diff --git a/spaces/1acneusushi/gradio-2dmoleculeeditor/data/Cleanfiles Downloader Exe.md b/spaces/1acneusushi/gradio-2dmoleculeeditor/data/Cleanfiles Downloader Exe.md
deleted file mode 100644
index a22e76be453d27780811408e9bbaf94ca2e4e3be..0000000000000000000000000000000000000000
--- a/spaces/1acneusushi/gradio-2dmoleculeeditor/data/Cleanfiles Downloader Exe.md
+++ /dev/null
@@ -1,27 +0,0 @@
-
-
How to Use CleanFiles Downloader to Download Files from CleanFiles.net
-CleanFiles Downloader is a software program that allows you to download files from CleanFiles.net, a file hosting service that requires you to complete a survey before accessing the download link. CleanFiles Downloader bypasses the survey and lets you download the file directly. Here is how to use CleanFiles Downloader to download files from CleanFiles.net:
-Cleanfiles Downloader Exe
Download Zip ✶ https://byltly.com/2uKvR3
-
-- Download CleanFiles Downloader from https://cleanfiles-downloader.software.informer.com/. This is the official website of the program and it is safe and virus-free[^1^]. You can also check other related programs such as µTorrent, Internet Download Manager, Creevity Mp3 Cover Downloader and MetaProducts Mass Downloader at the "download" section.
-- Install CleanFiles Downloader on your computer. The installation process is simple and straightforward. Just follow the instructions on the screen and accept the terms and conditions. The name of the program executable file is CleanFiles Downloader v5.1.exe.
-- Run CleanFiles Downloader on your computer. You will see a simple interface with a text box where you can enter the URL of the file you want to download from CleanFiles.net.
-- Copy and paste the URL of the file you want to download from CleanFiles.net into the text box. For example, if you want to download a file called example.exe, the URL might look like this: https://cleanfiles.net/?id=1234567890
-- Click on the "Download" button. CleanFiles Downloader will automatically bypass the survey and start downloading the file to your computer. You can see the progress of the download on the status bar.
-- Wait for the download to finish. Once the download is complete, you can find the file in your default download folder or in the folder you specified during the installation. You can then open or run the file as you wish.
-
-CleanFiles Downloader is a useful tool for downloading files from CleanFiles.net without completing surveys. However, you should be careful about what files you download from CleanFiles.net, as some of them might contain viruses or malware. You should always scan your files with a reliable antivirus program before opening or running them. You should also respect the intellectual property rights of the file owners and only download files that you have permission to use.
-
-How to Remove CleanFiles Downloader from Your Computer
-If you no longer need CleanFiles Downloader or you want to uninstall it for any reason, you can easily remove it from your computer. Here is how to remove CleanFiles Downloader from your computer:
-
-- Go to the Start menu and click on Control Panel.
-- Click on Programs and Features or Add/Remove Programs, depending on your version of Windows.
-- Find CleanFiles Downloader in the list of programs and click on it.
-- Click on the Uninstall button and follow the instructions on the screen.
-- Restart your computer if prompted.
-
-CleanFiles Downloader should be completely removed from your computer. You can also delete any files that you downloaded from CleanFiles.net using CleanFiles Downloader if you don't need them anymore. You should also scan your computer with a reliable antivirus program to make sure that there are no traces of viruses or malware left by CleanFiles Downloader or the files you downloaded from CleanFiles.net.
- cec2833e83
-
-
\ No newline at end of file
diff --git a/spaces/1acneusushi/gradio-2dmoleculeeditor/data/El Inolvidable Simon Birch [DVDRIP][.Spanish.].por.GammaRay.avi.md b/spaces/1acneusushi/gradio-2dmoleculeeditor/data/El Inolvidable Simon Birch [DVDRIP][.Spanish.].por.GammaRay.avi.md
deleted file mode 100644
index e40ebfd9ed512b128fbb4ab27c12f7ad00f83968..0000000000000000000000000000000000000000
--- a/spaces/1acneusushi/gradio-2dmoleculeeditor/data/El Inolvidable Simon Birch [DVDRIP][.Spanish.].por.GammaRay.avi.md
+++ /dev/null
@@ -1,110 +0,0 @@
-
-El Inolvidable Simon Birch: A Heartwarming Story of Faith and Friendship
-Have you ever watched a movie that made you laugh, cry, and think at the same time? A movie that touched your heart and inspired your soul? A movie that showed you the beauty of life and the power of faith? If not, then you should definitely watch El Inolvidable Simon Birch, a 1998 American comedy-drama film based on the novel A Prayer for Owen Meany by John Irving. In this article, I will tell you what this movie is about, who are the main characters, what are the themes and messages, and why you should watch it.
-El Inolvidable Simon Birch [DVDRIP][.Spanish.].por.GammaRay.avi
Download Zip 🆗 https://byltly.com/2uKvIB
-Introduction
-What is the movie about?
-El Inolvidable Simon Birch is a movie about a boy named Simon Birch who was born with a rare condition that made him very small and weak. Despite his physical limitations, he has a strong spirit and a firm belief that God has a special plan for him. He lives in a small town in New Hampshire in the 1960s with his parents who don't care much about him. His only friend is Joe Wenteworth, a boy who was born out of wedlock and doesn't know who his father is. Together, they go through many adventures and challenges as they try to find their purpose in life.
-Who are the main characters?
-The main characters of the movie are:
-
-- Simon Birch (played by Ian Michael Smith): The protagonist of the movie. He is a 12-year-old boy who suffers from Morquio syndrome, a rare genetic disorder that affects his growth and development. He is very smart, witty, and courageous. He believes that he is God's instrument and that he has a destiny to fulfill.
-- Joe Wenteworth (played by Joseph Mazzello): The narrator and deuteragonist of the movie. He is Simon's best friend and confidant. He is an illegitimate child who lives with his single mother Rebecca. He is loyal, kind, and protective of Simon. He is also curious about his father's identity.
-- Rebecca Wenteworth (played by Ashley Judd): Joe's mother and Simon's surrogate mother. She is a beautiful, loving, and independent woman who works as a librarian. She loves her son unconditionally and supports his friendship with Simon. She also has a secret affair with Ben Goodrich, the town's baseball coach.
-- Ben Goodrich (played by Oliver Platt): Rebecca's lover and Joe's potential father. He is a friendly, funny, and caring man who works as a baseball coach at the local school. He has a good relationship with Joe and Simon and treats them like his own sons.
-- Reverend Russell (played by David Strathairn): The town's minister and antagonist of the movie. He is a strict, stern, and hypocritical man who dislikes Simon for his unconventional views on religion. He tries to prevent Simon from participating in the church activities and often clashes with him.
-
-Why is it called El Inolvidable Simon Birch?
-The movie is called El Inolvidable Simon Birch because it is the Spanish title of the film. The original title was Simon Birch, but it was changed to El Inolvidable Simon Birch for the Spanish-speaking markets. The word "inolvidable" means "unforgettable" in Spanish, which reflects how Simon left a lasting impression on everyone who knew him.
-Plot Summary
-Simon's birth and childhood
-The movie begins with a flashback of Simon's birth in 1952. He was born prematurely and weighed less than two pounds. The doctors told his parents that he would not survive long, but he miraculously did. However, they also said that he would never grow beyond three feet tall and that he would have many health problems throughout his life.
-Simon grew up feeling different from everyone else. He was often bullied by other kids for his size and appearance. He also had trouble breathing and had to use an oxygen tank sometimes. His parents were ashamed of him and neglected him. They never celebrated his birthday or gave him any presents.
-The only person who cared for him was Rebecca Wenteworth, Joe's mother. She treated him like her own son and gave him love and attention. She also encouraged him to join the church choir and the Christmas pageant, where he met Joe.
-Simon's friendship with Joe
-Simon and Joe became best friends since they were both outsiders in their own way. They shared everything with each other and supported each other through thick and thin. They also had fun together by playing baseball, watching movies, reading comics, and exploring the town.
-One day, they decided to sneak into Rebecca's bedroom to look for clues about Joe's father. They found a locket with a picture of Rebecca and a man they didn't recognize. They also found a baseball signed by Mickey Mantle, which they assumed belonged to Joe's father.
-Descargar El Inolvidable Simon Birch DVDRIP en español por GammaRay
-Ver online El Inolvidable Simon Birch película completa español DVDRIP GammaRay
-El Inolvidable Simon Birch DVDRIP español torrent por GammaRay
-El Inolvidable Simon Birch DVDRIP español mega por GammaRay
-El Inolvidable Simon Birch DVDRIP español gratis por GammaRay
-El Inolvidable Simon Birch DVDRIP español calidad por GammaRay
-El Inolvidable Simon Birch DVDRIP español subtitulos por GammaRay
-El Inolvidable Simon Birch DVDRIP español 1 link por GammaRay
-El Inolvidable Simon Birch DVDRIP español full HD por GammaRay
-El Inolvidable Simon Birch DVDRIP español sin cortes por GammaRay
-El Inolvidable Simon Birch DVDRIP español descargar directa por GammaRay
-El Inolvidable Simon Birch DVDRIP español ver pelicula online por GammaRay
-El Inolvidable Simon Birch DVDRIP español descargar pelicula gratis por GammaRay
-El Inolvidable Simon Birch DVDRIP español descargar pelicula torrent por GammaRay
-El Inolvidable Simon Birch DVDRIP español descargar pelicula mega por GammaRay
-El Inolvidable Simon Birch DVDRIP español ver pelicula completa por GammaRay
-El Inolvidable Simon Birch DVDRIP español ver pelicula HD por GammaRay
-El Inolvidable Simon Birch DVDRIP español ver pelicula sin cortes por GammaRay
-El Inolvidable Simon Birch DVDRIP español ver pelicula subtitulada por GammaRay
-El Inolvidable Simon Birch DVDRIP español ver pelicula 1 link por GammaRay
-El Inolvidable Simon Birch película completa en español DVDRIP por GammaRay
-El Inolvidable Simon Birch película en español DVDRIP descargar por GammaRay
-El Inolvidable Simon Birch película en español DVDRIP online por GammaRay
-El Inolvidable Simon Birch película en español DVDRIP torrent por GammaRay
-El Inolvidable Simon Birch película en español DVDRIP mega por GammaRay
-El Inolvidable Simon Birch película en español DVDRIP gratis por GammaRay
-El Inolvidable Simon Birch película en español DVDRIP calidad por GammaRay
-El Inolvidable Simon Birch película en español DVDRIP subtitulos por GammaRay
-El Inolvidable Simon Birch película en español DVDRIP 1 link por GammaRay
-El Inolvidable Simon Birch película en español DVDRIP full HD por GammaRay
-El Inolvidable Simon Birch película en español DVDRIP sin cortes por GammaRay
-El Inolvidable Simon Birch película en español DVDRIP descargar directa por GammaRay
-El Inolvidable Simon Birch película en español DVDRIP ver online gratis por GammaRay
-El Inolvidable Simon Birch película en español DVDRIP ver online HD por GammaRay
-El Inolvidable Simon Birch película en español DVDRIP ver online sin cortes por GammaRay
-El Inolvidable Simon Birch película en español DVDRIP ver online subtitulada por GammaRay
-El Inolvidable Simon Birch película en español DVDRIP ver online 1 link por GammaRay
-Descarga directa de la película El Inolvidable Simon Birch en español DVDRIP por GammaRay
-Ver la película completa de El Inolvidable Simon Birch en español DVDRIP online gratis por GammaRay
-Torrent de la película El Inolvidable Simon Birch en español DVDRIP descargar gratis por GammaRay
-Mega de la película El Inolvidable Simon Birch en español DVDRIP descargar gratis por GammaRay
-Película completa de El Inolvidable Simon Birch en español DVDRIP online HD por GammaRay
-Película de El Inolvidable Simon Birch en español DVDRIP online sin cortes por GammaRay
-Película de El Inolvidable Simon Birch en español DVDRIP online subtitulada por GammaRay
-Película de El Inolvidable Simon Birch en español DVDRIP online 1 link por GammaRay
-They took the baseball with them to play catch at the lake. However, when Simon threw the ball to Joe, he missed it and hit Rebecca instead, who was on a boat with Ben Goodrich. The ball caused Rebecca to fall into the water and drown.
-Simon felt guilty for killing Rebecca and wondered if it was part of God's plan for him. Joe was devastated by losing his mother and blamed Simon for her death. He also learned that Ben Goodrich was his father after finding out that he had the same locket as Rebecca.
-Simon's quest to find his destiny
-After Rebecca's funeral, Joe moved in with Ben Goodrich while Simon stayed with his parents. They drifted apart for a while until Ben invited Simon to join them on a camping trip. There, they reconciled their friendship and decided to run away together to find Joe's real father.
-They boarded a bus that took them to another town where they met Miss Leavey (played by Jan Hooks), an old friend of Rebecca who ran an orphanage. She recognized Joe from Rebecca's pictures and offered to help them find Joe's father.
-She took them to a diner where she introduced them to Mr. Baines (played by Jim Carrey), an adult version of Joe who narrated the story from the beginning. He told them that he never found out who his father was but that he didn't care anymore because he had Ben as his father figure.
-He also told them that he became a successful writer because of Simon's influence on him. He said that Simon taught him how to see the world differently and how to appreciate life more.
-Simon's heroic act and death
-The next day, they went back to their hometown on another bus that was carrying some children from Miss Leavey's orphanage. On their way, they encountered an accident where a truck hit their bus and caused it to plunge into a frozen lake.
-Simon managed to escape from the bus through a window but saw that many children were still trapped inside. He decided to go back into the water to rescue them one by one using his oxygen tank as an air supply.
-He saved all the children except one girl named Marjorie (played by Sam Morton), who was too scared to leave her seatbelt. Simon tried to calm her down but ran out of air before he could free her.
-Joe saw what happened from outside and dived into the water to help them. He reached them just in time before they drowned but couldn't pull them out because they were too heavy.
-Luckily, Ben arrived at the scene with some firefighters who cut open the bus roof using chainsaws. They pulled out Joe, Simon, Marjorie out of the water along with other survivors.
-However, it was too late for Simon who died from hypothermia in Joe's arms. Before he died, he told Joe that he finally found his destiny: saving those children from drowning.
- Themes and Messages
-The power of faith and belief
-One of the main themes of the movie is the power of faith and belief. Simon is a character who has a strong faith in God and believes that he has a special mission in life. He doesn't let his physical condition or the negative opinions of others stop him from pursuing his dreams. He also inspires others to have faith and hope in themselves and in a higher purpose.
-For example, he convinces Joe to believe that his father is someone important and that he can find him someday. He also helps Marjorie overcome her fear of water by telling her that God loves her and that he will protect her. He also shows Reverend Russell that he is wrong about judging him and that he is a true believer.
-The value of friendship and loyalty
-Another theme of the movie is the value of friendship and loyalty. Simon and Joe are best friends who share a bond that transcends their differences and circumstances. They are always there for each other and support each other through good times and bad times. They also have fun together and enjoy each other's company.
-For example, they play baseball together even though Simon is not good at it. They also watch movies together and laugh at the funny scenes. They also run away together to find Joe's father and have an adventure. They also risk their lives for each other when they face danger.
-The meaning of life and death
-A third theme of the movie is the meaning of life and death. Simon is a character who has a different perspective on life and death than most people. He doesn't fear death because he believes that it is part of God's plan for him. He also thinks that life is a gift that should be cherished and lived fully.
-For example, he celebrates his birthday every day because he doesn't know when he will die. He also makes a list of things he wants to do before he dies, such as kissing a girl, seeing the ocean, and being a hero. He also sacrifices his life to save others because he thinks that it is his destiny.
-Conclusion
-Why you should watch this movie
-El Inolvidable Simon Birch is a movie that will make you laugh, cry, and think. It is a movie that will touch your heart and inspire your soul. It is a movie that will show you the beauty of life and the power of faith.
-You should watch this movie because it will teach you some valuable lessons about friendship, loyalty, courage, belief, purpose, and destiny. You should watch this movie because it will make you appreciate what you have and what you can do. You should watch this movie because it will make you remember Simon Birch, an unforgettable boy who changed the lives of many people.
-FAQs
-
-- Q: Is El Inolvidable Simon Birch based on a true story?
A: No, El Inolvidable Simon Birch is not based on a true story. It is based on a novel called A Prayer for Owen Meany by John Irving. However, some aspects of the movie are inspired by real events or people, such as the bus accident or the actor who played Simon.
-- Q: Who played Simon Birch?
A: Simon Birch was played by Ian Michael Smith, a boy who was born with Morquio syndrome, the same condition as Simon's character. He was discovered by the director Mark Steven Johnson after seeing his picture in an article about children with rare diseases. He was 11 years old when he made his debut in the movie.
-- Q: What happened to Ian Michael Smith after the movie?
A: Ian Michael Smith continued his acting career after the movie. He appeared in several TV shows and movies, such as The Secret Agent Club (1996), The Final Season (2007), and The Lurking Man (2017). He also graduated from MIT with a degree in computer science and became a software engineer.
-- Q: Why did John Irving dislike the movie?
A: John Irving, the author of the novel A Prayer for Owen Meany, disliked the movie adaptation because he felt that it changed too many things from his original story. He didn't like how the characters' names were changed, how the setting was moved from New England to New Hampshire, how some scenes were added or deleted, and how some themes were altered or omitted. He also didn't like how the movie used his title without his permission.
-- Q: Where can I watch El Inolvidable Simon Birch?
A: You can watch El Inolvidable Simon Birch on various streaming platforms, such as Amazon Prime Video, YouTube, Google Play Movies & TV, iTunes, Vudu, or Hulu. You can also buy or rent it on DVD or Blu-ray.
-
- 0a6ba089eb
-
-
\ No newline at end of file
diff --git a/spaces/1acneusushi/gradio-2dmoleculeeditor/data/GTA 3 A Masterpiece or a Menace?.md b/spaces/1acneusushi/gradio-2dmoleculeeditor/data/GTA 3 A Masterpiece or a Menace?.md
deleted file mode 100644
index d5d92c75973cdb58e399cb483a377211baa1c260..0000000000000000000000000000000000000000
--- a/spaces/1acneusushi/gradio-2dmoleculeeditor/data/GTA 3 A Masterpiece or a Menace?.md
+++ /dev/null
@@ -1,12 +0,0 @@
-
-Is GTA 3 Worth It?
-Grand Theft Auto III, or GTA 3, is a 2001 action-adventure game developed by DMA Design and published by Rockstar Games. It is the third main entry in the Grand Theft Auto series, and the fifth instalment overall. It is set in a fictional city called Liberty City, loosely based on New York City, and follows the story of Claude, a silent protagonist who seeks revenge after being betrayed by his girlfriend during a robbery.
-GTA 3 is widely considered as one of the most influential and groundbreaking games of its time, as it was the first game in the series to feature a fully 3D open world that players can explore freely. The game offers a variety of missions, activities, vehicles, weapons, and characters to interact with, as well as a darkly comic storyline and a stellar voice acting. The game also features a stunning soundtrack that includes licensed music from various genres and radio stations.
-is gta 3 worth it
DOWNLOAD ★★★ https://byltly.com/2uKA8T
-GTA 3 has received critical acclaim from critics and gamers alike, and has won several awards, including Game of the Year from various publications. It has also sold over 14.5 million copies worldwide, making it one of the best-selling games of all time. The game has been ported to many different platforms, including Windows, Xbox, Mac OS X, Android, iOS, and Fire OS. The game also received an enhanced version for its tenth anniversary in 2011, and another one for its twentieth anniversary in 2021.
-So, is GTA 3 worth it? The answer depends on what you are looking for in a game. If you are looking for a classic game that defined the open world genre and offers a lot of fun and freedom, then GTA 3 is definitely worth it. However, if you are looking for a game that has modern graphics, gameplay mechanics, and features, then you might find GTA 3 outdated and clunky compared to newer games in the series or genre. Ultimately, GTA 3 is a game that deserves respect and appreciation for its legacy and impact on gaming history.
Here are some more paragraphs for the article:
-GTA 3 is not only a game, but also a cultural phenomenon that has influenced many other games, movies, music, and art. The game has been referenced and parodied in various media, such as The Simpsons, Family Guy, South Park, Robot Chicken, and The Office. The game has also inspired many real-life events and controversies, such as lawsuits, crimes, protests, and bans. For example, in 2003, a teenager named Devin Moore killed three people and stole a police car in Alabama, and claimed that he was influenced by GTA 3. He was later sentenced to death.
-GTA 3 is also a game that has sparked many debates and discussions about the role of violence, sex, morality, and ethics in video games. The game has been criticized by many groups and individuals for its depiction of violence, especially towards women, minorities, and law enforcement. The game has also been accused of promoting crime, drug use, racism, sexism, and misogyny. Some critics have argued that GTA 3 is a satire and a critique of American society and culture, while others have argued that it is a glorification and a celebration of it.
-GTA 3 is a game that has left a lasting impression on the gaming industry and the gaming community. It is a game that has challenged the boundaries of what video games can do and be. It is a game that has given players a sense of freedom and empowerment that few games can match. It is a game that has made history and changed the world.
ddb901b051
-
-
\ No newline at end of file
diff --git a/spaces/1gistliPinn/ChatGPT4/Examples/Civil 3D 2015 Keygen Xforce Rar Free Download !EXCLUSIVE!.md b/spaces/1gistliPinn/ChatGPT4/Examples/Civil 3D 2015 Keygen Xforce Rar Free Download !EXCLUSIVE!.md
deleted file mode 100644
index 4eb9eb4d5af3d31b4be9b9cd40180f188b460215..0000000000000000000000000000000000000000
--- a/spaces/1gistliPinn/ChatGPT4/Examples/Civil 3D 2015 Keygen Xforce Rar Free Download !EXCLUSIVE!.md
+++ /dev/null
@@ -1,90 +0,0 @@
-
-Civil 3D 2015 Keygen Xforce Rar Free Download - A Guide to Activate Autodesk Civil 3D 2015 and Other Products
-Autodesk Civil 3D 2015 is a powerful software that allows civil engineers and designers to create, analyze, and document civil engineering projects. It offers features such as dynamic modeling, geospatial analysis, stormwater management, site grading, and more. However, to use Autodesk Civil 3D 2015 and other Autodesk products of the 2015 version, you need to have a valid product key that can activate the software and unlock all its features and options.
-Civil 3D 2015 keygen xforce rar free download
Download ->->->-> https://imgfil.com/2uy0yW
-One way to get a product key for Autodesk Civil 3D 2015 and other products is to purchase it from the official website or an authorized dealer. However, this can be expensive and not affordable for everyone. Another way to get a product key for Autodesk Civil 3D 2015 and other products is to use the Civil 3D 2015 keygen xforce rar free download. This is a file that contains a program called X-Force 2015 that can generate product keys for all Autodesk products of the 2015 version, including Civil 3D 2015. In this article, we will explain what is the Civil 3D 2015 keygen xforce rar free download, how to use it, and what are the benefits and risks of using it.
-What is the Civil 3D 2015 Keygen Xforce Rar Free Download?
-The Civil 3D 2015 keygen xforce rar free download is a file that contains a program called X-Force 2015. X-Force 2015 is a jailbreak software that can generate product keys for all Autodesk products of the 2015 version, such as Civil 3D 2015, AutoCAD 2015, Revit 2015, etc. The product key is required when you install an Autodesk product as a point product or from a product suite. It allows you to activate the product and use all its features and options without any limitations or restrictions.
-The Civil 3D 2015 keygen xforce rar free download is available on various websites that provide cracks, patches, mods, and tools for different software and games. You can download it for free from these websites and use it to activate your Autodesk products of the 2015 version.
-How to Use the Civil 3D 2015 Keygen Xforce Rar Free Download?
-To use the Civil 3D 2015 keygen xforce rar free download, you need to follow these steps:
-
-- Download the Civil 3D 2015 keygen xforce rar free download from a reliable source.
-- Extract the rar file using a program like WinRAR or 7-Zip.
-- Run the X-Force 2015 program as administrator.
-- Select your Autodesk product from the list and click on Generate.
-- Copy the generated product key and paste it in the installation window of your Autodesk product.
-- Click on Next and follow the instructions to complete the installation.
-- Restart your Autodesk product and enjoy its full features and options.
-
-What are the Benefits and Risks of Using the Civil 3D 2015 Keygen Xforce Rar Free Download?
-The Civil 3D 2015 keygen xforce rar free download has some benefits and risks for users who want to activate their Autodesk products of the 2015 version. Some of these benefits and risks are:
-Benefits
-
-- You can activate any Autodesk product of the 2015 version, such as Civil 3D 2015, without paying any fees or charges.
-- You can use all the features and options of your Autodesk product without any limitations or restrictions.
-- You can use various trainers, cheat codes, mods, and tools that can modify or enhance your Autodesk product's graphics, gameplay, sound, interface, etc.
-- You can use the map editor and create your own custom maps for your Autodesk product.
-
-Risks
-
-- You may violate the terms and conditions of Autodesk and face legal consequences or penalties.
-- You may encounter compatibility, stability, performance, or security issues with your Autodesk product or your PC.
-- You may not be able to access some features or options in your Autodesk product that require online activation or verification.
-- You may expose your PC to viruses, malware, or fake files that can harm your PC or your data.
-
-Conclusion
-The Civil 3D 2015 keygen xforce rar free download is a file that can help you activate your Autodesk products of the 2015 version, such as Civil 3D 2015. It can generate product keys for all Autodesk products of the 2015 version and allow you to use them without any limitations or restrictions. However, it also has some risks and challenges that you should be aware of and prepared for. The Civil 3D 2015 keygen xforce rar free download is not a perfect solution for activating your Autodesk products of the
-
-
-If you are interested in using the Civil 3D 2015 keygen xforce rar free download, you can download it from the links below. However, we recommend that you use it at your own risk and discretion. We are not responsible for any damages or losses that may occur from using the Civil 3D 2015 keygen xforce rar free download.
-Download Links for Civil 3D 2015 Keygen Xforce Rar Free Download
-Here are some of the websites that offer the Civil 3D 2015 keygen xforce rar free download:
-
-Final Words
-We hope that this article has helped you understand what is the Civil 3D 2015 keygen xforce rar free download, how to use it, and what are the benefits and risks of using it. If you have any questions or comments, please feel free to leave them below. Thank you for reading and have a great day!
-
-If you are interested in using the Civil 3D 2015 keygen xforce rar free download, you can download it from the links below. However, we recommend that you use it at your own risk and discretion. We are not responsible for any damages or losses that may occur from using the Civil 3D 2015 keygen xforce rar free download.
-Download Links for Civil 3D 2015 Keygen Xforce Rar Free Download
-Here are some of the websites that offer the Civil 3D 2015 keygen xforce rar free download:
-
-Final Words
-We hope that this article has helped you understand what is the Civil 3D 2015 keygen xforce rar free download, how to use it, and what are the benefits and risks of using it. If you have any questions or comments, please feel free to leave them below. Thank you for reading and have a great day!
-How to Use Autodesk Civil 3D 2015 After Activation
-After you have activated your Autodesk Civil 3D 2015 using the Civil 3D 2015 keygen xforce rar free download, you can start using the software and enjoy its features and options. Here are some of the things you can do with Autodesk Civil 3D 2015:
-
-- You can create, edit, and manage civil engineering projects using dynamic modeling, geospatial analysis, stormwater management, site grading, and more.
-- You can collaborate with other civil engineers and designers using data sharing, design review, and project management tools.
-- You can generate documentation, reports, and presentations for your civil engineering projects using annotation, layout, and visualization tools.
-- You can customize your Autodesk Civil 3D 2015 using various add-ons, plug-ins, extensions, and libraries that can enhance your workflow and productivity.
-
-Tips and Tricks for Using Autodesk Civil 3D 2015
-To make the most out of your Autodesk Civil 3D 2015, here are some tips and tricks that can help you improve your skills and efficiency:
-
-- Use keyboard shortcuts to access commands and tools faster and easier.
-- Use templates and styles to create consistent and standardized civil engineering projects.
-- Use data shortcuts to link data between different drawings and projects.
-- Use labels and tables to display dynamic information about your civil engineering objects.
-- Use data extraction to export data from your civil engineering projects to other formats and applications.
-
-Conclusion
-In this article, we have discussed what is the Civil 3D 2015 keygen xforce rar free download, how to use it, what are the benefits and risks of using it, how to use Autodesk Civil 3D 2015 after activation, and some tips and tricks for using Autodesk Civil 3D 2015. We hope that this article has been informative and helpful for you. If you have any feedback or suggestions, please let us know in the comments section below. Thank you for reading and have a wonderful day!
-Conclusion
-In this article, we have discussed what is the Civil 3D 2015 keygen xforce rar free download, how to use it, what are the benefits and risks of using it, how to use Autodesk Civil 3D 2015 after activation, and some tips and tricks for using Autodesk Civil 3D 2015. We hope that this article has been informative and helpful for you. If you have any feedback or suggestions, please let us know in the comments section below.
-If you are interested in using the Civil 3D 2015 keygen xforce rar free download, you can download it from the links we have provided in this article. However, we recommend that you use it at your own risk and discretion. We are not responsible for any damages or losses that may occur from using the Civil 3D 2015 keygen xforce rar free download.
-If you want to learn more about Autodesk Civil 3D 2015 and other Autodesk products of the 2015 version, you can visit the official website or check out some of the online tutorials and courses that are available on various platforms. You can also join some of the online communities and forums that are dedicated to Autodesk Civil 3D 2015 and other Autodesk products of the 2015 version. You can share your experiences, ask questions, get answers, and learn from other civil engineers and designers who use Autodesk Civil 3D 2015 and other Autodesk products of the 2015 version.
-Thank you for reading and have a wonderful day!
3cee63e6c2
-
-
\ No newline at end of file
diff --git a/spaces/1gistliPinn/ChatGPT4/Examples/Db Bot 1.3a Crack [PATCHED] Download.md b/spaces/1gistliPinn/ChatGPT4/Examples/Db Bot 1.3a Crack [PATCHED] Download.md
deleted file mode 100644
index ba932094dbb7ecca080794af82d338219e7475c5..0000000000000000000000000000000000000000
--- a/spaces/1gistliPinn/ChatGPT4/Examples/Db Bot 1.3a Crack [PATCHED] Download.md
+++ /dev/null
@@ -1,6 +0,0 @@
-Db Bot 1.3a Crack Download
DOWNLOAD ✦✦✦ https://imgfil.com/2uy0s5
-
- 1fdad05405
-
-
-
diff --git a/spaces/1gistliPinn/ChatGPT4/Examples/Download [BEST] Ta Ra Rum Pum Mp4 Download [BEST].md b/spaces/1gistliPinn/ChatGPT4/Examples/Download [BEST] Ta Ra Rum Pum Mp4 Download [BEST].md
deleted file mode 100644
index 2d137a6e9500d15d392518244d826cae7f8ddfdc..0000000000000000000000000000000000000000
--- a/spaces/1gistliPinn/ChatGPT4/Examples/Download [BEST] Ta Ra Rum Pum Mp4 Download [BEST].md
+++ /dev/null
@@ -1,6 +0,0 @@
-Download Ta Ra Rum Pum Mp4 Download
Download ○○○ https://imgfil.com/2uxWZr
-
- 1fdad05405
-
-
-
diff --git a/spaces/1gistliPinn/ChatGPT4/Examples/Engineering Metrology And Measurements By Vijayaraghavan Pdf Free Download.md b/spaces/1gistliPinn/ChatGPT4/Examples/Engineering Metrology And Measurements By Vijayaraghavan Pdf Free Download.md
deleted file mode 100644
index 687bd233e42f5c80b62420436adccfcd739f86dc..0000000000000000000000000000000000000000
--- a/spaces/1gistliPinn/ChatGPT4/Examples/Engineering Metrology And Measurements By Vijayaraghavan Pdf Free Download.md
+++ /dev/null
@@ -1,6 +0,0 @@
-Engineering Metrology And Measurements By Vijayaraghavan Pdf Free Download
DOWNLOAD ✔✔✔ https://imgfil.com/2uy05T
-
-April 25th, 2018 - Engineering Metrology and Measurements pdf Download as ... and measurement vijayaraghavan pdf FREE PDF DOWNLOAD NOW Source 2Â ... 1fdad05405
-
-
-
diff --git a/spaces/1line/AutoGPT/tests/unit/test_commands.py b/spaces/1line/AutoGPT/tests/unit/test_commands.py
deleted file mode 100644
index ecbac9b73bd9ad872931d77e144dd853b3d8ef64..0000000000000000000000000000000000000000
--- a/spaces/1line/AutoGPT/tests/unit/test_commands.py
+++ /dev/null
@@ -1,22 +0,0 @@
-"""Unit tests for the commands module"""
-from unittest.mock import MagicMock, patch
-
-import pytest
-
-import autogpt.agent.agent_manager as agent_manager
-from autogpt.app import execute_command, list_agents, start_agent
-
-
-@pytest.mark.integration_test
-def test_make_agent() -> None:
- """Test the make_agent command"""
- with patch("openai.ChatCompletion.create") as mock:
- obj = MagicMock()
- obj.response.choices[0].messages[0].content = "Test message"
- mock.return_value = obj
- start_agent("Test Agent", "chat", "Hello, how are you?", "gpt2")
- agents = list_agents()
- assert "List of agents:\n0: chat" == agents
- start_agent("Test Agent 2", "write", "Hello, how are you?", "gpt2")
- agents = list_agents()
- assert "List of agents:\n0: chat\n1: write" == agents
diff --git a/spaces/1pelhydcardo/ChatGPT-prompt-generator/assets/Download Blue Orchid Mod Apk and Experience a Gripping Story.md b/spaces/1pelhydcardo/ChatGPT-prompt-generator/assets/Download Blue Orchid Mod Apk and Experience a Gripping Story.md
deleted file mode 100644
index bcca4bb7a67139fa0a0c9c668359d81be2e4994c..0000000000000000000000000000000000000000
--- a/spaces/1pelhydcardo/ChatGPT-prompt-generator/assets/Download Blue Orchid Mod Apk and Experience a Gripping Story.md
+++ /dev/null
@@ -1,139 +0,0 @@
-
-Blue Orchid Mod APK: A Guide for Interactive Story Lovers
-If you are a fan of interactive stories, you might have heard of Blue Orchid, a game that lets you create your own character and live your own adventure. But did you know that there is a modded version of the game that gives you unlimited gems, coins, and choices? In this article, we will tell you everything you need to know about Blue Orchid Mod APK, including what it is, why you should download it, how to play it, and what are its pros and cons. Let's get started!
- What is Blue Orchid?
-A brief introduction to the game
-Blue Orchid is an interactive story game developed by Elia Games. It is available for Android devices and can be downloaded from Google Play Store. The game is set in a fictional city called Blue Orchid, where you can choose from different genres of stories, such as romance, drama, mystery, fantasy, and more. You can customize your character's appearance, name, personality, and preferences. You can also interact with other characters, make decisions that affect the outcome of the story, and enjoy various mini-games and activities.
-blue orchid mod apk
Download Zip »»» https://urlin.us/2uSSob
- The main features of the game
-Some of the features that make Blue Orchid stand out from other interactive story games are:
-
-- It has high-quality graphics and sound effects that create an immersive atmosphere.
-- It has a diverse and inclusive cast of characters that represent different backgrounds, cultures, orientations, and identities.
-- It has multiple storylines and endings that depend on your choices and actions.
-- It has a user-friendly interface and easy-to-use controls that make the game accessible and enjoyable.
-- It has regular updates and new content that keep the game fresh and exciting.
-
- Why download Blue Orchid Mod APK?
-The benefits of using the modded version
-While Blue Orchid is a free-to-play game, it also has some in-app purchases that require real money. For example, you need gems to unlock premium choices and outfits, coins to buy gifts and items, and tickets to access new chapters. These resources are limited and can run out quickly if you play frequently. This can limit your options and enjoyment of the game.
-That's why some players prefer to use Blue Orchid Mod APK, which is a modified version of the game that gives you unlimited gems, coins, and tickets. With this modded version, you can enjoy the following benefits:
-
-- You can make any choice you want without worrying about the cost or consequences.
-- You can dress up your character in any outfit you like without spending any money.
-- You can play any chapter you want without waiting for tickets to refill.
-- You can explore all the stories and genres without missing any content.
-- You can have more fun and freedom in the game without any restrictions or limitations.
-
- How to download and install Blue Orchid Mod APK
-If you want to try Blue Orchid Mod APK, you need to follow these steps:
-
-- Uninstall the original version of Blue Orchid from your device if you have it installed.
-- Download Blue Orchid Mod APK from a reliable source such as [PlayMods](^1^).
-- Enable unknown sources on your device settings to allow the installation of third-party apps.
-- Locate the downloaded file on your device storage and tap on it to start the installation process.
-- Follow the instructions on the screen to complete the installation.
- Launch the game and enjoy the modded features.
-
-Note: You may need to grant some permissions to the app to run properly. Also, make sure to download the modded version from a trusted source to avoid any malware or viruses.
- How to play Blue Orchid: Interactive Story
-The basic gameplay mechanics
-Playing Blue Orchid is simple and intuitive. Here are the basic steps you need to follow:
-
-- Choose a story genre that interests you from the main menu. You can browse through different categories such as romance, drama, mystery, fantasy, and more.
-- Create your character by selecting their gender, appearance, name, and personality. You can also change their outfit and accessories later in the game.
-- Start the story and read the dialogue and narration. You can tap on the screen to proceed or swipe left or right to go back or forward.
-- Make choices that affect the plot and your relationships with other characters. Some choices are free, while others require gems or coins. You can also use tickets to unlock new chapters.
-- Enjoy the mini-games and activities that are part of the story. For example, you can play match-3 puzzles, trivia quizzes, dress-up games, and more.
-- Earn rewards such as gems, coins, tickets, and items by completing achievements, watching ads, or spinning the wheel.
-
- The tips and tricks for a better experience
-If you want to have more fun and success in Blue Orchid, here are some tips and tricks you can use:
-blue orchid interactive story mod apk
-blue orchid mod apk unlimited diamonds
-blue orchid mod apk latest version
-blue orchid mod apk download for android
-blue orchid mod apk free shopping
-blue orchid mod apk 1.0.1
-blue orchid mod apk choices
-blue orchid mod apk offline
-blue orchid mod apk no ads
-blue orchid mod apk unlocked everything
-blue orchid mod apk android 1
-blue orchid mod apk revdl
-blue orchid mod apk happymod
-blue orchid mod apk rexdl
-blue orchid mod apk apkpure
-blue orchid mod apk 2023
-blue orchid mod apk update
-blue orchid mod apk premium
-blue orchid mod apk vip
-blue orchid mod apk pro
-blue orchid mod apk full version
-blue orchid mod apk hack
-blue orchid mod apk cheat
-blue orchid mod apk cracked
-blue orchid mod apk unlimited money
-blue orchid romance game mod apk
-blue orchid love story mod apk
-blue orchid dating sim mod apk
-blue orchid visual novel mod apk
-blue orchid otome game mod apk
-download game blue orchid mod apk
-download aplikasi blue orchid mod apk
-cara download blue orchid mod apk
-link download blue orchid mod apk
-how to install blue orchid mod apk
-how to play blue orchid mod apk
-how to get blue orchid mod apk
-how to update blue orchid mod apk
-how to hack blue orchid mod apk
-how to cheat in blue orchid mod apk
-is there a blue orchid mod apk
-where can i find blue orchid mod apk
-where to download blue orchid mod apk
-what is the best site for downloading the latest version of the Blue Orchids Mod Apk?
-
-- Pay attention to the hints and clues that are given in the story. They can help you make better choices and solve mysteries.
-- Explore different options and outcomes by replaying the chapters or stories. You can also use the modded version to access all the choices without spending any resources.
-- Interact with different characters and build your relationships with them. You can also romance them if you want. You can use gifts and items to increase your affection level with them.
-- Check out the shop and the wardrobe for new outfits and accessories. You can also use the modded version to get unlimited coins and gems to buy anything you want.
-- Follow the official social media accounts of Blue Orchid for news, updates, sneak peeks, and giveaways. You can also join the community of other players and share your opinions and feedback.
-
- The pros and cons of Blue Orchid Mod APK
-The advantages of the modded version
-Using Blue Orchid Mod APK has some advantages that make it appealing for many players. Some of them are:
-
-- You can enjoy unlimited resources such as gems, coins, and tickets that allow you to access all the content and features of the game.
-- You can have more control and flexibility over your choices and actions in the game without worrying about the cost or consequences.
-- You can have more fun and satisfaction in the game without any restrictions or limitations.
-- You can save your time and money by not having to wait for tickets to refill or spend real money on in-app purchases.
-
- The disadvantages of the modded version
-However, using Blue Orchid Mod APK also has some disadvantages that you should be aware of before downloading it. Some of them are:
-
-- You may face some technical issues or errors while playing the game such as crashes, glitches, or bugs.
-- You may lose your progress or data if you uninstall the game or switch devices.
-- You may get banned or suspended from the game if you are detected by the developers or reported by other players.
-- You may miss out on some of the original features or content of the game that are not included in the modded version.
-
- Conclusion
-A summary of the main points
-In conclusion, Blue Orchid is an interactive story game that lets you create your own character and live your own adventure in a fictional city. You can choose from different genres of stories, customize your character's appearance and personality, interact with other characters, make decisions that affect the outcome of the story, and enjoy various mini-games and activities. The game is free-to-play but also has some in-app purchases that require real money. If you want to have unlimited resources such as gems, coins, and tickets, you can download Blue Orchid Mod APK, which is a modified version of the game that gives you these benefits. However, you should also be aware of the potential risks and drawbacks of using this modded version such as technical issues, banned or suspended from the game, or missing out on some of the original features or content of the game.
- A call to action for the readers
-Now that you know everything about Blue Orchid Mod APK, you can decide whether you want to download it or not. If you do, make sure to follow the instructions we provided and enjoy the game with unlimited resources. If you don't, you can still play the original version of Blue Orchid and have a great time with the interactive stories. Either way, we hope you have fun and share your thoughts and experiences with us in the comments section below. Happy gaming!
- FAQs
-Here are some of the frequently asked questions about Blue Orchid Mod APK:
-
-- Is Blue Orchid Mod APK safe to use?
-Blue Orchid Mod APK is generally safe to use as long as you download it from a reliable source such as [PlayMods]. However, you should always be careful when installing third-party apps on your device and scan them for any malware or viruses.
-- How do I update Blue Orchid Mod APK?
-Blue Orchid Mod APK is usually updated automatically when the original version of the game is updated. However, if you encounter any problems or errors, you can check the source where you downloaded the modded version and see if there is a newer version available. You can also follow the official social media accounts of Blue Orchid for any news or updates.
-- Can I play Blue Orchid Mod APK offline?
-No, you cannot play Blue Orchid Mod APK offline. You need an internet connection to access the game and its features. However, you can play some of the mini-games and activities offline once you have downloaded them.
-- Can I transfer my progress from Blue Orchid to Blue Orchid Mod APK or vice versa?
-No, you cannot transfer your progress from Blue Orchid to Blue Orchid Mod APK or vice versa. The two versions of the game are not compatible and have different data and files. If you want to switch from one version to another, you will have to start from scratch.
-- Can I play Blue Orchid Mod APK with my friends?
-Yes, you can play Blue Orchid Mod APK with your friends. You can connect your game account to your Facebook account and invite your friends to join you in the game. You can also chat with them, send them gifts, and compete with them in the leaderboards.
-
197e85843d
-
-
\ No newline at end of file
diff --git a/spaces/1phancelerku/anime-remove-background/APK5-30 .md b/spaces/1phancelerku/anime-remove-background/APK5-30 .md
deleted file mode 100644
index f94e9385b69aaee76083bf2ff5dfb074e1111ac4..0000000000000000000000000000000000000000
--- a/spaces/1phancelerku/anime-remove-background/APK5-30 .md
+++ /dev/null
@@ -1,129 +0,0 @@
-
-What is APK5-30 and Why You Need It
-If you are looking for a reliable, efficient, and cost-effective axial fan for your cooling and ventilation needs, you might want to consider APK5-30. This is a product from Teral, a leading manufacturer of pumps and fans in Japan. In this article, we will explain what APK5-30 is, what are its features and benefits, how to use it, and how it compares with other axial fans in the market.
- Introduction
-Cooling and ventilation are essential for many industrial applications, such as machinery, equipment, exhaust, and air conditioning. However, not all fans are created equal. Some fans may not be able to deliver the required airflow and pressure, some may consume too much energy and generate too much noise, and some may not be durable or easy to install and maintain. That's why you need a fan that can meet your specific needs and expectations.
-apk5-30
Download →→→ https://jinyurl.com/2uNPSM
- What is APK5-30?
-APK5-30 is a type of axial fan that uses an aluminum impeller and a belt drive system to create a high-efficiency airflow. It has a circular shape that can be directly mounted on a duct or suspended from a ceiling. It can handle air temperatures from 0 to 40 degrees Celsius and has a frequency of 50Hz or 60Hz depending on the region. It has a size of 300mm, an output of 0.4kW, a voltage of 200V, and a speed of 4P.
- What are the features and benefits of APK5-30?
-APK5-30 has many features and benefits that make it a superior choice for cooling and ventilation purposes. Here are some of them:
-
-- It uses a top-runner efficiency motor (IE3 equivalent) that reduces energy consumption and carbon emissions. (Except for 0.2 to 0.4kW models)
-- It has a simple structure with few components, which makes it cheaper and easier to install and maintain than centrifugal fans.
-- It has an internal support leg that acts as a static blade, which increases the static pressure and improves the performance.
-- It has a wide range of models with different capacities, speeds, voltages, and frequencies to suit various applications.
-- It has a low noise level and vibration level due to its smooth operation and balanced impeller.
-
- How to use APK5-30 for your cooling and ventilation needs
-Now that you know what APK5-30 is and what it can do for you, let's see how you can use it for your cooling and ventilation needs. Here are some tips on how to install, operate, and maintain APK5-30.
- How to install APK5-30
-To install APK5-30, you need to follow these steps:
-
-- Select a suitable location for the fan that has enough space, ventilation, and accessibility.
-- Prepare the duct or ceiling where the fan will be mounted or suspended.
-- Connect the fan to the power supply according to the wiring diagram provided by the manufacturer.
-- Secure the fan with bolts or nuts on the duct or ceiling.
-- Check the rotation direction of the impeller by turning on the power briefly.
-- If the rotation direction is incorrect, reverse the wiring connection.
-
- How to operate APK5-30
-To operate To operate APK5-30, you need to follow these steps:
-- Turn on the power switch and adjust the speed controller if needed.
-- Monitor the fan operation and check for any abnormal sounds, vibrations, or smells.
-- If the fan stops working or malfunctions, turn off the power immediately and contact the manufacturer or a qualified technician.
-
- How to maintain APK5-30
-To maintain APK5-30, you need to follow these steps:
-
-- Turn off the power and disconnect the fan from the power supply before cleaning or inspecting.
-- Clean the fan regularly with a soft cloth or a brush to remove any dust or dirt.
-- Check the fan for any signs of wear, damage, or corrosion and replace any defective parts as soon as possible.
-- Lubricate the bearings and belts periodically with the recommended oil or grease.
-- Store the fan in a dry and cool place when not in use.
-
- Comparison of APK5-30 with other axial fans
-Now that you know how to use APK5-30, let's see how it compares with other axial fans in the market. Here are some aspects that you can use to evaluate different axial fans:
- How APK5-30 differs from other axial fans
-APK5-30 differs from other axial fans in several ways, such as:
-APK5-30 axial fan price
-APK5-30 axial fan specifications
-APK5-30 axial fan installation manual
-APK5-30 axial fan performance curve
-APK5-30 axial fan noise level
-APK5-30 axial fan maintenance
-APK5-30 axial fan replacement parts
-APK5-30 axial fan reviews
-APK5-30 axial fan dimensions
-APK5-30 axial fan weight
-APK5-30 axial fan power consumption
-APK5-30 axial fan airflow rate
-APK5-30 axial fan static pressure
-APK5-30 axial fan speed
-APK5-30 axial fan efficiency
-APK5-30 axial fan vs centrifugal fan
-APK5-30 axial fan applications
-APK5-30 axial fan advantages and disadvantages
-APK5-30 axial fan suppliers
-APK5-30 axial fan distributors
-APK5-30 axial fan online purchase
-APK5-30 axial fan warranty
-APK5-30 axial fan troubleshooting
-APK5-30 axial fan vibration analysis
-APK5-30 axial fan blade design
-APK5-30 axial fan motor type
-APK5-30 axial fan belt tension
-APK5-30 axial fan bearing lubrication
-APK5-30 axial fan impeller material
-APK5-30 axial fan casing material
-
-- It uses an aluminum impeller instead of a steel or plastic one, which makes it lighter and more resistant to corrosion.
-- It uses a belt drive system instead of a direct drive system, which allows it to adjust the speed and torque more easily.
-- It uses an internal support leg instead of an external one, which reduces the air resistance and increases the efficiency.
-
- How APK5-30 performs better than other axial fans
-APK5-30 performs better than other axial fans in several ways, such as:
-
-- It has a higher airflow rate and pressure than other axial fans of the same size and power.
-- It has a lower noise level and vibration level than other axial fans of the same size and power.
-- It has a longer service life and lower maintenance cost than other axial fans of the same size and power.
-
- How APK5-30 saves energy and costs than other axial fans
-APK5-30 saves energy and costs than other axial fans in several ways, such as:
-
-- It uses a top-runner efficiency motor (IE3 equivalent) that consumes less electricity and emits less carbon dioxide. (Except for 0.2 to 0.4kW models)
-- It has a simple structure with few components, which reduces the initial purchase price and installation cost.
-- It has a low operating cost due to its high efficiency and low maintenance requirements.
-
- Conclusion
- Summary of the main points
-In conclusion, APK5-30 is a type of axial fan that uses an aluminum impeller and a belt drive system to create a high-efficiency airflow. It has many features and benefits that make it a superior choice for cooling and ventilation purposes. It is easy to install, operate, and maintain, and it performs better than other axial fans in terms of airflow, pressure, noise, vibration, service life, and maintenance cost. It also saves energy and costs by using a top-runner efficiency motor (IE3 equivalent) that reduces electricity consumption and carbon emissions. (Except for 0.2 to 0.4kW models)
- Call to action
-If you are interested in purchasing APK5-30 or learning more about it, please visit our website or contact us today. We will be happy to assist you with any questions or inquiries you may have. Don't miss this opportunity to get your hands on this amazing product that will improve your cooling and ventilation needs.
- Frequently Asked Questions
- What is the warranty period for APK5-30?
-The warranty period for APK5-30 is one year from the date of purchase. If you encounter any problems with the product during this period, please contact us for repair or replacement.
- What are the dimensions and weight of APK5-30?
-The dimensions of APK5-30 are 300mm x 300mm x 300mm (L x W x H) and the weight is 9kg.
- What are the applications of What are the applications of APK5-30?
-APK5-30 can be used for various cooling and ventilation applications, such as:
-
-- Machinery and equipment cooling
-- Exhaust and smoke removal
-- Air conditioning and dehumidification
-- Greenhouse and farm ventilation
-- Warehouse and factory ventilation
-
- How can I order APK5-30 online?
-You can order APK5-30 online by visiting our website and filling out the order form. You will need to provide your name, address, phone number, email, and payment method. We will confirm your order and ship the product to you as soon as possible.
- What are the safety precautions for using APK5-30?
-When using APK5-30, you should follow these safety precautions:
-
-- Do not touch the fan or the impeller when it is running or hot.
-- Do not insert any objects or fingers into the fan or the duct.
-- Do not use the fan in wet, dusty, or flammable environments.
-- Do not overload the fan or the power supply.
-- Do not modify or repair the fan without authorization.
-
197e85843d
-
-
\ No newline at end of file
diff --git a/spaces/1phancelerku/anime-remove-background/Bubble Shooter Enjoy the Original Bubble Pop Game on Your iOS Device.md b/spaces/1phancelerku/anime-remove-background/Bubble Shooter Enjoy the Original Bubble Pop Game on Your iOS Device.md
deleted file mode 100644
index 584b2e976c4a31ab3e9229c6e3fa81699d23d168..0000000000000000000000000000000000000000
--- a/spaces/1phancelerku/anime-remove-background/Bubble Shooter Enjoy the Original Bubble Pop Game on Your iOS Device.md
+++ /dev/null
@@ -1,154 +0,0 @@
-
-Bubble Shooter for iPhone Free Download: How to Play the Classic and Addictive Game on Your iOS Device
- If you are looking for a fun and relaxing game to play on your iPhone, you might want to try Bubble Shooter. Bubble Shooter is a classic and addictive game that has been around for decades and is still popular among millions of players worldwide. In this article, we will tell you everything you need to know about Bubble Shooter, including what it is, how to download it for free, and how to play it on your iOS device. Let's get started!
- What is Bubble Shooter?
- Bubble Shooter is a puzzle game that involves shooting bubbles of the same color to make them pop and clear the board. The game is simple to learn but challenging to master, as you need to aim carefully and plan your moves ahead. The game has many variations and versions, but the basic concept remains the same: match 3 or more bubbles of the same color to burst them and score points.
-bubble shooter for iphone free download
Download ✅ https://jinyurl.com/2uNJP2
- The history of Bubble Shooter
- Bubble Shooter was originally developed by a company called Taito in 1994 as an arcade game called Puzzle Bobble. The game was a spin-off of the popular platformer game Bubble Bobble, which featured two cute dragons named Bub and Bob. Puzzle Bobble was later ported to various home consoles and computers, and became a huge hit worldwide. The game spawned several sequels and clones, and inspired many other bubble shooting games over the years.
- The gameplay of Bubble Shooter
- The gameplay of Bubble Shooter is very simple: you have a cannon at the bottom of the screen that shoots bubbles of different colors. You can aim the cannon by moving your finger or mouse cursor on the screen, and tap or click to fire a bubble. Your goal is to match 3 or more bubbles of the same color to make them pop and clear them from the board. If you clear all the bubbles, you win the level and move on to the next one. If the bubbles reach the bottom of the screen, you lose the game and have to start over.
- The benefits of playing Bubble Shooter
- Bubble Shooter is not only a fun and entertaining game, but also a beneficial one. Playing Bubble Shooter can help you improve your skills in various ways, such as:
-
-- Enhancing your concentration and focus
-- Boosting your memory and cognitive abilities
-- Developing your hand-eye coordination and reaction speed
-- Reducing your stress and anxiety levels
-- Increasing your creativity and problem-solving skills
-
- Besides, playing Bubble Shooter can also make you happy and relaxed, as popping bubbles can release endorphins in your brain that make you feel good.
- How to download Bubble Shooter for iPhone for free?
- If you want to play Bubble Shooter on your iPhone, you have plenty of options to choose from. There are many free apps that offer different versions and variations of Bubble Shooter on the App Store. Here are some of the best ones that we recommend:
- The best Bubble Shooter apps on the App Store
- Bubble Shooter - Pop Bubbles
- This app is one of the most popular and highly rated Bubble Shooter games on the App Store. It offers a classic and addictive gameplay with thousands of fun levels, amazing graphics and sounds, and various challenges and rewards. You can also play with your friends and family online and compete for the highest score. The app is free to download and play, but it contains ads and in-app purchases. You can download it from here: [Bubble Shooter - Pop Bubbles].
-free bubble shooter games download for iphone
-bubble shooter app for iphone free download
-bubble shooter classic for iphone free download
-bubble shooter puzzle for iphone free download
-bubble shooter adventure for iphone free download
-bubble shooter legend for iphone free download
-bubble shooter deluxe for iphone free download
-bubble shooter blast for iphone free download
-bubble shooter pop for iphone free download
-bubble shooter fun for iphone free download
-bubble shooter saga for iphone free download
-bubble shooter mania for iphone free download
-bubble shooter frenzy for iphone free download
-bubble shooter magic for iphone free download
-bubble shooter galaxy for iphone free download
-bubble shooter candy for iphone free download
-bubble shooter fruit for iphone free download
-bubble shooter animal for iphone free download
-bubble shooter dragon for iphone free download
-bubble shooter unicorn for iphone free download
-bubble shooter rainbow for iphone free download
-bubble shooter garden for iphone free download
-bubble shooter farm for iphone free download
-bubble shooter jungle for iphone free download
-bubble shooter forest for iphone free download
-bubble shooter ocean for iphone free download
-bubble shooter beach for iphone free download
-bubble shooter island for iphone free download
-bubble shooter pirate for iphone free download
-bubble shooter treasure for iphone free download
-bubble shooter gold for iphone free download
-bubble shooter diamond for iphone free download
-bubble shooter jewel for iphone free download
-bubble shooter crystal for iphone free download
-bubble shooter star for iphone free download
-bubble shooter space for iphone free download
-bubble shooter planet for iphone free download
-bubble shooter solar for iphone free download
-bubble shooter lunar for iphone free download
-bubble shooter halloween for iphone free download
-bubble shooter christmas for iphone free download
-bubble shooter winter for iphone free download
-bubble shooter spring for iphone free download
-bubble shooter summer for iphone free download
-bubble shooter autumn for iphone free download
-best bubble shooter game for iphone free download
-new bubble shooter game for iphone free download
-top bubble shooter game for iphone free download
-cool bubble shooter game for iphone free download
- Bubble Shooter - Addictive!
- This app is another great option for Bubble Shooter fans. It features a smooth and easy gameplay with over 3000 exciting levels, stunning graphics and effects, and a relaxing soundtrack. You can also customize your bubble shooter with different skins and themes, and enjoy daily bonuses and gifts. The app is free to download and play, but it contains ads and in-app purchases. You can download it from here: [Bubble Shooter - Addictive!].
- Bobble Shooter
- This app is a unique and innovative take on the Bubble Shooter genre. It combines the classic bubble popping gameplay with a physics-based puzzle element. You have to shoot bobbles of different shapes and sizes to create clusters of the same color and make them explode. The game has hundreds of challenging levels, colorful graphics and animations, and a catchy music. The app is free to download and play, but it contains ads and in-app purchases. You can download it from here: [Bobble Shooter].
- How to install and launch Bubble Shooter on your iPhone
- Installing and launching Bubble Shooter on your iPhone is very easy. Just follow these simple steps:
-
-- Open the App Store on your iPhone and search for the Bubble Shooter app that you want to download.
-- Tap on the app icon and then tap on the Get button to start the download process.
-- Wait for the app to finish downloading and then tap on the Open button to launch it.
-- Alternatively, you can also find the app icon on your home screen and tap on it to launch it.
-
- How to update and delete Bubble Shooter on your iPhone
- Updating and deleting Bubble Shooter on your iPhone is also very simple. Just follow these simple steps:
-
-- To update Bubble Shooter, open the App Store on your iPhone and tap on the Updates tab at the bottom.
-- Find the Bubble Shooter app that you want to update and tap on the Update button next to it.
-- Wait for the app to finish updating and then launch it as usual.
-- To delete Bubble Shooter, press and hold the app icon on your home screen until it starts to wiggle.
-- Tap on the X button on the top left corner of the app icon and then tap on Delete to confirm.
-
- How to play Bubble Shooter on your iPhone?
- Playing Bubble Shooter on your iPhone is very fun and easy. Here are some tips and tricks that will help you enjoy the game more:
- The basic rules and tips of Bubble Shooter
- The basic rules of Bubble Shooter are as follows:
-
-- You have a limited number of bubbles to shoot in each level.
-- You have to match 3 or more bubbles of the same color to pop them and clear them from the board.
-- You can bounce the bubbles off the walls to reach difficult spots.
-- You can see the next bubble that you are going to shoot at the bottom of the screen.
-- You can swap the current bubble with the next one by tapping on it.
-- You can use special bubbles that have different effects, such as bombs, rainbows, stars, etc.
-- You can earn coins and gems by popping bubbles, completing levels, and achieving goals.
-- You can use coins and gems to buy power-ups, boosters, lives, etc.
-
- Some tips that will help you improve your performance are:
-
-- Aim carefully before you shoot a bubble.
-- Try to pop as many bubbles as possible with one shot.
-- Try to create chain reactions by popping bubbles that are connected to other bubbles of the same color.
-- Try to clear the top rows of bubbles first, as they will drop all the bubbles below them when they pop.
-- Try to avoid leaving isolated bubbles that are hard to reach or match.
-- Use power-ups and boosters wisely, as they can help you clear difficult levels or get out of tricky situations.
-
- The different game modes and levels of Bubble Shooter
- Bubble Shooter offers a variety of game modes and levels that will keep you entertained for hours. Some of them are:
-
--
- Classic mode: This is the original and most popular mode of Bubble Shooter. It has hundreds of levels that range from easy to hard, and each level has a different layout and goal. You can play this mode offline or online, and you can also choose the difficulty level and the bubble design.
-- Arcade mode: This is a fast-paced and exciting mode of Bubble Shooter. It has endless levels that get harder and harder as you progress, and each level has a time limit and a score target. You have to pop as many bubbles as you can before the time runs out, and you can also use power-ups and boosters to speed up your progress.
-- Puzzle mode: This is a challenging and brain-teasing mode of Bubble Shooter. It has hundreds of levels that require logic and strategy to solve, and each level has a unique puzzle and goal. You have to pop all the bubbles using the least number of shots, and you can also use hints and skips to help you out.
-- Adventure mode: This is a fun and adventurous mode of Bubble Shooter. It has hundreds of levels that are based on different themes and stories, such as pirates, fairies, dinosaurs, etc. You have to pop bubbles and collect items to complete the levels, and you can also encounter obstacles and enemies along the way.
-
- The features and settings of Bubble Shooter
- Bubble Shooter also has many features and settings that will enhance your gaming experience. Some of them are:
-
-- You can connect your Facebook account to Bubble Shooter and share your progress, achievements, and scores with your friends.
-- You can play with other players from around the world in the multiplayer mode and compete for the highest score.
-- You can join or create a team with other players and chat, cooperate, and exchange gifts with them.
-- You can participate in various events, tournaments, and challenges that offer special rewards and prizes.
-- You can customize your bubble shooter with different skins, themes, backgrounds, sounds, etc.
-- You can adjust the game settings according to your preferences, such as the volume, the language, the notifications, etc.
-
- Conclusion
- Bubble Shooter is a classic and addictive game that you can play on your iPhone for free. It offers a simple but challenging gameplay with thousands of fun levels, amazing graphics and sounds, and various game modes and features. It also helps you improve your skills, reduce your stress, and have fun with your friends. If you are looking for a game that will keep you entertained for hours, download Bubble Shooter today and enjoy popping bubbles!
- FAQs
- Here are some frequently asked questions about Bubble Shooter:
-
-- How do I get more coins and gems in Bubble Shooter?
-You can get more coins and gems in Bubble Shooter by popping bubbles, completing levels, achieving goals, watching ads, spinning the wheel, opening chests, collecting daily bonuses, joining events, buying them with real money, etc.
-- How do I use power-ups and boosters in Bubble Shooter?
-You can use power-ups and boosters in Bubble Shooter by tapping on them before or during the game. Power-ups are special bubbles that have different effects, such as bombs, rainbows, stars, etc. Boosters are items that help you in various ways, such as extra moves, fireballs, magnets, etc.
-- How do I unlock new levels in Bubble Shooter?
-You can unlock new levels in Bubble Shooter by completing the previous levels or by paying coins or gems. You can also unlock new levels by joining events or teams that offer exclusive levels.
-- How do I reset my progress in Bubble Shooter?
-You can reset your progress in Bubble Shooter by deleting the app from your iPhone and reinstalling it. However, this will also erase all your coins, gems, power-ups, boosters, lives, etc. If you want to keep them, you can connect your Facebook account to Bubble Shooter and sync your progress across different devices.
-- How do I contact the support team of Bubble Shooter?
-You can contact the support team of Bubble Shooter by tapping on the settings icon on the main screen and then tapping on the help button. You can also email them at support@bubbleshooter.com or visit their website at www.bubbleshooter.com.
-
197e85843d
-
-
\ No newline at end of file
diff --git a/spaces/1phancelerku/anime-remove-background/Crafting and Building 1.18 APK A Free Game with Amazing Graphics and Multiplayer Mode.md b/spaces/1phancelerku/anime-remove-background/Crafting and Building 1.18 APK A Free Game with Amazing Graphics and Multiplayer Mode.md
deleted file mode 100644
index a72384daf227db3aa90dac1be1aabb55fb0587a6..0000000000000000000000000000000000000000
--- a/spaces/1phancelerku/anime-remove-background/Crafting and Building 1.18 APK A Free Game with Amazing Graphics and Multiplayer Mode.md
+++ /dev/null
@@ -1,121 +0,0 @@
-
-Crafting and Building 1.18 APK: A Free Game for Creative Minds
-Do you like building games? Do you want to create your own world with your own rules? If yes, then you should try crafting and building 1.18 apk, a new free game that lets you unleash your imagination and show your skills. Crafting and building 1.18 apk is a sandbox game that allows you to build anything you want, from houses and castles to farms and cities. You can also play with your friends online, explore their creations, and have fun together. Crafting and building 1.18 apk is a game for the whole family, suitable for kids, boys, girls, and adults.
-Features of Crafting and Building 1.18 APK
-Crafting and building 1.18 apk has many features that make it an enjoyable and addictive game. Here are some of them:
-crafting and building 1.18 apk
Download File ★★★★★ https://jinyurl.com/2uNLwa
-
-- Easy to use interface: The game has a simple and user-friendly interface that lets you access all the tools and options easily. You can drag and drop blocks, rotate them, change their colors, and customize them as you wish.
-- Many block types: The game offers a variety of block types, from grass and wood to stone and metal. You can also find special blocks, such as furniture, animals, plants, and even vehicles.
-- Multiplayer mode: The game supports online multiplayer mode, where you can join or create a server and play with your friends or other players from around the world. You can chat with them, visit their worlds, help them build, or compete with them.
-- Creative mode: The game has a creative mode, where you have unlimited resources and no enemies or dangers. You can build whatever you want without any limitations or restrictions.
-- Survival mode: The game also has a survival mode, where you have to gather resources, craft items, fight enemies, and survive in a hostile environment. You can also tame animals, farm crops, mine ores, and explore dungeons.
-
-Tips and Tricks for Crafting and Building 1.18 APK
-If you want to master crafting and building 1.18 apk, here are some tips and tricks that can help you:
-
-- Use trapdoors as walls: A clever way to make a pen for animals or a fence for your garden is to use trapdoors as walls. Animals can climb into the pen but not out of it, and you can easily access it by opening the trapdoors.
-- Find diamonds under clay patches: A useful tip to find diamonds easily is to dig under clay patches in rivers. Diamonds are often found below clay patches that have a star shape.
-- Use torches to breathe underwater: A handy trick to breathe underwater is to place torches on the wall or floor near your head. The torches will create air bubbles that will replenish your oxygen.
-- Use beds as explosives: A fun way to blow up things is to use beds as explosives. Beds will explode when placed in the Nether or the End dimensions, creating a large blast radius.
-- Use pistons to move blocks: A smart way to move blocks around is to use pistons. Pistons can push or pull blocks up to 12 blocks away, allowing you to create doors, bridges, elevators, traps, and more.
-
-Reviews of Crafting and Building 1.18 APK
-Crafting and building 1.18 apk has received many positive reviews from users who have played the game. Here are some of them:
-
-
-User |
-Rating |
-Comment |
-
-
-Amy |
-5 stars |
-I love this game! It's so fun and creative. I can build anything I want and play with my friends online. It's like Minecraft but better. |
-
-
-Jack |
-4 stars |
-This game is awesome, but it has some bugs and glitches. Sometimes the game crashes or freezes, and sometimes the blocks disappear or change color. Please fix these issues. |
-
-
-Lisa |
-3 stars |
-This game is good, but it needs more content and features. I wish there were more block types, more animals, more items, more modes, and more customization options. It gets boring after a while. |
-
-
-Tom |
-2 stars |
-This game is okay, but it's too similar to other games. It's like a copy of Minecraft or Roblox. It doesn't have anything original or unique. It's just another building game. |
-
-
-Anna |
-1 star |
-This game is terrible. It's full of ads and pop-ups that ruin the gameplay. It's also very laggy and slow. It takes forever to load and connect to the servers. It's a waste of time and space. |
-
-
- Conclusion: Download Crafting and Building 1.18 APK Now!
- Crafting and building 1.18 apk is a free game that lets you create your own world with your own rules. You can build anything you want, from houses and castles to farms and cities. You can also play with your friends online, explore their creations, and have fun together. Crafting and building 1.18 apk is a game for the whole family, suitable for kids, boys, girls, and adults.
-crafting and building 1.18 apk download free
-crafting and building 1.18 apk mod unlimited money
-crafting and building 1.18 apk latest version
-crafting and building 1.18 apk for android
-crafting and building 1.18 apk offline
-crafting and building 1.18 apk no ads
-crafting and building 1.18 apk update
-crafting and building 1.18 apk hack
-crafting and building 1.18 apk full version
-crafting and building 1.18 apk premium
-crafting and building 1.18 apk gameplay
-crafting and building 1.18 apk review
-crafting and building 1.18 apk features
-crafting and building 1.18 apk tips and tricks
-crafting and building 1.18 apk cheats
-crafting and building 1.18 apk guide
-crafting and building 1.18 apk tutorial
-crafting and building 1.18 apk best settings
-crafting and building 1.18 apk how to play
-crafting and building 1.18 apk requirements
-crafting and building 1.18 apk size
-crafting and building 1.18 apk screenshots
-crafting and building 1.18 apk video
-crafting and building 1.18 apk online multiplayer
-crafting and building 1.18 apk new features
-crafting and building 1.18 apk bugs fixes
-crafting and building 1.18 apk installation
-crafting and building 1.18 apk alternatives
-crafting and building 1.18 apk similar games
-crafting and building 1.18 apk comparison
-crafting and building 1.18 apk pros and cons
-crafting and building 1.18 apk ratings
-crafting and building 1.18 apk feedbacks
-crafting and building 1.18 apk comments
-crafting and building 1.18 apk questions and answers
-crafting and building 1.18 apk support
-crafting and building 1.18 apk developer contact
-crafting and building 1.18 apk official website
-crafting and building 1.18 apk social media links
-crafting and building 1.18 apk news and updates
-crafting and building 1.18 apk release date
-crafting and building 1.18 apk changelog
-crafting and building 1.18 apk download link
-crafting and building 1.18 apk mirror link
-crafting and building 1.18 apk direct link
-crafting and building 1.18 apk file information
-crafting and building 1.18 apk virus scan report
-crafting and building 1.18 apk safe to download
- If you are looking for a game that will challenge your creativity and imagination, then you should download crafting and building 1.18 apk now! You will not regret it!
- FAQs: Frequently Asked Questions About Crafting and Building 1.18 APK
- Here are some of the most common questions and answers about crafting and building 1.18 apk:
- Q: How can I download crafting and building 1.18 apk?
- A: You can download crafting and building 1.18 apk from the Google Play Store or from other websites that offer apk files. However, be careful when downloading from unknown sources, as they may contain viruses or malware.
- Q: How can I update crafting and building 1.18 apk?
- A: You can update crafting and building 1.18 apk from the Google Play Store or from the app itself. The app will notify you when there is a new version available and ask you to update it.
- Q: How can I play crafting and building 1.18 apk offline?
- A: You can play crafting and building 1.18 apk offline by choosing the single-player mode or the creative mode. You will not be able to access the multiplayer mode or the survival mode without an internet connection.
- Q: How can I play crafting and building 1.18 apk with my friends?
- A: You can play crafting and building 1.18 apk with your friends by choosing the multiplayer mode or the survival mode. You will need an internet connection and a valid account to join or create a server.
- Q: How can I contact the developers of crafting and building 1.18 apk?
- A: You can contact the developers of crafting and building 1.18 apk by sending them an email at genere@gmail.com or by leaving them a feedback on the Google Play Store or on their social media pages.
401be4b1e0
-
-
\ No newline at end of file
diff --git a/spaces/1phancelerku/anime-remove-background/Download MuksOS AI Launcher 2.0 Mod APK for Android - Latest Version with Voice Gesture and Text Control.md b/spaces/1phancelerku/anime-remove-background/Download MuksOS AI Launcher 2.0 Mod APK for Android - Latest Version with Voice Gesture and Text Control.md
deleted file mode 100644
index 1e86d3427be2261fc902ec063e266ed91a00d0a4..0000000000000000000000000000000000000000
--- a/spaces/1phancelerku/anime-remove-background/Download MuksOS AI Launcher 2.0 Mod APK for Android - Latest Version with Voice Gesture and Text Control.md
+++ /dev/null
@@ -1,108 +0,0 @@
-
-MuksOS AI Launcher 2.0: A Smart and Interactive Android Launcher
-If you are looking for a new and innovative way to interact with your phone, you might want to check out MuksOS AI Launcher 2.0. This is a unique android launcher that combines the features of an app launcher, a virtual assistant, and an AI tool for your DIY automation projects. In this article, we will tell you what MuksOS AI Launcher 2.0 is, what are its features, how to download it, and answer some frequently asked questions.
-muksos ai launcher 2.0 mod apk download
Download File 🌟 https://jinyurl.com/2uNMrY
- What is MuksOS AI Launcher 2.0?
-MuksOS AI Launcher 2.0 is an android app developed by Dr. Mukesh Bangar, a computer engineer and researcher in artificial intelligence. It is designed to make your phone smarter and more responsive by using voice, gestures, or text commands. You can use MuksOS AI Launcher 2.0 to open apps, make calls, search the web, set alarms, reminders, and more. You can also use it as a virtual assistant that can assist you anytime, anywhere with its cool and unique features like JARVIS has in Iron Man movie. And if you are into DIY automation projects, you can use MuksOS AI Launcher 2.0 as an easy AI tool to create amazing things using object recognition and smart connect features.
- Features of MuksOS AI Launcher 2.0
-MuksOS AI Launcher 2.0 has many features that make it stand out from other android launchers. Here are some of them:
- Teachable
-MuksOS AI Launcher 2.0 is not just a passive launcher that does what you say. It is also a teachable launcher that learns from you and adapts to your preferences. You can teach it voice commands, object recognition, and actions that suit your needs.
- Fast and smooth
-MuksOS AI Launcher 2.0 is designed to be fast and smooth, so you can get more done in less time. It has voice access that makes it faster than any other launcher and saves time. You can also use gestures or text commands if you prefer.
- Multiple voice options
-MuksOS AI Launcher 2.0 has six different voice options that you can choose from, depending on your mood and preference. You can switch between male and female voices, as well as different accents and languages.
- 100 % privacy
-MuksOS AI Launcher 2.0 respects your privacy and does not store your personal data on cloud servers. All your data is stored locally on your device and encrypted for security.
-muksos ai launcher 2.0 apk free download
-muksos ai launcher 2.0 latest version
-muksos ai launcher 2.0 android app
-muksos ai launcher 2.0 for pc
-muksos ai launcher 2.0 features
-muksos ai launcher 2.0 review
-muksos ai launcher 2.0 offline mode
-muksos ai launcher 2.0 voice access
-muksos ai launcher 2.0 smart connect
-muksos ai launcher 2.0 vision ability
-muksos ai launcher 2.0 write on home screen
-muksos ai launcher 2.0 speech reminders and alarm
-muksos ai launcher 2.0 dark and light theme
-muksos ai launcher 2.0 hide apps
-muksos ai launcher 2.0 power saver
-muksos ai launcher 2.0 teachable commands
-muksos ai launcher 2.0 object recognition
-muksos ai launcher 2.0 diy automation tool
-muksos ai launcher 2.0 virtual assistant
-muksos ai launcher 2.0 neon glow icons theme
-muksos ai launcher 2.0 apkcombo download
-muksos ai launcher 2.0 appbrain download
-muksos ai launcher 2.0 gameloop download
-muksos ai launcher 2.0 apk size and version
-muksos ai launcher 2.0 content rating and developer
-muksos ai launcher 2.0 install and update
-muksos ai launcher 2.0 google play id and category
-muksos ai launcher 2.0 interact with phone in natural way
-muksos ai launcher 2.0 open apps and contacts with voice or text or gestures
-muksos ai launcher 2.0 web search wikipedia or google or youtube with voice or text or gestures
-muksos ai launcher 2.0 create amazing AI projects with smart connect feature
-muksos ai launcher 2.0 train your mobile for object recognition and actions with vision ability feature
-muksos ai launcher 2.0 works without internet with offline mode feature
-muksos ai launcher 2.0 change theme in a single tap with dark and light theme feature
-muksos ai launcher 2.0 hide unwanted and distracting bloatware with hide apps feature
-muksos ai launcher 2.0 save phone battery and optimize battery usage with power saver feature
-muksos ai launcher 2.0 teach voice commands, object recognition and actions with teachable feature
-muksos ai launcher 2.0 get direct access to your favorite app from home screen with favorite apps feature
-muksos ai launcher 2.0 write on home screen to open apps, make a call or web search with write on home screen feature
-muksos ai launcher 2.0 quickly access all your apps, contacts, web searches, reminders, alarm etc with voice access feature
- User friendly
-MuksOS AI Launcher 2.0 is user friendly and easy to use. You don't need to scroll pages to find contacts, apps, alarms, reminders, etc. You can access them directly from the home screen with simple commands.
- Power saver
-MuksOS AI Launcher 2.0 saves your phone battery and optimizes battery usage by using minimal resources and background processes.
- Esthetic theme
-MuksOS AI Launcher 2.0 comes with a cool neon glow icons theme that's sure to stand out on your device. You can also customize the theme according to your liking by changing the colors, icons, fonts, and wallpapers.
- Dark and Light theme
-MuksOS AI Launcher 2.0 supports both dark and light themes that you can switch between depending on the time of the day or your preference. The dark theme is ideal for night time or low-light conditions, while the light theme is suitable for daytime or bright conditions.
- Works offline
-MuksOS AI Launcher 2.0 works offline as well as online, so you don't need to worry about internet connectivity or data usage. You can use most of the features without any internet connection, such as opening apps, making calls, setting alarms, reminders, etc.
- Favorite apps
-MuksOS AI Launcher 2.0 lets you add your favorite apps to the home screen for quick and easy access. You can also create folders and categories to organize your apps according to your needs.
- Hide apps
-MuksOS AI Launcher 2.0 allows you to hide apps that you don't want others to see or access. You can use a password or a fingerprint to lock and unlock the hidden apps.
- Premium Features of MuksOS AI Launcher 2.0
-MuksOS AI Launcher 2.0 also has some premium features that you can unlock by purchasing the mod apk version of the app. These features include:
- Write on Home Screen
-This feature lets you write anything on your home screen using your finger or a stylus. You can use this feature to take notes, draw sketches, make lists, etc.
- Voice Access
-This feature lets you control your phone with your voice without touching it. You can use voice commands to open apps, make calls, search the web, play music, etc.
- Speech reminders and Speech alarm
-This feature lets you set reminders and alarms with your voice. You can also choose what you want to hear when the reminder or alarm goes off, such as a song, a quote, a joke, etc.
- Smart connect
-This feature lets you connect your phone with other devices using Bluetooth or Wi-Fi. You can use this feature to transfer files, share photos, play games, etc.
- Vision ability
-This feature lets you use your phone's camera as an AI tool for object recognition and detection. You can use this feature to identify objects, faces, colors, text, etc.
- How to download MuksOS AI Launcher 2.0 mod apk?
-If you want to download MuksOS AI Launcher 2.0 mod apk and enjoy its premium features for free, you can follow these steps:
-
-- Go to the official website of MuksOS AI Launcher 2.0 and click on the download button.
-- Allow unknown sources in your device settings to install the app from outside the Google Play Store.
-- Locate the downloaded file in your file manager and tap on it to install it.
-- Launch the app and grant it the necessary permissions to access your device features.
-- Enjoy using MuksOS AI Launcher 2.0 mod apk with all its features unlocked.
-
- Conclusion
-MuksOS AI Launcher 2.0 is a smart and interactive android launcher that offers you a new and innovative way to interact with your phone. It has many features that make it stand out from other android launchers, such as teachable, fast and smooth, multiple voice options, 100 % privacy, user friendly, power saver, esthetic theme, dark and light theme, works offline, favorite apps, hide apps, etc. It also has some premium features that you can unlock by downloading the mod apk version of the app, such as write on home screen, voice access, speech reminders and speech alarm, smart connect, vision ability etc. If you are looking for a smart and interactive android launcher that combines the features of an app launcher, a virtual assistant and an AI tool for your DIY automation projects then MuksOS AI Launcher 2.0 is the perfect choice for you.
- FAQs
-
-- Q: Is MuksOS AI Launcher 2.0 safe to use?
-- A: Yes, MuksOS AI Launcher 2.0 is safe to use as it does not store your personal data on cloud servers and encrypts it locally on your device.
-- Q: How much does MuksOS AI Launcher 2.0 cost?
-- A: MuksOS AI Launcher 2.0 is free to download and use, but it has some premium features that require a one-time payment of $4.99 to unlock.
-- Q: What are the minimum requirements to run MuksOS AI Launcher 2.0?
-- A: MuksOS AI Launcher 2.0 requires Android 5.0 or higher and at least 1 GB of RAM to run smoothly.
-- Q: How can I contact the developer of MuksOS AI Launcher 2.0?
-- A: You can contact the developer of MuksOS AI Launcher 2.0 by sending an email to muksosailauncher@gmail.com or by visiting the official website of MuksOS AI Launcher 2.0.
-- Q: How can I support the development of MuksOS AI Launcher 2.0?
-- A: You can support the development of MuksOS AI Launcher 2.0 by rating and reviewing the app on the Google Play Store, sharing it with your friends and family, and providing feedback and suggestions to the developer.
-
401be4b1e0
-
-
\ No newline at end of file
diff --git a/spaces/1phancelerku/anime-remove-background/Experience GTA V Like Never Before with Online RP Launcher.md b/spaces/1phancelerku/anime-remove-background/Experience GTA V Like Never Before with Online RP Launcher.md
deleted file mode 100644
index 9fc3106bb31166039865714b288cd531cd8965a4..0000000000000000000000000000000000000000
--- a/spaces/1phancelerku/anime-remove-background/Experience GTA V Like Never Before with Online RP Launcher.md
+++ /dev/null
@@ -1,130 +0,0 @@
-
-What is an online rp launcher and why you need one
-If you are a fan of Grand Theft Auto (GTA) Online, you might have heard of online rp launchers. These are software applications that allow you to play GTA Online on customized dedicated servers, with different game modes, maps, vehicles, weapons, and more. Online rp launchers are also known as multiplayer modifications or frameworks, and they enable you to create or join your own GTA Online community.
-Online rp launchers work by modifying the game files of GTA V, but without affecting your original installation or your access to GTA Online. This means that you can switch between GTA Online and online rp launchers without getting banned by Rockstar. Online rp launchers also use Rockstar's network code with improvements, so you can enjoy the best synchronization and performance possible.
-online rp launcher
Download Zip ✅ https://jinyurl.com/2uNMx6
-Online rp launchers are not only fun and exciting, but also creative and innovative. You can make anything you wish with online rp launchers, such as roleplay, drifting, racing, deathmatch, or something completely original. You can also use different programming languages to create your own scripts and resources for your server. Online rp launchers give you total control over your GTA Online experience.
-How to choose the best online rp launcher for your needs
-There are many online rp launchers available for GTA Online, but not all of them are created equal. Some online rp launchers may have more features, compatibility, or popularity than others. Here are some factors to consider when choosing the best online rp launcher for your needs:
-Features
-The features of an online rp launcher determine what you can do with it. Some online rp launchers may have more options for customization, streaming, AI, scripting, or hosting than others. For example, some online rp launchers may allow you to use custom cars, maps, weapons, and more dynamically, while others may require you to download them manually. Some online rp launchers may also have more support for different programming languages or tools than others.
-Compatibility
-The compatibility of an online rp launcher determines how well it works with your system and your game version. Some online rp launchers may have higher or lower system requirements than others. For example, some online rp launchers may require Windows 10 or a certain CPU or GPU to run smoothly. Some online rp launchers may also be more compatible with the latest updates or patches of GTA V than others.
-Popularity
-The popularity of an online rp launcher determines how many players and servers are using it. Some online rp launchers may have more active and diverse communities than others. For example, some online rp launchers may have more players or servers in your region or language than others. Some online rp launchers may also have more famous or reputable servers or streamers than others.
-FiveM - the GTA V multiplayer modification you have dreamt of
-One of the most popular and well-known online rp launchers is FiveM. FiveM is a modification for GTA V that enables you to play multiplayer on customized dedicated servers powered by Cfx.re. FiveM has been around since 2014 and has over 178k players playing right now.
-online rp launcher for GTA V multiplayer
-online rp launcher for GTA SAMP on Android
-online rp launcher for RAGE MP mod
-online rp launcher for FiveM server hosting
-online rp launcher for GTA real life roleplay
-online rp launcher for GTA drifting and racing
-online rp launcher for GTA deathmatch and PvP
-online rp launcher for GTA open world sandbox
-online rp launcher for GTA custom cars and maps
-online rp launcher for GTA AI and sync quality
-online rp launcher for GTA source-available platform
-online rp launcher for GTA community-driven project
-online rp launcher for GTA Cfx.re framework
-online rp launcher for GTA multiple programming languages
-online rp launcher for GTA developer tools and resources
-online rp launcher for GTA net energy gain experiment
-online rp launcher for GTA holy grail fusion project
-online rp launcher for GTA mini Sun creation
-online rp launcher for GTA 100 million°C reactor
-online rp launcher for GTA Korea Superconducting Tokamak Advanced Research facility
-online rp launcher for GTA Korea Institute of Fusion Energy
-online rp launcher for GTA nuclear fusion reaction
-online rp launcher for GTA physics and engineering problem
-online rp launcher for GTA contributor program and rewards
-online rp launcher for GTA Rockstar Online Services validation
-online rp launcher for GTA game copy protection
-online rp launcher for GTA installation switcher
-online rp launcher for GTA ban prevention
-online rp launcher for GTA login information security
-online rp launcher for GTA multiplayer modification framework
-online rp launcher for GTA advanced and unique features
-online rp launcher for GTA creativity and personalization
-online rp launcher for GTA streaming and dynamic content
-online rp launcher for GTA Lua, C#, and JavaScript support
-online rp launcher for GTA web development knowledge and ecosystem
-online rp launcher for GTA solar core temperature comparison
-online rp launcher for GTA seven times hotter than the Sun achievement
-online rp launcher for GTA 15 million degrees kelvins measurement
-online rp launcher for GTA radiative zone and convection zone layers
-online rp launcher for GTA photosphere and chromosphere thicknesses
-online rp launcher for GTA sun spot cycle duration
-online rp launcher for GTA photosphere composition and elements
-online rp launcher for GTA solar atmosphere and surface gas pressure
-online rp launcher for GTA optical depth and effective temperature
-online rp launcher for GTA system requirements and specifications
-online rp launcher for GTA Intel Core CPU and NVIDIA GPU models
-online rapuncher.com domain name availability
-best online rapuncher reviews and ratings
-FiveM has many features that make it stand out from other online rp launchers. Some of these features are:
-
-- Streaming: FiveM allows servers to use custom cars, maps, weapons, and more without requiring the players to download them manually. This means that you can join any server and enjoy its custom content instantly.
-- AI: FiveM allows servers to use custom AI scripts and scenarios, such as traffic, pedestrians, animals, and more. This means that you can have a more realistic and immersive experience in GTA Online.
-- Scripting: FiveM allows servers to use different programming languages and frameworks, such as Lua, C#, JavaScript, and more. This means that you can create or join servers with different game modes, features, and mechanics.
-- Hosting: FiveM allows anyone to host their own server with their own rules and settings. This means that you can create or join your own GTA Online community with your friends or other players.
-
-FiveM is compatible with Windows 7 or higher and the latest version of GTA V. FiveM also has a large and active community of players, servers, developers, and streamers. You can find more information about FiveM on their website or their Discord.
-RAGE Multiplayer - fun, free and easy
-Another popular and well-known online rp launcher is RAGE Multiplayer. RAGE Multiplayer is a modification for GTA V that enables you to play multiplayer on customized dedicated servers powered by RAGE Technology Group. RAGE Multiplayer has been around since 2017 and has over 15k players playing right now.
-RAGE Multiplayer has many features that make it stand out from other online rp launchers. Some of these features are:
-
-- Free: RAGE Multiplayer is completely free to use and does not require any registration or activation. This means that you can download and play RAGE Multiplayer without any hassle or cost.
