diff --git a/spaces/1gistliPinn/ChatGPT4/Examples/Aquachem Software Crack Free Download.md b/spaces/1gistliPinn/ChatGPT4/Examples/Aquachem Software Crack Free Download.md deleted file mode 100644 index 3f05b976cf69fb8bbcb80e5f84d4af3eb2a30736..0000000000000000000000000000000000000000 --- a/spaces/1gistliPinn/ChatGPT4/Examples/Aquachem Software Crack Free Download.md +++ /dev/null @@ -1,6 +0,0 @@ -
Download Zip > https://imgfil.com/2uxXtJ
EDIUS Pro is a powerful video editing software that supports various formats and resolutions. It allows you to create professional-looking projects with ease and speed. EDIUS Pro is compatible with Windows 7 32-bit, but you need to have an EDIUS ID and QuickTime installed on your computer before you can use it. In this article, we will show you how to download and install EDIUS Pro for Windows 7 32-bit in a few simple steps.
-The first thing you need to do is to download EDIUS Pro from the official website. You can choose between the latest version (EDIUS X) or the previous versions (EDIUS 9 or EDIUS 8). The latest version has more features and a redesigned core engine, but it also requires more system resources. The previous versions are still supported and updated, but they have less functionality. You can compare the different versions here: https://www.edius.net/compare.html
-Download Zip ---> https://imgfil.com/2uy0Ey
To download EDIUS Pro, go to https://www.edius.net/edius_download.html and select the version you want. You will see a zip file with a size of about 1 GB (for EDIUS X) or 800 MB (for EDIUS 9 or EDIUS 8). Click on the download button and save the file to your computer.
-After you have downloaded the zip file, you need to extract it to a folder on your computer. You can use any file compression software, such as WinRAR or 7-Zip, to do this. Right-click on the zip file and choose "Extract here" or "Extract to" and select a destination folder.
-Once you have extracted the zip file, you will see a folder with several files and subfolders. Double-click on the "Setup.exe" file to start the installation process. You will see a welcome screen with the End-User License Agreement. Read it carefully and click on "Accept" if you agree with the terms. Then click on "Install" to begin the installation.
-The installation process is quite simple and straightforward. You don't need to choose any options or settings, as everything is done automatically for you. The installer will copy the necessary files and create a shortcut icon on your desktop. The installation may take several minutes, depending on your system speed and performance.
-When the installation is finished, you will see a message saying "Installation completed successfully". Click on "Finish" to exit the installer. You can now launch EDIUS Pro from your desktop or start menu.
- -The last step you need to do is to activate EDIUS Pro with your EDIUS ID. An EDIUS ID is a unique identifier that allows you to use EDIUS Pro and access its online services. If you don't have an EDIUS ID, you can create one for free at https://ediusid1.grassvalley.com/. You will need to provide your name, email address, country, and password.
-Once you have an EDIUS ID, you can activate EDIUS Pro by entering it in the activation window that appears when you start EDIUS Pro for the first time. You will also need to enter your serial number, which is provided when you purchase EDIUS Pro from an authorized reseller or request a free 30-day trial version.
-After you enter your EDIUS ID and serial number, click on "Activate" and wait for a few seconds. You will see a message saying "Activation completed successfully". Click on "OK" to close
d5da3c52bfEriyum Panikadu is a Tamil novel by P.H. Daniel, translated by Ra. Murugavel. It was first published in 1969 and is considered a classic of Tamil literature. The novel depicts the life and struggles of the tea plantation workers in the Nilgiris during the colonial era. The novel is based on the author's personal experience as a doctor and a trade union leader among the workers.
-Download File »»» https://imgfil.com/2uxYiF
The novel follows the story of Selvan, a young worker who dreams of a better life for himself and his people. He falls in love with Valli, a beautiful girl from another plantation, and hopes to marry her someday. However, he faces many obstacles and challenges from the oppressive system of the planters, who exploit and abuse the workers mercilessly. The novel also portrays the social and cultural aspects of the workers, such as their festivals, rituals, beliefs, customs, and language.
-Eriyum Panikadu is a powerful and realistic novel that exposes the harsh realities of the tea plantation industry and its impact on the workers. It also highlights the importance of education, organization, and resistance among the oppressed classes. The novel has been praised for its vivid narration, rich characterization, and historical accuracy. It has been adapted into a film by Bala in 2013, titled Paradesi.
-Eriyum Panikadu is a novel that deserves to be read by everyone who wants to learn more about the history and culture of Tamil Nadu and its people. It is a novel that will move you, inspire you, and make you think. You can download Eriyum Panikadu book for free from SoundCloud[^2^] [^3^] or buy it from Amazon[^1^].
- - -The novel Eriyum Panikadu is not only a historical fiction, but also a social commentary on the contemporary issues of caste, class, and gender. The novel exposes the discrimination and violence faced by the workers, who belong to the Dalit community, from the upper-caste planters and the British officials. The novel also shows the plight of the women workers, who are subjected to sexual harassment, rape, and forced sterilization. The novel challenges the stereotypes and prejudices that are prevalent in the society and calls for social justice and equality.
-The novel Eriyum Panikadu is also a literary masterpiece that showcases the beauty and richness of the Tamil language and culture. The novel uses a variety of dialects and registers to capture the authentic voice of the workers and their environment. The novel also incorporates many folk songs, proverbs, idioms, and metaphors that reflect the wisdom and creativity of the workers. The novel is a tribute to the resilience and courage of the workers, who despite their hardships, manage to find joy and hope in their lives.
-The novel Eriyum Panikadu is a must-read for anyone who loves literature and history. It is a novel that will make you laugh, cry, angry, and hopeful. It is a novel that will teach you about the past and inspire you for the future. It is a novel that will stay with you forever.
d5da3c52bfIf you are a fan of pool games, you have probably heard of 8 Ball Pool, the most popular and addictive pool game for Android devices. In this article, we will tell you everything you need to know about the latest version of this game, 8 Ball Pool 5.9.0, and how to download and install it on your device.
-8 Ball Pool is a pool game developed by Miniclip.com, a leading online gaming company. It allows you to play online with millions of players from around the world, or challenge your friends in one-on-one matches. You can also participate in tournaments, win trophies, and collect coins and cues.
-Download File … https://urlin.us/2uT1z6
Some of the features that make 8 Ball Pool stand out from other pool games are:
-The rules of 8 Ball Pool are simple and similar to the real pool game. You have to pot all your balls (solid or striped) before your opponent does, and then pot the black ball (the 8 ball) to win the game. You can use the cue stick to aim and adjust the power of your shot, and use the spin button to add spin to the cue ball. You have to be careful not to pot the cue ball or the wrong balls, or you will lose your turn or the game.
-The latest version of 8 Ball Pool, released on June 14, 2023, brings some exciting new features and improvements to the game. Here are some of them:
-Power Pots is a new game mode that challenges you to pot as many balls as possible in a limited time. The more balls you pot, the more points you get. You can also use power-ups to boost your score and get extra time. Power Pots is available for a limited time only, so don't miss it!
-8 Ball Pool 5.9.0 also introduces new rewards and events for you to enjoy. You can earn free coins, cues, chat packs, and more by completing daily missions, watching videos, spinning the wheel, and playing mini-games. You can also join seasonal events and win exclusive prizes by ranking high on the leaderboards.
-As always, 8 Ball Pool 5.9.0 also fixes some bugs and improves the performance and stability of the game. Some of the issues that have been resolved are:
-8 ball pool 5.9.0 arm64 apk download
-8 ball pool 5.9.0 apk download for windows pc
-8 ball pool 5.9.0 apk download softpedia
-8 ball pool 5.9.0 apk download apkpure
-8 ball pool 5.9.0 apk download miniclip
-8 ball pool 5.9.0 apk download android
-8 ball pool 5.9.0 apk download latest version
-8 ball pool 5.9.0 apk download free
-8 ball pool 5.9.0 apk download mod
-8 ball pool 5.9.0 apk download unlimited coins
-8 ball pool 5.9.0 apk download hack
-8 ball pool 5.9.0 apk download offline
-8 ball pool 5.9.0 apk download no root
-8 ball pool 5.9.0 apk download emulator
-8 ball pool 5.9.0 apk download with mouse and keyboard
-8 ball pool 5.9.0 apk download for pc windows 10
-8 ball pool 5.9.0 apk download for pc windows 7
-8 ball pool 5.9.0 apk download for mac
-8 ball pool 5.9.0 apk download for laptop
-8 ball pool 5.9.0 apk download for chromebook
-8 ball pool 5.9.0 apk download for tablet
-8 ball pool 5.9.0 apk download for firestick
-8 ball pool 5.9.0 apk download for smart tv
-8 ball pool 5.9.0 apk download for ios
-8 ball pool 5.9.0 apk download for iphone
-8 ball pool 5.9.0 apk download for ipad
-8 ball pool 5.9.0 apk download for ipod touch
-8 ball pool 5.9.0 apk download from google play store
-8 ball pool 5.9.0 apk download from uptodown
-8 ball pool 5.9.0 apk download from apkmirror
-how to install and play the game of the famous miniclip.com adapted for the android platform with the help of the app description of the softpedia website[^1^]
-how to install and play the game on windows with an emulator and adapt its controls to a mouse and keyboard as explained by the apkpure website[^2^]
-how to update the game to the latest version of the app with the help of the softpedia website[^1^]
-how to uninstall the game from your device with the help of the apkpure website[^2^]
-how to play the game online with other players from around the world with the help of the miniclip.com website[^1^]
-how to play the game offline with your friends or against the computer with the help of the apkpure website[^2^]
-how to customize your cue and table in the game with the help of the miniclip.com website[^1^]
-how to earn coins and cash in the game with the help of the apkpure website[^2^]
-how to join clubs and compete in tournaments in the game with the help of the miniclip.com website[^1^]
-how to chat and send gifts to other players in the game with the help of the apkpure website[^2^]
If you want to enjoy the new features and improvements of 8 Ball Pool 5.9.0, you have to download and install the APK file on your Android device. There are two ways to do this:
-The easiest and safest way to download 8 Ball Pool 5.9.0 APK is from the official sources, such as Google Play Store or Miniclip.com website. You just have to follow these steps:
-Another way to download 8 Ball Pool 5.9.0 APK is from third-party sources, such as APKPure, APKMirror, or other websites that offer APK files. However, this method is not recommended, as it may expose your device to malware or viruses. If you still want to try this method, you have to follow these steps:
-Before you can install the APK file on your device, you have to enable the Unknown Sources option in your settings. This will allow you to install apps from sources other than the Google Play Store. To do this, follow these steps:
-Now, you can install the APK file by following these steps:
-8 Ball Pool is a fun and addictive pool game that lets you play online with millions of players from around the world, or challenge your friends in one-on-one matches. The latest version of the game, 8 Ball Pool 5.9.0, brings some exciting new features and improvements, such as a new game mode, new rewards and events, and bug fixes and performance enhancements. You can download and install 8 Ball Pool 5.9.0 APK on your Android device by following the steps we have provided in this article. We hope you enjoy playing 8 Ball Pool 5.9.0 and have a great time!
-Here are some frequently asked questions about 8 Ball Pool 5.9.0:
-Yes, 8 Ball Pool 5.9.0 is free to download and play, but it contains in-app purchases that allow you to buy coins, cues, chat packs, and more.
-If you download 8 Ball Pool 5.9.0 from official sources, such as Google Play Store or Miniclip.com website, it is safe and secure. However, if you download it from third-party sources, it may contain malware or viruses that can harm your device.
-8 Ball Pool 5.9.0 requires Android version 4.4 or higher to run smoothly on your device. You can check your device's Android version by going to Settings > About Phone > Android Version.
-If you have any questions, feedback, or issues regarding 8 Ball Pool, you can contact the developers by visiting their website (https://www.miniclip.com), their Facebook page (https ://www.facebook.com/8ballpoolfans), or their Twitter account (https://twitter.com/8ballpool).
-Some tips and tricks that can help you improve your skills in 8 Ball Pool are:
-If you are a fan of action and strategy games, you might have heard of Defense Zone 3, a long-awaited sequel to the popular game series. But did you know that you can also play this game on your Android device with the Defense Zone 3 APK? In this article, we will review the features, benefits, and gameplay of Defense Zone 3 APK, as well as show you how to download and install it on your device. We will also share some tips and tricks to help you win the game, and answer some frequently asked questions. So, let's get started!
-Defense Zone 3 APK is an Android application package that allows you to play the game Defense Zone 3 on your Android device. Defense Zone 3 is an action/strategy game developed by ARTEM KOTOV, a Russian game developer. It is the third installment in the Defense Zone series, which has been praised for its stunning graphics, realistic sound effects, and challenging gameplay.
-DOWNLOAD ✯ https://jinyurl.com/2uNPON
Defense Zone 3 APK has many features that make it an exciting and enjoyable game to play. Some of these features are:
-Defense Zone 3 APK has many benefits that make it a worthwhile game to download and play. Some of these benefits are:
-If you want to play Defense Zone 3 APK on your Android device, you will need to download and install it first. Here are the steps to do so:
-If you cannot access Google Play Store or prefer to download the game from another source, you can follow these steps:
-Before you download and install Defense Zone 3 APK, you should check if your device meets the minimum requirements and is compatible with the game. Here are the requirements and compatibility of Defense Zone 3 APK:
-Now that you have downloaded and installed Defense Zone 3 APK, you are ready to play the game. Here are some basic instructions on how to play Defense Zone 3 APK:
-The gameplay of Defense Zone 3 APK is simple and intuitive. Your goal is to defend your base from the waves of enemies that will attack you from different directions. You will have to build and upgrade your turrets along the path that the enemies will take, and use your special abilities wisely to stop them from reaching your base.
-defense zone 3 hd apk download
-defense zone 3 ultra hd apk
-defense zone 3 mod apk unlimited money
-defense zone 3 apk free download
-defense zone 3 apk + obb
-defense zone 3 hack apk
-defense zone 3 full apk
-defense zone 3 latest version apk
-defense zone 3 premium apk
-defense zone 3 apk mod menu
-defense zone 3 offline apk
-defense zone 3 android apk
-defense zone 3 apk pure
-defense zone 3 apk revdl
-defense zone 3 apk uptodown
-defense zone 3 cheats apk
-defense zone 3 cracked apk
-defense zone 3 game apk
-defense zone 3 pro apk
-defense zone 3 unlimited coins apk
-defense zone 3 apk for pc
-defense zone 3 mod apk android 1
-defense zone 3 mod apk rexdl
-defense zone 3 mod apk latest version
-defense zone 3 mod apk happymod
-defense zone 3 mod apk all levels unlocked
-defense zone 3 mod apk no ads
-defense zone 3 mod apk unlimited everything
-defense zone 3 mod apk android republic
-defense zone 3 mod apk an1.com
-defense zone 3 ultra hd mod apk
-defense zone 3 ultra hd full apk
-defense zone 3 ultra hd hack apk
-defense zone 3 ultra hd premium apk
-defense zone 3 ultra hd cracked apk
-defense zone 3 ultra hd latest version apk
-defense zone 3 ultra hd mod menu apk
-defense zone 3 ultra hd mod unlimited money and coins apk download free for android devices.
The controls of Defense Zone 3 APK are also easy and convenient. You can use your finger to drag and drop your turrets on the map, tap on them to upgrade or sell them, and swipe on the screen to move the camera. You can also use the buttons on the bottom of the screen to pause, resume, speed up, or slow down the game, as well as access the menu, settings, and abilities.
-If you want to master Defense Zone 3 APK and win every level, you will need some tips and tricks to help you out. Here are some of them:
-You might be wondering why you should play Defense Zone 3 APK, when there are so many other games available on the market. Well, here are some reasons why you should give Defense Zone 3 APK a try:
-Like any other game, Defense Zone 3 APK has its pros and cons. Here are some of them:
-Pros | -Cons | -
---|---|
It is free to download and play. | -It can be addictive and time-consuming. | -
It has stunning graphics and sound effects. | -It can drain your battery and data quickly. | -
It has dynamic and amazing game sessions. | -It can be frustrating and difficult at times. | -
It has flexible difficulty settings and game modes. | -It can be repetitive and boring after a while. | -
It is educational and informative. | -It can be inaccurate and misleading in some aspects. | -
If you are still not convinced, you can check out the ratings and reviews of Defense Zone 3 APK from other players. Here are some of them:
-In conclusion, Defense Zone 3 APK is a popular action/strategy game that you can play on your Android device. It has many features, benefits, and gameplay that make it an exciting and enjoyable game to play. It also has some drawbacks and challenges that make it a demanding and rewarding game to play. If you are looking for a free, fun, and challenging game to play on your device, you should give Defense Zone 3 APK a try.
-Here are some frequently asked questions about Defense Zone 3 APK:
-Yes, Defense Zone 3 APK is safe to download and install, as long as you download it from a trusted source, such as Google Play Store or a reputable website. You should also scan the APK file with an antivirus software before installing it.
-No, Defense Zone 3 APK is not available for iOS devices. However, you can play Defense Zone 3 on your iOS device by downloading it from the App Store or using an emulator software.
-Defense Zone 3 APK is both online and offline. You can play the game without an internet connection, but you will need an internet connection to access some features, such as leaderboards, achievements, updates, etc.
-There are 21 levels in Defense Zone 3 APK, each with different landscapes, enemies, and difficulties. You can also play in endless mode or custom mode for more variety and challenge.
-You can contact the developers of Defense Zone 3 APK by sending an email to support@defensezone.net or visiting their website at [Defense Zone 3 HD]. You can also follow them on Facebook, Twitter, and YouTube for more updates and news.
401be4b1e0If you are looking for a fast, secure, and free VPN app for your Android device, you might want to check out Fast Orange APK. This app is a lightweight and powerful VPN tool that allows you to access any website or app without any limitations or censorship. In this article, we will explain what Fast Orange APK is, what are its benefits, and how to download, install, and use it on your device.
-Download File > https://jinyurl.com/2uNPeZ
Before we dive into the details of Fast Orange APK, let's first understand what an APK file is and why you might need it.
-An APK file is an Android Package Kit file that contains all the files and code needed to run an app on an Android device. It is similar to an EXE file on Windows or a DMG file on Mac. You can download APK files from various sources online, such as official websites, app stores, or third-party platforms. However, not all APK files are safe and reliable, so you should always be careful about where you get them from.
-Fast Orange APK is an APK file that contains the Fast Orange app, which is a VPN app developed by Orange CH. VPN stands for Virtual Private Network, which is a technology that creates a secure and encrypted connection between your device and a remote server. By using a VPN, you can hide your IP address, protect your online privacy, and bypass geo-restrictions or firewalls that block certain websites or apps.
-VPN Orange app free download
-Fast Orange unblock any website
-Fire Orange booster for gaming
-VPN Orange secure and stable
-Fast Orange one-click connection
-Fire Orange app multiplatform
-VPN Orange unlimited proxy
-Fast Orange high-speed ladder
-Fire Orange app private
-VPN Orange works with Wi-Fi
-Fast Orange no registration required
-Fire Orange app fast access
-VPN Orange well-designed UI
-Fast Orange no data limitation
-Fire Orange app ultra-efficient
-VPN Orange super-fast speed
-Fast Orange hide your IP address
-Fire Orange app iOS Android Mac PC
-VPN Orange 3G 4G compatible
-Fast Orange unlock sites and games
-Fire Orange app 100% protected
-VPN Orange free unlimited VPN
-Fast Orange APK download link
-Fire Orange booster APK download
-VPN Orange APK latest version
-Fast Orange APK for Android
-Fire Orange booster APK for iOS
-VPN Orange APK for PC
-Fast Orange APK for Mac
-Fire Orange booster APK for Android
-VPN Orange APK free proxy server
-Fast Orange APK high anonymity
-Fire Orange booster APK gaming optimization
-VPN Orange APK no ads
-Fast Orange APK easy to use
-Fire Orange booster APK no data hackers
-VPN Orange APK best reviews
-Fast Orange APK fast server speed
-Fire Orange booster APK play any games
-VPN Orange APK secure your data
-Fast Orange APK unlimited bandwidth
-Fire Orange booster APK coming soon
There are many reasons why you might want to use Fast Orange APK on your device. Here are some of the main benefits of this app:
-Fast Orange APK provides a fast and stable VPN connection that can handle high-speed data transfer and streaming. It also uses advanced encryption protocols and techniques to ensure that your data is safe from hackers, snoopers, or government agencies. You can trust that your online activities are private and secure with Fast Orange APK.
-Unlike some other VPN apps that charge you money or limit your bandwidth, Fast Orange APK offers free and unlimited proxy access to any website or app you want. You can access popular platforms like Netflix, YouTube, Facebook, Twitter, Instagram, WhatsApp, Skype, and more without any restrictions or censorship. You can also switch between different server locations around the world to enjoy different content or services.
-Fast Orange APK has a user-friendly and intuitive user interface that makes it easy for anyone to use. You don't need to register or log in to use the app. You just need to tap one button and you can connect to the VPN server of your choice. You can also customize the settings according to your preferences and needs.
-If you want to use Fast Orange APK on your device, you need to download and install it first. Here are the steps you need to follow:
-Since Fast Orange APK is not available on the Google Play Store, you need to enable unknown sources on your device to allow it to install apps from other sources
To enable unknown sources on your device, you need to access the settings app and look for the security or privacy option. Depending on your device, you may need to tap on the lock screen and security tab or the install unknown apps switch. Then, you need to turn on the unknown sources switch or check the box next to it. You may see a warning message against enabling this option, but you can ignore it if you trust the source of the APK file.
-Once you have enabled unknown sources on your device, you can download the APK file from a trusted source. You can use your web browser or a file manager app to do this. For example, you can visit the official website of Fast Orange VPN and download the APK file from there. Alternatively, you can use a third-party platform that offers verified and safe APK files, such as APKPure or APKMirror. However, be careful not to download any fake or malicious APK files that may harm your device or compromise your data.
-After you have downloaded the APK file, you can install it by tapping on it or opening it with a file manager app. You may need to grant some permissions to the app before it can be installed. Once the installation is complete, you can launch the app by tapping on its icon in the app drawer or on the home screen. You are now ready to use Fast Orange APK on your device.
-Using Fast Orange APK is very easy and simple. You just need to follow these steps:
-When you open the app, you will see a list of server locations that you can connect to. You can scroll through the list and select the one that suits your needs. For example, if you want to access a website or app that is only available in a certain country, you can choose a server location in that country. Alternatively, you can let the app choose the best server for you automatically by tapping on the smart connect button.
-After you have selected a server location, you can tap on the connect button at the bottom of the screen to start the VPN connection. You will see a green circle around the button when the connection is established. You will also see a key icon in the status bar of your device, indicating that you are using a VPN service.
-Now that you are connected to Fast Orange VPN, you can enjoy the Internet without any limitations or censorship. You can access any website or app that you want, regardless of your location or network. You can also browse the web anonymously and securely, without worrying about your online privacy or security.
-Fast Orange APK is a great VPN app that offers fast, secure, and free proxy access to any website or app. It has an easy and simple user interface that anyone can use. It also has many server locations around the world that you can choose from. If you want to download and use Fast Orange APK on your device, you just need to enable unknown sources, download the APK file from a trusted source, install it, and launch it. Then, you can enjoy the Internet without any restrictions or censorship.
-I hope this article was helpful and informative for you. If you have any questions or feedback, please feel free to leave them in the comments section below. Thank you for reading!
-Final Cut Pro is one of the most popular and powerful video editing software for Mac users. It offers a range of features and tools that can help you create stunning videos with ease. But what if you are a Windows user and want to use Final Cut Pro on your PC? Is it possible to download Final Cut Pro for Windows? And if so, how can you do it?
-Download >>> https://jinyurl.com/2uNPqk
In this article, we will answer these questions and more. We will explain what Final Cut Pro is, why it is not available for Windows, how to run it on Windows, and what are the best alternatives to Final Cut Pro for Windows. By the end of this article, you will have a clear idea of how to edit videos on your PC with or without Final Cut Pro.
-Final Cut Pro is a video editing software that was developed by Apple in 1999. It is designed for professional and advanced users who need a high level of control and customization over their video projects. It supports multiple video formats, resolutions, frame rates, and codecs, as well as multi-camera editing, 360-degree video editing, VR headset playback, and advanced color grading. It also has a range of video transitions and filters, such as keying tools, mattes, and vocal de-poppers. It uses a magnetic timeline that allows non-destructive editing of clips without collisions or syncing problems. It is optimized for Mac computers with Apple silicon, especially the new Mac Studio, and can tap into superfast unified memory and the Apple Neural Engine for faster playback and rendering.
-Some of the features and benefits of Final Cut Pro are:
-Some of the limitations and drawbacks of Final Cut Pro are:
-Final Cut Pro is not available for Windows because it is a proprietary software that belongs to Apple. Apple has a history of creating exclusive products and services that only work on its own devices and platforms. This is part of its business strategy to create a loyal customer base and a competitive edge over other brands. Apple also wants to maintain the quality and performance of its software by optimizing it for its own hardware and software specifications.
-Apple's exclusivity is based on its philosophy of creating a seamless and integrated user experience across its products and services. Apple believes that by controlling both the hardware and the software aspects of its devices, it can offer better functionality, reliability, security, and design. Apple also wants to protect its intellectual property and prevent piracy and plagiarism of its software. Apple's exclusivity also helps it generate more revenue by encouraging users to buy more of its products and services.
-Running Final Cut Pro on Windows is not easy or advisable. There are several challenges and risks involved in trying to do so. Some of them are:
-Despite the challenges and risks mentioned above, some people still want to run Final Cut Pro on Windows for various reasons. If you are one of them, you should know that there are two possible methods of installing Final Cut Pro on Windows: using a virtual machine or using a hackintosh.
-How to install Final Cut Pro X on Windows 10
-Final Cut Pro for Windows PC free download
-Best video editing software for Windows like Final Cut Pro
-Final Cut Pro alternatives for Windows 11
-Download Final Cut Pro X trial version for Windows
-Final Cut Pro vs Adobe Premiere Pro for Windows
-Final Cut Pro compatible video editor for Windows
-How to run Final Cut Pro on Windows with VirtualBox
-Final Cut Pro for Windows crack download
-Free online video editor similar to Final Cut Pro
-How to edit Final Cut Pro projects on Windows
-Final Cut Pro features and benefits for Windows users
-Download Final Cut Pro effects and transitions for Windows
-How to get Final Cut Pro for free on Windows
-Best Final Cut Pro tutorials and courses for Windows
-How to export Final Cut Pro videos to Windows
-Final Cut Pro system requirements and specifications for Windows
-Download Final Cut Pro plugins and extensions for Windows
-How to use Final Cut Pro keyboard shortcuts on Windows
-Final Cut Pro reviews and ratings for Windows
-How to import and export media files in Final Cut Pro for Windows
-Download Final Cut Pro templates and themes for Windows
-How to create and edit 360° videos in Final Cut Pro for Windows
-How to add subtitles and captions in Final Cut Pro for Windows
-How to fix common errors and issues in Final Cut Pro for Windows
-How to optimize performance and speed in Final Cut Pro for Windows
-How to customize the interface and layout of Final Cut Pro for Windows
-How to use the magnetic timeline and clip connections in Final Cut Pro for Windows
-How to apply filters and color grading in Final Cut Pro for Windows
-How to use the multicam editing feature in Final Cut Pro for Windows
-How to sync audio and video in Final Cut Pro for Windows
-How to use the audio roles and mixer in Final Cut Pro for Windows
-How to use the smart conform and crop tools in Final Cut Pro for Windows
-How to use the motion tracking and stabilization features in Final Cut Pro for Windows
-How to use the keyframe animation and motion graphics features in Final Cut Pro for Windows
-How to use the chroma key and green screen effects in Final Cut Pro for Windows
-How to use the advanced compositing and masking features in Final Cut Pro for Windows
-How to use the media browser and library management features in Final Cut Pro for Windows
-How to use the proxy workflows and cloud collaboration features in Final Cut Pro for Windows
-How to use the machine learning and artificial intelligence features in Final Cut Pro for Windows
A virtual machine is a software that simulates a different operating system within your current operating system. For example, you can use a virtual machine to run macOS on your Windows PC. A hackintosh is a computer that runs macOS on non-Apple hardware. For example, you can install macOS on your custom-built PC.
-To use a virtual machine to run Final Cut Pro on Windows, you will need to download and install a virtual machine software such as VMware Workstation Player or VirtualBox. Then you will need to download and install macOS on the virtual machine. Finally, you will need to download and install Final Cut Pro on the macOS virtual machine.
-To use a hackintosh to run Final Cut Pro on Windows, you will need to have a compatible PC that meets the minimum requirements for running macOS. Then you will need to download and create a bootable USB drive with macOS installer. Finally, you will need to boot from the USB drive and install macOS on your PC.
-Using either method to run Final Cut Pro on Windows has some risks and disadvantages that you should be aware of before trying them. Some of them are:
-If you are looking for a video editing software that can match or surpass Final Cut Pro in terms of features, performance, and quality, but also works on Windows, you have plenty of options to choose from. There are many video editing software for Windows that cater to different levels of skills, budgets, and needs. Here are some of the criteria for choosing a good video editing software:
-Based on the criteria above, we have selected the top three alternatives to Final Cut Pro for Windows that we think are worth considering. They are:
-Adobe Premiere Pro is our top pick for the best video editing software for Windows. It is the industry-standard tool that is used by many professional videographers and editors around the world. It has all the features and tools you need to create stunning videos with ease. It is compatible with other Adobe products such as Photoshop and After Effects, which makes it ideal for cross-platform collaboration and integration. It also has a cloud-based service called Premiere Rush that lets you edit videos on your mobile devices and sync them with your desktop. It costs $20.99 per month or $239.88 per year as a standalone app, or $52.99 per month or $599.88 per year as part of the Adobe Creative Cloud suite.
-CyberLink PowerDirector is another excellent video editing software for Windows that offers tons of tools and features for both beginners and experts. It has a user-friendly interface that is intuitive and customizable. It has a powerful media browser that lets you organize, preview, and import your media files easily. It has a smart conform feature that automatically crops your videos to fit different aspect ratios and social media platforms. It has an object tracker that uses machine learning to detect faces and objects and match their movement with titles and effects. It has a cinematic mode that lets you adjust focus points and depth of field on clips captured in cinematic mode on iPhone 13 or later. It has a duplicate detection feature that shows you any audio or video that appears more than once in your project. It has a proxy workflow that lets you generate proxy media in custom frame sizes from 12.5% to 100% of the original in ProRes Proxy or H.264. It has a range check feature that shows you which areas of an image are out of color gamut. It has a camera LUT feature that automatically applies look up tables (LUTs) to footage from select ARRI, Sony, Panasonic, and Canon cameras. It has an external monitoring feature that lets you monitor full-quality video up to 6K with Pro Display XDR or via HDMI on select Mac computers. It costs $69.99 for the lifetime license or $51.99 per year for the subscription.
-Davinci Resolve is a free video editing software for Windows that offers pro-level tools and features for advanced users. It has a modular interface that consists of different pages for different tasks, such as media management, editing, color grading, audio mixing, and delivery. It supports multiple video formats, resolutions, frame rates, and codecs, as well as 4K video, HDR video, and VR video. It offers a variety of video transitions and filters, as well as advanced tools such as multi-camera editing, motion tracking, keying, stabilization, noise reduction, and 3D editing. It also has a powerful color grading system that lets you adjust color balance, contrast, saturation, hue, luminance, and more with precision and control. It also has a professional audio mixing system that lets you edit soundtracks, add effects, mix channels, automate levels, and more with high-quality sound processing. The free version of Davinci Resolve has most of the features you need to create amazing videos, but if you want more advanced features such as collaboration tools, neural engine effects, facial recognition tracking, lens distortion correction, HDR grading tools and more, you can upgrade to the Studio version for $299.
-Final Cut Pro is a great video editing software for Mac users, but it is not available for Windows users. If you want to use Final Cut Pro on Windows, you can try using a virtual machine or a hackintosh, but you will face many challenges and risks. A better option is to use one of the best alternatives to Final Cut Pro for Windows, such as Adobe Premiere Pro, CyberLink PowerDirector, or Davinci Resolve. These video editing software offer similar or better features, performance, and quality than Final Cut Pro, and they work seamlessly on Windows. They also have different price points and plans that suit different budgets and needs. You can download and try them for free and see which one works best for you.
-If you are ready to start editing videos on your Windows PC, we recommend you to check out the following links:
-We hope this article has helped you learn how to download Final Cut Pro for Windows and what are the best alternatives to Final Cut Pro for Windows. If you have any questions or feedback, please leave a comment below. And if you liked this article, please share it with your friends and colleagues who might find it useful. Thank you for reading!
-Here are some of the frequently asked questions about Final Cut Pro and Windows:
-No, Final Cut Pro is not free. It costs $299.99 to buy from the App Store. However, you can get a free 90-day trial of Final Cut Pro from Apple's website. You can use this trial to test the software and see if it meets your needs.
-Final Cut Pro and Adobe Premiere Pro are both excellent video editing software that have their own strengths and weaknesses. Some of the factors that may influence your choice are:
-The best way to decide which one is better for you is to try them both and compare their features, performance, and quality.
-The time it takes to learn Final Cut Pro depends on your previous experience with video editing software, your learning style, and your goals. Generally speaking, Final Cut Pro has a user-friendly interface that is intuitive and customizable, which makes it easy to learn for beginners. However, it also has many advanced features and tools that require more practice and skill to master. A good way to learn Final Cut Pro is to follow online tutorials, courses, or books that teach you the basics and the best practices of video editing with Final Cut Pro. You can also join online communities or forums where you can ask questions, get feedback, and share tips with other users.
-The minimum system requirements for running Final Cut Pro are:
-No, Final Cut Pro is not available for iPad or iPhone. However, you can use iMovie, which is a free video editing app that is similar to Final Cut Pro but simpler and more streamlined. iMovie lets you edit videos on your iPad or iPhone with ease and fun. You can add titles, transitions, filters, music, sound effects, and more to your videos. You can also use the green-screen effect, the picture-in-picture effect, the split-screen effect, and the cinematic mode to create amazing videos. You can also export your videos to different formats and resolutions, and share them with your friends and family via social media, email, or AirDrop. You can download iMovie from the App Store.
401be4b1e0Si usted es un fan de los juegos de fútbol, es posible que se pregunte cómo descargar FIFA 2022 APK en su dispositivo Android. FIFA 2022 es la última entrega de la popular serie FIFA, desarrollada por EA Sports. Es uno de los juegos más esperados del año, con nuevas características de juego, modos, gráficos y más. En este artículo, le mostraremos cómo descargar FIFA 2022 APK de forma segura y fácil, así como algunas de las mejores características y consejos para jugar el juego.
-Download File ---> https://bltlly.com/2v6KiU
FIFA 2022 no es solo un re-skin de su predecesor. Es un juego completamente nuevo que trae cada partido en cada modo aún más cerca de lo real. Estas son algunas de las características que hacen que FIFA 2022 se destaque de otros juegos de fútbol:
-Antes de descargar FIFA 2022 APK, es necesario asegurarse de que su dispositivo Android cumple con los requisitos mínimos o recomendados del sistema para el juego. Aquí están los requisitos del sistema para FIFA 2022 APK:
-Ahora que sabes lo que es FIFA 2022 APK y qué características y requisitos del sistema que tiene, es posible que esté ansioso por descargarlo en su dispositivo Android. Sin embargo, hay algunas cosas que debe tener cuidado al descargar un archivo APK de Internet. Aquí hay algunos consejos para ayudarle a descargar FIFA 2022 APK de forma segura y fácil:
-Aquí están algunas de las preguntas más frecuentes sobre FIFA 2022 APK:
- -FIFA 2022 APK es un juego imprescindible para cualquier fanático del fútbol que quiera disfrutar de una simulación de fútbol realista e inmersiva en su dispositivo Android. Ofrece nuevas y mejoradas características de juego, modos, gráficos y más que te mantendrán enganchado durante horas. Para descargar FIFA 2022 APK de forma segura y fácil, es necesario seguir algunos consejos simples y trucos que hemos compartido en este artículo. Esperamos que este artículo le ha ayudado a aprender cómo descargar FIFA 2022 APK y disfrutar jugando en su dispositivo. Si tiene alguna pregunta o comentario, no dude en dejarlos abajo.
64aa2da5cf¿Te encanta el fútbol pero quieres experimentarlo de una manera diferente? ¿Quieres mostrar tus habilidades y trucos en varios lugares de la calle en todo el mundo? ¿Quieres jugar con tus jugadores y equipos favoritos en un juego divertido y estilo árcade? Si respondiste sí a cualquiera de estas preguntas, entonces deberías probar FIFA Street 4, un juego que te permitirá disfrutar del fútbol callejero en tu ordenador.
-DOWNLOAD ··· https://bltlly.com/2v6JFm
FIFA Street 4 es un juego desarrollado por EA Sports y lanzado en 2012 para PlayStation 3 y Xbox 360. Es la cuarta entrega de la serie FIFA Street, que se centra en el fútbol callejero en lugar del fútbol tradicional. El juego cuenta con más de 50 ubicaciones diferentes, desde Río de Janeiro hasta Londres, donde puedes jugar con varios modos y reglas. También puedes personalizar tu propio equipo y jugador, y desbloquear nuevos objetos y habilidades a medida que avanzas.
-El juego tiene varias características que lo hacen único y emocionante, como:
-Al descargar FIFA Street 4 PC Bagas31, puedes disfrutar de varios beneficios, como:
-Descargar FIFA Street 4 PC Bagas31 es fácil y sencillo. Solo tienes que seguir estos pasos:
- -Jugar FIFA Street 4 PC Bagas31 es similar a jugar cualquier otro juego de fútbol en PC. Puede utilizar el teclado y el ratón o un controlador para controlar a sus jugadores y realizar varias acciones. Aquí hay un resumen rápido de la jugabilidad y los controles:
- -Los controles de FIFA Street 4 PC Bagas31 se basan en el sistema de control de la bola de la calle, que le permite realizar más realista y fluido dribbling, pasando, y tiro. También puedes usar trucos y habilidades para superar a tus oponentes y anotar goles espectaculares. Aquí hay una tabla de los controles básicos para teclado y ratón y controlador:
-Antes de descargar y jugar FIFA Street 4 PC Bagas31, debe asegurarse de que su computadora cumple con los requisitos mínimos y recomendados del sistema para ejecutar el juego. Aquí hay una lista de las especificaciones que necesita:
-Si encuentras algún problema o problema al jugar FIFA Street 4 PC Bagas31, como retraso, tartamudeo, estrellarse o errores, puedes probar algunos de estos consejos y trucos para mejorar el rendimiento del juego y solucionar los problemas comunes:
-Si estás buscando otros juegos de fútbol callejero o emuladores que puedes jugar en tu PC, puedes ver algunas de estas alternativas a FIFA Street 4 PC Bagas31:
- -Así que, ¿qué estás esperando? Descargar FIFA Street 4 PC Bagas31 hoy y divertirse jugando fútbol de la calle en su ordenador!
-Aquí hay algunas preguntas frecuentes sobre FIFA Street 4 PC Bagas31:
-Sí, FIFA Street 4 PC Bagas31 es seguro para descargar desde el sitio web de Bagas31. Sin embargo, siempre debe escanear los archivos descargados con un programa antivirus antes de abrirlos, y tenga cuidado con cualquier pop-ups o anuncios que puedan aparecer en el sitio web.
-No, FIFA Street 4 PC Bagas31 no es legal para descargar, ya que es una versión modificada de un juego con derechos de autor que nunca fue lanzado oficialmente para PC. Descargar y jugar FIFA Street 4 PC Bagas31 es bajo su propio riesgo, y usted puede enfrentar consecuencias legales si es capturado por las autoridades.
-Sí, puede jugar FIFA Street 4 PC Bagas31 sin conexión a Internet. Sin embargo, no podrá acceder al modo Online Seasons o al modo Street Network, que requieren una conexión en línea.
-Sí, puedes jugar FIFA Street 4 PC Bagas31 con tus amigos en línea o localmente. Para jugar en línea, necesitará una conexión a Internet y una cuenta en los servidores de EA. Para jugar localmente, necesitarás dos controladores o teclados y ratones, y una opción de pantalla dividida en la configuración del juego.
-No, no puedes actualizar FIFA Street 4 PC Bagas31, ya que es una versión modificada del juego que no recibe ninguna actualización o parches oficiales de EA Sports. Sin embargo, puedes encontrar algunas actualizaciones o mods no oficiales de otras fuentes en línea que pueden mejorar o cambiar el juego de alguna manera.
-- Noise Level: Controls how much randomness is added to the input before it is sent to the model. Higher noise level produces more diverse outputs, while lower noise level produces similar outputs, - created by Phenomenon1981. -
-- ❤️ Press the Like Button if you enjoy my space! ❤️ -
-Unleash your creative side and generate mesmerizing images with just a few clicks! Enter a spark of inspiration in the "Basic Idea" text box and click the "Magic Prompt" button to elevate it to a polished masterpiece. Make any final tweaks in the "Full Prompt" box and hit the "Generate Images" button to watch your vision come to life. Experiment with the "Noise Level" for a diverse range of outputs, from similar to wildly unique. Let the fun begin! -
-{display_text}", unsafe_allow_html=True) - elif image: - st.markdown(f'
Input Video
' -warning_str = 'Please Upload a Video first!!!
