diff --git a/spaces/1acneusushi/gradio-2dmoleculeeditor/data/Free Download Internet Download Manager with Crack.rar How to Install and Use IDM with Crack.md b/spaces/1acneusushi/gradio-2dmoleculeeditor/data/Free Download Internet Download Manager with Crack.rar How to Install and Use IDM with Crack.md
deleted file mode 100644
index 43a784c3e0e64c690f44e00d93d4e896bc0397bc..0000000000000000000000000000000000000000
--- a/spaces/1acneusushi/gradio-2dmoleculeeditor/data/Free Download Internet Download Manager with Crack.rar How to Install and Use IDM with Crack.md
+++ /dev/null
@@ -1,151 +0,0 @@
-
-
If you are a devotee of Goddess Lalitha, the Divine Mother, you might be interested in downloading Sri Lalitha Sahasranamam lyrics in Tamil pdf. Sri Lalitha Sahasranamam is a sacred Hindu text that contains the thousand names of Goddess Lalitha, who is also known as Lalita Devi, Tripura Sundari, Shodashi, Rajarajeshwari, and many other names. In this article, we will tell you what is Sri Lalitha Sahasranamam, how to download it in Tamil pdf format, and how to chant it for maximum benefits.
- Sri Lalitha Sahasranamam is a part of the Brahmanda Purana, one of the 18 major Puranas in Hinduism. It is a hymn that praises Goddess Lalitha as the supreme power and creator of the universe. It describes her various attributes, qualities, forms, manifestations, and deeds. It also reveals her secret names that can grant various boons and blessings to her devotees.
-According to the legend, Sri Lalitha Sahasranamam was revealed by Lord Hayagriva, an incarnation of Lord Vishnu, to Sage Agastya, one of the seven great sages in Hinduism. Lord Hayagriva told Sage Agastya the story of how Goddess Lalitha incarnated as the daughter of Himalaya, the king of mountains, and married Lord Shiva, the destroyer of evil. He also narrated how she fought and killed a powerful demon named Bhandasura, who was created from the ashes of Kamadeva, the god of love. He then taught him the thousand names of Goddess Lalitha that can please her and invoke her grace.
- The meaning of Sri Lalitha Sahasranamam is "the thousand names of Sri Lalitha". The word "Sri" means auspiciousness, wealth, beauty, grace, and respect. The word "Lalitha" means playful, charming, delightful, graceful, and lovely. The word "Sahasranama" means thousand names. Each name of Goddess Lalitha has a deep meaning and significance that reflects her various aspects and powers. Some of her names are:
- And so on...
- Sri Lalitha Sahasranamam is not just a hymn but a powerful mantra that can bestow various benefits to those who recite it with devotion and faith. Some of the benefits are:
-The significance of Sri Lalitha Sahasranamam is that it reveals the true nature and glory of Goddess Lalitha as the supreme reality and source of everything. It also teaches us how to worship her with love and devotion. It also helps us to understand ourselves better as we are reflections of her divine attributes. It also guides us to attain liberation from the cycle of birth and death by merging with her supreme self.
- If you want to download Sri Lalitha Sahasranamam lyrics in Tamil pdf format for your convenience and ease of reading, you can follow these steps:
- Chanting Sri Lalitha Sahasranamam is a simple and effective way to worship Goddess Lalitha and receive her grace and blessings. However, there are some guidelines and rules that one should follow to chant it properly and correctly. Here are some of them:
- Here are some frequently asked questions about Sri Lalitha Sahasranama and their answers.
- take the latest episodes, select the episode you want to download and click on the download button. you have to seek tips from the airport staff to make your travel to have a safe and memorable holiday. carmen serban cu el numai cu el zippy flight floyd aerea unlimited ->>> download 100 movies with complete script.
- carmen serban cu el numai cu el zippy a fost foarte populară pe ultima lui perioadă, pentru că era un aeroport funcţional, dar necesită personal şi oameni care să rezolve probleme. dacă eşti o zonă dificilă, un loc de muncă, să puteţi merge într-o zonă dificilă, la volan. eu am fost pe la volan la volan de mai multe ori, aşa că aş fi bine să mă duc în această zonă şi să ştiu ce se întâmplă de la una la alta. dar a fost o mare plăcere, pentru că erau mulţi oameni de la care puteai să-ţi aduci aminte şi ei vorbeau de la cine ştia ce să facă, de ce aveau nevoie, dacă aveau nevoie. dar foarte bine, în general. carmen serban se afla în acel moment în aeroportul din iaşi, iar eu aş fi bine să-l întâlnesc aici şi să-l întâlnesc cu el înainte de mers pe jos. pentru că aşa era comunitatea, era un fel de prietenie şi voia să afle de ce te aştepţi când vorbeşti cu el. dacă vorbeşti cu oamenii că este zbor ce l-a nedepărtuit pe el, în timp ce e un avion care s-ar putea să plouă sau să zboare, sau de la cine ştie cine. unii zboară nespus de aşa, pentru că aşa ceva nu se întâmplă de atâţia ani. dacă se întâmplă sau se întâmplă, e un fel de aventură. unii dintre aceşti oameni nu ştiau să preia un avion, dacă nu aveau tehnologie, dacă nu aveau tehnologie de a preia avionul.
899543212bIf you are a fan of detective stories and hidden object games, you might have heard of Criminal Case, one of the most popular and addictive games on Facebook. But did you know that there is a mod apk version of the game that gives you unlimited energy and hints, as well as access to all the cases and features? In this article, we will tell you everything you need to know about Criminal Case World Mod Apk, including what it is, how to download and install it, why you should play it, and some tips and tricks to help you solve crimes faster and easier.
- Criminal Case is a hidden object game that puts you in the role of a detective who investigates murder cases in different locations around the world. You have to search for clues in crime scenes, examine evidence in the lab, interrogate suspects and witnesses, and bring the killer to justice. Along the way, you will also meet various characters, such as your partner, your boss, your forensic team, and other police officers.
-Criminal Case World Mod Apk is a modified version of the game that gives you some advantages over the original one. For example, you will have unlimited energy and hints, which means you can play as long as you want without waiting for them to refill. You will also be able to unlock all the cases and features in the game, such as new locations, new outfits, new pets, new trophies, and more. You will also be able to play with your friends who are also using the mod apk version.
- To download and install Criminal Case World Mod Apk, you will need an Android device that meets the minimum requirements of the game. You will also need to enable unknown sources in your device settings, so that you can install apps from outside the Google Play Store. Here are the steps to follow:
-One of the main reasons why you should play Criminal Case World Mod Apk is that you will never run out of energy or hints while playing. Energy is used to enter crime scenes and mini-games, while hints are used to highlight objects or areas that are relevant to the investigation. In the original game, both energy and hints are limited and take time to regenerate. This can be frustrating if you want to play more or if you are stuck on a difficult scene. With the mod apk version, you can play without any interruptions or limitations. You can also use hints more freely to help you find clues faster and easier.
- Another reason why you should play Criminal Case World Mod Apk is that you will experience the thrill and satisfaction of solving murder cases. Each case has a unique story, a different setting, and a diverse cast of characters. You will have to use your observation skills, your logic, and your intuition to find the evidence, analyze it, and deduce the killer. You will also have to face some twists and turns along the way, such as false leads, red herrings, and unexpected revelations. Solving cases will not only test your intelligence, but also your morality and your empathy.
-As you solve cases, you will also earn rewards, such as stars, coins, cash, and experience points. Stars are used to unlock new scenes and mini-games, as well as to perform certain actions, such as examining evidence or interrogating suspects. Coins are used to buy items in the shop, such as clothes, accessories, pets, and boosters. Cash is used to buy premium items, such as energy refills, hints, or special outfits. Experience points are used to level up and unlock new features and cases.
- A third reason why you should play Criminal Case World Mod Apk is that you will have more fun and excitement by playing with your friends. You can connect your game account to your Facebook account and invite your friends who are also using the mod apk version to join you. You can then team up with them to solve cases together, or compete with them to see who can score higher or rank higher in the leaderboards. You can also chat with them, send them gifts, ask them for help, or help them in return.
-Playing with friends will not only make the game more enjoyable, but also more social and interactive. You can share your opinions, your theories, your strategies, and your emotions with your friends. You can also learn from them, challenge them, support them, and congratulate them. Playing with friends will also motivate you to play more and improve your skills.
- If you want to rank up and earn stars faster in Criminal Case World Mod Apk, here are some tips and tricks that you can follow:
-Boosters and power-ups are very useful items that can help you solve cases faster and easier in Criminal Case World Mod Apk. However, they are also limited and costly, so you need to use them wisely. Here are some tips on how to use boosters and power-ups effectively:
-Clues and evidence are essential items that can help you solve cases and identify the killer in Criminal Case World Mod Apk. However, they are not always easy to find or recognize in the scenes or the mini-games. Here are some tips on how to find clues and evidence easily:
-Criminal Case World Mod Apk is a great game for anyone who loves detective stories and hidden object games. It offers unlimited energy and hints, as well as access to all the cases and features in the game. It also allows you to play with your friends who are also using the mod apk version. It is a fun and exciting way to test your intelligence, your morality, and your empathy as you solve murder cases around the world. If you want to download and install Criminal Case World Mod Apk, just follow the steps that we have provided in this article. And if you want to rank up and earn stars faster, use boosters and power-ups effectively, and find clues and evidence easily, just follow the tips and tricks that we have shared with you. We hope that this article has been helpful and informative for you. Now, what are you waiting for? Grab your magnifying glass and your badge, and start solving crimes with Criminal Case World Mod Apk!
- A1: Yes, Criminal Case World Mod Apk is safe to use as long as you download it from a trusted source and scan it with an antivirus program before installing it. However, you should be aware that using mod apk versions of games may violate their terms of service and may result in your account being banned or suspended by the developers. Therefore, use it at your own risk and discretion.
- A2: To update Criminal Case World Mod Apk, you will need to download the latest version of the mod apk file from [this link] and install it over the existing one. You do not need to uninstall the previous version first. However, you should back up your game data before updating, in case something goes wrong during the process.
- A3: To get more friends to play with in Criminal Case World Mod Apk, you can invite your existing Facebook friends who are also using the mod apk version to join you. You can also join online communities and groups of Criminal Case players who are looking for new friends and partners. You can also add random players who appear in your game as potential friends.
- A4: To report a bug or a problem with Criminal Case World Mod Apk, you can contact the developers or the support team through their official website [here]. You can also leave a comment or a review on [this page] where you downloaded the mod apk file. Please provide as much detail as possible about the issue that you encountered, such as when it happened, what you were doing, what device you were using, what error message you received, etc.
- A5: To contact the developers or the support team of Criminal Case World Mod Apk, you can use one of the following methods:
-We hope that this article has answered all your questions about Criminal Case World Mod Apk. If you have any other questions, feel free to contact us through any of the methods above. Thank you for reading and happy crime solving!
197e85843dHave you ever wondered how you can pay your salary and suppliers online without hassle or fees? If so, you might want to check out 1o5 version, a new way to make payments with your phone using Google Pay or WhatsApp.
-1o5 version is a solution that integrates directly with your Sage software, allowing you to send and receive money instantly, securely, and conveniently. You can also earn rewards, discover offers, and understand your spending with Google Pay. Or you can enjoy private messaging, voice and video calls, and group chats with WhatsApp.
-In this article, we will show you how to download 1o5 version on your device, how to open it via salary*, and what benefits you can get from using it. We will also answer some frequently asked questions about this innovative payment method.
- Downloading 1o5 version is easy and free. All you need is a smartphone or tablet that supports Google Pay or WhatsApp. Here are the steps to follow:
-Congratulations! You have successfully downloaded 1o5 version on your device. Now you are ready to open it via salary*.
- Opening 1o5 version via salary* is simple and fast. All you need is a Sage account that supports salary and supplier payments. Here are the steps to follow:
-That's it! You have successfully opened 1o5 version via salary* and made a payment with your phone. You will receive a confirmation message and a receipt for your transaction.
-Using 1o5 version via salary* has many benefits for you and your business. Here are some of them:
-As you can see, using 1o5 version via salary* can help you save money and time, improve your cash flow, and streamline your operations.
- You may have some questions about 1o5 version via salary*. Here are some of the most common ones:
-Salary* is a service that allows you to pay your salary and suppliers online with Sage. You can choose from various payment methods, such as bank transfer, debit card, credit card, PayPal, Google Pay, or WhatsApp. You can also access real-time reports, analytics, and insights on your payments.
-Yes, 1o5 version is safe to use. Google Pay and WhatsApp use advanced encryption and security features to protect your personal and financial information. They also comply with the Payment Card Industry Data Security Standard (PCI DSS) and the General Data Protection Regulation (GDPR). Sage also uses secure servers and encryption to safeguard your data.
-You can track your payments with 1o5 version by logging in to your Sage account and going to the "Salary and Supplier Payments" section. There you can see the status, date, amount, recipient, and reference of each payment. You can also download or print receipts for your records.
-If you have a problem with your payment, you can contact the customer support team of Google Pay or WhatsApp, depending on which app you used. They will help you resolve the issue as soon as possible. You can also contact Sage support if you need assistance with your Sage account or software.
-If you want to get more information about 1o5 version, you can visit the official websites of Google Pay or WhatsApp, or read their FAQs . You can also visit the Sage website or read their blog for more tips and insights on how to use salary* effectively.
- In conclusion, 1o5 version is a new way to pay your salary and suppliers online with your phone using Google Pay or WhatsApp. It is easy to download, simple to open via salary*, and beneficial for your business. It can help you save money and time, improve your cash flow, and streamline your operations.
-Why not give it a try today? Download 1o5 version on your device and open it via salary*. You will be amazed by how convenient and rewarding it is to make payments with your phone.
197e85843dIf you are a fan of racing games, you might have heard of CarX Street, a dynamic open world game that lets you become a street racer the way you want. In this game, you can customize your car, challenge other racers, and explore the city of Sunset City. But what if you want to enjoy the game with more features and unlimited resources? That's where CarX Street Mod APK 0.8.6 comes in handy.
-In this article, we will tell you what CarX Street is, how to download and install CarX Street Mod APK 0.8.6 on your Android device, why you should play it, and some tips and tricks to help you become a legend of the streets.
- CarX Street is a racing game developed by CarX Technologies, the creators of CarX Drift Racing and CarX Highway Racing. It was released in February 2022 for Android and iOS devices, and has received positive reviews from players and critics alike.
-CarX Street is different from other racing games because it gives you more freedom and control over your car and your racing style. You can choose from over 50 cars, each with its own characteristics and customization options. You can also tune your car's performance, appearance, and sound to suit your preferences.
-But CarX Street is not just about racing. It's also about exploring the vast and vibrant city of Sunset City, where you can find various events, challenges, and secrets. You can also interact with other racers, join clubs, or create your own club and invite your friends.
- If you want to play CarX Street with more features and unlimited resources, you can download and install CarX Street Mod APK 0.8.6 on your Android device. Here are the steps to do so:
-CarX Street Mod APK 0.8.6 is not just a regular racing game. It's a game that offers you more fun,
CarX Street Mod APK 0.8.6 is not just a regular racing game. It's a game that offers you more fun, excitement, and customization than ever before. Here are some of the benefits of playing CarX Street Mod APK 0.8.6:
- If you want to become a legend of the streets, you need to master the skills and strategies of racing in CarX Street Mod APK 0.8.6. Here are some tips and tricks to help you out:
-CarX Street Mod APK 0.8.6 is a game that will make you feel the thrill of street racing like never before. You can customize your car, challenge other racers, and explore the city of Sunset City with unlimited resources and features. If you are looking for a racing game that is realistic, dynamic, and fun, you should download and install CarX Street Mod APK 0.8.6 on your Android device today.
- A PUBG Mobile emulator is a software application that allows you to run PUBG Mobile on your PC. It simulates the Android environment and lets you access the Google Play Store and download the game. However, not all emulators are created equal. Some are faster, smoother, and more compatible than others. So, how do you choose the best PUBG Mobile emulator for your PC? And how do you download and install it? In this article, we will answer these questions and more. We will also review some of the best emulators available in the market and compare their features and performance.
- There are many reasons why you might want to use a PUBG Mobile emulator. For instance, you might have a low-end or old smartphone that cannot run the game smoothly or at all. Or, you might prefer playing on a larger screen with higher resolution and frame rate. Or, you might want to use a keyboard and mouse instead of touch controls for more accuracy and responsiveness. Whatever your reason, a PUBG Mobile emulator can help you achieve it.
- Using a PUBG Mobile emulator has many benefits and advantages over playing on your smartphone. Here are some of them:
-As you can see, using a PUBG Mobile emulator can enhance your gaming experience and make it more fun and enjoyable. However, not all emulators are the same. Some are better than others in terms of compatibility, performance, stability, and features. Therefore, you need to choose the best PUBG Mobile emulator for your PC.
- There are many factors and criteria that you need to consider when choosing the best PUBG Mobile emulator for your PC. Here are some of them:
-Based on these criteria, we have selected the best PUBG Mobile emulator for your PC: GameLoop.
- GameLoop has many advantages over other emulators for PUBG Mobile. Here are some of them:
- Downloading and installing GameLoop emulator is very easy and straightforward. Here are the steps that you need to follow:
-Playing PUBG Mobile on GameLoop emulator is very similar to playing it on your smartphone. However, there are some tips and tricks that you can use to optimize your gameplay and make it more enjoyable. Here are some of them:
-GameLoop emulator has many features that make it one of the best emulators for PUBG Mobile. Here are some of them:
-As you can see, GameLoop is a powerful and versatile emulator that can provide you with the best PUBG Mobile experience on your PC. However, if you want to try other emulators, there are some alternatives that you can consider.
- GameLoop is not the only emulator that can run PUBG Mobile on your PC. There are other emulators that have their own strengths and weaknesses. Here are some of them:
- However, BlueStacks is not very optimized for PUBG Mobile. It can run the game, but not as smoothly or as fast as GameLoop. It also has higher CPU and memory usage and lower graphics quality. Moreover, BlueStacks is not officially supported by Tencent Games or PUBG Corporation, which means that it might have compatibility or security issues in the future.
- Tencent Gaming Buddy is similar to GameLoop in many aspects, such as compatibility, performance, stability, and features. However, it is not as advanced or as refined as GameLoop. It also does not support the latest version of PUBG Mobile or its new modes and features. Therefore, it is recommended to use GameLoop instead of Tencent Gaming Buddy for PUBG Mobile.
- To help you compare and choose the best PUBG Mobile emulator for your PC, here is a table that summarizes and compares the main features and performance of GameLoop, BlueStacks, and Tencent Gaming Buddy:
- PUBG Mobile is one of the most popular and exciting mobile games in the world. It offers a thrilling and immersive battle royale experience that you can enjoy with your friends or solo. However, playing on a mobile device might not be the best way to experience PUBG Mobile. You might face issues such as low graphics quality, small screen size, poor controls, battery drain, overheating, etc.
-That is why using a PUBG Mobile emulator can be a great solution. A PUBG Mobile emulator allows you to play PUBG Mobile on your PC, which can improve your gameplay and convenience. You can enjoy better graphics and performance, bigger screen, better controls, more features and options, and more.
-However, not all PUBG Mobile emulators are the same. Some are better than others in terms of compatibility, performance, stability, features, user-friendliness, and reputation. Therefore, you need to choose the best PUBG Mobile emulator for your PC.
-In this article, we have reviewed and compared some of the best PUBG Mobile emulators available in the market. We have also provided a step-by-step guide on how to download and install GameLoop emulator, which is the official and best emulator for PUBG Mobile. We have also given some tips and tricks on how to play PUBG Mobile on GameLoop emulator and optimize your gameplay.
-We hope that this article has helped you learn how to download PUBG Mobile emulator for PC and enjoy PUBG Mobile on a bigger and better platform. If you have any questions or feedback, please feel free to leave a comment below. Happy gaming!
- Here are some frequently asked questions and answers about PUBG Mobile emulator for PC:
-` ().
-
- Example::
-
- num = Word(nums).set_parse_action(lambda toks: int(toks[0]))
- na = one_of("N/A NA").set_parse_action(replace_with(math.nan))
- term = na | num
-
- term[1, ...].parse_string("324 234 N/A 234") # -> [324, 234, nan, 234]
- """
- return lambda s, l, t: [repl_str]
-
-
-def remove_quotes(s, l, t):
- """
- Helper parse action for removing quotation marks from parsed
- quoted strings.
-
- Example::
-
- # by default, quotation marks are included in parsed results
- quoted_string.parse_string("'Now is the Winter of our Discontent'") # -> ["'Now is the Winter of our Discontent'"]
-
- # use remove_quotes to strip quotation marks from parsed results
- quoted_string.set_parse_action(remove_quotes)
- quoted_string.parse_string("'Now is the Winter of our Discontent'") # -> ["Now is the Winter of our Discontent"]
- """
- return t[0][1:-1]
-
-
-def with_attribute(*args, **attr_dict):
- """
- Helper to create a validating parse action to be used with start
- tags created with :class:`make_xml_tags` or
- :class:`make_html_tags`. Use ``with_attribute`` to qualify
- a starting tag with a required attribute value, to avoid false
- matches on common tags such as ```` or ````.
-
- Call ``with_attribute`` with a series of attribute names and
- values. Specify the list of filter attributes names and values as:
-
- - keyword arguments, as in ``(align="right")``, or
- - as an explicit dict with ``**`` operator, when an attribute
- name is also a Python reserved word, as in ``**{"class":"Customer", "align":"right"}``
- - a list of name-value tuples, as in ``(("ns1:class", "Customer"), ("ns2:align", "right"))``
-
- For attribute names with a namespace prefix, you must use the second
- form. Attribute names are matched insensitive to upper/lower case.
-
- If just testing for ``class`` (with or without a namespace), use
- :class:`with_class`.
-
- To verify that the attribute exists, but without specifying a value,
- pass ``with_attribute.ANY_VALUE`` as the value.
-
- Example::
-
- html = '''
-
- Some text
- 1 4 0 1 0
- 1,3 2,3 1,1
- this has no type
-
-
- '''
- div,div_end = make_html_tags("div")
-
- # only match div tag having a type attribute with value "grid"
- div_grid = div().set_parse_action(with_attribute(type="grid"))
- grid_expr = div_grid + SkipTo(div | div_end)("body")
- for grid_header in grid_expr.search_string(html):
- print(grid_header.body)
-
- # construct a match with any div tag having a type attribute, regardless of the value
- div_any_type = div().set_parse_action(with_attribute(type=with_attribute.ANY_VALUE))
- div_expr = div_any_type + SkipTo(div | div_end)("body")
- for div_header in div_expr.search_string(html):
- print(div_header.body)
-
- prints::
-
- 1 4 0 1 0
-
- 1 4 0 1 0
- 1,3 2,3 1,1
- """
- if args:
- attrs = args[:]
- else:
- attrs = attr_dict.items()
- attrs = [(k, v) for k, v in attrs]
-
- def pa(s, l, tokens):
- for attrName, attrValue in attrs:
- if attrName not in tokens:
- raise ParseException(s, l, "no matching attribute " + attrName)
- if attrValue != with_attribute.ANY_VALUE and tokens[attrName] != attrValue:
- raise ParseException(
- s,
- l,
- "attribute {!r} has value {!r}, must be {!r}".format(
- attrName, tokens[attrName], attrValue
- ),
- )
-
- return pa
-
-
-with_attribute.ANY_VALUE = object()
-
-
-def with_class(classname, namespace=""):
- """
- Simplified version of :class:`with_attribute` when
- matching on a div class - made difficult because ``class`` is
- a reserved word in Python.
-
- Example::
-
- html = '''
-
- Some text
- 1 4 0 1 0
- 1,3 2,3 1,1
- this <div> has no class
-
-
- '''
- div,div_end = make_html_tags("div")
- div_grid = div().set_parse_action(with_class("grid"))
-
- grid_expr = div_grid + SkipTo(div | div_end)("body")
- for grid_header in grid_expr.search_string(html):
- print(grid_header.body)
-
- div_any_type = div().set_parse_action(with_class(withAttribute.ANY_VALUE))
- div_expr = div_any_type + SkipTo(div | div_end)("body")
- for div_header in div_expr.search_string(html):
- print(div_header.body)
-
- prints::
-
- 1 4 0 1 0
-
- 1 4 0 1 0
- 1,3 2,3 1,1
- """
- classattr = "{}:class".format(namespace) if namespace else "class"
- return with_attribute(**{classattr: classname})
-
-
-# pre-PEP8 compatibility symbols
-replaceWith = replace_with
-removeQuotes = remove_quotes
-withAttribute = with_attribute
-withClass = with_class
-matchOnlyAtCol = match_only_at_col
diff --git a/spaces/Awesimo/jojogan/e4e/models/encoders/psp_encoders.py b/spaces/Awesimo/jojogan/e4e/models/encoders/psp_encoders.py
deleted file mode 100644
index dc49acd11f062cbd29f839ee3c04bce7fa84f479..0000000000000000000000000000000000000000
--- a/spaces/Awesimo/jojogan/e4e/models/encoders/psp_encoders.py
+++ /dev/null
@@ -1,200 +0,0 @@
-from enum import Enum
-import math
-import numpy as np
-import torch
-from torch import nn
-from torch.nn import Conv2d, BatchNorm2d, PReLU, Sequential, Module
-
-from e4e.models.encoders.helpers import get_blocks, bottleneck_IR, bottleneck_IR_SE, _upsample_add
-from e4e.models.stylegan2.model import EqualLinear
-
-
-class ProgressiveStage(Enum):
- WTraining = 0
- Delta1Training = 1
- Delta2Training = 2
- Delta3Training = 3
- Delta4Training = 4
- Delta5Training = 5
- Delta6Training = 6
- Delta7Training = 7
- Delta8Training = 8
- Delta9Training = 9
- Delta10Training = 10
- Delta11Training = 11
- Delta12Training = 12
- Delta13Training = 13
- Delta14Training = 14
- Delta15Training = 15
- Delta16Training = 16
- Delta17Training = 17
- Inference = 18
-
-
-class GradualStyleBlock(Module):
- def __init__(self, in_c, out_c, spatial):
- super(GradualStyleBlock, self).__init__()
- self.out_c = out_c
- self.spatial = spatial
- num_pools = int(np.log2(spatial))
- modules = []
- modules += [Conv2d(in_c, out_c, kernel_size=3, stride=2, padding=1),
- nn.LeakyReLU()]
- for i in range(num_pools - 1):
- modules += [
- Conv2d(out_c, out_c, kernel_size=3, stride=2, padding=1),
- nn.LeakyReLU()
- ]
- self.convs = nn.Sequential(*modules)
- self.linear = EqualLinear(out_c, out_c, lr_mul=1)
-
- def forward(self, x):
- x = self.convs(x)
- x = x.view(-1, self.out_c)
- x = self.linear(x)
- return x
-
-
-class GradualStyleEncoder(Module):
- def __init__(self, num_layers, mode='ir', opts=None):
- super(GradualStyleEncoder, self).__init__()
- assert num_layers in [50, 100, 152], 'num_layers should be 50,100, or 152'
- assert mode in ['ir', 'ir_se'], 'mode should be ir or ir_se'
- blocks = get_blocks(num_layers)
- if mode == 'ir':
- unit_module = bottleneck_IR
- elif mode == 'ir_se':
- unit_module = bottleneck_IR_SE
- self.input_layer = Sequential(Conv2d(3, 64, (3, 3), 1, 1, bias=False),
- BatchNorm2d(64),
- PReLU(64))
- modules = []
- for block in blocks:
- for bottleneck in block:
- modules.append(unit_module(bottleneck.in_channel,
- bottleneck.depth,
- bottleneck.stride))
- self.body = Sequential(*modules)
-
- self.styles = nn.ModuleList()
- log_size = int(math.log(opts.stylegan_size, 2))
- self.style_count = 2 * log_size - 2
- self.coarse_ind = 3
- self.middle_ind = 7
- for i in range(self.style_count):
- if i < self.coarse_ind:
- style = GradualStyleBlock(512, 512, 16)
- elif i < self.middle_ind:
- style = GradualStyleBlock(512, 512, 32)
- else:
- style = GradualStyleBlock(512, 512, 64)
- self.styles.append(style)
- self.latlayer1 = nn.Conv2d(256, 512, kernel_size=1, stride=1, padding=0)
- self.latlayer2 = nn.Conv2d(128, 512, kernel_size=1, stride=1, padding=0)
-
- def forward(self, x):
- x = self.input_layer(x)
-
- latents = []
- modulelist = list(self.body._modules.values())
- for i, l in enumerate(modulelist):
- x = l(x)
- if i == 6:
- c1 = x
- elif i == 20:
- c2 = x
- elif i == 23:
- c3 = x
-
- for j in range(self.coarse_ind):
- latents.append(self.styles[j](c3))
-
- p2 = _upsample_add(c3, self.latlayer1(c2))
- for j in range(self.coarse_ind, self.middle_ind):
- latents.append(self.styles[j](p2))
-
- p1 = _upsample_add(p2, self.latlayer2(c1))
- for j in range(self.middle_ind, self.style_count):
- latents.append(self.styles[j](p1))
-
- out = torch.stack(latents, dim=1)
- return out
-
-
-class Encoder4Editing(Module):
- def __init__(self, num_layers, mode='ir', opts=None):
- super(Encoder4Editing, self).__init__()
- assert num_layers in [50, 100, 152], 'num_layers should be 50,100, or 152'
- assert mode in ['ir', 'ir_se'], 'mode should be ir or ir_se'
- blocks = get_blocks(num_layers)
- if mode == 'ir':
- unit_module = bottleneck_IR
- elif mode == 'ir_se':
- unit_module = bottleneck_IR_SE
- self.input_layer = Sequential(Conv2d(3, 64, (3, 3), 1, 1, bias=False),
- BatchNorm2d(64),
- PReLU(64))
- modules = []
- for block in blocks:
- for bottleneck in block:
- modules.append(unit_module(bottleneck.in_channel,
- bottleneck.depth,
- bottleneck.stride))
- self.body = Sequential(*modules)
-
- self.styles = nn.ModuleList()
- log_size = int(math.log(opts.stylegan_size, 2))
- self.style_count = 2 * log_size - 2
- self.coarse_ind = 3
- self.middle_ind = 7
-
- for i in range(self.style_count):
- if i < self.coarse_ind:
- style = GradualStyleBlock(512, 512, 16)
- elif i < self.middle_ind:
- style = GradualStyleBlock(512, 512, 32)
- else:
- style = GradualStyleBlock(512, 512, 64)
- self.styles.append(style)
-
- self.latlayer1 = nn.Conv2d(256, 512, kernel_size=1, stride=1, padding=0)
- self.latlayer2 = nn.Conv2d(128, 512, kernel_size=1, stride=1, padding=0)
-
- self.progressive_stage = ProgressiveStage.Inference
-
- def get_deltas_starting_dimensions(self):
- ''' Get a list of the initial dimension of every delta from which it is applied '''
- return list(range(self.style_count)) # Each dimension has a delta applied to it
-
- def set_progressive_stage(self, new_stage: ProgressiveStage):
- self.progressive_stage = new_stage
- print('Changed progressive stage to: ', new_stage)
-
- def forward(self, x):
- x = self.input_layer(x)
-
- modulelist = list(self.body._modules.values())
- for i, l in enumerate(modulelist):
- x = l(x)
- if i == 6:
- c1 = x
- elif i == 20:
- c2 = x
- elif i == 23:
- c3 = x
-
- # Infer main W and duplicate it
- w0 = self.styles[0](c3)
- w = w0.repeat(self.style_count, 1, 1).permute(1, 0, 2)
- stage = self.progressive_stage.value
- features = c3
- for i in range(1, min(stage + 1, self.style_count)): # Infer additional deltas
- if i == self.coarse_ind:
- p2 = _upsample_add(c3, self.latlayer1(c2)) # FPN's middle features
- features = p2
- elif i == self.middle_ind:
- p1 = _upsample_add(p2, self.latlayer2(c1)) # FPN's fine features
- features = p1
- delta_i = self.styles[i](features)
- w[:, i] += delta_i
- return w
diff --git a/spaces/BAAI/AltDiffusion/js/index.js b/spaces/BAAI/AltDiffusion/js/index.js
deleted file mode 100644
index 2afe2db8da0b7305eb88a46a31d1f309ee9d0793..0000000000000000000000000000000000000000
--- a/spaces/BAAI/AltDiffusion/js/index.js
+++ /dev/null
@@ -1,186 +0,0 @@
-window.SD = (() => {
- /*
- * Painterro is made a field of the SD global object
- * To provide convinience when using w() method in css_and_js.py
- */
- class PainterroClass {
- static isOpen = false;
- static async init ({ x, toId }) {
- console.log(x)
-
- const originalImage = x[2] === 'Mask' ? x[1]?.image : x[0];
-
- if (window.Painterro === undefined) {
- try {
- await this.load();
- } catch (e) {
- SDClass.error(e);
-
- return this.fallback(originalImage);
- }
- }
-
- if (this.isOpen) {
- return this.fallback(originalImage);
- }
- this.isOpen = true;
-
- let resolveResult;
- const paintClient = Painterro({
- hiddenTools: ['arrow'],
- onHide: () => {
- resolveResult?.(null);
- },
- saveHandler: (image, done) => {
- const data = image.asDataURL();
-
- // ensures stable performance even
- // when the editor is in interactive mode
- SD.clearImageInput(SD.el.get(`#${toId}`));
-
- resolveResult(data);
-
- done(true);
- paintClient.hide();
- },
- });
-
- const result = await new Promise((resolve) => {
- resolveResult = resolve;
- paintClient.show(originalImage);
- });
- this.isOpen = false;
-
- return result ? this.success(result) : this.fallback(originalImage);
- }
- static success (result) { return [result, { image: result, mask: result }] };
- static fallback (image) { return [image, { image: image, mask: image }] };
- static load () {
- return new Promise((resolve, reject) => {
- const scriptId = '__painterro-script';
- if (document.getElementById(scriptId)) {
- reject(new Error('Tried to load painterro script, but script tag already exists.'));
- return;
- }
-
- const styleId = '__painterro-css-override';
- if (!document.getElementById(styleId)) {
- /* Ensure Painterro window is always on top */
- const style = document.createElement('style');
- style.id = styleId;
- style.setAttribute('type', 'text/css');
- style.appendChild(document.createTextNode(`
- .ptro-holder-wrapper {
- z-index: 100;
- }
- `));
- document.head.appendChild(style);
- }
-
- const script = document.createElement('script');
- script.id = scriptId;
- script.src = 'https://unpkg.com/painterro@1.2.78/build/painterro.min.js';
- script.onload = () => resolve(true);
- script.onerror = (e) => {
- // remove self on error to enable reattempting load
- document.head.removeChild(script);
- reject(e);
- };
- document.head.appendChild(script);
- });
- }
- }
-
- /*
- * Turns out caching elements doesn't actually work in gradio
- * As elements in tabs might get recreated
- */
- class ElementCache {
- #el;
- constructor () {
- this.root = document.querySelector('gradio-app').shadowRoot;
- }
- get (selector) {
- return this.root.querySelector(selector);
- }
- }
-
- /*
- * The main helper class to incapsulate functions
- * that change gradio ui functionality
- */
- class SDClass {
- el = new ElementCache();
- Painterro = PainterroClass;
- moveImageFromGallery ({ x, fromId, toId }) {
- x = x[0];
- if (!Array.isArray(x) || x.length === 0) return;
-
- this.clearImageInput(this.el.get(`#${toId}`));
-
- const i = this.#getGallerySelectedIndex(this.el.get(`#${fromId}`));
-
- return [x[i].replace('data:;','data:image/png;')];
- }
- async copyImageFromGalleryToClipboard ({ x, fromId }) {
- x = x[0];
- if (!Array.isArray(x) || x.length === 0) return;
-
- const i = this.#getGallerySelectedIndex(this.el.get(`#${fromId}`));
-
- const data = x[i];
- const blob = await (await fetch(data.replace('data:;','data:image/png;'))).blob();
- const item = new ClipboardItem({'image/png': blob});
-
- await this.copyToClipboard([item]);
- }
- clickFirstVisibleButton({ rowId }) {
- const generateButtons = this.el.get(`#${rowId}`).querySelectorAll('.gr-button-primary');
-
- if (!generateButtons) return;
-
- for (let i = 0, arr = [...generateButtons]; i < arr.length; i++) {
- const cs = window.getComputedStyle(arr[i]);
-
- if (cs.display !== 'none' && cs.visibility !== 'hidden') {
- console.log(arr[i]);
-
- arr[i].click();
- break;
- }
- }
- }
- async gradioInputToClipboard ({ x }) { return this.copyToClipboard(x[0]); }
- async copyToClipboard (value) {
- if (!value || typeof value === 'boolean') return;
- try {
- if (Array.isArray(value) &&
- value.length &&
- value[0] instanceof ClipboardItem) {
- await navigator.clipboard.write(value);
- } else {
- await navigator.clipboard.writeText(value);
- }
- } catch (e) {
- SDClass.error(e);
- }
- }
- static error (e) {
- console.error(e);
- if (typeof e === 'string') {
- alert(e);
- } else if(typeof e === 'object' && Object.hasOwn(e, 'message')) {
- alert(e.message);
- }
- }
- clearImageInput (imageEditor) {
- imageEditor?.querySelector('.modify-upload button:last-child')?.click();
- }
- #getGallerySelectedIndex (gallery) {
- const selected = gallery.querySelector(`.\\!ring-2`);
- return selected ? [...selected.parentNode.children].indexOf(selected) : 0;
- }
- }
-
- return new SDClass();
-})();
diff --git a/spaces/Benson/text-generation/Examples/Cmo Descargar Y Jugar Entre Nosotros En El PC.md b/spaces/Benson/text-generation/Examples/Cmo Descargar Y Jugar Entre Nosotros En El PC.md
deleted file mode 100644
index 76e3fec427295bced637664c0d537ab8cc335e1e..0000000000000000000000000000000000000000
--- a/spaces/Benson/text-generation/Examples/Cmo Descargar Y Jugar Entre Nosotros En El PC.md
+++ /dev/null
@@ -1,101 +0,0 @@
-
- Cómo descargar Brawl Stars para iPhone
- Si estás buscando un juego de ritmo rápido, lleno de acción y lleno de diversión para jugar en tu iPhone, definitivamente deberías ver Brawl Stars. Brawl Stars es un juego multijugador de arena de batalla en línea (MOBA) desarrollado por Supercell, los creadores de Clash of Clans y Clash Royale. En este juego, puedes elegir entre docenas de personajes únicos llamados Brawlers, cada uno con sus propias habilidades, armas y personalidades. Puedes hacer equipo con tus amigos o jugar solo en varios modos de juego, como Gem Grab, Showdown, Brawl Ball, Bounty, Heist y más. También puedes desbloquear nuevos skins, gadgets, poderes estelares y pines para personalizar tus Brawlers y mostrar tu estilo.
- cómo descargar y jugar entre nosotros en el PCDownload File … https://bltlly.com/2v6ILh
- Brawl Stars es uno de los juegos más populares para dispositivos móviles en este momento, con más de 100 millones de descargas solo en Google Play. Pero ¿qué pasa si quieres jugar en tu iPhone? No te preocupes, tenemos todo cubierto. En este artículo, te mostraremos cómo descargar Brawl Stars para iPhone en solo unos sencillos pasos. También te daremos algunos consejos y trucos para jugar Brawl Stars en el iPhone y responder a algunas preguntas frecuentes sobre el juego. Así que sin más preámbulos, ¡empecemos!
- ¿Qué es Brawl Stars?
- Brawl Stars es un juego MOBA 3v3 que combina elementos de tiro, lucha, estrategia y trabajo en equipo. El juego cuenta con varios modos que requieren diferentes objetivos y habilidades. Por ejemplo, en el modo Gem Grab, tienes que recoger y guardar 10 gemas para ganar; en el modo Showdown, tienes que sobrevivir el mayor tiempo posible en un battle royale; en el modo Brawl Ball, tienes que anotar dos goles antes que el otro equipo; y así sucesivamente.
-
- Brawl Stars es un juego que es fácil de aprender pero difícil de dominar. Tienes que usar tus habilidades, estrategia y trabajo en equipo para ganar partidos y subir de rango. También puede unirse o crear un club para chatear con otros jugadores, compartir consejos y jugar juntos. Brawl Stars es un juego que se actualiza constantemente con nuevos contenidos, como nuevos luchadores, skins, mapas, eventos y características. También puedes participar en desafíos especiales y torneos para ganar recompensas y fama.
- ¿Por qué jugar Brawl estrellas en el iPhone?
- Brawl Stars es un juego diseñado para dispositivos móviles, y jugarlo en iPhone tiene muchas ventajas. Estas son algunas de las razones por las que deberías jugar Brawl Stars en iPhone:
-
-
-- Compatibilidad: Brawl Stars es compatible con la mayoría de modelos de iPhone, desde iPhone 6S y versiones posteriores. No necesitas un dispositivo de alta gama para disfrutar del juego, ya que funciona sin problemas y de manera eficiente en la mayoría de los iPhones.
-- Rendimiento: Brawl Stars tiene una alta velocidad de fotogramas y baja latencia en el iPhone, lo que significa que puedes jugar el juego sin retrasos ni tartamudeos. También puede ajustar la calidad gráfica y el modo de ahorro de batería para optimizar el rendimiento de acuerdo con su preferencia.
-- Gráficos: Brawl Stars tiene gráficos coloridos y vibrantes que se ven muy bien en la pantalla de retina del iPhone. El juego tiene un estilo caricaturesco y encantador que atrae a jugadores de todas las edades. También puede apreciar los detalles y animaciones de los luchadores, pieles, mapas y efectos en la pantalla del iPhone.
-- Controles: Brawl Stars tiene controles simples e intuitivos que son fáciles de usar en la pantalla táctil del iPhone. Puede mover su brawler con el joystick izquierdo y apuntar y disparar con el joystick derecho. También puedes tocar para usar tu súper habilidad, deslizar el dedo para usar tu gadget y pellizcar para acercar o alejar. También puede personalizar los controles para adaptarse a su estilo de juego y comodidad.
-
-
- ¿Cómo obtener estrellas de pelea en el iPhone?
- Ahora que sabes por qué deberías jugar Brawl Stars en el iPhone, vamos a ver cómo puedes conseguirlo en tu dispositivo. El proceso es muy simple y sencillo, y solo toma unos minutos. Estos son los pasos que debes seguir:
- Paso 1: Abra la aplicación App Store
- Lo primero que tienes que hacer es abrir la aplicación App Store en tu iPhone. Puedes encontrarla en tu pantalla de inicio o en tu biblioteca de aplicaciones. La aplicación App Store tiene un icono azul con una letra blanca A dentro.
- 
- Paso 2: Buscar estrellas de pelea
- Una vez que abra la aplicación App Store, debe buscar Brawl Stars en la pestaña de búsqueda. Puede encontrar la pestaña de búsqueda en la esquina inferior derecha de la pantalla. Tiene un icono de lupa.
- 
- Toque en la pestaña de búsqueda y escriba "Brawl Stars" en la barra de búsqueda. Verá una lista de resultados que coinciden con su consulta. Busca el que dice "Brawl Stars" de Supercell y tiene un icono rojo con tres estrellas dentro.
- 
- Paso 3: Toque Obtener o el precio
- Cuando encuentre Brawl Stars en los resultados, toque en él para abrir su página en el App Store. Verás información sobre el juego, como su descripción, capturas de pantalla, valoraciones, reseñas y más.
- Para descargar Brawl Stars, necesitas tocar el botón Obtener o el precio si no es gratis en tu región. El botón Obtener o el precio se encuentra en la esquina superior derecha de la pantalla, junto al icono y nombre del juego.
- 
- Paso 4: Confirmar la descarga
-
- 
- Introduzca su contraseña o utilice su huella digital o su cara para confirmar la descarga. Verá un mensaje de confirmación que dice "Descargar..." o "Comprar".
- Paso 5: Espera a que termine la descarga
- Ahora solo tienes que esperar a que termine la descarga. Puedes comprobar el progreso de la descarga mirando el círculo alrededor del icono del juego. El círculo se llenará a medida que avance la descarga. También puede ver el estado de descarga en la pestaña Actualizaciones de la aplicación App Store.
