_char = $ht{UPPER_TO_LOWER_CASE}->{$char};
- if (defined($lc_char)) {
- $result .= $lc_char;
- } elsif ($norm_punct_p && defined($new_char = $ht{NORM_PUNCT}->{$char})) {
- $result .= $new_char;
- } else {
- $result .= $char;
- }
- $s = $rest;
- }
- return $result;
-}
-
-sub round_to_n_decimal_places {
- local($this, $x, $n, $fill_decimals_p) = @_;
-
- $fill_decimals_p = 0 unless defined($fill_decimals_p);
- unless (defined($x)) {
- return $x;
- }
- if (($x =~ /^-?\d+$/) && (! $fill_decimals_p)) {
- return $x;
- }
- $factor = 1;
- foreach $i ((1 .. $n)) {
- $factor *= 10;
- }
- my $rounded_number;
- if ($x > 0) {
- $rounded_number = (int(($factor * $x) + 0.5) / $factor);
- } else {
- $rounded_number = (int(($factor * $x) - 0.5) / $factor);
- }
- if ($fill_decimals_p) {
- ($period, $decimals) = ($rounded_number =~ /^-?\d+(\.?)(\d*)$/);
- $rounded_number .= "." unless $period || ($n == 0);
- foreach ((1 .. ($n - length($decimals)))) {
- $rounded_number .= 0;
- }
- }
- return $rounded_number;
-}
-
-sub commify {
- local($caller,$number) = @_;
-
- my $text = reverse $number;
- $text =~ s/(\d\d\d)(?=\d)(?!\d*\.)/$1,/g;
- return scalar reverse $text;
-}
-
-sub add_javascript_functions {
- local($caller,@function_names) = @_;
-
- $add_javascript_function_s = "";
- foreach $function_name (@function_names) {
-
- if ($function_name eq "highlight_elems") {
- $add_javascript_function_s .= "
- function highlight_elems(group_id, value) {
- if (group_id != '') {
- i = 1;
- id = group_id + '-' + i;
- while ((s = document.getElementById(id)) != null) {
- if (! s.origColor) {
- if (s.style.color) {
- s.origColor = s.style.color;
- } else {
- s.origColor = '#000000';
- }
- }
- if (value == '1') {
- s.style.color = '#0000FF';
- if (s.innerHTML == '-') {
- s.style.innerHtml = s.innerHTML;
- s.innerHTML = '- ← here';
- s.style.fontWeight = 900;
- } else {
- s.style.fontWeight = 'bold';
- }
- } else {
- s.style.fontWeight = 'normal';
- s.style.color = s.origColor;
- if (s.style.innerHtml != null) {
- s.innerHTML = s.style.innerHtml;
- }
- }
- i = i + 1;
- id = group_id + '-' + i;
- }
- }
- }
-";
- } elsif ($function_name eq "set_style_for_ids") {
- $add_javascript_function_s .= "
- function set_style_for_ids(style,id_list) {
- var ids = id_list.split(/\\s+/);
- var len = ids.length;
- var s;
- for (var i=0; i>$filename")) {
- print OUT $s;
- close(OUT);
- $result = "Appended";
- } else {
- $result = "Can't append";
- }
- } else {
- if (open(OUT, ">$filename")) {
- print OUT $s;
- close(OUT);
- $result = "Wrote";
- } else {
- $result = "Can't write";
- }
- }
- chmod($mod, $filename) if defined($mod) && -e $filename;
- return $result;
-}
-
-sub square {
- local($caller, $x) = @_;
-
- return $x * $x;
-}
-
-sub mutual_info {
- local($caller, $ab_count, $a_count, $b_count, $total_count, $smoothing) = @_;
-
- $smoothing = 1 unless defined($smoothing);
- $ab_count = 0 unless defined($ab_count);
- return 0 unless $a_count && $b_count && $total_count;
-
- my $p_ab = $ab_count / $total_count;
- my $p_a = $a_count / $total_count;
- my $p_b = $b_count / $total_count;
- my $expected_ab = $p_a * $p_b * $total_count;
-
- return -99 unless $expected_ab || $smoothing;
-
- return CORE::log(($ab_count + $smoothing) / ($expected_ab + $smoothing));
-}
-
-sub mutual_info_multi {
- local($caller, $multi_count, $total_count, $smoothing, @counts) = @_;
-
- return 0 unless $total_count;
- my $p_indivuals = 1;
- foreach $count (@counts) {
- return 0 unless $count;
- $p_indivuals *= ($count / $total_count);
- }
- my $expected_multi_count = $p_indivuals * $total_count;
- # print STDERR "actual vs. expected multi_count($multi_count, $total_count, $smoothing, @counts) = $multi_count vs. $expected_multi_count\n";
-
- return -99 unless $expected_multi_count || $smoothing;
-
- return CORE::log(($multi_count + $smoothing) / ($expected_multi_count + $smoothing));
-}
-
-sub precision_recall_fmeasure {
- local($caller, $n_gold, $n_test, $n_shared, $pretty_print_p) = @_;
-
- unless (($n_gold =~ /^[1-9]\d*$/) && ($n_test =~ /^[1-9]\d*$/)) {
- $zero = ($pretty_print_p) ? "0%" : 0;
- if ($n_gold =~ /^[1-9]\d*$/) {
- return ("n/a", $zero, $zero);
- } elsif ($n_test =~ /^[1-9]\d*$/) {
- return ($zero, "n/a", $zero);
- } else {
- return ("n/a", "n/a", "n/a");
- }
- }
- my $precision = $n_shared / $n_test;
- my $recall = $n_shared / $n_gold;
- my $f_measure = ($precision * $recall * 2) / ($precision + $recall);
-
- return ($precision, $recall, $f_measure) unless $pretty_print_p;
-
- my $pretty_precision = $caller->round_to_n_decimal_places(100*$precision, 1) . "%";
- my $pretty_recall = $caller->round_to_n_decimal_places(100*$recall, 1) . "%";
- my $pretty_f_measure = $caller->round_to_n_decimal_places(100*$f_measure, 1) . "%";
-
- return ($pretty_precision, $pretty_recall, $pretty_f_measure);
-}
-
-sub recapitalize_named_entity {
- local($caller, $s) = @_;
-
- my @comps = ();
- foreach $comp (split(/\s+/, $s)) {
- if ($comp =~ /^(and|da|for|of|on|the|van|von)$/) {
- push(@comps, $comp);
- } elsif ($comp =~ /^[a-z]/) {
- push(@comps, ucfirst $comp);
- } else {
- push(@comps, $comp);
- }
- }
- return join(" ", @comps);
-}
-
-sub slot_value_in_double_colon_del_list {
- local($this, $s, $slot, $default) = @_;
-
- $default = "" unless defined($default);
- if (($value) = ($s =~ /::$slot\s+(\S.*\S|\S)\s*$/)) {
- $value =~ s/\s*::\S.*\s*$//;
- return $value;
- } else {
- return $default;
- }
-}
-
-sub synt_in_double_colon_del_list {
- local($this, $s) = @_;
-
- ($value) = ($s =~ /::synt\s+(\S+|\S.*?\S)(?:\s+::.*)?$/);
- return (defined($value)) ? $value : "";
-}
-
-sub form_in_double_colon_del_list {
- local($this, $s) = @_;
-
- ($value) = ($s =~ /::form\s+(\S+|\S.*?\S)(?:\s+::.*)?$/);
- return (defined($value)) ? $value : "";
-}
-
-sub lex_in_double_colon_del_list {
- local($this, $s) = @_;
-
- ($value) = ($s =~ /::lex\s+(\S+|\S.*?\S)(?:\s+::.*)?$/);
- return (defined($value)) ? $value : "";
-}
-
-sub multi_slot_value_in_double_colon_del_list {
- # e.g. when there are multiple slot/value pairs in a line, e.g. ::eng ... :eng ...
- local($this, $s, $slot) = @_;
-
- @values = ();
- while (($value, $rest) = ($s =~ /::$slot\s+(\S|\S.*?\S)(\s+::\S.*|\s*)$/)) {
- push(@values, $value);
- $s = $rest;
- }
- return @values;
-}
-
-sub remove_slot_in_double_colon_del_list {
- local($this, $s, $slot) = @_;
-
- $s =~ s/::$slot(?:|\s+\S|\s+\S.*?\S)(\s+::\S.*|\s*)$/$1/;
- $s =~ s/^\s*//;
- return $s;
-}
-
-sub extract_split_info_from_split_dir {
- local($this, $dir, *ht) = @_;
-
- my $n_files = 0;
- my $n_snt_ids = 0;
- if (opendir(DIR, $dir)) {
- my @filenames = sort readdir(DIR);
- closedir(DIR);
- foreach $filename (@filenames) {
- next unless $filename =~ /\.txt$/;
- my $split_class;
- if (($split_class) = ($filename =~ /-(dev|training|test)-/)) {
- my $full_filename = "$dir/$filename";
- if (open(IN, $full_filename)) {
- my $old_n_snt_ids = $n_snt_ids;
- while () {
- if (($snt_id) = ($_ =~ /^#\s*::id\s+(\S+)/)) {
- if ($old_split_class = $ht{SPLIT_CLASS}->{$snt_id}) {
- unless ($old_split_class eq $split_class) {
- print STDERR "Conflicting split class for $snt_id: $old_split_class $split_class\n";
- }
- } else {
- $ht{SPLIT_CLASS}->{$snt_id} = $split_class;
- $ht{SPLIT_CLASS_COUNT}->{$split_class} = ($ht{SPLIT_CLASS_COUNT}->{$split_class} || 0) + 1;
- $n_snt_ids++;
- }
- }
- }
- $n_files++ unless $n_snt_ids == $old_n_snt_ids;
- close(IN);
- } else {
- print STDERR "Can't open file $full_filename";
- }
- } else {
- print STDERR "Skipping file $filename when extracting split info from $dir\n";
- }
- }
- print STDERR "Extracted $n_snt_ids split classes from $n_files files.\n";
- } else {
- print STDERR "Can't open directory $dir to extract split info.\n";
- }
-}
-
-sub extract_toks_for_split_class_from_dir {
- local($this, $dir, *ht, $split_class, $control) = @_;
-
- $control = "" unless defined($control);
- $print_snt_id_p = ($control =~ /\bwith-snt-id\b/);
- my $n_files = 0;
- my $n_snts = 0;
- if (opendir(DIR, $dir)) {
- my @filenames = sort readdir(DIR);
- closedir(DIR);
- foreach $filename (@filenames) {
- next unless $filename =~ /^alignment-release-.*\.txt$/;
- my $full_filename = "$dir/$filename";
- if (open(IN, $full_filename)) {
- my $old_n_snts = $n_snts;
- my $snt_id = "";
- while () {
- if (($s_value) = ($_ =~ /^#\s*::id\s+(\S+)/)) {
- $snt_id = $s_value;
- $proper_split_class_p
- = ($this_split_class = $ht{SPLIT_CLASS}->{$snt_id})
- && ($this_split_class eq $split_class);
- } elsif (($tok) = ($_ =~ /^#\s*::tok\s+(\S|\S.*\S)\s*$/)) {
- if ($proper_split_class_p) {
- print "$snt_id " if $print_snt_id_p;
- print "$tok\n";
- $n_snts++;
- }
- }
- }
- $n_files++ unless $n_snts == $old_n_snts;
- close(IN);
- } else {
- print STDERR "Can't open file $full_filename";
- }
- }
- print STDERR "Extracted $n_snts tokenized sentences ($split_class) from $n_files files.\n";
- } else {
- print STDERR "Can't open directory $dir to extract tokens.\n";
- }
-}
-
-sub load_relevant_tok_ngram_corpus {
- local($this, $filename, *ht, $max_lex_rule_span, $ngram_count_min, $optional_ngram_output_filename) = @_;
-
- $ngram_count_min = 1 unless $ngram_count_min;
- $max_lex_rule_span = 10 unless $max_lex_rule_span;
- my $n_ngram_instances = 0;
- my $n_ngram_types = 0;
- if (open(IN, $filename)) {
- while () {
- s/\s*$//;
- @tokens = split(/\s+/, $_);
- foreach $from_token_index ((0 .. $#tokens)) {
- foreach $to_token_index (($from_token_index .. ($from_token_index + $max_lex_rule_span -1))) {
- last if $to_token_index > $#tokens;
- my $ngram = join(" ", @tokens[$from_token_index .. $to_token_index]);
- $ht{RELEVANT_NGRAM}->{$ngram} = ($ht{RELEVANT_NGRAM}->{$ngram} || 0) + 1;
- }
- }
- }
- close(IN);
- if ($optional_ngram_output_filename && open(OUT, ">$optional_ngram_output_filename")) {
- foreach $ngram (sort keys %{$ht{RELEVANT_NGRAM}}) {
- $count = $ht{RELEVANT_NGRAM}->{$ngram};
- next unless $count >= $ngram_count_min;
- print OUT "($count) $ngram\n";
- $n_ngram_types++;
- $n_ngram_instances += $count;
- }
- close(OUT);
- print STDERR "Extracted $n_ngram_types ngram types, $n_ngram_instances ngram instances.\n";
- print STDERR "Wrote ngram stats to $optional_ngram_output_filename\n";
- }
- } else {
- print STDERR "Can't open relevant tok ngram corpus $filename\n";
- }
-}
-
-sub load_relevant_tok_ngrams {
- local($this, $filename, *ht) = @_;
-
- my $n_entries = 0;
- if (open(IN, $filename)) {
- while () {
- s/\s*$//;
- if (($count, $ngram) = ($_ =~ /^\((\d+)\)\s+(\S|\S.*\S)\s*$/)) {
- $lc_ngram = lc $ngram;
- $ht{RELEVANT_NGRAM}->{$lc_ngram} = ($ht{RELEVANT_NGRAM}->{$lc_ngram} || 0) + $count;
- $ht{RELEVANT_LC_NGRAM}->{$lc_ngram} = ($ht{RELEVANT_LC_NGRAM}->{$lc_ngram} || 0) + $count;
- $n_entries++;
- }
- }
- close(IN);
- print STDERR "Read in $n_entries entries from $filename\n";
- } else {
- print STDERR "Can't open relevant tok ngrams from $filename\n";
- }
-}
-
-sub snt_id_sort_function {
- local($this, $a, $b) = @_;
-
- if ((($core_a, $index_a) = ($a =~ /^(\S+)\.(\d+)$/))
- && (($core_b, $index_b) = ($b =~ /^(\S+)\.(\d+)$/))) {
- return ($core_a cmp $core_b) || ($index_a <=> $index_b);
- } else {
- return $a cmp $b;
- }
-}
-
-sub count_value_sort_function {
- local($this, $a_count, $b_count, $a_value, $b_value, $control) = @_;
-
- # normalize fractions such as "1/2"
- if ($a_count > $b_count) {
- return ($control eq "decreasing") ? -1 : 1;
- } elsif ($b_count > $a_count) {
- return ($control eq "decreasing") ? 1 : -1;
- }
- $a_value = $num / $den if ($num, $den) = ($a_value =~ /^([1-9]\d*)\/([1-9]\d*)$/);
- $b_value = $num / $den if ($num, $den) = ($b_value =~ /^([1-9]\d*)\/([1-9]\d*)$/);
- $a_value =~ s/:/\./ if $a_value =~ /^\d+:\d+$/;
- $b_value =~ s/:/\./ if $b_value =~ /^\d+:\d+$/;
- if (($a_value =~ /^-?\d+(\.\d+)?$/)
- && ($b_value =~ /^-?\d+(\.\d+)?$/)) {
- return $a_value <=> $b_value;
- } elsif ($a_value =~ /^-?\d+(\.\d+)?$/) {
- return 1;
- } elsif ($b_value =~ /^-?\d+(\.\d+)?$/) {
- return -1;
- } else {
- return $a_value cmp $b_value;
- }
-}
-
-sub undef_to_blank {
- local($this, $x) = @_;
-
- return (defined($x)) ? $x : "";
-}
-
-sub en_lex_amr_list {
- local($this, $s) = @_;
-
- $bpe = qr{ \( (?: (?> [^()]+ ) | (??{ $bpe }))* \) }x; # see Perl Cookbook 2nd ed. p. 218
- @en_lex_amr_list = ();
- my $amr_s;
- my $lex;
- my $test;
- while ($s =~ /\S/) {
- $s =~ s/^\s*//;
- if (($s =~ /^\([a-z]\d* .*\)/)
- && (($amr_s, $rest) = ($s =~ /^($bpe)(\s.*|)$/))) {
- push(@en_lex_amr_list, $amr_s);
- $s = $rest;
- } elsif (($lex, $rest) = ($s =~ /^\s*(\S+)(\s.*|)$/)) {
- push(@en_lex_amr_list, $lex);
- $s = $rest;
- } else {
- print STDERR "en_lex_amr_list can't process: $s\n";
- $s = "";
- }
- }
- return @en_lex_amr_list;
-}
-
-sub make_sure_dir_exists {
- local($this, $dir, $umask) = @_;
-
- mkdir($dir, $umask) unless -d $dir;
- chmod($umask, $dir);
-}
-
-sub pretty_percentage {
- local($this, $numerator, $denominator) = @_;
-
- return ($denominator == 0) ? "n/a" : ($this->round_to_n_decimal_places(100*$numerator/$denominator, 2) . "%");
-}
-
-sub html_color_nth_line {
- local($this, $s, $n, $color, $delimiter) = @_;
-
- $delimiter = " " unless defined($delimiter);
- @lines = split($delimiter, $s);
- $lines[$n] = "" . $lines[$n] . "" if ($n =~ /^\d+$/) && ($n <= $#lines);
- return join($delimiter, @lines);
-}
-
-sub likely_valid_url_format {
- local($this, $url) = @_;
-
- $url = lc $url;
- return 0 if $url =~ /\s/;
- return 0 if $url =~ /[@]/;
- return 1 if $url =~ /^https?:\/\/.+\.[a-z]+(\?.+)?$/;
- return 1 if $url =~ /[a-z].+\.(com|edu|gov|net|org)$/;
- return 0;
-}
-
-# see also EnglMorph->special_token_type
-$common_file_suffixes = "aspx?|bmp|cgi|docx?|gif|html?|jpeg|jpg|mp3|mp4|pdf|php|png|pptx?|stm|svg|txt|xml";
-$common_top_domain_suffixes = "museum|info|cat|com|edu|gov|int|mil|net|org|ar|at|au|be|bg|bi|br|ca|ch|cn|co|cz|de|dk|es|eu|fi|fr|gr|hk|hu|id|ie|il|in|ir|is|it|jp|ke|kr|lu|mg|mx|my|nl|no|nz|ph|pl|pt|ro|rs|ru|rw|se|sg|sk|so|tr|tv|tw|tz|ua|ug|uk|us|za";
-
-sub token_is_url_p {
- local($this, $token) = @_;
-
- return 1 if $token =~ /^www(\.[a-z0-9]([-a-z0-9_]|\xC3[\x80-\x96\x98-\xB6\xB8-\xBF])+)+\.([a-z]{2,2}|$common_top_domain_suffixes)(\/(\.{1,3}|[a-z0-9]([-a-z0-9_%]|\xC3[\x80-\x96\x98-\xB6\xB8-\xBF])+))*(\/[a-z0-9_][-a-z0-9_]+\.($common_file_suffixes))?$/i;
- return 1 if $token =~ /^https?:\/\/([a-z]\.)?([a-z0-9]([-a-z0-9_]|\xC3[\x80-\x96\x98-\xB6\xB8-\xBF])+\.)+[a-z]{2,}(\/(\.{1,3}|([-a-z0-9_%]|\xC3[\x80-\x96\x98-\xB6\xB8-\xBF])+))*(\/[a-z_][-a-z0-9_]+\.($common_file_suffixes))?$/i;
- return 1 if $token =~ /^[a-z][-a-z0-9_]+(\.[a-z][-a-z0-9_]+)*\.($common_top_domain_suffixes)(\/[a-z0-9]([-a-z0-9_%]|\xC3[\x80-\x96\x98-\xB6\xB8-\xBF])+)*(\/[a-z][-a-z0-9_]+\.($common_file_suffixes))?$/i;
- return 0;
-}
-
-sub token_is_email_p {
- local($this, $token) = @_;
-
- return ($token =~ /^[a-z][-a-z0-9_]+(\.[a-z][-a-z0-9_]+)*\@[a-z][-a-z0-9_]+(\.[a-z][-a-z0-9_]+)*\.($common_top_domain_suffixes)$/i);
-}
-
-sub token_is_filename_p {
- local($this, $token) = @_;
-
- return 1 if $token =~ /\.($common_file_suffixes)$/;
- return 0;
-}
-
-sub token_is_xml_token_p {
- local($this, $token) = @_;
-
- return ($token =~ /^&(amp|apos|gt|lt|nbsp|quot|\d+|[0-9A-F]+);$/i);
-}
-
-sub token_is_handle_p {
- local($this, $token) = @_;
-
- return ($token =~ /^\@[a-z][_a-z0-9]*[a-z0-9]$/i);
-}
-
-sub min {
- local($this, @list) = @_;
-
- my $min = "";
- foreach $item (@list) {
- $min = $item if ($item =~ /^-?\d+(?:\.\d*)?$/) && (($min eq "") || ($item < $min));
- }
- return $min;
-}
-
-sub max {
- local($this, @list) = @_;
-
- my $max = "";
- foreach $item (@list) {
- $max = $item if defined($item) && ($item =~ /^-?\d+(?:\.\d*)?(e[-+]\d+)?$/) && (($max eq "") || ($item > $max));
- }
- return $max;
-}
-
-sub split_tok_s_into_tokens {
- local($this, $tok_s) = @_;
-
- @token_list = ();
- while (($pre, $link_token, $post) = ($tok_s =~ /^(.*?)\s*(\@?<[^<>]+>\@?)\s*(.*)$/)) {
- # generate dummy token for leading blank(s)
- if (($tok_s =~ /^\s/) && ($pre eq "") && ($#token_list < 0)) {
- push(@token_list, "");
- } else {
- push(@token_list, split(/\s+/, $pre));
- }
- push(@token_list, $link_token);
- $tok_s = $post;
- }
- push(@token_list, split(/\s+/, $tok_s));
- return @token_list;
-}
-
-sub shuffle {
- local($this, @list) = @_;
-
- @shuffle_list = ();
- while (@list) {
- $len = $#list + 1;
- $rand_position = int(rand($len));
- push(@shuffle_list, $list[$rand_position]);
- splice(@list, $rand_position, 1);
- }
- $s = join(" ", @shuffle_list);
- return @shuffle_list;
-}
-
-sub timestamp_to_seconds {
- local($this, $timestamp) = @_;
-
- my $epochtime;
- if (($year, $month, $day, $hour, $minute, $second) = ($timestamp =~ /^(\d\d\d\d)-(\d\d)-(\d\d)T(\d\d):(\d\d):(\d\d)$/)) {
- $epochtime = timelocal($second, $minute, $hour, $day, $month-1, $year);
- } elsif (($year, $month, $day) = ($timestamp =~ /^(\d\d\d\d)-(\d\d)-(\d\d)$/)) {
- $epochtime = timelocal(0, 0, 0, $day, $month-1, $year);
- } elsif (($year, $month, $day, $hour, $minute, $second, $second_fraction) = ($timestamp =~ /^(\d\d\d\d)-(\d\d)-(\d\d)T(\d\d):(\d\d):(\d\d)\.(\d+)$/)) {
- $epochtime = timelocal($second, $minute, $hour, $day, $month-1, $year) + ($second_fraction / (10 ** length($second_fraction)));
- } else {
- $epochtime = 0;
- }
- return $epochtime;
-}
-
-sub timestamp_diff_in_seconds {
- local($this, $timestamp1, $timestamp2) = @_;
-
- my $epochtime1 = $this->timestamp_to_seconds($timestamp1);
- my $epochtime2 = $this->timestamp_to_seconds($timestamp2);
- return $epochtime2 - $epochtime1;
-}
-
-sub dirhash {
- # maps string to hash of length 4 with characters [a-z2-8] (shorter acc. to $len)
- local($this, $s, $len) = @_;
-
- $hash = 9999;
- $mega = 2 ** 20;
- $mega1 = $mega - 1;
- $giga = 2 ** 26;
- foreach $c (split //, $s) {
- $hash = $hash*33 + ord($c);
- $hash = ($hash >> 20) ^ ($hash & $mega1) if $hash >= $giga;
- }
- while ($hash >= $mega) {
- $hash = ($hash >> 20) ^ ($hash & $mega1);
- }
- $result = "";
- while ($hash) {
- $c = $hash & 31;
- $result .= CORE::chr($c + (($c >= 26) ? 24 : 97));
- $hash = $hash >> 5;
- }
- while (length($result) < 4) {
- $result .= "8";
- }
- return substr($result, 0, $len) if $len;
- return $result;
-}
-
-sub full_path_python {
-
- foreach $bin_path (split(":", "/usr/sbin:/usr/bin:/bin:/usr/local/bin")) {
- return $python if -x ($python = "$bin_path/python");
- }
- return "python";
-}
-
-sub string_contains_unbalanced_paras {
- local($this, $s) = @_;
-
- return 0 unless $s =~ /[(){}\[\]]/;
- $rest = $s;
- while (($pre,$left,$right,$post) = ($rest =~ /^(.*)([({\[]).*?([\]})])(.*)$/)) {
- return 1 unless (($left eq "(") && ($right eq ")"))
- || (($left eq "[") && ($right eq "]"))
- || (($left eq "{") && ($right eq "}"));
- $rest = "$pre$post";
- }
- return 1 if $rest =~ /[(){}\[\]]/;
- return 0;
-}
-
-sub dequote_string {
- local($this, $s) = @_;
-
- if ($s =~ /^".*"$/) {
- $s = substr($s, 1, -1);
- $s =~ s/\\"/"/g;
- return $s;
- } elsif ($s =~ /^'.*'$/) {
- $s = substr($s, 1, -1);
- $s =~ s/\\'/'/g;
- return $s;
- } else {
- return $s;
- }
-}
-
-sub defined_non_space {
- local($this, $s) = @_;
-
- return (defined($s) && ($s =~ /\S/));
-}
-
-sub default_if_undefined {
- local($this, $s, $default) = @_;
-
- return (defined($s) ? $s : $default);
-}
-
-sub remove_empties {
- local($this, @list) = @_;
-
- @filtered_list = ();
- foreach $elem (@list) {
- push(@filtered_list, $elem) if defined($elem) && (! ($elem =~ /^\s*$/)) && (! $this->member($elem, @filtered_list));
- }
-
- return @filtered_list;
-}
-
-# copied from AMRexp.pm
-sub new_var_for_surf_amr {
- local($this, $amr_s, $s) = @_;
-
- my $letter = ($s =~ /^[a-z]/i) ? lc substr($s, 0, 1) : "x";
- return $letter unless ($amr_s =~ /:\S+\s+\($letter\s+\//)
- || ($amr_s =~ /\s\($letter\s+\//)
- || ($amr_s =~ /^\s*\($letter\s+\//); # )))
- my $i = 2;
- while (($amr_s =~ /:\S+\s+\($letter$i\s+\//)
- || ($amr_s =~ /\s+\($letter$i\s+\//)
- || ($amr_s =~ /^\s*\($letter$i\s+\//)) { # )))
- $i++;
- }
- return "$letter$i";
-}
-
-# copied from AMRexp.pm
-sub new_vars_for_surf_amr {
- local($this, $amr_s, $ref_amr_s) = @_;
-
- my $new_amr_s = "";
- my %new_var_ht = ();
- my $remaining_amr_s = $amr_s;
- my $pre; my $var; my $concept; my $post;
- while (($pre, $var, $concept, $post) = ($remaining_amr_s =~ /^(.*?\()([a-z]\d*)\s+\/\s+([^ ()\s]+)(.*)$/s)) {
- $new_var = $this->new_var_for_surf_amr("$ref_amr_s $new_amr_s", $concept);
- $new_var_ht{$var} = $new_var;
- $new_amr_s .= "$pre$new_var / $concept";
- $remaining_amr_s = $post;
- }
- $new_amr_s .= $remaining_amr_s;
-
- # also update any reentrancy variables
- $remaining_amr_s = $new_amr_s;
- $new_amr_s2 = "";
- while (($pre, $var, $post) = ($remaining_amr_s =~ /^(.*?:\S+\s+)([a-z]\d*)([ ()\s].*)$/s)) {
- $new_var = $new_var_ht{$var} || $var;
- $new_amr_s2 .= "$pre$new_var";
- $remaining_amr_s = $post;
- }
- $new_amr_s2 .= $remaining_amr_s;
-
- return $new_amr_s2;
-}
-
-sub update_inner_span_for_id {
- local($this, $html_line, $slot, $new_value) = @_;
- # e.g. slot: workset-language-name value: Uyghur
-
- if (defined($new_value)
- && (($pre, $old_value, $post) = ($html_line =~ /^(.*]* id="$slot"[^<>]*>)([^<>]*)(<\/span\b[^<>]*>.*)$/i))
- && ($old_value ne $new_value)) {
- # print STDERR "Inserting new $slot $old_value -> $new_value\n";
- return $pre . $new_value . $post . "\n";
- } else {
- # no change
- return $html_line;
- }
-}
-
-sub levenshtein_distance {
- local($this, $s1, $s2) = @_;
-
- my $i;
- my $j;
- my @distance;
- my @s1_chars = $utf8->split_into_utf8_characters($s1, "return only chars", *empty_ht);
- my $s1_length = $#s1_chars + 1;
- my @s2_chars = $utf8->split_into_utf8_characters($s2, "return only chars", *empty_ht);
- my $s2_length = $#s2_chars + 1;
- for ($i = 0; $i <= $s1_length; $i++) {
- $distance[$i][0] = $i;
- }
- for ($j = 1; $j <= $s2_length; $j++) {
- $distance[0][$j] = $j;
- }
- for ($j = 1; $j <= $s2_length; $j++) {
- for ($i = 1; $i <= $s1_length; $i++) {
- my $substitution_cost = ($s1_chars[$i-1] eq $s2_chars[$j-1]) ? 0 : 1;
- $distance[$i][$j] = $this->min($distance[$i-1][$j] + 1,
- $distance[$i][$j-1] + 1,
- $distance[$i-1][$j-1] + $substitution_cost);
- # print STDERR "SC($i,$j) = $substitution_cost\n";
- # $d = $distance[$i][$j];
- # print STDERR "D($i,$j) = $d\n";
- }
- }
- return $distance[$s1_length][$s2_length];
-}
-
-sub markup_parts_of_string_in_common_with_ref {
- local($this, $s, $ref, $start_markup, $end_markup, $deletion_markup, $verbose) = @_;
-
- # \x01 temporary start-markup
- # \x02 temporary end-markup
- # \x03 temporary deletion-markup
- $s =~ s/[\x01-\x03]//g;
- $ref =~ s/[\x01-\x03]//g;
- my $i;
- my $j;
- my @distance;
- my @s_chars = $utf8->split_into_utf8_characters($s, "return only chars", *empty_ht);
- my $s_length = $#s_chars + 1;
- my @ref_chars = $utf8->split_into_utf8_characters($ref, "return only chars", *empty_ht);
- my $ref_length = $#ref_chars + 1;
- $distance[0][0] = 0;
- $del_ins_subst_op[0][0] = "-";
- for ($i = 1; $i <= $s_length; $i++) {
- $distance[$i][0] = $i;
- $del_ins_subst_op[$i][0] = 0;
- }
- for ($j = 1; $j <= $ref_length; $j++) {
- $distance[0][$j] = $j;
- $del_ins_subst_op[0][$j] = 1;
- }
- for ($j = 1; $j <= $ref_length; $j++) {
- for ($i = 1; $i <= $s_length; $i++) {
- my $substitution_cost = (($s_chars[$i-1] eq $ref_chars[$j-1])) ? 0 : 1;
- my @del_ins_subst_list = ($distance[$i-1][$j] + 1,
- $distance[$i][$j-1] + 1,
- $distance[$i-1][$j-1] + $substitution_cost);
- my $min = $this->min(@del_ins_subst_list);
- my $del_ins_subst_position = $this->position($min, @del_ins_subst_list);
- $distance[$i][$j] = $min;
- $del_ins_subst_op[$i][$j] = $del_ins_subst_position;
- }
- }
- $d = $distance[$s_length][$ref_length];
- print STDERR "markup_parts_of_string_in_common_with_ref LD($s,$ref) = $d\n" if $verbose;
- for ($j = 0; $j <= $ref_length; $j++) {
- for ($i = 0; $i <= $s_length; $i++) {
- $d = $distance[$i][$j];
- $op = $del_ins_subst_op[$i][$j];
- print STDERR "$d($op) " if $verbose;
- }
- print STDERR "\n" if $verbose;
- }
- my $result = "";
- my $i_end = $s_length;
- my $j_end = $ref_length;
- my $cost = $distance[$i_end][$j_end];
- $i = $i_end;
- $j = $j_end;
- while (1) {
- $result2 = $result;
- $result2 =~ s/\x01/$start_markup/g;
- $result2 =~ s/\x02/$end_markup/g;
- $result2 =~ s/\x03/$deletion_markup/g;
- print STDERR "i:$i i-end:$i_end j:$j j-end:$j_end r: $result2\n" if $verbose;
- # matching characters
- if ($i && $j && ($del_ins_subst_op[$i][$j] == 2) && ($distance[$i-1][$j-1] == $distance[$i][$j])) {
- $i--;
- $j--;
- } else {
- # previously matching characters
- if (($i < $i_end) && ($j < $j_end)) {
- my $sub_s = join("", @s_chars[$i .. $i_end-1]);
- $result = "\x01" . $sub_s . "\x02" . $result;
- }
- # character substitution
- if ($i && $j && ($del_ins_subst_op[$i][$j] == 2)) {
- $i--;
- $j--;
- $result = $s_chars[$i] . $result;
- } elsif ($i && ($del_ins_subst_op[$i][$j] == 0)) {
- $i--;
- $result = $s_chars[$i] . $result;
- } elsif ($j && ($del_ins_subst_op[$i][$j] == 1)) {
- $j--;
- $result = "\x03" . $result;
- } else {
- last;
- }
- $i_end = $i;
- $j_end = $j;
- }
- }
- $result2 = $result;
- $result2 =~ s/\x01/$start_markup/g;
- $result2 =~ s/\x02/$end_markup/g;
- $result2 =~ s/\x03/$deletion_markup/g;
- print STDERR "i:$i i-end:$i_end j:$j j-end:$j_end r: $result2 *\n" if $verbose;
- $result =~ s/(\x02)\x03+(\x01)/$1$deletion_markup$2/g;
- $result =~ s/(\x02)\x03+$/$1$deletion_markup/g;
- $result =~ s/^\x03+(\x01)/$deletion_markup$1/g;
- $result =~ s/\x03//g;
- $result =~ s/\x01/$start_markup/g;
- $result =~ s/\x02/$end_markup/g;
- return $result;
-}
-
-sub env_https {
- my $https = $ENV{'HTTPS'};
- return 1 if $https && ($https eq "on");
-
- my $http_via = $ENV{'HTTP_VIA'};
- return 1 if $http_via && ($http_via =~ /\bHTTPS\b.* \d+(?:\.\d+){3,}:443\b/); # tmp for beta.isi.edu
-
- return 0;
-}
-
-sub env_http_host {
- return $ENV{'HTTP_HOST'} || "";
-}
-
-sub env_script_filename {
- return $ENV{'SCRIPT_FILENAME'} || "";
-}
-
-sub cgi_mt_app_root_dir {
- local($this, $target) = @_;
- my $s;
- if ($target =~ /filename/i) {
- $s = $ENV{'SCRIPT_FILENAME'} || "";
- } else {
- $s = $ENV{'SCRIPT_NAME'} || "";
- }
- return "" unless $s;
- return $d if ($d) = ($s =~ /^(.*?\/(?:amr-editor|chinese-room-editor|utools|romanizer\/version\/[-.a-z0-9]+|romanizer))\//);
- return $d if ($d) = ($s =~ /^(.*)\/(?:bin|src|scripts?)\/[^\/]*$/);
- return $d if ($d) = ($s =~ /^(.*)\/[^\/]*$/);
- return "";
-}
-
-sub parent_dir {
- local($this, $dir) = @_;
-
- $dir =~ s/\/[^\/]+\/?$//;
- return $dir || "/";
-}
-
-sub span_start {
- local($this, $span, $default) = @_;
-
- $default = "" unless defined($default);
- return (($start) = ($span =~ /^(\d+)-\d+$/)) ? $start : $default;
-}
-
-sub span_end {
- local($this, $span, $default) = @_;
-
- $default = "" unless defined($default);
- return (($end) = ($span =~ /^\d+-(\d+)$/)) ? $end : $default;
-}
-
-sub oct_mode {
- local($this, $filename) = @_;
-
- @stat = stat($filename);
- return "" unless @stat;
- $mode = $stat[2];
- $oct_mode = sprintf("%04o", $mode & 07777);
- return $oct_mode;
-}
-
-sub csv_to_list {
- local($this, $s, $control_string) = @_;
- # Allow quoted string such as "Wait\, what?" as element with escaped comma inside.
-
- $control_string = "" unless defined($control_string);
- $strip_p = ($control_string =~ /\bstrip\b/);
- $allow_simple_commas_in_quote = ($control_string =~ /\bsimple-comma-ok\b/);
- $ignore_empty_elem_p = ($control_string =~ /\bno-empty\b/);
- @cvs_list = ();
- while ($s ne "") {
- if ((($elem, $rest) = ($s =~ /^"((?:\\[,\"]|[^,\"][\x80-\xBF]*)*)"(,.*|)$/))
- || ($allow_simple_commas_in_quote
- && (($elem, $rest) = ($s =~ /^"((?:\\[,\"]|[^\"][\x80-\xBF]*)*)"(,.*|)$/)))
- || (($elem, $rest) = ($s =~ /^([^,]*)(,.*|\s*)$/))
- || (($elem, $rest) = ($s =~ /^(.*)()$/))) {
- if ($strip_p) {
- $elem =~ s/^\s*//;
- $elem =~ s/\s*$//;
- }
- push(@cvs_list, $elem) unless $ignore_empty_elem_p && ($elem eq "");
- $rest =~ s/^,//;
- $s = $rest;
- } else {
- print STDERR "Error in csv_to_list processing $s\n";
- last;
- }
- }
- return @cvs_list;
-}
-
-sub kl_divergence {
- local($this, $distribution_id, $gold_distribution_id, *ht, $smoothing) = @_;
-
- my $total_count = $ht{DISTRIBUTION_TOTAL_COUNT}->{$distribution_id};
- my $total_gold_count = $ht{DISTRIBUTION_TOTAL_COUNT}->{$gold_distribution_id};
- return unless $total_count && $total_gold_count;
-
- my @values = keys %{$ht{DISTRIBUTION_VALUE_COUNT}->{$gold_distribution_id}};
- my $n_values = $#values + 1;
-
- my $min_total_count = $this->min($total_count, $total_gold_count);
- $smoothing = 1 - (10000/((100+$min_total_count)**2)) unless defined($smoothing);
- return unless $smoothing;
- my $smoothed_n_values = $smoothing * $n_values;
- my $divergence = 0;
- foreach $value (@values) {
- my $count = $ht{DISTRIBUTION_VALUE_COUNT}->{$distribution_id}->{$value} || 0;
- my $gold_count = $ht{DISTRIBUTION_VALUE_COUNT}->{$gold_distribution_id}->{$value};
- my $p = ($count + $smoothing) / ($total_count + $smoothed_n_values);
- my $q = ($gold_count + $smoothing) / ($total_gold_count + $smoothed_n_values);
- if ($p == 0) {
- # no impact on divergence
- } elsif ($q) {
- my $incr = $p * CORE::log($p/$q);
- $divergence += $incr;
- my $incr2 = $this->round_to_n_decimal_places($incr, 5);
- my $p2 = $this->round_to_n_decimal_places($p, 5);
- my $q2 = $this->round_to_n_decimal_places($q, 5);
- $incr2 = "+" . $incr2 if $incr > 0;
- $log = " value: $value count: $count gold_count: $gold_count p: $p2 q: $q2 $incr2\n";
- $ht{KL_DIVERGENCE_LOG}->{$distribution_id}->{$gold_distribution_id}->{$value} = $log;
- $ht{KL_DIVERGENCE_INCR}->{$distribution_id}->{$gold_distribution_id}->{$value} = $incr;
- } else {
- $divergence += 999;
- }
- }
- return $divergence;
-}
-
-sub read_ISO_8859_named_entities {
- local($this, *ht, $filename, $verbose) = @_;
- # e.g. from /nfs/isd/ulf/arabic/data/ISO-8859-1-HTML-named-entities.txt
- #
- #
- #
- #
- #
- #
-
- my $n = 0;
- if (open(IN, $filename)) {
- while () {
- s/^\xEF\xBB\xBF//;
- if (($name, $dec_unicode) = ($_ =~ /^{$name} = $dec_unicode;
- $ht{HTML_ENTITY_DECUNICODE_TO_NAME}->{$dec_unicode} = $name;
- $ht{HTML_ENTITY_NAME_TO_UTF8}->{$name} = $utf8->unicode2string($dec_unicode);
- $n++;
- # print STDERR "read_ISO_8859_named_entities $name $dec_unicode .\n" if $name =~ /dash/;
- }
- }
- close(IN);
- print STDERR "Loaded $n entries from $filename\n" if $verbose;
- } else {
- print STDERR "Could not open $filename\n" if $verbose;
- }
-}
-
-sub neg {
- local($this, $x) = @_;
-
- # robust
- return (defined($x) && ($x =~ /^-?\d+(?:\.\d+)?$/)) ? (- $x) : $x;
-}
-
-sub read_ttable_gloss_data {
- local($this, $filename, $lang_code, *ht, $direction) = @_;
- # e.g. /nfs/isd/ulf/croom/oov-lanpairs/som-eng/som-eng-ttable-glosses.txt
-
- $direction = "f to e" unless defined($direction);
- if (open(IN, $filename)) {
- while () {
- if (($headword, $gloss) = ($_ =~ /^(.*?)\t(.*?)\s*$/)) {
- if ($direction eq "e to f") {
- $ht{TTABLE_E_GLOSS}->{$lang_code}->{$headword} = $gloss;
- } else {
- $ht{TTABLE_F_GLOSS}->{$lang_code}->{$headword} = $gloss;
- }
- }
- }
- close(IN);
- }
-}
-
-sub format_gloss_for_tooltop {
- local($this, $gloss) = @_;
-
- $gloss =~ s/^\s*/\t/;
- $gloss =~ s/\s*$//;
- $gloss =~ s/ / /g;
- $gloss =~ s/\t/
/g;
- return $gloss;
-}
-
-sub obsolete_tooltip {
- local($this, $s, $lang_code, *ht) = @_;
-
- return $gloss if defined($gloss = $ht{TTABLE_F_GLOSS}->{$lang_code}->{$s});
- @e_s = sort { $ht{T_TABLE_F_E_C}->{$lang_code}->{$s}->{$b}
- <=> $ht{T_TABLE_F_E_C}->{$lang_code}->{$s}->{$a} }
- keys %{$ht{T_TABLE_F_E_C}->{$lang_code}->{$s}};
- if (@e_s) {
- $e = shift @e_s;
- $count = $ht{T_TABLE_F_E_C}->{$lang_code}->{$s}->{$e};
- $min_count = $this->max($count * 0.01, 1.0);
- $count =~ s/(\.\d\d)\d*$/$1/;
- $result = "$s:
$e ($count)";
- $n = 1;
- while (@e_s) {
- $e = shift @e_s;
- $count = $ht{T_TABLE_F_E_C}->{$lang_code}->{$s}->{$e};
- last if $count < $min_count;
- $count =~ s/(\.\d\d)\d*$/$1/;
- $result .= "
$e ($count)";
- $n++;
- last if $n >= 10;
- }
- $ht{TTABLE_F_GLOSS}->{$lang_code}->{$s} = $result;
- return $result;
- } else {
- return "";
- }
-}
-
-sub markup_html_line_init {
- local($this, $s, *ht, $id) = @_;
-
- my @chars = $utf8->split_into_utf8_characters($s, "return only chars", *empty_ht);
- $ht{S}->{$id} = $s;
-}
-
-sub markup_html_line_regex {
- local($this, $id, *ht, $regex, $m_slot, $m_value, *LOG) = @_;
-
- unless ($regex eq "") {
- my $s = $ht{S}->{$id};
- my $current_pos = 0;
- while (($pre, $match_s, $post) = ($s =~ /^(.*?)($regex)(.*)$/)) {
- $current_pos += $utf8->length_in_utf8_chars($pre);
- my $match_len = $utf8->length_in_utf8_chars($match_s);
- $ht{START}->{$id}->{$current_pos}->{$m_slot}->{$m_value} = 1;
- $ht{STOP}->{$id}->{($current_pos+$match_len)}->{$m_slot}->{$m_value} = 1;
- $current_pos += $match_len;
- $s = $post;
- }
- }
-}
-
-sub html_markup_line {
- local($this, $id, *ht, *LOG) = @_;
-
- my @titles = ();
- my @colors = ();
- my @text_decorations = ();
-
- my $s = $ht{S}->{$id};
- # print LOG "html_markup_line $id: $s\n";
- my @chars = $utf8->split_into_utf8_characters($s, "return only chars", *empty_ht);
- my $markedup_s = "";
-
- my $new_title = "";
- my $new_color = "";
- my $new_text_decoration = "";
- my $n_spans = 0;
- my $i;
- foreach $i ((0 .. ($#chars+1))) {
- my $stop_span_p = 0;
- foreach $m_slot (keys %{$ht{STOP}->{$id}->{$i}}) {
- foreach $m_value (keys %{$ht{STOP}->{$id}->{$i}->{$m_slot}}) {
- if ($m_slot eq "title") {
- my $last_positition = $this->last_position($m_value, @titles);
- splice(@titles, $last_positition, 1) if $last_positition >= 0;
- $stop_span_p = 1;
- } elsif ($m_slot eq "color") {
- my $last_positition = $this->last_position($m_value, @colors);
- splice(@colors, $last_positition, 1) if $last_positition >= 0;
- $stop_span_p = 1;
- } elsif ($m_slot eq "text-decoration") {
- my $last_positition = $this->last_position($m_value, @text_decorations);
- splice(@text_decorations, $last_positition, 1) if $last_positition >= 0;
- $stop_span_p = 1;
- }
- }
- }
- if ($stop_span_p) {
- $markedup_s .= "";
- $n_spans--;
- }
- my $start_span_p = 0;
- foreach $m_slot (keys %{$ht{START}->{$id}->{$i}}) {
- foreach $m_value (keys %{$ht{START}->{$id}->{$i}->{$m_slot}}) {
- if ($m_slot eq "title") {
- push(@titles, $m_value);
- $start_span_p = 1;
- } elsif ($m_slot eq "color") {
- push(@colors, $m_value);
- $start_span_p = 1;
- } elsif ($m_slot eq "text-decoration") {
- push(@text_decorations, $m_value);
- $start_span_p = 1;
- }
- }
- }
- if ($stop_span_p || $start_span_p) {
- my $new_title = (@titles) ? $titles[$#titles] : "";
- my $new_color = (@colors) ? $colors[$#colors] : "";
- my $new_text_decoration = (@text_decorations) ? $text_decorations[$#text_decorations] : "";
- if ($new_title || $new_color || $new_text_decoration) {
- my $args = "";
- if ($new_title) {
- $g_title = $this->guard_html_quote($new_title);
- $args .= " title=\"$g_title\"";
- }
- if ($new_color || $new_text_decoration) {
- $g_color = $this->guard_html_quote($new_color);
- $g_text_decoration = $this->guard_html_quote($new_text_decoration);
- $color_clause = ($new_color) ? "color:$g_color;" : "";
- $text_decoration_clause = ($new_text_decoration) ? "text-decoration:$g_text_decoration;" : "";
- $text_decoration_clause =~ s/text-decoration:(border-bottom:)/$1/g;
- $args .= " style=\"$color_clause$text_decoration_clause\"";
- }
- if ($n_spans) {
- $markedup_s .= "";
- $n_spans--;
- }
- $markedup_s .= "";
- $n_spans++;
- }
- }
- $markedup_s .= $chars[$i] if $i <= $#chars;
- }
- print LOG "Error in html_markup_line $id final no. of open spans: $n_spans\n" if $n_spans && $tokenization_log_verbose;
- return $markedup_s;
-}
-
-sub offset_adjustment {
- local($this, $g, $s, $offset, $snt_id, *ht, *LOG, $control) = @_;
- # s(tring) e.g. "can't"
- # g(old string) e.g. "can not"
- # Typically when s is a slight variation of g (e.g. with additional tokenization spaces in s)
- # returns mapping 0->0, 1->1, 2->2, 3->3, 6->4, 7->5
-
- $control = "" unless defined($control);
- my $verbose = ($control =~ /\bverbose\b/);
- my $s_offset = 0;
- my $g_offset = 0;
- my @s_chars = $utf8->split_into_utf8_characters($s, "return only chars", *ht);
- my @g_chars = $utf8->split_into_utf8_characters($g, "return only chars", *ht);
- my $s_len = $#s_chars + 1;
- my $g_len = $#g_chars + 1;
- $ht{OFFSET_MAP}->{$snt_id}->{$offset}->{$s_offset} = $g_offset;
- $ht{OFFSET_MAP}->{$snt_id}->{$offset}->{($s_offset+$s_len)} = $g_offset+$g_len;
-
- while (($s_offset < $s_len) && ($g_offset < $g_len)) {
- if ($s_chars[$s_offset] eq $g_chars[$g_offset]) {
- $s_offset++;
- $g_offset++;
- $ht{OFFSET_MAP}->{$snt_id}->{$offset}->{$s_offset} = $g_offset;
- } else {
- my $best_gm = 0;
- my $best_sm = 0;
- my $best_match_len = 0;
- foreach $max_m ((1 .. 4)) {
- foreach $sm ((0 .. $max_m)) {
- $max_match_len = 0;
- while ((($s_index = $s_offset+$sm+$max_match_len) < $s_len)
- && (($g_index = $g_offset+$max_m+$max_match_len) < $g_len)) {
- if ($s_chars[$s_index] eq $g_chars[$g_index]) {
- $max_match_len++;
- } else {
- last;
- }
- }
- if ($max_match_len > $best_match_len) {
- $best_match_len = $max_match_len;
- $best_sm = $sm;
- $best_gm = $max_m;
- }
- }
- foreach $gm ((0 .. $max_m)) {
- $max_match_len = 0;
- while ((($s_index = $s_offset+$max_m+$max_match_len) < $s_len)
- && (($g_index = $g_offset+$gm+$max_match_len) < $g_len)) {
- if ($s_chars[$s_index] eq $g_chars[$g_index]) {
- $max_match_len++;
- } else {
- last;
- }
- }
- if ($max_match_len > $best_match_len) {
- $best_match_len = $max_match_len;
- $best_sm = $max_m;
- $best_gm = $gm;
- }
- }
- }
- if ($best_match_len) {
- $s_offset += $best_sm;
- $g_offset += $best_gm;
- $ht{OFFSET_MAP}->{$snt_id}->{$offset}->{$s_offset} = $g_offset;
- } else {
- last;
- }
- }
- }
- if ($verbose) {
- foreach $s_offset (sort { $a <=> $b }
- keys %{$ht{OFFSET_MAP}->{$snt_id}->{$offset}}) {
- my $g_offset = $ht{OFFSET_MAP}->{$snt_id}->{$offset}->{$s_offset};
- print LOG " OFFSET_MAP $snt_id.$offset $s/$g $s_offset -> $g_offset\n" if $tokenization_log_verbose;
- }
- }
-}
-
-sub length_in_utf8_chars {
- local($this, $s) = @_;
-
- $s =~ s/[\x80-\xBF]//g;
- $s =~ s/[\x00-\x7F\xC0-\xFF]/c/g;
- return length($s);
-}
-
-sub split_into_utf8_characters {
- local($this, $text) = @_;
- # "return only chars; return trailing whitespaces"
-
- @characters = ();
- while (($char, $rest) = ($text =~ /^(.[\x80-\xBF]*)(.*)$/)) {
- push(@characters, $char);
- $text = $rest;
- }
- return @characters;
-}
-
-sub first_char_of_string {
- local($this, $s) = @_;
-
- $s =~ s/^(.[\x80-\xBF]*).*$/$1/;
- return $s;
-}
-
-sub last_char_of_string {
- local($this, $s) = @_;
-
- $s =~ s/^.*([^\x80-\xBF][\x80-\xBF]*)$/$1/;
- return $s;
-}
-
-sub first_n_chars_of_string {
- local($this, $s, $n) = @_;
-
- $s =~ s/^((?:.[\x80-\xBF]*){$n,$n}).*$/$1/;
- return $s;
-}
-
-sub last_n_chars_of_string {
- local($this, $s, $n) = @_;
-
- $s =~ s/^.*((?:[^\x80-\xBF][\x80-\xBF]*){$n,$n})$/$1/;
- return $s;
-}
-
-
-1;
diff --git a/spaces/alsrbdni/MagicPrompt-Stable-Diffusion/app.py b/spaces/alsrbdni/MagicPrompt-Stable-Diffusion/app.py
deleted file mode 100644
index 27f42add923bb600b1a709aeb94ec4845f946c37..0000000000000000000000000000000000000000
--- a/spaces/alsrbdni/MagicPrompt-Stable-Diffusion/app.py
+++ /dev/null
@@ -1,54 +0,0 @@
-from transformers import pipeline, set_seed
-import gradio as grad, random, re
-
-
-gpt2_pipe = pipeline('text-generation', model='Gustavosta/MagicPrompt-Stable-Diffusion', tokenizer='gpt2')
-with open("ideas.txt", "r") as f:
- line = f.readlines()
-
-
-def generate(starting_text):
- seed = random.randint(100, 1000000)
- set_seed(seed)
-
- if starting_text == "":
- starting_text: str = line[random.randrange(0, len(line))].replace("\n", "").lower().capitalize()
- starting_text: str = re.sub(r"[,:\-–.!;?_]", '', starting_text)
-
- response = gpt2_pipe(starting_text, max_length=(len(starting_text) + random.randint(60, 90)), num_return_sequences=4)
- response_list = []
- for x in response:
- resp = x['generated_text'].strip()
- if resp != starting_text and len(resp) > (len(starting_text) + 4) and resp.endswith((":", "-", "—")) is False:
- response_list.append(resp+'\n')
-
- response_end = "\n".join(response_list)
- response_end = re.sub('[^ ]+\.[^ ]+','', response_end)
- response_end = response_end.replace("<", "").replace(">", "")
-
- if response_end != "":
- return response_end
-
-
-txt = grad.Textbox(lines=1, label="Initial Text", placeholder="English Text here")
-out = grad.Textbox(lines=4, label="Generated Prompts")
-
-examples = []
-for x in range(8):
- examples.append(line[random.randrange(0, len(line))].replace("\n", "").lower().capitalize())
-
-title = "Prompt Generator"
-# description = 'This is a To use it, simply submit your text or click on one of the examples. To learn more about the model, [click here](https://huggingface.co/Gustavosta/MagicPrompt-Stable-Diffusion). '
-
-grad.Interface(fn=generate,
- inputs=txt,
- outputs=out,
- examples=examples,
- title=title,
- article='',
- allow_flagging='never',
- cache_examples=False,
- css="footer {visibility: hidden}",
- theme="default").launch(enable_queue=True, debug=True)
-
-
diff --git a/spaces/amarchheda/ChordDuplicate/portaudio/src/hostapi/wmme/pa_win_wmme.c b/spaces/amarchheda/ChordDuplicate/portaudio/src/hostapi/wmme/pa_win_wmme.c
deleted file mode 100644
index f8b1d7e4ec958210551020bd09630521c7027259..0000000000000000000000000000000000000000
--- a/spaces/amarchheda/ChordDuplicate/portaudio/src/hostapi/wmme/pa_win_wmme.c
+++ /dev/null
@@ -1,4033 +0,0 @@
-/*
- * $Id$
- * pa_win_wmme.c
- * Implementation of PortAudio for Windows MultiMedia Extensions (WMME)
- *
- * PortAudio Portable Real-Time Audio Library
- * Latest Version at: http://www.portaudio.com
- *
- * Authors: Ross Bencina and Phil Burk
- * Copyright (c) 1999-2000 Ross Bencina and Phil Burk
- *
- * Permission is hereby granted, free of charge, to any person obtaining
- * a copy of this software and associated documentation files
- * (the "Software"), to deal in the Software without restriction,
- * including without limitation the rights to use, copy, modify, merge,
- * publish, distribute, sublicense, and/or sell copies of the Software,
- * and to permit persons to whom the Software is furnished to do so,
- * subject to the following conditions:
- *
- * The above copyright notice and this permission notice shall be
- * included in all copies or substantial portions of the Software.
- *
- * THE SOFTWARE IS PROVIDED "AS IS", WITHOUT WARRANTY OF ANY KIND,
- * EXPRESS OR IMPLIED, INCLUDING BUT NOT LIMITED TO THE WARRANTIES OF
- * MERCHANTABILITY, FITNESS FOR A PARTICULAR PURPOSE AND NONINFRINGEMENT.
- * IN NO EVENT SHALL THE AUTHORS OR COPYRIGHT HOLDERS BE LIABLE FOR
- * ANY CLAIM, DAMAGES OR OTHER LIABILITY, WHETHER IN AN ACTION OF
- * CONTRACT, TORT OR OTHERWISE, ARISING FROM, OUT OF OR IN CONNECTION
- * WITH THE SOFTWARE OR THE USE OR OTHER DEALINGS IN THE SOFTWARE.
- */
-
-/*
- * The text above constitutes the entire PortAudio license; however,
- * the PortAudio community also makes the following non-binding requests:
- *
- * Any person wishing to distribute modifications to the Software is
- * requested to send the modifications to the original developer so that
- * they can be incorporated into the canonical version. It is also
- * requested that these non-binding requests be included along with the
- * license above.
- */
-
-/* Modification History:
- PLB = Phil Burk
- JM = Julien Maillard
- RDB = Ross Bencina
- PLB20010402 - sDevicePtrs now allocates based on sizeof(pointer)
- PLB20010413 - check for excessive numbers of channels
- PLB20010422 - apply Mike Berry's changes for CodeWarrior on PC
- including conditional inclusion of memory.h,
- and explicit typecasting on memory allocation
- PLB20010802 - use GlobalAlloc for sDevicesPtr instead of PaHost_AllocFastMemory
- PLB20010816 - pass process instead of thread to SetPriorityClass()
- PLB20010927 - use number of frames instead of real-time for CPULoad calculation.
- JM20020118 - prevent hung thread when buffers underflow.
- PLB20020321 - detect Win XP versus NT, 9x; fix DBUG typo; removed init of CurrentCount
- RDB20020411 - various renaming cleanups, factored streamData alloc and cpu usage init
- RDB20020417 - stopped counting WAVE_MAPPER when there were no real devices
- refactoring, renaming and fixed a few edge case bugs
- RDB20020531 - converted to V19 framework
- ** NOTE maintenance history is now stored in CVS **
-*/
-
-/** @file
- @ingroup hostapi_src
-
- @brief Win32 host API implementation for the Windows MultiMedia Extensions (WMME) audio API.
-*/
-
-/*
- How it works:
-
- For both callback and blocking read/write streams we open the MME devices
- in CALLBACK_EVENT mode. In this mode, MME signals an Event object whenever
- it has finished with a buffer (either filled it for input, or played it
- for output). Where necessary, we block waiting for Event objects using
- WaitMultipleObjects().
-
- When implementing a PA callback stream, we set up a high priority thread
- which waits on the MME buffer Events and drains/fills the buffers when
- they are ready.
-
- When implementing a PA blocking read/write stream, we simply wait on these
- Events (when necessary) inside the ReadStream() and WriteStream() functions.
-*/
-
-#include
-#include
-#include
-#include
-#include
-#include
-#ifndef UNDER_CE
-#include
-#endif
-#include
-/* PLB20010422 - "memory.h" doesn't work on CodeWarrior for PC. Thanks Mike Berry for the mod. */
-#ifndef __MWERKS__
-#include
-#include
-#endif /* __MWERKS__ */
-
-#include "portaudio.h"
-#include "pa_trace.h"
-#include "pa_util.h"
-#include "pa_allocation.h"
-#include "pa_hostapi.h"
-#include "pa_stream.h"
-#include "pa_cpuload.h"
-#include "pa_process.h"
-#include "pa_debugprint.h"
-
-#include "pa_win_wmme.h"
-#include "pa_win_waveformat.h"
-
-#ifdef PAWIN_USE_WDMKS_DEVICE_INFO
-#include "pa_win_wdmks_utils.h"
-#ifndef DRV_QUERYDEVICEINTERFACE
-#define DRV_QUERYDEVICEINTERFACE (DRV_RESERVED + 12)
-#endif
-#ifndef DRV_QUERYDEVICEINTERFACESIZE
-#define DRV_QUERYDEVICEINTERFACESIZE (DRV_RESERVED + 13)
-#endif
-#endif /* PAWIN_USE_WDMKS_DEVICE_INFO */
-
-/* use CreateThread for CYGWIN, _beginthreadex for all others */
-#if !defined(__CYGWIN__) && !defined(_WIN32_WCE)
-#define CREATE_THREAD (HANDLE)_beginthreadex( 0, 0, ProcessingThreadProc, stream, 0, &stream->processingThreadId )
-#define PA_THREAD_FUNC static unsigned WINAPI
-#define PA_THREAD_ID unsigned
-#else
-#define CREATE_THREAD CreateThread( 0, 0, ProcessingThreadProc, stream, 0, &stream->processingThreadId )
-#define PA_THREAD_FUNC static DWORD WINAPI
-#define PA_THREAD_ID DWORD
-#endif
-#if (defined(_WIN32_WCE))
-#pragma comment(lib, "Coredll.lib")
-#elif (defined(WIN32) && (defined(_MSC_VER) && (_MSC_VER >= 1200))) /* MSC version 6 and above */
-#pragma comment(lib, "winmm.lib")
-#endif
-
-/*
- provided in newer platform sdks
- */
-#ifndef DWORD_PTR
- #if defined(_WIN64)
- #define DWORD_PTR unsigned __int64
- #else
- #define DWORD_PTR unsigned long
- #endif
-#endif
-
-/************************************************* Constants ********/
-
-#define PA_MME_USE_HIGH_DEFAULT_LATENCY_ (0) /* For debugging glitches. */
-
-#if PA_MME_USE_HIGH_DEFAULT_LATENCY_
- #define PA_MME_WIN_9X_DEFAULT_LATENCY_ (0.4)
- #define PA_MME_MIN_HOST_OUTPUT_BUFFER_COUNT_ (4)
- #define PA_MME_MIN_HOST_INPUT_BUFFER_COUNT_FULL_DUPLEX_ (4)
- #define PA_MME_MIN_HOST_INPUT_BUFFER_COUNT_HALF_DUPLEX_ (4)
- #define PA_MME_HOST_BUFFER_GRANULARITY_FRAMES_WHEN_UNSPECIFIED_ (16)
- #define PA_MME_MAX_HOST_BUFFER_SECS_ (0.3) /* Do not exceed unless user buffer exceeds */
- #define PA_MME_MAX_HOST_BUFFER_BYTES_ (32 * 1024) /* Has precedence over PA_MME_MAX_HOST_BUFFER_SECS_, some drivers are known to crash with buffer sizes > 32k */
-#else
- #define PA_MME_WIN_9X_DEFAULT_LATENCY_ (0.2)
- #define PA_MME_MIN_HOST_OUTPUT_BUFFER_COUNT_ (2)
- #define PA_MME_MIN_HOST_INPUT_BUFFER_COUNT_FULL_DUPLEX_ (3) /* always use at least 3 input buffers for full duplex */
- #define PA_MME_MIN_HOST_INPUT_BUFFER_COUNT_HALF_DUPLEX_ (2)
- #define PA_MME_HOST_BUFFER_GRANULARITY_FRAMES_WHEN_UNSPECIFIED_ (16)
- #define PA_MME_MAX_HOST_BUFFER_SECS_ (0.1) /* Do not exceed unless user buffer exceeds */
- #define PA_MME_MAX_HOST_BUFFER_BYTES_ (32 * 1024) /* Has precedence over PA_MME_MAX_HOST_BUFFER_SECS_, some drivers are known to crash with buffer sizes > 32k */
-#endif
-
-/* Use higher latency for NT because it is even worse at real-time
- operation than Win9x.
-*/
-#define PA_MME_WIN_NT_DEFAULT_LATENCY_ (0.4)
-
-/* Default low latency for WDM based systems. This is based on a rough
- survey of workable latency settings using patest_wmme_find_best_latency_params.c.
- See pdf attached to ticket 185 for a graph of the survey results:
- http://www.portaudio.com/trac/ticket/185
-
- Workable latencies varied between 40ms and ~80ms on different systems (different
- combinations of hardware, 32 and 64 bit, WinXP, Vista and Win7. We didn't
- get enough Vista results to know if Vista has systemically worse latency.
- For now we choose a safe value across all Windows versions here.
-*/
-#define PA_MME_WIN_WDM_DEFAULT_LATENCY_ (0.090)
-
-
-/* When client suggestedLatency could result in many host buffers, we aim to have around 8,
- based off Windows documentation that suggests that the kmixer uses 8 buffers. This choice
- is somewhat arbitrary here, since we haven't observed significant stability degradation
- with using either more, or less buffers.
-*/
-#define PA_MME_TARGET_HOST_BUFFER_COUNT_ 8
-
-#define PA_MME_MIN_TIMEOUT_MSEC_ (1000)
-
-static const char constInputMapperSuffix_[] = " - Input";
-static const char constOutputMapperSuffix_[] = " - Output";
-
-/********************************************************************/
-
-/* Copy null-terminated WCHAR string to explicit char string using UTF8 encoding */
-static char *CopyWCharStringToUtf8CString(char *destination, size_t destLengthBytes, const WCHAR *source)
-{
- /* The cbMultiByte parameter ["destLengthBytes" below] is:
- """
- Size, in bytes, of the buffer indicated by lpMultiByteStr ["destination" below].
- If this parameter is set to 0, the function returns the required buffer
- size for lpMultiByteStr and makes no use of the output parameter itself.
- """
- Source: WideCharToMultiByte at MSDN:
- http://msdn.microsoft.com/en-us/library/windows/desktop/dd374130(v=vs.85).aspx
- */
- int intDestLengthBytes; /* cbMultiByte */
- /* intDestLengthBytes is an int, destLengthBytes is a size_t. Ensure that we don't overflow
- intDestLengthBytes by only using at most INT_MAX bytes of destination buffer.
- */
- if (destLengthBytes < INT_MAX)
- {
-#pragma warning (disable : 4267) /* "conversion from 'size_t' to 'int', possible loss of data" */
- intDestLengthBytes = (int)destLengthBytes; /* destLengthBytes is guaranteed < INT_MAX here */
-#pragma warning (default : 4267)
- }
- else
- {
- intDestLengthBytes = INT_MAX;
- }
-
- if (WideCharToMultiByte(CP_UTF8, 0, source, -1, destination, /*cbMultiByte=*/intDestLengthBytes, NULL, NULL) == 0)
- return NULL;
- return destination;
-}
-
-/* returns required length (in bytes) of destination buffer when
- converting WCHAR string to UTF8 bytes, not including the terminating null. */
-static size_t WCharStringLen(const WCHAR *str)
-{
- return WideCharToMultiByte(CP_UTF8, 0, str, -1, NULL, 0, NULL, NULL);
-}
-
-/********************************************************************/
-
-typedef struct PaWinMmeStream PaWinMmeStream; /* forward declaration */
-
-/* prototypes for functions declared in this file */
-
-#ifdef __cplusplus
-extern "C"
-{
-#endif /* __cplusplus */
-
-PaError PaWinMme_Initialize( PaUtilHostApiRepresentation **hostApi, PaHostApiIndex index );
-
-#ifdef __cplusplus
-}
-#endif /* __cplusplus */
-
-static void Terminate( struct PaUtilHostApiRepresentation *hostApi );
-static PaError OpenStream( struct PaUtilHostApiRepresentation *hostApi,
- PaStream** stream,
- const PaStreamParameters *inputParameters,
- const PaStreamParameters *outputParameters,
- double sampleRate,
- unsigned long framesPerBuffer,
- PaStreamFlags streamFlags,
- PaStreamCallback *streamCallback,
- void *userData );
-static PaError IsFormatSupported( struct PaUtilHostApiRepresentation *hostApi,
- const PaStreamParameters *inputParameters,
- const PaStreamParameters *outputParameters,
- double sampleRate );
-static PaError CloseStream( PaStream* stream );
-static PaError StartStream( PaStream *stream );
-static PaError StopStream( PaStream *stream );
-static PaError AbortStream( PaStream *stream );
-static PaError IsStreamStopped( PaStream *s );
-static PaError IsStreamActive( PaStream *stream );
-static PaTime GetStreamTime( PaStream *stream );
-static double GetStreamCpuLoad( PaStream* stream );
-static PaError ReadStream( PaStream* stream, void *buffer, unsigned long frames );
-static PaError WriteStream( PaStream* stream, const void *buffer, unsigned long frames );
-static signed long GetStreamReadAvailable( PaStream* stream );
-static signed long GetStreamWriteAvailable( PaStream* stream );
-
-
-/* macros for setting last host error information */
-
-#define PA_MME_SET_LAST_WAVEIN_ERROR( mmresult ) \
- { \
- wchar_t mmeErrorTextWide[ MAXERRORLENGTH ]; \
- char mmeErrorText[ MAXERRORLENGTH ]; \
- waveInGetErrorTextW( mmresult, mmeErrorTextWide, MAXERRORLENGTH ); \
- WideCharToMultiByte( CP_UTF8, 0, mmeErrorTextWide, -1, \
- mmeErrorText, MAXERRORLENGTH, NULL, NULL ); \
- PaUtil_SetLastHostErrorInfo( paMME, mmresult, mmeErrorText ); \
- }
-
-#define PA_MME_SET_LAST_WAVEOUT_ERROR( mmresult ) \
- { \
- wchar_t mmeErrorTextWide[ MAXERRORLENGTH ]; \
- char mmeErrorText[ MAXERRORLENGTH ]; \
- waveOutGetErrorTextW( mmresult, mmeErrorTextWide, MAXERRORLENGTH ); \
- WideCharToMultiByte( CP_UTF8, 0, mmeErrorTextWide, -1, \
- mmeErrorText, MAXERRORLENGTH, NULL, NULL ); \
- PaUtil_SetLastHostErrorInfo( paMME, mmresult, mmeErrorText ); \
- }
-
-
-static void PaMme_SetLastSystemError( DWORD errorCode )
-{
- char *lpMsgBuf;
- FormatMessage(
- FORMAT_MESSAGE_ALLOCATE_BUFFER | FORMAT_MESSAGE_FROM_SYSTEM,
- NULL,
- errorCode,
- MAKELANGID(LANG_NEUTRAL, SUBLANG_DEFAULT),
- (LPTSTR) &lpMsgBuf,
- 0,
- NULL
- );
- PaUtil_SetLastHostErrorInfo( paMME, errorCode, lpMsgBuf );
- LocalFree( lpMsgBuf );
-}
-
-#define PA_MME_SET_LAST_SYSTEM_ERROR( errorCode ) \
- PaMme_SetLastSystemError( errorCode )
-
-
-/* PaError returning wrappers for some commonly used win32 functions
- note that we allow passing a null ptr to have no effect.
-*/
-
-static PaError CreateEventWithPaError( HANDLE *handle,
- LPSECURITY_ATTRIBUTES lpEventAttributes,
- BOOL bManualReset,
- BOOL bInitialState,
- LPCTSTR lpName )
-{
- PaError result = paNoError;
-
- *handle = NULL;
-
- *handle = CreateEvent( lpEventAttributes, bManualReset, bInitialState, lpName );
- if( *handle == NULL )
- {
- result = paUnanticipatedHostError;
- PA_MME_SET_LAST_SYSTEM_ERROR( GetLastError() );
- }
-
- return result;
-}
-
-
-static PaError ResetEventWithPaError( HANDLE handle )
-{
- PaError result = paNoError;
-
- if( handle )
- {
- if( ResetEvent( handle ) == 0 )
- {
- result = paUnanticipatedHostError;
- PA_MME_SET_LAST_SYSTEM_ERROR( GetLastError() );
- }
- }
-
- return result;
-}
-
-
-static PaError CloseHandleWithPaError( HANDLE handle )
-{
- PaError result = paNoError;
-
- if( handle )
- {
- if( CloseHandle( handle ) == 0 )
- {
- result = paUnanticipatedHostError;
- PA_MME_SET_LAST_SYSTEM_ERROR( GetLastError() );
- }
- }
-
- return result;
-}
-
-
-/* PaWinMmeHostApiRepresentation - host api datastructure specific to this implementation */
-
-typedef struct
-{
- PaUtilHostApiRepresentation inheritedHostApiRep;
- PaUtilStreamInterface callbackStreamInterface;
- PaUtilStreamInterface blockingStreamInterface;
-
- PaUtilAllocationGroup *allocations;
-
- int inputDeviceCount, outputDeviceCount;
-
- /** winMmeDeviceIds is an array of WinMme device ids.
- fields in the range [0, inputDeviceCount) are input device ids,
- and [inputDeviceCount, inputDeviceCount + outputDeviceCount) are output
- device ids.
- */
- UINT *winMmeDeviceIds;
-}
-PaWinMmeHostApiRepresentation;
-
-
-typedef struct
-{
- PaDeviceInfo inheritedDeviceInfo;
- DWORD dwFormats; /**<< standard formats bitmask from the WAVEINCAPS and WAVEOUTCAPS structures */
- char deviceInputChannelCountIsKnown; /**<< if the system returns 0xFFFF then we don't really know the number of supported channels (1=>known, 0=>unknown)*/
- char deviceOutputChannelCountIsKnown; /**<< if the system returns 0xFFFF then we don't really know the number of supported channels (1=>known, 0=>unknown)*/
-}
-PaWinMmeDeviceInfo;
-
-
-/*************************************************************************
- * Returns recommended device ID.
- * On the PC, the recommended device can be specified by the user by
- * setting an environment variable. For example, to use device #1.
- *
- * set PA_RECOMMENDED_OUTPUT_DEVICE=1
- *
- * The user should first determine the available device ID by using
- * the supplied application "pa_devs".
- */
-#define PA_ENV_BUF_SIZE_ (32)
-#define PA_REC_IN_DEV_ENV_NAME_ ("PA_RECOMMENDED_INPUT_DEVICE")
-#define PA_REC_OUT_DEV_ENV_NAME_ ("PA_RECOMMENDED_OUTPUT_DEVICE")
-static PaDeviceIndex GetEnvDefaultDeviceID( char *envName )
-{
- PaDeviceIndex recommendedIndex = paNoDevice;
- DWORD hresult;
- char envbuf[PA_ENV_BUF_SIZE_];
-
-#ifndef WIN32_PLATFORM_PSPC /* no GetEnvironmentVariable on PocketPC */
-
- /* Let user determine default device by setting environment variable. */
- hresult = GetEnvironmentVariableA( envName, envbuf, PA_ENV_BUF_SIZE_ );
- if( (hresult > 0) && (hresult < PA_ENV_BUF_SIZE_) )
- {
- recommendedIndex = atoi( envbuf );
- }
-#endif
-
- return recommendedIndex;
-}
-
-
-static void InitializeDefaultDeviceIdsFromEnv( PaWinMmeHostApiRepresentation *hostApi )
-{
- PaDeviceIndex device;
-
- /* input */
- device = GetEnvDefaultDeviceID( PA_REC_IN_DEV_ENV_NAME_ );
- if( device != paNoDevice &&
- ( device >= 0 && device < hostApi->inheritedHostApiRep.info.deviceCount ) &&
- hostApi->inheritedHostApiRep.deviceInfos[ device ]->maxInputChannels > 0 )
- {
- hostApi->inheritedHostApiRep.info.defaultInputDevice = device;
- }
-
- /* output */
- device = GetEnvDefaultDeviceID( PA_REC_OUT_DEV_ENV_NAME_ );
- if( device != paNoDevice &&
- ( device >= 0 && device < hostApi->inheritedHostApiRep.info.deviceCount ) &&
- hostApi->inheritedHostApiRep.deviceInfos[ device ]->maxOutputChannels > 0 )
- {
- hostApi->inheritedHostApiRep.info.defaultOutputDevice = device;
- }
-}
-
-
-/** Convert external PA ID to a windows multimedia device ID
-*/
-static UINT LocalDeviceIndexToWinMmeDeviceId( PaWinMmeHostApiRepresentation *hostApi, PaDeviceIndex device )
-{
- assert( device >= 0 && device < hostApi->inputDeviceCount + hostApi->outputDeviceCount );
-
- return hostApi->winMmeDeviceIds[ device ];
-}
-
-
-static int SampleFormatAndWinWmmeSpecificFlagsToLinearWaveFormatTag( PaSampleFormat sampleFormat, unsigned long winMmeSpecificFlags )
-{
- int waveFormatTag = 0;
-
- if( winMmeSpecificFlags & paWinMmeWaveFormatDolbyAc3Spdif )
- waveFormatTag = PAWIN_WAVE_FORMAT_DOLBY_AC3_SPDIF;
- else if( winMmeSpecificFlags & paWinMmeWaveFormatWmaSpdif )
- waveFormatTag = PAWIN_WAVE_FORMAT_WMA_SPDIF;
- else
- waveFormatTag = PaWin_SampleFormatToLinearWaveFormatTag( sampleFormat );
-
- return waveFormatTag;
-}
-
-
-static PaError QueryInputWaveFormatEx( int deviceId, WAVEFORMATEX *waveFormatEx )
-{
- MMRESULT mmresult;
-
- switch( mmresult = waveInOpen( NULL, deviceId, waveFormatEx, 0, 0, WAVE_FORMAT_QUERY ) )
- {
- case MMSYSERR_NOERROR:
- return paNoError;
- case MMSYSERR_ALLOCATED: /* Specified resource is already allocated. */
- return paDeviceUnavailable;
- case MMSYSERR_NODRIVER: /* No device driver is present. */
- return paDeviceUnavailable;
- case MMSYSERR_NOMEM: /* Unable to allocate or lock memory. */
- return paInsufficientMemory;
- case WAVERR_BADFORMAT: /* Attempted to open with an unsupported waveform-audio format. */
- return paSampleFormatNotSupported;
-
- case MMSYSERR_BADDEVICEID: /* Specified device identifier is out of range. */
- /* falls through */
- default:
- PA_MME_SET_LAST_WAVEIN_ERROR( mmresult );
- return paUnanticipatedHostError;
- }
-}
-
-
-static PaError QueryOutputWaveFormatEx( int deviceId, WAVEFORMATEX *waveFormatEx )
-{
- MMRESULT mmresult;
-
- switch( mmresult = waveOutOpen( NULL, deviceId, waveFormatEx, 0, 0, WAVE_FORMAT_QUERY ) )
- {
- case MMSYSERR_NOERROR:
- return paNoError;
- case MMSYSERR_ALLOCATED: /* Specified resource is already allocated. */
- return paDeviceUnavailable;
- case MMSYSERR_NODRIVER: /* No device driver is present. */
- return paDeviceUnavailable;
- case MMSYSERR_NOMEM: /* Unable to allocate or lock memory. */
- return paInsufficientMemory;
- case WAVERR_BADFORMAT: /* Attempted to open with an unsupported waveform-audio format. */
- return paSampleFormatNotSupported;
-
- case MMSYSERR_BADDEVICEID: /* Specified device identifier is out of range. */
- /* falls through */
- default:
- PA_MME_SET_LAST_WAVEOUT_ERROR( mmresult );
- return paUnanticipatedHostError;
- }
-}
-
-
-static PaError QueryFormatSupported( PaDeviceInfo *deviceInfo,
- PaError (*waveFormatExQueryFunction)(int, WAVEFORMATEX*),
- int winMmeDeviceId, int channels, double sampleRate, unsigned long winMmeSpecificFlags )
-{
- PaWinMmeDeviceInfo *winMmeDeviceInfo = (PaWinMmeDeviceInfo*)deviceInfo;
- PaWinWaveFormat waveFormat;
- PaSampleFormat sampleFormat;
- int waveFormatTag;
-
- /* @todo at the moment we only query with 16 bit sample format and directout speaker config*/
-
- sampleFormat = paInt16;
- waveFormatTag = SampleFormatAndWinWmmeSpecificFlagsToLinearWaveFormatTag( sampleFormat, winMmeSpecificFlags );
-
- if( waveFormatTag == PaWin_SampleFormatToLinearWaveFormatTag( paInt16 ) ){
-
- /* attempt bypass querying the device for linear formats */
-
- if( sampleRate == 11025.0
- && ( (channels == 1 && (winMmeDeviceInfo->dwFormats & WAVE_FORMAT_1M16))
- || (channels == 2 && (winMmeDeviceInfo->dwFormats & WAVE_FORMAT_1S16)) ) ){
-
- return paNoError;
- }
-
- if( sampleRate == 22050.0
- && ( (channels == 1 && (winMmeDeviceInfo->dwFormats & WAVE_FORMAT_2M16))
- || (channels == 2 && (winMmeDeviceInfo->dwFormats & WAVE_FORMAT_2S16)) ) ){
-
- return paNoError;
- }
-
- if( sampleRate == 44100.0
- && ( (channels == 1 && (winMmeDeviceInfo->dwFormats & WAVE_FORMAT_4M16))
- || (channels == 2 && (winMmeDeviceInfo->dwFormats & WAVE_FORMAT_4S16)) ) ){
-
- return paNoError;
- }
- }
-
-
- /* first, attempt to query the device using WAVEFORMATEXTENSIBLE,
- if this fails we fall back to WAVEFORMATEX */
-
- PaWin_InitializeWaveFormatExtensible( &waveFormat, channels, sampleFormat, waveFormatTag,
- sampleRate, PAWIN_SPEAKER_DIRECTOUT );
-
- if( waveFormatExQueryFunction( winMmeDeviceId, (WAVEFORMATEX*)&waveFormat ) == paNoError )
- return paNoError;
-
- PaWin_InitializeWaveFormatEx( &waveFormat, channels, sampleFormat, waveFormatTag, sampleRate );
-
- return waveFormatExQueryFunction( winMmeDeviceId, (WAVEFORMATEX*)&waveFormat );
-}
-
-
-#define PA_DEFAULTSAMPLERATESEARCHORDER_COUNT_ (13) /* must match array length below */
-static double defaultSampleRateSearchOrder_[] =
- { 44100.0, 48000.0, 32000.0, 24000.0, 22050.0, 88200.0, 96000.0, 192000.0,
- 16000.0, 12000.0, 11025.0, 9600.0, 8000.0 };
-
-static void DetectDefaultSampleRate( PaWinMmeDeviceInfo *winMmeDeviceInfo, int winMmeDeviceId,
- PaError (*waveFormatExQueryFunction)(int, WAVEFORMATEX*), int maxChannels )
-{
- PaDeviceInfo *deviceInfo = &winMmeDeviceInfo->inheritedDeviceInfo;
- int i;
-
- deviceInfo->defaultSampleRate = 0.;
-
- for( i=0; i < PA_DEFAULTSAMPLERATESEARCHORDER_COUNT_; ++i )
- {
- double sampleRate = defaultSampleRateSearchOrder_[ i ];
- PaError paerror = QueryFormatSupported( deviceInfo, waveFormatExQueryFunction, winMmeDeviceId, maxChannels, sampleRate, 0 );
- if( paerror == paNoError )
- {
- deviceInfo->defaultSampleRate = sampleRate;
- break;
- }
- }
-}
-
-
-#ifdef PAWIN_USE_WDMKS_DEVICE_INFO
-static int QueryWaveInKSFilterMaxChannels( UINT waveInDeviceId, int *maxChannels )
-{
- void *devicePath;
- DWORD devicePathSize;
- int result = 0;
-
- /* pass UINT ID via punned HWAVEIN, as per DRV_QUERYDEVICEINTERFACESIZE documentation */
- HWAVEIN hDeviceId = (HWAVEIN)((UINT_PTR)waveInDeviceId);
-
- if( waveInMessage(hDeviceId, DRV_QUERYDEVICEINTERFACESIZE,
- (DWORD_PTR)&devicePathSize, 0 ) != MMSYSERR_NOERROR )
- return 0;
-
- devicePath = PaUtil_AllocateMemory( devicePathSize );
- if( !devicePath )
- return 0;
-
- /* apparently DRV_QUERYDEVICEINTERFACE returns a unicode interface path, although this is undocumented */
- if( waveInMessage(hDeviceId, DRV_QUERYDEVICEINTERFACE,
- (DWORD_PTR)devicePath, devicePathSize ) == MMSYSERR_NOERROR )
- {
- int count = PaWin_WDMKS_QueryFilterMaximumChannelCount( devicePath, /* isInput= */ 1 );
- if( count > 0 )
- {
- *maxChannels = count;
- result = 1;
- }
- }
-
- PaUtil_FreeMemory( devicePath );
-
- return result;
-}
-#endif /* PAWIN_USE_WDMKS_DEVICE_INFO */
-
-
-static PaError InitializeInputDeviceInfo( PaWinMmeHostApiRepresentation *winMmeHostApi,
- PaWinMmeDeviceInfo *winMmeDeviceInfo, UINT winMmeInputDeviceId, int *success )
-{
- PaError result = paNoError;
- char *deviceName; /* non-const ptr */
- MMRESULT mmresult;
- WAVEINCAPSW wic;
- PaDeviceInfo *deviceInfo = &winMmeDeviceInfo->inheritedDeviceInfo;
- size_t len;
-
- *success = 0;
-
- mmresult = waveInGetDevCapsW( winMmeInputDeviceId, &wic, sizeof( WAVEINCAPSW ) );
- if( mmresult == MMSYSERR_NOMEM )
- {
- result = paInsufficientMemory;
- goto error;
- }
- else if( mmresult != MMSYSERR_NOERROR )
- {
- /* instead of returning paUnanticipatedHostError we return
- paNoError, but leave success set as 0. This allows
- Pa_Initialize to just ignore this device, without failing
- the entire initialisation process.
- */
- return paNoError;
- }
-
- /* NOTE: the WAVEOUTCAPS.szPname is a null-terminated array of 32 characters,
- so we are limited to displaying only the first 31 characters of the device name. */
- if( winMmeInputDeviceId == WAVE_MAPPER )
- {
- len = WCharStringLen( wic.szPname ) + 1 + sizeof(constInputMapperSuffix_);
- /* Append I/O suffix to WAVE_MAPPER device. */
- deviceName = (char*)PaUtil_GroupAllocateMemory(
- winMmeHostApi->allocations,
- (long)len );
- if( !deviceName )
- {
- result = paInsufficientMemory;
- goto error;
- }
- CopyWCharStringToUtf8CString( deviceName, len, wic.szPname );
- strcat( deviceName, constInputMapperSuffix_ );
- }
- else
- {
- len = WCharStringLen( wic.szPname ) + 1;
- deviceName = (char*)PaUtil_GroupAllocateMemory(
- winMmeHostApi->allocations,
- (long)len );
- if( !deviceName )
- {
- result = paInsufficientMemory;
- goto error;
- }
- CopyWCharStringToUtf8CString( deviceName, len, wic.szPname );
- }
- deviceInfo->name = deviceName;
-
- if( wic.wChannels == 0xFFFF || wic.wChannels < 1 || wic.wChannels > 255 ){
- /* For Windows versions using WDM (possibly Windows 98 ME and later)
- * the kernel mixer sits between the application and the driver. As a result,
- * wave*GetDevCaps often kernel mixer channel counts, which are unlimited.
- * When this happens we assume the device is stereo and set a flag
- * so that other channel counts can be tried with OpenStream -- i.e. when
- * device*ChannelCountIsKnown is false, OpenStream will try whatever
- * channel count you supply.
- * see also InitializeOutputDeviceInfo() below.
- */
-
- PA_DEBUG(("Pa_GetDeviceInfo: Num input channels reported as %d! Changed to 2.\n", wic.wChannels ));
- deviceInfo->maxInputChannels = 2;
- winMmeDeviceInfo->deviceInputChannelCountIsKnown = 0;
- }else{
- deviceInfo->maxInputChannels = wic.wChannels;
- winMmeDeviceInfo->deviceInputChannelCountIsKnown = 1;
- }
-
-#ifdef PAWIN_USE_WDMKS_DEVICE_INFO
- winMmeDeviceInfo->deviceInputChannelCountIsKnown =
- QueryWaveInKSFilterMaxChannels( winMmeInputDeviceId, &deviceInfo->maxInputChannels );
-#endif /* PAWIN_USE_WDMKS_DEVICE_INFO */
-
- winMmeDeviceInfo->dwFormats = wic.dwFormats;
-
- DetectDefaultSampleRate( winMmeDeviceInfo, winMmeInputDeviceId,
- QueryInputWaveFormatEx, deviceInfo->maxInputChannels );
-
- *success = 1;
-
-error:
- return result;
-}
-
-
-#ifdef PAWIN_USE_WDMKS_DEVICE_INFO
-static int QueryWaveOutKSFilterMaxChannels( UINT waveOutDeviceId, int *maxChannels )
-{
- void *devicePath;
- DWORD devicePathSize;
- int result = 0;
-
- /* pass UINT ID via punned HWAVEOUT, as per DRV_QUERYDEVICEINTERFACESIZE documentation */
- HWAVEOUT hDeviceId = (HWAVEOUT)((UINT_PTR)waveOutDeviceId);
-
- if( waveOutMessage(hDeviceId, DRV_QUERYDEVICEINTERFACESIZE,
- (DWORD_PTR)&devicePathSize, 0 ) != MMSYSERR_NOERROR )
- return 0;
-
- devicePath = PaUtil_AllocateMemory( devicePathSize );
- if( !devicePath )
- return 0;
-
- /* apparently DRV_QUERYDEVICEINTERFACE returns a unicode interface path, although this is undocumented */
- if( waveOutMessage(hDeviceId, DRV_QUERYDEVICEINTERFACE,
- (DWORD_PTR)devicePath, devicePathSize ) == MMSYSERR_NOERROR )
- {
- int count = PaWin_WDMKS_QueryFilterMaximumChannelCount( devicePath, /* isInput= */ 0 );
- if( count > 0 )
- {
- *maxChannels = count;
- result = 1;
- }
- }
-
- PaUtil_FreeMemory( devicePath );
-
- return result;
-}
-#endif /* PAWIN_USE_WDMKS_DEVICE_INFO */
-
-
-static PaError InitializeOutputDeviceInfo( PaWinMmeHostApiRepresentation *winMmeHostApi,
- PaWinMmeDeviceInfo *winMmeDeviceInfo, UINT winMmeOutputDeviceId, int *success )
-{
- PaError result = paNoError;
- char *deviceName; /* non-const ptr */
- MMRESULT mmresult;
- WAVEOUTCAPSW woc;
- PaDeviceInfo *deviceInfo = &winMmeDeviceInfo->inheritedDeviceInfo;
- size_t len;
-#ifdef PAWIN_USE_WDMKS_DEVICE_INFO
- int wdmksDeviceOutputChannelCountIsKnown;
-#endif
-
- *success = 0;
-
- mmresult = waveOutGetDevCapsW( winMmeOutputDeviceId, &woc, sizeof( WAVEOUTCAPSW ) );
- if( mmresult == MMSYSERR_NOMEM )
- {
- result = paInsufficientMemory;
- goto error;
- }
- else if( mmresult != MMSYSERR_NOERROR )
- {
- /* instead of returning paUnanticipatedHostError we return
- paNoError, but leave success set as 0. This allows
- Pa_Initialize to just ignore this device, without failing
- the entire initialisation process.
- */
- return paNoError;
- }
-
- /* NOTE: the WAVEOUTCAPS.szPname is a null-terminated array of 32 characters,
- so we are limited to displaying only the first 31 characters of the device name. */
- if( winMmeOutputDeviceId == WAVE_MAPPER )
- {
- /* Append I/O suffix to WAVE_MAPPER device. */
- len = WCharStringLen( woc.szPname ) + 1 + sizeof(constOutputMapperSuffix_);
- deviceName = (char*)PaUtil_GroupAllocateMemory(
- winMmeHostApi->allocations,
- (long)len );
- if( !deviceName )
- {
- result = paInsufficientMemory;
- goto error;
- }
- CopyWCharStringToUtf8CString( deviceName, len, woc.szPname );
- strcat( deviceName, constOutputMapperSuffix_ );
- }
- else
- {
- len = WCharStringLen( woc.szPname ) + 1;
- deviceName = (char*)PaUtil_GroupAllocateMemory(
- winMmeHostApi->allocations,
- (long)len );
- if( !deviceName )
- {
- result = paInsufficientMemory;
- goto error;
- }
- CopyWCharStringToUtf8CString( deviceName, len, woc.szPname );
- }
- deviceInfo->name = deviceName;
-
- if( woc.wChannels == 0xFFFF || woc.wChannels < 1 || woc.wChannels > 255 ){
- /* For Windows versions using WDM (possibly Windows 98 ME and later)
- * the kernel mixer sits between the application and the driver. As a result,
- * wave*GetDevCaps often kernel mixer channel counts, which are unlimited.
- * When this happens we assume the device is stereo and set a flag
- * so that other channel counts can be tried with OpenStream -- i.e. when
- * device*ChannelCountIsKnown is false, OpenStream will try whatever
- * channel count you supply.
- * see also InitializeInputDeviceInfo() above.
- */
-
- PA_DEBUG(("Pa_GetDeviceInfo: Num output channels reported as %d! Changed to 2.\n", woc.wChannels ));
- deviceInfo->maxOutputChannels = 2;
- winMmeDeviceInfo->deviceOutputChannelCountIsKnown = 0;
- }else{
- deviceInfo->maxOutputChannels = woc.wChannels;
- winMmeDeviceInfo->deviceOutputChannelCountIsKnown = 1;
- }
-
-#ifdef PAWIN_USE_WDMKS_DEVICE_INFO
- wdmksDeviceOutputChannelCountIsKnown = QueryWaveOutKSFilterMaxChannels(
- winMmeOutputDeviceId, &deviceInfo->maxOutputChannels );
- if( wdmksDeviceOutputChannelCountIsKnown && !winMmeDeviceInfo->deviceOutputChannelCountIsKnown )
- winMmeDeviceInfo->deviceOutputChannelCountIsKnown = 1;
-#endif /* PAWIN_USE_WDMKS_DEVICE_INFO */
-
- winMmeDeviceInfo->dwFormats = woc.dwFormats;
-
- DetectDefaultSampleRate( winMmeDeviceInfo, winMmeOutputDeviceId,
- QueryOutputWaveFormatEx, deviceInfo->maxOutputChannels );
-
- *success = 1;
-
-error:
- return result;
-}
-
-
-static void GetDefaultLatencies( PaTime *defaultLowLatency, PaTime *defaultHighLatency )
-{
-/*
-NOTE: GetVersionEx() is deprecated as of Windows 8.1 and can not be used to reliably detect
-versions of Windows higher than Windows 8 (due to manifest requirements for reporting higher versions).
-Microsoft recommends switching to VerifyVersionInfo (available on Win 2k and later), however GetVersionEx
-is faster, for now we just disable the deprecation warning.
-See: https://msdn.microsoft.com/en-us/library/windows/desktop/ms724451(v=vs.85).aspx
-See: http://www.codeproject.com/Articles/678606/Part-Overcoming-Windows-s-deprecation-of-GetVe
-*/
-#pragma warning (disable : 4996) /* use of GetVersionEx */
-
- OSVERSIONINFO osvi;
- osvi.dwOSVersionInfoSize = sizeof( osvi );
- GetVersionEx( &osvi );
-
- /* Check for NT */
- if( (osvi.dwMajorVersion == 4) && (osvi.dwPlatformId == 2) )
- {
- *defaultLowLatency = PA_MME_WIN_NT_DEFAULT_LATENCY_;
- }
- else if(osvi.dwMajorVersion >= 5)
- {
- *defaultLowLatency = PA_MME_WIN_WDM_DEFAULT_LATENCY_;
- }
- else
- {
- *defaultLowLatency = PA_MME_WIN_9X_DEFAULT_LATENCY_;
- }
-
- *defaultHighLatency = *defaultLowLatency * 2;
-
-#pragma warning (default : 4996)
-}
-
-
-PaError PaWinMme_Initialize( PaUtilHostApiRepresentation **hostApi, PaHostApiIndex hostApiIndex )
-{
- PaError result = paNoError;
- int i;
- PaWinMmeHostApiRepresentation *winMmeHostApi;
- int inputDeviceCount, outputDeviceCount, maximumPossibleDeviceCount;
- PaWinMmeDeviceInfo *deviceInfoArray;
- int deviceInfoInitializationSucceeded;
- PaTime defaultLowLatency, defaultHighLatency;
- DWORD waveInPreferredDevice, waveOutPreferredDevice;
- DWORD preferredDeviceStatusFlags;
-
- winMmeHostApi = (PaWinMmeHostApiRepresentation*)PaUtil_AllocateMemory( sizeof(PaWinMmeHostApiRepresentation) );
- if( !winMmeHostApi )
- {
- result = paInsufficientMemory;
- goto error;
- }
-
- winMmeHostApi->allocations = PaUtil_CreateAllocationGroup();
- if( !winMmeHostApi->allocations )
- {
- result = paInsufficientMemory;
- goto error;
- }
-
- *hostApi = &winMmeHostApi->inheritedHostApiRep;
- (*hostApi)->info.structVersion = 1;
- (*hostApi)->info.type = paMME;
- (*hostApi)->info.name = "MME";
-
-
- /* initialise device counts and default devices under the assumption that
- there are no devices. These values are incremented below if and when
- devices are successfully initialized.
- */
- (*hostApi)->info.deviceCount = 0;
- (*hostApi)->info.defaultInputDevice = paNoDevice;
- (*hostApi)->info.defaultOutputDevice = paNoDevice;
- winMmeHostApi->inputDeviceCount = 0;
- winMmeHostApi->outputDeviceCount = 0;
-
-#if !defined(DRVM_MAPPER_PREFERRED_GET)
-/* DRVM_MAPPER_PREFERRED_GET is defined in mmddk.h but we avoid a dependency on the DDK by defining it here */
-#define DRVM_MAPPER_PREFERRED_GET (0x2000+21)
-#endif
-
- /* the following calls assume that if wave*Message fails the preferred device parameter won't be modified */
- preferredDeviceStatusFlags = 0;
- waveInPreferredDevice = -1;
- waveInMessage( (HWAVEIN)((UINT_PTR)WAVE_MAPPER), DRVM_MAPPER_PREFERRED_GET, (DWORD_PTR)&waveInPreferredDevice, (DWORD_PTR)&preferredDeviceStatusFlags );
-
- preferredDeviceStatusFlags = 0;
- waveOutPreferredDevice = -1;
- waveOutMessage( (HWAVEOUT)((UINT_PTR)WAVE_MAPPER), DRVM_MAPPER_PREFERRED_GET, (DWORD_PTR)&waveOutPreferredDevice, (DWORD_PTR)&preferredDeviceStatusFlags );
-
- maximumPossibleDeviceCount = 0;
-
- inputDeviceCount = waveInGetNumDevs();
- if( inputDeviceCount > 0 )
- maximumPossibleDeviceCount += inputDeviceCount + 1; /* assume there is a WAVE_MAPPER */
-
- outputDeviceCount = waveOutGetNumDevs();
- if( outputDeviceCount > 0 )
- maximumPossibleDeviceCount += outputDeviceCount + 1; /* assume there is a WAVE_MAPPER */
-
-
- if( maximumPossibleDeviceCount > 0 ){
-
- (*hostApi)->deviceInfos = (PaDeviceInfo**)PaUtil_GroupAllocateMemory(
- winMmeHostApi->allocations, sizeof(PaDeviceInfo*) * maximumPossibleDeviceCount );
- if( !(*hostApi)->deviceInfos )
- {
- result = paInsufficientMemory;
- goto error;
- }
-
- /* allocate all device info structs in a contiguous block */
- deviceInfoArray = (PaWinMmeDeviceInfo*)PaUtil_GroupAllocateMemory(
- winMmeHostApi->allocations, sizeof(PaWinMmeDeviceInfo) * maximumPossibleDeviceCount );
- if( !deviceInfoArray )
- {
- result = paInsufficientMemory;
- goto error;
- }
-
- winMmeHostApi->winMmeDeviceIds = (UINT*)PaUtil_GroupAllocateMemory(
- winMmeHostApi->allocations, sizeof(int) * maximumPossibleDeviceCount );
- if( !winMmeHostApi->winMmeDeviceIds )
- {
- result = paInsufficientMemory;
- goto error;
- }
-
- GetDefaultLatencies( &defaultLowLatency, &defaultHighLatency );
-
- if( inputDeviceCount > 0 ){
- /* -1 is the WAVE_MAPPER */
- for( i = -1; i < inputDeviceCount; ++i ){
- UINT winMmeDeviceId = (UINT)((i==-1) ? WAVE_MAPPER : i);
- PaWinMmeDeviceInfo *wmmeDeviceInfo = &deviceInfoArray[ (*hostApi)->info.deviceCount ];
- PaDeviceInfo *deviceInfo = &wmmeDeviceInfo->inheritedDeviceInfo;
- deviceInfo->structVersion = 2;
- deviceInfo->hostApi = hostApiIndex;
-
- deviceInfo->maxInputChannels = 0;
- wmmeDeviceInfo->deviceInputChannelCountIsKnown = 1;
- deviceInfo->maxOutputChannels = 0;
- wmmeDeviceInfo->deviceOutputChannelCountIsKnown = 1;
-
- deviceInfo->defaultLowInputLatency = defaultLowLatency;
- deviceInfo->defaultLowOutputLatency = defaultLowLatency;
- deviceInfo->defaultHighInputLatency = defaultHighLatency;
- deviceInfo->defaultHighOutputLatency = defaultHighLatency;
-
- result = InitializeInputDeviceInfo( winMmeHostApi, wmmeDeviceInfo,
- winMmeDeviceId, &deviceInfoInitializationSucceeded );
- if( result != paNoError )
- goto error;
-
- if( deviceInfoInitializationSucceeded ){
- if( (*hostApi)->info.defaultInputDevice == paNoDevice ){
- /* if there is currently no default device, use the first one available */
- (*hostApi)->info.defaultInputDevice = (*hostApi)->info.deviceCount;
-
- }else if( winMmeDeviceId == waveInPreferredDevice ){
- /* set the default device to the system preferred device */
- (*hostApi)->info.defaultInputDevice = (*hostApi)->info.deviceCount;
- }
-
- winMmeHostApi->winMmeDeviceIds[ (*hostApi)->info.deviceCount ] = winMmeDeviceId;
- (*hostApi)->deviceInfos[ (*hostApi)->info.deviceCount ] = deviceInfo;
-
- winMmeHostApi->inputDeviceCount++;
- (*hostApi)->info.deviceCount++;
- }
- }
- }
-
- if( outputDeviceCount > 0 ){
- /* -1 is the WAVE_MAPPER */
- for( i = -1; i < outputDeviceCount; ++i ){
- UINT winMmeDeviceId = (UINT)((i==-1) ? WAVE_MAPPER : i);
- PaWinMmeDeviceInfo *wmmeDeviceInfo = &deviceInfoArray[ (*hostApi)->info.deviceCount ];
- PaDeviceInfo *deviceInfo = &wmmeDeviceInfo->inheritedDeviceInfo;
- deviceInfo->structVersion = 2;
- deviceInfo->hostApi = hostApiIndex;
-
- deviceInfo->maxInputChannels = 0;
- wmmeDeviceInfo->deviceInputChannelCountIsKnown = 1;
- deviceInfo->maxOutputChannels = 0;
- wmmeDeviceInfo->deviceOutputChannelCountIsKnown = 1;
-
- deviceInfo->defaultLowInputLatency = defaultLowLatency;
- deviceInfo->defaultLowOutputLatency = defaultLowLatency;
- deviceInfo->defaultHighInputLatency = defaultHighLatency;
- deviceInfo->defaultHighOutputLatency = defaultHighLatency;
-
- result = InitializeOutputDeviceInfo( winMmeHostApi, wmmeDeviceInfo,
- winMmeDeviceId, &deviceInfoInitializationSucceeded );
- if( result != paNoError )
- goto error;
-
- if( deviceInfoInitializationSucceeded ){
- if( (*hostApi)->info.defaultOutputDevice == paNoDevice ){
- /* if there is currently no default device, use the first one available */
- (*hostApi)->info.defaultOutputDevice = (*hostApi)->info.deviceCount;
-
- }else if( winMmeDeviceId == waveOutPreferredDevice ){
- /* set the default device to the system preferred device */
- (*hostApi)->info.defaultOutputDevice = (*hostApi)->info.deviceCount;
- }
-
- winMmeHostApi->winMmeDeviceIds[ (*hostApi)->info.deviceCount ] = winMmeDeviceId;
- (*hostApi)->deviceInfos[ (*hostApi)->info.deviceCount ] = deviceInfo;
-
- winMmeHostApi->outputDeviceCount++;
- (*hostApi)->info.deviceCount++;
- }
- }
- }
- }
-
- InitializeDefaultDeviceIdsFromEnv( winMmeHostApi );
-
- (*hostApi)->Terminate = Terminate;
- (*hostApi)->OpenStream = OpenStream;
- (*hostApi)->IsFormatSupported = IsFormatSupported;
-
- PaUtil_InitializeStreamInterface( &winMmeHostApi->callbackStreamInterface, CloseStream, StartStream,
- StopStream, AbortStream, IsStreamStopped, IsStreamActive,
- GetStreamTime, GetStreamCpuLoad,
- PaUtil_DummyRead, PaUtil_DummyWrite,
- PaUtil_DummyGetReadAvailable, PaUtil_DummyGetWriteAvailable );
-
- PaUtil_InitializeStreamInterface( &winMmeHostApi->blockingStreamInterface, CloseStream, StartStream,
- StopStream, AbortStream, IsStreamStopped, IsStreamActive,
- GetStreamTime, PaUtil_DummyGetCpuLoad,
- ReadStream, WriteStream, GetStreamReadAvailable, GetStreamWriteAvailable );
-
- return result;
-
-error:
- if( winMmeHostApi )
- {
- if( winMmeHostApi->allocations )
- {
- PaUtil_FreeAllAllocations( winMmeHostApi->allocations );
- PaUtil_DestroyAllocationGroup( winMmeHostApi->allocations );
- }
-
- PaUtil_FreeMemory( winMmeHostApi );
- }
-
- return result;
-}
-
-
-static void Terminate( struct PaUtilHostApiRepresentation *hostApi )
-{
- PaWinMmeHostApiRepresentation *winMmeHostApi = (PaWinMmeHostApiRepresentation*)hostApi;
-
- if( winMmeHostApi->allocations )
- {
- PaUtil_FreeAllAllocations( winMmeHostApi->allocations );
- PaUtil_DestroyAllocationGroup( winMmeHostApi->allocations );
- }
-
- PaUtil_FreeMemory( winMmeHostApi );
-}
-
-
-static PaError IsInputChannelCountSupported( PaWinMmeDeviceInfo* deviceInfo, int channelCount )
-{
- PaError result = paNoError;
-
- if( channelCount > 0
- && deviceInfo->deviceInputChannelCountIsKnown
- && channelCount > deviceInfo->inheritedDeviceInfo.maxInputChannels ){
-
- result = paInvalidChannelCount;
- }
-
- return result;
-}
-
-static PaError IsOutputChannelCountSupported( PaWinMmeDeviceInfo* deviceInfo, int channelCount )
-{
- PaError result = paNoError;
-
- if( channelCount > 0
- && deviceInfo->deviceOutputChannelCountIsKnown
- && channelCount > deviceInfo->inheritedDeviceInfo.maxOutputChannels ){
-
- result = paInvalidChannelCount;
- }
-
- return result;
-}
-
-static PaError IsFormatSupported( struct PaUtilHostApiRepresentation *hostApi,
- const PaStreamParameters *inputParameters,
- const PaStreamParameters *outputParameters,
- double sampleRate )
-{
- PaWinMmeHostApiRepresentation *winMmeHostApi = (PaWinMmeHostApiRepresentation*)hostApi;
- PaDeviceInfo *inputDeviceInfo, *outputDeviceInfo;
- int inputChannelCount, outputChannelCount;
- int inputMultipleDeviceChannelCount, outputMultipleDeviceChannelCount;
- PaSampleFormat inputSampleFormat, outputSampleFormat;
- PaWinMmeStreamInfo *inputStreamInfo, *outputStreamInfo;
- UINT winMmeInputDeviceId, winMmeOutputDeviceId;
- unsigned int i;
- PaError paerror;
-
- /* The calls to QueryFormatSupported below are intended to detect invalid
- sample rates. If we assume that the channel count and format are OK,
- then the only thing that could fail is the sample rate. This isn't
- strictly true, but I can't think of a better way to test that the
- sample rate is valid.
- */
-
- if( inputParameters )
- {
- inputChannelCount = inputParameters->channelCount;
- inputSampleFormat = inputParameters->sampleFormat;
- inputStreamInfo = inputParameters->hostApiSpecificStreamInfo;
-
- /* all standard sample formats are supported by the buffer adapter,
- this implementation doesn't support any custom sample formats */
- if( inputSampleFormat & paCustomFormat )
- return paSampleFormatNotSupported;
-
- if( inputParameters->device == paUseHostApiSpecificDeviceSpecification
- && inputStreamInfo && (inputStreamInfo->flags & paWinMmeUseMultipleDevices) )
- {
- inputMultipleDeviceChannelCount = 0;
- for( i=0; i< inputStreamInfo->deviceCount; ++i )
- {
- inputMultipleDeviceChannelCount += inputStreamInfo->devices[i].channelCount;
-
- inputDeviceInfo = hostApi->deviceInfos[ inputStreamInfo->devices[i].device ];
-
- /* check that input device can support inputChannelCount */
- if( inputStreamInfo->devices[i].channelCount < 1 )
- return paInvalidChannelCount;
-
- paerror = IsInputChannelCountSupported( (PaWinMmeDeviceInfo*)inputDeviceInfo,
- inputStreamInfo->devices[i].channelCount );
- if( paerror != paNoError )
- return paerror;
-
- /* test for valid sample rate, see comment above */
- winMmeInputDeviceId = LocalDeviceIndexToWinMmeDeviceId( winMmeHostApi, inputStreamInfo->devices[i].device );
- paerror = QueryFormatSupported( inputDeviceInfo, QueryInputWaveFormatEx,
- winMmeInputDeviceId, inputStreamInfo->devices[i].channelCount, sampleRate,
- ((inputStreamInfo) ? inputStreamInfo->flags : 0) );
- if( paerror != paNoError )
- return paInvalidSampleRate;
- }
-
- if( inputMultipleDeviceChannelCount != inputChannelCount )
- return paIncompatibleHostApiSpecificStreamInfo;
- }
- else
- {
- if( inputStreamInfo && (inputStreamInfo->flags & paWinMmeUseMultipleDevices) )
- return paIncompatibleHostApiSpecificStreamInfo; /* paUseHostApiSpecificDeviceSpecification was not supplied as the input device */
-
- inputDeviceInfo = hostApi->deviceInfos[ inputParameters->device ];
-
- /* check that input device can support inputChannelCount */
- paerror = IsInputChannelCountSupported( (PaWinMmeDeviceInfo*)inputDeviceInfo, inputChannelCount );
- if( paerror != paNoError )
- return paerror;
-
- /* test for valid sample rate, see comment above */
- winMmeInputDeviceId = LocalDeviceIndexToWinMmeDeviceId( winMmeHostApi, inputParameters->device );
- paerror = QueryFormatSupported( inputDeviceInfo, QueryInputWaveFormatEx,
- winMmeInputDeviceId, inputChannelCount, sampleRate,
- ((inputStreamInfo) ? inputStreamInfo->flags : 0) );
- if( paerror != paNoError )
- return paInvalidSampleRate;
- }
- }
-
- if( outputParameters )
- {
- outputChannelCount = outputParameters->channelCount;
- outputSampleFormat = outputParameters->sampleFormat;
- outputStreamInfo = outputParameters->hostApiSpecificStreamInfo;
-
- /* all standard sample formats are supported by the buffer adapter,
- this implementation doesn't support any custom sample formats */
- if( outputSampleFormat & paCustomFormat )
- return paSampleFormatNotSupported;
-
- if( outputParameters->device == paUseHostApiSpecificDeviceSpecification
- && outputStreamInfo && (outputStreamInfo->flags & paWinMmeUseMultipleDevices) )
- {
- outputMultipleDeviceChannelCount = 0;
- for( i=0; i< outputStreamInfo->deviceCount; ++i )
- {
- outputMultipleDeviceChannelCount += outputStreamInfo->devices[i].channelCount;
-
- outputDeviceInfo = hostApi->deviceInfos[ outputStreamInfo->devices[i].device ];
-
- /* check that output device can support outputChannelCount */
- if( outputStreamInfo->devices[i].channelCount < 1 )
- return paInvalidChannelCount;
-
- paerror = IsOutputChannelCountSupported( (PaWinMmeDeviceInfo*)outputDeviceInfo,
- outputStreamInfo->devices[i].channelCount );
- if( paerror != paNoError )
- return paerror;
-
- /* test for valid sample rate, see comment above */
- winMmeOutputDeviceId = LocalDeviceIndexToWinMmeDeviceId( winMmeHostApi, outputStreamInfo->devices[i].device );
- paerror = QueryFormatSupported( outputDeviceInfo, QueryOutputWaveFormatEx,
- winMmeOutputDeviceId, outputStreamInfo->devices[i].channelCount, sampleRate,
- ((outputStreamInfo) ? outputStreamInfo->flags : 0) );
- if( paerror != paNoError )
- return paInvalidSampleRate;
- }
-
- if( outputMultipleDeviceChannelCount != outputChannelCount )
- return paIncompatibleHostApiSpecificStreamInfo;
- }
- else
- {
- if( outputStreamInfo && (outputStreamInfo->flags & paWinMmeUseMultipleDevices) )
- return paIncompatibleHostApiSpecificStreamInfo; /* paUseHostApiSpecificDeviceSpecification was not supplied as the output device */
-
- outputDeviceInfo = hostApi->deviceInfos[ outputParameters->device ];
-
- /* check that output device can support outputChannelCount */
- paerror = IsOutputChannelCountSupported( (PaWinMmeDeviceInfo*)outputDeviceInfo, outputChannelCount );
- if( paerror != paNoError )
- return paerror;
-
- /* test for valid sample rate, see comment above */
- winMmeOutputDeviceId = LocalDeviceIndexToWinMmeDeviceId( winMmeHostApi, outputParameters->device );
- paerror = QueryFormatSupported( outputDeviceInfo, QueryOutputWaveFormatEx,
- winMmeOutputDeviceId, outputChannelCount, sampleRate,
- ((outputStreamInfo) ? outputStreamInfo->flags : 0) );
- if( paerror != paNoError )
- return paInvalidSampleRate;
- }
- }
-
- /*
- - if a full duplex stream is requested, check that the combination
- of input and output parameters is supported
-
- - check that the device supports sampleRate
-
- for mme all we can do is test that the input and output devices
- support the requested sample rate and number of channels. we
- cannot test for full duplex compatibility.
- */
-
- return paFormatIsSupported;
-}
-
-
-static unsigned long ComputeHostBufferCountForFixedBufferSizeFrames(
- unsigned long suggestedLatencyFrames,
- unsigned long hostBufferSizeFrames,
- unsigned long minimumBufferCount )
-{
- /* Calculate the number of buffers of length hostFramesPerBuffer
- that fit in suggestedLatencyFrames, rounding up to the next integer.
-
- The value (hostBufferSizeFrames - 1) below is to ensure the buffer count is rounded up.
- */
- unsigned long resultBufferCount = ((suggestedLatencyFrames + (hostBufferSizeFrames - 1)) / hostBufferSizeFrames);
-
- /* We always need one extra buffer for processing while the rest are queued/playing.
- i.e. latency is framesPerBuffer * (bufferCount - 1)
- */
- resultBufferCount += 1;
-
- if( resultBufferCount < minimumBufferCount ) /* clamp to minimum buffer count */
- resultBufferCount = minimumBufferCount;
-
- return resultBufferCount;
-}
-
-
-static unsigned long ComputeHostBufferSizeGivenHardUpperLimit(
- unsigned long userFramesPerBuffer,
- unsigned long absoluteMaximumBufferSizeFrames )
-{
- static unsigned long primes_[] = { 2, 3, 5, 7, 11, 13, 17, 19, 23,
- 29, 31, 37, 41, 43, 47, 53, 59, 61, 67, 0 }; /* zero terminated */
-
- unsigned long result = userFramesPerBuffer;
- int i;
-
- assert( absoluteMaximumBufferSizeFrames > 67 ); /* assume maximum is large and we're only factoring by small primes */
-
- /* search for the largest integer factor of userFramesPerBuffer less
- than or equal to absoluteMaximumBufferSizeFrames */
-
- /* repeatedly divide by smallest prime factors until a buffer size
- smaller than absoluteMaximumBufferSizeFrames is found */
- while( result > absoluteMaximumBufferSizeFrames ){
-
- /* search for the smallest prime factor of result */
- for( i=0; primes_[i] != 0; ++i )
- {
- unsigned long p = primes_[i];
- unsigned long divided = result / p;
- if( divided*p == result )
- {
- result = divided;
- break; /* continue with outer while loop */
- }
- }
- if( primes_[i] == 0 )
- { /* loop failed to find a prime factor, return an approximate result */
- unsigned long d = (userFramesPerBuffer + (absoluteMaximumBufferSizeFrames-1))
- / absoluteMaximumBufferSizeFrames;
- return userFramesPerBuffer / d;
- }
- }
-
- return result;
-}
-
-
-static PaError SelectHostBufferSizeFramesAndHostBufferCount(
- unsigned long suggestedLatencyFrames,
- unsigned long userFramesPerBuffer,
- unsigned long minimumBufferCount,
- unsigned long preferredMaximumBufferSizeFrames, /* try not to exceed this. for example, don't exceed when coalescing buffers */
- unsigned long absoluteMaximumBufferSizeFrames, /* never exceed this, a hard limit */
- unsigned long *hostBufferSizeFrames,
- unsigned long *hostBufferCount )
-{
- unsigned long effectiveUserFramesPerBuffer;
- unsigned long numberOfUserBuffersPerHostBuffer;
-
-
- if( userFramesPerBuffer == paFramesPerBufferUnspecified ){
-
- effectiveUserFramesPerBuffer = PA_MME_HOST_BUFFER_GRANULARITY_FRAMES_WHEN_UNSPECIFIED_;
-
- }else{
-
- if( userFramesPerBuffer > absoluteMaximumBufferSizeFrames ){
-
- /* user has requested a user buffer that's larger than absoluteMaximumBufferSizeFrames.
- try to choose a buffer size that is equal or smaller than absoluteMaximumBufferSizeFrames
- but is also an integer factor of userFramesPerBuffer, so as to distribute computation evenly.
- the buffer processor will handle the block adaption between host and user buffer sizes.
- see http://www.portaudio.com/trac/ticket/189 for discussion.
- */
-
- effectiveUserFramesPerBuffer = ComputeHostBufferSizeGivenHardUpperLimit( userFramesPerBuffer, absoluteMaximumBufferSizeFrames );
- assert( effectiveUserFramesPerBuffer <= absoluteMaximumBufferSizeFrames );
-
- /* try to ensure that duration of host buffering is at least as
- large as duration of user buffer. */
- if( suggestedLatencyFrames < userFramesPerBuffer )
- suggestedLatencyFrames = userFramesPerBuffer;
-
- }else{
-
- effectiveUserFramesPerBuffer = userFramesPerBuffer;
- }
- }
-
- /* compute a host buffer count based on suggestedLatencyFrames and our granularity */
-
- *hostBufferSizeFrames = effectiveUserFramesPerBuffer;
-
- *hostBufferCount = ComputeHostBufferCountForFixedBufferSizeFrames(
- suggestedLatencyFrames, *hostBufferSizeFrames, minimumBufferCount );
-
- if( *hostBufferSizeFrames >= userFramesPerBuffer )
- {
- /*
- If there are too many host buffers we would like to coalesce
- them by packing an integer number of user buffers into each host buffer.
- We try to coalesce such that hostBufferCount will lie between
- PA_MME_TARGET_HOST_BUFFER_COUNT_ and (PA_MME_TARGET_HOST_BUFFER_COUNT_*2)-1.
- We limit coalescing to avoid exceeding either absoluteMaximumBufferSizeFrames and
- preferredMaximumBufferSizeFrames.
-
- First, compute a coalescing factor: the number of user buffers per host buffer.
- The goal is to achieve PA_MME_TARGET_HOST_BUFFER_COUNT_ total buffer count.
- Since our latency is computed based on (*hostBufferCount - 1) we compute a
- coalescing factor based on (*hostBufferCount - 1) and (PA_MME_TARGET_HOST_BUFFER_COUNT_-1).
-
- The + (PA_MME_TARGET_HOST_BUFFER_COUNT_-2) term below is intended to round up.
- */
- numberOfUserBuffersPerHostBuffer = ((*hostBufferCount - 1) + (PA_MME_TARGET_HOST_BUFFER_COUNT_-2)) / (PA_MME_TARGET_HOST_BUFFER_COUNT_ - 1);
-
- if( numberOfUserBuffersPerHostBuffer > 1 )
- {
- unsigned long maxCoalescedBufferSizeFrames = (absoluteMaximumBufferSizeFrames < preferredMaximumBufferSizeFrames) /* minimum of our limits */
- ? absoluteMaximumBufferSizeFrames
- : preferredMaximumBufferSizeFrames;
-
- unsigned long maxUserBuffersPerHostBuffer = maxCoalescedBufferSizeFrames / effectiveUserFramesPerBuffer; /* don't coalesce more than this */
-
- if( numberOfUserBuffersPerHostBuffer > maxUserBuffersPerHostBuffer )
- numberOfUserBuffersPerHostBuffer = maxUserBuffersPerHostBuffer;
-
- *hostBufferSizeFrames = effectiveUserFramesPerBuffer * numberOfUserBuffersPerHostBuffer;
-
- /* recompute hostBufferCount to approximate suggestedLatencyFrames now that hostBufferSizeFrames is larger */
- *hostBufferCount = ComputeHostBufferCountForFixedBufferSizeFrames(
- suggestedLatencyFrames, *hostBufferSizeFrames, minimumBufferCount );
- }
- }
-
- return paNoError;
-}
-
-
-static PaError CalculateMaxHostSampleFrameSizeBytes(
- int channelCount,
- PaSampleFormat hostSampleFormat,
- const PaWinMmeStreamInfo *streamInfo,
- int *hostSampleFrameSizeBytes )
-{
- unsigned int i;
- /* PA WMME streams may aggregate multiple WMME devices. When the stream addresses
- more than one device in a single direction, maxDeviceChannelCount is the maximum
- number of channels used by a single device.
- */
- int maxDeviceChannelCount = channelCount;
- int hostSampleSizeBytes = Pa_GetSampleSize( hostSampleFormat );
- if( hostSampleSizeBytes < 0 )
- {
- return hostSampleSizeBytes; /* the value of hostSampleSize here is an error code, not a sample size */
- }
-
- if( streamInfo && ( streamInfo->flags & paWinMmeUseMultipleDevices ) )
- {
- maxDeviceChannelCount = streamInfo->devices[0].channelCount;
- for( i=1; i< streamInfo->deviceCount; ++i )
- {
- if( streamInfo->devices[i].channelCount > maxDeviceChannelCount )
- maxDeviceChannelCount = streamInfo->devices[i].channelCount;
- }
- }
-
- *hostSampleFrameSizeBytes = hostSampleSizeBytes * maxDeviceChannelCount;
-
- return paNoError;
-}
-
-
-/* CalculateBufferSettings() fills the framesPerHostInputBuffer, hostInputBufferCount,
- framesPerHostOutputBuffer and hostOutputBufferCount parameters based on the values
- of the other parameters.
-*/
-
-static PaError CalculateBufferSettings(
- unsigned long *hostFramesPerInputBuffer, unsigned long *hostInputBufferCount,
- unsigned long *hostFramesPerOutputBuffer, unsigned long *hostOutputBufferCount,
- int inputChannelCount, PaSampleFormat hostInputSampleFormat,
- PaTime suggestedInputLatency, const PaWinMmeStreamInfo *inputStreamInfo,
- int outputChannelCount, PaSampleFormat hostOutputSampleFormat,
- PaTime suggestedOutputLatency, const PaWinMmeStreamInfo *outputStreamInfo,
- double sampleRate, unsigned long userFramesPerBuffer )
-{
- PaError result = paNoError;
-
- if( inputChannelCount > 0 ) /* stream has input */
- {
- int hostInputFrameSizeBytes;
- result = CalculateMaxHostSampleFrameSizeBytes(
- inputChannelCount, hostInputSampleFormat, inputStreamInfo, &hostInputFrameSizeBytes );
- if( result != paNoError )
- goto error;
-
- if( inputStreamInfo
- && ( inputStreamInfo->flags & paWinMmeUseLowLevelLatencyParameters ) )
- {
- /* input - using low level latency parameters if provided */
-
- if( inputStreamInfo->bufferCount <= 0
- || inputStreamInfo->framesPerBuffer <= 0 )
- {
- result = paIncompatibleHostApiSpecificStreamInfo;
- goto error;
- }
-
- *hostFramesPerInputBuffer = inputStreamInfo->framesPerBuffer;
- *hostInputBufferCount = inputStreamInfo->bufferCount;
- }
- else
- {
- /* input - not using low level latency parameters, so compute
- hostFramesPerInputBuffer and hostInputBufferCount
- based on userFramesPerBuffer and suggestedInputLatency. */
-
- unsigned long minimumBufferCount = (outputChannelCount > 0)
- ? PA_MME_MIN_HOST_INPUT_BUFFER_COUNT_FULL_DUPLEX_
- : PA_MME_MIN_HOST_INPUT_BUFFER_COUNT_HALF_DUPLEX_;
-
- result = SelectHostBufferSizeFramesAndHostBufferCount(
- (unsigned long)(suggestedInputLatency * sampleRate), /* (truncate) */
- userFramesPerBuffer,
- minimumBufferCount,
- (unsigned long)(PA_MME_MAX_HOST_BUFFER_SECS_ * sampleRate), /* in frames. preferred maximum */
- (PA_MME_MAX_HOST_BUFFER_BYTES_ / hostInputFrameSizeBytes), /* in frames. a hard limit. note truncation due to
- division is intentional here to limit max bytes */
- hostFramesPerInputBuffer,
- hostInputBufferCount );
- if( result != paNoError )
- goto error;
- }
- }
- else
- {
- *hostFramesPerInputBuffer = 0;
- *hostInputBufferCount = 0;
- }
-
- if( outputChannelCount > 0 ) /* stream has output */
- {
- if( outputStreamInfo
- && ( outputStreamInfo->flags & paWinMmeUseLowLevelLatencyParameters ) )
- {
- /* output - using low level latency parameters */
-
- if( outputStreamInfo->bufferCount <= 0
- || outputStreamInfo->framesPerBuffer <= 0 )
- {
- result = paIncompatibleHostApiSpecificStreamInfo;
- goto error;
- }
-
- *hostFramesPerOutputBuffer = outputStreamInfo->framesPerBuffer;
- *hostOutputBufferCount = outputStreamInfo->bufferCount;
-
- if( inputChannelCount > 0 ) /* full duplex */
- {
- /* harmonize hostFramesPerInputBuffer and hostFramesPerOutputBuffer */
-
- if( *hostFramesPerInputBuffer != *hostFramesPerOutputBuffer )
- {
- if( inputStreamInfo
- && ( inputStreamInfo->flags & paWinMmeUseLowLevelLatencyParameters ) )
- {
- /* a custom StreamInfo was used for specifying both input
- and output buffer sizes. We require that the larger buffer size
- must be a multiple of the smaller buffer size */
-
- if( *hostFramesPerInputBuffer < *hostFramesPerOutputBuffer )
- {
- if( *hostFramesPerOutputBuffer % *hostFramesPerInputBuffer != 0 )
- {
- result = paIncompatibleHostApiSpecificStreamInfo;
- goto error;
- }
- }
- else
- {
- assert( *hostFramesPerInputBuffer > *hostFramesPerOutputBuffer );
- if( *hostFramesPerInputBuffer % *hostFramesPerOutputBuffer != 0 )
- {
- result = paIncompatibleHostApiSpecificStreamInfo;
- goto error;
- }
- }
- }
- else
- {
- /* a custom StreamInfo was not used for specifying the input buffer size,
- so use the output buffer size, and approximately the suggested input latency. */
-
- *hostFramesPerInputBuffer = *hostFramesPerOutputBuffer;
-
- *hostInputBufferCount = ComputeHostBufferCountForFixedBufferSizeFrames(
- (unsigned long)(suggestedInputLatency * sampleRate),
- *hostFramesPerInputBuffer,
- PA_MME_MIN_HOST_INPUT_BUFFER_COUNT_FULL_DUPLEX_ );
- }
- }
- }
- }
- else
- {
- /* output - no low level latency parameters, so compute hostFramesPerOutputBuffer and hostOutputBufferCount
- based on userFramesPerBuffer and suggestedOutputLatency. */
-
- int hostOutputFrameSizeBytes;
- result = CalculateMaxHostSampleFrameSizeBytes(
- outputChannelCount, hostOutputSampleFormat, outputStreamInfo, &hostOutputFrameSizeBytes );
- if( result != paNoError )
- goto error;
-
- /* compute the output buffer size and count */
-
- result = SelectHostBufferSizeFramesAndHostBufferCount(
- (unsigned long)(suggestedOutputLatency * sampleRate), /* (truncate) */
- userFramesPerBuffer,
- PA_MME_MIN_HOST_OUTPUT_BUFFER_COUNT_,
- (unsigned long)(PA_MME_MAX_HOST_BUFFER_SECS_ * sampleRate), /* in frames. preferred maximum */
- (PA_MME_MAX_HOST_BUFFER_BYTES_ / hostOutputFrameSizeBytes), /* in frames. a hard limit. note truncation due to
- division is intentional here to limit max bytes */
- hostFramesPerOutputBuffer,
- hostOutputBufferCount );
- if( result != paNoError )
- goto error;
-
- if( inputChannelCount > 0 ) /* full duplex */
- {
- /* harmonize hostFramesPerInputBuffer and hostFramesPerOutputBuffer */
-
- /* ensure that both input and output buffer sizes are the same.
- if they don't match at this stage, choose the smallest one
- and use that for input and output and recompute the corresponding
- buffer count accordingly.
- */
-
- if( *hostFramesPerOutputBuffer != *hostFramesPerInputBuffer )
- {
- if( hostFramesPerInputBuffer < hostFramesPerOutputBuffer )
- {
- *hostFramesPerOutputBuffer = *hostFramesPerInputBuffer;
-
- *hostOutputBufferCount = ComputeHostBufferCountForFixedBufferSizeFrames(
- (unsigned long)(suggestedOutputLatency * sampleRate),
- *hostOutputBufferCount,
- PA_MME_MIN_HOST_OUTPUT_BUFFER_COUNT_ );
- }
- else
- {
- *hostFramesPerInputBuffer = *hostFramesPerOutputBuffer;
-
- *hostInputBufferCount = ComputeHostBufferCountForFixedBufferSizeFrames(
- (unsigned long)(suggestedInputLatency * sampleRate),
- *hostFramesPerInputBuffer,
- PA_MME_MIN_HOST_INPUT_BUFFER_COUNT_FULL_DUPLEX_ );
- }
- }
- }
- }
- }
- else
- {
- *hostFramesPerOutputBuffer = 0;
- *hostOutputBufferCount = 0;
- }
-
-error:
- return result;
-}
-
-
-typedef struct
-{
- HANDLE bufferEvent;
- void *waveHandles;
- unsigned int deviceCount;
- /* unsigned int channelCount; */
- WAVEHDR **waveHeaders; /* waveHeaders[device][buffer] */
- unsigned int bufferCount;
- unsigned int currentBufferIndex;
- unsigned int framesPerBuffer;
- unsigned int framesUsedInCurrentBuffer;
-}PaWinMmeSingleDirectionHandlesAndBuffers;
-
-/* prototypes for functions operating on PaWinMmeSingleDirectionHandlesAndBuffers */
-
-static void InitializeSingleDirectionHandlesAndBuffers( PaWinMmeSingleDirectionHandlesAndBuffers *handlesAndBuffers );
-static PaError InitializeWaveHandles( PaWinMmeHostApiRepresentation *winMmeHostApi,
- PaWinMmeSingleDirectionHandlesAndBuffers *handlesAndBuffers,
- unsigned long winMmeSpecificFlags,
- unsigned long bytesPerHostSample,
- double sampleRate, PaWinMmeDeviceAndChannelCount *devices,
- unsigned int deviceCount, PaWinWaveFormatChannelMask channelMask, int isInput );
-static PaError TerminateWaveHandles( PaWinMmeSingleDirectionHandlesAndBuffers *handlesAndBuffers, int isInput, int currentlyProcessingAnError );
-static PaError InitializeWaveHeaders( PaWinMmeSingleDirectionHandlesAndBuffers *handlesAndBuffers,
- unsigned long hostBufferCount,
- PaSampleFormat hostSampleFormat,
- unsigned long framesPerHostBuffer,
- PaWinMmeDeviceAndChannelCount *devices,
- int isInput );
-static void TerminateWaveHeaders( PaWinMmeSingleDirectionHandlesAndBuffers *handlesAndBuffers, int isInput );
-
-
-static void InitializeSingleDirectionHandlesAndBuffers( PaWinMmeSingleDirectionHandlesAndBuffers *handlesAndBuffers )
-{
- handlesAndBuffers->bufferEvent = 0;
- handlesAndBuffers->waveHandles = 0;
- handlesAndBuffers->deviceCount = 0;
- handlesAndBuffers->waveHeaders = 0;
- handlesAndBuffers->bufferCount = 0;
-}
-
-static PaError InitializeWaveHandles( PaWinMmeHostApiRepresentation *winMmeHostApi,
- PaWinMmeSingleDirectionHandlesAndBuffers *handlesAndBuffers,
- unsigned long winMmeSpecificFlags,
- unsigned long bytesPerHostSample,
- double sampleRate, PaWinMmeDeviceAndChannelCount *devices,
- unsigned int deviceCount, PaWinWaveFormatChannelMask channelMask, int isInput )
-{
- PaError result;
- MMRESULT mmresult;
- signed int i, j;
- PaSampleFormat sampleFormat;
- int waveFormatTag;
-
- /* for error cleanup we expect that InitializeSingleDirectionHandlesAndBuffers()
- has already been called to zero some fields */
-
- result = CreateEventWithPaError( &handlesAndBuffers->bufferEvent, NULL, FALSE, FALSE, NULL );
- if( result != paNoError ) goto error;
-
- if( isInput )
- handlesAndBuffers->waveHandles = (void*)PaUtil_AllocateMemory( sizeof(HWAVEIN) * deviceCount );
- else
- handlesAndBuffers->waveHandles = (void*)PaUtil_AllocateMemory( sizeof(HWAVEOUT) * deviceCount );
- if( !handlesAndBuffers->waveHandles )
- {
- result = paInsufficientMemory;
- goto error;
- }
-
- handlesAndBuffers->deviceCount = deviceCount;
-
- for( i = 0; i < (signed int)deviceCount; ++i )
- {
- if( isInput )
- ((HWAVEIN*)handlesAndBuffers->waveHandles)[i] = 0;
- else
- ((HWAVEOUT*)handlesAndBuffers->waveHandles)[i] = 0;
- }
-
- /* @todo at the moment we only use 16 bit sample format */
- sampleFormat = paInt16;
- waveFormatTag = SampleFormatAndWinWmmeSpecificFlagsToLinearWaveFormatTag( sampleFormat, winMmeSpecificFlags );
-
- for( i = 0; i < (signed int)deviceCount; ++i )
- {
- PaWinWaveFormat waveFormat;
- UINT winMmeDeviceId = LocalDeviceIndexToWinMmeDeviceId( winMmeHostApi, devices[i].device );
-
- /* @todo: consider providing a flag or #define to not try waveformat extensible
- this could just initialize j to 1 the first time round. */
-
- for( j = 0; j < 2; ++j )
- {
- switch(j){
- case 0:
- /* first, attempt to open the device using WAVEFORMATEXTENSIBLE,
- if this fails we fall back to WAVEFORMATEX */
-
- PaWin_InitializeWaveFormatExtensible( &waveFormat, devices[i].channelCount,
- sampleFormat, waveFormatTag, sampleRate, channelMask );
- break;
-
- case 1:
- /* retry with WAVEFORMATEX */
-
- PaWin_InitializeWaveFormatEx( &waveFormat, devices[i].channelCount,
- sampleFormat, waveFormatTag, sampleRate );
- break;
- }
-
- /* REVIEW: consider not firing an event for input when a full duplex
- stream is being used. this would probably depend on the
- neverDropInput flag. */
-
- if( isInput )
- {
- mmresult = waveInOpen( &((HWAVEIN*)handlesAndBuffers->waveHandles)[i], winMmeDeviceId,
- (WAVEFORMATEX*)&waveFormat,
- (DWORD_PTR)handlesAndBuffers->bufferEvent, (DWORD_PTR)0, CALLBACK_EVENT );
- }
- else
- {
- mmresult = waveOutOpen( &((HWAVEOUT*)handlesAndBuffers->waveHandles)[i], winMmeDeviceId,
- (WAVEFORMATEX*)&waveFormat,
- (DWORD_PTR)handlesAndBuffers->bufferEvent, (DWORD_PTR)0, CALLBACK_EVENT );
- }
-
- if( mmresult == MMSYSERR_NOERROR )
- {
- break; /* success */
- }
- else if( j == 0 )
- {
- continue; /* try again with WAVEFORMATEX */
- }
- else
- {
- switch( mmresult )
- {
- case MMSYSERR_ALLOCATED: /* Specified resource is already allocated. */
- result = paDeviceUnavailable;
- break;
- case MMSYSERR_NODRIVER: /* No device driver is present. */
- result = paDeviceUnavailable;
- break;
- case MMSYSERR_NOMEM: /* Unable to allocate or lock memory. */
- result = paInsufficientMemory;
- break;
-
- case MMSYSERR_BADDEVICEID: /* Specified device identifier is out of range. */
- /* falls through */
-
- case WAVERR_BADFORMAT: /* Attempted to open with an unsupported waveform-audio format. */
- /* This can also occur if we try to open the device with an unsupported
- * number of channels. This is attempted when device*ChannelCountIsKnown is
- * set to 0.
- */
- /* falls through */
- default:
- result = paUnanticipatedHostError;
- if( isInput )
- {
- PA_MME_SET_LAST_WAVEIN_ERROR( mmresult );
- }
- else
- {
- PA_MME_SET_LAST_WAVEOUT_ERROR( mmresult );
- }
- }
- goto error;
- }
- }
- }
-
- return result;
-
-error:
- TerminateWaveHandles( handlesAndBuffers, isInput, 1 /* currentlyProcessingAnError */ );
-
- return result;
-}
-
-
-static PaError TerminateWaveHandles( PaWinMmeSingleDirectionHandlesAndBuffers *handlesAndBuffers, int isInput, int currentlyProcessingAnError )
-{
- PaError result = paNoError;
- MMRESULT mmresult;
- signed int i;
-
- if( handlesAndBuffers->waveHandles )
- {
- for( i = handlesAndBuffers->deviceCount-1; i >= 0; --i )
- {
- if( isInput )
- {
- if( ((HWAVEIN*)handlesAndBuffers->waveHandles)[i] )
- mmresult = waveInClose( ((HWAVEIN*)handlesAndBuffers->waveHandles)[i] );
- else
- mmresult = MMSYSERR_NOERROR;
- }
- else
- {
- if( ((HWAVEOUT*)handlesAndBuffers->waveHandles)[i] )
- mmresult = waveOutClose( ((HWAVEOUT*)handlesAndBuffers->waveHandles)[i] );
- else
- mmresult = MMSYSERR_NOERROR;
- }
-
- if( mmresult != MMSYSERR_NOERROR &&
- !currentlyProcessingAnError ) /* don't update the error state if we're already processing an error */
- {
- result = paUnanticipatedHostError;
- if( isInput )
- {
- PA_MME_SET_LAST_WAVEIN_ERROR( mmresult );
- }
- else
- {
- PA_MME_SET_LAST_WAVEOUT_ERROR( mmresult );
- }
- /* note that we don't break here, we try to continue closing devices */
- }
- }
-
- PaUtil_FreeMemory( handlesAndBuffers->waveHandles );
- handlesAndBuffers->waveHandles = 0;
- }
-
- if( handlesAndBuffers->bufferEvent )
- {
- result = CloseHandleWithPaError( handlesAndBuffers->bufferEvent );
- handlesAndBuffers->bufferEvent = 0;
- }
-
- return result;
-}
-
-
-static PaError InitializeWaveHeaders( PaWinMmeSingleDirectionHandlesAndBuffers *handlesAndBuffers,
- unsigned long hostBufferCount,
- PaSampleFormat hostSampleFormat,
- unsigned long framesPerHostBuffer,
- PaWinMmeDeviceAndChannelCount *devices,
- int isInput )
-{
- PaError result = paNoError;
- MMRESULT mmresult;
- WAVEHDR *deviceWaveHeaders;
- signed int i, j;
-
- /* for error cleanup we expect that InitializeSingleDirectionHandlesAndBuffers()
- has already been called to zero some fields */
-
-
- /* allocate an array of pointers to arrays of wave headers, one array of
- wave headers per device */
- handlesAndBuffers->waveHeaders = (WAVEHDR**)PaUtil_AllocateMemory( sizeof(WAVEHDR*) * handlesAndBuffers->deviceCount );
- if( !handlesAndBuffers->waveHeaders )
- {
- result = paInsufficientMemory;
- goto error;
- }
-
- for( i = 0; i < (signed int)handlesAndBuffers->deviceCount; ++i )
- handlesAndBuffers->waveHeaders[i] = 0;
-
- handlesAndBuffers->bufferCount = hostBufferCount;
-
- for( i = 0; i < (signed int)handlesAndBuffers->deviceCount; ++i )
- {
- int bufferBytes = Pa_GetSampleSize( hostSampleFormat ) *
- framesPerHostBuffer * devices[i].channelCount;
- if( bufferBytes < 0 )
- {
- result = paInternalError;
- goto error;
- }
-
- /* Allocate an array of wave headers for device i */
- deviceWaveHeaders = (WAVEHDR *) PaUtil_AllocateMemory( sizeof(WAVEHDR)*hostBufferCount );
- if( !deviceWaveHeaders )
- {
- result = paInsufficientMemory;
- goto error;
- }
-
- for( j=0; j < (signed int)hostBufferCount; ++j )
- deviceWaveHeaders[j].lpData = 0;
-
- handlesAndBuffers->waveHeaders[i] = deviceWaveHeaders;
-
- /* Allocate a buffer for each wave header */
- for( j=0; j < (signed int)hostBufferCount; ++j )
- {
- deviceWaveHeaders[j].lpData = (char *)PaUtil_AllocateMemory( bufferBytes );
- if( !deviceWaveHeaders[j].lpData )
- {
- result = paInsufficientMemory;
- goto error;
- }
- deviceWaveHeaders[j].dwBufferLength = bufferBytes;
- deviceWaveHeaders[j].dwUser = 0xFFFFFFFF; /* indicates that *PrepareHeader() has not yet been called, for error clean up code */
-
- if( isInput )
- {
- mmresult = waveInPrepareHeader( ((HWAVEIN*)handlesAndBuffers->waveHandles)[i], &deviceWaveHeaders[j], sizeof(WAVEHDR) );
- if( mmresult != MMSYSERR_NOERROR )
- {
- result = paUnanticipatedHostError;
- PA_MME_SET_LAST_WAVEIN_ERROR( mmresult );
- goto error;
- }
- }
- else /* output */
- {
- mmresult = waveOutPrepareHeader( ((HWAVEOUT*)handlesAndBuffers->waveHandles)[i], &deviceWaveHeaders[j], sizeof(WAVEHDR) );
- if( mmresult != MMSYSERR_NOERROR )
- {
- result = paUnanticipatedHostError;
- PA_MME_SET_LAST_WAVEIN_ERROR( mmresult );
- goto error;
- }
- }
- deviceWaveHeaders[j].dwUser = devices[i].channelCount;
- }
- }
-
- return result;
-
-error:
- TerminateWaveHeaders( handlesAndBuffers, isInput );
-
- return result;
-}
-
-
-static void TerminateWaveHeaders( PaWinMmeSingleDirectionHandlesAndBuffers *handlesAndBuffers, int isInput )
-{
- signed int i, j;
- WAVEHDR *deviceWaveHeaders;
-
- if( handlesAndBuffers->waveHeaders )
- {
- for( i = handlesAndBuffers->deviceCount-1; i >= 0 ; --i )
- {
- deviceWaveHeaders = handlesAndBuffers->waveHeaders[i]; /* wave headers for device i */
- if( deviceWaveHeaders )
- {
- for( j = handlesAndBuffers->bufferCount-1; j >= 0; --j )
- {
- if( deviceWaveHeaders[j].lpData )
- {
- if( deviceWaveHeaders[j].dwUser != 0xFFFFFFFF )
- {
- if( isInput )
- waveInUnprepareHeader( ((HWAVEIN*)handlesAndBuffers->waveHandles)[i], &deviceWaveHeaders[j], sizeof(WAVEHDR) );
- else
- waveOutUnprepareHeader( ((HWAVEOUT*)handlesAndBuffers->waveHandles)[i], &deviceWaveHeaders[j], sizeof(WAVEHDR) );
- }
-
- PaUtil_FreeMemory( deviceWaveHeaders[j].lpData );
- }
- }
-
- PaUtil_FreeMemory( deviceWaveHeaders );
- }
- }
-
- PaUtil_FreeMemory( handlesAndBuffers->waveHeaders );
- handlesAndBuffers->waveHeaders = 0;
- }
-}
-
-
-
-/* PaWinMmeStream - a stream data structure specifically for this implementation */
-/* note that struct PaWinMmeStream is typedeffed to PaWinMmeStream above. */
-struct PaWinMmeStream
-{
- PaUtilStreamRepresentation streamRepresentation;
- PaUtilCpuLoadMeasurer cpuLoadMeasurer;
- PaUtilBufferProcessor bufferProcessor;
-
- int primeStreamUsingCallback;
-
- PaWinMmeSingleDirectionHandlesAndBuffers input;
- PaWinMmeSingleDirectionHandlesAndBuffers output;
-
- /* Processing thread management -------------- */
- HANDLE abortEvent;
- HANDLE processingThread;
- PA_THREAD_ID processingThreadId;
-
- char throttleProcessingThreadOnOverload; /* 0 -> don't throttle, non-0 -> throttle */
- int processingThreadPriority;
- int highThreadPriority;
- int throttledThreadPriority;
- unsigned long throttledSleepMsecs;
-
- int isStopped;
- volatile int isActive;
- volatile int stopProcessing; /* stop thread once existing buffers have been returned */
- volatile int abortProcessing; /* stop thread immediately */
-
- DWORD allBuffersDurationMs; /* used to calculate timeouts */
-};
-
-/* updates deviceCount if PaWinMmeUseMultipleDevices is used */
-
-static PaError ValidateWinMmeSpecificStreamInfo(
- const PaStreamParameters *streamParameters,
- const PaWinMmeStreamInfo *streamInfo,
- unsigned long *winMmeSpecificFlags,
- char *throttleProcessingThreadOnOverload,
- unsigned long *deviceCount )
-{
- if( streamInfo )
- {
- if( streamInfo->size != sizeof( PaWinMmeStreamInfo )
- || streamInfo->version != 1 )
- {
- return paIncompatibleHostApiSpecificStreamInfo;
- }
-
- *winMmeSpecificFlags = streamInfo->flags;
-
- if( streamInfo->flags & paWinMmeDontThrottleOverloadedProcessingThread )
- *throttleProcessingThreadOnOverload = 0;
-
- if( streamInfo->flags & paWinMmeUseMultipleDevices )
- {
- if( streamParameters->device != paUseHostApiSpecificDeviceSpecification )
- return paInvalidDevice;
-
- *deviceCount = streamInfo->deviceCount;
- }
- }
-
- return paNoError;
-}
-
-static PaError RetrieveDevicesFromStreamParameters(
- struct PaUtilHostApiRepresentation *hostApi,
- const PaStreamParameters *streamParameters,
- const PaWinMmeStreamInfo *streamInfo,
- PaWinMmeDeviceAndChannelCount *devices,
- unsigned long deviceCount )
-{
- PaError result = paNoError;
- unsigned int i;
- int totalChannelCount;
- PaDeviceIndex hostApiDevice;
-
- if( streamInfo && streamInfo->flags & paWinMmeUseMultipleDevices )
- {
- totalChannelCount = 0;
- for( i=0; i < deviceCount; ++i )
- {
- /* validate that the device number is within range */
- result = PaUtil_DeviceIndexToHostApiDeviceIndex( &hostApiDevice,
- streamInfo->devices[i].device, hostApi );
- if( result != paNoError )
- return result;
-
- devices[i].device = hostApiDevice;
- devices[i].channelCount = streamInfo->devices[i].channelCount;
-
- totalChannelCount += devices[i].channelCount;
- }
-
- if( totalChannelCount != streamParameters->channelCount )
- {
- /* channelCount must match total channels specified by multiple devices */
- return paInvalidChannelCount; /* REVIEW use of this error code */
- }
- }
- else
- {
- devices[0].device = streamParameters->device;
- devices[0].channelCount = streamParameters->channelCount;
- }
-
- return result;
-}
-
-static PaError ValidateInputChannelCounts(
- struct PaUtilHostApiRepresentation *hostApi,
- PaWinMmeDeviceAndChannelCount *devices,
- unsigned long deviceCount )
-{
- unsigned int i;
- PaWinMmeDeviceInfo *inputDeviceInfo;
- PaError paerror;
-
- for( i=0; i < deviceCount; ++i )
- {
- if( devices[i].channelCount < 1 )
- return paInvalidChannelCount;
-
- inputDeviceInfo =
- (PaWinMmeDeviceInfo*)hostApi->deviceInfos[ devices[i].device ];
-
- paerror = IsInputChannelCountSupported( inputDeviceInfo, devices[i].channelCount );
- if( paerror != paNoError )
- return paerror;
- }
-
- return paNoError;
-}
-
-static PaError ValidateOutputChannelCounts(
- struct PaUtilHostApiRepresentation *hostApi,
- PaWinMmeDeviceAndChannelCount *devices,
- unsigned long deviceCount )
-{
- unsigned int i;
- PaWinMmeDeviceInfo *outputDeviceInfo;
- PaError paerror;
-
- for( i=0; i < deviceCount; ++i )
- {
- if( devices[i].channelCount < 1 )
- return paInvalidChannelCount;
-
- outputDeviceInfo =
- (PaWinMmeDeviceInfo*)hostApi->deviceInfos[ devices[i].device ];
-
- paerror = IsOutputChannelCountSupported( outputDeviceInfo, devices[i].channelCount );
- if( paerror != paNoError )
- return paerror;
- }
-
- return paNoError;
-}
-
-
-/* the following macros are intended to improve the readability of the following code */
-#define PA_IS_INPUT_STREAM_( stream ) ( stream ->input.waveHandles )
-#define PA_IS_OUTPUT_STREAM_( stream ) ( stream ->output.waveHandles )
-#define PA_IS_FULL_DUPLEX_STREAM_( stream ) ( stream ->input.waveHandles && stream ->output.waveHandles )
-#define PA_IS_HALF_DUPLEX_STREAM_( stream ) ( !(stream ->input.waveHandles && stream ->output.waveHandles) )
-
-static PaError OpenStream( struct PaUtilHostApiRepresentation *hostApi,
- PaStream** s,
- const PaStreamParameters *inputParameters,
- const PaStreamParameters *outputParameters,
- double sampleRate,
- unsigned long framesPerBuffer,
- PaStreamFlags streamFlags,
- PaStreamCallback *streamCallback,
- void *userData )
-{
- PaError result;
- PaWinMmeHostApiRepresentation *winMmeHostApi = (PaWinMmeHostApiRepresentation*)hostApi;
- PaWinMmeStream *stream = 0;
- int bufferProcessorIsInitialized = 0;
- int streamRepresentationIsInitialized = 0;
- PaSampleFormat hostInputSampleFormat, hostOutputSampleFormat;
- int inputChannelCount, outputChannelCount;
- PaSampleFormat inputSampleFormat, outputSampleFormat;
- double suggestedInputLatency, suggestedOutputLatency;
- PaWinMmeStreamInfo *inputStreamInfo, *outputStreamInfo;
- PaWinWaveFormatChannelMask inputChannelMask, outputChannelMask;
- unsigned long framesPerHostInputBuffer;
- unsigned long hostInputBufferCount;
- unsigned long framesPerHostOutputBuffer;
- unsigned long hostOutputBufferCount;
- unsigned long framesPerBufferProcessorCall;
- PaWinMmeDeviceAndChannelCount *inputDevices = 0; /* contains all devices and channel counts as local host api ids, even when PaWinMmeUseMultipleDevices is not used */
- unsigned long winMmeSpecificInputFlags = 0;
- unsigned long inputDeviceCount = 0;
- PaWinMmeDeviceAndChannelCount *outputDevices = 0;
- unsigned long winMmeSpecificOutputFlags = 0;
- unsigned long outputDeviceCount = 0; /* contains all devices and channel counts as local host api ids, even when PaWinMmeUseMultipleDevices is not used */
- char throttleProcessingThreadOnOverload = 1;
-
-
- if( inputParameters )
- {
- inputChannelCount = inputParameters->channelCount;
- inputSampleFormat = inputParameters->sampleFormat;
- suggestedInputLatency = inputParameters->suggestedLatency;
-
- inputDeviceCount = 1;
-
- /* validate input hostApiSpecificStreamInfo */
- inputStreamInfo = (PaWinMmeStreamInfo*)inputParameters->hostApiSpecificStreamInfo;
- result = ValidateWinMmeSpecificStreamInfo( inputParameters, inputStreamInfo,
- &winMmeSpecificInputFlags,
- &throttleProcessingThreadOnOverload,
- &inputDeviceCount );
- if( result != paNoError ) return result;
-
- inputDevices = (PaWinMmeDeviceAndChannelCount*)alloca( sizeof(PaWinMmeDeviceAndChannelCount) * inputDeviceCount );
- if( !inputDevices ) return paInsufficientMemory;
-
- result = RetrieveDevicesFromStreamParameters( hostApi, inputParameters, inputStreamInfo, inputDevices, inputDeviceCount );
- if( result != paNoError ) return result;
-
- result = ValidateInputChannelCounts( hostApi, inputDevices, inputDeviceCount );
- if( result != paNoError ) return result;
-
- hostInputSampleFormat =
- PaUtil_SelectClosestAvailableFormat( paInt16 /* native formats */, inputSampleFormat );
-
- if( inputDeviceCount != 1 ){
- /* always use direct speakers when using multi-device multichannel mode */
- inputChannelMask = PAWIN_SPEAKER_DIRECTOUT;
- }
- else
- {
- if( inputStreamInfo && inputStreamInfo->flags & paWinMmeUseChannelMask )
- inputChannelMask = inputStreamInfo->channelMask;
- else
- inputChannelMask = PaWin_DefaultChannelMask( inputDevices[0].channelCount );
- }
- }
- else
- {
- inputChannelCount = 0;
- inputSampleFormat = 0;
- suggestedInputLatency = 0.;
- inputStreamInfo = 0;
- hostInputSampleFormat = 0;
- }
-
-
- if( outputParameters )
- {
- outputChannelCount = outputParameters->channelCount;
- outputSampleFormat = outputParameters->sampleFormat;
- suggestedOutputLatency = outputParameters->suggestedLatency;
-
- outputDeviceCount = 1;
-
- /* validate output hostApiSpecificStreamInfo */
- outputStreamInfo = (PaWinMmeStreamInfo*)outputParameters->hostApiSpecificStreamInfo;
- result = ValidateWinMmeSpecificStreamInfo( outputParameters, outputStreamInfo,
- &winMmeSpecificOutputFlags,
- &throttleProcessingThreadOnOverload,
- &outputDeviceCount );
- if( result != paNoError ) return result;
-
- outputDevices = (PaWinMmeDeviceAndChannelCount*)alloca( sizeof(PaWinMmeDeviceAndChannelCount) * outputDeviceCount );
- if( !outputDevices ) return paInsufficientMemory;
-
- result = RetrieveDevicesFromStreamParameters( hostApi, outputParameters, outputStreamInfo, outputDevices, outputDeviceCount );
- if( result != paNoError ) return result;
-
- result = ValidateOutputChannelCounts( hostApi, outputDevices, outputDeviceCount );
- if( result != paNoError ) return result;
-
- hostOutputSampleFormat =
- PaUtil_SelectClosestAvailableFormat( paInt16 /* native formats */, outputSampleFormat );
-
- if( outputDeviceCount != 1 ){
- /* always use direct speakers when using multi-device multichannel mode */
- outputChannelMask = PAWIN_SPEAKER_DIRECTOUT;
- }
- else
- {
- if( outputStreamInfo && outputStreamInfo->flags & paWinMmeUseChannelMask )
- outputChannelMask = outputStreamInfo->channelMask;
- else
- outputChannelMask = PaWin_DefaultChannelMask( outputDevices[0].channelCount );
- }
- }
- else
- {
- outputChannelCount = 0;
- outputSampleFormat = 0;
- outputStreamInfo = 0;
- hostOutputSampleFormat = 0;
- suggestedOutputLatency = 0.;
- }
-
-
- /*
- IMPLEMENT ME:
- - alter sampleRate to a close allowable rate if possible / necessary
- */
-
-
- /* validate platform specific flags */
- if( (streamFlags & paPlatformSpecificFlags) != 0 )
- return paInvalidFlag; /* unexpected platform specific flag */
-
-
- /* always disable clipping and dithering if we are outputting a raw spdif stream */
- if( (winMmeSpecificOutputFlags & paWinMmeWaveFormatDolbyAc3Spdif)
- || (winMmeSpecificOutputFlags & paWinMmeWaveFormatWmaSpdif) ){
-
- streamFlags = streamFlags | paClipOff | paDitherOff;
- }
-
-
- result = CalculateBufferSettings( &framesPerHostInputBuffer, &hostInputBufferCount,
- &framesPerHostOutputBuffer, &hostOutputBufferCount,
- inputChannelCount, hostInputSampleFormat, suggestedInputLatency, inputStreamInfo,
- outputChannelCount, hostOutputSampleFormat, suggestedOutputLatency, outputStreamInfo,
- sampleRate, framesPerBuffer );
- if( result != paNoError ) goto error;
-
-
- stream = (PaWinMmeStream*)PaUtil_AllocateMemory( sizeof(PaWinMmeStream) );
- if( !stream )
- {
- result = paInsufficientMemory;
- goto error;
- }
-
- InitializeSingleDirectionHandlesAndBuffers( &stream->input );
- InitializeSingleDirectionHandlesAndBuffers( &stream->output );
-
- stream->abortEvent = 0;
- stream->processingThread = 0;
-
- stream->throttleProcessingThreadOnOverload = throttleProcessingThreadOnOverload;
-
- PaUtil_InitializeStreamRepresentation( &stream->streamRepresentation,
- ( (streamCallback)
- ? &winMmeHostApi->callbackStreamInterface
- : &winMmeHostApi->blockingStreamInterface ),
- streamCallback, userData );
- streamRepresentationIsInitialized = 1;
-
- PaUtil_InitializeCpuLoadMeasurer( &stream->cpuLoadMeasurer, sampleRate );
-
-
- if( inputParameters && outputParameters ) /* full duplex */
- {
- if( framesPerHostInputBuffer < framesPerHostOutputBuffer )
- {
- assert( (framesPerHostOutputBuffer % framesPerHostInputBuffer) == 0 ); /* CalculateBufferSettings() should guarantee this condition */
-
- framesPerBufferProcessorCall = framesPerHostInputBuffer;
- }
- else
- {
- assert( (framesPerHostInputBuffer % framesPerHostOutputBuffer) == 0 ); /* CalculateBufferSettings() should guarantee this condition */
-
- framesPerBufferProcessorCall = framesPerHostOutputBuffer;
- }
- }
- else if( inputParameters )
- {
- framesPerBufferProcessorCall = framesPerHostInputBuffer;
- }
- else if( outputParameters )
- {
- framesPerBufferProcessorCall = framesPerHostOutputBuffer;
- }
-
- stream->input.framesPerBuffer = framesPerHostInputBuffer;
- stream->output.framesPerBuffer = framesPerHostOutputBuffer;
-
- result = PaUtil_InitializeBufferProcessor( &stream->bufferProcessor,
- inputChannelCount, inputSampleFormat, hostInputSampleFormat,
- outputChannelCount, outputSampleFormat, hostOutputSampleFormat,
- sampleRate, streamFlags, framesPerBuffer,
- framesPerBufferProcessorCall, paUtilFixedHostBufferSize,
- streamCallback, userData );
- if( result != paNoError ) goto error;
-
- bufferProcessorIsInitialized = 1;
-
- /* stream info input latency is the minimum buffering latency (unlike suggested and default which are *maximums*) */
- stream->streamRepresentation.streamInfo.inputLatency =
- (double)(PaUtil_GetBufferProcessorInputLatencyFrames(&stream->bufferProcessor)
- + framesPerHostInputBuffer) / sampleRate;
- stream->streamRepresentation.streamInfo.outputLatency =
- (double)(PaUtil_GetBufferProcessorOutputLatencyFrames(&stream->bufferProcessor)
- + (framesPerHostOutputBuffer * (hostOutputBufferCount-1))) / sampleRate;
- stream->streamRepresentation.streamInfo.sampleRate = sampleRate;
-
- stream->primeStreamUsingCallback = ( (streamFlags&paPrimeOutputBuffersUsingStreamCallback) && streamCallback ) ? 1 : 0;
-
- /* time to sleep when throttling due to >100% cpu usage.
- -a quarter of a buffer's duration */
- stream->throttledSleepMsecs =
- (unsigned long)(stream->bufferProcessor.framesPerHostBuffer *
- stream->bufferProcessor.samplePeriod * .25 * 1000);
-
- stream->isStopped = 1;
- stream->isActive = 0;
-
-
- /* for maximum compatibility with multi-device multichannel drivers,
- we first open all devices, then we prepare all buffers, finally
- we start all devices ( in StartStream() ). teardown in reverse order.
- */
-
- if( inputParameters )
- {
- result = InitializeWaveHandles( winMmeHostApi, &stream->input,
- winMmeSpecificInputFlags,
- stream->bufferProcessor.bytesPerHostInputSample, sampleRate,
- inputDevices, inputDeviceCount, inputChannelMask, 1 /* isInput */ );
- if( result != paNoError ) goto error;
- }
-
- if( outputParameters )
- {
- result = InitializeWaveHandles( winMmeHostApi, &stream->output,
- winMmeSpecificOutputFlags,
- stream->bufferProcessor.bytesPerHostOutputSample, sampleRate,
- outputDevices, outputDeviceCount, outputChannelMask, 0 /* isInput */ );
- if( result != paNoError ) goto error;
- }
-
- if( inputParameters )
- {
- result = InitializeWaveHeaders( &stream->input, hostInputBufferCount,
- hostInputSampleFormat, framesPerHostInputBuffer, inputDevices, 1 /* isInput */ );
- if( result != paNoError ) goto error;
- }
-
- if( outputParameters )
- {
- result = InitializeWaveHeaders( &stream->output, hostOutputBufferCount,
- hostOutputSampleFormat, framesPerHostOutputBuffer, outputDevices, 0 /* not isInput */ );
- if( result != paNoError ) goto error;
-
- stream->allBuffersDurationMs = (DWORD) (1000.0 * (framesPerHostOutputBuffer * stream->output.bufferCount) / sampleRate);
- }
- else
- {
- stream->allBuffersDurationMs = (DWORD) (1000.0 * (framesPerHostInputBuffer * stream->input.bufferCount) / sampleRate);
- }
-
-
- if( streamCallback )
- {
- /* abort event is only needed for callback streams */
- result = CreateEventWithPaError( &stream->abortEvent, NULL, TRUE, FALSE, NULL );
- if( result != paNoError ) goto error;
- }
-
- *s = (PaStream*)stream;
-
- return result;
-
-error:
-
- if( stream )
- {
- if( stream->abortEvent )
- CloseHandle( stream->abortEvent );
-
- TerminateWaveHeaders( &stream->output, 0 /* not isInput */ );
- TerminateWaveHeaders( &stream->input, 1 /* isInput */ );
-
- TerminateWaveHandles( &stream->output, 0 /* not isInput */, 1 /* currentlyProcessingAnError */ );
- TerminateWaveHandles( &stream->input, 1 /* isInput */, 1 /* currentlyProcessingAnError */ );
-
- if( bufferProcessorIsInitialized )
- PaUtil_TerminateBufferProcessor( &stream->bufferProcessor );
-
- if( streamRepresentationIsInitialized )
- PaUtil_TerminateStreamRepresentation( &stream->streamRepresentation );
-
- PaUtil_FreeMemory( stream );
- }
-
- return result;
-}
-
-
-/* return non-zero if all current buffers are done */
-static int BuffersAreDone( WAVEHDR **waveHeaders, unsigned int deviceCount, int bufferIndex )
-{
- unsigned int i;
-
- for( i=0; i < deviceCount; ++i )
- {
- if( !(waveHeaders[i][ bufferIndex ].dwFlags & WHDR_DONE) )
- {
- return 0;
- }
- }
-
- return 1;
-}
-
-static int CurrentInputBuffersAreDone( PaWinMmeStream *stream )
-{
- return BuffersAreDone( stream->input.waveHeaders, stream->input.deviceCount, stream->input.currentBufferIndex );
-}
-
-static int CurrentOutputBuffersAreDone( PaWinMmeStream *stream )
-{
- return BuffersAreDone( stream->output.waveHeaders, stream->output.deviceCount, stream->output.currentBufferIndex );
-}
-
-
-/* return non-zero if any buffers are queued */
-static int NoBuffersAreQueued( PaWinMmeSingleDirectionHandlesAndBuffers *handlesAndBuffers )
-{
- unsigned int i, j;
-
- if( handlesAndBuffers->waveHandles )
- {
- for( i=0; i < handlesAndBuffers->bufferCount; ++i )
- {
- for( j=0; j < handlesAndBuffers->deviceCount; ++j )
- {
- if( !( handlesAndBuffers->waveHeaders[ j ][ i ].dwFlags & WHDR_DONE) )
- {
- return 0;
- }
- }
- }
- }
-
- return 1;
-}
-
-
-#define PA_CIRCULAR_INCREMENT_( current, max )\
- ( (((current) + 1) >= (max)) ? (0) : (current+1) )
-
-#define PA_CIRCULAR_DECREMENT_( current, max )\
- ( ((current) == 0) ? ((max)-1) : (current-1) )
-
-
-static signed long GetAvailableFrames( PaWinMmeSingleDirectionHandlesAndBuffers *handlesAndBuffers )
-{
- signed long result = 0;
- unsigned int i;
-
- if( BuffersAreDone( handlesAndBuffers->waveHeaders, handlesAndBuffers->deviceCount, handlesAndBuffers->currentBufferIndex ) )
- {
- /* we could calculate the following in O(1) if we kept track of the
- last done buffer */
- result = handlesAndBuffers->framesPerBuffer - handlesAndBuffers->framesUsedInCurrentBuffer;
-
- i = PA_CIRCULAR_INCREMENT_( handlesAndBuffers->currentBufferIndex, handlesAndBuffers->bufferCount );
- while( i != handlesAndBuffers->currentBufferIndex )
- {
- if( BuffersAreDone( handlesAndBuffers->waveHeaders, handlesAndBuffers->deviceCount, i ) )
- {
- result += handlesAndBuffers->framesPerBuffer;
- i = PA_CIRCULAR_INCREMENT_( i, handlesAndBuffers->bufferCount );
- }
- else
- break;
- }
- }
-
- return result;
-}
-
-
-static PaError AdvanceToNextInputBuffer( PaWinMmeStream *stream )
-{
- PaError result = paNoError;
- MMRESULT mmresult;
- unsigned int i;
-
- for( i=0; i < stream->input.deviceCount; ++i )
- {
- stream->input.waveHeaders[i][ stream->input.currentBufferIndex ].dwFlags &= ~WHDR_DONE;
- mmresult = waveInAddBuffer( ((HWAVEIN*)stream->input.waveHandles)[i],
- &stream->input.waveHeaders[i][ stream->input.currentBufferIndex ],
- sizeof(WAVEHDR) );
- if( mmresult != MMSYSERR_NOERROR )
- {
- result = paUnanticipatedHostError;
- PA_MME_SET_LAST_WAVEIN_ERROR( mmresult );
- }
- }
-
- stream->input.currentBufferIndex =
- PA_CIRCULAR_INCREMENT_( stream->input.currentBufferIndex, stream->input.bufferCount );
-
- stream->input.framesUsedInCurrentBuffer = 0;
-
- return result;
-}
-
-
-static PaError AdvanceToNextOutputBuffer( PaWinMmeStream *stream )
-{
- PaError result = paNoError;
- MMRESULT mmresult;
- unsigned int i;
-
- for( i=0; i < stream->output.deviceCount; ++i )
- {
- mmresult = waveOutWrite( ((HWAVEOUT*)stream->output.waveHandles)[i],
- &stream->output.waveHeaders[i][ stream->output.currentBufferIndex ],
- sizeof(WAVEHDR) );
- if( mmresult != MMSYSERR_NOERROR )
- {
- result = paUnanticipatedHostError;
- PA_MME_SET_LAST_WAVEOUT_ERROR( mmresult );
- }
- }
-
- stream->output.currentBufferIndex =
- PA_CIRCULAR_INCREMENT_( stream->output.currentBufferIndex, stream->output.bufferCount );
-
- stream->output.framesUsedInCurrentBuffer = 0;
-
- return result;
-}
-
-
-/* requeue all but the most recent input with the driver. Used for catching
- up after a total input buffer underrun */
-static PaError CatchUpInputBuffers( PaWinMmeStream *stream )
-{
- PaError result = paNoError;
- unsigned int i;
-
- for( i=0; i < stream->input.bufferCount - 1; ++i )
- {
- result = AdvanceToNextInputBuffer( stream );
- if( result != paNoError )
- break;
- }
-
- return result;
-}
-
-
-/* take the most recent output and duplicate it to all other output buffers
- and requeue them. Used for catching up after a total output buffer underrun.
