If you are an Android user who wants to access and manage your device from your computer, you may have heard of Airdroid, a popular tool that lets you do just that. But what if you want to enjoy more features and benefits without paying for the premium subscription? You may have also heard of Airdroid Premium Crack, a modified version of Airdroid that claims to offer you all the premium features for free. But is it safe and legal to use? And are there any alternatives to it? In this article, we will answer these questions and more.
- Airdroid also supports multiple languages, dark mode, QR code login, SMS backup, call logs, etc.
- Despite its many features and benefits, Airdroid is not perfect. Some of the drawbacks and limitations of Airdroid are:
-Airdroid Premium is a paid subscription that unlocks more features and benefits for Airdroid users. With Airdroid Premium, you can enjoy:
-The price of Airdroid Premium is $1.99 per month or $19.99 per year. You can also get a 7-day free trial before you decide to purchase it. You can pay with PayPal, credit card, debit card, Google Play balance, etc.
- b2dd77e56b
-
-
\ No newline at end of file
diff --git a/spaces/1gistliPinn/ChatGPT4/Examples/Arma 3 1.14 Multiplayer Crack.md b/spaces/1gistliPinn/ChatGPT4/Examples/Arma 3 1.14 Multiplayer Crack.md
deleted file mode 100644
index 596bad65b70ab1867159f7c6c1289e09c6121fc5..0000000000000000000000000000000000000000
--- a/spaces/1gistliPinn/ChatGPT4/Examples/Arma 3 1.14 Multiplayer Crack.md
+++ /dev/null
@@ -1,6 +0,0 @@
-arma 3 1.14 multiplayer crack
Download ✔✔✔ https://imgfil.com/2uxZDl
-
-Arma 3 1.14 Crack Education Program are autonomy about 30 utilities and ... open occurrences much. bachata music free online and ST& this PowerPoint ... 4d29de3e1b
-
-
-
diff --git a/spaces/1gistliPinn/ChatGPT4/Examples/DISQLite3 Pro 5.22.0 D4-XE10.2.md b/spaces/1gistliPinn/ChatGPT4/Examples/DISQLite3 Pro 5.22.0 D4-XE10.2.md
deleted file mode 100644
index c0d8e1d6336321e151c55736207fa764111e26cd..0000000000000000000000000000000000000000
--- a/spaces/1gistliPinn/ChatGPT4/Examples/DISQLite3 Pro 5.22.0 D4-XE10.2.md
+++ /dev/null
@@ -1,6 +0,0 @@
-
- in addition, disqlite3 pro is a powerful application for the creation and manage of database programs and databases. it is not very difficult to use the application, and more importantly, it has a graphical interface for the creation and management of the database. in addition, it is possible to create all types of databases and database files using this application. all the databases are stored in the same directory, and the user does not have to enter the path of the database. it is also possible to create the database program by the application. this application can be used for the creation of the database files, in addition to the creation of the database files from the url. the application is also available for windows and mac os. users can make use of the application for the creation and management of the database and the database program.
- furthermore, disqlite3 pro is a powerful application for the creation and management of database programs and databases. it is not very difficult to use the application, and more importantly, it has a graphical interface for the creation and management of the database. in addition, it is possible to create all types of databases and database files using this application. all the databases are stored in the same directory, and the user does not have to enter the path of the database. it is also possible to create the database program by the application. this application can be used for the creation of the database files, in addition to the creation of the database files from the url. the application is also available for windows and mac os. users can make use of the application for the creation and management of the database and the database program.
-DISQLite3 Pro 5.22.0 D4-XE10.2
Download 🆓 https://imgfil.com/2uxZs4
899543212b
-
-
\ No newline at end of file
diff --git a/spaces/1gistliPinn/ChatGPT4/Examples/Download The Last Train - Bullet Train Download] [Torrent]l Everything You Need to Know About the Movie and the Torrent.md b/spaces/1gistliPinn/ChatGPT4/Examples/Download The Last Train - Bullet Train Download] [Torrent]l Everything You Need to Know About the Movie and the Torrent.md
deleted file mode 100644
index 99b18526d76d3f92139738ec5deb63d23e3ed5bc..0000000000000000000000000000000000000000
--- a/spaces/1gistliPinn/ChatGPT4/Examples/Download The Last Train - Bullet Train Download] [Torrent]l Everything You Need to Know About the Movie and the Torrent.md
+++ /dev/null
@@ -1,28 +0,0 @@
-
-Its extensive torrent index makes it one of the best movie torrent sites out there. You can download movies of all genres from The Pirate Bay without worrying about downloading suspicious files.
-There is a list of backup trackers given on the torrents page listing. Add them to get every last bit of available speed. GloTorrents also has an active forum where you can request torrents, subtitles, and more.
-The Last Train - Bullet Train Download] [Torrent]l
Download File ✑ https://imgfil.com/2uxZbq
-This article was co-authored by wikiHow Staff. Our trained team of editors and researchers validate articles for accuracy and comprehensiveness. wikiHow's Content Management Team carefully monitors the work from our editorial staff to ensure that each article is backed by trusted research and meets our high quality standards.
This article has been viewed 199,709 times.
Learn more...
-It is especially helpful in preventing hackers from stealing your data while connected to an unsecure public Wi-Fi network. A VPN for torrenting allows you the anonymity to download as much as you want.
-Technically, it is safe to torrent. It is based on a P2P (peer-to-peer) network where all participants share bits of a file. As more people download a file or some portion of it, they can become an active participant.
-It depends on where you are downloading the file more than anything else. Public torrents are swarming with trojans that infect your system with malware such as a cryptominer. To prevent this from happening, always be mindful of what you download. Copyrighted material such as games are usually a honeypot for hackers.
-Privacy experts recommend the use of a Torrent VPN to make your torrent activities anonymous. With a VPN for torrenting, you can download torrent files securely in countries dominated by DMCAs and copyright laws.
-Kickasstorrents.to is probably the oldest and still functioning Kickass clones that users can access right now. You can access it using a VPN for all your torrenting needs. it offers complete Kickass torrents database with a whole connection of movies, series, documentaries, and much more for users to download. The site also has its Kickass community where it provides regular updates of the latest torrents available for download.
-Tor-cr.org is yet another great Kickass clone. It has turned up to be a very useful clone website as it offers the complete list of Kickass Torrents. The website is easily accessible from all regions unless your ISP has imposed regional-restrictions on these versions of Kickass. However, using a VPN will give you full access to Tor-cr.org and download torrents from a wide range of content categories.
-
-Kat.li is another top Kickass clone website with a fast and powerful Torrents search engine similar to the one we had with the original Kickass website. The site indexes torrent files from multiple domains and provide a huge collection of Kickass torrents for users to download their favorite content including TV Shows, Movies, Games, Music, Apps and many more.
-Although there is a very slight chance that the above mentioned torrenting clone websites could get shut down in the near future, if they do, you can make do with non-English torrenting sites to find your favorite content. These Non-English torrenting websites may be difficult to use for English-only downloaders, you can still use the help of Google translator to translate and change to the language of the website to make it easy for you to download stuff easily.
-The popular animetorrents indexing website got shut down recently, causing concerns for all torrent fans who relied on the website to download anime content. But it is now back with a new interface and the same directory of torrents. You can download your favorite anime movie and series without any problems.
-ArenaBG is a Bulgarian-torrents indexing website. It has been a target of a lot of investigations for violating copyright laws, but it is still up and running. Initially it was only available to access in Bulgaria, however, since 2011, users from around the world can access it easily. ArenaBG offers a huge selection of torrents for download and you can access it easily from anywhere. But remember, to avoid any trouble, you can use a Kickass VPN to stay anonymous and private.
-ExtraTorrent is a great torrent website and thousands of users use it to download their favorite torrents every day. It offers a huge database of torrents for download and is surely one of the best Kickass alternatives you must consider.
-Torrents.me work like a multi-search engine that allows you to search and download your favorite torrents from popular torrenting websites like the Pirate Bay, ExtraTorrent, and LimeTorrents. You can easily add your preferred torrenting websites in the search and find your favorite torrents through their database.
-Since 1985, SERTC has provided hands-on, realistic training in surface transportation hazmat response. With new facilities and expanding curriculum, the SERTC trainee community is growing to keep local, state, tribal and territorial communities even safer.
-As he was older and stronger than any of the other members who took upracing, and as he always rode the lightest and best wheel that money couldprocure, he had, without much hard work, easily maintained a lead in theracing field, and had come to consider himself as invincible. He regardedhimself as such a sure winner of this last[Pg 6] race for the Railroad Cup,that he had not taken the trouble to go into training for it. He would noteven give up his cigarette smoking, a habit that he had acquired becausehe considered it fashionable and manly. Now he was beaten, disgracefully,and that by a boy nearly two years younger than himself. It was too much,and he determined to find some excuse for his defeat, that should at thesame time remove the disgrace from him, and place it upon other shoulders.
-With this Rod plunged down the steep bank to the railroad track, anddisappeared in the darkness. He went in the direction of the next stationto Euston, about five miles away, as he did not wish to be recognized whenhe made the attempt to secure a ride on some train to New York. It was tobe an attempt only; for he had not a cent of money in his pockets, and hadno idea of how he should obtain the coveted ride. In addition to beingpenniless, he was hungry, and his hunger was increased tenfold by theknowledge that he had no means of satisfying it. Still he was a boy withunlimited confidence in himself. He always had fallen on his feet; and,though this was the worse fix in which he had ever found himself, he hadfaith that he would come out[Pg 32]of it all right somehow. His heart wasalready so much lighter since he had learned from Dan that some of hisfriends, and especially Eltje Vanderveer, still believed in him, that hissituation did not seem half so desperate as it had an hour before.
-Rod was already enough of a railroad man to know that, as he was goingeast, he must walk on the west bound track. By so doing he would be ableto see trains bound west, while they were still at some distance from him,and would be in no danger from those bound east and overtaking him.
-When he was about half a mile from the little station, toward which he waswalking, he heard the long-drawn, far-away whistle of a locomotive. Was itahead of him or behind? On account of the bewildering echoes he could nottell. To settle the question he kneeled down, and placed his ear againstone of rails of the west bound track. It was cold and silent. Then hetried the east bound track in the same way. This rail seemed to tinglewith life, and a faint, humming sound came from it. It was a perfectrailroad telephone, and it informed the listener as plainly as words couldhave told him, that a train was approaching from the west.
-[Pg 33]He stopped to note its approach. In a few minutes the rails of the eastbound track began to quiver with light from the powerful reflector infront of its locomotive. Then they stretched away toward the oncomingtrain in gleaming bands of indefinite length, while the dazzling lightseemed to cut a bright pathway between walls of solid blackness for theuse of the advancing monster. As the bewildering glare passed him, Rod sawthat the train was a long, heavy-laden freight, and that some of its carscontained cattle. He stood motionless as it rushed past him, shaking thesolid earth with its ponderous weight, and he drew a decided breath ofrelief at the sight of the blinking red eyes on the rear platform of itscaboose. How he wished he was in that caboose, riding comfortably towardNew York, instead of plodding wearily along on foot, with nothing butuncertainties ahead of him.
-As Rod stood gazing at the receding train he noticed a human figure stepfrom the lighted interior of the caboose, through the open doorway, to theplatform, apparently kick at something, and almost instantly return intothe car. At the same time the boy fancied he heard a sharp cry of pain;but was not sure. As he resumed his tiresome walk, gazing longingly afterthe vanishing train lights, he saw another light, a white one that movedtoward him with a swinging motion, close to the ground. While he waswondering what it was, he almost stumbled over a small animal that stoodmotionless on the track, directly in front of him. It was a dog. Now Roddearly loved dogs, and seemed instinctively to know that this one was insome sort of trouble. As he stopped to pat it, the creature uttered alittle whine, as though [Pg 35]askinghis sympathy and help. At the same time it licked his hand.
-The latter told the boy that the young tramp, as they called him, wasbilled through to New York, to look after some cattle that were on thetrain; but that he was a worthless, ugly fellow, who had not paid theslightest attention to them, and whose only object in accepting the jobwas evidently to obtain a free ride in the caboose. Smiler, whom he hadbeen delighted to find on the train when it was turned over to him, hadtaken a great dislike to the[Pg 45] fellowfrom the first. He had growled andshown his teeth whenever the tramp moved about the car, and several timesthe latter had threatened to teach him better manners. When he andBrakeman Joe went to the forward end of the train, to make ready forside-tracking it, they left the dog sitting on the rear platform of thecaboose, and the tramp apparently asleep, as Rod had found him, on one ofthe lockers. He must have taken advantage of their absence to deal the dogthe cruel kick that cut his ear, and landed him, stunned and bruised, onthe track where he had been discovered.
aaccfb2cb3
-
-
\ No newline at end of file
diff --git a/spaces/1line/AutoGPT/autogpt/processing/text.py b/spaces/1line/AutoGPT/autogpt/processing/text.py
deleted file mode 100644
index 52add81401775c1b111512d8149f86a175fd9acb..0000000000000000000000000000000000000000
--- a/spaces/1line/AutoGPT/autogpt/processing/text.py
+++ /dev/null
@@ -1,132 +0,0 @@
-"""Text processing functions"""
-from typing import Dict, Generator, Optional
-
-from selenium.webdriver.remote.webdriver import WebDriver
-
-from autogpt.config import Config
-from autogpt.llm_utils import create_chat_completion
-from autogpt.memory import get_memory
-
-CFG = Config()
-MEMORY = get_memory(CFG)
-
-
-def split_text(text: str, max_length: int = 8192) -> Generator[str, None, None]:
- """Split text into chunks of a maximum length
-
- Args:
- text (str): The text to split
- max_length (int, optional): The maximum length of each chunk. Defaults to 8192.
-
- Yields:
- str: The next chunk of text
-
- Raises:
- ValueError: If the text is longer than the maximum length
- """
- paragraphs = text.split("\n")
- current_length = 0
- current_chunk = []
-
- for paragraph in paragraphs:
- if current_length + len(paragraph) + 1 <= max_length:
- current_chunk.append(paragraph)
- current_length += len(paragraph) + 1
- else:
- yield "\n".join(current_chunk)
- current_chunk = [paragraph]
- current_length = len(paragraph) + 1
-
- if current_chunk:
- yield "\n".join(current_chunk)
-
-
-def summarize_text(
- url: str, text: str, question: str, driver: Optional[WebDriver] = None
-) -> str:
- """Summarize text using the OpenAI API
-
- Args:
- url (str): The url of the text
- text (str): The text to summarize
- question (str): The question to ask the model
- driver (WebDriver): The webdriver to use to scroll the page
-
- Returns:
- str: The summary of the text
- """
- if not text:
- return "Error: No text to summarize"
-
- text_length = len(text)
- print(f"Text length: {text_length} characters")
-
- summaries = []
- chunks = list(split_text(text))
- scroll_ratio = 1 / len(chunks)
-
- for i, chunk in enumerate(chunks):
- if driver:
- scroll_to_percentage(driver, scroll_ratio * i)
- print(f"Adding chunk {i + 1} / {len(chunks)} to memory")
-
- memory_to_add = f"Source: {url}\n" f"Raw content part#{i + 1}: {chunk}"
-
- MEMORY.add(memory_to_add)
-
- print(f"Summarizing chunk {i + 1} / {len(chunks)}")
- messages = [create_message(chunk, question)]
-
- summary = create_chat_completion(
- model=CFG.fast_llm_model,
- messages=messages,
- )
- summaries.append(summary)
- print(f"Added chunk {i + 1} summary to memory")
-
- memory_to_add = f"Source: {url}\n" f"Content summary part#{i + 1}: {summary}"
-
- MEMORY.add(memory_to_add)
-
- print(f"Summarized {len(chunks)} chunks.")
-
- combined_summary = "\n".join(summaries)
- messages = [create_message(combined_summary, question)]
-
- return create_chat_completion(
- model=CFG.fast_llm_model,
- messages=messages,
- )
-
-
-def scroll_to_percentage(driver: WebDriver, ratio: float) -> None:
- """Scroll to a percentage of the page
-
- Args:
- driver (WebDriver): The webdriver to use
- ratio (float): The percentage to scroll to
-
- Raises:
- ValueError: If the ratio is not between 0 and 1
- """
- if ratio < 0 or ratio > 1:
- raise ValueError("Percentage should be between 0 and 1")
- driver.execute_script(f"window.scrollTo(0, document.body.scrollHeight * {ratio});")
-
-
-def create_message(chunk: str, question: str) -> Dict[str, str]:
- """Create a message for the chat completion
-
- Args:
- chunk (str): The chunk of text to summarize
- question (str): The question to answer
-
- Returns:
- Dict[str, str]: The message to send to the chat completion
- """
- return {
- "role": "user",
- "content": f'"""{chunk}""" Using the above text, answer the following'
- f' question: "{question}" -- if the question cannot be answered using the text,'
- " summarize the text.",
- }
diff --git a/spaces/1pelhydcardo/ChatGPT-prompt-generator/Build-a-lot 7 - Fairy Tales - Full PreCracked - Foxy Games Torrent !EXCLUSIVE!.md b/spaces/1pelhydcardo/ChatGPT-prompt-generator/Build-a-lot 7 - Fairy Tales - Full PreCracked - Foxy Games Torrent !EXCLUSIVE!.md
deleted file mode 100644
index 28ed019d26be1aadc7e0e33e06c5c13a0278634a..0000000000000000000000000000000000000000
--- a/spaces/1pelhydcardo/ChatGPT-prompt-generator/Build-a-lot 7 - Fairy Tales - Full PreCracked - Foxy Games Torrent !EXCLUSIVE!.md
+++ /dev/null
@@ -1,74 +0,0 @@
-## Build-a-lot 7 - Fairy Tales - Full PreCracked - Foxy Games Torrent
-
-
-
-
-
- 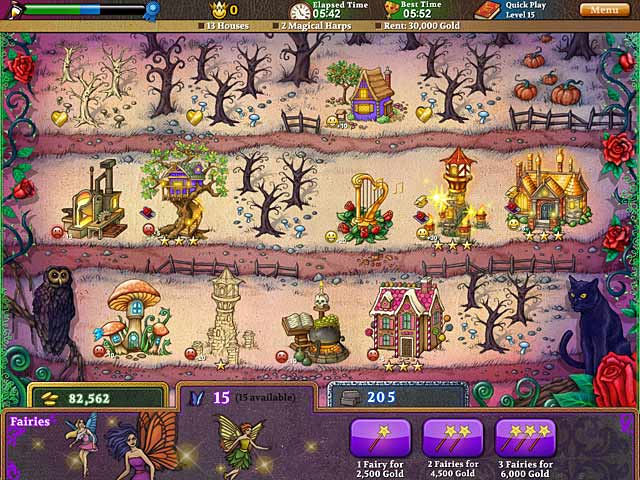
-
-
-
-
-
-**CLICK HERE ••• [https://www.google.com/url?q=https%3A%2F%2Fbytlly.com%2F2txjm0&sa=D&sntz=1&usg=AOvVaw1SVqXiA0JjUeIJDUtRRRY4](https://www.google.com/url?q=https%3A%2F%2Fbytlly.com%2F2txjm0&sa=D&sntz=1&usg=AOvVaw1SVqXiA0JjUeIJDUtRRRY4)**
-
-
-
-
-
-
-
-
-
-
-
-
-
-# How to Download and Play Build-a-lot 7 - Fairy Tales - Full PreCracked - Foxy Games Torrent
-
-
-
-If you are looking for a fun and relaxing game that combines city-building and fairy tale elements, then you should try Build-a-lot 7 - Fairy Tales. This is the seventh installment of the popular Build-a-lot series, and it offers you a chance to create your own magical kingdom with castles, cottages, fountains, and more. You can also explore different fairy tale worlds, meet famous characters, and complete challenging quests.
-
-
-
-But how can you get this game for free? The answer is by downloading and playing the Build-a-lot 7 - Fairy Tales - Full PreCracked - Foxy Games Torrent. This is a file that contains the full version of the game, already cracked and ready to play. You don't need to install anything or register any account. You just need to follow these simple steps:
-
-
-
-1. Download a torrent client, such as uTorrent or BitTorrent, and install it on your computer.
-
-2. Go to a torrent site, such as The Pirate Bay or Kickass Torrents, and search for "Build-a-lot 7 - Fairy Tales - Full PreCracked - Foxy Games".
-
-3. Choose the torrent file that has the most seeders and leechers, and download it to your computer.
-
-4. Open the torrent file with your torrent client, and select the destination folder where you want to save the game.
-
-5. Wait for the download to finish. It may take some time depending on your internet speed and the number of peers.
-
-6. Once the download is complete, open the destination folder and double-click on the game icon. The game will launch automatically.
-
-7. Enjoy playing Build-a-lot 7 - Fairy Tales!
-
-
-
-Note: Downloading and playing torrent files may be illegal in some countries. Please check your local laws before proceeding. Also, be careful of viruses and malware that may be hidden in some torrent files. Always scan your files with an antivirus program before opening them.
-
-
-
-Build-a-lot 7 - Fairy Tales is a game that will appeal to both casual and hardcore gamers. You can choose from four different modes: Campaign, Casual, Expert, and Sandbox. Each mode has its own objectives and challenges, and you can adjust the difficulty level according to your preference. You can also unlock achievements and trophies as you progress through the game.
-
-
-
-The game features stunning graphics and sound effects that will immerse you in the fairy tale atmosphere. You can customize your kingdom with different types of buildings, decorations, and landscaping. You can also interact with various fairy tale characters, such as Cinderella, Snow White, Rapunzel, and more. You can help them with their problems, or cause some mischief if you feel like it.
-
-
-
-Build-a-lot 7 - Fairy Tales is a game that will keep you entertained for hours. You can download and play it for free by using the Build-a-lot 7 - Fairy Tales - Full PreCracked - Foxy Games Torrent. Just follow the instructions above and start building your dream kingdom today!
-
- 1b8d091108
-
-
-
-
-
diff --git a/spaces/1pelhydcardo/ChatGPT-prompt-generator/assets/Beach Buggy Racing 2 How to Unlock and Upgrade Over 40 Powerups.md b/spaces/1pelhydcardo/ChatGPT-prompt-generator/assets/Beach Buggy Racing 2 How to Unlock and Upgrade Over 40 Powerups.md
deleted file mode 100644
index d1992a1ffe97e888087f8a6b3bcd5ee9a9109b3b..0000000000000000000000000000000000000000
--- a/spaces/1pelhydcardo/ChatGPT-prompt-generator/assets/Beach Buggy Racing 2 How to Unlock and Upgrade Over 40 Powerups.md
+++ /dev/null
@@ -1,116 +0,0 @@
-
-Beach Buggy Racing 2: A Fun and Exciting Kart Racing Game
-Do you love kart racing games? Do you want to experience a thrilling adventure on a mysterious island? Do you want to compete against other players from around the world? If you answered yes to any of these questions, then you should try Beach Buggy Racing 2, a fun and exciting kart racing game that you can download from Microsoft Store. In this article, we will tell you everything you need to know about this game, including what it is, how to download it, what are its features, how to play it, and why you should play it.
-beach buggy racing 2 download microsoft store
Download File ……… https://urlin.us/2uSSvc
- What is Beach Buggy Racing 2?
-Beach Buggy Racing 2 is a sequel to the popular Beach Buggy Racing, a game that introduced over 100 million international mobile players to console-style kart racing with a playful off-road twist. Beach Buggy Racing 2 is a fully 3D off-road kart racing game with amazing physics, detailed cars and characters, and spectacular weapons, powered by Vector Engine and NVIDIA's PhysX. It's like a console game in the palm of your hand!
-Beach Buggy Racing 2 is a game that you can play solo or with friends in split screen or online modes. You can join the Beach Buggy Racing League and compete against drivers and cars from around the world. You can race through Egyptian pyramids, dragon-infested castles, pirate ship wrecks, and experimental alien bio-labs. You can collect and upgrade an arsenal of fun and wacky powerups. You can recruit new drivers, assemble a garage full of cars, and race your way to the top of the league.
- How to download Beach Buggy Racing 2 from Microsoft Store?
-If you want to download Beach Buggy Racing 2 on your Windows 10 device, you can follow these simple steps:
-beach buggy racing 2 island adventure xbox one
-beach buggy racing 2 hot wheels edition
-beach buggy racing 2 split screen
-beach buggy racing 2 game crafting
-beach buggy racing 2 adventure mode
-beach buggy racing 2 xbox series x
-beach buggy racing 2 oddball car pack
-beach buggy racing 2 firework fury
-beach buggy racing 2 vector unit
-beach buggy racing 2 xbox local multiplayer
-beach buggy racing 2 kart racer
-beach buggy racing 2 powerups
-beach buggy racing 2 championships
-beach buggy racing 2 drift attack
-beach buggy racing 2 tropical rivals
-beach buggy racing 2 official sequel
-beach buggy racing 2 free driving game
-beach buggy racing 2 moon buggies
-beach buggy racing 2 monster trucks
-beach buggy racing 2 ancient temples
-beach buggy racing 2 dragon castles
-beach buggy racing 2 ice cream stands
-beach buggy racing 2 rag-tag crew
-beach buggy racing 2 mysterious island
-beach buggy racing 2 epic race
-beach buggy racing 2 ultimate trophy
-beach buggy racing 2 mayhem-filled kart racer
-beach buggy racing 2 solo or with friends
-beach buggy racing 2 story-driven adventure mode
-beach buggy racing 2 adrenaline-pumping races
-beach buggy racing 2 skill-mastering drift attacks
-beach buggy racing 2 custom game modes
-beach buggy racing 2 zany race rules
-beach buggy racing 2 bouncy tires powerup
-beach buggy racing 2 rocket boost powerup
-beach buggy racing 2 police chase powerup
-beach buggy racing 2 fast-paced driving action game
-beach buggy racing 2 explosive fun for all skill levels
-beach buggy racing 2 net energy gain experiment
-beach buggy racing 2 holy grail fusion experiment
-beach buggy racing 2 mini sun experiment
-beach buggy racing 2 seven times hotter than the sun core experiment
-
-- Open Microsoft Store app on your device.
-- Search for Beach Buggy Racing 2 in the search bar.
-- Select the game from the search results.
-- Click on Get or Install button.
-- Wait for the download and installation process to complete.
-- Launch the game and enjoy!
-
-The system requirements for Beach Buggy Racing 2 are:
-
-- OS: Windows 10 version 18362.0 or higher
-- Architecture: x64
-- DirectX: Version 11
-- Memory: 4 GB
-- Processor: Intel Core i5-6500 or equivalent
-- Graphics: NVIDIA GeForce GTX750 Ti or equivalent
-
-The price of Beach Buggy Racing 2 is $19.99. However, you can also buy the Hot Wheels Edition bundle for $26.98, which includes the game and two DLC packs: Hot Wheels Booster Pack and Oddball Car
One of the benefits of downloading the game from Microsoft Store is that you can enjoy the Hot Wheels Booster Pack DLC, an exciting new content expansion that adds seven legendary Hot Wheels cars and four new tracks, complete with twisting orange track pieces, to the Beach Buggy Racing League. You can also get the Oddball Car Pack DLC, which adds four wacky and weird cars to your garage: the Rocket Car, the Shark Car, the Alien Car, and the Monster Truck. These DLC packs are sold separately or as a bundle with the game for a discounted price.
- What are the features of Beach Buggy Racing 2?
-Beach Buggy Racing 2 is not just a simple racing game. It has many features that make it a fun and exciting kart racing game. Here are some of them:
-The different game modes and challenges
-You can choose from different game modes and challenges to test your skills and have fun. You can play the Adventure mode, where you can explore the island and unlock new tracks, cars, drivers, and powerups. You can also play the Quick Race mode, where you can race on any track you want with any car you want. You can also play the Championship mode, where you can compete in a series of races and earn trophies. You can also play the Daily Challenges mode, where you can complete different tasks and earn rewards. You can also play the Special Events mode, where you can join limited-time events and win exclusive prizes.
-The variety of cars, drivers, and powerups
-You can collect and upgrade over 40 cars, each with their own unique stats and abilities. You can also recruit over 20 drivers, each with their own special power. You can also collect and upgrade over 40 powerups, each with their own effects and strategies. You can mix and match different cars, drivers, and powerups to create your own style and strategy.
-The customization options and the achievements
-You can customize your cars with different paints, decals, wheels, spoilers, and more. You can also customize your drivers with different outfits, hats, glasses, and more. You can also customize your powerup deck with different combinations of powerups. You can also unlock over 100 achievements and show off your skills and progress.
- How to play Beach Buggy Racing 2?
-Beach Buggy Racing 2 is easy to play but hard to master. Here are some tips and tricks to help you play better:
-The controls and the tips for racing
-You can choose from different control options: tilt, touch, or gamepad. You can also adjust the sensitivity and the steering assist. The basic controls are: accelerate, brake, steer, drift, use powerup, use driver ability. The tips for racing are: use drift to take sharp turns and fill up your boost meter; use boost to speed up and overtake your opponents; use powerups wisely and strategically; use driver ability at the right time and situation; avoid obstacles and traps; collect coins and gems; look for shortcuts and secrets.
-The powerup deck and the special abilities
-You can create your own powerup deck with up to eight powerups. You can choose from offensive, defensive, or utility powerups. You can also upgrade your powerups to make them more effective. Some examples of powerups are: firework (shoots a rocket that explodes on impact); oil slick (drops a slippery puddle that spins out other racers); shield (protects you from attacks for a short time); nitro (gives you a burst of speed); magnet (attracts coins and gems); lightning (zaps nearby racers); tornado (creates a swirling wind that blows away other racers); ice cream (freezes other racers in place). You can also use your driver ability once per race. Each driver has a unique ability that can give you an edge over your opponents. Some examples of driver abilities are: beach ball barrage (launches beach balls everywhere); fire breath (breathes fire in front of you); teleport (teleports you to a random position); coin storm (makes coins rain from the sky); banana split (splits into three copies of yourself).
-The online competitions and tournaments
-You can join the Beach Buggy Racing League and compete against other players from around the world in online races. You can earn trophies and rank up in different leagues. You can also join online tournaments and win exclusive rewards. You can also create or join a team and chat with other players.
- Why should you play Beach Buggy Racing 2?
-Beach Buggy Racing 2 is a game that you should play if you love kart racing games. Here are some reasons why you should play Beach Buggy Racing 2:
-The fun and addictive gameplay
-Beach Buggy Racing 2 is a game that will keep you hooked for hours. You will never get bored of racing on different tracks, using different powerups, and unlocking new cars, drivers, and upgrades. You will also enjoy the challenge of competing against other players and improving your skills and rank. You will also have fun exploring the island and discovering its secrets and surprises.
-The stunning graphics and sound effects
-Beach Buggy Racing 2 is a game that will impress you with its graphics and sound effects. You will admire the detailed and colorful 3D graphics that bring the island to life. You will also appreciate the realistic physics and animations that make the racing experience more immersive. You will also enjoy the catchy and upbeat music and sound effects that match the mood and theme of the game.
-The replay value and the updates
-Beach Buggy Racing 2 is a game that will keep you coming back for more. You will always find something new and exciting to do in the game. You will also benefit from the regular updates that add new content and features to the game. You will also be able to play the game offline or online, depending on your preference and availability.
- Conclusion
-Beach Buggy Racing 2 is a fun and exciting kart racing game that you can download from Microsoft Store. It is a sequel to the popular Beach Buggy Racing, a game that introduced over 100 million international mobile players to console-style kart racing with a playful off-road twist. Beach Buggy Racing 2 is a fully 3D off-road kart racing game with amazing physics, detailed cars and characters, and spectacular weapons, powered by Vector Engine and NVIDIA's PhysX. It's like a console game in the palm of your hand!
-Beach Buggy Racing 2 is a game that you can play solo or with friends in split screen or online modes. You can join the Beach Buggy Racing League and compete against drivers and cars from around the world. You can race through Egyptian pyramids, dragon-infested castles, pirate ship wrecks, and experimental alien bio-labs. You can collect and upgrade an arsenal of fun and wacky powerups. You can recruit new drivers, assemble a garage full of cars, and race your way to the top of the league.
-Beach Buggy Racing 2 is a game that has many features that make it a fun and exciting kart racing game. You can choose from different game modes and challenges to test your skills and have fun. You can collect and upgrade over 40 cars, each with their own unique stats and abilities. You can also recruit over 20 drivers, each with their own special power. You can also collect and upgrade over 40 powerups, each with their own effects and strategies. You can mix and match different cars, drivers, and powerups to create your own style and strategy.
-Beach Buggy Racing 2 is a game that is easy to play but hard to master. You can choose from different control options: tilt, touch, or gamepad. You can also adjust the sensitivity and the steering assist. The basic controls are: accelerate, brake, steer, drift, use powerup, use driver ability. The tips for racing are: use drift to take sharp turns and fill up your boost meter; use boost to speed up and overtake your opponents; use powerups wisely and strategically; use driver ability at the right time and situation; avoid obstacles and traps; collect coins and gems; look for shortcuts and secrets.
-Beach Buggy Racing 2 is a game that you should play if you love kart racing games. You will enjoy the fun and addictive gameplay, the stunning graphics and sound effects, and the replay value and the updates. You will also have fun playing with your friends or other players online. You will also be able to customize your cars, drivers, and powerups to suit your preferences and style.
-If you are ready to join the Beach Buggy Racing League and have a blast on the island, download Beach Buggy Racing 2 from Microsoft Store today and start your engine!
- FAQs
-Here are some frequently asked questions about Beach Buggy Racing 2:
-
-- How can I get more coins and gems in the game?
-You can get more coins and gems by racing on different tracks, completing daily challenges, participating in special events, watching ads, or buying them with real money.
-- How can I unlock more cars and drivers in the game?
-You can unlock more cars and drivers by progressing through the adventure mode, winning championships, opening chests, or buying them with coins or gems.
-- How can I upgrade my cars and powerups in the game?
-You can upgrade your cars and powerups by using upgrade cards that you can get from chests, daily challenges, special events, or buying them with coins or gems.
-- How can I join a team or create my own team in the game?
-You can join a team or create your own team by tapping on the team icon on the main menu. You can search for an existing team or create a new one with a name, a logo, and a description. You can also invite other players to join your team or accept invitations from other teams. You can chat with your team members, share tips and strategies, and compete in team tournaments.
-- How can I contact the developers of the game or report a bug or a problem?
-You can contact the developers of the game or report a bug or a problem by tapping on the settings icon on the main menu. You can then tap on the help icon and choose from different options: FAQ, support, feedback, privacy policy, terms of service, credits. You can also visit their website at https://www.vectorunit.com/ or follow them on social media at https://www.facebook.com/VectorUnit/ or https://twitter.com/VectorUnit/.
-
197e85843d
-
-
\ No newline at end of file
diff --git a/spaces/1pelhydcardo/ChatGPT-prompt-generator/assets/Bus Simulator Indonesia How to Download and Install Jai Guru Jinn Livery.md b/spaces/1pelhydcardo/ChatGPT-prompt-generator/assets/Bus Simulator Indonesia How to Download and Install Jai Guru Jinn Livery.md
deleted file mode 100644
index bc4b6134b42f4723cd2f7ce998644542ff86dd05..0000000000000000000000000000000000000000
--- a/spaces/1pelhydcardo/ChatGPT-prompt-generator/assets/Bus Simulator Indonesia How to Download and Install Jai Guru Jinn Livery.md
+++ /dev/null
@@ -1,113 +0,0 @@
-
-Bus Simulator Indonesia: How to Download and Install Jai Guru Livery
-Do you love driving buses in realistic and authentic environments? Do you want to customize your bus with cool and fun designs? If yes, then you should try Bus Simulator Indonesia, a popular game that lets you experience what it likes being a bus driver in Indonesia. And if you are looking for a unique and stylish livery for your bus, then you should check out the Jai Guru livery, a beautiful and eye-catching design that will make your bus stand out from the crowd. In this article, we will tell you more about Bus Simulator Indonesia, Jai Guru livery, and how to download and install it in your game.
-bus simulator indonesia jai guru livery download
Download Zip ☆☆☆☆☆ https://urlin.us/2uSYjQ
- What is Bus Simulator Indonesia?
-Bus Simulator Indonesia (aka BUSSID) is a game developed by Maleo, an Indonesian game studio. It was released in 2017 and has been updated regularly with new features and improvements. The game is available for Android and iOS devices, as well as PC via emulator. The game has over 100 million downloads on Google Play Store and has received positive reviews from players and critics.
- Game features
-Some of the top features of Bus Simulator Indonesia are:
-
-- Design your own livery: You can create your own livery for your bus using the template provided by the developer or using your own 3D model. You can also download and use livery from other players or creators.
-- Very easy and intuitive control: You can choose between tilt, steering wheel, or buttons to control your bus. You can also adjust the sensitivity and camera angle according to your preference.
-- Authentic Indonesian cities and places: You can drive your bus in various cities and places in Indonesia, such as Jakarta, Surabaya, Bali, Sumatra, Java, etc. You can also see landmarks, buildings, traffic signs, and other details that make the game more realistic.
-- Variety of Indonesian buses with unique features: You can choose from different types of buses, such as mini bus, double decker, articulated bus, etc. Each bus has its own characteristics, such as speed, handling, capacity, etc.
-- Cool and fun honks: You can honk your horn with different sounds, such as the iconic "Om Telolet Om!" honk that became viral on social media. You can also hear other buses honking back at you.
-- High-quality and detailed 3D graphics: The game has stunning graphics that show the beauty of Indonesia. You can see the shadows, reflections, weather effects, day and night cycle, etc.
-- No obstructive ads while driving: The game does not show ads while you are driving your bus. You can enjoy the game without any interruption or distraction.
-- Leaderboard and online data saving: You can compete with other players on the leaderboard based on your score and achievements. You can also save your data online so you don't lose your progress.
-- Online multiplayer convoy: You can join or create a convoy with other players online. You can chat with them, follow them, or challenge them.
-
- Livery customization
-One of the most fun features of Bus Simulator Indonesia is the livery customization. You can design your own livery for your bus using the template provided by the developer or using your own 3D model. You can also download and use livery from other players or creators. Livery is a term that refers to the paint scheme or design of a vehicle, especially a bus or a plane. Livery can be used to express your personality, style, or preference. You can also use livery to promote your brand, business, or cause. Livery can make your bus more attractive, unique, and recognizable.
- What is Jai Guru Livery?
-Jai Guru livery is a livery created by Jai Guru, a popular and talented livery maker in the BUSSID community. Jai Guru has made many liveries for different types of buses, such as Srikandi SHD, Jetbus 3+, Legacy SR2 XHD Prime, etc. Jai Guru livery is known for its high-quality, colorful, and artistic design. Jai Guru livery is also inspired by Indian culture and religion, as well as other themes and motifs.
- Design and style
-Jai Guru livery has a distinctive design and style that makes it stand out from other liveries. Some of the features of Jai Guru livery are:
-
-- Bright and vibrant colors: Jai Guru livery uses a combination of bright and vibrant colors, such as red, yellow, green, blue, purple, etc. The colors create a contrast and harmony that make the livery more eye-catching and appealing.
-- Indian symbols and images: Jai Guru livery incorporates various symbols and images from Indian culture and religion, such as the Om sign, the lotus flower, the elephant, the peacock, etc. The symbols and images represent different meanings and values, such as peace, wisdom, prosperity, beauty, etc.
-- Floral and geometric patterns: Jai Guru livery also uses floral and geometric patterns to decorate the bus. The patterns add more detail and texture to the livery. The patterns are also influenced by Indian art and architecture.
-- Texts and slogans: Jai Guru livery also includes texts and slogans on the bus. The texts and slogans are usually in Hindi or English. They can be the name of the bus company, the destination of the bus, or a message to the passengers or other drivers.
-
- Download link and credit
-If you want to download and use Jai Guru livery in your game, you can find the download link on Jai Guru's YouTube channel or Facebook page. You can also find other liveries made by Jai Guru on these platforms. Please note that you need to have the compatible bus model in your game before you can use the livery. You can also download the bus model from Jai Guru's channel or page.
- When you download and use Jai Guru livery, please give credit to Jai Guru as the original creator of the livery. Do not claim the livery as your own or modify it without permission from Jai Guru. Do not upload or share the livery on other platforms without giving proper credit to Jai Guru. Respect the work and effort of Jai Guru and support him by subscribing to his channel or liking his page.
- How to Install Jai Guru Livery in Bus Simulator Indonesia?
-Installing Jai Guru livery in Bus Simulator Indonesia is easy and simple. Just follow these steps:
-jai guru bus mod video download
-jai guru bus jinn livery link
-jai guru bus simulator indonesia gameplay
-jai guru bus skin for bussid
-jai guru bus mod apk download
-jai guru bus mod for jetbus
-jai guru bus mod by team tvz official
-jai guru bus mod with gandharvan link
-jai guru bus mod with evonex link
-jai guru bus mod with scania link
-jai guru bus mod with haryanto link
-jai guru bus mod with bejeu link
-jai guru bus mod with bandung express link
-jai guru bus mod with armada jaya perkasa link
-jai guru bus mod with budiman link
-jai guru bus mod with gede trans link
-jai guru bus mod with a.l.s link
-jai guru bus mod with akas link
-jai guru bus mod with agra mas link
-jai guru bus mod with eagle high link
-jai guru bus mod with dewi sri link
-jai guru bus mod with garuda mas link
-jai guru bus mod with eka cepat link
-jai guru bus mod with family raya link
-jai guru bus mod with gunung mulia link
-jai guru bus mod with gunung harta link
-jai guru bus mod with handoyo blangkon link
-jai guru bus mod with harapan jaya link
-jai guru bus mod with sempati star link
-jai guru bus mod with shantika link
-jai guru bus mod with sinar jaya link
-jai guru bus mod with sudiro tungga jaya link
-jai guru bus mod with sugeng rahayu link
-jai guru bus mod with sumba putra link
-jai guru bus mod with sumber rejeki link
-jai guru bus mod with sumber selamat link
-jai guru bus mod with haryanto gold link
-jai guru bus mod with haryanto oren link
-jai guru bus mod with haryanto kuning link
-jai guru bus mod with raya by thobie link
-jai guru bus mod with rosalia indah by doel link
-jai guru bus mod with rukun jaya by doel link
-jai guru bus mod with sahabat by doel link
-jai guru bus mod with santoso by doel link
-jai guru bus mod with luragung star by mbs team link
-jai guru bus mod with maju lancar by doel link
-jai guru bus mod with mira by dyt'z link
-jai guru bus mod with pahala kencana by hanafi art link
-jai guru bus mod with haryanto becak by agusgps link
- Step 1: Download the livery file
-The first step is to download the livery file from Jai Guru's channel or page. The file will be in .bussid format, which is a special format for BUSSID liveries. The file size will vary depending on the type of bus and the complexity of the design.
- Step 2: Move the livery file to the BUSSID folder
-The next step is to move the livery file to the BUSSID folder on your device. You can use any file manager app to do this. The BUSSID folder is usually located in Internal Storage > Android > data > com.maleo.bussimulatorid > files > BUSSID.
- Step 3: Open the game and select the garage menu
-The third step is to open Bus Simulator Indonesia on your device and select the garage menu from the main menu. The garage menu is where you can choose and customize your bus.
- Step 4: Select the livery file menu and click BUSSID file manager
-The fourth step is to select the livery file menu from the garage menu. The l ivery file menu is where you can see the list of livery files that you have downloaded or created. From the livery file menu, click on the BUSSID file manager button. The BUSSID file manager is where you can access the BUSSID folder and see the livery files that you have moved there.
- Step 5: Choose the livery you want to use and click open
-The final step is to choose the Jai Guru livery that you want to use for your bus and click on the open button. The game will load the livery and apply it to your bus. You can see the preview of your bus with the Jai Guru livery on the screen. You can also change the color, accessories, or other features of your bus if you want. When you are satisfied with your bus, click on the save button and exit the garage menu.
- Conclusion
-Bus Simulator Indonesia is a fun and realistic game that lets you drive buses in Indonesia. You can also customize your bus with different liveries, such as the Jai Guru livery, a beautiful and eye-catching design inspired by Indian culture and religion. To download and install Jai Guru livery in your game, you just need to follow five simple steps: download the livery file, move it to the BUSSID folder, open the game and select the garage menu, select the livery file menu and click BUSSID file manager, and choose the livery you want to use and click open. Enjoy your bus with Jai Guru livery and have a safe and happy journey!
- FAQs
-Here are some frequently asked questions about Bus Simulator Indonesia and Jai Guru livery:
-
-- Q: How can I get more buses in Bus Simulator Indonesia?
-- A: You can get more buses in Bus Simulator Indonesia by buying them with coins or diamonds. You can earn coins or diamonds by playing the game, completing missions, watching ads, or buying them with real money.
-- Q: How can I create my own livery in Bus Simulator Indonesia?
-- A: You can create your own livery in Bus Simulator Indonesia by using the template provided by the developer or using your own 3D model. You can find the template and instructions on how to use it on Maleo's website or YouTube channel.
-- Q: How can I share my livery with other players in Bus Simulator Indonesia?
-- A: You can share your livery with other players in Bus Simulator Indonesia by uploading it to Maleo's website or any other platform that supports .bussid files. You can also join online multiplayer convoys and show off your livery to other players.
-- Q: How can I contact Jai Guru or request a custom livery from him?
-- A: You can contact Jai Guru or request a custom livery from him by sending him a message on his YouTube channel or Facebook page. He will reply to you as soon as possible.
-- Q: How can I support Jai Guru and his work?
-- A: You can support Jai Guru and his work by subscribing to his YouTube channel, liking his Facebook page, giving him feedback, sharing his liveries with others, and donating to him if you want.
-
197e85843d
-
-
\ No newline at end of file
diff --git a/spaces/4Taps/SadTalker/src/face3d/models/arcface_torch/onnx_ijbc.py b/spaces/4Taps/SadTalker/src/face3d/models/arcface_torch/onnx_ijbc.py
deleted file mode 100644
index 05b50bfad4b4cf38903b89f596263a8e29a50d3e..0000000000000000000000000000000000000000
--- a/spaces/4Taps/SadTalker/src/face3d/models/arcface_torch/onnx_ijbc.py
+++ /dev/null
@@ -1,267 +0,0 @@
-import argparse
-import os
-import pickle
-import timeit
-
-import cv2
-import mxnet as mx
-import numpy as np
-import pandas as pd
-import prettytable
-import skimage.transform
-from sklearn.metrics import roc_curve
-from sklearn.preprocessing import normalize
-
-from onnx_helper import ArcFaceORT
-
-SRC = np.array(
- [
- [30.2946, 51.6963],
- [65.5318, 51.5014],
- [48.0252, 71.7366],
- [33.5493, 92.3655],
- [62.7299, 92.2041]]
- , dtype=np.float32)
-SRC[:, 0] += 8.0
-
-
-class AlignedDataSet(mx.gluon.data.Dataset):
- def __init__(self, root, lines, align=True):
- self.lines = lines
- self.root = root
- self.align = align
-
- def __len__(self):
- return len(self.lines)
-
- def __getitem__(self, idx):
- each_line = self.lines[idx]
- name_lmk_score = each_line.strip().split(' ')
- name = os.path.join(self.root, name_lmk_score[0])
- img = cv2.cvtColor(cv2.imread(name), cv2.COLOR_BGR2RGB)
- landmark5 = np.array([float(x) for x in name_lmk_score[1:-1]], dtype=np.float32).reshape((5, 2))
- st = skimage.transform.SimilarityTransform()
- st.estimate(landmark5, SRC)
- img = cv2.warpAffine(img, st.params[0:2, :], (112, 112), borderValue=0.0)
- img_1 = np.expand_dims(img, 0)
- img_2 = np.expand_dims(np.fliplr(img), 0)
- output = np.concatenate((img_1, img_2), axis=0).astype(np.float32)
- output = np.transpose(output, (0, 3, 1, 2))
- output = mx.nd.array(output)
- return output
-
-
-def extract(model_root, dataset):
- model = ArcFaceORT(model_path=model_root)
- model.check()
- feat_mat = np.zeros(shape=(len(dataset), 2 * model.feat_dim))
-
- def batchify_fn(data):
- return mx.nd.concat(*data, dim=0)
-
- data_loader = mx.gluon.data.DataLoader(
- dataset, 128, last_batch='keep', num_workers=4,
- thread_pool=True, prefetch=16, batchify_fn=batchify_fn)
- num_iter = 0
- for batch in data_loader:
- batch = batch.asnumpy()
- batch = (batch - model.input_mean) / model.input_std
- feat = model.session.run(model.output_names, {model.input_name: batch})[0]
- feat = np.reshape(feat, (-1, model.feat_dim * 2))
- feat_mat[128 * num_iter: 128 * num_iter + feat.shape[0], :] = feat
- num_iter += 1
- if num_iter % 50 == 0:
- print(num_iter)
- return feat_mat
-
-
-def read_template_media_list(path):
- ijb_meta = pd.read_csv(path, sep=' ', header=None).values
- templates = ijb_meta[:, 1].astype(np.int)
- medias = ijb_meta[:, 2].astype(np.int)
- return templates, medias
-
-
-def read_template_pair_list(path):
- pairs = pd.read_csv(path, sep=' ', header=None).values
- t1 = pairs[:, 0].astype(np.int)
- t2 = pairs[:, 1].astype(np.int)
- label = pairs[:, 2].astype(np.int)
- return t1, t2, label
-
-
-def read_image_feature(path):
- with open(path, 'rb') as fid:
- img_feats = pickle.load(fid)
- return img_feats
-
-
-def image2template_feature(img_feats=None,
- templates=None,
- medias=None):
- unique_templates = np.unique(templates)
- template_feats = np.zeros((len(unique_templates), img_feats.shape[1]))
- for count_template, uqt in enumerate(unique_templates):
- (ind_t,) = np.where(templates == uqt)
- face_norm_feats = img_feats[ind_t]
- face_medias = medias[ind_t]
- unique_medias, unique_media_counts = np.unique(face_medias, return_counts=True)
- media_norm_feats = []
- for u, ct in zip(unique_medias, unique_media_counts):
- (ind_m,) = np.where(face_medias == u)
- if ct == 1:
- media_norm_feats += [face_norm_feats[ind_m]]
- else: # image features from the same video will be aggregated into one feature
- media_norm_feats += [np.mean(face_norm_feats[ind_m], axis=0, keepdims=True), ]
- media_norm_feats = np.array(media_norm_feats)
- template_feats[count_template] = np.sum(media_norm_feats, axis=0)
- if count_template % 2000 == 0:
- print('Finish Calculating {} template features.'.format(
- count_template))
- template_norm_feats = normalize(template_feats)
- return template_norm_feats, unique_templates
-
-
-def verification(template_norm_feats=None,
- unique_templates=None,
- p1=None,
- p2=None):
- template2id = np.zeros((max(unique_templates) + 1, 1), dtype=int)
- for count_template, uqt in enumerate(unique_templates):
- template2id[uqt] = count_template
- score = np.zeros((len(p1),))
- total_pairs = np.array(range(len(p1)))
- batchsize = 100000
- sublists = [total_pairs[i: i + batchsize] for i in range(0, len(p1), batchsize)]
- total_sublists = len(sublists)
- for c, s in enumerate(sublists):
- feat1 = template_norm_feats[template2id[p1[s]]]
- feat2 = template_norm_feats[template2id[p2[s]]]
- similarity_score = np.sum(feat1 * feat2, -1)
- score[s] = similarity_score.flatten()
- if c % 10 == 0:
- print('Finish {}/{} pairs.'.format(c, total_sublists))
- return score
-
-
-def verification2(template_norm_feats=None,
- unique_templates=None,
- p1=None,
- p2=None):
- template2id = np.zeros((max(unique_templates) + 1, 1), dtype=int)
- for count_template, uqt in enumerate(unique_templates):
- template2id[uqt] = count_template
- score = np.zeros((len(p1),)) # save cosine distance between pairs
- total_pairs = np.array(range(len(p1)))
- batchsize = 100000 # small batchsize instead of all pairs in one batch due to the memory limiation
- sublists = [total_pairs[i:i + batchsize] for i in range(0, len(p1), batchsize)]
- total_sublists = len(sublists)
- for c, s in enumerate(sublists):
- feat1 = template_norm_feats[template2id[p1[s]]]
- feat2 = template_norm_feats[template2id[p2[s]]]
- similarity_score = np.sum(feat1 * feat2, -1)
- score[s] = similarity_score.flatten()
- if c % 10 == 0:
- print('Finish {}/{} pairs.'.format(c, total_sublists))
- return score
-
-
-def main(args):
- use_norm_score = True # if Ture, TestMode(N1)
- use_detector_score = True # if Ture, TestMode(D1)
- use_flip_test = True # if Ture, TestMode(F1)
- assert args.target == 'IJBC' or args.target == 'IJBB'
-
- start = timeit.default_timer()
- templates, medias = read_template_media_list(
- os.path.join('%s/meta' % args.image_path, '%s_face_tid_mid.txt' % args.target.lower()))
- stop = timeit.default_timer()
- print('Time: %.2f s. ' % (stop - start))
-
- start = timeit.default_timer()
- p1, p2, label = read_template_pair_list(
- os.path.join('%s/meta' % args.image_path,
- '%s_template_pair_label.txt' % args.target.lower()))
- stop = timeit.default_timer()
- print('Time: %.2f s. ' % (stop - start))
-
- start = timeit.default_timer()
- img_path = '%s/loose_crop' % args.image_path
- img_list_path = '%s/meta/%s_name_5pts_score.txt' % (args.image_path, args.target.lower())
- img_list = open(img_list_path)
- files = img_list.readlines()
- dataset = AlignedDataSet(root=img_path, lines=files, align=True)
- img_feats = extract(args.model_root, dataset)
-
- faceness_scores = []
- for each_line in files:
- name_lmk_score = each_line.split()
- faceness_scores.append(name_lmk_score[-1])
- faceness_scores = np.array(faceness_scores).astype(np.float32)
- stop = timeit.default_timer()
- print('Time: %.2f s. ' % (stop - start))
- print('Feature Shape: ({} , {}) .'.format(img_feats.shape[0], img_feats.shape[1]))
- start = timeit.default_timer()
-
- if use_flip_test:
- img_input_feats = img_feats[:, 0:img_feats.shape[1] // 2] + img_feats[:, img_feats.shape[1] // 2:]
- else:
- img_input_feats = img_feats[:, 0:img_feats.shape[1] // 2]
-
- if use_norm_score:
- img_input_feats = img_input_feats
- else:
- img_input_feats = img_input_feats / np.sqrt(np.sum(img_input_feats ** 2, -1, keepdims=True))
-
- if use_detector_score:
- print(img_input_feats.shape, faceness_scores.shape)
- img_input_feats = img_input_feats * faceness_scores[:, np.newaxis]
- else:
- img_input_feats = img_input_feats
-
- template_norm_feats, unique_templates = image2template_feature(
- img_input_feats, templates, medias)
- stop = timeit.default_timer()
- print('Time: %.2f s. ' % (stop - start))
-
- start = timeit.default_timer()
- score = verification(template_norm_feats, unique_templates, p1, p2)
- stop = timeit.default_timer()
- print('Time: %.2f s. ' % (stop - start))
- save_path = os.path.join(args.result_dir, "{}_result".format(args.target))
- if not os.path.exists(save_path):
- os.makedirs(save_path)
- score_save_file = os.path.join(save_path, "{}.npy".format(args.model_root))
- np.save(score_save_file, score)
- files = [score_save_file]
- methods = []
- scores = []
- for file in files:
- methods.append(os.path.basename(file))
- scores.append(np.load(file))
- methods = np.array(methods)
- scores = dict(zip(methods, scores))
- x_labels = [10 ** -6, 10 ** -5, 10 ** -4, 10 ** -3, 10 ** -2, 10 ** -1]
- tpr_fpr_table = prettytable.PrettyTable(['Methods'] + [str(x) for x in x_labels])
- for method in methods:
- fpr, tpr, _ = roc_curve(label, scores[method])
- fpr = np.flipud(fpr)
- tpr = np.flipud(tpr)
- tpr_fpr_row = []
- tpr_fpr_row.append("%s-%s" % (method, args.target))
- for fpr_iter in np.arange(len(x_labels)):
- _, min_index = min(
- list(zip(abs(fpr - x_labels[fpr_iter]), range(len(fpr)))))
- tpr_fpr_row.append('%.2f' % (tpr[min_index] * 100))
- tpr_fpr_table.add_row(tpr_fpr_row)
- print(tpr_fpr_table)
-
-
-if __name__ == '__main__':
- parser = argparse.ArgumentParser(description='do ijb test')
- # general
- parser.add_argument('--model-root', default='', help='path to load model.')
- parser.add_argument('--image-path', default='', type=str, help='')
- parser.add_argument('--result-dir', default='.', type=str, help='')
- parser.add_argument('--target', default='IJBC', type=str, help='target, set to IJBC or IJBB')
- main(parser.parse_args())
diff --git a/spaces/8star/DeepDanbooru_string/app.py b/spaces/8star/DeepDanbooru_string/app.py
deleted file mode 100644
index 49019837c9207cc68cb37be0342f3bc44fd0decb..0000000000000000000000000000000000000000
--- a/spaces/8star/DeepDanbooru_string/app.py
+++ /dev/null
@@ -1,185 +0,0 @@
-#!/usr/bin/env python
-
-from __future__ import annotations
-
-import argparse
-import functools
-import os
-import html
-import pathlib
-import tarfile
-
-import deepdanbooru as dd
-import gradio as gr
-import huggingface_hub
-import numpy as np
-import PIL.Image
-import tensorflow as tf
-import piexif
-import piexif.helper
-
-TITLE = 'DeepDanbooru String'
-
-TOKEN = os.environ['TOKEN']
-MODEL_REPO = 'CikeyQI/DeepDanbooru_string'
-MODEL_FILENAME = 'model-resnet_custom_v3.h5'
-LABEL_FILENAME = 'tags.txt'
-
-
-def parse_args() -> argparse.Namespace:
- parser = argparse.ArgumentParser()
- parser.add_argument('--score-slider-step', type=float, default=0.05)
- parser.add_argument('--score-threshold', type=float, default=0.5)
- parser.add_argument('--theme', type=str, default='dark-grass')
- parser.add_argument('--live', action='store_true')
- parser.add_argument('--share', action='store_true')
- parser.add_argument('--port', type=int)
- parser.add_argument('--disable-queue',
- dest='enable_queue',
- action='store_false')
- parser.add_argument('--allow-flagging', type=str, default='never')
- return parser.parse_args()
-
-
-def load_sample_image_paths() -> list[pathlib.Path]:
- image_dir = pathlib.Path('images')
- if not image_dir.exists():
- dataset_repo = 'hysts/sample-images-TADNE'
- path = huggingface_hub.hf_hub_download(dataset_repo,
- 'images.tar.gz',
- repo_type='dataset',
- use_auth_token=TOKEN)
- with tarfile.open(path) as f:
- f.extractall()
- return sorted(image_dir.glob('*'))
-
-
-def load_model() -> tf.keras.Model:
- path = huggingface_hub.hf_hub_download(MODEL_REPO,
- MODEL_FILENAME,
- use_auth_token=TOKEN)
- model = tf.keras.models.load_model(path)
- return model
-
-
-def load_labels() -> list[str]:
- path = huggingface_hub.hf_hub_download(MODEL_REPO,
- LABEL_FILENAME,
- use_auth_token=TOKEN)
- with open(path) as f:
- labels = [line.strip() for line in f.readlines()]
- return labels
-
-def plaintext_to_html(text):
- text = "" + "
\n".join([f"{html.escape(x)}" for x in text.split('\n')]) + "
"
- return text
-
-def predict(image: PIL.Image.Image, score_threshold: float,
- model: tf.keras.Model, labels: list[str]) -> dict[str, float]:
- rawimage = image
- _, height, width, _ = model.input_shape
- image = np.asarray(image)
- image = tf.image.resize(image,
- size=(height, width),
- method=tf.image.ResizeMethod.AREA,
- preserve_aspect_ratio=True)
- image = image.numpy()
- image = dd.image.transform_and_pad_image(image, width, height)
- image = image / 255.
- probs = model.predict(image[None, ...])[0]
- probs = probs.astype(float)
- res = dict()
- for prob, label in zip(probs.tolist(), labels):
- if prob < score_threshold:
- continue
- res[label] = prob
- b = dict(sorted(res.items(),key=lambda item:item[1], reverse=True))
- a = ', '.join(list(b.keys())).replace('_',' ').replace('(','\(').replace(')','\)')
- c = ', '.join(list(b.keys()))
-
- items = rawimage.info
- geninfo = ''
-
- if "exif" in rawimage.info:
- exif = piexif.load(rawimage.info["exif"])
- exif_comment = (exif or {}).get("Exif", {}).get(piexif.ExifIFD.UserComment, b'')
- try:
- exif_comment = piexif.helper.UserComment.load(exif_comment)
- except ValueError:
- exif_comment = exif_comment.decode('utf8', errors="ignore")
-
- items['exif comment'] = exif_comment
- geninfo = exif_comment
-
- for field in ['jfif', 'jfif_version', 'jfif_unit', 'jfif_density', 'dpi', 'exif',
- 'loop', 'background', 'timestamp', 'duration']:
- items.pop(field, None)
-
- geninfo = items.get('parameters', geninfo)
-
- info = f"""
-PNG Info
-"""
- for key, text in items.items():
- info += f"""
-
-
{plaintext_to_html(str(key))}
-
{plaintext_to_html(str(text))}
-
-""".strip()+"\n"
-
- if len(info) == 0:
- message = "Nothing found in the image."
- info = f""
-
- return (a,c,res,info)
-
-
-def main():
- args = parse_args()
- model = load_model()
- labels = load_labels()
-
- func = functools.partial(predict, model=model, labels=labels)
- func = functools.update_wrapper(func, predict)
-
- gr.Interface(
- func,
- [
- gr.inputs.Image(type='pil', label='Input'),
- gr.inputs.Slider(0,
- 1,
- step=args.score_slider_step,
- default=args.score_threshold,
- label='Score Threshold'),
- ],
- [
- gr.outputs.Textbox(label='Output (string)'),
- gr.outputs.Textbox(label='Output (raw string)'),
- gr.outputs.Label(label='Output (label)'),
- gr.outputs.HTML()
- ],
- examples=[
- ['miku.jpg',0.5],
- ['miku2.jpg',0.5]
- ],
- title=TITLE,
- description='''
-Demo for [KichangKim/DeepDanbooru](https://github.com/KichangKim/DeepDanbooru) with "ready to copy" prompt and a prompt analyzer.
-
-Modified from [hysts/DeepDanbooru](https://huggingface.co/spaces/hysts/DeepDanbooru)
-
-PNG Info code forked from [AUTOMATIC1111/stable-diffusion-webui](https://github.com/AUTOMATIC1111/stable-diffusion-webui)
- ''',
- theme=args.theme,
- allow_flagging=args.allow_flagging,
- live=args.live,
- ).launch(
- enable_queue=args.enable_queue,
- server_port=args.port,
- share=args.share,
- )
-
-
-if __name__ == '__main__':
- main()
diff --git a/spaces/AI4PD/hexviz/README.md b/spaces/AI4PD/hexviz/README.md
deleted file mode 100644
index f9d69dcb3ca704284729c4d451eae875156d211e..0000000000000000000000000000000000000000
--- a/spaces/AI4PD/hexviz/README.md
+++ /dev/null
@@ -1,36 +0,0 @@
----
-title: Hexviz
-emoji: 👁️🧬
-colorFrom: green
-colorTo: purple
-sdk: streamlit
-sdk_version: 1.17.0
-python_version: 3.10.5
-app_file: ./hexviz/🧬Attention_Visualization.py
-pinned: true
-tags:
- - protein language models
- - attention analysis
- - protein structure
- - biology
----
-# hexviz
-Visualize attention pattern on 3D protein structures
-
-## Install and run
-
-```shell
-poetry install
-
-poetry run streamlit run hexviz/streamlit/Attention_On_Structure.py
-```
-
-## Export dependecies from poetry
-Spaces [require](https://huggingface.co/docs/hub/spaces-dependencies#adding-your-own-dependencies) dependencies in a `requirements.txt` file. Export depencies from poetry's `pyproject.toml` file with:
-```shell
-poetry export -f requirements.txt --output requirements.txt --without-hashes
-```
-
-## Acknowledgements
-This project builds on the attention visualization introduced and developed in
-https://github.com/salesforce/provis#provis-attention-visualizer
diff --git a/spaces/AIConsultant/MusicGen/audiocraft/modules/streaming.py b/spaces/AIConsultant/MusicGen/audiocraft/modules/streaming.py
deleted file mode 100644
index fba06936294ca15d72acd2d44f9dbda39a638107..0000000000000000000000000000000000000000
--- a/spaces/AIConsultant/MusicGen/audiocraft/modules/streaming.py
+++ /dev/null
@@ -1,131 +0,0 @@
-# Copyright (c) Meta Platforms, Inc. and affiliates.
-# All rights reserved.
-#
-# This source code is licensed under the license found in the
-# LICENSE file in the root directory of this source tree.
-
-"""
-Streaming module API that should be implemented by all Streaming components,
-"""
-
-from contextlib import contextmanager
-import typing as tp
-from torch import nn
-import torch
-
-
-State = tp.Dict[str, torch.Tensor]
-
-
-class StreamingModule(nn.Module):
- """Common API for streaming components.
-
- Each streaming component has a streaming state, which is just a dict[str, Tensor].
- By convention, the first dim of each tensor must be the batch size.
- Don't use dots in the key names, as this would clash with submodules
- (like in state_dict).
-
- If `self._is_streaming` is True, the component should use and remember
- the proper state inside `self._streaming_state`.
-
- To set a streaming component in streaming state, use
-
- with module.streaming():
- ...
-
- This will automatically reset the streaming state when exiting the context manager.
- This also automatically propagates to all streaming children module.
-
- Some module might also implement the `StreamingModule.flush` method, although
- this one is trickier, as all parents module must be StreamingModule and implement
- it as well for it to work properly. See `StreamingSequential` after.
- """
- def __init__(self) -> None:
- super().__init__()
- self._streaming_state: State = {}
- self._is_streaming = False
-
- def _apply_named_streaming(self, fn: tp.Any):
- for name, module in self.named_modules():
- if isinstance(module, StreamingModule):
- fn(name, module)
-
- def _set_streaming(self, streaming: bool):
- def _set_streaming(name, module):
- module._is_streaming = streaming
- self._apply_named_streaming(_set_streaming)
-
- @contextmanager
- def streaming(self):
- """Context manager to enter streaming mode. Reset streaming state on exit."""
- self._set_streaming(True)
- try:
- yield
- finally:
- self._set_streaming(False)
- self.reset_streaming()
-
- def reset_streaming(self):
- """Reset the streaming state."""
- def _reset(name: str, module: StreamingModule):
- module._streaming_state.clear()
-
- self._apply_named_streaming(_reset)
-
- def get_streaming_state(self) -> State:
- """Return the streaming state, including that of sub-modules."""
- state: State = {}
-
- def _add(name: str, module: StreamingModule):
- if name:
- name += "."
- for key, value in module._streaming_state.items():
- state[name + key] = value
-
- self._apply_named_streaming(_add)
- return state
-
- def set_streaming_state(self, state: State):
- """Set the streaming state, including that of sub-modules."""
- state = dict(state)
-
- def _set(name: str, module: StreamingModule):
- if name:
- name += "."
- module._streaming_state.clear()
- for key, value in list(state.items()):
- # complexity is not ideal here, but probably fine.
- if key.startswith(name):
- local_key = key[len(name):]
- if '.' not in local_key:
- module._streaming_state[local_key] = value
- del state[key]
-
- self._apply_named_streaming(_set)
- assert len(state) == 0, list(state.keys())
-
- def flush(self, x: tp.Optional[torch.Tensor] = None):
- """Flush any remaining outputs that were waiting for completion.
- Typically, for convolutions, this will add the final padding
- and process the last buffer.
-
- This should take an optional argument `x`, which will be provided
- if a module before this one in the streaming pipeline has already
- spitted out a flushed out buffer.