-- Easy: RAGE Multiplayer is easy to install and use. You just need to download the launcher, select your GTA V folder, and start playing. You can also easily switch between GTA Online and RAGE Multiplayer without any problems.
-- Fast: RAGE Multiplayer is fast and optimized for performance and synchronization. You can enjoy smooth gameplay and low latency on any server.
-- Flexible: RAGE Multiplayer allows servers to use different programming languages and frameworks, such as C#, JavaScript, TypeScript, Node.js, and more. This means that you can create or join servers with different game modes, features, and mechanics.
-
-RAGE Multiplayer is compatible with Windows 7 or higher and the latest version of GTA V. RAGE Multiplayer also has a large and active community of players, servers, developers, and streamers. You can find more information about RAGE Multiplayer on their website or their Discord.
-How to play on GTA RP servers
-GTA RP servers are one of the most popular types of online rp launchers. GTA RP stands for Grand Theft Auto Roleplay, which is a game mode where you create a character and live a virtual life in the GTA world. You can interact with other players, follow the laws, get a job, join a gang, or do whatever you want.
-GTA RP servers are usually hosted by online rp launchers such as FiveM or RAGE Multiplayer. To play on GTA RP servers, you need to have GTA V installed on your PC and an online rp launcher of your choice. You also need to find a GTA RP server that suits your preferences and style. Some GTA RP servers may have different rules, themes, whitelists, applications, or requirements than others.
-To join a GTA RP server, you need to follow these steps:
-
-- Launch your online rp launcher and select the server browser.
-- Search for a GTA RP server that you like and click on it.
-- Read the server's description, rules, website, Discord, or any other information provided by the server owner.
-- If the server requires an application or a whitelist, follow the instructions given by the server owner to apply or register.
-- If the server does not require an application or a whitelist, or if you have been accepted or whitelisted, click on connect to join the server.
-- Create your character and start roleplaying.
-
-GTA RP servers are fun and immersive ways to enjoy GTA Online with other players. You can make friends, enemies, allies, rivals, lovers, or anything else you can imagine. You can also explore different aspects of the GTA world that you may not have seen before. GTA RP servers are like living in your own GTA movie or TV show.
Tips and tricks for online rp launcher users
-Online rp launchers are great ways to enhance your GTA Online experience, but they also come with some challenges and risks. Here are some tips and tricks for online rp launcher users to make the most out of their online rp launcher adventures:
-Backup your game files
-Before installing or using any online rp launcher, it is always a good idea to backup your game files. This way, you can restore your original GTA V installation in case something goes wrong or you want to play GTA Online again. You can backup your game files by copying the GTA V folder to another location on your PC or using a backup software.
-Follow the server rules
-When playing on any online rp launcher server, you should always follow the server rules and respect the other players. This is especially important for GTA RP servers, where you are expected to roleplay realistically and follow the server's theme and lore. Breaking the server rules or disrupting the roleplay can result in a kick, a ban, or a report from the server owner or the admins.
-Update your online rp launcher regularly
-Online rp launchers are constantly being updated and improved by their developers and communities. To enjoy the latest features, fixes, and enhancements, you should always update your online rp launcher regularly. You can check for updates on the online rp launcher's website, Discord, or launcher. You should also update your GTA V game whenever a new patch or update is released by Rockstar.
-Use a VPN
-Using a VPN (virtual private network) can help you protect your privacy and security when playing on online rp launcher servers. A VPN can hide your IP address and encrypt your data, making it harder for hackers, trackers, or malicious players to access your information or harm your PC. A VPN can also help you bypass geo-restrictions or firewalls that may prevent you from accessing certain online rp launcher servers.
-Have fun
-The most important tip for online rp launcher users is to have fun. Online rp launchers are meant to provide you with endless possibilities and opportunities to enjoy GTA Online in new and creative ways. You can explore different worlds, meet new people, create your own stories, or just have a blast. Online rp launchers are all about having fun.
-Conclusion
-Online rp launchers are software applications that allow you to play GTA Online on customized dedicated servers with different game modes, maps, vehicles, weapons, and more. Online rp launchers are also known as multiplayer modifications or frameworks, and they enable you to create or join your own GTA Online community.
-There are many online rp launchers available for GTA Online, but some of the most popular and well-known ones are FiveM and RAGE Multiplayer. These online rp launchers have many features, compatibility, and popularity that make them stand out from other online rp launchers.
-GTA RP servers are one of the most popular types of online rp launchers. GTA RP stands for Grand Theft Auto Roleplay, which is a game mode where you create a character and live a virtual life in the GTA world. You can interact with other players, follow the laws, get a job, join a gang, or do whatever you want.
-To play on GTA RP servers, you need to have GTA V installed on your PC and an online rp launcher of your choice. You also need to find a GTA RP server that suits your preferences and style. Some GTA RP servers may have different rules, themes, whitelists, applications, or requirements than others.
-To make the most out of your online rp launcher experience, you should follow some tips and tricks such as backing up your game files, following the server rules, updating your online rp launcher regularly, using a VPN, and having fun.
-If you are looking for a new way to enjoy GTA Online with more freedom, creativity, and fun, you should definitely try online rp launchers. They will change the way you play GTA Online forever.
- Frequently Asked Questions
-
-- What is an online rp launcher?
-- An online rp launcher is a software application that allows you to play GTA Online on customized dedicated servers with different game modes, maps, vehicles, weapons, and more.
-- How do I install an online rp launcher?
-- To install an online rp launcher, you need to download the launcher from its website or Discord and select your GTA V folder. You also need to have GTA V installed on your PC.
-- Can I play GTA Online with an online rp launcher?
-- You can still play GTA Online with an online rp launcher, but you need to switch back to your original GTA V installation. Online rp launchers do not affect your GTA Online access or progress.
-- What are some of the best online rp launchers?
-- Some of the best online rp launchers are FiveM and RAGE Multiplayer. These online rp launchers have many features, compatibility, and popularity that make them stand out from other online rp launchers.
-- What are GTA RP servers?
-- GTA RP servers are online rp launcher servers that use a game mode called Grand Theft Auto Roleplay, where you create a character and live a virtual life in the GTA world. You can interact with other players, follow the laws, get a job, join a gang, or do whatever you want.
-- How do I join a GTA RP server?
-- To join a GTA RP server, you need to launch your online rp launcher and select the server browser. Then, you need to search for a GTA RP server that you like and click on it. You may also need to apply or register for some GTA RP servers that have whitelists or applications.
-
401be4b1e0
-
-
\ No newline at end of file
diff --git a/spaces/A00001/bingothoo/src/lib/utils.ts b/spaces/A00001/bingothoo/src/lib/utils.ts
deleted file mode 100644
index 0a09ddc4aa5518f681a00a64ad48566516f35417..0000000000000000000000000000000000000000
--- a/spaces/A00001/bingothoo/src/lib/utils.ts
+++ /dev/null
@@ -1,158 +0,0 @@
-import { clsx, type ClassValue } from 'clsx'
-import { customAlphabet } from 'nanoid'
-import { twMerge } from 'tailwind-merge'
-
-export function cn(...inputs: ClassValue[]) {
- return twMerge(clsx(inputs))
-}
-
-export const nanoid = customAlphabet(
- '0123456789ABCDEFGHIJKLMNOPQRSTUVWXYZabcdefghijklmnopqrstuvwxyz',
- 7
-) // 7-character random string
-
-export function createChunkDecoder() {
- const decoder = new TextDecoder()
- return function (chunk: Uint8Array | undefined): string {
- if (!chunk) return ''
- return decoder.decode(chunk, { stream: true })
- }
-}
-
-export function random (start: number, end: number) {
- return start + Math.ceil(Math.random() * (end - start))
-}
-
-export function randomIP() {
- return `11.${random(104, 107)}.${random(1, 255)}.${random(1, 255)}`
-}
-
-export const defaultUID = Math.random().toString(36).slice(2)
-
-export function parseHeadersFromCurl(content: string) {
- const re = /-H '([^:]+):\s*([^']+)/mg
- const headers: HeadersInit = {}
- content = content.replaceAll('-H "', '-H \'').replaceAll('" ^', '\'\\').replaceAll('^\\^"', '"') // 将 cmd curl 转成 bash curl
- content.replace(re, (_: string, key: string, value: string) => {
- headers[key] = value
- return ''
- })
-
- return headers
-}
-
-export const ChunkKeys = ['BING_HEADER', 'BING_HEADER1', 'BING_HEADER2']
-export function encodeHeadersToCookie(content: string) {
- const base64Content = btoa(content)
- const contentChunks = base64Content.match(/.{1,4000}/g) || []
- return ChunkKeys.map((key, index) => `${key}=${contentChunks[index] ?? ''}`)
-}
-
-export function extraCurlFromCookie(cookies: Partial<{ [key: string]: string }>) {
- let base64Content = ''
- ChunkKeys.forEach((key) => {
- base64Content += (cookies[key] || '')
- })
- try {
- return atob(base64Content)
- } catch(e) {
- return ''
- }
-}
-
-export function extraHeadersFromCookie(cookies: Partial<{ [key: string]: string }>) {
- return parseHeadersFromCurl(extraCurlFromCookie(cookies))
-}
-
-export function formatDate(input: string | number | Date): string {
- const date = new Date(input)
- return date.toLocaleDateString('en-US', {
- month: 'long',
- day: 'numeric',
- year: 'numeric'
- })
-}
-
-export function parseCookie(cookie: string, cookieName: string) {
- const targetCookie = new RegExp(`(?:[; ]|^)${cookieName}=([^;]*)`).test(cookie) ? RegExp.$1 : cookie
- return targetCookie ? decodeURIComponent(targetCookie).trim() : cookie.indexOf('=') === -1 ? cookie.trim() : ''
-}
-
-export function setCookie(key: string, value: string) {
- const maxAge = 86400 * 30
- document.cookie = `${key}=${value || ''}; Path=/; Max-Age=${maxAge}; SameSite=None; Secure`
-}
-
-export function getCookie(cookieName: string) {
- const re = new RegExp(`(?:[; ]|^)${cookieName}=([^;]*)`)
- return re.test(document.cookie) ? RegExp.$1 : ''
-}
-
-export function parseCookies(cookie: string, cookieNames: string[]) {
- const cookies: { [key: string]: string } = {}
- cookieNames.forEach(cookieName => {
- cookies[cookieName] = parseCookie(cookie, cookieName)
- })
- return cookies
-}
-
-export const DEFAULT_UA = 'Mozilla/5.0 (Windows NT 10.0; Win64; x64) AppleWebKit/537.36 (KHTML, like Gecko) Chrome/115.0.0.0 Safari/537.36 Edg/115.0.0.0'
-export const DEFAULT_IP = process.env.BING_IP || randomIP()
-
-export function parseUA(ua?: string, default_ua = DEFAULT_UA) {
- return / EDGE?/i.test(decodeURIComponent(ua || '')) ? decodeURIComponent(ua!.trim()) : default_ua
-}
-
-export function createHeaders(cookies: Partial<{ [key: string]: string }>, defaultHeaders?: Partial<{ [key: string]: string }>, type?: string) {
- let {
- BING_COOKIE = process.env.BING_COOKIE,
- BING_UA = process.env.BING_UA,
- BING_IP = process.env.BING_IP,
- BING_HEADER = process.env.BING_HEADER,
- IMAGE_ONLY = process.env.IMAGE_ONLY ?? '1',
- } = cookies
-
- if (BING_HEADER) {
- const headers = extraHeadersFromCookie({
- BING_HEADER,
- ...cookies,
- }) || {}
- if (/^(1|true|yes)$/.test(String(IMAGE_ONLY)) && type !== 'image') {
- // 仅画图时设置 cookie
- headers.cookie = `_U=${defaultUID}`
- }
- if (headers['user-agent']) {
- return headers
- }
- }
-
- const ua = parseUA(BING_UA)
-
- if (!BING_COOKIE) {
- BING_COOKIE = defaultHeaders?.IMAGE_BING_COOKIE || defaultUID // hf 暂时不用 Cookie 也可以正常使用
- }
-
- const parsedCookie = parseCookie(BING_COOKIE, '_U')
- if (!parsedCookie) {
- throw new Error('Invalid Cookie')
- }
- return {
- 'x-forwarded-for': BING_IP || DEFAULT_IP,
- 'Accept-Encoding': 'gzip, deflate, br',
- 'Accept-Language': 'zh-CN,zh;q=0.9,en;q=0.8,en-GB;q=0.7,en-US;q=0.6',
- 'User-Agent': ua!,
- 'x-ms-useragent': 'azsdk-js-api-client-factory/1.0.0-beta.1 core-rest-pipeline/1.10.0 OS/Win32',
- cookie: `_U=${parsedCookie}` || '',
- }
-}
-
-export class WatchDog {
- private tid = 0
- watch(fn: Function, timeout = 2000) {
- clearTimeout(this.tid)
- this.tid = setTimeout(fn, timeout + Math.random() * 1000)
- }
- reset() {
- clearTimeout(this.tid)
- }
-}
diff --git a/spaces/AIConsultant/MusicGen/audiocraft/utils/deadlock.py b/spaces/AIConsultant/MusicGen/audiocraft/utils/deadlock.py
deleted file mode 100644
index 8abd1bbeea5909e664cf816c020bd7c37effdb66..0000000000000000000000000000000000000000
--- a/spaces/AIConsultant/MusicGen/audiocraft/utils/deadlock.py
+++ /dev/null
@@ -1,58 +0,0 @@
-# Copyright (c) Meta Platforms, Inc. and affiliates.
-# All rights reserved.
-#
-# This source code is licensed under the license found in the
-# LICENSE file in the root directory of this source tree.
-
-import logging
-import os
-from queue import Queue, Empty
-import signal
-import sys
-import threading
-import traceback
-
-logger = logging.getLogger(__name__)
-
-
-class DeadlockDetect:
- def __init__(self, use: bool = False, timeout: float = 120.):
- self.use = use
- self.timeout = timeout
- self._queue: Queue = Queue()
-
- def update(self, stage: str):
- if self.use:
- self._queue.put(stage)
-
- def __enter__(self):
- if self.use:
- self._thread = threading.Thread(target=self._detector_thread)
- self._thread.start()
-
- def __exit__(self, exc_type, exc_val, exc_tb):
- if self.use:
- self._queue.put(None)
- self._thread.join()
-
- def _detector_thread(self):
- logger.debug("Deadlock detector started")
- last_stage = "init"
- while True:
- try:
- stage = self._queue.get(timeout=self.timeout)
- except Empty:
- break
- if stage is None:
- logger.debug("Exiting deadlock detector thread")
- return
- else:
- last_stage = stage
- logger.error("Deadlock detector timed out, last stage was %s", last_stage)
- for th in threading.enumerate():
- print(th, file=sys.stderr)
- traceback.print_stack(sys._current_frames()[th.ident])
- print(file=sys.stderr)
- sys.stdout.flush()
- sys.stderr.flush()
- os.kill(os.getpid(), signal.SIGKILL)
diff --git a/spaces/AIZero2Hero4Health/5-ImageToLineDrawing-GR/app.py b/spaces/AIZero2Hero4Health/5-ImageToLineDrawing-GR/app.py
deleted file mode 100644
index 5680950b2b2e4a9d5659e952867fca474eb890c3..0000000000000000000000000000000000000000
--- a/spaces/AIZero2Hero4Health/5-ImageToLineDrawing-GR/app.py
+++ /dev/null
@@ -1,126 +0,0 @@
-import numpy as np
-import torch
-import torch.nn as nn
-import gradio as gr
-from PIL import Image
-import torchvision.transforms as transforms
-
-norm_layer = nn.InstanceNorm2d
-
-class ResidualBlock(nn.Module):
- def __init__(self, in_features):
- super(ResidualBlock, self).__init__()
-
- conv_block = [ nn.ReflectionPad2d(1),
- nn.Conv2d(in_features, in_features, 3),
- norm_layer(in_features),
- nn.ReLU(inplace=True),
- nn.ReflectionPad2d(1),
- nn.Conv2d(in_features, in_features, 3),
- norm_layer(in_features)
- ]
-
- self.conv_block = nn.Sequential(*conv_block)
-
- def forward(self, x):
- return x + self.conv_block(x)
-
-
-class Generator(nn.Module):
- def __init__(self, input_nc, output_nc, n_residual_blocks=9, sigmoid=True):
- super(Generator, self).__init__()
-
- # Initial convolution block
- model0 = [ nn.ReflectionPad2d(3),
- nn.Conv2d(input_nc, 64, 7),
- norm_layer(64),
- nn.ReLU(inplace=True) ]
- self.model0 = nn.Sequential(*model0)
-
- # Downsampling
- model1 = []
- in_features = 64
- out_features = in_features*2
- for _ in range(2):
- model1 += [ nn.Conv2d(in_features, out_features, 3, stride=2, padding=1),
- norm_layer(out_features),
- nn.ReLU(inplace=True) ]
- in_features = out_features
- out_features = in_features*2
- self.model1 = nn.Sequential(*model1)
-
- model2 = []
- # Residual blocks
- for _ in range(n_residual_blocks):
- model2 += [ResidualBlock(in_features)]
- self.model2 = nn.Sequential(*model2)
-
- # Upsampling
- model3 = []
- out_features = in_features//2
- for _ in range(2):
- model3 += [ nn.ConvTranspose2d(in_features, out_features, 3, stride=2, padding=1, output_padding=1),
- norm_layer(out_features),
- nn.ReLU(inplace=True) ]
- in_features = out_features
- out_features = in_features//2
- self.model3 = nn.Sequential(*model3)
-
- # Output layer
- model4 = [ nn.ReflectionPad2d(3),
- nn.Conv2d(64, output_nc, 7)]
- if sigmoid:
- model4 += [nn.Sigmoid()]
-
- self.model4 = nn.Sequential(*model4)
-
- def forward(self, x, cond=None):
- out = self.model0(x)
- out = self.model1(out)
- out = self.model2(out)
- out = self.model3(out)
- out = self.model4(out)
-
- return out
-
-model1 = Generator(3, 1, 3)
-model1.load_state_dict(torch.load('model.pth', map_location=torch.device('cpu')))
-model1.eval()
-
-model2 = Generator(3, 1, 3)
-model2.load_state_dict(torch.load('model2.pth', map_location=torch.device('cpu')))
-model2.eval()
-
-def predict(input_img, ver):
- input_img = Image.open(input_img)
- transform = transforms.Compose([transforms.Resize(256, Image.BICUBIC), transforms.ToTensor()])
- input_img = transform(input_img)
- input_img = torch.unsqueeze(input_img, 0)
-
- drawing = 0
- with torch.no_grad():
- if ver == 'Simple Lines':
- drawing = model2(input_img)[0].detach()
- else:
- drawing = model1(input_img)[0].detach()
-
- drawing = transforms.ToPILImage()(drawing)
- return drawing
-
-title="Image to Line Drawings - Complex and Simple Portraits and Landscapes"
-examples=[
-['01.jpeg', 'Simple Lines'], ['02.jpeg', 'Simple Lines'], ['03.jpeg', 'Simple Lines'],
-['07.jpeg', 'Complex Lines'], ['08.jpeg', 'Complex Lines'], ['09.jpeg', 'Complex Lines'],
-['10.jpeg', 'Simple Lines'], ['11.jpeg', 'Simple Lines'], ['12.jpeg', 'Simple Lines'],
-['01.jpeg', 'Complex Lines'], ['02.jpeg', 'Complex Lines'], ['03.jpeg', 'Complex Lines'],
-['04.jpeg', 'Simple Lines'], ['05.jpeg', 'Simple Lines'], ['06.jpeg', 'Simple Lines'],
-['07.jpeg', 'Simple Lines'], ['08.jpeg', 'Simple Lines'], ['09.jpeg', 'Simple Lines'],
-['04.jpeg', 'Complex Lines'], ['05.jpeg', 'Complex Lines'], ['06.jpeg', 'Complex Lines'],
-['10.jpeg', 'Complex Lines'], ['11.jpeg', 'Complex Lines'], ['12.jpeg', 'Complex Lines']
-]
-
-iface = gr.Interface(predict, [gr.inputs.Image(type='filepath'),
- gr.inputs.Radio(['Complex Lines','Simple Lines'], type="value", default='Simple Lines', label='version')],
- gr.outputs.Image(type="pil"), title=title,examples=examples)
-
-iface.launch()
\ No newline at end of file
diff --git a/spaces/Abubakari/Sales_Prediction/README.md b/spaces/Abubakari/Sales_Prediction/README.md
deleted file mode 100644
index 6e43c21a356f1076322b51b0ff4b9761facde5db..0000000000000000000000000000000000000000
--- a/spaces/Abubakari/Sales_Prediction/README.md
+++ /dev/null
@@ -1,12 +0,0 @@
----
-title: Sales Prediction
-emoji: 💻
-colorFrom: blue
-colorTo: red
-sdk: streamlit
-sdk_version: 1.17.0
-app_file: app.py
-pinned: false
----
-
-Check out the configuration reference at https://huggingface.co/docs/hub/spaces-config-reference
diff --git a/spaces/AchyuthGamer/OpenGPT/g4f/Provider/ChatgptX.py b/spaces/AchyuthGamer/OpenGPT/g4f/Provider/ChatgptX.py
deleted file mode 100644
index 2944fb264ae78dd3502e20e28233da21799e467e..0000000000000000000000000000000000000000
--- a/spaces/AchyuthGamer/OpenGPT/g4f/Provider/ChatgptX.py
+++ /dev/null
@@ -1,97 +0,0 @@
-from __future__ import annotations
-
-import re
-import json
-
-from aiohttp import ClientSession
-from ..typing import AsyncResult, Messages
-from .base_provider import AsyncGeneratorProvider
-from .helper import format_prompt
-
-
-class ChatgptX(AsyncGeneratorProvider):
- url = "https://chatgptx.de"
- supports_gpt_35_turbo = True
- working = True
-
- @classmethod
- async def create_async_generator(
- cls,
- model: str,
- messages: Messages,
- **kwargs
- ) -> AsyncResult:
- headers = {
- 'accept-language': 'de-DE,de;q=0.9,en-DE;q=0.8,en;q=0.7,en-US',
- 'sec-ch-ua': '"Google Chrome";v="117", "Not;A=Brand";v="8", "Chromium";v="117"',
- 'sec-ch-ua-mobile': '?0',
- 'sec-ch-ua-platform': 'Linux',
- 'sec-fetch-dest': 'empty',
- 'sec-fetch-mode': 'cors',
- 'sec-fetch-site': 'same-origin',
- 'user-agent': 'Mozilla/5.0 (X11; Linux x86_64) AppleWebKit/537.36 (KHTML, like Gecko) Chrome/117.0.0.0 Safari/537.36',
- }
- async with ClientSession(headers=headers) as session:
- async with session.get(f"{cls.url}/") as response:
- response = await response.text()
- result = re.search(r'DDIM
-class DDIMSchedulerOutput(BaseOutput):
- """
- Output class for the scheduler's step function output.
-
- Args:
- prev_sample (`torch.FloatTensor` of shape `(batch_size, num_channels, height, width)` for images):
- Computed sample (x_{t-1}) of previous timestep. `prev_sample` should be used as next model input in the
- denoising loop.
- pred_original_sample (`torch.FloatTensor` of shape `(batch_size, num_channels, height, width)` for images):
- The predicted denoised sample (x_{0}) based on the model output from the current timestep.
- `pred_original_sample` can be used to preview progress or for guidance.
- """
-
- prev_sample: torch.FloatTensor
- pred_original_sample: Optional[torch.FloatTensor] = None
-
-
-# Copied from diffusers.schedulers.scheduling_ddpm.betas_for_alpha_bar
-def betas_for_alpha_bar(
- num_diffusion_timesteps,
- max_beta=0.999,
- alpha_transform_type="cosine",
-):
- """
- Create a beta schedule that discretizes the given alpha_t_bar function, which defines the cumulative product of
- (1-beta) over time from t = [0,1].
-
- Contains a function alpha_bar that takes an argument t and transforms it to the cumulative product of (1-beta) up
- to that part of the diffusion process.
-
-
- Args:
- num_diffusion_timesteps (`int`): the number of betas to produce.
- max_beta (`float`): the maximum beta to use; use values lower than 1 to
- prevent singularities.
- alpha_transform_type (`str`, *optional*, default to `cosine`): the type of noise schedule for alpha_bar.
- Choose from `cosine` or `exp`
-
- Returns:
- betas (`np.ndarray`): the betas used by the scheduler to step the model outputs
- """
- if alpha_transform_type == "cosine":
-
- def alpha_bar_fn(t):
- return math.cos((t + 0.008) / 1.008 * math.pi / 2) ** 2
-
- elif alpha_transform_type == "exp":
-
- def alpha_bar_fn(t):
- return math.exp(t * -12.0)
-
- else:
- raise ValueError(f"Unsupported alpha_tranform_type: {alpha_transform_type}")
-
- betas = []
- for i in range(num_diffusion_timesteps):
- t1 = i / num_diffusion_timesteps
- t2 = (i + 1) / num_diffusion_timesteps
- betas.append(min(1 - alpha_bar_fn(t2) / alpha_bar_fn(t1), max_beta))
- return torch.tensor(betas, dtype=torch.float32)
-
-
-# Copied from diffusers.schedulers.scheduling_ddim.rescale_zero_terminal_snr
-def rescale_zero_terminal_snr(betas):
- """
- Rescales betas to have zero terminal SNR Based on https://arxiv.org/pdf/2305.08891.pdf (Algorithm 1)
-
-
- Args:
- betas (`torch.FloatTensor`):
- the betas that the scheduler is being initialized with.
-
- Returns:
- `torch.FloatTensor`: rescaled betas with zero terminal SNR
- """
- # Convert betas to alphas_bar_sqrt
- alphas = 1.0 - betas
- alphas_cumprod = torch.cumprod(alphas, dim=0)
- alphas_bar_sqrt = alphas_cumprod.sqrt()
-
- # Store old values.
- alphas_bar_sqrt_0 = alphas_bar_sqrt[0].clone()
- alphas_bar_sqrt_T = alphas_bar_sqrt[-1].clone()
-
- # Shift so the last timestep is zero.
- alphas_bar_sqrt -= alphas_bar_sqrt_T
-
- # Scale so the first timestep is back to the old value.
- alphas_bar_sqrt *= alphas_bar_sqrt_0 / (alphas_bar_sqrt_0 - alphas_bar_sqrt_T)
-
- # Convert alphas_bar_sqrt to betas
- alphas_bar = alphas_bar_sqrt**2 # Revert sqrt
- alphas = alphas_bar[1:] / alphas_bar[:-1] # Revert cumprod
- alphas = torch.cat([alphas_bar[0:1], alphas])
- betas = 1 - alphas
-
- return betas
-
-
-class DDIMInverseScheduler(SchedulerMixin, ConfigMixin):
- """
- DDIMInverseScheduler is the reverse scheduler of [`DDIMScheduler`].
-
- [`~ConfigMixin`] takes care of storing all config attributes that are passed in the scheduler's `__init__`
- function, such as `num_train_timesteps`. They can be accessed via `scheduler.config.num_train_timesteps`.
- [`SchedulerMixin`] provides general loading and saving functionality via the [`SchedulerMixin.save_pretrained`] and
- [`~SchedulerMixin.from_pretrained`] functions.
-
- For more details, see the original paper: https://arxiv.org/abs/2010.02502
-
- Args:
- num_train_timesteps (`int`): number of diffusion steps used to train the model.
- beta_start (`float`): the starting `beta` value of inference.
- beta_end (`float`): the final `beta` value.
- beta_schedule (`str`):
- the beta schedule, a mapping from a beta range to a sequence of betas for stepping the model. Choose from
- `linear`, `scaled_linear`, or `squaredcos_cap_v2`.
- trained_betas (`np.ndarray`, optional):
- option to pass an array of betas directly to the constructor to bypass `beta_start`, `beta_end` etc.
- clip_sample (`bool`, default `True`):
- option to clip predicted sample for numerical stability.
- clip_sample_range (`float`, default `1.0`):
- the maximum magnitude for sample clipping. Valid only when `clip_sample=True`.
- set_alpha_to_zero (`bool`, default `True`):
- each diffusion step uses the value of alphas product at that step and at the previous one. For the final
- step there is no previous alpha. When this option is `True` the previous alpha product is fixed to `0`,
- otherwise it uses the value of alpha at step `num_train_timesteps - 1`.
- steps_offset (`int`, default `0`):
- an offset added to the inference steps. You can use a combination of `offset=1` and
- `set_alpha_to_zero=False`, to make the last step use step `num_train_timesteps - 1` for the previous alpha
- product.
- prediction_type (`str`, default `epsilon`, optional):
- prediction type of the scheduler function, one of `epsilon` (predicting the noise of the diffusion
- process), `sample` (directly predicting the noisy sample`) or `v_prediction` (see section 2.4
- https://imagen.research.google/video/paper.pdf)
- timestep_spacing (`str`, default `"leading"`):
- The way the timesteps should be scaled. Refer to Table 2. of [Common Diffusion Noise Schedules and Sample
- Steps are Flawed](https://arxiv.org/abs/2305.08891) for more information.
- rescale_betas_zero_snr (`bool`, default `False`):
- whether to rescale the betas to have zero terminal SNR (proposed by https://arxiv.org/pdf/2305.08891.pdf).
- This can enable the model to generate very bright and dark samples instead of limiting it to samples with
- medium brightness. Loosely related to
- [`--offset_noise`](https://github.com/huggingface/diffusers/blob/74fd735eb073eb1d774b1ab4154a0876eb82f055/examples/dreambooth/train_dreambooth.py#L506).
- """
-
- order = 1
- ignore_for_config = ["kwargs"]
- _deprecated_kwargs = ["set_alpha_to_zero"]
-
- @register_to_config
- def __init__(
- self,
- num_train_timesteps: int = 1000,
- beta_start: float = 0.0001,
- beta_end: float = 0.02,
- beta_schedule: str = "linear",
- trained_betas: Optional[Union[np.ndarray, List[float]]] = None,
- clip_sample: bool = True,
- set_alpha_to_one: bool = True,
- steps_offset: int = 0,
- prediction_type: str = "epsilon",
- clip_sample_range: float = 1.0,
- timestep_spacing: str = "leading",
- rescale_betas_zero_snr: bool = False,
- **kwargs,
- ):
- if kwargs.get("set_alpha_to_zero", None) is not None:
- deprecation_message = (
- "The `set_alpha_to_zero` argument is deprecated. Please use `set_alpha_to_one` instead."
- )
- deprecate("set_alpha_to_zero", "1.0.0", deprecation_message, standard_warn=False)
- set_alpha_to_one = kwargs["set_alpha_to_zero"]
- if trained_betas is not None:
- self.betas = torch.tensor(trained_betas, dtype=torch.float32)
- elif beta_schedule == "linear":
- self.betas = torch.linspace(beta_start, beta_end, num_train_timesteps, dtype=torch.float32)
- elif beta_schedule == "scaled_linear":
- # this schedule is very specific to the latent diffusion model.
- self.betas = (
- torch.linspace(beta_start**0.5, beta_end**0.5, num_train_timesteps, dtype=torch.float32) ** 2
- )
- elif beta_schedule == "squaredcos_cap_v2":
- # Glide cosine schedule
- self.betas = betas_for_alpha_bar(num_train_timesteps)
- else:
- raise NotImplementedError(f"{beta_schedule} does is not implemented for {self.__class__}")
-
- # Rescale for zero SNR
- if rescale_betas_zero_snr:
- self.betas = rescale_zero_terminal_snr(self.betas)
-
- self.alphas = 1.0 - self.betas
- self.alphas_cumprod = torch.cumprod(self.alphas, dim=0)
-
- # At every step in inverted ddim, we are looking into the next alphas_cumprod
- # For the initial step, there is no current alphas_cumprod, and the index is out of bounds
- # `set_alpha_to_one` decides whether we set this parameter simply to one
- # in this case, self.step() just output the predicted noise
- # or whether we use the initial alpha used in training the diffusion model.
- self.initial_alpha_cumprod = torch.tensor(1.0) if set_alpha_to_one else self.alphas_cumprod[0]
-
- # standard deviation of the initial noise distribution
- self.init_noise_sigma = 1.0
-
- # setable values
- self.num_inference_steps = None
- self.timesteps = torch.from_numpy(np.arange(0, num_train_timesteps).copy().astype(np.int64))
-
- # Copied from diffusers.schedulers.scheduling_ddim.DDIMScheduler.scale_model_input
- def scale_model_input(self, sample: torch.FloatTensor, timestep: Optional[int] = None) -> torch.FloatTensor:
- """
- Ensures interchangeability with schedulers that need to scale the denoising model input depending on the
- current timestep.
-
- Args:
- sample (`torch.FloatTensor`): input sample
- timestep (`int`, optional): current timestep
-
- Returns:
- `torch.FloatTensor`: scaled input sample
- """
- return sample
-
- def set_timesteps(self, num_inference_steps: int, device: Union[str, torch.device] = None):
- """
- Sets the discrete timesteps used for the diffusion chain. Supporting function to be run before inference.
-
- Args:
- num_inference_steps (`int`):
- the number of diffusion steps used when generating samples with a pre-trained model.
- """
-
- if num_inference_steps > self.config.num_train_timesteps:
- raise ValueError(
- f"`num_inference_steps`: {num_inference_steps} cannot be larger than `self.config.train_timesteps`:"
- f" {self.config.num_train_timesteps} as the unet model trained with this scheduler can only handle"
- f" maximal {self.config.num_train_timesteps} timesteps."
- )
-
- self.num_inference_steps = num_inference_steps
-
- # "leading" and "trailing" corresponds to annotation of Table 1. of https://arxiv.org/abs/2305.08891
- if self.config.timestep_spacing == "leading":
- step_ratio = self.config.num_train_timesteps // self.num_inference_steps
- # creates integer timesteps by multiplying by ratio
- # casting to int to avoid issues when num_inference_step is power of 3
- timesteps = (np.arange(0, num_inference_steps) * step_ratio).round().copy().astype(np.int64)
- timesteps += self.config.steps_offset
- elif self.config.timestep_spacing == "trailing":
- step_ratio = self.config.num_train_timesteps / self.num_inference_steps
- # creates integer timesteps by multiplying by ratio
- # casting to int to avoid issues when num_inference_step is power of 3
- timesteps = np.round(np.arange(self.config.num_train_timesteps, 0, -step_ratio)[::-1]).astype(np.int64)
- timesteps -= 1
- else:
- raise ValueError(
- f"{self.config.timestep_spacing} is not supported. Please make sure to choose one of 'leading' or 'trailing'."
- )
-
- # Roll timesteps array by one to reflect reversed origin and destination semantics for each step
- timesteps = np.roll(timesteps, 1)
- timesteps[0] = int(timesteps[1] - step_ratio)
- self.timesteps = torch.from_numpy(timesteps).to(device)
-
- def step(
- self,
- model_output: torch.FloatTensor,
- timestep: int,
- sample: torch.FloatTensor,
- eta: float = 0.0,
- use_clipped_model_output: bool = False,
- variance_noise: Optional[torch.FloatTensor] = None,
- return_dict: bool = True,
- ) -> Union[DDIMSchedulerOutput, Tuple]:
- # 1. get previous step value (=t+1)
- prev_timestep = timestep + self.config.num_train_timesteps // self.num_inference_steps
-
- # 2. compute alphas, betas
- # change original implementation to exactly match noise levels for analogous forward process
- alpha_prod_t = self.alphas_cumprod[timestep] if timestep >= 0 else self.initial_alpha_cumprod
- alpha_prod_t_prev = self.alphas_cumprod[prev_timestep]
-
- beta_prod_t = 1 - alpha_prod_t
-
- # 3. compute predicted original sample from predicted noise also called
- # "predicted x_0" of formula (12) from https://arxiv.org/pdf/2010.02502.pdf
- if self.config.prediction_type == "epsilon":
- pred_original_sample = (sample - beta_prod_t ** (0.5) * model_output) / alpha_prod_t ** (0.5)
- pred_epsilon = model_output
- elif self.config.prediction_type == "sample":
- pred_original_sample = model_output
- pred_epsilon = (sample - alpha_prod_t ** (0.5) * pred_original_sample) / beta_prod_t ** (0.5)
- elif self.config.prediction_type == "v_prediction":
- pred_original_sample = (alpha_prod_t**0.5) * sample - (beta_prod_t**0.5) * model_output
- pred_epsilon = (alpha_prod_t**0.5) * model_output + (beta_prod_t**0.5) * sample
- else:
- raise ValueError(
- f"prediction_type given as {self.config.prediction_type} must be one of `epsilon`, `sample`, or"
- " `v_prediction`"
- )
-
- # 4. Clip or threshold "predicted x_0"
- if self.config.clip_sample:
- pred_original_sample = pred_original_sample.clamp(
- -self.config.clip_sample_range, self.config.clip_sample_range
- )
-
- # 5. compute "direction pointing to x_t" of formula (12) from https://arxiv.org/pdf/2010.02502.pdf
- pred_sample_direction = (1 - alpha_prod_t_prev) ** (0.5) * pred_epsilon
-
- # 6. compute x_t without "random noise" of formula (12) from https://arxiv.org/pdf/2010.02502.pdf
- prev_sample = alpha_prod_t_prev ** (0.5) * pred_original_sample + pred_sample_direction
-
- if not return_dict:
- return (prev_sample, pred_original_sample)
- return DDIMSchedulerOutput(prev_sample=prev_sample, pred_original_sample=pred_original_sample)
-
- def __len__(self):
- return self.config.num_train_timesteps
diff --git a/spaces/Andy1621/uniformer_image_detection/configs/instaboost/README.md b/spaces/Andy1621/uniformer_image_detection/configs/instaboost/README.md
deleted file mode 100644
index 5ab74a1af13639fef753dbfd43f064400cba9129..0000000000000000000000000000000000000000
--- a/spaces/Andy1621/uniformer_image_detection/configs/instaboost/README.md
+++ /dev/null
@@ -1,44 +0,0 @@
-# InstaBoost for MMDetection
-
-[ALGORITHM]
-
-Configs in this directory is the implementation for ICCV2019 paper "InstaBoost: Boosting Instance Segmentation Via Probability Map Guided Copy-Pasting" and provided by the authors of the paper. InstaBoost is a data augmentation method for object detection and instance segmentation. The paper has been released on [`arXiv`](https://arxiv.org/abs/1908.07801).
-
-```latex
-@inproceedings{fang2019instaboost,
- title={Instaboost: Boosting instance segmentation via probability map guided copy-pasting},
- author={Fang, Hao-Shu and Sun, Jianhua and Wang, Runzhong and Gou, Minghao and Li, Yong-Lu and Lu, Cewu},
- booktitle={Proceedings of the IEEE International Conference on Computer Vision},
- pages={682--691},
- year={2019}
-}
-```
-
-## Usage
-
-### Requirements
-
-You need to install `instaboostfast` before using it.
-
-```shell
-pip install instaboostfast
-```
-
-The code and more details can be found [here](https://github.com/GothicAi/Instaboost).
-
-### Integration with MMDetection
-
-InstaBoost have been already integrated in the data pipeline, thus all you need is to add or change **InstaBoost** configurations after **LoadImageFromFile**. We have provided examples like [this](mask_rcnn_r50_fpn_instaboost_4x#L121). You can refer to [`InstaBoostConfig`](https://github.com/GothicAi/InstaBoost-pypi#instaboostconfig) for more details.
-
-## Results and Models
-
-- All models were trained on `coco_2017_train` and tested on `coco_2017_val` for conveinience of evaluation and comparison. In the paper, the results are obtained from `test-dev`.
-- To balance accuracy and training time when using InstaBoost, models released in this page are all trained for 48 Epochs. Other training and testing configs strictly follow the original framework.
-- For results and models in MMDetection V1.x, please refer to [Instaboost](https://github.com/GothicAi/Instaboost).
-
-| Network | Backbone | Lr schd | Mem (GB) | Inf time (fps) | box AP | mask AP | Config | Download |
-| :-------------: | :--------: | :-----: | :------: | :------------: | :------:| :-----: | :------: | :-----------------: |
-| Mask R-CNN | R-50-FPN | 4x | 4.4 | 17.5 | 40.6 | 36.6 | [config](https://github.com/open-mmlab/mmdetection/tree/master/configs/instaboost/mask_rcnn_r50_fpn_instaboost_4x_coco.py) | [model](http://download.openmmlab.com/mmdetection/v2.0/instaboost/mask_rcnn_r50_fpn_instaboost_4x_coco/mask_rcnn_r50_fpn_instaboost_4x_coco_20200307-d025f83a.pth) | [log](http://download.openmmlab.com/mmdetection/v2.0/instaboost/mask_rcnn_r50_fpn_instaboost_4x_coco/mask_rcnn_r50_fpn_instaboost_4x_coco_20200307_223635.log.json) |
-| Mask R-CNN | R-101-FPN | 4x | 6.4 | | 42.5 | 38.0 | [config](https://github.com/open-mmlab/mmdetection/tree/master/configs/instaboost/mask_rcnn_r101_fpn_instaboost_4x_coco.py) | [model](http://download.openmmlab.com/mmdetection/v2.0/instaboost/mask_rcnn_r101_fpn_instaboost_4x_coco/mask_rcnn_r101_fpn_instaboost_4x_coco_20200703_235738-f23f3a5f.pth) | [log](http://download.openmmlab.com/mmdetection/v2.0/instaboost/mask_rcnn_r101_fpn_instaboost_4x_coco/mask_rcnn_r101_fpn_instaboost_4x_coco_20200703_235738.log.json) |
-| Mask R-CNN | X-101-64x4d-FPN | 4x | 10.7 | | 44.7 | 39.7 | [config](https://github.com/open-mmlab/mmdetection/tree/master/configs/instaboost/mask_rcnn_x101_64x4d_fpn_instaboost_4x_coco.py) | [model](http://download.openmmlab.com/mmdetection/v2.0/instaboost/mask_rcnn_x101_64x4d_fpn_instaboost_4x_coco/mask_rcnn_x101_64x4d_fpn_instaboost_4x_coco_20200515_080947-8ed58c1b.pth) | [log](http://download.openmmlab.com/mmdetection/v2.0/instaboost/mask_rcnn_x101_64x4d_fpn_instaboost_4x_coco/mask_rcnn_x101_64x4d_fpn_instaboost_4x_coco_20200515_080947.log.json) |
-| Cascade R-CNN | R-101-FPN | 4x | 6.0 | 12.0 | 43.7 | 38.0 | [config](https://github.com/open-mmlab/mmdetection/tree/master/configs/instaboost/cascade_mask_rcnn_r50_fpn_instaboost_4x_coco.py) | [model](http://download.openmmlab.com/mmdetection/v2.0/instaboost/cascade_mask_rcnn_r50_fpn_instaboost_4x_coco/cascade_mask_rcnn_r50_fpn_instaboost_4x_coco_20200307-c19d98d9.pth) | [log](http://download.openmmlab.com/mmdetection/v2.0/instaboost/cascade_mask_rcnn_r50_fpn_instaboost_4x_coco/cascade_mask_rcnn_r50_fpn_instaboost_4x_coco_20200307_223646.log.json) |
diff --git a/spaces/Andy1621/uniformer_image_segmentation/configs/ann/ann_r101-d8_512x512_160k_ade20k.py b/spaces/Andy1621/uniformer_image_segmentation/configs/ann/ann_r101-d8_512x512_160k_ade20k.py
deleted file mode 100644
index 9e43af541f6e3df3f36479e736bb0c03fc916970..0000000000000000000000000000000000000000
--- a/spaces/Andy1621/uniformer_image_segmentation/configs/ann/ann_r101-d8_512x512_160k_ade20k.py
+++ /dev/null
@@ -1,2 +0,0 @@
-_base_ = './ann_r50-d8_512x512_160k_ade20k.py'
-model = dict(pretrained='open-mmlab://resnet101_v1c', backbone=dict(depth=101))
diff --git a/spaces/AnishKumbhar/ChatBot/text-generation-webui-main/update_windows.bat b/spaces/AnishKumbhar/ChatBot/text-generation-webui-main/update_windows.bat
deleted file mode 100644
index 0d8f815272c5eec8714ef1adc1a23d547d6bf62d..0000000000000000000000000000000000000000
--- a/spaces/AnishKumbhar/ChatBot/text-generation-webui-main/update_windows.bat
+++ /dev/null
@@ -1,37 +0,0 @@
-@echo off
-
-cd /D "%~dp0"
-
-set PATH=%PATH%;%SystemRoot%\system32
-
-echo "%CD%"| findstr /C:" " >nul && echo This script relies on Miniconda which can not be silently installed under a path with spaces. && goto end
-
-@rem fix failed install when installing to a separate drive
-set TMP=%cd%\installer_files
-set TEMP=%cd%\installer_files
-
-@rem deactivate existing conda envs as needed to avoid conflicts
-(call conda deactivate && call conda deactivate && call conda deactivate) 2>nul
-
-@rem config
-set CONDA_ROOT_PREFIX=%cd%\installer_files\conda
-set INSTALL_ENV_DIR=%cd%\installer_files\env
-
-@rem environment isolation
-set PYTHONNOUSERSITE=1
-set PYTHONPATH=
-set PYTHONHOME=
-set "CUDA_PATH=%INSTALL_ENV_DIR%"
-set "CUDA_HOME=%CUDA_PATH%"
-
-@rem activate installer env
-call "%CONDA_ROOT_PREFIX%\condabin\conda.bat" activate "%INSTALL_ENV_DIR%" || ( echo. && echo Miniconda hook not found. && goto end )
-
-@rem update installer env
-call python one_click.py --update && (
- echo.
- echo Done!
-)
-
-:end
-pause
diff --git a/spaces/Apex-X/ROOPOK/roop/core.py b/spaces/Apex-X/ROOPOK/roop/core.py
deleted file mode 100644
index ecde46e9747ca7bcfb7aca9499977b7b2aae88fd..0000000000000000000000000000000000000000
--- a/spaces/Apex-X/ROOPOK/roop/core.py
+++ /dev/null
@@ -1,215 +0,0 @@
-import os
-import sys
-# single thread doubles cuda performance - needs to be set before torch import
-if any(arg.startswith('--execution-provider') for arg in sys.argv):
- os.environ['OMP_NUM_THREADS'] = '1'
-# reduce tensorflow log level
-os.environ['TF_CPP_MIN_LOG_LEVEL'] = '2'
-import warnings
-from typing import List
-import platform
-import signal
-import shutil
-import argparse
-import torch
-import onnxruntime
-import tensorflow
-
-import roop.globals
-import roop.metadata
-import roop.ui as ui
-from roop.predicter import predict_image, predict_video
-from roop.processors.frame.core import get_frame_processors_modules
-from roop.utilities import has_image_extension, is_image, is_video, detect_fps, create_video, extract_frames, get_temp_frame_paths, restore_audio, create_temp, move_temp, clean_temp, normalize_output_path
-
-if 'ROCMExecutionProvider' in roop.globals.execution_providers:
- del torch
-
-warnings.filterwarnings('ignore', category=FutureWarning, module='insightface')
-warnings.filterwarnings('ignore', category=UserWarning, module='torchvision')
-
-
-def parse_args() -> None:
- signal.signal(signal.SIGINT, lambda signal_number, frame: destroy())
- program = argparse.ArgumentParser(formatter_class=lambda prog: argparse.HelpFormatter(prog, max_help_position=100))
- program.add_argument('-s', '--source', help='select an source image', dest='source_path')
- program.add_argument('-t', '--target', help='select an target image or video', dest='target_path')
- program.add_argument('-o', '--output', help='select output file or directory', dest='output_path')
- program.add_argument('--frame-processor', help='frame processors (choices: face_swapper, face_enhancer, ...)', dest='frame_processor', default=['face_swapper'], nargs='+')
- program.add_argument('--keep-fps', help='keep original fps', dest='keep_fps', action='store_true', default=False)
- program.add_argument('--keep-audio', help='keep original audio', dest='keep_audio', action='store_true', default=True)
- program.add_argument('--keep-frames', help='keep temporary frames', dest='keep_frames', action='store_true', default=False)
- program.add_argument('--many-faces', help='process every face', dest='many_faces', action='store_true', default=False)
- program.add_argument('--video-encoder', help='adjust output video encoder', dest='video_encoder', default='libx264', choices=['libx264', 'libx265', 'libvpx-vp9'])
- program.add_argument('--video-quality', help='adjust output video quality', dest='video_quality', type=int, default=18, choices=range(52), metavar='[0-51]')
- program.add_argument('--max-memory', help='maximum amount of RAM in GB', dest='max_memory', type=int, default=suggest_max_memory())
- program.add_argument('--execution-provider', help='available execution provider (choices: cpu, ...)', dest='execution_provider', default=['cpu'], choices=suggest_execution_providers(), nargs='+')
- program.add_argument('--execution-threads', help='number of execution threads', dest='execution_threads', type=int, default=suggest_execution_threads())
- program.add_argument('-v', '--version', action='version', version=f'{roop.metadata.name} {roop.metadata.version}')
-
- args = program.parse_args()
-
- roop.globals.source_path = args.source_path
- roop.globals.target_path = args.target_path
- roop.globals.output_path = normalize_output_path(roop.globals.source_path, roop.globals.target_path, args.output_path)
- roop.globals.frame_processors = args.frame_processor
- roop.globals.headless = args.source_path or args.target_path or args.output_path
- roop.globals.keep_fps = args.keep_fps
- roop.globals.keep_audio = args.keep_audio
- roop.globals.keep_frames = args.keep_frames
- roop.globals.many_faces = args.many_faces
- roop.globals.video_encoder = args.video_encoder
- roop.globals.video_quality = args.video_quality
- roop.globals.max_memory = args.max_memory
- roop.globals.execution_providers = decode_execution_providers(args.execution_provider)
- roop.globals.execution_threads = args.execution_threads
-
-
-def encode_execution_providers(execution_providers: List[str]) -> List[str]:
- return [execution_provider.replace('ExecutionProvider', '').lower() for execution_provider in execution_providers]
-
-
-def decode_execution_providers(execution_providers: List[str]) -> List[str]:
- return [provider for provider, encoded_execution_provider in zip(onnxruntime.get_available_providers(), encode_execution_providers(onnxruntime.get_available_providers()))
- if any(execution_provider in encoded_execution_provider for execution_provider in execution_providers)]
-
-
-def suggest_max_memory() -> int:
- if platform.system().lower() == 'darwin':
- return 4
- return 16
-
-
-def suggest_execution_providers() -> List[str]:
- return encode_execution_providers(onnxruntime.get_available_providers())
-
-
-def suggest_execution_threads() -> int:
- if 'DmlExecutionProvider' in roop.globals.execution_providers:
- return 1
- if 'ROCMExecutionProvider' in roop.globals.execution_providers:
- return 1
- return 8
-
-
-def limit_resources() -> None:
- # prevent tensorflow memory leak
- gpus = tensorflow.config.experimental.list_physical_devices('GPU')
- for gpu in gpus:
- tensorflow.config.experimental.set_virtual_device_configuration(gpu, [
- tensorflow.config.experimental.VirtualDeviceConfiguration(memory_limit=1024)
- ])
- # limit memory usage
- if roop.globals.max_memory:
- memory = roop.globals.max_memory * 1024 ** 3
- if platform.system().lower() == 'darwin':
- memory = roop.globals.max_memory * 1024 ** 6
- if platform.system().lower() == 'windows':
- import ctypes
- kernel32 = ctypes.windll.kernel32
- kernel32.SetProcessWorkingSetSize(-1, ctypes.c_size_t(memory), ctypes.c_size_t(memory))
- else:
- import resource
- resource.setrlimit(resource.RLIMIT_DATA, (memory, memory))
-
-
-def release_resources() -> None:
- if 'CUDAExecutionProvider' in roop.globals.execution_providers:
- torch.cuda.empty_cache()
-
-
-def pre_check() -> bool:
- if sys.version_info < (3, 9):
- update_status('Python version is not supported - please upgrade to 3.9 or higher.')
- return False
- if not shutil.which('ffmpeg'):
- update_status('ffmpeg is not installed.')
- return False
- return True
-
-
-def update_status(message: str, scope: str = 'ROOP.CORE') -> None:
- print(f'[{scope}] {message}')
- if not roop.globals.headless:
- ui.update_status(message)
-
-
-def start() -> None:
- for frame_processor in get_frame_processors_modules(roop.globals.frame_processors):
- if not frame_processor.pre_start():
- return
- # process image to image
- if has_image_extension(roop.globals.target_path):
- if predict_image(roop.globals.target_path):
- destroy()
- shutil.copy2(roop.globals.target_path, roop.globals.output_path)
- for frame_processor in get_frame_processors_modules(roop.globals.frame_processors):
- update_status('Progressing...', frame_processor.NAME)
- frame_processor.process_image(roop.globals.source_path, roop.globals.output_path, roop.globals.output_path)
- frame_processor.post_process()
- release_resources()
- if is_image(roop.globals.target_path):
- update_status('Processing to image succeed!')
- else:
- update_status('Processing to image failed!')
- return
- # process image to videos
- if predict_video(roop.globals.target_path):
- destroy()
- update_status('Creating temp resources...')
- create_temp(roop.globals.target_path)
- update_status('Extracting frames...')
- extract_frames(roop.globals.target_path)
- temp_frame_paths = get_temp_frame_paths(roop.globals.target_path)
- for frame_processor in get_frame_processors_modules(roop.globals.frame_processors):
- update_status('Progressing...', frame_processor.NAME)
- frame_processor.process_video(roop.globals.source_path, temp_frame_paths)
- frame_processor.post_process()
- release_resources()
- # handles fps
- if roop.globals.keep_fps:
- update_status('Detecting fps...')
- fps = detect_fps(roop.globals.target_path)
- update_status(f'Creating video with {fps} fps...')
- create_video(roop.globals.target_path, fps)
- else:
- update_status('Creating video with 30.0 fps...')
- create_video(roop.globals.target_path)
- # handle audio
- if roop.globals.keep_audio:
- if roop.globals.keep_fps:
- update_status('Restoring audio...')
- else:
- update_status('Restoring audio might cause issues as fps are not kept...')
- restore_audio(roop.globals.target_path, roop.globals.output_path)
- else:
- move_temp(roop.globals.target_path, roop.globals.output_path)
- # clean and validate
- clean_temp(roop.globals.target_path)
- if is_video(roop.globals.target_path):
- update_status('Processing to video succeed!')
- else:
- update_status('Processing to video failed!')
-
-
-def destroy() -> None:
- if roop.globals.target_path:
- clean_temp(roop.globals.target_path)
- quit()
-
-
-def run() -> None:
- parse_args()
- if not pre_check():
- return
- for frame_processor in get_frame_processors_modules(roop.globals.frame_processors):
- if not frame_processor.pre_check():
- return
- limit_resources()
- if roop.globals.headless:
- start()
- else:
- window = ui.init(start, destroy)
- window.mainloop()
-
-
\ No newline at end of file
diff --git a/spaces/Aristo/trafficsign/README.md b/spaces/Aristo/trafficsign/README.md
deleted file mode 100644
index a6e364cf875766a02d8083ff51ce45b846106c80..0000000000000000000000000000000000000000
--- a/spaces/Aristo/trafficsign/README.md
+++ /dev/null
@@ -1,13 +0,0 @@
----
-title: Trafficsign
-emoji: 🏃
-colorFrom: red
-colorTo: pink
-sdk: gradio
-sdk_version: 2.9.4
-app_file: app.py
-pinned: false
-license: afl-3.0
----
-
-Check out the configuration reference at https://huggingface.co/docs/hub/spaces#reference
diff --git a/spaces/Ataturk-Chatbot/HuggingFaceChat/venv/lib/python3.11/site-packages/setuptools/_distutils/dir_util.py b/spaces/Ataturk-Chatbot/HuggingFaceChat/venv/lib/python3.11/site-packages/setuptools/_distutils/dir_util.py
deleted file mode 100644
index 6f0bb8ad76a064dad843db670c91e493d0e19a0c..0000000000000000000000000000000000000000
--- a/spaces/Ataturk-Chatbot/HuggingFaceChat/venv/lib/python3.11/site-packages/setuptools/_distutils/dir_util.py
+++ /dev/null
@@ -1,243 +0,0 @@
-"""distutils.dir_util
-
-Utility functions for manipulating directories and directory trees."""
-
-import os
-import errno
-from distutils.errors import DistutilsInternalError, DistutilsFileError
-from distutils import log
-
-# cache for by mkpath() -- in addition to cheapening redundant calls,
-# eliminates redundant "creating /foo/bar/baz" messages in dry-run mode
-_path_created = {}
-
-
-def mkpath(name, mode=0o777, verbose=1, dry_run=0): # noqa: C901
- """Create a directory and any missing ancestor directories.
-
- If the directory already exists (or if 'name' is the empty string, which
- means the current directory, which of course exists), then do nothing.
- Raise DistutilsFileError if unable to create some directory along the way
- (eg. some sub-path exists, but is a file rather than a directory).
- If 'verbose' is true, print a one-line summary of each mkdir to stdout.
- Return the list of directories actually created.
-
- os.makedirs is not used because:
-
- a) It's new to Python 1.5.2, and
- b) it blows up if the directory already exists (in which case it should
- silently succeed).
- """
-
- global _path_created
-
- # Detect a common bug -- name is None
- if not isinstance(name, str):
- raise DistutilsInternalError(
- "mkpath: 'name' must be a string (got {!r})".format(name)
- )
-
- # XXX what's the better way to handle verbosity? print as we create
- # each directory in the path (the current behaviour), or only announce
- # the creation of the whole path? (quite easy to do the latter since
- # we're not using a recursive algorithm)
-
- name = os.path.normpath(name)
- created_dirs = []
- if os.path.isdir(name) or name == '':
- return created_dirs
- if _path_created.get(os.path.abspath(name)):
- return created_dirs
-
- (head, tail) = os.path.split(name)
- tails = [tail] # stack of lone dirs to create
-
- while head and tail and not os.path.isdir(head):
- (head, tail) = os.path.split(head)
- tails.insert(0, tail) # push next higher dir onto stack
-
- # now 'head' contains the deepest directory that already exists
- # (that is, the child of 'head' in 'name' is the highest directory
- # that does *not* exist)
- for d in tails:
- # print "head = %s, d = %s: " % (head, d),
- head = os.path.join(head, d)
- abs_head = os.path.abspath(head)
-
- if _path_created.get(abs_head):
- continue
-
- if verbose >= 1:
- log.info("creating %s", head)
-
- if not dry_run:
- try:
- os.mkdir(head, mode)
- except OSError as exc:
- if not (exc.errno == errno.EEXIST and os.path.isdir(head)):
- raise DistutilsFileError(
- "could not create '{}': {}".format(head, exc.args[-1])
- )
- created_dirs.append(head)
-
- _path_created[abs_head] = 1
- return created_dirs
-
-
-def create_tree(base_dir, files, mode=0o777, verbose=1, dry_run=0):
- """Create all the empty directories under 'base_dir' needed to put 'files'
- there.
-
- 'base_dir' is just the name of a directory which doesn't necessarily
- exist yet; 'files' is a list of filenames to be interpreted relative to
- 'base_dir'. 'base_dir' + the directory portion of every file in 'files'
- will be created if it doesn't already exist. 'mode', 'verbose' and
- 'dry_run' flags are as for 'mkpath()'.
- """
- # First get the list of directories to create
- need_dir = set()
- for file in files:
- need_dir.add(os.path.join(base_dir, os.path.dirname(file)))
-
- # Now create them
- for dir in sorted(need_dir):
- mkpath(dir, mode, verbose=verbose, dry_run=dry_run)
-
-
-def copy_tree( # noqa: C901
- src,
- dst,
- preserve_mode=1,
- preserve_times=1,
- preserve_symlinks=0,
- update=0,
- verbose=1,
- dry_run=0,
-):
- """Copy an entire directory tree 'src' to a new location 'dst'.
-
- Both 'src' and 'dst' must be directory names. If 'src' is not a
- directory, raise DistutilsFileError. If 'dst' does not exist, it is
- created with 'mkpath()'. The end result of the copy is that every
- file in 'src' is copied to 'dst', and directories under 'src' are
- recursively copied to 'dst'. Return the list of files that were
- copied or might have been copied, using their output name. The
- return value is unaffected by 'update' or 'dry_run': it is simply
- the list of all files under 'src', with the names changed to be
- under 'dst'.
-
- 'preserve_mode' and 'preserve_times' are the same as for
- 'copy_file'; note that they only apply to regular files, not to
- directories. If 'preserve_symlinks' is true, symlinks will be
- copied as symlinks (on platforms that support them!); otherwise
- (the default), the destination of the symlink will be copied.
- 'update' and 'verbose' are the same as for 'copy_file'.
- """
- from distutils.file_util import copy_file
-
- if not dry_run and not os.path.isdir(src):
- raise DistutilsFileError("cannot copy tree '%s': not a directory" % src)
- try:
- names = os.listdir(src)
- except OSError as e:
- if dry_run:
- names = []
- else:
- raise DistutilsFileError(
- "error listing files in '{}': {}".format(src, e.strerror)
- )
-
- if not dry_run:
- mkpath(dst, verbose=verbose)
-
- outputs = []
-
- for n in names:
- src_name = os.path.join(src, n)
- dst_name = os.path.join(dst, n)
-
- if n.startswith('.nfs'):
- # skip NFS rename files
- continue
-
- if preserve_symlinks and os.path.islink(src_name):
- link_dest = os.readlink(src_name)
- if verbose >= 1:
- log.info("linking %s -> %s", dst_name, link_dest)
- if not dry_run:
- os.symlink(link_dest, dst_name)
- outputs.append(dst_name)
-
- elif os.path.isdir(src_name):
- outputs.extend(
- copy_tree(
- src_name,
- dst_name,
- preserve_mode,
- preserve_times,
- preserve_symlinks,
- update,
- verbose=verbose,
- dry_run=dry_run,
- )
- )
- else:
- copy_file(
- src_name,
- dst_name,
- preserve_mode,
- preserve_times,
- update,
- verbose=verbose,
- dry_run=dry_run,
- )
- outputs.append(dst_name)
-
- return outputs
-
-
-def _build_cmdtuple(path, cmdtuples):
- """Helper for remove_tree()."""
- for f in os.listdir(path):
- real_f = os.path.join(path, f)
- if os.path.isdir(real_f) and not os.path.islink(real_f):
- _build_cmdtuple(real_f, cmdtuples)
- else:
- cmdtuples.append((os.remove, real_f))
- cmdtuples.append((os.rmdir, path))
-
-
-def remove_tree(directory, verbose=1, dry_run=0):
- """Recursively remove an entire directory tree.
-
- Any errors are ignored (apart from being reported to stdout if 'verbose'
- is true).
- """
- global _path_created
-
- if verbose >= 1:
- log.info("removing '%s' (and everything under it)", directory)
- if dry_run:
- return
- cmdtuples = []
- _build_cmdtuple(directory, cmdtuples)
- for cmd in cmdtuples:
- try:
- cmd[0](cmd[1])
- # remove dir from cache if it's already there
- abspath = os.path.abspath(cmd[1])
- if abspath in _path_created:
- del _path_created[abspath]
- except OSError as exc:
- log.warn("error removing %s: %s", directory, exc)
-
-
-def ensure_relative(path):
- """Take the full path 'path', and make it a relative path.
-
- This is useful to make 'path' the second argument to os.path.join().
- """
- drive, path = os.path.splitdrive(path)
- if path[0:1] == os.sep:
- path = drive + path[1:]
- return path
diff --git a/spaces/Ataturk-Chatbot/HuggingFaceChat/venv/lib/python3.11/site-packages/setuptools/_path.py b/spaces/Ataturk-Chatbot/HuggingFaceChat/venv/lib/python3.11/site-packages/setuptools/_path.py
deleted file mode 100644
index 3767523b784bb93b5b79890eff359628fcfcaa34..0000000000000000000000000000000000000000
--- a/spaces/Ataturk-Chatbot/HuggingFaceChat/venv/lib/python3.11/site-packages/setuptools/_path.py
+++ /dev/null
@@ -1,29 +0,0 @@
-import os
-from typing import Union
-
-_Path = Union[str, os.PathLike]
-
-
-def ensure_directory(path):
- """Ensure that the parent directory of `path` exists"""
- dirname = os.path.dirname(path)
- os.makedirs(dirname, exist_ok=True)
-
-
-def same_path(p1: _Path, p2: _Path) -> bool:
- """Differs from os.path.samefile because it does not require paths to exist.
- Purely string based (no comparison between i-nodes).
- >>> same_path("a/b", "./a/b")
- True
- >>> same_path("a/b", "a/./b")
- True
- >>> same_path("a/b", "././a/b")
- True
- >>> same_path("a/b", "./a/b/c/..")
- True
- >>> same_path("a/b", "../a/b/c")
- False
- >>> same_path("a", "a/b")
- False
- """
- return os.path.normpath(p1) == os.path.normpath(p2)
diff --git a/spaces/Ataturk-Chatbot/HuggingFaceChat/venv/lib/python3.11/site-packages/setuptools/_vendor/__init__.py b/spaces/Ataturk-Chatbot/HuggingFaceChat/venv/lib/python3.11/site-packages/setuptools/_vendor/__init__.py
deleted file mode 100644
index e69de29bb2d1d6434b8b29ae775ad8c2e48c5391..0000000000000000000000000000000000000000
diff --git a/spaces/Ataturk-Chatbot/HuggingFaceChat/venv/lib/python3.11/site-packages/setuptools/_vendor/typing_extensions.py b/spaces/Ataturk-Chatbot/HuggingFaceChat/venv/lib/python3.11/site-packages/setuptools/_vendor/typing_extensions.py
deleted file mode 100644
index 9f1c7aa31e20a7d0ef2e6877ea325c068d50e406..0000000000000000000000000000000000000000
--- a/spaces/Ataturk-Chatbot/HuggingFaceChat/venv/lib/python3.11/site-packages/setuptools/_vendor/typing_extensions.py
+++ /dev/null
@@ -1,2296 +0,0 @@
-import abc
-import collections
-import collections.abc
-import operator
-import sys
-import typing
-
-# After PEP 560, internal typing API was substantially reworked.
-# This is especially important for Protocol class which uses internal APIs
-# quite extensively.
-PEP_560 = sys.version_info[:3] >= (3, 7, 0)
-
-if PEP_560:
- GenericMeta = type
-else:
- # 3.6
- from typing import GenericMeta, _type_vars # noqa
-
-# The two functions below are copies of typing internal helpers.
-# They are needed by _ProtocolMeta
-
-
-def _no_slots_copy(dct):
- dict_copy = dict(dct)
- if '__slots__' in dict_copy:
- for slot in dict_copy['__slots__']:
- dict_copy.pop(slot, None)
- return dict_copy
-
-
-def _check_generic(cls, parameters):
- if not cls.__parameters__:
- raise TypeError(f"{cls} is not a generic class")
- alen = len(parameters)
- elen = len(cls.__parameters__)
- if alen != elen:
- raise TypeError(f"Too {'many' if alen > elen else 'few'} arguments for {cls};"
- f" actual {alen}, expected {elen}")
-
-
-# Please keep __all__ alphabetized within each category.
-__all__ = [
- # Super-special typing primitives.
- 'ClassVar',
- 'Concatenate',
- 'Final',
- 'ParamSpec',
- 'Self',
- 'Type',
-
- # ABCs (from collections.abc).
- 'Awaitable',
- 'AsyncIterator',
- 'AsyncIterable',
- 'Coroutine',
- 'AsyncGenerator',
- 'AsyncContextManager',
- 'ChainMap',
-
- # Concrete collection types.
- 'ContextManager',
- 'Counter',
- 'Deque',
- 'DefaultDict',
- 'OrderedDict',
- 'TypedDict',
-
- # Structural checks, a.k.a. protocols.
- 'SupportsIndex',
-
- # One-off things.
- 'Annotated',
- 'final',
- 'IntVar',
- 'Literal',
- 'NewType',
- 'overload',
- 'Protocol',
- 'runtime',
- 'runtime_checkable',
- 'Text',
- 'TypeAlias',
- 'TypeGuard',
- 'TYPE_CHECKING',
-]
-
-if PEP_560:
- __all__.extend(["get_args", "get_origin", "get_type_hints"])
-
-# 3.6.2+
-if hasattr(typing, 'NoReturn'):
- NoReturn = typing.NoReturn
-# 3.6.0-3.6.1
-else:
- class _NoReturn(typing._FinalTypingBase, _root=True):
- """Special type indicating functions that never return.
- Example::
-
- from typing import NoReturn
-
- def stop() -> NoReturn:
- raise Exception('no way')
-
- This type is invalid in other positions, e.g., ``List[NoReturn]``
- will fail in static type checkers.
- """
- __slots__ = ()
-
- def __instancecheck__(self, obj):
- raise TypeError("NoReturn cannot be used with isinstance().")
-
- def __subclasscheck__(self, cls):
- raise TypeError("NoReturn cannot be used with issubclass().")
-
- NoReturn = _NoReturn(_root=True)
-
-# Some unconstrained type variables. These are used by the container types.
-# (These are not for export.)
-T = typing.TypeVar('T') # Any type.
-KT = typing.TypeVar('KT') # Key type.
-VT = typing.TypeVar('VT') # Value type.
-T_co = typing.TypeVar('T_co', covariant=True) # Any type covariant containers.
-T_contra = typing.TypeVar('T_contra', contravariant=True) # Ditto contravariant.
-
-ClassVar = typing.ClassVar
-
-# On older versions of typing there is an internal class named "Final".
-# 3.8+
-if hasattr(typing, 'Final') and sys.version_info[:2] >= (3, 7):
- Final = typing.Final
-# 3.7
-elif sys.version_info[:2] >= (3, 7):
- class _FinalForm(typing._SpecialForm, _root=True):
-
- def __repr__(self):
- return 'typing_extensions.' + self._name
-
- def __getitem__(self, parameters):
- item = typing._type_check(parameters,
- f'{self._name} accepts only single type')
- return typing._GenericAlias(self, (item,))
-
- Final = _FinalForm('Final',
- doc="""A special typing construct to indicate that a name
- cannot be re-assigned or overridden in a subclass.
- For example:
-
- MAX_SIZE: Final = 9000
- MAX_SIZE += 1 # Error reported by type checker
-
- class Connection:
- TIMEOUT: Final[int] = 10
- class FastConnector(Connection):
- TIMEOUT = 1 # Error reported by type checker
-
- There is no runtime checking of these properties.""")
-# 3.6
-else:
- class _Final(typing._FinalTypingBase, _root=True):
- """A special typing construct to indicate that a name
- cannot be re-assigned or overridden in a subclass.
- For example:
-
- MAX_SIZE: Final = 9000
- MAX_SIZE += 1 # Error reported by type checker
-
- class Connection:
- TIMEOUT: Final[int] = 10
- class FastConnector(Connection):
- TIMEOUT = 1 # Error reported by type checker
-
- There is no runtime checking of these properties.
- """
-
- __slots__ = ('__type__',)
-
- def __init__(self, tp=None, **kwds):
- self.__type__ = tp
-
- def __getitem__(self, item):
- cls = type(self)
- if self.__type__ is None:
- return cls(typing._type_check(item,
- f'{cls.__name__[1:]} accepts only single type.'),
- _root=True)
- raise TypeError(f'{cls.__name__[1:]} cannot be further subscripted')
-
- def _eval_type(self, globalns, localns):
- new_tp = typing._eval_type(self.__type__, globalns, localns)
- if new_tp == self.__type__:
- return self
- return type(self)(new_tp, _root=True)
-
- def __repr__(self):
- r = super().__repr__()
- if self.__type__ is not None:
- r += f'[{typing._type_repr(self.__type__)}]'
- return r
-
- def __hash__(self):
- return hash((type(self).__name__, self.__type__))
-
- def __eq__(self, other):
- if not isinstance(other, _Final):
- return NotImplemented
- if self.__type__ is not None:
- return self.__type__ == other.__type__
- return self is other
-
- Final = _Final(_root=True)
-
-
-# 3.8+
-if hasattr(typing, 'final'):
- final = typing.final
-# 3.6-3.7
-else:
- def final(f):
- """This decorator can be used to indicate to type checkers that
- the decorated method cannot be overridden, and decorated class
- cannot be subclassed. For example:
-
- class Base:
- @final
- def done(self) -> None:
- ...
- class Sub(Base):
- def done(self) -> None: # Error reported by type checker
- ...
- @final
- class Leaf:
- ...
- class Other(Leaf): # Error reported by type checker
- ...
-
- There is no runtime checking of these properties.
- """
- return f
-
-
-def IntVar(name):
- return typing.TypeVar(name)
-
-
-# 3.8+:
-if hasattr(typing, 'Literal'):
- Literal = typing.Literal
-# 3.7:
-elif sys.version_info[:2] >= (3, 7):
- class _LiteralForm(typing._SpecialForm, _root=True):
-
- def __repr__(self):
- return 'typing_extensions.' + self._name
-
- def __getitem__(self, parameters):
- return typing._GenericAlias(self, parameters)
-
- Literal = _LiteralForm('Literal',
- doc="""A type that can be used to indicate to type checkers
- that the corresponding value has a value literally equivalent
- to the provided parameter. For example:
-
- var: Literal[4] = 4
-
- The type checker understands that 'var' is literally equal to
- the value 4 and no other value.
-
- Literal[...] cannot be subclassed. There is no runtime
- checking verifying that the parameter is actually a value
- instead of a type.""")
-# 3.6:
-else:
- class _Literal(typing._FinalTypingBase, _root=True):
- """A type that can be used to indicate to type checkers that the
- corresponding value has a value literally equivalent to the
- provided parameter. For example:
-
- var: Literal[4] = 4
-
- The type checker understands that 'var' is literally equal to the
- value 4 and no other value.
-
- Literal[...] cannot be subclassed. There is no runtime checking
- verifying that the parameter is actually a value instead of a type.
- """
-
- __slots__ = ('__values__',)
-
- def __init__(self, values=None, **kwds):
- self.__values__ = values
-
- def __getitem__(self, values):
- cls = type(self)
- if self.__values__ is None:
- if not isinstance(values, tuple):
- values = (values,)
- return cls(values, _root=True)
- raise TypeError(f'{cls.__name__[1:]} cannot be further subscripted')
-
- def _eval_type(self, globalns, localns):
- return self
-
- def __repr__(self):
- r = super().__repr__()
- if self.__values__ is not None:
- r += f'[{", ".join(map(typing._type_repr, self.__values__))}]'
- return r
-
- def __hash__(self):
- return hash((type(self).__name__, self.__values__))
-
- def __eq__(self, other):
- if not isinstance(other, _Literal):
- return NotImplemented
- if self.__values__ is not None:
- return self.__values__ == other.__values__
- return self is other
-
- Literal = _Literal(_root=True)
-
-
-_overload_dummy = typing._overload_dummy # noqa
-overload = typing.overload
-
-
-# This is not a real generic class. Don't use outside annotations.
-Type = typing.Type
-
-# Various ABCs mimicking those in collections.abc.
-# A few are simply re-exported for completeness.
-
-
-class _ExtensionsGenericMeta(GenericMeta):
- def __subclasscheck__(self, subclass):
- """This mimics a more modern GenericMeta.__subclasscheck__() logic
- (that does not have problems with recursion) to work around interactions
- between collections, typing, and typing_extensions on older
- versions of Python, see https://github.com/python/typing/issues/501.
- """
- if self.__origin__ is not None:
- if sys._getframe(1).f_globals['__name__'] not in ['abc', 'functools']:
- raise TypeError("Parameterized generics cannot be used with class "
- "or instance checks")
- return False
- if not self.__extra__:
- return super().__subclasscheck__(subclass)
- res = self.__extra__.__subclasshook__(subclass)
- if res is not NotImplemented:
- return res
- if self.__extra__ in subclass.__mro__:
- return True
- for scls in self.__extra__.__subclasses__():
- if isinstance(scls, GenericMeta):
- continue
- if issubclass(subclass, scls):
- return True
- return False
-
-
-Awaitable = typing.Awaitable
-Coroutine = typing.Coroutine
-AsyncIterable = typing.AsyncIterable
-AsyncIterator = typing.AsyncIterator
-
-# 3.6.1+
-if hasattr(typing, 'Deque'):
- Deque = typing.Deque
-# 3.6.0
-else:
- class Deque(collections.deque, typing.MutableSequence[T],
- metaclass=_ExtensionsGenericMeta,
- extra=collections.deque):
- __slots__ = ()
-
- def __new__(cls, *args, **kwds):
- if cls._gorg is Deque:
- return collections.deque(*args, **kwds)
- return typing._generic_new(collections.deque, cls, *args, **kwds)
-
-ContextManager = typing.ContextManager
-# 3.6.2+
-if hasattr(typing, 'AsyncContextManager'):
- AsyncContextManager = typing.AsyncContextManager
-# 3.6.0-3.6.1
-else:
- from _collections_abc import _check_methods as _check_methods_in_mro # noqa
-
- class AsyncContextManager(typing.Generic[T_co]):
- __slots__ = ()
-
- async def __aenter__(self):
- return self
-
- @abc.abstractmethod
- async def __aexit__(self, exc_type, exc_value, traceback):
- return None
-
- @classmethod
- def __subclasshook__(cls, C):
- if cls is AsyncContextManager:
- return _check_methods_in_mro(C, "__aenter__", "__aexit__")
- return NotImplemented
-
-DefaultDict = typing.DefaultDict
-
-# 3.7.2+
-if hasattr(typing, 'OrderedDict'):
- OrderedDict = typing.OrderedDict
-# 3.7.0-3.7.2
-elif (3, 7, 0) <= sys.version_info[:3] < (3, 7, 2):
- OrderedDict = typing._alias(collections.OrderedDict, (KT, VT))
-# 3.6
-else:
- class OrderedDict(collections.OrderedDict, typing.MutableMapping[KT, VT],
- metaclass=_ExtensionsGenericMeta,
- extra=collections.OrderedDict):
-
- __slots__ = ()
-
- def __new__(cls, *args, **kwds):
- if cls._gorg is OrderedDict:
- return collections.OrderedDict(*args, **kwds)
- return typing._generic_new(collections.OrderedDict, cls, *args, **kwds)
-
-# 3.6.2+
-if hasattr(typing, 'Counter'):
- Counter = typing.Counter
-# 3.6.0-3.6.1
-else:
- class Counter(collections.Counter,
- typing.Dict[T, int],
- metaclass=_ExtensionsGenericMeta, extra=collections.Counter):
-
- __slots__ = ()
-
- def __new__(cls, *args, **kwds):
- if cls._gorg is Counter:
- return collections.Counter(*args, **kwds)
- return typing._generic_new(collections.Counter, cls, *args, **kwds)
-
-# 3.6.1+
-if hasattr(typing, 'ChainMap'):
- ChainMap = typing.ChainMap
-elif hasattr(collections, 'ChainMap'):
- class ChainMap(collections.ChainMap, typing.MutableMapping[KT, VT],
- metaclass=_ExtensionsGenericMeta,
- extra=collections.ChainMap):
-
- __slots__ = ()
-
- def __new__(cls, *args, **kwds):
- if cls._gorg is ChainMap:
- return collections.ChainMap(*args, **kwds)
- return typing._generic_new(collections.ChainMap, cls, *args, **kwds)
-
-# 3.6.1+
-if hasattr(typing, 'AsyncGenerator'):
- AsyncGenerator = typing.AsyncGenerator
-# 3.6.0
-else:
- class AsyncGenerator(AsyncIterator[T_co], typing.Generic[T_co, T_contra],
- metaclass=_ExtensionsGenericMeta,
- extra=collections.abc.AsyncGenerator):
- __slots__ = ()
-
-NewType = typing.NewType
-Text = typing.Text
-TYPE_CHECKING = typing.TYPE_CHECKING
-
-
-def _gorg(cls):
- """This function exists for compatibility with old typing versions."""
- assert isinstance(cls, GenericMeta)
- if hasattr(cls, '_gorg'):
- return cls._gorg
- while cls.__origin__ is not None:
- cls = cls.__origin__
- return cls
-
-
-_PROTO_WHITELIST = ['Callable', 'Awaitable',
- 'Iterable', 'Iterator', 'AsyncIterable', 'AsyncIterator',
- 'Hashable', 'Sized', 'Container', 'Collection', 'Reversible',
- 'ContextManager', 'AsyncContextManager']
-
-
-def _get_protocol_attrs(cls):
- attrs = set()
- for base in cls.__mro__[:-1]: # without object
- if base.__name__ in ('Protocol', 'Generic'):
- continue
- annotations = getattr(base, '__annotations__', {})
- for attr in list(base.__dict__.keys()) + list(annotations.keys()):
- if (not attr.startswith('_abc_') and attr not in (
- '__abstractmethods__', '__annotations__', '__weakref__',
- '_is_protocol', '_is_runtime_protocol', '__dict__',
- '__args__', '__slots__',
- '__next_in_mro__', '__parameters__', '__origin__',
- '__orig_bases__', '__extra__', '__tree_hash__',
- '__doc__', '__subclasshook__', '__init__', '__new__',
- '__module__', '_MutableMapping__marker', '_gorg')):
- attrs.add(attr)
- return attrs
-
-
-def _is_callable_members_only(cls):
- return all(callable(getattr(cls, attr, None)) for attr in _get_protocol_attrs(cls))
-
-
-# 3.8+
-if hasattr(typing, 'Protocol'):
- Protocol = typing.Protocol
-# 3.7
-elif PEP_560:
- from typing import _collect_type_vars # noqa
-
- def _no_init(self, *args, **kwargs):
- if type(self)._is_protocol:
- raise TypeError('Protocols cannot be instantiated')
-
- class _ProtocolMeta(abc.ABCMeta):
- # This metaclass is a bit unfortunate and exists only because of the lack
- # of __instancehook__.
- def __instancecheck__(cls, instance):
- # We need this method for situations where attributes are
- # assigned in __init__.
- if ((not getattr(cls, '_is_protocol', False) or
- _is_callable_members_only(cls)) and
- issubclass(instance.__class__, cls)):
- return True
- if cls._is_protocol:
- if all(hasattr(instance, attr) and
- (not callable(getattr(cls, attr, None)) or
- getattr(instance, attr) is not None)
- for attr in _get_protocol_attrs(cls)):
- return True
- return super().__instancecheck__(instance)
-
- class Protocol(metaclass=_ProtocolMeta):
- # There is quite a lot of overlapping code with typing.Generic.
- # Unfortunately it is hard to avoid this while these live in two different
- # modules. The duplicated code will be removed when Protocol is moved to typing.
- """Base class for protocol classes. Protocol classes are defined as::
-
- class Proto(Protocol):
- def meth(self) -> int:
- ...
-
- Such classes are primarily used with static type checkers that recognize
- structural subtyping (static duck-typing), for example::
-
- class C:
- def meth(self) -> int:
- return 0
-
- def func(x: Proto) -> int:
- return x.meth()
-
- func(C()) # Passes static type check
-
- See PEP 544 for details. Protocol classes decorated with
- @typing_extensions.runtime act as simple-minded runtime protocol that checks
- only the presence of given attributes, ignoring their type signatures.
-
- Protocol classes can be generic, they are defined as::
-
- class GenProto(Protocol[T]):
- def meth(self) -> T:
- ...
- """
- __slots__ = ()
- _is_protocol = True
-
- def __new__(cls, *args, **kwds):
- if cls is Protocol:
- raise TypeError("Type Protocol cannot be instantiated; "
- "it can only be used as a base class")
- return super().__new__(cls)
-
- @typing._tp_cache
- def __class_getitem__(cls, params):
- if not isinstance(params, tuple):
- params = (params,)
- if not params and cls is not typing.Tuple:
- raise TypeError(
- f"Parameter list to {cls.__qualname__}[...] cannot be empty")
- msg = "Parameters to generic types must be types."
- params = tuple(typing._type_check(p, msg) for p in params) # noqa
- if cls is Protocol:
- # Generic can only be subscripted with unique type variables.
- if not all(isinstance(p, typing.TypeVar) for p in params):
- i = 0
- while isinstance(params[i], typing.TypeVar):
- i += 1
- raise TypeError(
- "Parameters to Protocol[...] must all be type variables."
- f" Parameter {i + 1} is {params[i]}")
- if len(set(params)) != len(params):
- raise TypeError(
- "Parameters to Protocol[...] must all be unique")
- else:
- # Subscripting a regular Generic subclass.
- _check_generic(cls, params)
- return typing._GenericAlias(cls, params)
-
- def __init_subclass__(cls, *args, **kwargs):
- tvars = []
- if '__orig_bases__' in cls.__dict__:
- error = typing.Generic in cls.__orig_bases__
- else:
- error = typing.Generic in cls.__bases__
- if error:
- raise TypeError("Cannot inherit from plain Generic")
- if '__orig_bases__' in cls.__dict__:
- tvars = _collect_type_vars(cls.__orig_bases__)
- # Look for Generic[T1, ..., Tn] or Protocol[T1, ..., Tn].
- # If found, tvars must be a subset of it.
- # If not found, tvars is it.
- # Also check for and reject plain Generic,
- # and reject multiple Generic[...] and/or Protocol[...].
- gvars = None
- for base in cls.__orig_bases__:
- if (isinstance(base, typing._GenericAlias) and
- base.__origin__ in (typing.Generic, Protocol)):
- # for error messages
- the_base = base.__origin__.__name__
- if gvars is not None:
- raise TypeError(
- "Cannot inherit from Generic[...]"
- " and/or Protocol[...] multiple types.")
- gvars = base.__parameters__
- if gvars is None:
- gvars = tvars
- else:
- tvarset = set(tvars)
- gvarset = set(gvars)
- if not tvarset <= gvarset:
- s_vars = ', '.join(str(t) for t in tvars if t not in gvarset)
- s_args = ', '.join(str(g) for g in gvars)
- raise TypeError(f"Some type variables ({s_vars}) are"
- f" not listed in {the_base}[{s_args}]")
- tvars = gvars
- cls.__parameters__ = tuple(tvars)
-
- # Determine if this is a protocol or a concrete subclass.
- if not cls.__dict__.get('_is_protocol', None):
- cls._is_protocol = any(b is Protocol for b in cls.__bases__)
-
- # Set (or override) the protocol subclass hook.
- def _proto_hook(other):
- if not cls.__dict__.get('_is_protocol', None):
- return NotImplemented
- if not getattr(cls, '_is_runtime_protocol', False):
- if sys._getframe(2).f_globals['__name__'] in ['abc', 'functools']:
- return NotImplemented
- raise TypeError("Instance and class checks can only be used with"
- " @runtime protocols")
- if not _is_callable_members_only(cls):
- if sys._getframe(2).f_globals['__name__'] in ['abc', 'functools']:
- return NotImplemented
- raise TypeError("Protocols with non-method members"
- " don't support issubclass()")
- if not isinstance(other, type):
- # Same error as for issubclass(1, int)
- raise TypeError('issubclass() arg 1 must be a class')
- for attr in _get_protocol_attrs(cls):
- for base in other.__mro__:
- if attr in base.__dict__:
- if base.__dict__[attr] is None:
- return NotImplemented
- break
- annotations = getattr(base, '__annotations__', {})
- if (isinstance(annotations, typing.Mapping) and
- attr in annotations and
- isinstance(other, _ProtocolMeta) and
- other._is_protocol):
- break
- else:
- return NotImplemented
- return True
- if '__subclasshook__' not in cls.__dict__:
- cls.__subclasshook__ = _proto_hook
-
- # We have nothing more to do for non-protocols.
- if not cls._is_protocol:
- return
-
- # Check consistency of bases.
- for base in cls.__bases__:
- if not (base in (object, typing.Generic) or
- base.__module__ == 'collections.abc' and
- base.__name__ in _PROTO_WHITELIST or
- isinstance(base, _ProtocolMeta) and base._is_protocol):
- raise TypeError('Protocols can only inherit from other'
- f' protocols, got {repr(base)}')
- cls.__init__ = _no_init
-# 3.6
-else:
- from typing import _next_in_mro, _type_check # noqa
-
- def _no_init(self, *args, **kwargs):
- if type(self)._is_protocol:
- raise TypeError('Protocols cannot be instantiated')
-
- class _ProtocolMeta(GenericMeta):
- """Internal metaclass for Protocol.