-*/
-static PaError CatchUpOutputBuffers( PaWinMmeStream *stream )
-{
- PaError result = paNoError;
- unsigned int i, j;
- unsigned int previousBufferIndex =
- PA_CIRCULAR_DECREMENT_( stream->output.currentBufferIndex, stream->output.bufferCount );
-
- for( i=0; i < stream->output.bufferCount - 1; ++i )
- {
- for( j=0; j < stream->output.deviceCount; ++j )
- {
- if( stream->output.waveHeaders[j][ stream->output.currentBufferIndex ].lpData
- != stream->output.waveHeaders[j][ previousBufferIndex ].lpData )
- {
- CopyMemory( stream->output.waveHeaders[j][ stream->output.currentBufferIndex ].lpData,
- stream->output.waveHeaders[j][ previousBufferIndex ].lpData,
- stream->output.waveHeaders[j][ stream->output.currentBufferIndex ].dwBufferLength );
- }
- }
-
- result = AdvanceToNextOutputBuffer( stream );
- if( result != paNoError )
- break;
- }
-
- return result;
-}
-
-
-PA_THREAD_FUNC ProcessingThreadProc( void *pArg )
-{
- PaWinMmeStream *stream = (PaWinMmeStream *)pArg;
- HANDLE events[3];
- int eventCount = 0;
- DWORD result = paNoError;
- DWORD waitResult;
- DWORD timeout = (unsigned long)(stream->allBuffersDurationMs * 0.5);
- int hostBuffersAvailable;
- signed int hostInputBufferIndex, hostOutputBufferIndex;
- PaStreamCallbackFlags statusFlags;
- int callbackResult;
- int done = 0;
- unsigned int channel, i;
- unsigned long framesProcessed;
-
- /* prepare event array for call to WaitForMultipleObjects() */
- if( stream->input.bufferEvent )
- events[eventCount++] = stream->input.bufferEvent;
- if( stream->output.bufferEvent )
- events[eventCount++] = stream->output.bufferEvent;
- events[eventCount++] = stream->abortEvent;
-
- statusFlags = 0; /** @todo support paInputUnderflow, paOutputOverflow and paNeverDropInput */
-
- /* loop until something causes us to stop */
- do{
- /* wait for MME to signal that a buffer is available, or for
- the PA abort event to be signaled.
-
- When this indicates that one or more buffers are available
- NoBuffersAreQueued() and Current*BuffersAreDone are used below to
- poll for additional done buffers. NoBuffersAreQueued() will fail
- to identify an underrun/overflow if the driver doesn't mark all done
- buffers prior to signalling the event. Some drivers do this
- (eg RME Digi96, and others don't eg VIA PC 97 input). This isn't a
- huge problem, it just means that we won't always be able to detect
- underflow/overflow.
- */
- waitResult = WaitForMultipleObjects( eventCount, events, FALSE /* wait all = FALSE */, timeout );
- if( waitResult == WAIT_FAILED )
- {
- result = paUnanticipatedHostError;
- /** @todo FIXME/REVIEW: can't return host error info from an asynchronous thread. see http://www.portaudio.com/trac/ticket/143 */
- done = 1;
- }
- else if( waitResult == WAIT_TIMEOUT )
- {
- /* if a timeout is encountered, continue */
- }
-
- if( stream->abortProcessing )
- {
- /* Pa_AbortStream() has been called, stop processing immediately */
- done = 1;
- }
- else if( stream->stopProcessing )
- {
- /* Pa_StopStream() has been called or the user callback returned
- non-zero, processing will continue until all output buffers
- are marked as done. The stream will stop immediately if it
- is input-only.
- */
-
- if( PA_IS_OUTPUT_STREAM_(stream) )
- {
- if( NoBuffersAreQueued( &stream->output ) )
- done = 1; /* Will cause thread to return. */
- }
- else
- {
- /* input only stream */
- done = 1; /* Will cause thread to return. */
- }
- }
- else
- {
- hostBuffersAvailable = 1;
-
- /* process all available host buffers */
- do
- {
- hostInputBufferIndex = -1;
- hostOutputBufferIndex = -1;
-
- if( PA_IS_INPUT_STREAM_(stream) )
- {
- if( CurrentInputBuffersAreDone( stream ) )
- {
- if( NoBuffersAreQueued( &stream->input ) )
- {
- /** @todo
- if all of the other buffers are also ready then
- we discard all but the most recent. This is an
- input buffer overflow. FIXME: these buffers should
- be passed to the callback in a paNeverDropInput
- stream. http://www.portaudio.com/trac/ticket/142
-
- note that it is also possible for an input overflow
- to happen while the callback is processing a buffer.
- that is handled further down.
- */
- result = CatchUpInputBuffers( stream );
- if( result != paNoError )
- done = 1;
-
- statusFlags |= paInputOverflow;
- }
-
- hostInputBufferIndex = stream->input.currentBufferIndex;
- }
- }
-
- if( PA_IS_OUTPUT_STREAM_(stream) )
- {
- if( CurrentOutputBuffersAreDone( stream ) )
- {
- /* ok, we have an output buffer */
-
- if( NoBuffersAreQueued( &stream->output ) )
- {
- /*
- if all of the other buffers are also ready, catch up by copying
- the most recently generated buffer into all but one of the output
- buffers.
-
- note that this catch up code only handles the case where all
- buffers have been played out due to this thread not having
- woken up at all. a more common case occurs when this thread
- is woken up, processes one buffer, but takes too long, and as
- a result all the other buffers have become un-queued. that
- case is handled further down.
- */
-
- result = CatchUpOutputBuffers( stream );
- if( result != paNoError )
- done = 1;
-
- statusFlags |= paOutputUnderflow;
- }
-
- hostOutputBufferIndex = stream->output.currentBufferIndex;
- }
- }
-
-
- if( (PA_IS_FULL_DUPLEX_STREAM_(stream) && hostInputBufferIndex != -1 && hostOutputBufferIndex != -1) ||
- (PA_IS_HALF_DUPLEX_STREAM_(stream) && ( hostInputBufferIndex != -1 || hostOutputBufferIndex != -1 ) ) )
- {
- PaStreamCallbackTimeInfo timeInfo = {0,0,0}; /** @todo implement inputBufferAdcTime */
-
-
- if( PA_IS_OUTPUT_STREAM_(stream) )
- {
- /* set timeInfo.currentTime and calculate timeInfo.outputBufferDacTime
- from the current wave out position */
- MMTIME mmtime;
- double timeBeforeGetPosition, timeAfterGetPosition;
- double time;
- long framesInBufferRing;
- long writePosition;
- long playbackPosition;
- HWAVEOUT firstWaveOutDevice = ((HWAVEOUT*)stream->output.waveHandles)[0];
-
- mmtime.wType = TIME_SAMPLES;
- timeBeforeGetPosition = PaUtil_GetTime();
- waveOutGetPosition( firstWaveOutDevice, &mmtime, sizeof(MMTIME) );
- timeAfterGetPosition = PaUtil_GetTime();
-
- timeInfo.currentTime = timeAfterGetPosition;
-
- /* approximate time at which wave out position was measured
- as half way between timeBeforeGetPosition and timeAfterGetPosition */
- time = timeBeforeGetPosition + (timeAfterGetPosition - timeBeforeGetPosition) * .5;
-
- framesInBufferRing = stream->output.bufferCount * stream->bufferProcessor.framesPerHostBuffer;
- playbackPosition = mmtime.u.sample % framesInBufferRing;
-
- writePosition = stream->output.currentBufferIndex * stream->bufferProcessor.framesPerHostBuffer
- + stream->output.framesUsedInCurrentBuffer;
-
- if( playbackPosition >= writePosition ){
- timeInfo.outputBufferDacTime =
- time + ((double)( writePosition + (framesInBufferRing - playbackPosition) ) * stream->bufferProcessor.samplePeriod );
- }else{
- timeInfo.outputBufferDacTime =
- time + ((double)( writePosition - playbackPosition ) * stream->bufferProcessor.samplePeriod );
- }
- }
-
-
- PaUtil_BeginCpuLoadMeasurement( &stream->cpuLoadMeasurer );
-
- PaUtil_BeginBufferProcessing( &stream->bufferProcessor, &timeInfo, statusFlags );
-
- /* reset status flags once they have been passed to the buffer processor */
- statusFlags = 0;
-
- if( PA_IS_INPUT_STREAM_(stream) )
- {
- PaUtil_SetInputFrameCount( &stream->bufferProcessor, 0 /* default to host buffer size */ );
-
- channel = 0;
- for( i=0; iinput.deviceCount; ++i )
- {
- /* we have stored the number of channels in the buffer in dwUser */
- int channelCount = (int)stream->input.waveHeaders[i][ hostInputBufferIndex ].dwUser;
-
- PaUtil_SetInterleavedInputChannels( &stream->bufferProcessor, channel,
- stream->input.waveHeaders[i][ hostInputBufferIndex ].lpData +
- stream->input.framesUsedInCurrentBuffer * channelCount *
- stream->bufferProcessor.bytesPerHostInputSample,
- channelCount );
-
-
- channel += channelCount;
- }
- }
-
- if( PA_IS_OUTPUT_STREAM_(stream) )
- {
- PaUtil_SetOutputFrameCount( &stream->bufferProcessor, 0 /* default to host buffer size */ );
-
- channel = 0;
- for( i=0; ioutput.deviceCount; ++i )
- {
- /* we have stored the number of channels in the buffer in dwUser */
- int channelCount = (int)stream->output.waveHeaders[i][ hostOutputBufferIndex ].dwUser;
-
- PaUtil_SetInterleavedOutputChannels( &stream->bufferProcessor, channel,
- stream->output.waveHeaders[i][ hostOutputBufferIndex ].lpData +
- stream->output.framesUsedInCurrentBuffer * channelCount *
- stream->bufferProcessor.bytesPerHostOutputSample,
- channelCount );
-
- channel += channelCount;
- }
- }
-
- callbackResult = paContinue;
- framesProcessed = PaUtil_EndBufferProcessing( &stream->bufferProcessor, &callbackResult );
-
- stream->input.framesUsedInCurrentBuffer += framesProcessed;
- stream->output.framesUsedInCurrentBuffer += framesProcessed;
-
- PaUtil_EndCpuLoadMeasurement( &stream->cpuLoadMeasurer, framesProcessed );
-
- if( callbackResult == paContinue )
- {
- /* nothing special to do */
- }
- else if( callbackResult == paAbort )
- {
- stream->abortProcessing = 1;
- done = 1;
- /** @todo FIXME: should probably reset the output device immediately once the callback returns paAbort
- see: http://www.portaudio.com/trac/ticket/141
- */
- result = paNoError;
- }
- else
- {
- /* User callback has asked us to stop with paComplete or other non-zero value */
- stream->stopProcessing = 1; /* stop once currently queued audio has finished */
- result = paNoError;
- }
-
-
- if( PA_IS_INPUT_STREAM_(stream)
- && stream->stopProcessing == 0 && stream->abortProcessing == 0
- && stream->input.framesUsedInCurrentBuffer == stream->input.framesPerBuffer )
- {
- if( NoBuffersAreQueued( &stream->input ) )
- {
- /** @todo need to handle PaNeverDropInput here where necessary */
- result = CatchUpInputBuffers( stream );
- if( result != paNoError )
- done = 1;
-
- statusFlags |= paInputOverflow;
- }
-
- result = AdvanceToNextInputBuffer( stream );
- if( result != paNoError )
- done = 1;
- }
-
-
- if( PA_IS_OUTPUT_STREAM_(stream) && !stream->abortProcessing )
- {
- if( stream->stopProcessing &&
- stream->output.framesUsedInCurrentBuffer < stream->output.framesPerBuffer )
- {
- /* zero remaining samples in output output buffer and flush */
-
- stream->output.framesUsedInCurrentBuffer += PaUtil_ZeroOutput( &stream->bufferProcessor,
- stream->output.framesPerBuffer - stream->output.framesUsedInCurrentBuffer );
-
- /* we send the entire buffer to the output devices, but we could
- just send a partial buffer, rather than zeroing the unused
- samples.
- */
- }
-
- if( stream->output.framesUsedInCurrentBuffer == stream->output.framesPerBuffer )
- {
- /* check for underflow before enquing the just-generated buffer,
- but recover from underflow after enquing it. This ensures
- that the most recent audio segment is repeated */
- int outputUnderflow = NoBuffersAreQueued( &stream->output );
-
- result = AdvanceToNextOutputBuffer( stream );
- if( result != paNoError )
- done = 1;
-
- if( outputUnderflow && !done && !stream->stopProcessing )
- {
- /* Recover from underflow in the case where the
- underflow occurred while processing the buffer
- we just finished */
-
- result = CatchUpOutputBuffers( stream );
- if( result != paNoError )
- done = 1;
-
- statusFlags |= paOutputUnderflow;
- }
- }
- }
-
- if( stream->throttleProcessingThreadOnOverload != 0 )
- {
- if( stream->stopProcessing || stream->abortProcessing )
- {
- if( stream->processingThreadPriority != stream->highThreadPriority )
- {
- SetThreadPriority( stream->processingThread, stream->highThreadPriority );
- stream->processingThreadPriority = stream->highThreadPriority;
- }
- }
- else if( PaUtil_GetCpuLoad( &stream->cpuLoadMeasurer ) > 1. )
- {
- if( stream->processingThreadPriority != stream->throttledThreadPriority )
- {
- SetThreadPriority( stream->processingThread, stream->throttledThreadPriority );
- stream->processingThreadPriority = stream->throttledThreadPriority;
- }
-
- /* sleep to give other processes a go */
- Sleep( stream->throttledSleepMsecs );
- }
- else
- {
- if( stream->processingThreadPriority != stream->highThreadPriority )
- {
- SetThreadPriority( stream->processingThread, stream->highThreadPriority );
- stream->processingThreadPriority = stream->highThreadPriority;
- }
- }
- }
- }
- else
- {
- hostBuffersAvailable = 0;
- }
- }
- while( hostBuffersAvailable &&
- stream->stopProcessing == 0 &&
- stream->abortProcessing == 0 &&
- !done );
- }
- }
- while( !done );
-
- stream->isActive = 0;
-
- if( stream->streamRepresentation.streamFinishedCallback != 0 )
- stream->streamRepresentation.streamFinishedCallback( stream->streamRepresentation.userData );
-
- PaUtil_ResetCpuLoadMeasurer( &stream->cpuLoadMeasurer );
-
- return result;
-}
-
-
-/*
- When CloseStream() is called, the multi-api layer ensures that
- the stream has already been stopped or aborted.
-*/
-static PaError CloseStream( PaStream* s )
-{
- PaError result;
- PaWinMmeStream *stream = (PaWinMmeStream*)s;
-
- result = CloseHandleWithPaError( stream->abortEvent );
- if( result != paNoError ) goto error;
-
- TerminateWaveHeaders( &stream->output, 0 /* not isInput */ );
- TerminateWaveHeaders( &stream->input, 1 /* isInput */ );
-
- TerminateWaveHandles( &stream->output, 0 /* not isInput */, 0 /* not currentlyProcessingAnError */ );
- TerminateWaveHandles( &stream->input, 1 /* isInput */, 0 /* not currentlyProcessingAnError */ );
-
- PaUtil_TerminateBufferProcessor( &stream->bufferProcessor );
- PaUtil_TerminateStreamRepresentation( &stream->streamRepresentation );
- PaUtil_FreeMemory( stream );
-
-error:
- /** @todo REVIEW: what is the best way to clean up a stream if an error is detected? */
- return result;
-}
-
-
-static PaError StartStream( PaStream *s )
-{
- PaError result;
- PaWinMmeStream *stream = (PaWinMmeStream*)s;
- MMRESULT mmresult;
- unsigned int i, j;
- int callbackResult;
- unsigned int channel;
- unsigned long framesProcessed;
- PaStreamCallbackTimeInfo timeInfo = {0,0,0}; /** @todo implement this for stream priming */
-
- PaUtil_ResetBufferProcessor( &stream->bufferProcessor );
-
- if( PA_IS_INPUT_STREAM_(stream) )
- {
- for( i=0; iinput.bufferCount; ++i )
- {
- for( j=0; jinput.deviceCount; ++j )
- {
- stream->input.waveHeaders[j][i].dwFlags &= ~WHDR_DONE;
- mmresult = waveInAddBuffer( ((HWAVEIN*)stream->input.waveHandles)[j], &stream->input.waveHeaders[j][i], sizeof(WAVEHDR) );
- if( mmresult != MMSYSERR_NOERROR )
- {
- result = paUnanticipatedHostError;
- PA_MME_SET_LAST_WAVEIN_ERROR( mmresult );
- goto error;
- }
- }
- }
- stream->input.currentBufferIndex = 0;
- stream->input.framesUsedInCurrentBuffer = 0;
- }
-
- if( PA_IS_OUTPUT_STREAM_(stream) )
- {
- for( i=0; ioutput.deviceCount; ++i )
- {
- if( (mmresult = waveOutPause( ((HWAVEOUT*)stream->output.waveHandles)[i] )) != MMSYSERR_NOERROR )
- {
- result = paUnanticipatedHostError;
- PA_MME_SET_LAST_WAVEOUT_ERROR( mmresult );
- goto error;
- }
- }
-
- for( i=0; ioutput.bufferCount; ++i )
- {
- if( stream->primeStreamUsingCallback )
- {
-
- stream->output.framesUsedInCurrentBuffer = 0;
- do{
-
- PaUtil_BeginBufferProcessing( &stream->bufferProcessor,
- &timeInfo,
- paPrimingOutput | ((stream->input.bufferCount > 0 ) ? paInputUnderflow : 0));
-
- if( stream->input.bufferCount > 0 )
- PaUtil_SetNoInput( &stream->bufferProcessor );
-
- PaUtil_SetOutputFrameCount( &stream->bufferProcessor, 0 /* default to host buffer size */ );
-
- channel = 0;
- for( j=0; joutput.deviceCount; ++j )
- {
- /* we have stored the number of channels in the buffer in dwUser */
- int channelCount = (int)stream->output.waveHeaders[j][i].dwUser;
-
- PaUtil_SetInterleavedOutputChannels( &stream->bufferProcessor, channel,
- stream->output.waveHeaders[j][i].lpData +
- stream->output.framesUsedInCurrentBuffer * channelCount *
- stream->bufferProcessor.bytesPerHostOutputSample,
- channelCount );
-
- /* we have stored the number of channels in the buffer in dwUser */
- channel += channelCount;
- }
-
- callbackResult = paContinue;
- framesProcessed = PaUtil_EndBufferProcessing( &stream->bufferProcessor, &callbackResult );
- stream->output.framesUsedInCurrentBuffer += framesProcessed;
-
- if( callbackResult != paContinue )
- {
- /** @todo fix this, what do we do if callback result is non-zero during stream
- priming?
-
- for complete: play out primed waveHeaders as usual
- for abort: clean up immediately.
- */
- }
-
- }while( stream->output.framesUsedInCurrentBuffer != stream->output.framesPerBuffer );
-
- }
- else
- {
- for( j=0; joutput.deviceCount; ++j )
- {
- ZeroMemory( stream->output.waveHeaders[j][i].lpData, stream->output.waveHeaders[j][i].dwBufferLength );
- }
- }
-
- /* we queue all channels of a single buffer frame (across all
- devices, because some multidevice multichannel drivers work
- better this way */
- for( j=0; joutput.deviceCount; ++j )
- {
- mmresult = waveOutWrite( ((HWAVEOUT*)stream->output.waveHandles)[j], &stream->output.waveHeaders[j][i], sizeof(WAVEHDR) );
- if( mmresult != MMSYSERR_NOERROR )
- {
- result = paUnanticipatedHostError;
- PA_MME_SET_LAST_WAVEOUT_ERROR( mmresult );
- goto error;
- }
- }
- }
- stream->output.currentBufferIndex = 0;
- stream->output.framesUsedInCurrentBuffer = 0;
- }
-
-
- stream->isStopped = 0;
- stream->isActive = 1;
- stream->stopProcessing = 0;
- stream->abortProcessing = 0;
-
- result = ResetEventWithPaError( stream->input.bufferEvent );
- if( result != paNoError ) goto error;
-
- result = ResetEventWithPaError( stream->output.bufferEvent );
- if( result != paNoError ) goto error;
-
-
- if( stream->streamRepresentation.streamCallback )
- {
- /* callback stream */
-
- result = ResetEventWithPaError( stream->abortEvent );
- if( result != paNoError ) goto error;
-
- /* Create thread that waits for audio buffers to be ready for processing. */
- stream->processingThread = CREATE_THREAD;
- if( !stream->processingThread )
- {
- result = paUnanticipatedHostError;
- PA_MME_SET_LAST_SYSTEM_ERROR( GetLastError() );
- goto error;
- }
-
- /** @todo could have mme specific stream parameters to allow the user
- to set the callback thread priorities */
- stream->highThreadPriority = THREAD_PRIORITY_TIME_CRITICAL;
- stream->throttledThreadPriority = THREAD_PRIORITY_NORMAL;
-
- if( !SetThreadPriority( stream->processingThread, stream->highThreadPriority ) )
- {
- result = paUnanticipatedHostError;
- PA_MME_SET_LAST_SYSTEM_ERROR( GetLastError() );
- goto error;
- }
- stream->processingThreadPriority = stream->highThreadPriority;
- }
- else
- {
- /* blocking read/write stream */
-
- }
-
- if( PA_IS_INPUT_STREAM_(stream) )
- {
- for( i=0; i < stream->input.deviceCount; ++i )
- {
- mmresult = waveInStart( ((HWAVEIN*)stream->input.waveHandles)[i] );
- PA_DEBUG(("Pa_StartStream: waveInStart returned = 0x%X.\n", mmresult));
- if( mmresult != MMSYSERR_NOERROR )
- {
- result = paUnanticipatedHostError;
- PA_MME_SET_LAST_WAVEIN_ERROR( mmresult );
- goto error;
- }
- }
- }
-
- if( PA_IS_OUTPUT_STREAM_(stream) )
- {
- for( i=0; i < stream->output.deviceCount; ++i )
- {
- if( (mmresult = waveOutRestart( ((HWAVEOUT*)stream->output.waveHandles)[i] )) != MMSYSERR_NOERROR )
- {
- result = paUnanticipatedHostError;
- PA_MME_SET_LAST_WAVEOUT_ERROR( mmresult );
- goto error;
- }
- }
- }
-
- return result;
-
-error:
- /** @todo FIXME: implement recovery as best we can
- This should involve rolling back to a state as-if this function had never been called
- */
- return result;
-}
-
-
-static PaError StopStream( PaStream *s )
-{
- PaError result = paNoError;
- PaWinMmeStream *stream = (PaWinMmeStream*)s;
- int timeout;
- DWORD waitResult;
- MMRESULT mmresult;
- signed int hostOutputBufferIndex;
- unsigned int channel, waitCount, i;
-
- /** @todo
- REVIEW: the error checking in this function needs review. the basic
- idea is to return from this function in a known state - for example
- there is no point avoiding calling waveInReset just because
- the thread times out.
- */
-
- if( stream->processingThread )
- {
- /* callback stream */
-
- /* Tell processing thread to stop generating more data and to let current data play out. */
- stream->stopProcessing = 1;
-
- /* Calculate timeOut longer than longest time it could take to return all buffers. */
- timeout = (int)(stream->allBuffersDurationMs * 1.5);
- if( timeout < PA_MME_MIN_TIMEOUT_MSEC_ )
- timeout = PA_MME_MIN_TIMEOUT_MSEC_;
-
- PA_DEBUG(("WinMME StopStream: waiting for background thread.\n"));
-
- waitResult = WaitForSingleObject( stream->processingThread, timeout );
- if( waitResult == WAIT_TIMEOUT )
- {
- /* try to abort */
- stream->abortProcessing = 1;
- SetEvent( stream->abortEvent );
- waitResult = WaitForSingleObject( stream->processingThread, timeout );
- if( waitResult == WAIT_TIMEOUT )
- {
- PA_DEBUG(("WinMME StopStream: timed out while waiting for background thread to finish.\n"));
- result = paTimedOut;
- }
- }
-
- CloseHandle( stream->processingThread );
- stream->processingThread = NULL;
- }
- else
- {
- /* blocking read / write stream */
-
- if( PA_IS_OUTPUT_STREAM_(stream) )
- {
- if( stream->output.framesUsedInCurrentBuffer > 0 )
- {
- /* there are still unqueued frames in the current buffer, so flush them */
-
- hostOutputBufferIndex = stream->output.currentBufferIndex;
-
- PaUtil_SetOutputFrameCount( &stream->bufferProcessor,
- stream->output.framesPerBuffer - stream->output.framesUsedInCurrentBuffer );
-
- channel = 0;
- for( i=0; ioutput.deviceCount; ++i )
- {
- /* we have stored the number of channels in the buffer in dwUser */
- int channelCount = (int)stream->output.waveHeaders[i][ hostOutputBufferIndex ].dwUser;
-
- PaUtil_SetInterleavedOutputChannels( &stream->bufferProcessor, channel,
- stream->output.waveHeaders[i][ hostOutputBufferIndex ].lpData +
- stream->output.framesUsedInCurrentBuffer * channelCount *
- stream->bufferProcessor.bytesPerHostOutputSample,
- channelCount );
-
- channel += channelCount;
- }
-
- PaUtil_ZeroOutput( &stream->bufferProcessor,
- stream->output.framesPerBuffer - stream->output.framesUsedInCurrentBuffer );
-
- /* we send the entire buffer to the output devices, but we could
- just send a partial buffer, rather than zeroing the unused
- samples.
- */
- AdvanceToNextOutputBuffer( stream );
- }
-
-
- timeout = (stream->allBuffersDurationMs / stream->output.bufferCount) + 1;
- if( timeout < PA_MME_MIN_TIMEOUT_MSEC_ )
- timeout = PA_MME_MIN_TIMEOUT_MSEC_;
-
- waitCount = 0;
- while( !NoBuffersAreQueued( &stream->output ) && waitCount <= stream->output.bufferCount )
- {
- /* wait for MME to signal that a buffer is available */
- waitResult = WaitForSingleObject( stream->output.bufferEvent, timeout );
- if( waitResult == WAIT_FAILED )
- {
- break;
- }
- else if( waitResult == WAIT_TIMEOUT )
- {
- /* keep waiting */
- }
-
- ++waitCount;
- }
- }
- }
-
- if( PA_IS_OUTPUT_STREAM_(stream) )
- {
- for( i =0; i < stream->output.deviceCount; ++i )
- {
- mmresult = waveOutReset( ((HWAVEOUT*)stream->output.waveHandles)[i] );
- if( mmresult != MMSYSERR_NOERROR )
- {
- result = paUnanticipatedHostError;
- PA_MME_SET_LAST_WAVEOUT_ERROR( mmresult );
- }
- }
- }
-
- if( PA_IS_INPUT_STREAM_(stream) )
- {
- for( i=0; i < stream->input.deviceCount; ++i )
- {
- mmresult = waveInReset( ((HWAVEIN*)stream->input.waveHandles)[i] );
- if( mmresult != MMSYSERR_NOERROR )
- {
- result = paUnanticipatedHostError;
- PA_MME_SET_LAST_WAVEIN_ERROR( mmresult );
- }
- }
- }
-
- stream->isStopped = 1;
- stream->isActive = 0;
-
- return result;
-}
-
-
-static PaError AbortStream( PaStream *s )
-{
- PaError result = paNoError;
- PaWinMmeStream *stream = (PaWinMmeStream*)s;
- int timeout;
- DWORD waitResult;
- MMRESULT mmresult;
- unsigned int i;
-
- /** @todo
- REVIEW: the error checking in this function needs review. the basic
- idea is to return from this function in a known state - for example
- there is no point avoiding calling waveInReset just because
- the thread times out.
- */
-
- if( stream->processingThread )
- {
- /* callback stream */
-
- /* Tell processing thread to abort immediately */
- stream->abortProcessing = 1;
- SetEvent( stream->abortEvent );
- }
-
-
- if( PA_IS_OUTPUT_STREAM_(stream) )
- {
- for( i =0; i < stream->output.deviceCount; ++i )
- {
- mmresult = waveOutReset( ((HWAVEOUT*)stream->output.waveHandles)[i] );
- if( mmresult != MMSYSERR_NOERROR )
- {
- PA_MME_SET_LAST_WAVEOUT_ERROR( mmresult );
- return paUnanticipatedHostError;
- }
- }
- }
-
- if( PA_IS_INPUT_STREAM_(stream) )
- {
- for( i=0; i < stream->input.deviceCount; ++i )
- {
- mmresult = waveInReset( ((HWAVEIN*)stream->input.waveHandles)[i] );
- if( mmresult != MMSYSERR_NOERROR )
- {
- PA_MME_SET_LAST_WAVEIN_ERROR( mmresult );
- return paUnanticipatedHostError;
- }
- }
- }
-
-
- if( stream->processingThread )
- {
- /* callback stream */
-
- PA_DEBUG(("WinMME AbortStream: waiting for background thread.\n"));
-
- /* Calculate timeOut longer than longest time it could take to return all buffers. */
- timeout = (int)(stream->allBuffersDurationMs * 1.5);
- if( timeout < PA_MME_MIN_TIMEOUT_MSEC_ )
- timeout = PA_MME_MIN_TIMEOUT_MSEC_;
-
- waitResult = WaitForSingleObject( stream->processingThread, timeout );
- if( waitResult == WAIT_TIMEOUT )
- {
- PA_DEBUG(("WinMME AbortStream: timed out while waiting for background thread to finish.\n"));
- return paTimedOut;
- }
-
- CloseHandle( stream->processingThread );
- stream->processingThread = NULL;
- }
-
- stream->isStopped = 1;
- stream->isActive = 0;
-
- return result;
-}
-
-
-static PaError IsStreamStopped( PaStream *s )
-{
- PaWinMmeStream *stream = (PaWinMmeStream*)s;
-
- return stream->isStopped;
-}
-
-
-static PaError IsStreamActive( PaStream *s )
-{
- PaWinMmeStream *stream = (PaWinMmeStream*)s;
-
- return stream->isActive;
-}
-
-
-static PaTime GetStreamTime( PaStream *s )
-{
- (void) s; /* unused parameter */
-
- return PaUtil_GetTime();
-}
-
-
-static double GetStreamCpuLoad( PaStream* s )
-{
- PaWinMmeStream *stream = (PaWinMmeStream*)s;
-
- return PaUtil_GetCpuLoad( &stream->cpuLoadMeasurer );
-}
-
-
-/*
- As separate stream interfaces are used for blocking and callback
- streams, the following functions can be guaranteed to only be called
- for blocking streams.
-*/
-
-static PaError ReadStream( PaStream* s,
- void *buffer,
- unsigned long frames )
-{
- PaError result = paNoError;
- PaWinMmeStream *stream = (PaWinMmeStream*)s;
- void *userBuffer;
- unsigned long framesRead = 0;
- unsigned long framesProcessed;
- signed int hostInputBufferIndex;
- DWORD waitResult;
- DWORD timeout = (unsigned long)(stream->allBuffersDurationMs * 0.5);
- unsigned int channel, i;
-
- if( PA_IS_INPUT_STREAM_(stream) )
- {
- /* make a local copy of the user buffer pointer(s). this is necessary
- because PaUtil_CopyInput() advances these pointers every time
- it is called.
- */
- if( stream->bufferProcessor.userInputIsInterleaved )
- {
- userBuffer = buffer;
- }
- else
- {
- userBuffer = (void*)alloca( sizeof(void*) * stream->bufferProcessor.inputChannelCount );
- if( !userBuffer )
- return paInsufficientMemory;
- for( i = 0; ibufferProcessor.inputChannelCount; ++i )
- ((void**)userBuffer)[i] = ((void**)buffer)[i];
- }
-
- do{
- if( CurrentInputBuffersAreDone( stream ) )
- {
- if( NoBuffersAreQueued( &stream->input ) )
- {
- /** @todo REVIEW: consider what to do if the input overflows.
- do we requeue all of the buffers? should we be running
- a thread to make sure they are always queued?
- see: http://www.portaudio.com/trac/ticket/117
- */
-
- result = paInputOverflowed;
- }
-
- hostInputBufferIndex = stream->input.currentBufferIndex;
-
- PaUtil_SetInputFrameCount( &stream->bufferProcessor,
- stream->input.framesPerBuffer - stream->input.framesUsedInCurrentBuffer );
-
- channel = 0;
- for( i=0; iinput.deviceCount; ++i )
- {
- /* we have stored the number of channels in the buffer in dwUser */
- int channelCount = (int)stream->input.waveHeaders[i][ hostInputBufferIndex ].dwUser;
-
- PaUtil_SetInterleavedInputChannels( &stream->bufferProcessor, channel,
- stream->input.waveHeaders[i][ hostInputBufferIndex ].lpData +
- stream->input.framesUsedInCurrentBuffer * channelCount *
- stream->bufferProcessor.bytesPerHostInputSample,
- channelCount );
-
- channel += channelCount;
- }
-
- framesProcessed = PaUtil_CopyInput( &stream->bufferProcessor, &userBuffer, frames - framesRead );
-
- stream->input.framesUsedInCurrentBuffer += framesProcessed;
- if( stream->input.framesUsedInCurrentBuffer == stream->input.framesPerBuffer )
- {
- result = AdvanceToNextInputBuffer( stream );
- if( result != paNoError )
- break;
- }
-
- framesRead += framesProcessed;
-
- }else{
- /* wait for MME to signal that a buffer is available */
- waitResult = WaitForSingleObject( stream->input.bufferEvent, timeout );
- if( waitResult == WAIT_FAILED )
- {
- result = paUnanticipatedHostError;
- break;
- }
- else if( waitResult == WAIT_TIMEOUT )
- {
- /* if a timeout is encountered, continue,
- perhaps we should give up eventually
- */
- }
- }
- }while( framesRead < frames );
- }
- else
- {
- result = paCanNotReadFromAnOutputOnlyStream;
- }
-
- return result;
-}
-
-
-static PaError WriteStream( PaStream* s,
- const void *buffer,
- unsigned long frames )
-{
- PaError result = paNoError;
- PaWinMmeStream *stream = (PaWinMmeStream*)s;
- const void *userBuffer;
- unsigned long framesWritten = 0;
- unsigned long framesProcessed;
- signed int hostOutputBufferIndex;
- DWORD waitResult;
- DWORD timeout = (unsigned long)(stream->allBuffersDurationMs * 0.5);
- unsigned int channel, i;
-
-
- if( PA_IS_OUTPUT_STREAM_(stream) )
- {
- /* make a local copy of the user buffer pointer(s). this is necessary
- because PaUtil_CopyOutput() advances these pointers every time
- it is called.
- */
- if( stream->bufferProcessor.userOutputIsInterleaved )
- {
- userBuffer = buffer;
- }
- else
- {
- userBuffer = (const void*)alloca( sizeof(void*) * stream->bufferProcessor.outputChannelCount );
- if( !userBuffer )
- return paInsufficientMemory;
- for( i = 0; ibufferProcessor.outputChannelCount; ++i )
- ((const void**)userBuffer)[i] = ((const void**)buffer)[i];
- }
-
- do{
- if( CurrentOutputBuffersAreDone( stream ) )
- {
- if( NoBuffersAreQueued( &stream->output ) )
- {
- /** @todo REVIEW: consider what to do if the output
- underflows. do we requeue all the existing buffers with
- zeros? should we run a separate thread to keep the buffers
- enqueued at all times?
- see: http://www.portaudio.com/trac/ticket/117
- */
-
- result = paOutputUnderflowed;
- }
-
- hostOutputBufferIndex = stream->output.currentBufferIndex;
-
- PaUtil_SetOutputFrameCount( &stream->bufferProcessor,
- stream->output.framesPerBuffer - stream->output.framesUsedInCurrentBuffer );
-
- channel = 0;
- for( i=0; ioutput.deviceCount; ++i )
- {
- /* we have stored the number of channels in the buffer in dwUser */
- int channelCount = (int)stream->output.waveHeaders[i][ hostOutputBufferIndex ].dwUser;
-
- PaUtil_SetInterleavedOutputChannels( &stream->bufferProcessor, channel,
- stream->output.waveHeaders[i][ hostOutputBufferIndex ].lpData +
- stream->output.framesUsedInCurrentBuffer * channelCount *
- stream->bufferProcessor.bytesPerHostOutputSample,
- channelCount );
-
- channel += channelCount;
- }
-
- framesProcessed = PaUtil_CopyOutput( &stream->bufferProcessor, &userBuffer, frames - framesWritten );
-
- stream->output.framesUsedInCurrentBuffer += framesProcessed;
- if( stream->output.framesUsedInCurrentBuffer == stream->output.framesPerBuffer )
- {
- result = AdvanceToNextOutputBuffer( stream );
- if( result != paNoError )
- break;
- }
-
- framesWritten += framesProcessed;
- }
- else
- {
- /* wait for MME to signal that a buffer is available */
- waitResult = WaitForSingleObject( stream->output.bufferEvent, timeout );
- if( waitResult == WAIT_FAILED )
- {
- result = paUnanticipatedHostError;
- break;
- }
- else if( waitResult == WAIT_TIMEOUT )
- {
- /* if a timeout is encountered, continue,
- perhaps we should give up eventually
- */
- }
- }
- }while( framesWritten < frames );
- }
- else
- {
- result = paCanNotWriteToAnInputOnlyStream;
- }
-
- return result;
-}
-
-
-static signed long GetStreamReadAvailable( PaStream* s )
-{
- PaWinMmeStream *stream = (PaWinMmeStream*)s;
-
- if( PA_IS_INPUT_STREAM_(stream) )
- return GetAvailableFrames( &stream->input );
- else
- return paCanNotReadFromAnOutputOnlyStream;
-}
-
-
-static signed long GetStreamWriteAvailable( PaStream* s )
-{
- PaWinMmeStream *stream = (PaWinMmeStream*)s;
-
- if( PA_IS_OUTPUT_STREAM_(stream) )
- return GetAvailableFrames( &stream->output );
- else
- return paCanNotWriteToAnInputOnlyStream;
-}
-
-
-/* NOTE: the following functions are MME-stream specific, and are called directly
- by client code. We need to check for many more error conditions here because
- we don't have the benefit of pa_front.c's parameter checking.
-*/
-
-static PaError GetWinMMEStreamPointer( PaWinMmeStream **stream, PaStream *s )
-{
- PaError result;
- PaUtilHostApiRepresentation *hostApi;
- PaWinMmeHostApiRepresentation *winMmeHostApi;
-
- result = PaUtil_ValidateStreamPointer( s );
- if( result != paNoError )
- return result;
-
- result = PaUtil_GetHostApiRepresentation( &hostApi, paMME );
- if( result != paNoError )
- return result;
-
- winMmeHostApi = (PaWinMmeHostApiRepresentation*)hostApi;
-
- /* note, the following would be easier if there was a generic way of testing
- that a stream belongs to a specific host API */
-
- if( PA_STREAM_REP( s )->streamInterface == &winMmeHostApi->callbackStreamInterface
- || PA_STREAM_REP( s )->streamInterface == &winMmeHostApi->blockingStreamInterface )
- {
- /* s is a WinMME stream */
- *stream = (PaWinMmeStream *)s;
- return paNoError;
- }
- else
- {
- return paIncompatibleStreamHostApi;
- }
-}
-
-
-int PaWinMME_GetStreamInputHandleCount( PaStream* s )
-{
- PaWinMmeStream *stream;
- PaError result = GetWinMMEStreamPointer( &stream, s );
-
- if( result == paNoError )
- return (PA_IS_INPUT_STREAM_(stream)) ? stream->input.deviceCount : 0;
- else
- return result;
-}
-
-
-HWAVEIN PaWinMME_GetStreamInputHandle( PaStream* s, int handleIndex )
-{
- PaWinMmeStream *stream;
- PaError result = GetWinMMEStreamPointer( &stream, s );
-
- if( result == paNoError
- && PA_IS_INPUT_STREAM_(stream)
- && handleIndex >= 0
- && (unsigned int)handleIndex < stream->input.deviceCount )
- return ((HWAVEIN*)stream->input.waveHandles)[handleIndex];
- else
- return 0;
-}
-
-
-int PaWinMME_GetStreamOutputHandleCount( PaStream* s)
-{
- PaWinMmeStream *stream;
- PaError result = GetWinMMEStreamPointer( &stream, s );
-
- if( result == paNoError )
- return (PA_IS_OUTPUT_STREAM_(stream)) ? stream->output.deviceCount : 0;
- else
- return result;
-}
-
-
-HWAVEOUT PaWinMME_GetStreamOutputHandle( PaStream* s, int handleIndex )
-{
- PaWinMmeStream *stream;
- PaError result = GetWinMMEStreamPointer( &stream, s );
-
- if( result == paNoError
- && PA_IS_OUTPUT_STREAM_(stream)
- && handleIndex >= 0
- && (unsigned int)handleIndex < stream->output.deviceCount )
- return ((HWAVEOUT*)stream->output.waveHandles)[handleIndex];
- else
- return 0;
-}
diff --git a/spaces/anastasiablackwood/Anastasiablackwood/Dockerfile b/spaces/anastasiablackwood/Anastasiablackwood/Dockerfile
deleted file mode 100644
index 6c01c09373883afcb4ea34ae2d316cd596e1737b..0000000000000000000000000000000000000000
--- a/spaces/anastasiablackwood/Anastasiablackwood/Dockerfile
+++ /dev/null
@@ -1,21 +0,0 @@
-FROM node:18-bullseye-slim
-
-RUN apt-get update && \
-
-apt-get install -y git
-
-RUN git clone https://gitgud.io/khanon/oai-reverse-proxy.git /app
-
-WORKDIR /app
-
-RUN npm install
-
-COPY Dockerfile greeting.md* .env* ./
-
-RUN npm run build
-
-EXPOSE 7860
-
-ENV NODE_ENV=production
-
-CMD [ "npm", "start" ]
\ No newline at end of file
diff --git a/spaces/annt/mrc_uit_squadv2/retro_reader/models/__init__.py b/spaces/annt/mrc_uit_squadv2/retro_reader/models/__init__.py
deleted file mode 100644
index 59af9979021edef1770c9043774cbb43bf8b297d..0000000000000000000000000000000000000000
--- a/spaces/annt/mrc_uit_squadv2/retro_reader/models/__init__.py
+++ /dev/null
@@ -1,16 +0,0 @@
-from .modeling_albert import (
- AlbertConfig,
- AlbertForSequenceClassification,
- AlbertForQuestionAnsweringAVPool,
- AlbertForQuestionAnsweringAVPoolBCEv3
-)
-from .modeling_electra import (
- ElectraConfig,
- ElectraForSequenceClassification,
- ElectraForQuestionAnsweringAVPool
-)
-from .modeling_roberta import (
- RobertaConfig,
- RobertaForSequenceClassification,
- RobertaForQuestionAnsweringAVPool
-)
\ No newline at end of file
diff --git a/spaces/antonovmaxim/text-generation-webui-space/css/chat.css b/spaces/antonovmaxim/text-generation-webui-space/css/chat.css
deleted file mode 100644
index b5102e9a72ca0b066b12d52ab371d8a24774ac19..0000000000000000000000000000000000000000
--- a/spaces/antonovmaxim/text-generation-webui-space/css/chat.css
+++ /dev/null
@@ -1,43 +0,0 @@
-.h-\[40vh\], .wrap.svelte-byatnx.svelte-byatnx.svelte-byatnx {
- height: 66.67vh
-}
-
-.gradio-container {
- margin-left: auto !important;
- margin-right: auto !important;
-}
-
-.w-screen {
- width: unset
-}
-
-div.svelte-362y77>*, div.svelte-362y77>.form>* {
- flex-wrap: nowrap
-}
-
-/* fixes the API documentation in chat mode */
-.api-docs.svelte-1iguv9h.svelte-1iguv9h.svelte-1iguv9h {
- display: grid;
-}
-
-.pending.svelte-1ed2p3z {
- opacity: 1;
-}
-
-#extensions {
- padding: 0;
- padding: 0;
-}
-
-#gradio-chatbot {
- height: 66.67vh;
-}
-
-.wrap.svelte-6roggh.svelte-6roggh {
- max-height: 92.5%;
-}
-
-/* This is for the microphone button in the whisper extension */
-.sm.svelte-1ipelgc {
- width: 100%;
-}
diff --git a/spaces/aodianyun/stable-diffusion-webui/scripts/xyz_grid.py b/spaces/aodianyun/stable-diffusion-webui/scripts/xyz_grid.py
deleted file mode 100644
index e457d53de2ade37a53cc200d6902323d3d6f25ce..0000000000000000000000000000000000000000
--- a/spaces/aodianyun/stable-diffusion-webui/scripts/xyz_grid.py
+++ /dev/null
@@ -1,620 +0,0 @@
-from collections import namedtuple
-from copy import copy
-from itertools import permutations, chain
-import random
-import csv
-from io import StringIO
-from PIL import Image
-import numpy as np
-
-import modules.scripts as scripts
-import gradio as gr
-
-from modules import images, paths, sd_samplers, processing, sd_models, sd_vae
-from modules.processing import process_images, Processed, StableDiffusionProcessingTxt2Img
-from modules.shared import opts, cmd_opts, state
-import modules.shared as shared
-import modules.sd_samplers
-import modules.sd_models
-import modules.sd_vae
-import glob
-import os
-import re
-
-from modules.ui_components import ToolButton
-
-fill_values_symbol = "\U0001f4d2" # 📒
-
-AxisInfo = namedtuple('AxisInfo', ['axis', 'values'])
-
-
-def apply_field(field):
- def fun(p, x, xs):
- setattr(p, field, x)
-
- return fun
-
-
-def apply_prompt(p, x, xs):
- if xs[0] not in p.prompt and xs[0] not in p.negative_prompt:
- raise RuntimeError(f"Prompt S/R did not find {xs[0]} in prompt or negative prompt.")
-
- p.prompt = p.prompt.replace(xs[0], x)
- p.negative_prompt = p.negative_prompt.replace(xs[0], x)
-
-
-def apply_order(p, x, xs):
- token_order = []
-
- # Initally grab the tokens from the prompt, so they can be replaced in order of earliest seen
- for token in x:
- token_order.append((p.prompt.find(token), token))
-
- token_order.sort(key=lambda t: t[0])
-
- prompt_parts = []
-
- # Split the prompt up, taking out the tokens
- for _, token in token_order:
- n = p.prompt.find(token)
- prompt_parts.append(p.prompt[0:n])
- p.prompt = p.prompt[n + len(token):]
-
- # Rebuild the prompt with the tokens in the order we want
- prompt_tmp = ""
- for idx, part in enumerate(prompt_parts):
- prompt_tmp += part
- prompt_tmp += x[idx]
- p.prompt = prompt_tmp + p.prompt
-
-
-def apply_sampler(p, x, xs):
- sampler_name = sd_samplers.samplers_map.get(x.lower(), None)
- if sampler_name is None:
- raise RuntimeError(f"Unknown sampler: {x}")
-
- p.sampler_name = sampler_name
-
-
-def confirm_samplers(p, xs):
- for x in xs:
- if x.lower() not in sd_samplers.samplers_map:
- raise RuntimeError(f"Unknown sampler: {x}")
-
-
-def apply_checkpoint(p, x, xs):
- info = modules.sd_models.get_closet_checkpoint_match(x)
- if info is None:
- raise RuntimeError(f"Unknown checkpoint: {x}")
- modules.sd_models.reload_model_weights(shared.sd_model, info)
-
-
-def confirm_checkpoints(p, xs):
- for x in xs:
- if modules.sd_models.get_closet_checkpoint_match(x) is None:
- raise RuntimeError(f"Unknown checkpoint: {x}")
-
-
-def apply_clip_skip(p, x, xs):
- opts.data["CLIP_stop_at_last_layers"] = x
-
-
-def apply_upscale_latent_space(p, x, xs):
- if x.lower().strip() != '0':
- opts.data["use_scale_latent_for_hires_fix"] = True
- else:
- opts.data["use_scale_latent_for_hires_fix"] = False
-
-
-def find_vae(name: str):
- if name.lower() in ['auto', 'automatic']:
- return modules.sd_vae.unspecified
- if name.lower() == 'none':
- return None
- else:
- choices = [x for x in sorted(modules.sd_vae.vae_dict, key=lambda x: len(x)) if name.lower().strip() in x.lower()]
- if len(choices) == 0:
- print(f"No VAE found for {name}; using automatic")
- return modules.sd_vae.unspecified
- else:
- return modules.sd_vae.vae_dict[choices[0]]
-
-
-def apply_vae(p, x, xs):
- modules.sd_vae.reload_vae_weights(shared.sd_model, vae_file=find_vae(x))
-
-
-def apply_styles(p: StableDiffusionProcessingTxt2Img, x: str, _):
- p.styles.extend(x.split(','))
-
-
-def format_value_add_label(p, opt, x):
- if type(x) == float:
- x = round(x, 8)
-
- return f"{opt.label}: {x}"
-
-
-def format_value(p, opt, x):
- if type(x) == float:
- x = round(x, 8)
- return x
-
-
-def format_value_join_list(p, opt, x):
- return ", ".join(x)
-
-
-def do_nothing(p, x, xs):
- pass
-
-
-def format_nothing(p, opt, x):
- return ""
-
-
-def str_permutations(x):
- """dummy function for specifying it in AxisOption's type when you want to get a list of permutations"""
- return x
-
-
-class AxisOption:
- def __init__(self, label, type, apply, format_value=format_value_add_label, confirm=None, cost=0.0, choices=None):
- self.label = label
- self.type = type
- self.apply = apply
- self.format_value = format_value
- self.confirm = confirm
- self.cost = cost
- self.choices = choices
-
-
-class AxisOptionImg2Img(AxisOption):
- def __init__(self, *args, **kwargs):
- super().__init__(*args, **kwargs)
- self.is_img2img = True
-
-class AxisOptionTxt2Img(AxisOption):
- def __init__(self, *args, **kwargs):
- super().__init__(*args, **kwargs)
- self.is_img2img = False
-
-
-axis_options = [
- AxisOption("Nothing", str, do_nothing, format_value=format_nothing),
- AxisOption("Seed", int, apply_field("seed")),
- AxisOption("Var. seed", int, apply_field("subseed")),
- AxisOption("Var. strength", float, apply_field("subseed_strength")),
- AxisOption("Steps", int, apply_field("steps")),
- AxisOptionTxt2Img("Hires steps", int, apply_field("hr_second_pass_steps")),
- AxisOption("CFG Scale", float, apply_field("cfg_scale")),
- AxisOptionImg2Img("Image CFG Scale", float, apply_field("image_cfg_scale")),
- AxisOption("Prompt S/R", str, apply_prompt, format_value=format_value),
- AxisOption("Prompt order", str_permutations, apply_order, format_value=format_value_join_list),
- AxisOptionTxt2Img("Sampler", str, apply_sampler, format_value=format_value, confirm=confirm_samplers, choices=lambda: [x.name for x in sd_samplers.samplers]),
- AxisOptionImg2Img("Sampler", str, apply_sampler, format_value=format_value, confirm=confirm_samplers, choices=lambda: [x.name for x in sd_samplers.samplers_for_img2img]),
- AxisOption("Checkpoint name", str, apply_checkpoint, format_value=format_value, confirm=confirm_checkpoints, cost=1.0, choices=lambda: list(sd_models.checkpoints_list)),
- AxisOption("Sigma Churn", float, apply_field("s_churn")),
- AxisOption("Sigma min", float, apply_field("s_tmin")),
- AxisOption("Sigma max", float, apply_field("s_tmax")),
- AxisOption("Sigma noise", float, apply_field("s_noise")),
- AxisOption("Eta", float, apply_field("eta")),
- AxisOption("Clip skip", int, apply_clip_skip),
- AxisOption("Denoising", float, apply_field("denoising_strength")),
- AxisOptionTxt2Img("Hires upscaler", str, apply_field("hr_upscaler"), choices=lambda: [*shared.latent_upscale_modes, *[x.name for x in shared.sd_upscalers]]),
- AxisOptionImg2Img("Cond. Image Mask Weight", float, apply_field("inpainting_mask_weight")),
- AxisOption("VAE", str, apply_vae, cost=0.7, choices=lambda: list(sd_vae.vae_dict)),
- AxisOption("Styles", str, apply_styles, choices=lambda: list(shared.prompt_styles.styles)),
-]
-
-
-def draw_xyz_grid(p, xs, ys, zs, x_labels, y_labels, z_labels, cell, draw_legend, include_lone_images, include_sub_grids, first_axes_processed, second_axes_processed, margin_size):
- hor_texts = [[images.GridAnnotation(x)] for x in x_labels]
- ver_texts = [[images.GridAnnotation(y)] for y in y_labels]
- title_texts = [[images.GridAnnotation(z)] for z in z_labels]
-
- # Temporary list of all the images that are generated to be populated into the grid.
- # Will be filled with empty images for any individual step that fails to process properly
- image_cache = [None] * (len(xs) * len(ys) * len(zs))
-
- processed_result = None
- cell_mode = "P"
- cell_size = (1, 1)
-
- state.job_count = len(xs) * len(ys) * len(zs) * p.n_iter
-
- def process_cell(x, y, z, ix, iy, iz):
- nonlocal image_cache, processed_result, cell_mode, cell_size
-
- def index(ix, iy, iz):
- return ix + iy * len(xs) + iz * len(xs) * len(ys)
-
- state.job = f"{index(ix, iy, iz) + 1} out of {len(xs) * len(ys) * len(zs)}"
-
- processed: Processed = cell(x, y, z)
-
- try:
- # this dereference will throw an exception if the image was not processed
- # (this happens in cases such as if the user stops the process from the UI)
- processed_image = processed.images[0]
-
- if processed_result is None:
- # Use our first valid processed result as a template container to hold our full results
- processed_result = copy(processed)
- cell_mode = processed_image.mode
- cell_size = processed_image.size
- processed_result.images = [Image.new(cell_mode, cell_size)]
- processed_result.all_prompts = [processed.prompt]
- processed_result.all_seeds = [processed.seed]
- processed_result.infotexts = [processed.infotexts[0]]
-
- image_cache[index(ix, iy, iz)] = processed_image
- if include_lone_images:
- processed_result.images.append(processed_image)
- processed_result.all_prompts.append(processed.prompt)
- processed_result.all_seeds.append(processed.seed)
- processed_result.infotexts.append(processed.infotexts[0])
- except:
- image_cache[index(ix, iy, iz)] = Image.new(cell_mode, cell_size)
-
- if first_axes_processed == 'x':
- for ix, x in enumerate(xs):
- if second_axes_processed == 'y':
- for iy, y in enumerate(ys):
- for iz, z in enumerate(zs):
- process_cell(x, y, z, ix, iy, iz)
- else:
- for iz, z in enumerate(zs):
- for iy, y in enumerate(ys):
- process_cell(x, y, z, ix, iy, iz)
- elif first_axes_processed == 'y':
- for iy, y in enumerate(ys):
- if second_axes_processed == 'x':
- for ix, x in enumerate(xs):
- for iz, z in enumerate(zs):
- process_cell(x, y, z, ix, iy, iz)
- else:
- for iz, z in enumerate(zs):
- for ix, x in enumerate(xs):
- process_cell(x, y, z, ix, iy, iz)
- elif first_axes_processed == 'z':
- for iz, z in enumerate(zs):
- if second_axes_processed == 'x':
- for ix, x in enumerate(xs):
- for iy, y in enumerate(ys):
- process_cell(x, y, z, ix, iy, iz)
- else:
- for iy, y in enumerate(ys):
- for ix, x in enumerate(xs):
- process_cell(x, y, z, ix, iy, iz)
-
- if not processed_result:
- print("Unexpected error: draw_xyz_grid failed to return even a single processed image")
- return Processed(p, [])
-
- sub_grids = [None] * len(zs)
- for i in range(len(zs)):
- start_index = i * len(xs) * len(ys)
- end_index = start_index + len(xs) * len(ys)
- grid = images.image_grid(image_cache[start_index:end_index], rows=len(ys))
- if draw_legend:
- grid = images.draw_grid_annotations(grid, cell_size[0], cell_size[1], hor_texts, ver_texts, margin_size)
- sub_grids[i] = grid
- if include_sub_grids and len(zs) > 1:
- processed_result.images.insert(i+1, grid)
-
- sub_grid_size = sub_grids[0].size
- z_grid = images.image_grid(sub_grids, rows=1)
- if draw_legend:
- z_grid = images.draw_grid_annotations(z_grid, sub_grid_size[0], sub_grid_size[1], title_texts, [[images.GridAnnotation()]])
- processed_result.images[0] = z_grid
-
- return processed_result, sub_grids
-
-
-class SharedSettingsStackHelper(object):
- def __enter__(self):
- self.CLIP_stop_at_last_layers = opts.CLIP_stop_at_last_layers
- self.vae = opts.sd_vae
-
- def __exit__(self, exc_type, exc_value, tb):
- opts.data["sd_vae"] = self.vae
- modules.sd_models.reload_model_weights()
- modules.sd_vae.reload_vae_weights()
-
- opts.data["CLIP_stop_at_last_layers"] = self.CLIP_stop_at_last_layers
-
-
-re_range = re.compile(r"\s*([+-]?\s*\d+)\s*-\s*([+-]?\s*\d+)(?:\s*\(([+-]\d+)\s*\))?\s*")
-re_range_float = re.compile(r"\s*([+-]?\s*\d+(?:.\d*)?)\s*-\s*([+-]?\s*\d+(?:.\d*)?)(?:\s*\(([+-]\d+(?:.\d*)?)\s*\))?\s*")
-
-re_range_count = re.compile(r"\s*([+-]?\s*\d+)\s*-\s*([+-]?\s*\d+)(?:\s*\[(\d+)\s*\])?\s*")
-re_range_count_float = re.compile(r"\s*([+-]?\s*\d+(?:.\d*)?)\s*-\s*([+-]?\s*\d+(?:.\d*)?)(?:\s*\[(\d+(?:.\d*)?)\s*\])?\s*")
-
-
-class Script(scripts.Script):
- def title(self):
- return "X/Y/Z plot"
-
- def ui(self, is_img2img):
- self.current_axis_options = [x for x in axis_options if type(x) == AxisOption or x.is_img2img == is_img2img]
-
- with gr.Row():
- with gr.Column(scale=19):
- with gr.Row():
- x_type = gr.Dropdown(label="X type", choices=[x.label for x in self.current_axis_options], value=self.current_axis_options[1].label, type="index", elem_id=self.elem_id("x_type"))
- x_values = gr.Textbox(label="X values", lines=1, elem_id=self.elem_id("x_values"))
- fill_x_button = ToolButton(value=fill_values_symbol, elem_id="xyz_grid_fill_x_tool_button", visible=False)
-
- with gr.Row():
- y_type = gr.Dropdown(label="Y type", choices=[x.label for x in self.current_axis_options], value=self.current_axis_options[0].label, type="index", elem_id=self.elem_id("y_type"))
- y_values = gr.Textbox(label="Y values", lines=1, elem_id=self.elem_id("y_values"))
- fill_y_button = ToolButton(value=fill_values_symbol, elem_id="xyz_grid_fill_y_tool_button", visible=False)
-
- with gr.Row():
- z_type = gr.Dropdown(label="Z type", choices=[x.label for x in self.current_axis_options], value=self.current_axis_options[0].label, type="index", elem_id=self.elem_id("z_type"))
- z_values = gr.Textbox(label="Z values", lines=1, elem_id=self.elem_id("z_values"))
- fill_z_button = ToolButton(value=fill_values_symbol, elem_id="xyz_grid_fill_z_tool_button", visible=False)
-
- with gr.Row(variant="compact", elem_id="axis_options"):
- with gr.Column():
- draw_legend = gr.Checkbox(label='Draw legend', value=True, elem_id=self.elem_id("draw_legend"))
- no_fixed_seeds = gr.Checkbox(label='Keep -1 for seeds', value=False, elem_id=self.elem_id("no_fixed_seeds"))
- with gr.Column():
- include_lone_images = gr.Checkbox(label='Include Sub Images', value=False, elem_id=self.elem_id("include_lone_images"))
- include_sub_grids = gr.Checkbox(label='Include Sub Grids', value=False, elem_id=self.elem_id("include_sub_grids"))
- with gr.Column():
- margin_size = gr.Slider(label="Grid margins (px)", minimum=0, maximum=500, value=0, step=2, elem_id=self.elem_id("margin_size"))
-
- with gr.Row(variant="compact", elem_id="swap_axes"):
- swap_xy_axes_button = gr.Button(value="Swap X/Y axes", elem_id="xy_grid_swap_axes_button")
- swap_yz_axes_button = gr.Button(value="Swap Y/Z axes", elem_id="yz_grid_swap_axes_button")
- swap_xz_axes_button = gr.Button(value="Swap X/Z axes", elem_id="xz_grid_swap_axes_button")
-
- def swap_axes(axis1_type, axis1_values, axis2_type, axis2_values):
- return self.current_axis_options[axis2_type].label, axis2_values, self.current_axis_options[axis1_type].label, axis1_values
-
- xy_swap_args = [x_type, x_values, y_type, y_values]
- swap_xy_axes_button.click(swap_axes, inputs=xy_swap_args, outputs=xy_swap_args)
- yz_swap_args = [y_type, y_values, z_type, z_values]
- swap_yz_axes_button.click(swap_axes, inputs=yz_swap_args, outputs=yz_swap_args)
- xz_swap_args = [x_type, x_values, z_type, z_values]
- swap_xz_axes_button.click(swap_axes, inputs=xz_swap_args, outputs=xz_swap_args)
-
- def fill(x_type):
- axis = self.current_axis_options[x_type]
- return ", ".join(axis.choices()) if axis.choices else gr.update()
-
- fill_x_button.click(fn=fill, inputs=[x_type], outputs=[x_values])
- fill_y_button.click(fn=fill, inputs=[y_type], outputs=[y_values])
- fill_z_button.click(fn=fill, inputs=[z_type], outputs=[z_values])
-
- def select_axis(x_type):
- return gr.Button.update(visible=self.current_axis_options[x_type].choices is not None)
-
- x_type.change(fn=select_axis, inputs=[x_type], outputs=[fill_x_button])
- y_type.change(fn=select_axis, inputs=[y_type], outputs=[fill_y_button])
- z_type.change(fn=select_axis, inputs=[z_type], outputs=[fill_z_button])
-
- self.infotext_fields = (
- (x_type, "X Type"),
- (x_values, "X Values"),
- (y_type, "Y Type"),
- (y_values, "Y Values"),
- (z_type, "Z Type"),
- (z_values, "Z Values"),
- )
-
- return [x_type, x_values, y_type, y_values, z_type, z_values, draw_legend, include_lone_images, include_sub_grids, no_fixed_seeds, margin_size]
-
- def run(self, p, x_type, x_values, y_type, y_values, z_type, z_values, draw_legend, include_lone_images, include_sub_grids, no_fixed_seeds, margin_size):
- if not no_fixed_seeds:
- modules.processing.fix_seed(p)
-
- if not opts.return_grid:
- p.batch_size = 1
-
- def process_axis(opt, vals):
- if opt.label == 'Nothing':
- return [0]
-
- valslist = [x.strip() for x in chain.from_iterable(csv.reader(StringIO(vals)))]
-
- if opt.type == int:
- valslist_ext = []
-
- for val in valslist:
- m = re_range.fullmatch(val)
- mc = re_range_count.fullmatch(val)
- if m is not None:
- start = int(m.group(1))
- end = int(m.group(2))+1
- step = int(m.group(3)) if m.group(3) is not None else 1
-
- valslist_ext += list(range(start, end, step))
- elif mc is not None:
- start = int(mc.group(1))
- end = int(mc.group(2))
- num = int(mc.group(3)) if mc.group(3) is not None else 1
-
- valslist_ext += [int(x) for x in np.linspace(start=start, stop=end, num=num).tolist()]
- else:
- valslist_ext.append(val)
-
- valslist = valslist_ext
- elif opt.type == float:
- valslist_ext = []
-
- for val in valslist:
- m = re_range_float.fullmatch(val)
- mc = re_range_count_float.fullmatch(val)
- if m is not None:
- start = float(m.group(1))
- end = float(m.group(2))
- step = float(m.group(3)) if m.group(3) is not None else 1
-
- valslist_ext += np.arange(start, end + step, step).tolist()
- elif mc is not None:
- start = float(mc.group(1))
- end = float(mc.group(2))
- num = int(mc.group(3)) if mc.group(3) is not None else 1
-
- valslist_ext += np.linspace(start=start, stop=end, num=num).tolist()
- else:
- valslist_ext.append(val)
-
- valslist = valslist_ext
- elif opt.type == str_permutations:
- valslist = list(permutations(valslist))
-
- valslist = [opt.type(x) for x in valslist]
-
- # Confirm options are valid before starting
- if opt.confirm:
- opt.confirm(p, valslist)
-
- return valslist
-
- x_opt = self.current_axis_options[x_type]
- xs = process_axis(x_opt, x_values)
-
- y_opt = self.current_axis_options[y_type]
- ys = process_axis(y_opt, y_values)
-
- z_opt = self.current_axis_options[z_type]
- zs = process_axis(z_opt, z_values)
-
- def fix_axis_seeds(axis_opt, axis_list):
- if axis_opt.label in ['Seed', 'Var. seed']:
- return [int(random.randrange(4294967294)) if val is None or val == '' or val == -1 else val for val in axis_list]
- else:
- return axis_list
-
- if not no_fixed_seeds:
- xs = fix_axis_seeds(x_opt, xs)
- ys = fix_axis_seeds(y_opt, ys)
- zs = fix_axis_seeds(z_opt, zs)
-
- if x_opt.label == 'Steps':
- total_steps = sum(xs) * len(ys) * len(zs)
- elif y_opt.label == 'Steps':
- total_steps = sum(ys) * len(xs) * len(zs)
- elif z_opt.label == 'Steps':
- total_steps = sum(zs) * len(xs) * len(ys)
- else:
- total_steps = p.steps * len(xs) * len(ys) * len(zs)
-
- if isinstance(p, StableDiffusionProcessingTxt2Img) and p.enable_hr:
- if x_opt.label == "Hires steps":
- total_steps += sum(xs) * len(ys) * len(zs)
- elif y_opt.label == "Hires steps":
- total_steps += sum(ys) * len(xs) * len(zs)
- elif z_opt.label == "Hires steps":
- total_steps += sum(zs) * len(xs) * len(ys)
- elif p.hr_second_pass_steps:
- total_steps += p.hr_second_pass_steps * len(xs) * len(ys) * len(zs)
- else:
- total_steps *= 2
-
- total_steps *= p.n_iter
-
- image_cell_count = p.n_iter * p.batch_size
- cell_console_text = f"; {image_cell_count} images per cell" if image_cell_count > 1 else ""
- plural_s = 's' if len(zs) > 1 else ''
- print(f"X/Y/Z plot will create {len(xs) * len(ys) * len(zs) * image_cell_count} images on {len(zs)} {len(xs)}x{len(ys)} grid{plural_s}{cell_console_text}. (Total steps to process: {total_steps})")
- shared.total_tqdm.updateTotal(total_steps)
-
- grid_infotext = [None]
-
- state.xyz_plot_x = AxisInfo(x_opt, xs)
- state.xyz_plot_y = AxisInfo(y_opt, ys)
- state.xyz_plot_z = AxisInfo(z_opt, zs)
-
- # If one of the axes is very slow to change between (like SD model
- # checkpoint), then make sure it is in the outer iteration of the nested
- # `for` loop.
- first_axes_processed = 'x'
- second_axes_processed = 'y'
- if x_opt.cost > y_opt.cost and x_opt.cost > z_opt.cost:
- first_axes_processed = 'x'
- if y_opt.cost > z_opt.cost:
- second_axes_processed = 'y'
- else:
- second_axes_processed = 'z'
- elif y_opt.cost > x_opt.cost and y_opt.cost > z_opt.cost:
- first_axes_processed = 'y'
- if x_opt.cost > z_opt.cost:
- second_axes_processed = 'x'
- else:
- second_axes_processed = 'z'
- elif z_opt.cost > x_opt.cost and z_opt.cost > y_opt.cost:
- first_axes_processed = 'z'
- if x_opt.cost > y_opt.cost:
- second_axes_processed = 'x'
- else:
- second_axes_processed = 'y'
-
- def cell(x, y, z):
- if shared.state.interrupted:
- return Processed(p, [], p.seed, "")
-
- pc = copy(p)
- pc.styles = pc.styles[:]
- x_opt.apply(pc, x, xs)
- y_opt.apply(pc, y, ys)
- z_opt.apply(pc, z, zs)
-
- res = process_images(pc)
-
- if grid_infotext[0] is None:
- pc.extra_generation_params = copy(pc.extra_generation_params)
- pc.extra_generation_params['Script'] = self.title()
-
- if x_opt.label != 'Nothing':
- pc.extra_generation_params["X Type"] = x_opt.label
- pc.extra_generation_params["X Values"] = x_values
- if x_opt.label in ["Seed", "Var. seed"] and not no_fixed_seeds:
- pc.extra_generation_params["Fixed X Values"] = ", ".join([str(x) for x in xs])
-
- if y_opt.label != 'Nothing':
- pc.extra_generation_params["Y Type"] = y_opt.label
- pc.extra_generation_params["Y Values"] = y_values
- if y_opt.label in ["Seed", "Var. seed"] and not no_fixed_seeds:
- pc.extra_generation_params["Fixed Y Values"] = ", ".join([str(y) for y in ys])
-
- if z_opt.label != 'Nothing':
- pc.extra_generation_params["Z Type"] = z_opt.label
- pc.extra_generation_params["Z Values"] = z_values
- if z_opt.label in ["Seed", "Var. seed"] and not no_fixed_seeds:
- pc.extra_generation_params["Fixed Z Values"] = ", ".join([str(z) for z in zs])
-
- grid_infotext[0] = processing.create_infotext(pc, pc.all_prompts, pc.all_seeds, pc.all_subseeds)
-
- return res
-
- with SharedSettingsStackHelper():
- processed, sub_grids = draw_xyz_grid(
- p,
- xs=xs,
- ys=ys,
- zs=zs,
- x_labels=[x_opt.format_value(p, x_opt, x) for x in xs],
- y_labels=[y_opt.format_value(p, y_opt, y) for y in ys],
- z_labels=[z_opt.format_value(p, z_opt, z) for z in zs],
- cell=cell,
- draw_legend=draw_legend,
- include_lone_images=include_lone_images,
- include_sub_grids=include_sub_grids,
- first_axes_processed=first_axes_processed,
- second_axes_processed=second_axes_processed,
- margin_size=margin_size
- )
-
- if opts.grid_save and len(sub_grids) > 1:
- for sub_grid in sub_grids:
- images.save_image(sub_grid, p.outpath_grids, "xyz_grid", info=grid_infotext[0], extension=opts.grid_format, prompt=p.prompt, seed=processed.seed, grid=True, p=p)
-
- if opts.grid_save:
- images.save_image(processed.images[0], p.outpath_grids, "xyz_grid", info=grid_infotext[0], extension=opts.grid_format, prompt=p.prompt, seed=processed.seed, grid=True, p=p)
-
- return processed
diff --git a/spaces/aquaaaaaaaaaaaa/AI-minato_aqua/inference/__init__.py b/spaces/aquaaaaaaaaaaaa/AI-minato_aqua/inference/__init__.py
deleted file mode 100644
index e69de29bb2d1d6434b8b29ae775ad8c2e48c5391..0000000000000000000000000000000000000000
diff --git a/spaces/arxify/RVC-beta-v2-0618/runtime/Lib/site-packages/Crypto/SelfTest/Hash/test_SHA256.py b/spaces/arxify/RVC-beta-v2-0618/runtime/Lib/site-packages/Crypto/SelfTest/Hash/test_SHA256.py
deleted file mode 100644
index bb99326dbfb25979f51eabda58ac9248d3dd0b87..0000000000000000000000000000000000000000
--- a/spaces/arxify/RVC-beta-v2-0618/runtime/Lib/site-packages/Crypto/SelfTest/Hash/test_SHA256.py
+++ /dev/null
@@ -1,94 +0,0 @@
-# -*- coding: utf-8 -*-
-#
-# SelfTest/Hash/test_SHA256.py: Self-test for the SHA-256 hash function
-#
-# Written in 2008 by Dwayne C. Litzenberger
-#
-# ===================================================================
-# The contents of this file are dedicated to the public domain. To
-# the extent that dedication to the public domain is not available,
-# everyone is granted a worldwide, perpetual, royalty-free,
-# non-exclusive license to exercise all rights associated with the
-# contents of this file for any purpose whatsoever.
-# No rights are reserved.
-#
-# THE SOFTWARE IS PROVIDED "AS IS", WITHOUT WARRANTY OF ANY KIND,
-# EXPRESS OR IMPLIED, INCLUDING BUT NOT LIMITED TO THE WARRANTIES OF
-# MERCHANTABILITY, FITNESS FOR A PARTICULAR PURPOSE AND
-# NONINFRINGEMENT. IN NO EVENT SHALL THE AUTHORS OR COPYRIGHT HOLDERS
-# BE LIABLE FOR ANY CLAIM, DAMAGES OR OTHER LIABILITY, WHETHER IN AN
-# ACTION OF CONTRACT, TORT OR OTHERWISE, ARISING FROM, OUT OF OR IN
-# CONNECTION WITH THE SOFTWARE OR THE USE OR OTHER DEALINGS IN THE
-# SOFTWARE.
-# ===================================================================
-
-"""Self-test suite for Crypto.Hash.SHA256"""
-
-import unittest
-from Crypto.Util.py3compat import *
-
-class LargeSHA256Test(unittest.TestCase):
- def runTest(self):
- """SHA256: 512/520 MiB test"""
- from Crypto.Hash import SHA256
- zeros = bchr(0x00) * (1024*1024)
-
- h = SHA256.new(zeros)
- for i in range(511):
- h.update(zeros)
-
- # This test vector is from PyCrypto's old testdata.py file.
- self.assertEqual('9acca8e8c22201155389f65abbf6bc9723edc7384ead80503839f49dcc56d767', h.hexdigest()) # 512 MiB
-
- for i in range(8):
- h.update(zeros)
-
- # This test vector is from PyCrypto's old testdata.py file.
- self.assertEqual('abf51ad954b246009dfe5a50ecd582fd5b8f1b8b27f30393853c3ef721e7fa6e', h.hexdigest()) # 520 MiB
-
-def get_tests(config={}):
- # Test vectors from FIPS PUB 180-2
- # This is a list of (expected_result, input[, description]) tuples.
- test_data = [
- # FIPS PUB 180-2, B.1 - "One-Block Message"
- ('ba7816bf8f01cfea414140de5dae2223b00361a396177a9cb410ff61f20015ad',
- 'abc'),
-
- # FIPS PUB 180-2, B.2 - "Multi-Block Message"
- ('248d6a61d20638b8e5c026930c3e6039a33ce45964ff2167f6ecedd419db06c1',
- 'abcdbcdecdefdefgefghfghighijhijkijkljklmklmnlmnomnopnopq'),
-
- # FIPS PUB 180-2, B.3 - "Long Message"
- ('cdc76e5c9914fb9281a1c7e284d73e67f1809a48a497200e046d39ccc7112cd0',
- 'a' * 10**6,
- '"a" * 10**6'),
-
- # Test for an old PyCrypto bug.
- ('f7fd017a3c721ce7ff03f3552c0813adcc48b7f33f07e5e2ba71e23ea393d103',
- 'This message is precisely 55 bytes long, to test a bug.',
- 'Length = 55 (mod 64)'),
-
- # Example from http://de.wikipedia.org/wiki/Secure_Hash_Algorithm
- ('e3b0c44298fc1c149afbf4c8996fb92427ae41e4649b934ca495991b7852b855', ''),
-
- ('d32b568cd1b96d459e7291ebf4b25d007f275c9f13149beeb782fac0716613f8',
- 'Franz jagt im komplett verwahrlosten Taxi quer durch Bayern'),
- ]
-
- from Crypto.Hash import SHA256
- from .common import make_hash_tests
- tests = make_hash_tests(SHA256, "SHA256", test_data,
- digest_size=32,
- oid="2.16.840.1.101.3.4.2.1")
-
- if config.get('slow_tests'):
- tests += [LargeSHA256Test()]
-
- return tests
-
-if __name__ == '__main__':
- import unittest
- suite = lambda: unittest.TestSuite(get_tests())
- unittest.main(defaultTest='suite')
-
-# vim:set ts=4 sw=4 sts=4 expandtab:
diff --git a/spaces/arxify/RVC-beta-v2-0618/runtime/Lib/site-packages/PySimpleGUI/PySimpleGUI.py b/spaces/arxify/RVC-beta-v2-0618/runtime/Lib/site-packages/PySimpleGUI/PySimpleGUI.py
deleted file mode 100644
index 7cf10cf64148c16f2631f3373c9ce415c5ccd2b8..0000000000000000000000000000000000000000
--- a/spaces/arxify/RVC-beta-v2-0618/runtime/Lib/site-packages/PySimpleGUI/PySimpleGUI.py
+++ /dev/null
@@ -1,24879 +0,0 @@
-#!/usr/bin/python3
-
-version = __version__ = "4.60.4 Released 10-Oct-2022"
-
-_change_log = """
- Changelog since 4.60.0 released to PyPI on 8-May-2022. These are "Dot" releases....
-
- 4.60.1
- A "dot-release" / patch for crash that occurs if the horizontal_scroll parm is set in Listbox element
- Was created when the ttk scrollbars were added
- 4.60.2
- A "dot-release" for Mac 12.3+ "Invisible window" problem. Adds option in Mac control panel to set Alpha to 0.99 as default
- 4.60.3
- Another shot at the 12.3+ Mac OS problem. Had bug in the version check code
- 4.60.4
- Dot release to quickly fix the Trinket detection which stopped working recently
- """
-
-__version__ = version.split()[0] # For PEP 396 and PEP 345
-
-# The shortened version of version
-try:
- ver = version.split(' ')[0]
-except:
- ver = ''
-
-# __version__ = version
-
-port = 'PySimpleGUI'
-
-# 8""""8 8""""8 8""""8 8 8 8
-# 8 8 e e 8 e eeeeeee eeeee e eeee 8 " 8 8 8
-# 8eeee8 8 8 8eeeee 8 8 8 8 8 8 8 8 8e 8e 8 8e
-# 88 8eeee8 88 8e 8e 8 8 8eee8 8e 8eee 88 ee 88 8 88
-# 88 88 e 88 88 88 8 8 88 88 88 88 8 88 8 88
-# 88 88 8eee88 88 88 8 8 88 88eee 88ee 88eee8 88ee8 88
-
-
-"""
- Copyright 2018, 2019, 2020, 2021, 2022 PySimpleGUI(tm)
-
- Before getting into the details, let's talk about the high level goals of the PySimpleGUI project.
-
- From the inception these have been the project principals upon which it is all built
- 1. Fun - it's a serious goal of the project. If we're not having FUN while making stuff, then something's not right
- 2. Successful - you need to be successful or it's all for naught
- 3. You are the important party - It's your success that determines the success of PySimpleGUI
-
- If these 3 things are kept at the forefront, then the rest tends to fall into place.
-
- PySimpleGUI is a "system", not just a program. There are 4 components of the "PySimpleGUI system"
- 1. This software - PySimpleGUI.com
- 2. The documentation - PySimpleGUI.org
- * PySimpleGUI.org
- * Calls.PySimpleGUI.org
- * Cookbook.PySimpleGUI.org
- 3. Demo Programs - Demos.PySimpleGUI.org
- 4. Support - Issues.PySimpleGUI.org
- 5. eCookbook - eCookbook.PySimpleGUI.org
-
-
- Now available - "The Official PySimpleGUI Course" on Udemy!
- https://www.udemy.com/pysimplegui
-
- Watch for a coupon codes in the documentation on PySimpleGUI.org
-
- Please consider sponsoring all open source developers that make software you or your business use. They need your help.
-
-
- This software is available for your use under a LGPL3+ license
-
- This notice, these first 150 lines of code shall remain unchanged
-
-
-
- 888 .d8888b. 8888888b. 888 .d8888b.
- 888 d88P Y88b 888 Y88b 888 d88P Y88b
- 888 888 888 888 888 888 .d88P
- 888 888 888 d88P 888 8888" 888
- 888 888 88888 8888888P" 888 "Y8b. 8888888
- 888 888 888 888 888 888 888 888
- 888 Y88b d88P 888 888 Y88b d88P
- 88888888 "Y8888P88 888 88888888 "Y8888P"
-
-
- In addition to the normal publishing requirements of LGPL3+, these also apply:
- 1. These and all comments are to remain in the source code
- 2. The "Official" version of PySimpleGUI and the associated documentation lives on two (and **only** two) places:
- 1. GitHub - (http://www.PySimpleGUI.com) currently pointing at:
- https://github.com/PySimpleGUI/PySimpleGUI
- 2. PyPI - pip install PySimpleGUI is the customary way of obtaining the latest release
-
- THE official documentation location is:
- Read the Docs (via http://www.PySimpleGUI.org). Currently is pointed at:
- https://pysimplegui.readthedocs.io/en/latest/
- If you've obtained this software in any other way, then those listed here, then SUPPORT WILL NOT BE PROVIDED.
- 3. If you use PySimpleGUI in your project/product, a notice of its use needs to be displayed in your readme file as per the license agreement
-
- -----------------------------------------------------------------------------------------------------------------
-
-
- The first bit of good news for you is that literally 100s of pages of documentation await you.
- 300 Demo Programs have been written as a "jump start" mechanism to get your running as quickly as possible.
-
- Some general bits of advice:
- Upgrade your software! python -m pip install --upgrade --no-cache-dir PySimpleGUI
- If you're thinking of filing an Issue or posting a problem, Upgrade your software first
- There are constantly something new and interesting coming out of this project so stay current if you can
-
- The FASTEST WAY to learn PySimpleGUI is to begin to play with it, and to read the documentation.
- http://www.PySimpleGUI.org
- http://Calls.PySimpleGUI.org
- http://Cookbook.PySimpleGUI.org
-
- The User Manual and the Cookbook are both designed to paint some nice looking GUIs on your screen within 5 minutes of you deciding to PySimpleGUI out.
-
- A final note from mike...
-
- “Don’t aim at success. The more you aim at it and make it a target, the more you are going to miss it.
- For success, like happiness, cannot be pursued; it must ensue, and it only does so as the unintended side effect of one’s personal dedication to a cause greater.”
- — Viktor Frankl
-
- I first saw this quote in a truncated format:
- "Happiness, cannot be pursued; it must ensue, and it only does so as the unintended side effect of one’s personal dedication to a cause greater."
-
- Everyone is different, but my experience with the PySimpleGUI project matches this theory. It's taken a lifetime of trying and "failing" and trying
- to find happiness before I finally figured this truth-for-me out. If I do a long list of things, and live life in a kind & loving way, then the
- result is happiness. It's a biproduct, not a directly produced thing. This should be taught in school. Or maybe it can't.
- I hope you find happiness, but more importantly, or maybe first, I hope you find that bigger-than-you thing. For me it's always been programming. It seems to be
- the giving back part, not just the calling, that makes the happiness fusion-reactor operate.
-
- "Thank you" has fueled this project. I'm incredibly grateful to have users that are in turn grateful. It's a feedback loop of gratitude. What a fantastic thing!
-"""
-
-# all of the tkinter involved imports
-import tkinter as tk
-from tkinter import filedialog
-from tkinter.colorchooser import askcolor
-from tkinter import ttk
-# import tkinter.scrolledtext as tkst
-import tkinter.font
-from uuid import uuid4
-
-# end of tkinter specific imports
-# get the tkinter detailed version
-tclversion_detailed = tkinter.Tcl().eval('info patchlevel')
-framework_version = tclversion_detailed
-import time
-import pickle
-import calendar
-import datetime
-import textwrap
-
-import inspect
-import traceback
-import difflib
-import copy
-import pprint
-try: # Because Raspberry Pi is still on 3.4....it's not critical if this module isn't imported on the Pi
- from typing import List, Any, Union, Tuple, Dict, SupportsAbs, Optional # because this code has to run on 2.7 can't use real type hints. Must do typing only in comments
-except:
- print('*** Skipping import of Typing module. "pip3 install typing" to remove this warning ***')
-import random
-import warnings
-from math import floor
-from math import fabs
-from functools import wraps
-
-try: # Because Raspberry Pi is still on 3.4....
- # from subprocess import run, PIPE, Popen
- import subprocess
-except Exception as e:
- print('** Import error {} **'.format(e))
-
-import threading
-import itertools
-import json
-import configparser
-import queue
-
-try:
- import webbrowser
-
- webbrowser_available = True
-except:
- webbrowser_available = False
-# used for github upgrades
-import urllib.request
-import urllib.error
-import urllib.parse
-import pydoc
-from urllib import request
-import os
-import sys
-import re
-import tempfile
-import ctypes
-import platform
-import socket
-
-pil_import_attempted = pil_imported = False
-
-warnings.simplefilter('always', UserWarning)
-
-g_time_start = 0
-g_time_end = 0
-g_time_delta = 0
-
-
-# These timer routines are to help you quickly time portions of code. Place the timer_start call at the point
-# you want to start timing and the timer_stop at the end point. The delta between the start and stop calls
-# is returned from calling timer_stop
-
-def timer_start():
- """
- Time your code easily.... starts the timer.
- Uses the time.time value, a technique known to not be terribly accurage, but tis' gclose enough for our purposes
- """
- global g_time_start
-
- g_time_start = time.time()
-
-
-def timer_stop():
- """
- Time your code easily.... stop the timer and print the number of milliseconds since the timer start
-
- :return: delta in milliseconds from timer_start was called
- :rtype: int
- """
- global g_time_delta, g_time_end
-
- g_time_end = time.time()
- g_time_delta = g_time_end - g_time_start
- return int(g_time_delta * 1000)
-
-
-def _timeit(func):
- """
- Put @_timeit as a decorator to a function to get the time spent in that function printed out
-
- :param func: Decorated function
- :type func:
- :return: Execution time for the decorated function
- :rtype:
- """
-
- @wraps(func)
- def wrapper(*args, **kwargs):
- start = time.time()
- result = func(*args, **kwargs)
- end = time.time()
- print('{} executed in {:.4f} seconds'.format(func.__name__, end - start))
- return result
-
- return wrapper
-
-
-_timeit_counter = 0
-MAX_TIMEIT_COUNT = 1000
-_timeit_total = 0
-
-
-def _timeit_summary(func):
- """
- Same as the timeit decorator except that the value is shown as an averave
- Put @_timeit_summary as a decorator to a function to get the time spent in that function printed out
-
- :param func: Decorated function
- :type func:
- :return: Execution time for the decorated function
- :rtype:
- """
-
- @wraps(func)
- def wrapper(*args, **kwargs):
- global _timeit_counter, _timeit_total
-
- start = time.time()
- result = func(*args, **kwargs)
- end = time.time()
- _timeit_counter += 1
- _timeit_total += end - start
- if _timeit_counter > MAX_TIMEIT_COUNT:
- print('{} executed in {:.4f} seconds'.format(func.__name__, _timeit_total / MAX_TIMEIT_COUNT))
- _timeit_counter = 0
- _timeit_total = 0
- return result
-
- return wrapper
-
-
-def running_linux():
- """
- Determines the OS is Linux by using sys.platform
-
- Returns True if Linux
-
- :return: True if sys.platform indicates running Linux
- :rtype: (bool)
- """
- return sys.platform.startswith('linux')
-
-
-def running_mac():
- """
- Determines the OS is Mac by using sys.platform
-
- Returns True if Mac
-
- :return: True if sys.platform indicates running Mac
- :rtype: (bool)
- """
- return sys.platform.startswith('darwin')
-
-
-def running_windows():
- """
- Determines the OS is Windows by using sys.platform
-
- Returns True if Windows
-
- :return: True if sys.platform indicates running Windows
- :rtype: (bool)
- """
- return sys.platform.startswith('win')
-
-
-
-def running_trinket():
- """
- A special case for Trinket. Uses the hostname an platform together to determine if running on Trinket
-
- Returns True if "Trinket" (in theory)
-
- :return: True if sys.platform indicates Linux and hostname starts with 'pygame-'
- :rtype: (bool)
- """
- if sys.platform.startswith('linux') and socket.gethostname().startswith('pygame-'):
- return True
- return False
-
-
-
-def running_replit():
- """
- A special case for REPLIT. Checks both the OS and for the existance of the number of environment variable REPL_OWNER
- Currently, Trinket only has ONE environment variable. This fact is used to figure out if Trinket is being used.
-
- Returns True if running on "replit"
-
- :return: True if sys.platform indicates Linux and setting REPL_OWNER is found in the environment variables
- :rtype: (bool)
- """
- if 'REPL_OWNER' in os.environ and sys.platform.startswith('linux'):
- return True
- return False
-
-
-
-
-# Handy python statements to increment and decrement with wrapping that I don't want to forget
-# count = (count + (MAX - 1)) % MAX # Decrement - roll over to MAX from 0
-# count = (count + 1) % MAX # Increment to MAX then roll over to 0
-
-"""
- Welcome to the "core" PySimpleGUI code....
-
- It's a mess.... really... it's a mess internally... it's the external-facing interfaces that
- are not a mess. The Elements and the methods for them are well-designed.
- PEP8 - this code is far far from PEP8 compliant.
- It was written PRIOR to learning that PEP8 existed.
-
- I'll be honest.... started learning Python in Nov 2017, started writing PySimpleGUI in Feb 2018.
- Released PySimpleGUI in July 2018. I knew so little about Python that my parameters were all named
- using CamelCase. DOH! Someone on Reddit set me straight on that. So overnight I renamed all of the
- parameters to lower case. Unfortunately, the internal naming conventions have been set. Mixing them
- with PEP8 at this moment would be even MORE confusing.
-
- Code I write now, outside PySimpleGUI, IS PEP8 compliant.
-
- The variable and function naming in particular are not compliant. There is
- liberal use of CamelVariableAndFunctionNames, but for anything externally facing, there are aliases
- available for all functions. If you've got a serious enough problem with 100% PEP8 compliance
- that you'll pass on this package, then that's your right and I invite you to do so. However, if
- perhaps you're a practical thinker where it's the results that matter, then you'll have no
- trouble with this code base. There is consisency however.
-
- I truly hope you get a lot of enjoyment out of using PySimpleGUI. It came from good intentions.
-"""
-
-# ----====----====----==== Constants the user CAN safely change ====----====----====----#
-
-# Base64 encoded GIF file
-DEFAULT_BASE64_ICON = b'R0lGODlhIQAgAPcAAAAAADBpmDBqmTFqmjJrmzJsnDNtnTRrmTZtmzZumzRtnTdunDRunTRunjVvnzdwnzhwnjlxnzVwoDZxoTdyojhzozl0ozh0pDp1pjp2pjp2pzx0oj12pD52pTt3qD54pjt4qDx4qDx5qTx5qj16qj57qz57rD58rT98rkB4pkJ7q0J9rEB9rkF+rkB+r0d9qkZ/rEl7o0h8p0x9pk5/p0l+qUB+sEyBrE2Crk2Er0KAsUKAskSCtEeEtUWEtkaGuEiHuEiHukiIu0qKu0mJvEmKvEqLvk2Nv1GErVGFr1SFrVGHslaHsFCItFSIs1COvlaPvFiJsVyRuWCNsWSPsWeQs2SQtGaRtW+Wt2qVuGmZv3GYuHSdv3ievXyfvV2XxGWZwmScx2mfyXafwHikyP7TPP/UO//UPP/UPf/UPv7UP//VQP/WQP/WQf/WQv/XQ//WRP7XSf/XSv/YRf/YRv/YR//YSP/YSf/YSv/ZS//aSv/aS/7YTv/aTP/aTf/bTv/bT//cT/7aUf/cUP/cUf/cUv/cU//dVP/dVf7dVv/eVv/eV//eWP/eWf/fWv/fW/7cX/7cYf7cZP7eZf7dav7eb//gW//gXP/gXf/gXv/gX//gYP/hYf/hYv/iYf/iYv7iZP7iZf/iZv/kZv7iaP/kaP/ka//ma//lbP/lbv/mbP/mbv7hdP7lcP/ncP/nc//ndv7gef7gev7iff7ke/7kfv7lf//ocf/ocv/odP/odv/peP/pe//ofIClw4Ory4GszoSszIqqxI+vyoSv0JGvx5OxyZSxyZSzzJi0y5m2zpC10pi715++16C6z6a/05/A2qHC3aXB2K3I3bLH2brP4P7jgv7jh/7mgf7lhP7mhf7liv/qgP7qh/7qiP7rjf7sjP7nkv7nlv7nmP7pkP7qkP7rkv7rlv7slP7sl/7qmv7rnv7snv7sn/7un/7sqv7vq/7vrf7wpv7wqf7wrv7wsv7wtv7ytv7zvP7zv8LU48LV5c3a5f70wP7z0AAAACH5BAEAAP8ALAAAAAAhACAAAAj/AP8JHEiwoMGDCA1uoYIF4bhK1vwlPOjlQICLApwVpFTGzBk1siYSrCLgoskFyQZKMsOypRyR/GKYnBkgQbF/s8603KnmWkIaNIMaw6lzZ8tYB2cIWMo0KIJj/7YV9XgGDRo14gpOIUBggNevXpkKGCDsXySradSoZcMmDsFnDxpEKEC3bl2uXCFQ+7emjV83bt7AgTNroJINAq0wWBxBgYHHdgt0+cdnMJw5c+jQqYNnoARkAx04kPEvS4PTqBswuPIPUp06duzcuYMHT55wAjkwEahsQgqBNSQIHy582D9BePTs2dOnjx8/f1gJ9GXhRpTqApFQoDChu3cOAps///9D/g+gQvYGjrlw4cU/fUnYX6hAn34HgZMABQo0iJB/Qoe8UxAXOQiEg3wIXvCBQLUU4mAhh0R4SCLqJOSEBhhqkAEGHIYgUDaGICIiIoossogj6yBUTQ4htNgiCCB4oIJAtJTIyI2MOOLIIxMtQQIJIwQZpAgwCKRNI43o6Igll1ySSTsI7dOECSaUYOWVKwhkiyVMYuJlJpp0IpA6oJRTkBQopHnCmmu2IBA2mmQi5yZ0fgJKPP+0IwoooZwzkDQ2uCCoCywUyoIW/5DDyaKefOLoJ6LU8w87pJgDTzqmDNSMDpzqYMOnn/7yTyiglBqKKKOMUopA7JgCy0DdeMEjUDM71GqrrcH8QwqqqpbiayqToqJKLwN5g45A0/TAw7LL2krGP634aoopp5yiiiqrZLuKK+jg444uBIHhw7g+MMsDFP/k4wq22rririu4xItLLriAUxAQ5ObrwzL/0PPKu7fIK3C8uxz0w8EIIwzMP/cM7HC88hxEzBBCBGGxxT8AwQzDujws7zcJQVMEEUKUbPITAt1D78OSivSFEUXEXATKA+HTscC80CPSQNGEccQRYhjUDzfxcjPPzkgnLVBAADs='
-
-DEFAULT_BASE64_ICON_16_BY_16 = b'iVBORw0KGgoAAAANSUhEUgAAABAAAAAQCAYAAAAf8/9hAAAAAXNSR0IArs4c6QAAAARnQU1BAACxjwv8YQUAAAAJcEhZcwAADsMAAA7DAcdvqGQAAAKCSURBVDhPVZNbSFRRFIb35YwXItBIGtDsiqENEUTRjJlZkJggPSUYBD0UhULElE6hBY6ID/ZSpD1IDxaCEPhUaFLRQyWRNxIJe8syMxCjMCbB07fOsaMt+GftvWf//7/2Whyt1sTei/fCpDqQBTrGOi9Myrk7URwhnQUfQLeOvErJuUQgADlK6gObvAOl5sHx0doHljwARFRiCpxG5J1sjPxALiYNgn9kiQ3gafdYUYzseCd+FICX7sShw7LR++q6cl3XHaXQHFdOJLxFsJtvKHnbUr1nqp01hhStpXAzo7TZZXOjJ+9orT9pY74aY3ZobZZYW8D/GpjM19Ob088fmJxW2tkC4AJt17Oeg2MLrHX6jXWes16w1sbBkrFWBTB2nTLpv5VJg7wGNhRDwCS0tR1cbECkidwMQohAdoScqiz8/FCZUKlPCgSWlQ71elOI1fcco9hCXp1kS7dX3u+qVOm2L4nW8qE4Neetvl8v83NOb++9703BcUI/cU3imuWV7JedKtv5LdFaMRzHLW+N+zJoVDZzRLj6SFNfPlMYwy5bDiRcCojmz15tKx+6hKPv7LvjrG/Q2RoOwjSyzNDlahyzA2dAJeNtFcMHA2cfLn24STNr6P4I728jJ7hvf/lEGuaXLnkRAp0PyFK+hlyLSJGyGWnKyeBi2oJU0IPIjNd15uuL2f2PJgueQBKhVRETCgNeYU+xaeEpnWaw8cQPRM7g/McT8eF0De9u7P+49TqXF7no98BDEEkdvvXem8LAtfJniFRB/A5XeiAiG2+/icgHVQUW5d5KyAhl3M2y+U+ysv1FDukyKGQW3Y+vHJWvU7mz8RJSPZgDd3H2RqiUUn8BSQuaBvGjGpsAAAAASUVORK5CYII='
-
-DEFAULT_BASE64_LOADING_GIF = b'R0lGODlhQABAAKUAAAQCBJyenERCRNTS1CQiJGRmZLS2tPTy9DQyNHR2dAwODKyqrFRSVNze3GxubMzKzPz6/Dw6PAwKDKSmpExKTNza3CwqLLy+vHx+fBQWFLSytAQGBKSipERGRNTW1CQmJGxqbLy6vPT29DQ2NHx6fBQSFKyurFRWVOTi5HRydPz+/Dw+PP7+/gAAAAAAAAAAAAAAAAAAAAAAAAAAAAAAAAAAAAAAAAAAAAAAAAAAAAAAAAAAAAAAAAAAAAAAAAAAACH/C05FVFNDQVBFMi4wAwEAAAAh+QQJCQAsACwAAAAAQABAAAAG/kCWcEgsGo/IpHLJbDqf0CjxwEmkJgepdrvIAL6A0mJLdi7AaMC4zD4eSmlwKduuCwNxdMDOfEw4D0oOeWAOfEkmBGgEJkgphF8ph0cYhCRHeJB7SCgJAgIJKFpnkGtTCoQKdEYGEmgSBlEqipAEEEakcROcqGkSok8PkGCBRhNwcrtICYQJUJnDm0YHASkpAatHK4Qrz8Nf0mTbed3B3wDFZY95kk8QtIS2bQ29r8BPE8PKbRquYBuxpJCwdKhBghUrQpFZAA8AgX2T7DwIACiixYsYM2rc+OSAhwrZOEa5QGHDlw0dLoiEAqEAoQK3VjJxCQmEzCUhzgXciOKE/gIFJ+4NEXBOAEcPyL6UqEBExLkvIjYyiMOAyICnAAZs9IdGgVWsWjWaTON1yAGsUTVOTUOhyLhh5TQi7cqUyIVzKjmiYCBBQtAjNAnZvKmk5cuYhJVc6DAWZd7ETTx6CAm5suXLRQY4sPDTQoqwmIlAADE2DYi0oUUQhbQC8WUQ5wZf9oDVA58KdaPAflqgTgMEXxA0iPIB64c6I9AgiFL624Y2FeLkbtJ82HM2tNPYfmLBOHLlUQJ/6z0POADhUa4+3V7HA/vw58gfEaFBA+qMIt6Su9/UPAL+F4mwWxwwJZGLGitp9kFfHzgAGhIHmhKaESIkB8AIrk1YBAQmDJiQoYYghijiiFAEAQAh+QQJCQApACwAAAAAQABAAIUEAgSEgoREQkTU0tRkYmQ0MjSkpqTs6ux0cnQUEhSMjozc3ty0trT09vRUUlRsamw8OjwMCgxMSkx8fnwcGhyUlpTk5uS8vrz8/vwEBgSMioxERkTc2txkZmQ0NjS0srT08vR0dnQUFhSUkpTk4uS8urz8+vxsbmw8Pjz+/v4AAAAAAAAAAAAAAAAAAAAAAAAAAAAAAAAAAAAAAAAAAAAAAAAAAAAAAAAAAAAAAAAAAAAAAAAAAAAAAAAAAAAAAAAG/sCUcEgsGo/IpHLJbDqf0Kh0Sl0aPACAx1DtOh/ZMODhLSMNYjHXzBZi01lPm42BizHz5CAk2YQGSSYZdll4eUUYCHAhJkhvcAWHRiGECGeEa0gNAR4QEw1TA4RZgEcdcB1KBwViBQdSiqOWZ6wABZlIE3ATUhujAAJsj2FyUQK/wWbDcVInvydsumm8UaKjpWWrra+whNBtDRMeHp9UJs5pJ4aSXgMnGxsI2Oz09fb3+Pn6+/xEJh8KRjBo1M/JiARiEowoyIQAIQIMk1T4tXAfBw6aEI5KAArfgjcFFhj58CsLg3zDIhXRUBKABnwc4GAkoqDly3vWxMxLQbLk/kl8tbKoJAJCIyGO+RbUCnlkxC8F/DjsLOLQDsSISRREEBMBKlYlDRgoUMCg49ezaNOqVQJCqtm1Qy5IGAQgw4YLcFOYOGWnA8G0fAmRSVui5c+zx0omM2NBgwYLUhq0zPKWSIMFHCojsUAhiwjIUHKWnPpBAF27H5YEEBOg2mQA80A4ICQBRBJpWVpDAfHabAMUv1BoFkJChGcSUoCXREGEUslZRxoHAB3lQku8Qg7Q/ZWB26HAdgYLmTi5Aru9hPwSqdryKrsLG07fNTJ7soN7IAZwsH2EfUn3ETk1WUVYWbDdKBlQh1Usv0D3VQPLpOHBcAyBIAFt/K31AQrbBqGQWhtBAAAh+QQJCQAyACwAAAAAQABAAIUEAgSEgoTEwsREQkTk4uQsLiykoqRkYmQUEhTU0tRUUlT08vS0srSMjox8enwMCgzMysw8OjwcGhxcWlz8+vy8urxMSkzs6uysqqxsamzc2tyUlpQEBgSMiozExsTk5uQ0NjSkpqRkZmQUFhRUVlT09vS0trSUkpR8fnwMDgzMzsw8PjwcHhxcXlz8/vy8vrxMTkzc3tz+/v4AAAAAAAAAAAAAAAAAAAAAAAAAAAAAAAAAAAAAAAAAAAAAAAAAAAAG/kCZcEgsGo/IpHLJbDqf0Kh0Sq1ar8nEgMOxqLBgZCIFKAMeibB6aDGbB2u1i+Muc1xxJSWmoSwpdHUcfnlGJSgIZSkoJUptdXCFRRQrdQArhEcqD24PX0wUmVMOlmUOSiqPXkwLLQ8PLQtTFCOlAAiiVyRuJFMatmVpYIB1jVEJwADCWCWBdsZQtLa4artmvaO2p2oXrhyxVCWVdSvQahR4ViUOZAApDuaSVhQaGvHy+Pn6+/z9/v8AAzrxICJCBBEeBII6YOnAPYVDWthqAfGIgGQC/H3o0OEDEonAKPL7IKHMCI9GQCQD0S+AmwBHVAJjyQ/FyyMgJ/YjUAvA/ggCFjFqDNAxSc46IitOOlqmRS6lQwSIABHhwAuoWLNq3cq1ogcHLVqgyFiFAoMGJ0w8teJBphsQCaWcaFcGwYkwITiV4hAiCsNSB7B4cLYXwpMNye5WcVEgWZkC6ZaUSAQMwUMnFRybqdCEgWYTVUhpBrBtSQfNHZC48BDCgIfIRKxpxrakAWojLjaUNCNhA2wZsh3TVuLZMWgiJRTYgiFKtObSShbQLZUinohkIohkHs25yYnERVRo/iSDQmPHBdYi+Wsp6ZDrjrNH1Uz2SYPpKRocOZ+sQJEQhLnBgQFTlHBWAyZcxoJmEhjRliVw4cMfMP4ZQYEADpDQggMvJ/yWB3zYYQWBZnFBxV4p8mFVAgzLqacQBSf0ZNIJLla0mgGu1ThFEAAh+QQJCQAqACwAAAAAQABAAIUEAgSUkpRERkTMyswkIiTs6uy0trRkZmQ0MjTU1tQcGhykpqRUVlT09vTEwsQsKix8enwMCgycnpzU0tS8vrw8Ojzc3txcXlz8/vwEBgSUlpRMSkzMzswkJiT08vS8urxsamw0NjTc2twcHhysqqz8+vzExsQsLix8fnxkYmT+/v4AAAAAAAAAAAAAAAAAAAAAAAAAAAAAAAAAAAAAAAAAAAAAAAAAAAAAAAAAAAAAAAAAAAAAAAAAAAAAAAAAAAAG/kCVcEgsGo/IpHLJbDqf0Kh0Sq1ar8tEAstdWk4AwMnSLRfBYbF5nUint+tu2w2Ax5OFghMdPt2TBg9hDwZMImgnIn9HH3QAhUxaTw0LCw1WHY4dax6CAA8eVAWOYXplEm4SoqQApl2oaapUmXSbZgW0HaFUBo6QZpQLu1UGub+LWHnIy8zNzs/Q0dLTzSYQFxcoDtRMAwiOCCZJDRwDl88kGawZC0YlEOoAGRDnywPx6wNEHnxpJ8N/SvRjdaLEkAOsDiyjwMrRByEe8NHJADAOhIZ0IAgZgFHcIgYY3TAQYqIjMpAhw4xUEXFdxTUXUwLQKAQhKYXIGsl8CHGg/piXa0p4wvgAA5EG8MLMq4esZEiPRRoMMMGU2QKJbthxQ2LiG51wW5NgcACBwQUIFIyGXcu2bdgGGjZ06LBBQ1UoJg5UqHAAKhcTBByN8OukRApHKe5OcYA1TQbCTC6wuoClQeCGIxQjcYBxm5UAKQM8kdyQshUBKQU8CYERwZURKUc88crKNZIJZRlAmIAEdkjZTkhPPtLAppsDd1GHVO2Ec0PPREoodyTAIBHQIUWPHm5EA0btQxoowKgAaJISwtNcsF7ENyvgRCg0Vgq5iYMDISqkoIDEQkoyRZjgXhojQHcHRyHpYwRcAhBAgAB2LeNfSACyNaBgbqngXUPgGLElHSvVZahCA4fRcYFma3GQGwQciAhNEAAh+QQJCQAwACwAAAAAQABAAIUEAgSEgoTEwsRERkTk4uQkIiSkpqRsamwUEhTU0tT08vSUkpRUUlQ0MjS0trQMCgzMyszs6ux8enwcGhzc2tz8+vyMioxMTkysrqw8OjwEBgSEhoTExsRMSkzk5uQkJiSsqqxsbmwUFhTU1tT09vSUlpRUVlQ0NjS8vrwMDgzMzszs7ux8fnwcHhzc3tz8/vz+/v4AAAAAAAAAAAAAAAAAAAAAAAAAAAAAAAAAAAAAAAAAAAAAAAAAAAAAAAAAAAAG/kCYcEgsGo/IpHLJbDqf0Kh0Sq1ar9hs1sNiebRgowsBACBczJcKA1K9wkxWucxSVgKTOUC0qcCTcnN1SBEnenoZX39iZAApaEcVhod6J35SFSgoJE4EXYpHFpSUAVIqBWUFKlkVIqOHIpdOJHlzE5xXEK+UHFAClChYBruHBlAowMLEesZPtHoiuFa6y2W9UBAtZS2rWK3VsVIkmtJYosuDi1Ekk68n5epPhe4R8VR3rnN8svZTLxAg2vDrR7CgwYMItZAo0eHDhw4l4CVMwgHVoRbXjrygMOLNQQEaXmnISARErQnNCFbQtqsFPBCUUtpbUG0BkRe19EzwaG9A/rUBREa8GkHQIrEWRCgMJcjyKJFvsHjG87kMaMmYBWkus1nEwEmZ9p7tmqBA44gRA/uhCDlq5MQlHJrOaSHgLZOFAwoUGBDRrt+/gAMLhkMiwYiyV0iogCARCwUTbDWYoHBPQmQJjak4eEDpgQMpKxpQarAiCwXOox4QhXLg1YEsDIgxgKKALSUNiKvUXpb5CLVXJKeoqNatCQdiwY2QyH0kAfEnu9syJ0Jiw4dUGxorqNb7SOtRr4+saDeH9BETsqOEHl36yIVXF46MQN15NRQSlstowIzk+K7kMGzW2WdUKAABB90FQEwp8l1g2wX2xfOda0oolkB3YWyw4GBCIfgHHIdCvDdKByAKsd4h5pUIAwkBsNRCdioWoUB7MRoUBAAh+QQJCQAuACwAAAAAQABAAIUEAgSEhoTMzsxMSkykpqQcHhz08vRkYmQUEhSUlpS0trTc3twsLixsbmwMCgzU1tSsrqz8+vycnpyMjoxUUlQkJiRsamwcGhy8vrw0NjR0dnQEBgTU0tSsqqz09vRkZmQUFhScmpy8urzk5uQ0MjR0cnQMDgzc2ty0srT8/vykoqSUkpRUVlQsKiz+/v4AAAAAAAAAAAAAAAAAAAAAAAAAAAAAAAAAAAAAAAAAAAAAAAAAAAAAAAAAAAAAAAAAAAAG/kCXcEgsGo8RRWlAaSgix6h0Sp2KKoCstiKqer/fkHasTYDP6KFoQ25303BqBNsmV6DxvBFSr0P0gEMNfW0WgYEDhGQDRwsTFhYTC4dTiYpajEQeB2xjBx6URxaXWoZDHiR9JKChRHykAH9DB4oHcQIlJQJRc6R3Qwukk2gcnRscUSKkb0ITpBNpo6VSCZ11ZkS0l7Zo0lmmUQp0YxUKRtq1aQLGyFNJDUxOeEXOl9DqDbqhJ6QnrYDo6nD7l8cDgz4MWBHMYyBglgMGFh46MeHDhwn+JGrcyLGjx48gO3rg8CBiSDQnWBhjkfFkFQUO2jgwF8UACgUmPz6IWcfB/oMjGBBkQYABJAVFFIwYMDEGQc6NBqz1USjk1RhZHAWQ2kUERRsUHrVe4jpk6RgTTzV6IEVVCAamAEwU/XiUUNIjNlGk5bizj0+XVGDKpAl4yoO6WSj8LOzFgwAObRlLnky5suXLEg2o0FCCwF40KU48SEGwg1AtCDrk6XAhywUCrTr0UZ1GNhnYhwycbuMUdGsyF0gHkqBIApoHfRYDKqGoAcrkhzQoKoEmAog2IIRHSSEiQAAR84wQJ2Qcje0xuKOcaDGmhfIiZuughUPg9+spI66TATEiyvnbeaTwwAPhidLHB1IQsBsACKS3kX7YTWGABLlI8BlBEShSIGUQIO6HmRDekIHgh/lh19+HLjzA3hbvfZiEdwpoh+KMjAUBACH5BAkJACYALAAAAABAAEAAhQQCBISGhMzKzERCRDQyNKSmpOzq7GRiZBQSFHRydJyanNTW1LS2tPz6/Dw6PAwODLSytPTy9GxubBweHHx6fKSipNze3AQGBIyKjMzOzExOTDQ2NKyqrOzu7GRmZBQWFHR2dJyenNza3Ly+vPz+/Dw+PP7+/gAAAAAAAAAAAAAAAAAAAAAAAAAAAAAAAAAAAAAAAAAAAAAAAAAAAAAAAAAAAAAAAAAAAAAAAAAAAAAAAAAAAAAAAAAAAAAAAAAAAAb+QJNwSCwaj8ikcslsmjoYx+fjwHSc2KyS8QF4vwiGdjxmXL5or5jMXnYQ6TTi2q4bA/F4wM60UDZTGxQWRw55aRt8SSQUhyAkRQ+HaA+KRw0akwAaDUSSmgCVRg0hA1MDCp1ZIKAACUQbrYlFBrGIBlgirV4LQ3ige0QNtnEbqkwSuwASQ2+aD3RDCpoKTgTKBEQMmmtEhpMlTp+tokMMcGkP3UToh+VL46DvQh0BGwgIGwHRkc/W2HW+HQrXJNkuZm2mTarWZIGyXm2GHTKGhRWoV3ZqFcOFBZMmTooaKCiBr0SqMQ0sxgFxzJIiESAI4CMAQoTLmzhz6tzJs6f+z59Ah0SoACJBgQhByXDoAoZD0iwcDjlFIuDAAQFPOzCNM+dIhjMALmRIGkJTiCMe0BxIavAQwiIH1CZNoAljka9exJI1iySDVaxJneV5gPQpk6h5Chh2UqAdAASKFzvpEKJoCH6SM2vezLmz58+gQ7fhsOHCBQeR20SAwKDwzbZf3o4ZgQ7BiJsFDqXOEiFeV0sCEZGBEGcqHxKaIGkhngaCJRJg41xQnkWwF8IuiQknM+LTg9tMBAQIADhJ7sRtOrDGfIRE3C8HWhqB7UV2Twx6lhQofWHDbp8TxDGBaEIgl4d8nwWYxoAEmvALGsEQ6J5aCIYmHnkNZqghgUEBAAAh+QQJCQAnACwAAAAAQABAAIUEAgSEgoRERkTEwsTk4uRkYmQ0MjQUFhRUVlTU1tT08vSkpqQMCgxMTkzMysxsbmz8+vzs6uwcHhxcXlzc3tysrqwEBgSEhoRMSkzExsRkZmQ8OjwcGhxcWlzc2tz09vSsqqwMDgxUUlTMzsx0dnT8/vzs7uz+/v4AAAAAAAAAAAAAAAAAAAAAAAAAAAAAAAAAAAAAAAAAAAAAAAAAAAAAAAAAAAAAAAAAAAAAAAAAAAAAAAAAAAAAAAAAAAAAAAAG/sCTcEgsGo/IpHLJbA5NjozJSa02RxiAFiAYWb/g08Ky3VoW4TRzxCiXLV613Jh1lwVzJ4RCgCQjdnZTeUkZImQAFiIZRxmBbgOERyUkjyQlRQOPZZFIFCAVHmGVmyRFgJtag0UUAncUVpqpAJ1Drpt4RhQHdgewVHWpGEUOiHZwR7d2uU0fbbMWfkRjx2hGHqkJTtizWqLEylwOSAup1kzc3d9GERlSShWpIE4fxpvRaumB2k7BuHPh7lSRlapWml29flEhZYkQARF31lGBwNANCWmEPIAAwS9MhgaILDQwKEnSHgoYS6pcqRJCSpZzMhTgBeBAAZIwrXzo8AjB/oecXxQYSGVgFdAmCLohODoEhAELFjacE+KoGy2mD+w8IJLU6lKgIB6d42C15tENjwwMKatFQc4SqTCdYAvALcwS9t7IpdntwNGhgdQK4en1aNhA5wjOwrkyq5utXJUyFbLgqQUDU4UIJWp3MhMFXe0gMOqZyYAJZAFwmMC4dBMIP13Lnk27tu3buHPnSYABKoaOYRwUKMBIZYJnWhgAtzIiZBxJ/rQw+6KhTIGSEPImkvulgPWSeI+9pNJcC7KS0bmoGTFhwnNJx8sod10BAYIKTRLcErD86IUyAeiGhAn2WECagCeMYMd7CJ5A4BsHIhgAgA0eUd99FWao4YYcAy4RBAA7OEloRWRqYW9jdzhOTjdUeHV4MTVCcmpRRWxDKzdGSWtiWnV5UUlCY0t5QTlKYmUzU25OM3ArSDd0K3JOMEtOTw=='
-
-# Old debugger logo
-# PSG_DEBUGGER_LOGO = b'R0lGODlhMgAtAPcAAAAAADD/2akK/4yz0pSxyZWyy5u3zZ24zpW30pG52J250J+60aC60KS90aDC3a3E163F2K3F2bPI2bvO3rzP3qvJ4LHN4rnR5P/zuf/zuv/0vP/0vsDS38XZ6cnb6f/xw//zwv/yxf/1w//zyP/1yf/2zP/3z//30wAAAAAAAAAAAAAAAAAAAAAAAAAAAAAAAAAAAAAAAAAAAAAAAAAAAAAAAAAAAAAAAAAAAAAAAAAAAAAAAAAAAAAAAAAAAAAAAAAAAAAAAAAAAAAAAAAAAAAAAAAAAAAAAAAAAAAAAAAAAAAAAAAAAAAAAAAAAAAAAAAAAAAAAAAAAAAAAAAAAAAAAAAAAAAAAAAAAAAAAAAAAAAAAAAAAAAAAAAAAAAAAAAAAAAAAAAAAAAAAAAAAAAAAAAAAAAAAAAAAAAAAAAAAAAAAAAAAAAAAAAAAAAAAAAAAAAAAAAAAAAAAAAAAAAAAAAAAAAAAAAAAAAAAAAAAAAAAAAAAAAAAAAAAAAAAAAAAAAAAAAAAAAAAAAAAAAAAAAAAAAAAAAAAAAAAAAAAAAAAAAAAAAAAAAAAAAAAAAAAAAAAAAAAAAAAAAAAAAAAAAAAAAAAAAAAAAAAAAAAAAAAAAAAAAAAAAAAAAAAAAAAAAAAAAAAAAAAAAAAAAAAAAAAAAAAAAAAAAAAAAAAAAAAAAAAAAAAAAAAAAAAAAAAAAAAAAAAAAAAAAAAAAAAAAAAAAAAAAAAAAAAAAAAAAAAAAAAAAAAAAAAAAAAAAAAAAAAAAAAAAAAAAAAAAAAAAAAAAAAAAAAAAAAAAAAAAAAAAAAAAAAAAAAAAAAAAAAAAAAAAAAAAAAAAAAAAAAAAAAAAAAAAAAAAAAAAAAAAAAAAAAAAAAAAAAAAAAAAAAAAAAAAAAAAAAAAAAAAAAAAAAAAAAAAAAAAAAAAAAAAAAAAAAAAAAAAAAAAAAAAAAAAAAAAAAAAAAAAAAAAAAAAAAAAAAAAAAAAAAAAAAAAAAAAAAAAAAAAAAAAAACH5BAEAAP8ALAAAAAAyAC0AAAj/AP8JHEiwoMGDCBMqXMiwoUOFAiJGXBigYoAPDxlK3CigwUGLIAOEyIiQI8cCBUOqJFnQpEkGA1XKZPlPgkuXBATK3JmRws2bB3TuXNmQw8+jQoeCbHj0qIGkSgNobNoUqlKIVJs++BfV4oiEWalaHVpyosCwJidw7Sr1YMQFBDn+y4qSbUW3AiDElXiWqoK1bPEKGLixr1jAXQ9GuGn4sN22Bl02roo4Kla+c8OOJbsQM9rNPJlORlr5asbPpTk/RP2YJGu7rjWnDm2RIQLZrSt3zgp6ZmqwmkHAng3ccWDEMe8Kpnw8JEHlkXnPdh6SxHPILaU/dp60LFUP07dfRq5aYntohAO0m+c+nvT6pVMPZ3jv8AJu8xktyNbw+ATJDtKFBx9NlA20gWU0DVQBYwZhsJMICRrkwEYJJGRCSBtEqGGCAQEAOw=='
-
-PSG_DEBUGGER_LOGO = b'iVBORw0KGgoAAAANSUhEUgAAAEAAAABACAYAAACqaXHeAAAAAXNSR0IArs4c6QAAAARnQU1BAACxjwv8YQUAAAAJcEhZcwAALiIAAC4iAari3ZIAAA2CSURBVHhe7VtplBXFGe03qBiN+RGJJjEGFGZYXWMETDhhZFEGDaA4KCbnmOTo0UQx7AwgMIDs+4ggGlAjI/BERxY3loggHpGdgRkGJlFQzxFzNCd6NC6hc28tXVXd/XrevBnyI/HC7ar6vuru735V1a9f9xvvG/yfI6XKBuO+QYN/hKIT+H1h8Lz3wG1lC+Z+KJu5obDrtc1QtAVPB98Ha/7y6uaTKBsFDUoARHP/m8BhYEcwfLyvwTQ4Gol4W1iyBIRfhmIa2ANsQpvCR+Cz4EIkYq+wNAA5JwDiL0TxJNhVGJLxMdgPSdgim8mA+GIUPHZTYYiHDz4PjkAijghLDsgpARDfC8VT4HeFITt8DvZBEjbIZjyU+OXgacJQN/4FcqZMRSK+FJZ6oF4JUFN+JDgZtKdltkhMQg7ibewH70AS9shmdsg6ARDPoJaAvxGG3BGbhAaK1/gCHAry+iAMdSGrBED8t1CsBG8UhobDSQLE34KiHGyIeBvLwLuzWRJ5qswIJf45sLHEEzzm8zg2r/AEE/JvWW0UcJauQWJ5nkQkzgAEeAaKNeB1wtD4CGYCgr0B9WfApCt/ffEy2A8zgeeJRcYZMOj+IUwOp9KpEk8EMwFBrkO9P8h13Fi4zvP9ZV1/UZhxoDMmIJVKTc3LyxsIeiTaiWwAGj8Jvo//ip43ABXeqMUiNvLBQ4YPRMHP+RQPkoQkfz33rf9ykAJj4R7b/xIdr9qydcsBZQgQScDQYSPbo3gTBzhbWuLRiMJtiCTMnzebSeiL+mowL0loRp86h/H5O2DqvHXba873COdmZviIUbjopV7ElP5xeIprEnF2MslHZuE/HWX/Tp2veXnFiuWbWzRvcT5sP6UjcxJglf9DMEZVXIBj1Bw7fsyZBc4MGDFy9AQU42XLHFIl04JriPpd5DAj3gE77HprBz+FjoGYjegj/0eh9nd90c44Tw2K9tu2b+OXNIHgIjiqZGwLXOxGmhHhhU8yeiE0Ptufl5dyqPvH+c2xbH/A5uDvt7z26kcIegUTRI1iDoh6PLGx/LK/08fzClD+UkkWCBKAQCj+TB0E6v8Ex4BFYAn4sfaFCZ9ifGLi/GZ/k5RQYu5gXAj4JUcEiI0lFAwLtWn5sGF5vxCsIJbAmLHjebXlg4tz2EYnXih+PuXBiW+wTZSMfoDfz99EYMGVWRzUAto+/MGyCvttJPkIdaxzt299rRl6cupKhM9pbXWhEfgsO1OAzcVvvPmGeD4hZgAyfyV4jjUS22zxxNQpk/ZhxNbQT42kGUUxysdRdkS5O86vmeQjLT+K1PeQhw9EzIInKUDVJbHhf8fm+kBrH1RTqBUpWToBeRfKk+vp2eRT4Q0BfU7ETV/EC/GpQiTtLdgX2z7TJ2vhtu2rk77f1IjJXqjxIfCIzb9KKlIJwIneDgnrOqF08gWih8KE0km8PvRWfkUR5HHsWzh5UmntuPETb4H9Ye2Tfp3U4NgOo8ID+2dov4tgL7ICF6X4p+uKgdAYn6Bj974jValrAMTy85dr4odsK1SCvwV3gi3Ah7BzMHUk/OM4WGHphAdqkSDnKy3sIbiGJL/0+RWTJk7o17lj5z+iMZcWA8oRRQjSED02AaP8TzyxY+cOcZEVM2DC+LFfIQHjQqPQAdwBfgFfLVhk/GbkKb504oPFqJeDp4VHHP0UzWyw/epcqq+m6D+r09WdIMa/1YycITYQ49qkWfniKDIg6sGzyeBjEEEsxYmf1sFYAZ2OesoEyuDkmh8/bkztpMlTi+FfjvZpbh9Jfawwtd+IdvwLJpaOex2BFiLijiJ0R0zWQqP0/PfgXKFkm1vhzZs3ed2691iHoK5AMAUmQHGNCAgch6XwgbEltQ9OmY6R95bDjpHXftNXMrx/nT4+6b3z808+PQsl63wvgJjFfwuqFbETxmcKseUdYN+du3cdZYPgWR1MnTaTn/OrEU9vaZFA8rgVa350yYha9CtGO3iGJ/02XIPrj/dhhCqwHbC2gg+g+Ow/hRhM34zncIpQJzSVheIH7tqzi+8pAkQSQEyfMUskQQYggeAw8l7hqJHDauEPHmAmCa9PUnB8jLZfXLGaXwC9VWAfViRUR7cA7APYRcQuxe/d7YgnYhNAzJg5W82EVG+KR7CFI0cMrZ0xc44S7zsPMKNibbjOcF8tfvWqVQyImz7cxXSzdlDViM/pYjUo3vcG7t63JyKeyJgAYuasuU2xFPDx500bPmxw7azZ85xpT7hinEZMUuL8FO8Vp59+mtGYkVddzR4RA6pWg4j6xMjv2bc3VjyRmAAbc+bOd57bN1w4SznyK8t5WL5DTOGbmnbKQsMR61QjHRV8KX7/voziiawSMG9+WVZrnkjy2z4tvvzPfAXorcL1X4x8DkKtLSArQvzeA8niiTpfby0oW4iPupQQrz+u4shcujZYVD3sA55HUbz8iSdYD13wQmKThSpYPl+K31e5P31p+0vO+ODDE4nvGxITUPbQonp/ztskoraUEP/k0qV0p3E4Z81LWCnIJJSIVpT4AxDfQXx9P++88ypPfHjir8IbAxllDBY+vDhhzROuwfVn8vkVmPoDlj32KBuY9l4f41KlgGxEfaaTqJkmINf8/oOV6Uvataf4jZCHmyj/c/Trc6DqYOwL2dgELFq8JMc1n9mn1/yfHlnMJqa9XPPcJ+gWrQhkOoeoySbE+wMPHDqY7tBWiocwPkgBxFYkobL6UCQJkQQ8suSxK1FsR8DBk58w6pcUtv212PZf8vBCtFLxNzmAqAXNuu0Cas1jhNMd2rSTI5+yb5+D/iIJBw9XOUlwEvDoY0ubINhdqPJAEcCnavGI88PG++4rFpWV8U3tKqx/Oe2Dru4+5hChY6FpLEFNiK+sOpRu36atmvZKvIbYL+j/GU7Q5VDN4d2qbb4NErhI9cU3scusb2WC+gIWtmvW4R96z913fYowpoB9RJJA8Y9liNioOquWjyLstu9/DQrx7Vq3uRz1jWAz5XOIja6fhaK8bX4Bf3Al4CQAwd5ufz0NC3N9UX+Y8PE5wlpclNrh5IN1QKQJqk6hhsqHQog/WF2VblfQ+nLYOK2b0Wf1/zu4Afwbd6FP+D2/NWx8/ygQJGDZ408i1lQX+zu9ESJpxMX7DWViwOfuuvN3OJ+PjZeH0g4wG6FxPiH+0OHqdNv81hh5bwO6qZGHEG58vxxsXlVzuCesreAbFewv+3WXqq0EQMjZYDMtSgrTIxxmdn7wLR4bJ+3Cs7pBgMlCRYmNbZfia6rTbfILLocF4iPT/h8o7q46UvMZz119pOZk9dGa6bBtoh8d2KclfUSQAAhpGhUWCHGY5Nc+Rf5YkrhAnjxroRaxt2kvwKimW7fK55rfAIM77cWxvGoI/kSe1gD+rbofWsHdoT0DPkLAfP4XEaWphWXra9KkCc9mBZe1UEm1D4kNy3tbt8wfjgrE62kfPubJlgUXt+Q7RQe0y66iH989CgQJ+NXtt/FNzF4pJsz6CbcoHq3jhMdMgMLgBh0Vauj6IMyfgVrkao+NrHseX6ZMzb/o4kBbqxYXdYGtmF7Vf7tymQQQCHiNFBOmFKTF2jS+MIVfvNrGCbeIE1tiIhQ+0VeIISN9bFr9NZUBHm8I2jshfCa4Eu1NCKOp8GEqgC8wLsK5EVqxMs33AvzoOlNa5AmSUIefN0EFpWPHtESvKtTlgxSxi9kvqIXshDG5dkKao3Yiwbem9p23gztRZwbcOuCW9zGai+zR1iMcZpb+VmBR9dEjRxHMAiYrjthEbJrYQIxrc30s4n0ZMEuVAk4CCAQ8Hnw3ThSphMX6yBj/nFXp1d9GUCUIar0IMEYQNo0tNA4c/a2qLhD5MkSsfraCr8DWUYu01H0eEUxmVIDFJcOGMuF87MsHrbRHIKz1E5Ut+PujS5GA4J0AEZkBxM039X0Bo7jMvqiFRzhMM+KsS1r+vmD5tNlzeAG6GVxPiUxCmNjIIBofk8PiidgEEBAzCEFXhoUboS61PyFp/cHymfPmiyRA6Hp1qv8GXgdnyKqL2CWgsWbt+nwU/Mx0v2IqiBFLQAY/l8BtQwfdFywHGk8hPgB/gtHXd6UOEhNArF33wjUo+NO54J16jsIDwP8Mjjdw8L1/ONVJ4C1xN4gX30nikHEJaNx4Q9F2rOdemMX80ZSYzmbqm/Vur3njd2n5uRweR2D8SezN4KlYDvxLkuIk8USdCSB6F/XajjXdFUGrj0ctWgtz17ydFNISLoj61yA/GbxTlAT+jVIPHPsl2cyMOpeAjRdfeuV8BM6Hpd2kxUVdUx892Ec8xirqdb3z0qJl8xbqhWyDlwN/CXoTxEeu+HGoVwKIl1/ZyFkzBJyIZIg/SMj2mqDF97q+Z+wbmwYmgT/tKwNLID7j3weEUe8EaGzYuLkAxSLwWmEIIZwULf66nt0TX1flmAQ+5BwE4fy4qxdyTgCxcRP/MCnF9YvbZ+8S2qKTgdNe/Pb31z26X+vchmaCSgLfmw0Qhsw4BPJP5sohPqc/uWlQAjQ2bX6Vx/kZktAPYq9G/VyQqTiCAvf/3lPduxVmPS0JJIFFT/AekMf8AciPNa7tbSBnyVYIT15//ytAQlKkan6DxoHn/QdmVLZzVZokoAAAAABJRU5ErkJggg=='
-
-UDEMY_ICON = b'iVBORw0KGgoAAAANSUhEUgAAAGcAAAAxCAIAAABI9CBEAAATn0lEQVR4nO2aaYxlx3Xf/+ecqvu23peZ7p6tZ4bTnOEMh6S4iKJtSCRlUZIlG7Hs2BIMx5ITJE6gGEn0xUAABfAnG4gRw7DhJIhhB3Zg2I6tSJYsypRDSlxEaTQaDmcjZ++e3vfl9Xv33jrn5ENT0gTqdsABiEDG/D883Fv1bqHqV+fWOXVu0fv/YhZ39TbF/7878EOpu9TuRHep3YnuUrsT3aV2J7pL7U50l9qdKOxUoZ6cxVwIqYKYbdLo6370QrhxuLjwgK93eebBo7GpewYyctu2nZJcHMxMDlX1gKCBiAz6jg3qHdeO1OAucHdrNHnvhI+95r0zEhMfXZED1+n8Qz55MG02kpET5eJVw/bUAtzdCHAnYyHiBBVzML1TY3rntSO1jGJSOTDOR85x35TXV72SQvJUQ6hMpZMtGb0W3jiR5vdaq6IQ3QEazACwG7m7wJHMCSxitsMDPwzakVrB/Ojf0fAN9K4458ktbjZ0ratsNLlzGZ3L2rPBHQvx2tH8yglf69uxISJxIksgdhHS0ihYMmX8Q7Q1Ub/v26CcaqTrdaz34eZBXRpC16rteZOGppydeud0X/CFIWl2+04QhBiUnDg117Rsl3lBtRAbPQiVd2pM77x2pOaELA955ssdGB/FrRMyM9JWwhylyf10/Du8a1LqK0lKJhNwG769O3ZNLkLwxYvfaF58qcxbtQPHdj/6AekdeccG9Y5rR2rBKW/Ywoi+/mCa2+95rc1kTlwkK3bZNz4gIzd87JRHAyS5gnaOYQzMpKvXzsx89U+KzbXuh3686553Vf5BUkuSvv6kzI1ZXg9OidWd2BAju2qpQaZGfWq43L1c2YzIJdU827YdZia4k4tBHHBkTvGHeE0D/p4o16x982Rq1ZKWyUvXTATu5IWXIkKuCUkymhosN3ZZ3bJSU0nuAQwxuFKAJxLetGayEh7gqeAIBCFVYkVZoG0cSViROxRMAZSjbGvOiI7ASgAMJK5tzw1ODjZnRGjw4AHU9hbQtpAlBpu7ewCRBGdNDGUzSm6iFIjInUot1JNRYspc4WROzCCQFjBFCUApvkVHtl98d6QWqMeD5cKeEUngUp3ApkRmcFN2p5LEY8wtGTwTYiMqU9I2KQnIAC+pSl1OQkRCTuRE2rIENQYHVMBkWggqasidcxRVlowbJZeiWlLhkoE5d6uhxuQJyYmSKgmBuQ1UuQLUQ5GLpZyjhNpmKopkpIhlEq+glMgJlhwKVop1kQo53Ioo5O7syeCle4AIKmamZkxOJFo2t4ezEzXVtHjqCyaVzLmx73g2OAKSwFx4SUy2cmvz1ptaJu7orgzulb4RI2J3h5St1fbEm8sTF3T5lnMt69k9cPAE9h9PqWDN1b1CkYgCxQKopLztli+Ml+OXFm+eS0ur5KV39vQeebDj4LFK7zCjMFPmrL0yu3bzImkiotrYw7SxvHbj9eLWdXetjBzuHHswDgxl4NbarfzypfXxy+20GGO1uvdE1/77daBPXFYvvtxurUdHbWBURkZjDPBA5GqJOIiTrS40Jy4W+QbHmIYOV/v2McvbowYurv/pb2sCER342KcH+j4UUVUrhYMrli+fGf/yH5Sry409hw9+8J9k/fsE7qTN89+c/OYXl984lW8uU6ul0JDFhb59A2OPtRZmCg4gJLh5Kr0kjWXRXjjzwsI3Prc2cUk3lvNmK2O3am3+W8/17B8dfuqXuo88xNUqYCsTb078z/+EjZUU4shTH29efGV5/Fx7czOYVhs9tcPv2vv0T4eQTf7vP1u9caG9PMepiJWq1Hs6j75n/0/8Eg0cnD313NrFb5aba30n3jvyU7/Cg8MKh7oxR1NyWzz/0vizf9BaXakNDhz6hc/WxcEVePE2qBHi+o1zQAB5aq4wEYOcncAQTvlmc/JquTQllqfWunrysr1y/dzE5393+eJL1toEKTwAKRG1F2+tXnmFG7sqeZ4cJEG44i6amkuvfu7a//qv7akrrDnHeqWzw5lobTVfnp29+XprYf7wx36189gTUpFYNPPpm63lmRh96kvrm/PTTCXMCrO0slyuzF1emQwcl86/ymnDGGwhNwuYWJ+/WabW4V/8bG3P4blXvtCeG2dI38n3NPoHg4iBPAinVKwtzp19fu3it0Qi+gd7hg9BRN22NbadqQlcAAXcyT2EUJiJs1MiEFMQkZLggUsnccsXZ6Y/97uLp58nb9fq3TK4P3b3x1o9X1/XjcWN6Yu6shAARXBNSm1L5cbNczf+8j+2piY8SLb/eM/+Y42RA0ZoTt5YvXq2mLm2funlG5/3g127Og7cU6TSvQVKZYLPXqvuOVobHEaSYv5qc+Zaq1m0zn4tc3Cj2nHg0Y7e3c12a3PyDVua8Y3W4stf3ve+n+u99/GZ7qG0OJMvTiy/9rXuk09KhQFxLUpUVsffaF45A7PY0zX4yAel2qWaExmwDbcdqZVlDgczYA64u6uV4MgwuMMSuW7RdbBurq6ce3n+1Ffghhjq9z02+qF/3n3Pca/25htL5eVTl/7y9zeuvJwAMgAcUUlrs3N/+6fNqQkGN/Yd2ffznxk8/iQ36tGotbG6fOa5K//t37fWljZeP730xoude0aIMw1VEMRD3D129JO/3nHPCWeaf+XZa3/+W8XsBCgVLIPHnhz9+GcaI/ekjbWZF/74yl/9HjWbnq8unn/x4DO/3D32YGvyctFcXr9xsZwbzw6MAUQeqMwXzryYz004SxjcN/TQ++ClSDQtt93z7OhDq9IpBvNk7EamyesUGIlQAaCqZVkSBZgIKF+dnnv5S25GsJ5DDx35hV/rfeC90uhWTvXOXV0PPXPiX/1GZWg/U3QiwIqU2+Li6vmvg0iZRj/6b/uPvCdUstRsp9yyLPTd/6OdT3w0xkbpefPsK625KWgRNYkBEoae+Vhj7P6s1lPNegdP/Ojuhz9ISCAw9PA//nede46jUm30DQ2efKp39DgTK1k+N67JBx58umPkMBDa85MLZ18ozM2gxPnizfzK2bK1Fur1vkP314cPJXhyI98+8tjZ1rCiCOTJEYgEktqgClc20wqkwyWFENQSAgW4b6SVmdedjQx7nv6n1Z69RoVQvZZSkzYJqI6M7Xv8H41/5Q/z5mpkE64sr1xvLt2CO4stvfrlubPPZ4GCVDatcFcxaU1eUkoAp8UZX11xmBIpIIKBe38scEMdzPBavTYw4iREnu06GPqHSDiYl1Cr9mS7Duj5bwTPvCzU8s5jT9DwPlw+XazMLJz68p5nPsVMAK+cf3lt7gKAOHyo77GPhEQqIHKWYL5NHnBnH4qMKRkRjMicjQmsZA3qcHiwTAkOoGy1JXFat831YEhAx+jBUO0wZ7NSzepULYTZrb73qGU1NNeTuhet1G5BA6BmPnv6i84RjASuJtNI6kxoI+UAynxjM9/8wf4ROQAiuq2Evvv7VtVWiftbt8w8PPbIxpun84Wb+fzMxqVTXQ+82/Lm9CvP5qtrYO46cF/fgaMlBxZoSm45becO/p7IIzMwIEJEDqPIFIDCJUtWFixEBOIQKqwcJUPSxBHm5jlCNIYkoiBKBZGzS7E1DIdQVpJXitw9d2ZIxoNDMXZRaqcItkwoEczdyIWIGrsOVju685X2tt2k7+v74G6v2rreAsfMjXsf7Trzwvzczdbq7PSrn+88+djGm6+V05eoLKuDI/33PS5du0szKo2D7JTI2TnnoaUwmZYAkrbZS4KakbGJU8xbSMqwQs1DJQZUOwbbixMO5JPX8t1jWUcHKDpSNAHM3dtTlyxvBgIzB8nQtRsCmGUxHvzIpzv6B00qxsk9C55KZ+IQA6uWoVqT3Qfzle+frLgdxw9iIiL32+0O7u7uW1W1oUN9Yw8vnH8JxebK618vFmanX/5i2twgou7DD3YfftDgwmbGCoBYtsvs75zzAEu9T9cXlaw5c721NFMZPMBKpQNJm1fPa6vpQMhqUqto1lkfGW0tTDhlsy/9TWP0RKodJQE5KdVTuVROvzH77edtYx0shZVMRF29tZ6RzaW5Ml+vhWrt2OPc1RM1JUAcBMmb65yxh6wSKl56079H5/sezLdbdG6H+IMlUqt3Hnq4PnS4deNsuTQ9+/U/Xz3zQru5ESq13qOPhf49MCVhCTFp+wdb+H9QU4mNA/eVV095u7ly7tWlobHeR58Otc4iX0u3rsy89lVKhRNV+4Zrnf3SOdT94NPLF15V8/nXXpBde4Z/5KezwYEQujbb4+2Fq9Nf/ePW9HWBJzOHKqHS1dU59njxzS+Zp2tf+cPRns6OA8fR6BaRslVifXbxzdPc6OgePZr690SpE9HtKTxi9x16vmVZIPLt/uLu1T339B979/j1s1qU08/+UTk/C0qd+492HHqAKw24l45IEDhBfbswY0dquRVDDz/VnrrUauWtW1dvfeH3V668Uttzb7E0s/Ct54qVWRBRtd419q5a/6hk3v/ujy6+/Fdr117TpJPP/fe1N0933nO80hhI81PTV8+mqUsAEkAwMY6xUuvbv/f9n1i+8GJaTatXv/3GH3124OSHOg4fi7V6a3566cLXVk8/b1lt73t/dv+HPykj9323X7ePwb536+7YgdHt12bGJNnAYM+Jd0+98LnUXN6cu+WASNZ7//uqw4cDMxwiQTUnsMO2NbYdqTXIO575Z8uXz6bvPFvmG83FyeaLE44vAWCQI1Lwoff8RN8D70e1UlLZMbB77FO/efZ3/qUtTZVFq3X1zObV7ygDngkVEqpuyQG3pISiKKox6z76yMGf+zcTf/Ibm3mrmL45Pfl7Co4IhqRkwQHOrEyJxTzfqZ/bMnJ3+P+F7HvvmkoUWDawr/vQscVzX3OPIOPunt57H896d3NKLoFNS20T10gC6TaTsWOUW1hqV3HkU7+298P/oto3GgIYiGCQGan09B945pfHfurTjdGjCRQMGiw7ct9Dn/nPvY98OFQ6TYKyCAAprLNvz499ZNcTP+n1LiZUJBOIs6Us2//en7/vX/9O1/EfMY5gEFHJhbOBwKP33/up/3Dvxz/Ts+tgxVndghcEg0twBZw5i06goiUEd6EACcIGGIBEqkgRAYAxYM6mJMG8pUQdA0f6HvlxACCI6/DJJ2nvQdGCRFRLg0qoMTNtb2qgHU/9eXCUYG+vr+YLt9qTV9bGL3trAyF27j3UdehE6N8bGl0i0RXCkYCkm8zUXlvO5280Jy625uc8obJruP/Q/egftuY6rS4W2ubO/urQnlDtJVeYe1mUzeXW9M2NyUv5wi12SPdgx9576sOHY/cuzqpbZtJcXdGpC+aiWvYeeQTVyA6LMRbF6vy8Ll6zMlG91nX4MZZIpqAyJW1Nj9vqnMUo9c7GnjFheIyptKCtxTdOv/7rn6DU1lg98av/pefk4416pzqrGwl/106ZsY2t7UgtcQiaC6hNIHPJ83ZrNZFXuGoSQr1BEqEQEJESe4lMrOVQcMUtlc01WCpVqjWuxB7l6CjdEtSE2DNYAnFwgjORqaYytVsolcXUY7XSCNVagik0EAMwSzAyMzBFEhWJToUasUoiAG5FChSl6kaRoKpBaNOUzZxYgsMDsZqZI+jq7PhX/sfUn/1mAes+/tjYr/xWz9AhZkn6Vli39bn2bftQsdxcHBJYwRqq1UatUbIGj2YJQGBSdzMlZtWSObFkaiVciYN09GchVMtSyctkgdxdnMAZJ3M1qURPpltxr7tHiVmjamZEshX0qydiCQjkFuAqosQScoYADrMkEVSYe6wHVzcLpMHdjawlCmSgkgIFp6ROROpqrpHrSmVr+vr8t/66gIEw8MhPdjZ2EUc12wrrvreXoB0c8Y7UmIISuzk7g6UEiB1J1ZUQiMRATk4CZgPIKairGnPI3J08pVQoczT1KAoFgZwMROKBihLCRO5EEOfgDsBIQCKaHACDoArAyQtCSIiQRBXy4IIK5aWXDA2oFSmZGXGWMTlZBZaciNicAmVMKuSc2DnpyvzK7OT6wo2NMy+WUzfAle7de3a/62nv6E7mgDPzlqu9fSv2NqiZIUZTTUnNQSIiJoFqBSdoigBUzWFwV1SEC2tvzY9aTqaBGMYuUiAXo8BR2ZJqBAuxubIrSIgIVoqbwZXFwcEMbswgdlV1d2ERFw0FAygpkRJJ7jmoRlRR23qnPCNPVBiCwdgANmMSmHpp5EQZmDZmbk3+xW+vT1xqbUxT0lDv7nvqFyv9Q0zJPBBhy7jcnUjUFVtfwd8GNdFcnYwYkQFXTa5EVUrskDKIu5obcwC4hBjWSapsFAlmRBwMTqxZqKRkltShIQjciuQBGcvWTBpIDEzkgchdSYmYwO7gINHdDdqmUrxi2Eota4VC0kCQxBy1JABBzMCIgaKzqOZiKu5OCSzODDiSlWtL60sT+dIUZcRdu/uOPHLoA5+0rK6k7EKkIHMoEYMJxltrxduglqFWpsSEwJZIjcTMAvJQyVTJXYWYWQyKYDnymtZFKU+lRnKGwJjM3FCKkARCEWBE4kysbrmgqnAzIyRhJpAZuwYRSqbuRIRkJZEzc0RgY3dXIZjlyClEWPLSkkQ4W2kUzEvlDCl3EzGiCDipe0jukgFGBiVzCrHWu6/niZ85+OFPUK1GsOisW1aGt7wBMbs7WGDbbNp2jjzuamfdPSt5J7pL7U50l9qd6C61O9Fdaneiu9TuRHep3YnuUrsT/R/W77z2m0J2SQAAAABJRU5ErkJggg=='
-
-BLANK_BASE64 = b'iVBORw0KGgoAAAANSUhEUgAAAAEAAAABCAYAAAAfFcSJAAAAAXNSR0IArs4c6QAAAARnQU1BAACxjwv8YQUAAAAJcEhZcwAADsMAAA7DAcdvqGQAAAANSURBVBhXY2BgYGAAAAAFAAGKM+MAAAAAAElFTkSuQmCC'
-BLANK_BASE64 = b'iVBORw0KGgoAAAANSUhEUgAAAAEAAAABCAQAAAC1HAwCAAAAC0lEQVR42mNkYAAAAAYAAjCB0C8AAAAASUVORK5CYII='
-
-
-DEFAULT_WINDOW_ICON = DEFAULT_BASE64_ICON
-
-DEFAULT_ELEMENT_SIZE = (45, 1) # In CHARACTERS
-DEFAULT_BUTTON_ELEMENT_SIZE = (10, 1) # In CHARACTERS
-DEFAULT_MARGINS = (10, 5) # Margins for each LEFT/RIGHT margin is first term
-DEFAULT_ELEMENT_PADDING = (5, 3) # Padding between elements (row, col) in pixels
-DEFAULT_AUTOSIZE_TEXT = True
-DEFAULT_AUTOSIZE_BUTTONS = True
-DEFAULT_FONT = ("Helvetica", 10)
-DEFAULT_TEXT_JUSTIFICATION = 'left'
-DEFAULT_BORDER_WIDTH = 1
-DEFAULT_AUTOCLOSE_TIME = 3 # time in seconds to show an autoclose form
-DEFAULT_DEBUG_WINDOW_SIZE = (80, 20)
-DEFAULT_WINDOW_LOCATION = (None, None)
-MAX_SCROLLED_TEXT_BOX_HEIGHT = 50
-DEFAULT_TOOLTIP_TIME = 400
-DEFAULT_TOOLTIP_OFFSET = (0, -20)
-DEFAULT_KEEP_ON_TOP = None
-DEFAULT_SCALING = None
-DEFAULT_ALPHA_CHANNEL = 1.0
-TOOLTIP_BACKGROUND_COLOR = "#ffffe0"
-TOOLTIP_FONT = None
-#################### COLOR STUFF ####################
-BLUES = ("#082567", "#0A37A3", "#00345B")
-PURPLES = ("#480656", "#4F2398", "#380474")
-GREENS = ("#01826B", "#40A860", "#96D2AB", "#00A949", "#003532")
-YELLOWS = ("#F3FB62", "#F0F595")
-TANS = ("#FFF9D5", "#F4EFCF", "#DDD8BA")
-NICE_BUTTON_COLORS = ((GREENS[3], TANS[0]),
- ('#000000', '#FFFFFF'),
- ('#FFFFFF', '#000000'),
- (YELLOWS[0], PURPLES[1]),
- (YELLOWS[0], GREENS[3]),
- (YELLOWS[0], BLUES[2]))
-
-COLOR_SYSTEM_DEFAULT = '1234567890' # A Magic Number kind of signal to PySimpleGUI that the color should not be set at all
-DEFAULT_BUTTON_COLOR = ('white', BLUES[0]) # Foreground, Background (None, None) == System Default
-OFFICIAL_PYSIMPLEGUI_BUTTON_COLOR = ('white', BLUES[0])
-
-# The "default PySimpleGUI theme"
-OFFICIAL_PYSIMPLEGUI_THEME = CURRENT_LOOK_AND_FEEL = 'Dark Blue 3'
-
-DEFAULT_ERROR_BUTTON_COLOR = ("#FFFFFF", "#FF0000")
-DEFAULT_BACKGROUND_COLOR = None
-DEFAULT_ELEMENT_BACKGROUND_COLOR = None
-DEFAULT_ELEMENT_TEXT_COLOR = COLOR_SYSTEM_DEFAULT
-DEFAULT_TEXT_ELEMENT_BACKGROUND_COLOR = None
-DEFAULT_TEXT_COLOR = COLOR_SYSTEM_DEFAULT
-DEFAULT_INPUT_ELEMENTS_COLOR = COLOR_SYSTEM_DEFAULT
-DEFAULT_INPUT_TEXT_COLOR = COLOR_SYSTEM_DEFAULT
-DEFAULT_SCROLLBAR_COLOR = None
-# DEFAULT_BUTTON_COLOR = (YELLOWS[0], PURPLES[0]) # (Text, Background) or (Color "on", Color) as a way to remember
-# DEFAULT_BUTTON_COLOR = (GREENS[3], TANS[0]) # Foreground, Background (None, None) == System Default
-# DEFAULT_BUTTON_COLOR = (YELLOWS[0], GREENS[4]) # Foreground, Background (None, None) == System Default
-# DEFAULT_BUTTON_COLOR = ('white', 'black') # Foreground, Background (None, None) == System Default
-# DEFAULT_BUTTON_COLOR = (YELLOWS[0], PURPLES[2]) # Foreground, Background (None, None) == System Default
-# DEFAULT_PROGRESS_BAR_COLOR = (GREENS[2], GREENS[0]) # a nice green progress bar
-# DEFAULT_PROGRESS_BAR_COLOR = (BLUES[1], BLUES[1]) # a nice green progress bar
-# DEFAULT_PROGRESS_BAR_COLOR = (BLUES[0], BLUES[0]) # a nice green progress bar
-# DEFAULT_PROGRESS_BAR_COLOR = (PURPLES[1],PURPLES[0]) # a nice purple progress bar
-
-
-# A transparent button is simply one that matches the background
-# TRANSPARENT_BUTTON = 'This constant has been depricated. You must set your button background = background it is on for it to be transparent appearing'
-
-
-# --------------------------------------------------------------------------------
-# Progress Bar Relief Choices
-RELIEF_RAISED = 'raised'
-RELIEF_SUNKEN = 'sunken'
-RELIEF_FLAT = 'flat'
-RELIEF_RIDGE = 'ridge'
-RELIEF_GROOVE = 'groove'
-RELIEF_SOLID = 'solid'
-RELIEF_LIST = (RELIEF_RAISED, RELIEF_FLAT, RELIEF_SUNKEN, RELIEF_RIDGE, RELIEF_SOLID, RELIEF_GROOVE)
-
-# These are the spepific themes that tkinter offers
-THEME_DEFAULT = 'default' # this is a TTK theme, not a PSG theme!!!
-THEME_WINNATIVE = 'winnative'
-THEME_CLAM = 'clam'
-THEME_ALT = 'alt'
-THEME_CLASSIC = 'classic'
-THEME_VISTA = 'vista'
-THEME_XPNATIVE = 'xpnative'
-
-# The theme to use by default for all windows
-DEFAULT_TTK_THEME = THEME_DEFAULT
-ttk_theme_in_use = None
-# TTK_THEME_LIST = ('default', 'winnative', 'clam', 'alt', 'classic', 'vista', 'xpnative')
-
-
-USE_TTK_BUTTONS = None
-
-DEFAULT_PROGRESS_BAR_COLOR = ("#01826B", '#D0D0D0') # a nice green progress bar
-DEFAULT_PROGRESS_BAR_COMPUTE = ('#000000', '#000000') # Means that the progress bar colors should be computed from other colors
-DEFAULT_PROGRESS_BAR_COLOR_OFFICIAL = ("#01826B", '#D0D0D0') # a nice green progress bar
-DEFAULT_PROGRESS_BAR_SIZE = (20, 20) # Size of Progress Bar (characters for length, pixels for width)
-DEFAULT_PROGRESS_BAR_BORDER_WIDTH = 1
-DEFAULT_PROGRESS_BAR_RELIEF = RELIEF_GROOVE
-# PROGRESS_BAR_STYLES = ('default', 'winnative', 'clam', 'alt', 'classic', 'vista', 'xpnative')
-DEFAULT_PROGRESS_BAR_STYLE = DEFAULT_TTK_THEME
-DEFAULT_METER_ORIENTATION = 'Horizontal'
-DEFAULT_SLIDER_ORIENTATION = 'vertical'
-DEFAULT_SLIDER_BORDER_WIDTH = 1
-DEFAULT_SLIDER_RELIEF = tk.FLAT
-DEFAULT_FRAME_RELIEF = tk.GROOVE
-
-DEFAULT_LISTBOX_SELECT_MODE = tk.SINGLE
-SELECT_MODE_MULTIPLE = tk.MULTIPLE
-LISTBOX_SELECT_MODE_MULTIPLE = 'multiple'
-SELECT_MODE_BROWSE = tk.BROWSE
-LISTBOX_SELECT_MODE_BROWSE = 'browse'
-SELECT_MODE_EXTENDED = tk.EXTENDED
-LISTBOX_SELECT_MODE_EXTENDED = 'extended'
-SELECT_MODE_SINGLE = tk.SINGLE
-LISTBOX_SELECT_MODE_SINGLE = 'single'
-
-TABLE_SELECT_MODE_NONE = tk.NONE
-TABLE_SELECT_MODE_BROWSE = tk.BROWSE
-TABLE_SELECT_MODE_EXTENDED = tk.EXTENDED
-DEFAULT_TABLE_SELECT_MODE = TABLE_SELECT_MODE_EXTENDED
-TABLE_CLICKED_INDICATOR = '+CLICKED+' # Part of the tuple returned as an event when a Table element has click events enabled
-DEFAULT_MODAL_WINDOWS_ENABLED = True
-DEFAULT_MODAL_WINDOWS_FORCED = False
-
-TAB_LOCATION_TOP = 'top'
-TAB_LOCATION_TOP_LEFT = 'topleft'
-TAB_LOCATION_TOP_RIGHT = 'topright'
-TAB_LOCATION_LEFT = 'left'
-TAB_LOCATION_LEFT_TOP = 'lefttop'
-TAB_LOCATION_LEFT_BOTTOM = 'leftbottom'
-TAB_LOCATION_RIGHT = 'right'
-TAB_LOCATION_RIGHT_TOP = 'righttop'
-TAB_LOCATION_RIGHT_BOTTOM = 'rightbottom'
-TAB_LOCATION_BOTTOM = 'bottom'
-TAB_LOCATION_BOTTOM_LEFT = 'bottomleft'
-TAB_LOCATION_BOTTOM_RIGHT = 'bottomright'
-
-
-TITLE_LOCATION_TOP = tk.N
-TITLE_LOCATION_BOTTOM = tk.S
-TITLE_LOCATION_LEFT = tk.W
-TITLE_LOCATION_RIGHT = tk.E
-TITLE_LOCATION_TOP_LEFT = tk.NW
-TITLE_LOCATION_TOP_RIGHT = tk.NE
-TITLE_LOCATION_BOTTOM_LEFT = tk.SW
-TITLE_LOCATION_BOTTOM_RIGHT = tk.SE
-
-TEXT_LOCATION_TOP = tk.N
-TEXT_LOCATION_BOTTOM = tk.S
-TEXT_LOCATION_LEFT = tk.W
-TEXT_LOCATION_RIGHT = tk.E
-TEXT_LOCATION_TOP_LEFT = tk.NW
-TEXT_LOCATION_TOP_RIGHT = tk.NE
-TEXT_LOCATION_BOTTOM_LEFT = tk.SW
-TEXT_LOCATION_BOTTOM_RIGHT = tk.SE
-TEXT_LOCATION_CENTER = tk.CENTER
-
-GRAB_ANYWHERE_IGNORE_THESE_WIDGETS = (ttk.Sizegrip, tk.Scale, ttk.Scrollbar, tk.Scrollbar, tk.Entry, tk.Text, tk.PanedWindow, tk.Listbox, tk.OptionMenu, ttk.Treeview)
-
-# ----====----====----==== Constants the user should NOT f-with ====----====----====----#
-ThisRow = 555666777 # magic number
-
-# DEFAULT_WINDOW_ICON = ''
-MESSAGE_BOX_LINE_WIDTH = 60
-
-# "Special" Key Values.. reserved
-# Key representing a Read timeout
-EVENT_TIMEOUT = TIMEOUT_EVENT = TIMEOUT_KEY = '__TIMEOUT__'
-WIN_CLOSED = WINDOW_CLOSED = None
-WINDOW_CLOSE_ATTEMPTED_EVENT = WIN_X_EVENT = WIN_CLOSE_ATTEMPTED_EVENT = '-WINDOW CLOSE ATTEMPTED-'
-
-TITLEBAR_MINIMIZE_KEY = '__TITLEBAR MINIMIZE__'
-TITLEBAR_MAXIMIZE_KEY = '__TITLEBAR MAXIMIZE__'
-TITLEBAR_CLOSE_KEY = '__TITLEBAR CLOSE__'
-TITLEBAR_IMAGE_KEY = '__TITLEBAR IMAGE__'
-TITLEBAR_DO_NOT_USE_AN_ICON = '__TITLEBAR_NO_ICON__'
-
-# Key indicating should not create any return values for element
-WRITE_ONLY_KEY = '__WRITE ONLY__'
-
-MENU_DISABLED_CHARACTER = '!'
-MENU_SHORTCUT_CHARACTER = '&'
-MENU_KEY_SEPARATOR = '::'
-MENU_SEPARATOR_LINE = '---'
-MENU_RIGHT_CLICK_EDITME_EXIT = ['', ['Edit Me', 'Exit']]
-MENU_RIGHT_CLICK_EDITME_VER_EXIT = ['', ['Edit Me', 'Version', 'Exit']]
-MENU_RIGHT_CLICK_EDITME_VER_LOC_EXIT = ['', ['Edit Me', 'Version', 'File Location', 'Exit']]
-MENU_RIGHT_CLICK_EDITME_VER_SETTINGS_EXIT = ['', ['Edit Me', 'Settings', 'Version', 'Exit']]
-MENU_RIGHT_CLICK_EXIT = ['', ['Exit']]
-MENU_RIGHT_CLICK_DISABLED = ['', []]
-_MENU_RIGHT_CLICK_TABGROUP_DEFAULT = ['TABGROUP DEFAULT', []]
-ENABLE_TK_WINDOWS = False
-
-USE_CUSTOM_TITLEBAR = None
-CUSTOM_TITLEBAR_BACKGROUND_COLOR = None
-CUSTOM_TITLEBAR_TEXT_COLOR = None
-CUSTOM_TITLEBAR_ICON = None
-CUSTOM_TITLEBAR_FONT = None
-TITLEBAR_METADATA_MARKER = 'This window has a titlebar'
-
-CUSTOM_MENUBAR_METADATA_MARKER = 'This is a custom menubar'
-
-SUPPRESS_ERROR_POPUPS = False
-SUPPRESS_RAISE_KEY_ERRORS = True
-SUPPRESS_KEY_GUESSING = False
-SUPPRESS_WIDGET_NOT_FINALIZED_WARNINGS = False
-ENABLE_TREEVIEW_869_PATCH = True
-
-# These are now set based on the global settings file
-ENABLE_MAC_NOTITLEBAR_PATCH = False
-ENABLE_MAC_MODAL_DISABLE_PATCH = False
-ENABLE_MAC_DISABLE_GRAB_ANYWHERE_WITH_TITLEBAR = True
-ENABLE_MAC_ALPHA_99_PATCH= False
-
-OLD_TABLE_TREE_SELECTED_ROW_COLORS = ('#FFFFFF', '#4A6984')
-ALTERNATE_TABLE_AND_TREE_SELECTED_ROW_COLORS = ('SystemHighlightText', 'SystemHighlight')
-
-# Some handy unicode symbols
-SYMBOL_SQUARE = '█'
-SYMBOL_CIRCLE = '⚫'
-SYMBOL_CIRCLE_OUTLINE = '◯'
-SYMBOL_UP = '▲'
-SYMBOL_RIGHT = '►'
-SYMBOL_LEFT = '◄'
-SYMBOL_DOWN = '▼'
-SYMBOL_X = '❎'
-SYMBOL_CHECK = '✅'
-SYMBOL_CHECK_SMALL = '✓'
-SYMBOL_X_SMALL = '✗'
-SYMBOL_BALLOT_X = '☒'
-SYMBOL_BALLOT_CHECK = '☑'
-SYMBOL_LEFT_DOUBLE = '«'
-SYMBOL_RIGHT_DOUBLE = '»'
-SYMBOL_LEFT_ARROWHEAD = '⮜'
-SYMBOL_RIGHT_ARROWHEAD = '⮞'
-SYMBOL_UP_ARROWHEAD = '⮝'
-SYMBOL_DOWN_ARROWHEAD = '⮟'
-
-if sum([int(i) for i in tclversion_detailed.split('.')]) > 19:
- SYMBOL_TITLEBAR_MINIMIZE = '_'
- SYMBOL_TITLEBAR_MAXIMIZE = '◻'
- SYMBOL_TITLEBAR_CLOSE = 'X'
-else:
- SYMBOL_TITLEBAR_MINIMIZE = '_'
- SYMBOL_TITLEBAR_MAXIMIZE = 'O'
- SYMBOL_TITLEBAR_CLOSE = 'X'
-
-#################### PATHS for user_settings APIs ####################
-# These paths are passed to os.path.expanduser to get the default path for user_settings
-# They can be changed using set_options
-
-DEFAULT_USER_SETTINGS_WIN_PATH = r'~\AppData\Local\PySimpleGUI\settings'
-DEFAULT_USER_SETTINGS_LINUX_PATH = r'~/.config/PySimpleGUI/settings'
-DEFAULT_USER_SETTINGS_MAC_PATH = r'~/Library/Application Support/PySimpleGUI/settings'
-DEFAULT_USER_SETTINGS_TRINKET_PATH = r'.'
-DEFAULT_USER_SETTINGS_REPLIT_PATH = r'.'
-DEFAULT_USER_SETTINGS_UNKNOWN_OS_PATH = r'~/Library/Application Support/PySimpleGUI/settings'
-DEFAULT_USER_SETTINGS_PATH = None # value set by user to override all paths above
-DEFAULT_USER_SETTINGS_PYSIMPLEGUI_PATH = None # location of the global PySimpleGUI settings
-DEFAULT_USER_SETTINGS_PYSIMPLEGUI_FILENAME = '_PySimpleGUI_settings_global_.json' # location of the global PySimpleGUI settings
-
-
-# ====================================================================== #
-# One-liner functions that are handy as f_ck #
-# ====================================================================== #
-def rgb(red, green, blue):
- """
- Given integer values of Red, Green, Blue, return a color string "#RRGGBB"
- :param red: Red portion from 0 to 255
- :type red: (int)
- :param green: Green portion from 0 to 255
- :type green: (int)
- :param blue: Blue portion from 0 to 255
- :type blue: (int)
- :return: A single RGB String in the format "#RRGGBB" where each pair is a hex number.
- :rtype: (str)
- """
- red = min(int(red), 255) if red > 0 else 0
- blue = min(int(blue), 255) if blue > 0 else 0
- green = min(int(green), 255) if green > 0 else 0
- return '#%02x%02x%02x' % (red, green, blue)
-
-
-# ====================================================================== #
-# Enums for types #
-# ====================================================================== #
-# ------------------------- Button types ------------------------- #
-# uncomment this line and indent to go back to using Enums
-BUTTON_TYPE_BROWSE_FOLDER = 1
-BUTTON_TYPE_BROWSE_FILE = 2
-BUTTON_TYPE_BROWSE_FILES = 21
-BUTTON_TYPE_SAVEAS_FILE = 3
-BUTTON_TYPE_CLOSES_WIN = 5
-BUTTON_TYPE_CLOSES_WIN_ONLY = 6
-BUTTON_TYPE_READ_FORM = 7
-BUTTON_TYPE_REALTIME = 9
-BUTTON_TYPE_CALENDAR_CHOOSER = 30
-BUTTON_TYPE_COLOR_CHOOSER = 40
-BUTTON_TYPE_SHOW_DEBUGGER = 50
-
-BROWSE_FILES_DELIMITER = ';' # the delimiter to be used between each file in the returned string
-
-FILE_TYPES_ALL_FILES = (("ALL Files", "*.* *"),)
-
-BUTTON_DISABLED_MEANS_IGNORE = 'ignore'
-
-# ------------------------- Element types ------------------------- #
-
-ELEM_TYPE_TEXT = 'text'
-ELEM_TYPE_INPUT_TEXT = 'input'
-ELEM_TYPE_INPUT_COMBO = 'combo'
-ELEM_TYPE_INPUT_OPTION_MENU = 'option menu'
-ELEM_TYPE_INPUT_RADIO = 'radio'
-ELEM_TYPE_INPUT_MULTILINE = 'multiline'
-ELEM_TYPE_INPUT_CHECKBOX = 'checkbox'
-ELEM_TYPE_INPUT_SPIN = 'spind'
-ELEM_TYPE_BUTTON = 'button'
-ELEM_TYPE_IMAGE = 'image'
-ELEM_TYPE_CANVAS = 'canvas'
-ELEM_TYPE_FRAME = 'frame'
-ELEM_TYPE_GRAPH = 'graph'
-ELEM_TYPE_TAB = 'tab'
-ELEM_TYPE_TAB_GROUP = 'tabgroup'
-ELEM_TYPE_INPUT_SLIDER = 'slider'
-ELEM_TYPE_INPUT_LISTBOX = 'listbox'
-ELEM_TYPE_OUTPUT = 'output'
-ELEM_TYPE_COLUMN = 'column'
-ELEM_TYPE_MENUBAR = 'menubar'
-ELEM_TYPE_PROGRESS_BAR = 'progressbar'
-ELEM_TYPE_BLANK = 'blank'
-ELEM_TYPE_TABLE = 'table'
-ELEM_TYPE_TREE = 'tree'
-ELEM_TYPE_ERROR = 'error'
-ELEM_TYPE_SEPARATOR = 'separator'
-ELEM_TYPE_STATUSBAR = 'statusbar'
-ELEM_TYPE_PANE = 'pane'
-ELEM_TYPE_BUTTONMENU = 'buttonmenu'
-ELEM_TYPE_TITLEBAR = 'titlebar'
-ELEM_TYPE_SIZEGRIP = 'sizegrip'
-
-# STRETCH == ERROR ELEMENT as a filler
-
-# ------------------------- Popup Buttons Types ------------------------- #
-POPUP_BUTTONS_YES_NO = 1
-POPUP_BUTTONS_CANCELLED = 2
-POPUP_BUTTONS_ERROR = 3
-POPUP_BUTTONS_OK_CANCEL = 4
-POPUP_BUTTONS_OK = 0
-POPUP_BUTTONS_NO_BUTTONS = 5
-
-
-
-PSG_THEME_PART_BUTTON_TEXT = 'Button Text Color'
-PSG_THEME_PART_BUTTON_BACKGROUND = 'Button Background Color'
-PSG_THEME_PART_BACKGROUND = 'Background Color'
-PSG_THEME_PART_INPUT_BACKGROUND = 'Input Element Background Color'
-PSG_THEME_PART_INPUT_TEXT = 'Input Element Text Color'
-PSG_THEME_PART_TEXT = 'Text Color'
-PSG_THEME_PART_SLIDER = 'Slider Color'
-PSG_THEME_PART_LIST = [PSG_THEME_PART_BACKGROUND, PSG_THEME_PART_BUTTON_BACKGROUND, PSG_THEME_PART_BUTTON_TEXT,PSG_THEME_PART_INPUT_BACKGROUND, PSG_THEME_PART_INPUT_TEXT, PSG_THEME_PART_TEXT, PSG_THEME_PART_SLIDER ]
-
-# theme_button
-
-TTK_SCROLLBAR_PART_TROUGH_COLOR = 'Trough Color'
-TTK_SCROLLBAR_PART_BACKGROUND_COLOR = 'Background Color'
-TTK_SCROLLBAR_PART_ARROW_BUTTON_ARROW_COLOR = 'Arrow Button Arrow Color'
-TTK_SCROLLBAR_PART_FRAME_COLOR = 'Frame Color'
-TTK_SCROLLBAR_PART_SCROLL_WIDTH = 'Frame Width'
-TTK_SCROLLBAR_PART_ARROW_WIDTH = 'Arrow Width'
-TTK_SCROLLBAR_PART_RELIEF = 'Relief'
-TTK_SCROLLBAR_PART_LIST = [TTK_SCROLLBAR_PART_TROUGH_COLOR, TTK_SCROLLBAR_PART_BACKGROUND_COLOR, TTK_SCROLLBAR_PART_ARROW_BUTTON_ARROW_COLOR,
- TTK_SCROLLBAR_PART_FRAME_COLOR, TTK_SCROLLBAR_PART_SCROLL_WIDTH, TTK_SCROLLBAR_PART_ARROW_WIDTH, TTK_SCROLLBAR_PART_RELIEF]
-TTK_SCROLLBAR_PART_THEME_BASED_LIST = [TTK_SCROLLBAR_PART_TROUGH_COLOR, TTK_SCROLLBAR_PART_BACKGROUND_COLOR, TTK_SCROLLBAR_PART_ARROW_BUTTON_ARROW_COLOR,
- TTK_SCROLLBAR_PART_FRAME_COLOR]
-DEFAULT_TTK_PART_MAPPING_DICT = {TTK_SCROLLBAR_PART_TROUGH_COLOR: PSG_THEME_PART_SLIDER,
- TTK_SCROLLBAR_PART_BACKGROUND_COLOR : PSG_THEME_PART_BUTTON_BACKGROUND,
- TTK_SCROLLBAR_PART_ARROW_BUTTON_ARROW_COLOR :PSG_THEME_PART_BUTTON_TEXT,
- TTK_SCROLLBAR_PART_FRAME_COLOR : PSG_THEME_PART_BACKGROUND,
- TTK_SCROLLBAR_PART_SCROLL_WIDTH : 12,
- TTK_SCROLLBAR_PART_ARROW_WIDTH: 12,
- TTK_SCROLLBAR_PART_RELIEF: RELIEF_RAISED}
-
-ttk_part_mapping_dict = copy.copy(DEFAULT_TTK_PART_MAPPING_DICT)
-
-class TTKPartOverrides():
- """
- This class contains "overrides" to the defaults for ttk scrollbars that are defined in the global settings file.
- This class is used in every element, in the Window class and there's a global one that is used by set_options.