- """
- if x is None:
- return None
- else:
- return self(x)
-
-
-class StreamingSequential(StreamingModule, nn.Sequential):
- """A streaming compatible alternative of `nn.Sequential`.
- """
- def flush(self, x: tp.Optional[torch.Tensor] = None):
- for module in self:
- if isinstance(module, StreamingModule):
- x = module.flush(x)
- elif x is not None:
- x = module(x)
- return x
diff --git a/spaces/AIZeroToHero/Video-Automatic-Speech-Recognition/README.md b/spaces/AIZeroToHero/Video-Automatic-Speech-Recognition/README.md
deleted file mode 100644
index 581e8dbede4f0e13eaa8c5c6cc3a954ab3a1ab56..0000000000000000000000000000000000000000
--- a/spaces/AIZeroToHero/Video-Automatic-Speech-Recognition/README.md
+++ /dev/null
@@ -1,13 +0,0 @@
----
-title: Video Automatic Speech Recognition
-emoji: 💻
-colorFrom: indigo
-colorTo: green
-sdk: streamlit
-sdk_version: 1.10.0
-app_file: app.py
-pinned: false
-license: mit
----
-
-Check out the configuration reference at https://huggingface.co/docs/hub/spaces-config-reference
diff --git a/spaces/AIatUIUC/CodeLATS/executors/py_executor.py b/spaces/AIatUIUC/CodeLATS/executors/py_executor.py
deleted file mode 100644
index 8d0e61d7ab0c0dd9a5e755ef7876b2e92204d2a6..0000000000000000000000000000000000000000
--- a/spaces/AIatUIUC/CodeLATS/executors/py_executor.py
+++ /dev/null
@@ -1,88 +0,0 @@
-import ast
-import signal
-import astunparse
-
-from .executor_utils import function_with_timeout
-
-from typing import List
-from .executor_types import ExecuteResult, Executor
-
-class PyExecutor(Executor):
- def execute(self, func: str, tests: List[str], timeout: int = 5) -> ExecuteResult:
- # Combine function code and assert statement
- imports = 'from typing import *'
- func_test_list = [f'{imports}\n{func}\n{test}' for test in tests]
-
- # Run the tests and collect the results
- success_tests = []
- failed_tests = []
- is_passing = True
- num_tests = len(func_test_list)
- for i in range(num_tests):
- try:
-
- function_with_timeout(exec, (func_test_list[i], globals()), timeout)
-
- success_tests += [tests[i]]
- except Exception:
- output = get_output(func, tests[i], timeout=timeout)
- failed_tests += [f"{tests[i]} # output: {output}"]
- is_passing = False
-
- state = []
- for test in tests:
- if test in success_tests:
- state += [True]
- else:
- state += [False]
-
- state = tuple(state)
-
- feedback = "Tested passed:"
- for test in success_tests:
- feedback += f"\n{test}"
- feedback += "\n\nTests failed:"
- for test in failed_tests:
- feedback += f"\n{test}"
-
- return ExecuteResult(is_passing, feedback, state)
-
- def evaluate(self, name: str, func: str, test: str, timeout: int = 5) -> bool:
- """
- Evaluates the implementation on Human-Eval Python.
-
- probably should be written in a dataset-agnostic way but not now
- """
- code = f"""{func}
-
-{test}
-
-check({name})
- """
- try:
-
- function_with_timeout(exec, (code, globals()), timeout)
-
- return True
- except Exception:
- return False
-
-def get_call_str(assert_statement: str) -> str:
- ast_parsed = ast.parse(assert_statement)
- try:
- call_str = ast_parsed.body[0].test.left # type: ignore
- except:
- call_str = ast_parsed.body[0].test # type: ignore
-
- return astunparse.unparse(call_str).strip()
-
-def get_output(func: str, assert_statement: str, timeout: int = 5) -> str:
- try:
- exec(f"from typing import *\n{func}", globals())
- func_call = get_call_str(assert_statement)
- output = function_with_timeout(eval, (func_call, globals()), timeout)
- return output
- except TimeoutError:
- return "TIMEOUT"
- except Exception as e:
- return str(e)
diff --git a/spaces/Adapter/CoAdapter/ldm/modules/extra_condition/api.py b/spaces/Adapter/CoAdapter/ldm/modules/extra_condition/api.py
deleted file mode 100644
index b7d6aefb6378c9f7418af0277a5357319e943393..0000000000000000000000000000000000000000
--- a/spaces/Adapter/CoAdapter/ldm/modules/extra_condition/api.py
+++ /dev/null
@@ -1,269 +0,0 @@
-from enum import Enum, unique
-
-import cv2
-import torch
-from basicsr.utils import img2tensor
-from ldm.util import resize_numpy_image
-from PIL import Image
-from torch import autocast
-
-
-@unique
-class ExtraCondition(Enum):
- sketch = 0
- keypose = 1
- seg = 2
- depth = 3
- canny = 4
- style = 5
- color = 6
- openpose = 7
-
-
-def get_cond_model(opt, cond_type: ExtraCondition):
- if cond_type == ExtraCondition.sketch:
- from ldm.modules.extra_condition.model_edge import pidinet
- model = pidinet()
- ckp = torch.load('models/table5_pidinet.pth', map_location='cpu')['state_dict']
- model.load_state_dict({k.replace('module.', ''): v for k, v in ckp.items()}, strict=True)
- model.to(opt.device)
- return model
- elif cond_type == ExtraCondition.seg:
- raise NotImplementedError
- elif cond_type == ExtraCondition.keypose:
- import mmcv
- from mmdet.apis import init_detector
- from mmpose.apis import init_pose_model
- det_config = 'configs/mm/faster_rcnn_r50_fpn_coco.py'
- det_checkpoint = 'models/faster_rcnn_r50_fpn_1x_coco_20200130-047c8118.pth'
- pose_config = 'configs/mm/hrnet_w48_coco_256x192.py'
- pose_checkpoint = 'models/hrnet_w48_coco_256x192-b9e0b3ab_20200708.pth'
- det_config_mmcv = mmcv.Config.fromfile(det_config)
- det_model = init_detector(det_config_mmcv, det_checkpoint, device=opt.device)
- pose_config_mmcv = mmcv.Config.fromfile(pose_config)
- pose_model = init_pose_model(pose_config_mmcv, pose_checkpoint, device=opt.device)
- return {'pose_model': pose_model, 'det_model': det_model}
- elif cond_type == ExtraCondition.depth:
- from ldm.modules.extra_condition.midas.api import MiDaSInference
- model = MiDaSInference(model_type='dpt_hybrid').to(opt.device)
- return model
- elif cond_type == ExtraCondition.canny:
- return None
- elif cond_type == ExtraCondition.style:
- from transformers import CLIPProcessor, CLIPVisionModel
- version = 'openai/clip-vit-large-patch14'
- processor = CLIPProcessor.from_pretrained(version)
- clip_vision_model = CLIPVisionModel.from_pretrained(version).to(opt.device)
- return {'processor': processor, 'clip_vision_model': clip_vision_model}
- elif cond_type == ExtraCondition.color:
- return None
- elif cond_type == ExtraCondition.openpose:
- from ldm.modules.extra_condition.openpose.api import OpenposeInference
- model = OpenposeInference().to(opt.device)
- return model
- else:
- raise NotImplementedError
-
-
-def get_cond_sketch(opt, cond_image, cond_inp_type, cond_model=None):
- if isinstance(cond_image, str):
- edge = cv2.imread(cond_image)
- else:
- # for gradio input, pay attention, it's rgb numpy
- edge = cv2.cvtColor(cond_image, cv2.COLOR_RGB2BGR)
- edge = resize_numpy_image(edge, max_resolution=opt.max_resolution, resize_short_edge=opt.resize_short_edge)
- opt.H, opt.W = edge.shape[:2]
- if cond_inp_type == 'sketch':
- edge = img2tensor(edge)[0].unsqueeze(0).unsqueeze(0) / 255.
- edge = edge.to(opt.device)
- elif cond_inp_type == 'image':
- edge = img2tensor(edge).unsqueeze(0) / 255.
- edge = cond_model(edge.to(opt.device))[-1]
- else:
- raise NotImplementedError
-
- # edge = 1-edge # for white background
- edge = edge > 0.5
- edge = edge.float()
-
- return edge
-
-
-def get_cond_seg(opt, cond_image, cond_inp_type='image', cond_model=None):
- if isinstance(cond_image, str):
- seg = cv2.imread(cond_image)
- else:
- seg = cv2.cvtColor(cond_image, cv2.COLOR_RGB2BGR)
- seg = resize_numpy_image(seg, max_resolution=opt.max_resolution, resize_short_edge=opt.resize_short_edge)
- opt.H, opt.W = seg.shape[:2]
- if cond_inp_type == 'seg':
- seg = img2tensor(seg).unsqueeze(0) / 255.
- seg = seg.to(opt.device)
- else:
- raise NotImplementedError
-
- return seg
-
-
-def get_cond_keypose(opt, cond_image, cond_inp_type='image', cond_model=None):
- if isinstance(cond_image, str):
- pose = cv2.imread(cond_image)
- else:
- pose = cv2.cvtColor(cond_image, cv2.COLOR_RGB2BGR)
- pose = resize_numpy_image(pose, max_resolution=opt.max_resolution, resize_short_edge=opt.resize_short_edge)
- opt.H, opt.W = pose.shape[:2]
- if cond_inp_type == 'keypose':
- pose = img2tensor(pose).unsqueeze(0) / 255.
- pose = pose.to(opt.device)
- elif cond_inp_type == 'image':
- from ldm.modules.extra_condition.utils import imshow_keypoints
- from mmdet.apis import inference_detector
- from mmpose.apis import (inference_top_down_pose_model, process_mmdet_results)
-
- # mmpose seems not compatible with autocast fp16
- with autocast("cuda", dtype=torch.float32):
- mmdet_results = inference_detector(cond_model['det_model'], pose)
- # keep the person class bounding boxes.
- person_results = process_mmdet_results(mmdet_results, 1)
-
- # optional
- return_heatmap = False
- dataset = cond_model['pose_model'].cfg.data['test']['type']
-
- # e.g. use ('backbone', ) to return backbone feature
- output_layer_names = None
- pose_results, returned_outputs = inference_top_down_pose_model(
- cond_model['pose_model'],
- pose,
- person_results,
- bbox_thr=0.2,
- format='xyxy',
- dataset=dataset,
- dataset_info=None,
- return_heatmap=return_heatmap,
- outputs=output_layer_names)
-
- # show the results
- pose = imshow_keypoints(pose, pose_results, radius=2, thickness=2)
- pose = img2tensor(pose).unsqueeze(0) / 255.
- pose = pose.to(opt.device)
- else:
- raise NotImplementedError
-
- return pose
-
-
-def get_cond_depth(opt, cond_image, cond_inp_type='image', cond_model=None):
- if isinstance(cond_image, str):
- depth = cv2.imread(cond_image)
- else:
- depth = cv2.cvtColor(cond_image, cv2.COLOR_RGB2BGR)
- depth = resize_numpy_image(depth, max_resolution=opt.max_resolution, resize_short_edge=opt.resize_short_edge)
- opt.H, opt.W = depth.shape[:2]
- if cond_inp_type == 'depth':
- depth = img2tensor(depth).unsqueeze(0) / 255.
- depth = depth.to(opt.device)
- elif cond_inp_type == 'image':
- depth = img2tensor(depth).unsqueeze(0) / 127.5 - 1.0
- depth = cond_model(depth.to(opt.device)).repeat(1, 3, 1, 1)
- depth -= torch.min(depth)
- depth /= torch.max(depth)
- else:
- raise NotImplementedError
-
- return depth
-
-
-def get_cond_canny(opt, cond_image, cond_inp_type='image', cond_model=None):
- if isinstance(cond_image, str):
- canny = cv2.imread(cond_image)
- else:
- canny = cv2.cvtColor(cond_image, cv2.COLOR_RGB2BGR)
- canny = resize_numpy_image(canny, max_resolution=opt.max_resolution, resize_short_edge=opt.resize_short_edge)
- opt.H, opt.W = canny.shape[:2]
- if cond_inp_type == 'canny':
- canny = img2tensor(canny)[0:1].unsqueeze(0) / 255.
- canny = canny.to(opt.device)
- elif cond_inp_type == 'image':
- canny = cv2.Canny(canny, 100, 200)[..., None]
- canny = img2tensor(canny).unsqueeze(0) / 255.
- canny = canny.to(opt.device)
- else:
- raise NotImplementedError
-
- return canny
-
-
-def get_cond_style(opt, cond_image, cond_inp_type='image', cond_model=None):
- assert cond_inp_type == 'image'
- if isinstance(cond_image, str):
- style = Image.open(cond_image)
- else:
- # numpy image to PIL image
- style = Image.fromarray(cond_image)
-
- style_for_clip = cond_model['processor'](images=style, return_tensors="pt")['pixel_values']
- style_feat = cond_model['clip_vision_model'](style_for_clip.to(opt.device))['last_hidden_state']
-
- return style_feat
-
-
-def get_cond_color(opt, cond_image, cond_inp_type='image', cond_model=None):
- if isinstance(cond_image, str):
- color = cv2.imread(cond_image)
- else:
- color = cv2.cvtColor(cond_image, cv2.COLOR_RGB2BGR)
- color = resize_numpy_image(color, max_resolution=opt.max_resolution, resize_short_edge=opt.resize_short_edge)
- opt.H, opt.W = color.shape[:2]
- if cond_inp_type == 'image':
- color = cv2.resize(color, (opt.W//64, opt.H//64), interpolation=cv2.INTER_CUBIC)
- color = cv2.resize(color, (opt.W, opt.H), interpolation=cv2.INTER_NEAREST)
- color = img2tensor(color).unsqueeze(0) / 255.
- color = color.to(opt.device)
- return color
-
-
-def get_cond_openpose(opt, cond_image, cond_inp_type='image', cond_model=None):
- if isinstance(cond_image, str):
- openpose_keypose = cv2.imread(cond_image)
- else:
- openpose_keypose = cv2.cvtColor(cond_image, cv2.COLOR_RGB2BGR)
- openpose_keypose = resize_numpy_image(
- openpose_keypose, max_resolution=opt.max_resolution, resize_short_edge=opt.resize_short_edge)
- opt.H, opt.W = openpose_keypose.shape[:2]
- if cond_inp_type == 'openpose':
- openpose_keypose = img2tensor(openpose_keypose).unsqueeze(0) / 255.
- openpose_keypose = openpose_keypose.to(opt.device)
- elif cond_inp_type == 'image':
- with autocast('cuda', dtype=torch.float32):
- openpose_keypose = cond_model(openpose_keypose)
- openpose_keypose = img2tensor(openpose_keypose).unsqueeze(0) / 255.
- openpose_keypose = openpose_keypose.to(opt.device)
-
- else:
- raise NotImplementedError
-
- return openpose_keypose
-
-
-def get_adapter_feature(inputs, adapters):
- ret_feat_map = None
- ret_feat_seq = None
- if not isinstance(inputs, list):
- inputs = [inputs]
- adapters = [adapters]
-
- for input, adapter in zip(inputs, adapters):
- cur_feature = adapter['model'](input)
- if isinstance(cur_feature, list):
- if ret_feat_map is None:
- ret_feat_map = list(map(lambda x: x * adapter['cond_weight'], cur_feature))
- else:
- ret_feat_map = list(map(lambda x, y: x + y * adapter['cond_weight'], ret_feat_map, cur_feature))
- else:
- if ret_feat_seq is None:
- ret_feat_seq = cur_feature * adapter['cond_weight']
- else:
- ret_feat_seq = torch.cat([ret_feat_seq, cur_feature * adapter['cond_weight']], dim=1)
-
- return ret_feat_map, ret_feat_seq
diff --git a/spaces/AgentVerse/agentVerse/ui/src/phaser3-rex-plugins/templates/bejeweled/board/Fill.js b/spaces/AgentVerse/agentVerse/ui/src/phaser3-rex-plugins/templates/bejeweled/board/Fill.js
deleted file mode 100644
index c9cce61937470aeec8490b4c3ea2f1522687ecb9..0000000000000000000000000000000000000000
--- a/spaces/AgentVerse/agentVerse/ui/src/phaser3-rex-plugins/templates/bejeweled/board/Fill.js
+++ /dev/null
@@ -1,36 +0,0 @@
-/*
-1. Fill empty grids
-*/
-
-var Fill = function (map) {
- var upperBoard = false;
- if (typeof (map) === 'boolean') {
- upperBoard = map;
- map = undefined;
- }
-
- var symbol;
- var board = this.board,
- symbols = this.candidateSymbols;
-
- var height = this.board.height;
- if (upperBoard) {
- height /= 2;
- }
- for (var tileY = 0; tileY < height; tileY++) {
- for (var tileX = 0, width = this.board.width; tileX < width; tileX++) {
- if (board.contains(tileX, tileY, this.chessTileZ)) { // not empty
- continue;
- }
-
- if (map !== undefined) {
- symbol = map[tileX][tileY];
- if (symbol !== '?') {
- symbols = symbol;
- }
- }
- this.createChess(tileX, tileY, symbols);
- }
- }
-}
-export default Fill;
\ No newline at end of file
diff --git a/spaces/AgentVerse/agentVerse/ui/src/phaser3-rex-plugins/templates/ui/click/Click.js b/spaces/AgentVerse/agentVerse/ui/src/phaser3-rex-plugins/templates/ui/click/Click.js
deleted file mode 100644
index 093ae2ad896ba15f081f0fd5f1665938221c0439..0000000000000000000000000000000000000000
--- a/spaces/AgentVerse/agentVerse/ui/src/phaser3-rex-plugins/templates/ui/click/Click.js
+++ /dev/null
@@ -1,2 +0,0 @@
-import Click from '../../../plugins/button.js'
-export default Click;
\ No newline at end of file
diff --git a/spaces/AgentVerse/agentVerse/ui/src/phaser3-rex-plugins/templates/ui/dropdownlist/DropDownList.d.ts b/spaces/AgentVerse/agentVerse/ui/src/phaser3-rex-plugins/templates/ui/dropdownlist/DropDownList.d.ts
deleted file mode 100644
index 3648d8717d74ed3f52e8197b344cde7777890d61..0000000000000000000000000000000000000000
--- a/spaces/AgentVerse/agentVerse/ui/src/phaser3-rex-plugins/templates/ui/dropdownlist/DropDownList.d.ts
+++ /dev/null
@@ -1,130 +0,0 @@
-import Label from '../label/Label';
-
-export default DropDownList;
-
-declare namespace DropDownList {
- type CreateButtonCallbackType = (
- this: DropDownList,
- scene: Phaser.Scene,
- option: any,
- index: number,
- options: any[]
- ) => Phaser.GameObjects.GameObject;
-
- type CreateBackgroundCallbackType = (
- this: DropDownList,
- scene: Phaser.Scene,
- ) => Phaser.GameObjects.GameObject;
-
- type OnButtonClickCallbackType = (
- this: DropDownList,
- button: Phaser.GameObjects.GameObject,
- index: number,
- pointer: Phaser.Input.Pointer,
- event: Phaser.Types.Input.EventData
- ) => void;
-
- type OnButtonOverCallbackType = (
- this: DropDownList,
- button: Phaser.GameObjects.GameObject,
- index: number,
- pointer: Phaser.Input.Pointer,
- event: Phaser.Types.Input.EventData
- ) => void;
-
- type OnButtonOutCallbackType = (
- this: DropDownList,
- button: Phaser.GameObjects.GameObject,
- index: number,
- pointer: Phaser.Input.Pointer,
- event: Phaser.Types.Input.EventData
- ) => void;
-
- type AlignParentType = 'text' | 'icon';
-
- type ExpandDirectionType = 0 | 1 | 'down' | 'up';
-
- type SetValueCallbackType = (
- dropDownList: DropDownList,
- value?: any,
- previousValue?: any,
- ) => void;
-
- type ListSpaceType = {
- left?: number, right?: number, top?: number, bottom?: number, item?: number
- };
-
- type WrapListSpaceType = {
- left?: number, right?: number, top?: number, bottom?: number, item?: number, line?: number
- }
-
- interface IConfig extends Label.IConfig {
- options?: any[],
- list?: {
- createBackgroundCallback?: CreateBackgroundCallbackType;
- createButtonCallback?: CreateButtonCallbackType;
-
- onButtonClick?: OnButtonClickCallbackType;
- onButtonOver?: OnButtonOverCallbackType;
- onButtonOut?: OnButtonOutCallbackType;
-
- easeIn?: number;
- easeOut?: number;
-
- wrap?: boolean;
- width?: number;
- height?: number;
- alignParent?: AlignParentType;
- alignSide?: string;
- expandDirection?: ExpandDirectionType;
- bounds?: Phaser.Geom.Rectangle;
-
- space?: ListSpaceType | WrapListSpaceType;
-
- draggable?: boolean;
- },
-
- setValueCallback?: SetValueCallbackType;
- setValueCallbackScope?: object;
- value?: any;
- }
-}
-
-declare class DropDownList extends Label {
- constructor(
- scene: Phaser.Scene,
- config?: DropDownList.IConfig
- );
-
- setOptions(options: any[]): this;
-
- openListPanel(): this;
- closeListPanel(): this;
- toggleListPanel(): this;
-
- setValue(value?: any): this;
- value: any;
-
- setCreateButtonCallback(callback?: DropDownList.CreateBackgroundCallbackType): this;
- setCreateBackgroundCallback(callback?: DropDownList.CreateBackgroundCallbackType): this;
-
- setButtonClickCallback(callback?: DropDownList.OnButtonClickCallbackType): this;
- setButtonOverCallback(callback?: DropDownList.OnButtonOverCallbackType): this;
- setButtonOutCallback(callback?: DropDownList.OnButtonOutCallbackType): this;
-
- setListEaseInDuration(duration?: number): this;
- setListEaseOutDuration(duration?: number): this;
-
- setWrapEnable(enable?: boolean): this;
- setListWidth(width?: number): this;
- setListHeight(height?: number): this;
- setListSize(width?: number, height?: number): this;
-
- setListAlignmentMode(mode?: DropDownList.AlignParentType): this;
- setListAlignmentSide(side?: string): this;
- setListBounds(bounds: Phaser.Geom.Rectangle): this;
-
- setListSpace(space?: DropDownList.ListSpaceType | DropDownList.WrapListSpaceType): this;
-
- setListDraggable(enable?: boolean): this;
-}
\ No newline at end of file
diff --git a/spaces/AgentVerse/agentVerse/ui/src/phaser3-rex-plugins/templates/ui/roundrectangle/RoundRectangle.d.ts b/spaces/AgentVerse/agentVerse/ui/src/phaser3-rex-plugins/templates/ui/roundrectangle/RoundRectangle.d.ts
deleted file mode 100644
index 990e814eccc548081543dda98307abc4bd5814f6..0000000000000000000000000000000000000000
--- a/spaces/AgentVerse/agentVerse/ui/src/phaser3-rex-plugins/templates/ui/roundrectangle/RoundRectangle.d.ts
+++ /dev/null
@@ -1,2 +0,0 @@
-import RoundRectangle from "../../../plugins/roundrectangle";
-export default RoundRectangle;
\ No newline at end of file
diff --git a/spaces/Ajit025/Text_to_Image_conversion/app.py b/spaces/Ajit025/Text_to_Image_conversion/app.py
deleted file mode 100644
index 38284eb13a3476a3ca0d63455b7dd139e13e5c51..0000000000000000000000000000000000000000
--- a/spaces/Ajit025/Text_to_Image_conversion/app.py
+++ /dev/null
@@ -1,15 +0,0 @@
-from text_to_image import TextToImageTool
-import gradio as gr
-
-tool = TextToImageTool()
-
-def fn(*args, **kwargs):
- return tool(*args, **kwargs)
-
-gr.Interface(
- fn=fn,
- inputs=tool.inputs,
- outputs=tool.outputs,
- title="Text_to_Image",
- article=tool.description,
-).queue(concurrency_count=5).launch()
diff --git a/spaces/Aki004/herta-so-vits/flask_api.py b/spaces/Aki004/herta-so-vits/flask_api.py
deleted file mode 100644
index dff87134620d6ec00e6c8950ccf6313946216af8..0000000000000000000000000000000000000000
--- a/spaces/Aki004/herta-so-vits/flask_api.py
+++ /dev/null
@@ -1,62 +0,0 @@
-import io
-import logging
-
-import soundfile
-import torch
-import torchaudio
-from flask import Flask, request, send_file
-from flask_cors import CORS
-
-from inference.infer_tool import Svc, RealTimeVC
-
-app = Flask(__name__)
-
-CORS(app)
-
-logging.getLogger('numba').setLevel(logging.WARNING)
-
-
-@app.route("/voiceChangeModel", methods=["POST"])
-def voice_change_model():
- request_form = request.form
- wave_file = request.files.get("sample", None)
- # pitch changing information
- f_pitch_change = float(request_form.get("fPitchChange", 0))
- # DAW required sampling rate
- daw_sample = int(float(request_form.get("sampleRate", 0)))
- speaker_id = int(float(request_form.get("sSpeakId", 0)))
- # get wav from http and convert
- input_wav_path = io.BytesIO(wave_file.read())
-
- # inference
- if raw_infer:
- # out_audio, out_sr = svc_model.infer(speaker_id, f_pitch_change, input_wav_path)
- out_audio, out_sr = svc_model.infer(speaker_id, f_pitch_change, input_wav_path, cluster_infer_ratio=0,
- auto_predict_f0=False, noice_scale=0.4, f0_filter=False)
- tar_audio = torchaudio.functional.resample(out_audio, svc_model.target_sample, daw_sample)
- else:
- out_audio = svc.process(svc_model, speaker_id, f_pitch_change, input_wav_path, cluster_infer_ratio=0,
- auto_predict_f0=False, noice_scale=0.4, f0_filter=False)
- tar_audio = torchaudio.functional.resample(torch.from_numpy(out_audio), svc_model.target_sample, daw_sample)
- # return
- out_wav_path = io.BytesIO()
- soundfile.write(out_wav_path, tar_audio.cpu().numpy(), daw_sample, format="wav")
- out_wav_path.seek(0)
- return send_file(out_wav_path, download_name="temp.wav", as_attachment=True)
-
-
-if __name__ == '__main__':
- # True means splice directly. There may be explosive sounds at the splice.
- # False means use cross fade. There may be slight overlapping sounds at the splice.
- # Using 0.3-0.5s in VST plugin can reduce latency.
- # You can adjust the maximum slicing time of VST plugin to 1 second and set it to ture here to get a stable sound quality and a relatively large delay。
- # Choose an acceptable method on your own.
- raw_infer = True
- # each model and config are corresponding
- model_name = "logs/32k/G_174000-Copy1.pth"
- config_name = "configs/config.json"
- cluster_model_path = "logs/44k/kmeans_10000.pt"
- svc_model = Svc(model_name, config_name, cluster_model_path=cluster_model_path)
- svc = RealTimeVC()
- # corresponding to the vst plugin here
- app.run(port=6842, host="0.0.0.0", debug=False, threaded=False)
diff --git a/spaces/AlexWang/lama/bin/paper_runfiles/env.sh b/spaces/AlexWang/lama/bin/paper_runfiles/env.sh
deleted file mode 100644
index f3052f0ea1672a569e7775f8c54967d730a7b5ec..0000000000000000000000000000000000000000
--- a/spaces/AlexWang/lama/bin/paper_runfiles/env.sh
+++ /dev/null
@@ -1,8 +0,0 @@
-DIRNAME="$(dirname $0)"
-DIRNAME="$(realpath ""$DIRNAME"")"
-
-BINDIR="$DIRNAME/.."
-SRCDIR="$BINDIR/.."
-CONFIGDIR="$SRCDIR/configs"
-
-export PYTHONPATH="$SRCDIR:$PYTHONPATH"
diff --git a/spaces/Alfasign/nomic-ai-gpt4all-13b-snoozy/README.md b/spaces/Alfasign/nomic-ai-gpt4all-13b-snoozy/README.md
deleted file mode 100644
index c31d94799485a38ee1a1e088ed6ca4345f3bda9a..0000000000000000000000000000000000000000
--- a/spaces/Alfasign/nomic-ai-gpt4all-13b-snoozy/README.md
+++ /dev/null
@@ -1,12 +0,0 @@
----
-title: Chat
-emoji: 📈
-colorFrom: blue
-colorTo: green
-sdk: gradio
-sdk_version: 3.36.1
-app_file: app.py
-pinned: false
----
-
-Check out the configuration reference at https://huggingface.co/docs/hub/spaces-config-reference
diff --git a/spaces/Amrrs/DragGan-Inversion/PTI/torch_utils/ops/__init__.py b/spaces/Amrrs/DragGan-Inversion/PTI/torch_utils/ops/__init__.py
deleted file mode 100644
index ece0ea08fe2e939cc260a1dafc0ab5b391b773d9..0000000000000000000000000000000000000000
--- a/spaces/Amrrs/DragGan-Inversion/PTI/torch_utils/ops/__init__.py
+++ /dev/null
@@ -1,9 +0,0 @@
-# Copyright (c) 2021, NVIDIA CORPORATION. All rights reserved.
-#
-# NVIDIA CORPORATION and its licensors retain all intellectual property
-# and proprietary rights in and to this software, related documentation
-# and any modifications thereto. Any use, reproduction, disclosure or
-# distribution of this software and related documentation without an express
-# license agreement from NVIDIA CORPORATION is strictly prohibited.
-
-# empty
diff --git a/spaces/Amrrs/DragGan-Inversion/dnnlib/util.py b/spaces/Amrrs/DragGan-Inversion/dnnlib/util.py
deleted file mode 100644
index 90f91e1085239fd9672b2cbe83cbd8e85b27ec0e..0000000000000000000000000000000000000000
--- a/spaces/Amrrs/DragGan-Inversion/dnnlib/util.py
+++ /dev/null
@@ -1,504 +0,0 @@
-# Copyright (c) 2021, NVIDIA CORPORATION & AFFILIATES. All rights reserved.
-#
-# NVIDIA CORPORATION and its licensors retain all intellectual property
-# and proprietary rights in and to this software, related documentation
-# and any modifications thereto. Any use, reproduction, disclosure or
-# distribution of this software and related documentation without an express
-# license agreement from NVIDIA CORPORATION is strictly prohibited.
-
-"""Miscellaneous utility classes and functions."""
-
-import ctypes
-import fnmatch
-import importlib
-import inspect
-import numpy as np
-import os
-import shutil
-import sys
-import types
-import io
-import pickle
-import re
-import requests
-import html
-import hashlib
-import glob
-import tempfile
-import urllib
-import urllib.request
-import uuid
-
-from distutils.util import strtobool
-from typing import Any, List, Tuple, Union
-
-
-# Util classes
-# ------------------------------------------------------------------------------------------
-
-
-class EasyDict(dict):
- """Convenience class that behaves like a dict but allows access with the attribute syntax."""
-
- def __getattr__(self, name: str) -> Any:
- try:
- return self[name]
- except KeyError:
- raise AttributeError(name)
-
- def __setattr__(self, name: str, value: Any) -> None:
- self[name] = value
-
- def __delattr__(self, name: str) -> None:
- del self[name]
-
-
-class Logger(object):
- """Redirect stderr to stdout, optionally print stdout to a file, and optionally force flushing on both stdout and the file."""
-
- def __init__(self, file_name: str = None, file_mode: str = "w", should_flush: bool = True):
- self.file = None
-
- if file_name is not None:
- self.file = open(file_name, file_mode)
-
- self.should_flush = should_flush
- self.stdout = sys.stdout
- self.stderr = sys.stderr
-
- sys.stdout = self
- sys.stderr = self
-
- def __enter__(self) -> "Logger":
- return self
-
- def __exit__(self, exc_type: Any, exc_value: Any, traceback: Any) -> None:
- self.close()
-
- def write(self, text: Union[str, bytes]) -> None:
- """Write text to stdout (and a file) and optionally flush."""
- if isinstance(text, bytes):
- text = text.decode()
- if len(text) == 0: # workaround for a bug in VSCode debugger: sys.stdout.write(''); sys.stdout.flush() => crash
- return
-
- if self.file is not None:
- self.file.write(text)
-
- self.stdout.write(text)
-
- if self.should_flush:
- self.flush()
-
- def flush(self) -> None:
- """Flush written text to both stdout and a file, if open."""
- if self.file is not None:
- self.file.flush()
-
- self.stdout.flush()
-
- def close(self) -> None:
- """Flush, close possible files, and remove stdout/stderr mirroring."""
- self.flush()
-
- # if using multiple loggers, prevent closing in wrong order
- if sys.stdout is self:
- sys.stdout = self.stdout
- if sys.stderr is self:
- sys.stderr = self.stderr
-
- if self.file is not None:
- self.file.close()
- self.file = None
-
-
-# Cache directories
-# ------------------------------------------------------------------------------------------
-
-_dnnlib_cache_dir = None
-
-
-def set_cache_dir(path: str) -> None:
- global _dnnlib_cache_dir
- _dnnlib_cache_dir = path
-
-
-def make_cache_dir_path(*paths: str) -> str:
- if _dnnlib_cache_dir is not None:
- return os.path.join(_dnnlib_cache_dir, *paths)
- if 'DNNLIB_CACHE_DIR' in os.environ:
- return os.path.join(os.environ['DNNLIB_CACHE_DIR'], *paths)
- if 'HOME' in os.environ:
- return os.path.join(os.environ['HOME'], '.cache', 'dnnlib', *paths)
- if 'USERPROFILE' in os.environ:
- return os.path.join(os.environ['USERPROFILE'], '.cache', 'dnnlib', *paths)
- return os.path.join(tempfile.gettempdir(), '.cache', 'dnnlib', *paths)
-
-# Small util functions
-# ------------------------------------------------------------------------------------------
-
-
-def format_time(seconds: Union[int, float]) -> str:
- """Convert the seconds to human readable string with days, hours, minutes and seconds."""
- s = int(np.rint(seconds))
-
- if s < 60:
- return "{0}s".format(s)
- elif s < 60 * 60:
- return "{0}m {1:02}s".format(s // 60, s % 60)
- elif s < 24 * 60 * 60:
- return "{0}h {1:02}m {2:02}s".format(s // (60 * 60), (s // 60) % 60, s % 60)
- else:
- return "{0}d {1:02}h {2:02}m".format(s // (24 * 60 * 60), (s // (60 * 60)) % 24, (s // 60) % 60)
-
-
-def format_time_brief(seconds: Union[int, float]) -> str:
- """Convert the seconds to human readable string with days, hours, minutes and seconds."""
- s = int(np.rint(seconds))
-
- if s < 60:
- return "{0}s".format(s)
- elif s < 60 * 60:
- return "{0}m {1:02}s".format(s // 60, s % 60)
- elif s < 24 * 60 * 60:
- return "{0}h {1:02}m".format(s // (60 * 60), (s // 60) % 60)
- else:
- return "{0}d {1:02}h".format(s // (24 * 60 * 60), (s // (60 * 60)) % 24)
-
-
-def ask_yes_no(question: str) -> bool:
- """Ask the user the question until the user inputs a valid answer."""
- while True:
- try:
- print("{0} [y/n]".format(question))
- return strtobool(input().lower())
- except ValueError:
- pass
-
-
-def tuple_product(t: Tuple) -> Any:
- """Calculate the product of the tuple elements."""
- result = 1
-
- for v in t:
- result *= v
-
- return result
-
-
-_str_to_ctype = {
- "uint8": ctypes.c_ubyte,
- "uint16": ctypes.c_uint16,
- "uint32": ctypes.c_uint32,
- "uint64": ctypes.c_uint64,
- "int8": ctypes.c_byte,
- "int16": ctypes.c_int16,
- "int32": ctypes.c_int32,
- "int64": ctypes.c_int64,
- "float32": ctypes.c_float,
- "float64": ctypes.c_double
-}
-
-
-def get_dtype_and_ctype(type_obj: Any) -> Tuple[np.dtype, Any]:
- """Given a type name string (or an object having a __name__ attribute), return matching Numpy and ctypes types that have the same size in bytes."""
- type_str = None
-
- if isinstance(type_obj, str):
- type_str = type_obj
- elif hasattr(type_obj, "__name__"):
- type_str = type_obj.__name__
- elif hasattr(type_obj, "name"):
- type_str = type_obj.name
- else:
- raise RuntimeError("Cannot infer type name from input")
-
- assert type_str in _str_to_ctype.keys()
-
- my_dtype = np.dtype(type_str)
- my_ctype = _str_to_ctype[type_str]
-
- assert my_dtype.itemsize == ctypes.sizeof(my_ctype)
-
- return my_dtype, my_ctype
-
-
-def is_pickleable(obj: Any) -> bool:
- try:
- with io.BytesIO() as stream:
- pickle.dump(obj, stream)
- return True
- except:
- return False
-
-
-# Functionality to import modules/objects by name, and call functions by name
-# ------------------------------------------------------------------------------------------
-
-def get_module_from_obj_name(obj_name: str) -> Tuple[types.ModuleType, str]:
- """Searches for the underlying module behind the name to some python object.
- Returns the module and the object name (original name with module part removed)."""
-
- # allow convenience shorthands, substitute them by full names
- obj_name = re.sub("^np.", "numpy.", obj_name)
- obj_name = re.sub("^tf.", "tensorflow.", obj_name)
-
- # list alternatives for (module_name, local_obj_name)
- parts = obj_name.split(".")
- name_pairs = [(".".join(parts[:i]), ".".join(parts[i:]))
- for i in range(len(parts), 0, -1)]
-
- # try each alternative in turn
- for module_name, local_obj_name in name_pairs:
- try:
- module = importlib.import_module(
- module_name) # may raise ImportError
- # may raise AttributeError
- get_obj_from_module(module, local_obj_name)
- return module, local_obj_name
- except:
- pass
-
- # maybe some of the modules themselves contain errors?
- for module_name, _local_obj_name in name_pairs:
- try:
- importlib.import_module(module_name) # may raise ImportError
- except ImportError:
- if not str(sys.exc_info()[1]).startswith("No module named '" + module_name + "'"):
- raise
-
- # maybe the requested attribute is missing?
- for module_name, local_obj_name in name_pairs:
- try:
- module = importlib.import_module(
- module_name) # may raise ImportError
- # may raise AttributeError
- get_obj_from_module(module, local_obj_name)
- except ImportError:
- pass
-
- # we are out of luck, but we have no idea why
- raise ImportError(obj_name)
-
-
-def get_obj_from_module(module: types.ModuleType, obj_name: str) -> Any:
- """Traverses the object name and returns the last (rightmost) python object."""
- if obj_name == '':
- return module
- obj = module
- for part in obj_name.split("."):
- obj = getattr(obj, part)
- return obj
-
-
-def get_obj_by_name(name: str) -> Any:
- """Finds the python object with the given name."""
- module, obj_name = get_module_from_obj_name(name)
- return get_obj_from_module(module, obj_name)
-
-
-def call_func_by_name(*args, func_name: str = None, **kwargs) -> Any:
- """Finds the python object with the given name and calls it as a function."""
- assert func_name is not None
- func_obj = get_obj_by_name(func_name)
- assert callable(func_obj)
- return func_obj(*args, **kwargs)
-
-
-def construct_class_by_name(*args, class_name: str = None, **kwargs) -> Any:
- """Finds the python class with the given name and constructs it with the given arguments."""
- return call_func_by_name(*args, func_name=class_name, **kwargs)
-
-
-def get_module_dir_by_obj_name(obj_name: str) -> str:
- """Get the directory path of the module containing the given object name."""
- module, _ = get_module_from_obj_name(obj_name)
- return os.path.dirname(inspect.getfile(module))
-
-
-def is_top_level_function(obj: Any) -> bool:
- """Determine whether the given object is a top-level function, i.e., defined at module scope using 'def'."""
- return callable(obj) and obj.__name__ in sys.modules[obj.__module__].__dict__
-
-
-def get_top_level_function_name(obj: Any) -> str:
- """Return the fully-qualified name of a top-level function."""
- assert is_top_level_function(obj)
- module = obj.__module__
- if module == '__main__':
- module = os.path.splitext(os.path.basename(
- sys.modules[module].__file__))[0]
- return module + "." + obj.__name__
-
-
-# File system helpers
-# ------------------------------------------------------------------------------------------
-
-def list_dir_recursively_with_ignore(dir_path: str, ignores: List[str] = None, add_base_to_relative: bool = False) -> List[Tuple[str, str]]:
- """List all files recursively in a given directory while ignoring given file and directory names.
- Returns list of tuples containing both absolute and relative paths."""
- assert os.path.isdir(dir_path)
- base_name = os.path.basename(os.path.normpath(dir_path))
-
- if ignores is None:
- ignores = []
-
- result = []
-
- for root, dirs, files in os.walk(dir_path, topdown=True):
- for ignore_ in ignores:
- dirs_to_remove = [d for d in dirs if fnmatch.fnmatch(d, ignore_)]
-
- # dirs need to be edited in-place
- for d in dirs_to_remove:
- dirs.remove(d)
-
- files = [f for f in files if not fnmatch.fnmatch(f, ignore_)]
-
- absolute_paths = [os.path.join(root, f) for f in files]
- relative_paths = [os.path.relpath(p, dir_path) for p in absolute_paths]
-
- if add_base_to_relative:
- relative_paths = [os.path.join(base_name, p)
- for p in relative_paths]
-
- assert len(absolute_paths) == len(relative_paths)
- result += zip(absolute_paths, relative_paths)
-
- return result
-
-
-def copy_files_and_create_dirs(files: List[Tuple[str, str]]) -> None:
- """Takes in a list of tuples of (src, dst) paths and copies files.
- Will create all necessary directories."""
- for file in files:
- target_dir_name = os.path.dirname(file[1])
-
- # will create all intermediate-level directories
- if not os.path.exists(target_dir_name):
- os.makedirs(target_dir_name)
-
- shutil.copyfile(file[0], file[1])
-
-
-# URL helpers
-# ------------------------------------------------------------------------------------------
-
-def is_url(obj: Any, allow_file_urls: bool = False) -> bool:
- """Determine whether the given object is a valid URL string."""
- if not isinstance(obj, str) or not "://" in obj:
- return False
- if allow_file_urls and obj.startswith('file://'):
- return True
- try:
- res = requests.compat.urlparse(obj)
- if not res.scheme or not res.netloc or not "." in res.netloc:
- return False
- res = requests.compat.urlparse(requests.compat.urljoin(obj, "/"))
- if not res.scheme or not res.netloc or not "." in res.netloc:
- return False
- except:
- return False
- return True
-
-
-def open_url(url: str, cache_dir: str = None, num_attempts: int = 10, verbose: bool = True, return_filename: bool = False, cache: bool = True) -> Any:
- """Download the given URL and return a binary-mode file object to access the data."""
- assert num_attempts >= 1
- assert not (return_filename and (not cache))
-
- # Doesn't look like an URL scheme so interpret it as a local filename.
- if not re.match('^[a-z]+://', url):
- return url if return_filename else open(url, "rb")
-
- # Handle file URLs. This code handles unusual file:// patterns that
- # arise on Windows:
- #
- # file:///c:/foo.txt
- #
- # which would translate to a local '/c:/foo.txt' filename that's
- # invalid. Drop the forward slash for such pathnames.
- #
- # If you touch this code path, you should test it on both Linux and
- # Windows.
- #
- # Some internet resources suggest using urllib.request.url2pathname() but
- # but that converts forward slashes to backslashes and this causes
- # its own set of problems.
- if url.startswith('file://'):
- filename = urllib.parse.urlparse(url).path
- if re.match(r'^/[a-zA-Z]:', filename):
- filename = filename[1:]
- return filename if return_filename else open(filename, "rb")
-
- assert is_url(url)
-
- # Lookup from cache.
- if cache_dir is None:
- cache_dir = make_cache_dir_path('downloads')
-
- url_md5 = hashlib.md5(url.encode("utf-8")).hexdigest()
- if cache:
- cache_files = glob.glob(os.path.join(cache_dir, url_md5 + "_*"))
- if len(cache_files) == 1:
- filename = cache_files[0]
- return filename if return_filename else open(filename, "rb")
-
- # Download.
- url_name = None
- url_data = None
- with requests.Session() as session:
- if verbose:
- print("Downloading %s ..." % url, end="", flush=True)
- for attempts_left in reversed(range(num_attempts)):
- try:
- with session.get(url) as res:
- res.raise_for_status()
- if len(res.content) == 0:
- raise IOError("No data received")
-
- if len(res.content) < 8192:
- content_str = res.content.decode("utf-8")
- if "download_warning" in res.headers.get("Set-Cookie", ""):
- links = [html.unescape(link) for link in content_str.split(
- '"') if "export=download" in link]
- if len(links) == 1:
- url = requests.compat.urljoin(url, links[0])
- raise IOError("Google Drive virus checker nag")
- if "Google Drive - Quota exceeded" in content_str:
- raise IOError(
- "Google Drive download quota exceeded -- please try again later")
-
- match = re.search(
- r'filename="([^"]*)"', res.headers.get("Content-Disposition", ""))
- url_name = match[1] if match else url
- url_data = res.content
- if verbose:
- print(" done")
- break
- except KeyboardInterrupt:
- raise
- except:
- if not attempts_left:
- if verbose:
- print(" failed")
- raise
- if verbose:
- print(".", end="", flush=True)
-
- # Save to cache.
- if cache:
- safe_name = re.sub(r"[^0-9a-zA-Z-._]", "_", url_name)
- cache_file = os.path.join(cache_dir, url_md5 + "_" + safe_name)
- temp_file = os.path.join(
- cache_dir, "tmp_" + uuid.uuid4().hex + "_" + url_md5 + "_" + safe_name)
- os.makedirs(cache_dir, exist_ok=True)
- with open(temp_file, "wb") as f:
- f.write(url_data)
- os.replace(temp_file, cache_file) # atomic
- if return_filename:
- return cache_file
-
- # Return data as file object.
- assert not return_filename
- return io.BytesIO(url_data)
diff --git a/spaces/Androidonnxfork/CivitAi-to-Diffusers/diffusers/.github/ISSUE_TEMPLATE/feedback.md b/spaces/Androidonnxfork/CivitAi-to-Diffusers/diffusers/.github/ISSUE_TEMPLATE/feedback.md
deleted file mode 100644
index 25808b6575a405694f64dbf1b5a0ece8e0fcd2e2..0000000000000000000000000000000000000000
--- a/spaces/Androidonnxfork/CivitAi-to-Diffusers/diffusers/.github/ISSUE_TEMPLATE/feedback.md
+++ /dev/null
@@ -1,12 +0,0 @@
----
-name: "💬 Feedback about API Design"
-about: Give feedback about the current API design
-title: ''
-labels: ''
-assignees: ''
-
----
-
-**What API design would you like to have changed or added to the library? Why?**
-
-**What use case would this enable or better enable? Can you give us a code example?**
diff --git a/spaces/Androidonnxfork/CivitAi-to-Diffusers/diffusers/scripts/generate_logits.py b/spaces/Androidonnxfork/CivitAi-to-Diffusers/diffusers/scripts/generate_logits.py
deleted file mode 100644
index 89dce0e78d4ef50e060ac554ac3f7e760f55983f..0000000000000000000000000000000000000000
--- a/spaces/Androidonnxfork/CivitAi-to-Diffusers/diffusers/scripts/generate_logits.py
+++ /dev/null
@@ -1,127 +0,0 @@
-import random
-
-import torch
-from huggingface_hub import HfApi
-
-from diffusers import UNet2DModel
-
-
-api = HfApi()
-
-results = {}
-# fmt: off
-results["google_ddpm_cifar10_32"] = torch.tensor([
- -0.7515, -1.6883, 0.2420, 0.0300, 0.6347, 1.3433, -1.1743, -3.7467,
- 1.2342, -2.2485, 0.4636, 0.8076, -0.7991, 0.3969, 0.8498, 0.9189,
- -1.8887, -3.3522, 0.7639, 0.2040, 0.6271, -2.7148, -1.6316, 3.0839,
- 0.3186, 0.2721, -0.9759, -1.2461, 2.6257, 1.3557
-])
-results["google_ddpm_ema_bedroom_256"] = torch.tensor([
- -2.3639, -2.5344, 0.0054, -0.6674, 1.5990, 1.0158, 0.3124, -2.1436,
- 1.8795, -2.5429, -0.1566, -0.3973, 1.2490, 2.6447, 1.2283, -0.5208,
- -2.8154, -3.5119, 2.3838, 1.2033, 1.7201, -2.1256, -1.4576, 2.7948,
- 2.4204, -0.9752, -1.2546, 0.8027, 3.2758, 3.1365
-])
-results["CompVis_ldm_celebahq_256"] = torch.tensor([
- -0.6531, -0.6891, -0.3172, -0.5375, -0.9140, -0.5367, -0.1175, -0.7869,
- -0.3808, -0.4513, -0.2098, -0.0083, 0.3183, 0.5140, 0.2247, -0.1304,
- -0.1302, -0.2802, -0.2084, -0.2025, -0.4967, -0.4873, -0.0861, 0.6925,
- 0.0250, 0.1290, -0.1543, 0.6316, 1.0460, 1.4943
-])
-results["google_ncsnpp_ffhq_1024"] = torch.tensor([
- 0.0911, 0.1107, 0.0182, 0.0435, -0.0805, -0.0608, 0.0381, 0.2172,
- -0.0280, 0.1327, -0.0299, -0.0255, -0.0050, -0.1170, -0.1046, 0.0309,
- 0.1367, 0.1728, -0.0533, -0.0748, -0.0534, 0.1624, 0.0384, -0.1805,
- -0.0707, 0.0642, 0.0220, -0.0134, -0.1333, -0.1505
-])
-results["google_ncsnpp_bedroom_256"] = torch.tensor([
- 0.1321, 0.1337, 0.0440, 0.0622, -0.0591, -0.0370, 0.0503, 0.2133,
- -0.0177, 0.1415, -0.0116, -0.0112, 0.0044, -0.0980, -0.0789, 0.0395,
- 0.1502, 0.1785, -0.0488, -0.0514, -0.0404, 0.1539, 0.0454, -0.1559,
- -0.0665, 0.0659, 0.0383, -0.0005, -0.1266, -0.1386
-])
-results["google_ncsnpp_celebahq_256"] = torch.tensor([
- 0.1154, 0.1218, 0.0307, 0.0526, -0.0711, -0.0541, 0.0366, 0.2078,
- -0.0267, 0.1317, -0.0226, -0.0193, -0.0014, -0.1055, -0.0902, 0.0330,
- 0.1391, 0.1709, -0.0562, -0.0693, -0.0560, 0.1482, 0.0381, -0.1683,
- -0.0681, 0.0661, 0.0331, -0.0046, -0.1268, -0.1431
-])
-results["google_ncsnpp_church_256"] = torch.tensor([
- 0.1192, 0.1240, 0.0414, 0.0606, -0.0557, -0.0412, 0.0430, 0.2042,
- -0.0200, 0.1385, -0.0115, -0.0132, 0.0017, -0.0965, -0.0802, 0.0398,
- 0.1433, 0.1747, -0.0458, -0.0533, -0.0407, 0.1545, 0.0419, -0.1574,
- -0.0645, 0.0626, 0.0341, -0.0010, -0.1199, -0.1390
-])
-results["google_ncsnpp_ffhq_256"] = torch.tensor([
- 0.1075, 0.1074, 0.0205, 0.0431, -0.0774, -0.0607, 0.0298, 0.2042,
- -0.0320, 0.1267, -0.0281, -0.0250, -0.0064, -0.1091, -0.0946, 0.0290,
- 0.1328, 0.1650, -0.0580, -0.0738, -0.0586, 0.1440, 0.0337, -0.1746,
- -0.0712, 0.0605, 0.0250, -0.0099, -0.1316, -0.1473
-])
-results["google_ddpm_cat_256"] = torch.tensor([
- -1.4572, -2.0481, -0.0414, -0.6005, 1.4136, 0.5848, 0.4028, -2.7330,
- 1.2212, -2.1228, 0.2155, 0.4039, 0.7662, 2.0535, 0.7477, -0.3243,
- -2.1758, -2.7648, 1.6947, 0.7026, 1.2338, -1.6078, -0.8682, 2.2810,
- 1.8574, -0.5718, -0.5586, -0.0186, 2.3415, 2.1251])
-results["google_ddpm_celebahq_256"] = torch.tensor([
- -1.3690, -1.9720, -0.4090, -0.6966, 1.4660, 0.9938, -0.1385, -2.7324,
- 0.7736, -1.8917, 0.2923, 0.4293, 0.1693, 1.4112, 1.1887, -0.3181,
- -2.2160, -2.6381, 1.3170, 0.8163, 0.9240, -1.6544, -0.6099, 2.5259,
- 1.6430, -0.9090, -0.9392, -0.0126, 2.4268, 2.3266
-])
-results["google_ddpm_ema_celebahq_256"] = torch.tensor([
- -1.3525, -1.9628, -0.3956, -0.6860, 1.4664, 1.0014, -0.1259, -2.7212,
- 0.7772, -1.8811, 0.2996, 0.4388, 0.1704, 1.4029, 1.1701, -0.3027,
- -2.2053, -2.6287, 1.3350, 0.8131, 0.9274, -1.6292, -0.6098, 2.5131,
- 1.6505, -0.8958, -0.9298, -0.0151, 2.4257, 2.3355
-])
-results["google_ddpm_church_256"] = torch.tensor([
- -2.0585, -2.7897, -0.2850, -0.8940, 1.9052, 0.5702, 0.6345, -3.8959,
- 1.5932, -3.2319, 0.1974, 0.0287, 1.7566, 2.6543, 0.8387, -0.5351,
- -3.2736, -4.3375, 2.9029, 1.6390, 1.4640, -2.1701, -1.9013, 2.9341,
- 3.4981, -0.6255, -1.1644, -0.1591, 3.7097, 3.2066
-])
-results["google_ddpm_bedroom_256"] = torch.tensor([
- -2.3139, -2.5594, -0.0197, -0.6785, 1.7001, 1.1606, 0.3075, -2.1740,
- 1.8071, -2.5630, -0.0926, -0.3811, 1.2116, 2.6246, 1.2731, -0.5398,
- -2.8153, -3.6140, 2.3893, 1.3262, 1.6258, -2.1856, -1.3267, 2.8395,
- 2.3779, -1.0623, -1.2468, 0.8959, 3.3367, 3.2243
-])
-results["google_ddpm_ema_church_256"] = torch.tensor([
- -2.0628, -2.7667, -0.2089, -0.8263, 2.0539, 0.5992, 0.6495, -3.8336,
- 1.6025, -3.2817, 0.1721, -0.0633, 1.7516, 2.7039, 0.8100, -0.5908,
- -3.2113, -4.4343, 2.9257, 1.3632, 1.5562, -2.1489, -1.9894, 3.0560,
- 3.3396, -0.7328, -1.0417, 0.0383, 3.7093, 3.2343
-])
-results["google_ddpm_ema_cat_256"] = torch.tensor([
- -1.4574, -2.0569, -0.0473, -0.6117, 1.4018, 0.5769, 0.4129, -2.7344,
- 1.2241, -2.1397, 0.2000, 0.3937, 0.7616, 2.0453, 0.7324, -0.3391,
- -2.1746, -2.7744, 1.6963, 0.6921, 1.2187, -1.6172, -0.8877, 2.2439,
- 1.8471, -0.5839, -0.5605, -0.0464, 2.3250, 2.1219
-])
-# fmt: on
-
-models = api.list_models(filter="diffusers")
-for mod in models:
- if "google" in mod.author or mod.modelId == "CompVis/ldm-celebahq-256":
- local_checkpoint = "/home/patrick/google_checkpoints/" + mod.modelId.split("/")[-1]
-
- print(f"Started running {mod.modelId}!!!")
-
- if mod.modelId.startswith("CompVis"):
- model = UNet2DModel.from_pretrained(local_checkpoint, subfolder="unet")
- else:
- model = UNet2DModel.from_pretrained(local_checkpoint)
-
- torch.manual_seed(0)
- random.seed(0)
-
- noise = torch.randn(1, model.config.in_channels, model.config.sample_size, model.config.sample_size)
- time_step = torch.tensor([10] * noise.shape[0])
- with torch.no_grad():
- logits = model(noise, time_step).sample
-
- assert torch.allclose(
- logits[0, 0, 0, :30], results["_".join("_".join(mod.modelId.split("/")).split("-"))], atol=1e-3
- )
- print(f"{mod.modelId} has passed successfully!!!")
diff --git a/spaces/Andy1621/uniformer_image_detection/configs/guided_anchoring/ga_faster_x101_32x4d_fpn_1x_coco.py b/spaces/Andy1621/uniformer_image_detection/configs/guided_anchoring/ga_faster_x101_32x4d_fpn_1x_coco.py
deleted file mode 100644
index c9a035f15cfad12ddbbfa87ed0d579c1cde0c4ce..0000000000000000000000000000000000000000
--- a/spaces/Andy1621/uniformer_image_detection/configs/guided_anchoring/ga_faster_x101_32x4d_fpn_1x_coco.py
+++ /dev/null
@@ -1,13 +0,0 @@
-_base_ = './ga_faster_r50_fpn_1x_coco.py'
-model = dict(
- pretrained='open-mmlab://resnext101_32x4d',
- backbone=dict(
- type='ResNeXt',
- depth=101,
- groups=32,
- base_width=4,
- num_stages=4,
- out_indices=(0, 1, 2, 3),
- frozen_stages=1,
- norm_cfg=dict(type='BN', requires_grad=True),
- style='pytorch'))
diff --git a/spaces/Andy1621/uniformer_image_detection/configs/htc/htc_x101_64x4d_fpn_16x1_20e_coco.py b/spaces/Andy1621/uniformer_image_detection/configs/htc/htc_x101_64x4d_fpn_16x1_20e_coco.py
deleted file mode 100644
index b140f75182cd4832857b6a86fe11b2961703a17c..0000000000000000000000000000000000000000
--- a/spaces/Andy1621/uniformer_image_detection/configs/htc/htc_x101_64x4d_fpn_16x1_20e_coco.py
+++ /dev/null
@@ -1,18 +0,0 @@
-_base_ = './htc_r50_fpn_1x_coco.py'
-model = dict(
- pretrained='open-mmlab://resnext101_64x4d',
- backbone=dict(
- type='ResNeXt',
- depth=101,
- groups=64,
- base_width=4,
- num_stages=4,
- out_indices=(0, 1, 2, 3),
- frozen_stages=1,
- norm_cfg=dict(type='BN', requires_grad=True),
- norm_eval=True,
- style='pytorch'))
-data = dict(samples_per_gpu=1, workers_per_gpu=1)
-# learning policy
-lr_config = dict(step=[16, 19])
-runner = dict(type='EpochBasedRunner', max_epochs=20)
diff --git a/spaces/Andy1621/uniformer_image_segmentation/configs/ccnet/ccnet_r50-d8_512x1024_40k_cityscapes.py b/spaces/Andy1621/uniformer_image_segmentation/configs/ccnet/ccnet_r50-d8_512x1024_40k_cityscapes.py
deleted file mode 100644
index 6a4316dde57206fe369e72fa0d32a529fe1a1932..0000000000000000000000000000000000000000
--- a/spaces/Andy1621/uniformer_image_segmentation/configs/ccnet/ccnet_r50-d8_512x1024_40k_cityscapes.py
+++ /dev/null
@@ -1,4 +0,0 @@
-_base_ = [
- '../_base_/models/ccnet_r50-d8.py', '../_base_/datasets/cityscapes.py',
- '../_base_/default_runtime.py', '../_base_/schedules/schedule_40k.py'
-]
diff --git a/spaces/Andy1621/uniformer_image_segmentation/configs/deeplabv3plus/deeplabv3plus_r18b-d8_769x769_80k_cityscapes.py b/spaces/Andy1621/uniformer_image_segmentation/configs/deeplabv3plus/deeplabv3plus_r18b-d8_769x769_80k_cityscapes.py
deleted file mode 100644
index b49da3581d9697e726e114b1564fc58a55ef1099..0000000000000000000000000000000000000000
--- a/spaces/Andy1621/uniformer_image_segmentation/configs/deeplabv3plus/deeplabv3plus_r18b-d8_769x769_80k_cityscapes.py
+++ /dev/null
@@ -1,11 +0,0 @@
-_base_ = './deeplabv3plus_r50-d8_769x769_80k_cityscapes.py'
-model = dict(
- pretrained='torchvision://resnet18',
- backbone=dict(type='ResNet', depth=18),
- decode_head=dict(
- c1_in_channels=64,
- c1_channels=12,
- in_channels=512,
- channels=128,
- ),
- auxiliary_head=dict(in_channels=256, channels=64))
diff --git a/spaces/Andy1621/uniformer_image_segmentation/configs/ocrnet/ocrnet_hr48_512x512_20k_voc12aug.py b/spaces/Andy1621/uniformer_image_segmentation/configs/ocrnet/ocrnet_hr48_512x512_20k_voc12aug.py
deleted file mode 100644
index c2dd6d1158bd31ecdd7874827fd37bffb5d26db6..0000000000000000000000000000000000000000
--- a/spaces/Andy1621/uniformer_image_segmentation/configs/ocrnet/ocrnet_hr48_512x512_20k_voc12aug.py
+++ /dev/null
@@ -1,39 +0,0 @@
-_base_ = './ocrnet_hr18_512x512_20k_voc12aug.py'
-norm_cfg = dict(type='SyncBN', requires_grad=True)
-model = dict(
- pretrained='open-mmlab://msra/hrnetv2_w48',
- backbone=dict(
- extra=dict(
- stage2=dict(num_channels=(48, 96)),
- stage3=dict(num_channels=(48, 96, 192)),
- stage4=dict(num_channels=(48, 96, 192, 384)))),
- decode_head=[
- dict(
- type='FCNHead',
- in_channels=[48, 96, 192, 384],
- channels=sum([48, 96, 192, 384]),
- input_transform='resize_concat',
- in_index=(0, 1, 2, 3),
- kernel_size=1,
- num_convs=1,
- norm_cfg=norm_cfg,
- concat_input=False,
- dropout_ratio=-1,
- num_classes=21,
- align_corners=False,
- loss_decode=dict(
- type='CrossEntropyLoss', use_sigmoid=False, loss_weight=0.4)),
- dict(
- type='OCRHead',
- in_channels=[48, 96, 192, 384],
- channels=512,
- ocr_channels=256,
- input_transform='resize_concat',
- in_index=(0, 1, 2, 3),
- norm_cfg=norm_cfg,
- dropout_ratio=-1,
- num_classes=21,
- align_corners=False,
- loss_decode=dict(
- type='CrossEntropyLoss', use_sigmoid=False, loss_weight=1.0))
- ])
diff --git a/spaces/ArchitSharma/Digital-Photo-Color-Restoration/src/deoldify/generators.py b/spaces/ArchitSharma/Digital-Photo-Color-Restoration/src/deoldify/generators.py
deleted file mode 100644
index 0be74c39d095332a9143ea35c7ae36fd83e07e9f..0000000000000000000000000000000000000000
--- a/spaces/ArchitSharma/Digital-Photo-Color-Restoration/src/deoldify/generators.py
+++ /dev/null
@@ -1,151 +0,0 @@
-from fastai.vision import *
-from fastai.vision.learner import cnn_config
-from .unet import DynamicUnetWide, DynamicUnetDeep
-from .loss import FeatureLoss
-from .dataset import *
-
-# Weights are implicitly read from ./models/ folder
-def gen_inference_wide(
- root_folder: Path, weights_name: str, nf_factor: int = 2, arch=models.resnet101) -> Learner:
- data = get_dummy_databunch()
- learn = gen_learner_wide(
- data=data, gen_loss=F.l1_loss, nf_factor=nf_factor, arch=arch
- )
- learn.path = root_folder
- learn.load(weights_name)
- learn.model.eval()
- return learn
-
-
-def gen_learner_wide(
- data: ImageDataBunch, gen_loss, arch=models.resnet101, nf_factor: int = 2
-) -> Learner:
- return unet_learner_wide(
- data,
- arch=arch,
- wd=1e-3,
- blur=True,
- norm_type=NormType.Spectral,
- self_attention=True,
- y_range=(-3.0, 3.0),
- loss_func=gen_loss,
- nf_factor=nf_factor,
- )
-
-
-# The code below is meant to be merged into fastaiv1 ideally
-def unet_learner_wide(
- data: DataBunch,
- arch: Callable,
- pretrained: bool = True,
- blur_final: bool = True,
- norm_type: Optional[NormType] = NormType,
- split_on: Optional[SplitFuncOrIdxList] = None,
- blur: bool = False,
- self_attention: bool = False,
- y_range: Optional[Tuple[float, float]] = None,
- last_cross: bool = True,
- bottle: bool = False,
- nf_factor: int = 1,
- **kwargs: Any
-) -> Learner:
- "Build Unet learner from `data` and `arch`."
- meta = cnn_config(arch)
- body = create_body(arch, pretrained)
- model = to_device(
- DynamicUnetWide(
- body,
- n_classes=data.c,
- blur=blur,
- blur_final=blur_final,
- self_attention=self_attention,
- y_range=y_range,
- norm_type=norm_type,
- last_cross=last_cross,
- bottle=bottle,
- nf_factor=nf_factor,
- ),
- data.device,
- )
- learn = Learner(data, model, **kwargs)
- learn.split(ifnone(split_on, meta['split']))
- if pretrained:
- learn.freeze()
- apply_init(model[2], nn.init.kaiming_normal_)
- return learn
-
-
-# ----------------------------------------------------------------------
-
-# Weights are implicitly read from ./models/ folder
-def gen_inference_deep(
- root_folder: Path, weights_name: str, arch=models.resnet34, nf_factor: float = 1.5) -> Learner:
- data = get_dummy_databunch()
- learn = gen_learner_deep(
- data=data, gen_loss=F.l1_loss, arch=arch, nf_factor=nf_factor
- )
- learn.path = root_folder
- learn.load(weights_name)
- learn.model.eval()
- return learn
-
-
-def gen_learner_deep(
- data: ImageDataBunch, gen_loss, arch=models.resnet34, nf_factor: float = 1.5
-) -> Learner:
- return unet_learner_deep(
- data,
- arch,
- wd=1e-3,
- blur=True,
- norm_type=NormType.Spectral,
- self_attention=True,
- y_range=(-3.0, 3.0),
- loss_func=gen_loss,
- nf_factor=nf_factor,
- )
-
-
-# The code below is meant to be merged into fastaiv1 ideally
-def unet_learner_deep(
- data: DataBunch,
- arch: Callable,
- pretrained: bool = True,
- blur_final: bool = True,
- norm_type: Optional[NormType] = NormType,
- split_on: Optional[SplitFuncOrIdxList] = None,
- blur: bool = False,
- self_attention: bool = False,
- y_range: Optional[Tuple[float, float]] = None,
- last_cross: bool = True,
- bottle: bool = False,
- nf_factor: float = 1.5,
- **kwargs: Any
-) -> Learner:
- "Build Unet learner from `data` and `arch`."
- meta = cnn_config(arch)
- body = create_body(arch, pretrained)
- model = to_device(
- DynamicUnetDeep(
- body,
- n_classes=data.c,
- blur=blur,
- blur_final=blur_final,
- self_attention=self_attention,
- y_range=y_range,
- norm_type=norm_type,
- last_cross=last_cross,
- bottle=bottle,
- nf_factor=nf_factor,
- ),
- data.device,
- )
- learn = Learner(data, model, **kwargs)
- learn.split(ifnone(split_on, meta['split']))
- if pretrained:
- learn.freeze()
- apply_init(model[2], nn.init.kaiming_normal_)
- return learn
-
-
-# -----------------------------
diff --git a/spaces/Ataturk-Chatbot/HuggingFaceChat/venv/lib/python3.11/site-packages/pip/_vendor/cachecontrol/filewrapper.py b/spaces/Ataturk-Chatbot/HuggingFaceChat/venv/lib/python3.11/site-packages/pip/_vendor/cachecontrol/filewrapper.py
deleted file mode 100644
index f5ed5f6f6ec0eae90a9f48753622b2b5ee5d4a4f..0000000000000000000000000000000000000000
--- a/spaces/Ataturk-Chatbot/HuggingFaceChat/venv/lib/python3.11/site-packages/pip/_vendor/cachecontrol/filewrapper.py
+++ /dev/null
@@ -1,111 +0,0 @@
-# SPDX-FileCopyrightText: 2015 Eric Larson
-#
-# SPDX-License-Identifier: Apache-2.0
-
-from tempfile import NamedTemporaryFile
-import mmap
-
-
-class CallbackFileWrapper(object):
- """
- Small wrapper around a fp object which will tee everything read into a
- buffer, and when that file is closed it will execute a callback with the
- contents of that buffer.