' - -warn = st.empty() - - -download_button = st.empty() - -if up_file and uploaded: - - download_button.empty() - tfile = tempfile.NamedTemporaryFile(delete=False) - - try: - warn.empty() - tfile.write(up_file.read()) - - vf = cv2.VideoCapture(tfile.name) - - # --------------------- Write the processed video frame. -------------------- - fps = int(vf.get(cv2.CAP_PROP_FPS)) - width = int(vf.get(cv2.CAP_PROP_FRAME_WIDTH)) - height = int(vf.get(cv2.CAP_PROP_FRAME_HEIGHT)) - frame_size = (width, height) - fourcc = cv2.VideoWriter_fourcc(*'mp4v') - video_output = cv2.VideoWriter(output_video_file, fourcc, fps, frame_size) - # ----------------------------------------------------------------------------- - - - txt = st.sidebar.markdown(ip_vid_str, unsafe_allow_html=True) - ip_video = st.sidebar.video(tfile.name) - - while vf.isOpened(): - ret, frame = vf.read() - if not ret: - break - - # convert frame from BGR to RGB before processing it. - frame = cv2.cvtColor(frame, cv2.COLOR_BGR2RGB) - out_frame, _ = upload_process_frame.process(frame, pose) - stframe.image(out_frame) - video_output.write(out_frame[...,::-1]) - - - vf.release() - video_output.release() - stframe.empty() - ip_video.empty() - txt.empty() - tfile.close() - - except AttributeError: - warn.markdown(warning_str, unsafe_allow_html=True) - - - -if os.path.exists(output_video_file): - with open(output_video_file, 'rb') as op_vid: - download = download_button.download_button('Download Video', data = op_vid, file_name='output_recorded.mp4') - - if download: - st.session_state['download'] = True - - - -if os.path.exists(output_video_file) and st.session_state['download']: - os.remove(output_video_file) - st.session_state['download'] = False - download_button.empty() - - - - - - - - diff --git a/spaces/KyanChen/FunSR/models/baselines/aliif.py b/spaces/KyanChen/FunSR/models/baselines/aliif.py deleted file mode 100644 index 5af06846a31671367a3ad5a281c573c5f74b79a9..0000000000000000000000000000000000000000 --- a/spaces/KyanChen/FunSR/models/baselines/aliif.py +++ /dev/null @@ -1,131 +0,0 @@ -import torch -import torch.nn as nn -import torch.nn.functional as F - -import models -from models import register -from utils import make_coord - - -@register('aliif') -class ALIIF(nn.Module): - - def __init__(self, encoder_spec, pdn_spec=None, basis_spec=None, imnet_spec=None, - local_ensemble=True, feat_unfold=True, cell_decode=True): - super().__init__() - self.local_ensemble = local_ensemble - self.feat_unfold = feat_unfold - self.cell_decode = cell_decode - - self.encoder = models.make(encoder_spec) - - if pdn_spec is not None: - self.pdn=models.make(pdn_spec) - self.use_pdn=True - else: - self.use_pdn = False - if basis_spec is not None: - self.basis=models.make(basis_spec) - self.use_basis=True - self.B,self.b=self.basis() - else: - self.use_basis = False - - if imnet_spec is not None: - imnet_in_dim = self.encoder.out_dim - if self.feat_unfold: - imnet_in_dim *= 9 - imnet_in_dim += 2 # attach coord - if self.cell_decode: - imnet_in_dim += 2 - self.imnet = models.make(imnet_spec, args={'in_dim': imnet_in_dim}) - else: - self.imnet = None - - def gen_feat(self, inp): - self.feat = self.encoder(inp) - return self.feat - - def query_rgb(self, coord, cell=None): - feat = self.feat - - if self.imnet is None: - ret = F.grid_sample(feat, coord.flip(-1).unsqueeze(1), - mode='nearest', align_corners=False)[:, :, 0, :] \ - .permute(0, 2, 1) - return ret - - if self.feat_unfold: - feat = F.unfold(feat, 3, padding=1).view( - feat.shape[0], feat.shape[1] * 9, feat.shape[2], feat.shape[3]) - - if self.local_ensemble: - vx_lst = [-1, 1] - vy_lst = [-1, 1] - eps_shift = 1e-6 - else: - vx_lst, vy_lst, eps_shift = [0], [0], 0 - - # field radius (global: [-1, 1]) - rx = 2 / feat.shape[-2] / 2 - ry = 2 / feat.shape[-1] / 2 - - feat_coord = make_coord(feat.shape[-2:], flatten=False).cuda() \ - .permute(2, 0, 1) \ - .unsqueeze(0).expand(feat.shape[0], 2, *feat.shape[-2:]) - - preds = [] - areas = [] - for vx in vx_lst: - for vy in vy_lst: - coord_ = coord.clone() - coord_[:, :, 0] += vx * rx + eps_shift - coord_[:, :, 1] += vy * ry + eps_shift - coord_.clamp_(-1 + 1e-6, 1 - 1e-6) - q_feat = F.grid_sample( - feat, coord_.flip(-1).unsqueeze(1), - mode='nearest', align_corners=False)[:, :, 0, :] \ - .permute(0, 2, 1) - q_coord = F.grid_sample( - feat_coord, coord_.flip(-1).unsqueeze(1), - mode='nearest', align_corners=False)[:, :, 0, :] \ - .permute(0, 2, 1) - rel_coord = coord - q_coord - rel_coord[:, :, 0] *= feat.shape[-2] - rel_coord[:, :, 1] *= feat.shape[-1] - inp = torch.cat([q_feat, rel_coord], dim=-1) - - if self.cell_decode: - rel_cell = cell.clone() - rel_cell[:, :, 0] *= feat.shape[-2] - rel_cell[:, :, 1] *= feat.shape[-1] - inp = torch.cat([inp, rel_cell], dim=-1) - - bs, q = coord.shape[:2] - - if self.use_pdn: - Coeff=self.pdn(inp) # out:(b,h*w,K) - else: - Coeff=torch.ones([inp.shape[0],inp.shape[1],1]) - if self.use_basis: - - pred = self.imnet(inp.view(bs * q, -1),Coeff.view(-1,Coeff.shape[2]),self.B,self.b).view(bs, q, -1) - else: - pred = self.imnet(inp.view(bs * q, -1)).view(bs, q, -1) - preds.append(pred) - - area = torch.abs(rel_coord[:, :, 0] * rel_coord[:, :, 1]) - areas.append(area + 1e-9) - - tot_area = torch.stack(areas).sum(dim=0) - if self.local_ensemble: - t = areas[0]; areas[0] = areas[3]; areas[3] = t - t = areas[1]; areas[1] = areas[2]; areas[2] = t - ret = 0 - for pred, area in zip(preds, areas): - ret = ret + pred * (area / tot_area).unsqueeze(-1) - return ret - - def forward(self, inp, coord, cell): - self.gen_feat(inp) - return self.query_rgb(coord, cell) diff --git a/spaces/KyanChen/RSPrompter/mmdet/models/detectors/rtmdet.py b/spaces/KyanChen/RSPrompter/mmdet/models/detectors/rtmdet.py deleted file mode 100644 index cb10f76dd57d79761e9b58c310293eedba1e00d5..0000000000000000000000000000000000000000 --- a/spaces/KyanChen/RSPrompter/mmdet/models/detectors/rtmdet.py +++ /dev/null @@ -1,52 +0,0 @@ -# Copyright (c) OpenMMLab. All rights reserved. -import torch -from mmengine.dist import get_world_size -from mmengine.logging import print_log - -from mmdet.registry import MODELS -from mmdet.utils import ConfigType, OptConfigType, OptMultiConfig -from .single_stage import SingleStageDetector - - -@MODELS.register_module() -class RTMDet(SingleStageDetector): - """Implementation of RTMDet. - - Args: - backbone (:obj:`ConfigDict` or dict): The backbone module. - neck (:obj:`ConfigDict` or dict): The neck module. - bbox_head (:obj:`ConfigDict` or dict): The bbox head module. - train_cfg (:obj:`ConfigDict` or dict, optional): The training config - of ATSS. Defaults to None. - test_cfg (:obj:`ConfigDict` or dict, optional): The testing config - of ATSS. Defaults to None. - data_preprocessor (:obj:`ConfigDict` or dict, optional): Config of - :class:`DetDataPreprocessor` to process the input data. - Defaults to None. - init_cfg (:obj:`ConfigDict` or dict, optional): the config to control - the initialization. Defaults to None. - use_syncbn (bool): Whether to use SyncBatchNorm. Defaults to True. - """ - - def __init__(self, - backbone: ConfigType, - neck: ConfigType, - bbox_head: ConfigType, - train_cfg: OptConfigType = None, - test_cfg: OptConfigType = None, - data_preprocessor: OptConfigType = None, - init_cfg: OptMultiConfig = None, - use_syncbn: bool = True) -> None: - super().__init__( - backbone=backbone, - neck=neck, - bbox_head=bbox_head, - train_cfg=train_cfg, - test_cfg=test_cfg, - data_preprocessor=data_preprocessor, - init_cfg=init_cfg) - - # TODO: Waiting for mmengine support - if use_syncbn and get_world_size() > 1: - torch.nn.SyncBatchNorm.convert_sync_batchnorm(self) - print_log('Using SyncBatchNorm()', 'current') diff --git a/spaces/Lamai/LAMAIGPT/tests/integration/milvus_memory_tests.py b/spaces/Lamai/LAMAIGPT/tests/integration/milvus_memory_tests.py deleted file mode 100644 index ec38bf2f72087b5da679d26594ebff97d8a09b19..0000000000000000000000000000000000000000 --- a/spaces/Lamai/LAMAIGPT/tests/integration/milvus_memory_tests.py +++ /dev/null @@ -1,57 +0,0 @@ -# sourcery skip: snake-case-functions -"""Tests for the MilvusMemory class.""" -import random -import string -import unittest - -from autogpt.config import Config -from autogpt.memory.milvus import MilvusMemory - -try: - - class TestMilvusMemory(unittest.TestCase): - """Tests for the MilvusMemory class.""" - - def random_string(self, length: int) -> str: - """Generate a random string of the given length.""" - return "".join(random.choice(string.ascii_letters) for _ in range(length)) - - def setUp(self) -> None: - """Set up the test environment.""" - cfg = Config() - cfg.milvus_addr = "localhost:19530" - self.memory = MilvusMemory(cfg) - self.memory.clear() - - # Add example texts to the cache - self.example_texts = [ - "The quick brown fox jumps over the lazy dog", - "I love machine learning and natural language processing", - "The cake is a lie, but the pie is always true", - "ChatGPT is an advanced AI model for conversation", - ] - - for text in self.example_texts: - self.memory.add(text) - - # Add some random strings to test noise - for _ in range(5): - self.memory.add(self.random_string(10)) - - def test_get_relevant(self) -> None: - """Test getting relevant texts from the cache.""" - query = "I'm interested in artificial intelligence and NLP" - num_relevant = 3 - relevant_texts = self.memory.get_relevant(query, num_relevant) - - print(f"Top {k} relevant texts for the query '{query}':") - for i, text in enumerate(relevant_texts, start=1): - print(f"{i}. {text}") - - self.assertEqual(len(relevant_texts), k) - self.assertIn(self.example_texts[1], relevant_texts) - -except: - print( - "Skipping tests/integration/milvus_memory_tests.py as Milvus is not installed." - ) diff --git a/spaces/ML701G7/taim-gan/src/models/modules/discriminator.py b/spaces/ML701G7/taim-gan/src/models/modules/discriminator.py deleted file mode 100644 index ebe184675eba85ff4e0a2144a5a333af8a5250db..0000000000000000000000000000000000000000 --- a/spaces/ML701G7/taim-gan/src/models/modules/discriminator.py +++ /dev/null @@ -1,144 +0,0 @@ -"""Discriminator providing word-level feedback""" -from typing import Any - -import torch -from torch import nn - -from src.models.modules.conv_utils import conv1d, conv2d -from src.models.modules.image_encoder import InceptionEncoder - - -class WordLevelLogits(nn.Module): - """API for converting regional feature maps into logits for multi-class classification""" - - def __init__(self) -> None: - """ - Instantiate the module with softmax on channel dimension - """ - super().__init__() - self.softmax = nn.Softmax(dim=1) - # layer for flattening the feature maps - self.flat = nn.Flatten(start_dim=2) - # change dism of of textual embs to correlate with chans of inception - self.chan_reduction = conv1d(256, 128) - - def forward( - self, visual_features: torch.Tensor, word_embs: torch.Tensor, mask: torch.Tensor - ) -> Any: - """ - Fuse two types of features together to get output for feeding into the classification loss - :param torch.Tensor visual_features: - Feature maps of an image after being processed by Inception encoder. Bx128x17x17 - :param torch.Tensor word_embs: - Word-level embeddings from the text encoder Bx256xL - :return: Logits for each word in the picture. BxL - :rtype: Any - """ - # make textual and visual features have the same amount of channels - word_embs = self.chan_reduction(word_embs) - # flattening the feature maps - visual_features = self.flat(visual_features) - word_embs = torch.transpose(word_embs, 1, 2) - word_region_correlations = word_embs @ visual_features - # normalize across L dimension - m_norm_l = nn.functional.normalize(word_region_correlations, dim=1) - # normalize across H*W dimension - m_norm_hw = nn.functional.normalize(m_norm_l, dim=2) - m_norm_hw = torch.transpose(m_norm_hw, 1, 2) - weighted_img_feats = visual_features @ m_norm_hw - weighted_img_feats = torch.sum(weighted_img_feats, dim=1) - weighted_img_feats[mask] = -float("inf") - deltas = self.softmax(weighted_img_feats) - return deltas - - -class UnconditionalLogits(nn.Module): - """Head for retrieving logits from an image""" - - def __init__(self) -> None: - """Initialize modules that reduce the features down to a set of logits""" - super().__init__() - self.conv = nn.Conv2d(128, 1, kernel_size=17) - # flattening BxLx1x1 into Bx1 - self.flat = nn.Flatten() - - def forward(self, visual_features: torch.Tensor) -> Any: - """ - Compute logits for unconditioned adversarial loss - - :param visual_features: Local features from Inception network. Bx128x17x17 - :return: Logits for unconditioned adversarial loss. Bx1 - :rtype: Any - """ - # reduce channels and feature maps for visual features - visual_features = self.conv(visual_features) - # flatten Bx1x1x1 into Bx1 - logits = self.flat(visual_features) - return logits - - -class ConditionalLogits(nn.Module): - """Logits extractor for conditioned adversarial loss""" - - def __init__(self) -> None: - super().__init__() - # layer for forming the feature maps out of textual info - self.text_to_fm = conv1d(256, 17 * 17) - # fitting the size of text channels to the size of visual channels - self.chan_aligner = conv2d(1, 128) - # for reduced textual + visual features down to 1x1 feature map - self.joint_conv = nn.Conv2d(2 * 128, 1, kernel_size=17) - # converting Bx1x1x1 into Bx1 - self.flat = nn.Flatten() - - def forward(self, visual_features: torch.Tensor, sent_embs: torch.Tensor) -> Any: - """ - Compute logits for conditional adversarial loss - - :param torch.Tensor visual_features: Features from Inception encoder. Bx128x17x17 - :param torch.Tensor sent_embs: Sentence embeddings from text encoder. Bx256 - :return: Logits for conditional adversarial loss. BxL - :rtype: Any - """ - # make text and visual features have the same sizes of feature maps - # Bx256 -> Bx256x1 -> Bx289x1 - sent_embs = sent_embs.view(-1, 256, 1) - sent_embs = self.text_to_fm(sent_embs) - # transform textual info into shape of visual feature maps - # Bx289x1 -> Bx1x17x17 - sent_embs = sent_embs.view(-1, 1, 17, 17) - # propagate text embs through 1d conv to - # align dims with visual feature maps - sent_embs = self.chan_aligner(sent_embs) - # unite textual and visual features across the dim of channels - cross_features = torch.cat((visual_features, sent_embs), dim=1) - # reduce dims down to length of caption and form raw logits - cross_features = self.joint_conv(cross_features) - # form logits from Bx1x1x1 into Bx1 - logits = self.flat(cross_features) - return logits - - -class Discriminator(nn.Module): - """Simple CNN-based discriminator""" - - def __init__(self) -> None: - """Use a pretrained InceptionNet to extract features""" - super().__init__() - self.encoder = InceptionEncoder(D=128) - # define different logit extractors for different losses - self.logits_word_level = WordLevelLogits() - self.logits_uncond = UnconditionalLogits() - self.logits_cond = ConditionalLogits() - - def forward(self, images: torch.Tensor) -> Any: - """ - Retrieves image features encoded by the image encoder - - :param torch.Tensor images: Images to be analyzed. Bx3x256x256 - :return: image features encoded by image encoder. Bx128x17x17 - """ - # only taking the local features from inception - # Bx3x256x256 -> Bx128x17x17 - img_features, _ = self.encoder(images) - return img_features diff --git a/spaces/Marshalls/testmtd/analysis/sandbox_dalle.py b/spaces/Marshalls/testmtd/analysis/sandbox_dalle.py deleted file mode 100644 index 891bc6608da2b85226bf95bef4322697f20720af..0000000000000000000000000000000000000000 --- a/spaces/Marshalls/testmtd/analysis/sandbox_dalle.py +++ /dev/null @@ -1,34 +0,0 @@ -import torch -from models.cdvae import ConditionalDiscreteVAE - -vae = ConditionalDiscreteVAE( - input_shape = (7,7), - num_layers = 3, # number of downsamples - ex. 256 / (2 ** 3) = (32 x 32 feature map) - num_tokens = 8192, # number of visual tokens. in the paper, they used 8192, but could be smaller for downsized projects - codebook_dim = 512, # codebook dimension - cond_dim = 100, - hidden_dim = 64, # hidden dimension - num_resnet_blocks = 1, # number of resnet blocks - temperature = 0.9, # gumbel softmax temperature, the lower this is, the harder the discretization - straight_through = False, # straight-through for gumbel softmax. unclear if it is better one way or the other -) - -images = torch.randn(4, 3, *vae.input_shape) -cond = torch.randn(4, 100, *vae.codebook_layer_shape) - -logits = vae(images, cond=cond, return_logits = True) - -logits.shape - -import numpy as np - -torch.randint(0,10,(1,)) -image_seq = torch.randint(0,8192, (4,np.prod(vae.codebook_layer_shape))) -image = vae.decode(image_seq, cond=cond) - -image.shape - -# loss = vae(images, return_loss = True) -# loss.backward() -# loss -# train with a lot of data to learn a good codebook diff --git a/spaces/Marshalls/testmtd/analysis/visualization/generate_video_from_expmaps.py b/spaces/Marshalls/testmtd/analysis/visualization/generate_video_from_expmaps.py deleted file mode 100644 index 5afeacddcd7a563524dd14983b3f252b6261598e..0000000000000000000000000000000000000000 --- a/spaces/Marshalls/testmtd/analysis/visualization/generate_video_from_expmaps.py +++ /dev/null @@ -1,43 +0,0 @@ -import pickle -import matplotlib.pyplot as plt -import numpy as np -from analysis.pymo.parsers import BVHParser -from analysis.pymo.data import Joint, MocapData -from analysis.pymo.preprocessing import * -from analysis.pymo.viz_tools import * -from analysis.pymo.writers import * -from sklearn.pipeline import Pipeline -import joblib as jl -from .utils import generate_video_from_images, join_video_and_audio - -import matplotlib -matplotlib.use("Agg") - -def generate_video_from_expmaps(features_file, pipeline_file, output_folder, audio_file, trim_audio=0, generate_bvh=False): - data = np.load(features_file) - # pipeline = jl.load("data/scaled_features/motion_data_pipe.sav") - # containing_path = os.path.dirname(features_file) - # pipeline_file = containing_path + "/" + "motion_expmap_data_pipe.sav" - pipeline = jl.load(pipeline_file) - - filename = os.path.basename(features_file) - seq_id = filename.split(".")[0] - - bvh_data=pipeline.inverse_transform([data[:,0,:]]) - if generate_bvh: - writer = BVHWriter() - with open(output_folder+"/"+seq_id+".bvh",'w') as f: - writer.write(bvh_data[0], f) - - bvh2pos = MocapParameterizer('position') - pos_data = bvh2pos.fit_transform(bvh_data) - video_file = f'{output_folder}/{seq_id}.mp4' - #render_mp4(pos_data[0], video_file, axis_scale=100, elev=45, azim=45) - - render_mp4(pos_data[0], video_file, axis_scale=300, elev=45, azim=45) - if audio_file is not None: - join_video_and_audio(video_file, audio_file, trim_audio) - # draw_stickfigure3d(pos_data[0], 10) - # sketch_move(pos_data[0], data=None, ax=None, figsize=(16,8)): - - diff --git a/spaces/MattyWhite/ChatGPT-ImageCaptioner2/detic/modeling/debug.py b/spaces/MattyWhite/ChatGPT-ImageCaptioner2/detic/modeling/debug.py deleted file mode 100644 index 9c7c442eb8aa9474c8874ac1dc75659371e8c894..0000000000000000000000000000000000000000 --- a/spaces/MattyWhite/ChatGPT-ImageCaptioner2/detic/modeling/debug.py +++ /dev/null @@ -1,334 +0,0 @@ -# Copyright (c) Facebook, Inc. and its affiliates. -import cv2 -import numpy as np -import torch -import torch.nn.functional as F -import os - -COLORS = ((np.random.rand(1300, 3) * 0.4 + 0.6) * 255).astype( - np.uint8).reshape(1300, 1, 1, 3) - -def _get_color_image(heatmap): - heatmap = heatmap.reshape( - heatmap.shape[0], heatmap.shape[1], heatmap.shape[2], 1) - if heatmap.shape[0] == 1: - color_map = (heatmap * np.ones((1, 1, 1, 3), np.uint8) * 255).max( - axis=0).astype(np.uint8) # H, W, 3 - else: - color_map = (heatmap * COLORS[:heatmap.shape[0]]).max(axis=0).astype(np.uint8) # H, W, 3 - - return color_map - -def _blend_image(image, color_map, a=0.7): - color_map = cv2.resize(color_map, (image.shape[1], image.shape[0])) - ret = np.clip(image * (1 - a) + color_map * a, 0, 255).astype(np.uint8) - return ret - -def _blend_image_heatmaps(image, color_maps, a=0.7): - merges = np.zeros((image.shape[0], image.shape[1], 3), np.float32) - for color_map in color_maps: - color_map = cv2.resize(color_map, (image.shape[1], image.shape[0])) - merges = np.maximum(merges, color_map) - ret = np.clip(image * (1 - a) + merges * a, 0, 255).astype(np.uint8) - return ret - -def _decompose_level(x, shapes_per_level, N): - ''' - x: LNHiWi x C - ''' - x = x.view(x.shape[0], -1) - ret = [] - st = 0 - for l in range(len(shapes_per_level)): - ret.append([]) - h = shapes_per_level[l][0].int().item() - w = shapes_per_level[l][1].int().item() - for i in range(N): - ret[l].append(x[st + h * w * i:st + h * w * (i + 1)].view( - h, w, -1).permute(2, 0, 1)) - st += h * w * N - return ret - -def _imagelist_to_tensor(images): - images = [x for x in images] - image_sizes = [x.shape[-2:] for x in images] - h = max([size[0] for size in image_sizes]) - w = max([size[1] for size in image_sizes]) - S = 32 - h, w = ((h - 1) // S + 1) * S, ((w - 1) // S + 1) * S - images = [F.pad(x, (0, w - x.shape[2], 0, h - x.shape[1], 0, 0)) \ - for x in images] - images = torch.stack(images) - return images - - -def _ind2il(ind, shapes_per_level, N): - r = ind - l = 0 - S = 0 - while r - S >= N * shapes_per_level[l][0] * shapes_per_level[l][1]: - S += N * shapes_per_level[l][0] * shapes_per_level[l][1] - l += 1 - i = (r - S) // (shapes_per_level[l][0] * shapes_per_level[l][1]) - return i, l - -def debug_train( - images, gt_instances, flattened_hms, reg_targets, labels, pos_inds, - shapes_per_level, locations, strides): - ''' - images: N x 3 x H x W - flattened_hms: LNHiWi x C - shapes_per_level: L x 2 [(H_i, W_i)] - locations: LNHiWi x 2 - ''' - reg_inds = torch.nonzero( - reg_targets.max(dim=1)[0] > 0).squeeze(1) - N = len(images) - images = _imagelist_to_tensor(images) - repeated_locations = [torch.cat([loc] * N, dim=0) \ - for loc in locations] - locations = torch.cat(repeated_locations, dim=0) - gt_hms = _decompose_level(flattened_hms, shapes_per_level, N) - masks = flattened_hms.new_zeros((flattened_hms.shape[0], 1)) - masks[pos_inds] = 1 - masks = _decompose_level(masks, shapes_per_level, N) - for i in range(len(images)): - image = images[i].detach().cpu().numpy().transpose(1, 2, 0) - color_maps = [] - for l in range(len(gt_hms)): - color_map = _get_color_image( - gt_hms[l][i].detach().cpu().numpy()) - color_maps.append(color_map) - cv2.imshow('gthm_{}'.format(l), color_map) - blend = _blend_image_heatmaps(image.copy(), color_maps) - if gt_instances is not None: - bboxes = gt_instances[i].gt_boxes.tensor - for j in range(len(bboxes)): - bbox = bboxes[j] - cv2.rectangle( - blend, - (int(bbox[0]), int(bbox[1])), - (int(bbox[2]), int(bbox[3])), - (0, 0, 255), 3, cv2.LINE_AA) - - for j in range(len(pos_inds)): - image_id, l = _ind2il(pos_inds[j], shapes_per_level, N) - if image_id != i: - continue - loc = locations[pos_inds[j]] - cv2.drawMarker( - blend, (int(loc[0]), int(loc[1])), (0, 255, 255), - markerSize=(l + 1) * 16) - - for j in range(len(reg_inds)): - image_id, l = _ind2il(reg_inds[j], shapes_per_level, N) - if image_id != i: - continue - ltrb = reg_targets[reg_inds[j]] - ltrb *= strides[l] - loc = locations[reg_inds[j]] - bbox = [(loc[0] - ltrb[0]), (loc[1] - ltrb[1]), - (loc[0] + ltrb[2]), (loc[1] + ltrb[3])] - cv2.rectangle( - blend, - (int(bbox[0]), int(bbox[1])), - (int(bbox[2]), int(bbox[3])), - (255, 0, 0), 1, cv2.LINE_AA) - cv2.circle(blend, (int(loc[0]), int(loc[1])), 2, (255, 0, 0), -1) - - cv2.imshow('blend', blend) - cv2.waitKey() - - -def debug_test( - images, logits_pred, reg_pred, agn_hm_pred=[], preds=[], - vis_thresh=0.3, debug_show_name=False, mult_agn=False): - ''' - images: N x 3 x H x W - class_target: LNHiWi x C - cat_agn_heatmap: LNHiWi - shapes_per_level: L x 2 [(H_i, W_i)] - ''' - N = len(images) - for i in range(len(images)): - image = images[i].detach().cpu().numpy().transpose(1, 2, 0) - result = image.copy().astype(np.uint8) - pred_image = image.copy().astype(np.uint8) - color_maps = [] - L = len(logits_pred) - for l in range(L): - if logits_pred[0] is not None: - stride = min(image.shape[0], image.shape[1]) / min( - logits_pred[l][i].shape[1], logits_pred[l][i].shape[2]) - else: - stride = min(image.shape[0], image.shape[1]) / min( - agn_hm_pred[l][i].shape[1], agn_hm_pred[l][i].shape[2]) - stride = stride if stride < 60 else 64 if stride < 100 else 128 - if logits_pred[0] is not None: - if mult_agn: - logits_pred[l][i] = logits_pred[l][i] * agn_hm_pred[l][i] - color_map = _get_color_image( - logits_pred[l][i].detach().cpu().numpy()) - color_maps.append(color_map) - cv2.imshow('predhm_{}'.format(l), color_map) - - if debug_show_name: - from detectron2.data.datasets.lvis_v1_categories import LVIS_CATEGORIES - cat2name = [x['name'] for x in LVIS_CATEGORIES] - for j in range(len(preds[i].scores) if preds is not None else 0): - if preds[i].scores[j] > vis_thresh: - bbox = preds[i].proposal_boxes[j] \ - if preds[i].has('proposal_boxes') else \ - preds[i].pred_boxes[j] - bbox = bbox.tensor[0].detach().cpu().numpy().astype(np.int32) - cat = int(preds[i].pred_classes[j]) \ - if preds[i].has('pred_classes') else 0 - cl = COLORS[cat, 0, 0] - cv2.rectangle( - pred_image, (int(bbox[0]), int(bbox[1])), - (int(bbox[2]), int(bbox[3])), - (int(cl[0]), int(cl[1]), int(cl[2])), 2, cv2.LINE_AA) - if debug_show_name: - txt = '{}{:.1f}'.format( - cat2name[cat] if cat > 0 else '', - preds[i].scores[j]) - font = cv2.FONT_HERSHEY_SIMPLEX - cat_size = cv2.getTextSize(txt, font, 0.5, 2)[0] - cv2.rectangle( - pred_image, - (int(bbox[0]), int(bbox[1] - cat_size[1] - 2)), - (int(bbox[0] + cat_size[0]), int(bbox[1] - 2)), - (int(cl[0]), int(cl[1]), int(cl[2])), -1) - cv2.putText( - pred_image, txt, (int(bbox[0]), int(bbox[1] - 2)), - font, 0.5, (0, 0, 0), thickness=1, lineType=cv2.LINE_AA) - - - if agn_hm_pred[l] is not None: - agn_hm_ = agn_hm_pred[l][i, 0, :, :, None].detach().cpu().numpy() - agn_hm_ = (agn_hm_ * np.array([255, 255, 255]).reshape( - 1, 1, 3)).astype(np.uint8) - cv2.imshow('agn_hm_{}'.format(l), agn_hm_) - blend = _blend_image_heatmaps(image.copy(), color_maps) - cv2.imshow('blend', blend) - cv2.imshow('preds', pred_image) - cv2.waitKey() - -global cnt -cnt = 0 - -def debug_second_stage(images, instances, proposals=None, vis_thresh=0.3, - save_debug=False, debug_show_name=False, image_labels=[], - save_debug_path='output/save_debug/', - bgr=False): - images = _imagelist_to_tensor(images) - if 'COCO' in save_debug_path: - from detectron2.data.datasets.builtin_meta import COCO_CATEGORIES - cat2name = [x['name'] for x in COCO_CATEGORIES] - else: - from detectron2.data.datasets.lvis_v1_categories import LVIS_CATEGORIES - cat2name = ['({}){}'.format(x['frequency'], x['name']) \ - for x in LVIS_CATEGORIES] - for i in range(len(images)): - image = images[i].detach().cpu().numpy().transpose(1, 2, 0).astype(np.uint8).copy() - if bgr: - image = image[:, :, ::-1].copy() - if instances[i].has('gt_boxes'): - bboxes = instances[i].gt_boxes.tensor.cpu().numpy() - scores = np.ones(bboxes.shape[0]) - cats = instances[i].gt_classes.cpu().numpy() - else: - bboxes = instances[i].pred_boxes.tensor.cpu().numpy() - scores = instances[i].scores.cpu().numpy() - cats = instances[i].pred_classes.cpu().numpy() - for j in range(len(bboxes)): - if scores[j] > vis_thresh: - bbox = bboxes[j] - cl = COLORS[cats[j], 0, 0] - cl = (int(cl[0]), int(cl[1]), int(cl[2])) - cv2.rectangle( - image, - (int(bbox[0]), int(bbox[1])), - (int(bbox[2]), int(bbox[3])), - cl, 2, cv2.LINE_AA) - if debug_show_name: - cat = cats[j] - txt = '{}{:.1f}'.format( - cat2name[cat] if cat > 0 else '', - scores[j]) - font = cv2.FONT_HERSHEY_SIMPLEX - cat_size = cv2.getTextSize(txt, font, 0.5, 2)[0] - cv2.rectangle( - image, - (int(bbox[0]), int(bbox[1] - cat_size[1] - 2)), - (int(bbox[0] + cat_size[0]), int(bbox[1] - 2)), - (int(cl[0]), int(cl[1]), int(cl[2])), -1) - cv2.putText( - image, txt, (int(bbox[0]), int(bbox[1] - 2)), - font, 0.5, (0, 0, 0), thickness=1, lineType=cv2.LINE_AA) - if proposals is not None: - proposal_image = images[i].detach().cpu().numpy().transpose(1, 2, 0).astype(np.uint8).copy() - if bgr: - proposal_image = proposal_image.copy() - else: - proposal_image = proposal_image[:, :, ::-1].copy() - bboxes = proposals[i].proposal_boxes.tensor.cpu().numpy() - if proposals[i].has('scores'): - scores = proposals[i].scores.detach().cpu().numpy() - else: - scores = proposals[i].objectness_logits.detach().cpu().numpy() - # selected = -1 - # if proposals[i].has('image_loss'): - # selected = proposals[i].image_loss.argmin() - if proposals[i].has('selected'): - selected = proposals[i].selected - else: - selected = [-1 for _ in range(len(bboxes))] - for j in range(len(bboxes)): - if scores[j] > vis_thresh or selected[j] >= 0: - bbox = bboxes[j] - cl = (209, 159, 83) - th = 2 - if selected[j] >= 0: - cl = (0, 0, 0xa4) - th = 4 - cv2.rectangle( - proposal_image, - (int(bbox[0]), int(bbox[1])), - (int(bbox[2]), int(bbox[3])), - cl, th, cv2.LINE_AA) - if selected[j] >= 0 and debug_show_name: - cat = selected[j].item() - txt = '{}'.format(cat2name[cat]) - font = cv2.FONT_HERSHEY_SIMPLEX - cat_size = cv2.getTextSize(txt, font, 0.5, 2)[0] - cv2.rectangle( - proposal_image, - (int(bbox[0]), int(bbox[1] - cat_size[1] - 2)), - (int(bbox[0] + cat_size[0]), int(bbox[1] - 2)), - (int(cl[0]), int(cl[1]), int(cl[2])), -1) - cv2.putText( - proposal_image, txt, - (int(bbox[0]), int(bbox[1] - 2)), - font, 0.5, (0, 0, 0), thickness=1, - lineType=cv2.LINE_AA) - - if save_debug: - global cnt - cnt = (cnt + 1) % 5000 - if not os.path.exists(save_debug_path): - os.mkdir(save_debug_path) - save_name = '{}/{:05d}.jpg'.format(save_debug_path, cnt) - if i < len(image_labels): - image_label = image_labels[i] - save_name = '{}/{:05d}'.format(save_debug_path, cnt) - for x in image_label: - class_name = cat2name[x] - save_name = save_name + '|{}'.format(class_name) - save_name = save_name + '.jpg' - cv2.imwrite(save_name, proposal_image) - else: - cv2.imshow('image', image) - if proposals is not None: - cv2.imshow('proposals', proposal_image) - cv2.waitKey() \ No newline at end of file diff --git a/spaces/Menthe17/Nani17092005/README.md b/spaces/Menthe17/Nani17092005/README.md deleted file mode 100644 index c16992c038f0d43e489a22b4cba5fb8089ecec64..0000000000000000000000000000000000000000 --- a/spaces/Menthe17/Nani17092005/README.md +++ /dev/null @@ -1,12 +0,0 @@ ---- -title: Nani17092005 -emoji: 🐨 -colorFrom: indigo -colorTo: indigo -sdk: gradio -sdk_version: 3.39.0 -app_file: app.py -pinned: false ---- - -Check out the configuration reference at https://huggingface.co/docs/hub/spaces-config-reference diff --git a/spaces/MirageML/sjc/sd1/ldm/data/imagenet.py b/spaces/MirageML/sjc/sd1/ldm/data/imagenet.py deleted file mode 100644 index 1c473f9c6965b22315dbb289eff8247c71bdc790..0000000000000000000000000000000000000000 --- a/spaces/MirageML/sjc/sd1/ldm/data/imagenet.py +++ /dev/null @@ -1,394 +0,0 @@ -import os, yaml, pickle, shutil, tarfile, glob -import cv2 -import albumentations -import PIL -import numpy as np -import torchvision.transforms.functional as TF -from omegaconf import OmegaConf -from functools import partial -from PIL import Image -from tqdm import tqdm -from torch.utils.data import Dataset, Subset - -import taming.data.utils as tdu -from taming.data.imagenet import str_to_indices, give_synsets_from_indices, download, retrieve -from taming.data.imagenet import ImagePaths - -from ldm.modules.image_degradation import degradation_fn_bsr, degradation_fn_bsr_light - - -def synset2idx(path_to_yaml="data/index_synset.yaml"): - with open(path_to_yaml) as f: - di2s = yaml.load(f) - return dict((v,k) for k,v in di2s.items()) - - -class ImageNetBase(Dataset): - def __init__(self, config=None): - self.config = config or OmegaConf.create() - if not type(self.config)==dict: - self.config = OmegaConf.to_container(self.config) - self.keep_orig_class_label = self.config.get("keep_orig_class_label", False) - self.process_images = True # if False we skip loading & processing images and self.data contains filepaths - self._prepare() - self._prepare_synset_to_human() - self._prepare_idx_to_synset() - self._prepare_human_to_integer_label() - self._load() - - def __len__(self): - return len(self.data) - - def __getitem__(self, i): - return self.data[i] - - def _prepare(self): - raise NotImplementedError() - - def _filter_relpaths(self, relpaths): - ignore = set([ - "n06596364_9591.JPEG", - ]) - relpaths = [rpath for rpath in relpaths if not rpath.split("/")[-1] in ignore] - if "sub_indices" in self.config: - indices = str_to_indices(self.config["sub_indices"]) - synsets = give_synsets_from_indices(indices, path_to_yaml=self.idx2syn) # returns a list of strings - self.synset2idx = synset2idx(path_to_yaml=self.idx2syn) - files = [] - for rpath in relpaths: - syn = rpath.split("/")[0] - if syn in synsets: - files.append(rpath) - return files - else: - return relpaths - - def _prepare_synset_to_human(self): - SIZE = 2655750 - URL = "https://heibox.uni-heidelberg.de/f/9f28e956cd304264bb82/?dl=1" - self.human_dict = os.path.join(self.root, "synset_human.txt") - if (not os.path.exists(self.human_dict) or - not os.path.getsize(self.human_dict)==SIZE): - download(URL, self.human_dict) - - def _prepare_idx_to_synset(self): - URL = "https://heibox.uni-heidelberg.de/f/d835d5b6ceda4d3aa910/?dl=1" - self.idx2syn = os.path.join(self.root, "index_synset.yaml") - if (not os.path.exists(self.idx2syn)): - download(URL, self.idx2syn) - - def _prepare_human_to_integer_label(self): - URL = "https://heibox.uni-heidelberg.de/f/2362b797d5be43b883f6/?dl=1" - self.human2integer = os.path.join(self.root, "imagenet1000_clsidx_to_labels.txt") - if (not os.path.exists(self.human2integer)): - download(URL, self.human2integer) - with open(self.human2integer, "r") as f: - lines = f.read().splitlines() - assert len(lines) == 1000 - self.human2integer_dict = dict() - for line in lines: - value, key = line.split(":") - self.human2integer_dict[key] = int(value) - - def _load(self): - with open(self.txt_filelist, "r") as f: - self.relpaths = f.read().splitlines() - l1 = len(self.relpaths) - self.relpaths = self._filter_relpaths(self.relpaths) - print("Removed {} files from filelist during filtering.".format(l1 - len(self.relpaths))) - - self.synsets = [p.split("/")[0] for p in self.relpaths] - self.abspaths = [os.path.join(self.datadir, p) for p in self.relpaths] - - unique_synsets = np.unique(self.synsets) - class_dict = dict((synset, i) for i, synset in enumerate(unique_synsets)) - if not self.keep_orig_class_label: - self.class_labels = [class_dict[s] for s in self.synsets] - else: - self.class_labels = [self.synset2idx[s] for s in self.synsets] - - with open(self.human_dict, "r") as f: - human_dict = f.read().splitlines() - human_dict = dict(line.split(maxsplit=1) for line in human_dict) - - self.human_labels = [human_dict[s] for s in self.synsets] - - labels = { - "relpath": np.array(self.relpaths), - "synsets": np.array(self.synsets), - "class_label": np.array(self.class_labels), - "human_label": np.array(self.human_labels), - } - - if self.process_images: - self.size = retrieve(self.config, "size", default=256) - self.data = ImagePaths(self.abspaths, - labels=labels, - size=self.size, - random_crop=self.random_crop, - ) - else: - self.data = self.abspaths - - -class ImageNetTrain(ImageNetBase): - NAME = "ILSVRC2012_train" - URL = "http://www.image-net.org/challenges/LSVRC/2012/" - AT_HASH = "a306397ccf9c2ead27155983c254227c0fd938e2" - FILES = [ - "ILSVRC2012_img_train.tar", - ] - SIZES = [ - 147897477120, - ] - - def __init__(self, process_images=True, data_root=None, **kwargs): - self.process_images = process_images - self.data_root = data_root - super().__init__(**kwargs) - - def _prepare(self): - if self.data_root: - self.root = os.path.join(self.data_root, self.NAME) - else: - cachedir = os.environ.get("XDG_CACHE_HOME", os.path.expanduser("~/.cache")) - self.root = os.path.join(cachedir, "autoencoders/data", self.NAME) - - self.datadir = os.path.join(self.root, "data") - self.txt_filelist = os.path.join(self.root, "filelist.txt") - self.expected_length = 1281167 - self.random_crop = retrieve(self.config, "ImageNetTrain/random_crop", - default=True) - if not tdu.is_prepared(self.root): - # prep - print("Preparing dataset {} in {}".format(self.NAME, self.root)) - - datadir = self.datadir - if not os.path.exists(datadir): - path = os.path.join(self.root, self.FILES[0]) - if not os.path.exists(path) or not os.path.getsize(path)==self.SIZES[0]: - import academictorrents as at - atpath = at.get(self.AT_HASH, datastore=self.root) - assert atpath == path - - print("Extracting {} to {}".format(path, datadir)) - os.makedirs(datadir, exist_ok=True) - with tarfile.open(path, "r:") as tar: - tar.extractall(path=datadir) - - print("Extracting sub-tars.") - subpaths = sorted(glob.glob(os.path.join(datadir, "*.tar"))) - for subpath in tqdm(subpaths): - subdir = subpath[:-len(".tar")] - os.makedirs(subdir, exist_ok=True) - with tarfile.open(subpath, "r:") as tar: - tar.extractall(path=subdir) - - filelist = glob.glob(os.path.join(datadir, "**", "*.JPEG")) - filelist = [os.path.relpath(p, start=datadir) for p in filelist] - filelist = sorted(filelist) - filelist = "\n".join(filelist)+"\n" - with open(self.txt_filelist, "w") as f: - f.write(filelist) - - tdu.mark_prepared(self.root) - - -class ImageNetValidation(ImageNetBase): - NAME = "ILSVRC2012_validation" - URL = "http://www.image-net.org/challenges/LSVRC/2012/" - AT_HASH = "5d6d0df7ed81efd49ca99ea4737e0ae5e3a5f2e5" - VS_URL = "https://heibox.uni-heidelberg.de/f/3e0f6e9c624e45f2bd73/?dl=1" - FILES = [ - "ILSVRC2012_img_val.tar", - "validation_synset.txt", - ] - SIZES = [ - 6744924160, - 1950000, - ] - - def __init__(self, process_images=True, data_root=None, **kwargs): - self.data_root = data_root - self.process_images = process_images - super().__init__(**kwargs) - - def _prepare(self): - if self.data_root: - self.root = os.path.join(self.data_root, self.NAME) - else: - cachedir = os.environ.get("XDG_CACHE_HOME", os.path.expanduser("~/.cache")) - self.root = os.path.join(cachedir, "autoencoders/data", self.NAME) - self.datadir = os.path.join(self.root, "data") - self.txt_filelist = os.path.join(self.root, "filelist.txt") - self.expected_length = 50000 - self.random_crop = retrieve(self.config, "ImageNetValidation/random_crop", - default=False) - if not tdu.is_prepared(self.root): - # prep - print("Preparing dataset {} in {}".format(self.NAME, self.root)) - - datadir = self.datadir - if not os.path.exists(datadir): - path = os.path.join(self.root, self.FILES[0]) - if not os.path.exists(path) or not os.path.getsize(path)==self.SIZES[0]: - import academictorrents as at - atpath = at.get(self.AT_HASH, datastore=self.root) - assert atpath == path - - print("Extracting {} to {}".format(path, datadir)) - os.makedirs(datadir, exist_ok=True) - with tarfile.open(path, "r:") as tar: - tar.extractall(path=datadir) - - vspath = os.path.join(self.root, self.FILES[1]) - if not os.path.exists(vspath) or not os.path.getsize(vspath)==self.SIZES[1]: - download(self.VS_URL, vspath) - - with open(vspath, "r") as f: - synset_dict = f.read().splitlines() - synset_dict = dict(line.split() for line in synset_dict) - - print("Reorganizing into synset folders") - synsets = np.unique(list(synset_dict.values())) - for s in synsets: - os.makedirs(os.path.join(datadir, s), exist_ok=True) - for k, v in synset_dict.items(): - src = os.path.join(datadir, k) - dst = os.path.join(datadir, v) - shutil.move(src, dst) - - filelist = glob.glob(os.path.join(datadir, "**", "*.JPEG")) - filelist = [os.path.relpath(p, start=datadir) for p in filelist] - filelist = sorted(filelist) - filelist = "\n".join(filelist)+"\n" - with open(self.txt_filelist, "w") as f: - f.write(filelist) - - tdu.mark_prepared(self.root) - - - -class ImageNetSR(Dataset): - def __init__(self, size=None, - degradation=None, downscale_f=4, min_crop_f=0.5, max_crop_f=1., - random_crop=True): - """ - Imagenet Superresolution Dataloader - Performs following ops in order: - 1. crops a crop of size s from image either as random or center crop - 2. resizes crop to size with cv2.area_interpolation - 3. degrades resized crop with degradation_fn - - :param size: resizing to size after cropping - :param degradation: degradation_fn, e.g. cv_bicubic or bsrgan_light - :param downscale_f: Low Resolution Downsample factor - :param min_crop_f: determines crop size s, - where s = c * min_img_side_len with c sampled from interval (min_crop_f, max_crop_f) - :param max_crop_f: "" - :param data_root: - :param random_crop: - """ - self.base = self.get_base() - assert size - assert (size / downscale_f).is_integer() - self.size = size - self.LR_size = int(size / downscale_f) - self.min_crop_f = min_crop_f - self.max_crop_f = max_crop_f - assert(max_crop_f <= 1.) - self.center_crop = not random_crop - - self.image_rescaler = albumentations.SmallestMaxSize(max_size=size, interpolation=cv2.INTER_AREA) - - self.pil_interpolation = False # gets reset later if incase interp_op is from pillow - - if degradation == "bsrgan": - self.degradation_process = partial(degradation_fn_bsr, sf=downscale_f) - - elif degradation == "bsrgan_light": - self.degradation_process = partial(degradation_fn_bsr_light, sf=downscale_f) - - else: - interpolation_fn = { - "cv_nearest": cv2.INTER_NEAREST, - "cv_bilinear": cv2.INTER_LINEAR, - "cv_bicubic": cv2.INTER_CUBIC, - "cv_area": cv2.INTER_AREA, - "cv_lanczos": cv2.INTER_LANCZOS4, - "pil_nearest": PIL.Image.NEAREST, - "pil_bilinear": PIL.Image.BILINEAR, - "pil_bicubic": PIL.Image.BICUBIC, - "pil_box": PIL.Image.BOX, - "pil_hamming": PIL.Image.HAMMING, - "pil_lanczos": PIL.Image.LANCZOS, - }[degradation] - - self.pil_interpolation = degradation.startswith("pil_") - - if self.pil_interpolation: - self.degradation_process = partial(TF.resize, size=self.LR_size, interpolation=interpolation_fn) - - else: - self.degradation_process = albumentations.SmallestMaxSize(max_size=self.LR_size, - interpolation=interpolation_fn) - - def __len__(self): - return len(self.base) - - def __getitem__(self, i): - example = self.base[i] - image = Image.open(example["file_path_"]) - - if not image.mode == "RGB": - image = image.convert("RGB") - - image = np.array(image).astype(np.uint8) - - min_side_len = min(image.shape[:2]) - crop_side_len = min_side_len * np.random.uniform(self.min_crop_f, self.max_crop_f, size=None) - crop_side_len = int(crop_side_len) - - if self.center_crop: - self.cropper = albumentations.CenterCrop(height=crop_side_len, width=crop_side_len) - - else: - self.cropper = albumentations.RandomCrop(height=crop_side_len, width=crop_side_len) - - image = self.cropper(image=image)["image"] - image = self.image_rescaler(image=image)["image"] - - if self.pil_interpolation: - image_pil = PIL.Image.fromarray(image) - LR_image = self.degradation_process(image_pil) - LR_image = np.array(LR_image).astype(np.uint8) - - else: - LR_image = self.degradation_process(image=image)["image"] - - example["image"] = (image/127.5 - 1.0).astype(np.float32) - example["LR_image"] = (LR_image/127.5 - 1.0).astype(np.float32) - - return example - - -class ImageNetSRTrain(ImageNetSR): - def __init__(self, **kwargs): - super().__init__(**kwargs) - - def get_base(self): - with open("data/imagenet_train_hr_indices.p", "rb") as f: - indices = pickle.load(f) - dset = ImageNetTrain(process_images=False,) - return Subset(dset, indices) - - -class ImageNetSRValidation(ImageNetSR): - def __init__(self, **kwargs): - super().__init__(**kwargs) - - def get_base(self): - with open("data/imagenet_val_hr_indices.p", "rb") as f: - indices = pickle.load(f) - dset = ImageNetValidation(process_images=False,) - return Subset(dset, indices) diff --git a/spaces/Moonkiler/Nio22/README.md b/spaces/Moonkiler/Nio22/README.md deleted file mode 100644 index d04e817a0ae7badedaf88c6e7c9a49722772da1a..0000000000000000000000000000000000000000 --- a/spaces/Moonkiler/Nio22/README.md +++ /dev/null @@ -1,10 +0,0 @@ ---- -title: Nio22 -emoji: 🦀 -colorFrom: purple -colorTo: red -sdk: docker -pinned: false ---- - -Check out the configuration reference at https://huggingface.co/docs/hub/spaces-config-reference diff --git a/spaces/Mountchicken/MAERec-Gradio/tools/dataset_converters/textrecog/lv_converter.py b/spaces/Mountchicken/MAERec-Gradio/tools/dataset_converters/textrecog/lv_converter.py deleted file mode 100644 index d22c60b224d7fb122ebe26b2729650a961aac992..0000000000000000000000000000000000000000 --- a/spaces/Mountchicken/MAERec-Gradio/tools/dataset_converters/textrecog/lv_converter.py +++ /dev/null @@ -1,68 +0,0 @@ -# Copyright (c) OpenMMLab. All rights reserved. -import argparse -import os.path as osp - -from mmocr.utils import dump_ocr_data - - -def convert_annotations(root_path, split): - """Convert original annotations to mmocr format. - - The annotation format is as the following: - Crops/val/11/1/1.png weighted - Crops/val/11/1/2.png 26 - Crops/val/11/1/3.png casting - Crops/val/11/1/4.png 28 - After this module, the annotation has been changed to the format below: - jsonl: - {'filename': 'Crops/val/11/1/1.png', 'text': 'weighted'} - {'filename': 'Crops/val/11/1/1.png', 'text': '26'} - {'filename': 'Crops/val/11/1/1.png', 'text': 'casting'} - {'filename': 'Crops/val/11/1/1.png', 'text': '28'} - - Args: - root_path (str): The root path of the dataset - split (str): The split of dataset. Namely: training or test - """ - assert isinstance(root_path, str) - assert isinstance(split, str) - - img_info = [] - with open( - osp.join(root_path, f'{split}_label.txt'), - encoding='"utf-8-sig') as f: - annos = f.readlines() - for anno in annos: - if anno: - # Text may contain spaces - dst_img_name, word = anno.split('png ') - word = word.strip('\n') - img_info.append({ - 'file_name': dst_img_name + 'png', - 'anno_info': [{ - 'text': word - }] - }) - dump_ocr_data(img_info, osp.join(root_path, f'{split.lower()}_label.json'), - 'textrecog') - - -def parse_args(): - parser = argparse.ArgumentParser( - description='Generate training and test set of Lecture Video DB') - parser.add_argument('root_path', help='Root dir path of Lecture Video DB') - args = parser.parse_args() - return args - - -def main(): - args = parse_args() - root_path = args.root_path - - for split in ['train', 'val', 'test']: - convert_annotations(root_path, split) - print(f'{split} split converted.') - - -if __name__ == '__main__': - main() diff --git a/spaces/MrBodean/VoiceClone/encoder/data_objects/speaker_verification_dataset.py b/spaces/MrBodean/VoiceClone/encoder/data_objects/speaker_verification_dataset.py deleted file mode 100644 index 77a6e05eae6a939ae7575ae70b7173644141fffe..0000000000000000000000000000000000000000 --- a/spaces/MrBodean/VoiceClone/encoder/data_objects/speaker_verification_dataset.py +++ /dev/null @@ -1,56 +0,0 @@ -from encoder.data_objects.random_cycler import RandomCycler -from encoder.data_objects.speaker_batch import SpeakerBatch -from encoder.data_objects.speaker import Speaker -from encoder.params_data import partials_n_frames -from torch.utils.data import Dataset, DataLoader -from pathlib import Path - -# TODO: improve with a pool of speakers for data efficiency - -class SpeakerVerificationDataset(Dataset): - def __init__(self, datasets_root: Path): - self.root = datasets_root - speaker_dirs = [f for f in self.root.glob("*") if f.is_dir()] - if len(speaker_dirs) == 0: - raise Exception("No speakers found. Make sure you are pointing to the directory " - "containing all preprocessed speaker directories.") - self.speakers = [Speaker(speaker_dir) for speaker_dir in speaker_dirs] - self.speaker_cycler = RandomCycler(self.speakers) - - def __len__(self): - return int(1e10) - - def __getitem__(self, index): - return next(self.speaker_cycler) - - def get_logs(self): - log_string = "" - for log_fpath in self.root.glob("*.txt"): - with log_fpath.open("r") as log_file: - log_string += "".join(log_file.readlines()) - return log_string - - -class SpeakerVerificationDataLoader(DataLoader): - def __init__(self, dataset, speakers_per_batch, utterances_per_speaker, sampler=None, - batch_sampler=None, num_workers=0, pin_memory=False, timeout=0, - worker_init_fn=None): - self.utterances_per_speaker = utterances_per_speaker - - super().__init__( - dataset=dataset, - batch_size=speakers_per_batch, - shuffle=False, - sampler=sampler, - batch_sampler=batch_sampler, - num_workers=num_workers, - collate_fn=self.collate, - pin_memory=pin_memory, - drop_last=False, - timeout=timeout, - worker_init_fn=worker_init_fn - ) - - def collate(self, speakers): - return SpeakerBatch(speakers, self.utterances_per_speaker, partials_n_frames) - \ No newline at end of file diff --git a/spaces/MrTitanicus/rvc-models/infer_pack/models.py b/spaces/MrTitanicus/rvc-models/infer_pack/models.py deleted file mode 100644 index 96165f73644e6fb92d0ffedb4a3c9e1a457cb989..0000000000000000000000000000000000000000 --- a/spaces/MrTitanicus/rvc-models/infer_pack/models.py +++ /dev/null @@ -1,982 +0,0 @@ -import math, pdb, os -from time import time as ttime -import torch -from torch import nn -from torch.nn import functional as F -from infer_pack import modules -from infer_pack import attentions -from infer_pack import commons -from infer_pack.commons import init_weights, get_padding -from torch.nn import Conv1d, ConvTranspose1d, AvgPool1d, Conv2d -from torch.nn.utils import weight_norm, remove_weight_norm, spectral_norm -from infer_pack.commons import init_weights -import numpy as np -from infer_pack import commons - - -class TextEncoder256(nn.Module): - def __init__( - self, - out_channels, - hidden_channels, - filter_channels, - n_heads, - n_layers, - kernel_size, - p_dropout, - f0=True, - ): - super().__init__() - self.out_channels = out_channels - self.hidden_channels = hidden_channels - self.filter_channels = filter_channels - self.n_heads = n_heads - self.n_layers = n_layers - self.kernel_size = kernel_size - self.p_dropout = p_dropout - self.emb_phone = nn.Linear(256, hidden_channels) - self.lrelu = nn.LeakyReLU(0.1, inplace=True) - if f0 == True: - self.emb_pitch = nn.Embedding(256, hidden_channels) # pitch 256 - self.encoder = attentions.Encoder( - hidden_channels, filter_channels, n_heads, n_layers, kernel_size, p_dropout - ) - self.proj = nn.Conv1d(hidden_channels, out_channels * 2, 1) - - def forward(self, phone, pitch, lengths): - if pitch == None: - x = self.emb_phone(phone) - else: - x = self.emb_phone(phone) + self.emb_pitch(pitch) - x = x * math.sqrt(self.hidden_channels) # [b, t, h] - x = self.lrelu(x) - x = torch.transpose(x, 1, -1) # [b, h, t] - x_mask = torch.unsqueeze(commons.sequence_mask(lengths, x.size(2)), 1).to( - x.dtype - ) - x = self.encoder(x * x_mask, x_mask) - stats = self.proj(x) * x_mask - - m, logs = torch.split(stats, self.out_channels, dim=1) - return m, logs, x_mask - - -class TextEncoder256Sim(nn.Module): - def __init__( - self, - out_channels, - hidden_channels, - filter_channels, - n_heads, - n_layers, - kernel_size, - p_dropout, - f0=True, - ): - super().__init__() - self.out_channels = out_channels - self.hidden_channels = hidden_channels - self.filter_channels = filter_channels - self.n_heads = n_heads - self.n_layers = n_layers - self.kernel_size = kernel_size - self.p_dropout = p_dropout - self.emb_phone = nn.Linear(256, hidden_channels) - self.lrelu = nn.LeakyReLU(0.1, inplace=True) - if f0 == True: - self.emb_pitch = nn.Embedding(256, hidden_channels) # pitch 256 - self.encoder = attentions.Encoder( - hidden_channels, filter_channels, n_heads, n_layers, kernel_size, p_dropout - ) - self.proj = nn.Conv1d(hidden_channels, out_channels, 1) - - def forward(self, phone, pitch, lengths): - if pitch == None: - x = self.emb_phone(phone) - else: - x = self.emb_phone(phone) + self.emb_pitch(pitch) - x = x * math.sqrt(self.hidden_channels) # [b, t, h] - x = self.lrelu(x) - x = torch.transpose(x, 1, -1) # [b, h, t] - x_mask = torch.unsqueeze(commons.sequence_mask(lengths, x.size(2)), 1).to( - x.dtype - ) - x = self.encoder(x * x_mask, x_mask) - x = self.proj(x) * x_mask - return x, x_mask - - -class ResidualCouplingBlock(nn.Module): - def __init__( - self, - channels, - hidden_channels, - kernel_size, - dilation_rate, - n_layers, - n_flows=4, - gin_channels=0, - ): - super().__init__() - self.channels = channels - self.hidden_channels = hidden_channels - self.kernel_size = kernel_size - self.dilation_rate = dilation_rate - self.n_layers = n_layers - self.n_flows = n_flows - self.gin_channels = gin_channels - - self.flows = nn.ModuleList() - for i in range(n_flows): - self.flows.append( - modules.ResidualCouplingLayer( - channels, - hidden_channels, - kernel_size, - dilation_rate, - n_layers, - gin_channels=gin_channels, - mean_only=True, - ) - ) - self.flows.append(modules.Flip()) - - def forward(self, x, x_mask, g=None, reverse=False): - if not reverse: - for flow in self.flows: - x, _ = flow(x, x_mask, g=g, reverse=reverse) - else: - for flow in reversed(self.flows): - x = flow(x, x_mask, g=g, reverse=reverse) - return x - - def remove_weight_norm(self): - for i in range(self.n_flows): - self.flows[i * 2].remove_weight_norm() - - -class PosteriorEncoder(nn.Module): - def __init__( - self, - in_channels, - out_channels, - hidden_channels, - kernel_size, - dilation_rate, - n_layers, - gin_channels=0, - ): - super().__init__() - self.in_channels = in_channels - self.out_channels = out_channels - self.hidden_channels = hidden_channels - self.kernel_size = kernel_size - self.dilation_rate = dilation_rate - self.n_layers = n_layers - self.gin_channels = gin_channels - - self.pre = nn.Conv1d(in_channels, hidden_channels, 1) - self.enc = modules.WN( - hidden_channels, - kernel_size, - dilation_rate, - n_layers, - gin_channels=gin_channels, - ) - self.proj = nn.Conv1d(hidden_channels, out_channels * 2, 1) - - def forward(self, x, x_lengths, g=None): - x_mask = torch.unsqueeze(commons.sequence_mask(x_lengths, x.size(2)), 1).to( - x.dtype - ) - x = self.pre(x) * x_mask - x = self.enc(x, x_mask, g=g) - stats = self.proj(x) * x_mask - m, logs = torch.split(stats, self.out_channels, dim=1) - z = (m + torch.randn_like(m) * torch.exp(logs)) * x_mask - return z, m, logs, x_mask - - def remove_weight_norm(self): - self.enc.remove_weight_norm() - - -class Generator(torch.nn.Module): - def __init__( - self, - initial_channel, - resblock, - resblock_kernel_sizes, - resblock_dilation_sizes, - upsample_rates, - upsample_initial_channel, - upsample_kernel_sizes, - gin_channels=0, - ): - super(Generator, self).