- """
- def __init__(self, sbar_trough_color=None, sbar_background_color=None, sbar_arrow_color=None, sbar_width=None, sbar_arrow_width=None, sbar_frame_color=None, sbar_relief=None):
- self.sbar_trough_color = sbar_trough_color
- self.sbar_background_color = sbar_background_color
- self.sbar_arrow_color = sbar_arrow_color
- self.sbar_width = sbar_width
- self.sbar_arrow_width = sbar_arrow_width
- self.sbar_frame_color = sbar_frame_color
- self.sbar_relief = sbar_relief
-
-ttk_part_overrides_from_options = TTKPartOverrides()
-
-# ------------------------- tkinter key codes for bindings ------------------------- #
-
-# The keycode that when pressed will take a snapshot of the current window
-DEFAULT_WINDOW_SNAPSHOT_KEY_CODE = None
-DEFAULT_WINDOW_SNAPSHOT_KEY = '--SCREENSHOT THIS WINDOW--'
-
-tkinter_keysyms = ('space', 'exclam', 'quotedbl', 'numbersign', 'dollar', 'percent', 'ampersand', 'quoteright', 'parenleft', 'parenright', 'asterisk', 'plus', 'comma', 'minus', 'period', 'slash', '0', '1', '2', '3', '4', '5', '6', '7', '8', '9', 'colon', 'semicolon', 'less', 'equal', 'greater', 'question', 'at', 'A', 'B', 'C', 'D', 'E', 'F', 'G', 'H', 'I', 'J', 'K', 'L', 'M', 'N', 'O', 'P', 'Q', 'R', 'S', 'T', 'U', 'V', 'W', 'X', 'Y', 'Z', 'bracketleft', 'backslash', 'bracketright', 'asciicircum', 'underscore', 'quoteleft', 'a', 'b', 'c', 'd', 'e', 'f', 'g', 'h', 'i', 'j', 'k', 'l', 'm', 'n', 'o', 'p', 'q', 'r', 's', 't', 'u', 'v', 'w', 'x', 'y', 'z', 'braceleft', 'bar', 'braceright', 'asciitilde', 'nobreakspace', 'exclamdown', 'cent', 'sterling', 'currency', 'yen', 'brokenbar', 'section', 'diaeresis', 'copyright', 'ordfeminine', 'guillemotleft', 'notsign', 'hyphen', 'registered', 'macron', 'degree', 'plusminus', 'twosuperior', 'threesuperior', 'acute', 'mu', 'paragraph', 'periodcentered', 'cedilla', 'onesuperior', 'masculine', 'guillemotright', 'onequarter', 'onehalf', 'threequarters', 'questiondown', 'Agrave', 'Aacute', 'Acircumflex', 'Atilde', 'Adiaeresis', 'Aring', 'AE', 'Ccedilla', 'Egrave', 'Eacute', 'Ecircumflex', 'Ediaeresis', 'Igrave', 'Iacute', 'Icircumflex', 'Idiaeresis', 'Eth', 'Ntilde', 'Ograve', 'Oacute', 'Ocircumflex', 'Otilde', 'Odiaeresis', 'multiply', 'Ooblique', 'Ugrave', 'Uacute', 'Ucircumflex', 'Udiaeresis', 'Yacute', 'Thorn', 'ssharp', 'agrave', 'aacute', 'acircumflex', 'atilde', 'adiaeresis', 'aring', 'ae', 'ccedilla', 'egrave', 'eacute', 'ecircumflex', 'ediaeresis', 'igrave', 'iacute', 'icircumflex', 'idiaeresis', 'eth', 'ntilde', 'ograve', 'oacute', 'ocircumflex', 'otilde', 'odiaeresis', 'division', 'oslash', 'ugrave', 'uacute', 'ucircumflex', 'udiaeresis', 'yacute', 'thorn', 'ydiaeresis', 'Aogonek', 'breve', 'Lstroke', 'Lcaron', 'Sacute', 'Scaron', 'Scedilla', 'Tcaron', 'Zacute', 'Zcaron', 'Zabovedot', 'aogonek', 'ogonek', 'lstroke', 'lcaron', 'sacute', 'caron', 'scaron', 'scedilla', 'tcaron', 'zacute', 'doubleacute', 'zcaron', 'zabovedot', 'Racute', 'Abreve', 'Cacute', 'Ccaron', 'Eogonek', 'Ecaron', 'Dcaron', 'Nacute', 'Ncaron', 'Odoubleacute', 'Rcaron', 'Uring', 'Udoubleacute', 'Tcedilla', 'racute', 'abreve', 'cacute', 'ccaron', 'eogonek', 'ecaron', 'dcaron', 'nacute', 'ncaron', 'odoubleacute', 'rcaron', 'uring', 'udoubleacute', 'tcedilla', 'abovedot', 'Hstroke', 'Hcircumflex', 'Iabovedot', 'Gbreve', 'Jcircumflex', 'hstroke', 'hcircumflex', 'idotless', 'gbreve', 'jcircumflex', 'Cabovedot', 'Ccircumflex', 'Gabovedot', 'Gcircumflex', 'Ubreve', 'Scircumflex', 'cabovedot', 'ccircumflex', 'gabovedot', 'gcircumflex', 'ubreve', 'scircumflex', 'kappa', 'Rcedilla', 'Itilde', 'Lcedilla', 'Emacron', 'Gcedilla', 'Tslash', 'rcedilla', 'itilde', 'lcedilla', 'emacron', 'gacute', 'tslash', 'ENG', 'eng', 'Amacron', 'Iogonek', 'Eabovedot', 'Imacron', 'Ncedilla', 'Omacron', 'Kcedilla', 'Uogonek', 'Utilde', 'Umacron', 'amacron', 'iogonek', 'eabovedot', 'imacron', 'ncedilla', 'omacron', 'kcedilla', 'uogonek', 'utilde', 'umacron', 'overline', 'kana_fullstop', 'kana_openingbracket', 'kana_closingbracket', 'kana_comma', 'kana_middledot', 'kana_WO', 'kana_a', 'kana_i', 'kana_u', 'kana_e', 'kana_o', 'kana_ya', 'kana_yu', 'kana_yo', 'kana_tu', 'prolongedsound', 'kana_A', 'kana_I', 'kana_U', 'kana_E', 'kana_O', 'kana_KA', 'kana_KI', 'kana_KU', 'kana_KE', 'kana_KO', 'kana_SA', 'kana_SHI', 'kana_SU', 'kana_SE', 'kana_SO', 'kana_TA', 'kana_TI', 'kana_TU', 'kana_TE', 'kana_TO', 'kana_NA', 'kana_NI', 'kana_NU', 'kana_NE', 'kana_NO', 'kana_HA', 'kana_HI', 'kana_HU', 'kana_HE', 'kana_HO', 'kana_MA', 'kana_MI', 'kana_MU', 'kana_ME', 'kana_MO', 'kana_YA', 'kana_YU', 'kana_YO', 'kana_RA', 'kana_RI', 'kana_RU', 'kana_RE', 'kana_RO', 'kana_WA', 'kana_N', 'voicedsound', 'semivoicedsound', 'Arabic_comma', 'Arabic_semicolon', 'Arabic_question_mark', 'Arabic_hamza', 'Arabic_maddaonalef', 'Arabic_hamzaonalef', 'Arabic_hamzaonwaw', 'Arabic_hamzaunderalef', 'Arabic_hamzaonyeh', 'Arabic_alef', 'Arabic_beh', 'Arabic_tehmarbuta', 'Arabic_teh', 'Arabic_theh', 'Arabic_jeem', 'Arabic_hah', 'Arabic_khah', 'Arabic_dal', 'Arabic_thal', 'Arabic_ra', 'Arabic_zain', 'Arabic_seen', 'Arabic_sheen', 'Arabic_sad', 'Arabic_dad', 'Arabic_tah', 'Arabic_zah', 'Arabic_ain', 'Arabic_ghain', 'Arabic_tatweel', 'Arabic_feh', 'Arabic_qaf', 'Arabic_kaf', 'Arabic_lam', 'Arabic_meem', 'Arabic_noon', 'Arabic_heh', 'Arabic_waw', 'Arabic_alefmaksura', 'Arabic_yeh', 'Arabic_fathatan', 'Arabic_dammatan', 'Arabic_kasratan', 'Arabic_fatha', 'Arabic_damma', 'Arabic_kasra', 'Arabic_shadda', 'Arabic_sukun', 'Serbian_dje', 'Macedonia_gje', 'Cyrillic_io', 'Ukranian_je', 'Macedonia_dse', 'Ukranian_i', 'Ukranian_yi', 'Serbian_je', 'Serbian_lje', 'Serbian_nje', 'Serbian_tshe', 'Macedonia_kje', 'Byelorussian_shortu', 'Serbian_dze', 'numerosign', 'Serbian_DJE', 'Macedonia_GJE', 'Cyrillic_IO', 'Ukranian_JE', 'Macedonia_DSE', 'Ukranian_I', 'Ukranian_YI', 'Serbian_JE', 'Serbian_LJE', 'Serbian_NJE', 'Serbian_TSHE', 'Macedonia_KJE', 'Byelorussian_SHORTU', 'Serbian_DZE', 'Cyrillic_yu', 'Cyrillic_a', 'Cyrillic_be', 'Cyrillic_tse', 'Cyrillic_de', 'Cyrillic_ie', 'Cyrillic_ef', 'Cyrillic_ghe', 'Cyrillic_ha', 'Cyrillic_i', 'Cyrillic_shorti', 'Cyrillic_ka', 'Cyrillic_el', 'Cyrillic_em', 'Cyrillic_en', 'Cyrillic_o', 'Cyrillic_pe', 'Cyrillic_ya', 'Cyrillic_er', 'Cyrillic_es', 'Cyrillic_te', 'Cyrillic_u', 'Cyrillic_zhe', 'Cyrillic_ve', 'Cyrillic_softsign', 'Cyrillic_yeru', 'Cyrillic_ze', 'Cyrillic_sha', 'Cyrillic_e', 'Cyrillic_shcha', 'Cyrillic_che', 'Cyrillic_hardsign', 'Cyrillic_YU', 'Cyrillic_A', 'Cyrillic_BE', 'Cyrillic_TSE', 'Cyrillic_DE', 'Cyrillic_IE', 'Cyrillic_EF', 'Cyrillic_GHE', 'Cyrillic_HA', 'Cyrillic_I', 'Cyrillic_SHORTI', 'Cyrillic_KA', 'Cyrillic_EL', 'Cyrillic_EM', 'Cyrillic_EN', 'Cyrillic_O', 'Cyrillic_PE', 'Cyrillic_YA', 'Cyrillic_ER', 'Cyrillic_ES', 'Cyrillic_TE', 'Cyrillic_U', 'Cyrillic_ZHE', 'Cyrillic_VE', 'Cyrillic_SOFTSIGN', 'Cyrillic_YERU', 'Cyrillic_ZE', 'Cyrillic_SHA', 'Cyrillic_E', 'Cyrillic_SHCHA', 'Cyrillic_CHE', 'Cyrillic_HARDSIGN', 'Greek_ALPHAaccent', 'Greek_EPSILONaccent', 'Greek_ETAaccent', 'Greek_IOTAaccent', 'Greek_IOTAdiaeresis', 'Greek_IOTAaccentdiaeresis', 'Greek_OMICRONaccent', 'Greek_UPSILONaccent', 'Greek_UPSILONdieresis', 'Greek_UPSILONaccentdieresis', 'Greek_OMEGAaccent', 'Greek_alphaaccent', 'Greek_epsilonaccent', 'Greek_etaaccent', 'Greek_iotaaccent', 'Greek_iotadieresis', 'Greek_iotaaccentdieresis', 'Greek_omicronaccent', 'Greek_upsilonaccent', 'Greek_upsilondieresis', 'Greek_upsilonaccentdieresis', 'Greek_omegaaccent', 'Greek_ALPHA', 'Greek_BETA', 'Greek_GAMMA', 'Greek_DELTA', 'Greek_EPSILON', 'Greek_ZETA', 'Greek_ETA', 'Greek_THETA', 'Greek_IOTA', 'Greek_KAPPA', 'Greek_LAMBDA', 'Greek_MU', 'Greek_NU', 'Greek_XI', 'Greek_OMICRON', 'Greek_PI', 'Greek_RHO', 'Greek_SIGMA', 'Greek_TAU', 'Greek_UPSILON', 'Greek_PHI', 'Greek_CHI', 'Greek_PSI', 'Greek_OMEGA', 'Greek_alpha', 'Greek_beta', 'Greek_gamma', 'Greek_delta', 'Greek_epsilon', 'Greek_zeta', 'Greek_eta', 'Greek_theta', 'Greek_iota', 'Greek_kappa', 'Greek_lambda', 'Greek_mu', 'Greek_nu', 'Greek_xi', 'Greek_omicron', 'Greek_pi', 'Greek_rho', 'Greek_sigma', 'Greek_finalsmallsigma', 'Greek_tau', 'Greek_upsilon', 'Greek_phi', 'Greek_chi', 'Greek_psi', 'Greek_omega', 'leftradical', 'topleftradical', 'horizconnector', 'topintegral', 'botintegral', 'vertconnector', 'topleftsqbracket', 'botleftsqbracket', 'toprightsqbracket', 'botrightsqbracket', 'topleftparens', 'botleftparens', 'toprightparens', 'botrightparens', 'leftmiddlecurlybrace', 'rightmiddlecurlybrace', 'topleftsummation', 'botleftsummation', 'topvertsummationconnector', 'botvertsummationconnector', 'toprightsummation', 'botrightsummation', 'rightmiddlesummation', 'lessthanequal', 'notequal', 'greaterthanequal', 'integral', 'therefore', 'variation', 'infinity', 'nabla', 'approximate', 'similarequal', 'ifonlyif', 'implies', 'identical', 'radical', 'includedin', 'includes', 'intersection', 'union', 'logicaland', 'logicalor', 'partialderivative', 'function', 'leftarrow', 'uparrow', 'rightarrow', 'downarrow', 'blank', 'soliddiamond', 'checkerboard', 'ht', 'ff', 'cr', 'lf', 'nl', 'vt', 'lowrightcorner', 'uprightcorner', 'upleftcorner', 'lowleftcorner', 'crossinglines', 'horizlinescan1', 'horizlinescan3', 'horizlinescan5', 'horizlinescan7', 'horizlinescan9', 'leftt', 'rightt', 'bott', 'topt', 'vertbar', 'emspace', 'enspace', 'em3space', 'em4space', 'digitspace', 'punctspace', 'thinspace', 'hairspace', 'emdash', 'endash', 'signifblank', 'ellipsis', 'doubbaselinedot', 'onethird', 'twothirds', 'onefifth', 'twofifths', 'threefifths', 'fourfifths', 'onesixth', 'fivesixths', 'careof', 'figdash', 'leftanglebracket', 'decimalpoint', 'rightanglebracket', 'marker', 'oneeighth', 'threeeighths', 'fiveeighths', 'seveneighths', 'trademark', 'signaturemark', 'trademarkincircle', 'leftopentriangle', 'rightopentriangle', 'emopencircle', 'emopenrectangle', 'leftsinglequotemark', 'rightsinglequotemark', 'leftdoublequotemark', 'rightdoublequotemark', 'prescription', 'minutes', 'seconds', 'latincross', 'hexagram', 'filledrectbullet', 'filledlefttribullet', 'filledrighttribullet', 'emfilledcircle', 'emfilledrect', 'enopencircbullet', 'enopensquarebullet', 'openrectbullet', 'opentribulletup', 'opentribulletdown', 'openstar', 'enfilledcircbullet', 'enfilledsqbullet', 'filledtribulletup', 'filledtribulletdown', 'leftpointer', 'rightpointer', 'club', 'diamond', 'heart', 'maltesecross', 'dagger', 'doubledagger', 'checkmark', 'ballotcross', 'musicalsharp', 'musicalflat', 'malesymbol', 'femalesymbol', 'telephone', 'telephonerecorder', 'phonographcopyright', 'caret', 'singlelowquotemark', 'doublelowquotemark', 'cursor', 'leftcaret', 'rightcaret', 'downcaret', 'upcaret', 'overbar', 'downtack', 'upshoe', 'downstile', 'underbar', 'jot', 'quad', 'uptack', 'circle', 'upstile', 'downshoe', 'rightshoe', 'leftshoe', 'lefttack', 'righttack', 'hebrew_aleph', 'hebrew_beth', 'hebrew_gimmel', 'hebrew_daleth', 'hebrew_he', 'hebrew_waw', 'hebrew_zayin', 'hebrew_het', 'hebrew_teth', 'hebrew_yod', 'hebrew_finalkaph', 'hebrew_kaph', 'hebrew_lamed', 'hebrew_finalmem', 'hebrew_mem', 'hebrew_finalnun', 'hebrew_nun', 'hebrew_samekh', 'hebrew_ayin', 'hebrew_finalpe', 'hebrew_pe', 'hebrew_finalzadi', 'hebrew_zadi', 'hebrew_kuf', 'hebrew_resh', 'hebrew_shin', 'hebrew_taf', 'BackSpace', 'Tab', 'Linefeed', 'Clear', 'Return', 'Pause', 'Scroll_Lock', 'Sys_Req', 'Escape', 'Multi_key', 'Kanji', 'Home', 'Left', 'Up', 'Right', 'Down', 'Prior', 'Next', 'End', 'Begin', 'Win_L', 'Win_R', 'App', 'Select', 'Print', 'Execute', 'Insert', 'Undo', 'Redo', 'Menu', 'Find', 'Cancel', 'Help', 'Break', 'Hebrew_switch', 'Num_Lock', 'KP_Space', 'KP_Tab', 'KP_Enter', 'KP_F1', 'KP_F2', 'KP_F3', 'KP_F4', 'KP_Multiply', 'KP_Add', 'KP_Separator', 'KP_Subtract', 'KP_Decimal', 'KP_Divide', 'KP_0', 'KP_1', 'KP_2', 'KP_3', 'KP_4', 'KP_5', 'KP_6', 'KP_7', 'KP_8', 'KP_9', 'KP_Equal', 'F1', 'F2', 'F3', 'F4', 'F5', 'F6', 'F7', 'F8', 'F9', 'F10', 'F11', 'F12', 'L1', 'L2', 'L3', 'L4', 'L5', 'L6', 'L7', 'L8', 'L9', 'L10', 'R1', 'R2', 'R3', 'R4', 'R5', 'R6', 'R7', 'R8', 'R9', 'R10', 'R11', 'R12', 'F33', 'R14', 'R15', 'Shift_L', 'Shift_R', 'Control_L', 'Control_R', 'Caps_Lock', 'Shift_Lock', 'Meta_L', 'Meta_R', 'Alt_L', 'Alt_R', 'Super_L', 'Super_R', 'Hyper_L', 'Hyper_R', 'Delete')
-
-
-
-
-
-# ------------------------------------------------------------------------- #
-# ToolTip used by the Elements #
-# ------------------------------------------------------------------------- #
-
-class ToolTip:
- """
- Create a tooltip for a given widget
- (inspired by https://stackoverflow.com/a/36221216)
- This is an INTERNALLY USED only class. Users should not refer to this class at all.
- """
-
- def __init__(self, widget, text, timeout=DEFAULT_TOOLTIP_TIME):
- """
- :param widget: The tkinter widget
- :type widget: widget type varies
- :param text: text for the tooltip. It can inslude \n
- :type text: (str)
- :param timeout: Time in milliseconds that mouse must remain still before tip is shown
- :type timeout: (int)
- """
- self.widget = widget
- self.text = text
- self.timeout = timeout
- # self.wraplength = wraplength if wraplength else widget.winfo_screenwidth() // 2
- self.tipwindow = None
- self.id = None
- self.x = self.y = 0
- self.widget.bind("", self.enter)
- self.widget.bind("", self.leave)
- self.widget.bind("", self.leave)
-
- def enter(self, event=None):
- """
- Called by tkinter when mouse enters a widget
- :param event: from tkinter. Has x,y coordinates of mouse
- :type event:
-
- """
- self.x = event.x
- self.y = event.y
- self.schedule()
-
- def leave(self, event=None):
- """
- Called by tktiner when mouse exits a widget
- :param event: from tkinter. Event info that's not used by function.
- :type event:
-
- """
- self.unschedule()
- self.hidetip()
-
- def schedule(self):
- """
- Schedule a timer to time how long mouse is hovering
- """
- self.unschedule()
- self.id = self.widget.after(self.timeout, self.showtip)
-
- def unschedule(self):
- """
- Cancel timer used to time mouse hover
- """
- if self.id:
- self.widget.after_cancel(self.id)
- self.id = None
-
- def showtip(self):
- """
- Creates a topoltip window with the tooltip text inside of it
- """
- if self.tipwindow:
- return
- x = self.widget.winfo_rootx() + self.x + DEFAULT_TOOLTIP_OFFSET[0]
- y = self.widget.winfo_rooty() + self.y + DEFAULT_TOOLTIP_OFFSET[1]
- self.tipwindow = tk.Toplevel(self.widget)
- # if not sys.platform.startswith('darwin'):
- try:
- self.tipwindow.wm_overrideredirect(True)
- # if running_mac() and ENABLE_MAC_NOTITLEBAR_PATCH:
- if _mac_should_apply_notitlebar_patch():
- self.tipwindow.wm_overrideredirect(False)
- except Exception as e:
- print('* Error performing wm_overrideredirect in showtip *', e)
- self.tipwindow.wm_geometry("+%d+%d" % (x, y))
- self.tipwindow.wm_attributes("-topmost", 1)
-
- label = ttk.Label(self.tipwindow, text=self.text, justify=tk.LEFT,
- background=TOOLTIP_BACKGROUND_COLOR, relief=tk.SOLID, borderwidth=1)
- if TOOLTIP_FONT is not None:
- label.config(font=TOOLTIP_FONT)
- label.pack()
-
- def hidetip(self):
- """
- Destroy the tooltip window
- """
- if self.tipwindow:
- self.tipwindow.destroy()
- self.tipwindow = None
-
-
-# ---------------------------------------------------------------------- #
-# Cascading structure.... Objects get larger #
-# Button #
-# Element #
-# Row #
-# Form #
-# ---------------------------------------------------------------------- #
-# ------------------------------------------------------------------------- #
-# Element CLASS #
-# ------------------------------------------------------------------------- #
-class Element():
- """ The base class for all Elements. Holds the basic description of an Element like size and colors """
-
- def __init__(self, type, size=(None, None), auto_size_text=None, font=None, background_color=None, text_color=None, key=None, pad=None, tooltip=None,
- visible=True, metadata=None,
- sbar_trough_color=None, sbar_background_color=None, sbar_arrow_color=None, sbar_width=None, sbar_arrow_width=None, sbar_frame_color=None, sbar_relief=None):
- """
- Element base class. Only used internally. User will not create an Element object by itself
-
- :param type: The type of element. These constants all start with "ELEM_TYPE_"
- :type type: (int) (could be enum)
- :param size: w=characters-wide, h=rows-high. If an int instead of a tuple is supplied, then height is auto-set to 1
- :type size: (int, int) | (None, None) | int
- :param auto_size_text: True if the Widget should be shrunk to exactly fit the number of chars to show
- :type auto_size_text: bool
- :param font: specifies the font family, size. Tuple or Single string format 'name size styles'. Styles: italic * roman bold normal underline overstrike
- :type font: (str or (str, int[, str]) or None)
- :param background_color: color of background. Can be in #RRGGBB format or a color name "black"
- :type background_color: (str)
- :param text_color: element's text color. Can be in #RRGGBB format or a color name "black"
- :type text_color: (str)
- :param key: Identifies an Element. Should be UNIQUE to this window.
- :type key: str | int | tuple | object
- :param pad: Amount of padding to put around element in pixels (left/right, top/bottom). If an int is given, then auto-converted to tuple (int, int)
- :type pad: (int, int) or ((int, int),(int,int)) or (int,(int,int)) or ((int, int),int) | int
- :param tooltip: text, that will appear when mouse hovers over the element
- :type tooltip: (str)
- :param visible: set visibility state of the element (Default = True)
- :type visible: (bool)
- :param metadata: User metadata that can be set to ANYTHING
- :type metadata: (Any)
- :param sbar_trough_color: Scrollbar color of the trough
- :type sbar_trough_color: (str)
- :param sbar_background_color: Scrollbar color of the background of the arrow buttons at the ends AND the color of the "thumb" (the thing you grab and slide). Switches to arrow color when mouse is over
- :type sbar_background_color: (str)
- :param sbar_arrow_color: Scrollbar color of the arrow at the ends of the scrollbar (it looks like a button). Switches to background color when mouse is over
- :type sbar_arrow_color: (str)
- :param sbar_width: Scrollbar width in pixels
- :type sbar_width: (int)
- :param sbar_arrow_width: Scrollbar width of the arrow on the scrollbar. It will potentially impact the overall width of the scrollbar
- :type sbar_arrow_width: (int)
- :param sbar_frame_color: Scrollbar Color of frame around scrollbar (available only on some ttk themes)
- :type sbar_frame_color: (str)
- :param sbar_relief: Scrollbar relief that will be used for the "thumb" of the scrollbar (the thing you grab that slides). Should be a constant that is defined at starting with "RELIEF_" - RELIEF_RAISED, RELIEF_SUNKEN, RELIEF_FLAT, RELIEF_RIDGE, RELIEF_GROOVE, RELIEF_SOLID
- :type sbar_relief: (str)
- """
-
- if size is not None and size != (None, None):
- if isinstance(size, int):
- size = (size, 1)
- if isinstance(size, tuple) and len(size) == 1:
- size = (size[0], 1)
-
- if pad is not None and pad != (None, None):
- if isinstance(pad, int):
- pad = (pad, pad)
-
-
- self.Size = size
- self.Type = type
- self.AutoSizeText = auto_size_text
-
- self.Pad = pad
- self.Font = font
-
- self.TKStringVar = None
- self.TKIntVar = None
- self.TKText = None
- self.TKEntry = None
- self.TKImage = None
- self.ttk_style_name = '' # set in the packer function
-
- self._metadata = None # type: Any
-
- self.ParentForm = None # type: Window
- self.ParentContainer = None # will be a Form, Column, or Frame element # UNBIND
- self.TextInputDefault = None
- self.Position = (0, 0) # Default position Row 0, Col 0
- self.BackgroundColor = background_color if background_color is not None else DEFAULT_ELEMENT_BACKGROUND_COLOR
- self.TextColor = text_color if text_color is not None else DEFAULT_ELEMENT_TEXT_COLOR
- self.Key = key # dictionary key for return values
- self.Tooltip = tooltip
- self.TooltipObject = None
- self._visible = visible
- self.TKRightClickMenu = None
- self.Widget = None # Set when creating window. Has the main tkinter widget for element
- self.Tearoff = False # needed because of right click menu code
- self.ParentRowFrame = None # type tk.Frame
- self.metadata = metadata
- self.user_bind_dict = {} # Used when user defines a tkinter binding using bind method - convert bind string to key modifier
- self.user_bind_event = None # Used when user defines a tkinter binding using bind method - event data from tkinter
- # self.pad_used = (0, 0) # the amount of pad used when was inserted into the layout
- self._popup_menu_location = (None, None)
- self.pack_settings = None
- ## TTK Scrollbar Settings
- self.ttk_part_overrides = TTKPartOverrides(sbar_trough_color=sbar_trough_color, sbar_background_color=sbar_background_color, sbar_arrow_color=sbar_arrow_color, sbar_width=sbar_width, sbar_arrow_width=sbar_arrow_width, sbar_frame_color=sbar_frame_color, sbar_relief=sbar_relief)
-
- PSG_THEME_PART_FUNC_MAP = {PSG_THEME_PART_BACKGROUND: theme_background_color,
- PSG_THEME_PART_BUTTON_BACKGROUND: theme_button_color_background,
- PSG_THEME_PART_BUTTON_TEXT: theme_button_color_text,
- PSG_THEME_PART_INPUT_BACKGROUND: theme_input_background_color,
- PSG_THEME_PART_INPUT_TEXT: theme_input_text_color,
- PSG_THEME_PART_TEXT: theme_text_color,
- PSG_THEME_PART_SLIDER: theme_slider_color}
-
- # class Theme_Parts():
- # PSG_THEME_PART_FUNC_MAP = {PSG_THEME_PART_BACKGROUND: theme_background_color,
- if sbar_trough_color is not None:
- self.scroll_trough_color = sbar_trough_color
- else:
- self.scroll_trough_color = PSG_THEME_PART_FUNC_MAP.get(ttk_part_mapping_dict[TTK_SCROLLBAR_PART_TROUGH_COLOR], ttk_part_mapping_dict[TTK_SCROLLBAR_PART_TROUGH_COLOR])
- if callable(self.scroll_trough_color):
- self.scroll_trough_color = self.scroll_trough_color()
-
-
- if sbar_background_color is not None:
- self.scroll_background_color = sbar_background_color
- else:
- self.scroll_background_color = PSG_THEME_PART_FUNC_MAP.get(ttk_part_mapping_dict[TTK_SCROLLBAR_PART_BACKGROUND_COLOR], ttk_part_mapping_dict[TTK_SCROLLBAR_PART_BACKGROUND_COLOR])
- if callable(self.scroll_background_color):
- self.scroll_background_color = self.scroll_background_color()
-
-
- if sbar_arrow_color is not None:
- self.scroll_arrow_color = sbar_arrow_color
- else:
- self.scroll_arrow_color = PSG_THEME_PART_FUNC_MAP.get(ttk_part_mapping_dict[TTK_SCROLLBAR_PART_ARROW_BUTTON_ARROW_COLOR], ttk_part_mapping_dict[TTK_SCROLLBAR_PART_ARROW_BUTTON_ARROW_COLOR])
- if callable(self.scroll_arrow_color):
- self.scroll_arrow_color = self.scroll_arrow_color()
-
-
- if sbar_frame_color is not None:
- self.scroll_frame_color = sbar_frame_color
- else:
- self.scroll_frame_color = PSG_THEME_PART_FUNC_MAP.get(ttk_part_mapping_dict[TTK_SCROLLBAR_PART_FRAME_COLOR], ttk_part_mapping_dict[TTK_SCROLLBAR_PART_FRAME_COLOR])
- if callable(self.scroll_frame_color):
- self.scroll_frame_color = self.scroll_frame_color()
-
- if sbar_relief is not None:
- self.scroll_relief = sbar_relief
- else:
- self.scroll_relief = ttk_part_mapping_dict[TTK_SCROLLBAR_PART_RELIEF]
-
- if sbar_width is not None:
- self.scroll_width = sbar_width
- else:
- self.scroll_width = ttk_part_mapping_dict[TTK_SCROLLBAR_PART_SCROLL_WIDTH]
-
- if sbar_arrow_width is not None:
- self.scroll_arrow_width = sbar_arrow_width
- else:
- self.scroll_arrow_width = ttk_part_mapping_dict[TTK_SCROLLBAR_PART_ARROW_WIDTH]
-
-
- if not hasattr(self, 'DisabledTextColor'):
- self.DisabledTextColor = None
- if not hasattr(self, 'ItemFont'):
- self.ItemFont = None
- if not hasattr(self, 'RightClickMenu'):
- self.RightClickMenu = None
- if not hasattr(self, 'Disabled'):
- self.Disabled = None # in case the element hasn't defined this, add it here
-
- @property
- def visible(self):
- """
- Returns visibility state for the element. This is a READONLY property
- :return: Visibility state for element
- :rtype: (bool)
- """
- return self._visible
-
- @property
- def metadata(self):
- """
- Metadata is an Element property that you can use at any time to hold any value
- :return: the current metadata value
- :rtype: (Any)
- """
- return self._metadata
-
- @metadata.setter
- def metadata(self, value):
- """
- Metadata is an Element property that you can use at any time to hold any value
- :param value: Anything you want it to be
- :type value: (Any)
- """
- self._metadata = value
-
- @property
- def key(self):
- """
- Returns key for the element. This is a READONLY property.
- Keys can be any hashable object (basically anything except a list... tuples are ok, but not lists)
- :return: The window's Key
- :rtype: (Any)
- """
- return self.Key
-
-
-
- @property
- def widget(self):
- """
- Returns tkinter widget for the element. This is a READONLY property.
- The implementation is that the Widget member variable is returned. This is a backward compatible addition
- :return: The element's underlying tkinter widget
- :rtype: (tkinter.Widget)
- """
- return self.Widget
-
-
-
-
- def _RightClickMenuCallback(self, event):
- """
- Callback function that's called when a right click happens. Shows right click menu as result
-
- :param event: information provided by tkinter about the event including x,y location of click
- :type event:
-
- """
- if self.Type == ELEM_TYPE_TAB_GROUP:
- try:
- index = self.Widget.index('@{},{}'.format(event.x,event.y))
- tab = self.Widget.tab(index, 'text')
- key = self.find_key_from_tab_name(tab)
- tab_element = self.ParentForm.key_dict[key]
- if tab_element.RightClickMenu is None: # if this tab didn't explicitly have a menu, then don't show anything
- return
- tab_element.TKRightClickMenu.tk_popup(event.x_root, event.y_root, 0)
- self.TKRightClickMenu.grab_release()
- except:
- pass
- return
- self.TKRightClickMenu.tk_popup(event.x_root, event.y_root, 0)
- self.TKRightClickMenu.grab_release()
- if self.Type == ELEM_TYPE_GRAPH:
- self._update_position_for_returned_values(event)
-
- def _tearoff_menu_callback(self, parent, menu):
- """
- Callback function that's called when a right click menu is torn off.
- The reason for this function is to relocate the torn-off menu. It will default to 0,0 otherwise
- This callback moves the right click menu window to the location of the current window
-
- :param parent: information provided by tkinter - the parent of the Meny
- :type parent:
- :param menu: information provided by tkinter - the menu window
- :type menu:
-
- """
- if self._popup_menu_location == (None, None):
- winx, winy = self.ParentForm.current_location()
- else:
- winx, winy = self._popup_menu_location
- # self.ParentForm.TKroot.update()
- self.ParentForm.TKroot.tk.call('wm', 'geometry', menu, "+{}+{}".format(winx, winy))
-
- def _MenuItemChosenCallback(self, item_chosen): # TEXT Menu item callback
- """
- Callback function called when user chooses a menu item from menubar, Button Menu or right click menu
-
- :param item_chosen: String holding the value chosen.
- :type item_chosen: str
-
- """
- # print('IN MENU ITEM CALLBACK', item_chosen)
- self.MenuItemChosen = item_chosen
- self.ParentForm.LastButtonClicked = self.MenuItemChosen
- self.ParentForm.FormRemainedOpen = True
- _exit_mainloop(self.ParentForm)
- # Window._window_that_exited = self.ParentForm
- # self.ParentForm.TKroot.quit() # kick the users out of the mainloop
-
- def _FindReturnKeyBoundButton(self, form):
- """
- Searches for which Button has the flag Button.BindReturnKey set. It is called recursively when a
- "Container Element" is encountered. Func has to walk entire window including these "sub-forms"
-
- :param form: the Window object to search
- :type form:
- :return: Button Object if a button is found, else None
- :rtype: Button | None
- """
- for row in form.Rows:
- for element in row:
- if element.Type == ELEM_TYPE_BUTTON:
- if element.BindReturnKey:
- return element
- if element.Type == ELEM_TYPE_COLUMN:
- rc = self._FindReturnKeyBoundButton(element)
- if rc is not None:
- return rc
- if element.Type == ELEM_TYPE_FRAME:
- rc = self._FindReturnKeyBoundButton(element)
- if rc is not None:
- return rc
- if element.Type == ELEM_TYPE_TAB_GROUP:
- rc = self._FindReturnKeyBoundButton(element)
- if rc is not None:
- return rc
- if element.Type == ELEM_TYPE_TAB:
- rc = self._FindReturnKeyBoundButton(element)
- if rc is not None:
- return rc
- if element.Type == ELEM_TYPE_PANE:
- rc = self._FindReturnKeyBoundButton(element)
- if rc is not None:
- return rc
- return None
-
- def _TextClickedHandler(self, event):
- """
- Callback that's called when a text element is clicked on with events enabled on the Text Element.
- Result is that control is returned back to user (quits mainloop).
-
- :param event:
- :type event:
-
- """
- # If this is a minimize button for a custom titlebar, then minimize the window
- if self.Key == TITLEBAR_MINIMIZE_KEY:
- if running_linux():
- self.ParentForm.TKroot.wm_attributes("-type", "normal")
- # self.ParentForm.TKroot.state('icon')
- # return
- # self.ParentForm.maximize()
- self.ParentForm.TKroot.wm_overrideredirect(False)
- # self.ParentForm.minimize()
- # self.ParentForm.TKroot.wm_overrideredirect(False)
- self.ParentForm.TKroot.iconify()
- # self._skip_first_restore_callback = True
- self.ParentForm.TKroot.bind('', self._titlebar_restore)
- else:
- self.ParentForm.TKroot.wm_overrideredirect(False)
- self.ParentForm.Minimize()
- self.ParentForm.TKroot.bind('', self._titlebar_restore)
- elif self.Key == TITLEBAR_MAXIMIZE_KEY:
- if self.ParentForm.maximized:
- self.ParentForm.normal()
- else:
- self.ParentForm.maximize()
- elif self.Key == TITLEBAR_CLOSE_KEY:
- self.ParentForm._OnClosingCallback()
- else:
- self._generic_callback_handler(self.DisplayText)
- return
-
- def _titlebar_restore(self, event):
- if running_linux():
- # if self._skip_first_restore_callback:
- # self._skip_first_restore_callback = False
- # return
- self.ParentForm.TKroot.unbind('')
- self.ParentForm.TKroot.deiconify()
-
- # self.ParentForm.TKroot.wm_overrideredirect(True)
- self.ParentForm.TKroot.wm_attributes("-type", 'dock')
-
- else:
- self.ParentForm.TKroot.unbind('')
- self.ParentForm.TKroot.wm_overrideredirect(True)
- self.ParentForm.normal()
-
- def _ReturnKeyHandler(self, event):
- """
- Internal callback for the ENTER / RETURN key. Results in calling the ButtonCallBack for element that has the return key bound to it, just as if button was clicked.
-
- :param event:
- :type event:
-
- """
- # if the element is disabled, ignore the event
- if self.Disabled:
- return
-
- MyForm = self.ParentForm
- button_element = self._FindReturnKeyBoundButton(MyForm)
- if button_element is not None:
- button_element.ButtonCallBack()
-
- def _generic_callback_handler(self, alternative_to_key=None, force_key_to_be=None):
- """
- Peforms the actions that were in many of the callback functions previously. Combined so that it's
- easier to modify and is in 1 place now
-
- :param event: From tkinter and is not used
- :type event: Any
- :param alternate_to_key: If key is None, then use this value instead
- :type alternate_to_key: Any
- """
- if force_key_to_be is not None:
- self.ParentForm.LastButtonClicked = force_key_to_be
- elif self.Key is not None:
- self.ParentForm.LastButtonClicked = self.Key
- else:
- self.ParentForm.LastButtonClicked = alternative_to_key
- self.ParentForm.FormRemainedOpen = True
-
- _exit_mainloop(self.ParentForm)
- # if self.ParentForm.CurrentlyRunningMainloop:
- # Window._window_that_exited = self.ParentForm
- # self.ParentForm.TKroot.quit() # kick the users out of the mainloop
-
- def _ListboxSelectHandler(self, event):
- """
- Internal callback function for when a listbox item is selected
-
- :param event: Information from tkinter about the callback
- :type event:
-
- """
- self._generic_callback_handler('')
-
- def _ComboboxSelectHandler(self, event):
- """
- Internal callback function for when an entry is selected in a Combobox.
- :param event: Event data from tkinter (not used)
- :type event:
-
- """
- self._generic_callback_handler('')
-
-
- def _SpinboxSelectHandler(self, event=None):
- """
- Internal callback function for when an entry is selected in a Spinbox.
- Note that the parm is optional because it's not used if arrows are used to change the value
- but if the return key is pressed, it will include the event parm
- :param event: Event data passed in by tkinter (not used)
- :type event:
- """
- self._generic_callback_handler('')
-
- def _RadioHandler(self):
- """
- Internal callback for when a radio button is selected and enable events was set for radio
- """
- self._generic_callback_handler('')
-
- def _CheckboxHandler(self):
- """
- Internal callback for when a checkbnox is selected and enable events was set for checkbox
- """
- self._generic_callback_handler('')
-
- def _TabGroupSelectHandler(self, event):
- """
- Internal callback for when a Tab is selected and enable events was set for TabGroup
-
- :param event: Event data passed in by tkinter (not used)
- :type event:
- """
- self._generic_callback_handler('')
-
- def _KeyboardHandler(self, event):
- """
- Internal callback for when a key is pressed andd return keyboard events was set for window
-
- :param event: Event data passed in by tkinter (not used)
- :type event:
- """
-
- # if the element is disabled, ignore the event
- if self.Disabled:
- return
- self._generic_callback_handler('')
-
- def _ClickHandler(self, event):
- """
- Internal callback for when a mouse was clicked... I think.
-
- :param event: Event data passed in by tkinter (not used)
- :type event:
- """
- self._generic_callback_handler('')
-
- def _user_bind_callback(self, bind_string, event, propagate=True):
- """
- Used when user binds a tkinter event directly to an element
-
- :param bind_string: The event that was bound so can lookup the key modifier
- :type bind_string: (str)
- :param event: Event data passed in by tkinter (not used)
- :type event: (Any)
- :param propagate: If True then tkinter will be told to propagate the event to the element
- :type propagate: (bool)
- """
- key_suffix = self.user_bind_dict.get(bind_string, '')
- self.user_bind_event = event
- if self.Type == ELEM_TYPE_GRAPH:
- self._update_position_for_returned_values(event)
- if self.Key is not None:
- if isinstance(self.Key, str):
- key = self.Key + str(key_suffix)
- else:
- key = (self.Key, key_suffix) # old way (pre 2021) was to make a brand new tuple
- # key = self.Key + (key_suffix,) # in 2021 tried this. It will break existing applications though - if key is a tuple, add one more item
- else:
- key = bind_string
-
- self._generic_callback_handler(force_key_to_be=key)
-
- return 'break' if propagate is not True else None
-
-
- def bind(self, bind_string, key_modifier, propagate=True):
- """
- Used to add tkinter events to an Element.
- The tkinter specific data is in the Element's member variable user_bind_event
- :param bind_string: The string tkinter expected in its bind function
- :type bind_string: (str)
- :param key_modifier: Additional data to be added to the element's key when event is returned
- :type key_modifier: (str)
- :param propagate: If True then tkinter will be told to propagate the event to the element
- :type propagate: (bool)
- """
- if not self._widget_was_created(): # if widget hasn't been created yet, then don't allow
- return
-
- try:
- self.Widget.bind(bind_string, lambda evt: self._user_bind_callback(bind_string, evt, propagate))
- except Exception as e:
- self.Widget.unbind_all(bind_string)
- return
-
- self.user_bind_dict[bind_string] = key_modifier
-
- def unbind(self, bind_string):
- """
- Removes a previously bound tkinter event from an Element.
- :param bind_string: The string tkinter expected in its bind function
- :type bind_string: (str)
- """
- if not self._widget_was_created(): # if widget hasn't been created yet, then don't allow
- return
- self.Widget.unbind(bind_string)
- self.user_bind_dict.pop(bind_string, None)
-
- def set_tooltip(self, tooltip_text):
- """
- Called by application to change the tooltip text for an Element. Normally invoked using the Element Object such as: window.Element('key').SetToolTip('New tip').
-
- :param tooltip_text: the text to show in tooltip.
- :type tooltip_text: (str)
- """
-
- if self.TooltipObject:
- try:
- self.TooltipObject.leave()
- except:
- pass
-
- self.TooltipObject = ToolTip(self.Widget, text=tooltip_text, timeout=DEFAULT_TOOLTIP_TIME)
-
- def set_focus(self, force=False):
- """
- Sets the current focus to be on this element
-
- :param force: if True will call focus_force otherwise calls focus_set
- :type force: bool
- """
- if not self._widget_was_created(): # if widget hasn't been created yet, then don't allow
- return
- try:
- if force:
- self.Widget.focus_force()
- else:
- self.Widget.focus_set()
- except Exception as e:
- _error_popup_with_traceback("Exception blocking focus. Check your element's Widget", e)
-
-
- def block_focus(self, block=True):
- """
- Enable or disable the element from getting focus by using the keyboard.
- If the block parameter is True, then this element will not be given focus by using
- the keyboard to go from one element to another.
- You CAN click on the element and utilize it.
-
- :param block: if True the element will not get focus via the keyboard
- :type block: bool
- """
- if not self._widget_was_created(): # if widget hasn't been created yet, then don't allow
- return
- try:
- self.ParentForm.TKroot.focus_force()
- if block:
- self.Widget.configure(takefocus=0)
- else:
- self.Widget.configure(takefocus=1)
- except Exception as e:
- _error_popup_with_traceback("Exception blocking focus. Check your element's Widget", e)
-
-
- def get_next_focus(self):
- """
- Gets the next element that should get focus after this element.
-
- :return: Element that will get focus after this one
- :rtype: (Element)
- """
- if not self._widget_was_created(): # if widget hasn't been created yet, then don't allow
- return None
-
- try:
- next_widget_focus = self.widget.tk_focusNext()
- return self.ParentForm.widget_to_element(next_widget_focus)
- except Exception as e:
- _error_popup_with_traceback("Exception getting next focus. Check your element's Widget", e)
-
-
- def get_previous_focus(self):
- """
- Gets the element that should get focus previous to this element.
-
- :return: Element that should get the focus before this one
- :rtype: (Element)
- """
- if not self._widget_was_created(): # if widget hasn't been created yet, then don't allow
- return None
- try:
- next_widget_focus = self.widget.tk_focusPrev() # tkinter.Widget
- return self.ParentForm.widget_to_element(next_widget_focus)
- except Exception as e:
- _error_popup_with_traceback("Exception getting previous focus. Check your element's Widget", e)
-
-
- def set_size(self, size=(None, None)):
- """
- Changes the size of an element to a specific size.
- It's possible to specify None for one of sizes so that only 1 of the element's dimensions are changed.
-
- :param size: The size in characters, rows typically. In some cases they are pixels
- :type size: (int, int)
- """
- try:
- if size[0] != None:
- self.Widget.config(width=size[0])
- except:
- print('Warning, error setting width on element with key=', self.Key)
- try:
- if size[1] != None:
- self.Widget.config(height=size[1])
- except:
- try:
- self.Widget.config(length=size[1])
- except:
- print('Warning, error setting height on element with key=', self.Key)
-
- if self.Type == ELEM_TYPE_GRAPH:
- self.CanvasSize = size
-
-
- def get_size(self):
- """
- Return the size of an element in Pixels. Care must be taken as some elements use characters to specify their size but will return pixels when calling this get_size method.
- :return: width and height of the element
- :rtype: (int, int)
- """
- try:
- w = self.Widget.winfo_width()
- h = self.Widget.winfo_height()
- except:
- print('Warning, error getting size of element', self.Key)
- w = h = None
- return w, h
-
- def hide_row(self):
- """
- Hide the entire row an Element is located on.
- Use this if you must have all space removed when you are hiding an element, including the row container
- """
- try:
- self.ParentRowFrame.pack_forget()
- except:
- print('Warning, error hiding element row for key =', self.Key)
-
- def unhide_row(self):
- """
- Unhides (makes visible again) the row container that the Element is located on.
- Note that it will re-appear at the bottom of the window / container, most likely.
- """
- try:
- self.ParentRowFrame.pack()
- except:
- print('Warning, error hiding element row for key =', self.Key)
-
- def expand(self, expand_x=False, expand_y=False, expand_row=True):
- """
- Causes the Element to expand to fill available space in the X and Y directions. Can specify which or both directions
-
- :param expand_x: If True Element will expand in the Horizontal directions
- :type expand_x: (bool)
- :param expand_y: If True Element will expand in the Vertical directions
- :type expand_y: (bool)
- :param expand_row: If True the row containing the element will also expand. Without this your element is "trapped" within the row
- :type expand_row: (bool)
- """
- if expand_x and expand_y:
- fill = tk.BOTH
- elif expand_x:
- fill = tk.X
- elif expand_y:
- fill = tk.Y
- else:
- return
-
- if not self._widget_was_created():
- return
- self.Widget.pack(expand=True, fill=fill)
- self.ParentRowFrame.pack(expand=expand_row, fill=fill)
- if self.element_frame is not None:
- self.element_frame.pack(expand=True, fill=fill)
-
- def set_cursor(self, cursor=None, cursor_color=None):
- """
- Sets the cursor for the current Element.
- "Cursor" is used in 2 different ways in this call.
- For the parameter "cursor" it's actually the mouse pointer.
- If you do not want any mouse pointer, then use the string "none"
- For the parameter "cursor_color" it's the color of the beam used when typing into an input element
-
- :param cursor: The tkinter cursor name
- :type cursor: (str)
- :param cursor_color: color to set the "cursor" to
- :type cursor_color: (str)
- """
- if not self._widget_was_created():
- return
- if cursor is not None:
- try:
- self.Widget.config(cursor=cursor)
- except Exception as e:
- print('Warning bad cursor specified ', cursor)
- print(e)
- if cursor_color is not None:
- try:
- self.Widget.config(insertbackground=cursor_color)
- except Exception as e:
- print('Warning bad cursor color', cursor_color)
- print(e)
-
- def set_vscroll_position(self, percent_from_top):
- """
- Attempts to set the vertical scroll postition for an element's Widget
- :param percent_from_top: From 0 to 1.0, the percentage from the top to move scrollbar to
- :type percent_from_top: (float)
- """
- try:
- self.Widget.yview_moveto(percent_from_top)
- except Exception as e:
- print('Warning setting the vertical scroll (yview_moveto failed)')
- print(e)
-
- def _widget_was_created(self):
- """
- Determines if a Widget was created for this element.
-
- :return: True if a Widget has been created previously (Widget is not None)
- :rtype: (bool)
- """
- if self.Widget is not None:
- return True
- else:
- if SUPPRESS_WIDGET_NOT_FINALIZED_WARNINGS:
- return False
-
- warnings.warn('You cannot Update element with key = {} until the window.read() is called or finalized=True when creating window'.format(self.Key), UserWarning)
- if not SUPPRESS_ERROR_POPUPS:
- _error_popup_with_traceback('Unable to complete operation on element with key {}'.format(self.Key),
- 'You cannot perform operations (such as calling update) on an Element until:',
- ' window.read() is called or finalize=True when Window created.',
- 'Adding a "finalize=True" parameter to your Window creation will likely fix this.',
- _create_error_message(),
- )
- return False
-
-
- def _grab_anywhere_on_using_control_key(self):
- """
- Turns on Grab Anywhere functionality AFTER a window has been created. Don't try on a window that's not yet
- been Finalized or Read.
- """
- self.Widget.bind("", self.ParentForm._StartMove)
- self.Widget.bind("", self.ParentForm._StopMove)
- self.Widget.bind("", self.ParentForm._OnMotion)
-
-
- def _grab_anywhere_on(self):
- """
- Turns on Grab Anywhere functionality AFTER a window has been created. Don't try on a window that's not yet
- been Finalized or Read.
- """
- self.Widget.bind("", self.ParentForm._StartMove)
- self.Widget.bind("", self.ParentForm._StopMove)
- self.Widget.bind("", self.ParentForm._OnMotion)
-
- def _grab_anywhere_off(self):
- """
- Turns off Grab Anywhere functionality AFTER a window has been created. Don't try on a window that's not yet
- been Finalized or Read.
- """
- self.Widget.unbind("")
- self.Widget.unbind("")
- self.Widget.unbind("")
-
- def grab_anywhere_exclude(self):
- """
- Excludes this element from being used by the grab_anywhere feature
- Handy for elements like a Graph element when dragging is enabled. You want the Graph element to get the drag events instead of the window dragging.
- """
- self.ParentForm._grab_anywhere_ignore_these_list.append(self.Widget)
-
- def grab_anywhere_include(self):
- """
- Includes this element in the grab_anywhere feature
- This will allow you to make a Multline element drag a window for example
- """
- self.ParentForm._grab_anywhere_include_these_list.append(self.Widget)
-
-
-
- def set_right_click_menu(self, menu=None):
- if menu == MENU_RIGHT_CLICK_DISABLED:
- return
- if menu is None:
- menu = self.ParentForm.RightClickMenu
- if menu is None:
- return
- if menu:
- top_menu = tk.Menu(self.ParentForm.TKroot, tearoff=self.ParentForm.right_click_menu_tearoff, tearoffcommand=self._tearoff_menu_callback)
-
- if self.ParentForm.right_click_menu_background_color not in (COLOR_SYSTEM_DEFAULT, None):
- top_menu.config(bg=self.ParentForm.right_click_menu_background_color)
- if self.ParentForm.right_click_menu_text_color not in (COLOR_SYSTEM_DEFAULT, None):
- top_menu.config(fg=self.ParentForm.right_click_menu_text_color)
- if self.ParentForm.right_click_menu_disabled_text_color not in (COLOR_SYSTEM_DEFAULT, None):
- top_menu.config(disabledforeground=self.ParentForm.right_click_menu_disabled_text_color)
- if self.ParentForm.right_click_menu_font is not None:
- top_menu.config(font=self.ParentForm.right_click_menu_font)
-
- if self.ParentForm.right_click_menu_selected_colors[0] not in (COLOR_SYSTEM_DEFAULT, None):
- top_menu.config(activeforeground=self.ParentForm.right_click_menu_selected_colors[0])
- if self.ParentForm.right_click_menu_selected_colors[1] not in (COLOR_SYSTEM_DEFAULT, None):
- top_menu.config(activebackground=self.ParentForm.right_click_menu_selected_colors[1])
- AddMenuItem(top_menu, menu[1], self, right_click_menu=True)
- self.TKRightClickMenu = top_menu
- if self.ParentForm.RightClickMenu: # if the top level has a right click menu, then setup a callback for the Window itself
- if self.ParentForm.TKRightClickMenu is None:
- self.ParentForm.TKRightClickMenu = top_menu
- if (running_mac()):
- self.ParentForm.TKroot.bind('', self.ParentForm._RightClickMenuCallback)
- else:
- self.ParentForm.TKroot.bind('', self.ParentForm._RightClickMenuCallback)
- if (running_mac()):
- self.Widget.bind('', self._RightClickMenuCallback)
- else:
- self.Widget.bind('', self._RightClickMenuCallback)
-
-
-
- def _pack_forget_save_settings(self, alternate_widget=None):
- """
- Performs a pack_forget which will make a widget invisible.
- This method saves the pack settings so that they can be restored if the element is made visible again
-
- :param alternate_widget: Widget to use that's different than the one defined in Element.Widget. These are usually Frame widgets
- :type alternate_widget: (tk.Widget)
- """
-
- if alternate_widget is not None and self.Widget is None:
- return
-
- widget = alternate_widget if alternate_widget is not None else self.Widget
- # if the widget is already invisible (i.e. not packed) then will get an error
- try:
- pack_settings = widget.pack_info()
- self.pack_settings = pack_settings
- widget.pack_forget()
- except:
- pass
-
- def _pack_restore_settings(self, alternate_widget=None):
- """
- Restores a previously packated widget which will make it visible again.
- If no settings were saved, then the widget is assumed to have not been unpacked and will not try to pack it again
-
- :param alternate_widget: Widget to use that's different than the one defined in Element.Widget. These are usually Frame widgets
- :type alternate_widget: (tk.Widget)
- """
-
- # if there are no saved pack settings, then assume it hasnb't been packaed before. The request will be ignored
- if self.pack_settings is None:
- return
-
- widget = alternate_widget if alternate_widget is not None else self.Widget
- if widget is not None:
- widget.pack(**self.pack_settings)
-
-
- def update(self, *args, **kwargs):
- """
- A dummy update call. This will only be called if an element hasn't implemented an update method
- It is provided here for docstring purposes. If you got here by browing code via PyCharm, know
- that this is not the function that will be called. Your actual element's update method will be called.
-
- If you call update, you must call window.refresh if you want the change to happen prior to your next
- window.read() call. Normally uou don't do this as the window.read call is likely going to happen next.
- """
- print('* Base Element Class update was called. Your element does not seem to have an update method')
-
-
- def __call__(self, *args, **kwargs):
- """
- Makes it possible to "call" an already existing element. When you do make the "call", it actually calls
- the Update method for the element.
- Example: If this text element was in yoiur layout:
- sg.Text('foo', key='T')
- Then you can call the Update method for that element by writing:
- window.find_element('T')('new text value')
- """
- return self.update(*args, **kwargs)
-
-
-
-
-
-
- SetTooltip = set_tooltip
- SetFocus = set_focus
-
-
-# ---------------------------------------------------------------------- #
-# Input Class #
-# ---------------------------------------------------------------------- #
-class Input(Element):
- """
- Display a single text input field. Based on the tkinter Widget `Entry`
- """
-
- def __init__(self, default_text='', size=(None, None), s=(None, None), disabled=False, password_char='',
- justification=None, background_color=None, text_color=None, font=None, tooltip=None, border_width=None,
- change_submits=False, enable_events=False, do_not_clear=True, key=None, k=None, focus=False, pad=None, p=None,
- use_readonly_for_disable=True, readonly=False, disabled_readonly_background_color=None, disabled_readonly_text_color=None, expand_x=False, expand_y=False,
- right_click_menu=None, visible=True, metadata=None):
- """
- :param default_text: Text initially shown in the input box as a default value(Default value = ''). Will automatically be converted to string
- :type default_text: (Any)
- :param size: w=characters-wide, h=rows-high. If an int is supplied rather than a tuple, then a tuple is created width=int supplied and heigh=1
- :type size: (int, int) | (int, None) | int
- :param s: Same as size parameter. It's an alias. If EITHER of them are set, then the one that's set will be used. If BOTH are set, size will be used
- :type s: (int, int) | (None, None) | int
- :param disabled: set disable state for element (Default = False)
- :type disabled: (bool)
- :param password_char: Password character if this is a password field (Default value = '')
- :type password_char: (char)
- :param justification: justification for data display. Valid choices - left, right, center
- :type justification: (str)
- :param background_color: color of background in one of the color formats
- :type background_color: (str)
- :param text_color: color of the text
- :type text_color: (str)
- :param font: specifies the font family, size. Tuple or Single string format 'name size styles'. Styles: italic * roman bold normal underline overstrike
- :type font: (str or (str, int[, str]) or None)
- :param tooltip: text, that will appear when mouse hovers over the element
- :type tooltip: (str)
- :param border_width: width of border around element in pixels
- :type border_width: (int)
- :param change_submits: * DEPRICATED DO NOT USE. Use `enable_events` instead
- :type change_submits: (bool)
- :param enable_events: If True then changes to this element are immediately reported as an event. Use this instead of change_submits (Default = False)
- :type enable_events: (bool)
- :param do_not_clear: If False then the field will be set to blank after ANY event (button, any event) (Default = True)
- :type do_not_clear: (bool)
- :param key: Value that uniquely identifies this element from all other elements. Used when Finding an element or in return values. Must be unique to the window
- :type key: str | int | tuple | object
- :param k: Same as the Key. You can use either k or key. Which ever is set will be used.
- :type k: str | int | tuple | object
- :param focus: Determines if initial focus should go to this element.
- :type focus: (bool)
- :param pad: Amount of padding to put around element. Normally (horizontal pixels, vertical pixels) but can be split apart further into ((horizontal left, horizontal right), (vertical above, vertical below)). If int is given, then converted to tuple (int, int) with the value provided duplicated
- :type pad: (int, int) or ((int, int),(int,int)) or (int,(int,int)) or ((int, int),int) | int
- :param p: Same as pad parameter. It's an alias. If EITHER of them are set, then the one that's set will be used. If BOTH are set, pad will be used
- :type p: (int, int) or ((int, int),(int,int)) or (int,(int,int)) or ((int, int),int) | int
- :param use_readonly_for_disable: If True (the default) tkinter state set to 'readonly'. Otherwise state set to 'disabled'
- :type use_readonly_for_disable: (bool)
- :param readonly: If True tkinter state set to 'readonly'. Use this in place of use_readonly_for_disable as another way of achieving readonly. Note cannot set BOTH readonly and disabled as tkinter only supplies a single flag
- :type readonly: (bool)
- :param disabled_readonly_background_color: If state is set to readonly or disabled, the color to use for the background
- :type disabled_readonly_background_color: (str)
- :param disabled_readonly_text_color: If state is set to readonly or disabled, the color to use for the text
- :type disabled_readonly_text_color: (str)
- :param expand_x: If True the element will automatically expand in the X direction to fill available space
- :type expand_x: (bool)
- :param expand_y: If True the element will automatically expand in the Y direction to fill available space
- :type expand_y: (bool)
- :param right_click_menu: A list of lists of Menu items to show when this element is right clicked. See user docs for exact format.
- :type right_click_menu: List[List[ List[str] | str ]]
- :param visible: set visibility state of the element (Default = True)
- :type visible: (bool)
- :param metadata: User metadata that can be set to ANYTHING
- :type metadata: (Any)
- """
-
-
- self.DefaultText = default_text if default_text is not None else ''
- self.PasswordCharacter = password_char
- bg = background_color if background_color is not None else DEFAULT_INPUT_ELEMENTS_COLOR
- fg = text_color if text_color is not None else DEFAULT_INPUT_TEXT_COLOR
- self.Focus = focus
- self.do_not_clear = do_not_clear
- self.Justification = justification
- self.Disabled = disabled
- self.ChangeSubmits = change_submits or enable_events
- self.RightClickMenu = right_click_menu
- self.UseReadonlyForDisable = use_readonly_for_disable
- self.disabled_readonly_background_color = disabled_readonly_background_color
- self.disabled_readonly_text_color = disabled_readonly_text_color
- self.ReadOnly = readonly
- self.BorderWidth = border_width if border_width is not None else DEFAULT_BORDER_WIDTH
- self.TKEntry = self.Widget = None # type: tk.Entry
- key = key if key is not None else k
- sz = size if size != (None, None) else s
- pad = pad if pad is not None else p
- self.expand_x = expand_x
- self.expand_y = expand_y
-
- super().__init__(ELEM_TYPE_INPUT_TEXT, size=sz, background_color=bg, text_color=fg, key=key, pad=pad,
- font=font, tooltip=tooltip, visible=visible, metadata=metadata)
-
- def update(self, value=None, disabled=None, select=None, visible=None, text_color=None, background_color=None, move_cursor_to='end', password_char=None, paste=None):
- """
- Changes some of the settings for the Input Element. Must call `Window.Read` or `Window.Finalize` prior.
- Changes will not be visible in your window until you call window.read or window.refresh.
-
- If you change visibility, your element may MOVE. If you want it to remain stationary, use the "layout helper"
- function "pin" to ensure your element is "pinned" to that location in your layout so that it returns there
- when made visible.
-
- :param value: new text to display as default text in Input field
- :type value: (str)
- :param disabled: disable or enable state of the element (sets Entry Widget to readonly or normal)
- :type disabled: (bool)
- :param select: if True, then the text will be selected
- :type select: (bool)
- :param visible: change visibility of element
- :type visible: (bool)
- :param text_color: change color of text being typed
- :type text_color: (str)
- :param background_color: change color of the background
- :type background_color: (str)
- :param move_cursor_to: Moves the cursor to a particular offset. Defaults to 'end'
- :type move_cursor_to: int | str
- :param password_char: Password character if this is a password field
- :type password_char: str
- :param paste: If True "Pastes" the value into the element rather than replacing the entire element. If anything is selected it is replaced. The text is inserted at the current cursor location.
- :type paste: bool
- """
- if not self._widget_was_created(): # if widget hasn't been created yet, then don't allow
- return
- if disabled is True:
- self.TKEntry['state'] = 'readonly' if self.UseReadonlyForDisable else 'disabled'
- elif disabled is False:
- self.TKEntry['state'] = 'readonly' if self.ReadOnly else 'normal'
- self.Disabled = disabled if disabled is not None else self.Disabled
-
- if background_color not in (None, COLOR_SYSTEM_DEFAULT):
- self.TKEntry.configure(background=background_color)
- if text_color not in (None, COLOR_SYSTEM_DEFAULT):
- self.TKEntry.configure(fg=text_color)
- if value is not None:
- if paste is not True:
- try:
- self.TKStringVar.set(value)
- except:
- pass
- self.DefaultText = value
- if paste is True:
- try:
- self.TKEntry.delete('sel.first', 'sel.last')
- except:
- pass
- self.TKEntry.insert("insert", value)
- if move_cursor_to == 'end':
- self.TKEntry.icursor(tk.END)
- elif move_cursor_to is not None:
- self.TKEntry.icursor(move_cursor_to)
- if select:
- self.TKEntry.select_range(0, 'end')
- if visible is False:
- self._pack_forget_save_settings()
- # self.TKEntry.pack_forget()
- elif visible is True:
- self._pack_restore_settings()
- # self.TKEntry.pack(padx=self.pad_used[0], pady=self.pad_used[1])
- # self.TKEntry.pack(padx=self.pad_used[0], pady=self.pad_used[1], in_=self.ParentRowFrame)
- if visible is not None:
- self._visible = visible
- if password_char is not None:
- self.TKEntry.configure(show=password_char)
- self.PasswordCharacter = password_char
-
- def get(self):
- """
- Read and return the current value of the input element. Must call `Window.Read` or `Window.Finalize` prior
-
- :return: current value of Input field or '' if error encountered
- :rtype: (str)
- """
- try:
- text = self.TKStringVar.get()
- except:
- text = ''
- return text
-
- Get = get
- Update = update
-
-
-# ------------------------- INPUT TEXT Element lazy functions ------------------------- #
-In = Input
-InputText = Input
-I = Input
-
-
-# ---------------------------------------------------------------------- #
-# Combo #
-# ---------------------------------------------------------------------- #
-class Combo(Element):
- """
- ComboBox Element - A combination of a single-line input and a drop-down menu. User can type in their own value or choose from list.
- """
-
- def __init__(self, values, default_value=None, size=(None, None), s=(None, None), auto_size_text=None, background_color=None, text_color=None, button_background_color=None, button_arrow_color=None, bind_return_key=False, change_submits=False, enable_events=False, disabled=False, key=None, k=None, pad=None, p=None, expand_x=False, expand_y=False, tooltip=None, readonly=False, font=None, visible=True, metadata=None):
- """
- :param values: values to choose. While displayed as text, the items returned are what the caller supplied, not text
- :type values: List[Any] or Tuple[Any]
- :param default_value: Choice to be displayed as initial value. Must match one of values variable contents
- :type default_value: (Any)
- :param size: width, height. Width = characters-wide, height = NOTE it's the number of entries to show in the list. If an Int is passed rather than a tuple, then height is auto-set to 1 and width is value of the int
- :type size: (int, int) | (None, None) | int
- :param s: Same as size parameter. It's an alias. If EITHER of them are set, then the one that's set will be used. If BOTH are set, size will be used
- :type s: (int, int) | (None, None) | int
- :param auto_size_text: True if element should be the same size as the contents
- :type auto_size_text: (bool)
- :param background_color: color of background
- :type background_color: (str)
- :param text_color: color of the text
- :type text_color: (str)
- :param button_background_color: The color of the background of the button on the combo box
- :type button_background_color: (str)
- :param button_arrow_color: The color of the arrow on the button on the combo box
- :type button_arrow_color: (str)
- :param bind_return_key: If True, then the return key will cause a the Combo to generate an event
- :type bind_return_key: (bool)
- :param change_submits: DEPRICATED DO NOT USE. Use `enable_events` instead
- :type change_submits: (bool)
- :param enable_events: Turns on the element specific events. Combo event is when a choice is made
- :type enable_events: (bool)
- :param disabled: set disable state for element
- :type disabled: (bool)
- :param key: Used with window.find_element and with return values to uniquely identify this element
- :type key: str | int | tuple | object
- :param k: Same as the Key. You can use either k or key. Which ever is set will be used.
- :type k: str | int | tuple | object
- :param pad: Amount of padding to put around element in pixels (left/right, top/bottom) or ((left, right), (top, bottom)) or an int. If an int, then it's converted into a tuple (int, int)
- :type pad: (int, int) or ((int, int),(int,int)) or (int,(int,int)) or ((int, int),int) | int
- :param p: Same as pad parameter. It's an alias. If EITHER of them are set, then the one that's set will be used. If BOTH are set, pad will be used
- :type p: (int, int) or ((int, int),(int,int)) or (int,(int,int)) or ((int, int),int) | int
- :param expand_x: If True the element will automatically expand in the X direction to fill available space
- :type expand_x: (bool)
- :param expand_y: If True the element will automatically expand in the Y direction to fill available space
- :type expand_y: (bool)
- :param tooltip: text that will appear when mouse hovers over this element
- :type tooltip: (str)
- :param readonly: make element readonly (user can't change). True means user cannot change
- :type readonly: (bool)
- :param font: specifies the font family, size, etc. Tuple or Single string format 'name size styles'. Styles: italic * roman bold normal underline overstrike
- :type font: (str or (str, int[, str]) or None)
- :param visible: set visibility state of the element
- :type visible: (bool)
- :param metadata: User metadata that can be set to ANYTHING
- :type metadata: (Any)
- """
-
-
- self.Values = values
- self.DefaultValue = default_value
- self.ChangeSubmits = change_submits or enable_events
- self.Widget = self.TKCombo = None # type: ttk.Combobox
- self.Disabled = disabled
- self.Readonly = readonly
- self.BindReturnKey = bind_return_key
- bg = background_color if background_color else DEFAULT_INPUT_ELEMENTS_COLOR
- fg = text_color if text_color is not None else DEFAULT_INPUT_TEXT_COLOR
- key = key if key is not None else k
- sz = size if size != (None, None) else s
- pad = pad if pad is not None else p
- self.expand_x = expand_x
- self.expand_y = expand_y
- if button_background_color is None:
- self.button_background_color = theme_button_color()[1]
- else:
- self.button_background_color = button_background_color
- if button_arrow_color is None:
- self.button_arrow_color = theme_button_color()[0]
- else:
- self.button_arrow_color = button_arrow_color
-
-
- super().__init__(ELEM_TYPE_INPUT_COMBO, size=sz, auto_size_text=auto_size_text, background_color=bg,
- text_color=fg, key=key, pad=pad, tooltip=tooltip, font=font or DEFAULT_FONT, visible=visible, metadata=metadata)
-
- def update(self, value=None, values=None, set_to_index=None, disabled=None, readonly=None, font=None, visible=None, size=(None, None)):
- """
- Changes some of the settings for the Combo Element. Must call `Window.Read` or `Window.Finalize` prior.
- Note that the state can be in 3 states only.... enabled, disabled, readonly even
- though more combinations are available. The easy way to remember is that if you
- change the readonly parameter then you are enabling the element.
-
- Changes will not be visible in your window until you call window.read or window.refresh.
-
- If you change visibility, your element may MOVE. If you want it to remain stationary, use the "layout helper"
- function "pin" to ensure your element is "pinned" to that location in your layout so that it returns there
- when made visible.
-
- :param value: change which value is current selected based on new list of previous list of choices
- :type value: (Any)
- :param values: change list of choices
- :type values: List[Any]
- :param set_to_index: change selection to a particular choice starting with index = 0
- :type set_to_index: (int)
- :param disabled: disable or enable state of the element
- :type disabled: (bool)
- :param readonly: if True make element readonly (user cannot change any choices). Enables the element if either choice are made.
- :type readonly: (bool)
- :param font: specifies the font family, size, etc. Tuple or Single string format 'name size styles'. Styles: italic * roman bold normal underline overstrike
- :type font: (str or (str, int[, str]) or None)
- :param visible: control visibility of element
- :type visible: (bool)
- :param size: width, height. Width = characters-wide, height = NOTE it's the number of entries to show in the list
- :type size: (int, int)
- """
- if size != (None, None):
- if isinstance(size, int):
- size = (size, 1)
- if isinstance(size, tuple) and len(size) == 1:
- size = (size[0], 1)
-
- if not self._widget_was_created(): # if widget hasn't been created yet, then don't allow
- return
- if values is not None:
- try:
- self.TKCombo['values'] = values
- # self.TKCombo.current(0) # don't set any value if a new set of values was made
- except:
- pass
- self.Values = values
- if value is None:
- self.TKCombo.set('')
- if size == (None, None):
- max_line_len = max([len(str(l)) for l in self.Values]) if len(self.Values) else 0
- if self.AutoSizeText is False:
- width = self.Size[0]
- else:
- width = max_line_len + 1
- # self.TKCombo.configure(width=width)
- else:
- self.TKCombo.configure(height=size[1])
- self.TKCombo.configure(width=size[0])
- if value is not None:
- if value not in self.Values:
- self.TKCombo.set(value)
- else:
- for index, v in enumerate(self.Values):
- if v == value:
- try:
- self.TKCombo.current(index)
- except:
- pass
- self.DefaultValue = value
- break
- if set_to_index is not None:
- try:
- self.TKCombo.current(set_to_index)
- self.DefaultValue = self.Values[set_to_index]
- except:
- pass
- if readonly:
- self.Readonly = True
- self.TKCombo['state'] = 'readonly'
- elif readonly is False:
- self.Readonly = False
- self.TKCombo['state'] = 'enable'
- if disabled is True:
- self.TKCombo['state'] = 'disable'
- elif disabled is False and self.Readonly is True:
- self.TKCombo['state'] = 'readonly'
- elif disabled is False and self.Readonly is False:
- self.TKCombo['state'] = 'enable'
- self.Disabled = disabled if disabled is not None else self.Disabled
- if font is not None:
- self.TKCombo.configure(font=font)
- if visible is False:
- self._pack_forget_save_settings()
- # self.TKCombo.pack_forget()
- elif visible is True:
- self._pack_restore_settings()
- # self.TKCombo.pack(padx=self.pad_used[0], pady=self.pad_used[1])
- if visible is not None:
- self._visible = visible
-
- def get(self):
- """
- Returns the current (right now) value of the Combo. DO NOT USE THIS AS THE NORMAL WAY OF READING A COMBO!
- You should be using values from your call to window.read instead. Know what you're doing if you use it.
-
- :return: Returns the value of what is currently chosen
- :rtype: Any | None
- """
- try:
- if self.TKCombo.current() == -1: # if the current value was not in the original list
- value = self.TKCombo.get() # then get the value typed in by user
- else:
- value = self.Values[self.TKCombo.current()] # get value from original list given index
- except:
- value = None # only would happen if user closes window
- return value
-
- Get = get
- Update = update
-
-
-# ------------------------- INPUT COMBO Element lazy functions ------------------------- #
-InputCombo = Combo
-DropDown = InputCombo
-Drop = InputCombo
-DD = Combo
-
-
-# ---------------------------------------------------------------------- #
-# Option Menu #
-# ---------------------------------------------------------------------- #
-class OptionMenu(Element):
- """
- Option Menu is an Element available ONLY on the tkinter port of PySimpleGUI. It's is a widget that is unique
- to tkinter. However, it looks much like a ComboBox. Instead of an arrow to click to pull down the list of
- choices, another little graphic is shown on the widget to indicate where you click. After clicking to activate,
- it looks like a Combo Box that you scroll to select a choice.
- """
-
- def __init__(self, values, default_value=None, size=(None, None), s=(None, None), disabled=False, auto_size_text=None, expand_x=False, expand_y=False,
- background_color=None, text_color=None, key=None, k=None, pad=None, p=None, tooltip=None, visible=True, metadata=None):
- """
- :param values: Values to be displayed
- :type values: List[Any] or Tuple[Any]
- :param default_value: the value to choose by default
- :type default_value: (Any)
- :param size: (width, height) size in characters (wide), height is ignored and present to be consistent with other elements
- :type size: (int, int) (width, UNUSED)
- :param s: Same as size parameter. It's an alias. If EITHER of them are set, then the one that's set will be used. If BOTH are set, size will be used
- :type s: (int, int) | (None, None) | int
- :param disabled: control enabled / disabled
- :type disabled: (bool)
- :param auto_size_text: True if size of Element should match the contents of the items
- :type auto_size_text: (bool)
- :param expand_x: If True the element will automatically expand in the X direction to fill available space
- :type expand_x: (bool)
- :param expand_y: If True the element will automatically expand in the Y direction to fill available space
- :type expand_y: (bool)
- :param background_color: color of background
- :type background_color: (str)
- :param text_color: color of the text
- :type text_color: (str)
- :param key: Used with window.find_element and with return values to uniquely identify this element
- :type key: str | int | tuple | object
- :param k: Same as the Key. You can use either k or key. Which ever is set will be used.
- :type k: str | int | tuple | object
- :param pad: Amount of padding to put around element in pixels (left/right, top/bottom) or ((left, right), (top, bottom)) or an int. If an int, then it's converted into a tuple (int, int)
- :type pad: (int, int) or ((int, int),(int,int)) or (int,(int,int)) or ((int, int),int) | int
- :param p: Same as pad parameter. It's an alias. If EITHER of them are set, then the one that's set will be used. If BOTH are set, pad will be used
- :type p: (int, int) or ((int, int),(int,int)) or (int,(int,int)) or ((int, int),int) | int
- :param tooltip: text that will appear when mouse hovers over this element
- :type tooltip: (str)
- :param visible: set visibility state of the element
- :type visible: (bool)
- :param metadata: User metadata that can be set to ANYTHING
- :type metadata: (Any)
- """
-
- self.Values = values
- self.DefaultValue = default_value
- self.Widget = self.TKOptionMenu = None # type: tk.OptionMenu
- self.Disabled = disabled
- bg = background_color if background_color else DEFAULT_INPUT_ELEMENTS_COLOR
- fg = text_color if text_color is not None else DEFAULT_INPUT_TEXT_COLOR
- key = key if key is not None else k
- sz = size if size != (None, None) else s
- pad = pad if pad is not None else p
- self.expand_x = expand_x
- self.expand_y = expand_y
-
-
- super().__init__(ELEM_TYPE_INPUT_OPTION_MENU, size=sz, auto_size_text=auto_size_text, background_color=bg,
- text_color=fg, key=key, pad=pad, tooltip=tooltip, visible=visible, metadata=metadata)
-
- def update(self, value=None, values=None, disabled=None, visible=None, size=(None, None)):
- """
- Changes some of the settings for the OptionMenu Element. Must call `Window.Read` or `Window.Finalize` prior
-
- Changes will not be visible in your window until you call window.read or window.refresh.
-
- If you change visibility, your element may MOVE. If you want it to remain stationary, use the "layout helper"
- function "pin" to ensure your element is "pinned" to that location in your layout so that it returns there
- when made visible.
-
- :param value: the value to choose by default
- :type value: (Any)
- :param values: Values to be displayed
- :type values: List[Any]
- :param disabled: disable or enable state of the element
- :type disabled: (bool)
- :param visible: control visibility of element
- :type visible: (bool)
- :param size: (width, height) size in characters (wide), height is ignored and present to be consistent with other elements
- :type size: (int, int) (width, UNUSED)
- """
- if not self._widget_was_created(): # if widget hasn't been created yet, then don't allow
- return
-
- if values is not None:
- self.Values = values
- self.TKOptionMenu['menu'].delete(0, 'end')
-
- # Insert list of new options (tk._setit hooks them up to var)
- # self.TKStringVar.set(self.Values[0])
- for new_value in self.Values:
- self.TKOptionMenu['menu'].add_command(label=new_value, command=tk._setit(self.TKStringVar, new_value))
- if value is None:
- self.TKStringVar.set('')
-
- if size == (None, None):
- max_line_len = max([len(str(l)) for l in self.Values]) if len(self.Values) else 0
- if self.AutoSizeText is False:
- width = self.Size[0]
- else:
- width = max_line_len + 1
- self.TKOptionMenu.configure(width=width)
- else:
- self.TKOptionMenu.configure(width=size[0])
-
- if value is not None:
- self.DefaultValue = value
- self.TKStringVar.set(value)
-
- if disabled is True:
- self.TKOptionMenu['state'] = 'disabled'
- elif disabled is False:
- self.TKOptionMenu['state'] = 'normal'
- self.Disabled = disabled if disabled is not None else self.Disabled
- if visible is False:
- self._pack_forget_save_settings()
- # self.TKOptionMenu.pack_forget()
- elif visible is True:
- self._pack_restore_settings()
- # self.TKOptionMenu.pack(padx=self.pad_used[0], pady=self.pad_used[1])
- if visible is not None:
- self._visible = visible
-
- Update = update
-
-
-# ------------------------- OPTION MENU Element lazy functions ------------------------- #
-InputOptionMenu = OptionMenu
-
-
-# ---------------------------------------------------------------------- #
-# Listbox #
-# ---------------------------------------------------------------------- #
-class Listbox(Element):
- """
- A List Box. Provide a list of values for the user to choose one or more of. Returns a list of selected rows
- when a window.read() is executed.
- """
-
- def __init__(self, values, default_values=None, select_mode=None, change_submits=False, enable_events=False,
- bind_return_key=False, size=(None, None), s=(None, None), disabled=False, auto_size_text=None, font=None, no_scrollbar=False, horizontal_scroll=False,
- background_color=None, text_color=None, highlight_background_color=None, highlight_text_color=None,
- sbar_trough_color=None, sbar_background_color=None, sbar_arrow_color=None, sbar_width=None, sbar_arrow_width=None, sbar_frame_color=None, sbar_relief=None,
- key=None, k=None, pad=None, p=None, tooltip=None, expand_x=False, expand_y=False,right_click_menu=None, visible=True, metadata=None):
- """
- :param values: list of values to display. Can be any type including mixed types as long as they have __str__ method
- :type values: List[Any] or Tuple[Any]
- :param default_values: which values should be initially selected
- :type default_values: List[Any]
- :param select_mode: Select modes are used to determine if only 1 item can be selected or multiple and how they can be selected. Valid choices begin with "LISTBOX_SELECT_MODE_" and include: LISTBOX_SELECT_MODE_SINGLE LISTBOX_SELECT_MODE_MULTIPLE LISTBOX_SELECT_MODE_BROWSE LISTBOX_SELECT_MODE_EXTENDED
- :type select_mode: [enum]
- :param change_submits: DO NOT USE. Only listed for backwards compat - Use enable_events instead
- :type change_submits: (bool)
- :param enable_events: Turns on the element specific events. Listbox generates events when an item is clicked
- :type enable_events: (bool)
- :param bind_return_key: If True, then the return key will cause a the Listbox to generate an event
- :type bind_return_key: (bool)
- :param size: w=characters-wide, h=rows-high. If an int instead of a tuple is supplied, then height is auto-set to 1
- :type size: (int, int) | (int, None) | int
- :param s: Same as size parameter. It's an alias. If EITHER of them are set, then the one that's set will be used. If BOTH are set, size will be used
- :type s: (int, int) | (None, None) | int
- :param disabled: set disable state for element
- :type disabled: (bool)
- :param auto_size_text: True if element should be the same size as the contents
- :type auto_size_text: (bool)
- :param font: specifies the font family, size, etc. Tuple or Single string format 'name size styles'. Styles: italic * roman bold normal underline overstrike
- :type font: (str or (str, int[, str]) or None)
- :param no_scrollbar: Controls if a scrollbar should be shown. If True, no scrollbar will be shown
- :type no_scrollbar: (bool)
- :param horizontal_scroll: Controls if a horizontal scrollbar should be shown. If True a horizontal scrollbar will be shown in addition to vertical
- :type horizontal_scroll: (bool)
- :param background_color: color of background
- :type background_color: (str)
- :param text_color: color of the text
- :type text_color: (str)
- :param highlight_background_color: color of the background when an item is selected. Defaults to normal text color (a reverse look)
- :type highlight_background_color: (str)
- :param highlight_text_color: color of the text when an item is selected. Defaults to the normal background color (a rerverse look)
- :type highlight_text_color: (str)
- :param sbar_trough_color: Scrollbar color of the trough
- :type sbar_trough_color: (str)
- :param sbar_background_color: Scrollbar color of the background of the arrow buttons at the ends AND the color of the "thumb" (the thing you grab and slide). Switches to arrow color when mouse is over
- :type sbar_background_color: (str)
- :param sbar_arrow_color: Scrollbar color of the arrow at the ends of the scrollbar (it looks like a button). Switches to background color when mouse is over
- :type sbar_arrow_color: (str)
- :param sbar_width: Scrollbar width in pixels
- :type sbar_width: (int)
- :param sbar_arrow_width: Scrollbar width of the arrow on the scrollbar. It will potentially impact the overall width of the scrollbar
- :type sbar_arrow_width: (int)
- :param sbar_frame_color: Scrollbar Color of frame around scrollbar (available only on some ttk themes)
- :type sbar_frame_color: (str)
- :param sbar_relief: Scrollbar relief that will be used for the "thumb" of the scrollbar (the thing you grab that slides). Should be a constant that is defined at starting with "RELIEF_" - RELIEF_RAISED, RELIEF_SUNKEN, RELIEF_FLAT, RELIEF_RIDGE, RELIEF_GROOVE, RELIEF_SOLID
- :type sbar_relief: (str)
- :param key: Used with window.find_element and with return values to uniquely identify this element
- :type key: str | int | tuple | object
- :param k: Same as the Key. You can use either k or key. Which ever is set will be used.
- :type k: str | int | tuple | object
- :param pad: Amount of padding to put around element in pixels (left/right, top/bottom) or ((left, right), (top, bottom)) or an int. If an int, then it's converted into a tuple (int, int)
- :type pad: (int, int) or ((int, int),(int,int)) or (int,(int,int)) or ((int, int),int) | int
- :param p: Same as pad parameter. It's an alias. If EITHER of them are set, then the one that's set will be used. If BOTH are set, pad will be used
- :type p: (int, int) or ((int, int),(int,int)) or (int,(int,int)) or ((int, int),int) | int
- :param tooltip: text, that will appear when mouse hovers over the element
- :type tooltip: (str)
- :param expand_x: If True the element will automatically expand in the X direction to fill available space
- :type expand_x: (bool)
- :param expand_y: If True the element will automatically expand in the Y direction to fill available space
- :type expand_y: (bool)
- :param right_click_menu: A list of lists of Menu items to show when this element is right clicked. See user docs for exact format.
- :type right_click_menu: List[List[ List[str] | str ]]
- :param visible: set visibility state of the element
- :type visible: (bool)
- :param metadata: User metadata that can be set to ANYTHING
- :type metadata: (Any)
- """
-
- self.Values = values
- self.DefaultValues = default_values
- self.TKListbox = None
- self.ChangeSubmits = change_submits or enable_events
- self.BindReturnKey = bind_return_key
- self.Disabled = disabled
- if select_mode == LISTBOX_SELECT_MODE_BROWSE:
- self.SelectMode = SELECT_MODE_BROWSE
- elif select_mode == LISTBOX_SELECT_MODE_EXTENDED:
- self.SelectMode = SELECT_MODE_EXTENDED
- elif select_mode == LISTBOX_SELECT_MODE_MULTIPLE:
- self.SelectMode = SELECT_MODE_MULTIPLE
- elif select_mode == LISTBOX_SELECT_MODE_SINGLE:
- self.SelectMode = SELECT_MODE_SINGLE
- else:
- self.SelectMode = DEFAULT_LISTBOX_SELECT_MODE
- bg = background_color if background_color is not None else theme_input_background_color()
- fg = text_color if text_color is not None else theme_input_text_color()
- self.HighlightBackgroundColor = highlight_background_color if highlight_background_color is not None else fg
- self.HighlightTextColor = highlight_text_color if highlight_text_color is not None else bg
- self.RightClickMenu = right_click_menu
- self.vsb = None # type: tk.Scrollbar or None
- self.hsb = None # type: tk.Scrollbar | None
- self.TKListbox = self.Widget = None # type: tk.Listbox
- self.element_frame = None # type: tk.Frame
- self.NoScrollbar = no_scrollbar
- self.HorizontalScroll = horizontal_scroll
- key = key if key is not None else k
- sz = size if size != (None, None) else s
- pad = pad if pad is not None else p
- self.expand_x = expand_x
- self.expand_y = expand_y
-
- super().__init__(ELEM_TYPE_INPUT_LISTBOX, size=sz, auto_size_text=auto_size_text, font=font,
- background_color=bg, text_color=fg, key=key, pad=pad, tooltip=tooltip, visible=visible, metadata=metadata,
- sbar_trough_color=sbar_trough_color, sbar_background_color=sbar_background_color, sbar_arrow_color=sbar_arrow_color, sbar_width=sbar_width, sbar_arrow_width=sbar_arrow_width, sbar_frame_color=sbar_frame_color, sbar_relief=sbar_relief)
-
- def update(self, values=None, disabled=None, set_to_index=None, scroll_to_index=None, select_mode=None, visible=None):
- """
- Changes some of the settings for the Listbox Element. Must call `Window.Read` or `Window.Finalize` prior
- Changes will not be visible in your window until you call window.read or window.refresh.
-
- If you change visibility, your element may MOVE. If you want it to remain stationary, use the "layout helper"
- function "pin" to ensure your element is "pinned" to that location in your layout so that it returns there
- when made visible.
-
- :param values: new list of choices to be shown to user
- :type values: List[Any]
- :param disabled: disable or enable state of the element
- :type disabled: (bool)
- :param set_to_index: highlights the item(s) indicated. If parm is an int one entry will be set. If is a list, then each entry in list is highlighted
- :type set_to_index: int | list | tuple
- :param scroll_to_index: scroll the listbox so that this index is the first shown
- :type scroll_to_index: (int)
- :param select_mode: changes the select mode according to tkinter's listbox widget
- :type select_mode: (str)
- :param visible: control visibility of element
- :type visible: (bool)
- """
-
- if not self._widget_was_created(): # if widget hasn't been created yet, then don't allow
- return
-
- if disabled is True:
- self.TKListbox.configure(state='disabled')
- elif disabled is False:
- self.TKListbox.configure(state='normal')
- self.Disabled = disabled if disabled is not None else self.Disabled
-
- if values is not None:
- self.TKListbox.delete(0, 'end')
- for item in list(values):
- self.TKListbox.insert(tk.END, item)
- # self.TKListbox.selection_set(0, 0)
- self.Values = list(values)
- if set_to_index is not None:
- self.TKListbox.selection_clear(0, len(self.Values)) # clear all listbox selections
- if type(set_to_index) in (tuple, list):
- for i in set_to_index:
- try:
- self.TKListbox.selection_set(i, i)
- except:
- warnings.warn('* Listbox Update selection_set failed with index {}*'.format(set_to_index))
- else:
- try:
- self.TKListbox.selection_set(set_to_index, set_to_index)
- except:
- warnings.warn('* Listbox Update selection_set failed with index {}*'.format(set_to_index))
- if visible is False:
- self._pack_forget_save_settings(self.element_frame)
- elif visible is True:
- self._pack_restore_settings(self.element_frame)
- if scroll_to_index is not None and len(self.Values):
- self.TKListbox.yview_moveto(scroll_to_index / len(self.Values))
- if select_mode is not None:
- try:
- self.TKListbox.config(selectmode=select_mode)
- except:
- print('Listbox.update error trying to change mode to: ', select_mode)
- if visible is not None:
- self._visible = visible
-
- def set_value(self, values):
- """
- Set listbox highlighted choices
-
- :param values: new values to choose based on previously set values
- :type values: List[Any] | Tuple[Any]
-
- """
- for index, item in enumerate(self.Values):
- try:
- if item in values:
- self.TKListbox.selection_set(index)
- else:
- self.TKListbox.selection_clear(index)
- except:
- pass
- self.DefaultValues = values
-
- def get_list_values(self):
- # type: (Listbox) -> List[Any]
- """
- Returns list of Values provided by the user in the user's format
-
- :return: List of values. Can be any / mixed types -> []
- :rtype: List[Any]
- """
- return self.Values
-
- def get_indexes(self):
- """
- Returns the items currently selected as a list of indexes
-
- :return: A list of offsets into values that is currently selected
- :rtype: List[int]
- """
- return self.TKListbox.curselection()
-
- def get(self):
- """
- Returns the list of items currently selected in this listbox. It should be identical
- to the value you would receive when performing a window.read() call.
-
- :return: The list of currently selected items. The actual items are returned, not the indexes
- :rtype: List[Any]
- """
- try:
- items = self.TKListbox.curselection()
- value = [self.Values[int(item)] for item in items]
- except:
- value = []
- return value
-
- GetIndexes = get_indexes
- GetListValues = get_list_values
- SetValue = set_value
- Update = update
-
-
-LBox = Listbox
-LB = Listbox
-
-
-# ---------------------------------------------------------------------- #
-# Radio #
-# ---------------------------------------------------------------------- #
-class Radio(Element):
- """
- Radio Button Element - Used in a group of other Radio Elements to provide user with ability to select only
- 1 choice in a list of choices.
- """
-
- def __init__(self, text, group_id, default=False, disabled=False, size=(None, None), s=(None, None), auto_size_text=None,
- background_color=None, text_color=None, circle_color=None, font=None, key=None, k=None, pad=None, p=None, tooltip=None,
- change_submits=False, enable_events=False, right_click_menu=None, expand_x=False, expand_y=False, visible=True, metadata=None):
- """
- :param text: Text to display next to button
- :type text: (str)
- :param group_id: Groups together multiple Radio Buttons. Any type works
- :type group_id: (Any)
- :param default: Set to True for the one element of the group you want initially selected
- :type default: (bool)
- :param disabled: set disable state
- :type disabled: (bool)
- :param size: (w, h) w=characters-wide, h=rows-high. If an int instead of a tuple is supplied, then height is auto-set to 1
- :type size: (int, int) | (None, None) | int
- :param s: Same as size parameter. It's an alias. If EITHER of them are set, then the one that's set will be used. If BOTH are set, size will be used
- :type s: (int, int) | (None, None) | int
- :param auto_size_text: if True will size the element to match the length of the text
- :type auto_size_text: (bool)
- :param background_color: color of background
- :type background_color: (str)
- :param text_color: color of the text
- :type text_color: (str)
- :param circle_color: color of background of the circle that has the dot selection indicator in it
- :type circle_color: (str)
- :param font: specifies the font family, size, etc. Tuple or Single string format 'name size styles'. Styles: italic * roman bold normal underline overstrike
- :type font: (str or (str, int[, str]) or None)
- :param key: Used with window.find_element and with return values to uniquely identify this element
- :type key: str | int | tuple | object
- :param k: Same as the Key. You can use either k or key. Which ever is set will be used.
- :type k: str | int | tuple | object
- :param pad: Amount of padding to put around element in pixels (left/right, top/bottom) or ((left, right), (top, bottom)) or an int. If an int, then it's converted into a tuple (int, int)
- :type pad: (int, int) or ((int, int),(int,int)) or (int,(int,int)) or ((int, int),int) | int
- :param p: Same as pad parameter. It's an alias. If EITHER of them are set, then the one that's set will be used. If BOTH are set, pad will be used
- :type p: (int, int) or ((int, int),(int,int)) or (int,(int,int)) or ((int, int),int) | int
- :param tooltip: text, that will appear when mouse hovers over the element
- :type tooltip: (str)
- :param change_submits: DO NOT USE. Only listed for backwards compat - Use enable_events instead
- :type change_submits: (bool)
- :param enable_events: Turns on the element specific events. Radio Button events happen when an item is selected
- :type enable_events: (bool)
- :param right_click_menu: A list of lists of Menu items to show when this element is right clicked. See user docs for exact format.
- :type right_click_menu: List[List[ List[str] | str ]]
- :param expand_x: If True the element will automatically expand in the X direction to fill available space
- :type expand_x: (bool)
- :param expand_y: If True the element will automatically expand in the Y direction to fill available space
- :type expand_y: (bool)
- :param visible: set visibility state of the element
- :type visible: (bool)
- :param metadata: User metadata that can be set to ANYTHING
- :type metadata: (Any)
- """
-
-
- self.InitialState = default
- self.Text = text
- self.Widget = self.TKRadio = None # type: tk.Radiobutton
- self.GroupID = group_id
- self.Value = None
- self.Disabled = disabled
- self.TextColor = text_color if text_color else theme_text_color()
- self.RightClickMenu = right_click_menu
-
- if circle_color is None:
- # ---- compute color of circle background ---
- try: # something in here will fail if a color is not specified in Hex
- text_hsl = _hex_to_hsl(self.TextColor)
- background_hsl = _hex_to_hsl(background_color if background_color else theme_background_color())
- l_delta = abs(text_hsl[2] - background_hsl[2]) / 10
- if text_hsl[2] > background_hsl[2]: # if the text is "lighter" than the background then make background darker
- bg_rbg = _hsl_to_rgb(background_hsl[0], background_hsl[1], background_hsl[2] - l_delta)
- else:
- bg_rbg = _hsl_to_rgb(background_hsl[0], background_hsl[1], background_hsl[2] + l_delta)
- self.CircleBackgroundColor = rgb(*bg_rbg)
- except:
- self.CircleBackgroundColor = background_color if background_color else theme_background_color()
- else:
- self.CircleBackgroundColor = circle_color
- self.ChangeSubmits = change_submits or enable_events
- self.EncodedRadioValue = None
- key = key if key is not None else k
- sz = size if size != (None, None) else s
- pad = pad if pad is not None else p
- self.expand_x = expand_x
- self.expand_y = expand_y
-
- super().__init__(ELEM_TYPE_INPUT_RADIO, size=sz, auto_size_text=auto_size_text, font=font,
- background_color=background_color, text_color=self.TextColor, key=key, pad=pad,
- tooltip=tooltip, visible=visible, metadata=metadata)
-
- def update(self, value=None, text=None, background_color=None, text_color=None, circle_color=None, disabled=None, visible=None):
- """
- Changes some of the settings for the Radio Button Element. Must call `Window.read` or `Window.finalize` prior
-
- Changes will not be visible in your window until you call window.read or window.refresh.
-
- If you change visibility, your element may MOVE. If you want it to remain stationary, use the "layout helper"
- function "pin" to ensure your element is "pinned" to that location in your layout so that it returns there
- when made visible.
-
- :param value: if True change to selected and set others in group to unselected
- :type value: (bool)
- :param text: Text to display next to radio button
- :type text: (str)
- :param background_color: color of background
- :type background_color: (str)
- :param text_color: color of the text. Note this also changes the color of the selection dot
- :type text_color: (str)
- :param circle_color: color of background of the circle that has the dot selection indicator in it
- :type circle_color: (str)
- :param disabled: disable or enable state of the element
- :type disabled: (bool)
- :param visible: control visibility of element
- :type visible: (bool)
- """
-
- if not self._widget_was_created(): # if widget hasn't been created yet, then don't allow
- return
-
- if value is not None:
- try:
- if value is True:
- self.TKIntVar.set(self.EncodedRadioValue)
- elif value is False:
- if self.TKIntVar.get() == self.EncodedRadioValue:
- self.TKIntVar.set(0)
- except:
- print('Error updating Radio')
- self.InitialState = value
- if text is not None:
- self.Text = str(text)
- self.TKRadio.configure(text=self.Text)
- if background_color not in (None, COLOR_SYSTEM_DEFAULT):
- self.TKRadio.configure(background=background_color)
- self.BackgroundColor = background_color
- if text_color not in (None, COLOR_SYSTEM_DEFAULT):
- self.TKRadio.configure(fg=text_color)
- self.TextColor = text_color
-
- if circle_color not in (None, COLOR_SYSTEM_DEFAULT):
- self.CircleBackgroundColor = circle_color
- self.TKRadio.configure(selectcolor=self.CircleBackgroundColor) # The background of the radio button
- elif text_color or background_color:
- if self.TextColor not in (None, COLOR_SYSTEM_DEFAULT) and self.BackgroundColor not in (None, COLOR_SYSTEM_DEFAULT) and self.TextColor.startswith(
- '#') and self.BackgroundColor.startswith('#'):
- # ---- compute color of circle background ---
- text_hsl = _hex_to_hsl(self.TextColor)
- background_hsl = _hex_to_hsl(self.BackgroundColor if self.BackgroundColor else theme_background_color())
- l_delta = abs(text_hsl[2] - background_hsl[2]) / 10
- if text_hsl[2] > background_hsl[2]: # if the text is "lighter" than the background then make background darker
- bg_rbg = _hsl_to_rgb(background_hsl[0], background_hsl[1], background_hsl[2] - l_delta)
- else:
- bg_rbg = _hsl_to_rgb(background_hsl[0], background_hsl[1], background_hsl[2] + l_delta)
- self.CircleBackgroundColor = rgb(*bg_rbg)
- self.TKRadio.configure(selectcolor=self.CircleBackgroundColor) # The background of the checkbox
-
- if disabled is True:
- self.TKRadio['state'] = 'disabled'
- elif disabled is False:
- self.TKRadio['state'] = 'normal'
- self.Disabled = disabled if disabled is not None else self.Disabled
-
- if visible is False:
- self._pack_forget_save_settings()
- elif visible is True:
- self._pack_restore_settings()
- if visible is not None:
- self._visible = visible
-
- def reset_group(self):
- """
- Sets all Radio Buttons in the group to not selected
- """
- self.TKIntVar.set(0)
-
- def get(self):
- # type: (Radio) -> bool
- """
- A snapshot of the value of Radio Button -> (bool)
-
- :return: True if this radio button is selected
- :rtype: (bool)
- """
- return self.TKIntVar.get() == self.EncodedRadioValue
-
- Get = get
- ResetGroup = reset_group
- Update = update
-
-
-R = Radio
-Rad = Radio
-
-
-# ---------------------------------------------------------------------- #
-# Checkbox #
-# ---------------------------------------------------------------------- #
-class Checkbox(Element):
- """
- Checkbox Element - Displays a checkbox and text next to it
- """
-
- def __init__(self, text, default=False, size=(None, None), s=(None, None), auto_size_text=None, font=None, background_color=None,
- text_color=None, checkbox_color=None, change_submits=False, enable_events=False, disabled=False, key=None, k=None, pad=None, p=None, tooltip=None,
- right_click_menu=None, expand_x=False, expand_y=False, visible=True, metadata=None):
- """
- :param text: Text to display next to checkbox
- :type text: (str)
- :param default: Set to True if you want this checkbox initially checked
- :type default: (bool)
- :param size: (w, h) w=characters-wide, h=rows-high. If an int instead of a tuple is supplied, then height is auto-set to 1
- :type size: (int, int) | (None, None) | int
- :param s: Same as size parameter. It's an alias. If EITHER of them are set, then the one that's set will be used. If BOTH are set, size will be used
- :type s: (int, int) | (None, None) | int
- :param auto_size_text: if True will size the element to match the length of the text
- :type auto_size_text: (bool)
- :param font: specifies the font family, size, etc. Tuple or Single string format 'name size styles'. Styles: italic * roman bold normal underline overstrike
- :type font: (str or (str, int[, str]) or None)
- :param background_color: color of background
- :type background_color: (str)
- :param text_color: color of the text
- :type text_color: (str)
- :param checkbox_color: color of background of the box that has the check mark in it. The checkmark is the same color as the text
- :type checkbox_color: (str)
- :param change_submits: DO NOT USE. Only listed for backwards compat - Use enable_events instead
- :type change_submits: (bool)
- :param enable_events: Turns on the element specific events. Checkbox events happen when an item changes
- :type enable_events: (bool)
- :param disabled: set disable state
- :type disabled: (bool)
- :param key: Used with window.find_element and with return values to uniquely identify this element
- :type key: str | int | tuple | object
- :param k: Same as the Key. You can use either k or key. Which ever is set will be used.
- :type k: str | int | tuple | object
- :param pad: Amount of padding to put around element in pixels (left/right, top/bottom) or ((left, right), (top, bottom)) or an int. If an int, then it's converted into a tuple (int, int)
- :type pad: (int, int) or ((int, int),(int,int)) or (int,(int,int)) or ((int, int),int) | int
- :param p: Same as pad parameter. It's an alias. If EITHER of them are set, then the one that's set will be used. If BOTH are set, pad will be used
- :type p: (int, int) or ((int, int),(int,int)) or (int,(int,int)) or ((int, int),int) | int
- :param tooltip: text, that will appear when mouse hovers over the element
- :type tooltip: (str)
- :param right_click_menu: A list of lists of Menu items to show when this element is right clicked. See user docs for exact format.
- :type right_click_menu: List[List[ List[str] | str ]]
- :param expand_x: If True the element will automatically expand in the X direction to fill available space
- :type expand_x: (bool)
- :param expand_y: If True the element will automatically expand in the Y direction to fill available space
- :type expand_y: (bool)
- :param visible: set visibility state of the element
- :type visible: (bool)
- :param metadata: User metadata that can be set to ANYTHING
- :type metadata: (Any)
- """
-
-
- self.Text = text
- self.InitialState = bool(default)
- self.Value = None
- self.TKCheckbutton = self.Widget = None # type: tk.Checkbutton
- self.Disabled = disabled
- self.TextColor = text_color if text_color else theme_text_color()
- self.RightClickMenu = right_click_menu
-
- # ---- compute color of circle background ---
- if checkbox_color is None:
- try: # something in here will fail if a color is not specified in Hex
- text_hsl = _hex_to_hsl(self.TextColor)
- background_hsl = _hex_to_hsl(background_color if background_color else theme_background_color())
- l_delta = abs(text_hsl[2] - background_hsl[2]) / 10
- if text_hsl[2] > background_hsl[2]: # if the text is "lighter" than the background then make background darker
- bg_rbg = _hsl_to_rgb(background_hsl[0], background_hsl[1], background_hsl[2] - l_delta)
- else:
- bg_rbg = _hsl_to_rgb(background_hsl[0], background_hsl[1], background_hsl[2] + l_delta)
- self.CheckboxBackgroundColor = rgb(*bg_rbg)
- except:
- self.CheckboxBackgroundColor = background_color if background_color else theme_background_color()
- else:
- self.CheckboxBackgroundColor = checkbox_color
- self.ChangeSubmits = change_submits or enable_events
- key = key if key is not None else k
- sz = size if size != (None, None) else s
- pad = pad if pad is not None else p
- self.expand_x = expand_x
- self.expand_y = expand_y
-
- super().__init__(ELEM_TYPE_INPUT_CHECKBOX, size=sz, auto_size_text=auto_size_text, font=font,
- background_color=background_color, text_color=self.TextColor, key=key, pad=pad,
- tooltip=tooltip, visible=visible, metadata=metadata)
-
- def get(self):
- # type: (Checkbox) -> bool
- """
- Return the current state of this checkbox
-
- :return: Current state of checkbox
- :rtype: (bool)
- """
- return self.TKIntVar.get() != 0
-
- def update(self, value=None, text=None, background_color=None, text_color=None, checkbox_color=None, disabled=None, visible=None):
- """
- Changes some of the settings for the Checkbox Element. Must call `Window.Read` or `Window.Finalize` prior.
- Note that changing visibility may cause element to change locations when made visible after invisible
-
- Changes will not be visible in your window until you call window.read or window.refresh.
-
- If you change visibility, your element may MOVE. If you want it to remain stationary, use the "layout helper"
- function "pin" to ensure your element is "pinned" to that location in your layout so that it returns there
- when made visible.
-
- :param value: if True checks the checkbox, False clears it
- :type value: (bool)
- :param text: Text to display next to checkbox
- :type text: (str)
- :param background_color: color of background
- :type background_color: (str)
- :param text_color: color of the text. Note this also changes the color of the checkmark
- :type text_color: (str)
- :param disabled: disable or enable element
- :type disabled: (bool)
- :param visible: control visibility of element
- :type visible: (bool)
- """
-
- if not self._widget_was_created(): # if widget hasn't been created yet, then don't allow
- return
-
- if value is not None:
- value = bool(value)
- try:
- self.TKIntVar.set(value)
- self.InitialState = value
- except:
- print('Checkbox update failed')
- if disabled is True:
- self.TKCheckbutton.configure(state='disabled')
- elif disabled is False:
- self.TKCheckbutton.configure(state='normal')
- self.Disabled = disabled if disabled is not None else self.Disabled
-
- if text is not None:
- self.Text = str(text)
- self.TKCheckbutton.configure(text=self.Text)
- if background_color not in (None, COLOR_SYSTEM_DEFAULT):
- self.TKCheckbutton.configure(background=background_color)
- self.BackgroundColor = background_color
- if text_color not in (None, COLOR_SYSTEM_DEFAULT):
- self.TKCheckbutton.configure(fg=text_color)
- self.TextColor = text_color
- # Color the checkbox itself
- if checkbox_color not in (None, COLOR_SYSTEM_DEFAULT):
- self.CheckboxBackgroundColor = checkbox_color
- self.TKCheckbutton.configure(selectcolor=self.CheckboxBackgroundColor) # The background of the checkbox
- elif text_color or background_color:
- if self.CheckboxBackgroundColor is not None and self.TextColor is not None and self.BackgroundColor is not None and self.TextColor.startswith(
- '#') and self.BackgroundColor.startswith('#'):
- # ---- compute color of checkbox background ---
- text_hsl = _hex_to_hsl(self.TextColor)
- background_hsl = _hex_to_hsl(self.BackgroundColor if self.BackgroundColor else theme_background_color())
- l_delta = abs(text_hsl[2] - background_hsl[2]) / 10
- if text_hsl[2] > background_hsl[2]: # if the text is "lighter" than the background then make background darker
- bg_rbg = _hsl_to_rgb(background_hsl[0], background_hsl[1], background_hsl[2] - l_delta)
- else:
- bg_rbg = _hsl_to_rgb(background_hsl[0], background_hsl[1], background_hsl[2] + l_delta)
- self.CheckboxBackgroundColor = rgb(*bg_rbg)
- self.TKCheckbutton.configure(selectcolor=self.CheckboxBackgroundColor) # The background of the checkbox
-
- if visible is False:
- self._pack_forget_save_settings()
- elif visible is True:
- self._pack_restore_settings()
-
- if visible is not None:
- self._visible = visible
-
- Get = get
- Update = update
-
-
-# ------------------------- CHECKBOX Element lazy functions ------------------------- #
-CB = Checkbox
-CBox = Checkbox
-Check = Checkbox
-
-
-# ---------------------------------------------------------------------- #
-# Spin #
-# ---------------------------------------------------------------------- #
-
-class Spin(Element):
- """
- A spinner with up/down buttons and a single line of text. Choose 1 values from list
- """
-
- def __init__(self, values, initial_value=None, disabled=False, change_submits=False, enable_events=False, readonly=False,
- size=(None, None), s=(None, None), auto_size_text=None, bind_return_key=None, font=None, background_color=None, text_color=None, key=None, k=None, pad=None, p=None,
- tooltip=None, right_click_menu=None, expand_x=False, expand_y=False, visible=True, metadata=None):
- """
- :param values: List of valid values
- :type values: Tuple[Any] or List[Any]
- :param initial_value: Initial item to show in window. Choose from list of values supplied
- :type initial_value: (Any)
- :param disabled: set disable state
- :type disabled: (bool)
- :param change_submits: DO NOT USE. Only listed for backwards compat - Use enable_events instead
- :type change_submits: (bool)
- :param enable_events: Turns on the element specific events. Spin events happen when an item changes
- :type enable_events: (bool)
- :param readonly: Turns on the element specific events. Spin events happen when an item changes
- :type readonly: (bool)
- :param size: (w, h) w=characters-wide, h=rows-high. If an int instead of a tuple is supplied, then height is auto-set to 1
- :type size: (int, int) | (None, None) | int
- :param s: Same as size parameter. It's an alias. If EITHER of them are set, then the one that's set will be used. If BOTH are set, size will be used
- :type s: (int, int) | (None, None) | int
- :param auto_size_text: if True will size the element to match the length of the text
- :type auto_size_text: (bool)
- :param bind_return_key: If True, then the return key will cause a the element to generate an event
- :type bind_return_key: (bool)
- :param font: specifies the font family, size, etc. Tuple or Single string format 'name size styles'. Styles: italic * roman bold normal underline overstrike
- :type font: (str or (str, int[, str]) or None)
- :param background_color: color of background
- :type background_color: (str)
- :param text_color: color of the text
- :type text_color: (str)
- :param key: Used with window.find_element and with return values to uniquely identify this element
- :type key: str | int | tuple | object
- :param k: Same as the Key. You can use either k or key. Which ever is set will be used.
- :type k: str | int | tuple | object
- :param pad: Amount of padding to put around element in pixels (left/right, top/bottom) or ((left, right), (top, bottom)) or an int. If an int, then it's converted into a tuple (int, int)
- :type pad: (int, int) or ((int, int),(int,int)) or (int,(int,int)) or ((int, int),int) | int
- :param p: Same as pad parameter. It's an alias. If EITHER of them are set, then the one that's set will be used. If BOTH are set, pad will be used
- :type p: (int, int) or ((int, int),(int,int)) or (int,(int,int)) or ((int, int),int) | int
- :param tooltip: text, that will appear when mouse hovers over the element
- :type tooltip: (str)
- :param right_click_menu: A list of lists of Menu items to show when this element is right clicked. See user docs for exact format.
- :type right_click_menu: List[List[ List[str] | str ]]
- :param expand_x: If True the element will automatically expand in the X direction to fill available space
- :type expand_x: (bool)
- :param expand_y: If True the element will automatically expand in the Y direction to fill available space
- :type expand_y: (bool)
- :param visible: set visibility state of the element
- :type visible: (bool)
- :param metadata: User metadata that can be set to ANYTHING
- :type metadata: (Any)
- """
-
-
- self.Values = values
- self.DefaultValue = initial_value
- self.ChangeSubmits = change_submits or enable_events
- self.TKSpinBox = self.Widget = None # type: tk.Spinbox
- self.Disabled = disabled
- self.Readonly = readonly
- self.RightClickMenu = right_click_menu
- self.BindReturnKey = bind_return_key
-
- bg = background_color if background_color else DEFAULT_INPUT_ELEMENTS_COLOR
- fg = text_color if text_color is not None else DEFAULT_INPUT_TEXT_COLOR
- key = key if key is not None else k
- sz = size if size != (None, None) else s
- pad = pad if pad is not None else p
- self.expand_x = expand_x
- self.expand_y = expand_y
-
-
- super().__init__(ELEM_TYPE_INPUT_SPIN, size=sz, auto_size_text=auto_size_text, font=font, background_color=bg, text_color=fg,
- key=key, pad=pad, tooltip=tooltip, visible=visible, metadata=metadata)
- return
-
- def update(self, value=None, values=None, disabled=None, readonly=None, visible=None):
- """
- Changes some of the settings for the Spin Element. Must call `Window.Read` or `Window.Finalize` prior
- Note that the state can be in 3 states only.... enabled, disabled, readonly even
- though more combinations are available. The easy way to remember is that if you
- change the readonly parameter then you are enabling the element.
-
- Changes will not be visible in your window until you call window.read or window.refresh.
-
- If you change visibility, your element may MOVE. If you want it to remain stationary, use the "layout helper"
- function "pin" to ensure your element is "pinned" to that location in your layout so that it returns there
- when made visible.
-
- :param value: set the current value from list of choices
- :type value: (Any)
- :param values: set available choices
- :type values: List[Any]
- :param disabled: disable. Note disabled and readonly cannot be mixed. It must be one OR the other
- :type disabled: (bool)
- :param readonly: make element readonly. Note disabled and readonly cannot be mixed. It must be one OR the other
- :type readonly: (bool)
- :param visible: control visibility of element
- :type visible: (bool)
- """
-
- if not self._widget_was_created(): # if widget hasn't been created yet, then don't allow
- return
-
- if values != None:
- old_value = self.TKStringVar.get()
- self.Values = values
- self.TKSpinBox.configure(values=values)
- self.TKStringVar.set(old_value)
- if value is not None:
- try:
- self.TKStringVar.set(value)
- self.DefaultValue = value
- except:
- pass
-
- if readonly is True:
- self.Readonly = True
- self.TKSpinBox['state'] = 'readonly'
- elif readonly is False:
- self.Readonly = False
- self.TKSpinBox['state'] = 'normal'
- if disabled is True:
- self.TKSpinBox['state'] = 'disable'
- elif disabled is False:
- if self.Readonly:
- self.TKSpinBox['state'] = 'readonly'
- else:
- self.TKSpinBox['state'] = 'normal'
- self.Disabled = disabled if disabled is not None else self.Disabled
-
- if visible is False:
- self._pack_forget_save_settings()
- elif visible is True:
- self._pack_restore_settings()
- if visible is not None:
- self._visible = visible
-
-
- def _SpinChangedHandler(self, event):
- """
- Callback function. Used internally only. Called by tkinter when Spinbox Widget changes. Results in Window.Read() call returning
-
- :param event: passed in from tkinter
- :type event:
- """
- # first, get the results table built
- if self.Key is not None:
- self.ParentForm.LastButtonClicked = self.Key
- else:
- self.ParentForm.LastButtonClicked = ''
- self.ParentForm.FormRemainedOpen = True
- _exit_mainloop(self.ParentForm)
- # if self.ParentForm.CurrentlyRunningMainloop:
- # Window._window_that_exited = self.ParentForm
- # self.ParentForm.TKroot.quit() # kick the users out of the mainloop
-
- def get(self):
- """
- Return the current chosen value showing in spinbox.
- This value will be the same as what was provided as list of choices. If list items are ints, then the
- item returned will be an int (not a string)
-
- :return: The currently visible entry
- :rtype: (Any)
- """
- value = self.TKStringVar.get()
- for v in self.Values:
- if str(v) == value:
- value = v
- break
- return value
-
- Get = get
- Update = update
-
-
-Sp = Spin # type: Spin
-
-
-# ---------------------------------------------------------------------- #
-# Multiline #
-# ---------------------------------------------------------------------- #
-class Multiline(Element):
- """
- Multiline Element - Display and/or read multiple lines of text. This is both an input and output element.
- Other PySimpleGUI ports have a separate MultilineInput and MultilineOutput elements. May want to split this
- one up in the future too.
- """
-
- def __init__(self, default_text='', enter_submits=False, disabled=False, autoscroll=False, border_width=None,
- size=(None, None), s=(None, None), auto_size_text=None, background_color=None, text_color=None, horizontal_scroll=False, change_submits=False,
- enable_events=False, do_not_clear=True, key=None, k=None, write_only=False, auto_refresh=False, reroute_stdout=False, reroute_stderr=False, reroute_cprint=False, echo_stdout_stderr=False, focus=False, font=None, pad=None, p=None, tooltip=None, justification=None, no_scrollbar=False,
- sbar_trough_color=None, sbar_background_color=None, sbar_arrow_color=None, sbar_width=None, sbar_arrow_width=None, sbar_frame_color=None, sbar_relief=None,
- expand_x=False, expand_y=False, rstrip=True, right_click_menu=None, visible=True, metadata=None):
- """
- :param default_text: Initial text to show
- :type default_text: (Any)
- :param enter_submits: if True, the Window.Read call will return is enter key is pressed in this element
- :type enter_submits: (bool)
- :param disabled: set disable state
- :type disabled: (bool)
- :param autoscroll: If True the contents of the element will automatically scroll as more data added to the end
- :type autoscroll: (bool)
- :param border_width: width of border around element in pixels
- :type border_width: (int)
- :param size: (w, h) w=characters-wide, h=rows-high. If an int instead of a tuple is supplied, then height is auto-set to 1
- :type size: (int, int) | (None, None) | int
- :param s: Same as size parameter. It's an alias. If EITHER of them are set, then the one that's set will be used. If BOTH are set, size will be used
- :type s: (int, int) | (None, None) | int
- :param auto_size_text: if True will size the element to match the length of the text
- :type auto_size_text: (bool)
- :param background_color: color of background
- :type background_color: (str)
- :param text_color: color of the text
- :type text_color: (str)
- :param horizontal_scroll: Controls if a horizontal scrollbar should be shown. If True a horizontal scrollbar will be shown in addition to vertical
- :type horizontal_scroll: (bool)
- :param change_submits: DO NOT USE. Only listed for backwards compat - Use enable_events instead
- :type change_submits: (bool)
- :param enable_events: Turns on the element specific events. Spin events happen when an item changes
- :type enable_events: (bool)
- :param do_not_clear: if False the element will be cleared any time the Window.Read call returns
- :type do_not_clear: (bool)
- :param key: Used with window.find_element and with return values to uniquely identify this element to uniquely identify this element
- :type key: str | int | tuple | object
- :param k: Same as the Key. You can use either k or key. Which ever is set will be used.
- :type k: str | int | tuple | object
- :param write_only: If True then no entry will be added to the values dictionary when the window is read
- :type write_only: bool
- :param auto_refresh: If True then anytime the element is updated, the window will be refreshed so that the change is immediately displayed
- :type auto_refresh: (bool)
- :param reroute_stdout: If True then all output to stdout will be output to this element
- :type reroute_stdout: (bool)
- :param reroute_stderr: If True then all output to stderr will be output to this element
- :type reroute_stderr: (bool)
- :param reroute_cprint: If True your cprint calls will output to this element. It's the same as you calling cprint_set_output_destination
- :type reroute_cprint: (bool)
- :param echo_stdout_stderr: If True then output to stdout and stderr will be output to this element AND also to the normal console location
- :type echo_stdout_stderr: (bool)
- :param focus: if True initial focus will go to this element
- :type focus: (bool)
- :param font: specifies the font family, size, etc. Tuple or Single string format 'name size styles'. Styles: italic * roman bold normal underline overstrike
- :type font: (str or (str, int[, str]) or None)
- :param pad: Amount of padding to put around element in pixels (left/right, top/bottom) or ((left, right), (top, bottom)) or an int. If an int, then it's converted into a tuple (int, int)
- :type pad: (int, int) or ((int, int),(int,int)) or (int,(int,int)) or ((int, int),int) | int
- :param p: Same as pad parameter. It's an alias. If EITHER of them are set, then the one that's set will be used. If BOTH are set, pad will be used
- :type p: (int, int) or ((int, int),(int,int)) or (int,(int,int)) or ((int, int),int) | int
- :param tooltip: text, that will appear when mouse hovers over the element
- :type tooltip: (str)
- :param justification: text justification. left, right, center. Can use single characters l, r, c.
- :type justification: (str)
- :param no_scrollbar: If False then a vertical scrollbar will be shown (the default)
- :type no_scrollbar: (bool)
- :param sbar_trough_color: Scrollbar color of the trough
- :type sbar_trough_color: (str)
- :param sbar_background_color: Scrollbar color of the background of the arrow buttons at the ends AND the color of the "thumb" (the thing you grab and slide). Switches to arrow color when mouse is over
- :type sbar_background_color: (str)
- :param sbar_arrow_color: Scrollbar color of the arrow at the ends of the scrollbar (it looks like a button). Switches to background color when mouse is over
- :type sbar_arrow_color: (str)
- :param sbar_width: Scrollbar width in pixels
- :type sbar_width: (int)
- :param sbar_arrow_width: Scrollbar width of the arrow on the scrollbar. It will potentially impact the overall width of the scrollbar
- :type sbar_arrow_width: (int)
- :param sbar_frame_color: Scrollbar Color of frame around scrollbar (available only on some ttk themes)
- :type sbar_frame_color: (str)
- :param sbar_relief: Scrollbar relief that will be used for the "thumb" of the scrollbar (the thing you grab that slides). Should be a constant that is defined at starting with "RELIEF_" - RELIEF_RAISED, RELIEF_SUNKEN, RELIEF_FLAT, RELIEF_RIDGE, RELIEF_GROOVE, RELIEF_SOLID
- :type sbar_relief: (str)
- :param expand_x: If True the element will automatically expand in the X direction to fill available space
- :type expand_x: (bool)
- :param expand_y: If True the element will automatically expand in the Y direction to fill available space
- :type expand_y: (bool)
- :param rstrip: If True the value returned in will have whitespace stripped from the right side
- :type rstrip: (bool)
- :param right_click_menu: A list of lists of Menu items to show when this element is right clicked. See user docs for exact format.
- :type right_click_menu: List[List[ List[str] | str ]]
- :param visible: set visibility state of the element
- :type visible: (bool)
- :param metadata: User metadata that can be set to ANYTHING
- :type metadata: (Any)
- """
-
-
- self.DefaultText = str(default_text)
- self.EnterSubmits = enter_submits
- bg = background_color if background_color else DEFAULT_INPUT_ELEMENTS_COLOR
- self.Focus = focus
- self.do_not_clear = do_not_clear
- fg = text_color if text_color is not None else DEFAULT_INPUT_TEXT_COLOR
- self.Autoscroll = autoscroll
- self.Disabled = disabled
- self.ChangeSubmits = change_submits or enable_events
- self.RightClickMenu = right_click_menu
- self.BorderWidth = border_width if border_width is not None else DEFAULT_BORDER_WIDTH
- self.TagCounter = 0
- self.TKText = self.Widget = None # type: tk.Text
- self.element_frame = None # type: tk.Frame
- self.HorizontalScroll = horizontal_scroll
- self.tags = set()
- self.WriteOnly = write_only
- self.AutoRefresh = auto_refresh
- key = key if key is not None else k
- self.previous_stdout = None
- self.previous_stderr = None
- self.reroute_cprint = reroute_cprint
- self.echo_stdout_stderr = echo_stdout_stderr
- self.Justification = 'left' if justification is None else justification
- self.justification_tag = self.just_center_tag = self.just_left_tag = self.just_right_tag = None
- pad = pad if pad is not None else p
- self.expand_x = expand_x
- self.expand_y = expand_y
- self.rstrip = rstrip
- if reroute_stdout:
- self.reroute_stdout_to_here()
- if reroute_stderr:
- self.reroute_stderr_to_here()
- self.no_scrollbar = no_scrollbar
- self.hscrollbar = None # The horizontal scrollbar
- sz = size if size != (None, None) else s
-
- super().__init__(ELEM_TYPE_INPUT_MULTILINE, size=sz, auto_size_text=auto_size_text, background_color=bg,
- text_color=fg, key=key, pad=pad, tooltip=tooltip, font=font or DEFAULT_FONT, visible=visible, metadata=metadata,
- sbar_trough_color=sbar_trough_color, sbar_background_color=sbar_background_color, sbar_arrow_color=sbar_arrow_color, sbar_width=sbar_width, sbar_arrow_width=sbar_arrow_width, sbar_frame_color=sbar_frame_color, sbar_relief=sbar_relief)
- return
-
- def update(self, value=None, disabled=None, append=False, font=None, text_color=None, background_color=None, text_color_for_value=None,
- background_color_for_value=None, visible=None, autoscroll=None, justification=None, font_for_value=None):
- """
- Changes some of the settings for the Multiline Element. Must call `Window.Read` or `Window.Finalize` prior
-
- Changes will not be visible in your window until you call window.read or window.refresh.
-
- If you change visibility, your element may MOVE. If you want it to remain stationary, use the "layout helper"
- function "pin" to ensure your element is "pinned" to that location in your layout so that it returns there
- when made visible.
-
- :param value: new text to display
- :type value: (Any)
- :param disabled: disable or enable state of the element
- :type disabled: (bool)
- :param append: if True then new value will be added onto the end of the current value. if False then contents will be replaced.
- :type append: (bool)
- :param font: specifies the font family, size, etc. Tuple or Single string format 'name size styles'. Styles: italic * roman bold normal underline overstrike for the entire element
- :type font: (str or (str, int[, str]) or None)
- :param text_color: color of the text
- :type text_color: (str)
- :param background_color: color of background
- :type background_color: (str)
- :param text_color_for_value: color of the new text being added (the value paramter)
- :type text_color_for_value: (str)
- :param background_color_for_value: color of the new background of the text being added (the value paramter)
- :type background_color_for_value: (str)
- :param visible: set visibility state of the element
- :type visible: (bool)
- :param autoscroll: if True then contents of element are scrolled down when new text is added to the end
- :type autoscroll: (bool)
- :param justification: text justification. left, right, center. Can use single characters l, r, c. Sets only for this value, not entire element
- :type justification: (str)
- :param font_for_value: specifies the font family, size, etc. Tuple or Single string format 'name size styles'. Styles: italic * roman bold normal underline overstrike for the value being updated
- :type font_for_value: str | (str, int)
- """
-
- if not self._widget_was_created(): # if widget hasn't been created yet, then don't allow
- return
-
- if autoscroll is not None:
- self.Autoscroll = autoscroll
-
- if justification is not None:
- if justification.startswith('l'):
- just_tag = 'left'
- if justification.startswith('r'):
- just_tag = 'right'
- if justification.startswith('c'):
- just_tag = 'center'
- else:
- just_tag = self.justification_tag
-
- starting_point = self.Widget.index(tk.INSERT)
- tag = None
- if value is not None:
- value = str(value)
- if background_color_for_value is not None or text_color_for_value is not None or font_for_value is not None:
- try:
- tag = 'Multiline(' + str(text_color_for_value) + ',' + str(background_color_for_value) + ',' + str(font_for_value) + ')'
- if tag not in self.tags:
- self.tags.add(tag)
- if background_color_for_value is not None:
- self.TKText.tag_configure(tag, background=background_color_for_value)
- if text_color_for_value is not None:
- self.TKText.tag_configure(tag, foreground=text_color_for_value)
- if font_for_value is not None:
- self.TKText.tag_configure(tag, font=font_for_value)
- except Exception as e:
- print('* Multiline.update - bad color likely specified:', e)
- if self.Disabled:
- self.TKText.configure(state='normal')
- try:
- if not append:
- self.TKText.delete('1.0', tk.END)
- if tag is not None or just_tag is not None:
- self.TKText.insert(tk.END, value, (just_tag, tag))
- else:
- self.TKText.insert(tk.END, value)
-
- # self.TKText.tag_add(just_tag, starting_point, starting_point)
-
- except Exception as e:
- print("* Error setting multiline *", e)
- if self.Disabled:
- self.TKText.configure(state='disabled')
- self.DefaultText = value
-
- if self.Autoscroll:
- self.TKText.see(tk.END)
- if disabled is True:
- self.TKText.configure(state='disabled')
- elif disabled is False:
- self.TKText.configure(state='normal')
- self.Disabled = disabled if disabled is not None else self.Disabled
-
- if background_color not in (None, COLOR_SYSTEM_DEFAULT):
- self.TKText.configure(background=background_color)
- if text_color not in (None, COLOR_SYSTEM_DEFAULT):
- self.TKText.configure(fg=text_color)
- if font is not None:
- self.TKText.configure(font=font)
-
-
- if visible is False:
- self._pack_forget_save_settings(alternate_widget=self.element_frame)
- # self.element_frame.pack_forget()
- elif visible is True:
- self._pack_restore_settings(alternate_widget=self.element_frame)
- # self.element_frame.pack(padx=self.pad_used[0], pady=self.pad_used[1])
-
- if self.AutoRefresh and self.ParentForm:
- try: # in case the window was destroyed
- self.ParentForm.refresh()
- except:
- pass
- if visible is not None:
- self._visible = visible
-
- def get(self):
- """
- Return current contents of the Multiline Element
-
- :return: current contents of the Multiline Element (used as an input type of Multiline
- :rtype: (str)
- """
- value = str(self.TKText.get(1.0, tk.END))
- if self.rstrip:
- return value.rstrip()
- return value
-
-
- def print(self, *args, end=None, sep=None, text_color=None, background_color=None, justification=None, font=None, colors=None, t=None, b=None, c=None,
- autoscroll=True):
- """
- Print like Python normally prints except route the output to a multiline element and also add colors if desired
-
- colors -(str, str) or str. A combined text/background color definition in a single parameter
-
- There are also "aliases" for text_color, background_color and colors (t, b, c)
- t - An alias for color of the text (makes for shorter calls)
- b - An alias for the background_color parameter
- c - (str, str) - "shorthand" way of specifying color. (foreground, backgrouned)
- c - str - can also be a string of the format "foreground on background" ("white on red")
-
- With the aliases it's possible to write the same print but in more compact ways:
- cprint('This will print white text on red background', c=('white', 'red'))
- cprint('This will print white text on red background', c='white on red')
- cprint('This will print white text on red background', text_color='white', background_color='red')
- cprint('This will print white text on red background', t='white', b='red')
-
- :param args: The arguments to print
- :type args: (Any)
- :param end: The end char to use just like print uses
- :type end: (str)
- :param sep: The separation character like print uses
- :type sep: (str)
- :param text_color: The color of the text
- :type text_color: (str)
- :param background_color: The background color of the line
- :type background_color: (str)
- :param justification: text justification. left, right, center. Can use single characters l, r, c. Sets only for this value, not entire element
- :type justification: (str)
- :param font: specifies the font family, size, etc. Tuple or Single string format 'name size styles'. Styles: italic * roman bold normal underline overstrike for the args being printed
- :type font: (str or (str, int[, str]) or None)
- :param colors: Either a tuple or a string that has both the text and background colors. Or just the text color
- :type colors: (str) or (str, str)
- :param t: Color of the text
- :type t: (str)
- :param b: The background color of the line
- :type b: (str)
- :param c: Either a tuple or a string that has both the text and background colors or just tex color (same as the color parm)
- :type c: (str) or (str, str)
- :param autoscroll: If True the contents of the element will automatically scroll as more data added to the end
- :type autoscroll: (bool)
- """
-
- kw_text_color = text_color or t
- kw_background_color = background_color or b
- dual_color = colors or c
- try:
- if isinstance(dual_color, tuple):
- kw_text_color = dual_color[0]
- kw_background_color = dual_color[1]
- elif isinstance(dual_color, str):
- if ' on ' in dual_color: # if has "on" in the string, then have both text and background
- kw_text_color = dual_color.split(' on ')[0]
- kw_background_color = dual_color.split(' on ')[1]
- else: # if no "on" then assume the color string is just the text color
- kw_text_color = dual_color
- except Exception as e:
- print('* multiline print warning * you messed up with color formatting', e)
-
- _print_to_element(self, *args, end=end, sep=sep, text_color=kw_text_color, background_color=kw_background_color, justification=justification,
- autoscroll=autoscroll, font=font)
-
- def reroute_stdout_to_here(self):
- """
- Sends stdout (prints) to this element
- """
- self.previous_stdout = sys.stdout
- sys.stdout = self
-
- def reroute_stderr_to_here(self):
- """
- Sends stderr to this element
- """
- self.previous_stderr = sys.stderr
- sys.stderr = self
-
- def restore_stdout(self):
- """
- Restore a previously re-reouted stdout back to the original destination
- """
- if self.previous_stdout:
- sys.stdout = self.previous_stdout
- self.previous_stdout = None # indicate no longer routed here
-
- def restore_stderr(self):
- """
- Restore a previously re-reouted stderr back to the original destination
- """
- if self.previous_stderr:
- sys.stderr = self.previous_stderr
- self.previous_stderr = None # indicate no longer routed here
-
- def write(self, txt):
- """
- Called by Python (not tkinter?) when stdout or stderr wants to write
-
- :param txt: text of output
- :type txt: (str)
- """
- try:
- self.update(txt, append=True)
- if self.echo_stdout_stderr:
- self.previous_stdout.write(txt)
- except:
- pass
-
- def flush(self):
- """
- Flush parameter was passed into a print statement.
- For now doing nothing. Not sure what action should be taken to ensure a flush happens regardless.
- """
- try:
- self.previous_stdout.flush()
- except:
- pass
-
- def __del__(self):
- """
- If this Widget is deleted, be sure and restore the old stdout, stderr
- """
- # These trys are here because found that if the init fails, then
- # the variables holding the old stdout won't exist and will get an error
- try:
- self.restore_stdout()
- except Exception as e:
- pass
- try:
- self.restore_stderr()
- except:
- pass
-
- Get = get
- Update = update
-
-
-ML = Multiline
-MLine = Multiline
-
-
-# ---------------------------------------------------------------------- #
-# Text #
-# ---------------------------------------------------------------------- #
-class Text(Element):
- """
- Text - Display some text in the window. Usually this means a single line of text. However, the text can also be multiple lines. If multi-lined there are no scroll bars.
- """
-
- def __init__(self, text='', size=(None, None), s=(None, None), auto_size_text=None, click_submits=False, enable_events=False, relief=None, font=None,
- text_color=None, background_color=None, border_width=None, justification=None, pad=None, p=None, key=None, k=None, right_click_menu=None, expand_x=False, expand_y=False, grab=None,
- tooltip=None, visible=True, metadata=None):
- """
- :param text: The text to display. Can include /n to achieve multiple lines. Will convert (optional) parameter into a string
- :type text: Any
- :param size: (w, h) w=characters-wide, h=rows-high. If an int instead of a tuple is supplied, then height is auto-set to 1
- :type size: (int, int) | (int, None) | (None, None) | (int, ) | int
- :param s: Same as size parameter. It's an alias. If EITHER of them are set, then the one that's set will be used. If BOTH are set, size will be used
- :type s: (int, int) | (int, None) | (None, None) | (int, ) | int
- :param auto_size_text: if True size of the Text Element will be sized to fit the string provided in 'text' parm
- :type auto_size_text: (bool)
- :param click_submits: DO NOT USE. Only listed for backwards compat - Use enable_events instead
- :type click_submits: (bool)
- :param enable_events: Turns on the element specific events. Text events happen when the text is clicked
- :type enable_events: (bool)
- :param relief: relief style around the text. Values are same as progress meter relief values. Should be a constant that is defined at starting with "RELIEF_" - RELIEF_RAISED, RELIEF_SUNKEN, RELIEF_FLAT, RELIEF_RIDGE, RELIEF_GROOVE, RELIEF_SOLID
- :type relief: (str/enum)
- :param font: specifies the font family, size, etc. Tuple or Single string format 'name size styles'. Styles: italic * roman bold normal underline overstrike
- :type font: (str or (str, int[, str]) or None)
- :param text_color: color of the text
- :type text_color: (str)
- :param background_color: color of background
- :type background_color: (str)
- :param border_width: number of pixels for the border (if using a relief)
- :type border_width: (int)
- :param justification: how string should be aligned within space provided by size. Valid choices = `left`, `right`, `center`
- :type justification: (str)
- :param pad: Amount of padding to put around element in pixels (left/right, top/bottom) or ((left, right), (top, bottom)) or an int. If an int, then it's converted into a tuple (int, int)
- :type pad: (int, int) or ((int, int),(int,int)) or (int,(int,int)) or ((int, int),int) | int
- :param p: Same as pad parameter. It's an alias. If EITHER of them are set, then the one that's set will be used. If BOTH are set, pad will be used
- :type p: (int, int) or ((int, int),(int,int)) or (int,(int,int)) or ((int, int),int) | int
- :param key: Used with window.find_element and with return values to uniquely identify this element to uniquely identify this element
- :type key: str or int or tuple or object
- :param k: Same as the Key. You can use either k or key. Which ever is set will be used.
- :type k: str | int | tuple | object
- :param right_click_menu: A list of lists of Menu items to show when this element is right clicked. See user docs for exact format.
- :type right_click_menu: List[List[ List[str] | str ]]
- :param expand_x: If True the element will automatically expand in the X direction to fill available space
- :type expand_x: (bool)
- :param expand_y: If True the element will automatically expand in the Y direction to fill available space
- :type expand_y: (bool)
- :param grab: If True can grab this element and move the window around. Default is False
- :type grab: (bool)
- :param tooltip: text, that will appear when mouse hovers over the element
- :type tooltip: (str)
- :param visible: set visibility state of the element
- :type visible: (bool)
- :param metadata: User metadata that can be set to ANYTHING
- :type metadata: (Any)
- """
-
- self.DisplayText = str(text)
- self.TextColor = text_color if text_color else DEFAULT_TEXT_COLOR
- self.Justification = justification
- self.Relief = relief
- self.ClickSubmits = click_submits or enable_events
- if background_color is None:
- bg = DEFAULT_TEXT_ELEMENT_BACKGROUND_COLOR
- else:
- bg = background_color
- self.RightClickMenu = right_click_menu
- self.TKRightClickMenu = None
- self.BorderWidth = border_width
- self.Grab = grab
- key = key if key is not None else k
- sz = size if size != (None, None) else s
- pad = pad if pad is not None else p
- self.expand_x = expand_x
- self.expand_y = expand_y
-
- super().__init__(ELEM_TYPE_TEXT, auto_size_text=auto_size_text, size=sz, background_color=bg, font=font if font else DEFAULT_FONT,
- text_color=self.TextColor, pad=pad, key=key, tooltip=tooltip, visible=visible, metadata=metadata)
-
- def update(self, value=None, background_color=None, text_color=None, font=None, visible=None):
- """
- Changes some of the settings for the Text Element. Must call `Window.Read` or `Window.Finalize` prior
-
- Changes will not be visible in your window until you call window.read or window.refresh.
-
- If you change visibility, your element may MOVE. If you want it to remain stationary, use the "layout helper"
- function "pin" to ensure your element is "pinned" to that location in your layout so that it returns there
- when made visible.
-
- :param value: new text to show
- :type value: (str)
- :param background_color: color of background
- :type background_color: (str)
- :param text_color: color of the text
- :type text_color: (str)
- :param font: specifies the font family, size, etc. Tuple or Single string format 'name size styles'. Styles: italic * roman bold normal underline overstrike
- :type font: (str or (str, int[, str]) or None)
- :param visible: set visibility state of the element
- :type visible: (bool)
- """
-
- if not self._widget_was_created(): # if widget hasn't been created yet, then don't allow
- return
- if value is not None:
- self.DisplayText = str(value)
- self.TKStringVar.set(str(value))
- if background_color not in (None, COLOR_SYSTEM_DEFAULT):
- self.TKText.configure(background=background_color)
- if text_color not in (None, COLOR_SYSTEM_DEFAULT):
- self.TKText.configure(fg=text_color)
- if font is not None:
- self.TKText.configure(font=font)
- if visible is False:
- self._pack_forget_save_settings()
- # self.TKText.pack_forget()
- elif visible is True:
- self._pack_restore_settings()
- # self.TKText.pack(padx=self.pad_used[0], pady=self.pad_used[1])
- if visible is not None:
- self._visible = visible
-
- def get(self):
- """
- Gets the current value of the displayed text
-
- :return: The current value
- :rtype: (str)
- """
- try:
- text = self.TKStringVar.get()
- except:
- text = ''
- return text
-
-
- @classmethod
- def fonts_installed_list(cls):
- """
- Returns a list of strings that tkinter reports as the installed fonts
-
- :return: List of the installed font names
- :rtype: List[str]
- """
- # A window must exist before can perform this operation. Create the hidden master root if it doesn't exist
- _get_hidden_master_root()
-
- fonts = list(tkinter.font.families())
- fonts.sort()
-
- return fonts
-
-
- @classmethod
- def char_width_in_pixels(cls, font, character='W'):
- """
- Get the with of the character "W" in pixels for the font being passed in or
- the character of your choosing if "W" is not a good representative character.
- Cannot be used until a window has been created.
- If an error occurs, 0 will be returned
- :param font: specifies the font family, size, etc. Tuple or Single string format 'name size styles'. Styles: italic * roman bold normal underline overstrike, to be measured
- :type font: (str or (str, int[, str]) or None)
- :param character: specifies a SINGLE CHARACTER character to measure
- :type character: (str)
- :return: Width in pixels of "A"
- :rtype: (int)
- """
- # A window must exist before can perform this operation. Create the hidden master root if it doesn't exist
- _get_hidden_master_root()
-
- size = 0
- try:
- size = tkinter.font.Font(font=font).measure(character) # single character width
- except Exception as e:
- _error_popup_with_traceback('Exception retrieving char width in pixels', e)
-
- return size
-
- @classmethod
- def char_height_in_pixels(cls, font):
- """
- Get the height of a string if using the supplied font in pixels.
- Cannot be used until a window has been created.
- If an error occurs, 0 will be returned
- :param font: specifies the font family, size, etc. Tuple or Single string format 'name size styles'. Styles: italic * roman bold normal underline overstrike, to be measured
- :type font: (str or (str, int[, str]) or None)
- :return: Height in pixels of "A"
- :rtype: (int)
- """
-
- # A window must exist before can perform this operation. Create the hidden master root if it doesn't exist
- _get_hidden_master_root()
-
-
- size = 0
- try:
- size = tkinter.font.Font(font=font).metrics('linespace')
- except Exception as e:
- _error_popup_with_traceback('Exception retrieving char height in pixels', e)
-
- return size
-
- @classmethod
- def string_width_in_pixels(cls, font, string):
- """
- Get the with of the supplied string in pixels for the font being passed in.
- If an error occurs, 0 will be returned
- :param font: specifies the font family, size, etc. Tuple or Single string format 'name size styles'. Styles: italic * roman bold normal underline overstrike, to be measured
- :type font: (str or (str, int[, str]) or None)
- :param string: the string to measure
- :type string: str
- :return: Width in pixels of string
- :rtype: (int)
- """
-
- # A window must exist before can perform this operation. Create the hidden master root if it doesn't exist
- _get_hidden_master_root()
-
- size = 0
- try:
- size = tkinter.font.Font(font=font).measure(string) # string's width
- except Exception as e:
- _error_popup_with_traceback('Exception retrieving string width in pixels', e)
-
- return size
-
- def _print_to_element(self, *args, end=None, sep=None, text_color=None, background_color=None, autoscroll=None, justification=None, font=None, append=None):
- """
- Print like Python normally prints except route the output to a multiline element and also add colors if desired
-
- :param multiline_element: The multiline element to be output to
- :type multiline_element: (Multiline)
- :param args: The arguments to print
- :type args: List[Any]
- :param end: The end char to use just like print uses
- :type end: (str)
- :param sep: The separation character like print uses
- :type sep: (str)
- :param text_color: color of the text
- :type text_color: (str)
- :param background_color: The background color of the line
- :type background_color: (str)
- :param autoscroll: If True (the default), the element will scroll to bottom after updating
- :type autoscroll: (bool)
- :param font: specifies the font family, size, etc. Tuple or Single string format 'name size styles'. Styles: italic * roman bold normal underline overstrike for the value being updated
- :type font: str | (str, int)
- """
- end_str = str(end) if end is not None else '\n'
- sep_str = str(sep) if sep is not None else ' '
-
- outstring = ''
- num_args = len(args)
- for i, arg in enumerate(args):
- outstring += str(arg)
- if i != num_args - 1:
- outstring += sep_str
- outstring += end_str
- if append:
- outstring = self.get() + outstring
-
- self.update(outstring, text_color=text_color, background_color=background_color, font=font)
-
- try: # if the element is set to autorefresh, then refresh the parent window
- if self.AutoRefresh:
- self.ParentForm.refresh()
- except:
- pass
-
- def print(self, *args, end=None, sep=None, text_color=None, background_color=None, justification=None, font=None, colors=None, t=None, b=None, c=None, autoscroll=True, append=True):
- """
- Print like Python normally prints except route the output to a multiline element and also add colors if desired
-
- colors -(str, str) or str. A combined text/background color definition in a single parameter
-
- There are also "aliases" for text_color, background_color and colors (t, b, c)
- t - An alias for color of the text (makes for shorter calls)
- b - An alias for the background_color parameter
- c - (str, str) - "shorthand" way of specifying color. (foreground, backgrouned)
- c - str - can also be a string of the format "foreground on background" ("white on red")
-
- With the aliases it's possible to write the same print but in more compact ways:
- cprint('This will print white text on red background', c=('white', 'red'))
- cprint('This will print white text on red background', c='white on red')
- cprint('This will print white text on red background', text_color='white', background_color='red')
- cprint('This will print white text on red background', t='white', b='red')
-
- :param args: The arguments to print
- :type args: (Any)
- :param end: The end char to use just like print uses
- :type end: (str)
- :param sep: The separation character like print uses
- :type sep: (str)
- :param text_color: The color of the text
- :type text_color: (str)
- :param background_color: The background color of the line
- :type background_color: (str)
- :param justification: text justification. left, right, center. Can use single characters l, r, c. Sets only for this value, not entire element
- :type justification: (str)
- :param font: specifies the font family, size, etc. Tuple or Single string format 'name size styles'. Styles: italic * roman bold normal underline overstrike for the args being printed
- :type font: (str or (str, int[, str]) or None)
- :param colors: Either a tuple or a string that has both the text and background colors. Or just the text color
- :type colors: (str) or (str, str)
- :param t: Color of the text
- :type t: (str)
- :param b: The background color of the line
- :type b: (str)
- :param c: Either a tuple or a string that has both the text and background colors or just tex color (same as the color parm)
- :type c: (str) or (str, str)
- :param autoscroll: If True the contents of the element will automatically scroll as more data added to the end
- :type autoscroll: (bool)
- """
-
- kw_text_color = text_color or t
- kw_background_color = background_color or b
- dual_color = colors or c
- try:
- if isinstance(dual_color, tuple):
- kw_text_color = dual_color[0]
- kw_background_color = dual_color[1]
- elif isinstance(dual_color, str):
- if ' on ' in dual_color: # if has "on" in the string, then have both text and background
- kw_text_color = dual_color.split(' on ')[0]
- kw_background_color = dual_color.split(' on ')[1]
- else: # if no "on" then assume the color string is just the text color
- kw_text_color = dual_color
- except Exception as e:
- print('* multiline print warning * you messed up with color formatting', e)
-
- self._print_to_element( *args, end=end, sep=sep, text_color=kw_text_color, background_color=kw_background_color, justification=justification, autoscroll=autoscroll, font=font, append=append)
-
-
- Get = get
- Update = update
-
-
-# ------------------------- Text Element lazy functions ------------------------- #
-
-Txt = Text # type: Text
-T = Text # type: Text
-
-
-# ---------------------------------------------------------------------- #
-# StatusBar #
-# ---------------------------------------------------------------------- #
-class StatusBar(Element):
- """
- A StatusBar Element creates the sunken text-filled strip at the bottom. Many Windows programs have this line
- """
-
- def __init__(self, text, size=(None, None), s=(None, None), auto_size_text=None, click_submits=None, enable_events=False,
- relief=RELIEF_SUNKEN, font=None, text_color=None, background_color=None, justification=None, pad=None, p=None,
- key=None, k=None, right_click_menu=None, expand_x=False, expand_y=False, tooltip=None, visible=True, metadata=None):
- """
- :param text: Text that is to be displayed in the widget
- :type text: (str)
- :param size: (w, h) w=characters-wide, h=rows-high. If an int instead of a tuple is supplied, then height is auto-set to 1
- :type size: (int, int) | (int, None) | int
- :param s: Same as size parameter. It's an alias. If EITHER of them are set, then the one that's set will be used. If BOTH are set, size will be used
- :type s: (int, int) | (None, None) | int
- :param auto_size_text: True if size should fit the text length
- :type auto_size_text: (bool)
- :param click_submits: DO NOT USE. Only listed for backwards compat - Use enable_events instead
- :type click_submits: (bool)
- :param enable_events: Turns on the element specific events. StatusBar events occur when the bar is clicked
- :type enable_events: (bool)
- :param relief: relief style. Values are same as progress meter relief values. Can be a constant or a string: `RELIEF_RAISED RELIEF_SUNKEN RELIEF_FLAT RELIEF_RIDGE RELIEF_GROOVE RELIEF_SOLID`
- :type relief: (enum)
- :param font: specifies the font family, size, etc. Tuple or Single string format 'name size styles'. Styles: italic * roman bold normal underline overstrike
- :type font: (str or (str, int[, str]) or None)
- :param text_color: color of the text
- :type text_color: (str)
- :param background_color: color of background
- :type background_color: (str)
- :param justification: how string should be aligned within space provided by size. Valid choices = `left`, `right`, `center`
- :type justification: (str)
- :param pad: Amount of padding to put around element in pixels (left/right, top/bottom) or ((left, right), (top, bottom)) or an int. If an int, then it's converted into a tuple (int, int)
- :type pad: (int, int) or ((int, int),(int,int)) or (int,(int,int)) or ((int, int),int) | int
- :param p: Same as pad parameter. It's an alias. If EITHER of them are set, then the one that's set will be used. If BOTH are set, pad will be used
- :type p: (int, int) or ((int, int),(int,int)) or (int,(int,int)) or ((int, int),int) | int
- :param key: Used with window.find_element and with return values to uniquely identify this element to uniquely identify this element
- :type key: str | int | tuple | object
- :param k: Same as the Key. You can use either k or key. Which ever is set will be used.
- :type k: str | int | tuple | object
- :param right_click_menu: A list of lists of Menu items to show when this element is right clicked. See user docs for exact format.
- :type right_click_menu: List[List[ List[str] | str ]]
- :param expand_x: If True the element will automatically expand in the X direction to fill available space
- :type expand_x: (bool)
- :param expand_y: If True the element will automatically expand in the Y direction to fill available space
- :type expand_y: (bool)
- :param tooltip: text, that will appear when mouse hovers over the element
- :type tooltip: (str)
- :param visible: set visibility state of the element
- :type visible: (bool)
- :param metadata: User metadata that can be set to ANYTHING
- :type metadata: (Any)
- """
-
-
- self.DisplayText = text
- self.TextColor = text_color if text_color else DEFAULT_TEXT_COLOR
- self.Justification = justification
- self.Relief = relief
- self.ClickSubmits = click_submits or enable_events
- if background_color is None:
- bg = DEFAULT_TEXT_ELEMENT_BACKGROUND_COLOR
- else:
- bg = background_color
- self.TKText = self.Widget = None # type: tk.Label
- key = key if key is not None else k
- self.RightClickMenu = right_click_menu
- sz = size if size != (None, None) else s
- pad = pad if pad is not None else p
- self.expand_x = expand_x
- self.expand_y = expand_y
-
- super().__init__(ELEM_TYPE_STATUSBAR, size=sz, auto_size_text=auto_size_text, background_color=bg,
- font=font or DEFAULT_FONT, text_color=self.TextColor, pad=pad, key=key, tooltip=tooltip,
- visible=visible, metadata=metadata)
- return
-
- def update(self, value=None, background_color=None, text_color=None, font=None, visible=None):
- """
- Changes some of the settings for the Status Bar Element. Must call `Window.Read` or `Window.Finalize` prior
-
- Changes will not be visible in your window until you call window.read or window.refresh.
-
- If you change visibility, your element may MOVE. If you want it to remain stationary, use the "layout helper"
- function "pin" to ensure your element is "pinned" to that location in your layout so that it returns there
- when made visible.
-
- :param value: new text to show
- :type value: (str)
- :param background_color: color of background
- :type background_color: (str)
- :param text_color: color of the text
- :type text_color: (str)
- :param font: specifies the font family, size, etc. Tuple or Single string format 'name size styles'. Styles: italic * roman bold normal underline overstrike
- :type font: (str or (str, int[, str]) or None)
- :param visible: set visibility state of the element
- :type visible: (bool)
- """
-
- if not self._widget_was_created(): # if widget hasn't been created yet, then don't allow
- return
-
- if value is not None:
- self.DisplayText = value
- stringvar = self.TKStringVar
- stringvar.set(value)
- if background_color not in (None, COLOR_SYSTEM_DEFAULT):
- self.TKText.configure(background=background_color)
- if text_color not in (None, COLOR_SYSTEM_DEFAULT):
- self.TKText.configure(fg=text_color)
- if font is not None:
- self.TKText.configure(font=font)
- if visible is False:
- self._pack_forget_save_settings()
- # self.TKText.pack_forget()
- elif visible is True:
- self._pack_restore_settings()
- # self.TKText.pack(padx=self.pad_used[0], pady=self.pad_used[1])
- if visible is not None:
- self._visible = visible
-
- Update = update
-
-
-SBar = StatusBar
-
-
-# ---------------------------------------------------------------------- #
-# TKProgressBar #
-# Emulate the TK ProgressBar using canvas and rectangles
-# ---------------------------------------------------------------------- #
-
-class TKProgressBar():
- uniqueness_counter = 0
-
- def __init__(self, root, max, length=400, width=DEFAULT_PROGRESS_BAR_SIZE[1], ttk_theme=DEFAULT_TTK_THEME, style_name='',
- relief=DEFAULT_PROGRESS_BAR_RELIEF, border_width=DEFAULT_PROGRESS_BAR_BORDER_WIDTH,
- orientation='horizontal', BarColor=(None, None), key=None):
- """
- :param root: The root window bar is to be shown in
- :type root: tk.Tk | tk.TopLevel
- :param max: Maximum value the bar will be measuring
- :type max: (int)
- :param length: length in pixels of the bar
- :type length: (int)
- :param width: width in pixels of the bar
- :type width: (int)
- :param style_name: Progress bar style to use. Set in the packer function
- :type style_name: (str)
- :param ttk_theme: Progress bar style defined as one of these 'default', 'winnative', 'clam', 'alt', 'classic', 'vista', 'xpnative'
- :type ttk_theme: (str)
- :param relief: relief style. Values are same as progress meter relief values. Can be a constant or a string: `RELIEF_RAISED RELIEF_SUNKEN RELIEF_FLAT RELIEF_RIDGE RELIEF_GROOVE RELIEF_SOLID` (Default value = DEFAULT_PROGRESS_BAR_RELIEF)
- :type relief: (str)
- :param border_width: The amount of pixels that go around the outside of the bar
- :type border_width: (int)
- :param orientation: 'horizontal' or 'vertical' ('h' or 'v' work) (Default value = 'vertical')
- :type orientation: (str)
- :param BarColor: The 2 colors that make up a progress bar. One is the background, the other is the bar
- :type BarColor: (str, str)
- :param key: Used with window.find_element and with return values to uniquely identify this element to uniquely identify this element
- :type key: str | int | tuple | object
- """
-
- self.Length = length
- self.Width = width
- self.Max = max
- self.Orientation = orientation
- self.Count = None
- self.PriorCount = 0
- self.style_name = style_name
-
- TKProgressBar.uniqueness_counter += 1
-
- if orientation.lower().startswith('h'):
- s = ttk.Style()
- _change_ttk_theme(s, ttk_theme)
-
- # self.style_name = str(key) + str(TKProgressBar.uniqueness_counter) + "my.Horizontal.TProgressbar"
- if BarColor != COLOR_SYSTEM_DEFAULT and BarColor[0] != COLOR_SYSTEM_DEFAULT:
- s.configure(self.style_name, background=BarColor[0], troughcolor=BarColor[1],
- troughrelief=relief, borderwidth=border_width, thickness=width)
- else:
- s.configure(self.style_name, troughrelief=relief, borderwidth=border_width, thickness=width)
-
- self.TKProgressBarForReal = ttk.Progressbar(root, maximum=self.Max, style=self.style_name, length=length, orient=tk.HORIZONTAL, mode='determinate')
- else:
- s = ttk.Style()
- _change_ttk_theme(s, ttk_theme)
- # self.style_name = str(key) + str(TKProgressBar.uniqueness_counter) + "my.Vertical.TProgressbar"
- if BarColor != COLOR_SYSTEM_DEFAULT and BarColor[0] != COLOR_SYSTEM_DEFAULT:
-
- s.configure(self.style_name, background=BarColor[0],
- troughcolor=BarColor[1], troughrelief=relief, borderwidth=border_width, thickness=width)
- else:
- s.configure(self.style_name, troughrelief=relief, borderwidth=border_width, thickness=width)
-
- self.TKProgressBarForReal = ttk.Progressbar(root, maximum=self.Max, style=self.style_name, length=length, orient=tk.VERTICAL, mode='determinate')
-
- def Update(self, count=None, max=None):
- """
- Update the current value of the bar and/or update the maximum value the bar can reach
- :param count: current value
- :type count: (int)
- :param max: the maximum value
- :type max: (int)
- """
- if max is not None:
- self.Max = max
- try:
- self.TKProgressBarForReal.config(maximum=max)
- except:
- return False
- if count is not None:
- try:
- self.TKProgressBarForReal['value'] = count
- except:
- return False
- return True
-
-#
-# # ---------------------------------------------------------------------- #
-# # TKOutput #
-# # New Type of TK Widget that's a Text Widget in disguise #
-# # Note that it's inherited from the TKFrame class so that the #
-# # Scroll bar will span the length of the frame #
-# # ---------------------------------------------------------------------- #
-# class TKOutput(tk.Frame):
-# """
-# tkinter style class. Inherits Frame class from tkinter. Adds a tk.Text and a scrollbar together.
-# Note - This is NOT a user controlled class. Users should NOT be directly using it unless making an extention
-# to PySimpleGUI by directly manipulating tkinter.
-# """
-#
-# def __init__(self, parent, width, height, bd, background_color=None, text_color=None, echo_stdout_stderr=False, font=None, pad=None):
-# """
-# :param parent: The "Root" that the Widget will be in
-# :type parent: tk.Tk | tk.Toplevel
-# :param width: Width in characters
-# :type width: (int)
-# :param height: height in rows
-# :type height: (int)
-# :param bd: Border Depth. How many pixels of border to show
-# :type bd: (int)
-# :param background_color: color of background
-# :type background_color: (str)
-# :param text_color: color of the text
-# :type text_color: (str)
-# :param echo_stdout_stderr: If True then output to stdout will be output to this element AND also to the normal console location
-# :type echo_stdout_stderr: (bool)
-# :param font: specifies the font family, size, etc. Tuple or Single string format 'name size styles'. Styles: italic * roman bold normal underline overstrike
-# :type font: (str or (str, int[, str]) or None)
-# :param pad: Amount of padding to put around element in pixels (left/right, top/bottom) or ((left, right), (top, bottom)) or an int. If an int, then it's converted into a tuple (int, int)
-# :type pad: (int, int) or ((int, int),(int,int)) or (int,(int,int)) or ((int, int),int) | int
-# """
-# self.frame = tk.Frame(parent)
-# tk.Frame.__init__(self, self.frame)
-# self.output = tk.Text(self.frame, width=width, height=height, bd=bd, font=font)
-# if background_color and background_color != COLOR_SYSTEM_DEFAULT:
-# self.output.configure(background=background_color)
-# self.frame.configure(background=background_color)
-# if text_color and text_color != COLOR_SYSTEM_DEFAULT:
-# self.output.configure(fg=text_color)
-# self.output.configure(insertbackground=text_color)
-# self.vsb = tk.Scrollbar(self.frame, orient="vertical", command=self.output.yview)
-# self.output.configure(yscrollcommand=self.vsb.set)
-# self.output.pack(side="left", fill="both", expand=True)
-# self.vsb.pack(side="left", fill="y", expand=False)
-# self.frame.pack(side="left", padx=pad[0], pady=pad[1], expand=True, fill='y')
-# self.previous_stdout = sys.stdout
-# self.previous_stderr = sys.stderr
-# self.parent = parent
-# self.echo_stdout_stderr = echo_stdout_stderr
-#
-# sys.stdout = self
-# sys.stderr = self
-# self.pack()
-#
-# def write(self, txt):
-# """
-# Called by Python (not tkinter?) when stdout or stderr wants to write
-# Refreshes the window after the write so that the change is immediately displayed
-#
-# :param txt: text of output
-# :type txt: (str)
-# """
-# try:
-# self.output.insert(tk.END, str(txt))
-# self.output.see(tk.END)
-# self.parent.update()
-# except:
-# pass
-#
-# try:
-# if self.echo_stdout_stderr:
-# self.previous_stdout.write(txt)
-# except:
-# pass
-#
-# def Close(self):
-# """
-# Called when wanting to restore the old stdout/stderr
-# """
-# sys.stdout = self.previous_stdout
-# sys.stderr = self.previous_stderr
-#
-# def flush(self):
-# """
-# Flush parameter was passed into a print statement.
-# For now doing nothing. Not sure what action should be taken to ensure a flush happens regardless.
-# """
-# try:
-# if self.echo_stdout_stderr:
-# self.previous_stdout.flush()
-# except:
-# pass
-#
-# def __del__(self):
-# """
-# If this Widget is deleted, be sure and restore the old stdout, stderr
-# """
-# sys.stdout = self.previous_stdout
-# sys.stderr = self.previous_stderr
-
-
-# ---------------------------------------------------------------------- #
-# Output #
-# Routes stdout, stderr to a scrolled window #
-# ---------------------------------------------------------------------- #
-class Output(Multiline):
- """
- ** NOTE - It's recommended to use Multiline Element instead **
-
- Output Element - a multi-lined text area where stdout and stderr are re-routed to.
-
- The Multiline Element is the superior and recommended method for showing the output of stdout.
- The Multiline Element has been added to significantly while the Output element has not.
- If you choose to use a Multiline element to replace an Output element, be sure an turn on the write_only paramter in the Multline
-
- Of course, Output Element continues to operate and be backwards compatible, but you're missing out on
- features such as routing the cprint output to the element.
-
- In Apr 2022, the Output Element was switched to be a subclass of the Multiline so that more code will be in common. Nowever
- you will not get all of the parms unless you switch to the Multline Specifically
- """
-
- def __init__(self, size=(None, None), s=(None, None), background_color=None, text_color=None, pad=None, p=None, echo_stdout_stderr=False, font=None, tooltip=None,
- key=None, k=None, right_click_menu=None, expand_x=False, expand_y=False, visible=True, metadata=None,
- sbar_trough_color=None, sbar_background_color=None, sbar_arrow_color=None, sbar_width=None, sbar_arrow_width=None, sbar_frame_color=None, sbar_relief=None):
- """
- :param size: (w, h) w=characters-wide, h=rows-high. If an int instead of a tuple is supplied, then height is auto-set to 1
- :type size: (int, int) | (None, None) | int
- :param s: Same as size parameter. It's an alias. If EITHER of them are set, then the one that's set will be used. If BOTH are set, size will be used
- :type s: (int, int) | (None, None) | int
- :param background_color: color of background
- :type background_color: (str)
- :param text_color: color of the text
- :type text_color: (str)
- :param pad: Amount of padding to put around element in pixels (left/right, top/bottom) or ((left, right), (top, bottom)) or an int. If an int, then it's converted into a tuple (int, int)
- :type pad: (int, int) or ((int, int),(int,int)) or (int,(int,int)) or ((int, int),int) | int
- :param p: Same as pad parameter. It's an alias. If EITHER of them are set, then the one that's set will be used. If BOTH are set, pad will be used
- :type p: (int, int) or ((int, int),(int,int)) or (int,(int,int)) or ((int, int),int) | int
- :param echo_stdout_stderr: If True then output to stdout will be output to this element AND also to the normal console location
- :type echo_stdout_stderr: (bool)
- :param font: specifies the font family, size, etc. Tuple or Single string format 'name size styles'. Styles: italic * roman bold normal underline overstrike
- :type font: (str or (str, int[, str]) or None)
- :param tooltip: text, that will appear when mouse hovers over the element
- :type tooltip: (str)
- :param key: Used with window.find_element and with return values to uniquely identify this element to uniquely identify this element
- :type key: str | int | tuple | object
- :param k: Same as the Key. You can use either k or key. Which ever is set will be used.
- :type k: str | int | tuple | object
- :param right_click_menu: A list of lists of Menu items to show when this element is right clicked. See user docs for exact format.
- :type right_click_menu: List[List[ List[str] | str ]]
- :param expand_x: If True the element will automatically expand in the X direction to fill available space
- :type expand_x: (bool)
- :param expand_y: If True the element will automatically expand in the Y direction to fill available space
- :type expand_y: (bool)
- :param visible: set visibility state of the element
- :type visible: (bool)
- :param metadata: User metadata that can be set to ANYTHING
- :type metadata: (Any)
- :param sbar_trough_color: Scrollbar color of the trough
- :type sbar_trough_color: (str)
- :param sbar_background_color: Scrollbar color of the background of the arrow buttons at the ends AND the color of the "thumb" (the thing you grab and slide). Switches to arrow color when mouse is over
- :type sbar_background_color: (str)
- :param sbar_arrow_color: Scrollbar color of the arrow at the ends of the scrollbar (it looks like a button). Switches to background color when mouse is over
- :type sbar_arrow_color: (str)
- :param sbar_width: Scrollbar width in pixels
- :type sbar_width: (int)
- :param sbar_arrow_width: Scrollbar width of the arrow on the scrollbar. It will potentially impact the overall width of the scrollbar
- :type sbar_arrow_width: (int)
- :param sbar_frame_color: Scrollbar Color of frame around scrollbar (available only on some ttk themes)
- :type sbar_frame_color: (str)
- :param sbar_relief: Scrollbar relief that will be used for the "thumb" of the scrollbar (the thing you grab that slides). Should be a constant that is defined at starting with "RELIEF_" - RELIEF_RAISED, RELIEF_SUNKEN, RELIEF_FLAT, RELIEF_RIDGE, RELIEF_GROOVE, RELIEF_SOLID
- :type sbar_relief: (str)
- """
-
-
- # self._TKOut = self.Widget = None # type: TKOutput
- # bg = background_color if background_color else DEFAULT_INPUT_ELEMENTS_COLOR
- # fg = text_color if text_color is not None else DEFAULT_INPUT_TEXT_COLOR
- # self.RightClickMenu = right_click_menu
- # key = key if key is not None else k
- # self.echo_stdout_stderr = echo_stdout_stderr
- # sz = size if size != (None, None) else s
- # pad = pad if pad is not None else p
- # self.expand_x = expand_x
- # self.expand_y = expand_y
-
- super().__init__(size=size, s=s, background_color=background_color, text_color=text_color, pad=pad, p=p, echo_stdout_stderr=echo_stdout_stderr, font=font, tooltip=tooltip,
- key=key, k=k, right_click_menu=right_click_menu, write_only=True, reroute_stdout=True, reroute_stderr=True, autoscroll=True, expand_x=expand_x, expand_y=expand_y, visible=visible, metadata=metadata,
- sbar_trough_color=sbar_trough_color, sbar_background_color=sbar_background_color, sbar_arrow_color=sbar_arrow_color, sbar_width=sbar_width, sbar_arrow_width=sbar_arrow_width, sbar_frame_color=sbar_frame_color, sbar_relief=sbar_relief)
- #
- # @property
- # def tk_out(self):
- # """
- # Returns the TKOutput object used to create the element
- #
- # :return: The TKOutput object
- # :rtype: (TKOutput)
- # """
- # if self._TKOut is None:
- # print('*** Did you forget to call Finalize()? Your code should look something like: ***')
- # print('*** form = sg.Window("My Form").Layout(layout).Finalize() ***')
- # return self._TKOut
- #
- # def update(self, value=None, visible=None):
- # """
- # Changes some of the settings for the Output Element. Must call `Window.Read` or `Window.Finalize` prior
- #
- # Changes will not be visible in your window until you call window.read or window.refresh.
- #
- # If you change visibility, your element may MOVE. If you want it to remain stationary, use the "layout helper"
- # function "pin" to ensure your element is "pinned" to that location in your layout so that it returns there
- # when made visible.
- #
- # :param value: string that will replace current contents of the output area
- # :type value: (str)
- # :param visible: control visibility of element
- # :type visible: (bool)
- # """
- # if not self._widget_was_created(): # if widget hasn't been created yet, then don't allow
- # return
- #
- # if value is not None:
- # self._TKOut.output.delete('1.0', tk.END)
- # self._TKOut.output.insert(tk.END, value)
- # if visible is False:
- # self._pack_forget_save_settings(self._TKOut.frame)
- # elif visible is True:
- # self._pack_restore_settings(self._TKOut.frame)
- #
- # if visible is not None:
- # self._visible = visible
- #
- # def get(self):
- # """
- # Returns the current contents of the output. Similar to Get method other Elements
- # :return: the current value of the output
- # :rtype: (str)
- # """
- # return self._TKOut.output.get(1.0, tk.END)
- #
- # def expand(self, expand_x=False, expand_y=False, expand_row=True):
- # """
- # Causes the Element to expand to fill available space in the X and Y directions. Can specify which or both directions
- #
- # :param expand_x: If True Element will expand in the Horizontal directions
- # :type expand_x: (bool)
- # :param expand_y: If True Element will expand in the Vertical directions
- # :type expand_y: (bool)
- # """
- #
- # if expand_x and expand_y:
- # fill = tk.BOTH
- # elif expand_x:
- # fill = tk.X
- # elif expand_y:
- # fill = tk.Y
- # else:
- # return
- #
- # self._TKOut.output.pack(expand=True, fill=fill)
- # self._TKOut.frame.pack(expand=True, fill=fill)
- # self.ParentRowFrame.pack(expand=expand_row, fill=fill)
- #
- # def __del__(self):
- # """
- # Delete this element. Normally Elements do not have their delete method specified, but for this one
- # it's important that the underlying TKOut object get deleted so that the stdout will get restored properly
- # """
- # self._TKOut.__del__()
-
- # TKOut = tk_out
- # Update = update
- # Get = get
-
-
-# ---------------------------------------------------------------------- #
-# Button Class #
-# ---------------------------------------------------------------------- #
-class Button(Element):
- """
- Button Element - Defines all possible buttons. The shortcuts such as Submit, FileBrowse, ... each create a Button
- """
-
- def __init__(self, button_text='', button_type=BUTTON_TYPE_READ_FORM, target=(None, None), tooltip=None,
- file_types=FILE_TYPES_ALL_FILES, initial_folder=None, default_extension='', disabled=False, change_submits=False,
- enable_events=False, image_filename=None, image_data=None, image_size=(None, None),
- image_subsample=None, image_source=None, border_width=None, size=(None, None), s=(None, None), auto_size_button=None, button_color=None,
- disabled_button_color=None,
- highlight_colors=None, mouseover_colors=(None, None), use_ttk_buttons=None, font=None, bind_return_key=False, focus=False, pad=None, p=None, key=None,
- k=None, right_click_menu=None, expand_x=False, expand_y=False, visible=True, metadata=None):
- """
- :param button_text: Text to be displayed on the button
- :type button_text: (str)
- :param button_type: You should NOT be setting this directly. ONLY the shortcut functions set this
- :type button_type: (int)
- :param target: key or (row,col) target for the button. Note that -1 for column means 1 element to the left of this one. The constant ThisRow is used to indicate the current row. The Button itself is a valid target for some types of button
- :type target: str | (int, int)
- :param tooltip: text, that will appear when mouse hovers over the element
- :type tooltip: (str)
- :param file_types: the filetypes that will be used to match files. To indicate all files: (("ALL Files", "*.* *"),). NOT avoilable on the MAC
- :type file_types: Tuple[(str, str), ...]