- 
- Brawl Stars es de unos 300 MB de tamaño, por lo que puede tardar unos minutos en descargarse dependiendo de su velocidad y conexión a Internet. Asegúrate de tener suficiente espacio de almacenamiento en tu iPhone y una conexión Wi-Fi o datos móviles estable.
- Paso 6: Estrellas de pelea abierta y disfrutar
- Enhorabuena, ¡has descargado con éxito Brawl Stars para iPhone! Ahora puedes abrir el juego y empezar a jugar. Puedes encontrar Brawl Stars en tu pantalla de inicio o en tu biblioteca de aplicaciones. El icono del juego es rojo con tres estrellas dentro.
- 
-
- 
- ¡Ahora estás listo para pelear! ¡Diviértete y disfruta de Brawl Stars en tu iPhone!
- Consejos y trucos para jugar Brawl estrellas en el iPhone
- Brawl Stars es un juego que requiere habilidad, estrategia y trabajo en equipo para ganar. Estos son algunos consejos y trucos que pueden ayudarte a mejorar tu experiencia de juego y convertirte en un mejor luchador:
-
-- Ajusta tus ajustes: Puedes personalizar tus ajustes para adaptarlos a tu estilo de juego y comodidad. Por ejemplo, puede cambiar el tamaño y la posición de los joysticks, activar o desactivar el objetivo automático, cambiar entre el modo vertical o horizontal y elegir entre tocar o deslizar para disparar. También puede activar o desactivar la vibración, los efectos de sonido, la música, el chat de voz y las notificaciones.
-- Únete a un club: Un club es un grupo de jugadores que pueden chatear, jugar juntos y compartir consejos. Unirte a un club puede ayudarte a hacer amigos, aprender de otros jugadores y divertirte más. Puede unirse a un club existente o crear su propio club con sus amigos. También puede participar en eventos del club y guerras para ganar recompensas y fama.
-- Usa gadgets y poderes estelares: Los gadgets y poderes estelares son habilidades especiales que pueden mejorar el rendimiento de tus luchadores. Los gadgets se activan deslizando sobre la pantalla y tienen un número limitado de usos por partido. Los poderes estelares son habilidades pasivas que siempre están activas una vez desbloqueadas. Puedes desbloquear gadgets y poderes estelares abriendo cajas o alcanzando ciertos hitos de trofeos. También puedes comprar gadgets y poderes estrella con monedas en la tienda. Los gadgets y los poderes de las estrellas pueden darte una ventaja en la batalla, pero tienes que usarlos sabiamente y estratégicamente.
-
-- Aprende de los pros: Si quieres mejorar tus habilidades y conocimientos, puedes ver vídeos y secuencias de reproductores profesionales y creadores de contenido. Puedes aprender de sus consejos, trucos, estrategias y errores. También puedes interactuar con ellos y hacer preguntas en el chat o comentarios. Puedes encontrar muchos videos y transmisiones de Brawl Stars en YouTube, Twitch, Reddit y otras plataformas.
-
- Preguntas frecuentes sobre Brawl Stars en iPhone
- Aquí están algunas de las preguntas y respuestas más comunes sobre Brawl Stars en iPhone:
- ¿Cómo actualizo Brawl Stars en el iPhone?
- Para actualizar Brawl Stars en el iPhone, debe abrir la aplicación App Store e ir a la pestaña Actualizaciones. Verá una lista de aplicaciones que tienen actualizaciones disponibles. Busque Brawl Stars y toque en el botón Actualizar junto a él. También puede habilitar las actualizaciones automáticas en la configuración de la aplicación App Store.
- ¿Cómo puedo restaurar mis compras en Brawl Stars en iPhone?
- Si has comprado gemas u otros artículos en Brawl Stars con dinero real y los has perdido debido a un cambio de dispositivo o un problema de juego, puedes restaurar tus compras siguiendo estos pasos:
-
-- Abrir Brawl Stars e ir al icono de configuración en la esquina superior derecha de la pantalla.
-- Toque en Ayuda y Soporte.
-- Toque en Contáctenos.
-- Escriba un mensaje explicando su situación y proporcione su etiqueta de jugador, número de recibo, fecha de compra y cantidad de compra.
-- Enviar el mensaje y esperar una respuesta del equipo de soporte.
-
- ¿Cómo puedo contactar al equipo de soporte de Brawl Stars en el iPhone?
- Si tienes algún problema, preguntas o comentarios sobre Brawl Stars en el iPhone, puedes ponerte en contacto con el equipo de soporte siguiendo estos pasos:
-
-- Abrir Brawl Stars e ir al icono de configuración en la esquina superior derecha de la pantalla.
-- Toque en Ayuda y Soporte.
-- Toque en Contáctenos.
-
-- Enviar el mensaje y esperar una respuesta del equipo de soporte.
-
- ¿Cómo puedo jugar con mis amigos en Brawl Stars en iPhone?
- Si quieres jugar con tus amigos en Brawl Stars en iPhone, tienes dos opciones:
-
-- Crear o unirse a una habitación amigable: Una habitación amigable es una habitación privada donde puedes invitar a tus amigos o miembros del club a jugar juntos. Puedes crear o unirte a una sala amigable tocando el botón de juego amigable en la esquina inferior izquierda del menú principal. Puedes elegir el modo de juego y el mapa que quieras, y también puedes habilitar modificadores y bots. Puedes invitar a tus amigos o miembros del club tocando el botón de invitación en la esquina inferior derecha de la pantalla de la habitación. También puede compartir un código de habitación con sus amigos o miembros del club pulsando en el botón compartir en la esquina superior derecha de la pantalla de la habitación amigable.
-- Crear o unirse a un código de equipo: Un código de equipo es un código que se puede utilizar para unirse a un equipo con otros jugadores que quieren jugar juntos. Puede crear o unirse a un código de equipo pulsando en el botón de reproducción en la esquina inferior derecha del menú principal. Verás una lista de modos de juego que están disponibles para emparejar. Elige uno y pulsa sobre él. Verá una pantalla donde puede seleccionar su luchador y ver a sus compañeros de equipo. En la esquina superior izquierda de esta pantalla, verá un botón que dice "Crear/ Unirse al código de equipo". Toque en él para crear o unirse a un código de equipo. Puedes compartir tu código de equipo con tus amigos o miembros del club pulsando en el botón de compartir al lado. También puede introducir un código de equipo que alguien más ha compartido con usted pulsando en el botón entrar junto a él.
-
- ¿Cómo puedo canjear códigos en Brawl Stars en iPhone?
-
-
-- Abre Brawl Stars y ve al icono de la tienda en la esquina superior izquierda del menú principal.
-- Desplácese hacia abajo hasta la parte inferior de la pantalla de la tienda y busque un botón que diga "Canjear código". Toque en él para abrir una ventana emergente.
-- Introduzca el código que ha recibido en el cuadro de texto y toque en el botón confirmar.
-- Verá un mensaje que dice "Código redimido" y las recompensas que ha recibido. Toque en el botón de reclamación para recoger sus recompensas.
-
- Tenga en cuenta que los códigos distinguen entre mayúsculas y minúsculas y tienen una fecha de vencimiento. Solo puede usar un código por cuenta. Si introduce un código inválido o caducado, verá un mensaje de error que dice "Código inválido" o "Código caducado".
- Conclusión
- Brawl Stars es un juego divertido y emocionante que puedes jugar en tu iPhone. Puedes descargarlo desde la App Store en unos sencillos pasos y disfrutar de sus características, modos, personajes y jugabilidad. También puedes mejorar tus habilidades, unirte a un club, participar en eventos y canjear códigos para obtener más recompensas y diversión. Brawl Stars es un juego que se actualiza constantemente con nuevos contenidos y mejoras, por lo que nunca te aburrirás de él.
- ¿Qué estás esperando? Descargar Brawl Stars para iPhone hoy y unirse a los millones de jugadores que están luchando su camino a la gloria!
- 64aa2da5cf
-
-
\ No newline at end of file
diff --git a/spaces/Big-Web/MMSD/env/Lib/site-packages/pip/_vendor/distro/__main__.py b/spaces/Big-Web/MMSD/env/Lib/site-packages/pip/_vendor/distro/__main__.py
deleted file mode 100644
index 0c01d5b08b6b44379b931d54d7fcf5221fdc9fde..0000000000000000000000000000000000000000
--- a/spaces/Big-Web/MMSD/env/Lib/site-packages/pip/_vendor/distro/__main__.py
+++ /dev/null
@@ -1,4 +0,0 @@
-from .distro import main
-
-if __name__ == "__main__":
- main()
diff --git a/spaces/Big-Web/MMSD/env/Lib/site-packages/pip/_vendor/pygments/formatters/pangomarkup.py b/spaces/Big-Web/MMSD/env/Lib/site-packages/pip/_vendor/pygments/formatters/pangomarkup.py
deleted file mode 100644
index bd00866b8b95a98edc8956608e895a6329a944a0..0000000000000000000000000000000000000000
--- a/spaces/Big-Web/MMSD/env/Lib/site-packages/pip/_vendor/pygments/formatters/pangomarkup.py
+++ /dev/null
@@ -1,83 +0,0 @@
-"""
- pygments.formatters.pangomarkup
- ~~~~~~~~~~~~~~~~~~~~~~~~~~~~~~~
-
- Formatter for Pango markup output.
-
- :copyright: Copyright 2006-2022 by the Pygments team, see AUTHORS.
- :license: BSD, see LICENSE for details.
-"""
-
-from pip._vendor.pygments.formatter import Formatter
-
-
-__all__ = ['PangoMarkupFormatter']
-
-
-_escape_table = {
- ord('&'): '&',
- ord('<'): '<',
-}
-
-
-def escape_special_chars(text, table=_escape_table):
- """Escape & and < for Pango Markup."""
- return text.translate(table)
-
-
-class PangoMarkupFormatter(Formatter):
- """
- Format tokens as Pango Markup code. It can then be rendered to an SVG.
-
- .. versionadded:: 2.9
- """
-
- name = 'Pango Markup'
- aliases = ['pango', 'pangomarkup']
- filenames = []
-
- def __init__(self, **options):
- Formatter.__init__(self, **options)
-
- self.styles = {}
-
- for token, style in self.style:
- start = ''
- end = ''
- if style['color']:
- start += ' ' % style['color']
- end = '' + end
- if style['bold']:
- start += ' '
- end = '' + end
- if style['italic']:
- start += ' '
- end = '' + end
- if style['underline']:
- start += ' '
- end = '' + end
- self.styles[token] = (start, end)
-
- def format_unencoded(self, tokensource, outfile):
- lastval = ''
- lasttype = None
-
- outfile.write(' ')
-
- for ttype, value in tokensource:
- while ttype not in self.styles:
- ttype = ttype.parent
- if ttype == lasttype:
- lastval += escape_special_chars(value)
- else:
- if lastval:
- stylebegin, styleend = self.styles[lasttype]
- outfile.write(stylebegin + lastval + styleend)
- lastval = escape_special_chars(value)
- lasttype = ttype
-
- if lastval:
- stylebegin, styleend = self.styles[lasttype]
- outfile.write(stylebegin + lastval + styleend)
-
- outfile.write('')
diff --git a/spaces/Big-Web/MMSD/env/Lib/site-packages/pip/_vendor/rich/style.py b/spaces/Big-Web/MMSD/env/Lib/site-packages/pip/_vendor/rich/style.py
deleted file mode 100644
index 313c889496d90cef94d5537c122e5c5e898e3bb4..0000000000000000000000000000000000000000
--- a/spaces/Big-Web/MMSD/env/Lib/site-packages/pip/_vendor/rich/style.py
+++ /dev/null
@@ -1,796 +0,0 @@
-import sys
-from functools import lru_cache
-from marshal import dumps, loads
-from random import randint
-from typing import Any, Dict, Iterable, List, Optional, Type, Union, cast
-
-from . import errors
-from .color import Color, ColorParseError, ColorSystem, blend_rgb
-from .repr import Result, rich_repr
-from .terminal_theme import DEFAULT_TERMINAL_THEME, TerminalTheme
-
-# Style instances and style definitions are often interchangeable
-StyleType = Union[str, "Style"]
-
-
-class _Bit:
- """A descriptor to get/set a style attribute bit."""
-
- __slots__ = ["bit"]
-
- def __init__(self, bit_no: int) -> None:
- self.bit = 1 << bit_no
-
- def __get__(self, obj: "Style", objtype: Type["Style"]) -> Optional[bool]:
- if obj._set_attributes & self.bit:
- return obj._attributes & self.bit != 0
- return None
-
-
-@rich_repr
-class Style:
- """A terminal style.
-
- A terminal style consists of a color (`color`), a background color (`bgcolor`), and a number of attributes, such
- as bold, italic etc. The attributes have 3 states: they can either be on
- (``True``), off (``False``), or not set (``None``).
-
- Args:
- color (Union[Color, str], optional): Color of terminal text. Defaults to None.
- bgcolor (Union[Color, str], optional): Color of terminal background. Defaults to None.
- bold (bool, optional): Enable bold text. Defaults to None.
- dim (bool, optional): Enable dim text. Defaults to None.
- italic (bool, optional): Enable italic text. Defaults to None.
- underline (bool, optional): Enable underlined text. Defaults to None.
- blink (bool, optional): Enabled blinking text. Defaults to None.
- blink2 (bool, optional): Enable fast blinking text. Defaults to None.
- reverse (bool, optional): Enabled reverse text. Defaults to None.
- conceal (bool, optional): Enable concealed text. Defaults to None.
- strike (bool, optional): Enable strikethrough text. Defaults to None.
- underline2 (bool, optional): Enable doubly underlined text. Defaults to None.
- frame (bool, optional): Enable framed text. Defaults to None.
- encircle (bool, optional): Enable encircled text. Defaults to None.
- overline (bool, optional): Enable overlined text. Defaults to None.
- link (str, link): Link URL. Defaults to None.
-
- """
-
- _color: Optional[Color]
- _bgcolor: Optional[Color]
- _attributes: int
- _set_attributes: int
- _hash: Optional[int]
- _null: bool
- _meta: Optional[bytes]
-
- __slots__ = [
- "_color",
- "_bgcolor",
- "_attributes",
- "_set_attributes",
- "_link",
- "_link_id",
- "_ansi",
- "_style_definition",
- "_hash",
- "_null",
- "_meta",
- ]
-
- # maps bits on to SGR parameter
- _style_map = {
- 0: "1",
- 1: "2",
- 2: "3",
- 3: "4",
- 4: "5",
- 5: "6",
- 6: "7",
- 7: "8",
- 8: "9",
- 9: "21",
- 10: "51",
- 11: "52",
- 12: "53",
- }
-
- STYLE_ATTRIBUTES = {
- "dim": "dim",
- "d": "dim",
- "bold": "bold",
- "b": "bold",
- "italic": "italic",
- "i": "italic",
- "underline": "underline",
- "u": "underline",
- "blink": "blink",
- "blink2": "blink2",
- "reverse": "reverse",
- "r": "reverse",
- "conceal": "conceal",
- "c": "conceal",
- "strike": "strike",
- "s": "strike",
- "underline2": "underline2",
- "uu": "underline2",
- "frame": "frame",
- "encircle": "encircle",
- "overline": "overline",
- "o": "overline",
- }
-
- def __init__(
- self,
- *,
- color: Optional[Union[Color, str]] = None,
- bgcolor: Optional[Union[Color, str]] = None,
- bold: Optional[bool] = None,
- dim: Optional[bool] = None,
- italic: Optional[bool] = None,
- underline: Optional[bool] = None,
- blink: Optional[bool] = None,
- blink2: Optional[bool] = None,
- reverse: Optional[bool] = None,
- conceal: Optional[bool] = None,
- strike: Optional[bool] = None,
- underline2: Optional[bool] = None,
- frame: Optional[bool] = None,
- encircle: Optional[bool] = None,
- overline: Optional[bool] = None,
- link: Optional[str] = None,
- meta: Optional[Dict[str, Any]] = None,
- ):
- self._ansi: Optional[str] = None
- self._style_definition: Optional[str] = None
-
- def _make_color(color: Union[Color, str]) -> Color:
- return color if isinstance(color, Color) else Color.parse(color)
-
- self._color = None if color is None else _make_color(color)
- self._bgcolor = None if bgcolor is None else _make_color(bgcolor)
- self._set_attributes = sum(
- (
- bold is not None,
- dim is not None and 2,
- italic is not None and 4,
- underline is not None and 8,
- blink is not None and 16,
- blink2 is not None and 32,
- reverse is not None and 64,
- conceal is not None and 128,
- strike is not None and 256,
- underline2 is not None and 512,
- frame is not None and 1024,
- encircle is not None and 2048,
- overline is not None and 4096,
- )
- )
- self._attributes = (
- sum(
- (
- bold and 1 or 0,
- dim and 2 or 0,
- italic and 4 or 0,
- underline and 8 or 0,
- blink and 16 or 0,
- blink2 and 32 or 0,
- reverse and 64 or 0,
- conceal and 128 or 0,
- strike and 256 or 0,
- underline2 and 512 or 0,
- frame and 1024 or 0,
- encircle and 2048 or 0,
- overline and 4096 or 0,
- )
- )
- if self._set_attributes
- else 0
- )
-
- self._link = link
- self._meta = None if meta is None else dumps(meta)
- self._link_id = (
- f"{randint(0, 999999)}{hash(self._meta)}" if (link or meta) else ""
- )
- self._hash: Optional[int] = None
- self._null = not (self._set_attributes or color or bgcolor or link or meta)
-
- @classmethod
- def null(cls) -> "Style":
- """Create an 'null' style, equivalent to Style(), but more performant."""
- return NULL_STYLE
-
- @classmethod
- def from_color(
- cls, color: Optional[Color] = None, bgcolor: Optional[Color] = None
- ) -> "Style":
- """Create a new style with colors and no attributes.
-
- Returns:
- color (Optional[Color]): A (foreground) color, or None for no color. Defaults to None.
- bgcolor (Optional[Color]): A (background) color, or None for no color. Defaults to None.
- """
- style: Style = cls.__new__(Style)
- style._ansi = None
- style._style_definition = None
- style._color = color
- style._bgcolor = bgcolor
- style._set_attributes = 0
- style._attributes = 0
- style._link = None
- style._link_id = ""
- style._meta = None
- style._null = not (color or bgcolor)
- style._hash = None
- return style
-
- @classmethod
- def from_meta(cls, meta: Optional[Dict[str, Any]]) -> "Style":
- """Create a new style with meta data.
-
- Returns:
- meta (Optional[Dict[str, Any]]): A dictionary of meta data. Defaults to None.
- """
- style: Style = cls.__new__(Style)
- style._ansi = None
- style._style_definition = None
- style._color = None
- style._bgcolor = None
- style._set_attributes = 0
- style._attributes = 0
- style._link = None
- style._meta = dumps(meta)
- style._link_id = f"{randint(0, 999999)}{hash(style._meta)}"
- style._hash = None
- style._null = not (meta)
- return style
-
- @classmethod
- def on(cls, meta: Optional[Dict[str, Any]] = None, **handlers: Any) -> "Style":
- """Create a blank style with meta information.
-
- Example:
- style = Style.on(click=self.on_click)
-
- Args:
- meta (Optional[Dict[str, Any]], optional): An optional dict of meta information.
- **handlers (Any): Keyword arguments are translated in to handlers.
-
- Returns:
- Style: A Style with meta information attached.
- """
- meta = {} if meta is None else meta
- meta.update({f"@{key}": value for key, value in handlers.items()})
- return cls.from_meta(meta)
-
- bold = _Bit(0)
- dim = _Bit(1)
- italic = _Bit(2)
- underline = _Bit(3)
- blink = _Bit(4)
- blink2 = _Bit(5)
- reverse = _Bit(6)
- conceal = _Bit(7)
- strike = _Bit(8)
- underline2 = _Bit(9)
- frame = _Bit(10)
- encircle = _Bit(11)
- overline = _Bit(12)
-
- @property
- def link_id(self) -> str:
- """Get a link id, used in ansi code for links."""
- return self._link_id
-
- def __str__(self) -> str:
- """Re-generate style definition from attributes."""
- if self._style_definition is None:
- attributes: List[str] = []
- append = attributes.append
- bits = self._set_attributes
- if bits & 0b0000000001111:
- if bits & 1:
- append("bold" if self.bold else "not bold")
- if bits & (1 << 1):
- append("dim" if self.dim else "not dim")
- if bits & (1 << 2):
- append("italic" if self.italic else "not italic")
- if bits & (1 << 3):
- append("underline" if self.underline else "not underline")
- if bits & 0b0000111110000:
- if bits & (1 << 4):
- append("blink" if self.blink else "not blink")
- if bits & (1 << 5):
- append("blink2" if self.blink2 else "not blink2")
- if bits & (1 << 6):
- append("reverse" if self.reverse else "not reverse")
- if bits & (1 << 7):
- append("conceal" if self.conceal else "not conceal")
- if bits & (1 << 8):
- append("strike" if self.strike else "not strike")
- if bits & 0b1111000000000:
- if bits & (1 << 9):
- append("underline2" if self.underline2 else "not underline2")
- if bits & (1 << 10):
- append("frame" if self.frame else "not frame")
- if bits & (1 << 11):
- append("encircle" if self.encircle else "not encircle")
- if bits & (1 << 12):
- append("overline" if self.overline else "not overline")
- if self._color is not None:
- append(self._color.name)
- if self._bgcolor is not None:
- append("on")
- append(self._bgcolor.name)
- if self._link:
- append("link")
- append(self._link)
- self._style_definition = " ".join(attributes) or "none"
- return self._style_definition
-
- def __bool__(self) -> bool:
- """A Style is false if it has no attributes, colors, or links."""
- return not self._null
-
- def _make_ansi_codes(self, color_system: ColorSystem) -> str:
- """Generate ANSI codes for this style.
-
- Args:
- color_system (ColorSystem): Color system.
-
- Returns:
- str: String containing codes.
- """
-
- if self._ansi is None:
- sgr: List[str] = []
- append = sgr.append
- _style_map = self._style_map
- attributes = self._attributes & self._set_attributes
- if attributes:
- if attributes & 1:
- append(_style_map[0])
- if attributes & 2:
- append(_style_map[1])
- if attributes & 4:
- append(_style_map[2])
- if attributes & 8:
- append(_style_map[3])
- if attributes & 0b0000111110000:
- for bit in range(4, 9):
- if attributes & (1 << bit):
- append(_style_map[bit])
- if attributes & 0b1111000000000:
- for bit in range(9, 13):
- if attributes & (1 << bit):
- append(_style_map[bit])
- if self._color is not None:
- sgr.extend(self._color.downgrade(color_system).get_ansi_codes())
- if self._bgcolor is not None:
- sgr.extend(
- self._bgcolor.downgrade(color_system).get_ansi_codes(
- foreground=False
- )
- )
- self._ansi = ";".join(sgr)
- return self._ansi
-
- @classmethod
- @lru_cache(maxsize=1024)
- def normalize(cls, style: str) -> str:
- """Normalize a style definition so that styles with the same effect have the same string
- representation.
-
- Args:
- style (str): A style definition.
-
- Returns:
- str: Normal form of style definition.
- """
- try:
- return str(cls.parse(style))
- except errors.StyleSyntaxError:
- return style.strip().lower()
-
- @classmethod
- def pick_first(cls, *values: Optional[StyleType]) -> StyleType:
- """Pick first non-None style."""
- for value in values:
- if value is not None:
- return value
- raise ValueError("expected at least one non-None style")
-
- def __rich_repr__(self) -> Result:
- yield "color", self.color, None
- yield "bgcolor", self.bgcolor, None
- yield "bold", self.bold, None,
- yield "dim", self.dim, None,
- yield "italic", self.italic, None
- yield "underline", self.underline, None,
- yield "blink", self.blink, None
- yield "blink2", self.blink2, None
- yield "reverse", self.reverse, None
- yield "conceal", self.conceal, None
- yield "strike", self.strike, None
- yield "underline2", self.underline2, None
- yield "frame", self.frame, None
- yield "encircle", self.encircle, None
- yield "link", self.link, None
- if self._meta:
- yield "meta", self.meta
-
- def __eq__(self, other: Any) -> bool:
- if not isinstance(other, Style):
- return NotImplemented
- return self.__hash__() == other.__hash__()
-
- def __ne__(self, other: Any) -> bool:
- if not isinstance(other, Style):
- return NotImplemented
- return self.__hash__() != other.__hash__()
-
- def __hash__(self) -> int:
- if self._hash is not None:
- return self._hash
- self._hash = hash(
- (
- self._color,
- self._bgcolor,
- self._attributes,
- self._set_attributes,
- self._link,
- self._meta,
- )
- )
- return self._hash
-
- @property
- def color(self) -> Optional[Color]:
- """The foreground color or None if it is not set."""
- return self._color
-
- @property
- def bgcolor(self) -> Optional[Color]:
- """The background color or None if it is not set."""
- return self._bgcolor
-
- @property
- def link(self) -> Optional[str]:
- """Link text, if set."""
- return self._link
-
- @property
- def transparent_background(self) -> bool:
- """Check if the style specified a transparent background."""
- return self.bgcolor is None or self.bgcolor.is_default
-
- @property
- def background_style(self) -> "Style":
- """A Style with background only."""
- return Style(bgcolor=self.bgcolor)
-
- @property
- def meta(self) -> Dict[str, Any]:
- """Get meta information (can not be changed after construction)."""
- return {} if self._meta is None else cast(Dict[str, Any], loads(self._meta))
-
- @property
- def without_color(self) -> "Style":
- """Get a copy of the style with color removed."""
- if self._null:
- return NULL_STYLE
- style: Style = self.__new__(Style)
- style._ansi = None
- style._style_definition = None
- style._color = None
- style._bgcolor = None
- style._attributes = self._attributes
- style._set_attributes = self._set_attributes
- style._link = self._link
- style._link_id = f"{randint(0, 999999)}" if self._link else ""
- style._null = False
- style._meta = None
- style._hash = None
- return style
-
- @classmethod
- @lru_cache(maxsize=4096)
- def parse(cls, style_definition: str) -> "Style":
- """Parse a style definition.
-
- Args:
- style_definition (str): A string containing a style.
-
- Raises:
- errors.StyleSyntaxError: If the style definition syntax is invalid.
-
- Returns:
- `Style`: A Style instance.
- """
- if style_definition.strip() == "none" or not style_definition:
- return cls.null()
-
- STYLE_ATTRIBUTES = cls.STYLE_ATTRIBUTES
- color: Optional[str] = None
- bgcolor: Optional[str] = None
- attributes: Dict[str, Optional[Any]] = {}
- link: Optional[str] = None
-
- words = iter(style_definition.split())
- for original_word in words:
- word = original_word.lower()
- if word == "on":
- word = next(words, "")
- if not word:
- raise errors.StyleSyntaxError("color expected after 'on'")
- try:
- Color.parse(word) is None
- except ColorParseError as error:
- raise errors.StyleSyntaxError(
- f"unable to parse {word!r} as background color; {error}"
- ) from None
- bgcolor = word
-
- elif word == "not":
- word = next(words, "")
- attribute = STYLE_ATTRIBUTES.get(word)
- if attribute is None:
- raise errors.StyleSyntaxError(
- f"expected style attribute after 'not', found {word!r}"
- )
- attributes[attribute] = False
-
- elif word == "link":
- word = next(words, "")
- if not word:
- raise errors.StyleSyntaxError("URL expected after 'link'")
- link = word
-
- elif word in STYLE_ATTRIBUTES:
- attributes[STYLE_ATTRIBUTES[word]] = True
-
- else:
- try:
- Color.parse(word)
- except ColorParseError as error:
- raise errors.StyleSyntaxError(
- f"unable to parse {word!r} as color; {error}"
- ) from None
- color = word
- style = Style(color=color, bgcolor=bgcolor, link=link, **attributes)
- return style
-
- @lru_cache(maxsize=1024)
- def get_html_style(self, theme: Optional[TerminalTheme] = None) -> str:
- """Get a CSS style rule."""
- theme = theme or DEFAULT_TERMINAL_THEME
- css: List[str] = []
- append = css.append
-
- color = self.color
- bgcolor = self.bgcolor
- if self.reverse:
- color, bgcolor = bgcolor, color
- if self.dim:
- foreground_color = (
- theme.foreground_color if color is None else color.get_truecolor(theme)
- )
- color = Color.from_triplet(
- blend_rgb(foreground_color, theme.background_color, 0.5)
- )
- if color is not None:
- theme_color = color.get_truecolor(theme)
- append(f"color: {theme_color.hex}")
- append(f"text-decoration-color: {theme_color.hex}")
- if bgcolor is not None:
- theme_color = bgcolor.get_truecolor(theme, foreground=False)
- append(f"background-color: {theme_color.hex}")
- if self.bold:
- append("font-weight: bold")
- if self.italic:
- append("font-style: italic")
- if self.underline:
- append("text-decoration: underline")
- if self.strike:
- append("text-decoration: line-through")
- if self.overline:
- append("text-decoration: overline")
- return "; ".join(css)
-
- @classmethod
- def combine(cls, styles: Iterable["Style"]) -> "Style":
- """Combine styles and get result.
-
- Args:
- styles (Iterable[Style]): Styles to combine.
-
- Returns:
- Style: A new style instance.
- """
- iter_styles = iter(styles)
- return sum(iter_styles, next(iter_styles))
-
- @classmethod
- def chain(cls, *styles: "Style") -> "Style":
- """Combine styles from positional argument in to a single style.
-
- Args:
- *styles (Iterable[Style]): Styles to combine.
-
- Returns:
- Style: A new style instance.
- """
- iter_styles = iter(styles)
- return sum(iter_styles, next(iter_styles))
-
- def copy(self) -> "Style":
- """Get a copy of this style.
-
- Returns:
- Style: A new Style instance with identical attributes.
- """
- if self._null:
- return NULL_STYLE
- style: Style = self.__new__(Style)
- style._ansi = self._ansi
- style._style_definition = self._style_definition
- style._color = self._color
- style._bgcolor = self._bgcolor
- style._attributes = self._attributes
- style._set_attributes = self._set_attributes
- style._link = self._link
- style._link_id = f"{randint(0, 999999)}" if self._link else ""
- style._hash = self._hash
- style._null = False
- style._meta = self._meta
- return style
-
- @lru_cache(maxsize=128)
- def clear_meta_and_links(self) -> "Style":
- """Get a copy of this style with link and meta information removed.
-
- Returns:
- Style: New style object.
- """
- if self._null:
- return NULL_STYLE
- style: Style = self.__new__(Style)
- style._ansi = self._ansi
- style._style_definition = self._style_definition
- style._color = self._color
- style._bgcolor = self._bgcolor
- style._attributes = self._attributes
- style._set_attributes = self._set_attributes
- style._link = None
- style._link_id = ""
- style._hash = self._hash
- style._null = False
- style._meta = None
- return style
-
- def update_link(self, link: Optional[str] = None) -> "Style":
- """Get a copy with a different value for link.
-
- Args:
- link (str, optional): New value for link. Defaults to None.
-
- Returns:
- Style: A new Style instance.
- """
- style: Style = self.__new__(Style)
- style._ansi = self._ansi
- style._style_definition = self._style_definition
- style._color = self._color
- style._bgcolor = self._bgcolor
- style._attributes = self._attributes
- style._set_attributes = self._set_attributes
- style._link = link
- style._link_id = f"{randint(0, 999999)}" if link else ""
- style._hash = None
- style._null = False
- style._meta = self._meta
- return style
-
- def render(
- self,
- text: str = "",
- *,
- color_system: Optional[ColorSystem] = ColorSystem.TRUECOLOR,
- legacy_windows: bool = False,
- ) -> str:
- """Render the ANSI codes for the style.
-
- Args:
- text (str, optional): A string to style. Defaults to "".
- color_system (Optional[ColorSystem], optional): Color system to render to. Defaults to ColorSystem.TRUECOLOR.
-
- Returns:
- str: A string containing ANSI style codes.
- """
- if not text or color_system is None:
- return text
- attrs = self._ansi or self._make_ansi_codes(color_system)
- rendered = f"\x1b[{attrs}m{text}\x1b[0m" if attrs else text
- if self._link and not legacy_windows:
- rendered = (
- f"\x1b]8;id={self._link_id};{self._link}\x1b\\{rendered}\x1b]8;;\x1b\\"
- )
- return rendered
-
- def test(self, text: Optional[str] = None) -> None:
- """Write text with style directly to terminal.
-
- This method is for testing purposes only.
-
- Args:
- text (Optional[str], optional): Text to style or None for style name.
-
- """
- text = text or str(self)
- sys.stdout.write(f"{self.render(text)}\n")
-
- @lru_cache(maxsize=1024)
- def _add(self, style: Optional["Style"]) -> "Style":
- if style is None or style._null:
- return self
- if self._null:
- return style
- new_style: Style = self.__new__(Style)
- new_style._ansi = None
- new_style._style_definition = None
- new_style._color = style._color or self._color
- new_style._bgcolor = style._bgcolor or self._bgcolor
- new_style._attributes = (self._attributes & ~style._set_attributes) | (
- style._attributes & style._set_attributes
- )
- new_style._set_attributes = self._set_attributes | style._set_attributes
- new_style._link = style._link or self._link
- new_style._link_id = style._link_id or self._link_id
- new_style._null = style._null
- if self._meta and style._meta:
- new_style._meta = dumps({**self.meta, **style.meta})
- else:
- new_style._meta = self._meta or style._meta
- new_style._hash = None
- return new_style
-
- def __add__(self, style: Optional["Style"]) -> "Style":
- combined_style = self._add(style)
- return combined_style.copy() if combined_style.link else combined_style
-
-
-NULL_STYLE = Style()
-
-
-class StyleStack:
- """A stack of styles."""
-
- __slots__ = ["_stack"]
-
- def __init__(self, default_style: "Style") -> None:
- self._stack: List[Style] = [default_style]
-
- def __repr__(self) -> str:
- return f" "
-
- @property
- def current(self) -> Style:
- """Get the Style at the top of the stack."""
- return self._stack[-1]
-
- def push(self, style: Style) -> None:
- """Push a new style on to the stack.
-
- Args:
- style (Style): New style to combine with current style.
- """
- self._stack.append(self._stack[-1] + style)
-
- def pop(self) -> Style:
- """Pop last style and discard.
-
- Returns:
- Style: New current style (also available as stack.current)
- """
- self._stack.pop()
- return self._stack[-1]
diff --git a/spaces/BraydenMoore/MARCI-NFL-Betting/Source/Test/xgboost_ML.py b/spaces/BraydenMoore/MARCI-NFL-Betting/Source/Test/xgboost_ML.py
deleted file mode 100644
index 0196886ed85201ec82142e45a7231de19e2f7afd..0000000000000000000000000000000000000000
--- a/spaces/BraydenMoore/MARCI-NFL-Betting/Source/Test/xgboost_ML.py
+++ /dev/null
@@ -1,59 +0,0 @@
-import xgboost as xgb
-import pandas as pd
-import pickle as pkl
-import numpy as np
-import os
-
-model = 'xgboost_ML_no_odds_71.4%'
-
-current_directory = os.path.dirname(os.path.abspath(__file__))
-parent_directory = os.path.dirname(current_directory)
-data_directory = os.path.join(parent_directory, 'Data')
-model_directory = os.path.join(parent_directory, 'Models')
-pickle_directory = os.path.join(parent_directory, 'Pickles')
-
-file_path = os.path.join(model_directory, f'{model}.json')
-xgb_ml = xgb.Booster()
-xgb_ml.load_model(file_path)
-
-file_path = os.path.join(pickle_directory, 'test_games_ML_no_odds.pkl')
-with open(file_path,'rb') as f:
- test_games = pkl.load(f).tolist()
-
-file_path = os.path.join(data_directory, 'gbg_and_odds.csv')
-gbg_and_odds = pd.read_csv(file_path)
-test_data = gbg_and_odds.loc[gbg_and_odds['game_id'].isin(test_games)]
-test_data_matrix = xgb.DMatrix(test_data.drop(columns=['game_id','Over','Home-Team-Win','Season','home_team','away_team','game_date','Key','Home Score','Away Score','Home Odds Close','Away Odds Close','Home Winnings','Away Winnings','Away Odds','Home Odds']).astype(float).values)
-
-predicted_probas = xgb_ml.predict(test_data_matrix)
-predictions = np.argmax(predicted_probas, axis=1)
-test_data['predicted_proba'] = [i[1] for i in predicted_probas]
-test_data['prediction'] = (test_data['predicted_proba']>0.5).astype(int)
-test_data['correct'] = test_data['Home-Team-Win']==test_data['prediction']
-
-bets = test_data.loc[(test_data['predicted_proba']>0.6) | (test_data['predicted_proba']<0.4)]
-bets['winnings'] = [h if p==1 else a for h,a,p in bets[['Home Winnings','Away Winnings','prediction']].values]
-
-import matplotlib.pyplot as plt
-fig = plt.figure(facecolor='black')
-ax = fig.add_subplot(1, 1, 1, facecolor='black')
-
-# Plot data with line color as RGB(0, 128, 0)
-ax.plot(bets['winnings'].cumsum().values*100, linewidth=3, color=(0/255, 128/255, 0/255))
-
-# Set title and labels
-ax.set_title('MARCI 3.0 - MoneyLine w/ 60% Confidence Threshold', color='white')
-ax.set_xlabel('Games Bet On', color='white')
-ax.set_ylabel('Return (%)', color='white')
-
-# Change tick colors to white
-ax.tick_params(axis='x', colors='white')
-ax.tick_params(axis='y', colors='white')
-
-# Change axis edge colors
-ax.spines['bottom'].set_color('white')
-ax.spines['top'].set_color('white')
-ax.spines['left'].set_color('white')
-ax.spines['right'].set_color('white')
-
-plt.savefig(f'{model}_dark.png', facecolor='black')
\ No newline at end of file
diff --git a/spaces/BrianL/CoE197-Fil-DialectTranslator/app.py b/spaces/BrianL/CoE197-Fil-DialectTranslator/app.py
deleted file mode 100644
index e5e8dbc5f7a38b444c21399167b84ed0d5a3253a..0000000000000000000000000000000000000000
--- a/spaces/BrianL/CoE197-Fil-DialectTranslator/app.py
+++ /dev/null
@@ -1,36 +0,0 @@
-import gradio as gr
-from transformers import pipeline
-
-
-def trnslt(TagalogText,Language):
- txt_inp = gr.Interface.load("huggingface/Helsinki-NLP/opus-mt-tl-en")
- if Language=="Cebuano":
- ceb1 = gr.Interface.load("huggingface/Helsinki-NLP/opus-mt-en-ceb")
- out_ceb = gr.Series(txt_inp,ceb1)
- return out_ceb(TagalogText)
- elif Language=="Ilocano":
- ilo1 = gr.Interface.load("huggingface/Helsinki-NLP/opus-mt-en-ilo")
- out_ilo = gr.Series(txt_inp,ilo1)
- return out_ilo(TagalogText)
- elif Language=="Hiligaynon":
- hil1 = gr.Interface.load("huggingface/Helsinki-NLP/opus-mt-en-hil")
- out_hil = gr.Series(txt_inp,hil1)
- return out_hil(TagalogText)
-
-iface = gr.Interface(
- fn=trnslt,
- inputs=[gr.inputs.Textbox(label="Input Tagalog Text"),
- gr.inputs.Radio(["Cebuano","Ilocano","Hiligaynon"],label="Translate to",optional=False)],
- outputs='text',
- examples=[["Magandang Umaga","Cebuano"],["Magandang gabi","Ilocano"],["Masarap ang Adobo","Hiligaynon"],
- ["Kumusta Ka Na","Cebuano"],["Bumibili si Juan ng manok","Ilocano"],["Magandang umaga","Hiligaynon"]],
- live=True,
- theme="dark-seafoam",
- title="Basic Filipino Dialect Translator",
- description=" This application uses Helsinki-NLP models to translate Tagalog texts to 3 other dialects of the Filipino language",
- css=".footer{display:none !important}",
-)
-
-iface.launch()
-
-
diff --git a/spaces/CVPR/LIVE/thrust/thrust/binary_search.h b/spaces/CVPR/LIVE/thrust/thrust/binary_search.h
deleted file mode 100644
index 127be16aab996b03e7290bac5ae3d1d1fce27588..0000000000000000000000000000000000000000
--- a/spaces/CVPR/LIVE/thrust/thrust/binary_search.h
+++ /dev/null
@@ -1,1902 +0,0 @@
-/*
- * Copyright 2008-2013 NVIDIA Corporation
- *
- * Licensed under the Apache License, Version 2.0 (the "License");
- * you may not use this file except in compliance with the License.
- * You may obtain a copy of the License at
- *
- * http://www.apache.org/licenses/LICENSE-2.0
- *
- * Unless required by applicable law or agreed to in writing, software
- * distributed under the License is distributed on an "AS IS" BASIS,
- * WITHOUT WARRANTIES OR CONDITIONS OF ANY KIND, either express or implied.
- * See the License for the specific language governing permissions and
- * limitations under the License.
- */
-
-
-/*! \file binary_search.h
- * \brief Search for values in sorted ranges.
- */
-
-#pragma once
-
-#include
-#include
-#include
-
-namespace thrust
-{
-
-
-/*! \addtogroup algorithms
- */
-
-
-/*! \addtogroup searching
- * \ingroup algorithms
- * \{
- */
-
-
-/*! \addtogroup binary_search Binary Search
- * \ingroup searching
- * \{
- */
-
-
-//////////////////////
-// Scalar Functions //
-//////////////////////
-
-
-/*! \p lower_bound is a version of binary search: it attempts to find
- * the element value in an ordered range [first, last).
- * Specifically, it returns the first position where value could be
- * inserted without violating the ordering. This version of
- * \p lower_bound uses operator< for comparison and returns
- * the furthermost iterator \c i in [first, last) such that,
- * for every iterator \c j in [first, i), *j < value.
- *
- * The algorithm's execution is parallelized as determined by \p exec.
- *
- * \param exec The execution policy to use for parallelization.
- * \param first The beginning of the ordered sequence.
- * \param last The end of the ordered sequence.
- * \param value The value to be searched.
- * \return The furthermost iterator \c i, such that *i < value.
- *
- * \tparam DerivedPolicy The name of the derived execution policy.
- * \tparam ForwardIterator is a model of Forward Iterator.
- * \tparam LessThanComparable is a model of LessThanComparable.
- *
- * The following code snippet demonstrates how to use \p lower_bound
- * to search for values in a ordered range using the \p thrust::device execution policy for parallelization:
- *
- * \code
- * #include
- * #include
- * #include
- * ...
- * thrust::device_vector input(5);
- *
- * input[0] = 0;
- * input[1] = 2;
- * input[2] = 5;
- * input[3] = 7;
- * input[4] = 8;
- *
- * thrust::lower_bound(thrust::device, input.begin(), input.end(), 0); // returns input.begin()
- * thrust::lower_bound(thrust::device, input.begin(), input.end(), 1); // returns input.begin() + 1
- * thrust::lower_bound(thrust::device, input.begin(), input.end(), 2); // returns input.begin() + 1
- * thrust::lower_bound(thrust::device, input.begin(), input.end(), 3); // returns input.begin() + 2
- * thrust::lower_bound(thrust::device, input.begin(), input.end(), 8); // returns input.begin() + 4
- * thrust::lower_bound(thrust::device, input.begin(), input.end(), 9); // returns input.end()
- * \endcode
- *
- * \see http://www.sgi.com/tech/stl/lower_bound.html
- * \see \p upper_bound
- * \see \p equal_range
- * \see \p binary_search
- */
-template
-__host__ __device__
-ForwardIterator lower_bound(const thrust::detail::execution_policy_base &exec,
- ForwardIterator first,
- ForwardIterator last,
- const LessThanComparable &value);
-
-
-/*! \p lower_bound is a version of binary search: it attempts to find
- * the element value in an ordered range [first, last).
- * Specifically, it returns the first position where value could be
- * inserted without violating the ordering. This version of
- * \p lower_bound uses operator< for comparison and returns
- * the furthermost iterator \c i in [first, last) such that,
- * for every iterator \c j in [first, i), *j < value.
- *
- * \param first The beginning of the ordered sequence.
- * \param last The end of the ordered sequence.
- * \param value The value to be searched.
- * \return The furthermost iterator \c i, such that *i < value.
- *
- * \tparam ForwardIterator is a model of Forward Iterator.
- * \tparam LessThanComparable is a model of LessThanComparable.