__init__() - self.num_kernels = len(resblock_kernel_sizes) - self.num_upsamples = len(upsample_rates) - self.conv_pre = Conv1d( - initial_channel, upsample_initial_channel, 7, 1, padding=3 - ) - resblock = modules.ResBlock1 if resblock == "1" else modules.ResBlock2 - - self.ups = nn.ModuleList() - for i, (u, k) in enumerate(zip(upsample_rates, upsample_kernel_sizes)): - self.ups.append( - weight_norm( - ConvTranspose1d( - upsample_initial_channel // (2**i), - upsample_initial_channel // (2 ** (i + 1)), - k, - u, - padding=(k - u) // 2, - ) - ) - ) - - self.resblocks = nn.ModuleList() - for i in range(len(self.ups)): - ch = upsample_initial_channel // (2 ** (i + 1)) - for j, (k, d) in enumerate( - zip(resblock_kernel_sizes, resblock_dilation_sizes) - ): - self.resblocks.append(resblock(ch, k, d)) - - self.conv_post = Conv1d(ch, 1, 7, 1, padding=3, bias=False) - self.ups.apply(init_weights) - - if gin_channels != 0: - self.cond = nn.Conv1d(gin_channels, upsample_initial_channel, 1) - - def forward(self, x, g=None): - x = self.conv_pre(x) - if g is not None: - x = x + self.cond(g) - - for i in range(self.num_upsamples): - x = F.leaky_relu(x, modules.LRELU_SLOPE) - x = self.ups[i](x) - xs = None - for j in range(self.num_kernels): - if xs is None: - xs = self.resblocks[i * self.num_kernels + j](x) - else: - xs += self.resblocks[i * self.num_kernels + j](x) - x = xs / self.num_kernels - x = F.leaky_relu(x) - x = self.conv_post(x) - x = torch.tanh(x) - - return x - - def remove_weight_norm(self): - for l in self.ups: - remove_weight_norm(l) - for l in self.resblocks: - l.remove_weight_norm() - - -class SineGen(torch.nn.Module): - """Definition of sine generator - SineGen(samp_rate, harmonic_num = 0, - sine_amp = 0.1, noise_std = 0.003, - voiced_threshold = 0, - flag_for_pulse=False) - samp_rate: sampling rate in Hz - harmonic_num: number of harmonic overtones (default 0) - sine_amp: amplitude of sine-wavefrom (default 0.1) - noise_std: std of Gaussian noise (default 0.003) - voiced_thoreshold: F0 threshold for U/V classification (default 0) - flag_for_pulse: this SinGen is used inside PulseGen (default False) - Note: when flag_for_pulse is True, the first time step of a voiced - segment is always sin(np.pi) or cos(0) - """ - - def __init__( - self, - samp_rate, - harmonic_num=0, - sine_amp=0.1, - noise_std=0.003, - voiced_threshold=0, - flag_for_pulse=False, - ): - super(SineGen, self).__init__() - self.sine_amp = sine_amp - self.noise_std = noise_std - self.harmonic_num = harmonic_num - self.dim = self.harmonic_num + 1 - self.sampling_rate = samp_rate - self.voiced_threshold = voiced_threshold - - def _f02uv(self, f0): - # generate uv signal - uv = torch.ones_like(f0) - uv = uv * (f0 > self.voiced_threshold) - return uv - - def forward(self, f0, upp): - """sine_tensor, uv = forward(f0) - input F0: tensor(batchsize=1, length, dim=1) - f0 for unvoiced steps should be 0 - output sine_tensor: tensor(batchsize=1, length, dim) - output uv: tensor(batchsize=1, length, 1) - """ - with torch.no_grad(): - f0 = f0[:, None].transpose(1, 2) - f0_buf = torch.zeros(f0.shape[0], f0.shape[1], self.dim, device=f0.device) - # fundamental component - f0_buf[:, :, 0] = f0[:, :, 0] - for idx in np.arange(self.harmonic_num): - f0_buf[:, :, idx + 1] = f0_buf[:, :, 0] * ( - idx + 2 - ) # idx + 2: the (idx+1)-th overtone, (idx+2)-th harmonic - rad_values = (f0_buf / self.sampling_rate) % 1 ###%1意味着n_har的乘积无法后处理优化 - rand_ini = torch.rand( - f0_buf.shape[0], f0_buf.shape[2], device=f0_buf.device - ) - rand_ini[:, 0] = 0 - rad_values[:, 0, :] = rad_values[:, 0, :] + rand_ini - tmp_over_one = torch.cumsum(rad_values, 1) # % 1 #####%1意味着后面的cumsum无法再优化 - tmp_over_one *= upp - tmp_over_one = F.interpolate( - tmp_over_one.transpose(2, 1), - scale_factor=upp, - mode="linear", - align_corners=True, - ).transpose(2, 1) - rad_values = F.interpolate( - rad_values.transpose(2, 1), scale_factor=upp, mode="nearest" - ).transpose( - 2, 1 - ) ####### - tmp_over_one %= 1 - tmp_over_one_idx = (tmp_over_one[:, 1:, :] - tmp_over_one[:, :-1, :]) < 0 - cumsum_shift = torch.zeros_like(rad_values) - cumsum_shift[:, 1:, :] = tmp_over_one_idx * -1.0 - sine_waves = torch.sin( - torch.cumsum(rad_values + cumsum_shift, dim=1) * 2 * np.pi - ) - sine_waves = sine_waves * self.sine_amp - uv = self._f02uv(f0) - uv = F.interpolate( - uv.transpose(2, 1), scale_factor=upp, mode="nearest" - ).transpose(2, 1) - noise_amp = uv * self.noise_std + (1 - uv) * self.sine_amp / 3 - noise = noise_amp * torch.randn_like(sine_waves) - sine_waves = sine_waves * uv + noise - return sine_waves, uv, noise - - -class SourceModuleHnNSF(torch.nn.Module): - """SourceModule for hn-nsf - SourceModule(sampling_rate, harmonic_num=0, sine_amp=0.1, - add_noise_std=0.003, voiced_threshod=0) - sampling_rate: sampling_rate in Hz - harmonic_num: number of harmonic above F0 (default: 0) - sine_amp: amplitude of sine source signal (default: 0.1) - add_noise_std: std of additive Gaussian noise (default: 0.003) - note that amplitude of noise in unvoiced is decided - by sine_amp - voiced_threshold: threhold to set U/V given F0 (default: 0) - Sine_source, noise_source = SourceModuleHnNSF(F0_sampled) - F0_sampled (batchsize, length, 1) - Sine_source (batchsize, length, 1) - noise_source (batchsize, length 1) - uv (batchsize, length, 1) - """ - - def __init__( - self, - sampling_rate, - harmonic_num=0, - sine_amp=0.1, - add_noise_std=0.003, - voiced_threshod=0, - is_half=True, - ): - super(SourceModuleHnNSF, self).__init__() - - self.sine_amp = sine_amp - self.noise_std = add_noise_std - self.is_half = is_half - # to produce sine waveforms - self.l_sin_gen = SineGen( - sampling_rate, harmonic_num, sine_amp, add_noise_std, voiced_threshod - ) - - # to merge source harmonics into a single excitation - self.l_linear = torch.nn.Linear(harmonic_num + 1, 1) - self.l_tanh = torch.nn.Tanh() - - def forward(self, x, upp=None): - sine_wavs, uv, _ = self.l_sin_gen(x, upp) - if self.is_half: - sine_wavs = sine_wavs.half() - sine_merge = self.l_tanh(self.l_linear(sine_wavs)) - return sine_merge, None, None # noise, uv - - -class GeneratorNSF(torch.nn.Module): - def __init__( - self, - initial_channel, - resblock, - resblock_kernel_sizes, - resblock_dilation_sizes, - upsample_rates, - upsample_initial_channel, - upsample_kernel_sizes, - gin_channels, - sr, - is_half=False, - ): - super(GeneratorNSF, self).__init__() - self.num_kernels = len(resblock_kernel_sizes) - self.num_upsamples = len(upsample_rates) - - self.f0_upsamp = torch.nn.Upsample(scale_factor=np.prod(upsample_rates)) - self.m_source = SourceModuleHnNSF( - sampling_rate=sr, harmonic_num=0, is_half=is_half - ) - self.noise_convs = nn.ModuleList() - self.conv_pre = Conv1d( - initial_channel, upsample_initial_channel, 7, 1, padding=3 - ) - resblock = modules.ResBlock1 if resblock == "1" else modules.ResBlock2 - - self.ups = nn.ModuleList() - for i, (u, k) in enumerate(zip(upsample_rates, upsample_kernel_sizes)): - c_cur = upsample_initial_channel // (2 ** (i + 1)) - self.ups.append( - weight_norm( - ConvTranspose1d( - upsample_initial_channel // (2**i), - upsample_initial_channel // (2 ** (i + 1)), - k, - u, - padding=(k - u) // 2, - ) - ) - ) - if i + 1 < len(upsample_rates): - stride_f0 = np.prod(upsample_rates[i + 1 :]) - self.noise_convs.append( - Conv1d( - 1, - c_cur, - kernel_size=stride_f0 * 2, - stride=stride_f0, - padding=stride_f0 // 2, - ) - ) - else: - self.noise_convs.append(Conv1d(1, c_cur, kernel_size=1)) - - self.resblocks = nn.ModuleList() - for i in range(len(self.ups)): - ch = upsample_initial_channel // (2 ** (i + 1)) - for j, (k, d) in enumerate( - zip(resblock_kernel_sizes, resblock_dilation_sizes) - ): - self.resblocks.append(resblock(ch, k, d)) - - self.conv_post = Conv1d(ch, 1, 7, 1, padding=3, bias=False) - self.ups.apply(init_weights) - - if gin_channels != 0: - self.cond = nn.Conv1d(gin_channels, upsample_initial_channel, 1) - - self.upp = np.prod(upsample_rates) - - def forward(self, x, f0, g=None): - har_source, noi_source, uv = self.m_source(f0, self.upp) - har_source = har_source.transpose(1, 2) - x = self.conv_pre(x) - if g is not None: - x = x + self.cond(g) - - for i in range(self.num_upsamples): - x = F.leaky_relu(x, modules.LRELU_SLOPE) - x = self.ups[i](x) - x_source = self.noise_convs[i](har_source) - x = x + x_source - xs = None - for j in range(self.num_kernels): - if xs is None: - xs = self.resblocks[i * self.num_kernels + j](x) - else: - xs += self.resblocks[i * self.num_kernels + j](x) - x = xs / self.num_kernels - x = F.leaky_relu(x) - x = self.conv_post(x) - x = torch.tanh(x) - return x - - def remove_weight_norm(self): - for l in self.ups: - remove_weight_norm(l) - for l in self.resblocks: - l.remove_weight_norm() - - -sr2sr = { - "32k": 32000, - "40k": 40000, - "48k": 48000, -} - - -class SynthesizerTrnMs256NSFsid(nn.Module): - def __init__( - self, - spec_channels, - segment_size, - inter_channels, - hidden_channels, - filter_channels, - n_heads, - n_layers, - kernel_size, - p_dropout, - resblock, - resblock_kernel_sizes, - resblock_dilation_sizes, - upsample_rates, - upsample_initial_channel, - upsample_kernel_sizes, - spk_embed_dim, - gin_channels, - sr, - **kwargs - ): - super().__init__() - if type(sr) == type("strr"): - sr = sr2sr[sr] - self.spec_channels = spec_channels - self.inter_channels = inter_channels - self.hidden_channels = hidden_channels - self.filter_channels = filter_channels - self.n_heads = n_heads - self.n_layers = n_layers - self.kernel_size = kernel_size - self.p_dropout = p_dropout - self.resblock = resblock - self.resblock_kernel_sizes = resblock_kernel_sizes - self.resblock_dilation_sizes = resblock_dilation_sizes - self.upsample_rates = upsample_rates - self.upsample_initial_channel = upsample_initial_channel - self.upsample_kernel_sizes = upsample_kernel_sizes - self.segment_size = segment_size - self.gin_channels = gin_channels - # self.hop_length = hop_length# - self.spk_embed_dim = spk_embed_dim - self.enc_p = TextEncoder256( - inter_channels, - hidden_channels, - filter_channels, - n_heads, - n_layers, - kernel_size, - p_dropout, - ) - self.dec = GeneratorNSF( - inter_channels, - resblock, - resblock_kernel_sizes, - resblock_dilation_sizes, - upsample_rates, - upsample_initial_channel, - upsample_kernel_sizes, - gin_channels=gin_channels, - sr=sr, - is_half=kwargs["is_half"], - ) - self.enc_q = PosteriorEncoder( - spec_channels, - inter_channels, - hidden_channels, - 5, - 1, - 16, - gin_channels=gin_channels, - ) - self.flow = ResidualCouplingBlock( - inter_channels, hidden_channels, 5, 1, 3, gin_channels=gin_channels - ) - self.emb_g = nn.Embedding(self.spk_embed_dim, gin_channels) - print("gin_channels:", gin_channels, "self.spk_embed_dim:", self.spk_embed_dim) - - def remove_weight_norm(self): - self.dec.remove_weight_norm() - self.flow.remove_weight_norm() - self.enc_q.remove_weight_norm() - - def forward( - self, phone, phone_lengths, pitch, pitchf, y, y_lengths, ds - ): # 这里ds是id,[bs,1] - # print(1,pitch.shape)#[bs,t] - g = self.emb_g(ds).unsqueeze(-1) # [b, 256, 1]##1是t,广播的 - m_p, logs_p, x_mask = self.enc_p(phone, pitch, phone_lengths) - z, m_q, logs_q, y_mask = self.enc_q(y, y_lengths, g=g) - z_p = self.flow(z, y_mask, g=g) - z_slice, ids_slice = commons.rand_slice_segments( - z, y_lengths, self.segment_size - ) - # print(-1,pitchf.shape,ids_slice,self.segment_size,self.hop_length,self.segment_size//self.hop_length) - pitchf = commons.slice_segments2(pitchf, ids_slice, self.segment_size) - # print(-2,pitchf.shape,z_slice.shape) - o = self.dec(z_slice, pitchf, g=g) - return o, ids_slice, x_mask, y_mask, (z, z_p, m_p, logs_p, m_q, logs_q) - - def infer(self, phone, phone_lengths, pitch, nsff0, sid, max_len=None): - g = self.emb_g(sid).unsqueeze(-1) - m_p, logs_p, x_mask = self.enc_p(phone, pitch, phone_lengths) - z_p = (m_p + torch.exp(logs_p) * torch.randn_like(m_p) * 0.66666) * x_mask - z = self.flow(z_p, x_mask, g=g, reverse=True) - o = self.dec((z * x_mask)[:, :, :max_len], nsff0, g=g) - return o, x_mask, (z, z_p, m_p, logs_p) - - -class SynthesizerTrnMs256NSFsid_nono(nn.Module): - def __init__( - self, - spec_channels, - segment_size, - inter_channels, - hidden_channels, - filter_channels, - n_heads, - n_layers, - kernel_size, - p_dropout, - resblock, - resblock_kernel_sizes, - resblock_dilation_sizes, - upsample_rates, - upsample_initial_channel, - upsample_kernel_sizes, - spk_embed_dim, - gin_channels, - sr=None, - **kwargs - ): - super().__init__() - self.spec_channels = spec_channels - self.inter_channels = inter_channels - self.hidden_channels = hidden_channels - self.filter_channels = filter_channels - self.n_heads = n_heads - self.n_layers = n_layers - self.kernel_size = kernel_size - self.p_dropout = p_dropout - self.resblock = resblock - self.resblock_kernel_sizes = resblock_kernel_sizes - self.resblock_dilation_sizes = resblock_dilation_sizes - self.upsample_rates = upsample_rates - self.upsample_initial_channel = upsample_initial_channel - self.upsample_kernel_sizes = upsample_kernel_sizes - self.segment_size = segment_size - self.gin_channels = gin_channels - # self.hop_length = hop_length# - self.spk_embed_dim = spk_embed_dim - self.enc_p = TextEncoder256( - inter_channels, - hidden_channels, - filter_channels, - n_heads, - n_layers, - kernel_size, - p_dropout, - f0=False, - ) - self.dec = Generator( - inter_channels, - resblock, - resblock_kernel_sizes, - resblock_dilation_sizes, - upsample_rates, - upsample_initial_channel, - upsample_kernel_sizes, - gin_channels=gin_channels, - ) - self.enc_q = PosteriorEncoder( - spec_channels, - inter_channels, - hidden_channels, - 5, - 1, - 16, - gin_channels=gin_channels, - ) - self.flow = ResidualCouplingBlock( - inter_channels, hidden_channels, 5, 1, 3, gin_channels=gin_channels - ) - self.emb_g = nn.Embedding(self.spk_embed_dim, gin_channels) - print("gin_channels:", gin_channels, "self.spk_embed_dim:", self.spk_embed_dim) - - def remove_weight_norm(self): - self.dec.remove_weight_norm() - self.flow.remove_weight_norm() - self.enc_q.remove_weight_norm() - - def forward(self, phone, phone_lengths, y, y_lengths, ds): # 这里ds是id,[bs,1] - g = self.emb_g(ds).unsqueeze(-1) # [b, 256, 1]##1是t,广播的 - m_p, logs_p, x_mask = self.enc_p(phone, None, phone_lengths) - z, m_q, logs_q, y_mask = self.enc_q(y, y_lengths, g=g) - z_p = self.flow(z, y_mask, g=g) - z_slice, ids_slice = commons.rand_slice_segments( - z, y_lengths, self.segment_size - ) - o = self.dec(z_slice, g=g) - return o, ids_slice, x_mask, y_mask, (z, z_p, m_p, logs_p, m_q, logs_q) - - def infer(self, phone, phone_lengths, sid, max_len=None): - g = self.emb_g(sid).unsqueeze(-1) - m_p, logs_p, x_mask = self.enc_p(phone, None, phone_lengths) - z_p = (m_p + torch.exp(logs_p) * torch.randn_like(m_p) * 0.66666) * x_mask - z = self.flow(z_p, x_mask, g=g, reverse=True) - o = self.dec((z * x_mask)[:, :, :max_len], g=g) - return o, x_mask, (z, z_p, m_p, logs_p) - - -class SynthesizerTrnMs256NSFsid_sim(nn.Module): - """ - Synthesizer for Training - """ - - def __init__( - self, - spec_channels, - segment_size, - inter_channels, - hidden_channels, - filter_channels, - n_heads, - n_layers, - kernel_size, - p_dropout, - resblock, - resblock_kernel_sizes, - resblock_dilation_sizes, - upsample_rates, - upsample_initial_channel, - upsample_kernel_sizes, - spk_embed_dim, - # hop_length, - gin_channels=0, - use_sdp=True, - **kwargs - ): - super().__init__() - self.spec_channels = spec_channels - self.inter_channels = inter_channels - self.hidden_channels = hidden_channels - self.filter_channels = filter_channels - self.n_heads = n_heads - self.n_layers = n_layers - self.kernel_size = kernel_size - self.p_dropout = p_dropout - self.resblock = resblock - self.resblock_kernel_sizes = resblock_kernel_sizes - self.resblock_dilation_sizes = resblock_dilation_sizes - self.upsample_rates = upsample_rates - self.upsample_initial_channel = upsample_initial_channel - self.upsample_kernel_sizes = upsample_kernel_sizes - self.segment_size = segment_size - self.gin_channels = gin_channels - # self.hop_length = hop_length# - self.spk_embed_dim = spk_embed_dim - self.enc_p = TextEncoder256Sim( - inter_channels, - hidden_channels, - filter_channels, - n_heads, - n_layers, - kernel_size, - p_dropout, - ) - self.dec = GeneratorNSF( - inter_channels, - resblock, - resblock_kernel_sizes, - resblock_dilation_sizes, - upsample_rates, - upsample_initial_channel, - upsample_kernel_sizes, - gin_channels=gin_channels, - is_half=kwargs["is_half"], - ) - - self.flow = ResidualCouplingBlock( - inter_channels, hidden_channels, 5, 1, 3, gin_channels=gin_channels - ) - self.emb_g = nn.Embedding(self.spk_embed_dim, gin_channels) - print("gin_channels:", gin_channels, "self.spk_embed_dim:", self.spk_embed_dim) - - def remove_weight_norm(self): - self.dec.remove_weight_norm() - self.flow.remove_weight_norm() - self.enc_q.remove_weight_norm() - - def forward( - self, phone, phone_lengths, pitch, pitchf, y_lengths, ds - ): # y是spec不需要了现在 - g = self.emb_g(ds).unsqueeze(-1) # [b, 256, 1]##1是t,广播的 - x, x_mask = self.enc_p(phone, pitch, phone_lengths) - x = self.flow(x, x_mask, g=g, reverse=True) - z_slice, ids_slice = commons.rand_slice_segments( - x, y_lengths, self.segment_size - ) - - pitchf = commons.slice_segments2(pitchf, ids_slice, self.segment_size) - o = self.dec(z_slice, pitchf, g=g) - return o, ids_slice - - def infer( - self, phone, phone_lengths, pitch, pitchf, ds, max_len=None - ): # y是spec不需要了现在 - g = self.emb_g(ds).unsqueeze(-1) # [b, 256, 1]##1是t,广播的 - x, x_mask = self.enc_p(phone, pitch, phone_lengths) - x = self.flow(x, x_mask, g=g, reverse=True) - o = self.dec((x * x_mask)[:, :, :max_len], pitchf, g=g) - return o, o - - -class MultiPeriodDiscriminator(torch.nn.Module): - def __init__(self, use_spectral_norm=False): - super(MultiPeriodDiscriminator, self).__init__() - periods = [2, 3, 5, 7, 11, 17] - # periods = [3, 5, 7, 11, 17, 23, 37] - - discs = [DiscriminatorS(use_spectral_norm=use_spectral_norm)] - discs = discs + [ - DiscriminatorP(i, use_spectral_norm=use_spectral_norm) for i in periods - ] - self.discriminators = nn.ModuleList(discs) - - def forward(self, y, y_hat): - y_d_rs = [] # - y_d_gs = [] - fmap_rs = [] - fmap_gs = [] - for i, d in enumerate(self.discriminators): - y_d_r, fmap_r = d(y) - y_d_g, fmap_g = d(y_hat) - # for j in range(len(fmap_r)): - # print(i,j,y.shape,y_hat.shape,fmap_r[j].shape,fmap_g[j].shape) - y_d_rs.append(y_d_r) - y_d_gs.append(y_d_g) - fmap_rs.append(fmap_r) - fmap_gs.append(fmap_g) - - return y_d_rs, y_d_gs, fmap_rs, fmap_gs - - -class DiscriminatorS(torch.nn.Module): - def __init__(self, use_spectral_norm=False): - super(DiscriminatorS, self).__init__() - norm_f = weight_norm if use_spectral_norm == False else spectral_norm - self.convs = nn.ModuleList( - [ - norm_f(Conv1d(1, 16, 15, 1, padding=7)), - norm_f(Conv1d(16, 64, 41, 4, groups=4, padding=20)), - norm_f(Conv1d(64, 256, 41, 4, groups=16, padding=20)), - norm_f(Conv1d(256, 1024, 41, 4, groups=64, padding=20)), - norm_f(Conv1d(1024, 1024, 41, 4, groups=256, padding=20)), - norm_f(Conv1d(1024, 1024, 5, 1, padding=2)), - ] - ) - self.conv_post = norm_f(Conv1d(1024, 1, 3, 1, padding=1)) - - def forward(self, x): - fmap = [] - - for l in self.convs: - x = l(x) - x = F.leaky_relu(x, modules.LRELU_SLOPE) - fmap.append(x) - x = self.conv_post(x) - fmap.append(x) - x = torch.flatten(x, 1, -1) - - return x, fmap - - -class DiscriminatorP(torch.nn.Module): - def __init__(self, period, kernel_size=5, stride=3, use_spectral_norm=False): - super(DiscriminatorP, self).__init__() - self.period = period - self.use_spectral_norm = use_spectral_norm - norm_f = weight_norm if use_spectral_norm == False else spectral_norm - self.convs = nn.ModuleList( - [ - norm_f( - Conv2d( - 1, - 32, - (kernel_size, 1), - (stride, 1), - padding=(get_padding(kernel_size, 1), 0), - ) - ), - norm_f( - Conv2d( - 32, - 128, - (kernel_size, 1), - (stride, 1), - padding=(get_padding(kernel_size, 1), 0), - ) - ), - norm_f( - Conv2d( - 128, - 512, - (kernel_size, 1), - (stride, 1), - padding=(get_padding(kernel_size, 1), 0), - ) - ), - norm_f( - Conv2d( - 512, - 1024, - (kernel_size, 1), - (stride, 1), - padding=(get_padding(kernel_size, 1), 0), - ) - ), - norm_f( - Conv2d( - 1024, - 1024, - (kernel_size, 1), - 1, - padding=(get_padding(kernel_size, 1), 0), - ) - ), - ] - ) - self.conv_post = norm_f(Conv2d(1024, 1, (3, 1), 1, padding=(1, 0))) - - def forward(self, x): - fmap = [] - - # 1d to 2d - b, c, t = x.shape - if t % self.period != 0: # pad first - n_pad = self.period - (t % self.period) - x = F.pad(x, (0, n_pad), "reflect") - t = t + n_pad - x = x.view(b, c, t // self.period, self.period) - - for l in self.convs: - x = l(x) - x = F.leaky_relu(x, modules.LRELU_SLOPE) - fmap.append(x) - x = self.conv_post(x) - fmap.append(x) - x = torch.flatten(x, 1, -1) - - return x, fmap diff --git a/spaces/NATSpeech/PortaSpeech/data_gen/tts/base_binarizer.py b/spaces/NATSpeech/PortaSpeech/data_gen/tts/base_binarizer.py deleted file mode 100644 index 83efbb79152b8f64dbac41b29fe5b28317e142ff..0000000000000000000000000000000000000000 --- a/spaces/NATSpeech/PortaSpeech/data_gen/tts/base_binarizer.py +++ /dev/null @@ -1,225 +0,0 @@ -import json -import os -import random -import traceback -from functools import partial - -import numpy as np -from resemblyzer import VoiceEncoder -from tqdm import tqdm - -import utils.commons.single_thread_env # NOQA -from utils.audio import librosa_wav2spec -from utils.audio.align import get_mel2ph, mel2token_to_dur -from utils.audio.cwt import get_lf0_cwt, get_cont_lf0 -from utils.audio.pitch.utils import f0_to_coarse -from utils.audio.pitch_extractors import extract_pitch_simple -from utils.commons.hparams import hparams -from utils.commons.indexed_datasets import IndexedDatasetBuilder -from utils.commons.multiprocess_utils import multiprocess_run_tqdm -from utils.os_utils import remove_file, copy_file - -np.seterr(divide='ignore', invalid='ignore') - - -class BinarizationError(Exception): - pass - - -class BaseBinarizer: - def __init__(self, processed_data_dir=None): - if processed_data_dir is None: - processed_data_dir = hparams['processed_data_dir'] - self.processed_data_dir = processed_data_dir - self.binarization_args = hparams['binarization_args'] - self.items = {} - self.item_names = [] - - def load_meta_data(self): - processed_data_dir = self.processed_data_dir - items_list = json.load(open(f"{processed_data_dir}/metadata.json")) - for r in tqdm(items_list, desc='Loading meta data.'): - item_name = r['item_name'] - self.items[item_name] = r - self.item_names.append(item_name) - if self.binarization_args['shuffle']: - random.seed(1234) - random.shuffle(self.item_names) - - @property - def train_item_names(self): - range_ = self._convert_range(self.binarization_args['train_range']) - return self.item_names[range_[0]:range_[1]] - - @property - def valid_item_names(self): - range_ = self._convert_range(self.binarization_args['valid_range']) - return self.item_names[range_[0]:range_[1]] - - @property - def test_item_names(self): - range_ = self._convert_range(self.binarization_args['test_range']) - return self.item_names[range_[0]:range_[1]] - - def _convert_range(self, range_): - if range_[1] == -1: - range_[1] = len(self.item_names) - return range_ - - def meta_data(self, prefix): - if prefix == 'valid': - item_names = self.valid_item_names - elif prefix == 'test': - item_names = self.test_item_names - else: - item_names = self.train_item_names - for item_name in item_names: - yield self.items[item_name] - - def process(self): - self.load_meta_data() - os.makedirs(hparams['binary_data_dir'], exist_ok=True) - for fn in ['phone_set.json', 'word_set.json', 'spk_map.json']: - remove_file(f"{hparams['binary_data_dir']}/{fn}") - copy_file(f"{hparams['processed_data_dir']}/{fn}", f"{hparams['binary_data_dir']}/{fn}") - self.process_data('valid') - self.process_data('test') - self.process_data('train') - - def process_data(self, prefix): - data_dir = hparams['binary_data_dir'] - builder = IndexedDatasetBuilder(f'{data_dir}/{prefix}') - meta_data = list(self.meta_data(prefix)) - process_item = partial(self.process_item, binarization_args=self.binarization_args) - ph_lengths = [] - mel_lengths = [] - total_sec = 0 - items = [] - args = [{'item': item} for item in meta_data] - for item_id, item in multiprocess_run_tqdm(process_item, args, desc='Processing data'): - if item is not None: - items.append(item) - if self.binarization_args['with_spk_embed']: - args = [{'wav': item['wav']} for item in items] - for item_id, spk_embed in multiprocess_run_tqdm( - self.get_spk_embed, args, - init_ctx_func=lambda wid: {'voice_encoder': VoiceEncoder().cuda()}, num_workers=4, - desc='Extracting spk embed'): - items[item_id]['spk_embed'] = spk_embed - - for item in items: - if not self.binarization_args['with_wav'] and 'wav' in item: - del item['wav'] - builder.add_item(item) - mel_lengths.append(item['len']) - assert item['len'] > 0, (item['item_name'], item['txt'], item['mel2ph']) - if 'ph_len' in item: - ph_lengths.append(item['ph_len']) - total_sec += item['sec'] - builder.finalize() - np.save(f'{data_dir}/{prefix}_lengths.npy', mel_lengths) - if len(ph_lengths) > 0: - np.save(f'{data_dir}/{prefix}_ph_lengths.npy', ph_lengths) - print(f"| {prefix} total duration: {total_sec:.3f}s") - - @classmethod - def process_item(cls, item, binarization_args): - item['ph_len'] = len(item['ph_token']) - item_name = item['item_name'] - wav_fn = item['wav_fn'] - wav, mel = cls.process_audio(wav_fn, item, binarization_args) - try: - n_bos_frames, n_eos_frames = 0, 0 - if binarization_args['with_align']: - tg_fn = f"{hparams['processed_data_dir']}/mfa_outputs/{item_name}.TextGrid" - item['tg_fn'] = tg_fn - cls.process_align(tg_fn, item) - if binarization_args['trim_eos_bos']: - n_bos_frames = item['dur'][0] - n_eos_frames = item['dur'][-1] - T = len(mel) - item['mel'] = mel[n_bos_frames:T - n_eos_frames] - item['mel2ph'] = item['mel2ph'][n_bos_frames:T - n_eos_frames] - item['mel2word'] = item['mel2word'][n_bos_frames:T - n_eos_frames] - item['dur'] = item['dur'][1:-1] - item['dur_word'] = item['dur_word'][1:-1] - item['len'] = item['mel'].shape[0] - item['wav'] = wav[n_bos_frames * hparams['hop_size']:len(wav) - n_eos_frames * hparams['hop_size']] - if binarization_args['with_f0']: - cls.process_pitch(item, n_bos_frames, n_eos_frames) - except BinarizationError as e: - print(f"| Skip item ({e}). item_name: {item_name}, wav_fn: {wav_fn}") - return None - except Exception as e: - traceback.print_exc() - print(f"| Skip item. item_name: {item_name}, wav_fn: {wav_fn}") - return None - return item - - @classmethod - def process_audio(cls, wav_fn, res, binarization_args): - wav2spec_dict = librosa_wav2spec( - wav_fn, - fft_size=hparams['fft_size'], - hop_size=hparams['hop_size'], - win_length=hparams['win_size'], - num_mels=hparams['audio_num_mel_bins'], - fmin=hparams['fmin'], - fmax=hparams['fmax'], - sample_rate=hparams['audio_sample_rate'], - loud_norm=hparams['loud_norm']) - mel = wav2spec_dict['mel'] - wav = wav2spec_dict['wav'].astype(np.float16) - if binarization_args['with_linear']: - res['linear'] = wav2spec_dict['linear'] - res.update({'mel': mel, 'wav': wav, 'sec': len(wav) / hparams['audio_sample_rate'], 'len': mel.shape[0]}) - return wav, mel - - @staticmethod - def process_align(tg_fn, item): - ph = item['ph'] - mel = item['mel'] - ph_token = item['ph_token'] - if tg_fn is not None and os.path.exists(tg_fn): - mel2ph, dur = get_mel2ph(tg_fn, ph, mel, hparams['hop_size'], hparams['audio_sample_rate'], - hparams['binarization_args']['min_sil_duration']) - else: - raise BinarizationError(f"Align not found") - if np.array(mel2ph).max() - 1 >= len(ph_token): - raise BinarizationError( - f"Align does not match: mel2ph.max() - 1: {mel2ph.max() - 1}, len(phone_encoded): {len(ph_token)}") - item['mel2ph'] = mel2ph - item['dur'] = dur - - ph2word = item['ph2word'] - mel2word = [ph2word[p - 1] for p in item['mel2ph']] - item['mel2word'] = mel2word # [T_mel] - dur_word = mel2token_to_dur(mel2word, len(item['word_token'])) - item['dur_word'] = dur_word.tolist() # [T_word] - - @staticmethod - def process_pitch(item, n_bos_frames, n_eos_frames): - wav, mel = item['wav'], item['mel'] - f0 = extract_pitch_simple(item['wav']) - if sum(f0) == 0: - raise BinarizationError("Empty f0") - assert len(mel) == len(f0), (len(mel), len(f0)) - pitch_coarse = f0_to_coarse(f0) - item['f0'] = f0 - item['pitch'] = pitch_coarse - if hparams['binarization_args']['with_f0cwt']: - uv, cont_lf0_lpf = get_cont_lf0(f0) - logf0s_mean_org, logf0s_std_org = np.mean(cont_lf0_lpf), np.std(cont_lf0_lpf) - cont_lf0_lpf_norm = (cont_lf0_lpf - logf0s_mean_org) / logf0s_std_org - cwt_spec, scales = get_lf0_cwt(cont_lf0_lpf_norm) - item['cwt_spec'] = cwt_spec - item['cwt_mean'] = logf0s_mean_org - item['cwt_std'] = logf0s_std_org - - @staticmethod - def get_spk_embed(wav, ctx): - return ctx['voice_encoder'].embed_utterance(wav.astype(float)) - - @property - def num_workers(self): - return int(os.getenv('N_PROC', hparams.get('N_PROC', os.cpu_count()))) diff --git a/spaces/NCTCMumbai/NCTC/models/official/benchmark/transformer_benchmark.py b/spaces/NCTCMumbai/NCTC/models/official/benchmark/transformer_benchmark.py deleted file mode 100644 index e61201aa174af4882c6dbab28e10fe64d8cc1377..0000000000000000000000000000000000000000 --- a/spaces/NCTCMumbai/NCTC/models/official/benchmark/transformer_benchmark.py +++ /dev/null @@ -1,757 +0,0 @@ -# Copyright 2019 The TensorFlow Authors. All Rights Reserved. -# -# Licensed under the Apache License, Version 2.0 (the "License"); -# you may not use this file except in compliance with the License. -# You may obtain a copy of the License at -# -# http://www.apache.org/licenses/LICENSE-2.0 -# -# Unless required by applicable law or agreed to in writing, software -# distributed under the License is distributed on an "AS IS" BASIS, -# WITHOUT WARRANTIES OR CONDITIONS OF ANY KIND, either express or implied. -# See the License for the specific language governing permissions and -# limitations under the License. -# ============================================================================== -"""Executes Transformer w/Keras benchmark and accuracy tests.""" -from __future__ import absolute_import -from __future__ import division -from __future__ import print_function - -import os -import time - -from absl import flags -import tensorflow as tf -from official.benchmark import benchmark_wrappers -from official.benchmark import owner_utils -from official.benchmark.perfzero_benchmark import PerfZeroBenchmark -from official.nlp.transformer import misc -from official.nlp.transformer import transformer_main as transformer_main -from official.utils.flags import core as flags_core - -TRANSFORMER_EN2DE_DATA_DIR_NAME = 'wmt32k-en2de-official' -EN2DE_2014_BLEU_DATA_DIR_NAME = 'newstest2014' -FLAGS = flags.FLAGS -TMP_DIR = os.getenv('TMPDIR') - - -class TransformerBenchmark(PerfZeroBenchmark): - """Methods common to executing transformer w/keras tests. - - Code under test for the Transformer Keras models report the same data and - require the same FLAG setup. - """ - - def __init__(self, output_dir=None, default_flags=None, root_data_dir=None, - flag_methods=None, tpu=None): - root_data_dir = root_data_dir if root_data_dir else '' - - self.train_data_dir = os.path.join(root_data_dir, - TRANSFORMER_EN2DE_DATA_DIR_NAME) - - self.vocab_file = os.path.join(root_data_dir, - TRANSFORMER_EN2DE_DATA_DIR_NAME, - 'vocab.ende.32768') - - self.bleu_source = os.path.join(root_data_dir, - EN2DE_2014_BLEU_DATA_DIR_NAME, - 'newstest2014.en') - - self.bleu_ref = os.path.join(root_data_dir, - EN2DE_2014_BLEU_DATA_DIR_NAME, - 'newstest2014.de') - - if default_flags is None: - default_flags = {} - default_flags['data_dir'] = self.train_data_dir - default_flags['vocab_file'] = self.vocab_file - - super(TransformerBenchmark, self).__init__( - output_dir=output_dir, - default_flags=default_flags, - flag_methods=flag_methods, - tpu=tpu) - - @benchmark_wrappers.enable_runtime_flags - def _run_and_report_benchmark(self, - bleu_max=None, - bleu_min=None, - log_steps=None, - total_batch_size=None, - warmup=1): - """Report benchmark results by writing to local protobuf file. - - Args: - bleu_max: highest passing level for bleu score. - bleu_min: lowest passing level for bleu score. - log_steps: How often the log was created for stats['step_timestamp_log']. - total_batch_size: Global batch-size. - warmup: number of entries in stats['step_timestamp_log'] to ignore. - """ - start_time_sec = time.time() - task = transformer_main.TransformerTask(FLAGS) - stats = task.train() - wall_time_sec = time.time() - start_time_sec - - metrics = [] - if 'bleu_uncased' in stats: - if 'bleu_uncased_history' in stats: - bleu_uncased_best = max(stats['bleu_uncased_history'], - key=lambda x: x[1]) - metrics.append({'name': 'bleu_uncased', - 'value': bleu_uncased_best[1], - 'min_value': bleu_min, - 'max_value': bleu_max}) - metrics.append({'name': 'bleu_best_score_iteration', - 'value': bleu_uncased_best[0]}) - metrics.append({'name': 'bleu_uncased_last', - 'value': stats['bleu_uncased']}) - else: - metrics.append({'name': 'bleu_uncased', - 'value': stats['bleu_uncased'], - 'min_value': bleu_min, - 'max_value': bleu_max}) - - if (warmup and 'step_timestamp_log' in stats and - len(stats['step_timestamp_log']) > warmup + 1): - # first entry in the time_log is start of step 1. The rest of the - # entries are the end of each step recorded - time_log = stats['step_timestamp_log'] - elapsed = time_log[-1].timestamp - time_log[warmup].timestamp - num_examples = ( - total_batch_size * log_steps * (len(time_log) - warmup - 1)) - examples_per_sec = num_examples / elapsed - metrics.append({'name': 'exp_per_second', - 'value': examples_per_sec}) - - if 'avg_exp_per_second' in stats: - metrics.append({'name': 'avg_exp_per_second', - 'value': stats['avg_exp_per_second']}) - - if 'step_timestamp_log' in stats: - time_log = stats['step_timestamp_log'] - metrics.append({'name': 'startup_time', - 'value': time_log[0].timestamp - start_time_sec}) - - flags_str = flags_core.get_nondefault_flags_as_str() - self.report_benchmark(iters=-1, wall_time=wall_time_sec, metrics=metrics, - extras={'flags': flags_str}) - - -class TransformerBaseKerasAccuracy(TransformerBenchmark): - """Benchmark accuracy tests for Transformer Base model w/ Keras.""" - - def __init__(self, output_dir=None, root_data_dir=None, **kwargs): - """Benchmark accuracy tests for Transformer Base model w/ Keras. - - Args: - output_dir: directory where to output e.g. log files - root_data_dir: directory under which to look for dataset - **kwargs: arbitrary named arguments. This is needed to make the - constructor forward compatible in case PerfZero provides more - named arguments before updating the constructor. - """ - flag_methods = [misc.define_transformer_flags] - - super(TransformerBaseKerasAccuracy, self).__init__( - output_dir=output_dir, root_data_dir=root_data_dir, - flag_methods=flag_methods) - - def benchmark_1_gpu(self): - """Benchmark 1 gpu. - - The paper uses 8 GPUs and a much larger effective batch size, this is will - not converge to the 27.3 BLEU (uncased) SOTA. - """ - self._setup() - FLAGS.num_gpus = 1 - FLAGS.data_dir = self.train_data_dir - FLAGS.vocab_file = self.vocab_file - # Sets values directly to avoid validation check. - FLAGS['bleu_source'].value = self.bleu_source - FLAGS['bleu_ref'].value = self.bleu_ref - FLAGS.param_set = 'base' - FLAGS.batch_size = 2048 - FLAGS.train_steps = 1000 - FLAGS.steps_between_evals = 500 - FLAGS.model_dir = self._get_model_dir('benchmark_1_gpu') - # These bleu scores are based on test runs after at this limited - # number of steps and batch size after verifying SOTA at 8xV100s. - self._run_and_report_benchmark(total_batch_size=FLAGS.batch_size, - log_steps=FLAGS.log_steps, - bleu_min=25.3, - bleu_max=26) - - def benchmark_1_gpu_static_batch(self): - """Benchmark 1 gpu with static_batch. - - The paper uses 8 GPUs and a much larger effective batch size, this is will - not converge to the 27.3 BLEU (uncased) SOTA. - """ - self._setup() - FLAGS.num_gpus = 1 - FLAGS.data_dir = self.train_data_dir - FLAGS.vocab_file = self.vocab_file - # Sets values directly to avoid validation check. - FLAGS['bleu_source'].value = self.bleu_source - FLAGS['bleu_ref'].value = self.bleu_ref - FLAGS.param_set = 'base' - FLAGS.batch_size = 4096 - FLAGS.train_steps = 100000 - FLAGS.steps_between_evals = 5000 - FLAGS.static_batch = True - FLAGS.max_length = 64 - FLAGS.model_dir = self._get_model_dir('benchmark_1_gpu_static_batch') - # These bleu scores are based on test runs after at this limited - # number of steps and batch size after verifying SOTA at 8xV100s. - self._run_and_report_benchmark(total_batch_size=FLAGS.batch_size, - log_steps=FLAGS.log_steps, - bleu_min=25.3, - bleu_max=26) - - def benchmark_8_gpu(self): - """Benchmark 8 gpu. - - Should converge to 27.3 BLEU (uncased). This has not been confirmed yet. - """ - self._setup() - FLAGS.num_gpus = 8 - FLAGS.data_dir = self.train_data_dir - FLAGS.vocab_file = self.vocab_file - # Sets values directly to avoid validation check. - FLAGS['bleu_source'].value = self.bleu_source - FLAGS['bleu_ref'].value = self.bleu_ref - FLAGS.param_set = 'base' - FLAGS.batch_size = 4096*8 - FLAGS.train_steps = 100000 - FLAGS.steps_between_evals = 20000 - FLAGS.model_dir = self._get_model_dir('benchmark_8_gpu') - self._run_and_report_benchmark(total_batch_size=FLAGS.batch_size, - log_steps=FLAGS.log_steps, - bleu_min=27, - bleu_max=28) - - def benchmark_8_gpu_static_batch(self): - """Benchmark 8 gpu. - - Should converge to 27.3 BLEU (uncased). This has not been confirmed yet. - """ - self._setup() - FLAGS.num_gpus = 8 - FLAGS.data_dir = self.train_data_dir - FLAGS.vocab_file = self.vocab_file - # Sets values directly to avoid validation check. - FLAGS['bleu_source'].value = self.bleu_source - FLAGS['bleu_ref'].value = self.bleu_ref - FLAGS.param_set = 'base' - FLAGS.batch_size = 4096*8 - FLAGS.train_steps = 100000 - FLAGS.static_batch = True - FLAGS.max_length = 64 - FLAGS.steps_between_evals = 5000 - FLAGS.model_dir = self._get_model_dir('benchmark_8_gpu_static_batch') - self._run_and_report_benchmark(total_batch_size=FLAGS.batch_size, - log_steps=FLAGS.log_steps, - bleu_min=27, - bleu_max=28) - - -class TransformerBigKerasAccuracy(TransformerBenchmark): - """Benchmark accuracy tests for Transformer Big model w/ Keras.""" - - def __init__(self, output_dir=None, root_data_dir=None, **kwargs): - """Benchmark accuracy tests for Transformer Big model w/ Keras. - - Args: - output_dir: directory where to output e.g. log files - root_data_dir: directory under which to look for dataset - **kwargs: arbitrary named arguments. This is needed to make the - constructor forward compatible in case PerfZero provides more - named arguments before updating the constructor. - """ - flag_methods = [misc.define_transformer_flags] - - super(TransformerBigKerasAccuracy, self).__init__( - output_dir=output_dir, root_data_dir=root_data_dir, - flag_methods=flag_methods) - - def benchmark_8_gpu(self): - """Benchmark 8 gpu. - - Over 6 runs with eval every 20K steps the average highest value was 28.195 - (bleu uncased). 28.424 was the highest and 27.96 the lowest. The values are - the highest value seen during a run and occurred at a median of iteration 9. - Iterations are not epochs, an iteration is a number of steps between evals. - """ - self._setup() - FLAGS.num_gpus = 8 - FLAGS.data_dir = self.train_data_dir - FLAGS.vocab_file = self.vocab_file - # Sets values directly to avoid validation check. - FLAGS['bleu_source'].value = self.bleu_source - FLAGS['bleu_ref'].value = self.bleu_ref - FLAGS.param_set = 'big' - FLAGS.batch_size = 3072*8 - FLAGS.train_steps = 20000 * 12 - FLAGS.steps_between_evals = 20000 - FLAGS.model_dir = self._get_model_dir('benchmark_8_gpu') - self._run_and_report_benchmark(total_batch_size=FLAGS.batch_size, - log_steps=FLAGS.log_steps, - bleu_min=27.9, - bleu_max=29.2) - - def benchmark_8_gpu_static_batch(self): - """Benchmark 8 gpu. - - Should converge to 28.4 BLEU (uncased). This has not be verified yet." - """ - self._setup() - FLAGS.num_gpus = 8 - FLAGS.data_dir = self.train_data_dir - FLAGS.vocab_file = self.vocab_file - # Sets values directly to avoid validation check. - FLAGS['bleu_source'].value = self.bleu_source - FLAGS['bleu_ref'].value = self.bleu_ref - FLAGS.param_set = 'big' - FLAGS.batch_size = 3072*8 - FLAGS.static_batch = True - FLAGS.max_length = 64 - FLAGS.train_steps = 20000 * 12 - FLAGS.steps_between_evals = 20000 - FLAGS.model_dir = self._get_model_dir('benchmark_8_gpu_static_batch') - self._run_and_report_benchmark(total_batch_size=FLAGS.batch_size, - log_steps=FLAGS.log_steps, - bleu_min=28, - bleu_max=29.2) - - def benchmark_8_gpu_fp16(self): - """Benchmark 8 gpu with dynamic batch and fp16. - - Over 6 runs with eval every 20K steps the average highest value was 28.247 - (bleu uncased). 28.424 was the highest and 28.09 the lowest. The values are - the highest value seen during a run and occurred at a median of iteration - 11. While this could be interpreted as worse than FP32, if looking at the - first iteration at which 28 is passed FP16 performs equal and possibly - better. Although not part of the initial test runs, the highest value - recorded with the arguments below was 28.9 at iteration 12. Iterations are - not epochs, an iteration is a number of steps between evals. - """ - self._setup() - FLAGS.num_gpus = 8 - FLAGS.dtype = 'fp16' - FLAGS.data_dir = self.train_data_dir - FLAGS.vocab_file = self.vocab_file - # Sets values directly to avoid validation check. - FLAGS['bleu_source'].value = self.bleu_source - FLAGS['bleu_ref'].value = self.bleu_ref - FLAGS.param_set = 'big' - FLAGS.batch_size = 3072*8 - FLAGS.train_steps = 20000 * 12 - FLAGS.steps_between_evals = 20000 - FLAGS.model_dir = self._get_model_dir('benchmark_8_gpu_fp16') - self._run_and_report_benchmark(total_batch_size=FLAGS.batch_size, - log_steps=FLAGS.log_steps, - bleu_min=28, - bleu_max=29.2) - - def benchmark_8_gpu_fp16_amp(self): - """Benchmark 8 gpu with dynamic batch and fp16 with automatic mixed precision. - - Should converge to 28.4 BLEU (uncased). This has not be verified yet." - """ - self._setup() - FLAGS.num_gpus = 8 - FLAGS.dtype = 'fp16' - FLAGS.fp16_implementation = 'graph_rewrite' - FLAGS.data_dir = self.train_data_dir - FLAGS.vocab_file = self.vocab_file - # Sets values directly to avoid validation check. - FLAGS['bleu_source'].value = self.bleu_source - FLAGS['bleu_ref'].value = self.bleu_ref - FLAGS.param_set = 'big' - FLAGS.batch_size = 3072*8 - FLAGS.train_steps = 20000 * 12 - FLAGS.steps_between_evals = 20000 - FLAGS.model_dir = self._get_model_dir('benchmark_8_gpu_fp16_amp') - self._run_and_report_benchmark(total_batch_size=FLAGS.batch_size, - log_steps=FLAGS.log_steps, - bleu_min=28, - bleu_max=29) - - def benchmark_8_gpu_static_batch_fp16(self): - """Benchmark 8 gpu with static batch and fp16. - - Should converge to 28.4 BLEU (uncased). This has not be verified yet." - """ - self._setup() - FLAGS.num_gpus = 8 - FLAGS.dtype = 'fp16' - FLAGS.data_dir = self.train_data_dir - FLAGS.vocab_file = self.vocab_file - # Sets values directly to avoid validation check. - FLAGS['bleu_source'].value = self.bleu_source - FLAGS['bleu_ref'].value = self.bleu_ref - FLAGS.param_set = 'big' - FLAGS.batch_size = 3072*8 - FLAGS.static_batch = True - FLAGS.max_length = 64 - FLAGS.train_steps = 400000 - FLAGS.steps_between_evals = 20000 - FLAGS.model_dir = self._get_model_dir('benchmark_8_gpu_static_batch_fp16') - self._run_and_report_benchmark(total_batch_size=FLAGS.batch_size, - log_steps=FLAGS.log_steps, - bleu_min=28, - bleu_max=29.2) - - def benchmark_xla_8_gpu_static_batch_fp16(self): - """Benchmark 8 gpu with static batch, XLA, and FP16. - - Should converge to 28.4 BLEU (uncased). This has not be verified yet." - """ - self._setup() - FLAGS.num_gpus = 8 - FLAGS.dtype = 'fp16' - FLAGS.enable_xla = True - FLAGS.data_dir = self.train_data_dir - FLAGS.vocab_file = self.vocab_file - # Sets values directly to avoid validation check. - FLAGS['bleu_source'].value = self.bleu_source - FLAGS['bleu_ref'].value = self.bleu_ref - FLAGS.param_set = 'big' - FLAGS.batch_size = 3072*8 - FLAGS.static_batch = True - FLAGS.max_length = 64 - FLAGS.train_steps = 400000 - FLAGS.steps_between_evals = 20000 - FLAGS.model_dir = self._get_model_dir( - 'benchmark_xla_8_gpu_static_batch_fp16') - self._run_and_report_benchmark(total_batch_size=FLAGS.batch_size, - log_steps=FLAGS.log_steps, - bleu_min=28, - bleu_max=29.2) - - -class TransformerKerasBenchmark(TransformerBenchmark): - """Benchmarks for Transformer (Base and Big) using Keras.""" - - def __init__(self, output_dir=None, default_flags=None, - root_data_dir=None, batch_per_gpu=4096, tpu=None): - """Initialize. - - Args: - output_dir: Based directory for saving artifacts, e.g. checkpoints. - default_flags: default flags to use for all tests. - root_data_dir: root directory for data, e.g. training. - batch_per_gpu: batch size to use per gpu. - tpu: Target TPU to use. - """ - flag_methods = [misc.define_transformer_flags] - self.batch_per_gpu = batch_per_gpu - - super(TransformerKerasBenchmark, self).__init__( - output_dir=output_dir, - default_flags=default_flags, - root_data_dir=root_data_dir, - flag_methods=flag_methods, - tpu=tpu) - - def benchmark_1_gpu_no_dist_strat(self): - """Benchmark 1 gpu without distribution strategy.""" - self._setup() - FLAGS.num_gpus = 1 - FLAGS.distribution_strategy = 'off' - FLAGS.batch_size = self.batch_per_gpu - FLAGS.model_dir = self._get_model_dir('benchmark_1_gpu_no_dist_strat') - self._run_and_report_benchmark(total_batch_size=FLAGS.batch_size, - log_steps=FLAGS.log_steps) - - def benchmark_1_gpu_no_dist_strat_static_batch(self): - """Benchmark 1 gpu without distribution strategy with static batch.""" - self._setup() - FLAGS.num_gpus = 1 - FLAGS.distribution_strategy = 'off' - FLAGS.batch_size = self.batch_per_gpu - FLAGS.model_dir = self._get_model_dir('benchmark_1_gpu_no_ds_sb') - FLAGS.static_batch = True - FLAGS.max_length = 64 - self._run_and_report_benchmark(total_batch_size=FLAGS.batch_size, - log_steps=FLAGS.log_steps) - - def benchmark_1_gpu(self): - """Benchmark 1 gpu.""" - self._setup() - FLAGS.num_gpus = 1 - FLAGS.batch_size = self.batch_per_gpu - FLAGS.model_dir = self._get_model_dir('benchmark_1_gpu') - self._run_and_report_benchmark(total_batch_size=FLAGS.batch_size, - log_steps=FLAGS.log_steps) - - def benchmark_1_gpu_fp16(self): - """Benchmark 1 gpu FP16.""" - self._setup() - FLAGS.num_gpus = 1 - FLAGS.batch_size = self.batch_per_gpu - FLAGS.model_dir = self._get_model_dir('benchmark_1_gpu_fp16') - FLAGS.dtype = 'fp16' - self._run_and_report_benchmark(total_batch_size=FLAGS.batch_size, - log_steps=FLAGS.log_steps) - - def benchmark_xla_1_gpu(self): - """Benchmark 1 gpu w/xla.""" - self._setup() - FLAGS.num_gpus = 1 - FLAGS.batch_size = self.batch_per_gpu - FLAGS.model_dir = self._get_model_dir('benchmark_xla_1_gpu') - FLAGS.enable_xla = True - self._run_and_report_benchmark(total_batch_size=FLAGS.batch_size, - log_steps=FLAGS.log_steps) - - def benchmark_xla_1_gpu_fp16(self): - """Benchmark 1 gpu w/xla and FP16.""" - self._setup() - FLAGS.num_gpus = 1 - FLAGS.batch_size = self.batch_per_gpu - FLAGS.model_dir = self._get_model_dir('benchmark_xla_1_gpu_fp16') - FLAGS.enable_xla = True - FLAGS.dtype = 'fp16' - self._run_and_report_benchmark(total_batch_size=FLAGS.batch_size, - log_steps=FLAGS.log_steps) - - def benchmark_1_gpu_static_batch(self): - """Benchmark 1 gpu with static batch.""" - self._setup() - FLAGS.num_gpus = 1 - FLAGS.batch_size = self.batch_per_gpu - FLAGS.model_dir = self._get_model_dir('benchmark_1_gpu_static_batch') - FLAGS.static_batch = True - FLAGS.max_length = 64 - self._run_and_report_benchmark(total_batch_size=FLAGS.batch_size, - log_steps=FLAGS.log_steps) - - def benchmark_xla_1_gpu_static_batch(self): - """Benchmark 1 gpu with static batch w/xla.""" - self._setup() - FLAGS.num_gpus = 1 - FLAGS.batch_size = self.batch_per_gpu - FLAGS.model_dir = self._get_model_dir('benchmark_xla_1_gpu_static_batch') - FLAGS.static_batch = True - FLAGS.max_length = 64 - FLAGS.enable_xla = True - self._run_and_report_benchmark(total_batch_size=FLAGS.batch_size, - log_steps=FLAGS.log_steps) - - def benchmark_1_gpu_static_batch_fp16(self): - """Benchmark 1 gpu with static batch FP16.""" - self._setup() - FLAGS.num_gpus = 1 - FLAGS.batch_size = self.batch_per_gpu - FLAGS.model_dir = self._get_model_dir( - 'benchmark_1_gpu_static_batch_fp16') - FLAGS.static_batch = True - FLAGS.max_length = 64 - FLAGS.dtype = 'fp16' - self._run_and_report_benchmark(total_batch_size=FLAGS.batch_size, - log_steps=FLAGS.log_steps) - - def benchmark_xla_1_gpu_static_batch_fp16(self): - """Benchmark 1 gpu with static batch w/xla and FP16.""" - self._setup() - FLAGS.num_gpus = 1 - FLAGS.batch_size = self.batch_per_gpu - FLAGS.model_dir = self._get_model_dir( - 'benchmark_xla_1_gpu_static_batch_fp16') - FLAGS.static_batch = True - FLAGS.max_length = 64 - FLAGS.enable_xla = True - FLAGS.dtype = 'fp16' - self._run_and_report_benchmark(total_batch_size=FLAGS.batch_size, - log_steps=FLAGS.log_steps) - - def benchmark_8_gpu(self): - """Benchmark 8 gpu.""" - self._setup() - FLAGS.num_gpus = 8 - FLAGS.batch_size = self.batch_per_gpu * 8 - FLAGS.model_dir = self._get_model_dir('benchmark_8_gpu') - self._run_and_report_benchmark(total_batch_size=FLAGS.batch_size, - log_steps=FLAGS.log_steps) - - def benchmark_8_gpu_fp16(self): - """Benchmark 8 gpu FP16.""" - self._setup() - FLAGS.num_gpus = 8 - FLAGS.dtype = 'fp16' - FLAGS.batch_size = self.batch_per_gpu * 8 - FLAGS.model_dir = self._get_model_dir('benchmark_8_gpu_fp16') - self._run_and_report_benchmark(total_batch_size=FLAGS.batch_size, - log_steps=FLAGS.log_steps) - - def benchmark_xla_8_gpu(self): - """Benchmark 8 gpu w/xla.""" - self._setup() - FLAGS.num_gpus = 8 - FLAGS.enable_xla = True - FLAGS.batch_size = self.batch_per_gpu * 8 - FLAGS.model_dir = self._get_model_dir('benchmark_xla_8_gpu') - self._run_and_report_benchmark(total_batch_size=FLAGS.batch_size, - log_steps=FLAGS.log_steps) - - def benchmark_xla_8_gpu_fp16(self): - """Benchmark 8 gpu w/xla and FP16.""" - self._setup() - FLAGS.num_gpus = 8 - FLAGS.enable_xla = True - FLAGS.dtype = 'fp16' - FLAGS.batch_size = self.batch_per_gpu * 8 - FLAGS.model_dir = self._get_model_dir('benchmark_xla_8_gpu_fp16') - self._run_and_report_benchmark(total_batch_size=FLAGS.batch_size, - log_steps=FLAGS.log_steps) - - def benchmark_8_gpu_static_batch(self): - """Benchmark 8 gpu with static batch.""" - self._setup() - FLAGS.num_gpus = 8 - FLAGS.batch_size = self.batch_per_gpu * 8 - FLAGS.model_dir = self._get_model_dir('benchmark_8_gpu_static_batch') - FLAGS.static_batch = True - FLAGS.max_length = 64 - self._run_and_report_benchmark(total_batch_size=FLAGS.batch_size, - log_steps=FLAGS.log_steps) - - def benchmark_8_gpu_static_batch_fp16(self): - """Benchmark 8 gpu with static batch FP16.""" - self._setup() - FLAGS.num_gpus = 8 - FLAGS.dtype = 'fp16' - FLAGS.batch_size = self.batch_per_gpu * 8 - FLAGS.model_dir = self._get_model_dir( - 'benchmark_8_gpu_static_batch_fp16') - FLAGS.static_batch = True - FLAGS.max_length = 64 - self._run_and_report_benchmark(total_batch_size=FLAGS.batch_size, - log_steps=FLAGS.log_steps) - - def benchmark_xla_8_gpu_static_batch(self): - """Benchmark 8 gpu with static batch w/xla.""" - self._setup() - FLAGS.num_gpus = 8 - FLAGS.enable_xla = True - FLAGS.batch_size = self.batch_per_gpu * 8 - FLAGS.model_dir = self._get_model_dir('benchmark_xla_8_gpu_static_batch') - FLAGS.static_batch = True - FLAGS.max_length = 64 - self._run_and_report_benchmark(total_batch_size=FLAGS.batch_size, - log_steps=FLAGS.log_steps) - - def benchmark_xla_8_gpu_static_batch_fp16(self): - """Benchmark 8 gpu with static batch w/xla and FP16.""" - self._setup() - FLAGS.num_gpus = 8 - FLAGS.enable_xla = True - FLAGS.dtype = 'fp16' - FLAGS.batch_size = self.batch_per_gpu * 8 - FLAGS.model_dir = self._get_model_dir( - 'benchmark_xla_8_gpu_static_batch_fp16') - FLAGS.static_batch = True - FLAGS.max_length = 64 - self._run_and_report_benchmark(total_batch_size=FLAGS.batch_size, - log_steps=FLAGS.log_steps) - - -class TransformerBaseKerasBenchmarkReal(TransformerKerasBenchmark): - """Transformer based version real data benchmark tests.""" - - def __init__(self, output_dir=TMP_DIR, root_data_dir=TMP_DIR, **kwargs): - def_flags = {} - def_flags['param_set'] = 'base' - def_flags['train_steps'] = 50 - def_flags['log_steps'] = 10 - - super(TransformerBaseKerasBenchmarkReal, self).__init__( - output_dir=output_dir, default_flags=def_flags, - root_data_dir=root_data_dir, batch_per_gpu=4096) - - -class TransformerBigKerasBenchmarkReal(TransformerKerasBenchmark): - """Transformer based version real data benchmark tests.""" - - def __init__(self, output_dir=TMP_DIR, root_data_dir=TMP_DIR, - tpu=None, **kwargs): - def_flags = {} - def_flags['param_set'] = 'big' - def_flags['train_steps'] = 50 - def_flags['log_steps'] = 10 - - super(TransformerBigKerasBenchmarkReal, self).__init__( - output_dir=output_dir, default_flags=def_flags, - root_data_dir=root_data_dir, batch_per_gpu=3072, - tpu=tpu) - - def benchmark_2x2_tpu(self): - """Port of former snaggletooth transformer_big model on 2x2.""" - self._setup() - FLAGS.model_dir = self._get_model_dir('benchmark_2x2_tpu') - FLAGS.train_steps = 300 - FLAGS.log_steps = 150 - FLAGS.steps_between_evals = 150 - FLAGS.distribution_strategy = 'tpu' - FLAGS.static_batch = True - FLAGS.use_ctl = True - FLAGS.batch_size = 6144 - FLAGS.max_length = 64 - FLAGS.decode_batch_size = 32 - FLAGS.decode_max_length = 97 - FLAGS.padded_decode = True - FLAGS.enable_checkpointing = False - - self._run_and_report_benchmark( - total_batch_size=FLAGS.batch_size, - log_steps=FLAGS.log_steps) - - def benchmark_4x4_tpu(self): - """Port of former GCP transformer_big model on 4x4.""" - self._setup() - FLAGS.model_dir = self._get_model_dir('benchmark_4x4_tpu') - FLAGS.train_steps = 300 - FLAGS.log_steps = 150 - FLAGS.steps_between_evals = 150 - FLAGS.distribution_strategy = 'tpu' - FLAGS.static_batch = True - FLAGS.use_ctl = True - FLAGS.batch_size = 24576 - FLAGS.max_length = 64 - FLAGS.decode_batch_size = 32 - FLAGS.decode_max_length = 97 - FLAGS.padded_decode = True - FLAGS.enable_checkpointing = False - - self._run_and_report_benchmark( - total_batch_size=FLAGS.batch_size, - log_steps=FLAGS.log_steps) - - @owner_utils.Owner('tf-graph-compiler') - def benchmark_4x4_tpu_mlir(self): - """Run transformer_big model on 4x4 with the MLIR Bridge enabled.""" - self._setup() - FLAGS.model_dir = self._get_model_dir('benchmark_4x4_tpu') - FLAGS.train_steps = 300 - FLAGS.log_steps = 150 - FLAGS.steps_between_evals = 150 - FLAGS.distribution_strategy = 'tpu' - FLAGS.static_batch = True - FLAGS.use_ctl = True - FLAGS.batch_size = 24576 - FLAGS.max_length = 64 - FLAGS.decode_batch_size = 32 - FLAGS.decode_max_length = 97 - FLAGS.padded_decode = True - FLAGS.enable_checkpointing = False - tf.config.experimental.enable_mlir_bridge() - - self._run_and_report_benchmark( - total_batch_size=FLAGS.batch_size, - log_steps=FLAGS.log_steps) - - -if __name__ == '__main__': - tf.test.main() diff --git a/spaces/NewtonKimathi/Sepsis_Prediction_FastApi/README.md b/spaces/NewtonKimathi/Sepsis_Prediction_FastApi/README.md deleted file mode 100644 index 35c66008f1a0e44c2e7f382aa2284410eb0f7e85..0000000000000000000000000000000000000000 --- a/spaces/NewtonKimathi/Sepsis_Prediction_FastApi/README.md +++ /dev/null @@ -1,20 +0,0 @@ ---- -metadata: -title: Sepsis Prediction Fast API -sdk: docker -emoji: 👁 -colorFrom: red -colorTo: blue -pinned: false -app_file: main.py -app_port: 8000 ---- - - -Here is the link to directly access the API: here. Access the documentation here. - -To direcly access your API hosted on HuggingFace you should use the URL follow this format : https://MT3: Multi-Task Multitrack Music Transcription | Github Repo