- :param initial_folder: starting path for folders and files
- :type initial_folder: (str)
- :param default_extension: If no extension entered by user, add this to filename (only used in saveas dialogs)
- :type default_extension: (str)
- :param disabled: If True button will be created disabled. If BUTTON_DISABLED_MEANS_IGNORE then the button will be ignored rather than disabled using tkinter
- :type disabled: (bool | str)
- :param change_submits: DO NOT USE. Only listed for backwards compat - Use enable_events instead
- :type change_submits: (bool)
- :param enable_events: Turns on the element specific events. If this button is a target, should it generate an event when filled in
- :type enable_events: (bool)
- :param image_source: Image to place on button. Use INSTEAD of the image_filename and image_data. Unifies these into 1 easier to use parm
- :type image_source: (str | bytes)
- :param image_filename: image filename if there is a button image. GIFs and PNGs only.
- :type image_filename: (str)
- :param image_data: Raw or Base64 representation of the image to put on button. Choose either filename or data
- :type image_data: bytes | str
- :param image_size: Size of the image in pixels (width, height)
- :type image_size: (int, int)
- :param image_subsample: amount to reduce the size of the image. Divides the size by this number. 2=1/2, 3=1/3, 4=1/4, etc
- :type image_subsample: (int)
- :param border_width: width of border around button in pixels
- :type border_width: (int)
- :param size: (w, h) w=characters-wide, h=rows-high. If an int instead of a tuple is supplied, then height is auto-set to 1
- :type size: (int | None, int | None) | (None, None) | int
- :param s: Same as size parameter. It's an alias. If EITHER of them are set, then the one that's set will be used. If BOTH are set, size will be used
- :type s: (int | None, int | None) | (None, None) | int
- :param auto_size_button: if True the button size is sized to fit the text
- :type auto_size_button: (bool)
- :param button_color: Color of button. default is from theme or the window. Easy to remember which is which if you say "ON" between colors. "red" on "green". Normally a tuple, but can be a simplified-button-color-string "foreground on background". Can be a single color if want to set only the background.
- :type button_color: (str, str) | str | (int, int) | None
- :param disabled_button_color: colors to use when button is disabled (text, background). Use None for a color if don't want to change. Only ttk buttons support both text and background colors. tk buttons only support changing text color
- :type disabled_button_color: (str, str) | str
- :param highlight_colors: colors to use when button has focus (has focus, does not have focus). None will use colors based on theme. Only used by Linux and only for non-TTK button
- :type highlight_colors: (str, str)
- :param mouseover_colors: Important difference between Linux & Windows! Linux - Colors when mouse moved over button. Windows - colors when button is pressed. The default is to switch the text and background colors (an inverse effect)
- :type mouseover_colors: (str, str) | str
- :param use_ttk_buttons: True = use ttk buttons. False = do not use ttk buttons. None (Default) = use ttk buttons only if on a Mac and not with button images
- :type use_ttk_buttons: (bool)
- :param font: specifies the font family, size, etc. Tuple or Single string format 'name size styles'. Styles: italic * roman bold normal underline overstrike
- :type font: (str or (str, int[, str]) or None)
- :param bind_return_key: If True the return key will cause this button to be pressed
- :type bind_return_key: (bool)
- :param focus: if True, initial focus will be put on this button
- :type focus: (bool)
- :param pad: Amount of padding to put around element in pixels (left/right, top/bottom) or ((left, right), (top, bottom)) or an int. If an int, then it's converted into a tuple (int, int)
- :type pad: (int, int) or ((int, int),(int,int)) or (int,(int,int)) or ((int, int),int) | int
- :param p: Same as pad parameter. It's an alias. If EITHER of them are set, then the one that's set will be used. If BOTH are set, pad will be used
- :type p: (int, int) or ((int, int),(int,int)) or (int,(int,int)) or ((int, int),int) | int
- :param key: Used with window.find_element and with return values to uniquely identify this element to uniquely identify this element
- :type key: str | int | tuple | object
- :param k: Same as the Key. You can use either k or key. Which ever is set will be used.
- :type k: str | int | tuple | object
- :param right_click_menu: A list of lists of Menu items to show when this element is right clicked. See user docs for exact format.
- :type right_click_menu: List[List[ List[str] | str ]]
- :param expand_x: If True the element will automatically expand in the X direction to fill available space
- :type expand_x: (bool)
- :param expand_y: If True the element will automatically expand in the Y direction to fill available space
- :type expand_y: (bool)
- :param visible: set visibility state of the element
- :type visible: (bool)
- :param metadata: User metadata that can be set to ANYTHING
- :type metadata: (Any)
- """
-
-
- self.AutoSizeButton = auto_size_button
- self.BType = button_type
- if file_types is not None and len(file_types) == 2 and isinstance(file_types[0], str) and isinstance(file_types[1], str):
- warnings.warn('file_types parameter not correctly specified. This parameter is a LIST of TUPLES. You have passed (str,str) rather than ((str, str),). Fixing it for you this time.\nchanging {} to {}\nPlease correct your code'.format(file_types, ((file_types[0], file_types[1]),)), UserWarning)
- file_types = ((file_types[0], file_types[1]),)
- self.FileTypes = file_types
- self.Widget = self.TKButton = None # type: tk.Button
- self.Target = target
- self.ButtonText = str(button_text)
- self.RightClickMenu = right_click_menu
- # Button colors can be a tuple (text, background) or a string with format "text on background"
- # bc = button_color
- # if button_color is None:
- # bc = DEFAULT_BUTTON_COLOR
- # else:
- # try:
- # if isinstance(button_color,str):
- # bc = button_color.split(' on ')
- # except Exception as e:
- # print('* cprint warning * you messed up with color formatting', e)
- # if bc[1] is None:
- # bc = (bc[0], theme_button_color()[1])
- # self.ButtonColor = bc
- self.ButtonColor = button_color_to_tuple(button_color)
-
- # experimental code to compute disabled button text color
- # if disabled_button_color is None:
- # try:
- # disabled_button_color = (get_complimentary_hex(theme_button_color()[0]), theme_button_color()[1])
- # # disabled_button_color = disabled_button_color
- # except:
- # print('* Problem computing disabled button color *')
- self.DisabledButtonColor = button_color_to_tuple(disabled_button_color) if disabled_button_color is not None else (None, None)
- if image_source is not None:
- if isinstance(image_source, bytes):
- image_data = image_source
- elif isinstance(image_source, str):
- image_filename = image_source
- self.ImageFilename = image_filename
- self.ImageData = image_data
- self.ImageSize = image_size
- self.ImageSubsample = image_subsample
- self.UserData = None
- self.BorderWidth = border_width if border_width is not None else DEFAULT_BORDER_WIDTH
- self.BindReturnKey = bind_return_key
- self.Focus = focus
- self.TKCal = None
- self.calendar_default_date_M_D_Y = (None, None, None)
- self.calendar_close_when_chosen = False
- self.calendar_locale = None
- self.calendar_format = None
- self.calendar_location = (None, None)
- self.calendar_no_titlebar = True
- self.calendar_begin_at_sunday_plus = 0
- self.calendar_month_names = None
- self.calendar_day_abbreviations = None
- self.calendar_title = ''
- self.calendar_selection = ''
- self.InitialFolder = initial_folder
- self.DefaultExtension = default_extension
- self.Disabled = disabled
- self.ChangeSubmits = change_submits or enable_events
- self.UseTtkButtons = use_ttk_buttons
- self.ttk_style_name = '' # set in the packer function
- self._files_delimiter = BROWSE_FILES_DELIMITER # used by the file browse button. used when multiple files are selected by user
- if use_ttk_buttons is None and running_mac():
- self.UseTtkButtons = True
- # if image_filename or image_data:
- # self.UseTtkButtons = False # if an image is to be displayed, then force the button to not be a TTK Button
- if key is None and k is None:
- _key = self.ButtonText
- else:
- _key = key if key is not None else k
- if highlight_colors is not None:
- self.HighlightColors = highlight_colors
- else:
- self.HighlightColors = self._compute_highlight_colors()
-
- if mouseover_colors != (None, None):
- self.MouseOverColors = button_color_to_tuple(mouseover_colors)
- elif button_color != None:
- self.MouseOverColors = (self.ButtonColor[1], self.ButtonColor[0])
- else:
- self.MouseOverColors = (theme_button_color()[1], theme_button_color()[0])
- pad = pad if pad is not None else p
- self.expand_x = expand_x
- self.expand_y = expand_y
-
- sz = size if size != (None, None) else s
- super().__init__(ELEM_TYPE_BUTTON, size=sz, font=font, pad=pad, key=_key, tooltip=tooltip, visible=visible, metadata=metadata)
- return
-
- def _compute_highlight_colors(self):
- """
- Determines the color to use to indicate the button has focus. This setting is only used by Linux.
- :return: Pair of colors. (Highlight, Highlight Background)
- :rtype: (str, str)
- """
- highlight_color = highlight_background = COLOR_SYSTEM_DEFAULT
- if self.ButtonColor != COLOR_SYSTEM_DEFAULT and theme_background_color() != COLOR_SYSTEM_DEFAULT:
- highlight_background = theme_background_color()
- if self.ButtonColor != COLOR_SYSTEM_DEFAULT and self.ButtonColor[0] != COLOR_SYSTEM_DEFAULT:
- if self.ButtonColor[0] != theme_background_color():
- highlight_color = self.ButtonColor[0]
- else:
- highlight_color = 'red'
- return (highlight_color, highlight_background)
-
- # Realtime button release callback
-
- def ButtonReleaseCallBack(self, parm):
- """
- Not a user callable function. Called by tkinter when a "realtime" button is released
-
- :param parm: the event info from tkinter
- :type parm:
-
- """
- self.LastButtonClickedWasRealtime = False
- self.ParentForm.LastButtonClicked = None
-
- # Realtime button callback
- def ButtonPressCallBack(self, parm):
- """
- Not a user callable method. Callback called by tkinter when a "realtime" button is pressed
-
- :param parm: Event info passed in by tkinter
- :type parm:
-
- """
- self.ParentForm.LastButtonClickedWasRealtime = True
- if self.Key is not None:
- self.ParentForm.LastButtonClicked = self.Key
- else:
- self.ParentForm.LastButtonClicked = self.ButtonText
- # if self.ParentForm.CurrentlyRunningMainloop:
- # Window._window_that_exited = self.ParentForm
- # self.ParentForm.TKroot.quit() # kick out of loop if read was called
- _exit_mainloop(self.ParentForm)
-
- def _find_target(self):
- target = self.Target
- target_element = None
- if target[0] == ThisRow:
- target = [self.Position[0], target[1]]
- if target[1] < 0:
- target[1] = self.Position[1] + target[1]
- strvar = None
- should_submit_window = False
- if target == (None, None):
- strvar = self.TKStringVar
- else:
- if not isinstance(target, str):
- if target[0] < 0:
- target = [self.Position[0] + target[0], target[1]]
- target_element = self.ParentContainer._GetElementAtLocation(target)
- else:
- target_element = self.ParentForm.find_element(target)
- try:
- strvar = target_element.TKStringVar
- except:
- pass
- try:
- if target_element.ChangeSubmits:
- should_submit_window = True
- except:
- pass
- return target_element, strvar, should_submit_window
-
- # ------- Button Callback ------- #
- def ButtonCallBack(self):
- """
- Not user callable! Called by tkinter when a button is clicked. This is where all the fun begins!
- """
-
- if self.Disabled == BUTTON_DISABLED_MEANS_IGNORE:
- return
- target_element, strvar, should_submit_window = self._find_target()
-
- filetypes = FILE_TYPES_ALL_FILES if self.FileTypes is None else self.FileTypes
-
- if self.BType == BUTTON_TYPE_BROWSE_FOLDER:
- if running_mac(): # macs don't like seeing the parent window (go firgure)
- folder_name = tk.filedialog.askdirectory(initialdir=self.InitialFolder) # show the 'get folder' dialog box
- else:
- folder_name = tk.filedialog.askdirectory(initialdir=self.InitialFolder, parent=self.ParentForm.TKroot) # show the 'get folder' dialog box
- if folder_name:
- try:
- strvar.set(folder_name)
- self.TKStringVar.set(folder_name)
- except:
- pass
- else: # if "cancel" button clicked, don't generate an event
- should_submit_window = False
- elif self.BType == BUTTON_TYPE_BROWSE_FILE:
- if running_mac():
- file_name = tk.filedialog.askopenfilename(initialdir=self.InitialFolder) # show the 'get file' dialog box
- else:
- file_name = tk.filedialog.askopenfilename(filetypes=filetypes, initialdir=self.InitialFolder, parent=self.ParentForm.TKroot) # show the 'get file' dialog box
-
- if file_name:
- strvar.set(file_name)
- self.TKStringVar.set(file_name)
- else: # if "cancel" button clicked, don't generate an event
- should_submit_window = False
- elif self.BType == BUTTON_TYPE_COLOR_CHOOSER:
- color = tk.colorchooser.askcolor(parent=self.ParentForm.TKroot) # show the 'get file' dialog box
- color = color[1] # save only the #RRGGBB portion
- strvar.set(color)
- self.TKStringVar.set(color)
- elif self.BType == BUTTON_TYPE_BROWSE_FILES:
- if running_mac():
- file_name = tk.filedialog.askopenfilenames(initialdir=self.InitialFolder)
- else:
- file_name = tk.filedialog.askopenfilenames(filetypes=filetypes, initialdir=self.InitialFolder, parent=self.ParentForm.TKroot)
-
- if file_name:
- file_name = self._files_delimiter.join(file_name) # normally a ';'
- strvar.set(file_name)
- self.TKStringVar.set(file_name)
- else: # if "cancel" button clicked, don't generate an event
- should_submit_window = False
- elif self.BType == BUTTON_TYPE_SAVEAS_FILE:
- # show the 'get file' dialog box
- if running_mac():
- file_name = tk.filedialog.asksaveasfilename(defaultextension=self.DefaultExtension, initialdir=self.InitialFolder)
- else:
- file_name = tk.filedialog.asksaveasfilename(filetypes=filetypes, defaultextension=self.DefaultExtension, initialdir=self.InitialFolder, parent=self.ParentForm.TKroot)
-
- if file_name:
- strvar.set(file_name)
- self.TKStringVar.set(file_name)
- else: # if "cancel" button clicked, don't generate an event
- should_submit_window = False
- elif self.BType == BUTTON_TYPE_CLOSES_WIN: # this is a return type button so GET RESULTS and destroy window
- # first, get the results table built
- # modify the Results table in the parent FlexForm object
- if self.Key is not None:
- self.ParentForm.LastButtonClicked = self.Key
- else:
- self.ParentForm.LastButtonClicked = self.ButtonText
- self.ParentForm.FormRemainedOpen = False
- self.ParentForm._Close()
- _exit_mainloop(self.ParentForm)
-
- if self.ParentForm.NonBlocking:
- self.ParentForm.TKroot.destroy()
- Window._DecrementOpenCount()
- elif self.BType == BUTTON_TYPE_READ_FORM: # LEAVE THE WINDOW OPEN!! DO NOT CLOSE
- # This is a PLAIN BUTTON
- # first, get the results table built
- # modify the Results table in the parent FlexForm object
- if self.Key is not None:
- self.ParentForm.LastButtonClicked = self.Key
- else:
- self.ParentForm.LastButtonClicked = self.ButtonText
- self.ParentForm.FormRemainedOpen = True
- _exit_mainloop(self.ParentForm)
- elif self.BType == BUTTON_TYPE_CLOSES_WIN_ONLY: # special kind of button that does not exit main loop
- self.ParentForm._Close(without_event=True)
- self.ParentForm.TKroot.destroy() # close the window with tkinter
- Window._DecrementOpenCount()
- elif self.BType == BUTTON_TYPE_CALENDAR_CHOOSER: # this is a return type button so GET RESULTS and destroy window
- # ------------ new chooser code -------------
- self.ParentForm.LastButtonClicked = self.Key # key should have been generated already if not set by user
- self.ParentForm.FormRemainedOpen = True
- should_submit_window = False
- _exit_mainloop(self.ParentForm)
- # elif self.BType == BUTTON_TYPE_SHOW_DEBUGGER:
- # **** DEPRICATED *****
- # if self.ParentForm.DebuggerEnabled:
- # show_debugger_popout_window()
-
- if should_submit_window:
- self.ParentForm.LastButtonClicked = target_element.Key
- self.ParentForm.FormRemainedOpen = True
- _exit_mainloop(self.ParentForm)
-
- return
-
- def update(self, text=None, button_color=(None, None), disabled=None, image_data=None, image_filename=None,
- visible=None, image_subsample=None, disabled_button_color=(None, None), image_size=None):
- """
- Changes some of the settings for the Button Element. Must call `Window.Read` or `Window.Finalize` prior
-
- Changes will not be visible in your window until you call window.read or window.refresh.
-
- If you change visibility, your element may MOVE. If you want it to remain stationary, use the "layout helper"
- function "pin" to ensure your element is "pinned" to that location in your layout so that it returns there
- when made visible.
-
- :param text: sets button text
- :type text: (str)
- :param button_color: Color of button. default is from theme or the window. Easy to remember which is which if you say "ON" between colors. "red" on "green". Normally a tuple, but can be a simplified-button-color-string "foreground on background". Can be a single color if want to set only the background.
- :type button_color: (str, str) | str | (int, int) | None
- :param disabled: True/False to enable/disable at the GUI level. Use BUTTON_DISABLED_MEANS_IGNORE to ignore clicks (won't change colors)
- :type disabled: (bool | str)
- :param image_data: Raw or Base64 representation of the image to put on button. Choose either filename or data
- :type image_data: bytes | str
- :param image_filename: image filename if there is a button image. GIFs and PNGs only.
- :type image_filename: (str)
- :param disabled_button_color: colors to use when button is disabled (text, background). Use None for a color if don't want to change. Only ttk buttons support both text and background colors. tk buttons only support changing text color
- :type disabled_button_color: (str, str)
- :param visible: control visibility of element
- :type visible: (bool)
- :param image_subsample: amount to reduce the size of the image. Divides the size by this number. 2=1/2, 3=1/3, 4=1/4, etc
- :type image_subsample: (int)
- :param image_size: Size of the image in pixels (width, height)
- :type image_size: (int, int)
- """
-
- if not self._widget_was_created(): # if widget hasn't been created yet, then don't allow
- return
-
- if self.UseTtkButtons:
- style_name = self.ttk_style_name # created when made initial window (in the pack)
- # style_name = str(self.Key) + 'custombutton.TButton'
- button_style = ttk.Style()
- if text is not None:
- self.TKButton.configure(text=text)
- self.ButtonText = text
- if button_color != (None, None) and button_color != COLOR_SYSTEM_DEFAULT:
- bc = button_color_to_tuple(button_color, self.ButtonColor)
- # if isinstance(button_color, str):
- # try:
- # button_color = button_color.split(' on ')
- # except Exception as e:
- # print('** Error in formatting your button color **', button_color, e)
- if self.UseTtkButtons:
- if bc[0] not in (None, COLOR_SYSTEM_DEFAULT):
- button_style.configure(style_name, foreground=bc[0])
- if bc[1] not in (None, COLOR_SYSTEM_DEFAULT):
- button_style.configure(style_name, background=bc[1])
- else:
- if bc[0] not in (None, COLOR_SYSTEM_DEFAULT):
- self.TKButton.config(foreground=bc[0], activeforeground=bc[0])
- if bc[1] not in (None, COLOR_SYSTEM_DEFAULT):
- self.TKButton.config(background=bc[1], activebackground=bc[1])
- self.ButtonColor = bc
- if disabled is True:
- self.TKButton['state'] = 'disabled'
- elif disabled is False:
- self.TKButton['state'] = 'normal'
- elif disabled == BUTTON_DISABLED_MEANS_IGNORE:
- self.TKButton['state'] = 'normal'
- self.Disabled = disabled if disabled is not None else self.Disabled
-
- if image_data is not None:
- image = tk.PhotoImage(data=image_data)
- if image_subsample:
- image = image.subsample(image_subsample)
- if image_size is not None:
- width, height = image_size
- else:
- width, height = image.width(), image.height()
- if self.UseTtkButtons:
- button_style.configure(style_name, image=image, width=width, height=height)
- else:
- self.TKButton.config(image=image, width=width, height=height)
- self.TKButton.image = image
- if image_filename is not None:
- image = tk.PhotoImage(file=image_filename)
- if image_subsample:
- image = image.subsample(image_subsample)
- if image_size is not None:
- width, height = image_size
- else:
- width, height = image.width(), image.height()
- if self.UseTtkButtons:
- button_style.configure(style_name, image=image, width=width, height=height)
- else:
- self.TKButton.config(highlightthickness=0, image=image, width=width, height=height)
- self.TKButton.image = image
- if visible is False:
- self._pack_forget_save_settings()
- elif visible is True:
- self._pack_restore_settings()
- if disabled_button_color != (None, None) and disabled_button_color != COLOR_SYSTEM_DEFAULT:
- if not self.UseTtkButtons:
- self.TKButton['disabledforeground'] = disabled_button_color[0]
- else:
- if disabled_button_color[0] is not None:
- button_style.map(style_name, foreground=[('disabled', disabled_button_color[0])])
- if disabled_button_color[1] is not None:
- button_style.map(style_name, background=[('disabled', disabled_button_color[1])])
- self.DisabledButtonColor = (disabled_button_color[0] if disabled_button_color[0] is not None else self.DisabledButtonColor[0],
- disabled_button_color[1] if disabled_button_color[1] is not None else self.DisabledButtonColor[1])
-
- if visible is not None:
- self._visible = visible
-
- def get_text(self):
- """
- Returns the current text shown on a button
-
- :return: The text currently displayed on the button
- :rtype: (str)
- """
- return self.ButtonText
-
- def click(self):
- """
- Generates a click of the button as if the user clicked the button
- Calls the tkinter invoke method for the button
- """
- try:
- self.TKButton.invoke()
- except:
- print('Exception clicking button')
-
- Click = click
- GetText = get_text
- Update = update
-
-
-# ------------------------- Button lazy functions ------------------------- #
-B = Button
-Btn = Button
-
-
-# ---------------------------------------------------------------------- #
-# ButtonMenu Class #
-# ---------------------------------------------------------------------- #
-class ButtonMenu(Element):
- """
- The Button Menu Element. Creates a button that when clicked will show a menu similar to right click menu
- """
-
- def __init__(self, button_text, menu_def, tooltip=None, disabled=False, image_source=None,
- image_filename=None, image_data=None, image_size=(None, None), image_subsample=None, border_width=None,
- size=(None, None), s=(None, None), auto_size_button=None, button_color=None, text_color=None, background_color=None, disabled_text_color=None,
- font=None, item_font=None, pad=None, p=None, expand_x=False, expand_y=False, key=None, k=None, tearoff=False, visible=True, metadata=None):
- """
- :param button_text: Text to be displayed on the button
- :type button_text: (str)
- :param menu_def: A list of lists of Menu items to show when this element is clicked. See docs for format as they are the same for all menu types
- :type menu_def: List[List[str]]
- :param tooltip: text, that will appear when mouse hovers over the element
- :type tooltip: (str)
- :param disabled: If True button will be created disabled
- :type disabled: (bool)
- :param image_source: Image to place on button. Use INSTEAD of the image_filename and image_data. Unifies these into 1 easier to use parm
- :type image_source: (str | bytes)
- :param image_filename: image filename if there is a button image. GIFs and PNGs only.
- :type image_filename: (str)
- :param image_data: Raw or Base64 representation of the image to put on button. Choose either filename or data
- :type image_data: bytes | str
- :param image_size: Size of the image in pixels (width, height)
- :type image_size: (int, int)
- :param image_subsample: amount to reduce the size of the image. Divides the size by this number. 2=1/2, 3=1/3, 4=1/4, etc
- :type image_subsample: (int)
- :param border_width: width of border around button in pixels
- :type border_width: (int)
- :param size: (w, h) w=characters-wide, h=rows-high. If an int instead of a tuple is supplied, then height is auto-set to 1
- :type size: (int, int) | (None, None) | int
- :param s: Same as size parameter. It's an alias. If EITHER of them are set, then the one that's set will be used. If BOTH are set, size will be used
- :type s: (int, int) | (None, None) | int
- :param auto_size_button: if True the button size is sized to fit the text
- :type auto_size_button: (bool)
- :param button_color: of button. Easy to remember which is which if you say "ON" between colors. "red" on "green"
- :type button_color: (str, str) or str
- :param background_color: color of the background
- :type background_color: (str)
- :param text_color: element's text color. Can be in #RRGGBB format or a color name "black"
- :type text_color: (str)
- :param disabled_text_color: color to use for text when item is disabled. Can be in #RRGGBB format or a color name "black"
- :type disabled_text_color: (str)
- :param font: specifies the font family, size, etc. Tuple or Single string format 'name size styles'. Styles: italic * roman bold normal underline overstrike
- :type font: (str or (str, int[, str]) or None)
- :param item_font: specifies the font family, size, etc. Tuple or Single string format 'name size styles'. Styles: italic * roman bold normal underline overstrike, for the menu items
- :type item_font: (str or (str, int[, str]) or None)
- :param pad: Amount of padding to put around element in pixels (left/right, top/bottom) or ((left, right), (top, bottom)) or an int. If an int, then it's converted into a tuple (int, int)
- :type pad: (int, int) or ((int, int),(int,int)) or (int,(int,int)) or ((int, int),int) | int
- :param p: Same as pad parameter. It's an alias. If EITHER of them are set, then the one that's set will be used. If BOTH are set, pad will be used
- :type p: (int, int) or ((int, int),(int,int)) or (int,(int,int)) or ((int, int),int) | int
- :param expand_x: If True the element will automatically expand in the X direction to fill available space
- :type expand_x: (bool)
- :param expand_y: If True the element will automatically expand in the Y direction to fill available space
- :type expand_y: (bool)
- :param key: Used with window.find_element and with return values to uniquely identify this element to uniquely identify this element
- :type key: str | int | tuple | object
- :param k: Same as the Key. You can use either k or key. Which ever is set will be used.
- :type k: str | int | tuple | object
- :param tearoff: Determines if menus should allow them to be torn off
- :type tearoff: (bool)
- :param visible: set visibility state of the element
- :type visible: (bool)
- :param metadata: User metadata that can be set to ANYTHING
- :type metadata: (Any)
- """
-
- self.MenuDefinition = copy.deepcopy(menu_def)
-
- self.AutoSizeButton = auto_size_button
- self.ButtonText = button_text
- self.ButtonColor = button_color_to_tuple(button_color)
- # self.TextColor = self.ButtonColor[0]
- # self.BackgroundColor = self.ButtonColor[1]
- self.BackgroundColor = background_color if background_color is not None else theme_input_background_color()
- self.TextColor = text_color if text_color is not None else theme_input_text_color()
- self.DisabledTextColor = disabled_text_color if disabled_text_color is not None else COLOR_SYSTEM_DEFAULT
- self.ItemFont = item_font
- self.BorderWidth = border_width if border_width is not None else DEFAULT_BORDER_WIDTH
- if image_source is not None:
- if isinstance(image_source, str):
- image_filename = image_source
- elif isinstance(image_source, bytes):
- image_data = image_source
- else:
- warnings.warn('ButtonMenu element - image_source is not a valid type: {}'.format(type(image_source)), UserWarning)
-
- self.ImageFilename = image_filename
- self.ImageData = image_data
- self.ImageSize = image_size
- self.ImageSubsample = image_subsample
- self.Disabled = disabled
- self.IsButtonMenu = True
- self.MenuItemChosen = None
- self.Widget = self.TKButtonMenu = None # type: tk.Menubutton
- self.TKMenu = None # type: tk.Menu
- self.part_of_custom_menubar = False
- self.custom_menubar_key = None
- # self.temp_size = size if size != (NONE, NONE) else
- key = key if key is not None else k
- sz = size if size != (None, None) else s
- pad = pad if pad is not None else p
- self.expand_x = expand_x
- self.expand_y = expand_y
-
- super().__init__(ELEM_TYPE_BUTTONMENU, size=sz, font=font, pad=pad, key=key, tooltip=tooltip,
- text_color=self.TextColor, background_color=self.BackgroundColor, visible=visible, metadata=metadata)
- self.Tearoff = tearoff
-
-
- def _MenuItemChosenCallback(self, item_chosen): # ButtonMenu Menu Item Chosen Callback
- """
- Not a user callable function. Called by tkinter when an item is chosen from the menu.
-
- :param item_chosen: The menu item chosen.
- :type item_chosen: (str)
- """
- # print('IN MENU ITEM CALLBACK', item_chosen)
- self.MenuItemChosen = item_chosen
- self.ParentForm.LastButtonClicked = self.Key
- self.ParentForm.FormRemainedOpen = True
- # if self.ParentForm.CurrentlyRunningMainloop:
- # self.ParentForm.TKroot.quit() # kick the users out of the mainloop
- _exit_mainloop(self.ParentForm)
-
-
- def update(self, menu_definition=None, visible=None, image_source=None, image_size=(None, None), image_subsample=None, button_text=None):
- """
- Changes some of the settings for the ButtonMenu Element. Must call `Window.Read` or `Window.Finalize` prior
-
- Changes will not be visible in your window until you call window.read or window.refresh.
-
- If you change visibility, your element may MOVE. If you want it to remain stationary, use the "layout helper"
- function "pin" to ensure your element is "pinned" to that location in your layout so that it returns there
- when made visible.
-
- :param menu_definition: (New menu definition (in menu definition format)
- :type menu_definition: List[List]
- :param visible: control visibility of element
- :type visible: (bool)
- :param image_source: new image if image is to be changed. Can be a filename or a base64 encoded byte-string
- :type image_source: (str | bytes)
- :param image_size: Size of the image in pixels (width, height)
- :type image_size: (int, int)
- :param image_subsample: amount to reduce the size of the image. Divides the size by this number. 2=1/2, 3=1/3, 4=1/4, etc
- :type image_subsample: (int)
- :param button_text: Text to be shown on the button
- :type button_text: (str)
- """
-
- if not self._widget_was_created(): # if widget hasn't been created yet, then don't allow
- return
-
- if menu_definition is not None:
- self.MenuDefinition = copy.deepcopy(menu_definition)
- top_menu = self.TKMenu = tk.Menu(self.TKButtonMenu, tearoff=self.Tearoff, font=self.ItemFont, tearoffcommand=self._tearoff_menu_callback)
-
- if self.BackgroundColor not in (COLOR_SYSTEM_DEFAULT, None):
- top_menu.config(bg=self.BackgroundColor)
- if self.TextColor not in (COLOR_SYSTEM_DEFAULT, None):
- top_menu.config(fg=self.TextColor)
- if self.DisabledTextColor not in (COLOR_SYSTEM_DEFAULT, None):
- top_menu.config(disabledforeground=self.DisabledTextColor)
- if self.ItemFont is not None:
- top_menu.config(font=self.ItemFont)
- AddMenuItem(self.TKMenu, self.MenuDefinition[1], self)
- self.TKButtonMenu.configure(menu=self.TKMenu)
- if image_source is not None:
- filename = data = None
- if image_source is not None:
- if isinstance(image_source, bytes):
- data = image_source
- elif isinstance(image_source, str):
- filename = image_source
- else:
- warnings.warn('ButtonMenu element - image_source is not a valid type: {}'.format(type(image_source)), UserWarning)
- image = None
- if filename is not None:
- image = tk.PhotoImage(file=filename)
- if image_subsample is not None:
- image = image.subsample(image_subsample)
- elif data is not None:
- # if type(data) is bytes:
- try:
- image = tk.PhotoImage(data=data)
- if image_subsample is not None:
- image = image.subsample(image_subsample)
- except Exception as e:
- image = data
-
- if image is not None:
- if type(image) is not bytes:
- width, height = image_size[0] if image_size[0] is not None else image.width(), image_size[1] if image_size[1] is not None else image.height()
- else:
- width, height = image_size
-
- self.TKButtonMenu.config(image=image, compound=tk.CENTER, width=width, height=height)
- self.TKButtonMenu.image = image
- if button_text is not None:
- self.TKButtonMenu.configure(text=button_text)
- self.ButtonText = button_text
- if visible is False:
- self._pack_forget_save_settings()
- elif visible is True:
- self._pack_restore_settings()
- if visible is not None:
- self._visible = visible
-
- def click(self):
- """
- Generates a click of the button as if the user clicked the button
- Calls the tkinter invoke method for the button
- """
- try:
- self.TKMenu.invoke(1)
- except:
- print('Exception clicking button')
-
- Update = update
- Click = click
-
-BMenu = ButtonMenu
-BM = ButtonMenu
-
-
-# ---------------------------------------------------------------------- #
-# ProgreessBar #
-# ---------------------------------------------------------------------- #
-class ProgressBar(Element):
- """
- Progress Bar Element - Displays a colored bar that is shaded as progress of some operation is made
- """
-
- def __init__(self, max_value, orientation=None, size=(None, None), s=(None, None), size_px=(None, None), auto_size_text=None, bar_color=None, style=None, border_width=None,
- relief=None, key=None, k=None, pad=None, p=None, right_click_menu=None, expand_x=False, expand_y=False, visible=True, metadata=None):
- """
- :param max_value: max value of progressbar
- :type max_value: (int)
- :param orientation: 'horizontal' or 'vertical'
- :type orientation: (str)
- :param size: Size of the bar. If horizontal (chars long, pixels wide), vert (chars high, pixels wide). Vert height measured using horizontal chars units.
- :type size: (int, int) | (int, None)
- :param s: Same as size parameter. It's an alias. If EITHER of them are set, then the one that's set will be used. If BOTH are set, size will be used
- :type s: (int, int) | (None, None)
- :param size_px: Size in pixels (length, width). Will be used in place of size parm if specified
- :type size_px: (int, int) | (None, None)
- :param auto_size_text: Not sure why this is here
- :type auto_size_text: (bool)
- :param bar_color: The 2 colors that make up a progress bar. Easy to remember which is which if you say "ON" between colors. "red" on "green".
- :type bar_color: (str, str) or str
- :param style: Progress bar style defined as one of these 'default', 'winnative', 'clam', 'alt', 'classic', 'vista', 'xpnative'
- :type style: (str)
- :param border_width: The amount of pixels that go around the outside of the bar
- :type border_width: (int)
- :param relief: relief style. Values are same as progress meter relief values. Can be a constant or a string: `RELIEF_RAISED RELIEF_SUNKEN RELIEF_FLAT RELIEF_RIDGE RELIEF_GROOVE RELIEF_SOLID` (Default value = DEFAULT_PROGRESS_BAR_RELIEF)
- :type relief: (str)
- :param key: Used with window.find_element and with return values to uniquely identify this element to uniquely identify this element
- :type key: str | int | tuple | object
- :param k: Same as the Key. You can use either k or key. Which ever is set will be used.
- :type k: str | int | tuple | object
- :param pad: Amount of padding to put around element in pixels (left/right, top/bottom) or ((left, right), (top, bottom)) or an int. If an int, then it's converted into a tuple (int, int)
- :type pad: (int, int) or ((int, int),(int,int)) or (int,(int,int)) or ((int, int),int) | int
- :param p: Same as pad parameter. It's an alias. If EITHER of them are set, then the one that's set will be used. If BOTH are set, pad will be used
- :type p: (int, int) or ((int, int),(int,int)) or (int,(int,int)) or ((int, int),int) | int
- :param right_click_menu: A list of lists of Menu items to show when this element is right clicked. See user docs for exact format.
- :type right_click_menu: List[List[ List[str] | str ]]
- :param expand_x: If True the element will automatically expand in the X direction to fill available space
- :type expand_x: (bool)
- :param expand_y: If True the element will automatically expand in the Y direction to fill available space
- :type expand_y: (bool)
- :param visible: set visibility state of the element
- :type visible: (bool)
- :param metadata: User metadata that can be set to ANYTHING
- :type metadata: (Any)
- """
-
-
- self.MaxValue = max_value
- self.TKProgressBar = None # type: TKProgressBar
- self.Cancelled = False
- self.NotRunning = True
- self.Orientation = orientation if orientation else DEFAULT_METER_ORIENTATION
- self.RightClickMenu = right_click_menu
- self.ttk_style_name = None # set in the pack function so can use in the update
- # Progress Bar colors can be a tuple (text, background) or a string with format "bar on background" - examples "red on white" or ("red", "white")
- if bar_color is None:
- bar_color = DEFAULT_PROGRESS_BAR_COLOR
- else:
- bar_color = _simplified_dual_color_to_tuple(bar_color, default=DEFAULT_PROGRESS_BAR_COLOR)
-
- self.BarColor = bar_color # should be a tuple at this point
- self.BarStyle = style if style else DEFAULT_TTK_THEME
- self.BorderWidth = border_width if border_width else DEFAULT_PROGRESS_BAR_BORDER_WIDTH
- self.Relief = relief if relief else DEFAULT_PROGRESS_BAR_RELIEF
- self.BarExpired = False
- key = key if key is not None else k
- sz = size if size != (None, None) else s
- pad = pad if pad is not None else p
- self.expand_x = expand_x
- self.expand_y = expand_y
- self.size_px = size_px
-
- super().__init__(ELEM_TYPE_PROGRESS_BAR, size=sz, auto_size_text=auto_size_text, key=key, pad=pad,
- visible=visible, metadata=metadata)
-
- # returns False if update failed
- def update_bar(self, current_count, max=None):
- """
- DEPRECATED BUT STILL USABLE - has been combined with the normal ProgressBar.update method.
- Change what the bar shows by changing the current count and optionally the max count
-
- :param current_count: sets the current value
- :type current_count: (int)
- :param max: changes the max value
- :type max: (int)
- """
-
- if self.ParentForm.TKrootDestroyed:
- return False
- self.TKProgressBar.Update(current_count, max=max)
- try:
- self.ParentForm.TKroot.update()
- except:
- Window._DecrementOpenCount()
- # _my_windows.Decrement()
- return False
- return True
-
- def update(self, current_count=None, max=None, bar_color=None, visible=None):
- """
- Changes some of the settings for the ProgressBar Element. Must call `Window.Read` or `Window.Finalize` prior
- Now has the ability to modify the count so that the update_bar method is not longer needed separately
-
- Changes will not be visible in your window until you call window.read or window.refresh.
-
- If you change visibility, your element may MOVE. If you want it to remain stationary, use the "layout helper"
- function "pin" to ensure your element is "pinned" to that location in your layout so that it returns there
- when made visible.
-
- :param current_count: sets the current value
- :type current_count: (int)
- :param max: changes the max value
- :type max: (int)
- :param bar_color: The 2 colors that make up a progress bar. Easy to remember which is which if you say "ON" between colors. "red" on "green".
- :type bar_color: (str, str) or str
- :param visible: control visibility of element
- :type visible: (bool)
- :return: Returns True if update was OK. False means something wrong with window or it was closed
- :rtype: (bool)
- """
- if not self._widget_was_created(): # if widget hasn't been created yet, then don't allow
- return False
-
- if self.ParentForm.TKrootDestroyed:
- return False
-
- if visible is False:
- self._pack_forget_save_settings()
- elif visible is True:
- self._pack_restore_settings()
-
- if visible is not None:
- self._visible = visible
- if bar_color is not None:
- bar_color = _simplified_dual_color_to_tuple(bar_color, default=DEFAULT_PROGRESS_BAR_COLOR)
- self.BarColor = bar_color
- style = ttk.Style()
- style.configure(self.ttk_style_name, background=bar_color[0], troughcolor=bar_color[1])
- if current_count is not None:
- self.TKProgressBar.Update(current_count, max=max)
-
- try:
- self.ParentForm.TKroot.update()
- except:
- # Window._DecrementOpenCount()
- # _my_windows.Decrement()
- return False
- return True
-
- Update = update
- UpdateBar = update_bar
-
-
-PBar = ProgressBar
-Prog = ProgressBar
-Progress = ProgressBar
-
-
-# ---------------------------------------------------------------------- #
-# Image #
-# ---------------------------------------------------------------------- #
-class Image(Element):
- """
- Image Element - show an image in the window. Should be a GIF or a PNG only
- """
-
- def __init__(self, source=None, filename=None, data=None, background_color=None, size=(None, None), s=(None, None), pad=None, p=None, key=None, k=None, tooltip=None, subsample=None, right_click_menu=None, expand_x=False, expand_y=False, visible=True, enable_events=False, metadata=None):
- """
- :param source: A filename or a base64 bytes. Will automatically detect the type and fill in filename or data for you.
- :type source: str | bytes | None
- :param filename: image filename if there is a button image. GIFs and PNGs only.
- :type filename: str | None
- :param data: Raw or Base64 representation of the image to put on button. Choose either filename or data
- :type data: bytes | str | None
- :param background_color: color of background
- :type background_color:
- :param size: (width, height) size of image in pixels
- :type size: (int, int)
- :param s: Same as size parameter. It's an alias. If EITHER of them are set, then the one that's set will be used. If BOTH are set, size will be used
- :type s: (int, int) | (None, None) | int
- :param pad: Amount of padding to put around element in pixels (left/right, top/bottom) or ((left, right), (top, bottom)) or an int. If an int, then it's converted into a tuple (int, int)
- :type pad: (int, int) or ((int, int),(int,int)) or (int,(int,int)) or ((int, int),int) | int
- :param p: Same as pad parameter. It's an alias. If EITHER of them are set, then the one that's set will be used. If BOTH are set, pad will be used
- :type p: (int, int) or ((int, int),(int,int)) or (int,(int,int)) or ((int, int),int) | int
- :param key: Used with window.find_element and with return values to uniquely identify this element to uniquely identify this element
- :type key: str | int | tuple | object
- :param k: Same as the Key. You can use either k or key. Which ever is set will be used.
- :type k: str | int | tuple | object
- :param tooltip: text, that will appear when mouse hovers over the element
- :type tooltip: (str)
- :param subsample: amount to reduce the size of the image. Divides the size by this number. 2=1/2, 3=1/3, 4=1/4, etc
- :type subsample: (int)
- :param right_click_menu: A list of lists of Menu items to show when this element is right clicked. See user docs for exact format.
- :type right_click_menu: List[List[ List[str] | str ]]
- :param expand_x: If True the element will automatically expand in the X direction to fill available space
- :type expand_x: (bool)
- :param expand_y: If True the element will automatically expand in the Y direction to fill available space
- :type expand_y: (bool)
- :param visible: set visibility state of the element
- :type visible: (bool)
- :param enable_events: Turns on the element specific events. For an Image element, the event is "image clicked"
- :type enable_events: (bool)
- :param metadata: User metadata that can be set to ANYTHING
- :type metadata: (Any)
- """
-
- if source is not None:
- if isinstance(source, bytes):
- data = source
- elif isinstance(source, str):
- filename = source
- else:
- warnings.warn('Image element - source is not a valid type: {}'.format(type(source)), UserWarning)
-
- self.Filename = filename
- self.Data = data
- self.Widget = self.tktext_label = None # type: tk.Label
- self.BackgroundColor = background_color
- if data is None and filename is None:
- self.Filename = ''
- self.EnableEvents = enable_events
- self.RightClickMenu = right_click_menu
- self.AnimatedFrames = None
- self.CurrentFrameNumber = 0
- self.TotalAnimatedFrames = 0
- self.LastFrameTime = 0
- self.ImageSubsample = subsample
-
- self.Source = filename if filename is not None else data
- key = key if key is not None else k
- sz = size if size != (None, None) else s
- pad = pad if pad is not None else p
- self.expand_x = expand_x
- self.expand_y = expand_y
-
- super().__init__(ELEM_TYPE_IMAGE, size=sz, background_color=background_color, pad=pad, key=key,
- tooltip=tooltip, visible=visible, metadata=metadata)
- return
-
- def update(self, source=None, filename=None, data=None, size=(None, None), subsample=None, visible=None):
- """
- Changes some of the settings for the Image Element. Must call `Window.Read` or `Window.Finalize` prior.
- To clear an image that's been displayed, call with NONE of the options set. A blank update call will
- delete the previously shown image.
-
- Changes will not be visible in your window until you call window.read or window.refresh.
-
- If you change visibility, your element may MOVE. If you want it to remain stationary, use the "layout helper"
- function "pin" to ensure your element is "pinned" to that location in your layout so that it returns there
- when made visible.
-
- :param source: A filename or a base64 bytes. Will automatically detect the type and fill in filename or data for you.
- :type source: str | bytes | None
- :param filename: filename to the new image to display.
- :type filename: (str)
- :param data: Base64 encoded string OR a tk.PhotoImage object
- :type data: str | tkPhotoImage
- :param size: (width, height) size of image in pixels
- :type size: Tuple[int,int]
- :param subsample: amount to reduce the size of the image. Divides the size by this number. 2=1/2, 3=1/3, 4=1/4, etc
- :type subsample: (int)
- :param visible: control visibility of element
- :type visible: (bool)
- """
-
- if not self._widget_was_created(): # if widget hasn't been created yet, then don't allow
- return
-
- if source is not None:
- if isinstance(source, bytes):
- data = source
- elif isinstance(source, str):
- filename = source
- else:
- warnings.warn('Image element - source is not a valid type: {}'.format(type(source)), UserWarning)
-
- image = None
- if filename is not None:
- try:
- image = tk.PhotoImage(file=filename)
- if subsample is not None:
- image = image.subsample(subsample)
- except Exception as e:
- _error_popup_with_traceback('Exception updating Image element', e)
-
- elif data is not None:
- # if type(data) is bytes:
- try:
- image = tk.PhotoImage(data=data)
- if subsample is not None:
- image = image.subsample(subsample)
- except Exception as e:
- image = data
- # return # an error likely means the window has closed so exit
-
- if image is not None:
- if type(image) is not bytes:
- width, height = size[0] if size[0] is not None else image.width(), size[1] if size[1] is not None else image.height()
- else:
- width, height = size
- try: # sometimes crashes if user closed with X
- self.tktext_label.configure(image=image, width=width, height=height)
- except Exception as e:
- _error_popup_with_traceback('Exception updating Image element', e)
- self.tktext_label.image = image
- if visible is False:
- self._pack_forget_save_settings()
- elif visible is True:
- self._pack_restore_settings()
-
- # if everything is set to None, then delete the image
- if filename is None and image is None and visible is None and size == (None, None):
- # Using a try because the image may have been previously deleted and don't want an error if that's happened
- try:
- self.tktext_label.configure(width=1, height=1, bd=0)
- self.tktext_label.image = None
- except:
- pass
-
- if visible is not None:
- self._visible = visible
-
- def update_animation(self, source, time_between_frames=0):
- """
- Show an Animated GIF. Call the function as often as you like. The function will determine when to show the next frame and will automatically advance to the next frame at the right time.
- NOTE - does NOT perform a sleep call to delay
- :param source: Filename or Base64 encoded string containing Animated GIF
- :type source: str | bytes | None
- :param time_between_frames: Number of milliseconds to wait between showing frames
- :type time_between_frames: (int)
- """
-
- if self.Source != source:
- self.AnimatedFrames = None
- self.Source = source
-
- if self.AnimatedFrames is None:
- self.TotalAnimatedFrames = 0
- self.AnimatedFrames = []
- # Load up to 1000 frames of animation. stops when a bad frame is returns by tkinter
- for i in range(1000):
- if type(source) is not bytes:
- try:
- self.AnimatedFrames.append(tk.PhotoImage(file=source, format='gif -index %i' % (i)))
- except Exception as e:
- break
- else:
- try:
- self.AnimatedFrames.append(tk.PhotoImage(data=source, format='gif -index %i' % (i)))
- except Exception as e:
- break
- self.TotalAnimatedFrames = len(self.AnimatedFrames)
- self.LastFrameTime = time.time()
- self.CurrentFrameNumber = -1 # start at -1 because it is incremented before every frame is shown
- # show the frame
-
- now = time.time()
-
- if time_between_frames:
- if (now - self.LastFrameTime) * 1000 > time_between_frames:
- self.LastFrameTime = now
- self.CurrentFrameNumber = (self.CurrentFrameNumber + 1) % self.TotalAnimatedFrames
- else: # don't reshow the frame again if not time for new frame
- return
- else:
- self.CurrentFrameNumber = (self.CurrentFrameNumber + 1) % self.TotalAnimatedFrames
- image = self.AnimatedFrames[self.CurrentFrameNumber]
- try: # needed in case the window was closed with an "X"
- self.tktext_label.configure(image=image, width=image.width(), heigh=image.height())
- except Exception as e:
- print('Exception in update_animation', e)
-
-
- def update_animation_no_buffering(self, source, time_between_frames=0):
- """
- Show an Animated GIF. Call the function as often as you like. The function will determine when to show the next frame and will automatically advance to the next frame at the right time.
- NOTE - does NOT perform a sleep call to delay
-
- :param source: Filename or Base64 encoded string containing Animated GIF
- :type source: str | bytes
- :param time_between_frames: Number of milliseconds to wait between showing frames
- :type time_between_frames: (int)
- """
-
- if self.Source != source:
- self.AnimatedFrames = None
- self.Source = source
- self.frame_num = 0
-
- now = time.time()
-
- if time_between_frames:
- if (now - self.LastFrameTime) * 1000 > time_between_frames:
- self.LastFrameTime = now
- else: # don't reshow the frame again if not time for new frame
- return
-
- # read a frame
- while True:
- if type(source) is not bytes:
- try:
- self.image = tk.PhotoImage(file=source, format='gif -index %i' % (self.frame_num))
- self.frame_num += 1
- except:
- self.frame_num = 0
- else:
- try:
- self.image = tk.PhotoImage(data=source, format='gif -index %i' % (self.frame_num))
- self.frame_num += 1
- except:
- self.frame_num = 0
- if self.frame_num:
- break
-
- try: # needed in case the window was closed with an "X"
- self.tktext_label.configure(image=self.image, width=self.image.width(), heigh=self.image.height())
-
- except:
- pass
-
- Update = update
- UpdateAnimation = update_animation
-
-
-Im = Image
-
-
-# ---------------------------------------------------------------------- #
-# Canvas #
-# ---------------------------------------------------------------------- #
-class Canvas(Element):
-
- def __init__(self, canvas=None, background_color=None, size=(None, None), s=(None, None), pad=None, p=None, key=None, k=None, tooltip=None,
- right_click_menu=None, expand_x=False, expand_y=False, visible=True, border_width=0, metadata=None):
- """
- :param canvas: Your own tk.Canvas if you already created it. Leave blank to create a Canvas
- :type canvas: (tk.Canvas)
- :param background_color: color of background
- :type background_color: (str)
- :param size: (width in char, height in rows) size in pixels to make canvas
- :type size: (int,int) | (None, None)
- :param s: Same as size parameter. It's an alias. If EITHER of them are set, then the one that's set will be used. If BOTH are set, size will be used
- :type s: (int, int) | (None, None) | int
- :param pad: Amount of padding to put around element in pixels (left/right, top/bottom) or ((left, right), (top, bottom)) or an int. If an int, then it's converted into a tuple (int, int)
- :type pad: (int, int) or ((int, int),(int,int)) or (int,(int,int)) or ((int, int),int) | int
- :param p: Same as pad parameter. It's an alias. If EITHER of them are set, then the one that's set will be used. If BOTH are set, pad will be used
- :type p: (int, int) or ((int, int),(int,int)) or (int,(int,int)) or ((int, int),int) | int
- :param key: Used with window.find_element and with return values to uniquely identify this element
- :type key: str | int | tuple | object
- :param k: Same as the Key. You can use either k or key. Which ever is set will be used.
- :type k: str | int | tuple | object
- :param tooltip: text, that will appear when mouse hovers over the element
- :type tooltip: (str)
- :param right_click_menu: A list of lists of Menu items to show when this element is right clicked. See user docs for exact format.
- :type right_click_menu: List[List[ List[str] | str ]]
- :param expand_x: If True the element will automatically expand in the X direction to fill available space
- :type expand_x: (bool)
- :param expand_y: If True the element will automatically expand in the Y direction to fill available space
- :type expand_y: (bool)
- :param visible: set visibility state of the element
- :type visible: (bool)
- :param border_width: width of border around element in pixels. Not normally used with Canvas element
- :type border_width: (int)
- :param metadata: User metadata that can be set to ANYTHING
- :type metadata: (Any)
- """
-
-
- self.BackgroundColor = background_color if background_color is not None else DEFAULT_BACKGROUND_COLOR
- self._TKCanvas = self.Widget = canvas
- self.RightClickMenu = right_click_menu
- self.BorderWidth = border_width
- key = key if key is not None else k
- sz = size if size != (None, None) else s
- pad = pad if pad is not None else p
- self.expand_x = expand_x
- self.expand_y = expand_y
-
- super().__init__(ELEM_TYPE_CANVAS, background_color=background_color, size=sz, pad=pad, key=key,
- tooltip=tooltip, visible=visible, metadata=metadata)
- return
-
-
- def update(self, background_color=None, visible=None):
- """
-
- :param background_color: color of background
- :type background_color: (str)
- :param visible: set visibility state of the element
- :type visible: (bool)
- """
-
- if not self._widget_was_created(): # if widget hasn't been created yet, then don't allow
- return
-
- if background_color not in (None, COLOR_SYSTEM_DEFAULT):
- self._TKCanvas.configure(background=background_color)
- if visible is False:
- self._pack_forget_save_settings()
- elif visible is True:
- self._pack_restore_settings()
- if visible is not None:
- self._visible = visible
-
-
- @property
- def tk_canvas(self):
- """
- Returns the underlying tkiner Canvas widget
-
- :return: The tkinter canvas widget
- :rtype: (tk.Canvas)
- """
- if self._TKCanvas is None:
- print('*** Did you forget to call Finalize()? Your code should look something like: ***')
- print('*** window = sg.Window("My Form", layout, finalize=True) ***')
- return self._TKCanvas
-
- TKCanvas = tk_canvas
-
-
-# ---------------------------------------------------------------------- #
-# Graph #
-# ---------------------------------------------------------------------- #
-class Graph(Element):
- """
- Creates an area for you to draw on. The MAGICAL property this Element has is that you interact
- with the element using your own coordinate system. This is an important point!! YOU define where the location
- is for (0,0). Want (0,0) to be in the middle of the graph like a math 4-quadrant graph? No problem! Set your
- lower left corner to be (-100,-100) and your upper right to be (100,100) and you've got yourself a graph with
- (0,0) at the center.
- One of THE coolest of the Elements.
- You can also use float values. To do so, be sure and set the float_values parameter.
- Mouse click and drag events are possible and return the (x,y) coordinates of the mouse
- Drawing primitives return an "id" that is referenced when you want to operation on that item (e.g. to erase it)
- """
-
- def __init__(self, canvas_size, graph_bottom_left, graph_top_right, background_color=None, pad=None, p=None,
- change_submits=False, drag_submits=False, enable_events=False, motion_events=False, key=None, k=None, tooltip=None,
- right_click_menu=None, expand_x=False, expand_y=False, visible=True, float_values=False, border_width=0, metadata=None):
- """
- :param canvas_size: size of the canvas area in pixels
- :type canvas_size: (int, int)
- :param graph_bottom_left: (x,y) The bottoms left corner of your coordinate system
- :type graph_bottom_left: (int, int)
- :param graph_top_right: (x,y) The top right corner of your coordinate system
- :type graph_top_right: (int, int)
- :param background_color: background color of the drawing area
- :type background_color: (str)
- :param pad: Amount of padding to put around element in pixels (left/right, top/bottom) or ((left, right), (top, bottom)) or an int. If an int, then it's converted into a tuple (int, int)
- :type pad: (int, int) or ((int, int),(int,int)) or (int,(int,int)) or ((int, int),int) | int
- :param p: Same as pad parameter. It's an alias. If EITHER of them are set, then the one that's set will be used. If BOTH are set, pad will be used
- :type p: (int, int) or ((int, int),(int,int)) or (int,(int,int)) or ((int, int),int) | int
- :param change_submits: * DEPRICATED DO NOT USE. Use `enable_events` instead
- :type change_submits: (bool)
- :param drag_submits: if True and Events are enabled for the Graph, will report Events any time the mouse moves while button down. When the mouse button is released, you'll get an event = graph key + '+UP' (if key is a string.. if not a string, it'll be made into a tuple)
- :type drag_submits: (bool)
- :param enable_events: If True then clicks on the Graph are immediately reported as an event. Use this instead of change_submits
- :type enable_events: (bool)
- :param motion_events: If True then if no button is down and the mouse is moved, an event is generated with key = graph key + '+MOVE' (if key is a string, it not a string then a tuple is returned)
- :type motion_events: (bool)
- :param key: Value that uniquely identifies this element from all other elements. Used when Finding an element or in return values. Must be unique to the window
- :type key: str | int | tuple | object
- :param k: Same as the Key. You can use either k or key. Which ever is set will be used.
- :type k: str | int | tuple | object
- :param tooltip: text, that will appear when mouse hovers over the element
- :type tooltip: (str)
- :param right_click_menu: A list of lists of Menu items to show when this element is right clicked. See user docs for exact format.
- :type right_click_menu: List[List[ List[str] | str ]]
- :param expand_x: If True the element will automatically expand in the X direction to fill available space
- :type expand_x: (bool)
- :param expand_y: If True the element will automatically expand in the Y direction to fill available space
- :type expand_y: (bool)
- :param visible: set visibility state of the element (Default = True)
- :type visible: (bool)
- :param float_values: If True x,y coordinates are returned as floats, not ints
- :type float_values: (bool)
- :param border_width: width of border around element in pixels. Not normally used for Graph Elements
- :type border_width: (int)
- :param metadata: User metadata that can be set to ANYTHING
- :type metadata: (Any)
- """
-
- self.CanvasSize = canvas_size
- self.BottomLeft = graph_bottom_left
- self.TopRight = graph_top_right
- # self._TKCanvas = None # type: tk.Canvas
- self._TKCanvas2 = self.Widget = None # type: tk.Canvas
- self.ChangeSubmits = change_submits or enable_events
- self.DragSubmits = drag_submits
- self.ClickPosition = (None, None)
- self.MouseButtonDown = False
- self.Images = {}
- self.RightClickMenu = right_click_menu
- self.FloatValues = float_values
- self.BorderWidth = border_width
- key = key if key is not None else k
- pad = pad if pad is not None else p
- self.expand_x = expand_x
- self.expand_y = expand_y
- self.motion_events = motion_events
-
- super().__init__(ELEM_TYPE_GRAPH, background_color=background_color, size=canvas_size, pad=pad, key=key,
- tooltip=tooltip, visible=visible, metadata=metadata)
- return
-
- def _convert_xy_to_canvas_xy(self, x_in, y_in):
- """
- Not user callable. Used to convert user's coordinates into the ones used by tkinter
- :param x_in: The x coordinate to convert
- :type x_in: int | float
- :param y_in: The y coordinate to convert
- :type y_in: int | float
- :return: (int, int) The converted canvas coordinates
- :rtype: (int, int)
- """
- if None in (x_in, y_in):
- return None, None
- try:
- scale_x = (self.CanvasSize[0] - 0) / (self.TopRight[0] - self.BottomLeft[0])
- scale_y = (0 - self.CanvasSize[1]) / (self.TopRight[1] - self.BottomLeft[1])
- except:
- scale_x = scale_y = 0
-
- new_x = 0 + scale_x * (x_in - self.BottomLeft[0])
- new_y = self.CanvasSize[1] + scale_y * (y_in - self.BottomLeft[1])
- return new_x, new_y
-
- def _convert_canvas_xy_to_xy(self, x_in, y_in):
- """
- Not user callable. Used to convert tkinter Canvas coords into user's coordinates
-
- :param x_in: The x coordinate in canvas coordinates
- :type x_in: (int)
- :param y_in: (int) The y coordinate in canvas coordinates
- :type y_in:
- :return: The converted USER coordinates
- :rtype: (int, int) | Tuple[float, float]
- """
- if None in (x_in, y_in):
- return None, None
- scale_x = (self.CanvasSize[0] - 0) / (self.TopRight[0] - self.BottomLeft[0])
- scale_y = (0 - self.CanvasSize[1]) / (self.TopRight[1] - self.BottomLeft[1])
-
- new_x = x_in / scale_x + self.BottomLeft[0]
- new_y = (y_in - self.CanvasSize[1]) / scale_y + self.BottomLeft[1]
- if self.FloatValues:
- return new_x, new_y
- else:
- return floor(new_x), floor(new_y)
-
- def draw_line(self, point_from, point_to, color='black', width=1):
- """
- Draws a line from one point to another point using USER'S coordinates. Can set the color and width of line
- :param point_from: Starting point for line
- :type point_from: (int, int) | Tuple[float, float]
- :param point_to: Ending point for line
- :type point_to: (int, int) | Tuple[float, float]
- :param color: Color of the line
- :type color: (str)
- :param width: width of line in pixels
- :type width: (int)
- :return: id returned from tktiner or None if user closed the window. id is used when you
- :rtype: int | None
- """
- if point_from == (None, None):
- return
- converted_point_from = self._convert_xy_to_canvas_xy(point_from[0], point_from[1])
- converted_point_to = self._convert_xy_to_canvas_xy(point_to[0], point_to[1])
- if self._TKCanvas2 is None:
- print('*** WARNING - The Graph element has not been finalized and cannot be drawn upon ***')
- print('Call Window.Finalize() prior to this operation')
- return None
- try: # in case window was closed with an X
- id = self._TKCanvas2.create_line(converted_point_from, converted_point_to, width=width, fill=color)
- except:
- id = None
- return id
-
- def draw_lines(self, points, color='black', width=1):
- """
- Draw a series of lines given list of points
-
- :param points: list of points that define the polygon
- :type points: List[(int, int) | Tuple[float, float]]
- :param color: Color of the line
- :type color: (str)
- :param width: width of line in pixels
- :type width: (int)
- :return: id returned from tktiner or None if user closed the window. id is used when you
- :rtype: int | None
- """
- converted_points = [self._convert_xy_to_canvas_xy(point[0], point[1]) for point in points]
-
- try: # in case window was closed with an X
- id = self._TKCanvas2.create_line(*converted_points, width=width, fill=color)
- except:
- if self._TKCanvas2 is None:
- print('*** WARNING - The Graph element has not been finalized and cannot be drawn upon ***')
- print('Call Window.Finalize() prior to this operation')
- id = None
- return id
-
- def draw_point(self, point, size=2, color='black'):
- """
- Draws a "dot" at the point you specify using the USER'S coordinate system
- :param point: Center location using USER'S coordinate system
- :type point: (int, int) | Tuple[float, float]
- :param size: Radius? (Or is it the diameter?) in user's coordinate values.
- :type size: int | float
- :param color: color of the point to draw
- :type color: (str)
- :return: id returned from tkinter that you'll need if you want to manipulate the point
- :rtype: int | None
- """
- if point == (None, None):
- return
- converted_point = self._convert_xy_to_canvas_xy(point[0], point[1])
- size_converted = self._convert_xy_to_canvas_xy(point[0] + size, point[1])
- size = size_converted[0] - converted_point[0]
- if self._TKCanvas2 is None:
- print('*** WARNING - The Graph element has not been finalized and cannot be drawn upon ***')
- print('Call Window.Finalize() prior to this operation')
- return None
- try: # needed in case window was closed with an X
- point1 = converted_point[0] - size // 2, converted_point[1] - size // 2
- point2 = converted_point[0] + size // 2, converted_point[1] + size // 2
- id = self._TKCanvas2.create_oval(point1[0], point1[1],
- point2[0], point2[1],
- width=0,
- fill=color,
- outline=color)
- except:
- id = None
- return id
-
- def draw_circle(self, center_location, radius, fill_color=None, line_color='black', line_width=1):
- """
- Draws a circle, cenetered at the location provided. Can set the fill and outline colors
- :param center_location: Center location using USER'S coordinate system
- :type center_location: (int, int) | Tuple[float, float]
- :param radius: Radius in user's coordinate values.
- :type radius: int | float
- :param fill_color: color of the point to draw
- :type fill_color: (str)
- :param line_color: color of the outer line that goes around the circle (sorry, can't set thickness)
- :type line_color: (str)
- :param line_width: width of the line around the circle, the outline, in pixels
- :type line_width: (int)
- :return: id returned from tkinter that you'll need if you want to manipulate the circle
- :rtype: int | None
- """
- if center_location == (None, None):
- return
- converted_point = self._convert_xy_to_canvas_xy(center_location[0], center_location[1])
- radius_converted = self._convert_xy_to_canvas_xy(center_location[0] + radius, center_location[1])
- radius = radius_converted[0] - converted_point[0]
- # radius = radius_converted[1]-5
- if self._TKCanvas2 is None:
- print('*** WARNING - The Graph element has not been finalized and cannot be drawn upon ***')
- print('Call Window.Finalize() prior to this operation')
- return None
- # print('Oval parms', int(converted_point[0]) - int(radius), int(converted_point[1]) - int(radius),
- # int(converted_point[0]) + int(radius), int(converted_point[1]) + int(radius))
- try: # needed in case the window was closed with an X
- id = self._TKCanvas2.create_oval(int(converted_point[0]) - int(radius), int(converted_point[1]) - int(radius),
- int(converted_point[0]) + int(radius), int(converted_point[1]) + int(radius), fill=fill_color,
- outline=line_color, width=line_width)
- except:
- id = None
- return id
-
- def draw_oval(self, top_left, bottom_right, fill_color=None, line_color=None, line_width=1):
- """
- Draws an oval based on coordinates in user coordinate system. Provide the location of a "bounding rectangle"
- :param top_left: the top left point of bounding rectangle
- :type top_left: (int, int) | Tuple[float, float]
- :param bottom_right: the bottom right point of bounding rectangle
- :type bottom_right: (int, int) | Tuple[float, float]
- :param fill_color: color of the interrior
- :type fill_color: (str)
- :param line_color: color of outline of oval
- :type line_color: (str)
- :param line_width: width of the line around the oval, the outline, in pixels
- :type line_width: (int)
- :return: id returned from tkinter that you'll need if you want to manipulate the oval
- :rtype: int | None
- """
- converted_top_left = self._convert_xy_to_canvas_xy(top_left[0], top_left[1])
- converted_bottom_right = self._convert_xy_to_canvas_xy(bottom_right[0], bottom_right[1])
- if self._TKCanvas2 is None:
- print('*** WARNING - The Graph element has not been finalized and cannot be drawn upon ***')
- print('Call Window.Finalize() prior to this operation')
- return None
- try: # in case windows close with X
- id = self._TKCanvas2.create_oval(converted_top_left[0], converted_top_left[1], converted_bottom_right[0],
- converted_bottom_right[1], fill=fill_color, outline=line_color, width=line_width)
- except:
- id = None
-
- return id
-
- def draw_arc(self, top_left, bottom_right, extent, start_angle, style=None, arc_color='black', line_width=1, fill_color=None):
- """
- Draws different types of arcs. Uses a "bounding box" to define location
- :param top_left: the top left point of bounding rectangle
- :type top_left: (int, int) | Tuple[float, float]
- :param bottom_right: the bottom right point of bounding rectangle
- :type bottom_right: (int, int) | Tuple[float, float]
- :param extent: Andle to end drawing. Used in conjunction with start_angle
- :type extent: (float)
- :param start_angle: Angle to begin drawing. Used in conjunction with extent
- :type start_angle: (float)
- :param style: Valid choices are One of these Style strings- 'pieslice', 'chord', 'arc', 'first', 'last', 'butt', 'projecting', 'round', 'bevel', 'miter'
- :type style: (str)
- :param arc_color: color to draw arc with
- :type arc_color: (str)
- :param fill_color: color to fill the area
- :type fill_color: (str)
- :return: id returned from tkinter that you'll need if you want to manipulate the arc
- :rtype: int | None
- """
- converted_top_left = self._convert_xy_to_canvas_xy(top_left[0], top_left[1])
- converted_bottom_right = self._convert_xy_to_canvas_xy(bottom_right[0], bottom_right[1])
- tkstyle = tk.PIESLICE if style is None else style
- if self._TKCanvas2 is None:
- print('*** WARNING - The Graph element has not been finalized and cannot be drawn upon ***')
- print('Call Window.Finalize() prior to this operation')
- return None
- try: # in case closed with X
- id = self._TKCanvas2.create_arc(converted_top_left[0], converted_top_left[1], converted_bottom_right[0],
- converted_bottom_right[1], extent=extent, start=start_angle, style=tkstyle,
- outline=arc_color, width=line_width, fill=fill_color)
- except Exception as e:
- print('Error encountered drawing arc.', e)
- id = None
- return id
-
- def draw_rectangle(self, top_left, bottom_right, fill_color=None, line_color=None, line_width=None):
- """
- Draw a rectangle given 2 points. Can control the line and fill colors
-
- :param top_left: the top left point of rectangle
- :type top_left: (int, int) | Tuple[float, float]
- :param bottom_right: the bottom right point of rectangle
- :type bottom_right: (int, int) | Tuple[float, float]
- :param fill_color: color of the interior
- :type fill_color: (str)
- :param line_color: color of outline
- :type line_color: (str)
- :param line_width: width of the line in pixels
- :type line_width: (int)
- :return: int | None id returned from tkinter that you'll need if you want to manipulate the rectangle
- :rtype: int | None
- """
-
- converted_top_left = self._convert_xy_to_canvas_xy(top_left[0], top_left[1])
- converted_bottom_right = self._convert_xy_to_canvas_xy(bottom_right[0], bottom_right[1])
- if self._TKCanvas2 is None:
- print('*** WARNING - The Graph element has not been finalized and cannot be drawn upon ***')
- print('Call Window.Finalize() prior to this operation')
- return None
- if line_width is None:
- line_width = 1
- try: # in case closed with X
- id = self._TKCanvas2.create_rectangle(converted_top_left[0], converted_top_left[1],
- converted_bottom_right[0],
- converted_bottom_right[1], fill=fill_color, outline=line_color, width=line_width)
- except:
- id = None
- return id
-
- def draw_polygon(self, points, fill_color=None, line_color=None, line_width=None):
- """
- Draw a polygon given list of points
-
- :param points: list of points that define the polygon
- :type points: List[(int, int) | Tuple[float, float]]
- :param fill_color: color of the interior
- :type fill_color: (str)
- :param line_color: color of outline
- :type line_color: (str)
- :param line_width: width of the line in pixels
- :type line_width: (int)
- :return: id returned from tkinter that you'll need if you want to manipulate the rectangle
- :rtype: int | None
- """
-
- converted_points = [self._convert_xy_to_canvas_xy(point[0], point[1]) for point in points]
- if self._TKCanvas2 is None:
- print('*** WARNING - The Graph element has not been finalized and cannot be drawn upon ***')
- print('Call Window.Finalize() prior to this operation')
- return None
- try: # in case closed with X
- id = self._TKCanvas2.create_polygon(converted_points, fill=fill_color, outline=line_color, width=line_width)
- except:
- id = None
- return id
-
- def draw_text(self, text, location, color='black', font=None, angle=0, text_location=TEXT_LOCATION_CENTER):
- """
- Draw some text on your graph. This is how you label graph number lines for example
-
- :param text: text to display
- :type text: (Any)
- :param location: location to place first letter
- :type location: (int, int) | Tuple[float, float]
- :param color: text color
- :type color: (str)
- :param font: specifies the font family, size, etc. Tuple or Single string format 'name size styles'. Styles: italic * roman bold normal underline overstrike
- :type font: (str or (str, int[, str]) or None)
- :param angle: Angle 0 to 360 to draw the text. Zero represents horizontal text
- :type angle: (float)
- :param text_location: "anchor" location for the text. Values start with TEXT_LOCATION_
- :type text_location: (enum)
- :return: id returned from tkinter that you'll need if you want to manipulate the text
- :rtype: int | None
- """
- text = str(text)
- if location == (None, None):
- return
- converted_point = self._convert_xy_to_canvas_xy(location[0], location[1])
- if self._TKCanvas2 is None:
- print('*** WARNING - The Graph element has not been finalized and cannot be drawn upon ***')
- print('Call Window.Finalize() prior to this operation')
- return None
- try: # in case closed with X
- id = self._TKCanvas2.create_text(converted_point[0], converted_point[1], text=text, font=font, fill=color, angle=angle, anchor=text_location)
- except:
- id = None
- return id
-
- def draw_image(self, filename=None, data=None, location=(None, None)):
- """
- Places an image onto your canvas. It's a really important method for this element as it enables so much
-
- :param filename: if image is in a file, path and filename for the image. (GIF and PNG only!)
- :type filename: (str)
- :param data: if image is in Base64 format or raw? format then use instead of filename
- :type data: str | bytes
- :param location: the (x,y) location to place image's top left corner
- :type location: (int, int) | Tuple[float, float]
- :return: id returned from tkinter that you'll need if you want to manipulate the image
- :rtype: int | None
- """
- if location == (None, None):
- return
- if filename is not None:
- image = tk.PhotoImage(file=filename)
- elif data is not None:
- # if type(data) is bytes:
- try:
- image = tk.PhotoImage(data=data)
- except:
- return None # an error likely means the window has closed so exit
- converted_point = self._convert_xy_to_canvas_xy(location[0], location[1])
- if self._TKCanvas2 is None:
- print('*** WARNING - The Graph element has not been finalized and cannot be drawn upon ***')
- print('Call Window.Finalize() prior to this operation')
- return None
- try: # in case closed with X
- id = self._TKCanvas2.create_image(converted_point, image=image, anchor=tk.NW)
- self.Images[id] = image
- except:
- id = None
- return id
-
- def erase(self):
- """
- Erase the Graph - Removes all figures previously "drawn" using the Graph methods (e.g. DrawText)
- """
- if self._TKCanvas2 is None:
- print('*** WARNING - The Graph element has not been finalized and cannot be drawn upon ***')
- print('Call Window.Finalize() prior to this operation')
- return None
- self.Images = {}
- try: # in case window was closed with X
- self._TKCanvas2.delete('all')
- except:
- pass
-
- def delete_figure(self, id):
- """
- Remove from the Graph the figure represented by id. The id is given to you anytime you call a drawing primitive
-
- :param id: the id returned to you when calling one of the drawing methods
- :type id: (int)
- """
- try:
- self._TKCanvas2.delete(id)
- except:
- print('DeleteFigure - bad ID {}'.format(id))
- try:
- del self.Images[id] # in case was an image. If wasn't an image, then will get exception
- except:
- pass
-
- def update(self, background_color=None, visible=None):
- """
- Changes some of the settings for the Graph Element. Must call `Window.Read` or `Window.Finalize` prior
-
- Changes will not be visible in your window until you call window.read or window.refresh.
-
- If you change visibility, your element may MOVE. If you want it to remain stationary, use the "layout helper"
- function "pin" to ensure your element is "pinned" to that location in your layout so that it returns there
- when made visible.
-
- :param background_color: color of background
- :type background_color: ???
- :param visible: control visibility of element
- :type visible: (bool)
- """
- if not self._widget_was_created(): # if widget hasn't been created yet, then don't allow
- return
-
- if background_color is not None and background_color != COLOR_SYSTEM_DEFAULT:
- self._TKCanvas2.configure(background=background_color)
-
- if visible is False:
- self._pack_forget_save_settings()
- elif visible is True:
- self._pack_restore_settings()
-
- if visible is not None:
- self._visible = visible
-
- def move(self, x_direction, y_direction):
- """
- Moves the entire drawing area (the canvas) by some delta from the current position. Units are indicated in your coordinate system indicated number of ticks in your coordinate system
-
- :param x_direction: how far to move in the "X" direction in your coordinates
- :type x_direction: int | float
- :param y_direction: how far to move in the "Y" direction in your coordinates
- :type y_direction: int | float
- """
- zero_converted = self._convert_xy_to_canvas_xy(0, 0)
- shift_converted = self._convert_xy_to_canvas_xy(x_direction, y_direction)
- shift_amount = (shift_converted[0] - zero_converted[0], shift_converted[1] - zero_converted[1])
- if self._TKCanvas2 is None:
- print('*** WARNING - The Graph element has not been finalized and cannot be drawn upon ***')
- print('Call Window.Finalize() prior to this operation')
- return None
- self._TKCanvas2.move('all', shift_amount[0], shift_amount[1])
-
- def move_figure(self, figure, x_direction, y_direction):
- """
- Moves a previously drawn figure using a "delta" from current position
-
- :param figure: Previously obtained figure-id. These are returned from all Draw methods
- :type figure: (id)
- :param x_direction: delta to apply to position in the X direction
- :type x_direction: int | float
- :param y_direction: delta to apply to position in the Y direction
- :type y_direction: int | float
- """
- zero_converted = self._convert_xy_to_canvas_xy(0, 0)
- shift_converted = self._convert_xy_to_canvas_xy(x_direction, y_direction)
- shift_amount = (shift_converted[0] - zero_converted[0], shift_converted[1] - zero_converted[1])
- if figure is None:
- print('* move_figure warning - your figure is None *')
- return None
- self._TKCanvas2.move(figure, shift_amount[0], shift_amount[1])
-
- def relocate_figure(self, figure, x, y):
- """
- Move a previously made figure to an arbitrary (x,y) location. This differs from the Move methods because it
- uses absolute coordinates versus relative for Move
-
- :param figure: Previously obtained figure-id. These are returned from all Draw methods
- :type figure: (id)
- :param x: location on X axis (in user coords) to move the upper left corner of the figure
- :type x: int | float
- :param y: location on Y axis (in user coords) to move the upper left corner of the figure
- :type y: int | float
- """
-
- zero_converted = self._convert_xy_to_canvas_xy(0, 0)
- shift_converted = self._convert_xy_to_canvas_xy(x, y)
- shift_amount = (shift_converted[0] - zero_converted[0], shift_converted[1] - zero_converted[1])
- if figure is None:
- print('*** WARNING - Your figure is None. It most likely means your did not Finalize your Window ***')
- print('Call Window.Finalize() prior to all graph operations')
- return None
- xy = self._TKCanvas2.coords(figure)
- self._TKCanvas2.move(figure, shift_converted[0] - xy[0], shift_converted[1] - xy[1])
-
- def send_figure_to_back(self, figure):
- """
- Changes Z-order of figures on the Graph. Sends the indicated figure to the back of all other drawn figures
-
- :param figure: value returned by tkinter when creating the figure / drawing
- :type figure: (int)
- """
- self.TKCanvas.tag_lower(figure) # move figure to the "bottom" of all other figure
-
- def bring_figure_to_front(self, figure):
- """
- Changes Z-order of figures on the Graph. Brings the indicated figure to the front of all other drawn figures
-
- :param figure: value returned by tkinter when creating the figure / drawing
- :type figure: (int)
- """
- self.TKCanvas.tag_raise(figure) # move figure to the "top" of all other figures
-
- def get_figures_at_location(self, location):
- """
- Returns a list of figures located at a particular x,y location within the Graph
-
- :param location: point to check
- :type location: (int, int) | Tuple[float, float]
- :return: a list of previously drawn "Figures" (returned from the drawing primitives)
- :rtype: List[int]
- """
- x, y = self._convert_xy_to_canvas_xy(location[0], location[1])
- ids = self.TKCanvas.find_overlapping(x, y, x, y)
- return ids
-
- def get_bounding_box(self, figure):
- """
- Given a figure, returns the upper left and lower right bounding box coordinates
-
- :param figure: a previously drawing figure
- :type figure: object
- :return: upper left x, upper left y, lower right x, lower right y
- :rtype: Tuple[int, int, int, int] | Tuple[float, float, float, float]
- """
- box = self.TKCanvas.bbox(figure)
- top_left = self._convert_canvas_xy_to_xy(box[0], box[1])
- bottom_right = self._convert_canvas_xy_to_xy(box[2], box[3])
- return top_left, bottom_right
-
- def change_coordinates(self, graph_bottom_left, graph_top_right):
- """
- Changes the corrdinate system to a new one. The same 2 points in space are used to define the coorinate
- system - the bottom left and the top right values of your graph.
-
- :param graph_bottom_left: The bottoms left corner of your coordinate system
- :type graph_bottom_left: (int, int) (x,y)
- :param graph_top_right: The top right corner of your coordinate system
- :type graph_top_right: (int, int) (x,y)
- """
- self.BottomLeft = graph_bottom_left
- self.TopRight = graph_top_right
-
- @property
- def tk_canvas(self):
- """
- Returns the underlying tkiner Canvas widget
-
- :return: The tkinter canvas widget
- :rtype: (tk.Canvas)
- """
- if self._TKCanvas2 is None:
- print('*** Did you forget to call Finalize()? Your code should look something like: ***')
- print('*** form = sg.Window("My Form").Layout(layout).Finalize() ***')
- return self._TKCanvas2
-
- # button release callback
- def button_release_call_back(self, event):
- """
- Not a user callable method. Used to get Graph click events. Called by tkinter when button is released
-
- :param event: (event) event info from tkinter. Note not used in this method
- :type event:
- """
- if not self.DragSubmits: # only report mouse up for drag operations
- return
- self.ClickPosition = self._convert_canvas_xy_to_xy(event.x, event.y)
- self.LastButtonClickedWasRealtime = not self.DragSubmits
- if self.Key is not None:
- self.ParentForm.LastButtonClicked = self.Key
- else:
- self.ParentForm.LastButtonClicked = '__GRAPH__' # need to put something rather than None
- # if self.ParentForm.CurrentlyRunningMainloop:
- # self.ParentForm.TKroot.quit()
- _exit_mainloop(self.ParentForm)
- if self.DragSubmits:
- if isinstance(self.ParentForm.LastButtonClicked, str):
- self.ParentForm.LastButtonClicked = self.ParentForm.LastButtonClicked + '+UP'
- else:
- self.ParentForm.LastButtonClicked = (self.ParentForm.LastButtonClicked, '+UP')
- # self.ParentForm.LastButtonClicked += '+UP' # this is the old method that required string key
- self.MouseButtonDown = False
-
- # button callback
- def button_press_call_back(self, event):
- """
- Not a user callable method. Used to get Graph click events. Called by tkinter when button is released
-
- :param event: (event) event info from tkinter. Contains the x and y coordinates of a click
- :type event:
- """
-
- self.ClickPosition = self._convert_canvas_xy_to_xy(event.x, event.y)
- self.ParentForm.LastButtonClickedWasRealtime = self.DragSubmits
- if self.Key is not None:
- self.ParentForm.LastButtonClicked = self.Key
- else:
- self.ParentForm.LastButtonClicked = '__GRAPH__' # need to put something rather than None
- # if self.ParentForm.CurrentlyRunningMainloop:
- # self.ParentForm.TKroot.quit() # kick out of loop if read was called
- _exit_mainloop(self.ParentForm)
- self.MouseButtonDown = True
-
- def _update_position_for_returned_values(self, event):
- """
- Updates the variable that's used when the values dictionary is returned from a window read.
-
- Not called by the user. It's called from another method/function that tkinter calledback
-
- :param event: (event) event info from tkinter. Contains the x and y coordinates of a click
- :type event:
- """
- """
- Updates the variable that's used when the values dictionary is returned from a window read.
-
- Not called by the user. It's called from another method/function that tkinter calledback
-
- :param event: (event) event info from tkinter. Contains the x and y coordinates of a click
- :type event:
- """
-
- self.ClickPosition = self._convert_canvas_xy_to_xy(event.x, event.y)
-
- # button callback
- def motion_call_back(self, event):
- """
- Not a user callable method. Used to get Graph mouse motion events. Called by tkinter when mouse moved
-
- :param event: (event) event info from tkinter. Contains the x and y coordinates of a mouse
- :type event:
- """
-
- if not self.MouseButtonDown and not self.motion_events:
- return
- self.ClickPosition = self._convert_canvas_xy_to_xy(event.x, event.y)
- self.ParentForm.LastButtonClickedWasRealtime = self.DragSubmits
- if self.Key is not None:
- self.ParentForm.LastButtonClicked = self.Key
- else:
- self.ParentForm.LastButtonClicked = '__GRAPH__' # need to put something rather than None
- # if self.ParentForm.CurrentlyRunningMainloop:
- # self.ParentForm.TKroot.quit() # kick out of loop if read was called
- if self.motion_events and not self.MouseButtonDown:
- if isinstance(self.ParentForm.LastButtonClicked, str):
- self.ParentForm.LastButtonClicked = self.ParentForm.LastButtonClicked + '+MOVE'
- else:
- self.ParentForm.LastButtonClicked = (self.ParentForm.LastButtonClicked, '+MOVE')
- _exit_mainloop(self.ParentForm)
-
- BringFigureToFront = bring_figure_to_front
- ButtonPressCallBack = button_press_call_back
- ButtonReleaseCallBack = button_release_call_back
- DeleteFigure = delete_figure
- DrawArc = draw_arc
- DrawCircle = draw_circle
- DrawImage = draw_image
- DrawLine = draw_line
- DrawOval = draw_oval
- DrawPoint = draw_point
- DrawPolygon = draw_polygon
- DrawLines = draw_lines
- DrawRectangle = draw_rectangle
- DrawText = draw_text
- GetFiguresAtLocation = get_figures_at_location
- GetBoundingBox = get_bounding_box
- Erase = erase
- MotionCallBack = motion_call_back
- Move = move
- MoveFigure = move_figure
- RelocateFigure = relocate_figure
- SendFigureToBack = send_figure_to_back
- TKCanvas = tk_canvas
- Update = update
-
-
-G = Graph
-
-
-# ---------------------------------------------------------------------- #
-# Frame #
-# ---------------------------------------------------------------------- #
-class Frame(Element):
- """
- A Frame Element that contains other Elements. Encloses with a line around elements and a text label.
- """
-
- def __init__(self, title, layout, title_color=None, background_color=None, title_location=None,
- relief=DEFAULT_FRAME_RELIEF, size=(None, None), s=(None, None), font=None, pad=None, p=None, border_width=None, key=None, k=None,
- tooltip=None, right_click_menu=None, expand_x=False, expand_y=False, grab=None, visible=True, element_justification='left', vertical_alignment=None, metadata=None):
- """
- :param title: text that is displayed as the Frame's "label" or title
- :type title: (str)
- :param layout: The layout to put inside the Frame
- :type layout: List[List[Elements]]
- :param title_color: color of the title text
- :type title_color: (str)
- :param background_color: background color of the Frame
- :type background_color: (str)
- :param title_location: location to place the text title. Choices include: TITLE_LOCATION_TOP TITLE_LOCATION_BOTTOM TITLE_LOCATION_LEFT TITLE_LOCATION_RIGHT TITLE_LOCATION_TOP_LEFT TITLE_LOCATION_TOP_RIGHT TITLE_LOCATION_BOTTOM_LEFT TITLE_LOCATION_BOTTOM_RIGHT
- :type title_location: (enum)
- :param relief: relief style. Values are same as other elements with reliefs. Choices include RELIEF_RAISED RELIEF_SUNKEN RELIEF_FLAT RELIEF_RIDGE RELIEF_GROOVE RELIEF_SOLID
- :type relief: (enum)
- :param size: (width, height) Sets an initial hard-coded size for the Frame. This used to be a problem, but was fixed in 4.53.0 and works better than Columns when using the size paramter
- :type size: (int, int)
- :param s: Same as size parameter. It's an alias. If EITHER of them are set, then the one that's set will be used. If BOTH are set, size will be used
- :type s: (int, int) | (None, None) | int
- :param font: specifies the font family, size, etc. for the TITLE. Tuple or Single string format 'name size styles'. Styles: italic * roman bold normal underline overstrike
- :type font: (str or (str, int[, str]) or None)
- :param pad: Amount of padding to put around element in pixels (left/right, top/bottom) or ((left, right), (top, bottom)) or an int. If an int, then it's converted into a tuple (int, int)
- :type pad: (int, int) or ((int, int),(int,int)) or (int,(int,int)) or ((int, int),int) | int
- :param p: Same as pad parameter. It's an alias. If EITHER of them are set, then the one that's set will be used. If BOTH are set, pad will be used
- :type p: (int, int) or ((int, int),(int,int)) or (int,(int,int)) or ((int, int),int) | int
- :param border_width: width of border around element in pixels
- :type border_width: (int)
- :param key: Value that uniquely identifies this element from all other elements. Used when Finding an element or in return values. Must be unique to the window
- :type key: str | int | tuple | object
- :param k: Same as the Key. You can use either k or key. Which ever is set will be used.
- :type k: str | int | tuple | object
- :param tooltip: text, that will appear when mouse hovers over the element
- :type tooltip: (str)
- :param right_click_menu: A list of lists of Menu items to show when this element is right clicked. See user docs for exact format.
- :type right_click_menu: List[List[ List[str] | str ]]
- :param expand_x: If True the element will automatically expand in the X direction to fill available space
- :type expand_x: (bool)
- :param expand_y: If True the element will automatically expand in the Y direction to fill available space
- :type expand_y: (bool)
- :param grab: If True can grab this element and move the window around. Default is False
- :type grab: (bool)
- :param visible: set visibility state of the element
- :type visible: (bool)
- :param element_justification: All elements inside the Frame will have this justification 'left', 'right', 'center' are valid values
- :type element_justification: (str)
- :param vertical_alignment: Place the Frame at the 'top', 'center', 'bottom' of the row (can also use t,c,r). Defaults to no setting (tkinter decides)
- :type vertical_alignment: (str)
- :param metadata: User metadata that can be set to ANYTHING
- :type metadata: (Any)
- """
-
-
- self.UseDictionary = False
- self.ReturnValues = None
- self.ReturnValuesList = []
- self.ReturnValuesDictionary = {}
- self.DictionaryKeyCounter = 0
- self.ParentWindow = None
- self.Rows = []
- # self.ParentForm = None
- self.TKFrame = None
- self.Title = title
- self.Relief = relief
- self.TitleLocation = title_location
- self.BorderWidth = border_width
- self.BackgroundColor = background_color if background_color is not None else DEFAULT_BACKGROUND_COLOR
- self.RightClickMenu = right_click_menu
- self.ContainerElemementNumber = Window._GetAContainerNumber()
- self.ElementJustification = element_justification
- self.VerticalAlignment = vertical_alignment
- self.Widget = None # type: tk.LabelFrame
- self.Grab = grab
- self.Layout(layout)
- key = key if key is not None else k
- sz = size if size != (None, None) else s
- pad = pad if pad is not None else p
- self.expand_x = expand_x
- self.expand_y = expand_y
-
- super().__init__(ELEM_TYPE_FRAME, background_color=background_color, text_color=title_color, size=sz,
- font=font, pad=pad, key=key, tooltip=tooltip, visible=visible, metadata=metadata)
- return
-
-
- def add_row(self, *args):
- """
- Not recommended user call. Used to add rows of Elements to the Frame Element.
-
- :param *args: The list of elements for this row
- :type *args: List[Element]
- """
- NumRows = len(self.Rows) # number of existing rows is our row number
- CurrentRowNumber = NumRows # this row's number
- CurrentRow = [] # start with a blank row and build up
- # ------------------------- Add the elements to a row ------------------------- #
- for i, element in enumerate(args): # Loop through list of elements and add them to the row
- if type(element) == list:
- PopupError('Error creating Frame layout',
- 'Layout has a LIST instead of an ELEMENT',
- 'This means you have a badly placed ]',
- 'The offensive list is:',
- element,
- 'This list will be stripped from your layout',
- keep_on_top=True
- )
- continue
- elif callable(element) and not isinstance(element, Element):
- PopupError('Error creating Frame layout',
- 'Layout has a FUNCTION instead of an ELEMENT',
- 'This likely means you are missing () from your layout',
- 'The offensive list is:',
- element,
- 'This item will be stripped from your layout',
- keep_on_top=True)
- continue
- if element.ParentContainer is not None:
- warnings.warn(
- '*** YOU ARE ATTEMPTING TO RESUSE AN ELEMENT IN YOUR LAYOUT! Once placed in a layout, an element cannot be used in another layout. ***',
- UserWarning)
- _error_popup_with_traceback('Error creating Frame layout',
- 'The layout specified has already been used',
- 'You MUST start witha "clean", unused layout every time you create a window',
- 'The offensive Element = ',
- element,
- 'and has a key = ', element.Key,
- 'This item will be stripped from your layout',
- 'Hint - try printing your layout and matching the IDs "print(layout)"',
- )
- continue
- element.Position = (CurrentRowNumber, i)
- element.ParentContainer = self
- CurrentRow.append(element)
- if element.Key is not None:
- self.UseDictionary = True
- # ------------------------- Append the row to list of Rows ------------------------- #
- self.Rows.append(CurrentRow)
-
- def layout(self, rows):
- """
- Can use like the Window.Layout method, but it's better to use the layout parameter when creating
-
- :param rows: The rows of Elements
- :type rows: List[List[Element]]
- :return: Used for chaining
- :rtype: (Frame)
- """
-
- for row in rows:
- try:
- iter(row)
- except TypeError:
- PopupError('Error creating Frame layout',
- 'Your row is not an iterable (e.g. a list)',
- 'Instead of a list, the type found was {}'.format(type(row)),
- 'The offensive row = ',
- row,
- 'This item will be stripped from your layout', keep_on_top=True, image=_random_error_emoji())
- continue
- self.AddRow(*row)
- return self
-
- def _GetElementAtLocation(self, location):
- """
- Not user callable. Used to find the Element at a row, col position within the layout
-
- :param location: (row, column) position of the element to find in layout
- :type location: (int, int)
- :return: (Element) The element found at the location
- :rtype: (Element)
- """
-
- (row_num, col_num) = location
- row = self.Rows[row_num]
- element = row[col_num]
- return element
-
- def update(self, value=None, visible=None):
- """
- Changes some of the settings for the Frame Element. Must call `Window.Read` or `Window.Finalize` prior
-
- Changes will not be visible in your window until you call window.read or window.refresh.
-
- If you change visibility, your element may MOVE. If you want it to remain stationary, use the "layout helper"
- function "pin" to ensure your element is "pinned" to that location in your layout so that it returns there
- when made visible.
-
- :param value: New text value to show on frame
- :type value: (Any)
- :param visible: control visibility of element
- :type visible: (bool)
- """
- if not self._widget_was_created(): # if widget hasn't been created yet, then don't allow
- return
-
- if visible is False:
- self._pack_forget_save_settings()
- # self.TKFrame.pack_forget()
- elif visible is True:
- self._pack_restore_settings()
- # self.TKFrame.pack(padx=self.pad_used[0], pady=self.pad_used[1])
- if value is not None:
- self.TKFrame.config(text=str(value))
- if visible is not None:
- self._visible = visible
-
- AddRow = add_row
- Layout = layout
- Update = update
-
-
-Fr = Frame
-
-
-# ---------------------------------------------------------------------- #
-# Vertical Separator #
-# ---------------------------------------------------------------------- #
-class VerticalSeparator(Element):
- """
- Vertical Separator Element draws a vertical line at the given location. It will span 1 "row". Usually paired with
- Column Element if extra height is needed
- """
-
- def __init__(self, color=None, pad=None, p=None, key=None, k=None):
- """
- :param color: Color of the line. Defaults to theme's text color. Can be name or #RRGGBB format
- :type color: (str)
- :param pad: Amount of padding to put around element in pixels (left/right, top/bottom) or ((left, right), (top, bottom)) or an int. If an int, then it's converted into a tuple (int, int)
- :type pad: (int, int) or ((int, int),(int,int)) or (int,(int,int)) or ((int, int),int) | int
- :param p: Same as pad parameter. It's an alias. If EITHER of them are set, then the one that's set will be used. If BOTH are set, pad will be used
- :type p: (int, int) or ((int, int),(int,int)) or (int,(int,int)) or ((int, int),int) | int
- :param key: Value that uniquely identifies this element from all other elements. Used when Finding an element or in return values. Must be unique to the window
- :type key: str | int | tuple | object
- :param k: Same as the Key. You can use either k or key. Which ever is set will be used.
- :type k: str | int | tuple | object
- """
- key = key if key is not None else k
- pad = pad if pad is not None else p
- self.expand_x = None
- self.expand_y = None
- self.Orientation = 'vertical' # for now only vertical works
- self.color = color if color is not None else theme_text_color()
- super().__init__(ELEM_TYPE_SEPARATOR, pad=pad, key=key)
-
-
-VSeperator = VerticalSeparator
-VSeparator = VerticalSeparator
-VSep = VerticalSeparator
-
-
-# ---------------------------------------------------------------------- #
-# Horizontal Separator #
-# ---------------------------------------------------------------------- #
-class HorizontalSeparator(Element):
- """
- Horizontal Separator Element draws a Horizontal line at the given location.
- """
-
- def __init__(self, color=None, pad=None, p=None, key=None, k=None):
- """
- :param color: Color of the line. Defaults to theme's text color. Can be name or #RRGGBB format
- :type color: (str)
- :param pad: Amount of padding to put around element in pixels (left/right, top/bottom) or ((left, right), (top, bottom)) or an int. If an int, then it's converted into a tuple (int, int)
- :type pad: (int, int) or ((int, int),(int,int)) or (int,(int,int)) or ((int, int),int) | int
- :param p: Same as pad parameter. It's an alias. If EITHER of them are set, then the one that's set will be used. If BOTH are set, pad will be used
- :type p: (int, int) or ((int, int),(int,int)) or (int,(int,int)) or ((int, int),int) | int
- :param key: Value that uniquely identifies this element from all other elements. Used when Finding an element or in return values. Must be unique to the window
- :type key: str | int | tuple | object
- :param k: Same as the Key. You can use either k or key. Which ever is set will be used.
- :type k: str | int | tuple | object
- """
-
- self.Orientation = 'horizontal' # for now only vertical works
- self.color = color if color is not None else theme_text_color()
- self.expand_x = True
- self.expand_y = None
- key = key if key is not None else k
- pad = pad if pad is not None else p
-
- super().__init__(ELEM_TYPE_SEPARATOR, pad=pad, key=key)
-
-
-HSeparator = HorizontalSeparator
-HSep = HorizontalSeparator
-
-
-# ---------------------------------------------------------------------- #
-# Sizegrip #
-# ---------------------------------------------------------------------- #
-class Sizegrip(Element):
- """
- Sizegrip element will be added to the bottom right corner of your window.
- It should be placed on the last row of your window along with any other elements on that row.
- The color will match the theme's background color.
- """
-
- def __init__(self, background_color=None, pad=None, p=(0,0), key=None, k=None):
- """
- Sizegrip Element
- :param background_color: color to use for the background of the grip
- :type background_color: str
- :param pad: Amount of padding to put around element in pixels (left/right, top/bottom) or ((left, right), (top, bottom)) or an int. If an int, then it's converted into a tuple (int, int)
- :type pad: (int, int) or ((int, int),(int,int)) or (int,(int,int)) or ((int, int),int) | int
- :param p: Same as pad parameter. It's an alias. If EITHER of them are set, then the one that's set will be used. If BOTH are set, pad will be used
- :type p: (int, int) or ((int, int),(int,int)) or (int,(int,int)) or ((int, int),int) | int
- :param key: Value that uniquely identifies this element from all other elements. Used when Finding an element or in return values. Must be unique to the window
- :type key: str | int | tuple | object
- :param k: Same as the Key. You can use either k or key. Which ever is set will be used.
- :type k: str | int | tuple | object
- """
-
- bg = background_color if background_color is not None else theme_background_color()
- pad = pad if pad is not None else p
- key = key if key is not None else k
-
-
- super().__init__(ELEM_TYPE_SIZEGRIP, background_color=bg,key=key, pad=pad)
-
-
-SGrip = Sizegrip
-
-
-# ---------------------------------------------------------------------- #
-# Tab #
-# ---------------------------------------------------------------------- #
-class Tab(Element):
- """
- Tab Element is another "Container" element that holds a layout and displays a tab with text. Used with TabGroup only
- Tabs are never placed directly into a layout. They are always "Contained" in a TabGroup layout
- """
-
- def __init__(self, title, layout, title_color=None, background_color=None, font=None, pad=None, p=None, disabled=False,
- border_width=None, key=None, k=None, tooltip=None, right_click_menu=None, expand_x=False, expand_y=False, visible=True, element_justification='left', image_source=None, image_subsample=None, metadata=None):
- """
- :param title: text to show on the tab
- :type title: (str)
- :param layout: The element layout that will be shown in the tab
- :type layout: List[List[Element]]
- :param title_color: color of the tab text (note not currently working on tkinter)
- :type title_color: (str)
- :param background_color: color of background of the entire layout
- :type background_color: (str)
- :param font: NOT USED in the tkinter port
- :type font: (str or (str, int[, str]) or None)
- :param pad: Amount of padding to put around element in pixels (left/right, top/bottom) or ((left, right), (top, bottom)) or an int. If an int, then it's converted into a tuple (int, int)
- :type pad: (int, int) or ((int, int),(int,int)) or (int,(int,int)) or ((int, int),int) | int
- :param p: Same as pad parameter. It's an alias. If EITHER of them are set, then the one that's set will be used. If BOTH are set, pad will be used
- :type p: (int, int) or ((int, int),(int,int)) or (int,(int,int)) or ((int, int),int) | int
- :param disabled: If True button will be created disabled
- :type disabled: (bool)
- :param border_width: NOT USED in tkinter port
- :type border_width: (int)
- :param key: Value that uniquely identifies this element from all other elements. Used when Finding an element or in return values. Must be unique to the window
- :type key: str | int | tuple | object
- :param k: Same as the Key. You can use either k or key. Which ever is set will be used.
- :type k: str | int | tuple | object
- :param tooltip: text, that will appear when mouse hovers over the element
- :type tooltip: (str)
- :param right_click_menu: A list of lists of Menu items to show when this element is right clicked. See user docs for exact format.
- :type right_click_menu: List[List[ List[str] | str ]]
- :param expand_x: If True the element will automatically expand in the X direction to fill available space
- :type expand_x: (bool)
- :param expand_y: If True the element will automatically expand in the Y direction to fill available space
- :type expand_y: (bool)
- :param visible: set visibility state of the element
- :type visible: (bool)
- :param element_justification: All elements inside the Tab will have this justification 'left', 'right', 'center' are valid values
- :type element_justification: (str)
- :param image_source: A filename or a base64 bytes of an image to place on the Tab
- :type image_source: str | bytes | None
- :param image_subsample: amount to reduce the size of the image. Divides the size by this number. 2=1/2, 3=1/3, 4=1/4, etc
- :type image_subsample: (int)
- :param metadata: User metadata that can be set to ANYTHING
- :type metadata: (Any)
- """
-
- filename = data = None
- if image_source is not None:
- if isinstance(image_source, bytes):
- data = image_source
- elif isinstance(image_source, str):
- filename = image_source
- else:
- warnings.warn('Image element - source is not a valid type: {}'.format(type(image_source)), UserWarning)
-
- self.Filename = filename
- self.Data = data
- self.ImageSubsample = image_subsample
- self.UseDictionary = False
- self.ReturnValues = None
- self.ReturnValuesList = []
- self.ReturnValuesDictionary = {}
- self.DictionaryKeyCounter = 0
- self.ParentWindow = None
- self.Rows = []
- self.TKFrame = None
- self.Widget = None # type: tk.Frame
- self.Title = title
- self.BorderWidth = border_width
- self.Disabled = disabled
- self.ParentNotebook = None
- self.TabID = None
- self.BackgroundColor = background_color if background_color is not None else DEFAULT_BACKGROUND_COLOR
- self.RightClickMenu = right_click_menu
- self.ContainerElemementNumber = Window._GetAContainerNumber()
- self.ElementJustification = element_justification
- key = key if key is not None else k
- pad = pad if pad is not None else p
- self.expand_x = expand_x
- self.expand_y = expand_y
-
- self.Layout(layout)
-
- super().__init__(ELEM_TYPE_TAB, background_color=background_color, text_color=title_color, font=font, pad=pad, key=key, tooltip=tooltip,
- visible=visible, metadata=metadata)
- return
-
- def add_row(self, *args):
- """
- Not recommended use call. Used to add rows of Elements to the Frame Element.
-
- :param *args: The list of elements for this row
- :type *args: List[Element]
- """
- NumRows = len(self.Rows) # number of existing rows is our row number
- CurrentRowNumber = NumRows # this row's number
- CurrentRow = [] # start with a blank row and build up
- # ------------------------- Add the elements to a row ------------------------- #
- for i, element in enumerate(args): # Loop through list of elements and add them to the row
- if type(element) == list:
- popup_error_with_traceback('Error creating Tab layout',
- 'Layout has a LIST instead of an ELEMENT',
- 'This means you have a badly placed ]',
- 'The offensive list is:',
- element,
- 'This list will be stripped from your layout')
- continue
- elif callable(element) and not isinstance(element, Element):
- popup_error_with_traceback('Error creating Tab layout',
- 'Layout has a FUNCTION instead of an ELEMENT',
- 'This likely means you are missing () from your layout',
- 'The offensive list is:',
- element,
- 'This item will be stripped from your layout')
- continue
- if element.ParentContainer is not None:
- warnings.warn(
- '*** YOU ARE ATTEMPTING TO RESUSE AN ELEMENT IN YOUR LAYOUT! Once placed in a layout, an element cannot be used in another layout. ***',
- UserWarning)
- popup_error_with_traceback('Error creating Tab layout',
- 'The layout specified has already been used',
- 'You MUST start witha "clean", unused layout every time you create a window',
- 'The offensive Element = ',
- element,
- 'and has a key = ', element.Key,
- 'This item will be stripped from your layout',
- 'Hint - try printing your layout and matching the IDs "print(layout)"')
- continue
- element.Position = (CurrentRowNumber, i)
- element.ParentContainer = self
- CurrentRow.append(element)
- if element.Key is not None:
- self.UseDictionary = True
- # ------------------------- Append the row to list of Rows ------------------------- #
- self.Rows.append(CurrentRow)
-
- def layout(self, rows):
- """
- Not user callable. Use layout parameter instead. Creates the layout using the supplied rows of Elements
-
- :param rows: List[List[Element]] The list of rows
- :type rows: List[List[Element]]
- :return: (Tab) used for chaining
- :rtype:
- """
-
- for row in rows:
- try:
- iter(row)
- except TypeError:
- PopupError('Error creating Tab layout',
- 'Your row is not an iterable (e.g. a list)',
- 'Instead of a list, the type found was {}'.format(type(row)),
- 'The offensive row = ',
- row,
- 'This item will be stripped from your layout', keep_on_top=True, image=_random_error_emoji())
- continue
- self.AddRow(*row)
- return self
-
- def update(self, title=None, disabled=None, visible=None):
- """
- Changes some of the settings for the Tab Element. Must call `Window.Read` or `Window.Finalize` prior
-
- Changes will not be visible in your window until you call window.read or window.refresh.
-
- If you change visibility, your element may MOVE. If you want it to remain stationary, use the "layout helper"
- function "pin" to ensure your element is "pinned" to that location in your layout so that it returns there
- when made visible.
-
- :param title: tab title
- :type title: (str)
- :param disabled: disable or enable state of the element
- :type disabled: (bool)
- :param visible: control visibility of element
- :type visible: (bool)
- """
- if not self._widget_was_created(): # if widget hasn't been created yet, then don't allow
- return
-
- state = 'normal'
- if disabled is not None:
- self.Disabled = disabled
- if disabled:
- state = 'disabled'
- if visible is False:
- state = 'hidden'
- if visible is not None:
- self._visible = visible
-
- self.ParentNotebook.tab(self.TabID, state=state)
-
- if title is not None:
- self.Title = str(title)
- self.ParentNotebook.tab(self.TabID, text=self.Title)
- # self.ParentNotebook.tab(self.ContainerElemementNumber-1, text=self.Title)
-
- # if visible is False:
- # self.ParentNotebook.pack_forget()
- # elif visible is True:
- # self.ParentNotebook.pack()
- return self
-
- def _GetElementAtLocation(self, location):
- """
- Not user callable. Used to find the Element at a row, col position within the layout
-
- :param location: (row, column) position of the element to find in layout
- :type location: (int, int)
- :return: The element found at the location
- :rtype: (Element)
- """
-
- (row_num, col_num) = location
- row = self.Rows[row_num]
- element = row[col_num]
- return element
-
- def select(self):
- """
- Create a tkinter event that mimics user clicking on a tab. Must have called window.Finalize / Read first!
-
- """
- # Use a try in case the window has been destoyed
- try:
- self.ParentNotebook.select(self.TabID)
- except Exception as e:
- print('Exception Selecting Tab {}'.format(e))
-
- AddRow = add_row
- Layout = layout
- Select = select
- Update = update
-
-
-# ---------------------------------------------------------------------- #
-# TabGroup #
-# ---------------------------------------------------------------------- #
-class TabGroup(Element):
- """
- TabGroup Element groups together your tabs into the group of tabs you see displayed in your window
- """
-
- def __init__(self, layout, tab_location=None, title_color=None, tab_background_color=None, selected_title_color=None, selected_background_color=None,
- background_color=None, focus_color=None, font=None, change_submits=False, enable_events=False, pad=None, p=None, border_width=None, tab_border_width=None, theme=None, key=None, k=None,
- size=(None, None), s=(None, None), tooltip=None, right_click_menu=None, expand_x=False, expand_y=False, visible=True, metadata=None):
- """
- :param layout: Layout of Tabs. Different than normal layouts. ALL Tabs should be on first row
- :type layout: List[List[Tab]]
- :param tab_location: location that tabs will be displayed. Choices are left, right, top, bottom, lefttop, leftbottom, righttop, rightbottom, bottomleft, bottomright, topleft, topright
- :type tab_location: (str)
- :param title_color: color of text on tabs
- :type title_color: (str)
- :param tab_background_color: color of all tabs that are not selected
- :type tab_background_color: (str)
- :param selected_title_color: color of tab text when it is selected
- :type selected_title_color: (str)
- :param selected_background_color: color of tab when it is selected
- :type selected_background_color: (str)
- :param background_color: color of background area that tabs are located on
- :type background_color: (str)
- :param focus_color: color of focus indicator on the tabs
- :type focus_color: (str)
- :param font: specifies the font family, size, etc. Tuple or Single string format 'name size styles'. Styles: italic * roman bold normal underline overstrike
- :type font: (str or (str, int[, str]) or None)
- :param change_submits: * DEPRICATED DO NOT USE. Use `enable_events` instead
- :type change_submits: (bool)
- :param enable_events: If True then switching tabs will generate an Event
- :type enable_events: (bool)
- :param pad: Amount of padding to put around element in pixels (left/right, top/bottom) or ((left, right), (top, bottom)) or an int. If an int, then it's converted into a tuple (int, int)
- :type pad: (int, int) or ((int, int),(int,int)) or (int,(int,int)) or ((int, int),int) | int
- :param p: Same as pad parameter. It's an alias. If EITHER of them are set, then the one that's set will be used. If BOTH are set, pad will be used
- :type p: (int, int) or ((int, int),(int,int)) or (int,(int,int)) or ((int, int),int) | int
- :param border_width: width of border around element in pixels
- :type border_width: (int)
- :param tab_border_width: width of border around the tabs
- :type tab_border_width: (int)
- :param theme: DEPRICATED - You can only specify themes using set options or when window is created. It's not possible to do it on an element basis
- :type theme: (enum)
- :param key: Value that uniquely identifies this element from all other elements. Used when Finding an element or in return values. Must be unique to the window
- :type key: str | int | tuple | object
- :param k: Same as the Key. You can use either k or key. Which ever is set will be used.
- :type k: str | int | tuple | object
- :param size: (width, height) w=pixels-wide, h=pixels-high. Either item in tuple can be None to indicate use the computed value and set only 1 direction
- :type size: (int|None, int|None)
- :param s: Same as size parameter. It's an alias. If EITHER of them are set, then the one that's set will be used. If BOTH are set, size will be used
- :type s: (int|None, int|None)
- :param tooltip: text, that will appear when mouse hovers over the element
- :type tooltip: (str)
- :param right_click_menu: A list of lists of Menu items to show when this element is right clicked. See user docs for exact format.
- :type right_click_menu: List[List[ List[str] | str ]]
- :param expand_x: If True the element will automatically expand in the X direction to fill available space
- :type expand_x: (bool)
- :param expand_y: If True the element will automatically expand in the Y direction to fill available space
- :type expand_y: (bool)
- :param visible: set visibility state of the element
- :type visible: (bool)
- :param metadata: User metadata that can be set to ANYTHING
- :type metadata: (Any)
- """
-
-
- self.UseDictionary = False
- self.ReturnValues = None
- self.ReturnValuesList = []
- self.ReturnValuesDictionary = {}
- self.DictionaryKeyCounter = 0
- self.ParentWindow = None
- self.SelectedTitleColor = selected_title_color if selected_title_color is not None else LOOK_AND_FEEL_TABLE[CURRENT_LOOK_AND_FEEL]['TEXT']
- self.SelectedBackgroundColor = selected_background_color if selected_background_color is not None else LOOK_AND_FEEL_TABLE[CURRENT_LOOK_AND_FEEL][
- 'BACKGROUND']
- title_color = title_color if title_color is not None else LOOK_AND_FEEL_TABLE[CURRENT_LOOK_AND_FEEL]['TEXT_INPUT']
- self.TabBackgroundColor = tab_background_color if tab_background_color is not None else LOOK_AND_FEEL_TABLE[CURRENT_LOOK_AND_FEEL]['INPUT']
- self.Rows = []
- self.TKNotebook = None # type: ttk.Notebook
- self.Widget = None # type: ttk.Notebook
- self.TabCount = 0
- self.BorderWidth = border_width
- self.BackgroundColor = background_color if background_color is not None else DEFAULT_BACKGROUND_COLOR
- self.ChangeSubmits = change_submits or enable_events
- self.TabLocation = tab_location
- self.ElementJustification = 'left'
- self.RightClickMenu = right_click_menu
- self.TabBorderWidth = tab_border_width
- self.FocusColor = focus_color
-
- key = key if key is not None else k
- sz = size if size != (None, None) else s
- pad = pad if pad is not None else p
- self.expand_x = expand_x
- self.expand_y = expand_y
-
- self.Layout(layout)
-
- super().__init__(ELEM_TYPE_TAB_GROUP, size=sz, background_color=background_color, text_color=title_color, font=font,
- pad=pad, key=key, tooltip=tooltip, visible=visible, metadata=metadata)
- return
-
- def add_row(self, *args):
- """
- Not recommended user call. Used to add rows of Elements to the Frame Element.
-
- :param *args: The list of elements for this row
- :type *args: List[Element]
- """
-
- NumRows = len(self.Rows) # number of existing rows is our row number
- CurrentRowNumber = NumRows # this row's number
- CurrentRow = [] # start with a blank row and build up
- # ------------------------- Add the elements to a row ------------------------- #
- for i, element in enumerate(args): # Loop through list of elements and add them to the row
- if type(element) == list:
- PopupError('Error creating Tab layout',
- 'Layout has a LIST instead of an ELEMENT',
- 'This means you have a badly placed ]',
- 'The offensive list is:',
- element,
- 'This list will be stripped from your layout', keep_on_top=True, image=_random_error_emoji()
- )
- continue
- elif callable(element) and not isinstance(element, Element):
- PopupError('Error creating Tab layout',
- 'Layout has a FUNCTION instead of an ELEMENT',
- 'This likely means you are missing () from your layout',
- 'The offensive list is:',
- element,
- 'This item will be stripped from your layout', keep_on_top=True, image=_random_error_emoji())
- continue
- if element.ParentContainer is not None:
- warnings.warn(
- '*** YOU ARE ATTEMPTING TO RESUSE AN ELEMENT IN YOUR LAYOUT! Once placed in a layout, an element cannot be used in another layout. ***',
- UserWarning)
- PopupError('Error creating Tab layout',
- 'The layout specified has already been used',
- 'You MUST start witha "clean", unused layout every time you create a window',
- 'The offensive Element = ',
- element,
- 'and has a key = ', element.Key,
- 'This item will be stripped from your layout',
- 'Hint - try printing your layout and matching the IDs "print(layout)"', keep_on_top=True, image=_random_error_emoji())
- continue
- element.Position = (CurrentRowNumber, i)
- element.ParentContainer = self
- CurrentRow.append(element)
- if element.Key is not None:
- self.UseDictionary = True
- # ------------------------- Append the row to list of Rows ------------------------- #
- self.Rows.append(CurrentRow)
-
- def layout(self, rows):
- """
- Can use like the Window.Layout method, but it's better to use the layout parameter when creating
-
- :param rows: The rows of Elements
- :type rows: List[List[Element]]
- :return: Used for chaining
- :rtype: (Frame)
- """
- for row in rows:
- try:
- iter(row)
- except TypeError:
- PopupError('Error creating Tab layout',
- 'Your row is not an iterable (e.g. a list)',
- 'Instead of a list, the type found was {}'.format(type(row)),
- 'The offensive row = ',
- row,
- 'This item will be stripped from your layout', keep_on_top=True, image=_random_error_emoji())
- continue
- self.AddRow(*row)
- return self
-
- def _GetElementAtLocation(self, location):
- """
- Not user callable. Used to find the Element at a row, col position within the layout
-
- :param location: (row, column) position of the element to find in layout
- :type location: (int, int)
- :return: The element found at the location
- :rtype: (Element)
- """
-
- (row_num, col_num) = location
- row = self.Rows[row_num]
- element = row[col_num]
- return element
-
- def find_key_from_tab_name(self, tab_name):
- """
- Searches through the layout to find the key that matches the text on the tab. Implies names should be unique
-
- :param tab_name: name of a tab
- :type tab_name: str
- :return: Returns the key or None if no key found
- :rtype: key | None
- """
- for row in self.Rows:
- for element in row:
- if element.Title == tab_name:
- return element.Key
- return None
-
- def get(self):
- """
- Returns the current value for the Tab Group, which will be the currently selected tab's KEY or the text on
- the tab if no key is defined. Returns None if an error occurs.
- Note that this is exactly the same data that would be returned from a call to Window.Read. Are you sure you
- are using this method correctly?
-
- :return: The key of the currently selected tab or the tab's text if it has no key
- :rtype: Any | None
- """
-
- try:
- value = self.TKNotebook.tab(self.TKNotebook.index('current'))['text']
- tab_key = self.FindKeyFromTabName(value)
- if tab_key is not None:
- value = tab_key
- except:
- value = None
- return value
-
- def add_tab(self, tab_element):
- """
- Add a new tab to an existing TabGroup
- This call was written so that tabs can be added at runtime as your user performs operations.
- Your Window should already be created and finalized.
-
- :param tab_element: A Tab Element that has a layout in it
- :type tab_element: Tab
- """
-
- self.add_row(tab_element)
- tab_element.TKFrame = tab_element.Widget = tk.Frame(self.TKNotebook)
- form = self.ParentForm
- form._BuildKeyDictForWindow(form, tab_element, form.AllKeysDict)
- form.AllKeysDict[tab_element.Key] = tab_element
- # Pack the tab's layout into the tab. NOTE - This does NOT pack the Tab itself... for that see below...
- PackFormIntoFrame(tab_element, tab_element.TKFrame, self.ParentForm)
-
- # - This is below - Perform the same operation that is performed when a Tab is packed into the window.
- # If there's an image in the tab, then do the imagey-stuff
- # ------------------- start of imagey-stuff -------------------
- try:
- if tab_element.Filename is not None:
- photo = tk.PhotoImage(file=tab_element.Filename)
- elif tab_element.Data is not None:
- photo = tk.PhotoImage(data=tab_element.Data)
- else:
- photo = None
-
- if tab_element.ImageSubsample and photo is not None:
- photo = photo.subsample(tab_element.ImageSubsample)
- # print('*ERROR laying out form.... Image Element has no image specified*')
- except Exception as e:
- photo = None
- _error_popup_with_traceback('Your Window has an Tab Element with an IMAGE problem',
- 'The traceback will show you the Window with the problem layout',
- 'Look in this Window\'s layout for an Image tab_element that has a key of {}'.format(tab_element.Key),
- 'The error occuring is:', e)
-
- tab_element.photo = photo
- # add the label
- if photo is not None:
- width, height = photo.width(), photo.height()
- tab_element.tktext_label = tk.Label(tab_element.ParentRowFrame, image=photo, width=width, height=height, bd=0)
- else:
- tab_element.tktext_label = tk.Label(tab_element.ParentRowFrame, bd=0)
- # ------------------- end of imagey-stuff -------------------
-
- state = 'normal'
- if tab_element.Disabled:
- state = 'disabled'
- if tab_element.visible is False:
- state = 'hidden'
- if photo is not None:
- self.TKNotebook.add(tab_element.TKFrame, text=tab_element.Title, compound=tk.LEFT, state=state, image=photo)
- else:
- self.TKNotebook.add(tab_element.TKFrame, text=tab_element.Title, state=state)
- tab_element.ParentNotebook = self.TKNotebook
- tab_element.TabID = self.TabCount
- tab_element.ParentForm = self.ParentForm
- self.TabCount += 1
- if tab_element.BackgroundColor != COLOR_SYSTEM_DEFAULT and tab_element.BackgroundColor is not None:
- tab_element.TKFrame.configure(background=tab_element.BackgroundColor, highlightbackground=tab_element.BackgroundColor,
- highlightcolor=tab_element.BackgroundColor)
- if tab_element.BorderWidth is not None:
- tab_element.TKFrame.configure(borderwidth=tab_element.BorderWidth)
- if tab_element.Tooltip is not None:
- tab_element.TooltipObject = ToolTip(tab_element.TKFrame, text=tab_element.Tooltip, timeout=DEFAULT_TOOLTIP_TIME)
- _add_right_click_menu(tab_element, form)
-
- AddRow = add_row
- FindKeyFromTabName = find_key_from_tab_name
- Get = get
- Layout = layout
-
-
-# ---------------------------------------------------------------------- #
-# Slider #
-# ---------------------------------------------------------------------- #
-class Slider(Element):
- """
- A slider, horizontal or vertical
- """
-
- def __init__(self, range=(None, None), default_value=None, resolution=None, tick_interval=None, orientation=None,
- disable_number_display=False, border_width=None, relief=None, change_submits=False,
- enable_events=False, disabled=False, size=(None, None), s=(None, None), font=None, background_color=None,
- text_color=None, trough_color=None, key=None, k=None, pad=None, p=None, expand_x=False, expand_y=False, tooltip=None, visible=True, metadata=None):
- """
- :param range: slider's range (min value, max value)
- :type range: (int, int) | Tuple[float, float]
- :param default_value: starting value for the slider
- :type default_value: int | float
- :param resolution: the smallest amount the slider can be moved
- :type resolution: int | float
- :param tick_interval: how often a visible tick should be shown next to slider
- :type tick_interval: int | float
- :param orientation: 'horizontal' or 'vertical' ('h' or 'v' also work)
- :type orientation: (str)
- :param disable_number_display: if True no number will be displayed by the Slider Element
- :type disable_number_display: (bool)
- :param border_width: width of border around element in pixels
- :type border_width: (int)
- :param relief: relief style. Use constants - RELIEF_RAISED RELIEF_SUNKEN RELIEF_FLAT RELIEF_RIDGE RELIEF_GROOVE RELIEF_SOLID
- :type relief: str | None
- :param change_submits: * DEPRICATED DO NOT USE. Use `enable_events` instead
- :type change_submits: (bool)
- :param enable_events: If True then moving the slider will generate an Event
- :type enable_events: (bool)
- :param disabled: set disable state for element
- :type disabled: (bool)
- :param size: (l=length chars/rows, w=width pixels)
- :type size: (int, int)
- :param s: Same as size parameter. It's an alias. If EITHER of them are set, then the one that's set will be used. If BOTH are set, size will be used
- :type s: (int, int) | (None, None)
- :param font: specifies the font family, size, etc. Tuple or Single string format 'name size styles'. Styles: italic * roman bold normal underline overstrike
- :type font: (str or (str, int[, str]) or None)
- :param background_color: color of slider's background
- :type background_color: (str)
- :param text_color: color of the slider's text
- :type text_color: (str)
- :param trough_color: color of the slider's trough
- :type trough_color: (str)
- :param key: Value that uniquely identifies this element from all other elements. Used when Finding an element or in return values. Must be unique to the window
- :type key: str | int | tuple | object
- :param k: Same as the Key. You can use either k or key. Which ever is set will be used.
- :type k: str | int | tuple | object
- :param pad: Amount of padding to put around element in pixels (left/right, top/bottom) or ((left, right), (top, bottom)) or an int. If an int, then it's converted into a tuple (int, int)
- :type pad: (int, int) or ((int, int),(int,int)) or (int,(int,int)) or ((int, int),int) | int
- :param p: Same as pad parameter. It's an alias. If EITHER of them are set, then the one that's set will be used. If BOTH are set, pad will be used
- :type p: (int, int) or ((int, int),(int,int)) or (int,(int,int)) or ((int, int),int) | int
- :param expand_x: If True the element will automatically expand in the X direction to fill available space
- :type expand_x: (bool)
- :param expand_y: If True the element will automatically expand in the Y direction to fill available space
- :type expand_y: (bool)
- :param tooltip: text, that will appear when mouse hovers over the element
- :type tooltip: (str)
- :param visible: set visibility state of the element
- :type visible: (bool)
- :param metadata: User metadata that can be set to ANYTHING
- :type metadata: (Any)
- """
-
- self.TKScale = self.Widget = None # type: tk.Scale
- self.Range = (1, 10) if range == (None, None) else range
- self.DefaultValue = self.Range[0] if default_value is None else default_value
- self.Orientation = orientation if orientation else DEFAULT_SLIDER_ORIENTATION
- self.BorderWidth = border_width if border_width else DEFAULT_SLIDER_BORDER_WIDTH
- self.Relief = relief if relief else DEFAULT_SLIDER_RELIEF
- self.Resolution = 1 if resolution is None else resolution
- self.ChangeSubmits = change_submits or enable_events
- self.Disabled = disabled
- self.TickInterval = tick_interval
- self.DisableNumericDisplay = disable_number_display
- self.TroughColor = trough_color or DEFAULT_SCROLLBAR_COLOR
- sz = size if size != (None, None) else s
- temp_size = sz
- if temp_size == (None, None):
- temp_size = (20, 20) if self.Orientation.startswith('h') else (8, 20)
- key = key if key is not None else k
- pad = pad if pad is not None else p
- self.expand_x = expand_x
- self.expand_y = expand_y
-
- super().__init__(ELEM_TYPE_INPUT_SLIDER, size=temp_size, font=font, background_color=background_color,
- text_color=text_color, key=key, pad=pad, tooltip=tooltip, visible=visible, metadata=metadata)
- return
-
- def update(self, value=None, range=(None, None), disabled=None, visible=None):
- """
- Changes some of the settings for the Slider Element. Must call `Window.Read` or `Window.Finalize` prior
-
- Changes will not be visible in your window until you call window.read or window.refresh.
-
- If you change visibility, your element may MOVE. If you want it to remain stationary, use the "layout helper"
- function "pin" to ensure your element is "pinned" to that location in your layout so that it returns there
- when made visible.
-
- :param value: sets current slider value
- :type value: int | float
- :param range: Sets a new range for slider
- :type range: (int, int) | Tuple[float, float
- :param disabled: disable or enable state of the element
- :type disabled: (bool)
- :param visible: control visibility of element
- :type visible: (bool)
- """
- if not self._widget_was_created(): # if widget hasn't been created yet, then don't allow
- return
-
- if range != (None, None):
- self.TKScale.config(from_=range[0], to_=range[1])
- if value is not None:
- try:
- self.TKIntVar.set(value)
- except:
- pass
- self.DefaultValue = value
- if disabled is True:
- self.TKScale['state'] = 'disabled'
- elif disabled is False:
- self.TKScale['state'] = 'normal'
- self.Disabled = disabled if disabled is not None else self.Disabled
-
- if visible is False:
- self._pack_forget_save_settings()
- elif visible is True:
- self._pack_restore_settings()
-
- if visible is not None:
- self._visible = visible
-
-
- def _SliderChangedHandler(self, event):
- """
- Not user callable. Callback function for when slider is moved.
-
- :param event: (event) the event data provided by tkinter. Unknown format. Not used.
- :type event:
- """
-
- if self.Key is not None:
- self.ParentForm.LastButtonClicked = self.Key
- else:
- self.ParentForm.LastButtonClicked = ''
- self.ParentForm.FormRemainedOpen = True
- # if self.ParentForm.CurrentlyRunningMainloop:
- # self.ParentForm.TKroot.quit() # kick the users out of the mainloop
- _exit_mainloop(self.ParentForm)
-
- Update = update
-
-
-Sl = Slider
-
-
-# ---------------------------------------------------------------------- #
-# TkFixedFrame (Used by Column) #
-# ---------------------------------------------------------------------- #
-class TkFixedFrame(tk.Frame):
- """
- A tkinter frame that is used with Column Elements that do not have a scrollbar
- """
-
- def __init__(self, master, **kwargs):
- """
- :param master: The parent widget
- :type master: (tk.Widget)
- :param **kwargs: The keyword args
- :type **kwargs:
- """
- tk.Frame.__init__(self, master, **kwargs)
-
- self.canvas = tk.Canvas(self)
-
- self.canvas.pack(side="left", fill="both", expand=True)
-
- # reset the view
- self.canvas.xview_moveto(0)
- self.canvas.yview_moveto(0)
-
- # create a frame inside the canvas which will be scrolled with it
- self.TKFrame = tk.Frame(self.canvas, **kwargs)
- self.frame_id = self.canvas.create_window(0, 0, window=self.TKFrame, anchor="nw")
- self.canvas.config(borderwidth=0, highlightthickness=0)
- self.TKFrame.config(borderwidth=0, highlightthickness=0)
- self.config(borderwidth=0, highlightthickness=0)
-
-
-# ---------------------------------------------------------------------- #
-# TkScrollableFrame (Used by Column) #
-# ---------------------------------------------------------------------- #
-class TkScrollableFrame(tk.Frame):
- """
- A frame with one or two scrollbars. Used to make Columns with scrollbars
- """
-
- def __init__(self, master, vertical_only, element, window, **kwargs):
- """
- :param master: The parent widget
- :type master: (tk.Widget)
- :param vertical_only: if True the only a vertical scrollbar will be shown
- :type vertical_only: (bool)
- :param element: The element containing this object
- :type element: (Column)
- """
- tk.Frame.__init__(self, master, **kwargs)
- # create a canvas object and a vertical scrollbar for scrolling it
-
- self.canvas = tk.Canvas(self)
- element.Widget = self.canvas
- # Okay, we're gonna make a list. Containing the y-min, x-min, y-max, and x-max of the frame
- element.element_frame = self
- _make_ttk_scrollbar(element, 'v', window)
- # element.vsb = tk.Scrollbar(self, orient=tk.VERTICAL)
- element.vsb.pack(side='right', fill="y", expand="false")
-
- if not vertical_only:
- _make_ttk_scrollbar(element, 'h', window)
- # self.hscrollbar = tk.Scrollbar(self, orient=tk.HORIZONTAL)
- element.hsb.pack(side='bottom', fill="x", expand="false")
- self.canvas.config(xscrollcommand=element.hsb.set)
- # self.canvas = tk.Canvas(self, )
- # else:
- # self.canvas = tk.Canvas(self)
-
- self.canvas.config(yscrollcommand=element.vsb.set)
- self.canvas.pack(side="left", fill="both", expand=True)
- element.vsb.config(command=self.canvas.yview)
- if not vertical_only:
- element.hsb.config(command=self.canvas.xview)
-
- # reset the view
- self.canvas.xview_moveto(0)
- self.canvas.yview_moveto(0)
-
- # create a frame inside the canvas which will be scrolled with it
- self.TKFrame = tk.Frame(self.canvas, **kwargs)
- self.frame_id = self.canvas.create_window(0, 0, window=self.TKFrame, anchor="nw")
- self.canvas.config(borderwidth=0, highlightthickness=0)
- self.TKFrame.config(borderwidth=0, highlightthickness=0)
- self.config(borderwidth=0, highlightthickness=0)
-
- # Canvas can be: master, canvas, TKFrame
-
- # Chr0nic
-
- # self.unhookMouseWheel(None)
- # self.TKFrame.bind("", self.hookMouseWheel)
- # self.TKFrame.bind("", self.unhookMouseWheel)
- # self.bind('', self.set_scrollregion)
-
-
- self.unhookMouseWheel(None)
- self.canvas.bind("", self.hookMouseWheel)
- self.canvas.bind("", self.unhookMouseWheel)
- self.bind('', self.set_scrollregion)
-
-
- # Chr0nic
- def hookMouseWheel(self, e):
- # print("enter")
- VarHolder.canvas_holder = self.canvas
- self.canvas.bind_all('<4>', self.yscroll, add='+')
- self.canvas.bind_all('<5>', self.yscroll, add='+')
- self.canvas.bind_all("", self.yscroll, add='+')
- self.canvas.bind_all("", self.xscroll, add='+')
-
- # Chr0nic
- def unhookMouseWheel(self, e):
- # print("leave")
- VarHolder.canvas_holder = None
- self.canvas.unbind_all('<4>')
- self.canvas.unbind_all('<5>')
- self.canvas.unbind_all("")
- self.canvas.unbind_all("")
-
- def resize_frame(self, e):
- self.canvas.itemconfig(self.frame_id, height=e.height, width=e.width)
-
- def yscroll(self, event):
- if self.canvas.yview() == (0.0, 1.0):
- return
- if event.num == 5 or event.delta < 0:
- self.canvas.yview_scroll(1, "unit")
- elif event.num == 4 or event.delta > 0:
- self.canvas.yview_scroll(-1, "unit")
-
- def xscroll(self, event):
- if event.num == 5 or event.delta < 0:
- self.canvas.xview_scroll(1, "unit")
- elif event.num == 4 or event.delta > 0:
- self.canvas.xview_scroll(-1, "unit")
-
- def bind_mouse_scroll(self, parent, mode):
- # ~~ Windows only
- parent.bind("", mode)
- # ~~ Unix only
- parent.bind("", mode)
- parent.bind("", mode)
-
- def set_scrollregion(self, event=None):
- """ Set the scroll region on the canvas """
- self.canvas.configure(scrollregion=self.canvas.bbox('all'))
-
-
-# ---------------------------------------------------------------------- #
-# Column #
-# ---------------------------------------------------------------------- #
-class Column(Element):
- """
- A container element that is used to create a layout within your window's layout
- """
-
- def __init__(self, layout, background_color=None, size=(None, None), s=(None, None), size_subsample_width=1, size_subsample_height=2, pad=None, p=None, scrollable=False,
- vertical_scroll_only=False, right_click_menu=None, key=None, k=None, visible=True, justification=None, element_justification=None,
- vertical_alignment=None, grab=None, expand_x=None, expand_y=None, metadata=None,
- sbar_trough_color=None, sbar_background_color=None, sbar_arrow_color=None, sbar_width=None, sbar_arrow_width=None,
- sbar_frame_color=None, sbar_relief=None):
- """
- :param layout: Layout that will be shown in the Column container
- :type layout: List[List[Element]]
- :param background_color: color of background of entire Column
- :type background_color: (str)
- :param size: (width, height) size in pixels (doesn't work quite right, sometimes only 1 dimension is set by tkinter. Use a Sizer Element to help set sizes
- :type size: (int | None, int | None)
- :param s: Same as size parameter. It's an alias. If EITHER of them are set, then the one that's set will be used. If BOTH are set, size will be used
- :type s: (int | None, int | None)
- :param size_subsample_width: Determines the size of a scrollable column width based on 1/size_subsample * required size. 1 = match the contents exactly, 2 = 1/2 contents size, 3 = 1/3. Can be a fraction to make larger than required.
- :type size_subsample_width: (float)
- :param size_subsample_height: Determines the size of a scrollable height based on 1/size_subsample * required size. 1 = match the contents exactly, 2 = 1/2 contents size, 3 = 1/3. Can be a fraction to make larger than required..
- :type size_subsample_height: (float)
- :param pad: Amount of padding to put around element in pixels (left/right, top/bottom) or ((left, right), (top, bottom)) or an int. If an int, then it's converted into a tuple (int, int)
- :type pad: (int, int) or ((int, int),(int,int)) or (int,(int,int)) or ((int, int),int) | int
- :param p: Same as pad parameter. It's an alias. If EITHER of them are set, then the one that's set will be used. If BOTH are set, pad will be used
- :type p: (int, int) or ((int, int),(int,int)) or (int,(int,int)) or ((int, int),int) | int
- :param scrollable: if True then scrollbars will be added to the column
- :type scrollable: (bool)
- :param vertical_scroll_only: if Truen then no horizontal scrollbar will be shown
- :type vertical_scroll_only: (bool)
- :param right_click_menu: A list of lists of Menu items to show when this element is right clicked. See user docs for exact format.
- :type right_click_menu: List[List[ List[str] | str ]]
- :param key: Value that uniquely identifies this element from all other elements. Used when Finding an element or in return values. Must be unique to the window
- :type key: str | int | tuple | object
- :param k: Same as the Key. You can use either k or key. Which ever is set will be used.
- :type k: str | int | tuple | object
- :param visible: set visibility state of the element
- :type visible: (bool)
- :param justification: set justification for the Column itself. Note entire row containing the Column will be affected
- :type justification: (str)
- :param element_justification: All elements inside the Column will have this justification 'left', 'right', 'center' are valid values
- :type element_justification: (str)
- :param vertical_alignment: Place the column at the 'top', 'center', 'bottom' of the row (can also use t,c,r). Defaults to no setting (tkinter decides)
- :type vertical_alignment: (str)
- :param grab: If True can grab this element and move the window around. Default is False
- :type grab: (bool)
- :param expand_x: If True the column will automatically expand in the X direction to fill available space
- :type expand_x: (bool)
- :param expand_y: If True the column will automatically expand in the Y direction to fill available space
- :type expand_y: (bool)
- :param metadata: User metadata that can be set to ANYTHING
- :type metadata: (Any)
- :param sbar_trough_color: Scrollbar color of the trough
- :type sbar_trough_color: (str)
- :param sbar_background_color: Scrollbar color of the background of the arrow buttons at the ends AND the color of the "thumb" (the thing you grab and slide). Switches to arrow color when mouse is over
- :type sbar_background_color: (str)
- :param sbar_arrow_color: Scrollbar color of the arrow at the ends of the scrollbar (it looks like a button). Switches to background color when mouse is over
- :type sbar_arrow_color: (str)
- :param sbar_width: Scrollbar width in pixels
- :type sbar_width: (int)
- :param sbar_arrow_width: Scrollbar width of the arrow on the scrollbar. It will potentially impact the overall width of the scrollbar
- :type sbar_arrow_width: (int)
- :param sbar_frame_color: Scrollbar Color of frame around scrollbar (available only on some ttk themes)
- :type sbar_frame_color: (str)
- :param sbar_relief: Scrollbar relief that will be used for the "thumb" of the scrollbar (the thing you grab that slides). Should be a constant that is defined at starting with "RELIEF_" - RELIEF_RAISED, RELIEF_SUNKEN, RELIEF_FLAT, RELIEF_RIDGE, RELIEF_GROOVE, RELIEF_SOLID
- :type sbar_relief: (str)
- """
-
-
- self.UseDictionary = False
- self.ReturnValues = None
- self.ReturnValuesList = []
- self.ReturnValuesDictionary = {}
- self.DictionaryKeyCounter = 0
- self.ParentWindow = None
- self.ParentPanedWindow = None
- self.Rows = []
- self.TKFrame = None
- self.TKColFrame = None # type: tk.Frame
- self.Scrollable = scrollable
- self.VerticalScrollOnly = vertical_scroll_only
-
- self.RightClickMenu = right_click_menu
- bg = background_color if background_color is not None else DEFAULT_BACKGROUND_COLOR
- self.ContainerElemementNumber = Window._GetAContainerNumber()
- self.ElementJustification = element_justification
- self.Justification = justification
- self.VerticalAlignment = vertical_alignment
- key = key if key is not None else k
- self.Grab = grab
- self.expand_x = expand_x
- self.expand_y = expand_y
- self.Layout(layout)
- sz = size if size != (None, None) else s
- pad = pad if pad is not None else p
- self.size_subsample_width = size_subsample_width
- self.size_subsample_height = size_subsample_height
-
- super().__init__(ELEM_TYPE_COLUMN, background_color=bg, size=sz, pad=pad, key=key, visible=visible, metadata=metadata,
- sbar_trough_color=sbar_trough_color, sbar_background_color=sbar_background_color, sbar_arrow_color=sbar_arrow_color, sbar_width=sbar_width, sbar_arrow_width=sbar_arrow_width, sbar_frame_color=sbar_frame_color, sbar_relief=sbar_relief)
- return
-
- def add_row(self, *args):
- """
- Not recommended user call. Used to add rows of Elements to the Column Element.
-
- :param *args: The list of elements for this row
- :type *args: List[Element]
- """
-
- NumRows = len(self.Rows) # number of existing rows is our row number
- CurrentRowNumber = NumRows # this row's number
- CurrentRow = [] # start with a blank row and build up
- # ------------------------- Add the elements to a row ------------------------- #
- for i, element in enumerate(args): # Loop through list of elements and add them to the row
- if type(element) == list:
- PopupError('Error creating Column layout',
- 'Layout has a LIST instead of an ELEMENT',
- 'This means you have a badly placed ]',
- 'The offensive list is:',
- element,
- 'This list will be stripped from your layout', keep_on_top=True, image=_random_error_emoji()
- )
- continue
- elif callable(element) and not isinstance(element, Element):
- PopupError('Error creating Column layout',
- 'Layout has a FUNCTION instead of an ELEMENT',
- 'This likely means you are missing () from your layout',
- 'The offensive list is:',
- element,
- 'This item will be stripped from your layout', keep_on_top=True, image=_random_error_emoji())
- continue
- if element.ParentContainer is not None:
- warnings.warn(
- '*** YOU ARE ATTEMPTING TO RESUSE AN ELEMENT IN YOUR LAYOUT! Once placed in a layout, an element cannot be used in another layout. ***',
- UserWarning)
- PopupError('Error creating Column layout',
- 'The layout specified has already been used',
- 'You MUST start witha "clean", unused layout every time you create a window',
- 'The offensive Element = ',
- element,
- 'and has a key = ', element.Key,
- 'This item will be stripped from your layout',
- 'Hint - try printing your layout and matching the IDs "print(layout)"', keep_on_top=True, image=_random_error_emoji())
- continue
- element.Position = (CurrentRowNumber, i)
- element.ParentContainer = self
- CurrentRow.append(element)
- if element.Key is not None:
- self.UseDictionary = True
- # ------------------------- Append the row to list of Rows ------------------------- #
- self.Rows.append(CurrentRow)
-
- def layout(self, rows):
- """
- Can use like the Window.Layout method, but it's better to use the layout parameter when creating
-
- :param rows: The rows of Elements
- :type rows: List[List[Element]]
- :return: Used for chaining
- :rtype: (Column)
- """
-
- for row in rows:
- try:
- iter(row)
- except TypeError:
- PopupError('Error creating Column layout',
- 'Your row is not an iterable (e.g. a list)',
- 'Instead of a list, the type found was {}'.format(type(row)),
- 'The offensive row = ',
- row,
- 'This item will be stripped from your layout', keep_on_top=True, image=_random_error_emoji())
- continue
- self.AddRow(*row)
- return self
-
- def _GetElementAtLocation(self, location):
- """
- Not user callable. Used to find the Element at a row, col position within the layout
-
- :param location: (row, column) position of the element to find in layout
- :type location: (int, int)
- :return: The element found at the location
- :rtype: (Element)
- """
-
- (row_num, col_num) = location
- row = self.Rows[row_num]
- element = row[col_num]
- return element
-
- def update(self, visible=None):
- """
- Changes some of the settings for the Column Element. Must call `Window.Read` or `Window.Finalize` prior
-
- Changes will not be visible in your window until you call window.read or window.refresh.
-
- If you change visibility, your element may MOVE. If you want it to remain stationary, use the "layout helper"
- function "pin" to ensure your element is "pinned" to that location in your layout so that it returns there
- when made visible.
-
- :param visible: control visibility of element
- :type visible: (bool)
- """
- if not self._widget_was_created(): # if widget hasn't been created yet, then don't allow
- return
-
- if self.expand_x and self.expand_y:
- expand = tk.BOTH
- elif self.expand_x:
- expand = tk.X
- elif self.expand_y:
- expand = tk.Y
- else:
- expand = None
-
- if visible is False:
- if self.TKColFrame:
- self._pack_forget_save_settings()
- # self.TKColFrame.pack_forget()
- if self.ParentPanedWindow:
- self.ParentPanedWindow.remove(self.TKColFrame)
- elif visible is True:
- if self.TKColFrame:
- self._pack_restore_settings()
- # self.TKColFrame.pack(padx=self.pad_used[0], pady=self.pad_used[1], fill=expand)
- if self.ParentPanedWindow:
- self.ParentPanedWindow.add(self.TKColFrame)
- if visible is not None:
- self._visible = visible
-
- def contents_changed(self):
- """
- When a scrollable column has part of its layout changed by making elements visible or invisible or the
- layout is extended for the Column, then this method needs to be called so that the new scroll area
- is computed to match the new contents.
- """
- self.TKColFrame.canvas.config(scrollregion=self.TKColFrame.canvas.bbox('all'))
-
- AddRow = add_row
- Layout = layout
- Update = update
-
-
-Col = Column
-
-
-# ---------------------------------------------------------------------- #
-# Pane #
-# ---------------------------------------------------------------------- #
-class Pane(Element):
- """
- A sliding Pane that is unique to tkinter. Uses Columns to create individual panes
- """
-
- def __init__(self, pane_list, background_color=None, size=(None, None), s=(None, None), pad=None, p=None, orientation='vertical',
- show_handle=True, relief=RELIEF_RAISED, handle_size=None, border_width=None, key=None, k=None, expand_x=None, expand_y=None, visible=True, metadata=None):
- """
- :param pane_list: Must be a list of Column Elements. Each Column supplied becomes one pane that's shown
- :type pane_list: List[Column] | Tuple[Column]
- :param background_color: color of background
- :type background_color: (str)
- :param size: (width, height) w=characters-wide, h=rows-high How much room to reserve for the Pane
- :type size: (int, int)
- :param s: Same as size parameter. It's an alias. If EITHER of them are set, then the one that's set will be used. If BOTH are set, size will be used
- :type s: (int, int) | (None, None)
- :param pad: Amount of padding to put around element in pixels (left/right, top/bottom) or ((left, right), (top, bottom)) or an int. If an int, then it's converted into a tuple (int, int)
- :type pad: (int, int) or ((int, int),(int,int)) or (int,(int,int)) or ((int, int),int) | int
- :param p: Same as pad parameter. It's an alias. If EITHER of them are set, then the one that's set will be used. If BOTH are set, pad will be used
- :type p: (int, int) or ((int, int),(int,int)) or (int,(int,int)) or ((int, int),int) | int
- :param orientation: 'horizontal' or 'vertical' or ('h' or 'v'). Direction the Pane should slide
- :type orientation: (str)
- :param show_handle: if True, the handle is drawn that makes it easier to grab and slide
- :type show_handle: (bool)
- :param relief: relief style. Values are same as other elements that use relief values. RELIEF_RAISED RELIEF_SUNKEN RELIEF_FLAT RELIEF_RIDGE RELIEF_GROOVE RELIEF_SOLID
- :type relief: (enum)
- :param handle_size: Size of the handle in pixels
- :type handle_size: (int)
- :param border_width: width of border around element in pixels
- :type border_width: (int)
- :param key: Value that uniquely identifies this element from all other elements. Used when Finding an element or in return values. Must be unique to the window
- :type key: str | int | tuple | object
- :param k: Same as the Key. You can use either k or key. Which ever is set will be used.
- :type k: str | int | tuple | object
- :param expand_x: If True the column will automatically expand in the X direction to fill available space
- :type expand_x: (bool)
- :param expand_y: If True the column will automatically expand in the Y direction to fill available space
- :type expand_y: (bool)
- :param visible: set visibility state of the element
- :type visible: (bool)
- :param metadata: User metadata that can be set to ANYTHING
- :type metadata: (Any)
- """
-
-
- self.UseDictionary = False
- self.ReturnValues = None
- self.ReturnValuesList = []
- self.ReturnValuesDictionary = {}
- self.DictionaryKeyCounter = 0
- self.ParentWindow = None
- self.Rows = []
- self.TKFrame = None
- self.PanedWindow = None
- self.Orientation = orientation
- self.PaneList = pane_list
- self.ShowHandle = show_handle
- self.Relief = relief
- self.HandleSize = handle_size or 8
- self.BorderDepth = border_width
- bg = background_color if background_color is not None else DEFAULT_BACKGROUND_COLOR
-
- self.Rows = [pane_list]
- key = key if key is not None else k
- sz = size if size != (None, None) else s
- pad = pad if pad is not None else p
- self.expand_x = expand_x
- self.expand_y = expand_y
-
- super().__init__(ELEM_TYPE_PANE, background_color=bg, size=sz, pad=pad, key=key, visible=visible, metadata=metadata)
- return
-
- def update(self, visible=None):
- """
- Changes some of the settings for the Pane Element. Must call `Window.Read` or `Window.Finalize` prior
-
- Changes will not be visible in your window until you call window.read or window.refresh.
-
- If you change visibility, your element may MOVE. If you want it to remain stationary, use the "layout helper"
- function "pin" to ensure your element is "pinned" to that location in your layout so that it returns there
- when made visible.
-
- :param visible: control visibility of element
- :type visible: (bool)
- """
- if not self._widget_was_created(): # if widget hasn't been created yet, then don't allow
- return
- if visible is False:
- self._pack_forget_save_settings()
- elif visible is True:
- self._pack_restore_settings()
-
- if visible is not None:
- self._visible = visible
-
- Update = update
-
-
-# ---------------------------------------------------------------------- #
-# Calendar #
-# ---------------------------------------------------------------------- #
-class TKCalendar(ttk.Frame):
- """
- This code was shamelessly lifted from moshekaplan's repository - moshekaplan/tkinter_components
- NONE of this code is user callable. Stay away!
- """
- # XXX ToDo: cget and configure
-
- datetime = calendar.datetime.datetime
- timedelta = calendar.datetime.timedelta
-
- def __init__(self, master=None, target_element=None, close_when_chosen=True, default_date=(None, None, None), **kw):
- """WIDGET-SPECIFIC OPTIONS: locale, firstweekday, year, month, selectbackground, selectforeground """
- self._TargetElement = target_element
- default_mon, default_day, default_year = default_date
- # remove custom options from kw before initializating ttk.Frame
- fwday = kw.pop('firstweekday', calendar.MONDAY)
- year = kw.pop('year', default_year or self.datetime.now().year)
- month = kw.pop('month', default_mon or self.datetime.now().month)
- locale = kw.pop('locale', None)
- sel_bg = kw.pop('selectbackground', '#ecffc4')
- sel_fg = kw.pop('selectforeground', '#05640e')
- self.format = kw.pop('format')
- if self.format is None:
- self.format = '%Y-%m-%d %H:%M:%S'
-
- self._date = self.datetime(year, month, default_day or 1)
- self._selection = None # no date selected
- self._master = master
- self.close_when_chosen = close_when_chosen
- ttk.Frame.__init__(self, master, **kw)
-
- # instantiate proper calendar class
- if locale is None:
- self._cal = calendar.TextCalendar(fwday)
- else:
- self._cal = calendar.LocaleTextCalendar(fwday, locale)
-
- self.__setup_styles() # creates custom styles
- self.__place_widgets() # pack/grid used widgets
- self.__config_calendar() # adjust calendar columns and setup tags
- # configure a canvas, and proper bindings, for selecting dates
- self.__setup_selection(sel_bg, sel_fg)
-
- # store items ids, used for insertion later
- self._items = [self._calendar.insert('', 'end', values='')
- for _ in range(6)]
- # insert dates in the currently empty calendar
- self._build_calendar()
-
- def __setitem__(self, item, value):
- if item in ('year', 'month'):
- raise AttributeError("attribute '%s' is not writeable" % item)
- elif item == 'selectbackground':
- self._canvas['background'] = value
- elif item == 'selectforeground':
- self._canvas.itemconfigure(self._canvas.text, item=value)
- else:
- ttk.Frame.__setitem__(self, item, value)
-
- def __getitem__(self, item):
- if item in ('year', 'month'):
- return getattr(self._date, item)
- elif item == 'selectbackground':
- return self._canvas['background']
- elif item == 'selectforeground':
- return self._canvas.itemcget(self._canvas.text, 'fill')
- else:
- r = ttk.tclobjs_to_py({item: ttk.Frame.__getitem__(self, item)})
- return r[item]
-
- def __setup_styles(self):
- # custom ttk styles
- style = ttk.Style(self.master)
- arrow_layout = lambda dir: (
- [('Button.focus', {'children': [('Button.%sarrow' % dir, None)]})]
- )
- style.layout('L.TButton', arrow_layout('left'))
- style.layout('R.TButton', arrow_layout('right'))
-
- def __place_widgets(self):
- # header frame and its widgets
- hframe = ttk.Frame(self)
- lbtn = ttk.Button(hframe, style='L.TButton', command=self._prev_month)
- rbtn = ttk.Button(hframe, style='R.TButton', command=self._next_month)
- self._header = ttk.Label(hframe, width=15, anchor='center')
- # the calendar
- self._calendar = ttk.Treeview(self, show='', selectmode='none', height=7)
-
- # pack the widgets
- hframe.pack(in_=self, side='top', pady=4, anchor='center')
- lbtn.grid(in_=hframe)
- self._header.grid(in_=hframe, column=1, row=0, padx=12)
- rbtn.grid(in_=hframe, column=2, row=0)
- self._calendar.pack(in_=self, expand=1, fill='both', side='bottom')
-
- def __config_calendar(self):
- cols = self._cal.formatweekheader(3).split()
- self._calendar['columns'] = cols
- self._calendar.tag_configure('header', background='grey90')
- self._calendar.insert('', 'end', values=cols, tag='header')
- # adjust its columns width
- font = tkinter.font.Font()
- maxwidth = max(font.measure(col) for col in cols)
- for col in cols:
- self._calendar.column(col, width=maxwidth, minwidth=maxwidth,
- anchor='e')
-
- def __setup_selection(self, sel_bg, sel_fg):
- self._font = tkinter.font.Font()
- self._canvas = canvas = tk.Canvas(self._calendar,
- background=sel_bg, borderwidth=0, highlightthickness=0)
- canvas.text = canvas.create_text(0, 0, fill=sel_fg, anchor='w')
-
- canvas.bind('', lambda evt: canvas.place_forget())
- self._calendar.bind('', lambda evt: canvas.place_forget())
- self._calendar.bind('', self._pressed)
-
- def __minsize(self, evt):
- width, height = self._calendar.master.geometry().split('x')
- height = height[:height.index('+')]
- self._calendar.master.minsize(width, height)
-
- def _build_calendar(self):
- year, month = self._date.year, self._date.month
-
- # update header text (Month, YEAR)
- header = self._cal.formatmonthname(year, month, 0)
- self._header['text'] = header.title()
-
- # update calendar shown dates
- cal = self._cal.monthdayscalendar(year, month)
- for indx, item in enumerate(self._items):
- week = cal[indx] if indx < len(cal) else []
- fmt_week = [('%02d' % day) if day else '' for day in week]
- self._calendar.item(item, values=fmt_week)
-
- def _show_selection(self, text, bbox):
- """ Configure canvas for a new selection. """
- x, y, width, height = bbox
-
- textw = self._font.measure(text)
-
- canvas = self._canvas
- canvas.configure(width=width, height=height)
- canvas.coords(canvas.text, width - textw, height / 2 - 1)
- canvas.itemconfigure(canvas.text, text=text)
- canvas.place(in_=self._calendar, x=x, y=y)
-
- # Callbacks
-
- def _pressed(self, evt):
- """ Clicked somewhere in the calendar. """
- x, y, widget = evt.x, evt.y, evt.widget
- item = widget.identify_row(y)
- column = widget.identify_column(x)
-
- if not column or not item in self._items:
- # clicked in the weekdays row or just outside the columns
- return
-
- item_values = widget.item(item)['values']
- if not len(item_values): # row is empty for this month
- return
-
- text = item_values[int(column[1]) - 1]
- if not text: # date is empty
- return
-
- bbox = widget.bbox(item, column)
- if not bbox: # calendar not visible yet
- return
-
- # update and then show selection
- text = '%02d' % text
- self._selection = (text, item, column)
- self._show_selection(text, bbox)
- year, month = self._date.year, self._date.month
- now = self.datetime.now()
- try:
- self._TargetElement.Update(
- self.datetime(year, month, int(self._selection[0]), now.hour, now.minute, now.second).strftime(
- self.format))
- if self._TargetElement.ChangeSubmits:
- self._TargetElement.ParentForm.LastButtonClicked = self._TargetElement.Key
- self._TargetElement.ParentForm.FormRemainedOpen = True
- self._TargetElement.ParentForm.TKroot.quit() # kick the users out of the mainloop
- except:
- pass
- if self.close_when_chosen:
- self._master.destroy()
-
- def _prev_month(self):
- """Updated calendar to show the previous month."""
- self._canvas.place_forget()
-
- self._date = self._date - self.timedelta(days=1)
- self._date = self.datetime(self._date.year, self._date.month, 1)
- self._build_calendar() # reconstuct calendar
-
- def _next_month(self):
- """Update calendar to show the next month."""
- self._canvas.place_forget()
-
- year, month = self._date.year, self._date.month
- self._date = self._date + self.timedelta(
- days=calendar.monthrange(year, month)[1] + 1)
- self._date = self.datetime(self._date.year, self._date.month, 1)
- self._build_calendar() # reconstruct calendar
-
- # Properties
-
- @property
- def selection(self):
- if not self._selection:
- return None
-
- year, month = self._date.year, self._date.month
- return self.datetime(year, month, int(self._selection[0]))
-
-
-# ---------------------------------------------------------------------- #
-# Menu #
-# ---------------------------------------------------------------------- #
-class Menu(Element):
- """
- Menu Element is the Element that provides a Menu Bar that goes across the top of the window, just below titlebar.
- Here is an example layout. The "&" are shortcut keys ALT+key.
- Is a List of - "Item String" + List
- Where Item String is what will be displayed on the Menubar itself.
- The List that follows the item represents the items that are shown then Menu item is clicked
- Notice how an "entry" in a mennu can be a list which means it branches out and shows another menu, etc. (resursive)
- menu_def = [['&File', ['!&Open', '&Save::savekey', '---', '&Properties', 'E&xit']],
- ['!&Edit', ['!&Paste', ['Special', 'Normal', ], 'Undo'], ],
- ['&Debugger', ['Popout', 'Launch Debugger']],
- ['&Toolbar', ['Command &1', 'Command &2', 'Command &3', 'Command &4']],
- ['&Help', '&About...'], ]
- Finally, "keys" can be added to entries so make them unique. The "Save" entry has a key associated with it. You
- can see it has a "::" which signifies the beginning of a key. The user will not see the key portion when the
- menu is shown. The key portion is returned as part of the event.
- """
-
- def __init__(self, menu_definition, background_color=None, text_color=None, disabled_text_color=None, size=(None, None), s=(None, None), tearoff=False,
- font=None, pad=None, p=None, key=None, k=None, visible=True, metadata=None):
- """
- :param menu_definition: The Menu definition specified using lists (docs explain the format)
- :type menu_definition: List[List[Tuple[str, List[str]]]
- :param background_color: color of the background
- :type background_color: (str)
- :param text_color: element's text color. Can be in #RRGGBB format or a color name "black"
- :type text_color: (str)
- :param disabled_text_color: color to use for text when item is disabled. Can be in #RRGGBB format or a color name "black"
- :type disabled_text_color: (str)
- :param size: Not used in the tkinter port
- :type size: (int, int)
- :param s: Same as size parameter. It's an alias. If EITHER of them are set, then the one that's set will be used. If BOTH are set, size will be used
- :type s: (int, int) | (None, None)
- :param tearoff: if True, then can tear the menu off from the window ans use as a floating window. Very cool effect
- :type tearoff: (bool)
- :param pad: Amount of padding to put around element in pixels (left/right, top/bottom) or ((left, right), (top, bottom)) or an int. If an int, then it's converted into a tuple (int, int)
- :type pad: (int, int) or ((int, int),(int,int)) or (int,(int,int)) or ((int, int),int) | int
- :param p: Same as pad parameter. It's an alias. If EITHER of them are set, then the one that's set will be used. If BOTH are set, pad will be used
- :type p: (int, int) or ((int, int),(int,int)) or (int,(int,int)) or ((int, int),int) | int
- :param font: specifies the font family, size, etc. Tuple or Single string format 'name size styles'. Styles: italic * roman bold normal underline overstrike
- :type font: (str or (str, int[, str]) or None)
- :param key: Value that uniquely identifies this element from all other elements. Used when Finding an element or in return values. Must be unique to the window
- :type key: str | int | tuple | object
- :param k: Same as the Key. You can use either k or key. Which ever is set will be used.
- :type k: str | int | tuple | object
- :param visible: set visibility state of the element
- :type visible: (bool)
- :param metadata: User metadata that can be set to ANYTHING
- :type metadata: (Any)
- """
- self.BackgroundColor = background_color if background_color is not None else theme_input_background_color()
- self.TextColor = text_color if text_color is not None else theme_input_text_color()
-
- self.DisabledTextColor = disabled_text_color if disabled_text_color is not None else COLOR_SYSTEM_DEFAULT
- self.MenuDefinition = copy.deepcopy(menu_definition)
- self.Widget = self.TKMenu = None # type: tk.Menu
- self.MenuItemChosen = None
- key = key if key is not None else k
- sz = size if size != (None, None) else s
- pad = pad if pad is not None else p
-
- super().__init__(ELEM_TYPE_MENUBAR, background_color=self.BackgroundColor, text_color=self.TextColor, size=sz, pad=pad, key=key, visible=visible,
- font=font, metadata=metadata)
- # super().__init__(ELEM_TYPE_MENUBAR, background_color=COLOR_SYSTEM_DEFAULT, text_color=COLOR_SYSTEM_DEFAULT, size=sz, pad=pad, key=key, visible=visible, font=None, metadata=metadata)
-
- self.Tearoff = tearoff
-
- return
-
- def _MenuItemChosenCallback(self, item_chosen): # Menu Menu Item Chosen Callback
- """
- Not user callable. Called when some end-point on the menu (an item) has been clicked. Send the information back to the application as an event. Before event can be sent
-
- :param item_chosen: the text that was clicked on / chosen from the menu
- :type item_chosen: (str)
- """
- # print('IN MENU ITEM CALLBACK', item_chosen)
- self.MenuItemChosen = item_chosen
- self.ParentForm.LastButtonClicked = item_chosen
- self.ParentForm.FormRemainedOpen = True
- # if self.ParentForm.CurrentlyRunningMainloop:
- # self.ParentForm.TKroot.quit() # kick the users out of the mainloop
- _exit_mainloop(self.ParentForm)
-
- def update(self, menu_definition=None, visible=None):
- """
- Update a menubar - can change the menu definition and visibility. The entire menu has to be specified
-
- Changes will not be visible in your window until you call window.read or window.refresh.
-
- If you change visibility, your element may MOVE. If you want it to remain stationary, use the "layout helper"
- function "pin" to ensure your element is "pinned" to that location in your layout so that it returns there
- when made visible.
-
- :param menu_definition: The menu definition list
- :type menu_definition: List[List[Tuple[str, List[str]]]
- :param visible: control visibility of element
- :type visible: (bool)
- """
- if not self._widget_was_created(): # if widget hasn't been created yet, then don't allow
- return
-
- if menu_definition is not None:
- self.MenuDefinition = copy.deepcopy(menu_definition)
-
- self.TKMenu = tk.Menu(self.ParentForm.TKroot, tearoff=self.Tearoff, tearoffcommand=self._tearoff_menu_callback) # create the menubar
- menubar = self.TKMenu
- for menu_entry in self.MenuDefinition:
- baritem = tk.Menu(menubar, tearoff=self.Tearoff, tearoffcommand=self._tearoff_menu_callback)
-
- if self.BackgroundColor not in (COLOR_SYSTEM_DEFAULT, None):
- baritem.config(bg=self.BackgroundColor)
- if self.TextColor not in (COLOR_SYSTEM_DEFAULT, None):
- baritem.config(fg=self.TextColor)
- if self.DisabledTextColor not in (COLOR_SYSTEM_DEFAULT, None):
- baritem.config(disabledforeground=self.DisabledTextColor)
- if self.Font is not None:
- baritem.config(font=self.Font)
-
- if self.Font is not None:
- baritem.config(font=self.Font)
- pos = menu_entry[0].find(MENU_SHORTCUT_CHARACTER)
- # print(pos)
- if pos != -1:
- if pos == 0 or menu_entry[0][pos - len(MENU_SHORTCUT_CHARACTER)] != "\\":
- menu_entry[0] = menu_entry[0][:pos] + menu_entry[0][pos + len(MENU_SHORTCUT_CHARACTER):]
- if menu_entry[0][0] == MENU_DISABLED_CHARACTER:
- menubar.add_cascade(label=menu_entry[0][len(MENU_DISABLED_CHARACTER):], menu=baritem, underline=pos)
- menubar.entryconfig(menu_entry[0][len(MENU_DISABLED_CHARACTER):], state='disabled')
- else:
- menubar.add_cascade(label=menu_entry[0], menu=baritem, underline=pos)
-
- if len(menu_entry) > 1:
- AddMenuItem(baritem, menu_entry[1], self)
-
- if visible is False:
- self.ParentForm.TKroot.configure(menu=[]) # this will cause the menubar to disappear
- elif self.TKMenu is not None:
- self.ParentForm.TKroot.configure(menu=self.TKMenu)
- if visible is not None:
- self._visible = visible
-
- Update = update
-
-
-MenuBar = Menu # another name for Menu to make it clear it's the Menu Bar
-Menubar = Menu # another name for Menu to make it clear it's the Menu Bar
-
-
-# ---------------------------------------------------------------------- #
-# Table #
-# ---------------------------------------------------------------------- #
-class Table(Element):
-
- def __init__(self, values, headings=None, visible_column_map=None, col_widths=None, def_col_width=10,
- auto_size_columns=True, max_col_width=20, select_mode=None, display_row_numbers=False, num_rows=None,
- row_height=None, font=None, justification='right', text_color=None, background_color=None,
- alternating_row_color=None, selected_row_colors=(None, None), header_text_color=None, header_background_color=None, header_font=None, header_border_width=None, header_relief=None,
- row_colors=None, vertical_scroll_only=True, hide_vertical_scroll=False, border_width=None,
- sbar_trough_color=None, sbar_background_color=None, sbar_arrow_color=None, sbar_width=None, sbar_arrow_width=None, sbar_frame_color=None, sbar_relief=None,
- size=(None, None), s=(None, None), change_submits=False, enable_events=False, enable_click_events=False, right_click_selects=False, bind_return_key=False, pad=None, p=None,
- key=None, k=None, tooltip=None, right_click_menu=None, expand_x=False, expand_y=False, visible=True, metadata=None):
- """
- :param values: Your table data represented as a 2-dimensions table... a list of rows, with each row representing a row in your table.
- :type values: List[List[str | int | float]]
- :param headings: The headings to show on the top line
- :type headings: List[str]
- :param visible_column_map: One entry for each column. False indicates the column is not shown
- :type visible_column_map: List[bool]
- :param col_widths: Number of characters that each column will occupy
- :type col_widths: List[int]
- :param def_col_width: Default column width in characters
- :type def_col_width: (int)
- :param auto_size_columns: if True columns will be sized automatically
- :type auto_size_columns: (bool)
- :param max_col_width: Maximum width for all columns in characters
- :type max_col_width: (int)
- :param select_mode: Select Mode. Valid values start with "TABLE_SELECT_MODE_". Valid values are: TABLE_SELECT_MODE_NONE TABLE_SELECT_MODE_BROWSE TABLE_SELECT_MODE_EXTENDED
- :type select_mode: (enum)
- :param display_row_numbers: if True, the first column of the table will be the row #
- :type display_row_numbers: (bool)
- :param num_rows: The number of rows of the table to display at a time
- :type num_rows: (int)
- :param row_height: height of a single row in pixels
- :type row_height: (int)
- :param font: specifies the font family, size, etc. Tuple or Single string format 'name size styles'. Styles: italic * roman bold normal underline overstrike
- :type font: (str or (str, int[, str]) or None)
- :param justification: 'left', 'right', 'center' are valid choices
- :type justification: (str)
- :param text_color: color of the text
- :type text_color: (str)
- :param background_color: color of background
- :type background_color: (str)
- :param alternating_row_color: if set then every other row will have this color in the background.