- *
- * The following code snippet demonstrates how to use \p lower_bound
- * to search for values in a ordered range.
- *
- * \code
- * #include
- * #include
- * ...
- * thrust::device_vector input(5);
- *
- * input[0] = 0;
- * input[1] = 2;
- * input[2] = 5;
- * input[3] = 7;
- * input[4] = 8;
- *
- * thrust::lower_bound(input.begin(), input.end(), 0); // returns input.begin()
- * thrust::lower_bound(input.begin(), input.end(), 1); // returns input.begin() + 1
- * thrust::lower_bound(input.begin(), input.end(), 2); // returns input.begin() + 1
- * thrust::lower_bound(input.begin(), input.end(), 3); // returns input.begin() + 2
- * thrust::lower_bound(input.begin(), input.end(), 8); // returns input.begin() + 4
- * thrust::lower_bound(input.begin(), input.end(), 9); // returns input.end()
- * \endcode
- *
- * \see http://www.sgi.com/tech/stl/lower_bound.html
- * \see \p upper_bound
- * \see \p equal_range
- * \see \p binary_search
- */
-template
-ForwardIterator lower_bound(ForwardIterator first,
- ForwardIterator last,
- const LessThanComparable& value);
-
-
-/*! \p lower_bound is a version of binary search: it attempts to find
- * the element value in an ordered range [first, last).
- * Specifically, it returns the first position where value could be
- * inserted without violating the ordering. This version of
- * \p lower_bound uses function object \c comp for comparison
- * and returns the furthermost iterator \c i in [first, last)
- * such that, for every iterator \c j in [first, i),
- * comp(*j, value) is \c true.
- *
- * The algorithm's execution is parallelized as determined by \p exec.
- *
- * \param exec The execution policy to use for parallelization.
- * \param first The beginning of the ordered sequence.
- * \param last The end of the ordered sequence.
- * \param value The value to be searched.
- * \param comp The comparison operator.
- * \return The furthermost iterator \c i, such that comp(*i, value) is \c true.
- *
- * \tparam DerivedPolicy The name of the derived execution policy.
- * \tparam ForwardIterator is a model of Forward Iterator.
- * \tparam T is comparable to \p ForwardIterator's \c value_type.
- * \tparam StrictWeakOrdering is a model of Strict Weak Ordering.
- *
- * The following code snippet demonstrates how to use \p lower_bound
- * to search for values in a ordered range using the \p thrust::device execution policy for parallelization:
- *
- * \code
- * #include
- * #include
- * #include
- * #include
- * ...
- * thrust::device_vector input(5);
- *
- * input[0] = 0;
- * input[1] = 2;
- * input[2] = 5;
- * input[3] = 7;
- * input[4] = 8;
- *
- * thrust::lower_bound(input.begin(), input.end(), 0, thrust::less()); // returns input.begin()
- * thrust::lower_bound(input.begin(), input.end(), 1, thrust::less()); // returns input.begin() + 1
- * thrust::lower_bound(input.begin(), input.end(), 2, thrust::less()); // returns input.begin() + 1
- * thrust::lower_bound(input.begin(), input.end(), 3, thrust::less()); // returns input.begin() + 2
- * thrust::lower_bound(input.begin(), input.end(), 8, thrust::less()); // returns input.begin() + 4
- * thrust::lower_bound(input.begin(), input.end(), 9, thrust::less()); // returns input.end()
- * \endcode
- *
- * \see http://www.sgi.com/tech/stl/lower_bound.html
- * \see \p upper_bound
- * \see \p equal_range
- * \see \p binary_search
- */
-template
-__host__ __device__
-ForwardIterator lower_bound(const thrust::detail::execution_policy_base &exec,
- ForwardIterator first,
- ForwardIterator last,
- const T &value,
- StrictWeakOrdering comp);
-
-
-/*! \p lower_bound is a version of binary search: it attempts to find
- * the element value in an ordered range [first, last).
- * Specifically, it returns the first position where value could be
- * inserted without violating the ordering. This version of
- * \p lower_bound uses function object \c comp for comparison
- * and returns the furthermost iterator \c i in [first, last)
- * such that, for every iterator \c j in [first, i),
- * comp(*j, value) is \c true.
- *
- * \param first The beginning of the ordered sequence.
- * \param last The end of the ordered sequence.
- * \param value The value to be searched.
- * \param comp The comparison operator.
- * \return The furthermost iterator \c i, such that comp(*i, value) is \c true.
- *
- * \tparam ForwardIterator is a model of Forward Iterator.
- * \tparam T is comparable to \p ForwardIterator's \c value_type.
- * \tparam StrictWeakOrdering is a model of Strict Weak Ordering.
- *
- * The following code snippet demonstrates how to use \p lower_bound
- * to search for values in a ordered range.
- *
- * \code
- * #include
- * #include
- * #include
- * ...
- * thrust::device_vector input(5);
- *
- * input[0] = 0;
- * input[1] = 2;
- * input[2] = 5;
- * input[3] = 7;
- * input[4] = 8;
- *
- * thrust::lower_bound(input.begin(), input.end(), 0, thrust::less()); // returns input.begin()
- * thrust::lower_bound(input.begin(), input.end(), 1, thrust::less()); // returns input.begin() + 1
- * thrust::lower_bound(input.begin(), input.end(), 2, thrust::less()); // returns input.begin() + 1
- * thrust::lower_bound(input.begin(), input.end(), 3, thrust::less()); // returns input.begin() + 2
- * thrust::lower_bound(input.begin(), input.end(), 8, thrust::less()); // returns input.begin() + 4
- * thrust::lower_bound(input.begin(), input.end(), 9, thrust::less()); // returns input.end()
- * \endcode
- *
- * \see http://www.sgi.com/tech/stl/lower_bound.html
- * \see \p upper_bound
- * \see \p equal_range
- * \see \p binary_search
- */
-template
-ForwardIterator lower_bound(ForwardIterator first,
- ForwardIterator last,
- const T& value,
- StrictWeakOrdering comp);
-
-
-/*! \p upper_bound is a version of binary search: it attempts to find
- * the element value in an ordered range [first, last).
- * Specifically, it returns the last position where value could be
- * inserted without violating the ordering. This version of
- * \p upper_bound uses operator< for comparison and returns
- * the furthermost iterator \c i in [first, last) such that,
- * for every iterator \c j in [first, i), value < *j
- * is \c false.
- *
- * The algorithm's execution is parallelized as determined by \p exec.
- *
- * \param exec The execution policy to use for parallelization.
- * \param first The beginning of the ordered sequence.
- * \param last The end of the ordered sequence.
- * \param value The value to be searched.
- * \return The furthermost iterator \c i, such that value < *i is \c false.
- *
- * \tparam DerivedPolicy The name of the derived execution policy.
- * \tparam ForwardIterator is a model of Forward Iterator.
- * \tparam LessThanComparable is a model of LessThanComparable.
- *
- * The following code snippet demonstrates how to use \p upper_bound
- * to search for values in a ordered range using the \p thrust::device execution policy for parallelism:
- *
- * \code
- * #include
- * #include
- * #include
- * ...
- * thrust::device_vector input(5);
- *
- * input[0] = 0;
- * input[1] = 2;
- * input[2] = 5;
- * input[3] = 7;
- * input[4] = 8;
- *
- * thrust::upper_bound(thrust::device, input.begin(), input.end(), 0); // returns input.begin() + 1
- * thrust::upper_bound(thrust::device, input.begin(), input.end(), 1); // returns input.begin() + 1
- * thrust::upper_bound(thrust::device, input.begin(), input.end(), 2); // returns input.begin() + 2
- * thrust::upper_bound(thrust::device, input.begin(), input.end(), 3); // returns input.begin() + 2
- * thrust::upper_bound(thrust::device, input.begin(), input.end(), 8); // returns input.end()
- * thrust::upper_bound(thrust::device, input.begin(), input.end(), 9); // returns input.end()
- * \endcode
- *
- * \see http://www.sgi.com/tech/stl/upper_bound.html
- * \see \p lower_bound
- * \see \p equal_range
- * \see \p binary_search
- */
-template
-__host__ __device__
-ForwardIterator upper_bound(const thrust::detail::execution_policy_base &exec,
- ForwardIterator first,
- ForwardIterator last,
- const LessThanComparable &value);
-
-
-/*! \p upper_bound is a version of binary search: it attempts to find
- * the element value in an ordered range [first, last).
- * Specifically, it returns the last position where value could be
- * inserted without violating the ordering. This version of
- * \p upper_bound uses operator< for comparison and returns
- * the furthermost iterator \c i in [first, last) such that,
- * for every iterator \c j in [first, i), value < *j
- * is \c false.
- *
- * \param first The beginning of the ordered sequence.
- * \param last The end of the ordered sequence.
- * \param value The value to be searched.
- * \return The furthermost iterator \c i, such that value < *i is \c false.
- *
- * \tparam ForwardIterator is a model of Forward Iterator.
- * \tparam LessThanComparable is a model of LessThanComparable.
- *
- * The following code snippet demonstrates how to use \p upper_bound
- * to search for values in a ordered range.
- *
- * \code
- * #include
- * #include
- * ...
- * thrust::device_vector input(5);
- *
- * input[0] = 0;
- * input[1] = 2;
- * input[2] = 5;
- * input[3] = 7;
- * input[4] = 8;
- *
- * thrust::upper_bound(input.begin(), input.end(), 0); // returns input.begin() + 1
- * thrust::upper_bound(input.begin(), input.end(), 1); // returns input.begin() + 1
- * thrust::upper_bound(input.begin(), input.end(), 2); // returns input.begin() + 2
- * thrust::upper_bound(input.begin(), input.end(), 3); // returns input.begin() + 2
- * thrust::upper_bound(input.begin(), input.end(), 8); // returns input.end()
- * thrust::upper_bound(input.begin(), input.end(), 9); // returns input.end()
- * \endcode
- *
- * \see http://www.sgi.com/tech/stl/upper_bound.html
- * \see \p lower_bound
- * \see \p equal_range
- * \see \p binary_search
- */
-template
-ForwardIterator upper_bound(ForwardIterator first,
- ForwardIterator last,
- const LessThanComparable& value);
-
-
-/*! \p upper_bound is a version of binary search: it attempts to find
- * the element value in an ordered range [first, last).
- * Specifically, it returns the last position where value could be
- * inserted without violating the ordering. This version of
- * \p upper_bound uses function object \c comp for comparison and returns
- * the furthermost iterator \c i in [first, last) such that,
- * for every iterator \c j in [first, i), comp(value, *j)
- * is \c false.
- *
- * The algorithm's execution is parallelized as determined by \p exec.
- *
- * \param exec The execution policy to use for parallelization.
- * \param first The beginning of the ordered sequence.
- * \param last The end of the ordered sequence.
- * \param value The value to be searched.
- * \param comp The comparison operator.
- * \return The furthermost iterator \c i, such that comp(value, *i) is \c false.
- *
- * \tparam DerivedPolicy The name of the derived execution policy.
- * \tparam ForwardIterator is a model of Forward Iterator.
- * \tparam T is comparable to \p ForwardIterator's \c value_type.
- * \tparam StrictWeakOrdering is a model of Strict Weak Ordering.
- *
- * The following code snippet demonstrates how to use \p upper_bound
- * to search for values in a ordered range using the \p thrust::device execution policy for parallelization:
- *
- * \code
- * #include
- * #include
- * #include
- * #include
- * ...
- * thrust::device_vector input(5);
- *
- * input[0] = 0;
- * input[1] = 2;
- * input[2] = 5;
- * input[3] = 7;
- * input[4] = 8;
- *
- * thrust::upper_bound(thrust::device, input.begin(), input.end(), 0, thrust::less()); // returns input.begin() + 1
- * thrust::upper_bound(thrust::device, input.begin(), input.end(), 1, thrust::less()); // returns input.begin() + 1
- * thrust::upper_bound(thrust::device, input.begin(), input.end(), 2, thrust::less()); // returns input.begin() + 2
- * thrust::upper_bound(thrust::device, input.begin(), input.end(), 3, thrust::less()); // returns input.begin() + 2
- * thrust::upper_bound(thrust::device, input.begin(), input.end(), 8, thrust::less()); // returns input.end()
- * thrust::upper_bound(thrust::device, input.begin(), input.end(), 9, thrust::less()); // returns input.end()
- * \endcode
- *
- * \see http://www.sgi.com/tech/stl/upper_bound.html
- * \see \p lower_bound
- * \see \p equal_range
- * \see \p binary_search
- */
-template
-__host__ __device__
-ForwardIterator upper_bound(const thrust::detail::execution_policy_base &exec,
- ForwardIterator first,
- ForwardIterator last,
- const T &value,
- StrictWeakOrdering comp);
-
-/*! \p upper_bound is a version of binary search: it attempts to find
- * the element value in an ordered range [first, last).
- * Specifically, it returns the last position where value could be
- * inserted without violating the ordering. This version of
- * \p upper_bound uses function object \c comp for comparison and returns
- * the furthermost iterator \c i in [first, last) such that,
- * for every iterator \c j in [first, i), comp(value, *j)
- * is \c false.
- *
- * \param first The beginning of the ordered sequence.
- * \param last The end of the ordered sequence.
- * \param value The value to be searched.
- * \param comp The comparison operator.
- * \return The furthermost iterator \c i, such that comp(value, *i) is \c false.
- *
- * \tparam ForwardIterator is a model of Forward Iterator.
- * \tparam T is comparable to \p ForwardIterator's \c value_type.
- * \tparam StrictWeakOrdering is a model of Strict Weak Ordering.
- *
- * The following code snippet demonstrates how to use \p upper_bound
- * to search for values in a ordered range.
- *
- * \code
- * #include
- * #include
- * #include
- * ...
- * thrust::device_vector input(5);
- *
- * input[0] = 0;
- * input[1] = 2;
- * input[2] = 5;
- * input[3] = 7;
- * input[4] = 8;
- *
- * thrust::upper_bound(input.begin(), input.end(), 0, thrust::less()); // returns input.begin() + 1
- * thrust::upper_bound(input.begin(), input.end(), 1, thrust::less()); // returns input.begin() + 1
- * thrust::upper_bound(input.begin(), input.end(), 2, thrust::less()); // returns input.begin() + 2
- * thrust::upper_bound(input.begin(), input.end(), 3, thrust::less()); // returns input.begin() + 2
- * thrust::upper_bound(input.begin(), input.end(), 8, thrust::less()); // returns input.end()
- * thrust::upper_bound(input.begin(), input.end(), 9, thrust::less()); // returns input.end()
- * \endcode
- *
- * \see http://www.sgi.com/tech/stl/upper_bound.html
- * \see \p lower_bound
- * \see \p equal_range
- * \see \p binary_search
- */
-template
-ForwardIterator upper_bound(ForwardIterator first,
- ForwardIterator last,
- const T& value,
- StrictWeakOrdering comp);
-
-
-/*! \p binary_search is a version of binary search: it attempts to find
- * the element value in an ordered range [first, last).
- * It returns \c true if an element that is equivalent to \c value
- * is present in [first, last) and \c false if no such element
- * exists. Specifically, this version returns \c true if and only if
- * there exists an iterator \c i in [first, last) such that
- * *i < value and value < *i are both \c false.
- *
- * The algorithm's execution is parallelized as determined by \p exec.
- *
- * \param exec The execution policy to use for parallelization.
- * \param first The beginning of the ordered sequence.
- * \param last The end of the ordered sequence.
- * \param value The value to be searched.
- * \return \c true if an equivalent element exists in [first, last), otherwise \c false.
- *
- * \tparam DerivedPolicy The name of the derived execution policy.
- * \tparam ForwardIterator is a model of Forward Iterator.
- * \tparam LessThanComparable is a model of LessThanComparable.
- *
- * The following code snippet demonstrates how to use \p binary_search
- * to search for values in a ordered range using the \p thrust::device execution policy for parallelization:
- *
- * \code
- * #include
- * #include
- * #include
- * ...
- * thrust::device_vector input(5);
- *
- * input[0] = 0;
- * input[1] = 2;
- * input[2] = 5;
- * input[3] = 7;
- * input[4] = 8;
- *
- * thrust::binary_search(thrust::device, input.begin(), input.end(), 0); // returns true
- * thrust::binary_search(thrust::device, input.begin(), input.end(), 1); // returns false
- * thrust::binary_search(thrust::device, input.begin(), input.end(), 2); // returns true
- * thrust::binary_search(thrust::device, input.begin(), input.end(), 3); // returns false
- * thrust::binary_search(thrust::device, input.begin(), input.end(), 8); // returns true
- * thrust::binary_search(thrust::device, input.begin(), input.end(), 9); // returns false
- * \endcode
- *
- * \see http://www.sgi.com/tech/stl/binary_search.html
- * \see \p lower_bound
- * \see \p upper_bound
- * \see \p equal_range
- */
-template
-__host__ __device__
-bool binary_search(const thrust::detail::execution_policy_base &exec,
- ForwardIterator first,
- ForwardIterator last,
- const LessThanComparable& value);
-
-
-/*! \p binary_search is a version of binary search: it attempts to find
- * the element value in an ordered range [first, last).
- * It returns \c true if an element that is equivalent to \c value
- * is present in [first, last) and \c false if no such element
- * exists. Specifically, this version returns \c true if and only if
- * there exists an iterator \c i in [first, last) such that
- * *i < value and value < *i are both \c false.
- *
- * \param first The beginning of the ordered sequence.
- * \param last The end of the ordered sequence.
- * \param value The value to be searched.
- * \return \c true if an equivalent element exists in [first, last), otherwise \c false.
- *
- * \tparam ForwardIterator is a model of Forward Iterator.
- * \tparam LessThanComparable is a model of LessThanComparable.
- *
- * The following code snippet demonstrates how to use \p binary_search
- * to search for values in a ordered range.
- *
- * \code
- * #include
- * #include
- * ...
- * thrust::device_vector input(5);
- *
- * input[0] = 0;
- * input[1] = 2;
- * input[2] = 5;
- * input[3] = 7;
- * input[4] = 8;
- *
- * thrust::binary_search(input.begin(), input.end(), 0); // returns true
- * thrust::binary_search(input.begin(), input.end(), 1); // returns false
- * thrust::binary_search(input.begin(), input.end(), 2); // returns true
- * thrust::binary_search(input.begin(), input.end(), 3); // returns false
- * thrust::binary_search(input.begin(), input.end(), 8); // returns true
- * thrust::binary_search(input.begin(), input.end(), 9); // returns false
- * \endcode
- *
- * \see http://www.sgi.com/tech/stl/binary_search.html
- * \see \p lower_bound
- * \see \p upper_bound
- * \see \p equal_range
- */
-template
-bool binary_search(ForwardIterator first,
- ForwardIterator last,
- const LessThanComparable& value);
-
-
-/*! \p binary_search is a version of binary search: it attempts to find
- * the element value in an ordered range [first, last).
- * It returns \c true if an element that is equivalent to \c value
- * is present in [first, last) and \c false if no such element
- * exists. Specifically, this version returns \c true if and only if
- * there exists an iterator \c i in [first, last) such that
- * comp(*i, value) and comp(value, *i) are both \c false.
- *
- * The algorithm's execution is parallelized as determined by \p exec.
- *
- * \param exec The execution policy to use for parallelization.
- * \param first The beginning of the ordered sequence.
- * \param last The end of the ordered sequence.
- * \param value The value to be searched.
- * \param comp The comparison operator.
- * \return \c true if an equivalent element exists in [first, last), otherwise \c false.
- *
- * \tparam DerivedPolicy The name of the derived execution policy.
- * \tparam ForwardIterator is a model of Forward Iterator.
- * \tparam T is comparable to \p ForwardIterator's \c value_type.
- * \tparam StrictWeakOrdering is a model of Strict Weak Ordering.
- *
- * The following code snippet demonstrates how to use \p binary_search
- * to search for values in a ordered range using the \p thrust::device execution policy for parallelization:
- *
- * \code
- * #include
- * #include
- * #include
- * #include
- * ...
- * thrust::device_vector input(5);
- *
- * input[0] = 0;
- * input[1] = 2;
- * input[2] = 5;
- * input[3] = 7;
- * input[4] = 8;
- *
- * thrust::binary_search(thrust::device, input.begin(), input.end(), 0, thrust::less()); // returns true
- * thrust::binary_search(thrust::device, input.begin(), input.end(), 1, thrust::less()); // returns false
- * thrust::binary_search(thrust::device, input.begin(), input.end(), 2, thrust::less()); // returns true
- * thrust::binary_search(thrust::device, input.begin(), input.end(), 3, thrust::less()); // returns false
- * thrust::binary_search(thrust::device, input.begin(), input.end(), 8, thrust::less()); // returns true
- * thrust::binary_search(thrust::device, input.begin(), input.end(), 9, thrust::less()); // returns false
- * \endcode
- *
- * \see http://www.sgi.com/tech/stl/binary_search.html
- * \see \p lower_bound
- * \see \p upper_bound
- * \see \p equal_range
- */
-template
-__host__ __device__
-bool binary_search(const thrust::detail::execution_policy_base &exec,
- ForwardIterator first,
- ForwardIterator last,
- const T& value,
- StrictWeakOrdering comp);
-
-
-/*! \p binary_search is a version of binary search: it attempts to find
- * the element value in an ordered range [first, last).
- * It returns \c true if an element that is equivalent to \c value
- * is present in [first, last) and \c false if no such element
- * exists. Specifically, this version returns \c true if and only if
- * there exists an iterator \c i in [first, last) such that
- * comp(*i, value) and comp(value, *i) are both \c false.
- *
- * \param first The beginning of the ordered sequence.
- * \param last The end of the ordered sequence.
- * \param value The value to be searched.
- * \param comp The comparison operator.
- * \return \c true if an equivalent element exists in [first, last), otherwise \c false.
- *
- * \tparam ForwardIterator is a model of Forward Iterator.
- * \tparam T is comparable to \p ForwardIterator's \c value_type.
- * \tparam StrictWeakOrdering is a model of Strict Weak Ordering.
- *
- * The following code snippet demonstrates how to use \p binary_search
- * to search for values in a ordered range.
- *
- * \code
- * #include
- * #include
- * #include
- * ...
- * thrust::device_vector input(5);
- *
- * input[0] = 0;
- * input[1] = 2;
- * input[2] = 5;
- * input[3] = 7;
- * input[4] = 8;
- *
- * thrust::binary_search(input.begin(), input.end(), 0, thrust::less()); // returns true
- * thrust::binary_search(input.begin(), input.end(), 1, thrust::less()); // returns false
- * thrust::binary_search(input.begin(), input.end(), 2, thrust::less()); // returns true
- * thrust::binary_search(input.begin(), input.end(), 3, thrust::less()); // returns false
- * thrust::binary_search(input.begin(), input.end(), 8, thrust::less()); // returns true
- * thrust::binary_search(input.begin(), input.end(), 9, thrust::less()); // returns false
- * \endcode
- *
- * \see http://www.sgi.com/tech/stl/binary_search.html
- * \see \p lower_bound
- * \see \p upper_bound
- * \see \p equal_range
- */
-template
-bool binary_search(ForwardIterator first,
- ForwardIterator last,
- const T& value,
- StrictWeakOrdering comp);
-
-
-/*! \p equal_range is a version of binary search: it attempts to find
- * the element value in an ordered range [first, last). The
- * value returned by \p equal_range is essentially a combination of
- * the values returned by \p lower_bound and \p upper_bound: it returns
- * a \p pair of iterators \c i and \c j such that \c i is the first
- * position where value could be inserted without violating the
- * ordering and \c j is the last position where value could be inserted
- * without violating the ordering. It follows that every element in the
- * range [i, j) is equivalent to value, and that
- * [i, j) is the largest subrange of [first, last) that
- * has this property.
- *
- * This version of \p equal_range returns a \p pair of iterators
- * [i, j), where \c i is the furthermost iterator in
- * [first, last) such that, for every iterator \c k in
- * [first, i), *k < value. \c j is the furthermost
- * iterator in [first, last) such that, for every iterator
- * \c k in [first, j), value < *k is \c false.
- * For every iterator \c k in [i, j), neither
- * value < *k nor *k < value is \c true.
- *
- * The algorithm's execution is parallelized as determined by \p exec.
- *
- * \param exec The execution policy to use for parallelization.
- * \param first The beginning of the ordered sequence.
- * \param last The end of the ordered sequence.
- * \param value The value to be searched.
- * \return A \p pair of iterators [i, j) that define the range of equivalent elements.
- *
- * \tparam DerivedPolicy The name of the derived execution policy.
- * \tparam ForwardIterator is a model of Forward Iterator.
- * \tparam LessThanComparable is a model of LessThanComparable.
- *
- * The following code snippet demonstrates how to use \p equal_range
- * to search for values in a ordered range using the \p thrust::device execution policy for parallelization:
- *
- * \code
- * #include
- * #include
- * #include
- * ...
- * thrust::device_vector input(5);
- *
- * input[0] = 0;
- * input[1] = 2;
- * input[2] = 5;
- * input[3] = 7;
- * input[4] = 8;
- *
- * thrust::equal_range(thrust::device, input.begin(), input.end(), 0); // returns [input.begin(), input.begin() + 1)
- * thrust::equal_range(thrust::device, input.begin(), input.end(), 1); // returns [input.begin() + 1, input.begin() + 1)
- * thrust::equal_range(thrust::device, input.begin(), input.end(), 2); // returns [input.begin() + 1, input.begin() + 2)
- * thrust::equal_range(thrust::device, input.begin(), input.end(), 3); // returns [input.begin() + 2, input.begin() + 2)
- * thrust::equal_range(thrust::device, input.begin(), input.end(), 8); // returns [input.begin() + 4, input.end)
- * thrust::equal_range(thrust::device, input.begin(), input.end(), 9); // returns [input.end(), input.end)
- * \endcode
- *
- * \see http://www.sgi.com/tech/stl/equal_range.html
- * \see \p lower_bound
- * \see \p upper_bound
- * \see \p binary_search
- */
-template
-__host__ __device__
-thrust::pair
-equal_range(const thrust::detail::execution_policy_base &exec,
- ForwardIterator first,
- ForwardIterator last,
- const LessThanComparable& value);
-
-
-/*! \p equal_range is a version of binary search: it attempts to find
- * the element value in an ordered range [first, last). The
- * value returned by \p equal_range is essentially a combination of
- * the values returned by \p lower_bound and \p upper_bound: it returns
- * a \p pair of iterators \c i and \c j such that \c i is the first
- * position where value could be inserted without violating the
- * ordering and \c j is the last position where value could be inserted
- * without violating the ordering. It follows that every element in the
- * range [i, j) is equivalent to value, and that
- * [i, j) is the largest subrange of [first, last) that
- * has this property.
- *
- * This version of \p equal_range returns a \p pair of iterators
- * [i, j), where \c i is the furthermost iterator in
- * [first, last) such that, for every iterator \c k in
- * [first, i), *k < value. \c j is the furthermost
- * iterator in [first, last) such that, for every iterator
- * \c k in [first, j), value < *k is \c false.
- * For every iterator \c k in [i, j), neither
- * value < *k nor *k < value is \c true.
- *
- * \param first The beginning of the ordered sequence.
- * \param last The end of the ordered sequence.
- * \param value The value to be searched.
- * \return A \p pair of iterators [i, j) that define the range of equivalent elements.
- *
- * \tparam ForwardIterator is a model of Forward Iterator.
- * \tparam LessThanComparable is a model of LessThanComparable.
- *
- * The following code snippet demonstrates how to use \p equal_range
- * to search for values in a ordered range.
- *
- * \code
- * #include
- * #include
- * ...
- * thrust::device_vector input(5);
- *
- * input[0] = 0;
- * input[1] = 2;
- * input[2] = 5;
- * input[3] = 7;
- * input[4] = 8;
- *
- * thrust::equal_range(input.begin(), input.end(), 0); // returns [input.begin(), input.begin() + 1)
- * thrust::equal_range(input.begin(), input.end(), 1); // returns [input.begin() + 1, input.begin() + 1)
- * thrust::equal_range(input.begin(), input.end(), 2); // returns [input.begin() + 1, input.begin() + 2)
- * thrust::equal_range(input.begin(), input.end(), 3); // returns [input.begin() + 2, input.begin() + 2)
- * thrust::equal_range(input.begin(), input.end(), 8); // returns [input.begin() + 4, input.end)
- * thrust::equal_range(input.begin(), input.end(), 9); // returns [input.end(), input.end)
- * \endcode
- *
- * \see http://www.sgi.com/tech/stl/equal_range.html
- * \see \p lower_bound
- * \see \p upper_bound
- * \see \p binary_search
- */
-template
-thrust::pair
-equal_range(ForwardIterator first,
- ForwardIterator last,
- const LessThanComparable& value);
-
-
-/*! \p equal_range is a version of binary search: it attempts to find
- * the element value in an ordered range [first, last). The
- * value returned by \p equal_range is essentially a combination of
- * the values returned by \p lower_bound and \p upper_bound: it returns
- * a \p pair of iterators \c i and \c j such that \c i is the first
- * position where value could be inserted without violating the
- * ordering and \c j is the last position where value could be inserted
- * without violating the ordering. It follows that every element in the
- * range [i, j) is equivalent to value, and that
- * [i, j) is the largest subrange of [first, last) that
- * has this property.
- *
- * This version of \p equal_range returns a \p pair of iterators
- * [i, j). \c i is the furthermost iterator in
- * [first, last) such that, for every iterator \c k in
- * [first, i), comp(*k, value) is \c true.
- * \c j is the furthermost iterator in [first, last) such
- * that, for every iterator \c k in [first, last),
- * comp(value, *k) is \c false. For every iterator \c k
- * in [i, j), neither comp(value, *k) nor
- * comp(*k, value) is \c true.
- *
- * The algorithm's execution is parallelized as determined by \p exec.
- *
- * \param exec The execution policy to use for parallelization.
- * \param first The beginning of the ordered sequence.
- * \param last The end of the ordered sequence.
- * \param value The value to be searched.
- * \param comp The comparison operator.
- * \return A \p pair of iterators [i, j) that define the range of equivalent elements.
- *
- * \tparam DerivedPolicy The name of the derived execution policy.
- * \tparam ForwardIterator is a model of Forward Iterator.
- * \tparam T is comparable to \p ForwardIterator's \c value_type.
- * \tparam StrictWeakOrdering is a model of Strict Weak Ordering.
- *
- * The following code snippet demonstrates how to use \p equal_range
- * to search for values in a ordered range using the \p thrust::device execution policy for parallelization:
- *
- * \code
- * #include
- * #include
- * #include
- * #include
- * ...
- * thrust::device_vector input(5);
- *
- * input[0] = 0;
- * input[1] = 2;
- * input[2] = 5;
- * input[3] = 7;
- * input[4] = 8;
- *
- * thrust::equal_range(thrust::device, input.begin(), input.end(), 0, thrust::less()); // returns [input.begin(), input.begin() + 1)
- * thrust::equal_range(thrust::device, input.begin(), input.end(), 1, thrust::less()); // returns [input.begin() + 1, input.begin() + 1)
- * thrust::equal_range(thrust::device, input.begin(), input.end(), 2, thrust::less()); // returns [input.begin() + 1, input.begin() + 2)
- * thrust::equal_range(thrust::device, input.begin(), input.end(), 3, thrust::less()); // returns [input.begin() + 2, input.begin() + 2)
- * thrust::equal_range(thrust::device, input.begin(), input.end(), 8, thrust::less()); // returns [input.begin() + 4, input.end)
- * thrust::equal_range(thrust::device, input.begin(), input.end(), 9, thrust::less()); // returns [input.end(), input.end)
- * \endcode
- *
- * \see http://www.sgi.com/tech/stl/equal_range.html
- * \see \p lower_bound
- * \see \p upper_bound
- * \see \p binary_search
- */
-template
-__host__ __device__
-thrust::pair
-equal_range(const thrust::detail::execution_policy_base &exec,
- ForwardIterator first,
- ForwardIterator last,
- const T& value,
- StrictWeakOrdering comp);
-
-
-/*! \p equal_range is a version of binary search: it attempts to find
- * the element value in an ordered range [first, last). The
- * value returned by \p equal_range is essentially a combination of
- * the values returned by \p lower_bound and \p upper_bound: it returns
- * a \p pair of iterators \c i and \c j such that \c i is the first
- * position where value could be inserted without violating the
- * ordering and \c j is the last position where value could be inserted
- * without violating the ordering. It follows that every element in the
- * range [i, j) is equivalent to value, and that
- * [i, j) is the largest subrange of [first, last) that
- * has this property.
- *
- * This version of \p equal_range returns a \p pair of iterators
- * [i, j). \c i is the furthermost iterator in
- * [first, last) such that, for every iterator \c k in
- * [first, i), comp(*k, value) is \c true.
- * \c j is the furthermost iterator in [first, last) such
- * that, for every iterator \c k in [first, last),
- * comp(value, *k) is \c false. For every iterator \c k
- * in [i, j), neither comp(value, *k) nor
- * comp(*k, value) is \c true.
- *
- * \param first The beginning of the ordered sequence.
- * \param last The end of the ordered sequence.
- * \param value The value to be searched.
- * \param comp The comparison operator.
- * \return A \p pair of iterators [i, j) that define the range of equivalent elements.
- *
- * \tparam ForwardIterator is a model of Forward Iterator.
- * \tparam T is comparable to \p ForwardIterator's \c value_type.
- * \tparam StrictWeakOrdering is a model of Strict Weak Ordering.
- *
- * The following code snippet demonstrates how to use \p equal_range
- * to search for values in a ordered range.
- *
- * \code
- * #include
- * #include
- * #include
- * ...
- * thrust::device_vector input(5);
- *
- * input[0] = 0;
- * input[1] = 2;
- * input[2] = 5;
- * input[3] = 7;
- * input[4] = 8;
- *
- * thrust::equal_range(input.begin(), input.end(), 0, thrust::less()); // returns [input.begin(), input.begin() + 1)
- * thrust::equal_range(input.begin(), input.end(), 1, thrust::less()); // returns [input.begin() + 1, input.begin() + 1)
- * thrust::equal_range(input.begin(), input.end(), 2, thrust::less()); // returns [input.begin() + 1, input.begin() + 2)
- * thrust::equal_range(input.begin(), input.end(), 3, thrust::less()); // returns [input.begin() + 2, input.begin() + 2)
- * thrust::equal_range(input.begin(), input.end(), 8, thrust::less()); // returns [input.begin() + 4, input.end)
- * thrust::equal_range(input.begin(), input.end(), 9, thrust::less()); // returns [input.end(), input.end)
- * \endcode
- *
- * \see http://www.sgi.com/tech/stl/equal_range.html
- * \see \p lower_bound
- * \see \p upper_bound
- * \see \p binary_search
- */
-template
-thrust::pair
-equal_range(ForwardIterator first,
- ForwardIterator last,
- const T& value,
- StrictWeakOrdering comp);
-
-
-/*! \addtogroup vectorized_binary_search Vectorized Searches
- * \ingroup binary_search
- * \{
- */
-
-
-//////////////////////
-// Vector Functions //
-//////////////////////
-
-
-/*! \p lower_bound is a vectorized version of binary search: for each
- * iterator \c v in [values_first, values_last) it attempts to
- * find the value *v in an ordered range [first, last).
- * Specifically, it returns the index of first position where value could
- * be inserted without violating the ordering.
- *
- * The algorithm's execution is parallelized as determined by \p exec.
- *
- * \param exec The execution policy to use for parallelization.
- * \param first The beginning of the ordered sequence.
- * \param last The end of the ordered sequence.
- * \param values_first The beginning of the search values sequence.
- * \param values_last The end of the search values sequence.
- * \param result The beginning of the output sequence.
- *
- * \tparam DerivedPolicy The name of the derived execution policy.
- * \tparam ForwardIterator is a model of Forward Iterator.
- * \tparam InputIterator is a model of Input Iterator.
- * and \c InputIterator's \c value_type is LessThanComparable.
- * \tparam OutputIterator is a model of Output Iterator.
- * and \c ForwardIterator's difference_type is convertible to \c OutputIterator's \c value_type.
- *
- * \pre The ranges [first,last) and [result, result + (last - first)) shall not overlap.
- *
- * The following code snippet demonstrates how to use \p lower_bound
- * to search for multiple values in a ordered range using the \p thrust::device execution policy for
- * parallelization:
- *
- * \code
- * #include
- * #include
- * #include
- * ...
- * thrust::device_vector input(5);
- *
- * input[0] = 0;
- * input[1] = 2;
- * input[2] = 5;
- * input[3] = 7;
- * input[4] = 8;
- *
- * thrust::device_vector values(6);
- * values[0] = 0;
- * values[1] = 1;
- * values[2] = 2;
- * values[3] = 3;
- * values[4] = 8;
- * values[5] = 9;
- *
- * thrust::device_vector output(6);
- *
- * thrust::lower_bound(thrust::device,
- * input.begin(), input.end(),
- * values.begin(), values.end(),
- * output.begin());
- *
- * // output is now [0, 1, 1, 2, 4, 5]
- * \endcode
- *
- * \see http://www.sgi.com/tech/stl/lower_bound.html
- * \see \p upper_bound
- * \see \p equal_range
- * \see \p binary_search
- */
-template
-__host__ __device__
-OutputIterator lower_bound(const thrust::detail::execution_policy_base &exec,
- ForwardIterator first,
- ForwardIterator last,
- InputIterator values_first,
- InputIterator values_last,
- OutputIterator result);
-
-
-/*! \p lower_bound is a vectorized version of binary search: for each
- * iterator \c v in [values_first, values_last) it attempts to
- * find the value *v in an ordered range [first, last).
- * Specifically, it returns the index of first position where value could
- * be inserted without violating the ordering.
- *
- * \param first The beginning of the ordered sequence.
- * \param last The end of the ordered sequence.
- * \param values_first The beginning of the search values sequence.
- * \param values_last The end of the search values sequence.
- * \param result The beginning of the output sequence.
- *
- * \tparam ForwardIterator is a model of Forward Iterator.
- * \tparam InputIterator is a model of Input Iterator.
- * and \c InputIterator's \c value_type is LessThanComparable.
- * \tparam OutputIterator is a model of Output Iterator.
- * and \c ForwardIterator's difference_type is convertible to \c OutputIterator's \c value_type.
- *
- * \pre The ranges [first,last) and [result, result + (last - first)) shall not overlap.
- *
- * The following code snippet demonstrates how to use \p lower_bound
- * to search for multiple values in a ordered range.
- *
- * \code
- * #include
- * #include
- * ...
- * thrust::device_vector input(5);
- *
- * input[0] = 0;
- * input[1] = 2;
- * input[2] = 5;
- * input[3] = 7;
- * input[4] = 8;
- *
- * thrust::device_vector values(6);
- * values[0] = 0;
- * values[1] = 1;
- * values[2] = 2;
- * values[3] = 3;
- * values[4] = 8;
- * values[5] = 9;
- *
- * thrust::device_vector output(6);
- *
- * thrust::lower_bound(input.begin(), input.end(),
- * values.begin(), values.end(),
- * output.begin());
- *
- * // output is now [0, 1, 1, 2, 4, 5]
- * \endcode
- *
- * \see http://www.sgi.com/tech/stl/lower_bound.html
- * \see \p upper_bound
- * \see \p equal_range
- * \see \p binary_search
- */
-template
-OutputIterator lower_bound(ForwardIterator first,
- ForwardIterator last,
- InputIterator values_first,
- InputIterator values_last,
- OutputIterator result);
-
-
-/*! \p lower_bound is a vectorized version of binary search: for each
- * iterator \c v in [values_first, values_last) it attempts to
- * find the value *v in an ordered range [first, last).
- * Specifically, it returns the index of first position where value could
- * be inserted without violating the ordering. This version of
- * \p lower_bound uses function object \c comp for comparison.
- *
- * The algorithm's execution is parallelized as determined by \p exec.
- *
- * \param exec The execution policy to use for parallelization.
- * \param first The beginning of the ordered sequence.
- * \param last The end of the ordered sequence.
- * \param values_first The beginning of the search values sequence.
- * \param values_last The end of the search values sequence.
- * \param result The beginning of the output sequence.
- * \param comp The comparison operator.
- *
- * \tparam DerivedPolicy The name of the derived execution policy.
- * \tparam ForwardIterator is a model of Forward Iterator.
- * \tparam InputIterator is a model of Input Iterator.
- * and \c InputIterator's \c value_type is comparable to \p ForwardIterator's \c value_type.
- * \tparam OutputIterator is a model of Output Iterator.
- * and \c ForwardIterator's difference_type is convertible to \c OutputIterator's \c value_type.
- * \tparam StrictWeakOrdering is a model of Strict Weak Ordering.
- *
- * \pre The ranges [first,last) and [result, result + (last - first)) shall not overlap.
- *
- * The following code snippet demonstrates how to use \p lower_bound
- * to search for multiple values in a ordered range.
- *
- * \code
- * #include
- * #include
- * #include
- * #include
- * ...
- * thrust::device_vector input(5);
- *
- * input[0] = 0;
- * input[1] = 2;
- * input[2] = 5;
- * input[3] = 7;
- * input[4] = 8;
- *
- * thrust::device_vector values(6);
- * values[0] = 0;
- * values[1] = 1;
- * values[2] = 2;
- * values[3] = 3;
- * values[4] = 8;
- * values[5] = 9;
- *
- * thrust::device_vector output(6);
- *
- * thrust::lower_bound(input.begin(), input.end(),
- * values.begin(), values.end(),
- * output.begin(),
- * thrust::less());
- *
- * // output is now [0, 1, 1, 2, 4, 5]
- * \endcode
- *
- * \see http://www.sgi.com/tech/stl/lower_bound.html
- * \see \p upper_bound
- * \see \p equal_range
- * \see \p binary_search
- */
-template
-__host__ __device__
-OutputIterator lower_bound(const thrust::detail::execution_policy_base &exec,
- ForwardIterator first,
- ForwardIterator last,
- InputIterator values_first,
- InputIterator values_last,
- OutputIterator result,
- StrictWeakOrdering comp);
-
-
-/*! \p lower_bound is a vectorized version of binary search: for each
- * iterator \c v in [values_first, values_last) it attempts to
- * find the value *v in an ordered range [first, last).
- * Specifically, it returns the index of first position where value could
- * be inserted without violating the ordering. This version of
- * \p lower_bound uses function object \c comp for comparison.
- *
- * \param first The beginning of the ordered sequence.
- * \param last The end of the ordered sequence.
- * \param values_first The beginning of the search values sequence.
- * \param values_last The end of the search values sequence.
- * \param result The beginning of the output sequence.
- * \param comp The comparison operator.
- *
- * \tparam ForwardIterator is a model of Forward Iterator.
- * \tparam InputIterator is a model of Input Iterator.
- * and \c InputIterator's \c value_type is comparable to \p ForwardIterator's \c value_type.
- * \tparam OutputIterator is a model of Output Iterator.
- * and \c ForwardIterator's difference_type is convertible to \c OutputIterator's \c value_type.
- * \tparam StrictWeakOrdering is a model of Strict Weak Ordering.
- *
- * \pre The ranges [first,last) and [result, result + (last - first)) shall not overlap.
- *
- * The following code snippet demonstrates how to use \p lower_bound
- * to search for multiple values in a ordered range.
- *
- * \code
- * #include
- * #include
- * #include
- * ...
- * thrust::device_vector input(5);
- *
- * input[0] = 0;
- * input[1] = 2;
- * input[2] = 5;
- * input[3] = 7;
- * input[4] = 8;
- *
- * thrust::device_vector values(6);
- * values[0] = 0;
- * values[1] = 1;
- * values[2] = 2;
- * values[3] = 3;
- * values[4] = 8;
- * values[5] = 9;
- *
- * thrust::device_vector output(6);
- *
- * thrust::lower_bound(input.begin(), input.end(),
- * values.begin(), values.end(),
- * output.begin(),
- * thrust::less());
- *
- * // output is now [0, 1, 1, 2, 4, 5]
- * \endcode
- *
- * \see http://www.sgi.com/tech/stl/lower_bound.html
- * \see \p upper_bound
- * \see \p equal_range
- * \see \p binary_search
- */
-template
-OutputIterator lower_bound(ForwardIterator first,
- ForwardIterator last,
- InputIterator values_first,
- InputIterator values_last,
- OutputIterator result,
- StrictWeakOrdering comp);
-
-
-/*! \p upper_bound is a vectorized version of binary search: for each
- * iterator \c v in [values_first, values_last) it attempts to
- * find the value *v in an ordered range [first, last).