-
- This exists so Protocol classes can be generic without deriving
- from Generic.
- """
- def __new__(cls, name, bases, namespace,
- tvars=None, args=None, origin=None, extra=None, orig_bases=None):
- # This is just a version copied from GenericMeta.__new__ that
- # includes "Protocol" special treatment. (Comments removed for brevity.)
- assert extra is None # Protocols should not have extra
- if tvars is not None:
- assert origin is not None
- assert all(isinstance(t, typing.TypeVar) for t in tvars), tvars
- else:
- tvars = _type_vars(bases)
- gvars = None
- for base in bases:
- if base is typing.Generic:
- raise TypeError("Cannot inherit from plain Generic")
- if (isinstance(base, GenericMeta) and
- base.__origin__ in (typing.Generic, Protocol)):
- if gvars is not None:
- raise TypeError(
- "Cannot inherit from Generic[...] or"
- " Protocol[...] multiple times.")
- gvars = base.__parameters__
- if gvars is None:
- gvars = tvars
- else:
- tvarset = set(tvars)
- gvarset = set(gvars)
- if not tvarset <= gvarset:
- s_vars = ", ".join(str(t) for t in tvars if t not in gvarset)
- s_args = ", ".join(str(g) for g in gvars)
- cls_name = "Generic" if any(b.__origin__ is typing.Generic
- for b in bases) else "Protocol"
- raise TypeError(f"Some type variables ({s_vars}) are"
- f" not listed in {cls_name}[{s_args}]")
- tvars = gvars
-
- initial_bases = bases
- if (extra is not None and type(extra) is abc.ABCMeta and
- extra not in bases):
- bases = (extra,) + bases
- bases = tuple(_gorg(b) if isinstance(b, GenericMeta) else b
- for b in bases)
- if any(isinstance(b, GenericMeta) and b is not typing.Generic for b in bases):
- bases = tuple(b for b in bases if b is not typing.Generic)
- namespace.update({'__origin__': origin, '__extra__': extra})
- self = super(GenericMeta, cls).__new__(cls, name, bases, namespace,
- _root=True)
- super(GenericMeta, self).__setattr__('_gorg',
- self if not origin else
- _gorg(origin))
- self.__parameters__ = tvars
- self.__args__ = tuple(... if a is typing._TypingEllipsis else
- () if a is typing._TypingEmpty else
- a for a in args) if args else None
- self.__next_in_mro__ = _next_in_mro(self)
- if orig_bases is None:
- self.__orig_bases__ = initial_bases
- elif origin is not None:
- self._abc_registry = origin._abc_registry
- self._abc_cache = origin._abc_cache
- if hasattr(self, '_subs_tree'):
- self.__tree_hash__ = (hash(self._subs_tree()) if origin else
- super(GenericMeta, self).__hash__())
- return self
-
- def __init__(cls, *args, **kwargs):
- super().__init__(*args, **kwargs)
- if not cls.__dict__.get('_is_protocol', None):
- cls._is_protocol = any(b is Protocol or
- isinstance(b, _ProtocolMeta) and
- b.__origin__ is Protocol
- for b in cls.__bases__)
- if cls._is_protocol:
- for base in cls.__mro__[1:]:
- if not (base in (object, typing.Generic) or
- base.__module__ == 'collections.abc' and
- base.__name__ in _PROTO_WHITELIST or
- isinstance(base, typing.TypingMeta) and base._is_protocol or
- isinstance(base, GenericMeta) and
- base.__origin__ is typing.Generic):
- raise TypeError(f'Protocols can only inherit from other'
- f' protocols, got {repr(base)}')
-
- cls.__init__ = _no_init
-
- def _proto_hook(other):
- if not cls.__dict__.get('_is_protocol', None):
- return NotImplemented
- if not isinstance(other, type):
- # Same error as for issubclass(1, int)
- raise TypeError('issubclass() arg 1 must be a class')
- for attr in _get_protocol_attrs(cls):
- for base in other.__mro__:
- if attr in base.__dict__:
- if base.__dict__[attr] is None:
- return NotImplemented
- break
- annotations = getattr(base, '__annotations__', {})
- if (isinstance(annotations, typing.Mapping) and
- attr in annotations and
- isinstance(other, _ProtocolMeta) and
- other._is_protocol):
- break
- else:
- return NotImplemented
- return True
- if '__subclasshook__' not in cls.__dict__:
- cls.__subclasshook__ = _proto_hook
-
- def __instancecheck__(self, instance):
- # We need this method for situations where attributes are
- # assigned in __init__.
- if ((not getattr(self, '_is_protocol', False) or
- _is_callable_members_only(self)) and
- issubclass(instance.__class__, self)):
- return True
- if self._is_protocol:
- if all(hasattr(instance, attr) and
- (not callable(getattr(self, attr, None)) or
- getattr(instance, attr) is not None)
- for attr in _get_protocol_attrs(self)):
- return True
- return super(GenericMeta, self).__instancecheck__(instance)
-
- def __subclasscheck__(self, cls):
- if self.__origin__ is not None:
- if sys._getframe(1).f_globals['__name__'] not in ['abc', 'functools']:
- raise TypeError("Parameterized generics cannot be used with class "
- "or instance checks")
- return False
- if (self.__dict__.get('_is_protocol', None) and
- not self.__dict__.get('_is_runtime_protocol', None)):
- if sys._getframe(1).f_globals['__name__'] in ['abc',
- 'functools',
- 'typing']:
- return False
- raise TypeError("Instance and class checks can only be used with"
- " @runtime protocols")
- if (self.__dict__.get('_is_runtime_protocol', None) and
- not _is_callable_members_only(self)):
- if sys._getframe(1).f_globals['__name__'] in ['abc',
- 'functools',
- 'typing']:
- return super(GenericMeta, self).__subclasscheck__(cls)
- raise TypeError("Protocols with non-method members"
- " don't support issubclass()")
- return super(GenericMeta, self).__subclasscheck__(cls)
-
- @typing._tp_cache
- def __getitem__(self, params):
- # We also need to copy this from GenericMeta.__getitem__ to get
- # special treatment of "Protocol". (Comments removed for brevity.)
- if not isinstance(params, tuple):
- params = (params,)
- if not params and _gorg(self) is not typing.Tuple:
- raise TypeError(
- f"Parameter list to {self.__qualname__}[...] cannot be empty")
- msg = "Parameters to generic types must be types."
- params = tuple(_type_check(p, msg) for p in params)
- if self in (typing.Generic, Protocol):
- if not all(isinstance(p, typing.TypeVar) for p in params):
- raise TypeError(
- f"Parameters to {repr(self)}[...] must all be type variables")
- if len(set(params)) != len(params):
- raise TypeError(
- f"Parameters to {repr(self)}[...] must all be unique")
- tvars = params
- args = params
- elif self in (typing.Tuple, typing.Callable):
- tvars = _type_vars(params)
- args = params
- elif self.__origin__ in (typing.Generic, Protocol):
- raise TypeError(f"Cannot subscript already-subscripted {repr(self)}")
- else:
- _check_generic(self, params)
- tvars = _type_vars(params)
- args = params
-
- prepend = (self,) if self.__origin__ is None else ()
- return self.__class__(self.__name__,
- prepend + self.__bases__,
- _no_slots_copy(self.__dict__),
- tvars=tvars,
- args=args,
- origin=self,
- extra=self.__extra__,
- orig_bases=self.__orig_bases__)
-
- class Protocol(metaclass=_ProtocolMeta):
- """Base class for protocol classes. Protocol classes are defined as::
-
- class Proto(Protocol):
- def meth(self) -> int:
- ...
-
- Such classes are primarily used with static type checkers that recognize
- structural subtyping (static duck-typing), for example::
-
- class C:
- def meth(self) -> int:
- return 0
-
- def func(x: Proto) -> int:
- return x.meth()
-
- func(C()) # Passes static type check
-
- See PEP 544 for details. Protocol classes decorated with
- @typing_extensions.runtime act as simple-minded runtime protocol that checks
- only the presence of given attributes, ignoring their type signatures.
-
- Protocol classes can be generic, they are defined as::
-
- class GenProto(Protocol[T]):
- def meth(self) -> T:
- ...
- """
- __slots__ = ()
- _is_protocol = True
-
- def __new__(cls, *args, **kwds):
- if _gorg(cls) is Protocol:
- raise TypeError("Type Protocol cannot be instantiated; "
- "it can be used only as a base class")
- return typing._generic_new(cls.__next_in_mro__, cls, *args, **kwds)
-
-
-# 3.8+
-if hasattr(typing, 'runtime_checkable'):
- runtime_checkable = typing.runtime_checkable
-# 3.6-3.7
-else:
- def runtime_checkable(cls):
- """Mark a protocol class as a runtime protocol, so that it
- can be used with isinstance() and issubclass(). Raise TypeError
- if applied to a non-protocol class.
-
- This allows a simple-minded structural check very similar to the
- one-offs in collections.abc such as Hashable.
- """
- if not isinstance(cls, _ProtocolMeta) or not cls._is_protocol:
- raise TypeError('@runtime_checkable can be only applied to protocol classes,'
- f' got {cls!r}')
- cls._is_runtime_protocol = True
- return cls
-
-
-# Exists for backwards compatibility.
-runtime = runtime_checkable
-
-
-# 3.8+
-if hasattr(typing, 'SupportsIndex'):
- SupportsIndex = typing.SupportsIndex
-# 3.6-3.7
-else:
- @runtime_checkable
- class SupportsIndex(Protocol):
- __slots__ = ()
-
- @abc.abstractmethod
- def __index__(self) -> int:
- pass
-
-
-if sys.version_info >= (3, 9, 2):
- # The standard library TypedDict in Python 3.8 does not store runtime information
- # about which (if any) keys are optional. See https://bugs.python.org/issue38834
- # The standard library TypedDict in Python 3.9.0/1 does not honour the "total"
- # keyword with old-style TypedDict(). See https://bugs.python.org/issue42059
- TypedDict = typing.TypedDict
-else:
- def _check_fails(cls, other):
- try:
- if sys._getframe(1).f_globals['__name__'] not in ['abc',
- 'functools',
- 'typing']:
- # Typed dicts are only for static structural subtyping.
- raise TypeError('TypedDict does not support instance and class checks')
- except (AttributeError, ValueError):
- pass
- return False
-
- def _dict_new(*args, **kwargs):
- if not args:
- raise TypeError('TypedDict.__new__(): not enough arguments')
- _, args = args[0], args[1:] # allow the "cls" keyword be passed
- return dict(*args, **kwargs)
-
- _dict_new.__text_signature__ = '($cls, _typename, _fields=None, /, **kwargs)'
-
- def _typeddict_new(*args, total=True, **kwargs):
- if not args:
- raise TypeError('TypedDict.__new__(): not enough arguments')
- _, args = args[0], args[1:] # allow the "cls" keyword be passed
- if args:
- typename, args = args[0], args[1:] # allow the "_typename" keyword be passed
- elif '_typename' in kwargs:
- typename = kwargs.pop('_typename')
- import warnings
- warnings.warn("Passing '_typename' as keyword argument is deprecated",
- DeprecationWarning, stacklevel=2)
- else:
- raise TypeError("TypedDict.__new__() missing 1 required positional "
- "argument: '_typename'")
- if args:
- try:
- fields, = args # allow the "_fields" keyword be passed
- except ValueError:
- raise TypeError('TypedDict.__new__() takes from 2 to 3 '
- f'positional arguments but {len(args) + 2} '
- 'were given')
- elif '_fields' in kwargs and len(kwargs) == 1:
- fields = kwargs.pop('_fields')
- import warnings
- warnings.warn("Passing '_fields' as keyword argument is deprecated",
- DeprecationWarning, stacklevel=2)
- else:
- fields = None
-
- if fields is None:
- fields = kwargs
- elif kwargs:
- raise TypeError("TypedDict takes either a dict or keyword arguments,"
- " but not both")
-
- ns = {'__annotations__': dict(fields)}
- try:
- # Setting correct module is necessary to make typed dict classes pickleable.
- ns['__module__'] = sys._getframe(1).f_globals.get('__name__', '__main__')
- except (AttributeError, ValueError):
- pass
-
- return _TypedDictMeta(typename, (), ns, total=total)
-
- _typeddict_new.__text_signature__ = ('($cls, _typename, _fields=None,'
- ' /, *, total=True, **kwargs)')
-
- class _TypedDictMeta(type):
- def __init__(cls, name, bases, ns, total=True):
- super().__init__(name, bases, ns)
-
- def __new__(cls, name, bases, ns, total=True):
- # Create new typed dict class object.
- # This method is called directly when TypedDict is subclassed,
- # or via _typeddict_new when TypedDict is instantiated. This way
- # TypedDict supports all three syntaxes described in its docstring.
- # Subclasses and instances of TypedDict return actual dictionaries
- # via _dict_new.
- ns['__new__'] = _typeddict_new if name == 'TypedDict' else _dict_new
- tp_dict = super().__new__(cls, name, (dict,), ns)
-
- annotations = {}
- own_annotations = ns.get('__annotations__', {})
- own_annotation_keys = set(own_annotations.keys())
- msg = "TypedDict('Name', {f0: t0, f1: t1, ...}); each t must be a type"
- own_annotations = {
- n: typing._type_check(tp, msg) for n, tp in own_annotations.items()
- }
- required_keys = set()
- optional_keys = set()
-
- for base in bases:
- annotations.update(base.__dict__.get('__annotations__', {}))
- required_keys.update(base.__dict__.get('__required_keys__', ()))
- optional_keys.update(base.__dict__.get('__optional_keys__', ()))
-
- annotations.update(own_annotations)
- if total:
- required_keys.update(own_annotation_keys)
- else:
- optional_keys.update(own_annotation_keys)
-
- tp_dict.__annotations__ = annotations
- tp_dict.__required_keys__ = frozenset(required_keys)
- tp_dict.__optional_keys__ = frozenset(optional_keys)
- if not hasattr(tp_dict, '__total__'):
- tp_dict.__total__ = total
- return tp_dict
-
- __instancecheck__ = __subclasscheck__ = _check_fails
-
- TypedDict = _TypedDictMeta('TypedDict', (dict,), {})
- TypedDict.__module__ = __name__
- TypedDict.__doc__ = \
- """A simple typed name space. At runtime it is equivalent to a plain dict.
-
- TypedDict creates a dictionary type that expects all of its
- instances to have a certain set of keys, with each key
- associated with a value of a consistent type. This expectation
- is not checked at runtime but is only enforced by type checkers.
- Usage::
-
- class Point2D(TypedDict):
- x: int
- y: int
- label: str
-
- a: Point2D = {'x': 1, 'y': 2, 'label': 'good'} # OK
- b: Point2D = {'z': 3, 'label': 'bad'} # Fails type check
-
- assert Point2D(x=1, y=2, label='first') == dict(x=1, y=2, label='first')
-
- The type info can be accessed via the Point2D.__annotations__ dict, and
- the Point2D.__required_keys__ and Point2D.__optional_keys__ frozensets.
- TypedDict supports two additional equivalent forms::
-
- Point2D = TypedDict('Point2D', x=int, y=int, label=str)
- Point2D = TypedDict('Point2D', {'x': int, 'y': int, 'label': str})
-
- The class syntax is only supported in Python 3.6+, while two other
- syntax forms work for Python 2.7 and 3.2+
- """
-
-
-# Python 3.9+ has PEP 593 (Annotated and modified get_type_hints)
-if hasattr(typing, 'Annotated'):
- Annotated = typing.Annotated
- get_type_hints = typing.get_type_hints
- # Not exported and not a public API, but needed for get_origin() and get_args()
- # to work.
- _AnnotatedAlias = typing._AnnotatedAlias
-# 3.7-3.8
-elif PEP_560:
- class _AnnotatedAlias(typing._GenericAlias, _root=True):
- """Runtime representation of an annotated type.
-
- At its core 'Annotated[t, dec1, dec2, ...]' is an alias for the type 't'
- with extra annotations. The alias behaves like a normal typing alias,
- instantiating is the same as instantiating the underlying type, binding
- it to types is also the same.
- """
- def __init__(self, origin, metadata):
- if isinstance(origin, _AnnotatedAlias):
- metadata = origin.__metadata__ + metadata
- origin = origin.__origin__
- super().__init__(origin, origin)
- self.__metadata__ = metadata
-
- def copy_with(self, params):
- assert len(params) == 1
- new_type = params[0]
- return _AnnotatedAlias(new_type, self.__metadata__)
-
- def __repr__(self):
- return (f"typing_extensions.Annotated[{typing._type_repr(self.__origin__)}, "
- f"{', '.join(repr(a) for a in self.__metadata__)}]")
-
- def __reduce__(self):
- return operator.getitem, (
- Annotated, (self.__origin__,) + self.__metadata__
- )
-
- def __eq__(self, other):
- if not isinstance(other, _AnnotatedAlias):
- return NotImplemented
- if self.__origin__ != other.__origin__:
- return False
- return self.__metadata__ == other.__metadata__
-
- def __hash__(self):
- return hash((self.__origin__, self.__metadata__))
-
- class Annotated:
- """Add context specific metadata to a type.
-
- Example: Annotated[int, runtime_check.Unsigned] indicates to the
- hypothetical runtime_check module that this type is an unsigned int.
- Every other consumer of this type can ignore this metadata and treat
- this type as int.
-
- The first argument to Annotated must be a valid type (and will be in
- the __origin__ field), the remaining arguments are kept as a tuple in
- the __extra__ field.
-
- Details:
-
- - It's an error to call `Annotated` with less than two arguments.
- - Nested Annotated are flattened::
-
- Annotated[Annotated[T, Ann1, Ann2], Ann3] == Annotated[T, Ann1, Ann2, Ann3]
-
- - Instantiating an annotated type is equivalent to instantiating the
- underlying type::
-
- Annotated[C, Ann1](5) == C(5)
-
- - Annotated can be used as a generic type alias::
-
- Optimized = Annotated[T, runtime.Optimize()]
- Optimized[int] == Annotated[int, runtime.Optimize()]
-
- OptimizedList = Annotated[List[T], runtime.Optimize()]
- OptimizedList[int] == Annotated[List[int], runtime.Optimize()]
- """
-
- __slots__ = ()
-
- def __new__(cls, *args, **kwargs):
- raise TypeError("Type Annotated cannot be instantiated.")
-
- @typing._tp_cache
- def __class_getitem__(cls, params):
- if not isinstance(params, tuple) or len(params) < 2:
- raise TypeError("Annotated[...] should be used "
- "with at least two arguments (a type and an "
- "annotation).")
- msg = "Annotated[t, ...]: t must be a type."
- origin = typing._type_check(params[0], msg)
- metadata = tuple(params[1:])
- return _AnnotatedAlias(origin, metadata)
-
- def __init_subclass__(cls, *args, **kwargs):
- raise TypeError(
- f"Cannot subclass {cls.__module__}.Annotated"
- )
-
- def _strip_annotations(t):
- """Strips the annotations from a given type.
- """
- if isinstance(t, _AnnotatedAlias):
- return _strip_annotations(t.__origin__)
- if isinstance(t, typing._GenericAlias):
- stripped_args = tuple(_strip_annotations(a) for a in t.__args__)
- if stripped_args == t.__args__:
- return t
- res = t.copy_with(stripped_args)
- res._special = t._special
- return res
- return t
-
- def get_type_hints(obj, globalns=None, localns=None, include_extras=False):
- """Return type hints for an object.
-
- This is often the same as obj.__annotations__, but it handles
- forward references encoded as string literals, adds Optional[t] if a
- default value equal to None is set and recursively replaces all
- 'Annotated[T, ...]' with 'T' (unless 'include_extras=True').
-
- The argument may be a module, class, method, or function. The annotations
- are returned as a dictionary. For classes, annotations include also
- inherited members.
-
- TypeError is raised if the argument is not of a type that can contain
- annotations, and an empty dictionary is returned if no annotations are
- present.
-
- BEWARE -- the behavior of globalns and localns is counterintuitive
- (unless you are familiar with how eval() and exec() work). The
- search order is locals first, then globals.
-
- - If no dict arguments are passed, an attempt is made to use the
- globals from obj (or the respective module's globals for classes),
- and these are also used as the locals. If the object does not appear
- to have globals, an empty dictionary is used.
-
- - If one dict argument is passed, it is used for both globals and
- locals.
-
- - If two dict arguments are passed, they specify globals and
- locals, respectively.
- """
- hint = typing.get_type_hints(obj, globalns=globalns, localns=localns)
- if include_extras:
- return hint
- return {k: _strip_annotations(t) for k, t in hint.items()}
-# 3.6
-else:
-
- def _is_dunder(name):
- """Returns True if name is a __dunder_variable_name__."""
- return len(name) > 4 and name.startswith('__') and name.endswith('__')
-
- # Prior to Python 3.7 types did not have `copy_with`. A lot of the equality
- # checks, argument expansion etc. are done on the _subs_tre. As a result we
- # can't provide a get_type_hints function that strips out annotations.
-
- class AnnotatedMeta(typing.GenericMeta):
- """Metaclass for Annotated"""
-
- def __new__(cls, name, bases, namespace, **kwargs):
- if any(b is not object for b in bases):
- raise TypeError("Cannot subclass " + str(Annotated))
- return super().__new__(cls, name, bases, namespace, **kwargs)
-
- @property
- def __metadata__(self):
- return self._subs_tree()[2]
-
- def _tree_repr(self, tree):
- cls, origin, metadata = tree
- if not isinstance(origin, tuple):
- tp_repr = typing._type_repr(origin)
- else:
- tp_repr = origin[0]._tree_repr(origin)
- metadata_reprs = ", ".join(repr(arg) for arg in metadata)
- return f'{cls}[{tp_repr}, {metadata_reprs}]'
-
- def _subs_tree(self, tvars=None, args=None): # noqa
- if self is Annotated:
- return Annotated
- res = super()._subs_tree(tvars=tvars, args=args)
- # Flatten nested Annotated
- if isinstance(res[1], tuple) and res[1][0] is Annotated:
- sub_tp = res[1][1]
- sub_annot = res[1][2]
- return (Annotated, sub_tp, sub_annot + res[2])
- return res
-
- def _get_cons(self):
- """Return the class used to create instance of this type."""
- if self.__origin__ is None:
- raise TypeError("Cannot get the underlying type of a "
- "non-specialized Annotated type.")
- tree = self._subs_tree()
- while isinstance(tree, tuple) and tree[0] is Annotated:
- tree = tree[1]
- if isinstance(tree, tuple):
- return tree[0]
- else:
- return tree
-
- @typing._tp_cache
- def __getitem__(self, params):
- if not isinstance(params, tuple):
- params = (params,)
- if self.__origin__ is not None: # specializing an instantiated type
- return super().__getitem__(params)
- elif not isinstance(params, tuple) or len(params) < 2:
- raise TypeError("Annotated[...] should be instantiated "
- "with at least two arguments (a type and an "
- "annotation).")
- else:
- msg = "Annotated[t, ...]: t must be a type."
- tp = typing._type_check(params[0], msg)
- metadata = tuple(params[1:])
- return self.__class__(
- self.__name__,
- self.__bases__,
- _no_slots_copy(self.__dict__),
- tvars=_type_vars((tp,)),
- # Metadata is a tuple so it won't be touched by _replace_args et al.
- args=(tp, metadata),
- origin=self,
- )
-
- def __call__(self, *args, **kwargs):
- cons = self._get_cons()
- result = cons(*args, **kwargs)
- try:
- result.__orig_class__ = self
- except AttributeError:
- pass
- return result
-
- def __getattr__(self, attr):
- # For simplicity we just don't relay all dunder names
- if self.__origin__ is not None and not _is_dunder(attr):
- return getattr(self._get_cons(), attr)
- raise AttributeError(attr)
-
- def __setattr__(self, attr, value):
- if _is_dunder(attr) or attr.startswith('_abc_'):
- super().__setattr__(attr, value)
- elif self.__origin__ is None:
- raise AttributeError(attr)
- else:
- setattr(self._get_cons(), attr, value)
-
- def __instancecheck__(self, obj):
- raise TypeError("Annotated cannot be used with isinstance().")
-
- def __subclasscheck__(self, cls):
- raise TypeError("Annotated cannot be used with issubclass().")
-
- class Annotated(metaclass=AnnotatedMeta):
- """Add context specific metadata to a type.
-
- Example: Annotated[int, runtime_check.Unsigned] indicates to the
- hypothetical runtime_check module that this type is an unsigned int.
- Every other consumer of this type can ignore this metadata and treat
- this type as int.
-
- The first argument to Annotated must be a valid type, the remaining
- arguments are kept as a tuple in the __metadata__ field.
-
- Details:
-
- - It's an error to call `Annotated` with less than two arguments.
- - Nested Annotated are flattened::
-
- Annotated[Annotated[T, Ann1, Ann2], Ann3] == Annotated[T, Ann1, Ann2, Ann3]
-
- - Instantiating an annotated type is equivalent to instantiating the
- underlying type::
-
- Annotated[C, Ann1](5) == C(5)
-
- - Annotated can be used as a generic type alias::
-
- Optimized = Annotated[T, runtime.Optimize()]
- Optimized[int] == Annotated[int, runtime.Optimize()]
-
- OptimizedList = Annotated[List[T], runtime.Optimize()]
- OptimizedList[int] == Annotated[List[int], runtime.Optimize()]
- """
-
-# Python 3.8 has get_origin() and get_args() but those implementations aren't
-# Annotated-aware, so we can't use those. Python 3.9's versions don't support
-# ParamSpecArgs and ParamSpecKwargs, so only Python 3.10's versions will do.
-if sys.version_info[:2] >= (3, 10):
- get_origin = typing.get_origin
- get_args = typing.get_args
-# 3.7-3.9
-elif PEP_560:
- try:
- # 3.9+
- from typing import _BaseGenericAlias
- except ImportError:
- _BaseGenericAlias = typing._GenericAlias
- try:
- # 3.9+
- from typing import GenericAlias
- except ImportError:
- GenericAlias = typing._GenericAlias
-
- def get_origin(tp):
- """Get the unsubscripted version of a type.
-
- This supports generic types, Callable, Tuple, Union, Literal, Final, ClassVar
- and Annotated. Return None for unsupported types. Examples::
-
- get_origin(Literal[42]) is Literal
- get_origin(int) is None
- get_origin(ClassVar[int]) is ClassVar
- get_origin(Generic) is Generic
- get_origin(Generic[T]) is Generic
- get_origin(Union[T, int]) is Union
- get_origin(List[Tuple[T, T]][int]) == list
- get_origin(P.args) is P
- """
- if isinstance(tp, _AnnotatedAlias):
- return Annotated
- if isinstance(tp, (typing._GenericAlias, GenericAlias, _BaseGenericAlias,
- ParamSpecArgs, ParamSpecKwargs)):
- return tp.__origin__
- if tp is typing.Generic:
- return typing.Generic
- return None
-
- def get_args(tp):
- """Get type arguments with all substitutions performed.
-
- For unions, basic simplifications used by Union constructor are performed.
- Examples::
- get_args(Dict[str, int]) == (str, int)
- get_args(int) == ()
- get_args(Union[int, Union[T, int], str][int]) == (int, str)
- get_args(Union[int, Tuple[T, int]][str]) == (int, Tuple[str, int])
- get_args(Callable[[], T][int]) == ([], int)
- """
- if isinstance(tp, _AnnotatedAlias):
- return (tp.__origin__,) + tp.__metadata__
- if isinstance(tp, (typing._GenericAlias, GenericAlias)):
- if getattr(tp, "_special", False):
- return ()
- res = tp.__args__
- if get_origin(tp) is collections.abc.Callable and res[0] is not Ellipsis:
- res = (list(res[:-1]), res[-1])
- return res
- return ()
-
-
-# 3.10+
-if hasattr(typing, 'TypeAlias'):
- TypeAlias = typing.TypeAlias
-# 3.9
-elif sys.version_info[:2] >= (3, 9):
- class _TypeAliasForm(typing._SpecialForm, _root=True):
- def __repr__(self):
- return 'typing_extensions.' + self._name
-
- @_TypeAliasForm
- def TypeAlias(self, parameters):
- """Special marker indicating that an assignment should
- be recognized as a proper type alias definition by type
- checkers.
-
- For example::
-
- Predicate: TypeAlias = Callable[..., bool]
-
- It's invalid when used anywhere except as in the example above.
- """
- raise TypeError(f"{self} is not subscriptable")
-# 3.7-3.8
-elif sys.version_info[:2] >= (3, 7):
- class _TypeAliasForm(typing._SpecialForm, _root=True):
- def __repr__(self):
- return 'typing_extensions.' + self._name
-
- TypeAlias = _TypeAliasForm('TypeAlias',
- doc="""Special marker indicating that an assignment should
- be recognized as a proper type alias definition by type
- checkers.
-
- For example::
-
- Predicate: TypeAlias = Callable[..., bool]
-
- It's invalid when used anywhere except as in the example
- above.""")
-# 3.6
-else:
- class _TypeAliasMeta(typing.TypingMeta):
- """Metaclass for TypeAlias"""
-
- def __repr__(self):
- return 'typing_extensions.TypeAlias'
-
- class _TypeAliasBase(typing._FinalTypingBase, metaclass=_TypeAliasMeta, _root=True):
- """Special marker indicating that an assignment should
- be recognized as a proper type alias definition by type
- checkers.
-
- For example::
-
- Predicate: TypeAlias = Callable[..., bool]
-
- It's invalid when used anywhere except as in the example above.
- """
- __slots__ = ()
-
- def __instancecheck__(self, obj):
- raise TypeError("TypeAlias cannot be used with isinstance().")
-
- def __subclasscheck__(self, cls):
- raise TypeError("TypeAlias cannot be used with issubclass().")
-
- def __repr__(self):
- return 'typing_extensions.TypeAlias'
-
- TypeAlias = _TypeAliasBase(_root=True)
-
-
-# Python 3.10+ has PEP 612
-if hasattr(typing, 'ParamSpecArgs'):
- ParamSpecArgs = typing.ParamSpecArgs
- ParamSpecKwargs = typing.ParamSpecKwargs
-# 3.6-3.9
-else:
- class _Immutable:
- """Mixin to indicate that object should not be copied."""
- __slots__ = ()
-
- def __copy__(self):
- return self
-
- def __deepcopy__(self, memo):
- return self
-
- class ParamSpecArgs(_Immutable):
- """The args for a ParamSpec object.
-
- Given a ParamSpec object P, P.args is an instance of ParamSpecArgs.
-
- ParamSpecArgs objects have a reference back to their ParamSpec:
-
- P.args.__origin__ is P
-
- This type is meant for runtime introspection and has no special meaning to
- static type checkers.
- """
- def __init__(self, origin):
- self.__origin__ = origin
-
- def __repr__(self):
- return f"{self.__origin__.__name__}.args"
-
- class ParamSpecKwargs(_Immutable):
- """The kwargs for a ParamSpec object.
-
- Given a ParamSpec object P, P.kwargs is an instance of ParamSpecKwargs.
-
- ParamSpecKwargs objects have a reference back to their ParamSpec:
-
- P.kwargs.__origin__ is P
-
- This type is meant for runtime introspection and has no special meaning to
- static type checkers.
- """
- def __init__(self, origin):
- self.__origin__ = origin
-
- def __repr__(self):
- return f"{self.__origin__.__name__}.kwargs"
-
-# 3.10+
-if hasattr(typing, 'ParamSpec'):
- ParamSpec = typing.ParamSpec
-# 3.6-3.9
-else:
-
- # Inherits from list as a workaround for Callable checks in Python < 3.9.2.
- class ParamSpec(list):
- """Parameter specification variable.
-
- Usage::
-
- P = ParamSpec('P')
-
- Parameter specification variables exist primarily for the benefit of static
- type checkers. They are used to forward the parameter types of one
- callable to another callable, a pattern commonly found in higher order
- functions and decorators. They are only valid when used in ``Concatenate``,
- or s the first argument to ``Callable``. In Python 3.10 and higher,
- they are also supported in user-defined Generics at runtime.
- See class Generic for more information on generic types. An
- example for annotating a decorator::
-
- T = TypeVar('T')
- P = ParamSpec('P')
-
- def add_logging(f: Callable[P, T]) -> Callable[P, T]:
- '''A type-safe decorator to add logging to a function.'''
- def inner(*args: P.args, **kwargs: P.kwargs) -> T:
- logging.info(f'{f.__name__} was called')
- return f(*args, **kwargs)
- return inner
-
- @add_logging
- def add_two(x: float, y: float) -> float:
- '''Add two numbers together.'''
- return x + y
-
- Parameter specification variables defined with covariant=True or
- contravariant=True can be used to declare covariant or contravariant
- generic types. These keyword arguments are valid, but their actual semantics
- are yet to be decided. See PEP 612 for details.
-
- Parameter specification variables can be introspected. e.g.:
-
- P.__name__ == 'T'
- P.__bound__ == None
- P.__covariant__ == False
- P.__contravariant__ == False
-
- Note that only parameter specification variables defined in global scope can
- be pickled.
- """
-
- # Trick Generic __parameters__.
- __class__ = typing.TypeVar
-
- @property
- def args(self):
- return ParamSpecArgs(self)
-
- @property
- def kwargs(self):
- return ParamSpecKwargs(self)
-
- def __init__(self, name, *, bound=None, covariant=False, contravariant=False):
- super().__init__([self])
- self.__name__ = name
- self.__covariant__ = bool(covariant)
- self.__contravariant__ = bool(contravariant)
- if bound:
- self.__bound__ = typing._type_check(bound, 'Bound must be a type.')
- else:
- self.__bound__ = None
-
- # for pickling:
- try:
- def_mod = sys._getframe(1).f_globals.get('__name__', '__main__')
- except (AttributeError, ValueError):
- def_mod = None
- if def_mod != 'typing_extensions':
- self.__module__ = def_mod
-
- def __repr__(self):
- if self.__covariant__:
- prefix = '+'
- elif self.__contravariant__:
- prefix = '-'
- else:
- prefix = '~'
- return prefix + self.__name__
-
- def __hash__(self):
- return object.__hash__(self)
-
- def __eq__(self, other):
- return self is other
-
- def __reduce__(self):
- return self.__name__
-
- # Hack to get typing._type_check to pass.
- def __call__(self, *args, **kwargs):
- pass
-
- if not PEP_560:
- # Only needed in 3.6.
- def _get_type_vars(self, tvars):
- if self not in tvars:
- tvars.append(self)
-
-
-# 3.6-3.9
-if not hasattr(typing, 'Concatenate'):
- # Inherits from list as a workaround for Callable checks in Python < 3.9.2.
- class _ConcatenateGenericAlias(list):
-
- # Trick Generic into looking into this for __parameters__.
- if PEP_560:
- __class__ = typing._GenericAlias
- else:
- __class__ = typing._TypingBase
-
- # Flag in 3.8.
- _special = False
- # Attribute in 3.6 and earlier.
- _gorg = typing.Generic
-
- def __init__(self, origin, args):
- super().__init__(args)
- self.__origin__ = origin
- self.__args__ = args
-
- def __repr__(self):
- _type_repr = typing._type_repr
- return (f'{_type_repr(self.__origin__)}'
- f'[{", ".join(_type_repr(arg) for arg in self.__args__)}]')
-
- def __hash__(self):
- return hash((self.__origin__, self.__args__))
-
- # Hack to get typing._type_check to pass in Generic.
- def __call__(self, *args, **kwargs):
- pass
-
- @property
- def __parameters__(self):
- return tuple(
- tp for tp in self.__args__ if isinstance(tp, (typing.TypeVar, ParamSpec))
- )
-
- if not PEP_560:
- # Only required in 3.6.
- def _get_type_vars(self, tvars):
- if self.__origin__ and self.__parameters__:
- typing._get_type_vars(self.__parameters__, tvars)
-
-
-# 3.6-3.9
-@typing._tp_cache
-def _concatenate_getitem(self, parameters):
- if parameters == ():
- raise TypeError("Cannot take a Concatenate of no types.")
- if not isinstance(parameters, tuple):
- parameters = (parameters,)
- if not isinstance(parameters[-1], ParamSpec):
- raise TypeError("The last parameter to Concatenate should be a "
- "ParamSpec variable.")
- msg = "Concatenate[arg, ...]: each arg must be a type."
- parameters = tuple(typing._type_check(p, msg) for p in parameters)
- return _ConcatenateGenericAlias(self, parameters)
-
-
-# 3.10+
-if hasattr(typing, 'Concatenate'):
- Concatenate = typing.Concatenate
- _ConcatenateGenericAlias = typing._ConcatenateGenericAlias # noqa
-# 3.9
-elif sys.version_info[:2] >= (3, 9):
- @_TypeAliasForm
- def Concatenate(self, parameters):
- """Used in conjunction with ``ParamSpec`` and ``Callable`` to represent a
- higher order function which adds, removes or transforms parameters of a
- callable.
-
- For example::
-
- Callable[Concatenate[int, P], int]
-
- See PEP 612 for detailed information.
- """
- return _concatenate_getitem(self, parameters)
-# 3.7-8
-elif sys.version_info[:2] >= (3, 7):
- class _ConcatenateForm(typing._SpecialForm, _root=True):
- def __repr__(self):
- return 'typing_extensions.' + self._name
-
- def __getitem__(self, parameters):
- return _concatenate_getitem(self, parameters)
-
- Concatenate = _ConcatenateForm(
- 'Concatenate',
- doc="""Used in conjunction with ``ParamSpec`` and ``Callable`` to represent a
- higher order function which adds, removes or transforms parameters of a
- callable.
-
- For example::
-
- Callable[Concatenate[int, P], int]
-
- See PEP 612 for detailed information.
- """)
-# 3.6
-else:
- class _ConcatenateAliasMeta(typing.TypingMeta):
- """Metaclass for Concatenate."""
-
- def __repr__(self):
- return 'typing_extensions.Concatenate'
-
- class _ConcatenateAliasBase(typing._FinalTypingBase,
- metaclass=_ConcatenateAliasMeta,
- _root=True):
- """Used in conjunction with ``ParamSpec`` and ``Callable`` to represent a
- higher order function which adds, removes or transforms parameters of a
- callable.
-
- For example::
-
- Callable[Concatenate[int, P], int]
-
- See PEP 612 for detailed information.
- """
- __slots__ = ()
-
- def __instancecheck__(self, obj):
- raise TypeError("Concatenate cannot be used with isinstance().")
-
- def __subclasscheck__(self, cls):
- raise TypeError("Concatenate cannot be used with issubclass().")
-
- def __repr__(self):
- return 'typing_extensions.Concatenate'
-
- def __getitem__(self, parameters):
- return _concatenate_getitem(self, parameters)
-
- Concatenate = _ConcatenateAliasBase(_root=True)
-
-# 3.10+
-if hasattr(typing, 'TypeGuard'):
- TypeGuard = typing.TypeGuard
-# 3.9
-elif sys.version_info[:2] >= (3, 9):
- class _TypeGuardForm(typing._SpecialForm, _root=True):
- def __repr__(self):
- return 'typing_extensions.' + self._name
-
- @_TypeGuardForm
- def TypeGuard(self, parameters):
- """Special typing form used to annotate the return type of a user-defined
- type guard function. ``TypeGuard`` only accepts a single type argument.
- At runtime, functions marked this way should return a boolean.
-
- ``TypeGuard`` aims to benefit *type narrowing* -- a technique used by static
- type checkers to determine a more precise type of an expression within a
- program's code flow. Usually type narrowing is done by analyzing
- conditional code flow and applying the narrowing to a block of code. The
- conditional expression here is sometimes referred to as a "type guard".
-
- Sometimes it would be convenient to use a user-defined boolean function
- as a type guard. Such a function should use ``TypeGuard[...]`` as its
- return type to alert static type checkers to this intention.
-
- Using ``-> TypeGuard`` tells the static type checker that for a given
- function:
-
- 1. The return value is a boolean.
- 2. If the return value is ``True``, the type of its argument
- is the type inside ``TypeGuard``.
-
- For example::
-
- def is_str(val: Union[str, float]):
- # "isinstance" type guard
- if isinstance(val, str):
- # Type of ``val`` is narrowed to ``str``
- ...
- else:
- # Else, type of ``val`` is narrowed to ``float``.
- ...
-
- Strict type narrowing is not enforced -- ``TypeB`` need not be a narrower
- form of ``TypeA`` (it can even be a wider form) and this may lead to
- type-unsafe results. The main reason is to allow for things like
- narrowing ``List[object]`` to ``List[str]`` even though the latter is not
- a subtype of the former, since ``List`` is invariant. The responsibility of
- writing type-safe type guards is left to the user.
-
- ``TypeGuard`` also works with type variables. For more information, see
- PEP 647 (User-Defined Type Guards).
- """
- item = typing._type_check(parameters, f'{self} accepts only single type.')
- return typing._GenericAlias(self, (item,))
-# 3.7-3.8
-elif sys.version_info[:2] >= (3, 7):
- class _TypeGuardForm(typing._SpecialForm, _root=True):
-
- def __repr__(self):
- return 'typing_extensions.' + self._name
-
- def __getitem__(self, parameters):
- item = typing._type_check(parameters,
- f'{self._name} accepts only a single type')
- return typing._GenericAlias(self, (item,))
-
- TypeGuard = _TypeGuardForm(
- 'TypeGuard',
- doc="""Special typing form used to annotate the return type of a user-defined
- type guard function. ``TypeGuard`` only accepts a single type argument.
- At runtime, functions marked this way should return a boolean.
-
- ``TypeGuard`` aims to benefit *type narrowing* -- a technique used by static
- type checkers to determine a more precise type of an expression within a
- program's code flow. Usually type narrowing is done by analyzing
- conditional code flow and applying the narrowing to a block of code. The
- conditional expression here is sometimes referred to as a "type guard".
-
- Sometimes it would be convenient to use a user-defined boolean function
- as a type guard. Such a function should use ``TypeGuard[...]`` as its
- return type to alert static type checkers to this intention.
-
- Using ``-> TypeGuard`` tells the static type checker that for a given
- function:
-
- 1. The return value is a boolean.
- 2. If the return value is ``True``, the type of its argument
- is the type inside ``TypeGuard``.
-
- For example::
-
- def is_str(val: Union[str, float]):
- # "isinstance" type guard
- if isinstance(val, str):
- # Type of ``val`` is narrowed to ``str``
- ...
- else:
- # Else, type of ``val`` is narrowed to ``float``.
- ...
-
- Strict type narrowing is not enforced -- ``TypeB`` need not be a narrower
- form of ``TypeA`` (it can even be a wider form) and this may lead to
- type-unsafe results. The main reason is to allow for things like
- narrowing ``List[object]`` to ``List[str]`` even though the latter is not
- a subtype of the former, since ``List`` is invariant. The responsibility of
- writing type-safe type guards is left to the user.
-
- ``TypeGuard`` also works with type variables. For more information, see
- PEP 647 (User-Defined Type Guards).
- """)
-# 3.6
-else:
- class _TypeGuard(typing._FinalTypingBase, _root=True):
- """Special typing form used to annotate the return type of a user-defined
- type guard function. ``TypeGuard`` only accepts a single type argument.
- At runtime, functions marked this way should return a boolean.
-
- ``TypeGuard`` aims to benefit *type narrowing* -- a technique used by static
- type checkers to determine a more precise type of an expression within a
- program's code flow. Usually type narrowing is done by analyzing
- conditional code flow and applying the narrowing to a block of code. The
- conditional expression here is sometimes referred to as a "type guard".
-
- Sometimes it would be convenient to use a user-defined boolean function
- as a type guard. Such a function should use ``TypeGuard[...]`` as its
- return type to alert static type checkers to this intention.
-
- Using ``-> TypeGuard`` tells the static type checker that for a given
- function:
-
- 1. The return value is a boolean.
- 2. If the return value is ``True``, the type of its argument
- is the type inside ``TypeGuard``.
-
- For example::
-
- def is_str(val: Union[str, float]):
- # "isinstance" type guard
- if isinstance(val, str):
- # Type of ``val`` is narrowed to ``str``
- ...
- else:
- # Else, type of ``val`` is narrowed to ``float``.
- ...
-
- Strict type narrowing is not enforced -- ``TypeB`` need not be a narrower
- form of ``TypeA`` (it can even be a wider form) and this may lead to
- type-unsafe results. The main reason is to allow for things like
- narrowing ``List[object]`` to ``List[str]`` even though the latter is not
- a subtype of the former, since ``List`` is invariant. The responsibility of
- writing type-safe type guards is left to the user.
-
- ``TypeGuard`` also works with type variables. For more information, see
- PEP 647 (User-Defined Type Guards).
- """
-
- __slots__ = ('__type__',)
-
- def __init__(self, tp=None, **kwds):
- self.__type__ = tp
-
- def __getitem__(self, item):
- cls = type(self)
- if self.__type__ is None:
- return cls(typing._type_check(item,
- f'{cls.__name__[1:]} accepts only a single type.'),
- _root=True)
- raise TypeError(f'{cls.__name__[1:]} cannot be further subscripted')
-
- def _eval_type(self, globalns, localns):
- new_tp = typing._eval_type(self.__type__, globalns, localns)
- if new_tp == self.__type__:
- return self
- return type(self)(new_tp, _root=True)
-
- def __repr__(self):
- r = super().__repr__()
- if self.__type__ is not None:
- r += f'[{typing._type_repr(self.__type__)}]'
- return r
-
- def __hash__(self):
- return hash((type(self).__name__, self.__type__))
-
- def __eq__(self, other):
- if not isinstance(other, _TypeGuard):
- return NotImplemented
- if self.__type__ is not None:
- return self.__type__ == other.__type__
- return self is other
-
- TypeGuard = _TypeGuard(_root=True)
-
-if hasattr(typing, "Self"):
- Self = typing.Self
-elif sys.version_info[:2] >= (3, 7):
- # Vendored from cpython typing._SpecialFrom
- class _SpecialForm(typing._Final, _root=True):
- __slots__ = ('_name', '__doc__', '_getitem')
-
- def __init__(self, getitem):
- self._getitem = getitem
- self._name = getitem.__name__
- self.__doc__ = getitem.__doc__
-
- def __getattr__(self, item):
- if item in {'__name__', '__qualname__'}:
- return self._name
-
- raise AttributeError(item)
-
- def __mro_entries__(self, bases):
- raise TypeError(f"Cannot subclass {self!r}")
-
- def __repr__(self):
- return f'typing_extensions.{self._name}'
-
- def __reduce__(self):
- return self._name
-
- def __call__(self, *args, **kwds):
- raise TypeError(f"Cannot instantiate {self!r}")
-
- def __or__(self, other):
- return typing.Union[self, other]
-
- def __ror__(self, other):
- return typing.Union[other, self]
-
- def __instancecheck__(self, obj):
- raise TypeError(f"{self} cannot be used with isinstance()")
-
- def __subclasscheck__(self, cls):
- raise TypeError(f"{self} cannot be used with issubclass()")
-
- @typing._tp_cache
- def __getitem__(self, parameters):
- return self._getitem(self, parameters)
-
- @_SpecialForm
- def Self(self, params):
- """Used to spell the type of "self" in classes.
-
- Example::
-
- from typing import Self
-
- class ReturnsSelf:
- def parse(self, data: bytes) -> Self:
- ...
- return self
-
- """
-
- raise TypeError(f"{self} is not subscriptable")
-else:
- class _Self(typing._FinalTypingBase, _root=True):
- """Used to spell the type of "self" in classes.
-
- Example::
-
- from typing import Self
-
- class ReturnsSelf:
- def parse(self, data: bytes) -> Self:
- ...
- return self
-
- """
-
- __slots__ = ()
-
- def __instancecheck__(self, obj):
- raise TypeError(f"{self} cannot be used with isinstance().")
-
- def __subclasscheck__(self, cls):
- raise TypeError(f"{self} cannot be used with issubclass().")
-
- Self = _Self(_root=True)
-
-
-if hasattr(typing, 'Required'):
- Required = typing.Required
- NotRequired = typing.NotRequired
-elif sys.version_info[:2] >= (3, 9):
- class _ExtensionsSpecialForm(typing._SpecialForm, _root=True):
- def __repr__(self):
- return 'typing_extensions.' + self._name
-
- @_ExtensionsSpecialForm
- def Required(self, parameters):
- """A special typing construct to mark a key of a total=False TypedDict
- as required. For example:
-
- class Movie(TypedDict, total=False):
- title: Required[str]
- year: int
-
- m = Movie(
- title='The Matrix', # typechecker error if key is omitted
- year=1999,
- )
-
- There is no runtime checking that a required key is actually provided
- when instantiating a related TypedDict.
- """
- item = typing._type_check(parameters, f'{self._name} accepts only single type')
- return typing._GenericAlias(self, (item,))
-
- @_ExtensionsSpecialForm
- def NotRequired(self, parameters):
- """A special typing construct to mark a key of a TypedDict as
- potentially missing. For example:
-
- class Movie(TypedDict):
- title: str
- year: NotRequired[int]
-
- m = Movie(
- title='The Matrix', # typechecker error if key is omitted
- year=1999,
- )
- """
- item = typing._type_check(parameters, f'{self._name} accepts only single type')
- return typing._GenericAlias(self, (item,))
-
-elif sys.version_info[:2] >= (3, 7):
- class _RequiredForm(typing._SpecialForm, _root=True):
- def __repr__(self):
- return 'typing_extensions.' + self._name
-
- def __getitem__(self, parameters):
- item = typing._type_check(parameters,
- '{} accepts only single type'.format(self._name))
- return typing._GenericAlias(self, (item,))
-
- Required = _RequiredForm(
- 'Required',
- doc="""A special typing construct to mark a key of a total=False TypedDict
- as required. For example:
-
- class Movie(TypedDict, total=False):
- title: Required[str]
- year: int
-
- m = Movie(
- title='The Matrix', # typechecker error if key is omitted
- year=1999,
- )
-
- There is no runtime checking that a required key is actually provided
- when instantiating a related TypedDict.
- """)
- NotRequired = _RequiredForm(
- 'NotRequired',
- doc="""A special typing construct to mark a key of a TypedDict as
- potentially missing. For example:
-
- class Movie(TypedDict):
- title: str
- year: NotRequired[int]
-
- m = Movie(
- title='The Matrix', # typechecker error if key is omitted
- year=1999,
- )
- """)
-else:
- # NOTE: Modeled after _Final's implementation when _FinalTypingBase available
- class _MaybeRequired(typing._FinalTypingBase, _root=True):
- __slots__ = ('__type__',)
-
- def __init__(self, tp=None, **kwds):
- self.__type__ = tp
-
- def __getitem__(self, item):
- cls = type(self)
- if self.__type__ is None:
- return cls(typing._type_check(item,
- '{} accepts only single type.'.format(cls.__name__[1:])),
- _root=True)
- raise TypeError('{} cannot be further subscripted'
- .format(cls.__name__[1:]))
-
- def _eval_type(self, globalns, localns):
- new_tp = typing._eval_type(self.__type__, globalns, localns)
- if new_tp == self.__type__:
- return self
- return type(self)(new_tp, _root=True)
-
- def __repr__(self):
- r = super().__repr__()
- if self.__type__ is not None:
- r += '[{}]'.format(typing._type_repr(self.__type__))
- return r
-
- def __hash__(self):
- return hash((type(self).__name__, self.__type__))
-
- def __eq__(self, other):
- if not isinstance(other, type(self)):
- return NotImplemented
- if self.__type__ is not None:
- return self.__type__ == other.__type__
- return self is other
-
- class _Required(_MaybeRequired, _root=True):
- """A special typing construct to mark a key of a total=False TypedDict
- as required. For example:
-
- class Movie(TypedDict, total=False):
- title: Required[str]
- year: int
-
- m = Movie(
- title='The Matrix', # typechecker error if key is omitted
- year=1999,
- )
-
- There is no runtime checking that a required key is actually provided
- when instantiating a related TypedDict.
- """
-
- class _NotRequired(_MaybeRequired, _root=True):
- """A special typing construct to mark a key of a TypedDict as
- potentially missing. For example:
-
- class Movie(TypedDict):
- title: str
- year: NotRequired[int]
-
- m = Movie(
- title='The Matrix', # typechecker error if key is omitted
- year=1999,
- )
- """
-
- Required = _Required(_root=True)
- NotRequired = _NotRequired(_root=True)
diff --git a/spaces/Atualli/node-media-server/app.js b/spaces/Atualli/node-media-server/app.js
deleted file mode 100644
index 56bd1b94edbd59824dbab8da12b0fc76afd50920..0000000000000000000000000000000000000000
--- a/spaces/Atualli/node-media-server/app.js
+++ /dev/null
@@ -1,18 +0,0 @@
-const NodeMediaServer = require('node-media-server');
-
-const config = {
- rtmp: {
- port: 7861,
- chunk_size: 60000,
- gop_cache: true,
- ping: 30,
- ping_timeout: 60
- },
- http: {
- port: 7860,
- allow_origin: '*'
- }
-};
-
-var nms = new NodeMediaServer(config)
-nms.run();
\ No newline at end of file
diff --git a/spaces/Awiny/Image2Paragraph/models/grit_src/third_party/CenterNet2/detectron2/config/instantiate.py b/spaces/Awiny/Image2Paragraph/models/grit_src/third_party/CenterNet2/detectron2/config/instantiate.py
deleted file mode 100644
index cbb32e19ea518eee84941b20f58d1054e84d1937..0000000000000000000000000000000000000000
--- a/spaces/Awiny/Image2Paragraph/models/grit_src/third_party/CenterNet2/detectron2/config/instantiate.py
+++ /dev/null
@@ -1,82 +0,0 @@
-# Copyright (c) Facebook, Inc. and its affiliates.
-import dataclasses
-import logging
-from collections import abc
-from typing import Any
-
-from detectron2.utils.registry import _convert_target_to_string, locate
-
-__all__ = ["dump_dataclass", "instantiate"]
-
-
-def dump_dataclass(obj: Any):
- """
- Dump a dataclass recursively into a dict that can be later instantiated.
-
- Args:
- obj: a dataclass object
-
- Returns:
- dict
- """
- assert dataclasses.is_dataclass(obj) and not isinstance(
- obj, type
- ), "dump_dataclass() requires an instance of a dataclass."
- ret = {"_target_": _convert_target_to_string(type(obj))}
- for f in dataclasses.fields(obj):
- v = getattr(obj, f.name)
- if dataclasses.is_dataclass(v):
- v = dump_dataclass(v)
- if isinstance(v, (list, tuple)):
- v = [dump_dataclass(x) if dataclasses.is_dataclass(x) else x for x in v]
- ret[f.name] = v
- return ret
-
-
-def instantiate(cfg):
- """
- Recursively instantiate objects defined in dictionaries by
- "_target_" and arguments.
-
- Args:
- cfg: a dict-like object with "_target_" that defines the caller, and
- other keys that define the arguments
-
- Returns:
- object instantiated by cfg
- """
- from omegaconf import ListConfig
-
- if isinstance(cfg, ListConfig):
- lst = [instantiate(x) for x in cfg]
- return ListConfig(lst, flags={"allow_objects": True})
- if isinstance(cfg, list):
- # Specialize for list, because many classes take
- # list[objects] as arguments, such as ResNet, DatasetMapper
- return [instantiate(x) for x in cfg]
-
- if isinstance(cfg, abc.Mapping) and "_target_" in cfg:
- # conceptually equivalent to hydra.utils.instantiate(cfg) with _convert_=all,
- # but faster: https://github.com/facebookresearch/hydra/issues/1200
- cfg = {k: instantiate(v) for k, v in cfg.items()}
- cls = cfg.pop("_target_")
- cls = instantiate(cls)
-
- if isinstance(cls, str):
- cls_name = cls
- cls = locate(cls_name)
- assert cls is not None, cls_name
- else:
- try:
- cls_name = cls.__module__ + "." + cls.__qualname__
- except Exception:
- # target could be anything, so the above could fail
- cls_name = str(cls)
- assert callable(cls), f"_target_ {cls} does not define a callable object"
- try:
- return cls(**cfg)
- except TypeError:
- logger = logging.getLogger(__name__)
- logger.error(f"Error when instantiating {cls_name}!")
- raise
- return cfg # return as-is if don't know what to do
diff --git a/spaces/Awiny/Image2Paragraph/models/grit_src/third_party/CenterNet2/detectron2/layers/mask_ops.py b/spaces/Awiny/Image2Paragraph/models/grit_src/third_party/CenterNet2/detectron2/layers/mask_ops.py
deleted file mode 100644
index e7a9f3a323ddbe75845b668ee6b40c5385d206c3..0000000000000000000000000000000000000000
--- a/spaces/Awiny/Image2Paragraph/models/grit_src/third_party/CenterNet2/detectron2/layers/mask_ops.py
+++ /dev/null
@@ -1,275 +0,0 @@
-# Copyright (c) Facebook, Inc. and its affiliates.
-import numpy as np
-from typing import Tuple
-import torch
-from PIL import Image
-from torch.nn import functional as F
-
-__all__ = ["paste_masks_in_image"]
-
-
-BYTES_PER_FLOAT = 4
-# TODO: This memory limit may be too much or too little. It would be better to
-# determine it based on available resources.
-GPU_MEM_LIMIT = 1024 ** 3 # 1 GB memory limit
-
-
-def _do_paste_mask(masks, boxes, img_h: int, img_w: int, skip_empty: bool = True):
- """
- Args:
- masks: N, 1, H, W
- boxes: N, 4
- img_h, img_w (int):
- skip_empty (bool): only paste masks within the region that
- tightly bound all boxes, and returns the results this region only.
- An important optimization for CPU.
-
- Returns:
- if skip_empty == False, a mask of shape (N, img_h, img_w)
- if skip_empty == True, a mask of shape (N, h', w'), and the slice
- object for the corresponding region.
- """
- # On GPU, paste all masks together (up to chunk size)
- # by using the entire image to sample the masks
- # Compared to pasting them one by one,
- # this has more operations but is faster on COCO-scale dataset.
- device = masks.device
-
- if skip_empty and not torch.jit.is_scripting():
- x0_int, y0_int = torch.clamp(boxes.min(dim=0).values.floor()[:2] - 1, min=0).to(
- dtype=torch.int32
- )
- x1_int = torch.clamp(boxes[:, 2].max().ceil() + 1, max=img_w).to(dtype=torch.int32)
- y1_int = torch.clamp(boxes[:, 3].max().ceil() + 1, max=img_h).to(dtype=torch.int32)
- else:
- x0_int, y0_int = 0, 0
- x1_int, y1_int = img_w, img_h
- x0, y0, x1, y1 = torch.split(boxes, 1, dim=1) # each is Nx1
-
- N = masks.shape[0]
-
- img_y = torch.arange(y0_int, y1_int, device=device, dtype=torch.float32) + 0.5
- img_x = torch.arange(x0_int, x1_int, device=device, dtype=torch.float32) + 0.5
- img_y = (img_y - y0) / (y1 - y0) * 2 - 1
- img_x = (img_x - x0) / (x1 - x0) * 2 - 1
- # img_x, img_y have shapes (N, w), (N, h)
-
- gx = img_x[:, None, :].expand(N, img_y.size(1), img_x.size(1))
- gy = img_y[:, :, None].expand(N, img_y.size(1), img_x.size(1))
- grid = torch.stack([gx, gy], dim=3)
-
- if not torch.jit.is_scripting():
- if not masks.dtype.is_floating_point:
- masks = masks.float()
- img_masks = F.grid_sample(masks, grid.to(masks.dtype), align_corners=False)
-
- if skip_empty and not torch.jit.is_scripting():
- return img_masks[:, 0], (slice(y0_int, y1_int), slice(x0_int, x1_int))
- else:
- return img_masks[:, 0], ()
-
-
-# Annotate boxes as Tensor (but not Boxes) in order to use scripting
-@torch.jit.script_if_tracing
-def paste_masks_in_image(
- masks: torch.Tensor, boxes: torch.Tensor, image_shape: Tuple[int, int], threshold: float = 0.5
-):
- """
- Paste a set of masks that are of a fixed resolution (e.g., 28 x 28) into an image.
- The location, height, and width for pasting each mask is determined by their
- corresponding bounding boxes in boxes.
-
- Note:
- This is a complicated but more accurate implementation. In actual deployment, it is
- often enough to use a faster but less accurate implementation.
- See :func:`paste_mask_in_image_old` in this file for an alternative implementation.
-
- Args:
- masks (tensor): Tensor of shape (Bimg, Hmask, Wmask), where Bimg is the number of
- detected object instances in the image and Hmask, Wmask are the mask width and mask
- height of the predicted mask (e.g., Hmask = Wmask = 28). Values are in [0, 1].
- boxes (Boxes or Tensor): A Boxes of length Bimg or Tensor of shape (Bimg, 4).
- boxes[i] and masks[i] correspond to the same object instance.
- image_shape (tuple): height, width
- threshold (float): A threshold in [0, 1] for converting the (soft) masks to
- binary masks.
-
- Returns:
- img_masks (Tensor): A tensor of shape (Bimg, Himage, Wimage), where Bimg is the
- number of detected object instances and Himage, Wimage are the image width
- and height. img_masks[i] is a binary mask for object instance i.
- """
-
- assert masks.shape[-1] == masks.shape[-2], "Only square mask predictions are supported"
- N = len(masks)
- if N == 0:
- return masks.new_empty((0,) + image_shape, dtype=torch.uint8)
- if not isinstance(boxes, torch.Tensor):
- boxes = boxes.tensor
- device = boxes.device
- assert len(boxes) == N, boxes.shape
-
- img_h, img_w = image_shape
-
- # The actual implementation split the input into chunks,
- # and paste them chunk by chunk.
- if device.type == "cpu" or torch.jit.is_scripting():
- # CPU is most efficient when they are pasted one by one with skip_empty=True
- # so that it performs minimal number of operations.
- num_chunks = N
- else:
- # GPU benefits from parallelism for larger chunks, but may have memory issue
- # int(img_h) because shape may be tensors in tracing
- num_chunks = int(np.ceil(N * int(img_h) * int(img_w) * BYTES_PER_FLOAT / GPU_MEM_LIMIT))
- assert (
- num_chunks <= N
- ), "Default GPU_MEM_LIMIT in mask_ops.py is too small; try increasing it"
- chunks = torch.chunk(torch.arange(N, device=device), num_chunks)
-
- img_masks = torch.zeros(
- N, img_h, img_w, device=device, dtype=torch.bool if threshold >= 0 else torch.uint8
- )
- for inds in chunks:
- masks_chunk, spatial_inds = _do_paste_mask(
- masks[inds, None, :, :], boxes[inds], img_h, img_w, skip_empty=device.type == "cpu"
- )
-
- if threshold >= 0:
- masks_chunk = (masks_chunk >= threshold).to(dtype=torch.bool)
- else:
- # for visualization and debugging
- masks_chunk = (masks_chunk * 255).to(dtype=torch.uint8)
-
- if torch.jit.is_scripting(): # Scripting does not use the optimized codepath
- img_masks[inds] = masks_chunk
- else:
- img_masks[(inds,) + spatial_inds] = masks_chunk
- return img_masks
-
-
-# The below are the original paste function (from Detectron1) which has
-# larger quantization error.
-# It is faster on CPU, while the aligned one is faster on GPU thanks to grid_sample.
-
-
-def paste_mask_in_image_old(mask, box, img_h, img_w, threshold):
- """
- Paste a single mask in an image.
- This is a per-box implementation of :func:`paste_masks_in_image`.
- This function has larger quantization error due to incorrect pixel
- modeling and is not used any more.
-
- Args:
- mask (Tensor): A tensor of shape (Hmask, Wmask) storing the mask of a single
- object instance. Values are in [0, 1].
- box (Tensor): A tensor of shape (4, ) storing the x0, y0, x1, y1 box corners
- of the object instance.
- img_h, img_w (int): Image height and width.
- threshold (float): Mask binarization threshold in [0, 1].
-
- Returns:
- im_mask (Tensor):
- The resized and binarized object mask pasted into the original
- image plane (a tensor of shape (img_h, img_w)).
- """
- # Conversion from continuous box coordinates to discrete pixel coordinates
- # via truncation (cast to int32). This determines which pixels to paste the
- # mask onto.
- box = box.to(dtype=torch.int32) # Continuous to discrete coordinate conversion
- # An example (1D) box with continuous coordinates (x0=0.7, x1=4.3) will map to
- # a discrete coordinates (x0=0, x1=4). Note that box is mapped to 5 = x1 - x0 + 1
- # pixels (not x1 - x0 pixels).
- samples_w = box[2] - box[0] + 1 # Number of pixel samples, *not* geometric width
- samples_h = box[3] - box[1] + 1 # Number of pixel samples, *not* geometric height
-
- # Resample the mask from it's original grid to the new samples_w x samples_h grid
- mask = Image.fromarray(mask.cpu().numpy())
- mask = mask.resize((samples_w, samples_h), resample=Image.BILINEAR)
- mask = np.array(mask, copy=False)
-
- if threshold >= 0:
- mask = np.array(mask > threshold, dtype=np.uint8)
- mask = torch.from_numpy(mask)
- else:
- # for visualization and debugging, we also
- # allow it to return an unmodified mask
- mask = torch.from_numpy(mask * 255).to(torch.uint8)
-
- im_mask = torch.zeros((img_h, img_w), dtype=torch.uint8)
- x_0 = max(box[0], 0)
- x_1 = min(box[2] + 1, img_w)
- y_0 = max(box[1], 0)
- y_1 = min(box[3] + 1, img_h)
-
- im_mask[y_0:y_1, x_0:x_1] = mask[
- (y_0 - box[1]) : (y_1 - box[1]), (x_0 - box[0]) : (x_1 - box[0])
- ]
- return im_mask
-
-
-# Our pixel modeling requires extrapolation for any continuous
-# coordinate < 0.5 or > length - 0.5. When sampling pixels on the masks,
-# we would like this extrapolation to be an interpolation between boundary values and zero,
-# instead of using absolute zero or boundary values.
-# Therefore `paste_mask_in_image_old` is often used with zero padding around the masks like this:
-# masks, scale = pad_masks(masks[:, 0, :, :], 1)
-# boxes = scale_boxes(boxes.tensor, scale)
-
-
-def pad_masks(masks, padding):
- """
- Args:
- masks (tensor): A tensor of shape (B, M, M) representing B masks.
- padding (int): Number of cells to pad on all sides.
-
- Returns:
- The padded masks and the scale factor of the padding size / original size.
- """
- B = masks.shape[0]
- M = masks.shape[-1]
- pad2 = 2 * padding
- scale = float(M + pad2) / M
- padded_masks = masks.new_zeros((B, M + pad2, M + pad2))
- padded_masks[:, padding:-padding, padding:-padding] = masks
- return padded_masks, scale
-
-
-def scale_boxes(boxes, scale):
- """
- Args:
- boxes (tensor): A tensor of shape (B, 4) representing B boxes with 4
- coords representing the corners x0, y0, x1, y1,
- scale (float): The box scaling factor.
-
- Returns:
- Scaled boxes.
- """
- w_half = (boxes[:, 2] - boxes[:, 0]) * 0.5
- h_half = (boxes[:, 3] - boxes[:, 1]) * 0.5
- x_c = (boxes[:, 2] + boxes[:, 0]) * 0.5
- y_c = (boxes[:, 3] + boxes[:, 1]) * 0.5
-
- w_half *= scale
- h_half *= scale
-
- scaled_boxes = torch.zeros_like(boxes)
- scaled_boxes[:, 0] = x_c - w_half
- scaled_boxes[:, 2] = x_c + w_half
- scaled_boxes[:, 1] = y_c - h_half
- scaled_boxes[:, 3] = y_c + h_half
- return scaled_boxes
-
-
-@torch.jit.script_if_tracing
-def _paste_masks_tensor_shape(
- masks: torch.Tensor,
- boxes: torch.Tensor,
- image_shape: Tuple[torch.Tensor, torch.Tensor],
- threshold: float = 0.5,
-):
- """
- A wrapper of paste_masks_in_image where image_shape is Tensor.
- During tracing, shapes might be tensors instead of ints. The Tensor->int
- conversion should be scripted rather than traced.
- """
- return paste_masks_in_image(masks, boxes, (int(image_shape[0]), int(image_shape[1])), threshold)
diff --git a/spaces/Awiny/Image2Paragraph/models/grit_src/third_party/CenterNet2/tests/modeling/test_model_e2e.py b/spaces/Awiny/Image2Paragraph/models/grit_src/third_party/CenterNet2/tests/modeling/test_model_e2e.py
deleted file mode 100644
index 5da35205eba60c739b8a919121f4e9a85a24138b..0000000000000000000000000000000000000000
--- a/spaces/Awiny/Image2Paragraph/models/grit_src/third_party/CenterNet2/tests/modeling/test_model_e2e.py
+++ /dev/null
@@ -1,223 +0,0 @@
-# Copyright (c) Facebook, Inc. and its affiliates.
-
-
-import itertools
-import unittest
-from contextlib import contextmanager
-from copy import deepcopy
-import torch
-
-from detectron2.structures import BitMasks, Boxes, ImageList, Instances
-from detectron2.utils.events import EventStorage
-from detectron2.utils.testing import get_model_no_weights
-
-
-@contextmanager
-def typecheck_hook(model, *, in_dtype=None, out_dtype=None):
- """
- Check that the model must be called with the given input/output dtype
- """
- if not isinstance(in_dtype, set):
- in_dtype = {in_dtype}
- if not isinstance(out_dtype, set):
- out_dtype = {out_dtype}
-
- def flatten(x):
- if isinstance(x, torch.Tensor):
- return [x]
- if isinstance(x, (list, tuple)):
- return list(itertools.chain(*[flatten(t) for t in x]))
- if isinstance(x, dict):
- return flatten(list(x.values()))
- return []
-
- def hook(module, input, output):
- if in_dtype is not None:
- dtypes = {x.dtype for x in flatten(input)}
- assert (
- dtypes == in_dtype
- ), f"Expected input dtype of {type(module)} is {in_dtype}. Got {dtypes} instead!"
-
- if out_dtype is not None:
- dtypes = {x.dtype for x in flatten(output)}
- assert (
- dtypes == out_dtype
- ), f"Expected output dtype of {type(module)} is {out_dtype}. Got {dtypes} instead!"
-
- with model.register_forward_hook(hook):
- yield
-
-
-def create_model_input(img, inst=None):
- if inst is not None:
- return {"image": img, "instances": inst}
- else:
- return {"image": img}
-
-
-def get_empty_instance(h, w):
- inst = Instances((h, w))
- inst.gt_boxes = Boxes(torch.rand(0, 4))
- inst.gt_classes = torch.tensor([]).to(dtype=torch.int64)
- inst.gt_masks = BitMasks(torch.rand(0, h, w))
- return inst
-
-
-def get_regular_bitmask_instances(h, w):
- inst = Instances((h, w))
- inst.gt_boxes = Boxes(torch.rand(3, 4))
- inst.gt_boxes.tensor[:, 2:] += inst.gt_boxes.tensor[:, :2]
- inst.gt_classes = torch.tensor([3, 4, 5]).to(dtype=torch.int64)
- inst.gt_masks = BitMasks((torch.rand(3, h, w) > 0.5))
- return inst
-
-
-class InstanceModelE2ETest:
- def setUp(self):
- torch.manual_seed(43)
- self.model = get_model_no_weights(self.CONFIG_PATH)
-
- def _test_eval(self, input_sizes):
- inputs = [create_model_input(torch.rand(3, s[0], s[1])) for s in input_sizes]
- self.model.eval()
- self.model(inputs)
-
- def _test_train(self, input_sizes, instances):
- assert len(input_sizes) == len(instances)
- inputs = [
- create_model_input(torch.rand(3, s[0], s[1]), inst)
- for s, inst in zip(input_sizes, instances)
- ]
- self.model.train()
- with EventStorage():
- losses = self.model(inputs)
- sum(losses.values()).backward()
- del losses
-
- def _inf_tensor(self, *shape):
- return 1.0 / torch.zeros(*shape, device=self.model.device)
-
- def _nan_tensor(self, *shape):
- return torch.zeros(*shape, device=self.model.device).fill_(float("nan"))
-
- def test_empty_data(self):
- instances = [get_empty_instance(200, 250), get_empty_instance(200, 249)]
- self._test_eval([(200, 250), (200, 249)])
- self._test_train([(200, 250), (200, 249)], instances)
-
- @unittest.skipIf(not torch.cuda.is_available(), "CUDA unavailable")
- def test_eval_tocpu(self):
- model = deepcopy(self.model).cpu()
- model.eval()
- input_sizes = [(200, 250), (200, 249)]
- inputs = [create_model_input(torch.rand(3, s[0], s[1])) for s in input_sizes]
- model(inputs)
-
-
-class MaskRCNNE2ETest(InstanceModelE2ETest, unittest.TestCase):
- CONFIG_PATH = "COCO-InstanceSegmentation/mask_rcnn_R_50_FPN_1x.yaml"
-
- def test_half_empty_data(self):
- instances = [get_empty_instance(200, 250), get_regular_bitmask_instances(200, 249)]
- self._test_train([(200, 250), (200, 249)], instances)
-
- # This test is flaky because in some environment the output features are zero due to relu
- # def test_rpn_inf_nan_data(self):
- # self.model.eval()
- # for tensor in [self._inf_tensor, self._nan_tensor]:
- # images = ImageList(tensor(1, 3, 512, 512), [(510, 510)])
- # features = {
- # "p2": tensor(1, 256, 256, 256),
- # "p3": tensor(1, 256, 128, 128),
- # "p4": tensor(1, 256, 64, 64),
- # "p5": tensor(1, 256, 32, 32),
- # "p6": tensor(1, 256, 16, 16),
- # }
- # props, _ = self.model.proposal_generator(images, features)
- # self.assertEqual(len(props[0]), 0)
-
- def test_roiheads_inf_nan_data(self):
- self.model.eval()
- for tensor in [self._inf_tensor, self._nan_tensor]:
- images = ImageList(tensor(1, 3, 512, 512), [(510, 510)])
- features = {
- "p2": tensor(1, 256, 256, 256),
- "p3": tensor(1, 256, 128, 128),
- "p4": tensor(1, 256, 64, 64),
- "p5": tensor(1, 256, 32, 32),
- "p6": tensor(1, 256, 16, 16),
- }
- props = [Instances((510, 510))]
- props[0].proposal_boxes = Boxes([[10, 10, 20, 20]]).to(device=self.model.device)
- props[0].objectness_logits = torch.tensor([1.0]).reshape(1, 1)
- det, _ = self.model.roi_heads(images, features, props)
- self.assertEqual(len(det[0]), 0)
-
- @unittest.skipIf(not torch.cuda.is_available(), "CUDA not available")
- def test_autocast(self):
- from torch.cuda.amp import autocast
-
- inputs = [{"image": torch.rand(3, 100, 100)}]
- self.model.eval()
- with autocast(), typecheck_hook(
- self.model.backbone, in_dtype=torch.float32, out_dtype=torch.float16
- ), typecheck_hook(
- self.model.roi_heads.box_predictor, in_dtype=torch.float16, out_dtype=torch.float16
- ):
- out = self.model.inference(inputs, do_postprocess=False)[0]
- self.assertEqual(out.pred_boxes.tensor.dtype, torch.float32)
- self.assertEqual(out.pred_masks.dtype, torch.float16)
- self.assertEqual(out.scores.dtype, torch.float32) # scores comes from softmax
-
-
-class RetinaNetE2ETest(InstanceModelE2ETest, unittest.TestCase):
- CONFIG_PATH = "COCO-Detection/retinanet_R_50_FPN_1x.yaml"
-
- def test_inf_nan_data(self):
- self.model.eval()
- self.model.score_threshold = -999999999
- for tensor in [self._inf_tensor, self._nan_tensor]:
- images = ImageList(tensor(1, 3, 512, 512), [(510, 510)])
- features = [
- tensor(1, 256, 128, 128),
- tensor(1, 256, 64, 64),
- tensor(1, 256, 32, 32),
- tensor(1, 256, 16, 16),
- tensor(1, 256, 8, 8),
- ]
- pred_logits, pred_anchor_deltas = self.model.head(features)
- pred_logits = [tensor(*x.shape) for x in pred_logits]
- pred_anchor_deltas = [tensor(*x.shape) for x in pred_anchor_deltas]
- det = self.model.forward_inference(images, features, [pred_logits, pred_anchor_deltas])
- # all predictions (if any) are infinite or nan
- if len(det[0]):
- self.assertTrue(torch.isfinite(det[0].pred_boxes.tensor).sum() == 0)
-
- @unittest.skipIf(not torch.cuda.is_available(), "CUDA not available")
- def test_autocast(self):
- from torch.cuda.amp import autocast
-
- inputs = [{"image": torch.rand(3, 100, 100)}]
- self.model.eval()
- with autocast(), typecheck_hook(
- self.model.backbone, in_dtype=torch.float32, out_dtype=torch.float16
- ), typecheck_hook(self.model.head, in_dtype=torch.float16, out_dtype=torch.float16):
- out = self.model(inputs)[0]["instances"]
- self.assertEqual(out.pred_boxes.tensor.dtype, torch.float32)
- self.assertEqual(out.scores.dtype, torch.float16)
-
-
-class SemSegE2ETest(unittest.TestCase):
- CONFIG_PATH = "Misc/semantic_R_50_FPN_1x.yaml"
-
- def setUp(self):
- torch.manual_seed(43)
- self.model = get_model_no_weights(self.CONFIG_PATH)
-
- def _test_eval(self, input_sizes):
- inputs = [create_model_input(torch.rand(3, s[0], s[1])) for s in input_sizes]
- self.model.eval()
- self.model(inputs)
-
- def test_forward(self):
- self._test_eval([(200, 250), (200, 249)])
diff --git a/spaces/Bart92/RVC_HF/julius/bands.py b/spaces/Bart92/RVC_HF/julius/bands.py
deleted file mode 100644
index ef2162440b69e960770aa7bf81b9aaec48a63243..0000000000000000000000000000000000000000
--- a/spaces/Bart92/RVC_HF/julius/bands.py
+++ /dev/null
@@ -1,119 +0,0 @@
-# File under the MIT license, see https://github.com/adefossez/julius/LICENSE for details.
-# Author: adefossez, 2020
-"""
-Decomposition of a signal over frequency bands in the waveform domain.
-"""
-from typing import Optional, Sequence
-import torch
-
-from .core import mel_frequencies
-from .lowpass import LowPassFilters
-from .utils import simple_repr
-
-
-class SplitBands(torch.nn.Module):
- """
- Decomposes a signal over the given frequency bands in the waveform domain using
- a cascade of low pass filters as implemented by `julius.lowpass.LowPassFilters`.
- You can either specify explicitely the frequency cutoffs, or just the number of bands,
- in which case the frequency cutoffs will be spread out evenly in mel scale.
-
- Args:
- sample_rate (float): Sample rate of the input signal in Hz.
- n_bands (int or None): number of bands, when not giving them explictely with `cutoffs`.
- In that case, the cutoff frequencies will be evenly spaced in mel-space.
- cutoffs (list[float] or None): list of frequency cutoffs in Hz.
- pad (bool): if True, appropriately pad the input with zero over the edge. If `stride=1`,
- the output will have the same length as the input.
- zeros (float): Number of zero crossings to keep. See `LowPassFilters` for more informations.
- fft (bool or None): See `LowPassFilters` for more info.
-
- ..note::
- The sum of all the bands will always be the input signal.
-
- ..warning::
- Unlike `julius.lowpass.LowPassFilters`, the cutoffs frequencies must be provided in Hz along
- with the sample rate.
-
- Shape:
-
- - Input: `[*, T]`
- - Output: `[B, *, T']`, with `T'=T` if `pad` is True.
- If `n_bands` was provided, `B = n_bands` otherwise `B = len(cutoffs) + 1`
-
- >>> bands = SplitBands(sample_rate=128, n_bands=10)
- >>> x = torch.randn(6, 4, 1024)
- >>> list(bands(x).shape)
- [10, 6, 4, 1024]
- """
-
- def __init__(self, sample_rate: float, n_bands: Optional[int] = None,
- cutoffs: Optional[Sequence[float]] = None, pad: bool = True,
- zeros: float = 8, fft: Optional[bool] = None):
- super().__init__()
- if (cutoffs is None) + (n_bands is None) != 1:
- raise ValueError("You must provide either n_bands, or cutoffs, but not boths.")
-
- self.sample_rate = sample_rate
- self.n_bands = n_bands
- self._cutoffs = list(cutoffs) if cutoffs is not None else None
- self.pad = pad
- self.zeros = zeros
- self.fft = fft
-
- if cutoffs is None:
- if n_bands is None:
- raise ValueError("You must provide one of n_bands or cutoffs.")
- if not n_bands >= 1:
- raise ValueError(f"n_bands must be greater than one (got {n_bands})")
- cutoffs = mel_frequencies(n_bands + 1, 0, sample_rate / 2)[1:-1]
- else:
- if max(cutoffs) > 0.5 * sample_rate:
- raise ValueError("A cutoff above sample_rate/2 does not make sense.")
- if len(cutoffs) > 0:
- self.lowpass = LowPassFilters(
- [c / sample_rate for c in cutoffs], pad=pad, zeros=zeros, fft=fft)
- else:
- # Here I cannot make both TorchScript and MyPy happy.
- # I miss the good old times, before all this madness was created.
- self.lowpass = None # type: ignore
-
- def forward(self, input):
- if self.lowpass is None:
- return input[None]
- lows = self.lowpass(input)
- low = lows[0]
- bands = [low]
- for low_and_band in lows[1:]:
- # Get a bandpass filter by substracting lowpasses
- band = low_and_band - low
- bands.append(band)
- low = low_and_band
- # Last band is whatever is left in the signal
- bands.append(input - low)
- return torch.stack(bands)
-
- @property
- def cutoffs(self):
- if self._cutoffs is not None:
- return self._cutoffs
- elif self.lowpass is not None:
- return [c * self.sample_rate for c in self.lowpass.cutoffs]
- else:
- return []
-
- def __repr__(self):
- return simple_repr(self, overrides={"cutoffs": self._cutoffs})
-
-
-def split_bands(signal: torch.Tensor, sample_rate: float, n_bands: Optional[int] = None,
- cutoffs: Optional[Sequence[float]] = None, pad: bool = True,
- zeros: float = 8, fft: Optional[bool] = None):
- """
- Functional version of `SplitBands`, refer to this class for more information.
-
- >>> x = torch.randn(6, 4, 1024)
- >>> list(split_bands(x, sample_rate=64, cutoffs=[12, 24]).shape)
- [3, 6, 4, 1024]
- """
- return SplitBands(sample_rate, n_bands, cutoffs, pad, zeros, fft).to(signal)(signal)
diff --git a/spaces/Bart92/RVC_HF/train/losses.py b/spaces/Bart92/RVC_HF/train/losses.py
deleted file mode 100644
index b89038f14d06d7fae43628183e9ffb465e4edafd..0000000000000000000000000000000000000000
--- a/spaces/Bart92/RVC_HF/train/losses.py
+++ /dev/null
@@ -1,59 +0,0 @@
-import torch
-from torch.nn import functional as F
-
-
-def feature_loss(fmap_r, fmap_g):
- loss = 0
- for dr, dg in zip(fmap_r, fmap_g):
- for rl, gl in zip(dr, dg):
- rl = rl.float().detach()
- gl = gl.float()
- loss += torch.mean(torch.abs(rl - gl))
-
- return loss * 2
-
-
-def discriminator_loss(disc_real_outputs, disc_generated_outputs):
- loss = 0
- r_losses = []
- g_losses = []
- for dr, dg in zip(disc_real_outputs, disc_generated_outputs):
- dr = dr.float()
- dg = dg.float()
- r_loss = torch.mean((1 - dr) ** 2)
- g_loss = torch.mean(dg**2)
- loss += r_loss + g_loss
- r_losses.append(r_loss.item())
- g_losses.append(g_loss.item())
-
- return loss, r_losses, g_losses
-
-
-def generator_loss(disc_outputs):
- loss = 0
- gen_losses = []
- for dg in disc_outputs:
- dg = dg.float()
- l = torch.mean((1 - dg) ** 2)
- gen_losses.append(l)
- loss += l
-
- return loss, gen_losses
-
-
-def kl_loss(z_p, logs_q, m_p, logs_p, z_mask):
- """
- z_p, logs_q: [b, h, t_t]
- m_p, logs_p: [b, h, t_t]
- """
- z_p = z_p.float()
- logs_q = logs_q.float()
- m_p = m_p.float()
- logs_p = logs_p.float()
- z_mask = z_mask.float()
-
- kl = logs_p - logs_q - 0.5
- kl += 0.5 * ((z_p - m_p) ** 2) * torch.exp(-2.0 * logs_p)
- kl = torch.sum(kl * z_mask)
- l = kl / torch.sum(z_mask)
- return l
diff --git a/spaces/Benson/text-generation/Examples/Descargar Garena Drifters Velocidad.md b/spaces/Benson/text-generation/Examples/Descargar Garena Drifters Velocidad.md
deleted file mode 100644
index 0fcad0177ac2d9480a2d4e840cbc4f03f82e05bb..0000000000000000000000000000000000000000
--- a/spaces/Benson/text-generation/Examples/Descargar Garena Drifters Velocidad.md
+++ /dev/null
@@ -1,73 +0,0 @@
-
-Descargar Garena AOV Mod dinero ilimitado: Cómo obtener la mejor experiencia MOBA en su dispositivo móvil
- Si eres un fan de los juegos multijugador online battle arena (MOBA), es posible que hayas oído hablar de Garena AOV, uno de los juegos más populares y emocionantes de este género. Pero ¿sabía usted que puede descargar Garena AOV mod dinero ilimitado y obtener acceso a características premium, contenido y recursos que mejorarán su experiencia de juego? En este artículo, te contaremos todo lo que necesitas saber sobre Garena AOV, por qué deberías descargar su versión mod y cómo hacerlo de forma segura y fácil.
- ¿Qué es Garena AOV?
- Garena AOV es un nuevo juego 5v5 MOBA que fue desarrollado por Tencent Games y publicado por Garena. También es conocida como Arena del Valor o Reino del Valor en algunas regiones. El juego cuenta con gráficos ultra-HD, jugabilidad suave, héroes equilibrados y varios modos para adaptarse a diferentes preferencias y niveles de habilidad. Puedes elegir entre más de 100 héroes, cada uno con sus propias habilidades, roles y estilos. También puedes hacer equipo con tus amigos u otros jugadores en línea y competir en partidos clasificados, partidos casuales o eventos especiales. El juego es gratis para descargar y jugar, pero también ofrece compras en la aplicación para algunos artículos y servicios.
-descargar garena drifters velocidad
Download Zip »»» https://bltlly.com/2v6ICS
- Características de Garena AOV
- Algunas de las características que hacen que Garena AOV se destaque de otros juegos de MOBA son:
-
-- Una diversa lista de héroes, incluyendo personajes originales y con licencia de DC Comics, como Batman, Superman, Wonder Woman, Joker, Harley Quinn y más.
-- Un sistema de juego justo y equilibrado que premia la habilidad y el trabajo en equipo, no la mecánica de pago a ganar.
-- Una variedad de modos de juego, como Grand Battle (5v5), Valley Skirmish (3v3), Abyssal Clash (5v5 héroes al azar), Solo Battle (1v1), Hook Wars (5v5 con ganchos), Death Match (5v5 con respawns ilimitados), y más.
-
-- Una plataforma social e interactiva que te permite chatear con tus amigos, unirte a gremios, ver transmisiones en vivo, compartir aspectos destacados y ganar recompensas.
-
- Beneficios de jugar Garena AOV
- Jugar a Garena AOV puede traerte muchos beneficios, como:
-
-- Mejorar tu pensamiento estratégico, toma de decisiones, comunicación y habilidades de trabajo en equipo.
-- Diviértete y diviértete con tus amigos u otros jugadores de todo el mundo.
-- Aprender cosas nuevas sobre diferentes culturas, mitos, leyendas e historias a través de los héroes y sus antecedentes.
-- Expresar su creatividad y personalidad a través de la personalización de sus héroes, pieles, emblemas, marcos, efectos, etc.
-- Obtener recompensas y reconocimiento por tus logros y rendimiento en el juego.