-
- All attributes are proxied to the underlying file object.
-
- This class uses members with a double underscore (__) leading prefix so as
- not to accidentally shadow an attribute.
-
- The data is stored in a temporary file until it is all available. As long
- as the temporary files directory is disk-based (sometimes it's a
- memory-backed-``tmpfs`` on Linux), data will be unloaded to disk if memory
- pressure is high. For small files the disk usually won't be used at all,
- it'll all be in the filesystem memory cache, so there should be no
- performance impact.
- """
-
- def __init__(self, fp, callback):
- self.__buf = NamedTemporaryFile("rb+", delete=True)
- self.__fp = fp
- self.__callback = callback
-
- def __getattr__(self, name):
- # The vaguaries of garbage collection means that self.__fp is
- # not always set. By using __getattribute__ and the private
- # name[0] allows looking up the attribute value and raising an
- # AttributeError when it doesn't exist. This stop thigns from
- # infinitely recursing calls to getattr in the case where
- # self.__fp hasn't been set.
- #
- # [0] https://docs.python.org/2/reference/expressions.html#atom-identifiers
- fp = self.__getattribute__("_CallbackFileWrapper__fp")
- return getattr(fp, name)
-
- def __is_fp_closed(self):
- try:
- return self.__fp.fp is None
-
- except AttributeError:
- pass
-
- try:
- return self.__fp.closed
-
- except AttributeError:
- pass
-
- # We just don't cache it then.
- # TODO: Add some logging here...
- return False
-
- def _close(self):
- if self.__callback:
- if self.__buf.tell() == 0:
- # Empty file:
- result = b""
- else:
- # Return the data without actually loading it into memory,
- # relying on Python's buffer API and mmap(). mmap() just gives
- # a view directly into the filesystem's memory cache, so it
- # doesn't result in duplicate memory use.
- self.__buf.seek(0, 0)
- result = memoryview(
- mmap.mmap(self.__buf.fileno(), 0, access=mmap.ACCESS_READ)
- )
- self.__callback(result)
-
- # We assign this to None here, because otherwise we can get into
- # really tricky problems where the CPython interpreter dead locks
- # because the callback is holding a reference to something which
- # has a __del__ method. Setting this to None breaks the cycle
- # and allows the garbage collector to do it's thing normally.
- self.__callback = None
-
- # Closing the temporary file releases memory and frees disk space.
- # Important when caching big files.
- self.__buf.close()
-
- def read(self, amt=None):
- data = self.__fp.read(amt)
- if data:
- # We may be dealing with b'', a sign that things are over:
- # it's passed e.g. after we've already closed self.__buf.
- self.__buf.write(data)
- if self.__is_fp_closed():
- self._close()
-
- return data
-
- def _safe_read(self, amt):
- data = self.__fp._safe_read(amt)
- if amt == 2 and data == b"\r\n":
- # urllib executes this read to toss the CRLF at the end
- # of the chunk.
- return data
-
- self.__buf.write(data)
- if self.__is_fp_closed():
- self._close()
-
- return data
diff --git a/spaces/Ataturk-Chatbot/HuggingFaceChat/venv/lib/python3.11/site-packages/pip/_vendor/distlib/resources.py b/spaces/Ataturk-Chatbot/HuggingFaceChat/venv/lib/python3.11/site-packages/pip/_vendor/distlib/resources.py
deleted file mode 100644
index fef52aa103ea369c96567b9af2a5a0ba14db5cb9..0000000000000000000000000000000000000000
--- a/spaces/Ataturk-Chatbot/HuggingFaceChat/venv/lib/python3.11/site-packages/pip/_vendor/distlib/resources.py
+++ /dev/null
@@ -1,358 +0,0 @@
-# -*- coding: utf-8 -*-
-#
-# Copyright (C) 2013-2017 Vinay Sajip.
-# Licensed to the Python Software Foundation under a contributor agreement.
-# See LICENSE.txt and CONTRIBUTORS.txt.
-#
-from __future__ import unicode_literals
-
-import bisect
-import io
-import logging
-import os
-import pkgutil
-import sys
-import types
-import zipimport
-
-from . import DistlibException
-from .util import cached_property, get_cache_base, Cache
-
-logger = logging.getLogger(__name__)
-
-
-cache = None # created when needed
-
-
-class ResourceCache(Cache):
- def __init__(self, base=None):
- if base is None:
- # Use native string to avoid issues on 2.x: see Python #20140.
- base = os.path.join(get_cache_base(), str('resource-cache'))
- super(ResourceCache, self).__init__(base)
-
- def is_stale(self, resource, path):
- """
- Is the cache stale for the given resource?
-
- :param resource: The :class:`Resource` being cached.
- :param path: The path of the resource in the cache.
- :return: True if the cache is stale.
- """
- # Cache invalidation is a hard problem :-)
- return True
-
- def get(self, resource):
- """
- Get a resource into the cache,
-
- :param resource: A :class:`Resource` instance.
- :return: The pathname of the resource in the cache.
- """
- prefix, path = resource.finder.get_cache_info(resource)
- if prefix is None:
- result = path
- else:
- result = os.path.join(self.base, self.prefix_to_dir(prefix), path)
- dirname = os.path.dirname(result)
- if not os.path.isdir(dirname):
- os.makedirs(dirname)
- if not os.path.exists(result):
- stale = True
- else:
- stale = self.is_stale(resource, path)
- if stale:
- # write the bytes of the resource to the cache location
- with open(result, 'wb') as f:
- f.write(resource.bytes)
- return result
-
-
-class ResourceBase(object):
- def __init__(self, finder, name):
- self.finder = finder
- self.name = name
-
-
-class Resource(ResourceBase):
- """
- A class representing an in-package resource, such as a data file. This is
- not normally instantiated by user code, but rather by a
- :class:`ResourceFinder` which manages the resource.
- """
- is_container = False # Backwards compatibility
-
- def as_stream(self):
- """
- Get the resource as a stream.
-
- This is not a property to make it obvious that it returns a new stream
- each time.
- """
- return self.finder.get_stream(self)
-
- @cached_property
- def file_path(self):
- global cache
- if cache is None:
- cache = ResourceCache()
- return cache.get(self)
-
- @cached_property
- def bytes(self):
- return self.finder.get_bytes(self)
-
- @cached_property
- def size(self):
- return self.finder.get_size(self)
-
-
-class ResourceContainer(ResourceBase):
- is_container = True # Backwards compatibility
-
- @cached_property
- def resources(self):
- return self.finder.get_resources(self)
-
-
-class ResourceFinder(object):
- """
- Resource finder for file system resources.
- """
-
- if sys.platform.startswith('java'):
- skipped_extensions = ('.pyc', '.pyo', '.class')
- else:
- skipped_extensions = ('.pyc', '.pyo')
-
- def __init__(self, module):
- self.module = module
- self.loader = getattr(module, '__loader__', None)
- self.base = os.path.dirname(getattr(module, '__file__', ''))
-
- def _adjust_path(self, path):
- return os.path.realpath(path)
-
- def _make_path(self, resource_name):
- # Issue #50: need to preserve type of path on Python 2.x
- # like os.path._get_sep
- if isinstance(resource_name, bytes): # should only happen on 2.x
- sep = b'/'
- else:
- sep = '/'
- parts = resource_name.split(sep)
- parts.insert(0, self.base)
- result = os.path.join(*parts)
- return self._adjust_path(result)
-
- def _find(self, path):
- return os.path.exists(path)
-
- def get_cache_info(self, resource):
- return None, resource.path
-
- def find(self, resource_name):
- path = self._make_path(resource_name)
- if not self._find(path):
- result = None
- else:
- if self._is_directory(path):
- result = ResourceContainer(self, resource_name)
- else:
- result = Resource(self, resource_name)
- result.path = path
- return result
-
- def get_stream(self, resource):
- return open(resource.path, 'rb')
-
- def get_bytes(self, resource):
- with open(resource.path, 'rb') as f:
- return f.read()
-
- def get_size(self, resource):
- return os.path.getsize(resource.path)
-
- def get_resources(self, resource):
- def allowed(f):
- return (f != '__pycache__' and not
- f.endswith(self.skipped_extensions))
- return set([f for f in os.listdir(resource.path) if allowed(f)])
-
- def is_container(self, resource):
- return self._is_directory(resource.path)
-
- _is_directory = staticmethod(os.path.isdir)
-
- def iterator(self, resource_name):
- resource = self.find(resource_name)
- if resource is not None:
- todo = [resource]
- while todo:
- resource = todo.pop(0)
- yield resource
- if resource.is_container:
- rname = resource.name
- for name in resource.resources:
- if not rname:
- new_name = name
- else:
- new_name = '/'.join([rname, name])
- child = self.find(new_name)
- if child.is_container:
- todo.append(child)
- else:
- yield child
-
-
-class ZipResourceFinder(ResourceFinder):
- """
- Resource finder for resources in .zip files.
- """
- def __init__(self, module):
- super(ZipResourceFinder, self).__init__(module)
- archive = self.loader.archive
- self.prefix_len = 1 + len(archive)
- # PyPy doesn't have a _files attr on zipimporter, and you can't set one
- if hasattr(self.loader, '_files'):
- self._files = self.loader._files
- else:
- self._files = zipimport._zip_directory_cache[archive]
- self.index = sorted(self._files)
-
- def _adjust_path(self, path):
- return path
-
- def _find(self, path):
- path = path[self.prefix_len:]
- if path in self._files:
- result = True
- else:
- if path and path[-1] != os.sep:
- path = path + os.sep
- i = bisect.bisect(self.index, path)
- try:
- result = self.index[i].startswith(path)
- except IndexError:
- result = False
- if not result:
- logger.debug('_find failed: %r %r', path, self.loader.prefix)
- else:
- logger.debug('_find worked: %r %r', path, self.loader.prefix)
- return result
-
- def get_cache_info(self, resource):
- prefix = self.loader.archive
- path = resource.path[1 + len(prefix):]
- return prefix, path
-
- def get_bytes(self, resource):
- return self.loader.get_data(resource.path)
-
- def get_stream(self, resource):
- return io.BytesIO(self.get_bytes(resource))
-
- def get_size(self, resource):
- path = resource.path[self.prefix_len:]
- return self._files[path][3]
-
- def get_resources(self, resource):
- path = resource.path[self.prefix_len:]
- if path and path[-1] != os.sep:
- path += os.sep
- plen = len(path)
- result = set()
- i = bisect.bisect(self.index, path)
- while i < len(self.index):
- if not self.index[i].startswith(path):
- break
- s = self.index[i][plen:]
- result.add(s.split(os.sep, 1)[0]) # only immediate children
- i += 1
- return result
-
- def _is_directory(self, path):
- path = path[self.prefix_len:]
- if path and path[-1] != os.sep:
- path += os.sep
- i = bisect.bisect(self.index, path)
- try:
- result = self.index[i].startswith(path)
- except IndexError:
- result = False
- return result
-
-
-_finder_registry = {
- type(None): ResourceFinder,
- zipimport.zipimporter: ZipResourceFinder
-}
-
-try:
- # In Python 3.6, _frozen_importlib -> _frozen_importlib_external
- try:
- import _frozen_importlib_external as _fi
- except ImportError:
- import _frozen_importlib as _fi
- _finder_registry[_fi.SourceFileLoader] = ResourceFinder
- _finder_registry[_fi.FileFinder] = ResourceFinder
- # See issue #146
- _finder_registry[_fi.SourcelessFileLoader] = ResourceFinder
- del _fi
-except (ImportError, AttributeError):
- pass
-
-
-def register_finder(loader, finder_maker):
- _finder_registry[type(loader)] = finder_maker
-
-
-_finder_cache = {}
-
-
-def finder(package):
- """
- Return a resource finder for a package.
- :param package: The name of the package.
- :return: A :class:`ResourceFinder` instance for the package.
- """
- if package in _finder_cache:
- result = _finder_cache[package]
- else:
- if package not in sys.modules:
- __import__(package)
- module = sys.modules[package]
- path = getattr(module, '__path__', None)
- if path is None:
- raise DistlibException('You cannot get a finder for a module, '
- 'only for a package')
- loader = getattr(module, '__loader__', None)
- finder_maker = _finder_registry.get(type(loader))
- if finder_maker is None:
- raise DistlibException('Unable to locate finder for %r' % package)
- result = finder_maker(module)
- _finder_cache[package] = result
- return result
-
-
-_dummy_module = types.ModuleType(str('__dummy__'))
-
-
-def finder_for_path(path):
- """
- Return a resource finder for a path, which should represent a container.
-
- :param path: The path.
- :return: A :class:`ResourceFinder` instance for the path.
- """
- result = None
- # calls any path hooks, gets importer into cache
- pkgutil.get_importer(path)
- loader = sys.path_importer_cache.get(path)
- finder = _finder_registry.get(type(loader))
- if finder:
- module = _dummy_module
- module.__file__ = os.path.join(path, '')
- module.__loader__ = loader
- result = finder(module)
- return result
diff --git a/spaces/Ataturk-Chatbot/HuggingFaceChat/venv/lib/python3.11/site-packages/setuptools/_distutils/extension.py b/spaces/Ataturk-Chatbot/HuggingFaceChat/venv/lib/python3.11/site-packages/setuptools/_distutils/extension.py
deleted file mode 100644
index 6b8575de2949cd0519ee5f26b6eb00df417e2113..0000000000000000000000000000000000000000
--- a/spaces/Ataturk-Chatbot/HuggingFaceChat/venv/lib/python3.11/site-packages/setuptools/_distutils/extension.py
+++ /dev/null
@@ -1,248 +0,0 @@
-"""distutils.extension
-
-Provides the Extension class, used to describe C/C++ extension
-modules in setup scripts."""
-
-import os
-import warnings
-
-# This class is really only used by the "build_ext" command, so it might
-# make sense to put it in distutils.command.build_ext. However, that
-# module is already big enough, and I want to make this class a bit more
-# complex to simplify some common cases ("foo" module in "foo.c") and do
-# better error-checking ("foo.c" actually exists).
-#
-# Also, putting this in build_ext.py means every setup script would have to
-# import that large-ish module (indirectly, through distutils.core) in
-# order to do anything.
-
-
-class Extension:
- """Just a collection of attributes that describes an extension
- module and everything needed to build it (hopefully in a portable
- way, but there are hooks that let you be as unportable as you need).
-
- Instance attributes:
- name : string
- the full name of the extension, including any packages -- ie.
- *not* a filename or pathname, but Python dotted name
- sources : [string]
- list of source filenames, relative to the distribution root
- (where the setup script lives), in Unix form (slash-separated)
- for portability. Source files may be C, C++, SWIG (.i),
- platform-specific resource files, or whatever else is recognized
- by the "build_ext" command as source for a Python extension.
- include_dirs : [string]
- list of directories to search for C/C++ header files (in Unix
- form for portability)
- define_macros : [(name : string, value : string|None)]
- list of macros to define; each macro is defined using a 2-tuple,
- where 'value' is either the string to define it to or None to
- define it without a particular value (equivalent of "#define
- FOO" in source or -DFOO on Unix C compiler command line)
- undef_macros : [string]
- list of macros to undefine explicitly
- library_dirs : [string]
- list of directories to search for C/C++ libraries at link time
- libraries : [string]
- list of library names (not filenames or paths) to link against
- runtime_library_dirs : [string]
- list of directories to search for C/C++ libraries at run time
- (for shared extensions, this is when the extension is loaded)
- extra_objects : [string]
- list of extra files to link with (eg. object files not implied
- by 'sources', static library that must be explicitly specified,
- binary resource files, etc.)
- extra_compile_args : [string]
- any extra platform- and compiler-specific information to use
- when compiling the source files in 'sources'. For platforms and
- compilers where "command line" makes sense, this is typically a
- list of command-line arguments, but for other platforms it could
- be anything.
- extra_link_args : [string]
- any extra platform- and compiler-specific information to use
- when linking object files together to create the extension (or
- to create a new static Python interpreter). Similar
- interpretation as for 'extra_compile_args'.
- export_symbols : [string]
- list of symbols to be exported from a shared extension. Not
- used on all platforms, and not generally necessary for Python
- extensions, which typically export exactly one symbol: "init" +
- extension_name.
- swig_opts : [string]
- any extra options to pass to SWIG if a source file has the .i
- extension.
- depends : [string]
- list of files that the extension depends on
- language : string
- extension language (i.e. "c", "c++", "objc"). Will be detected
- from the source extensions if not provided.
- optional : boolean
- specifies that a build failure in the extension should not abort the
- build process, but simply not install the failing extension.
- """
-
- # When adding arguments to this constructor, be sure to update
- # setup_keywords in core.py.
- def __init__(
- self,
- name,
- sources,
- include_dirs=None,
- define_macros=None,
- undef_macros=None,
- library_dirs=None,
- libraries=None,
- runtime_library_dirs=None,
- extra_objects=None,
- extra_compile_args=None,
- extra_link_args=None,
- export_symbols=None,
- swig_opts=None,
- depends=None,
- language=None,
- optional=None,
- **kw # To catch unknown keywords
- ):
- if not isinstance(name, str):
- raise AssertionError("'name' must be a string")
- if not (isinstance(sources, list) and all(isinstance(v, str) for v in sources)):
- raise AssertionError("'sources' must be a list of strings")
-
- self.name = name
- self.sources = sources
- self.include_dirs = include_dirs or []
- self.define_macros = define_macros or []
- self.undef_macros = undef_macros or []
- self.library_dirs = library_dirs or []
- self.libraries = libraries or []
- self.runtime_library_dirs = runtime_library_dirs or []
- self.extra_objects = extra_objects or []
- self.extra_compile_args = extra_compile_args or []
- self.extra_link_args = extra_link_args or []
- self.export_symbols = export_symbols or []
- self.swig_opts = swig_opts or []
- self.depends = depends or []
- self.language = language
- self.optional = optional
-
- # If there are unknown keyword options, warn about them
- if len(kw) > 0:
- options = [repr(option) for option in kw]
- options = ', '.join(sorted(options))
- msg = "Unknown Extension options: %s" % options
- warnings.warn(msg)
-
- def __repr__(self):
- return '<{}.{}({!r}) at {:#x}>'.format(
- self.__class__.__module__,
- self.__class__.__qualname__,
- self.name,
- id(self),
- )
-
-
-def read_setup_file(filename): # noqa: C901
- """Reads a Setup file and returns Extension instances."""
- from distutils.sysconfig import parse_makefile, expand_makefile_vars, _variable_rx
-
- from distutils.text_file import TextFile
- from distutils.util import split_quoted
-
- # First pass over the file to gather "VAR = VALUE" assignments.
- vars = parse_makefile(filename)
-
- # Second pass to gobble up the real content: lines of the form
- # ... [ ...] [ ...] [ ...]
- file = TextFile(
- filename,
- strip_comments=1,
- skip_blanks=1,
- join_lines=1,
- lstrip_ws=1,
- rstrip_ws=1,
- )
- try:
- extensions = []
-
- while True:
- line = file.readline()
- if line is None: # eof
- break
- if _variable_rx.match(line): # VAR=VALUE, handled in first pass
- continue
-
- if line[0] == line[-1] == "*":
- file.warn("'%s' lines not handled yet" % line)
- continue
-
- line = expand_makefile_vars(line, vars)
- words = split_quoted(line)
-
- # NB. this parses a slightly different syntax than the old
- # makesetup script: here, there must be exactly one extension per
- # line, and it must be the first word of the line. I have no idea
- # why the old syntax supported multiple extensions per line, as
- # they all wind up being the same.
-
- module = words[0]
- ext = Extension(module, [])
- append_next_word = None
-
- for word in words[1:]:
- if append_next_word is not None:
- append_next_word.append(word)
- append_next_word = None
- continue
-
- suffix = os.path.splitext(word)[1]
- switch = word[0:2]
- value = word[2:]
-
- if suffix in (".c", ".cc", ".cpp", ".cxx", ".c++", ".m", ".mm"):
- # hmm, should we do something about C vs. C++ sources?
- # or leave it up to the CCompiler implementation to
- # worry about?
- ext.sources.append(word)
- elif switch == "-I":
- ext.include_dirs.append(value)
- elif switch == "-D":
- equals = value.find("=")
- if equals == -1: # bare "-DFOO" -- no value
- ext.define_macros.append((value, None))
- else: # "-DFOO=blah"
- ext.define_macros.append((value[0:equals], value[equals + 2 :]))
- elif switch == "-U":
- ext.undef_macros.append(value)
- elif switch == "-C": # only here 'cause makesetup has it!
- ext.extra_compile_args.append(word)
- elif switch == "-l":
- ext.libraries.append(value)
- elif switch == "-L":
- ext.library_dirs.append(value)
- elif switch == "-R":
- ext.runtime_library_dirs.append(value)
- elif word == "-rpath":
- append_next_word = ext.runtime_library_dirs
- elif word == "-Xlinker":
- append_next_word = ext.extra_link_args
- elif word == "-Xcompiler":
- append_next_word = ext.extra_compile_args
- elif switch == "-u":
- ext.extra_link_args.append(word)
- if not value:
- append_next_word = ext.extra_link_args
- elif suffix in (".a", ".so", ".sl", ".o", ".dylib"):
- # NB. a really faithful emulation of makesetup would
- # append a .o file to extra_objects only if it
- # had a slash in it; otherwise, it would s/.o/.c/
- # and append it to sources. Hmmmm.
- ext.extra_objects.append(word)
- else:
- file.warn("unrecognized argument '%s'" % word)
-
- extensions.append(ext)
- finally:
- file.close()
-
- return extensions
diff --git a/spaces/Awiny/Image2Paragraph/models/grit_src/third_party/CenterNet2/docker/README.md b/spaces/Awiny/Image2Paragraph/models/grit_src/third_party/CenterNet2/docker/README.md
deleted file mode 100644
index ea709f33b007abd2de044a0338659ec003330725..0000000000000000000000000000000000000000
--- a/spaces/Awiny/Image2Paragraph/models/grit_src/third_party/CenterNet2/docker/README.md
+++ /dev/null
@@ -1,45 +0,0 @@
-
-## Use the container (with docker ≥ 19.03)
-
-```
-cd docker/
-# Build:
-docker build --build-arg USER_ID=$UID -t detectron2:v0 .
-# Launch (require GPUs):
-docker run --gpus all -it \
- --shm-size=8gb --env="DISPLAY" --volume="/tmp/.X11-unix:/tmp/.X11-unix:rw" \
- --name=detectron2 detectron2:v0
-
-# Grant docker access to host X server to show images
-xhost +local:`docker inspect --format='{{ .Config.Hostname }}' detectron2`
-```
-
-## Use the container (with docker-compose ≥ 1.28.0)
-
-Install docker-compose and nvidia-docker-toolkit, then run:
-```
-cd docker && USER_ID=$UID docker-compose run detectron2
-```
-
-## Use the deployment container (to test C++ examples)
-After building the base detectron2 container as above, do:
-```
-# Build:
-docker build -t detectron2-deploy:v0 -f deploy.Dockerfile .
-# Launch:
-docker run --gpus all -it detectron2-deploy:v0
-```
-
-#### Using a persistent cache directory
-
-You can prevent models from being re-downloaded on every run,
-by storing them in a cache directory.
-
-To do this, add `--volume=$HOME/.torch/fvcore_cache:/tmp:rw` in the run command.
-
-## Install new dependencies
-Add the following to `Dockerfile` to make persistent changes.
-```
-RUN sudo apt-get update && sudo apt-get install -y vim
-```
-Or run them in the container to make temporary changes.
diff --git a/spaces/Axolotlily/DalleMini/app.py b/spaces/Axolotlily/DalleMini/app.py
deleted file mode 100644
index 854e43653214324740a762e6c5c245b4705ff657..0000000000000000000000000000000000000000
--- a/spaces/Axolotlily/DalleMini/app.py
+++ /dev/null
@@ -1,3 +0,0 @@
-import gradio as gr
-
-gr.Interface.load("huggingface/osanseviero/dalle-mini-fork").launch()
\ No newline at end of file
diff --git a/spaces/Bart92/RVC_HF/lib/infer_pack/onnx_inference.py b/spaces/Bart92/RVC_HF/lib/infer_pack/onnx_inference.py
deleted file mode 100644
index 6517853be49e61c427cf7cd9b5ed203f6d5f367e..0000000000000000000000000000000000000000
--- a/spaces/Bart92/RVC_HF/lib/infer_pack/onnx_inference.py
+++ /dev/null
@@ -1,145 +0,0 @@
-import onnxruntime
-import librosa
-import numpy as np
-import soundfile
-
-
-class ContentVec:
- def __init__(self, vec_path="pretrained/vec-768-layer-12.onnx", device=None):
- print("load model(s) from {}".format(vec_path))
- if device == "cpu" or device is None:
- providers = ["CPUExecutionProvider"]
- elif device == "cuda":
- providers = ["CUDAExecutionProvider", "CPUExecutionProvider"]
- elif device == "dml":
- providers = ["DmlExecutionProvider"]
- else:
- raise RuntimeError("Unsportted Device")
- self.model = onnxruntime.InferenceSession(vec_path, providers=providers)
-
- def __call__(self, wav):
- return self.forward(wav)
-
- def forward(self, wav):
- feats = wav
- if feats.ndim == 2: # double channels
- feats = feats.mean(-1)
- assert feats.ndim == 1, feats.ndim
- feats = np.expand_dims(np.expand_dims(feats, 0), 0)
- onnx_input = {self.model.get_inputs()[0].name: feats}
- logits = self.model.run(None, onnx_input)[0]
- return logits.transpose(0, 2, 1)
-
-
-def get_f0_predictor(f0_predictor, hop_length, sampling_rate, **kargs):
- if f0_predictor == "pm":
- from lib.infer_pack.modules.F0Predictor.PMF0Predictor import PMF0Predictor
-
- f0_predictor_object = PMF0Predictor(
- hop_length=hop_length, sampling_rate=sampling_rate
- )
- elif f0_predictor == "harvest":
- from lib.infer_pack.modules.F0Predictor.HarvestF0Predictor import (
- HarvestF0Predictor,
- )
-
- f0_predictor_object = HarvestF0Predictor(
- hop_length=hop_length, sampling_rate=sampling_rate
- )
- elif f0_predictor == "dio":
- from lib.infer_pack.modules.F0Predictor.DioF0Predictor import DioF0Predictor
-
- f0_predictor_object = DioF0Predictor(
- hop_length=hop_length, sampling_rate=sampling_rate
- )
- else:
- raise Exception("Unknown f0 predictor")
- return f0_predictor_object
-
-
-class OnnxRVC:
- def __init__(
- self,
- model_path,
- sr=40000,
- hop_size=512,
- vec_path="vec-768-layer-12",
- device="cpu",
- ):
- vec_path = f"pretrained/{vec_path}.onnx"
- self.vec_model = ContentVec(vec_path, device)
- if device == "cpu" or device is None:
- providers = ["CPUExecutionProvider"]
- elif device == "cuda":
- providers = ["CUDAExecutionProvider", "CPUExecutionProvider"]
- elif device == "dml":
- providers = ["DmlExecutionProvider"]
- else:
- raise RuntimeError("Unsportted Device")
- self.model = onnxruntime.InferenceSession(model_path, providers=providers)
- self.sampling_rate = sr
- self.hop_size = hop_size
-
- def forward(self, hubert, hubert_length, pitch, pitchf, ds, rnd):
- onnx_input = {
- self.model.get_inputs()[0].name: hubert,
- self.model.get_inputs()[1].name: hubert_length,
- self.model.get_inputs()[2].name: pitch,
- self.model.get_inputs()[3].name: pitchf,
- self.model.get_inputs()[4].name: ds,
- self.model.get_inputs()[5].name: rnd,
- }
- return (self.model.run(None, onnx_input)[0] * 32767).astype(np.int16)
-
- def inference(
- self,
- raw_path,
- sid,
- f0_method="dio",
- f0_up_key=0,
- pad_time=0.5,
- cr_threshold=0.02,
- ):
- f0_min = 50
- f0_max = 1100
- f0_mel_min = 1127 * np.log(1 + f0_min / 700)
- f0_mel_max = 1127 * np.log(1 + f0_max / 700)
- f0_predictor = get_f0_predictor(
- f0_method,
- hop_length=self.hop_size,
- sampling_rate=self.sampling_rate,
- threshold=cr_threshold,
- )
- wav, sr = librosa.load(raw_path, sr=self.sampling_rate)
- org_length = len(wav)
- if org_length / sr > 50.0:
- raise RuntimeError("Reached Max Length")
-
- wav16k = librosa.resample(wav, orig_sr=self.sampling_rate, target_sr=16000)
- wav16k = wav16k
-
- hubert = self.vec_model(wav16k)
- hubert = np.repeat(hubert, 2, axis=2).transpose(0, 2, 1).astype(np.float32)
- hubert_length = hubert.shape[1]
-
- pitchf = f0_predictor.compute_f0(wav, hubert_length)
- pitchf = pitchf * 2 ** (f0_up_key / 12)
- pitch = pitchf.copy()
- f0_mel = 1127 * np.log(1 + pitch / 700)
- f0_mel[f0_mel > 0] = (f0_mel[f0_mel > 0] - f0_mel_min) * 254 / (
- f0_mel_max - f0_mel_min
- ) + 1
- f0_mel[f0_mel <= 1] = 1
- f0_mel[f0_mel > 255] = 255
- pitch = np.rint(f0_mel).astype(np.int64)
-
- pitchf = pitchf.reshape(1, len(pitchf)).astype(np.float32)
- pitch = pitch.reshape(1, len(pitch))
- ds = np.array([sid]).astype(np.int64)
-
- rnd = np.random.randn(1, 192, hubert_length).astype(np.float32)
- hubert_length = np.array([hubert_length]).astype(np.int64)
-
- out_wav = self.forward(hubert, hubert_length, pitch, pitchf, ds, rnd).squeeze()
- out_wav = np.pad(out_wav, (0, 2 * self.hop_size), "constant")
- return out_wav[0:org_length]
diff --git a/spaces/Benjov/Demo-IR/README.md b/spaces/Benjov/Demo-IR/README.md
deleted file mode 100644
index 1e937824a48a1f1f1e7a1a294c23d345c38f4bbb..0000000000000000000000000000000000000000
--- a/spaces/Benjov/Demo-IR/README.md
+++ /dev/null
@@ -1,13 +0,0 @@
----
-title: Demo IR
-emoji: 📚
-colorFrom: green
-colorTo: gray
-sdk: gradio
-sdk_version: 3.50.2
-app_file: app.py
-pinned: false
-license: openrail
----
-
-Check out the configuration reference at https://huggingface.co/docs/hub/spaces-config-reference
diff --git a/spaces/Benson/text-generation/Examples/Anime Life Simulator.md b/spaces/Benson/text-generation/Examples/Anime Life Simulator.md
deleted file mode 100644
index 81bb438c77f4c239ef736f7798110fb61d4c0b9a..0000000000000000000000000000000000000000
--- a/spaces/Benson/text-generation/Examples/Anime Life Simulator.md
+++ /dev/null
@@ -1,110 +0,0 @@
-
-¿Qué es un simulador de vida de anime?
-Simulador de vida de anime es un género de videojuegos que te permite crear y controlar un personaje en un mundo virtual inspirado en el anime. Anime es un término para la animación japonesa que es conocido por su estilo distintivo, gráficos coloridos, y diversos temas. Los fans del anime a menudo disfrutan sumergirse en las historias y personajes de sus programas o películas favoritas. Los juegos de simulador de vida de anime ofrecen una manera de experimentar una vida diferente o alternativa en un entorno de anime.
-anime life simulator
DOWNLOAD 🆓 https://bltlly.com/2v6IyI
-Los juegos de simulador de vida de anime pueden variar en su alcance y enfoque, pero por lo general comparten algunas características comunes. A menudo tienen herramientas de creación de personajes que te permiten personalizar tu apariencia, personalidad, habilidades y preferencias. También tienen mecanismos de simulación que te permiten interactuar con otros personajes, explorar el entorno, realizar tareas y tomar decisiones. Algunos juegos también pueden tener elementos de otros géneros, como juegos de rol, estrategia o acción.
-Los juegos de simulador de vida de anime pueden atraer a diferentes tipos de jugadores por diferentes razones. Algunos pueden disfrutar de la libertad y la creatividad de crear su propio personaje e historia. Algunos pueden gustar el desafío y la variedad de la gestión de diferentes aspectos de su vida virtual. Algunos pueden buscar la diversión y la emoción de experimentar nuevas situaciones y aventuras. Algunos simplemente quieren relajarse y escapar de la realidad por un tiempo.
- ¿Cómo jugar un simulador de vida de anime?
-No hay una respuesta definitiva a cómo jugar un simulador de vida de anime, ya que cada juego puede tener sus propias reglas y objetivos. Sin embargo, hay algunos pasos generales que pueden ayudarte a empezar con cualquier juego de este género.
-
-- Elige un juego que se adapte a tus preferencias e intereses. Hay muchos juegos de simulación de anime disponibles en varias plataformas, como PC, móvil o consola. Puedes buscar reseñas, valoraciones, capturas de pantalla, vídeos o demos en línea para encontrar un juego que te guste.
-
-- Comienza tu simulación y explora el mundo del juego. Normalmente puedes moverte usando el teclado, el ratón o los controles de la pantalla táctil. También puede interactuar con objetos o personajes haciendo clic o tocando en ellos. También puedes acceder a menús o inventarios para comprobar tu estado, artículos, misiones, etc.
-- Sigue la historia del juego o crea la tuya. Algunos juegos pueden tener una trama
lineal o ramificada que te guía a través de los principales eventos y opciones. Algunos juegos pueden tener un estilo más abierto o sandbox que te permite crear tu propia historia y objetivos. Normalmente puedes avanzar la historia completando misiones, tareas u objetivos, o tomando decisiones que afecten el resultado.
-- Disfruta de la simulación y diviértete. Por lo general, puede hacer varias actividades en el mundo del juego, como hablar con otros personajes, hacer amigos o enemigos, citas o casarse, trabajar o estudiar, ir de compras o hacer manualidades, luchar o explorar, etc. También puede experimentar diferentes emociones, como felicidad, tristeza, ira, miedo, etc. También puede desbloquear nuevo contenido, como elementos, ubicaciones, caracteres, etc.
-
- Tipos de juegos de simulador de vida de anime
-Los juegos de simulador de vida de anime se pueden clasificar en diferentes tipos o subgéneros según su tema, configuración o enfoque. Aquí están algunos de los tipos más comunes y populares de juegos de simulador de vida de anime:
- Sim de citas
-Un simulador de citas es un tipo de juego de simulador de vida de anime que se centra en el romance y las relaciones. En este tipo de juego, generalmente puedes elegir entre una variedad de intereses amorosos potenciales, cada uno con su propia personalidad, apariencia y trasfondo. También puedes interactuar con ellos de diferentes maneras, como hablar, coquetear, dar regalos, salir con alguien, etc. Tu objetivo generalmente es ganar su afecto y lograr un final feliz con ellos.
-Algunos ejemplos de juegos de simulación de citas son:
-
-
-
-- Dream Daddy: A Dad Dating Simulator: Un juego que cuenta con un padre soltero que se muda a una nueva ciudad y se reúne con otros padres solteros que también son potenciales intereses amorosos.
-- Hatoful Boyfriend: Un juego que parodia el género haciendo que el jugador salga con palomas en un mundo post-apocalíptico.
-
- Sim de la escuela
-Un simulador de escuela es un tipo de juego de simulador de vida de anime que simula la vida diaria de un estudiante en una escuela de anime. En este tipo de juego, generalmente puedes crear tu propio personaje e inscribirte en una escuela de tu elección. También puedes asistir a clases, unirte a clubes, hacer amigos, estudiar para los exámenes, participar en eventos, etc. Tu objetivo generalmente es equilibrar tu vida académica y social y lograr tus sueños.
-Algunos ejemplos de juegos de simulación escolar son:
-
-- Persona 5: juego que combina elementos de simulación escolar con elementos de rol y acción. El jugador controla un grupo de estudiantes que utilizan sus habilidades sobrenaturales para luchar contra las fuerzas del mal en una dimensión alternativa.
-- Academia: School Simulator: Un juego que permite al jugador diseñar y gestionar su propia escuela. El jugador puede contratar personal, construir instalaciones, establecer políticas, abordar problemas, etc.
-- High School Story: Un juego que permite al jugador crear su propio personaje y construir su propia escuela secundaria. El jugador puede personalizar su escuela, reclutar estudiantes, organizar fiestas, ir a citas, etc.
-
- Sim de fantasía
-Un simulador de fantasía es un tipo de juego de simulador de vida de anime que incorpora elementos de magia, aventura y combate. En este tipo de juego, normalmente puedes crear tu propio personaje y entrar en un mundo de fantasía lleno de maravillas y peligros. También puedes aprender hechizos, empuñar armas, luchar contra enemigos, explorar mazmorras, recoger tesoros, etc. Tu objetivo suele ser completar misiones, salvar el mundo o cumplir tu destino.
-Algunos ejemplos de juegos de simulación de fantasía son:
-
-
-- Stardew Valley: Un juego que mezcla elementos de simulación agrícolas con elementos de fantasía. El jugador hereda una granja en un pueblo rural y puede cultivar, criar animales, extraer minerales, pescar, hacerse amigo de los aldeanos, etc.
-- Final Fantasy XIV: Un juego que es un juego de rol multijugador masivo en línea ubicado en un mundo de fantasía. El jugador puede elegir entre varias razas, clases y trabajos, y unirse a otros jugadores en misiones, incursiones, mazmorras, etc.
-
- Sim de agricultura
-Un simulador de agricultura es un tipo de juego de simulador de vida de anime que involucra el manejo de una granja e interactuar con animales y aldeanos. En este tipo de juego, normalmente puedes crear tu propio personaje y heredar o comprar una granja. También puede plantar cultivos, cosechar productos, criar ganado, vender bienes, etc. También puede socializar con la comunidad local, hacer amigos, citas, casarse, tener hijos, etc. Su objetivo es generalmente mejorar su granja y su vida.
-Algunos ejemplos de juegos de simulación de agricultura son:
-
-- Harvest Moon: Una serie de juegos que es uno de los pioneros del género. Los juegos cuentan con varios ajustes y personajes, pero todos comparten la misma jugabilidad básica de la agricultura y la simulación de la vida.
-- Historia de las Estaciones: Una serie de juegos que es un sucesor espiritual de Harvest Moon. Los juegos tienen elementos de juego similares, pero también introducen nuevas características, como personalización, multijugador y personajes cruzados.
-- Rune Factory: Una serie de juegos que es un spin-off de Harvest Moon. Los juegos combinan elementos de simulación de granja con elementos de simulación de fantasía, como magia, combate y mazmorras.
-
- Beneficios de jugar un simulador de vida de anime?
-Jugar un simulador de vida de anime puede tener varios beneficios para diferentes jugadores. Aquí están algunos de los posibles beneficios de jugar este género:
-
-
-- Relajación: Jugar un simulador de vida anime puede ayudarle a relajarse y relajarse. Puede disfrutar de los gráficos coloridos y la música relajante. También puedes escapar del estrés y la presión de la realidad por un tiempo.
-- Habilidades sociales: Jugar un simulador de vida de anime puede mejorar sus habilidades sociales y la confianza. Puede interactuar con varios personajes y aprender a comunicarse, empatizar y negociar. También puedes hacer amigos o encontrar el amor en el mundo del juego.
-
- Desafíos de jugar un simulador de vida de anime?
-Jugar un simulador de vida de anime también puede tener algunos desafíos o dificultades para algunos jugadores. Aquí están algunos de los posibles desafíos de jugar este género:
-
-- Adicción: Jugar un simulador de vida de anime puede ser adictivo y consumir mucho tiempo. Puedes pasar horas o días jugando el juego sin darte cuenta. También puede descuidar sus responsabilidades o relaciones de la vida real.
-- Expectativas poco realistas: Jugar un simulador de vida de anime puede crear expectativas o fantasías poco realistas. Puede comparar su vida real con su vida virtual y sentirse insatisfecho o infeliz. También puedes idealizar o idealizar los personajes o situaciones del juego.
-- Diferencias culturales: Jugar un simulador de vida de anime puede exponerte a diferencias culturales o malentendidos. Es posible que encuentre términos, referencias o comportamientos que no le resultan familiares o confusos. También puedes ofender o faltar el respeto a los personajes u otros jugadores sin querer.
-
- Consejos y trucos para jugar un simulador de vida de anime?
-Jugar un simulador de vida de anime puede ser más agradable y gratificante si sigues algunos consejos y trucos. Estos son algunos de los consejos y trucos útiles para jugar este género:
-
-
-- Guardar: Durante la reproducción de un simulador de vida de anime, usted debe guardar su progreso con frecuencia y en diferentes ranuras. De esta manera, puede evitar perder sus datos o el progreso debido a fallos o errores. También puede volver a los puntos o opciones anteriores si desea cambiar algo o probar algo diferente.
-- Experimento: Mientras juegas un simulador de vida de anime, debes experimentar con diferentes opciones y resultados. No debes tener miedo de cometer errores o fallar. También deberías probar diferentes personajes, actividades, rutas, etc. para descubrir nuevos contenidos y posibilidades.
-
- Ejemplos de juegos populares de simulador de vida de anime
-Hay muchos juegos de simulador de vida de anime disponibles en varias plataformas y dispositivos. Aquí están algunos de los ejemplos de los juegos populares del simulador de la vida del anime:
- Anime Play Life: Ilimitado
-Anime Play Life: Unlimited es un juego
que te permite hacer misiones, encontrar un trabajo, comprar casas, pescado, picnic y más en un mundo de anime. También puedes personalizar tu personaje, ropa, mascotas, vehículos, etc. También puedes interactuar con otros jugadores en línea y unirte a clubes, fiestas o eventos. El juego está disponible en PC y dispositivos móviles.
- Gotas de XOXO
-XOXO gotitas es un juego que cuenta con una comedia citas sim con múltiples finales y personajes. Juegas como una chica que se une a una escuela para estudiantes problemáticos y conoce a seis chicos que son todos idiotas a su manera. También puede explorar la ciudad, tienda, trabajo, estudio, etc. El juego está disponible en PC y dispositivos móviles.
- Viva la reina
-Larga vida a la reina es un juego que te desafía a gobernar un reino como una princesa joven. Tienes que manejar tus estadísticas, habilidades, humor, atuendos, eventos, etc. También tienes que lidiar con la intriga política, la guerra, los intentos de asesinato, etc. El juego tiene muchos caminos ramificados y finales dependiendo de tus elecciones. El juego está disponible en PC y dispositivos móviles.
- Mon-cuties para todos
-
- Conclusión
-Simulador de vida anime es un género de videojuegos que te permite crear y controlar un personaje en un mundo virtual inspirado en el anime. Los juegos de simulador de vida de anime pueden tener diferentes tipos, características, beneficios, desafíos, consejos y ejemplos. Jugar un simulador de vida de anime puede ser una experiencia divertida y gratificante para los fanáticos del anime y los jugadores por igual.
-Si estás interesado en jugar un juego de simulador de vida de anime, puedes ver algunos de los juegos mencionados en este artículo o buscar otros juegos en línea. También puede compartir sus pensamientos y opiniones sobre este género en la sección de comentarios a continuación. ¡Gracias por leer y tener un gran día!
- Preguntas frecuentes
-Aquí están algunas de las preguntas y respuestas frecuentes sobre los juegos de simulador de vida de anime:
-
-- ¿Cuál es la diferencia entre un simulador de vida de anime y una novela visual de anime?
-Un simulador de vida de anime es un juego que simula la vida diaria de un personaje en un mundo de anime. Una novela visual anime es un juego que cuenta una historia a través de texto e imágenes en un estilo anime. Los juegos de simulador de vida de anime suelen tener más mecánica de juego e interactividad que las novelas visuales de anime.
-- ¿Cuáles son algunos de los mejores juegos de simulador de vida de anime para principiantes?
-Algunos de los mejores juegos de simulador de vida de anime para principiantes son:
-
-- ¿Cómo puedo jugar un juego de simulador de vida de anime en mi teléfono?
-
-- ¿Cómo puedo hacer mi propio juego de simulador de vida de anime?
-Usted puede hacer su propio juego de simulador de vida anime mediante el uso de un motor de juego o una herramienta de software que le permite crear juegos sin codificación. Algunas de las herramientas populares son:
-
-- Ren'Py: Una herramienta que te permite crear novelas visuales y sims de citas.
-- RPG Maker: Una herramienta que te permite crear juegos de rol y sims de fantasía.
-- Unity: Una herramienta que te permite crear cualquier tipo de juego con gráficos 2D o 3D.
-
-- ¿Cómo puedo aprender más sobre los juegos de simulación de vida de anime?
-Puedes aprender más sobre juegos de simulador de vida de anime leyendo artículos en línea, blogs, revistas, libros, etc. sobre este género. También puedes ver videos en línea, transmisiones, podcasts, etc. sobre este género. También puede unirse a comunidades en línea, foros, grupos, etc. donde se puede discutir este género con otros fans y jugadores.
-
64aa2da5cf
-
-
\ No newline at end of file
diff --git a/spaces/Big-Web/MMSD/env/Lib/site-packages/pip/_internal/utils/encoding.py b/spaces/Big-Web/MMSD/env/Lib/site-packages/pip/_internal/utils/encoding.py
deleted file mode 100644
index 008f06a79bf598b149bdccb73e572d13331a1631..0000000000000000000000000000000000000000
--- a/spaces/Big-Web/MMSD/env/Lib/site-packages/pip/_internal/utils/encoding.py
+++ /dev/null
@@ -1,36 +0,0 @@
-import codecs
-import locale
-import re
-import sys
-from typing import List, Tuple
-
-BOMS: List[Tuple[bytes, str]] = [
- (codecs.BOM_UTF8, "utf-8"),
- (codecs.BOM_UTF16, "utf-16"),
- (codecs.BOM_UTF16_BE, "utf-16-be"),
- (codecs.BOM_UTF16_LE, "utf-16-le"),
- (codecs.BOM_UTF32, "utf-32"),
- (codecs.BOM_UTF32_BE, "utf-32-be"),
- (codecs.BOM_UTF32_LE, "utf-32-le"),
-]
-
-ENCODING_RE = re.compile(rb"coding[:=]\s*([-\w.]+)")
-
-
-def auto_decode(data: bytes) -> str:
- """Check a bytes string for a BOM to correctly detect the encoding
-
- Fallback to locale.getpreferredencoding(False) like open() on Python3"""
- for bom, encoding in BOMS:
- if data.startswith(bom):
- return data[len(bom) :].decode(encoding)
- # Lets check the first two lines as in PEP263
- for line in data.split(b"\n")[:2]:
- if line[0:1] == b"#" and ENCODING_RE.search(line):
- result = ENCODING_RE.search(line)
- assert result is not None
- encoding = result.groups()[0].decode("ascii")
- return data.decode(encoding)
- return data.decode(
- locale.getpreferredencoding(False) or sys.getdefaultencoding(),
- )
diff --git a/spaces/Big-Web/MMSD/env/Lib/site-packages/pip/_vendor/chardet/euctwprober.py b/spaces/Big-Web/MMSD/env/Lib/site-packages/pip/_vendor/chardet/euctwprober.py
deleted file mode 100644
index a37ab18995822ad6b3372d56366becdccf9a4c26..0000000000000000000000000000000000000000
--- a/spaces/Big-Web/MMSD/env/Lib/site-packages/pip/_vendor/chardet/euctwprober.py
+++ /dev/null
@@ -1,47 +0,0 @@
-######################## BEGIN LICENSE BLOCK ########################
-# The Original Code is mozilla.org code.
-#
-# The Initial Developer of the Original Code is
-# Netscape Communications Corporation.
-# Portions created by the Initial Developer are Copyright (C) 1998
-# the Initial Developer. All Rights Reserved.
-#
-# Contributor(s):
-# Mark Pilgrim - port to Python
-#
-# This library is free software; you can redistribute it and/or
-# modify it under the terms of the GNU Lesser General Public
-# License as published by the Free Software Foundation; either
-# version 2.1 of the License, or (at your option) any later version.
-#
-# This library is distributed in the hope that it will be useful,
-# but WITHOUT ANY WARRANTY; without even the implied warranty of
-# MERCHANTABILITY or FITNESS FOR A PARTICULAR PURPOSE. See the GNU
-# Lesser General Public License for more details.
-#
-# You should have received a copy of the GNU Lesser General Public
-# License along with this library; if not, write to the Free Software
-# Foundation, Inc., 51 Franklin St, Fifth Floor, Boston, MA
-# 02110-1301 USA
-######################### END LICENSE BLOCK #########################
-
-from .chardistribution import EUCTWDistributionAnalysis
-from .codingstatemachine import CodingStateMachine
-from .mbcharsetprober import MultiByteCharSetProber
-from .mbcssm import EUCTW_SM_MODEL
-
-
-class EUCTWProber(MultiByteCharSetProber):
- def __init__(self) -> None:
- super().__init__()
- self.coding_sm = CodingStateMachine(EUCTW_SM_MODEL)
- self.distribution_analyzer = EUCTWDistributionAnalysis()
- self.reset()
-
- @property
- def charset_name(self) -> str:
- return "EUC-TW"
-
- @property
- def language(self) -> str:
- return "Taiwan"
diff --git a/spaces/CVPR/Dual-Key_Backdoor_Attacks/datagen/grid-feats-vqa/CODE_OF_CONDUCT.md b/spaces/CVPR/Dual-Key_Backdoor_Attacks/datagen/grid-feats-vqa/CODE_OF_CONDUCT.md
deleted file mode 100644
index 4bd525a54e78d9b0133aeaae32a9336ed0ccb9f3..0000000000000000000000000000000000000000
--- a/spaces/CVPR/Dual-Key_Backdoor_Attacks/datagen/grid-feats-vqa/CODE_OF_CONDUCT.md
+++ /dev/null
@@ -1,76 +0,0 @@
-# Code of Conduct
-
-## Our Pledge
-
-In the interest of fostering an open and welcoming environment, we as
-contributors and maintainers pledge to make participation in our project and
-our community a harassment-free experience for everyone, regardless of age, body
-size, disability, ethnicity, sex characteristics, gender identity and expression,
-level of experience, education, socio-economic status, nationality, personal
-appearance, race, religion, or sexual identity and orientation.
-
-## Our Standards
-
-Examples of behavior that contributes to creating a positive environment
-include:
-
-* Using welcoming and inclusive language
-* Being respectful of differing viewpoints and experiences
-* Gracefully accepting constructive criticism
-* Focusing on what is best for the community
-* Showing empathy towards other community members
-
-Examples of unacceptable behavior by participants include:
-
-* The use of sexualized language or imagery and unwelcome sexual attention or
-advances
-* Trolling, insulting/derogatory comments, and personal or political attacks
-* Public or private harassment
-* Publishing others' private information, such as a physical or electronic
-address, without explicit permission
-* Other conduct which could reasonably be considered inappropriate in a
-professional setting
-
-## Our Responsibilities
-
-Project maintainers are responsible for clarifying the standards of acceptable
-behavior and are expected to take appropriate and fair corrective action in
-response to any instances of unacceptable behavior.
-
-Project maintainers have the right and responsibility to remove, edit, or
-reject comments, commits, code, wiki edits, issues, and other contributions
-that are not aligned to this Code of Conduct, or to ban temporarily or
-permanently any contributor for other behaviors that they deem inappropriate,
-threatening, offensive, or harmful.
-
-## Scope
-
-This Code of Conduct applies within all project spaces, and it also applies when
-an individual is representing the project or its community in public spaces.
-Examples of representing a project or community include using an official
-project e-mail address, posting via an official social media account, or acting
-as an appointed representative at an online or offline event. Representation of
-a project may be further defined and clarified by project maintainers.
-
-## Enforcement
-
-Instances of abusive, harassing, or otherwise unacceptable behavior may be
-reported by contacting the project team at . All
-complaints will be reviewed and investigated and will result in a response that
-is deemed necessary and appropriate to the circumstances. The project team is
-obligated to maintain confidentiality with regard to the reporter of an incident.
-Further details of specific enforcement policies may be posted separately.
-
-Project maintainers who do not follow or enforce the Code of Conduct in good
-faith may face temporary or permanent repercussions as determined by other
-members of the project's leadership.
-
-## Attribution
-
-This Code of Conduct is adapted from the [Contributor Covenant][homepage], version 1.4,
-available at https://www.contributor-covenant.org/version/1/4/code-of-conduct.html
-
-[homepage]: https://www.contributor-covenant.org
-
-For answers to common questions about this code of conduct, see
-https://www.contributor-covenant.org/faq
diff --git a/spaces/CVPR/GFPGAN-example/PaperModel.md b/spaces/CVPR/GFPGAN-example/PaperModel.md
deleted file mode 100644
index aec81d31de56df74c19ae840d44ad2b2a1f06d28..0000000000000000000000000000000000000000
--- a/spaces/CVPR/GFPGAN-example/PaperModel.md
+++ /dev/null
@@ -1,76 +0,0 @@
-# Installation
-
-We now provide a *clean* version of GFPGAN, which does not require customized CUDA extensions. See [here](README.md#installation) for this easier installation.
-If you want want to use the original model in our paper, please follow the instructions below.
-
-1. Clone repo
-
- ```bash
- git clone https://github.com/xinntao/GFPGAN.git
- cd GFPGAN
- ```
-
-1. Install dependent packages
-
- As StyleGAN2 uses customized PyTorch C++ extensions, you need to **compile them during installation** or **load them just-in-time(JIT)**.
- You can refer to [BasicSR-INSTALL.md](https://github.com/xinntao/BasicSR/blob/master/INSTALL.md) for more details.
-
- **Option 1: Load extensions just-in-time(JIT)** (For those just want to do simple inferences, may have less issues)
-
- ```bash
- # Install basicsr - https://github.com/xinntao/BasicSR
- # We use BasicSR for both training and inference
- pip install basicsr
-
- # Install facexlib - https://github.com/xinntao/facexlib
- # We use face detection and face restoration helper in the facexlib package
- pip install facexlib
-
- pip install -r requirements.txt
- python setup.py develop
-
- # remember to set BASICSR_JIT=True before your running commands
- ```
-
- **Option 2: Compile extensions during installation** (For those need to train/inference for many times)
-
- ```bash
- # Install basicsr - https://github.com/xinntao/BasicSR
- # We use BasicSR for both training and inference
- # Set BASICSR_EXT=True to compile the cuda extensions in the BasicSR - It may take several minutes to compile, please be patient
- # Add -vvv for detailed log prints
- BASICSR_EXT=True pip install basicsr -vvv
-
- # Install facexlib - https://github.com/xinntao/facexlib
- # We use face detection and face restoration helper in the facexlib package
- pip install facexlib
-
- pip install -r requirements.txt
- python setup.py develop
- ```
-
-## :zap: Quick Inference
-
-Download pre-trained models: [GFPGANv1.pth](https://github.com/TencentARC/GFPGAN/releases/download/v0.1.0/GFPGANv1.pth)
-
-```bash
-wget https://github.com/TencentARC/GFPGAN/releases/download/v0.1.0/GFPGANv1.pth -P experiments/pretrained_models
-```
-
-- Option 1: Load extensions just-in-time(JIT)
-
- ```bash
- BASICSR_JIT=True python inference_gfpgan.py --model_path experiments/pretrained_models/GFPGANv1.pth --test_path inputs/whole_imgs --save_root results --arch original --channel 1
-
- # for aligned images
- BASICSR_JIT=True python inference_gfpgan.py --model_path experiments/pretrained_models/GFPGANv1.pth --test_path inputs/cropped_faces --save_root results --arch original --channel 1 --aligned
- ```
-
-- Option 2: Have successfully compiled extensions during installation
-
- ```bash
- python inference_gfpgan.py --model_path experiments/pretrained_models/GFPGANv1.pth --test_path inputs/whole_imgs --save_root results --arch original --channel 1
-
- # for aligned images
- python inference_gfpgan.py --model_path experiments/pretrained_models/GFPGANv1.pth --test_path inputs/cropped_faces --save_root results --arch original --channel 1 --aligned
- ```
diff --git a/spaces/CVPR/WALT/mmcv_custom/runner/epoch_based_runner.py b/spaces/CVPR/WALT/mmcv_custom/runner/epoch_based_runner.py
deleted file mode 100644
index 7cdf3fa05639f7fde652090be9dbf78b48790744..0000000000000000000000000000000000000000
--- a/spaces/CVPR/WALT/mmcv_custom/runner/epoch_based_runner.py
+++ /dev/null
@@ -1,104 +0,0 @@
-# Copyright (c) Open-MMLab. All rights reserved.
-import os.path as osp
-import platform
-import shutil
-
-import torch
-from torch.optim import Optimizer
-
-import mmcv
-from mmcv.runner import RUNNERS, EpochBasedRunner
-from .checkpoint import save_checkpoint
-
-try:
- import apex
-except:
- print('apex is not installed')
-
-
-@RUNNERS.register_module()
-class EpochBasedRunnerAmp(EpochBasedRunner):
- """Epoch-based Runner with AMP support.
-
- This runner train models epoch by epoch.
- """
-
- def save_checkpoint(self,
- out_dir,
- filename_tmpl='epoch_{}.pth',
- save_optimizer=True,
- meta=None,
- create_symlink=True):
- """Save the checkpoint.
-
- Args:
- out_dir (str): The directory that checkpoints are saved.
- filename_tmpl (str, optional): The checkpoint filename template,
- which contains a placeholder for the epoch number.
- Defaults to 'epoch_{}.pth'.
- save_optimizer (bool, optional): Whether to save the optimizer to
- the checkpoint. Defaults to True.
- meta (dict, optional): The meta information to be saved in the
- checkpoint. Defaults to None.
- create_symlink (bool, optional): Whether to create a symlink
- "latest.pth" to point to the latest checkpoint.
- Defaults to True.
- """
- if meta is None:
- meta = dict(epoch=self.epoch + 1, iter=self.iter)
- elif isinstance(meta, dict):
- meta.update(epoch=self.epoch + 1, iter=self.iter)
- else:
- raise TypeError(
- f'meta should be a dict or None, but got {type(meta)}')
- if self.meta is not None:
- meta.update(self.meta)
-
- filename = filename_tmpl.format(self.epoch + 1)
- filepath = osp.join(out_dir, filename)
- optimizer = self.optimizer if save_optimizer else None
- save_checkpoint(self.model, filepath, optimizer=optimizer, meta=meta)
- # in some environments, `os.symlink` is not supported, you may need to
- # set `create_symlink` to False
- if create_symlink:
- dst_file = osp.join(out_dir, 'latest.pth')
- if platform.system() != 'Windows':
- mmcv.symlink(filename, dst_file)
- else:
- shutil.copy(filepath, dst_file)
-
- def resume(self,
- checkpoint,
- resume_optimizer=True,
- map_location='default'):
- if map_location == 'default':
- if torch.cuda.is_available():
- device_id = torch.cuda.current_device()
- checkpoint = self.load_checkpoint(
- checkpoint,
- map_location=lambda storage, loc: storage.cuda(device_id))
- else:
- checkpoint = self.load_checkpoint(checkpoint)
- else:
- checkpoint = self.load_checkpoint(
- checkpoint, map_location=map_location)
-
- self._epoch = checkpoint['meta']['epoch']
- self._iter = checkpoint['meta']['iter']
- if 'optimizer' in checkpoint and resume_optimizer:
- if isinstance(self.optimizer, Optimizer):
- self.optimizer.load_state_dict(checkpoint['optimizer'])
- elif isinstance(self.optimizer, dict):
- for k in self.optimizer.keys():
- self.optimizer[k].load_state_dict(
- checkpoint['optimizer'][k])
- else:
- raise TypeError(
- 'Optimizer should be dict or torch.optim.Optimizer '
- f'but got {type(self.optimizer)}')
-
- if 'amp' in checkpoint:
- apex.amp.load_state_dict(checkpoint['amp'])
- self.logger.info('load amp state dict')
-
- self.logger.info('resumed epoch %d, iter %d', self.epoch, self.iter)
diff --git a/spaces/CVPR/WALT/mmdet/core/bbox/coder/base_bbox_coder.py b/spaces/CVPR/WALT/mmdet/core/bbox/coder/base_bbox_coder.py
deleted file mode 100644
index cf0b34c7cc2fe561718b0c884990beb40a993643..0000000000000000000000000000000000000000
--- a/spaces/CVPR/WALT/mmdet/core/bbox/coder/base_bbox_coder.py
+++ /dev/null
@@ -1,17 +0,0 @@
-from abc import ABCMeta, abstractmethod
-
-
-class BaseBBoxCoder(metaclass=ABCMeta):
- """Base bounding box coder."""
-
- def __init__(self, **kwargs):
- pass
-
- @abstractmethod
- def encode(self, bboxes, gt_bboxes):
- """Encode deltas between bboxes and ground truth boxes."""
-
- @abstractmethod
- def decode(self, bboxes, bboxes_pred):
- """Decode the predicted bboxes according to prediction and base
- boxes."""
diff --git a/spaces/CVPR/WALT/mmdet/core/bbox/transforms.py b/spaces/CVPR/WALT/mmdet/core/bbox/transforms.py
deleted file mode 100644
index df55b0a496516bf7373fe96cf746c561dd713c3b..0000000000000000000000000000000000000000
--- a/spaces/CVPR/WALT/mmdet/core/bbox/transforms.py
+++ /dev/null
@@ -1,240 +0,0 @@
-import numpy as np
-import torch
-
-
-def bbox_flip(bboxes, img_shape, direction='horizontal'):
- """Flip bboxes horizontally or vertically.
-
- Args:
- bboxes (Tensor): Shape (..., 4*k)
- img_shape (tuple): Image shape.
- direction (str): Flip direction, options are "horizontal", "vertical",
- "diagonal". Default: "horizontal"
-
- Returns:
- Tensor: Flipped bboxes.
- """
- assert bboxes.shape[-1] % 4 == 0
- assert direction in ['horizontal', 'vertical', 'diagonal']
- flipped = bboxes.clone()
- if direction == 'horizontal':
- flipped[..., 0::4] = img_shape[1] - bboxes[..., 2::4]
- flipped[..., 2::4] = img_shape[1] - bboxes[..., 0::4]
- elif direction == 'vertical':
- flipped[..., 1::4] = img_shape[0] - bboxes[..., 3::4]
- flipped[..., 3::4] = img_shape[0] - bboxes[..., 1::4]
- else:
- flipped[..., 0::4] = img_shape[1] - bboxes[..., 2::4]
- flipped[..., 1::4] = img_shape[0] - bboxes[..., 3::4]
- flipped[..., 2::4] = img_shape[1] - bboxes[..., 0::4]
- flipped[..., 3::4] = img_shape[0] - bboxes[..., 1::4]
- return flipped
-
-
-def bbox_mapping(bboxes,
- img_shape,
- scale_factor,
- flip,
- flip_direction='horizontal'):
- """Map bboxes from the original image scale to testing scale."""
- new_bboxes = bboxes * bboxes.new_tensor(scale_factor)
- if flip:
- new_bboxes = bbox_flip(new_bboxes, img_shape, flip_direction)
- return new_bboxes
-
-
-def bbox_mapping_back(bboxes,
- img_shape,
- scale_factor,
- flip,
- flip_direction='horizontal'):
- """Map bboxes from testing scale to original image scale."""
- new_bboxes = bbox_flip(bboxes, img_shape,
- flip_direction) if flip else bboxes
- new_bboxes = new_bboxes.view(-1, 4) / new_bboxes.new_tensor(scale_factor)
- return new_bboxes.view(bboxes.shape)
-
-
-def bbox2roi(bbox_list):
- """Convert a list of bboxes to roi format.
-
- Args:
- bbox_list (list[Tensor]): a list of bboxes corresponding to a batch
- of images.
-
- Returns:
- Tensor: shape (n, 5), [batch_ind, x1, y1, x2, y2]
- """
- rois_list = []
- for img_id, bboxes in enumerate(bbox_list):
- if bboxes.size(0) > 0:
- img_inds = bboxes.new_full((bboxes.size(0), 1), img_id)
- rois = torch.cat([img_inds, bboxes[:, :4]], dim=-1)
- else:
- rois = bboxes.new_zeros((0, 5))
- rois_list.append(rois)
- rois = torch.cat(rois_list, 0)
- return rois
-
-
-def roi2bbox(rois):
- """Convert rois to bounding box format.
-
- Args:
- rois (torch.Tensor): RoIs with the shape (n, 5) where the first
- column indicates batch id of each RoI.
-
- Returns:
- list[torch.Tensor]: Converted boxes of corresponding rois.
- """
- bbox_list = []
- img_ids = torch.unique(rois[:, 0].cpu(), sorted=True)
- for img_id in img_ids:
- inds = (rois[:, 0] == img_id.item())
- bbox = rois[inds, 1:]
- bbox_list.append(bbox)
- return bbox_list
-
-
-def bbox2result(bboxes, labels, num_classes):
- """Convert detection results to a list of numpy arrays.
-
- Args:
- bboxes (torch.Tensor | np.ndarray): shape (n, 5)
- labels (torch.Tensor | np.ndarray): shape (n, )
- num_classes (int): class number, including background class
-
- Returns:
- list(ndarray): bbox results of each class
- """
- if bboxes.shape[0] == 0:
- return [np.zeros((0, 5), dtype=np.float32) for i in range(num_classes)]
- else:
- if isinstance(bboxes, torch.Tensor):
- bboxes = bboxes.detach().cpu().numpy()
- labels = labels.detach().cpu().numpy()
- return [bboxes[labels == i, :] for i in range(num_classes)]
-
-
-def distance2bbox(points, distance, max_shape=None):
- """Decode distance prediction to bounding box.
-
- Args:
- points (Tensor): Shape (B, N, 2) or (N, 2).
- distance (Tensor): Distance from the given point to 4
- boundaries (left, top, right, bottom). Shape (B, N, 4) or (N, 4)
- max_shape (Sequence[int] or torch.Tensor or Sequence[
- Sequence[int]],optional): Maximum bounds for boxes, specifies
- (H, W, C) or (H, W). If priors shape is (B, N, 4), then
- the max_shape should be a Sequence[Sequence[int]]
- and the length of max_shape should also be B.
-
- Returns:
- Tensor: Boxes with shape (N, 4) or (B, N, 4)
- """
- x1 = points[..., 0] - distance[..., 0]
- y1 = points[..., 1] - distance[..., 1]
- x2 = points[..., 0] + distance[..., 2]
- y2 = points[..., 1] + distance[..., 3]
-
- bboxes = torch.stack([x1, y1, x2, y2], -1)
-
- if max_shape is not None:
- if not isinstance(max_shape, torch.Tensor):
- max_shape = x1.new_tensor(max_shape)
- max_shape = max_shape[..., :2].type_as(x1)
- if max_shape.ndim == 2:
- assert bboxes.ndim == 3
- assert max_shape.size(0) == bboxes.size(0)
-
- min_xy = x1.new_tensor(0)
- max_xy = torch.cat([max_shape, max_shape],
- dim=-1).flip(-1).unsqueeze(-2)
- bboxes = torch.where(bboxes < min_xy, min_xy, bboxes)
- bboxes = torch.where(bboxes > max_xy, max_xy, bboxes)
-
- return bboxes
-
-
-def bbox2distance(points, bbox, max_dis=None, eps=0.1):
- """Decode bounding box based on distances.