- :type alternating_row_color: (str)
- :param selected_row_colors: Sets the text color and background color for a selected row. Same format as button colors - tuple ('red', 'yellow') or string 'red on yellow'. Defaults to theme's button color
- :type selected_row_colors: str or (str, str)
- :param header_text_color: sets the text color for the header
- :type header_text_color: (str)
- :param header_background_color: sets the background color for the header
- :type header_background_color: (str)
- :param header_font: specifies the font family, size, etc. Tuple or Single string format 'name size styles'. Styles: italic * roman bold normal underline overstrike
- :type header_font: (str or (str, int[, str]) or None)
- :param header_border_width: Border width for the header portion
- :type header_border_width: (int | None)
- :param header_relief: Relief style for the header. Values are same as other elements that use relief. RELIEF_RAISED RELIEF_SUNKEN RELIEF_FLAT RELIEF_RIDGE RELIEF_GROOVE RELIEF_SOLID
- :type header_relief: (str | None)
- :param row_colors: list of tuples of (row, background color) OR (row, foreground color, background color). Sets the colors of listed rows to the color(s) provided (note the optional foreground color)
- :type row_colors: List[Tuple[int, str] | Tuple[Int, str, str]]
- :param vertical_scroll_only: if True only the vertical scrollbar will be visible
- :type vertical_scroll_only: (bool)
- :param hide_vertical_scroll: if True vertical scrollbar will be hidden
- :type hide_vertical_scroll: (bool)
- :param border_width: Border width/depth in pixels
- :type border_width: (int)
- :param sbar_trough_color: Scrollbar color of the trough
- :type sbar_trough_color: (str)
- :param sbar_background_color: Scrollbar color of the background of the arrow buttons at the ends AND the color of the "thumb" (the thing you grab and slide). Switches to arrow color when mouse is over
- :type sbar_background_color: (str)
- :param sbar_arrow_color: Scrollbar color of the arrow at the ends of the scrollbar (it looks like a button). Switches to background color when mouse is over
- :type sbar_arrow_color: (str)
- :param sbar_width: Scrollbar width in pixels
- :type sbar_width: (int)
- :param sbar_arrow_width: Scrollbar width of the arrow on the scrollbar. It will potentially impact the overall width of the scrollbar
- :type sbar_arrow_width: (int)
- :param sbar_frame_color: Scrollbar Color of frame around scrollbar (available only on some ttk themes)
- :type sbar_frame_color: (str)
- :param sbar_relief: Scrollbar relief that will be used for the "thumb" of the scrollbar (the thing you grab that slides). Should be a constant that is defined at starting with "RELIEF_" - RELIEF_RAISED, RELIEF_SUNKEN, RELIEF_FLAT, RELIEF_RIDGE, RELIEF_GROOVE, RELIEF_SOLID
- :type sbar_relief: (str)
- :param size: DO NOT USE! Use num_rows instead
- :type size: (int, int)
- :param change_submits: DO NOT USE. Only listed for backwards compat - Use enable_events instead
- :type change_submits: (bool)
- :param enable_events: Turns on the element specific events. Table events happen when row is clicked
- :type enable_events: (bool)
- :param enable_click_events: Turns on the element click events that will give you (row, col) click data when the table is clicked
- :type enable_click_events: (bool)
- :param right_click_selects: If True, then right clicking a row will select that row if multiple rows are not currently selected
- :type right_click_selects: (bool)
- :param bind_return_key: if True, pressing return key will cause event coming from Table, ALSO a left button double click will generate an event if this parameter is True
- :type bind_return_key: (bool)
- :param pad: Amount of padding to put around element in pixels (left/right, top/bottom) or ((left, right), (top, bottom)) or an int. If an int, then it's converted into a tuple (int, int)
- :type pad: (int, int) or ((int, int),(int,int)) or (int,(int,int)) or ((int, int),int) | int
- :param p: Same as pad parameter. It's an alias. If EITHER of them are set, then the one that's set will be used. If BOTH are set, pad will be used
- :type p: (int, int) or ((int, int),(int,int)) or (int,(int,int)) or ((int, int),int) | int
- :param key: Used with window.find_element and with return values to uniquely identify this element to uniquely identify this element
- :type key: str | int | tuple | object
- :param k: Same as the Key. You can use either k or key. Which ever is set will be used.
- :type k: str | int | tuple | object
- :param tooltip: text, that will appear when mouse hovers over the element
- :type tooltip: (str)
- :param right_click_menu: A list of lists of Menu items to show when this element is right clicked. See user docs for exact format.
- :type right_click_menu: List[List[ List[str] | str ]]
- :param expand_x: If True the element will automatically expand in the X direction to fill available space
- :type expand_x: (bool)
- :param expand_y: If True the element will automatically expand in the Y direction to fill available space
- :type expand_y: (bool)
- :param visible: set visibility state of the element
- :type visible: (bool)
- :param metadata: User metadata that can be set to ANYTHING
- :type metadata: (Any)
- """
-
-
- self.Values = values
- self.ColumnHeadings = headings
- self.ColumnsToDisplay = visible_column_map
- self.ColumnWidths = col_widths
- self.MaxColumnWidth = max_col_width
- self.DefaultColumnWidth = def_col_width
- self.AutoSizeColumns = auto_size_columns
- self.BackgroundColor = background_color if background_color is not None else DEFAULT_BACKGROUND_COLOR
- self.TextColor = text_color
- self.HeaderTextColor = header_text_color if header_text_color is not None else LOOK_AND_FEEL_TABLE[CURRENT_LOOK_AND_FEEL]['TEXT_INPUT']
- self.HeaderBackgroundColor = header_background_color if header_background_color is not None else LOOK_AND_FEEL_TABLE[CURRENT_LOOK_AND_FEEL]['INPUT']
- self.HeaderFont = header_font
- self.Justification = justification
- self.InitialState = None
- self.SelectMode = select_mode
- self.DisplayRowNumbers = display_row_numbers
- self.NumRows = num_rows if num_rows is not None else size[1]
- self.RowHeight = row_height
- self.Widget = self.TKTreeview = None # type: ttk.Treeview
- self.AlternatingRowColor = alternating_row_color
- self.VerticalScrollOnly = vertical_scroll_only
- self.HideVerticalScroll = hide_vertical_scroll
- self.SelectedRows = []
- self.ChangeSubmits = change_submits or enable_events
- self.BindReturnKey = bind_return_key
- self.StartingRowNumber = 0 # When displaying row numbers, where to start
- self.RowHeaderText = 'Row'
- self.enable_click_events = enable_click_events
- self.right_click_selects = right_click_selects
- self.last_clicked_position = (None, None)
- self.HeaderBorderWidth = header_border_width
- self.BorderWidth = border_width
- self.HeaderRelief = header_relief
- if selected_row_colors == (None, None):
- # selected_row_colors = DEFAULT_TABLE_AND_TREE_SELECTED_ROW_COLORS
- selected_row_colors = theme_button_color()
- else:
- try:
- if isinstance(selected_row_colors, str):
- selected_row_colors = selected_row_colors.split(' on ')
- except Exception as e:
- print('* Table Element Warning * you messed up with color formatting of Selected Row Color', e)
- self.SelectedRowColors = selected_row_colors
-
- self.RightClickMenu = right_click_menu
- self.RowColors = row_colors
- self.tree_ids = [] # ids returned when inserting items into table - will use to delete colors
- key = key if key is not None else k
- sz = size if size != (None, None) else s
- pad = pad if pad is not None else p
- self.expand_x = expand_x
- self.expand_y = expand_y
-
- super().__init__(ELEM_TYPE_TABLE, text_color=text_color, background_color=background_color, font=font,
- size=sz, pad=pad, key=key, tooltip=tooltip, visible=visible, metadata=metadata,
- sbar_trough_color=sbar_trough_color, sbar_background_color=sbar_background_color, sbar_arrow_color=sbar_arrow_color, sbar_width=sbar_width, sbar_arrow_width=sbar_arrow_width, sbar_frame_color=sbar_frame_color, sbar_relief=sbar_relief)
- return
-
- def update(self, values=None, num_rows=None, visible=None, select_rows=None, alternating_row_color=None, row_colors=None):
- """
- Changes some of the settings for the Table Element. Must call `Window.Read` or `Window.Finalize` prior
-
- Changes will not be visible in your window until you call window.read or window.refresh.
-
- If you change visibility, your element may MOVE. If you want it to remain stationary, use the "layout helper"
- function "pin" to ensure your element is "pinned" to that location in your layout so that it returns there
- when made visible.
-
- :param values: A new 2-dimensional table to show
- :type values: List[List[str | int | float]]
- :param num_rows: How many rows to display at a time
- :type num_rows: (int)
- :param visible: if True then will be visible
- :type visible: (bool)
- :param select_rows: List of rows to select as if user did
- :type select_rows: List[int]
- :param alternating_row_color: the color to make every other row
- :type alternating_row_color: (str)
- :param row_colors: list of tuples of (row, background color) OR (row, foreground color, background color). Changes the colors of listed rows to the color(s) provided (note the optional foreground color)
- :type row_colors: List[Tuple[int, str] | Tuple[Int, str, str]]
- """
- if not self._widget_was_created(): # if widget hasn't been created yet, then don't allow
- return
-
- if values is not None:
- for id in self.tree_ids:
- self.TKTreeview.item(id, tags=())
- if self.BackgroundColor is not None and self.BackgroundColor != COLOR_SYSTEM_DEFAULT:
- self.TKTreeview.tag_configure(id, background=self.BackgroundColor)
- else:
- self.TKTreeview.tag_configure(id, background='#FFFFFF', foreground='#000000')
- if self.TextColor is not None and self.TextColor != COLOR_SYSTEM_DEFAULT:
- self.TKTreeview.tag_configure(id, foreground=self.TextColor)
- else:
- self.TKTreeview.tag_configure(id, foreground='#000000')
-
- children = self.TKTreeview.get_children()
- for i in children:
- self.TKTreeview.detach(i)
- self.TKTreeview.delete(i)
- children = self.TKTreeview.get_children()
-
- self.tree_ids = []
- for i, value in enumerate(values):
- if self.DisplayRowNumbers:
- value = [i + self.StartingRowNumber] + value
- id = self.TKTreeview.insert('', 'end', text=value, iid=i + 1, values=value, tag=i)
- if self.BackgroundColor is not None and self.BackgroundColor != COLOR_SYSTEM_DEFAULT:
- self.TKTreeview.tag_configure(id, background=self.BackgroundColor)
- else:
- self.TKTreeview.tag_configure(id, background='#FFFFFF')
- self.tree_ids.append(id)
- self.Values = values
- self.SelectedRows = []
- if visible is False:
- self._pack_forget_save_settings(self.element_frame)
- elif visible is True:
- self._pack_restore_settings(self.element_frame)
-
- if num_rows is not None:
- self.TKTreeview.config(height=num_rows)
- if select_rows is not None:
- rows_to_select = [i + 1 for i in select_rows]
- self.TKTreeview.selection_set(rows_to_select)
-
- if alternating_row_color is not None: # alternating colors
- self.AlternatingRowColor = alternating_row_color
-
- if self.AlternatingRowColor is not None:
- for row in range(0, len(self.Values), 2):
- self.TKTreeview.tag_configure(row, background=self.AlternatingRowColor)
- if row_colors is not None: # individual row colors
- self.RowColors = row_colors
- for row_def in self.RowColors:
- if len(row_def) == 2: # only background is specified
- self.TKTreeview.tag_configure(row_def[0], background=row_def[1])
- else:
- self.TKTreeview.tag_configure(row_def[0], background=row_def[2], foreground=row_def[1])
- if visible is not None:
- self._visible = visible
-
- def _treeview_selected(self, event):
- """
- Not user callable. Callback function that is called when something is selected from Table.
- Stores the selected rows in Element as they are being selected. If events enabled, then returns from Read
-
- :param event: event information from tkinter
- :type event: (unknown)
- """
- # print('**-- in treeview selected --**')
- selections = self.TKTreeview.selection()
- self.SelectedRows = [int(x) - 1 for x in selections]
- if self.ChangeSubmits:
- if self.Key is not None:
- self.ParentForm.LastButtonClicked = self.Key
- else:
- self.ParentForm.LastButtonClicked = ''
- self.ParentForm.FormRemainedOpen = True
- # if self.ParentForm.CurrentlyRunningMainloop:
- # self.ParentForm.TKroot.quit()
- _exit_mainloop(self.ParentForm)
-
- def _treeview_double_click(self, event):
- """
- Not user callable. Callback function that is called when something is selected from Table.
- Stores the selected rows in Element as they are being selected. If events enabled, then returns from Read
-
- :param event: event information from tkinter
- :type event: (unknown)
- """
- selections = self.TKTreeview.selection()
- self.SelectedRows = [int(x) - 1 for x in selections]
- if self.BindReturnKey: # Signifies BOTH a return key AND a double click
- if self.Key is not None:
- self.ParentForm.LastButtonClicked = self.Key
- else:
- self.ParentForm.LastButtonClicked = ''
- self.ParentForm.FormRemainedOpen = True
- # if self.ParentForm.CurrentlyRunningMainloop:
- # self.ParentForm.TKroot.quit()
- _exit_mainloop(self.ParentForm)
-
-
- def _table_clicked(self, event):
- """
- Not user callable. Callback function that is called a click happens on a table.
- Stores the selected rows in Element as they are being selected. If events enabled, then returns from Read
-
- :param event: event information from tkinter
- :type event: (unknown)
- """
- if not self._widget_was_created(): # if widget hasn't been created yet, then don't allow
- return
- # popup(obj_to_string_single_obj(event))
- try:
- region = self.Widget.identify('region', event.x, event.y)
- if region == 'heading':
- row = -1
- elif region == 'cell':
- row = int(self.Widget.identify_row(event.y))-1
- elif region == 'separator':
- row = None
- else:
- row = None
- col_identified = self.Widget.identify_column(event.x)
- if col_identified: # Sometimes tkinter returns a value of '' which would cause an error if cast to an int
- column = int(self.Widget.identify_column(event.x)[1:])-1-int(self.DisplayRowNumbers is True)
- else:
- column = None
- except Exception as e:
- warnings.warn('Error getting table click data for table with key= {}\nError: {}'.format(self.Key, e), UserWarning)
- if not SUPPRESS_ERROR_POPUPS:
- _error_popup_with_traceback('Unable to complete operation getting the clicked event for table with key {}'.format(self.Key), _create_error_message(), e, 'Event data:', obj_to_string_single_obj(event))
- row = column = None
-
- self.last_clicked_position = (row, column)
-
- # update the rows being selected if appropriate
- self.ParentForm.TKroot.update()
- # self.TKTreeview.()
- selections = self.TKTreeview.selection()
- if self.right_click_selects and len(selections) <= 1:
- if (event.num == 3 and not running_mac()) or (event.num == 2 and running_mac()):
- if row != -1 and row is not None:
- selections = [row+1]
- self.TKTreeview.selection_set(selections)
- # print(selections)
- self.SelectedRows = [int(x) - 1 for x in selections]
- # print('The new selected rows = ', self.SelectedRows)
- if self.enable_click_events is True:
- if self.Key is not None:
- self.ParentForm.LastButtonClicked = (self.Key, TABLE_CLICKED_INDICATOR, (row, column))
- else:
- self.ParentForm.LastButtonClicked = ''
- self.ParentForm.FormRemainedOpen = True
- _exit_mainloop(self.ParentForm)
-
-
-
- def get(self):
- """
- Dummy function for tkinter port. In the Qt port you can read back the values in the table in case they were
- edited. Don't know yet how to enable editing of a Tree in tkinter so just returning the values provided by
- user when Table was created or Updated.
-
- :return: the current table values (for now what was originally provided up updated)
- :rtype: List[List[Any]]
- """
- return self.Values
-
-
- def get_last_clicked_position(self):
- """
- Returns a tuple with the row and column of the cell that was last clicked.
- Headers will have a row == -1 and the Row Number Column (if present) will have a column == -1
- :return: The (row,col) position of the last cell clicked in the table
- :rtype: (int | None, int | None)
- """
- return self.last_clicked_position
-
-
-
-
- Update = update
- Get = get
-
-
-# ---------------------------------------------------------------------- #
-# Tree #
-# ---------------------------------------------------------------------- #
-class Tree(Element):
- """
- Tree Element - Presents data in a tree-like manner, much like a file/folder browser. Uses the TreeData class
- to hold the user's data and pass to the element for display.
- """
-
- def __init__(self, data=None, headings=None, visible_column_map=None, col_widths=None, col0_width=10, col0_heading='',
- def_col_width=10, auto_size_columns=True, max_col_width=20, select_mode=None, show_expanded=False,
- change_submits=False, enable_events=False, font=None, justification='right', text_color=None, border_width=None,
- background_color=None, selected_row_colors=(None, None), header_text_color=None, header_background_color=None, header_font=None, header_border_width=None, header_relief=None, num_rows=None,
- sbar_trough_color=None, sbar_background_color=None, sbar_arrow_color=None, sbar_width=None, sbar_arrow_width=None, sbar_frame_color=None, sbar_relief=None,
- row_height=None, vertical_scroll_only=True, hide_vertical_scroll=False, pad=None, p=None, key=None, k=None, tooltip=None,
- right_click_menu=None, expand_x=False, expand_y=False, visible=True, metadata=None):
- """
- :param data: The data represented using a PySimpleGUI provided TreeData class
- :type data: (TreeData)
- :param headings: List of individual headings for each column
- :type headings: List[str]
- :param visible_column_map: Determines if a column should be visible. If left empty, all columns will be shown
- :type visible_column_map: List[bool]
- :param col_widths: List of column widths so that individual column widths can be controlled
- :type col_widths: List[int]
- :param col0_width: Size of Column 0 which is where the row numbers will be optionally shown
- :type col0_width: (int)
- :param col0_heading: Text to be shown in the header for the left-most column
- :type col0_heading: (str)
- :param def_col_width: default column width
- :type def_col_width: (int)
- :param auto_size_columns: if True, the size of a column is determined using the contents of the column
- :type auto_size_columns: (bool)
- :param max_col_width: the maximum size a column can be
- :type max_col_width: (int)
- :param select_mode: Use same values as found on Table Element. Valid values include: TABLE_SELECT_MODE_NONE TABLE_SELECT_MODE_BROWSE TABLE_SELECT_MODE_EXTENDED
- :type select_mode: (enum)
- :param show_expanded: if True then the tree will be initially shown with all nodes completely expanded
- :type show_expanded: (bool)
- :param change_submits: DO NOT USE. Only listed for backwards compat - Use enable_events instead
- :type change_submits: (bool)
- :param enable_events: Turns on the element specific events. Tree events happen when row is clicked
- :type enable_events: (bool)
- :param font: specifies the font family, size, etc. Tuple or Single string format 'name size styles'. Styles: italic * roman bold normal underline overstrike
- :type font: (str or (str, int[, str]) or None)
- :param justification: 'left', 'right', 'center' are valid choices
- :type justification: (str)
- :param text_color: color of the text
- :type text_color: (str)
- :param border_width: Border width/depth in pixels
- :type border_width: (int)
- :param background_color: color of background
- :type background_color: (str)
- :param selected_row_colors: Sets the text color and background color for a selected row. Same format as button colors - tuple ('red', 'yellow') or string 'red on yellow'. Defaults to theme's button color
- :type selected_row_colors: str or (str, str)
- :param header_text_color: sets the text color for the header
- :type header_text_color: (str)
- :param header_background_color: sets the background color for the header
- :type header_background_color: (str)
- :param header_font: specifies the font family, size, etc. Tuple or Single string format 'name size styles'. Styles: italic * roman bold normal underline overstrike
- :type header_font: (str or (str, int[, str]) or None)
- :param header_border_width: Border width for the header portion
- :type header_border_width: (int | None)
- :param header_relief: Relief style for the header. Values are same as other elements that use relief. RELIEF_RAISED RELIEF_SUNKEN RELIEF_FLAT RELIEF_RIDGE RELIEF_GROOVE RELIEF_SOLID
- :type header_relief: (str | None)
- :param num_rows: The number of rows of the table to display at a time
- :type num_rows: (int)
- :param row_height: height of a single row in pixels
- :type row_height: (int)
- :param vertical_scroll_only: if True only the vertical scrollbar will be visible
- :type vertical_scroll_only: (bool)
- :param hide_vertical_scroll: if True vertical scrollbar will be hidden
- :type hide_vertical_scroll: (bool)
- :param sbar_trough_color: Scrollbar color of the trough
- :type sbar_trough_color: (str)
- :param sbar_background_color: Scrollbar color of the background of the arrow buttons at the ends AND the color of the "thumb" (the thing you grab and slide). Switches to arrow color when mouse is over
- :type sbar_background_color: (str)
- :param sbar_arrow_color: Scrollbar color of the arrow at the ends of the scrollbar (it looks like a button). Switches to background color when mouse is over
- :type sbar_arrow_color: (str)
- :param sbar_width: Scrollbar width in pixels
- :type sbar_width: (int)
- :param sbar_arrow_width: Scrollbar width of the arrow on the scrollbar. It will potentially impact the overall width of the scrollbar
- :type sbar_arrow_width: (int)
- :param sbar_frame_color: Scrollbar Color of frame around scrollbar (available only on some ttk themes)
- :type sbar_frame_color: (str)
- :param sbar_relief: Scrollbar relief that will be used for the "thumb" of the scrollbar (the thing you grab that slides). Should be a constant that is defined at starting with "RELIEF_" - RELIEF_RAISED, RELIEF_SUNKEN, RELIEF_FLAT, RELIEF_RIDGE, RELIEF_GROOVE, RELIEF_SOLID
- :type sbar_relief: (str)
- :param pad: Amount of padding to put around element in pixels (left/right, top/bottom) or ((left, right), (top, bottom)) or an int. If an int, then it's converted into a tuple (int, int)
- :type pad: (int, int) or ((int, int),(int,int)) or (int,(int,int)) or ((int, int),int) | int
- :param p: Same as pad parameter. It's an alias. If EITHER of them are set, then the one that's set will be used. If BOTH are set, pad will be used
- :type p: (int, int) or ((int, int),(int,int)) or (int,(int,int)) or ((int, int),int) | int
- :param key: Used with window.find_element and with return values to uniquely identify this element to uniquely identify this element
- :type key: str | int | tuple | object
- :param k: Same as the Key. You can use either k or key. Which ever is set will be used.
- :type k: str | int | tuple | object
- :param tooltip: text, that will appear when mouse hovers over the element
- :type tooltip: (str)
- :param right_click_menu: A list of lists of Menu items to show when this element is right clicked. See user docs for exact format.
- :type right_click_menu: List[List[str] | str]]
- :param expand_x: If True the element will automatically expand in the X direction to fill available space
- :type expand_x: (bool)
- :param expand_y: If True the element will automatically expand in the Y direction to fill available space
- :type expand_y: (bool)
- :param visible: set visibility state of the element
- :type visible: (bool)
- :param metadata: User metadata that can be set to ANYTHING
- :type metadata: (Any)
- """
-
- self.image_dict = {}
-
- self.TreeData = data
- self.ColumnHeadings = headings
- self.ColumnsToDisplay = visible_column_map
- self.ColumnWidths = col_widths
- self.MaxColumnWidth = max_col_width
- self.DefaultColumnWidth = def_col_width
- self.AutoSizeColumns = auto_size_columns
- self.BackgroundColor = background_color if background_color is not None else DEFAULT_BACKGROUND_COLOR
- self.TextColor = text_color
- self.HeaderTextColor = header_text_color if header_text_color is not None else LOOK_AND_FEEL_TABLE[CURRENT_LOOK_AND_FEEL]['TEXT_INPUT']
- self.HeaderBackgroundColor = header_background_color if header_background_color is not None else LOOK_AND_FEEL_TABLE[CURRENT_LOOK_AND_FEEL]['INPUT']
- self.HeaderBorderWidth = header_border_width
- self.BorderWidth = border_width
- self.HeaderRelief = header_relief
-
- if selected_row_colors == (None, None):
- # selected_row_colors = DEFAULT_TABLE_AND_TREE_SELECTED_ROW_COLORS
- selected_row_colors = theme_button_color()
- else:
- try:
- if isinstance(selected_row_colors, str):
- selected_row_colors = selected_row_colors.split(' on ')
- except Exception as e:
- print('* Table Element Warning * you messed up with color formatting of Selected Row Color', e)
- self.SelectedRowColors = selected_row_colors
-
- self.HeaderFont = header_font
- self.Justification = justification
- self.InitialState = None
- self.SelectMode = select_mode
- self.ShowExpanded = show_expanded
- self.NumRows = num_rows
- self.Col0Width = col0_width
- self.col0_heading = col0_heading
- self.TKTreeview = None # type: ttk.Treeview
- self.element_frame = None # type: tk.Frame
- self.VerticalScrollOnly = vertical_scroll_only
- self.HideVerticalScroll = hide_vertical_scroll
- self.SelectedRows = []
- self.ChangeSubmits = change_submits or enable_events
- self.RightClickMenu = right_click_menu
- self.RowHeight = row_height
- self.IconList = {}
- self.IdToKey = {'': ''}
- self.KeyToID = {'': ''}
- key = key if key is not None else k
- pad = pad if pad is not None else p
- self.expand_x = expand_x
- self.expand_y = expand_y
-
- super().__init__(ELEM_TYPE_TREE, text_color=text_color, background_color=background_color, font=font, pad=pad, key=key, tooltip=tooltip,
- visible=visible, metadata=metadata,
- sbar_trough_color=sbar_trough_color, sbar_background_color=sbar_background_color, sbar_arrow_color=sbar_arrow_color, sbar_width=sbar_width, sbar_arrow_width=sbar_arrow_width, sbar_frame_color=sbar_frame_color, sbar_relief=sbar_relief)
- return
-
- def _treeview_selected(self, event):
- """
- Not a user function. Callback function that happens when an item is selected from the tree. In this
- method, it saves away the reported selections so they can be properly returned.
-
- :param event: An event parameter passed in by tkinter. Not used
- :type event: (Any)
- """
-
- selections = self.TKTreeview.selection()
- # self.SelectedRows = [x for x in selections]
- self.SelectedRows = [self.IdToKey[x] for x in selections]
- if self.ChangeSubmits:
- MyForm = self.ParentForm
- if self.Key is not None:
- self.ParentForm.LastButtonClicked = self.Key
- else:
- self.ParentForm.LastButtonClicked = ''
- self.ParentForm.FormRemainedOpen = True
- # if self.ParentForm.CurrentlyRunningMainloop:
- # self.ParentForm.TKroot.quit()
- _exit_mainloop(self.ParentForm)
-
- def add_treeview_data(self, node):
- """
- Not a user function. Recursive method that inserts tree data into the tkinter treeview widget.
-
- :param node: The node to insert. Will insert all nodes from starting point downward, recursively
- :type node: (TreeData)
- """
- if node.key != '':
- if node.icon:
- try:
- if node.icon not in self.image_dict:
- if type(node.icon) is bytes:
- photo = tk.PhotoImage(data=node.icon)
- else:
- photo = tk.PhotoImage(file=node.icon)
- self.image_dict[node.icon] = photo
- else:
- photo = self.image_dict.get(node.icon)
-
- node.photo = photo
- id = self.TKTreeview.insert(self.KeyToID[node.parent], 'end', iid=None, text=node.text,
- values=node.values, open=self.ShowExpanded, image=node.photo)
- self.IdToKey[id] = node.key
- self.KeyToID[node.key] = id
- except:
- self.photo = None
- else:
- id = self.TKTreeview.insert(self.KeyToID[node.parent], 'end', iid=None, text=node.text,
- values=node.values, open=self.ShowExpanded)
- self.IdToKey[id] = node.key
- self.KeyToID[node.key] = id
-
- for node in node.children:
- self.add_treeview_data(node)
-
- def update(self, values=None, key=None, value=None, text=None, icon=None, visible=None):
- """
- Changes some of the settings for the Tree Element. Must call `Window.Read` or `Window.Finalize` prior
-
- Changes will not be visible in your window until you call window.read or window.refresh.
-
- If you change visibility, your element may MOVE. If you want it to remain stationary, use the "layout helper"
- function "pin" to ensure your element is "pinned" to that location in your layout so that it returns there
- when made visible.
-
- :param values: Representation of the tree
- :type values: (TreeData)
- :param key: identifies a particular item in tree to update
- :type key: str | int | tuple | object
- :param value: sets the node identified by key to a particular value
- :type value: (Any)
- :param text: sets the node identified by key to this string
- :type text: (str)
- :param icon: can be either a base64 icon or a filename for the icon
- :type icon: bytes | str
- :param visible: control visibility of element
- :type visible: (bool)
- """
- if not self._widget_was_created(): # if widget hasn't been created yet, then don't allow
- return
-
- if values is not None:
- children = self.TKTreeview.get_children()
- for i in children:
- self.TKTreeview.detach(i)
- self.TKTreeview.delete(i)
- children = self.TKTreeview.get_children()
- self.TreeData = values
- self.IdToKey = {'': ''}
- self.KeyToID = {'': ''}
- self.add_treeview_data(self.TreeData.root_node)
- self.SelectedRows = []
- if key is not None:
- for id in self.IdToKey.keys():
- if key == self.IdToKey[id]:
- break
- else:
- id = None
- print('** Key not found **')
- else:
- id = None
- if id:
- # item = self.TKTreeview.item(id)
- if value is not None:
- self.TKTreeview.item(id, values=value)
- if text is not None:
- self.TKTreeview.item(id, text=text)
- if icon is not None:
- try:
- if type(icon) is bytes:
- photo = tk.PhotoImage(data=icon)
- else:
- photo = tk.PhotoImage(file=icon)
- self.TKTreeview.item(id, image=photo)
- self.IconList[key] = photo # save so that it's not deleted (save reference)
- except:
- pass
- # item = self.TKTreeview.item(id)
- if visible is False:
- self._pack_forget_save_settings(self.element_frame)
- elif visible is True:
- self._pack_restore_settings(self.element_frame)
-
- if visible is not None:
- self._visible = visible
-
- return self
-
- Update = update
-
-
-class TreeData(object):
- """
- Class that user fills in to represent their tree data. It's a very simple tree representation with a root "Node"
- with possibly one or more children "Nodes". Each Node contains a key, text to display, list of values to display
- and an icon. The entire tree is built using a single method, Insert. Nothing else is required to make the tree.
- """
-
- class Node(object):
- """
- Contains information about the individual node in the tree
- """
-
- def __init__(self, parent, key, text, values, icon=None):
- """
- Represents a node within the TreeData class
-
- :param parent: The parent Node
- :type parent: (TreeData.Node)
- :param key: Used to uniquely identify this node
- :type key: str | int | tuple | object
- :param text: The text that is displayed at this node's location
- :type text: (str)
- :param values: The list of values that are displayed at this node
- :type values: List[Any]
- :param icon: just a icon
- :type icon: str | bytes
- """
-
- self.parent = parent # type: TreeData.Node
- self.children = [] # type: List[TreeData.Node]
- self.key = key # type: str
- self.text = text # type: str
- self.values = values # type: List[Any]
- self.icon = icon # type: str | bytes
-
- def _Add(self, node):
- self.children.append(node)
-
- def __init__(self):
- """
- Instantiate the object, initializes the Tree Data, creates a root node for you
- """
- self.tree_dict = {} # type: Dict[str, TreeData.Node]
- self.root_node = self.Node("", "", 'root', [], None) # The root node
- self.tree_dict[""] = self.root_node # Start the tree out with the root node
-
- def _AddNode(self, key, node):
- """
- Adds a node to tree dictionary (not user callable)
-
- :param key: Uniquely identifies this Node
- :type key: (str)
- :param node: Node being added
- :type node: (TreeData.Node)
- """
- self.tree_dict[key] = node
-
- def insert(self, parent, key, text, values, icon=None):
- """
- Inserts a node into the tree. This is how user builds their tree, by Inserting Nodes
- This is the ONLY user callable method in the TreeData class
-
- :param parent: the parent Node
- :type parent: (Node)
- :param key: Used to uniquely identify this node
- :type key: str | int | tuple | object
- :param text: The text that is displayed at this node's location
- :type text: (str)
- :param values: The list of values that are displayed at this node
- :type values: List[Any]
- :param icon: icon
- :type icon: str | bytes
- """
-
- node = self.Node(parent, key, text, values, icon)
- self.tree_dict[key] = node
- parent_node = self.tree_dict[parent]
- parent_node._Add(node)
-
- def __repr__(self):
- """
- Converts the TreeData into a printable version, nicely formatted
-
- :return: (str) A formatted, text version of the TreeData
- :rtype:
- """
- return self._NodeStr(self.root_node, 1)
-
- def _NodeStr(self, node, level):
- """
- Does the magic of converting the TreeData into a nicely formatted string version
-
- :param node: The node to begin printing the tree
- :type node: (TreeData.Node)
- :param level: The indentation level for string formatting
- :type level: (int)
- """
- return '\n'.join(
- [str(node.key) + ' : ' + str(node.text) + ' [ ' + ', '.join([str(v) for v in node.values]) +' ]'] +
- [' ' * 4 * level + self._NodeStr(child, level + 1) for child in node.children])
-
- Insert = insert
-
-
-# ---------------------------------------------------------------------- #
-# Error Element #
-# ---------------------------------------------------------------------- #
-class ErrorElement(Element):
- """
- A "dummy Element" that is returned when there are error conditions, like trying to find an element that's invalid
- """
-
- def __init__(self, key=None, metadata=None):
- """
- :param key: Used with window.find_element and with return values to uniquely identify this element
- :type key:
- """
- self.Key = key
-
- super().__init__(ELEM_TYPE_ERROR, key=key, metadata=metadata)
-
- def update(self, silent_on_error=True, *args, **kwargs):
- """
- Update method for the Error Element, an element that should not be directly used by developer
-
- :param silent_on_error: if False, then a Popup window will be shown
- :type silent_on_error: (bool)
- :param *args: meant to "soak up" any normal parameters passed in
- :type *args: (Any)
- :param **kwargs: meant to "soak up" any keyword parameters that were passed in
- :type **kwargs: (Any)
- :return: returns 'self' so call can be chained
- :rtype: (ErrorElement)
- """
- print('** Your update is being ignored because you supplied a bad key earlier **')
- return self
-
- def get(self):
- """
- One of the method names found in other Elements. Used here to return an error string in case it's called
-
- :return: A warning text string.
- :rtype: (str)
- """
- return 'This is NOT a valid Element!\nSTOP trying to do things with it or I will have to crash at some point!'
-
- Get = get
- Update = update
-
-
-# ---------------------------------------------------------------------- #
-# Stretch Element #
-# ---------------------------------------------------------------------- #
-# This is for source code compatibility with tkinter version. No tkinter equivalent but you can fake it using a Text element that expands in the X direction
-def Stretch(background_color=None):
- """
- Acts like a Stretch element found in the Qt port.
- Used in a Horizontal fashion. Placing one on each side of an element will enter the element.
- Place one to the left and the element to the right will be right justified. See VStretch for vertical type
- :param background_color: color of background may be needed because of how this is implemented
- :type background_color: (str)
- :return: (Text)
- """
- return Text(font='_ 1', background_color=background_color, pad=(0,0), expand_x=True)
-
-Push = Stretch
-P = Stretch
-
-def VStretch(background_color=None):
- """
- Acts like a Stretch element found in the Qt port.
- Used in a Vertical fashion.
- :param background_color: color of background may be needed because of how this is implemented
- :type background_color: (str)
- :return: (Text)
- """
- return Text(font='_ 1', background_color=background_color, pad=(0,0), expand_y=True)
-
-
-VPush = VStretch
-VP = VStretch
-
-
-# ------------------------------------------------------------------------- #
-# Window CLASS #
-# ------------------------------------------------------------------------- #
-class Window:
- """
- Represents a single Window
- """
- NumOpenWindows = 0
- _user_defined_icon = None
- hidden_master_root = None # type: tk.Tk
- _animated_popup_dict = {} # type: Dict
- _active_windows = {} # type: Dict[Window, tk.Tk()]
- _move_all_windows = False # if one window moved, they will move
- _window_that_exited = None # type: Window
- _root_running_mainloop = None # type: tk.Tk() # (may be the hidden root or a window's root)
- _timeout_key = None
- _TKAfterID = None # timer that is used to run reads with timeouts
- _window_running_mainloop = None # The window that is running the mainloop
- _container_element_counter = 0 # used to get a number of Container Elements (Frame, Column, Tab)
- _read_call_from_debugger = False
- _timeout_0_counter = 0 # when timeout=0 then go through each window one at a time
- _counter_for_ttk_widgets = 0
- _floating_debug_window_build_needed = False
- _main_debug_window_build_needed = False
-
- def __init__(self, title, layout=None, default_element_size=None,
- default_button_element_size=(None, None),
- auto_size_text=None, auto_size_buttons=None, location=(None, None), relative_location=(None, None), size=(None, None),
- element_padding=None, margins=(None, None), button_color=None, font=None,
- progress_bar_color=(None, None), background_color=None, border_depth=None, auto_close=False,
- auto_close_duration=DEFAULT_AUTOCLOSE_TIME, icon=None, force_toplevel=False,
- alpha_channel=None, return_keyboard_events=False, use_default_focus=True, text_justification=None,
- no_titlebar=False, grab_anywhere=False, grab_anywhere_using_control=True, keep_on_top=None, resizable=False, disable_close=False,
- disable_minimize=False, right_click_menu=None, transparent_color=None, debugger_enabled=True,
- right_click_menu_background_color=None, right_click_menu_text_color=None, right_click_menu_disabled_text_color=None,
- right_click_menu_selected_colors=(None, None),
- right_click_menu_font=None, right_click_menu_tearoff=False,
- finalize=False, element_justification='left', ttk_theme=None, use_ttk_buttons=None, modal=False, enable_close_attempted_event=False,
- titlebar_background_color=None, titlebar_text_color=None, titlebar_font=None, titlebar_icon=None,
- use_custom_titlebar=None, scaling=None,
- sbar_trough_color=None, sbar_background_color=None, sbar_arrow_color=None, sbar_width=None, sbar_arrow_width=None, sbar_frame_color=None, sbar_relief=None,
- metadata=None):
- """
- :param title: The title that will be displayed in the Titlebar and on the Taskbar
- :type title: (str)
- :param layout: The layout for the window. Can also be specified in the Layout method
- :type layout: List[List[Element]] | Tuple[Tuple[Element]]
- :param default_element_size: size in characters (wide) and rows (high) for all elements in this window
- :type default_element_size: (int, int) - (width, height)
- :param default_button_element_size: (width, height) size in characters (wide) and rows (high) for all Button elements in this window
- :type default_button_element_size: (int, int)
- :param auto_size_text: True if Elements in Window should be sized to exactly fir the length of text
- :type auto_size_text: (bool)
- :param auto_size_buttons: True if Buttons in this Window should be sized to exactly fit the text on this.
- :type auto_size_buttons: (bool)
- :param relative_location: (x,y) location relative to the default location of the window, in pixels. Normally the window centers. This location is relative to the location the window would be created. Note they can be negative.
- :type relative_location: (int, int)
- :param location: (x,y) location, in pixels, to locate the upper left corner of the window on the screen. Default is to center on screen.
- :type location: (int, int)
- :param size: (width, height) size in pixels for this window. Normally the window is autosized to fit contents, not set to an absolute size by the user. Try not to set this value. You risk, the contents being cut off, etc. Let the layout determine the window size instead
- :type size: (int, int)
- :param element_padding: Default amount of padding to put around elements in window (left/right, top/bottom) or ((left, right), (top, bottom)), or an int. If an int, then it's converted into a tuple (int, int)
- :type element_padding: (int, int) or ((int, int),(int,int)) or int
- :param margins: (left/right, top/bottom) Amount of pixels to leave inside the window's frame around the edges before your elements are shown.
- :type margins: (int, int)
- :param button_color: Default button colors for all buttons in the window
- :type button_color: (str, str) or str
- :param font: specifies the font family, size, etc. Tuple or Single string format 'name size styles'. Styles: italic * roman bold normal underline overstrike
- :type font: (str or (str, int[, str]) or None)
- :param progress_bar_color: (bar color, background color) Sets the default colors for all progress bars in the window
- :type progress_bar_color: (str, str)
- :param background_color: color of background
- :type background_color: (str)
- :param border_depth: Default border depth (width) for all elements in the window
- :type border_depth: (int)
- :param auto_close: If True, the window will automatically close itself
- :type auto_close: (bool)
- :param auto_close_duration: Number of seconds to wait before closing the window
- :type auto_close_duration: (int)
- :param icon: Can be either a filename or Base64 value. For Windows if filename, it MUST be ICO format. For Linux, must NOT be ICO. Most portable is to use a Base64 of a PNG file. This works universally across all OS's
- :type icon: (str | bytes)
- :param force_toplevel: If True will cause this window to skip the normal use of a hidden master window
- :type force_toplevel: (bool)
- :param alpha_channel: Sets the opacity of the window. 0 = invisible 1 = completely visible. Values bewteen 0 & 1 will produce semi-transparent windows in SOME environments (The Raspberry Pi always has this value at 1 and cannot change.
- :type alpha_channel: (float)
- :param return_keyboard_events: if True key presses on the keyboard will be returned as Events from Read calls
- :type return_keyboard_events: (bool)
- :param use_default_focus: If True will use the default focus algorithm to set the focus to the "Correct" element
- :type use_default_focus: (bool)
- :param text_justification: Default text justification for all Text Elements in window
- :type text_justification: 'left' | 'right' | 'center'
- :param no_titlebar: If true, no titlebar nor frame will be shown on window. This means you cannot minimize the window and it will not show up on the taskbar
- :type no_titlebar: (bool)
- :param grab_anywhere: If True can use mouse to click and drag to move the window. Almost every location of the window will work except input fields on some systems
- :type grab_anywhere: (bool)
- :param grab_anywhere_using_control: If True can use CONTROL key + left mouse mouse to click and drag to move the window. DEFAULT is TRUE. Unlike normal grab anywhere, it works on all elements.
- :type grab_anywhere_using_control: (bool)
- :param keep_on_top: If True, window will be created on top of all other windows on screen. It can be bumped down if another window created with this parm
- :type keep_on_top: (bool)
- :param resizable: If True, allows the user to resize the window. Note the not all Elements will change size or location when resizing.
- :type resizable: (bool)
- :param disable_close: If True, the X button in the top right corner of the window will no work. Use with caution and always give a way out toyour users
- :type disable_close: (bool)
- :param disable_minimize: if True the user won't be able to minimize window. Good for taking over entire screen and staying that way.
- :type disable_minimize: (bool)
- :param right_click_menu: A list of lists of Menu items to show when this element is right clicked. See user docs for exact format.
- :type right_click_menu: List[List[ List[str] | str ]]
- :param transparent_color: Any portion of the window that has this color will be completely transparent. You can even click through these spots to the window under this window.
- :type transparent_color: (str)
- :param debugger_enabled: If True then the internal debugger will be enabled
- :type debugger_enabled: (bool)
- :param right_click_menu_background_color: Background color for right click menus
- :type right_click_menu_background_color: (str)
- :param right_click_menu_text_color: Text color for right click menus
- :type right_click_menu_text_color: (str)
- :param right_click_menu_disabled_text_color: Text color for disabled right click menu items
- :type right_click_menu_disabled_text_color: (str)
- :param right_click_menu_selected_colors: Text AND background colors for a selected item. Can be a Tuple OR a color string. simplified-button-color-string "foreground on background". Can be a single color if want to set only the background. Normally a tuple, but can be a simplified-dual-color-string "foreground on background". Can be a single color if want to set only the background.
- :type right_click_menu_selected_colors: (str, str) | str | Tuple
- :param right_click_menu_font: Font for right click menus
- :type right_click_menu_font: (str or (str, int[, str]) or None)
- :param right_click_menu_tearoff: If True then all right click menus can be torn off
- :type right_click_menu_tearoff: bool
- :param finalize: If True then the Finalize method will be called. Use this rather than chaining .Finalize for cleaner code
- :type finalize: (bool)
- :param element_justification: All elements in the Window itself will have this justification 'left', 'right', 'center' are valid values
- :type element_justification: (str)
- :param ttk_theme: Set the tkinter ttk "theme" of the window. Default = DEFAULT_TTK_THEME. Sets all ttk widgets to this theme as their default
- :type ttk_theme: (str)
- :param use_ttk_buttons: Affects all buttons in window. True = use ttk buttons. False = do not use ttk buttons. None = use ttk buttons only if on a Mac
- :type use_ttk_buttons: (bool)
- :param modal: If True then this window will be the only window a user can interact with until it is closed
- :type modal: (bool)
- :param enable_close_attempted_event: If True then the window will not close when "X" clicked. Instead an event WINDOW_CLOSE_ATTEMPTED_EVENT if returned from window.read
- :type enable_close_attempted_event: (bool)
- :param titlebar_background_color: If custom titlebar indicated by use_custom_titlebar, then use this as background color
- :type titlebar_background_color: (str | None)
- :param titlebar_text_color: If custom titlebar indicated by use_custom_titlebar, then use this as text color
- :type titlebar_text_color: (str | None)
- :param titlebar_font: If custom titlebar indicated by use_custom_titlebar, then use this as title font
- :type titlebar_font: (str or (str, int[, str]) or None)
- :param titlebar_icon: If custom titlebar indicated by use_custom_titlebar, then use this as the icon (file or base64 bytes)
- :type titlebar_icon: (bytes | str)
- :param use_custom_titlebar: If True, then a custom titlebar will be used instead of the normal titlebar
- :type use_custom_titlebar: bool
- :param scaling: Apply scaling to the elements in the window. Can be set on a global basis using set_options
- :type scaling: float
- :param sbar_trough_color: Scrollbar color of the trough
- :type sbar_trough_color: (str)
- :param sbar_background_color: Scrollbar color of the background of the arrow buttons at the ends AND the color of the "thumb" (the thing you grab and slide). Switches to arrow color when mouse is over
- :type sbar_background_color: (str)
- :param sbar_arrow_color: Scrollbar color of the arrow at the ends of the scrollbar (it looks like a button). Switches to background color when mouse is over
- :type sbar_arrow_color: (str)
- :param sbar_width: Scrollbar width in pixels
- :type sbar_width: (int)
- :param sbar_arrow_width: Scrollbar width of the arrow on the scrollbar. It will potentially impact the overall width of the scrollbar
- :type sbar_arrow_width: (int)
- :param sbar_frame_color: Scrollbar Color of frame around scrollbar (available only on some ttk themes)
- :type sbar_frame_color: (str)
- :param sbar_relief: Scrollbar relief that will be used for the "thumb" of the scrollbar (the thing you grab that slides). Should be a constant that is defined at starting with "RELIEF_" - RELIEF_RAISED, RELIEF_SUNKEN, RELIEF_FLAT, RELIEF_RIDGE, RELIEF_GROOVE, RELIEF_SOLID
- :type sbar_relief: (str)
- :param metadata: User metadata that can be set to ANYTHING
- :type metadata: (Any)
- """
-
-
- self._metadata = None # type: Any
- self.AutoSizeText = auto_size_text if auto_size_text is not None else DEFAULT_AUTOSIZE_TEXT
- self.AutoSizeButtons = auto_size_buttons if auto_size_buttons is not None else DEFAULT_AUTOSIZE_BUTTONS
- self.Title = str(title)
- self.Rows = [] # a list of ELEMENTS for this row
- self.DefaultElementSize = default_element_size if default_element_size is not None else DEFAULT_ELEMENT_SIZE
- self.DefaultButtonElementSize = default_button_element_size if default_button_element_size != (
- None, None) else DEFAULT_BUTTON_ELEMENT_SIZE
- self.Location = location
- self.RelativeLoction = relative_location
- self.ButtonColor = button_color_to_tuple(button_color)
- self.BackgroundColor = background_color if background_color else DEFAULT_BACKGROUND_COLOR
- self.ParentWindow = None
- self.Font = font if font else DEFAULT_FONT
- self.RadioDict = {}
- self.BorderDepth = border_depth
- if icon:
- self.WindowIcon = icon
- elif Window._user_defined_icon is not None:
- self.WindowIcon = Window._user_defined_icon
- else:
- self.WindowIcon = DEFAULT_WINDOW_ICON
- self.AutoClose = auto_close
- self.NonBlocking = False
- self.TKroot = None # type: tk.Tk
- self.TKrootDestroyed = False
- self.CurrentlyRunningMainloop = False
- self.FormRemainedOpen = False
- self.TKAfterID = None
- self.ProgressBarColor = progress_bar_color
- self.AutoCloseDuration = auto_close_duration
- self.RootNeedsDestroying = False
- self.Shown = False
- self.ReturnValues = None
- self.ReturnValuesList = []
- self.ReturnValuesDictionary = {}
- self.DictionaryKeyCounter = 0
- self.LastButtonClicked = None
- self.LastButtonClickedWasRealtime = False
- self.UseDictionary = False
- self.UseDefaultFocus = use_default_focus
- self.ReturnKeyboardEvents = return_keyboard_events
- self.LastKeyboardEvent = None
- self.TextJustification = text_justification
- self.NoTitleBar = no_titlebar
- self.Grab = grab_anywhere
- self.GrabAnywhere = grab_anywhere
- self.GrabAnywhereUsingControlKey = grab_anywhere_using_control
- if keep_on_top is None and DEFAULT_KEEP_ON_TOP is not None:
- keep_on_top = DEFAULT_KEEP_ON_TOP
- elif keep_on_top is None:
- keep_on_top = False
- self.KeepOnTop = keep_on_top
- self.ForceTopLevel = force_toplevel
- self.Resizable = resizable
- self._AlphaChannel = alpha_channel if alpha_channel is not None else DEFAULT_ALPHA_CHANNEL
- self.Timeout = None
- self.TimeoutKey = TIMEOUT_KEY
- self.TimerCancelled = False
- self.DisableClose = disable_close
- self.DisableMinimize = disable_minimize
- self._Hidden = False
- self._Size = size
- self.XFound = False
- if element_padding is not None:
- if isinstance(element_padding, int):
- element_padding = (element_padding, element_padding)
-
- if element_padding is None:
- self.ElementPadding = DEFAULT_ELEMENT_PADDING
- else:
- self.ElementPadding = element_padding
- self.RightClickMenu = right_click_menu
- self.Margins = margins if margins != (None, None) else DEFAULT_MARGINS
- self.ContainerElemementNumber = Window._GetAContainerNumber()
- # The dictionary containing all elements and keys for the window
- # The keys are the keys for the elements and the values are the elements themselves.
- self.AllKeysDict = {}
- self.TransparentColor = transparent_color
- self.UniqueKeyCounter = 0
- self.DebuggerEnabled = debugger_enabled
- self.WasClosed = False
- self.ElementJustification = element_justification
- self.FocusSet = False
- self.metadata = metadata
- self.TtkTheme = ttk_theme or DEFAULT_TTK_THEME
- self.UseTtkButtons = use_ttk_buttons if use_ttk_buttons is not None else USE_TTK_BUTTONS
- self.user_bind_dict = {} # Used when user defines a tkinter binding using bind method - convert bind string to key modifier
- self.user_bind_event = None # Used when user defines a tkinter binding using bind method - event data from tkinter
- self.modal = modal
- self.thread_queue = None # type: queue.Queue
- self.thread_lock = None # type: threading.Lock
- self.thread_timer = None # type: tk.Misc
- self.thread_strvar = None # type: tk.StringVar
- self.read_closed_window_count = 0
- self.config_last_size = (None, None)
- self.config_last_location = (None, None)
- self.starting_window_position = (None, None)
- self.not_completed_initial_movement = True
- self.config_count = 0
- self.saw_00 = False
- self.maximized = False
- self.right_click_menu_background_color = right_click_menu_background_color if right_click_menu_background_color is not None else theme_input_background_color()
- self.right_click_menu_text_color = right_click_menu_text_color if right_click_menu_text_color is not None else theme_input_text_color()
- self.right_click_menu_disabled_text_color = right_click_menu_disabled_text_color if right_click_menu_disabled_text_color is not None else COLOR_SYSTEM_DEFAULT
- self.right_click_menu_font = right_click_menu_font if right_click_menu_font is not None else self.Font
- self.right_click_menu_tearoff = right_click_menu_tearoff
- self.auto_close_timer_needs_starting = False
- self.finalize_in_progress = False
- self.close_destroys_window = not enable_close_attempted_event if enable_close_attempted_event is not None else None
- self.override_custom_titlebar = False
- self.use_custom_titlebar = use_custom_titlebar or theme_use_custom_titlebar()
- self.titlebar_background_color = titlebar_background_color
- self.titlebar_text_color = titlebar_text_color
- self.titlebar_font = titlebar_font
- self.titlebar_icon = titlebar_icon
- self.right_click_menu_selected_colors = _simplified_dual_color_to_tuple(right_click_menu_selected_colors,
- (self.right_click_menu_background_color, self.right_click_menu_text_color))
- self.TKRightClickMenu = None
- self._grab_anywhere_ignore_these_list = []
- self._grab_anywhere_include_these_list = []
- self._has_custom_titlebar = use_custom_titlebar
- self._mousex = self._mousey = 0
- self._startx = self._starty = 0
- self.scaling = scaling if scaling is not None else DEFAULT_SCALING
- if self.use_custom_titlebar:
- self.Margins = (0, 0)
- self.NoTitleBar = True
-
- self.ttk_part_overrides = TTKPartOverrides(sbar_trough_color=sbar_trough_color, sbar_background_color=sbar_background_color, sbar_arrow_color=sbar_arrow_color, sbar_width=sbar_width, sbar_arrow_width=sbar_arrow_width, sbar_frame_color=sbar_frame_color, sbar_relief=sbar_relief)
-
- if no_titlebar is True:
- self.override_custom_titlebar = True
-
- if layout is not None and type(layout) not in (list, tuple):
- warnings.warn('Your layout is not a list or tuple... this is not good!')
-
- if layout is not None:
- self.Layout(layout)
- if finalize:
- self.Finalize()
-
- if CURRENT_LOOK_AND_FEEL == 'Default':
- print("Window will be a boring gray. Try removing the theme call entirely\n",
- "You will get the default theme or the one set in global settings\n"
- "If you seriously want this gray window and no more nagging, add theme('DefaultNoMoreNagging') or theme('Gray Gray Gray') for completely gray/System Defaults")
-
-
- @classmethod
- def _GetAContainerNumber(cls):
- """
- Not user callable!
- :return: A simple counter that makes each container element unique
- :rtype:
- """
- cls._container_element_counter += 1
- return cls._container_element_counter
-
- @classmethod
- def _IncrementOpenCount(self):
- """
- Not user callable! Increments the number of open windows
- Note - there is a bug where this count easily gets out of sync. Issue has been opened already. No ill effects
- """
- self.NumOpenWindows += 1
- # print('+++++ INCREMENTING Num Open Windows = {} ---'.format(Window.NumOpenWindows))
-
- @classmethod
- def _DecrementOpenCount(self):
- """
- Not user callable! Decrements the number of open windows
- """
- self.NumOpenWindows -= 1 * (self.NumOpenWindows != 0) # decrement if not 0
- # print('----- DECREMENTING Num Open Windows = {} ---'.format(Window.NumOpenWindows))
-
- @classmethod
- def get_screen_size(self):
- """
- This is a "Class Method" meaning you call it by writing: width, height = Window.get_screen_size()
- Returns the size of the "screen" as determined by tkinter. This can vary depending on your operating system and the number of monitors installed on your system. For Windows, the primary monitor's size is returns. On some multi-monitored Linux systems, the monitors are combined and the total size is reported as if one screen.
-
- :return: Size of the screen in pixels as determined by tkinter
- :rtype: (int, int)
- """
- root = _get_hidden_master_root()
- screen_width = root.winfo_screenwidth()
- screen_height = root.winfo_screenheight()
- return screen_width, screen_height
-
- @property
- def metadata(self):
- """
- Metadata is available for all windows. You can set to any value.
- :return: the current metadata value
- :rtype: (Any)
- """
- return self._metadata
-
- @metadata.setter
- def metadata(self, value):
- """
- Metadata is available for all windows. You can set to any value.
- :param value: Anything you want it to be
- :type value: (Any)
- """
- self._metadata = value
-
- # ------------------------- Add ONE Row to Form ------------------------- #
- def add_row(self, *args):
- """
- Adds a single row of elements to a window's self.Rows variables.
- Generally speaking this is NOT how users should be building Window layouts.
- Users, create a single layout (a list of lists) and pass as a parameter to Window object, or call Window.Layout(layout)
-
- :param *args: List[Elements]
- :type *args:
- """
- NumRows = len(self.Rows) # number of existing rows is our row number
- CurrentRowNumber = NumRows # this row's number
- CurrentRow = [] # start with a blank row and build up
- # ------------------------- Add the elements to a row ------------------------- #
- for i, element in enumerate(args): # Loop through list of elements and add them to the row
-
- if isinstance(element, tuple) or isinstance(element, list):
- self.add_row(*element)
- continue
- _error_popup_with_traceback('Error creating Window layout',
- 'Layout has a LIST instead of an ELEMENT',
- 'This means you have a badly placed ]',
- 'The offensive list is:',
- element,
- 'This list will be stripped from your layout'
- )
- continue
- elif callable(element) and not isinstance(element, Element):
- _error_popup_with_traceback('Error creating Window layout',
- 'Layout has a FUNCTION instead of an ELEMENT',
- 'This likely means you are missing () from your layout',
- 'The offensive list is:',
- element,
- 'This item will be stripped from your layout')
- continue
- if element.ParentContainer is not None:
- warnings.warn(
- '*** YOU ARE ATTEMPTING TO RESUSE AN ELEMENT IN YOUR LAYOUT! Once placed in a layout, an element cannot be used in another layout. ***',
- UserWarning)
- _error_popup_with_traceback('Error creating Window layout',
- 'You have broken the layout reuse rule! Thou shall not reuse layouts.',
- 'The layout specified has already been used',
- 'You MUST start witha "clean", unused layout every time you create a window',
- 'The offensive Element = ',
- element,
- 'and has a key = ', element.Key,
- 'This item will be stripped from your layout',
- 'Hint - try printing your layout and matching the IDs "print(layout)"')
- continue
- element.Position = (CurrentRowNumber, i)
- element.ParentContainer = self
- CurrentRow.append(element)
- # if this element is a titlebar, then automatically set the window margins to (0,0) and turn off normal titlebar
- if element.metadata == TITLEBAR_METADATA_MARKER:
- self.Margins = (0, 0)
- self.NoTitleBar = True
- # ------------------------- Append the row to list of Rows ------------------------- #
- self.Rows.append(CurrentRow)
-
- # ------------------------- Add Multiple Rows to Form ------------------------- #
- def add_rows(self, rows):
- """
- Loops through a list of lists of elements and adds each row, list, to the layout.
- This is NOT the best way to go about creating a window. Sending the entire layout at one time and passing
- it as a parameter to the Window call is better.
-
- :param rows: A list of a list of elements
- :type rows: List[List[Elements]]
- """
- for row in rows:
- try:
- iter(row)
- except TypeError:
- _error_popup_with_traceback('Error Creating Window Layout', 'Error creating Window layout',
- 'Your row is not an iterable (e.g. a list)',
- 'Instead of a list, the type found was {}'.format(type(row)),
- 'The offensive row = ',
- row,
- 'This item will be stripped from your layout')
- continue
- self.add_row(*row)
-
- def layout(self, rows):
- """
- Second of two preferred ways of telling a Window what its layout is. The other way is to pass the layout as
- a parameter to Window object. The parameter method is the currently preferred method. This call to Layout
- has been removed from examples contained in documents and in the Demo Programs. Trying to remove this call
- from history and replace with sending as a parameter to Window.
-
- :param rows: Your entire layout
- :type rows: List[List[Elements]]
- :return: self so that you can chain method calls
- :rtype: (Window)
- """
- if self.use_custom_titlebar and not self.override_custom_titlebar:
- if self.titlebar_icon is not None:
- icon = self.titlebar_icon
- elif CUSTOM_TITLEBAR_ICON is not None:
- icon = CUSTOM_TITLEBAR_ICON
- elif self.titlebar_icon is not None:
- icon = self.titlebar_icon
- elif self.WindowIcon == DEFAULT_WINDOW_ICON:
- icon = DEFAULT_BASE64_ICON_16_BY_16
- else:
- icon = None
-
- new_rows = [[Titlebar(title=self.Title, icon=icon, text_color=self.titlebar_text_color, background_color=self.titlebar_background_color,
- font=self.titlebar_font)]] + rows
- else:
- new_rows = rows
- self.add_rows(new_rows)
- self._BuildKeyDict()
-
- if self._has_custom_titlebar_element():
- self.Margins = (0, 0)
- self.NoTitleBar = True
- self._has_custom_titlebar = True
- return self
-
- def extend_layout(self, container, rows):
- """
- Adds new rows to an existing container element inside of this window
- If the container is a scrollable Column, you need to also call the contents_changed() method
-
- :param container: The container Element the layout will be placed inside of
- :type container: Frame | Column | Tab
- :param rows: The layout to be added
- :type rows: (List[List[Element]])
- :return: (Window) self so could be chained
- :rtype: (Window)
- """
- column = Column(rows, pad=(0, 0))
- if self == container:
- frame = self.TKroot
- elif isinstance(container.Widget, TkScrollableFrame):
- frame = container.Widget.TKFrame
- else:
- frame = container.Widget
- PackFormIntoFrame(column, frame, self)
- # sg.PackFormIntoFrame(col, window.TKroot, window)
- self.AddRow(column)
- self.AllKeysDict = self._BuildKeyDictForWindow(self, column, self.AllKeysDict)
- return self
-
- def LayoutAndRead(self, rows, non_blocking=False):
- """
- Deprecated!! Now your layout your window's rows (layout) and then separately call Read.
-
- :param rows: The layout of the window
- :type rows: List[List[Element]]
- :param non_blocking: if True the Read call will not block
- :type non_blocking: (bool)
- """
- _error_popup_with_traceback('LayoutAndRead Depricated', 'Wow! You have been using PySimpleGUI for a very long time.',
- 'The Window.LayoutAndRead call is no longer supported')
-
- raise DeprecationWarning(
- 'LayoutAndRead is no longer supported... change your call window.Layout(layout).Read()\nor window(title, layout).Read()')
- # self.AddRows(rows)
- # self._Show(non_blocking=non_blocking)
- # return self.ReturnValues
-
- def LayoutAndShow(self, rows):
- """
- Deprecated - do not use any longer. Layout your window and then call Read. Or can add a Finalize call before the Read
- """
- raise DeprecationWarning('LayoutAndShow is no longer supported... ')
-
- def _Show(self, non_blocking=False):
- """
- NOT TO BE CALLED BY USERS. INTERNAL ONLY!
- It's this method that first shows the window to the user, collects results
-
- :param non_blocking: if True, this is a non-blocking call
- :type non_blocking: (bool)
- :return: Tuple[Any, Dict] The event, values turple that is returned from Read calls
- :rtype:
- """
- self.Shown = True
- # Compute num rows & num cols (it'll come in handy debugging)
- self.NumRows = len(self.Rows)
- self.NumCols = max(len(row) for row in self.Rows)
- self.NonBlocking = non_blocking
-
- # Search through entire form to see if any elements set the focus
- # if not, then will set the focus to the first input element
- found_focus = False
- for row in self.Rows:
- for element in row:
- try:
- if element.Focus:
- found_focus = True
- except:
- pass
- try:
- if element.Key is not None:
- self.UseDictionary = True
- except:
- pass
-
- if not found_focus and self.UseDefaultFocus:
- self.UseDefaultFocus = True
- else:
- self.UseDefaultFocus = False
- # -=-=-=-=-=-=-=-=- RUN the GUI -=-=-=-=-=-=-=-=- ##
- StartupTK(self)
- # If a button or keyboard event happened but no results have been built, build the results
- if self.LastKeyboardEvent is not None or self.LastButtonClicked is not None:
- return _BuildResults(self, False, self)
- return self.ReturnValues
-
- # ------------------------- SetIcon - set the window's fav icon ------------------------- #
- def set_icon(self, icon=None, pngbase64=None):
- """
- Changes the icon that is shown on the title bar and on the task bar.
- NOTE - The file type is IMPORTANT and depends on the OS!
- Can pass in:
- * filename which must be a .ICO icon file for windows, PNG file for Linux
- * bytes object
- * BASE64 encoded file held in a variable
-
- :param icon: Filename or bytes object
- :type icon: (str)
- :param pngbase64: Base64 encoded image
- :type pngbase64: (bytes)
- """
- if type(icon) is bytes or pngbase64 is not None:
- wicon = tkinter.PhotoImage(data=icon if icon is not None else pngbase64)
- try:
- self.TKroot.tk.call('wm', 'iconphoto', self.TKroot._w, wicon)
- except:
- wicon = tkinter.PhotoImage(data=DEFAULT_BASE64_ICON)
- try:
- self.TKroot.tk.call('wm', 'iconphoto', self.TKroot._w, wicon)
- except:
- pass
- self.WindowIcon = wicon
- return
-
- wicon = icon
- try:
- self.TKroot.iconbitmap(icon)
- except:
- try:
- wicon = tkinter.PhotoImage(file=icon)
- self.TKroot.tk.call('wm', 'iconphoto', self.TKroot._w, wicon)
- except:
- try:
- wicon = tkinter.PhotoImage(data=DEFAULT_BASE64_ICON)
- try:
- self.TKroot.tk.call('wm', 'iconphoto', self.TKroot._w, wicon)
- except:
- pass
- except:
- pass
- self.WindowIcon = wicon
-
- def _GetElementAtLocation(self, location):
- """
- Given a (row, col) location in a layout, return the element located at that position
-
- :param location: (int, int) Return the element located at (row, col) in layout
- :type location:
- :return: (Element) The Element located at that position in this window
- :rtype:
- """
-
- (row_num, col_num) = location
- row = self.Rows[row_num]
- element = row[col_num]
- return element
-
- def _GetDefaultElementSize(self):
- """
- Returns the default elementSize
-
- :return: (width, height) of the default element size
- :rtype: (int, int)
- """
-
- return self.DefaultElementSize
-
- def _AutoCloseAlarmCallback(self):
- """
- Function that's called by tkinter when autoclode timer expires. Closes the window
-
- """
- try:
- window = self
- if window:
- if window.NonBlocking:
- self.Close()
- else:
- window._Close()
- self.TKroot.quit()
- self.RootNeedsDestroying = True
- except:
- pass
-
- def _TimeoutAlarmCallback(self):
- """
- Read Timeout Alarm callback. Will kick a mainloop call out of the tkinter event loop and cause it to return
- """
- # first, get the results table built
- # modify the Results table in the parent FlexForm object
- # print('TIMEOUT CALLBACK')
- if self.TimerCancelled:
- # print('** timer was cancelled **')
- return
- self.LastButtonClicked = self.TimeoutKey
- self.FormRemainedOpen = True
- self.TKroot.quit() # kick the users out of the mainloop
-
- def _calendar_chooser_button_clicked(self, elem):
- """
-
- :param elem:
- :type elem:
- :return:
- :rtype:
- """
- target_element, strvar, should_submit_window = elem._find_target()
-
- if elem.calendar_default_date_M_D_Y == (None, None, None):
- now = datetime.datetime.now()
- cur_month, cur_day, cur_year = now.month, now.day, now.year
- else:
- cur_month, cur_day, cur_year = elem.calendar_default_date_M_D_Y
-
- date_chosen = popup_get_date(start_mon=cur_month, start_day=cur_day, start_year=cur_year, close_when_chosen=elem.calendar_close_when_chosen,
- no_titlebar=elem.calendar_no_titlebar, begin_at_sunday_plus=elem.calendar_begin_at_sunday_plus,
- locale=elem.calendar_locale, location=elem.calendar_location, month_names=elem.calendar_month_names,
- day_abbreviations=elem.calendar_day_abbreviations, title=elem.calendar_title)
- if date_chosen is not None:
- month, day, year = date_chosen
- now = datetime.datetime.now()
- hour, minute, second = now.hour, now.minute, now.second
- try:
- date_string = calendar.datetime.datetime(year, month, day, hour, minute, second).strftime(elem.calendar_format)
- except Exception as e:
- print('Bad format string in calendar chooser button', e)
- date_string = 'Bad format string'
-
- if target_element is not None and target_element != elem:
- target_element.update(date_string)
- elif target_element == elem:
- elem.calendar_selection = date_string
-
- strvar.set(date_string)
- elem.TKStringVar.set(date_string)
- if should_submit_window:
- self.LastButtonClicked = target_element.Key
- results = _BuildResults(self, False, self)
- else:
- should_submit_window = False
- return should_submit_window
-
- # @_timeit_summary
- def read(self, timeout=None, timeout_key=TIMEOUT_KEY, close=False):
- """
- THE biggest deal method in the Window class! This is how you get all of your data from your Window.
- Pass in a timeout (in milliseconds) to wait for a maximum of timeout milliseconds. Will return timeout_key
- if no other GUI events happen first.
-
- :param timeout: Milliseconds to wait until the Read will return IF no other GUI events happen first
- :type timeout: (int)
- :param timeout_key: The value that will be returned from the call if the timer expired
- :type timeout_key: (Any)
- :param close: if True the window will be closed prior to returning
- :type close: (bool)
- :return: (event, values)
- :rtype: Tuple[(Any), Dict[Any, Any], List[Any], None]
- """
-
- if Window._floating_debug_window_build_needed is True:
- Window._floating_debug_window_build_needed = False
- _Debugger.debugger._build_floating_window()
-
- if Window._main_debug_window_build_needed is True:
- Window._main_debug_window_build_needed = False
- _Debugger.debugger._build_main_debugger_window()
-
- # ensure called only 1 time through a single read cycle
- if not Window._read_call_from_debugger:
- _refresh_debugger()
-
- # if the user has not added timeout and a debug window is open, then set a timeout for them so the debugger continuously refreshes
- if _debugger_window_is_open() and not Window._read_call_from_debugger:
- if timeout is None or timeout > 3000:
- timeout = 200
-
-
- while True:
- Window._root_running_mainloop = self.TKroot
- results = self._read(timeout=timeout, timeout_key=timeout_key)
- if results is not None:
- if results[0] == DEFAULT_WINDOW_SNAPSHOT_KEY:
- self.save_window_screenshot_to_disk()
- popup_quick_message('Saved window screenshot to disk', background_color='#1c1e23', text_color='white', keep_on_top=True, font='_ 30')
- continue
- # Post processing for Calendar Chooser Button
- try:
- if results[0] == timeout_key: # if a timeout, then not a calendar button
- break
- elem = self.find_element(results[0], silent_on_error=True) # get the element that caused the event
- if elem.Type == ELEM_TYPE_BUTTON:
- if elem.BType == BUTTON_TYPE_CALENDAR_CHOOSER:
- if self._calendar_chooser_button_clicked(elem): # returns True if should break out
- # results[0] = self.LastButtonClicked
- results = self.ReturnValues
- break
- else:
- continue
- break
- except:
- break # wasn't a calendar button for sure
-
-
- if close:
- self.close()
-
- return results
-
- # @_timeit
- def _read(self, timeout=None, timeout_key=TIMEOUT_KEY):
- """
- THE biggest deal method in the Window class! This is how you get all of your data from your Window.
- Pass in a timeout (in milliseconds) to wait for a maximum of timeout milliseconds. Will return timeout_key
- if no other GUI events happen first.
-
- :param timeout: Milliseconds to wait until the Read will return IF no other GUI events happen first
- :type timeout: (int)
- :param timeout_key: The value that will be returned from the call if the timer expired
- :type timeout_key: (Any)
- :return: (event, values) (event or timeout_key or None, Dictionary of values or List of values from all elements in the Window)
- :rtype: Tuple[(Any), Dict[Any, Any], List[Any], None]
- """
-
- # if there are events in the thread event queue, then return those events before doing anything else.
- if self._queued_thread_event_available():
- self.ReturnValues = results = _BuildResults(self, False, self)
- return results
-
- if self.finalize_in_progress and self.auto_close_timer_needs_starting:
- self._start_autoclose_timer()
- self.auto_close_timer_needs_starting = False
-
- timeout = int(timeout) if timeout is not None else None
- if timeout == 0: # timeout of zero runs the old readnonblocking
- event, values = self._ReadNonBlocking()
- if event is None:
- event = timeout_key
- if values is None:
- event = None
- return event, values # make event None if values was None and return
- # Read with a timeout
- self.Timeout = timeout
- self.TimeoutKey = timeout_key
- self.NonBlocking = False
- if self.TKrootDestroyed:
- self.read_closed_window_count += 1
- if self.read_closed_window_count > 100:
- popup_error_with_traceback('Trying to read a closed window', 'You have tried 100 times to read a closed window.', 'You need to add a check for event == WIN_CLOSED',)
- return None, None
- if not self.Shown:
- self._Show()
- else:
- # if already have a button waiting, the return previously built results
- if self.LastButtonClicked is not None and not self.LastButtonClickedWasRealtime:
- results = _BuildResults(self, False, self)
- self.LastButtonClicked = None
- return results
- InitializeResults(self)
-
- if self._queued_thread_event_available():
- self.ReturnValues = results = _BuildResults(self, False, self)
- return results
-
- # if the last button clicked was realtime, emulate a read non-blocking
- # the idea is to quickly return realtime buttons without any blocks until released
- if self.LastButtonClickedWasRealtime:
- # clear the realtime flag if the element is not a button element (for example a graph element that is dragging)
- if self.AllKeysDict.get(self.LastButtonClicked, None):
- if self.AllKeysDict.get(self.LastButtonClicked).Type != ELEM_TYPE_BUTTON:
- self.LastButtonClickedWasRealtime = False # stops from generating events until something changes
- else: # it is possible for the key to not be in the dicitonary because it has a modifier. If so, then clear the realtime button flag
- self.LastButtonClickedWasRealtime = False # stops from generating events until something changes
-
- try:
- rc = self.TKroot.update()
- except:
- self.TKrootDestroyed = True
- Window._DecrementOpenCount()
- # _my_windows.Decrement()
- # print('ROOT Destroyed')
- results = _BuildResults(self, False, self)
- if results[0] != None and results[0] != timeout_key:
- return results
- else:
- pass
-
- # else:
- # print("** REALTIME PROBLEM FOUND **", results)
-
- if self.RootNeedsDestroying:
- # print('*** DESTROYING really late***')
- try:
- self.TKroot.destroy()
- except:
- pass
- # _my_windows.Decrement()
- self.LastButtonClicked = None
- return None, None
-
- # normal read blocking code....
- if timeout != None:
- self.TimerCancelled = False
- self.TKAfterID = self.TKroot.after(timeout, self._TimeoutAlarmCallback)
- self.CurrentlyRunningMainloop = True
- # self.TKroot.protocol("WM_DESTROY_WINDOW", self._OnClosingCallback)
- # self.TKroot.protocol("WM_DELETE_WINDOW", self._OnClosingCallback)
- Window._window_running_mainloop = self
- Window._root_running_mainloop.mainloop()
-
- # print('Out main')
- self.CurrentlyRunningMainloop = False
- # if self.LastButtonClicked != TIMEOUT_KEY:
- try:
- self.TKroot.after_cancel(self.TKAfterID)
- del self.TKAfterID
- except:
- pass
- # print('** tkafter cancel failed **')
- self.TimerCancelled = True
- if self.RootNeedsDestroying:
- # print('*** DESTROYING LATE ***')
- try:
- self.TKroot.destroy()
- except:
- pass
- Window._DecrementOpenCount()
- # _my_windows.Decrement()
- self.LastButtonClicked = None
- return None, None
- # if form was closed with X
- if self.LastButtonClicked is None and self.LastKeyboardEvent is None and self.ReturnValues[0] is None:
- Window._DecrementOpenCount()
- # _my_windows.Decrement()
- # Determine return values
- if self.LastKeyboardEvent is not None or self.LastButtonClicked is not None:
- results = _BuildResults(self, False, self)
- if not self.LastButtonClickedWasRealtime:
- self.LastButtonClicked = None
- return results
- else:
- if self._queued_thread_event_available():
- self.ReturnValues = results = _BuildResults(self, False, self)
- return results
- if not self.XFound and self.Timeout != 0 and self.Timeout is not None and self.ReturnValues[
- 0] is None: # Special Qt case because returning for no reason so fake timeout
- self.ReturnValues = self.TimeoutKey, self.ReturnValues[1] # fake a timeout
- elif not self.XFound and self.ReturnValues[0] is None: # Return a timeout event... can happen when autoclose used on another window
- # print("*** Faking timeout ***")
- self.ReturnValues = self.TimeoutKey, self.ReturnValues[1] # fake a timeout
- return self.ReturnValues
-
- def _ReadNonBlocking(self):
- """
- Should be NEVER called directly by the user. The user can call Window.Read(timeout=0) to get same effect
-
- :return: (event, values). (event or timeout_key or None, Dictionary of values or List of values from all elements in the Window)
- :rtype: Tuple[(Any), Dict[Any, Any] | List[Any] | None]
- """
- if self.TKrootDestroyed:
- try:
- self.TKroot.quit()
- self.TKroot.destroy()
- except:
- pass
- # print('DESTROY FAILED')
- return None, None
- if not self.Shown:
- self._Show(non_blocking=True)
- try:
- rc = self.TKroot.update()
- except:
- self.TKrootDestroyed = True
- Window._DecrementOpenCount()
- # _my_windows.Decrement()
- # print("read failed")
- # return None, None
- if self.RootNeedsDestroying:
- # print('*** DESTROYING LATE ***', self.ReturnValues)
- self.TKroot.destroy()
- Window._DecrementOpenCount()
- # _my_windows.Decrement()
- self.Values = None
- self.LastButtonClicked = None
- return None, None
- return _BuildResults(self, False, self)
-
- def _start_autoclose_timer(self):
- duration = DEFAULT_AUTOCLOSE_TIME if self.AutoCloseDuration is None else self.AutoCloseDuration
- self.TKAfterID = self.TKroot.after(int(duration * 1000), self._AutoCloseAlarmCallback)
-
- def finalize(self):
- """
- Use this method to cause your layout to built into a real tkinter window. In reality this method is like
- Read(timeout=0). It doesn't block and uses your layout to create tkinter widgets to represent the elements.
- Lots of action!
-
- :return: Returns 'self' so that method "Chaining" can happen (read up about it as it's very cool!)
- :rtype: (Window)
- """
-
- if self.TKrootDestroyed:
- return self
- self.finalize_in_progress = True
-
- self.Read(timeout=1)
-
- if self.AutoClose:
- self.auto_close_timer_needs_starting = True
- # add the window to the list of active windows
- Window._active_windows[self] = Window.hidden_master_root
- return self
- # OLD CODE FOLLOWS
- if not self.Shown:
- self._Show(non_blocking=True)
- try:
- rc = self.TKroot.update()
- except:
- self.TKrootDestroyed = True
- Window._DecrementOpenCount()
- print('** Finalize failed **')
- # _my_windows.Decrement()
- # return None, None
- return self
-
- def refresh(self):
- """
- Refreshes the window by calling tkroot.update(). Can sometimes get away with a refresh instead of a Read.
- Use this call when you want something to appear in your Window immediately (as soon as this function is called).
- If you change an element in a window, your change will not be visible until the next call to Window.read
- or a call to Window.refresh()
-
- :return: `self` so that method calls can be easily "chained"
- :rtype: (Window)
- """
-
- if self.TKrootDestroyed:
- return self
- try:
- rc = self.TKroot.update()
- except:
- pass
- return self
-
- def fill(self, values_dict):
- """
- Fill in elements that are input fields with data based on a 'values dictionary'
-
- :param values_dict: pairs
- :type values_dict: (Dict[Any, Any]) - {Element_key : value}
- :return: returns self so can be chained with other methods
- :rtype: (Window)
- """
-
- FillFormWithValues(self, values_dict)
- return self
-
- def _find_closest_key(self, search_key):
- if not isinstance(search_key, str):
- search_key = str(search_key)
- matches = difflib.get_close_matches(search_key, [str(k) for k in self.AllKeysDict.keys()])
- if not len(matches):
- return None
- for k in self.AllKeysDict.keys():
- if matches[0] == str(k):
- return k
- return matches[0] if len(matches) else None
-
- def FindElement(self, key, silent_on_error=False):
- """
- ** Warning ** This call will eventually be depricated. **
-
- It is suggested that you modify your code to use the recommended window[key] lookup or the PEP8 compliant window.find_element(key)
-
- For now, you'll only see a message printed and the call will continue to funcation as before.
-
- :param key: Used with window.find_element and with return values to uniquely identify this element
- :type key: str | int | tuple | object
- :param silent_on_error: If True do not display popup nor print warning of key errors
- :type silent_on_error: (bool)
- :return: Return value can be: the Element that matches the supplied key if found; an Error Element if silent_on_error is False; None if silent_on_error True;
- :rtype: Element | Error Element | None
- """
-
- warnings.warn('Use of FindElement is not recommended.\nEither switch to the recommended window[key] format\nor the PEP8 compliant find_element',
- UserWarning)
- print('** Warning - FindElement should not be used to look up elements. window[key] or window.find_element are recommended. **')
-
- return self.find_element(key, silent_on_error=silent_on_error)
-
- def find_element(self, key, silent_on_error=False):
- """
- Find element object associated with the provided key.
- THIS METHOD IS NO LONGER NEEDED to be called by the user
-
- You can perform the same operation by writing this statement:
- element = window[key]
-
- You can drop the entire "find_element" function name and use [ ] instead.
-
- However, if you wish to perform a lookup without error checking, and don't have error popups turned
- off globally, you'll need to make this call so that you can disable error checks on this call.
-
- find_element is yypically used in combination with a call to element's Update method (or any other element method!):
- window[key].update(new_value)
-
- Versus the "old way"
- window.FindElement(key).Update(new_value)
-
- This call can be abbreviated to any of these:
- find_element = FindElement == Element == Find
- With find_element being the PEP8 compliant call that should be used.
-
- Rememeber that this call will return None if no match is found which may cause your code to crash if not
- checked for.
-
- :param key: Used with window.find_element and with return values to uniquely identify this element
- :type key: str | int | tuple | object
- :param silent_on_error: If True do not display popup nor print warning of key errors
- :type silent_on_error: (bool)
- :return: Return value can be: the Element that matches the supplied key if found; an Error Element if silent_on_error is False; None if silent_on_error True;
- :rtype: Element | Error Element | None
- """
- try:
- element = self.AllKeysDict[key]
- key_error = False
- except KeyError:
- closest_key = self._find_closest_key(key)
- if not silent_on_error:
- print('** Error looking up your element using the key: ', key, 'The closest matching key: ', closest_key)
- _error_popup_with_traceback('Key Error', 'Problem finding your key ' + str(key), 'Closest match = ' + str(closest_key))
- if not SUPPRESS_RAISE_KEY_ERRORS:
- raise KeyError(key)
- element = ErrorElement(key=key)
- key_error = True
- else:
- element = ErrorElement(key=key)
- return element
- if key_error:
- if closest_key is not None and not SUPPRESS_KEY_GUESSING:
- element = self.AllKeysDict[closest_key]
- return element
- if not SUPPRESS_RAISE_KEY_ERRORS:
- raise KeyError(key)
- return element
-
- Element = find_element # Shortcut function
- Find = find_element # Shortcut function, most likely not used by many people.
- Elem = find_element # NEW for 2019! More laziness... Another shortcut
-
- def find_element_with_focus(self):
- """
- Returns the Element that currently has focus as reported by tkinter. If no element is found None is returned!
- :return: An Element if one has been found with focus or None if no element found
- :rtype: Element | None
- """
- element = _FindElementWithFocusInSubForm(self)
- return element
-
-
- def widget_to_element(self, widget):
- """
- Returns the element that matches a supplied tkinter widget.
- If no matching element is found, then None is returned.
-
-
- :return: Element that uses the specified widget
- :rtype: Element | None
- """
- if self.AllKeysDict is None or len(self.AllKeysDict) == 0:
- return None
- for key, element in self.AllKeysDict.items():
- if element.Widget == widget:
- return element
- return None
-
-
- def _BuildKeyDict(self):
- """
- Used internally only! Not user callable
- Builds a dictionary containing all elements with keys for this window.
- """
- dict = {}
- self.AllKeysDict = self._BuildKeyDictForWindow(self, self, dict)
-
- def _BuildKeyDictForWindow(self, top_window, window, key_dict):
- """
- Loop through all Rows and all Container Elements for this window and create the keys for all of them.
- Note that the calls are recursive as all pathes must be walked
-
- :param top_window: The highest level of the window
- :type top_window: (Window)
- :param window: The "sub-window" (container element) to be searched
- :type window: Column | Frame | TabGroup | Pane | Tab
- :param key_dict: The dictionary as it currently stands.... used as part of recursive call
- :type key_dict:
- :return: (dict) Dictionary filled with all keys in the window
- :rtype:
- """
- for row_num, row in enumerate(window.Rows):
- for col_num, element in enumerate(row):
- if element.Type == ELEM_TYPE_COLUMN:
- key_dict = self._BuildKeyDictForWindow(top_window, element, key_dict)
- if element.Type == ELEM_TYPE_FRAME:
- key_dict = self._BuildKeyDictForWindow(top_window, element, key_dict)
- if element.Type == ELEM_TYPE_TAB_GROUP:
- key_dict = self._BuildKeyDictForWindow(top_window, element, key_dict)
- if element.Type == ELEM_TYPE_PANE:
- key_dict = self._BuildKeyDictForWindow(top_window, element, key_dict)
- if element.Type == ELEM_TYPE_TAB:
- key_dict = self._BuildKeyDictForWindow(top_window, element, key_dict)
- if element.Key is None: # if no key has been assigned.... create one for input elements
- if element.Type == ELEM_TYPE_BUTTON:
- element.Key = element.ButtonText
- elif element.Type == ELEM_TYPE_TAB:
- element.Key = element.Title
- if element.Type in (ELEM_TYPE_MENUBAR, ELEM_TYPE_BUTTONMENU,
- ELEM_TYPE_INPUT_SLIDER, ELEM_TYPE_GRAPH, ELEM_TYPE_IMAGE,
- ELEM_TYPE_INPUT_CHECKBOX, ELEM_TYPE_INPUT_LISTBOX, ELEM_TYPE_INPUT_COMBO,
- ELEM_TYPE_INPUT_MULTILINE, ELEM_TYPE_INPUT_OPTION_MENU, ELEM_TYPE_INPUT_SPIN,
- ELEM_TYPE_INPUT_RADIO, ELEM_TYPE_INPUT_TEXT, ELEM_TYPE_PROGRESS_BAR,
- ELEM_TYPE_TABLE, ELEM_TYPE_TREE,
- ELEM_TYPE_TAB_GROUP, ELEM_TYPE_SEPARATOR):
- element.Key = top_window.DictionaryKeyCounter
- top_window.DictionaryKeyCounter += 1
- if element.Key is not None:
- if element.Key in key_dict.keys():
- if element.Type == ELEM_TYPE_BUTTON and WARN_DUPLICATE_BUTTON_KEY_ERRORS: # for Buttons see if should complain
- warnings.warn('*** Duplicate key found in your layout {} ***'.format(element.Key), UserWarning)
- warnings.warn('*** Replaced new key with {} ***'.format(str(element.Key) + str(self.UniqueKeyCounter)))
- if not SUPPRESS_ERROR_POPUPS:
- _error_popup_with_traceback('Duplicate key found in your layout', 'Dupliate key: {}'.format(element.Key),
- 'Is being replaced with: {}'.format(str(element.Key) + str(self.UniqueKeyCounter)),
- 'The line of code above shows you which layout, but does not tell you exactly where the element was defined',
- 'The element type is {}'.format(element.Type))
- element.Key = str(element.Key) + str(self.UniqueKeyCounter)
- self.UniqueKeyCounter += 1
- key_dict[element.Key] = element
- return key_dict
-
- def element_list(self):
- """
- Returns a list of all elements in the window
-
- :return: List of all elements in the window and container elements in the window
- :rtype: List[Element]
- """
- return self._build_element_list()
-
- def _build_element_list(self):
- """
- Used internally only! Not user callable
- Builds a dictionary containing all elements with keys for this window.
- """
- elem_list = []
- elem_list = self._build_element_list_for_form(self, self, elem_list)
- return elem_list
-
- def _build_element_list_for_form(self, top_window, window, elem_list):
- """
- Loop through all Rows and all Container Elements for this window and create a list
- Note that the calls are recursive as all pathes must be walked
-
- :param top_window: The highest level of the window
- :type top_window: (Window)
- :param window: The "sub-window" (container element) to be searched
- :type window: Column | Frame | TabGroup | Pane | Tab
- :param elem_list: The element list as it currently stands.... used as part of recursive call
- :type elem_list: ???
- :return: List of all elements in this sub-window
- :rtype: List[Element]
- """
- for row_num, row in enumerate(window.Rows):
- for col_num, element in enumerate(row):
- elem_list.append(element)
- if element.Type in (ELEM_TYPE_COLUMN, ELEM_TYPE_FRAME, ELEM_TYPE_TAB_GROUP, ELEM_TYPE_PANE, ELEM_TYPE_TAB):
- elem_list = self._build_element_list_for_form(top_window, element, elem_list)
- return elem_list
-
- def save_to_disk(self, filename):
- """
- Saves the values contained in each of the input areas of the form. Basically saves what would be returned from a call to Read. It takes these results and saves them to disk using pickle.
- Note that every element in your layout that is to be saved must have a key assigned to it.
-
- :param filename: Filename to save the values to in pickled form
- :type filename: str
- """
- try:
- event, values = _BuildResults(self, False, self)
- remove_these = []
- for key in values:
- if self.Element(key).Type == ELEM_TYPE_BUTTON:
- remove_these.append(key)
- for key in remove_these:
- del values[key]
- with open(filename, 'wb') as sf:
- pickle.dump(values, sf)
- except:
- print('*** Error saving Window contents to disk ***')
-
- def load_from_disk(self, filename):
- """
- Restore values from a previous call to SaveToDisk which saves the returned values dictionary in Pickle format
-
- :param filename: Pickle Filename to load
- :type filename: (str)
- """
- try:
- with open(filename, 'rb') as df:
- self.Fill(pickle.load(df))
- except:
- print('*** Error loading form to disk ***')
-
- def get_screen_dimensions(self):
- """
- Get the screen dimensions. NOTE - you must have a window already open for this to work (blame tkinter not me)
-
- :return: Tuple containing width and height of screen in pixels
- :rtype: Tuple[None, None] | Tuple[width, height]
- """
-
- if self.TKrootDestroyed or self.TKroot is None:
- return Window.get_screen_size()
- screen_width = self.TKroot.winfo_screenwidth() # get window info to move to middle of screen
- screen_height = self.TKroot.winfo_screenheight()
- return screen_width, screen_height
-
- def move(self, x, y):
- """
- Move the upper left corner of this window to the x,y coordinates provided
- :param x: x coordinate in pixels
- :type x: (int)
- :param y: y coordinate in pixels
- :type y: (int)
- """
- try:
- self.TKroot.geometry("+%s+%s" % (x, y))
- self.config_last_location = (int(x), (int(y)))
-
- except:
- pass
-
-
- def move_to_center(self):
- """
- Recenter your window after it's been moved or the size changed.
-
- This is a conveinence method. There are no tkinter calls involved, only pure PySimpleGUI API calls.
- """
- if not self._is_window_created('tried Window.move_to_center'):
- return
- screen_width, screen_height = self.get_screen_dimensions()
- win_width, win_height = self.size
- x, y = (screen_width - win_width)//2, (screen_height - win_height)//2
- self.move(x, y)
-
-
-
- def minimize(self):
- """
- Minimize this window to the task bar
- """
- if not self._is_window_created('tried Window.minimize'):
- return
- self.TKroot.iconify()
- self.maximized = False
-
- def maximize(self):
- """
- Maximize the window. This is done differently on a windows system versus a linux or mac one. For non-Windows
- the root attribute '-fullscreen' is set to True. For Windows the "root" state is changed to "zoomed"
- The reason for the difference is the title bar is removed in some cases when using fullscreen option
- """
-
- if not self._is_window_created('tried Window.maximize'):
- return
- if not running_linux():
- self.TKroot.state('zoomed')
- else:
- self.TKroot.attributes('-fullscreen', True)
- # this method removes the titlebar too
- # self.TKroot.attributes('-fullscreen', True)
- self.maximized = True
-
- def normal(self):
- """
- Restore a window to a non-maximized state. Does different things depending on platform. See Maximize for more.
- """
- if not self._is_window_created('tried Window.normal'):
- return
- if self.TKroot.state() == 'iconic':
- self.TKroot.deiconify()
- else:
- if not running_linux():
- self.TKroot.state('normal')
- else:
- self.TKroot.attributes('-fullscreen', False)
- self.maximized = False
-
-
- def _StartMoveUsingControlKey(self, event):
- """
- Used by "Grab Anywhere" style windows. This function is bound to mouse-down. It marks the beginning of a drag.
- :param event: event information passed in by tkinter. Contains x,y position of mouse
- :type event: (event)
- """
-
- self._StartMove(event)
-
-
- def _StartMoveGrabAnywhere(self, event):
-
-
- """
- Used by "Grab Anywhere" style windows. This function is bound to mouse-down. It marks the beginning of a drag.
- :param event: event information passed in by tkinter. Contains x,y position of mouse
- :type event: (event)
- """
- if (isinstance(event.widget, GRAB_ANYWHERE_IGNORE_THESE_WIDGETS) or event.widget in self._grab_anywhere_ignore_these_list) and event.widget not in self._grab_anywhere_include_these_list:
- # print('Found widget to ignore in grab anywhere...')
- return
-
- self._StartMove(event)
-
-
- def _StartMove(self, event):
- try:
- geometry = self.TKroot.geometry()
- location = geometry[geometry.find('+')+1:].split('+')
- self._startx = int(location[0])
- self._starty = int(location[1])
- self._mousex = event.x + event.widget.winfo_rootx()
- self._mousey = event.y + event.widget.winfo_rooty()
- # print(self._startx, self._starty)
- # print(self._mousex, self._mousey)
- # print(self.TKroot.geometry())
- except:
- pass
- # print('Start move {},{} widget {}'.format(event.x,event.y, event.widget))
-
- if Window._move_all_windows:
- for window in Window._active_windows:
- window._offsetx = event.x + event.widget.winfo_rootx() - window.TKroot.winfo_rootx()
- window._offsety = event.y + event.widget.winfo_rooty() - window.TKroot.winfo_rooty()
-
-
-
- def _StopMove(self, event):
- """
- Used by "Grab Anywhere" style windows. This function is bound to mouse-up. It marks the ending of a drag.
- Sets the position of the window to this final x,y coordinates
- :param event: event information passed in by tkinter. Contains x,y position of mouse
- :type event: (event)
- """
- return
-
-
- def _OnMotionUsingControlKey(self, event):
- self._OnMotion(event)
-
-
- def _OnMotionGrabAnywhere(self, event):
-
- """
- Used by "Grab Anywhere" style windows. This function is bound to mouse motion. It actually moves the window
- :param event: event information passed in by tkinter. Contains x,y position of mouse
- :type event: (event)
- """
- if (isinstance(event.widget, GRAB_ANYWHERE_IGNORE_THESE_WIDGETS) or event.widget in self._grab_anywhere_ignore_these_list) and event.widget not in self._grab_anywhere_include_these_list:
- # print('Found widget to ignore in grab anywhere...')
- return
-
- self._OnMotion(event)
-
-
- def _OnMotion(self, event):
- try:
-
- _mousex = event.x + event.widget.winfo_rootx()
- _mousey = event.y + event.widget.winfo_rooty()
- deltax = _mousex - self._mousex
- deltay = _mousey - self._mousey
- x = self._startx + deltax
- y = self._starty + deltay
- self.TKroot.geometry("+%s+%s" % (x, y)) # this is what really moves the window
- if Window._move_all_windows:
- for window in Window._active_windows:
- deltax = window._offsetx
- deltay = window._offsety
- x = window.TKroot.winfo_pointerx() - deltax
- y = window.TKroot.winfo_pointery() - deltay
- window.TKroot.geometry("+%s+%s" % (x, y)) # this is what really moves the window
- except Exception as e:
- print('on motion error', e)
-
- def _focus_callback(self, event):
- print('Focus event = {} window = {}'.format(event, self.Title))
-
- def _config_callback(self, event):
- print('Config event = {} window = {}'.format(event, self.Title))
- #
- # try:
- # deltax = event.x - self.TKroot.x
- # deltay = event.y - self.TKroot.y
- # x = self.TKroot.winfo_x() + deltax
- # y = self.TKroot.winfo_y() + deltay
- # self.TKroot.geometry("+%s+%s" % (x, y)) # this is what really moves the window
- # # print('{},{}'.format(x,y))
- #
- # if Window._move_all_windows:
- # for window in Window._active_windows:
- # x = window.TKroot.winfo_x() + deltax
- # y = window.TKroot.winfo_y() + deltay
- # window.TKroot.geometry("+%s+%s" % (x, y)) # this is what really moves the window
- # except Exception as e:
- # print('on motion error {}'.format(e), 'title = {}'.format(window.Title))
-
- """
- def _config_callback(self, event):
- new_x = event.x
- new_y = event.y
-
-
- if self.not_completed_initial_movement:
- if self.starting_window_position != (new_x, new_y):
- return
- self.not_completed_initial_movement = False
- return
-
- if not self.saw_00:
- if new_x == 0 and new_y == 0:
- self.saw_00 = True
-
- # self.config_count += 1
- # if self.config_count < 40:
- # return
-
- print('Move LOGIC')
-
- if self.config_last_size != (event.width, event.height):
- self.config_last_size = (event.width, event.height)
-
- if self.config_last_location[0] != new_x or self.config_last_location[1] != new_y:
- if self.config_last_location == (None, None):
- self.config_last_location = (new_x, new_y)
- return
-
- deltax = self.config_last_location[0] - event.x
- deltay = self.config_last_location[1] - event.y
- if deltax == 0 and deltay == 0:
- print('not moving so returning')
- return
- if Window._move_all_windows:
- print('checking all windows')
- for window in Window._active_windows:
- if window == self:
- continue
- x = window.TKroot.winfo_x() + deltax
- y = window.TKroot.winfo_y() + deltay
- # window.TKroot.geometry("+%s+%s" % (x, y)) # this is what really moves the window
- # window.config_last_location = (x,y)
- """
-
- def _KeyboardCallback(self, event):
- """
- Window keyboard callback. Called by tkinter. Will kick user out of the tkinter event loop. Should only be
- called if user has requested window level keyboard events
-
- :param event: object provided by tkinter that contains the key information
- :type event: (event)
- """
- self.LastButtonClicked = None
- self.FormRemainedOpen = True
- if event.char != '':
- self.LastKeyboardEvent = event.char
- else:
- self.LastKeyboardEvent = str(event.keysym) + ':' + str(event.keycode)
- # if not self.NonBlocking:
- # _BuildResults(self, False, self)
- _exit_mainloop(self)
-
- def _MouseWheelCallback(self, event):
- """
- Called by tkinter when a mouse wheel event has happened. Only called if keyboard events for the window
- have been enabled
-
- :param event: object sent in by tkinter that has the wheel direction
- :type event: (event)
- """
- self.LastButtonClicked = None
- self.FormRemainedOpen = True
- self.LastKeyboardEvent = 'MouseWheel:Down' if event.delta < 0 or event.num == 5 else 'MouseWheel:Up'
- # if not self.NonBlocking:
- # _BuildResults(self, False, self)
- _exit_mainloop(self)
-
- def _Close(self, without_event=False):
- """
- The internal close call that does the real work of building. This method basically sets up for closing
- but doesn't destroy the window like the User's version of Close does
-
- :parm without_event: if True, then do not cause an event to be generated, "silently" close the window
- :type without_event: (bool)
- """
- try:
- self.TKroot.update()
- except:
- pass
-
- if not self.NonBlocking or not without_event:
- _BuildResults(self, False, self)
- if self.TKrootDestroyed:
- return
- self.TKrootDestroyed = True
- self.RootNeedsDestroying = True
- return
-
- def close(self):
- """
- Closes window. Users can safely call even if window has been destroyed. Should always call when done with
- a window so that resources are properly freed up within your thread.
- """
- try:
- del Window._active_windows[self] # will only be in the list if window was explicitly finalized
- except:
- pass
-
- try:
- self.TKroot.update() # On Linux must call update if the user closed with X or else won't actually close the window
- except:
- pass
- if self.TKrootDestroyed:
- return
- try:
- self.TKroot.destroy()
- self.TKroot.update()
- Window._DecrementOpenCount()
- except:
- pass
- # if down to 1 window, try and destroy the hidden window, if there is one
- # if Window.NumOpenWindows == 1:
- # try:
- # Window.hidden_master_root.destroy()
- # Window.NumOpenWindows = 0 # if no hidden window, then this won't execute
- # except:
- # pass
- self.TKrootDestroyed = True
-
- # Free up anything that was held in the layout and the root variables
- self.Rows = None
- self.TKroot = None
-
- def is_closed(self):
- """
- Returns True is the window is maybe closed. Can be difficult to tell sometimes
-
- :return: True if the window was closed or destroyed
- :rtype: (bool)
- """
-
- if self.TKrootDestroyed or self.TKroot is None:
- return True
- # see if can do an update... if not, then it's been destroyed
- try:
- rc = self.TKroot.update()
- except:
- return True
- return False
-
-
- # IT FINALLY WORKED! 29-Oct-2018 was the first time this damned thing got called
- def _OnClosingCallback(self):
- """
- Internally used method ONLY. Not sure callable. tkinter calls this when the window is closed by clicking X
- """
- # global _my_windows
- # print('Got closing callback', self.DisableClose)
- if self.DisableClose:
- return
- if self.CurrentlyRunningMainloop: # quit if this is the current mainloop, otherwise don't quit!
- _exit_mainloop(self)
- if self.close_destroys_window:
- self.TKroot.destroy() # destroy this window
- self.TKrootDestroyed = True
- self.XFound = True
- else:
- self.LastButtonClicked = WINDOW_CLOSE_ATTEMPTED_EVENT
- elif Window._root_running_mainloop == Window.hidden_master_root:
- _exit_mainloop(self)
- else:
- if self.close_destroys_window:
- self.TKroot.destroy() # destroy this window
- self.XFound = True
- else:
- self.LastButtonClicked = WINDOW_CLOSE_ATTEMPTED_EVENT
- if self.close_destroys_window:
- self.RootNeedsDestroying = True
-
- def disable(self):
- """
- Disables window from taking any input from the user
- """
- if not self._is_window_created('tried Window.disable'):
- return
- self.TKroot.attributes('-disabled', 1)
- # self.TKroot.grab_set_global()
-
- def enable(self):
- """
- Re-enables window to take user input after having it be Disabled previously
- """
- if not self._is_window_created('tried Window.enable'):
- return
- self.TKroot.attributes('-disabled', 0)
- # self.TKroot.grab_release()
-
- def hide(self):
- """
- Hides the window from the screen and the task bar
- """
- if not self._is_window_created('tried Window.hide'):
- return
- self._Hidden = True
- self.TKroot.withdraw()
-
- def un_hide(self):
- """
- Used to bring back a window that was previously hidden using the Hide method
- """
- if not self._is_window_created('tried Window.un_hide'):
- return
- if self._Hidden:
- self.TKroot.deiconify()
- self._Hidden = False
-
- def disappear(self):
- """
- Causes a window to "disappear" from the screen, but remain on the taskbar. It does this by turning the alpha
- channel to 0. NOTE that on some platforms alpha is not supported. The window will remain showing on these
- platforms. The Raspberry Pi for example does not have an alpha setting
- """
- if not self._is_window_created('tried Window.disappear'):
- return
- self.TKroot.attributes('-alpha', 0)
-
- def reappear(self):
- """
- Causes a window previously made to "Disappear" (using that method). Does this by restoring the alpha channel
- """
- if not self._is_window_created('tried Window.reappear'):
- return
- self.TKroot.attributes('-alpha', 255)
-
- def set_alpha(self, alpha):
- """
- Sets the Alpha Channel for a window. Values are between 0 and 1 where 0 is completely transparent
-
- :param alpha: 0 to 1. 0 is completely transparent. 1 is completely visible and solid (can't see through)
- :type alpha: (float)
- """
- if not self._is_window_created('tried Window.set_alpha'):
- return
- self._AlphaChannel = alpha
- self.TKroot.attributes('-alpha', alpha)
-
- @property
- def alpha_channel(self):
- """
- A property that changes the current alpha channel value (internal value)
- :return: the current alpha channel setting according to self, not read directly from tkinter
- :rtype: (float)
- """
- return self._AlphaChannel
-
- @alpha_channel.setter
- def alpha_channel(self, alpha):
- """
- The setter method for this "property".
- Planning on depricating so that a Set call is always used by users. This is more in line with the SDK
- :param alpha: 0 to 1. 0 is completely transparent. 1 is completely visible and solid (can't see through)
- :type alpha: (float)
- """
- if not self._is_window_created('tried Window.alpha_channel'):
- return
- self._AlphaChannel = alpha
- self.TKroot.attributes('-alpha', alpha)
-
- def bring_to_front(self):
- """
- Brings this window to the top of all other windows (perhaps may not be brought before a window made to "stay
- on top")
- """
- if not self._is_window_created('tried Window.bring_to_front'):
- return
- if running_windows():
- try:
- self.TKroot.wm_attributes("-topmost", 0)
- self.TKroot.wm_attributes("-topmost", 1)
- if not self.KeepOnTop:
- self.TKroot.wm_attributes("-topmost", 0)
- except Exception as e:
- warnings.warn('Problem in Window.bring_to_front' + str(e), UserWarning)
- else:
- try:
- self.TKroot.lift()
- except:
- pass
-
- def send_to_back(self):
- """
- Pushes this window to the bottom of the stack of windows. It is the opposite of BringToFront
- """
- if not self._is_window_created('tried Window.send_to_back'):
- return
- try:
- self.TKroot.lower()
- except:
- pass
-
-
- def keep_on_top_set(self):
- """
- Sets keep_on_top after a window has been created. Effect is the same
- as if the window was created with this set. The Window is also brought
- to the front
- """
- if not self._is_window_created('tried Window.keep_on_top_set'):
- return
- self.KeepOnTop = True
- self.bring_to_front()
- try:
- self.TKroot.wm_attributes("-topmost", 1)
- except Exception as e:
- warnings.warn('Problem in Window.keep_on_top_set trying to set wm_attributes topmost' + str(e), UserWarning)
-
-
- def keep_on_top_clear(self):
- """
- Clears keep_on_top after a window has been created. Effect is the same
- as if the window was created with this set.
- """
- if not self._is_window_created('tried Window.keep_on_top_clear'):
- return
- self.KeepOnTop = False
- try:
- self.TKroot.wm_attributes("-topmost", 0)
- except Exception as e:
- warnings.warn('Problem in Window.keep_on_top_clear trying to clear wm_attributes topmost' + str(e), UserWarning)
-
-
-
- def current_location(self, more_accurate=False):
- """
- Get the current location of the window's top left corner.
- Sometimes, depending on the environment, the value returned does not include the titlebar,etc
- A new option, more_accurate, can be used to get the theoretical upper leftmost corner of the window.
- The titlebar and menubar are crated by the OS. It gets really confusing when running in a webpage (repl, trinket)
- Thus, the values can appear top be "off" due to the sometimes unpredictable way the location is calculated.
-
- :param more_accurate: If True, will use the window's geometry to get the topmost location with titlebar, menubar taken into account
- :type more_accurate: (bool)
- :return: The x and y location in tuple form (x,y)
- :rtype: Tuple[(int | None), (int | None)]
- """
-
- if not self._is_window_created('tried Window.current_location'):
- return (None, None)
- try:
- if more_accurate:
- geometry = self.TKroot.geometry()
- location = geometry[geometry.find('+') + 1:].split('+')
- x, y = int(location[0]), int(location[1])
- else:
- x, y = int(self.TKroot.winfo_x()), int(self.TKroot.winfo_y())
- except Exception as e:
- warnings.warn('Error in Window.current_location. Trouble getting x,y location\n' + str(e), UserWarning)
- x, y = (None, None)
- return (x,y)
-
- def current_size_accurate(self):
- """
- Get the current location of the window based on tkinter's geometry setting
-
- :return: The x and y size in tuple form (x,y)
- :rtype: Tuple[(int | None), (int | None)]
- """
-
- if not self._is_window_created('tried Window.current_location'):
- return (None, None)
- try:
- geometry = self.TKroot.geometry()
- geometry_tuple = geometry.split('+')
- window_size = geometry_tuple[0].split('x')
- x, y = int(window_size[0]), int(window_size[1])
- except Exception as e:
- warnings.warn('Error in Window.current_size_accurate. Trouble getting x,y size\n{} {}'.format(geometry, geometry_tuple) + str(e), UserWarning)
- x, y = (None, None)
- return (x,y)
-
- @property
- def size(self):
- """
- Return the current size of the window in pixels
-
- :return: (width, height) of the window
- :rtype: Tuple[(int), (int)] or Tuple[None, None]
- """
- if not self._is_window_created('Tried to use Window.size property'):
- return (None, None)
- win_width = self.TKroot.winfo_width()
- win_height = self.TKroot.winfo_height()
- return win_width, win_height
-
- @size.setter
- def size(self, size):
- """
- Changes the size of the window, if possible
-
- :param size: (width, height) of the desired window size
- :type size: (int, int)
- """
- try:
- self.TKroot.geometry("%sx%s" % (size[0], size[1]))
- self.TKroot.update_idletasks()
- except:
- pass
-
- def set_min_size(self, size):
- """
- Changes the minimum size of the window. Note Window must be read or finalized first.
-
- :param size: (width, height) tuple (int, int) of the desired window size in pixels
- :type size: (int, int)
- """
- if not self._is_window_created('tried Window.set_min_size'):
- return
- self.TKroot.minsize(size[0], size[1])
- self.TKroot.update_idletasks()
-
- def visibility_changed(self):
- """
- When making an element in a column or someplace that has a scrollbar, then you'll want to call this function
- prior to the column's contents_changed() method.
- """
- self.refresh()
-
- def set_transparent_color(self, color):
- """
- Set the color that will be transparent in your window. Areas with this color will be SEE THROUGH.
-
- :param color: Color string that defines the transparent color
- :type color: (str)
- """
- if not self._is_window_created('tried Window.set_transparent_color'):
- return
- try:
- self.TKroot.attributes('-transparentcolor', color)
- self.TransparentColor = color
- except:
- print('Transparent color not supported on this platform (windows only)')
-
- def mouse_location(self):
- """
- Return the (x,y) location of the mouse relative to the entire screen. It's the same location that
- you would use to create a window, popup, etc.
-
- :return: The location of the mouse pointer
- :rtype: (int, int)
- """
- if not self._is_window_created('tried Window.mouse_location'):
- return (0,0)
-
- return (self.TKroot.winfo_pointerx(), self.TKroot.winfo_pointery())
-
- def grab_any_where_on(self):
- """
- Turns on Grab Anywhere functionality AFTER a window has been created. Don't try on a window that's not yet
- been Finalized or Read.
- """
- if not self._is_window_created('tried Window.grab_any_where_on'):
- return
- self.TKroot.bind("", self._StartMoveGrabAnywhere)
- self.TKroot.bind("", self._StopMove)
- self.TKroot.bind("", self._OnMotionGrabAnywhere)
-
- def grab_any_where_off(self):
- """
- Turns off Grab Anywhere functionality AFTER a window has been created. Don't try on a window that's not yet
- been Finalized or Read.
- """
- if not self._is_window_created('tried Window.grab_any_where_off'):
- return
- self.TKroot.unbind("")
- self.TKroot.unbind("")
- self.TKroot.unbind("")
-
- def _user_bind_callback(self, bind_string, event, propagate=True):
- """
- Used when user binds a tkinter event directly to an element
-
- :param bind_string: The event that was bound so can lookup the key modifier
- :type bind_string: (str)
- :param event: Event data passed in by tkinter (not used)
- :type event:
- :param propagate: If True then tkinter will be told to propagate the event
- :type propagate: (bool)
- """
- # print('bind callback', bind_string, event)
- key = self.user_bind_dict.get(bind_string, '')
- self.user_bind_event = event
- if key is not None:
- self.LastButtonClicked = key
- else:
- self.LastButtonClicked = bind_string
- self.FormRemainedOpen = True
- # if self.CurrentlyRunningMainloop:
- # self.TKroot.quit()
- _exit_mainloop(self)
- return 'break' if propagate is not True else None
-
-
- def bind(self, bind_string, key, propagate=True):
- """
- Used to add tkinter events to a Window.
- The tkinter specific data is in the Window's member variable user_bind_event
- :param bind_string: The string tkinter expected in its bind function
- :type bind_string: (str)
- :param key: The event that will be generated when the tkinter event occurs
- :type key: str | int | tuple | object
- :param propagate: If True then tkinter will be told to propagate the event
- :type propagate: (bool)
- """
- if not self._is_window_created('tried Window.bind'):
- return
- try:
- self.TKroot.bind(bind_string, lambda evt: self._user_bind_callback(bind_string, evt, propagate))
- except Exception as e:
- self.TKroot.unbind_all(bind_string)
- return
- # _error_popup_with_traceback('Window.bind error', e)
- self.user_bind_dict[bind_string] = key
-
- def _callback_main_debugger_window_create_keystroke(self, event):
- """
- Called when user presses the key that creates the main debugger window
- March 2022 - now causes the user reads to return timeout events automatically
- :param event: (event) not used. Passed in event info
- :type event:
- """
- Window._main_debug_window_build_needed = True
- # exit the event loop in a way that resembles a timeout occurring
- self.LastButtonClicked = self.TimeoutKey
- self.FormRemainedOpen = True
- self.TKroot.quit() # kick the users out of the mainloop
-
- def _callback_popout_window_create_keystroke(self, event):
- """
- Called when user presses the key that creates the floating debugger window
- March 2022 - now causes the user reads to return timeout events automatically
- :param event: (event) not used. Passed in event info
- :type event:
- """
- Window._floating_debug_window_build_needed = True
- # exit the event loop in a way that resembles a timeout occurring
- self.LastButtonClicked = self.TimeoutKey
- self.FormRemainedOpen = True
- self.TKroot.quit() # kick the users out of the mainloop
-
- def enable_debugger(self):
- """
- Enables the internal debugger. By default, the debugger IS enabled
- """
- if not self._is_window_created('tried Window.enable_debugger'):
- return
- self.TKroot.bind('', self._callback_main_debugger_window_create_keystroke)
- self.TKroot.bind('', self._callback_popout_window_create_keystroke)
- self.DebuggerEnabled = True
-
- def disable_debugger(self):
- """
- Disable the internal debugger. By default the debugger is ENABLED
- """
- if not self._is_window_created('tried Window.disable_debugger'):
- return
- self.TKroot.unbind("")
- self.TKroot.unbind("")
- self.DebuggerEnabled = False
-
- def set_title(self, title):
- """
- Change the title of the window
-
- :param title: The string to set the title to
- :type title: (str)
- """
- if not self._is_window_created('tried Window.set_title'):
- return
- self.TKroot.wm_title(str(title))
-
- def make_modal(self):
- """
- Makes a window into a "Modal Window"
- This means user will not be able to interact with other windows until this one is closed
-
- NOTE - Sorry Mac users - you can't have modal windows.... lobby your tkinter Mac devs
- """
- if not self._is_window_created('tried Window.make_modal'):
- return
-
- if running_mac() and ENABLE_MAC_MODAL_DISABLE_PATCH:
- return
-
- # if modal windows have been disabled globally
- if not DEFAULT_MODAL_WINDOWS_ENABLED and not DEFAULT_MODAL_WINDOWS_FORCED:
- # if not DEFAULT_MODAL_WINDOWS_ENABLED:
- return
-
- try:
- self.TKroot.transient()
- self.TKroot.grab_set()
- self.TKroot.focus_force()
- except Exception as e:
- print('Exception trying to make modal', e)
-
- def force_focus(self):
- """
- Forces this window to take focus
- """
- if not self._is_window_created('tried Window.force_focus'):
- return
- self.TKroot.focus_force()
-
- def was_closed(self):
- """
- Returns True if the window was closed
-
- :return: True if the window is closed
- :rtype: bool
- """
- return self.TKrootDestroyed
-
- def set_cursor(self, cursor):
- """
- Sets the cursor for the window.
- If you do not want any mouse pointer, then use the string "none"
-
- :param cursor: The tkinter cursor name
- :type cursor: (str)
- """
-
- if not self._is_window_created('tried Window.set_cursor'):
- return
- try:
- self.TKroot.config(cursor=cursor)
- except Exception as e:
- print('Warning bad cursor specified ', cursor)
- print(e)
-
- def ding(self, display_number=0):
- """
- Make a "bell" sound. A capability provided by tkinter. Your window needs to be finalized prior to calling.
- Ring a display's bell is the tkinter description of the call.
- :param display_number: Passed to tkinter's bell method as parameter "displayof".
- :type display_number: int
- """
- if not self._is_window_created('tried Window.ding'):
- return
- try:
- self.TKroot.bell(display_number)
- except Exception as e:
- if not SUPPRESS_ERROR_POPUPS:
- _error_popup_with_traceback('Window.ding() - tkinter reported error from bell() call', e)
-
- def _window_tkvar_changed_callback(self, *args):
- """
- Internal callback function for when the thread
-
- :param event: Information from tkinter about the callback
- :type event:
-
- """
- # print('Thread callback info', threading.current_thread())
- # print(event)
- # trace_details = traceback.format_stack()
- # print(''.join(trace_details))
- # self.thread_lock.acquire()
- # if self.thread_timer:
- # self.TKroot.after_cancel(id=self.thread_timer)
- # self.thread_timer = None
- # self.thread_lock.release()
-
- if self._queued_thread_event_available():
- self.FormRemainedOpen = True
- _exit_mainloop(self)
-
- def _create_thread_queue(self):
- """
- Creates the queue used by threads to communicate with this window
- """
-
- if self.thread_queue is None:
- self.thread_queue = queue.Queue()
-
- if self.thread_lock is None:
- self.thread_lock = threading.Lock()
-
- if self.thread_strvar is None:
- self.thread_strvar = tk.StringVar()
- self.thread_strvar.trace('w', self._window_tkvar_changed_callback)
-
- def write_event_value(self, key, value):
- """
- Adds a key & value tuple to the queue that is used by threads to communicate with the window
-
- :param key: The key that will be returned as the event when reading the window
- :type key: Any
- :param value: The value that will be in the values dictionary
- :type value: Any
- """
-
- if self.thread_queue is None:
- print('*** Warning Window.write_event_value - no thread queue found ***')
- return
- # self.thread_lock.acquire() # first lock the critical section
- self.thread_queue.put(item=(key, value))
- self.TKroot.tk.willdispatch() # brilliant bit of code provided by Giuliano who I owe a million thank yous!
- self.thread_strvar.set('new item')
-
- # self.thread_queue.put(item=(key, value))
- # self.thread_strvar.set('new item')
- # March 28 2021 - finally found a solution! It needs a little more work and a lock
- # if no timer is running, then one should be started
- # if self.thread_timer is None:
- # print('Starting a timer')
- # self.thread_timer = self.TKroot.after(1, self._window_tkvar_changed_callback)
- # self.thread_lock.release()
-
- def _queued_thread_event_read(self):
- if self.thread_queue is None:
- return None
-
- try: # see if something has been posted to Queue
- message = self.thread_queue.get_nowait()
- except queue.Empty: # get_nowait() will get exception when Queue is empty
- return None
-
- return message
-
- def _queued_thread_event_available(self):
-
- if self.thread_queue is None:
- return False
- # self.thread_lock.acquire()
- qsize = self.thread_queue.qsize()
- if qsize == 0:
- self.thread_timer = None
- # self.thread_lock.release()
- return qsize != 0
-
-
-
- def _RightClickMenuCallback(self, event):
- """
- When a right click menu is specified for an entire window, then this callback catches right clicks
- that happen to the window itself, when there are no elements that are in that area.
-
- The only portion that is not currently covered correctly is the row frame itself. There will still
- be parts of the window, at the moment, that don't respond to a right click. It's getting there, bit
- by bit.
-
- Callback function that's called when a right click happens. Shows right click menu as result.
-
- :param event: information provided by tkinter about the event including x,y location of click
- :type event:
- """
- # if there are widgets under the mouse, then see if it's the root only. If not, then let the widget (element) show their menu instead
- x, y = self.TKroot.winfo_pointerxy()
- widget = self.TKroot.winfo_containing(x, y)
- if widget != self.TKroot:
- return
- self.TKRightClickMenu.tk_popup(event.x_root, event.y_root, 0)
- self.TKRightClickMenu.grab_release()
-
-
- def save_window_screenshot_to_disk(self, filename=None):
- """
- Saves an image of the PySimpleGUI window provided into the filename provided
-
- :param filename: Optional filename to save screenshot to. If not included, the User Settinds are used to get the filename
- :return: A PIL ImageGrab object that can be saved or manipulated
- :rtype: (PIL.ImageGrab | None)
- """
- global pil_import_attempted, pil_imported, PIL, ImageGrab, Image
-
- if not pil_import_attempted:
- try:
- import PIL as PIL
- from PIL import ImageGrab
- from PIL import Image
- pil_imported = True
- pil_import_attempted = True
- except:
- pil_imported = False
- pil_import_attempted = True
- print('FAILED TO IMPORT PIL!')
- return None
- try:
- # Get location of window to save
- pos = self.current_location()
- # Add a little to the X direction if window has a titlebar
- if not self.NoTitleBar:
- pos = (pos[0]+7, pos[1])
- # Get size of wiondow
- size = self.current_size_accurate()
- # Get size of the titlebar
- titlebar_height = self.TKroot.winfo_rooty() - self.TKroot.winfo_y()
- # Add titlebar to size of window so that titlebar and window will be saved
- size = (size[0], size[1] + titlebar_height)
- if not self.NoTitleBar:
- size_adjustment = (2,1)
- else:
- size_adjustment = (0,0)
- # Make the "Bounding rectangle" used by PLK to do the screen grap "operation
- rect = (pos[0], pos[1], pos[0] + size[0]+size_adjustment[0], pos[1] + size[1]+size_adjustment[1])
- # Grab the image
- grab = ImageGrab.grab(bbox=rect)
- # Save the grabbed image to disk
- except Exception as e:
- # print(e)
- popup_error_with_traceback('Screen capture failure', 'Error happened while trying to save screencapture', e)
-
- return None
- # return grab
- if filename is None:
- folder = pysimplegui_user_settings.get('-screenshots folder-', '')
- filename = pysimplegui_user_settings.get('-screenshots filename-', '')
- full_filename = os.path.join(folder, filename)
- else:
- full_filename = filename
- if full_filename:
- try:
- grab.save(full_filename)
- except Exception as e:
- popup_error_with_traceback('Screen capture failure', 'Error happened while trying to save screencapture', e)
- else:
- popup_error_with_traceback('Screen capture failure', 'You have attempted a screen capture but have not set up a good filename to save to')
- return grab
-
-
- def perform_long_operation(self, func, end_key):
- """
- Call your function that will take a long time to execute. When it's complete, send an event
- specified by the end_key.
-
- Starts a thread on your behalf.
-
- This is a way for you to "ease into" threading without learning the details of threading.
- Your function will run, and when it returns 2 things will happen:
- 1. The value you provide for end_key will be returned to you when you call window.read()
- 2. If your function returns a value, then the value returned will also be included in your windows.read call in the values dictionary
-
- IMPORTANT - This method uses THREADS... this means you CANNOT make any PySimpleGUI calls from
- the function you provide with the exception of one function, Window.write_event_value.
-
- :param func: A lambda or a function name with no parms
- :type func: Any
- :param end_key: The key that will be generated when the function returns
- :type end_key: (Any)
- :return: The id of the thread
- :rtype: threading.Thread
- """
-
- thread = threading.Thread(target=_long_func_thread, args=(self, end_key, func), daemon=True)
- thread.start()
- return thread
-
- @property
- def key_dict(self):
- """
- Returns a dictionary with all keys and their corresponding elements
- { key : Element }
- :return: Dictionary of keys and elements
- :rtype: Dict[Any, Element]
- """
- return self.AllKeysDict
-
- # def __enter__(self):
- # """
- # WAS used with context managers which are no longer needed nor advised. It is here for legacy support and
- # am afraid of removing right now
- # :return: (window)
- # :rtype:
- # """
- # return self
-
- def __getitem__(self, key):
- """
- Returns Element that matches the passed in key.
- This is "called" by writing code as thus:
- window['element key'].Update
-
- :param key: The key to find
- :type key: str | int | tuple | object
- :rtype: Element | Input | Combo | OptionMenu | Listbox | Radio | Checkbox | Spin | Multiline | Text | StatusBar | Output | Button | ButtonMenu | ProgressBar | Image | Canvas | Graph | Frame | VerticalSeparator | HorizontalSeparator | Tab | TabGroup | Slider | Column | Pane | Menu | Table | Tree | ErrorElement | None
- """
-
- return self.find_element(key)
-
- def __call__(self, *args, **kwargs):
- """
- Call window.read but without having to type it out.
- window() == window.read()
- window(timeout=50) == window.read(timeout=50)
-
- :return: The famous event, values that Read returns.
- :rtype: Tuple[Any, Dict[Any, Any]]
- """
- return self.read(*args, **kwargs)
-
- def _is_window_created(self, additional_message=''):
- msg = str(additional_message)
- if self.TKroot is None:
- warnings.warn(
- 'You cannot perform operations on a Window until it is read or finalized. Adding a "finalize=True" parameter to your Window creation will fix this. ' + msg,
- UserWarning)
- if not SUPPRESS_ERROR_POPUPS:
- _error_popup_with_traceback('You cannot perform operations on a Window until it is read or finalized.',
- 'Adding a "finalize=True" parameter to your Window creation will likely fix this', msg)
- return False
- return True
-
- def _has_custom_titlebar_element(self):
- for elem in self.AllKeysDict.values():
- if elem.Key in (TITLEBAR_MAXIMIZE_KEY, TITLEBAR_CLOSE_KEY, TITLEBAR_IMAGE_KEY):
- return True
- if elem.metadata == TITLEBAR_METADATA_MARKER:
- return True
- return False
-
- AddRow = add_row
- AddRows = add_rows
- AlphaChannel = alpha_channel
- BringToFront = bring_to_front
- Close = close
- CurrentLocation = current_location
- Disable = disable
- DisableDebugger = disable_debugger
- Disappear = disappear
- Enable = enable
- EnableDebugger = enable_debugger
- Fill = fill
- Finalize = finalize
- # FindElement = find_element
- FindElementWithFocus = find_element_with_focus
- GetScreenDimensions = get_screen_dimensions
- GrabAnyWhereOff = grab_any_where_off
- GrabAnyWhereOn = grab_any_where_on
- Hide = hide
- Layout = layout
- LoadFromDisk = load_from_disk
- Maximize = maximize
- Minimize = minimize
- Move = move
- Normal = normal
- Read = read
- Reappear = reappear
- Refresh = refresh
- SaveToDisk = save_to_disk
- SendToBack = send_to_back
- SetAlpha = set_alpha
- SetIcon = set_icon
- SetTransparentColor = set_transparent_color
- Size = size
- UnHide = un_hide
- VisibilityChanged = visibility_changed
- CloseNonBlocking = close
- CloseNonBlockingForm = close
- start_thread = perform_long_operation
- #
- # def __exit__(self, *a):
- # """
- # WAS used with context managers which are no longer needed nor advised. It is here for legacy support and
- # am afraid of removing right now
- # :param *a: (?) Not sure what's passed in.
- # :type *a:
- # :return: Always returns False which was needed for context manager to work
- # :rtype:
- # """
- # self.__del__()
- # return False
- #
- # def __del__(self):
- # # print('DELETING WINDOW')
- # for row in self.Rows:
- # for element in row:
- # element.__del__()
-
-
-# -------------------------------- PEP8-ify the Window Class USER Interfaces -------------------------------- #
-
-
-FlexForm = Window
-
-
-def _long_func_thread(window, end_key, original_func):
- """
- Used to run long operations on the user's behalf. Called by the window object
-
- :param window: The window that will get the event
- :type window: (Window)
- :param end_key: The event that will be sent when function returns
- :type end_key: (Any)
- :param original_func: The user's function that is called. Can be a function with no arguments or a lambda experession
- :type original_func: (Any)
- """
-
- return_value = original_func()
- window.write_event_value(end_key, return_value)
-
-
-def _exit_mainloop(exiting_window):
- if exiting_window == Window._window_running_mainloop or Window._root_running_mainloop == Window.hidden_master_root:
- Window._window_that_exited = exiting_window
- if Window._root_running_mainloop is not None:
- Window._root_running_mainloop.quit()
- # print('** Exited window mainloop **')
-
-
-def _timeout_alarm_callback_hidden():
- """
- Read Timeout Alarm callback. Will kick a mainloop call out of the tkinter event loop and cause it to return
- """
-
- del Window._TKAfterID
-
- # first, get the results table built
- # modify the Results table in the parent FlexForm object
- # print('TIMEOUT CALLBACK')
- Window._root_running_mainloop.quit() # kick the users out of the mainloop
-
- # Get window that caused return
- Window._window_that_exited = None
-
-
-def read_all_windows(timeout=None, timeout_key=TIMEOUT_KEY):
- """
- Reads all windows that are "active" when the call is made. "Active" means that it's been finalized or read.
- If a window has not been finalized then it will not be considered an "active window"
-
- If any of the active windows returns a value then the window and its event and values
- are returned.
-
- If no windows are open, then the value (None, WIN_CLOSED, None) will be returned
- Since WIN_CLOSED is None, it means (None, None, None) is what's returned when no windows remain opened
-
- :param timeout: Time in milliseconds to delay before a returning a timeout event
- :type timeout: (int)
- :param timeout_key: Key to return when a timeout happens. Defaults to the standard TIMEOUT_KEY
- :type timeout_key: (Any)
- :return: A tuple with the (Window, event, values dictionary/list)
- :rtype: (Window, Any, Dict | List)
- """
-
- if len(Window._active_windows) == 0:
- return None, WIN_CLOSED, None
-
- # first see if any queued events are waiting for any of the windows
- for window in Window._active_windows.keys():
- if window._queued_thread_event_available():
- _BuildResults(window, False, window)
- event, values = window.ReturnValues
- return window, event, values
-
- Window._root_running_mainloop = Window.hidden_master_root
- Window._timeout_key = timeout_key
-
- if timeout == 0:
- window = list(Window._active_windows.keys())[Window._timeout_0_counter]
- event, values = window._ReadNonBlocking()
- if event is None:
- event = timeout_key
- if values is None:
- event = None
- Window._timeout_0_counter = (Window._timeout_0_counter + 1) % len(Window._active_windows)
- return window, event, values
-
- Window._timeout_0_counter = 0 # reset value if not reading with timeout 0 so ready next time needed
-
- # setup timeout timer
- if timeout != None:
- try:
- Window.hidden_master_root.after_cancel(Window._TKAfterID)
- del Window._TKAfterID
- except:
- pass
-
- Window._TKAfterID = Window.hidden_master_root.after(timeout, _timeout_alarm_callback_hidden)
-
- # ------------ Call Mainloop ------------
- Window._root_running_mainloop.mainloop()
-
- try:
- Window.hidden_master_root.after_cancel(Window._TKAfterID)
- del Window._TKAfterID
- except:
- pass
- # print('** tkafter cancel failed **')
-
- # Get window that caused return
-
- window = Window._window_that_exited
-
- if window is None:
- return None, timeout_key, None
-
- if window.XFound:
- event, values = None, None
- window.close()
- try:
- del Window._active_windows[window]
- except:
- pass
- # print('Error deleting window, but OK')
- else:
- _BuildResults(window, False, window)
- event, values = window.ReturnValues
-
- return window, event, values
-
-# MP""""""`MM dP
-# M mmmmm..M 88
-# M. `YM dP dP .d8888b. d8888P .d8888b. 88d8b.d8b.
-# MMMMMMM. M 88 88 Y8ooooo. 88 88ooood8 88'`88'`88
-# M. .MMM' M 88. .88 88 88 88. ... 88 88 88
-# Mb. .dM `8888P88 `88888P' dP `88888P' dP dP dP
-# MMMMMMMMMMM .88
-# d8888P
-# M""""""""M
-# Mmmm mmmM
-# MMMM MMMM 88d888b. .d8888b. dP dP
-# MMMM MMMM 88' `88 88' `88 88 88
-# MMMM MMMM 88 88. .88 88. .88
-# MMMM MMMM dP `88888P8 `8888P88
-# MMMMMMMMMM .88
-# d8888P
-
-# ------------------------------------------------------------------------- #
-# SystemTray - class for implementing a psyeudo tray #
-# ------------------------------------------------------------------------- #
-
-# -------------------------------- System Tray Begins Here -------------------------------- #
-# Feb 2020 - Just starting on this so code commented out for now. Basing on PySimpleGUIQt's implementation / call format
-
-
-# -------------------------------------------------------------------
-# fade in/out info and default window alpha
-SYSTEM_TRAY_WIN_MARGINS = 160, 60 # from right edge of screen, from bottom of screen
-SYSTEM_TRAY_MESSAGE_MAX_LINE_LENGTH = 50
-# colors
-SYSTEM_TRAY_MESSAGE_WIN_COLOR = "#282828"
-SYSTEM_TRAY_MESSAGE_TEXT_COLOR = "#ffffff"
-
-SYSTEM_TRAY_MESSAGE_DISPLAY_DURATION_IN_MILLISECONDS = 3000 # how long to display the window
-SYSTEM_TRAY_MESSAGE_FADE_IN_DURATION = 1000 # how long to fade in / fade out the window
-
-EVENT_SYSTEM_TRAY_ICON_DOUBLE_CLICKED = '__DOUBLE_CLICKED__'
-EVENT_SYSTEM_TRAY_ICON_ACTIVATED = '__ACTIVATED__'
-EVENT_SYSTEM_TRAY_MESSAGE_CLICKED = '__MESSAGE_CLICKED__'
-
-# Base64 Images to use as icons in the window
-_tray_icon_error = b'iVBORw0KGgoAAAANSUhEUgAAACAAAAAgCAMAAABEpIrGAAAAA3NCSVQICAjb4U/gAAAACXBIWXMAAADlAAAA5QGP5Zs8AAAAGXRFWHRTb2Z0d2FyZQB3d3cuaW5rc2NhcGUub3Jnm+48GgAAAIpQTFRF////20lt30Bg30pg4FJc409g4FBe4E9f4U9f4U9g4U9f4E9g31Bf4E9f4E9f4E9f4E9f4E9f4FFh4Vdm4lhn42Bv5GNx5W575nJ/6HqH6HyI6YCM6YGM6YGN6oaR8Kev9MPI9cbM9snO9s3R+Nfb+dzg+d/i++vt/O7v/fb3/vj5//z8//7+////KofnuQAAABF0Uk5TAAcIGBktSYSXmMHI2uPy8/XVqDFbAAAA8UlEQVQ4y4VT15LCMBBTQkgPYem9d9D//x4P2I7vILN68kj2WtsAhyDO8rKuyzyLA3wjSnvi0Eujf3KY9OUP+kno651CvlB0Gr1byQ9UXff+py5SmRhhIS0oPj4SaUUCAJHxP9+tLb/ezU0uEYDUsCc+l5/T8smTIVMgsPXZkvepiMj0Tm5txQLENu7gSF7HIuMreRxYNkbmHI0u5Hk4PJOXkSMz5I3nyY08HMjbpOFylF5WswdJPmYeVaL28968yNfGZ2r9gvqFalJNUy2UWmq1Wa7di/3Kxl3tF1671YHRR04dWn3s9cXRV09f3vb1fwPD7z9j1WgeRgAAAABJRU5ErkJggg=='
-_tray_icon_success = b'iVBORw0KGgoAAAANSUhEUgAAACAAAAAgCAMAAABEpIrGAAAAA3NCSVQICAjb4U/gAAAACXBIWXMAAAEKAAABCgEWpLzLAAAAGXRFWHRTb2Z0d2FyZQB3d3cuaW5rc2NhcGUub3Jnm+48GgAAAHJQTFRF////ZsxmbbZJYL9gZrtVar9VZsJcbMRYaMZVasFYaL9XbMFbasRZaMFZacRXa8NYasFaasJaasFZasJaasNZasNYasJYasJZasJZasJZasJZasJZasJYasJZasJZasJZasJZasJaasJZasJZasJZasJZ2IAizQAAACV0Uk5TAAUHCA8YGRobHSwtPEJJUVtghJeYrbDByNjZ2tvj6vLz9fb3/CyrN0oAAADnSURBVDjLjZPbWoUgFIQnbNPBIgNKiwwo5v1fsQvMvUXI5oqPf4DFOgCrhLKjC8GNVgnsJY3nKm9kgTsduVHU3SU/TdxpOp15P7OiuV/PVzk5L3d0ExuachyaTWkAkLFtiBKAqZHPh/yuAYSv8R7XE0l6AVXnwBNJUsE2+GMOzWL8k3OEW7a/q5wOIS9e7t5qnGExvF5Bvlc4w/LEM4Abt+d0S5BpAHD7seMcf7+ZHfclp10TlYZc2y2nOqc6OwruxUWx0rDjNJtyp6HkUW4bJn0VWdf/a7nDpj1u++PBOR694+Ftj/8PKNdnDLn/V8YAAAAASUVORK5CYII='
-_tray_icon_halt = b'iVBORw0KGgoAAAANSUhEUgAAACAAAAAgCAMAAABEpIrGAAAAAXNSR0IArs4c6QAAAARnQU1BAACxjwv8YQUAAAMAUExURQAAANswNuMPDO8HBO8FCe0HCu4IBu4IB+oLDeoLDu8JC+wKCu4JDO4LDOwKEe4OEO4OEeUQDewQDe0QDucVEuYcG+ccHOsQFuwWHe4fH/EGAvMEBfMFBvAHBPMGBfEGBvYCAfYDAvcDA/cDBPcDBfUDBvYEAPYEAfYEAvYEA/QGAPQGAfQGAvYEBPUEBvYFB/QGBPQGBfQHB/EFCvIHCPMHCfIHC/IFDfMHDPQGCPQGCfQGCvEIBPIIBfAIB/UIB/QICPYICfoBAPoBAfoBAvsBA/kCAPkCAfkCAvkCA/oBBPkCBPkCBfkCBvgCB/gEAPkEAfgEAvkEA/gGAfkGAvkEBPgEBfkEBv0AAP0AAfwAAvwAA/wCAPwCAfwCAvwCA/wABP0ABfwCBfwEAPwFA/ASD/ESFPAUEvAUE/EXFvAdH+kbIOobIeofIfEfIOcmKOohIukgJOggJesiKuwiKewoLe0tLO0oMOQ3OO43Oew4OfAhIPAhIfAiIPEiI+dDRe9ES+lQTOdSWupSUOhTUehSV+hUVu1QUO1RUe1SV+tTWe5SWOxXWOpYV+pZWelYXexaW+xaXO9aX+lZYeNhYOxjZ+lna+psbOttbehsbupscepucuxtcuxucep3fet7e+p/ffB6gOmKiu2Iie2Sk+2Qle2QluySlOyTleuYmvKFivCOjgAAAAAAAAAAAAAAAAAAAAAAAAAAAAAAAAAAAAAAAAAAAAAAAAAAAAAAAAAAAAAAAAAAAAAAAAAAAAAAAAAAAAAAAAAAAAAAAAAAAAAAAAAAAAAAAAAAAAAAAAAAAAAAAAAAAAAAAAAAAAAAAAAAAAAAAAAAAAAAAAAAAAAAAAAAAAAAAAAAAAAAAAAAAAAAAAAAAAAAAAAAAAAAAAAAAAAAAAAAAAAAAAAAAAAAAAAAAAAAAAAAAAAAAAAAAAAAAAAAAAAAAAAAAAAAAAAAAAAAAAAAAAAAAAAAAAAAAAAAAAAAAAAAAAAAAAAAAAAAAAAAAAAAAAAAAAAAAIxGNZsAAAEAdFJOU////////////////////////////////////////////////////////////////////////////////////////////////////////////////////////////////////////////////////////////////////////////////////////////////////////////////////////////////////////////////////////////////////////////////////////////////////////////////////////////////////////////////////wBT9wclAAAACXBIWXMAAA7DAAAOwwHHb6hkAAACVElEQVQ4T22S93PTMBhADQdl791SSsuRARTKKHsn+STZBptAi6zIacous+w9yyxl7z1T1h8ptHLhrrzLD5+/987R2XZElZ/39tZsbGg42NdvF4pqcGMs4XEcozAB/oQeu6wGr5fkAZcKOUIIRgQXR723wgaXt/NSgcwlO1r3oARkATfhbmNMMCnlMZdz5J8RN9fVhglS5JA/pJUOJiYXoShCkz/flheDvpzlBCBmya5KcDG1sMSB+r/VQtG+YoFXlwN0Us4yeBXujPmWCOqNlVwX5zHntLH5iQ420YiqX9pqTZFSCrBGBc+InBUDAsbwLRlMC40fGJT8YLRwfnhY3v6/AUtDc9m5z0tRJBOAvHUaFchdY6+zDzEghHv1tUnrNCaIOw84Q2WQmkeO/Xopj1xFBREFr8ZZjuRhA++PEB+t05ggwBucpbH8i/n5C1ZU0EEEmRZnSMxoIYcarKigA0Cb1zpHAyZnGj21xqICAA9dcvo4UgEdZ41FBZSTzEOn30f6QeE3Vhl0gLN+2RGDzZPMHLHKoAO3MFy+ix4sDxFlvMXfrdNgFezy7qrXPaaJg0u27j5nneKrCjJ4pf4e3m4DVMcjNNNKxWnpo6jtnfnkunExB4GbuGKk5FNanpB1nJCjCsThJPAAJ8lVdSF5sSrklM2ZqmYdiC40G7Dfnhp57ZsQz6c3hylEO6ZoZQJxqiVgbhoQK3T6AIgU4rbjxthAPF6NAwAOAcS+ixlp/WBFJRDi0fj2RtcjWRwif8Qdu/w3EKLcu3/YslnrZzwo24UQQvwFCrp/iM1NnHwAAAAASUVORK5CYII='
-_tray_icon_notallowed = b'iVBORw0KGgoAAAANSUhEUgAAACAAAAAgCAMAAABEpIrGAAAAAXNSR0IArs4c6QAAAARnQU1BAACxjwv8YQUAAAMAUExURQAAAPcICPcLC/cMDPcQEPcSEvcXF/cYGPcaGvcbG/ccHPgxMfgyMvg0NPg5Ofg6Ovg7O/hBQfhCQvlFRflGRvljY/pkZPplZfpnZ/p2dgAAAAAAAAAAAAAAAAAAAAAAAAAAAAAAAAAAAAAAAAAAAAAAAAAAAAAAAAAAAAAAAAAAAAAAAAAAAAAAAAAAAAAAAAAAAAAAAAAAAAAAAAAAAAAAAAAAAAAAAAAAAAAAAAAAAAAAAAAAAAAAAAAAAAAAAAAAAAAAAAAAAAAAAAAAAAAAAAAAAAAAAAAAAAAAAAAAAAAAAAAAAAAAAAAAAAAAAAAAAAAAAAAAAAAAAAAAAAAAAAAAAAAAAAAAAAAAAAAAAAAAAAAAAAAAAAAAAAAAAAAAAAAAAAAAAAAAAAAAAAAAAAAAAAAAAAAAAAAAAAAAAAAAAAAAAAAAAAAAAAAAAAAAAAAAAAAAAAAAAAAAAAAAAAAAAAAAAAAAAAAAAAAAAAAAAAAAAAAAAAAAAAAAAAAAAAAAAAAAAAAAAAAAAAAAAAAAAAAAAAAAAAAAAAAAAAAAAAAAAAAAAAAAAAAAAAAAAAAAAAAAAAAAAAAAAAAAAAAAAAAAAAAAAAAAAAAAAAAAAAAAAAAAAAAAAAAAAAAAAAAAAAAAAAAAAAAAAAAAAAAAAAAAAAAAAAAAAAAAAAAAAAAAAAAAAAAAAAAAAAAAAAAAAAAAAAAAAAAAAAAAAAAAAAAAAAAAAAAAAAAAAAAAAAAAAAAAAAAAAAAAAAAAAAAAAAAAAAAAAAAAAAAAAAAAAAAAAAAAAAAAAAAAAAAAAAAAAAAAAAAAAAAAAAAAAAAAAAAAAAAAAAAAAAAAAAAAAAAAAAAAAAAAAAAAAAAAAAAAAAAAAAAAAAAAAAAAAAAAAAAAAAAAAAAAAAAAAAAAAAAAAAAAAAAAAAAAAAAAAAAAAAAAAAAAAAAAAAAAAAAAAAAAAAAAAAAAAAAAAAAAAAAAAAAAAAAAAAAAAAAAAAAAAAAAAAAAAAAAAMgEwNYAAAEAdFJOU////////////////////////////////////////////////////////////////////////////////////////////////////////////////////////////////////////////////////////////////////////////////////////////////////////////////////////////////////////////////////////////////////////////////////////////////////////////////////////////////////////////////////wBT9wclAAAACXBIWXMAAA7DAAAOwwHHb6hkAAABE0lEQVQ4T4WT65bDIAiExWbbtN0m3Uua+P4P6g4jGtN4NvNL4DuCCC5WWobe++uwmEmtwNxJUTebcwWCt5jJBwsYcKf3NE4hTOOJxj1FEnBTz4NH6qH2jUcCGr/QLLpkQgHe/6VWJXVqFgBB4yI/KVCkBCoFgPrPHw0CWbwCL8RibBFwzQDQH62/QeAtHQBeADUIDbkF/UnmnkB1ixtERrN3xCgyuF5kMntHTCJXh2vyv+wIdMhvgTeCQJ0C2hBMgSKfZlM1wSLXZ5oqgs8sjSpaCQ2VVlfKhLU6fdZGSvyWz9JMb+NE4jt/Nwfm0yJZSkBpYDg7TcJGrjm0Z7jK0B6P/fHiHK8e9Pp/eSmuf1+vf4x/ralnCN9IrncAAAAASUVORK5CYII='
-_tray_icon_stop = b'iVBORw0KGgoAAAANSUhEUgAAACAAAAAgCAMAAABEpIrGAAAAAXNSR0IArs4c6QAAAARnQU1BAACxjwv8YQUAAAMAUExURQAAANsAANsBAdsCAtwEBNwFBdwHB9wICNwLC90MDN0NDd0PD90REd0SEt4TE94UFN4WFt4XF94ZGeAjI+AlJeEnJ+EpKeEqKuErK+EsLOEuLuIvL+IyMuIzM+M1NeM2NuM3N+M6OuM8POQ9PeQ+PuQ/P+RAQOVISOVJSeVKSuZLS+ZOTuZQUOZRUedSUudVVehbW+lhYeljY+poaOtvb+twcOtxcetzc+t0dOx3d+x4eOx6eu19fe1+fu2AgO2Cgu6EhO6Ghu6Hh+6IiO6Jie+Kiu+Li++MjO+Nje+Oju+QkPCUlPCVlfKgoPKkpPKlpfKmpvOrq/SurvSxsfSysvW4uPW6uvW7u/W8vPa9vfa+vvbAwPbCwvfExPfFxffGxvfHx/fIyPfJyffKyvjLy/jNzfjQ0PjR0fnS0vnU1PnY2Pvg4Pvi4vvj4/vl5fvm5vzo6Pzr6/3u7v3v7/3x8f3z8/309P719f729v739/74+P75+f76+v77+//8/P/9/f/+/v///wAAAAAAAAAAAAAAAAAAAAAAAAAAAAAAAAAAAAAAAAAAAAAAAAAAAAAAAAAAAAAAAAAAAAAAAAAAAAAAAAAAAAAAAAAAAAAAAAAAAAAAAAAAAAAAAAAAAAAAAAAAAAAAAAAAAAAAAAAAAAAAAAAAAAAAAAAAAAAAAAAAAAAAAAAAAAAAAAAAAAAAAAAAAAAAAAAAAAAAAAAAAAAAAAAAAAAAAAAAAAAAAAAAAAAAAAAAAAAAAAAAAAAAAAAAAAAAAAAAAAAAAAAAAAAAAAAAAAAAAAAAAAAAAAAAAAAAAAAAAAAAAAAAAAAAAAAAAAAAAAAAAAAAAAAAAAAAAAAAAAAAAAAAAAAAAAAAAAAAAAAAAAAAAAAAAAAAAAAAAAAAAAAAAAAAAAAAAAAAAAAAAAAAAAAAAAAAAAAAAAAAAAAAAAAAAAAAAAAAAAAAAAAAAAAAAAAAAAAAAAAAAAAAAAAAAAAAAAAAAAAAAAAAAAAAAAAAAAAAAAAAAPHCyoUAAAEAdFJOU////////////////////////////////////////////////////////////////////////////////////////////////////////////////////////////////////////////////////////////////////////////////////////////////////////////////////////////////////////////////////////////////////////////////////////////////////////////////////////////////////////////////////wBT9wclAAAACXBIWXMAAA7DAAAOwwHHb6hkAAABnUlEQVQ4T33S50PTQBgG8D6lzLbsIUv2kD0FFWTvPWTvISDIUBGV1ecvj+8luZTR9P1wSe755XK5O4+hK4gn5bc7DcMBz/InQoMXeVjY4FXuCAtEyLUwQcTcFgq45JYQ4JqbwhMtV8IjeUJDjQ+5paqCyG9srEsGgoUlpeXpIjxA1nfyi2+Jqmo7Q9JeV+ODerpvBQTM8/ySzQ3t+xxoL7h7nJve5jd85M7wJq9McHaT8o6TwBTfIIfHQGzoAZ/YiSTSq8D5dSDQVqFADrJ5KFMLPaKLHQiQMQoscClezdgCB4CXD/jM90izR8g85UaKA3YAn4AejhV189acA5LX+DVOg00gnvfoVX/BRQsgbplNGqzLusgIffx1tDchiyRgdRbVHNdgRRZHQD9H1asm+PMzYyYMtoBU/sYgRxxgrmGtBRL/cnf5RL4zzCEHZF2QE14LoOWf6B9vMcJBG/iBxKo8dVtYnyStv6yuUq7FLfmqTzbLEOFest1GNGEemCjCPnKuwjm0LsLMbRBJWLkGr4WdO+Cl0HkYPBc6N4z//HcQqVwcOuIAAAAASUVORK5CYII='
-_tray_icon_exclamation = b'iVBORw0KGgoAAAANSUhEUgAAACAAAAAgCAMAAABEpIrGAAAAAXNSR0IArs4c6QAAAARnQU1BAACxjwv8YQUAAAMAUExURQAAAN0zM900NN01Nd02Nt03N944ON45Od46Ot47O98/P99BQd9CQt9DQ+FPT+JSUuJTU+JUVOJVVeJWVuNbW+ReXuVjY+Zra+dxceh4eOl7e+l8fOl+ful/f+qBgeqCguqDg+qFheuJieuLi+yPj+yQkO2Wlu+cnO+hofGqqvGtrfre3vrf3/ri4vvn5/75+f76+v/+/v///wAAAAAAAAAAAAAAAAAAAAAAAAAAAAAAAAAAAAAAAAAAAAAAAAAAAAAAAAAAAAAAAAAAAAAAAAAAAAAAAAAAAAAAAAAAAAAAAAAAAAAAAAAAAAAAAAAAAAAAAAAAAAAAAAAAAAAAAAAAAAAAAAAAAAAAAAAAAAAAAAAAAAAAAAAAAAAAAAAAAAAAAAAAAAAAAAAAAAAAAAAAAAAAAAAAAAAAAAAAAAAAAAAAAAAAAAAAAAAAAAAAAAAAAAAAAAAAAAAAAAAAAAAAAAAAAAAAAAAAAAAAAAAAAAAAAAAAAAAAAAAAAAAAAAAAAAAAAAAAAAAAAAAAAAAAAAAAAAAAAAAAAAAAAAAAAAAAAAAAAAAAAAAAAAAAAAAAAAAAAAAAAAAAAAAAAAAAAAAAAAAAAAAAAAAAAAAAAAAAAAAAAAAAAAAAAAAAAAAAAAAAAAAAAAAAAAAAAAAAAAAAAAAAAAAAAAAAAAAAAAAAAAAAAAAAAAAAAAAAAAAAAAAAAAAAAAAAAAAAAAAAAAAAAAAAAAAAAAAAAAAAAAAAAAAAAAAAAAAAAAAAAAAAAAAAAAAAAAAAAAAAAAAAAAAAAAAAAAAAAAAAAAAAAAAAAAAAAAAAAAAAAAAAAAAAAAAAAAAAAAAAAAAAAAAAAAAAAAAAAAAAAAAAAAAAAAAAAAAAAAAAAAAAAAAAAAAAAAAAAAAAAAAAAAAAAAAAAAAAAAAAAAAAAAAAAAAAAAAAAAAAAAAAAAAAAAAAAAAAAAAAAAAAAAAAAAAAAAAAAAAAAAAAAAAAAAAAAAAAAAAAAAAAAAAAAAAAAAAAAMQ8SQkAAAEAdFJOU////////////////////////////////////////////////////////////////////////////////////////////////////////////////////////////////////////////////////////////////////////////////////////////////////////////////////////////////////////////////////////////////////////////////////////////////////////////////////////////////////////////////////wBT9wclAAAACXBIWXMAAA7DAAAOwwHHb6hkAAABJElEQVQ4T4WS63KCMBBGsyBai62X0otY0aq90ZZa3v/dtpvsJwTijOfXt7tnILOJYY9tNonjNCtQOlqhuKKG0RrNVjgkmIHBHgMId+h7zHSiwg2a9FNVVYScupETmjkd67o+CWpYwft+R6CpCgeUlq5AOyf45+8JsRUKFI6eQLkI3n5CIREBUekLxGaLpATCymRISiAszARJCYSxiZGUQKDLQoqgnPnFhUPOTWeRoZD3FvVZlmVHkE2OEM9iV71GVoZDBGUpAg9QWN5/jx+Ilsi9hz0q4VHOWD+hEF70yc1QEr1a4Q0F0S3eJDfLuv8T4QEFXduZE1rj+et7g6hzCDxF08N+X4DAu+6lUSTnc5wE5tx73ckSTV8QVoux3N88Rykw/wP3i+vwPKk17AAAAABJRU5ErkJggg=='
-_tray_icon_none = None
-
-SYSTEM_TRAY_MESSAGE_ICON_INFORMATION = _tray_icon_success
-SYSTEM_TRAY_MESSAGE_ICON_WARNING = _tray_icon_exclamation
-SYSTEM_TRAY_MESSAGE_ICON_CRITICAL = _tray_icon_stop
-SYSTEM_TRAY_MESSAGE_ICON_NOICON = _tray_icon_none
-
-
-# ------------------------------------------------------------------------- #
-# Tray CLASS #
-# ------------------------------------------------------------------------- #
-class SystemTray:
- """
- A "Simulated System Tray" that duplicates the API calls available to PySimpleGUIWx and PySimpleGUIQt users.
-
- All of the functionality works. The icon is displayed ABOVE the system tray rather than inside of it.
- """
-
- def __init__(self, menu=None, filename=None, data=None, data_base64=None, tooltip=None, metadata=None):
- """
- SystemTray - create an icon in the system tray
- :param menu: Menu definition. Example - ['UNUSED', ['My', 'Simple', '---', 'Menu', 'Exit']]
- :type menu: List[List[List[str] or str]]
- :param filename: filename for icon
- :type filename: (str)
- :param data: in-ram image for icon (same as data_base64 parm)
- :type data: (bytes)
- :param data_base64: base-64 data for icon
- :type data_base64: (bytes)
- :param tooltip: tooltip string
- :type tooltip: (str)
- :param metadata: User metadata that can be set to ANYTHING
- :type metadata: (Any)
- """
- self._metadata = None
- self.Menu = menu
- self.TrayIcon = None
- self.Shown = False
- self.MenuItemChosen = TIMEOUT_KEY
- self.metadata = metadata
- self.last_message_event = None
-
- screen_size = Window.get_screen_size()
-
- if filename:
- image_elem = Image(filename=filename, background_color='red', enable_events=True, tooltip=tooltip, key='-IMAGE-')
- elif data_base64:
- image_elem = Image(data=data_base64, background_color='red', enable_events=True, tooltip=tooltip, key='-IMAGE-')
- elif data:
- image_elem = Image(data=data, background_color='red', enable_events=True, tooltip=tooltip, key='-IMAGE-')
- else:
- image_elem = Image(background_color='red', enable_events=True, tooltip=tooltip, key='-IMAGE-')
- layout = [
- [image_elem],
- ]
- self.window = Window('Window Title', layout, element_padding=(0, 0), margins=(0, 0), grab_anywhere=True, no_titlebar=True, transparent_color='red',
- keep_on_top=True, right_click_menu=menu, location=(screen_size[0] - 100, screen_size[1] - 100), finalize=True)
-
- self.window['-IMAGE-'].bind('', '+DOUBLE_CLICK')
-
- @property
- def metadata(self):
- """
- Metadata is an SystemTray property that you can use at any time to hold any value
- :return: the current metadata value
- :rtype: (Any)
- """
- return self._metadata
-
- @metadata.setter
- def metadata(self, value):
- """
- Metadata is an SystemTray property that you can use at any time to hold any value
- :param value: Anything you want it to be
- :type value: (Any)
- """
- self._metadata = value
-
- def read(self, timeout=None):
- """
- Reads the context menu
- :param timeout: Optional. Any value other than None indicates a non-blocking read
- :type timeout:
- :return:
- :rtype:
- """
- if self.last_message_event != TIMEOUT_KEY and self.last_message_event is not None:
- event = self.last_message_event
- self.last_message_event = None
- return event
- event, values = self.window.read(timeout=timeout)
- if event.endswith('DOUBLE_CLICK'):
- return EVENT_SYSTEM_TRAY_ICON_DOUBLE_CLICKED
- elif event == '-IMAGE-':
- return EVENT_SYSTEM_TRAY_ICON_ACTIVATED
-
- return event
-
- def hide(self):
- """
- Hides the icon
- """
- self.window.hide()
-
- def un_hide(self):
- """
- Restores a previously hidden icon
- """
- self.window.un_hide()
-
- def show_message(self, title, message, filename=None, data=None, data_base64=None, messageicon=None,
- time=(SYSTEM_TRAY_MESSAGE_FADE_IN_DURATION, SYSTEM_TRAY_MESSAGE_DISPLAY_DURATION_IN_MILLISECONDS)):
- """
- Shows a balloon above icon in system tray
- :param title: Title shown in balloon
- :type title: str
- :param message: Message to be displayed
- :type message: str
- :param filename: Optional icon filename
- :type filename: str
- :param data: Optional in-ram icon
- :type data: b''
- :param data_base64: Optional base64 icon
- :type data_base64: b''
- :param time: Amount of time to display message in milliseconds. If tuple, first item is fade in/out duration
- :type time: int | (int, int)
- :return: The event that happened during the display such as user clicked on message
- :rtype: Any
- """
-
- if isinstance(time, tuple):
- fade_duraction, display_duration = time
- else:
- fade_duration = SYSTEM_TRAY_MESSAGE_FADE_IN_DURATION
- display_duration = time
-
- user_icon = data_base64 or filename or data or messageicon
-
- event = self.notify(title, message, icon=user_icon, fade_in_duration=fade_duraction, display_duration_in_ms=display_duration)
- self.last_message_event = event
- return event
-
- def close(self):
- """
- Close the system tray window
- """
- self.window.close()
-
- def update(self, menu=None, tooltip=None, filename=None, data=None, data_base64=None, ):
- """
- Updates the menu, tooltip or icon
- :param menu: menu defintion
- :type menu: ???
- :param tooltip: string representing tooltip
- :type tooltip: ???
- :param filename: icon filename
- :type filename: ???
- :param data: icon raw image
- :type data: ???
- :param data_base64: icon base 64 image
- :type data_base64: ???
- """
- # Menu
- if menu is not None:
- top_menu = tk.Menu(self.window.TKroot, tearoff=False)
- AddMenuItem(top_menu, menu[1], self.window['-IMAGE-'])
- self.window['-IMAGE-'].TKRightClickMenu = top_menu
-
- if filename:
- self.window['-IMAGE-'].update(filename=filename)
- elif data_base64:
- self.window['-IMAGE-'].update(data=data_base64)
- elif data:
- self.window['-IMAGE-'].update(data=data)
-
- if tooltip:
- self.window['-IMAGE-'].set_tooltip(tooltip)
-
- @classmethod
- def notify(cls, title, message, icon=_tray_icon_success, display_duration_in_ms=SYSTEM_TRAY_MESSAGE_DISPLAY_DURATION_IN_MILLISECONDS,
- fade_in_duration=SYSTEM_TRAY_MESSAGE_FADE_IN_DURATION, alpha=0.9, location=None):
- """
- Displays a "notification window", usually in the bottom right corner of your display. Has an icon, a title, and a message
- The window will slowly fade in and out if desired. Clicking on the window will cause it to move through the end the current "phase". For example, if the window was fading in and it was clicked, then it would immediately stop fading in and instead be fully visible. It's a way for the user to quickly dismiss the window.
- :param title: Text to be shown at the top of the window in a larger font
- :type title: (str)
- :param message: Text message that makes up the majority of the window
- :type message: (str)
- :param icon: A base64 encoded PNG/GIF image or PNG/GIF filename that will be displayed in the window
- :type icon: bytes | str
- :param display_duration_in_ms: Number of milliseconds to show the window
- :type display_duration_in_ms: (int)
- :param fade_in_duration: Number of milliseconds to fade window in and out
- :type fade_in_duration: (int)
- :param alpha: Alpha channel. 0 - invisible 1 - fully visible
- :type alpha: (float)
- :param location: Location on the screen to display the window
- :type location: (int, int)
- :return: (int) reason for returning
- :rtype: (int)
- """
-
- messages = message.split('\n')
- full_msg = ''
- for m in messages:
- m_wrap = textwrap.fill(m, SYSTEM_TRAY_MESSAGE_MAX_LINE_LENGTH)
- full_msg += m_wrap + '\n'
- message = full_msg[:-1]
-
- win_msg_lines = message.count("\n") + 1
- max_line = max(message.split('\n'))
-
- screen_res_x, screen_res_y = Window.get_screen_size()
- win_margin = SYSTEM_TRAY_WIN_MARGINS # distance from screen edges
- win_width, win_height = 364, 66 + (14.8 * win_msg_lines)
-
- layout = [[Graph(canvas_size=(win_width, win_height), graph_bottom_left=(0, win_height), graph_top_right=(win_width, 0), key="-GRAPH-",
- background_color=SYSTEM_TRAY_MESSAGE_WIN_COLOR, enable_events=True)]]
-
- win_location = location if location is not None else (screen_res_x - win_width - win_margin[0], screen_res_y - win_height - win_margin[1])
- window = Window(title, layout, background_color=SYSTEM_TRAY_MESSAGE_WIN_COLOR, no_titlebar=True,
- location=win_location, keep_on_top=True, alpha_channel=0, margins=(0, 0), element_padding=(0, 0), grab_anywhere=True, finalize=True)
-
- window["-GRAPH-"].draw_rectangle((win_width, win_height), (-win_width, -win_height), fill_color=SYSTEM_TRAY_MESSAGE_WIN_COLOR,
- line_color=SYSTEM_TRAY_MESSAGE_WIN_COLOR)
- if type(icon) is bytes:
- window["-GRAPH-"].draw_image(data=icon, location=(20, 20))
- elif icon is not None:
- window["-GRAPH-"].draw_image(filename=icon, location=(20, 20))
- window["-GRAPH-"].draw_text(title, location=(64, 20), color=SYSTEM_TRAY_MESSAGE_TEXT_COLOR, font=("Helvetica", 12, "bold"),
- text_location=TEXT_LOCATION_TOP_LEFT)
- window["-GRAPH-"].draw_text(message, location=(64, 44), color=SYSTEM_TRAY_MESSAGE_TEXT_COLOR, font=("Helvetica", 9),
- text_location=TEXT_LOCATION_TOP_LEFT)
- window["-GRAPH-"].set_cursor('hand2')
-
- if fade_in_duration:
- for i in range(1, int(alpha * 100)): # fade in
- window.set_alpha(i / 100)
- event, values = window.read(timeout=fade_in_duration // 100)
- if event != TIMEOUT_KEY:
- window.set_alpha(1)
- break
- if event != TIMEOUT_KEY:
- window.close()
- return EVENT_SYSTEM_TRAY_MESSAGE_CLICKED if event == '-GRAPH-' else event
- event, values = window(timeout=display_duration_in_ms)
- if event == TIMEOUT_KEY:
- for i in range(int(alpha * 100), 1, -1): # fade out
- window.set_alpha(i / 100)
- event, values = window.read(timeout=fade_in_duration // 100)
- if event != TIMEOUT_KEY:
- break
- else:
- window.set_alpha(alpha)
- event, values = window(timeout=display_duration_in_ms)
- window.close()
-
- return EVENT_SYSTEM_TRAY_MESSAGE_CLICKED if event == '-GRAPH-' else event
-
- Close = close
- Hide = hide
- Read = read
- ShowMessage = show_message
- UnHide = un_hide
- Update = update
-
-
-# ################################################################################
-# ################################################################################
-# END OF ELEMENT DEFINITIONS
-# ################################################################################
-# ################################################################################
-
-
-# =========================================================================== #
-# Button Lazy Functions so the caller doesn't have to define a bunch of stuff #
-# =========================================================================== #
-
-# ------------------------- A fake Element... the Pad Element ------------------------- #
-def Sizer(h_pixels=0, v_pixels=0):
- """
- "Pushes" out the size of whatever it is placed inside of. This includes Columns, Frames, Tabs and Windows
-
- :param h_pixels: number of horizontal pixels
- :type h_pixels: (int)
- :param v_pixels: number of vertical pixels
- :type v_pixels: (int)
- :return: (Column) A column element that has a pad setting set according to parameters
- :rtype: (Column)
- """
-
- return Column([[]], pad=((h_pixels, 0), (v_pixels, 0)))
-
-
-def pin(elem, vertical_alignment=None, shrink=True, expand_x=None, expand_y=None):
- """
- Pin's an element provided into a layout so that when it's made invisible and visible again, it will
- be in the correct place. Otherwise it will be placed at the end of its containing window/column.
-
- :param elem: the element to put into the layout
- :type elem: Element
- :param vertical_alignment: Aligns elements vertically. 'top', 'center', 'bottom'. Can be shortened to 't', 'c', 'b'
- :type vertical_alignment: str | None
- :param shrink: If True, then the space will shrink down to a single pixel when hidden. False leaves the area large and blank
- :type shrink: bool
- :param expand_x: If True/False the value will be passed to the Column Elements used to make this feature
- :type expand_x: (bool)
- :param expand_y: If True/False the value will be passed to the Column Elements used to make this feature
- :type expand_y: (bool)
- :return: A column element containing the provided element
- :rtype: Column
- """
- if shrink:
- # return Column([[elem, Canvas(size=(0, 0),background_color=elem.BackgroundColor, pad=(0, 0))]], pad=(0, 0), vertical_alignment=vertical_alignment, expand_x=expand_x, expand_y=expand_y)
- return Column([[elem, Column([[]],pad=(0,0))]], pad=(0, 0), vertical_alignment=vertical_alignment, expand_x=expand_x, expand_y=expand_y)
- else:
- return Column([[elem]], pad=(0, 0), vertical_alignment=vertical_alignment, expand_x=expand_x, expand_y=expand_y)
-
-
-def vtop(elem_or_row, expand_x=None, expand_y=None):
- """
- Align an element or a row of elements to the top of the row that contains it
-
- :param elem_or_row: the element or row of elements
- :type elem_or_row: Element | List[Element] | Tuple[Element]
- :param expand_x: If True/False the value will be passed to the Column Elements used to make this feature
- :type expand_x: (bool)
- :param expand_y: If True/False the value will be passed to the Column Elements used to make this feature
- :type expand_y: (bool)
- :return: A column element containing the provided element aligned to the top or list of elements (a row)
- :rtype: Column | List[Column]
- """
-
- if isinstance(elem_or_row, list) or isinstance(elem_or_row, tuple):
- return [Column([[e]], pad=(0, 0), vertical_alignment='top', expand_x=expand_x, expand_y=expand_y) for e in elem_or_row]
-
- return Column([[elem_or_row]], pad=(0, 0), vertical_alignment='top', expand_x=expand_x, expand_y=expand_y)
-
-
-def vcenter(elem_or_row, expand_x=None, expand_y=None):
- """
- Align an element or a row of elements to the center of the row that contains it
-
- :param elem_or_row: the element or row of elements
- :type elem_or_row: Element | List[Element] | Tuple[Element]
- :param expand_x: If True/False the value will be passed to the Column Elements used to make this feature
- :type expand_x: (bool)
- :param expand_y: If True/False the value will be passed to the Column Elements used to make this feature
- :type expand_y: (bool)
- :return: A column element containing the provided element aligned to the center or list of elements (a row)
- :rtype: Column | List[Column]
- """
-
- if isinstance(elem_or_row, list) or isinstance(elem_or_row, tuple):
- return [Column([[e]], pad=(0, 0), vertical_alignment='center') for e in elem_or_row]
-
- return Column([[elem_or_row]], pad=(0, 0), vertical_alignment='center')
-
-
-def vbottom(elem_or_row, expand_x=None, expand_y=None):
- """
- Align an element or a row of elements to the bottom of the row that contains it
-
- :param elem_or_row: the element or row of elements
- :type elem_or_row: Element | List[Element] | Tuple[Element]
- :param expand_x: If True/False the value will be passed to the Column Elements used to make this feature
- :type expand_x: (bool)
- :param expand_y: If True/False the value will be passed to the Column Elements used to make this feature
- :type expand_y: (bool)
- :return: A column element containing the provided element aligned to the bottom or list of elements (a row)
- :rtype: Column | List[Column]
- """
-
- if isinstance(elem_or_row, list) or isinstance(elem_or_row, tuple):
- return [Column([[e]], pad=(0, 0), vertical_alignment='bottom') for e in elem_or_row]
-
- return Column([[elem_or_row]], pad=(0, 0), vertical_alignment='bottom')
-
-
-def Titlebar(title='', icon=None, text_color=None, background_color=None, font=None, key=None, k=None):
- """
- A custom titlebar that replaces the OS provided titlebar, thus giving you control
- the is not possible using the OS provided titlebar such as the color.
-
- NOTE LINUX USERS - at the moment the minimize function is not yet working. Windows users
- should have no problem and it should function as a normal window would.
-
- This titlebar is created from a row of elements that is then encapsulated into a
- one Column element which is what this Titlebar function returns to you.
-
- A custom titlebar removes the margins from your window. If you want the remainder
- of your Window to have margins, place the layout after the Titlebar into a Column and
- set the pad of that Column to the dimensions you would like your margins to have.
-
- The Titlebar is a COLUMN element. You can thus call the update method for the column and
- perform operations such as making the column visible/invisible
-
- :param icon: Can be either a filename or Base64 byte string of a PNG or GIF. This is used in an Image element to create the titlebar
- :type icon: str or bytes or None
- :param title: The "title" to show in the titlebar
- :type title: str
- :param text_color: Text color for titlebar
- :type text_color: str | None
- :param background_color: Background color for titlebar
- :type background_color: str | None
- :param font: Font to be used for the text and the symbols
- :type font: (str or (str, int[, str]) or None)
- :param key: Identifies an Element. Should be UNIQUE to this window.
- :type key: str | int | tuple | object | None
- :param k: Exactly the same as key. Choose one of them to use
- :type k: str | int | tuple | object | None
- :return: A single Column element that has eveything in 1 element
- :rtype: Column
- """
- bc = background_color or CUSTOM_TITLEBAR_BACKGROUND_COLOR or theme_button_color()[1]
- tc = text_color or CUSTOM_TITLEBAR_TEXT_COLOR or theme_button_color()[0]
- font = font or CUSTOM_TITLEBAR_FONT or ('Helvetica', 12)
- key = k or key
-
- if isinstance(icon, bytes):
- icon_and_text_portion = [Image(data=icon, background_color=bc, key=TITLEBAR_IMAGE_KEY)]
- elif icon == TITLEBAR_DO_NOT_USE_AN_ICON:
- icon_and_text_portion = []
- elif icon is not None:
- icon_and_text_portion = [Image(filename=icon, background_color=bc, key=TITLEBAR_IMAGE_KEY)]
- elif CUSTOM_TITLEBAR_ICON is not None:
- if isinstance(CUSTOM_TITLEBAR_ICON, bytes):
- icon_and_text_portion = [Image(data=CUSTOM_TITLEBAR_ICON, background_color=bc, key=TITLEBAR_IMAGE_KEY)]
- else:
- icon_and_text_portion = [Image(filename=CUSTOM_TITLEBAR_ICON, background_color=bc, key=TITLEBAR_IMAGE_KEY)]
- else:
- icon_and_text_portion = [Image(data=DEFAULT_BASE64_ICON_16_BY_16, background_color=bc, key=TITLEBAR_IMAGE_KEY)]
-
- icon_and_text_portion += [T(title, text_color=tc, background_color=bc, font=font, grab=True)]
-
- return Column([[Column([icon_and_text_portion], pad=(0, 0), background_color=bc),
- Column([[T(SYMBOL_TITLEBAR_MINIMIZE, text_color=tc, background_color=bc, enable_events=True, font=font, key=TITLEBAR_MINIMIZE_KEY),
- Text(SYMBOL_TITLEBAR_MAXIMIZE, text_color=tc, background_color=bc, enable_events=True, font=font, key=TITLEBAR_MAXIMIZE_KEY),
- Text(SYMBOL_TITLEBAR_CLOSE, text_color=tc, background_color=bc, font=font, enable_events=True, key=TITLEBAR_CLOSE_KEY), ]],
- element_justification='r', expand_x=True, grab=True, pad=(0, 0), background_color=bc)]], expand_x=True, grab=True,
- background_color=bc, pad=(0, 0), metadata=TITLEBAR_METADATA_MARKER, key=key)
-
-
-def MenubarCustom(menu_definition, disabled_text_color=None, bar_font=None, font=None, tearoff=False, pad=0, p=None, background_color=None, text_color=None,
- bar_background_color=None, bar_text_color=None, key=None, k=None):
- """
- A custom Menubar that replaces the OS provided Menubar
-
- Why?