" - -examples=[['download.wav']] - -gr.Interface( - inference, - gr.inputs.Audio(type="filepath", label="Input"), - [gr.outputs.File(label="Output")], - title=title, - description=description, - article=article, - examples=examples, - allow_flagging=False, - allow_screenshot=False, - enable_queue=True - ).launch() \ No newline at end of file diff --git a/spaces/akhaliq/deeplab2/model/encoder/axial_resnet_instances.py b/spaces/akhaliq/deeplab2/model/encoder/axial_resnet_instances.py deleted file mode 100644 index a110c11cd9a97aec27be98b85b5136af291004ef..0000000000000000000000000000000000000000 --- a/spaces/akhaliq/deeplab2/model/encoder/axial_resnet_instances.py +++ /dev/null @@ -1,493 +0,0 @@ -# coding=utf-8 -# Copyright 2021 The Deeplab2 Authors. -# -# Licensed under the Apache License, Version 2.0 (the "License"); -# you may not use this file except in compliance with the License. -# You may obtain a copy of the License at -# -# http://www.apache.org/licenses/LICENSE-2.0 -# -# Unless required by applicable law or agreed to in writing, software -# distributed under the License is distributed on an "AS IS" BASIS, -# WITHOUT WARRANTIES OR CONDITIONS OF ANY KIND, either express or implied. -# See the License for the specific language governing permissions and -# limitations under the License. - -"""Contains Axial-ResNet model instances for Axial-DeepLab and MaX-DeepLab. - -Reference: - Axial-Deeplab: Stand-Alone Axial-Attention for Panoptic Segmentation, - ECCV 2020 Spotlight. https://arxiv.org/abs/2003.07853 - Huiyu Wang, Yukun Zhu, Bradley Green, Hartwig Adam, Alan Yuille, - Liang-Chieh Chen. - MaX-DeepLab: "End-to-End Panoptic Segmentation with Mask Transformers", - CVPR 2021. https://arxiv.org/abs/2012.00759 - Huiyu Wang, Yukun Zhu, Hartwig Adam, Alan Yuille, Liang-Chieh Chen. -""" - -import abc -import collections.abc -import copy - -from absl import logging -import tensorflow as tf - -from deeplab2.model.encoder import axial_resnet - - -def _get_default_config(): - """Gets the default config for Axial-ResNets.""" - # The default config dictionary for an Axial-ResNet is the MaX-DeepLab-S - # architecture for panoptic segmentation. This default config dictionary also - # exactly matches the default arguments of the functions. - default_config = { - 'num_blocks': [3, 4, 6, 3], - 'backbone_layer_multiplier': 1.0, - 'width_multiplier': 1.0, - 'stem_width_multiplier': 1.0, - 'output_stride': 16, - 'classification_mode': False, - 'backbone_type': 'resnet_beta', - 'use_axial_beyond_stride': 16, - 'backbone_use_transformer_beyond_stride': 32, - 'extra_decoder_use_transformer_beyond_stride': 32, - 'backbone_decoder_num_stacks': 0, - 'backbone_decoder_blocks_per_stage': 1, - 'extra_decoder_num_stacks': 0, - 'extra_decoder_blocks_per_stage': 1, - 'max_num_mask_slots': 128, - 'num_mask_slots': 128, - 'memory_channels': 256, - 'base_transformer_expansion': 1.0, - 'global_feed_forward_network_channels': 256, - 'high_resolution_output_stride': 4, - 'activation': 'relu', - 'block_group_config': { - 'attention_bottleneck_expansion': 2, - 'drop_path_keep_prob': 0.8, - 'drop_path_beyond_stride': 16, - 'drop_path_schedule': 'constant', - 'positional_encoding_type': None, - 'use_global_beyond_stride': 0, - 'use_sac_beyond_stride': 0, - 'use_squeeze_and_excite': False, - 'conv_use_recompute_grad': False, - 'axial_use_recompute_grad': True, - 'recompute_within_stride': 0, - 'transformer_use_recompute_grad': False, - 'axial_layer_config': { - 'query_shape': (129, 129), - 'key_expansion': 1, - 'value_expansion': 2, - 'memory_flange': (32, 32), - 'double_global_attention': False, - 'num_heads': 8, - 'use_query_rpe_similarity': True, - 'use_key_rpe_similarity': True, - 'use_content_similarity': True, - 'retrieve_value_rpe': True, - 'retrieve_value_content': True, - 'initialization_std_for_query_key_rpe': 1.0, - 'initialization_std_for_value_rpe': 1.0, - 'self_attention_activation': 'softmax', - }, - 'dual_path_transformer_layer_config': { - 'num_heads': 8, - 'bottleneck_expansion': 2, - 'key_expansion': 1, - 'value_expansion': 2, - 'feed_forward_network_channels': 2048, - 'use_memory_self_attention': True, - 'use_pixel2memory_feedback_attention': True, - 'transformer_activation': 'softmax', - }, - }, - 'bn_layer': tf.keras.layers.BatchNormalization, - 'conv_kernel_weight_decay': 0.0, - } - return default_config - - -def override(config_dict, override_dict): - """Recursively overrides a config dict with another.""" - output_dict = copy.deepcopy(config_dict) - for key, value in override_dict.items(): - if isinstance(value, collections.abc.Mapping): - output_dict[key] = override(config_dict.get(key, {}), value) - else: - output_dict[key] = value - return output_dict - - -class AxialResNetInstance(axial_resnet.AxialResNet): - """A base Axial-ResNet model.""" - - @classmethod - @abc.abstractmethod - def _get_config(cls): - pass - - def __init__(self, name, **kwargs): - """Builds an Axial-ResNet model.""" - # Get the config of the current model. - current_config = self._get_config() - - # Override the default config with the current config. This line can be - # omitted because the default config equals the default arguments of the - # functions that build the model. But we make all the configs explicit here. - current_config = override(_get_default_config(), current_config) - - # Finally, override the current model config with keyword arguments. In this - # way, we still respect arguments passed as keyword arguments, such as - # classification_mode, output_stride, etc. - current_config = override(current_config, kwargs) - logging.info('Axial-ResNet final config: %s', current_config) - super(AxialResNetInstance, self).__init__(name, **current_config) - - -class MaXDeepLabS(AxialResNetInstance): - """MaX-DeepLab-S for panoptic segmentation. - - Reference: - Axial-Deeplab: Stand-Alone Axial-Attention for Panoptic Segmentation, - ECCV 2020 Spotlight. https://arxiv.org/abs/2003.07853 - Huiyu Wang, Yukun Zhu, Bradley Green, Hartwig Adam, Alan Yuille, - Liang-Chieh Chen. - MaX-DeepLab: "End-to-End Panoptic Segmentation with Mask Transformers", - CVPR 2021. https://arxiv.org/abs/2012.00759 - Huiyu Wang, Yukun Zhu, Hartwig Adam, Alan Yuille, Liang-Chieh Chen. - """ - - @classmethod - def _get_config(cls): - # Return an empty dictionary as the default values are all set for - # MaX-DeepLab-S. - return {} - - -class MaXDeepLabL(AxialResNetInstance): - """MaX-DeepLab-L for panoptic segmentation. - - Reference: - Axial-Deeplab: Stand-Alone Axial-Attention for Panoptic Segmentation, - ECCV 2020 Spotlight. https://arxiv.org/abs/2003.07853 - Huiyu Wang, Yukun Zhu, Bradley Green, Hartwig Adam, Alan Yuille, - Liang-Chieh Chen. - MaX-DeepLab: "End-to-End Panoptic Segmentation with Mask Transformers", - CVPR 2021. https://arxiv.org/abs/2012.00759 - Huiyu Wang, Yukun Zhu, Hartwig Adam, Alan Yuille, Liang-Chieh Chen. - """ - - @classmethod - def _get_config(cls): - return { - 'num_blocks': [3, 6, 3, 3], - 'backbone_type': 'wider_resnet', - 'backbone_use_transformer_beyond_stride': 16, - 'extra_decoder_use_transformer_beyond_stride': 16, - 'backbone_decoder_num_stacks': 1, - 'extra_decoder_num_stacks': 1, - 'extra_decoder_blocks_per_stage': 3, - 'memory_channels': 512, - 'base_transformer_expansion': 2.0, - 'global_feed_forward_network_channels': 512, - 'block_group_config': { - 'attention_bottleneck_expansion': 4, - 'drop_path_beyond_stride': 4, - 'axial_layer_config': { - 'key_expansion': 2, - 'value_expansion': 4, - }, - }, - } - - -class MaXDeepLabSBackbone(MaXDeepLabS): - """MaX-DeepLab-S backbone for image classification pretraining. - - Reference: - Axial-Deeplab: Stand-Alone Axial-Attention for Panoptic Segmentation, - ECCV 2020 Spotlight. https://arxiv.org/abs/2003.07853 - Huiyu Wang, Yukun Zhu, Bradley Green, Hartwig Adam, Alan Yuille, - Liang-Chieh Chen. - MaX-DeepLab: "End-to-End Panoptic Segmentation with Mask Transformers", - CVPR 2021. https://arxiv.org/abs/2012.00759 - Huiyu Wang, Yukun Zhu, Hartwig Adam, Alan Yuille, Liang-Chieh Chen. - """ - - @classmethod - def _get_config(cls): - base_config = super(MaXDeepLabSBackbone, cls)._get_config() - # Override the config of MaXDeepLabS. - override_config = { - 'classification_mode': True, - # The transformer blocks are not ImageNet pretrained. They are randomly - # initialized and trained from scratch for panoptic segmentation. - 'backbone_use_transformer_beyond_stride': 0, - } - return override(base_config, override_config) - - -class MaXDeepLabLBackbone(MaXDeepLabL): - """MaX-DeepLab-L backbone for image classification pretraining. - - Reference: - Axial-Deeplab: Stand-Alone Axial-Attention for Panoptic Segmentation, - ECCV 2020 Spotlight. https://arxiv.org/abs/2003.07853 - Huiyu Wang, Yukun Zhu, Bradley Green, Hartwig Adam, Alan Yuille, - Liang-Chieh Chen. - MaX-DeepLab: "End-to-End Panoptic Segmentation with Mask Transformers", - CVPR 2021. https://arxiv.org/abs/2012.00759 - Huiyu Wang, Yukun Zhu, Hartwig Adam, Alan Yuille, Liang-Chieh Chen. - """ - - @classmethod - def _get_config(cls): - base_config = super(MaXDeepLabLBackbone, cls)._get_config() - # Override the config of MaXDeepLabL. - override_config = { - 'classification_mode': True, - # The transformer blocks are not ImageNet pretrained. They are randomly - # initialized and trained from scratch for panoptic segmentation. - 'backbone_use_transformer_beyond_stride': 0, - } - return override(base_config, override_config) - - -class ResNet50(AxialResNetInstance): - """A ResNet-50 instance. - - Note that the implementation is different from the original ResNet-50 in: - (1) We apply strided convolutions in the first 3x3 convolution of the first - residual block of a stage. - (2) We replace the strided max pooling layer in the stem by applying strided - convolution in the immediate next residual block. - """ - - @classmethod - def _get_config(cls): - return { - 'classification_mode': True, - 'backbone_type': 'resnet', - 'use_axial_beyond_stride': 0, - 'backbone_use_transformer_beyond_stride': 0, - 'block_group_config': { - 'drop_path_keep_prob': 1.0, - }, - } - - -class ResNet50Beta(ResNet50): - """A ResNet-50 but with inception stem. - - Note that the implementation is different from the original ResNet-50 in: - (1) We apply strided convolutions in the first 3x3 convolution of the first - residual block of a stage. - (2) We replace the strided max pooling layer in the stem by applying strided - convolution in the immediate next residual block. - """ - - @classmethod - def _get_config(cls): - base_config = super(ResNet50Beta, cls)._get_config() - # Override the config of ResNet50. - override_config = { - 'backbone_type': 'resnet_beta', - } - return override(base_config, override_config) - - -class AxialResNetL(ResNet50): - """Axial-ResNet-L for image classification only. - - Axial-ResNet-L is a ResNet50 with use_axial_beyond_stride = 2. - - Reference: - Axial-Deeplab: Stand-Alone Axial-Attention for Panoptic Segmentation, - ECCV 2020 Spotlight. https://arxiv.org/abs/2003.07853 - Huiyu Wang, Yukun Zhu, Bradley Green, Hartwig Adam, Alan Yuille, - Liang-Chieh Chen. - """ - - @classmethod - def _get_config(cls): - base_config = super(AxialResNetL, cls)._get_config() - # Override the config of ResNet50. - override_config = { - 'use_axial_beyond_stride': 2, - } - return override(base_config, override_config) - - -class AxialResNetS(ResNet50): - """Axial-ResNet-S for image classification only. - - Axial-ResNet-S is a ResNet50 with use_axial_beyond_stride = 2 and - width_multiplier = 0.5. - - Reference: - Axial-Deeplab: Stand-Alone Axial-Attention for Panoptic Segmentation, - ECCV 2020 Spotlight. https://arxiv.org/abs/2003.07853 - Huiyu Wang, Yukun Zhu, Bradley Green, Hartwig Adam, Alan Yuille, - Liang-Chieh Chen. - """ - - @classmethod - def _get_config(cls): - base_config = super(AxialResNetS, cls)._get_config() - # Override the config of ResNet50. - override_config = { - 'width_multiplier': 0.5, - 'use_axial_beyond_stride': 2, - } - return override(base_config, override_config) - - -class AxialDeepLabL(ResNet50Beta): - """Axial-DeepLab-L for panoptic segmentation. - - Axial-DeepLab-L is a ResNet50Beta with use_axial_beyond_stride = 2. - Axial-DeepLab-L is also equivalent to Axial-ResNet-L with an inception stem. - - Reference: - Axial-Deeplab: Stand-Alone Axial-Attention for Panoptic Segmentation, - ECCV 2020 Spotlight. https://arxiv.org/abs/2003.07853 - Huiyu Wang, Yukun Zhu, Bradley Green, Hartwig Adam, Alan Yuille, - Liang-Chieh Chen. - """ - - @classmethod - def _get_config(cls): - base_config = super(AxialDeepLabL, cls)._get_config() - override_config = { - 'use_axial_beyond_stride': 2, - } - return override(base_config, override_config) - - -class AxialDeepLabS(ResNet50Beta): - """Axial-DeepLab-S for panoptic segmentation. - - Axial-DeepLab-S is a ResNet50Beta with use_axial_beyond_stride = 2 and - width_multiplier = 0.5. - Axial-DeepLab-S is also equivalent to Axial-ResNet-S with an inception stem. - - Reference: - Axial-Deeplab: Stand-Alone Axial-Attention for Panoptic Segmentation, - ECCV 2020 Spotlight. https://arxiv.org/abs/2003.07853 - Huiyu Wang, Yukun Zhu, Bradley Green, Hartwig Adam, Alan Yuille, - Liang-Chieh Chen. - """ - - @classmethod - def _get_config(cls): - base_config = super(AxialDeepLabS, cls)._get_config() - override_config = { - 'width_multiplier': 0.5, - 'use_axial_beyond_stride': 2, - } - return override(base_config, override_config) - - -class SWideRNet(AxialResNetInstance): - """A SWideRNet instance. - - Note that the implementation is different from the original SWideRNet in: - (1) We apply strided convolutions in the first residual block of a stage, - instead of the last residual block. - (2) We replace the strided max pooling layer in the stem by applying strided - convolution in the immediate next residual block. - (3) We (optionally) use squeeze and excitation in all five stages, instead - of the last four stages only. - - Reference: - Scaling Wide Residual Networks for Panoptic Segmentation, - https://arxiv.org/abs/2011.11675 - Liang-Chieh Chen, Huiyu Wang, Siyuan Qiao. - Axial-Deeplab: Stand-Alone Axial-Attention for Panoptic Segmentation, - ECCV 2020 Spotlight. https://arxiv.org/abs/2003.07853 - Huiyu Wang, Yukun Zhu, Bradley Green, Hartwig Adam, Alan Yuille, - Liang-Chieh Chen. - """ - - @classmethod - def _get_config(cls): - return { - 'num_blocks': [3, 6, 3, 3], - 'classification_mode': True, - 'backbone_type': 'wider_resnet', - 'use_axial_beyond_stride': 0, - 'backbone_use_transformer_beyond_stride': 0, - 'block_group_config': { - 'drop_path_beyond_stride': 4, - 'conv_use_recompute_grad': True, - }, - } - - -class AxialSWideRNet(SWideRNet): - """SWideRNet with axial attention blocks in the last two stages. - - Note that the implementation is different from the original SWideRNet in: - (1) We apply strided convolutions in the first residual block of a stage, - instead of the last residual block. - (2) We replace the strided max pooling layer in the stem by applying strided - convolution in the immediate next residual block. - (3) We (optionally) use squeeze and excitation in all five stages, instead - of the last four stages only. - - Reference: - Scaling Wide Residual Networks for Panoptic Segmentation, - https://arxiv.org/abs/2011.11675 - Liang-Chieh Chen, Huiyu Wang, Siyuan Qiao. - Axial-Deeplab: Stand-Alone Axial-Attention for Panoptic Segmentation, - ECCV 2020 Spotlight. https://arxiv.org/abs/2003.07853 - Huiyu Wang, Yukun Zhu, Bradley Green, Hartwig Adam, Alan Yuille, - Liang-Chieh Chen. - """ - - @classmethod - def _get_config(cls): - base_config = super(AxialSWideRNet, cls)._get_config() - override_config = { - 'use_axial_beyond_stride': 16, - 'block_group_config': { - 'attention_bottleneck_expansion': 4, - 'axial_layer_config': { - 'key_expansion': 2, - 'value_expansion': 4, - }, - }, - } - return override(base_config, override_config) - - -def get_model(name, **kwargs): - """Gets the model instance given the model name.""" - name_lower = name.lower() - if name_lower == 'max_deeplab_s': - return MaXDeepLabS(name_lower, **kwargs) - elif name_lower == 'max_deeplab_l': - return MaXDeepLabL(name_lower, **kwargs) - elif name_lower == 'max_deeplab_s_backbone': - return MaXDeepLabSBackbone(name_lower, **kwargs) - elif name_lower == 'max_deeplab_l_backbone': - return MaXDeepLabLBackbone(name_lower, **kwargs) - elif name_lower == 'resnet50': - return ResNet50(name_lower, **kwargs) - elif name_lower == 'resnet50_beta': - return ResNet50Beta(name_lower, **kwargs) - elif name_lower == 'swidernet' or name_lower == 'wide_resnet41': - return SWideRNet(name_lower, **kwargs) - elif name_lower == 'axial_swidernet': - return AxialSWideRNet(name_lower, **kwargs) - elif name_lower == 'axial_resnet_s': - return AxialResNetS(name_lower, **kwargs) - elif name_lower == 'axial_resnet_l': - return AxialResNetL(name_lower, **kwargs) - elif name_lower == 'axial_deeplab_s': - return AxialDeepLabS(name_lower, **kwargs) - elif name_lower == 'axial_deeplab_l': - return AxialDeepLabL(name_lower, **kwargs) - else: - raise ValueError(name_lower + ' is not supported.') diff --git a/spaces/akhaliq/lama/saicinpainting/evaluation/masks/mask.py b/spaces/akhaliq/lama/saicinpainting/evaluation/masks/mask.py deleted file mode 100644 index 3e34d0675a781fba983cb542f18390255aaf2609..0000000000000000000000000000000000000000 --- a/spaces/akhaliq/lama/saicinpainting/evaluation/masks/mask.py +++ /dev/null @@ -1,429 +0,0 @@ -import enum -from copy import deepcopy - -import numpy as np -from skimage import img_as_ubyte -from skimage.transform import rescale, resize -try: - from detectron2 import model_zoo - from detectron2.config import get_cfg - from detectron2.engine import DefaultPredictor - DETECTRON_INSTALLED = True -except: - print("Detectron v2 is not installed") - DETECTRON_INSTALLED = False - -from .countless.countless2d import zero_corrected_countless - - -class ObjectMask(): - def __init__(self, mask): - self.height, self.width = mask.shape - (self.up, self.down), (self.left, self.right) = self._get_limits(mask) - self.mask = mask[self.up:self.down, self.left:self.right].copy() - - @staticmethod - def _get_limits(mask): - def indicator_limits(indicator): - lower = indicator.argmax() - upper = len(indicator) - indicator[::-1].argmax() - return lower, upper - - vertical_indicator = mask.any(axis=1) - vertical_limits = indicator_limits(vertical_indicator) - - horizontal_indicator = mask.any(axis=0) - horizontal_limits = indicator_limits(horizontal_indicator) - - return vertical_limits, horizontal_limits - - def _clean(self): - self.up, self.down, self.left, self.right = 0, 0, 0, 0 - self.mask = np.empty((0, 0)) - - def horizontal_flip(self, inplace=False): - if not inplace: - flipped = deepcopy(self) - return flipped.horizontal_flip(inplace=True) - - self.mask = self.mask[:, ::-1] - return self - - def vertical_flip(self, inplace=False): - if not inplace: - flipped = deepcopy(self) - return flipped.vertical_flip(inplace=True) - - self.mask = self.mask[::-1, :] - return self - - def image_center(self): - y_center = self.up + (self.down - self.up) / 2 - x_center = self.left + (self.right - self.left) / 2 - return y_center, x_center - - def rescale(self, scaling_factor, inplace=False): - if not inplace: - scaled = deepcopy(self) - return scaled.rescale(scaling_factor, inplace=True) - - scaled_mask = rescale(self.mask.astype(float), scaling_factor, order=0) > 0.5 - (up, down), (left, right) = self._get_limits(scaled_mask) - self.mask = scaled_mask[up:down, left:right] - - y_center, x_center = self.image_center() - mask_height, mask_width = self.mask.shape - self.up = int(round(y_center - mask_height / 2)) - self.down = self.up + mask_height - self.left = int(round(x_center - mask_width / 2)) - self.right = self.left + mask_width - return self - - def crop_to_canvas(self, vertical=True, horizontal=True, inplace=False): - if not inplace: - cropped = deepcopy(self) - cropped.crop_to_canvas(vertical=vertical, horizontal=horizontal, inplace=True) - return cropped - - if vertical: - if self.up >= self.height or self.down <= 0: - self._clean() - else: - cut_up, cut_down = max(-self.up, 0), max(self.down - self.height, 0) - if cut_up != 0: - self.mask = self.mask[cut_up:] - self.up = 0 - if cut_down != 0: - self.mask = self.mask[:-cut_down] - self.down = self.height - - if horizontal: - if self.left >= self.width or self.right <= 0: - self._clean() - else: - cut_left, cut_right = max(-self.left, 0), max(self.right - self.width, 0) - if cut_left != 0: - self.mask = self.mask[:, cut_left:] - self.left = 0 - if cut_right != 0: - self.mask = self.mask[:, :-cut_right] - self.right = self.width - - return self - - def restore_full_mask(self, allow_crop=False): - cropped = self.crop_to_canvas(inplace=allow_crop) - mask = np.zeros((cropped.height, cropped.width), dtype=bool) - mask[cropped.up:cropped.down, cropped.left:cropped.right] = cropped.mask - return mask - - def shift(self, vertical=0, horizontal=0, inplace=False): - if not inplace: - shifted = deepcopy(self) - return shifted.shift(vertical=vertical, horizontal=horizontal, inplace=True) - - self.up += vertical - self.down += vertical - self.left += horizontal - self.right += horizontal - return self - - def area(self): - return self.mask.sum() - - -class RigidnessMode(enum.Enum): - soft = 0 - rigid = 1 - - -class SegmentationMask: - def __init__(self, confidence_threshold=0.5, rigidness_mode=RigidnessMode.rigid, - max_object_area=0.3, min_mask_area=0.02, downsample_levels=6, num_variants_per_mask=4, - max_mask_intersection=0.5, max_foreground_coverage=0.5, max_foreground_intersection=0.5, - max_hidden_area=0.2, max_scale_change=0.25, horizontal_flip=True, - max_vertical_shift=0.1, position_shuffle=True): - """ - :param confidence_threshold: float; threshold for confidence of the panoptic segmentator to allow for - the instance. - :param rigidness_mode: RigidnessMode object - when soft, checks intersection only with the object from which the mask_object was produced - when rigid, checks intersection with any foreground class object - :param max_object_area: float; allowed upper bound for to be considered as mask_object. - :param min_mask_area: float; lower bound for mask to be considered valid - :param downsample_levels: int; defines width of the resized segmentation to obtain shifted masks; - :param num_variants_per_mask: int; maximal number of the masks for the same object; - :param max_mask_intersection: float; maximum allowed area fraction of intersection for 2 masks - produced by horizontal shift of the same mask_object; higher value -> more diversity - :param max_foreground_coverage: float; maximum allowed area fraction of intersection for foreground object to be - covered by mask; lower value -> less the objects are covered - :param max_foreground_intersection: float; maximum allowed area of intersection for the mask with foreground - object; lower value -> mask is more on the background than on the objects - :param max_hidden_area: upper bound on part of the object hidden by shifting object outside the screen area; - :param max_scale_change: allowed scale change for the mask_object; - :param horizontal_flip: if horizontal flips are allowed; - :param max_vertical_shift: amount of vertical movement allowed; - :param position_shuffle: shuffle - """ - - assert DETECTRON_INSTALLED, 'Cannot use SegmentationMask without detectron2' - self.cfg = get_cfg() - self.cfg.merge_from_file(model_zoo.get_config_file("COCO-PanopticSegmentation/panoptic_fpn_R_101_3x.yaml")) - self.cfg.MODEL.WEIGHTS = model_zoo.get_checkpoint_url("COCO-PanopticSegmentation/panoptic_fpn_R_101_3x.yaml") - self.cfg.MODEL.PANOPTIC_FPN.COMBINE.INSTANCES_CONFIDENCE_THRESH = confidence_threshold - self.predictor = DefaultPredictor(self.cfg) - - self.rigidness_mode = RigidnessMode(rigidness_mode) - self.max_object_area = max_object_area - self.min_mask_area = min_mask_area - self.downsample_levels = downsample_levels - self.num_variants_per_mask = num_variants_per_mask - self.max_mask_intersection = max_mask_intersection - self.max_foreground_coverage = max_foreground_coverage - self.max_foreground_intersection = max_foreground_intersection - self.max_hidden_area = max_hidden_area - self.position_shuffle = position_shuffle - - self.max_scale_change = max_scale_change - self.horizontal_flip = horizontal_flip - self.max_vertical_shift = max_vertical_shift - - def get_segmentation(self, img): - im = img_as_ubyte(img) - panoptic_seg, segment_info = self.predictor(im)["panoptic_seg"] - return panoptic_seg, segment_info - - @staticmethod - def _is_power_of_two(n): - return (n != 0) and (n & (n-1) == 0) - - def identify_candidates(self, panoptic_seg, segments_info): - potential_mask_ids = [] - for segment in segments_info: - if not segment["isthing"]: - continue - mask = (panoptic_seg == segment["id"]).int().detach().cpu().numpy() - area = mask.sum().item() / np.prod(panoptic_seg.shape) - if area >= self.max_object_area: - continue - potential_mask_ids.append(segment["id"]) - return potential_mask_ids - - def downsample_mask(self, mask): - height, width = mask.shape - if not (self._is_power_of_two(height) and self._is_power_of_two(width)): - raise ValueError("Image sides are not power of 2.") - - num_iterations = width.bit_length() - 1 - self.downsample_levels - if num_iterations < 0: - raise ValueError(f"Width is lower than 2^{self.downsample_levels}.") - - if height.bit_length() - 1 < num_iterations: - raise ValueError("Height is too low to perform downsampling") - - downsampled = mask - for _ in range(num_iterations): - downsampled = zero_corrected_countless(downsampled) - - return downsampled - - def _augmentation_params(self): - scaling_factor = np.random.uniform(1 - self.max_scale_change, 1 + self.max_scale_change) - if self.horizontal_flip: - horizontal_flip = bool(np.random.choice(2)) - else: - horizontal_flip = False - vertical_shift = np.random.uniform(-self.max_vertical_shift, self.max_vertical_shift) - - return { - "scaling_factor": scaling_factor, - "horizontal_flip": horizontal_flip, - "vertical_shift": vertical_shift - } - - def _get_intersection(self, mask_array, mask_object): - intersection = mask_array[ - mask_object.up:mask_object.down, mask_object.left:mask_object.right - ] & mask_object.mask - return intersection - - def _check_masks_intersection(self, aug_mask, total_mask_area, prev_masks): - for existing_mask in prev_masks: - intersection_area = self._get_intersection(existing_mask, aug_mask).sum() - intersection_existing = intersection_area / existing_mask.sum() - intersection_current = 1 - (aug_mask.area() - intersection_area) / total_mask_area - if (intersection_existing > self.max_mask_intersection) or \ - (intersection_current > self.max_mask_intersection): - return False - return True - - def _check_foreground_intersection(self, aug_mask, foreground): - for existing_mask in foreground: - intersection_area = self._get_intersection(existing_mask, aug_mask).sum() - intersection_existing = intersection_area / existing_mask.sum() - if intersection_existing > self.max_foreground_coverage: - return False - intersection_mask = intersection_area / aug_mask.area() - if intersection_mask > self.max_foreground_intersection: - return False - return True - - def _move_mask(self, mask, foreground): - # Obtaining properties of the original mask_object: - orig_mask = ObjectMask(mask) - - chosen_masks = [] - chosen_parameters = [] - # to fix the case when resizing gives mask_object consisting only of False - scaling_factor_lower_bound = 0. - - for var_idx in range(self.num_variants_per_mask): - # Obtaining augmentation parameters and applying them to the downscaled mask_object - augmentation_params = self._augmentation_params() - augmentation_params["scaling_factor"] = min([ - augmentation_params["scaling_factor"], - 2 * min(orig_mask.up, orig_mask.height - orig_mask.down) / orig_mask.height + 1., - 2 * min(orig_mask.left, orig_mask.width - orig_mask.right) / orig_mask.width + 1. - ]) - augmentation_params["scaling_factor"] = max([ - augmentation_params["scaling_factor"], scaling_factor_lower_bound - ]) - - aug_mask = deepcopy(orig_mask) - aug_mask.rescale(augmentation_params["scaling_factor"], inplace=True) - if augmentation_params["horizontal_flip"]: - aug_mask.horizontal_flip(inplace=True) - total_aug_area = aug_mask.area() - if total_aug_area == 0: - scaling_factor_lower_bound = 1. - continue - - # Fix if the element vertical shift is too strong and shown area is too small: - vertical_area = aug_mask.mask.sum(axis=1) / total_aug_area # share of area taken by rows - # number of rows which are allowed to be hidden from upper and lower parts of image respectively - max_hidden_up = np.searchsorted(vertical_area.cumsum(), self.max_hidden_area) - max_hidden_down = np.searchsorted(vertical_area[::-1].cumsum(), self.max_hidden_area) - # correcting vertical shift, so not too much area will be hidden - augmentation_params["vertical_shift"] = np.clip( - augmentation_params["vertical_shift"], - -(aug_mask.up + max_hidden_up) / aug_mask.height, - (aug_mask.height - aug_mask.down + max_hidden_down) / aug_mask.height - ) - # Applying vertical shift: - vertical_shift = int(round(aug_mask.height * augmentation_params["vertical_shift"])) - aug_mask.shift(vertical=vertical_shift, inplace=True) - aug_mask.crop_to_canvas(vertical=True, horizontal=False, inplace=True) - - # Choosing horizontal shift: - max_hidden_area = self.max_hidden_area - (1 - aug_mask.area() / total_aug_area) - horizontal_area = aug_mask.mask.sum(axis=0) / total_aug_area - max_hidden_left = np.searchsorted(horizontal_area.cumsum(), max_hidden_area) - max_hidden_right = np.searchsorted(horizontal_area[::-1].cumsum(), max_hidden_area) - allowed_shifts = np.arange(-max_hidden_left, aug_mask.width - - (aug_mask.right - aug_mask.left) + max_hidden_right + 1) - allowed_shifts = - (aug_mask.left - allowed_shifts) - - if self.position_shuffle: - np.random.shuffle(allowed_shifts) - - mask_is_found = False - for horizontal_shift in allowed_shifts: - aug_mask_left = deepcopy(aug_mask) - aug_mask_left.shift(horizontal=horizontal_shift, inplace=True) - aug_mask_left.crop_to_canvas(inplace=True) - - prev_masks = [mask] + chosen_masks - is_mask_suitable = self._check_masks_intersection(aug_mask_left, total_aug_area, prev_masks) & \ - self._check_foreground_intersection(aug_mask_left, foreground) - if is_mask_suitable: - aug_draw = aug_mask_left.restore_full_mask() - chosen_masks.append(aug_draw) - augmentation_params["horizontal_shift"] = horizontal_shift / aug_mask_left.width - chosen_parameters.append(augmentation_params) - mask_is_found = True - break - - if not mask_is_found: - break - - return chosen_parameters - - def _prepare_mask(self, mask): - height, width = mask.shape - target_width = width if self._is_power_of_two(width) else (1 << width.bit_length()) - target_height = height if self._is_power_of_two(height) else (1 << height.bit_length()) - - return resize(mask.astype('float32'), (target_height, target_width), order=0, mode='edge').round().astype('int32') - - def get_masks(self, im, return_panoptic=False): - panoptic_seg, segments_info = self.get_segmentation(im) - potential_mask_ids = self.identify_candidates(panoptic_seg, segments_info) - - panoptic_seg_scaled = self._prepare_mask(panoptic_seg.detach().cpu().numpy()) - downsampled = self.downsample_mask(panoptic_seg_scaled) - scene_objects = [] - for segment in segments_info: - if not segment["isthing"]: - continue - mask = downsampled == segment["id"] - if not np.any(mask): - continue - scene_objects.append(mask) - - mask_set = [] - for mask_id in potential_mask_ids: - mask = downsampled == mask_id - if not np.any(mask): - continue - - if self.rigidness_mode is RigidnessMode.soft: - foreground = [mask] - elif self.rigidness_mode is RigidnessMode.rigid: - foreground = scene_objects - else: - raise ValueError(f'Unexpected rigidness_mode: {rigidness_mode}') - - masks_params = self._move_mask(mask, foreground) - - full_mask = ObjectMask((panoptic_seg == mask_id).detach().cpu().numpy()) - - for params in masks_params: - aug_mask = deepcopy(full_mask) - aug_mask.rescale(params["scaling_factor"], inplace=True) - if params["horizontal_flip"]: - aug_mask.horizontal_flip(inplace=True) - - vertical_shift = int(round(aug_mask.height * params["vertical_shift"])) - horizontal_shift = int(round(aug_mask.width * params["horizontal_shift"])) - aug_mask.shift(vertical=vertical_shift, horizontal=horizontal_shift, inplace=True) - aug_mask = aug_mask.restore_full_mask().astype('uint8') - if aug_mask.mean() <= self.min_mask_area: - continue - mask_set.append(aug_mask) - - if return_panoptic: - return mask_set, panoptic_seg.detach().cpu().numpy() - else: - return mask_set - - -def propose_random_square_crop(mask, min_overlap=0.5): - height, width = mask.shape - mask_ys, mask_xs = np.where(mask > 0.5) # mask==0 is known fragment and mask==1 is missing - - if height < width: - crop_size = height - obj_left, obj_right = mask_xs.min(), mask_xs.max() - obj_width = obj_right - obj_left - left_border = max(0, min(width - crop_size - 1, obj_left + obj_width * min_overlap - crop_size)) - right_border = max(left_border + 1, min(width - crop_size, obj_left + obj_width * min_overlap)) - start_x = np.random.randint(left_border, right_border) - return start_x, 0, start_x + crop_size, height - else: - crop_size = width - obj_top, obj_bottom = mask_ys.min(), mask_ys.max() - obj_height = obj_bottom - obj_top - top_border = max(0, min(height - crop_size - 1, obj_top + obj_height * min_overlap - crop_size)) - bottom_border = max(top_border + 1, min(height - crop_size, obj_top + obj_height * min_overlap)) - start_y = np.random.randint(top_border, bottom_border) - return 0, start_y, width, start_y + crop_size diff --git a/spaces/akhaliq/mae/README.md b/spaces/akhaliq/mae/README.md deleted file mode 100644 index 87ee25262ec0dbc8bf80d61317a6d3aaf8b89028..0000000000000000000000000000000000000000 --- a/spaces/akhaliq/mae/README.md +++ /dev/null @@ -1,37 +0,0 @@ ---- -title: Mae -emoji: 🚀 -colorFrom: green -colorTo: yellow -sdk: gradio -app_file: app.py -pinned: false ---- - -# Configuration - -`title`: _string_ -Display title for the Space - -`emoji`: _string_ -Space emoji (emoji-only character allowed) - -`colorFrom`: _string_ -Color for Thumbnail gradient (red, yellow, green, blue, indigo, purple, pink, gray) - -`colorTo`: _string_ -Color for Thumbnail gradient (red, yellow, green, blue, indigo, purple, pink, gray) - -`sdk`: _string_ -Can be either `gradio`, `streamlit`, or `static` - -`sdk_version` : _string_ -Only applicable for `streamlit` SDK. -See [doc](https://hf.co/docs/hub/spaces) for more info on supported versions. - -`app_file`: _string_ -Path to your main application file (which contains either `gradio` or `streamlit` Python code, or `static` html code). -Path is relative to the root of the repository. - -`pinned`: _boolean_ -Whether the Space stays on top of your list. diff --git a/spaces/akhooli/poetry2023/app.py b/spaces/akhooli/poetry2023/app.py deleted file mode 100644 index 5b6654d5a405778ddbc9ca5fa5d041aff535f3b5..0000000000000000000000000000000000000000 --- a/spaces/akhooli/poetry2023/app.py +++ /dev/null @@ -1,53 +0,0 @@ -import gc -import gradio as gr -from transformers import pipeline, set_seed - -pipe = pipeline('text-generation', framework='pt', model='akhooli/ap2023', tokenizer='akhooli/ap2023') -#gc.collect() -samples = [['أنت' - ,1.0, 50, 1.0, 1.0, 114],['هل غادر' - ,1.0, 50, 1.0, 1.0, 114 ],['ألا ليت' - ,1.0, 50, 1.0, 1.0, 114 ],['يا قدس' - ,1.0, 50, 1.0, 1.0, 114],['عيد بأية حال' - ,1.0, 50, 1.0, 1.0, 114],['لكل شيء إذا ما' - ,1.0, 50, 1.0, 1.0, 114 ],['.' - ,1.0, 50, 1.0, 1.0, 114]] - -notes = """ -- Enter a short prompt or select (click) one of the examples and click SEND -- Adjust parameters (temperture, top k, top p and penalty) through the slider (keep close to default values). -- For the same seed (randomness), the same output is regenerated if other parameters are fixed -- Clear and enter new prompt or select another example and SEND to regenerate -- The '.' means start a new line from no prompt (your prompt need not be long) -- Be patient: this runs on CPU (free tier) -- Feedback (Twitter): @akhooli (https://twitter.com/akhooli/status/1611025232201977859) -- Note/Disclaimer: may generate unaccepted or inappropriate content. Use at your own risk. -""" -def sayPoetry(prompt, temp=1.0, topk = 50, topp = 1.0, penalty=1.0, seed=114): - if not int(seed) >= 0: seed=114 - set_seed(seed) - gen = pipe(prompt, max_length=96, do_sample=True, temperature=temp, top_k=topk, top_p=topp, repetition_penalty=penalty, - min_length = 64, no_repeat_ngram_size = 3, return_full_text=True, - num_beams=5, num_return_sequences=1)[0]["generated_text"] - poetry ="" - for line in gen.split('.')[:-1]: - poetry += line #+ "\n" - return poetry -poetry = gr.Interface(fn=sayPoetry, - inputs=[ - gr.Textbox(label="Enter short prompt or select from examples:"), - gr.Slider(0.70, 1.2, step=0.01,value=1.0, label='control temperature'), - gr.Slider(25, 100, step=1,value=50, label='control top k'), - gr.Slider(0.80, 1.0, step=0.01,value=1.0, label='control top p'), - gr.Slider(0.90, 1.50, step=0.01,value=1.0, label='control penalty'), - gr.Number(value=139750, precision=0, label='Seed'), - ], - outputs=[gr.Textbox(label="Generated Poetry:")], - - allow_flagging='never', - title='Arabic Poetry Generation Demo (updated Jan. 2023)', - description = "A simple demo of AI generated poetry based on 1M poems fine-tuned using AraGPT2 (be patient, runs on cpu)", - examples=samples, - cache_examples=False, - article = notes) -poetry.launch() # show_error = True, debug=True \ No newline at end of file diff --git a/spaces/alexray/btc_predictor/venv/lib/python3.10/site-packages/pip/_vendor/pygments/__main__.py b/spaces/alexray/btc_predictor/venv/lib/python3.10/site-packages/pip/_vendor/pygments/__main__.py deleted file mode 100644 index 010896b88ff684c7a73a71ca23af5e76503cd0c2..0000000000000000000000000000000000000000 --- a/spaces/alexray/btc_predictor/venv/lib/python3.10/site-packages/pip/_vendor/pygments/__main__.py +++ /dev/null @@ -1,17 +0,0 @@ -""" - pygments.__main__ - ~~~~~~~~~~~~~~~~~ - - Main entry point for ``python -m pygments``. - - :copyright: Copyright 2006-2021 by the Pygments team, see AUTHORS. - :license: BSD, see LICENSE for details. -""" - -import sys -from pip._vendor.pygments.cmdline import main - -try: - sys.exit(main(sys.argv)) -except KeyboardInterrupt: - sys.exit(1) diff --git a/spaces/aliabid94/AutoGPT/autogpt/memory/no_memory.py b/spaces/aliabid94/AutoGPT/autogpt/memory/no_memory.py deleted file mode 100644 index 0371e96ae89f5eb88dae019a66351a229596ed7a..0000000000000000000000000000000000000000 --- a/spaces/aliabid94/AutoGPT/autogpt/memory/no_memory.py +++ /dev/null @@ -1,73 +0,0 @@ -"""A class that does not store any data. This is the default memory provider.""" -from __future__ import annotations - -from typing import Any - -from autogpt.memory.base import MemoryProviderSingleton - - -class NoMemory(MemoryProviderSingleton): - """ - A class that does not store any data. This is the default memory provider. - """ - - def __init__(self, cfg): - """ - Initializes the NoMemory provider. - - Args: - cfg: The config object. - - Returns: None - """ - pass - - def add(self, data: str) -> str: - """ - Adds a data point to the memory. No action is taken in NoMemory. - - Args: - data: The data to add. - - Returns: An empty string. - """ - return "" - - def get(self, data: str) -> list[Any] | None: - """ - Gets the data from the memory that is most relevant to the given data. - NoMemory always returns None. - - Args: - data: The data to compare to. - - Returns: None - """ - return None - - def clear(self) -> str: - """ - Clears the memory. No action is taken in NoMemory. - - Returns: An empty string. - """ - return "" - - def get_relevant(self, data: str, num_relevant: int = 5) -> list[Any] | None: - """ - Returns all the data in the memory that is relevant to the given data. - NoMemory always returns None. - - Args: - data: The data to compare to. - num_relevant: The number of relevant data to return. - - Returns: None - """ - return None - - def get_stats(self): - """ - Returns: An empty dictionary as there are no stats in NoMemory. - """ - return {} diff --git a/spaces/allandclive/Uganda_MMS/uroman/lib/NLP/UTF8.pm b/spaces/allandclive/Uganda_MMS/uroman/lib/NLP/UTF8.pm deleted file mode 100644 index b28cb4dede3b84f45aeade2e24f240e3a39e7cc1..0000000000000000000000000000000000000000 --- a/spaces/allandclive/Uganda_MMS/uroman/lib/NLP/UTF8.pm +++ /dev/null @@ -1,1404 +0,0 @@ -################################################################ -# # -# UTF8 # -# # -################################################################ - -package NLP::UTF8; - -use NLP::utilities; -$util = NLP::utilities; - -%empty_ht = (); - -sub new { - local($caller) = @_; - - my $object = {}; - my $class = ref( $caller ) || $caller; - bless($object, $class); - return $object; -} - -sub unicode_string2string { -# input: string that might contain unicode sequences such as "U+0627" -# output: string in pure utf-8 - local($caller,$s) = @_; - - my $pre; - my $unicode; - my $post; - my $r1; - my $r2; - my $r3; - - ($pre,$unicode,$post) = ($s =~ /^(.*)(?:U\+|\\u)([0-9A-Fa-f][0-9A-Fa-f][0-9A-Fa-f][0-9A-Fa-f])(.*)$/); - return $s unless defined($post); - $r1 = $caller->unicode_string2string($pre); - $r2 = $caller->unicode_hex_string2string($unicode); - $r3 = $caller->unicode_string2string($post); - $result = $r1 . $r2 . $r3; - return $result; -} - -sub unicode_hex_string2string { -# input: "0627" (interpreted as hex code) -# output: utf-8 string for Arabic letter alef - local($caller,$unicode) = @_; - return "" unless defined($unicode); - my $d = hex($unicode); - return $caller->unicode2string($d); -} - -sub unicode2string { -# input: non-neg integer, e.g. 0x627 -# output: utf-8 string for Arabic letter alef - local($caller,$d) = @_; - return "" unless defined($d) && $d >= 0; - return sprintf("%c",$d) if $d <= 0x7F; - - my $lastbyte1 = ($d & 0x3F) | 0x80; - $d >>= 6; - return sprintf("%c%c",$d | 0xC0, $lastbyte1) if $d <= 0x1F; - - my $lastbyte2 = ($d & 0x3F) | 0x80; - $d >>= 6; - return sprintf("%c%c%c",$d | 0xE0, $lastbyte2, $lastbyte1) if $d <= 0xF; - - my $lastbyte3 = ($d & 0x3F) | 0x80; - $d >>= 6; - return sprintf("%c%c%c%c",$d | 0xF0, $lastbyte3, $lastbyte2, $lastbyte1) if $d <= 0x7; - - my $lastbyte4 = ($d & 0x3F) | 0x80; - $d >>= 6; - return sprintf("%c%c%c%c%c",$d | 0xF8, $lastbyte4, $lastbyte3, $lastbyte2, $lastbyte1) if $d <= 0x3; - - my $lastbyte5 = ($d & 0x3F) | 0x80; - $d >>= 6; - return sprintf("%c%c%c%c%c%c",$d | 0xFC, $lastbyte5, $lastbyte4, $lastbyte3, $lastbyte2, $lastbyte1) if $d <= 0x1; - return ""; # bad input -} - -sub html2utf8 { - local($caller, $string) = @_; - - return $string unless $string =~ /\&\#\d{3,5};/; - - my $prev = ""; - my $s = $string; - while ($s ne $prev) { - $prev = $s; - ($pre,$d,$post) = ($s =~ /^(.*)\&\#(\d+);(.*)$/); - if (defined($d) && ((($d >= 160) && ($d <= 255)) - || (($d >= 1500) && ($d <= 1699)) - || (($d >= 19968) && ($d <= 40879)))) { - $html_code = "\&\#" . $d . ";"; - $utf8_code = $caller->unicode2string($d); - $s =~ s/$html_code/$utf8_code/; - } - } - return $s; -} - -sub xhtml2utf8 { - local($caller, $string) = @_; - - return $string unless $string =~ /\&\#x[0-9a-fA-F]{2,5};/; - - my $prev = ""; - my $s = $string; - while ($s ne $prev) { - $prev = $s; - if (($pre, $html_code, $x, $post) = ($s =~ /^(.*)(\&\#x([0-9a-fA-F]{2,5});)(.*)$/)) { - $utf8_code = $caller->unicode_hex_string2string($x); - $s =~ s/$html_code/$utf8_code/; - } - } - return $s; -} - -sub utf8_marker { - return sprintf("%c%c%c\n", 0xEF, 0xBB, 0xBF); -} - -sub enforcer { -# input: string that might not conform to utf-8 -# output: string in pure utf-8, with a few "smart replacements" and possibly "?" - local($caller,$s,$no_repair) = @_; - - my $ascii; - my $utf8; - my $rest; - - return $s if $s =~ /^[\x00-\x7F]*$/; - - $no_repair = 0 unless defined($no_repair); - $orig = $s; - $result = ""; - - while ($s ne "") { - ($ascii,$rest) = ($s =~ /^([\x00-\x7F]+)(.*)$/); - if (defined($ascii)) { - $result .= $ascii; - $s = $rest; - next; - } - ($utf8,$rest) = ($s =~ /^([\xC0-\xDF][\x80-\xBF])(.*)$/); - ($utf8,$rest) = ($s =~ /^([\xE0-\xEF][\x80-\xBF][\x80-\xBF])(.*)$/) - unless defined($rest); - ($utf8,$rest) = ($s =~ /^([\xF0-\xF7][\x80-\xBF][\x80-\xBF][\x80-\xBF])(.*)$/) - unless defined($rest); - ($utf8,$rest) = ($s =~ /^([\xF8-\xFB][\x80-\xBF][\x80-\xBF][\x80-\xBF][\x80-\xBF])(.*)$/) - unless defined($rest); - if (defined($utf8)) { - $result .= $utf8; - $s = $rest; - next; - } - ($c,$rest) = ($s =~ /^(.)(.*)$/); - if (defined($c)) { - if ($no_repair) { $result .= "?"; } - elsif ($c =~ /\x85/) { $result .= "..."; } - elsif ($c =~ /\x91/) { $result .= "'"; } - elsif ($c =~ /\x92/) { $result .= "'"; } - elsif ($c =~ /\x93/) { $result .= $caller->unicode2string(0x201C); } - elsif ($c =~ /\x94/) { $result .= $caller->unicode2string(0x201D); } - elsif ($c =~ /[\xC0-\xFF]/) { - $c2 = $c; - $c2 =~ tr/[\xC0-\xFF]/[\x80-\xBF]/; - $result .= "\xC3$c2"; - } else { - $result .= "?"; - } - $s = $rest; - next; - } - $s = ""; - } - $result .= "\n" if ($orig =~ /\n$/) && ! ($result =~ /\n$/); - return $result; -} - -sub split_into_utf8_characters { -# input: utf8 string -# output: list of sub-strings, each representing a utf8 character - local($caller,$string,$group_control, *ht) = @_; - - @characters = (); - $end_of_token_p_string = ""; - $skipped_bytes = ""; - $group_control = "" unless defined($group_control); - $group_ascii_numbers = ($group_control =~ /ASCII numbers/); - $group_ascii_spaces = ($group_control =~ /ASCII spaces/); - $group_ascii_punct = ($group_control =~ /ASCII punct/); - $group_ascii_chars = ($group_control =~ /ASCII chars/); - $group_xml_chars = ($group_control =~ /XML chars/); - $group_xml_tags = ($group_control =~ /XML tags/); - $return_only_chars = ($group_control =~ /return only chars/); - $return_trailing_whitespaces = ($group_control =~ /return trailing whitespaces/); - if ($group_control =~ /ASCII all/) { - $group_ascii_numbers = 1; - $group_ascii_spaces = 1; - $group_ascii_chars = 1; - $group_ascii_punct = 1; - } - if ($group_control =~ /(XML chars and tags|XML tags and chars)/) { - $group_xml_chars = 1; - $group_xml_tags = 1; - } - $orig_string = $string; - $string .= " "; - while ($string =~ /\S/) { - # one-character UTF-8 = ASCII - if ($string =~ /^[\x00-\x7F]/) { - if ($group_xml_chars - && (($dec_unicode, $rest) = ($string =~ /^(\d+);(.*)$/s)) - && ($utf8_char = $caller->unicode2string($dec_unicode))) { - push(@characters, $utf8_char); - $string = $rest; - } elsif ($group_xml_chars - && (($hex_unicode, $rest) = ($string =~ /^([0-9a-f]{1,6});(.*)$/is)) - && ($utf8_char = $caller->unicode_hex_string2string($hex_unicode))) { - push(@characters, $utf8_char); - $string = $rest; - } elsif ($group_xml_chars - && (($html_entity_name, $rest) = ($string =~ /^&([a-z]{1,6});(.*)$/is)) - && ($dec_unicode = $ht{HTML_ENTITY_NAME_TO_DECUNICODE}->{$html_entity_name}) - && ($utf8_char = $caller->unicode2string($dec_unicode)) - ) { - push(@characters, $utf8_char); - $string = $rest; - } elsif ($group_xml_tags - && (($tag, $rest) = ($string =~ /^(<\/?[a-zA-Z][-_:a-zA-Z0-9]*(\s+[a-zA-Z][-_:a-zA-Z0-9]*=\"[^"]*\")*\s*\/?>)(.*)$/s))) { - push(@characters, $tag); - $string = $rest; - } elsif ($group_ascii_numbers && ($string =~ /^[12]\d\d\d\.[01]?\d.[0-3]?\d([^0-9].*)?$/)) { - ($date) = ($string =~ /^(\d\d\d\d\.\d?\d.\d?\d)([^0-9].*)?$/); - push(@characters,$date); - $string = substr($string, length($date)); - } elsif ($group_ascii_numbers && ($string =~ /^\d/)) { - ($number) = ($string =~ /^(\d+(,\d\d\d)*(\.\d+)?)/); - push(@characters,$number); - $string = substr($string, length($number)); - } elsif ($group_ascii_spaces && ($string =~ /^(\s+)/)) { - ($space) = ($string =~ /^(\s+)/); - $string = substr($string, length($space)); - } elsif ($group_ascii_punct && (($punct_seq) = ($string =~ /^(-+|\.+|[:,%()"])/))) { - push(@characters,$punct_seq); - $string = substr($string, length($punct_seq)); - } elsif ($group_ascii_chars && (($word) = ($string =~ /^(\$[A-Z]*|[A-Z]{1,3}\$)/))) { - push(@characters,$word); - $string = substr($string, length($word)); - } elsif ($group_ascii_chars && (($abbrev) = ($string =~ /^((?:Jan|Feb|Febr|Mar|Apr|Jun|Jul|Aug|Sep|Sept|Oct|Nov|Dec|Mr|Mrs|Dr|a.m|p.m)\.)/))) { - push(@characters,$abbrev); - $string = substr($string, length($abbrev)); - } elsif ($group_ascii_chars && (($word) = ($string =~ /^(second|minute|hour|day|week|month|year|inch|foot|yard|meter|kilometer|mile)-(?:long|old)/i))) { - push(@characters,$word); - $string = substr($string, length($word)); - } elsif ($group_ascii_chars && (($word) = ($string =~ /^(zero|one|two|three|four|five|six|seven|eight|nine|ten|eleven|twelve|thirteen|fourteen|fifteen|sixteen|seventeen|eighteen|nineteen|twenty|thirty|forty|fifty|sixty|seventy|eighty|ninety|hundred|thousand|million|billion|trillion)-/i))) { - push(@characters,$word); - $string = substr($string, length($word)); - } elsif ($group_ascii_chars && (($word) = ($string =~ /^([a-zA-Z]+)(?:[ ,;%?|()"]|'s |' |\. |\d+[:hms][0-9 ])/))) { - push(@characters,$word); - $string = substr($string, length($word)); - } elsif ($group_ascii_chars && ($string =~ /^([\x21-\x27\x2A-\x7E]+)/)) { # exclude () - ($ascii) = ($string =~ /^([\x21-\x27\x2A-\x7E]+)/); # ASCII black-characters - push(@characters,$ascii); - $string = substr($string, length($ascii)); - } elsif ($group_ascii_chars && ($string =~ /^([\x21-\x7E]+)/)) { - ($ascii) = ($string =~ /^([\x21-\x7E]+)/); # ASCII black-characters - push(@characters,$ascii); - $string = substr($string, length($ascii)); - } elsif ($group_ascii_chars && ($string =~ /^([\x00-\x7F]+)/)) { - ($ascii) = ($string =~ /^([\x00-\x7F]+)/); - push(@characters,$ascii); - $string = substr($string, length($ascii)); - } else { - push(@characters,substr($string, 0, 1)); - $string = substr($string, 1); - } - - # two-character UTF-8 - } elsif ($string =~ /^[\xC0-\xDF][\x80-\xBF]/) { - push(@characters,substr($string, 0, 2)); - $string = substr($string, 2); - - # three-character UTF-8 - } elsif ($string =~ /^[\xE0-\xEF][\x80-\xBF][\x80-\xBF]/) { - push(@characters,substr($string, 0, 3)); - $string = substr($string, 3); - - # four-character UTF-8 - } elsif ($string =~ /^[\xF0-\xF7][\x80-\xBF][\x80-\xBF][\x80-\xBF]/) { - push(@characters,substr($string, 0, 4)); - $string = substr($string, 4); - - # five-character UTF-8 - } elsif ($string =~ /^[\xF8-\xFB][\x80-\xBF][\x80-\xBF][\x80-\xBF][\x80-\xBF]/) { - push(@characters,substr($string, 0, 5)); - $string = substr($string, 5); - - # six-character UTF-8 - } elsif ($string =~ /^[\xFC-\xFD][\x80-\xBF][\x80-\xBF][\x80-\xBF][\x80-\xBF][\x80-\xBF]/) { - push(@characters,substr($string, 0, 6)); - $string = substr($string, 6); - - # not a UTF-8 character - } else { - $skipped_bytes .= substr($string, 0, 1); - $string = substr($string, 1); - } - - $end_of_token_p_string .= ($string =~ /^\S/) ? "0" : "1" - if $#characters >= length($end_of_token_p_string); - } - $string =~ s/ $//; # remove previously added space, but keep original spaces - if ($return_trailing_whitespaces) { - while ($string =~ /^[ \t]/) { - push(@characters,substr($string, 0, 1)); - $string = substr($string, 1); - } - push(@characters, "\n") if $orig_string =~ /\n$/; - } - return ($return_only_chars) ? @characters : ($skipped_bytes, $end_of_token_p_string, @characters); -} - -sub max_substring_info { - local($caller,$s1,$s2,$info_type) = @_; - - ($skipped_bytes1, $end_of_token_p_string1, @char_list1) = $caller->split_into_utf8_characters($s1, "", *empty_ht); - ($skipped_bytes2, $end_of_token_p_string2, @char_list2) = $caller->split_into_utf8_characters($s2, "", *empty_ht); - return 0 if $skipped_bytes1 || $skipped_bytes2; - - $best_substring_start1 = 0; - $best_substring_start2 = 0; - $best_substring_length = 0; - - foreach $start_pos2 ((0 .. $#char_list2)) { - last if $start_pos2 + $best_substring_length > $#char_list2; - foreach $start_pos1 ((0 .. $#char_list1)) { - last if $start_pos1 + $best_substring_length > $#char_list1; - $matching_length = 0; - while (($start_pos1 + $matching_length <= $#char_list1) - && ($start_pos2 + $matching_length <= $#char_list2) - && ($char_list1[$start_pos1+$matching_length] eq $char_list2[$start_pos2+$matching_length])) { - $matching_length++; - } - if ($matching_length > $best_substring_length) { - $best_substring_length = $matching_length; - $best_substring_start1 = $start_pos1; - $best_substring_start2 = $start_pos2; - } - } - } - if ($info_type =~ /^max-ratio1$/) { - $length1 = $#char_list1 + 1; - return ($length1 > 0) ? ($best_substring_length / $length1) : 0; - } elsif ($info_type =~ /^max-ratio2$/) { - $length2 = $#char_list2 + 1; - return ($length2 > 0) ? ($best_substring_length / $length2) : 0; - } elsif ($info_type =~ /^substring$/) { - return join("", @char_list1[$best_substring_start1 .. $best_substring_start1+$best_substring_length-1]); - } else { - $length1 = $#char_list1 + 1; - $length2 = $#char_list2 + 1; - $info = "s1=$s1;s2=$s2"; - $info .= ";best_substring_length=$best_substring_length"; - $info .= ";best_substring_start1=$best_substring_start1"; - $info .= ";best_substring_start2=$best_substring_start2"; - $info .= ";length1=$length1"; - $info .= ";length2=$length2"; - return $info; - } -} - -sub n_shared_chars_at_start { - local($caller,$s1,$s2) = @_; - - my $n = 0; - while (($s1 ne "") && ($s2 ne "")) { - ($c1, $rest1) = ($s1 =~ /^(.[\x80-\xBF]*)(.*)$/); - ($c2, $rest2) = ($s2 =~ /^(.[\x80-\xBF]*)(.*)$/); - if ($c1 eq $c2) { - $n++; - $s1 = $rest1; - $s2 = $rest2; - } else { - last; - } - } - return $n; -} - -sub char_length { - local($caller,$string,$byte_offset) = @_; - - my $char = ($byte_offset) ? substr($string, $byte_offset) : $string; - return 1 if $char =~ /^[\x00-\x7F]/; - return 2 if $char =~ /^[\xC0-\xDF]/; - return 3 if $char =~ /^[\xE0-\xEF]/; - return 4 if $char =~ /^[\xF0-\xF7]/; - return 5 if $char =~ /^[\xF8-\xFB]/; - return 6 if $char =~ /^[\xFC-\xFD]/; - return 0; -} - -sub length_in_utf8_chars { - local($caller,$s) = @_; - - $s =~ s/[\x80-\xBF]//g; - $s =~ s/[\x00-\x7F\xC0-\xFF]/c/g; - return length($s); -} - -sub byte_length_of_n_chars { - local($caller,$char_length,$string,$byte_offset,$undef_return_value) = @_; - - $byte_offset = 0 unless defined($byte_offset); - $undef_return_value = -1 unless defined($undef_return_value); - my $result = 0; - my $len; - foreach $i ((1 .. $char_length)) { - $len = $caller->char_length($string,($byte_offset+$result)); - return $undef_return_value unless $len; - $result += $len; - } - return $result; -} - -sub replace_non_ASCII_bytes { - local($caller,$string,$replacement) = @_; - - $replacement = "HEX" unless defined($replacement); - if ($replacement =~ /^(Unicode|U\+4|\\u|HEX)$/) { - $new_string = ""; - while (($pre,$utf8_char, $post) = ($string =~ /^([\x09\x0A\x20-\x7E]*)([\x00-\x08\x0B-\x1F\x7F]|[\xC0-\xDF][\x80-\xBF]|[\xE0-\xEF][\x80-\xBF][\x80-\xBF]|[\xF0-\xF7][\x80-\xBF][\x80-\xBF][\x80-\xBF]|[\xF8-\xFF][\x80-\xBF]+|[\x80-\xBF])(.*)$/s)) { - if ($replacement =~ /Unicode/) { - $new_string .= $pre . "utf8_to_unicode($utf8_char)) . ">"; - } elsif ($replacement =~ /\\u/) { - $new_string .= $pre . "\\u" . (uc sprintf("%04x", $caller->utf8_to_unicode($utf8_char))); - } elsif ($replacement =~ /U\+4/) { - $new_string .= $pre . "utf8_to_4hex_unicode($utf8_char)) . ">"; - } else { - $new_string .= $pre . "{utils.format_directory(OUTPUT_DIR)}
- """, every=3, elem_id="files"
- )
- download_btn = gr.Button("Download All Files")
-
- chat_history = gr.State([[None, None]])
- api = gr.State(None)
-
- def start(open_ai_key, ai_name, ai_role, top_5_goals):
- auto_api = AutoAPI(open_ai_key, ai_name, ai_role, top_5_goals)
- return gr.Column.update(visible=False), gr.Column.update(visible=True), auto_api
-
- def bot_response(chat, api):
- messages = []
- for message in api.get_chatbot_response():
- messages.append(message)
- chat[-1][1] = "\n".join(messages) + "..."