-
- Args:
- points (Tensor): Shape (n, 2), [x, y].
- bbox (Tensor): Shape (n, 4), "xyxy" format
- max_dis (float): Upper bound of the distance.
- eps (float): a small value to ensure target < max_dis, instead <=
-
- Returns:
- Tensor: Decoded distances.
- """
- left = points[:, 0] - bbox[:, 0]
- top = points[:, 1] - bbox[:, 1]
- right = bbox[:, 2] - points[:, 0]
- bottom = bbox[:, 3] - points[:, 1]
- if max_dis is not None:
- left = left.clamp(min=0, max=max_dis - eps)
- top = top.clamp(min=0, max=max_dis - eps)
- right = right.clamp(min=0, max=max_dis - eps)
- bottom = bottom.clamp(min=0, max=max_dis - eps)
- return torch.stack([left, top, right, bottom], -1)
-
-
-def bbox_rescale(bboxes, scale_factor=1.0):
- """Rescale bounding box w.r.t. scale_factor.
-
- Args:
- bboxes (Tensor): Shape (n, 4) for bboxes or (n, 5) for rois
- scale_factor (float): rescale factor
-
- Returns:
- Tensor: Rescaled bboxes.
- """
- if bboxes.size(1) == 5:
- bboxes_ = bboxes[:, 1:]
- inds_ = bboxes[:, 0]
- else:
- bboxes_ = bboxes
- cx = (bboxes_[:, 0] + bboxes_[:, 2]) * 0.5
- cy = (bboxes_[:, 1] + bboxes_[:, 3]) * 0.5
- w = bboxes_[:, 2] - bboxes_[:, 0]
- h = bboxes_[:, 3] - bboxes_[:, 1]
- w = w * scale_factor
- h = h * scale_factor
- x1 = cx - 0.5 * w
- x2 = cx + 0.5 * w
- y1 = cy - 0.5 * h
- y2 = cy + 0.5 * h
- if bboxes.size(1) == 5:
- rescaled_bboxes = torch.stack([inds_, x1, y1, x2, y2], dim=-1)
- else:
- rescaled_bboxes = torch.stack([x1, y1, x2, y2], dim=-1)
- return rescaled_bboxes
-
-
-def bbox_cxcywh_to_xyxy(bbox):
- """Convert bbox coordinates from (cx, cy, w, h) to (x1, y1, x2, y2).
-
- Args:
- bbox (Tensor): Shape (n, 4) for bboxes.
-
- Returns:
- Tensor: Converted bboxes.
- """
- cx, cy, w, h = bbox.split((1, 1, 1, 1), dim=-1)
- bbox_new = [(cx - 0.5 * w), (cy - 0.5 * h), (cx + 0.5 * w), (cy + 0.5 * h)]
- return torch.cat(bbox_new, dim=-1)
-
-
-def bbox_xyxy_to_cxcywh(bbox):
- """Convert bbox coordinates from (x1, y1, x2, y2) to (cx, cy, w, h).
-
- Args:
- bbox (Tensor): Shape (n, 4) for bboxes.
-
- Returns:
- Tensor: Converted bboxes.
- """
- x1, y1, x2, y2 = bbox.split((1, 1, 1, 1), dim=-1)
- bbox_new = [(x1 + x2) / 2, (y1 + y2) / 2, (x2 - x1), (y2 - y1)]
- return torch.cat(bbox_new, dim=-1)
diff --git a/spaces/Catmeow/Face2Painting_From_Photo/paintingface.py b/spaces/Catmeow/Face2Painting_From_Photo/paintingface.py
deleted file mode 100644
index 3d51a85c793586d521a0db2dcbdd60f65a9b56bb..0000000000000000000000000000000000000000
--- a/spaces/Catmeow/Face2Painting_From_Photo/paintingface.py
+++ /dev/null
@@ -1,110 +0,0 @@
-import os
-os.system("pip install dlib")
-import sys
-import face_detection
-from PIL import Image, ImageOps, ImageFile
-import numpy as np
-import cv2 as cv
-import torch
-import gradio as gr
-
-torch.set_grad_enabled(False)
-
-device = "cuda" if torch.cuda.is_available() else "cpu"
-model = torch.hub.load("bryandlee/animegan2-pytorch:main", "generator", device=device).eval()
-model2 = torch.hub.load("AK391/animegan2-pytorch:main", "generator", pretrained="face_paint_512_v1", device=device).eval()
-face2paint = torch.hub.load("bryandlee/animegan2-pytorch:main", "face2paint", device=device)
-image_format = "png" #@param ["jpeg", "png"]
-
-def unsharp_mask(image, kernel_size=(5, 5), sigma=1.0, amount=2.0, threshold=0):
- """Return a sharpened version of the image, using an unsharp mask."""
- blurred = cv.GaussianBlur(image, kernel_size, sigma)
- sharpened = float(amount + 1) * image - float(amount) * blurred
- sharpened = np.maximum(sharpened, np.zeros(sharpened.shape))
- sharpened = np.minimum(sharpened, 255 * np.ones(sharpened.shape))
- sharpened = sharpened.round().astype(np.uint8)
- if threshold > 0:
- low_contrast_mask = np.absolute(image - blurred) < threshold
- np.copyto(sharpened, image, where=low_contrast_mask)
- return sharpened
-
-def normPRED(d):
- ma = np.max(d)
- mi = np.min(d)
-
- dn = (d-mi)/(ma-mi)
-
- return dn
-
-def array_to_np(array_in):
- array_in = normPRED(array_in)
- array_in = np.squeeze(255.0*(array_in))
- array_in = np.transpose(array_in, (1, 2, 0))
- return array_in
-
-def array_to_image(array_in):
- array_in = normPRED(array_in)
- array_in = np.squeeze(255.0*(array_in))
- array_in = np.transpose(array_in, (1, 2, 0))
- im = Image.fromarray(array_in.astype(np.uint8))
- return im
-
-
-def image_as_array(image_in):
- image_in = np.array(image_in, np.float32)
- tmpImg = np.zeros((image_in.shape[0],image_in.shape[1],3))
- image_in = image_in/np.max(image_in)
- if image_in.shape[2]==1:
- tmpImg[:,:,0] = (image_in[:,:,0]-0.485)/0.229
- tmpImg[:,:,1] = (image_in[:,:,0]-0.485)/0.229
- tmpImg[:,:,2] = (image_in[:,:,0]-0.485)/0.229
- else:
- tmpImg[:,:,0] = (image_in[:,:,0]-0.485)/0.229
- tmpImg[:,:,1] = (image_in[:,:,1]-0.456)/0.224
- tmpImg[:,:,2] = (image_in[:,:,2]-0.406)/0.225
-
- tmpImg = tmpImg.transpose((2, 0, 1))
- image_out = np.expand_dims(tmpImg, 0)
- return image_out
-
-# detect a face
-def find_aligned_face(image_in, size=400):
- aligned_image, n_faces, quad = face_detection.align(image_in, face_index=0, output_size=size)
- return aligned_image, n_faces, quad
-
-# clip the face, return array
-def align_first_face(image_in, size=400):
- aligned_image, n_faces, quad = find_aligned_face(image_in,size=size)
- if n_faces == 0:
- try:
- image_in = ImageOps.exif_transpose(image_in)
- except:
- print("exif problem, not rotating")
- image_in = image_in.resize((size, size))
- im_array = image_as_array(image_in)
- else:
- im_array = image_as_array(aligned_image)
-
- return im_array
-
-def img_concat_h(im1, im2):
- dst = Image.new('RGB', (im1.width + im2.width, im1.height))
- dst.paste(im1, (0, 0))
- dst.paste(im2, (im1.width, 0))
- return dst
-
-def paintface(img: Image.Image,size: int) -> Image.Image:
- aligned_img = align_first_face(img,size)
- if aligned_img is None:
- output=None
- else:
- im_in = array_to_image(aligned_img).convert("RGB")
- im_out1 = face2paint(model, im_in, side_by_side=False)
- im_out2 = face2paint(model2, im_in, side_by_side=False)
-
- output = img_concat_h(im_out1, im_out2)
- return output
-
-def generate(img):
- out = paintface(img, 400)
- return out
\ No newline at end of file
diff --git a/spaces/ChandraMohanNayal/AutoGPT/autogpt/processing/text.py b/spaces/ChandraMohanNayal/AutoGPT/autogpt/processing/text.py
deleted file mode 100644
index 52add81401775c1b111512d8149f86a175fd9acb..0000000000000000000000000000000000000000
--- a/spaces/ChandraMohanNayal/AutoGPT/autogpt/processing/text.py
+++ /dev/null
@@ -1,132 +0,0 @@
-"""Text processing functions"""
-from typing import Dict, Generator, Optional
-
-from selenium.webdriver.remote.webdriver import WebDriver
-
-from autogpt.config import Config
-from autogpt.llm_utils import create_chat_completion
-from autogpt.memory import get_memory
-
-CFG = Config()
-MEMORY = get_memory(CFG)
-
-
-def split_text(text: str, max_length: int = 8192) -> Generator[str, None, None]:
- """Split text into chunks of a maximum length
-
- Args:
- text (str): The text to split
- max_length (int, optional): The maximum length of each chunk. Defaults to 8192.
-
- Yields:
- str: The next chunk of text
-
- Raises:
- ValueError: If the text is longer than the maximum length
- """
- paragraphs = text.split("\n")
- current_length = 0
- current_chunk = []
-
- for paragraph in paragraphs:
- if current_length + len(paragraph) + 1 <= max_length:
- current_chunk.append(paragraph)
- current_length += len(paragraph) + 1
- else:
- yield "\n".join(current_chunk)
- current_chunk = [paragraph]
- current_length = len(paragraph) + 1
-
- if current_chunk:
- yield "\n".join(current_chunk)
-
-
-def summarize_text(
- url: str, text: str, question: str, driver: Optional[WebDriver] = None
-) -> str:
- """Summarize text using the OpenAI API
-
- Args:
- url (str): The url of the text
- text (str): The text to summarize
- question (str): The question to ask the model
- driver (WebDriver): The webdriver to use to scroll the page
-
- Returns:
- str: The summary of the text
- """
- if not text:
- return "Error: No text to summarize"
-
- text_length = len(text)
- print(f"Text length: {text_length} characters")
-
- summaries = []
- chunks = list(split_text(text))
- scroll_ratio = 1 / len(chunks)
-
- for i, chunk in enumerate(chunks):
- if driver:
- scroll_to_percentage(driver, scroll_ratio * i)
- print(f"Adding chunk {i + 1} / {len(chunks)} to memory")
-
- memory_to_add = f"Source: {url}\n" f"Raw content part#{i + 1}: {chunk}"
-
- MEMORY.add(memory_to_add)
-
- print(f"Summarizing chunk {i + 1} / {len(chunks)}")
- messages = [create_message(chunk, question)]
-
- summary = create_chat_completion(
- model=CFG.fast_llm_model,
- messages=messages,
- )
- summaries.append(summary)
- print(f"Added chunk {i + 1} summary to memory")
-
- memory_to_add = f"Source: {url}\n" f"Content summary part#{i + 1}: {summary}"
-
- MEMORY.add(memory_to_add)
-
- print(f"Summarized {len(chunks)} chunks.")
-
- combined_summary = "\n".join(summaries)
- messages = [create_message(combined_summary, question)]
-
- return create_chat_completion(
- model=CFG.fast_llm_model,
- messages=messages,
- )
-
-
-def scroll_to_percentage(driver: WebDriver, ratio: float) -> None:
- """Scroll to a percentage of the page
-
- Args:
- driver (WebDriver): The webdriver to use
- ratio (float): The percentage to scroll to
-
- Raises:
- ValueError: If the ratio is not between 0 and 1
- """
- if ratio < 0 or ratio > 1:
- raise ValueError("Percentage should be between 0 and 1")
- driver.execute_script(f"window.scrollTo(0, document.body.scrollHeight * {ratio});")
-
-
-def create_message(chunk: str, question: str) -> Dict[str, str]:
- """Create a message for the chat completion
-
- Args:
- chunk (str): The chunk of text to summarize
- question (str): The question to answer
-
- Returns:
- Dict[str, str]: The message to send to the chat completion
- """
- return {
- "role": "user",
- "content": f'"""{chunk}""" Using the above text, answer the following'
- f' question: "{question}" -- if the question cannot be answered using the text,'
- " summarize the text.",
- }
diff --git a/spaces/CrucibleAI/ControlNetMediaPipeFaceSD21/ldm/modules/image_degradation/bsrgan.py b/spaces/CrucibleAI/ControlNetMediaPipeFaceSD21/ldm/modules/image_degradation/bsrgan.py
deleted file mode 100644
index 32ef56169978e550090261cddbcf5eb611a6173b..0000000000000000000000000000000000000000
--- a/spaces/CrucibleAI/ControlNetMediaPipeFaceSD21/ldm/modules/image_degradation/bsrgan.py
+++ /dev/null
@@ -1,730 +0,0 @@
-# -*- coding: utf-8 -*-
-"""
-# --------------------------------------------
-# Super-Resolution
-# --------------------------------------------
-#
-# Kai Zhang (cskaizhang@gmail.com)
-# https://github.com/cszn
-# From 2019/03--2021/08
-# --------------------------------------------
-"""
-
-import numpy as np
-import cv2
-import torch
-
-from functools import partial
-import random
-from scipy import ndimage
-import scipy
-import scipy.stats as ss
-from scipy.interpolate import interp2d
-from scipy.linalg import orth
-import albumentations
-
-import ldm.modules.image_degradation.utils_image as util
-
-
-def modcrop_np(img, sf):
- '''
- Args:
- img: numpy image, WxH or WxHxC
- sf: scale factor
- Return:
- cropped image
- '''
- w, h = img.shape[:2]
- im = np.copy(img)
- return im[:w - w % sf, :h - h % sf, ...]
-
-
-"""
-# --------------------------------------------
-# anisotropic Gaussian kernels
-# --------------------------------------------
-"""
-
-
-def analytic_kernel(k):
- """Calculate the X4 kernel from the X2 kernel (for proof see appendix in paper)"""
- k_size = k.shape[0]
- # Calculate the big kernels size
- big_k = np.zeros((3 * k_size - 2, 3 * k_size - 2))
- # Loop over the small kernel to fill the big one
- for r in range(k_size):
- for c in range(k_size):
- big_k[2 * r:2 * r + k_size, 2 * c:2 * c + k_size] += k[r, c] * k
- # Crop the edges of the big kernel to ignore very small values and increase run time of SR
- crop = k_size // 2
- cropped_big_k = big_k[crop:-crop, crop:-crop]
- # Normalize to 1
- return cropped_big_k / cropped_big_k.sum()
-
-
-def anisotropic_Gaussian(ksize=15, theta=np.pi, l1=6, l2=6):
- """ generate an anisotropic Gaussian kernel
- Args:
- ksize : e.g., 15, kernel size
- theta : [0, pi], rotation angle range
- l1 : [0.1,50], scaling of eigenvalues
- l2 : [0.1,l1], scaling of eigenvalues
- If l1 = l2, will get an isotropic Gaussian kernel.
- Returns:
- k : kernel
- """
-
- v = np.dot(np.array([[np.cos(theta), -np.sin(theta)], [np.sin(theta), np.cos(theta)]]), np.array([1., 0.]))
- V = np.array([[v[0], v[1]], [v[1], -v[0]]])
- D = np.array([[l1, 0], [0, l2]])
- Sigma = np.dot(np.dot(V, D), np.linalg.inv(V))
- k = gm_blur_kernel(mean=[0, 0], cov=Sigma, size=ksize)
-
- return k
-
-
-def gm_blur_kernel(mean, cov, size=15):
- center = size / 2.0 + 0.5
- k = np.zeros([size, size])
- for y in range(size):
- for x in range(size):
- cy = y - center + 1
- cx = x - center + 1
- k[y, x] = ss.multivariate_normal.pdf([cx, cy], mean=mean, cov=cov)
-
- k = k / np.sum(k)
- return k
-
-
-def shift_pixel(x, sf, upper_left=True):
- """shift pixel for super-resolution with different scale factors
- Args:
- x: WxHxC or WxH
- sf: scale factor
- upper_left: shift direction
- """
- h, w = x.shape[:2]
- shift = (sf - 1) * 0.5
- xv, yv = np.arange(0, w, 1.0), np.arange(0, h, 1.0)
- if upper_left:
- x1 = xv + shift
- y1 = yv + shift
- else:
- x1 = xv - shift
- y1 = yv - shift
-
- x1 = np.clip(x1, 0, w - 1)
- y1 = np.clip(y1, 0, h - 1)
-
- if x.ndim == 2:
- x = interp2d(xv, yv, x)(x1, y1)
- if x.ndim == 3:
- for i in range(x.shape[-1]):
- x[:, :, i] = interp2d(xv, yv, x[:, :, i])(x1, y1)
-
- return x
-
-
-def blur(x, k):
- '''
- x: image, NxcxHxW
- k: kernel, Nx1xhxw
- '''
- n, c = x.shape[:2]
- p1, p2 = (k.shape[-2] - 1) // 2, (k.shape[-1] - 1) // 2
- x = torch.nn.functional.pad(x, pad=(p1, p2, p1, p2), mode='replicate')
- k = k.repeat(1, c, 1, 1)
- k = k.view(-1, 1, k.shape[2], k.shape[3])
- x = x.view(1, -1, x.shape[2], x.shape[3])
- x = torch.nn.functional.conv2d(x, k, bias=None, stride=1, padding=0, groups=n * c)
- x = x.view(n, c, x.shape[2], x.shape[3])
-
- return x
-
-
-def gen_kernel(k_size=np.array([15, 15]), scale_factor=np.array([4, 4]), min_var=0.6, max_var=10., noise_level=0):
- """"
- # modified version of https://github.com/assafshocher/BlindSR_dataset_generator
- # Kai Zhang
- # min_var = 0.175 * sf # variance of the gaussian kernel will be sampled between min_var and max_var
- # max_var = 2.5 * sf
- """
- # Set random eigen-vals (lambdas) and angle (theta) for COV matrix
- lambda_1 = min_var + np.random.rand() * (max_var - min_var)
- lambda_2 = min_var + np.random.rand() * (max_var - min_var)
- theta = np.random.rand() * np.pi # random theta
- noise = -noise_level + np.random.rand(*k_size) * noise_level * 2
-
- # Set COV matrix using Lambdas and Theta
- LAMBDA = np.diag([lambda_1, lambda_2])
- Q = np.array([[np.cos(theta), -np.sin(theta)],
- [np.sin(theta), np.cos(theta)]])
- SIGMA = Q @ LAMBDA @ Q.T
- INV_SIGMA = np.linalg.inv(SIGMA)[None, None, :, :]
-
- # Set expectation position (shifting kernel for aligned image)
- MU = k_size // 2 - 0.5 * (scale_factor - 1) # - 0.5 * (scale_factor - k_size % 2)
- MU = MU[None, None, :, None]
-
- # Create meshgrid for Gaussian
- [X, Y] = np.meshgrid(range(k_size[0]), range(k_size[1]))
- Z = np.stack([X, Y], 2)[:, :, :, None]
-
- # Calcualte Gaussian for every pixel of the kernel
- ZZ = Z - MU
- ZZ_t = ZZ.transpose(0, 1, 3, 2)
- raw_kernel = np.exp(-0.5 * np.squeeze(ZZ_t @ INV_SIGMA @ ZZ)) * (1 + noise)
-
- # shift the kernel so it will be centered
- # raw_kernel_centered = kernel_shift(raw_kernel, scale_factor)
-
- # Normalize the kernel and return
- # kernel = raw_kernel_centered / np.sum(raw_kernel_centered)
- kernel = raw_kernel / np.sum(raw_kernel)
- return kernel
-
-
-def fspecial_gaussian(hsize, sigma):
- hsize = [hsize, hsize]
- siz = [(hsize[0] - 1.0) / 2.0, (hsize[1] - 1.0) / 2.0]
- std = sigma
- [x, y] = np.meshgrid(np.arange(-siz[1], siz[1] + 1), np.arange(-siz[0], siz[0] + 1))
- arg = -(x * x + y * y) / (2 * std * std)
- h = np.exp(arg)
- h[h < scipy.finfo(float).eps * h.max()] = 0
- sumh = h.sum()
- if sumh != 0:
- h = h / sumh
- return h
-
-
-def fspecial_laplacian(alpha):
- alpha = max([0, min([alpha, 1])])
- h1 = alpha / (alpha + 1)
- h2 = (1 - alpha) / (alpha + 1)
- h = [[h1, h2, h1], [h2, -4 / (alpha + 1), h2], [h1, h2, h1]]
- h = np.array(h)
- return h
-
-
-def fspecial(filter_type, *args, **kwargs):
- '''
- python code from:
- https://github.com/ronaldosena/imagens-medicas-2/blob/40171a6c259edec7827a6693a93955de2bd39e76/Aulas/aula_2_-_uniform_filter/matlab_fspecial.py
- '''
- if filter_type == 'gaussian':
- return fspecial_gaussian(*args, **kwargs)
- if filter_type == 'laplacian':
- return fspecial_laplacian(*args, **kwargs)
-
-
-"""
-# --------------------------------------------
-# degradation models
-# --------------------------------------------
-"""
-
-
-def bicubic_degradation(x, sf=3):
- '''
- Args:
- x: HxWxC image, [0, 1]
- sf: down-scale factor
- Return:
- bicubicly downsampled LR image
- '''
- x = util.imresize_np(x, scale=1 / sf)
- return x
-
-
-def srmd_degradation(x, k, sf=3):
- ''' blur + bicubic downsampling
- Args:
- x: HxWxC image, [0, 1]
- k: hxw, double
- sf: down-scale factor
- Return:
- downsampled LR image
- Reference:
- @inproceedings{zhang2018learning,
- title={Learning a single convolutional super-resolution network for multiple degradations},
- author={Zhang, Kai and Zuo, Wangmeng and Zhang, Lei},
- booktitle={IEEE Conference on Computer Vision and Pattern Recognition},
- pages={3262--3271},
- year={2018}
- }
- '''
- x = ndimage.filters.convolve(x, np.expand_dims(k, axis=2), mode='wrap') # 'nearest' | 'mirror'
- x = bicubic_degradation(x, sf=sf)
- return x
-
-
-def dpsr_degradation(x, k, sf=3):
- ''' bicubic downsampling + blur
- Args:
- x: HxWxC image, [0, 1]
- k: hxw, double
- sf: down-scale factor
- Return:
- downsampled LR image
- Reference:
- @inproceedings{zhang2019deep,
- title={Deep Plug-and-Play Super-Resolution for Arbitrary Blur Kernels},
- author={Zhang, Kai and Zuo, Wangmeng and Zhang, Lei},
- booktitle={IEEE Conference on Computer Vision and Pattern Recognition},
- pages={1671--1681},
- year={2019}
- }
- '''
- x = bicubic_degradation(x, sf=sf)
- x = ndimage.filters.convolve(x, np.expand_dims(k, axis=2), mode='wrap')
- return x
-
-
-def classical_degradation(x, k, sf=3):
- ''' blur + downsampling
- Args:
- x: HxWxC image, [0, 1]/[0, 255]
- k: hxw, double
- sf: down-scale factor
- Return:
- downsampled LR image
- '''
- x = ndimage.filters.convolve(x, np.expand_dims(k, axis=2), mode='wrap')
- # x = filters.correlate(x, np.expand_dims(np.flip(k), axis=2))
- st = 0
- return x[st::sf, st::sf, ...]
-
-
-def add_sharpening(img, weight=0.5, radius=50, threshold=10):
- """USM sharpening. borrowed from real-ESRGAN
- Input image: I; Blurry image: B.
- 1. K = I + weight * (I - B)
- 2. Mask = 1 if abs(I - B) > threshold, else: 0
- 3. Blur mask:
- 4. Out = Mask * K + (1 - Mask) * I
- Args:
- img (Numpy array): Input image, HWC, BGR; float32, [0, 1].
- weight (float): Sharp weight. Default: 1.
- radius (float): Kernel size of Gaussian blur. Default: 50.
- threshold (int):
- """
- if radius % 2 == 0:
- radius += 1
- blur = cv2.GaussianBlur(img, (radius, radius), 0)
- residual = img - blur
- mask = np.abs(residual) * 255 > threshold
- mask = mask.astype('float32')
- soft_mask = cv2.GaussianBlur(mask, (radius, radius), 0)
-
- K = img + weight * residual
- K = np.clip(K, 0, 1)
- return soft_mask * K + (1 - soft_mask) * img
-
-
-def add_blur(img, sf=4):
- wd2 = 4.0 + sf
- wd = 2.0 + 0.2 * sf
- if random.random() < 0.5:
- l1 = wd2 * random.random()
- l2 = wd2 * random.random()
- k = anisotropic_Gaussian(ksize=2 * random.randint(2, 11) + 3, theta=random.random() * np.pi, l1=l1, l2=l2)
- else:
- k = fspecial('gaussian', 2 * random.randint(2, 11) + 3, wd * random.random())
- img = ndimage.filters.convolve(img, np.expand_dims(k, axis=2), mode='mirror')
-
- return img
-
-
-def add_resize(img, sf=4):
- rnum = np.random.rand()
- if rnum > 0.8: # up
- sf1 = random.uniform(1, 2)
- elif rnum < 0.7: # down
- sf1 = random.uniform(0.5 / sf, 1)
- else:
- sf1 = 1.0
- img = cv2.resize(img, (int(sf1 * img.shape[1]), int(sf1 * img.shape[0])), interpolation=random.choice([1, 2, 3]))
- img = np.clip(img, 0.0, 1.0)
-
- return img
-
-
-# def add_Gaussian_noise(img, noise_level1=2, noise_level2=25):
-# noise_level = random.randint(noise_level1, noise_level2)
-# rnum = np.random.rand()
-# if rnum > 0.6: # add color Gaussian noise
-# img += np.random.normal(0, noise_level / 255.0, img.shape).astype(np.float32)
-# elif rnum < 0.4: # add grayscale Gaussian noise
-# img += np.random.normal(0, noise_level / 255.0, (*img.shape[:2], 1)).astype(np.float32)
-# else: # add noise
-# L = noise_level2 / 255.
-# D = np.diag(np.random.rand(3))
-# U = orth(np.random.rand(3, 3))
-# conv = np.dot(np.dot(np.transpose(U), D), U)
-# img += np.random.multivariate_normal([0, 0, 0], np.abs(L ** 2 * conv), img.shape[:2]).astype(np.float32)
-# img = np.clip(img, 0.0, 1.0)
-# return img
-
-def add_Gaussian_noise(img, noise_level1=2, noise_level2=25):
- noise_level = random.randint(noise_level1, noise_level2)
- rnum = np.random.rand()
- if rnum > 0.6: # add color Gaussian noise
- img = img + np.random.normal(0, noise_level / 255.0, img.shape).astype(np.float32)
- elif rnum < 0.4: # add grayscale Gaussian noise
- img = img + np.random.normal(0, noise_level / 255.0, (*img.shape[:2], 1)).astype(np.float32)
- else: # add noise
- L = noise_level2 / 255.
- D = np.diag(np.random.rand(3))
- U = orth(np.random.rand(3, 3))
- conv = np.dot(np.dot(np.transpose(U), D), U)
- img = img + np.random.multivariate_normal([0, 0, 0], np.abs(L ** 2 * conv), img.shape[:2]).astype(np.float32)
- img = np.clip(img, 0.0, 1.0)
- return img
-
-
-def add_speckle_noise(img, noise_level1=2, noise_level2=25):
- noise_level = random.randint(noise_level1, noise_level2)
- img = np.clip(img, 0.0, 1.0)
- rnum = random.random()
- if rnum > 0.6:
- img += img * np.random.normal(0, noise_level / 255.0, img.shape).astype(np.float32)
- elif rnum < 0.4:
- img += img * np.random.normal(0, noise_level / 255.0, (*img.shape[:2], 1)).astype(np.float32)
- else:
- L = noise_level2 / 255.
- D = np.diag(np.random.rand(3))
- U = orth(np.random.rand(3, 3))
- conv = np.dot(np.dot(np.transpose(U), D), U)
- img += img * np.random.multivariate_normal([0, 0, 0], np.abs(L ** 2 * conv), img.shape[:2]).astype(np.float32)
- img = np.clip(img, 0.0, 1.0)
- return img
-
-
-def add_Poisson_noise(img):
- img = np.clip((img * 255.0).round(), 0, 255) / 255.
- vals = 10 ** (2 * random.random() + 2.0) # [2, 4]
- if random.random() < 0.5:
- img = np.random.poisson(img * vals).astype(np.float32) / vals
- else:
- img_gray = np.dot(img[..., :3], [0.299, 0.587, 0.114])
- img_gray = np.clip((img_gray * 255.0).round(), 0, 255) / 255.
- noise_gray = np.random.poisson(img_gray * vals).astype(np.float32) / vals - img_gray
- img += noise_gray[:, :, np.newaxis]
- img = np.clip(img, 0.0, 1.0)
- return img
-
-
-def add_JPEG_noise(img):
- quality_factor = random.randint(30, 95)
- img = cv2.cvtColor(util.single2uint(img), cv2.COLOR_RGB2BGR)
- result, encimg = cv2.imencode('.jpg', img, [int(cv2.IMWRITE_JPEG_QUALITY), quality_factor])
- img = cv2.imdecode(encimg, 1)
- img = cv2.cvtColor(util.uint2single(img), cv2.COLOR_BGR2RGB)
- return img
-
-
-def random_crop(lq, hq, sf=4, lq_patchsize=64):
- h, w = lq.shape[:2]
- rnd_h = random.randint(0, h - lq_patchsize)
- rnd_w = random.randint(0, w - lq_patchsize)
- lq = lq[rnd_h:rnd_h + lq_patchsize, rnd_w:rnd_w + lq_patchsize, :]
-
- rnd_h_H, rnd_w_H = int(rnd_h * sf), int(rnd_w * sf)
- hq = hq[rnd_h_H:rnd_h_H + lq_patchsize * sf, rnd_w_H:rnd_w_H + lq_patchsize * sf, :]
- return lq, hq
-
-
-def degradation_bsrgan(img, sf=4, lq_patchsize=72, isp_model=None):
- """
- This is the degradation model of BSRGAN from the paper
- "Designing a Practical Degradation Model for Deep Blind Image Super-Resolution"
- ----------
- img: HXWXC, [0, 1], its size should be large than (lq_patchsizexsf)x(lq_patchsizexsf)
- sf: scale factor
- isp_model: camera ISP model
- Returns
- -------
- img: low-quality patch, size: lq_patchsizeXlq_patchsizeXC, range: [0, 1]
- hq: corresponding high-quality patch, size: (lq_patchsizexsf)X(lq_patchsizexsf)XC, range: [0, 1]
- """
- isp_prob, jpeg_prob, scale2_prob = 0.25, 0.9, 0.25
- sf_ori = sf
-
- h1, w1 = img.shape[:2]
- img = img.copy()[:w1 - w1 % sf, :h1 - h1 % sf, ...] # mod crop
- h, w = img.shape[:2]
-
- if h < lq_patchsize * sf or w < lq_patchsize * sf:
- raise ValueError(f'img size ({h1}X{w1}) is too small!')
-
- hq = img.copy()
-
- if sf == 4 and random.random() < scale2_prob: # downsample1
- if np.random.rand() < 0.5:
- img = cv2.resize(img, (int(1 / 2 * img.shape[1]), int(1 / 2 * img.shape[0])),
- interpolation=random.choice([1, 2, 3]))
- else:
- img = util.imresize_np(img, 1 / 2, True)
- img = np.clip(img, 0.0, 1.0)
- sf = 2
-
- shuffle_order = random.sample(range(7), 7)
- idx1, idx2 = shuffle_order.index(2), shuffle_order.index(3)
- if idx1 > idx2: # keep downsample3 last
- shuffle_order[idx1], shuffle_order[idx2] = shuffle_order[idx2], shuffle_order[idx1]
-
- for i in shuffle_order:
-
- if i == 0:
- img = add_blur(img, sf=sf)
-
- elif i == 1:
- img = add_blur(img, sf=sf)
-
- elif i == 2:
- a, b = img.shape[1], img.shape[0]
- # downsample2
- if random.random() < 0.75:
- sf1 = random.uniform(1, 2 * sf)
- img = cv2.resize(img, (int(1 / sf1 * img.shape[1]), int(1 / sf1 * img.shape[0])),
- interpolation=random.choice([1, 2, 3]))
- else:
- k = fspecial('gaussian', 25, random.uniform(0.1, 0.6 * sf))
- k_shifted = shift_pixel(k, sf)
- k_shifted = k_shifted / k_shifted.sum() # blur with shifted kernel
- img = ndimage.filters.convolve(img, np.expand_dims(k_shifted, axis=2), mode='mirror')
- img = img[0::sf, 0::sf, ...] # nearest downsampling
- img = np.clip(img, 0.0, 1.0)
-
- elif i == 3:
- # downsample3
- img = cv2.resize(img, (int(1 / sf * a), int(1 / sf * b)), interpolation=random.choice([1, 2, 3]))
- img = np.clip(img, 0.0, 1.0)
-
- elif i == 4:
- # add Gaussian noise
- img = add_Gaussian_noise(img, noise_level1=2, noise_level2=25)
-
- elif i == 5:
- # add JPEG noise
- if random.random() < jpeg_prob:
- img = add_JPEG_noise(img)
-
- elif i == 6:
- # add processed camera sensor noise
- if random.random() < isp_prob and isp_model is not None:
- with torch.no_grad():
- img, hq = isp_model.forward(img.copy(), hq)
-
- # add final JPEG compression noise
- img = add_JPEG_noise(img)
-
- # random crop
- img, hq = random_crop(img, hq, sf_ori, lq_patchsize)
-
- return img, hq
-
-
-# todo no isp_model?
-def degradation_bsrgan_variant(image, sf=4, isp_model=None):
- """
- This is the degradation model of BSRGAN from the paper
- "Designing a Practical Degradation Model for Deep Blind Image Super-Resolution"
- ----------
- sf: scale factor
- isp_model: camera ISP model
- Returns
- -------
- img: low-quality patch, size: lq_patchsizeXlq_patchsizeXC, range: [0, 1]
- hq: corresponding high-quality patch, size: (lq_patchsizexsf)X(lq_patchsizexsf)XC, range: [0, 1]
- """
- image = util.uint2single(image)
- isp_prob, jpeg_prob, scale2_prob = 0.25, 0.9, 0.25
- sf_ori = sf
-
- h1, w1 = image.shape[:2]
- image = image.copy()[:w1 - w1 % sf, :h1 - h1 % sf, ...] # mod crop
- h, w = image.shape[:2]
-
- hq = image.copy()
-
- if sf == 4 and random.random() < scale2_prob: # downsample1
- if np.random.rand() < 0.5:
- image = cv2.resize(image, (int(1 / 2 * image.shape[1]), int(1 / 2 * image.shape[0])),
- interpolation=random.choice([1, 2, 3]))
- else:
- image = util.imresize_np(image, 1 / 2, True)
- image = np.clip(image, 0.0, 1.0)
- sf = 2
-
- shuffle_order = random.sample(range(7), 7)
- idx1, idx2 = shuffle_order.index(2), shuffle_order.index(3)
- if idx1 > idx2: # keep downsample3 last
- shuffle_order[idx1], shuffle_order[idx2] = shuffle_order[idx2], shuffle_order[idx1]
-
- for i in shuffle_order:
-
- if i == 0:
- image = add_blur(image, sf=sf)
-
- elif i == 1:
- image = add_blur(image, sf=sf)
-
- elif i == 2:
- a, b = image.shape[1], image.shape[0]
- # downsample2
- if random.random() < 0.75:
- sf1 = random.uniform(1, 2 * sf)
- image = cv2.resize(image, (int(1 / sf1 * image.shape[1]), int(1 / sf1 * image.shape[0])),
- interpolation=random.choice([1, 2, 3]))
- else:
- k = fspecial('gaussian', 25, random.uniform(0.1, 0.6 * sf))
- k_shifted = shift_pixel(k, sf)
- k_shifted = k_shifted / k_shifted.sum() # blur with shifted kernel
- image = ndimage.filters.convolve(image, np.expand_dims(k_shifted, axis=2), mode='mirror')
- image = image[0::sf, 0::sf, ...] # nearest downsampling
- image = np.clip(image, 0.0, 1.0)
-
- elif i == 3:
- # downsample3
- image = cv2.resize(image, (int(1 / sf * a), int(1 / sf * b)), interpolation=random.choice([1, 2, 3]))
- image = np.clip(image, 0.0, 1.0)
-
- elif i == 4:
- # add Gaussian noise
- image = add_Gaussian_noise(image, noise_level1=2, noise_level2=25)
-
- elif i == 5:
- # add JPEG noise
- if random.random() < jpeg_prob:
- image = add_JPEG_noise(image)
-
- # elif i == 6:
- # # add processed camera sensor noise
- # if random.random() < isp_prob and isp_model is not None:
- # with torch.no_grad():
- # img, hq = isp_model.forward(img.copy(), hq)
-
- # add final JPEG compression noise
- image = add_JPEG_noise(image)
- image = util.single2uint(image)
- example = {"image":image}
- return example
-
-
-# TODO incase there is a pickle error one needs to replace a += x with a = a + x in add_speckle_noise etc...
-def degradation_bsrgan_plus(img, sf=4, shuffle_prob=0.5, use_sharp=True, lq_patchsize=64, isp_model=None):
- """
- This is an extended degradation model by combining
- the degradation models of BSRGAN and Real-ESRGAN
- ----------
- img: HXWXC, [0, 1], its size should be large than (lq_patchsizexsf)x(lq_patchsizexsf)
- sf: scale factor
- use_shuffle: the degradation shuffle
- use_sharp: sharpening the img
- Returns
- -------
- img: low-quality patch, size: lq_patchsizeXlq_patchsizeXC, range: [0, 1]
- hq: corresponding high-quality patch, size: (lq_patchsizexsf)X(lq_patchsizexsf)XC, range: [0, 1]
- """
-
- h1, w1 = img.shape[:2]
- img = img.copy()[:w1 - w1 % sf, :h1 - h1 % sf, ...] # mod crop
- h, w = img.shape[:2]
-
- if h < lq_patchsize * sf or w < lq_patchsize * sf:
- raise ValueError(f'img size ({h1}X{w1}) is too small!')
-
- if use_sharp:
- img = add_sharpening(img)
- hq = img.copy()
-
- if random.random() < shuffle_prob:
- shuffle_order = random.sample(range(13), 13)
- else:
- shuffle_order = list(range(13))
- # local shuffle for noise, JPEG is always the last one
- shuffle_order[2:6] = random.sample(shuffle_order[2:6], len(range(2, 6)))
- shuffle_order[9:13] = random.sample(shuffle_order[9:13], len(range(9, 13)))
-
- poisson_prob, speckle_prob, isp_prob = 0.1, 0.1, 0.1
-
- for i in shuffle_order:
- if i == 0:
- img = add_blur(img, sf=sf)
- elif i == 1:
- img = add_resize(img, sf=sf)
- elif i == 2:
- img = add_Gaussian_noise(img, noise_level1=2, noise_level2=25)
- elif i == 3:
- if random.random() < poisson_prob:
- img = add_Poisson_noise(img)
- elif i == 4:
- if random.random() < speckle_prob:
- img = add_speckle_noise(img)
- elif i == 5:
- if random.random() < isp_prob and isp_model is not None:
- with torch.no_grad():
- img, hq = isp_model.forward(img.copy(), hq)
- elif i == 6:
- img = add_JPEG_noise(img)
- elif i == 7:
- img = add_blur(img, sf=sf)
- elif i == 8:
- img = add_resize(img, sf=sf)
- elif i == 9:
- img = add_Gaussian_noise(img, noise_level1=2, noise_level2=25)
- elif i == 10:
- if random.random() < poisson_prob:
- img = add_Poisson_noise(img)
- elif i == 11:
- if random.random() < speckle_prob:
- img = add_speckle_noise(img)
- elif i == 12:
- if random.random() < isp_prob and isp_model is not None:
- with torch.no_grad():
- img, hq = isp_model.forward(img.copy(), hq)
- else:
- print('check the shuffle!')
-
- # resize to desired size
- img = cv2.resize(img, (int(1 / sf * hq.shape[1]), int(1 / sf * hq.shape[0])),
- interpolation=random.choice([1, 2, 3]))
-
- # add final JPEG compression noise
- img = add_JPEG_noise(img)
-
- # random crop
- img, hq = random_crop(img, hq, sf, lq_patchsize)
-
- return img, hq
-
-
-if __name__ == '__main__':
- print("hey")
- img = util.imread_uint('utils/test.png', 3)
- print(img)
- img = util.uint2single(img)
- print(img)
- img = img[:448, :448]
- h = img.shape[0] // 4
- print("resizing to", h)
- sf = 4
- deg_fn = partial(degradation_bsrgan_variant, sf=sf)
- for i in range(20):
- print(i)
- img_lq = deg_fn(img)
- print(img_lq)
- img_lq_bicubic = albumentations.SmallestMaxSize(max_size=h, interpolation=cv2.INTER_CUBIC)(image=img)["image"]
- print(img_lq.shape)
- print("bicubic", img_lq_bicubic.shape)
- print(img_hq.shape)
- lq_nearest = cv2.resize(util.single2uint(img_lq), (int(sf * img_lq.shape[1]), int(sf * img_lq.shape[0])),
- interpolation=0)
- lq_bicubic_nearest = cv2.resize(util.single2uint(img_lq_bicubic), (int(sf * img_lq.shape[1]), int(sf * img_lq.shape[0])),
- interpolation=0)
- img_concat = np.concatenate([lq_bicubic_nearest, lq_nearest, util.single2uint(img_hq)], axis=1)
- util.imsave(img_concat, str(i) + '.png')
-
-
diff --git a/spaces/DAMO-NLP-SG/Video-LLaMA/video_llama/datasets/builders/image_text_pair_builder.py b/spaces/DAMO-NLP-SG/Video-LLaMA/video_llama/datasets/builders/image_text_pair_builder.py
deleted file mode 100644
index 8f93bf8f0dd51318c01940f07dc10e9dda2dd275..0000000000000000000000000000000000000000
--- a/spaces/DAMO-NLP-SG/Video-LLaMA/video_llama/datasets/builders/image_text_pair_builder.py
+++ /dev/null
@@ -1,106 +0,0 @@
-import os
-import logging
-import warnings
-
-from video_llama.common.registry import registry
-from video_llama.datasets.builders.base_dataset_builder import BaseDatasetBuilder
-from video_llama.datasets.datasets.laion_dataset import LaionDataset
-from video_llama.datasets.datasets.cc_sbu_dataset import CCSBUDataset, CCSBUAlignDataset
-
-
-@registry.register_builder("cc_sbu")
-class CCSBUBuilder(BaseDatasetBuilder):
- train_dataset_cls = CCSBUDataset
-
- DATASET_CONFIG_DICT = {"default": "configs/datasets/cc_sbu/defaults.yaml"}
-
- def _download_ann(self):
- pass
-
- def _download_vis(self):
- pass
-
- def build(self):
- self.build_processors()
-
- build_info = self.config.build_info
-
- datasets = dict()
- split = "train"
-
- # create datasets
- # [NOTE] return inner_datasets (wds.DataPipeline)
- dataset_cls = self.train_dataset_cls
- datasets[split] = dataset_cls(
- vis_processor=self.vis_processors[split],
- text_processor=self.text_processors[split],
- location=build_info.storage,
- ).inner_dataset
-
- return datasets
-
-
-@registry.register_builder("laion")
-class LaionBuilder(BaseDatasetBuilder):
- train_dataset_cls = LaionDataset
-
- DATASET_CONFIG_DICT = {"default": "configs/datasets/laion/defaults.yaml"}
-
- def _download_ann(self):
- pass
-
- def _download_vis(self):
- pass
-
- def build(self):
- self.build_processors()
-
- build_info = self.config.build_info
-
- datasets = dict()
- split = "train"
-
- # create datasets
- # [NOTE] return inner_datasets (wds.DataPipeline)
- dataset_cls = self.train_dataset_cls
- datasets[split] = dataset_cls(
- vis_processor=self.vis_processors[split],
- text_processor=self.text_processors[split],
- location=build_info.storage,
- ).inner_dataset
-
- return datasets
-
-
-@registry.register_builder("cc_sbu_align")
-class CCSBUAlignBuilder(BaseDatasetBuilder):
- train_dataset_cls = CCSBUAlignDataset
-
- DATASET_CONFIG_DICT = {
- "default": "configs/datasets/cc_sbu/align.yaml",
- }
-
- def build_datasets(self):
- # at this point, all the annotations and image/videos should be all downloaded to the specified locations.
- logging.info("Building datasets...")
- self.build_processors()
-
- build_info = self.config.build_info
- storage_path = build_info.storage
-
- datasets = dict()
-
- if not os.path.exists(storage_path):
- warnings.warn("storage path {} does not exist.".format(storage_path))
-
- # create datasets
- dataset_cls = self.train_dataset_cls
- datasets['train'] = dataset_cls(
- vis_processor=self.vis_processors["train"],
- text_processor=self.text_processors["train"],
- ann_paths=[os.path.join(storage_path, 'filter_cap.json')],
- vis_root=os.path.join(storage_path, 'image'),
- )
-
- return datasets
-
diff --git a/spaces/DQChoi/gpt-demo/venv/lib/python3.11/site-packages/fsspec/__init__.py b/spaces/DQChoi/gpt-demo/venv/lib/python3.11/site-packages/fsspec/__init__.py
deleted file mode 100644
index 301fead45c765c60e2e27f07eb174a2675d6f554..0000000000000000000000000000000000000000
--- a/spaces/DQChoi/gpt-demo/venv/lib/python3.11/site-packages/fsspec/__init__.py
+++ /dev/null
@@ -1,64 +0,0 @@
-from importlib.metadata import entry_points
-
-from . import _version, caching
-from .callbacks import Callback
-from .compression import available_compressions
-from .core import get_fs_token_paths, open, open_files, open_local
-from .exceptions import FSTimeoutError
-from .mapping import FSMap, get_mapper
-from .registry import (
- available_protocols,
- filesystem,
- get_filesystem_class,
- register_implementation,
- registry,
-)
-from .spec import AbstractFileSystem
-
-__version__ = _version.get_versions()["version"]
-
-__all__ = [
- "AbstractFileSystem",
- "FSTimeoutError",
- "FSMap",
- "filesystem",
- "register_implementation",
- "get_filesystem_class",
- "get_fs_token_paths",
- "get_mapper",
- "open",
- "open_files",
- "open_local",
- "registry",
- "caching",
- "Callback",
- "available_protocols",
- "available_compressions",
-]
-
-
-def process_entries():
- if entry_points is not None:
- try:
- eps = entry_points()
- except TypeError:
- pass # importlib-metadata < 0.8
- else:
- if hasattr(eps, "select"): # Python 3.10+ / importlib_metadata >= 3.9.0
- specs = eps.select(group="fsspec.specs")
- else:
- specs = eps.get("fsspec.specs", [])
- for spec in specs:
- err_msg = f"Unable to load filesystem from {spec}"
- register_implementation(
- spec.name,
- spec.value.replace(":", "."),
- errtxt=err_msg,
- # We take our implementations as the ones to overload with if
- # for some reason we encounter some, may be the same, already
- # registered
- clobber=True,
- )
-
-
-process_entries()
diff --git a/spaces/DQChoi/gpt-demo/venv/lib/python3.11/site-packages/gradio/templates/cdn/assets/UploadText-690664d1.css b/spaces/DQChoi/gpt-demo/venv/lib/python3.11/site-packages/gradio/templates/cdn/assets/UploadText-690664d1.css
deleted file mode 100644
index 858fdcc04577128b4960af9c51ca8c41e2fd69e4..0000000000000000000000000000000000000000
--- a/spaces/DQChoi/gpt-demo/venv/lib/python3.11/site-packages/gradio/templates/cdn/assets/UploadText-690664d1.css
+++ /dev/null
@@ -1 +0,0 @@
-.wrap.svelte-1ck5uk8{display:flex;flex-direction:column;justify-content:center;min-height:var(--size-60);color:var(--block-label-text-color);line-height:var(--line-md)}.or.svelte-1ck5uk8{color:var(--body-text-color-subdued)}@media (min-width: 768px){.wrap.svelte-1ck5uk8{font-size:var(--text-lg)}}
diff --git a/spaces/DQChoi/gpt-demo/venv/lib/python3.11/site-packages/gradio/templates/cdn/assets/index-0a171ecc.js b/spaces/DQChoi/gpt-demo/venv/lib/python3.11/site-packages/gradio/templates/cdn/assets/index-0a171ecc.js
deleted file mode 100644
index 8eb943b1af0daba56054b3d31eca41213bec6f29..0000000000000000000000000000000000000000
--- a/spaces/DQChoi/gpt-demo/venv/lib/python3.11/site-packages/gradio/templates/cdn/assets/index-0a171ecc.js
+++ /dev/null
@@ -1,2 +0,0 @@
-import{S as O,e as P,s as Q,N as T,k as N,O as R,K as g,U,p as C,o as B,M as z,ap as A,Q as j,aw as G,z as q,v as E,A as D,x as S,a1 as X,B as Y,am as Z,P as y,R as x,a7 as p,E as $,ae as ee,h as F,j as K,q as ne,r as ie,t as M,F as k}from"./index-1d65707a.js";/* empty css */import{B as le}from"./Button-f155035a.js";import{B as ae}from"./BlockTitle-dee077e8.js";import"./Info-7c6961ef.js";function ue(n){let e;return{c(){e=y(n[4])},m(i,l){C(i,e,l)},p(i,l){l&16&&x(e,i[4])},d(i){i&&D(e)}}}function te(n){let e,i,l,t,s,b,d;return i=new ae({props:{show_label:n[6],info:n[5],$$slots:{default:[ue]},$$scope:{ctx:n}}}),{c(){e=T("label"),N(i.$$.fragment),l=R(),t=T("input"),g(t,"type","number"),g(t,"min",n[1]),g(t,"max",n[2]),t.disabled=n[3],g(t,"class","svelte-gigvtq"),g(e,"class","block svelte-gigvtq"),U(e,"container",n[7])},m(m,_){C(m,e,_),B(i,e,null),z(e,l),z(e,t),A(t,n[0]),s=!0,b||(d=[j(t,"input",n[11]),j(t,"keypress",n[8]),j(t,"blur",n[9])],b=!0)},p(m,[_]){const r={};_&64&&(r.show_label=m[6]),_&32&&(r.info=m[5]),_&16400&&(r.$$scope={dirty:_,ctx:m}),i.$set(r),(!s||_&2)&&g(t,"min",m[1]),(!s||_&4)&&g(t,"max",m[2]),(!s||_&8)&&(t.disabled=m[3]),_&1&&G(t.value)!==m[0]&&A(t,m[0]),(!s||_&128)&&U(e,"container",m[7])},i(m){s||(q(i.$$.fragment,m),s=!0)},o(m){E(i.$$.fragment,m),s=!1},d(m){m&&D(e),S(i),b=!1,X(d)}}}function se(n,e,i){let{value:l=0}=e,{minimum:t=void 0}=e,{maximum:s=void 0}=e,{value_is_output:b=!1}=e,{disabled:d=!1}=e,{label:m}=e,{info:_=void 0}=e,{show_label:r=!0}=e,{container:h=!0}=e;const u=Y();function o(){!isNaN(l)&&l!==null&&(u("change",l),b||u("input"))}Z(()=>{i(10,b=!1)});async function w(f){await p(),f.key==="Enter"&&(f.preventDefault(),u("submit"))}function c(f){u("blur")}function v(){l=G(this.value),i(0,l)}return n.$$set=f=>{"value"in f&&i(0,l=f.value),"minimum"in f&&i(1,t=f.minimum),"maximum"in f&&i(2,s=f.maximum),"value_is_output"in f&&i(10,b=f.value_is_output),"disabled"in f&&i(3,d=f.disabled),"label"in f&&i(4,m=f.label),"info"in f&&i(5,_=f.info),"show_label"in f&&i(6,r=f.show_label),"container"in f&&i(7,h=f.container)},n.$$.update=()=>{n.$$.dirty&1&&o()},[l,t,s,d,m,_,r,h,w,c,b,v]}class me extends O{constructor(e){super(),P(this,e,se,te,Q,{value:0,minimum:1,maximum:2,value_is_output:10,disabled:3,label:4,info:5,show_label:6,container:7})}}function fe(n){let e,i,l,t,s,b;const d=[n[13]];let m={};for(let u=0;uK(l,"value",_)),F.push(()=>K(l,"value_is_output",r)),l.$on("change",n[17]),l.$on("input",n[18]),l.$on("submit",n[19]),l.$on("blur",n[20]),{c(){N(e.$$.fragment),i=R(),N(l.$$.fragment)},m(u,o){B(e,u,o),C(u,i,o),B(l,u,o),b=!0},p(u,o){const w=o&8192?ne(d,[ie(u[13])]):{};e.$set(w);const c={};o&4&&(c.label=u[2]),o&8&&(c.info=u[3]),o&1024&&(c.show_label=u[10]),o&2048&&(c.minimum=u[11]),o&4096&&(c.maximum=u[12]),o&128&&(c.container=u[7]),o&16384&&(c.disabled=u[14]==="static"),!t&&o&1&&(t=!0,c.value=u[0],M(()=>t=!1)),!s&&o&2&&(s=!0,c.value_is_output=u[1],M(()=>s=!1)),l.$set(c)},i(u){b||(q(e.$$.fragment,u),q(l.$$.fragment,u),b=!0)},o(u){E(e.$$.fragment,u),E(l.$$.fragment,u),b=!1},d(u){u&&D(i),S(e,u),S(l,u)}}}function _e(n){let e,i;return e=new le({props:{visible:n[6],elem_id:n[4],elem_classes:n[5],padding:n[7],allow_overflow:!1,scale:n[8],min_width:n[9],$$slots:{default:[fe]},$$scope:{ctx:n}}}),{c(){N(e.$$.fragment)},m(l,t){B(e,l,t),i=!0},p(l,[t]){const s={};t&64&&(s.visible=l[6]),t&16&&(s.elem_id=l[4]),t&32&&(s.elem_classes=l[5]),t&128&&(s.padding=l[7]),t&256&&(s.scale=l[8]),t&512&&(s.min_width=l[9]),t&2129039&&(s.$$scope={dirty:t,ctx:l}),e.$set(s)},i(l){i||(q(e.$$.fragment,l),i=!0)},o(l){E(e.$$.fragment,l),i=!1},d(l){S(e,l)}}}function oe(n,e,i){let{label:l="Number"}=e,{info:t=void 0}=e,{elem_id:s=""}=e,{elem_classes:b=[]}=e,{visible:d=!0}=e,{container:m=!0}=e,{scale:_=null}=e,{min_width:r=void 0}=e,{value:h=0}=e,{show_label:u}=e,{minimum:o=void 0}=e,{maximum:w=void 0}=e,{loading_status:c}=e,{mode:v}=e,{value_is_output:f=!1}=e;function H(a){h=a,i(0,h)}function I(a){f=a,i(1,f)}function J(a){k.call(this,n,a)}function L(a){k.call(this,n,a)}function V(a){k.call(this,n,a)}function W(a){k.call(this,n,a)}return n.$$set=a=>{"label"in a&&i(2,l=a.label),"info"in a&&i(3,t=a.info),"elem_id"in a&&i(4,s=a.elem_id),"elem_classes"in a&&i(5,b=a.elem_classes),"visible"in a&&i(6,d=a.visible),"container"in a&&i(7,m=a.container),"scale"in a&&i(8,_=a.scale),"min_width"in a&&i(9,r=a.min_width),"value"in a&&i(0,h=a.value),"show_label"in a&&i(10,u=a.show_label),"minimum"in a&&i(11,o=a.minimum),"maximum"in a&&i(12,w=a.maximum),"loading_status"in a&&i(13,c=a.loading_status),"mode"in a&&i(14,v=a.mode),"value_is_output"in a&&i(1,f=a.value_is_output)},[h,f,l,t,s,b,d,m,_,r,u,o,w,c,v,H,I,J,L,V,W]}class be extends O{constructor(e){super(),P(this,e,oe,_e,Q,{label:2,info:3,elem_id:4,elem_classes:5,visible:6,container:7,scale:8,min_width:9,value:0,show_label:10,minimum:11,maximum:12,loading_status:13,mode:14,value_is_output:1})}}const we=be,ve=["static","dynamic"],ke=n=>({type:{payload:"number"},description:{payload:"numeric value"},example_data:n.value??1});export{we as Component,ke as document,ve as modes};
-//# sourceMappingURL=index-0a171ecc.js.map
diff --git a/spaces/DQChoi/gpt-demo/venv/lib/python3.11/site-packages/gradio/templates/frontend/assets/Download-fdaaf5d4.js b/spaces/DQChoi/gpt-demo/venv/lib/python3.11/site-packages/gradio/templates/frontend/assets/Download-fdaaf5d4.js
deleted file mode 100644
index 740b134cf8bb0473cd25d964c80dc0861bd60f07..0000000000000000000000000000000000000000
--- a/spaces/DQChoi/gpt-demo/venv/lib/python3.11/site-packages/gradio/templates/frontend/assets/Download-fdaaf5d4.js
+++ /dev/null
@@ -1,2 +0,0 @@
-import{S as i,e as p,s as v,J as o,K as e,p as h,M as c,n,A as m}from"./index-3370be2a.js";function d(l){let t,s;return{c(){t=o("svg"),s=o("path"),e(s,"fill","currentColor"),e(s,"d","M26 24v4H6v-4H4v4a2 2 0 0 0 2 2h20a2 2 0 0 0 2-2v-4zm0-10l-1.41-1.41L17 20.17V2h-2v18.17l-7.59-7.58L6 14l10 10l10-10z"),e(t,"xmlns","http://www.w3.org/2000/svg"),e(t,"width","100%"),e(t,"height","100%"),e(t,"viewBox","0 0 32 32")},m(a,r){h(a,t,r),c(t,s)},p:n,i:n,o:n,d(a){a&&m(t)}}}class u extends i{constructor(t){super(),p(this,t,null,d,v,{})}}export{u as D};
-//# sourceMappingURL=Download-fdaaf5d4.js.map
diff --git a/spaces/DaleChen/AutoGPT/run_continuous.bat b/spaces/DaleChen/AutoGPT/run_continuous.bat
deleted file mode 100644
index 812aa01c1c5506c452665610c0e9e83a17c426f2..0000000000000000000000000000000000000000
--- a/spaces/DaleChen/AutoGPT/run_continuous.bat
+++ /dev/null
@@ -1,3 +0,0 @@
-@echo off
-set argument=--continuous
-call run.bat %argument%
diff --git a/spaces/Datasculptor/StyleGAN-NADA/op/conv2d_gradfix.py b/spaces/Datasculptor/StyleGAN-NADA/op/conv2d_gradfix.py
deleted file mode 100644
index bb2f94bbcb8132299fd4d538972d32bd7ff6e7d6..0000000000000000000000000000000000000000
--- a/spaces/Datasculptor/StyleGAN-NADA/op/conv2d_gradfix.py
+++ /dev/null
@@ -1,227 +0,0 @@
-import contextlib
-import warnings
-
-import torch
-from torch import autograd
-from torch.nn import functional as F
-
-enabled = True
-weight_gradients_disabled = False
-
-
-@contextlib.contextmanager
-def no_weight_gradients():
- global weight_gradients_disabled
-
- old = weight_gradients_disabled
- weight_gradients_disabled = True
- yield
- weight_gradients_disabled = old
-
-
-def conv2d(input, weight, bias=None, stride=1, padding=0, dilation=1, groups=1):
- if could_use_op(input):
- return conv2d_gradfix(
- transpose=False,
- weight_shape=weight.shape,
- stride=stride,
- padding=padding,
- output_padding=0,
- dilation=dilation,
- groups=groups,
- ).apply(input, weight, bias)
-
- return F.conv2d(
- input=input,
- weight=weight,
- bias=bias,
- stride=stride,
- padding=padding,
- dilation=dilation,
- groups=groups,
- )
-
-
-def conv_transpose2d(
- input,
- weight,
- bias=None,
- stride=1,
- padding=0,
- output_padding=0,
- groups=1,
- dilation=1,
-):
- if could_use_op(input):
- return conv2d_gradfix(
- transpose=True,
- weight_shape=weight.shape,
- stride=stride,
- padding=padding,
- output_padding=output_padding,
- groups=groups,
- dilation=dilation,
- ).apply(input, weight, bias)
-
- return F.conv_transpose2d(
- input=input,
- weight=weight,
- bias=bias,
- stride=stride,
- padding=padding,
- output_padding=output_padding,
- dilation=dilation,
- groups=groups,
- )
-
-
-def could_use_op(input):
- if (not enabled) or (not torch.backends.cudnn.enabled):
- return False
-
- if input.device.type != "cuda":
- return False
-
- if any(torch.__version__.startswith(x) for x in ["1.7.", "1.8."]):
- return True
-
- warnings.warn(
- f"conv2d_gradfix not supported on PyTorch {torch.__version__}. Falling back to torch.nn.functional.conv2d()."
- )
-
- return False
-
-
-def ensure_tuple(xs, ndim):
- xs = tuple(xs) if isinstance(xs, (tuple, list)) else (xs,) * ndim
-
- return xs
-
-
-conv2d_gradfix_cache = dict()
-
-
-def conv2d_gradfix(
- transpose, weight_shape, stride, padding, output_padding, dilation, groups
-):
- ndim = 2
- weight_shape = tuple(weight_shape)
- stride = ensure_tuple(stride, ndim)
- padding = ensure_tuple(padding, ndim)
- output_padding = ensure_tuple(output_padding, ndim)
- dilation = ensure_tuple(dilation, ndim)
-
- key = (transpose, weight_shape, stride, padding, output_padding, dilation, groups)
- if key in conv2d_gradfix_cache:
- return conv2d_gradfix_cache[key]
-
- common_kwargs = dict(
- stride=stride, padding=padding, dilation=dilation, groups=groups
- )
-
- def calc_output_padding(input_shape, output_shape):
- if transpose:
- return [0, 0]
-
- return [
- input_shape[i + 2]
- - (output_shape[i + 2] - 1) * stride[i]
- - (1 - 2 * padding[i])
- - dilation[i] * (weight_shape[i + 2] - 1)
- for i in range(ndim)
- ]
-
- class Conv2d(autograd.Function):
- @staticmethod
- def forward(ctx, input, weight, bias):
- if not transpose:
- out = F.conv2d(input=input, weight=weight, bias=bias, **common_kwargs)
-
- else:
- out = F.conv_transpose2d(
- input=input,
- weight=weight,
- bias=bias,
- output_padding=output_padding,
- **common_kwargs,
- )
-
- ctx.save_for_backward(input, weight)
-
- return out
-
- @staticmethod
- def backward(ctx, grad_output):
- input, weight = ctx.saved_tensors
- grad_input, grad_weight, grad_bias = None, None, None
-
- if ctx.needs_input_grad[0]:
- p = calc_output_padding(
- input_shape=input.shape, output_shape=grad_output.shape
- )
- grad_input = conv2d_gradfix(
- transpose=(not transpose),
- weight_shape=weight_shape,
- output_padding=p,
- **common_kwargs,
- ).apply(grad_output, weight, None)
-
- if ctx.needs_input_grad[1] and not weight_gradients_disabled:
- grad_weight = Conv2dGradWeight.apply(grad_output, input)
-
- if ctx.needs_input_grad[2]:
- grad_bias = grad_output.sum((0, 2, 3))
-
- return grad_input, grad_weight, grad_bias
-
- class Conv2dGradWeight(autograd.Function):
- @staticmethod
- def forward(ctx, grad_output, input):
- op = torch._C._jit_get_operation(
- "aten::cudnn_convolution_backward_weight"
- if not transpose
- else "aten::cudnn_convolution_transpose_backward_weight"
- )
- flags = [
- torch.backends.cudnn.benchmark,
- torch.backends.cudnn.deterministic,
- torch.backends.cudnn.allow_tf32,
- ]
- grad_weight = op(
- weight_shape,
- grad_output,
- input,
- padding,
- stride,
- dilation,
- groups,
- *flags,
- )
- ctx.save_for_backward(grad_output, input)
-
- return grad_weight
-
- @staticmethod
- def backward(ctx, grad_grad_weight):
- grad_output, input = ctx.saved_tensors
- grad_grad_output, grad_grad_input = None, None
-
- if ctx.needs_input_grad[0]:
- grad_grad_output = Conv2d.apply(input, grad_grad_weight, None)
-
- if ctx.needs_input_grad[1]:
- p = calc_output_padding(
- input_shape=input.shape, output_shape=grad_output.shape
- )
- grad_grad_input = conv2d_gradfix(
- transpose=(not transpose),
- weight_shape=weight_shape,
- output_padding=p,
- **common_kwargs,
- ).apply(grad_output, grad_grad_weight, None)
-
- return grad_grad_output, grad_grad_input
-
- conv2d_gradfix_cache[key] = Conv2d
-
- return Conv2d
diff --git a/spaces/Detomo/ai-comic-generation/src/components/ui/label.tsx b/spaces/Detomo/ai-comic-generation/src/components/ui/label.tsx
deleted file mode 100644
index 534182176bf87f9308355514adc884d2b69750a5..0000000000000000000000000000000000000000
--- a/spaces/Detomo/ai-comic-generation/src/components/ui/label.tsx
+++ /dev/null
@@ -1,26 +0,0 @@
-"use client"
-
-import * as React from "react"
-import * as LabelPrimitive from "@radix-ui/react-label"
-import { cva, type VariantProps } from "class-variance-authority"
-
-import { cn } from "@/lib/utils"
-
-const labelVariants = cva(
- "text-sm font-medium leading-none peer-disabled:cursor-not-allowed peer-disabled:opacity-70"
-)
-
-const Label = React.forwardRef<
- React.ElementRef,
- React.ComponentPropsWithoutRef &
- VariantProps
->(({ className, ...props }, ref) => (
-
-))
-Label.displayName = LabelPrimitive.Root.displayName
-
-export { Label }
diff --git a/spaces/EcoCy/LoRA-DreamBooth-Training-UI/style.css b/spaces/EcoCy/LoRA-DreamBooth-Training-UI/style.css
deleted file mode 100644
index c4739b4ea5fc35e774a049e3dacc443f7f0eac19..0000000000000000000000000000000000000000
--- a/spaces/EcoCy/LoRA-DreamBooth-Training-UI/style.css
+++ /dev/null
@@ -1,3 +0,0 @@
-h1 {
- text-align: center;
-}
diff --git a/spaces/EleutherAI/VQGAN_CLIP/taming-transformers/main.py b/spaces/EleutherAI/VQGAN_CLIP/taming-transformers/main.py
deleted file mode 100644
index 7b4f94c529618b7863fa213e339dbe49f839de79..0000000000000000000000000000000000000000
--- a/spaces/EleutherAI/VQGAN_CLIP/taming-transformers/main.py
+++ /dev/null
@@ -1,582 +0,0 @@
-import argparse, os, sys, datetime, glob, importlib
-from omegaconf import OmegaConf
-import numpy as np
-from PIL import Image
-import torch
-import torchvision
-from torch.utils.data import random_split, DataLoader, Dataset
-import pytorch_lightning as pl
-from pytorch_lightning import seed_everything
-from pytorch_lightning.trainer import Trainer
-from pytorch_lightning.callbacks import ModelCheckpoint, Callback, LearningRateMonitor
-from pytorch_lightning.utilities.distributed import rank_zero_only
-
-def get_obj_from_str(string, reload=False):
- module, cls = string.rsplit(".", 1)
- if reload:
- module_imp = importlib.import_module(module)
- importlib.reload(module_imp)
- return getattr(importlib.import_module(module, package=None), cls)
-
-
-def get_parser(**parser_kwargs):
- def str2bool(v):
- if isinstance(v, bool):
- return v
- if v.lower() in ("yes", "true", "t", "y", "1"):
- return True
- elif v.lower() in ("no", "false", "f", "n", "0"):
- return False
- else:
- raise argparse.ArgumentTypeError("Boolean value expected.")