- Two reasons - 1. they look great (see custom titlebar) 2. if you have a custom titlebar, then you have to use a custom menubar if you want a menubar
-
- :param menu_definition: The Menu definition specified using lists (docs explain the format)
- :type menu_definition: List[List[Tuple[str, List[str]]]
- :param disabled_text_color: color to use for text when item is disabled. Can be in #RRGGBB format or a color name "black"
- :type disabled_text_color: (str)
- :param bar_font: specifies the font family, size to be used for the chars in the bar itself
- :type bar_font: (str or (str, int[, str]) or None)
- :param font: specifies the font family, size to be used for the menu items
- :type font: (str or (str, int[, str]) or None)
- :param tearoff: if True, then can tear the menu off from the window ans use as a floating window. Very cool effect
- :type tearoff: (bool)
- :param pad: Amount of padding to put around element in pixels (left/right, top/bottom) or ((left, right), (top, bottom)) or an int. If an int, then it's converted into a tuple (int, int). TIP - 0 will make flush with titlebar
- :type pad: (int, int) or ((int, int),(int,int)) or (int,(int,int)) or ((int, int),int) | int
- :param p: Same as pad parameter. It's an alias. If EITHER of them are set, then the one that's set will be used. If BOTH are set, pad will be used
- :type p: (int, int) or ((int, int),(int,int)) or (int,(int,int)) or ((int, int),int) | int
- :param background_color: color to use for background of the menus that are displayed after making a section. Can be in #RRGGBB format or a color name "black". Defaults to the color of the bar text
- :type background_color: (str)
- :param text_color: color to use for the text of the many items in the displayed menus. Can be in #RRGGBB format or a color name "black". Defaults to the bar background
- :type text_color: (str)
- :param bar_background_color: color to use for the menubar. Can be in #RRGGBB format or a color name "black". Defaults to theme's button text color
- :type bar_background_color: (str)
- :param bar_text_color: color to use for the menu items text when item is disabled. Can be in #RRGGBB format or a color name "black". Defaults to theme's button background color
- :type bar_text_color: (str)
- :param key: Value that uniquely identifies this element from all other elements. Used when Finding an element or in return values. Must be unique to the window
- :type key: str | int | tuple | object
- :param k: Same as the Key. You can use either k or key. Which ever is set will be used.
- :type k: str | int | tuple | object
- :returns: A Column element that has a series of ButtonMenu elements
- :rtype: Column
- """
-
- bar_bg = bar_background_color if bar_background_color is not None else theme_button_color()[0]
- bar_text = bar_text_color if bar_text_color is not None else theme_button_color()[1]
- menu_bg = background_color if background_color is not None else bar_text
- menu_text = text_color if text_color is not None else bar_bg
- pad = pad if pad is not None else p
-
- row = []
- for menu in menu_definition:
- text = menu[0]
- if MENU_SHORTCUT_CHARACTER in text:
- text = text.replace(MENU_SHORTCUT_CHARACTER, '')
- if text.startswith(MENU_DISABLED_CHARACTER):
- disabled = True
- text = text[len(MENU_DISABLED_CHARACTER):]
- else:
- disabled = False
-
- button_menu = ButtonMenu(text, menu, border_width=0, button_color=(bar_text, bar_bg), key=text, pad=(0, 0), disabled=disabled, font=bar_font,
- item_font=font, disabled_text_color=disabled_text_color, text_color=menu_text, background_color=menu_bg, tearoff=tearoff)
- button_menu.part_of_custom_menubar = True
- button_menu.custom_menubar_key = key if key is not None else k
- row += [button_menu]
- return Column([row], pad=pad, background_color=bar_bg, expand_x=True, key=key if key is not None else k)
-
-
-# ------------------------- FOLDER BROWSE Element lazy function ------------------------- #
-def FolderBrowse(button_text='Browse', target=(ThisRow, -1), initial_folder=None, tooltip=None, size=(None, None), s=(None, None),
- auto_size_button=None, button_color=None, disabled=False, change_submits=False, enable_events=False,
- font=None, pad=None, p=None, key=None, k=None, visible=True, metadata=None):
- """
- :param button_text: text in the button (Default value = 'Browse')
- :type button_text: (str)
- :param target: target for the button (Default value = (ThisRow, -1))
- :type target: str | (int, int)
- :param initial_folder: starting path for folders and files
- :type initial_folder: (str)
- :param tooltip: text, that will appear when mouse hovers over the element
- :type tooltip: (str)
- :param size: (w,h) w=characters-wide, h=rows-high
- :type size: (int, int)
- :param s: Same as size parameter. It's an alias. If EITHER of them are set, then the one that's set will be used. If BOTH are set, size will be used
- :type s: (int, int) | (None, None) | int
- :param auto_size_button: True if button size is determined by button text
- :type auto_size_button: (bool)
- :param button_color: button color (foreground, background)
- :type button_color:
- :param disabled: set disable state for element (Default = False)
- :type disabled: (bool)
- :param change_submits: If True, pressing Enter key submits window (Default = False)
- :type enable_events: (bool)
- :param enable_events: Turns on the element specific events.(Default = False)
- :type enable_events: (bool)
- :param font: specifies the font family, size, etc. Tuple or Single string format 'name size styles'. Styles: italic * roman bold normal underline overstrike
- :type font: (str or (str, int[, str]) or None)
- :param pad: Amount of padding to put around element in pixels (left/right, top/bottom) or ((left, right), (top, bottom)) or an int. If an int, then it's converted into a tuple (int, int)
- :type pad: (int, int) or ((int, int),(int,int)) or (int,(int,int)) or ((int, int),int) | int
- :param p: Same as pad parameter. It's an alias. If EITHER of them are set, then the one that's set will be used. If BOTH are set, pad will be used
- :type p: (int, int) or ((int, int),(int,int)) or (int,(int,int)) or ((int, int),int) | int
- :param key: Used with window.find_element and with return values to uniquely identify this element
- :type key: str | int | tuple | object
- :param k: Same as the Key. You can use either k or key. Which ever is set will be used.
- :type k: str | int | tuple | object
- :param visible: set initial visibility state of the Button
- :type visible: (bool)
- :param metadata: Anything you want to store along with this button
- :type metadata: (Any)
- :return: The Button created
- :rtype: (Button)
- """
-
- return Button(button_text=button_text, button_type=BUTTON_TYPE_BROWSE_FOLDER, target=target,
- initial_folder=initial_folder, tooltip=tooltip, size=size, s=s, auto_size_button=auto_size_button,
- disabled=disabled, button_color=button_color, change_submits=change_submits,
- enable_events=enable_events, font=font, pad=pad, p=p, key=key, k=k, visible=visible, metadata=metadata)
-
-
-# ------------------------- FILE BROWSE Element lazy function ------------------------- #
-def FileBrowse(button_text='Browse', target=(ThisRow, -1), file_types=FILE_TYPES_ALL_FILES, initial_folder=None,
- tooltip=None, size=(None, None), s=(None, None), auto_size_button=None, button_color=None, change_submits=False,
- enable_events=False, font=None, disabled=False,
- pad=None, p=None, key=None, k=None, visible=True, metadata=None):
- """
-
- :param button_text: text in the button (Default value = 'Browse')
- :type button_text: (str)
- :param target: key or (row,col) target for the button (Default value = (ThisRow, -1))
- :type target: str | (int, int)
- :param file_types: filter file types Default value = (("ALL Files", "*.* *"),). NOT avoilable on the MAC
- :type file_types: Tuple[(str, str), ...]
- :param initial_folder: starting path for folders and files
- :type initial_folder:
- :param tooltip: text, that will appear when mouse hovers over the element
- :type tooltip: (str)
- :param size: (w,h) w=characters-wide, h=rows-high
- :type size: (int, int)
- :param s: Same as size parameter. It's an alias. If EITHER of them are set, then the one that's set will be used. If BOTH are set, size will be used
- :type s: (int, int) | (None, None) | int
- :param auto_size_button: True if button size is determined by button text
- :type auto_size_button: (bool)
- :param button_color: button color (foreground, background)
- :type button_color: (str, str) or str
- :param change_submits: If True, pressing Enter key submits window (Default = False)
- :type change_submits: (bool)
- :param enable_events: Turns on the element specific events.(Default = False)
- :type enable_events: (bool)
- :param font: specifies the font family, size, etc. Tuple or Single string format 'name size styles'. Styles: italic * roman bold normal underline overstrike
- :type font: (str or (str, int[, str]) or None)
- :param disabled: set disable state for element (Default = False)
- :type disabled: (bool)
- :param pad: Amount of padding to put around element in pixels (left/right, top/bottom) or ((left, right), (top, bottom)) or an int. If an int, then it's converted into a tuple (int, int)
- :type pad: (int, int) or ((int, int),(int,int)) or (int,(int,int)) or ((int, int),int) | int
- :param p: Same as pad parameter. It's an alias. If EITHER of them are set, then the one that's set will be used. If BOTH are set, pad will be used
- :type p: (int, int) or ((int, int),(int,int)) or (int,(int,int)) or ((int, int),int) | int
- :param key: key for uniquely identify this element (for window.find_element)
- :type key: str | int | tuple | object
- :param k: Same as the Key. You can use either k or key. Which ever is set will be used.
- :type k: str | int | tuple | object
- :param visible: set initial visibility state of the Button
- :type visible: (bool)
- :param metadata: Anything you want to store along with this button
- :type metadata: (Any)
- :return: returns a button
- :rtype: (Button)
- """
- return Button(button_text=button_text, button_type=BUTTON_TYPE_BROWSE_FILE, target=target, file_types=file_types,
- initial_folder=initial_folder, tooltip=tooltip, size=size, s=s, auto_size_button=auto_size_button,
- change_submits=change_submits, enable_events=enable_events, disabled=disabled,
- button_color=button_color, font=font, pad=pad, p=p, key=key, k=k, visible=visible, metadata=metadata)
-
-
-# ------------------------- FILES BROWSE Element (Multiple file selection) lazy function ------------------------- #
-def FilesBrowse(button_text='Browse', target=(ThisRow, -1), file_types=FILE_TYPES_ALL_FILES, disabled=False,
- initial_folder=None, tooltip=None, size=(None, None), s=(None, None), auto_size_button=None, button_color=None,
- change_submits=False, enable_events=False,
- font=None, pad=None, p=None, key=None, k=None, visible=True, files_delimiter=BROWSE_FILES_DELIMITER, metadata=None):
- """
- Allows browsing of multiple files. File list is returned as a single list with the delimiter defined using the files_delimiter parameter.
-
- :param button_text: text in the button (Default value = 'Browse')
- :type button_text: (str)
- :param target: key or (row,col) target for the button (Default value = (ThisRow, -1))
- :type target: str | (int, int)
- :param file_types: Default value = (("ALL Files", "*.* *"),). NOT avoilable on the MAC
- :type file_types: Tuple[(str, str), ...]
- :param disabled: set disable state for element (Default = False)
- :type disabled: (bool)
- :param initial_folder: starting path for folders and files
- :type initial_folder: (str)
- :param tooltip: text, that will appear when mouse hovers over the element
- :type tooltip: (str)
- :param size: (w,h) w=characters-wide, h=rows-high
- :type size: (int, int)
- :param s: Same as size parameter. It's an alias. If EITHER of them are set, then the one that's set will be used. If BOTH are set, size will be used
- :type s: (int, int) | (None, None) | int
- :param auto_size_button: True if button size is determined by button text
- :type auto_size_button: (bool)
- :param button_color: button color (foreground, background)
- :type button_color: (str, str) or str
- :param change_submits: If True, pressing Enter key submits window (Default = False)
- :type change_submits: (bool)
- :param enable_events: Turns on the element specific events.(Default = False)
- :type enable_events: (bool)
- :param font: specifies the font family, size, etc. Tuple or Single string format 'name size styles'. Styles: italic * roman bold normal underline overstrike
- :type font: (str or (str, int[, str]) or None)
- :param pad: Amount of padding to put around element in pixels (left/right, top/bottom) or ((left, right), (top, bottom)) or an int. If an int, then it's converted into a tuple (int, int)
- :type pad: (int, int) or ((int, int),(int,int)) or (int,(int,int)) or ((int, int),int) | int
- :param p: Same as pad parameter. It's an alias. If EITHER of them are set, then the one that's set will be used. If BOTH are set, pad will be used
- :type p: (int, int) or ((int, int),(int,int)) or (int,(int,int)) or ((int, int),int) | int
- :param key: key for uniquely identify this element (for window.find_element)
- :type key: str | int | tuple | object
- :param k: Same as the Key. You can use either k or key. Which ever is set will be used.
- :type k: str | int | tuple | object
- :param visible: set initial visibility state of the Button
- :type visible: (bool)
- :param files_delimiter: String to place between files when multiple files are selected. Normally a ;
- :type files_delimiter: str
- :param metadata: Anything you want to store along with this button
- :type metadata: (Any)
- :return: returns a button
- :rtype: (Button)
- """
- button = Button(button_text=button_text, button_type=BUTTON_TYPE_BROWSE_FILES, target=target, file_types=file_types,
- initial_folder=initial_folder, change_submits=change_submits, enable_events=enable_events,
- tooltip=tooltip, size=size, s=s, auto_size_button=auto_size_button,
- disabled=disabled, button_color=button_color, font=font, pad=pad, p=p, key=key, k=k, visible=visible, metadata=metadata)
- button._files_delimiter = files_delimiter
- return button
-
-
-# ------------------------- FILE BROWSE Element lazy function ------------------------- #
-def FileSaveAs(button_text='Save As...', target=(ThisRow, -1), file_types=FILE_TYPES_ALL_FILES, initial_folder=None,
- default_extension='', disabled=False, tooltip=None, size=(None, None), s=(None, None), auto_size_button=None, button_color=None,
- change_submits=False, enable_events=False, font=None,
- pad=None, p=None, key=None, k=None, visible=True, metadata=None):
- """
-
- :param button_text: text in the button (Default value = 'Save As...')
- :type button_text: (str)
- :param target: key or (row,col) target for the button (Default value = (ThisRow, -1))
- :type target: str | (int, int)
- :param file_types: Default value = (("ALL Files", "*.* *"),). NOT avoilable on the MAC
- :type file_types: Tuple[(str, str), ...]
- :param default_extension: If no extension entered by user, add this to filename (only used in saveas dialogs)
- :type default_extension: (str)
- :param initial_folder: starting path for folders and files
- :type initial_folder: (str)
- :param disabled: set disable state for element (Default = False)
- :type disabled: (bool)
- :param tooltip: text, that will appear when mouse hovers over the element
- :type tooltip: (str)
- :param size: (w,h) w=characters-wide, h=rows-high
- :type size: (int, int)
- :param s: Same as size parameter. It's an alias. If EITHER of them are set, then the one that's set will be used. If BOTH are set, size will be used
- :type s: (int, int) | (None, None) | int
- :param auto_size_button: True if button size is determined by button text
- :type auto_size_button: (bool)
- :param button_color: button color (foreground, background)
- :type button_color: (str, str) or str
- :param change_submits: If True, pressing Enter key submits window (Default = False)
- :type change_submits: (bool)
- :param enable_events: Turns on the element specific events.(Default = False)
- :type enable_events: (bool)
- :param font: specifies the font family, size, etc. Tuple or Single string format 'name size styles'. Styles: italic * roman bold normal underline overstrike
- :type font: (str or (str, int[, str]) or None)
- :param pad: Amount of padding to put around element in pixels (left/right, top/bottom) or ((left, right), (top, bottom)) or an int. If an int, then it's converted into a tuple (int, int)
- :type pad: (int, int) or ((int, int),(int,int)) or (int,(int,int)) or ((int, int),int) | int
- :param p: Same as pad parameter. It's an alias. If EITHER of them are set, then the one that's set will be used. If BOTH are set, pad will be used
- :type p: (int, int) or ((int, int),(int,int)) or (int,(int,int)) or ((int, int),int) | int
- :param key: key for uniquely identify this element (for window.find_element)
- :type key: str | int | tuple | object
- :param k: Same as the Key. You can use either k or key. Which ever is set will be used.
- :type k: str | int | tuple | object
- :param visible: set initial visibility state of the Button
- :type visible: (bool)
- :param metadata: Anything you want to store along with this button
- :type metadata: (Any)
- :return: returns a button
- :rtype: (Button)
- """
- return Button(button_text=button_text, button_type=BUTTON_TYPE_SAVEAS_FILE, target=target, file_types=file_types,
- initial_folder=initial_folder, default_extension=default_extension, tooltip=tooltip, size=size, s=s, disabled=disabled,
- auto_size_button=auto_size_button, button_color=button_color, change_submits=change_submits,
- enable_events=enable_events, font=font, pad=pad, p=p, key=key, k=k, visible=visible, metadata=metadata)
-
-
-# ------------------------- SAVE AS Element lazy function ------------------------- #
-def SaveAs(button_text='Save As...', target=(ThisRow, -1), file_types=FILE_TYPES_ALL_FILES, initial_folder=None, default_extension='',
- disabled=False, tooltip=None, size=(None, None), s=(None, None), auto_size_button=None, button_color=None,
- change_submits=False, enable_events=False, font=None,
- pad=None, p=None, key=None, k=None, visible=True, metadata=None):
- """
-
- :param button_text: text in the button (Default value = 'Save As...')
- :type button_text: (str)
- :param target: key or (row,col) target for the button (Default value = (ThisRow, -1))
- :type target: str | (int, int)
- :param file_types: Default value = (("ALL Files", "*.* *"),). NOT avoilable on the MAC
- :type file_types: Tuple[(str, str), ...]
- :param default_extension: If no extension entered by user, add this to filename (only used in saveas dialogs)
- :type default_extension: (str)
- :param initial_folder: starting path for folders and files
- :type initial_folder: (str)
- :param disabled: set disable state for element (Default = False)
- :type disabled: (bool)
- :param tooltip: text, that will appear when mouse hovers over the element
- :type tooltip: (str)
- :param size: (w,h) w=characters-wide, h=rows-high
- :type size: (int, int)
- :param s: Same as size parameter. It's an alias. If EITHER of them are set, then the one that's set will be used. If BOTH are set, size will be used
- :type s: (int, int) | (None, None) | int
- :param auto_size_button: True if button size is determined by button text
- :type auto_size_button: (bool)
- :param button_color: button color (foreground, background)
- :type button_color: (str, str) or str
- :param change_submits: If True, pressing Enter key submits window (Default = False)
- :type change_submits: (bool)
- :param enable_events: Turns on the element specific events.(Default = False)
- :type enable_events: (bool)
- :param font: specifies the font family, size, etc. Tuple or Single string format 'name size styles'. Styles: italic * roman bold normal underline overstrike
- :type font: (str or (str, int[, str]) or None)
- :param pad: Amount of padding to put around element in pixels (left/right, top/bottom) or ((left, right), (top, bottom)) or an int. If an int, then it's converted into a tuple (int, int)
- :type pad: (int, int) or ((int, int),(int,int)) or (int,(int,int)) or ((int, int),int) | int
- :param p: Same as pad parameter. It's an alias. If EITHER of them are set, then the one that's set will be used. If BOTH are set, pad will be used
- :type p: (int, int) or ((int, int),(int,int)) or (int,(int,int)) or ((int, int),int) | int :param key: key for uniquely identify this element (for window.find_element)
- :type key: str | int | tuple | object
- :param k: Same as the Key. You can use either k or key. Which ever is set will be used.
- :type k: str | int | tuple | object
- :param visible: set initial visibility state of the Button
- :type visible: (bool)
- :param metadata: Anything you want to store along with this button
- :type metadata: (Any)
- :return: returns a button
- :rtype: (Button)
- """
- return Button(button_text=button_text, button_type=BUTTON_TYPE_SAVEAS_FILE, target=target, file_types=file_types,
- initial_folder=initial_folder, default_extension=default_extension, tooltip=tooltip, size=size, s=s, disabled=disabled,
- auto_size_button=auto_size_button, button_color=button_color, change_submits=change_submits,
- enable_events=enable_events, font=font, pad=pad, p=p, key=key, k=k, visible=visible, metadata=metadata)
-
-
-# ------------------------- SAVE BUTTON Element lazy function ------------------------- #
-def Save(button_text='Save', size=(None, None), s=(None, None), auto_size_button=None, button_color=None, bind_return_key=True,
- disabled=False, tooltip=None, font=None, focus=False, pad=None, p=None, key=None, k=None, visible=True, metadata=None):
- """
-
- :param button_text: text in the button (Default value = 'Save')
- :type button_text: (str)
- :param size: (w,h) w=characters-wide, h=rows-high
- :type size: (int, int)
- :param s: Same as size parameter. It's an alias. If EITHER of them are set, then the one that's set will be used. If BOTH are set, size will be used
- :type s: (int, int) | (None, None) | int
- :param auto_size_button: True if button size is determined by button text
- :type auto_size_button: (bool)
- :param button_color: button color (foreground, background)
- :type button_color: (str, str) or str
- :param bind_return_key: (Default = True) If True, then the return key will cause a the Listbox to generate an event
- :type bind_return_key: (bool)
- :param disabled: set disable state for element (Default = False)
- :type disabled: (bool)
- :param tooltip: text, that will appear when mouse hovers over the element
- :type tooltip: (str)
- :param font: specifies the font family, size, etc. Tuple or Single string format 'name size styles'. Styles: italic * roman bold normal underline overstrike
- :type font: (str or (str, int[, str]) or None)
- :param focus: if focus should be set to this
- :type focus: idk_yetReally
- :param pad: Amount of padding to put around element in pixels (left/right, top/bottom) or ((left, right), (top, bottom)) or an int. If an int, then it's converted into a tuple (int, int)
- :type pad: (int, int) or ((int, int),(int,int)) or (int,(int,int)) or ((int, int),int) | int
- :param p: Same as pad parameter. It's an alias. If EITHER of them are set, then the one that's set will be used. If BOTH are set, pad will be used
- :type p: (int, int) or ((int, int),(int,int)) or (int,(int,int)) or ((int, int),int) | int
- :param key: key for uniquely identify this element (for window.find_element)
- :type key: str | int | tuple | object
- :param k: Same as the Key. You can use either k or key. Which ever is set will be used.
- :type k: str | int | tuple | object
- :param visible: set initial visibility state of the Button
- :type visible: (bool)
- :param metadata: Anything you want to store along with this button
- :type metadata: (Any)
- :return: returns a button
- :rtype: (Button)
- """
- return Button(button_text=button_text, button_type=BUTTON_TYPE_READ_FORM, tooltip=tooltip, size=size, s=s,
- auto_size_button=auto_size_button, button_color=button_color, font=font, disabled=disabled,
- bind_return_key=bind_return_key, focus=focus, pad=pad, p=p, key=key, k=k, visible=visible, metadata=metadata)
-
-
-# ------------------------- SUBMIT BUTTON Element lazy function ------------------------- #
-def Submit(button_text='Submit', size=(None, None), s=(None, None), auto_size_button=None, button_color=None, disabled=False,
- bind_return_key=True, tooltip=None, font=None, focus=False, pad=None, p=None, key=None, k=None, visible=True, metadata=None):
- """
-
- :param button_text: text in the button (Default value = 'Submit')
- :type button_text: (str)
- :param size: (w,h) w=characters-wide, h=rows-high
- :type size: (int, int)
- :param s: Same as size parameter. It's an alias. If EITHER of them are set, then the one that's set will be used. If BOTH are set, size will be used
- :type s: (int, int) | (None, None) | int
- :param auto_size_button: True if button size is determined by button text
- :type auto_size_button: (bool)
- :param button_color: button color (foreground, background)
- :type button_color: (str, str) or str
- :param disabled: set disable state for element (Default = False)
- :type disabled: (bool)
- :param bind_return_key: (Default = True) If True, then the return key will cause a the Listbox to generate an event
- :type bind_return_key: (bool)
- :param tooltip: text, that will appear when mouse hovers over the element
- :type tooltip: (str)
- :param font: specifies the font family, size, etc. Tuple or Single string format 'name size styles'. Styles: italic * roman bold normal underline overstrike
- :type font: (str or (str, int[, str]) or None)
- :param focus: if focus should be set to this
- :type focus: idk_yetReally
- :param pad: Amount of padding to put around element in pixels (left/right, top/bottom) or ((left, right), (top, bottom)) or an int. If an int, then it's converted into a tuple (int, int)
- :type pad: (int, int) or ((int, int),(int,int)) or (int,(int,int)) or ((int, int),int) | int
- :param p: Same as pad parameter. It's an alias. If EITHER of them are set, then the one that's set will be used. If BOTH are set, pad will be used
- :type p: (int, int) or ((int, int),(int,int)) or (int,(int,int)) or ((int, int),int) | int
- :param key: key for uniquely identify this element (for window.find_element)
- :type key: str | int | tuple | object
- :param k: Same as the Key. You can use either k or key. Which ever is set will be used.
- :type k: str | int | tuple | object
- :param visible: set initial visibility state of the Button
- :type visible: (bool)
- :param metadata: Anything you want to store along with this button
- :type metadata: (Any)
- :return: returns a button
- :rtype: (Button)
- """
- return Button(button_text=button_text, button_type=BUTTON_TYPE_READ_FORM, tooltip=tooltip, size=size, s=s,
- auto_size_button=auto_size_button, button_color=button_color, font=font, disabled=disabled,
- bind_return_key=bind_return_key, focus=focus, pad=pad, p=p, key=key, k=k, visible=visible, metadata=metadata)
-
-
-# ------------------------- OPEN BUTTON Element lazy function ------------------------- #
-# ------------------------- OPEN BUTTON Element lazy function ------------------------- #
-def Open(button_text='Open', size=(None, None), s=(None, None), auto_size_button=None, button_color=None, disabled=False,
- bind_return_key=True, tooltip=None, font=None, focus=False, pad=None, p=None, key=None, k=None, visible=True, metadata=None):
- """
-
- :param button_text: text in the button (Default value = 'Open')
- :type button_text: (str)
- :param size: (w,h) w=characters-wide, h=rows-high
- :type size: (int, int)
- :param s: Same as size parameter. It's an alias. If EITHER of them are set, then the one that's set will be used. If BOTH are set, size will be used
- :type s: (int, int) | (None, None) | int
- :param auto_size_button: True if button size is determined by button text
- :type auto_size_button: (bool)
- :param button_color: button color (foreground, background)
- :type button_color: (str, str) or str
- :param disabled: set disable state for element (Default = False)
- :type disabled: (bool)
- :param bind_return_key: (Default = True) If True, then the return key will cause a the Listbox to generate an event
- :type bind_return_key: (bool)
- :param tooltip: text, that will appear when mouse hovers over the element
- :type tooltip: (str)
- :param font: specifies the font family, size, etc. Tuple or Single string format 'name size styles'. Styles: italic * roman bold normal underline overstrike
- :type font: (str or (str, int[, str]) or None)
- :param focus: if focus should be set to this
- :type focus: idk_yetReally
- :param pad: Amount of padding to put around element in pixels (left/right, top/bottom) or ((left, right), (top, bottom)) or an int. If an int, then it's converted into a tuple (int, int)
- :type pad: (int, int) or ((int, int),(int,int)) or (int,(int,int)) or ((int, int),int) | int
- :param p: Same as pad parameter. It's an alias. If EITHER of them are set, then the one that's set will be used. If BOTH are set, pad will be used
- :type p: (int, int) or ((int, int),(int,int)) or (int,(int,int)) or ((int, int),int) | int
- :param key: key for uniquely identify this element (for window.find_element)
- :type key: str | int | tuple | object
- :param k: Same as the Key. You can use either k or key. Which ever is set will be used.
- :type k: str | int | tuple | object
- :param visible: set initial visibility state of the Button
- :type visible: (bool)
- :param metadata: Anything you want to store along with this button
- :type metadata: (Any)
- :return: returns a button
- :rtype: (Button)
- """
- return Button(button_text=button_text, button_type=BUTTON_TYPE_READ_FORM, tooltip=tooltip, size=size, s=s,
- auto_size_button=auto_size_button, button_color=button_color, font=font, disabled=disabled,
- bind_return_key=bind_return_key, focus=focus, pad=pad, p=p, key=key, k=k, visible=visible, metadata=metadata)
-
-
-# ------------------------- OK BUTTON Element lazy function ------------------------- #
-def OK(button_text='OK', size=(None, None), s=(None, None), auto_size_button=None, button_color=None, disabled=False,
- bind_return_key=True, tooltip=None, font=None, focus=False, pad=None, p=None, key=None, k=None, visible=True, metadata=None):
- """
-
- :param button_text: text in the button (Default value = 'OK')
- :type button_text: (str)
- :param size: (w,h) w=characters-wide, h=rows-high
- :type size: (int, int)
- :param s: Same as size parameter. It's an alias. If EITHER of them are set, then the one that's set will be used. If BOTH are set, size will be used
- :type s: (int, int) | (None, None) | int
- :param auto_size_button: True if button size is determined by button text
- :type auto_size_button: (bool)
- :param button_color: button color (foreground, background)
- :type button_color: (str, str) or str
- :param disabled: set disable state for element (Default = False)
- :type disabled: (bool)
- :param bind_return_key: (Default = True) If True, then the return key will cause a the Listbox to generate an event
- :type bind_return_key: (bool)
- :param tooltip: text, that will appear when mouse hovers over the element
- :type tooltip: (str)
- :param font: specifies the font family, size, etc. Tuple or Single string format 'name size styles'. Styles: italic * roman bold normal underline overstrike
- :type font: (str or (str, int[, str]) or None)
- :param focus: if focus should be set to this
- :type focus: idk_yetReally
- :param pad: Amount of padding to put around element in pixels (left/right, top/bottom) or ((left, right), (top, bottom)) or an int. If an int, then it's converted into a tuple (int, int)
- :type pad: (int, int) or ((int, int),(int,int)) or (int,(int,int)) or ((int, int),int) | int
- :param p: Same as pad parameter. It's an alias. If EITHER of them are set, then the one that's set will be used. If BOTH are set, pad will be used
- :type p: (int, int) or ((int, int),(int,int)) or (int,(int,int)) or ((int, int),int) | int
- :param key: key for uniquely identify this element (for window.find_element)
- :type key: str | int | tuple | object
- :param k: Same as the Key. You can use either k or key. Which ever is set will be used.
- :type k: str | int | tuple | object
- :param visible: set initial visibility state of the Button
- :type visible: (bool)
- :param metadata: Anything you want to store along with this button
- :type metadata: (Any)
- :return: returns a button
- :rtype: (Button)
- """
- return Button(button_text=button_text, button_type=BUTTON_TYPE_READ_FORM, tooltip=tooltip, size=size, s=s,
- auto_size_button=auto_size_button, button_color=button_color, font=font, disabled=disabled,
- bind_return_key=bind_return_key, focus=focus, pad=pad, p=p, key=key, k=k, visible=visible, metadata=metadata)
-
-
-# ------------------------- YES BUTTON Element lazy function ------------------------- #
-def Ok(button_text='Ok', size=(None, None), s=(None, None), auto_size_button=None, button_color=None, disabled=False,
- bind_return_key=True, tooltip=None, font=None, focus=False, pad=None, p=None, key=None, k=None, visible=True, metadata=None):
- """
-
- :param button_text: text in the button (Default value = 'Ok')
- :type button_text: (str)
- :param size: (w,h) w=characters-wide, h=rows-high
- :type size: (int, int)
- :param s: Same as size parameter. It's an alias. If EITHER of them are set, then the one that's set will be used. If BOTH are set, size will be used
- :type s: (int, int) | (None, None) | int
- :param auto_size_button: True if button size is determined by button text
- :type auto_size_button: (bool)
- :param button_color: button color (foreground, background)
- :type button_color: (str, str) or str
- :param disabled: set disable state for element (Default = False)
- :type disabled: (bool)
- :param bind_return_key: (Default = True) If True, then the return key will cause a the Listbox to generate an event
- :type bind_return_key: (bool)
- :param tooltip: text, that will appear when mouse hovers over the element
- :type tooltip: (str)
- :param font: specifies the font family, size, etc. Tuple or Single string format 'name size styles'. Styles: italic * roman bold normal underline overstrike
- :type font: (str or (str, int[, str]) or None)
- :param focus: if focus should be set to this
- :type focus: idk_yetReally
- :param pad: Amount of padding to put around element in pixels (left/right, top/bottom) or ((left, right), (top, bottom)) or an int. If an int, then it's converted into a tuple (int, int)
- :type pad: (int, int) or ((int, int),(int,int)) or (int,(int,int)) or ((int, int),int) | int
- :param p: Same as pad parameter. It's an alias. If EITHER of them are set, then the one that's set will be used. If BOTH are set, pad will be used
- :type p: (int, int) or ((int, int),(int,int)) or (int,(int,int)) or ((int, int),int) | int
- :param key: key for uniquely identify this element (for window.find_element)
- :type key: str | int | tuple | object
- :param k: Same as the Key. You can use either k or key. Which ever is set will be used.
- :type k: str | int | tuple | object
- :param visible: set initial visibility state of the Button
- :type visible: (bool)
- :param metadata: Anything you want to store along with this button
- :type metadata: (Any)
- :return: returns a button
- :rtype: (Button)
- """
- return Button(button_text=button_text, button_type=BUTTON_TYPE_READ_FORM, tooltip=tooltip, size=size, s=s,
- auto_size_button=auto_size_button, button_color=button_color, font=font, disabled=disabled,
- bind_return_key=bind_return_key, focus=focus, pad=pad, p=p, key=key, k=k, visible=visible, metadata=metadata)
-
-
-# ------------------------- CANCEL BUTTON Element lazy function ------------------------- #
-def Cancel(button_text='Cancel', size=(None, None), s=(None, None), auto_size_button=None, button_color=None, disabled=False,
- tooltip=None, font=None, bind_return_key=False, focus=False, pad=None, p=None, key=None, k=None, visible=True, metadata=None):
- """
-
- :param button_text: text in the button (Default value = 'Cancel')
- :type button_text: (str)
- :param size: (w,h) w=characters-wide, h=rows-high
- :type size: (int, int)
- :param s: Same as size parameter. It's an alias. If EITHER of them are set, then the one that's set will be used. If BOTH are set, size will be used
- :type s: (int, int) | (None, None) | int
- :param auto_size_button: True if button size is determined by button text
- :type auto_size_button: (bool)
- :param button_color: button color (foreground, background)
- :type button_color: (str, str) or str
- :param disabled: set disable state for element (Default = False)
- :type disabled: (bool)
- :param tooltip: text, that will appear when mouse hovers over the element
- :type tooltip: (str)
- :param font: specifies the font family, size, etc. Tuple or Single string format 'name size styles'. Styles: italic * roman bold normal underline overstrike
- :type font: (str or (str, int[, str]) or None)
- :param bind_return_key: (Default = False) If True, then the return key will cause a the Listbox to generate an event
- :type bind_return_key: (bool)
- :param focus: if focus should be set to this
- :type focus:
- :param pad: Amount of padding to put around element in pixels (left/right, top/bottom) or ((left, right), (top, bottom)) or an int. If an int, then it's converted into a tuple (int, int)
- :type pad: (int, int) or ((int, int),(int,int)) or (int,(int,int)) or ((int, int),int) | int
- :param p: Same as pad parameter. It's an alias. If EITHER of them are set, then the one that's set will be used. If BOTH are set, pad will be used
- :type p: (int, int) or ((int, int),(int,int)) or (int,(int,int)) or ((int, int),int) | int
- :param key: key for uniquely identify this element (for window.find_element)
- :type key: str | int | tuple | object
- :param k: Same as the Key. You can use either k or key. Which ever is set will be used.
- :type k: str | int | tuple | object
- :param visible: set initial visibility state of the Button
- :type visible: (bool)
- :param metadata: Anything you want to store along with this button
- :type metadata: (Any)
- :return: returns a button
- :rtype: (Button)
- """
- return Button(button_text=button_text, button_type=BUTTON_TYPE_READ_FORM, tooltip=tooltip, size=size, s=s,
- auto_size_button=auto_size_button, button_color=button_color, font=font, disabled=disabled,
- bind_return_key=bind_return_key, focus=focus, pad=pad, p=p, key=key, k=k, visible=visible, metadata=metadata)
-
-
-# ------------------------- QUIT BUTTON Element lazy function ------------------------- #
-def Quit(button_text='Quit', size=(None, None), s=(None, None), auto_size_button=None, button_color=None, disabled=False, tooltip=None,
- font=None, bind_return_key=False, focus=False, pad=None, p=None, key=None, k=None, visible=True, metadata=None):
- """
-
- :param button_text: text in the button (Default value = 'Quit')
- :type button_text: (str)
- :param size: (w,h) w=characters-wide, h=rows-high
- :type size: (int, int)
- :param s: Same as size parameter. It's an alias. If EITHER of them are set, then the one that's set will be used. If BOTH are set, size will be used
- :type s: (int, int) | (None, None) | int
- :param auto_size_button: True if button size is determined by button text
- :type auto_size_button: (bool)
- :param button_color: button color (foreground, background)
- :type button_color: (str, str) or str
- :param disabled: set disable state for element (Default = False)
- :type disabled: (bool)
- :param tooltip: text, that will appear when mouse hovers over the element
- :type tooltip: (str)
- :param font: specifies the font family, size, etc. Tuple or Single string format 'name size styles'. Styles: italic * roman bold normal underline overstrike
- :type font: (str or (str, int[, str]) or None)
- :param bind_return_key: (Default = False) If True, then the return key will cause a the Listbox to generate an event
- :type bind_return_key: (bool)
- :param focus: if focus should be set to this
- :type focus: (bool)
- :param pad: Amount of padding to put around element in pixels (left/right, top/bottom) or ((left, right), (top, bottom)) or an int. If an int, then it's converted into a tuple (int, int)
- :type pad: (int, int) or ((int, int),(int,int)) or (int,(int,int)) or ((int, int),int) | int
- :param p: Same as pad parameter. It's an alias. If EITHER of them are set, then the one that's set will be used. If BOTH are set, pad will be used
- :type p: (int, int) or ((int, int),(int,int)) or (int,(int,int)) or ((int, int),int) | int
- :param key: key for uniquely identify this element (for window.find_element)
- :type key: str | int | tuple | object
- :param k: Same as the Key. You can use either k or key. Which ever is set will be used.
- :type k: str | int | tuple | object
- :param visible: set initial visibility state of the Button
- :type visible: (bool)
- :param metadata: Anything you want to store along with this button
- :type metadata: (Any)
- :return: returns a button
- :rtype: (Button)
- """
- return Button(button_text=button_text, button_type=BUTTON_TYPE_READ_FORM, tooltip=tooltip, size=size, s=s,
- auto_size_button=auto_size_button, button_color=button_color, font=font, disabled=disabled,
- bind_return_key=bind_return_key, focus=focus, pad=pad, p=p, key=key, k=k, visible=visible, metadata=metadata)
-
-
-# ------------------------- Exit BUTTON Element lazy function ------------------------- #
-def Exit(button_text='Exit', size=(None, None), s=(None, None), auto_size_button=None, button_color=None, disabled=False, tooltip=None,
- font=None, bind_return_key=False, focus=False, pad=None, p=None, key=None, k=None, visible=True, metadata=None):
- """
-
- :param button_text: text in the button (Default value = 'Exit')
- :type button_text: (str)
- :param size: (w,h) w=characters-wide, h=rows-high
- :type size: (int, int)
- :param s: Same as size parameter. It's an alias. If EITHER of them are set, then the one that's set will be used. If BOTH are set, size will be used
- :type s: (int, int) | (None, None) | int
- :param auto_size_button: True if button size is determined by button text
- :type auto_size_button: (bool)
- :param button_color: button color (foreground, background)
- :type button_color: (str, str) or str
- :param disabled: set disable state for element (Default = False)
- :type disabled: (bool)
- :param tooltip: text, that will appear when mouse hovers over the element
- :type tooltip: (str)
- :param font: specifies the font family, size, etc. Tuple or Single string format 'name size styles'. Styles: italic * roman bold normal underline overstrike
- :type font: (str or (str, int[, str]) or None)
- :param bind_return_key: (Default = False) If True, then the return key will cause a the Listbox to generate an event
- :type bind_return_key: (bool)
- :param focus: if focus should be set to this
- :type focus:
- :param pad: Amount of padding to put around element in pixels (left/right, top/bottom) or ((left, right), (top, bottom)) or an int. If an int, then it's converted into a tuple (int, int)
- :type pad: (int, int) or ((int, int),(int,int)) or (int,(int,int)) or ((int, int),int) | int
- :param p: Same as pad parameter. It's an alias. If EITHER of them are set, then the one that's set will be used. If BOTH are set, pad will be used
- :type p: (int, int) or ((int, int),(int,int)) or (int,(int,int)) or ((int, int),int) | int
- :param key: key for uniquely identify this element (for window.find_element)
- :type key: str | int | tuple | object
- :param k: Same as the Key. You can use either k or key. Which ever is set will be used.
- :type k: str | int | tuple | object
- :param visible: set initial visibility state of the Button
- :type visible: (bool)
- :param metadata: Anything you want to store along with this button
- :type metadata: (Any)
- :return: returns a button
- :rtype: (Button)
- """
- return Button(button_text=button_text, button_type=BUTTON_TYPE_READ_FORM, tooltip=tooltip, size=size, s=s,
- auto_size_button=auto_size_button, button_color=button_color, font=font, disabled=disabled,
- bind_return_key=bind_return_key, focus=focus, pad=pad, p=p, key=key, k=k, visible=visible, metadata=metadata)
-
-
-# ------------------------- YES BUTTON Element lazy function ------------------------- #
-def Yes(button_text='Yes', size=(None, None), s=(None, None), auto_size_button=None, button_color=None, disabled=False, tooltip=None,
- font=None, bind_return_key=True, focus=False, pad=None, p=None, key=None, k=None, visible=True, metadata=None):
- """
-
- :param button_text: text in the button (Default value = 'Yes')
- :type button_text: (str)
- :param size: (w,h) w=characters-wide, h=rows-high
- :type size: (int, int)
- :param s: Same as size parameter. It's an alias. If EITHER of them are set, then the one that's set will be used. If BOTH are set, size will be used
- :type s: (int, int) | (None, None) | int
- :param auto_size_button: True if button size is determined by button text
- :type auto_size_button: (bool)
- :param button_color: button color (foreground, background)
- :type button_color: (str, str) or str
- :param disabled: set disable state for element (Default = False)
- :type disabled: (bool)
- :param tooltip: text, that will appear when mouse hovers over the element
- :type tooltip: (str)
- :param font: specifies the font family, size, etc. Tuple or Single string format 'name size styles'. Styles: italic * roman bold normal underline overstrike
- :type font: (str or (str, int[, str]) or None)
- :param bind_return_key: (Default = True) If True, then the return key will cause a the Listbox to generate an event
- :type bind_return_key: (bool)
- :param focus: if focus should be set to this
- :type focus:
- :param pad: Amount of padding to put around element in pixels (left/right, top/bottom) or ((left, right), (top, bottom)) or an int. If an int, then it's converted into a tuple (int, int)
- :type pad: (int, int) or ((int, int),(int,int)) or (int,(int,int)) or ((int, int),int) | int
- :param p: Same as pad parameter. It's an alias. If EITHER of them are set, then the one that's set will be used. If BOTH are set, pad will be used
- :type p: (int, int) or ((int, int),(int,int)) or (int,(int,int)) or ((int, int),int) | int
- :param key: key for uniquely identify this element (for window.find_element)
- :type key: str | int | tuple | object
- :param k: Same as the Key. You can use either k or key. Which ever is set will be used.
- :type k: str | int | tuple | object
- :param visible: set initial visibility state of the Button
- :type visible: (bool)
- :param metadata: Anything you want to store along with this button
- :type metadata: (Any)
- :return: returns a button
- :rtype: (Button)
- """
- return Button(button_text=button_text, button_type=BUTTON_TYPE_READ_FORM, tooltip=tooltip, size=size, s=s,
- auto_size_button=auto_size_button, button_color=button_color, font=font, disabled=disabled,
- bind_return_key=bind_return_key, focus=focus, pad=pad, p=p, key=key, k=k, visible=visible, metadata=metadata)
-
-
-# ------------------------- NO BUTTON Element lazy function ------------------------- #
-def No(button_text='No', size=(None, None), s=(None, None), auto_size_button=None, button_color=None, disabled=False, tooltip=None,
- font=None, bind_return_key=False, focus=False, pad=None, p=None, key=None, k=None, visible=True, metadata=None):
- """
-
- :param button_text: text in the button (Default value = 'No')
- :type button_text: (str)
- :param size: (w,h) w=characters-wide, h=rows-high
- :type size: (int, int)
- :param s: Same as size parameter. It's an alias. If EITHER of them are set, then the one that's set will be used. If BOTH are set, size will be used
- :type s: (int, int) | (None, None) | int
- :param auto_size_button: True if button size is determined by button text
- :type auto_size_button: (bool)
- :param button_color: button color (foreground, background)
- :type button_color: (str, str) or str
- :param disabled: set disable state for element (Default = False)
- :type disabled: (bool)
- :param tooltip: text, that will appear when mouse hovers over the element
- :type tooltip: (str)
- :param font: specifies the font family, size, etc. Tuple or Single string format 'name size styles'. Styles: italic * roman bold normal underline overstrike
- :type font: (str or (str, int[, str]) or None)
- :param bind_return_key: (Default = False) If True, then the return key will cause a the Listbox to generate an event
- :type bind_return_key: (bool)
- :param focus: if focus should be set to this
- :type focus:
- :param pad: Amount of padding to put around element in pixels (left/right, top/bottom) or ((left, right), (top, bottom)) or an int. If an int, then it's converted into a tuple (int, int)
- :type pad: (int, int) or ((int, int),(int,int)) or (int,(int,int)) or ((int, int),int) | int
- :param p: Same as pad parameter. It's an alias. If EITHER of them are set, then the one that's set will be used. If BOTH are set, pad will be used
- :type p: (int, int) or ((int, int),(int,int)) or (int,(int,int)) or ((int, int),int) | int
- :param key: key for uniquely identify this element (for window.find_element)
- :type key: str | int | tuple | object
- :param k: Same as the Key. You can use either k or key. Which ever is set will be used.
- :type k: str | int | tuple | object
- :param visible: set initial visibility state of the Button
- :type visible: (bool)
- :param metadata: Anything you want to store along with this button
- :type metadata: (Any)
- :return: returns a button
- :rtype: (Button)
- """
- return Button(button_text=button_text, button_type=BUTTON_TYPE_READ_FORM, tooltip=tooltip, size=size, s=s,
- auto_size_button=auto_size_button, button_color=button_color, font=font, disabled=disabled,
- bind_return_key=bind_return_key, focus=focus, pad=pad, p=p, key=key, k=k, visible=visible, metadata=metadata)
-
-
-# ------------------------- NO BUTTON Element lazy function ------------------------- #
-def Help(button_text='Help', size=(None, None), s=(None, None), auto_size_button=None, button_color=None, disabled=False, font=None,
- tooltip=None, bind_return_key=False, focus=False, pad=None, p=None, key=None, k=None, visible=True, metadata=None):
- """
-
- :param button_text: text in the button (Default value = 'Help')
- :type button_text: (str)
- :param size: (w,h) w=characters-wide, h=rows-high
- :type size: (int, int)
- :param s: Same as size parameter. It's an alias. If EITHER of them are set, then the one that's set will be used. If BOTH are set, size will be used
- :type s: (int, int) | (None, None) | int
- :param auto_size_button: True if button size is determined by button text
- :type auto_size_button: (bool)
- :param button_color: button color (foreground, background)
- :type button_color: (str, str) or str
- :param disabled: set disable state for element (Default = False)
- :type disabled: (bool)
- :param font: specifies the font family, size, etc. Tuple or Single string format 'name size styles'. Styles: italic * roman bold normal underline overstrike
- :type font: (str or (str, int[, str]) or None)
- :param tooltip: text, that will appear when mouse hovers over the element
- :type tooltip: (str)
- :param bind_return_key: (Default = False) If True, then the return key will cause a the Listbox to generate an event
- :type bind_return_key: (bool)
- :param focus: if focus should be set to this
- :type focus:
- :param pad: Amount of padding to put around element in pixels (left/right, top/bottom) or ((left, right), (top, bottom)) or an int. If an int, then it's converted into a tuple (int, int)
- :type pad: (int, int) or ((int, int),(int,int)) or (int,(int,int)) or ((int, int),int) | int
- :param p: Same as pad parameter. It's an alias. If EITHER of them are set, then the one that's set will be used. If BOTH are set, pad will be used
- :type p: (int, int) or ((int, int),(int,int)) or (int,(int,int)) or ((int, int),int) | int
- :param key: key for uniquely identify this element (for window.find_element)
- :type key: str | int | tuple | object
- :param k: Same as the Key. You can use either k or key. Which ever is set will be used.
- :type k: str | int | tuple | object
- :param visible: set initial visibility state of the Button
- :type visible: (bool)
- :param metadata: Anything you want to store along with this button
- :type metadata: (Any)
- :return: returns a button
- :rtype: (Button)
- """
- return Button(button_text=button_text, button_type=BUTTON_TYPE_READ_FORM, tooltip=tooltip, size=size, s=s,
- auto_size_button=auto_size_button, button_color=button_color, font=font, disabled=disabled,
- bind_return_key=bind_return_key, focus=focus, pad=pad, p=p, key=key, k=k, visible=visible, metadata=metadata)
-
-
-# ------------------------- NO BUTTON Element lazy function ------------------------- #
-def Debug(button_text='', size=(None, None), s=(None, None), auto_size_button=None, button_color=None, disabled=False, font=None,
- tooltip=None, bind_return_key=False, focus=False, pad=None, p=None, key=None, k=None, visible=True, metadata=None):
- """
- This Button has been changed in how it works!!
- Your button has been replaced with a normal button that has the PySimpleGUI Debugger buggon logo on it.
- In your event loop, you will need to check for the event of this button and then call:
- show_debugger_popout_window()
- :param button_text: text in the button (Default value = '')
- :type button_text: (str)
- :param size: (w,h) w=characters-wide, h=rows-high
- :type size: (int, int)
- :param s: Same as size parameter. It's an alias. If EITHER of them are set, then the one that's set will be used. If BOTH are set, size will be used
- :type s: (int, int) | (None, None) | int
- :param auto_size_button: True if button size is determined by button text
- :type auto_size_button: (bool)
- :param button_color: button color (foreground, background)
- :type button_color: (str, str) or str
- :param disabled: set disable state for element (Default = False)
- :type disabled: (bool)
- :param font: specifies the font family, size, etc. Tuple or Single string format 'name size styles'. Styles: italic * roman bold normal underline overstrike
- :type font: (str or (str, int[, str]) or None)
- :param tooltip: text, that will appear when mouse hovers over the element
- :type tooltip: (str)
- :param bind_return_key: (Default = False) If True, then the return key will cause a the Listbox to generate an event
- :type bind_return_key: (bool)
- :param focus: if focus should be set to this
- :type focus:
- :param pad: Amount of padding to put around element in pixels (left/right, top/bottom) or ((left, right), (top, bottom)) or an int. If an int, then it's converted into a tuple (int, int)
- :type pad: (int, int) or ((int, int),(int,int)) or (int,(int,int)) or ((int, int),int) | int
- :param p: Same as pad parameter. It's an alias. If EITHER of them are set, then the one that's set will be used. If BOTH are set, pad will be used
- :type p: (int, int) or ((int, int),(int,int)) or (int,(int,int)) or ((int, int),int) | int
- :param key: key for uniquely identify this element (for window.find_element)
- :type key: str | int | tuple | object
- :param k: Same as the Key. You can use either k or key. Which ever is set will be used.
- :type k: str | int | tuple | object
- :param visible: set initial visibility state of the Button
- :type visible: (bool)
- :param metadata: Anything you want to store along with this button
- :type metadata: (Any)
- :return: returns a button
- :rtype: (Button)
- """
-
-
- user_key = key if key is not None else k if k is not None else button_text
-
- return Button(button_text='', button_type=BUTTON_TYPE_READ_FORM, tooltip=tooltip, size=size, s=s,
- auto_size_button=auto_size_button, button_color=theme_button_color(), font=font, disabled=disabled,
- bind_return_key=bind_return_key, focus=focus, pad=pad, p=p, key=user_key, k=k, visible=visible, image_data=PSG_DEBUGGER_LOGO,
- image_subsample=2, border_width=0, metadata=metadata)
-
-
-# ------------------------- GENERIC BUTTON Element lazy function ------------------------- #
-def SimpleButton(button_text, image_filename=None, image_data=None, image_size=(None, None), image_subsample=None,
- border_width=None, tooltip=None, size=(None, None), s=(None, None), auto_size_button=None, button_color=None,
- font=None, bind_return_key=False, disabled=False, focus=False, pad=None, p=None, key=None, k=None, metadata=None):
- """
- DEPIRCATED
-
- This Button should not be used.
-
- :param button_text: text in the button
- :type button_text: (str)
- :param image_filename: image filename if there is a button image
- :type image_filename: image filename if there is a button image
- :param image_data: in-RAM image to be displayed on button
- :type image_data: in-RAM image to be displayed on button
- :param image_size: image size (O.K.)
- :type image_size: (Default = (None))
- :param image_subsample: amount to reduce the size of the image
- :type image_subsample: amount to reduce the size of the image
- :param tooltip: text, that will appear when mouse hovers over the element
- :type tooltip: (str)
- :param size: (w,h) w=characters-wide, h=rows-high
- :type size: (int, int)
- :param s: Same as size parameter. It's an alias. If EITHER of them are set, then the one that's set will be used. If BOTH are set, size will be used
- :type s: (int, int) | (None, None) | int
- :param auto_size_button: True if button size is determined by button text
- :type auto_size_button: (bool)
- :param button_color: button color (foreground, background)
- :type button_color: (str, str) or str
- :param font: specifies the font family, size, etc. Tuple or Single string format 'name size styles'. Styles: italic * roman bold normal underline overstrike
- :type font: (str or (str, int[, str]) or None)
- :param bind_return_key: (Default = False) If True, then the return key will cause a the Listbox to generate an event
- :type bind_return_key: (bool)
- :param disabled: set disable state for element (Default = False)
- :type disabled: (bool)
- :param focus: if focus should be set to this
- :type focus: idk_yetReally
- :param pad: Amount of padding to put around element in pixels (left/right, top/bottom) or ((left, right), (top, bottom)) or an int. If an int, then it's converted into a tuple (int, int)
- :type pad: (int, int) or ((int, int),(int,int)) or (int,(int,int)) or ((int, int),int) | int
- :param p: Same as pad parameter. It's an alias. If EITHER of them are set, then the one that's set will be used. If BOTH are set, pad will be used
- :type p: (int, int) or ((int, int),(int,int)) or (int,(int,int)) or ((int, int),int) | int
- :param key: key for uniquely identify this element (for window.find_element)
- :type key: str | int | tuple | object
- :param k: Same as the Key. You can use either k or key. Which ever is set will be used.
- :type k: str | int | tuple | object
- :param metadata: Anything you want to store along with this button
- :type metadata: (Any)
- :return: returns a button
- :rtype: (Button)
- """
- return Button(button_text=button_text, button_type=BUTTON_TYPE_CLOSES_WIN, image_filename=image_filename,
- image_data=image_data, image_size=image_size, image_subsample=image_subsample,
- border_width=border_width, tooltip=tooltip, disabled=disabled, size=size, s=s,
- auto_size_button=auto_size_button, button_color=button_color, font=font,
- bind_return_key=bind_return_key, focus=focus, pad=pad, p=p, key=key, k=k, metadata=metadata)
-
-
-# ------------------------- CLOSE BUTTON Element lazy function ------------------------- #
-def CloseButton(button_text, image_filename=None, image_data=None, image_size=(None, None), image_subsample=None,
- border_width=None, tooltip=None, size=(None, None), s=(None, None), auto_size_button=None, button_color=None, font=None,
- bind_return_key=False, disabled=False, focus=False, pad=None, p=None, key=None, k=None, metadata=None):
- """
- DEPRICATED
-
- This button should not be used. Instead explicitly close your windows by calling window.close() or by using
- the close parameter in window.read
-
- :param button_text: text in the button
- :type button_text: (str)
- :param image_filename: image filename if there is a button image
- :type image_filename: image filename if there is a button image
- :param image_data: in-RAM image to be displayed on button
- :type image_data: in-RAM image to be displayed on button
- :param image_size: image size (O.K.)
- :type image_size: (Default = (None))
- :param image_subsample: amount to reduce the size of the image
- :type image_subsample: amount to reduce the size of the image
- :param tooltip: text, that will appear when mouse hovers over the element
- :type tooltip: (str)
- :param size: (w,h) w=characters-wide, h=rows-high
- :type size: (int, int)
- :param s: Same as size parameter. It's an alias. If EITHER of them are set, then the one that's set will be used. If BOTH are set, size will be used
- :type s: (int, int) | (None, None) | int
- :param auto_size_button: True if button size is determined by button text
- :type auto_size_button: (bool)
- :param button_color: button color (foreground, background)
- :type button_color: (str, str) or str
- :param font: specifies the font family, size, etc. Tuple or Single string format 'name size styles'. Styles: italic * roman bold normal underline overstrike
- :type font: (str or (str, int[, str]) or None)
- :param bind_return_key: (Default = False) If True, then the return key will cause a the Listbox to generate an event
- :type bind_return_key: (bool)
- :param disabled: set disable state for element (Default = False)
- :type disabled: (bool)
- :param focus: if focus should be set to this
- :type focus: idk_yetReally
- :param pad: Amount of padding to put around element in pixels (left/right, top/bottom) or ((left, right), (top, bottom)) or an int. If an int, then it's converted into a tuple (int, int)
- :type pad: (int, int) or ((int, int),(int,int)) or (int,(int,int)) or ((int, int),int) | int
- :param p: Same as pad parameter. It's an alias. If EITHER of them are set, then the one that's set will be used. If BOTH are set, pad will be used
- :type p: (int, int) or ((int, int),(int,int)) or (int,(int,int)) or ((int, int),int) | int
- :param key: key for uniquely identify this element (for window.find_element)
- :type key: str | int | tuple | object
- :param k: Same as the Key. You can use either k or key. Which ever is set will be used.
- :type k: str | int | tuple | object
- :param metadata: Anything you want to store along with this button
- :type metadata: (Any)
- :return: returns a button
- :rtype: (Button)
- """
- return Button(button_text=button_text, button_type=BUTTON_TYPE_CLOSES_WIN, image_filename=image_filename,
- image_data=image_data, image_size=image_size, image_subsample=image_subsample,
- border_width=border_width, tooltip=tooltip, disabled=disabled, size=size, s=s,
- auto_size_button=auto_size_button, button_color=button_color, font=font,
- bind_return_key=bind_return_key, focus=focus, pad=pad, p=p, key=key, k=k, metadata=metadata)
-
-
-CButton = CloseButton
-
-
-# ------------------------- GENERIC BUTTON Element lazy function ------------------------- #
-def ReadButton(button_text, image_filename=None, image_data=None, image_size=(None, None), image_subsample=None,
- border_width=None, tooltip=None, size=(None, None), s=(None, None), auto_size_button=None, button_color=None, font=None,
- bind_return_key=False, disabled=False, focus=False, pad=None, p=None, key=None, k=None, metadata=None):
- """
- :param button_text: text in the button
- :type button_text: (str)
- :param image_filename: image filename if there is a button image
- :type image_filename: image filename if there is a button image
- :param image_data: in-RAM image to be displayed on button
- :type image_data: in-RAM image to be displayed on button
- :param image_size: image size (O.K.)
- :type image_size: (Default = (None))
- :param image_subsample: amount to reduce the size of the image
- :type image_subsample: amount to reduce the size of the image
- :param tooltip: text, that will appear when mouse hovers over the element
- :type tooltip: (str)
- :param size: (w,h) w=characters-wide, h=rows-high
- :type size: (int, int)
- :param s: Same as size parameter. It's an alias. If EITHER of them are set, then the one that's set will be used. If BOTH are set, size will be used
- :type s: (int, int) | (None, None) | int
- :param auto_size_button: True if button size is determined by button text
- :type auto_size_button: (bool)
- :param button_color: button color (foreground, background)
- :type button_color: (str, str) or str
- :param font: specifies the font family, size, etc. Tuple or Single string format 'name size styles'. Styles: italic * roman bold normal underline overstrike
- :type font: (str or (str, int[, str]) or None)
- :param bind_return_key: (Default = False) If True, then the return key will cause a the Listbox to generate an event
- :type bind_return_key: (bool)
- :param disabled: set disable state for element (Default = False)
- :type disabled: (bool)
- :param focus: if focus should be set to this
- :type focus: idk_yetReally
- :param pad: Amount of padding to put around element in pixels (left/right, top/bottom) or ((left, right), (top, bottom)) or an int. If an int, then it's converted into a tuple (int, int)
- :type pad: (int, int) or ((int, int),(int,int)) or (int,(int,int)) or ((int, int),int) | int
- :param p: Same as pad parameter. It's an alias. If EITHER of them are set, then the one that's set will be used. If BOTH are set, pad will be used
- :type p: (int, int) or ((int, int),(int,int)) or (int,(int,int)) or ((int, int),int) | int
- :param key: key for uniquely identify this element (for window.find_element)
- :type key: str | int | tuple | object
- :param k: Same as the Key. You can use either k or key. Which ever is set will be used.
- :type k: str | int | tuple | object
- :param border_width: width of border around element
- :type border_width: (int)
- :param metadata: Anything you want to store along with this button
- :type metadata: (Any)
- :return: Button created
- :rtype: (Button)
- """
-
- return Button(button_text=button_text, button_type=BUTTON_TYPE_READ_FORM, image_filename=image_filename,
- image_data=image_data, image_size=image_size, image_subsample=image_subsample,
- border_width=border_width, tooltip=tooltip, size=size, s=s, disabled=disabled,
- auto_size_button=auto_size_button, button_color=button_color, font=font,
- bind_return_key=bind_return_key, focus=focus, pad=pad, p=p, key=key, k=k, metadata=metadata)
-
-
-ReadFormButton = ReadButton
-RButton = ReadFormButton
-
-
-# ------------------------- Realtime BUTTON Element lazy function ------------------------- #
-def RealtimeButton(button_text, image_filename=None, image_data=None, image_size=(None, None), image_subsample=None,
- border_width=None, tooltip=None, size=(None, None), s=(None, None), auto_size_button=None, button_color=None,
- font=None, disabled=False, bind_return_key=False, focus=False, pad=None, p=None, key=None, k=None, visible=True, metadata=None):
- """
-
- :param button_text: text in the button
- :type button_text: (str)
- :param image_filename: image filename if there is a button image
- :type image_filename: image filename if there is a button image
- :param image_data: in-RAM image to be displayed on button
- :type image_data: in-RAM image to be displayed on button
- :param image_size: image size (O.K.)
- :type image_size: (Default = (None))
- :param image_subsample: amount to reduce the size of the image
- :type image_subsample: amount to reduce the size of the image
- :param border_width: width of border around element
- :type border_width: (int)
- :param tooltip: text, that will appear when mouse hovers over the element
- :type tooltip: (str)
- :param size: (w,h) w=characters-wide, h=rows-high
- :type size: (int, int)
- :param s: Same as size parameter. It's an alias. If EITHER of them are set, then the one that's set will be used. If BOTH are set, size will be used
- :type s: (int, int) | (None, None) | int
- :param auto_size_button: True if button size is determined by button text
- :type auto_size_button: (bool)
- :param button_color: button color (foreground, background)
- :type button_color: (str, str) or str
- :param font: specifies the font family, size, etc. Tuple or Single string format 'name size styles'. Styles: italic * roman bold normal underline overstrike
- :type font: (str or (str, int[, str]) or None)
- :param disabled: set disable state for element (Default = False)
- :type disabled: (bool)
- :param bind_return_key: (Default = False) If True, then the return key will cause a the Listbox to generate an event
- :type bind_return_key: (bool)
- :param focus: if focus should be set to this
- :type focus: (bool)
- :param pad: Amount of padding to put around element in pixels (left/right, top/bottom) or ((left, right), (top, bottom)) or an int. If an int, then it's converted into a tuple (int, int)
- :type pad: (int, int) or ((int, int),(int,int)) or (int,(int,int)) or ((int, int),int) | int
- :param p: Same as pad parameter. It's an alias. If EITHER of them are set, then the one that's set will be used. If BOTH are set, pad will be used
- :type p: (int, int) or ((int, int),(int,int)) or (int,(int,int)) or ((int, int),int) | int
- :param key: key for uniquely identify this element (for window.find_element)
- :type key: str | int | tuple | object
- :param k: Same as the Key. You can use either k or key. Which ever is set will be used.
- :type k: str | int | tuple | object
- :param visible: set initial visibility state of the Button
- :type visible: (bool)
- :param metadata: Anything you want to store along with this button
- :type metadata: (Any)
- :return: Button created
- :rtype: (Button)
- """
- return Button(button_text=button_text, button_type=BUTTON_TYPE_REALTIME, image_filename=image_filename,
- image_data=image_data, image_size=image_size, image_subsample=image_subsample,
- border_width=border_width, tooltip=tooltip, disabled=disabled, size=size, s=s,
- auto_size_button=auto_size_button, button_color=button_color, font=font,
- bind_return_key=bind_return_key, focus=focus, pad=pad, p=p, key=key, k=k, visible=visible, metadata=metadata)
-
-
-# ------------------------- Dummy BUTTON Element lazy function ------------------------- #
-def DummyButton(button_text, image_filename=None, image_data=None, image_size=(None, None), image_subsample=None,
- border_width=None, tooltip=None, size=(None, None), s=(None, None), auto_size_button=None, button_color=None, font=None,
- disabled=False, bind_return_key=False, focus=False, pad=None, p=None, key=None, k=None, visible=True, metadata=None):
- """
- This is a special type of Button.
-
- It will close the window but NOT send an event that the window has been closed.
-
- It's used in conjunction with non-blocking windows to silently close them. They are used to
- implement the non-blocking popup windows. They're also found in some Demo Programs, so look there for proper use.
-
- :param button_text: text in the button
- :type button_text: (str)
- :param image_filename: image filename if there is a button image
- :type image_filename: image filename if there is a button image
- :param image_data: in-RAM image to be displayed on button
- :type image_data: in-RAM image to be displayed on button
- :param image_size: image size (O.K.)
- :type image_size: (Default = (None))
- :param image_subsample: amount to reduce the size of the image
- :type image_subsample: amount to reduce the size of the image
- :param border_width: width of border around element
- :type border_width: (int)
- :param tooltip: text, that will appear when mouse hovers over the element
- :type tooltip: (str)
- :param size: (w,h) w=characters-wide, h=rows-high
- :type size: (int, int)
- :param s: Same as size parameter. It's an alias. If EITHER of them are set, then the one that's set will be used. If BOTH are set, size will be used
- :type s: (int, int) | (None, None) | int
- :param auto_size_button: True if button size is determined by button text
- :type auto_size_button: (bool)
- :param button_color: button color (foreground, background)
- :type button_color: (str, str) or str
- :param font: specifies the font family, size, etc. Tuple or Single string format 'name size styles'. Styles: italic * roman bold normal underline overstrike
- :type font: (str or (str, int[, str]) or None)
- :param disabled: set disable state for element (Default = False)
- :type disabled: (bool)
- :param bind_return_key: (Default = False) If True, then the return key will cause a the Listbox to generate an event
- :type bind_return_key: (bool)
- :param focus: if focus should be set to this
- :type focus: (bool)
- :param pad: Amount of padding to put around element in pixels (left/right, top/bottom) or ((left, right), (top, bottom)) or an int. If an int, then it's converted into a tuple (int, int)
- :type pad: (int, int) or ((int, int),(int,int)) or (int,(int,int)) or ((int, int),int) | int
- :param p: Same as pad parameter. It's an alias. If EITHER of them are set, then the one that's set will be used. If BOTH are set, pad will be used
- :type p: (int, int) or ((int, int),(int,int)) or (int,(int,int)) or ((int, int),int) | int
- :param key: key for uniquely identify this element (for window.find_element)
- :type key: str | int | tuple | object
- :param k: Same as the Key. You can use either k or key. Which ever is set will be used.
- :type k: str | int | tuple | object
- :param visible: set initial visibility state of the Button
- :type visible: (bool)
- :param metadata: Anything you want to store along with this button
- :type metadata: (Any)
- :return: returns a button
- :rtype: (Button)
- """
- return Button(button_text=button_text, button_type=BUTTON_TYPE_CLOSES_WIN_ONLY, image_filename=image_filename,
- image_data=image_data, image_size=image_size, image_subsample=image_subsample,
- border_width=border_width, tooltip=tooltip, size=size, s=s, auto_size_button=auto_size_button,
- button_color=button_color, font=font, disabled=disabled, bind_return_key=bind_return_key, focus=focus,
- pad=pad, p=p, key=key, k=k, visible=visible, metadata=metadata)
-
-
-# ------------------------- Calendar Chooser Button lazy function ------------------------- #
-def CalendarButton(button_text, target=(ThisRow, -1), close_when_date_chosen=True, default_date_m_d_y=(None, None, None),
- image_filename=None, image_data=None, image_size=(None, None),
- image_subsample=None, tooltip=None, border_width=None, size=(None, None), s=(None, None), auto_size_button=None,
- button_color=None, disabled=False, font=None, bind_return_key=False, focus=False, pad=None, p=None, enable_events=None,
- key=None, k=None, visible=True, locale=None, format='%Y-%m-%d %H:%M:%S', begin_at_sunday_plus=0, month_names=None, day_abbreviations=None,
- title='Choose Date',
- no_titlebar=True, location=(None, None), metadata=None):
- """
- Button that will show a calendar chooser window. Fills in the target element with result
-
- :param button_text: text in the button
- :type button_text: (str)
- :param target: Key or "coordinate" (see docs) of target element
- :type target: (int, int) | Any
- :param close_when_date_chosen: (Default = True)
- :type close_when_date_chosen: bool
- :param default_date_m_d_y: Beginning date to show
- :type default_date_m_d_y: (int, int or None, int)
- :param image_filename: image filename if there is a button image
- :type image_filename: image filename if there is a button image
- :param image_data: in-RAM image to be displayed on button
- :type image_data: in-RAM image to be displayed on button
- :param image_size: image size (O.K.)
- :type image_size: (Default = (None))
- :param image_subsample: amount to reduce the size of the image
- :type image_subsample: amount to reduce the size of the image
- :param tooltip: text, that will appear when mouse hovers over the element
- :type tooltip: (str)
- :param border_width: width of border around element
- :type border_width: width of border around element
- :param size: (w,h) w=characters-wide, h=rows-high
- :type size: (int, int)
- :param s: Same as size parameter. It's an alias. If EITHER of them are set, then the one that's set will be used. If BOTH are set, size will be used
- :type s: (int, int) | (None, None) | int
- :param auto_size_button: True if button size is determined by button text
- :type auto_size_button: (bool)
- :param button_color: button color (foreground, background)
- :type button_color: (str, str) or str
- :param disabled: set disable state for element (Default = False)
- :type disabled: (bool)
- :param font: specifies the font family, size, etc. Tuple or Single string format 'name size styles'. Styles: italic * roman bold normal underline overstrike
- :type font: (str or (str, int[, str]) or None)
- :param bind_return_key: (Default = False) If True, then the return key will cause a the Listbox to generate an event
- :type bind_return_key: bool
- :param focus: if focus should be set to this
- :type focus: bool
- :param pad: Amount of padding to put around element in pixels (left/right, top/bottom) or ((left, right), (top, bottom)) or an int. If an int, then it's converted into a tuple (int, int)
- :type pad: (int, int) or ((int, int),(int,int)) or (int,(int,int)) or ((int, int),int) | int
- :param p: Same as pad parameter. It's an alias. If EITHER of them are set, then the one that's set will be used. If BOTH are set, pad will be used
- :type p: (int, int) or ((int, int),(int,int)) or (int,(int,int)) or ((int, int),int) | int
- :param key: key for uniquely identify this element (for window.find_element)
- :type key: str | int | tuple | object
- :param k: Same as the Key. You can use either k or key. Which ever is set will be used.
- :type k: str | int | tuple | object
- :param locale: defines the locale used to get day names
- :type locale: str
- :param format: formats result using this strftime format
- :type format: str
- :param month_names: optional list of month names to use (should be 12 items)
- :type month_names: List[str]
- :param day_abbreviations: optional list of abbreviations to display as the day of week
- :type day_abbreviations: List[str]
- :param title: Title shown on the date chooser window
- :type title: (str)
- :param no_titlebar: if True no titlebar will be shown on the date chooser window
- :type no_titlebar: bool
- :param location: Location on the screen (x,y) to show the calendar popup window
- :type location: (int, int)
- :param visible: set initial visibility state of the Button
- :type visible: (bool)
- :param metadata: Anything you want to store along with this button
- :type metadata: (Any)
- :return: returns a button
- :rtype: (Button)
- """
- button = Button(button_text=button_text, button_type=BUTTON_TYPE_CALENDAR_CHOOSER, target=target,
- image_filename=image_filename, image_data=image_data, image_size=image_size,
- image_subsample=image_subsample, border_width=border_width, tooltip=tooltip, size=size, s=s,
- auto_size_button=auto_size_button, button_color=button_color, font=font, disabled=disabled, enable_events=enable_events,
- bind_return_key=bind_return_key, focus=focus, pad=pad, p=p, key=key, k=k, visible=visible, metadata=metadata)
- button.calendar_close_when_chosen = close_when_date_chosen
- button.calendar_default_date_M_D_Y = default_date_m_d_y
- button.calendar_locale = locale
- button.calendar_format = format
- button.calendar_no_titlebar = no_titlebar
- button.calendar_location = location
- button.calendar_begin_at_sunday_plus = begin_at_sunday_plus
- button.calendar_month_names = month_names
- button.calendar_day_abbreviations = day_abbreviations
- button.calendar_title = title
-
- return button
-
-
-# ------------------------- Calendar Chooser Button lazy function ------------------------- #
-def ColorChooserButton(button_text, target=(ThisRow, -1), image_filename=None, image_data=None, image_size=(None, None),
- image_subsample=None, tooltip=None, border_width=None, size=(None, None), s=(None, None), auto_size_button=None,
- button_color=None, disabled=False, font=None, bind_return_key=False, focus=False, pad=None, p=None,
- key=None, k=None, visible=True, metadata=None):
- """
-
- :param button_text: text in the button
- :type button_text: (str)
- :param target: key or (row,col) target for the button. Note that -1 for column means 1 element to the left of this one. The constant ThisRow is used to indicate the current row. The Button itself is a valid target for some types of button
- :type target: str | (int, int)
- :type image_filename: (str)
- :param image_filename: image filename if there is a button image. GIFs and PNGs only.
- :type image_filename: (str)
- :param image_data: Raw or Base64 representation of the image to put on button. Choose either filename or data
- :type image_data: bytes | str
- :param image_size: Size of the image in pixels (width, height)
- :type image_size: (int, int)
- :param image_subsample: amount to reduce the size of the image. Divides the size by this number. 2=1/2, 3=1/3, 4=1/4, etc
- :type image_subsample: (int)
- :param tooltip: text, that will appear when mouse hovers over the element
- :type tooltip: (str)
- :param border_width: width of border around element
- :type border_width: (int)
- :param size: (w,h) w=characters-wide, h=rows-high
- :type size: (int, int)
- :param s: Same as size parameter. It's an alias. If EITHER of them are set, then the one that's set will be used. If BOTH are set, size will be used
- :type s: (int, int) | (None, None) | int
- :param auto_size_button: True if button size is determined by button text
- :type auto_size_button: (bool)
- :param button_color: button color (foreground, background)
- :type button_color: (str, str) or str
- :param disabled: set disable state for element (Default = False)
- :type disabled: (bool)
- :param font: specifies the font family, size, etc. Tuple or Single string format 'name size styles'. Styles: italic * roman bold normal underline overstrike
- :type font: (str or (str, int[, str]) or None)
- :param bind_return_key: If True, then the return key will cause a the Listbox to generate an event
- :type bind_return_key: (bool)
- :param focus: Determines if initial focus should go to this element.
- :type focus: (bool)
- :param pad: Amount of padding to put around element in pixels (left/right, top/bottom) or ((left, right), (top, bottom)) or an int. If an int, then it's converted into a tuple (int, int)
- :type pad: (int, int) or ((int, int),(int,int)) or (int,(int,int)) or ((int, int),int) | int
- :param p: Same as pad parameter. It's an alias. If EITHER of them are set, then the one that's set will be used. If BOTH are set, pad will be used
- :type p: (int, int) or ((int, int),(int,int)) or (int,(int,int)) or ((int, int),int) | int
- :param key: key for uniquely identify this element (for window.find_element)
- :type key: str | int | tuple | object
- :param k: Same as the Key. You can use either k or key. Which ever is set will be used.
- :type k: str | int | tuple | object
- :param visible: set initial visibility state of the Button
- :type visible: (bool)
- :param metadata: User metadata that can be set to ANYTHING
- :type metadata: (Any)
- :return: returns a button
- :rtype: (Button)
- """
- return Button(button_text=button_text, button_type=BUTTON_TYPE_COLOR_CHOOSER, target=target,
- image_filename=image_filename, image_data=image_data, image_size=image_size,
- image_subsample=image_subsample, border_width=border_width, tooltip=tooltip, size=size, s=s,
- auto_size_button=auto_size_button, button_color=button_color, font=font, disabled=disabled,
- bind_return_key=bind_return_key, focus=focus, pad=pad, p=p, key=key, k=k, visible=visible, metadata=metadata)
-
-
-##################################### ----- BUTTON Functions ------ ##################################################
-
-def button_color_to_tuple(color_tuple_or_string, default=(None, None)):
- """
- Convert a color tuple or color string into 2 components and returns them as a tuple
- (Text Color, Button Background Color)
- If None is passed in as the first parameter, then the theme's button color is
- returned
-
- :param color_tuple_or_string: Button color - tuple or a simplied color string with word "on" between color
- :type color_tuple_or_string: str | (str, str)
- :param default: The 2 colors to use if there is a problem. Otherwise defaults to the theme's button color
- :type default: (str, str)
- :return: (str | (str, str)
- :rtype: str | (str, str)
- """
- if default == (None, None):
- color_tuple = _simplified_dual_color_to_tuple(color_tuple_or_string, default=theme_button_color())
- elif color_tuple_or_string == COLOR_SYSTEM_DEFAULT:
- color_tuple = (COLOR_SYSTEM_DEFAULT, COLOR_SYSTEM_DEFAULT)
- else:
- color_tuple = _simplified_dual_color_to_tuple(color_tuple_or_string, default=default)
-
- return color_tuple
-
-
-def _simplified_dual_color_to_tuple(color_tuple_or_string, default=(None, None)):
- """
- Convert a color tuple or color string into 2 components and returns them as a tuple
- (Text Color, Button Background Color)
- If None is passed in as the first parameter, theme_
-
- :param color_tuple_or_string: Button color - tuple or a simplied color string with word "on" between color
- :type color_tuple_or_string: str | (str, str} | (None, None)
- :param default: The 2 colors to use if there is a problem. Otherwise defaults to the theme's button color
- :type default: (str, str)
- :return: (str | (str, str)
- :rtype: str | (str, str)
- """
- if color_tuple_or_string is None or color_tuple_or_string == (None, None):
- color_tuple_or_string = default
- if color_tuple_or_string == COLOR_SYSTEM_DEFAULT:
- return (COLOR_SYSTEM_DEFAULT, COLOR_SYSTEM_DEFAULT)
- text_color = background_color = COLOR_SYSTEM_DEFAULT
- try:
- if isinstance(color_tuple_or_string, (tuple, list)):
- if len(color_tuple_or_string) >= 2:
- text_color = color_tuple_or_string[0] or default[0]
- background_color = color_tuple_or_string[1] or default[1]
- elif len(color_tuple_or_string) == 1:
- background_color = color_tuple_or_string[0] or default[1]
- elif isinstance(color_tuple_or_string, str):
- color_tuple_or_string = color_tuple_or_string.lower()
- split_colors = color_tuple_or_string.split(' on ')
- if len(split_colors) >= 2:
- text_color = split_colors[0].strip() or default[0]
- background_color = split_colors[1].strip() or default[1]
- elif len(split_colors) == 1:
- split_colors = color_tuple_or_string.split('on')
- if len(split_colors) == 1:
- text_color, background_color = default[0], split_colors[0].strip()
- else:
- split_colors = split_colors[0].strip(), split_colors[1].strip()
- text_color = split_colors[0] or default[0]
- background_color = split_colors[1] or default[1]
- # text_color, background_color = color_tuple_or_string, default[1]
- else:
- text_color, background_color = default
- else:
- if not SUPPRESS_ERROR_POPUPS:
- _error_popup_with_traceback('** Badly formatted dual-color... not a tuple nor string **', color_tuple_or_string)
- else:
- print('** Badly formatted dual-color... not a tuple nor string **', color_tuple_or_string)
- text_color, background_color = default
- except Exception as e:
- if not SUPPRESS_ERROR_POPUPS:
- _error_popup_with_traceback('** Badly formatted button color **', color_tuple_or_string, e)
- else:
- print('** Badly formatted button color... not a tuple nor string **', color_tuple_or_string, e)
- text_color, background_color = default
- if isinstance(text_color, int):
- text_color = "#%06X" % text_color
- if isinstance(background_color, int):
- background_color = "#%06X" % background_color
- # print('converted button color', color_tuple_or_string, 'to', (text_color, background_color))
-
- return (text_color, background_color)
-
-
-##################################### ----- RESULTS ------ ##################################################
-
-def AddToReturnDictionary(form, element, value):
- form.ReturnValuesDictionary[element.Key] = value
- # if element.Key is None:
- # form.ReturnValuesDictionary[form.DictionaryKeyCounter] = value
- # element.Key = form.DictionaryKeyCounter
- # form.DictionaryKeyCounter += 1
- # else:
- # form.ReturnValuesDictionary[element.Key] = value
-
-
-def AddToReturnList(form, value):
- form.ReturnValuesList.append(value)
-
-
-# ----------------------------------------------------------------------------#
-# ------- FUNCTION InitializeResults. Sets up form results matrix --------#
-def InitializeResults(form):
- _BuildResults(form, True, form)
- return
-
-
-# ===== Radio Button RadVar encoding and decoding =====#
-# ===== The value is simply the row * 1000 + col =====#
-def DecodeRadioRowCol(RadValue):
- container = RadValue // 100000
- row = RadValue // 1000
- col = RadValue % 1000
- return container, row, col
-
-
-def EncodeRadioRowCol(container, row, col):
- RadValue = container * 100000 + row * 1000 + col
- return RadValue
-
-
-# ------- FUNCTION BuildResults. Form exiting so build the results to pass back ------- #
-# format of return values is
-# (Button Pressed, input_values)
-def _BuildResults(form, initialize_only, top_level_form):
- # Results for elements are:
- # TEXT - Nothing
- # INPUT - Read value from TK
- # Button - Button Text and position as a Tuple
-
- # Get the initialized results so we don't have to rebuild
- # form.DictionaryKeyCounter = 0
- form.ReturnValuesDictionary = {}
- form.ReturnValuesList = []
- _BuildResultsForSubform(form, initialize_only, top_level_form)
- if not top_level_form.LastButtonClickedWasRealtime:
- top_level_form.LastButtonClicked = None
- return form.ReturnValues
-
-
-def _BuildResultsForSubform(form, initialize_only, top_level_form):
- event = top_level_form.LastButtonClicked
- for row_num, row in enumerate(form.Rows):
- for col_num, element in enumerate(row):
- if element.Key is not None and WRITE_ONLY_KEY in str(element.Key):
- continue
- value = None
- if element.Type == ELEM_TYPE_COLUMN:
- element.DictionaryKeyCounter = top_level_form.DictionaryKeyCounter
- element.ReturnValuesList = []
- element.ReturnValuesDictionary = {}
- _BuildResultsForSubform(element, initialize_only, top_level_form)
- for item in element.ReturnValuesList:
- AddToReturnList(top_level_form, item)
- if element.UseDictionary:
- top_level_form.UseDictionary = True
- if element.ReturnValues[0] is not None: # if a button was clicked
- event = element.ReturnValues[0]
-
- if element.Type == ELEM_TYPE_FRAME:
- element.DictionaryKeyCounter = top_level_form.DictionaryKeyCounter
- element.ReturnValuesList = []
- element.ReturnValuesDictionary = {}
- _BuildResultsForSubform(element, initialize_only, top_level_form)
- for item in element.ReturnValuesList:
- AddToReturnList(top_level_form, item)
- if element.UseDictionary:
- top_level_form.UseDictionary = True
- if element.ReturnValues[0] is not None: # if a button was clicked
- event = element.ReturnValues[0]
-
- if element.Type == ELEM_TYPE_PANE:
- element.DictionaryKeyCounter = top_level_form.DictionaryKeyCounter
- element.ReturnValuesList = []
- element.ReturnValuesDictionary = {}
- _BuildResultsForSubform(element, initialize_only, top_level_form)
- for item in element.ReturnValuesList:
- AddToReturnList(top_level_form, item)
- if element.UseDictionary:
- top_level_form.UseDictionary = True
- if element.ReturnValues[0] is not None: # if a button was clicked
- event = element.ReturnValues[0]
-
- if element.Type == ELEM_TYPE_TAB_GROUP:
- element.DictionaryKeyCounter = top_level_form.DictionaryKeyCounter
- element.ReturnValuesList = []
- element.ReturnValuesDictionary = {}
- _BuildResultsForSubform(element, initialize_only, top_level_form)
- for item in element.ReturnValuesList:
- AddToReturnList(top_level_form, item)
- if element.UseDictionary:
- top_level_form.UseDictionary = True
- if element.ReturnValues[0] is not None: # if a button was clicked
- event = element.ReturnValues[0]
-
- if element.Type == ELEM_TYPE_TAB:
- element.DictionaryKeyCounter = top_level_form.DictionaryKeyCounter
- element.ReturnValuesList = []
- element.ReturnValuesDictionary = {}
- _BuildResultsForSubform(element, initialize_only, top_level_form)
- for item in element.ReturnValuesList:
- AddToReturnList(top_level_form, item)
- if element.UseDictionary:
- top_level_form.UseDictionary = True
- if element.ReturnValues[0] is not None: # if a button was clicked
- event = element.ReturnValues[0]
-
- if not initialize_only:
- if element.Type == ELEM_TYPE_INPUT_TEXT:
- try:
- value = element.TKStringVar.get()
- except:
- value = ''
- if not top_level_form.NonBlocking and not element.do_not_clear and not top_level_form.ReturnKeyboardEvents:
- element.TKStringVar.set('')
- elif element.Type == ELEM_TYPE_INPUT_CHECKBOX:
- value = element.TKIntVar.get()
- value = (value != 0)
- elif element.Type == ELEM_TYPE_INPUT_RADIO:
- RadVar = element.TKIntVar.get()
- this_rowcol = EncodeRadioRowCol(form.ContainerElemementNumber, row_num, col_num)
- # this_rowcol = element.EncodedRadioValue # could use the saved one
- value = RadVar == this_rowcol
- elif element.Type == ELEM_TYPE_BUTTON:
- if top_level_form.LastButtonClicked == element.Key:
- event = top_level_form.LastButtonClicked
- if element.BType != BUTTON_TYPE_REALTIME: # Do not clear realtime buttons
- top_level_form.LastButtonClicked = None
- if element.BType == BUTTON_TYPE_CALENDAR_CHOOSER:
- # value = None
- value = element.calendar_selection
- else:
- try:
- value = element.TKStringVar.get()
- except:
- value = None
- elif element.Type == ELEM_TYPE_INPUT_COMBO:
- element = element # type: Combo
- # value = element.TKStringVar.get()
- try:
- if element.TKCombo.current() == -1: # if the current value was not in the original list
- value = element.TKCombo.get()
- else:
- value = element.Values[element.TKCombo.current()] # get value from original list given index
- except:
- value = '*Exception occurred*'
- elif element.Type == ELEM_TYPE_INPUT_OPTION_MENU:
- value = element.TKStringVar.get()
- elif element.Type == ELEM_TYPE_INPUT_LISTBOX:
- try:
- items = element.TKListbox.curselection()
- value = [element.Values[int(item)] for item in items]
- except Exception as e:
- value = ''
- elif element.Type == ELEM_TYPE_INPUT_SPIN:
- try:
- value = element.TKStringVar.get()
- for v in element.Values:
- if str(v) == value:
- value = v
- break
- except:
- value = 0
- elif element.Type == ELEM_TYPE_INPUT_SLIDER:
- try:
- value = float(element.TKScale.get())
- except:
- value = 0
- elif element.Type == ELEM_TYPE_INPUT_MULTILINE:
- if element.WriteOnly: # if marked as "write only" when created, then don't include with the values being returned
- continue
- try:
- value = element.TKText.get(1.0, tk.END)
- if element.rstrip:
- value = value.rstrip()
- if not top_level_form.NonBlocking and not element.do_not_clear and not top_level_form.ReturnKeyboardEvents:
- element.TKText.delete('1.0', tk.END)
- except:
- value = None
- elif element.Type == ELEM_TYPE_TAB_GROUP:
- try:
- value = element.TKNotebook.tab(element.TKNotebook.index('current'))['text']
- tab_key = element.FindKeyFromTabName(value)
- if tab_key is not None:
- value = tab_key
- except:
- value = None
- elif element.Type == ELEM_TYPE_TABLE:
- value = element.SelectedRows
- elif element.Type == ELEM_TYPE_TREE:
- value = element.SelectedRows
- elif element.Type == ELEM_TYPE_GRAPH:
- value = element.ClickPosition
- elif element.Type == ELEM_TYPE_MENUBAR:
- if element.MenuItemChosen is not None:
- event = top_level_form.LastButtonClicked = element.MenuItemChosen
- value = element.MenuItemChosen
- element.MenuItemChosen = None
- elif element.Type == ELEM_TYPE_BUTTONMENU:
- element = element # type: ButtonMenu
- value = element.MenuItemChosen
- if element.part_of_custom_menubar:
- if element.MenuItemChosen is not None:
- value = event = element.MenuItemChosen
- top_level_form.LastButtonClicked = element.MenuItemChosen
- if element.custom_menubar_key is not None:
- top_level_form.ReturnValuesDictionary[element.custom_menubar_key] = value
- element.MenuItemChosen = None
- else:
- if element.custom_menubar_key not in top_level_form.ReturnValuesDictionary:
- top_level_form.ReturnValuesDictionary[element.custom_menubar_key] = None
- value = None
-
- # if element.MenuItemChosen is not None:
- # button_pressed_text = top_level_form.LastButtonClicked = element.MenuItemChosen
- # value = element.MenuItemChosen
- # element.MenuItemChosen = None
- else:
- value = None
-
- # if an input type element, update the results
- if element.Type not in (
- ELEM_TYPE_BUTTON, ELEM_TYPE_TEXT, ELEM_TYPE_IMAGE, ELEM_TYPE_OUTPUT, ELEM_TYPE_PROGRESS_BAR, ELEM_TYPE_COLUMN, ELEM_TYPE_FRAME, ELEM_TYPE_SEPARATOR,
- ELEM_TYPE_TAB):
- if not (element.Type == ELEM_TYPE_BUTTONMENU and element.part_of_custom_menubar):
- AddToReturnList(form, value)
- AddToReturnDictionary(top_level_form, element, value)
- elif (element.Type == ELEM_TYPE_BUTTON and
- element.BType == BUTTON_TYPE_COLOR_CHOOSER and
- element.Target == (None, None)) or \
- (element.Type == ELEM_TYPE_BUTTON
- and element.Key is not None and
- (element.BType in (BUTTON_TYPE_SAVEAS_FILE, BUTTON_TYPE_BROWSE_FILE, BUTTON_TYPE_BROWSE_FILES,
- BUTTON_TYPE_BROWSE_FOLDER, BUTTON_TYPE_CALENDAR_CHOOSER))):
- AddToReturnList(form, value)
- AddToReturnDictionary(top_level_form, element, value)
-
- # if this is a column, then will fail so need to wrap with try
- try:
- if form.ReturnKeyboardEvents and form.LastKeyboardEvent is not None:
- event = form.LastKeyboardEvent
- form.LastKeyboardEvent = None
- except:
- pass
-
- try:
- form.ReturnValuesDictionary.pop(None, None) # clean up dictionary include None was included
- except:
- pass
-
- # if no event was found
- if not initialize_only and event is None and form == top_level_form:
- queued_event_value = form._queued_thread_event_read()
- if queued_event_value is not None:
- event, value = queued_event_value
- AddToReturnList(form, value)
- form.ReturnValuesDictionary[event] = value
-
- if not form.UseDictionary:
- form.ReturnValues = event, form.ReturnValuesList
- else:
- form.ReturnValues = event, form.ReturnValuesDictionary
-
- return form.ReturnValues
-
-
-def fill_form_with_values(window, values_dict):
- """
- Fills a window with values provided in a values dictionary { element_key : new_value }
-
- :param window: The window object to fill
- :type window: (Window)
- :param values_dict: A dictionary with element keys as key and value is values parm for Update call
- :type values_dict: (Dict[Any, Any])
- :return: None
- :rtype: None
- """
-
- for element_key in values_dict:
- try:
- window.AllKeysDict[element_key].Update(values_dict[element_key])
- except Exception as e:
- print('Problem filling form. Perhaps bad key? This is a suspected bad key: {}'.format(element_key))
-
-
-def _FindElementWithFocusInSubForm(form):
- """
- Searches through a "sub-form" (can be a window or container) for the current element with focus
-
- :param form: a Window, Column, Frame, or TabGroup (container elements)
- :type form: container elements
- :return: Element
- :rtype: Element | None
- """
- for row_num, row in enumerate(form.Rows):
- for col_num, element in enumerate(row):
- if element.Type == ELEM_TYPE_COLUMN:
- matching_elem = _FindElementWithFocusInSubForm(element)
- if matching_elem is not None:
- return matching_elem
- elif element.Type == ELEM_TYPE_FRAME:
- matching_elem = _FindElementWithFocusInSubForm(element)
- if matching_elem is not None:
- return matching_elem
- elif element.Type == ELEM_TYPE_TAB_GROUP:
- matching_elem = _FindElementWithFocusInSubForm(element)
- if matching_elem is not None:
- return matching_elem
- elif element.Type == ELEM_TYPE_TAB:
- matching_elem = _FindElementWithFocusInSubForm(element)
- if matching_elem is not None:
- return matching_elem
- elif element.Type == ELEM_TYPE_PANE:
- matching_elem = _FindElementWithFocusInSubForm(element)
- if matching_elem is not None:
- return matching_elem
- elif element.Type == ELEM_TYPE_INPUT_TEXT:
- if element.TKEntry is not None:
- if element.TKEntry is element.TKEntry.focus_get():
- return element
- elif element.Type == ELEM_TYPE_INPUT_MULTILINE:
- if element.TKText is not None:
- if element.TKText is element.TKText.focus_get():
- return element
- elif element.Type == ELEM_TYPE_BUTTON:
- if element.TKButton is not None:
- if element.TKButton is element.TKButton.focus_get():
- return element
- else: # The "Catch All" - if type isn't one of the above, try generic element.Widget
- try:
- if element.Widget is not None:
- if element.Widget is element.Widget.focus_get():
- return element
- except:
- return None
-
- return None
-
-
-def AddMenuItem(top_menu, sub_menu_info, element, is_sub_menu=False, skip=False, right_click_menu=False):
- """
- Only to be used internally. Not user callable
- :param top_menu: ???
- :type top_menu: ???
- :param sub_menu_info: ???
- :type sub_menu_info:
- :param element: ???
- :type element: idk_yetReally
- :param is_sub_menu: (Default = False)
- :type is_sub_menu: (bool)
- :param skip: (Default = False)
- :type skip: (bool)
-
- """
- return_val = None
- if type(sub_menu_info) is str:
- if not is_sub_menu and not skip:
- pos = sub_menu_info.find(MENU_SHORTCUT_CHARACTER)
- if pos != -1:
- if pos < len(MENU_SHORTCUT_CHARACTER) or sub_menu_info[pos - len(MENU_SHORTCUT_CHARACTER)] != "\\":
- sub_menu_info = sub_menu_info[:pos] + sub_menu_info[pos + len(MENU_SHORTCUT_CHARACTER):]
- if sub_menu_info == '---':
- top_menu.add('separator')
- else:
- try:
- item_without_key = sub_menu_info[:sub_menu_info.index(MENU_KEY_SEPARATOR)]
- except:
- item_without_key = sub_menu_info
-
- if item_without_key[0] == MENU_DISABLED_CHARACTER:
- top_menu.add_command(label=item_without_key[len(MENU_DISABLED_CHARACTER):], underline=pos - 1,
- command=lambda: element._MenuItemChosenCallback(sub_menu_info))
- top_menu.entryconfig(item_without_key[len(MENU_DISABLED_CHARACTER):], state='disabled')
- else:
- top_menu.add_command(label=item_without_key, underline=pos,
- command=lambda: element._MenuItemChosenCallback(sub_menu_info))
- else:
- i = 0
- while i < (len(sub_menu_info)):
- item = sub_menu_info[i]
- if i != len(sub_menu_info) - 1:
- if type(sub_menu_info[i + 1]) == list:
- new_menu = tk.Menu(top_menu, tearoff=element.Tearoff)
- # if a right click menu, then get styling from the top-level window
- if right_click_menu:
- window = element.ParentForm
- if window.right_click_menu_background_color not in (COLOR_SYSTEM_DEFAULT, None):
- new_menu.config(bg=window.right_click_menu_background_color)
- if window.right_click_menu_text_color not in (COLOR_SYSTEM_DEFAULT, None):
- new_menu.config(fg=window.right_click_menu_text_color)
- if window.right_click_menu_disabled_text_color not in (COLOR_SYSTEM_DEFAULT, None):
- new_menu.config(disabledforeground=window.right_click_menu_disabled_text_color)
- if window.right_click_menu_font is not None:
- new_menu.config(font=window.right_click_menu_font)
- else:
- if element.Font is not None:
- new_menu.config(font=element.Font)
- if element.BackgroundColor not in (COLOR_SYSTEM_DEFAULT, None):
- new_menu.config(bg=element.BackgroundColor)
- if element.TextColor not in (COLOR_SYSTEM_DEFAULT, None):
- new_menu.config(fg=element.TextColor)
- if element.DisabledTextColor not in (COLOR_SYSTEM_DEFAULT, None):
- new_menu.config(disabledforeground=element.DisabledTextColor)
- if element.ItemFont is not None:
- new_menu.config(font=element.ItemFont)
- return_val = new_menu
- pos = sub_menu_info[i].find(MENU_SHORTCUT_CHARACTER)
- if pos != -1:
- if pos < len(MENU_SHORTCUT_CHARACTER) or sub_menu_info[i][pos - len(MENU_SHORTCUT_CHARACTER)] != "\\":
- sub_menu_info[i] = sub_menu_info[i][:pos] + sub_menu_info[i][pos + len(MENU_SHORTCUT_CHARACTER):]
- if sub_menu_info[i][0] == MENU_DISABLED_CHARACTER:
- top_menu.add_cascade(label=sub_menu_info[i][len(MENU_DISABLED_CHARACTER):], menu=new_menu,
- underline=pos, state='disabled')
- else:
- top_menu.add_cascade(label=sub_menu_info[i], menu=new_menu, underline=pos)
- AddMenuItem(new_menu, sub_menu_info[i + 1], element, is_sub_menu=True, right_click_menu=right_click_menu)
- i += 1 # skip the next one
- else:
- AddMenuItem(top_menu, item, element, right_click_menu=right_click_menu)
- else:
- AddMenuItem(top_menu, item, element, right_click_menu=right_click_menu)
- i += 1
- return return_val
-
-
-# 888 888 d8b 888
-# 888 888 Y8P 888
-# 888 888 888
-# 888888 888 888 888 88888b. 888888 .d88b. 888d888
-# 888 888 .88P 888 888 "88b 888 d8P Y8b 888P"
-# 888 888888K 888 888 888 888 88888888 888
-# Y88b. 888 "88b 888 888 888 Y88b. Y8b. 888
-# "Y888 888 888 888 888 888 "Y888 "Y8888 888
-
-# My crappy tkinter code starts here. (search for "crappy" to get here quickly... that's the purpose if you hadn't caught on
-
-"""
- )
- (
- ,
- ___)\
- (_____)
- (_______)
-
-"""
-
-
-# Chr0nic || This is probably *very* bad practice. But it works. Simple, but it works...
-class VarHolder(object):
- canvas_holder = None
-
- def __init__(self):
- self.canvas_holder = None
-
-
-# Also, to get to the point in the code where each element's widget is created, look for element + "p lacement" (without the space)
-
-
-# ======================== TK CODE STARTS HERE ========================================= #
-def _fixed_map(style, style_name, option, highlight_colors=(None, None)):
- # Fix for setting text colour for Tkinter 8.6.9
- # From: https://core.tcl.tk/tk/info/509cafafae
-
- # default_map = [elm for elm in style.map("Treeview", query_opt=option) if '!' not in elm[0]]
- # custom_map = [elm for elm in style.map(style_name, query_opt=option) if '!' not in elm[0]]
- default_map = [elm for elm in style.map("Treeview", query_opt=option) if '!' not in elm[0] and 'selected' not in elm[0]]
- custom_map = [elm for elm in style.map(style_name, query_opt=option) if '!' not in elm[0] and 'selected' not in elm[0]]
- if option == 'background':
- custom_map.append(('selected', highlight_colors[1] if highlight_colors[1] is not None else ALTERNATE_TABLE_AND_TREE_SELECTED_ROW_COLORS[1]))
- elif option == 'foreground':
- custom_map.append(('selected', highlight_colors[0] if highlight_colors[0] is not None else ALTERNATE_TABLE_AND_TREE_SELECTED_ROW_COLORS[0]))
-
- new_map = custom_map + default_map
- return new_map
- #
- # new_map = [elm for elm in style.map(style_name, query_opt=option) if elm[:2] != ('!disabled', '!selected')]
- #
- # if option == 'background':
- # new_map.append(('selected', highlight_colors[1] if highlight_colors[1] is not None else ALTERNATE_TABLE_AND_TREE_SELECTED_ROW_COLORS[1]))
- # elif option == 'foreground':
- # new_map.append(('selected', highlight_colors[0] if highlight_colors[0] is not None else ALTERNATE_TABLE_AND_TREE_SELECTED_ROW_COLORS[0]))
- # return new_map
- #
-
-def _add_right_click_menu(element, toplevel_form):
- if element.RightClickMenu == MENU_RIGHT_CLICK_DISABLED:
- return
- if element.RightClickMenu or toplevel_form.RightClickMenu:
- menu = element.RightClickMenu or toplevel_form.RightClickMenu
- top_menu = tk.Menu(toplevel_form.TKroot, tearoff=toplevel_form.right_click_menu_tearoff, tearoffcommand=element._tearoff_menu_callback)
-
- if toplevel_form.right_click_menu_background_color not in (COLOR_SYSTEM_DEFAULT, None):
- top_menu.config(bg=toplevel_form.right_click_menu_background_color)
- if toplevel_form.right_click_menu_text_color not in (COLOR_SYSTEM_DEFAULT, None):
- top_menu.config(fg=toplevel_form.right_click_menu_text_color)
- if toplevel_form.right_click_menu_disabled_text_color not in (COLOR_SYSTEM_DEFAULT, None):
- top_menu.config(disabledforeground=toplevel_form.right_click_menu_disabled_text_color)
- if toplevel_form.right_click_menu_font is not None:
- top_menu.config(font=toplevel_form.right_click_menu_font)
-
- if toplevel_form.right_click_menu_selected_colors[0] not in (COLOR_SYSTEM_DEFAULT, None):
- top_menu.config(activeforeground=toplevel_form.right_click_menu_selected_colors[0])
- if toplevel_form.right_click_menu_selected_colors[1] not in (COLOR_SYSTEM_DEFAULT, None):
- top_menu.config(activebackground=toplevel_form.right_click_menu_selected_colors[1])
- AddMenuItem(top_menu, menu[1], element, right_click_menu=True)
- element.TKRightClickMenu = top_menu
- if (running_mac()):
- element.Widget.bind('', element._RightClickMenuCallback)
- else:
- element.Widget.bind('', element._RightClickMenuCallback)
-
-
-def _change_ttk_theme(style, theme_name):
- global ttk_theme_in_use
- if theme_name not in style.theme_names():
- _error_popup_with_traceback('You are trying to use TTK theme "{}"'.format(theme_name),
- 'This is not legal for your system',
- 'The valid themes to choose from are: {}'.format(', '.join(style.theme_names())))
- return False
-
- style.theme_use(theme_name)
- ttk_theme_in_use = theme_name
- return True
-
-# class Stylist:
-# """
-# A class to help get information about ttk styles
-# """
-# @staticmethod
-# def get_elements(layout):
-# """Return a list of elements contained in the style"""
-# elements = []
-# element = layout[0][0]
-# elements.append(element)
-# sublayout = layout[0][1]
-#
-# if 'children' in sublayout:
-# child_elements = Stylist.get_elements(sublayout['children'])
-# elements.extend(child_elements)
-# return elements
-#
-# @staticmethod
-# def get_options(ttkstyle, theme=None):
-# style = ttk.Style()
-# if theme is not None:
-# style.theme_use(theme)
-# layout = style.layout(ttkstyle)
-# elements = Stylist.get_elements(layout)
-# options = []
-# for e in elements:
-# _opts = style.element_options(e)
-# if _opts:
-# options.extend(list(_opts))
-# return list(set(options))
-#
-# @staticmethod
-# def create_style(base_style: str, theme=None, **kwargs):
-# style = ttk.Style()
-# if theme is not None:
-# style.theme_use(theme)
-# style_id = uuid4()
-# ttkstyle = '{}.{}'.format(style_id, base_style)
-# style.configure(ttkstyle, **kwargs)
-# return ttkstyle
-
-def _make_ttk_style_name(base_style, element):
- Window._counter_for_ttk_widgets += 1
- style_name = str(Window._counter_for_ttk_widgets) + '___' + str(element.Key) + base_style
- element.ttk_style_name = style_name
- return style_name
-
-
-def _make_ttk_scrollbar(element, orientation, window):
- """
- Creates a ttk scrollbar for elements as they are being added to the layout
-
- :param element: The element
- :type element: (Element)
- :param orientation: The orientation vertical ('v') or horizontal ('h')
- :type orientation: (str)
- :param window: The window containing the scrollbar
- :type window: (Window)
- """
-
- style = ttk.Style()
- _change_ttk_theme(style, window.TtkTheme)
- if orientation[0].lower() == 'v':
- orient = 'vertical'
- style_name = _make_ttk_style_name('.Vertical.TScrollbar', element)
- # style_name_thumb = _make_ttk_style_name('.Vertical.TScrollbar.thumb', element)
- element.vsb_style = style
- element.vsb = ttk.Scrollbar(element.element_frame, orient=orient, command=element.Widget.yview, style=style_name)
- else:
- orient = 'horizontal'
- style_name = _make_ttk_style_name('.Horizontal.TScrollbar', element)
- element.hsb_style = style
- element.hsb = ttk.Scrollbar(element.element_frame, orient=orient, command=element.Widget.xview, style=style_name)
-
-
- # ------------------ Get the colors using heirarchy of element, window, options, settings ------------------
- # Trough Color
- if element.ttk_part_overrides.sbar_trough_color is not None:
- trough_color = element.ttk_part_overrides.sbar_trough_color
- elif window.ttk_part_overrides.sbar_trough_color is not None:
- trough_color = window.ttk_part_overrides.sbar_trough_color
- elif ttk_part_overrides_from_options.sbar_trough_color is not None:
- trough_color = ttk_part_overrides_from_options.sbar_trough_color
- else:
- trough_color = element.scroll_trough_color
- # Relief
- if element.ttk_part_overrides.sbar_relief is not None:
- scroll_relief = element.ttk_part_overrides.sbar_relief
- elif window.ttk_part_overrides.sbar_relief is not None:
- scroll_relief = window.ttk_part_overrides.sbar_relief
- elif ttk_part_overrides_from_options.sbar_relief is not None:
- scroll_relief = ttk_part_overrides_from_options.sbar_relief
- else:
- scroll_relief = element.scroll_relief
- # Frame Color
- if element.ttk_part_overrides.sbar_frame_color is not None:
- frame_color = element.ttk_part_overrides.sbar_frame_color
- elif window.ttk_part_overrides.sbar_frame_color is not None:
- frame_color = window.ttk_part_overrides.sbar_frame_color
- elif ttk_part_overrides_from_options.sbar_frame_color is not None:
- frame_color = ttk_part_overrides_from_options.sbar_frame_color
- else:
- frame_color = element.scroll_frame_color
- # Background Color
- if element.ttk_part_overrides.sbar_background_color is not None:
- background_color = element.ttk_part_overrides.sbar_background_color
- elif window.ttk_part_overrides.sbar_background_color is not None:
- background_color = window.ttk_part_overrides.sbar_background_color
- elif ttk_part_overrides_from_options.sbar_background_color is not None:
- background_color = ttk_part_overrides_from_options.sbar_background_color
- else:
- background_color = element.scroll_background_color
- # Arrow Color
- if element.ttk_part_overrides.sbar_arrow_color is not None:
- arrow_color = element.ttk_part_overrides.sbar_arrow_color
- elif window.ttk_part_overrides.sbar_arrow_color is not None:
- arrow_color = window.ttk_part_overrides.sbar_arrow_color
- elif ttk_part_overrides_from_options.sbar_arrow_color is not None:
- arrow_color = ttk_part_overrides_from_options.sbar_arrow_color
- else:
- arrow_color = element.scroll_arrow_color
- # Arrow Width
- if element.ttk_part_overrides.sbar_arrow_width is not None:
- arrow_width = element.ttk_part_overrides.sbar_arrow_width
- elif window.ttk_part_overrides.sbar_arrow_width is not None:
- arrow_width = window.ttk_part_overrides.sbar_arrow_width
- elif ttk_part_overrides_from_options.sbar_arrow_width is not None:
- arrow_width = ttk_part_overrides_from_options.sbar_arrow_width
- else:
- arrow_width = element.scroll_arrow_width
- # Scroll Width
- if element.ttk_part_overrides.sbar_width is not None:
- scroll_width = element.ttk_part_overrides.sbar_width
- elif window.ttk_part_overrides.sbar_width is not None:
- scroll_width = window.ttk_part_overrides.sbar_width
- elif ttk_part_overrides_from_options.sbar_width is not None:
- scroll_width = ttk_part_overrides_from_options.sbar_width
- else:
- scroll_width = element.scroll_width
-
-
- if trough_color not in (None, COLOR_SYSTEM_DEFAULT):
- style.configure(style_name, troughcolor=trough_color)
-
- if frame_color not in (None, COLOR_SYSTEM_DEFAULT):
- style.configure(style_name, framecolor=frame_color)
- if frame_color not in (None, COLOR_SYSTEM_DEFAULT):
- style.configure(style_name, bordercolor=frame_color)
-
- if (background_color not in (None, COLOR_SYSTEM_DEFAULT)) and \
- (arrow_color not in (None, COLOR_SYSTEM_DEFAULT)):
- style.map(style_name, background=[("selected", background_color), ('active', arrow_color), ('background', background_color), ('!focus', background_color)])
- if (background_color not in (None, COLOR_SYSTEM_DEFAULT)) and \
- (arrow_color not in (None, COLOR_SYSTEM_DEFAULT)):
- style.map(style_name, arrowcolor=[("selected", arrow_color), ('active', background_color), ('background', background_color),('!focus', arrow_color)])
-
- if scroll_width not in (None, COLOR_SYSTEM_DEFAULT):
- style.configure(style_name, width=scroll_width)
- if arrow_width not in (None, COLOR_SYSTEM_DEFAULT):
- style.configure(style_name, arrowsize=arrow_width)
-
- if scroll_relief not in (None, COLOR_SYSTEM_DEFAULT):
- style.configure(style_name, relief=scroll_relief)
-
-# if __name__ == '__main__':
-# root = tk.Tk()
-#
-# # find out what options are available for the theme and widget style
-# options = Stylist.get_options('TFrame', 'default')
-# print('The options for this style and theme are', options)
-#
-# # create a new style
-# frame_style = Stylist.create_style('TFrame', 'alt', relief=tk.RAISED, borderwidth=1)
-#
-# # apply the new style
-# ttk.Frame(style=frame_style, width=100, height=100).pack(padx=10, pady=10)
-#
-# root.mainloop()
-
-# @_timeit
-def PackFormIntoFrame(form, containing_frame, toplevel_form):
- """
-
- :param form: a window class
- :type form: (Window)
- :param containing_frame: ???
- :type containing_frame: ???
- :param toplevel_form: ???
- :type toplevel_form: (Window)
-
- """
-
- # Old bindings
- def yscroll_old(event):
- try:
- if event.num == 5 or event.delta < 0:
- VarHolder.canvas_holder.yview_scroll(1, "unit")
- elif event.num == 4 or event.delta > 0:
- VarHolder.canvas_holder.yview_scroll(-1, "unit")
- except:
- pass
-
- def xscroll_old(event):
- try:
- if event.num == 5 or event.delta < 0:
- VarHolder.canvas_holder.xview_scroll(1, "unit")
- elif event.num == 4 or event.delta > 0:
- VarHolder.canvas_holder.xview_scroll(-1, "unit")
- except:
- pass
-
- # Chr0nic
- def testMouseHook2(em):
- combo = em.TKCombo
- combo.unbind_class("TCombobox", "")
- combo.unbind_class("TCombobox", "")
- combo.unbind_class("TCombobox", "")
- containing_frame.unbind_all('<4>')
- containing_frame.unbind_all('<5>')
- containing_frame.unbind_all("")
- containing_frame.unbind_all("")
-
- # Chr0nic
- def testMouseUnhook2(em):
- containing_frame.bind_all('<4>', yscroll_old, add="+")
- containing_frame.bind_all('<5>', yscroll_old, add="+")
- containing_frame.bind_all("", yscroll_old, add="+")
- containing_frame.bind_all("", xscroll_old, add="+")
-
- # Chr0nic
- def testMouseHook(em):
- containing_frame.unbind_all('<4>')
- containing_frame.unbind_all('<5>')
- containing_frame.unbind_all("")
- containing_frame.unbind_all("")
-
- # Chr0nic
- def testMouseUnhook(em):
- containing_frame.bind_all('<4>', yscroll_old, add="+")
- containing_frame.bind_all('<5>', yscroll_old, add="+")
- containing_frame.bind_all("", yscroll_old, add="+")
- containing_frame.bind_all("", xscroll_old, add="+")
-
- def _char_width_in_pixels(font):
- return tkinter.font.Font(font=font).measure('A') # single character width
-
- def _char_height_in_pixels(font):
- return tkinter.font.Font(font=font).metrics('linespace')
-
- def _string_width_in_pixels(font, string):
- return tkinter.font.Font(font=font).measure(string) # single character width
-
-
-
-
- # def _valid_theme(style, theme_name):
- # if theme_name in style.theme_names():
- # return True
- # _error_popup_with_traceback('Your Window has an invalid ttk theme specified',
- # 'The traceback will show you the Window with the problem layout',
- # '** Invalid ttk theme specified {} **'.format(theme_name),
- # '\nValid choices include: {}'.format(style.theme_names()))
- #
- # # print('** Invalid ttk theme specified {} **'.format(theme_name),
- # # '\nValid choices include: {}'.format(style.theme_names()))
- # return False
-
-
-
- def _add_grab(element):
-
- try:
- if form.Grab is True or element.Grab is True:
- # if something already about to the button, then don't do the grab stuff
- if '' not in element.Widget.bind():
- element.Widget.bind("", toplevel_form._StartMoveGrabAnywhere)
- element.Widget.bind("", toplevel_form._StopMove)
- element.Widget.bind("", toplevel_form._OnMotionGrabAnywhere)
- element.ParentRowFrame.bind("", toplevel_form._StartMoveGrabAnywhere)
- element.ParentRowFrame.bind("", toplevel_form._StopMove)
- element.ParentRowFrame.bind("", toplevel_form._OnMotionGrabAnywhere)
- if element.Type == ELEM_TYPE_COLUMN:
- element.TKColFrame.canvas.bind("", toplevel_form._StartMoveGrabAnywhere)
- element.TKColFrame.canvas.bind("", toplevel_form._StopMove)
- element.TKColFrame.canvas.bind("", toplevel_form._OnMotionGrabAnywhere)
- except Exception as e:
- pass
- # print(e)
-
- def _add_right_click_menu_and_grab(element):
- if element.RightClickMenu == MENU_RIGHT_CLICK_DISABLED:
- return
- if element.Type == ELEM_TYPE_TAB_GROUP: # unless everything disabled, then need to always set a right click menu for tabgroups
- if toplevel_form.RightClickMenu == MENU_RIGHT_CLICK_DISABLED:
- return
- menu = _MENU_RIGHT_CLICK_TABGROUP_DEFAULT
- else:
- menu = element.RightClickMenu or form.RightClickMenu or toplevel_form.RightClickMenu
-
- if menu:
- top_menu = tk.Menu(toplevel_form.TKroot, tearoff=toplevel_form.right_click_menu_tearoff, tearoffcommand=element._tearoff_menu_callback)
-
- if toplevel_form.right_click_menu_background_color not in (COLOR_SYSTEM_DEFAULT, None):
- top_menu.config(bg=toplevel_form.right_click_menu_background_color)
- if toplevel_form.right_click_menu_text_color not in (COLOR_SYSTEM_DEFAULT, None):
- top_menu.config(fg=toplevel_form.right_click_menu_text_color)
- if toplevel_form.right_click_menu_disabled_text_color not in (COLOR_SYSTEM_DEFAULT, None):
- top_menu.config(disabledforeground=toplevel_form.right_click_menu_disabled_text_color)
- if toplevel_form.right_click_menu_font is not None:
- top_menu.config(font=toplevel_form.right_click_menu_font)
-
- if toplevel_form.right_click_menu_selected_colors[0] not in (COLOR_SYSTEM_DEFAULT, None):
- top_menu.config(activeforeground=toplevel_form.right_click_menu_selected_colors[0])
- if toplevel_form.right_click_menu_selected_colors[1] not in (COLOR_SYSTEM_DEFAULT, None):
- top_menu.config(activebackground=toplevel_form.right_click_menu_selected_colors[1])
- AddMenuItem(top_menu, menu[1], element, right_click_menu=True)
- element.TKRightClickMenu = top_menu
- if toplevel_form.RightClickMenu: # if the top level has a right click menu, then setup a callback for the Window itself
- if toplevel_form.TKRightClickMenu is None:
- toplevel_form.TKRightClickMenu = top_menu
- if (running_mac()):
- toplevel_form.TKroot.bind('', toplevel_form._RightClickMenuCallback)
- else:
- toplevel_form.TKroot.bind('', toplevel_form._RightClickMenuCallback)
- if (running_mac()):
- element.Widget.bind('', element._RightClickMenuCallback)
- else:
- element.Widget.bind('', element._RightClickMenuCallback)
- try:
- if element.Type == ELEM_TYPE_COLUMN:
- element.TKColFrame.canvas.bind('', element._RightClickMenuCallback)
- except:
- pass
- _add_grab(element)
-
-
- def _add_expansion(element, row_should_expand, row_fill_direction):
- expand = True
- if element.expand_x and element.expand_y:
- fill = tk.BOTH
- row_fill_direction = tk.BOTH
- row_should_expand = True
- elif element.expand_x:
- fill = tk.X
- row_fill_direction = tk.X if row_fill_direction == tk.NONE else tk.BOTH if row_fill_direction == tk.Y else tk.X
- elif element.expand_y:
- fill = tk.Y
- row_fill_direction = tk.Y if row_fill_direction == tk.NONE else tk.BOTH if row_fill_direction == tk.X else tk.Y
- row_should_expand = True
- else:
- fill = tk.NONE
- expand = False
- return expand, fill, row_should_expand, row_fill_direction
-
- tclversion_detailed = tkinter.Tcl().eval('info patchlevel')
-
-
- # --------------------------------------------------------------------------- #
- # **************** Use FlexForm to build the tkinter window ********** ----- #
- # Building is done row by row. #
- # WARNING - You can't use print in this function. If the user has rerouted #
- # stdout then there will be an error saying the window isn't finalized #
- # --------------------------------------------------------------------------- #
- ######################### LOOP THROUGH ROWS #########################
- # *********** ------- Loop through ROWS ------- ***********#
- for row_num, flex_row in enumerate(form.Rows):
- ######################### LOOP THROUGH ELEMENTS ON ROW #########################
- # *********** ------- Loop through ELEMENTS ------- ***********#
- # *********** Make TK Row ***********#
- tk_row_frame = tk.Frame(containing_frame)
- row_should_expand = False
- row_fill_direction = tk.NONE
-
- if form.ElementJustification is not None:
- row_justify = form.ElementJustification
- else:
- row_justify = 'l'
-
- for col_num, element in enumerate(flex_row):
- element.ParentRowFrame = tk_row_frame
- element.element_frame = None # for elements that have a scrollbar too
- element.ParentForm = toplevel_form # save the button's parent form object
- if toplevel_form.Font and (element.Font == DEFAULT_FONT or element.Font is None):
- font = toplevel_form.Font
- elif element.Font is not None:
- font = element.Font
- else:
- font = DEFAULT_FONT
- # ------- Determine Auto-Size setting on a cascading basis ------- #
- if element.AutoSizeText is not None: # if element overide
- auto_size_text = element.AutoSizeText
- elif toplevel_form.AutoSizeText is not None: # if form override
- auto_size_text = toplevel_form.AutoSizeText
- else:
- auto_size_text = DEFAULT_AUTOSIZE_TEXT
- element_type = element.Type
- # Set foreground color
- text_color = element.TextColor
- elementpad = element.Pad if element.Pad is not None else toplevel_form.ElementPadding
- # element.pad_used = elementpad # store the value used back into the element
- # Determine Element size
- element_size = element.Size
- if (element_size == (None, None) and element_type not in (
- ELEM_TYPE_BUTTON, ELEM_TYPE_BUTTONMENU)): # user did not specify a size
- element_size = toplevel_form.DefaultElementSize
- elif (element_size == (None, None) and element_type in (ELEM_TYPE_BUTTON, ELEM_TYPE_BUTTONMENU)):
- element_size = toplevel_form.DefaultButtonElementSize
- else:
- auto_size_text = False # if user has specified a size then it shouldn't autosize
-
- border_depth = toplevel_form.BorderDepth if toplevel_form.BorderDepth is not None else DEFAULT_BORDER_WIDTH
- try:
- if element.BorderWidth is not None:
- border_depth = element.BorderWidth
- except:
- pass
-
- # ------------------------- COLUMN placement element ------------------------- #
- if element_type == ELEM_TYPE_COLUMN:
- element = element # type: Column
- # ----------------------- SCROLLABLE Column ----------------------
- if element.Scrollable:
- element.Widget = element.TKColFrame = TkScrollableFrame(tk_row_frame, element.VerticalScrollOnly, element, toplevel_form) # do not use yet! not working
- PackFormIntoFrame(element, element.TKColFrame.TKFrame, toplevel_form)
- element.TKColFrame.TKFrame.update()
- if element.Size == (None, None): # if no size specified, use column width x column height/2
- element.TKColFrame.canvas.config(width=element.TKColFrame.TKFrame.winfo_reqwidth() // element.size_subsample_width,
- height=element.TKColFrame.TKFrame.winfo_reqheight() // element.size_subsample_height)
- else:
- element.TKColFrame.canvas.config(width=element.TKColFrame.TKFrame.winfo_reqwidth() // element.size_subsample_width,
- height=element.TKColFrame.TKFrame.winfo_reqheight() // element.size_subsample_height)
- if None not in (element.Size[0], element.Size[1]):
- element.TKColFrame.canvas.config(width=element.Size[0], height=element.Size[1])
- elif element.Size[1] is not None:
- element.TKColFrame.canvas.config(height=element.Size[1])
- elif element.Size[0] is not None:
- element.TKColFrame.canvas.config(width=element.Size[0])
- if not element.BackgroundColor in (None, COLOR_SYSTEM_DEFAULT):
- element.TKColFrame.canvas.config(background=element.BackgroundColor)
- element.TKColFrame.TKFrame.config(background=element.BackgroundColor, borderwidth=0, highlightthickness=0)
- element.TKColFrame.config(background=element.BackgroundColor, borderwidth=0,
- highlightthickness=0)
- # ----------------------- PLAIN Column ----------------------
- else:
- if element.Size != (None, None):
- element.Widget = element.TKColFrame = TkFixedFrame(tk_row_frame)
- PackFormIntoFrame(element, element.TKColFrame.TKFrame, toplevel_form)
- element.TKColFrame.TKFrame.update()
- if None not in (element.Size[0], element.Size[1]):
- element.TKColFrame.canvas.config(width=element.Size[0], height=element.Size[1])
- elif element.Size[1] is not None:
- element.TKColFrame.canvas.config(height=element.Size[1])
- elif element.Size[0] is not None:
- element.TKColFrame.canvas.config(width=element.Size[0])
- if not element.BackgroundColor in (None, COLOR_SYSTEM_DEFAULT):
- element.TKColFrame.canvas.config(background=element.BackgroundColor)
- element.TKColFrame.TKFrame.config(background=element.BackgroundColor, borderwidth=0, highlightthickness=0)
- else:
- element.Widget = element.TKColFrame = tk.Frame(tk_row_frame)
- PackFormIntoFrame(element, element.TKColFrame, toplevel_form)
- if element.BackgroundColor not in (None, COLOR_SYSTEM_DEFAULT):
- element.TKColFrame.config(background=element.BackgroundColor, borderwidth=0, highlightthickness=0)
-
- if element.Justification is None:
- pass
- elif element.Justification.lower().startswith('l'):
- row_justify = 'l'
- elif element.Justification.lower().startswith('c'):
- row_justify = 'c'
- elif element.Justification.lower().startswith('r'):
- row_justify = 'r'
-
- # anchor=tk.NW
- # side = tk.LEFT
- # row_justify = element.Justification
-
- # element.Widget = element.TKColFrame
-
- expand = True
- if element.expand_x and element.expand_y:
- fill = tk.BOTH
- row_fill_direction = tk.BOTH
- row_should_expand = True
- elif element.expand_x:
- fill = tk.X
- row_fill_direction = tk.X
- elif element.expand_y:
- fill = tk.Y
- row_fill_direction = tk.Y
- row_should_expand = True
- else:
- fill = tk.NONE
- expand = False
-
- if element.VerticalAlignment is not None:
- anchor = tk.CENTER # Default to center if a bad choice is made
-
- if element.VerticalAlignment.lower().startswith('t'):
- anchor = tk.N
- if element.VerticalAlignment.lower().startswith('c'):
- anchor = tk.CENTER
- if element.VerticalAlignment.lower().startswith('b'):
- anchor = tk.S
- element.TKColFrame.pack(side=tk.LEFT, anchor=anchor, padx=elementpad[0], pady=elementpad[1], expand=expand, fill=fill)
- else:
- element.TKColFrame.pack(side=tk.LEFT, padx=elementpad[0], pady=elementpad[1], expand=expand, fill=fill)
-
- # element.TKColFrame.pack(side=side, padx=elementpad[0], pady=elementpad[1], expand=True, fill='both')
- if element.visible is False:
- element._pack_forget_save_settings()
- # element.TKColFrame.pack_forget()
-
- _add_right_click_menu_and_grab(element)
- # if element.Grab:
- # element._grab_anywhere_on()
- # row_should_expand = True
- # ------------------------- Pane placement element ------------------------- #
- if element_type == ELEM_TYPE_PANE:
- bd = element.BorderDepth if element.BorderDepth is not None else border_depth
- element.PanedWindow = element.Widget = tk.PanedWindow(tk_row_frame,
- orient=tk.VERTICAL if element.Orientation.startswith(
- 'v') else tk.HORIZONTAL,
- borderwidth=bd,
- bd=bd,
- )
- if element.Relief is not None:
- element.PanedWindow.configure(relief=element.Relief)
- element.PanedWindow.configure(handlesize=element.HandleSize)
- if element.ShowHandle:
- element.PanedWindow.config(showhandle=True)
- if element.Size != (None, None):
- element.PanedWindow.config(width=element.Size[0], height=element.Size[1])
- if element.BackgroundColor is not None and element.BackgroundColor != COLOR_SYSTEM_DEFAULT:
- element.PanedWindow.configure(background=element.BackgroundColor)
- for pane in element.PaneList:
- pane.Widget = pane.TKColFrame = tk.Frame(element.PanedWindow)
- pane.ParentPanedWindow = element.PanedWindow
- PackFormIntoFrame(pane, pane.TKColFrame, toplevel_form)
- if pane.visible:
- element.PanedWindow.add(pane.TKColFrame)
- if pane.BackgroundColor != COLOR_SYSTEM_DEFAULT and pane.BackgroundColor is not None:
- pane.TKColFrame.configure(background=pane.BackgroundColor,
- highlightbackground=pane.BackgroundColor,
- highlightcolor=pane.BackgroundColor)
- expand, fill, row_should_expand, row_fill_direction = _add_expansion(element, row_should_expand, row_fill_direction)
- element.PanedWindow.pack(side=tk.LEFT, padx=elementpad[0], pady=elementpad[1], expand=expand, fill=fill)
- # element.PanedWindow.pack(side=tk.LEFT, padx=elementpad[0], pady=elementpad[1], expand=True, fill='both')
- if element.visible is False:
- element._pack_forget_save_settings()
- # element.PanedWindow.pack_forget()
- # ------------------------- TEXT placement element ------------------------- #
- elif element_type == ELEM_TYPE_TEXT:
- # auto_size_text = element.AutoSizeText
- element = element # type: Text
- display_text = element.DisplayText # text to display
- if auto_size_text is False:
- width, height = element_size
- else:
- width, height = None, None
- # lines = display_text.split('\n')
- # max_line_len = max([len(l) for l in lines])
- # num_lines = len(lines)
- # if max_line_len > element_size[0]: # if text exceeds element size, the will have to wrap
- # width = element_size[0]
- # else:
- # width = max_line_len
- # height = num_lines
- # ---===--- LABEL widget create and place --- #
- element = element # type: Text
- bd = element.BorderWidth if element.BorderWidth is not None else border_depth
- stringvar = tk.StringVar()
- element.TKStringVar = stringvar
- stringvar.set(str(display_text))
- if auto_size_text:
- width = 0
- if element.Justification is not None:
- justification = element.Justification
- elif toplevel_form.TextJustification is not None:
- justification = toplevel_form.TextJustification
- else:
- justification = DEFAULT_TEXT_JUSTIFICATION
- justify = tk.LEFT if justification.startswith('l') else tk.CENTER if justification.startswith('c') else tk.RIGHT
- anchor = tk.NW if justification.startswith('l') else tk.N if justification.startswith('c') else tk.NE
- tktext_label = element.Widget = tk.Label(tk_row_frame, textvariable=stringvar, width=width,
- height=height, justify=justify, bd=bd, font=font)
- # Set wrap-length for text (in PIXELS) == PAIN IN THE ASS
- wraplen = tktext_label.winfo_reqwidth() # width of widget in Pixels
- if auto_size_text or (not auto_size_text and height == 1): # if just 1 line high, ensure no wrap happens
- wraplen = 0
- tktext_label.configure(anchor=anchor, wraplen=wraplen) # set wrap to width of widget
- if element.Relief is not None:
- tktext_label.configure(relief=element.Relief)
- if element.BackgroundColor is not None and element.BackgroundColor != COLOR_SYSTEM_DEFAULT:
- tktext_label.configure(background=element.BackgroundColor)
- if element.TextColor != COLOR_SYSTEM_DEFAULT and element.TextColor is not None:
- tktext_label.configure(fg=element.TextColor)
- expand, fill, row_should_expand, row_fill_direction = _add_expansion(element, row_should_expand, row_fill_direction)
- tktext_label.pack(side=tk.LEFT, padx=elementpad[0], pady=elementpad[1], expand=expand, fill=fill)
- if element.visible is False:
- element._pack_forget_save_settings()
- # tktext_label.pack_forget()
- element.TKText = tktext_label
- if element.ClickSubmits:
- tktext_label.bind('', element._TextClickedHandler)
- if element.Tooltip is not None:
- element.TooltipObject = ToolTip(element.TKText, text=element.Tooltip, timeout=DEFAULT_TOOLTIP_TIME)
- _add_right_click_menu_and_grab(element)
- if element.Grab:
- element._grab_anywhere_on()
- # ------------------------- BUTTON placement element non-ttk version ------------------------- #
- elif (element_type == ELEM_TYPE_BUTTON and element.UseTtkButtons is False) or \
- (element_type == ELEM_TYPE_BUTTON and element.UseTtkButtons is not True and toplevel_form.UseTtkButtons is not True):
- element = element # type: Button
- element.UseTtkButtons = False # indicate that ttk button was not used
- stringvar = tk.StringVar()
- element.TKStringVar = stringvar
- element.Location = (row_num, col_num)
- btext = element.ButtonText
- btype = element.BType
- if element.AutoSizeButton is not None:
- auto_size = element.AutoSizeButton
- else:
- auto_size = toplevel_form.AutoSizeButtons
- if auto_size is False or element.Size[0] is not None:
- width, height = element_size
- else:
- width = 0
- height = toplevel_form.DefaultButtonElementSize[1]
- if element.ButtonColor != (None, None) and element.ButtonColor != DEFAULT_BUTTON_COLOR:
- bc = element.ButtonColor
- elif toplevel_form.ButtonColor != (None, None) and toplevel_form.ButtonColor != DEFAULT_BUTTON_COLOR:
- bc = toplevel_form.ButtonColor
- else:
- bc = DEFAULT_BUTTON_COLOR
-
- bd = element.BorderWidth
-
- tkbutton = element.Widget = tk.Button(tk_row_frame, text=btext, width=width, height=height, justify=tk.CENTER, bd=bd, font=font)
- try:
- if btype != BUTTON_TYPE_REALTIME:
- tkbutton.config( command=element.ButtonCallBack)
-
- else:
- tkbutton.bind('', element.ButtonReleaseCallBack)
- tkbutton.bind('', element.ButtonPressCallBack)
- if bc != (None, None) and COLOR_SYSTEM_DEFAULT not in bc:
- tkbutton.config(foreground=bc[0], background=bc[1])
- else:
- if bc[0] != COLOR_SYSTEM_DEFAULT:
- tkbutton.config(foreground=bc[0])
- if bc[1] != COLOR_SYSTEM_DEFAULT:
- tkbutton.config(background=bc[1])
- except Exception as e:
- _error_popup_with_traceback('Button has a problem....',
- 'The traceback information will not show the line in your layout with the problem, but it does tell you which window.',
- 'Error {}'.format(e),
- # 'Button Text: {}'.format(btext),
- # 'Button key: {}'.format(element.Key),
- # 'Color string: {}'.format(bc),
- "Parent Window's Title: {}".format(toplevel_form.Title))
-
- if bd == 0 and not running_mac():
- tkbutton.config(relief=tk.FLAT)
-
- element.TKButton = tkbutton # not used yet but save the TK button in case
- if elementpad[0] == 0 or elementpad[1] == 0:
- tkbutton.config(highlightthickness=0)
-
- ## -------------- TK Button With Image -------------- ##
- if element.ImageFilename: # if button has an image on it
- tkbutton.config(highlightthickness=0)
- try:
- photo = tk.PhotoImage(file=element.ImageFilename)
- if element.ImageSubsample:
- photo = photo.subsample(element.ImageSubsample)
- if element.ImageSize != (None, None):
- width, height = element.ImageSize
- else:
- width, height = photo.width(), photo.height()
- except Exception as e:
- _error_popup_with_traceback('Button Element error {}'.format(e), 'Image filename: {}'.format(element.ImageFilename),
- 'Button element key: {}'.format(element.Key),
- "Parent Window's Title: {}".format(toplevel_form.Title))
- tkbutton.config(image=photo, compound=tk.CENTER, width=width, height=height)
- tkbutton.image = photo
- if element.ImageData: # if button has an image on it
- tkbutton.config(highlightthickness=0)
- try:
- photo = tk.PhotoImage(data=element.ImageData)
- if element.ImageSubsample:
- photo = photo.subsample(element.ImageSubsample)
- if element.ImageSize != (None, None):
- width, height = element.ImageSize
- else:
- width, height = photo.width(), photo.height()
- tkbutton.config(image=photo, compound=tk.CENTER, width=width, height=height)
- tkbutton.image = photo
- except Exception as e:
- _error_popup_with_traceback('Button Element error {}'.format(e),
- 'Problem using BASE64 Image data Image Susample',
- 'Buton element key: {}'.format(element.Key),
- "Parent Window's Title: {}".format(toplevel_form.Title))
-
- if width != 0:
- wraplen = width * _char_width_in_pixels(font)
- tkbutton.configure(wraplength=wraplen) # set wrap to width of widget
- expand, fill, row_should_expand, row_fill_direction = _add_expansion(element, row_should_expand, row_fill_direction)
-
- tkbutton.pack(side=tk.LEFT, padx=elementpad[0], pady=elementpad[1], expand=expand, fill=fill)
- if element.visible is False:
- element._pack_forget_save_settings()
- # tkbutton.pack_forget()
- if element.BindReturnKey:
- element.TKButton.bind('', element._ReturnKeyHandler)
- if element.Focus is True or (toplevel_form.UseDefaultFocus and not toplevel_form.FocusSet):
- toplevel_form.FocusSet = True
- element.TKButton.bind('', element._ReturnKeyHandler)
- element.TKButton.focus_set()
- toplevel_form.TKroot.focus_force()
- if element.Disabled is True:
- element.TKButton['state'] = 'disabled'
- if element.DisabledButtonColor != (None, None) and element.DisabledButtonColor != (COLOR_SYSTEM_DEFAULT, COLOR_SYSTEM_DEFAULT):
- if element.DisabledButtonColor[0] not in (None, COLOR_SYSTEM_DEFAULT):
- element.TKButton['disabledforeground'] = element.DisabledButtonColor[0]
- if element.MouseOverColors[1] not in (COLOR_SYSTEM_DEFAULT, None):
- tkbutton.config(activebackground=element.MouseOverColors[1])
- if element.MouseOverColors[0] not in (COLOR_SYSTEM_DEFAULT, None):
- tkbutton.config(activeforeground=element.MouseOverColors[0])
-
- if element.Tooltip is not None:
- element.TooltipObject = ToolTip(element.TKButton, text=element.Tooltip,
- timeout=DEFAULT_TOOLTIP_TIME)
- try:
- if element.HighlightColors[1] != COLOR_SYSTEM_DEFAULT:
- tkbutton.config(highlightbackground=element.HighlightColors[1])
- if element.HighlightColors[0] != COLOR_SYSTEM_DEFAULT:
- tkbutton.config(highlightcolor=element.HighlightColors[0])
- except Exception as e:
- _error_popup_with_traceback('Button Element error {}'.format(e),
- 'Button element key: {}'.format(element.Key),
- 'Button text: {}'.format(btext),
- 'Has a bad highlight color {}'.format(element.HighlightColors),
- "Parent Window's Title: {}".format(toplevel_form.Title))
- # print('Button with text: ', btext, 'has a bad highlight color', element.HighlightColors)
- _add_right_click_menu_and_grab(element)
-
- # ------------------------- BUTTON placement element ttk version ------------------------- #
- elif element_type == ELEM_TYPE_BUTTON:
- element = element # type: Button
- element.UseTtkButtons = True # indicate that ttk button was used
- stringvar = tk.StringVar()
- element.TKStringVar = stringvar
- element.Location = (row_num, col_num)
- btext = element.ButtonText
- btype = element.BType
- if element.AutoSizeButton is not None:
- auto_size = element.AutoSizeButton
- else:
- auto_size = toplevel_form.AutoSizeButtons
- if auto_size is False or element.Size[0] is not None:
- width, height = element_size
- else:
- width = 0
- height = toplevel_form.DefaultButtonElementSize[1]
- if element.ButtonColor != (None, None) and element.ButtonColor != COLOR_SYSTEM_DEFAULT:
- bc = element.ButtonColor
- elif toplevel_form.ButtonColor != (None, None) and toplevel_form.ButtonColor != COLOR_SYSTEM_DEFAULT:
- bc = toplevel_form.ButtonColor
- else:
- bc = DEFAULT_BUTTON_COLOR
- bd = element.BorderWidth
- tkbutton = element.Widget = ttk.Button(tk_row_frame, text=btext, width=width)
- if btype != BUTTON_TYPE_REALTIME:
- tkbutton.config(command=element.ButtonCallBack)
- else:
- tkbutton.bind('', element.ButtonReleaseCallBack)
- tkbutton.bind('', element.ButtonPressCallBack)
- style_name = _make_ttk_style_name('.custombutton.TButton', element)
- button_style = ttk.Style()
- _change_ttk_theme(button_style, toplevel_form.TtkTheme)
- button_style.configure(style_name, font=font)
-
- if bc != (None, None) and COLOR_SYSTEM_DEFAULT not in bc:
- button_style.configure(style_name, foreground=bc[0], background=bc[1])
- elif bc[0] != COLOR_SYSTEM_DEFAULT:
- button_style.configure(style_name, foreground=bc[0])
- elif bc[1] != COLOR_SYSTEM_DEFAULT:
- button_style.configure(style_name, background=bc[1])
-
- if bd == 0 and not running_mac():
- button_style.configure(style_name, relief=tk.FLAT)
- button_style.configure(style_name, borderwidth=0)
- else:
- button_style.configure(style_name, borderwidth=bd)
- button_style.configure(style_name, justify=tk.CENTER)
-
- if element.MouseOverColors[1] not in (COLOR_SYSTEM_DEFAULT, None):
- button_style.map(style_name, background=[('active', element.MouseOverColors[1])])
- if element.MouseOverColors[0] not in (COLOR_SYSTEM_DEFAULT, None):
- button_style.map(style_name, foreground=[('active', element.MouseOverColors[0])])
-
- if element.DisabledButtonColor[0] not in (COLOR_SYSTEM_DEFAULT, None):
- button_style.map(style_name, foreground=[('disabled', element.DisabledButtonColor[0])])
- if element.DisabledButtonColor[1] not in (COLOR_SYSTEM_DEFAULT, None):
- button_style.map(style_name, background=[('disabled', element.DisabledButtonColor[1])])
-
- if height > 1:
- button_style.configure(style_name, padding=height * _char_height_in_pixels(font)) # should this be height instead?
- if width != 0:
- wraplen = width * _char_width_in_pixels(font) # width of widget in Pixels
- button_style.configure(style_name, wraplength=wraplen) # set wrap to width of widget
-
- ## -------------- TTK Button With Image -------------- ##
- if element.ImageFilename: # if button has an image on it
- button_style.configure(style_name, borderwidth=0)
- # tkbutton.configure(highlightthickness=0)
- photo = tk.PhotoImage(file=element.ImageFilename)
- if element.ImageSubsample:
- photo = photo.subsample(element.ImageSubsample)
- if element.ImageSize != (None, None):
- width, height = element.ImageSize
- else:
- width, height = photo.width(), photo.height()
- button_style.configure(style_name, image=photo, compound=tk.CENTER, width=width, height=height)
- tkbutton.image = photo
- if element.ImageData: # if button has an image on it
- # tkbutton.configure(highlightthickness=0)
- button_style.configure(style_name, borderwidth=0)
-
- photo = tk.PhotoImage(data=element.ImageData)
- if element.ImageSubsample:
- photo = photo.subsample(element.ImageSubsample)
- if element.ImageSize != (None, None):
- width, height = element.ImageSize
- else:
- width, height = photo.width(), photo.height()
- button_style.configure(style_name, image=photo, compound=tk.CENTER, width=width, height=height)
- # tkbutton.configure(image=photo, compound=tk.CENTER, width=width, height=height)
- tkbutton.image = photo
-
- element.TKButton = tkbutton # not used yet but save the TK button in case
- expand, fill, row_should_expand, row_fill_direction = _add_expansion(element, row_should_expand, row_fill_direction)
- tkbutton.pack(side=tk.LEFT, padx=elementpad[0], pady=elementpad[1], expand=expand, fill=fill)
- if element.visible is False:
- element._pack_forget_save_settings()
- # tkbutton.pack_forget()
- if element.BindReturnKey:
- element.TKButton.bind('', element._ReturnKeyHandler)
- if element.Focus is True or (toplevel_form.UseDefaultFocus and not toplevel_form.FocusSet):
- toplevel_form.FocusSet = True
- element.TKButton.bind('', element._ReturnKeyHandler)
- element.TKButton.focus_set()
- toplevel_form.TKroot.focus_force()
- if element.Disabled is True:
- element.TKButton['state'] = 'disabled'
-
- tkbutton.configure(style=style_name) # IMPORTANT! Apply the style to the button!
- _add_right_click_menu_and_grab(element)
-
- if element.Tooltip is not None:
- element.TooltipObject = ToolTip(element.TKButton, text=element.Tooltip,
- timeout=DEFAULT_TOOLTIP_TIME)
- # ------------------------- BUTTONMENU placement element ------------------------- #
- elif element_type == ELEM_TYPE_BUTTONMENU:
- element = element # type: ButtonMenu
- element.Location = (row_num, col_num)
- btext = element.ButtonText
- if element.AutoSizeButton is not None:
- auto_size = element.AutoSizeButton
- else:
- auto_size = toplevel_form.AutoSizeButtons
- if auto_size is False or element.Size[0] is not None:
- width, height = element_size
- else:
- width = 0
- height = toplevel_form.DefaultButtonElementSize[1]
- if element.ButtonColor != (None, None) and element.ButtonColor != DEFAULT_BUTTON_COLOR:
- bc = element.ButtonColor
- elif toplevel_form.ButtonColor != (None, None) and toplevel_form.ButtonColor != DEFAULT_BUTTON_COLOR:
- bc = toplevel_form.ButtonColor
- else:
- bc = DEFAULT_BUTTON_COLOR
- bd = element.BorderWidth
- if element.ItemFont is None:
- element.ItemFont = font
- tkbutton = element.Widget = tk.Menubutton(tk_row_frame, text=btext, width=width, height=height, justify=tk.LEFT, bd=bd, font=font)
- element.TKButtonMenu = tkbutton
- if bc != (None, None) and bc != COLOR_SYSTEM_DEFAULT and bc[1] != COLOR_SYSTEM_DEFAULT:
- tkbutton.config(foreground=bc[0], background=bc[1])
- elif bc[0] != COLOR_SYSTEM_DEFAULT:
- tkbutton.config(foreground=bc[0])
- if bd == 0 and not running_mac():
- tkbutton.config(relief=RELIEF_FLAT)
- elif bd != 0:
- tkbutton.config(relief=RELIEF_RAISED)
-
- element.TKButton = tkbutton # not used yet but save the TK button in case
- wraplen = tkbutton.winfo_reqwidth() # width of widget in Pixels
- if element.ImageFilename: # if button has an image on it
- photo = tk.PhotoImage(file=element.ImageFilename)
- if element.ImageSize != (None, None):
- width, height = element.ImageSize
- if element.ImageSubsample:
- photo = photo.subsample(element.ImageSubsample)
- else:
- width, height = photo.width(), photo.height()
- tkbutton.config(image=photo, compound=tk.CENTER, width=width, height=height)
- tkbutton.image = photo
- if element.ImageData: # if button has an image on it
- photo = tk.PhotoImage(data=element.ImageData)
- if element.ImageSize != (None, None):
- width, height = element.ImageSize
- if element.ImageSubsample:
- photo = photo.subsample(element.ImageSubsample)
- else:
- width, height = photo.width(), photo.height()
- tkbutton.config(image=photo, compound=tk.CENTER, width=width, height=height)
- tkbutton.image = photo
- if width != 0:
- tkbutton.configure(wraplength=wraplen + 10) # set wrap to width of widget
- expand, fill, row_should_expand, row_fill_direction = _add_expansion(element, row_should_expand, row_fill_direction)
- tkbutton.pack(side=tk.LEFT, padx=elementpad[0], pady=elementpad[1], expand=expand, fill=fill)
-
- menu_def = element.MenuDefinition
-
- element.TKMenu = top_menu = tk.Menu(tkbutton, tearoff=element.Tearoff, font=element.ItemFont, tearoffcommand=element._tearoff_menu_callback)
-
- if element.BackgroundColor not in (COLOR_SYSTEM_DEFAULT, None):
- top_menu.config(bg=element.BackgroundColor)
- if element.TextColor not in (COLOR_SYSTEM_DEFAULT, None):
- top_menu.config(fg=element.TextColor)
- if element.DisabledTextColor not in (COLOR_SYSTEM_DEFAULT, None):
- top_menu.config(disabledforeground=element.DisabledTextColor)
- if element.ItemFont is not None:
- top_menu.config(font=element.ItemFont)
-
- AddMenuItem(top_menu, menu_def[1], element)
- if elementpad[0] == 0 or elementpad[1] == 0:
- tkbutton.config(highlightthickness=0)
- tkbutton.configure(menu=top_menu)
- element.TKMenu = top_menu
- if element.visible is False:
- element._pack_forget_save_settings()
- # tkbutton.pack_forget()
- if element.Disabled == True:
- element.TKButton['state'] = 'disabled'
- if element.Tooltip is not None:
- element.TooltipObject = ToolTip(element.TKButton, text=element.Tooltip, timeout=DEFAULT_TOOLTIP_TIME)
-
- # ------------------------- INPUT placement element ------------------------- #
- elif element_type == ELEM_TYPE_INPUT_TEXT:
- element = element # type: InputText
- default_text = element.DefaultText
- element.TKStringVar = tk.StringVar()
- element.TKStringVar.set(default_text)
- show = element.PasswordCharacter if element.PasswordCharacter else ""
- bd = border_depth
- if element.Justification is not None:
- justification = element.Justification
- else:
- justification = DEFAULT_TEXT_JUSTIFICATION
- justify = tk.LEFT if justification.startswith('l') else tk.CENTER if justification.startswith('c') else tk.RIGHT
- # anchor = tk.NW if justification == 'left' else tk.N if justification == 'center' else tk.NE
- element.TKEntry = element.Widget = tk.Entry(tk_row_frame, width=element_size[0],
- textvariable=element.TKStringVar, bd=bd,
- font=font, show=show, justify=justify)
- if element.ChangeSubmits:
- element.TKEntry.bind('', element._KeyboardHandler)
- element.TKEntry.bind('', element._ReturnKeyHandler)
-
- if element.BackgroundColor is not None and element.BackgroundColor != COLOR_SYSTEM_DEFAULT:
- element.TKEntry.configure(background=element.BackgroundColor, selectforeground=element.BackgroundColor)
- if text_color is not None and text_color != COLOR_SYSTEM_DEFAULT:
- element.TKEntry.configure(fg=text_color, selectbackground=text_color)
-
- if element.disabled_readonly_background_color is not None:
- element.TKEntry.config(readonlybackground=element.disabled_readonly_background_color)
- if element.disabled_readonly_text_color is not None:
- element.TKEntry.config(fg=element.disabled_readonly_text_color)
-
- element.Widget.config(highlightthickness=0)
- # element.pack_keywords = {'side':tk.LEFT, 'padx':elementpad[0], 'pady':elementpad[1], 'expand':False, 'fill':tk.NONE }
- # element.TKEntry.pack(**element.pack_keywords)
- expand, fill, row_should_expand, row_fill_direction = _add_expansion(element, row_should_expand, row_fill_direction)
- element.TKEntry.pack(side=tk.LEFT, padx=elementpad[0], pady=elementpad[1], expand=expand, fill=fill)
- if element.visible is False:
- element._pack_forget_save_settings()
- # element.TKEntry.pack_forget()
- if element.Focus is True or (toplevel_form.UseDefaultFocus and not toplevel_form.FocusSet):
- toplevel_form.FocusSet = True
- element.TKEntry.focus_set()
- if element.Disabled:
- element.TKEntry['state'] = 'readonly' if element.UseReadonlyForDisable else 'disabled'
- if element.ReadOnly:
- element.TKEntry['state'] = 'readonly'
-
- if element.Tooltip is not None:
- element.TooltipObject = ToolTip(element.TKEntry, text=element.Tooltip, timeout=DEFAULT_TOOLTIP_TIME)
- _add_right_click_menu_and_grab(element)
- if theme_input_text_color() not in (COLOR_SYSTEM_DEFAULT, None):
- element.Widget.config(insertbackground=theme_input_text_color())
-
- # row_should_expand = True
-
- # ------------------------- COMBO placement element ------------------------- #
- elif element_type == ELEM_TYPE_INPUT_COMBO:
- element = element # type: Combo
- max_line_len = max([len(str(l)) for l in element.Values]) if len(element.Values) else 0
- if auto_size_text is False:
- width = element_size[0]
- else:
- width = max_line_len + 1
- element.TKStringVar = tk.StringVar()
- style_name = _make_ttk_style_name('.TCombobox', element)
-
- combostyle = ttk.Style()
- element.ttk_style = combostyle
- _change_ttk_theme(combostyle, toplevel_form.TtkTheme)
-
- # Creates a unique name for each field element(Sure there is a better way to do this)
- unique_field = _make_ttk_style_name('.TCombobox.field', element)
-
-
- # Set individual widget options
- try:
- if element.TextColor not in (None, COLOR_SYSTEM_DEFAULT):
- combostyle.configure(style_name, foreground=element.TextColor)
- combostyle.configure(style_name, selectbackground=element.TextColor)
- combostyle.configure(style_name, insertcolor=element.TextColor)
- if element.BackgroundColor not in (None, COLOR_SYSTEM_DEFAULT):
- combostyle.configure(style_name, selectforeground=element.BackgroundColor)
- combostyle.map(style_name, fieldbackground=[('readonly', element.BackgroundColor)])
- combostyle.configure(style_name, fieldbackground=element.BackgroundColor)
-
- if element.button_arrow_color not in (None, COLOR_SYSTEM_DEFAULT):
- combostyle.configure(style_name, arrowcolor=element.button_arrow_color)
- if element.button_background_color not in (None, COLOR_SYSTEM_DEFAULT):
- combostyle.configure(style_name, background=element.button_background_color)
-
- except Exception as e:
- _error_popup_with_traceback('Combo Element error {}'.format(e),
- 'Combo element key: {}'.format(element.Key),
- 'One of your colors is bad. Check the text, background, button background and button arrow colors',
- "Parent Window's Title: {}".format(toplevel_form.Title))
-
- # Strange code that is needed to set the font for the drop-down list
- element._newfont = tkinter.font.Font(font=font)
- tk_row_frame.option_add("*TCombobox*Listbox*Font", element._newfont)
-
- element.TKCombo = element.Widget = ttk.Combobox(tk_row_frame, width=width, textvariable=element.TKStringVar, font=font, style=style_name)
-
- # make tcl call to deal with colors for the drop-down formatting
- try:
- if element.BackgroundColor not in (None, COLOR_SYSTEM_DEFAULT) and \
- element.TextColor not in (None, COLOR_SYSTEM_DEFAULT):
- element.Widget.tk.eval(
- '[ttk::combobox::PopdownWindow {}].f.l configure -foreground {} -background {} -selectforeground {} -selectbackground {}'.format(element.Widget,
- element.TextColor,
- element.BackgroundColor,
- element.BackgroundColor,
- element.TextColor))
- except Exception as e:
- pass # going to let this one slide
-
- # Chr0nic
- element.TKCombo.bind("", lambda event, em=element: testMouseHook2(em))
- element.TKCombo.bind("", lambda event, em=element: testMouseUnhook2(em))
-
- if toplevel_form.UseDefaultFocus and not toplevel_form.FocusSet:
- toplevel_form.FocusSet = True
- element.TKCombo.focus_set()
-
- if element.Size[1] != 1 and element.Size[1] is not None:
- element.TKCombo.configure(height=element.Size[1])
- element.TKCombo['values'] = element.Values
- expand, fill, row_should_expand, row_fill_direction = _add_expansion(element, row_should_expand, row_fill_direction)
- element.TKCombo.pack(side=tk.LEFT, padx=elementpad[0], pady=elementpad[1], expand=expand, fill=fill)
- if element.visible is False:
- element._pack_forget_save_settings()
- # element.TKCombo.pack_forget()
- if element.DefaultValue is not None:
- element.TKCombo.set(element.DefaultValue)
- # for i, v in enumerate(element.Values):
- # if v == element.DefaultValue:
- # element.TKCombo.current(i)
- # break
- # elif element.Values:
- # element.TKCombo.current(0)
- if element.ChangeSubmits:
- element.TKCombo.bind('<>', element._ComboboxSelectHandler)
- if element.BindReturnKey:
- element.TKCombo.bind('', element._ComboboxSelectHandler)
- if element.Readonly:
- element.TKCombo['state'] = 'readonly'
- if element.Disabled is True: # note overrides readonly if disabled
- element.TKCombo['state'] = 'disabled'
- if element.Tooltip is not None:
- element.TooltipObject = ToolTip(element.TKCombo, text=element.Tooltip, timeout=DEFAULT_TOOLTIP_TIME)
- _add_right_click_menu_and_grab(element)
-
- # ------------------------- OPTIONMENU placement Element (Like ComboBox but different) element ------------------------- #
- elif element_type == ELEM_TYPE_INPUT_OPTION_MENU:
- max_line_len = max([len(str(l)) for l in element.Values])
- if auto_size_text is False:
- width = element_size[0]
- else:
- width = max_line_len
- element.TKStringVar = tk.StringVar()
- if element.DefaultValue:
- element.TKStringVar.set(element.DefaultValue)
- element.TKOptionMenu = element.Widget = tk.OptionMenu(tk_row_frame, element.TKStringVar, *element.Values)
- element.TKOptionMenu.config(highlightthickness=0, font=font, width=width)
- element.TKOptionMenu['menu'].config(font=font)
- element.TKOptionMenu.config(borderwidth=border_depth)
- if element.BackgroundColor is not None and element.BackgroundColor != COLOR_SYSTEM_DEFAULT:
- element.TKOptionMenu.configure(background=element.BackgroundColor)
- element.TKOptionMenu['menu'].config(background=element.BackgroundColor)
- if element.TextColor != COLOR_SYSTEM_DEFAULT and element.TextColor is not None:
- element.TKOptionMenu.configure(fg=element.TextColor)
- element.TKOptionMenu['menu'].config(fg=element.TextColor)
- expand, fill, row_should_expand, row_fill_direction = _add_expansion(element, row_should_expand, row_fill_direction)
- element.TKOptionMenu.pack(side=tk.LEFT, padx=elementpad[0], pady=elementpad[1], expand=expand, fill=fill)
- if element.visible is False:
- element._pack_forget_save_settings()
- # element.TKOptionMenu.pack_forget()
- if element.Disabled == True:
- element.TKOptionMenu['state'] = 'disabled'
- if element.Tooltip is not None:
- element.TooltipObject = ToolTip(element.TKOptionMenu, text=element.Tooltip,
- timeout=DEFAULT_TOOLTIP_TIME)
- # ------------------------- LISTBOX placement element ------------------------- #
- elif element_type == ELEM_TYPE_INPUT_LISTBOX:
- element = element # type: Listbox
- max_line_len = max([len(str(l)) for l in element.Values]) if len(element.Values) else 0
- if auto_size_text is False:
- width = element_size[0]
- else:
- width = max_line_len
- element_frame = tk.Frame(tk_row_frame)
- element.element_frame = element_frame
-
- element.TKStringVar = tk.StringVar()
- element.TKListbox = element.Widget = tk.Listbox(element_frame, height=element_size[1], width=width,
- selectmode=element.SelectMode, font=font, exportselection=False)
- element.Widget.config(highlightthickness=0)
- for index, item in enumerate(element.Values):
- element.TKListbox.insert(tk.END, item)
- if element.DefaultValues is not None and item in element.DefaultValues:
- element.TKListbox.selection_set(index)
- if element.BackgroundColor is not None and element.BackgroundColor != COLOR_SYSTEM_DEFAULT:
- element.TKListbox.configure(background=element.BackgroundColor)
- if element.HighlightBackgroundColor is not None and element.HighlightBackgroundColor != COLOR_SYSTEM_DEFAULT:
- element.TKListbox.config(selectbackground=element.HighlightBackgroundColor)
- if text_color is not None and text_color != COLOR_SYSTEM_DEFAULT:
- element.TKListbox.configure(fg=text_color)
- if element.HighlightTextColor is not None and element.HighlightTextColor != COLOR_SYSTEM_DEFAULT:
- element.TKListbox.config(selectforeground=element.HighlightTextColor)
- if element.ChangeSubmits:
- element.TKListbox.bind('<>', element._ListboxSelectHandler)
-
-
-
-
- if not element.NoScrollbar:
- _make_ttk_scrollbar(element, 'v', toplevel_form)
- element.Widget.configure(yscrollcommand=element.vsb.set)
- element.vsb.pack(side=tk.RIGHT, fill='y')
-
- # Horizontal scrollbar
- if element.HorizontalScroll:
- _make_ttk_scrollbar(element, 'h', toplevel_form)
- element.hsb.pack(side=tk.BOTTOM, fill='x')
- element.Widget.configure(xscrollcommand=element.hsb.set)
-
- if not element.NoScrollbar or element.HorizontalScroll:
- # Chr0nic
- element.Widget.bind("", lambda event, em=element: testMouseHook(em))
- element.Widget.bind("", lambda event, em=element: testMouseUnhook(em))
-
- # else:
- # element.TKText.config(wrap='word')
-
- # if not element.NoScrollbar:
- # # Vertical scrollbar
- # element.vsb = tk.Scrollbar(element_frame, orient="vertical", command=element.TKListbox.yview)
- # element.TKListbox.configure(yscrollcommand=element.vsb.set)
- # element.vsb.pack(side=tk.RIGHT, fill='y')
-
- # Horizontal scrollbar
- # if element.HorizontalScroll:
- # hscrollbar = tk.Scrollbar(element_frame, orient=tk.HORIZONTAL)
- # hscrollbar.pack(side=tk.BOTTOM, fill='x')
- # hscrollbar.config(command=element.Widget.xview)
- # element.Widget.configure(xscrollcommand=hscrollbar.set)
- # element.hsb = hscrollbar
- #
- # # Chr0nic
- # element.TKListbox.bind("", lambda event, em=element: testMouseHook(em))
- # element.TKListbox.bind("", lambda event, em=element: testMouseUnhook(em))
- #
- #
-
-
-
- expand, fill, row_should_expand, row_fill_direction = _add_expansion(element, row_should_expand, row_fill_direction)
- element_frame.pack(side=tk.LEFT, padx=elementpad[0], pady=elementpad[1], fill=fill, expand=expand)
- element.TKListbox.pack(side=tk.LEFT, fill=fill, expand=expand)
- if element.visible is False:
- element._pack_forget_save_settings(alternate_widget=element_frame)
- # element_frame.pack_forget()
- if element.BindReturnKey:
- element.TKListbox.bind('', element._ListboxSelectHandler)
- element.TKListbox.bind('', element._ListboxSelectHandler)
- if element.Disabled is True:
- element.TKListbox['state'] = 'disabled'
- if element.Tooltip is not None:
- element.TooltipObject = ToolTip(element.TKListbox, text=element.Tooltip,
- timeout=DEFAULT_TOOLTIP_TIME)
- _add_right_click_menu_and_grab(element)
- # ------------------------- MULTILINE placement element ------------------------- #
- elif element_type == ELEM_TYPE_INPUT_MULTILINE:
- element = element # type: Multiline
- width, height = element_size
- bd = element.BorderWidth
- element.element_frame = element_frame = tk.Frame(tk_row_frame)
-
- # if element.no_scrollbar:
- element.TKText = element.Widget = tk.Text(element_frame, width=width, height=height, bd=bd, font=font, relief=RELIEF_SUNKEN)
- # else:
- # element.TKText = element.Widget = tk.scrolledtext.ScrolledText(element_frame, width=width, height=height, bd=bd, font=font, relief=RELIEF_SUNKEN)
-
- if not element.no_scrollbar:
- _make_ttk_scrollbar(element, 'v', toplevel_form)
-
- element.Widget.configure(yscrollcommand=element.vsb.set)
- element.vsb.pack(side=tk.RIGHT, fill='y')
-
- # Horizontal scrollbar
- if element.HorizontalScroll:
- element.TKText.config(wrap='none')
- _make_ttk_scrollbar(element, 'h', toplevel_form)
- element.hsb.pack(side=tk.BOTTOM, fill='x')
- element.Widget.configure(xscrollcommand=element.hsb.set)
- else:
- element.TKText.config(wrap='word')
-
- if not element.no_scrollbar or element.HorizontalScroll:
- # Chr0nic
- element.TKText.bind("", lambda event, em=element: testMouseHook(em))
- element.TKText.bind("", lambda event, em=element: testMouseUnhook(em))
-
- if element.DefaultText:
- element.TKText.insert(1.0, element.DefaultText) # set the default text
- element.TKText.config(highlightthickness=0)
- if element.BackgroundColor is not None and element.BackgroundColor != COLOR_SYSTEM_DEFAULT:
- element.TKText.configure(background=element.BackgroundColor, selectforeground=element.BackgroundColor)
-
- element.TKText.tag_configure("center", justify='center')
- element.TKText.tag_configure("left", justify='left')
- element.TKText.tag_configure("right", justify='right')
-
- if element.Justification.startswith('l'):
- element.TKText.tag_add("left", 1.0, "end")
- element.justification_tag = 'left'
- elif element.Justification.startswith('r'):
- element.TKText.tag_add("right", 1.0, "end")
- element.justification_tag = 'right'
- elif element.Justification.startswith('c'):
- element.TKText.tag_add("center", 1.0, "end")
- element.justification_tag = 'center'
- # if DEFAULT_SCROLLBAR_COLOR not in (None, COLOR_SYSTEM_DEFAULT): # only works on Linux so not including it
- # element.TKText.vbar.config(troughcolor=DEFAULT_SCROLLBAR_COLOR)
- expand, fill, row_should_expand, row_fill_direction = _add_expansion(element, row_should_expand, row_fill_direction)
-
- element.element_frame.pack(side=tk.LEFT, padx=elementpad[0], pady=elementpad[1], fill=fill, expand=expand)
- element.Widget.pack(side=tk.LEFT, fill=fill, expand=expand)
-
- if element.visible is False:
- element._pack_forget_save_settings(alternate_widget=element_frame)
- # element.element_frame.pack_forget()
- else:
- # Chr0nic
- element.TKText.bind("", lambda event, em=element: testMouseHook(em))
- element.TKText.bind("", lambda event, em=element: testMouseUnhook(em))
- if element.ChangeSubmits:
- element.TKText.bind('', element._KeyboardHandler)
- if element.EnterSubmits:
- element.TKText.bind('', element._ReturnKeyHandler)
- if element.Focus is True or (toplevel_form.UseDefaultFocus and not toplevel_form.FocusSet):
- toplevel_form.FocusSet = True
- element.TKText.focus_set()
- if text_color is not None and text_color != COLOR_SYSTEM_DEFAULT:
- element.TKText.configure(fg=text_color, selectbackground=text_color)
-
-
- if element.Disabled is True:
- element.TKText['state'] = 'disabled'
- if element.Tooltip is not None:
- element.TooltipObject = ToolTip(element.TKText, text=element.Tooltip, timeout=DEFAULT_TOOLTIP_TIME)
-
- if element.reroute_cprint:
- cprint_set_output_destination(toplevel_form, element.Key)
-
- _add_right_click_menu_and_grab(element)
- if theme_input_text_color() not in (COLOR_SYSTEM_DEFAULT, None):
- element.Widget.config(insertbackground=theme_input_text_color())
-
- # row_should_expand = True
- # ------------------------- CHECKBOX pleacement element ------------------------- #
- elif element_type == ELEM_TYPE_INPUT_CHECKBOX:
- element = element # type: Checkbox
- width = 0 if auto_size_text else element_size[0]
- default_value = element.InitialState
- element.TKIntVar = tk.IntVar()
- element.TKIntVar.set(default_value if default_value is not None else 0)
-
- element.TKCheckbutton = element.Widget = tk.Checkbutton(tk_row_frame, anchor=tk.NW,
- text=element.Text, width=width,
- variable=element.TKIntVar, bd=border_depth,
- font=font)
- if element.ChangeSubmits:
- element.TKCheckbutton.configure(command=element._CheckboxHandler)
- if element.Disabled:
- element.TKCheckbutton.configure(state='disable')
- if element.BackgroundColor is not None and element.BackgroundColor != COLOR_SYSTEM_DEFAULT:
- element.TKCheckbutton.configure(background=element.BackgroundColor)
- element.TKCheckbutton.configure(selectcolor=element.CheckboxBackgroundColor) # The background of the checkbox
- element.TKCheckbutton.configure(activebackground=element.BackgroundColor)
- if text_color is not None and text_color != COLOR_SYSTEM_DEFAULT:
- element.TKCheckbutton.configure(fg=text_color)
-
- element.Widget.configure(highlightthickness=1)
- if element.BackgroundColor != COLOR_SYSTEM_DEFAULT:
- element.TKCheckbutton.config(highlightbackground=element.BackgroundColor)
- if element.TextColor != COLOR_SYSTEM_DEFAULT:
- element.TKCheckbutton.config(highlightcolor=element.TextColor)
- expand, fill, row_should_expand, row_fill_direction = _add_expansion(element, row_should_expand, row_fill_direction)
- element.TKCheckbutton.pack(side=tk.LEFT, padx=elementpad[0], pady=elementpad[1], expand=expand, fill=fill)
- if element.visible is False:
- element._pack_forget_save_settings()
- # element.TKCheckbutton.pack_forget()
- if element.Tooltip is not None:
- element.TooltipObject = ToolTip(element.TKCheckbutton, text=element.Tooltip,
- timeout=DEFAULT_TOOLTIP_TIME)
- _add_right_click_menu_and_grab(element)
-
- # ------------------------- PROGRESS placement element ------------------------- #
- elif element_type == ELEM_TYPE_PROGRESS_BAR:
- element = element # type: ProgressBar
- if element.size_px != (None, None):
- progress_length, progress_width = element.size_px
- else:
- width = element_size[0]
- fnt = tkinter.font.Font()
- char_width = fnt.measure('A') # single character width
- progress_length = width * char_width
- progress_width = element_size[1]
- direction = element.Orientation
- if element.BarColor != (None, None): # if element has a bar color, use it
- bar_color = element.BarColor
- else:
- bar_color = DEFAULT_PROGRESS_BAR_COLOR
- if element.Orientation.lower().startswith('h'):
- base_style_name = ".Horizontal.TProgressbar"
- else:
- base_style_name = ".Vertical.TProgressbar"
- style_name = _make_ttk_style_name(base_style_name, element)
- element.TKProgressBar = TKProgressBar(tk_row_frame, element.MaxValue, progress_length, progress_width,
- orientation=direction, BarColor=bar_color,
- border_width=element.BorderWidth, relief=element.Relief,
- ttk_theme=toplevel_form.TtkTheme, key=element.Key, style_name=style_name)
- element.Widget = element.TKProgressBar.TKProgressBarForReal
- expand, fill, row_should_expand, row_fill_direction = _add_expansion(element, row_should_expand, row_fill_direction)
- element.TKProgressBar.TKProgressBarForReal.pack(side=tk.LEFT, padx=elementpad[0], pady=elementpad[1], expand=expand, fill=fill)
- if element.visible is False:
- element._pack_forget_save_settings(alternate_widget=element.TKProgressBar.TKProgressBarForReal)
- # element.TKProgressBar.TKProgressBarForReal.pack_forget()
- _add_right_click_menu_and_grab(element)
-
- # ------------------------- RADIO placement element ------------------------- #
- elif element_type == ELEM_TYPE_INPUT_RADIO:
- element = element # type: Radio
- width = 0 if auto_size_text else element_size[0]
- default_value = element.InitialState
- ID = element.GroupID
- # see if ID has already been placed
- value = EncodeRadioRowCol(form.ContainerElemementNumber, row_num,
- col_num) # value to set intvar to if this radio is selected
- element.EncodedRadioValue = value
- if ID in toplevel_form.RadioDict:
- RadVar = toplevel_form.RadioDict[ID]
- else:
- RadVar = tk.IntVar()
- toplevel_form.RadioDict[ID] = RadVar
- element.TKIntVar = RadVar # store the RadVar in Radio object
- if default_value: # if this radio is the one selected, set RadVar to match
- element.TKIntVar.set(value)
- element.TKRadio = element.Widget = tk.Radiobutton(tk_row_frame, anchor=tk.NW, text=element.Text,
- width=width, variable=element.TKIntVar, value=value,
- bd=border_depth, font=font)
- if element.ChangeSubmits:
- element.TKRadio.configure(command=element._RadioHandler)
- if not element.BackgroundColor in (None, COLOR_SYSTEM_DEFAULT):
- element.TKRadio.configure(background=element.BackgroundColor)
- element.TKRadio.configure(selectcolor=element.CircleBackgroundColor)
- element.TKRadio.configure(activebackground=element.BackgroundColor)
- if text_color is not None and text_color != COLOR_SYSTEM_DEFAULT:
- element.TKRadio.configure(fg=text_color)
-
- element.Widget.configure(highlightthickness=1)
- if element.BackgroundColor != COLOR_SYSTEM_DEFAULT:
- element.TKRadio.config(highlightbackground=element.BackgroundColor)
- if element.TextColor != COLOR_SYSTEM_DEFAULT:
- element.TKRadio.config(highlightcolor=element.TextColor)
-
- if element.Disabled:
- element.TKRadio['state'] = 'disabled'
- expand, fill, row_should_expand, row_fill_direction = _add_expansion(element, row_should_expand, row_fill_direction)
- element.TKRadio.pack(side=tk.LEFT, padx=elementpad[0], pady=elementpad[1], expand=expand, fill=fill)
- if element.visible is False:
- element._pack_forget_save_settings()
- # element.TKRadio.pack_forget()
- if element.Tooltip is not None:
- element.TooltipObject = ToolTip(element.TKRadio, text=element.Tooltip, timeout=DEFAULT_TOOLTIP_TIME)
- _add_right_click_menu_and_grab(element)
-
- # ------------------------- SPIN placement element ------------------------- #
- elif element_type == ELEM_TYPE_INPUT_SPIN:
- element = element # type: Spin
- width, height = element_size
- width = 0 if auto_size_text else element_size[0]
- element.TKStringVar = tk.StringVar()
- element.TKSpinBox = element.Widget = tk.Spinbox(tk_row_frame, values=element.Values, textvariable=element.TKStringVar, width=width, bd=border_depth)
- if element.DefaultValue is not None:
- element.TKStringVar.set(element.DefaultValue)
- element.TKSpinBox.configure(font=font) # set wrap to width of widget
- if element.BackgroundColor is not None and element.BackgroundColor != COLOR_SYSTEM_DEFAULT:
- element.TKSpinBox.configure(background=element.BackgroundColor)
- element.TKSpinBox.configure(buttonbackground=element.BackgroundColor)
- element.Widget.config(highlightthickness=0)
- expand, fill, row_should_expand, row_fill_direction = _add_expansion(element, row_should_expand, row_fill_direction)
- element.TKSpinBox.pack(side=tk.LEFT, padx=elementpad[0], pady=elementpad[1], expand=expand, fill=fill)
- if element.visible is False:
- element._pack_forget_save_settings()
- # element.TKSpinBox.pack_forget()
- if text_color is not None and text_color != COLOR_SYSTEM_DEFAULT:
- element.TKSpinBox.configure(fg=text_color)
- if element.ChangeSubmits:
- element.TKSpinBox.configure(command=element._SpinboxSelectHandler)
- # element.TKSpinBox.bind('', element._SpinChangedHandler)
- # element.TKSpinBox.bind('', element._SpinChangedHandler)
- # element.TKSpinBox.bind('', element._SpinChangedHandler)
- if element.Readonly:
- element.TKSpinBox['state'] = 'readonly'
- if element.Disabled is True: # note overrides readonly if disabled
- element.TKSpinBox['state'] = 'disabled'
- if element.Tooltip is not None:
- element.TooltipObject = ToolTip(element.TKSpinBox, text=element.Tooltip, timeout=DEFAULT_TOOLTIP_TIME)
- if element.BindReturnKey:
- element.TKSpinBox.bind('', element._SpinboxSelectHandler)
- if theme_input_text_color() not in (COLOR_SYSTEM_DEFAULT, None):
- element.Widget.config(insertbackground=theme_input_text_color())
- _add_right_click_menu_and_grab(element)
- # ------------------------- IMAGE placement element ------------------------- #
- elif element_type == ELEM_TYPE_IMAGE:
- element = element # type: Image
- try:
- if element.Filename is not None:
- photo = tk.PhotoImage(file=element.Filename)
- elif element.Data is not None:
- photo = tk.PhotoImage(data=element.Data)
- else:
- photo = None
-
- if element.ImageSubsample and photo is not None:
- photo = photo.subsample(element.ImageSubsample)
- # print('*ERROR laying out form.... Image Element has no image specified*')
- except Exception as e:
- photo = None
- _error_popup_with_traceback('Your Window has an Image Element with a problem',
- 'The traceback will show you the Window with the problem layout',
- 'Look in this Window\'s layout for an Image element that has a key of {}'.format(element.Key),
- 'The error occuring is:', e)
-
- element.tktext_label = element.Widget = tk.Label(tk_row_frame, bd=0)
-
- if photo is not None:
- if element_size == (None, None) or element_size is None or element_size == toplevel_form.DefaultElementSize:
- width, height = photo.width(), photo.height()
- else:
- width, height = element_size
- element.tktext_label.config(image=photo, width=width, height=height)
-
-
- if not element.BackgroundColor in (None, COLOR_SYSTEM_DEFAULT):
- element.tktext_label.config(background=element.BackgroundColor)
-
- element.tktext_label.image = photo
- # tktext_label.configure(anchor=tk.NW, image=photo)
- expand, fill, row_should_expand, row_fill_direction = _add_expansion(element, row_should_expand, row_fill_direction)
- element.tktext_label.pack(side=tk.LEFT, padx=elementpad[0], pady=elementpad[1], expand=expand, fill=fill)
-
- if element.visible is False:
- element._pack_forget_save_settings()
- # element.tktext_label.pack_forget()
- if element.Tooltip is not None:
- element.TooltipObject = ToolTip(element.tktext_label, text=element.Tooltip,
- timeout=DEFAULT_TOOLTIP_TIME)
- if element.EnableEvents and element.tktext_label is not None:
- element.tktext_label.bind('', element._ClickHandler)
-
- _add_right_click_menu_and_grab(element)
-
- # ------------------------- Canvas placement element ------------------------- #
- elif element_type == ELEM_TYPE_CANVAS:
- element = element # type: Canvas
- width, height = element_size
- if element._TKCanvas is None:
- element._TKCanvas = tk.Canvas(tk_row_frame, width=width, height=height, bd=border_depth)
- else:
- element._TKCanvas.master = tk_row_frame
- element.Widget = element._TKCanvas
-
- if element.BackgroundColor is not None and element.BackgroundColor != COLOR_SYSTEM_DEFAULT:
- element._TKCanvas.configure(background=element.BackgroundColor, highlightthickness=0)
- expand, fill, row_should_expand, row_fill_direction = _add_expansion(element, row_should_expand, row_fill_direction)
- element._TKCanvas.pack(side=tk.LEFT, padx=elementpad[0], pady=elementpad[1], expand=expand, fill=fill)
- if element.visible is False:
- element._pack_forget_save_settings()
- # element._TKCanvas.pack_forget()
- if element.Tooltip is not None:
- element.TooltipObject = ToolTip(element._TKCanvas, text=element.Tooltip,
- timeout=DEFAULT_TOOLTIP_TIME)
- _add_right_click_menu_and_grab(element)
-
- # ------------------------- Graph placement element ------------------------- #
- elif element_type == ELEM_TYPE_GRAPH:
- element = element # type: Graph
- width, height = element_size
- # I don't know why TWO canvases were being defined, on inside the other. Was it so entire canvas can move?