-
- ¿Por qué descargar Garena AOV mod dinero ilimitado?
- Como mencionamos anteriormente, Garena AOV es gratis para descargar y jugar, pero también tiene algunas compras en la aplicación que pueden mejorar su experiencia de juego. Por ejemplo, puedes comprar gemas, vales, monedas de oro, cofres de arcanos, cofres de héroes, cofres de piel, etc. Estos artículos pueden ayudarte a desbloquear nuevos héroes, pieles, conjuntos de arcanos, talentos, etc. Sin embargo, estos artículos no son baratos y pueden costar mucho dinero real. No todos pueden permitirse gastar tanto dinero en un juego, especialmente si tienen un presupuesto ajustado o tienen otras prioridades. Es por eso que algunas personas buscan maneras de obtener estos artículos de forma gratuita o a un costo más bajo. Una de las maneras de hacer eso es descargar Garena AOV mod ilimitado dinero.
- Ventajas de usar Garena AOV mod unlimited money
- Garena AOV mod unlimited money es una versión modificada del juego original que te da acceso a gemas ilimitadas, vales, monedas de oro y otros recursos. Con este mod, puedes:
-
-- Desbloquea todos los héroes y skins que quieras, sin tener que esperar eventos, misiones o sorteos.
-
-- Compre cualquier artículo de la tienda, como emblemas, marcos, efectos, etc., sin tener que preocuparse por quedarse sin joyas o cupones.
-- Disfruta del juego sin anuncios ni interrupciones.
-- Tener ventaja sobre tus oponentes en el juego, especialmente si están usando la versión normal.
-
- Los riesgos de usar Garena AOV mod dinero ilimitado
- Sin embargo, el uso de Garena AOV mod ilimitado dinero también viene con algunos riesgos y desventajas que usted debe ser consciente de antes de descargarlo. Algunos de ellos son:
-
-- El mod puede no ser compatible con la última versión del juego o con su dispositivo. Esto puede causar fallos, fallos, errores o un rendimiento deficiente.
-- El mod puede contener virus, malware, spyware u otros programas dañinos que pueden dañar su dispositivo o robar su información personal.
-- El mod puede violar los términos y condiciones del juego y conseguir que se le prohibió jugar. Esto puede resultar en la pérdida de su cuenta, progreso y datos.
-- El mod puede arruinar la diversión y el desafío del juego por lo que es demasiado fácil o aburrido. Puede perder interés en el juego o sentirse culpable por hacer trampa.
-- El mod puede no funcionar como se anuncia o tener algunos costos ocultos o limitaciones. Usted puede terminar perdiendo su tiempo y recursos en algo que no entrega lo que esperaba.
-
- Cómo descargar e instalar Garena AOV mod dinero ilimitado?
- Si todavía desea descargar e instalar Garena AOV mod dinero ilimitado a pesar de los riesgos, es necesario seguir algunos pasos con cuidado y cautela. Estos son los pasos que debes seguir:
- Paso 1: Encontrar una fuente confiable para el archivo apk mod
-
- Paso 2: Habilitar fuentes desconocidas en la configuración del dispositivo
- Lo siguiente que debe hacer es habilitar fuentes desconocidas en la configuración del dispositivo. Esto le permitirá instalar aplicaciones desde fuentes distintas de Google Play Store. Para hacer esto, vaya a la configuración del dispositivo > seguridad > fuentes desconocidas > activar. También es posible que tenga que desactivar cualquier software antivirus o firewall que pueda bloquear o interferir con el proceso de instalación.
-
- Paso 3: Descargar e instalar el archivo apk mod
- La tercera cosa que necesita hacer es descargar e instalar el archivo apk mod en su dispositivo. Para hacer esto, vaya a la página web donde encontró el mod y haga clic en el botón de descarga. Espere a que termine la descarga y luego localice el archivo en el almacenamiento del dispositivo. Toque en el archivo y siga las instrucciones en la pantalla para instalarlo. Es posible que necesite conceder algunos permisos o aceptar algunos términos y condiciones durante el proceso de instalación.
- Paso 4: Iniciar el juego y disfrutar del dinero ilimitado
- Lo último que tienes que hacer es lanzar el juego y disfrutar del dinero ilimitado. Para ello, abre el juego desde el cajón de la aplicación o la pantalla de inicio e inicia sesión con tu cuenta. Deberías ver que tienes gemas ilimitadas, vales, monedas de oro y otros recursos en tu cuenta. Ahora puedes usarlos para comprar lo que quieras de la tienda o desbloquear cualquier héroe o piel que te guste. Diviértete jugando Garena AOV con tus amigos u otros jugadores en línea!
- Conclusión
-
- Preguntas frecuentes
- Aquí hay algunas preguntas frecuentes sobre Garena AOV mod unlimited money:
-
-- ¿Es Garena AOV dinero ilimitado legal?
-No, Garena AOV mod dinero ilimitado no es legal. Es una versión modificada del juego original que viola los términos y condiciones del juego y sus desarrolladores. Usar este mod puede hacer que te prohíban jugar el juego o enfrentar acciones legales de las autoridades.
-- ¿Es seguro el dinero ilimitado Garena AOV mod?
-No necesariamente. Garena AOV mod dinero ilimitado puede contener virus, malware, spyware, u otros programas dañinos que pueden dañar su dispositivo o robar su información personal. Siempre debe escanear el archivo apk mod con un software antivirus de buena reputación antes de descargar e instalar. También debe hacer copias de seguridad de sus datos y utilizar una cuenta secundaria para jugar el juego con este mod.
-- ¿Garena AOV mod es dinero ilimitado gratis?
-Sí, Garena AOV mod dinero ilimitado es gratis para descargar y usar. Sin embargo, algunos sitios web pueden pedirle que complete encuestas, ofertas o tareas antes de darle el enlace de descarga. Usted debe evitar estos sitios web, ya que pueden ser estafas o intentos de phishing. También debe tener cuidado con los costos ocultos o limitaciones que pueden venir con este mod.
-- ¿Cómo puedo actualizar Garena AOV mod unlimited money?
-Puede actualizar Garena AOV mod dinero ilimitado siguiendo los mismos pasos que descargarlo e instalarlo. Sin embargo, siempre debes comprobar si el mod es compatible con la última versión del juego o con tu dispositivo antes de actualizarlo. También debe hacer una copia de seguridad de sus datos y desinstalar la versión anterior del mod antes de instalar el nuevo.
-- ¿Dónde puedo encontrar más información sobre Garena AOV?
-
-
64aa2da5cf
-
-
\ No newline at end of file
diff --git a/spaces/Big-Web/MMSD/env/Lib/site-packages/pip/_vendor/pygments/lexer.py b/spaces/Big-Web/MMSD/env/Lib/site-packages/pip/_vendor/pygments/lexer.py
deleted file mode 100644
index 74ab9b9088fa6af68976545ffc1ba94c3e9685ca..0000000000000000000000000000000000000000
--- a/spaces/Big-Web/MMSD/env/Lib/site-packages/pip/_vendor/pygments/lexer.py
+++ /dev/null
@@ -1,883 +0,0 @@
-"""
- pygments.lexer
- ~~~~~~~~~~~~~~
-
- Base lexer classes.
-
- :copyright: Copyright 2006-2022 by the Pygments team, see AUTHORS.
- :license: BSD, see LICENSE for details.
-"""
-
-import re
-import sys
-import time
-
-from pip._vendor.pygments.filter import apply_filters, Filter
-from pip._vendor.pygments.filters import get_filter_by_name
-from pip._vendor.pygments.token import Error, Text, Other, Whitespace, _TokenType
-from pip._vendor.pygments.util import get_bool_opt, get_int_opt, get_list_opt, \
- make_analysator, Future, guess_decode
-from pip._vendor.pygments.regexopt import regex_opt
-
-__all__ = ['Lexer', 'RegexLexer', 'ExtendedRegexLexer', 'DelegatingLexer',
- 'LexerContext', 'include', 'inherit', 'bygroups', 'using', 'this',
- 'default', 'words', 'line_re']
-
-line_re = re.compile('.*?\n')
-
-_encoding_map = [(b'\xef\xbb\xbf', 'utf-8'),
- (b'\xff\xfe\0\0', 'utf-32'),
- (b'\0\0\xfe\xff', 'utf-32be'),
- (b'\xff\xfe', 'utf-16'),
- (b'\xfe\xff', 'utf-16be')]
-
-_default_analyse = staticmethod(lambda x: 0.0)
-
-
-class LexerMeta(type):
- """
- This metaclass automagically converts ``analyse_text`` methods into
- static methods which always return float values.
- """
-
- def __new__(mcs, name, bases, d):
- if 'analyse_text' in d:
- d['analyse_text'] = make_analysator(d['analyse_text'])
- return type.__new__(mcs, name, bases, d)
-
-
-class Lexer(metaclass=LexerMeta):
- """
- Lexer for a specific language.
-
- Basic options recognized:
- ``stripnl``
- Strip leading and trailing newlines from the input (default: True).
- ``stripall``
- Strip all leading and trailing whitespace from the input
- (default: False).
- ``ensurenl``
- Make sure that the input ends with a newline (default: True). This
- is required for some lexers that consume input linewise.
-
- .. versionadded:: 1.3
-
- ``tabsize``
- If given and greater than 0, expand tabs in the input (default: 0).
- ``encoding``
- If given, must be an encoding name. This encoding will be used to
- convert the input string to Unicode, if it is not already a Unicode
- string (default: ``'guess'``, which uses a simple UTF-8 / Locale /
- Latin1 detection. Can also be ``'chardet'`` to use the chardet
- library, if it is installed.
- ``inencoding``
- Overrides the ``encoding`` if given.
- """
-
- #: Name of the lexer
- name = None
-
- #: URL of the language specification/definition
- url = None
-
- #: Shortcuts for the lexer
- aliases = []
-
- #: File name globs
- filenames = []
-
- #: Secondary file name globs
- alias_filenames = []
-
- #: MIME types
- mimetypes = []
-
- #: Priority, should multiple lexers match and no content is provided
- priority = 0
-
- def __init__(self, **options):
- self.options = options
- self.stripnl = get_bool_opt(options, 'stripnl', True)
- self.stripall = get_bool_opt(options, 'stripall', False)
- self.ensurenl = get_bool_opt(options, 'ensurenl', True)
- self.tabsize = get_int_opt(options, 'tabsize', 0)
- self.encoding = options.get('encoding', 'guess')
- self.encoding = options.get('inencoding') or self.encoding
- self.filters = []
- for filter_ in get_list_opt(options, 'filters', ()):
- self.add_filter(filter_)
-
- def __repr__(self):
- if self.options:
- return '' % (self.__class__.__name__,
- self.options)
- else:
- return '' % self.__class__.__name__
-
- def add_filter(self, filter_, **options):
- """
- Add a new stream filter to this lexer.
- """
- if not isinstance(filter_, Filter):
- filter_ = get_filter_by_name(filter_, **options)
- self.filters.append(filter_)
-
- def analyse_text(text):
- """
- Has to return a float between ``0`` and ``1`` that indicates
- if a lexer wants to highlight this text. Used by ``guess_lexer``.
- If this method returns ``0`` it won't highlight it in any case, if
- it returns ``1`` highlighting with this lexer is guaranteed.
-
- The `LexerMeta` metaclass automatically wraps this function so
- that it works like a static method (no ``self`` or ``cls``
- parameter) and the return value is automatically converted to
- `float`. If the return value is an object that is boolean `False`
- it's the same as if the return values was ``0.0``.
- """
-
- def get_tokens(self, text, unfiltered=False):
- """
- Return an iterable of (tokentype, value) pairs generated from
- `text`. If `unfiltered` is set to `True`, the filtering mechanism
- is bypassed even if filters are defined.
-
- Also preprocess the text, i.e. expand tabs and strip it if
- wanted and applies registered filters.
- """
- if not isinstance(text, str):
- if self.encoding == 'guess':
- text, _ = guess_decode(text)
- elif self.encoding == 'chardet':
- try:
- from pip._vendor import chardet
- except ImportError as e:
- raise ImportError('To enable chardet encoding guessing, '
- 'please install the chardet library '
- 'from http://chardet.feedparser.org/') from e
- # check for BOM first
- decoded = None
- for bom, encoding in _encoding_map:
- if text.startswith(bom):
- decoded = text[len(bom):].decode(encoding, 'replace')
- break
- # no BOM found, so use chardet
- if decoded is None:
- enc = chardet.detect(text[:1024]) # Guess using first 1KB
- decoded = text.decode(enc.get('encoding') or 'utf-8',
- 'replace')
- text = decoded
- else:
- text = text.decode(self.encoding)
- if text.startswith('\ufeff'):
- text = text[len('\ufeff'):]
- else:
- if text.startswith('\ufeff'):
- text = text[len('\ufeff'):]
-
- # text now *is* a unicode string
- text = text.replace('\r\n', '\n')
- text = text.replace('\r', '\n')
- if self.stripall:
- text = text.strip()
- elif self.stripnl:
- text = text.strip('\n')
- if self.tabsize > 0:
- text = text.expandtabs(self.tabsize)
- if self.ensurenl and not text.endswith('\n'):
- text += '\n'
-
- def streamer():
- for _, t, v in self.get_tokens_unprocessed(text):
- yield t, v
- stream = streamer()
- if not unfiltered:
- stream = apply_filters(stream, self.filters, self)
- return stream
-
- def get_tokens_unprocessed(self, text):
- """
- Return an iterable of (index, tokentype, value) pairs where "index"
- is the starting position of the token within the input text.
-
- In subclasses, implement this method as a generator to
- maximize effectiveness.
- """
- raise NotImplementedError
-
-
-class DelegatingLexer(Lexer):
- """
- This lexer takes two lexer as arguments. A root lexer and
- a language lexer. First everything is scanned using the language
- lexer, afterwards all ``Other`` tokens are lexed using the root
- lexer.
-
- The lexers from the ``template`` lexer package use this base lexer.
- """
-
- def __init__(self, _root_lexer, _language_lexer, _needle=Other, **options):
- self.root_lexer = _root_lexer(**options)
- self.language_lexer = _language_lexer(**options)
- self.needle = _needle
- Lexer.__init__(self, **options)
-
- def get_tokens_unprocessed(self, text):
- buffered = ''
- insertions = []
- lng_buffer = []
- for i, t, v in self.language_lexer.get_tokens_unprocessed(text):
- if t is self.needle:
- if lng_buffer:
- insertions.append((len(buffered), lng_buffer))
- lng_buffer = []
- buffered += v
- else:
- lng_buffer.append((i, t, v))
- if lng_buffer:
- insertions.append((len(buffered), lng_buffer))
- return do_insertions(insertions,
- self.root_lexer.get_tokens_unprocessed(buffered))
-
-
-# ------------------------------------------------------------------------------
-# RegexLexer and ExtendedRegexLexer
-#
-
-
-class include(str): # pylint: disable=invalid-name
- """
- Indicates that a state should include rules from another state.
- """
- pass
-
-
-class _inherit:
- """
- Indicates the a state should inherit from its superclass.
- """
- def __repr__(self):
- return 'inherit'
-
-inherit = _inherit() # pylint: disable=invalid-name
-
-
-class combined(tuple): # pylint: disable=invalid-name
- """
- Indicates a state combined from multiple states.
- """
-
- def __new__(cls, *args):
- return tuple.__new__(cls, args)
-
- def __init__(self, *args):
- # tuple.__init__ doesn't do anything
- pass
-
-
-class _PseudoMatch:
- """
- A pseudo match object constructed from a string.
- """
-
- def __init__(self, start, text):
- self._text = text
- self._start = start
-
- def start(self, arg=None):
- return self._start
-
- def end(self, arg=None):
- return self._start + len(self._text)
-
- def group(self, arg=None):
- if arg:
- raise IndexError('No such group')
- return self._text
-
- def groups(self):
- return (self._text,)
-
- def groupdict(self):
- return {}
-
-
-def bygroups(*args):
- """
- Callback that yields multiple actions for each group in the match.
- """
- def callback(lexer, match, ctx=None):
- for i, action in enumerate(args):
- if action is None:
- continue
- elif type(action) is _TokenType:
- data = match.group(i + 1)
- if data:
- yield match.start(i + 1), action, data
- else:
- data = match.group(i + 1)
- if data is not None:
- if ctx:
- ctx.pos = match.start(i + 1)
- for item in action(lexer,
- _PseudoMatch(match.start(i + 1), data), ctx):
- if item:
- yield item
- if ctx:
- ctx.pos = match.end()
- return callback
-
-
-class _This:
- """
- Special singleton used for indicating the caller class.
- Used by ``using``.
- """
-
-this = _This()
-
-
-def using(_other, **kwargs):
- """
- Callback that processes the match with a different lexer.
-
- The keyword arguments are forwarded to the lexer, except `state` which
- is handled separately.
-
- `state` specifies the state that the new lexer will start in, and can
- be an enumerable such as ('root', 'inline', 'string') or a simple
- string which is assumed to be on top of the root state.
-
- Note: For that to work, `_other` must not be an `ExtendedRegexLexer`.
- """
- gt_kwargs = {}
- if 'state' in kwargs:
- s = kwargs.pop('state')
- if isinstance(s, (list, tuple)):
- gt_kwargs['stack'] = s
- else:
- gt_kwargs['stack'] = ('root', s)
-
- if _other is this:
- def callback(lexer, match, ctx=None):
- # if keyword arguments are given the callback
- # function has to create a new lexer instance
- if kwargs:
- # XXX: cache that somehow
- kwargs.update(lexer.options)
- lx = lexer.__class__(**kwargs)
- else:
- lx = lexer
- s = match.start()
- for i, t, v in lx.get_tokens_unprocessed(match.group(), **gt_kwargs):
- yield i + s, t, v
- if ctx:
- ctx.pos = match.end()
- else:
- def callback(lexer, match, ctx=None):
- # XXX: cache that somehow
- kwargs.update(lexer.options)
- lx = _other(**kwargs)
-
- s = match.start()
- for i, t, v in lx.get_tokens_unprocessed(match.group(), **gt_kwargs):
- yield i + s, t, v
- if ctx:
- ctx.pos = match.end()
- return callback
-
-
-class default:
- """
- Indicates a state or state action (e.g. #pop) to apply.
- For example default('#pop') is equivalent to ('', Token, '#pop')
- Note that state tuples may be used as well.
-
- .. versionadded:: 2.0
- """
- def __init__(self, state):
- self.state = state
-
-
-class words(Future):
- """
- Indicates a list of literal words that is transformed into an optimized
- regex that matches any of the words.
-
- .. versionadded:: 2.0
- """
- def __init__(self, words, prefix='', suffix=''):
- self.words = words
- self.prefix = prefix
- self.suffix = suffix
-
- def get(self):
- return regex_opt(self.words, prefix=self.prefix, suffix=self.suffix)
-
-
-class RegexLexerMeta(LexerMeta):
- """
- Metaclass for RegexLexer, creates the self._tokens attribute from
- self.tokens on the first instantiation.
- """
-
- def _process_regex(cls, regex, rflags, state):
- """Preprocess the regular expression component of a token definition."""
- if isinstance(regex, Future):
- regex = regex.get()
- return re.compile(regex, rflags).match
-
- def _process_token(cls, token):
- """Preprocess the token component of a token definition."""
- assert type(token) is _TokenType or callable(token), \
- 'token type must be simple type or callable, not %r' % (token,)
- return token
-
- def _process_new_state(cls, new_state, unprocessed, processed):
- """Preprocess the state transition action of a token definition."""
- if isinstance(new_state, str):
- # an existing state
- if new_state == '#pop':
- return -1
- elif new_state in unprocessed:
- return (new_state,)
- elif new_state == '#push':
- return new_state
- elif new_state[:5] == '#pop:':
- return -int(new_state[5:])
- else:
- assert False, 'unknown new state %r' % new_state
- elif isinstance(new_state, combined):
- # combine a new state from existing ones
- tmp_state = '_tmp_%d' % cls._tmpname
- cls._tmpname += 1
- itokens = []
- for istate in new_state:
- assert istate != new_state, 'circular state ref %r' % istate
- itokens.extend(cls._process_state(unprocessed,
- processed, istate))
- processed[tmp_state] = itokens
- return (tmp_state,)
- elif isinstance(new_state, tuple):
- # push more than one state
- for istate in new_state:
- assert (istate in unprocessed or
- istate in ('#pop', '#push')), \
- 'unknown new state ' + istate
- return new_state
- else:
- assert False, 'unknown new state def %r' % new_state
-
- def _process_state(cls, unprocessed, processed, state):
- """Preprocess a single state definition."""
- assert type(state) is str, "wrong state name %r" % state
- assert state[0] != '#', "invalid state name %r" % state
- if state in processed:
- return processed[state]
- tokens = processed[state] = []
- rflags = cls.flags
- for tdef in unprocessed[state]:
- if isinstance(tdef, include):
- # it's a state reference
- assert tdef != state, "circular state reference %r" % state
- tokens.extend(cls._process_state(unprocessed, processed,
- str(tdef)))
- continue
- if isinstance(tdef, _inherit):
- # should be processed already, but may not in the case of:
- # 1. the state has no counterpart in any parent
- # 2. the state includes more than one 'inherit'
- continue
- if isinstance(tdef, default):
- new_state = cls._process_new_state(tdef.state, unprocessed, processed)
- tokens.append((re.compile('').match, None, new_state))
- continue
-
- assert type(tdef) is tuple, "wrong rule def %r" % tdef
-
- try:
- rex = cls._process_regex(tdef[0], rflags, state)
- except Exception as err:
- raise ValueError("uncompilable regex %r in state %r of %r: %s" %
- (tdef[0], state, cls, err)) from err
-
- token = cls._process_token(tdef[1])
-
- if len(tdef) == 2:
- new_state = None
- else:
- new_state = cls._process_new_state(tdef[2],
- unprocessed, processed)
-
- tokens.append((rex, token, new_state))
- return tokens
-
- def process_tokendef(cls, name, tokendefs=None):
- """Preprocess a dictionary of token definitions."""
- processed = cls._all_tokens[name] = {}
- tokendefs = tokendefs or cls.tokens[name]
- for state in list(tokendefs):
- cls._process_state(tokendefs, processed, state)
- return processed
-
- def get_tokendefs(cls):
- """
- Merge tokens from superclasses in MRO order, returning a single tokendef
- dictionary.
-
- Any state that is not defined by a subclass will be inherited
- automatically. States that *are* defined by subclasses will, by
- default, override that state in the superclass. If a subclass wishes to
- inherit definitions from a superclass, it can use the special value
- "inherit", which will cause the superclass' state definition to be
- included at that point in the state.
- """
- tokens = {}
- inheritable = {}
- for c in cls.__mro__:
- toks = c.__dict__.get('tokens', {})
-
- for state, items in toks.items():
- curitems = tokens.get(state)
- if curitems is None:
- # N.b. because this is assigned by reference, sufficiently
- # deep hierarchies are processed incrementally (e.g. for
- # A(B), B(C), C(RegexLexer), B will be premodified so X(B)
- # will not see any inherits in B).
- tokens[state] = items
- try:
- inherit_ndx = items.index(inherit)
- except ValueError:
- continue
- inheritable[state] = inherit_ndx
- continue
-
- inherit_ndx = inheritable.pop(state, None)
- if inherit_ndx is None:
- continue
-
- # Replace the "inherit" value with the items
- curitems[inherit_ndx:inherit_ndx+1] = items
- try:
- # N.b. this is the index in items (that is, the superclass
- # copy), so offset required when storing below.
- new_inh_ndx = items.index(inherit)
- except ValueError:
- pass
- else:
- inheritable[state] = inherit_ndx + new_inh_ndx
-
- return tokens
-
- def __call__(cls, *args, **kwds):
- """Instantiate cls after preprocessing its token definitions."""
- if '_tokens' not in cls.__dict__:
- cls._all_tokens = {}
- cls._tmpname = 0
- if hasattr(cls, 'token_variants') and cls.token_variants:
- # don't process yet
- pass
- else:
- cls._tokens = cls.process_tokendef('', cls.get_tokendefs())
-
- return type.__call__(cls, *args, **kwds)
-
-
-class RegexLexer(Lexer, metaclass=RegexLexerMeta):
- """
- Base for simple stateful regular expression-based lexers.
- Simplifies the lexing process so that you need only
- provide a list of states and regular expressions.
- """
-
- #: Flags for compiling the regular expressions.
- #: Defaults to MULTILINE.
- flags = re.MULTILINE
-
- #: At all time there is a stack of states. Initially, the stack contains
- #: a single state 'root'. The top of the stack is called "the current state".
- #:
- #: Dict of ``{'state': [(regex, tokentype, new_state), ...], ...}``
- #:
- #: ``new_state`` can be omitted to signify no state transition.
- #: If ``new_state`` is a string, it is pushed on the stack. This ensure
- #: the new current state is ``new_state``.
- #: If ``new_state`` is a tuple of strings, all of those strings are pushed
- #: on the stack and the current state will be the last element of the list.
- #: ``new_state`` can also be ``combined('state1', 'state2', ...)``
- #: to signify a new, anonymous state combined from the rules of two
- #: or more existing ones.
- #: Furthermore, it can be '#pop' to signify going back one step in
- #: the state stack, or '#push' to push the current state on the stack
- #: again. Note that if you push while in a combined state, the combined
- #: state itself is pushed, and not only the state in which the rule is
- #: defined.
- #:
- #: The tuple can also be replaced with ``include('state')``, in which
- #: case the rules from the state named by the string are included in the
- #: current one.
- tokens = {}
-
- def get_tokens_unprocessed(self, text, stack=('root',)):
- """
- Split ``text`` into (tokentype, text) pairs.
-
- ``stack`` is the initial stack (default: ``['root']``)
- """
- pos = 0
- tokendefs = self._tokens
- statestack = list(stack)
- statetokens = tokendefs[statestack[-1]]
- while 1:
- for rexmatch, action, new_state in statetokens:
- m = rexmatch(text, pos)
- if m:
- if action is not None:
- if type(action) is _TokenType:
- yield pos, action, m.group()
- else:
- yield from action(self, m)
- pos = m.end()
- if new_state is not None:
- # state transition
- if isinstance(new_state, tuple):
- for state in new_state:
- if state == '#pop':
- if len(statestack) > 1:
- statestack.pop()
- elif state == '#push':
- statestack.append(statestack[-1])
- else:
- statestack.append(state)
- elif isinstance(new_state, int):
- # pop, but keep at least one state on the stack
- # (random code leading to unexpected pops should
- # not allow exceptions)
- if abs(new_state) >= len(statestack):
- del statestack[1:]
- else:
- del statestack[new_state:]
- elif new_state == '#push':
- statestack.append(statestack[-1])
- else:
- assert False, "wrong state def: %r" % new_state
- statetokens = tokendefs[statestack[-1]]
- break
- else:
- # We are here only if all state tokens have been considered
- # and there was not a match on any of them.
- try:
- if text[pos] == '\n':
- # at EOL, reset state to "root"
- statestack = ['root']
- statetokens = tokendefs['root']
- yield pos, Whitespace, '\n'
- pos += 1
- continue
- yield pos, Error, text[pos]
- pos += 1
- except IndexError:
- break
-
-
-class LexerContext:
- """
- A helper object that holds lexer position data.
- """
-
- def __init__(self, text, pos, stack=None, end=None):
- self.text = text
- self.pos = pos
- self.end = end or len(text) # end=0 not supported ;-)
- self.stack = stack or ['root']
-
- def __repr__(self):
- return 'LexerContext(%r, %r, %r)' % (
- self.text, self.pos, self.stack)
-
-
-class ExtendedRegexLexer(RegexLexer):
- """
- A RegexLexer that uses a context object to store its state.
- """
-
- def get_tokens_unprocessed(self, text=None, context=None):
- """
- Split ``text`` into (tokentype, text) pairs.
- If ``context`` is given, use this lexer context instead.
- """
- tokendefs = self._tokens
- if not context:
- ctx = LexerContext(text, 0)
- statetokens = tokendefs['root']
- else:
- ctx = context
- statetokens = tokendefs[ctx.stack[-1]]
- text = ctx.text
- while 1:
- for rexmatch, action, new_state in statetokens:
- m = rexmatch(text, ctx.pos, ctx.end)
- if m:
- if action is not None:
- if type(action) is _TokenType:
- yield ctx.pos, action, m.group()
- ctx.pos = m.end()
- else:
- yield from action(self, m, ctx)
- if not new_state:
- # altered the state stack?
- statetokens = tokendefs[ctx.stack[-1]]
- # CAUTION: callback must set ctx.pos!
- if new_state is not None:
- # state transition
- if isinstance(new_state, tuple):
- for state in new_state:
- if state == '#pop':
- if len(ctx.stack) > 1:
- ctx.stack.pop()
- elif state == '#push':
- ctx.stack.append(ctx.stack[-1])
- else:
- ctx.stack.append(state)
- elif isinstance(new_state, int):
- # see RegexLexer for why this check is made
- if abs(new_state) >= len(ctx.stack):
- del ctx.stack[1:]
- else:
- del ctx.stack[new_state:]
- elif new_state == '#push':
- ctx.stack.append(ctx.stack[-1])
- else:
- assert False, "wrong state def: %r" % new_state
- statetokens = tokendefs[ctx.stack[-1]]
- break
- else:
- try:
- if ctx.pos >= ctx.end:
- break
- if text[ctx.pos] == '\n':
- # at EOL, reset state to "root"
- ctx.stack = ['root']
- statetokens = tokendefs['root']
- yield ctx.pos, Text, '\n'
- ctx.pos += 1
- continue
- yield ctx.pos, Error, text[ctx.pos]
- ctx.pos += 1
- except IndexError:
- break
-
-
-def do_insertions(insertions, tokens):
- """
- Helper for lexers which must combine the results of several
- sublexers.
-
- ``insertions`` is a list of ``(index, itokens)`` pairs.
- Each ``itokens`` iterable should be inserted at position
- ``index`` into the token stream given by the ``tokens``
- argument.
-
- The result is a combined token stream.
-
- TODO: clean up the code here.
- """
- insertions = iter(insertions)
- try:
- index, itokens = next(insertions)
- except StopIteration:
- # no insertions
- yield from tokens
- return
-
- realpos = None
- insleft = True
-
- # iterate over the token stream where we want to insert
- # the tokens from the insertion list.
- for i, t, v in tokens:
- # first iteration. store the position of first item
- if realpos is None:
- realpos = i
- oldi = 0
- while insleft and i + len(v) >= index:
- tmpval = v[oldi:index - i]
- if tmpval:
- yield realpos, t, tmpval
- realpos += len(tmpval)
- for it_index, it_token, it_value in itokens:
- yield realpos, it_token, it_value
- realpos += len(it_value)
- oldi = index - i
- try:
- index, itokens = next(insertions)
- except StopIteration:
- insleft = False
- break # not strictly necessary
- if oldi < len(v):
- yield realpos, t, v[oldi:]
- realpos += len(v) - oldi
-
- # leftover tokens
- while insleft:
- # no normal tokens, set realpos to zero
- realpos = realpos or 0
- for p, t, v in itokens:
- yield realpos, t, v
- realpos += len(v)
- try:
- index, itokens = next(insertions)
- except StopIteration:
- insleft = False
- break # not strictly necessary
-
-
-class ProfilingRegexLexerMeta(RegexLexerMeta):
- """Metaclass for ProfilingRegexLexer, collects regex timing info."""
-
- def _process_regex(cls, regex, rflags, state):
- if isinstance(regex, words):
- rex = regex_opt(regex.words, prefix=regex.prefix,
- suffix=regex.suffix)
- else:
- rex = regex
- compiled = re.compile(rex, rflags)
-
- def match_func(text, pos, endpos=sys.maxsize):
- info = cls._prof_data[-1].setdefault((state, rex), [0, 0.0])
- t0 = time.time()
- res = compiled.match(text, pos, endpos)
- t1 = time.time()
- info[0] += 1
- info[1] += t1 - t0
- return res
- return match_func
-
-
-class ProfilingRegexLexer(RegexLexer, metaclass=ProfilingRegexLexerMeta):
- """Drop-in replacement for RegexLexer that does profiling of its regexes."""
-
- _prof_data = []
- _prof_sort_index = 4 # defaults to time per call
-
- def get_tokens_unprocessed(self, text, stack=('root',)):
- # this needs to be a stack, since using(this) will produce nested calls
- self.__class__._prof_data.append({})
- yield from RegexLexer.get_tokens_unprocessed(self, text, stack)
- rawdata = self.__class__._prof_data.pop()
- data = sorted(((s, repr(r).strip('u\'').replace('\\\\', '\\')[:65],
- n, 1000 * t, 1000 * t / n)
- for ((s, r), (n, t)) in rawdata.items()),
- key=lambda x: x[self._prof_sort_index],
- reverse=True)
- sum_total = sum(x[3] for x in data)
-
- print()
- print('Profiling result for %s lexing %d chars in %.3f ms' %
- (self.__class__.__name__, len(text), sum_total))
- print('=' * 110)
- print('%-20s %-64s ncalls tottime percall' % ('state', 'regex'))
- print('-' * 110)
- for d in data:
- print('%-20s %-65s %5d %8.4f %8.4f' % d)
- print('=' * 110)
diff --git a/spaces/Big-Web/MMSD/env/Lib/site-packages/setuptools/_distutils/__init__.py b/spaces/Big-Web/MMSD/env/Lib/site-packages/setuptools/_distutils/__init__.py
deleted file mode 100644
index b3ac0146cb3f4cb1894f55fc09775875bc4e1177..0000000000000000000000000000000000000000
--- a/spaces/Big-Web/MMSD/env/Lib/site-packages/setuptools/_distutils/__init__.py
+++ /dev/null
@@ -1,24 +0,0 @@
-"""distutils
-
-The main package for the Python Module Distribution Utilities. Normally
-used from a setup script as
-
- from distutils.core import setup
-
- setup (...)
-"""
-
-import sys
-import importlib
-
-__version__ = sys.version[: sys.version.index(' ')]
-
-
-try:
- # Allow Debian and pkgsrc (only) to customize system
- # behavior. Ref pypa/distutils#2 and pypa/distutils#16.
- # This hook is deprecated and no other environments
- # should use it.
- importlib.import_module('_distutils_system_mod')
-except ImportError:
- pass
diff --git a/spaces/Big-Web/MMSD/env/Lib/site-packages/setuptools/build_meta.py b/spaces/Big-Web/MMSD/env/Lib/site-packages/setuptools/build_meta.py
deleted file mode 100644
index e8f1c72d598d6d5a03b75f68a6d567b1d6b1e9a2..0000000000000000000000000000000000000000
--- a/spaces/Big-Web/MMSD/env/Lib/site-packages/setuptools/build_meta.py
+++ /dev/null
@@ -1,511 +0,0 @@
-"""A PEP 517 interface to setuptools
-
-Previously, when a user or a command line tool (let's call it a "frontend")
-needed to make a request of setuptools to take a certain action, for
-example, generating a list of installation requirements, the frontend would
-would call "setup.py egg_info" or "setup.py bdist_wheel" on the command line.
-
-PEP 517 defines a different method of interfacing with setuptools. Rather
-than calling "setup.py" directly, the frontend should:
-
- 1. Set the current directory to the directory with a setup.py file
- 2. Import this module into a safe python interpreter (one in which
- setuptools can potentially set global variables or crash hard).
- 3. Call one of the functions defined in PEP 517.
-
-What each function does is defined in PEP 517. However, here is a "casual"
-definition of the functions (this definition should not be relied on for
-bug reports or API stability):
-
- - `build_wheel`: build a wheel in the folder and return the basename
- - `get_requires_for_build_wheel`: get the `setup_requires` to build
- - `prepare_metadata_for_build_wheel`: get the `install_requires`
- - `build_sdist`: build an sdist in the folder and return the basename
- - `get_requires_for_build_sdist`: get the `setup_requires` to build
-
-Again, this is not a formal definition! Just a "taste" of the module.
-"""
-
-import io
-import os
-import shlex
-import sys
-import tokenize
-import shutil
-import contextlib
-import tempfile
-import warnings
-from pathlib import Path
-from typing import Dict, Iterator, List, Optional, Union
-
-import setuptools
-import distutils
-from . import errors
-from ._path import same_path
-from ._reqs import parse_strings
-from ._deprecation_warning import SetuptoolsDeprecationWarning
-from distutils.util import strtobool
-
-
-__all__ = ['get_requires_for_build_sdist',
- 'get_requires_for_build_wheel',
- 'prepare_metadata_for_build_wheel',
- 'build_wheel',
- 'build_sdist',
- 'get_requires_for_build_editable',
- 'prepare_metadata_for_build_editable',
- 'build_editable',
- '__legacy__',
- 'SetupRequirementsError']
-
-SETUPTOOLS_ENABLE_FEATURES = os.getenv("SETUPTOOLS_ENABLE_FEATURES", "").lower()
-LEGACY_EDITABLE = "legacy-editable" in SETUPTOOLS_ENABLE_FEATURES.replace("_", "-")
-
-
-class SetupRequirementsError(BaseException):
- def __init__(self, specifiers):
- self.specifiers = specifiers
-
-
-class Distribution(setuptools.dist.Distribution):
- def fetch_build_eggs(self, specifiers):
- specifier_list = list(parse_strings(specifiers))
-
- raise SetupRequirementsError(specifier_list)
-
- @classmethod
- @contextlib.contextmanager
- def patch(cls):
- """
- Replace
- distutils.dist.Distribution with this class
- for the duration of this context.
- """
- orig = distutils.core.Distribution
- distutils.core.Distribution = cls
- try:
- yield
- finally:
- distutils.core.Distribution = orig
-
-
-@contextlib.contextmanager
-def no_install_setup_requires():
- """Temporarily disable installing setup_requires
-
- Under PEP 517, the backend reports build dependencies to the frontend,
- and the frontend is responsible for ensuring they're installed.
- So setuptools (acting as a backend) should not try to install them.
- """
- orig = setuptools._install_setup_requires
- setuptools._install_setup_requires = lambda attrs: None
- try:
- yield
- finally:
- setuptools._install_setup_requires = orig
-
-
-def _get_immediate_subdirectories(a_dir):
- return [name for name in os.listdir(a_dir)
- if os.path.isdir(os.path.join(a_dir, name))]
-
-
-def _file_with_extension(directory, extension):
- matching = (
- f for f in os.listdir(directory)
- if f.endswith(extension)
- )
- try:
- file, = matching
- except ValueError:
- raise ValueError(
- 'No distribution was found. Ensure that `setup.py` '
- 'is not empty and that it calls `setup()`.')
- return file
-
-
-def _open_setup_script(setup_script):
- if not os.path.exists(setup_script):
- # Supply a default setup.py
- return io.StringIO(u"from setuptools import setup; setup()")
-
- return getattr(tokenize, 'open', open)(setup_script)
-
-
-@contextlib.contextmanager
-def suppress_known_deprecation():
- with warnings.catch_warnings():
- warnings.filterwarnings('ignore', 'setup.py install is deprecated')
- yield
-
-
-_ConfigSettings = Optional[Dict[str, Union[str, List[str], None]]]
-"""
-Currently the user can run::
-
- pip install -e . --config-settings key=value
- python -m build -C--key=value -C key=value
-
-- pip will pass both key and value as strings and overwriting repeated keys
- (pypa/pip#11059).
-- build will accumulate values associated with repeated keys in a list.
- It will also accept keys with no associated value.
- This means that an option passed by build can be ``str | list[str] | None``.
-- PEP 517 specifies that ``config_settings`` is an optional dict.
-"""
-
-
-class _ConfigSettingsTranslator:
- """Translate ``config_settings`` into distutils-style command arguments.
- Only a limited number of options is currently supported.
- """
- # See pypa/setuptools#1928 pypa/setuptools#2491
-
- def _get_config(self, key: str, config_settings: _ConfigSettings) -> List[str]:
- """
- Get the value of a specific key in ``config_settings`` as a list of strings.
-
- >>> fn = _ConfigSettingsTranslator()._get_config
- >>> fn("--global-option", None)
- []
- >>> fn("--global-option", {})
- []
- >>> fn("--global-option", {'--global-option': 'foo'})
- ['foo']
- >>> fn("--global-option", {'--global-option': ['foo']})
- ['foo']
- >>> fn("--global-option", {'--global-option': 'foo'})
- ['foo']
- >>> fn("--global-option", {'--global-option': 'foo bar'})
- ['foo', 'bar']
- """
- cfg = config_settings or {}
- opts = cfg.get(key) or []
- return shlex.split(opts) if isinstance(opts, str) else opts
-
- def _valid_global_options(self):
- """Global options accepted by setuptools (e.g. quiet or verbose)."""
- options = (opt[:2] for opt in setuptools.dist.Distribution.global_options)
- return {flag for long_and_short in options for flag in long_and_short if flag}
-
- def _global_args(self, config_settings: _ConfigSettings) -> Iterator[str]:
- """
- Let the user specify ``verbose`` or ``quiet`` + escape hatch via
- ``--global-option``.
- Note: ``-v``, ``-vv``, ``-vvv`` have similar effects in setuptools,
- so we just have to cover the basic scenario ``-v``.
-
- >>> fn = _ConfigSettingsTranslator()._global_args
- >>> list(fn(None))
- []
- >>> list(fn({"verbose": "False"}))
- ['-q']
- >>> list(fn({"verbose": "1"}))
- ['-v']
- >>> list(fn({"--verbose": None}))
- ['-v']
- >>> list(fn({"verbose": "true", "--global-option": "-q --no-user-cfg"}))
- ['-v', '-q', '--no-user-cfg']
- >>> list(fn({"--quiet": None}))
- ['-q']
- """
- cfg = config_settings or {}
- falsey = {"false", "no", "0", "off"}
- if "verbose" in cfg or "--verbose" in cfg:
- level = str(cfg.get("verbose") or cfg.get("--verbose") or "1")
- yield ("-q" if level.lower() in falsey else "-v")
- if "quiet" in cfg or "--quiet" in cfg:
- level = str(cfg.get("quiet") or cfg.get("--quiet") or "1")
- yield ("-v" if level.lower() in falsey else "-q")
-
- valid = self._valid_global_options()
- args = self._get_config("--global-option", config_settings)
- yield from (arg for arg in args if arg.strip("-") in valid)
-
- def __dist_info_args(self, config_settings: _ConfigSettings) -> Iterator[str]:
- """
- The ``dist_info`` command accepts ``tag-date`` and ``tag-build``.
-
- .. warning::
- We cannot use this yet as it requires the ``sdist`` and ``bdist_wheel``
- commands run in ``build_sdist`` and ``build_wheel`` to re-use the egg-info
- directory created in ``prepare_metadata_for_build_wheel``.
-
- >>> fn = _ConfigSettingsTranslator()._ConfigSettingsTranslator__dist_info_args
- >>> list(fn(None))
- []
- >>> list(fn({"tag-date": "False"}))
- ['--no-date']
- >>> list(fn({"tag-date": None}))
- ['--no-date']
- >>> list(fn({"tag-date": "true", "tag-build": ".a"}))
- ['--tag-date', '--tag-build', '.a']
- """
- cfg = config_settings or {}
- if "tag-date" in cfg:
- val = strtobool(str(cfg["tag-date"] or "false"))
- yield ("--tag-date" if val else "--no-date")
- if "tag-build" in cfg:
- yield from ["--tag-build", str(cfg["tag-build"])]
-
- def _editable_args(self, config_settings: _ConfigSettings) -> Iterator[str]:
- """
- The ``editable_wheel`` command accepts ``editable-mode=strict``.
-
- >>> fn = _ConfigSettingsTranslator()._editable_args
- >>> list(fn(None))
- []
- >>> list(fn({"editable-mode": "strict"}))
- ['--mode', 'strict']
- """
- cfg = config_settings or {}
- mode = cfg.get("editable-mode") or cfg.get("editable_mode")
- if not mode:
- return
- yield from ["--mode", str(mode)]
-
- def _arbitrary_args(self, config_settings: _ConfigSettings) -> Iterator[str]:
- """
- Users may expect to pass arbitrary lists of arguments to a command
- via "--global-option" (example provided in PEP 517 of a "escape hatch").
-
- >>> fn = _ConfigSettingsTranslator()._arbitrary_args
- >>> list(fn(None))
- []
- >>> list(fn({}))
- []
- >>> list(fn({'--build-option': 'foo'}))
- ['foo']
- >>> list(fn({'--build-option': ['foo']}))
- ['foo']
- >>> list(fn({'--build-option': 'foo'}))
- ['foo']
- >>> list(fn({'--build-option': 'foo bar'}))
- ['foo', 'bar']
- >>> warnings.simplefilter('error', SetuptoolsDeprecationWarning)
- >>> list(fn({'--global-option': 'foo'})) # doctest: +IGNORE_EXCEPTION_DETAIL
- Traceback (most recent call last):
- SetuptoolsDeprecationWarning: ...arguments given via `--global-option`...
- """
- args = self._get_config("--global-option", config_settings)
- global_opts = self._valid_global_options()
- bad_args = []
-
- for arg in args:
- if arg.strip("-") not in global_opts:
- bad_args.append(arg)
- yield arg
-
- yield from self._get_config("--build-option", config_settings)
-
- if bad_args:
- msg = f"""
- The arguments {bad_args!r} were given via `--global-option`.
- Please use `--build-option` instead,
- `--global-option` is reserved to flags like `--verbose` or `--quiet`.
- """
- warnings.warn(msg, SetuptoolsDeprecationWarning)
-
-
-class _BuildMetaBackend(_ConfigSettingsTranslator):
- def _get_build_requires(self, config_settings, requirements):
- sys.argv = [
- *sys.argv[:1],
- *self._global_args(config_settings),
- "egg_info",
- *self._arbitrary_args(config_settings),
- ]
- try:
- with Distribution.patch():
- self.run_setup()
- except SetupRequirementsError as e:
- requirements += e.specifiers
-
- return requirements
-
- def run_setup(self, setup_script='setup.py'):
- # Note that we can reuse our build directory between calls
- # Correctness comes first, then optimization later
- __file__ = setup_script
- __name__ = '__main__'
-
- with _open_setup_script(__file__) as f:
- code = f.read().replace(r'\r\n', r'\n')
-
- exec(code, locals())
-
- def get_requires_for_build_wheel(self, config_settings=None):
- return self._get_build_requires(config_settings, requirements=['wheel'])
-
- def get_requires_for_build_sdist(self, config_settings=None):
- return self._get_build_requires(config_settings, requirements=[])
-
- def _bubble_up_info_directory(self, metadata_directory: str, suffix: str) -> str:
- """
- PEP 517 requires that the .dist-info directory be placed in the
- metadata_directory. To comply, we MUST copy the directory to the root.
-
- Returns the basename of the info directory, e.g. `proj-0.0.0.dist-info`.
- """
- info_dir = self._find_info_directory(metadata_directory, suffix)
- if not same_path(info_dir.parent, metadata_directory):
- shutil.move(str(info_dir), metadata_directory)
- # PEP 517 allow other files and dirs to exist in metadata_directory
- return info_dir.name
-
- def _find_info_directory(self, metadata_directory: str, suffix: str) -> Path:
- for parent, dirs, _ in os.walk(metadata_directory):
- candidates = [f for f in dirs if f.endswith(suffix)]
-
- if len(candidates) != 0 or len(dirs) != 1:
- assert len(candidates) == 1, f"Multiple {suffix} directories found"
- return Path(parent, candidates[0])
-
- msg = f"No {suffix} directory found in {metadata_directory}"
- raise errors.InternalError(msg)
-
- def prepare_metadata_for_build_wheel(self, metadata_directory,
- config_settings=None):
- sys.argv = [
- *sys.argv[:1],
- *self._global_args(config_settings),
- "dist_info",
- "--output-dir", metadata_directory,
- "--keep-egg-info",
- ]
- with no_install_setup_requires():
- self.run_setup()
-
- self._bubble_up_info_directory(metadata_directory, ".egg-info")
- return self._bubble_up_info_directory(metadata_directory, ".dist-info")
-
- def _build_with_temp_dir(self, setup_command, result_extension,
- result_directory, config_settings):
- result_directory = os.path.abspath(result_directory)
-
- # Build in a temporary directory, then copy to the target.
- os.makedirs(result_directory, exist_ok=True)
- with tempfile.TemporaryDirectory(dir=result_directory) as tmp_dist_dir:
- sys.argv = [
- *sys.argv[:1],
- *self._global_args(config_settings),
- *setup_command,
- "--dist-dir", tmp_dist_dir,
- *self._arbitrary_args(config_settings),
- ]
- with no_install_setup_requires():
- self.run_setup()
-
- result_basename = _file_with_extension(
- tmp_dist_dir, result_extension)
- result_path = os.path.join(result_directory, result_basename)
- if os.path.exists(result_path):
- # os.rename will fail overwriting on non-Unix.
- os.remove(result_path)
- os.rename(os.path.join(tmp_dist_dir, result_basename), result_path)
-
- return result_basename
-
- def build_wheel(self, wheel_directory, config_settings=None,
- metadata_directory=None):
- with suppress_known_deprecation():
- return self._build_with_temp_dir(['bdist_wheel'], '.whl',
- wheel_directory, config_settings)
-
- def build_sdist(self, sdist_directory, config_settings=None):
- return self._build_with_temp_dir(['sdist', '--formats', 'gztar'],
- '.tar.gz', sdist_directory,
- config_settings)
-
- def _get_dist_info_dir(self, metadata_directory: Optional[str]) -> Optional[str]:
- if not metadata_directory:
- return None
- dist_info_candidates = list(Path(metadata_directory).glob("*.dist-info"))
- assert len(dist_info_candidates) <= 1
- return str(dist_info_candidates[0]) if dist_info_candidates else None
-
- if not LEGACY_EDITABLE:
-
- # PEP660 hooks:
- # build_editable
- # get_requires_for_build_editable
- # prepare_metadata_for_build_editable
- def build_editable(
- self, wheel_directory, config_settings=None, metadata_directory=None
- ):
- # XXX can or should we hide our editable_wheel command normally?
- info_dir = self._get_dist_info_dir(metadata_directory)
- opts = ["--dist-info-dir", info_dir] if info_dir else []
- cmd = ["editable_wheel", *opts, *self._editable_args(config_settings)]
- with suppress_known_deprecation():
- return self._build_with_temp_dir(
- cmd, ".whl", wheel_directory, config_settings
- )
-
- def get_requires_for_build_editable(self, config_settings=None):
- return self.get_requires_for_build_wheel(config_settings)
-
- def prepare_metadata_for_build_editable(self, metadata_directory,
- config_settings=None):
- return self.prepare_metadata_for_build_wheel(
- metadata_directory, config_settings
- )
-
-
-class _BuildMetaLegacyBackend(_BuildMetaBackend):
- """Compatibility backend for setuptools
-
- This is a version of setuptools.build_meta that endeavors
- to maintain backwards
- compatibility with pre-PEP 517 modes of invocation. It
- exists as a temporary
- bridge between the old packaging mechanism and the new
- packaging mechanism,
- and will eventually be removed.
- """
- def run_setup(self, setup_script='setup.py'):
- # In order to maintain compatibility with scripts assuming that
- # the setup.py script is in a directory on the PYTHONPATH, inject
- # '' into sys.path. (pypa/setuptools#1642)
- sys_path = list(sys.path) # Save the original path
-
- script_dir = os.path.dirname(os.path.abspath(setup_script))
- if script_dir not in sys.path:
- sys.path.insert(0, script_dir)
-
- # Some setup.py scripts (e.g. in pygame and numpy) use sys.argv[0] to
- # get the directory of the source code. They expect it to refer to the
- # setup.py script.
- sys_argv_0 = sys.argv[0]
- sys.argv[0] = setup_script
-
- try:
- super(_BuildMetaLegacyBackend,
- self).run_setup(setup_script=setup_script)
- finally:
- # While PEP 517 frontends should be calling each hook in a fresh
- # subprocess according to the standard (and thus it should not be
- # strictly necessary to restore the old sys.path), we'll restore
- # the original path so that the path manipulation does not persist
- # within the hook after run_setup is called.
- sys.path[:] = sys_path
- sys.argv[0] = sys_argv_0
-
-
-# The primary backend
-_BACKEND = _BuildMetaBackend()
-
-get_requires_for_build_wheel = _BACKEND.get_requires_for_build_wheel
-get_requires_for_build_sdist = _BACKEND.get_requires_for_build_sdist
-prepare_metadata_for_build_wheel = _BACKEND.prepare_metadata_for_build_wheel
-build_wheel = _BACKEND.build_wheel
-build_sdist = _BACKEND.build_sdist
-
-if not LEGACY_EDITABLE:
- get_requires_for_build_editable = _BACKEND.get_requires_for_build_editable
- prepare_metadata_for_build_editable = _BACKEND.prepare_metadata_for_build_editable
- build_editable = _BACKEND.build_editable
-
-
-# The legacy backend
-__legacy__ = _BuildMetaLegacyBackend()
diff --git a/spaces/Bl1tzie/Jam/README.md b/spaces/Bl1tzie/Jam/README.md
deleted file mode 100644
index 6a2d56d43206a5674f8b936686f161211fec7c05..0000000000000000000000000000000000000000
--- a/spaces/Bl1tzie/Jam/README.md
+++ /dev/null
@@ -1,10 +0,0 @@
----
-title: Jam
-emoji: 😻
-colorFrom: green
-colorTo: gray
-sdk: docker
-pinned: false
----
-
-Check out the configuration reference at https://huggingface.co/docs/hub/spaces-config-reference
diff --git a/spaces/Boadiwaa/Recipes/openai/cli.py b/spaces/Boadiwaa/Recipes/openai/cli.py
deleted file mode 100644
index fd9c8469ad68affdb53220d816d011eea806120f..0000000000000000000000000000000000000000
--- a/spaces/Boadiwaa/Recipes/openai/cli.py
+++ /dev/null
@@ -1,1018 +0,0 @@
-import datetime
-import os
-import signal
-import sys
-import warnings
-from functools import partial
-from typing import Optional
-
-import requests
-
-import openai
-import openai.wandb_logger
-from openai.upload_progress import BufferReader
-from openai.validators import (
- apply_necessary_remediation,
- apply_validators,
- get_search_validators,
- get_validators,
- read_any_format,
- write_out_file,
- write_out_search_file,
-)
-
-
-class bcolors:
- HEADER = "\033[95m"
- OKBLUE = "\033[94m"
- OKGREEN = "\033[92m"
- WARNING = "\033[93m"
- FAIL = "\033[91m"
- ENDC = "\033[0m"
- BOLD = "\033[1m"
- UNDERLINE = "\033[4m"
-
-
-def organization_info(obj):
- organization = getattr(obj, "organization", None)
- if organization is not None:
- return "[organization={}] ".format(organization)
- else:
- return ""
-
-
-def display(obj):
- sys.stderr.write(organization_info(obj))
- sys.stderr.flush()
- print(obj)
-
-
-def display_error(e):
- extra = (
- " (HTTP status code: {})".format(e.http_status)
- if e.http_status is not None
- else ""
- )
- sys.stderr.write(
- "{}{}Error:{} {}{}\n".format(
- organization_info(e), bcolors.FAIL, bcolors.ENDC, e, extra
- )
- )
-
-
-class Engine:
- @classmethod
- def get(cls, args):
- engine = openai.Engine.retrieve(id=args.id)
- display(engine)
-
- @classmethod
- def update(cls, args):
- engine = openai.Engine.modify(args.id, replicas=args.replicas)
- display(engine)
-
- @classmethod
- def generate(cls, args):
- warnings.warn(
- "Engine.generate is deprecated, use Completion.create", DeprecationWarning
- )
- if args.completions and args.completions > 1 and args.stream:
- raise ValueError("Can't stream multiple completions with openai CLI")
-
- kwargs = {}
- if args.model is not None:
- kwargs["model"] = args.model
- resp = openai.Engine(id=args.id).generate(
- completions=args.completions,
- context=args.context,
- length=args.length,
- stream=args.stream,
- temperature=args.temperature,
- top_p=args.top_p,
- logprobs=args.logprobs,
- stop=args.stop,
- **kwargs,
- )
- if not args.stream:
- resp = [resp]
-
- for part in resp:
- completions = len(part["data"])
- for c_idx, c in enumerate(part["data"]):
- if completions > 1:
- sys.stdout.write("===== Completion {} =====\n".format(c_idx))
- sys.stdout.write("".join(c["text"]))
- if completions > 1:
- sys.stdout.write("\n")
- sys.stdout.flush()
-
- @classmethod
- def search(cls, args):
- params = {
- "query": args.query,
- "max_rerank": args.max_rerank,
- "return_metadata": args.return_metadata,
- }
- if args.documents:
- params["documents"] = args.documents
- if args.file:
- params["file"] = args.file
-
- if args.version:
- params["version"] = args.version
-
- resp = openai.Engine(id=args.id).search(**params)
- scores = [
- (search_result["score"], search_result["document"])
- for search_result in resp["data"]
- ]
- scores.sort(reverse=True)
- dataset = (
- args.documents if args.documents else [x["text"] for x in resp["data"]]
- )
- for score, document_idx in scores:
- print("=== score {:.3f} ===".format(score))
- print(dataset[document_idx])
- if (
- args.return_metadata
- and args.file
- and "metadata" in resp["data"][document_idx]
- ):
- print(f"METADATA: {resp['data'][document_idx]['metadata']}")
-
- @classmethod
- def list(cls, args):
- engines = openai.Engine.list()
- display(engines)
-
-
-class Completion:
- @classmethod
- def create(cls, args):
- if args.n is not None and args.n > 1 and args.stream:
- raise ValueError("Can't stream completions with n>1 with the current CLI")
-
- if args.engine and args.model:
- warnings.warn(
- "In most cases, you should not be specifying both engine and model."
- )
-
- resp = openai.Completion.create(
- engine=args.engine,
- model=args.model,
- n=args.n,
- max_tokens=args.max_tokens,
- logprobs=args.logprobs,
- prompt=args.prompt,
- stream=args.stream,
- temperature=args.temperature,
- top_p=args.top_p,
- stop=args.stop,
- echo=True,
- )
- if not args.stream:
- resp = [resp]
-
- for part in resp:
- choices = part["choices"]
- for c_idx, c in enumerate(sorted(choices, key=lambda s: s["index"])):
- if len(choices) > 1:
- sys.stdout.write("===== Completion {} =====\n".format(c_idx))
- sys.stdout.write(c["text"])
- if len(choices) > 1:
- sys.stdout.write("\n")
- sys.stdout.flush()
-
-
-class Model:
- @classmethod
- def get(cls, args):
- resp = openai.Model.retrieve(id=args.id)
- print(resp)
-
- @classmethod
- def delete(cls, args):
- model = openai.Model.delete(args.id)
- print(model)
-
- @classmethod
- def list(cls, args):
- models = openai.Model.list()
- print(models)
-
-
-class File:
- @classmethod
- def create(cls, args):
- with open(args.file, "rb") as file_reader:
- buffer_reader = BufferReader(file_reader.read(), desc="Upload progress")
- resp = openai.File.create(
- file=buffer_reader,
- purpose=args.purpose,
- model=args.model,
- user_provided_filename=args.file,
- )
- print(resp)
-
- @classmethod
- def get(cls, args):
- resp = openai.File.retrieve(id=args.id)
- print(resp)
-
- @classmethod
- def delete(cls, args):
- file = openai.File.delete(args.id)
- print(file)
-
- @classmethod
- def list(cls, args):
- file = openai.File.list()
- print(file)
-
-
-class Search:
- @classmethod
- def prepare_data(cls, args, purpose):
-
- sys.stdout.write("Analyzing...\n")
- fname = args.file
- auto_accept = args.quiet
-
- optional_fields = ["metadata"]
-
- if purpose == "classifications":
- required_fields = ["text", "label"]
- else:
- required_fields = ["text"]
-
- df, remediation = read_any_format(
- fname, fields=required_fields + optional_fields
- )
-
- if "metadata" not in df:
- df["metadata"] = None
-
- apply_necessary_remediation(None, remediation)
- validators = get_search_validators(required_fields, optional_fields)
-
- write_out_file_func = partial(
- write_out_search_file,
- purpose=purpose,
- fields=required_fields + optional_fields,
- )
-
- apply_validators(
- df, fname, remediation, validators, auto_accept, write_out_file_func
- )
-
- @classmethod
- def create(cls, args):
- resp = openai.Search.create(
- query=args.query,
- documents=args.documents,
- model=args.model,
- )
- print(resp)
-
-
-class FineTune:
- @classmethod
- def list(cls, args):
- resp = openai.FineTune.list()
- print(resp)
-
- @classmethod
- def _is_url(cls, file: str):
- return file.lower().startswith("http")
-
- @classmethod
- def _download_file_from_public_url(cls, url: str) -> Optional[bytes]:
- resp = requests.get(url)
- if resp.status_code == 200:
- return resp.content
- else:
- return None
-
- @classmethod
- def _maybe_upload_file(
- cls,
- file: Optional[str] = None,
- content: Optional[bytes] = None,
- user_provided_file: Optional[str] = None,
- check_if_file_exists: bool = True,
- ):
- # Exactly one of `file` or `content` must be provided
- if (file is None) == (content is None):
- raise ValueError("Exactly one of `file` or `content` must be provided")
-
- if content is None:
- assert file is not None
- with open(file, "rb") as f:
- content = f.read()
-
- if check_if_file_exists:
- bytes = len(content)
- matching_files = openai.File.find_matching_files(
- name=user_provided_file or f.name, bytes=bytes, purpose="fine-tune"
- )
- if len(matching_files) > 0:
- file_ids = [f["id"] for f in matching_files]
- sys.stdout.write(
- "Found potentially duplicated files with name '{name}', purpose 'fine-tune' and size {size} bytes\n".format(
- name=os.path.basename(matching_files[0]["filename"]),
- size=matching_files[0]["bytes"] if "bytes" in matching_files[0] else matching_files[0]["size"],
- )
- )
- sys.stdout.write("\n".join(file_ids))
- while True:
- sys.stdout.write(
- "\nEnter file ID to reuse an already uploaded file, or an empty string to upload this file anyway: "
- )
- inp = sys.stdin.readline().strip()
- if inp in file_ids:
- sys.stdout.write(
- "Reusing already uploaded file: {id}\n".format(id=inp)
- )
- return inp
- elif inp == "":
- break
- else:
- sys.stdout.write(
- "File id '{id}' is not among the IDs of the potentially duplicated files\n".format(
- id=inp
- )
- )
-
- buffer_reader = BufferReader(content, desc="Upload progress")
- resp = openai.File.create(
- file=buffer_reader,
- purpose="fine-tune",
- user_provided_filename=user_provided_file or file,
- )
- sys.stdout.write(
- "Uploaded file from {file}: {id}\n".format(
- file=user_provided_file or file, id=resp["id"]
- )
- )
- return resp["id"]
-
- @classmethod
- def _get_or_upload(cls, file, check_if_file_exists=True):
- try:
- # 1. If it's a valid file, use it
- openai.File.retrieve(file)
- return file
- except openai.error.InvalidRequestError:
- pass
- if os.path.isfile(file):
- # 2. If it's a file on the filesystem, upload it
- return cls._maybe_upload_file(
- file=file, check_if_file_exists=check_if_file_exists
- )
- if cls._is_url(file):
- # 3. If it's a URL, download it temporarily
- content = cls._download_file_from_public_url(file)
- if content is not None:
- return cls._maybe_upload_file(
- content=content,
- check_if_file_exists=check_if_file_exists,
- user_provided_file=file,
- )
- return file
-
- @classmethod
- def create(cls, args):
- create_args = {
- "training_file": cls._get_or_upload(
- args.training_file, args.check_if_files_exist
- ),
- }
- if args.validation_file:
- create_args["validation_file"] = cls._get_or_upload(
- args.validation_file, args.check_if_files_exist
- )
-
- for hparam in (
- "model",
- "suffix",
- "n_epochs",
- "batch_size",
- "learning_rate_multiplier",
- "prompt_loss_weight",
- "compute_classification_metrics",
- "classification_n_classes",
- "classification_positive_class",
- "classification_betas",
- ):
- attr = getattr(args, hparam)
- if attr is not None:
- create_args[hparam] = attr
-
- resp = openai.FineTune.create(**create_args)
-
- if args.no_follow:
- print(resp)
- return
-
- sys.stdout.write(
- "Created fine-tune: {job_id}\n"
- "Streaming events until fine-tuning is complete...\n\n"
- "(Ctrl-C will interrupt the stream, but not cancel the fine-tune)\n".format(
- job_id=resp["id"]
- )
- )
- cls._stream_events(resp["id"])
-
- @classmethod
- def get(cls, args):
- resp = openai.FineTune.retrieve(id=args.id)
- print(resp)
-
- @classmethod
- def results(cls, args):
- fine_tune = openai.FineTune.retrieve(id=args.id)
- if "result_files" not in fine_tune or len(fine_tune["result_files"]) == 0:
- raise openai.error.InvalidRequestError(
- f"No results file available for fine-tune {args.id}", "id"
- )
- result_file = openai.FineTune.retrieve(id=args.id)["result_files"][0]
- resp = openai.File.download(id=result_file["id"])
- print(resp.decode("utf-8"))
-
- @classmethod
- def events(cls, args):
- if args.stream:
- raise openai.error.OpenAIError(
- message=(
- "The --stream parameter is deprecated, use fine_tunes.follow "
- "instead:\n\n"
- " openai api fine_tunes.follow -i {id}\n".format(id=args.id)
- ),
- )
-
- resp = openai.FineTune.list_events(id=args.id) # type: ignore
- print(resp)
-
- @classmethod
- def follow(cls, args):
- cls._stream_events(args.id)
-
- @classmethod
- def _stream_events(cls, job_id):
- def signal_handler(sig, frame):
- status = openai.FineTune.retrieve(job_id).status
- sys.stdout.write(
- "\nStream interrupted. Job is still {status}.\n"
- "To resume the stream, run:\n\n"
- " openai api fine_tunes.follow -i {job_id}\n\n"
- "To cancel your job, run:\n\n"
- " openai api fine_tunes.cancel -i {job_id}\n\n".format(
- status=status, job_id=job_id
- )
- )
- sys.exit(0)
-
- signal.signal(signal.SIGINT, signal_handler)
-
- events = openai.FineTune.stream_events(job_id)
- # TODO(rachel): Add a nifty spinner here.
- try:
- for event in events:
- sys.stdout.write(
- "[%s] %s"
- % (
- datetime.datetime.fromtimestamp(event["created_at"]),
- event["message"],
- )
- )
- sys.stdout.write("\n")
- sys.stdout.flush()
- except Exception:
- sys.stdout.write(
- "\nStream interrupted (client disconnected).\n"
- "To resume the stream, run:\n\n"
- " openai api fine_tunes.follow -i {job_id}\n\n".format(job_id=job_id)
- )
- return
-
- resp = openai.FineTune.retrieve(id=job_id)
- status = resp["status"]
- if status == "succeeded":
- sys.stdout.write("\nJob complete! Status: succeeded 🎉")
- sys.stdout.write(
- "\nTry out your fine-tuned model:\n\n"
- "openai api completions.create -m {model} -p ".format(
- model=resp["fine_tuned_model"]
- )
- )
- elif status == "failed":
- sys.stdout.write(
- "\nJob failed. Please contact support@openai.com if you need assistance."
- )
- sys.stdout.write("\n")
-
- @classmethod
- def cancel(cls, args):
- resp = openai.FineTune.cancel(id=args.id)
- print(resp)
-
- @classmethod
- def prepare_data(cls, args):
-
- sys.stdout.write("Analyzing...\n")
- fname = args.file
- auto_accept = args.quiet
- df, remediation = read_any_format(fname)
- apply_necessary_remediation(None, remediation)
-
- validators = get_validators()
-
- apply_validators(
- df,
- fname,
- remediation,
- validators,
- auto_accept,
- write_out_file_func=write_out_file,
- )
-
-
-class WandbLogger:
- @classmethod
- def sync(cls, args):
- resp = openai.wandb_logger.WandbLogger.sync(
- id=args.id,
- n_fine_tunes=args.n_fine_tunes,
- project=args.project,
- entity=args.entity,
- force=args.force,
- )
- print(resp)
-
-
-def tools_register(parser):
- subparsers = parser.add_subparsers(
- title="Tools", help="Convenience client side tools"
- )
-
- def help(args):
- parser.print_help()
-
- parser.set_defaults(func=help)
-
- sub = subparsers.add_parser("fine_tunes.prepare_data")
- sub.add_argument(
- "-f",
- "--file",
- required=True,
- help="JSONL, JSON, CSV, TSV, TXT or XLSX file containing prompt-completion examples to be analyzed."
- "This should be the local file path.",
- )
- sub.add_argument(
- "-q",
- "--quiet",
- required=False,
- action="store_true",
- help="Auto accepts all suggestions, without asking for user input. To be used within scripts.",
- )
- sub.set_defaults(func=FineTune.prepare_data)
-
- sub = subparsers.add_parser("search.prepare_data")
- sub.add_argument(
- "-f",
- "--file",
- required=True,
- help="JSONL, JSON, CSV, TSV, TXT or XLSX file containing text examples to be analyzed."
- "This should be the local file path.",
- )
- sub.add_argument(
- "-q",
- "--quiet",
- required=False,
- action="store_true",
- help="Auto accepts all suggestions, without asking for user input. To be used within scripts.",
- )
- sub.set_defaults(func=partial(Search.prepare_data, purpose="search"))
-
- sub = subparsers.add_parser("classifications.prepare_data")
- sub.add_argument(
- "-f",
- "--file",
- required=True,
- help="JSONL, JSON, CSV, TSV, TXT or XLSX file containing text-label examples to be analyzed."
- "This should be the local file path.",
- )
- sub.add_argument(
- "-q",
- "--quiet",
- required=False,
- action="store_true",
- help="Auto accepts all suggestions, without asking for user input. To be used within scripts.",
- )
- sub.set_defaults(func=partial(Search.prepare_data, purpose="classifications"))
-
- sub = subparsers.add_parser("answers.prepare_data")
- sub.add_argument(
- "-f",
- "--file",
- required=True,
- help="JSONL, JSON, CSV, TSV, TXT or XLSX file containing text examples to be analyzed."
- "This should be the local file path.",
- )
- sub.add_argument(
- "-q",
- "--quiet",
- required=False,
- action="store_true",
- help="Auto accepts all suggestions, without asking for user input. To be used within scripts.",
- )
- sub.set_defaults(func=partial(Search.prepare_data, purpose="answer"))
-
-
-def api_register(parser):
- # Engine management
- subparsers = parser.add_subparsers(help="All API subcommands")
-
- def help(args):
- parser.print_help()
-
- parser.set_defaults(func=help)
-
- sub = subparsers.add_parser("engines.list")
- sub.set_defaults(func=Engine.list)
-
- sub = subparsers.add_parser("engines.get")
- sub.add_argument("-i", "--id", required=True)
- sub.set_defaults(func=Engine.get)
-
- sub = subparsers.add_parser("engines.update")
- sub.add_argument("-i", "--id", required=True)
- sub.add_argument("-r", "--replicas", type=int)
- sub.set_defaults(func=Engine.update)
-
- sub = subparsers.add_parser("engines.generate")
- sub.add_argument("-i", "--id", required=True)
- sub.add_argument(
- "--stream", help="Stream tokens as they're ready.", action="store_true"
- )
- sub.add_argument("-c", "--context", help="An optional context to generate from")
- sub.add_argument("-l", "--length", help="How many tokens to generate", type=int)
- sub.add_argument(
- "-t",
- "--temperature",
- help="""What sampling temperature to use. Higher values means the model will take more risks. Try 0.9 for more creative applications, and 0 (argmax sampling) for ones with a well-defined answer.
-
-Mutually exclusive with `top_p`.""",
- type=float,
- )
- sub.add_argument(
- "-p",
- "--top_p",
- help="""An alternative to sampling with temperature, called nucleus sampling, where the considers the results of the tokens with top_p probability mass. So 0.1 means only the tokens comprising the top 10%% probability mass are considered.
-
- Mutually exclusive with `temperature`.""",
- type=float,
- )
- sub.add_argument(
- "-n",
- "--completions",
- help="How many parallel completions to run on this context",
- type=int,
- )
- sub.add_argument(
- "--logprobs",
- help="Include the log probabilites on the `logprobs` most likely tokens. So for example, if `logprobs` is 10, the API will return a list of the 10 most likely tokens. If `logprobs` is supplied, the API will always return the logprob of the generated token, so there may be up to `logprobs+1` elements in the response.",
- type=int,
- )
- sub.add_argument(
- "--stop", help="A stop sequence at which to stop generating tokens."
- )
- sub.add_argument(
- "-m",
- "--model",
- required=False,
- help="A model (most commonly a model ID) to generate from. Defaults to the engine's default model.",
- )
- sub.set_defaults(func=Engine.generate)
-
- sub = subparsers.add_parser("engines.search")
- sub.add_argument("-i", "--id", required=True)
- sub.add_argument(
- "-d",
- "--documents",
- action="append",
- help="List of documents to search over. Only one of `documents` or `file` may be supplied.",
- required=False,
- )
- sub.add_argument(
- "-f",
- "--file",
- help="A file id to search over. Only one of `documents` or `file` may be supplied.",
- required=False,
- )
- sub.add_argument(
- "--max_rerank",
- help="The maximum number of documents to be re-ranked and returned by search. This flag only takes effect when `file` is set.",
- type=int,
- default=200,
- )
- sub.add_argument(
- "--return_metadata",
- help="A special boolean flag for showing metadata. If set `true`, each document entry in the returned json will contain a 'metadata' field. Default to be `false`. This flag only takes effect when `file` is set.",
- type=bool,
- default=False,
- )
- sub.add_argument(
- "--version",
- help="The version of the search routing to use",
- )
-
- sub.add_argument("-q", "--query", required=True, help="Search query")
- sub.set_defaults(func=Engine.search)
-
- # Completions
- sub = subparsers.add_parser("completions.create")
- sub.add_argument(
- "-e",
- "--engine",
- help="The engine to use. See https://beta.openai.com/docs/engines for more about what engines are available.",
- )
- sub.add_argument(
- "-m",
- "--model",
- help="The model to use. At most one of `engine` or `model` should be specified.",
- )
- sub.add_argument(
- "--stream", help="Stream tokens as they're ready.", action="store_true"
- )
- sub.add_argument("-p", "--prompt", help="An optional prompt to complete from")
- sub.add_argument(
- "-M", "--max-tokens", help="The maximum number of tokens to generate", type=int
- )
- sub.add_argument(
- "-t",
- "--temperature",
- help="""What sampling temperature to use. Higher values means the model will take more risks. Try 0.9 for more creative applications, and 0 (argmax sampling) for ones with a well-defined answer.
-
-Mutually exclusive with `top_p`.""",
- type=float,
- )
- sub.add_argument(
- "-P",
- "--top_p",
- help="""An alternative to sampling with temperature, called nucleus sampling, where the considers the results of the tokens with top_p probability mass. So 0.1 means only the tokens comprising the top 10%% probability mass are considered.
-
- Mutually exclusive with `temperature`.""",
- type=float,
- )
- sub.add_argument(
- "-n",
- "--n",
- help="How many sub-completions to generate for each prompt.",
- type=int,
- )
- sub.add_argument(
- "--logprobs",
- help="Include the log probabilites on the `logprobs` most likely tokens, as well the chosen tokens. So for example, if `logprobs` is 10, the API will return a list of the 10 most likely tokens. If `logprobs` is 0, only the chosen tokens will have logprobs returned.",
- type=int,
- )
- sub.add_argument(
- "--stop", help="A stop sequence at which to stop generating tokens."
- )
- sub.set_defaults(func=Completion.create)
-
- # Models
- sub = subparsers.add_parser("models.list")
- sub.set_defaults(func=Model.list)
-
- sub = subparsers.add_parser("models.get")
- sub.add_argument("-i", "--id", required=True, help="The model ID")
- sub.set_defaults(func=Model.get)
-
- sub = subparsers.add_parser("models.delete")
- sub.add_argument("-i", "--id", required=True, help="The model ID")
- sub.set_defaults(func=Model.delete)
-
- # Files
- sub = subparsers.add_parser("files.create")
-
- sub.add_argument(
- "-f",
- "--file",
- required=True,
- help="File to upload",
- )
- sub.add_argument(
- "-p",
- "--purpose",
- help="Why are you uploading this file? (see https://beta.openai.com/docs/api-reference/ for purposes)",
- required=True,
- )
- sub.add_argument(
- "-m",
- "--model",
- help="Model for search indexing (e.g. 'ada'). Only meaningful if --purpose is 'search'.",
- )
- sub.set_defaults(func=File.create)
-
- sub = subparsers.add_parser("files.get")
- sub.add_argument("-i", "--id", required=True, help="The files ID")
- sub.set_defaults(func=File.get)
-
- sub = subparsers.add_parser("files.delete")
- sub.add_argument("-i", "--id", required=True, help="The files ID")
- sub.set_defaults(func=File.delete)
-
- sub = subparsers.add_parser("files.list")
- sub.set_defaults(func=File.list)
-
- # Search
- sub = subparsers.add_parser("search.create")
-
- sub.add_argument(
- "-d",
- "--documents",
- help="Documents to search over",
- type=str,
- nargs="+",
- )
- sub.add_argument(
- "-q",
- "--query",
- required=True,
- help="Search query",
- )
- sub.add_argument(
- "-m",
- "--model",
- help="The model to search with",
- )
- sub.set_defaults(func=Search.create)
-
- # Finetune
- sub = subparsers.add_parser("fine_tunes.list")
- sub.set_defaults(func=FineTune.list)
-
- sub = subparsers.add_parser("fine_tunes.create")
- sub.add_argument(
- "-t",
- "--training_file",
- required=True,
- help="JSONL file containing prompt-completion examples for training. This can "
- "be the ID of a file uploaded through the OpenAI API (e.g. file-abcde12345), "
- 'a local file path, or a URL that starts with "http".',
- )
- sub.add_argument(
- "-v",
- "--validation_file",
- help="JSONL file containing prompt-completion examples for validation. This can "
- "be the ID of a file uploaded through the OpenAI API (e.g. file-abcde12345), "
- 'a local file path, or a URL that starts with "http".',
- )
- sub.add_argument(
- "--no_check_if_files_exist",
- dest="check_if_files_exist",
- action="store_false",
- help="If this argument is set and training_file or validation_file are file paths, immediately upload them. If this argument is not set, check if they may be duplicates of already uploaded files before uploading, based on file name and file size.",
- )
- sub.add_argument(
- "-m",
- "--model",
- help="The model to start fine-tuning from",
- )
- sub.add_argument(
- "--suffix",
- help="If set, this argument can be used to customize the generated fine-tuned model name."
- "All punctuation and whitespace in `suffix` will be replaced with a "
- "single dash, and the string will be lower cased. The max "
- "length of `suffix` is 40 chars. "
- "The generated name will match the form `{base_model}:ft-{org-title}:{suffix}-{timestamp}`. "
- 'For example, `openai api fine_tunes.create -t test.jsonl -m ada --suffix "custom model name" '
- "could generate a model with the name "
- "ada:ft-your-org:custom-model-name-2022-02-15-04-21-04",
- )
- sub.add_argument(
- "--no_follow",
- action="store_true",
- help="If set, returns immediately after creating the job. Otherwise, streams events and waits for the job to complete.",
- )
- sub.add_argument(
- "--n_epochs",
- type=int,
- help="The number of epochs to train the model for. An epoch refers to one "
- "full cycle through the training dataset.",
- )
- sub.add_argument(
- "--batch_size",
- type=int,
- help="The batch size to use for training. The batch size is the number of "
- "training examples used to train a single forward and backward pass.",
- )
- sub.add_argument(
- "--learning_rate_multiplier",
- type=float,
- help="The learning rate multiplier to use for training. The fine-tuning "
- "learning rate is determined by the original learning rate used for "
- "pretraining multiplied by this value.",
- )
- sub.add_argument(
- "--prompt_loss_weight",
- type=float,
- help="The weight to use for the prompt loss. The optimum value here depends "
- "depends on your use case. This determines how much the model prioritizes "
- "learning from prompt tokens vs learning from completion tokens.",
- )
- sub.add_argument(
- "--compute_classification_metrics",
- action="store_true",
- help="If set, we calculate classification-specific metrics such as accuracy "
- "and F-1 score using the validation set at the end of every epoch.",
- )
- sub.set_defaults(compute_classification_metrics=None)
- sub.add_argument(
- "--classification_n_classes",
- type=int,
- help="The number of classes in a classification task. This parameter is "
- "required for multiclass classification.",
- )
- sub.add_argument(
- "--classification_positive_class",
- help="The positive class in binary classification. This parameter is needed "
- "to generate precision, recall and F-1 metrics when doing binary "
- "classification.",
- )
- sub.add_argument(
- "--classification_betas",
- type=float,
- nargs="+",
- help="If this is provided, we calculate F-beta scores at the specified beta "
- "values. The F-beta score is a generalization of F-1 score. This is only "
- "used for binary classification.",
- )
- sub.set_defaults(func=FineTune.create)
-
- sub = subparsers.add_parser("fine_tunes.get")
- sub.add_argument("-i", "--id", required=True, help="The id of the fine-tune job")
- sub.set_defaults(func=FineTune.get)
-
- sub = subparsers.add_parser("fine_tunes.results")
- sub.add_argument("-i", "--id", required=True, help="The id of the fine-tune job")
- sub.set_defaults(func=FineTune.results)
-
- sub = subparsers.add_parser("fine_tunes.events")
- sub.add_argument("-i", "--id", required=True, help="The id of the fine-tune job")
-
- # TODO(rachel): Remove this in 1.0
- sub.add_argument(
- "-s",
- "--stream",
- action="store_true",
- help="[DEPRECATED] If set, events will be streamed until the job is done. Otherwise, "
- "displays the event history to date.",
- )
- sub.set_defaults(func=FineTune.events)
-
- sub = subparsers.add_parser("fine_tunes.follow")
- sub.add_argument("-i", "--id", required=True, help="The id of the fine-tune job")
- sub.set_defaults(func=FineTune.follow)
-
- sub = subparsers.add_parser("fine_tunes.cancel")
- sub.add_argument("-i", "--id", required=True, help="The id of the fine-tune job")
- sub.set_defaults(func=FineTune.cancel)
-
-
-def wandb_register(parser):
- subparsers = parser.add_subparsers(
- title="wandb", help="Logging with Weights & Biases"
- )
-
- def help(args):
- parser.print_help()
-
- parser.set_defaults(func=help)
-
- sub = subparsers.add_parser("sync")
- sub.add_argument("-i", "--id", help="The id of the fine-tune job (optional)")
- sub.add_argument(
- "-n",
- "--n_fine_tunes",
- type=int,
- default=None,
- help="Number of most recent fine-tunes to log when an id is not provided. By default, every fine-tune is synced.",
- )
- sub.add_argument(
- "--project",
- default="GPT-3",
- help="""Name of the project where you're sending runs. By default, it is "GPT-3".""",
- )
- sub.add_argument(
- "--entity",
- help="Username or team name where you're sending runs. By default, your default entity is used, which is usually your username.",
- )
- sub.add_argument(
- "--force",
- action="store_true",
- help="Forces logging and overwrite existing wandb run of the same fine-tune.",
- )
- sub.set_defaults(force=False)
- sub.set_defaults(func=WandbLogger.sync)
diff --git a/spaces/CVPR/LIVE/pybind11/tests/test_methods_and_attributes.cpp b/spaces/CVPR/LIVE/pybind11/tests/test_methods_and_attributes.cpp
deleted file mode 100644
index 11d4e7b3501a8bb37b829af6c4aa5d4a4e094f8e..0000000000000000000000000000000000000000
--- a/spaces/CVPR/LIVE/pybind11/tests/test_methods_and_attributes.cpp
+++ /dev/null
@@ -1,372 +0,0 @@
-/*
- tests/test_methods_and_attributes.cpp -- constructors, deconstructors, attribute access,
- __str__, argument and return value conventions
-
- Copyright (c) 2016 Wenzel Jakob
-
- All rights reserved. Use of this source code is governed by a
- BSD-style license that can be found in the LICENSE file.