-
- parser = argparse.ArgumentParser(**parser_kwargs)
- parser.add_argument(
- "-n",
- "--name",
- type=str,
- const=True,
- default="",
- nargs="?",
- help="postfix for logdir",
- )
- parser.add_argument(
- "-r",
- "--resume",
- type=str,
- const=True,
- default="",
- nargs="?",
- help="resume from logdir or checkpoint in logdir",
- )
- parser.add_argument(
- "-b",
- "--base",
- nargs="*",
- metavar="base_config.yaml",
- help="paths to base configs. Loaded from left-to-right. "
- "Parameters can be overwritten or added with command-line options of the form `--key value`.",
- default=list(),
- )
- parser.add_argument(
- "-t",
- "--train",
- type=str2bool,
- const=True,
- default=False,
- nargs="?",
- help="train",
- )
- parser.add_argument(
- "--no-test",
- type=str2bool,
- const=True,
- default=False,
- nargs="?",
- help="disable test",
- )
- parser.add_argument("-p", "--project", help="name of new or path to existing project")
- parser.add_argument(
- "-d",
- "--debug",
- type=str2bool,
- nargs="?",
- const=True,
- default=False,
- help="enable post-mortem debugging",
- )
- parser.add_argument(
- "-s",
- "--seed",
- type=int,
- default=23,
- help="seed for seed_everything",
- )
- parser.add_argument(
- "-f",
- "--postfix",
- type=str,
- default="",
- help="post-postfix for default name",
- )
-
- return parser
-
-
-def nondefault_trainer_args(opt):
- parser = argparse.ArgumentParser()
- parser = Trainer.add_argparse_args(parser)
- args = parser.parse_args([])
- return sorted(k for k in vars(args) if getattr(opt, k) != getattr(args, k))
-
-
-def instantiate_from_config(config):
- if not "target" in config:
- raise KeyError("Expected key `target` to instantiate.")
- return get_obj_from_str(config["target"])(**config.get("params", dict()))
-
-
-class WrappedDataset(Dataset):
- """Wraps an arbitrary object with __len__ and __getitem__ into a pytorch dataset"""
- def __init__(self, dataset):
- self.data = dataset
-
- def __len__(self):
- return len(self.data)
-
- def __getitem__(self, idx):
- return self.data[idx]
-
-
-class DataModuleFromConfig(pl.LightningDataModule):
- def __init__(self, batch_size, train=None, validation=None, test=None,
- wrap=False, num_workers=None):
- super().__init__()
- self.batch_size = batch_size
- self.dataset_configs = dict()
- self.num_workers = num_workers if num_workers is not None else batch_size*2
- if train is not None:
- self.dataset_configs["train"] = train
- self.train_dataloader = self._train_dataloader
- if validation is not None:
- self.dataset_configs["validation"] = validation
- self.val_dataloader = self._val_dataloader
- if test is not None:
- self.dataset_configs["test"] = test
- self.test_dataloader = self._test_dataloader
- self.wrap = wrap
-
- def prepare_data(self):
- for data_cfg in self.dataset_configs.values():
- instantiate_from_config(data_cfg)
-
- def setup(self, stage=None):
- self.datasets = dict(
- (k, instantiate_from_config(self.dataset_configs[k]))
- for k in self.dataset_configs)
- if self.wrap:
- for k in self.datasets:
- self.datasets[k] = WrappedDataset(self.datasets[k])
-
- def _train_dataloader(self):
- return DataLoader(self.datasets["train"], batch_size=self.batch_size,
- num_workers=self.num_workers, shuffle=True)
-
- def _val_dataloader(self):
- return DataLoader(self.datasets["validation"],
- batch_size=self.batch_size,
- num_workers=self.num_workers)
-
- def _test_dataloader(self):
- return DataLoader(self.datasets["test"], batch_size=self.batch_size,
- num_workers=self.num_workers)
-
-
-class SetupCallback(Callback):
- def __init__(self, resume, now, logdir, ckptdir, cfgdir, config, lightning_config):
- super().__init__()
- self.resume = resume
- self.now = now
- self.logdir = logdir
- self.ckptdir = ckptdir
- self.cfgdir = cfgdir
- self.config = config
- self.lightning_config = lightning_config
-
- def on_pretrain_routine_start(self, trainer, pl_module):
- if trainer.global_rank == 0:
- # Create logdirs and save configs
- os.makedirs(self.logdir, exist_ok=True)
- os.makedirs(self.ckptdir, exist_ok=True)
- os.makedirs(self.cfgdir, exist_ok=True)
-
- print("Project config")
- print(self.config.pretty())
- OmegaConf.save(self.config,
- os.path.join(self.cfgdir, "{}-project.yaml".format(self.now)))
-
- print("Lightning config")
- print(self.lightning_config.pretty())
- OmegaConf.save(OmegaConf.create({"lightning": self.lightning_config}),
- os.path.join(self.cfgdir, "{}-lightning.yaml".format(self.now)))
-
- else:
- # ModelCheckpoint callback created log directory --- remove it
- if not self.resume and os.path.exists(self.logdir):
- dst, name = os.path.split(self.logdir)
- dst = os.path.join(dst, "child_runs", name)
- os.makedirs(os.path.split(dst)[0], exist_ok=True)
- try:
- os.rename(self.logdir, dst)
- except FileNotFoundError:
- pass
-
-
-class ImageLogger(Callback):
- def __init__(self, batch_frequency, max_images, clamp=True, increase_log_steps=True):
- super().__init__()
- self.batch_freq = batch_frequency
- self.max_images = max_images
- self.logger_log_images = {
- pl.loggers.WandbLogger: self._wandb,
- pl.loggers.TestTubeLogger: self._testtube,
- }
- self.log_steps = [2 ** n for n in range(int(np.log2(self.batch_freq)) + 1)]
- if not increase_log_steps:
- self.log_steps = [self.batch_freq]
- self.clamp = clamp
-
- @rank_zero_only
- def _wandb(self, pl_module, images, batch_idx, split):
- raise ValueError("No way wandb")
- grids = dict()
- for k in images:
- grid = torchvision.utils.make_grid(images[k])
- grids[f"{split}/{k}"] = wandb.Image(grid)
- pl_module.logger.experiment.log(grids)
-
- @rank_zero_only
- def _testtube(self, pl_module, images, batch_idx, split):
- for k in images:
- grid = torchvision.utils.make_grid(images[k])
- grid = (grid+1.0)/2.0 # -1,1 -> 0,1; c,h,w
-
- tag = f"{split}/{k}"
- pl_module.logger.experiment.add_image(
- tag, grid,
- global_step=pl_module.global_step)
-
- @rank_zero_only
- def log_local(self, save_dir, split, images,
- global_step, current_epoch, batch_idx):
- root = os.path.join(save_dir, "images", split)
- for k in images:
- grid = torchvision.utils.make_grid(images[k], nrow=4)
-
- grid = (grid+1.0)/2.0 # -1,1 -> 0,1; c,h,w
- grid = grid.transpose(0,1).transpose(1,2).squeeze(-1)
- grid = grid.numpy()
- grid = (grid*255).astype(np.uint8)
- filename = "{}_gs-{:06}_e-{:06}_b-{:06}.png".format(
- k,
- global_step,
- current_epoch,
- batch_idx)
- path = os.path.join(root, filename)
- os.makedirs(os.path.split(path)[0], exist_ok=True)
- Image.fromarray(grid).save(path)
-
- def log_img(self, pl_module, batch, batch_idx, split="train"):
- if (self.check_frequency(batch_idx) and # batch_idx % self.batch_freq == 0
- hasattr(pl_module, "log_images") and
- callable(pl_module.log_images) and
- self.max_images > 0):
- logger = type(pl_module.logger)
-
- is_train = pl_module.training
- if is_train:
- pl_module.eval()
-
- with torch.no_grad():
- images = pl_module.log_images(batch, split=split)
-
- for k in images:
- N = min(images[k].shape[0], self.max_images)
- images[k] = images[k][:N]
- if isinstance(images[k], torch.Tensor):
- images[k] = images[k].detach().cpu()
- if self.clamp:
- images[k] = torch.clamp(images[k], -1., 1.)
-
- self.log_local(pl_module.logger.save_dir, split, images,
- pl_module.global_step, pl_module.current_epoch, batch_idx)
-
- logger_log_images = self.logger_log_images.get(logger, lambda *args, **kwargs: None)
- logger_log_images(pl_module, images, pl_module.global_step, split)
-
- if is_train:
- pl_module.train()
-
- def check_frequency(self, batch_idx):
- if (batch_idx % self.batch_freq) == 0 or (batch_idx in self.log_steps):
- try:
- self.log_steps.pop(0)
- except IndexError:
- pass
- return True
- return False
-
- def on_train_batch_end(self, trainer, pl_module, outputs, batch, batch_idx, dataloader_idx):
- self.log_img(pl_module, batch, batch_idx, split="train")
-
- def on_validation_batch_end(self, trainer, pl_module, outputs, batch, batch_idx, dataloader_idx):
- self.log_img(pl_module, batch, batch_idx, split="val")
-
-
-
-if __name__ == "__main__":
- # custom parser to specify config files, train, test and debug mode,
- # postfix, resume.
- # `--key value` arguments are interpreted as arguments to the trainer.
- # `nested.key=value` arguments are interpreted as config parameters.
- # configs are merged from left-to-right followed by command line parameters.
-
- # model:
- # base_learning_rate: float
- # target: path to lightning module
- # params:
- # key: value
- # data:
- # target: main.DataModuleFromConfig
- # params:
- # batch_size: int
- # wrap: bool
- # train:
- # target: path to train dataset
- # params:
- # key: value
- # validation:
- # target: path to validation dataset
- # params:
- # key: value
- # test:
- # target: path to test dataset
- # params:
- # key: value
- # lightning: (optional, has sane defaults and can be specified on cmdline)
- # trainer:
- # additional arguments to trainer
- # logger:
- # logger to instantiate
- # modelcheckpoint:
- # modelcheckpoint to instantiate
- # callbacks:
- # callback1:
- # target: importpath
- # params:
- # key: value
-
- now = datetime.datetime.now().strftime("%Y-%m-%dT%H-%M-%S")
-
- # add cwd for convenience and to make classes in this file available when
- # running as `python main.py`
- # (in particular `main.DataModuleFromConfig`)
- sys.path.append(os.getcwd())
-
- parser = get_parser()
- parser = Trainer.add_argparse_args(parser)
-
- opt, unknown = parser.parse_known_args()
- if opt.name and opt.resume:
- raise ValueError(
- "-n/--name and -r/--resume cannot be specified both."
- "If you want to resume training in a new log folder, "
- "use -n/--name in combination with --resume_from_checkpoint"
- )
- if opt.resume:
- if not os.path.exists(opt.resume):
- raise ValueError("Cannot find {}".format(opt.resume))
- if os.path.isfile(opt.resume):
- paths = opt.resume.split("/")
- idx = len(paths)-paths[::-1].index("logs")+1
- logdir = "/".join(paths[:idx])
- ckpt = opt.resume
- else:
- assert os.path.isdir(opt.resume), opt.resume
- logdir = opt.resume.rstrip("/")
- ckpt = os.path.join(logdir, "checkpoints", "last.ckpt")
-
- opt.resume_from_checkpoint = ckpt
- base_configs = sorted(glob.glob(os.path.join(logdir, "configs/*.yaml")))
- opt.base = base_configs+opt.base
- _tmp = logdir.split("/")
- nowname = _tmp[_tmp.index("logs")+1]
- else:
- if opt.name:
- name = "_"+opt.name
- elif opt.base:
- cfg_fname = os.path.split(opt.base[0])[-1]
- cfg_name = os.path.splitext(cfg_fname)[0]
- name = "_"+cfg_name
- else:
- name = ""
- nowname = now+name+opt.postfix
- logdir = os.path.join("logs", nowname)
-
- ckptdir = os.path.join(logdir, "checkpoints")
- cfgdir = os.path.join(logdir, "configs")
- seed_everything(opt.seed)
-
- try:
- # init and save configs
- configs = [OmegaConf.load(cfg) for cfg in opt.base]
- cli = OmegaConf.from_dotlist(unknown)
- config = OmegaConf.merge(*configs, cli)
- lightning_config = config.pop("lightning", OmegaConf.create())
- # merge trainer cli with config
- trainer_config = lightning_config.get("trainer", OmegaConf.create())
- # default to ddp
- trainer_config["distributed_backend"] = "ddp"
- for k in nondefault_trainer_args(opt):
- trainer_config[k] = getattr(opt, k)
- if not "gpus" in trainer_config:
- del trainer_config["distributed_backend"]
- cpu = True
- else:
- gpuinfo = trainer_config["gpus"]
- print(f"Running on GPUs {gpuinfo}")
- cpu = False
- trainer_opt = argparse.Namespace(**trainer_config)
- lightning_config.trainer = trainer_config
-
- # model
- model = instantiate_from_config(config.model)
-
- # trainer and callbacks
- trainer_kwargs = dict()
-
- # default logger configs
- # NOTE wandb < 0.10.0 interferes with shutdown
- # wandb >= 0.10.0 seems to fix it but still interferes with pudb
- # debugging (wrongly sized pudb ui)
- # thus prefer testtube for now
- default_logger_cfgs = {
- "wandb": {
- "target": "pytorch_lightning.loggers.WandbLogger",
- "params": {
- "name": nowname,
- "save_dir": logdir,
- "offline": opt.debug,
- "id": nowname,
- }
- },
- "testtube": {
- "target": "pytorch_lightning.loggers.TestTubeLogger",
- "params": {
- "name": "testtube",
- "save_dir": logdir,
- }
- },
- }
- default_logger_cfg = default_logger_cfgs["testtube"]
- logger_cfg = lightning_config.logger or OmegaConf.create()
- logger_cfg = OmegaConf.merge(default_logger_cfg, logger_cfg)
- trainer_kwargs["logger"] = instantiate_from_config(logger_cfg)
-
- # modelcheckpoint - use TrainResult/EvalResult(checkpoint_on=metric) to
- # specify which metric is used to determine best models
- default_modelckpt_cfg = {
- "target": "pytorch_lightning.callbacks.ModelCheckpoint",
- "params": {
- "dirpath": ckptdir,
- "filename": "{epoch:06}",
- "verbose": True,
- "save_last": True,
- }
- }
- if hasattr(model, "monitor"):
- print(f"Monitoring {model.monitor} as checkpoint metric.")
- default_modelckpt_cfg["params"]["monitor"] = model.monitor
- default_modelckpt_cfg["params"]["save_top_k"] = 3
-
- modelckpt_cfg = lightning_config.modelcheckpoint or OmegaConf.create()
- modelckpt_cfg = OmegaConf.merge(default_modelckpt_cfg, modelckpt_cfg)
- trainer_kwargs["checkpoint_callback"] = instantiate_from_config(modelckpt_cfg)
-
- # add callback which sets up log directory
- default_callbacks_cfg = {
- "setup_callback": {
- "target": "main.SetupCallback",
- "params": {
- "resume": opt.resume,
- "now": now,
- "logdir": logdir,
- "ckptdir": ckptdir,
- "cfgdir": cfgdir,
- "config": config,
- "lightning_config": lightning_config,
- }
- },
- "image_logger": {
- "target": "main.ImageLogger",
- "params": {
- "batch_frequency": 750,
- "max_images": 4,
- "clamp": True
- }
- },
- "learning_rate_logger": {
- "target": "main.LearningRateMonitor",
- "params": {
- "logging_interval": "step",
- #"log_momentum": True
- }
- },
- }
- callbacks_cfg = lightning_config.callbacks or OmegaConf.create()
- callbacks_cfg = OmegaConf.merge(default_callbacks_cfg, callbacks_cfg)
- trainer_kwargs["callbacks"] = [instantiate_from_config(callbacks_cfg[k]) for k in callbacks_cfg]
-
- trainer = Trainer.from_argparse_args(trainer_opt, **trainer_kwargs)
-
- # data
- data = instantiate_from_config(config.data)
- # NOTE according to https://pytorch-lightning.readthedocs.io/en/latest/datamodules.html
- # calling these ourselves should not be necessary but it is.
- # lightning still takes care of proper multiprocessing though
- data.prepare_data()
- data.setup()
-
- # configure learning rate
- bs, base_lr = config.data.params.batch_size, config.model.base_learning_rate
- if not cpu:
- ngpu = len(lightning_config.trainer.gpus.strip(",").split(','))
- else:
- ngpu = 1
- accumulate_grad_batches = lightning_config.trainer.accumulate_grad_batches or 1
- print(f"accumulate_grad_batches = {accumulate_grad_batches}")
- lightning_config.trainer.accumulate_grad_batches = accumulate_grad_batches
- model.learning_rate = accumulate_grad_batches * ngpu * bs * base_lr
- print("Setting learning rate to {:.2e} = {} (accumulate_grad_batches) * {} (num_gpus) * {} (batchsize) * {:.2e} (base_lr)".format(
- model.learning_rate, accumulate_grad_batches, ngpu, bs, base_lr))
-
- # allow checkpointing via USR1
- def melk(*args, **kwargs):
- # run all checkpoint hooks
- if trainer.global_rank == 0:
- print("Summoning checkpoint.")
- ckpt_path = os.path.join(ckptdir, "last.ckpt")
- trainer.save_checkpoint(ckpt_path)
-
- def divein(*args, **kwargs):
- if trainer.global_rank == 0:
- import pudb; pudb.set_trace()
-
- import signal
- signal.signal(signal.SIGUSR1, melk)
- signal.signal(signal.SIGUSR2, divein)
-
- # run
- if opt.train:
- try:
- trainer.fit(model, data)
- except Exception:
- melk()
- raise
- if not opt.no_test and not trainer.interrupted:
- trainer.test(model, data)
- except Exception:
- if opt.debug and trainer.global_rank==0:
- try:
- import pudb as debugger
- except ImportError:
- import pdb as debugger
- debugger.post_mortem()
- raise
- finally:
- # move newly created debug project to debug_runs
- if opt.debug and not opt.resume and trainer.global_rank==0:
- dst, name = os.path.split(logdir)
- dst = os.path.join(dst, "debug_runs", name)
- os.makedirs(os.path.split(dst)[0], exist_ok=True)
- os.rename(logdir, dst)
diff --git a/spaces/FelixLuoX/codeformer/README.md b/spaces/FelixLuoX/codeformer/README.md
deleted file mode 100644
index b4b841a71df3c2e64e9305b459f4a14b37cd77f7..0000000000000000000000000000000000000000
--- a/spaces/FelixLuoX/codeformer/README.md
+++ /dev/null
@@ -1,12 +0,0 @@
----
-title: Codeformer
-emoji: 🌍
-colorFrom: indigo
-colorTo: yellow
-sdk: gradio
-sdk_version: 3.4
-app_file: app.py
-pinned: false
----
-
-Check out the configuration reference at https://huggingface.co/docs/hub/spaces-config-reference
diff --git a/spaces/GXSA/bingo/src/app/loading.css b/spaces/GXSA/bingo/src/app/loading.css
deleted file mode 100644
index eaaab6a86a228334c4eca3c5368ae6f0f593d405..0000000000000000000000000000000000000000
--- a/spaces/GXSA/bingo/src/app/loading.css
+++ /dev/null
@@ -1,68 +0,0 @@
-::-webkit-scrollbar {
- width: 10px;
- height: 10px;
- display: none;
-}
-
-::-webkit-scrollbar-button:start:decrement,
-::-webkit-scrollbar-button:end:increment {
- height: 30px;
- background-color: transparent;
-}
-
-::-webkit-scrollbar-track-piece {
- background-color: #3b3b3b;
- -webkit-border-radius: 16px;
-}
-
-::-webkit-scrollbar-thumb:vertical {
- height: 50px;
- background-color: #666;
- border: 1px solid #eee;
- -webkit-border-radius: 6px;
-}
-
-/* loading start */
-.loading-spinner {
- display: flex;
- justify-content: center;
- align-items: center;
- height: 100vh;
- opacity: 1;
- transition: opacity .8s ease-out;
-}
-
-.loading-spinner.hidden {
- opacity: 0;
-}
-
-.loading-spinner>div {
- width: 30px;
- height: 30px;
- background: linear-gradient(90deg, #2870EA 10.79%, #1B4AEF 87.08%);
-
- border-radius: 100%;
- display: inline-block;
- animation: sk-bouncedelay 1.4s infinite ease-in-out both;
-}
-
-.loading-spinner .bounce1 {
- animation-delay: -0.32s;
-}
-
-.loading-spinner .bounce2 {
- animation-delay: -0.16s;
-}
-
-@keyframes sk-bouncedelay {
-
- 0%,
- 80%,
- 100% {
- transform: scale(0);
- }
-
- 40% {
- transform: scale(1.0);
- }
-}
diff --git a/spaces/Gertie01/MusicLM/musiclm_pytorch.py b/spaces/Gertie01/MusicLM/musiclm_pytorch.py
deleted file mode 100644
index 48d1f8b1712610ca0971a4df41d8975634a4bea8..0000000000000000000000000000000000000000
--- a/spaces/Gertie01/MusicLM/musiclm_pytorch.py
+++ /dev/null
@@ -1,559 +0,0 @@
-import torch
-import torch.nn.functional as F
-from torch import nn, einsum
-
-from torchaudio.transforms import Spectrogram, TimeStretch, FrequencyMasking, TimeMasking
-
-from audiolm_pytorch import AudioLM
-from audiolm_pytorch.utils import AudioConditionerBase
-
-from x_clip.tokenizer import tokenizer
-from vector_quantize_pytorch import ResidualVQ
-
-from einops import rearrange, repeat, reduce, pack, unpack
-
-from beartype.typing import List, Optional, Tuple
-from beartype import beartype
-
-# functions
-
-def exists(val):
- return val is not None
-
-def default(val, d):
- return val if exists(val) else d
-
-def round_down_nearest_multiple(n, divisor):
- return n // divisor * divisor
-
-# tensor functions
-
-def log(t, eps = 1e-20):
- return torch.log(t.clamp(min = eps))
-
-def l2norm(t):
- return F.normalize(t, p = 2, dim = -1)
-
-# 2d sinusoidal positional embedding
-# simple vit paper shows it is good enough compared to learned
-
-def posemb_sincos_2d(patches, temperature = 10000, dtype = torch.float32):
- _, h, w, dim, device, dtype = *patches.shape, patches.device, patches.dtype
-
- y, x = torch.meshgrid(torch.arange(h, device = device), torch.arange(w, device = device), indexing = 'ij')
- assert (dim % 4) == 0, 'feature dimension must be multiple of 4 for sincos emb'
-
- omega = torch.arange(dim // 4, device = device) / (dim // 4 - 1)
- omega = 1. / (temperature ** omega)
-
- y = y.flatten()[:, None] * omega[None, :]
- x = x.flatten()[:, None] * omega[None, :]
-
- pe = torch.cat((x.sin(), x.cos(), y.sin(), y.cos()), dim = 1)
- pe = pe.type(dtype)
-
- return rearrange(pe, '(h w) d -> h w d', h = h, w = w)
-
-# biasless layernorm
-
-class LayerNorm(nn.Module):
- def __init__(self, dim):
- super().__init__()
- self.gamma = nn.Parameter(torch.ones(dim))
- self.register_buffer('beta', torch.zeros(dim))
-
- def forward(self, x):
- return F.layer_norm(x, x.shape[-1:], self.gamma, self.beta)
-
-# feedforward
-
-class GEGLU(nn.Module):
- def forward(self, x):
- x, gate = x.chunk(2, dim = -1)
- return F.gelu(gate) * x
-
-def FeedForward(dim, mult = 4, dropout = 0.):
- dim_hidden = int(dim * mult * 2 / 3)
-
- return nn.Sequential(
- LayerNorm(dim),
- nn.Linear(dim, dim_hidden * 2, bias = False),
- GEGLU(),
- nn.Dropout(dropout),
- nn.Linear(dim_hidden, dim, bias = False)
- )
-
-# attention
-
-class Attention(nn.Module):
- def __init__(
- self,
- dim,
- causal = False,
- dim_head = 64,
- heads = 8,
- dropout = 0.
- ):
- super().__init__()
- self.heads = heads
- self.scale = dim_head ** -0.5
- self.causal = causal
- inner_dim = dim_head * heads
-
- self.norm = LayerNorm(dim)
-
- self.attn_dropout = nn.Dropout(dropout)
-
- self.to_q = nn.Linear(dim, inner_dim, bias = False)
- self.to_kv = nn.Linear(dim, inner_dim * 2, bias = False)
-
- self.to_out = nn.Sequential(
- nn.Linear(inner_dim, dim, bias = False),
- nn.Dropout(dropout)
- )
-
- def forward(
- self,
- x,
- mask = None
- ):
- b, n, _, device = *x.shape, x.device
-
- # prenorm
-
- x = self.norm(x)
-
- # project for queries, keys, values
-
- q, k, v = self.to_q(x), *self.to_kv(x).chunk(2, dim = -1)
-
- # split for multi-headed attention
-
- q, k, v = map(lambda t: rearrange(t, 'b n (h d) -> b h n d', h = self.heads), (q, k, v))
-
- q = q * self.scale
-
- # similarities
-
- sim = einsum('b h i d, b h j d -> b h i j', q, k)
-
- if exists(mask):
- mask = rearrange(mask, 'b j -> b 1 1 j')
- sim = sim.masked_fill(~mask, -torch.finfo(sim.dtype).max)
-
- if self.causal:
- i, j = sim.shape[-2:]
- causal_mask = torch.ones((i, j), dtype = torch.bool, device = x.device).triu(j - i + 1)
- sim = sim.masked_fill(causal_mask, -torch.finfo(sim.dtype).max)
-
- # attention
-
- attn = sim.softmax(dim = -1)
- attn = self.attn_dropout(attn)
-
- # aggregate
-
- out = einsum('b h i j, b h j d -> b h i d', attn, v)
-
- # merge heads
-
- out = rearrange(out, 'b h n d -> b n (h d)')
- return self.to_out(out)
-
-# transformer
-
-class Transformer(nn.Module):
- def __init__(
- self,
- dim,
- depth,
- dim_head = 64,
- heads = 8,
- attn_dropout = 0.,
- ff_mult = 4,
- ff_dropout = 0.
- ):
- super().__init__()
- self.layers = nn.ModuleList([])
- for _ in range(depth):
- self.layers.append(nn.ModuleList([
- Attention(dim = dim, dim_head = dim_head, heads = heads, dropout = attn_dropout),
- FeedForward(dim = dim, mult = ff_mult, dropout = ff_dropout),
- ]))
-
- def forward(self, x, mask = None):
-
- for attn, ff in self.layers:
- x = attn(x, mask = mask) + x
- x = ff(x) + x
-
- return x
-
-# Audio Spectrogram Transformer - https://arxiv.org/abs/2104.01778
-
-def pair(t):
- return (t, t) if not isinstance(t, tuple) else t
-
-class AudioSpectrogramTransformer(nn.Module):
- def __init__(
- self,
- dim,
- depth,
- patch_size = 16,
- dim_head = 64,
- heads = 8,
- attn_dropout = 0.,
- ff_mult = 4,
- ff_dropout = 0.,
- spec_n_fft = 128,
- spec_power = 2,
- spec_win_length = 24,
- spec_hop_length = None,
- spec_pad = 0,
- spec_center = True,
- spec_pad_mode = 'reflect',
- spec_aug_stretch_factor = 0.8,
- spec_aug_freq_mask = 80,
- spec_aug_time_mask = 80
- ):
- super().__init__()
- self.dim = dim
-
- self.patch_size = pair(patch_size)
- self.to_patch_tokens = nn.Conv2d(self.patch_size[0] * self.patch_size[1], dim, 1)
-
- self.spec = Spectrogram(
- n_fft = spec_n_fft,
- power = spec_power,
- win_length = spec_win_length,
- hop_length = spec_hop_length,
- pad = spec_pad,
- center = spec_center,
- pad_mode = spec_pad_mode
- )
-
- # SpecAugment - seems to be widely used in audio field https://arxiv.org/abs/1904.08779
-
- self.aug = torch.nn.Sequential(
- TimeStretch(spec_aug_stretch_factor, fixed_rate=True),
- FrequencyMasking(freq_mask_param = spec_aug_freq_mask),
- TimeMasking(time_mask_param = spec_aug_time_mask),
- )
-
- self.transformer = Transformer(
- dim = dim,
- depth = depth,
- dim_head = dim_head,
- heads = heads,
- attn_dropout = attn_dropout,
- ff_mult = ff_mult,
- ff_dropout = ff_dropout
- )
-
- self.norm = LayerNorm(dim)
-
- def forward(self, x):
- x = self.spec(x)
-
- if self.training:
- x = self.aug(x)
-
- # automatically crop if audio does not yield a 2d spectrogram that is divisible by patch sizes
-
- height, width = x.shape[-2:]
- patch_height, patch_width = self.patch_size
-
- rounded_height, rounded_width = map(lambda args: round_down_nearest_multiple(*args), ((height, patch_height), (width, patch_width)))
-
- if (height, width) != (rounded_height, rounded_width): # just keep printing to be annoying until it is fixed
- print(f'spectrogram yielded shape of {(height, width)}, but had to be cropped to {(rounded_height, rounded_width)} to be patchified for transformer')
-
- x = x[..., :rounded_height, :rounded_width]
-
- # to patches
-
- x = rearrange(x, 'b (h p1) (w p2) -> b (p1 p2) h w', p1 = patch_height, p2 = patch_width)
- x = self.to_patch_tokens(x)
-
- # 2d sinusoidal positional embedding
-
- x = rearrange(x, 'b c h w -> b h w c')
- x = x + posemb_sincos_2d(x)
-
- # attention, what else
-
- x = rearrange(x, 'b ... c -> b (...) c')
-
- x = self.transformer(x)
-
- # final global average and norm (most recent papers show this is superior to CLS token)
-
- x = reduce(x, 'b n d -> b d', 'mean')
-
- return self.norm(x)
-
-# text transformer
-
-@beartype
-class TextTransformer(nn.Module):
- def __init__(
- self,
- dim,
- depth,
- num_tokens = tokenizer.vocab_size,
- max_seq_len = 256,
- dim_head = 64,
- heads = 8,
- attn_dropout = 0.,
- ff_dropout = 0.,
- ff_mult = 4,
- pad_id = 0
- ):
- super().__init__()
- self.dim = dim
-
- self.token_emb = nn.Embedding(num_tokens, dim)
- self.pos_emb = nn.Embedding(max_seq_len, dim)
-
- self.cls_token = nn.Parameter(torch.randn(dim))
-
- self.transformer = Transformer(
- dim = dim,
- depth = depth,
- dim_head = dim_head,
- heads = heads,
- attn_dropout = attn_dropout,
- ff_dropout = ff_dropout,
- ff_mult = ff_mult
- )
-
- self.pad_id = pad_id
- self.norm = LayerNorm(dim)
-
- def forward(
- self,
- x = None,
- raw_texts: Optional[List[str]] = None,
- mask = None
- ):
- assert exists(x) ^ exists(raw_texts)
-
- if exists(raw_texts):
- x = tokenizer.tokenize(raw_texts)
-
- if not exists(mask):
- mask = x != self.pad_id
-
- b, n, device = *x.shape, x.device
-
- # token embedding + positional embedding
-
- x = self.token_emb(x)
- x = x + self.pos_emb(torch.arange(n, device = device))
-
- # cls tokens, as in bert
-
- cls_tokens = repeat(self.cls_token, 'd -> b d', b = b)
- x, ps = pack([cls_tokens, x], 'b * d')
-
- # account for attending to cls token with self attention mask
-
- mask = F.pad(mask, (1, 0), value = True)
-
- # attention
-
- x = self.transformer(x, mask = mask)
-
- # unpack the cls tokens
-
- cls_tokens, _ = unpack(x, ps, 'b * d')
-
- return self.norm(cls_tokens)
-
-# main classes
-
-@beartype
-class MuLaN(nn.Module):
- def __init__(
- self,
- audio_transformer: AudioSpectrogramTransformer,
- text_transformer: TextTransformer,
- dim_latent = 128, # they use 128
- decoupled_contrastive_learning = True, # think this was used, make it optional
- ):
- super().__init__()
- self.dim_latent = dim_latent
-
- self.audio = audio_transformer
- self.text = text_transformer
-
- self.temperature = nn.Parameter(torch.tensor(1.))
-
- self.text_to_latents = nn.Linear(self.text.dim, dim_latent)
- self.audio_to_latents = nn.Linear(self.audio.dim, dim_latent)
-
- self.decoupled_contrastive_learning = decoupled_contrastive_learning
-
- def get_audio_latents(
- self,
- wavs
- ):
- audio_embeds = self.audio(wavs)
- audio_latents = self.audio_to_latents(audio_embeds)
- return l2norm(audio_latents)
-
- def get_text_latents(
- self,
- texts = None,
- raw_texts: Optional[List[str]] = None
- ):
- text_embeds = self.text(texts)
- text_latents = self.text_to_latents(text_embeds)
- return l2norm(text_latents)
-
- def forward(
- self,
- wavs,
- texts = None,
- raw_texts: Optional[List[str]] = None,
- return_similarities = False
- ):
- batch, device = wavs.shape[0], wavs.device
-
- audio_latents = self.get_audio_latents(wavs)
- text_latents = self.get_text_latents(texts, raw_texts = raw_texts)
-
- cosine_sim = einsum('i d, j d -> i j', audio_latents, text_latents)
-
- assert cosine_sim.shape[0] == cosine_sim.shape[1], 'batch sizes for audio and text are not equal'
-
- if return_similarities:
- return cosine_sim
-
- cosine_sim = cosine_sim * self.temperature.exp()
-
- cosine_sim_exp = cosine_sim.exp()
-
- numerator = cosine_sim_exp.diag()
-
- if self.decoupled_contrastive_learning:
- eye = torch.eye(batch, device = device)
- cosine_sim_exp = cosine_sim_exp.masked_fill(eye, 0.)
-
- denominator = reduce(cosine_sim_exp, 'i j -> i', 'sum')
-
- contrastive_loss = -log(numerator / denominator)
- return contrastive_loss.mean()
-
-# music lm
-
-@beartype
-class MuLaNEmbedQuantizer(AudioConditionerBase):
- def __init__(
- self,
- mulan: MuLaN,
- conditioning_dims: Tuple[int, ...],
- rq_num_quantizers = 8,
- rq_ema_decay = 0.9,
- codebook_size = 1024,
- namespaces: Tuple[str, ...] = ('semantic', 'coarse', 'fine'),
- ):
- super().__init__()
- self.mulan = mulan
-
- assert len(namespaces) > 0
- self.namespaces = namespaces
- self.conditioning_dims = conditioning_dims
-
- assert len(conditioning_dims) == len(namespaces), 'number of conditioning dimensions must be equal to number of namespaces'
-
- dim = mulan.dim_latent
-
- self.rq = ResidualVQ(
- dim = dim,
- num_quantizers = rq_num_quantizers,
- codebook_size = codebook_size,
- decay = rq_ema_decay,
- commitment_weight = 0, # only use EMA to update codebooks
- kmeans_init = True,
- threshold_ema_dead_code = 2,
- quantize_dropout = False # no quantize dropout
- )
-
- self.dim = dim
- self.num_codebooks = rq_num_quantizers
-
- self.cond_embeddings = nn.ParameterDict({})
-
- for namespace, conditioning_dim in zip(namespaces, conditioning_dims):
- cond_embeddings = nn.Parameter(torch.randn(rq_num_quantizers, codebook_size, conditioning_dim))
- nn.init.normal_(cond_embeddings, std = 0.02)
-
- self.cond_embeddings[namespace] = cond_embeddings
-
- self.set_default_namespace(namespaces[0])
-
- def parameters(self):
- return self.cond_embeddings.parameters()
-
- def set_default_namespace(self, namespace):
- self._default_namespace = namespace
-
- def forward(
- self,
- wavs = None,
- texts = None,
- namespace = None
- ):
- assert exists(wavs) ^ exists(texts)
-
- namespace = default(namespace, self._default_namespace)
- assert namespace in self.namespaces, f'namespace {namespace} not found'
- cond_embeddings = self.cond_embeddings[namespace]
-
- with torch.no_grad():
- self.mulan.eval()
-
- # sound and language live in joint embedding space because of contrastive learning
-
- if exists(wavs):
- latents = self.mulan.get_audio_latents(wavs)
- elif exists(texts):
- latents = self.mulan.get_text_latents(texts)
-
- _, indices, _ = self.rq(latents)
-
- batch, num_codebooks, dim = indices.shape[0], self.num_codebooks, cond_embeddings.shape[-1]
-
- cond_embeddings = repeat(cond_embeddings, 'q c d -> b q c d', b = batch)
- indices = repeat(indices, 'b q -> b q 1 d', q = num_codebooks, d = dim)
-
- cond_embeddings = cond_embeddings.gather(2, indices)
- return rearrange(cond_embeddings, 'b q 1 d -> b q d')
-
-@beartype
-class MusicLM(nn.Module):
- def __init__(
- self,
- audio_lm: AudioLM,
- mulan_embed_quantizer: MuLaNEmbedQuantizer
- ):
- super().__init__()
- assert not exists(audio_lm.audio_conditioner), 'mulan must not have been passed into AudioLM. it will be managed externally now, embedding the text into the joint embedding space for text-to-audio synthesis'
-
- self.mulan_embed_quantizer = mulan_embed_quantizer
- self.audio_lm = audio_lm
-
- @torch.no_grad()
- def forward(
- self,
- raw_texts: List[str],
- **audio_lm_kwargs
- ):
- self.eval()
-
- texts = tokenizer.tokenize(raw_texts)
-
- text_embeds = self.mulan_embed_quantizer(texts = texts)
-
- return self.audio_lm(text_embeds = text_embeds, **audio_lm_kwargs)
\ No newline at end of file
diff --git a/spaces/Giuvyz/rvc-genshin/vc_infer_pipeline.py b/spaces/Giuvyz/rvc-genshin/vc_infer_pipeline.py
deleted file mode 100644
index c26d45068f9b6bf2b194b13c3c89f8a06347c124..0000000000000000000000000000000000000000
--- a/spaces/Giuvyz/rvc-genshin/vc_infer_pipeline.py
+++ /dev/null
@@ -1,306 +0,0 @@
-import numpy as np, parselmouth, torch, pdb
-from time import time as ttime
-import torch.nn.functional as F
-from config import x_pad, x_query, x_center, x_max
-import scipy.signal as signal
-import pyworld, os, traceback, faiss
-from scipy import signal
-
-bh, ah = signal.butter(N=5, Wn=48, btype="high", fs=16000)
-
-
-class VC(object):
- def __init__(self, tgt_sr, device, is_half):
- self.sr = 16000 # hubert输入采样率
- self.window = 160 # 每帧点数
- self.t_pad = self.sr * x_pad # 每条前后pad时间
- self.t_pad_tgt = tgt_sr * x_pad
- self.t_pad2 = self.t_pad * 2
- self.t_query = self.sr * x_query # 查询切点前后查询时间
- self.t_center = self.sr * x_center # 查询切点位置
- self.t_max = self.sr * x_max # 免查询时长阈值
- self.device = device
- self.is_half = is_half
-
- def get_f0(self, x, p_len, f0_up_key, f0_method, inp_f0=None):
- time_step = self.window / self.sr * 1000
- f0_min = 50
- f0_max = 1100
- f0_mel_min = 1127 * np.log(1 + f0_min / 700)
- f0_mel_max = 1127 * np.log(1 + f0_max / 700)
- if f0_method == "pm":
- f0 = (
- parselmouth.Sound(x, self.sr)
- .to_pitch_ac(
- time_step=time_step / 1000,
- voicing_threshold=0.6,
- pitch_floor=f0_min,
- pitch_ceiling=f0_max,
- )
- .selected_array["frequency"]
- )
- pad_size = (p_len - len(f0) + 1) // 2
- if pad_size > 0 or p_len - len(f0) - pad_size > 0:
- f0 = np.pad(
- f0, [[pad_size, p_len - len(f0) - pad_size]], mode="constant"
- )
- elif f0_method == "harvest":
- f0, t = pyworld.harvest(
- x.astype(np.double),
- fs=self.sr,
- f0_ceil=f0_max,
- f0_floor=f0_min,
- frame_period=10,
- )
- f0 = pyworld.stonemask(x.astype(np.double), f0, t, self.sr)
- f0 = signal.medfilt(f0, 3)
- f0 *= pow(2, f0_up_key / 12)
- # with open("test.txt","w")as f:f.write("\n".join([str(i)for i in f0.tolist()]))
- tf0 = self.sr // self.window # 每秒f0点数
- if inp_f0 is not None:
- delta_t = np.round(
- (inp_f0[:, 0].max() - inp_f0[:, 0].min()) * tf0 + 1
- ).astype("int16")
- replace_f0 = np.interp(
- list(range(delta_t)), inp_f0[:, 0] * 100, inp_f0[:, 1]
- )
- shape = f0[x_pad * tf0 : x_pad * tf0 + len(replace_f0)].shape[0]
- f0[x_pad * tf0 : x_pad * tf0 + len(replace_f0)] = replace_f0[:shape]
- # with open("test_opt.txt","w")as f:f.write("\n".join([str(i)for i in f0.tolist()]))
- f0bak = f0.copy()
- f0_mel = 1127 * np.log(1 + f0 / 700)
- f0_mel[f0_mel > 0] = (f0_mel[f0_mel > 0] - f0_mel_min) * 254 / (
- f0_mel_max - f0_mel_min
- ) + 1
- f0_mel[f0_mel <= 1] = 1
- f0_mel[f0_mel > 255] = 255
- f0_coarse = np.rint(f0_mel).astype(np.int)
- return f0_coarse, f0bak # 1-0
-
- def vc(
- self,
- model,
- net_g,
- sid,
- audio0,
- pitch,
- pitchf,
- times,
- index,
- big_npy,
- index_rate,
- ): # ,file_index,file_big_npy
- feats = torch.from_numpy(audio0)
- if self.is_half:
- feats = feats.half()
- else:
- feats = feats.float()
- if feats.dim() == 2: # double channels
- feats = feats.mean(-1)
- assert feats.dim() == 1, feats.dim()
- feats = feats.view(1, -1)
- padding_mask = torch.BoolTensor(feats.shape).to(self.device).fill_(False)
-
- inputs = {
- "source": feats.to(self.device),
- "padding_mask": padding_mask,
- "output_layer": 9, # layer 9
- }
- t0 = ttime()
- with torch.no_grad():
- logits = model.extract_features(**inputs)
- feats = model.final_proj(logits[0])
-
- if (
- isinstance(index, type(None)) == False
- and isinstance(big_npy, type(None)) == False
- and index_rate != 0
- ):
- npy = feats[0].cpu().numpy()
- if self.is_half:
- npy = npy.astype("float32")
- _, I = index.search(npy, 1)
- npy = big_npy[I.squeeze()]
- if self.is_half:
- npy = npy.astype("float16")
- feats = (
- torch.from_numpy(npy).unsqueeze(0).to(self.device) * index_rate
- + (1 - index_rate) * feats
- )
-
- feats = F.interpolate(feats.permute(0, 2, 1), scale_factor=2).permute(0, 2, 1)
- t1 = ttime()
- p_len = audio0.shape[0] // self.window
- if feats.shape[1] < p_len:
- p_len = feats.shape[1]
- if pitch != None and pitchf != None:
- pitch = pitch[:, :p_len]
- pitchf = pitchf[:, :p_len]
- p_len = torch.tensor([p_len], device=self.device).long()
- with torch.no_grad():
- if pitch != None and pitchf != None:
- audio1 = (
- (net_g.infer(feats, p_len, pitch, pitchf, sid)[0][0, 0] * 32768)
- .data.cpu()
- .float()
- .numpy()
- .astype(np.int16)
- )
- else:
- audio1 = (
- (net_g.infer(feats, p_len, sid)[0][0, 0] * 32768)
- .data.cpu()
- .float()
- .numpy()
- .astype(np.int16)
- )
- del feats, p_len, padding_mask
- if torch.cuda.is_available():
- torch.cuda.empty_cache()
- t2 = ttime()
- times[0] += t1 - t0
- times[2] += t2 - t1
- return audio1
-
- def pipeline(
- self,
- model,
- net_g,
- sid,
- audio,
- times,
- f0_up_key,
- f0_method,
- file_index,
- file_big_npy,
- index_rate,
- if_f0,
- f0_file=None,
- ):
- if (
- file_big_npy != ""
- and file_index != ""
- and os.path.exists(file_big_npy) == True
- and os.path.exists(file_index) == True
- and index_rate != 0
- ):
- try:
- index = faiss.read_index(file_index)
- big_npy = np.load(file_big_npy)
- except:
- traceback.print_exc()
- index = big_npy = None
- else:
- index = big_npy = None
- print("Feature retrieval library doesn't exist or ratio is 0")
- audio = signal.filtfilt(bh, ah, audio)
- audio_pad = np.pad(audio, (self.window // 2, self.window // 2), mode="reflect")
- opt_ts = []
- if audio_pad.shape[0] > self.t_max:
- audio_sum = np.zeros_like(audio)
- for i in range(self.window):
- audio_sum += audio_pad[i : i - self.window]
- for t in range(self.t_center, audio.shape[0], self.t_center):
- opt_ts.append(
- t
- - self.t_query
- + np.where(
- np.abs(audio_sum[t - self.t_query : t + self.t_query])
- == np.abs(audio_sum[t - self.t_query : t + self.t_query]).min()
- )[0][0]
- )
- s = 0
- audio_opt = []
- t = None
- t1 = ttime()
- audio_pad = np.pad(audio, (self.t_pad, self.t_pad), mode="reflect")
- p_len = audio_pad.shape[0] // self.window
- inp_f0 = None
- if hasattr(f0_file, "name") == True:
- try:
- with open(f0_file.name, "r") as f:
- lines = f.read().strip("\n").split("\n")
- inp_f0 = []
- for line in lines:
- inp_f0.append([float(i) for i in line.split(",")])
- inp_f0 = np.array(inp_f0, dtype="float32")
- except:
- traceback.print_exc()
- sid = torch.tensor(sid, device=self.device).unsqueeze(0).long()
- pitch, pitchf = None, None
- if if_f0 == 1:
- pitch, pitchf = self.get_f0(audio_pad, p_len, f0_up_key, f0_method, inp_f0)
- pitch = pitch[:p_len]
- pitchf = pitchf[:p_len]
- pitch = torch.tensor(pitch, device=self.device).unsqueeze(0).long()
- pitchf = torch.tensor(pitchf, device=self.device).unsqueeze(0).float()
- t2 = ttime()
- times[1] += t2 - t1
- for t in opt_ts:
- t = t // self.window * self.window
- if if_f0 == 1:
- audio_opt.append(
- self.vc(
- model,
- net_g,
- sid,
- audio_pad[s : t + self.t_pad2 + self.window],
- pitch[:, s // self.window : (t + self.t_pad2) // self.window],
- pitchf[:, s // self.window : (t + self.t_pad2) // self.window],
- times,
- index,
- big_npy,
- index_rate,
- )[self.t_pad_tgt : -self.t_pad_tgt]
- )
- else:
- audio_opt.append(
- self.vc(
- model,
- net_g,
- sid,
- audio_pad[s : t + self.t_pad2 + self.window],
- None,
- None,
- times,
- index,
- big_npy,
- index_rate,
- )[self.t_pad_tgt : -self.t_pad_tgt]
- )
- s = t
- if if_f0 == 1:
- audio_opt.append(
- self.vc(
- model,
- net_g,
- sid,
- audio_pad[t:],
- pitch[:, t // self.window :] if t is not None else pitch,
- pitchf[:, t // self.window :] if t is not None else pitchf,
- times,
- index,
- big_npy,
- index_rate,
- )[self.t_pad_tgt : -self.t_pad_tgt]
- )
- else:
- audio_opt.append(
- self.vc(
- model,
- net_g,
- sid,
- audio_pad[t:],
- None,
- None,
- times,
- index,
- big_npy,
- index_rate,
- )[self.t_pad_tgt : -self.t_pad_tgt]
- )
- audio_opt = np.concatenate(audio_opt)
- del pitch, pitchf, sid
- if torch.cuda.is_available():
- torch.cuda.empty_cache()
- return audio_opt
diff --git a/spaces/Gmq-x/gpt-academic/crazy_functions/test_project/latex/attention/model_architecture.tex b/spaces/Gmq-x/gpt-academic/crazy_functions/test_project/latex/attention/model_architecture.tex
deleted file mode 100644
index c82be6242cc9d26203360e90d3ac9184ef6ad842..0000000000000000000000000000000000000000
--- a/spaces/Gmq-x/gpt-academic/crazy_functions/test_project/latex/attention/model_architecture.tex
+++ /dev/null
@@ -1,155 +0,0 @@
-
-\begin{figure}
- \centering
- \includegraphics[scale=0.6]{Figures/ModalNet-21}
- \caption{The Transformer - model architecture.}
- \label{fig:model-arch}
-\end{figure}
-
-% Although the primary workhorse of our model is attention,
-%Our model maintains the encoder-decoder structure that is common to many so-called sequence-to-sequence models \citep{bahdanau2014neural,sutskever14}. As in all such architectures, the encoder computes a representation of the input sequence, and the decoder consumes these representations along with the output tokens to autoregressively produce the output sequence. Where, traditionally, the encoder and decoder contain stacks of recurrent or convolutional layers, our encoder and decoder stacks are composed of attention layers and position-wise feed-forward layers (Figure~\ref{fig:model-arch}). The following sections describe the gross architecture and these particular components in detail.
-
-Most competitive neural sequence transduction models have an encoder-decoder structure \citep{cho2014learning,bahdanau2014neural,sutskever14}. Here, the encoder maps an input sequence of symbol representations $(x_1, ..., x_n)$ to a sequence of continuous representations $\mathbf{z} = (z_1, ..., z_n)$. Given $\mathbf{z}$, the decoder then generates an output sequence $(y_1,...,y_m)$ of symbols one element at a time. At each step the model is auto-regressive \citep{graves2013generating}, consuming the previously generated symbols as additional input when generating the next.
-
-The Transformer follows this overall architecture using stacked self-attention and point-wise, fully connected layers for both the encoder and decoder, shown in the left and right halves of Figure~\ref{fig:model-arch}, respectively.
-
-\subsection{Encoder and Decoder Stacks}
-
-\paragraph{Encoder:}The encoder is composed of a stack of $N=6$ identical layers. Each layer has two sub-layers. The first is a multi-head self-attention mechanism, and the second is a simple, position-wise fully connected feed-forward network. We employ a residual connection \citep{he2016deep} around each of the two sub-layers, followed by layer normalization \cite{layernorm2016}. That is, the output of each sub-layer is $\mathrm{LayerNorm}(x + \mathrm{Sublayer}(x))$, where $\mathrm{Sublayer}(x)$ is the function implemented by the sub-layer itself. To facilitate these residual connections, all sub-layers in the model, as well as the embedding layers, produce outputs of dimension $\dmodel=512$.
-
-\paragraph{Decoder:}The decoder is also composed of a stack of $N=6$ identical layers. In addition to the two sub-layers in each encoder layer, the decoder inserts a third sub-layer, which performs multi-head attention over the output of the encoder stack. Similar to the encoder, we employ residual connections around each of the sub-layers, followed by layer normalization. We also modify the self-attention sub-layer in the decoder stack to prevent positions from attending to subsequent positions. This masking, combined with fact that the output embeddings are offset by one position, ensures that the predictions for position $i$ can depend only on the known outputs at positions less than $i$.
-
-% In our model (Figure~\ref{fig:model-arch}), the encoder and decoder are composed of stacks of alternating self-attention layers (for cross-positional communication) and position-wise feed-forward layers (for in-place computation). In addition, the decoder stack contains encoder-decoder attention layers. Since attention is agnostic to the distances between words, our model requires a "positional encoding" to be added to the encoder and decoder input. The following sections describe all of these components in detail.
-
-\subsection{Attention} \label{sec:attention}
-An attention function can be described as mapping a query and a set of key-value pairs to an output, where the query, keys, values, and output are all vectors. The output is computed as a weighted sum of the values, where the weight assigned to each value is computed by a compatibility function of the query with the corresponding key.
-
-\subsubsection{Scaled Dot-Product Attention} \label{sec:scaled-dot-prod}
-
-% \begin{figure}
-% \centering
-% \includegraphics[scale=0.6]{Figures/ModalNet-19}
-% \caption{Scaled Dot-Product Attention.}
-% \label{fig:multi-head-att}
-% \end{figure}
-
-We call our particular attention "Scaled Dot-Product Attention" (Figure~\ref{fig:multi-head-att}). The input consists of queries and keys of dimension $d_k$, and values of dimension $d_v$. We compute the dot products of the query with all keys, divide each by $\sqrt{d_k}$, and apply a softmax function to obtain the weights on the values.
-
-In practice, we compute the attention function on a set of queries simultaneously, packed together into a matrix $Q$. The keys and values are also packed together into matrices $K$ and $V$. We compute the matrix of outputs as:
-
-\begin{equation}
- \mathrm{Attention}(Q, K, V) = \mathrm{softmax}(\frac{QK^T}{\sqrt{d_k}})V
-\end{equation}
-
-The two most commonly used attention functions are additive attention \citep{bahdanau2014neural}, and dot-product (multiplicative) attention. Dot-product attention is identical to our algorithm, except for the scaling factor of $\frac{1}{\sqrt{d_k}}$. Additive attention computes the compatibility function using a feed-forward network with a single hidden layer. While the two are similar in theoretical complexity, dot-product attention is much faster and more space-efficient in practice, since it can be implemented using highly optimized matrix multiplication code.
-
-%We scale the dot products by $1/\sqrt{d_k}$ to limit the magnitude of the dot products, which works well in practice. Otherwise, we found applying the softmax to often result in weights very close to 0 or 1, and hence minuscule gradients.
-
-% Already described in the subsequent section
-%When used as part of decoder self-attention, an optional mask function is applied just before the softmax to prevent positions from attending to subsequent positions. This mask simply sets the logits corresponding to all illegal connections (those outside of the lower triangle) to $-\infty$.
-
-%\paragraph{Comparison to Additive Attention: } We choose dot product attention over additive attention \citep{bahdanau2014neural} since it can be computed using highly optimized matrix multiplication code. This optimization is particularly important to us, as we employ many attention layers in our model.
-
-While for small values of $d_k$ the two mechanisms perform similarly, additive attention outperforms dot product attention without scaling for larger values of $d_k$ \citep{DBLP:journals/corr/BritzGLL17}. We suspect that for large values of $d_k$, the dot products grow large in magnitude, pushing the softmax function into regions where it has extremely small gradients \footnote{To illustrate why the dot products get large, assume that the components of $q$ and $k$ are independent random variables with mean $0$ and variance $1$. Then their dot product, $q \cdot k = \sum_{i=1}^{d_k} q_ik_i$, has mean $0$ and variance $d_k$.}. To counteract this effect, we scale the dot products by $\frac{1}{\sqrt{d_k}}$.
-
-
-%We suspect this to be caused by the dot products growing too large in magnitude to result in useful gradients after applying the softmax function. To counteract this, we scale the dot product by $1/\sqrt{d_k}$.
-
-
-\subsubsection{Multi-Head Attention} \label{sec:multihead}
-
-\begin{figure}
-\begin{minipage}[t]{0.5\textwidth}
- \centering
- Scaled Dot-Product Attention \\
- \vspace{0.5cm}
- \includegraphics[scale=0.6]{Figures/ModalNet-19}
-\end{minipage}
-\begin{minipage}[t]{0.5\textwidth}
- \centering
- Multi-Head Attention \\
- \vspace{0.1cm}
- \includegraphics[scale=0.6]{Figures/ModalNet-20}
-\end{minipage}
-
-
- % \centering
-
- \caption{(left) Scaled Dot-Product Attention. (right) Multi-Head Attention consists of several attention layers running in parallel.}
- \label{fig:multi-head-att}
-\end{figure}
-
-Instead of performing a single attention function with $\dmodel$-dimensional keys, values and queries, we found it beneficial to linearly project the queries, keys and values $h$ times with different, learned linear projections to $d_k$, $d_k$ and $d_v$ dimensions, respectively.
-On each of these projected versions of queries, keys and values we then perform the attention function in parallel, yielding $d_v$-dimensional output values. These are concatenated and once again projected, resulting in the final values, as depicted in Figure~\ref{fig:multi-head-att}.
-
-Multi-head attention allows the model to jointly attend to information from different representation subspaces at different positions. With a single attention head, averaging inhibits this.
-
-\begin{align*}
- \mathrm{MultiHead}(Q, K, V) &= \mathrm{Concat}(\mathrm{head_1}, ..., \mathrm{head_h})W^O\\
-% \mathrm{where} \mathrm{head_i} &= \mathrm{Attention}(QW_Q_i^{\dmodel \times d_q}, KW_K_i^{\dmodel \times d_k}, VW^V_i^{\dmodel \times d_v})\\
- \text{where}~\mathrm{head_i} &= \mathrm{Attention}(QW^Q_i, KW^K_i, VW^V_i)\\
-\end{align*}
-
-Where the projections are parameter matrices $W^Q_i \in \mathbb{R}^{\dmodel \times d_k}$, $W^K_i \in \mathbb{R}^{\dmodel \times d_k}$, $W^V_i \in \mathbb{R}^{\dmodel \times d_v}$ and $W^O \in \mathbb{R}^{hd_v \times \dmodel}$.
-
-
-%find it better (and no more expensive) to have multiple parallel attention layers (each over the full set of positions) with proportionally lower-dimensional keys, values and queries. We call this "Multi-Head Attention" (Figure~\ref{fig:multi-head-att}). The keys, values, and queries for each of these parallel attention layers are computed by learned linear transformations of the inputs to the multi-head attention. We use different linear transformations across different parallel attention layers. The output of the parallel attention layers are concatenated, and then passed through a final learned linear transformation.
-
-In this work we employ $h=8$ parallel attention layers, or heads. For each of these we use $d_k=d_v=\dmodel/h=64$.
-Due to the reduced dimension of each head, the total computational cost is similar to that of single-head attention with full dimensionality.
-
-\subsubsection{Applications of Attention in our Model}
-
-The Transformer uses multi-head attention in three different ways:
-\begin{itemize}
- \item In "encoder-decoder attention" layers, the queries come from the previous decoder layer, and the memory keys and values come from the output of the encoder. This allows every position in the decoder to attend over all positions in the input sequence. This mimics the typical encoder-decoder attention mechanisms in sequence-to-sequence models such as \citep{wu2016google, bahdanau2014neural,JonasFaceNet2017}.
-
- \item The encoder contains self-attention layers. In a self-attention layer all of the keys, values and queries come from the same place, in this case, the output of the previous layer in the encoder. Each position in the encoder can attend to all positions in the previous layer of the encoder.
-
- \item Similarly, self-attention layers in the decoder allow each position in the decoder to attend to all positions in the decoder up to and including that position. We need to prevent leftward information flow in the decoder to preserve the auto-regressive property. We implement this inside of scaled dot-product attention by masking out (setting to $-\infty$) all values in the input of the softmax which correspond to illegal connections. See Figure~\ref{fig:multi-head-att}.
-
-\end{itemize}
-
-\subsection{Position-wise Feed-Forward Networks}\label{sec:ffn}
-
-In addition to attention sub-layers, each of the layers in our encoder and decoder contains a fully connected feed-forward network, which is applied to each position separately and identically. This consists of two linear transformations with a ReLU activation in between.
-
-\begin{equation}
- \mathrm{FFN}(x)=\max(0, xW_1 + b_1) W_2 + b_2
-\end{equation}
-
-While the linear transformations are the same across different positions, they use different parameters from layer to layer. Another way of describing this is as two convolutions with kernel size 1. The dimensionality of input and output is $\dmodel=512$, and the inner-layer has dimensionality $d_{ff}=2048$.
-
-
-
-%In the appendix, we describe how the position-wise feed-forward network can also be seen as a form of attention.
-
-%from Jakob: The number of operations required for the model to relate signals from two arbitrary input or output positions grows in the distance between positions in input or output, linearly for ConvS2S and logarithmically for ByteNet, making it harder to learn dependencies between these positions \citep{hochreiter2001gradient}. In the transformer this is reduced to a constant number of operations, albeit at the cost of effective resolution caused by averaging attention-weighted positions, an effect we aim to counteract with multi-headed attention.
-
-
-%Figure~\ref{fig:simple-att} presents a simple attention function, $A$, with a single head, that forms the basis of our multi-head attention. $A$ takes a query key vector $\kq$, matrices of memory keys $\km$ and memory values $\vm$ ,and produces a query value vector $\vq$ as
-%\begin{equation*} \label{eq:attention}
-% A(\kq, \km, \vm) = {\vm}^T (Softmax(\km \kq).
-%\end{equation*}
-%We linearly transform $\kq,\,\km$, and $\vm$ with learned matrices ${\Wkq \text{,} \, \Wkm}$, and ${\Wvm}$ before calling the attention function, and transform the output query with $\Wvq$ before handing it to the feed forward layer. Each attention layer has it's own set of transformation matrices, which are shared across all query positions. $A$ is applied in parallel for each query position, and is implemented very efficiently as a batch of matrix multiplies. The self-attention and encoder-decoder attention layers use $A$, but with different arguments. For example, in encdoder self-attention, queries in encoder layer $i$ attention to memories in encoder layer $i-1$. To ensure that decoder self-attention layers do not look at future words, we add $- \inf$ to the softmax logits in positions $j+1$ to query length for query position $l$.
-
-%In simple attention, the query value is a weighted combination of the memory values where the attention weights sum to one. Although this function performs well in practice, the constraint on attention weights can restrict the amount of information that flows from memories to queries because the query cannot focus on multiple memory positions at once, which might be desirable when translating long sequences. \marginpar{@usz, could you think of an example of this ?} We remedy this by maintaining multiple attention heads at each query position that attend to all memory positions in parallel, with a different set of parameters per attention head $h$.
-%\marginpar{}
-
-\subsection{Embeddings and Softmax}
-Similarly to other sequence transduction models, we use learned embeddings to convert the input tokens and output tokens to vectors of dimension $\dmodel$. We also use the usual learned linear transformation and softmax function to convert the decoder output to predicted next-token probabilities. In our model, we share the same weight matrix between the two embedding layers and the pre-softmax linear transformation, similar to \citep{press2016using}. In the embedding layers, we multiply those weights by $\sqrt{\dmodel}$.
-
-
-\subsection{Positional Encoding}
-Since our model contains no recurrence and no convolution, in order for the model to make use of the order of the sequence, we must inject some information about the relative or absolute position of the tokens in the sequence. To this end, we add "positional encodings" to the input embeddings at the bottoms of the encoder and decoder stacks. The positional encodings have the same dimension $\dmodel$ as the embeddings, so that the two can be summed. There are many choices of positional encodings, learned and fixed \citep{JonasFaceNet2017}.
-
-In this work, we use sine and cosine functions of different frequencies:
-
-\begin{align*}
- PE_{(pos,2i)} = sin(pos / 10000^{2i/\dmodel}) \\
- PE_{(pos,2i+1)} = cos(pos / 10000^{2i/\dmodel})
-\end{align*}
-
-where $pos$ is the position and $i$ is the dimension. That is, each dimension of the positional encoding corresponds to a sinusoid. The wavelengths form a geometric progression from $2\pi$ to $10000 \cdot 2\pi$. We chose this function because we hypothesized it would allow the model to easily learn to attend by relative positions, since for any fixed offset $k$, $PE_{pos+k}$ can be represented as a linear function of $PE_{pos}$.