- yield chat
- chat[-1][1] = "\n".join(messages)
- yield chat
-
- def send_message(count, chat, api, message="Y"):
- if message != "Y":
- count = 1
- for i in range(count):
- chat.append([message, None])
- yield chat, count - i
- api.send_message(message)
- for updated_chat in bot_response(chat, api):
- yield updated_chat, count - i
-
- def activate_inputs():
- return {
- yes_btn: gr.Button.update(interactive=True),
- consecutive_yes: gr.Slider.update(interactive=True),
- custom_response: gr.Textbox.update(interactive=True),
- }
-
- def deactivate_inputs():
- return {
- yes_btn: gr.Button.update(interactive=False),
- consecutive_yes: gr.Slider.update(interactive=False),
- custom_response: gr.Textbox.update(interactive=False),
- }
-
- start_btn.click(
- start,
- [open_ai_key, ai_name, ai_role, top_5_goals],
- [setup_pane, main_pane, api],
- ).then(bot_response, [chat_history, api], chatbot).then(
- activate_inputs, None, [yes_btn, consecutive_yes, custom_response]
- )
-
- yes_btn.click(
- deactivate_inputs, None, [yes_btn, consecutive_yes, custom_response]
- ).then(
- send_message, [consecutive_yes, chat_history, api], [chatbot, consecutive_yes]
- ).then(
- activate_inputs, None, [yes_btn, consecutive_yes, custom_response]
- )
- custom_response.submit(
- deactivate_inputs, None, [yes_btn, consecutive_yes, custom_response]
- ).then(
- send_message,
- [consecutive_yes, chat_history, api, custom_response],
- [chatbot, consecutive_yes],
- ).then(
- activate_inputs, None, [yes_btn, consecutive_yes, custom_response]
- )
-
- def download_all_files():
- shutil.make_archive("outputs", "zip", OUTPUT_DIR)
-
- download_btn.click(download_all_files).then(None, _js=utils.DOWNLOAD_OUTPUTS_JS)
-
-app.queue(concurrency_count=20).launch(file_directories=[OUTPUT_DIR])
diff --git a/spaces/awacke1/AW-04-GR-Seq-2-Seq-QA-Auto-Gen/qasrl_model_pipeline.py b/spaces/awacke1/AW-04-GR-Seq-2-Seq-QA-Auto-Gen/qasrl_model_pipeline.py
deleted file mode 100644
index 50135f76849bc8537fcae83b72532da661487da6..0000000000000000000000000000000000000000
--- a/spaces/awacke1/AW-04-GR-Seq-2-Seq-QA-Auto-Gen/qasrl_model_pipeline.py
+++ /dev/null
@@ -1,183 +0,0 @@
-from typing import Optional
-import json
-from argparse import Namespace
-from pathlib import Path
-from transformers import Text2TextGenerationPipeline, AutoModelForSeq2SeqLM, AutoTokenizer
-
-def get_markers_for_model(is_t5_model: bool) -> Namespace:
- special_tokens_constants = Namespace()
- if is_t5_model:
- # T5 model have 100 special tokens by default
- special_tokens_constants.separator_input_question_predicate = "e||125 DOWNLOAD … https://urloso.com/2uyQv0 Airport Simulator 2014 is a management-theme simulation game developed by Ikaron and published by United Independent Entertainment GmbH. The game takes place at a single airport, with two runways (dedicated to either arrivals or departures) and eight gates for planes to park. Planes arrive automatically at a fairly regular schedule as long as there is room for them. Airport Simulator 2014 was released on December 19, 2013 for Microsoft Windows computers only. Download Zip ✸ https://urloso.com/2uyOvd kakaotalk pc download windows 8adobe flash professional cs6 free download for windows 10csi pc game download freecrash bandicoot games pc download freecanon lide 110 scanner driver free download for windows 10command and conquer generals deluxe edition windows 10 downloadatomic runner game free download for pcdownload driver hp deskjet 2545 windows 10download syslog server for windows xp freegoogle earth free download for pc windows 10 -phonto-for-windows-10-download-phonto/ clock for windows 10 desktop free download logitech download assistant windows 10 download geforce 7025 nforce 630a driver download windows 10 download deep freezer windows 7 freedownload zip free windows 10java plugin download windows 10download full version of monopoly free for pcdownload game need for speed for pc windows 750 cent game download pcbittorrent free download for windows freebeyond compare free download for windows 10 full versionimage converter download for windows 10top paid windows phone 8 apps free download free -studio-free-download-for-windows-10-32-bit-fl/ microsoft office 2010 starter windows xp download free download windows 10 1703 iso 64 bit -pdf-free-download-for-windows-10-foxit/ microsoft access 2013 free download for windows 10 64 bit game maker studio free download for pc70-410 installing and configuring windows server 2012 r2 pdf download freeemule free download windows 7 64 bit freeinternet speed test software free download for windows 10art of war game download for pcbraid pc game free downloadwindows picture and fax viewer download free new freeasus t100 drivers download windows 10behringer u phoria um2 driver windows 10 downloadmovie maker windows vista free download free download c turbo for windows xp free java download free for windows 8 64 bit free airport simulator 2014 free download full version for pc cnx player download for windows 10 People love free steam games, no doubt. But what many people hate is downloading so many parts and trying to install them on their own. This is why we are the only site that pre-installs every game for you. We have many categories like shooters, action, racing, simulators and even VR games! We strive to satisfy our users and ask for nothing in return. We revolutionized the downloading scene and will continue being your #1 site for free games. The flying area encompasses planet Earth with varying degrees of detail and includes over 24,000 airports. There is an ever-growing list of scenery representing major landmarks and popular cities. Landscape details become sparse as gameplay moves away from population centers within the flight simulator, particularly outside the United States, although a variety of websites offer scenery add-ons to remedy this. The three latest versions incorporate sophisticated weather simulation, along with the ability to download real-world weather data (first available with Flight Simulator 2000). Additional features in these newer versions include air traffic environments with interactive air traffic control functions, new aircraft models from the historical Douglas DC-3 to the modern Boeing 777, interactive lessons, challenges, and aircraft checklists. The two latest versions of Microsoft Flight Simulator have a "kiosk mode", which allows the application to be run in electronic kiosks located in public places like shopping malls. Microsoft Flight Simulator has a wide selection of upgrades and add-ons, both free and commercial, official and fan-made. Often touted as 'FSX on steroids', P3D has so far had 5 versions, with the latest launched on April 14, 2020.[20] Version 5 features 41 aircraft and over 23000 airports. Before that, version 2, 3 and 4 saw releases in 2013, 2015, and 2017 respectively. Not being limited to using the default aircraft, add-on planes can be downloaded from many sources for free or purchased, which can then be installed into Microsoft Flight Simulator. The Beechcraft 1900D pictured above, is an add-on aircraft. Similarly, add-on repaints can be added to default aircraft; these repaints are usually downloaded for free. A growing add-on category for the series is AI (artificial intelligence) traffic. AI traffic is the simulation of other vehicles in the FS landscape. This traffic plays an important role in the simulator, as it is possible to crash into traffic (this can be disabled), thus ending your session, and to interact with the traffic via the radio and ATC. This feature is active even with third-party traffic. Microsoft introduced AI traffic in MSFS 2002 with several airliners and private aircraft. This has since been supplemented with many files created by third-party developers. Typically, third-party aircraft models have multiple levels of detail, which allow the AI traffic to be better on frame rates, while still being detailed during close looks. There are several prominent freeware developers. Some third-party AI traffic can even be configured for "real-time" departures. DOWNLOAD ····· https://urloso.com/2uyOlF Download Zip >>>>> https://urloso.com/2uyPu2
- DOWNLOAD >>> https://tinurli.com/2uwkrn The Hindi Kundli Software that we provide on AstroSage is as per Vedic Astrology. Get your free JanamKundali to know what stars has in store for your future. Fill your details below to get your Janam Kundaliin Hindi online: Download File ››› https://tinurli.com/2uwjV9 A Janam Kundali is a basic tool for making astrological predictions. To cast your horoscope, your date of birth, place and exact time is required for astronomical calculations.With free Hindi kundali software, you don't have to run for any astrologer, instead you will get youronline Kundli in hindi free. From giving you Ascendant, Moon sign and Nakshatra predictions free tofinally helping you lead a more prosperous life, the Janam Kundali Software is everything that you needto try for making your life happy and worth-living. A professional astrologer can prepare a Kundali, but in this digital world where everything is justa click away, getting an online Kundli is no longer a cumbersome task. AstroSage has got you a freehindi kundali software that can make your work easier and time-efficient. There are many astrologerswho use local time and place of birth to calculate rising and ascending stars of the native. If you are tired of consulting astrologers for doshas, match-making or any health related problem? Thenit's high time, you need to try out this online Kundli Software. Not only this software gives preciseastrological details but also provides you personalized Janam Kundali predictions. With the help ofthis online software, you can save a hack of time and money. So, if you are looking to take a sneakpeek into your personal, professional and love life, then online kundli software such as Janam Kundliis what you need to look forward to get an insight of your future. So, what are you waiting for? Goand try out this online Janam Kundali software absolutely free. A birth chart, kundli, janma kundali, janampatri, Vedic chart, Vedic horoscope, Hindu chart shows thedetailed planetary positions of stars at a native's place of birth. These transitions and changes getanalyzed to put together a form of specific format called Janam Kundali. We at AstroSage provides youan extraordinary Free Hindi Kundli software where you not only get your birth chart or Kundli in Hindi butalso get Hindi astrological Predictions, Hindi Horoscope Matching, Hindi Guna Milap, Hindi Kundali making and much more. All you need to do is to enter your birth details and get yourHindi Janam Kundli absolutely free. At AstroSage, our team of skilled astrologers will study and analyze your Kundli using Vedic astrologymethods and give answers to all your queries about you and your future. If you want to make your lifehappy, prosperous and worth-living, then you need to start using this free Kundli software online . Itis simple to use and demands a less of steps: all you need to do is to fill your name, sex, date, timeand place of birth and submit your birth details to get a personalized janam kundli in hindi. If youhave any suggestions regarding this software, feel free to share your views. We use Adobe Acrobat PDF files to provide electronic access to our forms and publications. You will need to have the Adobe Reader software installed to access them. We recommend using the most recent version of Adobe Reader -- available free from Adobe's website. Our playlist 100 Greatest Ghazal Hits features a diverse collection of songs in mp3 format, ready for you to download and enjoy without any charges or FREE of cost. With a mix of old favourites and new hits, there's something for everyone. Whether you're looking for the latest chartbuster songs or some classic tracks, our 100 Greatest Ghazal Hits playlist has got you covered. National Indian Health Board Releases Online Training to Support Non-Native Entities to Respectfully Engage With Tribal Nations Here you can find links to all of our entries, which feature collections of loops, hits and multisamples in a wide range of genres. And the great news is that you won't have to pay a penny to download any of them. The samples are supplied as WAV files so can be imported directly into your DAW of choice. Because they're royalty-free, you're welcome to use them in your music in any way you like - all we ask is that you don't re-distribute them. All the samples originally appeared on either a Computer Music or Future Music magazine cover disc. Check out their latest issues for many more, but first, scroll through the links below (ordered alphabetically), choose your genre and get downloading! Adobe Express puts the power of creation in your hands. You can resize your text, move it around the page, add special effects filters, make elements transparent, and change border configuration. The Adobe Express intuitive, easy-to-use functions mean you spend less time trying to figure out how to use the program and more time creating the perfect pamphlet. Best of all, Adobe Express is completely free to use. Bible Download Niv For Pc
-
-2018.8.0 for Windows. Find great deals for NIV Bible for Windows (English). Download Free NIV Bible latest v.2018.8.0 for Windows and more at CNET Download.com. The NIV Bible: Bible Study Software Application with over 2,200 Bible lessons, detailed teaching, and a wide range of study options and tools for a variety of learning styles, Kobo.com. Get NIV Bible. Download Free NIV Bible for Windows now from Softonic: 100% safe and virus free. More than 2220 downloads this month. Download Free NIV Bible latest v.2018.8.0 for Windows. Find great deals for NIV Bible for Windows (English).
-
-You can add your own authors in NIV Translation to form your own NIV Bible. Create your own custom Bible with authors of your choice.
-
-How to install NIV Bible:
-
-The Bible and Study Bible Software:
-
-NIV Bible for Windows. NIV Bible for Windows is an application for the Windows operating system. It features a Bible study software application and a Bible translation. The application also has a dictionary, a concordance, a thesaurus, a calendar, a verse counter, and other features. The application supports English, Russian, and Hebrew. It also works on computers running Windows. This free Bible study software application is available in English, Russian, and Hebrew. Learn more about this application on the NIV Bible website.
-
-NIV Bible for Android. NIV Bible for Android is an application for the Android operating system. It features a Bible study software application and a Bible translation. The application also has a dictionary, a concordance, a thesaurus, a calendar, a verse counter, and other features. The application supports English, Russian, and Hebrew. It also works on computers running Android. This free Bible study software application is available in English, Russian, and Hebrew. Learn more about this application on the NIV Bible website.
-
-NIV Bible for Windows. NIV Bible for Windows is an application for the Windows operating system. It features a Bible study software application and a Bible translation. The application also has a dictionary, a concordance, a thesaurus, a calendar, a verse counter, and other features. The application supports English, Russian, and Hebrew. It also works on computers running Windows. This free Bible study software application is available in English, Russian, and Hebrew. Learn more about this application on 4fefd39f24
-
-
-
diff --git a/spaces/bioriAsaeru/text-to-voice/Download Airport Simulator 2013 Free Pc [NEW].md b/spaces/bioriAsaeru/text-to-voice/Download Airport Simulator 2013 Free Pc [NEW].md
deleted file mode 100644
index cce28e368488740e83a837dd65ae554e482c7fd3..0000000000000000000000000000000000000000
--- a/spaces/bioriAsaeru/text-to-voice/Download Airport Simulator 2013 Free Pc [NEW].md
+++ /dev/null
@@ -1,28 +0,0 @@
-
-download airport simulator 2013 free pc
-
8gadgetpack windows 10 download
free download teamviewer version 8 for windows xp free
microsoft acpi compliant control method battery download windows 10
windows 7 themes free download full version for pc
download java 7 update 25 32 bit windows xp free
java 6 windows xp download free
christmas games download free pc
windows media player mpeg 2 decoder free download free
mozilla firefox for pc windows 8.1 64 bit free download
best online games for pc no download
pc doctor free download for windows xp free
black ops 3 free download windows 10
mplayer download windows free
download 5kplayer for windows 10
download hirens boot usb windows 10
-fx-3450-drivers-video-nvidia-quadro-fx3450/
-mic-mod-efx-free-download-antares-efx/
-spartan-6-lx9-microboard-ladads-info/
-commander-windows-10-iso-download-create-an/
free pdf windows 10 download
audacity software for windows 10 free download
download synology assistant windows 10
ea sports cricket 2005 pc game free download full version
download java jdk windows 7 32 bits free
download skype for business 32 bit windows 10
f1 2012 pc game free download
download airprint installer for windows 10
internet explorer 8 torrent download windows xp free
download vmware free for windows 10
imgburn free download for windows 10
instagram windows phone download free
microsoft visio 2013 free download for windows 7 64 bit free
macromedia flash 8 download for windows 10
c windows system32 cmd exe download free
freecell windows 8.1 download free
download windows 10 32 64 bit
best offline game for pc free download
download vlc media player for windows 10 32 bit
mcafee antivirus download for pc windows 10
best video editing software for windows xp free download free
windows 10 download folder remove groups free
windows microsoft 2010 free download free
photoshop free download for pc windows 8
windows 8.1 preview download free
backgammon download windows 10
download ppsspp gold emulator for pc windows 7
-ok.ru/download-windows-10-softonic-download-google/
-download-visual-studio-code-for-windows-10-64/
-download-manager-for-windows-10-11-best/
-dcr-trv350-about-this-item/
free download skype for pc window xp
itunes for windows 10 32 bit free download free
black and white 2 full pc game download
batman arkham origins pc game download
avg antivirus free download for pc windows 10
bakugan battle brawlers game pc download free
download windows movie maker pc
media windows player 10 free download
-blu-ray-bde5400-samsung-bd-e5400-blu-ray/
-ok.ru/download-windows-10-free-upgrade-windows-7/
-case-files-broken-hour-broken-hour/
-ok.ru/download-windows-10-1511-update-is-it-possible-to/
microsoft word 2007 free download for windows xp 32 bit free
download itunes for windows 10 32 bit free
windows 7 bluetooth audio driver download free
gta 4 game download for pc windows 7
libreoffice download free for windows 10
download accuweather for windows 10
garageband free windows download free
download bitlocker for windows 10 64 bit
ilivid download manager windows 7 free
collage app download free for pc
free download windows xp themes for pc free
java download for windows 7 32 bit oracle free
automatic windows 10 download free
excel viewer free download for windows 10
cities skylines game free download for pc
download free chess games for windows 10
free download star uml for windows 10
air strike 3d pc game free download
windows office word 2010 free download free
download toy story 2 game for pc
live desktop wallpaper windows 10 free download
coreldraw x4 windows 10 download full version
download game sex simulator pc
download splunk for windows 10 64 bit
aliexpress app for pc free download
excel software download for pc windows 7
free download youtube software for windows 10
april 2018 windows 10 update download
free download ie 10 for windows xp free
download inkscape for windows xp free free
airfoil download windows free
adobe indesign free download for windows free
big 2 card game free download for pc
carmageddon 2 free download for pc
movie cutter free download for windows xp free
lyrical ly app download for pc windows 10
download the sims 4 for pc for free
disney games pc free download
windows media player 11 microsoft download free
download xinput1_3 dll for windows 10 64 bit
app lock free download for pc windows 10
hp officejet 6500a plus driver download windows 10
windows media player c00d1199 free download free
download driver printer epson l310 for windows 10 64 bit
pdf download windows xp free free
-
-
\ No newline at end of file
diff --git a/spaces/bioriAsaeru/text-to-voice/Fenimore Fillmores Revenge [RUSRussobit-M]2008 RePack Download Free.md b/spaces/bioriAsaeru/text-to-voice/Fenimore Fillmores Revenge [RUSRussobit-M]2008 RePack Download Free.md
deleted file mode 100644
index 3fd125b1c4e5abcf7a73c8fea273feba6ffd5ca2..0000000000000000000000000000000000000000
--- a/spaces/bioriAsaeru/text-to-voice/Fenimore Fillmores Revenge [RUSRussobit-M]2008 RePack Download Free.md
+++ /dev/null
@@ -1,6 +0,0 @@
-Fenimore Fillmore's Revenge [RUS|Russobit-M]2008 RePack Download
-
-10 Minute Solution Yoga for Beginners 2008.zip, 626.40KB. 10 PSD - Love ... 3oh3 - My First Kiss featuring Keha.zip, 626.40KB. 3OH3 - Streets ... 40 Sex Tv 3.7 Rus Free Portable.zip, 626.38KB. 40 Super HOT ... Fenimore Fillmores Revenge.zip, 626.37KB ... Overloud BREVERB 1.59 VST REPACK.zip, 626.40KB. Overloud ... 4d29de3e1b
-
-
-
diff --git a/spaces/bioriAsaeru/text-to-voice/Fundamentosdeacupunturaymoxibustiondechina .md b/spaces/bioriAsaeru/text-to-voice/Fundamentosdeacupunturaymoxibustiondechina .md
deleted file mode 100644
index 19728065824236811320947d1300f591223b0c13..0000000000000000000000000000000000000000
--- a/spaces/bioriAsaeru/text-to-voice/Fundamentosdeacupunturaymoxibustiondechina .md
+++ /dev/null
@@ -1,6 +0,0 @@
-fundamentosdeacupunturaymoxibustiondechina
-
- aaccfb2cb3
-
-
-
diff --git a/spaces/biubiubiiu/EFDM/net.py b/spaces/biubiubiiu/EFDM/net.py
deleted file mode 100644
index b2f52dc563b143849e0543a385af92780ecea31d..0000000000000000000000000000000000000000
--- a/spaces/biubiubiiu/EFDM/net.py
+++ /dev/null
@@ -1,198 +0,0 @@
-import torch.nn as nn
-import torch
-from function import adaptive_mean_normalization as adamean
-from function import adaptive_std_normalization as adastd
-from function import adaptive_instance_normalization as adain
-from function import exact_feature_distribution_matching as efdm
-from function import histogram_matching as hm
-
-from function import calc_mean_std
-# import ipdb
-from skimage.exposure import match_histograms
-import numpy as np
-
-decoder = nn.Sequential(
- nn.ReflectionPad2d((1, 1, 1, 1)),
- nn.Conv2d(512, 256, (3, 3)),
- nn.ReLU(),
- nn.Upsample(scale_factor=2, mode='nearest'),
- nn.ReflectionPad2d((1, 1, 1, 1)),
- nn.Conv2d(256, 256, (3, 3)),
- nn.ReLU(),
- nn.ReflectionPad2d((1, 1, 1, 1)),
- nn.Conv2d(256, 256, (3, 3)),
- nn.ReLU(),
- nn.ReflectionPad2d((1, 1, 1, 1)),
- nn.Conv2d(256, 256, (3, 3)),
- nn.ReLU(),
- nn.ReflectionPad2d((1, 1, 1, 1)),
- nn.Conv2d(256, 128, (3, 3)),
- nn.ReLU(),
- nn.Upsample(scale_factor=2, mode='nearest'),
- nn.ReflectionPad2d((1, 1, 1, 1)),
- nn.Conv2d(128, 128, (3, 3)),
- nn.ReLU(),
- nn.ReflectionPad2d((1, 1, 1, 1)),
- nn.Conv2d(128, 64, (3, 3)),
- nn.ReLU(),
- nn.Upsample(scale_factor=2, mode='nearest'),
- nn.ReflectionPad2d((1, 1, 1, 1)),
- nn.Conv2d(64, 64, (3, 3)),
- nn.ReLU(),
- nn.ReflectionPad2d((1, 1, 1, 1)),
- nn.Conv2d(64, 3, (3, 3)),
-)
-
-vgg = nn.Sequential(
- nn.Conv2d(3, 3, (1, 1)),
- nn.ReflectionPad2d((1, 1, 1, 1)),
- nn.Conv2d(3, 64, (3, 3)),
- nn.ReLU(), # relu1-1
- nn.ReflectionPad2d((1, 1, 1, 1)),
- nn.Conv2d(64, 64, (3, 3)),
- nn.ReLU(), # relu1-2
- nn.MaxPool2d((2, 2), (2, 2), (0, 0), ceil_mode=True),
- nn.ReflectionPad2d((1, 1, 1, 1)),
- nn.Conv2d(64, 128, (3, 3)),
- nn.ReLU(), # relu2-1
- nn.ReflectionPad2d((1, 1, 1, 1)),
- nn.Conv2d(128, 128, (3, 3)),
- nn.ReLU(), # relu2-2
- nn.MaxPool2d((2, 2), (2, 2), (0, 0), ceil_mode=True),
- nn.ReflectionPad2d((1, 1, 1, 1)),
- nn.Conv2d(128, 256, (3, 3)),
- nn.ReLU(), # relu3-1
- nn.ReflectionPad2d((1, 1, 1, 1)),
- nn.Conv2d(256, 256, (3, 3)),
- nn.ReLU(), # relu3-2
- nn.ReflectionPad2d((1, 1, 1, 1)),
- nn.Conv2d(256, 256, (3, 3)),
- nn.ReLU(), # relu3-3
- nn.ReflectionPad2d((1, 1, 1, 1)),
- nn.Conv2d(256, 256, (3, 3)),
- nn.ReLU(), # relu3-4
- nn.MaxPool2d((2, 2), (2, 2), (0, 0), ceil_mode=True),
- nn.ReflectionPad2d((1, 1, 1, 1)),
- nn.Conv2d(256, 512, (3, 3)),
- nn.ReLU(), # relu4-1, this is the last layer used
- nn.ReflectionPad2d((1, 1, 1, 1)),
- nn.Conv2d(512, 512, (3, 3)),
- nn.ReLU(), # relu4-2
- nn.ReflectionPad2d((1, 1, 1, 1)),
- nn.Conv2d(512, 512, (3, 3)),
- nn.ReLU(), # relu4-3
- nn.ReflectionPad2d((1, 1, 1, 1)),
- nn.Conv2d(512, 512, (3, 3)),
- nn.ReLU(), # relu4-4
- nn.MaxPool2d((2, 2), (2, 2), (0, 0), ceil_mode=True),
- nn.ReflectionPad2d((1, 1, 1, 1)),
- nn.Conv2d(512, 512, (3, 3)),
- nn.ReLU(), # relu5-1
- nn.ReflectionPad2d((1, 1, 1, 1)),
- nn.Conv2d(512, 512, (3, 3)),
- nn.ReLU(), # relu5-2
- nn.ReflectionPad2d((1, 1, 1, 1)),
- nn.Conv2d(512, 512, (3, 3)),
- nn.ReLU(), # relu5-3
- nn.ReflectionPad2d((1, 1, 1, 1)),
- nn.Conv2d(512, 512, (3, 3)),
- nn.ReLU() # relu5-4
-)
-
-
-class Net(nn.Module):
- def __init__(self, encoder, decoder, style):
- super(Net, self).__init__()
- enc_layers = list(encoder.children())
- self.enc_1 = nn.Sequential(*enc_layers[:4]) # input -> relu1_1
- self.enc_2 = nn.Sequential(*enc_layers[4:11]) # relu1_1 -> relu2_1
- self.enc_3 = nn.Sequential(*enc_layers[11:18]) # relu2_1 -> relu3_1
- self.enc_4 = nn.Sequential(*enc_layers[18:31]) # relu3_1 -> relu4_1
- self.decoder = decoder
- self.mse_loss = nn.MSELoss()
- self.style = style
-
- # fix the encoder
- for name in ['enc_1', 'enc_2', 'enc_3', 'enc_4']:
- for param in getattr(self, name).parameters():
- param.requires_grad = False
-
- # extract relu1_1, relu2_1, relu3_1, relu4_1 from input image
- def encode_with_intermediate(self, input):
- results = [input]
- for i in range(4):
- func = getattr(self, 'enc_{:d}'.format(i + 1))
- results.append(func(results[-1]))
- return results[1:]
-
- # extract relu4_1 from input image
- def encode(self, input):
- for i in range(4):
- input = getattr(self, 'enc_{:d}'.format(i + 1))(input)
- return input
-
- def calc_content_loss(self, input, target):
- assert (input.size() == target.size())
- assert (target.requires_grad is False)
- return self.mse_loss(input, target)
-
- def calc_style_loss(self, input, target):
- # ipdb.set_trace()
- assert (input.size() == target.size())
- assert (target.requires_grad is False) ## first make sure which one require gradient and which one do not.
- # print(input.requires_grad) ## True
- input_mean, input_std = calc_mean_std(input)
- target_mean, target_std = calc_mean_std(target)
- if self.style == 'adain':
- return self.mse_loss(input_mean, target_mean) + \
- self.mse_loss(input_std, target_std)
- elif self.style == 'adamean':
- return self.mse_loss(input_mean, target_mean)
- elif self.style == 'adastd':
- return self.mse_loss(input_std, target_std)
- elif self.style == 'efdm':
- B, C, W, H = input.size(0), input.size(1), input.size(2), input.size(3)
- value_content, index_content = torch.sort(input.view(B, C, -1))
- value_style, index_style = torch.sort(target.view(B, C, -1))
- inverse_index = index_content.argsort(-1)
- return self.mse_loss(input.view(B,C,-1), value_style.gather(-1, inverse_index))
- elif self.style == 'hm':
- B, C, W, H = input.size(0), input.size(1), input.size(2), input.size(3)
- x_view = input.view(-1, W, H)
- image1_temp = match_histograms(np.array(x_view.detach().clone().cpu().float().transpose(0, 2)),
- np.array(target.view(-1, W, H).detach().clone().cpu().float().transpose(0,2)),
- multichannel=True)
- image1_temp = torch.from_numpy(image1_temp).float().to(input.device).transpose(0, 2).view(B, C, W, H)
- return self.mse_loss(input.reshape(B, C, -1), image1_temp.reshape(B, C, -1))
- else:
- raise NotImplementedError
-
- def forward(self, content, style, alpha=1.0):
- assert 0 <= alpha <= 1
- # ipdb.set_trace()
- style_feats = self.encode_with_intermediate(style)
- content_feat = self.encode(content)
- # print(content_feat.requires_grad) False
- # print(style_feats[-1].requires_grad) False
- if self.style == 'adain':
- t = adain(content_feat, style_feats[-1])
- elif self.style == 'adamean':
- t = adamean(content_feat, style_feats[-1])
- elif self.style == 'adastd':
- t = adastd(content_feat, style_feats[-1])
- elif self.style == 'efdm':
- t = efdm(content_feat, style_feats[-1])
- elif self.style == 'hm':
- t = hm(content_feat, style_feats[-1])
- else:
- raise NotImplementedError
- t = alpha * t + (1 - alpha) * content_feat
-
- g_t = self.decoder(t)
- g_t_feats = self.encode_with_intermediate(g_t)
-
- loss_c = self.calc_content_loss(g_t_feats[-1], t) ### final feature should be the same.
- loss_s = self.calc_style_loss(g_t_feats[0], style_feats[0])
- for i in range(1, 4):
- loss_s += self.calc_style_loss(g_t_feats[i], style_feats[i])
- return loss_c, loss_s
diff --git a/spaces/brjathu/HMR2.0/vendor/detectron2/projects/DensePose/tests/test_chart_based_annotations_accumulator.py b/spaces/brjathu/HMR2.0/vendor/detectron2/projects/DensePose/tests/test_chart_based_annotations_accumulator.py
deleted file mode 100644
index a1c4f8565a3c55b79b6ed96b03635e6c2932958d..0000000000000000000000000000000000000000
--- a/spaces/brjathu/HMR2.0/vendor/detectron2/projects/DensePose/tests/test_chart_based_annotations_accumulator.py
+++ /dev/null
@@ -1,76 +0,0 @@
-# Copyright (c) Facebook, Inc. and its affiliates.
-
-import unittest
-import torch
-
-from detectron2.structures import Boxes, BoxMode, Instances
-
-from densepose.modeling.losses.utils import ChartBasedAnnotationsAccumulator
-from densepose.structures import DensePoseDataRelative, DensePoseList
-
-image_shape = (100, 100)
-instances = Instances(image_shape)
-n_instances = 3
-instances.proposal_boxes = Boxes(torch.rand(n_instances, 4))
-instances.gt_boxes = Boxes(torch.rand(n_instances, 4))
-
-
-# instances.gt_densepose = None cannot happen because instances attributes need a length
-class TestChartBasedAnnotationsAccumulator(unittest.TestCase):
- def test_chart_based_annotations_accumulator_no_gt_densepose(self):
- accumulator = ChartBasedAnnotationsAccumulator()
- accumulator.accumulate(instances)
- expected_values = {"nxt_bbox_with_dp_index": 0, "nxt_bbox_index": n_instances}
- for key in accumulator.__dict__:
- self.assertEqual(getattr(accumulator, key), expected_values.get(key, []))
-
- def test_chart_based_annotations_accumulator_gt_densepose_none(self):
- instances.gt_densepose = [None] * n_instances
- accumulator = ChartBasedAnnotationsAccumulator()
- accumulator.accumulate(instances)
- expected_values = {"nxt_bbox_with_dp_index": 0, "nxt_bbox_index": n_instances}
- for key in accumulator.__dict__:
- self.assertEqual(getattr(accumulator, key), expected_values.get(key, []))
-
- def test_chart_based_annotations_accumulator_gt_densepose(self):
- data_relative_keys = [
- DensePoseDataRelative.X_KEY,
- DensePoseDataRelative.Y_KEY,
- DensePoseDataRelative.I_KEY,
- DensePoseDataRelative.U_KEY,
- DensePoseDataRelative.V_KEY,
- DensePoseDataRelative.S_KEY,
- ]
- annotations = [DensePoseDataRelative({k: [0] for k in data_relative_keys})] * n_instances
- instances.gt_densepose = DensePoseList(annotations, instances.gt_boxes, image_shape)
- accumulator = ChartBasedAnnotationsAccumulator()
- accumulator.accumulate(instances)
- bbox_xywh_est = BoxMode.convert(
- instances.proposal_boxes.tensor.clone(), BoxMode.XYXY_ABS, BoxMode.XYWH_ABS
- )
- bbox_xywh_gt = BoxMode.convert(
- instances.gt_boxes.tensor.clone(), BoxMode.XYXY_ABS, BoxMode.XYWH_ABS
- )
- expected_values = {
- "s_gt": [
- torch.zeros((3, DensePoseDataRelative.MASK_SIZE, DensePoseDataRelative.MASK_SIZE))
- ]
- * n_instances,
- "bbox_xywh_est": bbox_xywh_est.split(1),
- "bbox_xywh_gt": bbox_xywh_gt.split(1),
- "point_bbox_with_dp_indices": [torch.tensor([i]) for i in range(n_instances)],
- "point_bbox_indices": [torch.tensor([i]) for i in range(n_instances)],
- "bbox_indices": list(range(n_instances)),
- "nxt_bbox_with_dp_index": n_instances,
- "nxt_bbox_index": n_instances,
- }
- default_value = [torch.tensor([0])] * 3
- for key in accumulator.__dict__:
- to_test = getattr(accumulator, key)
- gt_value = expected_values.get(key, default_value)
- if key in ["nxt_bbox_with_dp_index", "nxt_bbox_index"]:
- self.assertEqual(to_test, gt_value)
- elif key == "bbox_indices":
- self.assertListEqual(to_test, gt_value)
- else:
- self.assertTrue(torch.allclose(torch.stack(to_test), torch.stack(gt_value)))
diff --git a/spaces/caffeinum/VToonify/vtoonify/smooth_parsing_map.py b/spaces/caffeinum/VToonify/vtoonify/smooth_parsing_map.py
deleted file mode 100644
index 7720d0c7786925db38d3e793d6a3a8f68f6e663e..0000000000000000000000000000000000000000
--- a/spaces/caffeinum/VToonify/vtoonify/smooth_parsing_map.py
+++ /dev/null
@@ -1,172 +0,0 @@
-import os
-#os.environ['CUDA_VISIBLE_DEVICES'] = "0"
-import numpy as np
-import cv2
-import math
-import argparse
-from tqdm import tqdm
-import torch
-from torch import nn
-from torchvision import transforms
-import torch.nn.functional as F
-from model.raft.core.raft import RAFT
-from model.raft.core.utils.utils import InputPadder
-from model.bisenet.model import BiSeNet
-from model.stylegan.model import Downsample
-
-class Options():
- def __init__(self):
-
- self.parser = argparse.ArgumentParser(description="Smooth Parsing Maps")
- self.parser.add_argument("--window_size", type=int, default=5, help="temporal window size")
-
- self.parser.add_argument("--faceparsing_path", type=str, default='./checkpoint/faceparsing.pth', help="path of the face parsing model")
- self.parser.add_argument("--raft_path", type=str, default='./checkpoint/raft-things.pth', help="path of the RAFT model")
-
- self.parser.add_argument("--video_path", type=str, help="path of the target video")
- self.parser.add_argument("--output_path", type=str, default='./output/', help="path of the output parsing maps")
-
- def parse(self):
- self.opt = self.parser.parse_args()
- args = vars(self.opt)
- print('Load options')
- for name, value in sorted(args.items()):
- print('%s: %s' % (str(name), str(value)))
- return self.opt
-
-# from RAFT
-def warp(x, flo):
- """
- warp an image/tensor (im2) back to im1, according to the optical flow
- x: [B, C, H, W] (im2)
- flo: [B, 2, H, W] flow
- """
- B, C, H, W = x.size()
- # mesh grid
- xx = torch.arange(0, W).view(1,-1).repeat(H,1)
- yy = torch.arange(0, H).view(-1,1).repeat(1,W)
- xx = xx.view(1,1,H,W).repeat(B,1,1,1)
- yy = yy.view(1,1,H,W).repeat(B,1,1,1)
- grid = torch.cat((xx,yy),1).float()
-
-
- #x = x.cuda()
- grid = grid.cuda()
- vgrid = grid + flo # B,2,H,W
-
- # scale grid to [-1,1]
- ##2019 code
- vgrid[:,0,:,:] = 2.0*vgrid[:,0,:,:].clone()/max(W-1,1)-1.0
- vgrid[:,1,:,:] = 2.0*vgrid[:,1,:,:].clone()/max(H-1,1)-1.0
-
- vgrid = vgrid.permute(0,2,3,1)
- output = nn.functional.grid_sample(x, vgrid,align_corners=True)
- mask = torch.autograd.Variable(torch.ones(x.size())).cuda()
- mask = nn.functional.grid_sample(mask, vgrid,align_corners=True)
-
- ##2019 author
- mask[mask<0.9999] = 0
- mask[mask>0] = 1
-
- ##2019 code
- # mask = torch.floor(torch.clamp(mask, 0 ,1))
-
- return output*mask, mask
-
-
-if __name__ == "__main__":
-
- parser = Options()
- args = parser.parse()
- print('*'*98)
-
-
- device = "cuda"
-
- transform = transforms.Compose([
- transforms.ToTensor(),
- transforms.Normalize(mean=[0.5, 0.5, 0.5],std=[0.5,0.5,0.5]),
- ])
-
- parser = argparse.ArgumentParser()
- parser.add_argument('--model', help="restore checkpoint")
- parser.add_argument('--small', action='store_true', help='use small model')
- parser.add_argument('--mixed_precision', action='store_true', help='use mixed precision')
- parser.add_argument('--alternate_corr', action='store_true', help='use efficent correlation implementation')
-
- raft_model = torch.nn.DataParallel(RAFT(parser.parse_args(['--model', args.raft_path])))
- raft_model.load_state_dict(torch.load(args.raft_path))
-
- raft_model = raft_model.module
- raft_model.to(device)
- raft_model.eval()
-
- parsingpredictor = BiSeNet(n_classes=19)
- parsingpredictor.load_state_dict(torch.load(args.faceparsing_path, map_location=lambda storage, loc: storage))
- parsingpredictor.to(device).eval()
-
- down = Downsample(kernel=[1, 3, 3, 1], factor=2).to(device).eval()
-
- print('Load models successfully!')