- * Specifically, it returns the index of last position where value could
- * be inserted without violating the ordering.
- *
- * The algorithm's execution is parallelized as determined by \p exec.
- *
- * \param exec The execution policy to use for parallelization.
- * \param first The beginning of the ordered sequence.
- * \param last The end of the ordered sequence.
- * \param values_first The beginning of the search values sequence.
- * \param values_last The end of the search values sequence.
- * \param result The beginning of the output sequence.
- *
- * \tparam DerivedPolicy The name of the derived execution policy.
- * \tparam ForwardIterator is a model of Forward Iterator.
- * \tparam InputIterator is a model of Input Iterator.
- * and \c InputIterator's \c value_type is LessThanComparable.
- * \tparam OutputIterator is a model of Output Iterator.
- * and \c ForwardIterator's difference_type is convertible to \c OutputIterator's \c value_type.
- *
- * \pre The ranges [first,last) and [result, result + (last - first)) shall not overlap.
- *
- * The following code snippet demonstrates how to use \p upper_bound
- * to search for multiple values in a ordered range using the \p thrust::device execution policy for
- * parallelization:
- *
- * \code
- * #include
- * #include
- * #include
- * ...
- * thrust::device_vector input(5);
- *
- * input[0] = 0;
- * input[1] = 2;
- * input[2] = 5;
- * input[3] = 7;
- * input[4] = 8;
- *
- * thrust::device_vector values(6);
- * values[0] = 0;
- * values[1] = 1;
- * values[2] = 2;
- * values[3] = 3;
- * values[4] = 8;
- * values[5] = 9;
- *
- * thrust::device_vector output(6);
- *
- * thrust::upper_bound(thrust::device,
- * input.begin(), input.end(),
- * values.begin(), values.end(),
- * output.begin());
- *
- * // output is now [1, 1, 2, 2, 5, 5]
- * \endcode
- *
- * \see http://www.sgi.com/tech/stl/upper_bound.html
- * \see \p upper_bound
- * \see \p equal_range
- * \see \p binary_search
- */
-template
-__host__ __device__
-OutputIterator upper_bound(const thrust::detail::execution_policy_base &exec,
- ForwardIterator first,
- ForwardIterator last,
- InputIterator values_first,
- InputIterator values_last,
- OutputIterator result);
-
-
-/*! \p upper_bound is a vectorized version of binary search: for each
- * iterator \c v in [values_first, values_last) it attempts to
- * find the value *v in an ordered range [first, last).
- * Specifically, it returns the index of last position where value could
- * be inserted without violating the ordering.
- *
- * \param first The beginning of the ordered sequence.
- * \param last The end of the ordered sequence.
- * \param values_first The beginning of the search values sequence.
- * \param values_last The end of the search values sequence.
- * \param result The beginning of the output sequence.
- *
- * \tparam ForwardIterator is a model of Forward Iterator.
- * \tparam InputIterator is a model of Input Iterator.
- * and \c InputIterator's \c value_type is LessThanComparable.
- * \tparam OutputIterator is a model of Output Iterator.
- * and \c ForwardIterator's difference_type is convertible to \c OutputIterator's \c value_type.
- *
- * \pre The ranges [first,last) and [result, result + (last - first)) shall not overlap.
- *
- * The following code snippet demonstrates how to use \p upper_bound
- * to search for multiple values in a ordered range.
- *
- * \code
- * #include
- * #include
- * ...
- * thrust::device_vector input(5);
- *
- * input[0] = 0;
- * input[1] = 2;
- * input[2] = 5;
- * input[3] = 7;
- * input[4] = 8;
- *
- * thrust::device_vector values(6);
- * values[0] = 0;
- * values[1] = 1;
- * values[2] = 2;
- * values[3] = 3;
- * values[4] = 8;
- * values[5] = 9;
- *
- * thrust::device_vector output(6);
- *
- * thrust::upper_bound(input.begin(), input.end(),
- * values.begin(), values.end(),
- * output.begin());
- *
- * // output is now [1, 1, 2, 2, 5, 5]
- * \endcode
- *
- * \see http://www.sgi.com/tech/stl/upper_bound.html
- * \see \p upper_bound
- * \see \p equal_range
- * \see \p binary_search
- */
-template
-OutputIterator upper_bound(ForwardIterator first,
- ForwardIterator last,
- InputIterator values_first,
- InputIterator values_last,
- OutputIterator result);
-
-
-/*! \p upper_bound is a vectorized version of binary search: for each
- * iterator \c v in [values_first, values_last) it attempts to
- * find the value *v in an ordered range [first, last).
- * Specifically, it returns the index of first position where value could
- * be inserted without violating the ordering. This version of
- * \p upper_bound uses function object \c comp for comparison.
- *
- * The algorithm's execution is parallelized as determined by \p exec.
- *
- * \param exec The execution policy to use for parallelization.
- * \param first The beginning of the ordered sequence.
- * \param last The end of the ordered sequence.
- * \param values_first The beginning of the search values sequence.
- * \param values_last The end of the search values sequence.
- * \param result The beginning of the output sequence.
- * \param comp The comparison operator.
- *
- * \tparam DerivedPolicy The name of the derived execution policy.
- * \tparam ForwardIterator is a model of Forward Iterator.
- * \tparam InputIterator is a model of Input Iterator.
- * and \c InputIterator's \c value_type is comparable to \p ForwardIterator's \c value_type.
- * \tparam OutputIterator is a model of Output Iterator.
- * and \c ForwardIterator's difference_type is convertible to \c OutputIterator's \c value_type.
- * \tparam StrictWeakOrdering is a model of Strict Weak Ordering.
- *
- * \pre The ranges [first,last) and [result, result + (last - first)) shall not overlap.
- *
- * The following code snippet demonstrates how to use \p upper_bound
- * to search for multiple values in a ordered range using the \p thrust::device execution policy for
- * parallelization:
- *
- * \code
- * #include
- * #include
- * #include
- * #include
- * ...
- * thrust::device_vector input(5);
- *
- * input[0] = 0;
- * input[1] = 2;
- * input[2] = 5;
- * input[3] = 7;
- * input[4] = 8;
- *
- * thrust::device_vector values(6);
- * values[0] = 0;
- * values[1] = 1;
- * values[2] = 2;
- * values[3] = 3;
- * values[4] = 8;
- * values[5] = 9;
- *
- * thrust::device_vector output(6);
- *
- * thrust::upper_bound(thrust::device,
- * input.begin(), input.end(),
- * values.begin(), values.end(),
- * output.begin(),
- * thrust::less());
- *
- * // output is now [1, 1, 2, 2, 5, 5]
- * \endcode
- *
- * \see http://www.sgi.com/tech/stl/upper_bound.html
- * \see \p lower_bound
- * \see \p equal_range
- * \see \p binary_search
- */
-template
-__host__ __device__
-OutputIterator upper_bound(const thrust::detail::execution_policy_base &exec,
- ForwardIterator first,
- ForwardIterator last,
- InputIterator values_first,
- InputIterator values_last,
- OutputIterator result,
- StrictWeakOrdering comp);
-
-
-/*! \p upper_bound is a vectorized version of binary search: for each
- * iterator \c v in [values_first, values_last) it attempts to
- * find the value *v in an ordered range [first, last).
- * Specifically, it returns the index of first position where value could
- * be inserted without violating the ordering. This version of
- * \p upper_bound uses function object \c comp for comparison.
- *
- * \param first The beginning of the ordered sequence.
- * \param last The end of the ordered sequence.
- * \param values_first The beginning of the search values sequence.
- * \param values_last The end of the search values sequence.
- * \param result The beginning of the output sequence.
- * \param comp The comparison operator.
- *
- * \tparam ForwardIterator is a model of Forward Iterator.
- * \tparam InputIterator is a model of Input Iterator.
- * and \c InputIterator's \c value_type is comparable to \p ForwardIterator's \c value_type.
- * \tparam OutputIterator is a model of Output Iterator.
- * and \c ForwardIterator's difference_type is convertible to \c OutputIterator's \c value_type.
- * \tparam StrictWeakOrdering is a model of Strict Weak Ordering.
- *
- * \pre The ranges [first,last) and [result, result + (last - first)) shall not overlap.
- *
- * The following code snippet demonstrates how to use \p upper_bound
- * to search for multiple values in a ordered range.
- *
- * \code
- * #include
- * #include
- * #include
- * ...
- * thrust::device_vector input(5);
- *
- * input[0] = 0;
- * input[1] = 2;
- * input[2] = 5;
- * input[3] = 7;
- * input[4] = 8;
- *
- * thrust::device_vector values(6);
- * values[0] = 0;
- * values[1] = 1;
- * values[2] = 2;
- * values[3] = 3;
- * values[4] = 8;
- * values[5] = 9;
- *
- * thrust::device_vector output(6);
- *
- * thrust::upper_bound(input.begin(), input.end(),
- * values.begin(), values.end(),
- * output.begin(),
- * thrust::less());
- *
- * // output is now [1, 1, 2, 2, 5, 5]
- * \endcode
- *
- * \see http://www.sgi.com/tech/stl/upper_bound.html
- * \see \p lower_bound
- * \see \p equal_range
- * \see \p binary_search
- */
-template
-OutputIterator upper_bound(ForwardIterator first,
- ForwardIterator last,
- InputIterator values_first,
- InputIterator values_last,
- OutputIterator result,
- StrictWeakOrdering comp);
-
-
-/*! \p binary_search is a vectorized version of binary search: for each
- * iterator \c v in [values_first, values_last) it attempts to
- * find the value *v in an ordered range [first, last).
- * It returns \c true if an element that is equivalent to \c value
- * is present in [first, last) and \c false if no such element
- * exists.
- *
- * The algorithm's execution is parallelized as determined by \p exec.
- *
- * \param exec The execution policy to use for parallelization.
- * \param first The beginning of the ordered sequence.
- * \param last The end of the ordered sequence.
- * \param values_first The beginning of the search values sequence.
- * \param values_last The end of the search values sequence.
- * \param result The beginning of the output sequence.
- *
- * \tparam DerivedPolicy The name of the derived execution policy.
- * \tparam ForwardIterator is a model of Forward Iterator.
- * \tparam InputIterator is a model of Input Iterator.
- * and \c InputIterator's \c value_type is LessThanComparable.
- * \tparam OutputIterator is a model of Output Iterator.
- * and bool is convertible to \c OutputIterator's \c value_type.
- *
- * \pre The ranges [first,last) and [result, result + (last - first)) shall not overlap.
- *
- * The following code snippet demonstrates how to use \p binary_search
- * to search for multiple values in a ordered range using the \p thrust::device execution policy for
- * parallelization:
- *
- * \code
- * #include
- * #include
- * #include
- * ...
- * thrust::device_vector input(5);
- *
- * input[0] = 0;
- * input[1] = 2;
- * input[2] = 5;
- * input[3] = 7;
- * input[4] = 8;
- *
- * thrust::device_vector values(6);
- * values[0] = 0;
- * values[1] = 1;
- * values[2] = 2;
- * values[3] = 3;
- * values[4] = 8;
- * values[5] = 9;
- *
- * thrust::device_vector output(6);
- *
- * thrust::binary_search(thrust::device,
- * input.begin(), input.end(),
- * values.begin(), values.end(),
- * output.begin());
- *
- * // output is now [true, false, true, false, true, false]
- * \endcode
- *
- * \see http://www.sgi.com/tech/stl/binary_search.html
- * \see \p lower_bound
- * \see \p upper_bound
- * \see \p equal_range
- */
-template
-__host__ __device__
-OutputIterator binary_search(const thrust::detail::execution_policy_base &exec,
- ForwardIterator first,
- ForwardIterator last,
- InputIterator values_first,
- InputIterator values_last,
- OutputIterator result);
-
-
-/*! \p binary_search is a vectorized version of binary search: for each
- * iterator \c v in [values_first, values_last) it attempts to
- * find the value *v in an ordered range [first, last).
- * It returns \c true if an element that is equivalent to \c value
- * is present in [first, last) and \c false if no such element
- * exists.
- *
- * \param first The beginning of the ordered sequence.
- * \param last The end of the ordered sequence.
- * \param values_first The beginning of the search values sequence.
- * \param values_last The end of the search values sequence.
- * \param result The beginning of the output sequence.
- *
- * \tparam ForwardIterator is a model of Forward Iterator.
- * \tparam InputIterator is a model of Input Iterator.
- * and \c InputIterator's \c value_type is LessThanComparable.
- * \tparam OutputIterator is a model of Output Iterator.
- * and bool is convertible to \c OutputIterator's \c value_type.
- *
- * \pre The ranges [first,last) and [result, result + (last - first)) shall not overlap.
- *
- * The following code snippet demonstrates how to use \p binary_search
- * to search for multiple values in a ordered range.
- *
- * \code
- * #include
- * #include
- * ...
- * thrust::device_vector input(5);
- *
- * input[0] = 0;
- * input[1] = 2;
- * input[2] = 5;
- * input[3] = 7;
- * input[4] = 8;
- *
- * thrust::device_vector values(6);
- * values[0] = 0;
- * values[1] = 1;
- * values[2] = 2;
- * values[3] = 3;
- * values[4] = 8;
- * values[5] = 9;
- *
- * thrust::device_vector output(6);
- *
- * thrust::binary_search(input.begin(), input.end(),
- * values.begin(), values.end(),
- * output.begin());
- *
- * // output is now [true, false, true, false, true, false]
- * \endcode
- *
- * \see http://www.sgi.com/tech/stl/binary_search.html
- * \see \p lower_bound
- * \see \p upper_bound
- * \see \p equal_range
- */
-template
-OutputIterator binary_search(ForwardIterator first,
- ForwardIterator last,
- InputIterator values_first,
- InputIterator values_last,
- OutputIterator result);
-
-
-/*! \p binary_search is a vectorized version of binary search: for each
- * iterator \c v in [values_first, values_last) it attempts to
- * find the value *v in an ordered range [first, last).
- * It returns \c true if an element that is equivalent to \c value
- * is present in [first, last) and \c false if no such element
- * exists. This version of \p binary_search uses function object
- * \c comp for comparison.
- *
- * The algorithm's execution is parallelized as determined by \p exec.
- *
- * \param exec The execution policy to use for parallelization.
- * \param first The beginning of the ordered sequence.
- * \param last The end of the ordered sequence.
- * \param values_first The beginning of the search values sequence.
- * \param values_last The end of the search values sequence.
- * \param result The beginning of the output sequence.
- * \param comp The comparison operator.
- *
- * \tparam DerivedPolicy The name of the derived execution policy.
- * \tparam ForwardIterator is a model of Forward Iterator.
- * \tparam InputIterator is a model of Input Iterator.
- * and \c InputIterator's \c value_type is LessThanComparable.
- * \tparam OutputIterator is a model of Output Iterator.
- * and bool is convertible to \c OutputIterator's \c value_type.
- * \tparam StrictWeakOrdering is a model of Strict Weak Ordering.
- *
- * \pre The ranges [first,last) and [result, result + (last - first)) shall not overlap.
- *
- * The following code snippet demonstrates how to use \p binary_search
- * to search for multiple values in a ordered range using the \p thrust::device execution policy for
- * parallelization:
- *
- * \code
- * #include
- * #include
- * #include
- * #include
- * ...
- * thrust::device_vector input(5);
- *
- * input[0] = 0;
- * input[1] = 2;
- * input[2] = 5;
- * input[3] = 7;
- * input[4] = 8;
- *
- * thrust::device_vector values(6);
- * values[0] = 0;
- * values[1] = 1;
- * values[2] = 2;
- * values[3] = 3;
- * values[4] = 8;
- * values[5] = 9;
- *
- * thrust::device_vector output(6);
- *
- * thrust::binary_search(thrust::device,
- * input.begin(), input.end(),
- * values.begin(), values.end(),
- * output.begin(),
- * thrust::less());
- *
- * // output is now [true, false, true, false, true, false]
- * \endcode
- *
- * \see http://www.sgi.com/tech/stl/binary_search.html
- * \see \p lower_bound
- * \see \p upper_bound
- * \see \p equal_range
- */
-template
-__host__ __device__
-OutputIterator binary_search(const thrust::detail::execution_policy_base &exec,
- ForwardIterator first,
- ForwardIterator last,
- InputIterator values_first,
- InputIterator values_last,
- OutputIterator result,
- StrictWeakOrdering comp);
-
-
-/*! \p binary_search is a vectorized version of binary search: for each
- * iterator \c v in [values_first, values_last) it attempts to
- * find the value *v in an ordered range [first, last).
- * It returns \c true if an element that is equivalent to \c value
- * is present in [first, last) and \c false if no such element
- * exists. This version of \p binary_search uses function object
- * \c comp for comparison.
- *
- * \param first The beginning of the ordered sequence.
- * \param last The end of the ordered sequence.
- * \param values_first The beginning of the search values sequence.
- * \param values_last The end of the search values sequence.
- * \param result The beginning of the output sequence.
- * \param comp The comparison operator.
- *
- * \tparam ForwardIterator is a model of Forward Iterator.
- * \tparam InputIterator is a model of Input Iterator.
- * and \c InputIterator's \c value_type is LessThanComparable.
- * \tparam OutputIterator is a model of Output Iterator.
- * and bool is convertible to \c OutputIterator's \c value_type.
- * \tparam StrictWeakOrdering is a model of Strict Weak Ordering.
- *
- * \pre The ranges [first,last) and [result, result + (last - first)) shall not overlap.
- *
- * The following code snippet demonstrates how to use \p binary_search
- * to search for multiple values in a ordered range.
- *
- * \code
- * #include
- * #include
- * #include
- * ...
- * thrust::device_vector input(5);
- *
- * input[0] = 0;
- * input[1] = 2;
- * input[2] = 5;
- * input[3] = 7;
- * input[4] = 8;
- *
- * thrust::device_vector values(6);
- * values[0] = 0;
- * values[1] = 1;
- * values[2] = 2;
- * values[3] = 3;
- * values[4] = 8;
- * values[5] = 9;
- *
- * thrust::device_vector output(6);
- *
- * thrust::binary_search(input.begin(), input.end(),
- * values.begin(), values.end(),
- * output.begin(),
- * thrust::less());
- *
- * // output is now [true, false, true, false, true, false]
- * \endcode
- *
- * \see http://www.sgi.com/tech/stl/binary_search.html
- * \see \p lower_bound
- * \see \p upper_bound
- * \see \p equal_range
- */
-template
-OutputIterator binary_search(ForwardIterator first,
- ForwardIterator last,
- InputIterator values_first,
- InputIterator values_last,
- OutputIterator result,
- StrictWeakOrdering comp);
-
-
-/*! \} // end vectorized_binary_search
- */
-
-
-/*! \} // end binary_search
- */
-
-
-/*! \} // end searching
- */
-
-
-} // end namespace thrust
-
-#include
-
diff --git a/spaces/ClearLove443/Robby-chatbot/modules/utils.py b/spaces/ClearLove443/Robby-chatbot/modules/utils.py
deleted file mode 100644
index d0b0288d3b65ea88bd4b6067be5c5af8804ee321..0000000000000000000000000000000000000000
--- a/spaces/ClearLove443/Robby-chatbot/modules/utils.py
+++ /dev/null
@@ -1,105 +0,0 @@
-import os
-import pandas as pd
-import streamlit as st
-import pdfplumber
-
-from modules.chatbot import Chatbot
-from modules.embedder import Embedder
-
-class Utilities:
-
- @staticmethod
- def load_api_key():
- """
- Loads the OpenAI API key from the .env file or
- from the user's input and returns it
- """
- if not hasattr(st.session_state, "api_key"):
- st.session_state.api_key = None
- #you can define your API key in .env directly
- if os.path.exists(".env") and os.environ.get("OPENAI_API_KEY") is not None:
- user_api_key = os.environ["OPENAI_API_KEY"]
- st.sidebar.success("API key loaded from .env", icon="🚀")
- else:
- if st.session_state.api_key is not None:
- user_api_key = st.session_state.api_key
- st.sidebar.success("API key loaded from previous input", icon="🚀")
- else:
- user_api_key = st.sidebar.text_input(
- label="#### Your OpenAI API key 👇", placeholder="sk-...", type="password"
- )
- if user_api_key:
- st.session_state.api_key = user_api_key
-
- return user_api_key
-
-
- @staticmethod
- def handle_upload(file_types):
- """
- Handles and display uploaded_file
- :param file_types: List of accepted file types, e.g., ["csv", "pdf", "txt"]
- """
- uploaded_file = st.sidebar.file_uploader("upload", type=file_types, label_visibility="collapsed")
- if uploaded_file is not None:
-
- def show_csv_file(uploaded_file):
- file_container = st.expander("Your CSV file :")
- uploaded_file.seek(0)
- shows = pd.read_csv(uploaded_file)
- file_container.write(shows)
-
- def show_pdf_file(uploaded_file):
- file_container = st.expander("Your PDF file :")
- with pdfplumber.open(uploaded_file) as pdf:
- pdf_text = ""
- for page in pdf.pages:
- pdf_text += page.extract_text() + "\n\n"
- file_container.write(pdf_text)
-
- def show_txt_file(uploaded_file):
- file_container = st.expander("Your TXT file:")
- uploaded_file.seek(0)
- content = uploaded_file.read().decode("utf-8")
- file_container.write(content)
-
- def get_file_extension(uploaded_file):
- return os.path.splitext(uploaded_file)[1].lower()
-
- file_extension = get_file_extension(uploaded_file.name)
-
- # Show the contents of the file based on its extension
- #if file_extension == ".csv" :
- # show_csv_file(uploaded_file)
- if file_extension== ".pdf" :
- show_pdf_file(uploaded_file)
- elif file_extension== ".txt" :
- show_txt_file(uploaded_file)
-
- else:
- st.session_state["reset_chat"] = True
-
- #print(uploaded_file)
- return uploaded_file
-
- @staticmethod
- def setup_chatbot(uploaded_file, model, temperature):
- """
- Sets up the chatbot with the uploaded file, model, and temperature
- """
- embeds = Embedder()
-
- with st.spinner("Processing..."):
- uploaded_file.seek(0)
- file = uploaded_file.read()
- # Get the document embeddings for the uploaded file
- vectors = embeds.getDocEmbeds(file, uploaded_file.name)
-
- # Create a Chatbot instance with the specified model and temperature
- chatbot = Chatbot(model, temperature,vectors)
- st.session_state["ready"] = True
-
- return chatbot
-
-
-
diff --git "a/spaces/Cong723/gpt-academic-public/crazy_functions/\346\200\273\347\273\223word\346\226\207\346\241\243.py" "b/spaces/Cong723/gpt-academic-public/crazy_functions/\346\200\273\347\273\223word\346\226\207\346\241\243.py"
deleted file mode 100644
index f1fe20171cc54aec0c79f4961e71b57845f252d5..0000000000000000000000000000000000000000
--- "a/spaces/Cong723/gpt-academic-public/crazy_functions/\346\200\273\347\273\223word\346\226\207\346\241\243.py"
+++ /dev/null
@@ -1,127 +0,0 @@
-from toolbox import update_ui
-from toolbox import CatchException, report_execption, write_results_to_file
-from .crazy_utils import request_gpt_model_in_new_thread_with_ui_alive
-fast_debug = False
-
-
-def 解析docx(file_manifest, project_folder, llm_kwargs, plugin_kwargs, chatbot, history, system_prompt):
- import time, os
- # pip install python-docx 用于docx格式,跨平台
- # pip install pywin32 用于doc格式,仅支持Win平台
- for index, fp in enumerate(file_manifest):
- if fp.split(".")[-1] == "docx":
- from docx import Document
- doc = Document(fp)
- file_content = "\n".join([para.text for para in doc.paragraphs])
- else:
- import win32com.client
- word = win32com.client.Dispatch("Word.Application")
- word.visible = False
- # 打开文件
- print('fp', os.getcwd())
- doc = word.Documents.Open(os.getcwd() + '/' + fp)
- # file_content = doc.Content.Text
- doc = word.ActiveDocument
- file_content = doc.Range().Text
- doc.Close()
- word.Quit()
-
- print(file_content)
- # private_upload里面的文件名在解压zip后容易出现乱码(rar和7z格式正常),故可以只分析文章内容,不输入文件名
- from .crazy_utils import breakdown_txt_to_satisfy_token_limit_for_pdf
- from request_llm.bridge_all import model_info
- max_token = model_info[llm_kwargs['llm_model']]['max_token']
- TOKEN_LIMIT_PER_FRAGMENT = max_token * 3 // 4
- paper_fragments = breakdown_txt_to_satisfy_token_limit_for_pdf(
- txt=file_content,
- get_token_fn=model_info[llm_kwargs['llm_model']]['token_cnt'],
- limit=TOKEN_LIMIT_PER_FRAGMENT
- )
- this_paper_history = []
- for i, paper_frag in enumerate(paper_fragments):
- i_say = f'请对下面的文章片段用中文做概述,文件名是{os.path.relpath(fp, project_folder)},文章内容是 ```{paper_frag}```'
- i_say_show_user = f'请对下面的文章片段做概述: {os.path.abspath(fp)}的第{i+1}/{len(paper_fragments)}个片段。'
- gpt_say = yield from request_gpt_model_in_new_thread_with_ui_alive(
- inputs=i_say,
- inputs_show_user=i_say_show_user,
- llm_kwargs=llm_kwargs,
- chatbot=chatbot,
- history=[],
- sys_prompt="总结文章。"
- )
-
- chatbot[-1] = (i_say_show_user, gpt_say)
- history.extend([i_say_show_user,gpt_say])
- this_paper_history.extend([i_say_show_user,gpt_say])
-
- # 已经对该文章的所有片段总结完毕,如果文章被切分了,
- if len(paper_fragments) > 1:
- i_say = f"根据以上的对话,总结文章{os.path.abspath(fp)}的主要内容。"
- gpt_say = yield from request_gpt_model_in_new_thread_with_ui_alive(
- inputs=i_say,
- inputs_show_user=i_say,
- llm_kwargs=llm_kwargs,
- chatbot=chatbot,
- history=this_paper_history,
- sys_prompt="总结文章。"
- )
-
- history.extend([i_say,gpt_say])
- this_paper_history.extend([i_say,gpt_say])
-
- res = write_results_to_file(history)
- chatbot.append(("完成了吗?", res))
- yield from update_ui(chatbot=chatbot, history=history) # 刷新界面
-
- res = write_results_to_file(history)
- chatbot.append(("所有文件都总结完成了吗?", res))
- yield from update_ui(chatbot=chatbot, history=history) # 刷新界面
-
-
-@CatchException
-def 总结word文档(txt, llm_kwargs, plugin_kwargs, chatbot, history, system_prompt, web_port):
- import glob, os
-
- # 基本信息:功能、贡献者
- chatbot.append([
- "函数插件功能?",
- "批量总结Word文档。函数插件贡献者: JasonGuo1"])
- yield from update_ui(chatbot=chatbot, history=history) # 刷新界面
-
- # 尝试导入依赖,如果缺少依赖,则给出安装建议
- try:
- from docx import Document
- except:
- report_execption(chatbot, history,
- a=f"解析项目: {txt}",
- b=f"导入软件依赖失败。使用该模块需要额外依赖,安装方法```pip install --upgrade python-docx pywin32```。")
- yield from update_ui(chatbot=chatbot, history=history) # 刷新界面
- return
-
- # 清空历史,以免输入溢出
- history = []
-
- # 检测输入参数,如没有给定输入参数,直接退出
- if os.path.exists(txt):
- project_folder = txt
- else:
- if txt == "": txt = '空空如也的输入栏'
- report_execption(chatbot, history, a=f"解析项目: {txt}", b=f"找不到本地项目或无权访问: {txt}")
- yield from update_ui(chatbot=chatbot, history=history) # 刷新界面
- return
-
- # 搜索需要处理的文件清单
- if txt.endswith('.docx') or txt.endswith('.doc'):
- file_manifest = [txt]
- else:
- file_manifest = [f for f in glob.glob(f'{project_folder}/**/*.docx', recursive=True)] + \
- [f for f in glob.glob(f'{project_folder}/**/*.doc', recursive=True)]
-
- # 如果没找到任何文件
- if len(file_manifest) == 0:
- report_execption(chatbot, history, a=f"解析项目: {txt}", b=f"找不到任何.docx或doc文件: {txt}")
- yield from update_ui(chatbot=chatbot, history=history) # 刷新界面
- return
-
- # 开始正式执行任务
- yield from 解析docx(file_manifest, project_folder, llm_kwargs, plugin_kwargs, chatbot, history, system_prompt)
diff --git a/spaces/Curranj/FlowerDiffusion/app.py b/spaces/Curranj/FlowerDiffusion/app.py
deleted file mode 100644
index 7b0b10379b315d319921da1edbe887397c29ff5a..0000000000000000000000000000000000000000
--- a/spaces/Curranj/FlowerDiffusion/app.py
+++ /dev/null
@@ -1,72 +0,0 @@
-import io
-import os
-import warnings
-
-from PIL import Image
-from stability_sdk import client
-import stability_sdk.interfaces.gooseai.generation.generation_pb2 as generation
-
-import gradio as gr
-stability_api = client.StabilityInference(
- key=os.environ["Secret"],
- verbose=True,
-)
-
-
-def infer(prompt):
- # the object returned is a python generator
- answers = stability_api.generate(
- prompt=f"Beautiful Portait of a {prompt} made out of flowers 💐 🌺 🌸 , artstation winner by Victo Ngai, Kilian Eng, vibrant colors, winning-award masterpiece, aesthetic octane render, 8K HD",
- height =640
- )
-
- # iterating over the generator produces the api response
- for resp in answers:
- for artifact in resp.artifacts:
- if artifact.finish_reason == generation.FILTER:
- warnings.warn(
- "Your request activated the API's safety filters and could not be processed."
- "Please modify the prompt and try again.")
- if artifact.type == generation.ARTIFACT_IMAGE:
- img = Image.open(io.BytesIO(artifact.binary))
- return img
-
-
-block = gr.Blocks(css=".container { max-width: 600px; margin: auto; }")
-
-num_samples = 1
-
-
-
-with block as demo:
- gr.Markdown("Flower Diffusion")
- gr.Markdown(
- "Get a pretty flowery image from any prompt - keep it simple!"
- )
- with gr.Group():
- with gr.Box():
- with gr.Row().style(mobile_collapse=False, equal_height=True):
-
- text = gr.Textbox(
- value = "Kitty cat",
- label="Enter your prompt", show_label=False, max_lines=1
- ).style(
- border=(True, False, True, True),
- rounded=(True, False, False, True),
- container=False,
- )
- btn = gr.Button("Run").style(
- margin=False,
- rounded=(False, True, True, False),
- )
-
-
- gallery = gr.Image()
- text.submit(infer, inputs=[text], outputs=gallery)
- btn.click(infer, inputs=[text], outputs=gallery)
-
-
-
-
-
-demo.launch(debug=True, enable_queue = True)
\ No newline at end of file
diff --git a/spaces/DQChoi/gpt-demo/venv/lib/python3.11/site-packages/PIL/BufrStubImagePlugin.py b/spaces/DQChoi/gpt-demo/venv/lib/python3.11/site-packages/PIL/BufrStubImagePlugin.py
deleted file mode 100644
index 0425bbd750eacf884ca1fc0ba8aa893a71ccdfc6..0000000000000000000000000000000000000000
--- a/spaces/DQChoi/gpt-demo/venv/lib/python3.11/site-packages/PIL/BufrStubImagePlugin.py
+++ /dev/null
@@ -1,73 +0,0 @@
-#
-# The Python Imaging Library
-# $Id$
-#
-# BUFR stub adapter
-#
-# Copyright (c) 1996-2003 by Fredrik Lundh
-#
-# See the README file for information on usage and redistribution.
-#
-
-from . import Image, ImageFile
-
-_handler = None
-
-
-def register_handler(handler):
- """
- Install application-specific BUFR image handler.
-
- :param handler: Handler object.
- """
- global _handler
- _handler = handler
-
-
-# --------------------------------------------------------------------
-# Image adapter
-
-
-def _accept(prefix):
- return prefix[:4] == b"BUFR" or prefix[:4] == b"ZCZC"
-
-
-class BufrStubImageFile(ImageFile.StubImageFile):
- format = "BUFR"
- format_description = "BUFR"
-
- def _open(self):
- offset = self.fp.tell()
-
- if not _accept(self.fp.read(4)):
- msg = "Not a BUFR file"
- raise SyntaxError(msg)
-
- self.fp.seek(offset)
-
- # make something up
- self.mode = "F"
- self._size = 1, 1
-
- loader = self._load()
- if loader:
- loader.open(self)
-
- def _load(self):
- return _handler
-
-
-def _save(im, fp, filename):
- if _handler is None or not hasattr(_handler, "save"):
- msg = "BUFR save handler not installed"
- raise OSError(msg)
- _handler.save(im, fp, filename)
-
-
-# --------------------------------------------------------------------
-# Registry
-
-Image.register_open(BufrStubImageFile.format, BufrStubImageFile, _accept)
-Image.register_save(BufrStubImageFile.format, _save)
-
-Image.register_extension(BufrStubImageFile.format, ".bufr")
diff --git a/spaces/DQChoi/gpt-demo/venv/lib/python3.11/site-packages/fontTools/subset/__main__.py b/spaces/DQChoi/gpt-demo/venv/lib/python3.11/site-packages/fontTools/subset/__main__.py
deleted file mode 100644
index decf9ee6e50a612c65a87ebeaa8be115f1d25242..0000000000000000000000000000000000000000
--- a/spaces/DQChoi/gpt-demo/venv/lib/python3.11/site-packages/fontTools/subset/__main__.py
+++ /dev/null
@@ -1,6 +0,0 @@
-import sys
-from fontTools.subset import main
-
-
-if __name__ == "__main__":
- sys.exit(main())
diff --git a/spaces/DQChoi/gpt-demo/venv/lib/python3.11/site-packages/gradio/templates/frontend/assets/Model3D-98fc2b2c.css b/spaces/DQChoi/gpt-demo/venv/lib/python3.11/site-packages/gradio/templates/frontend/assets/Model3D-98fc2b2c.css
deleted file mode 100644
index cee82ea831d77ca0e001baf10a07f84e176679f0..0000000000000000000000000000000000000000
--- a/spaces/DQChoi/gpt-demo/venv/lib/python3.11/site-packages/gradio/templates/frontend/assets/Model3D-98fc2b2c.css
+++ /dev/null
@@ -1 +0,0 @@
-.gallery.svelte-1ayixqk{padding:var(--size-1) var(--size-2)}
diff --git a/spaces/DaleChen/AutoGPT/autogpt/commands/web_playwright.py b/spaces/DaleChen/AutoGPT/autogpt/commands/web_playwright.py
deleted file mode 100644
index 4e388ded203cefb5e24f9116f7fe5b8a94893413..0000000000000000000000000000000000000000
--- a/spaces/DaleChen/AutoGPT/autogpt/commands/web_playwright.py
+++ /dev/null
@@ -1,80 +0,0 @@
-"""Web scraping commands using Playwright"""
-from __future__ import annotations
-
-try:
- from playwright.sync_api import sync_playwright
-except ImportError:
- print(
- "Playwright not installed. Please install it with 'pip install playwright' to use."
- )
-from bs4 import BeautifulSoup
-
-from autogpt.processing.html import extract_hyperlinks, format_hyperlinks
-
-
-def scrape_text(url: str) -> str:
- """Scrape text from a webpage
-
- Args:
- url (str): The URL to scrape text from
-
- Returns:
- str: The scraped text
- """
- with sync_playwright() as p:
- browser = p.chromium.launch()
- page = browser.new_page()
-
- try:
- page.goto(url)
- html_content = page.content()
- soup = BeautifulSoup(html_content, "html.parser")
-
- for script in soup(["script", "style"]):
- script.extract()
-
- text = soup.get_text()
- lines = (line.strip() for line in text.splitlines())
- chunks = (phrase.strip() for line in lines for phrase in line.split(" "))
- text = "\n".join(chunk for chunk in chunks if chunk)
-
- except Exception as e:
- text = f"Error: {str(e)}"
-
- finally:
- browser.close()
-
- return text
-
-
-def scrape_links(url: str) -> str | list[str]:
- """Scrape links from a webpage
-
- Args:
- url (str): The URL to scrape links from
-
- Returns:
- Union[str, List[str]]: The scraped links
- """
- with sync_playwright() as p:
- browser = p.chromium.launch()
- page = browser.new_page()
-
- try:
- page.goto(url)
- html_content = page.content()
- soup = BeautifulSoup(html_content, "html.parser")
-
- for script in soup(["script", "style"]):
- script.extract()
-
- hyperlinks = extract_hyperlinks(soup, url)
- formatted_links = format_hyperlinks(hyperlinks)
-
- except Exception as e:
- formatted_links = f"Error: {str(e)}"
-
- finally:
- browser.close()
-
- return formatted_links
diff --git a/spaces/DamianMH/Mlove/Dockerfile b/spaces/DamianMH/Mlove/Dockerfile
deleted file mode 100644
index 6c01c09373883afcb4ea34ae2d316cd596e1737b..0000000000000000000000000000000000000000
--- a/spaces/DamianMH/Mlove/Dockerfile
+++ /dev/null
@@ -1,21 +0,0 @@
-FROM node:18-bullseye-slim
-
-RUN apt-get update && \
-
-apt-get install -y git
-
-RUN git clone https://gitgud.io/khanon/oai-reverse-proxy.git /app
-
-WORKDIR /app
-
-RUN npm install
-
-COPY Dockerfile greeting.md* .env* ./
-
-RUN npm run build
-
-EXPOSE 7860
-
-ENV NODE_ENV=production
-
-CMD [ "npm", "start" ]
\ No newline at end of file
diff --git a/spaces/Datasculptor/DescriptionGPT/detic/modeling/meta_arch/d2_deformable_detr.py b/spaces/Datasculptor/DescriptionGPT/detic/modeling/meta_arch/d2_deformable_detr.py
deleted file mode 100644
index 47ff220fc3946d1bf68fad87076589e46b274ef3..0000000000000000000000000000000000000000
--- a/spaces/Datasculptor/DescriptionGPT/detic/modeling/meta_arch/d2_deformable_detr.py
+++ /dev/null
@@ -1,308 +0,0 @@
-# Copyright (c) Facebook, Inc. and its affiliates.
-import torch
-import torch.nn.functional as F
-from torch import nn
-import math
-
-from detectron2.modeling import META_ARCH_REGISTRY, build_backbone
-from detectron2.structures import Boxes, Instances
-from ..utils import load_class_freq, get_fed_loss_inds
-
-from models.backbone import Joiner
-from models.deformable_detr import DeformableDETR, SetCriterion, MLP
-from models.deformable_detr import _get_clones
-from models.matcher import HungarianMatcher
-from models.position_encoding import PositionEmbeddingSine
-from models.deformable_transformer import DeformableTransformer
-from models.segmentation import sigmoid_focal_loss
-from util.box_ops import box_cxcywh_to_xyxy, box_xyxy_to_cxcywh
-from util.misc import NestedTensor, accuracy
-
-
-__all__ = ["DeformableDetr"]
-
-class CustomSetCriterion(SetCriterion):
- def __init__(self, num_classes, matcher, weight_dict, losses, \
- focal_alpha=0.25, use_fed_loss=False):
- super().__init__(num_classes, matcher, weight_dict, losses, focal_alpha)
- self.use_fed_loss = use_fed_loss
- if self.use_fed_loss:
- self.register_buffer(
- 'fed_loss_weight', load_class_freq(freq_weight=0.5))
-
- def loss_labels(self, outputs, targets, indices, num_boxes, log=True):
- """Classification loss (NLL)
- targets dicts must contain the key "labels" containing a tensor of dim [nb_target_boxes]
- """
- assert 'pred_logits' in outputs
- src_logits = outputs['pred_logits']
-
- idx = self._get_src_permutation_idx(indices)
- target_classes_o = torch.cat([t["labels"][J] for t, (_, J) in zip(targets, indices)])
- target_classes = torch.full(src_logits.shape[:2], self.num_classes,
- dtype=torch.int64, device=src_logits.device)
- target_classes[idx] = target_classes_o
-
- target_classes_onehot = torch.zeros(
- [src_logits.shape[0], src_logits.shape[1], src_logits.shape[2] + 1],
- dtype=src_logits.dtype, layout=src_logits.layout,
- device=src_logits.device)
- target_classes_onehot.scatter_(2, target_classes.unsqueeze(-1), 1)
-
- target_classes_onehot = target_classes_onehot[:,:,:-1] # B x N x C
- if self.use_fed_loss:
- inds = get_fed_loss_inds(
- gt_classes=target_classes_o,
- num_sample_cats=50,
- weight=self.fed_loss_weight,
- C=target_classes_onehot.shape[2])
- loss_ce = sigmoid_focal_loss(
- src_logits[:, :, inds],
- target_classes_onehot[:, :, inds],
- num_boxes,
- alpha=self.focal_alpha,
- gamma=2) * src_logits.shape[1]
- else:
- loss_ce = sigmoid_focal_loss(
- src_logits, target_classes_onehot, num_boxes,
- alpha=self.focal_alpha,
- gamma=2) * src_logits.shape[1]
- losses = {'loss_ce': loss_ce}
-
- if log:
- # TODO this should probably be a separate loss, not hacked in this one here
- losses['class_error'] = 100 - accuracy(src_logits[idx], target_classes_o)[0]
- return losses
-
-
-class MaskedBackbone(nn.Module):
- """ This is a thin wrapper around D2's backbone to provide padding masking"""
-
- def __init__(self, cfg):
- super().__init__()
- self.backbone = build_backbone(cfg)
- backbone_shape = self.backbone.output_shape()
- self.feature_strides = [backbone_shape[f].stride for f in backbone_shape.keys()]
- self.strides = [backbone_shape[f].stride for f in backbone_shape.keys()]
- self.num_channels = [backbone_shape[x].channels for x in backbone_shape.keys()]
-
- def forward(self, tensor_list: NestedTensor):
- xs = self.backbone(tensor_list.tensors)
- out = {}
- for name, x in xs.items():
- m = tensor_list.mask
- assert m is not None
- mask = F.interpolate(m[None].float(), size=x.shape[-2:]).to(torch.bool)[0]
- out[name] = NestedTensor(x, mask)
- return out
-
-@META_ARCH_REGISTRY.register()
-class DeformableDetr(nn.Module):
- """
- Implement Deformable Detr
- """
-
- def __init__(self, cfg):
- super().__init__()
- self.with_image_labels = cfg.WITH_IMAGE_LABELS
- self.weak_weight = cfg.MODEL.DETR.WEAK_WEIGHT
-
- self.device = torch.device(cfg.MODEL.DEVICE)
- self.test_topk = cfg.TEST.DETECTIONS_PER_IMAGE
- self.num_classes = cfg.MODEL.DETR.NUM_CLASSES
- self.mask_on = cfg.MODEL.MASK_ON
- hidden_dim = cfg.MODEL.DETR.HIDDEN_DIM
- num_queries = cfg.MODEL.DETR.NUM_OBJECT_QUERIES
-
- # Transformer parameters:
- nheads = cfg.MODEL.DETR.NHEADS
- dropout = cfg.MODEL.DETR.DROPOUT
- dim_feedforward = cfg.MODEL.DETR.DIM_FEEDFORWARD
- enc_layers = cfg.MODEL.DETR.ENC_LAYERS
- dec_layers = cfg.MODEL.DETR.DEC_LAYERS
- num_feature_levels = cfg.MODEL.DETR.NUM_FEATURE_LEVELS
- two_stage = cfg.MODEL.DETR.TWO_STAGE
- with_box_refine = cfg.MODEL.DETR.WITH_BOX_REFINE
-
- # Loss parameters:
- giou_weight = cfg.MODEL.DETR.GIOU_WEIGHT
- l1_weight = cfg.MODEL.DETR.L1_WEIGHT
- deep_supervision = cfg.MODEL.DETR.DEEP_SUPERVISION
- cls_weight = cfg.MODEL.DETR.CLS_WEIGHT
- focal_alpha = cfg.MODEL.DETR.FOCAL_ALPHA
-
- N_steps = hidden_dim // 2
- d2_backbone = MaskedBackbone(cfg)
- backbone = Joiner(d2_backbone, PositionEmbeddingSine(N_steps, normalize=True))
-
- transformer = DeformableTransformer(
- d_model=hidden_dim,
- nhead=nheads,
- num_encoder_layers=enc_layers,
- num_decoder_layers=dec_layers,
- dim_feedforward=dim_feedforward,
- dropout=dropout,
- activation="relu",
- return_intermediate_dec=True,
- num_feature_levels=num_feature_levels,
- dec_n_points=4,
- enc_n_points=4,
- two_stage=two_stage,
- two_stage_num_proposals=num_queries)
-
- self.detr = DeformableDETR(
- backbone, transformer, num_classes=self.num_classes,
- num_queries=num_queries,
- num_feature_levels=num_feature_levels,
- aux_loss=deep_supervision,
- with_box_refine=with_box_refine,
- two_stage=two_stage,
- )
-
- if self.mask_on:
- assert 0, 'Mask is not supported yet :('
-
- matcher = HungarianMatcher(
- cost_class=cls_weight, cost_bbox=l1_weight, cost_giou=giou_weight)
- weight_dict = {"loss_ce": cls_weight, "loss_bbox": l1_weight}
- weight_dict["loss_giou"] = giou_weight
- if deep_supervision:
- aux_weight_dict = {}
- for i in range(dec_layers - 1):
- aux_weight_dict.update({k + f"_{i}": v for k, v in weight_dict.items()})
- weight_dict.update(aux_weight_dict)
- print('weight_dict', weight_dict)
- losses = ["labels", "boxes", "cardinality"]
- if self.mask_on:
- losses += ["masks"]
- self.criterion = CustomSetCriterion(
- self.num_classes, matcher=matcher, weight_dict=weight_dict,
- focal_alpha=focal_alpha,
- losses=losses,
- use_fed_loss=cfg.MODEL.DETR.USE_FED_LOSS
- )
- pixel_mean = torch.Tensor(cfg.MODEL.PIXEL_MEAN).to(self.device).view(3, 1, 1)
- pixel_std = torch.Tensor(cfg.MODEL.PIXEL_STD).to(self.device).view(3, 1, 1)
- self.normalizer = lambda x: (x - pixel_mean) / pixel_std
-
-
- def forward(self, batched_inputs):
- """
- Args:
- Returns:
- dict[str: Tensor]:
- mapping from a named loss to a tensor storing the loss. Used during training only.