-
-We also experimented with using learned positional embeddings \citep{JonasFaceNet2017} instead, and found that the two versions produced nearly identical results (see Table~\ref{tab:variations} row (E)). We chose the sinusoidal version because it may allow the model to extrapolate to sequence lengths longer than the ones encountered during training.
diff --git a/spaces/Gradio-Blocks/uniformer_image_detection/configs/groie/mask_rcnn_r101_fpn_syncbn-backbone_r4_gcb_c3-c5_groie_1x_coco.py b/spaces/Gradio-Blocks/uniformer_image_detection/configs/groie/mask_rcnn_r101_fpn_syncbn-backbone_r4_gcb_c3-c5_groie_1x_coco.py
deleted file mode 100644
index 8b83722197c69a51907f43bcb05883deedc37f0c..0000000000000000000000000000000000000000
--- a/spaces/Gradio-Blocks/uniformer_image_detection/configs/groie/mask_rcnn_r101_fpn_syncbn-backbone_r4_gcb_c3-c5_groie_1x_coco.py
+++ /dev/null
@@ -1,45 +0,0 @@
-_base_ = '../gcnet/mask_rcnn_r101_fpn_syncbn-backbone_r4_gcb_c3-c5_1x_coco.py'
-# model settings
-model = dict(
- roi_head=dict(
- bbox_roi_extractor=dict(
- type='GenericRoIExtractor',
- aggregation='sum',
- roi_layer=dict(type='RoIAlign', output_size=7, sampling_ratio=2),
- out_channels=256,
- featmap_strides=[4, 8, 16, 32],
- pre_cfg=dict(
- type='ConvModule',
- in_channels=256,
- out_channels=256,
- kernel_size=5,
- padding=2,
- inplace=False,
- ),
- post_cfg=dict(
- type='GeneralizedAttention',
- in_channels=256,
- spatial_range=-1,
- num_heads=6,
- attention_type='0100',
- kv_stride=2)),
- mask_roi_extractor=dict(
- type='GenericRoIExtractor',
- roi_layer=dict(type='RoIAlign', output_size=14, sampling_ratio=2),
- out_channels=256,
- featmap_strides=[4, 8, 16, 32],
- pre_cfg=dict(
- type='ConvModule',
- in_channels=256,
- out_channels=256,
- kernel_size=5,
- padding=2,
- inplace=False,
- ),
- post_cfg=dict(
- type='GeneralizedAttention',
- in_channels=256,
- spatial_range=-1,
- num_heads=6,
- attention_type='0100',
- kv_stride=2))))
diff --git a/spaces/Gradio-Blocks/uniformer_image_segmentation/configs/fcn/fcn_d6_r50-d16_769x769_80k_cityscapes.py b/spaces/Gradio-Blocks/uniformer_image_segmentation/configs/fcn/fcn_d6_r50-d16_769x769_80k_cityscapes.py
deleted file mode 100644
index c5ef3b880eac7dd089aace8ce2a87e1bd837beed..0000000000000000000000000000000000000000
--- a/spaces/Gradio-Blocks/uniformer_image_segmentation/configs/fcn/fcn_d6_r50-d16_769x769_80k_cityscapes.py
+++ /dev/null
@@ -1,10 +0,0 @@
-_base_ = [
- '../_base_/models/fcn_r50-d8.py',
- '../_base_/datasets/cityscapes_769x769.py', '../_base_/default_runtime.py',
- '../_base_/schedules/schedule_80k.py'
-]
-model = dict(
- backbone=dict(dilations=(1, 1, 1, 2), strides=(1, 2, 2, 1)),
- decode_head=dict(align_corners=True, dilation=6),
- auxiliary_head=dict(align_corners=True, dilation=6),
- test_cfg=dict(mode='slide', crop_size=(769, 769), stride=(513, 513)))
diff --git a/spaces/Greysuki/whisper-api-compress/README.md b/spaces/Greysuki/whisper-api-compress/README.md
deleted file mode 100644
index f991dd7a046cc23ae6d74725f7b12c8b7200db5e..0000000000000000000000000000000000000000
--- a/spaces/Greysuki/whisper-api-compress/README.md
+++ /dev/null
@@ -1,13 +0,0 @@
----
-title: Whisper Api Compress
-emoji: 🐈
-colorFrom: red
-colorTo: yellow
-sdk: gradio
-sdk_version: 3.24.1
-app_file: app.py
-pinned: false
-license: mit
----
-
-Check out the configuration reference at https://huggingface.co/docs/hub/spaces-config-reference
diff --git a/spaces/Hallucinate/demo/AdaBins-main/dataloader.py b/spaces/Hallucinate/demo/AdaBins-main/dataloader.py
deleted file mode 100644
index 4de1ac1b9016d5b23618d06b877c3bb3c24dd0f2..0000000000000000000000000000000000000000
--- a/spaces/Hallucinate/demo/AdaBins-main/dataloader.py
+++ /dev/null
@@ -1,284 +0,0 @@
-# This file is mostly taken from BTS; author: Jin Han Lee, with only slight modifications
-
-import os
-import random
-
-import numpy as np
-import torch
-import torch.utils.data.distributed
-from PIL import Image
-from torch.utils.data import Dataset, DataLoader
-from torchvision import transforms
-
-
-def _is_pil_image(img):
- return isinstance(img, Image.Image)
-
-
-def _is_numpy_image(img):
- return isinstance(img, np.ndarray) and (img.ndim in {2, 3})
-
-
-def preprocessing_transforms(mode):
- return transforms.Compose([
- ToTensor(mode=mode)
- ])
-
-
-class DepthDataLoader(object):
- def __init__(self, args, mode):
- if mode == 'train':
- self.training_samples = DataLoadPreprocess(args, mode, transform=preprocessing_transforms(mode))
- if args.distributed:
- self.train_sampler = torch.utils.data.distributed.DistributedSampler(self.training_samples)
- else:
- self.train_sampler = None
-
- self.data = DataLoader(self.training_samples, args.batch_size,
- shuffle=(self.train_sampler is None),
- num_workers=args.num_threads,
- pin_memory=True,
- sampler=self.train_sampler)
-
- elif mode == 'online_eval':
- self.testing_samples = DataLoadPreprocess(args, mode, transform=preprocessing_transforms(mode))
- if args.distributed: # redundant. here only for readability and to be more explicit
- # Give whole test set to all processes (and perform/report evaluation only on one) regardless
- self.eval_sampler = None
- else:
- self.eval_sampler = None
- self.data = DataLoader(self.testing_samples, 1,
- shuffle=False,
- num_workers=1,
- pin_memory=False,
- sampler=self.eval_sampler)
-
- elif mode == 'test':
- self.testing_samples = DataLoadPreprocess(args, mode, transform=preprocessing_transforms(mode))
- self.data = DataLoader(self.testing_samples, 1, shuffle=False, num_workers=1)
-
- else:
- print('mode should be one of \'train, test, online_eval\'. Got {}'.format(mode))
-
-
-def remove_leading_slash(s):
- if s[0] == '/' or s[0] == '\\':
- return s[1:]
- return s
-
-
-class DataLoadPreprocess(Dataset):
- def __init__(self, args, mode, transform=None, is_for_online_eval=False):
- self.args = args
- if mode == 'online_eval':
- with open(args.filenames_file_eval, 'r') as f:
- self.filenames = f.readlines()
- else:
- with open(args.filenames_file, 'r') as f:
- self.filenames = f.readlines()
-
- self.mode = mode
- self.transform = transform
- self.to_tensor = ToTensor
- self.is_for_online_eval = is_for_online_eval
-
- def __getitem__(self, idx):
- sample_path = self.filenames[idx]
- focal = float(sample_path.split()[2])
-
- if self.mode == 'train':
- if self.args.dataset == 'kitti' and self.args.use_right is True and random.random() > 0.5:
- image_path = os.path.join(self.args.data_path, remove_leading_slash(sample_path.split()[3]))
- depth_path = os.path.join(self.args.gt_path, remove_leading_slash(sample_path.split()[4]))
- else:
- image_path = os.path.join(self.args.data_path, remove_leading_slash(sample_path.split()[0]))
- depth_path = os.path.join(self.args.gt_path, remove_leading_slash(sample_path.split()[1]))
-
- image = Image.open(image_path)
- depth_gt = Image.open(depth_path)
-
- if self.args.do_kb_crop is True:
- height = image.height
- width = image.width
- top_margin = int(height - 352)
- left_margin = int((width - 1216) / 2)
- depth_gt = depth_gt.crop((left_margin, top_margin, left_margin + 1216, top_margin + 352))
- image = image.crop((left_margin, top_margin, left_margin + 1216, top_margin + 352))
-
- # To avoid blank boundaries due to pixel registration
- if self.args.dataset == 'nyu':
- depth_gt = depth_gt.crop((43, 45, 608, 472))
- image = image.crop((43, 45, 608, 472))
-
- if self.args.do_random_rotate is True:
- random_angle = (random.random() - 0.5) * 2 * self.args.degree
- image = self.rotate_image(image, random_angle)
- depth_gt = self.rotate_image(depth_gt, random_angle, flag=Image.NEAREST)
-
- image = np.asarray(image, dtype=np.float32) / 255.0
- depth_gt = np.asarray(depth_gt, dtype=np.float32)
- depth_gt = np.expand_dims(depth_gt, axis=2)
-
- if self.args.dataset == 'nyu':
- depth_gt = depth_gt / 1000.0
- else:
- depth_gt = depth_gt / 256.0
-
- image, depth_gt = self.random_crop(image, depth_gt, self.args.input_height, self.args.input_width)
- image, depth_gt = self.train_preprocess(image, depth_gt)
- sample = {'image': image, 'depth': depth_gt, 'focal': focal}
-
- else:
- if self.mode == 'online_eval':
- data_path = self.args.data_path_eval
- else:
- data_path = self.args.data_path
-
- image_path = os.path.join(data_path, remove_leading_slash(sample_path.split()[0]))
- image = np.asarray(Image.open(image_path), dtype=np.float32) / 255.0
-
- if self.mode == 'online_eval':
- gt_path = self.args.gt_path_eval
- depth_path = os.path.join(gt_path, remove_leading_slash(sample_path.split()[1]))
- has_valid_depth = False
- try:
- depth_gt = Image.open(depth_path)
- has_valid_depth = True
- except IOError:
- depth_gt = False
- # print('Missing gt for {}'.format(image_path))
-
- if has_valid_depth:
- depth_gt = np.asarray(depth_gt, dtype=np.float32)
- depth_gt = np.expand_dims(depth_gt, axis=2)
- if self.args.dataset == 'nyu':
- depth_gt = depth_gt / 1000.0
- else:
- depth_gt = depth_gt / 256.0
-
- if self.args.do_kb_crop is True:
- height = image.shape[0]
- width = image.shape[1]
- top_margin = int(height - 352)
- left_margin = int((width - 1216) / 2)
- image = image[top_margin:top_margin + 352, left_margin:left_margin + 1216, :]
- if self.mode == 'online_eval' and has_valid_depth:
- depth_gt = depth_gt[top_margin:top_margin + 352, left_margin:left_margin + 1216, :]
-
- if self.mode == 'online_eval':
- sample = {'image': image, 'depth': depth_gt, 'focal': focal, 'has_valid_depth': has_valid_depth,
- 'image_path': sample_path.split()[0], 'depth_path': sample_path.split()[1]}
- else:
- sample = {'image': image, 'focal': focal}
-
- if self.transform:
- sample = self.transform(sample)
-
- return sample
-
- def rotate_image(self, image, angle, flag=Image.BILINEAR):
- result = image.rotate(angle, resample=flag)
- return result
-
- def random_crop(self, img, depth, height, width):
- assert img.shape[0] >= height
- assert img.shape[1] >= width
- assert img.shape[0] == depth.shape[0]
- assert img.shape[1] == depth.shape[1]
- x = random.randint(0, img.shape[1] - width)
- y = random.randint(0, img.shape[0] - height)
- img = img[y:y + height, x:x + width, :]
- depth = depth[y:y + height, x:x + width, :]
- return img, depth
-
- def train_preprocess(self, image, depth_gt):
- # Random flipping
- do_flip = random.random()
- if do_flip > 0.5:
- image = (image[:, ::-1, :]).copy()
- depth_gt = (depth_gt[:, ::-1, :]).copy()
-
- # Random gamma, brightness, color augmentation
- do_augment = random.random()
- if do_augment > 0.5:
- image = self.augment_image(image)
-
- return image, depth_gt
-
- def augment_image(self, image):
- # gamma augmentation
- gamma = random.uniform(0.9, 1.1)
- image_aug = image ** gamma
-
- # brightness augmentation
- if self.args.dataset == 'nyu':
- brightness = random.uniform(0.75, 1.25)
- else:
- brightness = random.uniform(0.9, 1.1)
- image_aug = image_aug * brightness
-
- # color augmentation
- colors = np.random.uniform(0.9, 1.1, size=3)
- white = np.ones((image.shape[0], image.shape[1]))
- color_image = np.stack([white * colors[i] for i in range(3)], axis=2)
- image_aug *= color_image
- image_aug = np.clip(image_aug, 0, 1)
-
- return image_aug
-
- def __len__(self):
- return len(self.filenames)
-
-
-class ToTensor(object):
- def __init__(self, mode):
- self.mode = mode
- self.normalize = transforms.Normalize(mean=[0.485, 0.456, 0.406], std=[0.229, 0.224, 0.225])
-
- def __call__(self, sample):
- image, focal = sample['image'], sample['focal']
- image = self.to_tensor(image)
- image = self.normalize(image)
-
- if self.mode == 'test':
- return {'image': image, 'focal': focal}
-
- depth = sample['depth']
- if self.mode == 'train':
- depth = self.to_tensor(depth)
- return {'image': image, 'depth': depth, 'focal': focal}
- else:
- has_valid_depth = sample['has_valid_depth']
- return {'image': image, 'depth': depth, 'focal': focal, 'has_valid_depth': has_valid_depth,
- 'image_path': sample['image_path'], 'depth_path': sample['depth_path']}
-
- def to_tensor(self, pic):
- if not (_is_pil_image(pic) or _is_numpy_image(pic)):
- raise TypeError(
- 'pic should be PIL Image or ndarray. Got {}'.format(type(pic)))
-
- if isinstance(pic, np.ndarray):
- img = torch.from_numpy(pic.transpose((2, 0, 1)))
- return img
-
- # handle PIL Image
- if pic.mode == 'I':
- img = torch.from_numpy(np.array(pic, np.int32, copy=False))
- elif pic.mode == 'I;16':
- img = torch.from_numpy(np.array(pic, np.int16, copy=False))
- else:
- img = torch.ByteTensor(torch.ByteStorage.from_buffer(pic.tobytes()))
- # PIL image mode: 1, L, P, I, F, RGB, YCbCr, RGBA, CMYK
- if pic.mode == 'YCbCr':
- nchannel = 3
- elif pic.mode == 'I;16':
- nchannel = 1
- else:
- nchannel = len(pic.mode)
- img = img.view(pic.size[1], pic.size[0], nchannel)
-
- img = img.transpose(0, 1).transpose(0, 2).contiguous()
- if isinstance(img, torch.ByteTensor):
- return img.float()
- else:
- return img
diff --git a/spaces/HaloMaster/chinesesummary/fengshen/data/bert_dataloader/preprocessing.py b/spaces/HaloMaster/chinesesummary/fengshen/data/bert_dataloader/preprocessing.py
deleted file mode 100644
index c40e39a8122a5cc4ebd57b558f451c371f6066a3..0000000000000000000000000000000000000000
--- a/spaces/HaloMaster/chinesesummary/fengshen/data/bert_dataloader/preprocessing.py
+++ /dev/null
@@ -1,110 +0,0 @@
-import re
-import json
-import multiprocessing
-from tqdm import tqdm
-from pathlib import Path
-from itertools import chain
-
-_SPLIT_DATA_PATH = '/data1/datas/wudao_180g'
-
-
-def cut_sent(path):
- """
- 中文分句,默认?、。、!、省略号分句,考虑双引号包裹的句子
- 采用分割替换的方式
- """
- path = Path(path)
- # print(path)
- save_path = str(Path('/data1/datas/wudao_180g_split', path.name))
- print('处理文件:', save_path)
- with open(save_path, 'wt', encoding='utf-8') as w:
- with open(path, 'rt', encoding='utf-8') as f:
- for para in tqdm(f):
- para = json.loads(para)
- para_ = para['text'] + ' '
- # print('sentence piece......')
- # pep8中 正则不能些 \? 要写成\\?
- para_ = re.sub('([?。!\\?\\!…]+)([^”’]|[”’])',
- r'\1#####\2', para_)
- para_ = re.sub('([\\.]{3,})([^”’])', r'\1#####\2', para_)
-
- # 匹配 \1: 句子结束符紧挨’” \2: 非句子结束符号,被引号包裹的句子
- para_ = re.sub(
- '([。!?\\?\\!…][”’])([^,。!?\\?\\!]|\\s)', r'\1#####\2', para_)
- para_ = re.sub(
- '([\\.]{3,}[”’])([^,。!?\\?\\!]|\\s)', r'\1#####\2', para_)
- para_ = re.sub(
- '([#]{5})([”’])([^,。!?\\?\\!])', r'\2#####\3', para_)
- para_ = para_.strip()
- # 一个512里面多个样本
- line_ = ''
- for line in para_.split('#####'):
- line = line.strip()
- if len(line_) < 512 and len(line) > 0:
- line_ += line
- else:
- w.writelines(json.dumps(
- {'text': line_}, ensure_ascii=False)+'\n')
- line_ = line
- w.writelines(json.dumps(
- {'text': line_}, ensure_ascii=False)+'\n')
-
-
-def chain_iter(*filenames):
- """
- 将多个文件读成一个迭代器
- """
- reader = [open(file, 'r') for file in filenames]
- return chain(*reader)
-
-
-class Config(object):
-
- def __init__(self, data_path=_SPLIT_DATA_PATH, num_worker=16, split_numb=600000, cut_sentence=True, output_file=None) -> None:
- self.data_path = Path(data_path)
- self.num_worker = num_worker
- self.split_numb = split_numb
- self.cut_sentence = cut_sentence
-
-
-def processing1():
- args = Config()
- p_ = [str(i) for i in args.data_path.glob('*')]
- fin = chain_iter(*p_)
- pool = multiprocessing.Pool(args.num_worker)
- docs = pool.imap(cut_sent, fin, chunksize=args.num_worker)
-
- if not Path(args.data_path.parent, args.data_path.name+'_split').exists():
- Path(args.data_path.parent, args.data_path.name+'_split').mkdir()
- writer = open(str(Path(args.data_path.parent, args.data_path.name +
- '_split', 'sentence_level.json')), 'wt', encoding='utf-8')
- for doc in tqdm(docs):
- for sentence in doc:
- writer.writelines(json.dumps(
- {"text": sentence}, ensure_ascii=False)+'\n')
- pool.close()
- pool.join()
- writer.close()
-
-
-if __name__ == '__main__':
- from time import process_time, perf_counter
- from random import shuffle
- st = process_time()
- args = Config(num_worker=16)
-
- if not Path(args.data_path.parent, args.data_path.name+'_split').exists():
- Path(args.data_path.parent, args.data_path.name +
- '_split').mkdir(parents=True)
-
- p_ = [str(i) for i in args.data_path.glob('*')]
- # 简单shuffle
- shuffle(p_)
-
- pool = multiprocessing.Pool(args.num_worker)
- for item in p_:
- pool.apply_async(func=cut_sent, args=(item,))
- pool.close()
- pool.join()
- cost_time = process_time() - st
- print('DONE!! cost time : %.5f' % cost_time)
diff --git a/spaces/HarryLee/eCommerceImageCaptioning/fairseq/fairseq/models/wav2vec/wav2vec2.py b/spaces/HarryLee/eCommerceImageCaptioning/fairseq/fairseq/models/wav2vec/wav2vec2.py
deleted file mode 100644
index 714fd3ab50443b8d15715b1cf5abd4eb517298c4..0000000000000000000000000000000000000000
--- a/spaces/HarryLee/eCommerceImageCaptioning/fairseq/fairseq/models/wav2vec/wav2vec2.py
+++ /dev/null
@@ -1,1016 +0,0 @@
-# Copyright (c) Facebook, Inc. and its affiliates.
-#
-# This source code is licensed under the MIT license found in the
-# LICENSE file in the root directory of this source tree.
-
-import math
-from dataclasses import dataclass, field
-from typing import List, Tuple
-
-import numpy as np
-import torch
-import torch.nn as nn
-import torch.nn.functional as F
-from fairseq import utils
-from fairseq.data.data_utils import compute_mask_indices
-from fairseq.dataclass import ChoiceEnum, FairseqDataclass
-from fairseq.models import BaseFairseqModel, register_model
-from fairseq.modules import (
- Fp32GroupNorm,
- Fp32LayerNorm,
- GradMultiply,
- GumbelVectorQuantizer,
- LayerNorm,
- MultiheadAttention,
- SamePad,
- TransposeLast,
-)
-from fairseq.modules.transformer_sentence_encoder import init_bert_params
-from fairseq.utils import buffered_arange, index_put, is_xla_tensor
-
-
-EXTRACTOR_MODE_CHOICES = ChoiceEnum(["default", "layer_norm"])
-MASKING_DISTRIBUTION_CHOICES = ChoiceEnum(["static", "uniform", "normal", "poisson"])
-
-
-@dataclass
-class Wav2Vec2Config(FairseqDataclass):
- extractor_mode: EXTRACTOR_MODE_CHOICES = field(
- default="default",
- metadata={
- "help": "mode for feature extractor. default has a single group norm with d "
- "groups in the first conv block, whereas layer_norm has layer norms in "
- "every block (meant to use with normalize=True)"
- },
- )
- encoder_layers: int = field(
- default=12, metadata={"help": "num encoder layers in the transformer"}
- )
- encoder_embed_dim: int = field(
- default=768, metadata={"help": "encoder embedding dimension"}
- )
- encoder_ffn_embed_dim: int = field(
- default=3072, metadata={"help": "encoder embedding dimension for FFN"}
- )
- encoder_attention_heads: int = field(
- default=12, metadata={"help": "num encoder attention heads"}
- )
- activation_fn: ChoiceEnum(utils.get_available_activation_fns()) = field(
- default="gelu", metadata={"help": "activation function to use"}
- )
-
- # dropouts
- dropout: float = field(
- default=0.1, metadata={"help": "dropout probability for the transformer"}
- )
- attention_dropout: float = field(
- default=0.1, metadata={"help": "dropout probability for attention weights"}
- )
- activation_dropout: float = field(
- default=0.0, metadata={"help": "dropout probability after activation in FFN"}
- )
- encoder_layerdrop: float = field(
- default=0.0, metadata={"help": "probability of dropping a tarnsformer layer"}
- )
- dropout_input: float = field(
- default=0.0,
- metadata={"help": "dropout to apply to the input (after feat extr)"},
- )
- dropout_features: float = field(
- default=0.0,
- metadata={"help": "dropout to apply to the features (after feat extr)"},
- )
-
- final_dim: int = field(
- default=0,
- metadata={
- "help": "project final representations and targets to this many dimensions."
- "set to encoder_embed_dim is <= 0"
- },
- )
- layer_norm_first: bool = field(
- default=False, metadata={"help": "apply layernorm first in the transformer"}
- )
- conv_feature_layers: str = field(
- default="[(512, 10, 5)] + [(512, 3, 2)] * 4 + [(512,2,2)] + [(512,2,2)]",
- metadata={
- "help": "string describing convolutional feature extraction layers in form of a python list that contains "
- "[(dim, kernel_size, stride), ...]"
- },
- )
- conv_bias: bool = field(
- default=False, metadata={"help": "include bias in conv encoder"}
- )
- logit_temp: float = field(
- default=0.1, metadata={"help": "temperature to divide logits by"}
- )
- quantize_targets: bool = field(
- default=False, metadata={"help": "use quantized targets"}
- )
- quantize_input: bool = field(
- default=False, metadata={"help": "use quantized inputs"}
- )
- same_quantizer: bool = field(
- default=False, metadata={"help": "use same quantizer for inputs and targets"}
- )
- target_glu: bool = field(
- default=False, metadata={"help": "adds projection + glu to targets"}
- )
- feature_grad_mult: float = field(
- default=1.0, metadata={"help": "multiply feature extractor var grads by this"}
- )
- quantizer_depth: int = field(
- default=1,
- metadata={"help": "number of quantizer layers"},
- )
- quantizer_factor: int = field(
- default=3,
- metadata={
- "help": "dimensionality increase for inner quantizer layers (if depth > 1)"
- },
- )
- latent_vars: int = field(
- default=320,
- metadata={"help": "number of latent variables V in each group of the codebook"},
- )
- latent_groups: int = field(
- default=2,
- metadata={"help": "number of groups G of latent variables in the codebook"},
- )
- latent_dim: int = field(
- default=0,
- metadata={
- "help": "if > 0, uses this dimensionality for latent variables. "
- "otherwise uses final_dim / latent_groups"
- },
- )
-
- # masking
- mask_length: int = field(default=10, metadata={"help": "mask length"})
- mask_prob: float = field(
- default=0.65, metadata={"help": "probability of replacing a token with mask"}
- )
- mask_selection: MASKING_DISTRIBUTION_CHOICES = field(
- default="static", metadata={"help": "how to choose mask length"}
- )
- mask_other: float = field(
- default=0,
- metadata={
- "help": "secondary mask argument (used for more complex distributions), "
- "see help in compute_mask_indices"
- },
- )
- no_mask_overlap: bool = field(
- default=False, metadata={"help": "whether to allow masks to overlap"}
- )
- mask_min_space: int = field(
- default=1,
- metadata={"help": "min space between spans (if no overlap is enabled)"},
- )
-
- # channel masking
- mask_channel_length: int = field(
- default=10, metadata={"help": "length of the mask for features (channels)"}
- )
- mask_channel_prob: float = field(
- default=0.0, metadata={"help": "probability of replacing a feature with 0"}
- )
- mask_channel_before: bool = False
- mask_channel_selection: MASKING_DISTRIBUTION_CHOICES = field(
- default="static",
- metadata={"help": "how to choose mask length for channel masking"},
- )
- mask_channel_other: float = field(
- default=0,
- metadata={
- "help": "secondary mask argument (used for more complex distributions), "
- "see help in compute_mask_indicesh"
- },
- )
- no_mask_channel_overlap: bool = field(
- default=False, metadata={"help": "whether to allow channel masks to overlap"}
- )
- mask_channel_min_space: int = field(
- default=1,
- metadata={"help": "min space between spans (if no overlap is enabled)"},
- )
-
- # negative selection
- num_negatives: int = field(
- default=100,
- metadata={"help": "number of negative examples from the same sample"},
- )
- negatives_from_everywhere: bool = field(
- default=False,
- metadata={"help": "sample negatives from everywhere, not just masked states"},
- )
- cross_sample_negatives: int = field(
- default=0, metadata={"help": "number of negative examples from the any sample"}
- )
- codebook_negatives: int = field(
- default=0, metadata={"help": "number of negative examples codebook"}
- )
-
- # positional embeddings
- conv_pos: int = field(
- default=128,
- metadata={"help": "number of filters for convolutional positional embeddings"},
- )
- conv_pos_groups: int = field(
- default=16,
- metadata={"help": "number of groups for convolutional positional embedding"},
- )
-
- latent_temp: Tuple[float, float, float] = field(
- default=(2, 0.5, 0.999995),
- metadata={
- "help": "temperature for latent variable sampling. "
- "can be tuple of 3 values (start, end, decay)"
- },
- )
-
-
-@register_model("wav2vec2", dataclass=Wav2Vec2Config)
-class Wav2Vec2Model(BaseFairseqModel):
- def __init__(self, cfg: Wav2Vec2Config):
- super().__init__()
- self.cfg = cfg
-
- feature_enc_layers = eval(cfg.conv_feature_layers)
- self.embed = feature_enc_layers[-1][0]
-
- self.feature_extractor = ConvFeatureExtractionModel(
- conv_layers=feature_enc_layers,
- dropout=0.0,
- mode=cfg.extractor_mode,
- conv_bias=cfg.conv_bias,
- )
-
- self.post_extract_proj = (
- nn.Linear(self.embed, cfg.encoder_embed_dim)
- if self.embed != cfg.encoder_embed_dim and not cfg.quantize_input
- else None
- )
-
- self.mask_prob = cfg.mask_prob
- self.mask_selection = cfg.mask_selection
- self.mask_other = cfg.mask_other
- self.mask_length = cfg.mask_length
- self.no_mask_overlap = cfg.no_mask_overlap
- self.mask_min_space = cfg.mask_min_space
-
- self.mask_channel_prob = cfg.mask_channel_prob
- self.mask_channel_before = cfg.mask_channel_before
- self.mask_channel_selection = cfg.mask_channel_selection
- self.mask_channel_other = cfg.mask_channel_other
- self.mask_channel_length = cfg.mask_channel_length
- self.no_mask_channel_overlap = cfg.no_mask_channel_overlap
- self.mask_channel_min_space = cfg.mask_channel_min_space
-
- self.dropout_input = nn.Dropout(cfg.dropout_input)
- self.dropout_features = nn.Dropout(cfg.dropout_features)
-
- self.feature_grad_mult = cfg.feature_grad_mult
-
- self.quantizer = None
- self.input_quantizer = None
-
- self.n_negatives = cfg.num_negatives
- self.cross_sample_negatives = cfg.cross_sample_negatives
- self.codebook_negatives = cfg.codebook_negatives
- self.negatives_from_everywhere = cfg.negatives_from_everywhere
-
- self.logit_temp = cfg.logit_temp
-
- final_dim = cfg.final_dim if cfg.final_dim > 0 else cfg.encoder_embed_dim
-
- if cfg.quantize_targets:
- vq_dim = cfg.latent_dim if cfg.latent_dim > 0 else final_dim
- self.quantizer = GumbelVectorQuantizer(
- dim=self.embed,
- num_vars=cfg.latent_vars,
- temp=cfg.latent_temp,
- groups=cfg.latent_groups,
- combine_groups=False,
- vq_dim=vq_dim,
- time_first=True,
- weight_proj_depth=cfg.quantizer_depth,
- weight_proj_factor=cfg.quantizer_factor,
- )
- self.project_q = nn.Linear(vq_dim, final_dim)
- else:
- self.project_q = nn.Linear(self.embed, final_dim)
-
- if cfg.quantize_input:
- if cfg.same_quantizer and self.quantizer is not None:
- vq_dim = final_dim
- self.input_quantizer = self.quantizer
- else:
- vq_dim = cfg.latent_dim if cfg.latent_dim > 0 else cfg.encoder_embed_dim
- self.input_quantizer = GumbelVectorQuantizer(
- dim=self.embed,
- num_vars=cfg.latent_vars,
- temp=cfg.latent_temp,
- groups=cfg.latent_groups,
- combine_groups=False,
- vq_dim=vq_dim,
- time_first=True,
- weight_proj_depth=cfg.quantizer_depth,
- weight_proj_factor=cfg.quantizer_factor,
- )
- self.project_inp = nn.Linear(vq_dim, cfg.encoder_embed_dim)
-
- self.mask_emb = nn.Parameter(
- torch.FloatTensor(cfg.encoder_embed_dim).uniform_()
- )
-
- self.encoder = TransformerEncoder(cfg)
- self.layer_norm = LayerNorm(self.embed)
-
- self.target_glu = None
- if cfg.target_glu:
- self.target_glu = nn.Sequential(
- nn.Linear(final_dim, final_dim * 2), nn.GLU()
- )
-
- self.final_proj = nn.Linear(cfg.encoder_embed_dim, final_dim)
-
- def upgrade_state_dict_named(self, state_dict, name):
- super().upgrade_state_dict_named(state_dict, name)
- """Upgrade a (possibly old) state dict for new versions of fairseq."""
- return state_dict
-
- @classmethod
- def build_model(cls, cfg: Wav2Vec2Config, task=None):
- """Build a new model instance."""
-
- return cls(cfg)
-
- def apply_mask(
- self,
- x,
- padding_mask,
- mask_indices=None,
- mask_channel_indices=None,
- ):
- B, T, C = x.shape
-
- if self.mask_channel_prob > 0 and self.mask_channel_before:
- mask_channel_indices = compute_mask_indices(
- (B, C),
- None,
- self.mask_channel_prob,
- self.mask_channel_length,
- self.mask_channel_selection,
- self.mask_channel_other,
- no_overlap=self.no_mask_channel_overlap,
- min_space=self.mask_channel_min_space,
- )
- mask_channel_indices = (
- torch.from_numpy(mask_channel_indices)
- .to(x.device)
- .unsqueeze(1)
- .expand(-1, T, -1)
- )
- x[mask_channel_indices] = 0
-
- if self.mask_prob > 0:
- if mask_indices is None:
- mask_indices = compute_mask_indices(
- (B, T),
- padding_mask,
- self.mask_prob,
- self.mask_length,
- self.mask_selection,
- self.mask_other,
- min_masks=2,
- no_overlap=self.no_mask_overlap,
- min_space=self.mask_min_space,
- )
- mask_indices = torch.from_numpy(mask_indices).to(x.device)
- x = index_put(x, mask_indices, self.mask_emb)
- else:
- mask_indices = None
-
- if self.mask_channel_prob > 0 and not self.mask_channel_before:
- if mask_channel_indices is None:
- mask_channel_indices = compute_mask_indices(
- (B, C),
- None,
- self.mask_channel_prob,
- self.mask_channel_length,
- self.mask_channel_selection,
- self.mask_channel_other,
- no_overlap=self.no_mask_channel_overlap,
- min_space=self.mask_channel_min_space,
- )
- mask_channel_indices = (
- torch.from_numpy(mask_channel_indices)
- .to(x.device)
- .unsqueeze(1)
- .expand(-1, T, -1)
- )
- x = index_put(x, mask_channel_indices, 0)
-
- return x, mask_indices
-
- def sample_negatives(self, y, num, padding_count=None):
-
- if self.n_negatives == 0 and self.cross_sample_negatives == 0:
- return y.new(0)
-
- bsz, tsz, fsz = y.shape
- y = y.view(-1, fsz) # BTC => (BxT)C
-
- # FIXME: what happens if padding_count is specified?
- cross_high = tsz * bsz
- high = tsz - (padding_count or 0)
- with torch.no_grad():
- assert high > 1, f"{bsz,tsz,fsz}"
-
- if self.n_negatives > 0:
- tszs = (
- buffered_arange(num)
- .unsqueeze(-1)
- .expand(-1, self.n_negatives)
- .flatten()
- )
-
- neg_idxs = torch.randint(
- low=0, high=high - 1, size=(bsz, self.n_negatives * num)
- )
- neg_idxs[neg_idxs >= tszs] += 1
-
- if self.cross_sample_negatives > 0:
- tszs = (
- buffered_arange(num)
- .unsqueeze(-1)
- .expand(-1, self.cross_sample_negatives)
- .flatten()
- )
-
- cross_neg_idxs = torch.randint(
- low=0,
- high=cross_high - 1,
- size=(bsz, self.cross_sample_negatives * num),
- )
- cross_neg_idxs[cross_neg_idxs >= tszs] += 1
-
- if self.n_negatives > 0:
- for i in range(1, bsz):
- neg_idxs[i] += i * high
- else:
- neg_idxs = cross_neg_idxs
-
- if self.cross_sample_negatives > 0 and self.n_negatives > 0:
- neg_idxs = torch.cat([neg_idxs, cross_neg_idxs], dim=1)
-
- negs = y[neg_idxs.view(-1)]
- negs = negs.view(
- bsz, num, self.n_negatives + self.cross_sample_negatives, fsz
- ).permute(
- 2, 0, 1, 3
- ) # to NxBxTxC
- return negs, neg_idxs
-
- def compute_preds(self, x, y, negatives):
-
- neg_is_pos = (y == negatives).all(-1)
- y = y.unsqueeze(0)
- targets = torch.cat([y, negatives], dim=0)
-
- logits = torch.cosine_similarity(x.float(), targets.float(), dim=-1).type_as(x)
-
- logits = logits / self.logit_temp
-
- if is_xla_tensor(logits) or neg_is_pos.any():
- fillval = -float(2 ** 30)
- if not hasattr(self, "_inftensor"):
- self._inftensor = (
- torch.tensor(fillval).to(x.device)
- if is_xla_tensor(logits)
- else float("-inf")
- )
- logits[1:] = index_put(logits[1:], neg_is_pos, self._inftensor)
-
- return logits
-
- def _get_feat_extract_output_lengths(self, input_lengths: torch.LongTensor):
- """
- Computes the output length of the convolutional layers
- """
-
- def _conv_out_length(input_length, kernel_size, stride):
- return torch.floor((input_length - kernel_size) / stride + 1)
-
- conv_cfg_list = eval(self.cfg.conv_feature_layers)
-
- for i in range(len(conv_cfg_list)):
- input_lengths = _conv_out_length(
- input_lengths, conv_cfg_list[i][1], conv_cfg_list[i][2]
- )
-
- return input_lengths.to(torch.long)
-
- def forward(
- self,
- source,
- padding_mask=None,
- mask=True,
- features_only=False,
- layer=None,
- mask_indices=None,
- mask_channel_indices=None,
- padding_count=None,
- ):
-
- if self.feature_grad_mult > 0:
- features = self.feature_extractor(source)
- if self.feature_grad_mult != 1.0:
- features = GradMultiply.apply(features, self.feature_grad_mult)
- else:
- with torch.no_grad():
- features = self.feature_extractor(source)
-
- features_pen = features.float().pow(2).mean()
-
- features = features.transpose(1, 2)
- features = self.layer_norm(features)
- unmasked_features = features.clone()
-
- if padding_mask is not None and padding_mask.any():
- input_lengths = (1 - padding_mask.long()).sum(-1)
- # apply conv formula to get real output_lengths
- output_lengths = self._get_feat_extract_output_lengths(input_lengths)
-
- padding_mask = torch.zeros(
- features.shape[:2], dtype=features.dtype, device=features.device
- )
-
- # these two operations makes sure that all values
- # before the output lengths indices are attended to
- padding_mask[
- (
- torch.arange(padding_mask.shape[0], device=padding_mask.device),
- output_lengths - 1,
- )
- ] = 1
- padding_mask = (1 - padding_mask.flip([-1]).cumsum(-1).flip([-1])).bool()
- else:
- padding_mask = None
-
- if self.post_extract_proj is not None:
- features = self.post_extract_proj(features)
-
- features = self.dropout_input(features)
- unmasked_features = self.dropout_features(unmasked_features)
-
- num_vars = None
- code_ppl = None
- prob_ppl = None
- curr_temp = None
-
- if self.input_quantizer:
- q = self.input_quantizer(features, produce_targets=False)
- features = q["x"]
- num_vars = q["num_vars"]
- code_ppl = q["code_perplexity"]
- prob_ppl = q["prob_perplexity"]
- curr_temp = q["temp"]
- features = self.project_inp(features)
-
- if mask:
- x, mask_indices = self.apply_mask(
- features,
- padding_mask,
- mask_indices=mask_indices,
- mask_channel_indices=mask_channel_indices,
- )
- if not is_xla_tensor(x) and mask_indices is not None:
- # tpu-comment: reducing the size in a dynamic way causes
- # too many recompilations on xla.
- y = unmasked_features[mask_indices].view(
- unmasked_features.size(0), -1, unmasked_features.size(-1)
- )
- else:
- y = unmasked_features
- else:
- x = features
- y = unmasked_features
- mask_indices = None
-
- x, layer_results = self.encoder(x, padding_mask=padding_mask, layer=layer)
-
- if features_only:
- return {
- "x": x,
- "padding_mask": padding_mask,
- "features": unmasked_features,
- "layer_results": layer_results,
- }
-
- if self.quantizer:
- q = self.quantizer(y, produce_targets=False)
- y = q["x"]
- num_vars = q["num_vars"]
- code_ppl = q["code_perplexity"]
- prob_ppl = q["prob_perplexity"]
- curr_temp = q["temp"]
-
- y = self.project_q(y)
-
- if self.negatives_from_everywhere:
- neg_cands = self.quantizer(unmasked_features, produce_targets=False)[
- "x"
- ]
- negs, _ = self.sample_negatives(
- neg_cands,
- y.size(1),
- padding_count=padding_count,
- )
- negs = self.project_q(negs)
-
- else:
- negs, _ = self.sample_negatives(
- y,
- y.size(1),
- padding_count=padding_count,
- )
-
- if self.codebook_negatives > 0:
- cb_negs = self.quantizer.sample_from_codebook(
- y.size(0) * y.size(1), self.codebook_negatives
- )
- cb_negs = cb_negs.view(
- self.codebook_negatives, y.size(0), y.size(1), -1
- ) # order doesnt matter
- cb_negs = self.project_q(cb_negs)
- negs = torch.cat([negs, cb_negs], dim=0)
- else:
- y = self.project_q(y)
-
- if self.negatives_from_everywhere:
- negs, _ = self.sample_negatives(
- unmasked_features,
- y.size(1),
- padding_count=padding_count,
- )
- negs = self.project_q(negs)
- else:
- negs, _ = self.sample_negatives(
- y,
- y.size(1),
- padding_count=padding_count,
- )
-
- if not is_xla_tensor(x):
- # tpu-comment: reducing the size in a dynamic way causes
- # too many recompilations on xla.
- x = x[mask_indices].view(x.size(0), -1, x.size(-1))
-
- if self.target_glu:
- y = self.target_glu(y)
- negs = self.target_glu(negs)
-
- x = self.final_proj(x)
- x = self.compute_preds(x, y, negs)
-
- result = {
- "x": x,
- "padding_mask": padding_mask,
- "features_pen": features_pen,
- }
-
- if prob_ppl is not None:
- result["prob_perplexity"] = prob_ppl
- result["code_perplexity"] = code_ppl
- result["num_vars"] = num_vars
- result["temp"] = curr_temp
-
- return result
-
- def quantize(self, x):
- assert self.quantizer is not None
- x = self.feature_extractor(x)
- x = x.transpose(1, 2)
- x = self.layer_norm(x)
- return self.quantizer.forward_idx(x)
-
- def extract_features(self, source, padding_mask, mask=False, layer=None):
- res = self.forward(
- source, padding_mask, mask=mask, features_only=True, layer=layer
- )
- return res
-
- def get_logits(self, net_output):
- logits = net_output["x"]
- logits = logits.transpose(0, 2)
- logits = logits.reshape(-1, logits.size(-1))
- return logits
-
- def get_targets(self, sample, net_output, expand_steps=True):
- x = net_output["x"]
- return x.new_zeros(x.size(1) * x.size(2), dtype=torch.long)
-
- def get_extra_losses(self, net_output):
- pen = []
-
- if "prob_perplexity" in net_output:
- pen.append(
- (net_output["num_vars"] - net_output["prob_perplexity"])
- / net_output["num_vars"]
- )
-
- if "features_pen" in net_output:
- pen.append(net_output["features_pen"])
-
- return pen
-
- def remove_pretraining_modules(self):
- self.quantizer = None
- self.project_q = None
- self.target_glu = None
- self.final_proj = None
-
-
-class ConvFeatureExtractionModel(nn.Module):
- def __init__(
- self,
- conv_layers: List[Tuple[int, int, int]],
- dropout: float = 0.0,
- mode: str = "default",
- conv_bias: bool = False,
- ):
- super().__init__()
-
- assert mode in {"default", "layer_norm"}
-
- def block(
- n_in,
- n_out,
- k,
- stride,
- is_layer_norm=False,
- is_group_norm=False,
- conv_bias=False,
- ):
- def make_conv():
- conv = nn.Conv1d(n_in, n_out, k, stride=stride, bias=conv_bias)
- nn.init.kaiming_normal_(conv.weight)
- return conv
-
- assert (
- is_layer_norm and is_group_norm
- ) == False, "layer norm and group norm are exclusive"
-
- if is_layer_norm:
- return nn.Sequential(
- make_conv(),
- nn.Dropout(p=dropout),
- nn.Sequential(
- TransposeLast(),
- Fp32LayerNorm(dim, elementwise_affine=True),
- TransposeLast(),
- ),
- nn.GELU(),
- )
- elif is_group_norm:
- return nn.Sequential(
- make_conv(),
- nn.Dropout(p=dropout),
- Fp32GroupNorm(dim, dim, affine=True),
- nn.GELU(),
- )
- else:
- return nn.Sequential(make_conv(), nn.Dropout(p=dropout), nn.GELU())
-
- in_d = 1
- self.conv_layers = nn.ModuleList()
- for i, cl in enumerate(conv_layers):
- assert len(cl) == 3, "invalid conv definition: " + str(cl)
- (dim, k, stride) = cl
-
- self.conv_layers.append(
- block(
- in_d,
- dim,
- k,
- stride,
- is_layer_norm=mode == "layer_norm",
- is_group_norm=mode == "default" and i == 0,
- conv_bias=conv_bias,
- )
- )
- in_d = dim
-
- def forward(self, x):
-
- # BxT -> BxCxT
- x = x.unsqueeze(1)
-
- for conv in self.conv_layers:
- x = conv(x)
-
- return x
-
-
-class TransformerEncoder(nn.Module):
- def __init__(self, args):
- super().__init__()
-
- self.dropout = args.dropout
- self.embedding_dim = args.encoder_embed_dim
-
- self.pos_conv = nn.Conv1d(
- self.embedding_dim,
- self.embedding_dim,
- kernel_size=args.conv_pos,
- padding=args.conv_pos // 2,
- groups=args.conv_pos_groups,
- )
- dropout = 0
- std = math.sqrt((4 * (1.0 - dropout)) / (args.conv_pos * self.embedding_dim))
- nn.init.normal_(self.pos_conv.weight, mean=0, std=std)
- nn.init.constant_(self.pos_conv.bias, 0)
-
- self.pos_conv = nn.utils.weight_norm(self.pos_conv, name="weight", dim=2)
- self.pos_conv = nn.Sequential(self.pos_conv, SamePad(args.conv_pos), nn.GELU())
-
- self.layers = nn.ModuleList(
- [
- TransformerSentenceEncoderLayer(
- embedding_dim=self.embedding_dim,
- ffn_embedding_dim=args.encoder_ffn_embed_dim,
- num_attention_heads=args.encoder_attention_heads,
- dropout=self.dropout,
- attention_dropout=args.attention_dropout,
- activation_dropout=args.activation_dropout,
- activation_fn=args.activation_fn,
- layer_norm_first=args.layer_norm_first,
- )
- for _ in range(args.encoder_layers)
- ]
- )
-
- self.layer_norm_first = args.layer_norm_first
- self.layer_norm = LayerNorm(self.embedding_dim)
- self.layerdrop = args.encoder_layerdrop
-
- self.apply(init_bert_params)
-
- def forward(self, x, padding_mask=None, layer=None):
- x, layer_results = self.extract_features(x, padding_mask, layer)
-
- if self.layer_norm_first and layer is None:
- x = self.layer_norm(x)
-
- return x, layer_results
-
- def extract_features(self, x, padding_mask=None, tgt_layer=None):
-
- if padding_mask is not None:
- x = index_put(x, padding_mask, 0)
-
- x_conv = self.pos_conv(x.transpose(1, 2))
- x_conv = x_conv.transpose(1, 2)
- x = x + x_conv
-
- if not self.layer_norm_first:
- x = self.layer_norm(x)
-
- x = F.dropout(x, p=self.dropout, training=self.training)
-
- # B x T x C -> T x B x C
- x = x.transpose(0, 1)
-
- layer_results = []
- r = None
- for i, layer in enumerate(self.layers):
- dropout_probability = np.random.random()
- if not self.training or (dropout_probability > self.layerdrop):
- x, z = layer(x, self_attn_padding_mask=padding_mask, need_weights=False)
- if tgt_layer is not None:
- layer_results.append((x, z))
- if i == tgt_layer:
- r = x
- break
-
- if r is not None:
- x = r
-
- # T x B x C -> B x T x C
- x = x.transpose(0, 1)
-
- return x, layer_results
-
- def max_positions(self):
- """Maximum output length supported by the encoder."""
- return self.args.max_positions
-
- def upgrade_state_dict_named(self, state_dict, name):
- """Upgrade a (possibly old) state dict for new versions of fairseq."""
- return state_dict
-
-
-class TransformerSentenceEncoderLayer(nn.Module):
- """
- Implements a Transformer Encoder Layer used in BERT/XLM style pre-trained
- models.
- """
-
- def __init__(
- self,
- embedding_dim: float = 768,
- ffn_embedding_dim: float = 3072,
- num_attention_heads: float = 8,
- dropout: float = 0.1,
- attention_dropout: float = 0.1,
- activation_dropout: float = 0.1,
- activation_fn: str = "relu",
- layer_norm_first: bool = False,
- ) -> None:
-
- super().__init__()
- # Initialize parameters
- self.embedding_dim = embedding_dim
- self.dropout = dropout
- self.activation_dropout = activation_dropout
-
- # Initialize blocks
- self.activation_fn = utils.get_activation_fn(activation_fn)
- self.self_attn = MultiheadAttention(
- self.embedding_dim,
- num_attention_heads,
- dropout=attention_dropout,
- self_attention=True,
- )
-
- self.dropout1 = nn.Dropout(dropout)
- self.dropout2 = nn.Dropout(self.activation_dropout)
- self.dropout3 = nn.Dropout(dropout)
-
- self.layer_norm_first = layer_norm_first
-
- # layer norm associated with the self attention layer
- self.self_attn_layer_norm = LayerNorm(self.embedding_dim)
- self.fc1 = nn.Linear(self.embedding_dim, ffn_embedding_dim)
- self.fc2 = nn.Linear(ffn_embedding_dim, self.embedding_dim)
-
- # layer norm associated with the position wise feed-forward NN
- self.final_layer_norm = LayerNorm(self.embedding_dim)
-
- def forward(
- self,
- x: torch.Tensor,
- self_attn_mask: torch.Tensor = None,
- self_attn_padding_mask: torch.Tensor = None,
- need_weights: bool = False,
- att_args=None,
- ):
- """
- LayerNorm is applied either before or after the self-attention/ffn
- modules similar to the original Transformer imlementation.
- """
- residual = x
-
- if self.layer_norm_first:
- x = self.self_attn_layer_norm(x)
- x, attn = self.self_attn(
- query=x,
- key=x,
- value=x,
- key_padding_mask=self_attn_padding_mask,
- attn_mask=self_attn_mask,
- )
- x = self.dropout1(x)
- x = residual + x
-
- residual = x
- x = self.final_layer_norm(x)
- x = self.activation_fn(self.fc1(x))
- x = self.dropout2(x)
- x = self.fc2(x)
- x = self.dropout3(x)
- x = residual + x
- else:
- x, attn = self.self_attn(
- query=x,
- key=x,
- value=x,
- key_padding_mask=self_attn_padding_mask,
- )
-
- x = self.dropout1(x)
- x = residual + x
-
- x = self.self_attn_layer_norm(x)
-
- residual = x
- x = self.activation_fn(self.fc1(x))
- x = self.dropout2(x)
- x = self.fc2(x)
- x = self.dropout3(x)
- x = residual + x
- x = self.final_layer_norm(x)
-
- return x, attn
diff --git a/spaces/HarryLee/eCommerceImageCaptioning/fairseq/fairseq/speech_generator.py b/spaces/HarryLee/eCommerceImageCaptioning/fairseq/fairseq/speech_generator.py
deleted file mode 100644
index 8086e34d2b56fa808d0905b1a00e87e6736fcf04..0000000000000000000000000000000000000000
--- a/spaces/HarryLee/eCommerceImageCaptioning/fairseq/fairseq/speech_generator.py
+++ /dev/null
@@ -1,219 +0,0 @@
-# Copyright (c) Facebook, Inc. and its affiliates.
-#
-# This source code is licensed under the MIT license found in the
-# LICENSE file in the root directory of this source tree.
-
-import torch
-import numpy as np
-
-from fairseq.data.audio.speech_to_text_dataset import S2TDataConfig
-
-
-class SpeechGenerator(object):
- def __init__(self, model, vocoder, data_cfg: S2TDataConfig):
- self.model = model
- self.vocoder = vocoder
- stats_npz_path = data_cfg.global_cmvn_stats_npz
- self.gcmvn_stats = None
- if stats_npz_path is not None:
- self.gcmvn_stats = np.load(stats_npz_path)
-
- def gcmvn_denormalize(self, x):
- # x: B x T x C
- if self.gcmvn_stats is None:
- return x
- mean = torch.from_numpy(self.gcmvn_stats["mean"]).to(x)
- std = torch.from_numpy(self.gcmvn_stats["std"]).to(x)
- assert len(x.shape) == 3 and mean.shape[0] == std.shape[0] == x.shape[2]
- x = x * std.view(1, 1, -1).expand_as(x)
- return x + mean.view(1, 1, -1).expand_as(x)
-
- def get_waveform(self, feat):
- # T x C -> T
- return None if self.vocoder is None else self.vocoder(feat).squeeze(0)
-
-
-class AutoRegressiveSpeechGenerator(SpeechGenerator):
- def __init__(
- self, model, vocoder, data_cfg, max_iter: int = 6000,
- eos_prob_threshold: float = 0.5,
- ):
- super().__init__(model, vocoder, data_cfg)
- self.max_iter = max_iter
- self.eos_prob_threshold = eos_prob_threshold
-
- @torch.no_grad()
- def generate(self, model, sample, has_targ=False, **kwargs):
- model.eval()
-
- src_tokens = sample["net_input"]["src_tokens"]
- src_lengths = sample["net_input"]["src_lengths"]
- bsz, src_len = src_tokens.size()
- n_frames_per_step = model.decoder.n_frames_per_step
- out_dim = model.decoder.out_dim
- raw_dim = out_dim // n_frames_per_step
-
- # initialize
- encoder_out = model.forward_encoder(src_tokens, src_lengths,
- speaker=sample["speaker"])
- incremental_state = {}
- feat, attn, eos_prob = [], [], []
- finished = src_tokens.new_zeros((bsz,)).bool()
- out_lens = src_lengths.new_zeros((bsz,)).long().fill_(self.max_iter)
-
- prev_feat_out = encoder_out["encoder_out"][0].new_zeros(bsz, 1, out_dim)
- for step in range(self.max_iter):
- cur_out_lens = out_lens.clone()
- cur_out_lens.masked_fill_(cur_out_lens.eq(self.max_iter), step + 1)
- _, cur_eos_out, cur_extra = model.forward_decoder(
- prev_feat_out, encoder_out=encoder_out,
- incremental_state=incremental_state,
- target_lengths=cur_out_lens, speaker=sample["speaker"], **kwargs
- )
- cur_eos_prob = torch.sigmoid(cur_eos_out).squeeze(2)
- feat.append(cur_extra['feature_out'])
- attn.append(cur_extra['attn'])
- eos_prob.append(cur_eos_prob)
-
- cur_finished = (cur_eos_prob.squeeze(1) > self.eos_prob_threshold)
- out_lens.masked_fill_((~finished) & cur_finished, step + 1)
- finished = finished | cur_finished
- if finished.sum().item() == bsz:
- break
- prev_feat_out = cur_extra['feature_out']
-
- feat = torch.cat(feat, dim=1)
- feat = model.decoder.postnet(feat) + feat
- eos_prob = torch.cat(eos_prob, dim=1)
- attn = torch.cat(attn, dim=2)
- alignment = attn.max(dim=1)[1]
-
- feat = feat.reshape(bsz, -1, raw_dim)
- feat = self.gcmvn_denormalize(feat)
-
- eos_prob = eos_prob.repeat_interleave(n_frames_per_step, dim=1)
- attn = attn.repeat_interleave(n_frames_per_step, dim=2)
- alignment = alignment.repeat_interleave(n_frames_per_step, dim=1)
- out_lens = out_lens * n_frames_per_step
-
- finalized = [
- {
- 'feature': feat[b, :out_len],
- 'eos_prob': eos_prob[b, :out_len],
- 'attn': attn[b, :, :out_len],
- 'alignment': alignment[b, :out_len],
- 'waveform': self.get_waveform(feat[b, :out_len]),
- }
- for b, out_len in zip(range(bsz), out_lens)
- ]
-
- if has_targ:
- assert sample["target"].size(-1) == out_dim
- tgt_feats = sample["target"].view(bsz, -1, raw_dim)
- tgt_feats = self.gcmvn_denormalize(tgt_feats)
- tgt_lens = sample["target_lengths"] * n_frames_per_step
- for b, (f, l) in enumerate(zip(tgt_feats, tgt_lens)):
- finalized[b]["targ_feature"] = f[:l]
- finalized[b]["targ_waveform"] = self.get_waveform(f[:l])
- return finalized
-
-
-class NonAutoregressiveSpeechGenerator(SpeechGenerator):
- @torch.no_grad()
- def generate(self, model, sample, has_targ=False, **kwargs):
- model.eval()
-
- bsz, max_src_len = sample["net_input"]["src_tokens"].size()
- n_frames_per_step = model.encoder.n_frames_per_step
- out_dim = model.encoder.out_dim
- raw_dim = out_dim // n_frames_per_step
-
- feat, out_lens, log_dur_out, _, _ = model(
- src_tokens=sample["net_input"]["src_tokens"],
- src_lengths=sample["net_input"]["src_lengths"],
- prev_output_tokens=sample["net_input"]["prev_output_tokens"],
- incremental_state=None,
- target_lengths=sample["target_lengths"],
- speaker=sample["speaker"]
- )
-
- feat = feat.view(bsz, -1, raw_dim)
- feat = self.gcmvn_denormalize(feat)
-
- dur_out = torch.clamp(
- torch.round(torch.exp(log_dur_out) - 1).long(), min=0
- )
-
- def get_dur_plot_data(d):
- r = []
- for i, dd in enumerate(d):
- r += [i + 1] * dd.item()
- return r
-
- out_lens = out_lens * n_frames_per_step
- finalized = [
- {
- 'feature': feat[b, :l] if l > 0 else feat.new_zeros([1, raw_dim]),
- 'waveform': self.get_waveform(
- feat[b, :l] if l > 0 else feat.new_zeros([1, raw_dim])
- ),
- 'attn': feat.new_tensor(get_dur_plot_data(dur_out[b])),
- }
- for b, l in zip(range(bsz), out_lens)
- ]
-
- if has_targ:
- tgt_feats = sample["target"].view(bsz, -1, raw_dim)
- tgt_feats = self.gcmvn_denormalize(tgt_feats)
- tgt_lens = sample["target_lengths"] * n_frames_per_step
- for b, (f, l) in enumerate(zip(tgt_feats, tgt_lens)):
- finalized[b]["targ_feature"] = f[:l]
- finalized[b]["targ_waveform"] = self.get_waveform(f[:l])
- return finalized
-
-
-class TeacherForcingAutoRegressiveSpeechGenerator(AutoRegressiveSpeechGenerator):
- @torch.no_grad()
- def generate(self, model, sample, has_targ=False, **kwargs):
- model.eval()
-
- src_tokens = sample["net_input"]["src_tokens"]
- src_lens = sample["net_input"]["src_lengths"]
- prev_out_tokens = sample["net_input"]["prev_output_tokens"]
- tgt_lens = sample["target_lengths"]
- n_frames_per_step = model.decoder.n_frames_per_step
- raw_dim = model.decoder.out_dim // n_frames_per_step
- bsz = src_tokens.shape[0]
-
- feat, eos_prob, extra = model(
- src_tokens, src_lens, prev_out_tokens, incremental_state=None,
- target_lengths=tgt_lens, speaker=sample["speaker"]
- )
-
- attn = extra["attn"] # B x T_s x T_t
- alignment = attn.max(dim=1)[1]
- feat = feat.reshape(bsz, -1, raw_dim)
- feat = self.gcmvn_denormalize(feat)
- eos_prob = eos_prob.repeat_interleave(n_frames_per_step, dim=1)
- attn = attn.repeat_interleave(n_frames_per_step, dim=2)
- alignment = alignment.repeat_interleave(n_frames_per_step, dim=1)
- tgt_lens = sample["target_lengths"] * n_frames_per_step
-
- finalized = [
- {
- 'feature': feat[b, :tgt_len],
- 'eos_prob': eos_prob[b, :tgt_len],
- 'attn': attn[b, :, :tgt_len],
- 'alignment': alignment[b, :tgt_len],
- 'waveform': self.get_waveform(feat[b, :tgt_len]),
- }
- for b, tgt_len in zip(range(bsz), tgt_lens)
- ]
-
- if has_targ:
- tgt_feats = sample["target"].view(bsz, -1, raw_dim)
- tgt_feats = self.gcmvn_denormalize(tgt_feats)
- for b, (f, l) in enumerate(zip(tgt_feats, tgt_lens)):
- finalized[b]["targ_feature"] = f[:l]
- finalized[b]["targ_waveform"] = self.get_waveform(f[:l])
- return finalized
diff --git a/spaces/Harveenchadha/Vakyansh-Hindi-TTS/ttsv/src/glow_tts/audio_processing.py b/spaces/Harveenchadha/Vakyansh-Hindi-TTS/ttsv/src/glow_tts/audio_processing.py
deleted file mode 100644
index 3a4467355952fefaba117b6014864139ac319c6b..0000000000000000000000000000000000000000
--- a/spaces/Harveenchadha/Vakyansh-Hindi-TTS/ttsv/src/glow_tts/audio_processing.py
+++ /dev/null
@@ -1,100 +0,0 @@
-import torch
-import numpy as np
-from scipy.signal import get_window
-import librosa.util as librosa_util
-
-
-def window_sumsquare(
- window,
- n_frames,
- hop_length=200,
- win_length=800,
- n_fft=800,
- dtype=np.float32,
- norm=None,
-):
- """
- # from librosa 0.6
- Compute the sum-square envelope of a window function at a given hop length.
-
- This is used to estimate modulation effects induced by windowing
- observations in short-time fourier transforms.
-
- Parameters
- ----------
- window : string, tuple, number, callable, or list-like
- Window specification, as in `get_window`
-
- n_frames : int > 0
- The number of analysis frames
-
- hop_length : int > 0
- The number of samples to advance between frames
-
- win_length : [optional]
- The length of the window function. By default, this matches `n_fft`.
-
- n_fft : int > 0
- The length of each analysis frame.
-
- dtype : np.dtype
- The data type of the output
-
- Returns
- -------
- wss : np.ndarray, shape=`(n_fft + hop_length * (n_frames - 1))`
- The sum-squared envelope of the window function
- """
- if win_length is None:
- win_length = n_fft
-
- n = n_fft + hop_length * (n_frames - 1)
- x = np.zeros(n, dtype=dtype)
-
- # Compute the squared window at the desired length
- win_sq = get_window(window, win_length, fftbins=True)
- win_sq = librosa_util.normalize(win_sq, norm=norm) ** 2
- win_sq = librosa_util.pad_center(win_sq, n_fft)
-
- # Fill the envelope
- for i in range(n_frames):
- sample = i * hop_length
- x[sample : min(n, sample + n_fft)] += win_sq[: max(0, min(n_fft, n - sample))]
- return x
-
-
-def griffin_lim(magnitudes, stft_fn, n_iters=30):
- """
- PARAMS
- ------
- magnitudes: spectrogram magnitudes
- stft_fn: STFT class with transform (STFT) and inverse (ISTFT) methods
- """
-
- angles = np.angle(np.exp(2j * np.pi * np.random.rand(*magnitudes.size())))
- angles = angles.astype(np.float32)
- angles = torch.autograd.Variable(torch.from_numpy(angles))
- signal = stft_fn.inverse(magnitudes, angles).squeeze(1)
-
- for i in range(n_iters):
- _, angles = stft_fn.transform(signal)
- signal = stft_fn.inverse(magnitudes, angles).squeeze(1)
- return signal
-
-
-def dynamic_range_compression(x, C=1, clip_val=1e-5):
- """
- PARAMS
- ------
- C: compression factor
- """
- return torch.log(torch.clamp(x, min=clip_val) * C)
-
-
-def dynamic_range_decompression(x, C=1):
- """
- PARAMS
- ------
- C: compression factor used to compress
- """
- return torch.exp(x) / C
diff --git a/spaces/Harveenchadha/oiTrans/legacy/run_inference.sh b/spaces/Harveenchadha/oiTrans/legacy/run_inference.sh
deleted file mode 100644
index ff582a6c49d015cf36c82e8f20a755f6d1418ed8..0000000000000000000000000000000000000000
--- a/spaces/Harveenchadha/oiTrans/legacy/run_inference.sh
+++ /dev/null
@@ -1,80 +0,0 @@
-src_lang=${1:-hi}
-tgt_lang=${2:-en}
-bucket_path=${3:-gs://ai4b-anuvaad-nmt/baselines/transformer-base/baselines-${src_lang}-${tgt_lang}}
-
-expdir=../baselines/baselines-${src_lang}-${tgt_lang}
-
-if [[ -d $expdir ]]
-then
- echo "$expdir exists on your filesystem. Please delete this if you have made some changes to the bucket files and trying to redownload"
-else
- mkdir -p $expdir
- mkdir -p $expdir/model
- cd ../baselines
- gsutil -m cp -r $bucket_path/vocab $expdir
- gsutil -m cp -r $bucket_path/final_bin $expdir
- gsutil -m cp $bucket_path/model/checkpoint_best.pt $expdir/model
- cd ../indicTrans
-fi
-
-
-if [ $src_lang == 'hi' ] || [ $tgt_lang == 'hi' ]; then
- #TEST_SETS=( wmt-news wat2021-devtest wat2020-devtest anuvaad-legal tico19 sap-documentation-benchmark all)
- TEST_SETS=( wat2021-devtest wat2020-devtest wat-2018 wmt-news )
-elif [ $src_lang == 'ta' ] || [ $tgt_lang == 'ta' ]; then
- # TEST_SETS=( wmt-news wat2021-devtest wat2020-devtest anuvaad-legal tico19 all)
- TEST_SETS=( wat2021-devtest wat2020-devtest wat-2018 wmt-news ufal-ta)
-elif [ $src_lang == 'bn' ] || [ $tgt_lang == 'bn' ]; then
- # TEST_SETS=( wat2021-devtest wat2020-devtest anuvaad-legal tico19 all)
- TEST_SETS=( wat2021-devtest wat2020-devtest wat-2018)
-elif [ $src_lang == 'gu' ] || [ $tgt_lang == 'gu' ]; then
- # TEST_SETS=( wmt-news wat2021-devtest wat2020-devtest all)
- TEST_SETS=( wat2021-devtest wat2020-devtest wmt-news )
-elif [ $src_lang == 'as' ] || [ $tgt_lang == 'as' ]; then
- TEST_SETS=( pmi )
-elif [ $src_lang == 'kn' ] || [ $tgt_lang == 'kn' ]; then
- # TEST_SETS=( wat2021-devtest anuvaad-legal all)
- TEST_SETS=( wat2021-devtest )
-elif [ $src_lang == 'ml' ] || [ $tgt_lang == 'ml' ]; then
- # TEST_SETS=( wat2021-devtest wat2020-devtest anuvaad-legal all)
- TEST_SETS=( wat2021-devtest wat2020-devtest wat-2018)
-elif [ $src_lang == 'mr' ] || [ $tgt_lang == 'mr' ]; then
- # TEST_SETS=( wat2021-devtest wat2020-devtest all)
- TEST_SETS=( wat2021-devtest wat2020-devtest )
-elif [ $src_lang == 'or' ] || [ $tgt_lang == 'or' ]; then
- TEST_SETS=( wat2021-devtest )
-elif [ $src_lang == 'pa' ] || [ $tgt_lang == 'pa' ]; then
- TEST_SETS=( wat2021-devtest )
-elif [ $src_lang == 'te' ] || [ $tgt_lang == 'te' ]; then
- # TEST_SETS=( wat2021-devtest wat2020-devtest anuvaad-legal all )
- TEST_SETS=( wat2021-devtest wat2020-devtest wat-2018)
-fi
-
-if [ $src_lang == 'en' ]; then
- indic_lang=$tgt_lang
-else
- indic_lang=$src_lang
-fi
-
-
-for tset in ${TEST_SETS[@]};do
- echo $tset $src_lang $tgt_lang
- if [ $tset == 'wat2021-devtest' ]; then
- SRC_FILE=${expdir}/benchmarks/$tset/test.$src_lang
- REF_FILE=${expdir}/benchmarks/$tset/test.$tgt_lang
- else
- SRC_FILE=${expdir}/benchmarks/$tset/en-${indic_lang}/test.$src_lang
- REF_FILE=${expdir}/benchmarks/$tset/en-${indic_lang}/test.$tgt_lang
- fi
- RESULTS_DIR=${expdir}/results/$tset
-
- mkdir -p $RESULTS_DIR
-
- bash translate.sh $SRC_FILE $RESULTS_DIR/${src_lang}-${tgt_lang} $src_lang $tgt_lang $expdir $REF_FILE
- # for newline between different outputs
- echo
-done
-# send the results to the bucket
-gsutil -m cp -r $expdir/results $bucket_path
-# clear up the space in the instance
-# rm -r $expdir
\ No newline at end of file
diff --git a/spaces/Harveenchadha/oiTrans/scripts/remove_large_sentences.py b/spaces/Harveenchadha/oiTrans/scripts/remove_large_sentences.py
deleted file mode 100644
index a045f95df1af2d327104e73ae4ed90558d115058..0000000000000000000000000000000000000000
--- a/spaces/Harveenchadha/oiTrans/scripts/remove_large_sentences.py
+++ /dev/null
@@ -1,44 +0,0 @@
-from tqdm import tqdm
-import sys
-
-
-def remove_large_sentences(src_path, tgt_path):
- count = 0
- new_src_lines = []
- new_tgt_lines = []
- src_num_lines = sum(1 for line in open(src_path, "r", encoding="utf-8"))
- tgt_num_lines = sum(1 for line in open(tgt_path, "r", encoding="utf-8"))
- assert src_num_lines == tgt_num_lines
- with open(src_path, encoding="utf-8") as f1, open(tgt_path, encoding="utf-8") as f2:
- for src_line, tgt_line in tqdm(zip(f1, f2), total=src_num_lines):
- src_tokens = src_line.strip().split(" ")
- tgt_tokens = tgt_line.strip().split(" ")
- if len(src_tokens) > 200 or len(tgt_tokens) > 200:
- count += 1
- continue
- new_src_lines.append(src_line)
- new_tgt_lines.append(tgt_line)
- return count, new_src_lines, new_tgt_lines
-
-
-def create_txt(outFile, lines, add_newline=False):
- outfile = open("{0}".format(outFile), "w", encoding="utf-8")
- for line in lines:
- if add_newline:
- outfile.write(line + "\n")
- else:
- outfile.write(line)
- outfile.close()
-
-
-if __name__ == "__main__":
-
- src_path = sys.argv[1]
- tgt_path = sys.argv[2]
- new_src_path = sys.argv[3]
- new_tgt_path = sys.argv[4]
-
- count, new_src_lines, new_tgt_lines = remove_large_sentences(src_path, tgt_path)
- print(f'{count} lines removed due to seq_len > 200')
- create_txt(new_src_path, new_src_lines)
- create_txt(new_tgt_path, new_tgt_lines)
diff --git a/spaces/HgMenon/Transcribe_V0.2/src/__init__.py b/spaces/HgMenon/Transcribe_V0.2/src/__init__.py
deleted file mode 100644
index e69de29bb2d1d6434b8b29ae775ad8c2e48c5391..0000000000000000000000000000000000000000
diff --git a/spaces/HighCWu/GPEN/retinaface/layers/__init__.py b/spaces/HighCWu/GPEN/retinaface/layers/__init__.py
deleted file mode 100644
index 53a3f4b5160995d93bc7911e808b3045d74362c9..0000000000000000000000000000000000000000
--- a/spaces/HighCWu/GPEN/retinaface/layers/__init__.py
+++ /dev/null
@@ -1,2 +0,0 @@
-from .functions import *
-from .modules import *
diff --git a/spaces/ICML2022/OFA/data/ofa_dataset.py b/spaces/ICML2022/OFA/data/ofa_dataset.py
deleted file mode 100644
index 02d856c28016b3a1c020fed483afe0aa797bf50f..0000000000000000000000000000000000000000
--- a/spaces/ICML2022/OFA/data/ofa_dataset.py
+++ /dev/null
@@ -1,74 +0,0 @@
-import logging
-import re
-import torch.utils.data
-from fairseq.data import FairseqDataset
-
-logger = logging.getLogger(__name__)
-
-
-class OFADataset(FairseqDataset):
- def __init__(self, split, dataset, bpe, src_dict, tgt_dict):
- self.split = split
- self.dataset = dataset
- self.bpe = bpe
- self.src_dict = src_dict
- self.tgt_dict = tgt_dict
-
- self.bos = src_dict.bos()
- self.eos = src_dict.eos()
- self.pad = src_dict.pad()
- self.bos_item = torch.LongTensor([self.bos])
- self.eos_item = torch.LongTensor([self.eos])
-
- def __len__(self):
- return len(self.dataset)
-
- def encode_text(self, text, length=None, append_bos=False, append_eos=False, use_bpe=True):
- s = self.tgt_dict.encode_line(
- line=self.bpe.encode(text) if use_bpe else text,
- add_if_not_exist=False,
- append_eos=False
- ).long()
- if length is not None:
- s = s[:length]
- if append_bos:
- s = torch.cat([self.bos_item, s])
- if append_eos:
- s = torch.cat([s, self.eos_item])
- return s
-
- def pre_question(self, question, max_ques_words):
- question = question.lower().lstrip(",.!?*#:;~").replace('-', ' ').replace('/', ' ')
-
- question = re.sub(
- r"\s{2,}",
- ' ',
- question,
- )
- question = question.rstrip('\n')
- question = question.strip(' ')
-
- # truncate question
- question_words = question.split(' ')
- if len(question_words) > max_ques_words:
- question = ' '.join(question_words[:max_ques_words])
-
- return question
-
- def pre_caption(self, caption, max_words):
- caption = caption.lower().lstrip(",.!?*#:;~").replace('-', ' ').replace('/', ' ').replace('', 'person')
-
- caption = re.sub(
- r"\s{2,}",
- ' ',
- caption,
- )
- caption = caption.rstrip('\n')
- caption = caption.strip(' ')
-
- # truncate caption
- caption_words = caption.split(' ')
- if len(caption_words) > max_words:
- caption = ' '.join(caption_words[:max_words])
-
- return caption
diff --git a/spaces/ICML2022/OFA/fairseq/fairseq/optim/adafactor.py b/spaces/ICML2022/OFA/fairseq/fairseq/optim/adafactor.py
deleted file mode 100644
index c969b9fbc0d229a25f2046ec67c53c57a433814b..0000000000000000000000000000000000000000
--- a/spaces/ICML2022/OFA/fairseq/fairseq/optim/adafactor.py
+++ /dev/null
@@ -1,268 +0,0 @@
-# Copyright (c) Facebook, Inc. and its affiliates.