-*/
-
-#include "pybind11_tests.h"
-#include "constructor_stats.h"
-
-#if !defined(PYBIND11_OVERLOAD_CAST)
-template
-using overload_cast_ = pybind11::detail::overload_cast_impl;
-#endif
-
-class ExampleMandA {
-public:
- ExampleMandA() { print_default_created(this); }
- ExampleMandA(int value) : value(value) { print_created(this, value); }
- ExampleMandA(const ExampleMandA &e) : value(e.value) { print_copy_created(this); }
- ExampleMandA(std::string&&) {}
- ExampleMandA(ExampleMandA &&e) : value(e.value) { print_move_created(this); }
- ~ExampleMandA() { print_destroyed(this); }
-
- std::string toString() {
- return "ExampleMandA[value=" + std::to_string(value) + "]";
- }
-
- void operator=(const ExampleMandA &e) { print_copy_assigned(this); value = e.value; }
- void operator=(ExampleMandA &&e) { print_move_assigned(this); value = e.value; }
-
- void add1(ExampleMandA other) { value += other.value; } // passing by value
- void add2(ExampleMandA &other) { value += other.value; } // passing by reference
- void add3(const ExampleMandA &other) { value += other.value; } // passing by const reference
- void add4(ExampleMandA *other) { value += other->value; } // passing by pointer
- void add5(const ExampleMandA *other) { value += other->value; } // passing by const pointer
-
- void add6(int other) { value += other; } // passing by value
- void add7(int &other) { value += other; } // passing by reference
- void add8(const int &other) { value += other; } // passing by const reference
- void add9(int *other) { value += *other; } // passing by pointer
- void add10(const int *other) { value += *other; } // passing by const pointer
-
- void consume_str(std::string&&) {}
-
- ExampleMandA self1() { return *this; } // return by value
- ExampleMandA &self2() { return *this; } // return by reference
- const ExampleMandA &self3() { return *this; } // return by const reference
- ExampleMandA *self4() { return this; } // return by pointer
- const ExampleMandA *self5() { return this; } // return by const pointer
-
- int internal1() { return value; } // return by value
- int &internal2() { return value; } // return by reference
- const int &internal3() { return value; } // return by const reference
- int *internal4() { return &value; } // return by pointer
- const int *internal5() { return &value; } // return by const pointer
-
- py::str overloaded() { return "()"; }
- py::str overloaded(int) { return "(int)"; }
- py::str overloaded(int, float) { return "(int, float)"; }
- py::str overloaded(float, int) { return "(float, int)"; }
- py::str overloaded(int, int) { return "(int, int)"; }
- py::str overloaded(float, float) { return "(float, float)"; }
- py::str overloaded(int) const { return "(int) const"; }
- py::str overloaded(int, float) const { return "(int, float) const"; }
- py::str overloaded(float, int) const { return "(float, int) const"; }
- py::str overloaded(int, int) const { return "(int, int) const"; }
- py::str overloaded(float, float) const { return "(float, float) const"; }
-
- static py::str overloaded(float) { return "static float"; }
-
- int value = 0;
-};
-
-struct TestProperties {
- int value = 1;
- static int static_value;
-
- int get() const { return value; }
- void set(int v) { value = v; }
-
- static int static_get() { return static_value; }
- static void static_set(int v) { static_value = v; }
-};
-int TestProperties::static_value = 1;
-
-struct TestPropertiesOverride : TestProperties {
- int value = 99;
- static int static_value;
-};
-int TestPropertiesOverride::static_value = 99;
-
-struct TestPropRVP {
- UserType v1{1};
- UserType v2{1};
- static UserType sv1;
- static UserType sv2;
-
- const UserType &get1() const { return v1; }
- const UserType &get2() const { return v2; }
- UserType get_rvalue() const { return v2; }
- void set1(int v) { v1.set(v); }
- void set2(int v) { v2.set(v); }
-};
-UserType TestPropRVP::sv1(1);
-UserType TestPropRVP::sv2(1);
-
-// Test None-allowed py::arg argument policy
-class NoneTester { public: int answer = 42; };
-int none1(const NoneTester &obj) { return obj.answer; }
-int none2(NoneTester *obj) { return obj ? obj->answer : -1; }
-int none3(std::shared_ptr &obj) { return obj ? obj->answer : -1; }
-int none4(std::shared_ptr *obj) { return obj && *obj ? (*obj)->answer : -1; }
-int none5(std::shared_ptr obj) { return obj ? obj->answer : -1; }
-
-struct StrIssue {
- int val = -1;
-
- StrIssue() = default;
- StrIssue(int i) : val{i} {}
-};
-
-// Issues #854, #910: incompatible function args when member function/pointer is in unregistered base class
-class UnregisteredBase {
-public:
- void do_nothing() const {}
- void increase_value() { rw_value++; ro_value += 0.25; }
- void set_int(int v) { rw_value = v; }
- int get_int() const { return rw_value; }
- double get_double() const { return ro_value; }
- int rw_value = 42;
- double ro_value = 1.25;
-};
-class RegisteredDerived : public UnregisteredBase {
-public:
- using UnregisteredBase::UnregisteredBase;
- double sum() const { return rw_value + ro_value; }
-};
-
-// Test explicit lvalue ref-qualification
-struct RefQualified {
- int value = 0;
-
- void refQualified(int other) & { value += other; }
- int constRefQualified(int other) const & { return value + other; }
-};
-
-TEST_SUBMODULE(methods_and_attributes, m) {
- // test_methods_and_attributes
- py::class_ emna(m, "ExampleMandA");
- emna.def(py::init<>())
- .def(py::init())
- .def(py::init())
- .def(py::init())
- .def("add1", &ExampleMandA::add1)
- .def("add2", &ExampleMandA::add2)
- .def("add3", &ExampleMandA::add3)
- .def("add4", &ExampleMandA::add4)
- .def("add5", &ExampleMandA::add5)
- .def("add6", &ExampleMandA::add6)
- .def("add7", &ExampleMandA::add7)
- .def("add8", &ExampleMandA::add8)
- .def("add9", &ExampleMandA::add9)
- .def("add10", &ExampleMandA::add10)
- .def("consume_str", &ExampleMandA::consume_str)
- .def("self1", &ExampleMandA::self1)
- .def("self2", &ExampleMandA::self2)
- .def("self3", &ExampleMandA::self3)
- .def("self4", &ExampleMandA::self4)
- .def("self5", &ExampleMandA::self5)
- .def("internal1", &ExampleMandA::internal1)
- .def("internal2", &ExampleMandA::internal2)
- .def("internal3", &ExampleMandA::internal3)
- .def("internal4", &ExampleMandA::internal4)
- .def("internal5", &ExampleMandA::internal5)
-#if defined(PYBIND11_OVERLOAD_CAST)
- .def("overloaded", py::overload_cast<>(&ExampleMandA::overloaded))
- .def("overloaded", py::overload_cast(&ExampleMandA::overloaded))
- .def("overloaded", py::overload_cast(&ExampleMandA::overloaded))
- .def("overloaded", py::overload_cast(&ExampleMandA::overloaded))
- .def("overloaded", py::overload_cast(&ExampleMandA::overloaded))
- .def("overloaded", py::overload_cast(&ExampleMandA::overloaded))
- .def("overloaded_float", py::overload_cast(&ExampleMandA::overloaded))
- .def("overloaded_const", py::overload_cast(&ExampleMandA::overloaded, py::const_))
- .def("overloaded_const", py::overload_cast(&ExampleMandA::overloaded, py::const_))
- .def("overloaded_const", py::overload_cast(&ExampleMandA::overloaded, py::const_))
- .def("overloaded_const", py::overload_cast(&ExampleMandA::overloaded, py::const_))
- .def("overloaded_const", py::overload_cast(&ExampleMandA::overloaded, py::const_))
-#else
- // Use both the traditional static_cast method and the C++11 compatible overload_cast_
- .def("overloaded", overload_cast_<>()(&ExampleMandA::overloaded))
- .def("overloaded", overload_cast_()(&ExampleMandA::overloaded))
- .def("overloaded", overload_cast_()(&ExampleMandA::overloaded))
- .def("overloaded", static_cast(&ExampleMandA::overloaded))
- .def("overloaded", static_cast(&ExampleMandA::overloaded))
- .def("overloaded", static_cast(&ExampleMandA::overloaded))
- .def("overloaded_float", overload_cast_()(&ExampleMandA::overloaded))
- .def("overloaded_const", overload_cast_()(&ExampleMandA::overloaded, py::const_))
- .def("overloaded_const", overload_cast_()(&ExampleMandA::overloaded, py::const_))
- .def("overloaded_const", static_cast(&ExampleMandA::overloaded))
- .def("overloaded_const", static_cast(&ExampleMandA::overloaded))
- .def("overloaded_const", static_cast(&ExampleMandA::overloaded))
-#endif
- // test_no_mixed_overloads
- // Raise error if trying to mix static/non-static overloads on the same name:
- .def_static("add_mixed_overloads1", []() {
- auto emna = py::reinterpret_borrow>(py::module::import("pybind11_tests.methods_and_attributes").attr("ExampleMandA"));
- emna.def ("overload_mixed1", static_cast(&ExampleMandA::overloaded))
- .def_static("overload_mixed1", static_cast(&ExampleMandA::overloaded));
- })
- .def_static("add_mixed_overloads2", []() {
- auto emna = py::reinterpret_borrow>(py::module::import("pybind11_tests.methods_and_attributes").attr("ExampleMandA"));
- emna.def_static("overload_mixed2", static_cast(&ExampleMandA::overloaded))
- .def ("overload_mixed2", static_cast(&ExampleMandA::overloaded));
- })
- .def("__str__", &ExampleMandA::toString)
- .def_readwrite("value", &ExampleMandA::value);
-
- // test_copy_method
- // Issue #443: can't call copied methods in Python 3
- emna.attr("add2b") = emna.attr("add2");
-
- // test_properties, test_static_properties, test_static_cls
- py::class_(m, "TestProperties")
- .def(py::init<>())
- .def_readonly("def_readonly", &TestProperties::value)
- .def_readwrite("def_readwrite", &TestProperties::value)
- .def_property("def_writeonly", nullptr,
- [](TestProperties& s,int v) { s.value = v; } )
- .def_property("def_property_writeonly", nullptr, &TestProperties::set)
- .def_property_readonly("def_property_readonly", &TestProperties::get)
- .def_property("def_property", &TestProperties::get, &TestProperties::set)
- .def_property("def_property_impossible", nullptr, nullptr)
- .def_readonly_static("def_readonly_static", &TestProperties::static_value)
- .def_readwrite_static("def_readwrite_static", &TestProperties::static_value)
- .def_property_static("def_writeonly_static", nullptr,
- [](py::object, int v) { TestProperties::static_value = v; })
- .def_property_readonly_static("def_property_readonly_static",
- [](py::object) { return TestProperties::static_get(); })
- .def_property_static("def_property_writeonly_static", nullptr,
- [](py::object, int v) { return TestProperties::static_set(v); })
- .def_property_static("def_property_static",
- [](py::object) { return TestProperties::static_get(); },
- [](py::object, int v) { TestProperties::static_set(v); })
- .def_property_static("static_cls",
- [](py::object cls) { return cls; },
- [](py::object cls, py::function f) { f(cls); });
-
- py::class_(m, "TestPropertiesOverride")
- .def(py::init<>())
- .def_readonly("def_readonly", &TestPropertiesOverride::value)
- .def_readonly_static("def_readonly_static", &TestPropertiesOverride::static_value);
-
- auto static_get1 = [](py::object) -> const UserType & { return TestPropRVP::sv1; };
- auto static_get2 = [](py::object) -> const UserType & { return TestPropRVP::sv2; };
- auto static_set1 = [](py::object, int v) { TestPropRVP::sv1.set(v); };
- auto static_set2 = [](py::object, int v) { TestPropRVP::sv2.set(v); };
- auto rvp_copy = py::return_value_policy::copy;
-
- // test_property_return_value_policies
- py::class_(m, "TestPropRVP")
- .def(py::init<>())
- .def_property_readonly("ro_ref", &TestPropRVP::get1)
- .def_property_readonly("ro_copy", &TestPropRVP::get2, rvp_copy)
- .def_property_readonly("ro_func", py::cpp_function(&TestPropRVP::get2, rvp_copy))
- .def_property("rw_ref", &TestPropRVP::get1, &TestPropRVP::set1)
- .def_property("rw_copy", &TestPropRVP::get2, &TestPropRVP::set2, rvp_copy)
- .def_property("rw_func", py::cpp_function(&TestPropRVP::get2, rvp_copy), &TestPropRVP::set2)
- .def_property_readonly_static("static_ro_ref", static_get1)
- .def_property_readonly_static("static_ro_copy", static_get2, rvp_copy)
- .def_property_readonly_static("static_ro_func", py::cpp_function(static_get2, rvp_copy))
- .def_property_static("static_rw_ref", static_get1, static_set1)
- .def_property_static("static_rw_copy", static_get2, static_set2, rvp_copy)
- .def_property_static("static_rw_func", py::cpp_function(static_get2, rvp_copy), static_set2)
- // test_property_rvalue_policy
- .def_property_readonly("rvalue", &TestPropRVP::get_rvalue)
- .def_property_readonly_static("static_rvalue", [](py::object) { return UserType(1); });
-
- // test_metaclass_override
- struct MetaclassOverride { };
- py::class_(m, "MetaclassOverride", py::metaclass((PyObject *) &PyType_Type))
- .def_property_readonly_static("readonly", [](py::object) { return 1; });
-
-#if !defined(PYPY_VERSION)
- // test_dynamic_attributes
- class DynamicClass {
- public:
- DynamicClass() { print_default_created(this); }
- DynamicClass(const DynamicClass&) = delete;
- ~DynamicClass() { print_destroyed(this); }
- };
- py::class_(m, "DynamicClass", py::dynamic_attr())
- .def(py::init());
-
- class CppDerivedDynamicClass : public DynamicClass { };
- py::class_(m, "CppDerivedDynamicClass")
- .def(py::init());
-#endif
-
- // test_bad_arg_default
- // Issue/PR #648: bad arg default debugging output
-#if !defined(NDEBUG)
- m.attr("debug_enabled") = true;
-#else
- m.attr("debug_enabled") = false;
-#endif
- m.def("bad_arg_def_named", []{
- auto m = py::module::import("pybind11_tests");
- m.def("should_fail", [](int, UnregisteredType) {}, py::arg(), py::arg("a") = UnregisteredType());
- });
- m.def("bad_arg_def_unnamed", []{
- auto m = py::module::import("pybind11_tests");
- m.def("should_fail", [](int, UnregisteredType) {}, py::arg(), py::arg() = UnregisteredType());
- });
-
- // test_accepts_none
- py::class_>(m, "NoneTester")
- .def(py::init<>());
- m.def("no_none1", &none1, py::arg().none(false));
- m.def("no_none2", &none2, py::arg().none(false));
- m.def("no_none3", &none3, py::arg().none(false));
- m.def("no_none4", &none4, py::arg().none(false));
- m.def("no_none5", &none5, py::arg().none(false));
- m.def("ok_none1", &none1);
- m.def("ok_none2", &none2, py::arg().none(true));
- m.def("ok_none3", &none3);
- m.def("ok_none4", &none4, py::arg().none(true));
- m.def("ok_none5", &none5);
-
- // test_str_issue
- // Issue #283: __str__ called on uninitialized instance when constructor arguments invalid
- py::class_(m, "StrIssue")
- .def(py::init())
- .def(py::init<>())
- .def("__str__", [](const StrIssue &si) {
- return "StrIssue[" + std::to_string(si.val) + "]"; }
- );
-
- // test_unregistered_base_implementations
- //
- // Issues #854/910: incompatible function args when member function/pointer is in unregistered
- // base class The methods and member pointers below actually resolve to members/pointers in
- // UnregisteredBase; before this test/fix they would be registered via lambda with a first
- // argument of an unregistered type, and thus uncallable.
- py::class_(m, "RegisteredDerived")
- .def(py::init<>())
- .def("do_nothing", &RegisteredDerived::do_nothing)
- .def("increase_value", &RegisteredDerived::increase_value)
- .def_readwrite("rw_value", &RegisteredDerived::rw_value)
- .def_readonly("ro_value", &RegisteredDerived::ro_value)
- // These should trigger a static_assert if uncommented
- //.def_readwrite("fails", &UserType::value) // should trigger a static_assert if uncommented
- //.def_readonly("fails", &UserType::value) // should trigger a static_assert if uncommented
- .def_property("rw_value_prop", &RegisteredDerived::get_int, &RegisteredDerived::set_int)
- .def_property_readonly("ro_value_prop", &RegisteredDerived::get_double)
- // This one is in the registered class:
- .def("sum", &RegisteredDerived::sum)
- ;
-
- using Adapted = decltype(py::method_adaptor(&RegisteredDerived::do_nothing));
- static_assert(std::is_same::value, "");
-
- // test_methods_and_attributes
- py::class_(m, "RefQualified")
- .def(py::init<>())
- .def_readonly("value", &RefQualified::value)
- .def("refQualified", &RefQualified::refQualified)
- .def("constRefQualified", &RefQualified::constRefQualified);
-}
diff --git a/spaces/CVPR/LIVE/thrust/thrust/detail/complex/math_private.h b/spaces/CVPR/LIVE/thrust/thrust/detail/complex/math_private.h
deleted file mode 100644
index bc2d6357f2c169ee7e4e60f466dc09f4ed4b30d2..0000000000000000000000000000000000000000
--- a/spaces/CVPR/LIVE/thrust/thrust/detail/complex/math_private.h
+++ /dev/null
@@ -1,136 +0,0 @@
-/*
- * Copyright 2008-2013 NVIDIA Corporation
- * Copyright 2013 Filipe RNC Maia
- *
- * Licensed under the Apache License, Version 2.0 (the "License");
- * you may not use this file except in compliance with the License.
- * You may obtain a copy of the License at
- *
- * http://www.apache.org/licenses/LICENSE-2.0
- *
- * Unless required by applicable law or agreed to in writing, software
- * distributed under the License is distributed on an "AS IS" BASIS,
- * WITHOUT WARRANTIES OR CONDITIONS OF ANY KIND, either express or implied.
- * See the License for the specific language governing permissions and
- * limitations under the License.
- */
-
-/*
- * ====================================================
- * Copyright (C) 1993 by Sun Microsystems, Inc. All rights reserved.
- *
- * Developed at SunPro, a Sun Microsystems, Inc. business.
- * Permission to use, copy, modify, and distribute this
- * software is freely granted, provided that this notice
- * is preserved.
- * ====================================================
- */
-
-/* adapted from FreeBSD:
- * lib/msun/src/math_private.h
- */
-#pragma once
-
-#include
-#include
-#include
-
-namespace thrust{
-namespace detail{
-namespace complex{
-
-using thrust::complex;
-
-typedef union
-{
- float value;
- uint32_t word;
-} ieee_float_shape_type;
-
-__host__ __device__
-inline void get_float_word(uint32_t & i, float d){
- ieee_float_shape_type gf_u;
- gf_u.value = (d);
- (i) = gf_u.word;
-}
-
-__host__ __device__
-inline void get_float_word(int32_t & i, float d){
- ieee_float_shape_type gf_u;
- gf_u.value = (d);
- (i) = gf_u.word;
-}
-
-__host__ __device__
-inline void set_float_word(float & d, uint32_t i){
- ieee_float_shape_type sf_u;
- sf_u.word = (i);
- (d) = sf_u.value;
-}
-
-// Assumes little endian ordering
-typedef union
-{
- double value;
- struct
- {
- uint32_t lsw;
- uint32_t msw;
- } parts;
- struct
- {
- uint64_t w;
- } xparts;
-} ieee_double_shape_type;
-
-__host__ __device__ inline
-void get_high_word(uint32_t & i,double d){
- ieee_double_shape_type gh_u;
- gh_u.value = (d);
- (i) = gh_u.parts.msw;
-}
-
-/* Set the more significant 32 bits of a double from an int. */
-__host__ __device__ inline
-void set_high_word(double & d, uint32_t v){
- ieee_double_shape_type sh_u;
- sh_u.value = (d);
- sh_u.parts.msw = (v);
- (d) = sh_u.value;
-}
-
-
-__host__ __device__ inline
-void insert_words(double & d, uint32_t ix0, uint32_t ix1){
- ieee_double_shape_type iw_u;
- iw_u.parts.msw = (ix0);
- iw_u.parts.lsw = (ix1);
- (d) = iw_u.value;
-}
-
-/* Get two 32 bit ints from a double. */
-__host__ __device__ inline
-void extract_words(uint32_t & ix0,uint32_t & ix1, double d){
- ieee_double_shape_type ew_u;
- ew_u.value = (d);
- (ix0) = ew_u.parts.msw;
- (ix1) = ew_u.parts.lsw;
-}
-
-/* Get two 32 bit ints from a double. */
-__host__ __device__ inline
-void extract_words(int32_t & ix0,int32_t & ix1, double d){
- ieee_double_shape_type ew_u;
- ew_u.value = (d);
- (ix0) = ew_u.parts.msw;
- (ix1) = ew_u.parts.lsw;
-}
-
-} // namespace complex
-
-} // namespace detail
-
-} // namespace thrust
-
-
-#include
diff --git a/spaces/CVPR/LIVE/thrust/thrust/detail/functional/operators/arithmetic_operators.h b/spaces/CVPR/LIVE/thrust/thrust/detail/functional/operators/arithmetic_operators.h
deleted file mode 100644
index bd5b707e3ba163d7308b3d893a4f4b773af1933f..0000000000000000000000000000000000000000
--- a/spaces/CVPR/LIVE/thrust/thrust/detail/functional/operators/arithmetic_operators.h
+++ /dev/null
@@ -1,432 +0,0 @@
-/*
- * Copyright 2008-2013 NVIDIA Corporation
- *
- * Licensed under the Apache License, Version 2.0 (the "License");
- * you may not use this file except in compliance with the License.
- * You may obtain a copy of the License at
- *
- * http://www.apache.org/licenses/LICENSE-2.0
- *
- * Unless required by applicable law or agreed to in writing, software
- * distributed under the License is distributed on an "AS IS" BASIS,
- * WITHOUT WARRANTIES OR CONDITIONS OF ANY KIND, either express or implied.
- * See the License for the specific language governing permissions and
- * limitations under the License.
- */
-
-#pragma once
-
-#include
-#include
-#include
-#include
-#include
-
-namespace thrust
-{
-namespace detail
-{
-namespace functional
-{
-
-template
-__host__ __device__
-actor<
- composite<
- transparent_unary_operator>,
- actor
- >
->
-__host__ __device__
-operator-(const actor &_1)
-{
- return compose(transparent_unary_operator>(), _1);
-} // end operator-()
-
-// there's no standard unary_plus functional, so roll an ad hoc one here
-struct unary_plus
-{
- using is_transparent = void;
-
- __thrust_exec_check_disable__
- template
- __host__ __device__
- constexpr auto operator()(T1&& t1) const
- noexcept(noexcept(+THRUST_FWD(t1))) -> decltype(+THRUST_FWD(t1))
- {
- return +THRUST_FWD(t1);
- }
-};
-
-template
-__host__ __device__
-actor<
- composite<
- transparent_unary_operator,
- actor
- >
->
-operator+(const actor &_1)
-{
- return compose(transparent_unary_operator(), _1);
-} // end operator+()
-
-template
-__host__ __device__
-actor<
- composite<
- transparent_binary_operator>,
- actor,
- typename as_actor::type
- >
->
-operator+(const actor &_1, const T2 &_2)
-{
- return compose(transparent_binary_operator>(),
- make_actor(_1),
- make_actor(_2));
-} // end operator+()
-
-template
-__host__ __device__
-actor<
- composite<
- transparent_binary_operator>,
- typename as_actor::type,
- actor
- >
->
-operator+(const T1 &_1, const actor &_2)
-{
- return compose(transparent_binary_operator>(),
- make_actor(_1),
- make_actor(_2));
-} // end operator+()
-
-template
-__host__ __device__
-actor<
- composite<
- transparent_binary_operator>,
- actor,
- actor
- >
->
-operator+(const actor &_1, const actor &_2)
-{
- return compose(transparent_binary_operator>(),
- make_actor(_1),
- make_actor(_2));
-} // end operator+()
-
-template
-__host__ __device__
-actor<
- composite<
- transparent_binary_operator>,
- typename as_actor::type,
- actor
- >
->
-operator-(const T1 &_1, const actor &_2)
-{
- return compose(transparent_binary_operator>(),
- make_actor(_1),
- make_actor(_2));
-} // end operator-()
-
-template
-__host__ __device__
-actor<
- composite<
- transparent_binary_operator>,
- actor,
- typename as_actor::type
- >
->
-operator-(const actor &_1, const T2 &_2)
-{
- return compose(transparent_binary_operator>(),
- make_actor(_1),
- make_actor(_2));
-} // end operator-()
-
-template
-__host__ __device__
-actor<
- composite<
- transparent_binary_operator>,
- actor,
- actor
- >
->
-operator-(const actor &_1, const actor &_2)
-{
- return compose(transparent_binary_operator>(),
- make_actor(_1),
- make_actor(_2));
-} // end operator-()
-
-template
-__host__ __device__
-actor<
- composite<
- transparent_binary_operator>,
- typename as_actor::type,
- actor
- >
->
-operator*(const T1 &_1, const actor &_2)
-{
- return compose(transparent_binary_operator>(),
- make_actor(_1),
- make_actor(_2));
-} // end operator*()
-
-template
-__host__ __device__
-actor<
- composite<
- transparent_binary_operator>,
- actor,
- typename as_actor::type
- >
->
-operator*(const actor &_1, const T2 &_2)
-{
- return compose(transparent_binary_operator>(),
- make_actor(_1),
- make_actor(_2));
-} // end operator*()
-
-template
-__host__ __device__
-actor<
- composite<
- transparent_binary_operator>,
- actor,
- actor
- >
->
-operator*(const actor &_1, const actor &_2)
-{
- return compose(transparent_binary_operator>(),
- make_actor(_1),
- make_actor(_2));
-} // end operator*()
-
-template
-__host__ __device__
-actor<
- composite<
- transparent_binary_operator>,
- actor,
- typename as_actor::type
- >
->
-operator/(const actor &_1, const T2 &_2)
-{
- return compose(transparent_binary_operator>(),
- make_actor(_1),
- make_actor(_2));
-} // end operator/()
-
-template
-__host__ __device__
-actor<
- composite<
- transparent_binary_operator>,
- typename as_actor::type,
- actor
- >
->
-operator/(const T1 &_1, const actor &_2)
-{
- return compose(transparent_binary_operator>(),
- make_actor(_1),
- make_actor(_2));
-} // end operator/()
-
-template
-__host__ __device__
-actor<
- composite<
- transparent_binary_operator>,
- actor,
- actor
- >
->
-operator/(const actor &_1, const actor &_2)
-{
- return compose(transparent_binary_operator>(),
- make_actor(_1),
- make_actor(_2));
-} // end operator/()
-
-template
-__host__ __device__
-actor<
- composite<
- transparent_binary_operator>,
- actor,
- typename as_actor::type
- >
->
-operator%(const actor &_1, const T2 &_2)
-{
- return compose(transparent_binary_operator>(),
- make_actor(_1),
- make_actor(_2));
-} // end operator%()
-
-template
-__host__ __device__
-actor<
- composite<
- transparent_binary_operator>,
- typename as_actor::type,
- actor
- >
->
-operator%(const T1 &_1, const actor &_2)
-{
- return compose(transparent_binary_operator>(),
- make_actor(_1),
- make_actor(_2));
-} // end operator%()
-
-template
-__host__ __device__
-actor<
- composite<
- transparent_binary_operator>,
- actor,
- actor
- >
->
-operator%(const actor &_1, const actor &_2)
-{
- return compose(transparent_binary_operator>(),
- make_actor(_1),
- make_actor(_2));
-} // end operator%()
-
-// there's no standard prefix_increment functional, so roll an ad hoc one here
-struct prefix_increment
-{
- using is_transparent = void;
-
- __thrust_exec_check_disable__
- template
- __host__ __device__
- constexpr auto operator()(T1&& t1) const
- noexcept(noexcept(++THRUST_FWD(t1))) -> decltype(++THRUST_FWD(t1))
- {
- return ++THRUST_FWD(t1);
- }
-}; // end prefix_increment
-
-template
-__host__ __device__
-actor<
- composite<
- transparent_unary_operator,
- actor
- >
->
-operator++(const actor &_1)
-{
- return compose(transparent_unary_operator(), _1);
-} // end operator++()
-
-
-// there's no standard postfix_increment functional, so roll an ad hoc one here
-struct postfix_increment
-{
- using is_transparent = void;
-
- __thrust_exec_check_disable__
- template
- __host__ __device__
- constexpr auto operator()(T1&& t1) const
- noexcept(noexcept(THRUST_FWD(t1)++)) -> decltype(THRUST_FWD(t1)++)
- {
- return THRUST_FWD(t1)++;
- }
-}; // end postfix_increment
-
-template
-__host__ __device__
-actor<
- composite<
- transparent_unary_operator,
- actor
- >
->
-operator++(const actor &_1, int)
-{
- return compose(transparent_unary_operator(), _1);
-} // end operator++()
-
-
-// there's no standard prefix_decrement functional, so roll an ad hoc one here
-struct prefix_decrement
-{
- using is_transparent = void;
-
- __thrust_exec_check_disable__
- template
- __host__ __device__
- constexpr auto operator()(T1&& t1) const
- noexcept(noexcept(--THRUST_FWD(t1))) -> decltype(--THRUST_FWD(t1))
- {
- return --THRUST_FWD(t1);
- }
-}; // end prefix_decrement
-
-template
-__host__ __device__
-actor<
- composite<
- transparent_unary_operator,
- actor
- >
->
-operator--(const actor &_1)
-{
- return compose(transparent_unary_operator(), _1);
-} // end operator--()
-
-
-// there's no standard postfix_decrement functional, so roll an ad hoc one here
-struct postfix_decrement
-{
- using is_transparent = void;
-
- __thrust_exec_check_disable__
- template
- __host__ __device__
- constexpr auto operator()(T1&& t1) const
- noexcept(noexcept(THRUST_FWD(t1)--)) -> decltype(THRUST_FWD(t1)--)
- {
- return THRUST_FWD(t1)--;
- }
-}; // end prefix_increment
-
-template
-__host__ __device__
-actor<
- composite<
- transparent_unary_operator,
- actor
- >
->
-operator--(const actor &_1, int)
-{
- return compose(transparent_unary_operator(), _1);
-} // end operator--()
-
-} // end functional
-} // end detail
-} // end thrust
-
diff --git a/spaces/CVPR/Text2Human/Text2Human/models/archs/vqgan_arch.py b/spaces/CVPR/Text2Human/Text2Human/models/archs/vqgan_arch.py
deleted file mode 100644
index 51980ec048dc25e5c84ae26ba6bde384d1d2a94f..0000000000000000000000000000000000000000
--- a/spaces/CVPR/Text2Human/Text2Human/models/archs/vqgan_arch.py
+++ /dev/null
@@ -1,1203 +0,0 @@
-# pytorch_diffusion + derived encoder decoder
-import math
-from urllib.request import proxy_bypass
-
-import numpy as np
-import torch
-import torch.nn as nn
-import torch.nn.functional as F
-from einops import rearrange
-
-
-class VectorQuantizer(nn.Module):
- """
- Improved version over VectorQuantizer, can be used as a drop-in replacement. Mostly
- avoids costly matrix multiplications and allows for post-hoc remapping of indices.
- """
-
- # NOTE: due to a bug the beta term was applied to the wrong term. for
- # backwards compatibility we use the buggy version by default, but you can
- # specify legacy=False to fix it.
- def __init__(self,
- n_e,
- e_dim,
- beta,
- remap=None,
- unknown_index="random",
- sane_index_shape=False,
- legacy=True):
- super().__init__()
- self.n_e = n_e
- self.e_dim = e_dim
- self.beta = beta
- self.legacy = legacy
-
- self.embedding = nn.Embedding(self.n_e, self.e_dim)
- self.embedding.weight.data.uniform_(-1.0 / self.n_e, 1.0 / self.n_e)
-
- self.remap = remap
- if self.remap is not None:
- self.register_buffer("used", torch.tensor(np.load(self.remap)))
- self.re_embed = self.used.shape[0]
- self.unknown_index = unknown_index # "random" or "extra" or integer
- if self.unknown_index == "extra":
- self.unknown_index = self.re_embed
- self.re_embed = self.re_embed + 1
- print(f"Remapping {self.n_e} indices to {self.re_embed} indices. "
- f"Using {self.unknown_index} for unknown indices.")
- else:
- self.re_embed = n_e
-
- self.sane_index_shape = sane_index_shape
-
- def remap_to_used(self, inds):
- ishape = inds.shape
- assert len(ishape) > 1
- inds = inds.reshape(ishape[0], -1)
- used = self.used.to(inds)
- match = (inds[:, :, None] == used[None, None, ...]).long()
- new = match.argmax(-1)
- unknown = match.sum(2) < 1
- if self.unknown_index == "random":
- new[unknown] = torch.randint(
- 0, self.re_embed,
- size=new[unknown].shape).to(device=new.device)
- else:
- new[unknown] = self.unknown_index
- return new.reshape(ishape)
-
- def unmap_to_all(self, inds):
- ishape = inds.shape
- assert len(ishape) > 1
- inds = inds.reshape(ishape[0], -1)
- used = self.used.to(inds)
- if self.re_embed > self.used.shape[0]: # extra token
- inds[inds >= self.used.shape[0]] = 0 # simply set to zero
- back = torch.gather(used[None, :][inds.shape[0] * [0], :], 1, inds)
- return back.reshape(ishape)
-
- def forward(self, z, temp=None, rescale_logits=False, return_logits=False):
- assert temp is None or temp == 1.0, "Only for interface compatible with Gumbel"
- assert rescale_logits == False, "Only for interface compatible with Gumbel"
- assert return_logits == False, "Only for interface compatible with Gumbel"
- # reshape z -> (batch, height, width, channel) and flatten
- z = rearrange(z, 'b c h w -> b h w c').contiguous()
- z_flattened = z.view(-1, self.e_dim)
- # distances from z to embeddings e_j (z - e)^2 = z^2 + e^2 - 2 e * z
-
- d = torch.sum(z_flattened ** 2, dim=1, keepdim=True) + \
- torch.sum(self.embedding.weight**2, dim=1) - 2 * \
- torch.einsum('bd,dn->bn', z_flattened, rearrange(self.embedding.weight, 'n d -> d n'))
-
- min_encoding_indices = torch.argmin(d, dim=1)
- z_q = self.embedding(min_encoding_indices).view(z.shape)
- perplexity = None
- min_encodings = None
-
- # compute loss for embedding
- if not self.legacy:
- loss = self.beta * torch.mean((z_q.detach()-z)**2) + \
- torch.mean((z_q - z.detach()) ** 2)
- else:
- loss = torch.mean((z_q.detach()-z)**2) + self.beta * \
- torch.mean((z_q - z.detach()) ** 2)
-
- # preserve gradients
- z_q = z + (z_q - z).detach()
-
- # reshape back to match original input shape
- z_q = rearrange(z_q, 'b h w c -> b c h w').contiguous()
-
- if self.remap is not None:
- min_encoding_indices = min_encoding_indices.reshape(
- z.shape[0], -1) # add batch axis
- min_encoding_indices = self.remap_to_used(min_encoding_indices)
- min_encoding_indices = min_encoding_indices.reshape(-1,
- 1) # flatten
-
- if self.sane_index_shape:
- min_encoding_indices = min_encoding_indices.reshape(
- z_q.shape[0], z_q.shape[2], z_q.shape[3])
-
- return z_q, loss, (perplexity, min_encodings, min_encoding_indices)
-
- def get_codebook_entry(self, indices, shape):
- # shape specifying (batch, height, width, channel)
- if self.remap is not None:
- indices = indices.reshape(shape[0], -1) # add batch axis
- indices = self.unmap_to_all(indices)
- indices = indices.reshape(-1) # flatten again
-
- # get quantized latent vectors
- z_q = self.embedding(indices)
-
- if shape is not None:
- z_q = z_q.view(shape)
- # reshape back to match original input shape
- z_q = z_q.permute(0, 3, 1, 2).contiguous()
-
- return z_q
-
-
-class VectorQuantizerTexture(nn.Module):
- """
- Improved version over VectorQuantizer, can be used as a drop-in replacement. Mostly
- avoids costly matrix multiplications and allows for post-hoc remapping of indices.
- """
-
- # NOTE: due to a bug the beta term was applied to the wrong term. for
- # backwards compatibility we use the buggy version by default, but you can
- # specify legacy=False to fix it.
- def __init__(self,
- n_e,
- e_dim,
- beta,
- remap=None,
- unknown_index="random",
- sane_index_shape=False,
- legacy=True):
- super().__init__()
- self.n_e = n_e
- self.e_dim = e_dim
- self.beta = beta
- self.legacy = legacy
-
- # TODO: decide number of embeddings
- self.embedding_list = nn.ModuleList(
- [nn.Embedding(self.n_e, self.e_dim) for i in range(18)])
- for embedding in self.embedding_list:
- embedding.weight.data.uniform_(-1.0 / self.n_e, 1.0 / self.n_e)
-
- self.remap = remap
- if self.remap is not None:
- self.register_buffer("used", torch.tensor(np.load(self.remap)))
- self.re_embed = self.used.shape[0]
- self.unknown_index = unknown_index # "random" or "extra" or integer
- if self.unknown_index == "extra":
- self.unknown_index = self.re_embed
- self.re_embed = self.re_embed + 1
- print(f"Remapping {self.n_e} indices to {self.re_embed} indices. "
- f"Using {self.unknown_index} for unknown indices.")
- else:
- self.re_embed = n_e
-
- self.sane_index_shape = sane_index_shape
-
- def remap_to_used(self, inds):
- ishape = inds.shape
- assert len(ishape) > 1
- inds = inds.reshape(ishape[0], -1)
- used = self.used.to(inds)
- match = (inds[:, :, None] == used[None, None, ...]).long()
- new = match.argmax(-1)
- unknown = match.sum(2) < 1
- if self.unknown_index == "random":
- new[unknown] = torch.randint(
- 0, self.re_embed,
- size=new[unknown].shape).to(device=new.device)
- else:
- new[unknown] = self.unknown_index
- return new.reshape(ishape)
-
- def unmap_to_all(self, inds):
- ishape = inds.shape
- assert len(ishape) > 1
- inds = inds.reshape(ishape[0], -1)
- used = self.used.to(inds)
- if self.re_embed > self.used.shape[0]: # extra token
- inds[inds >= self.used.shape[0]] = 0 # simply set to zero
- back = torch.gather(used[None, :][inds.shape[0] * [0], :], 1, inds)
- return back.reshape(ishape)
-
- def forward(self,
- z,
- segm_map,
- temp=None,
- rescale_logits=False,
- return_logits=False):
- assert temp is None or temp == 1.0, "Only for interface compatible with Gumbel"
- assert rescale_logits == False, "Only for interface compatible with Gumbel"
- assert return_logits == False, "Only for interface compatible with Gumbel"
-
- segm_map = F.interpolate(segm_map, size=z.size()[2:], mode='nearest')
- # reshape z -> (batch, height, width, channel) and flatten
- z = rearrange(z, 'b c h w -> b h w c').contiguous()
- z_flattened = z.view(-1, self.e_dim)
-
- # flatten segm_map (b, h, w)
- segm_map_flatten = segm_map.view(-1)
-
- z_q = torch.zeros_like(z_flattened)
- min_encoding_indices_list = []
- min_encoding_indices_continual = torch.full(
- segm_map_flatten.size(),
- fill_value=-1,
- dtype=torch.long,
- device=segm_map_flatten.device)
- for codebook_idx in range(18):
- min_encoding_indices = torch.full(
- segm_map_flatten.size(),
- fill_value=-1,
- dtype=torch.long,
- device=segm_map_flatten.device)
- if torch.sum(segm_map_flatten == codebook_idx) > 0:
- z_selected = z_flattened[segm_map_flatten == codebook_idx]
- # distances from z to embeddings e_j (z - e)^2 = z^2 + e^2 - 2 e * z
- d_selected = torch.sum(
- z_selected**2, dim=1, keepdim=True) + torch.sum(
- self.embedding_list[codebook_idx].weight**2,
- dim=1) - 2 * torch.einsum(
- 'bd,dn->bn', z_selected,
- rearrange(self.embedding_list[codebook_idx].weight,
- 'n d -> d n'))
- min_encoding_indices_selected = torch.argmin(d_selected, dim=1)
- z_q_selected = self.embedding_list[codebook_idx](
- min_encoding_indices_selected)
- z_q[segm_map_flatten == codebook_idx] = z_q_selected
- min_encoding_indices[
- segm_map_flatten ==
- codebook_idx] = min_encoding_indices_selected
- min_encoding_indices_continual[
- segm_map_flatten ==
- codebook_idx] = min_encoding_indices_selected + 1024 * codebook_idx
- min_encoding_indices = min_encoding_indices.reshape(
- z.shape[0], z.shape[1], z.shape[2])
- min_encoding_indices_list.append(min_encoding_indices)
-
- min_encoding_indices_continual = min_encoding_indices_continual.reshape(
- z.shape[0], z.shape[1], z.shape[2])
- z_q = z_q.view(z.shape)
- perplexity = None
-
- # compute loss for embedding
- if not self.legacy:
- loss = self.beta * torch.mean((z_q.detach()-z)**2) + \
- torch.mean((z_q - z.detach()) ** 2)
- else:
- loss = torch.mean((z_q.detach()-z)**2) + self.beta * \
- torch.mean((z_q - z.detach()) ** 2)
-
- # preserve gradients
- z_q = z + (z_q - z).detach()
-
- # reshape back to match original input shape
- z_q = rearrange(z_q, 'b h w c -> b c h w').contiguous()
-
- return z_q, loss, (perplexity, min_encoding_indices_continual,
- min_encoding_indices_list)
-
- def get_codebook_entry(self, indices_list, segm_map, shape):
- # flatten segm_map (b, h, w)
- segm_map = F.interpolate(
- segm_map, size=(shape[1], shape[2]), mode='nearest')
- segm_map_flatten = segm_map.view(-1)
-
- z_q = torch.zeros((shape[0] * shape[1] * shape[2]),
- self.e_dim).to(segm_map.device)
- for codebook_idx in range(18):
- if torch.sum(segm_map_flatten == codebook_idx) > 0:
- min_encoding_indices_selected = indices_list[
- codebook_idx].view(-1)[segm_map_flatten == codebook_idx]
- z_q_selected = self.embedding_list[codebook_idx](
- min_encoding_indices_selected)
- z_q[segm_map_flatten == codebook_idx] = z_q_selected
-
- z_q = z_q.view(shape)
- # reshape back to match original input shape
- z_q = z_q.permute(0, 3, 1, 2).contiguous()
-
- return z_q
-
-
-def sample_patches(inputs, patch_size=3, stride=1):
- """Extract sliding local patches from an input feature tensor.
- The sampled pathes are row-major.
- Args:
- inputs (Tensor): the input feature maps, shape: (n, c, h, w).
- patch_size (int): the spatial size of sampled patches. Default: 3.
- stride (int): the stride of sampling. Default: 1.
- Returns:
- patches (Tensor): extracted patches, shape: (n, c * patch_size *
- patch_size, n_patches).
- """
-
- patches = F.unfold(inputs, (patch_size, patch_size), stride=stride)
-
- return patches
-
-
-class VectorQuantizerSpatialTextureAware(nn.Module):
- """
- Improved version over VectorQuantizer, can be used as a drop-in replacement. Mostly
- avoids costly matrix multiplications and allows for post-hoc remapping of indices.
- """
-
- # NOTE: due to a bug the beta term was applied to the wrong term. for
- # backwards compatibility we use the buggy version by default, but you can
- # specify legacy=False to fix it.
- def __init__(self,
- n_e,
- e_dim,
- beta,
- spatial_size,
- remap=None,
- unknown_index="random",
- sane_index_shape=False,
- legacy=True):
- super().__init__()
- self.n_e = n_e
- self.e_dim = e_dim * spatial_size * spatial_size
- self.beta = beta
- self.legacy = legacy
- self.spatial_size = spatial_size
-
- # TODO: decide number of embeddings
- self.embedding_list = nn.ModuleList(
- [nn.Embedding(self.n_e, self.e_dim) for i in range(18)])
- for embedding in self.embedding_list:
- embedding.weight.data.uniform_(-1.0 / self.n_e, 1.0 / self.n_e)
-
- self.remap = remap
- if self.remap is not None:
- self.register_buffer("used", torch.tensor(np.load(self.remap)))
- self.re_embed = self.used.shape[0]
- self.unknown_index = unknown_index # "random" or "extra" or integer
- if self.unknown_index == "extra":
- self.unknown_index = self.re_embed
- self.re_embed = self.re_embed + 1
- print(f"Remapping {self.n_e} indices to {self.re_embed} indices. "
- f"Using {self.unknown_index} for unknown indices.")
- else:
- self.re_embed = n_e
-
- self.sane_index_shape = sane_index_shape
-
- def forward(self,
- z,
- segm_map,
- temp=None,
- rescale_logits=False,
- return_logits=False):
- assert temp is None or temp == 1.0, "Only for interface compatible with Gumbel"
- assert rescale_logits == False, "Only for interface compatible with Gumbel"
- assert return_logits == False, "Only for interface compatible with Gumbel"
-
- segm_map = F.interpolate(
- segm_map,
- size=(z.size(2) // self.spatial_size,
- z.size(3) // self.spatial_size),
- mode='nearest')
-
- # reshape z -> (batch, height, width, channel) and flatten
- # z = rearrange(z, 'b c h w -> b h w c').contiguous() ?
- z_patches = sample_patches(
- z, patch_size=self.spatial_size,
- stride=self.spatial_size).permute(0, 2, 1)
- z_patches_flattened = z_patches.reshape(-1, self.e_dim)
- # distances from z to embeddings e_j (z - e)^2 = z^2 + e^2 - 2 e * z
-
- # flatten segm_map (b, h, w)
- segm_map_flatten = segm_map.view(-1)
-
- z_q = torch.zeros_like(z_patches_flattened)
- min_encoding_indices_list = []
- min_encoding_indices_continual = torch.full(
- segm_map_flatten.size(),
- fill_value=-1,
- dtype=torch.long,
- device=segm_map_flatten.device)
-
- for codebook_idx in range(18):
- min_encoding_indices = torch.full(
- segm_map_flatten.size(),
- fill_value=-1,
- dtype=torch.long,
- device=segm_map_flatten.device)
- if torch.sum(segm_map_flatten == codebook_idx) > 0:
- z_selected = z_patches_flattened[segm_map_flatten ==
- codebook_idx]
- # distances from z to embeddings e_j (z - e)^2 = z^2 + e^2 - 2 e * z
- d_selected = torch.sum(
- z_selected**2, dim=1, keepdim=True) + torch.sum(
- self.embedding_list[codebook_idx].weight**2,
- dim=1) - 2 * torch.einsum(
- 'bd,dn->bn', z_selected,
- rearrange(self.embedding_list[codebook_idx].weight,
- 'n d -> d n'))
- min_encoding_indices_selected = torch.argmin(d_selected, dim=1)
- z_q_selected = self.embedding_list[codebook_idx](
- min_encoding_indices_selected)
- z_q[segm_map_flatten == codebook_idx] = z_q_selected
- min_encoding_indices[
- segm_map_flatten ==
- codebook_idx] = min_encoding_indices_selected
- min_encoding_indices_continual[
- segm_map_flatten ==
- codebook_idx] = min_encoding_indices_selected + self.n_e * codebook_idx
- min_encoding_indices = min_encoding_indices.reshape(
- z_patches.shape[0], segm_map.shape[2], segm_map.shape[3])
- min_encoding_indices_list.append(min_encoding_indices)
-
- z_q = F.fold(
- z_q.view(z_patches.shape).permute(0, 2, 1),
- z.size()[2:],
- kernel_size=(self.spatial_size, self.spatial_size),
- stride=self.spatial_size)
-
- perplexity = None
-
- # compute loss for embedding
- if not self.legacy:
- loss = self.beta * torch.mean((z_q.detach()-z)**2) + \
- torch.mean((z_q - z.detach()) ** 2)
- else:
- loss = torch.mean((z_q.detach()-z)**2) + self.beta * \
- torch.mean((z_q - z.detach()) ** 2)
-
- # preserve gradients
- z_q = z + (z_q - z).detach()
-
- return z_q, loss, (perplexity, min_encoding_indices_continual,
- min_encoding_indices_list)
-
- def get_codebook_entry(self, indices_list, segm_map, shape):
- # flatten segm_map (b, h, w)
- segm_map = F.interpolate(
- segm_map, size=(shape[1], shape[2]), mode='nearest')
- segm_map_flatten = segm_map.view(-1)
-
- z_q = torch.zeros((shape[0] * shape[1] * shape[2]),
- self.e_dim).to(segm_map.device)
- for codebook_idx in range(18):
- if torch.sum(segm_map_flatten == codebook_idx) > 0:
- min_encoding_indices_selected = indices_list[
- codebook_idx].view(-1)[segm_map_flatten == codebook_idx]
- z_q_selected = self.embedding_list[codebook_idx](
- min_encoding_indices_selected)
- z_q[segm_map_flatten == codebook_idx] = z_q_selected
-
- z_q = F.fold(
- z_q.view(((shape[0], shape[1] * shape[2],
- self.e_dim))).permute(0, 2, 1),
- (shape[1] * self.spatial_size, shape[2] * self.spatial_size),
- kernel_size=(self.spatial_size, self.spatial_size),
- stride=self.spatial_size)
-
- return z_q
-
-
-def get_timestep_embedding(timesteps, embedding_dim):
- """
- This matches the implementation in Denoising Diffusion Probabilistic Models:
- From Fairseq.
- Build sinusoidal embeddings.
- This matches the implementation in tensor2tensor, but differs slightly
- from the description in Section 3.5 of "Attention Is All You Need".
- """
- assert len(timesteps.shape) == 1
-
- half_dim = embedding_dim // 2
- emb = math.log(10000) / (half_dim - 1)
- emb = torch.exp(torch.arange(half_dim, dtype=torch.float32) * -emb)
- emb = emb.to(device=timesteps.device)
- emb = timesteps.float()[:, None] * emb[None, :]
- emb = torch.cat([torch.sin(emb), torch.cos(emb)], dim=1)
- if embedding_dim % 2 == 1: # zero pad
- emb = torch.nn.functional.pad(emb, (0, 1, 0, 0))
- return emb
-
-
-def nonlinearity(x):
- # swish
- return x * torch.sigmoid(x)
-
-
-def Normalize(in_channels):
- return torch.nn.GroupNorm(
- num_groups=32, num_channels=in_channels, eps=1e-6, affine=True)
-
-
-class Upsample(nn.Module):
-
- def __init__(self, in_channels, with_conv):
- super().__init__()
- self.with_conv = with_conv
- if self.with_conv:
- self.conv = torch.nn.Conv2d(
- in_channels, in_channels, kernel_size=3, stride=1, padding=1)
-
- def forward(self, x):
- x = torch.nn.functional.interpolate(
- x, scale_factor=2.0, mode="nearest")
- if self.with_conv:
- x = self.conv(x)
- return x
-
-
-class Downsample(nn.Module):
-
- def __init__(self, in_channels, with_conv):
- super().__init__()
- self.with_conv = with_conv
- if self.with_conv:
- # no asymmetric padding in torch conv, must do it ourselves
- self.conv = torch.nn.Conv2d(
- in_channels, in_channels, kernel_size=3, stride=2, padding=0)
-
- def forward(self, x):
- if self.with_conv:
- pad = (0, 1, 0, 1)
- x = torch.nn.functional.pad(x, pad, mode="constant", value=0)
- x = self.conv(x)
- else:
- x = torch.nn.functional.avg_pool2d(x, kernel_size=2, stride=2)
- return x
-
-
-class ResnetBlock(nn.Module):
-
- def __init__(self,
- *,
- in_channels,
- out_channels=None,
- conv_shortcut=False,
- dropout,
- temb_channels=512):
- super().__init__()
- self.in_channels = in_channels
- out_channels = in_channels if out_channels is None else out_channels
- self.out_channels = out_channels
- self.use_conv_shortcut = conv_shortcut
-
- self.norm1 = Normalize(in_channels)
- self.conv1 = torch.nn.Conv2d(
- in_channels, out_channels, kernel_size=3, stride=1, padding=1)
- if temb_channels > 0:
- self.temb_proj = torch.nn.Linear(temb_channels, out_channels)
- self.norm2 = Normalize(out_channels)
- self.dropout = torch.nn.Dropout(dropout)
- self.conv2 = torch.nn.Conv2d(
- out_channels, out_channels, kernel_size=3, stride=1, padding=1)
- if self.in_channels != self.out_channels:
- if self.use_conv_shortcut:
- self.conv_shortcut = torch.nn.Conv2d(
- in_channels,
- out_channels,
- kernel_size=3,
- stride=1,
- padding=1)
- else:
- self.nin_shortcut = torch.nn.Conv2d(
- in_channels,
- out_channels,
- kernel_size=1,
- stride=1,
- padding=0)
-
- def forward(self, x, temb):
- h = x
- h = self.norm1(h)
- h = nonlinearity(h)
- h = self.conv1(h)
-
- if temb is not None:
- h = h + self.temb_proj(nonlinearity(temb))[:, :, None, None]
-
- h = self.norm2(h)
- h = nonlinearity(h)
- h = self.dropout(h)
- h = self.conv2(h)
-
- if self.in_channels != self.out_channels:
- if self.use_conv_shortcut:
- x = self.conv_shortcut(x)
- else:
- x = self.nin_shortcut(x)
-
- return x + h
-
-
-class AttnBlock(nn.Module):
-
- def __init__(self, in_channels):
- super().__init__()
- self.in_channels = in_channels
-
- self.norm = Normalize(in_channels)
- self.q = torch.nn.Conv2d(
- in_channels, in_channels, kernel_size=1, stride=1, padding=0)
- self.k = torch.nn.Conv2d(
- in_channels, in_channels, kernel_size=1, stride=1, padding=0)
- self.v = torch.nn.Conv2d(
- in_channels, in_channels, kernel_size=1, stride=1, padding=0)
- self.proj_out = torch.nn.Conv2d(
- in_channels, in_channels, kernel_size=1, stride=1, padding=0)
-
- def forward(self, x):
- h_ = x
- h_ = self.norm(h_)
- q = self.q(h_)
- k = self.k(h_)
- v = self.v(h_)
-
- # compute attention
- b, c, h, w = q.shape
- q = q.reshape(b, c, h * w)
- q = q.permute(0, 2, 1) # b,hw,c
- k = k.reshape(b, c, h * w) # b,c,hw
- w_ = torch.bmm(q, k) # b,hw,hw w[b,i,j]=sum_c q[b,i,c]k[b,c,j]
- w_ = w_ * (int(c)**(-0.5))
- w_ = torch.nn.functional.softmax(w_, dim=2)
-
- # attend to values
- v = v.reshape(b, c, h * w)
- w_ = w_.permute(0, 2, 1) # b,hw,hw (first hw of k, second of q)
- h_ = torch.bmm(
- v, w_) # b, c,hw (hw of q) h_[b,c,j] = sum_i v[b,c,i] w_[b,i,j]
- h_ = h_.reshape(b, c, h, w)
-
- h_ = self.proj_out(h_)
-
- return x + h_
-
-
-class Model(nn.Module):
-
- def __init__(self,
- *,
- ch,
- out_ch,
- ch_mult=(1, 2, 4, 8),
- num_res_blocks,
- attn_resolutions,
- dropout=0.0,
- resamp_with_conv=True,
- in_channels,
- resolution,
- use_timestep=True):
- super().__init__()
- self.ch = ch
- self.temb_ch = self.ch * 4
- self.num_resolutions = len(ch_mult)
- self.num_res_blocks = num_res_blocks
- self.resolution = resolution
- self.in_channels = in_channels
-
- self.use_timestep = use_timestep
- if self.use_timestep:
- # timestep embedding
- self.temb = nn.Module()
- self.temb.dense = nn.ModuleList([
- torch.nn.Linear(self.ch, self.temb_ch),
- torch.nn.Linear(self.temb_ch, self.temb_ch),
- ])
-
- # downsampling
- self.conv_in = torch.nn.Conv2d(
- in_channels, self.ch, kernel_size=3, stride=1, padding=1)
-
- curr_res = resolution
- in_ch_mult = (1, ) + tuple(ch_mult)
- self.down = nn.ModuleList()
- for i_level in range(self.num_resolutions):
- block = nn.ModuleList()
- attn = nn.ModuleList()
- block_in = ch * in_ch_mult[i_level]
- block_out = ch * ch_mult[i_level]
- for i_block in range(self.num_res_blocks):
- block.append(
- ResnetBlock(
- in_channels=block_in,
- out_channels=block_out,
- temb_channels=self.temb_ch,
- dropout=dropout))
- block_in = block_out
- if curr_res in attn_resolutions:
- attn.append(AttnBlock(block_in))
- down = nn.Module()
- down.block = block
- down.attn = attn
- if i_level != self.num_resolutions - 1:
- down.downsample = Downsample(block_in, resamp_with_conv)
- curr_res = curr_res // 2
- self.down.append(down)
-
- # middle
- self.mid = nn.Module()
- self.mid.block_1 = ResnetBlock(
- in_channels=block_in,
- out_channels=block_in,
- temb_channels=self.temb_ch,
- dropout=dropout)
- self.mid.attn_1 = AttnBlock(block_in)
- self.mid.block_2 = ResnetBlock(
- in_channels=block_in,
- out_channels=block_in,
- temb_channels=self.temb_ch,
- dropout=dropout)
-
- # upsampling
- self.up = nn.ModuleList()
- for i_level in reversed(range(self.num_resolutions)):
- block = nn.ModuleList()
- attn = nn.ModuleList()
- block_out = ch * ch_mult[i_level]
- skip_in = ch * ch_mult[i_level]
- for i_block in range(self.num_res_blocks + 1):
- if i_block == self.num_res_blocks:
- skip_in = ch * in_ch_mult[i_level]
- block.append(
- ResnetBlock(
- in_channels=block_in + skip_in,
- out_channels=block_out,
- temb_channels=self.temb_ch,
- dropout=dropout))
- block_in = block_out
- if curr_res in attn_resolutions:
- attn.append(AttnBlock(block_in))
- up = nn.Module()
- up.block = block
- up.attn = attn
- if i_level != 0:
- up.upsample = Upsample(block_in, resamp_with_conv)
- curr_res = curr_res * 2
- self.up.insert(0, up) # prepend to get consistent order
-
- # end
- self.norm_out = Normalize(block_in)
- self.conv_out = torch.nn.Conv2d(
- block_in, out_ch, kernel_size=3, stride=1, padding=1)
-
- def forward(self, x, t=None):
- #assert x.shape[2] == x.shape[3] == self.resolution
-
- if self.use_timestep:
- # timestep embedding
- assert t is not None
- temb = get_timestep_embedding(t, self.ch)
- temb = self.temb.dense[0](temb)
- temb = nonlinearity(temb)
- temb = self.temb.dense[1](temb)
- else:
- temb = None
-
- # downsampling
- hs = [self.conv_in(x)]
- for i_level in range(self.num_resolutions):
- for i_block in range(self.num_res_blocks):
- h = self.down[i_level].block[i_block](hs[-1], temb)
- if len(self.down[i_level].attn) > 0:
- h = self.down[i_level].attn[i_block](h)
- hs.append(h)
- if i_level != self.num_resolutions - 1:
- hs.append(self.down[i_level].downsample(hs[-1]))
-
- # middle
- h = hs[-1]
- h = self.mid.block_1(h, temb)
- h = self.mid.attn_1(h)
- h = self.mid.block_2(h, temb)
-
- # upsampling
- for i_level in reversed(range(self.num_resolutions)):
- for i_block in range(self.num_res_blocks + 1):
- h = self.up[i_level].block[i_block](torch.cat([h, hs.pop()],
- dim=1), temb)
- if len(self.up[i_level].attn) > 0:
- h = self.up[i_level].attn[i_block](h)
- if i_level != 0:
- h = self.up[i_level].upsample(h)
-
- # end
- h = self.norm_out(h)
- h = nonlinearity(h)
- h = self.conv_out(h)
- return h
-
-
-class Encoder(nn.Module):
-
- def __init__(self,
- ch,
- num_res_blocks,
- attn_resolutions,
- in_channels,
- resolution,
- z_channels,
- ch_mult=(1, 2, 4, 8),
- dropout=0.0,
- resamp_with_conv=True,
- double_z=True):
- super().__init__()
- self.ch = ch
- self.temb_ch = 0
- self.num_resolutions = len(ch_mult)
- self.num_res_blocks = num_res_blocks
- self.resolution = resolution
- self.in_channels = in_channels
-
- # downsampling
- self.conv_in = torch.nn.Conv2d(
- in_channels, self.ch, kernel_size=3, stride=1, padding=1)
-
- curr_res = resolution
- in_ch_mult = (1, ) + tuple(ch_mult)
- self.down = nn.ModuleList()
- for i_level in range(self.num_resolutions):
- block = nn.ModuleList()
- attn = nn.ModuleList()
- block_in = ch * in_ch_mult[i_level]
- block_out = ch * ch_mult[i_level]
- for i_block in range(self.num_res_blocks):
- block.append(
- ResnetBlock(
- in_channels=block_in,
- out_channels=block_out,
- temb_channels=self.temb_ch,
- dropout=dropout))
- block_in = block_out
- if curr_res in attn_resolutions:
- attn.append(AttnBlock(block_in))
- down = nn.Module()
- down.block = block
- down.attn = attn
- if i_level != self.num_resolutions - 1:
- down.downsample = Downsample(block_in, resamp_with_conv)
- curr_res = curr_res // 2
- self.down.append(down)
-
- # middle
- self.mid = nn.Module()
- self.mid.block_1 = ResnetBlock(
- in_channels=block_in,
- out_channels=block_in,
- temb_channels=self.temb_ch,
- dropout=dropout)
- self.mid.attn_1 = AttnBlock(block_in)
- self.mid.block_2 = ResnetBlock(
- in_channels=block_in,
- out_channels=block_in,
- temb_channels=self.temb_ch,
- dropout=dropout)
-
- # end
- self.norm_out = Normalize(block_in)
- self.conv_out = torch.nn.Conv2d(
- block_in,
- 2 * z_channels if double_z else z_channels,
- kernel_size=3,
- stride=1,
- padding=1)
-
- def forward(self, x):
- #assert x.shape[2] == x.shape[3] == self.resolution, "{}, {}, {}".format(x.shape[2], x.shape[3], self.resolution)
-
- # timestep embedding
- temb = None
-
- # downsampling
- hs = [self.conv_in(x)]
- for i_level in range(self.num_resolutions):
- for i_block in range(self.num_res_blocks):
- h = self.down[i_level].block[i_block](hs[-1], temb)
- if len(self.down[i_level].attn) > 0:
- h = self.down[i_level].attn[i_block](h)
- hs.append(h)
- if i_level != self.num_resolutions - 1:
- hs.append(self.down[i_level].downsample(hs[-1]))
-
- # middle
- h = hs[-1]
- h = self.mid.block_1(h, temb)
- h = self.mid.attn_1(h)
- h = self.mid.block_2(h, temb)
-
- # end
- h = self.norm_out(h)
- h = nonlinearity(h)
- h = self.conv_out(h)
- return h
-
-
-class Decoder(nn.Module):
-
- def __init__(self,
- in_channels,
- resolution,
- z_channels,
- ch,
- out_ch,
- num_res_blocks,
- attn_resolutions,
- ch_mult=(1, 2, 4, 8),
- dropout=0.0,
- resamp_with_conv=True,
- give_pre_end=False):
- super().__init__()
- self.ch = ch
- self.temb_ch = 0
- self.num_resolutions = len(ch_mult)
- self.num_res_blocks = num_res_blocks
- self.resolution = resolution
- self.in_channels = in_channels
- self.give_pre_end = give_pre_end
-
- # compute in_ch_mult, block_in and curr_res at lowest res
- in_ch_mult = (1, ) + tuple(ch_mult)
- block_in = ch * ch_mult[self.num_resolutions - 1]
- curr_res = resolution // 2**(self.num_resolutions - 1)
- self.z_shape = (1, z_channels, curr_res, curr_res // 2)
- print("Working with z of shape {} = {} dimensions.".format(
- self.z_shape, np.prod(self.z_shape)))
-
- # z to block_in
- self.conv_in = torch.nn.Conv2d(
- z_channels, block_in, kernel_size=3, stride=1, padding=1)
-
- # middle
- self.mid = nn.Module()
- self.mid.block_1 = ResnetBlock(
- in_channels=block_in,
- out_channels=block_in,
- temb_channels=self.temb_ch,
- dropout=dropout)
- self.mid.attn_1 = AttnBlock(block_in)
- self.mid.block_2 = ResnetBlock(
- in_channels=block_in,
- out_channels=block_in,
- temb_channels=self.temb_ch,
- dropout=dropout)
-
- # upsampling
- self.up = nn.ModuleList()
- for i_level in reversed(range(self.num_resolutions)):
- block = nn.ModuleList()
- attn = nn.ModuleList()
- block_out = ch * ch_mult[i_level]
- for i_block in range(self.num_res_blocks + 1):
- block.append(
- ResnetBlock(
- in_channels=block_in,
- out_channels=block_out,
- temb_channels=self.temb_ch,
- dropout=dropout))
- block_in = block_out
- if curr_res in attn_resolutions:
- attn.append(AttnBlock(block_in))
- up = nn.Module()
- up.block = block
- up.attn = attn
- if i_level != 0:
- up.upsample = Upsample(block_in, resamp_with_conv)
- curr_res = curr_res * 2
- self.up.insert(0, up) # prepend to get consistent order
-
- # end
- self.norm_out = Normalize(block_in)
- self.conv_out = torch.nn.Conv2d(
- block_in, out_ch, kernel_size=3, stride=1, padding=1)
-
- def forward(self, z, bot_h=None):
- #assert z.shape[1:] == self.z_shape[1:]
- self.last_z_shape = z.shape
-
- # timestep embedding
- temb = None
-
- # z to block_in
- h = self.conv_in(z)
-
- # middle
- h = self.mid.block_1(h, temb)
- h = self.mid.attn_1(h)
- h = self.mid.block_2(h, temb)
-
- # upsampling
- for i_level in reversed(range(self.num_resolutions)):
- for i_block in range(self.num_res_blocks + 1):
- h = self.up[i_level].block[i_block](h, temb)
- if len(self.up[i_level].attn) > 0:
- h = self.up[i_level].attn[i_block](h)
- if i_level != 0:
- h = self.up[i_level].upsample(h)
- if i_level == 4 and bot_h is not None:
- h += bot_h
-
- # end
- if self.give_pre_end:
- return h
-
- h = self.norm_out(h)
- h = nonlinearity(h)
- h = self.conv_out(h)
- return h
-
- def get_feature_top(self, z):
- #assert z.shape[1:] == self.z_shape[1:]
- self.last_z_shape = z.shape
-
- # timestep embedding
- temb = None
-
- # z to block_in
- h = self.conv_in(z)
-
- # middle
- h = self.mid.block_1(h, temb)
- h = self.mid.attn_1(h)
- h = self.mid.block_2(h, temb)
-
- # upsampling
- for i_level in reversed(range(self.num_resolutions)):
- for i_block in range(self.num_res_blocks + 1):
- h = self.up[i_level].block[i_block](h, temb)
- if len(self.up[i_level].attn) > 0:
- h = self.up[i_level].attn[i_block](h)
- if i_level != 0:
- h = self.up[i_level].upsample(h)
- if i_level == 4:
- return h
-
- def get_feature_middle(self, z, mid_h):
- #assert z.shape[1:] == self.z_shape[1:]
- self.last_z_shape = z.shape
-
- # timestep embedding
- temb = None
-
- # z to block_in
- h = self.conv_in(z)
-
- # middle
- h = self.mid.block_1(h, temb)
- h = self.mid.attn_1(h)
- h = self.mid.block_2(h, temb)
-
- # upsampling
- for i_level in reversed(range(self.num_resolutions)):
- for i_block in range(self.num_res_blocks + 1):
- h = self.up[i_level].block[i_block](h, temb)
- if len(self.up[i_level].attn) > 0:
- h = self.up[i_level].attn[i_block](h)
- if i_level != 0:
- h = self.up[i_level].upsample(h)
- if i_level == 4:
- h += mid_h
- if i_level == 3:
- return h
-
-
-class DecoderRes(nn.Module):
-
- def __init__(self,
- in_channels,
- resolution,
- z_channels,
- ch,
- num_res_blocks,
- ch_mult=(1, 2, 4, 8),
- dropout=0.0,
- give_pre_end=False):
- super().__init__()
- self.ch = ch
- self.temb_ch = 0
- self.num_resolutions = len(ch_mult)
- self.num_res_blocks = num_res_blocks
- self.resolution = resolution
- self.in_channels = in_channels
- self.give_pre_end = give_pre_end
-
- # compute in_ch_mult, block_in and curr_res at lowest res
- in_ch_mult = (1, ) + tuple(ch_mult)
- block_in = ch * ch_mult[self.num_resolutions - 1]
- curr_res = resolution // 2**(self.num_resolutions - 1)
- self.z_shape = (1, z_channels, curr_res, curr_res // 2)
- print("Working with z of shape {} = {} dimensions.".format(
- self.z_shape, np.prod(self.z_shape)))
-
- # z to block_in
- self.conv_in = torch.nn.Conv2d(
- z_channels, block_in, kernel_size=3, stride=1, padding=1)
-
- # middle
- self.mid = nn.Module()
- self.mid.block_1 = ResnetBlock(
- in_channels=block_in,
- out_channels=block_in,
- temb_channels=self.temb_ch,
- dropout=dropout)
- self.mid.attn_1 = AttnBlock(block_in)
- self.mid.block_2 = ResnetBlock(
- in_channels=block_in,
- out_channels=block_in,
- temb_channels=self.temb_ch,
- dropout=dropout)
-
- def forward(self, z):
- #assert z.shape[1:] == self.z_shape[1:]
- self.last_z_shape = z.shape
-
- # timestep embedding
- temb = None
-
- # z to block_in
- h = self.conv_in(z)
-
- # middle
- h = self.mid.block_1(h, temb)
- h = self.mid.attn_1(h)
- h = self.mid.block_2(h, temb)
-
- return h
-
-
-# patch based discriminator
-class Discriminator(nn.Module):
-
- def __init__(self, nc, ndf, n_layers=3):
- super().__init__()
-
- layers = [
- nn.Conv2d(nc, ndf, kernel_size=4, stride=2, padding=1),
- nn.LeakyReLU(0.2, True)
- ]
- ndf_mult = 1
- ndf_mult_prev = 1
- for n in range(1,
- n_layers): # gradually increase the number of filters
- ndf_mult_prev = ndf_mult
- ndf_mult = min(2**n, 8)
- layers += [
- nn.Conv2d(
- ndf * ndf_mult_prev,
- ndf * ndf_mult,
- kernel_size=4,
- stride=2,
- padding=1,
- bias=False),
- nn.BatchNorm2d(ndf * ndf_mult),
- nn.LeakyReLU(0.2, True)
- ]
-
- ndf_mult_prev = ndf_mult
- ndf_mult = min(2**n_layers, 8)
-
- layers += [
- nn.Conv2d(
- ndf * ndf_mult_prev,
- ndf * ndf_mult,
- kernel_size=4,
- stride=1,
- padding=1,
- bias=False),
- nn.BatchNorm2d(ndf * ndf_mult),
- nn.LeakyReLU(0.2, True)
- ]
-
- layers += [
- nn.Conv2d(ndf * ndf_mult, 1, kernel_size=4, stride=1, padding=1)
- ] # output 1 channel prediction map
- self.main = nn.Sequential(*layers)
-
- def forward(self, x):
- return self.main(x)
diff --git a/spaces/CVPR/WALT/mmdet/core/bbox/assigners/atss_assigner.py b/spaces/CVPR/WALT/mmdet/core/bbox/assigners/atss_assigner.py
deleted file mode 100644
index d4fe9d0e3c8704bd780d493eff20a5505dbe9580..0000000000000000000000000000000000000000
--- a/spaces/CVPR/WALT/mmdet/core/bbox/assigners/atss_assigner.py
+++ /dev/null
@@ -1,178 +0,0 @@
-import torch
-
-from ..builder import BBOX_ASSIGNERS
-from ..iou_calculators import build_iou_calculator
-from .assign_result import AssignResult
-from .base_assigner import BaseAssigner
-
-
-@BBOX_ASSIGNERS.register_module()
-class ATSSAssigner(BaseAssigner):
- """Assign a corresponding gt bbox or background to each bbox.
-
- Each proposals will be assigned with `0` or a positive integer
- indicating the ground truth index.
-
- - 0: negative sample, no assigned gt
- - positive integer: positive sample, index (1-based) of assigned gt
-
- Args:
- topk (float): number of bbox selected in each level
- """
-
- def __init__(self,
- topk,
- iou_calculator=dict(type='BboxOverlaps2D'),
- ignore_iof_thr=-1):
- self.topk = topk
- self.iou_calculator = build_iou_calculator(iou_calculator)
- self.ignore_iof_thr = ignore_iof_thr
-
- # https://github.com/sfzhang15/ATSS/blob/master/atss_core/modeling/rpn/atss/loss.py
-
- def assign(self,
- bboxes,
- num_level_bboxes,
- gt_bboxes,
- gt_bboxes_ignore=None,
- gt_labels=None):
- """Assign gt to bboxes.
-
- The assignment is done in following steps
-
- 1. compute iou between all bbox (bbox of all pyramid levels) and gt
- 2. compute center distance between all bbox and gt
- 3. on each pyramid level, for each gt, select k bbox whose center
- are closest to the gt center, so we total select k*l bbox as
- candidates for each gt
- 4. get corresponding iou for the these candidates, and compute the
- mean and std, set mean + std as the iou threshold
- 5. select these candidates whose iou are greater than or equal to
- the threshold as positive
- 6. limit the positive sample's center in gt
-
-
- Args:
- bboxes (Tensor): Bounding boxes to be assigned, shape(n, 4).
- num_level_bboxes (List): num of bboxes in each level
- gt_bboxes (Tensor): Groundtruth boxes, shape (k, 4).
- gt_bboxes_ignore (Tensor, optional): Ground truth bboxes that are
- labelled as `ignored`, e.g., crowd boxes in COCO.
- gt_labels (Tensor, optional): Label of gt_bboxes, shape (k, ).
-
- Returns:
- :obj:`AssignResult`: The assign result.
- """
- INF = 100000000
- bboxes = bboxes[:, :4]
- num_gt, num_bboxes = gt_bboxes.size(0), bboxes.size(0)
-
- # compute iou between all bbox and gt
- overlaps = self.iou_calculator(bboxes, gt_bboxes)
-
- # assign 0 by default
- assigned_gt_inds = overlaps.new_full((num_bboxes, ),
- 0,
- dtype=torch.long)
-
- if num_gt == 0 or num_bboxes == 0:
- # No ground truth or boxes, return empty assignment
- max_overlaps = overlaps.new_zeros((num_bboxes, ))
- if num_gt == 0:
- # No truth, assign everything to background
- assigned_gt_inds[:] = 0
- if gt_labels is None:
- assigned_labels = None
- else:
- assigned_labels = overlaps.new_full((num_bboxes, ),
- -1,
- dtype=torch.long)
- return AssignResult(
- num_gt, assigned_gt_inds, max_overlaps, labels=assigned_labels)
-
- # compute center distance between all bbox and gt
- gt_cx = (gt_bboxes[:, 0] + gt_bboxes[:, 2]) / 2.0
- gt_cy = (gt_bboxes[:, 1] + gt_bboxes[:, 3]) / 2.0
- gt_points = torch.stack((gt_cx, gt_cy), dim=1)
-
- bboxes_cx = (bboxes[:, 0] + bboxes[:, 2]) / 2.0
- bboxes_cy = (bboxes[:, 1] + bboxes[:, 3]) / 2.0
- bboxes_points = torch.stack((bboxes_cx, bboxes_cy), dim=1)
-
- distances = (bboxes_points[:, None, :] -
- gt_points[None, :, :]).pow(2).sum(-1).sqrt()
-
- if (self.ignore_iof_thr > 0 and gt_bboxes_ignore is not None
- and gt_bboxes_ignore.numel() > 0 and bboxes.numel() > 0):
- ignore_overlaps = self.iou_calculator(
- bboxes, gt_bboxes_ignore, mode='iof')
- ignore_max_overlaps, _ = ignore_overlaps.max(dim=1)
- ignore_idxs = ignore_max_overlaps > self.ignore_iof_thr
- distances[ignore_idxs, :] = INF
- assigned_gt_inds[ignore_idxs] = -1
-
- # Selecting candidates based on the center distance
- candidate_idxs = []
- start_idx = 0
- for level, bboxes_per_level in enumerate(num_level_bboxes):
- # on each pyramid level, for each gt,
- # select k bbox whose center are closest to the gt center
- end_idx = start_idx + bboxes_per_level
- distances_per_level = distances[start_idx:end_idx, :]
- selectable_k = min(self.topk, bboxes_per_level)
- _, topk_idxs_per_level = distances_per_level.topk(
- selectable_k, dim=0, largest=False)
- candidate_idxs.append(topk_idxs_per_level + start_idx)
- start_idx = end_idx
- candidate_idxs = torch.cat(candidate_idxs, dim=0)
-
- # get corresponding iou for the these candidates, and compute the
- # mean and std, set mean + std as the iou threshold
- candidate_overlaps = overlaps[candidate_idxs, torch.arange(num_gt)]
- overlaps_mean_per_gt = candidate_overlaps.mean(0)
- overlaps_std_per_gt = candidate_overlaps.std(0)
- overlaps_thr_per_gt = overlaps_mean_per_gt + overlaps_std_per_gt
-
- is_pos = candidate_overlaps >= overlaps_thr_per_gt[None, :]
-
- # limit the positive sample's center in gt
- for gt_idx in range(num_gt):
- candidate_idxs[:, gt_idx] += gt_idx * num_bboxes
- ep_bboxes_cx = bboxes_cx.view(1, -1).expand(
- num_gt, num_bboxes).contiguous().view(-1)
- ep_bboxes_cy = bboxes_cy.view(1, -1).expand(
- num_gt, num_bboxes).contiguous().view(-1)
- candidate_idxs = candidate_idxs.view(-1)
-
- # calculate the left, top, right, bottom distance between positive
- # bbox center and gt side
- l_ = ep_bboxes_cx[candidate_idxs].view(-1, num_gt) - gt_bboxes[:, 0]
- t_ = ep_bboxes_cy[candidate_idxs].view(-1, num_gt) - gt_bboxes[:, 1]
- r_ = gt_bboxes[:, 2] - ep_bboxes_cx[candidate_idxs].view(-1, num_gt)
- b_ = gt_bboxes[:, 3] - ep_bboxes_cy[candidate_idxs].view(-1, num_gt)
- is_in_gts = torch.stack([l_, t_, r_, b_], dim=1).min(dim=1)[0] > 0.01
- is_pos = is_pos & is_in_gts
-
- # if an anchor box is assigned to multiple gts,
- # the one with the highest IoU will be selected.
- overlaps_inf = torch.full_like(overlaps,
- -INF).t().contiguous().view(-1)
- index = candidate_idxs.view(-1)[is_pos.view(-1)]
- overlaps_inf[index] = overlaps.t().contiguous().view(-1)[index]
- overlaps_inf = overlaps_inf.view(num_gt, -1).t()
-
- max_overlaps, argmax_overlaps = overlaps_inf.max(dim=1)
- assigned_gt_inds[
- max_overlaps != -INF] = argmax_overlaps[max_overlaps != -INF] + 1
-
- if gt_labels is not None:
- assigned_labels = assigned_gt_inds.new_full((num_bboxes, ), -1)
- pos_inds = torch.nonzero(
- assigned_gt_inds > 0, as_tuple=False).squeeze()
- if pos_inds.numel() > 0:
- assigned_labels[pos_inds] = gt_labels[
- assigned_gt_inds[pos_inds] - 1]
- else:
- assigned_labels = None
- return AssignResult(
- num_gt, assigned_gt_inds, max_overlaps, labels=assigned_labels)
diff --git a/spaces/CVPR/WALT/mmdet/core/bbox/builder.py b/spaces/CVPR/WALT/mmdet/core/bbox/builder.py
deleted file mode 100644
index 682683b62ae55396f24e9f9eea0f8193e2e88de6..0000000000000000000000000000000000000000
--- a/spaces/CVPR/WALT/mmdet/core/bbox/builder.py
+++ /dev/null
@@ -1,20 +0,0 @@
-from mmcv.utils import Registry, build_from_cfg
-
-BBOX_ASSIGNERS = Registry('bbox_assigner')
-BBOX_SAMPLERS = Registry('bbox_sampler')
-BBOX_CODERS = Registry('bbox_coder')
-
-
-def build_assigner(cfg, **default_args):
- """Builder of box assigner."""
- return build_from_cfg(cfg, BBOX_ASSIGNERS, default_args)
-
-
-def build_sampler(cfg, **default_args):
- """Builder of box sampler."""
- return build_from_cfg(cfg, BBOX_SAMPLERS, default_args)
-
-
-def build_bbox_coder(cfg, **default_args):
- """Builder of box coder."""
- return build_from_cfg(cfg, BBOX_CODERS, default_args)
diff --git a/spaces/CVPR/lama-example/saicinpainting/training/visualizers/directory.py b/spaces/CVPR/lama-example/saicinpainting/training/visualizers/directory.py
deleted file mode 100644
index bc42e00500c7a5b70b2cef83b03e45b5bb471ff8..0000000000000000000000000000000000000000
--- a/spaces/CVPR/lama-example/saicinpainting/training/visualizers/directory.py
+++ /dev/null
@@ -1,36 +0,0 @@
-import os
-
-import cv2
-import numpy as np
-
-from saicinpainting.training.visualizers.base import BaseVisualizer, visualize_mask_and_images_batch
-from saicinpainting.utils import check_and_warn_input_range
-
-
-class DirectoryVisualizer(BaseVisualizer):
- DEFAULT_KEY_ORDER = 'image predicted_image inpainted'.split(' ')
-
- def __init__(self, outdir, key_order=DEFAULT_KEY_ORDER, max_items_in_batch=10,
- last_without_mask=True, rescale_keys=None):
- self.outdir = outdir
- os.makedirs(self.outdir, exist_ok=True)
- self.key_order = key_order
- self.max_items_in_batch = max_items_in_batch
- self.last_without_mask = last_without_mask
- self.rescale_keys = rescale_keys
-
- def __call__(self, epoch_i, batch_i, batch, suffix='', rank=None):
- check_and_warn_input_range(batch['image'], 0, 1, 'DirectoryVisualizer target image')
- vis_img = visualize_mask_and_images_batch(batch, self.key_order, max_items=self.max_items_in_batch,
- last_without_mask=self.last_without_mask,
- rescale_keys=self.rescale_keys)
-
- vis_img = np.clip(vis_img * 255, 0, 255).astype('uint8')
-
- curoutdir = os.path.join(self.outdir, f'epoch{epoch_i:04d}{suffix}')
- os.makedirs(curoutdir, exist_ok=True)
- rank_suffix = f'_r{rank}' if rank is not None else ''
- out_fname = os.path.join(curoutdir, f'batch{batch_i:07d}{rank_suffix}.jpg')
-
- vis_img = cv2.cvtColor(vis_img, cv2.COLOR_RGB2BGR)
- cv2.imwrite(out_fname, vis_img)
diff --git a/spaces/CVPR/v-doc_abstractive_mac/program_translator.py b/spaces/CVPR/v-doc_abstractive_mac/program_translator.py
deleted file mode 100644
index f790d9d9d44ba0b45c74a81f1c39a941730b6109..0000000000000000000000000000000000000000
--- a/spaces/CVPR/v-doc_abstractive_mac/program_translator.py
+++ /dev/null
@@ -1,104 +0,0 @@
-
-class ProgramTranslator(object):
- def __init__(self, programDict, maxArity):
- self.programDict = programDict
- self.maxArity = maxArity
-
- self.maxStack = 0
-
- def functionToKey(self, function, withValInputs = True):
- valInputs = ""
- if withValInputs:
- valInputs = "_" + ",".join(function["value_inputs"])
- functionKey = function["function"] if "_" in function["function"] else \
- "_".join([function["function"], function["function"]])
- return str(len(function["inputs"])) + "_" + functionKey + valInputs
-
- def typeToKey(self, function, withValInputs = True):
- valInputs = ""
- if withValInputs:
- valInputs = "_" + ",".join(function["value_inputs"])
- functionKey = function["type"] if "_" in function["type"] else \
- "_".join([function["type"], function["type"]])
- return str(len(function["inputs"])) + "_" + functionKey + valInputs
-
- def keyToFunction(self, key):
- assert key not in self.programDict.invalidSymbols
- function = {}
- parts = key.split("_")
- arity = int(parts[0])
- function["function"] = "_".join([parts[1], parts[2]])
- function["value_inputs"] = []
- if len(parts) == 4:
- function["value_inputs"] = parts[3].split(",")
- function["inputs"] = []
- return function, arity
-
- def keyToArity(self, key):
- if key in self.programDict.invalidSymbols:
- return 0
- return int(key.split("_")[0])
-
- def keyToType(self, key):
- if key in self.programDict.invalidSymbols:
- return ["0", "0", "0"]
- return ["0:" + key.split("_")[0], "1:" + key.split("_")[1], "2:" + key.split("_")[2]]
-
- def programToPostfixProgram(self, program):
- newProgram = []
-
- def programToPostfixAux(currIndex = -1):
- childrenIndices = program[currIndex]["inputs"]
- #[int(child) for child in program[currIndex]["inputs"]]
- childrenNewIndices = []
- for child in childrenIndices:
- programToPostfixAux(child)
- childrenNewIndices.append(len(newProgram) - 1)
- program[currIndex]["inputs"] = childrenNewIndices
- newProgram.append(program[currIndex])
-
- programToPostfixAux()
- return newProgram
-
- def programToSeq(self, program):
- return [self.functionToKey(function) for function in program]
-
- def pdfProgramToSeq(self, program):
- return [self.typeToKey(function) for function in program]
-
- def programToInputs(self, program, offset = 0):
- inputs = [function["inputs"] for function in program]
- offsetedInputs = [[FuncInput + offset for FuncInput in FuncInputs] for FuncInputs in inputs]
- return offsetedInputs
-
- # def seqToProgram(self, seq, enforceValidPrograms = True):
- # program = []
-
- # def seqToProgramAux(currIndex = len(seq) - 1):
- # if currIndex < 0:
- # program = None
- # return
- # currFunc, arity = self.keyToFunction(seq[currIndex])
- # nextIndex = currIndex - 1
- # program.append(currFunc)
- # for _ in arity:
- # currFunc["inputs"].append(nextIndex)
- # nextIndex = seqToProgramAux(nextIndex)
- # currFunc["inputs"].reverse()
- # return nextIndex
-
- # if enforceValidPrograms:
- # seqToProgramAux()
- # if program is not None:
- # program.reverse()
- # else:
- # stack = [0] * self.maxArity
- # for i in range(len(seq)):
- # func, arity = self.keyToFunction(seq[i])
- # func["inputs"] = stack[len(stack) - arity:]
- # newLength = max(len(stack) - arity, self.maxArity)
- # stack = stack[:newLength] + [i + self.maxArity]
- # self.maxStack = max(len(stack), self.maxStack)
- # program.append(func)
-
- # return program
diff --git a/spaces/Caoyunkang/Segment-Any-Anomaly/GroundingDINO/groundingdino/models/GroundingDINO/csrc/MsDeformAttn/ms_deform_attn_cuda.h b/spaces/Caoyunkang/Segment-Any-Anomaly/GroundingDINO/groundingdino/models/GroundingDINO/csrc/MsDeformAttn/ms_deform_attn_cuda.h
deleted file mode 100644
index ad1311a78f61303616504eb991aaa9c4a93d9948..0000000000000000000000000000000000000000
--- a/spaces/Caoyunkang/Segment-Any-Anomaly/GroundingDINO/groundingdino/models/GroundingDINO/csrc/MsDeformAttn/ms_deform_attn_cuda.h
+++ /dev/null
@@ -1,33 +0,0 @@
-/*!
-**************************************************************************************************
-* Deformable DETR
-* Copyright (c) 2020 SenseTime. All Rights Reserved.