- """
- images = self.preprocess_image(batched_inputs)
- output = self.detr(images)
- if self.training:
- gt_instances = [x["instances"].to(self.device) for x in batched_inputs]
- targets = self.prepare_targets(gt_instances)
- loss_dict = self.criterion(output, targets)
- weight_dict = self.criterion.weight_dict
- for k in loss_dict.keys():
- if k in weight_dict:
- loss_dict[k] *= weight_dict[k]
- if self.with_image_labels:
- if batched_inputs[0]['ann_type'] in ['image', 'captiontag']:
- loss_dict['loss_image'] = self.weak_weight * self._weak_loss(
- output, batched_inputs)
- else:
- loss_dict['loss_image'] = images[0].new_zeros(
- [1], dtype=torch.float32)[0]
- # import pdb; pdb.set_trace()
- return loss_dict
- else:
- image_sizes = output["pred_boxes"].new_tensor(
- [(t["height"], t["width"]) for t in batched_inputs])
- results = self.post_process(output, image_sizes)
- return results
-
-
- def prepare_targets(self, targets):
- new_targets = []
- for targets_per_image in targets:
- h, w = targets_per_image.image_size
- image_size_xyxy = torch.as_tensor([w, h, w, h], dtype=torch.float, device=self.device)
- gt_classes = targets_per_image.gt_classes
- gt_boxes = targets_per_image.gt_boxes.tensor / image_size_xyxy
- gt_boxes = box_xyxy_to_cxcywh(gt_boxes)
- new_targets.append({"labels": gt_classes, "boxes": gt_boxes})
- if self.mask_on and hasattr(targets_per_image, 'gt_masks'):
- assert 0, 'Mask is not supported yet :('
- gt_masks = targets_per_image.gt_masks
- gt_masks = convert_coco_poly_to_mask(gt_masks.polygons, h, w)
- new_targets[-1].update({'masks': gt_masks})
- return new_targets
-
-
- def post_process(self, outputs, target_sizes):
- """
- """
- out_logits, out_bbox = outputs['pred_logits'], outputs['pred_boxes']
- assert len(out_logits) == len(target_sizes)
- assert target_sizes.shape[1] == 2
-
- prob = out_logits.sigmoid()
- topk_values, topk_indexes = torch.topk(
- prob.view(out_logits.shape[0], -1), self.test_topk, dim=1)
- scores = topk_values
- topk_boxes = topk_indexes // out_logits.shape[2]
- labels = topk_indexes % out_logits.shape[2]
- boxes = box_cxcywh_to_xyxy(out_bbox)
- boxes = torch.gather(boxes, 1, topk_boxes.unsqueeze(-1).repeat(1,1,4))
-
- # and from relative [0, 1] to absolute [0, height] coordinates
- img_h, img_w = target_sizes.unbind(1)
- scale_fct = torch.stack([img_w, img_h, img_w, img_h], dim=1)
- boxes = boxes * scale_fct[:, None, :]
-
- results = []
- for s, l, b, size in zip(scores, labels, boxes, target_sizes):
- r = Instances((size[0], size[1]))
- r.pred_boxes = Boxes(b)
- r.scores = s
- r.pred_classes = l
- results.append({'instances': r})
- return results
-
-
- def preprocess_image(self, batched_inputs):
- """
- Normalize, pad and batch the input images.
- """
- images = [self.normalizer(x["image"].to(self.device)) for x in batched_inputs]
- return images
-
-
- def _weak_loss(self, outputs, batched_inputs):
- loss = 0
- for b, x in enumerate(batched_inputs):
- labels = x['pos_category_ids']
- pred_logits = [outputs['pred_logits'][b]]
- pred_boxes = [outputs['pred_boxes'][b]]
- for xx in outputs['aux_outputs']:
- pred_logits.append(xx['pred_logits'][b])
- pred_boxes.append(xx['pred_boxes'][b])
- pred_logits = torch.stack(pred_logits, dim=0) # L x N x C
- pred_boxes = torch.stack(pred_boxes, dim=0) # L x N x 4
- for label in labels:
- loss += self._max_size_loss(
- pred_logits, pred_boxes, label) / len(labels)
- loss = loss / len(batched_inputs)
- return loss
-
-
- def _max_size_loss(self, logits, boxes, label):
- '''
- Inputs:
- logits: L x N x C
- boxes: L x N x 4
- '''
- target = logits.new_zeros((logits.shape[0], logits.shape[2]))
- target[:, label] = 1.
- sizes = boxes[..., 2] * boxes[..., 3] # L x N
- ind = sizes.argmax(dim=1) # L
- loss = F.binary_cross_entropy_with_logits(
- logits[range(len(ind)), ind], target, reduction='sum')
- return loss
\ No newline at end of file
diff --git a/spaces/Datasculptor/StyleGAN-NADA/e4e/options/__init__.py b/spaces/Datasculptor/StyleGAN-NADA/e4e/options/__init__.py
deleted file mode 100644
index e69de29bb2d1d6434b8b29ae775ad8c2e48c5391..0000000000000000000000000000000000000000
diff --git a/spaces/Dinoking/Guccio-AI-Designer/decomposition.py b/spaces/Dinoking/Guccio-AI-Designer/decomposition.py
deleted file mode 100644
index 4819e3324707f15c33fba6f35ab6abdc66dea919..0000000000000000000000000000000000000000
--- a/spaces/Dinoking/Guccio-AI-Designer/decomposition.py
+++ /dev/null
@@ -1,402 +0,0 @@
-# Copyright 2020 Erik Härkönen. All rights reserved.
-# This file is licensed to you under the Apache License, Version 2.0 (the "License");
-# you may not use this file except in compliance with the License. You may obtain a copy
-# of the License at http://www.apache.org/licenses/LICENSE-2.0
-
-# Unless required by applicable law or agreed to in writing, software distributed under
-# the License is distributed on an "AS IS" BASIS, WITHOUT WARRANTIES OR REPRESENTATIONS
-# OF ANY KIND, either express or implied. See the License for the specific language
-# governing permissions and limitations under the License.
-
-# Patch for broken CTRL+C handler
-# https://github.com/ContinuumIO/anaconda-issues/issues/905
-import os
-os.environ['FOR_DISABLE_CONSOLE_CTRL_HANDLER'] = '1'
-
-import numpy as np
-import os
-from pathlib import Path
-import re
-import sys
-import datetime
-import argparse
-import torch
-import json
-from types import SimpleNamespace
-import scipy
-from scipy.cluster.vq import kmeans
-from tqdm import trange
-from netdissect.nethook import InstrumentedModel
-from config import Config
-from estimators import get_estimator
-from models import get_instrumented_model
-
-SEED_SAMPLING = 1
-SEED_RANDOM_DIRS = 2
-SEED_LINREG = 3
-SEED_VISUALIZATION = 5
-
-B = 20
-n_clusters = 500
-
-def get_random_dirs(components, dimensions):
- gen = np.random.RandomState(seed=SEED_RANDOM_DIRS)
- dirs = gen.normal(size=(components, dimensions))
- dirs /= np.sqrt(np.sum(dirs**2, axis=1, keepdims=True))
- return dirs.astype(np.float32)
-
-# Compute maximum batch size for given VRAM and network
-def get_max_batch_size(inst, device, layer_name=None):
- inst.remove_edits()
-
- # Reset statistics
- torch.cuda.reset_max_memory_cached(device)
- torch.cuda.reset_max_memory_allocated(device)
- total_mem = torch.cuda.get_device_properties(device).total_memory
-
- B_max = 20
-
- # Measure actual usage
- for i in range(2, B_max, 2):
- z = inst.model.sample_latent(n_samples=i)
- if layer_name:
- inst.model.partial_forward(z, layer_name)
- else:
- inst.model.forward(z)
-
- maxmem = torch.cuda.max_memory_allocated(device)
- del z
-
- if maxmem > 0.5*total_mem:
- print('Batch size {:d}: memory usage {:.0f}MB'.format(i, maxmem / 1e6))
- return i
-
- return B_max
-
-# Solve for directions in latent space that match PCs in activaiton space
-def linreg_lstsq(comp_np, mean_np, stdev_np, inst, config):
- print('Performing least squares regression', flush=True)
-
- torch.manual_seed(SEED_LINREG)
- np.random.seed(SEED_LINREG)
-
- comp = torch.from_numpy(comp_np).float().to(inst.model.device)
- mean = torch.from_numpy(mean_np).float().to(inst.model.device)
- stdev = torch.from_numpy(stdev_np).float().to(inst.model.device)
-
- n_samp = max(10_000, config.n) // B * B # make divisible
- n_comp = comp.shape[0]
- latent_dims = inst.model.get_latent_dims()
-
- # We're looking for M s.t. M*P*G'(Z) = Z => M*A = Z
- # Z = batch of latent vectors (n_samples x latent_dims)
- # G'(Z) = batch of activations at intermediate layer
- # A = P*G'(Z) = projected activations (n_samples x pca_coords)
- # M = linear mapping (pca_coords x latent_dims)
-
- # Minimization min_M ||MA - Z||_l2 rewritten as min_M.T ||A.T*M.T - Z.T||_l2
- # to match format expected by pytorch.lstsq
-
- # TODO: regression on pixel-space outputs? (using nonlinear optimizer)
- # min_M lpips(G_full(MA), G_full(Z))
-
- # Tensors to fill with data
- # Dimensions other way around, so these are actually the transposes
- A = np.zeros((n_samp, n_comp), dtype=np.float32)
- Z = np.zeros((n_samp, latent_dims), dtype=np.float32)
-
- # Project tensor X onto PCs, return coordinates
- def project(X, comp):
- N = X.shape[0]
- K = comp.shape[0]
- coords = torch.bmm(comp.expand([N]+[-1]*comp.ndim), X.view(N, -1, 1))
- return coords.reshape(N, K)
-
- for i in trange(n_samp // B, desc='Collecting samples', ascii=True):
- z = inst.model.sample_latent(B)
- inst.model.partial_forward(z, config.layer)
- act = inst.retained_features()[config.layer].reshape(B, -1)
-
- # Project onto basis
- act = act - mean
- coords = project(act, comp)
- coords_scaled = coords / stdev
-
- A[i*B:(i+1)*B] = coords_scaled.detach().cpu().numpy()
- Z[i*B:(i+1)*B] = z.detach().cpu().numpy().reshape(B, -1)
-
- # Solve least squares fit
-
- # gelsd = divide-and-conquer SVD; good default
- # gelsy = complete orthogonal factorization; sometimes faster
- # gelss = SVD; slow but less memory hungry
- M_t = scipy.linalg.lstsq(A, Z, lapack_driver='gelsd')[0] # torch.lstsq(Z, A)[0][:n_comp, :]
-
- # Solution given by rows of M_t
- Z_comp = M_t[:n_comp, :]
- Z_mean = np.mean(Z, axis=0, keepdims=True)
-
- return Z_comp, Z_mean
-
-def regression(comp, mean, stdev, inst, config):
- # Sanity check: verify orthonormality
- M = np.dot(comp, comp.T)
- if not np.allclose(M, np.identity(M.shape[0])):
- det = np.linalg.det(M)
- print(f'WARNING: Computed basis is not orthonormal (determinant={det})')
-
- return linreg_lstsq(comp, mean, stdev, inst, config)
-
-def compute(config, dump_name, instrumented_model):
- global B
-
- timestamp = lambda : datetime.datetime.now().strftime("%d.%m %H:%M")
- print(f'[{timestamp()}] Computing', dump_name.name)
-
- # Ensure reproducibility
- torch.manual_seed(0) # also sets cuda seeds
- np.random.seed(0)
-
- # Speed up backend
- torch.backends.cudnn.benchmark = True
-
- has_gpu = torch.cuda.is_available()
- device = torch.device('cuda' if has_gpu else 'cpu')
- layer_key = config.layer
-
- if instrumented_model is None:
- inst = get_instrumented_model(config.model, config.output_class, layer_key, device)
- model = inst.model
- else:
- print('Reusing InstrumentedModel instance')
- inst = instrumented_model
- model = inst.model
- inst.remove_edits()
- model.set_output_class(config.output_class)
-
- # Regress back to w space
- if config.use_w:
- print('Using W latent space')
- model.use_w()
-
- inst.retain_layer(layer_key)
- model.partial_forward(model.sample_latent(1), layer_key)
- sample_shape = inst.retained_features()[layer_key].shape
- sample_dims = np.prod(sample_shape)
- print('Feature shape:', sample_shape)
-
- input_shape = inst.model.get_latent_shape()
- input_dims = inst.model.get_latent_dims()
-
- config.components = min(config.components, sample_dims)
- transformer = get_estimator(config.estimator, config.components, config.sparsity)
-
- X = None
- X_global_mean = None
-
- # Figure out batch size if not provided
- B = config.batch_size or get_max_batch_size(inst, device, layer_key)
-
- # Divisible by B (ignored in output name)
- N = config.n // B * B
-
- # Compute maximum batch size based on RAM + pagefile budget
- target_bytes = 20 * 1_000_000_000 # GB
- feat_size_bytes = sample_dims * np.dtype('float64').itemsize
- N_limit_RAM = np.floor_divide(target_bytes, feat_size_bytes)
- if not transformer.batch_support and N > N_limit_RAM:
- print('WARNING: estimator does not support batching, ' \
- 'given config will use {:.1f} GB memory.'.format(feat_size_bytes / 1_000_000_000 * N))
-
- # 32-bit LAPACK gets very unhappy about huge matrices (in linalg.svd)
- if config.estimator == 'ica':
- lapack_max_N = np.floor_divide(np.iinfo(np.int32).max // 4, sample_dims) # 4x extra buffer
- if N > lapack_max_N:
- raise RuntimeError(f'Matrices too large for ICA, please use N <= {lapack_max_N}')
-
- print('B={}, N={}, dims={}, N/dims={:.1f}'.format(B, N, sample_dims, N/sample_dims), flush=True)
-
- # Must not depend on chosen batch size (reproducibility)
- NB = max(B, max(2_000, 3*config.components)) # ipca: as large as possible!
-
- samples = None
- if not transformer.batch_support:
- samples = np.zeros((N + NB, sample_dims), dtype=np.float32)
-
- torch.manual_seed(config.seed or SEED_SAMPLING)
- np.random.seed(config.seed or SEED_SAMPLING)
-
- # Use exactly the same latents regardless of batch size
- # Store in main memory, since N might be huge (1M+)
- # Run in batches, since sample_latent() might perform Z -> W mapping
- n_lat = ((N + NB - 1) // B + 1) * B
- latents = np.zeros((n_lat, *input_shape[1:]), dtype=np.float32)
- with torch.no_grad():
- for i in trange(n_lat // B, desc='Sampling latents'):
- latents[i*B:(i+1)*B] = model.sample_latent(n_samples=B).cpu().numpy()
-
- # Decomposition on non-Gaussian latent space
- samples_are_latents = layer_key in ['g_mapping', 'style'] and inst.model.latent_space_name() == 'W'
-
- canceled = False
- try:
- X = np.ones((NB, sample_dims), dtype=np.float32)
- action = 'Fitting' if transformer.batch_support else 'Collecting'
- for gi in trange(0, N, NB, desc=f'{action} batches (NB={NB})', ascii=True):
- for mb in range(0, NB, B):
- z = torch.from_numpy(latents[gi+mb:gi+mb+B]).to(device)
-
- if samples_are_latents:
- # Decomposition on latents directly (e.g. StyleGAN W)
- batch = z.reshape((B, -1))
- else:
- # Decomposition on intermediate layer
- with torch.no_grad():
- model.partial_forward(z, layer_key)
-
- # Permuted to place PCA dimensions last
- batch = inst.retained_features()[layer_key].reshape((B, -1))
-
- space_left = min(B, NB - mb)
- X[mb:mb+space_left] = batch.cpu().numpy()[:space_left]
-
- if transformer.batch_support:
- if not transformer.fit_partial(X.reshape(-1, sample_dims)):
- break
- else:
- samples[gi:gi+NB, :] = X.copy()
- except KeyboardInterrupt:
- if not transformer.batch_support:
- sys.exit(1) # no progress yet
-
- dump_name = dump_name.parent / dump_name.name.replace(f'n{N}', f'n{gi}')
- print(f'Saving current state to "{dump_name.name}" before exiting')
- canceled = True
-
- if not transformer.batch_support:
- X = samples # Use all samples
- X_global_mean = X.mean(axis=0, keepdims=True, dtype=np.float32) # TODO: activations surely multi-modal...!
- X -= X_global_mean
-
- print(f'[{timestamp()}] Fitting whole batch')
- t_start_fit = datetime.datetime.now()
-
- transformer.fit(X)
-
- print(f'[{timestamp()}] Done in {datetime.datetime.now() - t_start_fit}')
- assert np.all(transformer.transformer.mean_ < 1e-3), 'Mean of normalized data should be zero'
- else:
- X_global_mean = transformer.transformer.mean_.reshape((1, sample_dims))
- X = X.reshape(-1, sample_dims)
- X -= X_global_mean
-
- X_comp, X_stdev, X_var_ratio = transformer.get_components()
-
- assert X_comp.shape[1] == sample_dims \
- and X_comp.shape[0] == config.components \
- and X_global_mean.shape[1] == sample_dims \
- and X_stdev.shape[0] == config.components, 'Invalid shape'
-
- # 'Activations' are really latents in a secondary latent space
- if samples_are_latents:
- Z_comp = X_comp
- Z_global_mean = X_global_mean
- else:
- Z_comp, Z_global_mean = regression(X_comp, X_global_mean, X_stdev, inst, config)
-
- # Normalize
- Z_comp /= np.linalg.norm(Z_comp, axis=-1, keepdims=True)
-
- # Random projections
- # We expect these to explain much less of the variance
- random_dirs = get_random_dirs(config.components, np.prod(sample_shape))
- n_rand_samples = min(5000, X.shape[0])
- X_view = X[:n_rand_samples, :].T
- assert np.shares_memory(X_view, X), "Error: slice produced copy"
- X_stdev_random = np.dot(random_dirs, X_view).std(axis=1)
-
- # Inflate back to proper shapes (for easier broadcasting)
- X_comp = X_comp.reshape(-1, *sample_shape)
- X_global_mean = X_global_mean.reshape(sample_shape)
- Z_comp = Z_comp.reshape(-1, *input_shape)
- Z_global_mean = Z_global_mean.reshape(input_shape)
-
- # Compute stdev in latent space if non-Gaussian
- lat_stdev = np.ones_like(X_stdev)
- if config.use_w:
- samples = model.sample_latent(5000).reshape(5000, input_dims).detach().cpu().numpy()
- coords = np.dot(Z_comp.reshape(-1, input_dims), samples.T)
- lat_stdev = coords.std(axis=1)
-
- os.makedirs(dump_name.parent, exist_ok=True)
- np.savez_compressed(dump_name, **{
- 'act_comp': X_comp.astype(np.float32),
- 'act_mean': X_global_mean.astype(np.float32),
- 'act_stdev': X_stdev.astype(np.float32),
- 'lat_comp': Z_comp.astype(np.float32),
- 'lat_mean': Z_global_mean.astype(np.float32),
- 'lat_stdev': lat_stdev.astype(np.float32),
- 'var_ratio': X_var_ratio.astype(np.float32),
- 'random_stdevs': X_stdev_random.astype(np.float32),
- })
-
- if canceled:
- sys.exit(1)
-
- # Don't shutdown if passed as param
- if instrumented_model is None:
- inst.close()
- del inst
- del model
-
- del X
- del X_comp
- del random_dirs
- del batch
- del samples
- del latents
- torch.cuda.empty_cache()
-
-# Return cached results or commpute if needed
-# Pass existing InstrumentedModel instance to reuse it
-def get_or_compute(config, model=None, submit_config=None, force_recompute=False):
- if submit_config is None:
- wrkdir = str(Path(__file__).parent.resolve())
- submit_config = SimpleNamespace(run_dir_root = wrkdir, run_dir = wrkdir)
-
- # Called directly by run.py
- return _compute(submit_config, config, model, force_recompute)
-
-def _compute(submit_config, config, model=None, force_recompute=False):
- basedir = Path(submit_config.run_dir)
- outdir = basedir / 'out'
-
- if config.n is None:
- raise RuntimeError('Must specify number of samples with -n=XXX')
-
- if model and not isinstance(model, InstrumentedModel):
- raise RuntimeError('Passed model has to be wrapped in "InstrumentedModel"')
-
- if config.use_w and not 'StyleGAN' in config.model:
- raise RuntimeError(f'Cannot change latent space of non-StyleGAN model {config.model}')
-
- transformer = get_estimator(config.estimator, config.components, config.sparsity)
- dump_name = "{}-{}_{}_{}_n{}{}{}.npz".format(
- config.model.lower(),
- config.output_class.replace(' ', '_'),
- config.layer.lower(),
- transformer.get_param_str(),
- config.n,
- '_w' if config.use_w else '',
- f'_seed{config.seed}' if config.seed else ''
- )
-
- dump_path = basedir / 'cache' / 'components' / dump_name
-
- if not dump_path.is_file() or force_recompute:
- print('Not cached')
- t_start = datetime.datetime.now()
- compute(config, dump_path, model)
- print('Total time:', datetime.datetime.now() - t_start)
-
- return dump_path
\ No newline at end of file
diff --git a/spaces/Disguised/anime_character_recognizer/app.py b/spaces/Disguised/anime_character_recognizer/app.py
deleted file mode 100644
index 662b79156ca568397324ce9f05f54fd0284c47e7..0000000000000000000000000000000000000000
--- a/spaces/Disguised/anime_character_recognizer/app.py
+++ /dev/null
@@ -1,20 +0,0 @@
-import gradio as gr
-from fastai.vision.all import *
-import gradio as gr
-import re
-from glob import glob
-
-learn = load_learner('model_ft15(extra).pkl')
-
-categories = learn.dls.vocab
-
-def classify_image(img):
- pred,idx,probs = learn.predict(img)
- return dict(zip(categories, map(float,probs)))
-
-image = gr.inputs.Image(shape=(192, 192))
-label = gr.outputs.Label()
-
-
-intf = gr.Interface(fn=classify_image, inputs=image, outputs=label, examples='./examples')
-intf.launch(inline=False)
\ No newline at end of file
diff --git a/spaces/Dorado607/ChuanhuChatGPT/modules/config.py b/spaces/Dorado607/ChuanhuChatGPT/modules/config.py
deleted file mode 100644
index 115312dd2ec4e0bd99eb8b5869b2f0aeed649039..0000000000000000000000000000000000000000
--- a/spaces/Dorado607/ChuanhuChatGPT/modules/config.py
+++ /dev/null
@@ -1,269 +0,0 @@
-from collections import defaultdict
-from contextlib import contextmanager
-import os
-import logging
-import sys
-import commentjson as json
-
-from . import shared
-from . import presets
-
-
-__all__ = [
- "my_api_key",
- "sensitive_id",
- "authflag",
- "auth_list",
- "dockerflag",
- "retrieve_proxy",
- "log_level",
- "advance_docs",
- "update_doc_config",
- "usage_limit",
- "multi_api_key",
- "server_name",
- "server_port",
- "share",
- "check_update",
- "latex_delimiters_set",
- "hide_history_when_not_logged_in",
- "default_chuanhu_assistant_model",
- "show_api_billing"
-]
-
-# 添加一个统一的config文件,避免文件过多造成的疑惑(优先级最低)
-# 同时,也可以为后续支持自定义功能提供config的帮助
-if os.path.exists("config.json"):
- with open("config.json", "r", encoding='utf-8') as f:
- config = json.load(f)
-else:
- config = {}
-
-
-def load_config_to_environ(key_list):
- global config
- for key in key_list:
- if key in config:
- os.environ[key.upper()] = os.environ.get(key.upper(), config[key])
-
-
-lang_config = config.get("language", "auto")
-language = os.environ.get("LANGUAGE", lang_config)
-
-hide_history_when_not_logged_in = config.get(
- "hide_history_when_not_logged_in", False)
-check_update = config.get("check_update", True)
-show_api_billing = config.get("show_api_billing", False)
-show_api_billing = bool(os.environ.get("SHOW_API_BILLING", show_api_billing))
-
-if os.path.exists("api_key.txt"):
- logging.info("检测到api_key.txt文件,正在进行迁移...")
- with open("api_key.txt", "r", encoding="utf-8") as f:
- config["openai_api_key"] = f.read().strip()
- os.rename("api_key.txt", "api_key(deprecated).txt")
- with open("config.json", "w", encoding='utf-8') as f:
- json.dump(config, f, indent=4, ensure_ascii=False)
-
-if os.path.exists("auth.json"):
- logging.info("检测到auth.json文件,正在进行迁移...")
- auth_list = []
- with open("auth.json", "r", encoding='utf-8') as f:
- auth = json.load(f)
- for _ in auth:
- if auth[_]["username"] and auth[_]["password"]:
- auth_list.append((auth[_]["username"], auth[_]["password"]))
- else:
- logging.error("请检查auth.json文件中的用户名和密码!")
- sys.exit(1)
- config["users"] = auth_list
- os.rename("auth.json", "auth(deprecated).json")
- with open("config.json", "w", encoding='utf-8') as f:
- json.dump(config, f, indent=4, ensure_ascii=False)
-
-# 处理docker if we are running in Docker
-dockerflag = config.get("dockerflag", False)
-if os.environ.get("dockerrun") == "yes":
- dockerflag = True
-
-# 处理 api-key 以及 允许的用户列表
-my_api_key = config.get("openai_api_key", "")
-my_api_key = os.environ.get("OPENAI_API_KEY", my_api_key)
-os.environ["OPENAI_API_KEY"] = my_api_key
-os.environ["OPENAI_EMBEDDING_API_KEY"] = my_api_key
-
-if config.get("legacy_api_usage", False):
- sensitive_id = config.get("sensitive_id", "")
- sensitive_id = os.environ.get("SENSITIVE_ID", sensitive_id)
-else:
- sensitive_id = my_api_key
-
-google_palm_api_key = config.get("google_palm_api_key", "")
-google_palm_api_key = os.environ.get(
- "GOOGLE_PALM_API_KEY", google_palm_api_key)
-os.environ["GOOGLE_PALM_API_KEY"] = google_palm_api_key
-
-xmchat_api_key = config.get("xmchat_api_key", "")
-os.environ["XMCHAT_API_KEY"] = xmchat_api_key
-
-minimax_api_key = config.get("minimax_api_key", "")
-os.environ["MINIMAX_API_KEY"] = minimax_api_key
-minimax_group_id = config.get("minimax_group_id", "")
-os.environ["MINIMAX_GROUP_ID"] = minimax_group_id
-
-load_config_to_environ(["openai_api_type", "azure_openai_api_key", "azure_openai_api_base_url",
- "azure_openai_api_version", "azure_deployment_name", "azure_embedding_deployment_name", "azure_embedding_model_name"])
-
-
-usage_limit = os.environ.get("USAGE_LIMIT", config.get("usage_limit", 120))
-
-# 多账户机制
-multi_api_key = config.get("multi_api_key", False) # 是否开启多账户机制
-if multi_api_key:
- api_key_list = config.get("api_key_list", [])
- if len(api_key_list) == 0:
- logging.error("多账号模式已开启,但api_key_list为空,请检查config.json")
- sys.exit(1)
- shared.state.set_api_key_queue(api_key_list)
-
-auth_list = config.get("users", []) # 实际上是使用者的列表
-authflag = len(auth_list) > 0 # 是否开启认证的状态值,改为判断auth_list长度
-
-# 处理自定义的api_host,优先读环境变量的配置,如果存在则自动装配
-api_host = os.environ.get(
- "OPENAI_API_BASE", config.get("openai_api_base", None))
-if api_host is not None:
- shared.state.set_api_host(api_host)
- os.environ["OPENAI_API_BASE"] = f"{api_host}/v1"
- logging.info(f"OpenAI API Base set to: {os.environ['OPENAI_API_BASE']}")
-
-default_chuanhu_assistant_model = config.get(
- "default_chuanhu_assistant_model", "gpt-3.5-turbo")
-for x in ["GOOGLE_CSE_ID", "GOOGLE_API_KEY", "WOLFRAM_ALPHA_APPID", "SERPAPI_API_KEY"]:
- if config.get(x, None) is not None:
- os.environ[x] = config[x]
-
-
-@contextmanager
-def retrieve_openai_api(api_key=None):
- old_api_key = os.environ.get("OPENAI_API_KEY", "")
- if api_key is None:
- os.environ["OPENAI_API_KEY"] = my_api_key
- yield my_api_key
- else:
- os.environ["OPENAI_API_KEY"] = api_key
- yield api_key
- os.environ["OPENAI_API_KEY"] = old_api_key
-
-
-# 处理log
-log_level = config.get("log_level", "INFO")
-logging.basicConfig(
- level=log_level,
- format="%(asctime)s [%(levelname)s] [%(filename)s:%(lineno)d] %(message)s",
-)
-
-# 处理代理:
-http_proxy = os.environ.get("HTTP_PROXY", "")
-https_proxy = os.environ.get("HTTPS_PROXY", "")
-http_proxy = config.get("http_proxy", http_proxy)
-https_proxy = config.get("https_proxy", https_proxy)
-
-# 重置系统变量,在不需要设置的时候不设置环境变量,以免引起全局代理报错
-os.environ["HTTP_PROXY"] = ""
-os.environ["HTTPS_PROXY"] = ""
-
-local_embedding = config.get("local_embedding", False) # 是否使用本地embedding
-
-
-@contextmanager
-def retrieve_proxy(proxy=None):
- """
- 1, 如果proxy = NONE,设置环境变量,并返回最新设置的代理
- 2,如果proxy != NONE,更新当前的代理配置,但是不更新环境变量
- """
- global http_proxy, https_proxy
- if proxy is not None:
- http_proxy = proxy
- https_proxy = proxy
- yield http_proxy, https_proxy
- else:
- old_var = os.environ["HTTP_PROXY"], os.environ["HTTPS_PROXY"]
- os.environ["HTTP_PROXY"] = http_proxy
- os.environ["HTTPS_PROXY"] = https_proxy
- yield http_proxy, https_proxy # return new proxy
-
- # return old proxy
- os.environ["HTTP_PROXY"], os.environ["HTTPS_PROXY"] = old_var
-
-
-# 处理latex options
-user_latex_option = config.get("latex_option", "default")
-if user_latex_option == "default":
- latex_delimiters_set = [
- {"left": "$$", "right": "$$", "display": True},
- {"left": "$", "right": "$", "display": False},
- {"left": "\\(", "right": "\\)", "display": False},
- {"left": "\\[", "right": "\\]", "display": True},
- ]
-elif user_latex_option == "strict":
- latex_delimiters_set = [
- {"left": "$$", "right": "$$", "display": True},
- {"left": "\\(", "right": "\\)", "display": False},
- {"left": "\\[", "right": "\\]", "display": True},
- ]
-elif user_latex_option == "all":
- latex_delimiters_set = [
- {"left": "$$", "right": "$$", "display": True},
- {"left": "$", "right": "$", "display": False},
- {"left": "\\(", "right": "\\)", "display": False},
- {"left": "\\[", "right": "\\]", "display": True},
- {"left": "\\begin{equation}", "right": "\\end{equation}", "display": True},
- {"left": "\\begin{align}", "right": "\\end{align}", "display": True},
- {"left": "\\begin{alignat}", "right": "\\end{alignat}", "display": True},
- {"left": "\\begin{gather}", "right": "\\end{gather}", "display": True},
- {"left": "\\begin{CD}", "right": "\\end{CD}", "display": True},
- ]
-elif user_latex_option == "disabled":
- latex_delimiters_set = []
-else:
- latex_delimiters_set = [
- {"left": "$$", "right": "$$", "display": True},
- {"left": "$", "right": "$", "display": False},
- {"left": "\\(", "right": "\\)", "display": False},
- {"left": "\\[", "right": "\\]", "display": True},
- ]
-
-# 处理advance docs
-advance_docs = defaultdict(lambda: defaultdict(dict))
-advance_docs.update(config.get("advance_docs", {}))
-
-
-def update_doc_config(two_column_pdf):
- global advance_docs
- advance_docs["pdf"]["two_column"] = two_column_pdf
-
- logging.info(f"更新后的文件参数为:{advance_docs}")
-
-
-# 处理gradio.launch参数
-server_name = config.get("server_name", None)
-server_port = config.get("server_port", None)
-if server_name is None:
- if dockerflag:
- server_name = "0.0.0.0"
- else:
- server_name = "127.0.0.1"
-if server_port is None:
- if dockerflag:
- server_port = 7860
-
-assert server_port is None or type(server_port) == int, "要求port设置为int类型"
-
-# 设置默认model
-default_model = config.get("default_model", "")
-try:
- presets.DEFAULT_MODEL = presets.MODELS.index(default_model)
-except ValueError:
- pass
-
-share = config.get("share", False)
diff --git a/spaces/Drac77/hakurei-waifu-diffusion/app.py b/spaces/Drac77/hakurei-waifu-diffusion/app.py
deleted file mode 100644
index ccef706bf3035fe470bf6a4f5bd701b18bf59133..0000000000000000000000000000000000000000
--- a/spaces/Drac77/hakurei-waifu-diffusion/app.py
+++ /dev/null
@@ -1,3 +0,0 @@
-import gradio as gr
-
-gr.Interface.load("models/hakurei/waifu-diffusion").launch()
\ No newline at end of file
diff --git a/spaces/EDGAhab/Aatrox-Talking/models.py b/spaces/EDGAhab/Aatrox-Talking/models.py
deleted file mode 100644
index f5acdeb2bedd47897348407c0ae55c9a160da881..0000000000000000000000000000000000000000
--- a/spaces/EDGAhab/Aatrox-Talking/models.py
+++ /dev/null
@@ -1,534 +0,0 @@
-import copy
-import math
-import torch
-from torch import nn
-from torch.nn import functional as F
-
-import commons
-import modules
-import attentions
-import monotonic_align
-
-from torch.nn import Conv1d, ConvTranspose1d, AvgPool1d, Conv2d
-from torch.nn.utils import weight_norm, remove_weight_norm, spectral_norm
-from commons import init_weights, get_padding
-
-
-class StochasticDurationPredictor(nn.Module):
- def __init__(self, in_channels, filter_channels, kernel_size, p_dropout, n_flows=4, gin_channels=0):
- super().__init__()
- filter_channels = in_channels # it needs to be removed from future version.
- self.in_channels = in_channels
- self.filter_channels = filter_channels
- self.kernel_size = kernel_size
- self.p_dropout = p_dropout
- self.n_flows = n_flows
- self.gin_channels = gin_channels
-
- self.log_flow = modules.Log()
- self.flows = nn.ModuleList()
- self.flows.append(modules.ElementwiseAffine(2))
- for i in range(n_flows):
- self.flows.append(modules.ConvFlow(2, filter_channels, kernel_size, n_layers=3))
- self.flows.append(modules.Flip())
-
- self.post_pre = nn.Conv1d(1, filter_channels, 1)
- self.post_proj = nn.Conv1d(filter_channels, filter_channels, 1)
- self.post_convs = modules.DDSConv(filter_channels, kernel_size, n_layers=3, p_dropout=p_dropout)
- self.post_flows = nn.ModuleList()
- self.post_flows.append(modules.ElementwiseAffine(2))
- for i in range(4):
- self.post_flows.append(modules.ConvFlow(2, filter_channels, kernel_size, n_layers=3))
- self.post_flows.append(modules.Flip())
-
- self.pre = nn.Conv1d(in_channels, filter_channels, 1)
- self.proj = nn.Conv1d(filter_channels, filter_channels, 1)
- self.convs = modules.DDSConv(filter_channels, kernel_size, n_layers=3, p_dropout=p_dropout)
- if gin_channels != 0:
- self.cond = nn.Conv1d(gin_channels, filter_channels, 1)
-
- def forward(self, x, x_mask, w=None, g=None, reverse=False, noise_scale=1.0):
- x = torch.detach(x)
- x = self.pre(x)
- if g is not None:
- g = torch.detach(g)
- x = x + self.cond(g)
- x = self.convs(x, x_mask)
- x = self.proj(x) * x_mask
-
- if not reverse:
- flows = self.flows
- assert w is not None
-
- logdet_tot_q = 0
- h_w = self.post_pre(w)
- h_w = self.post_convs(h_w, x_mask)
- h_w = self.post_proj(h_w) * x_mask
- e_q = torch.randn(w.size(0), 2, w.size(2)).to(device=x.device, dtype=x.dtype) * x_mask
- z_q = e_q
- for flow in self.post_flows:
- z_q, logdet_q = flow(z_q, x_mask, g=(x + h_w))
- logdet_tot_q += logdet_q
- z_u, z1 = torch.split(z_q, [1, 1], 1)
- u = torch.sigmoid(z_u) * x_mask
- z0 = (w - u) * x_mask
- logdet_tot_q += torch.sum((F.logsigmoid(z_u) + F.logsigmoid(-z_u)) * x_mask, [1,2])
- logq = torch.sum(-0.5 * (math.log(2*math.pi) + (e_q**2)) * x_mask, [1,2]) - logdet_tot_q
-
- logdet_tot = 0
- z0, logdet = self.log_flow(z0, x_mask)
- logdet_tot += logdet
- z = torch.cat([z0, z1], 1)
- for flow in flows:
- z, logdet = flow(z, x_mask, g=x, reverse=reverse)
- logdet_tot = logdet_tot + logdet
- nll = torch.sum(0.5 * (math.log(2*math.pi) + (z**2)) * x_mask, [1,2]) - logdet_tot
- return nll + logq # [b]
- else:
- flows = list(reversed(self.flows))
- flows = flows[:-2] + [flows[-1]] # remove a useless vflow
- z = torch.randn(x.size(0), 2, x.size(2)).to(device=x.device, dtype=x.dtype) * noise_scale
- for flow in flows:
- z = flow(z, x_mask, g=x, reverse=reverse)
- z0, z1 = torch.split(z, [1, 1], 1)
- logw = z0
- return logw
-
-
-class DurationPredictor(nn.Module):
- def __init__(self, in_channels, filter_channels, kernel_size, p_dropout, gin_channels=0):
- super().__init__()
-
- self.in_channels = in_channels
- self.filter_channels = filter_channels
- self.kernel_size = kernel_size
- self.p_dropout = p_dropout
- self.gin_channels = gin_channels
-
- self.drop = nn.Dropout(p_dropout)
- self.conv_1 = nn.Conv1d(in_channels, filter_channels, kernel_size, padding=kernel_size//2)
- self.norm_1 = modules.LayerNorm(filter_channels)
- self.conv_2 = nn.Conv1d(filter_channels, filter_channels, kernel_size, padding=kernel_size//2)
- self.norm_2 = modules.LayerNorm(filter_channels)
- self.proj = nn.Conv1d(filter_channels, 1, 1)
-
- if gin_channels != 0:
- self.cond = nn.Conv1d(gin_channels, in_channels, 1)
-
- def forward(self, x, x_mask, g=None):
- x = torch.detach(x)
- if g is not None:
- g = torch.detach(g)
- x = x + self.cond(g)
- x = self.conv_1(x * x_mask)
- x = torch.relu(x)
- x = self.norm_1(x)
- x = self.drop(x)
- x = self.conv_2(x * x_mask)
- x = torch.relu(x)
- x = self.norm_2(x)
- x = self.drop(x)
- x = self.proj(x * x_mask)
- return x * x_mask
-
-
-class TextEncoder(nn.Module):
- def __init__(self,
- n_vocab,
- out_channels,
- hidden_channels,
- filter_channels,
- n_heads,
- n_layers,
- kernel_size,
- p_dropout):
- super().__init__()
- self.n_vocab = n_vocab
- self.out_channels = out_channels
- self.hidden_channels = hidden_channels
- self.filter_channels = filter_channels
- self.n_heads = n_heads
- self.n_layers = n_layers
- self.kernel_size = kernel_size
- self.p_dropout = p_dropout
-
- self.emb = nn.Embedding(n_vocab, hidden_channels)
- nn.init.normal_(self.emb.weight, 0.0, hidden_channels**-0.5)
-
- self.encoder = attentions.Encoder(
- hidden_channels,
- filter_channels,
- n_heads,
- n_layers,
- kernel_size,
- p_dropout)
- self.proj= nn.Conv1d(hidden_channels, out_channels * 2, 1)
-
- def forward(self, x, x_lengths):
- x = self.emb(x) * math.sqrt(self.hidden_channels) # [b, t, h]
- x = torch.transpose(x, 1, -1) # [b, h, t]
- x_mask = torch.unsqueeze(commons.sequence_mask(x_lengths, x.size(2)), 1).to(x.dtype)
-
- x = self.encoder(x * x_mask, x_mask)
- stats = self.proj(x) * x_mask
-
- m, logs = torch.split(stats, self.out_channels, dim=1)
- return x, m, logs, x_mask
-
-
-class ResidualCouplingBlock(nn.Module):
- def __init__(self,
- channels,
- hidden_channels,
- kernel_size,
- dilation_rate,
- n_layers,
- n_flows=4,
- gin_channels=0):
- super().__init__()
- self.channels = channels
- self.hidden_channels = hidden_channels
- self.kernel_size = kernel_size
- self.dilation_rate = dilation_rate
- self.n_layers = n_layers
- self.n_flows = n_flows
- self.gin_channels = gin_channels
-
- self.flows = nn.ModuleList()
- for i in range(n_flows):
- self.flows.append(modules.ResidualCouplingLayer(channels, hidden_channels, kernel_size, dilation_rate, n_layers, gin_channels=gin_channels, mean_only=True))
- self.flows.append(modules.Flip())
-
- def forward(self, x, x_mask, g=None, reverse=False):
- if not reverse:
- for flow in self.flows:
- x, _ = flow(x, x_mask, g=g, reverse=reverse)
- else:
- for flow in reversed(self.flows):
- x = flow(x, x_mask, g=g, reverse=reverse)
- return x
-
-
-class PosteriorEncoder(nn.Module):
- def __init__(self,
- in_channels,
- out_channels,
- hidden_channels,
- kernel_size,
- dilation_rate,
- n_layers,
- gin_channels=0):
- super().__init__()
- self.in_channels = in_channels
- self.out_channels = out_channels
- self.hidden_channels = hidden_channels
- self.kernel_size = kernel_size
- self.dilation_rate = dilation_rate
- self.n_layers = n_layers
- self.gin_channels = gin_channels
-
- self.pre = nn.Conv1d(in_channels, hidden_channels, 1)
- self.enc = modules.WN(hidden_channels, kernel_size, dilation_rate, n_layers, gin_channels=gin_channels)
- self.proj = nn.Conv1d(hidden_channels, out_channels * 2, 1)
-
- def forward(self, x, x_lengths, g=None):
- x_mask = torch.unsqueeze(commons.sequence_mask(x_lengths, x.size(2)), 1).to(x.dtype)
- x = self.pre(x) * x_mask
- x = self.enc(x, x_mask, g=g)
- stats = self.proj(x) * x_mask
- m, logs = torch.split(stats, self.out_channels, dim=1)
- z = (m + torch.randn_like(m) * torch.exp(logs)) * x_mask
- return z, m, logs, x_mask
-
-
-class Generator(torch.nn.Module):
- def __init__(self, initial_channel, resblock, resblock_kernel_sizes, resblock_dilation_sizes, upsample_rates, upsample_initial_channel, upsample_kernel_sizes, gin_channels=0):
- super(Generator, self).__init__()
- self.num_kernels = len(resblock_kernel_sizes)
- self.num_upsamples = len(upsample_rates)
- self.conv_pre = Conv1d(initial_channel, upsample_initial_channel, 7, 1, padding=3)
- resblock = modules.ResBlock1 if resblock == '1' else modules.ResBlock2
-
- self.ups = nn.ModuleList()
- for i, (u, k) in enumerate(zip(upsample_rates, upsample_kernel_sizes)):
- self.ups.append(weight_norm(
- ConvTranspose1d(upsample_initial_channel//(2**i), upsample_initial_channel//(2**(i+1)),
- k, u, padding=(k-u)//2)))
-
- self.resblocks = nn.ModuleList()
- for i in range(len(self.ups)):
- ch = upsample_initial_channel//(2**(i+1))
- for j, (k, d) in enumerate(zip(resblock_kernel_sizes, resblock_dilation_sizes)):
- self.resblocks.append(resblock(ch, k, d))
-
- self.conv_post = Conv1d(ch, 1, 7, 1, padding=3, bias=False)
- self.ups.apply(init_weights)
-
- if gin_channels != 0:
- self.cond = nn.Conv1d(gin_channels, upsample_initial_channel, 1)
-
- def forward(self, x, g=None):
- x = self.conv_pre(x)
- if g is not None:
- x = x + self.cond(g)
-
- for i in range(self.num_upsamples):
- x = F.leaky_relu(x, modules.LRELU_SLOPE)
- x = self.ups[i](x)
- xs = None
- for j in range(self.num_kernels):
- if xs is None:
- xs = self.resblocks[i*self.num_kernels+j](x)
- else:
- xs += self.resblocks[i*self.num_kernels+j](x)
- x = xs / self.num_kernels
- x = F.leaky_relu(x)
- x = self.conv_post(x)
- x = torch.tanh(x)
-
- return x
-
- def remove_weight_norm(self):
- print('Removing weight norm...')