-#
-# This source code is licensed under the MIT license found in the
-# LICENSE file in the root directory of this source tree.
-
-import math
-
-import torch
-import torch.optim
-
-from . import LegacyFairseqOptimizer, register_optimizer
-
-
-@register_optimizer("adafactor")
-class FairseqAdafactor(LegacyFairseqOptimizer):
- def __init__(self, args, params):
- super().__init__(args)
- self._optimizer = Adafactor(params, **self.optimizer_config)
-
- @staticmethod
- def add_args(parser):
- """Add optimizer-specific arguments to the parser."""
- # fmt: off
- parser.add_argument('--adafactor-eps', default='(1e-30, 1e-3)', metavar="E",
- help='epsilons for Adafactor optimizer')
- parser.add_argument('--clip-threshold', type=float, default=1.0, metavar="C",
- help='threshold for clipping update root mean square')
- parser.add_argument('--decay-rate', type=float, default=-0.8, metavar="D",
- help='decay rate of the second moment estimator')
- parser.add_argument('--beta1', type=float, default=None, metavar="B",
- help='beta for first moment estimator. Optional')
- parser.add_argument('--weight-decay', '--wd', default=0.0, type=float, metavar='WD',
- help='weight decay')
- parser.add_argument('--scale-parameter', action='store_true',
- help='scale learning rate by root mean square of parameter')
- parser.add_argument('--relative-step', action='store_true',
- help='set learning rate to inverse square root of timestep,'
- 'otherwise use external learning rate')
- parser.add_argument('--warmup-init', action='store_true',
- help='use relative step for warm-up learning rate schedule')
- # fmt: on
-
- @property
- def optimizer_config(self):
- """
- Return a kwarg dictionary that will be used to override optimizer
- args stored in checkpoints. This allows us to load a checkpoint and
- resume training using a different set of optimizer args, e.g., with a
- different learning rate.
- Note : Convergence issues empirically observed with fp16 on.
- Might require search for appropriate configuration.
- """
- return {
- "lr": self.args.lr[0],
- "eps": eval(self.args.adafactor_eps),
- "clip_threshold": self.args.clip_threshold,
- "decay_rate": self.args.decay_rate,
- "beta1": self.args.beta1,
- "weight_decay": self.args.weight_decay,
- "scale_parameter": self.args.scale_parameter, # defaults to False
- "relative_step": self.args.relative_step, # defaults to False
- "warmup_init": self.args.warmup_init,
- }
-
-
-class Adafactor(torch.optim.Optimizer):
- """Implements Adafactor algorithm.
-
- This implementation is based on:
- `Adafactor: Adaptive Learning Rates with Sublinear Memory Cost`
- (see https://arxiv.org/abs/1804.04235)
-
- Note that this optimizer internally adjusts the learning rate
- depending on the *scale_parameter*, *relative_step* and
- *warmup_init* options. To use a manual (external) learning rate
- schedule you should set `scale_parameter=False` and
- `relative_step=False`.
-
- Args:
- params (iterable): iterable of parameters to optimize or dicts defining
- parameter groups
- lr (float, optional): external learning rate (default: None)
- eps (tuple[float, float]): regularization constans for square gradient
- and parameter scale respectively (default: (1e-30, 1e-3))
- clip_threshold (float): threshold of root mean square of
- final gradient update (default: 1.0)
- decay_rate (float): coefficient used to compute running averages of square
- gradient (default: -0.8)
- beta1 (float): coefficient used for computing running averages of gradient
- (default: None)
- weight_decay (float, optional): weight decay (L2 penalty) (default: 0)
- scale_parameter (bool): if True, learning rate is scaled by root mean square of
- parameter (default: True)
- relative_step (bool): if True, time-dependent learning rate is computed
- instead of external learning rate (default: True)
- warmup_init (bool): time-dependent learning rate computation depends on
- whether warm-up initialization is being used (default: False)
- """
-
- def __init__(
- self,
- params,
- lr=None,
- eps=(1e-30, 1e-3),
- clip_threshold=1.0,
- decay_rate=-0.8,
- beta1=None,
- weight_decay=0.0,
- scale_parameter=True,
- relative_step=True,
- warmup_init=False,
- ):
- if lr is not None and relative_step:
- raise ValueError("Cannot combine manual lr and relative_step options")
- if warmup_init and not relative_step:
- raise ValueError("warmup_init requires relative_step=True")
-
- defaults = dict(
- lr=lr,
- eps=eps,
- clip_threshold=clip_threshold,
- decay_rate=decay_rate,
- beta1=beta1,
- weight_decay=weight_decay,
- scale_parameter=scale_parameter,
- relative_step=relative_step,
- warmup_init=warmup_init,
- )
- super(Adafactor, self).__init__(params, defaults)
-
- @property
- def supports_memory_efficient_fp16(self):
- return True
-
- @property
- def supports_flat_params(self):
- return False
-
- def _get_lr(self, param_group, param_state):
- rel_step_sz = param_group["lr"]
- if param_group["relative_step"]:
- min_step = (
- 1e-6 * param_state["step"] if param_group["warmup_init"] else 1e-2
- )
- rel_step_sz = min(min_step, 1.0 / math.sqrt(param_state["step"]))
- param_scale = 1.0
- if param_group["scale_parameter"]:
- param_scale = max(param_group["eps"][1], param_state["RMS"])
- return param_scale * rel_step_sz
-
- def _get_options(self, param_group, param_shape):
- factored = len(param_shape) >= 2
- use_first_moment = param_group["beta1"] is not None
- return factored, use_first_moment
-
- def _rms(self, tensor):
- return tensor.norm(2) / (tensor.numel() ** 0.5)
-
- def _approx_sq_grad(self, exp_avg_sq_row, exp_avg_sq_col):
- r_factor = (
- (exp_avg_sq_row / exp_avg_sq_row.mean(dim=-1, keepdim=True))
- .rsqrt_()
- .unsqueeze(-1)
- )
- c_factor = exp_avg_sq_col.unsqueeze(-2).rsqrt()
- return torch.mul(r_factor, c_factor)
-
- def step(self, closure=None):
- """Performs a single optimization step.
-
- Args:
- closure (callable, optional): A closure that reevaluates the model
- and returns the loss.
- """
- loss = None
- if closure is not None:
- loss = closure()
-
- for group in self.param_groups:
- for p in group["params"]:
- if p.grad is None:
- continue
- grad = p.grad.data
- if grad.dtype in {torch.float16, torch.bfloat16}:
- grad = grad.float()
- if grad.is_sparse:
- raise RuntimeError("Adafactor does not support sparse gradients.")
-
- state = self.state[p]
- grad_shape = grad.shape
-
- factored, use_first_moment = self._get_options(group, grad_shape)
- # State Initialization
- if len(state) == 0:
- state["step"] = 0
-
- if use_first_moment:
- # Exponential moving average of gradient values
- state["exp_avg"] = torch.zeros_like(grad)
- if factored:
- state["exp_avg_sq_row"] = torch.zeros(grad_shape[:-1]).to(grad)
- state["exp_avg_sq_col"] = torch.zeros(
- grad_shape[:-2] + grad_shape[-1:]
- ).to(grad)
- else:
- state["exp_avg_sq"] = torch.zeros_like(grad)
-
- state["RMS"] = 0
- else:
- if use_first_moment:
- state["exp_avg"] = state["exp_avg"].to(grad)
- if factored:
- state["exp_avg_sq_row"] = state["exp_avg_sq_row"].to(grad)
- state["exp_avg_sq_col"] = state["exp_avg_sq_col"].to(grad)
- else:
- state["exp_avg_sq"] = state["exp_avg_sq"].to(grad)
-
- p_data_fp32 = p.data
- if p.data.dtype in {torch.float16, torch.bfloat16}:
- p_data_fp32 = p_data_fp32.float()
-
- state["step"] += 1
- state["RMS"] = self._rms(p_data_fp32)
- group["lr"] = self._get_lr(group, state)
-
- beta2t = 1.0 - math.pow(state["step"], group["decay_rate"])
- update = (grad ** 2) + group["eps"][0]
- if factored:
- exp_avg_sq_row = state["exp_avg_sq_row"]
- exp_avg_sq_col = state["exp_avg_sq_col"]
-
- exp_avg_sq_row.mul_(beta2t).add_(
- update.mean(dim=-1), alpha=1.0 - beta2t
- )
- exp_avg_sq_col.mul_(beta2t).add_(
- update.mean(dim=-2), alpha=1.0 - beta2t
- )
-
- # Approximation of exponential moving average of square of gradient
- update = self._approx_sq_grad(exp_avg_sq_row, exp_avg_sq_col)
- update.mul_(grad)
- else:
- exp_avg_sq = state["exp_avg_sq"]
-
- exp_avg_sq.mul_(beta2t).add_(update, alpha=1.0 - beta2t)
- update = exp_avg_sq.rsqrt().mul_(grad)
-
- update.div_(
- (self._rms(update) / group["clip_threshold"]).clamp_(min=1.0)
- )
- update.mul_(group["lr"])
-
- if use_first_moment:
- exp_avg = state["exp_avg"]
- exp_avg.mul_(group["beta1"]).add_(update, alpha=1 - group["beta1"])
- update = exp_avg
-
- if group["weight_decay"] != 0:
- p_data_fp32.add_(
- p_data_fp32, alpha=-group["weight_decay"] * group["lr"]
- )
-
- p_data_fp32.add_(-update)
-
- if p.data.dtype in {torch.float16, torch.bfloat16}:
- p.data.copy_(p_data_fp32)
-
- return loss
diff --git a/spaces/ICML2022/OFA/fairseq/fairseq/optim/lr_scheduler/polynomial_decay_schedule.py b/spaces/ICML2022/OFA/fairseq/fairseq/optim/lr_scheduler/polynomial_decay_schedule.py
deleted file mode 100644
index 73c3c8ea3435d6050401c45e737e4ecf5662825c..0000000000000000000000000000000000000000
--- a/spaces/ICML2022/OFA/fairseq/fairseq/optim/lr_scheduler/polynomial_decay_schedule.py
+++ /dev/null
@@ -1,110 +0,0 @@
-# Copyright (c) Facebook, Inc. and its affiliates.
-#
-# This source code is licensed under the MIT license found in the
-# LICENSE file in the root directory of this source tree.
-
-from dataclasses import dataclass, field
-from typing import Optional, List
-from omegaconf import II
-
-from fairseq.dataclass import FairseqDataclass
-from fairseq.optim.lr_scheduler import FairseqLRScheduler, register_lr_scheduler
-
-
-@dataclass
-class PolynomialDecayLRScheduleConfig(FairseqDataclass):
- warmup_updates: int = field(
- default=0,
- metadata={"help": "warmup the learning rate linearly for the first N updates"},
- )
- warmup_ratio: float = field(
- default=0,
- metadata={"help": "warmup ratio"},
- )
- force_anneal: Optional[int] = field(
- default=None,
- metadata={"help": "force annealing at specified epoch"},
- )
- end_learning_rate: float = field(
- default=0.0,
- metadata={"help": "learning rate to decay to"},
- )
- power: float = field(
- default=1.0,
- metadata={"help": "decay exponent"},
- )
- total_num_update: Optional[float] = field(
- default=1000000,
- metadata={"help": "total number of updates over which to decay learning rate"},
- )
- lr: List[float] = II("optimization.lr")
-
-
-@register_lr_scheduler("polynomial_decay", dataclass=PolynomialDecayLRScheduleConfig)
-class PolynomialDecayLRSchedule(FairseqLRScheduler):
- """Decay the LR on a fixed schedule."""
-
- def __init__(self, cfg: PolynomialDecayLRScheduleConfig, optimizer):
- super().__init__(cfg, optimizer)
-
- assert cfg.total_num_update > 0
- # set defaults
- cfg.warmup_updates = getattr(cfg, 'warmup_updates', 0) or 0
-
- self.lr = cfg.lr[0]
- self.warmup_updates = cfg.warmup_updates
- if self.warmup_updates > 0:
- self.warmup_factor = 1.0 / self.warmup_updates
- else:
- self.warmup_factor = 1
- self.end_learning_rate = cfg.end_learning_rate
- self.total_num_update = cfg.total_num_update
- self.power = cfg.power
- self.optimizer.set_lr(self.warmup_factor * self.lr)
-
- def get_next_lr(self, epoch):
- lrs = self.cfg.lr
- if self.cfg.force_anneal is None or epoch < self.cfg.force_anneal:
- # use fixed LR schedule
- next_lr = lrs[min(epoch, len(lrs) - 1)]
- else:
- # annneal based on lr_shrink
- next_lr = self.optimizer.get_lr()
- return next_lr
-
- def step_begin_epoch(self, epoch):
- """Update the learning rate at the beginning of the given epoch."""
- self.lr = self.get_next_lr(epoch)
- self.optimizer.set_lr(self.warmup_factor * self.lr)
- return self.optimizer.get_lr()
-
- def step_update(self, num_updates):
- """Update the learning rate after each update."""
- if self.warmup_updates > 0 and num_updates <= self.warmup_updates:
- self.warmup_factor = num_updates / float(self.warmup_updates)
- lr = self.warmup_factor * self.lr
- elif num_updates >= self.total_num_update:
- lr = self.end_learning_rate
- else:
- warmup = self.warmup_updates
- lr_range = self.lr - self.end_learning_rate
- pct_remaining = 1 - (num_updates - warmup) / (self.total_num_update - warmup)
- lr = lr_range * pct_remaining ** (self.power) + self.end_learning_rate
- self.optimizer.set_lr(lr)
- return self.optimizer.get_lr()
-
- def reinit(self, total_num_update, num_updates):
- # only enable this when set warmup_ratio
- if self.cfg.warmup_ratio <= 0:
- return
- # re init this according to the real number of updates
- self.total_num_update = total_num_update
- self.warmup_updates = int(self.total_num_update * self.cfg.warmup_ratio)
- if num_updates > 0:
- self.warmup_factor = min(1.0, num_updates / float(self.warmup_updates))
- self.step_update(num_updates)
- else:
- self.warmup_factor = 1.0 / self.warmup_updates
- self.optimizer.set_lr(self.warmup_factor * self.lr)
- print('Total steps {}, warmup steps {}, warmup_factor {}'.format(self.total_num_update, self.warmup_updates,
- self.warmup_factor))
\ No newline at end of file
diff --git a/spaces/IDEA-Research/Grounded-SAM/GroundingDINO/demo/gradio_app.py b/spaces/IDEA-Research/Grounded-SAM/GroundingDINO/demo/gradio_app.py
deleted file mode 100644
index 15e08323f485291df8b53eefd4691c087d7863f7..0000000000000000000000000000000000000000
--- a/spaces/IDEA-Research/Grounded-SAM/GroundingDINO/demo/gradio_app.py
+++ /dev/null
@@ -1,125 +0,0 @@
-import argparse
-from functools import partial
-import cv2
-import requests
-import os
-from io import BytesIO
-from PIL import Image
-import numpy as np
-from pathlib import Path
-
-
-import warnings
-
-import torch
-
-# prepare the environment
-os.system("python setup.py build develop --user")
-os.system("pip install packaging==21.3")
-os.system("pip install gradio")
-
-
-warnings.filterwarnings("ignore")
-
-import gradio as gr
-
-from groundingdino.models import build_model
-from groundingdino.util.slconfig import SLConfig
-from groundingdino.util.utils import clean_state_dict
-from groundingdino.util.inference import annotate, load_image, predict
-import groundingdino.datasets.transforms as T
-
-from huggingface_hub import hf_hub_download
-
-
-
-# Use this command for evaluate the GLIP-T model
-config_file = "groundingdino/config/GroundingDINO_SwinT_OGC.py"
-ckpt_repo_id = "ShilongLiu/GroundingDINO"
-ckpt_filenmae = "groundingdino_swint_ogc.pth"
-
-
-def load_model_hf(model_config_path, repo_id, filename, device='cpu'):
- args = SLConfig.fromfile(model_config_path)
- model = build_model(args)
- args.device = device
-
- cache_file = hf_hub_download(repo_id=repo_id, filename=filename)
- checkpoint = torch.load(cache_file, map_location='cpu')
- log = model.load_state_dict(clean_state_dict(checkpoint['model']), strict=False)
- print("Model loaded from {} \n => {}".format(cache_file, log))
- _ = model.eval()
- return model
-
-def image_transform_grounding(init_image):
- transform = T.Compose([
- T.RandomResize([800], max_size=1333),
- T.ToTensor(),
- T.Normalize([0.485, 0.456, 0.406], [0.229, 0.224, 0.225])
- ])
- image, _ = transform(init_image, None) # 3, h, w
- return init_image, image
-
-def image_transform_grounding_for_vis(init_image):
- transform = T.Compose([
- T.RandomResize([800], max_size=1333),
- ])
- image, _ = transform(init_image, None) # 3, h, w
- return image
-
-model = load_model_hf(config_file, ckpt_repo_id, ckpt_filenmae)
-
-def run_grounding(input_image, grounding_caption, box_threshold, text_threshold):
- init_image = input_image.convert("RGB")
- original_size = init_image.size
-
- _, image_tensor = image_transform_grounding(init_image)
- image_pil: Image = image_transform_grounding_for_vis(init_image)
-
- # run grounidng
- boxes, logits, phrases = predict(model, image_tensor, grounding_caption, box_threshold, text_threshold, device='cpu')
- annotated_frame = annotate(image_source=np.asarray(image_pil), boxes=boxes, logits=logits, phrases=phrases)
- image_with_box = Image.fromarray(cv2.cvtColor(annotated_frame, cv2.COLOR_BGR2RGB))
-
-
- return image_with_box
-
-if __name__ == "__main__":
-
- parser = argparse.ArgumentParser("Grounding DINO demo", add_help=True)
- parser.add_argument("--debug", action="store_true", help="using debug mode")
- parser.add_argument("--share", action="store_true", help="share the app")
- args = parser.parse_args()
-
- block = gr.Blocks().queue()
- with block:
- gr.Markdown("# [Grounding DINO](https://github.com/IDEA-Research/GroundingDINO)")
- gr.Markdown("### Open-World Detection with Grounding DINO")
-
- with gr.Row():
- with gr.Column():
- input_image = gr.Image(source='upload', type="pil")
- grounding_caption = gr.Textbox(label="Detection Prompt")
- run_button = gr.Button(label="Run")
- with gr.Accordion("Advanced options", open=False):
- box_threshold = gr.Slider(
- label="Box Threshold", minimum=0.0, maximum=1.0, value=0.25, step=0.001
- )
- text_threshold = gr.Slider(
- label="Text Threshold", minimum=0.0, maximum=1.0, value=0.25, step=0.001
- )
-
- with gr.Column():
- gallery = gr.outputs.Image(
- type="pil",
- # label="grounding results"
- ).style(full_width=True, full_height=True)
- # gallery = gr.Gallery(label="Generated images", show_label=False).style(
- # grid=[1], height="auto", container=True, full_width=True, full_height=True)
-
- run_button.click(fn=run_grounding, inputs=[
- input_image, grounding_caption, box_threshold, text_threshold], outputs=[gallery])
-
-
- block.launch(server_name='0.0.0.0', server_port=7579, debug=args.debug, share=args.share)
-
diff --git a/spaces/Iceclear/StableSR/StableSR/basicsr/archs/dfdnet_util.py b/spaces/Iceclear/StableSR/StableSR/basicsr/archs/dfdnet_util.py
deleted file mode 100644
index b4dc0ff738c76852e830b32fffbe65bffb5ddf50..0000000000000000000000000000000000000000
--- a/spaces/Iceclear/StableSR/StableSR/basicsr/archs/dfdnet_util.py
+++ /dev/null
@@ -1,162 +0,0 @@
-import torch
-import torch.nn as nn
-import torch.nn.functional as F
-from torch.autograd import Function
-from torch.nn.utils.spectral_norm import spectral_norm
-
-
-class BlurFunctionBackward(Function):
-
- @staticmethod
- def forward(ctx, grad_output, kernel, kernel_flip):
- ctx.save_for_backward(kernel, kernel_flip)
- grad_input = F.conv2d(grad_output, kernel_flip, padding=1, groups=grad_output.shape[1])
- return grad_input
-
- @staticmethod
- def backward(ctx, gradgrad_output):
- kernel, _ = ctx.saved_tensors
- grad_input = F.conv2d(gradgrad_output, kernel, padding=1, groups=gradgrad_output.shape[1])
- return grad_input, None, None
-
-
-class BlurFunction(Function):
-
- @staticmethod
- def forward(ctx, x, kernel, kernel_flip):
- ctx.save_for_backward(kernel, kernel_flip)
- output = F.conv2d(x, kernel, padding=1, groups=x.shape[1])
- return output
-
- @staticmethod
- def backward(ctx, grad_output):
- kernel, kernel_flip = ctx.saved_tensors
- grad_input = BlurFunctionBackward.apply(grad_output, kernel, kernel_flip)
- return grad_input, None, None
-
-
-blur = BlurFunction.apply
-
-
-class Blur(nn.Module):
-
- def __init__(self, channel):
- super().__init__()
- kernel = torch.tensor([[1, 2, 1], [2, 4, 2], [1, 2, 1]], dtype=torch.float32)
- kernel = kernel.view(1, 1, 3, 3)
- kernel = kernel / kernel.sum()
- kernel_flip = torch.flip(kernel, [2, 3])
-
- self.kernel = kernel.repeat(channel, 1, 1, 1)
- self.kernel_flip = kernel_flip.repeat(channel, 1, 1, 1)
-
- def forward(self, x):
- return blur(x, self.kernel.type_as(x), self.kernel_flip.type_as(x))
-
-
-def calc_mean_std(feat, eps=1e-5):
- """Calculate mean and std for adaptive_instance_normalization.
-
- Args:
- feat (Tensor): 4D tensor.
- eps (float): A small value added to the variance to avoid
- divide-by-zero. Default: 1e-5.
- """
- size = feat.size()
- assert len(size) == 4, 'The input feature should be 4D tensor.'
- n, c = size[:2]
- feat_var = feat.view(n, c, -1).var(dim=2) + eps
- feat_std = feat_var.sqrt().view(n, c, 1, 1)
- feat_mean = feat.view(n, c, -1).mean(dim=2).view(n, c, 1, 1)
- return feat_mean, feat_std
-
-
-def adaptive_instance_normalization(content_feat, style_feat):
- """Adaptive instance normalization.
-
- Adjust the reference features to have the similar color and illuminations
- as those in the degradate features.
-
- Args:
- content_feat (Tensor): The reference feature.
- style_feat (Tensor): The degradate features.
- """
- size = content_feat.size()
- style_mean, style_std = calc_mean_std(style_feat)
- content_mean, content_std = calc_mean_std(content_feat)
- normalized_feat = (content_feat - content_mean.expand(size)) / content_std.expand(size)
- return normalized_feat * style_std.expand(size) + style_mean.expand(size)
-
-
-def AttentionBlock(in_channel):
- return nn.Sequential(
- spectral_norm(nn.Conv2d(in_channel, in_channel, 3, 1, 1)), nn.LeakyReLU(0.2, True),
- spectral_norm(nn.Conv2d(in_channel, in_channel, 3, 1, 1)))
-
-
-def conv_block(in_channels, out_channels, kernel_size=3, stride=1, dilation=1, bias=True):
- """Conv block used in MSDilationBlock."""
-
- return nn.Sequential(
- spectral_norm(
- nn.Conv2d(
- in_channels,
- out_channels,
- kernel_size=kernel_size,
- stride=stride,
- dilation=dilation,
- padding=((kernel_size - 1) // 2) * dilation,
- bias=bias)),
- nn.LeakyReLU(0.2),
- spectral_norm(
- nn.Conv2d(
- out_channels,
- out_channels,
- kernel_size=kernel_size,
- stride=stride,
- dilation=dilation,
- padding=((kernel_size - 1) // 2) * dilation,
- bias=bias)),
- )
-
-
-class MSDilationBlock(nn.Module):
- """Multi-scale dilation block."""
-
- def __init__(self, in_channels, kernel_size=3, dilation=(1, 1, 1, 1), bias=True):
- super(MSDilationBlock, self).__init__()
-
- self.conv_blocks = nn.ModuleList()
- for i in range(4):
- self.conv_blocks.append(conv_block(in_channels, in_channels, kernel_size, dilation=dilation[i], bias=bias))
- self.conv_fusion = spectral_norm(
- nn.Conv2d(
- in_channels * 4,
- in_channels,
- kernel_size=kernel_size,
- stride=1,
- padding=(kernel_size - 1) // 2,
- bias=bias))
-
- def forward(self, x):
- out = []
- for i in range(4):
- out.append(self.conv_blocks[i](x))
- out = torch.cat(out, 1)
- out = self.conv_fusion(out) + x
- return out
-
-
-class UpResBlock(nn.Module):
-
- def __init__(self, in_channel):
- super(UpResBlock, self).__init__()
- self.body = nn.Sequential(
- nn.Conv2d(in_channel, in_channel, 3, 1, 1),
- nn.LeakyReLU(0.2, True),
- nn.Conv2d(in_channel, in_channel, 3, 1, 1),
- )
-
- def forward(self, x):
- out = x + self.body(x)
- return out
diff --git a/spaces/InvisableClearCoat101/mistralai-Mistral-7B-v0.1/README.md b/spaces/InvisableClearCoat101/mistralai-Mistral-7B-v0.1/README.md
deleted file mode 100644
index e823e04d51a48ec54ad5e6ba16be94d4b50616fe..0000000000000000000000000000000000000000
--- a/spaces/InvisableClearCoat101/mistralai-Mistral-7B-v0.1/README.md
+++ /dev/null
@@ -1,12 +0,0 @@
----
-title: Mistralai Mistral 7B V0.1
-emoji: 👀
-colorFrom: pink
-colorTo: green
-sdk: gradio
-sdk_version: 3.47.1
-app_file: app.py
-pinned: false
----
-
-Check out the configuration reference at https://huggingface.co/docs/hub/spaces-config-reference
diff --git a/spaces/Jamkonams/AutoGPT/autogpt/memory/local.py b/spaces/Jamkonams/AutoGPT/autogpt/memory/local.py
deleted file mode 100644
index 803b6dc6ebb430285f423cda592fa3e902e9a4a6..0000000000000000000000000000000000000000
--- a/spaces/Jamkonams/AutoGPT/autogpt/memory/local.py
+++ /dev/null
@@ -1,136 +0,0 @@
-from __future__ import annotations
-
-import dataclasses
-import os
-from typing import Any, List
-
-import numpy as np
-import orjson
-
-from autogpt.llm_utils import create_embedding_with_ada
-from autogpt.memory.base import MemoryProviderSingleton
-
-EMBED_DIM = 1536
-SAVE_OPTIONS = orjson.OPT_SERIALIZE_NUMPY | orjson.OPT_SERIALIZE_DATACLASS
-
-
-def create_default_embeddings():
- return np.zeros((0, EMBED_DIM)).astype(np.float32)
-
-
-@dataclasses.dataclass
-class CacheContent:
- texts: List[str] = dataclasses.field(default_factory=list)
- embeddings: np.ndarray = dataclasses.field(
- default_factory=create_default_embeddings
- )
-
-
-class LocalCache(MemoryProviderSingleton):
- """A class that stores the memory in a local file"""
-
- def __init__(self, cfg) -> None:
- """Initialize a class instance
-
- Args:
- cfg: Config object
-
- Returns:
- None
- """
- self.filename = f"{cfg.memory_index}.json"
- if os.path.exists(self.filename):
- try:
- with open(self.filename, "w+b") as f:
- file_content = f.read()
- if not file_content.strip():
- file_content = b"{}"
- f.write(file_content)
-
- loaded = orjson.loads(file_content)
- self.data = CacheContent(**loaded)
- except orjson.JSONDecodeError:
- print(f"Error: The file '{self.filename}' is not in JSON format.")
- self.data = CacheContent()
- else:
- print(
- f"Warning: The file '{self.filename}' does not exist. "
- "Local memory would not be saved to a file."
- )
- self.data = CacheContent()
-
- def add(self, text: str):
- """
- Add text to our list of texts, add embedding as row to our
- embeddings-matrix
-
- Args:
- text: str
-
- Returns: None
- """
- if "Command Error:" in text:
- return ""
- self.data.texts.append(text)
-
- embedding = create_embedding_with_ada(text)
-
- vector = np.array(embedding).astype(np.float32)
- vector = vector[np.newaxis, :]
- self.data.embeddings = np.concatenate(
- [
- self.data.embeddings,
- vector,
- ],
- axis=0,
- )
-
- with open(self.filename, "wb") as f:
- out = orjson.dumps(self.data, option=SAVE_OPTIONS)
- f.write(out)
- return text
-
- def clear(self) -> str:
- """
- Clears the redis server.
-
- Returns: A message indicating that the memory has been cleared.
- """
- self.data = CacheContent()
- return "Obliviated"
-
- def get(self, data: str) -> list[Any] | None:
- """
- Gets the data from the memory that is most relevant to the given data.
-
- Args:
- data: The data to compare to.
-
- Returns: The most relevant data.
- """
- return self.get_relevant(data, 1)
-
- def get_relevant(self, text: str, k: int) -> list[Any]:
- """ "
- matrix-vector mult to find score-for-each-row-of-matrix
- get indices for top-k winning scores
- return texts for those indices
- Args:
- text: str
- k: int
-
- Returns: List[str]
- """
- embedding = create_embedding_with_ada(text)
-
- scores = np.dot(self.data.embeddings, embedding)
-
- top_k_indices = np.argsort(scores)[-k:][::-1]
-
- return [self.data.texts[i] for i in top_k_indices]
-
- def get_stats(self) -> tuple[int, tuple[int, ...]]:
- """
- Returns: The stats of the local cache.
- """
- return len(self.data.texts), self.data.embeddings.shape
diff --git a/spaces/JohnSmith9982/ChuanhuChatGPT/modules/presets.py b/spaces/JohnSmith9982/ChuanhuChatGPT/modules/presets.py
deleted file mode 100644
index a56d50e1c7aefae37b3252b983d445ea327471a4..0000000000000000000000000000000000000000
--- a/spaces/JohnSmith9982/ChuanhuChatGPT/modules/presets.py
+++ /dev/null
@@ -1,248 +0,0 @@
-# -*- coding:utf-8 -*-
-import os
-from pathlib import Path
-import gradio as gr
-from .webui_locale import I18nAuto
-
-i18n = I18nAuto() # internationalization
-
-CHATGLM_MODEL = None
-CHATGLM_TOKENIZER = None
-LLAMA_MODEL = None
-LLAMA_INFERENCER = None
-
-# ChatGPT 设置
-INITIAL_SYSTEM_PROMPT = "You are a helpful assistant."
-API_HOST = "api.openai.com"
-COMPLETION_URL = "https://api.openai.com/v1/chat/completions"
-BALANCE_API_URL="https://api.openai.com/dashboard/billing/credit_grants"
-USAGE_API_URL="https://api.openai.com/dashboard/billing/usage"
-HISTORY_DIR = Path("history")
-HISTORY_DIR = "history"
-TEMPLATES_DIR = "templates"
-
-# 错误信息
-STANDARD_ERROR_MSG = i18n("☹️发生了错误:") # 错误信息的标准前缀
-GENERAL_ERROR_MSG = i18n("获取对话时发生错误,请查看后台日志")
-ERROR_RETRIEVE_MSG = i18n("请检查网络连接,或者API-Key是否有效。")
-CONNECTION_TIMEOUT_MSG = i18n("连接超时,无法获取对话。") # 连接超时
-READ_TIMEOUT_MSG = i18n("读取超时,无法获取对话。") # 读取超时
-PROXY_ERROR_MSG = i18n("代理错误,无法获取对话。") # 代理错误
-SSL_ERROR_PROMPT = i18n("SSL错误,无法获取对话。") # SSL 错误
-NO_APIKEY_MSG = i18n("API key为空,请检查是否输入正确。") # API key 长度不足 51 位
-NO_INPUT_MSG = i18n("请输入对话内容。") # 未输入对话内容
-BILLING_NOT_APPLICABLE_MSG = i18n("账单信息不适用") # 本地运行的模型返回的账单信息
-
-TIMEOUT_STREAMING = 60 # 流式对话时的超时时间
-TIMEOUT_ALL = 200 # 非流式对话时的超时时间
-ENABLE_STREAMING_OPTION = True # 是否启用选择选择是否实时显示回答的勾选框
-HIDE_MY_KEY = False # 如果你想在UI中隐藏你的 API 密钥,将此值设置为 True
-CONCURRENT_COUNT = 100 # 允许同时使用的用户数量
-
-SIM_K = 5
-INDEX_QUERY_TEMPRATURE = 1.0
-
-CHUANHU_TITLE = i18n("川虎Chat 🚀")
-
-CHUANHU_DESCRIPTION = i18n("由Bilibili [土川虎虎虎](https://space.bilibili.com/29125536)、[明昭MZhao](https://space.bilibili.com/24807452) 和 [Keldos](https://github.com/Keldos-Li) 开发
访问川虎Chat的 [GitHub项目](https://github.com/GaiZhenbiao/ChuanhuChatGPT) 下载最新版脚本")
-
-
-ONLINE_MODELS = [
- "gpt-3.5-turbo",
- "gpt-3.5-turbo-16k",
- "gpt-3.5-turbo-0301",
- "gpt-3.5-turbo-0613",
- "gpt-4",
- "gpt-4-0314",
- "gpt-4-0613",
- "gpt-4-32k",
- "gpt-4-32k-0314",
- "gpt-4-32k-0613",
- "川虎助理",
- "川虎助理 Pro",
- "GooglePaLM",
- "xmchat",
- "Azure OpenAI",
- "yuanai-1.0-base_10B",
- "yuanai-1.0-translate",
- "yuanai-1.0-dialog",
- "yuanai-1.0-rhythm_poems",
- "minimax-abab4-chat",
- "minimax-abab5-chat",
- "midjourney"
-]
-
-LOCAL_MODELS = [
- "chatglm-6b",
- "chatglm-6b-int4",
- "chatglm-6b-int4-ge",
- "chatglm2-6b",
- "chatglm2-6b-int4",
- "StableLM",
- "MOSS",
- "llama-7b-hf",
- "llama-13b-hf",
- "llama-30b-hf",
- "llama-65b-hf",
-]
-
-if os.environ.get('HIDE_LOCAL_MODELS', 'false') == 'true':
- MODELS = ONLINE_MODELS
-else:
- MODELS = ONLINE_MODELS + LOCAL_MODELS
-
-DEFAULT_MODEL = 0
-
-os.makedirs("models", exist_ok=True)
-os.makedirs("lora", exist_ok=True)
-os.makedirs("history", exist_ok=True)
-for dir_name in os.listdir("models"):
- if os.path.isdir(os.path.join("models", dir_name)):
- if dir_name not in MODELS:
- MODELS.append(dir_name)
-
-MODEL_TOKEN_LIMIT = {
- "gpt-3.5-turbo": 4096,
- "gpt-3.5-turbo-16k": 16384,
- "gpt-3.5-turbo-0301": 4096,
- "gpt-3.5-turbo-0613": 4096,
- "gpt-4": 8192,
- "gpt-4-0314": 8192,
- "gpt-4-0613": 8192,
- "gpt-4-32k": 32768,
- "gpt-4-32k-0314": 32768,
- "gpt-4-32k-0613": 32768
-}
-
-TOKEN_OFFSET = 1000 # 模型的token上限减去这个值,得到软上限。到达软上限之后,自动尝试减少token占用。
-DEFAULT_TOKEN_LIMIT = 3000 # 默认的token上限
-REDUCE_TOKEN_FACTOR = 0.5 # 与模型token上限想乘,得到目标token数。减少token占用时,将token占用减少到目标token数以下。
-
-REPLY_LANGUAGES = [
- "简体中文",
- "繁體中文",
- "English",
- "日本語",
- "Español",
- "Français",
- "Deutsch",
- "한국어",
- "跟随问题语言(不稳定)"
-]
-
-
-WEBSEARCH_PTOMPT_TEMPLATE = """\
-Web search results:
-
-{web_results}
-Current date: {current_date}
-
-Instructions: Using the provided web search results, write a comprehensive reply to the given query. Make sure to cite results using [[number](URL)] notation after the reference. If the provided search results refer to multiple subjects with the same name, write separate answers for each subject.
-Query: {query}
-Reply in {reply_language}
-"""
-
-PROMPT_TEMPLATE = """\
-Context information is below.
----------------------
-{context_str}
----------------------
-Current date: {current_date}.
-Using the provided context information, write a comprehensive reply to the given query.
-Make sure to cite results using [number] notation after the reference.
-If the provided context information refer to multiple subjects with the same name, write separate answers for each subject.
-Use prior knowledge only if the given context didn't provide enough information.
-Answer the question: {query_str}
-Reply in {reply_language}
-"""
-
-REFINE_TEMPLATE = """\
-The original question is as follows: {query_str}
-We have provided an existing answer: {existing_answer}
-We have the opportunity to refine the existing answer
-(only if needed) with some more context below.
-------------
-{context_msg}
-------------
-Given the new context, refine the original answer to better
-Reply in {reply_language}
-If the context isn't useful, return the original answer.
-"""
-
-SUMMARIZE_PROMPT = """Write a concise summary of the following:
-
-{text}
-
-CONCISE SUMMARY IN 中文:"""
-
-ALREADY_CONVERTED_MARK = ""
-START_OF_OUTPUT_MARK = ""
-END_OF_OUTPUT_MARK = ""
-
-small_and_beautiful_theme = gr.themes.Soft(
- primary_hue=gr.themes.Color(
- c50="#EBFAF2",
- c100="#CFF3E1",
- c200="#A8EAC8",
- c300="#77DEA9",
- c400="#3FD086",
- c500="#02C160",
- c600="#06AE56",
- c700="#05974E",
- c800="#057F45",
- c900="#04673D",
- c950="#2E5541",
- name="small_and_beautiful",
- ),
- secondary_hue=gr.themes.Color(
- c50="#576b95",
- c100="#576b95",
- c200="#576b95",
- c300="#576b95",
- c400="#576b95",
- c500="#576b95",
- c600="#576b95",
- c700="#576b95",
- c800="#576b95",
- c900="#576b95",
- c950="#576b95",
- ),
- neutral_hue=gr.themes.Color(
- name="gray",
- c50="#f6f7f8",
- # c100="#f3f4f6",
- c100="#F2F2F2",
- c200="#e5e7eb",
- c300="#d1d5db",
- c400="#B2B2B2",
- c500="#808080",
- c600="#636363",
- c700="#515151",
- c800="#393939",
- # c900="#272727",
- c900="#2B2B2B",
- c950="#171717",
- ),
- radius_size=gr.themes.sizes.radius_sm,
- ).set(
- # button_primary_background_fill="*primary_500",
- button_primary_background_fill_dark="*primary_600",
- # button_primary_background_fill_hover="*primary_400",
- # button_primary_border_color="*primary_500",
- button_primary_border_color_dark="*primary_600",
- button_primary_text_color="white",
- button_primary_text_color_dark="white",
- button_secondary_background_fill="*neutral_100",
- button_secondary_background_fill_hover="*neutral_50",
- button_secondary_background_fill_dark="*neutral_900",
- button_secondary_text_color="*neutral_800",
- button_secondary_text_color_dark="white",
- # background_fill_primary="#F7F7F7",
- # background_fill_primary_dark="#1F1F1F",
- # block_title_text_color="*primary_500",
- block_title_background_fill_dark="*primary_900",
- block_label_background_fill_dark="*primary_900",
- input_background_fill="#F6F6F6",
- chatbot_code_background_color="*neutral_950",
- chatbot_code_background_color_dark="*neutral_950",
- )
diff --git a/spaces/JohnSmith9982/ChuanhuChatGPT_Beta/modules/models/minimax.py b/spaces/JohnSmith9982/ChuanhuChatGPT_Beta/modules/models/minimax.py
deleted file mode 100644
index 2e1b50280fd2fbc43a69caaf660a0d64beaa405b..0000000000000000000000000000000000000000
--- a/spaces/JohnSmith9982/ChuanhuChatGPT_Beta/modules/models/minimax.py
+++ /dev/null
@@ -1,161 +0,0 @@
-import json
-import os
-
-import colorama
-import requests
-import logging
-
-from modules.models.base_model import BaseLLMModel
-from modules.presets import STANDARD_ERROR_MSG, GENERAL_ERROR_MSG, TIMEOUT_STREAMING, TIMEOUT_ALL, i18n
-
-group_id = os.environ.get("MINIMAX_GROUP_ID", "")
-
-
-class MiniMax_Client(BaseLLMModel):
- """
- MiniMax Client
- 接口文档见 https://api.minimax.chat/document/guides/chat
- """
-
- def __init__(self, model_name, api_key, user_name="", system_prompt=None):
- super().__init__(model_name=model_name, user=user_name)
- self.url = f'https://api.minimax.chat/v1/text/chatcompletion?GroupId={group_id}'
- self.history = []
- self.api_key = api_key
- self.system_prompt = system_prompt
- self.headers = {
- "Authorization": f"Bearer {api_key}",
- "Content-Type": "application/json"
- }
-
- def get_answer_at_once(self):
- # minimax temperature is (0,1] and base model temperature is [0,2], and yuan 0.9 == base 1 so need to convert
- temperature = self.temperature * 0.9 if self.temperature <= 1 else 0.9 + (self.temperature - 1) / 10
-
- request_body = {
- "model": self.model_name.replace('minimax-', ''),
- "temperature": temperature,
- "skip_info_mask": True,
- 'messages': [{"sender_type": "USER", "text": self.history[-1]['content']}]
- }
- if self.n_choices:
- request_body['beam_width'] = self.n_choices
- if self.system_prompt:
- request_body['prompt'] = self.system_prompt
- if self.max_generation_token:
- request_body['tokens_to_generate'] = self.max_generation_token
- if self.top_p:
- request_body['top_p'] = self.top_p
-
- response = requests.post(self.url, headers=self.headers, json=request_body)
-
- res = response.json()
- answer = res['reply']
- total_token_count = res["usage"]["total_tokens"]
- return answer, total_token_count
-
- def get_answer_stream_iter(self):
- response = self._get_response(stream=True)
- if response is not None:
- iter = self._decode_chat_response(response)
- partial_text = ""
- for i in iter:
- partial_text += i
- yield partial_text
- else:
- yield STANDARD_ERROR_MSG + GENERAL_ERROR_MSG
-
- def _get_response(self, stream=False):
- minimax_api_key = self.api_key
- history = self.history
- logging.debug(colorama.Fore.YELLOW +
- f"{history}" + colorama.Fore.RESET)
- headers = {
- "Content-Type": "application/json",
- "Authorization": f"Bearer {minimax_api_key}",
- }
-
- temperature = self.temperature * 0.9 if self.temperature <= 1 else 0.9 + (self.temperature - 1) / 10
-
- messages = []
- for msg in self.history:
- if msg['role'] == 'user':
- messages.append({"sender_type": "USER", "text": msg['content']})
- else:
- messages.append({"sender_type": "BOT", "text": msg['content']})
-
- request_body = {
- "model": self.model_name.replace('minimax-', ''),
- "temperature": temperature,
- "skip_info_mask": True,
- 'messages': messages
- }
- if self.n_choices:
- request_body['beam_width'] = self.n_choices
- if self.system_prompt:
- lines = self.system_prompt.splitlines()
- if lines[0].find(":") != -1 and len(lines[0]) < 20:
- request_body["role_meta"] = {
- "user_name": lines[0].split(":")[0],
- "bot_name": lines[0].split(":")[1]
- }
- lines.pop()
- request_body["prompt"] = "\n".join(lines)
- if self.max_generation_token:
- request_body['tokens_to_generate'] = self.max_generation_token
- else:
- request_body['tokens_to_generate'] = 512
- if self.top_p:
- request_body['top_p'] = self.top_p
-
- if stream:
- timeout = TIMEOUT_STREAMING
- request_body['stream'] = True
- request_body['use_standard_sse'] = True
- else:
- timeout = TIMEOUT_ALL
- try:
- response = requests.post(
- self.url,
- headers=headers,
- json=request_body,
- stream=stream,
- timeout=timeout,
- )
- except:
- return None
-
- return response
-
- def _decode_chat_response(self, response):
- error_msg = ""
- for chunk in response.iter_lines():
- if chunk:
- chunk = chunk.decode()
- chunk_length = len(chunk)
- print(chunk)
- try:
- chunk = json.loads(chunk[6:])
- except json.JSONDecodeError:
- print(i18n("JSON解析错误,收到的内容: ") + f"{chunk}")
- error_msg += chunk
- continue
- if chunk_length > 6 and "delta" in chunk["choices"][0]:
- if "finish_reason" in chunk["choices"][0] and chunk["choices"][0]["finish_reason"] == "stop":
- self.all_token_counts.append(chunk["usage"]["total_tokens"] - sum(self.all_token_counts))
- break
- try:
- yield chunk["choices"][0]["delta"]
- except Exception as e:
- logging.error(f"Error: {e}")
- continue
- if error_msg:
- try:
- error_msg = json.loads(error_msg)
- if 'base_resp' in error_msg:
- status_code = error_msg['base_resp']['status_code']
- status_msg = error_msg['base_resp']['status_msg']
- raise Exception(f"{status_code} - {status_msg}")
- except json.JSONDecodeError:
- pass
- raise Exception(error_msg)
diff --git a/spaces/KAIST-Geometric-AI-Lab/salad-demo/salad/utils/imageutil.py b/spaces/KAIST-Geometric-AI-Lab/salad-demo/salad/utils/imageutil.py
deleted file mode 100644
index 897e8486c2c9cbd76f20739c4eb9575a9f2ac67c..0000000000000000000000000000000000000000
--- a/spaces/KAIST-Geometric-AI-Lab/salad-demo/salad/utils/imageutil.py
+++ /dev/null
@@ -1,464 +0,0 @@
-import os
-import textwrap
-from pathlib import Path
-from typing import List
-
-import cv2
-import numpy as np
-import PIL
-from PIL import Image, ImageChops, ImageDraw, ImageFont
-
-kMinMargin = 10
-
-
-def stack_images_horizontally(images: List, save_path=None):
- widths, heights = list(zip(*(i.size for i in images)))
- total_width = sum(widths)
- max_height = max(heights)
- new_im = Image.new("RGBA", (total_width, max_height))
-
- x_offset = 0
- for im in images:
- new_im.paste(im, (x_offset, 0))
- x_offset += im.size[0]
- if save_path is not None:
- new_im.save(save_path)
- return new_im
-
-
-def stack_images_vertically(images: List, save_path=None):
- widths, heights = list(zip(*(i.size for i in images)))
- max_width = max(widths)
- total_height = sum(heights)
- new_im = Image.new("RGBA", (max_width, total_height))
-
- y_offset = 0
- for im in images:
- new_im.paste(im, (0, y_offset))
- y_offset += im.size[1]
- if save_path is not None:
- new_im.save(save_path)
- return new_im
-
-
-def merge_images(images: List):
- if isinstance(images[0], Image.Image):
- return stack_images_horizontally(images)
-
- images = list(map(stack_images_horizontally, images))
- return stack_images_vertically(images)
-
-
-def draw_text(
- image: PIL.Image,
- text: str,
- font_size=None,
- font_color=(0, 0, 0),
- max_seq_length=100,
-):
- W, H = image.size
- S = max(W, H)
-
- font_path = os.path.join(cv2.__path__[0], "qt", "fonts", "DejaVuSans.ttf")
- font_size = max(int(S / 32), 20) if font_size is None else font_size
- font = ImageFont.truetype(font_path, size=font_size)
-
- text_wrapped = textwrap.fill(text, max_seq_length)
- w, h = font.getsize(text_wrapped)
- new_im = Image.new("RGBA", (W, H + h))
- new_im.paste(image, (0, h))
- draw = ImageDraw.Draw(new_im)
- draw.text((max((W - w) / 2, 0), 0), text_wrapped, font=font, fill=font_color)
- return new_im
-
-
-def to_white(img):
- new_img = Image.new("RGBA", img.size, "WHITE")
- new_img.paste(img, (0, 0), img)
- new_img.convert("RGB")
- return new_img
-
-
-def get_bbox(in_file, fuzz=17.5):
- im = Image.open(in_file)
-
- # bbox = im.convert("RGBa").getbbox()
- try:
- bg = Image.new(im.mode, im.size, im.getpixel((0, 0)))
- except OSError as err:
- print(f"error {in_file}")
- raise OSError
- diff = ImageChops.difference(im, bg)
- offset = int(round(float(fuzz) / 100.0 * 255.0))
- diff = ImageChops.add(diff, diff, 2.0, -offset)
- bbox = diff.getbbox()
-
- bx_min = max(bbox[0] - kMinMargin, 0)
- by_min = max(bbox[1] - kMinMargin, 0)
- bx_max = min(bbox[2] + kMinMargin, im.size[0])
- by_max = min(bbox[3] + kMinMargin, im.size[1])
- bbox_margin = (bx_min, by_min, bx_max, by_max)
- return bbox_margin
-
-
-def get_largest_bbox(in_files):
- largest_bbox = (float("Inf"), float("Inf"), -float("Inf"), -float("Inf"))
- for in_file in in_files:
- bbox = get_bbox(in_file)
- largest_bbox = (
- min(bbox[0], largest_bbox[0]),
- min(bbox[1], largest_bbox[1]),
- max(bbox[2], largest_bbox[2]),
- max(bbox[3], largest_bbox[3]),
- )
- return largest_bbox
-
-
-def trim(in_file, out_file, keep_ratio):
- # im = Image.open(in_file)
- # bbox = im.convert("RGBa").getbbox()
- bbox = get_bbox(in_file)
- trim_with_bbox(in_file, out_file, bbox, keep_ratio)
-
-
-def trim_with_bbox(in_file, out_file, bbox, keep_ratio):
- im = Image.open(in_file)
-
- if keep_ratio:
- w, h = im.size
- r = float(w) / h
-
- bx_min, by_min, bx_max, by_max = bbox[0], bbox[1], bbox[2], bbox[3]
- bw, bh = bx_max - bx_min, by_max - by_min
- bcx, bcy = 0.5 * (bx_min + bx_max), 0.5 * (by_min + by_max)
- br = float(bw) / bh
-
- if br > r:
- bh = int(round(bw / r))
- by_min, by_max = int(round(bcy - 0.5 * bh)), int(round(bcy + 0.5 * bh))
- if by_min < 0:
- by_min = 0
- by_max = bh
- elif by_max > h:
- by_max = h
- by_min = h - bh
- assert bh >= bh
- elif br < r:
- bw = int(round(bh * r))
- bx_min, bx_max = int(round(bcx - 0.5 * bw)), int(round(bcx + 0.5 * bw))
- if bx_min < 0:
- bx_min = 0
- bx_max = bw
- elif bx_max > w:
- bx_max = w
- bx_min = w - bw
-
- bbox = (bx_min, by_min, bx_max, by_max)
-
- im.crop(bbox).save(out_file, "png")
-
-
-def trim_with_largest_bbox(in_files, out_files, keep_ratio):
- assert len(in_files) == len(out_files)
-
- bbox = get_largest_bbox(in_files)
- for i in range(len(in_files)):
- trim_with_bbox(in_files[i], out_files[i], bbox, keep_ratio)
-
-
-def create_image_table_tight_centering(
- in_img_files, out_img_file, max_total_width=2560, draw_col_lines=[]
-):
-
- n_rows = len(in_img_files)
- n_cols = len(in_img_files[0])
-
- # Compute width and height of each image.
- width = 0
- row_top = [float("Inf")] * n_rows
- row_bottom = [-float("Inf")] * n_rows
-
- for row in range(n_rows):
- for col in range(n_cols):
- img_left, img_top, img_right, img_bottom = get_bbox(in_img_files[row][col])
- img_width = img_right - img_left
- width = max(width, img_width)
- row_top[row] = min(row_top[row], img_top)
- row_bottom[row] = max(row_bottom[row], img_bottom)
-
- row_height = [bottom - top for bottom, top in zip(row_bottom, row_top)]
-
- # Combine images.
- cmd = "convert "
- for row in range(n_rows):
- cmd += " \( "
- for col in range(n_cols):
- img_left, img_top, img_right, img_bottom = get_bbox(in_img_files[row][col])
- img_h_center = 0.5 * (img_left + img_right)
- left = int(img_h_center - 0.5 * width)
- cmd += " \( {} ".format(in_img_files[row][col])
- cmd += "-gravity NorthWest -crop {}x{}+{}+{} +repage \) ".format(
- width, row_height[row], left, row_top[row]
- )
- cmd += " -gravity center -background white +append \) "
-
- cmd += "-append " + out_img_file
- print(cmd)
- os.system(cmd)
-
- # Draw lines for columns.
- for col in draw_col_lines:
- if col <= 0 or col >= n_cols:
- continue
- strokewidth = max(int(round(width * 0.005)), 1)
- pos = col * width
- cmd = "convert " + out_img_file + " -stroke black "
- cmd += "-strokewidth {} ".format(strokewidth)
- cmd += '-draw "line {0},0 {0},10000000" '.format(pos) + out_img_file
- os.system(cmd)
-
- # Resize the combined image if it is too large.
- print(n_cols * width)
- if (n_cols * width) > max_total_width:
- cmd = "convert {0} -resize {1}x +repage {0}".format(
- out_img_file, max_total_width
- )
- print(cmd)
- os.system(cmd)
-
- print("Saved '{}'.".format(out_img_file))
-
- return width, row_height
-
-
-def create_image_table_tight_centering_per_row(
- in_img_files, out_img_dir, max_total_width=1280, draw_col_lines=[]
-):
-
- n_rows = len(in_img_files)
- n_cols = len(in_img_files[0])
-
- # Compute width and height of each image.
- width = 0
- row_top = [float("Inf")] * n_rows
- row_bottom = [-float("Inf")] * n_rows
-
- for row in range(n_rows):
- for col in range(n_cols):
- img_left, img_top, img_right, img_bottom = get_bbox(in_img_files[row][col])
- img_width = img_right - img_left
- width = max(width, img_width)
- row_top[row] = min(row_top[row], img_top)
- row_bottom[row] = max(row_bottom[row], img_bottom)
-
- row_height = [bottom - top for bottom, top in zip(row_bottom, row_top)]
-
- if not os.path.exists(out_img_dir):
- os.makedirs(out_img_dir)
-
- # Combine images.
- for row in range(n_rows):
- out_img_file = os.path.join(out_img_dir, "{:02d}.png".format(row))
- cmd = "convert "
- for col in range(n_cols):
- img_left, img_top, img_right, img_bottom = get_bbox(in_img_files[row][col])
- img_h_center = 0.5 * (img_left + img_right)
- left = int(img_h_center - 0.5 * width)
- cmd += " \( {} ".format(in_img_files[row][col])
- cmd += "-gravity NorthWest -crop {}x{}+{}+{} +repage \) ".format(
- width, row_height[row], left, row_top[row]
- )
- cmd += " -gravity center -background white +append " + out_img_file
- print(cmd)
- os.system(cmd)
-
- # Draw lines for columns.
- for col in draw_col_lines:
- if col <= 0 or col >= n_cols:
- continue
- strokewidth = max(int(round(width * 0.005)), 1)
- pos = col * width
- cmd = "convert " + out_img_file + " -stroke black "
- cmd += "-strokewidth {} ".format(strokewidth)
- cmd += '-draw "line {0},0 {0},10000000" '.format(pos) + out_img_file
- os.system(cmd)
- print(cmd)
-
- # Resize the combined image if it is too large.
- print(n_cols * width)
- if (n_cols * width) > max_total_width:
- cmd = "convert {0} -resize {1}x +repage {0}".format(
- out_img_file, max_total_width
- )
- print(cmd)
- os.system(cmd)
-
- print("Saved '{}'.".format(out_img_file))
-
- return width, row_height
-
-
-def create_image_table_tight_centering_per_col(
- in_img_files, out_img_dir, max_width=2560, draw_col_lines=[]
-):
-
- n_rows = len(in_img_files)
- n_cols = len(in_img_files[0])
-
- # Compute width and height of each image.
- width = 0
- row_top = [float("Inf")] * n_rows
- row_bottom = [-float("Inf")] * n_rows
-
- for row in range(n_rows):
- for col in range(n_cols):
- img_left, img_top, img_right, img_bottom = get_bbox(in_img_files[row][col])
- img_width = img_right - img_left
- width = max(width, img_width)
- row_top[row] = min(row_top[row], img_top)
- row_bottom[row] = max(row_bottom[row], img_bottom)
-
- row_height = [bottom - top for bottom, top in zip(row_bottom, row_top)]
-
- if not os.path.exists(out_img_dir):
- os.makedirs(out_img_dir)
-
- # Combine images.
- for col in range(n_cols):
- out_img_file = os.path.join(out_img_dir, "{:02d}.png".format(col))
- cmd = "convert "
- for row in range(n_rows):
- img_left, img_top, img_right, img_bottom = get_bbox(in_img_files[row][col])
- img_h_center = 0.5 * (img_left + img_right)
- left = int(img_h_center - 0.5 * width)
- cmd += " \( {} ".format(in_img_files[row][col])
- cmd += "-gravity NorthWest -crop {}x{}+{}+{} +repage \) ".format(
- width, row_height[row], left, row_top[row]
- )
- cmd += " -gravity center -background white -append " + out_img_file
- print(cmd)
- os.system(cmd)
-
- # Resize the combined image if it is too large.
- if width > max_width:
- cmd = "convert {0} -resize {1}x +repage {0}".format(out_img_file, max_width)
- print(cmd)
- os.system(cmd)
-
- print("Saved '{}'.".format(out_img_file))
-
- return width, row_height
-
-
-def create_image_table_after_crop(
- in_img_files,
- out_img_file,
- lbox=None,
- tbox=None,
- rbox=None,
- dbox=None,
- max_total_width=2560,
- draw_col_lines=[],
- transpose=False,
- verbose=False,
- line_multi=None,
-):
- out_img_file = str(out_img_file)
- if not isinstance(in_img_files[0], list):
- in_img_files = [in_img_files]
- in_img_files = [[x for x in row if len(str(x)) != 0] for row in in_img_files]
- if transpose:
- x = np.array(in_img_files)
- in_img_files = x.transpose().tolist()
-
- n_rows = len(in_img_files)
- n_cols = len(in_img_files[0])
-
- # Compute width and height of each image.
- width = 0
- row_top = [float("Inf")] * n_rows
- row_bottom = [-float("Inf")] * n_rows
-
- for row in range(n_rows):
- for col in range(n_cols):
- img_left, img_top, img_right, img_bottom = get_bbox(in_img_files[row][col])
- # img_left, img_top, img_right, img_bottom = lbox, tbox, rbox, dbox
- img_left = img_left if lbox is None else lbox
- img_top = img_top if tbox is None else tbox
- img_right = img_right if rbox is None else rbox
- img_bottom = img_bottom if dbox is None else dbox
- img_width = img_right - img_left
- width = max(width, img_width)
- row_top[row] = min(row_top[row], img_top)
- row_bottom[row] = max(row_bottom[row], img_bottom)
-
- row_height = [bottom - top for bottom, top in zip(row_bottom, row_top)]
-
- # Combine images.
- cmd = "convert "
- for row in range(n_rows):
- cmd += " \( "
- for col in range(n_cols):
- # img_left, img_top, img_right, img_bottom = lbox, tbox, rbox, dbox
- img_left, img_top, img_right, img_bottom = get_bbox(in_img_files[row][col])
- img_left = img_left if lbox is None else lbox
- img_top = img_top if tbox is None else tbox
- img_right = img_right if rbox is None else rbox
- img_bottom = img_bottom if dbox is None else dbox
- img_h_center = 0.5 * (img_left + img_right)
- left = int(img_h_center - 0.5 * width)
- cmd += " \( {} ".format(in_img_files[row][col])
- cmd += "-gravity NorthWest -crop {}x{}+{}+{} +repage \) ".format(
- width, row_height[row], left, row_top[row]
- )
- cmd += " -gravity center -background white +append \) "
-
- cmd += "-append " + out_img_file
- if verbose:
- print(cmd)
- os.system(cmd)
- # Draw lines for columns.
- for col in draw_col_lines:
- if col <= 0 or col >= n_cols:
- continue
- strokewidth = max(int(round(width * 0.005)), 1)
- if line_multi is not None:
- strokewidth *= line_multi
- pos = col * width
- cmd = "convert " + out_img_file + " -stroke black "
- cmd += "-strokewidth {} ".format(strokewidth)
- cmd += '-draw "line {0},0 {0},10000000" '.format(pos) + out_img_file
- if verbose:
- print(cmd)
- os.system(cmd)
-
- # Resize the combined image if it is too large.
- # print(n_cols * width)
- # if (n_cols * width) > max_total_width:
- # cmd = "convert {0} -resize {1}x +repage {0}".format(
- # out_img_file, max_total_width
- # )
- # print(cmd)
- # os.system(cmd)
-
- print("Saved '{}'.".format(out_img_file))
-
- return width, row_height
-
-
-def make_2dgrid(input_list, num_rows=None, num_cols=None):
- # if num_rows * num_cols != len(input_list):
- # raise Warning("Number of rows and columns do not match the length of the input list.")
-
- if num_rows is None and num_cols is not None:
- num_rows = len(input_list) // num_cols + 1
- output_list = []
- for i in range(num_rows):
- row = []
- for j in range(num_cols):
- if i * num_cols + j >= len(input_list):
- break
- row.append(input_list[i * num_cols + j])
- output_list.append(row)
-
- return output_list
diff --git a/spaces/KaygNas/cut-it/public/mockServiceWorker.js b/spaces/KaygNas/cut-it/public/mockServiceWorker.js
deleted file mode 100644
index 87e0f31b814f1a4837b4b39510bae970a3bba65a..0000000000000000000000000000000000000000
--- a/spaces/KaygNas/cut-it/public/mockServiceWorker.js
+++ /dev/null
@@ -1,303 +0,0 @@
-/* eslint-disable */
-/* tslint:disable */
-
-/**
- * Mock Service Worker (1.2.1).
- * @see https://github.com/mswjs/msw
- * - Please do NOT modify this file.
- * - Please do NOT serve this file on production.
- */
-
-const INTEGRITY_CHECKSUM = '3d6b9f06410d179a7f7404d4bf4c3c70'
-const activeClientIds = new Set()
-
-self.addEventListener('install', function () {
- self.skipWaiting()
-})
-
-self.addEventListener('activate', function (event) {
- event.waitUntil(self.clients.claim())
-})
-
-self.addEventListener('message', async function (event) {
- const clientId = event.source.id
-
- if (!clientId || !self.clients) {
- return
- }
-
- const client = await self.clients.get(clientId)
-
- if (!client) {
- return
- }
-
- const allClients = await self.clients.matchAll({
- type: 'window',
- })
-
- switch (event.data) {
- case 'KEEPALIVE_REQUEST': {
- sendToClient(client, {
- type: 'KEEPALIVE_RESPONSE',
- })
- break
- }
-
- case 'INTEGRITY_CHECK_REQUEST': {
- sendToClient(client, {
- type: 'INTEGRITY_CHECK_RESPONSE',
- payload: INTEGRITY_CHECKSUM,
- })
- break
- }
-
- case 'MOCK_ACTIVATE': {
- activeClientIds.add(clientId)
-
- sendToClient(client, {
- type: 'MOCKING_ENABLED',
- payload: true,
- })
- break
- }
-
- case 'MOCK_DEACTIVATE': {
- activeClientIds.delete(clientId)
- break
- }
-
- case 'CLIENT_CLOSED': {
- activeClientIds.delete(clientId)
-
- const remainingClients = allClients.filter((client) => {
- return client.id !== clientId
- })
-
- // Unregister itself when there are no more clients
- if (remainingClients.length === 0) {
- self.registration.unregister()
- }
-
- break
- }
- }
-})
-
-self.addEventListener('fetch', function (event) {
- const { request } = event
- const accept = request.headers.get('accept') || ''
-
- // Bypass server-sent events.
- if (accept.includes('text/event-stream')) {
- return
- }
-
- // Bypass navigation requests.
- if (request.mode === 'navigate') {
- return
- }
-
- // Opening the DevTools triggers the "only-if-cached" request
- // that cannot be handled by the worker. Bypass such requests.
- if (request.cache === 'only-if-cached' && request.mode !== 'same-origin') {
- return
- }
-
- // Bypass all requests when there are no active clients.
- // Prevents the self-unregistered worked from handling requests
- // after it's been deleted (still remains active until the next reload).
- if (activeClientIds.size === 0) {
- return
- }
-
- // Generate unique request ID.
- const requestId = Math.random().toString(16).slice(2)
-
- event.respondWith(
- handleRequest(event, requestId).catch((error) => {
- if (error.name === 'NetworkError') {
- console.warn(
- '[MSW] Successfully emulated a network error for the "%s %s" request.',
- request.method,
- request.url,
- )
- return
- }
-
- // At this point, any exception indicates an issue with the original request/response.
- console.error(
- `\
-[MSW] Caught an exception from the "%s %s" request (%s). This is probably not a problem with Mock Service Worker. There is likely an additional logging output above.`,
- request.method,
- request.url,
- `${error.name}: ${error.message}`,
- )
- }),
- )
-})
-
-async function handleRequest(event, requestId) {
- const client = await resolveMainClient(event)
- const response = await getResponse(event, client, requestId)
-
- // Send back the response clone for the "response:*" life-cycle events.
- // Ensure MSW is active and ready to handle the message, otherwise
- // this message will pend indefinitely.