-* Licensed under the Apache License, Version 2.0 [see LICENSE for details]
-**************************************************************************************************
-* Modified from https://github.com/chengdazhi/Deformable-Convolution-V2-PyTorch/tree/pytorch_1.0.0
-**************************************************************************************************
-*/
-
-#pragma once
-#include
-
-namespace groundingdino {
-
-at::Tensor ms_deform_attn_cuda_forward(
- const at::Tensor &value,
- const at::Tensor &spatial_shapes,
- const at::Tensor &level_start_index,
- const at::Tensor &sampling_loc,
- const at::Tensor &attn_weight,
- const int im2col_step);
-
-std::vector ms_deform_attn_cuda_backward(
- const at::Tensor &value,
- const at::Tensor &spatial_shapes,
- const at::Tensor &level_start_index,
- const at::Tensor &sampling_loc,
- const at::Tensor &attn_weight,
- const at::Tensor &grad_output,
- const int im2col_step);
-
-} // namespace groundingdino
\ No newline at end of file
diff --git a/spaces/Cecil8352/vits-models/utils.py b/spaces/Cecil8352/vits-models/utils.py
deleted file mode 100644
index ee4b01ddfbe8173965371b29f770f3e87615fe71..0000000000000000000000000000000000000000
--- a/spaces/Cecil8352/vits-models/utils.py
+++ /dev/null
@@ -1,225 +0,0 @@
-import os
-import sys
-import argparse
-import logging
-import json
-import subprocess
-import numpy as np
-import librosa
-import torch
-
-MATPLOTLIB_FLAG = False
-
-logging.basicConfig(stream=sys.stdout, level=logging.DEBUG)
-logger = logging
-
-
-def load_checkpoint(checkpoint_path, model, optimizer=None):
- assert os.path.isfile(checkpoint_path)
- checkpoint_dict = torch.load(checkpoint_path, map_location='cpu')
- iteration = checkpoint_dict['iteration']
- learning_rate = checkpoint_dict['learning_rate']
- if optimizer is not None:
- optimizer.load_state_dict(checkpoint_dict['optimizer'])
- saved_state_dict = checkpoint_dict['model']
- if hasattr(model, 'module'):
- state_dict = model.module.state_dict()
- else:
- state_dict = model.state_dict()
- new_state_dict= {}
- for k, v in state_dict.items():
- try:
- new_state_dict[k] = saved_state_dict[k]
- except:
- logger.info("%s is not in the checkpoint" % k)
- new_state_dict[k] = v
- if hasattr(model, 'module'):
- model.module.load_state_dict(new_state_dict)
- else:
- model.load_state_dict(new_state_dict)
- logger.info("Loaded checkpoint '{}' (iteration {})" .format(
- checkpoint_path, iteration))
- return model, optimizer, learning_rate, iteration
-
-
-def plot_spectrogram_to_numpy(spectrogram):
- global MATPLOTLIB_FLAG
- if not MATPLOTLIB_FLAG:
- import matplotlib
- matplotlib.use("Agg")
- MATPLOTLIB_FLAG = True
- mpl_logger = logging.getLogger('matplotlib')
- mpl_logger.setLevel(logging.WARNING)
- import matplotlib.pylab as plt
- import numpy as np
-
- fig, ax = plt.subplots(figsize=(10,2))
- im = ax.imshow(spectrogram, aspect="auto", origin="lower",
- interpolation='none')
- plt.colorbar(im, ax=ax)
- plt.xlabel("Frames")
- plt.ylabel("Channels")
- plt.tight_layout()
-
- fig.canvas.draw()
- data = np.fromstring(fig.canvas.tostring_rgb(), dtype=np.uint8, sep='')
- data = data.reshape(fig.canvas.get_width_height()[::-1] + (3,))
- plt.close()
- return data
-
-
-def plot_alignment_to_numpy(alignment, info=None):
- global MATPLOTLIB_FLAG
- if not MATPLOTLIB_FLAG:
- import matplotlib
- matplotlib.use("Agg")
- MATPLOTLIB_FLAG = True
- mpl_logger = logging.getLogger('matplotlib')
- mpl_logger.setLevel(logging.WARNING)
- import matplotlib.pylab as plt
- import numpy as np
-
- fig, ax = plt.subplots(figsize=(6, 4))
- im = ax.imshow(alignment.transpose(), aspect='auto', origin='lower',
- interpolation='none')
- fig.colorbar(im, ax=ax)
- xlabel = 'Decoder timestep'
- if info is not None:
- xlabel += '\n\n' + info
- plt.xlabel(xlabel)
- plt.ylabel('Encoder timestep')
- plt.tight_layout()
-
- fig.canvas.draw()
- data = np.fromstring(fig.canvas.tostring_rgb(), dtype=np.uint8, sep='')
- data = data.reshape(fig.canvas.get_width_height()[::-1] + (3,))
- plt.close()
- return data
-
-
-def load_audio_to_torch(full_path, target_sampling_rate):
- audio, sampling_rate = librosa.load(full_path, sr=target_sampling_rate, mono=True)
- return torch.FloatTensor(audio.astype(np.float32))
-
-
-def load_filepaths_and_text(filename, split="|"):
- with open(filename, encoding='utf-8') as f:
- filepaths_and_text = [line.strip().split(split) for line in f]
- return filepaths_and_text
-
-
-def get_hparams(init=True):
- parser = argparse.ArgumentParser()
- parser.add_argument('-c', '--config', type=str, default="./configs/base.json",
- help='JSON file for configuration')
- parser.add_argument('-m', '--model', type=str, required=True,
- help='Model name')
-
- args = parser.parse_args()
- model_dir = os.path.join("./logs", args.model)
-
- if not os.path.exists(model_dir):
- os.makedirs(model_dir)
-
- config_path = args.config
- config_save_path = os.path.join(model_dir, "config.json")
- if init:
- with open(config_path, "r") as f:
- data = f.read()
- with open(config_save_path, "w") as f:
- f.write(data)
- else:
- with open(config_save_path, "r") as f:
- data = f.read()
- config = json.loads(data)
-
- hparams = HParams(**config)
- hparams.model_dir = model_dir
- return hparams
-
-
-def get_hparams_from_dir(model_dir):
- config_save_path = os.path.join(model_dir, "config.json")
- with open(config_save_path, "r") as f:
- data = f.read()
- config = json.loads(data)
-
- hparams =HParams(**config)
- hparams.model_dir = model_dir
- return hparams
-
-
-def get_hparams_from_file(config_path):
- with open(config_path, "r") as f:
- data = f.read()
- config = json.loads(data)
-
- hparams =HParams(**config)
- return hparams
-
-
-def check_git_hash(model_dir):
- source_dir = os.path.dirname(os.path.realpath(__file__))
- if not os.path.exists(os.path.join(source_dir, ".git")):
- logger.warn("{} is not a git repository, therefore hash value comparison will be ignored.".format(
- source_dir
- ))
- return
-
- cur_hash = subprocess.getoutput("git rev-parse HEAD")
-
- path = os.path.join(model_dir, "githash")
- if os.path.exists(path):
- saved_hash = open(path).read()
- if saved_hash != cur_hash:
- logger.warn("git hash values are different. {}(saved) != {}(current)".format(
- saved_hash[:8], cur_hash[:8]))
- else:
- open(path, "w").write(cur_hash)
-
-
-def get_logger(model_dir, filename="train.log"):
- global logger
- logger = logging.getLogger(os.path.basename(model_dir))
- logger.setLevel(logging.DEBUG)
-
- formatter = logging.Formatter("%(asctime)s\t%(name)s\t%(levelname)s\t%(message)s")
- if not os.path.exists(model_dir):
- os.makedirs(model_dir)
- h = logging.FileHandler(os.path.join(model_dir, filename))
- h.setLevel(logging.DEBUG)
- h.setFormatter(formatter)
- logger.addHandler(h)
- return logger
-
-
-class HParams():
- def __init__(self, **kwargs):
- for k, v in kwargs.items():
- if type(v) == dict:
- v = HParams(**v)
- self[k] = v
-
- def keys(self):
- return self.__dict__.keys()
-
- def items(self):
- return self.__dict__.items()
-
- def values(self):
- return self.__dict__.values()
-
- def __len__(self):
- return len(self.__dict__)
-
- def __getitem__(self, key):
- return getattr(self, key)
-
- def __setitem__(self, key, value):
- return setattr(self, key, value)
-
- def __contains__(self, key):
- return key in self.__dict__
-
- def __repr__(self):
- return self.__dict__.__repr__()
diff --git a/spaces/ClipHamper/stable-diffusion-webui/app.py b/spaces/ClipHamper/stable-diffusion-webui/app.py
deleted file mode 100644
index 00c05986f7e088955e9aecbb5657c3be8dfce651..0000000000000000000000000000000000000000
--- a/spaces/ClipHamper/stable-diffusion-webui/app.py
+++ /dev/null
@@ -1,190 +0,0 @@
-"""
-Stable Diffusion Webui Version 1.6
-https://github.com/AUTOMATIC1111/stable-diffusion-webui/releases/tag/v1.6.0
-
-"""
-commit_id=r"5ef669de080814067961f28357256e8fe27544f4" #Version 1.3.0
-import os
-from sys import executable
-import subprocess
-import pathlib
-import gc
-
-def Gitclone(URI:str,ClonePath:pathlib.Path ) -> int :
- if pathlib.Path.exists(ClonePath):
- return 0
- for z in range(10):
- i=subprocess.run([r"git",r"clone",str(URI),str(ClonePath)])
- if(i.returncode == 0 ):
- del i
- return 0
- else :
- del i
- raise Exception(str.format("clone \'{0}\' failed",URI))
-
-
-def DownLoad(URI:str,DownloadPath:pathlib.Path,DownLoadFileName:str ) -> int:
- if (DownloadPath / DownLoadFileName).is_file(): return 0
- for z in range(10):
- i=subprocess.run([r"aria2c",r"-c",r"-x" ,r"16", r"-s",r"16", r"-k" ,r"1M" ,r"-m",r"0",r"--enable-mmap=false",r"--console-log-level=error",r"-d",str(DownloadPath),r"-o",DownLoadFileName,URI]);
- if(i.returncode == 0 ):
- del i
- gc.collect()
- return 0
- else :
- del i
- raise Exception(str.format("download \'{0}\' failed",URI))
-
-user_home =pathlib.Path.home().resolve()
-os.chdir(str(user_home))
-#clone stable-diffusion-webui repo
-print("cloning stable-diffusion-webui repo")
-Gitclone(r"https://github.com/AUTOMATIC1111/stable-diffusion-webui.git",user_home / r"stable-diffusion-webui")
-os.chdir(str(user_home / r"stable-diffusion-webui"))
-os.system("git reset --hard "+commit_id)
-#install extensions
-print("installing extensions")
-Gitclone(r"https://github.com/vorstcavry/embeddings",user_home / r"stable-diffusion-webui" / r"embeddings" / r"negative")
-Gitclone(r"https://github.com/vorstcavry/lora",user_home / r"stable-diffusion-webui" / r"models" / r"Lora" / r"positive")
-Gitclone(r"https://github.com/vorstcavry/Checkpoint-Model",user_home / r"stable-diffusion-webui" / r"models" / r"Stable-diffusion" / r"Checkpoint")
-
-DownLoad(r"https://huggingface.co/embed/upscale/resolve/main/4x-UltraSharp.pth",user_home / r"stable-diffusion-webui" / r"models" / r"ESRGAN" ,r"4x-UltraSharp.pth")
-while (True):
- i=subprocess.run([r"wget",r"https://raw.githubusercontent.com/camenduru/stable-diffusion-webui-scripts/main/run_n_times.py",r"-O",str(user_home / r"stable-diffusion-webui" / r"scripts" / r"run_n_times.py")])
- if(i.returncode == 0 ):
- del i
- gc.collect()
- break
- else :
- del i
-Gitclone(r"https://github.com/deforum-art/deforum-for-automatic1111-webui",user_home / r"stable-diffusion-webui" / r"extensions" / r"deforum-for-automatic1111-webui" )
-Gitclone(r"https://github.com/AlUlkesh/stable-diffusion-webui-images-browser",user_home / r"stable-diffusion-webui" / r"extensions"/ r"stable-diffusion-webui-images-browser")
-Gitclone(r"https://github.com/camenduru/stable-diffusion-webui-huggingface",user_home / r"stable-diffusion-webui" / r"extensions" / r"stable-diffusion-webui-huggingface")
-Gitclone(r"https://github.com/BlafKing/sd-civitai-browser-plus",user_home / r"stable-diffusion-webui" / r"extensions" / r"civitai-browser")
-Gitclone(r"https://github.com/kohya-ss/sd-webui-additional-networks",user_home / r"stable-diffusion-webui" / r"extensions" / r"sd-webui-additional-networks")
-Gitclone(r"https://github.com/Mikubill/sd-webui-controlnet",user_home / r"stable-diffusion-webui" / r"extensions" / r"sd-webui-controlnet")
-Gitclone(r"https://github.com/fkunn1326/openpose-editor",user_home / r"stable-diffusion-webui" / r"extensions" / r"openpose-editor")
-Gitclone(r"https://github.com/jexom/sd-webui-depth-lib",user_home / r"stable-diffusion-webui" / r"extensions" / r"sd-webui-depth-lib")
-Gitclone(r"https://github.com/hnmr293/posex",user_home / r"stable-diffusion-webui" / r"extensions" / r"posex")
-Gitclone(r"https://github.com/nonnonstop/sd-webui-3d-open-pose-editor",user_home / r"stable-diffusion-webui" / r"extensions" / r"sd-webui-3d-open-pose-editor")
-#中文本地化的请解除下一行的注释
-#Gitclone(r"https://github.com/dtlnor/stable-diffusion-webui-localization-zh_CN.git",user_home / r"stable-diffusion-webui" / r"extensions" / r"stable-diffusion-webui-localization-zh_CN")
-Gitclone(r"https://github.com/DominikDoom/a1111-sd-webui-tagcomplete.git" , user_home / r"stable-diffusion-webui" / r"extensions" / r"a1111-sd-webui-tagcomplete")
-Gitclone(r"https://github.com/camenduru/sd-webui-tunnels",user_home / r"stable-diffusion-webui" / r"extensions" / r"sd-webui-tunnels")
-Gitclone(r"https://github.com/etherealxx/batchlinks-webui",user_home / r"stable-diffusion-webui" / r"extensions" / r"batchlinks-webui")
-Gitclone(r"https://github.com/catppuccin/stable-diffusion-webui",user_home / r"stable-diffusion-webui" / r"extensions" / r"stable-diffusion-webui-catppuccin")
-Gitclone(r"https://github.com/AUTOMATIC1111/stable-diffusion-webui-rembg",user_home / r"stable-diffusion-webui" / r"extensions" / r"stable-diffusion-webui-rembg")
-Gitclone(r"https://tinyurl.com/aspect-ratio-v",user_home / r"stable-diffusion-webui" / r"extensions" / r"aspect-ratio")
-Gitclone(r"https://github.com/Iyashinouta/sd-model-downloader",user_home / r"stable-diffusion-webui" / r"extensions" / r"sd-model-downloader")
-Gitclone(r"https://github.com/AIrjen/OneButtonPrompt",user_home / r"stable-diffusion-webui" / r"extensions" / r"OneButtonPrompt")
-Gitclone(r"https://github.com/AUTOMATIC1111/stable-diffusion-webui-wildcards",user_home / r"stable-diffusion-webui" / r"extensions" / r"stable-diffusion-webui-wildcards")
-Gitclone(r"https://github.com/adieyal/sd-dynamic-prompts",user_home / r"stable-diffusion-webui" / r"extensions" / r"sd-dynamic-prompts")
-Gitclone(r"https://github.com/d8ahazard/sd_dreambooth_extension",user_home / r"stable-diffusion-webui" / r"extensions" / r"sd_dreambooth_extension")
-Gitclone(r"https://github.com/yfszzx/stable-diffusion-webui-inspiration",user_home / r"stable-diffusion-webui" / r"extensions" / r"stable-diffusion-webui-inspiration")
-Gitclone(r"https://github.com/Coyote-A/ultimate-upscale-for-automatic1111",user_home / r"stable-diffusion-webui" / r"extensions" / r"ultimate-upscale-for-automatic1111")
-os.chdir(user_home / r"stable-diffusion-webui")
-#download ControlNet models
-print("extensions dolwnload done .\ndownloading ControlNet models")
-dList =[r"https://huggingface.co/ckpt/ControlNet-v1-1/resolve/main/control_v11e_sd15_ip2p_fp16.safetensors",
- r"https://huggingface.co/ckpt/ControlNet-v1-1/resolve/main/control_v11e_sd15_shuffle_fp16.safetensors",
- r"https://huggingface.co/ckpt/ControlNet-v1-1/resolve/main/control_v11p_sd15_canny_fp16.safetensors",
- r"https://huggingface.co/ckpt/ControlNet-v1-1/resolve/main/control_v11f1p_sd15_depth_fp16.safetensors",
- r"https://huggingface.co/ckpt/ControlNet-v1-1/resolve/main/control_v11p_sd15_inpaint_fp16.safetensors",
- r"https://huggingface.co/ckpt/ControlNet-v1-1/resolve/main/control_v11p_sd15_lineart_fp16.safetensors",
- r"https://huggingface.co/ckpt/ControlNet-v1-1/resolve/main/control_v11p_sd15_mlsd_fp16.safetensors",
- r"https://huggingface.co/ckpt/ControlNet-v1-1/resolve/main/control_v11p_sd15_normalbae_fp16.safetensors",
- r"https://huggingface.co/ckpt/ControlNet-v1-1/resolve/main/control_v11p_sd15_openpose_fp16.safetensors",
- r"https://huggingface.co/ckpt/ControlNet-v1-1/resolve/main/control_v11p_sd15_scribble_fp16.safetensors",
- r"https://huggingface.co/ckpt/ControlNet-v1-1/resolve/main/control_v11p_sd15_seg_fp16.safetensors",
- r"https://huggingface.co/ckpt/ControlNet-v1-1/resolve/main/control_v11p_sd15_softedge_fp16.safetensors",
- r"https://huggingface.co/ckpt/ControlNet-v1-1/resolve/main/control_v11p_sd15s2_lineart_anime_fp16.safetensors",
- r"https://huggingface.co/ckpt/ControlNet-v1-1/resolve/main/control_v11f1e_sd15_tile_fp16.safetensors",
- r"https://huggingface.co/ckpt/ControlNet-v1-1/raw/main/control_v11e_sd15_ip2p_fp16.yaml",
- r"https://huggingface.co/ckpt/ControlNet-v1-1/raw/main/control_v11e_sd15_shuffle_fp16.yaml",
- r"https://huggingface.co/ckpt/ControlNet-v1-1/raw/main/control_v11p_sd15_canny_fp16.yaml",
- r"https://huggingface.co/ckpt/ControlNet-v1-1/raw/main/control_v11f1p_sd15_depth_fp16.yaml",
- r"https://huggingface.co/ckpt/ControlNet-v1-1/raw/main/control_v11p_sd15_inpaint_fp16.yaml",
- r"https://huggingface.co/ckpt/ControlNet-v1-1/raw/main/control_v11p_sd15_lineart_fp16.yaml",
- r"https://huggingface.co/ckpt/ControlNet-v1-1/raw/main/control_v11p_sd15_mlsd_fp16.yaml",
- r"https://huggingface.co/ckpt/ControlNet-v1-1/raw/main/control_v11p_sd15_normalbae_fp16.yaml",
- r"https://huggingface.co/ckpt/ControlNet-v1-1/raw/main/control_v11p_sd15_openpose_fp16.yaml",
- r"https://huggingface.co/ckpt/ControlNet-v1-1/raw/main/control_v11p_sd15_scribble_fp16.yaml",
- r"https://huggingface.co/ckpt/ControlNet-v1-1/raw/main/control_v11p_sd15_seg_fp16.yaml",
- r"https://huggingface.co/ckpt/ControlNet-v1-1/raw/main/control_v11p_sd15_softedge_fp16.yaml",
- r"https://huggingface.co/ckpt/ControlNet-v1-1/raw/main/control_v11p_sd15s2_lineart_anime_fp16.yaml",
- r"https://huggingface.co/ckpt/ControlNet-v1-1/raw/main/control_v11f1e_sd15_tile_fp16.yaml",
- r"https://huggingface.co/ckpt/ControlNet-v1-1/resolve/main/t2iadapter_style_sd14v1.pth",
- r"https://huggingface.co/ckpt/ControlNet-v1-1/resolve/main/t2iadapter_sketch_sd14v1.pth",
- r"https://huggingface.co/ckpt/ControlNet-v1-1/resolve/main/t2iadapter_seg_sd14v1.pth",
- r"https://huggingface.co/ckpt/ControlNet-v1-1/resolve/main/t2iadapter_openpose_sd14v1.pth",
- r"https://huggingface.co/ckpt/ControlNet-v1-1/resolve/main/t2iadapter_keypose_sd14v1.pth",
- r"https://huggingface.co/ckpt/ControlNet-v1-1/resolve/main/t2iadapter_depth_sd14v1.pth",
- r"https://huggingface.co/ckpt/ControlNet-v1-1/resolve/main/t2iadapter_canny_sd14v1.pth",
- r"https://huggingface.co/ckpt/ControlNet-v1-1/resolve/main/t2iadapter_canny_sd15v2.pth",
- r"https://huggingface.co/ckpt/ControlNet-v1-1/resolve/main/t2iadapter_depth_sd15v2.pth",
- r"https://huggingface.co/ckpt/ControlNet-v1-1/resolve/main/t2iadapter_sketch_sd15v2.pth",
- r"https://huggingface.co/ckpt/ControlNet-v1-1/resolve/main/t2iadapter_zoedepth_sd15v1.pth"]
-for i in range(0,len(dList)): DownLoad(dList[i],user_home / r"stable-diffusion-webui" / r"extensions" / "sd-webui-controlnet" / r"models",pathlib.Path(dList[i]).name)
-del dList
-#download model
-#you can change model download address here
-print("ControlNet models download done.\ndownloading model")
-#Stable Diffusion Checkpoint Model
-#anything version4.5
-#DownLoad(r"https://huggingface.co/ckpt/anything-v4.0/resolve/main/anything-v4.5-pruned.ckpt",user_home / r"stable-diffusion-webui" / r"models" / r"Stable-diffusion" / r"Checkpoint",r"anything-v4.5-pruned.ckpt")
-#DownLoad(r"https://huggingface.co/ckpt/anything-v4.0/resolve/main/anything-v4.0.vae.pt",user_home / r"stable-diffusion-webui" / r"models" / r"Stable-diffusion" / r"Checkpoint",r"anything-v4.0.vae.pt")
-#Counterfeit-V3.0
-#DownLoad(r"https://huggingface.co/gsdf/Counterfeit-V3.0/resolve/main/Counterfeit-V3.0_fp16.safetensors",user_home / r"stable-diffusion-webui" / r"models" / r"Stable-diffusion" / r"Checkpoint",r"Counterfeit-V3.0_fp16.safetensors")
-#AbyssOrangeMix2 sfw
-#DownLoad(r"https://huggingface.co/WarriorMama777/OrangeMixs/resolve/main/Models/AbyssOrangeMix2/AbyssOrangeMix2_sfw.safetensors",user_home / r"stable-diffusion-webui" / r"models" / r"Stable-diffusion" / r"Checkpoint",r"AbyssOrangeMix2_sfw.safetensors")
-#DownLoad(r"https://huggingface.co/WarriorMama777/OrangeMixs/resolve/main/VAEs/orangemix.vae.pt",user_home / r"stable-diffusion-webui" / r"models" / r"Stable-diffusion" / r"Checkpoint",r"orangemix.vae.pt")
-#MeinaPastelV5
-#DownLoad(r"https://huggingface.co/Meina/MeinaPastel/resolve/main/MeinaPastelV5%20-%20Baked%20VAE.safetensors",user_home / r"stable-diffusion-webui" / r"models" / r"Stable-diffusion" / r"Checkpoint",r"MeinaPastelV5_BakedVAE.safetensors")
-#DownLoad(r"https://huggingface.co/AnonPerson/ChilloutMix/resolve/main/ChilloutMix-ni-fp16.safetensors",user_home / r"stable-diffusion-webui" / r"models" / r"Stable-diffusion" / r"Checkpoint",r"ChilloutMix-ni-fp16.safetensors")
-#DownLoad(r"https://huggingface.co/Meina/MeinaPastel/resolve/main/MeinaPastelV4%20-%20Without%20VAE.safetensors",user_home / r"stable-diffusion-webui" / r"models" / r"Stable-diffusion" / r"Checkpoint",r"MeinaPastelV4%20-%20Without%20VAE.safetensors")
-#DownLoad(r"https://huggingface.co/ckpt/perfect_world/resolve/main/perfectWorld_v2Baked.safetensors",user_home / r"stable-diffusion-webui" / r"models" / r"Stable-diffusion" / r"Checkpoint",r"perfectWorld_v2Baked.safetensors")
-#DownLoad(r"https://huggingface.co/vorstcavry/figurestyle1/resolve/main/figure.safetensors",user_home / r"stable-diffusion-webui" / r"models" / r"Stable-diffusion" / r"Checkpoint",r"figure.safetensors")
-#DownLoad(r"https://huggingface.co/vorstcavry/dosmix/resolve/main/ddosmix_V2.safetensors",user_home / r"stable-diffusion-webui" / r"models" / r"Stable-diffusion" / r"Checkpoint",r"ddosmix_V2.safetensors")
-#DownLoad(r"https://huggingface.co/ckpt/rev-animated/resolve/main/revAnimated_v11.safetensors",user_home / r"stable-diffusion-webui" / r"models" / r"Stable-diffusion" / r"Checkpoint",r"revAnimated_v11.safetensors")
-#DownLoad(r"https://huggingface.co/ckpt/MeinaMix/resolve/main/Meina_V8_baked_VAE.safetensors",user_home / r"stable-diffusion-webui" / r"models" / r"Stable-diffusion" / r"Checkpoint",r"Meina_V8_baked_VAE.safetensors")
-#DownLoad(r"https://huggingface.co/ckpt/CyberRealistic/resolve/main/cyberrealistic_v13.safetensors",user_home / r"stable-diffusion-webui" / r"models" / r"Stable-diffusion" / r"Checkpoint",r"cyberrealistic_v13.safetensors")
-DownLoad(r"https://huggingface.co/vorstcavry/mymodel/resolve/main/Cavry_V2.safetensors",user_home / r"stable-diffusion-webui" / r"models" / r"Stable-diffusion" / r"Checkpoint",r"Cavry_V2.safetensors")
-#downloadvae
-DownLoad(r"https://huggingface.co/stabilityai/sd-vae-ft-mse-original/resolve/main/vae-ft-mse-840000-ema-pruned.safetensors",user_home / r"stable-diffusion-webui" / r"models" / r"VAE",r"vae-ft-mse-840000-ema-pruned.safetensors")
-
-#Lora Model
-#Better Light
-#DownLoad(r"https://civitai.com/api/download/models/39885",user_home / r"stable-diffusion-webui" / r"extensions" / r"sd-webui-additional-networks" / r"models"/ r"lora",r"Better_light.safetensors")
-#DownLoad(r"https://civitai.com/api/download/models/39885",user_home / r"stable-diffusion-webui" / r"models"/ r"lora",r"Better_light.safetensors")
-#LAS
-#DownLoad(r"https://civitai.com/api/download/models/21065",user_home / r"stable-diffusion-webui" / r"extensions" / r"sd-webui-additional-networks" / r"models"/ r"lora",r"LAS.safetensors")
-#DownLoad(r"https://civitai.com/api/download/models/21065",user_home / r"stable-diffusion-webui" / r"models"/ r"lora",r"LAS.safetensors")
-#Backlighting
-#DownLoad(r"https://civitai.com/api/download/models/39164",user_home / r"stable-diffusion-webui" / r"extensions" / r"sd-webui-additional-networks" / r"models"/ r"lora",r"backlighting.safetensors")
-#DownLoad(r"https://civitai.com/api/download/models/39164",user_home / r"stable-diffusion-webui" / r"models"/ r"lora",r"backlighting.safetensors")
-DownLoad(r"https://huggingface.co/vorstcavry/loraasia1/resolve/main/japaneseDollLikeness_v15.safetensors",user_home / r"stable-diffusion-webui" / r"models"/ r"lora",r"japaneseDollLikeness_v15.safetensors")
-DownLoad(r"https://huggingface.co/vorstcavry/loraasia1/resolve/main/koreanDollLikeness_v20.safetensors",user_home / r"stable-diffusion-webui" / r"models"/ r"lora",r"koreanDollLikeness_v20.safetensors")
-DownLoad(r"https://huggingface.co/vorstcavry/loraasia1/resolve/main/taiwanDollLikeness_v15.safetensors",user_home / r"stable-diffusion-webui" / r"models"/ r"lora",r"taiwanDollLikeness_v15.safetensors")
-
-
-
-
-#GFPGAN Model
-#detection Resnet50
-DownLoad(r"https://github.com/xinntao/facexlib/releases/download/v0.1.0/detection_Resnet50_Final.pth",user_home / r"stable-diffusion-webui"/r"models"/r"GFPGAN",r"detection_Resnet50_Final.pth")
-#parsing_parsenet
-DownLoad(r"https://github.com/xinntao/facexlib/releases/download/v0.2.2/parsing_parsenet.pth",user_home / r"stable-diffusion-webui"/r"models"/r"GFPGAN",r"parsing_parsenet.pth")
-#GFPGANv1.4
-DownLoad(r"https://github.com/TencentARC/GFPGAN/releases/download/v1.3.0/GFPGANv1.4.pth",user_home / r"stable-diffusion-webui"/r"models"/r"GFPGAN",r"GFPGANv1.4.pth")
-#strt Stable Diffusion Webui
-print("Done\nStarting Webui...")
-os.chdir(user_home / r"stable-diffusion-webui")
-gc.collect()
-while True:
- ret=subprocess.run([executable ,user_home / r"stable-diffusion-webui" / r"launch.py",r"--precision",r"full",r"--no-half",r"--no-half-vae",r"--enable-insecure-extension-access",r"--medvram",r"--skip-torch-cuda-test",r"--enable-console-prompts",r"--ui-settings-file="+str(pathlib.Path(__file__).parent /r"config.json")])
- if(ret.returncode == 0 ):
- del ret
- gc.collect()
- else :
- del ret
-del os ,user_home ,pyexecutable ,subprocess
\ No newline at end of file
diff --git a/spaces/Cran-May/SEA-orca/README.md b/spaces/Cran-May/SEA-orca/README.md
deleted file mode 100644
index 5e9a064b9c395116f8dfea377f9ac76ea847ef2c..0000000000000000000000000000000000000000
--- a/spaces/Cran-May/SEA-orca/README.md
+++ /dev/null
@@ -1,12 +0,0 @@
----
-title: Shi-CI Extensional Analyzer
-emoji: ⚡
-colorFrom: yellow
-colorTo: indigo
-sdk: gradio
-sdk_version: 3.45.2
-app_file: app.py
-pinned: true
----
-
-Check out the configuration reference at https://huggingface.co/docs/hub/spaces-config-reference
\ No newline at end of file
diff --git a/spaces/CrucibleAI/ControlNetMediaPipeFaceSD21/cldm/model.py b/spaces/CrucibleAI/ControlNetMediaPipeFaceSD21/cldm/model.py
deleted file mode 100644
index fed3c31ac145b78907c7f771d1d8db6fb32d92ed..0000000000000000000000000000000000000000
--- a/spaces/CrucibleAI/ControlNetMediaPipeFaceSD21/cldm/model.py
+++ /dev/null
@@ -1,28 +0,0 @@
-import os
-import torch
-
-from omegaconf import OmegaConf
-from ldm.util import instantiate_from_config
-
-
-def get_state_dict(d):
- return d.get('state_dict', d)
-
-
-def load_state_dict(ckpt_path, location='cpu'):
- _, extension = os.path.splitext(ckpt_path)
- if extension.lower() == ".safetensors":
- import safetensors.torch
- state_dict = safetensors.torch.load_file(ckpt_path, device=location)
- else:
- state_dict = get_state_dict(torch.load(ckpt_path, map_location=torch.device(location)))
- state_dict = get_state_dict(state_dict)
- print(f'Loaded state_dict from [{ckpt_path}]')
- return state_dict
-
-
-def create_model(config_path):
- config = OmegaConf.load(config_path)
- model = instantiate_from_config(config.model).cpu()
- print(f'Loaded model config from [{config_path}]')
- return model
diff --git a/spaces/DQChoi/gpt-demo/venv/lib/python3.11/site-packages/fontTools/ttLib/tables/otTraverse.py b/spaces/DQChoi/gpt-demo/venv/lib/python3.11/site-packages/fontTools/ttLib/tables/otTraverse.py
deleted file mode 100644
index bf22dcfdb500cd50525fce749562384a82b1cb0f..0000000000000000000000000000000000000000
--- a/spaces/DQChoi/gpt-demo/venv/lib/python3.11/site-packages/fontTools/ttLib/tables/otTraverse.py
+++ /dev/null
@@ -1,161 +0,0 @@
-"""Methods for traversing trees of otData-driven OpenType tables."""
-from collections import deque
-from typing import Callable, Deque, Iterable, List, Optional, Tuple
-from .otBase import BaseTable
-
-
-__all__ = [
- "bfs_base_table",
- "dfs_base_table",
- "SubTablePath",
-]
-
-
-class SubTablePath(Tuple[BaseTable.SubTableEntry, ...]):
- def __str__(self) -> str:
- path_parts = []
- for entry in self:
- path_part = entry.name
- if entry.index is not None:
- path_part += f"[{entry.index}]"
- path_parts.append(path_part)
- return ".".join(path_parts)
-
-
-# Given f(current frontier, new entries) add new entries to frontier
-AddToFrontierFn = Callable[[Deque[SubTablePath], List[SubTablePath]], None]
-
-
-def dfs_base_table(
- root: BaseTable,
- root_accessor: Optional[str] = None,
- skip_root: bool = False,
- predicate: Optional[Callable[[SubTablePath], bool]] = None,
- iter_subtables_fn: Optional[
- Callable[[BaseTable], Iterable[BaseTable.SubTableEntry]]
- ] = None,
-) -> Iterable[SubTablePath]:
- """Depth-first search tree of BaseTables.
-
- Args:
- root (BaseTable): the root of the tree.
- root_accessor (Optional[str]): attribute name for the root table, if any (mostly
- useful for debugging).
- skip_root (Optional[bool]): if True, the root itself is not visited, only its
- children.
- predicate (Optional[Callable[[SubTablePath], bool]]): function to filter out
- paths. If True, the path is yielded and its subtables are added to the
- queue. If False, the path is skipped and its subtables are not traversed.
- iter_subtables_fn (Optional[Callable[[BaseTable], Iterable[BaseTable.SubTableEntry]]]):
- function to iterate over subtables of a table. If None, the default
- BaseTable.iterSubTables() is used.
-
- Yields:
- SubTablePath: tuples of BaseTable.SubTableEntry(name, table, index) namedtuples
- for each of the nodes in the tree. The last entry in a path is the current
- subtable, whereas preceding ones refer to its parent tables all the way up to
- the root.
- """
- yield from _traverse_ot_data(
- root,
- root_accessor,
- skip_root,
- predicate,
- lambda frontier, new: frontier.extendleft(reversed(new)),
- iter_subtables_fn,
- )
-
-
-def bfs_base_table(
- root: BaseTable,
- root_accessor: Optional[str] = None,
- skip_root: bool = False,
- predicate: Optional[Callable[[SubTablePath], bool]] = None,
- iter_subtables_fn: Optional[
- Callable[[BaseTable], Iterable[BaseTable.SubTableEntry]]
- ] = None,
-) -> Iterable[SubTablePath]:
- """Breadth-first search tree of BaseTables.
-
- Args:
- the root of the tree.
- root_accessor (Optional[str]): attribute name for the root table, if any (mostly
- useful for debugging).
- skip_root (Optional[bool]): if True, the root itself is not visited, only its
- children.
- predicate (Optional[Callable[[SubTablePath], bool]]): function to filter out
- paths. If True, the path is yielded and its subtables are added to the
- queue. If False, the path is skipped and its subtables are not traversed.
- iter_subtables_fn (Optional[Callable[[BaseTable], Iterable[BaseTable.SubTableEntry]]]):
- function to iterate over subtables of a table. If None, the default
- BaseTable.iterSubTables() is used.
-
- Yields:
- SubTablePath: tuples of BaseTable.SubTableEntry(name, table, index) namedtuples
- for each of the nodes in the tree. The last entry in a path is the current
- subtable, whereas preceding ones refer to its parent tables all the way up to
- the root.
- """
- yield from _traverse_ot_data(
- root,
- root_accessor,
- skip_root,
- predicate,
- lambda frontier, new: frontier.extend(new),
- iter_subtables_fn,
- )
-
-
-def _traverse_ot_data(
- root: BaseTable,
- root_accessor: Optional[str],
- skip_root: bool,
- predicate: Optional[Callable[[SubTablePath], bool]],
- add_to_frontier_fn: AddToFrontierFn,
- iter_subtables_fn: Optional[
- Callable[[BaseTable], Iterable[BaseTable.SubTableEntry]]
- ] = None,
-) -> Iterable[SubTablePath]:
- # no visited because general otData cannot cycle (forward-offset only)
- if root_accessor is None:
- root_accessor = type(root).__name__
-
- if predicate is None:
-
- def predicate(path):
- return True
-
- if iter_subtables_fn is None:
-
- def iter_subtables_fn(table):
- return table.iterSubTables()
-
- frontier: Deque[SubTablePath] = deque()
-
- root_entry = BaseTable.SubTableEntry(root_accessor, root)
- if not skip_root:
- frontier.append((root_entry,))
- else:
- add_to_frontier_fn(
- frontier,
- [
- (root_entry, subtable_entry)
- for subtable_entry in iter_subtables_fn(root)
- ],
- )
-
- while frontier:
- # path is (value, attr_name) tuples. attr_name is attr of parent to get value
- path = frontier.popleft()
- current = path[-1].value
-
- if not predicate(path):
- continue
-
- yield SubTablePath(path)
-
- new_entries = [
- path + (subtable_entry,) for subtable_entry in iter_subtables_fn(current)
- ]
-
- add_to_frontier_fn(frontier, new_entries)
diff --git a/spaces/DQChoi/gpt-demo/venv/lib/python3.11/site-packages/gradio/templates/frontend/assets/Button-9b719f62.css b/spaces/DQChoi/gpt-demo/venv/lib/python3.11/site-packages/gradio/templates/frontend/assets/Button-9b719f62.css
deleted file mode 100644
index 1febd1de643feeadb668f5d0fc297f661ce47482..0000000000000000000000000000000000000000
--- a/spaces/DQChoi/gpt-demo/venv/lib/python3.11/site-packages/gradio/templates/frontend/assets/Button-9b719f62.css
+++ /dev/null
@@ -1 +0,0 @@
-.block.svelte-90oupt{position:relative;margin:0;box-shadow:var(--block-shadow);border-width:var(--block-border-width);border-color:var(--block-border-color);border-radius:var(--block-radius);background:var(--block-background-fill);width:100%;line-height:var(--line-sm)}.block.border_focus.svelte-90oupt{border-color:var(--color-accent)}.padded.svelte-90oupt{padding:var(--block-padding)}.hidden.svelte-90oupt{display:none}.hide-container.svelte-90oupt{margin:0;box-shadow:none;--block-border-width:0;background:transparent;padding:0;overflow:visible}div.svelte-e8n7p6{margin-bottom:var(--spacing-lg);color:var(--block-info-text-color);font-weight:var(--block-info-text-weight);font-size:var(--block-info-text-size);line-height:var(--line-sm)}span.has-info.svelte-1gfkn6j{margin-bottom:var(--spacing-xs)}span.svelte-1gfkn6j:not(.has-info){margin-bottom:var(--spacing-lg)}span.svelte-1gfkn6j{display:inline-block;position:relative;z-index:var(--layer-4);border:solid var(--block-title-border-width) var(--block-title-border-color);border-radius:var(--block-title-radius);background:var(--block-title-background-fill);padding:var(--block-title-padding);color:var(--block-title-text-color);font-weight:var(--block-title-text-weight);font-size:var(--block-title-text-size);line-height:var(--line-sm)}.hide.svelte-1gfkn6j{margin:0;height:0}div.svelte-1mwvhlq{display:inline-flex;align-items:center;z-index:var(--layer-2);box-shadow:var(--block-label-shadow);border:var(--block-label-border-width) solid var(--border-color-primary);border-top:none;border-left:none;border-radius:var(--block-label-radius);background:var(--block-label-background-fill);padding:var(--block-label-padding);pointer-events:none;color:var(--block-label-text-color);font-weight:var(--block-label-text-weight);font-size:var(--block-label-text-size);line-height:var(--line-sm)}.gr-group div.svelte-1mwvhlq{border-top-left-radius:0}div.float.svelte-1mwvhlq{position:absolute;top:var(--block-label-margin);left:var(--block-label-margin)}div.svelte-1mwvhlq:not(.float){position:static;margin-top:var(--block-label-margin);margin-left:var(--block-label-margin)}.hide.svelte-1mwvhlq{height:0}span.svelte-1mwvhlq{opacity:.8;margin-right:var(--size-2);width:calc(var(--block-label-text-size) - 1px);height:calc(var(--block-label-text-size) - 1px)}.hide-label.svelte-1mwvhlq{box-shadow:none;border-width:0;background:transparent;overflow:visible}button.svelte-1030q2h{display:flex;justify-content:center;align-items:center;gap:1px;z-index:var(--layer-1);box-shadow:var(--shadow-drop);border:1px solid var(--button-secondary-border-color);border-radius:var(--radius-sm);background:var(--background-fill-primary);padding:2px;color:var(--block-label-text-color)}button.svelte-1030q2h:hover{cursor:pointer;border:2px solid var(--button-secondary-border-color-hover);padding:1px;color:var(--block-label-text-color)}span.svelte-1030q2h{padding:0 1px;font-size:10px}div.svelte-1030q2h{padding:2px;width:14px;height:14px}.pending.svelte-1030q2h{animation:svelte-1030q2h-flash .5s infinite}@keyframes svelte-1030q2h-flash{0%{opacity:.5}50%{opacity:1}to{opacity:.5}}.empty.svelte-lk9eg8{display:flex;justify-content:center;align-items:center;margin-top:calc(0px - var(--size-6));height:var(--size-full)}.icon.svelte-lk9eg8{opacity:.5;height:var(--size-5);color:var(--body-text-color)}.small.svelte-lk9eg8{min-height:calc(var(--size-32) - 20px)}.large.svelte-lk9eg8{min-height:calc(var(--size-64) - 20px)}.unpadded_box.svelte-lk9eg8{margin-top:0}.small_parent.svelte-lk9eg8{min-height:100%!important}.dropdown-arrow.svelte-p5edak{fill:var(--body-text-color);margin-right:var(--size-2);width:var(--size-5)}button.svelte-1e89no8{display:inline-flex;justify-content:center;align-items:center;transition:var(--button-transition);box-shadow:var(--button-shadow);padding:var(--size-0-5) var(--size-2);text-align:center}button.svelte-1e89no8:hover,button[disabled].svelte-1e89no8{box-shadow:var(--button-shadow-hover)}button.svelte-1e89no8:active{box-shadow:var(--button-shadow-active)}button[disabled].svelte-1e89no8{opacity:.5;filter:grayscale(30%);cursor:not-allowed}.hidden.svelte-1e89no8{display:none}.primary.svelte-1e89no8{border:var(--button-border-width) solid var(--button-primary-border-color);background:var(--button-primary-background-fill);color:var(--button-primary-text-color)}.primary.svelte-1e89no8:hover,.primary[disabled].svelte-1e89no8{border-color:var(--button-primary-border-color-hover);background:var(--button-primary-background-fill-hover);color:var(--button-primary-text-color-hover)}.secondary.svelte-1e89no8{border:var(--button-border-width) solid var(--button-secondary-border-color);background:var(--button-secondary-background-fill);color:var(--button-secondary-text-color)}.secondary.svelte-1e89no8:hover,.secondary[disabled].svelte-1e89no8{border-color:var(--button-secondary-border-color-hover);background:var(--button-secondary-background-fill-hover);color:var(--button-secondary-text-color-hover)}.stop.svelte-1e89no8{border:var(--button-border-width) solid var(--button-cancel-border-color);background:var(--button-cancel-background-fill);color:var(--button-cancel-text-color)}.stop.svelte-1e89no8:hover,.stop[disabled].svelte-1e89no8{border-color:var(--button-cancel-border-color-hover);background:var(--button-cancel-background-fill-hover);color:var(--button-cancel-text-color-hover)}.sm.svelte-1e89no8{border-radius:var(--button-small-radius);padding:var(--button-small-padding);font-weight:var(--button-small-text-weight);font-size:var(--button-small-text-size)}.lg.svelte-1e89no8{border-radius:var(--button-large-radius);padding:var(--button-large-padding);font-weight:var(--button-large-text-weight);font-size:var(--button-large-text-size)}
diff --git a/spaces/DQChoi/gpt-demo/venv/lib/python3.11/site-packages/gradio/templates/frontend/assets/index-ae57ca19.js b/spaces/DQChoi/gpt-demo/venv/lib/python3.11/site-packages/gradio/templates/frontend/assets/index-ae57ca19.js
deleted file mode 100644
index 30c112e12695d0ee969a974e89b676b5aa8218ab..0000000000000000000000000000000000000000
--- a/spaces/DQChoi/gpt-demo/venv/lib/python3.11/site-packages/gradio/templates/frontend/assets/index-ae57ca19.js
+++ /dev/null
@@ -1,2 +0,0 @@
-import{P as j,N as G,c as E,D as U,e as w,T as b,I as H}from"./index-f90e1963.js";class P{constructor(t,e,s,i,h,r,n,a,l,f=0,u){this.p=t,this.stack=e,this.state=s,this.reducePos=i,this.pos=h,this.score=r,this.buffer=n,this.bufferBase=a,this.curContext=l,this.lookAhead=f,this.parent=u}toString(){return`[${this.stack.filter((t,e)=>e%3==0).concat(this.state)}]@${this.pos}${this.score?"!"+this.score:""}`}static start(t,e,s=0){let i=t.parser.context;return new P(t,[],e,s,s,0,[],0,i?new y(i,i.start):null,0,null)}get context(){return this.curContext?this.curContext.context:null}pushState(t,e){this.stack.push(this.state,e,this.bufferBase+this.buffer.length),this.state=t}reduce(t){var e;let s=t>>19,i=t&65535,{parser:h}=this.p,r=h.dynamicPrecedence(i);if(r&&(this.score+=r),s==0){this.pushState(h.getGoto(this.state,i,!0),this.reducePos),i=2e3&&!(!((e=this.p.parser.nodeSet.types[i])===null||e===void 0)&&e.isAnonymous)&&(a==this.p.lastBigReductionStart?(this.p.bigReductionCount++,this.p.lastBigReductionSize=l):this.p.lastBigReductionSizen;)this.stack.pop();this.reduceContext(i,a)}storeNode(t,e,s,i=4,h=!1){if(t==0&&(!this.stack.length||this.stack[this.stack.length-1]0&&r.buffer[n-4]==0&&r.buffer[n-1]>-1){if(e==s)return;if(r.buffer[n-2]>=e){r.buffer[n-2]=s;return}}}if(!h||this.pos==s)this.buffer.push(t,e,s,i);else{let r=this.buffer.length;if(r>0&&this.buffer[r-4]!=0)for(;r>0&&this.buffer[r-2]>s;)this.buffer[r]=this.buffer[r-4],this.buffer[r+1]=this.buffer[r-3],this.buffer[r+2]=this.buffer[r-2],this.buffer[r+3]=this.buffer[r-1],r-=4,i>4&&(i-=4);this.buffer[r]=t,this.buffer[r+1]=e,this.buffer[r+2]=s,this.buffer[r+3]=i}}shift(t,e,s){let i=this.pos;if(t&131072)this.pushState(t&65535,this.pos);else if(t&262144)this.pos=s,this.shiftContext(e,i),e<=this.p.parser.maxNode&&this.buffer.push(e,i,s,4);else{let h=t,{parser:r}=this.p;(s>this.pos||e<=r.maxNode)&&(this.pos=s,r.stateFlag(h,1)||(this.reducePos=s)),this.pushState(h,i),this.shiftContext(e,i),e<=r.maxNode&&this.buffer.push(e,i,s,4)}}apply(t,e,s){t&65536?this.reduce(t):this.shift(t,e,s)}useNode(t,e){let s=this.p.reused.length-1;(s<0||this.p.reused[s]!=t)&&(this.p.reused.push(t),s++);let i=this.pos;this.reducePos=this.pos=i+t.length,this.pushState(e,i),this.buffer.push(s,i,this.reducePos,-1),this.curContext&&this.updateContext(this.curContext.tracker.reuse(this.curContext.context,t,this,this.p.stream.reset(this.pos-t.length)))}split(){let t=this,e=t.buffer.length;for(;e>0&&t.buffer[e-2]>t.reducePos;)e-=4;let s=t.buffer.slice(e),i=t.bufferBase+e;for(;t&&i==t.bufferBase;)t=t.parent;return new P(this.p,this.stack.slice(),this.state,this.reducePos,this.pos,this.score,s,i,this.curContext,this.lookAhead,t)}recoverByDelete(t,e){let s=t<=this.p.parser.maxNode;s&&this.storeNode(t,this.pos,e,4),this.storeNode(0,this.pos,e,s?8:4),this.pos=this.reducePos=e,this.score-=190}canShift(t){for(let e=new W(this);;){let s=this.p.parser.stateSlot(e.state,4)||this.p.parser.hasAction(e.state,t);if(s==0)return!1;if(!(s&65536))return!0;e.reduce(s)}}recoverByInsert(t){if(this.stack.length>=300)return[];let e=this.p.parser.nextStates(this.state);if(e.length>4<<1||this.stack.length>=120){let i=[];for(let h=0,r;ha&1&&n==r)||i.push(e[h],r)}e=i}let s=[];for(let i=0;i>19,i=t&65535,h=this.stack.length-s*3;if(h<0||e.getGoto(this.stack[h],i,!1)<0)return!1;this.storeNode(0,this.reducePos,this.reducePos,4,!0),this.score-=100}return this.reducePos=this.pos,this.reduce(t),!0}forceAll(){for(;!this.p.parser.stateFlag(this.state,2);)if(!this.forceReduce()){this.storeNode(0,this.pos,this.pos,4,!0);break}return this}get deadEnd(){if(this.stack.length!=3)return!1;let{parser:t}=this.p;return t.data[t.stateSlot(this.state,1)]==65535&&!t.stateSlot(this.state,4)}restart(){this.state=this.stack[0],this.stack.length=0}sameState(t){if(this.state!=t.state||this.stack.length!=t.stack.length)return!1;for(let e=0;ethis.lookAhead&&(this.emitLookAhead(),this.lookAhead=t)}close(){this.curContext&&this.curContext.tracker.strict&&this.emitContext(),this.lookAhead>0&&this.emitLookAhead()}}class y{constructor(t,e){this.tracker=t,this.context=e,this.hash=t.strict?t.hash(e):0}}var N;(function(o){o[o.Insert=200]="Insert",o[o.Delete=190]="Delete",o[o.Reduce=100]="Reduce",o[o.MaxNext=4]="MaxNext",o[o.MaxInsertStackDepth=300]="MaxInsertStackDepth",o[o.DampenInsertStackDepth=120]="DampenInsertStackDepth",o[o.MinBigReduction=2e3]="MinBigReduction"})(N||(N={}));class W{constructor(t){this.start=t,this.state=t.state,this.stack=t.stack,this.base=this.stack.length}reduce(t){let e=t&65535,s=t>>19;s==0?(this.stack==this.start.stack&&(this.stack=this.stack.slice()),this.stack.push(this.state,0,0),this.base+=3):this.base-=(s-1)*3;let i=this.start.p.parser.getGoto(this.stack[this.base-3],e,!0);this.state=i}}class C{constructor(t,e,s){this.stack=t,this.pos=e,this.index=s,this.buffer=t.buffer,this.index==0&&this.maybeNext()}static create(t,e=t.bufferBase+t.buffer.length){return new C(t,e,e-t.bufferBase)}maybeNext(){let t=this.stack.parent;t!=null&&(this.index=this.stack.bufferBase-t.bufferBase,this.stack=t,this.buffer=t.buffer)}get id(){return this.buffer[this.index-4]}get start(){return this.buffer[this.index-3]}get end(){return this.buffer[this.index-2]}get size(){return this.buffer[this.index-1]}next(){this.index-=4,this.pos-=4,this.index==0&&this.maybeNext()}fork(){return new C(this.stack,this.pos,this.index)}}function x(o,t=Uint16Array){if(typeof o!="string")return o;let e=null;for(let s=0,i=0;s=92&&r--,r>=34&&r--;let a=r-32;if(a>=46&&(a-=46,n=!0),h+=a,n)break;h*=46}e?e[i++]=h:e=new t(h)}return e}class S{constructor(){this.start=-1,this.value=-1,this.end=-1,this.extended=-1,this.lookAhead=0,this.mask=0,this.context=0}}const D=new S;class q{constructor(t,e){this.input=t,this.ranges=e,this.chunk="",this.chunkOff=0,this.chunk2="",this.chunk2Pos=0,this.next=-1,this.token=D,this.rangeIndex=0,this.pos=this.chunkPos=e[0].from,this.range=e[0],this.end=e[e.length-1].to,this.readNext()}resolveOffset(t,e){let s=this.range,i=this.rangeIndex,h=this.pos+t;for(;hs.to:h>=s.to;){if(i==this.ranges.length-1)return null;let r=this.ranges[++i];h+=r.from-s.to,s=r}return h}clipPos(t){if(t>=this.range.from&&tt)return Math.max(t,e.from);return this.end}peek(t){let e=this.chunkOff+t,s,i;if(e>=0&&e=this.chunk2Pos&&sn.to&&(this.chunk2=this.chunk2.slice(0,n.to-s)),i=this.chunk2.charCodeAt(0)}}return s>=this.token.lookAhead&&(this.token.lookAhead=s+1),i}acceptToken(t,e=0){let s=e?this.resolveOffset(e,-1):this.pos;if(s==null||s=this.chunk2Pos&&this.posthis.range.to?t.slice(0,this.range.to-this.pos):t,this.chunkPos=this.pos,this.chunkOff=0}}readNext(){return this.chunkOff>=this.chunk.length&&(this.getChunk(),this.chunkOff==this.chunk.length)?this.next=-1:this.next=this.chunk.charCodeAt(this.chunkOff)}advance(t=1){for(this.chunkOff+=t;this.pos+t>=this.range.to;){if(this.rangeIndex==this.ranges.length-1)return this.setDone();t-=this.range.to-this.pos,this.range=this.ranges[++this.rangeIndex],this.pos=this.range.from}return this.pos+=t,this.pos>=this.token.lookAhead&&(this.token.lookAhead=this.pos+1),this.readNext()}setDone(){return this.pos=this.chunkPos=this.end,this.range=this.ranges[this.rangeIndex=this.ranges.length-1],this.chunk="",this.next=-1}reset(t,e){if(e?(this.token=e,e.start=t,e.lookAhead=t+1,e.value=e.extended=-1):this.token=D,this.pos!=t){if(this.pos=t,t==this.end)return this.setDone(),this;for(;t=this.range.to;)this.range=this.ranges[++this.rangeIndex];t>=this.chunkPos&&t=this.chunkPos&&e<=this.chunkPos+this.chunk.length)return this.chunk.slice(t-this.chunkPos,e-this.chunkPos);if(t>=this.chunk2Pos&&e<=this.chunk2Pos+this.chunk2.length)return this.chunk2.slice(t-this.chunk2Pos,e-this.chunk2Pos);if(t>=this.range.from&&e<=this.range.to)return this.input.read(t,e);let s="";for(let i of this.ranges){if(i.from>=e)break;i.to>t&&(s+=this.input.read(Math.max(i.from,t),Math.min(i.to,e)))}return s}}class m{constructor(t,e){this.data=t,this.id=e}token(t,e){let{parser:s}=e.p;F(this.data,t,e,this.id,s.data,s.tokenPrecTable)}}m.prototype.contextual=m.prototype.fallback=m.prototype.extend=!1;class J{constructor(t,e,s){this.precTable=e,this.elseToken=s,this.data=typeof t=="string"?x(t):t}token(t,e){let s=t.pos,i;for(;i=t.pos,F(this.data,t,e,0,this.data,this.precTable),!(t.token.value>-1);){if(this.elseToken==null)return;if(t.next<0)break;t.advance(),t.reset(i+1,t.token)}i>s&&(t.reset(s,t.token),t.acceptToken(this.elseToken,i-s))}}J.prototype.contextual=m.prototype.fallback=m.prototype.extend=!1;class tt{constructor(t,e={}){this.token=t,this.contextual=!!e.contextual,this.fallback=!!e.fallback,this.extend=!!e.extend}}function F(o,t,e,s,i,h){let r=0,n=1<0){let d=o[p];if(a.allows(d)&&(t.token.value==-1||t.token.value==d||K(d,t.token.value,i,h))){t.acceptToken(d);break}}let f=t.next,u=0,c=o[r+2];if(t.next<0&&c>u&&o[l+c*3-3]==65535&&o[l+c*3-3]==65535){r=o[l+c*3-1];continue t}for(;u>1,d=l+p+(p<<1),L=o[d],$=o[d+1]||65536;if(f=$)u=p+1;else{r=o[d+2],t.advance();continue t}}break}}function I(o,t,e){for(let s=t,i;(i=o[s])!=65535;s++)if(i==e)return s-t;return-1}function K(o,t,e,s){let i=I(e,s,t);return i<0||I(e,s,o)t)&&!s.type.isError)return e<0?Math.max(0,Math.min(s.to-1,t-25)):Math.min(o.length,Math.max(s.from+1,t+25));if(e<0?s.prevSibling():s.nextSibling())break;if(!s.parent())return e<0?0:o.length}}class Q{constructor(t,e){this.fragments=t,this.nodeSet=e,this.i=0,this.fragment=null,this.safeFrom=-1,this.safeTo=-1,this.trees=[],this.start=[],this.index=[],this.nextFragment()}nextFragment(){let t=this.fragment=this.i==this.fragments.length?null:this.fragments[this.i++];if(t){for(this.safeFrom=t.openStart?B(t.tree,t.from+t.offset,1)-t.offset:t.from,this.safeTo=t.openEnd?B(t.tree,t.to+t.offset,-1)-t.offset:t.to;this.trees.length;)this.trees.pop(),this.start.pop(),this.index.pop();this.trees.push(t.tree),this.start.push(-t.offset),this.index.push(0),this.nextStart=this.safeFrom}else this.nextStart=1e9}nodeAt(t){if(tt)return this.nextStart=r,null;if(h instanceof b){if(r==t){if(r=Math.max(this.safeFrom,t)&&(this.trees.push(h),this.start.push(r),this.index.push(0))}else this.index[e]++,this.nextStart=r+h.length}}}class V{constructor(t,e){this.stream=e,this.tokens=[],this.mainToken=null,this.actions=[],this.tokens=t.tokenizers.map(s=>new S)}getActions(t){let e=0,s=null,{parser:i}=t.p,{tokenizers:h}=i,r=i.stateSlot(t.state,3),n=t.curContext?t.curContext.hash:0,a=0;for(let l=0;lu.end+25&&(a=Math.max(u.lookAhead,a)),u.value!=0)){let c=e;if(u.extended>-1&&(e=this.addActions(t,u.extended,u.end,e)),e=this.addActions(t,u.value,u.end,e),!f.extend&&(s=u,e>c))break}}for(;this.actions.length>e;)this.actions.pop();return a&&t.setLookAhead(a),!s&&t.pos==this.stream.end&&(s=new S,s.value=t.p.parser.eofTerm,s.start=s.end=t.pos,e=this.addActions(t,s.value,s.end,e)),this.mainToken=s,this.actions}getMainToken(t){if(this.mainToken)return this.mainToken;let e=new S,{pos:s,p:i}=t;return e.start=s,e.end=Math.min(s+1,i.stream.end),e.value=s==i.stream.end?i.parser.eofTerm:0,e}updateCachedToken(t,e,s){let i=this.stream.clipPos(s.pos);if(e.token(this.stream.reset(i,t),s),t.value>-1){let{parser:h}=s.p;for(let r=0;r=0&&s.p.parser.dialect.allows(n>>1)){n&1?t.extended=n>>1:t.value=n>>1;break}}}else t.value=0,t.end=this.stream.clipPos(i+1)}putAction(t,e,s,i){for(let h=0;ht.bufferLength*4?new Q(s,t.nodeSet):null}get parsedPos(){return this.minStackPos}advance(){let t=this.stacks,e=this.minStackPos,s=this.stacks=[],i,h;if(this.bigReductionCount>300&&t.length==1){let[r]=t;for(;r.forceReduce()&&r.stack.length&&r.stack[r.stack.length-2]>=this.lastBigReductionStart;);this.bigReductionCount=this.lastBigReductionSize=0}for(let r=0;re)s.push(n);else{if(this.advanceStack(n,s,t))continue;{i||(i=[],h=[]),i.push(n);let a=this.tokens.getMainToken(n);h.push(a.value,a.end)}}break}}if(!s.length){let r=i&&Z(i);if(r)return this.stackToTree(r);if(this.parser.strict)throw g&&i&&console.log("Stuck with token "+(this.tokens.mainToken?this.parser.getName(this.tokens.mainToken.value):"none")),new SyntaxError("No parse at "+e);this.recovering||(this.recovering=5)}if(this.recovering&&i){let r=this.stoppedAt!=null&&i[0].pos>this.stoppedAt?i[0]:this.runRecovery(i,h,s);if(r)return this.stackToTree(r.forceAll())}if(this.recovering){let r=this.recovering==1?1:this.recovering*3;if(s.length>r)for(s.sort((n,a)=>a.score-n.score);s.length>r;)s.pop();s.some(n=>n.reducePos>e)&&this.recovering--}else if(s.length>1){t:for(let r=0;r500&&l.buffer.length>500)if((n.score-l.score||n.buffer.length-l.buffer.length)>0)s.splice(a--,1);else{s.splice(r--,1);continue t}}}s.length>12&&s.splice(12,s.length-12)}this.minStackPos=s[0].pos;for(let r=1;r ":"";if(this.stoppedAt!=null&&i>this.stoppedAt)return t.forceReduce()?t:null;if(this.fragments){let l=t.curContext&&t.curContext.tracker.strict,f=l?t.curContext.hash:0;for(let u=this.fragments.nodeAt(i);u;){let c=this.parser.nodeSet.types[u.type.id]==u.type?h.getGoto(t.state,u.type.id):-1;if(c>-1&&u.length&&(!l||(u.prop(w.contextHash)||0)==f))return t.useNode(u,c),g&&console.log(r+this.stackID(t)+` (via reuse of ${h.getName(u.type.id)})`),!0;if(!(u instanceof b)||u.children.length==0||u.positions[0]>0)break;let p=u.children[0];if(p instanceof b&&u.positions[0]==0)u=p;else break}}let n=h.stateSlot(t.state,4);if(n>0)return t.reduce(n),g&&console.log(r+this.stackID(t)+` (via always-reduce ${h.getName(n&65535)})`),!0;if(t.stack.length>=15e3)for(;t.stack.length>9e3&&t.forceReduce(););let a=this.tokens.getActions(t);for(let l=0;li?e.push(d):s.push(d)}return!1}advanceFully(t,e){let s=t.pos;for(;;){if(!this.advanceStack(t,null,null))return!1;if(t.pos>s)return R(t,e),!0}}runRecovery(t,e,s){let i=null,h=!1;for(let r=0;r ":"";if(n.deadEnd&&(h||(h=!0,n.restart(),g&&console.log(f+this.stackID(n)+" (restarted)"),this.advanceFully(n,s))))continue;let u=n.split(),c=f;for(let p=0;u.forceReduce()&&p<10&&(g&&console.log(c+this.stackID(u)+" (via force-reduce)"),!this.advanceFully(u,s));p++)g&&(c=this.stackID(u)+" -> ");for(let p of n.recoverByInsert(a))g&&console.log(f+this.stackID(p)+" (via recover-insert)"),this.advanceFully(p,s);this.stream.end>n.pos?(l==n.pos&&(l++,a=0),n.recoverByDelete(a,l),g&&console.log(f+this.stackID(n)+` (via recover-delete ${this.parser.getName(a)})`),R(n,s)):(!i||i.scoreo;class et{constructor(t){this.start=t.start,this.shift=t.shift||T,this.reduce=t.reduce||T,this.reuse=t.reuse||T,this.hash=t.hash||(()=>0),this.strict=t.strict!==!1}}class v extends j{constructor(t){if(super(),this.wrappers=[],t.version!=14)throw new RangeError(`Parser version (${t.version}) doesn't match runtime version (14)`);let e=t.nodeNames.split(" ");this.minRepeatTerm=e.length;for(let n=0;nt.topRules[n][1]),i=[];for(let n=0;n=0)h(f,a,n[l++]);else{let u=n[l+-f];for(let c=-f;c>0;c--)h(n[l++],a,u);l++}}}this.nodeSet=new G(e.map((n,a)=>E.define({name:a>=this.minRepeatTerm?void 0:n,id:a,props:i[a],top:s.indexOf(a)>-1,error:a==0,skipped:t.skippedNodes&&t.skippedNodes.indexOf(a)>-1}))),t.propSources&&(this.nodeSet=this.nodeSet.extend(...t.propSources)),this.strict=!1,this.bufferLength=U;let r=x(t.tokenData);this.context=t.context,this.specializerSpecs=t.specialized||[],this.specialized=new Uint16Array(this.specializerSpecs.length);for(let n=0;ntypeof n=="number"?new m(r,n):n),this.topRules=t.topRules,this.dialects=t.dialects||{},this.dynamicPrecedences=t.dynamicPrecedences||null,this.tokenPrecTable=t.tokenPrec,this.termNames=t.termNames||null,this.maxNode=this.nodeSet.types.length-1,this.dialect=this.parseDialect(),this.top=this.topRules[Object.keys(this.topRules)[0]]}createParse(t,e,s){let i=new X(this,t,e,s);for(let h of this.wrappers)i=h(i,t,e,s);return i}getGoto(t,e,s=!1){let i=this.goto;if(e>=i[0])return-1;for(let h=i[e+1];;){let r=i[h++],n=r&1,a=i[h++];if(n&&s)return a;for(let l=h+(r>>1);h0}validAction(t,e){if(e==this.stateSlot(t,4))return!0;for(let s=this.stateSlot(t,1);;s+=3){if(this.data[s]==65535)if(this.data[s+1]==1)s=k(this.data,s+2);else return!1;if(e==k(this.data,s+1))return!0}}nextStates(t){let e=[];for(let s=this.stateSlot(t,1);;s+=3){if(this.data[s]==65535)if(this.data[s+1]==1)s=k(this.data,s+2);else break;if(!(this.data[s+2]&1)){let i=this.data[s+1];e.some((h,r)=>r&1&&h==i)||e.push(this.data[s],i)}}return e}configure(t){let e=Object.assign(Object.create(v.prototype),this);if(t.props&&(e.nodeSet=this.nodeSet.extend(...t.props)),t.top){let s=this.topRules[t.top];if(!s)throw new RangeError(`Invalid top rule name ${t.top}`);e.top=s}return t.tokenizers&&(e.tokenizers=this.tokenizers.map(s=>{let i=t.tokenizers.find(h=>h.from==s);return i?i.to:s})),t.specializers&&(e.specializers=this.specializers.slice(),e.specializerSpecs=this.specializerSpecs.map((s,i)=>{let h=t.specializers.find(n=>n.from==s.external);if(!h)return s;let r=Object.assign(Object.assign({},s),{external:h.to});return e.specializers[i]=O(r),r})),t.contextTracker&&(e.context=t.contextTracker),t.dialect&&(e.dialect=this.parseDialect(t.dialect)),t.strict!=null&&(e.strict=t.strict),t.wrap&&(e.wrappers=e.wrappers.concat(t.wrap)),t.bufferLength!=null&&(e.bufferLength=t.bufferLength),e}hasWrappers(){return this.wrappers.length>0}getName(t){return this.termNames?this.termNames[t]:String(t<=this.maxNode&&this.nodeSet.types[t].name||t)}get eofTerm(){return this.maxNode+1}get topNode(){return this.nodeSet.types[this.top[1]]}dynamicPrecedence(t){let e=this.dynamicPrecedences;return e==null?0:e[t]||0}parseDialect(t){let e=Object.keys(this.dialects),s=e.map(()=>!1);if(t)for(let h of t.split(" ")){let r=e.indexOf(h);r>=0&&(s[r]=!0)}let i=null;for(let h=0;hs)&&e.p.parser.stateFlag(e.state,2)&&(!t||t.scoreo.external(e,s)<<1|t}return o.get}export{et as C,tt as E,v as L,J as a};
-//# sourceMappingURL=index-ae57ca19.js.map
diff --git a/spaces/DaleChen/AutoGPT/tests/test_image_gen.py b/spaces/DaleChen/AutoGPT/tests/test_image_gen.py
deleted file mode 100644
index 19c57e427d5c1b84aa7f72925733d0056ddf5268..0000000000000000000000000000000000000000
--- a/spaces/DaleChen/AutoGPT/tests/test_image_gen.py
+++ /dev/null
@@ -1,102 +0,0 @@
-import hashlib
-import os
-import unittest
-
-from PIL import Image
-
-from autogpt.commands.image_gen import generate_image, generate_image_with_sd_webui
-from autogpt.config import Config
-from autogpt.workspace import path_in_workspace
-
-
-def lst(txt):
- return txt.split(":")[1].strip()
-
-
-@unittest.skipIf(os.getenv("CI"), "Skipping image generation tests")
-class TestImageGen(unittest.TestCase):
- def setUp(self):
- self.config = Config()
-
- def test_dalle(self):
- self.config.image_provider = "dalle"
-
- # Test using size 256
- result = lst(generate_image("astronaut riding a horse", 256))
- image_path = path_in_workspace(result)
- self.assertTrue(image_path.exists())
- with Image.open(image_path) as img:
- self.assertEqual(img.size, (256, 256))
- image_path.unlink()
-
- # Test using size 512
- result = lst(generate_image("astronaut riding a horse", 512))
- image_path = path_in_workspace(result)
- with Image.open(image_path) as img:
- self.assertEqual(img.size, (512, 512))
- image_path.unlink()
-
- def test_huggingface(self):
- self.config.image_provider = "huggingface"
-
- # Test usin SD 1.4 model and size 512
- self.config.huggingface_image_model = "CompVis/stable-diffusion-v1-4"
- result = lst(generate_image("astronaut riding a horse", 512))
- image_path = path_in_workspace(result)
- self.assertTrue(image_path.exists())
- with Image.open(image_path) as img:
- self.assertEqual(img.size, (512, 512))
- image_path.unlink()
-
- # Test using SD 2.1 768 model and size 768
- self.config.huggingface_image_model = "stabilityai/stable-diffusion-2-1"
- result = lst(generate_image("astronaut riding a horse", 768))
- image_path = path_in_workspace(result)
- with Image.open(image_path) as img:
- self.assertEqual(img.size, (768, 768))
- image_path.unlink()
-
- def test_sd_webui(self):
- self.config.image_provider = "sd_webui"
- return
-
- # Test using size 128
- result = lst(generate_image_with_sd_webui("astronaut riding a horse", 128))
- image_path = path_in_workspace(result)
- self.assertTrue(image_path.exists())
- with Image.open(image_path) as img:
- self.assertEqual(img.size, (128, 128))
- image_path.unlink()
-
- # Test using size 64 and negative prompt
- result = lst(
- generate_image_with_sd_webui(
- "astronaut riding a horse",
- negative_prompt="horse",
- size=64,
- extra={"seed": 123},
- )
- )
- image_path = path_in_workspace(result)
- with Image.open(image_path) as img:
- self.assertEqual(img.size, (64, 64))
- neg_image_hash = hashlib.md5(img.tobytes()).hexdigest()
- image_path.unlink()
-
- # Same test as above but without the negative prompt
- result = lst(
- generate_image_with_sd_webui(
- "astronaut riding a horse", image_size=64, size=1, extra={"seed": 123}
- )
- )
- image_path = path_in_workspace(result)
- with Image.open(image_path) as img:
- self.assertEqual(img.size, (64, 64))
- image_hash = hashlib.md5(img.tobytes()).hexdigest()
- image_path.unlink()
-
- self.assertNotEqual(image_hash, neg_image_hash)
-
-
-if __name__ == "__main__":
- unittest.main()
diff --git a/spaces/Danielzero/GPT3.5/assets/custom.js b/spaces/Danielzero/GPT3.5/assets/custom.js
deleted file mode 100644
index b8071034f3618c541e3f4169c7fc6d6593d56f44..0000000000000000000000000000000000000000
--- a/spaces/Danielzero/GPT3.5/assets/custom.js
+++ /dev/null
@@ -1,224 +0,0 @@
-
-// custom javascript here
-
-const MAX_HISTORY_LENGTH = 32;
-
-var key_down_history = [];
-var currentIndex = -1;
-var user_input_ta;
-
-var gradioContainer = null;
-var user_input_ta = null;
-var user_input_tb = null;
-var userInfoDiv = null;
-var appTitleDiv = null;
-var chatbot = null;
-var apSwitch = null;
-
-var ga = document.getElementsByTagName("gradio-app");
-var targetNode = ga[0];
-var isInIframe = (window.self !== window.top);
-
-// gradio 页面加载好了么??? 我能动你的元素了么??
-function gradioLoaded(mutations) {
- for (var i = 0; i < mutations.length; i++) {
- if (mutations[i].addedNodes.length) {
- gradioContainer = document.querySelector(".gradio-container");
- user_input_tb = document.getElementById('user_input_tb');
- userInfoDiv = document.getElementById("user_info");
- appTitleDiv = document.getElementById("app_title");
- chatbot = document.querySelector('#chuanhu_chatbot');
- apSwitch = document.querySelector('.apSwitch input[type="checkbox"]');
-
- if (gradioContainer && apSwitch) { // gradioCainter 加载出来了没?
- adjustDarkMode();
- }
- if (user_input_tb) { // user_input_tb 加载出来了没?
- selectHistory();
- }
- if (userInfoDiv && appTitleDiv) { // userInfoDiv 和 appTitleDiv 加载出来了没?
- setTimeout(showOrHideUserInfo(), 2000);
- }
- if (chatbot) { // chatbot 加载出来了没?
- setChatbotHeight()
- }
- }
- }
-}
-
-function selectHistory() {
- user_input_ta = user_input_tb.querySelector("textarea");
- if (user_input_ta) {
- observer.disconnect(); // 停止监听
- // 在 textarea 上监听 keydown 事件
- user_input_ta.addEventListener("keydown", function (event) {
- var value = user_input_ta.value.trim();
- // 判断按下的是否为方向键
- if (event.code === 'ArrowUp' || event.code === 'ArrowDown') {
- // 如果按下的是方向键,且输入框中有内容,且历史记录中没有该内容,则不执行操作
- if (value && key_down_history.indexOf(value) === -1)
- return;
- // 对于需要响应的动作,阻止默认行为。
- event.preventDefault();
- var length = key_down_history.length;
- if (length === 0) {
- currentIndex = -1; // 如果历史记录为空,直接将当前选中的记录重置
- return;
- }
- if (currentIndex === -1) {
- currentIndex = length;
- }
- if (event.code === 'ArrowUp' && currentIndex > 0) {
- currentIndex--;
- user_input_ta.value = key_down_history[currentIndex];
- } else if (event.code === 'ArrowDown' && currentIndex < length - 1) {
- currentIndex++;
- user_input_ta.value = key_down_history[currentIndex];
- }
- user_input_ta.selectionStart = user_input_ta.value.length;
- user_input_ta.selectionEnd = user_input_ta.value.length;
- const input_event = new InputEvent("input", { bubbles: true, cancelable: true });
- user_input_ta.dispatchEvent(input_event);
- } else if (event.code === "Enter") {
- if (value) {
- currentIndex = -1;
- if (key_down_history.indexOf(value) === -1) {
- key_down_history.push(value);
- if (key_down_history.length > MAX_HISTORY_LENGTH) {
- key_down_history.shift();
- }
- }
- }
- }
- });
- }
-}
-
-function toggleUserInfoVisibility(shouldHide) {
- if (userInfoDiv) {
- if (shouldHide) {
- userInfoDiv.classList.add("hideK");
- } else {
- userInfoDiv.classList.remove("hideK");
- }
- }
-}
-function showOrHideUserInfo() {
- var sendBtn = document.getElementById("submit_btn");
-
- // Bind mouse/touch events to show/hide user info
- appTitleDiv.addEventListener("mouseenter", function () {
- toggleUserInfoVisibility(false);
- });
- userInfoDiv.addEventListener("mouseenter", function () {
- toggleUserInfoVisibility(false);
- });
- sendBtn.addEventListener("mouseenter", function () {
- toggleUserInfoVisibility(false);
- });
-
- appTitleDiv.addEventListener("mouseleave", function () {
- toggleUserInfoVisibility(true);
- });
- userInfoDiv.addEventListener("mouseleave", function () {
- toggleUserInfoVisibility(true);
- });
- sendBtn.addEventListener("mouseleave", function () {
- toggleUserInfoVisibility(true);
- });
-
- appTitleDiv.ontouchstart = function () {
- toggleUserInfoVisibility(false);
- };
- userInfoDiv.ontouchstart = function () {
- toggleUserInfoVisibility(false);
- };
- sendBtn.ontouchstart = function () {
- toggleUserInfoVisibility(false);
- };
-
- appTitleDiv.ontouchend = function () {
- setTimeout(function () {
- toggleUserInfoVisibility(true);
- }, 3000);
- };
- userInfoDiv.ontouchend = function () {
- setTimeout(function () {
- toggleUserInfoVisibility(true);
- }, 3000);
- };
- sendBtn.ontouchend = function () {
- setTimeout(function () {
- toggleUserInfoVisibility(true);
- }, 3000); // Delay 1 second to hide user info
- };
-
- // Hide user info after 2 second
- setTimeout(function () {
- toggleUserInfoVisibility(true);
- }, 2000);
-}
-
-function toggleDarkMode(isEnabled) {
- if (isEnabled) {
- gradioContainer.classList.add("dark");
- document.body.style.setProperty("background-color", "var(--neutral-950)", "important");
- } else {
- gradioContainer.classList.remove("dark");
- document.body.style.backgroundColor = "";
- }
-}
-function adjustDarkMode() {
- const darkModeQuery = window.matchMedia("(prefers-color-scheme: dark)");
-
- // 根据当前颜色模式设置初始状态
- apSwitch.checked = darkModeQuery.matches;
- toggleDarkMode(darkModeQuery.matches);
- // 监听颜色模式变化
- darkModeQuery.addEventListener("change", (e) => {
- apSwitch.checked = e.matches;
- toggleDarkMode(e.matches);
- });
- // apSwitch = document.querySelector('.apSwitch input[type="checkbox"]');
- apSwitch.addEventListener("change", (e) => {
- toggleDarkMode(e.target.checked);
- });
-}
-
-function setChatbotHeight() {
- const screenWidth = window.innerWidth;
- const statusDisplay = document.querySelector('#status_display');
- const statusDisplayHeight = statusDisplay ? statusDisplay.offsetHeight : 0;
- const wrap = chatbot.querySelector('.wrap');
- const vh = window.innerHeight * 0.01;
- document.documentElement.style.setProperty('--vh', `${vh}px`);
- if (isInIframe) {
- chatbot.style.height = `700px`;
- wrap.style.maxHeight = `calc(700px - var(--line-sm) * 1rem - 2 * var(--block-label-margin))`
- } else {
- if (screenWidth <= 320) {
- chatbot.style.height = `calc(var(--vh, 1vh) * 100 - ${statusDisplayHeight + 150}px)`;
- wrap.style.maxHeight = `calc(var(--vh, 1vh) * 100 - ${statusDisplayHeight + 150}px - var(--line-sm) * 1rem - 2 * var(--block-label-margin))`;
- } else if (screenWidth <= 499) {
- chatbot.style.height = `calc(var(--vh, 1vh) * 100 - ${statusDisplayHeight + 100}px)`;
- wrap.style.maxHeight = `calc(var(--vh, 1vh) * 100 - ${statusDisplayHeight + 100}px - var(--line-sm) * 1rem - 2 * var(--block-label-margin))`;
- } else {
- chatbot.style.height = `calc(var(--vh, 1vh) * 100 - ${statusDisplayHeight + 160}px)`;
- wrap.style.maxHeight = `calc(var(--vh, 1vh) * 100 - ${statusDisplayHeight + 160}px - var(--line-sm) * 1rem - 2 * var(--block-label-margin))`;
- }
- }
-}
-
-// 监视页面内部 DOM 变动
-var observer = new MutationObserver(function (mutations) {
- gradioLoaded(mutations);
-});
-observer.observe(targetNode, { childList: true, subtree: true });
-
-// 监视页面变化
-window.addEventListener("DOMContentLoaded", function () {
- isInIframe = (window.self !== window.top);
-});
-window.addEventListener('resize', setChatbotHeight);
-window.addEventListener('scroll', setChatbotHeight);
-window.matchMedia("(prefers-color-scheme: dark)").addEventListener("change", adjustDarkMode);
\ No newline at end of file
diff --git a/spaces/Devaholic/fruit-demo/app.py b/spaces/Devaholic/fruit-demo/app.py
deleted file mode 100644
index 97f28f20d80cec14fc1c4940b9b89f7102de756a..0000000000000000000000000000000000000000
--- a/spaces/Devaholic/fruit-demo/app.py
+++ /dev/null
@@ -1,43 +0,0 @@
-from tensorflow.keras.models import load_model
-import numpy as np
-import gradio as gr
-from utils import remove_number
-
-model = load_model('main_model.h5')
-
-labels = ['Apple Braeburn', 'Apple Crimson Snow', 'Apple Golden 1', 'Apple Golden 2', 'Apple Golden 3', 'Apple Granny Smith', 'Apple Pink Lady', 'Apple Red 1', 'Apple Red 2', 'Apple Red 3', 'Apple Red Delicious', 'Apple Red Yellow 1', 'Apple Red Yellow 2', 'Apricot', 'Avocado', 'Avocado ripe', 'Banana', 'Banana Lady Finger', 'Banana Red', 'Beetroot', 'Blueberry', 'Cactus fruit', 'Cantaloupe 1', 'Cantaloupe 2', 'Carambula', 'Cauliflower', 'Cherry 1', 'Cherry 2', 'Cherry Rainier', 'Cherry Wax Black', 'Cherry Wax Red', 'Cherry Wax Yellow', 'Chestnut', 'Clementine', 'Cocos', 'Corn', 'Corn Husk', 'Cucumber Ripe', 'Cucumber Ripe 2', 'Dates', 'Eggplant', 'Fig', 'Ginger Root', 'Granadilla', 'Grape Blue', 'Grape Pink', 'Grape White', 'Grape White 2', 'Grape White 3', 'Grape White 4', 'Grapefruit Pink', 'Grapefruit White', 'Guava', 'Hazelnut', 'Huckleberry', 'Kaki', 'Kiwi', 'Kohlrabi', 'Kumquats', 'Lemon', 'Lemon Meyer', 'Limes', 'Lychee', 'Mandarine', 'Mango', 'Mango Red', 'Mangostan', 'Maracuja', 'Melon Piel de Sapo', 'Mulberry', 'Nectarine', 'Nectarine Flat', 'Nut Forest', 'Nut Pecan', 'Onion Red', 'Onion Red Peeled', 'Onion White', 'Orange', 'Papaya', 'Passion Fruit', 'Peach', 'Peach 2', 'Peach Flat', 'Pear', 'Pear 2', 'Pear Abate', 'Pear Forelle', 'Pear Kaiser', 'Pear Monster', 'Pear Red', 'Pear Stone', 'Pear Williams', 'Pepino', 'Pepper Green', 'Pepper Orange', 'Pepper Red', 'Pepper Yellow', 'Physalis', 'Physalis with Husk', 'Pineapple', 'Pineapple Mini', 'Pitahaya Red', 'Plum', 'Plum 2', 'Plum 3', 'Pomegranate', 'Pomelo Sweetie', 'Potato Red', 'Potato Red Washed', 'Potato Sweet', 'Potato White', 'Quince', 'Rambutan', 'Raspberry', 'Redcurrant', 'Salak', 'Strawberry', 'Strawberry Wedge', 'Tamarillo', 'Tangelo', 'Tomato 1', 'Tomato 2', 'Tomato 3', 'Tomato 4', 'Tomato Cherry Red', 'Tomato Heart', 'Tomato Maroon', 'Tomato not Ripened', 'Tomato Yellow', 'Walnut', 'Watermelon']
-
-def get_prediction(image: np.ndarray) -> str:
- """
- Get the prediction of the image
- """
- image = image.reshape(1, 299, 299, 3)
- image = image / 255.0
-
- prediction = model.predict(image)
- prediction = np.argmax(prediction)
-
- predicted_label = remove_number(labels[int(prediction)])
- return predicted_label
-
-def get_predicted_labels(image) -> dict:
- """
- Get the labels
- """
- image = image.reshape(1, 299, 299, 3)
- image = image / 255.0
-
- prediction = model.predict(image)
- prediction = np.ravel(prediction)
-
- confidences = {label: float(prob) for label, prob in zip(labels, list(prediction))}
-
- return confidences
-
-if __name__ == '__main__':
- app = gr.Interface(
- fn=get_predicted_labels,
- inputs=gr.Image(shape=(299, 299), image_mode='RGB', tool='select'),
- outputs=gr.outputs.Label(num_top_classes=5)
- )
- app.launch(share=True)
\ No newline at end of file
diff --git a/spaces/DhruvShek/chatlm/utils.py b/spaces/DhruvShek/chatlm/utils.py
deleted file mode 100644
index 6fde4a947858dabce091ae59322cf01417eeb5f1..0000000000000000000000000000000000000000
--- a/spaces/DhruvShek/chatlm/utils.py
+++ /dev/null
@@ -1,91 +0,0 @@
-import torch
-import torch.nn as nn
-from torch.utils.data import Dataset
-import torch.utils.data
-import json
-
-device = torch.device("cuda" if torch.cuda.is_available() else "cpu")
-
-class Dataset(Dataset):
-
- def __init__(self):
-
- self.pairs = json.load(open('pairs_encoded.json'))
- self.dataset_size = len(self.pairs)
-
- def __getitem__(self, i):
-
- question = torch.LongTensor(self.pairs[i][0])
- reply = torch.LongTensor(self.pairs[i][1])
-
- return question, reply
-
- def __len__(self):
- return self.dataset_size
-
-
-def create_masks(question, reply_input, reply_target):
-
- def subsequent_mask(size):
- mask = torch.triu(torch.ones(size, size)).transpose(0, 1).type(dtype=torch.uint8)
- return mask.unsqueeze(0)
-
- question_mask = (question!=0).to(device)
- question_mask = question_mask.unsqueeze(1).unsqueeze(1) # (batch_size, 1, 1, max_words)
-
- reply_input_mask = reply_input!=0
- reply_input_mask = reply_input_mask.unsqueeze(1) # (batch_size, 1, max_words)
- reply_input_mask = reply_input_mask & subsequent_mask(reply_input.size(-1)).type_as(reply_input_mask.data)
- reply_input_mask = reply_input_mask.unsqueeze(1) # (batch_size, 1, max_words, max_words)
- reply_target_mask = reply_target!=0 # (batch_size, max_words)
-
- return question_mask, reply_input_mask, reply_target_mask
-
-
-class AdamWarmup:
-
- def __init__(self, model_size, warmup_steps, optimizer):
-
- self.model_size = model_size
- self.warmup_steps = warmup_steps
- self.optimizer = optimizer
- self.current_step = 0
- self.lr = 0
-
- def get_lr(self):
- return self.model_size ** (-0.5) * min(self.current_step ** (-0.5), self.current_step * self.warmup_steps ** (-1.5))
-
- def step(self):
- # Increment the number of steps each time we call the step function
- self.current_step += 1
- lr = self.get_lr()
- for param_group in self.optimizer.param_groups:
- param_group['lr'] = lr
- # update the learning rate
- self.lr = lr
- self.optimizer.step()
-
-class LossWithLS(nn.Module):
-
- def __init__(self, size, smooth):
- super(LossWithLS, self).__init__()
- self.criterion = nn.KLDivLoss(size_average=False, reduce=False)
- self.confidence = 1.0 - smooth
- self.smooth = smooth
- self.size = size
-
- def forward(self, prediction, target, mask):
- """
- prediction of shape: (batch_size, max_words, vocab_size)
- target and mask of shape: (batch_size, max_words)
- """
- prediction = prediction.view(-1, prediction.size(-1)) # (batch_size * max_words, vocab_size)
- target = target.contiguous().view(-1) # (batch_size * max_words)
- mask = mask.float()
- mask = mask.view(-1) # (batch_size * max_words)
- labels = prediction.data.clone()
- labels.fill_(self.smooth / (self.size - 1))
- labels.scatter_(1, target.data.unsqueeze(1), self.confidence)
- loss = self.criterion(prediction, labels) # (batch_size * max_words, vocab_size)
- loss = (loss.sum(1) * mask).sum() / mask.sum()
- return loss
diff --git a/spaces/Dorado607/ChuanhuChatGPT/Dockerfile b/spaces/Dorado607/ChuanhuChatGPT/Dockerfile
deleted file mode 100644
index 85d5045d5316ac160277af1e7d60afa823c0f953..0000000000000000000000000000000000000000
--- a/spaces/Dorado607/ChuanhuChatGPT/Dockerfile
+++ /dev/null
@@ -1,18 +0,0 @@
-FROM python:3.9-slim-buster as builder
-RUN apt-get update \
- && apt-get install -y build-essential \
- && apt-get clean \
- && rm -rf /var/lib/apt/lists/*
-COPY requirements.txt .
-COPY requirements_advanced.txt .
-RUN pip install --user --no-cache-dir -r requirements.txt
-# RUN pip install --user --no-cache-dir -r requirements_advanced.txt
-
-FROM python:3.9-slim-buster
-LABEL maintainer="iskoldt"
-COPY --from=builder /root/.local /root/.local
-ENV PATH=/root/.local/bin:$PATH
-COPY . /app
-WORKDIR /app
-ENV dockerrun=yes
-CMD ["python3", "-u", "ChuanhuChatbot.py","2>&1", "|", "tee", "/var/log/application.log"]
diff --git a/spaces/Dorado607/ChuanhuChatGPT/modules/pdf_func.py b/spaces/Dorado607/ChuanhuChatGPT/modules/pdf_func.py
deleted file mode 100644
index 1b1087f2687fd26c8676867dd45189c069dd56a5..0000000000000000000000000000000000000000
--- a/spaces/Dorado607/ChuanhuChatGPT/modules/pdf_func.py
+++ /dev/null
@@ -1,180 +0,0 @@
-from types import SimpleNamespace
-import pdfplumber
-import logging
-from langchain.docstore.document import Document
-
-def prepare_table_config(crop_page):
- """Prepare table查找边界, 要求page为原始page
-
- From https://github.com/jsvine/pdfplumber/issues/242
- """
- page = crop_page.root_page # root/parent
- cs = page.curves + page.edges
- def curves_to_edges():
- """See https://github.com/jsvine/pdfplumber/issues/127"""
- edges = []
- for c in cs:
- edges += pdfplumber.utils.rect_to_edges(c)
- return edges
- edges = curves_to_edges()
- return {
- "vertical_strategy": "explicit",
- "horizontal_strategy": "explicit",
- "explicit_vertical_lines": edges,
- "explicit_horizontal_lines": edges,
- "intersection_y_tolerance": 10,
- }
-
-def get_text_outside_table(crop_page):
- ts = prepare_table_config(crop_page)
- if len(ts["explicit_vertical_lines"]) == 0 or len(ts["explicit_horizontal_lines"]) == 0:
- return crop_page
-
- ### Get the bounding boxes of the tables on the page.