- for l in self.ups:
- remove_weight_norm(l)
- for l in self.resblocks:
- l.remove_weight_norm()
-
-
-class DiscriminatorP(torch.nn.Module):
- def __init__(self, period, kernel_size=5, stride=3, use_spectral_norm=False):
- super(DiscriminatorP, self).__init__()
- self.period = period
- self.use_spectral_norm = use_spectral_norm
- norm_f = weight_norm if use_spectral_norm == False else spectral_norm
- self.convs = nn.ModuleList([
- norm_f(Conv2d(1, 32, (kernel_size, 1), (stride, 1), padding=(get_padding(kernel_size, 1), 0))),
- norm_f(Conv2d(32, 128, (kernel_size, 1), (stride, 1), padding=(get_padding(kernel_size, 1), 0))),
- norm_f(Conv2d(128, 512, (kernel_size, 1), (stride, 1), padding=(get_padding(kernel_size, 1), 0))),
- norm_f(Conv2d(512, 1024, (kernel_size, 1), (stride, 1), padding=(get_padding(kernel_size, 1), 0))),
- norm_f(Conv2d(1024, 1024, (kernel_size, 1), 1, padding=(get_padding(kernel_size, 1), 0))),
- ])
- self.conv_post = norm_f(Conv2d(1024, 1, (3, 1), 1, padding=(1, 0)))
-
- def forward(self, x):
- fmap = []
-
- # 1d to 2d
- b, c, t = x.shape
- if t % self.period != 0: # pad first
- n_pad = self.period - (t % self.period)
- x = F.pad(x, (0, n_pad), "reflect")
- t = t + n_pad
- x = x.view(b, c, t // self.period, self.period)
-
- for l in self.convs:
- x = l(x)
- x = F.leaky_relu(x, modules.LRELU_SLOPE)
- fmap.append(x)
- x = self.conv_post(x)
- fmap.append(x)
- x = torch.flatten(x, 1, -1)
-
- return x, fmap
-
-
-class DiscriminatorS(torch.nn.Module):
- def __init__(self, use_spectral_norm=False):
- super(DiscriminatorS, self).__init__()
- norm_f = weight_norm if use_spectral_norm == False else spectral_norm
- self.convs = nn.ModuleList([
- norm_f(Conv1d(1, 16, 15, 1, padding=7)),
- norm_f(Conv1d(16, 64, 41, 4, groups=4, padding=20)),
- norm_f(Conv1d(64, 256, 41, 4, groups=16, padding=20)),
- norm_f(Conv1d(256, 1024, 41, 4, groups=64, padding=20)),
- norm_f(Conv1d(1024, 1024, 41, 4, groups=256, padding=20)),
- norm_f(Conv1d(1024, 1024, 5, 1, padding=2)),
- ])
- self.conv_post = norm_f(Conv1d(1024, 1, 3, 1, padding=1))
-
- def forward(self, x):
- fmap = []
-
- for l in self.convs:
- x = l(x)
- x = F.leaky_relu(x, modules.LRELU_SLOPE)
- fmap.append(x)
- x = self.conv_post(x)
- fmap.append(x)
- x = torch.flatten(x, 1, -1)
-
- return x, fmap
-
-
-class MultiPeriodDiscriminator(torch.nn.Module):
- def __init__(self, use_spectral_norm=False):
- super(MultiPeriodDiscriminator, self).__init__()
- periods = [2,3,5,7,11]
-
- discs = [DiscriminatorS(use_spectral_norm=use_spectral_norm)]
- discs = discs + [DiscriminatorP(i, use_spectral_norm=use_spectral_norm) for i in periods]
- self.discriminators = nn.ModuleList(discs)
-
- def forward(self, y, y_hat):
- y_d_rs = []
- y_d_gs = []
- fmap_rs = []
- fmap_gs = []
- for i, d in enumerate(self.discriminators):
- y_d_r, fmap_r = d(y)
- y_d_g, fmap_g = d(y_hat)
- y_d_rs.append(y_d_r)
- y_d_gs.append(y_d_g)
- fmap_rs.append(fmap_r)
- fmap_gs.append(fmap_g)
-
- return y_d_rs, y_d_gs, fmap_rs, fmap_gs
-
-
-
-class SynthesizerTrn(nn.Module):
- """
- Synthesizer for Training
- """
-
- def __init__(self,
- n_vocab,
- spec_channels,
- segment_size,
- inter_channels,
- hidden_channels,
- filter_channels,
- n_heads,
- n_layers,
- kernel_size,
- p_dropout,
- resblock,
- resblock_kernel_sizes,
- resblock_dilation_sizes,
- upsample_rates,
- upsample_initial_channel,
- upsample_kernel_sizes,
- n_speakers=0,
- gin_channels=0,
- use_sdp=True,
- **kwargs):
-
- super().__init__()
- self.n_vocab = n_vocab
- self.spec_channels = spec_channels
- self.inter_channels = inter_channels
- self.hidden_channels = hidden_channels
- self.filter_channels = filter_channels
- self.n_heads = n_heads
- self.n_layers = n_layers
- self.kernel_size = kernel_size
- self.p_dropout = p_dropout
- self.resblock = resblock
- self.resblock_kernel_sizes = resblock_kernel_sizes
- self.resblock_dilation_sizes = resblock_dilation_sizes
- self.upsample_rates = upsample_rates
- self.upsample_initial_channel = upsample_initial_channel
- self.upsample_kernel_sizes = upsample_kernel_sizes
- self.segment_size = segment_size
- self.n_speakers = n_speakers
- self.gin_channels = gin_channels
-
- self.use_sdp = use_sdp
-
- self.enc_p = TextEncoder(n_vocab,
- inter_channels,
- hidden_channels,
- filter_channels,
- n_heads,
- n_layers,
- kernel_size,
- p_dropout)
- self.dec = Generator(inter_channels, resblock, resblock_kernel_sizes, resblock_dilation_sizes, upsample_rates, upsample_initial_channel, upsample_kernel_sizes, gin_channels=gin_channels)
- self.enc_q = PosteriorEncoder(spec_channels, inter_channels, hidden_channels, 5, 1, 16, gin_channels=gin_channels)
- self.flow = ResidualCouplingBlock(inter_channels, hidden_channels, 5, 1, 4, gin_channels=gin_channels)
-
- if use_sdp:
- self.dp = StochasticDurationPredictor(hidden_channels, 192, 3, 0.5, 4, gin_channels=gin_channels)
- else:
- self.dp = DurationPredictor(hidden_channels, 256, 3, 0.5, gin_channels=gin_channels)
-
- if n_speakers > 1:
- self.emb_g = nn.Embedding(n_speakers, gin_channels)
-
- def forward(self, x, x_lengths, y, y_lengths, sid=None):
-
- x, m_p, logs_p, x_mask = self.enc_p(x, x_lengths)
- if self.n_speakers > 0:
- g = self.emb_g(sid).unsqueeze(-1) # [b, h, 1]
- else:
- g = None
-
- z, m_q, logs_q, y_mask = self.enc_q(y, y_lengths, g=g)
- z_p = self.flow(z, y_mask, g=g)
-
- with torch.no_grad():
- # negative cross-entropy
- s_p_sq_r = torch.exp(-2 * logs_p) # [b, d, t]
- neg_cent1 = torch.sum(-0.5 * math.log(2 * math.pi) - logs_p, [1], keepdim=True) # [b, 1, t_s]
- neg_cent2 = torch.matmul(-0.5 * (z_p ** 2).transpose(1, 2), s_p_sq_r) # [b, t_t, d] x [b, d, t_s] = [b, t_t, t_s]
- neg_cent3 = torch.matmul(z_p.transpose(1, 2), (m_p * s_p_sq_r)) # [b, t_t, d] x [b, d, t_s] = [b, t_t, t_s]
- neg_cent4 = torch.sum(-0.5 * (m_p ** 2) * s_p_sq_r, [1], keepdim=True) # [b, 1, t_s]
- neg_cent = neg_cent1 + neg_cent2 + neg_cent3 + neg_cent4
-
- attn_mask = torch.unsqueeze(x_mask, 2) * torch.unsqueeze(y_mask, -1)
- attn = monotonic_align.maximum_path(neg_cent, attn_mask.squeeze(1)).unsqueeze(1).detach()
-
- w = attn.sum(2)
- if self.use_sdp:
- l_length = self.dp(x, x_mask, w, g=g)
- l_length = l_length / torch.sum(x_mask)
- else:
- logw_ = torch.log(w + 1e-6) * x_mask
- logw = self.dp(x, x_mask, g=g)
- l_length = torch.sum((logw - logw_)**2, [1,2]) / torch.sum(x_mask) # for averaging
-
- # expand prior
- m_p = torch.matmul(attn.squeeze(1), m_p.transpose(1, 2)).transpose(1, 2)
- logs_p = torch.matmul(attn.squeeze(1), logs_p.transpose(1, 2)).transpose(1, 2)
-
- z_slice, ids_slice = commons.rand_slice_segments(z, y_lengths, self.segment_size)
- o = self.dec(z_slice, g=g)
- return o, l_length, attn, ids_slice, x_mask, y_mask, (z, z_p, m_p, logs_p, m_q, logs_q)
-
- def infer(self, x, x_lengths, sid=None, noise_scale=1, length_scale=1, noise_scale_w=1., max_len=None):
- x, m_p, logs_p, x_mask = self.enc_p(x, x_lengths)
- if self.n_speakers > 0:
- g = self.emb_g(sid).unsqueeze(-1) # [b, h, 1]
- else:
- g = None
-
- if self.use_sdp:
- logw = self.dp(x, x_mask, g=g, reverse=True, noise_scale=noise_scale_w)
- else:
- logw = self.dp(x, x_mask, g=g)
- w = torch.exp(logw) * x_mask * length_scale
- w_ceil = torch.ceil(w)
- y_lengths = torch.clamp_min(torch.sum(w_ceil, [1, 2]), 1).long()
- y_mask = torch.unsqueeze(commons.sequence_mask(y_lengths, None), 1).to(x_mask.dtype)
- attn_mask = torch.unsqueeze(x_mask, 2) * torch.unsqueeze(y_mask, -1)
- attn = commons.generate_path(w_ceil, attn_mask)
-
- m_p = torch.matmul(attn.squeeze(1), m_p.transpose(1, 2)).transpose(1, 2) # [b, t', t], [b, t, d] -> [b, d, t']
- logs_p = torch.matmul(attn.squeeze(1), logs_p.transpose(1, 2)).transpose(1, 2) # [b, t', t], [b, t, d] -> [b, d, t']
-
- z_p = m_p + torch.randn_like(m_p) * torch.exp(logs_p) * noise_scale
- z = self.flow(z_p, y_mask, g=g, reverse=True)
- o = self.dec((z * y_mask)[:,:,:max_len], g=g)
- return o, attn, y_mask, (z, z_p, m_p, logs_p)
-
- def voice_conversion(self, y, y_lengths, sid_src, sid_tgt):
- assert self.n_speakers > 0, "n_speakers have to be larger than 0."
- g_src = self.emb_g(sid_src).unsqueeze(-1)
- g_tgt = self.emb_g(sid_tgt).unsqueeze(-1)
- z, m_q, logs_q, y_mask = self.enc_q(y, y_lengths, g=g_src)
- z_p = self.flow(z, y_mask, g=g_src)
- z_hat = self.flow(z_p, y_mask, g=g_tgt, reverse=True)
- o_hat = self.dec(z_hat * y_mask, g=g_tgt)
- return o_hat, y_mask, (z, z_p, z_hat)
-
diff --git a/spaces/EDGAhab/Aatrox-Talking/text/cleaners.py b/spaces/EDGAhab/Aatrox-Talking/text/cleaners.py
deleted file mode 100644
index 759db477e3deb72a03ff65957419c3694781b5ef..0000000000000000000000000000000000000000
--- a/spaces/EDGAhab/Aatrox-Talking/text/cleaners.py
+++ /dev/null
@@ -1,138 +0,0 @@
-""" from https://github.com/keithito/tacotron """
-
-'''
-Cleaners are transformations that run over the input text at both training and eval time.
-
-Cleaners can be selected by passing a comma-delimited list of cleaner names as the "cleaners"
-hyperparameter. Some cleaners are English-specific. You'll typically want to use:
- 1. "english_cleaners" for English text
- 2. "transliteration_cleaners" for non-English text that can be transliterated to ASCII using
- the Unidecode library (https://pypi.python.org/pypi/Unidecode)
- 3. "basic_cleaners" if you do not want to transliterate (in this case, you should also update
- the symbols in symbols.py to match your data).
-'''
-
-import re
-from unidecode import unidecode
-from phonemizer import phonemize
-from pypinyin import Style, pinyin
-from pypinyin.style._utils import get_finals, get_initials
-# Regular expression matching whitespace:
-_whitespace_re = re.compile(r'\s+')
-
-# List of (regular expression, replacement) pairs for abbreviations:
-_abbreviations = [(re.compile('\\b%s\\.' % x[0], re.IGNORECASE), x[1]) for x in [
- ('mrs', 'misess'),
- ('mr', 'mister'),
- ('dr', 'doctor'),
- ('st', 'saint'),
- ('co', 'company'),
- ('jr', 'junior'),
- ('maj', 'major'),
- ('gen', 'general'),
- ('drs', 'doctors'),
- ('rev', 'reverend'),
- ('lt', 'lieutenant'),
- ('hon', 'honorable'),
- ('sgt', 'sergeant'),
- ('capt', 'captain'),
- ('esq', 'esquire'),
- ('ltd', 'limited'),
- ('col', 'colonel'),
- ('ft', 'fort'),
-]]
-
-
-def expand_abbreviations(text):
- for regex, replacement in _abbreviations:
- text = re.sub(regex, replacement, text)
- return text
-
-
-def expand_numbers(text):
- return normalize_numbers(text)
-
-
-def lowercase(text):
- return text.lower()
-
-
-def collapse_whitespace(text):
- return re.sub(_whitespace_re, ' ', text)
-
-
-def convert_to_ascii(text):
- return unidecode(text)
-
-
-def basic_cleaners(text):
- '''Basic pipeline that lowercases and collapses whitespace without transliteration.'''
- text = lowercase(text)
- text = collapse_whitespace(text)
- return text
-
-
-def transliteration_cleaners(text):
- '''Pipeline for non-English text that transliterates to ASCII.'''
- text = convert_to_ascii(text)
- text = lowercase(text)
- text = collapse_whitespace(text)
- return text
-
-
-def english_cleaners(text):
- '''Pipeline for English text, including abbreviation expansion.'''
- text = convert_to_ascii(text)
- text = lowercase(text)
- text = expand_abbreviations(text)
- phonemes = phonemize(text, language='en-us', backend='espeak', strip=True)
- phonemes = collapse_whitespace(phonemes)
- return phonemes
-
-
-def english_cleaners2(text):
- '''Pipeline for English text, including abbreviation expansion. + punctuation + stress'''
- text = convert_to_ascii(text)
- text = lowercase(text)
- text = expand_abbreviations(text)
- phonemes = phonemize(text, language='en-us', backend='espeak', strip=True, preserve_punctuation=True, with_stress=True)
- phonemes = collapse_whitespace(phonemes)
- return phonemes
-
-
-
-
-def chinese_cleaners1(text):
- from pypinyin import Style, pinyin
-
- phones = [phone[0] for phone in pinyin(text, style=Style.TONE3)]
- return ' '.join(phones)
-
-
-def chinese_cleaners2(text):
- phones = [
- p
- for phone in pinyin(text, style=Style.TONE3)
- for p in [
- get_initials(phone[0], strict=True),
- get_finals(phone[0][:-1], strict=True) + phone[0][-1]
- if phone[0][-1].isdigit()
- else get_finals(phone[0], strict=True)
- if phone[0][-1].isalnum()
- else phone[0],
- ]
- # Remove the case of individual tones as a phoneme
- if len(p) != 0 and not p.isdigit()
- ]
- return phones
- # return phonemes
-
-if __name__ == '__main__':
- res = chinese_cleaners2('这是语音测试!')
- print(res)
- res = chinese_cleaners1('"第一,南京不是发展的不行,是大家对他期望很高,')
- print(res)
-
-
- res = english_cleaners2('this is a club test for one train.GDP')
- print(res)
\ No newline at end of file
diff --git a/spaces/Eddycrack864/Applio-Inference/lib/infer_pack/onnx_inference.py b/spaces/Eddycrack864/Applio-Inference/lib/infer_pack/onnx_inference.py
deleted file mode 100644
index 6517853be49e61c427cf7cd9b5ed203f6d5f367e..0000000000000000000000000000000000000000
--- a/spaces/Eddycrack864/Applio-Inference/lib/infer_pack/onnx_inference.py
+++ /dev/null
@@ -1,145 +0,0 @@
-import onnxruntime
-import librosa
-import numpy as np
-import soundfile
-
-
-class ContentVec:
- def __init__(self, vec_path="pretrained/vec-768-layer-12.onnx", device=None):
- print("load model(s) from {}".format(vec_path))
- if device == "cpu" or device is None:
- providers = ["CPUExecutionProvider"]
- elif device == "cuda":
- providers = ["CUDAExecutionProvider", "CPUExecutionProvider"]
- elif device == "dml":
- providers = ["DmlExecutionProvider"]
- else:
- raise RuntimeError("Unsportted Device")
- self.model = onnxruntime.InferenceSession(vec_path, providers=providers)
-
- def __call__(self, wav):
- return self.forward(wav)
-
- def forward(self, wav):
- feats = wav
- if feats.ndim == 2: # double channels
- feats = feats.mean(-1)
- assert feats.ndim == 1, feats.ndim
- feats = np.expand_dims(np.expand_dims(feats, 0), 0)
- onnx_input = {self.model.get_inputs()[0].name: feats}
- logits = self.model.run(None, onnx_input)[0]
- return logits.transpose(0, 2, 1)
-
-
-def get_f0_predictor(f0_predictor, hop_length, sampling_rate, **kargs):
- if f0_predictor == "pm":
- from lib.infer_pack.modules.F0Predictor.PMF0Predictor import PMF0Predictor
-
- f0_predictor_object = PMF0Predictor(
- hop_length=hop_length, sampling_rate=sampling_rate
- )
- elif f0_predictor == "harvest":
- from lib.infer_pack.modules.F0Predictor.HarvestF0Predictor import (
- HarvestF0Predictor,
- )
-
- f0_predictor_object = HarvestF0Predictor(
- hop_length=hop_length, sampling_rate=sampling_rate
- )
- elif f0_predictor == "dio":
- from lib.infer_pack.modules.F0Predictor.DioF0Predictor import DioF0Predictor
-
- f0_predictor_object = DioF0Predictor(
- hop_length=hop_length, sampling_rate=sampling_rate
- )
- else:
- raise Exception("Unknown f0 predictor")
- return f0_predictor_object
-
-
-class OnnxRVC:
- def __init__(
- self,
- model_path,
- sr=40000,
- hop_size=512,
- vec_path="vec-768-layer-12",
- device="cpu",
- ):
- vec_path = f"pretrained/{vec_path}.onnx"
- self.vec_model = ContentVec(vec_path, device)
- if device == "cpu" or device is None:
- providers = ["CPUExecutionProvider"]
- elif device == "cuda":
- providers = ["CUDAExecutionProvider", "CPUExecutionProvider"]
- elif device == "dml":
- providers = ["DmlExecutionProvider"]
- else:
- raise RuntimeError("Unsportted Device")
- self.model = onnxruntime.InferenceSession(model_path, providers=providers)
- self.sampling_rate = sr
- self.hop_size = hop_size
-
- def forward(self, hubert, hubert_length, pitch, pitchf, ds, rnd):
- onnx_input = {
- self.model.get_inputs()[0].name: hubert,
- self.model.get_inputs()[1].name: hubert_length,
- self.model.get_inputs()[2].name: pitch,
- self.model.get_inputs()[3].name: pitchf,
- self.model.get_inputs()[4].name: ds,
- self.model.get_inputs()[5].name: rnd,
- }
- return (self.model.run(None, onnx_input)[0] * 32767).astype(np.int16)
-
- def inference(
- self,
- raw_path,
- sid,
- f0_method="dio",
- f0_up_key=0,
- pad_time=0.5,
- cr_threshold=0.02,
- ):
- f0_min = 50
- f0_max = 1100
- f0_mel_min = 1127 * np.log(1 + f0_min / 700)
- f0_mel_max = 1127 * np.log(1 + f0_max / 700)
- f0_predictor = get_f0_predictor(
- f0_method,
- hop_length=self.hop_size,
- sampling_rate=self.sampling_rate,
- threshold=cr_threshold,
- )
- wav, sr = librosa.load(raw_path, sr=self.sampling_rate)
- org_length = len(wav)
- if org_length / sr > 50.0:
- raise RuntimeError("Reached Max Length")
-
- wav16k = librosa.resample(wav, orig_sr=self.sampling_rate, target_sr=16000)
- wav16k = wav16k
-
- hubert = self.vec_model(wav16k)
- hubert = np.repeat(hubert, 2, axis=2).transpose(0, 2, 1).astype(np.float32)
- hubert_length = hubert.shape[1]
-
- pitchf = f0_predictor.compute_f0(wav, hubert_length)
- pitchf = pitchf * 2 ** (f0_up_key / 12)
- pitch = pitchf.copy()
- f0_mel = 1127 * np.log(1 + pitch / 700)
- f0_mel[f0_mel > 0] = (f0_mel[f0_mel > 0] - f0_mel_min) * 254 / (
- f0_mel_max - f0_mel_min
- ) + 1
- f0_mel[f0_mel <= 1] = 1
- f0_mel[f0_mel > 255] = 255
- pitch = np.rint(f0_mel).astype(np.int64)
-
- pitchf = pitchf.reshape(1, len(pitchf)).astype(np.float32)
- pitch = pitch.reshape(1, len(pitch))
- ds = np.array([sid]).astype(np.int64)
-
- rnd = np.random.randn(1, 192, hubert_length).astype(np.float32)
- hubert_length = np.array([hubert_length]).astype(np.int64)
-
- out_wav = self.forward(hubert, hubert_length, pitch, pitchf, ds, rnd).squeeze()
- out_wav = np.pad(out_wav, (0, 2 * self.hop_size), "constant")
- return out_wav[0:org_length]
diff --git a/spaces/EuroPython2022/mmocr-demo/configs/textdet/drrg/README.md b/spaces/EuroPython2022/mmocr-demo/configs/textdet/drrg/README.md
deleted file mode 100644
index 2f2beb1b757ccbf2dd2e41a70769d963b098264d..0000000000000000000000000000000000000000
--- a/spaces/EuroPython2022/mmocr-demo/configs/textdet/drrg/README.md
+++ /dev/null
@@ -1,37 +0,0 @@
-# DRRG
-
-> [Deep relational reasoning graph network for arbitrary shape text detection](https://arxiv.org/abs/2003.07493)
-
-
-
-## Abstract
-
-Arbitrary shape text detection is a challenging task due to the high variety and complexity of scenes texts. In this paper, we propose a novel unified relational reasoning graph network for arbitrary shape text detection. In our method, an innovative local graph bridges a text proposal model via Convolutional Neural Network (CNN) and a deep relational reasoning network via Graph Convolutional Network (GCN), making our network end-to-end trainable. To be concrete, every text instance will be divided into a series of small rectangular components, and the geometry attributes (e.g., height, width, and orientation) of the small components will be estimated by our text proposal model. Given the geometry attributes, the local graph construction model can roughly establish linkages between different text components. For further reasoning and deducing the likelihood of linkages between the component and its neighbors, we adopt a graph-based network to perform deep relational reasoning on local graphs. Experiments on public available datasets demonstrate the state-of-the-art performance of our method.
-
-
- 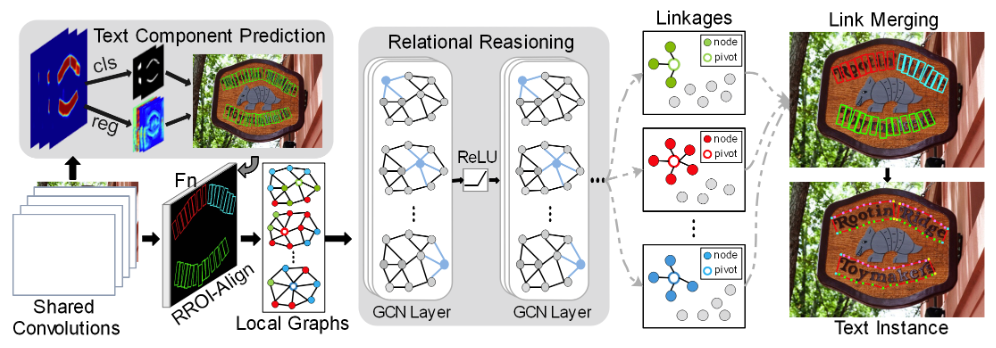
-
-
-## Results and models
-
-### CTW1500
-
-| Method | Pretrained Model | Training set | Test set | #epochs | Test size | Recall | Precision | Hmean | Download |
-| :-------------------------------------------------: | :--------------: | :-----------: | :----------: | :-----: | :-------: | :-----------: | :-----------: | :-----------: | :---------------------------------------------------: |
-| [DRRG](configs/textdet/drrg/drrg_r50_fpn_unet_1200e_ctw1500.py) | ImageNet | CTW1500 Train | CTW1500 Test | 1200 | 640 | 0.822 (0.791) | 0.858 (0.862) | 0.840 (0.825) | [model](https://download.openmmlab.com/mmocr/textdet/drrg/drrg_r50_fpn_unet_1200e_ctw1500_20211022-fb30b001.pth) \\ [log](https://download.openmmlab.com/mmocr/textdet/drrg/20210511_234719.log) |
-
-```{note}
-We've upgraded our IoU backend from `Polygon3` to `shapely`. There are some performance differences for some models due to the backends' different logics to handle invalid polygons (more info [here](https://github.com/open-mmlab/mmocr/issues/465)). **New evaluation result is presented in brackets** and new logs will be uploaded soon.
-```
-
-## Citation
-
-```bibtex
-@article{zhang2020drrg,
- title={Deep relational reasoning graph network for arbitrary shape text detection},
- author={Zhang, Shi-Xue and Zhu, Xiaobin and Hou, Jie-Bo and Liu, Chang and Yang, Chun and Wang, Hongfa and Yin, Xu-Cheng},
- booktitle={CVPR},
- pages={9699-9708},
- year={2020}
-}
-```
diff --git a/spaces/Ezi/Licences_check/read_extract.py b/spaces/Ezi/Licences_check/read_extract.py
deleted file mode 100644
index 7917e89b6d7d929b65a64c06d1bb71877b6ead30..0000000000000000000000000000000000000000
--- a/spaces/Ezi/Licences_check/read_extract.py
+++ /dev/null
@@ -1,271 +0,0 @@
-import os
-import re
-
-import nltk
-nltk.download('stopwords')
-nltk.download('punkt')
-
-
-from nltk.corpus import stopwords
-from nltk.tokenize import word_tokenize
-from nltk.util import ngrams
-import spacy
-# from gensim.summarization.summarizer import summarize
-# from gensim.summarization import keywords
-
-# Abstractive Summarisation
-from transformers import BartForConditionalGeneration
-from transformers import AutoTokenizer
-import torch
-
-# Keyword/Keyphrase Extraction
-from keybert import _highlight
-from keybert import KeyBERT
-from keyphrase_vectorizers import KeyphraseCountVectorizer, KeyphraseTfidfVectorizer
-from sklearn.feature_extraction.text import CountVectorizer
-
-import time
-import threading
-from collections import defaultdict
-
-class AbstractiveSummarizer:
-
- def __init__(self):
- self.nlp = spacy.load('en_core_web_lg')
- self.summary = ""
-
- def generate_batch(self, text, tokenizer):
- """
- Convert the text into multiple sentence parts of appropriate input size to feed to the model
-
- Arguments:
- text: The License text to summarise
- tokenizer: The tokenizer corresponding to the model used to convert the text into separate words(tokens)
-
- Returns:
- The text formatted into List of sentences to feed to the model
- """
- parsed = self.nlp(text)
- sents = [sent.text for sent in parsed.sents]
- max_size = tokenizer.model_max_length
-
- batch = tokenizer(sents, return_tensors='pt', return_length=True, padding='longest')
-
- inp_batch = []
- cur_batch = torch.empty((0,), dtype=torch.int64)
- for enc_sent, length in zip(batch['input_ids'], batch['length']):
- cur_size = cur_batch.shape[0]
- if (cur_size + length.item()) <= max_size:
- cur_batch = torch.cat((cur_batch,enc_sent[:length.item()]))
- else:
- inp_batch.append(torch.unsqueeze(cur_batch,0))
- cur_batch = enc_sent[:length.item()]
- inp_batch.append(torch.unsqueeze(cur_batch,0))
-
- return inp_batch
-
- def summarize(self, src, tokenizer, model):
- """
- Function to use the pre-trained model to generate the summary
- Arguments:
- src: License text to summarise
- tokenizer: The tokenizer corresponding to the model used to convert the text into separate words(tokens)
- model: The pre-trained Model object used to perform the summarization
-
- Returns:
- summary: The summarised texts
- """
- batch_texts = self.generate_batch(src, tokenizer)
-
- enc_summary_list = [model.generate(batch, max_length=512) for batch in batch_texts]
-
- summary_list = [tokenizer.batch_decode(enc_summ, skip_special_tokens=True) for enc_summ in enc_summary_list]
- # orig_list = [tokenizer.batch_decode(batch, skip_special_tokens=True) for batch in batch_texts]
-
- summary_texts = [summ[0] for summ in summary_list]
- summary = " ".join(summary_texts)
-
- self.summary = summary
-
-
- def bart(self, src):
- """
- Initialize the facebook BART pre-trained model and call necessary functions to summarize
- Arguments:
- src: The text to summarise
-
- Returns/Set as instance variable:
- The summarized text
- """
-
- start_time = time.time()
- model_name = 'facebook/bart-large-cnn'
- device = 'cuda' if torch.cuda.is_available() else 'cpu'
- tokenizer = AutoTokenizer.from_pretrained(model_name)
- model = BartForConditionalGeneration.from_pretrained(model_name).to(device)
-
- self.summarize(src, tokenizer, model)
-
-
-
-def get_summary(lic_txt):
- """
- Summarize the license and return it
- Arguments:
- spdx - Id of License to summarise
-
- Returns:
- pos_text: The part of the License containing information for permitted use
- neg_text: The part of the License containing information about usage restrictions
- lic_txt: The full license text
- summary - The generated summary of the license
- """
- print('Summarising...')
- absSum = AbstractiveSummarizer()
-
- # Generate summary
- thread = absSum.bart(lic_txt)
-
- return thread
-
-
-def extract_ngrams(phrase):
- phrase = re.sub('[^a-zA-Z0-9]',' ', phrase)
- tokens = word_tokenize(phrase)
- res = []
- for num in range(len(tokens)+1):
- temp = ngrams(tokens, num)
- res += [' '.join(grams) for grams in temp]
-
- return res
-
-
-def get_highlight_text(text, keywords):
- """
- Custom function to find exact position of keywords for highlighting
- """
-
- text = re.sub('[-/]',' ', text)
- # text = re.sub('(\n *){2,}','\n',text)
- text = re.sub(' {2,}', ' ', text)
-
- # Group keywords by length
- kw_len = defaultdict(list)
- for kw in keywords:
- kw_len[len(kw)].append(kw)
-
- # Use sliding window technique to check equal strings
- spans = []
- for length in kw_len:
- w_start, w_end = 0, length
-
- while w_end <= len(text):
-
- for kw in kw_len[length]:
- j = w_start
- eq = True
- for i in range(len(kw)):
- if text[j] != kw[i]:
- eq = False
- break
- j += 1
- if eq:
- spans.append([w_start, w_end])
- break
-
- w_start += 1
- w_end += 1
-
- if not spans:
- return text
-
- # merge spans
- spans.sort(key=lambda x: x[0])
- merged = []
-
- st, end = spans[0][0], spans[0][1]
-
- for i in range(1, len(spans)):
- s,e = spans[i]
-
- if st <= s <= end:
- end = max(e, end)
- else:
- merged.append([st, end])
- st, end = s,e
- merged.append([st,end])
-
- res = []
- sub_start = 0
- for s,e in merged:
- res.append(text[sub_start:s])
- res.append((text[s:e], "", "#f66"))
- sub_start = e
- res.append(text[sub_start:])
-
- return res
-
-
-
-def get_keywords(datatype, task, field, pos_text, neg_text):
- """
- Summarize the license and generate the good and bad use tags
- Arguments:
- datafield - Type of 'data' used under the license: Eg. Model, Data, Model Derivatives, Source Code
- task - The type of task the model is designed to do
- field - Which 'field' to use the data in: Eg. Medical, Commercial, Non-Commercial, Research
- pos_text: The part of the License containing information for permitted use
- neg_text: The part of the License containing information about usage restrictions
-
- Returns:
- p_keywords - List of Positive(Permitted use) keywords extracted from summary
- n_keywords - List of Negative(Restriction) keywords extracted from summary
- contrd - boolean flag to show if there is any contradiction or not
- hl_text - the license text formatted to display in a highlighted manner
- """
- print('Extracting keywords...')
-
- #[e.lower() for e in list_strings]
- datatype, task, field = datatype.lower(), task.lower(), field.lower()
- #datatype = [e.lower() for e in datatype]
- #task = [e.lower() for e in task]
- #field = [e.lower() for e in field]
- #datatype, task, field = datatype, task, str(field)
-
-
- stop_words = set(stopwords.words('english'))
- #stops = nltk.corpus.stopwords.words('english')
- #stop_words = set(stops)
- stop_words = stop_words.union({'license', 'licensing', 'licensor', 'copyright', 'copyrights', 'patent'})
-
- pos_kw_model = KeyBERT()
- neg_kw_model = KeyBERT()
-
- candidates = []
- for term in [datatype, task, field]:
- candidates += extract_ngrams(term)
-
- p_kw = pos_kw_model.extract_keywords(docs=pos_text, top_n=40, vectorizer=KeyphraseCountVectorizer(stop_words=stop_words))#, pos_pattern='+'))
- n_kw = neg_kw_model.extract_keywords(docs=neg_text, top_n=40, vectorizer=KeyphraseCountVectorizer(stop_words=stop_words))#, pos_pattern='+'))
-
- ngram_max = max([len(word_tokenize(x)) for x in [datatype, task, field]])
-
- pc_kw = pos_kw_model.extract_keywords(docs=pos_text ,candidates=candidates, keyphrase_ngram_range=(1,ngram_max))
- nc_kw = neg_kw_model.extract_keywords(docs=neg_text ,candidates=candidates, keyphrase_ngram_range=(1,ngram_max))
-
- # Check contradiction
- all_cont = [kw for (kw,_) in nc_kw]
- cont_terms = set(all_cont).intersection(set(extract_ngrams(field)))
- contrd = True if len(cont_terms) > 0 else False
- hl_text = "" if not contrd else get_highlight_text(neg_text, all_cont)
-
- p_kw += pc_kw
- n_kw += nc_kw
-
- p_kw.sort(key=lambda x: x[1], reverse=True)
- n_kw.sort(key=lambda x: x[1], reverse=True)
-
- p_keywords = [kw for (kw,score) in p_kw if score < 0.5]
- n_keywords = [kw for (kw,score) in n_kw if score < 0.5]
-
- return p_keywords, n_keywords, contrd, hl_text
\ No newline at end of file
diff --git a/spaces/FridaZuley/RVC_HFKawaii/infer/modules/vc/modules.py b/spaces/FridaZuley/RVC_HFKawaii/infer/modules/vc/modules.py
deleted file mode 100644
index 458cfbe860b23bdd8f07abc2934443e6b8b01c3a..0000000000000000000000000000000000000000
--- a/spaces/FridaZuley/RVC_HFKawaii/infer/modules/vc/modules.py
+++ /dev/null
@@ -1,526 +0,0 @@
-import os, sys
-import traceback
-import logging
-now_dir = os.getcwd()
-sys.path.append(now_dir)
-logger = logging.getLogger(__name__)
-import lib.globals.globals as rvc_globals
-import numpy as np
-import soundfile as sf
-import torch
-from io import BytesIO
-from infer.lib.audio import load_audio
-from infer.lib.audio import wav2
-from infer.lib.infer_pack.models import (
- SynthesizerTrnMs256NSFsid,
- SynthesizerTrnMs256NSFsid_nono,
- SynthesizerTrnMs768NSFsid,
- SynthesizerTrnMs768NSFsid_nono,
-)
-from infer.modules.vc.pipeline import Pipeline
-from infer.modules.vc.utils import *
-import time
-import scipy.io.wavfile as wavfile
-
-def note_to_hz(note_name):
- SEMITONES = {'C': -9, 'C#': -8, 'D': -7, 'D#': -6, 'E': -5, 'F': -4, 'F#': -3, 'G': -2, 'G#': -1, 'A': 0, 'A#': 1, 'B': 2}
- pitch_class, octave = note_name[:-1], int(note_name[-1])
- semitone = SEMITONES[pitch_class]
- note_number = 12 * (octave - 4) + semitone
- frequency = 440.0 * (2.0 ** (1.0/12)) ** note_number
- return frequency
-
-class VC:
- def __init__(self, config):
- self.n_spk = None
- self.tgt_sr = None
- self.net_g = None
- self.pipeline = None
- self.cpt = None
- self.version = None
- self.if_f0 = None
- self.version = None
- self.hubert_model = None
-
- self.config = config
-
- def get_vc(self, sid, *to_return_protect):
- logger.info("Get sid: " + sid)
-
- to_return_protect0 = {
- "visible": self.if_f0 != 0,
- "value": to_return_protect[0]
- if self.if_f0 != 0 and to_return_protect
- else 0.5,
- "__type__": "update",
- }
- to_return_protect1 = {
- "visible": self.if_f0 != 0,
- "value": to_return_protect[1]
- if self.if_f0 != 0 and to_return_protect
- else 0.33,
- "__type__": "update",
- }
-
- if not sid:
- if self.hubert_model is not None: # 考虑到轮询, 需要加个判断看是否 sid 是由有模型切换到无模型的
- logger.info("Clean model cache")
- del (
- self.net_g,
- self.n_spk,
- self.vc,
- self.hubert_model,
- self.tgt_sr,
- ) # ,cpt
- self.hubert_model = (
- self.net_g
- ) = self.n_spk = self.vc = self.hubert_model = self.tgt_sr = None
- if torch.cuda.is_available():
- torch.cuda.empty_cache()
- ###楼下不这么折腾清理不干净
- self.if_f0 = self.cpt.get("f0", 1)
- self.version = self.cpt.get("version", "v1")
- if self.version == "v1":
- if self.if_f0 == 1:
- self.net_g = SynthesizerTrnMs256NSFsid(
- *self.cpt["config"], is_half=self.config.is_half
- )
- else:
- self.net_g = SynthesizerTrnMs256NSFsid_nono(*self.cpt["config"])
- elif self.version == "v2":
- if self.if_f0 == 1:
- self.net_g = SynthesizerTrnMs768NSFsid(
- *self.cpt["config"], is_half=self.config.is_half
- )
- else:
- self.net_g = SynthesizerTrnMs768NSFsid_nono(*self.cpt["config"])
- del self.net_g, self.cpt
- if torch.cuda.is_available():
- torch.cuda.empty_cache()
- return (
- {"visible": False, "__type__": "update"},
- {
- "visible": True,
- "value": to_return_protect0,
- "__type__": "update",
- },
- {
- "visible": True,
- "value": to_return_protect1,
- "__type__": "update",
- },
- "",
- "",
- )
- #person = f'{os.getenv("weight_root")}/{sid}'
- person = f'{sid}'
- #logger.info(f"Loading: {person}")
- logger.info(f"Loading...")