- # if element._TKCanvas is None:
- # element._TKCanvas = tk.Canvas(tk_row_frame, width=width, height=height, bd=border_depth)
- # else:
- # element._TKCanvas.master = tk_row_frame
- element._TKCanvas2 = element.Widget = tk.Canvas(tk_row_frame, width=width, height=height,
- bd=border_depth)
- expand, fill, row_should_expand, row_fill_direction = _add_expansion(element, row_should_expand, row_fill_direction)
- element._TKCanvas2.pack(side=tk.LEFT, expand=expand, fill=fill)
- element._TKCanvas2.addtag_all('mytag')
- if element.BackgroundColor is not None and element.BackgroundColor != COLOR_SYSTEM_DEFAULT:
- element._TKCanvas2.configure(background=element.BackgroundColor, highlightthickness=0)
- # element._TKCanvas.configure(background=element.BackgroundColor, highlightthickness=0)
- element._TKCanvas2.pack(side=tk.LEFT, padx=elementpad[0], pady=elementpad[1], expand=expand, fill=fill)
- if element.visible is False:
- element._pack_forget_save_settings()
- # element._TKCanvas2.pack_forget()
- if element.Tooltip is not None:
- element.TooltipObject = ToolTip(element._TKCanvas2, text=element.Tooltip,
- timeout=DEFAULT_TOOLTIP_TIME)
- if element.ChangeSubmits:
- element._TKCanvas2.bind('', element.ButtonReleaseCallBack)
- element._TKCanvas2.bind('', element.ButtonPressCallBack)
- if element.DragSubmits:
- element._TKCanvas2.bind('', element.MotionCallBack)
- _add_right_click_menu_and_grab(element)
- # ------------------------- MENU placement element ------------------------- #
- elif element_type == ELEM_TYPE_MENUBAR:
- element = element # type: MenuBar
- menu_def = element.MenuDefinition
- element.TKMenu = element.Widget = tk.Menu(toplevel_form.TKroot, tearoff=element.Tearoff,
- tearoffcommand=element._tearoff_menu_callback) # create the menubar
- menubar = element.TKMenu
- if font is not None: # if a font is used, make sure it's saved in the element
- element.Font = font
- for menu_entry in menu_def:
- baritem = tk.Menu(menubar, tearoff=element.Tearoff, tearoffcommand=element._tearoff_menu_callback)
- if element.BackgroundColor not in (COLOR_SYSTEM_DEFAULT, None):
- baritem.config(bg=element.BackgroundColor)
- if element.TextColor not in (COLOR_SYSTEM_DEFAULT, None):
- baritem.config(fg=element.TextColor)
- if element.DisabledTextColor not in (COLOR_SYSTEM_DEFAULT, None):
- baritem.config(disabledforeground=element.DisabledTextColor)
- if font is not None:
- baritem.config(font=font)
- pos = menu_entry[0].find(MENU_SHORTCUT_CHARACTER)
- # print(pos)
- if pos != -1:
- if pos == 0 or menu_entry[0][pos - len(MENU_SHORTCUT_CHARACTER)] != "\\":
- menu_entry[0] = menu_entry[0][:pos] + menu_entry[0][pos + 1:]
- if menu_entry[0][0] == MENU_DISABLED_CHARACTER:
- menubar.add_cascade(label=menu_entry[0][len(MENU_DISABLED_CHARACTER):], menu=baritem,
- underline=pos - 1)
- menubar.entryconfig(menu_entry[0][len(MENU_DISABLED_CHARACTER):], state='disabled')
- else:
- menubar.add_cascade(label=menu_entry[0], menu=baritem, underline=pos)
-
- if len(menu_entry) > 1:
- AddMenuItem(baritem, menu_entry[1], element)
- toplevel_form.TKroot.configure(menu=element.TKMenu)
- # ------------------------- Frame placement element ------------------------- #
- elif element_type == ELEM_TYPE_FRAME:
- element = element # type: Frame
- labeled_frame = element.Widget = tk.LabelFrame(tk_row_frame, text=element.Title, relief=element.Relief)
- element.TKFrame = labeled_frame
- PackFormIntoFrame(element, labeled_frame, toplevel_form)
- expand, fill, row_should_expand, row_fill_direction = _add_expansion(element, row_should_expand, row_fill_direction)
- if element.VerticalAlignment is not None:
- anchor = tk.CENTER # Default to center if a bad choice is made
- if element.VerticalAlignment.lower().startswith('t'):
- anchor = tk.N
- if element.VerticalAlignment.lower().startswith('c'):
- anchor = tk.CENTER
- if element.VerticalAlignment.lower().startswith('b'):
- anchor = tk.S
- labeled_frame.pack(side=tk.LEFT, anchor=anchor, padx=elementpad[0], pady=elementpad[1], expand=expand, fill=fill)
- else:
- labeled_frame.pack(side=tk.LEFT, padx=elementpad[0], pady=elementpad[1], expand=expand, fill=fill)
-
- if element.Size != (None, None):
- labeled_frame.config(width=element.Size[0], height=element.Size[1])
- labeled_frame.pack_propagate(0)
- if not element.visible:
- element._pack_forget_save_settings()
- # labeled_frame.pack_forget()
- if element.BackgroundColor != COLOR_SYSTEM_DEFAULT and element.BackgroundColor is not None:
- labeled_frame.configure(background=element.BackgroundColor,
- highlightbackground=element.BackgroundColor,
- highlightcolor=element.BackgroundColor)
- if element.TextColor != COLOR_SYSTEM_DEFAULT and element.TextColor is not None:
- labeled_frame.configure(foreground=element.TextColor)
- if font is not None:
- labeled_frame.configure(font=font)
- if element.TitleLocation is not None:
- labeled_frame.configure(labelanchor=element.TitleLocation)
- if element.BorderWidth is not None:
- labeled_frame.configure(borderwidth=element.BorderWidth)
- if element.Tooltip is not None:
- element.TooltipObject = ToolTip(labeled_frame, text=element.Tooltip, timeout=DEFAULT_TOOLTIP_TIME)
- _add_right_click_menu_and_grab(element)
- # row_should_expand=True
- # ------------------------- Tab placement element ------------------------- #
- elif element_type == ELEM_TYPE_TAB:
- element = element # type: Tab
- element.TKFrame = element.Widget = tk.Frame(form.TKNotebook)
- PackFormIntoFrame(element, element.TKFrame, toplevel_form)
- state = 'normal'
- if element.Disabled:
- state = 'disabled'
- if element.visible is False:
- state = 'hidden'
- # this code will add an image to the tab. Use it when adding the image on a tab enhancement
- try:
- if element.Filename is not None:
- photo = tk.PhotoImage(file=element.Filename)
- elif element.Data is not None:
- photo = tk.PhotoImage(data=element.Data)
- else:
- photo = None
-
- if element.ImageSubsample and photo is not None:
- photo = photo.subsample(element.ImageSubsample)
- # print('*ERROR laying out form.... Image Element has no image specified*')
- except Exception as e:
- photo = None
- _error_popup_with_traceback('Your Window has an Tab Element with an IMAGE problem',
- 'The traceback will show you the Window with the problem layout',
- 'Look in this Window\'s layout for an Image element that has a key of {}'.format(element.Key),
- 'The error occuring is:', e)
-
- element.photo = photo
- if photo is not None:
- if element_size == (None, None) or element_size is None or element_size == toplevel_form.DefaultElementSize:
- width, height = photo.width(), photo.height()
- else:
- width, height = element_size
- element.tktext_label = tk.Label(tk_row_frame, image=photo, width=width, height=height, bd=0)
- else:
- element.tktext_label = tk.Label(tk_row_frame, bd=0)
- if photo is not None:
- form.TKNotebook.add(element.TKFrame, text=element.Title, compound=tk.LEFT, state=state,image=photo)
-
- # element.photo_image = tk.PhotoImage(data=DEFAULT_BASE64_ICON)
- # form.TKNotebook.add(element.TKFrame, text=element.Title, compound=tk.LEFT, state=state,image = element.photo_image)
- form.TKNotebook.add(element.TKFrame, text=element.Title, state=state)
- expand, fill, row_should_expand, row_fill_direction = _add_expansion(element, row_should_expand, row_fill_direction)
- form.TKNotebook.pack(side=tk.LEFT, padx=elementpad[0], pady=elementpad[1], fill=fill, expand=expand)
-
- element.ParentNotebook = form.TKNotebook
- element.TabID = form.TabCount
- form.TabCount += 1
- if element.BackgroundColor != COLOR_SYSTEM_DEFAULT and element.BackgroundColor is not None:
- element.TKFrame.configure(background=element.BackgroundColor,
- highlightbackground=element.BackgroundColor,
- highlightcolor=element.BackgroundColor)
-
- # if element.BorderWidth is not None:
- # element.TKFrame.configure(borderwidth=element.BorderWidth)
- if element.Tooltip is not None:
- element.TooltipObject = ToolTip(element.TKFrame, text=element.Tooltip, timeout=DEFAULT_TOOLTIP_TIME)
- _add_right_click_menu_and_grab(element)
- # row_should_expand = True
- # ------------------------- TabGroup placement element ------------------------- #
- elif element_type == ELEM_TYPE_TAB_GROUP:
- element = element # type: TabGroup
- # custom_style = str(element.Key) + 'customtab.TNotebook'
- custom_style = _make_ttk_style_name('.customtab.TNotebook', element)
- style = ttk.Style()
- _change_ttk_theme(style, toplevel_form.TtkTheme)
-
- if element.TabLocation is not None:
- position_dict = {'left': 'w', 'right': 'e', 'top': 'n', 'bottom': 's', 'lefttop': 'wn',
- 'leftbottom': 'ws', 'righttop': 'en', 'rightbottom': 'es', 'bottomleft': 'sw',
- 'bottomright': 'se', 'topleft': 'nw', 'topright': 'ne'}
- try:
- tab_position = position_dict[element.TabLocation]
- except:
- tab_position = position_dict['top']
- style.configure(custom_style, tabposition=tab_position)
-
- if element.BackgroundColor is not None and element.BackgroundColor != COLOR_SYSTEM_DEFAULT:
- style.configure(custom_style, background=element.BackgroundColor)
-
- # FINALLY the proper styling to get tab colors!
- if element.SelectedTitleColor is not None and element.SelectedTitleColor != COLOR_SYSTEM_DEFAULT:
- style.map(custom_style + '.Tab', foreground=[("selected", element.SelectedTitleColor)])
- if element.SelectedBackgroundColor is not None and element.SelectedBackgroundColor != COLOR_SYSTEM_DEFAULT:
- style.map(custom_style + '.Tab', background=[("selected", element.SelectedBackgroundColor)])
- if element.TabBackgroundColor is not None and element.TabBackgroundColor != COLOR_SYSTEM_DEFAULT:
- style.configure(custom_style + '.Tab', background=element.TabBackgroundColor)
- if element.TextColor is not None and element.TextColor != COLOR_SYSTEM_DEFAULT:
- style.configure(custom_style + '.Tab', foreground=element.TextColor)
- if element.BorderWidth is not None:
- style.configure(custom_style, borderwidth=element.BorderWidth)
- if element.TabBorderWidth is not None:
- style.configure(custom_style + '.Tab', borderwidth=element.TabBorderWidth) # if ever want to get rid of border around the TABS themselves
- if element.FocusColor is not None:
- style.configure(custom_style + '.Tab', focuscolor=element.FocusColor)
-
- style.configure(custom_style + '.Tab', font=font)
- element.Style = style
- element.StyleName = custom_style
- element.TKNotebook = element.Widget = ttk.Notebook(tk_row_frame, style=custom_style)
-
- PackFormIntoFrame(element, toplevel_form.TKroot, toplevel_form)
-
- expand, fill, row_should_expand, row_fill_direction = _add_expansion(element, row_should_expand, row_fill_direction)
- element.TKNotebook.pack(anchor=tk.SW, side=tk.LEFT, padx=elementpad[0], pady=elementpad[1], fill=fill, expand=expand)
-
- if element.ChangeSubmits:
- element.TKNotebook.bind('<>', element._TabGroupSelectHandler)
- if element.Tooltip is not None:
- element.TooltipObject = ToolTip(element.TKNotebook, text=element.Tooltip, timeout=DEFAULT_TOOLTIP_TIME)
- if element.Size != (None, None):
- element.TKNotebook.configure(width=element.Size[0], height=element.Size[1])
- _add_right_click_menu_and_grab(element)
-
- # row_should_expand = True
- # ------------------- SLIDER placement element ------------------------- #
- elif element_type == ELEM_TYPE_INPUT_SLIDER:
- element = element # type: Slider
- slider_length = element_size[0] * _char_width_in_pixels(font)
- slider_width = element_size[1]
- element.TKIntVar = tk.IntVar()
- element.TKIntVar.set(element.DefaultValue)
- if element.Orientation.startswith('v'):
- range_from = element.Range[1]
- range_to = element.Range[0]
- slider_length += DEFAULT_MARGINS[1] * (element_size[0] * 2) # add in the padding
- else:
- range_from = element.Range[0]
- range_to = element.Range[1]
- tkscale = element.Widget = tk.Scale(tk_row_frame, orient=element.Orientation,
- variable=element.TKIntVar,
- from_=range_from, to_=range_to, resolution=element.Resolution,
- length=slider_length, width=slider_width,
- bd=element.BorderWidth,
- relief=element.Relief, font=font,
- tickinterval=element.TickInterval)
- tkscale.config(highlightthickness=0)
- if element.ChangeSubmits:
- tkscale.config(command=element._SliderChangedHandler)
- if element.BackgroundColor is not None and element.BackgroundColor != COLOR_SYSTEM_DEFAULT:
- tkscale.configure(background=element.BackgroundColor)
- if element.TroughColor != COLOR_SYSTEM_DEFAULT:
- tkscale.config(troughcolor=element.TroughColor)
- if element.DisableNumericDisplay:
- tkscale.config(showvalue=0)
- if text_color is not None and text_color != COLOR_SYSTEM_DEFAULT:
- tkscale.configure(fg=text_color)
- expand, fill, row_should_expand, row_fill_direction = _add_expansion(element, row_should_expand, row_fill_direction)
- tkscale.pack(side=tk.LEFT, padx=elementpad[0], pady=elementpad[1], expand=expand, fill=fill)
- if element.visible is False:
- element._pack_forget_save_settings()
- # tkscale.pack_forget()
- element.TKScale = tkscale
- if element.Disabled == True:
- element.TKScale['state'] = 'disabled'
- if element.Tooltip is not None:
- element.TooltipObject = ToolTip(element.TKScale, text=element.Tooltip, timeout=DEFAULT_TOOLTIP_TIME)
- _add_right_click_menu_and_grab(element)
-
- # ------------------------- TABLE placement element ------------------------- #
- elif element_type == ELEM_TYPE_TABLE:
- element = element # type: Table
- element.element_frame = frame = tk.Frame(tk_row_frame)
- element.table_frame = frame
- height = element.NumRows
- if element.Justification.startswith('l'):
- anchor = tk.W
- elif element.Justification.startswith('r'):
- anchor = tk.E
- else:
- anchor = tk.CENTER
- column_widths = {}
- # create column width list
- for row in element.Values:
- for i, col in enumerate(row):
- col_width = min(len(str(col)), element.MaxColumnWidth)
- try:
- if col_width > column_widths[i]:
- column_widths[i] = col_width
- except:
- column_widths[i] = col_width
-
- if element.ColumnsToDisplay is None:
- displaycolumns = element.ColumnHeadings if element.ColumnHeadings is not None else element.Values[0]
- else:
- displaycolumns = []
- for i, should_display in enumerate(element.ColumnsToDisplay):
- if should_display:
- if element.ColumnHeadings is not None:
- displaycolumns.append(element.ColumnHeadings[i])
- else:
- displaycolumns.append(str(i))
-
- column_headings = element.ColumnHeadings if element.ColumnHeadings is not None else displaycolumns
- if element.DisplayRowNumbers: # if display row number, tack on the numbers to front of columns
- displaycolumns = [element.RowHeaderText, ] + displaycolumns
- if column_headings is not None:
- column_headings = [element.RowHeaderText, ] + element.ColumnHeadings
- else:
- column_headings = [element.RowHeaderText, ] + displaycolumns
- element.TKTreeview = element.Widget = ttk.Treeview(frame, columns=column_headings,
- displaycolumns=displaycolumns, show='headings',
- height=height,
- selectmode=element.SelectMode, )
- treeview = element.TKTreeview
- if element.DisplayRowNumbers:
- treeview.heading(element.RowHeaderText, text=element.RowHeaderText) # make a dummy heading
- row_number_header_width =_string_width_in_pixels(element.HeaderFont, element.RowHeaderText) + 10
- row_number_width = _string_width_in_pixels(font, str(len(element.Values))) + 10
- row_number_width = max(row_number_header_width, row_number_width)
- treeview.column(element.RowHeaderText, width=row_number_width, minwidth=10, anchor=anchor, stretch=0)
-
- headings = element.ColumnHeadings if element.ColumnHeadings is not None else element.Values[0]
- for i, heading in enumerate(headings):
- treeview.heading(heading, text=heading)
- if element.AutoSizeColumns:
- col_width = column_widths.get(i, len(heading)) # in case more headings than there are columns of data
- width = max(col_width * _char_width_in_pixels(font), len(heading)*_char_width_in_pixels(element.HeaderFont))
- else:
- try:
- width = element.ColumnWidths[i] * _char_width_in_pixels(font)
- except:
- width = element.DefaultColumnWidth * _char_width_in_pixels(font)
- treeview.column(heading, width=width, minwidth=10, anchor=anchor, stretch=element.expand_x)
- # Insert values into the tree
- for i, value in enumerate(element.Values):
- if element.DisplayRowNumbers:
- value = [i + element.StartingRowNumber] + value
- id = treeview.insert('', 'end', text=value, iid=i + 1, values=value, tag=i)
- element.tree_ids.append(id)
- if element.AlternatingRowColor is not None: # alternating colors
- for row in range(0, len(element.Values), 2):
- treeview.tag_configure(row, background=element.AlternatingRowColor)
- if element.RowColors is not None: # individual row colors
- for row_def in element.RowColors:
- if len(row_def) == 2: # only background is specified
- treeview.tag_configure(row_def[0], background=row_def[1])
- else:
- treeview.tag_configure(row_def[0], background=row_def[2], foreground=row_def[1])
- # ------ Do Styling of Colors -----
- # style_name = str(element.Key) + 'customtable.Treeview'
- style_name = _make_ttk_style_name( '.customtable.Treeview', element)
-
- table_style = ttk.Style()
- element.ttk_style = table_style
-
- _change_ttk_theme(table_style, toplevel_form.TtkTheme)
-
- if element.BackgroundColor is not None and element.BackgroundColor != COLOR_SYSTEM_DEFAULT:
- table_style.configure(style_name, background=element.BackgroundColor, fieldbackground=element.BackgroundColor, )
- if element.SelectedRowColors[1] is not None:
- table_style.map(style_name, background=_fixed_map(table_style, style_name, 'background', element.SelectedRowColors))
- if element.TextColor is not None and element.TextColor != COLOR_SYSTEM_DEFAULT:
- table_style.configure(style_name, foreground=element.TextColor)
- if element.SelectedRowColors[0] is not None:
- table_style.map(style_name, foreground=_fixed_map(table_style, style_name, 'foreground', element.SelectedRowColors))
- if element.RowHeight is not None:
- table_style.configure(style_name, rowheight=element.RowHeight)
- else:
- table_style.configure(style_name, rowheight=_char_height_in_pixels(font))
- if element.HeaderTextColor is not None and element.HeaderTextColor != COLOR_SYSTEM_DEFAULT:
- table_style.configure(style_name + '.Heading', foreground=element.HeaderTextColor)
- if element.HeaderBackgroundColor is not None and element.HeaderBackgroundColor != COLOR_SYSTEM_DEFAULT:
- table_style.configure(style_name + '.Heading', background=element.HeaderBackgroundColor)
- if element.HeaderFont is not None:
- table_style.configure(style_name + '.Heading', font=element.HeaderFont)
- else:
- table_style.configure(style_name + '.Heading', font=font)
- if element.HeaderBorderWidth is not None:
- table_style.configure(style_name + '.Heading', borderwidth=element.HeaderBorderWidth)
- if element.HeaderRelief is not None:
- table_style.configure(style_name + '.Heading', relief=element.HeaderRelief)
- table_style.configure(style_name, font=font)
- if element.BorderWidth is not None:
- table_style.configure(style_name, borderwidth=element.BorderWidth)
- treeview.configure(style=style_name)
- # scrollable_frame.pack(side=tk.LEFT, padx=elementpad[0], pady=elementpad[1], expand=True, fill='both')
- if element.enable_click_events is True:
- treeview.bind('', element._table_clicked)
- if element.right_click_selects:
- if running_mac():
- treeview.bind('', element._table_clicked)
- else:
- treeview.bind('', element._table_clicked)
- treeview.bind("<>", element._treeview_selected)
- if element.BindReturnKey:
- treeview.bind('', element._treeview_double_click)
- treeview.bind('', element._treeview_double_click)
-
-
-
-
- if not element.HideVerticalScroll:
- _make_ttk_scrollbar(element, 'v', toplevel_form)
-
- element.Widget.configure(yscrollcommand=element.vsb.set)
- element.vsb.pack(side=tk.RIGHT, fill='y')
-
- # Horizontal scrollbar
- if not element.VerticalScrollOnly:
- # element.Widget.config(wrap='none')
- _make_ttk_scrollbar(element, 'h', toplevel_form)
- element.hsb.pack(side=tk.BOTTOM, fill='x')
- element.Widget.configure(xscrollcommand=element.hsb.set)
-
- if not element.HideVerticalScroll or not element.VerticalScrollOnly:
- # Chr0nic
- element.Widget.bind("", lambda event, em=element: testMouseHook(em))
- element.Widget.bind("", lambda event, em=element: testMouseUnhook(em))
-
-
-
- # if not element.HideVerticalScroll:
- # scrollbar = tk.Scrollbar(frame)
- # scrollbar.pack(side=tk.RIGHT, fill='y')
- # scrollbar.config(command=treeview.yview)
- # treeview.configure(yscrollcommand=scrollbar.set)
-
- # if not element.VerticalScrollOnly:
- # hscrollbar = tk.Scrollbar(frame, orient=tk.HORIZONTAL)
- # hscrollbar.pack(side=tk.BOTTOM, fill='x')
- # hscrollbar.config(command=treeview.xview)
- # treeview.configure(xscrollcommand=hscrollbar.set)
-
-
-
-
-
-
- expand, fill, row_should_expand, row_fill_direction = _add_expansion(element, row_should_expand, row_fill_direction)
- element.TKTreeview.pack(side=tk.LEFT, padx=0, pady=0, expand=expand, fill=fill)
- frame.pack(side=tk.LEFT, padx=elementpad[0], pady=elementpad[1], expand=expand, fill=fill)
- if element.visible is False:
- element._pack_forget_save_settings(alternate_widget=element.element_frame) # seems like it should be the frame if following other elements conventions
- # element.TKTreeview.pack_forget()
- if element.Tooltip is not None:
- element.TooltipObject = ToolTip(element.TKTreeview, text=element.Tooltip,
- timeout=DEFAULT_TOOLTIP_TIME)
- _add_right_click_menu_and_grab(element)
-
- if tclversion_detailed == '8.6.9' and ENABLE_TREEVIEW_869_PATCH:
- # print('*** tk version 8.6.9 detected.... patching ttk treeview code ***')
- table_style.map(style_name,
- foreground=_fixed_map(table_style, style_name, 'foreground', element.SelectedRowColors),
- background=_fixed_map(table_style, style_name, 'background', element.SelectedRowColors))
- # ------------------------- Tree placement element ------------------------- #
- elif element_type == ELEM_TYPE_TREE:
- element = element # type: Tree
- element.element_frame = element_frame = tk.Frame(tk_row_frame)
-
- height = element.NumRows
- if element.Justification.startswith('l'): # justification
- anchor = tk.W
- elif element.Justification.startswith('r'):
- anchor = tk.E
- else:
- anchor = tk.CENTER
-
- if element.ColumnsToDisplay is None: # Which cols to display
- displaycolumns = element.ColumnHeadings
- else:
- displaycolumns = []
- for i, should_display in enumerate(element.ColumnsToDisplay):
- if should_display:
- displaycolumns.append(element.ColumnHeadings[i])
- column_headings = element.ColumnHeadings
- # ------------- GET THE TREEVIEW WIDGET -------------
- element.TKTreeview = element.Widget = ttk.Treeview(element_frame, columns=column_headings,
- displaycolumns=displaycolumns, show='tree headings',
- height=height,
- selectmode=element.SelectMode)
- treeview = element.TKTreeview
- max_widths = {}
- for key, node in element.TreeData.tree_dict.items():
- for i, value in enumerate(node.values):
- max_width = max_widths.get(i, 0)
- if len(str(value)) > max_width:
- max_widths[i] = len(str(value))
-
-
- for i, heading in enumerate(element.ColumnHeadings): # Configure cols + headings
- treeview.heading(heading, text=heading)
- if element.AutoSizeColumns:
- max_width = max_widths.get(i, 0)
- max_width = max(max_width, len(heading))
- width = min(element.MaxColumnWidth, max_width+1)
- else:
- try:
- width = element.ColumnWidths[i]
- except:
- width = element.DefaultColumnWidth
- treeview.column(heading, width=width * _char_width_in_pixels(font) + 10, anchor=anchor)
-
- def add_treeview_data(node):
- """
-
- :param node:
- :type node:
-
- """
- if node.key != '':
- if node.icon:
- if node.icon not in element.image_dict:
- if type(node.icon) is bytes:
- photo = tk.PhotoImage(data=node.icon)
- else:
- photo = tk.PhotoImage(file=node.icon)
- element.image_dict[node.icon] = photo
- else:
- photo = element.image_dict.get(node.icon)
-
- node.photo = photo
- try:
- id = treeview.insert(element.KeyToID[node.parent], 'end', iid=None, text=node.text, values=node.values, open=element.ShowExpanded, image=node.photo)
- element.IdToKey[id] = node.key
- element.KeyToID[node.key] = id
- except Exception as e:
- print('Error inserting image into tree', e)
- else:
- id = treeview.insert(element.KeyToID[node.parent], 'end', iid=None, text=node.text, values=node.values, open=element.ShowExpanded)
- element.IdToKey[id] = node.key
- element.KeyToID[node.key] = id
-
- for node in node.children:
- add_treeview_data(node)
-
- add_treeview_data(element.TreeData.root_node)
- treeview.column('#0', width=element.Col0Width * _char_width_in_pixels(font), anchor=tk.W)
- treeview.heading('#0', text=element.col0_heading)
-
- # ----- configure colors -----
- # style_name = str(element.Key) + '.Treeview'
- style_name = _make_ttk_style_name('.Treeview', element)
- tree_style = ttk.Style()
- _change_ttk_theme(tree_style, toplevel_form.TtkTheme)
-
- if element.BackgroundColor is not None and element.BackgroundColor != COLOR_SYSTEM_DEFAULT:
- tree_style.configure(style_name, background=element.BackgroundColor, fieldbackground=element.BackgroundColor)
- if element.SelectedRowColors[1] is not None:
- tree_style.map(style_name, background=_fixed_map(tree_style, style_name, 'background', element.SelectedRowColors))
- if element.TextColor is not None and element.TextColor != COLOR_SYSTEM_DEFAULT:
- tree_style.configure(style_name, foreground=element.TextColor)
- if element.SelectedRowColors[0] is not None:
- tree_style.map(style_name, foreground=_fixed_map(tree_style, style_name, 'foreground', element.SelectedRowColors))
- if element.HeaderTextColor is not None and element.HeaderTextColor != COLOR_SYSTEM_DEFAULT:
- tree_style.configure(style_name + '.Heading', foreground=element.HeaderTextColor)
- if element.HeaderBackgroundColor is not None and element.HeaderBackgroundColor != COLOR_SYSTEM_DEFAULT:
- tree_style.configure(style_name + '.Heading', background=element.HeaderBackgroundColor)
- if element.HeaderFont is not None:
- tree_style.configure(style_name + '.Heading', font=element.HeaderFont)
- else:
- tree_style.configure(style_name + '.Heading', font=font)
- if element.HeaderBorderWidth is not None:
- tree_style.configure(style_name + '.Heading', borderwidth=element.HeaderBorderWidth)
- if element.HeaderRelief is not None:
- tree_style.configure(style_name + '.Heading', relief=element.HeaderRelief)
- tree_style.configure(style_name, font=font)
- if element.RowHeight:
- tree_style.configure(style_name, rowheight=element.RowHeight)
- else:
- tree_style.configure(style_name, rowheight=_char_height_in_pixels(font))
- if element.BorderWidth is not None:
- tree_style.configure(style_name, borderwidth=element.BorderWidth)
-
- treeview.configure(style=style_name) # IMPORTANT! Be sure and set the style name for this widget
-
-
-
- if not element.HideVerticalScroll:
- _make_ttk_scrollbar(element, 'v', toplevel_form)
-
- element.Widget.configure(yscrollcommand=element.vsb.set)
- element.vsb.pack(side=tk.RIGHT, fill='y')
-
- # Horizontal scrollbar
- if not element.VerticalScrollOnly:
- # element.Widget.config(wrap='none')
- _make_ttk_scrollbar(element, 'h', toplevel_form)
- element.hsb.pack(side=tk.BOTTOM, fill='x')
- element.Widget.configure(xscrollcommand=element.hsb.set)
-
- if not element.HideVerticalScroll or not element.VerticalScrollOnly:
- # Chr0nic
- element.Widget.bind("", lambda event, em=element: testMouseHook(em))
- element.Widget.bind("", lambda event, em=element: testMouseUnhook(em))
-
-
- # Horizontal scrollbar
- # if not element.VerticalScrollOnly:
- # element.TKText.config(wrap='none')
- # _make_ttk_scrollbar(element, 'h')
- # element.hsb.pack(side=tk.BOTTOM, fill='x')
- # element.Widget.configure(xscrollcommand=element.hsb.set)
-
- # if not element.HideVerticalScroll or not element.VerticalScrollOnly:
- # Chr0nic
- # element.Widget.bind("", lambda event, em=element: testMouseHook(em))
- # element.Widget.bind("", lambda event, em=element: testMouseUnhook(em))
-
-
-
-
-
- # element.scrollbar = scrollbar = tk.Scrollbar(element_frame)
- # scrollbar.pack(side=tk.RIGHT, fill='y')
- # scrollbar.config(command=treeview.yview)
- # treeview.configure(yscrollcommand=scrollbar.set)
-
-
- expand, fill, row_should_expand, row_fill_direction = _add_expansion(element, row_should_expand, row_fill_direction)
- element.TKTreeview.pack(side=tk.LEFT, padx=0, pady=0, expand=expand, fill=fill)
- element_frame.pack(side=tk.LEFT, padx=elementpad[0], pady=elementpad[1], expand=expand, fill=fill)
- if element.visible is False:
- element._pack_forget_save_settings(alternate_widget=element.element_frame) # seems like it should be the frame if following other elements conventions
- # element.TKTreeview.pack_forget()
- treeview.bind("<>", element._treeview_selected)
- if element.Tooltip is not None: # tooltip
- element.TooltipObject = ToolTip(element.TKTreeview, text=element.Tooltip,
- timeout=DEFAULT_TOOLTIP_TIME)
- _add_right_click_menu_and_grab(element)
-
- if tclversion_detailed == '8.6.9' and ENABLE_TREEVIEW_869_PATCH:
- # print('*** tk version 8.6.9 detected.... patching ttk treeview code ***')
- tree_style.map(style_name,
- foreground=_fixed_map(tree_style, style_name, 'foreground', element.SelectedRowColors),
- background=_fixed_map(tree_style, style_name, 'background', element.SelectedRowColors))
-
- # ------------------------- Separator placement element ------------------------- #
- elif element_type == ELEM_TYPE_SEPARATOR:
- element = element # type: VerticalSeparator
- # style_name = str(element.Key) + "Line.TSeparator"
- style_name = _make_ttk_style_name(".Line.TSeparator", element)
- style = ttk.Style()
-
- _change_ttk_theme(style, toplevel_form.TtkTheme)
-
- if element.color is not None:
- style.configure(style_name, background=element.color)
- separator = element.Widget = ttk.Separator(tk_row_frame, orient=element.Orientation, )
-
- expand, fill, row_should_expand, row_fill_direction = _add_expansion(element, row_should_expand, row_fill_direction)
-
- if element.Orientation.startswith('h'):
- separator.pack(side=tk.LEFT, padx=elementpad[0], pady=elementpad[1], fill=tk.X, expand=True)
- else:
- separator.pack(side=tk.LEFT, padx=elementpad[0], pady=elementpad[1], fill=tk.Y, expand=False)
- element.Widget.configure(style=style_name) # IMPORTANT! Apply the style
- # ------------------------- SizeGrip placement element ------------------------- #
- elif element_type == ELEM_TYPE_SIZEGRIP:
- element = element # type: Sizegrip
- style_name = "Sizegrip.TSizegrip"
- style = ttk.Style()
-
- _change_ttk_theme(style, toplevel_form.TtkTheme)
-
- size_grip = element.Widget = ttk.Sizegrip(tk_row_frame)
- toplevel_form.sizegrip_widget = size_grip
- # if no size is specified, then use the background color for the window
- if element.BackgroundColor != COLOR_SYSTEM_DEFAULT:
- style.configure(style_name, background=element.BackgroundColor)
- else:
- style.configure(style_name, background=toplevel_form.TKroot['bg'])
- size_grip.configure(style=style_name)
-
- size_grip.pack(side=tk.BOTTOM, anchor='se', padx=elementpad[0], pady=elementpad[1], fill=tk.X, expand=True)
- # tricky part of sizegrip... it shouldn't cause the row to expand, but should expand and should add X axis if
- # not already filling in that direction. Otherwise, leaves things alone!
- # row_should_expand = True
- row_fill_direction = tk.BOTH if row_fill_direction in (tk.Y, tk.BOTH) else tk.X
- # ------------------------- StatusBar placement element ------------------------- #
- elif element_type == ELEM_TYPE_STATUSBAR:
- # auto_size_text = element.AutoSizeText
- display_text = element.DisplayText # text to display
- if auto_size_text is False:
- width, height = element_size
- else:
- lines = display_text.split('\n')
- max_line_len = max([len(l) for l in lines])
- num_lines = len(lines)
- if max_line_len > element_size[0]: # if text exceeds element size, the will have to wrap
- width = element_size[0]
- else:
- width = max_line_len
- height = num_lines
- # ---===--- LABEL widget create and place --- #
- stringvar = tk.StringVar()
- element.TKStringVar = stringvar
- stringvar.set(display_text)
- if auto_size_text:
- width = 0
- if element.Justification is not None:
- justification = element.Justification
- elif toplevel_form.TextJustification is not None:
- justification = toplevel_form.TextJustification
- else:
- justification = DEFAULT_TEXT_JUSTIFICATION
- justify = tk.LEFT if justification.startswith('l') else tk.CENTER if justification.startswith('c') else tk.RIGHT
- anchor = tk.NW if justification.startswith('l') else tk.N if justification.startswith('c') else tk.NE
- # tktext_label = tk.Label(tk_row_frame, textvariable=stringvar, width=width, height=height,
- # justify=justify, bd=border_depth, font=font)
- tktext_label = element.Widget = tk.Label(tk_row_frame, textvariable=stringvar, width=width,
- height=height,
- justify=justify, bd=border_depth, font=font)
- # Set wrap-length for text (in PIXELS) == PAIN IN THE ASS
- wraplen = tktext_label.winfo_reqwidth() + 40 # width of widget in Pixels
- if not auto_size_text and height == 1:
- wraplen = 0
- # print("wraplen, width, height", wraplen, width, height)
- tktext_label.configure(anchor=anchor, wraplen=wraplen) # set wrap to width of widget
- if element.Relief is not None:
- tktext_label.configure(relief=element.Relief)
- if element.BackgroundColor is not None and element.BackgroundColor != COLOR_SYSTEM_DEFAULT:
- tktext_label.configure(background=element.BackgroundColor)
- if element.TextColor != COLOR_SYSTEM_DEFAULT and element.TextColor is not None:
- tktext_label.configure(fg=element.TextColor)
- tktext_label.pack(side=tk.LEFT, padx=elementpad[0], pady=elementpad[1], fill=tk.X, expand=True)
- row_fill_direction = tk.X
- if element.visible is False:
- element._pack_forget_save_settings()
- # tktext_label.pack_forget()
- element.TKText = tktext_label
- if element.ClickSubmits:
- tktext_label.bind('', element._TextClickedHandler)
- if element.Tooltip is not None:
- element.TooltipObject = ToolTip(element.TKText, text=element.Tooltip, timeout=DEFAULT_TOOLTIP_TIME)
- _add_right_click_menu_and_grab(element)
-
- # ............................DONE WITH ROW pack the row of widgets ..........................#
- # done with row, pack the row of widgets
- # tk_row_frame.grid(row=row_num+2, sticky=tk.NW, padx=DEFAULT_MARGINS[0])
-
- anchor = 'nw'
-
- if row_justify.lower().startswith('c'):
- anchor = 'n'
- side = tk.LEFT
- elif row_justify.lower().startswith('r'):
- anchor = 'ne'
- side = tk.RIGHT
- elif row_justify.lower().startswith('l'):
- anchor = 'nw'
- side = tk.LEFT
- # elif toplevel_form.ElementJustification.lower().startswith('c'):
- # anchor = 'n'
- # side = tk.TOP
- # elif toplevel_form.ElementJustification.lower().startswith('r'):
- # anchor = 'ne'
- # side = tk.TOP
- # else:
- # anchor = 'nw'
- # side = tk.TOP
-
- # row_should_expand = False
-
- # if form.RightClickMenu:
- # menu = form.RightClickMenu
- # top_menu = tk.Menu(toplevel_form.TKroot, tearoff=False)
- # AddMenuItem(top_menu, menu[1], form)
- # tk_row_frame.bind('', form._RightClickMenuCallback)
-
- tk_row_frame.pack(side=tk.TOP, anchor=anchor, padx=0, pady=0, expand=row_should_expand, fill=row_fill_direction)
- if form.BackgroundColor is not None and form.BackgroundColor != COLOR_SYSTEM_DEFAULT:
- tk_row_frame.configure(background=form.BackgroundColor)
-
- return
-
-
-def _get_hidden_master_root():
- """
- Creates the hidden master root window. This window is never visible and represents the overall "application"
- """
-
- # if one is already made, then skip making another
- if Window.hidden_master_root is None:
- Window._IncrementOpenCount()
- Window.hidden_master_root = tk.Tk()
- Window.hidden_master_root.attributes('-alpha', 0) # HIDE this window really really really
- # if not running_mac():
- try:
- Window.hidden_master_root.wm_overrideredirect(True)
- except Exception as e:
- if not running_mac():
- print('* Error performing wm_overrideredirect while hiding the hidden master root*', e)
- Window.hidden_master_root.withdraw()
- return Window.hidden_master_root
-
-
-def _no_titlebar_setup(window):
- """
- Does the operations required to turn off the titlebar for the window.
- The Raspberry Pi required the settings to be make after the window's creation.
- Calling twice seems to have had better overall results so that's what's currently done.
- The MAC has been the problem with this feature. It's been a chronic problem on the Mac.
- :param window: window to turn off the titlebar if indicated in the settings
- :type window: Window
- """
- try:
- if window.NoTitleBar:
- if running_linux():
- # window.TKroot.wm_attributes("-type", 'splash')
- window.TKroot.wm_attributes("-type", 'dock')
- else:
- window.TKroot.wm_overrideredirect(True)
- # Special case for Mac. Need to clear flag again if not tkinter version 8.6.10+
- # Previously restricted patch to only certain tkinter versions. Now use the patch setting exclusively regardless of tk ver
- # if running_mac() and ENABLE_MAC_NOTITLEBAR_PATCH and (sum([int(i) for i in tclversion_detailed.split('.')]) < 24):
- # if running_mac() and ENABLE_MAC_NOTITLEBAR_PATCH:
- if _mac_should_apply_notitlebar_patch():
- print('* Applying Mac no_titlebar patch *')
- window.TKroot.wm_overrideredirect(False)
- except Exception as e:
- warnings.warn('** Problem setting no titlebar {} **'.format(e), UserWarning)
-
-
-def _convert_window_to_tk(window):
- """
-
- :type window: (Window)
-
- """
- master = window.TKroot
- master.title(window.Title)
- InitializeResults(window)
-
- PackFormIntoFrame(window, master, window)
-
- window.TKroot.configure(padx=window.Margins[0], pady=window.Margins[1])
-
- # ....................................... DONE creating and laying out window ..........................#
- if window._Size != (None, None):
- master.geometry("%sx%s" % (window._Size[0], window._Size[1]))
- screen_width = master.winfo_screenwidth() # get window info to move to middle of screen
- screen_height = master.winfo_screenheight()
- if window.Location != (None, None):
- x, y = window.Location
- elif DEFAULT_WINDOW_LOCATION != (None, None):
- x, y = DEFAULT_WINDOW_LOCATION
- else:
- master.update_idletasks() # don't forget to do updates or values are bad
- win_width = master.winfo_width()
- win_height = master.winfo_height()
- x = screen_width / 2 - win_width / 2
- y = screen_height / 2 - win_height / 2
- if y + win_height > screen_height:
- y = screen_height - win_height
- if x + win_width > screen_width:
- x = screen_width - win_width
-
- if window.RelativeLoction != (None, None):
- x += window.RelativeLoction[0]
- y += window.RelativeLoction[1]
-
- move_string = '+%i+%i' % (int(x), int(y))
- master.geometry(move_string)
- window.config_last_location = (int(x), (int(y)))
- window.TKroot.x = int(x)
- window.TKroot.y = int(y)
- window.starting_window_position = (int(x), (int(y)))
- master.update_idletasks() # don't forget
- master.geometry(move_string)
- master.update_idletasks() # don't forget
-
- _no_titlebar_setup(window)
-
- return
-
-
-# ----====----====----====----====----==== STARTUP TK ====----====----====----====----====----#
-def StartupTK(window):
- """
- NOT user callable
- Creates the window (for real) lays out all the elements, etc. It's a HUGE set of things it does. It's the basic
- "porting layer" that will change depending on the GUI framework PySimpleGUI is running on top of.
-
- :param window: you window object
- :type window: (Window)
-
- """
- window = window # type: Window
- # global _my_windows
- # ow = _my_windows.NumOpenWindows
- ow = Window.NumOpenWindows
- # print('Starting TK open Windows = {}'.format(ow))
- if ENABLE_TK_WINDOWS:
- root = tk.Tk()
- elif not ow and not window.ForceTopLevel:
- # if first window being created, make a throwaway, hidden master root. This stops one user
- # window from becoming the child of another user window. All windows are children of this hidden window
- _get_hidden_master_root()
- root = tk.Toplevel(class_=window.Title)
- else:
- root = tk.Toplevel(class_=window.Title)
- if window.DebuggerEnabled:
- root.bind('', window._callback_main_debugger_window_create_keystroke)
- root.bind('', window._callback_popout_window_create_keystroke)
-
- # root.bind('', Debugger._build_main_debugger_window)
- # root.bind('', Debugger._build_floating_window)
- try:
- if not running_mac() or \
- (running_mac() and not window.NoTitleBar) or \
- (running_mac() and window.NoTitleBar and not _mac_should_apply_notitlebar_patch()):
- root.attributes('-alpha', 0) # hide window while building it. makes for smoother 'paint'
- except Exception as e:
- print('*** Exception setting alpha channel to zero while creating window ***', e)
-
-
- if window.BackgroundColor is not None and window.BackgroundColor != COLOR_SYSTEM_DEFAULT:
- root.configure(background=window.BackgroundColor)
- Window._IncrementOpenCount()
-
- window.TKroot = root
-
- window._create_thread_queue()
-
- # for the Raspberry Pi. Need to set the attributes here, prior to the building of the window
- # so going ahead and doing it for all platforms, in addition to doing it after the window is packed
- _no_titlebar_setup(window)
-
- if not window.Resizable:
- root.resizable(False, False)
-
- if window.DisableMinimize:
- root.attributes("-toolwindow", 1)
-
- if window.KeepOnTop:
- root.wm_attributes("-topmost", 1)
-
- if window.TransparentColor is not None:
- window.SetTransparentColor(window.TransparentColor)
-
- if window.scaling is not None:
- root.tk.call('tk', 'scaling', window.scaling)
-
-
- # root.protocol("WM_DELETE_WINDOW", MyFlexForm.DestroyedCallback())
- # root.bind('', MyFlexForm.DestroyedCallback())
- _convert_window_to_tk(window)
-
- # Make moveable window
- if (window.GrabAnywhere is not False and not (
- window.NonBlocking and window.GrabAnywhere is not True)):
- if not (ENABLE_MAC_DISABLE_GRAB_ANYWHERE_WITH_TITLEBAR and running_mac() and not window.NoTitleBar):
- root.bind("", window._StartMoveGrabAnywhere)
- root.bind("", window._StopMove)
- root.bind("", window._OnMotionGrabAnywhere)
- if (window.GrabAnywhereUsingControlKey is not False and not (
- window.NonBlocking and window.GrabAnywhereUsingControlKey is not True)):
- root.bind("", window._StartMoveUsingControlKey)
- root.bind("", window._StopMove)
- root.bind("", window._OnMotionUsingControlKey)
- window.set_icon(window.WindowIcon)
-
- try:
- root.attributes('-alpha', 1 if window.AlphaChannel is None else window.AlphaChannel) # Make window visible again
- except:
- pass
-
- if window.ReturnKeyboardEvents and not window.NonBlocking:
- root.bind("", window._KeyboardCallback)
- root.bind("", window._MouseWheelCallback)
- root.bind("", window._MouseWheelCallback)
- root.bind("", window._MouseWheelCallback)
- elif window.ReturnKeyboardEvents:
- root.bind("", window._KeyboardCallback)
- root.bind("", window._MouseWheelCallback)
- root.bind("", window._MouseWheelCallback)
- root.bind("", window._MouseWheelCallback)
-
- DEFAULT_WINDOW_SNAPSHOT_KEY_CODE = main_global_get_screen_snapshot_symcode()
-
- if DEFAULT_WINDOW_SNAPSHOT_KEY_CODE:
- # print('**** BINDING THE SNAPSHOT!', DEFAULT_WINDOW_SNAPSHOT_KEY_CODE, DEFAULT_WINDOW_SNAPSHOT_KEY)
- window.bind(DEFAULT_WINDOW_SNAPSHOT_KEY_CODE, DEFAULT_WINDOW_SNAPSHOT_KEY, propagate=False)
- # window.bind('', DEFAULT_WINDOW_SNAPSHOT_KEY, )
-
- if window.NoTitleBar:
- window.TKroot.focus_force()
-
- if window.AutoClose:
- # if the window is being finalized, then don't start the autoclose timer
- if not window.finalize_in_progress:
- window._start_autoclose_timer()
- # duration = DEFAULT_AUTOCLOSE_TIME if window.AutoCloseDuration is None else window.AutoCloseDuration
- # window.TKAfterID = root.after(int(duration * 1000), window._AutoCloseAlarmCallback)
-
- if window.Timeout != None:
- window.TKAfterID = root.after(int(window.Timeout), window._TimeoutAlarmCallback)
- if window.NonBlocking:
- window.TKroot.protocol("WM_DESTROY_WINDOW", window._OnClosingCallback)
- window.TKroot.protocol("WM_DELETE_WINDOW", window._OnClosingCallback)
-
- else: # it's a blocking form
- # print('..... CALLING MainLoop')
- window.CurrentlyRunningMainloop = True
- window.TKroot.protocol("WM_DESTROY_WINDOW", window._OnClosingCallback)
- window.TKroot.protocol("WM_DELETE_WINDOW", window._OnClosingCallback)
-
- if window.modal or DEFAULT_MODAL_WINDOWS_FORCED:
- window.make_modal()
-
- # window.TKroot.bind("", window._config_callback)
-
- # ----------------------------------- tkinter mainloop call -----------------------------------
- Window._window_running_mainloop = window
- Window._root_running_mainloop = window.TKroot
- window.TKroot.mainloop()
- window.CurrentlyRunningMainloop = False
- window.TimerCancelled = True
- # print('..... BACK from MainLoop')
- if not window.FormRemainedOpen:
- Window._DecrementOpenCount()
- # _my_windows.Decrement()
- if window.RootNeedsDestroying:
- try:
- window.TKroot.destroy()
- except:
- pass
- window.RootNeedsDestroying = False
- return
-
-
-def _set_icon_for_tkinter_window(root, icon=None, pngbase64=None):
- """
- At the moment, this function is only used by the get_filename or folder with the no_window option set.
- Changes the icon that is shown on the title bar and on the task bar.
- NOTE - The file type is IMPORTANT and depends on the OS!
- Can pass in:
- * filename which must be a .ICO icon file for windows, PNG file for Linux
- * bytes object
- * BASE64 encoded file held in a variable
-
- :param root: The window being modified
- :type root: (tk.Tk or tk.TopLevel)
- :param icon: Filename or bytes object
- :type icon: (str | bytes)
- :param pngbase64: Base64 encoded image
- :type pngbase64: (bytes)
- """
-
- if type(icon) is bytes or pngbase64 is not None:
- wicon = tkinter.PhotoImage(data=icon if icon is not None else pngbase64)
- try:
- root.tk.call('wm', 'iconphoto', root._w, wicon)
- except:
- wicon = tkinter.PhotoImage(data=DEFAULT_BASE64_ICON)
- try:
- root.tk.call('wm', 'iconphoto', root._w, wicon)
- except Exception as e:
- print('Set icon exception', e)
- pass
- return
-
- wicon = icon
- try:
- root.iconbitmap(icon)
- except Exception as e:
- try:
- wicon = tkinter.PhotoImage(file=icon)
- root.tk.call('wm', 'iconphoto', root._w, wicon)
- except Exception as e:
- try:
- wicon = tkinter.PhotoImage(data=DEFAULT_BASE64_ICON)
- try:
- root.tk.call('wm', 'iconphoto', root._w, wicon)
- except Exception as e:
- print('Set icon exception', e)
- pass
- except:
- print('Set icon exception', e)
- pass
-
-
-# ==============================_GetNumLinesNeeded ==#
-# Helper function for determining how to wrap text #
-# ===================================================#
-def _GetNumLinesNeeded(text, max_line_width):
- if max_line_width == 0:
- return 1
- lines = text.split('\n')
- num_lines = len(lines) # number of original lines of text
- max_line_len = max([len(l) for l in lines]) # longest line
- lines_used = []
- for L in lines:
- lines_used.append(len(L) // max_line_width + (len(L) % max_line_width > 0)) # fancy math to round up
- total_lines_needed = sum(lines_used)
- return total_lines_needed
-
-
-# ============================== PROGRESS METER ========================================== #
-
-def convert_args_to_single_string(*args):
- """
-
- :param *args:
- :type *args:
-
- """
- max_line_total, width_used, total_lines, = 0, 0, 0
- single_line_message = ''
- # loop through args and built a SINGLE string from them
- for message in args:
- # fancy code to check if string and convert if not is not need. Just always convert to string :-)
- # if not isinstance(message, str): message = str(message)
- message = str(message)
- longest_line_len = max([len(l) for l in message.split('\n')])
- width_used = max(longest_line_len, width_used)
- max_line_total = max(max_line_total, width_used)
- lines_needed = _GetNumLinesNeeded(message, width_used)
- total_lines += lines_needed
- single_line_message += message + '\n'
- return single_line_message, width_used, total_lines
-
-
-METER_REASON_CANCELLED = 'cancelled'
-METER_REASON_CLOSED = 'closed'
-METER_REASON_REACHED_MAX = 'finished'
-METER_OK = True
-METER_STOPPED = False
-
-
-class QuickMeter(object):
- active_meters = {}
- exit_reasons = {}
-
- def __init__(self, title, current_value, max_value, key, *args, orientation='v', bar_color=(None, None), button_color=(None, None),
- size=DEFAULT_PROGRESS_BAR_SIZE, border_width=None, grab_anywhere=False, no_titlebar=False, keep_on_top=None, no_button=False):
- """
-
- :param title: text to display in element
- :type title: (str)
- :param current_value: current value
- :type current_value: (int)
- :param max_value: max value of QuickMeter
- :type max_value: (int)
- :param key: Used with window.find_element and with return values to uniquely identify this element
- :type key: str | int | tuple | object
- :param *args: stuff to output
- :type *args: (Any)
- :param orientation: 'horizontal' or 'vertical' ('h' or 'v' work) (Default value = 'vertical' / 'v')
- :type orientation: (str)
- :param bar_color: color of a bar line
- :type bar_color: (str, str)
- :param button_color: button color (foreground, background)
- :type button_color: (str, str) or str
- :param size: (w,h) w=characters-wide, h=rows-high (Default value = DEFAULT_PROGRESS_BAR_SIZE)
- :type size: (int, int)
- :param border_width: width of border around element
- :type border_width: (int)
- :param grab_anywhere: If True: can grab anywhere to move the window (Default = False)
- :type grab_anywhere: (bool)
- :param no_titlebar: If True: window will be created without a titlebar
- :type no_titlebar: (bool)
- :param keep_on_top: If True the window will remain above all current windows
- :type keep_on_top: (bool)
- :param no_button: If True: window will be created without a cancel button
- :type no_button: (bool)
- """
- self.start_time = datetime.datetime.utcnow()
- self.key = key
- self.orientation = orientation
- self.bar_color = bar_color
- self.size = size
- self.grab_anywhere = grab_anywhere
- self.button_color = button_color
- self.border_width = border_width
- self.no_titlebar = no_titlebar
- self.title = title
- self.current_value = current_value
- self.max_value = max_value
- self.close_reason = None
- self.keep_on_top = keep_on_top
- self.no_button = no_button
- self.window = self.BuildWindow(*args)
-
- def BuildWindow(self, *args):
- layout = []
- if self.orientation.lower().startswith('h'):
- col = []
- col += [[T(''.join(map(lambda x: str(x) + '\n', args)),
- key='-OPTMSG-')]] ### convert all *args into one string that can be updated
- col += [[T('', size=(30, 10), key='-STATS-')],
- [ProgressBar(max_value=self.max_value, orientation='h', key='-PROG-', size=self.size,
- bar_color=self.bar_color)]]
- if not self.no_button:
- col += [[Cancel(button_color=self.button_color), Stretch()]]
- layout = [Column(col)]
- else:
- col = [[ProgressBar(max_value=self.max_value, orientation='v', key='-PROG-', size=self.size,
- bar_color=self.bar_color)]]
- col2 = []
- col2 += [[T(''.join(map(lambda x: str(x) + '\n', args)),
- key='-OPTMSG-')]] ### convert all *args into one string that can be updated
- col2 += [[T('', size=(30, 10), key='-STATS-')]]
- if not self.no_button:
- col2 += [[Cancel(button_color=self.button_color), Stretch()]]
-
- layout = [Column(col), Column(col2)]
- self.window = Window(self.title, grab_anywhere=self.grab_anywhere, border_depth=self.border_width, no_titlebar=self.no_titlebar, disable_close=True, keep_on_top=self.keep_on_top)
- self.window.Layout([layout]).Finalize()
-
- return self.window
-
- def UpdateMeter(self, current_value, max_value, *args): ### support for *args when updating
-
- self.current_value = current_value
- self.max_value = max_value
- self.window.Element('-PROG-').UpdateBar(self.current_value, self.max_value)
- self.window.Element('-STATS-').Update('\n'.join(self.ComputeProgressStats()))
- self.window.Element('-OPTMSG-').Update(
- value=''.join(map(lambda x: str(x) + '\n', args))) ### update the string with the args
- event, values = self.window.read(timeout=0)
- if event in ('Cancel', None) or current_value >= max_value:
- exit_reason = METER_REASON_CANCELLED if event in ('Cancel', None) else METER_REASON_REACHED_MAX if current_value >= max_value else METER_STOPPED
- self.window.close()
- del (QuickMeter.active_meters[self.key])
- QuickMeter.exit_reasons[self.key] = exit_reason
- return QuickMeter.exit_reasons[self.key]
- return METER_OK
-
- def ComputeProgressStats(self):
- utc = datetime.datetime.utcnow()
- time_delta = utc - self.start_time
- total_seconds = time_delta.total_seconds()
- if not total_seconds:
- total_seconds = 1
- try:
- time_per_item = total_seconds / self.current_value
- except:
- time_per_item = 1
- seconds_remaining = (self.max_value - self.current_value) * time_per_item
- time_remaining = str(datetime.timedelta(seconds=seconds_remaining))
- time_remaining_short = (time_remaining).split(".")[0]
- time_delta_short = str(time_delta).split(".")[0]
- total_time = time_delta + datetime.timedelta(seconds=seconds_remaining)
- total_time_short = str(total_time).split(".")[0]
- self.stat_messages = [
- '{} of {}'.format(self.current_value, self.max_value),
- '{} %'.format(100 * self.current_value // self.max_value),
- '',
- ' {:6.2f} Iterations per Second'.format(self.current_value / total_seconds),
- ' {:6.2f} Seconds per Iteration'.format(total_seconds / (self.current_value if self.current_value else 1)),
- '',
- '{} Elapsed Time'.format(time_delta_short),
- '{} Time Remaining'.format(time_remaining_short),
- '{} Estimated Total Time'.format(total_time_short)]
- return self.stat_messages
-
-
-def one_line_progress_meter(title, current_value, max_value, *args, key='OK for 1 meter', orientation='v', bar_color=(None, None), button_color=None, size=DEFAULT_PROGRESS_BAR_SIZE, border_width=None, grab_anywhere=False, no_titlebar=False, keep_on_top=None, no_button=False):
- """
- :param title: text to display in eleemnt
- :type title: (str)
- :param current_value: current value
- :type current_value: (int)
- :param max_value: max value of QuickMeter
- :type max_value: (int)
- :param *args: stuff to output
- :type *args: (Any)
- :param key: Used to differentiate between mutliple meters. Used to cancel meter early. Now optional as there is a default value for single meters
- :type key: str | int | tuple | object
- :param orientation: 'horizontal' or 'vertical' ('h' or 'v' work) (Default value = 'vertical' / 'v')
- :type orientation: (str)
- :param bar_color: color of a bar line
- :type bar_color: Tuple(str, str)
- :param button_color: button color (foreground, background)
- :type button_color: (str, str) or str
- :param size: (w,h) w=characters-wide, h=rows-high (Default value = DEFAULT_PROGRESS_BAR_SIZE)
- :type size: (int, int)
- :param border_width: width of border around element
- :type border_width: (int)
- :param grab_anywhere: If True: can grab anywhere to move the window (Default = False)
- :type grab_anywhere: (bool)
- :param no_titlebar: If True: no titlebar will be shown on the window
- :type no_titlebar: (bool)
- :param keep_on_top: If True the window will remain above all current windows
- :type keep_on_top: (bool)
- :param no_button: If True: window will be created without a cancel button
- :type no_button: (bool)
- :return: True if updated successfully. False if user closed the meter with the X or Cancel button
- :rtype: (bool)
- """
- if key not in QuickMeter.active_meters:
- meter = QuickMeter(title, current_value, max_value, key, *args, orientation=orientation, bar_color=bar_color, button_color=button_color, size=size, border_width=border_width, grab_anywhere=grab_anywhere, no_titlebar=no_titlebar, keep_on_top=keep_on_top, no_button=no_button)
- QuickMeter.active_meters[key] = meter
- QuickMeter.exit_reasons[key] = None
-
- else:
- meter = QuickMeter.active_meters[key]
-
- rc = meter.UpdateMeter(current_value, max_value, *args) ### pass the *args to to UpdateMeter function
- OneLineProgressMeter.exit_reasons = getattr(OneLineProgressMeter, 'exit_reasons', QuickMeter.exit_reasons)
- exit_reason = OneLineProgressMeter.exit_reasons.get(key)
- return METER_OK if exit_reason in (None, METER_REASON_REACHED_MAX) else METER_STOPPED
-
-
-def one_line_progress_meter_cancel(key='OK for 1 meter'):
- """
- Cancels and closes a previously created One Line Progress Meter window
-
- :param key: Key used when meter was created
- :type key: (Any)
- :return: None
- :rtype: None
- """
- try:
- meter = QuickMeter.active_meters[key]
- meter.window.Close()
- del (QuickMeter.active_meters[key])
- QuickMeter.exit_reasons[key] = METER_REASON_CANCELLED
- except: # meter is already deleted
- return
-
-
-def get_complimentary_hex(color):
- """
- :param color: color string, like "#RRGGBB"
- :type color: (str)
- :return: color string, like "#RRGGBB"
- :rtype: (str)
- """
-
- # strip the # from the beginning
- color = color[1:]
- # convert the string into hex
- color = int(color, 16)
- # invert the three bytes
- # as good as substracting each of RGB component by 255(FF)
- comp_color = 0xFFFFFF ^ color
- # convert the color back to hex by prefixing a #
- comp_color = "#%06X" % comp_color
- return comp_color
-
-
-# ======================== EasyPrint =====#
-# ===================================================#
-class _DebugWin():
- debug_window = None
-
- def __init__(self, size=(None, None), location=(None, None), relative_location=(None, None), font=None, no_titlebar=False, no_button=False,
- grab_anywhere=False, keep_on_top=None, do_not_reroute_stdout=True, echo_stdout=False, resizable=True, blocking=False):
- """
-
- :param size: (w,h) w=characters-wide, h=rows-high
- :type size: (int, int)
- :param location: Location of upper left corner of the window
- :type location: (int, int)
- :param relative_location: (x,y) location relative to the default location of the window, in pixels. Normally the window centers. This location is relative to the location the window would be created. Note they can be negative.
- :type relative_location: (int, int)
- :param font: specifies the font family, size, etc. Tuple or Single string format 'name size styles'. Styles: italic * roman bold normal underline overstrike
- :type font: (str or (str, int[, str]) or None)
- :param no_titlebar: If True no titlebar will be shown
- :type no_titlebar: (bool)
- :param no_button: show button
- :type no_button: (bool)
- :param grab_anywhere: If True: can grab anywhere to move the window (Default = False)
- :type grab_anywhere: (bool)
- :param location: Location of upper left corner of the window
- :type location: (int, int)
- :param do_not_reroute_stdout: bool value
- :type do_not_reroute_stdout: (bool)
- :param echo_stdout: If True stdout is sent to both the console and the debug window
- :type echo_stdout: (bool)
- :param resizable: if True, makes the window resizble
- :type resizable: (bool)
- :param blocking: if True, makes the window block instead of returning immediately
- :type blocking: (bool)
- """
-
- # Show a form that's a running counter
- self.size = size
- self.location = location
- self.relative_location = relative_location
- self.font = font
- self.no_titlebar = no_titlebar
- self.no_button = no_button
- self.grab_anywhere = grab_anywhere
- self.keep_on_top = keep_on_top
- self.do_not_reroute_stdout = do_not_reroute_stdout
- self.echo_stdout = echo_stdout
- self.resizable = resizable
- self.blocking = blocking
-
- win_size = size if size != (None, None) else DEFAULT_DEBUG_WINDOW_SIZE
- self.output_element = Multiline(size=win_size, autoscroll=True, auto_refresh=True, reroute_stdout=False if do_not_reroute_stdout else True, echo_stdout_stderr=self.echo_stdout, reroute_stderr=False if do_not_reroute_stdout else True, expand_x=True, expand_y=True, key='-MULTILINE-')
- if no_button:
- self.layout = [[self.output_element]]
- else:
- if blocking:
- self.quit_button = Button('Quit', key='Quit')
- else:
- self.quit_button = DummyButton('Quit', key='Quit')
- self.layout = [[self.output_element],
- [pin(self.quit_button), pin(B('Pause', key='-PAUSE-')), Stretch()]]
-
- self.layout[-1] += [Sizegrip()]
-
- self.window = Window('Debug Window', self.layout, no_titlebar=no_titlebar, auto_size_text=True, location=location, relative_location=relative_location,
- font=font or ('Courier New', 10), grab_anywhere=grab_anywhere, keep_on_top=keep_on_top, finalize=True, resizable=resizable)
- return
-
- def reopen_window(self):
- if self.window is None or (self.window is not None and self.window.is_closed()):
- self.__init__(size=self.size, location=self.location, relative_location=self.relative_location, font=self.font, no_titlebar=self.no_titlebar,
- no_button=self.no_button, grab_anywhere=self.grab_anywhere, keep_on_top=self.keep_on_top,
- do_not_reroute_stdout=self.do_not_reroute_stdout, resizable=self.resizable, echo_stdout=self.echo_stdout)
-
-
- def Print(self, *args, end=None, sep=None, text_color=None, background_color=None, erase_all=False, font=None, blocking=None):
- global SUPPRESS_WIDGET_NOT_FINALIZED_WARNINGS
- suppress = SUPPRESS_WIDGET_NOT_FINALIZED_WARNINGS
- SUPPRESS_WIDGET_NOT_FINALIZED_WARNINGS = True
- sepchar = sep if sep is not None else ' '
- endchar = end if end is not None else '\n'
- self.reopen_window() # if needed, open the window again
-
- timeout = 0 if not blocking else None
- if erase_all:
- self.output_element.update('')
-
- if self.do_not_reroute_stdout:
- end_str = str(end) if end is not None else '\n'
- sep_str = str(sep) if sep is not None else ' '
-
- outstring = ''
- num_args = len(args)
- for i, arg in enumerate(args):
- outstring += str(arg)
- if i != num_args - 1:
- outstring += sep_str
- outstring += end_str
- try:
- self.output_element.update(outstring, append=True, text_color_for_value=text_color, background_color_for_value=background_color, font_for_value=font)
- except:
- self.window=None
- self.reopen_window()
- self.output_element.update(outstring, append=True, text_color_for_value=text_color, background_color_for_value=background_color, font_for_value=font)
-
- else:
- print(*args, sep=sepchar, end=endchar)
- # This is tricky....changing the button type depending on the blocking parm. If blocking, then the "Quit" button should become a normal button
- if blocking:
- self.quit_button.BType = BUTTON_TYPE_READ_FORM
- try: # The window may be closed by user at any time, so have to protect
- self.quit_button.update(text='Click to continue...')
- except:
- self.window = None
- else:
- self.quit_button.BType = BUTTON_TYPE_CLOSES_WIN_ONLY
- try: # The window may be closed by user at any time, so have to protect
- self.quit_button.update(text='Quit')
- except:
- self.window = None
-
- try: # The window may be closed by user at any time, so have to protect
- if blocking:
- self.window['-PAUSE-'].update(visible=False)
- else:
- self.window['-PAUSE-'].update(visible=True)
- except:
- self.window = None
-
- self.reopen_window() # if needed, open the window again
-
- paused = None
- while True:
- event, values = self.window.read(timeout=timeout)
-
- if event == WIN_CLOSED:
- self.Close()
- break
- elif blocking and event == 'Quit':
- break
- elif not paused and event == TIMEOUT_EVENT and not blocking:
- break
- elif event == '-PAUSE-':
- if blocking: # if blocking, ignore the pause button entirely
- continue
- if paused:
- self.window['-PAUSE-'].update(text='Pause')
- self.quit_button.update(visible=True)
- break
- paused = True
- self.window['-PAUSE-'].update(text='Resume')
- self.quit_button.update(visible=False)
- timeout = None
-
- SUPPRESS_WIDGET_NOT_FINALIZED_WARNINGS = suppress
-
- def Close(self):
- if self.window.XFound: # increment the number of open windows to get around a bug with debug windows
- Window._IncrementOpenCount()
- self.window.close()
- self.window = None
-
-
-def easy_print(*args, size=(None, None), end=None, sep=None, location=(None, None), relative_location=(None, None), font=None, no_titlebar=False,
- no_button=False, grab_anywhere=False, keep_on_top=None, do_not_reroute_stdout=True, echo_stdout=False, text_color=None, background_color=None, colors=None, c=None, erase_all=False, resizable=True, blocking=None, wait=None):
- """
- Works like a "print" statement but with windowing options. Routes output to the "Debug Window"
-
- In addition to the normal text and background colors, you can use a "colors" tuple/string
- The "colors" or "c" parameter defines both the text and background in a single parm.
- It can be a tuple or a single single. Both text and background colors need to be specified
- colors -(str, str) or str. A combined text/background color definition in a single parameter
- c - (str, str) - Colors tuple has format (foreground, backgrouned)
- c - str - can also be a string of the format "foreground on background" ("white on red")
-
- :param *args: stuff to output
- :type *args: (Any)
- :param size: (w,h) w=characters-wide, h=rows-high
- :type size: (int, int)
- :param end: end character
- :type end: (str)
- :param sep: separator character
- :type sep: (str)
- :param location: Location of upper left corner of the window
- :type location: (int, int)
- :param relative_location: (x,y) location relative to the default location of the window, in pixels. Normally the window centers. This location is relative to the location the window would be created. Note they can be negative.
- :type relative_location: (int, int)
- :param font: specifies the font family, size, etc. Tuple or Single string format 'name size styles'. Styles: italic * roman bold normal underline overstrike
- :type font: (str or (str, int[, str]) or None)
- :param no_titlebar: If True no titlebar will be shown
- :type no_titlebar: (bool)
- :param no_button: don't show button
- :type no_button: (bool)
- :param grab_anywhere: If True: can grab anywhere to move the window (Default = False)
- :type grab_anywhere: (bool)
- :param background_color: color of background
- :type background_color: (str)
- :param text_color: color of the text
- :type text_color: (str)
- :param keep_on_top: If True the window will remain above all current windows
- :type keep_on_top: (bool)
- :param location: Location of upper left corner of the window
- :type location: (int, int)
- :param do_not_reroute_stdout: do not reroute stdout and stderr. If False, both stdout and stderr will reroute to here
- :type do_not_reroute_stdout: (bool)
- :param echo_stdout: If True stdout is sent to both the console and the debug window
- :type echo_stdout: (bool)
- :param colors: Either a tuple or a string that has both the text and background colors
- :type colors: (str) or (str, str)
- :param c: Either a tuple or a string that has both the text and background colors
- :type c: (str) or (str, str)
- :param resizable: if True, the user can resize the debug window. Default is True
- :type resizable: (bool)
- :param erase_all: If True when erase the output before printing
- :type erase_all: (bool)
- :param blocking: if True, makes the window block instead of returning immediately. The "Quit" button changers to "More"
- :type blocking: (bool | None)
- :param wait: Same as the "blocking" parm. It's an alias. if True, makes the window block instead of returning immediately. The "Quit" button changes to "Click to Continue..."
- :type wait: (bool | None)
- :return:
- :rtype:
- """
-
- blocking = blocking or wait
- if _DebugWin.debug_window is None:
- _DebugWin.debug_window = _DebugWin(size=size, location=location, relative_location=relative_location, font=font, no_titlebar=no_titlebar,
- no_button=no_button, grab_anywhere=grab_anywhere, keep_on_top=keep_on_top,
- do_not_reroute_stdout=do_not_reroute_stdout, echo_stdout=echo_stdout, resizable=resizable, blocking=blocking)
- txt_color, bg_color = _parse_colors_parm(c or colors)
- _DebugWin.debug_window.Print(*args, end=end, sep=sep, text_color=text_color or txt_color, background_color=background_color or bg_color,
- erase_all=erase_all, font=font, blocking=blocking)
-
-
-def easy_print_close():
- """
- Close a previously opened EasyPrint window
-
- :return:
- :rtype:
- """
- if _DebugWin.debug_window is not None:
- _DebugWin.debug_window.Close()
- _DebugWin.debug_window = None
-
-
-# d8b 888
-# Y8P 888
-# 888
-# .d8888b 88888b. 888d888 888 88888b. 888888
-# d88P" 888 "88b 888P" 888 888 "88b 888
-# 888 888 888 888 888 888 888 888
-# Y88b. 888 d88P 888 888 888 888 Y88b.
-# "Y8888P 88888P" 888 888 888 888 "Y888
-# 888
-# 888
-# 888
-
-
-CPRINT_DESTINATION_WINDOW = None
-CPRINT_DESTINATION_MULTILINE_ELMENT_KEY = None
-
-
-def cprint_set_output_destination(window, multiline_key):
- """
- Sets up the color print (cprint) output destination
- :param window: The window that the cprint call will route the output to
- :type window: (Window)
- :param multiline_key: Key for the Multiline Element where output will be sent
- :type multiline_key: (Any)
- :return: None
- :rtype: None
- """
-
- global CPRINT_DESTINATION_WINDOW, CPRINT_DESTINATION_MULTILINE_ELMENT_KEY
-
- CPRINT_DESTINATION_WINDOW = window
- CPRINT_DESTINATION_MULTILINE_ELMENT_KEY = multiline_key
-
-
-def cprint(*args, end=None, sep=' ', text_color=None, font=None, t=None, background_color=None, b=None, colors=None, c=None, window=None, key=None,
- justification=None, autoscroll=True):
- """
- Color print to a multiline element in a window of your choice.
- Must have EITHER called cprint_set_output_destination prior to making this call so that the
- window and element key can be saved and used here to route the output, OR used the window
- and key parameters to the cprint function to specicy these items.
-
- args is a variable number of things you want to print.
-
- end - The end char to use just like print uses
- sep - The separation character like print uses
- text_color - The color of the text
- key - overrides the previously defined Multiline key
- window - overrides the previously defined window to output to
- background_color - The color of the background
- colors -(str, str) or str. A combined text/background color definition in a single parameter
-
- There are also "aliases" for text_color, background_color and colors (t, b, c)
- t - An alias for color of the text (makes for shorter calls)
- b - An alias for the background_color parameter
- c - (str, str) - "shorthand" way of specifying color. (foreground, backgrouned)
- c - str - can also be a string of the format "foreground on background" ("white on red")
-
- With the aliases it's possible to write the same print but in more compact ways:
- cprint('This will print white text on red background', c=('white', 'red'))
- cprint('This will print white text on red background', c='white on red')
- cprint('This will print white text on red background', text_color='white', background_color='red')
- cprint('This will print white text on red background', t='white', b='red')
-
- :param *args: stuff to output
- :type *args: (Any)
- :param text_color: Color of the text
- :type text_color: (str)
- :param font: specifies the font family, size, etc. Tuple or Single string format 'name size styles'. Styles: italic * roman bold normal underline overstrike for the value being updated
- :type font: (str or (str, int[, str]) or None)
- :param background_color: The background color of the line
- :type background_color: (str)
- :param colors: Either a tuple or a string that has both the text and background colors "text on background" or just the text color
- :type colors: (str) or (str, str)
- :param t: Color of the text
- :type t: (str)
- :param b: The background color of the line
- :type b: (str)
- :param c: Either a tuple or a string. Same as the color parm
- :type c: (str) or (str, str)
- :param end: end character
- :type end: (str)
- :param sep: separator character
- :type sep: (str)
- :param key: key of multiline to output to (if you want to override the one previously set)
- :type key: (Any)
- :param window: Window containing the multiline to output to (if you want to override the one previously set)
- :type window: (Window)
- :param justification: text justification. left, right, center. Can use single characters l, r, c. Sets only for this value, not entire element
- :type justification: (str)
- :param autoscroll: If True the contents of the element will automatically scroll as more data added to the end
- :type autoscroll: (bool)
- """
-
- destination_key = CPRINT_DESTINATION_MULTILINE_ELMENT_KEY if key is None else key
- destination_window = window or CPRINT_DESTINATION_WINDOW
-
- if (destination_window is None and window is None) or (destination_key is None and key is None):
- print('** Warning ** Attempting to perform a cprint without a valid window & key',
- 'Will instead print on Console',
- 'You can specify window and key in this cprint call, or set ahead of time using cprint_set_output_destination')
- print(*args)
- return
-
- kw_text_color = text_color or t
- kw_background_color = background_color or b
- dual_color = colors or c
- try:
- if isinstance(dual_color, tuple):
- kw_text_color = dual_color[0]
- kw_background_color = dual_color[1]
- elif isinstance(dual_color, str):
- if ' on ' in dual_color: # if has "on" in the string, then have both text and background
- kw_text_color = dual_color.split(' on ')[0]
- kw_background_color = dual_color.split(' on ')[1]
- else: # if no "on" then assume the color string is just the text color
- kw_text_color = dual_color
- except Exception as e:
- print('* cprint warning * you messed up with color formatting', e)
-
- mline = destination_window.find_element(destination_key, silent_on_error=True) # type: Multiline
- try:
- # mline = destination_window[destination_key] # type: Multiline
- if end is None:
- mline.print(*args, text_color=kw_text_color, background_color=kw_background_color, end='', sep=sep, justification=justification, font=font,
- autoscroll=autoscroll)
- mline.print('', justification=justification, autoscroll=autoscroll)
- else:
- mline.print(*args, text_color=kw_text_color, background_color=kw_background_color, end=end, sep=sep, justification=justification, font=font,
- autoscroll=autoscroll)
- except Exception as e:
- print('** cprint error trying to print to the multiline. Printing to console instead **', e)
- print(*args, end=end, sep=sep)
-
-
-# ------------------------------------------------------------------------------------------------ #
-# A print-like call that can be used to output to a multiline element as if it's an Output element #
-# ------------------------------------------------------------------------------------------------ #
-
-def _print_to_element(multiline_element, *args, end=None, sep=None, text_color=None, background_color=None, autoscroll=None, justification=None, font=None):
- """
- Print like Python normally prints except route the output to a multiline element and also add colors if desired
-
- :param multiline_element: The multiline element to be output to
- :type multiline_element: (Multiline)
- :param args: The arguments to print
- :type args: List[Any]
- :param end: The end char to use just like print uses
- :type end: (str)
- :param sep: The separation character like print uses
- :type sep: (str)
- :param text_color: color of the text
- :type text_color: (str)
- :param background_color: The background color of the line
- :type background_color: (str)
- :param autoscroll: If True (the default), the element will scroll to bottom after updating
- :type autoscroll: (bool)
- :param font: specifies the font family, size, etc. Tuple or Single string format 'name size styles'. Styles: italic * roman bold normal underline overstrike for the value being updated
- :type font: str | (str, int)
- """
- end_str = str(end) if end is not None else '\n'
- sep_str = str(sep) if sep is not None else ' '
-
- outstring = ''
- num_args = len(args)
- for i, arg in enumerate(args):
- outstring += str(arg)
- if i != num_args - 1:
- outstring += sep_str
- outstring += end_str
-
- multiline_element.update(outstring, append=True, text_color_for_value=text_color, background_color_for_value=background_color, autoscroll=autoscroll,
- justification=justification, font_for_value=font)
-
- try: # if the element is set to autorefresh, then refresh the parent window
- if multiline_element.AutoRefresh:
- multiline_element.ParentForm.refresh()
- except:
- pass
-
-
-def _parse_colors_parm(colors):
- """
- Parse a colors parameter into its separate colors.
- Some functions accept a dual colors string/tuple.
- This function parses the parameter into the component colors
-
- :param colors: Either a tuple or a string that has both the text and background colors
- :type colors: (str) or (str, str)
- :return: tuple with the individual text and background colors
- :rtype: (str, str)
- """
- if colors is None:
- return None, None
- dual_color = colors
- kw_text_color = kw_background_color = None
- try:
- if isinstance(dual_color, tuple):
- kw_text_color = dual_color[0]
- kw_background_color = dual_color[1]
- elif isinstance(dual_color, str):
- if ' on ' in dual_color: # if has "on" in the string, then have both text and background
- kw_text_color = dual_color.split(' on ')[0]
- kw_background_color = dual_color.split(' on ')[1]
- else: # if no "on" then assume the color string is just the text color
- kw_text_color = dual_color
- except Exception as e:
- print('* warning * you messed up with color formatting', e)
-
- return kw_text_color, kw_background_color
-
-
-# ============================== set_global_icon ====#
-# Sets the icon to be used by default #
-# ===================================================#
-def set_global_icon(icon):
- """
- Sets the icon which will be used any time a window is created if an icon is not provided when the
- window is created.
-
- :param icon: Either a Base64 byte string or a filename
- :type icon: bytes | str
- """
-
- Window._user_defined_icon = icon
-
-
-# ============================== set_options ========#
-# Sets the icon to be used by default #
-# ===================================================#
-def set_options(icon=None, button_color=None, element_size=(None, None), button_element_size=(None, None),
- margins=(None, None),
- element_padding=(None, None), auto_size_text=None, auto_size_buttons=None, font=None, border_width=None,
- slider_border_width=None, slider_relief=None, slider_orientation=None,
- autoclose_time=None, message_box_line_width=None,
- progress_meter_border_depth=None, progress_meter_style=None,
- progress_meter_relief=None, progress_meter_color=None, progress_meter_size=None,
- text_justification=None, background_color=None, element_background_color=None,
- text_element_background_color=None, input_elements_background_color=None, input_text_color=None,
- scrollbar_color=None, text_color=None, element_text_color=None, debug_win_size=(None, None),
- window_location=(None, None), error_button_color=(None, None), tooltip_time=None, tooltip_font=None, use_ttk_buttons=None, ttk_theme=None,
- suppress_error_popups=None, suppress_raise_key_errors=None, suppress_key_guessing=None,warn_button_key_duplicates=False, enable_treeview_869_patch=None,
- enable_mac_notitlebar_patch=None, use_custom_titlebar=None, titlebar_background_color=None, titlebar_text_color=None, titlebar_font=None,
- titlebar_icon=None, user_settings_path=None, pysimplegui_settings_path=None, pysimplegui_settings_filename=None, keep_on_top=None, dpi_awareness=None, scaling=None, disable_modal_windows=None, force_modal_windows=None, tooltip_offset=(None, None),
- sbar_trough_color=None, sbar_background_color=None, sbar_arrow_color=None, sbar_width=None, sbar_arrow_width=None, sbar_frame_color=None, sbar_relief=None, alpha_channel=None):
- """
- :param icon: Can be either a filename or Base64 value. For Windows if filename, it MUST be ICO format. For Linux, must NOT be ICO. Most portable is to use a Base64 of a PNG file. This works universally across all OS's
- :type icon: bytes | str
- :param button_color: Color of the button (text, background)
- :type button_color: (str, str) or str
- :param element_size: element size (width, height) in characters
- :type element_size: (int, int)
- :param button_element_size: Size of button
- :type button_element_size: (int, int)
- :param margins: (left/right, top/bottom) tkinter margins around outsize. Amount of pixels to leave inside the window's frame around the edges before your elements are shown.
- :type margins: (int, int)
- :param element_padding: Default amount of padding to put around elements in window (left/right, top/bottom) or ((left, right), (top, bottom))
- :type element_padding: (int, int) or ((int, int),(int,int))
- :param auto_size_text: True if the Widget should be shrunk to exactly fit the number of chars to show
- :type auto_size_text: bool
- :param auto_size_buttons: True if Buttons in this Window should be sized to exactly fit the text on this.
- :type auto_size_buttons: (bool)
- :param font: specifies the font family, size, etc. Tuple or Single string format 'name size styles'. Styles: italic * roman bold normal underline overstrike
- :type font: (str or (str, int[, str]) or None)
- :param border_width: width of border around element
- :type border_width: (int)
- :param slider_border_width: Width of the border around sliders
- :type slider_border_width: (int)
- :param slider_relief: Type of relief to use for sliders
- :type slider_relief: (str)
- :param slider_orientation: ???
- :type slider_orientation: ???
- :param autoclose_time: ???
- :type autoclose_time: ???
- :param message_box_line_width: ???
- :type message_box_line_width: ???
- :param progress_meter_border_depth: ???
- :type progress_meter_border_depth: ???
- :param progress_meter_style: You can no longer set a progress bar style. All ttk styles must be the same for the window
- :type progress_meter_style: ???
- :param progress_meter_relief:
- :type progress_meter_relief: ???
- :param progress_meter_color: ???
- :type progress_meter_color: ???
- :param progress_meter_size: ???
- :type progress_meter_size: ???
- :param text_justification: Default text justification for all Text Elements in window
- :type text_justification: 'left' | 'right' | 'center'
- :param background_color: color of background
- :type background_color: (str)
- :param element_background_color: element background color
- :type element_background_color: (str)
- :param text_element_background_color: text element background color
- :type text_element_background_color: (str)
- :param input_elements_background_color: Default color to use for the background of input elements
- :type input_elements_background_color: (str)
- :param input_text_color: Default color to use for the text for Input elements
- :type input_text_color: (str)
- :param scrollbar_color: Default color to use for the slider trough
- :type scrollbar_color: (str)
- :param text_color: color of the text
- :type text_color: (str)
- :param element_text_color: Default color to use for Text elements
- :type element_text_color: (str)
- :param debug_win_size: window size
- :type debug_win_size: (int, int)
- :param window_location: Default location to place windows. Not setting will center windows on the display
- :type window_location: (int, int) | None
- :param error_button_color: (Default = (None))
- :type error_button_color: ???
- :param tooltip_time: time in milliseconds to wait before showing a tooltip. Default is 400ms
- :type tooltip_time: (int)
- :param tooltip_font: font to use for all tooltips
- :type tooltip_font: str or Tuple[str, int] or Tuple[str, int, str]
- :param use_ttk_buttons: if True will cause all buttons to be ttk buttons
- :type use_ttk_buttons: (bool)
- :param ttk_theme: Theme to use with ttk widgets. Choices (on Windows) include - 'default', 'winnative', 'clam', 'alt', 'classic', 'vista', 'xpnative'
- :type ttk_theme: (str)
- :param suppress_error_popups: If True then error popups will not be shown if generated internally to PySimpleGUI
- :type suppress_error_popups: (bool)
- :param suppress_raise_key_errors: If True then key errors won't be raised (you'll still get popup error)
- :type suppress_raise_key_errors: (bool)
- :param suppress_key_guessing: If True then key errors won't try and find closest matches for you
- :type suppress_key_guessing: (bool)
- :param warn_button_key_duplicates: If True then duplicate Button Keys generate warnings (not recommended as they're expected)
- :type warn_button_key_duplicates: (bool)
- :param enable_treeview_869_patch: If True, then will use the treeview color patch for tk 8.6.9
- :type enable_treeview_869_patch: (bool)
- :param enable_mac_notitlebar_patch: If True then Windows with no titlebar use an alternative technique when tkinter version < 8.6.10
- :type enable_mac_notitlebar_patch: (bool)
- :param use_custom_titlebar: If True then a custom titlebar is used instead of the normal system titlebar
- :type use_custom_titlebar: (bool)
- :param titlebar_background_color: If custom titlebar indicated by use_custom_titlebar, then use this as background color
- :type titlebar_background_color: str | None
- :param titlebar_text_color: If custom titlebar indicated by use_custom_titlebar, then use this as text color
- :type titlebar_text_color: str | None
- :param titlebar_font: If custom titlebar indicated by use_custom_titlebar, then use this as title font
- :type titlebar_font: (str or (str, int[, str]) or None) | None
- :param titlebar_icon: If custom titlebar indicated by use_custom_titlebar, then use this as the icon (file or base64 bytes)
- :type titlebar_icon: bytes | str
- :param user_settings_path: default path for user_settings API calls. Expanded with os.path.expanduser so can contain ~ to represent user
- :type user_settings_path: (str)
- :param pysimplegui_settings_path: default path for the global PySimpleGUI user_settings
- :type pysimplegui_settings_path: (str)
- :param pysimplegui_settings_filename: default filename for the global PySimpleGUI user_settings
- :type pysimplegui_settings_filename: (str)
- :param keep_on_top: If True then all windows will automatically be set to keep_on_top=True
- :type keep_on_top: (bool)
- :param dpi_awareness: If True then will turn on DPI awareness (Windows only at the moment)
- :type dpi_awareness: (bool)
- :param scaling: Sets the default scaling for all windows including popups, etc.
- :type scaling: (float)
- :param disable_modal_windows: If True then all windows, including popups, will not be modal windows (unless they've been set to FORCED using another option)
- :type disable_modal_windows: (bool)
- :param force_modal_windows: If True then all windows will be modal (the disable option will be ignored... all windows will be forced to be modal)
- :type force_modal_windows: (bool)
- :param tooltip_offset: Offset to use for tooltips as a tuple. These values will be added to the mouse location when the widget was entered.
- :type tooltip_offset: ((None, None) | (int, int))
- :param sbar_trough_color: Scrollbar color of the trough
- :type sbar_trough_color: (str)
- :param sbar_background_color: Scrollbar color of the background of the arrow buttons at the ends AND the color of the "thumb" (the thing you grab and slide). Switches to arrow color when mouse is over
- :type sbar_background_color: (str)
- :param sbar_arrow_color: Scrollbar color of the arrow at the ends of the scrollbar (it looks like a button). Switches to background color when mouse is over
- :type sbar_arrow_color: (str)
- :param sbar_width: Scrollbar width in pixels
- :type sbar_width: (int)
- :param sbar_arrow_width: Scrollbar width of the arrow on the scrollbar. It will potentially impact the overall width of the scrollbar
- :type sbar_arrow_width: (int)
- :param sbar_frame_color: Scrollbar Color of frame around scrollbar (available only on some ttk themes)
- :type sbar_frame_color: (str)
- :param sbar_relief: Scrollbar relief that will be used for the "thumb" of the scrollbar (the thing you grab that slides). Should be a constant that is defined at starting with "RELIEF_" - RELIEF_RAISED, RELIEF_SUNKEN, RELIEF_FLAT, RELIEF_RIDGE, RELIEF_GROOVE, RELIEF_SOLID
- :param alpha_channel Default alpha channel to be used on all windows
- :type alpha_channel (float)
- :type sbar_relief: (str)
- :return: None
- :rtype: None
- """
-
- global DEFAULT_ELEMENT_SIZE
- global DEFAULT_BUTTON_ELEMENT_SIZE
- global DEFAULT_MARGINS # Margins for each LEFT/RIGHT margin is first term
- global DEFAULT_ELEMENT_PADDING # Padding between elements (row, col) in pixels
- global DEFAULT_AUTOSIZE_TEXT
- global DEFAULT_AUTOSIZE_BUTTONS
- global DEFAULT_FONT
- global DEFAULT_BORDER_WIDTH
- global DEFAULT_AUTOCLOSE_TIME
- global DEFAULT_BUTTON_COLOR
- global MESSAGE_BOX_LINE_WIDTH
- global DEFAULT_PROGRESS_BAR_BORDER_WIDTH
- global DEFAULT_PROGRESS_BAR_STYLE
- global DEFAULT_PROGRESS_BAR_RELIEF
- global DEFAULT_PROGRESS_BAR_COLOR
- global DEFAULT_PROGRESS_BAR_SIZE
- global DEFAULT_TEXT_JUSTIFICATION
- global DEFAULT_DEBUG_WINDOW_SIZE
- global DEFAULT_SLIDER_BORDER_WIDTH
- global DEFAULT_SLIDER_RELIEF
- global DEFAULT_SLIDER_ORIENTATION
- global DEFAULT_BACKGROUND_COLOR
- global DEFAULT_INPUT_ELEMENTS_COLOR
- global DEFAULT_ELEMENT_BACKGROUND_COLOR
- global DEFAULT_TEXT_ELEMENT_BACKGROUND_COLOR
- global DEFAULT_SCROLLBAR_COLOR
- global DEFAULT_TEXT_COLOR
- global DEFAULT_WINDOW_LOCATION
- global DEFAULT_ELEMENT_TEXT_COLOR
- global DEFAULT_INPUT_TEXT_COLOR
- global DEFAULT_TOOLTIP_TIME
- global DEFAULT_ERROR_BUTTON_COLOR
- global DEFAULT_TTK_THEME
- global USE_TTK_BUTTONS
- global TOOLTIP_FONT
- global SUPPRESS_ERROR_POPUPS
- global SUPPRESS_RAISE_KEY_ERRORS
- global SUPPRESS_KEY_GUESSING
- global WARN_DUPLICATE_BUTTON_KEY_ERRORS
- global ENABLE_TREEVIEW_869_PATCH
- global ENABLE_MAC_NOTITLEBAR_PATCH
- global USE_CUSTOM_TITLEBAR
- global CUSTOM_TITLEBAR_BACKGROUND_COLOR
- global CUSTOM_TITLEBAR_TEXT_COLOR
- global CUSTOM_TITLEBAR_ICON
- global CUSTOM_TITLEBAR_FONT
- global DEFAULT_USER_SETTINGS_PATH
- global DEFAULT_USER_SETTINGS_PYSIMPLEGUI_PATH
- global DEFAULT_USER_SETTINGS_PYSIMPLEGUI_FILENAME
- global DEFAULT_KEEP_ON_TOP
- global DEFAULT_SCALING
- global DEFAULT_MODAL_WINDOWS_ENABLED
- global DEFAULT_MODAL_WINDOWS_FORCED
- global DEFAULT_TOOLTIP_OFFSET
- global DEFAULT_ALPHA_CHANNEL
-
- global _pysimplegui_user_settings
- global ttk_part_overrides_from_options
- # global _my_windows
-
- if icon:
- Window._user_defined_icon = icon
- # _my_windows._user_defined_icon = icon
-
- if button_color != None:
- if button_color == COLOR_SYSTEM_DEFAULT:
- DEFAULT_BUTTON_COLOR = (COLOR_SYSTEM_DEFAULT, COLOR_SYSTEM_DEFAULT)
- else:
- DEFAULT_BUTTON_COLOR = button_color
-
- if element_size != (None, None):
- DEFAULT_ELEMENT_SIZE = element_size
-
- if button_element_size != (None, None):
- DEFAULT_BUTTON_ELEMENT_SIZE = button_element_size
-
- if margins != (None, None):
- DEFAULT_MARGINS = margins
-
- if element_padding != (None, None):
- DEFAULT_ELEMENT_PADDING = element_padding
-
- if auto_size_text != None:
- DEFAULT_AUTOSIZE_TEXT = auto_size_text
-
- if auto_size_buttons != None:
- DEFAULT_AUTOSIZE_BUTTONS = auto_size_buttons
-
- if font != None:
- DEFAULT_FONT = font
-
- if border_width != None:
- DEFAULT_BORDER_WIDTH = border_width
-
- if autoclose_time != None:
- DEFAULT_AUTOCLOSE_TIME = autoclose_time
-
- if message_box_line_width != None:
- MESSAGE_BOX_LINE_WIDTH = message_box_line_width
-
- if progress_meter_border_depth != None:
- DEFAULT_PROGRESS_BAR_BORDER_WIDTH = progress_meter_border_depth
-
- if progress_meter_style != None:
- warnings.warn('You can no longer set a progress bar style. All ttk styles must be the same for the window', UserWarning)
- # DEFAULT_PROGRESS_BAR_STYLE = progress_meter_style
-
- if progress_meter_relief != None:
- DEFAULT_PROGRESS_BAR_RELIEF = progress_meter_relief
-
- if progress_meter_color != None:
- DEFAULT_PROGRESS_BAR_COLOR = progress_meter_color
-
- if progress_meter_size != None:
- DEFAULT_PROGRESS_BAR_SIZE = progress_meter_size
-
- if slider_border_width != None:
- DEFAULT_SLIDER_BORDER_WIDTH = slider_border_width
-
- if slider_orientation != None:
- DEFAULT_SLIDER_ORIENTATION = slider_orientation
-
- if slider_relief != None:
- DEFAULT_SLIDER_RELIEF = slider_relief
-
- if text_justification != None:
- DEFAULT_TEXT_JUSTIFICATION = text_justification
-
- if background_color != None:
- DEFAULT_BACKGROUND_COLOR = background_color
-
- if text_element_background_color != None:
- DEFAULT_TEXT_ELEMENT_BACKGROUND_COLOR = text_element_background_color
-
- if input_elements_background_color != None:
- DEFAULT_INPUT_ELEMENTS_COLOR = input_elements_background_color
-
- if element_background_color != None:
- DEFAULT_ELEMENT_BACKGROUND_COLOR = element_background_color
-
- if window_location != (None, None):
- DEFAULT_WINDOW_LOCATION = window_location
-
- if debug_win_size != (None, None):
- DEFAULT_DEBUG_WINDOW_SIZE = debug_win_size
-
- if text_color != None:
- DEFAULT_TEXT_COLOR = text_color
-
- if scrollbar_color != None:
- DEFAULT_SCROLLBAR_COLOR = scrollbar_color
-
- if element_text_color != None:
- DEFAULT_ELEMENT_TEXT_COLOR = element_text_color
-
- if input_text_color is not None:
- DEFAULT_INPUT_TEXT_COLOR = input_text_color
-
- if tooltip_time is not None:
- DEFAULT_TOOLTIP_TIME = tooltip_time
-
- if error_button_color != (None, None):
- DEFAULT_ERROR_BUTTON_COLOR = error_button_color
-
- if ttk_theme is not None:
- DEFAULT_TTK_THEME = ttk_theme
-
- if use_ttk_buttons is not None:
- USE_TTK_BUTTONS = use_ttk_buttons
-
- if tooltip_font is not None:
- TOOLTIP_FONT = tooltip_font
-
- if suppress_error_popups is not None:
- SUPPRESS_ERROR_POPUPS = suppress_error_popups
-
- if suppress_raise_key_errors is not None:
- SUPPRESS_RAISE_KEY_ERRORS = suppress_raise_key_errors
-
- if suppress_key_guessing is not None:
- SUPPRESS_KEY_GUESSING = suppress_key_guessing
-
- if warn_button_key_duplicates is not None:
- WARN_DUPLICATE_BUTTON_KEY_ERRORS = warn_button_key_duplicates
-
- if enable_treeview_869_patch is not None:
- ENABLE_TREEVIEW_869_PATCH = enable_treeview_869_patch
-
- if enable_mac_notitlebar_patch is not None:
- ENABLE_MAC_NOTITLEBAR_PATCH = enable_mac_notitlebar_patch
-
- if use_custom_titlebar is not None:
- USE_CUSTOM_TITLEBAR = use_custom_titlebar
-
- if titlebar_background_color is not None:
- CUSTOM_TITLEBAR_BACKGROUND_COLOR = titlebar_background_color
-
- if titlebar_text_color is not None:
- CUSTOM_TITLEBAR_TEXT_COLOR = titlebar_text_color
-
- if titlebar_font is not None:
- CUSTOM_TITLEBAR_FONT = titlebar_font
-
- if titlebar_icon is not None:
- CUSTOM_TITLEBAR_ICON = titlebar_icon
-
- if user_settings_path is not None:
- DEFAULT_USER_SETTINGS_PATH = user_settings_path
-
- if pysimplegui_settings_path is not None:
- DEFAULT_USER_SETTINGS_PYSIMPLEGUI_PATH = pysimplegui_settings_path
-
- if pysimplegui_settings_filename is not None:
- DEFAULT_USER_SETTINGS_PYSIMPLEGUI_FILENAME = pysimplegui_settings_filename
-
- if pysimplegui_settings_filename is not None or pysimplegui_settings_filename is not None:
- _pysimplegui_user_settings = UserSettings(filename=DEFAULT_USER_SETTINGS_PYSIMPLEGUI_FILENAME,
- path=DEFAULT_USER_SETTINGS_PYSIMPLEGUI_PATH)
-
- if keep_on_top is not None:
- DEFAULT_KEEP_ON_TOP = keep_on_top
-
- if dpi_awareness is True:
- if running_windows():
- if platform.release() == "7":
- ctypes.windll.user32.SetProcessDPIAware()
- elif platform.release() == "8" or platform.release() == "10":
- ctypes.windll.shcore.SetProcessDpiAwareness(1)
-
- if scaling is not None:
- DEFAULT_SCALING = scaling
-
- if disable_modal_windows is not None:
- DEFAULT_MODAL_WINDOWS_ENABLED = not disable_modal_windows
-
- if force_modal_windows is not None:
- DEFAULT_MODAL_WINDOWS_FORCED = force_modal_windows
-
- if tooltip_offset != (None, None):
- DEFAULT_TOOLTIP_OFFSET = tooltip_offset
-
- if alpha_channel is not None:
- DEFAULT_ALPHA_CHANNEL = alpha_channel
-
- # ---------------- ttk scrollbar section ----------------
- if sbar_background_color is not None:
- ttk_part_overrides_from_options.sbar_background_color = sbar_background_color
-
- if sbar_trough_color is not None:
- ttk_part_overrides_from_options.sbar_trough_color = sbar_trough_color
-
- if sbar_arrow_color is not None:
- ttk_part_overrides_from_options.sbar_arrow_color = sbar_arrow_color
-
- if sbar_frame_color is not None:
- ttk_part_overrides_from_options.sbar_frame_color = sbar_frame_color
-
- if sbar_relief is not None:
- ttk_part_overrides_from_options.sbar_relief = sbar_relief
-
- if sbar_arrow_width is not None:
- ttk_part_overrides_from_options.sbar_arrow_width = sbar_arrow_width
-
- if sbar_width is not None:
- ttk_part_overrides_from_options.sbar_width = sbar_width
-
- return True
-
-
-# ----------------------------------------------------------------- #
-
-# .########.##.....##.########.##.....##.########..######.
-# ....##....##.....##.##.......###...###.##.......##....##
-# ....##....##.....##.##.......####.####.##.......##......
-# ....##....#########.######...##.###.##.######....######.
-# ....##....##.....##.##.......##.....##.##.............##
-# ....##....##.....##.##.......##.....##.##.......##....##
-# ....##....##.....##.########.##.....##.########..######.
-
-# ----------------------------------------------------------------- #
-
-# The official Theme code
-
-#################### ChangeLookAndFeel #######################
-# Predefined settings that will change the colors and styles #
-# of the elements. #
-##############################################################
-LOOK_AND_FEEL_TABLE = {
- "SystemDefault": {"BACKGROUND": COLOR_SYSTEM_DEFAULT, "TEXT": COLOR_SYSTEM_DEFAULT, "INPUT": COLOR_SYSTEM_DEFAULT, "TEXT_INPUT": COLOR_SYSTEM_DEFAULT,
- "SCROLL": COLOR_SYSTEM_DEFAULT, "BUTTON": OFFICIAL_PYSIMPLEGUI_BUTTON_COLOR, "PROGRESS": COLOR_SYSTEM_DEFAULT, "BORDER": 1,
- "SLIDER_DEPTH": 1, "PROGRESS_DEPTH": 0, },
- "SystemDefaultForReal": {"BACKGROUND": COLOR_SYSTEM_DEFAULT, "TEXT": COLOR_SYSTEM_DEFAULT, "INPUT": COLOR_SYSTEM_DEFAULT,
- "TEXT_INPUT": COLOR_SYSTEM_DEFAULT, "SCROLL": COLOR_SYSTEM_DEFAULT, "BUTTON": COLOR_SYSTEM_DEFAULT,
- "PROGRESS": COLOR_SYSTEM_DEFAULT, "BORDER": 1, "SLIDER_DEPTH": 1, "PROGRESS_DEPTH": 0, },
- "SystemDefault1": {"BACKGROUND": COLOR_SYSTEM_DEFAULT, "TEXT": COLOR_SYSTEM_DEFAULT, "INPUT": COLOR_SYSTEM_DEFAULT, "TEXT_INPUT": COLOR_SYSTEM_DEFAULT,
- "SCROLL": COLOR_SYSTEM_DEFAULT, "BUTTON": COLOR_SYSTEM_DEFAULT, "PROGRESS": COLOR_SYSTEM_DEFAULT, "BORDER": 1, "SLIDER_DEPTH": 1,
- "PROGRESS_DEPTH": 0, },
- "Material1": {"BACKGROUND": "#E3F2FD", "TEXT": "#000000", "INPUT": "#86A8FF", "TEXT_INPUT": "#000000", "SCROLL": "#86A8FF",
- "BUTTON": ("#FFFFFF", "#5079D3"), "PROGRESS": DEFAULT_PROGRESS_BAR_COMPUTE, "BORDER": 0, "SLIDER_DEPTH": 0, "PROGRESS_DEPTH": 0,
- "ACCENT1": "#FF0266", "ACCENT2": "#FF5C93", "ACCENT3": "#C5003C", },
- "Material2": {"BACKGROUND": "#FAFAFA", "TEXT": "#000000", "INPUT": "#004EA1", "TEXT_INPUT": "#FFFFFF", "SCROLL": "#5EA7FF",
- "BUTTON": ("#FFFFFF", "#0079D3"), "PROGRESS": DEFAULT_PROGRESS_BAR_COMPUTE, "BORDER": 0, "SLIDER_DEPTH": 0, "PROGRESS_DEPTH": 0,
- "ACCENT1": "#FF0266", "ACCENT2": "#FF5C93", "ACCENT3": "#C5003C", },
- "Reddit": {"BACKGROUND": "#ffffff", "TEXT": "#1a1a1b", "INPUT": "#dae0e6", "TEXT_INPUT": "#222222", "SCROLL": "#a5a4a4", "BUTTON": ("#FFFFFF", "#0079d3"),
- "PROGRESS": DEFAULT_PROGRESS_BAR_COMPUTE, "BORDER": 1, "SLIDER_DEPTH": 0, "PROGRESS_DEPTH": 0, "ACCENT1": "#ff5414", "ACCENT2": "#33a8ff",
- "ACCENT3": "#dbf0ff", },
- "Topanga": {"BACKGROUND": "#282923", "TEXT": "#E7DB74", "INPUT": "#393a32", "TEXT_INPUT": "#E7C855", "SCROLL": "#E7C855", "BUTTON": ("#E7C855", "#284B5A"),
- "PROGRESS": DEFAULT_PROGRESS_BAR_COMPUTE, "BORDER": 1, "SLIDER_DEPTH": 0, "PROGRESS_DEPTH": 0, "ACCENT1": "#c15226", "ACCENT2": "#7a4d5f",
- "ACCENT3": "#889743", },
- "GreenTan": {"BACKGROUND": "#9FB8AD", "TEXT": '#000000', "INPUT": "#F7F3EC", "TEXT_INPUT": "#000000", "SCROLL": "#F7F3EC", "BUTTON": ("#FFFFFF", "#475841"),
- "PROGRESS": DEFAULT_PROGRESS_BAR_COMPUTE, "BORDER": 1, "SLIDER_DEPTH": 0, "PROGRESS_DEPTH": 0, },
- "Dark": {"BACKGROUND": "#404040", "TEXT": "#FFFFFF", "INPUT": "#4D4D4D", "TEXT_INPUT": "#FFFFFF", "SCROLL": "#707070", "BUTTON": ("#FFFFFF", "#004F00"),
- "PROGRESS": DEFAULT_PROGRESS_BAR_COMPUTE, "BORDER": 1, "SLIDER_DEPTH": 0, "PROGRESS_DEPTH": 0, },
- "LightGreen": {"BACKGROUND": "#B7CECE", "TEXT": "#000000", "INPUT": "#FDFFF7", "TEXT_INPUT": "#000000", "SCROLL": "#FDFFF7",
- "BUTTON": ("#FFFFFF", "#658268"), "PROGRESS": DEFAULT_PROGRESS_BAR_COMPUTE, "BORDER": 1, "SLIDER_DEPTH": 0, "ACCENT1": "#76506d",
- "ACCENT2": "#5148f1", "ACCENT3": "#0a1c84", "PROGRESS_DEPTH": 0, },
- "Dark2": {"BACKGROUND": "#404040", "TEXT": "#FFFFFF", "INPUT": "#FFFFFF", "TEXT_INPUT": "#000000", "SCROLL": "#707070", "BUTTON": ("#FFFFFF", "#004F00"),
- "PROGRESS": DEFAULT_PROGRESS_BAR_COMPUTE, "BORDER": 1, "SLIDER_DEPTH": 0, "PROGRESS_DEPTH": 0, },
- "Black": {"BACKGROUND": "#000000", "TEXT": "#FFFFFF", "INPUT": "#4D4D4D", "TEXT_INPUT": "#FFFFFF", "SCROLL": "#707070", "BUTTON": ("#000000", "#FFFFFF"),
- "PROGRESS": DEFAULT_PROGRESS_BAR_COMPUTE, "BORDER": 1, "SLIDER_DEPTH": 0, "PROGRESS_DEPTH": 0, },
- "Tan": {"BACKGROUND": "#fdf6e3", "TEXT": "#268bd1", "INPUT": "#eee8d5", "TEXT_INPUT": "#6c71c3", "SCROLL": "#eee8d5", "BUTTON": ("#FFFFFF", "#063542"),
- "PROGRESS": DEFAULT_PROGRESS_BAR_COMPUTE, "BORDER": 1, "SLIDER_DEPTH": 0, "PROGRESS_DEPTH": 0, },
- "TanBlue": {"BACKGROUND": "#e5dece", "TEXT": "#063289", "INPUT": "#f9f8f4", "TEXT_INPUT": "#242834", "SCROLL": "#eee8d5", "BUTTON": ("#FFFFFF", "#063289"),
- "PROGRESS": DEFAULT_PROGRESS_BAR_COMPUTE, "BORDER": 1, "SLIDER_DEPTH": 0, "PROGRESS_DEPTH": 0, },
- "DarkTanBlue": {"BACKGROUND": "#242834", "TEXT": "#dfe6f8", "INPUT": "#97755c", "TEXT_INPUT": "#FFFFFF", "SCROLL": "#a9afbb",
- "BUTTON": ("#FFFFFF", "#063289"), "PROGRESS": DEFAULT_PROGRESS_BAR_COMPUTE, "BORDER": 1, "SLIDER_DEPTH": 0, "PROGRESS_DEPTH": 0, },
- "DarkAmber": {"BACKGROUND": "#2c2825", "TEXT": "#fdcb52", "INPUT": "#705e52", "TEXT_INPUT": "#fdcb52", "SCROLL": "#705e52",
- "BUTTON": ("#000000", "#fdcb52"), "PROGRESS": DEFAULT_PROGRESS_BAR_COMPUTE, "BORDER": 1, "SLIDER_DEPTH": 0, "PROGRESS_DEPTH": 0, },
- "DarkBlue": {"BACKGROUND": "#1a2835", "TEXT": "#d1ecff", "INPUT": "#335267", "TEXT_INPUT": "#acc2d0", "SCROLL": "#1b6497", "BUTTON": ("#000000", "#fafaf8"),
- "PROGRESS": DEFAULT_PROGRESS_BAR_COMPUTE, "BORDER": 1, "SLIDER_DEPTH": 0, "PROGRESS_DEPTH": 0, },
- "Reds": {"BACKGROUND": "#280001", "TEXT": "#FFFFFF", "INPUT": "#d8d584", "TEXT_INPUT": "#000000", "SCROLL": "#763e00", "BUTTON": ("#000000", "#daad28"),
- "PROGRESS": DEFAULT_PROGRESS_BAR_COMPUTE, "BORDER": 1, "SLIDER_DEPTH": 0, "PROGRESS_DEPTH": 0, },
- "Green": {"BACKGROUND": "#82a459", "TEXT": "#000000", "INPUT": "#d8d584", "TEXT_INPUT": "#000000", "SCROLL": "#e3ecf3", "BUTTON": ("#FFFFFF", "#517239"),
- "PROGRESS": DEFAULT_PROGRESS_BAR_COMPUTE, "BORDER": 1, "SLIDER_DEPTH": 0, "PROGRESS_DEPTH": 0, },
- "BluePurple": {"BACKGROUND": "#A5CADD", "TEXT": "#6E266E", "INPUT": "#E0F5FF", "TEXT_INPUT": "#000000", "SCROLL": "#E0F5FF",
- "BUTTON": ("#FFFFFF", "#303952"), "PROGRESS": DEFAULT_PROGRESS_BAR_COMPUTE, "BORDER": 1, "SLIDER_DEPTH": 0, "PROGRESS_DEPTH": 0, },
- "Purple": {"BACKGROUND": "#B0AAC2", "TEXT": "#000000", "INPUT": "#F2EFE8", "SCROLL": "#F2EFE8", "TEXT_INPUT": "#000000", "BUTTON": ("#000000", "#C2D4D8"),
- "PROGRESS": DEFAULT_PROGRESS_BAR_COMPUTE, "BORDER": 1, "SLIDER_DEPTH": 0, "PROGRESS_DEPTH": 0, },
- "BlueMono": {"BACKGROUND": "#AAB6D3", "TEXT": "#000000", "INPUT": "#F1F4FC", "SCROLL": "#F1F4FC", "TEXT_INPUT": "#000000", "BUTTON": ("#FFFFFF", "#7186C7"),
- "PROGRESS": DEFAULT_PROGRESS_BAR_COMPUTE, "BORDER": 1, "SLIDER_DEPTH": 0, "PROGRESS_DEPTH": 0, },
- "GreenMono": {"BACKGROUND": "#A8C1B4", "TEXT": "#000000", "INPUT": "#DDE0DE", "SCROLL": "#E3E3E3", "TEXT_INPUT": "#000000",
- "BUTTON": ("#FFFFFF", "#6D9F85"), "PROGRESS": DEFAULT_PROGRESS_BAR_COMPUTE, "BORDER": 1, "SLIDER_DEPTH": 0, "PROGRESS_DEPTH": 0, },
- "BrownBlue": {"BACKGROUND": "#64778d", "TEXT": "#FFFFFF", "INPUT": "#f0f3f7", "SCROLL": "#A6B2BE", "TEXT_INPUT": "#000000",
- "BUTTON": ("#FFFFFF", "#283b5b"), "PROGRESS": DEFAULT_PROGRESS_BAR_COMPUTE, "BORDER": 1, "SLIDER_DEPTH": 0, "PROGRESS_DEPTH": 0, },
- "BrightColors": {"BACKGROUND": "#b4ffb4", "TEXT": "#000000", "INPUT": "#ffff64", "SCROLL": "#ffb482", "TEXT_INPUT": "#000000",
- "BUTTON": ("#000000", "#ffa0dc"), "PROGRESS": DEFAULT_PROGRESS_BAR_COMPUTE, "BORDER": 1, "SLIDER_DEPTH": 0, "PROGRESS_DEPTH": 0, },
- "NeutralBlue": {"BACKGROUND": "#92aa9d", "TEXT": "#000000", "INPUT": "#fcfff6", "SCROLL": "#fcfff6", "TEXT_INPUT": "#000000",
- "BUTTON": ("#000000", "#d0dbbd"), "PROGRESS": DEFAULT_PROGRESS_BAR_COMPUTE, "BORDER": 1, "SLIDER_DEPTH": 0, "PROGRESS_DEPTH": 0, },
- "Kayak": {"BACKGROUND": "#a7ad7f", "TEXT": "#000000", "INPUT": "#e6d3a8", "SCROLL": "#e6d3a8", "TEXT_INPUT": "#000000", "BUTTON": ("#FFFFFF", "#5d907d"),
- "PROGRESS": DEFAULT_PROGRESS_BAR_COMPUTE, "BORDER": 1, "SLIDER_DEPTH": 0, "PROGRESS_DEPTH": 0, },
- "SandyBeach": {"BACKGROUND": "#efeccb", "TEXT": "#012f2f", "INPUT": "#e6d3a8", "SCROLL": "#e6d3a8", "TEXT_INPUT": "#012f2f",
- "BUTTON": ("#FFFFFF", "#046380"), "PROGRESS": DEFAULT_PROGRESS_BAR_COMPUTE, "BORDER": 1, "SLIDER_DEPTH": 0, "PROGRESS_DEPTH": 0, },
- "TealMono": {"BACKGROUND": "#a8cfdd", "TEXT": "#000000", "INPUT": "#dfedf2", "SCROLL": "#dfedf2", "TEXT_INPUT": "#000000", "BUTTON": ("#FFFFFF", "#183440"),
- "PROGRESS": DEFAULT_PROGRESS_BAR_COMPUTE, "BORDER": 1, "SLIDER_DEPTH": 0, "PROGRESS_DEPTH": 0, },
- "Default": {"BACKGROUND": COLOR_SYSTEM_DEFAULT, "TEXT": COLOR_SYSTEM_DEFAULT, "INPUT": COLOR_SYSTEM_DEFAULT, "TEXT_INPUT": COLOR_SYSTEM_DEFAULT,
- "SCROLL": COLOR_SYSTEM_DEFAULT, "BUTTON": OFFICIAL_PYSIMPLEGUI_BUTTON_COLOR, "PROGRESS": COLOR_SYSTEM_DEFAULT, "BORDER": 1, "SLIDER_DEPTH": 1,
- "PROGRESS_DEPTH": 0, },
- "Default1": {"BACKGROUND": COLOR_SYSTEM_DEFAULT, "TEXT": COLOR_SYSTEM_DEFAULT, "INPUT": COLOR_SYSTEM_DEFAULT, "TEXT_INPUT": COLOR_SYSTEM_DEFAULT,
- "SCROLL": COLOR_SYSTEM_DEFAULT, "BUTTON": COLOR_SYSTEM_DEFAULT, "PROGRESS": COLOR_SYSTEM_DEFAULT, "BORDER": 1, "SLIDER_DEPTH": 1,
- "PROGRESS_DEPTH": 0, },
- "DefaultNoMoreNagging": {"BACKGROUND": COLOR_SYSTEM_DEFAULT, "TEXT": COLOR_SYSTEM_DEFAULT, "INPUT": COLOR_SYSTEM_DEFAULT,
- "TEXT_INPUT": COLOR_SYSTEM_DEFAULT, "SCROLL": COLOR_SYSTEM_DEFAULT, "BUTTON": OFFICIAL_PYSIMPLEGUI_BUTTON_COLOR,
- "PROGRESS": COLOR_SYSTEM_DEFAULT, "BORDER": 1, "SLIDER_DEPTH": 1, "PROGRESS_DEPTH": 0, },
- "GrayGrayGray": {"BACKGROUND": COLOR_SYSTEM_DEFAULT, "TEXT": COLOR_SYSTEM_DEFAULT, "INPUT": COLOR_SYSTEM_DEFAULT, "TEXT_INPUT": COLOR_SYSTEM_DEFAULT,
- "SCROLL": COLOR_SYSTEM_DEFAULT, "BUTTON": COLOR_SYSTEM_DEFAULT, "PROGRESS": COLOR_SYSTEM_DEFAULT, "BORDER": 1, "SLIDER_DEPTH": 1,
- "PROGRESS_DEPTH": 0, },
- "LightBlue": {"BACKGROUND": "#E3F2FD", "TEXT": "#000000", "INPUT": "#86A8FF", "TEXT_INPUT": "#000000", "SCROLL": "#86A8FF",
- "BUTTON": ("#FFFFFF", "#5079D3"), "PROGRESS": DEFAULT_PROGRESS_BAR_COMPUTE, "BORDER": 0, "SLIDER_DEPTH": 0, "PROGRESS_DEPTH": 0,
- "ACCENT1": "#FF0266", "ACCENT2": "#FF5C93", "ACCENT3": "#C5003C", },
- "LightGrey": {"BACKGROUND": "#FAFAFA", "TEXT": "#000000", "INPUT": "#004EA1", "TEXT_INPUT": "#FFFFFF", "SCROLL": "#5EA7FF",
- "BUTTON": ("#FFFFFF", "#0079D3"), "PROGRESS": DEFAULT_PROGRESS_BAR_COMPUTE, "BORDER": 0, "SLIDER_DEPTH": 0, "PROGRESS_DEPTH": 0,
- "ACCENT1": "#FF0266", "ACCENT2": "#FF5C93", "ACCENT3": "#C5003C", },
- "LightGrey1": {"BACKGROUND": "#ffffff", "TEXT": "#1a1a1b", "INPUT": "#dae0e6", "TEXT_INPUT": "#222222", "SCROLL": "#a5a4a4",
- "BUTTON": ("#FFFFFF", "#0079d3"), "PROGRESS": DEFAULT_PROGRESS_BAR_COMPUTE, "BORDER": 1, "SLIDER_DEPTH": 0, "PROGRESS_DEPTH": 0,
- "ACCENT1": "#ff5414", "ACCENT2": "#33a8ff", "ACCENT3": "#dbf0ff", },
- "DarkBrown": {"BACKGROUND": "#282923", "TEXT": "#E7DB74", "INPUT": "#393a32", "TEXT_INPUT": "#E7C855", "SCROLL": "#E7C855",
- "BUTTON": ("#E7C855", "#284B5A"), "PROGRESS": DEFAULT_PROGRESS_BAR_COMPUTE, "BORDER": 1, "SLIDER_DEPTH": 0, "PROGRESS_DEPTH": 0,
- "ACCENT1": "#c15226", "ACCENT2": "#7a4d5f", "ACCENT3": "#889743", },
- "LightGreen1": {"BACKGROUND": "#9FB8AD", "TEXT": "#000000", "INPUT": "#F7F3EC", "TEXT_INPUT": "#000000", "SCROLL": "#F7F3EC",
- "BUTTON": ("#FFFFFF", "#475841"), "PROGRESS": DEFAULT_PROGRESS_BAR_COMPUTE, "BORDER": 1, "SLIDER_DEPTH": 0, "PROGRESS_DEPTH": 0, },
- "DarkGrey": {"BACKGROUND": "#404040", "TEXT": "#FFFFFF", "INPUT": "#4D4D4D", "TEXT_INPUT": "#FFFFFF", "SCROLL": "#707070", "BUTTON": ("#FFFFFF", "#004F00"),
- "PROGRESS": DEFAULT_PROGRESS_BAR_COMPUTE, "BORDER": 1, "SLIDER_DEPTH": 0, "PROGRESS_DEPTH": 0, },
- "LightGreen2": {"BACKGROUND": "#B7CECE", "TEXT": "#000000", "INPUT": "#FDFFF7", "TEXT_INPUT": "#000000", "SCROLL": "#FDFFF7",
- "BUTTON": ("#FFFFFF", "#658268"), "PROGRESS": DEFAULT_PROGRESS_BAR_COMPUTE, "BORDER": 1, "SLIDER_DEPTH": 0, "ACCENT1": "#76506d",
- "ACCENT2": "#5148f1", "ACCENT3": "#0a1c84", "PROGRESS_DEPTH": 0, },
- "DarkGrey1": {"BACKGROUND": "#404040", "TEXT": "#FFFFFF", "INPUT": "#FFFFFF", "TEXT_INPUT": "#000000", "SCROLL": "#707070",
- "BUTTON": ("#FFFFFF", "#004F00"), "PROGRESS": DEFAULT_PROGRESS_BAR_COMPUTE, "BORDER": 1, "SLIDER_DEPTH": 0, "PROGRESS_DEPTH": 0, },
- "DarkBlack": {"BACKGROUND": "#000000", "TEXT": "#FFFFFF", "INPUT": "#4D4D4D", "TEXT_INPUT": "#FFFFFF", "SCROLL": "#707070",
- "BUTTON": ("#000000", "#FFFFFF"), "PROGRESS": DEFAULT_PROGRESS_BAR_COMPUTE, "BORDER": 1, "SLIDER_DEPTH": 0, "PROGRESS_DEPTH": 0, },
- "LightBrown": {"BACKGROUND": "#fdf6e3", "TEXT": "#268bd1", "INPUT": "#eee8d5", "TEXT_INPUT": "#6c71c3", "SCROLL": "#eee8d5",
- "BUTTON": ("#FFFFFF", "#063542"), "PROGRESS": DEFAULT_PROGRESS_BAR_COMPUTE, "BORDER": 1, "SLIDER_DEPTH": 0, "PROGRESS_DEPTH": 0, },
- "LightBrown1": {"BACKGROUND": "#e5dece", "TEXT": "#063289", "INPUT": "#f9f8f4", "TEXT_INPUT": "#242834", "SCROLL": "#eee8d5",
- "BUTTON": ("#FFFFFF", "#063289"), "PROGRESS": DEFAULT_PROGRESS_BAR_COMPUTE, "BORDER": 1, "SLIDER_DEPTH": 0, "PROGRESS_DEPTH": 0, },
- "DarkBlue1": {"BACKGROUND": "#242834", "TEXT": "#dfe6f8", "INPUT": "#97755c", "TEXT_INPUT": "#FFFFFF", "SCROLL": "#a9afbb",
- "BUTTON": ("#FFFFFF", "#063289"), "PROGRESS": DEFAULT_PROGRESS_BAR_COMPUTE, "BORDER": 1, "SLIDER_DEPTH": 0, "PROGRESS_DEPTH": 0, },
- "DarkBrown1": {"BACKGROUND": "#2c2825", "TEXT": "#fdcb52", "INPUT": "#705e52", "TEXT_INPUT": "#fdcb52", "SCROLL": "#705e52",
- "BUTTON": ("#000000", "#fdcb52"), "PROGRESS": DEFAULT_PROGRESS_BAR_COMPUTE, "BORDER": 1, "SLIDER_DEPTH": 0, "PROGRESS_DEPTH": 0, },
- "DarkBlue2": {"BACKGROUND": "#1a2835", "TEXT": "#d1ecff", "INPUT": "#335267", "TEXT_INPUT": "#acc2d0", "SCROLL": "#1b6497",
- "BUTTON": ("#000000", "#fafaf8"), "PROGRESS": DEFAULT_PROGRESS_BAR_COMPUTE, "BORDER": 1, "SLIDER_DEPTH": 0, "PROGRESS_DEPTH": 0, },
- "DarkBrown2": {"BACKGROUND": "#280001", "TEXT": "#FFFFFF", "INPUT": "#d8d584", "TEXT_INPUT": "#000000", "SCROLL": "#763e00",
- "BUTTON": ("#000000", "#daad28"), "PROGRESS": DEFAULT_PROGRESS_BAR_COMPUTE, "BORDER": 1, "SLIDER_DEPTH": 0, "PROGRESS_DEPTH": 0, },
- "DarkGreen": {"BACKGROUND": "#82a459", "TEXT": "#000000", "INPUT": "#d8d584", "TEXT_INPUT": "#000000", "SCROLL": "#e3ecf3",
- "BUTTON": ("#FFFFFF", "#517239"), "PROGRESS": DEFAULT_PROGRESS_BAR_COMPUTE, "BORDER": 1, "SLIDER_DEPTH": 0, "PROGRESS_DEPTH": 0, },
- "LightBlue1": {"BACKGROUND": "#A5CADD", "TEXT": "#6E266E", "INPUT": "#E0F5FF", "TEXT_INPUT": "#000000", "SCROLL": "#E0F5FF",
- "BUTTON": ("#FFFFFF", "#303952"), "PROGRESS": DEFAULT_PROGRESS_BAR_COMPUTE, "BORDER": 1, "SLIDER_DEPTH": 0, "PROGRESS_DEPTH": 0, },
- "LightPurple": {"BACKGROUND": "#B0AAC2", "TEXT": "#000000", "INPUT": "#F2EFE8", "SCROLL": "#F2EFE8", "TEXT_INPUT": "#000000",
- "BUTTON": ("#000000", "#C2D4D8"), "PROGRESS": DEFAULT_PROGRESS_BAR_COMPUTE, "BORDER": 1, "SLIDER_DEPTH": 0, "PROGRESS_DEPTH": 0, },
- "LightBlue2": {"BACKGROUND": "#AAB6D3", "TEXT": "#000000", "INPUT": "#F1F4FC", "SCROLL": "#F1F4FC", "TEXT_INPUT": "#000000",
- "BUTTON": ("#FFFFFF", "#7186C7"), "PROGRESS": DEFAULT_PROGRESS_BAR_COMPUTE, "BORDER": 1, "SLIDER_DEPTH": 0, "PROGRESS_DEPTH": 0, },
- "LightGreen3": {"BACKGROUND": "#A8C1B4", "TEXT": "#000000", "INPUT": "#DDE0DE", "SCROLL": "#E3E3E3", "TEXT_INPUT": "#000000",
- "BUTTON": ("#FFFFFF", "#6D9F85"), "PROGRESS": DEFAULT_PROGRESS_BAR_COMPUTE, "BORDER": 1, "SLIDER_DEPTH": 0, "PROGRESS_DEPTH": 0, },
- "DarkBlue3": {"BACKGROUND": "#64778d", "TEXT": "#FFFFFF", "INPUT": "#f0f3f7", "SCROLL": "#A6B2BE", "TEXT_INPUT": "#000000",
- "BUTTON": ("#FFFFFF", "#283b5b"), "PROGRESS": DEFAULT_PROGRESS_BAR_COMPUTE, "BORDER": 1, "SLIDER_DEPTH": 0, "PROGRESS_DEPTH": 0, },
- "LightGreen4": {"BACKGROUND": "#b4ffb4", "TEXT": "#000000", "INPUT": "#ffff64", "SCROLL": "#ffb482", "TEXT_INPUT": "#000000",
- "BUTTON": ("#000000", "#ffa0dc"), "PROGRESS": DEFAULT_PROGRESS_BAR_COMPUTE, "BORDER": 1, "SLIDER_DEPTH": 0, "PROGRESS_DEPTH": 0, },
- "LightGreen5": {"BACKGROUND": "#92aa9d", "TEXT": "#000000", "INPUT": "#fcfff6", "SCROLL": "#fcfff6", "TEXT_INPUT": "#000000",
- "BUTTON": ("#000000", "#d0dbbd"), "PROGRESS": DEFAULT_PROGRESS_BAR_COMPUTE, "BORDER": 1, "SLIDER_DEPTH": 0, "PROGRESS_DEPTH": 0, },
- "LightBrown2": {"BACKGROUND": "#a7ad7f", "TEXT": "#000000", "INPUT": "#e6d3a8", "SCROLL": "#e6d3a8", "TEXT_INPUT": "#000000",
- "BUTTON": ("#FFFFFF", "#5d907d"), "PROGRESS": DEFAULT_PROGRESS_BAR_COMPUTE, "BORDER": 1, "SLIDER_DEPTH": 0, "PROGRESS_DEPTH": 0, },
- "LightBrown3": {"BACKGROUND": "#efeccb", "TEXT": "#012f2f", "INPUT": "#e6d3a8", "SCROLL": "#e6d3a8", "TEXT_INPUT": "#012f2f",
- "BUTTON": ("#FFFFFF", "#046380"), "PROGRESS": DEFAULT_PROGRESS_BAR_COMPUTE, "BORDER": 1, "SLIDER_DEPTH": 0, "PROGRESS_DEPTH": 0, },
- "LightBlue3": {"BACKGROUND": "#a8cfdd", "TEXT": "#000000", "INPUT": "#dfedf2", "SCROLL": "#dfedf2", "TEXT_INPUT": "#000000",
- "BUTTON": ("#FFFFFF", "#183440"), "PROGRESS": DEFAULT_PROGRESS_BAR_COMPUTE, "BORDER": 1, "SLIDER_DEPTH": 0, "PROGRESS_DEPTH": 0, },
- "LightBrown4": {"BACKGROUND": "#d7c79e", "TEXT": "#a35638", "INPUT": "#9dab86", "TEXT_INPUT": "#000000", "SCROLL": "#a35638",
- "BUTTON": ("#FFFFFF", "#a35638"), "PROGRESS": DEFAULT_PROGRESS_BAR_COMPUTE, "BORDER": 1, "SLIDER_DEPTH": 0, "PROGRESS_DEPTH": 0,
- "COLOR_LIST": ["#a35638", "#9dab86", "#e08f62", "#d7c79e"], },
- "DarkTeal": {"BACKGROUND": "#003f5c", "TEXT": "#fb5b5a", "INPUT": "#bc4873", "TEXT_INPUT": "#FFFFFF", "SCROLL": "#bc4873", "BUTTON": ("#FFFFFF", "#fb5b5a"),
- "PROGRESS": DEFAULT_PROGRESS_BAR_COMPUTE, "BORDER": 1, "SLIDER_DEPTH": 0, "PROGRESS_DEPTH": 0,
- "COLOR_LIST": ["#003f5c", "#472b62", "#bc4873", "#fb5b5a"], },
- "DarkPurple": {"BACKGROUND": "#472b62", "TEXT": "#fb5b5a", "INPUT": "#bc4873", "TEXT_INPUT": "#FFFFFF", "SCROLL": "#bc4873",
- "BUTTON": ("#FFFFFF", "#472b62"), "PROGRESS": DEFAULT_PROGRESS_BAR_COMPUTE, "BORDER": 1, "SLIDER_DEPTH": 0, "PROGRESS_DEPTH": 0,
- "COLOR_LIST": ["#003f5c", "#472b62", "#bc4873", "#fb5b5a"], },
- "LightGreen6": {"BACKGROUND": "#eafbea", "TEXT": "#1f6650", "INPUT": "#6f9a8d", "TEXT_INPUT": "#FFFFFF", "SCROLL": "#1f6650",
- "BUTTON": ("#FFFFFF", "#1f6650"), "PROGRESS": DEFAULT_PROGRESS_BAR_COMPUTE, "BORDER": 1, "SLIDER_DEPTH": 0, "PROGRESS_DEPTH": 0,
- "COLOR_LIST": ["#1f6650", "#6f9a8d", "#ea5e5e", "#eafbea"], },
- "DarkGrey2": {"BACKGROUND": "#2b2b28", "TEXT": "#f8f8f8", "INPUT": "#f1d6ab", "TEXT_INPUT": "#000000", "SCROLL": "#f1d6ab",
- "BUTTON": ("#2b2b28", "#e3b04b"), "PROGRESS": DEFAULT_PROGRESS_BAR_COMPUTE, "BORDER": 1, "SLIDER_DEPTH": 0, "PROGRESS_DEPTH": 0,
- "COLOR_LIST": ["#2b2b28", "#e3b04b", "#f1d6ab", "#f8f8f8"], },
- "LightBrown6": {"BACKGROUND": "#f9b282", "TEXT": "#8f4426", "INPUT": "#de6b35", "TEXT_INPUT": "#FFFFFF", "SCROLL": "#8f4426",
- "BUTTON": ("#FFFFFF", "#8f4426"), "PROGRESS": DEFAULT_PROGRESS_BAR_COMPUTE, "BORDER": 1, "SLIDER_DEPTH": 0, "PROGRESS_DEPTH": 0,
- "COLOR_LIST": ["#8f4426", "#de6b35", "#64ccda", "#f9b282"], },
- "DarkTeal1": {"BACKGROUND": "#396362", "TEXT": "#ffe7d1", "INPUT": "#f6c89f", "TEXT_INPUT": "#000000", "SCROLL": "#f6c89f",
- "BUTTON": ("#ffe7d1", "#4b8e8d"), "PROGRESS": DEFAULT_PROGRESS_BAR_COMPUTE, "BORDER": 1, "SLIDER_DEPTH": 0, "PROGRESS_DEPTH": 0,
- "COLOR_LIST": ["#396362", "#4b8e8d", "#f6c89f", "#ffe7d1"], },
- "LightBrown7": {"BACKGROUND": "#f6c89f", "TEXT": "#396362", "INPUT": "#4b8e8d", "TEXT_INPUT": "#FFFFFF", "SCROLL": "#396362",
- "BUTTON": ("#FFFFFF", "#396362"), "PROGRESS": DEFAULT_PROGRESS_BAR_COMPUTE, "BORDER": 1, "SLIDER_DEPTH": 0, "PROGRESS_DEPTH": 0,
- "COLOR_LIST": ["#396362", "#4b8e8d", "#f6c89f", "#ffe7d1"], },
- "DarkPurple1": {"BACKGROUND": "#0c093c", "TEXT": "#fad6d6", "INPUT": "#eea5f6", "TEXT_INPUT": "#000000", "SCROLL": "#eea5f6",
- "BUTTON": ("#FFFFFF", "#df42d1"), "PROGRESS": DEFAULT_PROGRESS_BAR_COMPUTE, "BORDER": 1, "SLIDER_DEPTH": 0, "PROGRESS_DEPTH": 0,
- "COLOR_LIST": ["#0c093c", "#df42d1", "#eea5f6", "#fad6d6"], },
- "DarkGrey3": {"BACKGROUND": "#211717", "TEXT": "#dfddc7", "INPUT": "#f58b54", "TEXT_INPUT": "#000000", "SCROLL": "#f58b54",
- "BUTTON": ("#dfddc7", "#a34a28"), "PROGRESS": DEFAULT_PROGRESS_BAR_COMPUTE, "BORDER": 1, "SLIDER_DEPTH": 0, "PROGRESS_DEPTH": 0,
- "COLOR_LIST": ["#211717", "#a34a28", "#f58b54", "#dfddc7"], },
- "LightBrown8": {"BACKGROUND": "#dfddc7", "TEXT": "#211717", "INPUT": "#a34a28", "TEXT_INPUT": "#dfddc7", "SCROLL": "#211717",
- "BUTTON": ("#dfddc7", "#a34a28"), "PROGRESS": DEFAULT_PROGRESS_BAR_COMPUTE, "BORDER": 1, "SLIDER_DEPTH": 0, "PROGRESS_DEPTH": 0,
- "COLOR_LIST": ["#211717", "#a34a28", "#f58b54", "#dfddc7"], },
- "DarkBlue4": {"BACKGROUND": "#494ca2", "TEXT": "#e3e7f1", "INPUT": "#c6cbef", "TEXT_INPUT": "#000000", "SCROLL": "#c6cbef",
- "BUTTON": ("#FFFFFF", "#8186d5"), "PROGRESS": DEFAULT_PROGRESS_BAR_COMPUTE, "BORDER": 1, "SLIDER_DEPTH": 0, "PROGRESS_DEPTH": 0,
- "COLOR_LIST": ["#494ca2", "#8186d5", "#c6cbef", "#e3e7f1"], },
- "LightBlue4": {"BACKGROUND": "#5c94bd", "TEXT": "#470938", "INPUT": "#1a3e59", "TEXT_INPUT": "#FFFFFF", "SCROLL": "#470938",
- "BUTTON": ("#FFFFFF", "#470938"), "PROGRESS": DEFAULT_PROGRESS_BAR_COMPUTE, "BORDER": 1, "SLIDER_DEPTH": 0, "PROGRESS_DEPTH": 0,
- "COLOR_LIST": ["#470938", "#1a3e59", "#5c94bd", "#f2d6eb"], },
- "DarkTeal2": {"BACKGROUND": "#394a6d", "TEXT": "#c0ffb3", "INPUT": "#52de97", "TEXT_INPUT": "#000000", "SCROLL": "#52de97",
- "BUTTON": ("#c0ffb3", "#394a6d"), "PROGRESS": DEFAULT_PROGRESS_BAR_COMPUTE, "BORDER": 1, "SLIDER_DEPTH": 0, "PROGRESS_DEPTH": 0,
- "COLOR_LIST": ["#394a6d", "#3c9d9b", "#52de97", "#c0ffb3"], },
- "DarkTeal3": {"BACKGROUND": "#3c9d9b", "TEXT": "#c0ffb3", "INPUT": "#52de97", "TEXT_INPUT": "#000000", "SCROLL": "#52de97",
- "BUTTON": ("#c0ffb3", "#394a6d"), "PROGRESS": DEFAULT_PROGRESS_BAR_COMPUTE, "BORDER": 1, "SLIDER_DEPTH": 0, "PROGRESS_DEPTH": 0,
- "COLOR_LIST": ["#394a6d", "#3c9d9b", "#52de97", "#c0ffb3"], },
- "DarkPurple5": {"BACKGROUND": "#730068", "TEXT": "#f6f078", "INPUT": "#01d28e", "TEXT_INPUT": "#000000", "SCROLL": "#01d28e",
- "BUTTON": ("#f6f078", "#730068"), "PROGRESS": DEFAULT_PROGRESS_BAR_COMPUTE, "BORDER": 1, "SLIDER_DEPTH": 0, "PROGRESS_DEPTH": 0,
- "COLOR_LIST": ["#730068", "#434982", "#01d28e", "#f6f078"], },
- "DarkPurple2": {"BACKGROUND": "#202060", "TEXT": "#b030b0", "INPUT": "#602080", "TEXT_INPUT": "#FFFFFF", "SCROLL": "#602080",
- "BUTTON": ("#FFFFFF", "#202040"), "PROGRESS": DEFAULT_PROGRESS_BAR_COMPUTE, "BORDER": 1, "SLIDER_DEPTH": 0, "PROGRESS_DEPTH": 0,
- "COLOR_LIST": ["#202040", "#202060", "#602080", "#b030b0"], },
- "DarkBlue5": {"BACKGROUND": "#000272", "TEXT": "#ff6363", "INPUT": "#a32f80", "TEXT_INPUT": "#FFFFFF", "SCROLL": "#a32f80",
- "BUTTON": ("#FFFFFF", "#341677"), "PROGRESS": DEFAULT_PROGRESS_BAR_COMPUTE, "BORDER": 1, "SLIDER_DEPTH": 0, "PROGRESS_DEPTH": 0,
- "COLOR_LIST": ["#000272", "#341677", "#a32f80", "#ff6363"], },
- "LightGrey2": {"BACKGROUND": "#f6f6f6", "TEXT": "#420000", "INPUT": "#d4d7dd", "TEXT_INPUT": "#420000", "SCROLL": "#420000",
- "BUTTON": ("#420000", "#d4d7dd"), "PROGRESS": DEFAULT_PROGRESS_BAR_COMPUTE, "BORDER": 1, "SLIDER_DEPTH": 0, "PROGRESS_DEPTH": 0,
- "COLOR_LIST": ["#420000", "#d4d7dd", "#eae9e9", "#f6f6f6"], },
- "LightGrey3": {"BACKGROUND": "#eae9e9", "TEXT": "#420000", "INPUT": "#d4d7dd", "TEXT_INPUT": "#420000", "SCROLL": "#420000",
- "BUTTON": ("#420000", "#d4d7dd"), "PROGRESS": DEFAULT_PROGRESS_BAR_COMPUTE, "BORDER": 1, "SLIDER_DEPTH": 0, "PROGRESS_DEPTH": 0,
- "COLOR_LIST": ["#420000", "#d4d7dd", "#eae9e9", "#f6f6f6"], },
- "DarkBlue6": {"BACKGROUND": "#01024e", "TEXT": "#ff6464", "INPUT": "#8b4367", "TEXT_INPUT": "#FFFFFF", "SCROLL": "#8b4367",
- "BUTTON": ("#FFFFFF", "#543864"), "PROGRESS": DEFAULT_PROGRESS_BAR_COMPUTE, "BORDER": 1, "SLIDER_DEPTH": 0, "PROGRESS_DEPTH": 0,
- "COLOR_LIST": ["#01024e", "#543864", "#8b4367", "#ff6464"], },
- "DarkBlue7": {"BACKGROUND": "#241663", "TEXT": "#eae7af", "INPUT": "#a72693", "TEXT_INPUT": "#eae7af", "SCROLL": "#a72693",
- "BUTTON": ("#eae7af", "#160f30"), "PROGRESS": DEFAULT_PROGRESS_BAR_COMPUTE, "BORDER": 1, "SLIDER_DEPTH": 0, "PROGRESS_DEPTH": 0,
- "COLOR_LIST": ["#160f30", "#241663", "#a72693", "#eae7af"], },
- "LightBrown9": {"BACKGROUND": "#f6d365", "TEXT": "#3a1f5d", "INPUT": "#c83660", "TEXT_INPUT": "#f6d365", "SCROLL": "#3a1f5d",
- "BUTTON": ("#f6d365", "#c83660"), "PROGRESS": DEFAULT_PROGRESS_BAR_COMPUTE, "BORDER": 1, "SLIDER_DEPTH": 0, "PROGRESS_DEPTH": 0,
- "COLOR_LIST": ["#3a1f5d", "#c83660", "#e15249", "#f6d365"], },
- "DarkPurple3": {"BACKGROUND": "#6e2142", "TEXT": "#ffd692", "INPUT": "#e16363", "TEXT_INPUT": "#ffd692", "SCROLL": "#e16363",
- "BUTTON": ("#ffd692", "#943855"), "PROGRESS": DEFAULT_PROGRESS_BAR_COMPUTE, "BORDER": 1, "SLIDER_DEPTH": 0, "PROGRESS_DEPTH": 0,
- "COLOR_LIST": ["#6e2142", "#943855", "#e16363", "#ffd692"], },
- "LightBrown10": {"BACKGROUND": "#ffd692", "TEXT": "#6e2142", "INPUT": "#943855", "TEXT_INPUT": "#FFFFFF", "SCROLL": "#6e2142",
- "BUTTON": ("#FFFFFF", "#6e2142"), "PROGRESS": DEFAULT_PROGRESS_BAR_COMPUTE, "BORDER": 1, "SLIDER_DEPTH": 0, "PROGRESS_DEPTH": 0,
- "COLOR_LIST": ["#6e2142", "#943855", "#e16363", "#ffd692"], },
- "DarkPurple4": {"BACKGROUND": "#200f21", "TEXT": "#f638dc", "INPUT": "#5a3d5c", "TEXT_INPUT": "#FFFFFF", "SCROLL": "#5a3d5c",
- "BUTTON": ("#FFFFFF", "#382039"), "PROGRESS": DEFAULT_PROGRESS_BAR_COMPUTE, "BORDER": 1, "SLIDER_DEPTH": 0, "PROGRESS_DEPTH": 0,
- "COLOR_LIST": ["#200f21", "#382039", "#5a3d5c", "#f638dc"], },
- "LightBlue5": {"BACKGROUND": "#b2fcff", "TEXT": "#3e64ff", "INPUT": "#5edfff", "TEXT_INPUT": "#000000", "SCROLL": "#3e64ff",
- "BUTTON": ("#FFFFFF", "#3e64ff"), "PROGRESS": DEFAULT_PROGRESS_BAR_COMPUTE, "BORDER": 1, "SLIDER_DEPTH": 0, "PROGRESS_DEPTH": 0,
- "COLOR_LIST": ["#3e64ff", "#5edfff", "#b2fcff", "#ecfcff"], },
- "DarkTeal4": {"BACKGROUND": "#464159", "TEXT": "#c7f0db", "INPUT": "#8bbabb", "TEXT_INPUT": "#000000", "SCROLL": "#8bbabb",
- "BUTTON": ("#FFFFFF", "#6c7b95"), "PROGRESS": DEFAULT_PROGRESS_BAR_COMPUTE, "BORDER": 1, "SLIDER_DEPTH": 0, "PROGRESS_DEPTH": 0,
- "COLOR_LIST": ["#464159", "#6c7b95", "#8bbabb", "#c7f0db"], },
- "LightTeal": {"BACKGROUND": "#c7f0db", "TEXT": "#464159", "INPUT": "#6c7b95", "TEXT_INPUT": "#FFFFFF", "SCROLL": "#464159",
- "BUTTON": ("#FFFFFF", "#464159"), "PROGRESS": DEFAULT_PROGRESS_BAR_COMPUTE, "BORDER": 1, "SLIDER_DEPTH": 0, "PROGRESS_DEPTH": 0,
- "COLOR_LIST": ["#464159", "#6c7b95", "#8bbabb", "#c7f0db"], },
- "DarkTeal5": {"BACKGROUND": "#8bbabb", "TEXT": "#464159", "INPUT": "#6c7b95", "TEXT_INPUT": "#FFFFFF", "SCROLL": "#464159",
- "BUTTON": ("#c7f0db", "#6c7b95"), "PROGRESS": DEFAULT_PROGRESS_BAR_COMPUTE, "BORDER": 1, "SLIDER_DEPTH": 0, "PROGRESS_DEPTH": 0,
- "COLOR_LIST": ["#464159", "#6c7b95", "#8bbabb", "#c7f0db"], },
- "LightGrey4": {"BACKGROUND": "#faf5ef", "TEXT": "#672f2f", "INPUT": "#99b19c", "TEXT_INPUT": "#672f2f", "SCROLL": "#672f2f",
- "BUTTON": ("#672f2f", "#99b19c"), "PROGRESS": DEFAULT_PROGRESS_BAR_COMPUTE, "BORDER": 1, "SLIDER_DEPTH": 0, "PROGRESS_DEPTH": 0,
- "COLOR_LIST": ["#672f2f", "#99b19c", "#d7d1c9", "#faf5ef"], },
- "LightGreen7": {"BACKGROUND": "#99b19c", "TEXT": "#faf5ef", "INPUT": "#d7d1c9", "TEXT_INPUT": "#000000", "SCROLL": "#d7d1c9",
- "BUTTON": ("#FFFFFF", "#99b19c"), "PROGRESS": DEFAULT_PROGRESS_BAR_COMPUTE, "BORDER": 1, "SLIDER_DEPTH": 0, "PROGRESS_DEPTH": 0,
- "COLOR_LIST": ["#672f2f", "#99b19c", "#d7d1c9", "#faf5ef"], },
- "LightGrey5": {"BACKGROUND": "#d7d1c9", "TEXT": "#672f2f", "INPUT": "#99b19c", "TEXT_INPUT": "#672f2f", "SCROLL": "#672f2f",
- "BUTTON": ("#FFFFFF", "#672f2f"), "PROGRESS": DEFAULT_PROGRESS_BAR_COMPUTE, "BORDER": 1, "SLIDER_DEPTH": 0, "PROGRESS_DEPTH": 0,
- "COLOR_LIST": ["#672f2f", "#99b19c", "#d7d1c9", "#faf5ef"], },
- "DarkBrown3": {"BACKGROUND": "#a0855b", "TEXT": "#f9f6f2", "INPUT": "#f1d6ab", "TEXT_INPUT": "#000000", "SCROLL": "#f1d6ab",
- "BUTTON": ("#FFFFFF", "#38470b"), "PROGRESS": DEFAULT_PROGRESS_BAR_COMPUTE, "BORDER": 1, "SLIDER_DEPTH": 0, "PROGRESS_DEPTH": 0,
- "COLOR_LIST": ["#38470b", "#a0855b", "#f1d6ab", "#f9f6f2"], },
- "LightBrown11": {"BACKGROUND": "#f1d6ab", "TEXT": "#38470b", "INPUT": "#a0855b", "TEXT_INPUT": "#FFFFFF", "SCROLL": "#38470b",
- "BUTTON": ("#f9f6f2", "#a0855b"), "PROGRESS": DEFAULT_PROGRESS_BAR_COMPUTE, "BORDER": 1, "SLIDER_DEPTH": 0, "PROGRESS_DEPTH": 0,
- "COLOR_LIST": ["#38470b", "#a0855b", "#f1d6ab", "#f9f6f2"], },
- "DarkRed": {"BACKGROUND": "#83142c", "TEXT": "#f9d276", "INPUT": "#ad1d45", "TEXT_INPUT": "#FFFFFF", "SCROLL": "#ad1d45", "BUTTON": ("#f9d276", "#ad1d45"),
- "PROGRESS": DEFAULT_PROGRESS_BAR_COMPUTE, "BORDER": 1, "SLIDER_DEPTH": 0, "PROGRESS_DEPTH": 0,
- "COLOR_LIST": ["#44000d", "#83142c", "#ad1d45", "#f9d276"], },
- "DarkTeal6": {"BACKGROUND": "#204969", "TEXT": "#fff7f7", "INPUT": "#dadada", "TEXT_INPUT": "#000000", "SCROLL": "#dadada",
- "BUTTON": ("#000000", "#fff7f7"), "PROGRESS": DEFAULT_PROGRESS_BAR_COMPUTE, "BORDER": 1, "SLIDER_DEPTH": 0, "PROGRESS_DEPTH": 0,
- "COLOR_LIST": ["#204969", "#08ffc8", "#dadada", "#fff7f7"], },
- "DarkBrown4": {"BACKGROUND": "#252525", "TEXT": "#ff0000", "INPUT": "#af0404", "TEXT_INPUT": "#FFFFFF", "SCROLL": "#af0404",
- "BUTTON": ("#FFFFFF", "#252525"), "PROGRESS": DEFAULT_PROGRESS_BAR_COMPUTE, "BORDER": 1, "SLIDER_DEPTH": 0, "PROGRESS_DEPTH": 0,
- "COLOR_LIST": ["#252525", "#414141", "#af0404", "#ff0000"], },
- "LightYellow": {"BACKGROUND": "#f4ff61", "TEXT": "#27aa80", "INPUT": "#32ff6a", "TEXT_INPUT": "#000000", "SCROLL": "#27aa80",
- "BUTTON": ("#f4ff61", "#27aa80"), "PROGRESS": DEFAULT_PROGRESS_BAR_COMPUTE, "BORDER": 1, "SLIDER_DEPTH": 0, "PROGRESS_DEPTH": 0,
- "COLOR_LIST": ["#27aa80", "#32ff6a", "#a8ff3e", "#f4ff61"], },
- "DarkGreen1": {"BACKGROUND": "#2b580c", "TEXT": "#fdef96", "INPUT": "#f7b71d", "TEXT_INPUT": "#000000", "SCROLL": "#f7b71d",
- "BUTTON": ("#fdef96", "#2b580c"), "PROGRESS": DEFAULT_PROGRESS_BAR_COMPUTE, "BORDER": 1, "SLIDER_DEPTH": 0, "PROGRESS_DEPTH": 0,
- "COLOR_LIST": ["#2b580c", "#afa939", "#f7b71d", "#fdef96"], },
- "LightGreen8": {"BACKGROUND": "#c8dad3", "TEXT": "#63707e", "INPUT": "#93b5b3", "TEXT_INPUT": "#000000", "SCROLL": "#63707e",
- "BUTTON": ("#FFFFFF", "#63707e"), "PROGRESS": DEFAULT_PROGRESS_BAR_COMPUTE, "BORDER": 1, "SLIDER_DEPTH": 0, "PROGRESS_DEPTH": 0,
- "COLOR_LIST": ["#63707e", "#93b5b3", "#c8dad3", "#f2f6f5"], },
- "DarkTeal7": {"BACKGROUND": "#248ea9", "TEXT": "#fafdcb", "INPUT": "#aee7e8", "TEXT_INPUT": "#000000", "SCROLL": "#aee7e8",
- "BUTTON": ("#000000", "#fafdcb"), "PROGRESS": DEFAULT_PROGRESS_BAR_COMPUTE, "BORDER": 1, "SLIDER_DEPTH": 0, "PROGRESS_DEPTH": 0,
- "COLOR_LIST": ["#248ea9", "#28c3d4", "#aee7e8", "#fafdcb"], },
- "DarkBlue8": {"BACKGROUND": "#454d66", "TEXT": "#d9d872", "INPUT": "#58b368", "TEXT_INPUT": "#000000", "SCROLL": "#58b368",
- "BUTTON": ("#000000", "#009975"), "PROGRESS": DEFAULT_PROGRESS_BAR_COMPUTE, "BORDER": 1, "SLIDER_DEPTH": 0, "PROGRESS_DEPTH": 0,
- "COLOR_LIST": ["#009975", "#454d66", "#58b368", "#d9d872"], },
- "DarkBlue9": {"BACKGROUND": "#263859", "TEXT": "#ff6768", "INPUT": "#6b778d", "TEXT_INPUT": "#FFFFFF", "SCROLL": "#6b778d",
- "BUTTON": ("#ff6768", "#263859"), "PROGRESS": DEFAULT_PROGRESS_BAR_COMPUTE, "BORDER": 1, "SLIDER_DEPTH": 0, "PROGRESS_DEPTH": 0,
- "COLOR_LIST": ["#17223b", "#263859", "#6b778d", "#ff6768"], },
- "DarkBlue10": {"BACKGROUND": "#0028ff", "TEXT": "#f1f4df", "INPUT": "#10eaf0", "TEXT_INPUT": "#000000", "SCROLL": "#10eaf0",
- "BUTTON": ("#f1f4df", "#24009c"), "PROGRESS": DEFAULT_PROGRESS_BAR_COMPUTE, "BORDER": 1, "SLIDER_DEPTH": 0, "PROGRESS_DEPTH": 0,
- "COLOR_LIST": ["#24009c", "#0028ff", "#10eaf0", "#f1f4df"], },
- "DarkBlue11": {"BACKGROUND": "#6384b3", "TEXT": "#e6f0b6", "INPUT": "#b8e9c0", "TEXT_INPUT": "#000000", "SCROLL": "#b8e9c0",
- "BUTTON": ("#e6f0b6", "#684949"), "PROGRESS": DEFAULT_PROGRESS_BAR_COMPUTE, "BORDER": 1, "SLIDER_DEPTH": 0, "PROGRESS_DEPTH": 0,
- "COLOR_LIST": ["#684949", "#6384b3", "#b8e9c0", "#e6f0b6"], },
- "DarkTeal8": {"BACKGROUND": "#71a0a5", "TEXT": "#212121", "INPUT": "#665c84", "TEXT_INPUT": "#FFFFFF", "SCROLL": "#212121",
- "BUTTON": ("#fab95b", "#665c84"), "PROGRESS": DEFAULT_PROGRESS_BAR_COMPUTE, "BORDER": 1, "SLIDER_DEPTH": 0, "PROGRESS_DEPTH": 0,
- "COLOR_LIST": ["#212121", "#665c84", "#71a0a5", "#fab95b"], },
- "DarkRed1": {"BACKGROUND": "#c10000", "TEXT": "#eeeeee", "INPUT": "#dedede", "TEXT_INPUT": "#000000", "SCROLL": "#dedede", "BUTTON": ("#c10000", "#eeeeee"),
- "PROGRESS": DEFAULT_PROGRESS_BAR_COMPUTE, "BORDER": 1, "SLIDER_DEPTH": 0, "PROGRESS_DEPTH": 0,
- "COLOR_LIST": ["#c10000", "#ff4949", "#dedede", "#eeeeee"], },
- "LightBrown5": {"BACKGROUND": "#fff591", "TEXT": "#e41749", "INPUT": "#f5587b", "TEXT_INPUT": "#000000", "SCROLL": "#e41749",
- "BUTTON": ("#fff591", "#e41749"), "PROGRESS": DEFAULT_PROGRESS_BAR_COMPUTE, "BORDER": 1, "SLIDER_DEPTH": 0, "PROGRESS_DEPTH": 0,
- "COLOR_LIST": ["#e41749", "#f5587b", "#ff8a5c", "#fff591"], },
- "LightGreen9": {"BACKGROUND": "#f1edb3", "TEXT": "#3b503d", "INPUT": "#4a746e", "TEXT_INPUT": "#f1edb3", "SCROLL": "#3b503d",
- "BUTTON": ("#f1edb3", "#3b503d"), "PROGRESS": DEFAULT_PROGRESS_BAR_COMPUTE, "BORDER": 1, "SLIDER_DEPTH": 0, "PROGRESS_DEPTH": 0,
- "COLOR_LIST": ["#3b503d", "#4a746e", "#c8cf94", "#f1edb3"], "DESCRIPTION": ["Green", "Turquoise", "Yellow"], },
- "DarkGreen2": {"BACKGROUND": "#3b503d", "TEXT": "#f1edb3", "INPUT": "#c8cf94", "TEXT_INPUT": "#000000", "SCROLL": "#c8cf94",
- "BUTTON": ("#f1edb3", "#3b503d"), "PROGRESS": DEFAULT_PROGRESS_BAR_COMPUTE, "BORDER": 1, "SLIDER_DEPTH": 0, "PROGRESS_DEPTH": 0,
- "COLOR_LIST": ["#3b503d", "#4a746e", "#c8cf94", "#f1edb3"], "DESCRIPTION": ["Green", "Turquoise", "Yellow"], },
- "LightGray1": {"BACKGROUND": "#f2f2f2", "TEXT": "#222831", "INPUT": "#393e46", "TEXT_INPUT": "#FFFFFF", "SCROLL": "#222831",
- "BUTTON": ("#f2f2f2", "#222831"), "PROGRESS": DEFAULT_PROGRESS_BAR_COMPUTE, "BORDER": 1, "SLIDER_DEPTH": 0, "PROGRESS_DEPTH": 0,
- "COLOR_LIST": ["#222831", "#393e46", "#f96d00", "#f2f2f2"], "DESCRIPTION": ["#000000", "Grey", "Orange", "Grey", "Autumn"], },
- "DarkGrey4": {"BACKGROUND": "#52524e", "TEXT": "#e9e9e5", "INPUT": "#d4d6c8", "TEXT_INPUT": "#000000", "SCROLL": "#d4d6c8",
- "BUTTON": ("#FFFFFF", "#9a9b94"), "PROGRESS": DEFAULT_PROGRESS_BAR_COMPUTE, "BORDER": 1, "SLIDER_DEPTH": 0, "PROGRESS_DEPTH": 0,
- "COLOR_LIST": ["#52524e", "#9a9b94", "#d4d6c8", "#e9e9e5"], "DESCRIPTION": ["Grey", "Pastel", "Winter"], },
- "DarkBlue12": {"BACKGROUND": "#324e7b", "TEXT": "#f8f8f8", "INPUT": "#86a6df", "TEXT_INPUT": "#000000", "SCROLL": "#86a6df",
- "BUTTON": ("#FFFFFF", "#5068a9"), "PROGRESS": DEFAULT_PROGRESS_BAR_COMPUTE, "BORDER": 1, "SLIDER_DEPTH": 0, "PROGRESS_DEPTH": 0,
- "COLOR_LIST": ["#324e7b", "#5068a9", "#86a6df", "#f8f8f8"], "DESCRIPTION": ["Blue", "Grey", "Cold", "Winter"], },
- "DarkPurple6": {"BACKGROUND": "#070739", "TEXT": "#e1e099", "INPUT": "#c327ab", "TEXT_INPUT": "#e1e099", "SCROLL": "#c327ab",
- "BUTTON": ("#e1e099", "#521477"), "PROGRESS": DEFAULT_PROGRESS_BAR_COMPUTE, "BORDER": 1, "SLIDER_DEPTH": 0, "PROGRESS_DEPTH": 0,
- "COLOR_LIST": ["#070739", "#521477", "#c327ab", "#e1e099"], "DESCRIPTION": ["#000000", "Purple", "Yellow", "Dark"], },
- "DarkPurple7": {"BACKGROUND": "#191930", "TEXT": "#B1B7C5", "INPUT": "#232B5C", "TEXT_INPUT": "#D0E3E7", "SCROLL": "#B1B7C5",
- "BUTTON": ("#272D38", "#B1B7C5"), "PROGRESS": DEFAULT_PROGRESS_BAR_COMPUTE, "BORDER": 1, "SLIDER_DEPTH": 0, "PROGRESS_DEPTH": 0, },
- "DarkBlue13": {"BACKGROUND": "#203562", "TEXT": "#e3e8f8", "INPUT": "#c0c5cd", "TEXT_INPUT": "#000000", "SCROLL": "#c0c5cd",
- "BUTTON": ("#FFFFFF", "#3e588f"), "PROGRESS": DEFAULT_PROGRESS_BAR_COMPUTE, "BORDER": 1, "SLIDER_DEPTH": 0, "PROGRESS_DEPTH": 0,
- "COLOR_LIST": ["#203562", "#3e588f", "#c0c5cd", "#e3e8f8"], "DESCRIPTION": ["Blue", "Grey", "Wedding", "Cold"], },
- "DarkBrown5": {"BACKGROUND": "#3c1b1f", "TEXT": "#f6e1b5", "INPUT": "#e2bf81", "TEXT_INPUT": "#000000", "SCROLL": "#e2bf81",
- "BUTTON": ("#3c1b1f", "#f6e1b5"), "PROGRESS": DEFAULT_PROGRESS_BAR_COMPUTE, "BORDER": 1, "SLIDER_DEPTH": 0, "PROGRESS_DEPTH": 0,
- "COLOR_LIST": ["#3c1b1f", "#b21e4b", "#e2bf81", "#f6e1b5"], "DESCRIPTION": ["Brown", "Red", "Yellow", "Warm"], },
- "DarkGreen3": {"BACKGROUND": "#062121", "TEXT": "#eeeeee", "INPUT": "#e4dcad", "TEXT_INPUT": "#000000", "SCROLL": "#e4dcad",
- "BUTTON": ("#eeeeee", "#181810"), "PROGRESS": DEFAULT_PROGRESS_BAR_COMPUTE, "BORDER": 1, "SLIDER_DEPTH": 0, "PROGRESS_DEPTH": 0,
- "COLOR_LIST": ["#062121", "#181810", "#e4dcad", "#eeeeee"], "DESCRIPTION": ["#000000", "#000000", "Brown", "Grey"], },
- "DarkBlack1": {"BACKGROUND": "#181810", "TEXT": "#eeeeee", "INPUT": "#e4dcad", "TEXT_INPUT": "#000000", "SCROLL": "#e4dcad",
- "BUTTON": ("#FFFFFF", "#062121"), "PROGRESS": DEFAULT_PROGRESS_BAR_COMPUTE, "BORDER": 1, "SLIDER_DEPTH": 0, "PROGRESS_DEPTH": 0,
- "COLOR_LIST": ["#062121", "#181810", "#e4dcad", "#eeeeee"], "DESCRIPTION": ["#000000", "#000000", "Brown", "Grey"], },
- "DarkGrey5": {"BACKGROUND": "#343434", "TEXT": "#f3f3f3", "INPUT": "#e9dcbe", "TEXT_INPUT": "#000000", "SCROLL": "#e9dcbe",
- "BUTTON": ("#FFFFFF", "#8e8b82"), "PROGRESS": DEFAULT_PROGRESS_BAR_COMPUTE, "BORDER": 1, "SLIDER_DEPTH": 0, "PROGRESS_DEPTH": 0,
- "COLOR_LIST": ["#343434", "#8e8b82", "#e9dcbe", "#f3f3f3"], "DESCRIPTION": ["Grey", "Brown"], },
- "LightBrown12": {"BACKGROUND": "#8e8b82", "TEXT": "#f3f3f3", "INPUT": "#e9dcbe", "TEXT_INPUT": "#000000", "SCROLL": "#e9dcbe",
- "BUTTON": ("#f3f3f3", "#8e8b82"), "PROGRESS": DEFAULT_PROGRESS_BAR_COMPUTE, "BORDER": 1, "SLIDER_DEPTH": 0, "PROGRESS_DEPTH": 0,
- "COLOR_LIST": ["#343434", "#8e8b82", "#e9dcbe", "#f3f3f3"], "DESCRIPTION": ["Grey", "Brown"], },
- "DarkTeal9": {"BACKGROUND": "#13445a", "TEXT": "#fef4e8", "INPUT": "#446878", "TEXT_INPUT": "#FFFFFF", "SCROLL": "#446878",
- "BUTTON": ("#fef4e8", "#446878"), "PROGRESS": DEFAULT_PROGRESS_BAR_COMPUTE, "BORDER": 1, "SLIDER_DEPTH": 0, "PROGRESS_DEPTH": 0,
- "COLOR_LIST": ["#13445a", "#970747", "#446878", "#fef4e8"], "DESCRIPTION": ["Red", "Grey", "Blue", "Wedding", "Retro"], },
- "DarkBlue14": {"BACKGROUND": "#21273d", "TEXT": "#f1f6f8", "INPUT": "#b9d4f1", "TEXT_INPUT": "#000000", "SCROLL": "#b9d4f1",
- "BUTTON": ("#FFFFFF", "#6a759b"), "PROGRESS": DEFAULT_PROGRESS_BAR_COMPUTE, "BORDER": 1, "SLIDER_DEPTH": 0, "PROGRESS_DEPTH": 0,
- "COLOR_LIST": ["#21273d", "#6a759b", "#b9d4f1", "#f1f6f8"], "DESCRIPTION": ["Blue", "#000000", "Grey", "Cold", "Winter"], },
- "LightBlue6": {"BACKGROUND": "#f1f6f8", "TEXT": "#21273d", "INPUT": "#6a759b", "TEXT_INPUT": "#FFFFFF", "SCROLL": "#21273d",
- "BUTTON": ("#f1f6f8", "#6a759b"), "PROGRESS": DEFAULT_PROGRESS_BAR_COMPUTE, "BORDER": 1, "SLIDER_DEPTH": 0, "PROGRESS_DEPTH": 0,
- "COLOR_LIST": ["#21273d", "#6a759b", "#b9d4f1", "#f1f6f8"], "DESCRIPTION": ["Blue", "#000000", "Grey", "Cold", "Winter"], },
- "DarkGreen4": {"BACKGROUND": "#044343", "TEXT": "#e4e4e4", "INPUT": "#045757", "TEXT_INPUT": "#e4e4e4", "SCROLL": "#045757",
- "BUTTON": ("#e4e4e4", "#045757"), "PROGRESS": DEFAULT_PROGRESS_BAR_COMPUTE, "BORDER": 1, "SLIDER_DEPTH": 0, "PROGRESS_DEPTH": 0,
- "COLOR_LIST": ["#222222", "#044343", "#045757", "#e4e4e4"], "DESCRIPTION": ["#000000", "Turquoise", "Grey", "Dark"], },
- "DarkGreen5": {"BACKGROUND": "#1b4b36", "TEXT": "#e0e7f1", "INPUT": "#aebd77", "TEXT_INPUT": "#000000", "SCROLL": "#aebd77",
- "BUTTON": ("#FFFFFF", "#538f6a"), "PROGRESS": DEFAULT_PROGRESS_BAR_COMPUTE, "BORDER": 1, "SLIDER_DEPTH": 0, "PROGRESS_DEPTH": 0,
- "COLOR_LIST": ["#1b4b36", "#538f6a", "#aebd77", "#e0e7f1"], "DESCRIPTION": ["Green", "Grey"], },
- "DarkTeal10": {"BACKGROUND": "#0d3446", "TEXT": "#d8dfe2", "INPUT": "#71adb5", "TEXT_INPUT": "#000000", "SCROLL": "#71adb5",
- "BUTTON": ("#FFFFFF", "#176d81"), "PROGRESS": DEFAULT_PROGRESS_BAR_COMPUTE, "BORDER": 1, "SLIDER_DEPTH": 0, "PROGRESS_DEPTH": 0,
- "COLOR_LIST": ["#0d3446", "#176d81", "#71adb5", "#d8dfe2"], "DESCRIPTION": ["Grey", "Turquoise", "Winter", "Cold"], },
- "DarkGrey6": {"BACKGROUND": "#3e3e3e", "TEXT": "#ededed", "INPUT": "#68868c", "TEXT_INPUT": "#ededed", "SCROLL": "#68868c",
- "BUTTON": ("#FFFFFF", "#405559"), "PROGRESS": DEFAULT_PROGRESS_BAR_COMPUTE, "BORDER": 1, "SLIDER_DEPTH": 0, "PROGRESS_DEPTH": 0,
- "COLOR_LIST": ["#3e3e3e", "#405559", "#68868c", "#ededed"], "DESCRIPTION": ["Grey", "Turquoise", "Winter"], },
- "DarkTeal11": {"BACKGROUND": "#405559", "TEXT": "#ededed", "INPUT": "#68868c", "TEXT_INPUT": "#ededed", "SCROLL": "#68868c",
- "BUTTON": ("#ededed", "#68868c"), "PROGRESS": DEFAULT_PROGRESS_BAR_COMPUTE, "BORDER": 1, "SLIDER_DEPTH": 0, "PROGRESS_DEPTH": 0,
- "COLOR_LIST": ["#3e3e3e", "#405559", "#68868c", "#ededed"], "DESCRIPTION": ["Grey", "Turquoise", "Winter"], },
- "LightBlue7": {"BACKGROUND": "#9ed0e0", "TEXT": "#19483f", "INPUT": "#5c868e", "TEXT_INPUT": "#FFFFFF", "SCROLL": "#19483f",
- "BUTTON": ("#FFFFFF", "#19483f"), "PROGRESS": DEFAULT_PROGRESS_BAR_COMPUTE, "BORDER": 1, "SLIDER_DEPTH": 0, "PROGRESS_DEPTH": 0,
- "COLOR_LIST": ["#19483f", "#5c868e", "#ff6a38", "#9ed0e0"], "DESCRIPTION": ["Orange", "Blue", "Turquoise"], },
- "LightGreen10": {"BACKGROUND": "#d8ebb5", "TEXT": "#205d67", "INPUT": "#639a67", "TEXT_INPUT": "#FFFFFF", "SCROLL": "#205d67",
- "BUTTON": ("#d8ebb5", "#205d67"), "PROGRESS": DEFAULT_PROGRESS_BAR_COMPUTE, "BORDER": 1, "SLIDER_DEPTH": 0, "PROGRESS_DEPTH": 0,
- "COLOR_LIST": ["#205d67", "#639a67", "#d9bf77", "#d8ebb5"], "DESCRIPTION": ["Blue", "Green", "Brown", "Vintage"], },
- "DarkBlue15": {"BACKGROUND": "#151680", "TEXT": "#f1fea4", "INPUT": "#375fc0", "TEXT_INPUT": "#f1fea4", "SCROLL": "#375fc0",
- "BUTTON": ("#f1fea4", "#1c44ac"), "PROGRESS": DEFAULT_PROGRESS_BAR_COMPUTE, "BORDER": 1, "SLIDER_DEPTH": 0, "PROGRESS_DEPTH": 0,
- "COLOR_LIST": ["#151680", "#1c44ac", "#375fc0", "#f1fea4"], "DESCRIPTION": ["Blue", "Yellow", "Cold"], },
- "DarkBlue16": {"BACKGROUND": "#1c44ac", "TEXT": "#f1fea4", "INPUT": "#375fc0", "TEXT_INPUT": "#f1fea4", "SCROLL": "#375fc0",
- "BUTTON": ("#f1fea4", "#151680"), "PROGRESS": DEFAULT_PROGRESS_BAR_COMPUTE, "BORDER": 1, "SLIDER_DEPTH": 0, "PROGRESS_DEPTH": 0,
- "COLOR_LIST": ["#151680", "#1c44ac", "#375fc0", "#f1fea4"], "DESCRIPTION": ["Blue", "Yellow", "Cold"], },
- "DarkTeal12": {"BACKGROUND": "#004a7c", "TEXT": "#fafafa", "INPUT": "#e8f1f5", "TEXT_INPUT": "#000000", "SCROLL": "#e8f1f5",
- "BUTTON": ("#fafafa", "#005691"), "PROGRESS": DEFAULT_PROGRESS_BAR_COMPUTE, "BORDER": 1, "SLIDER_DEPTH": 0, "PROGRESS_DEPTH": 0,
- "COLOR_LIST": ["#004a7c", "#005691", "#e8f1f5", "#fafafa"], "DESCRIPTION": ["Grey", "Blue", "Cold", "Winter"], },
- "LightBrown13": {"BACKGROUND": "#ebf5ee", "TEXT": "#921224", "INPUT": "#bdc6b8", "TEXT_INPUT": "#921224", "SCROLL": "#921224",
- "BUTTON": ("#FFFFFF", "#921224"), "PROGRESS": DEFAULT_PROGRESS_BAR_COMPUTE, "BORDER": 1, "SLIDER_DEPTH": 0, "PROGRESS_DEPTH": 0,
- "COLOR_LIST": ["#921224", "#bdc6b8", "#bce0da", "#ebf5ee"], "DESCRIPTION": ["Red", "Blue", "Grey", "Vintage", "Wedding"], },
- "DarkBlue17": {"BACKGROUND": "#21294c", "TEXT": "#f9f2d7", "INPUT": "#f2dea8", "TEXT_INPUT": "#000000", "SCROLL": "#f2dea8",
- "BUTTON": ("#f9f2d7", "#141829"), "PROGRESS": DEFAULT_PROGRESS_BAR_COMPUTE, "BORDER": 1, "SLIDER_DEPTH": 0, "PROGRESS_DEPTH": 0,
- "COLOR_LIST": ["#141829", "#21294c", "#f2dea8", "#f9f2d7"], "DESCRIPTION": ["#000000", "Blue", "Yellow"], },
- "DarkBrown6": {"BACKGROUND": "#785e4d", "TEXT": "#f2eee3", "INPUT": "#baaf92", "TEXT_INPUT": "#000000", "SCROLL": "#baaf92",
- "BUTTON": ("#FFFFFF", "#785e4d"), "PROGRESS": DEFAULT_PROGRESS_BAR_COMPUTE, "BORDER": 1, "SLIDER_DEPTH": 0, "PROGRESS_DEPTH": 0,
- "COLOR_LIST": ["#785e4d", "#ff8426", "#baaf92", "#f2eee3"], "DESCRIPTION": ["Grey", "Brown", "Orange", "Autumn"], },
- "DarkGreen6": {"BACKGROUND": "#5c715e", "TEXT": "#f2f9f1", "INPUT": "#ddeedf", "TEXT_INPUT": "#000000", "SCROLL": "#ddeedf",
- "BUTTON": ("#f2f9f1", "#5c715e"), "PROGRESS": DEFAULT_PROGRESS_BAR_COMPUTE, "BORDER": 1, "SLIDER_DEPTH": 0, "PROGRESS_DEPTH": 0,
- "COLOR_LIST": ["#5c715e", "#b6cdbd", "#ddeedf", "#f2f9f1"], "DESCRIPTION": ["Grey", "Green", "Vintage"], },
- "DarkGreen7": {"BACKGROUND": "#0C231E", "TEXT": "#efbe1c", "INPUT": "#153C33", "TEXT_INPUT": "#efbe1c", "SCROLL": "#153C33",
- "BUTTON": ("#efbe1c", "#153C33"), "PROGRESS": DEFAULT_PROGRESS_BAR_COMPUTE, "BORDER": 1, "SLIDER_DEPTH": 0, "PROGRESS_DEPTH": 0, },
- "DarkGrey7": {"BACKGROUND": "#4b586e", "TEXT": "#dddddd", "INPUT": "#574e6d", "TEXT_INPUT": "#dddddd", "SCROLL": "#574e6d",
- "BUTTON": ("#dddddd", "#43405d"), "PROGRESS": DEFAULT_PROGRESS_BAR_COMPUTE, "BORDER": 1, "SLIDER_DEPTH": 0, "PROGRESS_DEPTH": 0,
- "COLOR_LIST": ["#43405d", "#4b586e", "#574e6d", "#dddddd"], "DESCRIPTION": ["Grey", "Winter", "Cold"], },
- "DarkRed2": {"BACKGROUND": "#ab1212", "TEXT": "#f6e4b5", "INPUT": "#cd3131", "TEXT_INPUT": "#f6e4b5", "SCROLL": "#cd3131", "BUTTON": ("#f6e4b5", "#ab1212"),
- "PROGRESS": DEFAULT_PROGRESS_BAR_COMPUTE, "BORDER": 1, "SLIDER_DEPTH": 0, "PROGRESS_DEPTH": 0,
- "COLOR_LIST": ["#ab1212", "#1fad9f", "#cd3131", "#f6e4b5"], "DESCRIPTION": ["Turquoise", "Red", "Yellow"], },
- "LightGrey6": {"BACKGROUND": "#e3e3e3", "TEXT": "#233142", "INPUT": "#455d7a", "TEXT_INPUT": "#e3e3e3", "SCROLL": "#233142",
- "BUTTON": ("#e3e3e3", "#455d7a"), "PROGRESS": DEFAULT_PROGRESS_BAR_COMPUTE, "BORDER": 1, "SLIDER_DEPTH": 0, "PROGRESS_DEPTH": 0,
- "COLOR_LIST": ["#233142", "#455d7a", "#f95959", "#e3e3e3"], "DESCRIPTION": ["#000000", "Blue", "Red", "Grey"], },
- "HotDogStand": {"BACKGROUND": "red", "TEXT": "yellow", "INPUT": "yellow", "TEXT_INPUT": "#000000", "SCROLL": "yellow", "BUTTON": ("red", "yellow"),
- "PROGRESS": DEFAULT_PROGRESS_BAR_COMPUTE, "BORDER": 1, "SLIDER_DEPTH": 0, "PROGRESS_DEPTH": 0, },
- "DarkGrey8": {"BACKGROUND": "#19232D", "TEXT": "#ffffff", "INPUT": "#32414B", "TEXT_INPUT": "#ffffff", "SCROLL": "#505F69",
- "BUTTON": ("#ffffff", "#32414B"), "PROGRESS": ("#505F69", "#32414B"), "BORDER": 1, "SLIDER_DEPTH": 0, "PROGRESS_DEPTH": 0, },
- "DarkGrey9": {"BACKGROUND": "#36393F", "TEXT": "#DCDDDE", "INPUT": "#40444B", "TEXT_INPUT": "#ffffff", "SCROLL": "#202225",
- "BUTTON": ("#202225", "#B9BBBE"), "PROGRESS": ("#202225", "#40444B"), "BORDER": 1, "SLIDER_DEPTH": 0, "PROGRESS_DEPTH": 0, },
- "DarkGrey10": {"BACKGROUND": "#1c1e23", "TEXT": "#cccdcf", "INPUT": "#272a31", "TEXT_INPUT": "#8b9fde", "SCROLL": "#313641",
- "BUTTON": ("#f5f5f6", "#2e3d5a"), "PROGRESS": DEFAULT_PROGRESS_BAR_COMPUTE, "BORDER": 1, "SLIDER_DEPTH": 0, "PROGRESS_DEPTH": 0, },
- "DarkGrey11": {"BACKGROUND": "#1c1e23", "TEXT": "#cccdcf", "INPUT": "#313641", "TEXT_INPUT": "#cccdcf", "SCROLL": "#313641",
- "BUTTON": ("#f5f5f6", "#313641"), "PROGRESS": DEFAULT_PROGRESS_BAR_COMPUTE, "BORDER": 1, "SLIDER_DEPTH": 0, "PROGRESS_DEPTH": 0, },
- "DarkGrey12": {"BACKGROUND": "#1c1e23", "TEXT": "#8b9fde", "INPUT": "#313641", "TEXT_INPUT": "#8b9fde", "SCROLL": "#313641",
- "BUTTON": ("#cccdcf", "#2e3d5a"), "PROGRESS": DEFAULT_PROGRESS_BAR_COMPUTE, "BORDER": 1, "SLIDER_DEPTH": 0, "PROGRESS_DEPTH": 0, },
- "DarkGrey13": {"BACKGROUND": "#1c1e23", "TEXT": "#cccdcf", "INPUT": "#272a31", "TEXT_INPUT": "#cccdcf", "SCROLL": "#313641",
- "BUTTON": ("#8b9fde", "#313641"), "PROGRESS": ("#cccdcf", "#272a31"), "BORDER": 1, "SLIDER_DEPTH": 0, "PROGRESS_DEPTH": 0, },
- "DarkGrey14": {"BACKGROUND": "#24292e", "TEXT": "#fafbfc", "INPUT": "#1d2125", "TEXT_INPUT": "#fafbfc", "SCROLL": "#1d2125",
- "BUTTON": ("#fafbfc", "#155398"), "PROGRESS": ("#155398", "#1d2125"), "BORDER": 1, "SLIDER_DEPTH": 0, "PROGRESS_DEPTH": 0, },
- "DarkGrey15": {'BACKGROUND': '#121212', 'TEXT': '#dddddd', 'INPUT': '#1e1e1e', 'TEXT_INPUT': '#69b1ef', 'SCROLL': '#272727',
- 'BUTTON': ('#69b1ef', '#2e2e2e'), 'PROGRESS': ('#69b1ef', '#2e2e2e'), 'BORDER': 1, 'SLIDER_DEPTH': 0, 'PROGRESS_DEPTH': 0,},
- "DarkBrown7": {"BACKGROUND": "#2c2417", "TEXT": "#baa379", "INPUT": "#baa379", "TEXT_INPUT": "#000000", "SCROLL": "#392e1c",
- "BUTTON": ("#000000", "#baa379"), "PROGRESS": ("#baa379", "#453923"), "BORDER": 1, "SLIDER_DEPTH": 1, "PROGRESS_DEPTH": 0, },
- "Python": {"BACKGROUND": "#3d7aab", "TEXT": "#ffde56", "INPUT": "#295273", "TEXT_INPUT": "#ffde56", "SCROLL": "#295273",
- "BUTTON": ("#ffde56", "#295273"), "PROGRESS": ("#ffde56", "#295273"), "BORDER": 1, "SLIDER_DEPTH": 1, "PROGRESS_DEPTH": 0, },
- "PythonPlus": {"BACKGROUND": "#001d3c", "TEXT": "#ffffff", "INPUT": "#015bbb", "TEXT_INPUT": "#fed500", "SCROLL": "#015bbb",
- "BUTTON": ("#fed500", "#015bbb"), "PROGRESS": ("#015bbb", "#fed500"), "BORDER": 1, "SLIDER_DEPTH": 1, "PROGRESS_DEPTH": 0, },
-}
-
-
-
-def list_of_look_and_feel_values():
- """
- Get a list of the valid values to pass into your call to change_look_and_feel
-
- :return: list of valid string values
- :rtype: List[str]
- """
-
- return sorted(list(LOOK_AND_FEEL_TABLE.keys()))
-
-
-def theme(new_theme=None):
- """
- Sets / Gets the current Theme. If none is specified then returns the current theme.