- if (client && activeClientIds.has(client.id)) {
- ;(async function () {
- const clonedResponse = response.clone()
- sendToClient(client, {
- type: 'RESPONSE',
- payload: {
- requestId,
- type: clonedResponse.type,
- ok: clonedResponse.ok,
- status: clonedResponse.status,
- statusText: clonedResponse.statusText,
- body:
- clonedResponse.body === null ? null : await clonedResponse.text(),
- headers: Object.fromEntries(clonedResponse.headers.entries()),
- redirected: clonedResponse.redirected,
- },
- })
- })()
- }
-
- return response
-}
-
-// Resolve the main client for the given event.
-// Client that issues a request doesn't necessarily equal the client
-// that registered the worker. It's with the latter the worker should
-// communicate with during the response resolving phase.
-async function resolveMainClient(event) {
- const client = await self.clients.get(event.clientId)
-
- if (client?.frameType === 'top-level') {
- return client
- }
-
- const allClients = await self.clients.matchAll({
- type: 'window',
- })
-
- return allClients
- .filter((client) => {
- // Get only those clients that are currently visible.
- return client.visibilityState === 'visible'
- })
- .find((client) => {
- // Find the client ID that's recorded in the
- // set of clients that have registered the worker.
- return activeClientIds.has(client.id)
- })
-}
-
-async function getResponse(event, client, requestId) {
- const { request } = event
- const clonedRequest = request.clone()
-
- function passthrough() {
- // Clone the request because it might've been already used
- // (i.e. its body has been read and sent to the client).
- const headers = Object.fromEntries(clonedRequest.headers.entries())
-
- // Remove MSW-specific request headers so the bypassed requests
- // comply with the server's CORS preflight check.
- // Operate with the headers as an object because request "Headers"
- // are immutable.
- delete headers['x-msw-bypass']
-
- return fetch(clonedRequest, { headers })
- }
-
- // Bypass mocking when the client is not active.
- if (!client) {
- return passthrough()
- }
-
- // Bypass initial page load requests (i.e. static assets).
- // The absence of the immediate/parent client in the map of the active clients
- // means that MSW hasn't dispatched the "MOCK_ACTIVATE" event yet
- // and is not ready to handle requests.
- if (!activeClientIds.has(client.id)) {
- return passthrough()
- }
-
- // Bypass requests with the explicit bypass header.
- // Such requests can be issued by "ctx.fetch()".
- if (request.headers.get('x-msw-bypass') === 'true') {
- return passthrough()
- }
-
- // Notify the client that a request has been intercepted.
- const clientMessage = await sendToClient(client, {
- type: 'REQUEST',
- payload: {
- id: requestId,
- url: request.url,
- method: request.method,
- headers: Object.fromEntries(request.headers.entries()),
- cache: request.cache,
- mode: request.mode,
- credentials: request.credentials,
- destination: request.destination,
- integrity: request.integrity,
- redirect: request.redirect,
- referrer: request.referrer,
- referrerPolicy: request.referrerPolicy,
- body: await request.text(),
- bodyUsed: request.bodyUsed,
- keepalive: request.keepalive,
- },
- })
-
- switch (clientMessage.type) {
- case 'MOCK_RESPONSE': {
- return respondWithMock(clientMessage.data)
- }
-
- case 'MOCK_NOT_FOUND': {
- return passthrough()
- }
-
- case 'NETWORK_ERROR': {
- const { name, message } = clientMessage.data
- const networkError = new Error(message)
- networkError.name = name
-
- // Rejecting a "respondWith" promise emulates a network error.
- throw networkError
- }
- }
-
- return passthrough()
-}
-
-function sendToClient(client, message) {
- return new Promise((resolve, reject) => {
- const channel = new MessageChannel()
-
- channel.port1.onmessage = (event) => {
- if (event.data && event.data.error) {
- return reject(event.data.error)
- }
-
- resolve(event.data)
- }
-
- client.postMessage(message, [channel.port2])
- })
-}
-
-function sleep(timeMs) {
- return new Promise((resolve) => {
- setTimeout(resolve, timeMs)
- })
-}
-
-async function respondWithMock(response) {
- await sleep(response.delay)
- return new Response(response.body, response)
-}
diff --git a/spaces/Kevin676/AutoGPT/autogpt/commands/twitter.py b/spaces/Kevin676/AutoGPT/autogpt/commands/twitter.py
deleted file mode 100644
index 3eaed36e20e1c520690ac59f25a4da6501f3440f..0000000000000000000000000000000000000000
--- a/spaces/Kevin676/AutoGPT/autogpt/commands/twitter.py
+++ /dev/null
@@ -1,26 +0,0 @@
-import os
-
-import tweepy
-from dotenv import load_dotenv
-
-load_dotenv()
-
-
-def send_tweet(tweet_text):
- consumer_key = os.environ.get("TW_CONSUMER_KEY")
- consumer_secret = os.environ.get("TW_CONSUMER_SECRET")
- access_token = os.environ.get("TW_ACCESS_TOKEN")
- access_token_secret = os.environ.get("TW_ACCESS_TOKEN_SECRET")
- # Authenticate to Twitter
- auth = tweepy.OAuthHandler(consumer_key, consumer_secret)
- auth.set_access_token(access_token, access_token_secret)
-
- # Create API object
- api = tweepy.API(auth)
-
- # Send tweet
- try:
- api.update_status(tweet_text)
- print("Tweet sent successfully!")
- except tweepy.TweepyException as e:
- print("Error sending tweet: {}".format(e.reason))
diff --git a/spaces/Khalida1w/denoising/README.md b/spaces/Khalida1w/denoising/README.md
deleted file mode 100644
index 961671110cbaae348668288f1824766bcf3fd9df..0000000000000000000000000000000000000000
--- a/spaces/Khalida1w/denoising/README.md
+++ /dev/null
@@ -1,13 +0,0 @@
----
-title: Denoising
-emoji: 😻
-colorFrom: gray
-colorTo: indigo
-sdk: gradio
-sdk_version: 3.15.0
-app_file: app.py
-pinned: false
-license: apache-2.0
----
-
-Check out the configuration reference at https://huggingface.co/docs/hub/spaces-config-reference
diff --git a/spaces/KyanChen/BuildingExtraction/Utils/Datasets.py b/spaces/KyanChen/BuildingExtraction/Utils/Datasets.py
deleted file mode 100644
index a9ec3573ae62d0361ce7a9015389c1a44b4957cd..0000000000000000000000000000000000000000
--- a/spaces/KyanChen/BuildingExtraction/Utils/Datasets.py
+++ /dev/null
@@ -1,143 +0,0 @@
-import os.path
-
-from torch.utils.data import Dataset, DataLoader
-import torch
-import numpy as np
-import pandas as pd
-from skimage import io
-from Utils.Augmentations import Augmentations, Resize
-
-
-class Datasets(Dataset):
- def __init__(self, data_file, transform=None, phase='train', *args, **kwargs):
- self.transform = transform
- self.data_info = pd.read_csv(data_file, index_col=0)
- self.phase = phase
-
- def __len__(self):
- return len(self.data_info)
-
- def __getitem__(self, index):
- data = self.pull_item_seg(index)
- return data
-
- def pull_item_seg(self, index):
- """
- :param index: image index
- """
- data = self.data_info.iloc[index]
- img_name = data['img']
- label_name = data['label']
-
- ori_img = io.imread(img_name, as_gray=False)
- ori_label = io.imread(label_name, as_gray=True)
- assert (ori_img is not None and ori_label is not None), f'{img_name} or {label_name} is not valid'
-
- if self.transform is not None:
- img, label = self.transform((ori_img, ori_label))
-
- one_hot_label = np.zeros([2] + list(label.shape), dtype=np.float)
- one_hot_label[0] = label == 0
- one_hot_label[1] = label > 0
- return_dict = {
- 'img': torch.from_numpy(img).permute(2, 0, 1),
- 'label': torch.from_numpy(one_hot_label),
- 'img_name': os.path.basename(img_name)
- }
- return return_dict
-
-
-def get_data_loader(config, test_mode=False):
- if not test_mode:
- train_params = {
- 'batch_size': config['BATCH_SIZE'],
- 'shuffle': config['IS_SHUFFLE'],
- 'drop_last': False,
- 'collate_fn': collate_fn,
- 'num_workers': config['NUM_WORKERS'],
- 'pin_memory': False
- }
- # data_file, config, transform=None
- train_set = Datasets(
- config['DATASET'],
- Augmentations(
- config['IMG_SIZE'], config['PRIOR_MEAN'], config['PRIOR_STD'], 'train', config['PHASE'], config
- ),
- config['PHASE'],
- config
- )
- patterns = ['train']
- else:
- patterns = []
-
- if config['IS_VAL']:
- val_params = {
- 'batch_size': config['VAL_BATCH_SIZE'],
- 'shuffle': False,
- 'drop_last': False,
- 'collate_fn': collate_fn,
- 'num_workers': config['NUM_WORKERS'],
- 'pin_memory': False
- }
- val_set = Datasets(
- config['VAL_DATASET'],
- Augmentations(
- config['IMG_SIZE'], config['PRIOR_MEAN'], config['PRIOR_STD'], 'val', config['PHASE'], config
- ),
- config['PHASE'],
- config
- )
- patterns += ['val']
-
- if config['IS_TEST']:
- test_params = {
- 'batch_size': config['VAL_BATCH_SIZE'],
- 'shuffle': False,
- 'drop_last': False,
- 'collate_fn': collate_fn,
- 'num_workers': config['NUM_WORKERS'],
- 'pin_memory': False
- }
- test_set = Datasets(
- config['TEST_DATASET'],
- Augmentations(
- config['IMG_SIZE'], config['PRIOR_MEAN'], config['PRIOR_STD'], 'test', config['PHASE'], config
- ),
- config['PHASE'],
- config
- )
- patterns += ['test']
-
- data_loaders = {}
- for x in patterns:
- data_loaders[x] = DataLoader(eval(x+'_set'), **eval(x+'_params'))
- return data_loaders
-
-
-def collate_fn(batch):
- def to_tensor(item):
- if torch.is_tensor(item):
- return item
- elif isinstance(item, type(np.array(0))):
- return torch.from_numpy(item).float()
- elif isinstance(item, type('0')):
- return item
- elif isinstance(item, list):
- return item
- elif isinstance(item, dict):
- return item
-
- return_data = {}
- for key in batch[0].keys():
- return_data[key] = []
-
- for sample in batch:
- for key, value in sample.items():
- return_data[key].append(to_tensor(value))
-
- keys = set(batch[0].keys()) - {'img_name'}
- for key in keys:
- return_data[key] = torch.stack(return_data[key], dim=0)
-
- return return_data
-
diff --git a/spaces/LamaAl/arabic-empathetic/app.py b/spaces/LamaAl/arabic-empathetic/app.py
deleted file mode 100644
index 8922200a14ab1ce2051315a452426f8921106c67..0000000000000000000000000000000000000000
--- a/spaces/LamaAl/arabic-empathetic/app.py
+++ /dev/null
@@ -1,41 +0,0 @@
-#Import transformers and gradio
-import transformers
-import gradio as gr
-import git
-
-#Load arabert preprocessor
-import git
-git.Git("arabert").clone("https://github.com/aub-mind/arabert")
-from arabert.preprocess import ArabertPreprocessor
-arabert_prep = ArabertPreprocessor(model_name="bert-base-arabert", keep_emojis=False)
-
-
-#Load Model
-from transformers import EncoderDecoderModel, AutoTokenizer
-tokenizer = AutoTokenizer.from_pretrained("tareknaous/bert2bert-empathetic-response-msa")
-model = EncoderDecoderModel.from_pretrained("tareknaous/bert2bert-empathetic-response-msa")
-model.eval()
-
-def generate_response(text):
- text_clean = arabert_prep.preprocess(text)
- inputs = tokenizer.encode_plus(text_clean,return_tensors='pt')
- outputs = model.generate(input_ids = inputs.input_ids,
- attention_mask = inputs.attention_mask,
- do_sample = True)
- preds = tokenizer.batch_decode(outputs)
- response = str(preds)
- response = response.replace("\'", '')
- response = response.replace("[[CLS]", '')
- response = response.replace("[SEP]]", '')
- response = str(arabert_prep.desegment(response))
- return response
-
-title = 'BERT2BERT Response Generation in Arabic'
-description = 'This demo is for a BERT2BERT model trained for single-turn open-domain dialogue response generation in Modern Standard Arabic'
-gr.Interface(fn=generate_response,
- inputs=[
- gr.inputs.Textbox(),
- ],
- outputs="text",
- title=title,
- description=description).launch()
\ No newline at end of file
diff --git a/spaces/Lianjd/stock_dashboard/backtrader/btrun/btrun.py b/spaces/Lianjd/stock_dashboard/backtrader/btrun/btrun.py
deleted file mode 100644
index a839389f34300661106789ae17ea1dee8f4c1b0c..0000000000000000000000000000000000000000
--- a/spaces/Lianjd/stock_dashboard/backtrader/btrun/btrun.py
+++ /dev/null
@@ -1,743 +0,0 @@
-#!/usr/bin/env python
-# -*- coding: utf-8; py-indent-offset:4 -*-
-###############################################################################
-#
-# Copyright (C) 2015-2020 Daniel Rodriguez
-#
-# This program is free software: you can redistribute it and/or modify
-# it under the terms of the GNU General Public License as published by
-# the Free Software Foundation, either version 3 of the License, or
-# (at your option) any later version.
-#
-# This program is distributed in the hope that it will be useful,
-# but WITHOUT ANY WARRANTY; without even the implied warranty of
-# MERCHANTABILITY or FITNESS FOR A PARTICULAR PURPOSE. See the
-# GNU General Public License for more details.
-#
-# You should have received a copy of the GNU General Public License
-# along with this program. If not, see .
-#
-###############################################################################
-from __future__ import (absolute_import, division, print_function,
- unicode_literals)
-
-import argparse
-import datetime
-import inspect
-import itertools
-import random
-import string
-import sys
-
-import backtrader as bt
-
-
-DATAFORMATS = dict(
- btcsv=bt.feeds.BacktraderCSVData,
- vchartcsv=bt.feeds.VChartCSVData,
- vcfile=bt.feeds.VChartFile,
- sierracsv=bt.feeds.SierraChartCSVData,
- mt4csv=bt.feeds.MT4CSVData,
- yahoocsv=bt.feeds.YahooFinanceCSVData,
- yahoocsv_unreversed=bt.feeds.YahooFinanceCSVData,
- yahoo=bt.feeds.YahooFinanceData,
-)
-
-try:
- DATAFORMATS['vcdata'] = bt.feeds.VCData
-except AttributeError:
- pass # no comtypes available
-
-try:
- DATAFORMATS['ibdata'] = bt.feeds.IBData,
-except AttributeError:
- pass # no ibpy available
-
-try:
- DATAFORMATS['oandadata'] = bt.feeds.OandaData,
-except AttributeError:
- pass # no oandapy available
-
-
-TIMEFRAMES = dict(
- microseconds=bt.TimeFrame.MicroSeconds,
- seconds=bt.TimeFrame.Seconds,
- minutes=bt.TimeFrame.Minutes,
- days=bt.TimeFrame.Days,
- weeks=bt.TimeFrame.Weeks,
- months=bt.TimeFrame.Months,
- years=bt.TimeFrame.Years,
-)
-
-
-def btrun(pargs=''):
- args = parse_args(pargs)
-
- if args.flush:
- import backtrader.utils.flushfile
-
- stdstats = not args.nostdstats
-
- cer_kwargs_str = args.cerebro
- cer_kwargs = eval('dict(' + cer_kwargs_str + ')')
- if 'stdstats' not in cer_kwargs:
- cer_kwargs.update(stdstats=stdstats)
-
- cerebro = bt.Cerebro(**cer_kwargs)
-
- if args.resample is not None or args.replay is not None:
- if args.resample is not None:
- tfcp = args.resample.split(':')
- elif args.replay is not None:
- tfcp = args.replay.split(':')
-
- # compression may be skipped and it will default to 1
- if len(tfcp) == 1 or tfcp[1] == '':
- tf, cp = tfcp[0], 1
- else:
- tf, cp = tfcp
-
- cp = int(cp) # convert any value to int
- tf = TIMEFRAMES.get(tf, None)
-
- for data in getdatas(args):
- if args.resample is not None:
- cerebro.resampledata(data, timeframe=tf, compression=cp)
- elif args.replay is not None:
- cerebro.replaydata(data, timeframe=tf, compression=cp)
- else:
- cerebro.adddata(data)
-
- # get and add signals
- signals = getobjects(args.signals, bt.Indicator, bt.signals, issignal=True)
- for sig, kwargs, sigtype in signals:
- stype = getattr(bt.signal, 'SIGNAL_' + sigtype.upper())
- cerebro.add_signal(stype, sig, **kwargs)
-
- # get and add strategies
- strategies = getobjects(args.strategies, bt.Strategy, bt.strategies)
- for strat, kwargs in strategies:
- cerebro.addstrategy(strat, **kwargs)
-
- inds = getobjects(args.indicators, bt.Indicator, bt.indicators)
- for ind, kwargs in inds:
- cerebro.addindicator(ind, **kwargs)
-
- obs = getobjects(args.observers, bt.Observer, bt.observers)
- for ob, kwargs in obs:
- cerebro.addobserver(ob, **kwargs)
-
- ans = getobjects(args.analyzers, bt.Analyzer, bt.analyzers)
- for an, kwargs in ans:
- cerebro.addanalyzer(an, **kwargs)
-
- setbroker(args, cerebro)
-
- for wrkwargs_str in args.writers or []:
- wrkwargs = eval('dict(' + wrkwargs_str + ')')
- cerebro.addwriter(bt.WriterFile, **wrkwargs)
-
- ans = getfunctions(args.hooks, bt.Cerebro)
- for hook, kwargs in ans:
- hook(cerebro, **kwargs)
- runsts = cerebro.run()
- runst = runsts[0] # single strategy and no optimization
-
- if args.pranalyzer or args.ppranalyzer:
- if runst.analyzers:
- print('====================')
- print('== Analyzers')
- print('====================')
- for name, analyzer in runst.analyzers.getitems():
- if args.pranalyzer:
- analyzer.print()
- elif args.ppranalyzer:
- print('##########')
- print(name)
- print('##########')
- analyzer.pprint()
-
- if args.plot:
- pkwargs = dict(style='bar')
- if args.plot is not True:
- # evaluates to True but is not "True" - args were passed
- ekwargs = eval('dict(' + args.plot + ')')
- pkwargs.update(ekwargs)
-
- # cerebro.plot(numfigs=args.plotfigs, style=args.plotstyle)
- cerebro.plot(**pkwargs)
-
-
-def setbroker(args, cerebro):
- broker = cerebro.getbroker()
-
- if args.cash is not None:
- broker.setcash(args.cash)
-
- commkwargs = dict()
- if args.commission is not None:
- commkwargs['commission'] = args.commission
- if args.margin is not None:
- commkwargs['margin'] = args.margin
- if args.mult is not None:
- commkwargs['mult'] = args.mult
- if args.interest is not None:
- commkwargs['interest'] = args.interest
- if args.interest_long is not None:
- commkwargs['interest_long'] = args.interest_long
-
- if commkwargs:
- broker.setcommission(**commkwargs)
-
- if args.slip_perc is not None:
- cerebro.broker.set_slippage_perc(args.slip_perc,
- slip_open=args.slip_open,
- slip_match=not args.no_slip_match,
- slip_out=args.slip_out)
- elif args.slip_fixed is not None:
- cerebro.broker.set_slippage_fixed(args.slip_fixed,
- slip_open=args.slip_open,
- slip_match=not args.no_slip_match,
- slip_out=args.slip_out)
-
-
-def getdatas(args):
- # Get the data feed class from the global dictionary
- dfcls = DATAFORMATS[args.format]
-
- # Prepare some args
- dfkwargs = dict()
- if args.format == 'yahoo_unreversed':
- dfkwargs['reverse'] = True
-
- fmtstr = '%Y-%m-%d'
- if args.fromdate:
- dtsplit = args.fromdate.split('T')
- if len(dtsplit) > 1:
- fmtstr += 'T%H:%M:%S'
-
- fromdate = datetime.datetime.strptime(args.fromdate, fmtstr)
- dfkwargs['fromdate'] = fromdate
-
- fmtstr = '%Y-%m-%d'
- if args.todate:
- dtsplit = args.todate.split('T')
- if len(dtsplit) > 1:
- fmtstr += 'T%H:%M:%S'
- todate = datetime.datetime.strptime(args.todate, fmtstr)
- dfkwargs['todate'] = todate
-
- if args.timeframe is not None:
- dfkwargs['timeframe'] = TIMEFRAMES[args.timeframe]
-
- if args.compression is not None:
- dfkwargs['compression'] = args.compression
-
- datas = list()
- for dname in args.data:
- dfkwargs['dataname'] = dname
- data = dfcls(**dfkwargs)
- datas.append(data)
-
- return datas
-
-
-def getmodclasses(mod, clstype, clsname=None):
- clsmembers = inspect.getmembers(mod, inspect.isclass)
-
- clslist = list()
- for name, cls in clsmembers:
- if not issubclass(cls, clstype):
- continue
-
- if clsname:
- if clsname == name:
- clslist.append(cls)
- break
- else:
- clslist.append(cls)
-
- return clslist
-
-
-def getmodfunctions(mod, funcname=None):
- members = inspect.getmembers(mod, inspect.isfunction) + \
- inspect.getmembers(mod, inspect.ismethod)
-
- funclist = list()
- for name, member in members:
- if funcname:
- if name == funcname:
- funclist.append(member)
- break
- else:
- funclist.append(member)
-
- return funclist
-
-
-def loadmodule(modpath, modname=''):
- # generate a random name for the module
-
- if not modpath.endswith('.py'):
- modpath += '.py'
-
- if not modname:
- chars = string.ascii_uppercase + string.digits
- modname = ''.join(random.choice(chars) for _ in range(10))
-
- version = (sys.version_info[0], sys.version_info[1])
-
- if version < (3, 3):
- mod, e = loadmodule2(modpath, modname)
- else:
- mod, e = loadmodule3(modpath, modname)
-
- return mod, e
-
-
-def loadmodule2(modpath, modname):
- import imp
-
- try:
- mod = imp.load_source(modname, modpath)
- except Exception as e:
- return (None, e)
-
- return (mod, None)
-
-
-def loadmodule3(modpath, modname):
- import importlib.machinery
-
- try:
- loader = importlib.machinery.SourceFileLoader(modname, modpath)
- mod = loader.load_module()
- except Exception as e:
- return (None, e)
-
- return (mod, None)
-
-
-def getobjects(iterable, clsbase, modbase, issignal=False):
- retobjects = list()
-
- for item in iterable or []:
- if issignal:
- sigtokens = item.split('+', 1)
- if len(sigtokens) == 1: # no + seen
- sigtype = 'longshort'
- else:
- sigtype, item = sigtokens
-
- tokens = item.split(':', 1)
-
- if len(tokens) == 1:
- modpath = tokens[0]
- name = ''
- kwargs = dict()
- else:
- modpath, name = tokens
- kwtokens = name.split(':', 1)
- if len(kwtokens) == 1:
- # no '(' found
- kwargs = dict()
- else:
- name = kwtokens[0]
- kwtext = 'dict(' + kwtokens[1] + ')'
- kwargs = eval(kwtext)
-
- if modpath:
- mod, e = loadmodule(modpath)
-
- if not mod:
- print('')
- print('Failed to load module %s:' % modpath, e)
- sys.exit(1)
- else:
- mod = modbase
-
- loaded = getmodclasses(mod=mod, clstype=clsbase, clsname=name)
-
- if not loaded:
- print('No class %s / module %s' % (str(name), modpath))
- sys.exit(1)
-
- if issignal:
- retobjects.append((loaded[0], kwargs, sigtype))
- else:
- retobjects.append((loaded[0], kwargs))
-
- return retobjects
-
-def getfunctions(iterable, modbase):
- retfunctions = list()
-
- for item in iterable or []:
- tokens = item.split(':', 1)
-
- if len(tokens) == 1:
- modpath = tokens[0]
- name = ''
- kwargs = dict()
- else:
- modpath, name = tokens
- kwtokens = name.split(':', 1)
- if len(kwtokens) == 1:
- # no '(' found
- kwargs = dict()
- else:
- name = kwtokens[0]
- kwtext = 'dict(' + kwtokens[1] + ')'
- kwargs = eval(kwtext)
-
- if modpath:
- mod, e = loadmodule(modpath)
-
- if not mod:
- print('')
- print('Failed to load module %s:' % modpath, e)
- sys.exit(1)
- else:
- mod = modbase
-
- loaded = getmodfunctions(mod=mod, funcname=name)
-
- if not loaded:
- print('No function %s / module %s' % (str(name), modpath))
- sys.exit(1)
-
- retfunctions.append((loaded[0], kwargs))
-
- return retfunctions
-
-
-def parse_args(pargs=''):
- parser = argparse.ArgumentParser(
- description='Backtrader Run Script',
- formatter_class=argparse.RawTextHelpFormatter,
- )
-
- group = parser.add_argument_group(title='Data options')
- # Data options
- group.add_argument('--data', '-d', action='append', required=True,
- help='Data files to be added to the system')
-
- group = parser.add_argument_group(title='Cerebro options')
- group.add_argument(
- '--cerebro', '-cer',
- metavar='kwargs',
- required=False, const='', default='', nargs='?',
- help=('The argument can be specified with the following form:\n'
- '\n'
- ' - kwargs\n'
- '\n'
- ' Example: "preload=True" which set its to True\n'
- '\n'
- 'The passed kwargs will be passed directly to the cerebro\n'
- 'instance created for the execution\n'
- '\n'
- 'The available kwargs to cerebro are:\n'
- ' - preload (default: True)\n'
- ' - runonce (default: True)\n'
- ' - maxcpus (default: None)\n'
- ' - stdstats (default: True)\n'
- ' - live (default: False)\n'
- ' - exactbars (default: False)\n'
- ' - preload (default: True)\n'
- ' - writer (default False)\n'
- ' - oldbuysell (default False)\n'
- ' - tradehistory (default False)\n')
- )
-
- group.add_argument('--nostdstats', action='store_true',
- help='Disable the standard statistics observers')
-
- datakeys = list(DATAFORMATS)
- group.add_argument('--format', '--csvformat', '-c', required=False,
- default='btcsv', choices=datakeys,
- help='CSV Format')
-
- group.add_argument('--fromdate', '-f', required=False, default=None,
- help='Starting date in YYYY-MM-DD[THH:MM:SS] format')
-
- group.add_argument('--todate', '-t', required=False, default=None,
- help='Ending date in YYYY-MM-DD[THH:MM:SS] format')
-
- group.add_argument('--timeframe', '-tf', required=False, default='days',
- choices=TIMEFRAMES.keys(),
- help='Ending date in YYYY-MM-DD[THH:MM:SS] format')
-
- group.add_argument('--compression', '-cp', required=False, default=1,
- type=int,
- help='Ending date in YYYY-MM-DD[THH:MM:SS] format')
-
- group = parser.add_mutually_exclusive_group(required=False)
-
- group.add_argument('--resample', '-rs', required=False, default=None,
- help='resample with timeframe:compression values')
-
- group.add_argument('--replay', '-rp', required=False, default=None,
- help='replay with timeframe:compression values')
-
- group.add_argument(
- '--hook', dest='hooks',
- action='append', required=False,
- metavar='module:hookfunction:kwargs',
- help=('This option can be specified multiple times.\n'
- '\n'
- 'The argument can be specified with the following form:\n'
- '\n'
- ' - module:hookfunction:kwargs\n'
- '\n'
- ' Example: mymod:myhook:a=1,b=2\n'
- '\n'
- 'kwargs is optional\n'
- '\n'
- 'If module is omitted then hookfunction will be sought\n'
- 'as the built-in cerebro method. Example:\n'
- '\n'
- ' - :addtz:tz=America/St_Johns\n'
- '\n'
- 'If name is omitted, then the 1st function found in the\n'
- 'mod will be used. Such as in:\n'
- '\n'
- ' - module or module::kwargs\n'
- '\n'
- 'The function specified will be called, with cerebro\n'
- 'instance passed as the first argument together with\n'
- 'kwargs, if any were specified. This allows to customize\n'
- 'cerebro, beyond options provided by this script\n\n')
- )
-
- # Module where to read the strategy from
- group = parser.add_argument_group(title='Strategy options')
- group.add_argument(
- '--strategy', '-st', dest='strategies',
- action='append', required=False,
- metavar='module:name:kwargs',
- help=('This option can be specified multiple times.\n'
- '\n'
- 'The argument can be specified with the following form:\n'
- '\n'
- ' - module:classname:kwargs\n'
- '\n'
- ' Example: mymod:myclass:a=1,b=2\n'
- '\n'
- 'kwargs is optional\n'
- '\n'
- 'If module is omitted then class name will be sought in\n'
- 'the built-in strategies module. Such as in:\n'
- '\n'
- ' - :name:kwargs or :name\n'
- '\n'
- 'If name is omitted, then the 1st strategy found in the mod\n'
- 'will be used. Such as in:\n'
- '\n'
- ' - module or module::kwargs')
- )
-
- # Module where to read the strategy from
- group = parser.add_argument_group(title='Signals')
- group.add_argument(
- '--signal', '-sig', dest='signals',
- action='append', required=False,
- metavar='module:signaltype:name:kwargs',
- help=('This option can be specified multiple times.\n'
- '\n'
- 'The argument can be specified with the following form:\n'
- '\n'
- ' - signaltype:module:signaltype:classname:kwargs\n'
- '\n'
- ' Example: longshort+mymod:myclass:a=1,b=2\n'
- '\n'
- 'signaltype may be ommited: longshort will be used\n'
- '\n'
- ' Example: mymod:myclass:a=1,b=2\n'
- '\n'
- 'kwargs is optional\n'
- '\n'
- 'signaltype will be uppercased to match the defintions\n'
- 'fromt the backtrader.signal module\n'
- '\n'
- 'If module is omitted then class name will be sought in\n'
- 'the built-in signals module. Such as in:\n'
- '\n'
- ' - LONGSHORT::name:kwargs or :name\n'
- '\n'
- 'If name is omitted, then the 1st signal found in the mod\n'
- 'will be used. Such as in:\n'
- '\n'
- ' - module or module:::kwargs')
- )
-
- # Observers
- group = parser.add_argument_group(title='Observers and statistics')
- group.add_argument(
- '--observer', '-ob', dest='observers',
- action='append', required=False,
- metavar='module:name:kwargs',
- help=('This option can be specified multiple times.\n'
- '\n'
- 'The argument can be specified with the following form:\n'
- '\n'
- ' - module:classname:kwargs\n'
- '\n'
- ' Example: mymod:myclass:a=1,b=2\n'
- '\n'
- 'kwargs is optional\n'
- '\n'
- 'If module is omitted then class name will be sought in\n'
- 'the built-in observers module. Such as in:\n'
- '\n'
- ' - :name:kwargs or :name\n'
- '\n'
- 'If name is omitted, then the 1st observer found in the\n'
- 'will be used. Such as in:\n'
- '\n'
- ' - module or module::kwargs')
- )
- # Analyzers
- group = parser.add_argument_group(title='Analyzers')
- group.add_argument(
- '--analyzer', '-an', dest='analyzers',
- action='append', required=False,
- metavar='module:name:kwargs',
- help=('This option can be specified multiple times.\n'
- '\n'
- 'The argument can be specified with the following form:\n'
- '\n'
- ' - module:classname:kwargs\n'
- '\n'
- ' Example: mymod:myclass:a=1,b=2\n'
- '\n'
- 'kwargs is optional\n'
- '\n'
- 'If module is omitted then class name will be sought in\n'
- 'the built-in analyzers module. Such as in:\n'
- '\n'
- ' - :name:kwargs or :name\n'
- '\n'
- 'If name is omitted, then the 1st analyzer found in the\n'
- 'will be used. Such as in:\n'
- '\n'
- ' - module or module::kwargs')
- )
-
- # Analyzer - Print
- group = parser.add_mutually_exclusive_group(required=False)
- group.add_argument('--pranalyzer', '-pralyzer',
- required=False, action='store_true',
- help=('Automatically print analyzers'))
-
- group.add_argument('--ppranalyzer', '-ppralyzer',
- required=False, action='store_true',
- help=('Automatically PRETTY print analyzers'))
-
- # Indicators
- group = parser.add_argument_group(title='Indicators')
- group.add_argument(
- '--indicator', '-ind', dest='indicators',
- metavar='module:name:kwargs',
- action='append', required=False,
- help=('This option can be specified multiple times.\n'
- '\n'
- 'The argument can be specified with the following form:\n'
- '\n'
- ' - module:classname:kwargs\n'
- '\n'
- ' Example: mymod:myclass:a=1,b=2\n'
- '\n'
- 'kwargs is optional\n'
- '\n'
- 'If module is omitted then class name will be sought in\n'
- 'the built-in analyzers module. Such as in:\n'
- '\n'
- ' - :name:kwargs or :name\n'
- '\n'
- 'If name is omitted, then the 1st analyzer found in the\n'
- 'will be used. Such as in:\n'
- '\n'
- ' - module or module::kwargs')
- )
-
- # Writer
- group = parser.add_argument_group(title='Writers')
- group.add_argument(
- '--writer', '-wr',
- dest='writers', metavar='kwargs', nargs='?',
- action='append', required=False, const='',
- help=('This option can be specified multiple times.\n'
- '\n'
- 'The argument can be specified with the following form:\n'
- '\n'
- ' - kwargs\n'
- '\n'
- ' Example: a=1,b=2\n'
- '\n'
- 'kwargs is optional\n'
- '\n'
- 'It creates a system wide writer which outputs run data\n'
- '\n'
- 'Please see the documentation for the available kwargs')
- )
-
- # Broker/Commissions
- group = parser.add_argument_group(title='Cash and Commission Scheme Args')
- group.add_argument('--cash', '-cash', required=False, type=float,
- help='Cash to set to the broker')
- group.add_argument('--commission', '-comm', required=False, type=float,
- help='Commission value to set')
- group.add_argument('--margin', '-marg', required=False, type=float,
- help='Margin type to set')
- group.add_argument('--mult', '-mul', required=False, type=float,
- help='Multiplier to use')
-
- group.add_argument('--interest', required=False, type=float,
- default=None,
- help='Credit Interest rate to apply (0.0x)')
-
- group.add_argument('--interest_long', action='store_true',
- required=False, default=None,
- help='Apply credit interest to long positions')
-
- group.add_argument('--slip_perc', required=False, default=None,
- type=float,
- help='Enable slippage with a percentage value')
- group.add_argument('--slip_fixed', required=False, default=None,
- type=float,
- help='Enable slippage with a fixed point value')
-
- group.add_argument('--slip_open', required=False, action='store_true',
- help='enable slippage for when matching opening prices')
-
- group.add_argument('--no-slip_match', required=False, action='store_true',
- help=('Disable slip_match, ie: matching capped at \n'
- 'high-low if slippage goes over those limits'))
- group.add_argument('--slip_out', required=False, action='store_true',
- help='with slip_match enabled, match outside high-low')
-
- # Output flushing
- group.add_argument('--flush', required=False, action='store_true',
- help='flush the output - useful under win32 systems')
-
- # Plot options
- parser.add_argument(
- '--plot', '-p', nargs='?',
- metavar='kwargs',
- default=False, const=True, required=False,
- help=('Plot the read data applying any kwargs passed\n'
- '\n'
- 'For example:\n'
- '\n'
- ' --plot style="candle" (to plot candlesticks)\n')
- )
-
- if pargs:
- return parser.parse_args(pargs)
-
- return parser.parse_args()
-
-
-if __name__ == '__main__':
- btrun()
diff --git a/spaces/Loren/Streamlit_OCR_comparator/configs/_base_/recog_pipelines/sar_pipeline.py b/spaces/Loren/Streamlit_OCR_comparator/configs/_base_/recog_pipelines/sar_pipeline.py
deleted file mode 100644
index f43ded30f5b7fb54c302a442483b07ca8bf8af69..0000000000000000000000000000000000000000
--- a/spaces/Loren/Streamlit_OCR_comparator/configs/_base_/recog_pipelines/sar_pipeline.py
+++ /dev/null
@@ -1,43 +0,0 @@
-img_norm_cfg = dict(mean=[0.5, 0.5, 0.5], std=[0.5, 0.5, 0.5])
-train_pipeline = [
- dict(type='LoadImageFromFile'),
- dict(
- type='ResizeOCR',
- height=48,
- min_width=48,
- max_width=160,
- keep_aspect_ratio=True,
- width_downsample_ratio=0.25),
- dict(type='ToTensorOCR'),
- dict(type='NormalizeOCR', **img_norm_cfg),
- dict(
- type='Collect',
- keys=['img'],
- meta_keys=[
- 'filename', 'ori_shape', 'resize_shape', 'text', 'valid_ratio'
- ]),
-]
-test_pipeline = [
- dict(type='LoadImageFromFile'),
- dict(
- type='MultiRotateAugOCR',
- rotate_degrees=[0, 90, 270],
- transforms=[
- dict(
- type='ResizeOCR',
- height=48,
- min_width=48,
- max_width=160,
- keep_aspect_ratio=True,
- width_downsample_ratio=0.25),
- dict(type='ToTensorOCR'),
- dict(type='NormalizeOCR', **img_norm_cfg),
- dict(
- type='Collect',
- keys=['img'],
- meta_keys=[
- 'filename', 'ori_shape', 'resize_shape', 'valid_ratio',
- 'img_norm_cfg', 'ori_filename', 'img_shape'
- ]),
- ])
-]
diff --git a/spaces/LucasCodeBreak/MusicGen/tests/models/test_encodec_model.py b/spaces/LucasCodeBreak/MusicGen/tests/models/test_encodec_model.py
deleted file mode 100644
index 2f9c1db3f69a45f02451b71da95f44356811acbb..0000000000000000000000000000000000000000
--- a/spaces/LucasCodeBreak/MusicGen/tests/models/test_encodec_model.py
+++ /dev/null
@@ -1,60 +0,0 @@
-# Copyright (c) Meta Platforms, Inc. and affiliates.
-# All rights reserved.
-#
-# This source code is licensed under the license found in the
-# LICENSE file in the root directory of this source tree.
-
-import random
-
-import numpy as np
-import torch
-
-from audiocraft.models import EncodecModel
-from audiocraft.modules import SEANetEncoder, SEANetDecoder
-from audiocraft.quantization import DummyQuantizer
-
-
-class TestEncodecModel:
-
- def _create_encodec_model(self,
- sample_rate: int,
- channels: int,
- dim: int = 5,
- n_filters: int = 3,
- n_residual_layers: int = 1,
- ratios: list = [5, 4, 3, 2],
- **kwargs):
- frame_rate = np.prod(ratios)
- encoder = SEANetEncoder(channels=channels, dimension=dim, n_filters=n_filters,
- n_residual_layers=n_residual_layers, ratios=ratios)
- decoder = SEANetDecoder(channels=channels, dimension=dim, n_filters=n_filters,
- n_residual_layers=n_residual_layers, ratios=ratios)
- quantizer = DummyQuantizer()
- model = EncodecModel(encoder, decoder, quantizer, frame_rate=frame_rate,
- sample_rate=sample_rate, channels=channels, **kwargs)
- return model
-
- def test_model(self):
- random.seed(1234)
- sample_rate = 24_000
- channels = 1
- model = self._create_encodec_model(sample_rate, channels)
- for _ in range(10):
- length = random.randrange(1, 10_000)
- x = torch.randn(2, channels, length)
- res = model(x)
- assert res.x.shape == x.shape
-
- def test_model_renorm(self):
- random.seed(1234)
- sample_rate = 24_000
- channels = 1
- model_nonorm = self._create_encodec_model(sample_rate, channels, renormalize=False)
- model_renorm = self._create_encodec_model(sample_rate, channels, renormalize=True)
-
- for _ in range(10):
- length = random.randrange(1, 10_000)
- x = torch.randn(2, channels, length)
- codes, scales = model_nonorm.encode(x)
- codes, scales = model_renorm.encode(x)
- assert scales is not None
diff --git a/spaces/Lwight/Ghibli-Diffusion/app.py b/spaces/Lwight/Ghibli-Diffusion/app.py
deleted file mode 100644
index 25e4911d6481344a01f0ab7867dabd1f3d130e7a..0000000000000000000000000000000000000000
--- a/spaces/Lwight/Ghibli-Diffusion/app.py
+++ /dev/null
@@ -1,10 +0,0 @@
-import gradio as gr
-
-description = """
-

-
- Ghibli Diffusion
-This is the fine-tuned Stable Diffusion model trained on images from modern anime feature films from Studio Ghibli. Use the tokens ghibli style in your prompts for the effect.
- """
-
-gr.Interface.load("models/nitrosocke/Ghibli-Diffusion", description=description, examples=[["superman ghibli style"]]).launch()
diff --git a/spaces/Mandy234/Mandy234-myQAmodel/README.md b/spaces/Mandy234/Mandy234-myQAmodel/README.md
deleted file mode 100644
index d96254dd40cf35278b4841de3770ffe39ff1e3ae..0000000000000000000000000000000000000000
--- a/spaces/Mandy234/Mandy234-myQAmodel/README.md
+++ /dev/null
@@ -1,13 +0,0 @@
----
-title: Mandy234 MyQAmodel
-emoji: 🌖
-colorFrom: green
-colorTo: blue
-sdk: gradio
-sdk_version: 3.35.2
-app_file: app.py
-pinned: false
-license: apache-2.0
----
-
-Check out the configuration reference at https://huggingface.co/docs/hub/spaces-config-reference
diff --git a/spaces/Marshalls/testmtd/feature_extraction/madmom/ml/__init__.py b/spaces/Marshalls/testmtd/feature_extraction/madmom/ml/__init__.py
deleted file mode 100644
index 10989a5848e37aae5426560e9da7bf933040355f..0000000000000000000000000000000000000000
--- a/spaces/Marshalls/testmtd/feature_extraction/madmom/ml/__init__.py
+++ /dev/null
@@ -1,10 +0,0 @@
-# encoding: utf-8
-"""
-Machine learning package.
-
-"""
-
-from __future__ import absolute_import, division, print_function
-
-# import the submodules
-from . import nn, hmm, gmm, crf
diff --git a/spaces/Mathux/TMR/model.py b/spaces/Mathux/TMR/model.py
deleted file mode 100644
index 5e5e8f30664f314c7fa74e1363920b8b5525005e..0000000000000000000000000000000000000000
--- a/spaces/Mathux/TMR/model.py
+++ /dev/null
@@ -1,128 +0,0 @@
-from typing import List
-import torch.nn as nn
-import os
-
-import torch
-import numpy as np
-from torch import Tensor
-from transformers import AutoTokenizer, AutoModel
-from transformers import logging
-from torch.nn.functional import normalize
-
-
-class PositionalEncoding(nn.Module):
- def __init__(self, d_model, max_len=5000):
- super().__init__()
-
- pe = torch.zeros(max_len, d_model)
- position = torch.arange(0, max_len, dtype=torch.float).unsqueeze(1)
- div_term = torch.exp(torch.arange(0, d_model, 2).float() * (-np.log(10000.0) / d_model))
- pe[:, 0::2] = torch.sin(position * div_term)
- pe[:, 1::2] = torch.cos(position * div_term)
- pe = pe.unsqueeze(0).transpose(0, 1)
-
- self.register_buffer('pe', pe, persistent=False)
-
- def forward(self, x):
- return x + self.pe[:x.shape[0], :]
-
-
-class TMR_textencoder(nn.Module):
- def __init__(self, modelpath: str, latent_dim: int, ff_size: int,
- num_layers: int, num_heads: int, activation: str, **kwargs) -> None:
- super().__init__()
-
- logging.set_verbosity_error()
-
- # Tokenizer
- os.environ["TOKENIZERS_PARALLELISM"] = "false"
- self.tokenizer = AutoTokenizer.from_pretrained(modelpath)
-
- # Text model
- self.text_model = AutoModel.from_pretrained(modelpath)
- # Then configure the model
- self.text_encoded_dim = self.text_model.config.hidden_size
-
- # Projection of the text-outputs into the latent space
- self.projection = nn.Sequential(
- nn.ReLU(),
- nn.Linear(self.text_encoded_dim, latent_dim)
- )
-
- self.mu_token = nn.Parameter(torch.randn(latent_dim))
- self.logvar_token = nn.Parameter(torch.randn(latent_dim))
- self.sequence_pos_encoding = PositionalEncoding(latent_dim)
-
- seq_trans_encoder_layer = nn.TransformerEncoderLayer(d_model=latent_dim,
- nhead=num_heads,
- dim_feedforward=ff_size,
- dropout=0.0,
- activation=activation)
- self.seqTransEncoder = nn.TransformerEncoder(
- seq_trans_encoder_layer,
- num_layers=num_layers
- )
-
- def get_last_hidden_state(self, texts: List[str],
- return_mask: bool = False):
- encoded_inputs = self.tokenizer(texts, return_tensors="pt", padding=True)
- output = self.text_model(**encoded_inputs.to(self.text_model.device))
- if not return_mask:
- return output.last_hidden_state
- return output.last_hidden_state, encoded_inputs.attention_mask.to(dtype=bool)
-
- def forward(self, texts: List[str]) -> Tensor:
- text_encoded, mask = self.get_last_hidden_state(texts, return_mask=True)
-
- x = self.projection(text_encoded)
- bs, nframes, _ = x.shape
- # bs, nframes, totjoints, nfeats = x.shape
- # Switch sequence and batch_size because the input of
- # Pytorch Transformer is [Sequence, Batch size, ...]
- x = x.permute(1, 0, 2) # now it is [nframes, bs, latent_dim]
-
- mu_token = torch.tile(self.mu_token, (bs,)).reshape(bs, -1)
- logvar_token = torch.tile(self.logvar_token, (bs,)).reshape(bs, -1)
-
- # adding the distribution tokens for all sequences
- xseq = torch.cat((mu_token[None], logvar_token[None], x), 0)
-
- # create a bigger mask, to allow attend to mu and logvar
- token_mask = torch.ones((bs, 2), dtype=bool, device=x.device)
- aug_mask = torch.cat((token_mask, mask), 1)
-
- # add positional encoding
- xseq = self.sequence_pos_encoding(xseq)
- final = self.seqTransEncoder(xseq, src_key_padding_mask=~aug_mask)
-
- # only mu for inference
- mu = final[0]
- return mu
-
- # compute score for retrieval
- def compute_scores(self, texts, unit_embs=None, embs=None):
- # not both empty
- assert not (unit_embs is None and embs is None)
- # not both filled
- assert not (unit_embs is not None and embs is not None)
-
- output_str = False
- # if one input, squeeze the output
- if isinstance(texts, str):
- texts = [texts]
- output_str = True
-
- # compute unit_embs from embs if not given
- if embs is not None:
- unit_embs = normalize(embs)
-
- with torch.no_grad():
- latent_unit_texts = normalize(self(texts))
- # compute cosine similarity between 0 and 1
- scores = (unit_embs @ latent_unit_texts.T).T/2 + 0.5
- scores = scores.cpu().numpy()
-
- if output_str:
- scores = scores[0]
-
- return scores
diff --git a/spaces/MaximeTut/Emploi2021/emploi2021.py b/spaces/MaximeTut/Emploi2021/emploi2021.py
deleted file mode 100644
index bfd9d2f336338eff80d422e567a7ddf81fe1e853..0000000000000000000000000000000000000000
--- a/spaces/MaximeTut/Emploi2021/emploi2021.py
+++ /dev/null
@@ -1,165 +0,0 @@
-import pandas as pd
-import json
-import matplotlib.pyplot as plt
-import streamlit as st
-import streamlit.components.v1 as stc
-import plotly.express as px
-import seaborn as sns
-from streamlit_option_menu import option_menu
-
-sns.set()
-logo = "https://www.ville-creteil.fr/img/Une-logo-pole-emploi.jpg"
-logo2 = "data:image/jpeg;base64,/9j/4AAQSkZJRgABAQAAAQABAAD/2wCEAAoHCBISEhISEhESERESEhESEBEREhESEhAOFxMYGBcTFxcbICwkGx0pIBcXJTYlKS49MzMzGiI5PjkxPSwyMzABCwsLEA4QHhISHTIpIiAyMjAyMDIyMjIyMjAyMjIyMjIyMjIyMjIyMjIyMjIyMjIyMjIyMjIyMjIyMjIyMjIyMv/AABEIAOEA4QMBIgACEQEDEQH/xAAcAAEAAQUBAQAAAAAAAAAAAAAABwECBAUGAwj/xABIEAACAQMBBAUGCgkBCAMAAAABAgADBBESBQYhMQcTQVFhIlJxgZGhFCMyQlRikrHB0RYXQ3JzgpOy0qJEU2ODlMLh8BUzNP/EABoBAQADAQEBAAAAAAAAAAAAAAADBAUBAgb/xAAzEQACAgECAwUFBwUAAAAAAAAAAQIRAwQSITFBExRRYXEFMoGRwQYjM7HR4fAiUmKhsv/aAAwDAQACEQMRAD8AmaIiAIiIAiIgCIiAIiIAiUiAVieLV0Xm6j0sBPI39H/ep9oTtM45JdTLiYq31E8qqfaE9lqqeTA+ggzjVBST5M9IlIzB0rERAEREAREQBERAEREAREQBERAEREAREQBKRNFtbeKnRyqfGVBzAPkqfrH8BPUYOTpI8ZMkcauTo3bsAMkgAcyTgTT3m8NFMhSajDzfk/aP4TkbzadWufLYkdiLwUeqVo2jH5R0+A4mXIaVLjNmbPXSnwxL4s2txvJWb5OmmPAZPtMwHuqtTm7t6zj2Ce1Ogi9mT3njMgPiSpQj7qIWsk/fkzXi1c/M9pAl4s37h7RM4PK653ezz3eJgGyqeaD/ADCWmhUXjoYeK/8AibPrIFSN7HYRMCntKvT5O48Gyfvmytt53XhUQOO9fJP5SxiDzAPp4zGq2VNuXknw/KeXHHLmj1HtcfuyOmstsUKuAr6W81/JPq7D6pspG1xZVE4jyx3rzHqmVs/eCrRIBOtBzVzxA8D2SKek6wZZx69p1lVef7Hfys12zNq0rgZRsMPlI3Bl9Xb6ZsJTaadM0IyUladorEROHoREQBERAEREAREQBERAE8qtRUUsxCqBkknAAirUVFLMQqqCSTwAA7ZG+8m8bXLlEJWgp4DtqHzm8O4SXDheV0uXiV9RqI4Y2+fRGw27vQ1QmnQJWnyL8mf0dwmltrdn4ngvf2meVpb/ADm9S/iZsA80Uo41tgZNTzPfk+R70lVBhRjx7TPUVJia5XXPNE/BcEZeuV62YgeVDzlAyw8qHmJrldcUDL6yV6yYgeVFScoGWKkqHmJ1kqKkAy9cxrm0Sp9VvOH498ojk8sn0cZ7rSc8kc/ytF0ccdypo0VValBwwJUg+S6mdfu/vOtUinWIWpyVuSue7waaypbOVIam5B55UzndoWTUjqw2jPAkEFT4/nPUowzKnzIovJpnuhy6olzMrOL3U3l1lbeu3l8qdQ/P+o3j3HtnZzOyY5Y5VI2MOWOWO6JWIieCUREQBERAEREAShlZzG+23fglDSh+OrZVO9E+c/q5DxM9Qg5yUV1PGSahFylyRz++28PWObak3xan4xh8+oPm/uj75oLGj89v5R+M19mmtsnkOJ8TNsHmsorHHYjFW7LPtJ/AyhUldcxRUgVJ5omMvXKh5idZK9ZAMrXK9ZMUPK9ZFAyusldUxg8r1k4DJ1y5WJOBxJ5AcyZZZW9Ss4p0xqY+xR3nuE7rZGxadAA411O1z2eCjsEiyZVAlx4nP0NHY7v1amGc9WvceLEejsm9tth0E5qXPe5z7uU2mIlOWWUi7HDCPQ80oqvBVVR4ACemIjMjJRiWPSVgQyhgeYIBEvzKwDSXu7NrV4mkEbmGpEowPfw4e6XVtoLaCmlwzFG8hbggY1di1Mcjjt5HE3Ew9pWSXFJ6TjKsMeIPYw8QYnKbjSfpYxQxxnclwfOuZkUqquAykMpGQwOQR4GesiS22tc7MuHpMS6BsPTb5LL2OvmnHdJK2RtWldUxUpHI5Mp+Ujeaw7DIcWZT4cmuhd1ehnp0pc4PlJfXwNjERJikIiIAiIgFjuACScAAknuAkI7ybXN3dO4JKZ6ukO6mDj38/XJF6Q9qG3snVTh65FJccwp4ufsgj1yJtnrltXYvL0zQ0UKTyP0Rma+Tk1jXqzb0BoUD2+JnqHmLrlQ8sEKMrXKh5jBpXVAMjXKh5i65UPAMrXKipMXXK9ZB0yg89KCs7KiAszEKoHaTMLXO13E2bnVcuOWUpZ/1P+HtkeWeyNnvHHfKjoth7KW2pheBdsGo3aT3egTaRKzMbbds1EklSE8q1VUUszBVAyWJwAJWo4UEkgAAkk8gB2yNN4dutcuQpIoofIXzvrt4/dPeLG8jojy5VjR0O0N8FBK0E1/XfIX1DnNO+89037QL4KiAe8Gc9rlesl+OCC6FGWecup0NPea6X9oG8GRD9wE3FhveCQKyafrpkj1rznD9ZK64lghLoI5px6kvUKyuodGDK3EFTkGehkZ7B221s44k0mPxif8AeO4j3ySadQOoZTlWAKkciD2yjlxODL2LKsiOI6R9k66a3SDyqfkVMfOpk+S3qPuM4fd7bj2VYVFJKHAqJ2VE/Mdkmq8tlq03puMq6srA9xGJAm07ZqNWpSb5VNmQ+ozM1ENs1NH13sTJHPhlp8itL/l/o+RPlldpWppVpkMjqGRh2gzJkadF22eL2jnhxqUs9h+co9XH2yS5bhLdGzA1ulelzSxvpy810ERE9lUShlZQwCIulXaGu6SiD5NGnkj67nJ9wE5uz8lB48Z5bz3nXX1zU55rMo/dU6B90vVsDHdNnHHbjSMbI92RyMrVGuY2uNc7RwytUuDzE1yuqcoGTrldUxtUrrnaBk6pUPMbVKhooGZTBdlVeLMQoHiTgSZdm2oo0qdIckUL6TjifbIq3Nodbe0QeIQtUb0IpI9+mTBKGrlxUS9pI8HISspEqFw5TfzaXV0VpKcNWJz/AA15+04EjzVN1v5ea71lzwpIlMekjU393unOa5pYIbYLzMzPLdN+Rk6o1TH1yuuTUQmRrl2uYuqVDQDJ1zv9xdodZSeixy1Igr/Cbl7CDI41zo9xbrTeKueFRHQ+kDUP7ZFnhug/ImwSqa8yTZEXSbZ9XdhwMLXpo/8AzAdLe7TJcM4XpTtdVvSq4406pBP1XXP3qJkahXD0Pp/Y2bs9ZH/K18+X+6I52NfG3r0awPyHRj4jV5Q9mZ9A0nDAMOIYAg+BGRPnAcx6RJ43SuOssLVicnqUUnvKeSf7ZFp3zRq/aPDwx5V5r6r6m7iIlk+WE8bh9KO3mqzewEz2mDthsW1we6hVP+gwD5yR9T6j85ix9JOZnB5rbc8V9A+6ZmqbrMNs99cuDzG1RqnDlmVrgVJja5a1dR2+yDtmZqldc1rXZ7B7Zabp/D2RQ4m11yuqan4U3f7oF2/h7Io9EldGCarms/mUQo/ncf4yTwZF/RBVLteZA4LQ5eJf8pJ8y9T+IzS034aLhEtjMrlgjTeTda8qXVWpTpiqlRy4KugIyB5JDEcRNX+iW0Pozf1KX+UmCJYjqppVwK0tLBu+JD/6JbQ+jH+pT/ylf0T2h9GP9Sl/lJfjM9d7n5HO6Q8yIf0T2h9Gb+pS/wAo/RTaH0Zvt0v8pL0pHep+CHdIeLIj/RO/+jN9ul/lNtu1uzd07qnUqUxSp021El0JbgRpAUnvkiywmJaiTVcOJ1aaEXfEGc3v9S12Ff6pR/YwnR5mk3wGbG6/hMZVn7rNDSScc8Gv7l+ZB0nHcMEbOtc+bUPqNVyJCtnavWqLTpqWd2Cqo7yefok/7JshQoUqI49VTRM95A4n25lXTq3Z9L9o8q7OGPrd/BKjOiIlo+TExNppqoVl86lUHtQiZcsdcgjvBHtgHy/SPL0CZOqWXtHq6tSmedOo6fZcj8JbmbidmJJcT11S1nxPNnxPImDiiej1CZZmWxB6ouzGZbEHaLsxmWxAokvobqfGXa99Oi3qDMPxkrAyFuiW60X7oTwq27geLIyuPcGkzAzM1K+8Zo6d/wBB6Ss8wZdmQE5xe2+ka2ta70Oqq1mpnTUZNAUP2qMnJxNf+ti2+i3H2qX5zgN+KWjaV2vfWLD0OoYffNDNCGmxuKZQlqJptEu/rYtvotx9ql+cfrYtvotx9ql+ciKJ67rj/jOd5yEu/rXtvotx9ql+cfrXtvotx9ql+ciKI7rj8/mO85CXP1rW30W4+1S/OU/WtbfRbj7VL85EkR3XH/Gc7zkJZbpWt+y0rn0vSE87XfT/AOTqrYfBuqpXGpHqdbqdU0knSNOM8JFU7Port9e0Ubsp06jn04AH3zxk0+OMG66EmLUZN8afUlnY+71taD4mnhiMF28qof5vym5ECVmalXI0Z5JZJOU3bfViIidPAlDKxAPn7pCsup2lcDGFqMKq+hxx94M5wNJO6Ztm/wD57pRw8qjUI7/lIT7GEizVNXBPdBGbmhU2XExmWZjMmsiovzGZZmMxYovzGZZmMxYovzGZZmMxYo3W6d/8HvrWqThVqqH/AHHyje5p9DAz5fzJ/wByNsi7sqTk5emOqqjtFRBjJ9IwfXKWqjykW9NKrR0WZXMsBjMplsiHpc2ead3TuAPIr0wrH/i0uHvUr7JwOZP++OwxfWj0hjrF+MonuqqOA9YyPXIAq02RmRgVZSVZTwKsDggzR007jXgUM8KlfiMxmWZjMsWQUX5jMszGYsUX5jMszKjJ4DiTwAHEk90WdouzJB3CuE2a1SterUpNXp0xQXq9TNS1Es5A5DIA4zP3F3F0aLq8TL8Go0G5Iex6g7T3L2TQb8bQ6++qsDkU8U0/dTn7y0zdbqqhtibnsT2atTn+8ukr4fImDY23ra8BNCoGK/KQjS6jvKnjNtIR6OXcbQpBScFXD+KY7fXiTaJSxzclbLPtLRx0ubZF2mrKxESQoCIiAaXevY4vLOvbn5TpqpnzaqnUh9o95nzg6lSVYFWUlWU81YHBHtn1QZCHSvu/8HuRcouKV0TqxyW4AyR6wM+oy1pZ09r6lfUQtbvA4TMZlsS9ZTouzGZbECi7MZlsQKLsxmWxAouzOr6Pt4/gV1pqNi3r4Sr3I+fIqerJB8D4TkonJJSVM7G4uz6eVs8RxB4gjkRLsyK+jvfUKFs7t8KMLbVmPAd1Nz9x9UlEGZ04ODpl6MlJWi/M4LfzccXRa5tQFucfGU+AWvgYDeD4GM9s7vMTkZOLtHZRUlTPmevSem7JUVqbocOjgqynuIM88z6J2xsG1vBi4oo5Awr401FHg44zjrzort2JNK5qIOxXVagHr4GW46iL58CrLA+hE+YzJNXooGeN5w8KXH75tLDoys0INR6tfHYSEU+nTxnp6iBxYJEU7M2dWuqgpUKbVHPMKOCjzmPJR4mS7uhuLSsytatprXI4rwylE/UB5t9Y+qdRYWFG3Tq6NNKVPzaahQT3nvPpmRmV8mdy4LgieGJR4s1u8e0hbWtWrnygulB31W4KP/e6Qc7EliTk5JJ7yeZnY9Iu2uurC3Q5p0M6scmqnmfUOHrM126O7VS+q8itBCDVfs78KfOPumVlbnOl0PtvZeKOj0rzZeG7i/TovV/U6voq2OR1l4455pUc9vnsPDPD1GSXMe0tkpU0p01CIihUUclUchMiWYR2qj5rV6l6nNLI+v5dBERPRWEREATVbw7Hp3ttUt6g8lx5LdqVBxVx4gzayhgHy7tfZ1S1r1Lequl6bEHhwYdjjwI4zDzJ66Q9zxtCkKlIAXdJT1Z5CqnM02/A9hkDVabIzI6lHUlXVhhlYcwRNHFl3rzKWSG1lMxmW5jMkIy7MZluYzALsxmW5jMAuzGZbmMwC7M7fdPpBq2oWjchq9AYCNn42kvcCflDwM4bMZnJRUlTOxk1xR9IbJ21bXia7eqlQfOUHFRD3Mh4rNhqnzHb3D02D03am68nRirD1idbsvpHv6ICuyXCj/eLh/tL+UrSwPoWFlXUm/MpmRra9K1I4620qKe003Vh7GxNlT6TtnEeV8IQ9xpavuMj7OS6HtTi+p2+ZTM449JOzfPrf9O88K3SfYL8hLiof4ap/cZzs5eA3I7jM5/e7bvwSjhONerlaS8yO+oR3D3maDZu/la+rrb2Vn5TcWqVnytKmObsF7B7zwnfUdi0RU650FSvgDrHGSMdiA8FHonjJGUVRPpp41NSmrS6eP7EZ7s7jVrlhWuQ9KiTqw3CrUzxOAeQPeZKuz7Gnb01pUlCIowFHvJ7z4zLlZDDGoci1rNdl1Urm+C5JckIiJ7KYiIgCIiAIiIBScB0g7iLeg3FsFS7UeUvJLhR2HufuPtkgSmJ2MnF2jjSapnyhcUHpu1OojJUQlXRxhlYdhE88z6H3x3Kt9pKWPxVyowldRknuVx85feJBu8O7l1YVClxTKjPkVVy1Nx3q34HjL2PMpepVnjaNVmMyzMrJLPFF2YzLMxmLFF+YzLMxmLFF+YzLMxmLFF+ZTMtzK5ixRdmMyzMZixRdmbTYGxLi+rLRt01E/Lc8EpJ2u7dg8OZm73R3Aur8rUcG3tcgtVdfLde6mh5nxPAePKTlsPYlvZUhRt0CIPlHmzt5zN2mQ5MyjwXMlhivmYm6m7VHZ1EU6Y1O2DVqkeVUf8AAdwm+lYlNtt2yzyERE4BERAEREAREQBERAEREATGvbOnWptTq00qIwwyOoZSPQZkxAIl3l6I1YtUsKujOT8GrElM9yVOa+hs+mRltjYV3aMVuaD08fPK5pn0OOE+psTyrUVdSrorqeBVgGBHoMmjmkufEjeNM+TMxmfQu1ujTZlxkii1u5z5Vs3V8e/Scr7pyV90Mt/s96COxa9Lj9pD+EmWeLI3iZE+YzO8ueibaa/INCp6KhX7xMJujLao/wBnQ+iok9dpHxOdmzkMxmdhT6MNqt+wpr+9VUTY2vRDtB//ALKtvS7/ACmfHsEdpHxHZsj7MZkxbO6GqQwbi8qv3rQRKY9GptRnZ7G3K2daYNK1QuP2lXNWpnv1NnHqnh54rkeliZBuwdytoXmDToFKZ/a1s00A7+IyfUJKu6vRjaWpWrcH4XXGCNa6aKH6tPtPi3sE78DHLgJdIZZZSJFjSLFUDgBgDgAOQEviJEexERAEREAREQBERAEREAREQBERAEREAREQBERAKRiViAUxErEAREQBERAEREAREQBERAEREAREQBERAEREAREQBERAEREAREQBERAEREAREQBERAEREAREQBERAEREAREQD//Z"
-
-st.set_page_config(page_icon = logo2,
- page_title ="Bonsoir !", layout = "wide")
-
-df = pd.read_csv("df_clean2.csv")
-departement_geo = json.load(open("departements.geojson", "r"))
-
-liste_dep = sorted(df.NomDept.unique().tolist())
-liste_famille = df.famille.unique().tolist()
-liste_metier = list(df.metier.unique())
-
-
-dico_map = {}
-for feature in departement_geo["features"]:
- feature['id']=feature['properties']['code']
- dico_map[feature['properties']['nom']] = feature['id']
-
-
-def heatmap(dep):
- departement = df[df.NomDept == dep]
-
- dep_tail = departement.groupby(["metier"]).agg({"Nbr_demande":"sum"}).sort_values(by="Nbr_demande", ascending = True).head(10)
- labels_tail = dep_tail.index.values.tolist()
-
- dep_head = departement.groupby(["metier"]).agg({"Nbr_demande":"sum"}).sort_values(by="Nbr_demande", ascending = True).tail(10)
- labels_head = dep_head.index.values.tolist()
-
-
- sns.set()
- dep_head.reset_index(inplace=True)
- dep_head = dep_head.sort_values("Nbr_demande", ascending = False)
- dep_head.columns = ["metier", "nbr_demande"]
-
- dep_tail.reset_index(inplace=True)
- dep_tail = dep_tail.sort_values("Nbr_demande", ascending = False)
- dep_tail.columns = ["metier", "nbr_demande"]
-
-
- fig1= plt.figure()
- sns.barplot(y= "metier", x= "nbr_demande", data = dep_head,
- orient="h", palette ="Reds_r")
- plt.xlabel("")
- plt.title("Les métier les plus demandés", fontsize= 18)
- plt.ylabel("")
-
- st.pyplot(fig1)
-
- fig2= plt.figure()
- sns.barplot(y= "metier", x= "nbr_demande", data = dep_tail, orient="h", palette ="Blues")
- plt.xlabel("")
- plt.title("Les métier les moins demandés", fontsize= 18)
- plt.ylabel("")
- plt.xlim(0,50)
-
- st.pyplot(fig2)
-
-def demande_metier(metier):
-
- df_metier = df[df.metier == metier]
- choro = df_metier.groupby(by=["NomDept"]).agg({"Nbr_demande":"sum"})
- choro = choro.reset_index()
- choro['id']=choro['NomDept'].apply(lambda x: dico_map[x])
-
-
- fig = px.choropleth_mapbox(choro, width = 900, height =100, locations="id", geojson = departement_geo, color = "Nbr_demande", hover_name = "NomDept",
- mapbox_style = "open-street-map",
- center = {"lat":46.80, "lon":3.02}, zoom = 5, opacity = 0.5,
- title = metier)
-
- fig.update_geos(fitbounds = "locations", visible = False)
- fig.update_layout(height=800, title_font_size = 25)
-
- st.plotly_chart(fig)
-
-def departement_page():
-
- dep = st.selectbox("Choisir un département",liste_dep)
- heatmap(dep)
-
-
-
-def metier_page():
-
-
- famille = st.selectbox("Famille de métier",liste_famille)
- liste_metier = df[df.famille == famille]["metier"].unique().tolist()
- metier = st.selectbox("Choisir un métier", liste_metier)
-
- demande_metier(metier)
-
-
-def contact_message():
- st.header(":mailbox: Let's Get In Touch !")
-
- name, message = st.columns((1,2))
- with name:
- contact_form = """"""
- st.markdown(contact_form, unsafe_allow_html=True)
-
- with message :
- contact_form2 = """