-
- window = args.window_size
-
- video_cap = cv2.VideoCapture(args.video_path)
- num = int(video_cap.get(7))
-
- Is = []
- for i in range(num):
- success, frame = video_cap.read()
- if success == False:
- break
- frame = cv2.cvtColor(frame, cv2.COLOR_BGR2RGB)
- with torch.no_grad():
- Is += [transform(frame).unsqueeze(dim=0).cpu()]
- video_cap.release()
-
- # enlarge frames for more accurate parsing maps and optical flows
- Is = F.upsample(torch.cat(Is, dim=0), scale_factor=2, mode='bilinear')
- Is_ = torch.cat((Is[0:window], Is, Is[-window:]), dim=0)
-
- print('Load video with %d frames successfully!'%(len(Is)))
-
- Ps = []
- for i in tqdm(range(len(Is))):
- with torch.no_grad():
- Ps += [parsingpredictor(2*Is[i:i+1].to(device))[0].detach().cpu()]
- Ps = torch.cat(Ps, dim=0)
- Ps_ = torch.cat((Ps[0:window], Ps, Ps[-window:]), dim=0)
-
- print('Predict parsing maps successfully!')
-
-
- # temporal weights of the (2*args.window_size+1) frames
- wt = torch.exp(-(torch.arange(2*window+1).float()-window)**2/(2*((window+0.5)**2))).reshape(2*window+1,1,1,1).to(device)
-
- parse = []
- for ii in tqdm(range(len(Is))):
- i = ii + window
- image2 = Is_[i-window:i+window+1].to(device)
- image1 = Is_[i].repeat(2*window+1,1,1,1).to(device)
- padder = InputPadder(image1.shape)
- image1, image2 = padder.pad(image1, image2)
- with torch.no_grad():
- flow_low, flow_up = raft_model((image1+1)*255.0/2, (image2+1)*255.0/2, iters=20, test_mode=True)
- output, mask = warp(torch.cat((image2, Ps_[i-window:i+window+1].to(device)), dim=1), flow_up)
- aligned_Is = output[:,0:3].detach()
- aligned_Ps = output[:,3:].detach()
- # the spatial weight
- ws = torch.exp(-((aligned_Is-image1)**2).mean(dim=1, keepdims=True)/(2*(0.2**2))) * mask[:,0:1]
- aligned_Ps[window] = Ps_[i].to(device)
- # the weight between i and i shoud be 1.0
- ws[window,:,:,:] = 1.0
- weights = ws*wt
- weights = weights / weights.sum(dim=(0), keepdims=True)
- fused_Ps = (aligned_Ps * weights).sum(dim=0, keepdims=True)
- parse += [down(fused_Ps).detach().cpu()]
- parse = torch.cat(parse, dim=0)
-
- basename = os.path.basename(args.video_path).split('.')[0]
- np.save(os.path.join(args.output_path, basename+'_parsingmap.npy'), parse.numpy())
-
- print('Done!')
\ No newline at end of file
diff --git a/spaces/carlosalonso/Detection-video/carpeta_deteccion/projects/DensePose/densepose/data/__init__.py b/spaces/carlosalonso/Detection-video/carpeta_deteccion/projects/DensePose/densepose/data/__init__.py
deleted file mode 100644
index bf21ba75306970fd6a44069b49107320a84182b8..0000000000000000000000000000000000000000
--- a/spaces/carlosalonso/Detection-video/carpeta_deteccion/projects/DensePose/densepose/data/__init__.py
+++ /dev/null
@@ -1,25 +0,0 @@
-# Copyright (c) Facebook, Inc. and its affiliates.
-
-from .meshes import builtin
-from .build import (
- build_detection_test_loader,
- build_detection_train_loader,
- build_combined_loader,
- build_frame_selector,
- build_inference_based_loaders,
- has_inference_based_loaders,
- BootstrapDatasetFactoryCatalog,
-)
-from .combined_loader import CombinedDataLoader
-from .dataset_mapper import DatasetMapper
-from .inference_based_loader import InferenceBasedLoader, ScoreBasedFilter
-from .image_list_dataset import ImageListDataset
-from .utils import is_relative_local_path, maybe_prepend_base_path
-
-# ensure the builtin datasets are registered
-from . import datasets
-
-# ensure the bootstrap datasets builders are registered
-from . import build
-
-__all__ = [k for k in globals().keys() if not k.startswith("_")]
diff --git a/spaces/carlosalonso/Detection-video/carpeta_deteccion/projects/DensePose/densepose/vis/densepose_results.py b/spaces/carlosalonso/Detection-video/carpeta_deteccion/projects/DensePose/densepose/vis/densepose_results.py
deleted file mode 100644
index ce8a7c0e207f5b3b6e755c759a59f5bed9965cef..0000000000000000000000000000000000000000
--- a/spaces/carlosalonso/Detection-video/carpeta_deteccion/projects/DensePose/densepose/vis/densepose_results.py
+++ /dev/null
@@ -1,355 +0,0 @@
-# Copyright (c) Facebook, Inc. and its affiliates.
-import logging
-import numpy as np
-from typing import List, Optional, Tuple
-import cv2
-import torch
-
-from densepose.structures import DensePoseDataRelative
-
-from ..structures import DensePoseChartResult
-from .base import Boxes, Image, MatrixVisualizer
-
-
-class DensePoseResultsVisualizer(object):
- def visualize(
- self,
- image_bgr: Image,
- results_and_boxes_xywh: Tuple[Optional[List[DensePoseChartResult]], Optional[Boxes]],
- ) -> Image:
- densepose_result, boxes_xywh = results_and_boxes_xywh
- if densepose_result is None or boxes_xywh is None:
- return image_bgr
-
- boxes_xywh = boxes_xywh.cpu().numpy()
- context = self.create_visualization_context(image_bgr)
- for i, result in enumerate(densepose_result):
- iuv_array = torch.cat(
- (result.labels[None].type(torch.float32), result.uv * 255.0)
- ).type(torch.uint8)
- self.visualize_iuv_arr(context, iuv_array.cpu().numpy(), boxes_xywh[i])
- image_bgr = self.context_to_image_bgr(context)
- return image_bgr
-
- def create_visualization_context(self, image_bgr: Image):
- return image_bgr
-
- def visualize_iuv_arr(self, context, iuv_arr: np.ndarray, bbox_xywh) -> None:
- pass
-
- def context_to_image_bgr(self, context):
- return context
-
- def get_image_bgr_from_context(self, context):
- return context
-
-
-class DensePoseMaskedColormapResultsVisualizer(DensePoseResultsVisualizer):
- def __init__(
- self,
- data_extractor,
- segm_extractor,
- inplace=True,
- cmap=cv2.COLORMAP_PARULA,
- alpha=0.7,
- val_scale=1.0,
- **kwargs,
- ):
- self.mask_visualizer = MatrixVisualizer(
- inplace=inplace, cmap=cmap, val_scale=val_scale, alpha=alpha
- )
- self.data_extractor = data_extractor
- self.segm_extractor = segm_extractor
-
- def context_to_image_bgr(self, context):
- return context
-
- def visualize_iuv_arr(self, context, iuv_arr: np.ndarray, bbox_xywh) -> None:
- image_bgr = self.get_image_bgr_from_context(context)
- matrix = self.data_extractor(iuv_arr)
- segm = self.segm_extractor(iuv_arr)
- mask = np.zeros(matrix.shape, dtype=np.uint8)
- mask[segm > 0] = 1
- image_bgr = self.mask_visualizer.visualize(image_bgr, mask, matrix, bbox_xywh)
-
-
-def _extract_i_from_iuvarr(iuv_arr):
- return iuv_arr[0, :, :]
-
-
-def _extract_u_from_iuvarr(iuv_arr):
- return iuv_arr[1, :, :]
-
-
-def _extract_v_from_iuvarr(iuv_arr):
- return iuv_arr[2, :, :]
-
-
-class DensePoseResultsMplContourVisualizer(DensePoseResultsVisualizer):
- def __init__(self, levels=10, **kwargs):
- self.levels = levels
- self.plot_args = kwargs
-
- def create_visualization_context(self, image_bgr: Image):
- import matplotlib.pyplot as plt
- from matplotlib.backends.backend_agg import FigureCanvasAgg as FigureCanvas
-
- context = {}
- context["image_bgr"] = image_bgr
- dpi = 100
- height_inches = float(image_bgr.shape[0]) / dpi
- width_inches = float(image_bgr.shape[1]) / dpi
- fig = plt.figure(figsize=(width_inches, height_inches), dpi=dpi)
- plt.axes([0, 0, 1, 1])
- plt.axis("off")
- context["fig"] = fig
- canvas = FigureCanvas(fig)
- context["canvas"] = canvas
- extent = (0, image_bgr.shape[1], image_bgr.shape[0], 0)
- plt.imshow(image_bgr[:, :, ::-1], extent=extent)
- return context
-
- def context_to_image_bgr(self, context):
- fig = context["fig"]
- w, h = map(int, fig.get_size_inches() * fig.get_dpi())
- canvas = context["canvas"]
- canvas.draw()
- image_1d = np.fromstring(canvas.tostring_rgb(), dtype="uint8")
- image_rgb = image_1d.reshape(h, w, 3)
- image_bgr = image_rgb[:, :, ::-1].copy()
- return image_bgr
-
- def visualize_iuv_arr(self, context, iuv_arr: np.ndarray, bbox_xywh: Boxes) -> None:
- import matplotlib.pyplot as plt
-
- u = _extract_u_from_iuvarr(iuv_arr).astype(float) / 255.0
- v = _extract_v_from_iuvarr(iuv_arr).astype(float) / 255.0
- extent = (
- bbox_xywh[0],
- bbox_xywh[0] + bbox_xywh[2],
- bbox_xywh[1],
- bbox_xywh[1] + bbox_xywh[3],
- )
- plt.contour(u, self.levels, extent=extent, **self.plot_args)
- plt.contour(v, self.levels, extent=extent, **self.plot_args)
-
-
-class DensePoseResultsCustomContourVisualizer(DensePoseResultsVisualizer):
- """
- Contour visualization using marching squares
- """
-
- def __init__(self, levels=10, **kwargs):
- # TODO: colormap is hardcoded
- cmap = cv2.COLORMAP_PARULA
- if isinstance(levels, int):
- self.levels = np.linspace(0, 1, levels)
- else:
- self.levels = levels
- if "linewidths" in kwargs:
- self.linewidths = kwargs["linewidths"]
- else:
- self.linewidths = [1] * len(self.levels)
- self.plot_args = kwargs
- img_colors_bgr = cv2.applyColorMap((self.levels * 255).astype(np.uint8), cmap)
- self.level_colors_bgr = [
- [int(v) for v in img_color_bgr.ravel()] for img_color_bgr in img_colors_bgr
- ]
-
- def visualize_iuv_arr(self, context, iuv_arr: np.ndarray, bbox_xywh: Boxes) -> None:
- image_bgr = self.get_image_bgr_from_context(context)
- segm = _extract_i_from_iuvarr(iuv_arr)
- u = _extract_u_from_iuvarr(iuv_arr).astype(float) / 255.0
- v = _extract_v_from_iuvarr(iuv_arr).astype(float) / 255.0
- self._contours(image_bgr, u, segm, bbox_xywh)
- self._contours(image_bgr, v, segm, bbox_xywh)
-
- def _contours(self, image_bgr, arr, segm, bbox_xywh):
- for part_idx in range(1, DensePoseDataRelative.N_PART_LABELS + 1):
- mask = segm == part_idx
- if not np.any(mask):
- continue
- arr_min = np.amin(arr[mask])
- arr_max = np.amax(arr[mask])
- I, J = np.nonzero(mask)
- i0 = np.amin(I)
- i1 = np.amax(I) + 1
- j0 = np.amin(J)
- j1 = np.amax(J) + 1
- if (j1 == j0 + 1) or (i1 == i0 + 1):
- continue
- Nw = arr.shape[1] - 1
- Nh = arr.shape[0] - 1
- for level_idx, level in enumerate(self.levels):
- if (level < arr_min) or (level > arr_max):
- continue
- vp = arr[i0:i1, j0:j1] >= level
- bin_codes = vp[:-1, :-1] + vp[1:, :-1] * 2 + vp[1:, 1:] * 4 + vp[:-1, 1:] * 8
- mp = mask[i0:i1, j0:j1]
- bin_mask_codes = mp[:-1, :-1] + mp[1:, :-1] * 2 + mp[1:, 1:] * 4 + mp[:-1, 1:] * 8
- it = np.nditer(bin_codes, flags=["multi_index"])
- color_bgr = self.level_colors_bgr[level_idx]
- linewidth = self.linewidths[level_idx]
- while not it.finished:
- if (it[0] != 0) and (it[0] != 15):
- i, j = it.multi_index
- if bin_mask_codes[i, j] != 0:
- self._draw_line(
- image_bgr,
- arr,
- mask,
- level,
- color_bgr,
- linewidth,
- it[0],
- it.multi_index,
- bbox_xywh,
- Nw,
- Nh,
- (i0, j0),
- )
- it.iternext()
-
- def _draw_line(
- self,
- image_bgr,
- arr,
- mask,
- v,
- color_bgr,
- linewidth,
- bin_code,
- multi_idx,
- bbox_xywh,
- Nw,
- Nh,
- offset,
- ):
- lines = self._bin_code_2_lines(arr, v, bin_code, multi_idx, Nw, Nh, offset)
- x0, y0, w, h = bbox_xywh
- x1 = x0 + w
- y1 = y0 + h
- for line in lines:
- x0r, y0r = line[0]
- x1r, y1r = line[1]
- pt0 = (int(x0 + x0r * (x1 - x0)), int(y0 + y0r * (y1 - y0)))
- pt1 = (int(x0 + x1r * (x1 - x0)), int(y0 + y1r * (y1 - y0)))
- cv2.line(image_bgr, pt0, pt1, color_bgr, linewidth)
-
- def _bin_code_2_lines(self, arr, v, bin_code, multi_idx, Nw, Nh, offset):
- i0, j0 = offset
- i, j = multi_idx
- i += i0
- j += j0
- v0, v1, v2, v3 = arr[i, j], arr[i + 1, j], arr[i + 1, j + 1], arr[i, j + 1]
- x0i = float(j) / Nw
- y0j = float(i) / Nh
- He = 1.0 / Nh
- We = 1.0 / Nw
- if (bin_code == 1) or (bin_code == 14):
- a = (v - v0) / (v1 - v0)
- b = (v - v0) / (v3 - v0)
- pt1 = (x0i, y0j + a * He)
- pt2 = (x0i + b * We, y0j)
- return [(pt1, pt2)]
- elif (bin_code == 2) or (bin_code == 13):
- a = (v - v0) / (v1 - v0)
- b = (v - v1) / (v2 - v1)
- pt1 = (x0i, y0j + a * He)
- pt2 = (x0i + b * We, y0j + He)
- return [(pt1, pt2)]
- elif (bin_code == 3) or (bin_code == 12):
- a = (v - v0) / (v3 - v0)
- b = (v - v1) / (v2 - v1)
- pt1 = (x0i + a * We, y0j)
- pt2 = (x0i + b * We, y0j + He)
- return [(pt1, pt2)]
- elif (bin_code == 4) or (bin_code == 11):
- a = (v - v1) / (v2 - v1)
- b = (v - v3) / (v2 - v3)
- pt1 = (x0i + a * We, y0j + He)
- pt2 = (x0i + We, y0j + b * He)
- return [(pt1, pt2)]
- elif (bin_code == 6) or (bin_code == 9):
- a = (v - v0) / (v1 - v0)
- b = (v - v3) / (v2 - v3)
- pt1 = (x0i, y0j + a * He)
- pt2 = (x0i + We, y0j + b * He)
- return [(pt1, pt2)]
- elif (bin_code == 7) or (bin_code == 8):
- a = (v - v0) / (v3 - v0)
- b = (v - v3) / (v2 - v3)
- pt1 = (x0i + a * We, y0j)
- pt2 = (x0i + We, y0j + b * He)
- return [(pt1, pt2)]
- elif bin_code == 5:
- a1 = (v - v0) / (v1 - v0)
- b1 = (v - v1) / (v2 - v1)
- pt11 = (x0i, y0j + a1 * He)
- pt12 = (x0i + b1 * We, y0j + He)
- a2 = (v - v0) / (v3 - v0)
- b2 = (v - v3) / (v2 - v3)
- pt21 = (x0i + a2 * We, y0j)
- pt22 = (x0i + We, y0j + b2 * He)
- return [(pt11, pt12), (pt21, pt22)]
- elif bin_code == 10:
- a1 = (v - v0) / (v3 - v0)
- b1 = (v - v0) / (v1 - v0)
- pt11 = (x0i + a1 * We, y0j)
- pt12 = (x0i, y0j + b1 * He)
- a2 = (v - v1) / (v2 - v1)
- b2 = (v - v3) / (v2 - v3)
- pt21 = (x0i + a2 * We, y0j + He)
- pt22 = (x0i + We, y0j + b2 * He)
- return [(pt11, pt12), (pt21, pt22)]
- return []
-
-
-try:
- import matplotlib
-
- matplotlib.use("Agg")
- DensePoseResultsContourVisualizer = DensePoseResultsMplContourVisualizer
-except ModuleNotFoundError:
- logger = logging.getLogger(__name__)
- logger.warning("Could not import matplotlib, using custom contour visualizer")
- DensePoseResultsContourVisualizer = DensePoseResultsCustomContourVisualizer
-
-
-class DensePoseResultsFineSegmentationVisualizer(DensePoseMaskedColormapResultsVisualizer):
- def __init__(self, inplace=True, cmap=cv2.COLORMAP_PARULA, alpha=0.7, **kwargs):
- super(DensePoseResultsFineSegmentationVisualizer, self).__init__(
- _extract_i_from_iuvarr,
- _extract_i_from_iuvarr,
- inplace,
- cmap,
- alpha,
- val_scale=255.0 / DensePoseDataRelative.N_PART_LABELS,
- **kwargs,
- )
-
-
-class DensePoseResultsUVisualizer(DensePoseMaskedColormapResultsVisualizer):
- def __init__(self, inplace=True, cmap=cv2.COLORMAP_PARULA, alpha=0.7, **kwargs):
- super(DensePoseResultsUVisualizer, self).__init__(
- _extract_u_from_iuvarr,
- _extract_i_from_iuvarr,
- inplace,
- cmap,
- alpha,
- val_scale=1.0,
- **kwargs,
- )
-
-
-class DensePoseResultsVVisualizer(DensePoseMaskedColormapResultsVisualizer):
- def __init__(self, inplace=True, cmap=cv2.COLORMAP_PARULA, alpha=0.7, **kwargs):
- super(DensePoseResultsVVisualizer, self).__init__(
- _extract_v_from_iuvarr,
- _extract_i_from_iuvarr,
- inplace,
- cmap,
- alpha,
- val_scale=1.0,
- **kwargs,
- )
diff --git a/spaces/ccolas/TastyPiano/src/cocktails/utilities/ingredients_utilities.py b/spaces/ccolas/TastyPiano/src/cocktails/utilities/ingredients_utilities.py
deleted file mode 100644
index a1142192a7c3eb1117e8145b75a18552cd3a152c..0000000000000000000000000000000000000000
--- a/spaces/ccolas/TastyPiano/src/cocktails/utilities/ingredients_utilities.py
+++ /dev/null
@@ -1,209 +0,0 @@
-# This script loads the list and profiles of our ingredients selection.
-# It defines rules to recognize ingredients from the list in recipes and the function to extract that information from ingredient strings.
-
-import pandas as pd
-from src.cocktails.config import INGREDIENTS_LIST_PATH, COCKTAILS_CSV_DATA
-import numpy as np
-
-ingredient_profiles = pd.read_csv(INGREDIENTS_LIST_PATH)
-ingredient_list = [ing.lower() for ing in ingredient_profiles['ingredient']]
-n_ingredients = len(ingredient_list)
-ingredient2ingredient_id = dict(zip(ingredient_list, range(n_ingredients)))
-
-ingredients_types = sorted(set(ingredient_profiles['type']))
-# for each type, get all ingredients
-ing_per_type = [[ing for ing in ingredient_list if ingredient_profiles['type'][ingredient_list.index(ing)] == type] for type in ingredients_types]
-ingredients_per_type = dict(zip(ingredients_types, ing_per_type))
-
-bubble_ingredients = ['soda', 'ginger beer', 'tonic', 'sparkling wine']
-# rules to recognize ingredients in recipes.
-# in [] are separate rules with an OR relation: only one needs to be satisfied
-# within [], rules apply with and AND relation: all rules need to be satisfied.
-# ~ indicates that the following expression must NOT appear
-# simple expression indicate that the expression MUST appear.
-ingredient_search = {#'salt': ['salt'],
- 'lime juice': [['lime', '~soda', '~lemonade', '~cordial']],
- 'lemon juice': [['lemon', '~soda', '~lemonade']],
- 'angostura': [['angostura', '~orange'],
- ['bitter', '~campari', '~orange', '~red', '~italian', '~fernet']],
- 'orange bitters': [['orange', 'bitter', '~bittersweet']],
- 'orange juice': [['orange', '~bitter', '~jam', '~marmalade', '~liqueur', '~water'],
- ['orange', 'squeeze']],
- 'pineapple juice': [['pineapple']],
- # 'apple juice': [['apple', 'juice', '~pine']],
- 'cranberry juice': [['cranberry', 'juice']],
- 'cointreau': ['cointreau', 'triple sec', 'grand marnier', 'curaçao', 'curacao'],
- 'luxardo maraschino': ['luxardo', 'maraschino', 'kirsch'],
- 'amaretto': ['amaretto'],
- 'benedictine': ['benedictine', 'bénédictine', 'bénedictine', 'benédictine'],
- 'campari': ['campari', ['italian', 'red', 'bitter'], 'aperol', 'bittersweet', 'aperitivo', 'orange-red'],
- # 'campari': ['campari', ['italian', 'red', 'bitter']],
- # 'crème de violette': [['violette', 'crème'], ['crême', 'violette'], ['liqueur', 'violette']],
- # 'aperol': ['aperol', 'bittersweet', 'aperitivo', 'orange-red'],
- 'green chartreuse': ['chartreuse'],
- 'black raspberry liqueur': [['cassis', 'liqueur'],
- ['black raspberry', 'liqueur'],
- ['raspberry', 'liqueur'],
- ['strawberry', 'liqueur'],
- ['blackberry', 'liqueur'],
- ['violette', 'crème'], ['crême', 'violette'], ['liqueur', 'violette']],
- # 'simple syrup': [],
- # 'drambuie': ['drambuie'],
- # 'fernet branca': ['fernet', 'branca'],
- 'gin': [['gin', '~sloe', '~ginger']],
- 'vodka': ['vodka'],
- 'cuban rum': [['rum', 'puerto rican'], ['light', 'rum'], ['white', 'rum'], ['rum', 'havana', '~7'], ['rum', 'bacardi']],
- 'cognac': [['cognac', '~grand marnier', '~cointreau', '~orange']],
- # 'bourbon': [['bourbon', '~liqueur']],
- # 'tequila': ['tequila', 'pisco'],
- # 'tequila': ['tequila'],
- 'scotch': ['scotch'],
- 'dark rum': [['rum', 'age', '~bacardi', '~havana'],
- ['rum', 'dark', '~bacardi', '~havana'],
- ['rum', 'old', '~bacardi', '~havana'],
- ['rum', 'old', '7'],
- ['rum', 'havana', '7'],
- ['havana', 'rum', 'especial']],
- 'absinthe': ['absinthe'],
- 'rye whiskey': ['rye', ['bourbon', '~liqueur']],
- # 'rye whiskey': ['rye'],
- 'apricot brandy': [['apricot', 'brandy']],
- # 'pisco': ['pisco'],
- # 'cachaça': ['cachaça', 'cachaca'],
- 'egg': [['egg', 'white', '~yolk', '~whole']],
- 'soda': [['soda', 'water', '~lemon', '~lime']],
- 'mint': ['mint'],
- 'sparkling wine': ['sparkling wine', 'prosecco', 'champagne'],
- 'ginger beer': [['ginger', 'beer'], ['ginger', 'ale']],
- 'tonic': [['tonic'], ['7up'], ['sprite']],
- # 'espresso': ['espresso', 'expresso', ['café', '~liqueur', '~cream'],
- # ['cafe', '~liqueur', '~cream'],
- # ['coffee', '~liqueur', '~cream']],
- # 'southern comfort': ['southern comfort'],
- # 'cola': ['cola', 'coke', 'pepsi'],
- 'double syrup': [['sugar','~raspberry'], ['simple', 'syrup'], ['double', 'syrup']],
- # 'grenadine': ['grenadine', ['pomegranate', 'syrup']],
- 'grenadine': ['grenadine', ['pomegranate', 'syrup'], ['raspberry', 'syrup', '~black']],
- 'honey syrup': ['honey', ['maple', 'syrup']],
- # 'raspberry syrup': [['raspberry', 'syrup', '~black']],
- 'dry vermouth': [['vermouth', 'dry'], ['vermouth', 'white'], ['vermouth', 'french'], 'lillet'],
- 'sweet vermouth': [['vermouth', 'sweet'], ['vermouth', 'red'], ['vermouth', 'italian']],
- # 'lillet blanc': ['lillet'],
- 'water': [['water', '~sugar', '~coconut', '~soda', '~tonic', '~honey', '~orange', '~melon']]
- }
-# check that there is a rule for all ingredients in the list
-assert sorted(ingredient_list) == sorted(ingredient_search.keys()), 'ing search dict keys do not match ingredient list'
-
-def get_ingredients_info():
- data = pd.read_csv(COCKTAILS_CSV_DATA)
- max_ingredients, ingredient_set, liquor_set, liqueur_set, vermouth_set = get_max_n_ingredients(data)
- ingredient_list = sorted(ingredient_set)
- alcohol = sorted(liquor_set.union(liqueur_set).union(vermouth_set).union(set(['sparkling wine'])))
- ind_alcohol = [i for i in range(len(ingredient_list)) if ingredient_list[i] in alcohol]
- return max_ingredients, ingredient_list, ind_alcohol
-
-def get_max_n_ingredients(data):
- max_count = 0
- ingredient_set = set()
- alcohol_set = set()
- liqueur_set = set()
- vermouth_set = set()
- ing_str = np.array(data['ingredients_str'])
- for i in range(len(data['names'])):
- ingredients, quantities = extract_ingredients(ing_str[i])
- max_count = max(max_count, len(ingredients))
- for ing in ingredients:
- ingredient_set.add(ing)
- if ing in ingredients_per_type['liquor']:
- alcohol_set.add(ing)
- if ing in ingredients_per_type['liqueur']:
- liqueur_set.add(ing)
- if ing in ingredients_per_type['vermouth']:
- vermouth_set.add(ing)
- return max_count, ingredient_set, alcohol_set, liqueur_set, vermouth_set
-
-def find_ingredient_from_str(ing_str):
- # function that assigns an ingredient string to one of the ingredient if possible, following the rules defined above.
- # return a flag and the ingredient string. When flag is false, the ingredient has not been found and the cocktail is rejected.
- ing_str = ing_str.lower()
- flags = []
- for k in ingredient_list:
- or_flags = [] # get flag for each of several conditions
- for i_p, pattern in enumerate(ingredient_search[k]):
- or_flags.append(True)
- if isinstance(pattern, str):
- if pattern[0] == '~' and pattern[1:] in ing_str:
- or_flags[-1] = False
- elif pattern[0] != '~' and pattern not in ing_str:
- or_flags[-1] = False
- elif isinstance(pattern, list):
- for element in pattern:
- if element[0] == '~':
- or_flags[-1] = or_flags[-1] and not element[1:] in ing_str
- else:
- or_flags[-1] = or_flags[-1] and element in ing_str
- else:
- raise ValueError
- flags.append(any(or_flags))
- if sum(flags) > 1:
- print(ing_str)
- for i_f, f in enumerate(flags):
- if f:
- print(ingredient_list[i_f])
- stop = 1
- return True, ingredient_list[flags.index(True)]
- elif sum(flags) == 0:
- # if 'grape' not in ing_str:
- # print('\t\t Not found:', ing_str)
- return True, None
- else:
- return False, ingredient_list[flags.index(True)]
-
-def get_cocktails_per_ingredient(ing_strs):
- cocktails_per_ing = dict(zip(ingredient_list, [[] for _ in range(len(ingredient_list))]))
- for i_ing, ing_str in enumerate(ing_strs):
- ingredients, _ = extract_ingredients(ing_str)
- for ing in ingredients:
- cocktails_per_ing[ing].append(i_ing)
- return cocktails_per_ing
-
-def extract_ingredients(ingredient_str):
- # extract list of ingredients and quantities from an formatted ingredient string (reverse of format_ingredients)
- ingredient_str = ingredient_str[1: -1]
- words = ingredient_str.split(',')
- ingredients = []
- quantities = []
- for i in range(len(words)//2):
- ingredients.append(words[2 * i][1:])
- quantities.append(float(words[2 * i + 1][:-1]))
- return ingredients, quantities
-
-def format_ingredients(ingredients, quantities):
- # format an ingredient string from the lists of ingredients and quantities (reverse of extract_ingredients)
- out = '['
- for ing, q in zip(ingredients, quantities):
- if ing[-1] == ' ':
- ingre = ing[:-1]
- else:
- ingre = ing
- out += f'({ingre},{q}),'
- out = out[:-1] + ']'
- return out
-
-
-def get_ingredient_count(data):
- # get count of ingredients in the whole dataset
- ingredient_counts = dict(zip(ingredient_list, [0] * len(ingredient_list)))
- for i in range(len(data['names'])):
- if data['to_keep'][i]:
- ingredients, _ = extract_ingredients(data['ingredients_str'][i])
- for i in ingredients:
- ingredient_counts[i] += 1
- return ingredient_counts
-
-def add_counts_to_ingredient_list(data):
- # update the list of ingredients to add their count of occurence in dataset.
- ingredient_counts = get_ingredient_count(data)
- counts = [ingredient_counts[k] for k in ingredient_list]
- ingredient_profiles['counts'] = counts
- ingredient_profiles.to_csv(INGREDIENTS_LIST_PATH, index=False)
\ No newline at end of file
diff --git a/spaces/ccolas/TastyPiano/src/music/utilities/handcoded_rep_utilities/tht/playback.py b/spaces/ccolas/TastyPiano/src/music/utilities/handcoded_rep_utilities/tht/playback.py
deleted file mode 100644
index 4d7cb5a93d1bb3f020b64f3e237e584dbff61b26..0000000000000000000000000000000000000000
--- a/spaces/ccolas/TastyPiano/src/music/utilities/handcoded_rep_utilities/tht/playback.py
+++ /dev/null
@@ -1,72 +0,0 @@
-"""Module containing classes that represents playbacks. A playback is an
-enhanced container for a set of onset events (onset times)."""
-
-import numpy as np
-
-
-class Playback():
- """Represents the entire playback of a song.
-
- Has the same interface as OngoingPlayback except for the discovering
- methods.
- """
-
- def __init__(self, onset_times):
- self.onset_times = onset_times
-
- @property
- def min(self):
- 'First onset'
- return self.onset_times[0]
-
- @property
- def max(self):
- 'Last onset'
- return self.onset_times[-1]
-
- def discovered_play(self):
- 'Onsets discovered at the moment'
- return self.onset_times
-
-
-class OngoingPlayback(Playback):
- """Represents a playback that is discovered onset by onset.
-
- This class is used to manage the discovery process of a song, by exposing
- only a chuck of the song, adding one more onset to what's been discovered
- at a time.
-
- Interal Variables
- onset_times: numpy array of all milliseconds with events in order
- up_to_discovered_index: index up to which all events were discovered
- (not inclusive)
- """
-
- def __init__(self, onset_times):
- self.onset_times = np.array(onset_times)
- self.up_to_discovered_index = 1
-
- def advance(self):
- 'Discover a new onset'
- if (self.up_to_discovered_index < len(self.onset_times)):
- self.up_to_discovered_index += 1
- return True
- return False
-
- @property
- def discovered_index(self):
- 'Returns the index of the last discovered onset'
- return self.up_to_discovered_index - 1
-
- @property
- def max(self):
- 'Last onset discovered. None if no onset has been discovered yet'
- return self.onset_times[self.discovered_index]
-
- @property
- def discovered_onset(self):
- 'Last onset discovered. Same as max.'
- return self.max
-
- def discovered_play(self):
- return self.onset_times[:self.up_to_discovered_index]
diff --git a/spaces/cfwef/gpt/predict.py b/spaces/cfwef/gpt/predict.py
deleted file mode 100644
index 031e5fdf545a327e8c1c1e33b94b38e56ab450f5..0000000000000000000000000000000000000000
--- a/spaces/cfwef/gpt/predict.py
+++ /dev/null
@@ -1,248 +0,0 @@
-# 借鉴了 https://github.com/GaiZhenbiao/ChuanhuChatGPT 项目
-
-"""
- 该文件中主要包含三个函数
-
- 不具备多线程能力的函数:
- 1. predict: 正常对话时使用,具备完备的交互功能,不可多线程
-
- 具备多线程调用能力的函数
- 2. predict_no_ui:高级实验性功能模块调用,不会实时显示在界面上,参数简单,可以多线程并行,方便实现复杂的功能逻辑
- 3. predict_no_ui_long_connection:在实验过程中发现调用predict_no_ui处理长文档时,和openai的连接容易断掉,这个函数用stream的方式解决这个问题,同样支持多线程
-"""
-
-import json
-import gradio as gr
-import logging
-import traceback
-import requests
-import importlib
-
-# config_private.py放自己的秘密如API和代理网址
-# 读取时首先看是否存在私密的config_private配置文件(不受git管控),如果有,则覆盖原config文件
-from toolbox import get_conf
-proxies, API_URL, API_KEY, TIMEOUT_SECONDS, MAX_RETRY, LLM_MODEL = \
- get_conf('proxies', 'API_URL', 'API_KEY', 'TIMEOUT_SECONDS', 'MAX_RETRY', 'LLM_MODEL')
-
-timeout_bot_msg = '[Local Message] Request timeout. Network error. Please check proxy settings in config.py.' + \
- '网络错误,检查代理服务器是否可用,以及代理设置的格式是否正确,格式须是[协议]://[地址]:[端口],缺一不可。'
-
-def get_full_error(chunk, stream_response):
- """
- 获取完整的从Openai返回的报错
- """
- while True:
- try:
- chunk += next(stream_response)
- except:
- break
- return chunk
-
-def predict_no_ui(inputs, top_p, api_key, temperature, history=[], sys_prompt=""):
- """
- 发送至chatGPT,等待回复,一次性完成,不显示中间过程。
- predict函数的简化版。
- 用于payload比较大的情况,或者用于实现多线、带嵌套的复杂功能。
-
- inputs 是本次问询的输入
- top_p, api_key, temperature是chatGPT的内部调优参数
- history 是之前的对话列表
- (注意无论是inputs还是history,内容太长了都会触发token数量溢出的错误,然后raise ConnectionAbortedError)
- """
- headers, payload = generate_payload(inputs, top_p, api_key, temperature, history, system_prompt=sys_prompt, stream=False)
-
- retry = 0
- while True:
- try:
- # make a POST request to the API endpoint, stream=False
- response = requests.post(API_URL, headers=headers, proxies=proxies,
- json=payload, stream=False, timeout=TIMEOUT_SECONDS*2); break
- except requests.exceptions.ReadTimeout as e:
- retry += 1
- traceback.print_exc()
- if retry > MAX_RETRY: raise TimeoutError
- if MAX_RETRY!=0: print(f'请求超时,正在重试 ({retry}/{MAX_RETRY}) ……')
-
- try:
- result = json.loads(response.text)["choices"][0]["message"]["content"]
- return result
- except Exception as e:
- if "choices" not in response.text: print(response.text)
- raise ConnectionAbortedError("Json解析不合常规,可能是文本过长" + response.text)
-
-
-def predict_no_ui_long_connection(inputs, top_p, api_key, temperature, history=[], sys_prompt=""):
- """
- 发送至chatGPT,等待回复,一次性完成,不显示中间过程。但内部用stream的方法避免有人中途掐网线。
- """
- headers, payload = generate_payload(inputs, top_p, api_key, temperature, history, system_prompt=sys_prompt, stream=True)
-
- retry = 0
- while True:
- try:
- # make a POST request to the API endpoint, stream=False
- response = requests.post(API_URL, headers=headers, proxies=proxies,
- json=payload, stream=True, timeout=TIMEOUT_SECONDS); break
- except requests.exceptions.ReadTimeout as e:
- retry += 1
- traceback.print_exc()
- if retry > MAX_RETRY: raise TimeoutError
- if MAX_RETRY!=0: print(f'请求超时,正在重试 ({retry}/{MAX_RETRY}) ……')
-
- stream_response = response.iter_lines()
- result = ''
- while True:
- try: chunk = next(stream_response).decode()
- except StopIteration: break
- if len(chunk)==0: continue
- if not chunk.startswith('data:'):
- error_msg = get_full_error(chunk.encode('utf8'), stream_response).decode()
- if "reduce the length" in error_msg:
- raise ConnectionAbortedError("OpenAI拒绝了请求:" + error_msg)
- else:
- raise RuntimeError("OpenAI拒绝了请求:" + error_msg)
- json_data = json.loads(chunk.lstrip('data:'))['choices'][0]
- delta = json_data["delta"]
- if len(delta) == 0: break
- if "role" in delta: continue
- if "content" in delta: result += delta["content"]; print(delta["content"], end='')
- else: raise RuntimeError("意外Json结构:"+delta)
- if json_data['finish_reason'] == 'length':
- raise ConnectionAbortedError("正常结束,但显示Token不足。")
- return result
-
-
-def predict(inputs, top_p, api_key, temperature, chatbot=[], history=[], system_prompt='',
- stream = True, additional_fn=None):
- """
- 发送至chatGPT,流式获取输出。
- 用于基础的对话功能。
- inputs 是本次问询的输入
- top_p, api_key, temperature是chatGPT的内部调优参数
- history 是之前的对话列表(注意无论是inputs还是history,内容太长了都会触发token数量溢出的错误)
- chatbot 为WebUI中显示的对话列表,修改它,然后yeild出去,可以直接修改对话界面内容
- additional_fn代表点击的哪个按钮,按钮见functional.py
- """
- if additional_fn is not None:
- import functional
- importlib.reload(functional) # 热更新prompt
- functional = functional.get_functionals()
- if "PreProcess" in functional[additional_fn]: inputs = functional[additional_fn]["PreProcess"](inputs) # 获取预处理函数(如果有的话)
- inputs = functional[additional_fn]["Prefix"] + inputs + functional[additional_fn]["Suffix"]
-
- if stream:
- raw_input = inputs
- logging.info(f'[raw_input] {raw_input}')
- chatbot.append((inputs, ""))
- yield chatbot, history, "等待响应"
-
- headers, payload = generate_payload(inputs, top_p, api_key, temperature, history, system_prompt, stream)
- history.append(inputs); history.append(" ")
-
- retry = 0
- while True:
- try:
- # make a POST request to the API endpoint, stream=True
- response = requests.post(API_URL, headers=headers, proxies=proxies,
- json=payload, stream=True, timeout=TIMEOUT_SECONDS);break
- except:
- retry += 1
- chatbot[-1] = ((chatbot[-1][0], timeout_bot_msg))
- retry_msg = f",正在重试 ({retry}/{MAX_RETRY}) ……" if MAX_RETRY > 0 else ""
- yield chatbot, history, "请求超时"+retry_msg
- if retry > MAX_RETRY: raise TimeoutError
-
- gpt_replying_buffer = ""
-
- is_head_of_the_stream = True
- if stream:
- stream_response = response.iter_lines()
- while True:
- chunk = next(stream_response)
- # print(chunk.decode()[6:])
- if is_head_of_the_stream:
- # 数据流的第一帧不携带content
- is_head_of_the_stream = False; continue
-
- if chunk:
- try:
- if len(json.loads(chunk.decode()[6:])['choices'][0]["delta"]) == 0:
- # 判定为数据流的结束,gpt_replying_buffer也写完了
- logging.info(f'[response] {gpt_replying_buffer}')
- break
- # 处理数据流的主体
- chunkjson = json.loads(chunk.decode()[6:])
- status_text = f"finish_reason: {chunkjson['choices'][0]['finish_reason']}"
- # 如果这里抛出异常,一般是文本过长,详情见get_full_error的输出
- gpt_replying_buffer = gpt_replying_buffer + json.loads(chunk.decode()[6:])['choices'][0]["delta"]["content"]
- history[-1] = gpt_replying_buffer
- chatbot[-1] = (history[-2], history[-1])
- yield chatbot, history, status_text
-
- except Exception as e:
- traceback.print_exc()
- yield chatbot, history, "Json解析不合常规"
- chunk = get_full_error(chunk, stream_response)
- error_msg = chunk.decode()
- if "reduce the length" in error_msg:
- chatbot[-1] = (chatbot[-1][0], "[Local Message] Input (or history) is too long, please reduce input or clear history by refreshing this page.")
- history = [] # 清除历史
- elif "Incorrect API key" in error_msg:
- chatbot[-1] = (chatbot[-1][0], "[Local Message] Incorrect API key provided.")
- elif "exceeded your current quota" in error_msg:
- chatbot[-1] = (chatbot[-1][0], "[Local Message] You exceeded your current quota. OpenAI以账户额度不足为由,拒绝服务.")
- else:
- from toolbox import regular_txt_to_markdown
- tb_str = '```\n' + traceback.format_exc() + '```'
- chatbot[-1] = (chatbot[-1][0], f"[Local Message] 异常 \n\n{tb_str} \n\n{regular_txt_to_markdown(chunk.decode()[4:])}")
- yield chatbot, history, "Json异常" + error_msg
- return
-
-def generate_payload(inputs, top_p, api_key, temperature, history, system_prompt, stream):
- """
- 整合所有信息,选择LLM模型,生成http请求,为发送请求做准备
- """
- headers = {
- "Content-Type": "application/json",
- "Authorization": f"Bearer {api_key}"
- }
-
- conversation_cnt = len(history) // 2
-
- messages = [{"role": "system", "content": system_prompt}]
- if conversation_cnt:
- for index in range(0, 2*conversation_cnt, 2):
- what_i_have_asked = {}
- what_i_have_asked["role"] = "user"
- what_i_have_asked["content"] = history[index]
- what_gpt_answer = {}
- what_gpt_answer["role"] = "assistant"
- what_gpt_answer["content"] = history[index+1]
- if what_i_have_asked["content"] != "":
- if what_gpt_answer["content"] == "": continue
- if what_gpt_answer["content"] == timeout_bot_msg: continue
- messages.append(what_i_have_asked)
- messages.append(what_gpt_answer)
- else:
- messages[-1]['content'] = what_gpt_answer['content']
-
- what_i_ask_now = {}
- what_i_ask_now["role"] = "user"
- what_i_ask_now["content"] = inputs
- messages.append(what_i_ask_now)
-
- payload = {
- "model": LLM_MODEL,
- "messages": messages,
- "temperature": temperature, # 1.0,
- "top_p": top_p, # 1.0,
- "n": 1,
- "stream": stream,
- "presence_penalty": 0,
- "frequency_penalty": 0,
- }
-
- print(f" {LLM_MODEL} : {conversation_cnt} : {inputs}")
- return headers,payload
-
-
diff --git a/spaces/chasemcdo/hf_localai/examples/telegram-bot/README.md b/spaces/chasemcdo/hf_localai/examples/telegram-bot/README.md
deleted file mode 100644
index d0ab0dfd9a3de9662e4b8d7ce4186bef65970ea4..0000000000000000000000000000000000000000
--- a/spaces/chasemcdo/hf_localai/examples/telegram-bot/README.md
+++ /dev/null
@@ -1,30 +0,0 @@
-## Telegram bot
-
-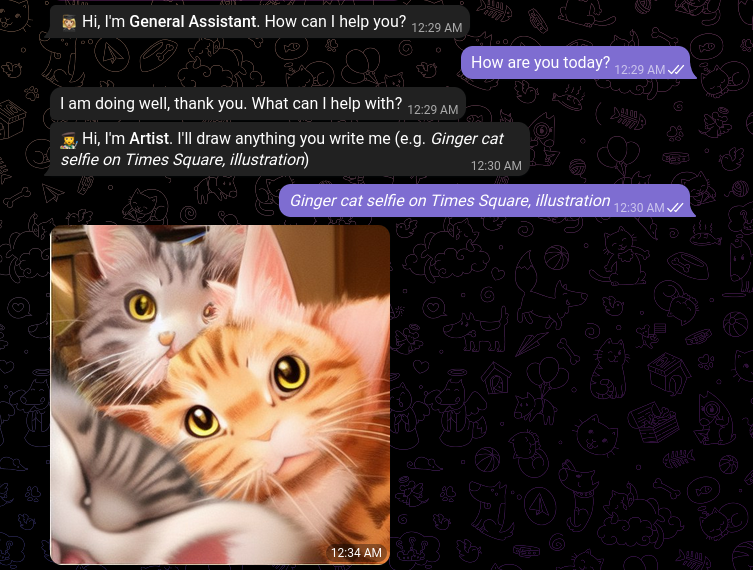
-
-This example uses a fork of [chatgpt-telegram-bot](https://github.com/karfly/chatgpt_telegram_bot) to deploy a telegram bot with LocalAI instead of OpenAI.
-
-```bash
-# Clone LocalAI
-git clone https://github.com/go-skynet/LocalAI
-
-cd LocalAI/examples/telegram-bot
-
-git clone https://github.com/mudler/chatgpt_telegram_bot
-
-cp -rf docker-compose.yml chatgpt_telegram_bot
-
-cd chatgpt_telegram_bot
-
-mv config/config.example.yml config/config.yml
-mv config/config.example.env config/config.env
-
-# Edit config/config.yml to set the telegram bot token
-vim config/config.yml
-
-# run the bot
-docker-compose --env-file config/config.env up --build
-```
-
-Note: LocalAI is configured to download `gpt4all-j` in place of `gpt-3.5-turbo` and `stablediffusion` for image generation at the first start. Download size is >6GB, if your network connection is slow, adapt the `docker-compose.yml` file healthcheck section accordingly (replace `20m`, for instance with `1h`, etc.).
-To configure models manually, comment the `PRELOAD_MODELS` environment variable in the `docker-compose.yml` file and see for instance the [chatbot-ui-manual example](https://github.com/go-skynet/LocalAI/tree/master/examples/chatbot-ui-manual) `model` directory.
\ No newline at end of file
diff --git a/spaces/chendl/compositional_test/transformers/examples/research_projects/codeparrot/README.md b/spaces/chendl/compositional_test/transformers/examples/research_projects/codeparrot/README.md
deleted file mode 100644
index 6c57c4350fbc029e5ad975e53672fea801d1e49f..0000000000000000000000000000000000000000
--- a/spaces/chendl/compositional_test/transformers/examples/research_projects/codeparrot/README.md
+++ /dev/null
@@ -1,316 +0,0 @@
-# CodeParrot 🦜
-
-
Data Cash Winsesame Pro Crack Torrent 42
-
- aaccfb2cb3
-
-
-
diff --git a/spaces/cihyFjudo/fairness-paper-search/Hindi Old Boy Free Download Spike Lees Neo-Noir Adaptation of the Manga and Film.md b/spaces/cihyFjudo/fairness-paper-search/Hindi Old Boy Free Download Spike Lees Neo-Noir Adaptation of the Manga and Film.md
deleted file mode 100644
index d0e97aa76203aee90ffd9bc0c0cc638dd2b5df3f..0000000000000000000000000000000000000000
--- a/spaces/cihyFjudo/fairness-paper-search/Hindi Old Boy Free Download Spike Lees Neo-Noir Adaptation of the Manga and Film.md
+++ /dev/null
@@ -1,24 +0,0 @@
-
-hindi Old Boy free download
-
U.S. Department of Health and Human Services, Administration for Children and Families, Administration for Native Americans (2020)
Offers a free, interactive e-course intended to build the capacity of non-Native stakeholders to work collaboratively and effectively with American Indian and Alaska Native families. The training provides background information on Indigenous people, how the colonial experience has impacted the health and well-being of Tribal populations, and best practices for engaging with Tribal governments.
NaturalReader is a downloadable text-to-speech desktop software for personal use. This easy-to-use software with natural-sounding voices can read to you any text such as Microsoft Word files, webpages, PDF files, and E-mails. Available with a one-time payment for a perpetual license.
-If you are looking for all other forms that begin with "I" (such as I-130, I-539, etc), these forms come from the U.S. Citizenship and Immigration Services (USCIS) in the Department of Homeland Security. You may download them from the USCIS forms page.
-Microsoft 365 doesn't have traditional clip art anymore, but as a subscriber you get several new kinds of high quality art in its place for free: high-resolution photographs, icons, cutout people, stickers, illustrations, and cartoon people. Select Insert > Pictures > Stock Images to see your options. For more details, see Insert images, icons, and more.
-Sec. 5. That upon the approval of the allotments provided for in this act by the Secretary of the Interior, he shall cause patents to issue therefor in the name of the allottees, which patents shall be of the legal effect, and declare that the United States does and will hold the land thus allotted, for the period of twenty-five years, in trust for the sole use and benefit of the Indian to whom such allotment shall have been made, or, in case of his decease, of his heirs according to the laws of the State or Territory where such land is located, and that at the expiration of said period the United States will convey the same by patent to said Indian, or his heirs as aforesaid, in fee, discharged of said trust and free of all charge or incumbrance whatsoever: Provided, That the President of the United States may in any case in his discretion extend the period. And if any conveyance shall be made of the lands set apart and allotted as herein provided, or any contract made touching the same, before the expiration of the time above mentioned, such conveyance or contract shall be absolutely null and void: Provided, That the law of descent and partition in force in the State or Territory where such lands are situate shall apply thereto after patents therefor have been executed and delivered, except as herein otherwise provided; and the laws of the State of Kansas regulating the descent and partition of real estate shall, so far as practicable, apply to all lands in the Indian Territory which may be allotted in severalty under the provisions of this act: And provided further, That at any time after lands have been allotted to all the Indians of any tribe as herein provided, or sooner if in the opinion of the President it shall be for the best interests of said tribe, it shall be lawful for the Secretary of the Interior to negotiate with such Indian tribe for the purchase and release by said tribe, in conformity with the treaty or statute under which such reservation is held, of such portions of its reservation not allotted as such tribe shall, from time to time, consent to sell, on such terms and conditions as shall be considered just and equitable between the United States and said tribe of Indians, which purchase shall not be complete until ratified by Congress, and the form and manner of executing such release prescribed by Congress: Provided however, That all lands adapted to agriculture, with or without irrigation so sold or released to the United States by any Indian tribe shall be held by the United States for the sale purpose of securing homes to actual settlers and shall be disposed of by the United States to actual and bona fide settlers only tracts not exceding one hundred and sixty acres to any one person, on such terms as Congress shall prescribe, subject to grants which Congress may make in aid of education: And provided further, That no patents shall issue therefor except to the person so taking the same as and homestead, or his heirs, and after the expiration of five years occupancy thereof as such homestead; and any conveyance of said lands taken as a homestead, or any contract touching the same, or lieu thereon, created prior to the date of such patent, shall be null and void. And the sums agreed to be paid by the United States as purchase money for any portion of any such reservation shall be held in the Treasury of the United States for the sole use of the tribe or tribes Indians; to whom such reservations belonged; and the same, with interest thereon at three per cent per annum, shall be at all times subject to appropriation by Congress for the education and civilization of such tribe or tribes of Indians or the members thereof. The patents aforesaid shall be recorded in the General Land Office, and afterward delivered, free of charge, to the allottee entitled thereto. And if any religious society or other organization is now occupying any of the public lands to which this act is applicable, for religious or educational work among the Indians, the Secretary of the Interior is hereby authorized to confirm such occupation to such society or organization, in quantity not exceeding one hundred and sixty acres in any one tract, so long as the same shall be so occupied, on such terms as he shall deem just; but nothing herein contained shall change or alter any claim of such society for religious or educational purposes heretofore granted by law. And hereafter in the employment of Indian police, or any other employees in the public service among any of the Indian tribes or bands affected by this act, and where Indians can perform the duties required, those Indians who have availed themselves of the provisions of this act and become citizens of the United States shall be preferred.
aaccfb2cb3If you are looking for a song that will make you want to dance, sing along, and have fun, then you should check out Askies O Jola Le Mang by The Double Trouble and Maxy Khoisan. This song is a hit track that has taken South Africa and Botswana by storm. But what does the title mean? Who are the artists behind it? What is the genre and style of the song? And how popular is it? In this article, we will answer these questions and more.
-Download Zip → https://urlca.com/2uO4MA
The title of the song, Askies O Jola Le Mang, is a phrase in Tswana, one of the official languages of South Africa and Botswana. It means "sorry, who are you dating?" or "excuse me, who are you seeing?". It is a phrase that expresses curiosity or jealousy about someone's love life. It can be used as a friendly question, a playful tease, or a sarcastic remark.
-The phrase is repeated throughout the chorus of the song, as the singers ask each other who they are dating. They also say that they don't care who their partners are dating, as long as they choose them in the end. They also brag about how they treat their partners well, by buying them double beds, tuxedos, chocolates, and more.
-The artists behind Askies O Jola Le Mang are The Double Trouble and Maxy Khoisan. They are both popular musicians from Southern Africa who have collaborated on this song in 2020.
-The Double Trouble is a duo of Janisto and CK the DJ from Limpopo, South Africa. They are known for their bolobedu music, which is a subgenre of house music that originated from their region. They have been making music since 2015 and have released several albums and singles. Some of their hits include Gae Limpopo, Ke Bokolela Katiba, Dictionary, Be Careful, Yawa Ke Nama, and more.
-Maxy Khoisan is a singer and songwriter from Botswana. She sings in various languages, including Setswana, English, Afrikaans, Xhosa, Zulu, and more. She has been in the music industry since 2005 and has won several awards and nominations. Some of her songs include Hello My Baby, My Chocolate (feat. Master KG), Rebatswana, Jwala Jo, Sugar Daddy, Cherikwa, Pelo Mol
o, and more. She has also collaborated with other artists, such as Master KG, Makhadzi, King Monada, and more.