- This call replaces the ChangeLookAndFeel / change_look_and_feel call which only sets the theme.
-
- :param new_theme: the new theme name to use
- :type new_theme: (str)
- :return: the currently selected theme
- :rtype: (str)
- """
- global TRANSPARENT_BUTTON
-
- if new_theme is not None:
- change_look_and_feel(new_theme)
- TRANSPARENT_BUTTON = (theme_background_color(), theme_background_color())
- return CURRENT_LOOK_AND_FEEL
-
-
-def theme_background_color(color=None):
- """
- Sets/Returns the background color currently in use
- Used for Windows and containers (Column, Frame, Tab) and tables
-
- :param color: new background color to use (optional)
- :type color: (str)
- :return: color string of the background color currently in use
- :rtype: (str)
- """
- if color is not None:
- set_options(background_color=color)
- return DEFAULT_BACKGROUND_COLOR
-
-
-# This "constant" is misleading but rather than remove and break programs, will try this method instead
-TRANSPARENT_BUTTON = (theme_background_color(), theme_background_color()) # replaces an older version that had hardcoded numbers
-
-
-def theme_element_background_color(color=None):
- """
- Sets/Returns the background color currently in use for all elements except containers
-
- :return: (str) - color string of the element background color currently in use
- :rtype: (str)
- """
- if color is not None:
- set_options(element_background_color=color)
- return DEFAULT_ELEMENT_BACKGROUND_COLOR
-
-
-def theme_text_color(color=None):
- """
- Sets/Returns the text color currently in use
-
- :return: (str) - color string of the text color currently in use
- :rtype: (str)
- """
- if color is not None:
- set_options(text_color=color)
- return DEFAULT_TEXT_COLOR
-
-
-def theme_text_element_background_color(color=None):
- """
- Sets/Returns the background color for text elements
-
- :return: (str) - color string of the text background color currently in use
- :rtype: (str)
- """
- if color is not None:
- set_options(text_element_background_color=color)
- return DEFAULT_TEXT_ELEMENT_BACKGROUND_COLOR
-
-
-def theme_input_background_color(color=None):
- """
- Sets/Returns the input element background color currently in use
-
- :return: (str) - color string of the input element background color currently in use
- :rtype: (str)
- """
- if color is not None:
- set_options(input_elements_background_color=color)
- return DEFAULT_INPUT_ELEMENTS_COLOR
-
-
-def theme_input_text_color(color=None):
- """
- Sets/Returns the input element entry color (not the text but the thing that's displaying the text)
-
- :return: (str) - color string of the input element color currently in use
- :rtype: (str)
- """
- if color is not None:
- set_options(input_text_color=color)
- return DEFAULT_INPUT_TEXT_COLOR
-
-
-def theme_button_color(color=None):
- """
- Sets/Returns the button color currently in use
-
- :return: (str, str) - TUPLE with color strings of the button color currently in use (button text color, button background color)
- :rtype: (str, str)
- """
- if color is not None:
- if color == COLOR_SYSTEM_DEFAULT:
- color_tuple = (COLOR_SYSTEM_DEFAULT, COLOR_SYSTEM_DEFAULT)
- else:
- color_tuple = button_color_to_tuple(color, (None, None))
- if color_tuple == (None, None):
- if not SUPPRESS_ERROR_POPUPS:
- popup_error('theme_button_color - bad color string passed in', color)
- else:
- print('** Badly formatted button color... not a tuple nor string **', color)
- set_options(button_color=color) # go ahead and try with their string
- else:
- set_options(button_color=color_tuple)
- return DEFAULT_BUTTON_COLOR
-
-
-def theme_button_color_background():
- """
- Returns the button color background currently in use. Note this function simple calls the theme_button_color
- function and splits apart the tuple
-
- :return: color string of the button color background currently in use
- :rtype: (str)
- """
- return theme_button_color()[1]
-
-
-def theme_button_color_text():
- """
- Returns the button color text currently in use. Note this function simple calls the theme_button_color
- function and splits apart the tuple
-
- :return: color string of the button color text currently in use
- :rtype: (str)
- """
- return theme_button_color()[0]
-
-
-def theme_progress_bar_color(color=None):
- """
- Sets/Returns the progress bar colors by the current color theme
-
- :return: (str, str) - TUPLE with color strings of the ProgressBar color currently in use(button text color, button background color)
- :rtype: (str, str)
- """
- if color is not None:
- set_options(progress_meter_color=color)
- return DEFAULT_PROGRESS_BAR_COLOR
-
-
-def theme_slider_color(color=None):
- """
- Sets/Returns the slider color (used for sliders)
-
- :return: color string of the slider color currently in use
- :rtype: (str)
- """
- if color is not None:
- set_options(scrollbar_color=color)
- return DEFAULT_SCROLLBAR_COLOR
-
-
-def theme_border_width(border_width=None):
- """
- Sets/Returns the border width currently in use
- Used by non ttk elements at the moment
-
- :return: border width currently in use
- :rtype: (int)
- """
- if border_width is not None:
- set_options(border_width=border_width)
- return DEFAULT_BORDER_WIDTH
-
-
-def theme_slider_border_width(border_width=None):
- """
- Sets/Returns the slider border width currently in use
-
- :return: border width currently in use for sliders
- :rtype: (int)
- """
- if border_width is not None:
- set_options(slider_border_width=border_width)
- return DEFAULT_SLIDER_BORDER_WIDTH
-
-
-def theme_progress_bar_border_width(border_width=None):
- """
- Sets/Returns the progress meter border width currently in use
-
- :return: border width currently in use for progress meters
- :rtype: (int)
- """
- if border_width is not None:
- set_options(progress_meter_border_depth=border_width)
- return DEFAULT_PROGRESS_BAR_BORDER_WIDTH
-
-
-def theme_element_text_color(color=None):
- """
- Sets/Returns the text color used by elements that have text as part of their display (Tables, Trees and Sliders)
-
- :return: color string currently in use
- :rtype: (str)
- """
- if color is not None:
- set_options(element_text_color=color)
- return DEFAULT_ELEMENT_TEXT_COLOR
-
-
-def theme_list():
- """
- Returns a sorted list of the currently available color themes
-
- :return: A sorted list of the currently available color themes
- :rtype: List[str]
- """
- return list_of_look_and_feel_values()
-
-
-def theme_add_new(new_theme_name, new_theme_dict):
- """
- Add a new theme to the dictionary of themes
-
- :param new_theme_name: text to display in element
- :type new_theme_name: (str)
- :param new_theme_dict: text to display in element
- :type new_theme_dict: (dict)
- """
- global LOOK_AND_FEEL_TABLE
- try:
- LOOK_AND_FEEL_TABLE[new_theme_name] = new_theme_dict
- except Exception as e:
- print('Exception during adding new theme {}'.format(e))
-
-
-def theme_use_custom_titlebar():
- """
- Returns True if a custom titlebar will be / should be used.
- The setting is in the Global Settings window and can be overwridden
- using set_options call
-
- :return: True if a custom titlebar / custom menubar should be used
- :rtype: (bool)
- """
- if USE_CUSTOM_TITLEBAR is False:
- return False
-
- return USE_CUSTOM_TITLEBAR or pysimplegui_user_settings.get('-custom titlebar-', False)
-
-
-def theme_global(new_theme=None):
- """
- Sets / Gets the global PySimpleGUI Theme. If none is specified then returns the global theme from user settings.
- Note the theme must be a standard, built-in PySimpleGUI theme... not a user-created theme.
-
- :param new_theme: the new theme name to use
- :type new_theme: (str)
- :return: the currently selected theme
- :rtype: (str)
- """
- if new_theme is not None:
- if new_theme not in theme_list():
- popup_error_with_traceback('Cannot use custom themes with theme_global call',
- 'Your request to use theme {} cannot be performed.'.format(new_theme),
- 'The PySimpleGUI Global User Settings are meant for PySimpleGUI standard items, not user config items',
- 'You can use any of the many built-in themes instead or use your own UserSettings file to store your custom theme')
- return pysimplegui_user_settings.get('-theme-', CURRENT_LOOK_AND_FEEL)
- pysimplegui_user_settings.set('-theme-', new_theme)
- theme(new_theme)
- return new_theme
- else:
- return pysimplegui_user_settings.get('-theme-', CURRENT_LOOK_AND_FEEL)
-
-
-def theme_previewer(columns=12, scrollable=False, scroll_area_size=(None, None), search_string=None, location=(None, None)):
- """
- Displays a "Quick Reference Window" showing all of the different Look and Feel settings that are available.
- They are sorted alphabetically. The legacy color names are mixed in, but otherwise they are sorted into Dark and Light halves
-
- :param columns: The number of themes to display per row
- :type columns: int
- :param scrollable: If True then scrollbars will be added
- :type scrollable: bool
- :param scroll_area_size: Size of the scrollable area (The Column Element used to make scrollable)
- :type scroll_area_size: (int, int)
- :param search_string: If specified then only themes containing this string will be shown
- :type search_string: str
- :param location: Location on the screen to place the window. Defaults to the center like all windows
- :type location: (int, int)
- """
-
- current_theme = theme()
-
- # Show a "splash" type message so the user doesn't give up waiting
- popup_quick_message('Hang on for a moment, this will take a bit to create....', keep_on_top=True, background_color='red', text_color='#FFFFFF',
- auto_close=True, non_blocking=True)
-
- web = False
-
- win_bg = 'black'
-
- def sample_layout():
- return [[Text('Text element'), InputText('Input data here', size=(10, 1))],
- [Button('Ok'), Button('Disabled', disabled=True), Slider((1, 10), orientation='h', size=(5, 15))]]
-
- names = list_of_look_and_feel_values()
- names.sort()
- if search_string not in (None, ''):
- names = [name for name in names if search_string.lower().replace(" ", "") in name.lower().replace(" ", "")]
-
- if search_string not in (None, ''):
- layout = [[Text('Themes containing "{}"'.format(search_string), font='Default 18', background_color=win_bg)]]
- else:
- layout = [[Text('List of all themes', font='Default 18', background_color=win_bg)]]
-
- col_layout = []
- row = []
- for count, theme_name in enumerate(names):
- theme(theme_name)
- if not count % columns:
- col_layout += [row]
- row = []
- row += [Frame(theme_name, sample_layout() if not web else [[T(theme_name)]] + sample_layout(), pad=(2, 2))]
- if row:
- col_layout += [row]
-
- layout += [[Column(col_layout, scrollable=scrollable, size=scroll_area_size, pad=(0, 0), background_color=win_bg, key='-COL-')]]
- window = Window('Preview of Themes', layout, background_color=win_bg, resizable=True, location=location, keep_on_top=True, finalize=True, modal=True)
- window['-COL-'].expand(True, True, True) # needed so that col will expand with the window
- window.read(close=True)
- theme(current_theme)
-
-
-preview_all_look_and_feel_themes = theme_previewer
-
-
-def _theme_preview_window_swatches():
- # Begin the layout with a header
- layout = [[Text('Themes as color swatches', text_color='white', background_color='black', font='Default 25')],
- [Text('Tooltip and right click a color to get the value', text_color='white', background_color='black', font='Default 15')],
- [Text('Left click a color to copy to clipboard', text_color='white', background_color='black', font='Default 15')]]
- layout = [[Column(layout, element_justification='c', background_color='black')]]
- # Create the pain part, the rows of Text with color swatches
- for i, theme_name in enumerate(theme_list()):
- theme(theme_name)
- colors = [theme_background_color(), theme_text_color(), theme_input_background_color(),
- theme_input_text_color()]
- if theme_button_color() != COLOR_SYSTEM_DEFAULT:
- colors.append(theme_button_color()[0])
- colors.append(theme_button_color()[1])
- colors = list(set(colors)) # de-duplicate items
- row = [T(theme(), background_color='black', text_color='white', size=(20, 1), justification='r')]
- for color in colors:
- if color != COLOR_SYSTEM_DEFAULT:
- row.append(T(SYMBOL_SQUARE, text_color=color, background_color='black', pad=(0, 0), font='DEFAUlT 20', right_click_menu=['Nothing', [color]],
- tooltip=color, enable_events=True, key=(i, color)))
- layout += [row]
- # place layout inside of a Column so that it's scrollable
- layout = [[Column(layout, size=(500, 900), scrollable=True, vertical_scroll_only=True, background_color='black')]]
- # finish the layout by adding an exit button
- layout += [[B('Exit')]]
-
- # create and return Window that uses the layout
- return Window('Theme Color Swatches', layout, background_color='black', finalize=True, keep_on_top=True)
-
-
-def theme_previewer_swatches():
- """
- Display themes in a window as color swatches.
- Click on a color swatch to see the hex value printed on the console.
- If you hover over a color or right click it you'll also see the hext value.
- """
- current_theme = theme()
- popup_quick_message('This is going to take a minute...', text_color='white', background_color='red', font='Default 20', keep_on_top=True)
- window = _theme_preview_window_swatches()
- theme(OFFICIAL_PYSIMPLEGUI_THEME)
- # col_height = window.get_screen_size()[1]-200
- # if window.size[1] > 100:
- # window.size = (window.size[0], col_height)
- # window.move(window.get_screen_size()[0] // 2 - window.size[0] // 2, 0)
-
- while True: # Event Loop
- event, values = window.read()
- if event == WIN_CLOSED or event == 'Exit':
- break
- if isinstance(event, tuple): # someone clicked a swatch
- chosen_color = event[1]
- else:
- if event[0] == '#': # someone right clicked
- chosen_color = event
- else:
- chosen_color = ''
- print('Copied to clipboard color = ', chosen_color)
- clipboard_set(chosen_color)
- # window.TKroot.clipboard_clear()
- # window.TKroot.clipboard_append(chosen_color)
- window.close()
- theme(current_theme)
-
-
-def change_look_and_feel(index, force=False):
- """
- Change the "color scheme" of all future PySimpleGUI Windows.
- The scheme are string names that specify a group of colors. Background colors, text colors, button colors.
- There are 13 different color settings that are changed at one time using a single call to ChangeLookAndFeel
- The look and feel table itself has these indexes into the dictionary LOOK_AND_FEEL_TABLE.
- The original list was (prior to a major rework and renaming)... these names still work...
- In Nov 2019 a new Theme Formula was devised to make choosing a theme easier:
- The "Formula" is:
- ["Dark" or "Light"] Color Number
- Colors can be Blue Brown Grey Green Purple Red Teal Yellow Black
- The number will vary for each pair. There are more DarkGrey entries than there are LightYellow for example.
- Default = The default settings (only button color is different than system default)
- Default1 = The full system default including the button (everything's gray... how sad... don't be all gray... please....)
- :param index: the name of the index into the Look and Feel table (does not have to be exact, can be "fuzzy")
- :type index: (str)
- :param force: no longer used
- :type force: (bool)
- :return: None
- :rtype: None
- """
- global CURRENT_LOOK_AND_FEEL
-
- # if running_mac() and not force:
- # print('*** Changing look and feel is not supported on Mac platform ***')
- # return
-
- requested_theme_name = index
- theme_names_list = list_of_look_and_feel_values()
- # normalize available l&f values by setting all to lower case
- lf_values_lowercase = [item.lower() for item in theme_names_list]
- # option 1
- opt1 = requested_theme_name.replace(' ', '').lower()
- # option 3 is option 1 with gray replaced with grey
- opt3 = opt1.replace('gray', 'grey')
- # option 2 (reverse lookup)
- optx = requested_theme_name.lower().split(' ')
- optx.reverse()
- opt2 = ''.join(optx)
-
- # search for valid l&f name
- if requested_theme_name in theme_names_list:
- ix = theme_names_list.index(requested_theme_name)
- elif opt1 in lf_values_lowercase:
- ix = lf_values_lowercase.index(opt1)
- elif opt2 in lf_values_lowercase:
- ix = lf_values_lowercase.index(opt2)
- elif opt3 in lf_values_lowercase:
- ix = lf_values_lowercase.index(opt3)
- else:
- ix = random.randint(0, len(lf_values_lowercase) - 1)
- print('** Warning - {} Theme is not a valid theme. Change your theme call. **'.format(index))
- print('valid values are', list_of_look_and_feel_values())
- print('Instead, please enjoy a random Theme named {}'.format(list_of_look_and_feel_values()[ix]))
-
- selection = theme_names_list[ix]
- CURRENT_LOOK_AND_FEEL = selection
- try:
- colors = LOOK_AND_FEEL_TABLE[selection]
-
- # Color the progress bar using button background and input colors...unless they're the same
- if colors['PROGRESS'] != COLOR_SYSTEM_DEFAULT:
- if colors['PROGRESS'] == DEFAULT_PROGRESS_BAR_COMPUTE:
- if colors['BUTTON'][1] != colors['INPUT'] and colors['BUTTON'][1] != colors['BACKGROUND']:
- colors['PROGRESS'] = colors['BUTTON'][1], colors['INPUT']
- else: # if the same, then use text input on top of input color
- colors['PROGRESS'] = (colors['TEXT_INPUT'], colors['INPUT'])
- else:
- colors['PROGRESS'] = DEFAULT_PROGRESS_BAR_COLOR_OFFICIAL
- # call to change all the colors
- SetOptions(background_color=colors['BACKGROUND'],
- text_element_background_color=colors['BACKGROUND'],
- element_background_color=colors['BACKGROUND'],
- text_color=colors['TEXT'],
- input_elements_background_color=colors['INPUT'],
- # button_color=colors['BUTTON'] if not running_mac() else None,
- button_color=colors['BUTTON'],
- progress_meter_color=colors['PROGRESS'],
- border_width=colors['BORDER'],
- slider_border_width=colors['SLIDER_DEPTH'],
- progress_meter_border_depth=colors['PROGRESS_DEPTH'],
- scrollbar_color=(colors['SCROLL']),
- element_text_color=colors['TEXT'],
- input_text_color=colors['TEXT_INPUT'])
- except: # most likely an index out of range
- print('** Warning - Theme value not valid. Change your theme call. **')
- print('valid values are', list_of_look_and_feel_values())
-
-
-# ------------------------ Color processing functions ------------------------
-
-def _hex_to_hsl(hex):
- r, g, b = _hex_to_rgb(hex)
- return _rgb_to_hsl(r, g, b)
-
-
-def _hex_to_rgb(hex):
- hex = hex.lstrip('#')
- hlen = len(hex)
- return tuple(int(hex[i:i + hlen // 3], 16) for i in range(0, hlen, hlen // 3))
-
-
-def _rgb_to_hsl(r, g, b):
- r = float(r)
- g = float(g)
- b = float(b)
- high = max(r, g, b)
- low = min(r, g, b)
- h, s, v = ((high + low) / 2,) * 3
- if high == low:
- h = s = 0.0
- else:
- d = high - low
- l = (high + low) / 2
- s = d / (2 - high - low) if l > 0.5 else d / (high + low)
- h = {
- r: (g - b) / d + (6 if g < b else 0),
- g: (b - r) / d + 2,
- b: (r - g) / d + 4,
- }[high]
- h /= 6
- return h, s, v
-
-
-def _hsl_to_rgb(h, s, l):
- def hue_to_rgb(p, q, t):
- t += 1 if t < 0 else 0
- t -= 1 if t > 1 else 0
- if t < 1 / 6: return p + (q - p) * 6 * t
- if t < 1 / 2: return q
- if t < 2 / 3: p + (q - p) * (2 / 3 - t) * 6
- return p
-
- if s == 0:
- r, g, b = l, l, l
- else:
- q = l * (1 + s) if l < 0.5 else l + s - l * s
- p = 2 * l - q
- r = hue_to_rgb(p, q, h + 1 / 3)
- g = hue_to_rgb(p, q, h)
- b = hue_to_rgb(p, q, h - 1 / 3)
-
- return r, g, b
-
-
-def _hsv_to_hsl(h, s, v):
- l = 0.5 * v * (2 - s)
- s = v * s / (1 - fabs(2 * l - 1))
- return h, s, l
-
-
-def _hsl_to_hsv(h, s, l):
- v = (2 * l + s * (1 - fabs(2 * l - 1))) / 2
- s = 2 * (v - l) / v
- return h, s, v
-
-
-# Converts an object's contents into a nice printable string. Great for dumping debug data
-def obj_to_string_single_obj(obj):
- """
- Dumps an Object's values as a formatted string. Very nicely done. Great way to display an object's member variables in human form
- Returns only the top-most object's variables instead of drilling down to dispolay more
- :param obj: The object to display
- :type obj: (Any)
- :return: Formatted output of the object's values
- :rtype: (str)
- """
- if obj is None:
- return 'None'
- return str(obj.__class__) + '\n' + '\n'.join(
- (repr(item) + ' = ' + repr(obj.__dict__[item]) for item in sorted(obj.__dict__)))
-
-
-def obj_to_string(obj, extra=' '):
- """
- Dumps an Object's values as a formatted string. Very nicely done. Great way to display an object's member variables in human form
- :param obj: The object to display
- :type obj: (Any)
- :param extra: extra stuff (Default value = ' ')
- :type extra: (str)
- :return: Formatted output of the object's values
- :rtype: (str)
- """
- if obj is None:
- return 'None'
- return str(obj.__class__) + '\n' + '\n'.join(
- (extra + (str(item) + ' = ' +
- (ObjToString(obj.__dict__[item], extra + ' ') if hasattr(obj.__dict__[item], '__dict__') else str(
- obj.__dict__[item])))
- for item in sorted(obj.__dict__)))
-
-
-# ...######..##.......####.########..########...#######.....###....########..########.
-# ..##....##.##........##..##.....##.##.....##.##.....##...##.##...##.....##.##.....##
-# ..##.......##........##..##.....##.##.....##.##.....##..##...##..##.....##.##.....##
-# ..##.......##........##..########..########..##.....##.##.....##.########..##.....##
-# ..##.......##........##..##........##.....##.##.....##.#########.##...##...##.....##
-# ..##....##.##........##..##........##.....##.##.....##.##.....##.##....##..##.....##
-# ...######..########.####.##........########...#######..##.....##.##.....##.########.
-# ................................................................................
-# ..########.##.....##.##....##..######..########.####..#######..##....##..######.
-# ..##.......##.....##.###...##.##....##....##.....##..##.....##.###...##.##....##
-# ..##.......##.....##.####..##.##..........##.....##..##.....##.####..##.##......
-# ..######...##.....##.##.##.##.##..........##.....##..##.....##.##.##.##..######.
-# ..##.......##.....##.##..####.##..........##.....##..##.....##.##..####.......##
-# ..##.......##.....##.##...###.##....##....##.....##..##.....##.##...###.##....##
-# ..##........#######..##....##..######.....##....####..#######..##....##..######.
-
-def clipboard_set(new_value):
- """
- Sets the clipboard to a specific value.
- IMPORTANT NOTE - Your PySimpleGUI application needs to remain running until you've pasted
- your clipboard. This is a tkinter limitation. A workaround was found for Windows, but you still
- need to stay running for Linux systems.
-
- :param new_value: value to set the clipboard to. Will be converted to a string
- :type new_value: (str | bytes)
- """
- root = _get_hidden_master_root()
- root.clipboard_clear()
- root.clipboard_append(str(new_value))
- root.update()
-
-def clipboard_get():
- """
- Gets the clipboard current value.
-
- :return: The current value of the clipboard
- :rtype: (str)
- """
- root = _get_hidden_master_root()
-
- try:
- value = root.clipboard_get()
- except:
- value = ''
- root.update()
- return value
-
-
-# MM"""""""`YM
-# MM mmmmm M
-# M' .M .d8888b. 88d888b. dP dP 88d888b. .d8888b.
-# MM MMMMMMMM 88' `88 88' `88 88 88 88' `88 Y8ooooo.
-# MM MMMMMMMM 88. .88 88. .88 88. .88 88. .88 88
-# MM MMMMMMMM `88888P' 88Y888P' `88888P' 88Y888P' `88888P'
-# MMMMMMMMMMMM 88 88
-# dP dP
-# ------------------------------------------------------------------------------------------------------------------ #
-# ===================================== Upper PySimpleGUI ======================================================== #
-# ------------------------------------------------------------------------------------------------------------------ #
-# ----------------------------------- The mighty Popup! ------------------------------------------------------------ #
-
-def popup(*args, title=None, button_color=None, background_color=None, text_color=None, button_type=POPUP_BUTTONS_OK, auto_close=False,
- auto_close_duration=None, custom_text=(None, None), non_blocking=False, icon=None, line_width=None, font=None, no_titlebar=False, grab_anywhere=False,
- keep_on_top=None, location=(None, None), relative_location=(None, None), any_key_closes=False, image=None, modal=True):
- """
- Popup - Display a popup Window with as many parms as you wish to include. This is the GUI equivalent of the
- "print" statement. It's also great for "pausing" your program's flow until the user can read some error messages.
-
- If this popup doesn't have the features you want, then you can easily make your own. Popups can be accomplished in 1 line of code:
- choice, _ = sg.Window('Continue?', [[sg.T('Do you want to continue?')], [sg.Yes(s=10), sg.No(s=10)]], disable_close=True).read(close=True)
-
-
- :param *args: Variable number of your arguments. Load up the call with stuff to see!
- :type *args: (Any)
- :param title: Optional title for the window. If none provided, the first arg will be used instead.
- :type title: (str)
- :param button_color: Color of the buttons shown (text color, button color)
- :type button_color: (str, str) | None
- :param background_color: Window's background color
- :type background_color: (str)
- :param text_color: text color
- :type text_color: (str)
- :param button_type: NOT USER SET! Determines which pre-defined buttons will be shown (Default value = POPUP_BUTTONS_OK). There are many Popup functions and they call Popup, changing this parameter to get the desired effect.
- :type button_type: (int)
- :param auto_close: If True the window will automatically close
- :type auto_close: (bool)
- :param auto_close_duration: time in seconds to keep window open before closing it automatically
- :type auto_close_duration: (int)
- :param custom_text: A string or pair of strings that contain the text to display on the buttons
- :type custom_text: (str, str) | str
- :param non_blocking: If True then will immediately return from the function without waiting for the user's input.
- :type non_blocking: (bool)
- :param icon: icon to display on the window. Same format as a Window call
- :type icon: str | bytes
- :param line_width: Width of lines in characters. Defaults to MESSAGE_BOX_LINE_WIDTH
- :type line_width: (int)
- :param font: specifies the font family, size, etc. Tuple or Single string format 'name size styles'. Styles: italic * roman bold normal underline overstrike
- :type font: str | Tuple[font_name, size, modifiers]
- :param no_titlebar: If True will not show the frame around the window and the titlebar across the top
- :type no_titlebar: (bool)
- :param grab_anywhere: If True can grab anywhere to move the window. If no_titlebar is True, grab_anywhere should likely be enabled too
- :type grab_anywhere: (bool)
- :param location: Location on screen to display the top left corner of window. Defaults to window centered on screen
- :type location: (int, int)
- :param relative_location: (x,y) location relative to the default location of the window, in pixels. Normally the window centers. This location is relative to the location the window would be created. Note they can be negative.
- :type relative_location: (int, int)
- :param keep_on_top: If True the window will remain above all current windows
- :type keep_on_top: (bool)
- :param any_key_closes: If True then will turn on return_keyboard_events for the window which will cause window to close as soon as any key is pressed. Normally the return key only will close the window. Default is false.
- :type any_key_closes: (bool)
- :param image: Image to include at the top of the popup window
- :type image: (str) or (bytes)
- :param modal: If True then makes the popup will behave like a Modal window... all other windows are non-operational until this one is closed. Default = True
- :type modal: bool
- :return: Returns text of the button that was pressed. None will be returned if user closed window with X
- :rtype: str | None
- """
-
- if not args:
- args_to_print = ['']
- else:
- args_to_print = args
- if line_width != None:
- local_line_width = line_width
- else:
- local_line_width = MESSAGE_BOX_LINE_WIDTH
- _title = title if title is not None else args_to_print[0]
-
- layout = [[]]
- max_line_total, total_lines = 0, 0
- if image is not None:
- if isinstance(image, str):
- layout += [[Image(filename=image)]]
- else:
- layout += [[Image(data=image)]]
-
- for message in args_to_print:
- # fancy code to check if string and convert if not is not need. Just always convert to string :-)
- # if not isinstance(message, str): message = str(message)
- message = str(message)
- if message.count('\n'): # if there are line breaks, then wrap each segment separately
- # message_wrapped = message # used to just do this, but now breaking into smaller pieces
- message_wrapped = ''
- msg_list = message.split('\n') # break into segments that will each be wrapped
- message_wrapped = '\n'.join([textwrap.fill(msg, local_line_width) for msg in msg_list])
- else:
- message_wrapped = textwrap.fill(message, local_line_width)
- message_wrapped_lines = message_wrapped.count('\n') + 1
- longest_line_len = max([len(l) for l in message.split('\n')])
- width_used = min(longest_line_len, local_line_width)
- max_line_total = max(max_line_total, width_used)
- # height = _GetNumLinesNeeded(message, width_used)
- height = message_wrapped_lines
- layout += [[
- Text(message_wrapped, auto_size_text=True, text_color=text_color, background_color=background_color)]]
- total_lines += height
-
- if non_blocking:
- PopupButton = DummyButton # important to use or else button will close other windows too!
- else:
- PopupButton = Button
- # show either an OK or Yes/No depending on paramater
- if custom_text != (None, None):
- if type(custom_text) is not tuple:
- layout += [[PopupButton(custom_text, size=(len(custom_text), 1), button_color=button_color, focus=True,
- bind_return_key=True)]]
- elif custom_text[1] is None:
- layout += [[
- PopupButton(custom_text[0], size=(len(custom_text[0]), 1), button_color=button_color, focus=True,
- bind_return_key=True)]]
- else:
- layout += [[PopupButton(custom_text[0], button_color=button_color, focus=True, bind_return_key=True,
- size=(len(custom_text[0]), 1)),
- PopupButton(custom_text[1], button_color=button_color, size=(len(custom_text[1]), 1))]]
- elif button_type is POPUP_BUTTONS_YES_NO:
- layout += [[PopupButton('Yes', button_color=button_color, focus=True, bind_return_key=True, pad=((20, 5), 3),
- size=(5, 1)), PopupButton('No', button_color=button_color, size=(5, 1))]]
- elif button_type is POPUP_BUTTONS_CANCELLED:
- layout += [[
- PopupButton('Cancelled', button_color=button_color, focus=True, bind_return_key=True, pad=((20, 0), 3))]]
- elif button_type is POPUP_BUTTONS_ERROR:
- layout += [[PopupButton('Error', size=(6, 1), button_color=button_color, focus=True, bind_return_key=True,
- pad=((20, 0), 3))]]
- elif button_type is POPUP_BUTTONS_OK_CANCEL:
- layout += [[PopupButton('OK', size=(6, 1), button_color=button_color, focus=True, bind_return_key=True),
- PopupButton('Cancel', size=(6, 1), button_color=button_color)]]
- elif button_type is POPUP_BUTTONS_NO_BUTTONS:
- pass
- else:
- layout += [[PopupButton('OK', size=(5, 1), button_color=button_color, focus=True, bind_return_key=True,
- pad=((20, 0), 3))]]
-
- window = Window(_title, layout, auto_size_text=True, background_color=background_color, button_color=button_color,
- auto_close=auto_close, auto_close_duration=auto_close_duration, icon=icon, font=font,
- no_titlebar=no_titlebar, grab_anywhere=grab_anywhere, keep_on_top=keep_on_top, location=location, relative_location=relative_location, return_keyboard_events=any_key_closes,
- modal=modal)
-
-
- if non_blocking:
- button, values = window.read(timeout=0)
- else:
- button, values = window.read()
- window.close()
- del window
-
- return button
-
-
-# ============================== MsgBox============#
-# Lazy function. Same as calling Popup with parms #
-# This function WILL Disappear perhaps today #
-# ==================================================#
-# MsgBox is the legacy call and should not be used any longer
-def MsgBox(*args):
- """
- Do not call this anymore it will raise exception. Use Popups instead
- :param *args:
- :type *args:
-
- """
- raise DeprecationWarning('MsgBox is no longer supported... change your call to Popup')
-
-
-# ======================== Scrolled Text Box =====#
-# ===================================================#
-def popup_scrolled(*args, title=None, button_color=None, background_color=None, text_color=None, yes_no=False, auto_close=False, auto_close_duration=None,
- size=(None, None), location=(None, None), relative_location=(None, None), non_blocking=False, no_titlebar=False, grab_anywhere=False, keep_on_top=None, font=None,
- image=None, icon=None, modal=True, no_sizegrip=False):
- """
- Show a scrolled Popup window containing the user's text that was supplied. Use with as many items to print as you
- want, just like a print statement.
-
- :param *args: Variable number of items to display
- :type *args: (Any)
- :param title: Title to display in the window.
- :type title: (str)
- :param button_color: button color (foreground, background)
- :type button_color: (str, str) or str
- :param yes_no: If True, displays Yes and No buttons instead of Ok
- :type yes_no: (bool)
- :param auto_close: if True window will close itself
- :type auto_close: (bool)
- :param auto_close_duration: Older versions only accept int. Time in seconds until window will close
- :type auto_close_duration: int | float
- :param size: (w,h) w=characters-wide, h=rows-high
- :type size: (int, int)
- :param location: Location on the screen to place the upper left corner of the window
- :type location: (int, int)
- :param relative_location: (x,y) location relative to the default location of the window, in pixels. Normally the window centers. This location is relative to the location the window would be created. Note they can be negative.
- :type relative_location: (int, int)
- :param non_blocking: if True the call will immediately return rather than waiting on user input
- :type non_blocking: (bool)
- :param background_color: color of background
- :type background_color: (str)
- :param text_color: color of the text
- :type text_color: (str)
- :param no_titlebar: If True no titlebar will be shown
- :type no_titlebar: (bool)
- :param grab_anywhere: If True, than can grab anywhere to move the window (Default = False)
- :type grab_anywhere: (bool)
- :param keep_on_top: If True the window will remain above all current windows
- :type keep_on_top: (bool)
- :param font: specifies the font family, size, etc. Tuple or Single string format 'name size styles'. Styles: italic * roman bold normal underline overstrike
- :type font: (str or (str, int[, str]) or None)
- :param image: Image to include at the top of the popup window
- :type image: (str) or (bytes)
- :param icon: filename or base64 string to be used for the window's icon
- :type icon: bytes | str
- :param modal: If True then makes the popup will behave like a Modal window... all other windows are non-operational until this one is closed. Default = True
- :type modal: bool
- :param no_sizegrip: If True no Sizegrip will be shown when there is no titlebar. It's only shown if there is no titlebar
- :type no_sizegrip: (bool)
- :return: Returns text of the button that was pressed. None will be returned if user closed window with X
- :rtype: str | None | TIMEOUT_KEY
- """
- if not args: return
- width, height = size
- width = width if width else MESSAGE_BOX_LINE_WIDTH
-
- layout = [[]]
-
- if image is not None:
- if isinstance(image, str):
- layout += [[Image(filename=image)]]
- else:
- layout += [[Image(data=image)]]
- max_line_total, max_line_width, total_lines, height_computed = 0, 0, 0, 0
- complete_output = ''
- for message in args:
- # fancy code to check if string and convert if not is not need. Just always convert to string :-)
- # if not isinstance(message, str): message = str(message)
- message = str(message)
- longest_line_len = max([len(l) for l in message.split('\n')])
- width_used = min(longest_line_len, width)
- max_line_total = max(max_line_total, width_used)
- max_line_width = width
- lines_needed = _GetNumLinesNeeded(message, width_used)
- height_computed += lines_needed + 1
- complete_output += message + '\n'
- total_lines += lines_needed
- height_computed = MAX_SCROLLED_TEXT_BOX_HEIGHT if height_computed > MAX_SCROLLED_TEXT_BOX_HEIGHT else height_computed
- if height:
- height_computed = height
- layout += [[Multiline(complete_output, size=(max_line_width, height_computed), background_color=background_color, text_color=text_color, expand_x=True,
- expand_y=True, k='-MLINE-')]]
- pad = max_line_total - 15 if max_line_total > 15 else 1
- # show either an OK or Yes/No depending on paramater
- button = DummyButton if non_blocking else Button
- if yes_no:
- layout += [[Text('', size=(pad, 1), auto_size_text=False, background_color=background_color), button('Yes'), button('No')]]
- else:
- layout += [[Text('', size=(pad, 1), auto_size_text=False, background_color=background_color),
- button('OK', size=(5, 1), button_color=button_color)]]
- if no_titlebar and no_sizegrip is not True:
- layout += [[Sizegrip()]]
-
- window = Window(title or args[0], layout, auto_size_text=True, button_color=button_color, auto_close=auto_close,
- auto_close_duration=auto_close_duration, location=location, relative_location=relative_location, resizable=True, font=font, background_color=background_color,
- no_titlebar=no_titlebar, grab_anywhere=grab_anywhere, keep_on_top=keep_on_top, modal=modal, icon=icon)
- if non_blocking:
- button, values = window.read(timeout=0)
- else:
- button, values = window.read()
- window.close()
- del window
- return button
-
-
-# ============================== sprint ======#
-# Is identical to the Scrolled Text Box #
-# Provides a crude 'print' mechanism but in a #
-# GUI environment #
-# This is in addition to the Print function #
-# which routes output to a "Debug Window" #
-# ============================================#
-
-
-# --------------------------- popup_no_buttons ---------------------------
-def popup_no_buttons(*args, title=None, background_color=None, text_color=None, auto_close=False,
- auto_close_duration=None, non_blocking=False, icon=None, line_width=None, font=None,
- no_titlebar=False, grab_anywhere=False, keep_on_top=None, location=(None, None), relative_location=(None, None), image=None, modal=True):
- """Show a Popup but without any buttons
-
- :param *args: Variable number of items to display
- :type *args: (Any)
- :param title: Title to display in the window.
- :type title: (str)
- :param background_color: color of background
- :type background_color: (str)
- :param text_color: color of the text
- :type text_color: (str)
- :param auto_close: if True window will close itself
- :type auto_close: (bool)
- :param auto_close_duration: Older versions only accept int. Time in seconds until window will close
- :type auto_close_duration: int | float
- :param non_blocking: If True then will immediately return from the function without waiting for the user's input. (Default = False)
- :type non_blocking: (bool)
- :param icon: filename or base64 string to be used for the window's icon
- :type icon: bytes | str
- :param line_width: Width of lines in characters
- :type line_width: (int)
- :param font: specifies the font family, size, etc. Tuple or Single string format 'name size styles'. Styles: italic * roman bold normal underline overstrike
- :type font: (str or (str, int[, str]) or None)
- :param no_titlebar: If True no titlebar will be shown
- :type no_titlebar: (bool)
- :param grab_anywhere: If True, than can grab anywhere to move the window (Default = False)
- :type grab_anywhere: (bool)
- :param location: Location of upper left corner of the window
- :type location: (int, int)
- :param relative_location: (x,y) location relative to the default location of the window, in pixels. Normally the window centers. This location is relative to the location the window would be created. Note they can be negative.
- :type relative_location: (int, int)
- :param image: Image to include at the top of the popup window
- :type image: (str) or (bytes)
- :param modal: If True then makes the popup will behave like a Modal window... all other windows are non-operational until this one is closed. Default = True
- :type modal: bool
- :return: Returns text of the button that was pressed. None will be returned if user closed window with X
- :rtype: str | None | TIMEOUT_KEY """
- Popup(*args, title=title, background_color=background_color, text_color=text_color,
- button_type=POPUP_BUTTONS_NO_BUTTONS,
- auto_close=auto_close, auto_close_duration=auto_close_duration, non_blocking=non_blocking, icon=icon,
- line_width=line_width,
- font=font, no_titlebar=no_titlebar, grab_anywhere=grab_anywhere, keep_on_top=keep_on_top, location=location, relative_location=relative_location, image=image, modal=modal)
-
-
-# --------------------------- popup_non_blocking ---------------------------
-def popup_non_blocking(*args, title=None, button_type=POPUP_BUTTONS_OK, button_color=None, background_color=None,
- text_color=None, auto_close=False, auto_close_duration=None, non_blocking=True, icon=None,
- line_width=None, font=None, no_titlebar=False, grab_anywhere=False, keep_on_top=None,
- location=(None, None), relative_location=(None, None), image=None, modal=False):
- """
- Show Popup window and immediately return (does not block)
-
- :param *args: Variable number of items to display
- :type *args: (Any)
- :param title: Title to display in the window.
- :type title: (str)
- :param button_type: Determines which pre-defined buttons will be shown (Default value = POPUP_BUTTONS_OK).
- :type button_type: (int)
- :param button_color: button color (foreground, background)
- :type button_color: (str, str) or str
- :param background_color: color of background
- :type background_color: (str)
- :param text_color: color of the text
- :type text_color: (str)
- :param auto_close: if True window will close itself
- :type auto_close: (bool)
- :param auto_close_duration: Older versions only accept int. Time in seconds until window will close
- :type auto_close_duration: int | float
- :param non_blocking: if True the call will immediately return rather than waiting on user input
- :type non_blocking: (bool)
- :param icon: filename or base64 string to be used for the window's icon
- :type icon: bytes | str
- :param line_width: Width of lines in characters
- :type line_width: (int)
- :param font: specifies the font family, size, etc. Tuple or Single string format 'name size styles'. Styles: italic * roman bold normal underline overstrike
- :type font: (str or (str, int[, str]) or None)
- :param no_titlebar: If True no titlebar will be shown
- :type no_titlebar: (bool)
- :param grab_anywhere: If True: can grab anywhere to move the window (Default = False)
- :type grab_anywhere: (bool)
- :param location: Location of upper left corner of the window
- :type location: (int, int)
- :param relative_location: (x,y) location relative to the default location of the window, in pixels. Normally the window centers. This location is relative to the location the window would be created. Note they can be negative.
- :type relative_location: (int, int)
- :param image: Image to include at the top of the popup window
- :type image: (str) or (bytes)
- :param modal: If True then makes the popup will behave like a Modal window... all other windows are non-operational until this one is closed. Default = False
- :type modal: bool
- :return: Reason for popup closing
- :rtype: str | None
- """
-
- return popup(*args, title=title, button_color=button_color, background_color=background_color, text_color=text_color,
- button_type=button_type,
- auto_close=auto_close, auto_close_duration=auto_close_duration, non_blocking=non_blocking, icon=icon,
- line_width=line_width,
- font=font, no_titlebar=no_titlebar, grab_anywhere=grab_anywhere, keep_on_top=keep_on_top, location=location, relative_location=relative_location, image=image, modal=modal)
-
-
-# --------------------------- popup_quick - a NonBlocking, Self-closing Popup ---------------------------
-def popup_quick(*args, title=None, button_type=POPUP_BUTTONS_OK, button_color=None, background_color=None,
- text_color=None, auto_close=True, auto_close_duration=2, non_blocking=True, icon=None, line_width=None,
- font=None, no_titlebar=False, grab_anywhere=False, keep_on_top=None, location=(None, None), relative_location=(None, None), image=None, modal=False):
- """
- Show Popup box that doesn't block and closes itself
-
- :param *args: Variable number of items to display
- :type *args: (Any)
- :param title: Title to display in the window.
- :type title: (str)
- :param button_type: Determines which pre-defined buttons will be shown (Default value = POPUP_BUTTONS_OK).
- :type button_type: (int)
- :param button_color: button color (foreground, background)
- :type button_color: (str, str) or str
- :param background_color: color of background
- :type background_color: (str)
- :param text_color: color of the text
- :type text_color: (str)
- :param auto_close: if True window will close itself
- :type auto_close: (bool)
- :param auto_close_duration: Older versions only accept int. Time in seconds until window will close
- :type auto_close_duration: int | float
- :param non_blocking: if True the call will immediately return rather than waiting on user input
- :type non_blocking: (bool)
- :param icon: filename or base64 string to be used for the window's icon
- :type icon: bytes | str
- :param line_width: Width of lines in characters
- :type line_width: (int)
- :param font: specifies the font family, size, etc. Tuple or Single string format 'name size styles'. Styles: italic * roman bold normal underline overstrike
- :type font: (str or (str, int[, str]) or None)
- :param no_titlebar: If True no titlebar will be shown
- :type no_titlebar: (bool)
- :param grab_anywhere: If True: can grab anywhere to move the window (Default = False)
- :type grab_anywhere: (bool)
- :param keep_on_top: If True the window will remain above all current windows
- :type keep_on_top: (bool)
- :param location: Location of upper left corner of the window
- :type location: (int, int)
- :param relative_location: (x,y) location relative to the default location of the window, in pixels. Normally the window centers. This location is relative to the location the window would be created. Note they can be negative.
- :type relative_location: (int, int)
- :param image: Image to include at the top of the popup window
- :type image: (str) or (bytes)
- :param modal: If True then makes the popup will behave like a Modal window... all other windows are non-operational until this one is closed. Default = False
- :type modal: bool
- :return: Returns text of the button that was pressed. None will be returned if user closed window with X
- :rtype: str | None | TIMEOUT_KEY
- """
-
- return popup(*args, title=title, button_color=button_color, background_color=background_color, text_color=text_color,
- button_type=button_type,
- auto_close=auto_close, auto_close_duration=auto_close_duration, non_blocking=non_blocking, icon=icon,
- line_width=line_width,
- font=font, no_titlebar=no_titlebar, grab_anywhere=grab_anywhere, keep_on_top=keep_on_top, location=location, relative_location=relative_location, image=image, modal=modal)
-
-
-# --------------------------- popup_quick_message - a NonBlocking, Self-closing Popup with no titlebar and no buttons ---------------------------
-def popup_quick_message(*args, title=None, button_type=POPUP_BUTTONS_NO_BUTTONS, button_color=None, background_color=None,
- text_color=None, auto_close=True, auto_close_duration=2, non_blocking=True, icon=None, line_width=None,
- font=None, no_titlebar=True, grab_anywhere=False, keep_on_top=None, location=(None, None), relative_location=(None, None), image=None, modal=False):
- """
- Show Popup window with no titlebar, doesn't block, and auto closes itself.
-
- :param *args: Variable number of items to display
- :type *args: (Any)
- :param title: Title to display in the window.
- :type title: (str)
- :param button_type: Determines which pre-defined buttons will be shown (Default value = POPUP_BUTTONS_OK).
- :type button_type: (int)
- :param button_color: button color (foreground, background)
- :type button_color: (str, str) or str
- :param keep_on_top: If True the window will remain above all current windows
- :type keep_on_top: (bool)
- :param background_color: color of background
- :type background_color: (str)
- :param text_color: color of the text
- :type text_color: (str)
- :param auto_close: if True window will close itself
- :type auto_close: (bool)
- :param auto_close_duration: Older versions only accept int. Time in seconds until window will close
- :type auto_close_duration: int | float
- :param non_blocking: if True the call will immediately return rather than waiting on user input
- :type non_blocking: (bool)
- :param icon: filename or base64 string to be used for the window's icon
- :type icon: bytes | str
- :param line_width: Width of lines in characters
- :type line_width: (int)
- :param font: specifies the font family, size, etc. Tuple or Single string format 'name size styles'. Styles: italic * roman bold normal underline overstrike
- :type font: (str or (str, int[, str]) or None)
- :param no_titlebar: If True no titlebar will be shown
- :type no_titlebar: (bool)
- :param grab_anywhere: If True: can grab anywhere to move the window (Default = False)
- :type grab_anywhere: (bool)
- :param location: Location of upper left corner of the window
- :type location: (int, int)
- :param relative_location: (x,y) location relative to the default location of the window, in pixels. Normally the window centers. This location is relative to the location the window would be created. Note they can be negative.
- :type relative_location: (int, int)
- :param image: Image to include at the top of the popup window
- :type image: (str) or (bytes)
- :param modal: If True then makes the popup will behave like a Modal window... all other windows are non-operational until this one is closed. Default = False
- :type modal: bool
- :return: Returns text of the button that was pressed. None will be returned if user closed window with X
- :rtype: str | None | TIMEOUT_KEY
- """
- return popup(*args, title=title, button_color=button_color, background_color=background_color, text_color=text_color,
- button_type=button_type,
- auto_close=auto_close, auto_close_duration=auto_close_duration, non_blocking=non_blocking, icon=icon,
- line_width=line_width,
- font=font, no_titlebar=no_titlebar, grab_anywhere=grab_anywhere, keep_on_top=keep_on_top, location=location, relative_location=relative_location, image=image, modal=modal)
-
-
-# --------------------------- PopupNoTitlebar ---------------------------
-def popup_no_titlebar(*args, title=None, button_type=POPUP_BUTTONS_OK, button_color=None, background_color=None,
- text_color=None, auto_close=False, auto_close_duration=None, non_blocking=False, icon=None,
- line_width=None, font=None, grab_anywhere=True, keep_on_top=None, location=(None, None), relative_location=(None, None), image=None, modal=True):
- """
- Display a Popup without a titlebar. Enables grab anywhere so you can move it
-
- :param *args: Variable number of items to display
- :type *args: (Any)
- :param title: Title to display in the window.
- :type title: (str)
- :param button_type: Determines which pre-defined buttons will be shown (Default value = POPUP_BUTTONS_OK).
- :type button_type: (int)
- :param button_color: button color (foreground, background)
- :type button_color: (str, str) or str
- :param background_color: color of background
- :type background_color: (str)
- :param text_color: color of the text
- :type text_color: (str)
- :param auto_close: if True window will close itself
- :type auto_close: (bool)
- :param auto_close_duration: Older versions only accept int. Time in seconds until window will close
- :type auto_close_duration: int | float
- :param non_blocking: if True the call will immediately return rather than waiting on user input
- :type non_blocking: (bool)
- :param icon: filename or base64 string to be used for the window's icon
- :type icon: bytes | str
- :param line_width: Width of lines in characters
- :type line_width: (int)
- :param font: specifies the font family, size, etc. Tuple or Single string format 'name size styles'. Styles: italic * roman bold normal underline overstrike
- :type font: (str or (str, int[, str]) or None)
- :param grab_anywhere: If True: can grab anywhere to move the window (Default = False)
- :type grab_anywhere: (bool)
- :param keep_on_top: If True the window will remain above all current windows
- :type keep_on_top: (bool)
- :param location: Location of upper left corner of the window
- :type location: (int, int)
- :param relative_location: (x,y) location relative to the default location of the window, in pixels. Normally the window centers. This location is relative to the location the window would be created. Note they can be negative.
- :type relative_location: (int, int)
- :param image: Image to include at the top of the popup window
- :type image: (str) or (bytes)
- :param modal: If True then makes the popup will behave like a Modal window... all other windows are non-operational until this one is closed. Default = True
- :type modal: bool
- :return: Returns text of the button that was pressed. None will be returned if user closed window with X
- :rtype: str | None | TIMEOUT_KEY
- """
- return popup(*args, title=title, button_color=button_color, background_color=background_color, text_color=text_color,
- button_type=button_type,
- auto_close=auto_close, auto_close_duration=auto_close_duration, non_blocking=non_blocking, icon=icon,
- line_width=line_width,
- font=font, no_titlebar=True, grab_anywhere=grab_anywhere, keep_on_top=keep_on_top, location=location, relative_location=relative_location, image=image, modal=modal)
-
-
-# --------------------------- PopupAutoClose ---------------------------
-def popup_auto_close(*args, title=None, button_type=POPUP_BUTTONS_OK, button_color=None, background_color=None, text_color=None,
- auto_close=True, auto_close_duration=None, non_blocking=False, icon=None,
- line_width=None, font=None, no_titlebar=False, grab_anywhere=False, keep_on_top=None,
- location=(None, None), relative_location=(None, None), image=None, modal=True):
- """Popup that closes itself after some time period
-
- :param *args: Variable number of items to display
- :type *args: (Any)
- :param title: Title to display in the window.
- :type title: (str)
- :param button_type: Determines which pre-defined buttons will be shown (Default value = POPUP_BUTTONS_OK).
- :type button_type: (int)
- :param button_color: button color (foreground, background)
- :type button_color: (str, str) or str
- :param background_color: color of background
- :type background_color: (str)
- :param text_color: color of the text
- :type text_color: (str)
- :param auto_close: if True window will close itself
- :type auto_close: (bool)
- :param auto_close_duration: Older versions only accept int. Time in seconds until window will close
- :type auto_close_duration: int | float
- :param non_blocking: if True the call will immediately return rather than waiting on user input
- :type non_blocking: (bool)
- :param icon: filename or base64 string to be used for the window's icon
- :type icon: bytes | str
- :param line_width: Width of lines in characters
- :type line_width: (int)
- :param font: specifies the font family, size, etc. Tuple or Single string format 'name size styles'. Styles: italic * roman bold normal underline overstrike
- :type font: (str or (str, int[, str]) or None)
- :param no_titlebar: If True no titlebar will be shown
- :type no_titlebar: (bool)
- :param grab_anywhere: If True: can grab anywhere to move the window (Default = False)
- :type grab_anywhere: (bool)
- :param keep_on_top: If True the window will remain above all current windows
- :type keep_on_top: (bool)
- :param location: Location of upper left corner of the window
- :type location: (int, int)
- :param relative_location: (x,y) location relative to the default location of the window, in pixels. Normally the window centers. This location is relative to the location the window would be created. Note they can be negative.
- :type relative_location: (int, int)
- :param image: Image to include at the top of the popup window
- :type image: (str) or (bytes)
- :param modal: If True then makes the popup will behave like a Modal window... all other windows are non-operational until this one is closed. Default = True
- :type modal: bool
- :return: Returns text of the button that was pressed. None will be returned if user closed window with X
- :rtype: str | None | TIMEOUT_KEY
- """
-
- return popup(*args, title=title, button_color=button_color, background_color=background_color, text_color=text_color,
- button_type=button_type,
- auto_close=auto_close, auto_close_duration=auto_close_duration, non_blocking=non_blocking, icon=icon,
- line_width=line_width,
- font=font, no_titlebar=no_titlebar, grab_anywhere=grab_anywhere, keep_on_top=keep_on_top, location=location, relative_location=relative_location, image=image, modal=modal)
-
-
-# --------------------------- popup_error ---------------------------
-def popup_error(*args, title=None, button_color=(None, None), background_color=None, text_color=None, auto_close=False,
- auto_close_duration=None, non_blocking=False, icon=None, line_width=None, font=None,
- no_titlebar=False, grab_anywhere=False, keep_on_top=None, location=(None, None), relative_location=(None, None), image=None, modal=True):
- """
- Popup with colored button and 'Error' as button text
-
- :param *args: Variable number of items to display
- :type *args: (Any)
- :param title: Title to display in the window.
- :type title: (str)
- :param button_color: button color (foreground, background)
- :type button_color: (str, str) or str
- :param background_color: color of background
- :type background_color: (str)
- :param text_color: color of the text
- :type text_color: (str)
- :param auto_close: if True window will close itself
- :type auto_close: (bool)
- :param auto_close_duration: Older versions only accept int. Time in seconds until window will close
- :type auto_close_duration: int | float
- :param non_blocking: if True the call will immediately return rather than waiting on user input
- :type non_blocking: (bool)
- :param icon: filename or base64 string to be used for the window's icon
- :type icon: bytes | str
- :param line_width: Width of lines in characters
- :type line_width: (int)
- :param font: specifies the font family, size, etc. Tuple or Single string format 'name size styles'. Styles: italic * roman bold normal underline overstrike
- :type font: (str or (str, int[, str]) or None)
- :param no_titlebar: If True no titlebar will be shown
- :type no_titlebar: (bool)
- :param grab_anywhere: If True: can grab anywhere to move the window (Default = False)
- :type grab_anywhere: (bool)
- :param keep_on_top: If True the window will remain above all current windows
- :type keep_on_top: (bool)
- :param location: Location of upper left corner of the window
- :type location: (int, int)
- :param relative_location: (x,y) location relative to the default location of the window, in pixels. Normally the window centers. This location is relative to the location the window would be created. Note they can be negative.
- :type relative_location: (int, int)
- :param image: Image to include at the top of the popup window
- :type image: (str) or (bytes)
- :param modal: If True then makes the popup will behave like a Modal window... all other windows are non-operational until this one is closed. Default = True
- :type modal: bool
- :return: Returns text of the button that was pressed. None will be returned if user closed window with X
- :rtype: str | None | TIMEOUT_KEY
- """
- tbutton_color = DEFAULT_ERROR_BUTTON_COLOR if button_color == (None, None) else button_color
- return popup(*args, title=title, button_type=POPUP_BUTTONS_ERROR, background_color=background_color, text_color=text_color,
- non_blocking=non_blocking, icon=icon, line_width=line_width, button_color=tbutton_color,
- auto_close=auto_close,
- auto_close_duration=auto_close_duration, font=font, no_titlebar=no_titlebar, grab_anywhere=grab_anywhere,
- keep_on_top=keep_on_top, location=location, relative_location=relative_location, image=image, modal=modal)
-
-
-# --------------------------- popup_cancel ---------------------------
-def popup_cancel(*args, title=None, button_color=None, background_color=None, text_color=None, auto_close=False,
- auto_close_duration=None, non_blocking=False, icon=None, line_width=None, font=None,
- no_titlebar=False, grab_anywhere=False, keep_on_top=None, location=(None, None), relative_location=(None, None), image=None, modal=True):
- """
- Display Popup with "cancelled" button text
-
- :param *args: Variable number of items to display
- :type *args: (Any)
- :param title: Title to display in the window.
- :type title: (str)
- :param button_color: button color (foreground, background)
- :type button_color: (str, str) or str
- :param background_color: color of background
- :type background_color: (str)
- :param text_color: color of the text
- :type text_color: (str)
- :param auto_close: if True window will close itself
- :type auto_close: (bool)
- :param auto_close_duration: Older versions only accept int. Time in seconds until window will close
- :type auto_close_duration: int | float
- :param non_blocking: if True the call will immediately return rather than waiting on user input
- :type non_blocking: (bool)
- :param icon: filename or base64 string to be used for the window's icon
- :type icon: bytes | str
- :param line_width: Width of lines in characters
- :type line_width: (int)
- :param font: specifies the font family, size, etc. Tuple or Single string format 'name size styles'. Styles: italic * roman bold normal underline overstrike
- :type font: (str or (str, int[, str]) or None)
- :param no_titlebar: If True no titlebar will be shown
- :type no_titlebar: (bool)
- :param grab_anywhere: If True: can grab anywhere to move the window (Default = False)
- :type grab_anywhere: (bool)
- :param keep_on_top: If True the window will remain above all current windows
- :type keep_on_top: (bool)
- :param location: Location of upper left corner of the window
- :type location: (int, int)
- :param relative_location: (x,y) location relative to the default location of the window, in pixels. Normally the window centers. This location is relative to the location the window would be created. Note they can be negative.
- :type relative_location: (int, int)
- :param image: Image to include at the top of the popup window
- :type image: (str) or (bytes)
- :param modal: If True then makes the popup will behave like a Modal window... all other windows are non-operational until this one is closed. Default = True
- :type modal: bool
- :return: Returns text of the button that was pressed. None will be returned if user closed window with X
- :rtype: str | None | TIMEOUT_KEY
- """
- return popup(*args, title=title, button_type=POPUP_BUTTONS_CANCELLED, background_color=background_color,
- text_color=text_color,
- non_blocking=non_blocking, icon=icon, line_width=line_width, button_color=button_color, auto_close=auto_close,
- auto_close_duration=auto_close_duration, font=font, no_titlebar=no_titlebar, grab_anywhere=grab_anywhere,
- keep_on_top=keep_on_top, location=location, relative_location=relative_location, image=image, modal=modal)
-
-
-# --------------------------- popup_ok ---------------------------
-def popup_ok(*args, title=None, button_color=None, background_color=None, text_color=None, auto_close=False,
- auto_close_duration=None, non_blocking=False, icon=None, line_width=None, font=None,
- no_titlebar=False, grab_anywhere=False, keep_on_top=None, location=(None, None), relative_location=(None, None), image=None, modal=True):
- """
- Display Popup with OK button only
-
- :param *args: Variable number of items to display
- :type *args: (Any)
- :param title: Title to display in the window.
- :type title: (str)
- :param button_color: button color (foreground, background)
- :type button_color: (str, str) or str
- :param background_color: color of background
- :type background_color: (str)
- :param text_color: color of the text
- :type text_color: (str)
- :param auto_close: if True window will close itself
- :type auto_close: (bool)
- :param auto_close_duration: Older versions only accept int. Time in seconds until window will close
- :type auto_close_duration: int | float
- :param non_blocking: if True the call will immediately return rather than waiting on user input
- :type non_blocking: (bool)
- :param icon: filename or base64 string to be used for the window's icon
- :type icon: bytes | str
- :param line_width: Width of lines in characters
- :type line_width: (int)
- :param font: specifies the font family, size, etc. Tuple or Single string format 'name size styles'. Styles: italic * roman bold normal underline overstrike
- :type font: (str or (str, int[, str]) or None)
- :param no_titlebar: If True no titlebar will be shown
- :type no_titlebar: (bool)
- :param grab_anywhere: If True: can grab anywhere to move the window (Default = False)
- :type grab_anywhere: (bool)
- :param keep_on_top: If True the window will remain above all current windows
- :type keep_on_top: (bool)
- :param location: Location of upper left corner of the window
- :type location: (int, int)
- :param relative_location: (x,y) location relative to the default location of the window, in pixels. Normally the window centers. This location is relative to the location the window would be created. Note they can be negative.
- :type relative_location: (int, int)
- :param image: Image to include at the top of the popup window
- :type image: (str) or (bytes)
- :param modal: If True then makes the popup will behave like a Modal window... all other windows are non-operational until this one is closed. Default = True
- :type modal: bool
- :return: Returns text of the button that was pressed. None will be returned if user closed window with X
- :rtype: str | None | TIMEOUT_KEY
- """
- return popup(*args, title=title, button_type=POPUP_BUTTONS_OK, background_color=background_color, text_color=text_color,
- non_blocking=non_blocking, icon=icon, line_width=line_width, button_color=button_color, auto_close=auto_close,
- auto_close_duration=auto_close_duration, font=font, no_titlebar=no_titlebar, grab_anywhere=grab_anywhere,
- keep_on_top=keep_on_top, location=location, relative_location=relative_location, image=image, modal=modal)
-
-
-# --------------------------- popup_ok_cancel ---------------------------
-def popup_ok_cancel(*args, title=None, button_color=None, background_color=None, text_color=None, auto_close=False,
- auto_close_duration=None, non_blocking=False, icon=DEFAULT_WINDOW_ICON, line_width=None, font=None,
- no_titlebar=False, grab_anywhere=False, keep_on_top=None, location=(None, None), relative_location=(None, None), image=None, modal=True):
- """
- Display popup with OK and Cancel buttons
-
- :param *args: Variable number of items to display
- :type *args: (Any)
- :param title: Title to display in the window.
- :type title: (str)
- :param button_color: button color (foreground, background)
- :type button_color: (str, str) or str
- :param background_color: color of background
- :type background_color: (str)
- :param text_color: color of the text
- :type text_color: (str)
- :param auto_close: if True window will close itself
- :type auto_close: (bool)
- :param auto_close_duration: Older versions only accept int. Time in seconds until window will close
- :type auto_close_duration: int | float
- :param non_blocking: if True the call will immediately return rather than waiting on user input
- :type non_blocking: (bool)
- :param icon: filename or base64 string to be used for the window's icon
- :type icon: bytes | str
- :param line_width: Width of lines in characters
- :type line_width: (int)
- :param font: specifies the font family, size, etc. Tuple or Single string format 'name size styles'. Styles: italic * roman bold normal underline overstrike
- :type font: (str or (str, int[, str]) or None)
- :param no_titlebar: If True no titlebar will be shown
- :type no_titlebar: (bool)
- :param grab_anywhere: If True: can grab anywhere to move the window (Default = False)
- :type grab_anywhere: (bool)
- :param keep_on_top: If True the window will remain above all current windows
- :type keep_on_top: (bool)
- :param location: Location of upper left corner of the window
- :type location: (int, int)
- :param relative_location: (x,y) location relative to the default location of the window, in pixels. Normally the window centers. This location is relative to the location the window would be created. Note they can be negative.
- :type relative_location: (int, int)
- :param image: Image to include at the top of the popup window
- :type image: (str) or (bytes)
- :param modal: If True then makes the popup will behave like a Modal window... all other windows are non-operational until this one is closed. Default = True
- :type modal: bool
- :return: clicked button
- :rtype: "OK" | "Cancel" | None
- """
- return popup(*args, title=title, button_type=POPUP_BUTTONS_OK_CANCEL, background_color=background_color,
- text_color=text_color,
- non_blocking=non_blocking, icon=icon, line_width=line_width, button_color=button_color,
- auto_close=auto_close, auto_close_duration=auto_close_duration, font=font, no_titlebar=no_titlebar,
- grab_anywhere=grab_anywhere, keep_on_top=keep_on_top, location=location, relative_location=relative_location, image=image, modal=modal)
-
-
-# --------------------------- popup_yes_no ---------------------------
-def popup_yes_no(*args, title=None, button_color=None, background_color=None, text_color=None, auto_close=False,
- auto_close_duration=None, non_blocking=False, icon=None, line_width=None, font=None,
- no_titlebar=False, grab_anywhere=False, keep_on_top=None, location=(None, None), relative_location=(None, None), image=None, modal=True):
- """
- Display Popup with Yes and No buttons
-
- :param *args: Variable number of items to display
- :type *args: (Any)
- :param title: Title to display in the window.
- :type title: (str)
- :param button_color: button color (foreground, background)
- :type button_color: (str, str) or str
- :param background_color: color of background
- :type background_color: (str)
- :param text_color: color of the text
- :type text_color: (str)
- :param auto_close: if True window will close itself
- :type auto_close: (bool)
- :param auto_close_duration: Older versions only accept int. Time in seconds until window will close
- :type auto_close_duration: int | float
- :param non_blocking: if True the call will immediately return rather than waiting on user input
- :type non_blocking: (bool)
- :param icon: filename or base64 string to be used for the window's icon
- :type icon: bytes | str
- :param line_width: Width of lines in characters
- :type line_width: (int)
- :param font: specifies the font family, size, etc. Tuple or Single string format 'name size styles'. Styles: italic * roman bold normal underline overstrike
- :type font: (str or (str, int[, str]) or None)
- :param no_titlebar: If True no titlebar will be shown
- :type no_titlebar: (bool)
- :param grab_anywhere: If True: can grab anywhere to move the window (Default = False)
- :type grab_anywhere: (bool)
- :param keep_on_top: If True the window will remain above all current windows
- :type keep_on_top: (bool)
- :param location: Location of upper left corner of the window
- :type location: (int, int)
- :param relative_location: (x,y) location relative to the default location of the window, in pixels. Normally the window centers. This location is relative to the location the window would be created. Note they can be negative.
- :type relative_location: (int, int)
- :param image: Image to include at the top of the popup window
- :type image: (str) or (bytes)
- :param modal: If True then makes the popup will behave like a Modal window... all other windows are non-operational until this one is closed. Default = True
- :type modal: bool
- :return: clicked button
- :rtype: "Yes" | "No" | None
- """
- return popup(*args, title=title, button_type=POPUP_BUTTONS_YES_NO, background_color=background_color,
- text_color=text_color,
- non_blocking=non_blocking, icon=icon, line_width=line_width, button_color=button_color,
- auto_close=auto_close, auto_close_duration=auto_close_duration, font=font, no_titlebar=no_titlebar,
- grab_anywhere=grab_anywhere, keep_on_top=keep_on_top, location=location, relative_location=relative_location, image=image, modal=modal)
-
-
-##############################################################################
-# The popup_get_____ functions - Will return user input #
-##############################################################################
-
-# --------------------------- popup_get_folder ---------------------------
-
-
-def popup_get_folder(message, title=None, default_path='', no_window=False, size=(None, None), button_color=None,
- background_color=None, text_color=None, icon=None, font=None, no_titlebar=False,
- grab_anywhere=False, keep_on_top=None, location=(None, None), relative_location=(None, None), initial_folder=None, image=None, modal=True, history=False,
- history_setting_filename=None):
- """
- Display popup with text entry field and browse button so that a folder can be chosen.
-
- :param message: message displayed to user
- :type message: (str)
- :param title: Window title
- :type title: (str)
- :param default_path: path to display to user as starting point (filled into the input field)
- :type default_path: (str)
- :param no_window: if True, no PySimpleGUI window will be shown. Instead just the tkinter dialog is shown
- :type no_window: (bool)
- :param size: (width, height) of the InputText Element
- :type size: (int, int)
- :param button_color: button color (foreground, background)
- :type button_color: (str, str) or str
- :param background_color: color of background
- :type background_color: (str)
- :param text_color: color of the text
- :type text_color: (str)
- :param icon: filename or base64 string to be used for the window's icon
- :type icon: bytes | str
- :param font: specifies the font family, size, etc. Tuple or Single string format 'name size styles'. Styles: italic * roman bold normal underline overstrike
- :type font: (str or (str, int[, str]) or None)
- :param no_titlebar: If True no titlebar will be shown
- :type no_titlebar: (bool)
- :param grab_anywhere: If True: can grab anywhere to move the window (Default = False)
- :type grab_anywhere: (bool)
- :param keep_on_top: If True the window will remain above all current windows
- :type keep_on_top: (bool)
- :param location: Location of upper left corner of the window
- :type location: (int, int)
- :param relative_location: (x,y) location relative to the default location of the window, in pixels. Normally the window centers. This location is relative to the location the window would be created. Note they can be negative.
- :type relative_location: (int, int)
- :param initial_folder: location in filesystem to begin browsing
- :type initial_folder: (str)
- :param image: Image to include at the top of the popup window
- :type image: (str) or (bytes)
- :param modal: If True then makes the popup will behave like a Modal window... all other windows are non-operational until this one is closed. Default = True
- :type modal: bool
- :param history: If True then enable a "history" feature that will display previous entries used. Uses settings filename provided or default if none provided
- :type history: bool
- :param history_setting_filename: Filename to use for the User Settings. Will store list of previous entries in this settings file
- :type history_setting_filename: (str)
- :return: string representing the path chosen, None if cancelled or window closed with X
- :rtype: str | None
- """
-
- # First setup the history settings file if history feature is enabled
- if history and history_setting_filename is not None:
- try:
- history_settings = UserSettings(history_setting_filename)
- except Exception as e:
- _error_popup_with_traceback('popup_get_folder - Something is wrong with your supplied history settings filename',
- 'Exception: {}'.format(e))
- return None
- elif history:
- history_settings_filename = os.path.basename(inspect.stack()[1].filename)
- history_settings_filename = os.path.splitext(history_settings_filename)[0] + '.json'
- history_settings = UserSettings(history_settings_filename)
- else:
- history_settings = None
-
- # global _my_windows
- if no_window:
- _get_hidden_master_root()
- root = tk.Toplevel()
-
- try:
- root.attributes('-alpha', 0) # hide window while building it. makes for smoother 'paint'
- # if not running_mac():
- try:
- root.wm_overrideredirect(True)
- except Exception as e:
- print('* Error performing wm_overrideredirect while hiding the window during creation in get folder *', e)
- root.withdraw()
- except:
- pass
- folder_name = tk.filedialog.askdirectory(initialdir=initial_folder) # show the 'get folder' dialog box
-
- root.destroy()
-
-
- return folder_name
-
- browse_button = FolderBrowse(initial_folder=initial_folder)
-
- if image is not None:
- if isinstance(image, str):
- layout = [[Image(filename=image)]]
- else:
- layout = [[Image(data=image)]]
- else:
- layout = [[]]
-
- layout += [[Text(message, auto_size_text=True, text_color=text_color, background_color=background_color)]]
-
- if not history:
- layout += [[InputText(default_text=default_path, size=size, key='-INPUT-'), browse_button]]
- else:
- file_list = history_settings.get('-PSG folder list-', [])
- last_entry = file_list[0] if file_list else ''
- layout += [[Combo(file_list, default_value=last_entry, key='-INPUT-', size=size if size != (None, None) else (80, 1), bind_return_key=True),
- browse_button, Button('Clear History', tooltip='Clears the list of folders shown in the combobox')]]
-
- layout += [[Button('Ok', size=(6, 1), bind_return_key=True), Button('Cancel', size=(6, 1))]]
-
- window = Window(title=title or message, layout=layout, icon=icon, auto_size_text=True, button_color=button_color,
- font=font, background_color=background_color, no_titlebar=no_titlebar, grab_anywhere=grab_anywhere, keep_on_top=keep_on_top,
- location=location, relative_location=relative_location, modal=modal)
-
- while True:
- event, values = window.read()
- if event in ('Cancel', WIN_CLOSED):
- break
- elif event == 'Clear History':
- history_settings.set('-PSG folder list-', [])
- window['-INPUT-'].update('', [])
- popup_quick_message('History of Previous Choices Cleared', background_color='red', text_color='white', font='_ 20', keep_on_top=True)
- elif event in ('Ok', '-INPUT-'):
- if values['-INPUT-'] != '':
- if history_settings is not None:
- list_of_entries = history_settings.get('-PSG folder list-', [])
- if values['-INPUT-'] in list_of_entries:
- list_of_entries.remove(values['-INPUT-'])
- list_of_entries.insert(0, values['-INPUT-'])
- history_settings.set('-PSG folder list-', list_of_entries)
- break
-
- window.close()
- del window
- if event in ('Cancel', WIN_CLOSED):
- return None
-
- return values['-INPUT-']
-
-
-# --------------------------- popup_get_file ---------------------------
-
-def popup_get_file(message, title=None, default_path='', default_extension='', save_as=False, multiple_files=False,
- file_types=FILE_TYPES_ALL_FILES,
- no_window=False, size=(None, None), button_color=None, background_color=None, text_color=None,
- icon=None, font=None, no_titlebar=False, grab_anywhere=False, keep_on_top=None,
- location=(None, None), relative_location=(None, None), initial_folder=None, image=None, files_delimiter=BROWSE_FILES_DELIMITER, modal=True, history=False, show_hidden=True,
- history_setting_filename=None):
- """
- Display popup window with text entry field and browse button so that a file can be chosen by user.
-
- :param message: message displayed to user
- :type message: (str)
- :param title: Window title
- :type title: (str)
- :param default_path: path to display to user as starting point (filled into the input field)
- :type default_path: (str)
- :param default_extension: If no extension entered by user, add this to filename (only used in saveas dialogs)
- :type default_extension: (str)
- :param save_as: if True, the "save as" dialog is shown which will verify before overwriting
- :type save_as: (bool)
- :param multiple_files: if True, then allows multiple files to be selected that are returned with ';' between each filename
- :type multiple_files: (bool)
- :param file_types: List of extensions to show using wildcards. All files (the default) = (("ALL Files", "*.* *"),). NOT avoilable on the MAC
- :type file_types: Tuple[Tuple[str,str]]
- :param no_window: if True, no PySimpleGUI window will be shown. Instead just the tkinter dialog is shown
- :type no_window: (bool)
- :param size: (width, height) of the InputText Element or Combo element if using history feature
- :type size: (int, int)
- :param button_color: Color of the button (text, background)
- :type button_color: (str, str) or str
- :param background_color: background color of the entire window
- :type background_color: (str)
- :param text_color: color of the text
- :type text_color: (str)
- :param icon: filename or base64 string to be used for the window's icon
- :type icon: bytes | str
- :param font: specifies the font family, size, etc. Tuple or Single string format 'name size styles'. Styles: italic * roman bold normal underline overstrike
- :type font: (str or (str, int[, str]) or None)
- :param no_titlebar: If True no titlebar will be shown
- :type no_titlebar: (bool)
- :param grab_anywhere: If True: can grab anywhere to move the window (Default = False)
- :type grab_anywhere: (bool)
- :param keep_on_top: If True the window will remain above all current windows
- :type keep_on_top: (bool)
- :param location: Location of upper left corner of the window
- :type location: (int, int)
- :param relative_location: (x,y) location relative to the default location of the window, in pixels. Normally the window centers. This location is relative to the location the window would be created. Note they can be negative.
- :type relative_location: (int, int)
- :param initial_folder: location in filesystem to begin browsing
- :type initial_folder: (str)
- :param image: Image to include at the top of the popup window
- :type image: (str) or (bytes)
- :param files_delimiter: String to place between files when multiple files are selected. Normally a ;
- :type files_delimiter: str
- :param modal: If True then makes the popup will behave like a Modal window... all other windows are non-operational until this one is closed. Default = True
- :type modal: bool
- :param history: If True then enable a "history" feature that will display previous entries used. Uses settings filename provided or default if none provided
- :type history: bool
- :param show_hidden: If True then enables the checkbox in the system dialog to select hidden files to be shown
- :type show_hidden: bool
- :param history_setting_filename: Filename to use for the User Settings. Will store list of previous entries in this settings file
- :type history_setting_filename: (str)
- :return: string representing the file(s) chosen, None if cancelled or window closed with X
- :rtype: str | None
- """
-
- # First setup the history settings file if history feature is enabled
- if history and history_setting_filename is not None:
- try:
- history_settings = UserSettings(history_setting_filename)
- except Exception as e:
- _error_popup_with_traceback('popup_get_file - Something is wrong with your supplied history settings filename',
- 'Exception: {}'.format(e))
- return None
- elif history:
- history_settings_filename = os.path.basename(inspect.stack()[1].filename)
- history_settings_filename = os.path.splitext(history_settings_filename)[0] + '.json'
- history_settings = UserSettings(history_settings_filename)
- else:
- history_settings = None
-
- if icon is None:
- icon = Window._user_defined_icon or DEFAULT_BASE64_ICON
- if no_window:
- _get_hidden_master_root()
- root = tk.Toplevel()
-
- try:
- root.attributes('-alpha', 0) # hide window while building it. makes for smoother 'paint'
- # if not running_mac():
- try:
- root.wm_overrideredirect(True)
- except Exception as e:
- print('* Error performing wm_overrideredirect in get file *', e)
- root.withdraw()
- except:
- pass
-
- if show_hidden is False:
- try:
- # call a dummy dialog with an impossible option to initialize the file
- # dialog without really getting a dialog window; this will throw a
- # TclError, so we need a try...except :
- try:
- root.tk.call('tk_getOpenFile', '-foobarbaz')
- except tk.TclError:
- pass
- # now set the magic variables accordingly
- root.tk.call('set', '::tk::dialog::file::showHiddenBtn', '1')
- root.tk.call('set', '::tk::dialog::file::showHiddenVar', '0')
- except:
- pass
-
- if root and icon is not None:
- _set_icon_for_tkinter_window(root, icon=icon)
- # for Macs, setting parent=None fixes a warning problem.
- if save_as:
- if running_mac():
- filename = tk.filedialog.asksaveasfilename(initialdir=initial_folder,
- initialfile=default_path,
- defaultextension=default_extension) # show the 'get file' dialog box
- else:
- filename = tk.filedialog.asksaveasfilename(filetypes=file_types,
- initialdir=initial_folder,
- initialfile=default_path,
- parent=root,
- defaultextension=default_extension) # show the 'get file' dialog box
- elif multiple_files:
- if running_mac():
- filename = tk.filedialog.askopenfilenames(initialdir=initial_folder,
- initialfile=default_path,
- defaultextension=default_extension) # show the 'get file' dialog box
- else:
- filename = tk.filedialog.askopenfilenames(filetypes=file_types,
- initialdir=initial_folder,
- initialfile=default_path,
- parent=root,
- defaultextension=default_extension) # show the 'get file' dialog box
- else:
- if running_mac():
- filename = tk.filedialog.askopenfilename(initialdir=initial_folder,
- initialfile=default_path,
- defaultextension=default_extension) # show the 'get files' dialog box
- else:
- filename = tk.filedialog.askopenfilename(filetypes=file_types,
- initialdir=initial_folder,
- initialfile=default_path,
- parent=root,
- defaultextension=default_extension) # show the 'get files' dialog box
- root.destroy()
-
- if not multiple_files and type(filename) in (tuple, list):
- if len(filename): # only if not 0 length, otherwise will get an error
- filename = filename[0]
- return filename
-
- if save_as:
- browse_button = SaveAs(file_types=file_types, initial_folder=initial_folder, default_extension=default_extension)
- elif multiple_files:
- browse_button = FilesBrowse(file_types=file_types, initial_folder=initial_folder, files_delimiter=files_delimiter)
- else:
- browse_button = FileBrowse(file_types=file_types, initial_folder=initial_folder)
-
- if image is not None:
- if isinstance(image, str):
- layout = [[Image(filename=image)]]
- else:
- layout = [[Image(data=image)]]
- else:
- layout = [[]]
-
- layout += [[Text(message, auto_size_text=True, text_color=text_color, background_color=background_color)]]
-
- if not history:
- layout += [[InputText(default_text=default_path, size=size, key='-INPUT-'), browse_button]]
- else:
- file_list = history_settings.get("-PSG file list-", [])
- last_entry = file_list[0] if file_list else ''
- layout += [[Combo(file_list, default_value=last_entry, key='-INPUT-', size=size if size != (None, None) else (80, 1), bind_return_key=True),
- browse_button, Button('Clear History', tooltip='Clears the list of files shown in the combobox')]]
-
- layout += [[Button('Ok', size=(6, 1), bind_return_key=True), Button('Cancel', size=(6, 1))]]
-
- window = Window(title=title or message, layout=layout, icon=icon, auto_size_text=True, button_color=button_color,
- font=font, background_color=background_color, no_titlebar=no_titlebar, grab_anywhere=grab_anywhere, keep_on_top=keep_on_top, location=location, relative_location=relative_location, modal=modal, finalize=True)
-
- if running_linux() and show_hidden is True:
- window.TKroot.tk.eval('catch {tk_getOpenFile -badoption}') # dirty hack to force autoloading of Tk's file dialog code
- window.TKroot.setvar('::tk::dialog::file::showHiddenBtn', 1) # enable the "show hidden files" checkbox (it's necessary)
- window.TKroot.setvar('::tk::dialog::file::showHiddenVar', 0) # start with the hidden files... well... hidden
-
- while True:
- event, values = window.read()
- if event in ('Cancel', WIN_CLOSED):
- break
- elif event == 'Clear History':
- history_settings.set('-PSG file list-', [])
- window['-INPUT-'].update('', [])
- popup_quick_message('History of Previous Choices Cleared', background_color='red', text_color='white', font='_ 20', keep_on_top=True)
- elif event in ('Ok', '-INPUT-'):
- if values['-INPUT-'] != '':
- if history_settings is not None:
- list_of_entries = history_settings.get('-PSG file list-', [])
- if values['-INPUT-'] in list_of_entries:
- list_of_entries.remove(values['-INPUT-'])
- list_of_entries.insert(0, values['-INPUT-'])
- history_settings.set('-PSG file list-', list_of_entries)
- break
-
- window.close()
- del window
- if event in ('Cancel', WIN_CLOSED):
- return None
-
- return values['-INPUT-']
-
-
-# --------------------------- popup_get_text ---------------------------
-
-def popup_get_text(message, title=None, default_text='', password_char='', size=(None, None), button_color=None,
- background_color=None, text_color=None, icon=None, font=None, no_titlebar=False,
- grab_anywhere=False, keep_on_top=None, location=(None, None), relative_location=(None, None), image=None, modal=True):
- """
- Display Popup with text entry field. Returns the text entered or None if closed / cancelled
-
- :param message: message displayed to user
- :type message: (str)
- :param title: Window title
- :type title: (str)
- :param default_text: default value to put into input area
- :type default_text: (str)
- :param password_char: character to be shown instead of actually typed characters
- :type password_char: (str)
- :param size: (width, height) of the InputText Element
- :type size: (int, int)
- :param button_color: Color of the button (text, background)
- :type button_color: (str, str) or str
- :param background_color: background color of the entire window
- :type background_color: (str)
- :param text_color: color of the message text
- :type text_color: (str)
- :param icon: filename or base64 string to be used for the window's icon
- :type icon: bytes | str
- :param font: specifies the font family, size, etc. Tuple or Single string format 'name size styles'. Styles: italic * roman bold normal underline overstrike
- :type font: (str or (str, int[, str]) or None)
- :param no_titlebar: If True no titlebar will be shown
- :type no_titlebar: (bool)
- :param grab_anywhere: If True can click and drag anywhere in the window to move the window
- :type grab_anywhere: (bool)
- :param keep_on_top: If True the window will remain above all current windows
- :type keep_on_top: (bool)
- :param location: (x,y) Location on screen to display the upper left corner of window
- :type location: (int, int)
- :param relative_location: (x,y) location relative to the default location of the window, in pixels. Normally the window centers. This location is relative to the location the window would be created. Note they can be negative.
- :type relative_location: (int, int)
- :param image: Image to include at the top of the popup window
- :type image: (str) or (bytes)
- :param modal: If True then makes the popup will behave like a Modal window... all other windows are non-operational until this one is closed. Default = True
- :type modal: bool
- :return: Text entered or None if window was closed or cancel button clicked
- :rtype: str | None
- """
-
- if image is not None:
- if isinstance(image, str):
- layout = [[Image(filename=image)]]
- else:
- layout = [[Image(data=image)]]
- else:
- layout = [[]]
-
- layout += [[Text(message, auto_size_text=True, text_color=text_color, background_color=background_color)],
- [InputText(default_text=default_text, size=size, key='-INPUT-', password_char=password_char)],
- [Button('Ok', size=(6, 1), bind_return_key=True), Button('Cancel', size=(6, 1))]]
-
- window = Window(title=title or message, layout=layout, icon=icon, auto_size_text=True, button_color=button_color, no_titlebar=no_titlebar,
- background_color=background_color, grab_anywhere=grab_anywhere, keep_on_top=keep_on_top, location=location, relative_location=relative_location, finalize=True, modal=modal, font=font)
-
- button, values = window.read()
- window.close()
- del window
- if button != 'Ok':
- return None
- else:
- path = values['-INPUT-']
- return path
-
-
-def popup_get_date(start_mon=None, start_day=None, start_year=None, begin_at_sunday_plus=0, no_titlebar=True, title='Choose Date', keep_on_top=True,
- location=(None, None), relative_location=(None, None), close_when_chosen=False, icon=None, locale=None, month_names=None, day_abbreviations=None, modal=True):
- """
- Display a calendar window, get the user's choice, return as a tuple (mon, day, year)
-
- :param start_mon: The starting month
- :type start_mon: (int)
- :param start_day: The starting day - optional. Set to None or 0 if no date to be chosen at start
- :type start_day: int | None
- :param start_year: The starting year
- :type start_year: (int)
- :param begin_at_sunday_plus: Determines the left-most day in the display. 0=sunday, 1=monday, etc
- :type begin_at_sunday_plus: (int)
- :param icon: Same as Window icon parameter. Can be either a filename or Base64 value. For Windows if filename, it MUST be ICO format. For Linux, must NOT be ICO
- :type icon: (str | bytes)
- :param location: (x,y) location on the screen to place the top left corner of your window. Default is to center on screen
- :type location: (int, int)
- :param relative_location: (x,y) location relative to the default location of the window, in pixels. Normally the window centers. This location is relative to the location the window would be created. Note they can be negative.
- :type relative_location: (int, int)
- :param title: Title that will be shown on the window
- :type title: (str)
- :param close_when_chosen: If True, the window will close and function return when a day is clicked
- :type close_when_chosen: (bool)
- :param locale: locale used to get the day names
- :type locale: (str)
- :param no_titlebar: If True no titlebar will be shown
- :type no_titlebar: (bool)
- :param keep_on_top: If True the window will remain above all current windows
- :type keep_on_top: (bool)
- :param month_names: optional list of month names to use (should be 12 items)
- :type month_names: List[str]
- :param day_abbreviations: optional list of abbreviations to display as the day of week
- :type day_abbreviations: List[str]
- :param modal: If True then makes the popup will behave like a Modal window... all other windows are non-operational until this one is closed. Default = True
- :type modal: bool
- :return: Tuple containing (month, day, year) of chosen date or None if was cancelled
- :rtype: None | (int, int, int)
- """
-
- if month_names is not None and len(month_names) != 12:
- if not SUPPRESS_ERROR_POPUPS:
- popup_error('Incorrect month names list specified. Must have 12 entries.', 'Your list:', month_names)
-
- if day_abbreviations is not None and len(day_abbreviations) != 7:
- if not SUPPRESS_ERROR_POPUPS:
- popup_error('Incorrect day abbreviation list. Must have 7 entries.', 'Your list:', day_abbreviations)
-
- day_font = 'TkFixedFont 9'
- mon_year_font = 'TkFixedFont 10'
- arrow_font = 'TkFixedFont 7'
-
- now = datetime.datetime.now()
- cur_month, cur_day, cur_year = now.month, now.day, now.year
- cur_month = start_mon or cur_month
- if start_mon is not None:
- cur_day = start_day
- else:
- cur_day = cur_day
- cur_year = start_year or cur_year
-
- def update_days(window, month, year, begin_at_sunday_plus):
- [window[(week, day)].update('') for day in range(7) for week in range(6)]
- weeks = calendar.monthcalendar(year, month)
- month_days = list(itertools.chain.from_iterable([[0 for _ in range(8 - begin_at_sunday_plus)]] + weeks))
- if month_days[6] == 0:
- month_days = month_days[7:]
- if month_days[6] == 0:
- month_days = month_days[7:]
- for i, day in enumerate(month_days):
- offset = i
- if offset >= 6 * 7:
- break
- window[(offset // 7, offset % 7)].update(str(day) if day else '')
-
- def make_days_layout():
- days_layout = []
- for week in range(6):
- row = []
- for day in range(7):
- row.append(T('', size=(4, 1), justification='c', font=day_font, key=(week, day), enable_events=True, pad=(0, 0)))
- days_layout.append(row)
- return days_layout
-
- # Create table of month names and week day abbreviations
-
- if day_abbreviations is None or len(day_abbreviations) != 7:
- fwday = calendar.SUNDAY
- try:
- if locale is not None:
- _cal = calendar.LocaleTextCalendar(fwday, locale)
- else:
- _cal = calendar.TextCalendar(fwday)
- day_names = _cal.formatweekheader(3).split()
- except Exception as e:
- print('Exception building day names from locale', locale, e)
- day_names = ('Sun', 'Mon', 'Tue', 'Wed', 'Th', 'Fri', 'Sat')
- else:
- day_names = day_abbreviations
-
- mon_names = month_names if month_names is not None and len(month_names) == 12 else [calendar.month_name[i] for i in range(1, 13)]
- days_layout = make_days_layout()
-
- layout = [[B('◄◄', font=arrow_font, border_width=0, key='-YEAR-DOWN-', pad=((10, 2), 2)),
- B('◄', font=arrow_font, border_width=0, key='-MON-DOWN-', pad=(0, 2)),
- Text('{} {}'.format(mon_names[cur_month - 1], cur_year), size=(16, 1), justification='c', font=mon_year_font, key='-MON-YEAR-', pad=(0, 2)),
- B('►', font=arrow_font, border_width=0, key='-MON-UP-', pad=(0, 2)),
- B('►►', font=arrow_font, border_width=0, key='-YEAR-UP-', pad=(2, 2))]]
- layout += [[Col([[T(day_names[i - (7 - begin_at_sunday_plus) % 7], size=(4, 1), font=day_font, background_color=theme_text_color(),
- text_color=theme_background_color(), pad=(0, 0)) for i in range(7)]], background_color=theme_text_color(), pad=(0, 0))]]
- layout += days_layout
- if not close_when_chosen:
- layout += [[Button('Ok', border_width=0, font='TkFixedFont 8'), Button('Cancel', border_width=0, font='TkFixedFont 8')]]
-
- window = Window(title, layout, no_titlebar=no_titlebar, grab_anywhere=True, keep_on_top=keep_on_top, font='TkFixedFont 12', use_default_focus=False,
- location=location, relative_location=relative_location, finalize=True, icon=icon)
-
- update_days(window, cur_month, cur_year, begin_at_sunday_plus)
-
- prev_choice = chosen_mon_day_year = None
-
- if cur_day:
- chosen_mon_day_year = cur_month, cur_day, cur_year
- for week in range(6):
- for day in range(7):
- if window[(week, day)].DisplayText == str(cur_day):
- window[(week, day)].update(background_color=theme_text_color(), text_color=theme_background_color())
- prev_choice = (week, day)
- break
-
- if modal or DEFAULT_MODAL_WINDOWS_FORCED:
- window.make_modal()
-
- while True: # Event Loop
- event, values = window.read()
- if event in (None, 'Cancel'):
- chosen_mon_day_year = None
- break
- if event == 'Ok':
- break
- if event in ('-MON-UP-', '-MON-DOWN-', '-YEAR-UP-', '-YEAR-DOWN-'):
- cur_month += (event == '-MON-UP-')
- cur_month -= (event == '-MON-DOWN-')
- cur_year += (event == '-YEAR-UP-')
- cur_year -= (event == '-YEAR-DOWN-')
- if cur_month > 12:
- cur_month = 1
- cur_year += 1
- elif cur_month < 1:
- cur_month = 12
- cur_year -= 1
- window['-MON-YEAR-'].update('{} {}'.format(mon_names[cur_month - 1], cur_year))
- update_days(window, cur_month, cur_year, begin_at_sunday_plus)
- if prev_choice:
- window[prev_choice].update(background_color=theme_background_color(), text_color=theme_text_color())
- elif type(event) is tuple:
- if window[event].DisplayText != "":
- chosen_mon_day_year = cur_month, int(window[event].DisplayText), cur_year
- if prev_choice:
- window[prev_choice].update(background_color=theme_background_color(), text_color=theme_text_color())
- window[event].update(background_color=theme_text_color(), text_color=theme_background_color())
- prev_choice = event
- if close_when_chosen:
- break
- window.close()
- return chosen_mon_day_year
-
-
-# --------------------------- PopupAnimated ---------------------------
-
-def popup_animated(image_source, message=None, background_color=None, text_color=None, font=None, no_titlebar=True, grab_anywhere=True, keep_on_top=True,
- location=(None, None), relative_location=(None, None), alpha_channel=None, time_between_frames=0, transparent_color=None, title='', icon=None):
- """
- Show animation one frame at a time. This function has its own internal clocking meaning you can call it at any frequency
- and the rate the frames of video is shown remains constant. Maybe your frames update every 30 ms but your
- event loop is running every 10 ms. You don't have to worry about delaying, just call it every time through the
- loop.
-
- :param image_source: Either a filename or a base64 string. Use None to close the window.
- :type image_source: str | bytes | None
- :param message: An optional message to be shown with the animation
- :type message: (str)
- :param background_color: color of background
- :type background_color: (str)
- :param text_color: color of the text
- :type text_color: (str)
- :param font: specifies the font family, size, etc. Tuple or Single string format 'name size styles'. Styles: italic * roman bold normal underline overstrike
- :type font: str | tuple
- :param no_titlebar: If True then the titlebar and window frame will not be shown
- :type no_titlebar: (bool)
- :param grab_anywhere: If True then you can move the window just clicking anywhere on window, hold and drag
- :type grab_anywhere: (bool)
- :param keep_on_top: If True then Window will remain on top of all other windows currently shownn
- :type keep_on_top: (bool)
- :param location: (x,y) location on the screen to place the top left corner of your window. Default is to center on screen
- :type location: (int, int)
- :param relative_location: (x,y) location relative to the default location of the window, in pixels. Normally the window centers. This location is relative to the location the window would be created. Note they can be negative.
- :type relative_location: (int, int)
- :param alpha_channel: Window transparency 0 = invisible 1 = completely visible. Values between are see through
- :type alpha_channel: (float)
- :param time_between_frames: Amount of time in milliseconds between each frame
- :type time_between_frames: (int)
- :param transparent_color: This color will be completely see-through in your window. Can even click through
- :type transparent_color: (str)
- :param title: Title that will be shown on the window
- :type title: (str)
- :param icon: Same as Window icon parameter. Can be either a filename or Base64 byte string. For Windows if filename, it MUST be ICO format. For Linux, must NOT be ICO
- :type icon: str | bytes
- :return: True if the window updated OK. False if the window was closed
- :rtype: bool
- """
- if image_source is None:
- for image in Window._animated_popup_dict:
- window = Window._animated_popup_dict[image]
- window.close()
- Window._animated_popup_dict = {}
- return
-
- if image_source not in Window._animated_popup_dict:
- if type(image_source) is bytes or len(image_source) > 300:
- layout = [[Image(data=image_source, background_color=background_color, key='-IMAGE-')], ]
- else:
- layout = [[Image(filename=image_source, background_color=background_color, key='-IMAGE-', )], ]
- if message:
- layout.append([Text(message, background_color=background_color, text_color=text_color, font=font)])
-
- window = Window(title, layout, no_titlebar=no_titlebar, grab_anywhere=grab_anywhere,
- keep_on_top=keep_on_top, background_color=background_color, location=location,
- alpha_channel=alpha_channel, element_padding=(0, 0), margins=(0, 0),
- transparent_color=transparent_color, finalize=True, element_justification='c', icon=icon, relative_location=relative_location)
- Window._animated_popup_dict[image_source] = window
- else:
- window = Window._animated_popup_dict[image_source]
- window['-IMAGE-'].update_animation(image_source, time_between_frames=time_between_frames)
- event, values = window.read(1)
- if event == WIN_CLOSED:
- return False
- # window.refresh() # call refresh instead of Read to save significant CPU time
- return True
-
-
-# Popup Notify
-def popup_notify(*args, title='', icon=SYSTEM_TRAY_MESSAGE_ICON_INFORMATION, display_duration_in_ms=SYSTEM_TRAY_MESSAGE_DISPLAY_DURATION_IN_MILLISECONDS,
- fade_in_duration=SYSTEM_TRAY_MESSAGE_FADE_IN_DURATION, alpha=0.9, location=None):
- """
- Displays a "notification window", usually in the bottom right corner of your display. Has an icon, a title, and a message. It is more like a "toaster" window than the normal popups.
-
- The window will slowly fade in and out if desired. Clicking on the window will cause it to move through the end the current "phase". For example, if the window was fading in and it was clicked, then it would immediately stop fading in and instead be fully visible. It's a way for the user to quickly dismiss the window.
-
- The return code specifies why the call is returning (e.g. did the user click the message to dismiss it)
-
- :param title: Text to be shown at the top of the window in a larger font
- :type title: (str)
- :param message: Text message that makes up the majority of the window
- :type message: (str)
- :param icon: A base64 encoded PNG/GIF image or PNG/GIF filename that will be displayed in the window
- :type icon: bytes | str
- :param display_duration_in_ms: Number of milliseconds to show the window
- :type display_duration_in_ms: (int)
- :param fade_in_duration: Number of milliseconds to fade window in and out
- :type fade_in_duration: (int)
- :param alpha: Alpha channel. 0 - invisible 1 - fully visible
- :type alpha: (float)
- :param location: Location on the screen to display the window
- :type location: (int, int)
- :return: reason for returning
- :rtype: (int)
- """
-
- if not args:
- args_to_print = ['']
- else:
- args_to_print = args
- output = ''
- max_line_total, total_lines, local_line_width = 0, 0, SYSTEM_TRAY_MESSAGE_MAX_LINE_LENGTH
- for message in args_to_print:
- # fancy code to check if string and convert if not is not need. Just always convert to string :-)
- # if not isinstance(message, str): message = str(message)
- message = str(message)
- if message.count('\n'):
- message_wrapped = message
- else:
- message_wrapped = textwrap.fill(message, local_line_width)
- message_wrapped_lines = message_wrapped.count('\n') + 1
- longest_line_len = max([len(l) for l in message.split('\n')])
- width_used = min(longest_line_len, local_line_width)
- max_line_total = max(max_line_total, width_used)
- # height = _GetNumLinesNeeded(message, width_used)
- height = message_wrapped_lines
- output += message_wrapped + '\n'
- total_lines += height
-
- message = output
-
- # def __init__(self, menu=None, filename=None, data=None, data_base64=None, tooltip=None, metadata=None):
- return SystemTray.notify(title=title, message=message, icon=icon, display_duration_in_ms=display_duration_in_ms, fade_in_duration=fade_in_duration,
- alpha=alpha, location=location)
-
-
-def popup_menu(window, element, menu_def, title=None, location=(None, None)):
- """
- Makes a "popup menu"
- This type of menu is what you get when a normal menu or a right click menu is torn off
- The settings for the menu are obtained from the window parameter's Window
-
-
- :param window: The window associated with the popup menu. The theme and right click menu settings for this window will be used
- :type window: Window
- :param element: An element in your window to associate the menu to. It can be any element
- :type element: Element
- :param menu_def: A menu definition. This will be the same format as used for Right Click Menus1
- :type menu_def: List[List[ List[str] | str ]]
- :param title: The title that will be shown on the torn off menu window. Defaults to window titlr
- :type title: str
- :param location: The location on the screen to place the window
- :type location: (int, int) | (None, None)
- """
-
- element._popup_menu_location = location
- top_menu = tk.Menu(window.TKroot, tearoff=True, tearoffcommand=element._tearoff_menu_callback)
- if window.right_click_menu_background_color not in (COLOR_SYSTEM_DEFAULT, None):
- top_menu.config(bg=window.right_click_menu_background_color)
- if window.right_click_menu_text_color not in (COLOR_SYSTEM_DEFAULT, None):
- top_menu.config(fg=window.right_click_menu_text_color)
- if window.right_click_menu_disabled_text_color not in (COLOR_SYSTEM_DEFAULT, None):
- top_menu.config(disabledforeground=window.right_click_menu_disabled_text_color)
- if window.right_click_menu_font is not None:
- top_menu.config(font=window.right_click_menu_font)
- if window.right_click_menu_selected_colors[0] != COLOR_SYSTEM_DEFAULT:
- top_menu.config(activeforeground=window.right_click_menu_selected_colors[0])
- if window.right_click_menu_selected_colors[1] != COLOR_SYSTEM_DEFAULT:
- top_menu.config(activebackground=window.right_click_menu_selected_colors[1])
- top_menu.config(title=window.Title if title is None else title)
- AddMenuItem(top_menu, menu_def[1], element, right_click_menu=True)
- # element.Widget.bind('', element._RightClickMenuCallback)
- top_menu.invoke(0)
-
-
-def popup_error_with_traceback(title, *messages):
- """
- Show an error message and as many additoinal lines of messages as you want.
- Will show the same error window as PySimpleGUI uses internally. Has a button to
- take the user to the line of code you called this popup from
-
- :param title: The title that will be shown in the popup's titlebar and in the first line of the window
- :type title: str
- :param messages: A variable number of lines of messages you wish to show your user
- :type messages: *Any
- """
-
- # For now, call the function that PySimpleGUI uses internally
- _error_popup_with_traceback(str(title), *messages)
-
-
-def _error_popup_with_traceback(title, *args):
- if SUPPRESS_ERROR_POPUPS:
- return
- trace_details = traceback.format_stack()
- error_message = ''
- file_info_pysimplegui = None
- for line in reversed(trace_details):
- if __file__ not in line:
- file_info_pysimplegui = line.split(",")[0]
- error_message = line
- break
- if file_info_pysimplegui is None:
- _error_popup_with_code(title, None, None, 'Did not find your traceback info', *args)
- return
-
- error_parts = None
- if error_message != '':
- error_parts = error_message.split(', ')
- if len(error_parts) < 4:
- error_message = error_parts[0] + '\n' + error_parts[1] + '\n' + ''.join(error_parts[2:])
- if error_parts is None:
- print('*** Error popup attempted but unable to parse error details ***')
- print(trace_details)
- return
- filename = error_parts[0][error_parts[0].index('File ') + 5:]
- line_num = error_parts[1][error_parts[1].index('line ') + 5:]
- _error_popup_with_code(title, filename, line_num, error_message, *args)
-
-
-def _error_popup_with_code(title, filename=None, line_num=None, *args):
- layout = [[Text('ERROR'), Text(title)],
- [Image(data=_random_error_emoji())]]
- # make max length of line be 90 chars and allow the height to vary based on number of needed lines
- lines = [str(msg).split('\n') for msg in args]
- max_line_len = 0
- for line in lines:
- max_line_len = max(max_line_len, max([len(s) for s in line]))
-
- layout += [[Text(str(msg), size=(min(max_line_len, 90), None))] for msg in args]
-
- layout += [[Button('Close'), Button('Take me to error'), Button('Kill Application', button_color='white on red')]]
-
- window = Window(title, layout, keep_on_top=True)
-
- while True:
- event, values = window.read()
- if event in ('Close', WIN_CLOSED):
- break
- if event == 'Kill Application':
- window.close()
- popup_quick_message('KILLING APP! BYE!', font='_ 18', keep_on_top=True, text_color='white', background_color='red', non_blocking=False)
- sys.exit()
- if event == 'Take me to error' and filename is not None and line_num is not None:
- execute_editor(filename, line_num)
-
- window.close()
-
-
-#####################################################################
-# Animated window while shell command is executed
-#####################################################################
-
-def _process_thread(*args):
- global __shell_process__
-
- # start running the command with arugments
- try:
- __shell_process__ = subprocess.run(args, shell=True, stdout=subprocess.PIPE)
- except Exception as e:
- print('Exception running process args = {}'.format(args))
- __shell_process__ = None
-
-
-def shell_with_animation(command, args=None, image_source=DEFAULT_BASE64_LOADING_GIF, message=None, background_color=None, text_color=None, font=None,
- no_titlebar=True, grab_anywhere=True, keep_on_top=True, location=(None, None), alpha_channel=None, time_between_frames=100,
- transparent_color=None):
- """
- Execute a "shell command" (anything capable of being launched using subprocess.run) and
- while the command is running, show an animated popup so that the user knows that a long-running
- command is being executed. Without this mechanism, the GUI appears locked up.
-
- :param command: The command to run
- :type command: (str)
- :param args: List of arguments
- :type args: List[str]
- :param image_source: Either a filename or a base64 string.
- :type image_source: str | bytes
- :param message: An optional message to be shown with the animation
- :type message: (str)
- :param background_color: color of background
- :type background_color: (str)
- :param text_color: color of the text
- :type text_color: (str)
- :param font: specifies the font family, size, etc. Tuple or Single string format 'name size styles'. Styles: italic * roman bold normal underline overstrike
- :type font: str | tuple
- :param no_titlebar: If True then the titlebar and window frame will not be shown
- :type no_titlebar: (bool)
- :param grab_anywhere: If True then you can move the window just clicking anywhere on window, hold and drag
- :type grab_anywhere: (bool)
- :param keep_on_top: If True then Window will remain on top of all other windows currently shownn
- :type keep_on_top: (bool)
- :param location: (x,y) location on the screen to place the top left corner of your window. Default is to center on screen
- :type location: (int, int)
- :param alpha_channel: Window transparency 0 = invisible 1 = completely visible. Values between are see through
- :type alpha_channel: (float)
- :param time_between_frames: Amount of time in milliseconds between each frame
- :type time_between_frames: (int)
- :param transparent_color: This color will be completely see-through in your window. Can even click through
- :type transparent_color: (str)
- :return: The resulting string output from stdout
- :rtype: (str)
- """
-
- global __shell_process__
-
- real_args = [command]
- if args is not None:
- for arg in args:
- real_args.append(arg)
- # real_args.append(args)
- thread = threading.Thread(target=_process_thread, args=real_args, daemon=True)
- thread.start()
-
- # Poll to see if the thread is still running. If so, then continue showing the animation
- while True:
- popup_animated(image_source=image_source, message=message, time_between_frames=time_between_frames, transparent_color=transparent_color,
- text_color=text_color, background_color=background_color, font=font, no_titlebar=no_titlebar, grab_anywhere=grab_anywhere,
- keep_on_top=keep_on_top, location=location, alpha_channel=alpha_channel)
- thread.join(timeout=time_between_frames / 1000)
- if not thread.is_alive():
- break
- popup_animated(None) # stop running the animation
-
- output = __shell_process__.__str__().replace('\\r\\n', '\n') # fix up the output string
- output = output[output.index("stdout=b'") + 9:-2]
- return output
-
-
-#######################################################################
-# 8888888888
-# 888
-# 888
-# 8888888 888d888 888d888 .d88b. 888d888
-# 888 888P" 888P" d88""88b 888P"
-# 888 888 888 888 888 888
-# 888 888 888 Y88..88P 888
-# 8888888888 888 888 "Y88P" 888
-#
-#
-#
-# 888b d888
-# 8888b d8888
-# 88888b.d88888
-# 888Y88888P888 .d88b. .d8888b .d8888b 8888b. .d88b. .d88b.
-# 888 Y888P 888 d8P Y8b 88K 88K "88b d88P"88b d8P Y8b
-# 888 Y8P 888 88888888 "Y8888b. "Y8888b. .d888888 888 888 88888888
-# 888 " 888 Y8b. X88 X88 888 888 Y88b 888 Y8b.
-# 888 888 "Y8888 88888P' 88888P' "Y888888 "Y88888 "Y8888
-# 888
-# Y8b d88P
-# "Y88P"
-# Code to make messages to help user find errors in their code
-#######################################################################
-
-def _create_error_message():
- """
- Creates an error message containing the filename and line number of the users
- code that made the call into PySimpleGUI
- :return: Error string to display with file, line number, and line of code
- :rtype: str
- """
-
- called_func = inspect.stack()[1].function
- trace_details = traceback.format_stack()
- error_message = ''
- file_info_pysimplegui = trace_details[-1].split(",")[0]
- for line in reversed(trace_details):
- if line.split(",")[0] != file_info_pysimplegui:
- error_message = line
- break
- if error_message != '':
- error_parts = error_message.split(', ')
- if len(error_parts) < 4:
- error_message = error_parts[0] + '\n' + error_parts[1] + '\n' + ''.join(error_parts[2:])
- return 'The PySimpleGUI internal reporting function is ' + called_func + '\n' + \
- 'The error originated from:\n' + error_message
-
-
-# .d8888b. 888 888 d8b
-# d88P Y88b 888 888 Y8P
-# Y88b. 888 888
-# "Y888b. .d88b. 888888 888888 888 88888b. .d88b. .d8888b
-# "Y88b. d8P Y8b 888 888 888 888 "88b d88P"88b 88K
-# "888 88888888 888 888 888 888 888 888 888 "Y8888b.
-# Y88b d88P Y8b. Y88b. Y88b. 888 888 888 Y88b 888 X88
-# "Y8888P" "Y8888 "Y888 "Y888 888 888 888 "Y88888 88888P'
-# 888
-# Y8b d88P
-# "Y88P"
-
-# Interface to saving / loading user program settings in json format
-# This is a new set of APIs supplied by PySimpleGUI that enables users to easily set/save/load individual
-# settings. They are automatically saved to a JSON file. If no file/path is specified then a filename is
-# created from the source file filename.
-
-class UserSettings:
- # A reserved settings object for use by the setting functions. It's a way for users
- # to access the user settings without diarectly using the UserSettings class
- _default_for_function_interface = None # type: UserSettings
-
- def __init__(self, filename=None, path=None, silent_on_error=False, autosave=True, use_config_file=None, convert_bools_and_none=True):
- """
- User Settings
-
- :param filename: The name of the file to use. Can be a full path and filename or just filename
- :type filename: (str or None)
- :param path: The folder that the settings file will be stored in. Do not include the filename.
- :type path: (str or None)
- :param silent_on_error: If True errors will not be reported
- :type silent_on_error: (bool)
- :param autosave: If True the settings file is saved after every update
- :type autosave: (bool)
- :param use_config_file: If True then the file format will be a config.ini rather than json
- :type use_config_file: (bool)
- :param convert_bools_and_none: If True then "True", "False", "None" will be converted to the Python values True, False, None when using INI files. Default is TRUE
- :type convert_bools_and_none: (bool)
- """
-
- self.path = path
- self.filename = filename
- self.full_filename = None
- self.dict = {}
- self.default_value = None
- self.silent_on_error = silent_on_error
- self.autosave = autosave
- if filename is not None and filename.endswith('.ini') and use_config_file is None:
- warnings.warn('[UserSettings] You have specified a filename with .ini extension but did not set use_config_file. Setting use_config_file for you.', UserWarning)
- use_config_file = True
- self.use_config_file = use_config_file
- # self.retain_config_comments = retain_config_comments
- self.convert_bools = convert_bools_and_none
- if use_config_file:
- self.config = configparser.ConfigParser()
- self.config.optionxform = str
- # self.config_dict = {}
- self.section_class_dict = {} # type: dict[_SectionDict]
- if filename is not None or path is not None:
- self.load(filename=filename, path=path)
-
-
- ########################################################################################################
- ## FIRST is the _SectionDict helper class
- ## It is typically not directly accessed, although it is possible to call delete_section, get, set
- ########################################################################################################
-
- class _SectionDict:
- item_count = 0
- def __init__(self, section_name, section_dict, config, user_settings_parent): # (str, Dict, configparser.ConfigParser)
- """
- The Section Dictionary. It holds the values for a section.
-
- :param section_name: Name of the section
- :type section_name: str
- :param section_dict: Dictionary of values for the section
- :type section_dict: dict
- :param config: The configparser object
- :type config: configparser.ConfigParser
- :param user_settings_parent: The parent UserSettings object that hdas this section
- :type user_settings_parent: UserSettings
- """
- self.section_name = section_name
- self.section_dict = section_dict # type: Dict
- self.new_section = False
- self.config = config # type: configparser.ConfigParser
- self.user_settings_parent = user_settings_parent # type: UserSettings
- UserSettings._SectionDict.item_count += 1
-
- if self.user_settings_parent.convert_bools:
- for key, value in self.section_dict.items():
- if value == 'True':
- value = True
- self.section_dict[key] = value
- elif value == 'False':
- value = False
- self.section_dict[key] = value
- elif value == 'None':
- value = None
- self.section_dict[key] = value
- # print(f'++++++ making a new SectionDict with name = {section_name}')
-
-
- def __repr__(self):
- """
- Converts the settings dictionary into a string for easy display
-
- :return: the dictionary as a string
- :rtype: (str)
- """
- return_string = '{}:\n'.format(self.section_name)
- for entry in self.section_dict.keys():
- return_string += ' {} : {}\n'.format(entry, self.section_dict[entry])
-
- return return_string
-
-
- def get(self, key, default=None):
- """
- Returns the value of a specified setting. If the setting is not found in the settings dictionary, then
- the user specified default value will be returned. It no default is specified and nothing is found, then
- the "default value" is returned. This default can be specified in this call, or previously defined
- by calling set_default. If nothing specified now or previously, then None is returned as default.
-
- :param key: Key used to lookup the setting in the settings dictionary
- :type key: (Any)
- :param default: Value to use should the key not be found in the dictionary
- :type default: (Any)
- :return: Value of specified settings
- :rtype: (Any)
- """
- value = self.section_dict.get(key, default)
- if self.user_settings_parent.convert_bools:
- if value == 'True':
- value = True
- elif value == 'False':
- value = False
- return value
-
- def set(self, key, value):
- value = str(value) # all values must be strings
- if self.new_section:
- self.config.add_section(self.section_name)
- self.new_section = False
- self.config.set(section=self.section_name, option=key, value=value)
- self.section_dict[key] = value
- if self.user_settings_parent.autosave:
- self.user_settings_parent.save()
-
- def delete_section(self):
- # print(f'** Section Dict deleting section = {self.section_name}')
- self.config.remove_section(section=self.section_name)
- del self.user_settings_parent.section_class_dict[self.section_name]
- if self.user_settings_parent.autosave:
- self.user_settings_parent.save()
-
- def __getitem__(self, item):
- # print('*** In SectionDict Get ***')
- return self.get(item)
-
- def __setitem__(self, item, value):
- """
- Enables setting a setting by using [ ] notation like a dictionary.
- Your code will have this kind of design pattern:
- settings = sg.UserSettings()
- settings[item] = value
-
- :param item: The key for the setting to change. Needs to be a hashable type. Basically anything but a list
- :type item: Any
- :param value: The value to set the setting to
- :type value: Any
- """
- # print(f'*** In SectionDict SET *** item = {item} value = {value}')
- self.set(item, value)
- self.section_dict[item] = value
-
- def __delitem__(self, item):
- """
- Delete an individual user setting. This is the same as calling delete_entry. The syntax
- for deleting the item using this manner is:
- del settings['entry']
- :param item: The key for the setting to delete
- :type item: Any
- """
- # print(f'** In SectionDict delete! section name = {self.section_name} item = {item} ')
- self.config.remove_option(section=self.section_name, option=item)
- try:
- del self.section_dict[item]
- except Exception as e:
- pass
- # print(e)
- if self.user_settings_parent.autosave:
- self.user_settings_parent.save()
-
-
- ########################################################################################################
-
- def __repr__(self):
- """
- Converts the settings dictionary into a string for easy display
-
- :return: the dictionary as a string
- :rtype: (str)
- """
- if not self.use_config_file:
- return pprint.pformat(self.dict)
- else:
- # rvalue = '-------------------- Settings ----------------------\n'
- rvalue = ''
- for name, section in self.section_class_dict.items():
- rvalue += str(section)
-
- # rvalue += '\n-------------------- Settings End----------------------\n'
- rvalue += '\n'
- return rvalue
- # return str(self.dict) # previouisly returned just a string version of the dictionary
-
-
- def set_default_value(self, default):
- """
- Set the value that will be returned if a requested setting is not found
-
- :param default: value to be returned if a setting is not found in the settings dictionary
- :type default: Any
- """
- self.default_value = default
-
- def _compute_filename(self, filename=None, path=None):
- """
- Creates the full filename given the path or the filename or both.
-
- :param filename: The name of the file to use. Can be a full path and filename or just filename
- :type filename: (str or None)
- :param path: The folder that the settings file will be stored in. Do not include the filename.
- :type path: (str or None)
- :return: Tuple with (full filename, path, filename)
- :rtype: Tuple[str, str, str]
- """
- if filename is not None:
- dirname_from_filename = os.path.dirname(filename) # see if a path was provided as part of filename
- if dirname_from_filename:
- path = dirname_from_filename
- filename = os.path.basename(filename)
- elif self.filename is not None:
- filename = self.filename
- else:
- if not self.use_config_file:
- filename = os.path.splitext(os.path.basename(sys.modules["__main__"].__file__))[0] + '.json'
- else:
- filename = os.path.splitext(os.path.basename(sys.modules["__main__"].__file__))[0] + '.ini'
-
- if path is None:
- if self.path is not None:
- # path = self.path
- path = os.path.expanduser(self.path) # expand user provided path in case it has user ~ in it. Don't think it'll hurt
- elif DEFAULT_USER_SETTINGS_PATH is not None: # if user set the path manually system-wide using set options
- path = os.path.expanduser(DEFAULT_USER_SETTINGS_PATH)
- elif running_trinket():
- path = os.path.expanduser(DEFAULT_USER_SETTINGS_TRINKET_PATH)
- elif running_replit():
- path = os.path.expanduser(DEFAULT_USER_SETTINGS_REPLIT_PATH)
- elif running_windows():
- path = os.path.expanduser(DEFAULT_USER_SETTINGS_WIN_PATH)
- elif running_linux():
- path = os.path.expanduser(DEFAULT_USER_SETTINGS_LINUX_PATH)
- elif running_mac():
- path = os.path.expanduser(DEFAULT_USER_SETTINGS_MAC_PATH)
- else:
- path = '.'
-
- full_filename = os.path.join(path, filename)
- return (full_filename, path, filename)
-
- def set_location(self, filename=None, path=None):
- """
- Sets the location of the settings file
-
- :param filename: The name of the file to use. Can be a full path and filename or just filename
- :type filename: (str or None)
- :param path: The folder that the settings file will be stored in. Do not include the filename.
- :type path: (str or None)
- """
- cfull_filename, cpath, cfilename = self._compute_filename(filename=filename, path=path)
-
- self.filename = cfilename
- self.path = cpath
- self.full_filename = cfull_filename
-
- def get_filename(self, filename=None, path=None):
- """
- Sets the filename and path for your settings file. Either paramter can be optional.
-
- If you don't choose a path, one is provided for you that is OS specific
- Windows path default = users/name/AppData/Local/PySimpleGUI/settings.
-
- If you don't choose a filename, your application's filename + '.json' will be used.
-
- Normally the filename and path are split in the user_settings calls. However for this call they
- can be combined so that the filename contains both the path and filename.
-
- :param filename: The name of the file to use. Can be a full path and filename or just filename
- :type filename: (str or None)
- :param path: The folder that the settings file will be stored in. Do not include the filename.
- :type path: (str or None)
- :return: The full pathname of the settings file that has both the path and filename combined.
- :rtype: (str)
- """
- if filename is not None or path is not None or (filename is None and path is None and self.full_filename is None):
- self.set_location(filename=filename, path=path)
- self.read()
- return self.full_filename
-
- #
- # def merge_comments_from_file(self, full_filename):
- # print('--- merging comments -----')
- # merged_lines = []
- # with open(full_filename, 'r') as f:
- # new_file_contents = f.readlines()
- # current_section = ''
- # for line in new_file_contents:
- # if len(line) == 0: # skip blank lines
- # merged_lines.append(line)
- # continue
- # if line[0] == '[': # if a new section
- # current_section = line[:line.index(']')]
- # merged_lines.append(line)
- # continue
- # if len(line.lstrip()):
- # if line.lstrip()[0] == '#': # if a comment line, save it
- # merged_lines.append(line)
- # # Process a line with an = in it
- # try:
- # key = line[:line.index('=')]
- # merged_lines.append(line)
- # except:
- # merged_lines.append(line)
- # print('--- merging complete ----')
- # print(*merged_lines)
- #
-
-
- def save(self, filename=None, path=None):
- """
- Saves the current settings dictionary. If a filename or path is specified in the call, then it will override any
- previously specitfied filename to create a new settings file. The settings dictionary is then saved to the newly defined file.
-
- :param filename: The fFilename to save to. Can specify a path or just the filename. If no filename specified, then the caller's filename will be used.
- :type filename: (str or None)
- :param path: The (optional) path to use to save the file.
- :type path: (str or None)
- :return: The full path and filename used to save the settings
- :rtype: (str)
- """
- if filename is not None or path is not None:
- self.set_location(filename=filename, path=path)
- try:
- if not os.path.exists(self.path):
- os.makedirs(self.path)
- with open(self.full_filename, 'w') as f:
- if not self.use_config_file:
- json.dump(self.dict, f)
- else:
- self.config.write(f)
- except Exception as e:
- if not self.silent_on_error:
- _error_popup_with_traceback('UserSettings.save error', '*** UserSettings.save() Error saving settings to file:***\n', self.full_filename, e)
-
- # if self.use_config_file and self.retain_config_comments:
- # self.merge_comments_from_file(self.full_filename)
-
- return self.full_filename
-
-
-
- def load(self, filename=None, path=None):
- """
- Specifies the path and filename to use for the settings and reads the contents of the file.
- The filename can be a full filename including a path, or the path can be specified separately.
- If no filename is specified, then the caller's filename will be used with the extension ".json"
-
- :param filename: Filename to load settings from (and save to in the future)
- :type filename: (str or None)
- :param path: Path to the file. Defaults to a specific folder depending on the operating system
- :type path: (str or None)
- :return: The settings dictionary (i.e. all settings)
- :rtype: (dict)
- """
- if filename is not None or path is not None or self.full_filename is None:
- self.set_location(filename, path)
- self.read()
- return self.dict
-
- def delete_file(self, filename=None, path=None, report_error=False):
- """
- Deltes the filename and path for your settings file. Either paramter can be optional.
- If you don't choose a path, one is provided for you that is OS specific
- Windows path default = users/name/AppData/Local/PySimpleGUI/settings.
- If you don't choose a filename, your application's filename + '.json' will be used
- Also sets your current dictionary to a blank one.
-
- :param filename: The name of the file to use. Can be a full path and filename or just filename
- :type filename: (str or None)
- :param path: The folder that the settings file will be stored in. Do not include the filename.
- :type path: (str or None)
- :param report_error: Determines if an error should be shown if a delete error happen (i.e. file isn't present)
- :type report_error: (bool)
- """
-
- if filename is not None or path is not None or (filename is None and path is None):
- self.set_location(filename=filename, path=path)
- try:
- os.remove(self.full_filename)
- except Exception as e:
- if report_error:
- _error_popup_with_traceback('UserSettings delete_file warning ***', 'Exception trying to perform os.remove', e)
- self.dict = {}
-
- def write_new_dictionary(self, settings_dict):
- """
- Writes a specified dictionary to the currently defined settings filename.
-
- :param settings_dict: The dictionary to be written to the currently defined settings file
- :type settings_dict: (dict)
- """
- if self.full_filename is None:
- self.set_location()
- self.dict = settings_dict
- self.save()
-
- # def as_dict(config):
- # """
- # Converts a ConfigParser object into a dictionary.
- #
- # The resulting dictionary has sections as keys which point to a dict of the
- # sections options as key => value pairs.
- # """
- # the_dict = {}
- # for section in config.sections():
- # the_dict[section] = {}
- # for key, val in config.items(section):
- # the_dict[section][key] = val
- # return the_dict
-
- def read(self):
- """
- Reads settings file and returns the dictionary.
- If you have anything changed in an existing settings dictionary, you will lose your changes.
- :return: settings dictionary
- :rtype: (dict)
- """
- if self.full_filename is None:
- return {}
- try:
- if os.path.exists(self.full_filename):
- with open(self.full_filename, 'r') as f:
- if not self.use_config_file: # if using json
- self.dict = json.load(f)
- else: # if using a config file
- self.config.read_file(f)
- # Make a dictionary of SectionDict classses. Keys are the config.sections().
- self.section_class_dict = {}
- for section in self.config.sections():
- section_dict = dict(self.config[section])
- self.section_class_dict[section] = self._SectionDict(section, section_dict, self.config, self)
-
- self.dict = self.section_class_dict
- self.config_sections = self.config.sections()
- # self.config_dict = {section_name : dict(self.config[section_name]) for section_name in self.config.sections()}
- # if self.retain_config_comments:
- # self.config_file_contents = f.readlines()
- except Exception as e:
- if not self.silent_on_error:
- _error_popup_with_traceback('User Settings read warning', 'Error reading settings from file', self.full_filename, e)
- # print('*** UserSettings.read - Error reading settings from file: ***\n', self.full_filename, e)
- # print(_create_error_message())
-
- return self.dict
-
- def exists(self, filename=None, path=None):
- """
- Check if a particular settings file exists. Returns True if file exists
-
- :param filename: The name of the file to use. Can be a full path and filename or just filename
- :type filename: (str or None)
- :param path: The folder that the settings file will be stored in. Do not include the filename.
- :type path: (str or None)
- """
- cfull_filename, cpath, cfilename = self._compute_filename(filename=filename, path=path)
- if os.path.exists(cfull_filename):
- return True
- return False
-
- def delete_entry(self, key, section=None):
- """
- Deletes an individual entry. If no filename has been specified up to this point,
- then a default filename will be used.
- After value has been deleted, the settings file is written to disk.
-
- :param key: Setting to be deleted. Can be any valid dictionary key type (i.e. must be hashable)
- :type key: (Any)
- """
- if self.full_filename is None:
- self.set_location()
- self.read()
- if not self.use_config_file: # Is using JSON file
- if key in self.dict:
- del self.dict[key]
- if self.autosave:
- self.save()
- else:
- if not self.silent_on_error:
- _error_popup_with_traceback('User Settings delete_entry Warning - key', key, ' not found in settings')
-
- else:
- if section is not None:
- section_dict = self.get(section)
- # print(f'** Trying to delete an entry with a config file in use ** id of section_dict = {id(section_dict)}')
- # section_dict = self.section_class_dict[section]
- del self.get(section)[key]
- # del section_dict[key]
- # del section_dict[key]
-
- def delete_section(self, section):
- """
- Deletes a section with the name provided in the section parameter. Your INI file will be saved afterwards if auto-save enabled (default is ON)
- :param section: Name of the section to delete
- :type section: str
- """
- if not self.use_config_file:
- return
-
- section_dict = self.section_class_dict.get(section, None)
- section_dict.delete_section()
- del self.section_class_dict[section]
- if self.autosave:
- self.save()
-
- def set(self, key, value):
- """
- Sets an individual setting to the specified value. If no filename has been specified up to this point,
- then a default filename will be used.
- After value has been modified, the settings file is written to disk.
- Note that this call is not value for a config file normally. If it is, then the key is assumed to be the
- Section key and the value written will be the default value.
- :param key: Setting to be saved. Can be any valid dictionary key type
- :type key: (Any)
- :param value: Value to save as the setting's value. Can be anything
- :type value: (Any)
- :return: value that key was set to
- :rtype: (Any)
- """
-
- if self.full_filename is None:
- self.set_location()
- # if not autosaving, then don't read the file or else will lose changes
- if not self.use_config_file:
- if self.autosave or self.dict == {}:
- self.read()
- self.dict[key] = value
- else:
- self.section_class_dict[key].set(value, self.default_value)
-
- if self.autosave:
- self.save()
- return value
-
- def get(self, key, default=None):
- """
- Returns the value of a specified setting. If the setting is not found in the settings dictionary, then
- the user specified default value will be returned. It no default is specified and nothing is found, then
- the "default value" is returned. This default can be specified in this call, or previously defined
- by calling set_default. If nothing specified now or previously, then None is returned as default.
-
- :param key: Key used to lookup the setting in the settings dictionary
- :type key: (Any)
- :param default: Value to use should the key not be found in the dictionary
- :type default: (Any)
- :return: Value of specified settings
- :rtype: (Any)
- """
- if self.default_value is not None:
- default = self.default_value
-
- if self.full_filename is None:
- self.set_location()
- if self.autosave or self.dict == {}:
- self.read()
- if not self.use_config_file:
- value = self.dict.get(key, default)
- else:
- value = self.section_class_dict.get(key, None)
- if key not in list(self.section_class_dict.keys()):
- self.section_class_dict[key] = self._SectionDict(key, {}, self.config, self)
- value = self.section_class_dict[key]
- value.new_section = True
- return value
-
- def get_dict(self):
- """
- Returns the current settings dictionary. If you've not setup the filename for the
- settings, a default one will be used and then read.
-
- Note that you can display the dictionary in text format by printing the object itself.
-
- :return: The current settings dictionary
- :rtype: Dict
- """
- if self.full_filename is None:
- self.set_location()
- if self.autosave or self.dict == {}:
- self.read()
- self.save()
- return self.dict
-
- def __setitem__(self, item, value):
- """
- Enables setting a setting by using [ ] notation like a dictionary.
- Your code will have this kind of design pattern:
- settings = sg.UserSettings()
- settings[item] = value
-
- :param item: The key for the setting to change. Needs to be a hashable type. Basically anything but a list
- :type item: Any
- :param value: The value to set the setting to
- :type value: Any
- """
- return self.set(item, value)
-
- def __getitem__(self, item):
- """
- Enables accessing a setting using [ ] notation like a dictionary.
- If the entry does not exist, then the default value will be returned. This default
- value is None unless user sets by calling UserSettings.set_default_value(default_value)
-
- :param item: The key for the setting to change. Needs to be a hashable type. Basically anything but a list
- :type item: Any
- :return: The setting value
- :rtype: Any
- """
- return self.get(item, self.default_value)
-
- def __delitem__(self, item):
- """
- Delete an individual user setting. This is the same as calling delete_entry. The syntax
- for deleting the item using this manner is:
- del settings['entry']
- :param item: The key for the setting to delete
- :type item: Any
- """
- if self.use_config_file:
- return self.get(item)
- else:
- self.delete_entry(key=item)
-
-
-# Create a singleton for the settings information so that the settings functions can be used
-if UserSettings._default_for_function_interface is None:
- UserSettings._default_for_function_interface = UserSettings()
-
-
-def user_settings_filename(filename=None, path=None):
- """
- Sets the filename and path for your settings file. Either paramter can be optional.
-
- If you don't choose a path, one is provided for you that is OS specific
- Windows path default = users/name/AppData/Local/PySimpleGUI/settings.
-
- If you don't choose a filename, your application's filename + '.json' will be used.
-
- Normally the filename and path are split in the user_settings calls. However for this call they
- can be combined so that the filename contains both the path and filename.
-
- :param filename: The name of the file to use. Can be a full path and filename or just filename
- :type filename: (str)
- :param path: The folder that the settings file will be stored in. Do not include the filename.
- :type path: (str)
- :return: The full pathname of the settings file that has both the path and filename combined.
- :rtype: (str)
- """
- settings = UserSettings._default_for_function_interface
- return settings.get_filename(filename, path)
-
-
-def user_settings_delete_filename(filename=None, path=None, report_error=False):
- """
- Deltes the filename and path for your settings file. Either paramter can be optional.
- If you don't choose a path, one is provided for you that is OS specific
- Windows path default = users/name/AppData/Local/PySimpleGUI/settings.
- If you don't choose a filename, your application's filename + '.json' will be used
- Also sets your current dictionary to a blank one.
-
- :param filename: The name of the file to use. Can be a full path and filename or just filename
- :type filename: (str)
- :param path: The folder that the settings file will be stored in. Do not include the filename.
- :type path: (str)
- """
- settings = UserSettings._default_for_function_interface
- settings.delete_file(filename, path, report_error=report_error)
-
-
-def user_settings_set_entry(key, value):
- """
- Sets an individual setting to the specified value. If no filename has been specified up to this point,
- then a default filename will be used.
- After value has been modified, the settings file is written to disk.
-
- :param key: Setting to be saved. Can be any valid dictionary key type
- :type key: (Any)
- :param value: Value to save as the setting's value. Can be anything
- :type value: (Any)
- """
- settings = UserSettings._default_for_function_interface
- settings.set(key, value)
-
-
-def user_settings_delete_entry(key):
- """
- Deletes an individual entry. If no filename has been specified up to this point,
- then a default filename will be used.
- After value has been deleted, the settings file is written to disk.
-
- :param key: Setting to be saved. Can be any valid dictionary key type (hashable)
- :type key: (Any)
- """
- settings = UserSettings._default_for_function_interface
- settings.delete_entry(key)
-
-
-def user_settings_get_entry(key, default=None):
- """
- Returns the value of a specified setting. If the setting is not found in the settings dictionary, then
- the user specified default value will be returned. It no default is specified and nothing is found, then
- None is returned. If the key isn't in the dictionary, then it will be added and the settings file saved.
- If no filename has been specified up to this point, then a default filename will be assigned and used.
- The settings are SAVED prior to returning.
-
- :param key: Key used to lookup the setting in the settings dictionary
- :type key: (Any)
- :param default: Value to use should the key not be found in the dictionary
- :type default: (Any)
- :return: Value of specified settings
- :rtype: (Any)
- """
- settings = UserSettings._default_for_function_interface
- return settings.get(key, default)
-
-
-def user_settings_save(filename=None, path=None):
- """
- Saves the current settings dictionary. If a filename or path is specified in the call, then it will override any
- previously specitfied filename to create a new settings file. The settings dictionary is then saved to the newly defined file.
-
- :param filename: The fFilename to save to. Can specify a path or just the filename. If no filename specified, then the caller's filename will be used.
- :type filename: (str)
- :param path: The (optional) path to use to save the file.
- :type path: (str)
- :return: The full path and filename used to save the settings
- :rtype: (str)
- """
- settings = UserSettings._default_for_function_interface
- return settings.save(filename, path)
-
-
-def user_settings_load(filename=None, path=None):
- """
- Specifies the path and filename to use for the settings and reads the contents of the file.
- The filename can be a full filename including a path, or the path can be specified separately.
- If no filename is specified, then the caller's filename will be used with the extension ".json"
-
- :param filename: Filename to load settings from (and save to in the future)
- :type filename: (str)
- :param path: Path to the file. Defaults to a specific folder depending on the operating system
- :type path: (str)
- :return: The settings dictionary (i.e. all settings)
- :rtype: (dict)
- """
- settings = UserSettings._default_for_function_interface
- return settings.load(filename, path)
-
-
-def user_settings_file_exists(filename=None, path=None):
- """
- Determines if a settings file exists. If so a boolean True is returned.
- If either a filename or a path is not included, then the appropriate default
- will be used.
-
- :param filename: Filename to check
- :type filename: (str)
- :param path: Path to the file. Defaults to a specific folder depending on the operating system
- :type path: (str)
- :return: True if the file exists
- :rtype: (bool)
- """
- settings = UserSettings._default_for_function_interface
- return settings.exists(filename=filename, path=path)
-
-
-def user_settings_write_new_dictionary(settings_dict):
- """
- Writes a specified dictionary to the currently defined settings filename.
-
- :param settings_dict: The dictionary to be written to the currently defined settings file
- :type settings_dict: (dict)
- """
- settings = UserSettings._default_for_function_interface
- settings.write_new_dictionary(settings_dict)
-
-
-def user_settings_silent_on_error(silent_on_error=False):
- """
- Used to control the display of error messages. By default, error messages are displayed to stdout.
-
- :param silent_on_error: If True then all error messages are silenced (not displayed on the console)
- :type silent_on_error: (bool)
- """
- settings = UserSettings._default_for_function_interface
- settings.silent_on_error = silent_on_error
-
-
-def user_settings():
- """
- Returns the current settings dictionary. If you've not setup the filename for the
- settings, a default one will be used and then read.
- :return: The current settings dictionary as a dictionary or a nicely formatted string representing it
- :rtype: (dict or str)
- """
- settings = UserSettings._default_for_function_interface
- return settings.get_dict()
-
-
-def user_settings_object():
- """
- Returns the object that is used for the function version of this API.
- With this object you can use the object interface, print it out in a nice format, etc.
-
- :return: The UserSettings obect used for the function level interface
- :rtype: (UserSettings)
- """
- return UserSettings._default_for_function_interface
-
-
-'''
-'########:'##::::'##:'########::'######::'##::::'##:'########:'########:
- ##.....::. ##::'##:: ##.....::'##... ##: ##:::: ##:... ##..:: ##.....::
- ##::::::::. ##'##::: ##::::::: ##:::..:: ##:::: ##:::: ##:::: ##:::::::
- ######:::::. ###:::: ######::: ##::::::: ##:::: ##:::: ##:::: ######:::
- ##...:::::: ## ##::: ##...:::: ##::::::: ##:::: ##:::: ##:::: ##...::::
- ##:::::::: ##:. ##:: ##::::::: ##::: ##: ##:::: ##:::: ##:::: ##:::::::
- ########: ##:::. ##: ########:. ######::. #######::::: ##:::: ########:
-........::..:::::..::........:::......::::.......::::::..:::::........::
-:::'###::::'########::'####::'######::
-::'## ##::: ##.... ##:. ##::'##... ##:
-:'##:. ##:: ##:::: ##:: ##:: ##:::..::
-'##:::. ##: ########::: ##::. ######::
- #########: ##.....:::: ##:::..... ##:
- ##.... ##: ##::::::::: ##::'##::: ##:
- ##:::: ##: ##::::::::'####:. ######::
-..:::::..::..:::::::::....:::......:::
-
-
-
-These are the functions used to implement the subprocess APIs (Exec APIs) of PySimpleGUI
-
-'''
-
-
-def execute_command_subprocess(command, *args, wait=False, cwd=None, pipe_output=False, merge_stderr_with_stdout=True, stdin=None):
- """
- Runs the specified command as a subprocess.
- By default the call is non-blocking.
- The function will immediately return without waiting for the process to complete running. You can use the returned Popen object to communicate with the subprocess and get the results.
- Returns a subprocess Popen object.
-
- :param command: Filename to load settings from (and save to in the future)
- :type command: (str)
- :param *args: Variable number of arguments that are passed to the program being started as command line parms
- :type *args: (Any)
- :param wait: If True then wait for the subprocess to finish
- :type wait: (bool)
- :param cwd: Working directory to use when executing the subprocess
- :type cwd: (str))
- :param pipe_output: If True then output from the subprocess will be piped. You MUST empty the pipe by calling execute_get_results or your subprocess will block until no longer full
- :type pipe_output: (bool)
- :param merge_stderr_with_stdout: If True then output from the subprocess stderr will be merged with stdout. The result is ALL output will be on stdout.
- :type merge_stderr_with_stdout: (bool)
- :param stdin: Value passed to the Popen call. Defaults to subprocess.DEVNULL so that the pyinstaller created executable work correctly
- :type stdin: (bool)
- :return: Popen object
- :rtype: (subprocess.Popen)
- """
- if stdin is None:
- stdin = subprocess.DEVNULL
- try:
- if args is not None:
- expanded_args = ' '.join(args)
- # print('executing subprocess command:',command, 'args:',expanded_args)
- if command[0] != '"' and ' ' in command:
- command = '"' + command + '"'
- # print('calling popen with:', command +' '+ expanded_args)
- # sp = subprocess.Popen(command +' '+ expanded_args, shell=True, stdout=subprocess.DEVNULL, stderr=subprocess.DEVNULL, cwd=cwd)
- if pipe_output:
- if merge_stderr_with_stdout:
- sp = subprocess.Popen(command + ' ' + expanded_args, shell=True, stdout=subprocess.PIPE, stderr=subprocess.STDOUT, cwd=cwd, stdin=stdin)
- else:
- sp = subprocess.Popen(command + ' ' + expanded_args, shell=True, stdout=subprocess.PIPE, stderr=subprocess.PIPE, cwd=cwd, stdin=stdin)
- else:
- sp = subprocess.Popen(command + ' ' + expanded_args, shell=True, stdout=subprocess.DEVNULL, stderr=subprocess.DEVNULL, cwd=cwd, stdin=stdin)
- else:
- sp = subprocess.Popen(command, shell=True, stdout=subprocess.PIPE, stderr=subprocess.PIPE, cwd=cwd, stdin=stdin)
- if wait:
- out, err = sp.communicate()
- if out:
- print(out.decode("utf-8"))
- if err:
- print(err.decode("utf-8"))
- except Exception as e:
- warnings.warn('Error in execute_command_subprocess {}'.format(e), UserWarning)
- _error_popup_with_traceback('Error in execute_command_subprocess', e, 'command={}'.format(command), 'args={}'.format(args), 'cwd={}'.format(cwd))
- sp = None
- return sp
-
-
-def execute_py_file(pyfile, parms=None, cwd=None, interpreter_command=None, wait=False, pipe_output=False, merge_stderr_with_stdout=True):
- """
- Executes a Python file.
- The interpreter to use is chosen based on this priority order:
- 1. interpreter_command paramter
- 2. global setting "-python command-"
- 3. the interpreter running running PySimpleGUI
- :param pyfile: the file to run
- :type pyfile: (str)
- :param parms: parameters to pass on the command line
- :type parms: (str)
- :param cwd: the working directory to use
- :type cwd: (str)
- :param interpreter_command: the command used to invoke the Python interpreter
- :type interpreter_command: (str)
- :param wait: the working directory to use
- :type wait: (bool)
- :param pipe_output: If True then output from the subprocess will be piped. You MUST empty the pipe by calling execute_get_results or your subprocess will block until no longer full
- :type pipe_output: (bool)
- :param merge_stderr_with_stdout: If True then output from the subprocess stderr will be merged with stdout. The result is ALL output will be on stdout.
- :type merge_stderr_with_stdout: (bool)
- :return: Popen object
- :rtype: (subprocess.Popen) | None
- """
-
- if cwd is None:
- # if the specific file is not found (not an absolute path) then assume it's relative to '.'
- if not os.path.exists(pyfile):
- cwd = '.'
-
- if pyfile[0] != '"' and ' ' in pyfile:
- pyfile = '"' + pyfile + '"'
- if interpreter_command is not None:
- python_program = interpreter_command
- else:
- # use the version CURRENTLY RUNNING if nothing is specified. Previously used the one from the settings file
- pysimplegui_user_settings.load() # Refresh the settings just in case they've changed via another program
- python_program = pysimplegui_user_settings.get('-python command-', '')
- if python_program == '': # if no interpreter set in the settings, then use the current one
- python_program = sys.executable
- # python_program = 'python' if running_windows() else 'python3'
- if parms is not None and python_program:
- sp = execute_command_subprocess(python_program, pyfile, parms, wait=wait, cwd=cwd, pipe_output=pipe_output, merge_stderr_with_stdout=merge_stderr_with_stdout)
- elif python_program:
- sp = execute_command_subprocess(python_program, pyfile, wait=wait, cwd=cwd, pipe_output=pipe_output, merge_stderr_with_stdout=merge_stderr_with_stdout)
- else:
- print('execute_py_file - No interpreter has been configured')
- sp = None
- return sp
-
-
-def execute_py_get_interpreter():
- """
- Returns the command that is currently running. Previously returned the one from the system settings, but
- have determined that the one currently running is the better choice.
-
- :return: Full path to python interpreter (uses sys.executable)
- :rtype: (str)
- """
- pysimplegui_user_settings.load() # Refresh the settings just in case they've changed via another program
- interpreter = pysimplegui_user_settings.get('-python command-', '')
- if interpreter == '':
- interpreter = sys.executable
- return interpreter
-
-
-def execute_editor(file_to_edit, line_number=None):
- """
- Runs the editor that was configured in the global settings and opens the file to a specific line number.
- Two global settings keys are used.
- '-editor program-' the command line used to startup your editor. It's set
- in the global settings window or by directly manipulating the PySimpleGUI settings object
- '-editor format string-' a string containing 3 "tokens" that describes the command that is executed
-
- :param file_to_edit: the full path to the file to edit
- :type file_to_edit: (str)
- :param line_number: optional line number to place the cursor
- :type line_number: (int)
- :return: Popen object
- :rtype: (subprocess.Popen) | None
- """
- if file_to_edit is not None and len(file_to_edit) != 0 and file_to_edit[0] not in ('\"', "\'") and ' ' in file_to_edit:
- file_to_edit = '"' + file_to_edit + '"'
- pysimplegui_user_settings.load() # Refresh the settings just in case they've changed via another program
- editor_program = pysimplegui_user_settings.get('-editor program-', None)
- if editor_program is not None:
- format_string = pysimplegui_user_settings.get('-editor format string-', None)
- # if no format string, then just launch the editor with the filename
- if not format_string or line_number is None:
- sp = execute_command_subprocess(editor_program, file_to_edit)
- else:
- command = _create_full_editor_command(file_to_edit, line_number, format_string)
- # print('final command line = ', command)
- sp = execute_command_subprocess(editor_program, command)
- else:
- print('No editor has been configured in the global settings')
- sp = None
- return sp
-
-
-def execute_get_results(subprocess_id, timeout=None):
- """
- Get the text results of a previously executed execute call
- Returns a tuple of the strings (stdout, stderr)
- :param subprocess_id: a Popen subprocess ID returned from a previous execute call
- :type subprocess_id: (subprocess.Popen)
- :param timeout: Time in fractions of a second to wait. Returns '','' if timeout. Default of None means wait forever
- :type timeout: (None | float)
- :returns: Tuple with 2 strings (stdout, stderr)
- :rtype: (str | None , str | None)
- """
-
- out_decoded = err_decoded = None
- if subprocess_id is not None:
- try:
- out, err = subprocess_id.communicate(timeout=timeout)
- if out:
- out_decoded = out.decode("utf-8")
- if err:
- err_decoded = err.decode("utf-8")
- except ValueError:
- # will get an error if stdout and stderr are combined and attempt to read stderr
- # so ignore the error that would be generated
- pass
- except subprocess.TimeoutExpired:
- # a Timeout error is not actually an error that needs to be reported
- pass
- except Exception as e:
- popup_error('Error in execute_get_results', e)
- return out_decoded, err_decoded
-
-
-def execute_subprocess_still_running(subprocess_id):
- """
- Returns True is the subprocess ID provided is for a process that is still running
-
- :param subprocess_id: ID previously returned from Exec API calls that indicate this value is returned
- :type subprocess_id: (subprocess.Popen)
- :return: True if the subproces is running
- :rtype: bool
- """
- if subprocess_id.poll() == 0:
- return False
- return True
-
-
-def execute_file_explorer(folder_to_open=''):
- """
- The global settings has a setting called - "-explorer program-"
- It defines the program to run when this function is called.
- The optional folder paramter specified which path should be opened.
-
- :param folder_to_open: The path to open in the explorer program
- :type folder_to_open: str
- :return: Popen object
- :rtype: (subprocess.Popen) | None
- """
- pysimplegui_user_settings.load() # Refresh the settings just in case they've changed via another program
- explorer_program = pysimplegui_user_settings.get('-explorer program-', None)
- if explorer_program is not None:
- sp = execute_command_subprocess(explorer_program, folder_to_open)
- else:
- print('No file explorer has been configured in the global settings')
- sp = None
- return sp
-
-
-def execute_find_callers_filename():
- """
- Returns the first filename found in a traceback that is not the nsame of this file (__file__)
- Used internally with the debugger for example.
-
- :return: filename of the caller, asseumed to be the first non PySimpleGUI file
- :rtype: str
- """
- try: # lots can go wrong so wrapping the entire thing
- trace_details = traceback.format_stack()
- file_info_pysimplegui, error_message = None, ''
- for line in reversed(trace_details):
- if __file__ not in line:
- file_info_pysimplegui = line.split(",")[0]
- error_message = line
- break
- if file_info_pysimplegui is None:
- return ''
- error_parts = None
- if error_message != '':
- error_parts = error_message.split(', ')
- if len(error_parts) < 4:
- error_message = error_parts[0] + '\n' + error_parts[1] + '\n' + ''.join(error_parts[2:])
- if error_parts is None:
- print('*** Error popup attempted but unable to parse error details ***')
- print(trace_details)
- return ''
- filename = error_parts[0][error_parts[0].index('File ') + 5:]
- return filename
- except:
- return ''
-
-
-def _create_full_editor_command(file_to_edit, line_number, edit_format_string):
- """
- The global settings has a setting called - "-editor format string-"
- It uses 3 "tokens" to describe how to invoke the editor in a way that starts at a specific line #
-