- bboxes = [table.bbox for table in crop_page.root_page.find_tables(table_settings=ts)]
- def not_within_bboxes(obj):
- """Check if the object is in any of the table's bbox."""
- def obj_in_bbox(_bbox):
- """See https://github.com/jsvine/pdfplumber/blob/stable/pdfplumber/table.py#L404"""
- v_mid = (obj["top"] + obj["bottom"]) / 2
- h_mid = (obj["x0"] + obj["x1"]) / 2
- x0, top, x1, bottom = _bbox
- return (h_mid >= x0) and (h_mid < x1) and (v_mid >= top) and (v_mid < bottom)
- return not any(obj_in_bbox(__bbox) for __bbox in bboxes)
-
- return crop_page.filter(not_within_bboxes)
-# 请使用 LaTeX 表达公式,行内公式以 $ 包裹,行间公式以 $$ 包裹
-
-extract_words = lambda page: page.extract_words(keep_blank_chars=True, y_tolerance=0, x_tolerance=1, extra_attrs=["fontname", "size", "object_type"])
-# dict_keys(['text', 'x0', 'x1', 'top', 'doctop', 'bottom', 'upright', 'direction', 'fontname', 'size'])
-
-def get_title_with_cropped_page(first_page):
- title = [] # 处理标题
- x0,top,x1,bottom = first_page.bbox # 获取页面边框
-
- for word in extract_words(first_page):
- word = SimpleNamespace(**word)
-
- if word.size >= 14:
- title.append(word.text)
- title_bottom = word.bottom
- elif word.text == "Abstract": # 获取页面abstract
- top = word.top
-
- user_info = [i["text"] for i in extract_words(first_page.within_bbox((x0,title_bottom,x1,top)))]
- # 裁剪掉上半部分, within_bbox: full_included; crop: partial_included
- return title, user_info, first_page.within_bbox((x0,top,x1,bottom))
-
-def get_column_cropped_pages(pages, two_column=True):
- new_pages = []
- for page in pages:
- if two_column:
- left = page.within_bbox((0, 0, page.width/2, page.height),relative=True)
- right = page.within_bbox((page.width/2, 0, page.width, page.height), relative=True)
- new_pages.append(left)
- new_pages.append(right)
- else:
- new_pages.append(page)
-
- return new_pages
-
-def parse_pdf(filename, two_column = True):
- level = logging.getLogger().level
- if level == logging.getLevelName("DEBUG"):
- logging.getLogger().setLevel("INFO")
-
- with pdfplumber.open(filename) as pdf:
- title, user_info, first_page = get_title_with_cropped_page(pdf.pages[0])
- new_pages = get_column_cropped_pages([first_page] + pdf.pages[1:], two_column)
-
- chapters = []
- # tuple (chapter_name, [pageid] (start,stop), chapter_text)
- create_chapter = lambda page_start,name_top,name_bottom: SimpleNamespace(
- name=[],
- name_top=name_top,
- name_bottom=name_bottom,
- record_chapter_name = True,
-
- page_start=page_start,
- page_stop=None,
-
- text=[],
- )
- cur_chapter = None
-
- # 按页遍历PDF文档
- for idx, page in enumerate(new_pages):
- page = get_text_outside_table(page)
-
- # 按行遍历页面文本
- for word in extract_words(page):
- word = SimpleNamespace(**word)
-
- # 检查行文本是否以12号字体打印,如果是,则将其作为新章节开始
- if word.size >= 11: # 出现chapter name
- if cur_chapter is None:
- cur_chapter = create_chapter(page.page_number, word.top, word.bottom)
- elif not cur_chapter.record_chapter_name or (cur_chapter.name_bottom != cur_chapter.name_bottom and cur_chapter.name_top != cur_chapter.name_top):
- # 不再继续写chapter name
- cur_chapter.page_stop = page.page_number # stop id
- chapters.append(cur_chapter)
- # 重置当前chapter信息
- cur_chapter = create_chapter(page.page_number, word.top, word.bottom)
-
- # print(word.size, word.top, word.bottom, word.text)
- cur_chapter.name.append(word.text)
- else:
- cur_chapter.record_chapter_name = False # chapter name 结束
- cur_chapter.text.append(word.text)
- else:
- # 处理最后一个章节
- cur_chapter.page_stop = page.page_number # stop id
- chapters.append(cur_chapter)
-
- for i in chapters:
- logging.info(f"section: {i.name} pages:{i.page_start, i.page_stop} word-count:{len(i.text)}")
- logging.debug(" ".join(i.text))
-
- title = " ".join(title)
- user_info = " ".join(user_info)
- text = f"Article Title: {title}, Information:{user_info}\n"
- for idx, chapter in enumerate(chapters):
- chapter.name = " ".join(chapter.name)
- text += f"The {idx}th Chapter {chapter.name}: " + " ".join(chapter.text) + "\n"
-
- logging.getLogger().setLevel(level)
- return Document(page_content=text, metadata={"title": title})
-
-BASE_POINTS = """
-1. Who are the authors?
-2. What is the process of the proposed method?
-3. What is the performance of the proposed method? Please note down its performance metrics.
-4. What are the baseline models and their performances? Please note down these baseline methods.
-5. What dataset did this paper use?
-"""
-
-READING_PROMPT = """
-You are a researcher helper bot. You can help the user with research paper reading and summarizing. \n
-Now I am going to send you a paper. You need to read it and summarize it for me part by part. \n
-When you are reading, You need to focus on these key points:{}
-"""
-
-READING_PROMT_V2 = """
-You are a researcher helper bot. You can help the user with research paper reading and summarizing. \n
-Now I am going to send you a paper. You need to read it and summarize it for me part by part. \n
-When you are reading, You need to focus on these key points:{},
-
-And You need to generate a brief but informative title for this part.
-Your return format:
-- title: '...'
-- summary: '...'
-"""
-
-SUMMARY_PROMPT = "You are a researcher helper bot. Now you need to read the summaries of a research paper."
-
-
-if __name__ == '__main__':
- # Test code
- z = parse_pdf("./build/test.pdf")
- print(z["user_info"])
- print(z["title"])
\ No newline at end of file
diff --git a/spaces/DragGan/DragGan-Inversion/PTI/models/StyleCLIP/mapper/training/__init__.py b/spaces/DragGan/DragGan-Inversion/PTI/models/StyleCLIP/mapper/training/__init__.py
deleted file mode 100644
index e69de29bb2d1d6434b8b29ae775ad8c2e48c5391..0000000000000000000000000000000000000000
diff --git a/spaces/ECCV2022/bytetrack/exps/example/mot/yolox_s_mix_det.py b/spaces/ECCV2022/bytetrack/exps/example/mot/yolox_s_mix_det.py
deleted file mode 100644
index 95f1810872b9cefd4a4d5c21c45df7b9747a24aa..0000000000000000000000000000000000000000
--- a/spaces/ECCV2022/bytetrack/exps/example/mot/yolox_s_mix_det.py
+++ /dev/null
@@ -1,138 +0,0 @@
-# encoding: utf-8
-import os
-import random
-import torch
-import torch.nn as nn
-import torch.distributed as dist
-
-from yolox.exp import Exp as MyExp
-from yolox.data import get_yolox_datadir
-
-class Exp(MyExp):
- def __init__(self):
- super(Exp, self).__init__()
- self.num_classes = 1
- self.depth = 0.33
- self.width = 0.50
- self.exp_name = os.path.split(os.path.realpath(__file__))[1].split(".")[0]
- self.train_ann = "train.json"
- self.val_ann = "train.json"
- self.input_size = (608, 1088)
- self.test_size = (608, 1088)
- self.random_size = (12, 26)
- self.max_epoch = 80
- self.print_interval = 20
- self.eval_interval = 5
- self.test_conf = 0.001
- self.nmsthre = 0.7
- self.no_aug_epochs = 10
- self.basic_lr_per_img = 0.001 / 64.0
- self.warmup_epochs = 1
-
- def get_data_loader(self, batch_size, is_distributed, no_aug=False):
- from yolox.data import (
- MOTDataset,
- TrainTransform,
- YoloBatchSampler,
- DataLoader,
- InfiniteSampler,
- MosaicDetection,
- )
-
- dataset = MOTDataset(
- data_dir=os.path.join(get_yolox_datadir(), "mix_det"),
- json_file=self.train_ann,
- name='',
- img_size=self.input_size,
- preproc=TrainTransform(
- rgb_means=(0.485, 0.456, 0.406),
- std=(0.229, 0.224, 0.225),
- max_labels=500,
- ),
- )
-
- dataset = MosaicDetection(
- dataset,
- mosaic=not no_aug,
- img_size=self.input_size,
- preproc=TrainTransform(
- rgb_means=(0.485, 0.456, 0.406),
- std=(0.229, 0.224, 0.225),
- max_labels=1000,
- ),
- degrees=self.degrees,
- translate=self.translate,
- scale=self.scale,
- shear=self.shear,
- perspective=self.perspective,
- enable_mixup=self.enable_mixup,
- )
-
- self.dataset = dataset
-
- if is_distributed:
- batch_size = batch_size // dist.get_world_size()
-
- sampler = InfiniteSampler(
- len(self.dataset), seed=self.seed if self.seed else 0
- )
-
- batch_sampler = YoloBatchSampler(
- sampler=sampler,
- batch_size=batch_size,
- drop_last=False,
- input_dimension=self.input_size,
- mosaic=not no_aug,
- )
-
- dataloader_kwargs = {"num_workers": self.data_num_workers, "pin_memory": True}
- dataloader_kwargs["batch_sampler"] = batch_sampler
- train_loader = DataLoader(self.dataset, **dataloader_kwargs)
-
- return train_loader
-
- def get_eval_loader(self, batch_size, is_distributed, testdev=False):
- from yolox.data import MOTDataset, ValTransform
-
- valdataset = MOTDataset(
- data_dir=os.path.join(get_yolox_datadir(), "mot"),
- json_file=self.val_ann,
- img_size=self.test_size,
- name='train',
- preproc=ValTransform(
- rgb_means=(0.485, 0.456, 0.406),
- std=(0.229, 0.224, 0.225),
- ),
- )
-
- if is_distributed:
- batch_size = batch_size // dist.get_world_size()
- sampler = torch.utils.data.distributed.DistributedSampler(
- valdataset, shuffle=False
- )
- else:
- sampler = torch.utils.data.SequentialSampler(valdataset)
-
- dataloader_kwargs = {
- "num_workers": self.data_num_workers,
- "pin_memory": True,
- "sampler": sampler,
- }
- dataloader_kwargs["batch_size"] = batch_size
- val_loader = torch.utils.data.DataLoader(valdataset, **dataloader_kwargs)
-
- return val_loader
-
- def get_evaluator(self, batch_size, is_distributed, testdev=False):
- from yolox.evaluators import COCOEvaluator
-
- val_loader = self.get_eval_loader(batch_size, is_distributed, testdev=testdev)
- evaluator = COCOEvaluator(
- dataloader=val_loader,
- img_size=self.test_size,
- confthre=self.test_conf,
- nmsthre=self.nmsthre,
- num_classes=self.num_classes,
- testdev=testdev,
- )
- return evaluator
diff --git a/spaces/Egrt/GCycleGAN/utils/utils_fit.py b/spaces/Egrt/GCycleGAN/utils/utils_fit.py
deleted file mode 100644
index c57a55ffa174d24a1d2b99b5a50d0f668fe176df..0000000000000000000000000000000000000000
--- a/spaces/Egrt/GCycleGAN/utils/utils_fit.py
+++ /dev/null
@@ -1,249 +0,0 @@
-import os
-import torch
-import torch.nn.functional as F
-from tqdm import tqdm
-from nets.cyclegan import compute_gradient_penalty
-from utils.utils import get_lr, show_result
-
-
-def fit_one_epoch(G_model_A2B_train, G_model_B2A_train, D_model_A_train, D_model_B_train, G_model_A2B, G_model_B2A, D_model_A, D_model_B, VGG_feature_model, ResNeSt_model, loss_history,
- G_optimizer, D_optimizer_A, D_optimizer_B, BCE_loss, L1_loss, Face_loss, epoch, epoch_step, gen, Epoch, cuda, fp16, scaler, save_period, save_dir, photo_save_step, local_rank=0):
- G_total_loss = 0
- D_total_loss_A = 0
- D_total_loss_B = 0
-
- if local_rank == 0:
- print('Start Train')
- pbar = tqdm(total=epoch_step,desc=f'Epoch {epoch + 1}/{Epoch}',postfix=dict,mininterval=0.3)
- for iteration, batch in enumerate(gen):
- if iteration >= epoch_step:
- break
-
- images_A, images_B = batch[0], batch[1]
- batch_size = images_A.size()[0]
- y_real = torch.ones(batch_size)
- y_fake = torch.zeros(batch_size)
-
- with torch.no_grad():
- if cuda:
- images_A, images_B, y_real, y_fake = images_A.cuda(local_rank), images_B.cuda(local_rank), y_real.cuda(local_rank), y_fake.cuda(local_rank)
-
- if not fp16:
- #---------------------------------#
- # 训练生成器A2B和B2A
- #---------------------------------#
- G_optimizer.zero_grad()
-
- Same_B = G_model_A2B_train(images_B)
- loss_identity_B = L1_loss(Same_B, images_B)
-
- Same_A = G_model_B2A_train(images_A)
- loss_identity_A = L1_loss(Same_A, images_A)
-
- fake_B = G_model_A2B_train(images_A)
- pred_real = D_model_B_train(images_B)
- pred_fake = D_model_B_train(fake_B)
- pred_rf = pred_real - pred_fake.mean()
- pred_fr = pred_fake - pred_real.mean()
- D_train_loss_rf = BCE_loss(pred_rf, y_fake)
- D_train_loss_fr = BCE_loss(pred_fr, y_real)
- loss_GAN_A2B = (D_train_loss_rf + D_train_loss_fr) / 2
-
- fake_A = G_model_B2A_train(images_B)
- pred_real = D_model_A_train(images_A)
- pred_fake = D_model_A_train(fake_A)
- pred_rf = pred_real - pred_fake.mean()
- pred_fr = pred_fake - pred_real.mean()
- D_train_loss_rf = BCE_loss(pred_rf, y_fake)
- D_train_loss_fr = BCE_loss(pred_fr, y_real)
- loss_GAN_B2A = (D_train_loss_rf + D_train_loss_fr) / 2
-
- recovered_A = G_model_B2A_train(fake_B)
- loss_cycle_ABA = L1_loss(recovered_A, images_A)
-
- loss_per_ABA = L1_loss(VGG_feature_model(recovered_A), VGG_feature_model(images_A))
-
- recovered_A_face = F.interpolate(recovered_A, size=(112, 112), mode='bicubic', align_corners=True)
- images_A_face = F.interpolate(images_A, size=(112, 112), mode='bicubic', align_corners=True)
- loss_face_ABA = torch.mean(1. - Face_loss(ResNeSt_model(recovered_A_face), ResNeSt_model(images_A_face)))
-
- recovered_B = G_model_A2B_train(fake_A)
- loss_cycle_BAB = L1_loss(recovered_B, images_B)
-
- loss_per_BAB = L1_loss(VGG_feature_model(recovered_B), VGG_feature_model(images_B))
-
- recovered_B_face = F.interpolate(recovered_B, size=(112, 112), mode='bicubic', align_corners=True)
- images_B_face = F.interpolate(images_B, size=(112, 112), mode='bicubic', align_corners=True)
- loss_face_BAB = torch.mean(1. - Face_loss(ResNeSt_model(recovered_B_face), ResNeSt_model(images_B_face)))
-
- G_loss = loss_identity_A * 5.0 + loss_identity_B * 5.0 + loss_GAN_A2B + loss_GAN_B2A + loss_per_ABA * 2.5 \
- + loss_per_BAB *2.5 + loss_cycle_ABA * 10.0 + loss_cycle_BAB * 10.0 + loss_face_ABA * 5 + loss_face_BAB * 5
- G_loss.backward()
- G_optimizer.step()
-
- #---------------------------------#
- # 训练评价器A
- #---------------------------------#
- D_optimizer_A.zero_grad()
- pred_real = D_model_A_train(images_A)
- pred_fake = D_model_A_train(fake_A.detach())
- pred_rf = pred_real - pred_fake.mean()
- pred_fr = pred_fake - pred_real.mean()
- D_train_loss_rf = BCE_loss(pred_rf, y_real)
- D_train_loss_fr = BCE_loss(pred_fr, y_fake)
- gradient_penalty = compute_gradient_penalty(D_model_A_train, images_A, fake_A.detach())
-
- D_loss_A = 10 * gradient_penalty + (D_train_loss_rf + D_train_loss_fr) / 2
- D_loss_A.backward()
- D_optimizer_A.step()
-
- #---------------------------------#
- # 训练评价器B
- #---------------------------------#
- D_optimizer_B.zero_grad()
-
- pred_real = D_model_B_train(images_B)
- pred_fake = D_model_B_train(fake_B.detach())
- pred_rf = pred_real - pred_fake.mean()
- pred_fr = pred_fake - pred_real.mean()
- D_train_loss_rf = BCE_loss(pred_rf, y_real)
- D_train_loss_fr = BCE_loss(pred_fr, y_fake)
- gradient_penalty = compute_gradient_penalty(D_model_B_train, images_B, fake_B.detach())
-
- D_loss_B = 10 * gradient_penalty + (D_train_loss_rf + D_train_loss_fr) / 2
- D_loss_B.backward()
- D_optimizer_B.step()
-
- else:
- from torch.cuda.amp import autocast
-
- #---------------------------------#
- # 训练生成器A2B和B2A
- #---------------------------------#
- with autocast():
- G_optimizer.zero_grad()
- Same_B = G_model_A2B_train(images_B)
- loss_identity_B = L1_loss(Same_B, images_B)
-
- Same_A = G_model_B2A_train(images_A)
- loss_identity_A = L1_loss(Same_A, images_A)
-
- fake_B = G_model_A2B_train(images_A)
- pred_real = D_model_B_train(images_B)
- pred_fake = D_model_B_train(fake_B)
- pred_rf = pred_real - pred_fake.mean()
- pred_fr = pred_fake - pred_real.mean()
- D_train_loss_rf = BCE_loss(pred_rf, y_fake)
- D_train_loss_fr = BCE_loss(pred_fr, y_real)
- loss_GAN_A2B = (D_train_loss_rf + D_train_loss_fr) / 2
-
- fake_A = G_model_B2A_train(images_B)
- pred_real = D_model_A_train(images_A)
- pred_fake = D_model_A_train(fake_A)
- pred_rf = pred_real - pred_fake.mean()
- pred_fr = pred_fake - pred_real.mean()
- D_train_loss_rf = BCE_loss(pred_rf, y_fake)
- D_train_loss_fr = BCE_loss(pred_fr, y_real)
- loss_GAN_B2A = (D_train_loss_rf + D_train_loss_fr) / 2
-
- recovered_A = G_model_B2A_train(fake_B)
- loss_cycle_ABA = L1_loss(recovered_A, images_A)
- recovered_A_face = F.interpolate(recovered_A, size=(112, 112), mode='bicubic', align_corners=True)
- images_A_face = F.interpolate(images_A, size=(112, 112), mode='bicubic', align_corners=True)
- loss_face_ABA = torch.mean(1. - Face_loss(ResNeSt_model(recovered_A_face), ResNeSt_model(images_A_face)))
-
- recovered_B = G_model_A2B_train(fake_A)
- loss_cycle_BAB = L1_loss(recovered_B, images_B)
- recovered_B_face = F.interpolate(recovered_B, size=(112, 112), mode='bicubic', align_corners=True)
- images_B_face = F.interpolate(images_B, size=(112, 112), mode='bicubic', align_corners=True)
- loss_face_BAB = torch.mean(1. - Face_loss(ResNeSt_model(recovered_B_face), ResNeSt_model(images_B_face)))
-
- G_loss = loss_identity_A * 5.0 + loss_identity_B * 5.0 + loss_GAN_A2B + loss_GAN_B2A \
- + loss_cycle_ABA * 10.0 + loss_cycle_BAB * 10.0 + loss_face_ABA * 5 + loss_face_BAB * 5
- #----------------------#
- # 反向传播
- #----------------------#
- scaler.scale(G_loss).backward()
- scaler.step(G_optimizer)
- scaler.update()
-
- #---------------------------------#
- # 训练评价器A
- #---------------------------------#
- with autocast():
- D_optimizer_A.zero_grad()
- pred_real = D_model_A_train(images_A)
- pred_fake = D_model_A_train(fake_A.detach())
- pred_rf = pred_real - pred_fake.mean()
- pred_fr = pred_fake - pred_real.mean()
- D_train_loss_rf = BCE_loss(pred_rf, y_real)
- D_train_loss_fr = BCE_loss(pred_fr, y_fake)
- gradient_penalty = compute_gradient_penalty(D_model_A_train, images_A, fake_A.detach())
-
- D_loss_A = 10 * gradient_penalty + (D_train_loss_rf + D_train_loss_fr) / 2
- #----------------------#
- # 反向传播
- #----------------------#
- scaler.scale(D_loss_A).backward()
- scaler.step(D_optimizer_A)
- scaler.update()
-
- #---------------------------------#
- # 训练评价器B
- #---------------------------------#
- with autocast():
- D_optimizer_B.zero_grad()
-
- pred_real = D_model_B_train(images_B)
- pred_fake = D_model_B_train(fake_B.detach())
- pred_rf = pred_real - pred_fake.mean()
- pred_fr = pred_fake - pred_real.mean()
- D_train_loss_rf = BCE_loss(pred_rf, y_real)
- D_train_loss_fr = BCE_loss(pred_fr, y_fake)
- gradient_penalty = compute_gradient_penalty(D_model_B_train, images_B, fake_B.detach())
-
- D_loss_B = 10 * gradient_penalty + (D_train_loss_rf + D_train_loss_fr) / 2
- #----------------------#
- # 反向传播
- #----------------------#
- scaler.scale(D_loss_B).backward()
- scaler.step(D_optimizer_B)
- scaler.update()
-
- G_total_loss += G_loss.item()
- D_total_loss_A += D_loss_A.item()
- D_total_loss_B += D_loss_B.item()
-
- if local_rank == 0:
- pbar.set_postfix(**{'G_loss' : G_total_loss / (iteration + 1),
- 'D_loss_A' : D_total_loss_A / (iteration + 1),
- 'D_loss_B' : D_total_loss_B / (iteration + 1),
- 'lr' : get_lr(G_optimizer)})
- pbar.update(1)
-
- if iteration % photo_save_step == 0:
- show_result(epoch + 1, G_model_A2B, G_model_B2A, images_A, images_B)
-
- G_total_loss = G_total_loss / epoch_step
- D_total_loss_A = D_total_loss_A / epoch_step
- D_total_loss_B = D_total_loss_B / epoch_step
-
- if local_rank == 0:
- pbar.close()
- print('Epoch:'+ str(epoch + 1) + '/' + str(Epoch))
- print('G Loss: %.4f || D Loss A: %.4f || D Loss B: %.4f ' % (G_total_loss, D_total_loss_A, D_total_loss_B))
- loss_history.append_loss(epoch + 1, G_total_loss = G_total_loss, D_total_loss_A = D_total_loss_A, D_total_loss_B = D_total_loss_B)
-
- #-----------------------------------------------#
- # 保存权值
- #-----------------------------------------------#
- if (epoch + 1) % save_period == 0 or epoch + 1 == Epoch:
- torch.save(G_model_A2B.state_dict(), os.path.join(save_dir, 'G_model_A2B_Epoch%d-GLoss%.4f-DALoss%.4f-DBLoss%.4f.pth'%(epoch + 1, G_total_loss, D_total_loss_A, D_total_loss_B)))
- torch.save(G_model_B2A.state_dict(), os.path.join(save_dir, 'G_model_B2A_Epoch%d-GLoss%.4f-DALoss%.4f-DBLoss%.4f.pth'%(epoch + 1, G_total_loss, D_total_loss_A, D_total_loss_B)))
- torch.save(D_model_A.state_dict(), os.path.join(save_dir, 'D_model_A_Epoch%d-GLoss%.4f-DALoss%.4f-DBLoss%.4f.pth'%(epoch + 1, G_total_loss, D_total_loss_A, D_total_loss_B)))
- torch.save(D_model_B.state_dict(), os.path.join(save_dir, 'D_model_B_Epoch%d-GLoss%.4f-DALoss%.4f-DBLoss%.4f.pth'%(epoch + 1, G_total_loss, D_total_loss_A, D_total_loss_B)))
-
- torch.save(G_model_A2B.state_dict(), os.path.join(save_dir, "G_model_A2B_last_epoch_weights.pth"))
- torch.save(G_model_B2A.state_dict(), os.path.join(save_dir, "G_model_B2A_last_epoch_weights.pth"))
- torch.save(D_model_A.state_dict(), os.path.join(save_dir, "D_model_A_last_epoch_weights.pth"))
- torch.save(D_model_B.state_dict(), os.path.join(save_dir, "D_model_B_last_epoch_weights.pth"))
\ No newline at end of file
diff --git a/spaces/EnigmaOfTheWorld/GenZBot/README.md b/spaces/EnigmaOfTheWorld/GenZBot/README.md
deleted file mode 100644
index d08c4ce8c09c3e0deebb7b5637f61c34c227b466..0000000000000000000000000000000000000000
--- a/spaces/EnigmaOfTheWorld/GenZBot/README.md
+++ /dev/null
@@ -1,12 +0,0 @@
----
-title: GenZBot
-emoji: 📚
-colorFrom: gray
-colorTo: gray
-sdk: gradio
-sdk_version: 3.27.0
-app_file: app.py
-pinned: false
----
-
-Check out the configuration reference at https://huggingface.co/docs/hub/spaces-config-reference
diff --git a/spaces/EronSamez/RVC_HFmeu/lib/infer_pack/modules/F0Predictor/__init__.py b/spaces/EronSamez/RVC_HFmeu/lib/infer_pack/modules/F0Predictor/__init__.py
deleted file mode 100644
index e69de29bb2d1d6434b8b29ae775ad8c2e48c5391..0000000000000000000000000000000000000000
diff --git a/spaces/FrankZxShen/vits-fast-finetuning-umamusume/models.py b/spaces/FrankZxShen/vits-fast-finetuning-umamusume/models.py
deleted file mode 100644
index 65f9ae5255616efa19a4f28bc0a840d4c453a060..0000000000000000000000000000000000000000
--- a/spaces/FrankZxShen/vits-fast-finetuning-umamusume/models.py
+++ /dev/null
@@ -1,722 +0,0 @@
-import copy
-import math
-import torch
-from torch import nn
-from torch.nn import functional as F
-
-import commons
-import modules
-import attentions
-import monotonic_align
-
-from torch.nn import Conv1d, ConvTranspose1d, AvgPool1d, Conv2d
-from torch.nn.utils import weight_norm, remove_weight_norm, spectral_norm
-from commons import init_weights, get_padding
-
-
-class StochasticDurationPredictor(nn.Module):
- def __init__(self, in_channels, filter_channels, kernel_size, p_dropout, n_flows=4, gin_channels=0):
- super().__init__()
- filter_channels = in_channels # it needs to be removed from future version.
- self.in_channels = in_channels
- self.filter_channels = filter_channels
- self.kernel_size = kernel_size
- self.p_dropout = p_dropout
- self.n_flows = n_flows
- self.gin_channels = gin_channels
-
- self.log_flow = modules.Log()
- self.flows = nn.ModuleList()
- self.flows.append(modules.ElementwiseAffine(2))
- for i in range(n_flows):
- self.flows.append(modules.ConvFlow(2, filter_channels, kernel_size, n_layers=3))
- self.flows.append(modules.Flip())
-
- self.post_pre = nn.Conv1d(1, filter_channels, 1)
- self.post_proj = nn.Conv1d(filter_channels, filter_channels, 1)
- self.post_convs = modules.DDSConv(filter_channels, kernel_size, n_layers=3, p_dropout=p_dropout)
- self.post_flows = nn.ModuleList()
- self.post_flows.append(modules.ElementwiseAffine(2))
- for i in range(4):
- self.post_flows.append(modules.ConvFlow(2, filter_channels, kernel_size, n_layers=3))
- self.post_flows.append(modules.Flip())
-
- self.pre = nn.Conv1d(in_channels, filter_channels, 1)
- self.proj = nn.Conv1d(filter_channels, filter_channels, 1)
- self.convs = modules.DDSConv(filter_channels, kernel_size, n_layers=3, p_dropout=p_dropout)
- if gin_channels != 0:
- self.cond = nn.Conv1d(gin_channels, filter_channels, 1)
-
- def forward(self, x, x_mask, w=None, g=None, reverse=False, noise_scale=1.0):
- x = torch.detach(x)
- x = self.pre(x)
- if g is not None:
- g = torch.detach(g)
- x = x + self.cond(g)
- x = self.convs(x, x_mask)
- x = self.proj(x) * x_mask
-
- if not reverse:
- flows = self.flows
- assert w is not None
-
- logdet_tot_q = 0
- h_w = self.post_pre(w)
- h_w = self.post_convs(h_w, x_mask)
- h_w = self.post_proj(h_w) * x_mask
- e_q = torch.randn(w.size(0), 2, w.size(2)).to(device=x.device, dtype=x.dtype) * x_mask
- z_q = e_q
- for flow in self.post_flows:
- z_q, logdet_q = flow(z_q, x_mask, g=(x + h_w))
- logdet_tot_q += logdet_q
- z_u, z1 = torch.split(z_q, [1, 1], 1)
- u = torch.sigmoid(z_u) * x_mask
- z0 = (w - u) * x_mask
- logdet_tot_q += torch.sum((F.logsigmoid(z_u) + F.logsigmoid(-z_u)) * x_mask, [1,2])
- logq = torch.sum(-0.5 * (math.log(2*math.pi) + (e_q**2)) * x_mask, [1,2]) - logdet_tot_q
-
- logdet_tot = 0
- z0, logdet = self.log_flow(z0, x_mask)
- logdet_tot += logdet
- z = torch.cat([z0, z1], 1)
- for flow in flows:
- z, logdet = flow(z, x_mask, g=x, reverse=reverse)
- logdet_tot = logdet_tot + logdet
- nll = torch.sum(0.5 * (math.log(2*math.pi) + (z**2)) * x_mask, [1,2]) - logdet_tot
- return nll + logq # [b]
- else:
- flows = list(reversed(self.flows))
- flows = flows[:-2] + [flows[-1]] # remove a useless vflow
- z = torch.randn(x.size(0), 2, x.size(2)).to(device=x.device, dtype=x.dtype) * noise_scale
- for flow in flows:
- z = flow(z, x_mask, g=x, reverse=reverse)
- z0, z1 = torch.split(z, [1, 1], 1)
- logw = z0
- return logw
-
-
-class DurationPredictor(nn.Module):
- def __init__(self, in_channels, filter_channels, kernel_size, p_dropout, gin_channels=0):
- super().__init__()
-
- self.in_channels = in_channels
- self.filter_channels = filter_channels
- self.kernel_size = kernel_size
- self.p_dropout = p_dropout
- self.gin_channels = gin_channels
-
- self.drop = nn.Dropout(p_dropout)
- self.conv_1 = nn.Conv1d(in_channels, filter_channels, kernel_size, padding=kernel_size//2)
- self.norm_1 = modules.LayerNorm(filter_channels)
- self.conv_2 = nn.Conv1d(filter_channels, filter_channels, kernel_size, padding=kernel_size//2)
- self.norm_2 = modules.LayerNorm(filter_channels)
- self.proj = nn.Conv1d(filter_channels, 1, 1)
-
- if gin_channels != 0:
- self.cond = nn.Conv1d(gin_channels, in_channels, 1)
-
- def forward(self, x, x_mask, g=None):
- x = torch.detach(x)
- if g is not None:
- g = torch.detach(g)
- x = x + self.cond(g)
- x = self.conv_1(x * x_mask)
- x = torch.relu(x)
- x = self.norm_1(x)
- x = self.drop(x)
- x = self.conv_2(x * x_mask)
- x = torch.relu(x)
- x = self.norm_2(x)
- x = self.drop(x)
- x = self.proj(x * x_mask)
- return x * x_mask
-
-
-class TextEncoder(nn.Module):
- def __init__(self,
- n_vocab,
- out_channels,
- hidden_channels,
- filter_channels,
- n_heads,
- n_layers,
- kernel_size,
- p_dropout):
- super().__init__()
- self.n_vocab = n_vocab
- self.out_channels = out_channels
- self.hidden_channels = hidden_channels
- self.filter_channels = filter_channels
- self.n_heads = n_heads
- self.n_layers = n_layers
- self.kernel_size = kernel_size
- self.p_dropout = p_dropout
-
- self.emb = nn.Embedding(n_vocab, hidden_channels)
- nn.init.normal_(self.emb.weight, 0.0, hidden_channels**-0.5)
-
- self.encoder = attentions.Encoder(
- hidden_channels,
- filter_channels,
- n_heads,
- n_layers,
- kernel_size,
- p_dropout)
- self.proj= nn.Conv1d(hidden_channels, out_channels * 2, 1)
-
- def forward(self, x, x_lengths):
- x = self.emb(x) * math.sqrt(self.hidden_channels) # [b, t, h]
- x = torch.transpose(x, 1, -1) # [b, h, t]
- x_mask = torch.unsqueeze(commons.sequence_mask(x_lengths, x.size(2)), 1).to(x.dtype)
-
- x = self.encoder(x * x_mask, x_mask)
- stats = self.proj(x) * x_mask
-
- m, logs = torch.split(stats, self.out_channels, dim=1)
- return x, m, logs, x_mask
-
-
-class TextEncoder_lora(nn.Module):
- def __init__(self,
- n_vocab,
- out_channels,
- hidden_channels,
- filter_channels,
- n_heads,
- n_layers,
- kernel_size,
- p_dropout):
- super().__init__()
- self.n_vocab = n_vocab
- self.out_channels = out_channels
- self.hidden_channels = hidden_channels
- self.filter_channels = filter_channels
- self.n_heads = n_heads
- self.n_layers = n_layers
- self.kernel_size = kernel_size
- self.p_dropout = p_dropout
-
- self.emb = nn.Embedding(n_vocab, hidden_channels, r=4)
- nn.init.normal_(self.emb.weight, 0.0, hidden_channels**-0.5)
-
- self.encoder = attentions.Encoder_lora(
- hidden_channels,
- filter_channels,
- n_heads,
- n_layers,
- kernel_size,
- p_dropout)
- self.proj= nn.Conv1d(hidden_channels, out_channels * 2, 1)
-
- def forward(self, x, x_lengths):
- x = self.emb(x) * math.sqrt(self.hidden_channels) # [b, t, h]
- x = torch.transpose(x, 1, -1) # [b, h, t]
- x_mask = torch.unsqueeze(commons.sequence_mask(x_lengths, x.size(2)), 1).to(x.dtype)
-
- x = self.encoder(x * x_mask, x_mask)
- stats = self.proj(x) * x_mask
-
- m, logs = torch.split(stats, self.out_channels, dim=1)
- return x, m, logs, x_mask
-
-class ResidualCouplingBlock(nn.Module):
- def __init__(self,
- channels,
- hidden_channels,
- kernel_size,
- dilation_rate,
- n_layers,
- n_flows=4,
- gin_channels=0):
- super().__init__()
- self.channels = channels
- self.hidden_channels = hidden_channels
- self.kernel_size = kernel_size
- self.dilation_rate = dilation_rate
- self.n_layers = n_layers
- self.n_flows = n_flows
- self.gin_channels = gin_channels
-
- self.flows = nn.ModuleList()
- for i in range(n_flows):
- self.flows.append(modules.ResidualCouplingLayer(channels, hidden_channels, kernel_size, dilation_rate, n_layers, gin_channels=gin_channels, mean_only=True))
- self.flows.append(modules.Flip())
-
- def forward(self, x, x_mask, g=None, reverse=False):
- if not reverse:
- for flow in self.flows:
- x, _ = flow(x, x_mask, g=g, reverse=reverse)
- else:
- for flow in reversed(self.flows):
- x = flow(x, x_mask, g=g, reverse=reverse)
- return x
-
-
-class PosteriorEncoder(nn.Module):
- def __init__(self,
- in_channels,
- out_channels,
- hidden_channels,
- kernel_size,
- dilation_rate,
- n_layers,
- gin_channels=0):
- super().__init__()
- self.in_channels = in_channels
- self.out_channels = out_channels
- self.hidden_channels = hidden_channels
- self.kernel_size = kernel_size
- self.dilation_rate = dilation_rate
- self.n_layers = n_layers
- self.gin_channels = gin_channels
-
- self.pre = nn.Conv1d(in_channels, hidden_channels, 1)
- self.enc = modules.WN(hidden_channels, kernel_size, dilation_rate, n_layers, gin_channels=gin_channels)
- self.proj = nn.Conv1d(hidden_channels, out_channels * 2, 1)
-
- def forward(self, x, x_lengths, g=None):
- x_mask = torch.unsqueeze(commons.sequence_mask(x_lengths, x.size(2)), 1).to(x.dtype)
- x = self.pre(x) * x_mask
- x = self.enc(x, x_mask, g=g)
- stats = self.proj(x) * x_mask
- m, logs = torch.split(stats, self.out_channels, dim=1)
- z = (m + torch.randn_like(m) * torch.exp(logs)) * x_mask
- return z, m, logs, x_mask
-
-
-class Generator(torch.nn.Module):
- def __init__(self, initial_channel, resblock, resblock_kernel_sizes, resblock_dilation_sizes, upsample_rates, upsample_initial_channel, upsample_kernel_sizes, gin_channels=0):
- super(Generator, self).__init__()
- self.num_kernels = len(resblock_kernel_sizes)
- self.num_upsamples = len(upsample_rates)
- self.conv_pre = Conv1d(initial_channel, upsample_initial_channel, 7, 1, padding=3)
- resblock = modules.ResBlock1 if resblock == '1' else modules.ResBlock2
-
- self.ups = nn.ModuleList()
- for i, (u, k) in enumerate(zip(upsample_rates, upsample_kernel_sizes)):
- self.ups.append(weight_norm(
- ConvTranspose1d(upsample_initial_channel//(2**i), upsample_initial_channel//(2**(i+1)),
- k, u, padding=(k-u)//2)))
-
- self.resblocks = nn.ModuleList()
- for i in range(len(self.ups)):
- ch = upsample_initial_channel//(2**(i+1))
- for j, (k, d) in enumerate(zip(resblock_kernel_sizes, resblock_dilation_sizes)):
- self.resblocks.append(resblock(ch, k, d))
-
- self.conv_post = Conv1d(ch, 1, 7, 1, padding=3, bias=False)
- self.ups.apply(init_weights)
-
- if gin_channels != 0:
- self.cond = nn.Conv1d(gin_channels, upsample_initial_channel, 1)
-
- def forward(self, x, g=None):
- x = self.conv_pre(x)
- if g is not None:
- x = x + self.cond(g)
-
- for i in range(self.num_upsamples):
- x = F.leaky_relu(x, modules.LRELU_SLOPE)
- x = self.ups[i](x)
- xs = None
- for j in range(self.num_kernels):
- if xs is None:
- xs = self.resblocks[i*self.num_kernels+j](x)
- else:
- xs += self.resblocks[i*self.num_kernels+j](x)
- x = xs / self.num_kernels
- x = F.leaky_relu(x)
- x = self.conv_post(x)
- x = torch.tanh(x)
-
- return x
-
- def remove_weight_norm(self):
- print('Removing weight norm...')
- for l in self.ups:
- remove_weight_norm(l)
- for l in self.resblocks:
- l.remove_weight_norm()
-
-
-class DiscriminatorP(torch.nn.Module):
- def __init__(self, period, kernel_size=5, stride=3, use_spectral_norm=False):
- super(DiscriminatorP, self).__init__()
- self.period = period
- self.use_spectral_norm = use_spectral_norm
- norm_f = weight_norm if use_spectral_norm == False else spectral_norm
- self.convs = nn.ModuleList([
- norm_f(Conv2d(1, 32, (kernel_size, 1), (stride, 1), padding=(get_padding(kernel_size, 1), 0))),
- norm_f(Conv2d(32, 128, (kernel_size, 1), (stride, 1), padding=(get_padding(kernel_size, 1), 0))),
- norm_f(Conv2d(128, 512, (kernel_size, 1), (stride, 1), padding=(get_padding(kernel_size, 1), 0))),
- norm_f(Conv2d(512, 1024, (kernel_size, 1), (stride, 1), padding=(get_padding(kernel_size, 1), 0))),
- norm_f(Conv2d(1024, 1024, (kernel_size, 1), 1, padding=(get_padding(kernel_size, 1), 0))),
- ])
- self.conv_post = norm_f(Conv2d(1024, 1, (3, 1), 1, padding=(1, 0)))
-
- def forward(self, x):
- fmap = []
-
- # 1d to 2d
- b, c, t = x.shape
- if t % self.period != 0: # pad first
- n_pad = self.period - (t % self.period)
- x = F.pad(x, (0, n_pad), "reflect")
- t = t + n_pad
- x = x.view(b, c, t // self.period, self.period)
-
- for l in self.convs:
- x = l(x)
- x = F.leaky_relu(x, modules.LRELU_SLOPE)
- fmap.append(x)
- x = self.conv_post(x)
- fmap.append(x)
- x = torch.flatten(x, 1, -1)
-
- return x, fmap
-
-
-class DiscriminatorS(torch.nn.Module):
- def __init__(self, use_spectral_norm=False):
- super(DiscriminatorS, self).__init__()
- norm_f = weight_norm if use_spectral_norm == False else spectral_norm
- self.convs = nn.ModuleList([
- norm_f(Conv1d(1, 16, 15, 1, padding=7)),
- norm_f(Conv1d(16, 64, 41, 4, groups=4, padding=20)),
- norm_f(Conv1d(64, 256, 41, 4, groups=16, padding=20)),
- norm_f(Conv1d(256, 1024, 41, 4, groups=64, padding=20)),
- norm_f(Conv1d(1024, 1024, 41, 4, groups=256, padding=20)),
- norm_f(Conv1d(1024, 1024, 5, 1, padding=2)),
- ])
- self.conv_post = norm_f(Conv1d(1024, 1, 3, 1, padding=1))
-
- def forward(self, x):
- fmap = []
-
- for l in self.convs:
- x = l(x)
- x = F.leaky_relu(x, modules.LRELU_SLOPE)
- fmap.append(x)
- x = self.conv_post(x)
- fmap.append(x)
- x = torch.flatten(x, 1, -1)
-
- return x, fmap
-
-
-class MultiPeriodDiscriminator(torch.nn.Module):
- def __init__(self, use_spectral_norm=False):
- super(MultiPeriodDiscriminator, self).__init__()
- periods = [2,3,5,7,11]
-
- discs = [DiscriminatorS(use_spectral_norm=use_spectral_norm)]
- discs = discs + [DiscriminatorP(i, use_spectral_norm=use_spectral_norm) for i in periods]
- self.discriminators = nn.ModuleList(discs)
-
- def forward(self, y, y_hat):
- y_d_rs = []
- y_d_gs = []
- fmap_rs = []
- fmap_gs = []
- for i, d in enumerate(self.discriminators):
- y_d_r, fmap_r = d(y)
- y_d_g, fmap_g = d(y_hat)
- y_d_rs.append(y_d_r)
- y_d_gs.append(y_d_g)
- fmap_rs.append(fmap_r)
- fmap_gs.append(fmap_g)
-
- return y_d_rs, y_d_gs, fmap_rs, fmap_gs
-
-
-
-class SynthesizerTrn(nn.Module):
- """
- Synthesizer for Training
- """
-
- def __init__(self,
- n_vocab,
- spec_channels,
- segment_size,
- inter_channels,
- hidden_channels,
- filter_channels,
- n_heads,
- n_layers,
- kernel_size,
- p_dropout,
- resblock,
- resblock_kernel_sizes,
- resblock_dilation_sizes,
- upsample_rates,
- upsample_initial_channel,
- upsample_kernel_sizes,
- n_speakers=0,
- gin_channels=0,
- use_sdp=True,
- **kwargs):
-
- super().__init__()
- self.n_vocab = n_vocab
- self.spec_channels = spec_channels
- self.inter_channels = inter_channels
- self.hidden_channels = hidden_channels
- self.filter_channels = filter_channels
- self.n_heads = n_heads
- self.n_layers = n_layers
- self.kernel_size = kernel_size
- self.p_dropout = p_dropout
- self.resblock = resblock
- self.resblock_kernel_sizes = resblock_kernel_sizes
- self.resblock_dilation_sizes = resblock_dilation_sizes
- self.upsample_rates = upsample_rates
- self.upsample_initial_channel = upsample_initial_channel
- self.upsample_kernel_sizes = upsample_kernel_sizes
- self.segment_size = segment_size
- self.n_speakers = n_speakers
- self.gin_channels = gin_channels
-
- self.use_sdp = use_sdp
-
- self.enc_p = TextEncoder(n_vocab,
- inter_channels,
- hidden_channels,
- filter_channels,
- n_heads,
- n_layers,
- kernel_size,
- p_dropout)
- self.dec = Generator(inter_channels, resblock, resblock_kernel_sizes, resblock_dilation_sizes, upsample_rates, upsample_initial_channel, upsample_kernel_sizes, gin_channels=gin_channels)
- self.enc_q = PosteriorEncoder(spec_channels, inter_channels, hidden_channels, 5, 1, 16, gin_channels=gin_channels)
- self.flow = ResidualCouplingBlock(inter_channels, hidden_channels, 5, 1, 4, gin_channels=gin_channels)
-
- if use_sdp:
- self.dp = StochasticDurationPredictor(hidden_channels, 192, 3, 0.5, 4, gin_channels=gin_channels)
- else:
- self.dp = DurationPredictor(hidden_channels, 256, 3, 0.5, gin_channels=gin_channels)
-
- if n_speakers >= 1:
- self.emb_g = nn.Embedding(n_speakers, gin_channels)
-
- def forward(self, x, x_lengths, y, y_lengths, sid=None):
-
- x, m_p, logs_p, x_mask = self.enc_p(x, x_lengths)
- if self.n_speakers > 0:
- g = self.emb_g(sid).unsqueeze(-1) # [b, h, 1]
- else:
- g = None
-
- z, m_q, logs_q, y_mask = self.enc_q(y, y_lengths, g=g)
- z_p = self.flow(z, y_mask, g=g)
-
- with torch.no_grad():
- # negative cross-entropy
- s_p_sq_r = torch.exp(-2 * logs_p) # [b, d, t]
- neg_cent1 = torch.sum(-0.5 * math.log(2 * math.pi) - logs_p, [1], keepdim=True) # [b, 1, t_s]
- neg_cent2 = torch.matmul(-0.5 * (z_p ** 2).transpose(1, 2), s_p_sq_r) # [b, t_t, d] x [b, d, t_s] = [b, t_t, t_s]
- neg_cent3 = torch.matmul(z_p.transpose(1, 2), (m_p * s_p_sq_r)) # [b, t_t, d] x [b, d, t_s] = [b, t_t, t_s]
- neg_cent4 = torch.sum(-0.5 * (m_p ** 2) * s_p_sq_r, [1], keepdim=True) # [b, 1, t_s]
- neg_cent = neg_cent1 + neg_cent2 + neg_cent3 + neg_cent4
-
- attn_mask = torch.unsqueeze(x_mask, 2) * torch.unsqueeze(y_mask, -1)
- attn = monotonic_align.maximum_path(neg_cent, attn_mask.squeeze(1)).unsqueeze(1).detach()
-
- w = attn.sum(2)
- if self.use_sdp:
- l_length = self.dp(x, x_mask, w, g=g)
- l_length = l_length / torch.sum(x_mask)
- else:
- logw_ = torch.log(w + 1e-6) * x_mask
- logw = self.dp(x, x_mask, g=g)
- l_length = torch.sum((logw - logw_)**2, [1,2]) / torch.sum(x_mask) # for averaging
-
- # expand prior
- m_p = torch.matmul(attn.squeeze(1), m_p.transpose(1, 2)).transpose(1, 2)
- logs_p = torch.matmul(attn.squeeze(1), logs_p.transpose(1, 2)).transpose(1, 2)
-
- z_slice, ids_slice = commons.rand_slice_segments(z, y_lengths, self.segment_size)
- o = self.dec(z_slice, g=g)
- return o, l_length, attn, ids_slice, x_mask, y_mask, (z, z_p, m_p, logs_p, m_q, logs_q)
-
- def infer(self, x, x_lengths, sid=None, noise_scale=1, length_scale=1, noise_scale_w=1., max_len=None):
- x, m_p, logs_p, x_mask = self.enc_p(x, x_lengths)
- if self.n_speakers > 0:
- g = self.emb_g(sid).unsqueeze(-1) # [b, h, 1]
- else:
- g = None
-
- if self.use_sdp:
- logw = self.dp(x, x_mask, g=g, reverse=True, noise_scale=noise_scale_w)
- else:
- logw = self.dp(x, x_mask, g=g)
- w = torch.exp(logw) * x_mask * length_scale
- w_ceil = torch.ceil(w)
- y_lengths = torch.clamp_min(torch.sum(w_ceil, [1, 2]), 1).long()
- y_mask = torch.unsqueeze(commons.sequence_mask(y_lengths, None), 1).to(x_mask.dtype)
- attn_mask = torch.unsqueeze(x_mask, 2) * torch.unsqueeze(y_mask, -1)
- attn = commons.generate_path(w_ceil, attn_mask)
-
- m_p = torch.matmul(attn.squeeze(1), m_p.transpose(1, 2)).transpose(1, 2) # [b, t', t], [b, t, d] -> [b, d, t']
- logs_p = torch.matmul(attn.squeeze(1), logs_p.transpose(1, 2)).transpose(1, 2) # [b, t', t], [b, t, d] -> [b, d, t']
-
- z_p = m_p + torch.randn_like(m_p) * torch.exp(logs_p) * noise_scale
- z = self.flow(z_p, y_mask, g=g, reverse=True)
- o = self.dec((z * y_mask)[:,:,:max_len], g=g)
- return o, attn, y_mask, (z, z_p, m_p, logs_p)
-
- def voice_conversion(self, y, y_lengths, sid_src, sid_tgt):
- assert self.n_speakers > 0, "n_speakers have to be larger than 0."
- g_src = self.emb_g(sid_src).unsqueeze(-1)
- g_tgt = self.emb_g(sid_tgt).unsqueeze(-1)
- z, m_q, logs_q, y_mask = self.enc_q(y, y_lengths, g=g_src)
- z_p = self.flow(z, y_mask, g=g_src)
- z_hat = self.flow(z_p, y_mask, g=g_tgt, reverse=True)
- o_hat = self.dec(z_hat * y_mask, g=g_tgt)
- return o_hat, y_mask, (z, z_p, z_hat)
-
-
-class SynthesizerTrn_lora(nn.Module):
- """
- Synthesizer for Training
- """
-
- def __init__(self,
- n_vocab,
- spec_channels,
- segment_size,
- inter_channels,
- hidden_channels,
- filter_channels,
- n_heads,
- n_layers,
- kernel_size,
- p_dropout,
- resblock,
- resblock_kernel_sizes,
- resblock_dilation_sizes,
- upsample_rates,
- upsample_initial_channel,
- upsample_kernel_sizes,
- n_speakers=0,
- gin_channels=0,
- use_sdp=True,
- **kwargs):
-
- super().__init__()
- self.n_vocab = n_vocab
- self.spec_channels = spec_channels
- self.inter_channels = inter_channels
- self.hidden_channels = hidden_channels
- self.filter_channels = filter_channels
- self.n_heads = n_heads
- self.n_layers = n_layers
- self.kernel_size = kernel_size
- self.p_dropout = p_dropout
- self.resblock = resblock
- self.resblock_kernel_sizes = resblock_kernel_sizes
- self.resblock_dilation_sizes = resblock_dilation_sizes
- self.upsample_rates = upsample_rates
- self.upsample_initial_channel = upsample_initial_channel
- self.upsample_kernel_sizes = upsample_kernel_sizes
- self.segment_size = segment_size
- self.n_speakers = n_speakers
- self.gin_channels = gin_channels
-
- self.use_sdp = use_sdp
-
- self.enc_p = TextEncoder_lora(n_vocab,
- inter_channels,
- hidden_channels,
- filter_channels,
- n_heads,
- n_layers,
- kernel_size,
- p_dropout)
- self.dec = Generator(inter_channels, resblock, resblock_kernel_sizes, resblock_dilation_sizes, upsample_rates, upsample_initial_channel, upsample_kernel_sizes, gin_channels=gin_channels)
- self.enc_q = PosteriorEncoder(spec_channels, inter_channels, hidden_channels, 5, 1, 16, gin_channels=gin_channels)
- self.flow = ResidualCouplingBlock(inter_channels, hidden_channels, 5, 1, 4, gin_channels=gin_channels)
-
- if use_sdp:
- self.dp = StochasticDurationPredictor(hidden_channels, 192, 3, 0.5, 4, gin_channels=gin_channels)
- else:
- self.dp = DurationPredictor(hidden_channels, 256, 3, 0.5, gin_channels=gin_channels)
-
- if n_speakers >= 1:
- self.emb_g = nn.Embedding(n_speakers, gin_channels)
-
- def forward(self, x, x_lengths, y, y_lengths, sid=None):
-
- x, m_p, logs_p, x_mask = self.enc_p(x, x_lengths)
- if self.n_speakers > 0:
- g = self.emb_g(sid).unsqueeze(-1) # [b, h, 1]
- else:
- g = None
-
- z, m_q, logs_q, y_mask = self.enc_q(y, y_lengths, g=g)
- z_p = self.flow(z, y_mask, g=g)
-
- with torch.no_grad():
- # negative cross-entropy
- s_p_sq_r = torch.exp(-2 * logs_p) # [b, d, t]
- neg_cent1 = torch.sum(-0.5 * math.log(2 * math.pi) - logs_p, [1], keepdim=True) # [b, 1, t_s]
- neg_cent2 = torch.matmul(-0.5 * (z_p ** 2).transpose(1, 2), s_p_sq_r) # [b, t_t, d] x [b, d, t_s] = [b, t_t, t_s]
- neg_cent3 = torch.matmul(z_p.transpose(1, 2), (m_p * s_p_sq_r)) # [b, t_t, d] x [b, d, t_s] = [b, t_t, t_s]
- neg_cent4 = torch.sum(-0.5 * (m_p ** 2) * s_p_sq_r, [1], keepdim=True) # [b, 1, t_s]
- neg_cent = neg_cent1 + neg_cent2 + neg_cent3 + neg_cent4
-
- attn_mask = torch.unsqueeze(x_mask, 2) * torch.unsqueeze(y_mask, -1)
- attn = monotonic_align.maximum_path(neg_cent, attn_mask.squeeze(1)).unsqueeze(1).detach()
-
- w = attn.sum(2)
- if self.use_sdp:
- l_length = self.dp(x, x_mask, w, g=g)
- l_length = l_length / torch.sum(x_mask)
- else:
- logw_ = torch.log(w + 1e-6) * x_mask
- logw = self.dp(x, x_mask, g=g)
- l_length = torch.sum((logw - logw_)**2, [1,2]) / torch.sum(x_mask) # for averaging
-
- # expand prior
- m_p = torch.matmul(attn.squeeze(1), m_p.transpose(1, 2)).transpose(1, 2)
- logs_p = torch.matmul(attn.squeeze(1), logs_p.transpose(1, 2)).transpose(1, 2)
-
- z_slice, ids_slice = commons.rand_slice_segments(z, y_lengths, self.segment_size)
- o = self.dec(z_slice, g=g)
- return o, l_length, attn, ids_slice, x_mask, y_mask, (z, z_p, m_p, logs_p, m_q, logs_q)
-
- def infer(self, x, x_lengths, sid=None, noise_scale=1, length_scale=1, noise_scale_w=1., max_len=None):
- x, m_p, logs_p, x_mask = self.enc_p(x, x_lengths)
- if self.n_speakers > 0:
- g = self.emb_g(sid).unsqueeze(-1) # [b, h, 1]
- else:
- g = None
-
- if self.use_sdp:
- logw = self.dp(x, x_mask, g=g, reverse=True, noise_scale=noise_scale_w)
- else:
- logw = self.dp(x, x_mask, g=g)
- w = torch.exp(logw) * x_mask * length_scale
- w_ceil = torch.ceil(w)
- y_lengths = torch.clamp_min(torch.sum(w_ceil, [1, 2]), 1).long()
- y_mask = torch.unsqueeze(commons.sequence_mask(y_lengths, None), 1).to(x_mask.dtype)
- attn_mask = torch.unsqueeze(x_mask, 2) * torch.unsqueeze(y_mask, -1)
- attn = commons.generate_path(w_ceil, attn_mask)
-
- m_p = torch.matmul(attn.squeeze(1), m_p.transpose(1, 2)).transpose(1, 2) # [b, t', t], [b, t, d] -> [b, d, t']
- logs_p = torch.matmul(attn.squeeze(1), logs_p.transpose(1, 2)).transpose(1, 2) # [b, t', t], [b, t, d] -> [b, d, t']
-
- z_p = m_p + torch.randn_like(m_p) * torch.exp(logs_p) * noise_scale
- z = self.flow(z_p, y_mask, g=g, reverse=True)
- o = self.dec((z * y_mask)[:,:,:max_len], g=g)
- return o, attn, y_mask, (z, z_p, m_p, logs_p)
-
- def voice_conversion(self, y, y_lengths, sid_src, sid_tgt):
- assert self.n_speakers > 0, "n_speakers have to be larger than 0."
- g_src = self.emb_g(sid_src).unsqueeze(-1)
- g_tgt = self.emb_g(sid_tgt).unsqueeze(-1)
- z, m_q, logs_q, y_mask = self.enc_q(y, y_lengths, g=g_src)
- z_p = self.flow(z, y_mask, g=g_src)
- z_hat = self.flow(z_p, y_mask, g=g_tgt, reverse=True)
- o_hat = self.dec(z_hat * y_mask, g=g_tgt)
- return o_hat, y_mask, (z, z_p, z_hat)
\ No newline at end of file
diff --git a/spaces/FridaZuley/RVC_HFKawaii/infer/lib/audio.py b/spaces/FridaZuley/RVC_HFKawaii/infer/lib/audio.py
deleted file mode 100644
index 9ad4ff74218957cf18782fa71add40a734b47e78..0000000000000000000000000000000000000000
--- a/spaces/FridaZuley/RVC_HFKawaii/infer/lib/audio.py
+++ /dev/null
@@ -1,197 +0,0 @@
-import librosa
-import numpy as np
-import av
-from io import BytesIO
-import ffmpeg
-import os
-import sys
-
-import random
-from infer.lib.csvutil import CSVutil
-#import csv
-
-platform_stft_mapping = {
- 'linux': 'stftpitchshift',
- 'darwin': 'stftpitchshift',
- 'win32': 'stftpitchshift.exe',
-}
-
-stft = platform_stft_mapping.get(sys.platform)
-
-def wav2(i, o, format):
- inp = av.open(i, 'rb')
- if format == "m4a": format = "mp4"
- out = av.open(o, 'wb', format=format)
- if format == "ogg": format = "libvorbis"
- if format == "mp4": format = "aac"
-
- ostream = out.add_stream(format)
-
- for frame in inp.decode(audio=0):
- for p in ostream.encode(frame): out.mux(p)
-
- for p in ostream.encode(None): out.mux(p)
-
- out.close()
- inp.close()
-
-def audio2(i, o, format, sr):
- inp = av.open(i, 'rb')
- out = av.open(o, 'wb', format=format)
- if format == "ogg": format = "libvorbis"
- if format == "f32le": format = "pcm_f32le"
-
- ostream = out.add_stream(format, channels=1)
- ostream.sample_rate = sr
-
- for frame in inp.decode(audio=0):
- for p in ostream.encode(frame): out.mux(p)
-
- out.close()
- inp.close()
-
-def load_audion(file, sr):
- try:
- file = (
- file.strip(" ").strip('"').strip("\n").strip('"').strip(" ")
- ) # 防止小白拷路径头尾带了空格和"和回车
- with open(file, "rb") as f:
- with BytesIO() as out:
- audio2(f, out, "f32le", sr)
- return np.frombuffer(out.getvalue(), np.float32).flatten()
-
- except AttributeError:
- audio = file[1] / 32768.0
- if len(audio.shape) == 2:
- audio = np.mean(audio, -1)
- return librosa.resample(audio, orig_sr=file[0], target_sr=16000)
-
- except Exception as e:
- raise RuntimeError(f"Failed to load audio: {e}")
-
-
-
-
-def load_audio(file, sr, DoFormant=False, Quefrency=1.0, Timbre=1.0):
- converted = False
- DoFormant, Quefrency, Timbre = CSVutil("csvdb/formanting.csv", "r", "formanting")
- try:
- # https://github.com/openai/whisper/blob/main/whisper/audio.py#L26
- # This launches a subprocess to decode audio while down-mixing and resampling as necessary.
- # Requires the ffmpeg CLI and `ffmpeg-python` package to be installed.
- file = (
- file.strip(" ").strip('"').strip("\n").strip('"').strip(" ")
- ) # 防止小白拷路径头尾带了空格和"和回车
- file_formanted = file.strip(" ").strip('"').strip("\n").strip('"').strip(" ")
-
- # print(f"dofor={bool(DoFormant)} timbr={Timbre} quef={Quefrency}\n")
-
- if (
- lambda DoFormant: True
- if DoFormant.lower() == "true"
- else (False if DoFormant.lower() == "false" else DoFormant)
- )(DoFormant):
- numerator = round(random.uniform(1, 4), 4)
- # os.system(f"stftpitchshift -i {file} -q {Quefrency} -t {Timbre} -o {file_formanted}")
- # print('stftpitchshift -i "%s" -p 1.0 --rms -w 128 -v 8 -q %s -t %s -o "%s"' % (file, Quefrency, Timbre, file_formanted))
-
- if not file.endswith(".wav"):
- if not os.path.isfile(f"{file_formanted}.wav"):
- converted = True
- # print(f"\nfile = {file}\n")
- # print(f"\nfile_formanted = {file_formanted}\n")
- converting = (
- ffmpeg.input(file_formanted, threads=0)
- .output(f"{file_formanted}.wav")
- .run(
- cmd=["ffmpeg", "-nostdin"],
- capture_stdout=True,
- capture_stderr=True,
- )
- )
- else:
- pass
-
- file_formanted = (
- f"{file_formanted}.wav"
- if not file_formanted.endswith(".wav")
- else file_formanted
- )
-
- print(f" · Formanting {file_formanted}...\n")
-
- os.system(
- '%s -i "%s" -q "%s" -t "%s" -o "%sFORMANTED_%s.wav"'
- % (
- stft,
- file_formanted,
- Quefrency,
- Timbre,
- file_formanted,
- str(numerator),
- )
- )
-
- print(f" · Formanted {file_formanted}!\n")
-
- # filepraat = (os.path.abspath(os.getcwd()) + '\\' + file).replace('/','\\')
- # file_formantedpraat = ('"' + os.path.abspath(os.getcwd()) + '/' + 'formanted'.join(file_formanted) + '"').replace('/','\\')
- # print("%sFORMANTED_%s.wav" % (file_formanted, str(numerator)))
-
- out, _ = (
- ffmpeg.input(
- "%sFORMANTED_%s.wav" % (file_formanted, str(numerator)), threads=0
- )
- .output("-", format="f32le", acodec="pcm_f32le", ac=1, ar=sr)
- .run(
- cmd=["ffmpeg", "-nostdin"], capture_stdout=True, capture_stderr=True
- )
- )
-
- try:
- os.remove("%sFORMANTED_%s.wav" % (file_formanted, str(numerator)))
- except Exception:
- pass
- print("couldn't remove formanted type of file")
-
- else:
- out, _ = (
- ffmpeg.input(file, threads=0)
- .output("-", format="f32le", acodec="pcm_f32le", ac=1, ar=sr)
- .run(
- cmd=["ffmpeg", "-nostdin"], capture_stdout=True, capture_stderr=True
- )
- )
- except Exception as e:
- raise RuntimeError(f"Failed to load audio: {e}")
-
- if converted:
- try:
- os.remove(file_formanted)
- except Exception:
- pass
- print("couldn't remove converted type of file")
- converted = False
-
- return np.frombuffer(out, np.float32).flatten()
-
-
-def check_audio_duration(file):
- try:
- file = file.strip(" ").strip('"').strip("\n").strip('"').strip(" ")
-
- probe = ffmpeg.probe(file)
-
- duration = float(probe['streams'][0]['duration'])
-
- if duration < 0.76:
- print(
- f"\n------------\n"
- f"Audio file, {file.split('/')[-1]}, under ~0.76s detected - file is too short. Target at least 1-2s for best results."
- f"\n------------\n\n"
- )
- return False
-
- return True
- except Exception as e:
- raise RuntimeError(f"Failed to check audio duration: {e}")
\ No newline at end of file
diff --git a/spaces/Gen-Sim/Gen-Sim/cliport/generated_tasks/cylinder_stand_alignment.py b/spaces/Gen-Sim/Gen-Sim/cliport/generated_tasks/cylinder_stand_alignment.py
deleted file mode 100644
index 7c5bda6db24e6541249a47894f7c3ae6d17a0df1..0000000000000000000000000000000000000000
--- a/spaces/Gen-Sim/Gen-Sim/cliport/generated_tasks/cylinder_stand_alignment.py
+++ /dev/null
@@ -1,58 +0,0 @@
-import numpy as np
-import os
-import pybullet as p
-import random
-from cliport.tasks import primitives
-from cliport.tasks.grippers import Spatula
-from cliport.tasks.task import Task
-from cliport.utils import utils
-import numpy as np
-from cliport.tasks.task import Task
-from cliport.utils import utils
-
-class CylinderStandAlignment(Task):
- """Arrange four colored cylinders (red, blue, green, yellow) in order of their colors on four stands of matching color."""
-
- def __init__(self):
- super().__init__()
- self.max_steps = 20
- self.lang_template = "Arrange the {color} cylinder on the {color} stand"
- self.task_completed_desc = "done arranging cylinders."
- self.additional_reset()
-
- def reset(self, env):
- super().reset(env)
-
- # Define colors and corresponding names
- colors = [utils.COLORS['red'], utils.COLORS['blue'], utils.COLORS['green'], utils.COLORS['yellow']]
- color_names = ['red', 'blue', 'green', 'yellow']
-
- # Add cylinders.
- # x, y, z dimensions for the asset size
- cylinder_size = (0.04, 0.04, 0.04)
- cylinder_urdf = 'cylinder/cylinder-template.urdf'
- cylinders = []
- for i in range(4):
- cylinder_pose = self.get_random_pose(env, cylinder_size)
- replace = {'DIM': cylinder_size, 'HALF': (cylinder_size[0] / 2, cylinder_size[1] / 2, cylinder_size[2] / 2),
- 'COLOR': colors[i]}
- # IMPORTANT: REPLACE THE TEMPLATE URDF
- urdf = self.fill_template(cylinder_urdf, replace)
- cylinder_id = env.add_object(urdf, cylinder_pose)
- cylinders.append(cylinder_id)
-
- # Add stands.
- # x, y, z dimensions for the asset size
- stand_size = (0.05, 0.05, 0.005)
- stand_urdf = 'stacking/stand.urdf'
- stands = []
- for i in range(4):
- stand_pose = self.get_random_pose(env, stand_size)
- env.add_object(stand_urdf, stand_pose, color=colors[i], category='fixed')
- stands.append(stand_pose)
-
- # Goal: each cylinder is on a stand of the same color.
- for i in range(4):
- self.add_goal(objs=[cylinders[i]], matches=np.ones((1, 1)), targ_poses=[stands[i]], replace=False,
- rotations=True, metric='pose', params=None, step_max_reward=1 / 4,
- language_goal=self.lang_template.format(color=color_names[i]))
\ No newline at end of file
diff --git a/spaces/Gradio-Blocks/uniformer_image_detection/mmdet/core/mask/mask_target.py b/spaces/Gradio-Blocks/uniformer_image_detection/mmdet/core/mask/mask_target.py
deleted file mode 100644
index 15d26a88bbf3710bd92813335918407db8c4e053..0000000000000000000000000000000000000000
--- a/spaces/Gradio-Blocks/uniformer_image_detection/mmdet/core/mask/mask_target.py
+++ /dev/null
@@ -1,122 +0,0 @@
-import numpy as np
-import torch
-from torch.nn.modules.utils import _pair
-
-
-def mask_target(pos_proposals_list, pos_assigned_gt_inds_list, gt_masks_list,
- cfg):
- """Compute mask target for positive proposals in multiple images.
-
- Args:
- pos_proposals_list (list[Tensor]): Positive proposals in multiple
- images.
- pos_assigned_gt_inds_list (list[Tensor]): Assigned GT indices for each
- positive proposals.
- gt_masks_list (list[:obj:`BaseInstanceMasks`]): Ground truth masks of
- each image.
- cfg (dict): Config dict that specifies the mask size.
-
- Returns:
- list[Tensor]: Mask target of each image.
-
- Example:
- >>> import mmcv
- >>> import mmdet
- >>> from mmdet.core.mask import BitmapMasks
- >>> from mmdet.core.mask.mask_target import *
- >>> H, W = 17, 18
- >>> cfg = mmcv.Config({'mask_size': (13, 14)})
- >>> rng = np.random.RandomState(0)
- >>> # Positive proposals (tl_x, tl_y, br_x, br_y) for each image
- >>> pos_proposals_list = [
- >>> torch.Tensor([
- >>> [ 7.2425, 5.5929, 13.9414, 14.9541],
- >>> [ 7.3241, 3.6170, 16.3850, 15.3102],
- >>> ]),
- >>> torch.Tensor([
- >>> [ 4.8448, 6.4010, 7.0314, 9.7681],
- >>> [ 5.9790, 2.6989, 7.4416, 4.8580],
- >>> [ 0.0000, 0.0000, 0.1398, 9.8232],
- >>> ]),
- >>> ]
- >>> # Corresponding class index for each proposal for each image
- >>> pos_assigned_gt_inds_list = [
- >>> torch.LongTensor([7, 0]),
- >>> torch.LongTensor([5, 4, 1]),
- >>> ]
- >>> # Ground truth mask for each true object for each image
- >>> gt_masks_list = [
- >>> BitmapMasks(rng.rand(8, H, W), height=H, width=W),
- >>> BitmapMasks(rng.rand(6, H, W), height=H, width=W),
- >>> ]
- >>> mask_targets = mask_target(
- >>> pos_proposals_list, pos_assigned_gt_inds_list,
- >>> gt_masks_list, cfg)
- >>> assert mask_targets.shape == (5,) + cfg['mask_size']
- """
- cfg_list = [cfg for _ in range(len(pos_proposals_list))]
- mask_targets = map(mask_target_single, pos_proposals_list,
- pos_assigned_gt_inds_list, gt_masks_list, cfg_list)
- mask_targets = list(mask_targets)
- if len(mask_targets) > 0:
- mask_targets = torch.cat(mask_targets)
- return mask_targets
-
-
-def mask_target_single(pos_proposals, pos_assigned_gt_inds, gt_masks, cfg):
- """Compute mask target for each positive proposal in the image.
-
- Args:
- pos_proposals (Tensor): Positive proposals.
- pos_assigned_gt_inds (Tensor): Assigned GT inds of positive proposals.
- gt_masks (:obj:`BaseInstanceMasks`): GT masks in the format of Bitmap
- or Polygon.
- cfg (dict): Config dict that indicate the mask size.
-
- Returns:
- Tensor: Mask target of each positive proposals in the image.
-
- Example:
- >>> import mmcv
- >>> import mmdet
- >>> from mmdet.core.mask import BitmapMasks
- >>> from mmdet.core.mask.mask_target import * # NOQA
- >>> H, W = 32, 32
- >>> cfg = mmcv.Config({'mask_size': (7, 11)})
- >>> rng = np.random.RandomState(0)
- >>> # Masks for each ground truth box (relative to the image)
- >>> gt_masks_data = rng.rand(3, H, W)
- >>> gt_masks = BitmapMasks(gt_masks_data, height=H, width=W)
- >>> # Predicted positive boxes in one image
- >>> pos_proposals = torch.FloatTensor([
- >>> [ 16.2, 5.5, 19.9, 20.9],
- >>> [ 17.3, 13.6, 19.3, 19.3],
- >>> [ 14.8, 16.4, 17.0, 23.7],
- >>> [ 0.0, 0.0, 16.0, 16.0],
- >>> [ 4.0, 0.0, 20.0, 16.0],
- >>> ])
- >>> # For each predicted proposal, its assignment to a gt mask
- >>> pos_assigned_gt_inds = torch.LongTensor([0, 1, 2, 1, 1])
- >>> mask_targets = mask_target_single(
- >>> pos_proposals, pos_assigned_gt_inds, gt_masks, cfg)
- >>> assert mask_targets.shape == (5,) + cfg['mask_size']
- """
- device = pos_proposals.device
- mask_size = _pair(cfg.mask_size)
- num_pos = pos_proposals.size(0)
- if num_pos > 0:
- proposals_np = pos_proposals.cpu().numpy()
- maxh, maxw = gt_masks.height, gt_masks.width
- proposals_np[:, [0, 2]] = np.clip(proposals_np[:, [0, 2]], 0, maxw)
- proposals_np[:, [1, 3]] = np.clip(proposals_np[:, [1, 3]], 0, maxh)
- pos_assigned_gt_inds = pos_assigned_gt_inds.cpu().numpy()
-
- mask_targets = gt_masks.crop_and_resize(
- proposals_np, mask_size, device=device,
- inds=pos_assigned_gt_inds).to_ndarray()
-
- mask_targets = torch.from_numpy(mask_targets).float().to(device)
- else:
- mask_targets = pos_proposals.new_zeros((0, ) + mask_size)
-
- return mask_targets
diff --git a/spaces/Hakim571/Food-Classification/app.py b/spaces/Hakim571/Food-Classification/app.py
deleted file mode 100644
index 25892462aa1a926e359404b844c0ab0d8c36ba41..0000000000000000000000000000000000000000
--- a/spaces/Hakim571/Food-Classification/app.py
+++ /dev/null
@@ -1,22 +0,0 @@
-import tensorflow as tf
-from tensorflow.keras.utils import load_img, img_to_array
-import numpy as np
-import gradio as gr
-
-class_names=['Ayam Goreng','Bakso','Bubur Ayam','Ikan Lele Goreng','Mi Goreng','Nasi','Sate','Soto','Telur dadar','Telur mata sapi','Ikan mujahir goreng','Lontong','Pempek telur','Singkong Goreng','Tempe kedelai murni, goreng']
-
-model=tf.keras.models.load_model('./my_model')
-
-def import_and_predict(image_data):
- x = image_data.reshape((-1, 224, 224, 3))
- x = tf.keras.applications.imagenet_utils.preprocess_input(x, mode="tf")
- prediction = model.predict(x)
- labels=class_names
- confidences = {labels[i]: float(prediction[0][i]) for i in range(15)}
- return confidences
-#test
-gr.Interface(fn=import_and_predict,
- inputs=gr.inputs.Image(shape=(224, 224)),
- outputs=gr.outputs.Label(num_top_classes=3),
- cache_examples=False,
- examples=["Bakso.jpeg", "Sate.jpeg"]).launch()
\ No newline at end of file
diff --git a/spaces/HaloMaster/chinesesummary/fengshen/models/longformer/modeling_longformer.py b/spaces/HaloMaster/chinesesummary/fengshen/models/longformer/modeling_longformer.py
deleted file mode 100644
index 697782a467a212926bba68e8a6791545f3c9f6e2..0000000000000000000000000000000000000000
--- a/spaces/HaloMaster/chinesesummary/fengshen/models/longformer/modeling_longformer.py
+++ /dev/null
@@ -1,2485 +0,0 @@
-# coding=utf-8
-# Copyright 2020 The Allen Institute for AI team and The HuggingFace Inc. team.
-#
-# Licensed under the Apache License, Version 2.0 (the "License");
-# you may not use this file except in compliance with the License.
-# You may obtain a copy of the License at
-#
-# http://www.apache.org/licenses/LICENSE-2.0
-#
-# Unless required by applicable law or agreed to in writing, software
-# distributed under the License is distributed on an "AS IS" BASIS,
-# WITHOUT WARRANTIES OR CONDITIONS OF ANY KIND, either express or implied.
-# See the License for the specific language governing permissions and
-# limitations under the License.
-"""PyTorch Longformer model. """
-
-import math
-from dataclasses import dataclass
-from typing import Optional, Tuple
-from numpy.lib.function_base import kaiser
-
-import torch
-import torch.utils.checkpoint
-from torch import nn
-from torch.nn import BCEWithLogitsLoss, CrossEntropyLoss, MSELoss
-
-from transformers.activations import ACT2FN, gelu
-from transformers.file_utils import (
- ModelOutput,
- add_code_sample_docstrings,
- add_start_docstrings,
- add_start_docstrings_to_model_forward,
- replace_return_docstrings,
-)
-from transformers.modeling_utils import (
- PreTrainedModel,
- apply_chunking_to_forward,
- find_pruneable_heads_and_indices,
- prune_linear_layer,
-)
-from transformers.utils import logging
-from transformers import LongformerConfig
-
-logger = logging.get_logger(__name__)
-
-_CHECKPOINT_FOR_DOC = "allenai/longformer-base-4096"
-_CONFIG_FOR_DOC = "LongformerConfig"
-_TOKENIZER_FOR_DOC = "LongformerTokenizer"
-
-LONGFORMER_PRETRAINED_MODEL_ARCHIVE_LIST = [
- "allenai/longformer-base-4096",
- "allenai/longformer-large-4096",
- "allenai/longformer-large-4096-finetuned-triviaqa",
- "allenai/longformer-base-4096-extra.pos.embd.only",
- "allenai/longformer-large-4096-extra.pos.embd.only",
- # See all Longformer models at https://huggingface.co/models?filter=longformer
-]
-
-
-@dataclass
-class LongformerBaseModelOutput(ModelOutput):
- """
- Base class for Longformer's outputs, with potential hidden states, local and global attentions.
-
- Args:
- last_hidden_state (:obj:`torch.FloatTensor` of shape :obj:`(batch_size, sequence_length, hidden_size)`):
- Sequence of hidden-states at the output of the last layer of the model.
- hidden_states (:obj:`tuple(torch.FloatTensor)`, `optional`, returned when ``output_hidden_states=True`` is passed or when ``config.output_hidden_states=True``):
- Tuple of :obj:`torch.FloatTensor` (one for the output of the embeddings + one for the output of each layer)
- of shape :obj:`(batch_size, sequence_length, hidden_size)`.
-
- Hidden-states of the model at the output of each layer plus the initial embedding outputs.
- attentions (:obj:`tuple(torch.FloatTensor)`, `optional`, returned when ``output_attentions=True`` is passed or when ``config.output_attentions=True``):
- Tuple of :obj:`torch.FloatTensor` (one for each layer) of shape :obj:`(batch_size, num_heads,
- sequence_length, x + attention_window + 1)`, where ``x`` is the number of tokens with global attention
- mask.
-
- Local attentions weights after the attention softmax, used to compute the weighted average in the
- self-attention heads. Those are the attention weights from every token in the sequence to every token with
- global attention (first ``x`` values) and to every token in the attention window (remaining
- ``attention_window + 1`` values). Note that the first ``x`` values refer to tokens with fixed positions in
- the text, but the remaining ``attention_window + 1`` values refer to tokens with relative positions: the
- attention weight of a token to itself is located at index ``x + attention_window / 2`` and the
- ``attention_window / 2`` preceding (succeeding) values are the attention weights to the ``attention_window
- / 2`` preceding (succeeding) tokens. If the attention window contains a token with global attention, the
- attention weight at the corresponding index is set to 0; the value should be accessed from the first ``x``
- attention weights. If a token has global attention, the attention weights to all other tokens in
- :obj:`attentions` is set to 0, the values should be accessed from :obj:`global_attentions`.
- global_attentions (:obj:`tuple(torch.FloatTensor)`, `optional`, returned when ``output_attentions=True`` is passed or when ``config.output_attentions=True``):
- Tuple of :obj:`torch.FloatTensor` (one for each layer) of shape :obj:`(batch_size, num_heads,
- sequence_length, x)`, where ``x`` is the number of tokens with global attention mask.
-
- Global attentions weights after the attention softmax, used to compute the weighted average in the
- self-attention heads. Those are the attention weights from every token with global attention to every token
- in the sequence.
- """
-
- last_hidden_state: torch.FloatTensor
- hidden_states: Optional[Tuple[torch.FloatTensor]] = None
- attentions: Optional[Tuple[torch.FloatTensor]] = None
- global_attentions: Optional[Tuple[torch.FloatTensor]] = None
-
-
-@dataclass
-class LongformerBaseModelOutputWithPooling(ModelOutput):
- """
- Base class for Longformer's outputs that also contains a pooling of the last hidden states.
-
- Args:
- last_hidden_state (:obj:`torch.FloatTensor` of shape :obj:`(batch_size, sequence_length, hidden_size)`):
- Sequence of hidden-states at the output of the last layer of the model.
- pooler_output (:obj:`torch.FloatTensor` of shape :obj:`(batch_size, hidden_size)`):
- Last layer hidden-state of the first token of the sequence (classification token) further processed by a
- Linear layer and a Tanh activation function. The Linear layer weights are trained from the next sentence
- prediction (classification) objective during pretraining.
- hidden_states (:obj:`tuple(torch.FloatTensor)`, `optional`, returned when ``output_hidden_states=True`` is passed or when ``config.output_hidden_states=True``):
- Tuple of :obj:`torch.FloatTensor` (one for the output of the embeddings + one for the output of each layer)
- of shape :obj:`(batch_size, sequence_length, hidden_size)`.
-
- Hidden-states of the model at the output of each layer plus the initial embedding outputs.
- attentions (:obj:`tuple(torch.FloatTensor)`, `optional`, returned when ``output_attentions=True`` is passed or when ``config.output_attentions=True``):
- Tuple of :obj:`torch.FloatTensor` (one for each layer) of shape :obj:`(batch_size, num_heads,
- sequence_length, x + attention_window + 1)`, where ``x`` is the number of tokens with global attention
- mask.
-
- Local attentions weights after the attention softmax, used to compute the weighted average in the
- self-attention heads. Those are the attention weights from every token in the sequence to every token with
- global attention (first ``x`` values) and to every token in the attention window (remaining
- ``attention_window + 1`` values). Note that the first ``x`` values refer to tokens with fixed positions in
- the text, but the remaining ``attention_window + 1`` values refer to tokens with relative positions: the
- attention weight of a token to itself is located at index ``x + attention_window / 2`` and the
- ``attention_window / 2`` preceding (succeeding) values are the attention weights to the ``attention_window
- / 2`` preceding (succeeding) tokens. If the attention window contains a token with global attention, the
- attention weight at the corresponding index is set to 0; the value should be accessed from the first ``x``
- attention weights. If a token has global attention, the attention weights to all other tokens in
- :obj:`attentions` is set to 0, the values should be accessed from :obj:`global_attentions`.
- global_attentions (:obj:`tuple(torch.FloatTensor)`, `optional`, returned when ``output_attentions=True`` is passed or when ``config.output_attentions=True``):
- Tuple of :obj:`torch.FloatTensor` (one for each layer) of shape :obj:`(batch_size, num_heads,
- sequence_length, x)`, where ``x`` is the number of tokens with global attention mask.
-
- Global attentions weights after the attention softmax, used to compute the weighted average in the
- self-attention heads. Those are the attention weights from every token with global attention to every token
- in the sequence.
- """
-
- last_hidden_state: torch.FloatTensor
- pooler_output: torch.FloatTensor = None
- hidden_states: Optional[Tuple[torch.FloatTensor]] = None
- attentions: Optional[Tuple[torch.FloatTensor]] = None
- global_attentions: Optional[Tuple[torch.FloatTensor]] = None
-
-
-@dataclass
-class LongformerMaskedLMOutput(ModelOutput):
- """
- Base class for masked language models outputs.
-
- Args:
- loss (:obj:`torch.FloatTensor` of shape :obj:`(1,)`, `optional`, returned when :obj:`labels` is provided):
- Masked language modeling (MLM) loss.
- logits (:obj:`torch.FloatTensor` of shape :obj:`(batch_size, sequence_length, config.vocab_size)`):
- Prediction scores of the language modeling head (scores for each vocabulary token before SoftMax).
- hidden_states (:obj:`tuple(torch.FloatTensor)`, `optional`, returned when ``output_hidden_states=True`` is passed or when ``config.output_hidden_states=True``):
- Tuple of :obj:`torch.FloatTensor` (one for the output of the embeddings + one for the output of each layer)
- of shape :obj:`(batch_size, sequence_length, hidden_size)`.
-
- Hidden-states of the model at the output of each layer plus the initial embedding outputs.
- attentions (:obj:`tuple(torch.FloatTensor)`, `optional`, returned when ``output_attentions=True`` is passed or when ``config.output_attentions=True``):
- Tuple of :obj:`torch.FloatTensor` (one for each layer) of shape :obj:`(batch_size, num_heads,
- sequence_length, x + attention_window + 1)`, where ``x`` is the number of tokens with global attention
- mask.
-
- Local attentions weights after the attention softmax, used to compute the weighted average in the
- self-attention heads. Those are the attention weights from every token in the sequence to every token with
- global attention (first ``x`` values) and to every token in the attention window (remaining
- ``attention_window + 1`` values). Note that the first ``x`` values refer to tokens with fixed positions in
- the text, but the remaining ``attention_window + 1`` values refer to tokens with relative positions: the
- attention weight of a token to itself is located at index ``x + attention_window / 2`` and the
- ``attention_window / 2`` preceding (succeeding) values are the attention weights to the ``attention_window
- / 2`` preceding (succeeding) tokens. If the attention window contains a token with global attention, the
- attention weight at the corresponding index is set to 0; the value should be accessed from the first ``x``
- attention weights. If a token has global attention, the attention weights to all other tokens in
- :obj:`attentions` is set to 0, the values should be accessed from :obj:`global_attentions`.
- global_attentions (:obj:`tuple(torch.FloatTensor)`, `optional`, returned when ``output_attentions=True`` is passed or when ``config.output_attentions=True``):
- Tuple of :obj:`torch.FloatTensor` (one for each layer) of shape :obj:`(batch_size, num_heads,
- sequence_length, x)`, where ``x`` is the number of tokens with global attention mask.
-
- Global attentions weights after the attention softmax, used to compute the weighted average in the
- self-attention heads. Those are the attention weights from every token with global attention to every token
- in the sequence.
- """
-
- loss: Optional[torch.FloatTensor] = None
- logits: torch.FloatTensor = None
- hidden_states: Optional[Tuple[torch.FloatTensor]] = None
- attentions: Optional[Tuple[torch.FloatTensor]] = None
- global_attentions: Optional[Tuple[torch.FloatTensor]] = None
-
-
-@dataclass
-class LongformerQuestionAnsweringModelOutput(ModelOutput):
- """
- Base class for outputs of question answering Longformer models.
-
- Args:
- loss (:obj:`torch.FloatTensor` of shape :obj:`(1,)`, `optional`, returned when :obj:`labels` is provided):
- Total span extraction loss is the sum of a Cross-Entropy for the start and end positions.
- start_logits (:obj:`torch.FloatTensor` of shape :obj:`(batch_size, sequence_length)`):
- Span-start scores (before SoftMax).
- end_logits (:obj:`torch.FloatTensor` of shape :obj:`(batch_size, sequence_length)`):
- Span-end scores (before SoftMax).
- hidden_states (:obj:`tuple(torch.FloatTensor)`, `optional`, returned when ``output_hidden_states=True`` is passed or when ``config.output_hidden_states=True``):
- Tuple of :obj:`torch.FloatTensor` (one for the output of the embeddings + one for the output of each layer)
- of shape :obj:`(batch_size, sequence_length, hidden_size)`.