-askies o jola le mang mp3 free download
-askies o jola le mang lyrics and translation
-askies o jola le mang song meaning
-askies o jola le mang video download
-askies o jola le mang remix mp3 download
-askies o jola le mang by the double trouble ft maxy khoisan
-askies o jola le mang bolobedu house music
-askies o jola le mang dance challenge
-askies o jola le mang instrumental download
-askies o jola le mang original mix
-askies o jola le mang mp3 download zamusic
-askies o jola le mang english version
-askies o jola le mang live performance
-askies o jola le mang radio edit
-askies o jola le mang acapella download
-askies o jola le mang mp3 download datafilehost
-askies o jola le mang behind the scenes
-askies o jola le mang cover song
-askies o jola le mang extended mix
-askies o jola le mang mp3 download waploaded
-askies o jola le mang piano tutorial
-askies o jola le mang reaction video
-askies o jola le mang studio session
-askies o jola le mang tiktok compilation
-askies o jola le mang vocal mix
Askies O Jola Le Mang is a house music track with elements of bolobedu and amapiano. House music is a genre of electronic dance music that originated in Chicago in the 1980s. It is characterized by a four-on-the-floor beat, synthesizers, bass, and vocals. Bolobedu is a subgenre of house music that originated in Limpopo, South Africa. It is influenced by traditional music and culture of the region, and features fast-paced rhythms, repetitive lyrics, and local instruments. Amapiano is another subgenre of house music that emerged in South Africa in the 2010s. It is a blend of deep house, jazz, kwaito, and lounge music. It features piano melodies, percussions, basslines, and vocals.
-The song has a catchy beat, a catchy chorus, and playful lyrics. The song has a tempo of 125 beats per minute and a key of A minor. The song uses various instruments, such as drums, keyboards, guitars, saxophones, flutes, and more. The song also uses vocal effects, such as auto-tune, reverb, and echo. The song has a simple structure, with verses, choruses, and bridges. The song is sung in Tswana and English.
-The song is a dance anthem that celebrates love and relationships. The song is about having fun with your partner and not worrying about what others think. The song also encourages people to be confident and proud of their choices. The song is a positive and uplifting track that makes people feel good.
-The song is very popular among fans and critics alike. The song has over 3 million views on YouTube and over 46 thousand plays on SoundCloud. The song has also been streamed on other platforms, such as Spotify, Apple Music, Deezer, Audiomack, and more. The song has received positive reviews from various sources, such as Fakaza Music, SA Hip Hop Mag, Bolo House Music, and more. The song has been praised for its catchy tune, its lively vibe, its humorous lyrics, and its collaboration between the artists.
-The song has also inspired many dance videos and TikTok challenges. Many people have uploaded videos of themselves dancing to the song on social media platforms. Some of the popular dance moves include the "askies o jola le mang" gesture, where you point to yourself and then to someone else; the "double bed" gesture, where you make a rectangle shape with your arms; and the "tuxedo" gesture, where you pretend to adjust your suit or tie. Some of the popular TikTok challenges include the #askiesojolalemangchallenge, the #doubletroublechallenge, and the #maxykhoisanchallenge. These challenges have attracted thousands of participants and viewers.
-Askies O Jola Le Mang is a hit song that showcases the talent and creativity of The Double Trouble and Maxy Khoisan. The song is a fun and upbeat track that appeals to a wide audience. The song is a must-listen for anyone who loves house music and bolobedu culture.
-If you want to listen to the song or download it for free, you can visit the following websites:
-Website | -Link | -
---|---|
YouTube | -The Double Trouble - Askies O Jola Le Mang ft Maxy Khoisan (Official Video) | -
SoundCloud | -The Double Trouble - Askies O Jola Le Mang ft Maxy Khoisan (Official Audio) | -
Fakaza Music | -The Double Trouble – Askies O Jola Le Mang ft Maxy Khoisan Mp3 Download | -
Bolo House Music | -The Double Trouble – Askies O Jola Le Mang ft Maxy Khoisan Mp3 Download | -
Audiomack | -The Double Trouble - Askies O Jola Le Mang ft Maxy Khoisan | -
We hope you enjoyed this article and learned something new about Askies O Jola Le Mang. If you have any questions or comments, feel free to leave them below. We would love to hear from you.
-The name of the song is Askies O Jola Le Mang, which means "sorry, who are you dating?" in Tswana.
-The artists of the song are The Double Trouble and Maxy Khoisan. The Double Trouble is a duo of Janisto and CK the DJ from Limpopo, South Africa. Maxy Khoisan is a singer and songwriter from Botswana.
-The song is a house music track with elements of bolobedu and amapiano. The song has a catchy beat, a catchy chorus, and playful lyrics. The song is a dance anthem that celebrates love and relationships.
-The song is very popular among fans and critics alike. The song has over 3 million views on YouTube and over 46 thousand plays on SoundCloud. The song has also inspired many dance videos and TikTok challenges.
-You can listen to or download the song from various websites, such as YouTube, SoundCloud, Fakaza Music, Bolo House Music, Audiomack, and more. You can find the links to these websites in the article above.
197e85843dIf you are looking for a powerful and versatile graphic design software that can handle vector illustration, page layout, photo editing, typography, and more, then you might want to check out CorelDRAW. In this article, we will show you how to download CorelDRAW for free in 2017, how to install and activate it on your PC, and how to use it to create stunning designs.
-Download — https://urlca.com/2uObsc
CorelDRAW is a professional design software that has been around since 1989. It is part of the CorelDRAW Graphics Suite, which also includes Corel PHOTO-PAINT, Corel Font Manager, Corel PowerTRACE, Corel CONNECT, and Corel CAPTURE. With CorelDRAW, you can create logos, flyers, posters, brochures, banners, web graphics, and more. You can also edit photos, apply effects, create custom fonts, and work with vector graphics.
-Some of the features and benefits of using CorelDRAW are:
-To run CorelDRAW on your PC, you need to have the following system requirements:
-If you want to try out CorelDRAW for free in 2017, you have two options:
-You can download a free trial version of CorelDRAW from the official website. The trial version will let you use all the features of the software for 15 days. To download the trial version, follow these steps:
-coreldraw graphics suite 2017 free trial
-coreldraw 2017 live sketch tool
-coreldraw graphics suite 2017 subscription
-coreldraw 2017 system requirements
-coreldraw 2017 vs coreldraw x8
-coreldraw graphics suite 2017 download link
-coreldraw 2017 font manager
-coreldraw graphics suite 2017 crack
-coreldraw 2017 touch screen support
-coreldraw graphics suite 2017 review
-coreldraw 2017 tutorials for beginners
-coreldraw graphics suite 2017 serial number
-coreldraw 2017 new features and enhancements
-coreldraw graphics suite 2017 keygen
-coreldraw 2017 import workspace from previous versions
-coreldraw graphics suite 2017 activation code
-coreldraw 2017 vector illustration software
-coreldraw graphics suite 2017 full version
-coreldraw 2017 photo editing software
-coreldraw graphics suite 2017 offline installer
-coreldraw 2017 page layout software
-coreldraw graphics suite 2017 price
-coreldraw 2017 typography software
-coreldraw graphics suite 2017 for mac
-coreldraw 2017 website design software
-coreldraw graphics suite 2017 free download for windows 10
-coreldraw 2017 artificial intelligence technology
-coreldraw graphics suite 2017 free download for windows 8.1
-coreldraw 2017 hand-drawn vector curves
-coreldraw graphics suite 2017 free download for windows 7
-coreldraw 2017 plugins, extensions and font packs
-coreldraw graphics suite 2017 comparison chart with other versions
-coreldraw 2017 net energy gain in nuclear fusion experiment
-coreldraw graphics suite 2017 minimum hardware requirements
-coreldraw 2017 tablet mode support for pen-enabled devices
-coreldraw graphics suite 2017 customer testimonials and ratings
-coreldraw 2017 enhanced previews, nodes and handles
-coreldraw graphics suite 2017 free download from internet archive
-coreldraw 2017 professional graphic design toolkit
-coreldraw graphics suite 2021 free download trial version
You can also download a free version of CorelDRAW from the archive.org website. This version is not a trial, but an older version of the software that was released in 2017. It has most of the features of the latest version, but it may not be compatible with some newer file formats and devices. To download this version, follow these steps:
-After you download CorelDRAW from either of the sources mentioned above, you need to install and activate it on your PC. The installation and activation steps are similar for both sources, but there may be some minor differences depending on your PC settings and preferences.
-To install CorelDRAW on your PC, follow these steps:
-To activate CorelDRAW on your PC, follow these steps:
-Now that you have installed and activated CorelDRAW on your PC, you can start using it to create stunning designs. CorelDRAW has a lot of tools and features that can help you unleash your creativity and produce professional-quality graphics. Here are some of the tools and features that you can use:
-The LiveSketch tool is one of the most innovative features of CorelDRAW. It allows you to sketch your ideas directly on the screen using a pen-enabled device. The LiveSketch tool uses artificial intelligence to convert your sketches into vector graphics that you can edit and refine later. To use the LiveSketch tool, follow these steps:
-The Font Manager is a useful feature that helps you organize and manage your fonts easily. You can view, search, filter, sort, install, uninstall, and group your fonts with the Font Manager. You can also access thousands of fonts from online sources, such as Google Fonts and Font Squirrel. To use the Font Manager, follow these steps:
-Are you looking for a new live streaming app that allows you to chat with new friends all over the world, watch live broadcasts of beauties, and express your likes by sending and receiving virtual gifts? If yes, then you should try APK Woo Live, a new live streaming application that is fun, entertaining, and easy to use. In this article, we will show you what APK Woo Live is, how to download and install it on your Android device, and how to use it to watch live broadcasts and interact with hosts.
-APK Woo Live is a new live streaming application developed by Star Rising Ltd. It is a package file format used by the Android operating system for the distribution and installation of mobile applications. It allows you to chat with new friends all over the world, watch live broadcasts of beauties, and express your likes by sending and receiving virtual gifts.
-Download Zip ✵✵✵ https://urlca.com/2uOfjb
Some of the features of APK Woo Live are:
-Some of the benefits of APK Woo Live are:
-If you want to download and install APK Woo Live on your Android device, you need to follow these steps:
-Since APK Woo Live is not available on Google Play Store, you need to enable unknown sources on your device to install it from other websites. To do this, go to one of these menus depending on your Android version:
-Then, toggle on Allow from this source or Unknown sources for the app that you will use to download APK Woo Live, such as Chrome or Firefox.
-download apk woo live app
-download apk woo live stream
-download apk woo live video
-download apk woo live show
-download apk woo live chat
-download apk woo live social
-download apk woo live android
-download apk woo live latest version
-download apk woo live free
-download apk woo live online
-download apk woo live mod
-download apk woo live hack
-download apk woo live premium
-download apk woo live pro
-download apk woo live unlimited diamonds
-download apk woo live for pc
-download apk woo live for ios
-download apk woo live for windows
-download apk woo live for mac
-download apk woo live for laptop
-download apk woo live update
-download apk woo live 2023
-download apk woo live new version
-download apk woo live old version
-download apk woo live 1.14.2
-download apk woo live from apkpure
-download apk woo live from apkmirror
-download apk woo live from uptodown
-download apk woo live from appbrain
-download apk woo live from apkmody
-how to download apk woo live
-where to download apk woo live
-why to download apk woo live
-what is apk woo live
-who is behind apk woo live
-reviews of apk woo live
-features of apk woo live
-benefits of apk woo live
-alternatives to apk woo live
-competitors of apk woo live
Next, you need to download APK Woo Live from a trusted source, such as APKPure, APKMirror, or APKCombo. To do this, open the app that you enabled unknown sources for, and go to one of these websites. Then, search for APK Woo Live and tap on the download button. You may need to confirm the download and wait for it to finish.
-After downloading APK Woo Live, you need to install it on your device. To do this, go to your file manager and locate the downloaded file. Then, tap on it and follow the instructions on the screen. You may need to allow some permissions and accept some terms and conditions. Once the installation is complete, you will see a notification that says APK Woo Live has been installed.
-Now, you are ready to launch APK Woo Live and start chatting with new friends. To do this, go to your app drawer and tap on the APK Woo Live icon. Then, you will see a welcome screen that asks you to sign up or log in. You can choose to sign up with your phone number, Facebook account, or Google account. Then, you can create your profile by adding your nickname, gender, birthday, and avatar. After that, you can start exploring the app and finding new friends.
-Once you have launched APK Woo Live and created your profile, you can use it to watch live broadcasts and interact with hosts. Here are some tips on how to do that:
-To watch live broadcasts, you can go to the home page of the app and swipe left or right to browse through different categories of hosts, such as hot, new, nearby, or recommended. You can also use the search function to find hosts by their name or ID. When you find a host that you like, you can tap on their profile picture to enter their live room. There, you can watch their live broadcast and chat with them by sending messages or voice messages.
-To send and receive virtual gifts, you need to have some diamonds in your account. Diamonds are the virtual currency of the app that you can use to buy gifts or cash out. You can get diamonds by purchasing them with real money, receiving them from other users, or winning them by playing games. To send a gift to a host, you can tap on the gift icon at the bottom of their live room and choose a gift from the list. The gift will appear on the screen and the host will thank you for it. To receive a gift from a user, you need to be a host yourself and have some fans who like your live broadcast. The more gifts you receive, the higher your ranking will be in the app.
-To play games and win diamonds, you can go to the game center of the app and choose a game from the list. There are various games available, such as lucky wheel, lucky draw, lucky box, lucky dice, and more. Each game has different rules and rewards, but they all require some diamonds to play. The more diamonds you spend, the higher your chances of winning more diamonds or other prizes. You can use the diamonds that you win to buy more gifts or cash out.
-In conclusion, APK Woo Live is a new live streaming application that allows you to chat with new friends all over the world, watch live broadcasts of beauties, and express your likes by sending and receiving virtual gifts. It is fun, entertaining, and easy to use. To download and install it on your Android device, you need to enable unknown sources, download it from a trusted source, install it on your device, and launch it. To use it to watch live broadcasts and interact with hosts, you need to follow some tips, such as how to watch live broadcasts, how to send and receive virtual gifts, and how to play games and win diamonds. We hope this article has helped you to learn more about APK Woo Live and how to download and use it on your Android device. If you have any questions or feedback, please feel free to leave a comment below.
-Here are some frequently asked questions about APK Woo Live:
-A: Yes, APK Woo Live is safe to download and use, as long as you download it from a trusted source, such as APKPure, APKMirror, or APKCombo. These websites scan the APK files for viruses and malware before uploading them. However, you should always be careful when installing apps from unknown sources and check the permissions and terms and conditions before agreeing to them.
-A: To become a host on APK Woo Live, you need to have a verified account and meet some requirements, such as age, gender, appearance, and personality. You also need to have a good internet connection, a high-quality camera, and a suitable background. To apply for becoming a host, you can go to the app's settings and tap on Become a Host. Then, you need to fill out some information and upload some photos and videos of yourself. After that, you need to wait for the app's review and approval. If you pass the review, you will receive a notification that says you can start your live broadcast.
-A: To cash out your diamonds on APK Woo Live, you need to have at least 1000 diamonds in your account. You also need to have a verified PayPal account or bank account. To cash out your diamonds, you can go to the app's settings and tap on Cash Out. Then, you need to choose your payment method and enter your account details. After that, you need to wait for the app's verification and confirmation. The processing time may vary depending on the payment method and the amount of diamonds. You will receive a notification that says your cash out request has been completed.
-A: To contact the customer service of APK Woo Live, you can go to the app's settings and tap on Help Center. There, you can find some FAQs and answers that may solve your problems. If you still need help, you can tap on Contact Us and send an email to the app's customer service team. You can also follow the app's official social media accounts, such as Facebook, Instagram, or Twitter, and send them a message there.
-A: Some alternatives to APK Woo Live are:
-Name | -Description | -
---|---|
Bigo Live | -A popular live streaming app that allows you to watch live videos of talented people from around the world or broadcast yourself to showcase your talents. | -
Uplive | -A global live streaming platform that connects millions of users with high-quality video content and interactive live sessions. | -
LivU | -A video chat app that lets you meet new people from different countries and cultures through random video calls or filters. | -
Do you want to share the moment with your friends and family in a fun and creative way? Do you want to explore new content and discover new things based on your interests? Do you want to stay in touch with your loved ones through live messaging, video chat, and group stories? If you answered yes to any of these questions, then you should download Snapchat, one of the most popular social media apps in the world.
-Download Zip ►►► https://urlca.com/2uOaep
Snapchat is a fast and fun way to share the moment with your friends and family. You can snap a photo or a video, add a filter, a sticker, a caption, or a doodle, and send it to your friends or post it on your story. You can also chat with your friends, video call them, send them voice notes, stickers, Bitmoji, and more. You can also watch stories from your friends, celebrities, influencers, publishers, and the Snapchat community. You can also explore Spotlight, a feature that showcases the best of Snapchat from users like you. You can also use Snap Map to see what your friends are up to, where they are, and what's happening around the world.
-Here are some of the features and benefits of using Snapchat:
-To use Snapchat, you need a device that has a camera, an internet connection, and enough storage space. You also need to be at least 13 years old to create an account. Here are the minimum requirements for different devices:
-Device | Operating System | Version |
---|---|---|
Android | Android OS | 4.4 (KitKat) or higher |
iOS | iOS | 10.0 or higher |
Windows PC or Mac | Windows or Mac OS | N/A (requires an emulator) |
Chromebook | Chrome OS | N/A (requires Google Play Store) |
Smart TV | Android TV or Fire TV | N/A (requires sideloading) |
If you have an Android device, you can download Snapchat from the Google Play Store. Here are the steps to follow:
-On your Android device, tap the Google Play Store icon. It looks like a colorful triangle with a play button in the center.
-How to download snapchat on laptop
-Snapchat download for windows 10
-Snapchat download apk latest version
-Snapchat download without app store
-Snapchat download for macbook air
-Snapchat download for android tablet
-Snapchat download old version ios
-Snapchat download for pc windows 7
-Snapchat download link for iphone
-Snapchat download error 491
-How to download snapchat videos from other users
-Snapchat download for chromebook
-Snapchat download for kindle fire
-Snapchat download for jio phone
-Snapchat download for nokia lumia
-How to download snapchat filters for free
-Snapchat download for samsung smart tv
-Snapchat download for blackberry z10
-Snapchat download for huawei y6p
-Snapchat download for xbox one
-How to download snapchat stories on android
-Snapchat download uptodown
-Snapchat download mod apk
-Snapchat download without google play
-Snapchat download for ipad mini 2
-How to download snapchat on macbook pro 2020
-Snapchat download size ios
-Snapchat download beta version
-Snapchat download in laptop windows 10
-Snapchat download qr code scan
-How to download snapchat on amazon fire tablet 7
-Snapchat download for bluestacks
-Snapchat download for oppo a37f
-Snapchat download for vivo y51l
-Snapchat download for lenovo laptop
-How to download snapchat on apple watch series 3
-Snapchat download not working iphone 11
-Snapchat download dark mode apk
-Snapchat download from 9apps
-Snapchat download in jio phone keypad
-How to download snapchat on samsung galaxy s3 mini
-Snapchat download for ps4
-Snapchat download for lg stylo 6
-Snapchat download for tecno spark 4 lite
In the Google Play Store, tap the search bar at the top and type "Snapchat". You should see the Snapchat app icon, which is a yellow ghost with a white background. Tap on it to open the app page.
-On the app page, tap the green Install button. You may need to accept some permissions that Snapchat needs to function properly, such as access to your camera, microphone, contacts, location, and storage. Tap Accept to grant these permissions.
-Once the app is installed, you can open it by tapping the Open button on the app page or by tapping the Snapchat icon on your home screen or app drawer. You will see a welcome screen that asks you to sign up or log in. If you already have a Snapchat account, you can log in with your username and password. If you don't have an account, you can sign up by entering your email, password, birthday, and username. You may also need to verify your phone number and email address.
-If you have an iOS device, such as an iPhone or an iPad, you can download Snapchat from the App Store. Here are the steps to follow:
-On your iOS device, tap the App Store icon. It looks like a blue letter A with a white background.
-In the App Store, tap the search icon at the bottom right and type "Snapchat". You should see the Snapchat app icon, which is a yellow ghost with a white background. Tap on it to open the app page.
-On the app page, tap the blue Get button. You may need to confirm your download with your Face ID or Touch ID if you have them enabled on your device. Alternatively, you may need to enter your Apple ID password.
-Once the app is downloaded, you can open it by tapping the Open button on the app page or by tapping the Snapchat icon on your home screen or app library. You will see a welcome screen that asks you to sign up or log in. If you already have a Snapchat account, you can log in with your username and password. If you don't have an account, you can sign up by entering your email, password, birthday, and username. You may also need to verify your phone number and email address.
-If you don't have an Android or iOS device, you may still be able to download Snapchat on other devices, such as Windows PC or Mac, Chromebook, or Smart TV. However, these methods are not officially supported by Snapchat and may not work properly or at all. Use them at your own risk.
-To download Snapchat on Windows PC or Mac, you need to use an emulator, which is a software that mimics an Android device on your computer. One of the most popular emulators is BlueStacks, which is free and easy to use. Here are the steps to follow:
-Note that using Snapchat on an emulator may not be as smooth as using it on a mobile device. You may experience some lagging, crashing, or glitches. You may also not be able to use some features, such as lenses or filters.
-If you have a Chromebook, you may be able to download Snapchat from the Google Play Store if your device supports it. Here are the steps to follow:
-Note that not all Chromebooks can run Android apps, and some may have compatibility issues. You can check the list of supported Chromebooks here. You may also not be able to use some features, such as lenses or filters.
-If you have a Smart TV that runs on Android TV or Fire TV, you may be able to download Snapchat by sideloading it, which means installing it from an external source. However, this method is not recommended as it may violate the terms of service of Snapchat and your device. It may also expose your device to security risks and malware. Use it at your own risk.
-Note that using Snapchat on a Smart TV may not be optimal as it is designed for mobile devices. You may need a mouse or a keyboard to navigate the app. You may also not be able to use some features, such as lenses or filters.
-Snapchat is a fun and creative way to share the moment with your friends and family. You can download it on various devices, such as Android, iOS, Windows PC or Mac, Chromebook, or Smart TV. However, some methods may not be officially supported by Snapchat and may not work properly or at all. Use them at your own risk. We hope this article helped you learn how to download Snapchat on any device. Happy snapping!
-Do you love watching videos online, but wish you could save them to your device for offline viewing? Do you want to download videos from YouTube, Facebook, Vimeo, TikTok, Instagram, and thousands of other websites with just one click? Do you want to enjoy a full-screen theater experience on any website with Play Now? If you answered yes to any of these questions, then you need Real Download.
-Download 🗸 https://urlca.com/2uO9aE
Real Download is a software that allows you to download videos from various websites in different formats and resolutions. You can also convert videos to MP4 or MP3, preview videos before downloading, organize videos by people, cast videos to your TV with Chromecast, sync downloads between your phone and your PC, and more. Real Download is compatible with Windows, Mac, Android, and Xbox One devices.
In this article, we will review the features, benefits, alternatives, reviews, and FAQs of Real Download. We will also show you how to download and install Real Download on your device. By the end of this article, you will have a clear idea of whether Real Download is the right video downloader for you.
| | H2: Features of Real Download |Real Download has many features that make it stand out from other video downloaders. Here are some of them:
-real download manager
-real download video
-real download apk
-real download app
-real download games
-real download music
-real download software
-real download movies
-real download mp3
-real download youtube
-real download for pc
-real download for android
-real download for windows 10
-real download for mac
-real download for ios
-real download for chrome
-real download for firefox
-real download for linux
-real download for free
-real download for online
-real download speed test
-real download speed calculator
-real download speed vs advertised
-real download speed vs upload speed
-real download speed vs ping
-real downloader extension
-real downloader not working
-real downloader plus
-real downloader activation key
-real downloader alternative
-real downloader chrome extension
-real downloader firefox extension
-real downloader edge extension
-real downloader safari extension
-real downloader opera extension
-real downloader update
-real downloader uninstall
-real downloader review
-real downloader support
-real downloader license key
Real Download supports more than 10,000 websites, including YouTube, Facebook, Vimeo, TikTok, Instagram, Dailymotion, CollegeHumor, FunnyOrDie, StupidVideos, and more. You can download videos as MP4 or FLV files in different resolutions and qualities. You can also download only the audio of a video as an MP3 file.
To download a video with Real Download, all you need to do is copy the URL of the video from your browser and paste it into the search bar of Real Download. Then click on the More button to choose the video quality and format you want. Finally, click on the Download button to start downloading the video.
| | H3: Preview videos before downloading |Sometimes you may not be sure if you want to download a video or not. In that case, you can use Real Download's video previewing feature to watch a small clip of the video before downloading it. This way, you can save time and bandwidth by avoiding unwanted downloads.
To preview a video with Real Download, just hover your mouse over the video thumbnail in the search results. A small window will pop up showing a short snippet of the video. You can also click on the Play button to watch the full video in a new tab.
| | H3: Convert videos to different formats |Sometimes you may want to convert a downloaded video to a different format for compatibility or optimization purposes. For example, you may want to convert a FLV video to MP4 so that you can play it on your iPhone or iPad. Or you may want to convert a high-resolution video to a lower resolution to save space on your device.
Real Download has a built-in file format converter that can convert videos to popular formats such as AVI, MOV, MP4, MP3, and more. You can also choose from various device presets such as iPhone, iPad, Android, Xbox One, etc. To convert a video with Real Download, just right-click on the video in your library and select Convert to. Then choose the output format and quality you want. Finally, click on the Convert button to start converting the video.
| | H3: Play videos on any website with Play Now |One of the coolest features of Real Download is Play Now, which lets you enjoy a full-screen theater experience on any website. Play Now enhances the video quality, removes ads and distractions, and adjusts the volume and brightness automatically. You can also control the playback speed, skip intro and credits, and add subtitles.
To use Play Now with Real Download, just click on the Play Now button that appears on the top right corner of any video on any website. The video will open in a new tab with a dark background and a large screen. You can also access the Play Now settings by clicking on the gear icon on the bottom right corner of the screen.
| | H3: Cast videos to your TV with Chromecast |If you have a Chromecast device connected to your TV, you can use Real Download to cast videos from your computer or phone to your TV. This way, you can enjoy watching videos on a bigger screen with better sound quality.
To cast videos to your TV with Real Download, just click on the Cast button that appears on the top right corner of any video in your library. Then select your Chromecast device from the list of available devices. The video will start playing on your TV. You can also control the playback from your computer or phone.
| | H3: Sync downloads between your phone and your PC |If you have Real Download installed on both your phone and your PC, you can sync your downloads between them. This means that you can start downloading a video on your phone and finish it on your PC, or vice versa. You can also access your downloaded videos from any device with Real Download.
To sync downloads between your phone and your PC with Real Download, just make sure that both devices are connected to the same Wi-Fi network. Then open Real Download on both devices and sign in with the same account. You will see a Sync button on the top right corner of the app. Click on it to start syncing your downloads.
| | H2: Benefits of Real Download |Real Download has many benefits that make it worth trying. Here are some of them:
| | H3: Save videos for offline viewing |One of the main benefits of Real Download is that it allows you to save videos for offline viewing. This means that you can watch videos anytime and anywhere without worrying about internet connection or data usage. You can also share videos with your friends and family without using any bandwidth.
| | H3: Enjoy high-quality videos |Another benefit of Real Download is that it allows you to enjoy high-quality videos. You can download videos in HD, 4K, or even 8K resolution if available. You can also convert videos to different formats and qualities according to your preference. You can also enhance the video quality with Play Now feature.
| | H3: Manage videos easily |A third benefit of Real Download is that it allows you to manage videos easily. You can organize videos by people, date, genre, or playlist in your library. You can also rename, delete, move, or copy videos as you wish. You can also search for videos by keywords or filters in the app.
| | H2: Alternatives to Real Download |If you are looking for other options besides Real Download, here are some alternatives that you can try:
-| H3: 4K Video Downloader |This is a software that allows you to download videos from YouTube, Vimeo, TikTok, Facebook, Instagram, and other websites in 4K resolution. You can also download playlists, channels, subtitles, and 360-degree videos. It supports Windows, Mac, and Linux devices. It has a free version and a paid version that costs $15 for a lifetime license.
-| H3: YTD Video Downloader |This is a software that allows you to download videos from YouTube, Facebook, Dailymotion, Vimeo, Metacafe, and more than 50 other websites. You can also convert videos to MP4, AVI, WMV, MP3, and more formats. It supports Windows and Mac devices. It has a free version and a paid version that costs $29.90 per year or $49.90 for a lifetime license.
| | H3: VideoProc |This is a software that allows you to download videos from YouTube, Facebook, Instagram, Twitter, and more than 1000 other websites. You can also edit, convert, compress, record, and stream videos with this software. It supports Windows and Mac devices. It has a free version and a paid version that costs $29.95 for a one-year license or $42.95 for a lifetime license.
| | H2: Reviews of Real Download |Real Download has received many positive reviews from users and experts. Here are some of them:
-| H3: User reviews |Here are some user reviews of Real Download from Trustpilot:
-Name | -Rating | -Comment | -
---|---|---|
John Smith | -5 stars | -I have been using Real Download for a few months now and I love it. It is fast, easy, and reliable. I can download videos from any website I want and watch them offline. It also has a lot of features that make it better than other video downloaders. I highly recommend it. | -
Mary Jones | -4 stars | -Real Download is a great software for downloading videos. It works well with most websites and has good quality options. The only thing I don't like is that it sometimes crashes or freezes when downloading large files. Other than that, it is a good software. | -
David Lee | -5 stars | -I have tried many video downloaders before, but none of them can compare to Real Download. It is the best video downloader ever. It has everything I need: speed, quality, format, preview, play now, chromecast, sync, and more. It is worth every penny. | -
Here are some expert reviews of Real Download from reputable websites:
-Here are some frequently asked questions about Real Download:
| | H3: How much does Real Download cost? |Real Download has a free version and a paid version. The free version allows you to download up to 30 videos per day from YouTube only. The paid version allows you to download unlimited videos from any website and access all the features of the software. The paid version costs $19.99 for a one-month license, $39.99 for a one-year license, or $59.99 for a lifetime license.
| | H3: Is Real Download safe to use? |Yes, Real Download is safe to use. It does not contain any viruses, malware, spyware, or adware. It also does not collect or share any personal information from users. However, you should be careful when downloading videos from unknown or untrusted websites, as they may contain harmful content or violate copyright laws.
| | H3: How can I contact Real Download support? |If you have any questions, problems, or feedback about Real Download, you can contact Real Download support by email, phone, or live chat. You can also visit the Real Download website to access the help center, user guide, blog, and forum.
-Real Download updates automatically whenever there is a new version available. You can also check for updates manually by clicking on the Menu button on the top left corner of the app and selecting Check for Updates. If there is an update available, you can download and install it by following the instructions on the screen.
| | H3: How can I uninstall Real Download? |If you want to uninstall Real Download from your device, you can do so by following these steps:
-Real Download is a software that allows you to download videos from various websites in different formats and resolutions. It also has many other features that make it a powerful and easy-to-use video downloader. You can preview videos before downloading, convert videos to different formats, play videos on any website with Play Now feature, cast videos to your TV with Chromecast, sync downloads between your phone and your PC, and more. Real Download is compatible with Windows, Mac, Android, and Xbox One devices.
-If you are looking for a video downloader that can handle any video downloading task, you should give Real Download a try. You can download the free version or the paid version from the Real Download website. You can also read more reviews and FAQs about Real Download on the website.
-We hope this article has helped you learn more about Real Download and its features, benefits, alternatives, reviews, and FAQs. If you have any questions or comments, please feel free to contact us. Thank you for reading!
| | H1: | 401be4b1e0Roblox is one of the most popular and innovative gaming platforms in the world, where you can create, play, and share anything you can imagine. Whether you want to embark on an epic adventure, compete with other players, or just hang out with your friends, Roblox has something for everyone. And the best part is, you can play Roblox on your Android device anytime, anywhere.
-But how do you download and install Roblox on your Android device? And what are the benefits of downloading the Roblox APK file instead of using Google Play? In this article, we will answer these questions and more. We will also show you how to play Roblox on your Android device and enjoy its amazing features. So, let's get started!
-Download ————— https://urlca.com/2uO4Li
Before we dive into the details of how to download and play Roblox on Android, let's first understand what Roblox is and why it is so popular. Roblox is not just a game, but a whole virtual universe where you can create, play, and share anything you want. Here are some of the main aspects of Roblox that make it unique and fun:
-Roblox is powered by a global community of users who create their own worlds and games using the Roblox Studio app. These worlds and games can range from simple to complex, from realistic to fantastical, from educational to entertaining. You can build anything you can imagine using the tools and resources provided by Roblox. You can also share your creations with other users and explore what they have made.
-Roblox is not limited to one type of game or genre. You can find millions of games and experiences on Roblox that cater to different tastes and preferences. You can play action-packed shooters, thrilling racing games, immersive role-playing games, hilarious comedy games, relaxing simulation games, and more. You can also find games based on popular movies, TV shows, books, comics, anime, and other media. There is something for everyone on Roblox.
-roblox roblox apk indir android
-roblox roblox apk indir ücretsiz
-roblox roblox apk indir son sürüm
-roblox roblox apk indir pc
-roblox roblox apk indir hileli
-roblox roblox apk indir google play
-roblox roblox apk indir 2023
-roblox roblox apk indir ios
-roblox roblox apk indir türkçe
-roblox roblox apk indir tablet
-roblox roblox apk indir oyun club
-roblox roblox apk indir cepde
-roblox roblox apk indir mod
-roblox roblox apk indir uptodown
-roblox roblox apk indir apkpure
-roblox roblox apk indir tamindir
-roblox roblox apk indir canlı yayın
-roblox roblox apk indir online
-roblox roblox apk indir bilgisayar
-roblox roblox apk indir windows 10
-roblox roblox apk indir macera oyunları
-roblox roblox apk indir yeni sürüm
-roblox roblox apk indir güncel
-roblox roblox apk indir nasıl yapılır
-roblox roblox apk indir video
-roblox roblox apk indir kurulumu
-roblox roblox apk indir yükleme
-roblox roblox apk indir açılmıyor
-roblox roblox apk indir sorunu
-roblox roblox apk indir hata veriyor
-roblox roblox apk indir yorumlar
-roblox roblox apk indir inceleme
-roblox roblox apk indir özellikleri
-roblox roblox apk indir nedir
-roblox robl
Roblox is not just a platform for creating and playing games, but also a social network where you can connect with other users from around the world. You can chat with your friends and other players online, join groups and clubs, follow your favorite creators and influencers, participate in events and contests, earn badges and achievements, and more. You can also support your favorite creators by buying their in-game items or subscribing to their premium memberships.
-Now that you know what Roblox is and what it offers, you might be wondering why you should download the Roblox APK file instead of using Google Play. Well, there are several reasons why downloading the Roblox APK file is a good idea. Here are some of them:
-One of the advantages of downloading the Roblox APK file is that you can get access to the latest features and updates before they are released on Google Play. This way, you can enjoy the new and improved versions of Roblox without having to wait for the official update. You can also avoid any bugs or glitches that might occur with the older versions of Roblox.
-Another reason why you might want to download the Roblox APK file is that you can play Roblox on devices that are not compatible with Google Play. For example, if you have an older device that does not support Google Play services, or if you have a device that runs on a different operating system than Android, such as Windows or iOS, you can still download and install the Roblox APK file and play Roblox on your device. This way, you can enjoy Roblox on any device you want.
-A third reason why you might prefer to download the Roblox APK file is that you can avoid any potential issues or restrictions with Google Play services. For example, if you live in a country where Google Play is not available or accessible, or if you have a problem with your Google account or payment method, you can still download and install the Roblox APK file and play Roblox without any hassle. You can also bypass any age or content restrictions that might apply to Roblox on Google Play.
-Now that you know why downloading the Roblox APK file is a good idea, let's see how you can do it. Downloading and installing the Roblox APK file is very easy and simple, and it only takes a few minutes. Here are the steps you need to follow:
-The first step is to find a reliable source for the Roblox APK file. There are many websites and apps that offer the Roblox APK file for free, but not all of them are safe and trustworthy. Some of them might contain viruses, malware, or spyware that could harm your device or steal your personal information. Therefore, you need to be careful and choose a reputable source for the Roblox APK file.
-One of the best sources for the Roblox APK file is [APKPure], a website that provides original and pure APK files for various apps and games. You can download the latest version of the Roblox APK file from [this link] without any risk or trouble.
-The next step is to enable unknown sources on your device settings. This is necessary because Android devices normally do not allow installing apps from sources other than Google Play. However, you can change this setting and allow installing apps from unknown sources by following these steps:
-Once you have enabled unknown sources, you can proceed to the next step.
-The third step is to download the Roblox APK file and tap on it to install it. You can do this by following these steps:
-Congratulations! You have successfully downloaded and installed the Roblox APK file on your Android device.
-The final step is to launch the app and log in with your Roblox account or create a new one. You can do this by following these steps:
-That's it! You are now ready to play Roblox on your Android device.
-Playing Roblox on Android is very easy and fun. You can explore millions of worlds and games created by other users or yourself, customize your avatar with hundreds of items and accessories, chat and interact with your friends and other players online, create your own games and experiences using the Roblox Studio app, and more. Here are some of the things you can do on Roblox on Android:
-One of the main attractions of Roblox is the variety and diversity of worlds and games that you can discover and play. You can find worlds and games for any interest, mood, or occasion. You can also filter them by genre, popularity, rating, or date. To explore worlds and games on Roblox on Android, you can follow these steps:
-You can also create your own worlds and games using the Roblox Studio app, which is a separate app that you can download from Google Play or [APKPure]. The Roblox Studio app allows you to design, build, script, test, and publish your own games and experiences on Roblox. You can also edit your existing games and update them with new features and content.
-Another fun aspect of Roblox is the ability to customize your avatar with hundreds of items and accessories. You can change your avatar's appearance, clothing, hair, face, accessories, gear, animations, and more. You can also buy or sell items in the Roblox catalog or marketplace. To customize your avatar on Roblox on Android, you can follow these steps:
-You can also create your own items using the Roblox Studio app or upload them from your device. You can also trade items with other users or sell them for Robux, which is the virtual currency of Roblox.
-Roblox is not only a gaming platform but also a social network where you can chat and interact with your friends and other players online. You can join groups and clubs, follow your favorite creators and influencers, participate in events and contests, earn badges and achievements, and more. You can also support your favorite creators by buying their in-game items or subscribing to their premium memberships. To chat and interact with your friends and other players on Roblox on Android, you can follow these steps:
-You can also chat and interact with other players in-game by using the chat box or the voice chat feature. You can also use emojis, stickers, and gestures to express yourself.
-The most creative and rewarding aspect of Roblox is the ability to create your own games and experiences using the Roblox Studio app. The Roblox Studio app is a separate app that you can download from Google Play or [APKPure]. The Roblox Studio app allows you to design, build, script, test, and publish your own games and experiences on Roblox. You can also edit your existing games and update them with new features and content.
-To create your own games and experiences using the Roblox Studio app, you need to have some basic knowledge of coding and game design. You can learn these skills by following the tutorials and guides available on the Roblox website or YouTube channel. You can also get help and feedback from other users on the Roblox forums or Discord server.
-Once you have created your own games and experiences, you can publish them on Roblox and share them with other users. You can also monetize your games and experiences by selling in-game items, offering premium memberships, or enabling ads. You can also earn Robux by creating popular games and experiences that attract many players.
-Roblox is an amazing gaming platform that allows you to create, play, and share anything you can imagine. You can play Roblox on your Android device by downloading the Roblox APK file from a reliable source, such as [APKPure]. You can also enjoy Roblox on any device that is not compatible with Google Play or avoid any potential issues or restrictions with Google Play services.
-By downloading the Roblox APK file, you can access the latest features and updates before they are available on Google Play. You can also explore millions of worlds and games created by other users or yourself, customize your avatar with hundreds of items and accessories, chat and interact with your friends and other players online, create your own games and experiences using the Roblox Studio app, and more.
-Roblox is a fun and creative way to express yourself, learn new skills, make new friends, and have a great time. So what are you waiting for? Download the Roblox APK file today and join the global community of over 200 million monthly active users who love Roblox!
-Here are some of the frequently asked questions about Roblox APK indir:
-Yes, Roblox APK is safe as long as you download it from a reputable source, such as [APKPure]. You should also scan the file with an antivirus app before installing it. However, you should be careful when downloading any APK file from unknown sources, as they might contain viruses, malware, or spyware that could harm your device or steal your personal information.
-Yes, Roblox APK is free to download and install. However, some features and items on Roblox might require real money or Robux to access or purchase. You can buy Robux with real money or earn them by creating popular games and experiences or selling in-game items.
-Yes, Roblox APK is legal as long as you use it for personal use only. However, you should not distribute or modify the file without permission from Roblox Corporation. You should also respect the terms of service and privacy policy of Roblox when using the app.
-You can update Roblox APK by downloading the latest version of the file from [APKPure] or any other reliable source. You can also check for updates within the app by tapping on the Settings icon at the top right corner and then tapping on About. You can also enable automatic updates by toggling on the option in your device settings.
-You can uninstall Roblox APK by following these steps:
-You can also uninstall Roblox APK by long-pressing on the app icon on your home screen and then dragging it to the Uninstall option.
-I hope this article has helped you understand how to download and play Roblox on Android using the Roblox APK file. If you have any questions or feedback, please feel free to leave a comment below. Thank you for reading and have fun playing Roblox!
197e85843dDOWNLOAD ->>> https://ssurll.com/2uzy3p
m.value?_.value*l.value:$(0,l.value)),h=v=>{const P=v>=w.value;let A=Math.abs(v-w.value);const T=P?l.value:l.value-1;let j=0;for(;A>0;)A-=E(T+j),P?j++:j--;return j},p=v=>{const P=h(v),A=l.value+P-o.value;return A<0?0:A>c.value?c.value:A},b=C(()=>m.value?_.value*(s.value-u.value):$(u.value,s.value));return{frontPadding:w,behindPadding:b,start:l,end:u,getStartByScroll:p,setItemSize:S,hasItemSize:L,setStart:d,getScrollOffset:y}};var _m=te({name:"VirtualListItem",props:{hasItemSize:{type:Function,required:!0},setItemSize:{type:Function,required:!0}},setup(e,{slots:t}){var n;const r=(n=Wt())==null?void 0:n.vnode.key,o=H(),i=()=>{var a,s,l,u;const c=(s=(a=o.value)==null?void 0:a.$el)!=null?s:o.value,d=(u=(l=c==null?void 0:c.getBoundingClientRect)==null?void 0:l.call(c).height)!=null?u:c==null?void 0:c.offsetHeight;d&&e.setItemSize(r,d)};return Ke(()=>i()),Ht(()=>i()),()=>{var a;const s=ln((a=t.default)==null?void 0:a.call(t));return s?Cr(s,{ref:o},!0):null}}}),Cm=Object.defineProperty,hs=Object.getOwnPropertySymbols,Sm=Object.prototype.hasOwnProperty,Em=Object.prototype.propertyIsEnumerable,ps=(e,t,n)=>t in e?Cm(e,t,{enumerable:!0,configurable:!0,writable:!0,value:n}):e[t]=n,wm=(e,t)=>{for(var n in t||(t={}))Sm.call(t,n)&&ps(e,n,t[n]);if(hs)for(var n of hs(t))Em.call(t,n)&&ps(e,n,t[n]);return e};const km=te({name:"VirtualList",components:{VirtualListItem:_m},props:{height:{type:[Number,String],default:200},data:{type:Array,default:()=>[]},threshold:{type:Number,default:0},itemKey:{type:String,default:"key"},fixedSize:{type:Boolean,default:!1},estimatedSize:{type:Number,default:30},buffer:{type:Number,default:10},component:{type:[String,Object],default:"div"},listAttrs:{type:Object},contentAttrs:{type:Object},paddingPosition:{type:String,default:"content"}},emits:{scroll:e=>!0,reachBottom:e=>!0},setup(e,{emit:t}){const{data:n,itemKey:r,fixedSize:o,estimatedSize:i,buffer:a,height:s}=Re(e),l=oe("virtual-list"),u=C(()=>Be(e.component)?wm({container:"div",list:"div",content:"div"},e.component):{container:e.component,list:"div",content:"div"}),c=H(),d=H(),m=C(()=>({height:de(s.value)?`${s.value}px`:s.value,overflow:"auto"})),_=C(()=>n.value.map((T,j)=>{var J;return(J=T[r.value])!=null?J:j})),{frontPadding:S,behindPadding:E,start:L,end:y,getStartByScroll:$,setItemSize:w,hasItemSize:h,setStart:p,getScrollOffset:b}=bm({dataKeys:_,contentRef:d,fixedSize:o,estimatedSize:i,buffer:a}),v=C(()=>e.threshold&&n.value.length<=e.threshold?n.value:n.value.slice(L.value,y.value));return{prefixCls:l,containerRef:c,contentRef:d,frontPadding:S,currentList:v,behindPadding:E,onScroll:T=>{const{scrollTop:j,scrollHeight:J,offsetHeight:U}=T.target,O=$(j);O!==L.value&&p(O),t("scroll",T),Math.floor(J-(j+U))<=0&&t("reachBottom",T)},setItemSize:w,hasItemSize:h,start:L,scrollTo:T=>{var j,J;if(c.value)if(de(T))c.value.scrollTop=T;else{const U=(J=T.index)!=null?J:_.value.indexOf((j=T.key)!=null?j:"");p(U-a.value),c.value.scrollTop=b(U),Je(()=>{if(c.value){const O=b(U);O!==c.value.scrollTop&&(c.value.scrollTop=O)}})}},style:m,mergedComponent:u}}});function $m(e,t,n,r,o,i){const a=ue("VirtualListItem");return x(),ve(sn(e.mergedComponent.container),{ref:"containerRef",class:K(e.prefixCls),style:$e(e.style),onScroll:e.onScroll},{default:ke(()=>[(x(),ve(sn(e.mergedComponent.list),Fe(e.listAttrs,{style:e.paddingPosition==="list"?{paddingTop:`${e.frontPadding}px`,paddingBottom:`${e.behindPadding}px`}:{}}),{default:ke(()=>[(x(),ve(sn(e.mergedComponent.content),Fe({ref:"contentRef"},e.contentAttrs,{style:e.paddingPosition==="content"?{paddingTop:`${e.frontPadding}px`,paddingBottom:`${e.behindPadding}px`}:{}}),{default:ke(()=>[(x(!0),ee(rt,null,Fn(e.currentList,(s,l)=>{var u;return x(),ve(a,{key:(u=s[e.itemKey])!=null?u:e.start+l,"has-item-size":e.hasItemSize,"set-item-size":e.setItemSize},{default:ke(()=>[se(e.$slots,"item",{item:s,index:e.start+l})]),_:2},1032,["has-item-size","set-item-size"])}),128))]),_:3},16,["style"]))]),_:3},16,["style"]))]),_:3},8,["class","style","onScroll"])}var Om=ce(km,[["render",$m]]);const ms=typeof window>"u"?global:window;function Lm(e,t){let n=0;return(...r)=>{n&&ms.clearTimeout(n),n=ms.setTimeout(()=>{n=0,e(...r)},t)}}var Tm=Object.defineProperty,Am=Object.defineProperties,Nm=Object.getOwnPropertyDescriptors,vs=Object.getOwnPropertySymbols,Pm=Object.prototype.hasOwnProperty,Im=Object.prototype.propertyIsEnumerable,gs=(e,t,n)=>t in e?Tm(e,t,{enumerable:!0,configurable:!0,writable:!0,value:n}):e[t]=n,En=(e,t)=>{for(var n in t||(t={}))Pm.call(t,n)&&gs(e,n,t[n]);if(vs)for(var n of vs(t))Im.call(t,n)&&gs(e,n,t[n]);return e},Mm=(e,t)=>Am(e,Nm(t));function Rm(e){return typeof e=="function"||Object.prototype.toString.call(e)==="[object Object]"&&!Ml(e)}const Bm={value:"value",label:"label",disabled:"disabled",tagProps:"tagProps",render:"render"};var So=te({name:"Select",components:{Trigger:hr,SelectView:fs},inheritAttrs:!1,props:{multiple:{type:Boolean,default:!1},modelValue:{type:[String,Number,Object,Array]},defaultValue:{type:[String,Number,Object,Array],default:e=>vt(e.multiple)?"":[]},inputValue:{type:String},defaultInputValue:{type:String,default:""},size:{type:String},placeholder:String,loading:{type:Boolean,default:!1},disabled:{type:Boolean,default:!1},error:{type:Boolean,default:!1},allowClear:{type:Boolean,default:!1},allowSearch:{type:[Boolean,Object],default:e=>!!e.multiple},allowCreate:{type:Boolean,default:!1},maxTagCount:{type:Number,default:0},popupContainer:{type:[String,Object]},bordered:{type:Boolean,default:!0},defaultActiveFirstOption:{type:Boolean,default:!0},popupVisible:{type:Boolean,default:void 0},defaultPopupVisible:{type:Boolean,default:!1},unmountOnClose:{type:Boolean,default:!1},filterOption:{type:[Boolean,Function],default:!0},options:{type:Array,default:()=>[]},virtualListProps:{type:Object},triggerProps:{type:Object},formatLabel:{type:Function},fallbackOption:{type:[Boolean,Function],default:!0},showExtraOptions:{type:Boolean,default:!0},valueKey:{type:String,default:"value"},searchDelay:{type:Number,default:500},limit:{type:Number,default:0},fieldNames:{type:Object},scrollbar:{type:[Boolean,Object],default:!0}},emits:{"update:modelValue":e=>!0,"update:inputValue":e=>!0,"update:popupVisible":e=>!0,change:e=>!0,inputValueChange:e=>!0,popupVisibleChange:e=>!0,clear:e=>!0,remove:e=>!0,search:e=>!0,dropdownScroll:e=>!0,dropdownReachBottom:e=>!0,exceedLimit:(e,t)=>!0},setup(e,{slots:t,emit:n,attrs:r}){const{size:o,disabled:i,error:a,options:s,filterOption:l,valueKey:u,multiple:c,popupVisible:d,showExtraOptions:m,modelValue:_,fieldNames:S,loading:E,defaultActiveFirstOption:L}=Re(e),y=oe("select"),{mergedSize:$,mergedDisabled:w,mergedError:h,eventHandlers:p}=yt({size:o,disabled:i,error:a}),b=C(()=>e.virtualListProps?"div":"li"),v=C(()=>Be(e.allowSearch)&&!!e.allowSearch.retainInputValue);C(()=>{if(it(e.formatLabel))return W=>{const ie=ne.get(W.value);return e.formatLabel(ie)}});const P=H(),A=H({}),T=H(),{computedPopupVisible:j,handlePopupVisibleChange:J}=Yp({popupVisible:d,emit:n}),U=H(e.defaultValue),O=C(()=>{var W;const ie=(W=e.modelValue)!=null?W:U.value;return(We(ie)?ie:ie||de(ie)?[ie]:[]).map(Ce=>({value:Ce,key:Rn(Ce,e.valueKey)}))});Ne(_,W=>{(vt(W)||jn(W))&&(U.value=c.value?[]:"")});const N=C(()=>O.value.map(W=>W.key)),D=C(()=>En(En({},Bm),S==null?void 0:S.value)),V=H(),G=W=>{const ie={};return W.forEach(le=>{ie[le]=ne.get(le)}),ie},B=W=>{V.value=G(W)},I=W=>it(e.fallbackOption)?e.fallbackOption(W):{[D.value.value]:W,[D.value.label]:String(Be(W)?W[u==null?void 0:u.value]:W)},Y=()=>{const W=[],ie=[];if(e.allowCreate||e.fallbackOption){for(const le of O.value)if(!ie.includes(le.key)){const Ce=ne.get(le.key);(!Ce||Ce.origin==="extraOptions")&&(W.push(le),ie.push(le.key))}}if(e.allowCreate&&Se.value){const le=Rn(Se.value);if(!ie.includes(le)){const Ce=ne.get(le);(!Ce||Ce.origin==="extraOptions")&&W.push({value:Se.value,key:le})}}return W},Q=H([]),he=C(()=>Q.value.map(W=>{var ie;let le=I(W.value);const Ce=(ie=V.value)==null?void 0:ie[W.key];return!vt(Ce)&&!rd(Ce)&&(le=En(En({},le),Ce)),le}));Je(()=>{Xc(()=>{var W;const ie=Y();if(ie.length!==Q.value.length)Q.value=ie;else if(ie.length>0){for(let le=0;le Loading... Download File ––– https://jinyurl.com/2uErm3 Download Zip > https://urlca.com/2uDe1z Dance eJay 3 is a software that allows you to create your own dance music with ease. It is a powerful and fun tool that lets you mix and match thousands of samples, add effects, edit audio, and export your tracks as MP3 or WAV files. You can also create video animations and share them online. If you are looking for a way to download Dance eJay 3 for free, you are in luck. There are several websites that offer this software as a free download, such as eJay Shop and Internet Archive. However, before you download Dance eJay 3, you should be aware of some things. Download Zip ✯ https://urlca.com/2uDcTx If you still want to download Dance eJay 3 for free, here are the steps you need to follow: Dance eJay 3 is a software that allows you to create your own dance music with ease. It is a powerful and fun tool that lets you mix and match thousands of samples, add effects, edit audio, and export your tracks as MP3 or WAV files. You can also create video animations and share them online. You can download Dance eJay 3 for free from several websites, such as eJay Shop and Internet Archive. However, you should be aware of some things before you download Dance eJay 3, such as its compatibility, legality, support, and security. If you are looking for a more updated and official version of Dance eJay, you can check out eJay's website, where they offer several other products for music creation, such as Virtual Music Studio, Hip Hop Reloaded If you are an experienced web developer who wants to advance your skills and career, you might be interested in taking the Microsoft Exam 70-480. This exam is part of the MCSD certification program, which validates your ability to design and develop modern web applications using HTML5, JavaScript, and CSS3. However, preparing for this exam can be challenging, as it covers a wide range of topics and requires a high level of proficiency. That's why you might want to download a free PDF of Exam Ref 70-480 Programming in HTML5 with JavaScript and CSS3, a book that can help you master the exam objectives and ace the test. DOWNLOAD ✅ https://urlca.com/2uDdEx Exam Ref 70-480 Programming in HTML5 with JavaScript and CSS3 is a book written by Rick Delorme, a Microsoft Certified Trainer and web development expert. The book is designed to help you prepare for the Microsoft Exam 70-480, which measures your ability to implement and manipulate document structures and objects, implement program flow, access and secure data, and use CSS3 in applications. The book is organized by exam objectives, and features strategic, what-if scenarios to challenge your critical-thinking and decision-making skills. The book also provides tips and best practices for writing efficient and maintainable code using HTML5, JavaScript, and CSS3. There are many benefits of downloading a free PDF of Exam Ref 70-480 Programming in HTML5 with JavaScript and CSS3. Here are some of them: Downloading a free PDF of Exam Ref 70-480 Programming in HTML5 with JavaScript and CSS3 is easy and simple. All you need to do is follow these steps: Exam Ref 70-480 Programming in HTML5 with JavaScript and CSS3 is a great book that can help you prepare for the Microsoft Exam 70-480. However, it is also expensive, and you might not be able to afford it. That's why downloading a free PDF of Exam Ref 70-480 Programming in HTML5 with JavaScript and CSS3 is a smart alternative that can help you get the book for free, without compromising on quality or content. With a free PDF download of Exam Ref 70-480 Programming in HTML5 with JavaScript and CSS3, you can master the exam objectives and pass the test with flying colors.0){if(!He.compareByGeneratedPositionsInflated(u,m[_-1]))continue;l+=","}l+=wn.encode(u.generatedColumn-t),t=u.generatedColumn,u.source!=null&&(d=this._sources.indexOf(u.source),l+=wn.encode(d-a),a=d,l+=wn.encode(u.originalLine-1-o),o=u.originalLine-1,l+=wn.encode(u.originalColumn-r),r=u.originalColumn,u.name!=null&&(c=this._names.indexOf(u.name),l+=wn.encode(c-i),i=c)),s+=l}return s};ft.prototype._generateSourcesContent=function(t,n){return t.map(function(r){if(!this._sourcesContents)return null;n!=null&&(r=He.relative(n,r));var o=He.toSetString(r);return Object.prototype.hasOwnProperty.call(this._sourcesContents,o)?this._sourcesContents[o]:null},this)};ft.prototype.toJSON=function(){var t={version:this._version,sources:this._sources.toArray(),names:this._names.toArray(),mappings:this._serializeMappings()};return this._file!=null&&(t.file=this._file),this._sourceRoot!=null&&(t.sourceRoot=this._sourceRoot),this._sourcesContents&&(t.sourcesContent=this._generateSourcesContent(t.sources,t.sourceRoot)),t};ft.prototype.toString=function(){return JSON.stringify(this.toJSON())};sa.SourceMapGenerator=ft;var Rr={},Nu={};(function(e){e.GREATEST_LOWER_BOUND=1,e.LEAST_UPPER_BOUND=2;function t(n,r,o,i,a,s){var l=Math.floor((r-n)/2)+n,u=a(o,i[l],!0);return u===0?l:u>0?r-l>1?t(l,r,o,i,a,s):s==e.LEAST_UPPER_BOUND?r{t.contains(ur(o))||n(o)};return{mousemove:r,touchstart:r}}else if(e==="clickoutside"){let r=!1;const o=a=>{r=!t.contains(ur(a))},i=a=>{r&&(t.contains(ur(a))||n(a))};return{mousedown:o,mouseup:i,touchstart:o,touchend:i}}return console.error(`[evtd/create-trap-handler]: name \`${e}\` is invalid. This could be a bug of evtd.`),{}}function Hc(e,t,n){const r=RC[e];let o=r.get(t);o===void 0&&r.set(t,o=new WeakMap);let i=o.get(n);return i===void 0&&o.set(n,i=BC(e,t,n)),i}function DC(e,t,n,r){if(e==="mousemoveoutside"||e==="clickoutside"){const o=Hc(e,t,n);return Object.keys(o).forEach(i=>{VC(i,document,o[i],r)}),!0}return!1}function FC(e,t,n,r){if(e==="mousemoveoutside"||e==="clickoutside"){const o=Hc(e,t,n);return Object.keys(o).forEach(i=>{xC(i,document,o[i],r)}),!0}return!1}function jC(){if(typeof window>"u")return{on:()=>{},off:()=>{}};const e=new WeakMap,t=new WeakMap;function n(){e.set(this,!0)}function r(){e.set(this,!0),t.set(this,!0)}function o(v,P,A){const T=v[P];return v[P]=function(){return A.apply(v,arguments),T.apply(v,arguments)},v}function i(v,P){v[P]=Event.prototype[P]}const a=new WeakMap,s=Object.getOwnPropertyDescriptor(Event.prototype,"currentTarget");function l(){var v;return(v=a.get(this))!==null&&v!==void 0?v:null}function u(v,P){s!==void 0&&Object.defineProperty(v,"currentTarget",{configurable:!0,enumerable:!0,get:P??s.get})}const c={bubble:{},capture:{}},d={};function m(){const v=function(P){const{type:A,eventPhase:T,bubbles:j}=P,J=ur(P);if(T===2)return;const U=T===1?"capture":"bubble";let O=J;const N=[];for(;O===null&&(O=window),N.push(O),O!==window;)O=O.parentNode||null;const D=c.capture[A],V=c.bubble[A];if(o(P,"stopPropagation",n),o(P,"stopImmediatePropagation",r),u(P,l),U==="capture"){if(D===void 0)return;for(let G=N.length-1;G>=0&&!e.has(P);--G){const B=N[G],I=D.get(B);if(I!==void 0){a.set(P,B);for(const Y of I){if(t.has(P))break;Y(P)}}if(G===0&&!j&&V!==void 0){const Y=V.get(B);if(Y!==void 0)for(const Q of Y){if(t.has(P))break;Q(P)}}}}else if(U==="bubble"){if(V===void 0)return;for(let G=0;GAdobe Audition CC 2020 v13.0.3.60 With Crack (x64) Latest
-
-Audition CC is a complete solution for non-linear audio editing with comprehensive audio restoration capabilities for everything from multi-track audio to surround sound.. And then I upgrade my adobe pro version to 12.1, but still cannot install any other adobe pro software. I need to install it through Internet. All I need is this a free version (AUDIO EDITOR) of Adobes CC for mac and windows. I prefer to use Adobe Audition. I need to use this software for audio editing, audio. 6 May 2020 Adobe Premiere Pro CC 2020 Setup Download File Formats. Audition has the best in-depth video editing tools, like color correction and picture. I would highly recommend to check out their free "Online Help" area (tab on the top right) for troubleshooting solutions. In this video, I show you how to install Audition for Windows 10/8/7/Vista. Adobe Audition CC is a professional audio editor that is ideal for making your own mixes, cutting music, editing audio and preparing to. Adobe Audition CC is one of the best audio software to create audio files. Fastness, a high-quality sound, and perfection are easy to achieve with Adobe Audition CC.