- self.cpt = torch.load(person, map_location="cpu")
- self.tgt_sr = self.cpt["config"][-1]
- self.cpt["config"][-3] = self.cpt["weight"]["emb_g.weight"].shape[0] # n_spk
- self.if_f0 = self.cpt.get("f0", 1)
- self.version = self.cpt.get("version", "v1")
-
- synthesizer_class = {
- ("v1", 1): SynthesizerTrnMs256NSFsid,
- ("v1", 0): SynthesizerTrnMs256NSFsid_nono,
- ("v2", 1): SynthesizerTrnMs768NSFsid,
- ("v2", 0): SynthesizerTrnMs768NSFsid_nono,
- }
-
- self.net_g = synthesizer_class.get(
- (self.version, self.if_f0), SynthesizerTrnMs256NSFsid
- )(*self.cpt["config"], is_half=self.config.is_half)
-
- del self.net_g.enc_q
-
- self.net_g.load_state_dict(self.cpt["weight"], strict=False)
- self.net_g.eval().to(self.config.device)
- if self.config.is_half:
- self.net_g = self.net_g.half()
- else:
- self.net_g = self.net_g.float()
-
- self.pipeline = Pipeline(self.tgt_sr, self.config)
- n_spk = self.cpt["config"][-3]
- index = {"value": get_index_path_from_model(sid), "__type__": "update"}
- logger.info("Select index: " + index["value"])
-
- return (
- (
- {"visible": False, "maximum": n_spk, "__type__": "update"},
- to_return_protect0,
- to_return_protect1
- )
- if to_return_protect
- else {"visible": False, "maximum": n_spk, "__type__": "update"}
- )
-
-
- def vc_single(
- self,
- sid,
- input_audio_path0,
- input_audio_path1,
- f0_up_key,
- f0_file,
- f0_method,
- file_index,
- file_index2,
- index_rate,
- filter_radius,
- resample_sr,
- rms_mix_rate,
- protect,
- crepe_hop_length,
- f0_min,
- note_min,
- f0_max,
- note_max,
- f0_autotune,
- ):
- global total_time
- total_time = 0
- start_time = time.time()
- if not input_audio_path0 and not input_audio_path1:
- return "You need to upload an audio", None
-
- if (not os.path.exists(input_audio_path0)) and (not os.path.exists(os.path.join(now_dir, input_audio_path0))):
- return "Audio was not properly selected or doesn't exist", None
-
- input_audio_path1 = input_audio_path1 or input_audio_path0
- print(f"\nStarting inference for '{os.path.basename(input_audio_path1)}'")
- print("-------------------")
- f0_up_key = int(f0_up_key)
- if rvc_globals.NotesOrHertz and f0_method != 'rmvpe':
- f0_min = note_to_hz(note_min) if note_min else 50
- f0_max = note_to_hz(note_max) if note_max else 1100
- print(f"Converted Min pitch: freq - {f0_min}\n"
- f"Converted Max pitch: freq - {f0_max}")
- else:
- f0_min = f0_min or 50
- f0_max = f0_max or 1100
- try:
- input_audio_path1 = input_audio_path1 or input_audio_path0
- print(f"Attempting to load {input_audio_path1}....")
- audio = load_audio(file=input_audio_path1,
- sr=16000,
- DoFormant=rvc_globals.DoFormant,
- Quefrency=rvc_globals.Quefrency,
- Timbre=rvc_globals.Timbre)
-
- audio_max = np.abs(audio).max() / 0.95
- if audio_max > 1:
- audio /= audio_max
- times = [0, 0, 0]
-
- if self.hubert_model is None:
- self.hubert_model = load_hubert(self.config)
-
- try:
- self.if_f0 = self.cpt.get("f0", 1)
- except NameError:
- message = "Model was not properly selected"
- print(message)
- return message, None
-
- file_index = (
- (
- file_index.strip(" ")
- .strip('"')
- .strip("\n")
- .strip('"')
- .strip(" ")
- .replace("trained", "added")
- )
- if file_index != ""
- else file_index2
- ) # 防止小白写错,自动帮他替换掉
-
- try:
- audio_opt = self.pipeline.pipeline(
- self.hubert_model,
- self.net_g,
- sid,
- audio,
- input_audio_path1,
- times,
- f0_up_key,
- f0_method,
- file_index,
- index_rate,
- self.if_f0,
- filter_radius,
- self.tgt_sr,
- resample_sr,
- rms_mix_rate,
- self.version,
- protect,
- crepe_hop_length,
- f0_autotune,
- f0_file=f0_file,
- f0_min=f0_min,
- f0_max=f0_max
- )
- except AssertionError:
- message = "Mismatching index version detected (v1 with v2, or v2 with v1)."
- print(message)
- return message, None
- except NameError:
- message = "RVC libraries are still loading. Please try again in a few seconds."
- print(message)
- return message, None
-
- if self.tgt_sr != resample_sr >= 16000:
- self.tgt_sr = resample_sr
- index_info = (
- "Index:\n%s." % file_index
- if os.path.exists(file_index)
- else "Index not used."
- )
- end_time = time.time()
- total_time = end_time - start_time
-
- output_folder = "audio-outputs"
- os.makedirs(output_folder, exist_ok=True)
- output_filename = "generated_audio_{}.wav"
- output_count = 1
- while True:
- current_output_path = os.path.join(output_folder, output_filename.format(output_count))
- if not os.path.exists(current_output_path):
- break
- output_count += 1
-
- wavfile.write(current_output_path, self.tgt_sr, audio_opt)
- print(f"Generated audio saved to: {current_output_path}")
- return f"Success.\n {index_info}\nTime:\n npy:{times[0]}, f0:{times[1]}, infer:{times[2]}\nTotal Time: {total_time} seconds", (self.tgt_sr, audio_opt)
- except:
- info = traceback.format_exc()
- logger.warn(info)
- return info, (None, None)
-
- def vc_single_dont_save(
- self,
- sid,
- input_audio_path0,
- input_audio_path1,
- f0_up_key,
- f0_file,
- f0_method,
- file_index,
- file_index2,
- index_rate,
- filter_radius,
- resample_sr,
- rms_mix_rate,
- protect,
- crepe_hop_length,
- f0_min,
- note_min,
- f0_max,
- note_max,
- f0_autotune,
- ):
- global total_time
- total_time = 0
- start_time = time.time()
- if not input_audio_path0 and not input_audio_path1:
- return "You need to upload an audio", None
-
- if (not os.path.exists(input_audio_path0)) and (not os.path.exists(os.path.join(now_dir, input_audio_path0))):
- return "Audio was not properly selected or doesn't exist", None
-
- input_audio_path1 = input_audio_path1 or input_audio_path0
- print(f"\nStarting inference for '{os.path.basename(input_audio_path1)}'")
- print("-------------------")
- f0_up_key = int(f0_up_key)
- if rvc_globals.NotesOrHertz and f0_method != 'rmvpe':
- f0_min = note_to_hz(note_min) if note_min else 50
- f0_max = note_to_hz(note_max) if note_max else 1100
- print(f"Converted Min pitch: freq - {f0_min}\n"
- f"Converted Max pitch: freq - {f0_max}")
- else:
- f0_min = f0_min or 50
- f0_max = f0_max or 1100
- try:
- input_audio_path1 = input_audio_path1 or input_audio_path0
- print(f"Attempting to load {input_audio_path1}....")
- audio = load_audio(file=input_audio_path1,
- sr=16000,
- DoFormant=rvc_globals.DoFormant,
- Quefrency=rvc_globals.Quefrency,
- Timbre=rvc_globals.Timbre)
-
- audio_max = np.abs(audio).max() / 0.95
- if audio_max > 1:
- audio /= audio_max
- times = [0, 0, 0]
-
- if self.hubert_model is None:
- self.hubert_model = load_hubert(self.config)
-
- try:
- self.if_f0 = self.cpt.get("f0", 1)
- except NameError:
- message = "Model was not properly selected"
- print(message)
- return message, None
-
- file_index = (
- (
- file_index.strip(" ")
- .strip('"')
- .strip("\n")
- .strip('"')
- .strip(" ")
- .replace("trained", "added")
- )
- if file_index != ""
- else file_index2
- ) # 防止小白写错,自动帮他替换掉
-
- try:
- audio_opt = self.pipeline.pipeline(
- self.hubert_model,
- self.net_g,
- sid,
- audio,
- input_audio_path1,
- times,
- f0_up_key,
- f0_method,
- file_index,
- index_rate,
- self.if_f0,
- filter_radius,
- self.tgt_sr,
- resample_sr,
- rms_mix_rate,
- self.version,
- protect,
- crepe_hop_length,
- f0_autotune,
- f0_file=f0_file,
- f0_min=f0_min,
- f0_max=f0_max
- )
- except AssertionError:
- message = "Mismatching index version detected (v1 with v2, or v2 with v1)."
- print(message)
- return message, None
- except NameError:
- message = "RVC libraries are still loading. Please try again in a few seconds."
- print(message)
- return message, None
-
- if self.tgt_sr != resample_sr >= 16000:
- self.tgt_sr = resample_sr
- index_info = (
- "Index:\n%s." % file_index
- if os.path.exists(file_index)
- else "Index not used."
- )
- end_time = time.time()
- total_time = end_time - start_time
-
- return f"Success.\n {index_info}\nTime:\n npy:{times[0]}, f0:{times[1]}, infer:{times[2]}\nTotal Time: {total_time} seconds", (self.tgt_sr, audio_opt)
- except:
- info = traceback.format_exc()
- logger.warn(info)
- return info, (None, None)
-
-
- def vc_multi(
- self,
- sid,
- dir_path,
- opt_root,
- paths,
- f0_up_key,
- f0_method,
- file_index,
- file_index2,
- index_rate,
- filter_radius,
- resample_sr,
- rms_mix_rate,
- protect,
- format1,
- crepe_hop_length,
- f0_min,
- note_min,
- f0_max,
- note_max,
- f0_autotune,
- ):
- if rvc_globals.NotesOrHertz and f0_method != 'rmvpe':
- f0_min = note_to_hz(note_min) if note_min else 50
- f0_max = note_to_hz(note_max) if note_max else 1100
- print(f"Converted Min pitch: freq - {f0_min}\n"
- f"Converted Max pitch: freq - {f0_max}")
- else:
- f0_min = f0_min or 50
- f0_max = f0_max or 1100
- try:
- dir_path = (
- dir_path.strip(" ").strip('"').strip("\n").strip('"').strip(" ")
- ) # 防止小白拷路径头尾带了空格和"和回车
- opt_root = opt_root.strip(" ").strip('"').strip("\n").strip('"').strip(" ")
- os.makedirs(opt_root, exist_ok=True)
- try:
- if dir_path != "":
- paths = [
- os.path.join(dir_path, name) for name in os.listdir(dir_path)
- ]
- else:
- paths = [path.name for path in paths]
- except:
- traceback.print_exc()
- paths = [path.name for path in paths]
- infos = []
- for path in paths:
- info, opt = self.vc_single(
- sid,
- path,
- f0_up_key,
- None,
- f0_method,
- file_index,
- file_index2,
- # file_big_npy,
- index_rate,
- filter_radius,
- resample_sr,
- rms_mix_rate,
- protect,
- )
- if "Success" in info:
- try:
- tgt_sr, audio_opt = opt
- if format1 in ["wav", "flac"]:
- sf.write(
- "%s/%s.%s"
- % (opt_root, os.path.basename(path), format1),
- audio_opt,
- tgt_sr,
- )
- else:
- path = "%s/%s.%s" % (opt_root, os.path.basename(path), format1)
- with BytesIO() as wavf:
- sf.write(
- wavf,
- audio_opt,
- tgt_sr,
- format="wav"
- )
- wavf.seek(0, 0)
- with open(path, "wb") as outf:
- wav2(wavf, outf, format1)
- except:
- info += traceback.format_exc()
- infos.append("%s->%s" % (os.path.basename(path), info))
- yield "\n".join(infos)
- yield "\n".join(infos)
- except:
- yield traceback.format_exc()
diff --git a/spaces/Froleptan/stablediffusion-infinity/PyPatchMatch/csrc/pyinterface.cpp b/spaces/Froleptan/stablediffusion-infinity/PyPatchMatch/csrc/pyinterface.cpp
deleted file mode 100644
index 612ccba6544ff111a2da0dce9adc4019858ebded..0000000000000000000000000000000000000000
--- a/spaces/Froleptan/stablediffusion-infinity/PyPatchMatch/csrc/pyinterface.cpp
+++ /dev/null
@@ -1,107 +0,0 @@
-#include "pyinterface.h"
-#include "inpaint.h"
-
-static unsigned int PM_seed = 1212;
-static bool PM_verbose = false;
-
-int _dtype_py_to_cv(int dtype_py);
-int _dtype_cv_to_py(int dtype_cv);
-cv::Mat _py_to_cv2(PM_mat_t pymat);
-PM_mat_t _cv2_to_py(cv::Mat cvmat);
-
-void PM_set_random_seed(unsigned int seed) {
- PM_seed = seed;
-}
-
-void PM_set_verbose(int value) {
- PM_verbose = static_cast(value);
-}
-
-void PM_free_pymat(PM_mat_t pymat) {
- free(pymat.data_ptr);
-}
-
-PM_mat_t PM_inpaint(PM_mat_t source_py, PM_mat_t mask_py, int patch_size) {
- cv::Mat source = _py_to_cv2(source_py);
- cv::Mat mask = _py_to_cv2(mask_py);
- auto metric = PatchSSDDistanceMetric(patch_size);
- cv::Mat result = Inpainting(source, mask, &metric).run(PM_verbose, false, PM_seed);
- return _cv2_to_py(result);
-}
-
-PM_mat_t PM_inpaint_regularity(PM_mat_t source_py, PM_mat_t mask_py, PM_mat_t ijmap_py, int patch_size, float guide_weight) {
- cv::Mat source = _py_to_cv2(source_py);
- cv::Mat mask = _py_to_cv2(mask_py);
- cv::Mat ijmap = _py_to_cv2(ijmap_py);
-
- auto metric = RegularityGuidedPatchDistanceMetricV2(patch_size, ijmap, guide_weight);
- cv::Mat result = Inpainting(source, mask, &metric).run(PM_verbose, false, PM_seed);
- return _cv2_to_py(result);
-}
-
-PM_mat_t PM_inpaint2(PM_mat_t source_py, PM_mat_t mask_py, PM_mat_t global_mask_py, int patch_size) {
- cv::Mat source = _py_to_cv2(source_py);
- cv::Mat mask = _py_to_cv2(mask_py);
- cv::Mat global_mask = _py_to_cv2(global_mask_py);
-
- auto metric = PatchSSDDistanceMetric(patch_size);
- cv::Mat result = Inpainting(source, mask, global_mask, &metric).run(PM_verbose, false, PM_seed);
- return _cv2_to_py(result);
-}
-
-PM_mat_t PM_inpaint2_regularity(PM_mat_t source_py, PM_mat_t mask_py, PM_mat_t global_mask_py, PM_mat_t ijmap_py, int patch_size, float guide_weight) {
- cv::Mat source = _py_to_cv2(source_py);
- cv::Mat mask = _py_to_cv2(mask_py);
- cv::Mat global_mask = _py_to_cv2(global_mask_py);
- cv::Mat ijmap = _py_to_cv2(ijmap_py);
-
- auto metric = RegularityGuidedPatchDistanceMetricV2(patch_size, ijmap, guide_weight);
- cv::Mat result = Inpainting(source, mask, global_mask, &metric).run(PM_verbose, false, PM_seed);
- return _cv2_to_py(result);
-}
-
-int _dtype_py_to_cv(int dtype_py) {
- switch (dtype_py) {
- case PM_UINT8: return CV_8U;
- case PM_INT8: return CV_8S;
- case PM_UINT16: return CV_16U;
- case PM_INT16: return CV_16S;
- case PM_INT32: return CV_32S;
- case PM_FLOAT32: return CV_32F;
- case PM_FLOAT64: return CV_64F;
- }
-
- return CV_8U;
-}
-
-int _dtype_cv_to_py(int dtype_cv) {
- switch (dtype_cv) {
- case CV_8U: return PM_UINT8;
- case CV_8S: return PM_INT8;
- case CV_16U: return PM_UINT16;
- case CV_16S: return PM_INT16;
- case CV_32S: return PM_INT32;
- case CV_32F: return PM_FLOAT32;
- case CV_64F: return PM_FLOAT64;
- }
-
- return PM_UINT8;
-}
-
-cv::Mat _py_to_cv2(PM_mat_t pymat) {
- int dtype = _dtype_py_to_cv(pymat.dtype);
- dtype = CV_MAKETYPE(pymat.dtype, pymat.shape.channels);
- return cv::Mat(cv::Size(pymat.shape.width, pymat.shape.height), dtype, pymat.data_ptr).clone();
-}
-
-PM_mat_t _cv2_to_py(cv::Mat cvmat) {
- PM_shape_t shape = {cvmat.size().width, cvmat.size().height, cvmat.channels()};
- int dtype = _dtype_cv_to_py(cvmat.depth());
- size_t dsize = cvmat.total() * cvmat.elemSize();
-
- void *data_ptr = reinterpret_cast(malloc(dsize));
- memcpy(data_ptr, reinterpret_cast(cvmat.data), dsize);
-
- return PM_mat_t {data_ptr, shape, dtype};
-}
-
diff --git a/spaces/GlimmeringStars/Testing/README.md b/spaces/GlimmeringStars/Testing/README.md
deleted file mode 100644
index 3fe16448835a66b015adc61b6dc7170c0eafc66b..0000000000000000000000000000000000000000
--- a/spaces/GlimmeringStars/Testing/README.md
+++ /dev/null
@@ -1,10 +0,0 @@
----
-title: Testing
-emoji: 🏃
-colorFrom: yellow
-colorTo: green
-sdk: docker
-pinned: false
----
-
-Check out the configuration reference at https://huggingface.co/docs/hub/spaces-config-reference
diff --git a/spaces/Gradio-Blocks/StyleGAN-NADA/e4e/models/encoders/model_irse.py b/spaces/Gradio-Blocks/StyleGAN-NADA/e4e/models/encoders/model_irse.py
deleted file mode 100644
index 6a94d67542f961ff6533f0335cf4cb0fa54024fb..0000000000000000000000000000000000000000
--- a/spaces/Gradio-Blocks/StyleGAN-NADA/e4e/models/encoders/model_irse.py
+++ /dev/null
@@ -1,84 +0,0 @@
-from torch.nn import Linear, Conv2d, BatchNorm1d, BatchNorm2d, PReLU, Dropout, Sequential, Module
-from e4e.models.encoders.helpers import get_blocks, Flatten, bottleneck_IR, bottleneck_IR_SE, l2_norm
-
-"""
-Modified Backbone implementation from [TreB1eN](https://github.com/TreB1eN/InsightFace_Pytorch)
-"""
-
-
-class Backbone(Module):
- def __init__(self, input_size, num_layers, mode='ir', drop_ratio=0.4, affine=True):
- super(Backbone, self).__init__()
- assert input_size in [112, 224], "input_size should be 112 or 224"
- assert num_layers in [50, 100, 152], "num_layers should be 50, 100 or 152"
- assert mode in ['ir', 'ir_se'], "mode should be ir or ir_se"
- blocks = get_blocks(num_layers)
- if mode == 'ir':
- unit_module = bottleneck_IR
- elif mode == 'ir_se':
- unit_module = bottleneck_IR_SE
- self.input_layer = Sequential(Conv2d(3, 64, (3, 3), 1, 1, bias=False),
- BatchNorm2d(64),
- PReLU(64))
- if input_size == 112:
- self.output_layer = Sequential(BatchNorm2d(512),
- Dropout(drop_ratio),
- Flatten(),
- Linear(512 * 7 * 7, 512),
- BatchNorm1d(512, affine=affine))
- else:
- self.output_layer = Sequential(BatchNorm2d(512),
- Dropout(drop_ratio),
- Flatten(),
- Linear(512 * 14 * 14, 512),
- BatchNorm1d(512, affine=affine))
-
- modules = []
- for block in blocks:
- for bottleneck in block:
- modules.append(unit_module(bottleneck.in_channel,
- bottleneck.depth,
- bottleneck.stride))
- self.body = Sequential(*modules)
-
- def forward(self, x):
- x = self.input_layer(x)
- x = self.body(x)
- x = self.output_layer(x)
- return l2_norm(x)
-
-
-def IR_50(input_size):
- """Constructs a ir-50 model."""
- model = Backbone(input_size, num_layers=50, mode='ir', drop_ratio=0.4, affine=False)
- return model
-
-
-def IR_101(input_size):
- """Constructs a ir-101 model."""
- model = Backbone(input_size, num_layers=100, mode='ir', drop_ratio=0.4, affine=False)
- return model
-
-
-def IR_152(input_size):
- """Constructs a ir-152 model."""
- model = Backbone(input_size, num_layers=152, mode='ir', drop_ratio=0.4, affine=False)
- return model
-
-
-def IR_SE_50(input_size):
- """Constructs a ir_se-50 model."""
- model = Backbone(input_size, num_layers=50, mode='ir_se', drop_ratio=0.4, affine=False)
- return model
-
-
-def IR_SE_101(input_size):
- """Constructs a ir_se-101 model."""
- model = Backbone(input_size, num_layers=100, mode='ir_se', drop_ratio=0.4, affine=False)
- return model
-
-
-def IR_SE_152(input_size):
- """Constructs a ir_se-152 model."""
- model = Backbone(input_size, num_layers=152, mode='ir_se', drop_ratio=0.4, affine=False)
- return model
diff --git a/spaces/Gradio-Blocks/protGPT2_gradioFold/alphafold/alphafold/common/__init__.py b/spaces/Gradio-Blocks/protGPT2_gradioFold/alphafold/alphafold/common/__init__.py
deleted file mode 100644
index d3c65d69d5f61b7b9547153c47d84e7f545e2636..0000000000000000000000000000000000000000
--- a/spaces/Gradio-Blocks/protGPT2_gradioFold/alphafold/alphafold/common/__init__.py
+++ /dev/null
@@ -1,14 +0,0 @@
-# Copyright 2021 DeepMind Technologies Limited
-#
-# Licensed under the Apache License, Version 2.0 (the "License");
-# you may not use this file except in compliance with the License.
-# You may obtain a copy of the License at
-#
-# http://www.apache.org/licenses/LICENSE-2.0
-#
-# Unless required by applicable law or agreed to in writing, software
-# distributed under the License is distributed on an "AS IS" BASIS,
-# WITHOUT WARRANTIES OR CONDITIONS OF ANY KIND, either express or implied.
-# See the License for the specific language governing permissions and
-# limitations under the License.
-"""Common data types and constants used within Alphafold."""
diff --git a/spaces/Gradio-Blocks/protGPT2_gradioFold/alphafold/alphafold/model/tf/utils.py b/spaces/Gradio-Blocks/protGPT2_gradioFold/alphafold/alphafold/model/tf/utils.py
deleted file mode 100644
index fc40a2ceb2de1c2d56c17697393713804d7da350..0000000000000000000000000000000000000000
--- a/spaces/Gradio-Blocks/protGPT2_gradioFold/alphafold/alphafold/model/tf/utils.py
+++ /dev/null
@@ -1,47 +0,0 @@
-# Copyright 2021 DeepMind Technologies Limited
-#
-# Licensed under the Apache License, Version 2.0 (the "License");
-# you may not use this file except in compliance with the License.
-# You may obtain a copy of the License at
-#
-# http://www.apache.org/licenses/LICENSE-2.0
-#
-# Unless required by applicable law or agreed to in writing, software
-# distributed under the License is distributed on an "AS IS" BASIS,
-# WITHOUT WARRANTIES OR CONDITIONS OF ANY KIND, either express or implied.
-# See the License for the specific language governing permissions and
-# limitations under the License.
-
-"""Shared utilities for various components."""
-import tensorflow.compat.v1 as tf
-
-
-def tf_combine_mask(*masks):
- """Take the intersection of float-valued masks."""
- ret = 1
- for m in masks:
- ret *= m
- return ret
-
-
-class SeedMaker(object):
- """Return unique seeds."""
-
- def __init__(self, initial_seed=0):
- self.next_seed = initial_seed
-
- def __call__(self):
- i = self.next_seed
- self.next_seed += 1
- return i
-
-seed_maker = SeedMaker()
-
-
-def make_random_seed():
- return tf.random.uniform([2],
- tf.int32.min,
- tf.int32.max,
- tf.int32,
- seed=seed_maker())
-
diff --git a/spaces/Gradio-Blocks/uniformer_image_detection/configs/_base_/models/rpn_r50_caffe_c4.py b/spaces/Gradio-Blocks/uniformer_image_detection/configs/_base_/models/rpn_r50_caffe_c4.py
deleted file mode 100644
index 9c32a55ddaa88812c8020872c33502122c409041..0000000000000000000000000000000000000000
--- a/spaces/Gradio-Blocks/uniformer_image_detection/configs/_base_/models/rpn_r50_caffe_c4.py
+++ /dev/null
@@ -1,56 +0,0 @@
-# model settings
-model = dict(
- type='RPN',
- pretrained='open-mmlab://detectron2/resnet50_caffe',
- backbone=dict(
- type='ResNet',
- depth=50,
- num_stages=3,
- strides=(1, 2, 2),
- dilations=(1, 1, 1),
- out_indices=(2, ),
- frozen_stages=1,
- norm_cfg=dict(type='BN', requires_grad=False),
- norm_eval=True,
- style='caffe'),
- neck=None,
- rpn_head=dict(
- type='RPNHead',
- in_channels=1024,
- feat_channels=1024,
- anchor_generator=dict(
- type='AnchorGenerator',
- scales=[2, 4, 8, 16, 32],
- ratios=[0.5, 1.0, 2.0],
- strides=[16]),
- bbox_coder=dict(
- type='DeltaXYWHBBoxCoder',
- target_means=[.0, .0, .0, .0],
- target_stds=[1.0, 1.0, 1.0, 1.0]),
- loss_cls=dict(
- type='CrossEntropyLoss', use_sigmoid=True, loss_weight=1.0),
- loss_bbox=dict(type='L1Loss', loss_weight=1.0)),
- # model training and testing settings
- train_cfg=dict(
- rpn=dict(
- assigner=dict(
- type='MaxIoUAssigner',
- pos_iou_thr=0.7,
- neg_iou_thr=0.3,
- min_pos_iou=0.3,
- ignore_iof_thr=-1),
- sampler=dict(
- type='RandomSampler',
- num=256,
- pos_fraction=0.5,
- neg_pos_ub=-1,
- add_gt_as_proposals=False),
- allowed_border=0,
- pos_weight=-1,
- debug=False)),
- test_cfg=dict(
- rpn=dict(
- nms_pre=12000,
- max_per_img=2000,
- nms=dict(type='nms', iou_threshold=0.7),
- min_bbox_size=0)))
diff --git a/spaces/Gradio-Blocks/uniformer_image_detection/configs/fcos/fcos_x101_64x4d_fpn_gn-head_mstrain_640-800_2x_coco.py b/spaces/Gradio-Blocks/uniformer_image_detection/configs/fcos/fcos_x101_64x4d_fpn_gn-head_mstrain_640-800_2x_coco.py
deleted file mode 100644
index fc576f6a674ee61b7332dc2085c488bebf972030..0000000000000000000000000000000000000000
--- a/spaces/Gradio-Blocks/uniformer_image_detection/configs/fcos/fcos_x101_64x4d_fpn_gn-head_mstrain_640-800_2x_coco.py
+++ /dev/null
@@ -1,59 +0,0 @@
-_base_ = './fcos_r50_caffe_fpn_gn-head_1x_coco.py'
-model = dict(
- pretrained='open-mmlab://resnext101_64x4d',
- backbone=dict(
- type='ResNeXt',
- depth=101,
- groups=64,
- base_width=4,
- num_stages=4,
- out_indices=(0, 1, 2, 3),
- frozen_stages=1,
- norm_cfg=dict(type='BN', requires_grad=True),
- norm_eval=True,
- style='pytorch'))
-img_norm_cfg = dict(
- mean=[123.675, 116.28, 103.53], std=[58.395, 57.12, 57.375], to_rgb=True)
-train_pipeline = [
- dict(type='LoadImageFromFile'),
- dict(type='LoadAnnotations', with_bbox=True),
- dict(
- type='Resize',
- img_scale=[(1333, 640), (1333, 800)],
- multiscale_mode='value',
- keep_ratio=True),
- dict(type='RandomFlip', flip_ratio=0.5),
- dict(type='Normalize', **img_norm_cfg),
- dict(type='Pad', size_divisor=32),
- dict(type='DefaultFormatBundle'),
- dict(type='Collect', keys=['img', 'gt_bboxes', 'gt_labels']),
-]
-test_pipeline = [
- dict(type='LoadImageFromFile'),
- dict(
- type='MultiScaleFlipAug',
- img_scale=(1333, 800),
- flip=False,
- transforms=[
- dict(type='Resize', keep_ratio=True),
- dict(type='RandomFlip'),
- dict(type='Normalize', **img_norm_cfg),
- dict(type='Pad', size_divisor=32),
- dict(type='ImageToTensor', keys=['img']),
- dict(type='Collect', keys=['img']),
- ])
-]
-data = dict(
- samples_per_gpu=2,
- workers_per_gpu=2,
- train=dict(pipeline=train_pipeline),
- val=dict(pipeline=test_pipeline),
- test=dict(pipeline=test_pipeline))
-# optimizer
-optimizer = dict(
- lr=0.01, paramwise_cfg=dict(bias_lr_mult=2., bias_decay_mult=0.))
-optimizer_config = dict(
- _delete_=True, grad_clip=dict(max_norm=35, norm_type=2))
-# learning policy
-lr_config = dict(step=[16, 22])
-runner = dict(type='EpochBasedRunner', max_epochs=24)
diff --git a/spaces/Gradio-Blocks/uniformer_image_detection/mmdet/core/evaluation/__init__.py b/spaces/Gradio-Blocks/uniformer_image_detection/mmdet/core/evaluation/__init__.py
deleted file mode 100644
index d11ef15b9db95166b4427ad4d08debbd0630a741..0000000000000000000000000000000000000000
--- a/spaces/Gradio-Blocks/uniformer_image_detection/mmdet/core/evaluation/__init__.py
+++ /dev/null
@@ -1,15 +0,0 @@
-from .class_names import (cityscapes_classes, coco_classes, dataset_aliases,
- get_classes, imagenet_det_classes,
- imagenet_vid_classes, voc_classes)
-from .eval_hooks import DistEvalHook, EvalHook
-from .mean_ap import average_precision, eval_map, print_map_summary
-from .recall import (eval_recalls, plot_iou_recall, plot_num_recall,
- print_recall_summary)
-
-__all__ = [
- 'voc_classes', 'imagenet_det_classes', 'imagenet_vid_classes',
- 'coco_classes', 'cityscapes_classes', 'dataset_aliases', 'get_classes',
- 'DistEvalHook', 'EvalHook', 'average_precision', 'eval_map',
- 'print_map_summary', 'eval_recalls', 'print_recall_summary',
- 'plot_num_recall', 'plot_iou_recall'
-]
diff --git a/spaces/Gradio-Blocks/uniformer_image_segmentation/configs/resnest/deeplabv3plus_s101-d8_512x1024_80k_cityscapes.py b/spaces/Gradio-Blocks/uniformer_image_segmentation/configs/resnest/deeplabv3plus_s101-d8_512x1024_80k_cityscapes.py
deleted file mode 100644
index 69bef7238345cf6aabb126012af992602f910287..0000000000000000000000000000000000000000
--- a/spaces/Gradio-Blocks/uniformer_image_segmentation/configs/resnest/deeplabv3plus_s101-d8_512x1024_80k_cityscapes.py
+++ /dev/null
@@ -1,9 +0,0 @@
-_base_ = '../deeplabv3plus/deeplabv3plus_r101-d8_512x1024_80k_cityscapes.py'
-model = dict(
- pretrained='open-mmlab://resnest101',
- backbone=dict(
- type='ResNeSt',
- stem_channels=128,
- radix=2,
- reduction_factor=4,
- avg_down_stride=True))
diff --git a/spaces/HaloMaster/chinesesummary/fengshen/README.md b/spaces/HaloMaster/chinesesummary/fengshen/README.md
deleted file mode 100644
index 45f7b3579c36a68f899a9a02cfcfbe1330d413d8..0000000000000000000000000000000000000000
--- a/spaces/HaloMaster/chinesesummary/fengshen/README.md
+++ /dev/null
@@ -1,105 +0,0 @@
-## 最新发布
-
-* \[2022.09.13\] [更新ErLangShen系列DeBERTa预训练代码](https://huggingface.co/IDEA-CCNL/Erlangshen-DeBERTa-v2-97M-Chinese)
-* \[2022.09.13\] [更新RanDeng系列Bart预训练代码](https://huggingface.co/IDEA-CCNL/Randeng-BART-139M)
-* \[2022.09.13\] [更新ErLangShen系列Bert预训练代码](https://huggingface.co/IDEA-CCNL/Erlangshen-MegatronBert-1.3B)
-* \[2022.05.11\] [更新TaiYi系列VIT多模态模型及下游任务示例](https://fengshenbang-doc.readthedocs.io/zh/latest/docs/太乙系列/Taiyi-vit-87M-D.html)
-* \[2022.05.11\] [更新BiGan系列Transformer-XL去噪模型及下游任务示例](https://fengshenbang-doc.readthedocs.io/zh/latest/docs/比干系列/Bigan-Transformer-XL-denoise-1.1B.html)
-* \[2022.05.11\] [更新ErLangShen系列下游任务示例](https://fengshenbang-doc.readthedocs.io/zh/latest/docs/二郎神系列/Erlangshen-Roberta-110M-NLI.html)
-
-# 导航
-
-- [导航](#导航)
- - [框架简介](#框架简介)
- - [依赖环境](#依赖环境)
- - [项目结构](#项目结构)
- - [设计思路](#设计思路)
- - [分类下游任务](#分类下游任务)
-
-## 框架简介
-
-FengShen训练框架是封神榜大模型开源计划的重要一环,在大模型的生产和应用中起到至关重要的作用。FengShen可以应用在基于海量数据的预训练以及各种下游任务的finetune中。封神榜专注于NLP大模型开源,然而模型的增大带来不仅仅是训练的问题,在使用上也存在诸多不便。为了解决训练和使用的问题,FengShen参考了目前开源的优秀方案并且重新设计了Pipeline,用户可以根据自己的需求,从封神榜中选取丰富的预训练模型,同时利用FengShen快速微调下游任务。
-
-目前所有实例以及文档可以查看我们的[Wiki](https://fengshenbang-doc.readthedocs.io/zh/latest/index.html)
-所有的模型可以在[Huggingface主页](https://huggingface.co/IDEA-CCNL)找到
-
-通过我们的框架,你可以快速享受到:
-
-1. 比原生torch更强的性能,训练速度提升**300%**
-2. 支持更大的模型,支持**百亿级别**内模型训练及微调
-3. 支持**TB级以上**的数据集,在家用主机上即可享受预训练模型带来的效果提升
-3. 丰富的预训练、下游任务示例,一键开始训练
-4. 适应各种设备环境,支持在CPU、GPU、TPU等不同设备上运行
-5. 集成主流的分布式训练逻辑,无需修改代码即可支持DDP、Zero Optimizer等分布式优化技术
-
-
-
-## 依赖环境
-
-* Python >= 3.8
-* torch >= 1.8
-* transformers >= 3.2.0
-* pytorch-lightning >= 1.5.10
-
-在Fengshenbang-LM根目录下
-pip install --editable ./
-
-## 项目结构
-
-```
-├── data # 支持多种数据处理方式以及数据集
-│ ├── cbart_dataloader
-| ├── fs_datasets # 基于transformers datasets的封装,新增中文数据集(开源计划中)
-| ├── universal_datamodule # 打通fs_datasets与lightning datamodule,减少重复开发工作量
-│ ├── megatron_dataloader # 支持基于Megatron实现的TB级别数据集处理、训练
-│ ├── mmap_dataloader # 通用的Memmap形式的数据加载
-│ └── task_dataloader # 支持多种下游任务
-├── examples # 丰富的示例,从预训练到下游任务,应有尽有。
-├── metric # 提供各种metric计算,支持用户自定义metric
-├── losses # 同样支持loss自定义,满足定制化需求
-├── tokenizer # 支持自定义tokenizer,比如我们使用的SentencePiece训练代码等
-├── models # 模型库
-│ ├── auto # 支持自动导入对应的模型
-│ ├── bart
-│ ├── longformer
-│ ├── megatron_t5
-│ └── roformer
-└── utils # 实用函数
-```
-
-## 设计思路
-
-FengShen框架目前整体基于Pytorch-Lightning & Transformer进行开发,在底层框架上不断开源基于中文的预训练模型,同时提供丰富的examples,每一个封神榜的模型都能找到对应的预训练、下游任务代码。
-
-在FengShen上开发,整体可以按照下面的三个步骤进行:
-
-1. 封装数据处理流程 -> pytorch_lightning.LightningDataModule
-2. 封装模型结构 -> pytorch_lightning.LightningModule
-3. 配置一些插件,比如log_monitor,checkpoint_callback等等。
-
-一个完整的DEMO可以看Randeng-BART系列实例 -> [文档](https://fengshenbang-doc.readthedocs.io/zh/latest/docs/燃灯系列/BART-139M.html) [代码](https://github.com/IDEA-CCNL/Fengshenbang-LM/tree/hf-ds/fengshen/examples/pretrain_bart)
-
-## 分类下游任务
-
- 在examples/classification目录下,我们提供丰富的分类任务的示例,其中我们提供三个一键式运行的示例。
-
-* demo_classification_afqmc_roberta.sh 使用DDP微调roberta
-* demo_classification_afqmc_roberta_deepspeed.sh 结合deepspeed微调roberta,获得更快的运算速度
-* demo_classification_afqmc_erlangshen_offload.sh 仅需7G显存即可微调我们效果最好的二郎神系列模型
-
- 上述示例均采用AFQMC的数据集,关于数据集的介绍可以在[这里](https://www.cluebenchmarks.com/introduce.html)找到。
- 同时我们处理过的数据文件已经放在Huggingface上,点击[这里](https://huggingface.co/datasets/IDEA-CCNL/AFQMC)直达源文件。
- 仅需要按我们的格式稍微处理一下数据集,即可适配下游不同的分类任务。
- 在脚本示例中,仅需要修改如下参数即可适配本地文件
-
- ```
- --dataset_name IDEA-CCNL/AFQMC \
-
- -------> 修改为
-
- --data_dir $DATA_DIR \ # 数据目录
- --train_data train.json \ # 数据文件
- --valid_data dev.json \
- --test_data test.json \
-
- ```
diff --git a/spaces/HaloMaster/chinesesummary/fengshen/examples/zen2_finetune/ner_zen2_base_cmeee.sh b/spaces/HaloMaster/chinesesummary/fengshen/examples/zen2_finetune/ner_zen2_base_cmeee.sh
deleted file mode 100644
index 46f27f142891c62587f6c7184c372f4883215bbf..0000000000000000000000000000000000000000
--- a/spaces/HaloMaster/chinesesummary/fengshen/examples/zen2_finetune/ner_zen2_base_cmeee.sh
+++ /dev/null
@@ -1,91 +0,0 @@
-#!/bin/bash
-#SBATCH --job-name=zen2_base_cmeee # create a short name for your job
-#SBATCH --nodes=1 # node count
-#SBATCH --ntasks=1 # total number of tasks across all nodes
-#SBATCH --cpus-per-task=30 # cpu-cores per task (>1 if multi-threaded tasks)
-#SBATCH --gres=gpu:1 # number of gpus per node
-#SBATCH --mail-type=ALL # send email when job begins, ends or failed etc.
-#SBATCH -o /cognitive_comp/ganruyi/experiments/ner_finetune/zen2_base_cmeee/%x-%j.log # output and error file name (%x=job name, %j=job id)
-
-
-# export CUDA_VISIBLE_DEVICES='2'
-export TORCH_EXTENSIONS_DIR=/cognitive_comp/ganruyi/tmp/torch_extendsions
-
-MODEL_NAME=zen2_base
-
-TASK=cmeee
-
-ZERO_STAGE=1
-STRATEGY=deepspeed_stage_${ZERO_STAGE}
-
-ROOT_DIR=/cognitive_comp/ganruyi/experiments/ner_finetune/${MODEL_NAME}_${TASK}
-if [ ! -d ${ROOT_DIR} ];then
- mkdir -p ${ROOT_DIR}
- echo ${ROOT_DIR} created!!!!!!!!!!!!!!
-else
- echo ${ROOT_DIR} exist!!!!!!!!!!!!!!!
-fi
-
-DATA_DIR=/cognitive_comp/lujunyu/data_zh/NER_Aligned/CMeEE/
-PRETRAINED_MODEL_PATH=/cognitive_comp/ganruyi/hf_models/zen/zh_zen_base_2.0
-
-CHECKPOINT_PATH=${ROOT_DIR}/ckpt/
-OUTPUT_PATH=${ROOT_DIR}/predict.json
-
-DATA_ARGS="\
- --data_dir $DATA_DIR \
- --train_data train.char.bio \
- --valid_data dev.char.bio \
- --test_data dev.char.bio \
- --train_batchsize 32 \
- --valid_batchsize 16 \
- --max_seq_length 256 \
- --task_name cmeee \
- "
-
-MODEL_ARGS="\
- --learning_rate 3e-5 \
- --weight_decay 0.1 \
- --warmup_ratio 0.01 \
- --markup bio \
- --middle_prefix I- \
- "
-
-MODEL_CHECKPOINT_ARGS="\
- --monitor val_f1 \
- --save_top_k 3 \
- --mode max \
- --every_n_train_steps 100 \
- --save_weights_only True \
- --dirpath $CHECKPOINT_PATH \
- --filename model-{epoch:02d}-{val_f1:.4f} \
- "
-
-TRAINER_ARGS="\
- --max_epochs 30 \
- --gpus 1 \
- --check_val_every_n_epoch 1 \
- --val_check_interval 100 \
- --default_root_dir $ROOT_DIR \
- "
-
-
-options=" \
- --pretrained_model_path $PRETRAINED_MODEL_PATH \
- --vocab_file $PRETRAINED_MODEL_PATH/vocab.txt \
- --do_lower_case \
- --output_save_path $OUTPUT_PATH \
- $DATA_ARGS \
- $MODEL_ARGS \
- $MODEL_CHECKPOINT_ARGS \
- $TRAINER_ARGS \
-"
-SCRIPT_PATH=/cognitive_comp/ganruyi/Fengshenbang-LM/fengshen/examples/zen2_finetune/fengshen_token_level_ft_task.py
-/home/ganruyi/anaconda3/bin/python $SCRIPT_PATH $options
-
-# SINGULARITY_PATH=/cognitive_comp/ganruyi/pytorch21_06_py3_docker_image_v2.sif
-# python3 $SCRIPT_PATH $options
-# source activate base
-# singularity exec --nv -B /cognitive_comp/:/cognitive_comp/ $SINGULARITY_PATH /home/ganruyi/anaconda3/bin/python $SCRIPT_PATH $options
-# /home/ganruyi/anaconda3/bin/python $SCRIPT_PATH $options
-
diff --git a/spaces/HarryLee/eCommerceImageCaptioning/fairseq/examples/megatron_11b/detok.py b/spaces/HarryLee/eCommerceImageCaptioning/fairseq/examples/megatron_11b/detok.py
deleted file mode 100644
index 49921b28a1f35c6216b5ed85729453524e7a049d..0000000000000000000000000000000000000000
--- a/spaces/HarryLee/eCommerceImageCaptioning/fairseq/examples/megatron_11b/detok.py
+++ /dev/null
@@ -1,32 +0,0 @@
-#!/usr/bin/env python3 -u
-# Copyright (c) Facebook, Inc. and its affiliates.
-#
-# This source code is licensed under the MIT license found in the
-# LICENSE file in the root directory of this source tree.