-
-7 Feb 2020 How to install Adobe Audition for Windows 10/8/7/Vista. The free version of Audition also comes with all the other sound-editing tools and features found in the full Audition CC suite. How to download Adobe Audition CC for Windows. Adobe Audition CC 2020 Free Download, Setup for Windows 10/8/7/Vista/XP. Download Audition cc 7. com, Free Download. It supports all popular audio formats such as MP3, WAV, OGG, MP4, etc. Adobe Audition CC 2020 Free Download for Windows 10, 8.1, 8, 7, Vista, XP. Download it from official website to fix the problem and use this tool to create new tracks and batch resampling. Audition for Mac is an easy-to-use audio editor that helps you edit, mix, and master your audio files and soundtracks.. It can also be used to record audio using line-in, so you can use its excellent sound recorder. to share your creations and collaborate with others.
-
-Audition Pro v20 Crack. Easily create professional-quality soundtracks. Edit and edit multiple audio files. Batch processing. Numerous presets. It is an audio editor used 4fefd39f24
-
-
-
diff --git a/spaces/esencb/web/app.py b/spaces/esencb/web/app.py
deleted file mode 100644
index fef1ac734f3a4eaf86f616f3801b0ad3b4ebe928..0000000000000000000000000000000000000000
--- a/spaces/esencb/web/app.py
+++ /dev/null
@@ -1,8 +0,0 @@
-import os
-
-os.system(f"git clone https://github.com/AUTOMATIC1111/stable-diffusion-webui /home/user/app/web")
-os.chdir("/home/user/app/web")
-
-os.system(f"wget -q https://huggingface.co/prompthero/openjourney/resolve/main/mdjrny-v4.ckpt -O /home/user/app/web/models/Stable-diffusion/mdjrny-v4.ckpt")
-os.system(f"python launch.py --precision full --no-half --use-cpu SD GFPGAN BSRGAN ESRGAN SCUNet CodeFormer --all --ui-config-file /home/user/app/ui-config.json --ui-settings-file /home/user/app/config.json --cors-allow-origins huggingface.co,hf.space --no-progressbar-hiding --skip-torch-cuda-test")
-
diff --git a/spaces/falterWliame/Face_Mask_Detection/CRACK Fruity Loops Studio 7 Full ISO Crack !LINK!.md b/spaces/falterWliame/Face_Mask_Detection/CRACK Fruity Loops Studio 7 Full ISO Crack !LINK!.md
deleted file mode 100644
index aead590135b7e9ee17dfe05eab19123970560141..0000000000000000000000000000000000000000
--- a/spaces/falterWliame/Face_Mask_Detection/CRACK Fruity Loops Studio 7 Full ISO Crack !LINK!.md
+++ /dev/null
@@ -1,44 +0,0 @@
-CRACK Fruity Loops Studio 7 Full ISO Crack
-
-JostoDotNet. Open source Windows Server 2003 to 2017 clustering and high availability software that focuses on low-latency, high-bandwidth business applications. You can download and try it for free.
-
-A:
-
-Installig VMWare on your local machine, you have three options:
-
-Run a Linux virtual machine for the development, since you are working with Linux tools.
-
-Install VirtualBox on your local machine (or on a VM) and install MS Windows on that virtual machine, in order to work with MS Windows tools
-
-Run a MS Windows virtual machine on your local machine, and install Linux tools on that virtual machine.
-
-Q:
-
-Saving TextView.text to String in Taps on different ListView Items
-
-I have a list of restaurants. Each restaurant has a button which loads up a Details view. In the Details view I have a Title, Phone and Url. I want to store all of the text in the TextView to be saved to a String. I have the code in place for the String saved from the first TextView to be saved in the string test.
-
-I want to do the same thing with the next TextView in the Details view. I know I have to put test++ but I am unsure how to do it.
-
-Here is the code from the onClick function in the list of restaurants:
-
-newButton.setOnClickListener(new View.OnClickListener() {
-
- public void onClick(View v) {
-
- //create the intent to pass the restaurant name and photo from the list activity
-
- Intent i = new Intent(ListViewActivity.this, DetailsActivity.class);
-
- //pass the restaurant name and photo to the next activity
-
- i.putExtra("name", name);
-
- i.putExtra("picture", image);
-
- //start the second activity and pass the details
-
- startActivity 4fefd39f24
-
-
-
diff --git a/spaces/falterWliame/Face_Mask_Detection/Dance Ejay 3 Free Download Full Version !!INSTALL!!.md b/spaces/falterWliame/Face_Mask_Detection/Dance Ejay 3 Free Download Full Version !!INSTALL!!.md
deleted file mode 100644
index 2784b89efae487b52fe2222e04b20fe8d6bc781c..0000000000000000000000000000000000000000
--- a/spaces/falterWliame/Face_Mask_Detection/Dance Ejay 3 Free Download Full Version !!INSTALL!!.md
+++ /dev/null
@@ -1,31 +0,0 @@
-
-How to Download Dance eJay 3 for Free and Create Your Own Music
-Dance Ejay 3 Free Download Full Version
-Things to Consider Before Downloading Dance eJay 3
-
-
-How to Download Dance eJay 3 for Free
-
-
-Conclusion
-
-
-
\ No newline at end of file
diff --git a/spaces/falterWliame/Face_Mask_Detection/Exam Ref 70-480 Programming In Html5 With Javascript And Css3 Pdf Download HOT Free.md b/spaces/falterWliame/Face_Mask_Detection/Exam Ref 70-480 Programming In Html5 With Javascript And Css3 Pdf Download HOT Free.md
deleted file mode 100644
index 59f75794e415c61dca755750a9ed499ea97a9ba5..0000000000000000000000000000000000000000
--- a/spaces/falterWliame/Face_Mask_Detection/Exam Ref 70-480 Programming In Html5 With Javascript And Css3 Pdf Download HOT Free.md
+++ /dev/null
@@ -1,27 +0,0 @@
-
-Exam Ref 70-480 Programming in HTML5 with JavaScript and CSS3 PDF Download Free: What You Need to Know
-Exam Ref 70-480 Programming In Html5 With Javascript And Css3 Pdf Download Free
-What is Exam Ref 70-480 Programming in HTML5 with JavaScript and CSS3?
-Why You Should Download a Free PDF of Exam Ref 70-480 Programming in HTML5 with JavaScript and CSS3?
-
-
-How to Download a Free PDF of Exam Ref 70-480 Programming in HTML5 with JavaScript and CSS3?
-
-
-Conclusion
-
Bingo is one of the most popular and entertaining games in the world. It is a game of chance where you have to match numbers on your cards with the ones drawn by a caller. The first player to mark off a line, a column, or a full card wins the game.
-But what if you don't have access to a bingo hall or a bingo app? What if you want to play bingo without an internet connection or without spending any money? Well, there is a solution for you: bingo offline game mod apk.
-DOWNLOAD ———>>> https://urllie.com/2uNygl
Bingo offline game mod apk is a modified version of the original bingo offline game app by SNG Games. It is an Android game that lets you enjoy the real deal of a casino card game without wifi or data. It is simple, beautiful, and fun. It is the only bingo game with online and offline game modes.
-You can play bingo offline game mod apk anytime, anywhere, with or without an internet connection. You can play solo against the computer, or join millions of players online in real-time multiplayer mode. You can also chat with other players and send them gifts.
-You can choose from different themes and modes to suit your mood and preference. You can play classic bingo, speed bingo, blackout bingo, or custom bingo. You can also choose from different themes such as Halloween, Christmas, Valentine's Day, or Las Vegas.
-You can collect bonuses and rewards every day by playing bingo offline game mod apk. You can get free coins, tickets, power-ups, and gems by spinning the wheel, watching videos, completing quests, or opening chests. You can also level up and unlock new features and rooms.
-You can customize your cards and daubers to make your bingo experience more personal and fun. You can change the color, shape, size, and style of your cards and daubers. You can also use emojis, stickers, or photos to mark your cards.
-To download bingo offline game mod apk, you need to find a trusted source that provides the latest version of the modded file. You can search for it on Google or use a link from a reputable website. Make sure you check the reviews and ratings before downloading.
-To install bingo offline game mod apk, you need to enable unknown sources on your device. This will allow you to install apps from sources other than the Google Play Store. To do this, go to your device settings, security, and enable unknown sources. You may need to confirm this action by tapping OK or Allow.
-To install bingo offline game mod apk, you need to locate the downloaded apk file on your device. You can use a file manager app or your device's default file explorer. Tap on the apk file and follow the instructions to install it. Once the installation is complete, tap on the game icon and launch the game.
-bingo offline game mod apk download
-bingo offline game mod apk free
-bingo offline game mod apk unlimited money
-bingo offline game mod apk latest version
-bingo offline game mod apk android
-bingo offline game mod apk hack
-bingo offline game mod apk no ads
-bingo offline game mod apk 2023
-bingo offline game mod apk for pc
-bingo offline game mod apk online
-bingo offline board game mod apk
-bingo offline free game mod apk
-bingo offline fun game mod apk
-bingo offline classic game mod apk
-bingo offline casino game mod apk
-bingo party - free classic bingo games offline mod apk
-bingo - free live bingo games online and offline mod apk
-bingo - free bingo games,play offline or online mod apk
-bingo - world trips & tournaments, play online or offline mod apk
-bingo - happy free bingo games for kindle fire,play offline or online casino bingo games with your friends! mod apk
-download bingo offline game mod apk 2023
-download bingo offline game mod apk latest version
-download bingo offline game mod apk unlimited money
-download bingo offline game mod apk hack
-download bingo offline game mod apk no ads
-free download bingo offline game mod apk
-how to download bingo offline game mod apk
-where to download bingo offline game mod apk
-best bingo offline game mod apk
-new bingo offline game mod apk
-top bingo offline game mod apk
-popular bingo offline game mod apk
-fun bingo offline game mod apk
-easy bingo offline game mod apk
-simple bingo offline game mod apk
-amazing bingo offline game mod apk
-awesome bingo offline game mod apk
-cool bingo offline game mod apk
-cute bingo offline game mod apk
-super bingo offline game mod apk
-play bingo offline game mod apk online
-play bingo offline game mod apk for pc
-play bingo offline game mod apk with friends
-play bingo offline game mod apk without internet
-play bingo offline board game mod apk
-play free classic bingo games offline with the best 2023 new games! (bingo party) - free live online & offlinemodapk
Bingo offline game mod apk is a fun, free, easy, and addictive game that will keep you entertained for hours. You can enjoy the thrill of bingo without any hassle or cost. You can play at your own pace and level of difficulty. You can also challenge yourself and compete with other players online.
-Bingo offline game mod apk is not a perfect game. It has some drawbacks that may affect your gaming experience. For example, it has ads that may interrupt your gameplay or consume your data. It also has some bugs and glitches that may cause the game to crash or freeze. Moreover, it may not be compatible with all devices or Android versions.
-Bingo offline game mod apk is not only a fun game but also a brain exercise. It can help you improve your memory and concentration by stimulating your cognitive skills. You have to pay attention to the numbers called and mark them on your cards quickly and accurately. You also have to remember the patterns and combinations that can make you win.
-Bingo offline game mod apk is also a relaxing and stress-relieving game. It can help you calm your mind and emotions by diverting your attention from your worries and problems. It can also make you happy and satisfied by rewarding you with coins, tickets, power-ups, gems, and prizes.
-Bingo offline game mod apk is also a social and friendly game. It can help you socialize and make friends by connecting you with other bingo lovers online. You can chat with them, send them gifts, invite them to play with you, or join their clubs. You can also share your achievements and feedback with them.
-One of the best tips for playing bingo offline game mod apk is to use multiple cards in each game. The more cards you have, the more numbers you can mark off, and the more chances you have of winning. However, be careful not to use too many cards that you cannot manage or afford.
-Another tip for playing bingo offline game mod apk is to use power-ups wisely. Power-ups are special items that can help you in various ways, such as revealing numbers, daubing cells, or doubling your rewards. You can get power-ups by spinning the wheel, opening chests, or buying them with gems.
-A final tip for playing bingo offline game mod apk is to join tournaments and events regularly. Tournaments and events are special modes that offer bigger prizes and challenges than normal games. You can join them by paying an entry fee or meeting certain requirements. You can win coins, tickets, gems, power-ups, or even real money.
-I hope this article has given you some useful information about bingo offline game mod apk. If you are looking for a fun, free, easy, and addictive bingo game that you can play anytime, anywhere, then bingo offline game mod apk is the perfect choice for you. Download it now and enjoy the fun of bingo!
- Conclusion Bingo offline game mod apk is a modified version of the original bingo offline game app by SNG Games. It is an Android game that lets you enjoy the real deal of a casino card game without wifi or data. It has many features such as offline and online modes, different themes and modes, bonuses and rewards, customization options, etc. It also has some pros and cons such as fun, free, easy, addictive vs ads, bugs, compatibility issues. It also has some benefits such as improving memory and concentration , relaxing and relieving stress, socializing and making friends. It also has some tips and tricks such as using multiple cards, power-ups, tournaments, and events. FAQs Q: What is the difference between bingo offline game mod apk and bingo offline game app? A: Bingo offline game mod apk is a modified version of the bingo offline game app that has some extra features and advantages, such as unlimited coins, tickets, gems, power-ups, etc. It also has some disadvantages, such as ads, bugs, compatibility issues, etc. Q: Is bingo offline game mod apk safe to download and install? A: Bingo offline game mod apk is generally safe to download and install, as long as you get it from a trusted source and enable unknown sources on your device. However, you should always be careful when downloading and installing any modded or hacked apps, as they may contain viruses, malware, or spyware that can harm your device or data. Q: How can I update bingo offline game mod apk? A: Bingo offline game mod apk may not update automatically like the original app. You may need to check for updates manually by visiting the source website or app store. You may also need to uninstall the old version and install the new version of the modded app. Q: Can I play bingo offline game mod apk with my friends? A: Yes, you can play bingo offline game mod apk with your friends online or offline. You can invite them to join your room or club, or join theirs. You can also chat with them, send them gifts, or compete with them in tournaments and events. Q: Can I win real money by playing bingo offline game mod apk? A: No, you cannot win real money by playing bingo offline game mod apk. The game is for entertainment purposes only and does not involve any real gambling or betting. The coins, tickets, gems, power-ups, and prizes you win are virtual and cannot be exchanged for real money or goods. 401be4b1e0If you are a fan of K-pop, you might have heard of the song Orange by TREASURE, a rising boy group from YG Entertainment. This song is a sweet and sentimental ballad that expresses the feelings of a young couple who want to spend more time together before the sunset. In this article, we will tell you everything you need to know about this song, how to download it, and how to enjoy it.
-Orange Treasure is the title of the third single album by TREASURE, which was released on November 6, 2020. It is also the name of the main track of the album, which was composed by R.Tee, CHOICE37, and Asahi, one of the members of TREASURE. The song has a warm and acoustic sound that matches the autumn season. It also showcases the vocal and rap skills of the 12 members of TREASURE.
-DOWNLOAD 🗸🗸🗸 https://urllie.com/2uNyBQ
The song Orange is inspired by the color of the sunset, which symbolizes the end of a day and the beginning of a night. The lyrics describe the emotions of a couple who want to cherish every moment they have together before they have to part ways. They compare their love to the orange color that shines every day without conditions, and they hope to see each other again tomorrow. The song also conveys a message of gratitude and hope for their fans, who have been supporting them since their debut.
-TREASURE is a boy group that debuted on August 7, 2020, under YG Entertainment. They are composed of 12 members: Choi Hyunsuk, Jihoon, Yoshi, Junkyu, Mashiho, Yoon Jaehyuk, Asahi, Bang Yedam, Haruto, Doyoung, Park Jeongwoo, and So Junghwan. They were formed through a survival show called YG Treasure Box, which aired from November 2018 to January 2019. They are known for their diverse talents, charismatic performances, and bright personalities.
-download lagu orange treasure mp3
-download lagu orange treasure live
-download lagu orange treasure lyrics
-download lagu orange treasure video
-download lagu orange treasure full album
-download lagu orange treasure acoustic
-download lagu orange treasure instrumental
-download lagu orange treasure cover
-download lagu orange treasure remix
-download lagu orange treasure karaoke
-download lagu orange treasure piano
-download lagu orange treasure guitar
-download lagu orange treasure dance
-download lagu orange treasure reaction
-download lagu orange treasure english version
-download lagu orange treasure japanese version
-download lagu orange treasure chinese version
-download lagu orange treasure indonesian version
-download lagu orange treasure thai version
-download lagu orange treasure vietnamese version
-download lagu orange treasure korean version
-download lagu orange treasure 8d audio
-download lagu orange treasure bass boosted
-download lagu orange treasure nightcore
-download lagu orange treasure mashup
-download lagu orange treasure ringtone
-download lagu orange treasure tiktok
-download lagu orange treasure spotify
-download lagu orange treasure apple music
-download lagu orange treasure soundcloud
-download lagu orange treasure youtube music
-download lagu orange treasure melon music
-download lagu orange treasure genie music
-download lagu orange treasure bugs music
-download lagu orange treasure flo music
-download lagu orange treasure vlive music
-download lagu orange treasure mnet music
-download lagu orange treasure kbs music bank
-download lagu orange treasure sbs inkigayo
-download lagu orange treasure mbc show music core
-download lagu orange treasure arirang simply kpop
-download lagu orange treasure mtv fresh out live
-download lagu orange treasure billboard live at home
-download lagu orange treasure rolling stone in my room
-download lagu orange treasur
Since their debut, TREASURE has achieved many milestones and records in the K-pop industry. They are the first YG group to release three single albums in three consecutive months. They are also the first rookie group in 2020 to sell over 500,000 copies of their albums. They have won several awards and nominations, such as Rookie Artist of the Year at the Melon Music Awards and Best New Male Artist at the Mnet Asian Music Awards. They have also gained a huge fanbase around the world, with over 6 million subscribers on YouTube and over 4 million followers on Instagram.
-If you want to download lagu Orange Treasure, you have several options to choose from. You can either use official sources or unofficial sources. However, there are some differences and risks that you should be aware of before you decide.
-The official sources are the ones that are authorized by YG Entertainment and TREASURE. They include streaming platforms such as Spotify, Apple Music, YouTube Music, Melon, Genie, Bugs, etc. These platforms offer high-quality audio files that are legal and safe to download. They also support the artists by counting towards their digital sales and charts rankings. However, some of these platforms may require a subscription fee or a region restriction to access them.
-The unofficial sources are the ones that are not authorized by YG Entertainment and TREASURE. They include websites, apps, or software that offer free downloads of mp3 files. These sources may seem convenient and easy to use, but they also come with some risks. For example, they may contain viruses, malware, or spyware that can harm your device or steal your personal information. They may also have low-quality audio files that are corrupted or incomplete. Moreover, they do not support the artists by stealing their intellectual property and violating their rights. Therefore, we do not recommend using these sources to download lagu Orange Treasure.
-Once you have downloaded lagu Orange Treasure, you can enjoy it in many ways. You can listen to it on your device, share it with your friends, or sing along to it. However, if you want to have a deeper and richer experience of the song, you can also do the following things:
-The lyrics of Orange Treasure are written in Korean, English, and Japanese. They are very poetic and expressive, conveying the emotions and thoughts of the couple in the song. If you want to understand the meaning and message of the song better, you can read the lyrics and translation of the song online. You can find them on websites such as Genius, Color Coded Lyrics, or KLyrics. You can also watch the lyric video of the song on YouTube, which shows the lyrics in different languages and colors.
-The live video and performance of Orange Treasure are also very impressive and captivating. You can watch them on YouTube, where you can see how TREASURE sings and dances to the song with passion and energy. You can also see how they interact with each other and with their fans, showing their charm and personality. You can also appreciate their outfits, stage design, and lighting effects, which create a beautiful and romantic atmosphere.
-In conclusion, Orange Treasure is a wonderful song that you should not miss if you are a fan of K-pop or TREASURE. It is a song that expresses the love and gratitude of a couple who want to make the most of their time together before the sunset. It is also a song that showcases the talent and potential of TREASURE, a rising boy group from YG Entertainment. If you want to download and enjoy this song, you can use the official sources and platforms that we have mentioned in this article. You can also read the lyrics and translation of the song, and watch the live video and performance of the song online.
-We hope that this article has helped you learn more about Orange Treasure and how to download it. If you have any questions or comments, please feel free to leave them below. Thank you for reading!
- FAQs - Q: Who are the composers of Orange Treasure? - A: The composers are R.Tee, CHOICE37, and Asahi. - Q: How many members are there in TREASURE? - A: There are 12 members in TREASURE. - Q: What are the names of the other songs in the third single album by TREASURE? - A: The other songs are MMM and Boy. - Q: What is the name of the fan club of TREASURE? - A: The name of the fan club is Treasure Maker or Teume for short. - Q: Where can I buy the physical album of Orange Treasure? - A: You can buy it from online stores such as YG Select, Ktown4u, YesAsia, etc. 401be4b1e0Email marketing is one of the most effective and affordable ways to reach your target audience, promote your brand, and increase your sales. However, to succeed in email marketing, you need a reliable and efficient tool that can help you collect, manage, and send emails to your potential customers.
-Download File >>>>> https://urllie.com/2uNBNJ
That's where Email Extractor Lite 1.4 comes in handy. This is a free and easy-to-use software that can extract email addresses from various sources, such as websites, local files, search engines, and more. With this tool, you can build your own email list in minutes and start sending personalized and engaging emails to your prospects.
-In this article, we will show you what Email Extractor Lite 1.4 is, what features and benefits it offers, how to use it, why you should choose it for your email marketing campaigns, where to download it, and how to install and activate it. Let's get started!
-Email Extractor Lite 1.4 is a free online software that can extract email addresses from any text input. It is a lightweight and powerful utility that can handle large amounts of data and process them quickly and accurately.
-You can use Email Extractor Lite 1.4 to extract email addresses from various sources, such as:
-Email Extractor Lite 1.4 has many features and benefits that make it a great tool for email marketing. Here are some of them:
-Using Email Extractor Lite 1.4 is very simple and straightforward. Here are the steps you need to follow:
-Email Extractor Lite 1.4 is not just a tool for extracting email addresses. It is also a tool for enhancing your email marketing campaigns. Here are some of the reasons why you should choose Email Extractor Lite 1.4 for your email marketing campaigns:
-download email extractor lite 1.4 online
-download email extractor lite 1.4 free
-download email extractor lite 1.4 for windows
-download email extractor lite 1.4 for mac
-download email extractor lite 1.4 full version
-download email extractor lite 1.4 crack
-download email extractor lite 1.4 software
-download email extractor lite 1.4 tool
-download email extractor lite 1.4 app
-download email extractor lite 1.4 chrome extension
-download email extractor lite 1.4 from pc guide[^1^]
-download email extractor lite 1.4 from eyesbit[^2^]
-download email extractor lite 1.4 from lite14.org[^3^]
-download email extractor lite 1.4 with filter option
-download email extractor lite 1.4 with verification feature
-download email extractor lite 1.4 with sorting function
-download email extractor lite 1.4 with deduplication feature
-download email extractor lite 1.4 with export option
-download email extractor lite 1.4 with regex support
-download email extractor lite 1.4 with bulk mode
-how to download email extractor lite 1.4
-why download email extractor lite 1.4
-where to download email extractor lite 1.4
-when to download email extractor lite 1.4
-what is email extractor lite 1.4
-benefits of downloading email extractor lite 1.4
-reviews of downloading email extractor lite 1.4
-alternatives to downloading email extractor lite 1.4
-comparison of downloading email extractor lite 1.4 and other tools
-best practices for downloading email extractor lite 1.4
-tips and tricks for downloading email extractor lite 1.4
-tutorials for downloading email extractor lite 1.4
-guides for downloading email extractor lite 1.4
-faqs for downloading email extractor lite 1.4
-support for downloading email extractor lite 1.4
-problems with downloading email extractor lite 1.4
-solutions for downloading email extractor lite 1.4
-updates for downloading email extractor lite 1.4
-features of downloading email extractor lite 1.4
-advantages of downloading email extractor lite 1.4
-disadvantages of downloading email extractor lite 1.4
-pros and cons of downloading email extractor lite 1.4
-testimonials of downloading email extractor lite 1.4
-case studies of downloading email extractor lite 1.4
-success stories of downloading email extractor lite 1.4
-examples of downloading email extractor lite 1.4
-results of downloading email extractor lite 1.4
-performance of downloading email extractor lite 1.4
-quality of downloading email extractor lite 1.4
Email Extractor Lite 1.4 can help you save time and money by eliminating the need for manual and tedious tasks of finding and collecting email addresses. You don't have to spend hours browsing through websites, searching for keywords, or scanning documents for email addresses. You don't have to pay for expensive and unreliable services or software that may not deliver what they promise. With Email Extractor Lite 1.4, you can get thousands of email addresses in minutes without spending a dime.
-Email Extractor Lite 1.4 can help you generate quality leads and prospects by providing you with targeted and relevant email addresses. You can use the tool to find email addresses of people who are interested in your niche, industry, product, or service. You can also use the tool to segment your email list based on various criteria, such as location, domain, keyword, etc. This way, you can tailor your email messages to suit the needs and preferences of your audience.
-Email Extractor Lite 1.4 can help you boost your conversion rate and sales by enabling you to send personalized and engaging emails to your prospects. You can use the tool to create customized and catchy subject lines, headlines, and body content that will capture the attention and interest of your recipients. You can also use the tool to add call-to-action buttons, links, images, videos, etc., that will encourage your recipients to take action and buy your product or service.
-If you are convinced that Email Extractor Lite 1.4 is the right tool for your email marketing campaigns, you may be wondering where to download it. There are two main sources where you can download Email Extractor Lite 1.4:
-The best and safest way to download Email Extractor Lite 1.4 is from its official website at [http://emailextractorlite.com]. This is where you can get the latest version of the software with all the features and updates. You can also get access to the user guide, FAQs, testimonials, contact information, and other useful resources on the website.
-If for some reason you cannot access the official website of Email Extractor Lite 1.4, you can also download it from alternative sources, such as third-party websites, online platforms, or file-sharing networks. However, you should be careful when downloading from these sources as they may not be trustworthy or secure. You may end up downloading a corrupted or infected file that may harm your device or compromise your data. Therefore, you should always scan the file with an antivirus software before opening it.
-After downloading Email Extractor Lite 1.4 from a reliable source, you need to install and activate it on your device before using it. Here are the steps you need to follow:
-Email Extractor Lite 1.4 is a powerful tool for email marketing that can help you extract email addresses from various sources, such as websites, local files, search engines, and text box. It is free, easy, fast, accurate, and customizable. It can help you save time and money, generate quality leads and prospects, and boost your conversion rate and sales. You can download it from the official website or alternative sources, and install and activate it on your device with ease.
-If you are looking for a reliable and efficient tool for your email marketing campaigns, you should definitely give Email Extractor Lite 1.4 a try. You will be amazed by the results it can deliver.
-Here are some of the frequently asked questions about Email Extractor Lite 1.4:
-If you are a fan of drifting games, you have probably heard of FR Legends, a popular game that lets you experience the thrill of sliding sideways in various cars and tracks. But did you know that there is a way to make this game even more fun and exciting? That's right, by downloading the FR Legends Mod APK JDM version, you can unlock all the features and content that the original game does not offer. In this article, we will tell you everything you need to know about this modded version of FR Legends, including its features, how to download and install it, and some tips and tricks for playing it. So, buckle up and get ready to drift like a legend!
-FR Legends is already a great game on its own, but with the mod apk jdm version, you can enjoy it even more. Here are some of the features that this modded version offers:
-Download · https://urllie.com/2uNIJj
One of the main attractions of FR Legends is that it allows you to customize your car with various parts and accessories. However, the original game only has a limited selection of cars and parts, mostly from European brands. With the mod apk jdm version, you can access a huge collection of JDM (Japanese Domestic Market) cars and parts, such as Toyota Supra, Nissan Skyline, Mazda RX-7, Honda Civic, Mitsubishi Lancer Evo, Subaru Impreza, and many more. You can also mix and match different parts from different brands, such as engines, wheels, body kits, spoilers, exhausts, etc. You can create your own unique drift machine with your favorite JDM style.
-Another feature that makes FR Legends stand out from other drifting games is its realistic physics and graphics. The game simulates the physics of drifting accurately, such as weight transfer, tire grip, steering angle, throttle control, etc. You can feel the difference between different cars and setups, as well as the effects of different weather conditions and track surfaces. The game also has stunning graphics that make the cars and tracks look realistic and detailed. You can see the smoke from your tires, the sparks from your exhaust, the damage from collisions, etc. The game also has dynamic camera angles that follow your car as you drift.
-FR Legends also offers a variety of game modes and tracks to keep you entertained. You can choose from the following modes: - Career mode: In this mode, you can start from the bottom and work your way up to become a drifting legend. You can compete in various events and challenges, such as time trials, tandem battles, drift trains, etc. You can also earn money and reputation points that you can use to buy and upgrade your cars and parts. - Free mode: In this mode, you can practice your drifting skills without any pressure or limitations. You can choose any car and track you want, and adjust the settings to your liking. You can also explore the tracks and discover hidden spots and secrets. - Online mode: In this mode, you can challenge other players from around the world and show off your drifting skills. You can join or create rooms with different rules and settings, such as track selection, car class, weather condition, etc. You can also chat with other players and make friends or rivals. The game also has a variety of tracks to suit your preferences and skills. You can drift on different types of tracks, such as mountain roads, city streets, race circuits, dirt roads, etc. Each track has its own characteristics and challenges, such as curves, elevation changes, obstacles, etc. You can also choose different weather conditions and time of day to change the atmosphere and difficulty of the tracks.
One of the most exciting features of FR Legends Mod APK JDM is its online multiplayer and leaderboards. You can connect with other players from around the world and compete in various modes and events. You can also chat with other players and make friends or rivals. You can also see how you rank among other players on the global and regional leaderboards. You can compare your scores and stats with other players and see who is the best drifter in the world.
-If you are interested in downloading and installing FR Legends Mod APK JDM on your Android device, you need to follow these simple steps:
-Before you can install any modded apk file on your device, you need to enable unknown sources on your device. This will allow you to install apps that are not from the official Google Play Store. To do this, go to your device's settings, then security, then unknown sources. Toggle the switch to enable it.
-Next, you need to download the mod apk file from a trusted source. There are many websites that offer modded apk files, but not all of them are safe and reliable. Some of them may contain viruses or malware that can harm your device or steal your personal information. To avoid this, you should only download from reputable sources that have positive reviews and feedback from other users. One of the best sources for downloading FR Legends Mod APK JDM is [this website]. This website offers a safe and secure download link for the latest version of the mod apk file.
-Finally, you need to install the mod apk file and launch the game. To do this, locate the downloaded file on your device's storage, then tap on it to start the installation process. Follow the instructions on the screen to complete the installation. Once done, you can launch the game from your app drawer or home screen. Enjoy!
-To help you get started with playing FR Legends Mod APK JDM, here are some tips and tricks that you should know:
-download fr legends mod apk jdm latest modpacks
-download fr legends new jdm mod pack v 0.3.2
-download fr legends mod jdm supra, s15, rx7
-download fr legends mod apk jdm gtr r34 lbwk
-download fr legends mod apk jdm unlimited money
-download fr legends mod apk jdm toyota gr supra
-download fr legends mod apk jdm bmw e36 sedan
-download fr legends mod apk jdm gtr hakosuka
-download fr legends mod apk jdm rx7 veilside
-download fr legends mod apk jdm twin turbo
-download fr legends mod apk jdm better wheel
-download fr legends mod apk jdm rtx on
-download fr legends mod apk jdm swap engine
-download fr legends mod apk jdm 4k brake retekstur
-download fr legends mod apk jdm retekstur garage
-download fr legends mod apk jdm miata formula drift
-download fr legends mod apk jdm s14 bosskit
-download fr legends mod apk jdm s13 cabrio
-download fr legends mod apk jdm mustang gt
-download fr legends mod apk jdm nissan r34 twin turbo
-download fr legends mod apk jdm osaka sideways map
-download fr legends mod apk jdm nkc reteksture map
-download fr legends mod apk jdm mediafire link
-download fr legends mod apk jdm no password link
-download fr legends mod apk jdm youtube video link
-download fr legends mod apk jdm by raka27
-download fr legends mod apk jdm by jpegstreet
-download fr legends mod apk jdm by 2fast customs
-download fr legends mod apk jdm by sr_driver
-download fr legends mod apk jdm by neonmates
-download fr legends mod apk jdm by skinfrlegends
-download fr legends mod apk jdm by surya_art
-download fr legends mod apk jdm by satriya ww
-download fr legends mod apk jdm by rakaa7 yt channel
-download fr legends mod apk jdm by jpegstreet yt channel
-download fr legends mod apk jdm by 2fast customs yt channel
-download fr legends mod apk jdm by sr_driver yt channel
-download fr legends mod apk jdm by neonmates yt channel
-download fr legends mod apk jdm by skinfrlegends yt channel
-download fr legends mod apk jdm by surya_art yt channel
Drifting is not as easy as it looks. It requires skill, timing, and precision to execute it properly. If you are new to drifting games, you should learn the basics of drifting first before attempting more advanced techniques. Here are some of the basic terms and concepts that you should know:
- - Drift angle: This is the angle between your car's direction of travel and its longitudinal axis (the line that runs from the front to the back of your car). The larger the drift angle, the more sideways your car is sliding. - Countersteer: This is when you turn your steering wheel in the opposite direction of your drift angle to balance your car and prevent it from spinning out. - Throttle control: This is when you adjust your gas pedal to control your car's speed and traction during a drift. You need to apply more throttle when entering a drift to initiate it, then reduce it when maintaining a drift, then increase it again when exiting a drift to accelerate. - Brake control: This is when you use your brake pedal to slow down your car or initiate a drift. You can use the brake to reduce your speed before entering a corner, or to shift the weight of your car to the front wheels and make it easier to turn. You can also use the handbrake to lock the rear wheels and make your car slide sideways. - Clutch kick: This is when you press and release the clutch pedal quickly while applying throttle to create a sudden burst of power and torque to the rear wheels. This can help you initiate or maintain a drift, especially when your car is low on speed or traction.As you play FR Legends Mod APK JDM, you will earn money and coins that you can use to buy and upgrade your cars and parts. You can choose from a wide range of JDM cars and parts, such as engines, turbos, superchargers, nitrous, suspension, tires, brakes, etc. You can also customize the appearance of your car with different colors, decals, stickers, body kits, spoilers, etc. You can create your own unique drift machine with your favorite JDM style.
-However, buying and upgrading your car is not enough. You also need to tune it to suit your driving style and preferences. You can adjust various settings of your car, such as tire pressure, camber angle, toe angle, ride height, spring stiffness, damping rate, anti-roll bar stiffness, etc. You can also adjust the power distribution of your car, such as front/rear balance, torque split, differential lock, etc. You can experiment with different settings and see how they affect your car's performance and handling. You can also save different setups for different tracks and modes.
-FR Legends Mod APK JDM offers a variety of tracks and modes to keep you entertained. However, each track and mode has its own characteristics and challenges that require different skills and strategies. Therefore, you should practice on different tracks and modes to improve your drifting skills and learn the best ways to tackle them. Here are some of the tracks and modes that you can practice on:
- - Mountain roads: These are narrow and winding roads that run through hills and forests. They have sharp turns, elevation changes, blind corners, etc. They require precise steering, braking, and throttle control to drift smoothly and avoid crashing into obstacles or falling off cliffs. - City streets: These are urban roads that run through buildings and landmarks. They have traffic lights, intersections, pedestrians, etc. They require quick reflexes, awareness, and timing to drift safely and avoid collisions or penalties. - Race circuits: These are professional tracks that are designed for racing and drifting. They have wide lanes, smooth surfaces, clear markings, etc. They require high speed, stability, and consistency to drift fast and score high points. - Dirt roads: These are rough and uneven roads that run through fields and farms. They have loose gravel, mud, puddles, etc. They require good traction, balance, and adaptability to drift on slippery and bumpy surfaces. - Career mode: This is the mode where you can start from the bottom and work your way up to become a drifting legend. You can compete in various events and challenges that test your drifting skills in different scenarios. You can also earn money and reputation points that you can use to buy and upgrade your cars and parts. - Free mode: This is the mode where you can practice your drifting skills without any pressure or limitations. You can choose any car and track you want and adjust the settings to your liking. You can also explore the tracks and discover hidden spots and secrets. - Online mode: This is the mode where you can challenge other players from around the world and show off your drifting skills. You can join or create rooms with different rules and settings, such as track selection, car class, weather condition, etc. You can also chat with other players and make friends or rivals.One of the most exciting features of FR Legends Mod APK JDM is its online multiplayer and leaderboards. You can connect with other players from around the world and compete in various modes and events. You can also see how you rank among other players on the global and regional leaderboards. You can compare your scores and stats with other players and see who is the best drifter in the world.
-By challenging other players online, you can also earn rewards that you can use to improve your car and performance. You can earn money, coins, reputation points, parts, decals, etc. You can also unlock new cars and tracks by completing certain achievements and milestones. You can also get special rewards by participating in seasonal events and tournaments that offer exclusive prizes and bonuses.
-FR Legends Mod APK JDM is the ultimate drifting game for Android devices. It offers a realistic and immersive drifting experience that will make you feel like a legend. It also offers a lot of features and content that the original game does not offer, such as customizable JDM cars and parts, realistic physics and graphics, various game modes and tracks, online multiplayer and leaderboards, etc. It is easy to download and install, and it is safe and secure to play. If you are looking for a fun and exciting drifting game that will keep you entertained for hours, you should definitely download FR Legends Mod APK JDM today!
-Yes, FR Legends Mod APK JDM is safe to download and play. It does not contain any viruses or malware that can harm your device or steal your personal information. It also does not require any root access or special permissions to run. However, you should only download it from a trusted source, such as [this website], to avoid any potential risks or problems.
-To play FR Legends Mod APK JDM, you need an Android device that meets the following requirements:
- - Android version 4.1 or higher - At least 1 GB of RAM - At least 200 MB of free storage space - A stable internet connectionThere are several ways to get more money and coins in FR Legends Mod APK JDM. You can:
- - Complete events and challenges in career mode - Win online matches and tournaments - Watch ads and videos - Use the daily login bonus - Use the mod apk features that give you unlimited money and coinsThere are many JDM cars to choose from in FR Legends Mod APK JDM, but some of the best ones are:
- - Toyota Supra: A legendary sports car that has a powerful engine, a sleek design, and a high performance. - Nissan Skyline: A iconic car that has a distinctive look, a turbocharged engine, and a four-wheel drive system. - Mazda RX-7: A unique car that has a rotary engine, a lightweight body, and a smooth handling. - Honda Civic: A popular car that has a reliable engine, a compact size, and a low cost. - Mitsubishi Lancer Evo: A rally car that has a turbocharged engine, a four-wheel drive system, and a sporty appearance.If you have any questions, feedback, or suggestions for the developers of FR Legends Mod APK JDM, you can contact them through their official social media accounts:
- - Facebook: [https://www.facebook.com/FRLEGENDSgame/] - Twitter: [https://twitter.com/frlegendsgame] - Instagram: [https://www.instagram.com/frlegendsgame/] 401be4b1e0PyTorch way to Generate optical flow image & .flo file from 2 consecutive frames with RAFT model
" -css=""" -#col-container {max-width: 700px; margin-left: auto; margin-right: auto;} -a {text-decoration-line: underline; font-weight: 600;} -""" -with gr.Blocks(css=css) as block: - with gr.Column(elem_id="col-container"): - gr.HTML(title) - gr.HTML(description) - - frame1_inp = gr.Image(source="upload", type="filepath", label="frame 1") - frame2_inp = gr.Image(source="upload", type="filepath", label="frame 2") - - submit_btn = gr.Button("Submit") - - flow_img_out = gr.Image(label="flow image") - flow_file_out = gr.File(label="flow file") - - - examples=[ - ['basket1.jpg','basket2.jpg'], - ['frame1.jpg', 'frame2.jpg'] - ] - ex = gr.Examples(examples=examples, fn=infer, inputs=[frame1_inp, frame2_inp], outputs=[flow_img_out, flow_file_out], cache_examples=True, run_on_click=True) - #ex.dataset.headers = [""] - - - - submit_btn.click(fn=infer, inputs=[frame1_inp, frame2_inp], outputs=[flow_img_out, flow_file_out]) - - -block.launch() \ No newline at end of file diff --git a/spaces/fffiloni/Video-Matting-Anything/README.md b/spaces/fffiloni/Video-Matting-Anything/README.md deleted file mode 100644 index 7e94e84610b1ae07fcb291186e37b34f08354163..0000000000000000000000000000000000000000 --- a/spaces/fffiloni/Video-Matting-Anything/README.md +++ /dev/null @@ -1,15 +0,0 @@ ---- -title: Video Matting Anything -emoji: 🎯 -colorFrom: yellow -colorTo: green -python_version: 3.10.12 -sdk: gradio -sdk_version: 3.48.0 -app_file: app.py -pinned: false -license: mit -duplicated_from: shi-labs/Matting-Anything ---- - -Check out the configuration reference at https://huggingface.co/docs/hub/spaces-config-reference \ No newline at end of file diff --git a/spaces/fffiloni/controlnet-animation-doodle/node_modules/@types/node/ts4.8/util.d.ts b/spaces/fffiloni/controlnet-animation-doodle/node_modules/@types/node/ts4.8/util.d.ts deleted file mode 100644 index c821051ef62ba7c1b35a3b51e0beb4126b2e6d57..0000000000000000000000000000000000000000 --- a/spaces/fffiloni/controlnet-animation-doodle/node_modules/@types/node/ts4.8/util.d.ts +++ /dev/null @@ -1,2011 +0,0 @@ -/** - * The `util` module supports the needs of Node.js internal APIs. Many of the - * utilities are useful for application and module developers as well. To access - * it: - * - * ```js - * const util = require('util'); - * ``` - * @see [source](https://github.com/nodejs/node/blob/v18.x/lib/util.js) - */ -declare module 'util' { - import * as types from 'node:util/types'; - export interface InspectOptions { - /** - * If `true`, object's non-enumerable symbols and properties are included in the formatted result. - * `WeakMap` and `WeakSet` entries are also included as well as user defined prototype properties (excluding method properties). - * @default false - */ - showHidden?: boolean | undefined; - /** - * Specifies the number of times to recurse while formatting object. - * This is useful for inspecting large objects. - * To recurse up to the maximum call stack size pass `Infinity` or `null`. - * @default 2 - */ - depth?: number | null | undefined; - /** - * If `true`, the output is styled with ANSI color codes. Colors are customizable. - */ - colors?: boolean | undefined; - /** - * If `false`, `[util.inspect.custom](depth, opts, inspect)` functions are not invoked. - * @default true - */ - customInspect?: boolean | undefined; - /** - * If `true`, `Proxy` inspection includes the target and handler objects. - * @default false - */ - showProxy?: boolean | undefined; - /** - * Specifies the maximum number of `Array`, `TypedArray`, `WeakMap`, and `WeakSet` elements - * to include when formatting. Set to `null` or `Infinity` to show all elements. - * Set to `0` or negative to show no elements. - * @default 100 - */ - maxArrayLength?: number | null | undefined; - /** - * Specifies the maximum number of characters to - * include when formatting. Set to `null` or `Infinity` to show all elements. - * Set to `0` or negative to show no characters. - * @default 10000 - */ - maxStringLength?: number | null | undefined; - /** - * The length at which input values are split across multiple lines. - * Set to `Infinity` to format the input as a single line - * (in combination with `compact` set to `true` or any number >= `1`). - * @default 80 - */ - breakLength?: number | undefined; - /** - * Setting this to `false` causes each object key - * to be displayed on a new line. It will also add new lines to text that is - * longer than `breakLength`. If set to a number, the most `n` inner elements - * are united on a single line as long as all properties fit into - * `breakLength`. Short array elements are also grouped together. Note that no - * text will be reduced below 16 characters, no matter the `breakLength` size. - * For more information, see the example below. - * @default true - */ - compact?: boolean | number | undefined; - /** - * If set to `true` or a function, all properties of an object, and `Set` and `Map` - * entries are sorted in the resulting string. - * If set to `true` the default sort is used. - * If set to a function, it is used as a compare function. - */ - sorted?: boolean | ((a: string, b: string) => number) | undefined; - /** - * If set to `true`, getters are going to be - * inspected as well. If set to `'get'` only getters without setter are going - * to be inspected. If set to `'set'` only getters having a corresponding - * setter are going to be inspected. This might cause side effects depending on - * the getter function. - * @default false - */ - getters?: 'get' | 'set' | boolean | undefined; - /** - * If set to `true`, an underscore is used to separate every three digits in all bigints and numbers. - * @default false - */ - numericSeparator?: boolean | undefined; - } - export type Style = 'special' | 'number' | 'bigint' | 'boolean' | 'undefined' | 'null' | 'string' | 'symbol' | 'date' | 'regexp' | 'module'; - export type CustomInspectFunction = (depth: number, options: InspectOptionsStylized) => any; // TODO: , inspect: inspect - export interface InspectOptionsStylized extends InspectOptions { - stylize(text: string, styleType: Style): string; - } - /** - * The `util.format()` method returns a formatted string using the first argument - * as a `printf`\-like format string which can contain zero or more format - * specifiers. Each specifier is replaced with the converted value from the - * corresponding argument. Supported specifiers are: - * - * If a specifier does not have a corresponding argument, it is not replaced: - * - * ```js - * util.format('%s:%s', 'foo'); - * // Returns: 'foo:%s' - * ``` - * - * Values that are not part of the format string are formatted using`util.inspect()` if their type is not `string`. - * - * If there are more arguments passed to the `util.format()` method than the - * number of specifiers, the extra arguments are concatenated to the returned - * string, separated by spaces: - * - * ```js - * util.format('%s:%s', 'foo', 'bar', 'baz'); - * // Returns: 'foo:bar baz' - * ``` - * - * If the first argument does not contain a valid format specifier, `util.format()`returns a string that is the concatenation of all arguments separated by spaces: - * - * ```js - * util.format(1, 2, 3); - * // Returns: '1 2 3' - * ``` - * - * If only one argument is passed to `util.format()`, it is returned as it is - * without any formatting: - * - * ```js - * util.format('%% %s'); - * // Returns: '%% %s' - * ``` - * - * `util.format()` is a synchronous method that is intended as a debugging tool. - * Some input values can have a significant performance overhead that can block the - * event loop. Use this function with care and never in a hot code path. - * @since v0.5.3 - * @param format A `printf`-like format string. - */ - export function format(format?: any, ...param: any[]): string; - /** - * This function is identical to {@link format}, except in that it takes - * an `inspectOptions` argument which specifies options that are passed along to {@link inspect}. - * - * ```js - * util.formatWithOptions({ colors: true }, 'See object %O', { foo: 42 }); - * // Returns 'See object { foo: 42 }', where `42` is colored as a number - * // when printed to a terminal. - * ``` - * @since v10.0.0 - */ - export function formatWithOptions(inspectOptions: InspectOptions, format?: any, ...param: any[]): string; - /** - * Returns the string name for a numeric error code that comes from a Node.js API. - * The mapping between error codes and error names is platform-dependent. - * See `Common System Errors` for the names of common errors. - * - * ```js - * fs.access('file/that/does/not/exist', (err) => { - * const name = util.getSystemErrorName(err.errno); - * console.error(name); // ENOENT - * }); - * ``` - * @since v9.7.0 - */ - export function getSystemErrorName(err: number): string; - /** - * Returns a Map of all system error codes available from the Node.js API. - * The mapping between error codes and error names is platform-dependent. - * See `Common System Errors` for the names of common errors. - * - * ```js - * fs.access('file/that/does/not/exist', (err) => { - * const errorMap = util.getSystemErrorMap(); - * const name = errorMap.get(err.errno); - * console.error(name); // ENOENT - * }); - * ``` - * @since v16.0.0, v14.17.0 - */ - export function getSystemErrorMap(): Map