-
-import argparse
-import fileinput
-
-import sacremoses
-
-
-def main():
- parser = argparse.ArgumentParser(description="")
- parser.add_argument("files", nargs="*", help="input files")
- args = parser.parse_args()
-
- detok = sacremoses.MosesDetokenizer()
-
- for line in fileinput.input(args.files, openhook=fileinput.hook_compressed):
- print(
- detok.detokenize(line.strip().split(" "))
- .replace(" @", "")
- .replace("@ ", "")
- .replace(" =", "=")
- .replace("= ", "=")
- .replace(" – ", "–")
- )
-
-
-if __name__ == "__main__":
- main()
diff --git a/spaces/HarryLee/eCommerceImageCaptioning/fairseq/examples/textless_nlp/gslm/unit2speech/tacotron2/utils.py b/spaces/HarryLee/eCommerceImageCaptioning/fairseq/examples/textless_nlp/gslm/unit2speech/tacotron2/utils.py
deleted file mode 100644
index 66a426d2223ce75ffae6cee2131770556c5949bc..0000000000000000000000000000000000000000
--- a/spaces/HarryLee/eCommerceImageCaptioning/fairseq/examples/textless_nlp/gslm/unit2speech/tacotron2/utils.py
+++ /dev/null
@@ -1,167 +0,0 @@
-import collections
-import io
-import json
-import librosa
-import numpy as np
-import soundfile as sf
-import time
-import torch
-from scipy.io.wavfile import read
-from .text import SOS_TOK, EOS_TOK
-
-
-def get_mask_from_lengths(lengths):
- max_len = torch.max(lengths).item()
- ids = torch.arange(0, max_len, out=torch.cuda.LongTensor(max_len))
- mask = (ids < lengths.unsqueeze(1))
- return mask
-
-
-def load_wav_to_torch(full_path, sr=None):
- data, sr = librosa.load(full_path, sr=sr)
- data = np.clip(data, -1, 1) # potentially out of [-1, 1] due to resampling
- data = data * 32768.0 # match values loaded by scipy
- return torch.FloatTensor(data.astype(np.float32)), sr
-
-
-def read_binary_audio(bin_data, tar_sr=None):
- """
- read binary audio (`bytes` or `uint8` `numpy.ndarray`) to `float32`
- `numpy.ndarray`
-
- RETURNS:
- data (np.ndarray) : audio of shape (n,) or (2, n)
- tar_sr (int) : sample rate
- """
- data, ori_sr = sf.read(io.BytesIO(bin_data), dtype='float32')
- data = data.T
- if (tar_sr is not None) and (ori_sr != tar_sr):
- data = librosa.resample(data, ori_sr, tar_sr)
- else:
- tar_sr = ori_sr
- data = np.clip(data, -1, 1)
- data = data * 32768.0
- return torch.FloatTensor(data.astype(np.float32)), tar_sr
-
-
-def load_filepaths_and_text(filename):
- with open(filename, encoding='utf-8') as f:
- data = [json.loads(line.rstrip()) for line in f]
- return data
-
-
-def to_gpu(x):
- x = x.contiguous()
-
- if torch.cuda.is_available():
- x = x.cuda(non_blocking=True)
- return torch.autograd.Variable(x)
-
-
-def load_code_dict(path, add_sos=False, add_eos=False):
- if not path:
- return {}
-
- with open(path, 'r') as f:
- codes = ['_'] + [line.rstrip() for line in f] # '_' for pad
- code_dict = {c: i for i, c in enumerate(codes)}
-
- if add_sos:
- code_dict[SOS_TOK] = len(code_dict)
- if add_eos:
- code_dict[EOS_TOK] = len(code_dict)
- assert(set(code_dict.values()) == set(range(len(code_dict))))
-
- return code_dict
-
-
-def load_obs_label_dict(path):
- if not path:
- return {}
- with open(path, 'r') as f:
- obs_labels = [line.rstrip() for line in f]
- return {c: i for i, c in enumerate(obs_labels)}
-
-
-# A simple timer class inspired from `tnt.TimeMeter`
-class CudaTimer:
- def __init__(self, keys):
- self.keys = keys
- self.reset()
-
- def start(self, key):
- s = torch.cuda.Event(enable_timing=True)
- s.record()
- self.start_events[key].append(s)
- return self
-
- def stop(self, key):
- e = torch.cuda.Event(enable_timing=True)
- e.record()
- self.end_events[key].append(e)
- return self
-
- def reset(self):
- self.start_events = collections.defaultdict(list)
- self.end_events = collections.defaultdict(list)
- self.running_times = collections.defaultdict(float)
- self.n = collections.defaultdict(int)
- return self
-
- def value(self):
- self._synchronize()
- return {k: self.running_times[k] / self.n[k] for k in self.keys}
-
- def _synchronize(self):
- torch.cuda.synchronize()
- for k in self.keys:
- starts = self.start_events[k]
- ends = self.end_events[k]
- if len(starts) == 0:
- raise ValueError("Trying to divide by zero in TimeMeter")
- if len(ends) != len(starts):
- raise ValueError("Call stop before checking value!")
- time = 0
- for start, end in zip(starts, ends):
- time += start.elapsed_time(end)
- self.running_times[k] += time * 1e-3
- self.n[k] += len(starts)
- self.start_events = collections.defaultdict(list)
- self.end_events = collections.defaultdict(list)
-
-
-# Used to measure the time taken for multiple events
-class Timer:
- def __init__(self, keys):
- self.keys = keys
- self.n = {}
- self.running_time = {}
- self.total_time = {}
- self.reset()
-
- def start(self, key):
- self.running_time[key] = time.time()
- return self
-
- def stop(self, key):
- self.total_time[key] = time.time() - self.running_time[key]
- self.n[key] += 1
- self.running_time[key] = None
- return self
-
- def reset(self):
- for k in self.keys:
- self.total_time[k] = 0
- self.running_time[k] = None
- self.n[k] = 0
- return self
-
- def value(self):
- vals = {}
- for k in self.keys:
- if self.n[k] == 0:
- raise ValueError("Trying to divide by zero in TimeMeter")
- else:
- vals[k] = self.total_time[k] / self.n[k]
- return vals
-
diff --git a/spaces/Harveenchadha/Vakyansh-Hindi-TTS/ttsv/scripts/glow/prepare_data.sh b/spaces/Harveenchadha/Vakyansh-Hindi-TTS/ttsv/scripts/glow/prepare_data.sh
deleted file mode 100644
index 2357eeebd0fb7e6fba858242af44e8b8aa87fdf9..0000000000000000000000000000000000000000
--- a/spaces/Harveenchadha/Vakyansh-Hindi-TTS/ttsv/scripts/glow/prepare_data.sh
+++ /dev/null
@@ -1,12 +0,0 @@
-input_text_path='/home/harveen/en/iitm_data/english/txt.done.data'
-input_wav_path='/home/harveen/en/iitm_data/english/wav_22k'
-gender='male'
-
-
-output_data_path='../../data/glow/'$gender
-
-valid_samples=100
-test_samples=10
-
-mkdir -p $output_data_path
-python ../../utils/glow/prepare_iitm_data_glow_en.py -i $input_text_path -o $output_data_path -w $input_wav_path -v $valid_samples -t $test_samples
diff --git a/spaces/ICML2022/OFA/fairseq/examples/roberta/README.custom_classification.md b/spaces/ICML2022/OFA/fairseq/examples/roberta/README.custom_classification.md
deleted file mode 100644
index 7254bb7d178760ef5b847901bbcac3711af33ca2..0000000000000000000000000000000000000000
--- a/spaces/ICML2022/OFA/fairseq/examples/roberta/README.custom_classification.md
+++ /dev/null
@@ -1,168 +0,0 @@
-# Finetuning RoBERTa on a custom classification task
-
-This example shows how to finetune RoBERTa on the IMDB dataset, but should illustrate the process for most classification tasks.
-
-### 1) Get the data
-
-```bash
-wget http://ai.stanford.edu/~amaas/data/sentiment/aclImdb_v1.tar.gz
-tar zxvf aclImdb_v1.tar.gz
-```
-
-
-### 2) Format data
-
-`IMDB` data has one data-sample in each file, below python code-snippet converts it one file for train and valid each for ease of processing.
-```python
-import argparse
-import os
-import random
-from glob import glob
-
-random.seed(0)
-
-def main(args):
- for split in ['train', 'test']:
- samples = []
- for class_label in ['pos', 'neg']:
- fnames = glob(os.path.join(args.datadir, split, class_label) + '/*.txt')
- for fname in fnames:
- with open(fname) as fin:
- line = fin.readline()
- samples.append((line, 1 if class_label == 'pos' else 0))
- random.shuffle(samples)
- out_fname = 'train' if split == 'train' else 'dev'
- f1 = open(os.path.join(args.datadir, out_fname + '.input0'), 'w')
- f2 = open(os.path.join(args.datadir, out_fname + '.label'), 'w')
- for sample in samples:
- f1.write(sample[0] + '\n')
- f2.write(str(sample[1]) + '\n')
- f1.close()
- f2.close()
-
-if __name__ == '__main__':
- parser = argparse.ArgumentParser()
- parser.add_argument('--datadir', default='aclImdb')
- args = parser.parse_args()
- main(args)
-```
-
-
-### 3) BPE encode
-
-Run `multiprocessing_bpe_encoder`, you can also do this in previous step for each sample but that might be slower.
-```bash
-# Download encoder.json and vocab.bpe
-wget -N 'https://dl.fbaipublicfiles.com/fairseq/gpt2_bpe/encoder.json'
-wget -N 'https://dl.fbaipublicfiles.com/fairseq/gpt2_bpe/vocab.bpe'
-
-for SPLIT in train dev; do
- python -m examples.roberta.multiprocessing_bpe_encoder \
- --encoder-json encoder.json \
- --vocab-bpe vocab.bpe \
- --inputs "aclImdb/$SPLIT.input0" \
- --outputs "aclImdb/$SPLIT.input0.bpe" \
- --workers 60 \
- --keep-empty
-done
-```
-
-
-### 4) Preprocess data
-
-```bash
-# Download fairseq dictionary.
-wget -N 'https://dl.fbaipublicfiles.com/fairseq/gpt2_bpe/dict.txt'
-
-fairseq-preprocess \
- --only-source \
- --trainpref "aclImdb/train.input0.bpe" \
- --validpref "aclImdb/dev.input0.bpe" \
- --destdir "IMDB-bin/input0" \
- --workers 60 \
- --srcdict dict.txt
-
-fairseq-preprocess \
- --only-source \
- --trainpref "aclImdb/train.label" \
- --validpref "aclImdb/dev.label" \
- --destdir "IMDB-bin/label" \
- --workers 60
-
-```
-
-
-### 5) Run training
-
-```bash
-TOTAL_NUM_UPDATES=7812 # 10 epochs through IMDB for bsz 32
-WARMUP_UPDATES=469 # 6 percent of the number of updates
-LR=1e-05 # Peak LR for polynomial LR scheduler.
-HEAD_NAME=imdb_head # Custom name for the classification head.
-NUM_CLASSES=2 # Number of classes for the classification task.
-MAX_SENTENCES=8 # Batch size.
-ROBERTA_PATH=/path/to/roberta.large/model.pt
-
-CUDA_VISIBLE_DEVICES=0 fairseq-train IMDB-bin/ \
- --restore-file $ROBERTA_PATH \
- --max-positions 512 \
- --batch-size $MAX_SENTENCES \
- --max-tokens 4400 \
- --task sentence_prediction \
- --reset-optimizer --reset-dataloader --reset-meters \
- --required-batch-size-multiple 1 \
- --init-token 0 --separator-token 2 \
- --arch roberta_large \
- --criterion sentence_prediction \
- --classification-head-name $HEAD_NAME \
- --num-classes $NUM_CLASSES \
- --dropout 0.1 --attention-dropout 0.1 \
- --weight-decay 0.1 --optimizer adam --adam-betas "(0.9, 0.98)" --adam-eps 1e-06 \
- --clip-norm 0.0 \
- --lr-scheduler polynomial_decay --lr $LR --total-num-update $TOTAL_NUM_UPDATES --warmup-updates $WARMUP_UPDATES \
- --fp16 --fp16-init-scale 4 --threshold-loss-scale 1 --fp16-scale-window 128 \
- --max-epoch 10 \
- --best-checkpoint-metric accuracy --maximize-best-checkpoint-metric \
- --shorten-method "truncate" \
- --find-unused-parameters \
- --update-freq 4
-```
-
-The above command will finetune RoBERTa-large with an effective batch-size of 32
-sentences (`--batch-size=8 --update-freq=4`). The expected
-`best-validation-accuracy` after 10 epochs is ~96.5%.
-
-If you run out of GPU memory, try decreasing `--batch-size` and increase
-`--update-freq` to compensate.
-
-
-### 6) Load model using hub interface
-
-Now we can load the trained model checkpoint using the RoBERTa hub interface.
-
-Assuming your checkpoints are stored in `checkpoints/`:
-```python
-from fairseq.models.roberta import RobertaModel
-roberta = RobertaModel.from_pretrained(
- 'checkpoints',
- checkpoint_file='checkpoint_best.pt',
- data_name_or_path='IMDB-bin'
-)
-roberta.eval() # disable dropout
-```
-
-Finally you can make predictions using the `imdb_head` (or whatever you set
-`--classification-head-name` to during training):
-```python
-label_fn = lambda label: roberta.task.label_dictionary.string(
- [label + roberta.task.label_dictionary.nspecial]
-)
-
-tokens = roberta.encode('Best movie this year')
-pred = label_fn(roberta.predict('imdb_head', tokens).argmax().item())
-assert pred == '1' # positive
-
-tokens = roberta.encode('Worst movie ever')
-pred = label_fn(roberta.predict('imdb_head', tokens).argmax().item())
-assert pred == '0' # negative
-```
diff --git a/spaces/IISRFactCheck/claim_detection/code/prediction.py b/spaces/IISRFactCheck/claim_detection/code/prediction.py
deleted file mode 100644
index 7cbe68fe78c2e77d60371195d6cd175362bd0f64..0000000000000000000000000000000000000000
--- a/spaces/IISRFactCheck/claim_detection/code/prediction.py
+++ /dev/null
@@ -1,102 +0,0 @@
-import torch
-from args import args, config
-from tqdm import tqdm
-from items_dataset import items_dataset
-
-def test_predict(test_loader, device, model, min_label=1, max_label=3):
- model.eval()
- result = []
-
- for i, test_batch in enumerate(tqdm(test_loader)):
- batch_text = test_batch['batch_text']
- input_ids = test_batch['input_ids'].to(device)
- token_type_ids = test_batch['token_type_ids'].to(device)
- attention_mask = test_batch['attention_mask'].to(device)
- #labels = test_batch['labels'].to(device)
- crf_mask = test_batch["crf_mask"].to(device)
- sample_mapping = test_batch["overflow_to_sample_mapping"]
- output = model(input_ids=input_ids, token_type_ids=token_type_ids, attention_mask=attention_mask, labels=None, crf_mask=crf_mask)
- if args.use_crf:
- prediction = model.crf.decode(output[0], crf_mask)
- else:
- prediction = torch.max(output[0], -1).indices
-
- #make result of every sample
- sample_id = -1
- sample_result= {"text_a" : test_batch['batch_text'][0]}
- for batch_id in range(len(sample_mapping)):
- change_sample = False
- if sample_id != sample_mapping[batch_id]: change_sample = True
- #print(i, id)
- if change_sample:
- sample_id = sample_mapping[batch_id]
- sample_result= {"text_a" : test_batch['batch_text'][sample_id]}
- decode_span_table = torch.zeros(len(test_batch['batch_text'][sample_id]))
-
- spans = items_dataset.cal_agreement_span(None, agreement_table=prediction[batch_id], min_agree=min_label, max_agree=max_label)
- #decode spans
- for span in spans:
- #print(span)
- if span[0]==0: span[0]+=1
- if span[1]==1: span[1]+=1
-
- while(True):
- start = test_batch[batch_id].token_to_chars(span[0])
- if start != None or span[0]>=span[1]:
- break
- span[0]+=1
-
- while(True):
- end = test_batch[batch_id].token_to_chars(span[1])
- if end != None or span[0]>=span[1]:
- break
- span[1]-=1
-
- if span[0]512): print(de_start, de_end)
- decode_span_table[de_start:de_end]=2 #insite
- decode_span_table[de_start]=1 #begin
- if change_sample:
- sample_result["predict_span_table"] = decode_span_table
- #sample_result["boundary"] = test_batch["boundary"][id]
- result.append(sample_result)
- model.train()
- return result
-
-def add_sentence_table(result, pattern =":;。,,?!~!: ", threshold_num=5, threshold_rate=0.5):
- for sample in result:
- boundary_list = []
- for i, char in enumerate(sample['text_a']):
- if char in pattern:
- boundary_list.append(i)
- boundary_list.append(len(sample['text_a'])+1)
- start=0
- end =0
- fist_sentence = True
- sample["predict_sentence_table"] = torch.zeros(len(sample["predict_span_table"]))
- for boundary in boundary_list:
- end = boundary
- predict_num = sum(sample["predict_span_table"][start:end]>0)
- sentence_num = len(sample["predict_span_table"][start:end])
- if(predict_num > threshold_num) or (predict_num > sentence_num*threshold_rate):
- if fist_sentence:
- sample["predict_sentence_table"][start:end] = 2
- sample["predict_sentence_table"][start] = 1
- fist_sentence=False
- else:
- sample["predict_sentence_table"][start-1:end] = 2
- else: fist_sentence =True
- start = end+1
-
-def add_doc_id(result, test_data):
- #make dict {'text_a':"docid"}
- text_to_id = dict()
- for sample in test_data:
- text_to_id[sample["text_a"]] = sample["docid"]
-
- #add doc_id
- for sample in result:
- sample["docid"] = text_to_id[sample["text_a"]]
\ No newline at end of file
diff --git a/spaces/Iceclear/StableSR/StableSR/basicsr/data/vimeo90k_dataset.py b/spaces/Iceclear/StableSR/StableSR/basicsr/data/vimeo90k_dataset.py
deleted file mode 100644
index e5e33e1082667aeee61fecf2436fb287e82e0936..0000000000000000000000000000000000000000
--- a/spaces/Iceclear/StableSR/StableSR/basicsr/data/vimeo90k_dataset.py
+++ /dev/null
@@ -1,199 +0,0 @@
-import random
-import torch
-from pathlib import Path
-from torch.utils import data as data
-
-from basicsr.data.transforms import augment, paired_random_crop
-from basicsr.utils import FileClient, get_root_logger, imfrombytes, img2tensor
-from basicsr.utils.registry import DATASET_REGISTRY
-
-
-@DATASET_REGISTRY.register()
-class Vimeo90KDataset(data.Dataset):
- """Vimeo90K dataset for training.
-
- The keys are generated from a meta info txt file.
- basicsr/data/meta_info/meta_info_Vimeo90K_train_GT.txt
-
- Each line contains the following items, separated by a white space.
-
- 1. clip name;
- 2. frame number;
- 3. image shape
-
- Examples:
-
- ::
-
- 00001/0001 7 (256,448,3)
- 00001/0002 7 (256,448,3)
-
- - Key examples: "00001/0001"
- - GT (gt): Ground-Truth;
- - LQ (lq): Low-Quality, e.g., low-resolution/blurry/noisy/compressed frames.
-
- The neighboring frame list for different num_frame:
-
- ::
-
- num_frame | frame list
- 1 | 4
- 3 | 3,4,5
- 5 | 2,3,4,5,6
- 7 | 1,2,3,4,5,6,7
-
- Args:
- opt (dict): Config for train dataset. It contains the following keys:
- dataroot_gt (str): Data root path for gt.
- dataroot_lq (str): Data root path for lq.
- meta_info_file (str): Path for meta information file.
- io_backend (dict): IO backend type and other kwarg.
- num_frame (int): Window size for input frames.
- gt_size (int): Cropped patched size for gt patches.
- random_reverse (bool): Random reverse input frames.
- use_hflip (bool): Use horizontal flips.
- use_rot (bool): Use rotation (use vertical flip and transposing h and w for implementation).
- scale (bool): Scale, which will be added automatically.
- """
-
- def __init__(self, opt):
- super(Vimeo90KDataset, self).__init__()
- self.opt = opt
- self.gt_root, self.lq_root = Path(opt['dataroot_gt']), Path(opt['dataroot_lq'])
-
- with open(opt['meta_info_file'], 'r') as fin:
- self.keys = [line.split(' ')[0] for line in fin]
-
- # file client (io backend)
- self.file_client = None
- self.io_backend_opt = opt['io_backend']
- self.is_lmdb = False
- if self.io_backend_opt['type'] == 'lmdb':
- self.is_lmdb = True
- self.io_backend_opt['db_paths'] = [self.lq_root, self.gt_root]
- self.io_backend_opt['client_keys'] = ['lq', 'gt']
-
- # indices of input images
- self.neighbor_list = [i + (9 - opt['num_frame']) // 2 for i in range(opt['num_frame'])]
-
- # temporal augmentation configs
- self.random_reverse = opt['random_reverse']
- logger = get_root_logger()
- logger.info(f'Random reverse is {self.random_reverse}.')
-
- def __getitem__(self, index):
- if self.file_client is None:
- self.file_client = FileClient(self.io_backend_opt.pop('type'), **self.io_backend_opt)
-
- # random reverse
- if self.random_reverse and random.random() < 0.5:
- self.neighbor_list.reverse()
-
- scale = self.opt['scale']
- gt_size = self.opt['gt_size']
- key = self.keys[index]
- clip, seq = key.split('/') # key example: 00001/0001
-
- # get the GT frame (im4.png)
- if self.is_lmdb:
- img_gt_path = f'{key}/im4'
- else:
- img_gt_path = self.gt_root / clip / seq / 'im4.png'
- img_bytes = self.file_client.get(img_gt_path, 'gt')
- img_gt = imfrombytes(img_bytes, float32=True)
-
- # get the neighboring LQ frames
- img_lqs = []
- for neighbor in self.neighbor_list:
- if self.is_lmdb:
- img_lq_path = f'{clip}/{seq}/im{neighbor}'
- else:
- img_lq_path = self.lq_root / clip / seq / f'im{neighbor}.png'
- img_bytes = self.file_client.get(img_lq_path, 'lq')
- img_lq = imfrombytes(img_bytes, float32=True)
- img_lqs.append(img_lq)
-
- # randomly crop
- img_gt, img_lqs = paired_random_crop(img_gt, img_lqs, gt_size, scale, img_gt_path)
-
- # augmentation - flip, rotate
- img_lqs.append(img_gt)
- img_results = augment(img_lqs, self.opt['use_hflip'], self.opt['use_rot'])
-
- img_results = img2tensor(img_results)
- img_lqs = torch.stack(img_results[0:-1], dim=0)
- img_gt = img_results[-1]
-
- # img_lqs: (t, c, h, w)
- # img_gt: (c, h, w)
- # key: str
- return {'lq': img_lqs, 'gt': img_gt, 'key': key}
-
- def __len__(self):
- return len(self.keys)
-
-
-@DATASET_REGISTRY.register()
-class Vimeo90KRecurrentDataset(Vimeo90KDataset):
-
- def __init__(self, opt):
- super(Vimeo90KRecurrentDataset, self).__init__(opt)
-
- self.flip_sequence = opt['flip_sequence']
- self.neighbor_list = [1, 2, 3, 4, 5, 6, 7]
-
- def __getitem__(self, index):
- if self.file_client is None:
- self.file_client = FileClient(self.io_backend_opt.pop('type'), **self.io_backend_opt)
-
- # random reverse
- if self.random_reverse and random.random() < 0.5:
- self.neighbor_list.reverse()
-
- scale = self.opt['scale']
- gt_size = self.opt['gt_size']
- key = self.keys[index]
- clip, seq = key.split('/') # key example: 00001/0001
-
- # get the neighboring LQ and GT frames
- img_lqs = []
- img_gts = []
- for neighbor in self.neighbor_list:
- if self.is_lmdb:
- img_lq_path = f'{clip}/{seq}/im{neighbor}'
- img_gt_path = f'{clip}/{seq}/im{neighbor}'
- else:
- img_lq_path = self.lq_root / clip / seq / f'im{neighbor}.png'
- img_gt_path = self.gt_root / clip / seq / f'im{neighbor}.png'
- # LQ
- img_bytes = self.file_client.get(img_lq_path, 'lq')
- img_lq = imfrombytes(img_bytes, float32=True)
- # GT
- img_bytes = self.file_client.get(img_gt_path, 'gt')
- img_gt = imfrombytes(img_bytes, float32=True)
-
- img_lqs.append(img_lq)
- img_gts.append(img_gt)
-
- # randomly crop
- img_gts, img_lqs = paired_random_crop(img_gts, img_lqs, gt_size, scale, img_gt_path)
-
- # augmentation - flip, rotate
- img_lqs.extend(img_gts)
- img_results = augment(img_lqs, self.opt['use_hflip'], self.opt['use_rot'])
-
- img_results = img2tensor(img_results)
- img_lqs = torch.stack(img_results[:7], dim=0)
- img_gts = torch.stack(img_results[7:], dim=0)
-
- if self.flip_sequence: # flip the sequence: 7 frames to 14 frames
- img_lqs = torch.cat([img_lqs, img_lqs.flip(0)], dim=0)
- img_gts = torch.cat([img_gts, img_gts.flip(0)], dim=0)
-
- # img_lqs: (t, c, h, w)
- # img_gt: (c, h, w)
- # key: str
- return {'lq': img_lqs, 'gt': img_gts, 'key': key}
-
- def __len__(self):
- return len(self.keys)
diff --git a/spaces/Iceclear/StableSR/StableSR/scripts/sr_val_ddpm_text_T_vqganfin_oldcanvas_tile.py b/spaces/Iceclear/StableSR/StableSR/scripts/sr_val_ddpm_text_T_vqganfin_oldcanvas_tile.py
deleted file mode 100644
index d8d0671f9c059edb00a32773d6a5fe9deb1014d9..0000000000000000000000000000000000000000
--- a/spaces/Iceclear/StableSR/StableSR/scripts/sr_val_ddpm_text_T_vqganfin_oldcanvas_tile.py
+++ /dev/null
@@ -1,422 +0,0 @@
-"""make variations of input image"""
-
-import argparse, os, sys, glob
-import PIL
-import torch
-import numpy as np
-import torchvision
-from omegaconf import OmegaConf
-from PIL import Image
-from tqdm import tqdm, trange
-from itertools import islice
-from einops import rearrange, repeat
-from torchvision.utils import make_grid
-from torch import autocast
-from contextlib import nullcontext
-import time
-from pytorch_lightning import seed_everything
-
-from ldm.util import instantiate_from_config
-from ldm.models.diffusion.ddim import DDIMSampler
-from ldm.models.diffusion.plms import PLMSSampler
-import math
-import copy
-import torch.nn.functional as F
-import cv2
-from util_image import ImageSpliterTh
-from pathlib import Path
-from scripts.wavelet_color_fix import wavelet_reconstruction, adaptive_instance_normalization
-
-def space_timesteps(num_timesteps, section_counts):
- """
- Create a list of timesteps to use from an original diffusion process,
- given the number of timesteps we want to take from equally-sized portions
- of the original process.
- For example, if there's 300 timesteps and the section counts are [10,15,20]
- then the first 100 timesteps are strided to be 10 timesteps, the second 100
- are strided to be 15 timesteps, and the final 100 are strided to be 20.
- If the stride is a string starting with "ddim", then the fixed striding
- from the DDIM paper is used, and only one section is allowed.
- :param num_timesteps: the number of diffusion steps in the original
- process to divide up.
- :param section_counts: either a list of numbers, or a string containing
- comma-separated numbers, indicating the step count
- per section. As a special case, use "ddimN" where N
- is a number of steps to use the striding from the
- DDIM paper.
- :return: a set of diffusion steps from the original process to use.
- """
- if isinstance(section_counts, str):
- if section_counts.startswith("ddim"):
- desired_count = int(section_counts[len("ddim"):])
- for i in range(1, num_timesteps):
- if len(range(0, num_timesteps, i)) == desired_count:
- return set(range(0, num_timesteps, i))
- raise ValueError(
- f"cannot create exactly {num_timesteps} steps with an integer stride"
- )
- section_counts = [int(x) for x in section_counts.split(",")] #[250,]
- size_per = num_timesteps // len(section_counts)
- extra = num_timesteps % len(section_counts)
- start_idx = 0
- all_steps = []
- for i, section_count in enumerate(section_counts):
- size = size_per + (1 if i < extra else 0)
- if size < section_count:
- raise ValueError(
- f"cannot divide section of {size} steps into {section_count}"
- )
- if section_count <= 1:
- frac_stride = 1
- else:
- frac_stride = (size - 1) / (section_count - 1)
- cur_idx = 0.0
- taken_steps = []
- for _ in range(section_count):
- taken_steps.append(start_idx + round(cur_idx))
- cur_idx += frac_stride
- all_steps += taken_steps
- start_idx += size
- return set(all_steps)
-
-def chunk(it, size):
- it = iter(it)
- return iter(lambda: tuple(islice(it, size)), ())
-
-
-def load_model_from_config(config, ckpt, verbose=False):
- print(f"Loading model from {ckpt}")
- pl_sd = torch.load(ckpt, map_location="cpu")
- if "global_step" in pl_sd:
- print(f"Global Step: {pl_sd['global_step']}")
- sd = pl_sd["state_dict"]
- model = instantiate_from_config(config.model)
- m, u = model.load_state_dict(sd, strict=False)
- if len(m) > 0 and verbose:
- print("missing keys:")
- print(m)
- if len(u) > 0 and verbose:
- print("unexpected keys:")
- print(u)
-
- model.cuda()
- model.eval()
- return model
-
-def load_img(path):
- image = Image.open(path).convert("RGB")
- w, h = image.size
- print(f"loaded input image of size ({w}, {h}) from {path}")
- w, h = map(lambda x: x - x % 8, (w, h)) # resize to integer multiple of 32
- image = image.resize((w, h), resample=PIL.Image.LANCZOS)
- image = np.array(image).astype(np.float32) / 255.0
- image = image[None].transpose(0, 3, 1, 2)
- image = torch.from_numpy(image)
- return 2.*image - 1.
-
-def read_image(im_path):
- im = np.array(Image.open(im_path).convert("RGB"))
- im = im.astype(np.float32)/255.0
- im = im[None].transpose(0,3,1,2)
- im = (torch.from_numpy(im) - 0.5) / 0.5
-
- return im.cuda()
-
-def main():
- parser = argparse.ArgumentParser()
-
- parser.add_argument(
- "--init-img",
- type=str,
- nargs="?",
- help="path to the input image",
- default="inputs/user_upload"
- )
- parser.add_argument(
- "--outdir",
- type=str,
- nargs="?",
- help="dir to write results to",
- default="outputs/user_upload"
- )
- parser.add_argument(
- "--ddpm_steps",
- type=int,
- default=1000,
- help="number of ddpm sampling steps",
- )
- parser.add_argument(
- "--n_iter",
- type=int,
- default=1,
- help="sample this often",
- )
- parser.add_argument(
- "--C",
- type=int,
- default=4,
- help="latent channels",
- )
- parser.add_argument(
- "--f",
- type=int,
- default=8,
- help="downsampling factor, most often 8 or 16",
- )
- parser.add_argument(
- "--n_samples",
- type=int,
- default=1,
- help="how many samples to produce for each given prompt. A.k.a batch size",
- )
- parser.add_argument(
- "--config",
- type=str,
- default="configs/stable-diffusion/v1-inference.yaml",
- help="path to config which constructs model",
- )
- parser.add_argument(
- "--ckpt",
- type=str,
- default="./stablesr_000117.ckpt",
- help="path to checkpoint of model",
- )
- parser.add_argument(
- "--vqgan_ckpt",
- type=str,
- default="./vqgan_cfw_00011.ckpt",
- help="path to checkpoint of VQGAN model",
- )
- parser.add_argument(
- "--seed",
- type=int,
- default=42,
- help="the seed (for reproducible sampling)",
- )
- parser.add_argument(
- "--precision",
- type=str,
- help="evaluate at this precision",
- choices=["full", "autocast"],
- default="autocast"
- )
- parser.add_argument(
- "--dec_w",
- type=float,
- default=0.5,
- help="weight for combining VQGAN and Diffusion",
- )
- parser.add_argument(
- "--tile_overlap",
- type=int,
- default=32,
- help="tile overlap size (in latent)",
- )
- parser.add_argument(
- "--upscale",
- type=float,
- default=4.0,
- help="upsample scale",
- )
- parser.add_argument(
- "--colorfix_type",
- type=str,
- default="nofix",
- help="Color fix type to adjust the color of HR result according to LR input: adain (used in paper); wavelet; nofix",
- )
- parser.add_argument(
- "--vqgantile_stride",
- type=int,
- default=1000,
- help="the stride for tile operation before VQGAN decoder (in pixel)",
- )
- parser.add_argument(
- "--vqgantile_size",
- type=int,
- default=1280,
- help="the size for tile operation before VQGAN decoder (in pixel)",
- )
- parser.add_argument(
- "--input_size",
- type=int,
- default=512,
- help="input size",
- )
-
- opt = parser.parse_args()
- seed_everything(opt.seed)
-
- print('>>>>>>>>>>color correction>>>>>>>>>>>')
- if opt.colorfix_type == 'adain':
- print('Use adain color correction')
- elif opt.colorfix_type == 'wavelet':
- print('Use wavelet color correction')
- else:
- print('No color correction')
- print('>>>>>>>>>>>>>>>>>>>>>>>>>>>>>>>>>>>>>')
-
- config = OmegaConf.load(f"{opt.config}")
- model = load_model_from_config(config, f"{opt.ckpt}")
- device = torch.device("cuda") if torch.cuda.is_available() else torch.device("cpu")
- model = model.to(device)
-
- model.configs = config
-
- vqgan_config = OmegaConf.load("configs/autoencoder/autoencoder_kl_64x64x4_resi.yaml")
- vq_model = load_model_from_config(vqgan_config, opt.vqgan_ckpt)
- vq_model = vq_model.to(device)
- vq_model.decoder.fusion_w = opt.dec_w
-
- os.makedirs(opt.outdir, exist_ok=True)
- outpath = opt.outdir
-
- batch_size = opt.n_samples
-
- images_path_ori = sorted(glob.glob(os.path.join(opt.init_img, "*")))
- images_path = copy.deepcopy(images_path_ori)
- for item in images_path_ori:
- img_name = item.split('/')[-1]
- if os.path.exists(os.path.join(outpath, img_name)):
- images_path.remove(item)
- print(f"Found {len(images_path)} inputs.")
-
- model.register_schedule(given_betas=None, beta_schedule="linear", timesteps=1000,
- linear_start=0.00085, linear_end=0.0120, cosine_s=8e-3)
- model.num_timesteps = 1000
-
- sqrt_alphas_cumprod = copy.deepcopy(model.sqrt_alphas_cumprod)
- sqrt_one_minus_alphas_cumprod = copy.deepcopy(model.sqrt_one_minus_alphas_cumprod)
-
- use_timesteps = set(space_timesteps(1000, [opt.ddpm_steps]))
- last_alpha_cumprod = 1.0
- new_betas = []
- timestep_map = []
- for i, alpha_cumprod in enumerate(model.alphas_cumprod):
- if i in use_timesteps:
- new_betas.append(1 - alpha_cumprod / last_alpha_cumprod)
- last_alpha_cumprod = alpha_cumprod
- timestep_map.append(i)
- new_betas = [beta.data.cpu().numpy() for beta in new_betas]
- model.register_schedule(given_betas=np.array(new_betas), timesteps=len(new_betas))
- model.num_timesteps = 1000
- model.ori_timesteps = list(use_timesteps)
- model.ori_timesteps.sort()
- model = model.to(device)
-
- precision_scope = autocast if opt.precision == "autocast" else nullcontext
- niqe_list = []
- with torch.no_grad():
- with precision_scope("cuda"):
- with model.ema_scope():
- tic = time.time()
- all_samples = list()
- for n in trange(len(images_path), desc="Sampling"):
- if (n + 1) % opt.n_samples == 1 or opt.n_samples == 1:
- cur_image = read_image(images_path[n])
- size_min = min(cur_image.size(-1), cur_image.size(-2))
- upsample_scale = max(opt.input_size/size_min, opt.upscale)
- cur_image = F.interpolate(
- cur_image,
- size=(int(cur_image.size(-2)*upsample_scale),
- int(cur_image.size(-1)*upsample_scale)),
- mode='bicubic',
- )
- cur_image = cur_image.clamp(-1, 1)
- im_lq_bs = [cur_image, ] # 1 x c x h x w, [-1, 1]
- im_path_bs = [images_path[n], ]
- else:
- cur_image = read_image(images_path[n])
- size_min = min(cur_image.size(-1), cur_image.size(-2))
- upsample_scale = max(opt.input_size/size_min, opt.upscale)
- cur_image = F.interpolate(
- cur_image,
- size=(int(cur_image.size(-2)*upsample_scale),
- int(cur_image.size(-1)*upsample_scale)),
- mode='bicubic',
- )
- cur_image = cur_image.clamp(-1, 1)
- im_lq_bs.append(cur_image) # 1 x c x h x w, [-1, 1]
- im_path_bs.append(images_path[n]) # 1 x c x h x w, [-1, 1]
-
- if (n + 1) % opt.n_samples == 0 or (n+1) == len(images_path):
- im_lq_bs = torch.cat(im_lq_bs, dim=0)
- ori_h, ori_w = im_lq_bs.shape[2:]
- ref_patch=None
- if not (ori_h % 32 == 0 and ori_w % 32 == 0):
- flag_pad = True
- pad_h = ((ori_h // 32) + 1) * 32 - ori_h
- pad_w = ((ori_w // 32) + 1) * 32 - ori_w
- im_lq_bs = F.pad(im_lq_bs, pad=(0, pad_w, 0, pad_h), mode='reflect')
- else:
- flag_pad = False
-
- if im_lq_bs.shape[2] > opt.vqgantile_size or im_lq_bs.shape[3] > opt.vqgantile_size:
- im_spliter = ImageSpliterTh(im_lq_bs, opt.vqgantile_size, opt.vqgantile_stride, sf=1)
- for im_lq_pch, index_infos in im_spliter:
- seed_everything(opt.seed)
- init_latent = model.get_first_stage_encoding(model.encode_first_stage(im_lq_pch)) # move to latent space
- text_init = ['']*opt.n_samples
- semantic_c = model.cond_stage_model(text_init)
- noise = torch.randn_like(init_latent)
- # If you would like to start from the intermediate steps, you can add noise to LR to the specific steps.
- t = repeat(torch.tensor([999]), '1 -> b', b=im_lq_bs.size(0))
- t = t.to(device).long()
- x_T = model.q_sample_respace(x_start=init_latent, t=t, sqrt_alphas_cumprod=sqrt_alphas_cumprod, sqrt_one_minus_alphas_cumprod=sqrt_one_minus_alphas_cumprod, noise=noise)
- # x_T = noise
- samples, _ = model.sample_canvas(cond=semantic_c, struct_cond=init_latent, batch_size=im_lq_pch.size(0), timesteps=opt.ddpm_steps, time_replace=opt.ddpm_steps, x_T=x_T, return_intermediates=True, tile_size=int(opt.input_size/8), tile_overlap=opt.tile_overlap, batch_size_sample=opt.n_samples)
- _, enc_fea_lq = vq_model.encode(im_lq_pch)
- x_samples = vq_model.decode(samples * 1. / model.scale_factor, enc_fea_lq)
- if opt.colorfix_type == 'adain':
- x_samples = adaptive_instance_normalization(x_samples, im_lq_pch)
- elif opt.colorfix_type == 'wavelet':
- x_samples = wavelet_reconstruction(x_samples, im_lq_pch)
- im_spliter.update(x_samples, index_infos)
- im_sr = im_spliter.gather()
- im_sr = torch.clamp((im_sr+1.0)/2.0, min=0.0, max=1.0)
- else:
- init_latent = model.get_first_stage_encoding(model.encode_first_stage(im_lq_bs)) # move to latent space
- text_init = ['']*opt.n_samples
- semantic_c = model.cond_stage_model(text_init)
- noise = torch.randn_like(init_latent)
- # If you would like to start from the intermediate steps, you can add noise to LR to the specific steps.
- t = repeat(torch.tensor([999]), '1 -> b', b=im_lq_bs.size(0))
- t = t.to(device).long()
- x_T = model.q_sample_respace(x_start=init_latent, t=t, sqrt_alphas_cumprod=sqrt_alphas_cumprod, sqrt_one_minus_alphas_cumprod=sqrt_one_minus_alphas_cumprod, noise=noise)
- # x_T = noise
- samples, _ = model.sample_canvas(cond=semantic_c, struct_cond=init_latent, batch_size=im_lq_bs.size(0), timesteps=opt.ddpm_steps, time_replace=opt.ddpm_steps, x_T=x_T, return_intermediates=True, tile_size=int(opt.input_size/8), tile_overlap=opt.tile_overlap, batch_size_sample=opt.n_samples)
- _, enc_fea_lq = vq_model.encode(im_lq_bs)
- x_samples = vq_model.decode(samples * 1. / model.scale_factor, enc_fea_lq)
- if opt.colorfix_type == 'adain':
- x_samples = adaptive_instance_normalization(x_samples, im_lq_bs)
- elif opt.colorfix_type == 'wavelet':
- x_samples = wavelet_reconstruction(x_samples, im_lq_bs)
- im_sr = torch.clamp((x_samples+1.0)/2.0, min=0.0, max=1.0)
-
- if upsample_scale > opt.upscale:
- im_sr = F.interpolate(
- im_sr,
- size=(int(im_lq_bs.size(-2)*opt.upscale/upsample_scale),
- int(im_lq_bs.size(-1)*opt.upscale/upsample_scale)),
- mode='bicubic',
- )
- im_sr = torch.clamp(im_sr, min=0.0, max=1.0)
-
- im_sr = im_sr.cpu().numpy().transpose(0,2,3,1)*255 # b x h x w x c
-
- if flag_pad:
- im_sr = im_sr[:, :ori_h, :ori_w, ]
-
- for jj in range(im_lq_bs.shape[0]):
- img_name = str(Path(im_path_bs[jj]).name)
- basename = os.path.splitext(os.path.basename(img_name))[0]
- outpath = str(Path(opt.outdir)) + '/' + basename + '.png'
- Image.fromarray(im_sr[jj, ].astype(np.uint8)).save(outpath)
-
- toc = time.time()
-
- print(f"Your samples are ready and waiting for you here: \n{outpath} \n"
- f" \nEnjoy.")
-
-
-if __name__ == "__main__":
- main()
diff --git a/spaces/Illumotion/Koboldcpp/include/CL/Utils/OpenCLUtilsCpp_Export.h b/spaces/Illumotion/Koboldcpp/include/CL/Utils/OpenCLUtilsCpp_Export.h
deleted file mode 100644
index b063c9fe11a1ecd5959feb5a30562f052012f8a2..0000000000000000000000000000000000000000
--- a/spaces/Illumotion/Koboldcpp/include/CL/Utils/OpenCLUtilsCpp_Export.h
+++ /dev/null
@@ -1,42 +0,0 @@
-
-#ifndef UTILSCPP_EXPORT_H
-#define UTILSCPP_EXPORT_H
-
-#ifdef OPENCLUTILSCPP_STATIC_DEFINE
-# define UTILSCPP_EXPORT
-# define OPENCLUTILSCPP_NO_EXPORT
-#else
-# ifndef UTILSCPP_EXPORT
-# ifdef OpenCLUtilsCpp_EXPORTS
- /* We are building this library */
-# define UTILSCPP_EXPORT
-# else
- /* We are using this library */
-# define UTILSCPP_EXPORT
-# endif
-# endif
-
-# ifndef OPENCLUTILSCPP_NO_EXPORT
-# define OPENCLUTILSCPP_NO_EXPORT
-# endif
-#endif
-
-#ifndef OPENCLUTILSCPP_DEPRECATED
-# define OPENCLUTILSCPP_DEPRECATED __declspec(deprecated)
-#endif
-
-#ifndef OPENCLUTILSCPP_DEPRECATED_EXPORT
-# define OPENCLUTILSCPP_DEPRECATED_EXPORT UTILSCPP_EXPORT OPENCLUTILSCPP_DEPRECATED
-#endif
-
-#ifndef OPENCLUTILSCPP_DEPRECATED_NO_EXPORT
-# define OPENCLUTILSCPP_DEPRECATED_NO_EXPORT OPENCLUTILSCPP_NO_EXPORT OPENCLUTILSCPP_DEPRECATED
-#endif
-
-#if 0 /* DEFINE_NO_DEPRECATED */
-# ifndef OPENCLUTILSCPP_NO_DEPRECATED
-# define OPENCLUTILSCPP_NO_DEPRECATED
-# endif
-#endif
-
-#endif /* UTILSCPP_EXPORT_H */
diff --git a/spaces/Jeff2323/ai-comic-factory/src/components/ui/table.tsx b/spaces/Jeff2323/ai-comic-factory/src/components/ui/table.tsx
deleted file mode 100644
index 953fb3c003bc0cd9d93059c373bc23e6aecbded8..0000000000000000000000000000000000000000
--- a/spaces/Jeff2323/ai-comic-factory/src/components/ui/table.tsx
+++ /dev/null
@@ -1,114 +0,0 @@
-import * as React from "react"
-
-import { cn } from "@/lib/utils"
-
-const Table = React.forwardRef<
- HTMLTableElement,
- React.HTMLAttributes
->(({ className, ...props }, ref) => (
-
-))
-Table.displayName = "Table"
-
-const TableHeader = React.forwardRef<
- HTMLTableSectionElement,
- React.HTMLAttributes
->(({ className, ...props }, ref) => (
-
-))
-TableHeader.displayName = "TableHeader"
-
-const TableBody = React.forwardRef<
- HTMLTableSectionElement,
- React.HTMLAttributes
->(({ className, ...props }, ref) => (
-
-))
-TableBody.displayName = "TableBody"
-
-const TableFooter = React.forwardRef<
- HTMLTableSectionElement,
- React.HTMLAttributes
->(({ className, ...props }, ref) => (
- |