-
- Hidden-states of the model at the output of each layer plus the initial embedding outputs.
- attentions (:obj:`tuple(torch.FloatTensor)`, `optional`, returned when ``output_attentions=True`` is passed or when ``config.output_attentions=True``):
- Tuple of :obj:`torch.FloatTensor` (one for each layer) of shape :obj:`(batch_size, num_heads,
- sequence_length, x + attention_window + 1)`, where ``x`` is the number of tokens with global attention
- mask.
-
- Local attentions weights after the attention softmax, used to compute the weighted average in the
- self-attention heads. Those are the attention weights from every token in the sequence to every token with
- global attention (first ``x`` values) and to every token in the attention window (remaining
- ``attention_window + 1`` values). Note that the first ``x`` values refer to tokens with fixed positions in
- the text, but the remaining ``attention_window + 1`` values refer to tokens with relative positions: the
- attention weight of a token to itself is located at index ``x + attention_window / 2`` and the
- ``attention_window / 2`` preceding (succeeding) values are the attention weights to the ``attention_window
- / 2`` preceding (succeeding) tokens. If the attention window contains a token with global attention, the
- attention weight at the corresponding index is set to 0; the value should be accessed from the first ``x``
- attention weights. If a token has global attention, the attention weights to all other tokens in
- :obj:`attentions` is set to 0, the values should be accessed from :obj:`global_attentions`.
- global_attentions (:obj:`tuple(torch.FloatTensor)`, `optional`, returned when ``output_attentions=True`` is passed or when ``config.output_attentions=True``):
- Tuple of :obj:`torch.FloatTensor` (one for each layer) of shape :obj:`(batch_size, num_heads,
- sequence_length, x)`, where ``x`` is the number of tokens with global attention mask.
-
- Global attentions weights after the attention softmax, used to compute the weighted average in the
- self-attention heads. Those are the attention weights from every token with global attention to every token
- in the sequence.
- """
-
- loss: Optional[torch.FloatTensor] = None
- start_logits: torch.FloatTensor = None
- end_logits: torch.FloatTensor = None
- hidden_states: Optional[Tuple[torch.FloatTensor]] = None
- attentions: Optional[Tuple[torch.FloatTensor]] = None
- global_attentions: Optional[Tuple[torch.FloatTensor]] = None
-
-
-@dataclass
-class LongformerSequenceClassifierOutput(ModelOutput):
- """
- Base class for outputs of sentence classification models.
-
- Args:
- loss (:obj:`torch.FloatTensor` of shape :obj:`(1,)`, `optional`, returned when :obj:`labels` is provided):
- Classification (or regression if config.num_labels==1) loss.
- logits (:obj:`torch.FloatTensor` of shape :obj:`(batch_size, config.num_labels)`):
- Classification (or regression if config.num_labels==1) scores (before SoftMax).
- hidden_states (:obj:`tuple(torch.FloatTensor)`, `optional`, returned when ``output_hidden_states=True`` is passed or when ``config.output_hidden_states=True``):
- Tuple of :obj:`torch.FloatTensor` (one for the output of the embeddings + one for the output of each layer)
- of shape :obj:`(batch_size, sequence_length, hidden_size)`.
-
- Hidden-states of the model at the output of each layer plus the initial embedding outputs.
- attentions (:obj:`tuple(torch.FloatTensor)`, `optional`, returned when ``output_attentions=True`` is passed or when ``config.output_attentions=True``):
- Tuple of :obj:`torch.FloatTensor` (one for each layer) of shape :obj:`(batch_size, num_heads,
- sequence_length, x + attention_window + 1)`, where ``x`` is the number of tokens with global attention
- mask.
-
- Local attentions weights after the attention softmax, used to compute the weighted average in the
- self-attention heads. Those are the attention weights from every token in the sequence to every token with
- global attention (first ``x`` values) and to every token in the attention window (remaining
- ``attention_window + 1`` values). Note that the first ``x`` values refer to tokens with fixed positions in
- the text, but the remaining ``attention_window + 1`` values refer to tokens with relative positions: the
- attention weight of a token to itself is located at index ``x + attention_window / 2`` and the
- ``attention_window / 2`` preceding (succeeding) values are the attention weights to the ``attention_window
- / 2`` preceding (succeeding) tokens. If the attention window contains a token with global attention, the
- attention weight at the corresponding index is set to 0; the value should be accessed from the first ``x``
- attention weights. If a token has global attention, the attention weights to all other tokens in
- :obj:`attentions` is set to 0, the values should be accessed from :obj:`global_attentions`.
- global_attentions (:obj:`tuple(torch.FloatTensor)`, `optional`, returned when ``output_attentions=True`` is passed or when ``config.output_attentions=True``):
- Tuple of :obj:`torch.FloatTensor` (one for each layer) of shape :obj:`(batch_size, num_heads,
- sequence_length, x)`, where ``x`` is the number of tokens with global attention mask.
-
- Global attentions weights after the attention softmax, used to compute the weighted average in the
- self-attention heads. Those are the attention weights from every token with global attention to every token
- in the sequence.
- """
-
- loss: Optional[torch.FloatTensor] = None
- logits: torch.FloatTensor = None
- hidden_states: Optional[Tuple[torch.FloatTensor]] = None
- attentions: Optional[Tuple[torch.FloatTensor]] = None
- global_attentions: Optional[Tuple[torch.FloatTensor]] = None
-
-
-@dataclass
-class LongformerMultipleChoiceModelOutput(ModelOutput):
- """
- Base class for outputs of multiple choice Longformer models.
-
- Args:
- loss (:obj:`torch.FloatTensor` of shape `(1,)`, `optional`, returned when :obj:`labels` is provided):
- Classification loss.
- logits (:obj:`torch.FloatTensor` of shape :obj:`(batch_size, num_choices)`):
- `num_choices` is the second dimension of the input tensors. (see `input_ids` above).
-
- Classification scores (before SoftMax).
- hidden_states (:obj:`tuple(torch.FloatTensor)`, `optional`, returned when ``output_hidden_states=True`` is passed or when ``config.output_hidden_states=True``):
- Tuple of :obj:`torch.FloatTensor` (one for the output of the embeddings + one for the output of each layer)
- of shape :obj:`(batch_size, sequence_length, hidden_size)`.
-
- Hidden-states of the model at the output of each layer plus the initial embedding outputs.
- attentions (:obj:`tuple(torch.FloatTensor)`, `optional`, returned when ``output_attentions=True`` is passed or when ``config.output_attentions=True``):
- Tuple of :obj:`torch.FloatTensor` (one for each layer) of shape :obj:`(batch_size, num_heads,
- sequence_length, x + attention_window + 1)`, where ``x`` is the number of tokens with global attention
- mask.
-
- Local attentions weights after the attention softmax, used to compute the weighted average in the
- self-attention heads. Those are the attention weights from every token in the sequence to every token with
- global attention (first ``x`` values) and to every token in the attention window (remaining
- ``attention_window + 1`` values). Note that the first ``x`` values refer to tokens with fixed positions in
- the text, but the remaining ``attention_window + 1`` values refer to tokens with relative positions: the
- attention weight of a token to itself is located at index ``x + attention_window / 2`` and the
- ``attention_window / 2`` preceding (succeeding) values are the attention weights to the ``attention_window
- / 2`` preceding (succeeding) tokens. If the attention window contains a token with global attention, the
- attention weight at the corresponding index is set to 0; the value should be accessed from the first ``x``
- attention weights. If a token has global attention, the attention weights to all other tokens in
- :obj:`attentions` is set to 0, the values should be accessed from :obj:`global_attentions`.
- global_attentions (:obj:`tuple(torch.FloatTensor)`, `optional`, returned when ``output_attentions=True`` is passed or when ``config.output_attentions=True``):
- Tuple of :obj:`torch.FloatTensor` (one for each layer) of shape :obj:`(batch_size, num_heads,
- sequence_length, x)`, where ``x`` is the number of tokens with global attention mask.
-
- Global attentions weights after the attention softmax, used to compute the weighted average in the
- self-attention heads. Those are the attention weights from every token with global attention to every token
- in the sequence.
- """
-
- loss: Optional[torch.FloatTensor] = None
- logits: torch.FloatTensor = None
- hidden_states: Optional[Tuple[torch.FloatTensor]] = None
- attentions: Optional[Tuple[torch.FloatTensor]] = None
- global_attentions: Optional[Tuple[torch.FloatTensor]] = None
-
-
-@dataclass
-class LongformerTokenClassifierOutput(ModelOutput):
- """
- Base class for outputs of token classification models.
-
- Args:
- loss (:obj:`torch.FloatTensor` of shape :obj:`(1,)`, `optional`, returned when ``labels`` is provided) :
- Classification loss.
- logits (:obj:`torch.FloatTensor` of shape :obj:`(batch_size, sequence_length, config.num_labels)`):
- Classification scores (before SoftMax).
- hidden_states (:obj:`tuple(torch.FloatTensor)`, `optional`, returned when ``output_hidden_states=True`` is passed or when ``config.output_hidden_states=True``):
- Tuple of :obj:`torch.FloatTensor` (one for the output of the embeddings + one for the output of each layer)
- of shape :obj:`(batch_size, sequence_length, hidden_size)`.
-
- Hidden-states of the model at the output of each layer plus the initial embedding outputs.
- attentions (:obj:`tuple(torch.FloatTensor)`, `optional`, returned when ``output_attentions=True`` is passed or when ``config.output_attentions=True``):
- Tuple of :obj:`torch.FloatTensor` (one for each layer) of shape :obj:`(batch_size, num_heads,
- sequence_length, x + attention_window + 1)`, where ``x`` is the number of tokens with global attention
- mask.
-
- Local attentions weights after the attention softmax, used to compute the weighted average in the
- self-attention heads. Those are the attention weights from every token in the sequence to every token with
- global attention (first ``x`` values) and to every token in the attention window (remaining
- ``attention_window + 1`` values). Note that the first ``x`` values refer to tokens with fixed positions in
- the text, but the remaining ``attention_window + 1`` values refer to tokens with relative positions: the
- attention weight of a token to itself is located at index ``x + attention_window / 2`` and the
- ``attention_window / 2`` preceding (succeeding) values are the attention weights to the ``attention_window
- / 2`` preceding (succeeding) tokens. If the attention window contains a token with global attention, the
- attention weight at the corresponding index is set to 0; the value should be accessed from the first ``x``
- attention weights. If a token has global attention, the attention weights to all other tokens in
- :obj:`attentions` is set to 0, the values should be accessed from :obj:`global_attentions`.
- global_attentions (:obj:`tuple(torch.FloatTensor)`, `optional`, returned when ``output_attentions=True`` is passed or when ``config.output_attentions=True``):
- Tuple of :obj:`torch.FloatTensor` (one for each layer) of shape :obj:`(batch_size, num_heads,
- sequence_length, x)`, where ``x`` is the number of tokens with global attention mask.
-
- Global attentions weights after the attention softmax, used to compute the weighted average in the
- self-attention heads. Those are the attention weights from every token with global attention to every token
- in the sequence.
- """
-
- loss: Optional[torch.FloatTensor] = None
- logits: torch.FloatTensor = None
- hidden_states: Optional[Tuple[torch.FloatTensor]] = None
- attentions: Optional[Tuple[torch.FloatTensor]] = None
- global_attentions: Optional[Tuple[torch.FloatTensor]] = None
-
-
-def _get_question_end_index(input_ids, sep_token_id):
- """
- Computes the index of the first occurrence of `sep_token_id`.
- """
-
- sep_token_indices = (input_ids == sep_token_id).nonzero()
- batch_size = input_ids.shape[0]
-
- assert sep_token_indices.shape[1] == 2, "`input_ids` should have two dimensions"
- assert (
- sep_token_indices.shape[0] == 3 * batch_size
- ), f"There should be exactly three separator tokens: {sep_token_id} in every sample for questions answering. You might also consider to set `global_attention_mask` manually in the forward function to avoid this error."
- return sep_token_indices.view(batch_size, 3, 2)[:, 0, 1]
-
-
-def _compute_global_attention_mask(input_ids, sep_token_id, before_sep_token=True):
- """
- Computes global attention mask by putting attention on all tokens before `sep_token_id` if `before_sep_token is
- True` else after `sep_token_id`.
- """
- question_end_index = _get_question_end_index(input_ids, sep_token_id)
- question_end_index = question_end_index.unsqueeze(
- dim=1) # size: batch_size x 1
- # bool attention mask with True in locations of global attention
- attention_mask = torch.arange(input_ids.shape[1], device=input_ids.device)
- if before_sep_token is True:
- attention_mask = (attention_mask.expand_as(input_ids)
- < question_end_index).to(torch.uint8)
- else:
- # last token is separation token and should not be counted and in the middle are two separation tokens
- attention_mask = (attention_mask.expand_as(input_ids) > (question_end_index + 1)).to(torch.uint8) * (
- attention_mask.expand_as(input_ids) < input_ids.shape[-1]
- ).to(torch.uint8)
-
- return attention_mask
-
-
-def create_position_ids_from_input_ids(input_ids, padding_idx):
- """
- Replace non-padding symbols with their position numbers. Position numbers begin at padding_idx+1. Padding symbols
- are ignored. This is modified from fairseq's `utils.make_positions`.
-
- Args:
- x: torch.Tensor x:
-
- Returns: torch.Tensor
- """
- # The series of casts and type-conversions here are carefully balanced to both work with ONNX export and XLA.
- mask = input_ids.ne(padding_idx).int()
- incremental_indices = torch.cumsum(mask, dim=1).type_as(mask) * mask
- return incremental_indices.long() + padding_idx
-
-
-class LongformerEmbeddings(nn.Module):
- """
- Same as BertEmbeddings with a tiny tweak for positional embeddings indexing.
- """
-
- def __init__(self, config):
- super().__init__()
- self.word_embeddings = nn.Embedding(
- config.vocab_size, config.hidden_size, padding_idx=config.pad_token_id)
- self.position_embeddings = nn.Embedding(
- config.max_position_embeddings, config.hidden_size)
- self.token_type_embeddings = nn.Embedding(
- config.type_vocab_size, config.hidden_size)
-
- # self.LayerNorm is not snake-cased to stick with TensorFlow model variable name and be able to load
- # any TensorFlow checkpoint file
- self.LayerNorm = nn.LayerNorm(
- config.hidden_size, eps=config.layer_norm_eps)
- self.dropout = nn.Dropout(config.hidden_dropout_prob)
-
- # position_ids (1, len position emb) is contiguous in memory and exported when serialized
-
- # Modify
- # self.register_buffer("position_ids", torch.arange(config.max_position_embeddings).expand((1, -1)))
- # self.position_embedding_type = getattr(config, "position_embedding_type", "absolute")
-
- # self.padding_idx = config.pad_token_id
- # self.position_embeddings = nn.Embedding(
- # config.max_position_embeddings, config.hidden_size, padding_idx=self.padding_idx
- # )
-
- def forward(self, input_ids=None, token_type_ids=None, position_ids=None, inputs_embeds=None):
-
- # if position_ids is None:
- # if input_ids is not None:
- # # Create the position ids from the input token ids. Any padded tokens remain padded.
- # position_ids = create_position_ids_from_input_ids(input_ids, self.padding_idx).to(input_ids.device)
- # else:
- # position_ids = self.create_position_ids_from_inputs_embeds(inputs_embeds)
-
- if input_ids is not None:
- input_shape = input_ids.size()
- else:
- input_shape = inputs_embeds.size()[:-1]
-
- seq_length = input_shape[1]
-
- # if position_ids is None:
- # position_ids = self.position_ids[:, :seq_length]
-
- if token_type_ids is None:
- token_type_ids = torch.zeros(
- input_shape, dtype=torch.long, device=self.position_ids.device)
-
- if inputs_embeds is None:
- inputs_embeds = self.word_embeddings(input_ids)
-
- # Modify
- # position_embeddings = self.position_embeddings(position_ids)
-
- token_type_embeddings = self.token_type_embeddings(token_type_ids)
-
- embeddings = inputs_embeds + token_type_embeddings
- embeddings = self.LayerNorm(embeddings)
- embeddings = self.dropout(embeddings)
- return embeddings
-
- def create_position_ids_from_inputs_embeds(self, inputs_embeds):
- """
- We are provided embeddings directly. We cannot infer which are padded so just generate sequential position ids.
-
- Args:
- inputs_embeds: torch.Tensor inputs_embeds:
-
- Returns: torch.Tensor
- """
- input_shape = inputs_embeds.size()[:-1]
- sequence_length = input_shape[1]
-
- position_ids = torch.arange(
- self.padding_idx + 1, sequence_length + self.padding_idx + 1, dtype=torch.long, device=inputs_embeds.device
- )
- return position_ids.unsqueeze(0).expand(input_shape)
-
-
-class RoPEmbedding(nn.Module):
- def __init__(self, d_model):
- super(RoPEmbedding, self).__init__()
- self.d_model = d_model
- div_term = torch.exp(torch.arange(
- 0, d_model, 2).float() * (-math.log(10000.0) / d_model))
- self.register_buffer('div_term', div_term)
-
- def forward(self, x, seq_dim=0):
- x = x # [seq_len,num_head,batch_size,per_head_hidden_size]
- t = torch.arange(x.size(seq_dim), device=x.device).type_as(
- self.div_term)
- sinusoid_inp = torch.outer(t, self.div_term)
- sin, cos = sinusoid_inp.sin(), sinusoid_inp.cos() # [s, hn]
- o_shape = (sin.size(0), 1, 1, sin.size(1))
- sin, cos = sin.view(*o_shape), cos.view(*o_shape) # [s, 1, 1, hn]
- sin = torch.repeat_interleave(sin, 2, dim=-1)
- cos = torch.repeat_interleave(cos, 2, dim=-1)
- x2 = torch.stack([-x[..., 1::2], x[..., ::2]], dim=-1).reshape_as(x)
- x = cos * x + sin * x2
- return x
-
-
-class LongformerSelfAttention(nn.Module):
- def __init__(self, config, layer_id):
- super().__init__()
- if config.hidden_size % config.num_attention_heads != 0:
- raise ValueError(
- f"The hidden size ({config.hidden_size}) is not a multiple of the number of attention "
- f"heads ({config.num_attention_heads})"
- )
- self.config = config
- self.num_heads = config.num_attention_heads
- self.head_dim = int(config.hidden_size / config.num_attention_heads)
- self.embed_dim = config.hidden_size
-
- self.query = nn.Linear(config.hidden_size, self.embed_dim)
- self.key = nn.Linear(config.hidden_size, self.embed_dim)
- self.value = nn.Linear(config.hidden_size, self.embed_dim)
-
- # separate projection layers for tokens with global attention
- # self.query_global = nn.Linear(config.hidden_size, self.embed_dim)
- # self.key_global = nn.Linear(config.hidden_size, self.embed_dim)
- # self.value_global = nn.Linear(config.hidden_size, self.embed_dim)
-
- self.dropout = config.attention_probs_dropout_prob
-
- self.layer_id = layer_id
- attention_window = config.attention_window[self.layer_id]
- assert (
- attention_window % 2 == 0
- ), f"`attention_window` for layer {self.layer_id} has to be an even value. Given {attention_window}"
- assert (
- attention_window > 0
- ), f"`attention_window` for layer {self.layer_id} has to be positive. Given {attention_window}"
-
- self.one_sided_attn_window_size = attention_window // 2
- self.rope_emb = RoPEmbedding(self.head_dim)
-
- def forward(
- self,
- hidden_states,
- attention_mask=None,
- layer_head_mask=None,
- is_index_masked=None,
- is_index_global_attn=None,
- is_global_attn=None,
- output_attentions=False,
- ):
- """
- :class:`LongformerSelfAttention` expects `len(hidden_states)` to be multiple of `attention_window`. Padding to
- `attention_window` happens in :meth:`LongformerModel.forward` to avoid redoing the padding on each layer.
-
- The `attention_mask` is changed in :meth:`LongformerModel.forward` from 0, 1, 2 to:
-
- * -10000: no attention
- * 0: local attention
- * +10000: global attention
- """
-
- # print(attention_mask.shape)
- if not self.config.use_sparse_attention: # 如果不使用稀疏attention,则使用标准的attention
- hidden_states = hidden_states.transpose(0, 1)
- # project hidden states
- query_vectors = self.query(hidden_states)
- key_vectors = self.key(hidden_states)
- value_vectors = self.value(hidden_states)
-
- seq_len, batch_size, embed_dim = hidden_states.size()
- assert (
- embed_dim == self.embed_dim
- ), f"hidden_states should have embed_dim = {self.embed_dim}, but has {embed_dim}"
-
- # normalize query
-
- # query_vectors = query_vectors.view(seq_len, batch_size, self.num_heads, self.head_dim).transpose(0, 1)
- # key_vectors = key_vectors.view(seq_len, batch_size, self.num_heads, self.head_dim).transpose(0, 1)
-
- # print('query_vectors',query_vectors.shape)
-
- query_vectors = query_vectors.view(
- seq_len, batch_size, self.num_heads, self.head_dim).transpose(1, 2)
- key_vectors = key_vectors.view(
- seq_len, batch_size, self.num_heads, self.head_dim).transpose(1, 2)
-
- query_vectors = self.rope_emb(query_vectors)
- key_vectors = self.rope_emb(key_vectors)
-
- query_vectors = query_vectors.transpose(0, 2) # [b,mh,s,hd]
- key_vectors = key_vectors.transpose(0, 2).transpose(2, 3)
-
- # print('query_vectors',query_vectors.shape)
-
- query_vectors /= math.sqrt(self.head_dim)
-
- attention_mask = self.get_extended_attention_mask(
- attention_mask, attention_mask.shape, attention_mask.device)
- attn_scores = torch.matmul(
- query_vectors, key_vectors)+attention_mask
-
- attn_scores = torch.nn.functional.softmax(attn_scores, dim=-1)
-
- value_vectors = value_vectors.view(
- seq_len, batch_size, self.num_heads, self.head_dim).transpose(0, 1).transpose(1, 2)
- outputs = torch.matmul(attn_scores, value_vectors).transpose(
- 1, 2).contiguous().view(batch_size, seq_len, self.num_heads*self.head_dim)
-
- # print('output',outputs.shape)
- outputs = (outputs,)
- return outputs+(attn_scores,)
-
- # print('hidden.shape',hidden_states.shape)
- # print('attention_mask.shape',attention_mask.shape)
- # print('att_mask:',attention_mask)
-
- hidden_states = hidden_states.transpose(0, 1)
-
- # project hidden states
- query_vectors = self.query(hidden_states)
- key_vectors = self.key(hidden_states)
- value_vectors = self.value(hidden_states)
-
- seq_len, batch_size, embed_dim = hidden_states.size()
- assert (
- embed_dim == self.embed_dim
- ), f"hidden_states should have embed_dim = {self.embed_dim}, but has {embed_dim}"
-
- # normalize query
-
- # query_vectors = query_vectors.view(seq_len, batch_size, self.num_heads, self.head_dim).transpose(0, 1)
- # key_vectors = key_vectors.view(seq_len, batch_size, self.num_heads, self.head_dim).transpose(0, 1)
-
- query_vectors = query_vectors.view(
- seq_len, batch_size, self.num_heads, self.head_dim).transpose(1, 2)
- key_vectors = key_vectors.view(
- seq_len, batch_size, self.num_heads, self.head_dim).transpose(1, 2)
-
- query_vectors = self.rope_emb(query_vectors)
- key_vectors = self.rope_emb(key_vectors)
-
- query_vectors = query_vectors.transpose(1, 2).transpose(0, 1)
- key_vectors = key_vectors.transpose(1, 2).transpose(0, 1)
-
- query_vectors /= math.sqrt(self.head_dim)
-
- attn_scores = self._sliding_chunks_query_key_matmul(
- query_vectors, key_vectors, self.one_sided_attn_window_size
- )
- # print('att:',attn_scores.shape)
- # values to pad for attention probs
- remove_from_windowed_attention_mask = (
- attention_mask != 0)[:, :, None, None]
-
- # cast to fp32/fp16 then replace 1's with -inf
- float_mask = remove_from_windowed_attention_mask.type_as(query_vectors).masked_fill(
- remove_from_windowed_attention_mask, -10000.0
- )
- # diagonal mask with zeros everywhere and -inf inplace of padding
- diagonal_mask = self._sliding_chunks_query_key_matmul(
- float_mask.new_ones(size=float_mask.size()
- ), float_mask, self.one_sided_attn_window_size
- )
-
- # pad local attention probs
- attn_scores += diagonal_mask
-
- assert list(attn_scores.size()) == [
- batch_size,
- seq_len,
- self.num_heads,
- self.one_sided_attn_window_size * 2 + 1,
- ], f"local_attn_probs should be of size ({batch_size}, {seq_len}, {self.num_heads}, {self.one_sided_attn_window_size * 2 + 1}), but is of size {attn_scores.size()}"
-
- # compute local attention probs from global attention keys and contact over window dim
- if is_global_attn:
- # compute global attn indices required through out forward fn
- (
- max_num_global_attn_indices,
- is_index_global_attn_nonzero,
- is_local_index_global_attn_nonzero,
- is_local_index_no_global_attn_nonzero,
- ) = self._get_global_attn_indices(is_index_global_attn)
- # calculate global attn probs from global key
-
- global_key_attn_scores = self._concat_with_global_key_attn_probs(
- query_vectors=query_vectors,
- key_vectors=key_vectors,
- max_num_global_attn_indices=max_num_global_attn_indices,
- is_index_global_attn_nonzero=is_index_global_attn_nonzero,
- is_local_index_global_attn_nonzero=is_local_index_global_attn_nonzero,
- is_local_index_no_global_attn_nonzero=is_local_index_no_global_attn_nonzero,
- )
- # concat to local_attn_probs
- # (batch_size, seq_len, num_heads, extra attention count + 2*window+1)
- attn_scores = torch.cat(
- (global_key_attn_scores, attn_scores), dim=-1)
-
- # free memory
- del global_key_attn_scores
-
- attn_probs = nn.functional.softmax(
- attn_scores, dim=-1, dtype=torch.float32
- ) # use fp32 for numerical stability
-
- if layer_head_mask is not None:
- assert layer_head_mask.size() == (
- self.num_heads,
- ), f"Head mask for a single layer should be of size {(self.num_heads,)}, but is {layer_head_mask.size()}"
- attn_probs = layer_head_mask.view(1, 1, -1, 1) * attn_probs
-
- # softmax sometimes inserts NaN if all positions are masked, replace them with 0
- attn_probs = torch.masked_fill(
- attn_probs, is_index_masked[:, :, None, None], 0.0)
- attn_probs = attn_probs.type_as(attn_scores)
-
- # free memory
- del attn_scores
-
- # apply dropout
- attn_probs = nn.functional.dropout(
- attn_probs, p=self.dropout, training=self.training)
-
- value_vectors = value_vectors.view(
- seq_len, batch_size, self.num_heads, self.head_dim).transpose(0, 1)
-
- # compute local attention output with global attention value and add
- if is_global_attn:
- # compute sum of global and local attn
- attn_output = self._compute_attn_output_with_global_indices(
- value_vectors=value_vectors,
- attn_probs=attn_probs,
- max_num_global_attn_indices=max_num_global_attn_indices,
- is_index_global_attn_nonzero=is_index_global_attn_nonzero,
- is_local_index_global_attn_nonzero=is_local_index_global_attn_nonzero,
- )
- else:
- # compute local attn only
- attn_output = self._sliding_chunks_matmul_attn_probs_value(
- attn_probs, value_vectors, self.one_sided_attn_window_size
- )
-
- assert attn_output.size() == (batch_size, seq_len, self.num_heads,
- self.head_dim), "Unexpected size"
- attn_output = attn_output.transpose(0, 1).reshape(
- seq_len, batch_size, embed_dim).contiguous()
-
- # compute value for global attention and overwrite to attention output
- # TODO: remove the redundant computation
- if is_global_attn:
- global_attn_output, global_attn_probs = self._compute_global_attn_output_from_hidden(
- global_query_vectors=query_vectors,
- global_key_vectors=key_vectors,
- global_value_vectors=value_vectors,
- max_num_global_attn_indices=max_num_global_attn_indices,
- layer_head_mask=layer_head_mask,
- is_local_index_global_attn_nonzero=is_local_index_global_attn_nonzero,
- is_index_global_attn_nonzero=is_index_global_attn_nonzero,
- is_local_index_no_global_attn_nonzero=is_local_index_no_global_attn_nonzero,
- is_index_masked=is_index_masked,
- )
- # print('global_attn_output',global_attn_output.shape)
- # get only non zero global attn output
- nonzero_global_attn_output = global_attn_output[
- is_local_index_global_attn_nonzero[0], :, is_local_index_global_attn_nonzero[1]
- ]
- # print('nonzero_global_attn_output',nonzero_global_attn_output.shape)
- # overwrite values with global attention
- attn_output[is_index_global_attn_nonzero[::-1]] = nonzero_global_attn_output.view(
- len(is_local_index_global_attn_nonzero[0]), -1
- )
- # The attention weights for tokens with global attention are
- # just filler values, they were never used to compute the output.
- # Fill with 0 now, the correct values are in 'global_attn_probs'.
- attn_probs[is_index_global_attn_nonzero] = 0
-
- outputs = (attn_output.transpose(0, 1),)
-
- if output_attentions:
- outputs += (attn_probs,)
-
- return outputs + (global_attn_probs,) if (is_global_attn and output_attentions) else outputs
-
- @staticmethod
- def _pad_and_transpose_last_two_dims(hidden_states_padded, padding):
- """pads rows and then flips rows and columns"""
- hidden_states_padded = nn.functional.pad(
- hidden_states_padded, padding
- ) # padding value is not important because it will be overwritten
- hidden_states_padded = hidden_states_padded.view(
- *hidden_states_padded.size()[:-2], hidden_states_padded.size(-1), hidden_states_padded.size(-2)
- )
- return hidden_states_padded
-
- @staticmethod
- def _pad_and_diagonalize(chunked_hidden_states):
- """
- shift every row 1 step right, converting columns into diagonals.
-
- Example::
-
- chunked_hidden_states: [ 0.4983, 2.6918, -0.0071, 1.0492,
- -1.8348, 0.7672, 0.2986, 0.0285,
- -0.7584, 0.4206, -0.0405, 0.1599,
- 2.0514, -1.1600, 0.5372, 0.2629 ]
- window_overlap = num_rows = 4
- (pad & diagonalize) =>
- [ 0.4983, 2.6918, -0.0071, 1.0492, 0.0000, 0.0000, 0.0000
- 0.0000, -1.8348, 0.7672, 0.2986, 0.0285, 0.0000, 0.0000
- 0.0000, 0.0000, -0.7584, 0.4206, -0.0405, 0.1599, 0.0000
- 0.0000, 0.0000, 0.0000, 2.0514, -1.1600, 0.5372, 0.2629 ]
- """
- total_num_heads, num_chunks, window_overlap, hidden_dim = chunked_hidden_states.size()
- chunked_hidden_states = nn.functional.pad(
- chunked_hidden_states, (0, window_overlap + 1)
- ) # total_num_heads x num_chunks x window_overlap x (hidden_dim+window_overlap+1). Padding value is not important because it'll be overwritten
- chunked_hidden_states = chunked_hidden_states.view(
- total_num_heads, num_chunks, -1
- ) # total_num_heads x num_chunks x window_overlap*window_overlap+window_overlap
- chunked_hidden_states = chunked_hidden_states[
- :, :, :-window_overlap
- ] # total_num_heads x num_chunks x window_overlap*window_overlap
- chunked_hidden_states = chunked_hidden_states.view(
- total_num_heads, num_chunks, window_overlap, window_overlap + hidden_dim
- )
- chunked_hidden_states = chunked_hidden_states[:, :, :, :-1]
- return chunked_hidden_states
-
- @staticmethod
- def _chunk(hidden_states, window_overlap):
- """convert into overlapping chunks. Chunk size = 2w, overlap size = w"""
-
- # non-overlapping chunks of size = 2w
- hidden_states = hidden_states.view(
- hidden_states.size(0),
- hidden_states.size(1) // (window_overlap * 2),
- window_overlap * 2,
- hidden_states.size(2),
- )
-
- # use `as_strided` to make the chunks overlap with an overlap size = window_overlap
- chunk_size = list(hidden_states.size())
- chunk_size[1] = chunk_size[1] * 2 - 1
-
- chunk_stride = list(hidden_states.stride())
- chunk_stride[1] = chunk_stride[1] // 2
- return hidden_states.as_strided(size=chunk_size, stride=chunk_stride)
-
- @staticmethod
- def _mask_invalid_locations(input_tensor, affected_seq_len) -> torch.Tensor:
- beginning_mask_2d = input_tensor.new_ones(
- affected_seq_len, affected_seq_len + 1).tril().flip(dims=[0])
- beginning_mask = beginning_mask_2d[None, :, None, :]
- ending_mask = beginning_mask.flip(dims=(1, 3))
- beginning_input = input_tensor[:,
- :affected_seq_len, :, : affected_seq_len + 1]
- beginning_mask = beginning_mask.expand(beginning_input.size())
- # `== 1` converts to bool or uint8
- beginning_input.masked_fill_(beginning_mask == 1, -float("inf"))
- ending_input = input_tensor[:, -
- affected_seq_len:, :, -(affected_seq_len + 1):]
- ending_mask = ending_mask.expand(ending_input.size())
- # `== 1` converts to bool or uint8
- ending_input.masked_fill_(ending_mask == 1, -float("inf"))
-
- def _sliding_chunks_query_key_matmul(self, query: torch.Tensor, key: torch.Tensor, window_overlap: int):
- """
- Matrix multiplication of query and key tensors using with a sliding window attention pattern. This
- implementation splits the input into overlapping chunks of size 2w (e.g. 512 for pretrained Longformer) with an
- overlap of size window_overlap
- """
- batch_size, seq_len, num_heads, head_dim = query.size()
- assert (
- seq_len % (window_overlap * 2) == 0
- ), f"Sequence length should be multiple of {window_overlap * 2}. Given {seq_len}"
- assert query.size() == key.size()
-
- chunks_count = seq_len // window_overlap - 1
-
- # group batch_size and num_heads dimensions into one, then chunk seq_len into chunks of size window_overlap * 2
- query = query.transpose(1, 2).reshape(
- batch_size * num_heads, seq_len, head_dim)
- key = key.transpose(1, 2).reshape(
- batch_size * num_heads, seq_len, head_dim)
-
- query = self._chunk(query, window_overlap)
- key = self._chunk(key, window_overlap)
-
- # matrix multiplication
- # bcxd: batch_size * num_heads x chunks x 2window_overlap x head_dim
- # bcyd: batch_size * num_heads x chunks x 2window_overlap x head_dim
- # bcxy: batch_size * num_heads x chunks x 2window_overlap x 2window_overlap
- diagonal_chunked_attention_scores = torch.einsum(
- "bcxd,bcyd->bcxy", (query, key)) # multiply
-
- # convert diagonals into columns
- diagonal_chunked_attention_scores = self._pad_and_transpose_last_two_dims(
- diagonal_chunked_attention_scores, padding=(0, 0, 0, 1)
- )
-
- # allocate space for the overall attention matrix where the chunks are combined. The last dimension
- # has (window_overlap * 2 + 1) columns. The first (window_overlap) columns are the window_overlap lower triangles (attention from a word to
- # window_overlap previous words). The following column is attention score from each word to itself, then
- # followed by window_overlap columns for the upper triangle.
-
- diagonal_attention_scores = diagonal_chunked_attention_scores.new_empty(
- (batch_size * num_heads, chunks_count + 1,
- window_overlap, window_overlap * 2 + 1)
- )
-
- # copy parts from diagonal_chunked_attention_scores into the combined matrix of attentions
- # - copying the main diagonal and the upper triangle
- diagonal_attention_scores[:, :-1, :, window_overlap:] = diagonal_chunked_attention_scores[
- :, :, :window_overlap, : window_overlap + 1
- ]
- diagonal_attention_scores[:, -1, :, window_overlap:] = diagonal_chunked_attention_scores[
- :, -1, window_overlap:, : window_overlap + 1
- ]
- # - copying the lower triangle
- diagonal_attention_scores[:, 1:, :, :window_overlap] = diagonal_chunked_attention_scores[
- :, :, -(window_overlap + 1): -1, window_overlap + 1:
- ]
-
- diagonal_attention_scores[:, 0, 1:window_overlap, 1:window_overlap] = diagonal_chunked_attention_scores[
- :, 0, : window_overlap - 1, 1 - window_overlap:
- ]
-
- # separate batch_size and num_heads dimensions again
- diagonal_attention_scores = diagonal_attention_scores.view(
- batch_size, num_heads, seq_len, 2 * window_overlap + 1
- ).transpose(2, 1)
-
- self._mask_invalid_locations(diagonal_attention_scores, window_overlap)
- return diagonal_attention_scores
-
- def _sliding_chunks_matmul_attn_probs_value(
- self, attn_probs: torch.Tensor, value: torch.Tensor, window_overlap: int
- ):
- """
- Same as _sliding_chunks_query_key_matmul but for attn_probs and value tensors. Returned tensor will be of the
- same shape as `attn_probs`
- """
- batch_size, seq_len, num_heads, head_dim = value.size()
-
- assert seq_len % (window_overlap * 2) == 0
- assert attn_probs.size()[:3] == value.size()[:3]
- assert attn_probs.size(3) == 2 * window_overlap + 1
- chunks_count = seq_len // window_overlap - 1
- # group batch_size and num_heads dimensions into one, then chunk seq_len into chunks of size 2 window overlap
-
- chunked_attn_probs = attn_probs.transpose(1, 2).reshape(
- batch_size * num_heads, seq_len // window_overlap, window_overlap, 2 * window_overlap + 1
- )
-
- # group batch_size and num_heads dimensions into one
- value = value.transpose(1, 2).reshape(
- batch_size * num_heads, seq_len, head_dim)
-
- # pad seq_len with w at the beginning of the sequence and another window overlap at the end
- padded_value = nn.functional.pad(
- value, (0, 0, window_overlap, window_overlap), value=-1)
-
- # chunk padded_value into chunks of size 3 window overlap and an overlap of size window overlap
- chunked_value_size = (batch_size * num_heads,
- chunks_count + 1, 3 * window_overlap, head_dim)
- chunked_value_stride = padded_value.stride()
- chunked_value_stride = (
- chunked_value_stride[0],
- window_overlap * chunked_value_stride[1],
- chunked_value_stride[1],
- chunked_value_stride[2],
- )
- chunked_value = padded_value.as_strided(
- size=chunked_value_size, stride=chunked_value_stride)
-
- chunked_attn_probs = self._pad_and_diagonalize(chunked_attn_probs)
-
- context = torch.einsum(
- "bcwd,bcdh->bcwh", (chunked_attn_probs, chunked_value))
- return context.view(batch_size, num_heads, seq_len, head_dim).transpose(1, 2)
-
- @staticmethod
- def _get_global_attn_indices(is_index_global_attn):
- """compute global attn indices required throughout forward pass"""
- # helper variable
- num_global_attn_indices = is_index_global_attn.long().sum(dim=1)
-
- # max number of global attn indices in batch
- max_num_global_attn_indices = num_global_attn_indices.max()
-
- # indices of global attn
- is_index_global_attn_nonzero = is_index_global_attn.nonzero(
- as_tuple=True)
-
- # helper variable
- is_local_index_global_attn = torch.arange(
- max_num_global_attn_indices, device=is_index_global_attn.device
- ) < num_global_attn_indices.unsqueeze(dim=-1)
-
- # location of the non-padding values within global attention indices
- is_local_index_global_attn_nonzero = is_local_index_global_attn.nonzero(
- as_tuple=True)
-
- # location of the padding values within global attention indices
- is_local_index_no_global_attn_nonzero = (
- is_local_index_global_attn == 0).nonzero(as_tuple=True)
- return (
- max_num_global_attn_indices,
- is_index_global_attn_nonzero,
- is_local_index_global_attn_nonzero,
- is_local_index_no_global_attn_nonzero,
- )
-
- def _concat_with_global_key_attn_probs(
- self,
- key_vectors,
- query_vectors,
- max_num_global_attn_indices,
- is_index_global_attn_nonzero,
- is_local_index_global_attn_nonzero,
- is_local_index_no_global_attn_nonzero,
- ):
- batch_size = key_vectors.shape[0]
-
- # create only global key vectors
- key_vectors_only_global = key_vectors.new_zeros(
- batch_size, max_num_global_attn_indices, self.num_heads, self.head_dim
- )
-
- key_vectors_only_global[is_local_index_global_attn_nonzero] = key_vectors[is_index_global_attn_nonzero]
-
- # (batch_size, seq_len, num_heads, max_num_global_attn_indices)
- attn_probs_from_global_key = torch.einsum(
- "blhd,bshd->blhs", (query_vectors, key_vectors_only_global))
-
- attn_probs_from_global_key[
- is_local_index_no_global_attn_nonzero[0], :, :, is_local_index_no_global_attn_nonzero[1]
- ] = -10000.0
-
- return attn_probs_from_global_key
-
- def _compute_attn_output_with_global_indices(
- self,
- value_vectors,
- attn_probs,
- max_num_global_attn_indices,
- is_index_global_attn_nonzero,
- is_local_index_global_attn_nonzero,
- ):
- batch_size = attn_probs.shape[0]
-
- # cut local attn probs to global only
- attn_probs_only_global = attn_probs.narrow(
- -1, 0, max_num_global_attn_indices)
- # get value vectors for global only
- value_vectors_only_global = value_vectors.new_zeros(
- batch_size, max_num_global_attn_indices, self.num_heads, self.head_dim
- )
- value_vectors_only_global[is_local_index_global_attn_nonzero] = value_vectors[is_index_global_attn_nonzero]
-
- # use `matmul` because `einsum` crashes sometimes with fp16
- # attn = torch.einsum('blhs,bshd->blhd', (selected_attn_probs, selected_v))
- # compute attn output only global
- attn_output_only_global = torch.matmul(
- attn_probs_only_global.transpose(
- 1, 2), value_vectors_only_global.transpose(1, 2)
- ).transpose(1, 2)
-
- # reshape attn probs
- attn_probs_without_global = attn_probs.narrow(
- -1, max_num_global_attn_indices, attn_probs.size(-1) - max_num_global_attn_indices
- ).contiguous()
-
- # compute attn output with global
- attn_output_without_global = self._sliding_chunks_matmul_attn_probs_value(
- attn_probs_without_global, value_vectors, self.one_sided_attn_window_size
- )
- return attn_output_only_global + attn_output_without_global
-
- def _compute_global_attn_output_from_hidden(
- self,
- global_query_vectors,
- global_key_vectors,
- global_value_vectors,
- max_num_global_attn_indices,
- layer_head_mask,
- is_local_index_global_attn_nonzero,
- is_index_global_attn_nonzero,
- is_local_index_no_global_attn_nonzero,
- is_index_masked,
- ):
-
- global_query_vectors = global_query_vectors.transpose(0, 1)
- seq_len, batch_size, _, _ = global_query_vectors.shape
- global_query_vectors_only_global = global_query_vectors.new_zeros(
- max_num_global_attn_indices, batch_size, self.num_heads, self.head_dim)
- global_query_vectors_only_global[is_local_index_global_attn_nonzero[::-1]] = global_query_vectors[
- is_index_global_attn_nonzero[::-1]
- ]
-
- seq_len_q, batch_size_q, _, _ = global_query_vectors_only_global.shape
-
- # print('global_query_vectors_only_global',global_query_vectors_only_global.shape)
-
- global_query_vectors_only_global = global_query_vectors_only_global.view(
- seq_len_q, batch_size_q, self.num_heads, self.head_dim)
- global_key_vectors = global_key_vectors.transpose(0, 1)
- global_value_vectors = global_value_vectors.transpose(0, 1)
-
- # reshape
- global_query_vectors_only_global = (
- global_query_vectors_only_global.contiguous()
- .view(max_num_global_attn_indices, batch_size * self.num_heads, self.head_dim)
- .transpose(0, 1)
- ) # (batch_size * self.num_heads, max_num_global_attn_indices, head_dim)
- global_key_vectors = (
- global_key_vectors.contiguous().view(-1, batch_size * self.num_heads,
- self.head_dim).transpose(0, 1)
- ) # batch_size * self.num_heads, seq_len, head_dim)
- global_value_vectors = (
- global_value_vectors.contiguous().view(-1, batch_size * self.num_heads,
- self.head_dim).transpose(0, 1)
- ) # batch_size * self.num_heads, seq_len, head_dim)
-
- # compute attn scores
-
- global_attn_scores = torch.bmm(
- global_query_vectors_only_global, global_key_vectors.transpose(1, 2))
-
- assert list(global_attn_scores.size()) == [
- batch_size * self.num_heads,
- max_num_global_attn_indices,
- seq_len,
- ], f"global_attn_scores have the wrong size. Size should be {(batch_size * self.num_heads, max_num_global_attn_indices, seq_len)}, but is {global_attn_scores.size()}."
-
- global_attn_scores = global_attn_scores.view(
- batch_size, self.num_heads, max_num_global_attn_indices, seq_len)
-
- global_attn_scores[
- is_local_index_no_global_attn_nonzero[0], :, is_local_index_no_global_attn_nonzero[1], :
- ] = -10000.0
-
- global_attn_scores = global_attn_scores.masked_fill(
- is_index_masked[:, None, None, :],
- -10000.0,
- )
-
- global_attn_scores = global_attn_scores.view(
- batch_size * self.num_heads, max_num_global_attn_indices, seq_len)
-
- # compute global attn probs
- global_attn_probs_float = nn.functional.softmax(
- global_attn_scores, dim=-1, dtype=torch.float32
- ) # use fp32 for numerical stability
-
- # apply layer head masking
- if layer_head_mask is not None:
- assert layer_head_mask.size() == (
- self.num_heads,
- ), f"Head mask for a single layer should be of size {(self.num_heads,)}, but is {layer_head_mask.size()}"
- global_attn_probs_float = layer_head_mask.view(1, -1, 1, 1) * global_attn_probs_float.view(
- batch_size, self.num_heads, max_num_global_attn_indices, seq_len
- )
- global_attn_probs_float = global_attn_probs_float.view(
- batch_size * self.num_heads, max_num_global_attn_indices, seq_len
- )
-
- global_attn_probs = nn.functional.dropout(
- global_attn_probs_float.type_as(global_attn_scores), p=self.dropout, training=self.training
- )
-
- # global attn output
- global_attn_output = torch.bmm(global_attn_probs, global_value_vectors)
-
- assert list(global_attn_output.size()) == [
- batch_size * self.num_heads,
- max_num_global_attn_indices,
- self.head_dim,
- ], f"global_attn_output tensor has the wrong size. Size should be {(batch_size * self.num_heads, max_num_global_attn_indices, self.head_dim)}, but is {global_attn_output.size()}."
-
- global_attn_probs = global_attn_probs.view(
- batch_size, self.num_heads, max_num_global_attn_indices, seq_len)
- global_attn_output = global_attn_output.view(
- batch_size, self.num_heads, max_num_global_attn_indices, self.head_dim
- )
- return global_attn_output, global_attn_probs
-
- def get_extended_attention_mask(self, attention_mask, input_shape, device):
- """
- Makes broadcastable attention and causal masks so that future and masked tokens are ignored.
-
- Arguments:
- attention_mask (:obj:`torch.Tensor`):
- Mask with ones indicating tokens to attend to, zeros for tokens to ignore.
- input_shape (:obj:`Tuple[int]`):
- The shape of the input to the model.
- device: (:obj:`torch.device`):
- The device of the input to the model.
-
- Returns:
- :obj:`torch.Tensor` The extended attention mask, with a the same dtype as :obj:`attention_mask.dtype`.
- """
- # We can provide a self-attention mask of dimensions [batch_size, from_seq_length, to_seq_length]
- # ourselves in which case we just need to make it broadcastable to all heads.
-
- ones = torch.ones_like(attention_mask)
- zero = torch.zeros_like(attention_mask)
- attention_mask = torch.where(attention_mask < 0, zero, ones)
-
- if attention_mask.dim() == 3:
- extended_attention_mask = attention_mask[:, None, :, :]
- elif attention_mask.dim() == 2:
- extended_attention_mask = attention_mask[:, None, None, :]
- else:
- raise ValueError(
- f"Wrong shape for input_ids (shape {input_shape}) or attention_mask (shape {attention_mask.shape})"
- )
-
- # Since attention_mask is 1.0 for positions we want to attend and 0.0 for
- # masked positions, this operation will create a tensor which is 0.0 for
- # positions we want to attend and -10000.0 for masked positions.
- # Since we are adding it to the raw scores before the softmax, this is
- # effectively the same as removing these entirely.
- # extended_attention_mask = extended_attention_mask.to(dtype=self.dtype) # fp16 compatibility
- extended_attention_mask = (1.0 - extended_attention_mask) * -10000.0
- return extended_attention_mask
-
-
-# Copied from transformers.models.bert.modeling_bert.BertSelfOutput
-class LongformerSelfOutput(nn.Module):
- def __init__(self, config):
- super().__init__()
- self.dense = nn.Linear(config.hidden_size, config.hidden_size)
- self.LayerNorm = nn.LayerNorm(
- config.hidden_size, eps=config.layer_norm_eps)
- self.dropout = nn.Dropout(config.hidden_dropout_prob)
-
- def forward(self, hidden_states, input_tensor):
- hidden_states = self.dense(hidden_states)
- hidden_states = self.dropout(hidden_states)
- hidden_states = self.LayerNorm(hidden_states + input_tensor)
- return hidden_states
-
-
-class LongformerAttention(nn.Module):
- def __init__(self, config, layer_id=0):
- super().__init__()
- self.self = LongformerSelfAttention(config, layer_id)
- self.output = LongformerSelfOutput(config)
- self.pruned_heads = set()
-
- def prune_heads(self, heads):
- if len(heads) == 0:
- return
- heads, index = find_pruneable_heads_and_indices(
- heads, self.self.num_attention_heads, self.self.attention_head_size, self.pruned_heads
- )
-
- # Prune linear layers
- self.self.query = prune_linear_layer(self.self.query, index)
- self.self.key = prune_linear_layer(self.self.key, index)
- self.self.value = prune_linear_layer(self.self.value, index)
- self.output.dense = prune_linear_layer(self.output.dense, index, dim=1)
-
- # Update hyper params and store pruned heads
- self.self.num_attention_heads = self.self.num_attention_heads - \
- len(heads)
- self.self.all_head_size = self.self.attention_head_size * \
- self.self.num_attention_heads
- self.pruned_heads = self.pruned_heads.union(heads)
-
- def forward(
- self,
- hidden_states,
- attention_mask=None,
- layer_head_mask=None,
- is_index_masked=None,
- is_index_global_attn=None,
- is_global_attn=None,
- output_attentions=False,
- ):
- self_outputs = self.self(
- hidden_states,
- attention_mask=attention_mask,
- layer_head_mask=layer_head_mask,
- is_index_masked=is_index_masked,
- is_index_global_attn=is_index_global_attn,
- is_global_attn=is_global_attn,
- output_attentions=output_attentions,
- )
- attn_output = self.output(self_outputs[0], hidden_states)
- outputs = (attn_output,) + self_outputs[1:]
- return outputs
-
-
-# Copied from transformers.models.bert.modeling_bert.BertIntermediate
-class LongformerIntermediate(nn.Module):
- def __init__(self, config):
- super().__init__()
- self.dense = nn.Linear(config.hidden_size, config.intermediate_size)
- if isinstance(config.hidden_act, str):
- self.intermediate_act_fn = ACT2FN[config.hidden_act]
- else:
- self.intermediate_act_fn = config.hidden_act
-
- def forward(self, hidden_states):
- hidden_states = self.dense(hidden_states)
- hidden_states = self.intermediate_act_fn(hidden_states)
- return hidden_states
-
-
-# Copied from transformers.models.bert.modeling_bert.BertOutput
-class LongformerOutput(nn.Module):
- def __init__(self, config):
- super().__init__()
- self.dense = nn.Linear(config.intermediate_size, config.hidden_size)
- self.LayerNorm = nn.LayerNorm(
- config.hidden_size, eps=config.layer_norm_eps)
- self.dropout = nn.Dropout(config.hidden_dropout_prob)
-
- def forward(self, hidden_states, input_tensor):
- hidden_states = self.dense(hidden_states)
- hidden_states = self.dropout(hidden_states)
- hidden_states = self.LayerNorm(hidden_states + input_tensor)
- return hidden_states
-
-
-class LongformerLayer(nn.Module):
- def __init__(self, config, layer_id=0):
- super().__init__()
- self.attention = LongformerAttention(config, layer_id)
- self.intermediate = LongformerIntermediate(config)
- self.output = LongformerOutput(config)
- self.chunk_size_feed_forward = config.chunk_size_feed_forward
- self.seq_len_dim = 1
-
- def forward(
- self,
- hidden_states,
- attention_mask=None,
- layer_head_mask=None,
- is_index_masked=None,
- is_index_global_attn=None,
- is_global_attn=None,
- output_attentions=False,
- ):
- self_attn_outputs = self.attention(
- hidden_states,
- attention_mask=attention_mask,
- layer_head_mask=layer_head_mask,
- is_index_masked=is_index_masked,
- is_index_global_attn=is_index_global_attn,
- is_global_attn=is_global_attn,
- output_attentions=output_attentions,
- )
- attn_output = self_attn_outputs[0]
- outputs = self_attn_outputs[1:]
-
- layer_output = apply_chunking_to_forward(
- self.ff_chunk, self.chunk_size_feed_forward, self.seq_len_dim, attn_output
- )
- outputs = (layer_output,) + outputs
- return outputs
-
- def ff_chunk(self, attn_output):
- intermediate_output = self.intermediate(attn_output)
- layer_output = self.output(intermediate_output, attn_output)
- return layer_output
-
-
-class LongformerEncoder(nn.Module):
- def __init__(self, config):
- super().__init__()
- self.config = config
- self.layer = nn.ModuleList(
- [LongformerLayer(config, layer_id=i) for i in range(config.num_hidden_layers)])
-
- def forward(
- self,
- hidden_states,
- attention_mask=None,
- head_mask=None,
- output_attentions=False,
- output_hidden_states=False,
- return_dict=True,
- ):
-
- is_index_masked = attention_mask < 0
- is_index_global_attn = attention_mask > 0
- is_global_attn = is_index_global_attn.flatten().any().item()
-
- all_hidden_states = () if output_hidden_states else None
- # All local attentions.
- all_attentions = () if output_attentions else None
- all_global_attentions = () if (output_attentions and is_global_attn) else None
-
- # check if head_mask has a correct number of layers specified if desired
- if head_mask is not None:
- assert head_mask.size()[0] == (
- len(self.layer)
- ), f"The head_mask should be specified for {len(self.layer)} layers, but it is for {head_mask.size()[0]}."
- for idx, layer_module in enumerate(self.layer):
- if output_hidden_states:
- all_hidden_states = all_hidden_states + (hidden_states,)
-
- if getattr(self.config, "gradient_checkpointing", False) and self.training:
-
- def create_custom_forward(module):
- def custom_forward(*inputs):
- return module(*inputs, is_global_attn, output_attentions)
-
- return custom_forward
-
- layer_outputs = torch.utils.checkpoint.checkpoint(
- create_custom_forward(layer_module),
- hidden_states,
- attention_mask,
- head_mask[idx] if head_mask is not None else None,
- is_index_masked,
- is_index_global_attn,
- )
- else:
- layer_outputs = layer_module(
- hidden_states,
- attention_mask=attention_mask,
- layer_head_mask=head_mask[idx] if head_mask is not None else None,
- is_index_masked=is_index_masked,
- is_index_global_attn=is_index_global_attn,
- is_global_attn=is_global_attn,
- output_attentions=output_attentions,
- )
- hidden_states = layer_outputs[0]
-
- if output_attentions:
- # bzs x seq_len x num_attn_heads x (num_global_attn + attention_window_len + 1) => bzs x num_attn_heads x seq_len x (num_global_attn + attention_window_len + 1)
- all_attentions = all_attentions + \
- (layer_outputs[1].transpose(1, 2),)
-
- if is_global_attn:
- # bzs x num_attn_heads x num_global_attn x seq_len => bzs x num_attn_heads x seq_len x num_global_attn
- all_global_attentions = all_global_attentions + \
- (layer_outputs[2].transpose(2, 3),)
-
- # Add last layer
- if output_hidden_states:
- all_hidden_states = all_hidden_states + (hidden_states,)
-
- if not return_dict:
- return tuple(
- v for v in [hidden_states, all_hidden_states, all_attentions, all_global_attentions] if v is not None
- )
- return LongformerBaseModelOutput(
- last_hidden_state=hidden_states,
- hidden_states=all_hidden_states,
- attentions=all_attentions,
- global_attentions=all_global_attentions,
- )
-
-
-# Copied from transformers.models.bert.modeling_bert.BertPooler
-class LongformerPooler(nn.Module):
- def __init__(self, config):
- super().__init__()
- self.dense = nn.Linear(config.hidden_size, config.hidden_size)
- self.activation = nn.Tanh()
-
- def forward(self, hidden_states):
- # We "pool" the model by simply taking the hidden state corresponding
- # to the first token.
- first_token_tensor = hidden_states[:, 0]
- pooled_output = self.dense(first_token_tensor)
- pooled_output = self.activation(pooled_output)
- return pooled_output
-
-
-# Copied from transformers.models.roberta.modeling_roberta.RobertaLMHead with Roberta->Longformer
-class LongformerLMHead(nn.Module):
- """Longformer Head for masked language modeling."""
-
- def __init__(self, config):
- super().__init__()
- self.dense = nn.Linear(config.hidden_size, config.hidden_size)
- self.layer_norm = nn.LayerNorm(
- config.hidden_size, eps=config.layer_norm_eps)
-
- self.decoder = nn.Linear(config.hidden_size, config.vocab_size)
- self.bias = nn.Parameter(torch.zeros(config.vocab_size))
- self.decoder.bias = self.bias
-
- def forward(self, features, **kwargs):
- x = self.dense(features)
- x = gelu(x)
- x = self.layer_norm(x)
-
- # project back to size of vocabulary with bias
- x = self.decoder(x)
-
- return x
-
- def _tie_weights(self):
- # To tie those two weights if they get disconnected (on TPU or when the bias is resized)
- self.bias = self.decoder.bias
-
-
-class LongformerPreTrainedModel(PreTrainedModel):
- """
- An abstract class to handle weights initialization and a simple interface for downloading and loading pretrained
- models.
- """
-
- config_class = LongformerConfig
- base_model_prefix = "longformer"
- _keys_to_ignore_on_load_missing = [r"position_ids"]
-
- def _init_weights(self, module):
- """Initialize the weights"""
- if isinstance(module, nn.Linear):
- # Slightly different from the TF version which uses truncated_normal for initialization
- # cf https://github.com/pytorch/pytorch/pull/5617
- module.weight.data.normal_(
- mean=0.0, std=self.config.initializer_range)
- if module.bias is not None:
- module.bias.data.zero_()
- elif isinstance(module, nn.Embedding):
- module.weight.data.normal_(
- mean=0.0, std=self.config.initializer_range)
- if module.padding_idx is not None:
- module.weight.data[module.padding_idx].zero_()
- elif isinstance(module, nn.LayerNorm):
- module.bias.data.zero_()
- module.weight.data.fill_(1.0)
-
-
-LONGFORMER_START_DOCSTRING = r"""
-
- This model inherits from :class:`~transformers.PreTrainedModel`. Check the superclass documentation for the generic
- methods the library implements for all its model (such as downloading or saving, resizing the input embeddings,
- pruning heads etc.)
-
- This model is also a PyTorch `torch.nn.Module `__
- subclass. Use it as a regular PyTorch Module and refer to the PyTorch documentation for all matter related to
- general usage and behavior.
-
- Parameters:
- config (:class:`~transformers.LongformerConfig`): Model configuration class with all the parameters of the
- model. Initializing with a config file does not load the weights associated with the model, only the
- configuration. Check out the :meth:`~transformers.PreTrainedModel.from_pretrained` method to load the model
- weights.
-"""
-
-LONGFORMER_INPUTS_DOCSTRING = r"""
- Args:
- input_ids (:obj:`torch.LongTensor` of shape :obj:`({0})`):
- Indices of input sequence tokens in the vocabulary.
-
- Indices can be obtained using :class:`~transformers.LongformerTokenizer`. See
- :meth:`transformers.PreTrainedTokenizer.encode` and :meth:`transformers.PreTrainedTokenizer.__call__` for
- details.
-
- `What are input IDs? <../glossary.html#input-ids>`__
- attention_mask (:obj:`torch.FloatTensor` of shape :obj:`({0})`, `optional`):
- Mask to avoid performing attention on padding token indices. Mask values selected in ``[0, 1]``:
-
- - 1 for tokens that are **not masked**,
- - 0 for tokens that are **masked**.
-
- `What are attention masks? <../glossary.html#attention-mask>`__
- global_attention_mask (:obj:`torch.FloatTensor` of shape :obj:`({0})`, `optional`):
- Mask to decide the attention given on each token, local attention or global attention. Tokens with global
- attention attends to all other tokens, and all other tokens attend to them. This is important for
- task-specific finetuning because it makes the model more flexible at representing the task. For example,
- for classification, the token should be given global attention. For QA, all question tokens should also
- have global attention. Please refer to the `Longformer paper `__ for more
- details. Mask values selected in ``[0, 1]``:
-
- - 0 for local attention (a sliding window attention),
- - 1 for global attention (tokens that attend to all other tokens, and all other tokens attend to them).
-
- head_mask (:obj:`torch.Tensor` of shape :obj:`(num_layers, num_heads)`, `optional`):
- Mask to nullify selected heads of the attention modules in the encoder. Mask values selected in ``[0, 1]``:
-
- - 1 indicates the head is **not masked**,
- - 0 indicates the head is **masked**.
-
- decoder_head_mask (:obj:`torch.Tensor` of shape :obj:`(num_layers, num_heads)`, `optional`):
- Mask to nullify selected heads of the attention modules in the decoder. Mask values selected in ``[0, 1]``:
-
- - 1 indicates the head is **not masked**,
- - 0 indicates the head is **masked**.
-
- token_type_ids (:obj:`torch.LongTensor` of shape :obj:`({0})`, `optional`):
- Segment token indices to indicate first and second portions of the inputs. Indices are selected in ``[0,
- 1]``:
-
- - 0 corresponds to a `sentence A` token,
- - 1 corresponds to a `sentence B` token.
-
- `What are token type IDs? <../glossary.html#token-type-ids>`_
- position_ids (:obj:`torch.LongTensor` of shape :obj:`({0})`, `optional`):
- Indices of positions of each input sequence tokens in the position embeddings. Selected in the range ``[0,
- config.max_position_embeddings - 1]``.
-
- `What are position IDs? <../glossary.html#position-ids>`_
- inputs_embeds (:obj:`torch.FloatTensor` of shape :obj:`({0}, hidden_size)`, `optional`):
- Optionally, instead of passing :obj:`input_ids` you can choose to directly pass an embedded representation.
- This is useful if you want more control over how to convert :obj:`input_ids` indices into associated
- vectors than the model's internal embedding lookup matrix.
- output_attentions (:obj:`bool`, `optional`):
- Whether or not to return the attentions tensors of all attention layers. See ``attentions`` under returned
- tensors for more detail.
- output_hidden_states (:obj:`bool`, `optional`):
- Whether or not to return the hidden states of all layers. See ``hidden_states`` under returned tensors for
- more detail.
- return_dict (:obj:`bool`, `optional`):
- Whether or not to return a :class:`~transformers.file_utils.ModelOutput` instead of a plain tuple.
-"""
-
-
-@add_start_docstrings(
- "The bare Longformer Model outputting raw hidden-states without any specific head on top.",
- LONGFORMER_START_DOCSTRING,
-)
-class LongformerModel(LongformerPreTrainedModel):
- """
- This class copied code from :class:`~transformers.RobertaModel` and overwrote standard self-attention with
- longformer self-attention to provide the ability to process long sequences following the self-attention approach
- described in `Longformer: the Long-Document Transformer `__ by Iz Beltagy,
- Matthew E. Peters, and Arman Cohan. Longformer self-attention combines a local (sliding window) and global
- attention to extend to long documents without the O(n^2) increase in memory and compute.
-
- The self-attention module :obj:`LongformerSelfAttention` implemented here supports the combination of local and
- global attention but it lacks support for autoregressive attention and dilated attention. Autoregressive and
- dilated attention are more relevant for autoregressive language modeling than finetuning on downstream tasks.
- Future release will add support for autoregressive attention, but the support for dilated attention requires a
- custom CUDA kernel to be memory and compute efficient.
-
- """
-
- def __init__(self, config, add_pooling_layer=True):
- super().__init__(config)
- self.config = config
-
- if isinstance(config.attention_window, int):
- assert config.attention_window % 2 == 0, "`config.attention_window` has to be an even value"
- assert config.attention_window > 0, "`config.attention_window` has to be positive"
- config.attention_window = [
- config.attention_window] * config.num_hidden_layers # one value per layer
- else:
- assert len(config.attention_window) == config.num_hidden_layers, (
- "`len(config.attention_window)` should equal `config.num_hidden_layers`. "
- f"Expected {config.num_hidden_layers}, given {len(config.attention_window)}"
- )
-
- self.embeddings = LongformerEmbeddings(config)
- self.encoder = LongformerEncoder(config)
- self.pooler = LongformerPooler(config) if add_pooling_layer else None
-
- self.init_weights()
-
- def get_input_embeddings(self):
- return self.embeddings.word_embeddings
-
- def set_input_embeddings(self, value):
- self.embeddings.word_embeddings = value
-
- def _prune_heads(self, heads_to_prune):
- """
- Prunes heads of the model. heads_to_prune: dict of {layer_num: list of heads to prune in this layer} See base
- class PreTrainedModel
- """
- for layer, heads in heads_to_prune.items():
- self.encoder.layer[layer].attention.prune_heads(heads)
-
- def _pad_to_window_size(
- self,
- input_ids: torch.Tensor,
- attention_mask: torch.Tensor,
- token_type_ids: torch.Tensor,
- position_ids: torch.Tensor,
- inputs_embeds: torch.Tensor,
- pad_token_id: int,
- ):
- """A helper function to pad tokens and mask to work with implementation of Longformer self-attention."""
- # padding
- attention_window = (
- self.config.attention_window
- if isinstance(self.config.attention_window, int)
- else max(self.config.attention_window)
- )
-
- assert attention_window % 2 == 0, f"`attention_window` should be an even value. Given {attention_window}"
- input_shape = input_ids.shape if input_ids is not None else inputs_embeds.shape
- batch_size, seq_len = input_shape[:2]
-
- padding_len = (attention_window - seq_len %
- attention_window) % attention_window
- if padding_len > 0:
- logger.info(
- f"Input ids are automatically padded from {seq_len} to {seq_len + padding_len} to be a multiple of "
- f"`config.attention_window`: {attention_window}"
- )
- if input_ids is not None:
- input_ids = nn.functional.pad(
- input_ids, (0, padding_len), value=pad_token_id)
- if position_ids is not None:
- # pad with position_id = pad_token_id as in modeling_roberta.RobertaEmbeddings
- position_ids = nn.functional.pad(
- position_ids, (0, padding_len), value=pad_token_id)
- if inputs_embeds is not None:
- input_ids_padding = inputs_embeds.new_full(
- (batch_size, padding_len),
- self.config.pad_token_id,
- dtype=torch.long,
- )
- inputs_embeds_padding = self.embeddings(input_ids_padding)
- inputs_embeds = torch.cat(
- [inputs_embeds, inputs_embeds_padding], dim=-2)
-
- attention_mask = nn.functional.pad(
- attention_mask, (0, padding_len), value=False
- ) # no attention on the padding tokens
- token_type_ids = nn.functional.pad(
- token_type_ids, (0, padding_len), value=0) # pad with token_type_id = 0
-
- return padding_len, input_ids, attention_mask, token_type_ids, position_ids, inputs_embeds
-
- def _merge_to_attention_mask(self, attention_mask: torch.Tensor, global_attention_mask: torch.Tensor):
- # longformer self attention expects attention mask to have 0 (no attn), 1 (local attn), 2 (global attn)
- # (global_attention_mask + 1) => 1 for local attention, 2 for global attention
- # => final attention_mask => 0 for no attention, 1 for local attention 2 for global attention
- if attention_mask is not None:
- attention_mask = attention_mask * (global_attention_mask + 1)
- else:
- # simply use `global_attention_mask` as `attention_mask`
- # if no `attention_mask` is given
- attention_mask = global_attention_mask + 1
- return attention_mask
-
- @add_start_docstrings_to_model_forward(LONGFORMER_INPUTS_DOCSTRING.format("batch_size, sequence_length"))
- @replace_return_docstrings(output_type=LongformerBaseModelOutputWithPooling, config_class=_CONFIG_FOR_DOC)
- def forward(
- self,
- input_ids=None,
- attention_mask=None,
- global_attention_mask=None,
- head_mask=None,
- token_type_ids=None,
- position_ids=None,
- inputs_embeds=None,
- output_attentions=None,
- output_hidden_states=None,
- return_dict=None,
- ):
- r"""
-
- Returns:
-
- Examples::
-
- >>> import torch
- >>> from transformers import LongformerModel, LongformerTokenizer
-
- >>> model = LongformerModel.from_pretrained('allenai/longformer-base-4096')
- >>> tokenizer = LongformerTokenizer.from_pretrained('allenai/longformer-base-4096')
-
- >>> SAMPLE_TEXT = ' '.join(['Hello world! '] * 1000) # long input document
- >>> input_ids = torch.tensor(tokenizer.encode(SAMPLE_TEXT)).unsqueeze(0) # batch of size 1
-
- >>> attention_mask = torch.ones(input_ids.shape, dtype=torch.long, device=input_ids.device) # initialize to local attention
- >>> global_attention_mask = torch.zeros(input_ids.shape, dtype=torch.long, device=input_ids.device) # initialize to global attention to be deactivated for all tokens
- >>> global_attention_mask[:, [1, 4, 21,]] = 1 # Set global attention to random tokens for the sake of this example
- ... # Usually, set global attention based on the task. For example,
- ... # classification: the token
- ... # QA: question tokens
- ... # LM: potentially on the beginning of sentences and paragraphs
- >>> outputs = model(input_ids, attention_mask=attention_mask, global_attention_mask=global_attention_mask)
- >>> sequence_output = outputs.last_hidden_state
- >>> pooled_output = outputs.pooler_output
- """
-
- output_attentions = output_attentions if output_attentions is not None else self.config.output_attentions
- output_hidden_states = (
- output_hidden_states if output_hidden_states is not None else self.config.output_hidden_states
- )
- return_dict = return_dict if return_dict is not None else self.config.use_return_dict
-
- if input_ids is not None and inputs_embeds is not None:
- raise ValueError(
- "You cannot specify both input_ids and inputs_embeds at the same time")
- elif input_ids is not None:
- input_shape = input_ids.size()
- elif inputs_embeds is not None:
- input_shape = inputs_embeds.size()[:-1]
- else:
- raise ValueError(
- "You have to specify either input_ids or inputs_embeds")
-
- device = input_ids.device if input_ids is not None else inputs_embeds.device
-
- if attention_mask is None:
- attention_mask = torch.ones(input_shape, device=device)
- if token_type_ids is None:
- token_type_ids = torch.zeros(
- input_shape, dtype=torch.long, device=device)
-
- # merge `global_attention_mask` and `attention_mask`
- if global_attention_mask is not None:
- attention_mask = self._merge_to_attention_mask(
- attention_mask, global_attention_mask)
-
- if self.config.use_sparse_attention:
- padding_len, input_ids, attention_mask, token_type_ids, position_ids, inputs_embeds = self._pad_to_window_size(
- input_ids=input_ids,
- attention_mask=attention_mask,
- token_type_ids=token_type_ids,
- position_ids=position_ids,
- inputs_embeds=inputs_embeds,
- pad_token_id=self.config.pad_token_id,
- )
-
- # We can provide a self-attention mask of dimensions [batch_size, from_seq_length, to_seq_length]
- # ourselves in which case we just need to make it broadcastable to all heads.
- extended_attention_mask: torch.Tensor = self.get_extended_attention_mask(attention_mask, input_shape, device)[
- :, 0, 0, :
- ]
-
- embedding_output = self.embeddings(
- input_ids=input_ids, position_ids=position_ids, token_type_ids=token_type_ids, inputs_embeds=inputs_embeds
- )
-
- encoder_outputs = self.encoder(
- embedding_output,
- attention_mask=extended_attention_mask,
- head_mask=head_mask,
- output_attentions=output_attentions,
- output_hidden_states=output_hidden_states,
- return_dict=return_dict,
- )
- sequence_output = encoder_outputs[0]
- pooled_output = self.pooler(
- sequence_output) if self.pooler is not None else None
-
- # undo padding
- if self.config.use_sparse_attention:
- if padding_len > 0:
- # unpad `sequence_output` because the calling function is expecting a length == input_ids.size(1)
- sequence_output = sequence_output[:, :-padding_len]
-
- if not return_dict:
- return (sequence_output, pooled_output) + encoder_outputs[1:]
-
- return LongformerBaseModelOutputWithPooling(
- last_hidden_state=sequence_output,
- pooler_output=pooled_output,
- hidden_states=encoder_outputs.hidden_states,
- attentions=encoder_outputs.attentions,
- global_attentions=encoder_outputs.global_attentions,
- )
-
-
-@add_start_docstrings("""Longformer Model with a `language modeling` head on top. """, LONGFORMER_START_DOCSTRING)
-class LongformerForMaskedLM(LongformerPreTrainedModel):
-
- _keys_to_ignore_on_load_unexpected = [r"pooler"]
-
- def __init__(self, config):
- super().__init__(config)
-
- self.longformer = LongformerModel(config, add_pooling_layer=False)
- self.lm_head = LongformerLMHead(config)
-
- self.init_weights()
-
- def get_output_embeddings(self):
- return self.lm_head.decoder
-
- def set_output_embeddings(self, new_embeddings):
- self.lm_head.decoder = new_embeddings
-
- @add_start_docstrings_to_model_forward(LONGFORMER_INPUTS_DOCSTRING.format("batch_size, sequence_length"))
- @replace_return_docstrings(output_type=LongformerMaskedLMOutput, config_class=_CONFIG_FOR_DOC)
- def forward(
- self,
- input_ids=None,
- attention_mask=None,
- global_attention_mask=None,
- head_mask=None,
- token_type_ids=None,
- position_ids=None,
- inputs_embeds=None,
- labels=None,
- output_attentions=None,
- output_hidden_states=None,
- return_dict=None,
- ):
- r"""
- labels (:obj:`torch.LongTensor` of shape :obj:`(batch_size, sequence_length)`, `optional`):
- Labels for computing the masked language modeling loss. Indices should be in ``[-100, 0, ...,
- config.vocab_size]`` (see ``input_ids`` docstring) Tokens with indices set to ``-100`` are ignored
- (masked), the loss is only computed for the tokens with labels in ``[0, ..., config.vocab_size]``
- kwargs (:obj:`Dict[str, any]`, optional, defaults to `{}`):
- Used to hide legacy arguments that have been deprecated.
-
- Returns:
-
- Examples::
-
- >>> import torch
- >>> from transformers import LongformerForMaskedLM, LongformerTokenizer
-
- >>> model = LongformerForMaskedLM.from_pretrained('allenai/longformer-base-4096')
- >>> tokenizer = LongformerTokenizer.from_pretrained('allenai/longformer-base-4096')
-
- >>> SAMPLE_TEXT = ' '.join(['Hello world! '] * 1000) # long input document
- >>> input_ids = torch.tensor(tokenizer.encode(SAMPLE_TEXT)).unsqueeze(0) # batch of size 1
-
- >>> attention_mask = None # default is local attention everywhere, which is a good choice for MaskedLM
- ... # check ``LongformerModel.forward`` for more details how to set `attention_mask`
- >>> outputs = model(input_ids, attention_mask=attention_mask, labels=input_ids)
- >>> loss = outputs.loss
- >>> prediction_logits = output.logits
- """
- return_dict = return_dict if return_dict is not None else self.config.use_return_dict
-
- outputs = self.longformer(
- input_ids,
- attention_mask=attention_mask,
- global_attention_mask=global_attention_mask,
- head_mask=head_mask,
- token_type_ids=token_type_ids,
- position_ids=position_ids,
- inputs_embeds=inputs_embeds,
- output_attentions=output_attentions,
- output_hidden_states=output_hidden_states,
- return_dict=return_dict,
- )
- sequence_output = outputs[0]
- prediction_scores = self.lm_head(sequence_output)
-
- masked_lm_loss = None
- if labels is not None:
- loss_fct = CrossEntropyLoss()
- masked_lm_loss = loss_fct(
- prediction_scores.view(-1, self.config.vocab_size), labels.view(-1))
-
- if not return_dict:
- output = (prediction_scores,) + outputs[2:]
- return ((masked_lm_loss,) + output) if masked_lm_loss is not None else output
-
- return LongformerMaskedLMOutput(
- loss=masked_lm_loss,
- logits=prediction_scores,
- hidden_states=outputs.hidden_states,
- attentions=outputs.attentions,
- global_attentions=outputs.global_attentions,
- )
-
-
-@add_start_docstrings(
- """
- Longformer Model transformer with a sequence classification/regression head on top (a linear layer on top of the
- pooled output) e.g. for GLUE tasks.
- """,
- LONGFORMER_START_DOCSTRING,
-)
-class LongformerForSequenceClassification(LongformerPreTrainedModel):
-
- _keys_to_ignore_on_load_unexpected = [r"pooler"]
-
- def __init__(self, config):
- super().__init__(config)
- self.num_labels = config.num_labels
- self.config = config
-
- self.longformer = LongformerModel(config, add_pooling_layer=False)
- self.classifier = LongformerClassificationHead(config)
-
- self.init_weights()
-
- @add_start_docstrings_to_model_forward(LONGFORMER_INPUTS_DOCSTRING.format("batch_size, sequence_length"))
- @add_code_sample_docstrings(
- processor_class=_TOKENIZER_FOR_DOC,
- checkpoint=_CHECKPOINT_FOR_DOC,
- output_type=LongformerSequenceClassifierOutput,
- config_class=_CONFIG_FOR_DOC,
- )
- def forward(
- self,
- input_ids=None,
- attention_mask=None,
- global_attention_mask=None,
- head_mask=None,
- token_type_ids=None,
- position_ids=None,
- inputs_embeds=None,
- labels=None,
- output_attentions=None,
- output_hidden_states=None,
- return_dict=None,
- ):
- r"""
- labels (:obj:`torch.LongTensor` of shape :obj:`(batch_size,)`, `optional`):
- Labels for computing the sequence classification/regression loss. Indices should be in :obj:`[0, ...,
- config.num_labels - 1]`. If :obj:`config.num_labels == 1` a regression loss is computed (Mean-Square loss),
- If :obj:`config.num_labels > 1` a classification loss is computed (Cross-Entropy).
- """
- return_dict = return_dict if return_dict is not None else self.config.use_return_dict
-
- if global_attention_mask is None:
- logger.info("Initializing global attention on CLS token...")
- global_attention_mask = torch.zeros_like(input_ids)
- # global attention on cls token
- global_attention_mask[:, 0] = 1
-
- outputs = self.longformer(
- input_ids,
- attention_mask=attention_mask,
- global_attention_mask=global_attention_mask,
- head_mask=head_mask,
- token_type_ids=token_type_ids,
- position_ids=position_ids,
- inputs_embeds=inputs_embeds,
- output_attentions=output_attentions,
- output_hidden_states=output_hidden_states,
- return_dict=return_dict,
- )
- sequence_output = outputs[0]
- logits = self.classifier(sequence_output)
-
- loss = None
- if labels is not None:
- if self.config.problem_type is None:
- if self.num_labels == 1:
- self.config.problem_type = "regression"
- elif self.num_labels > 1 and (labels.dtype == torch.long or labels.dtype == torch.int):
- self.config.problem_type = "single_label_classification"
- else:
- self.config.problem_type = "multi_label_classification"
-
- if self.config.problem_type == "regression":
- loss_fct = MSELoss()
- if self.num_labels == 1:
- loss = loss_fct(logits.squeeze(), labels.squeeze())
- else:
- loss = loss_fct(logits, labels)
- elif self.config.problem_type == "single_label_classification":
- loss_fct = CrossEntropyLoss()
- loss = loss_fct(
- logits.view(-1, self.num_labels), labels.view(-1))
- elif self.config.problem_type == "multi_label_classification":
- loss_fct = BCEWithLogitsLoss()
- loss = loss_fct(logits, labels)
-
- if not return_dict:
- output = (logits,) + outputs[2:]
- return ((loss,) + output) if loss is not None else output
-
- return LongformerSequenceClassifierOutput(
- loss=loss,
- logits=logits,
- hidden_states=outputs.hidden_states,
- attentions=outputs.attentions,
- global_attentions=outputs.global_attentions,
- )
-
-
-class LongformerClassificationHead(nn.Module):
- """Head for sentence-level classification tasks."""
-
- def __init__(self, config):
- super().__init__()
- self.dense = nn.Linear(config.hidden_size, config.hidden_size)
- self.dropout = nn.Dropout(config.hidden_dropout_prob)
- self.out_proj = nn.Linear(config.hidden_size, config.num_labels)
-
- def forward(self, hidden_states, **kwargs):
- # take token (equiv. to [CLS])
- hidden_states = hidden_states[:, 0, :]
- hidden_states = self.dropout(hidden_states)
- hidden_states = self.dense(hidden_states)
- hidden_states = torch.tanh(hidden_states)
- hidden_states = self.dropout(hidden_states)
- output = self.out_proj(hidden_states)
- return output
-
-
-@add_start_docstrings(
- """
- Longformer Model with a span classification head on top for extractive question-answering tasks like SQuAD /
- TriviaQA (a linear layers on top of the hidden-states output to compute `span start logits` and `span end logits`).
- """,
- LONGFORMER_START_DOCSTRING,
-)
-class LongformerForQuestionAnswering(LongformerPreTrainedModel):
-
- _keys_to_ignore_on_load_unexpected = [r"pooler"]
-
- def __init__(self, config):
- super().__init__(config)
- self.num_labels = config.num_labels
-
- self.longformer = LongformerModel(config, add_pooling_layer=False)
- self.qa_outputs = nn.Linear(config.hidden_size, config.num_labels)
-
- self.init_weights()
-
- @add_start_docstrings_to_model_forward(LONGFORMER_INPUTS_DOCSTRING.format("batch_size, sequence_length"))
- @replace_return_docstrings(output_type=LongformerQuestionAnsweringModelOutput, config_class=_CONFIG_FOR_DOC)
- def forward(
- self,
- input_ids=None,
- attention_mask=None,
- global_attention_mask=None,
- head_mask=None,
- token_type_ids=None,
- position_ids=None,
- inputs_embeds=None,
- start_positions=None,
- end_positions=None,
- output_attentions=None,
- output_hidden_states=None,
- return_dict=None,
- ):
- r"""
- start_positions (:obj:`torch.LongTensor` of shape :obj:`(batch_size,)`, `optional`):
- Labels for position (index) of the start of the labelled span for computing the token classification loss.
- Positions are clamped to the length of the sequence (:obj:`sequence_length`). Position outside of the
- sequence are not taken into account for computing the loss.
- end_positions (:obj:`torch.LongTensor` of shape :obj:`(batch_size,)`, `optional`):
- Labels for position (index) of the end of the labelled span for computing the token classification loss.
- Positions are clamped to the length of the sequence (:obj:`sequence_length`). Position outside of the
- sequence are not taken into account for computing the loss.
-
- Returns:
-
- Examples::
-
- >>> from transformers import LongformerTokenizer, LongformerForQuestionAnswering
- >>> import torch
-
- >>> tokenizer = LongformerTokenizer.from_pretrained("allenai/longformer-large-4096-finetuned-triviaqa")
- >>> model = LongformerForQuestionAnswering.from_pretrained("allenai/longformer-large-4096-finetuned-triviaqa")
-
- >>> question, text = "Who was Jim Henson?", "Jim Henson was a nice puppet"
- >>> encoding = tokenizer(question, text, return_tensors="pt")
- >>> input_ids = encoding["input_ids"]
-
- >>> # default is local attention everywhere
- >>> # the forward method will automatically set global attention on question tokens
- >>> attention_mask = encoding["attention_mask"]
-
- >>> outputs = model(input_ids, attention_mask=attention_mask)
- >>> start_logits = outputs.start_logits
- >>> end_logits = outputs.end_logits
- >>> all_tokens = tokenizer.convert_ids_to_tokens(input_ids[0].tolist())
-
- >>> answer_tokens = all_tokens[torch.argmax(start_logits) :torch.argmax(end_logits)+1]
- >>> answer = tokenizer.decode(tokenizer.convert_tokens_to_ids(answer_tokens)) # remove space prepending space token
-
- """
- return_dict = return_dict if return_dict is not None else self.config.use_return_dict
-
- if global_attention_mask is None:
- if input_ids is None:
- logger.warning(
- "It is not possible to automatically generate the `global_attention_mask` because input_ids is None. Please make sure that it is correctly set."
- )
- else:
- # set global attention on question tokens automatically
- global_attention_mask = _compute_global_attention_mask(
- input_ids, self.config.sep_token_id)
-
- outputs = self.longformer(
- input_ids,
- attention_mask=attention_mask,
- global_attention_mask=global_attention_mask,
- head_mask=head_mask,
- token_type_ids=token_type_ids,
- position_ids=position_ids,
- inputs_embeds=inputs_embeds,
- output_attentions=output_attentions,
- output_hidden_states=output_hidden_states,
- return_dict=return_dict,
- )
-
- sequence_output = outputs[0]
-
- logits = self.qa_outputs(sequence_output)
- start_logits, end_logits = logits.split(1, dim=-1)
- start_logits = start_logits.squeeze(-1).contiguous()
- end_logits = end_logits.squeeze(-1).contiguous()
-
- total_loss = None
- if start_positions is not None and end_positions is not None:
- # If we are on multi-GPU, split add a dimension
- if len(start_positions.size()) > 1:
- start_positions = start_positions.squeeze(-1)
- if len(end_positions.size()) > 1:
- end_positions = end_positions.squeeze(-1)
- # sometimes the start/end positions are outside our model inputs, we ignore these terms
- ignored_index = start_logits.size(1)
- start_positions = start_positions.clamp(0, ignored_index)
- end_positions = end_positions.clamp(0, ignored_index)
-
- loss_fct = CrossEntropyLoss(ignore_index=ignored_index)
- start_loss = loss_fct(start_logits, start_positions)
- end_loss = loss_fct(end_logits, end_positions)
- total_loss = (start_loss + end_loss) / 2
-
- if not return_dict:
- output = (start_logits, end_logits) + outputs[2:]
- return ((total_loss,) + output) if total_loss is not None else output
-
- return LongformerQuestionAnsweringModelOutput(
- loss=total_loss,
- start_logits=start_logits,
- end_logits=end_logits,
- hidden_states=outputs.hidden_states,
- attentions=outputs.attentions,
- global_attentions=outputs.global_attentions,
- )
-
-
-@add_start_docstrings(
- """
- Longformer Model with a token classification head on top (a linear layer on top of the hidden-states output) e.g.
- for Named-Entity-Recognition (NER) tasks.
- """,
- LONGFORMER_START_DOCSTRING,
-)
-class LongformerForTokenClassification(LongformerPreTrainedModel):
-
- _keys_to_ignore_on_load_unexpected = [r"pooler"]
-
- def __init__(self, config):
- super().__init__(config)
- self.num_labels = config.num_labels
-
- self.longformer = LongformerModel(config, add_pooling_layer=False)
- self.dropout = nn.Dropout(config.hidden_dropout_prob)
- self.classifier = nn.Linear(config.hidden_size, config.num_labels)
-
- self.init_weights()
-
- @add_start_docstrings_to_model_forward(LONGFORMER_INPUTS_DOCSTRING.format("batch_size, sequence_length"))
- @add_code_sample_docstrings(
- processor_class=_TOKENIZER_FOR_DOC,
- checkpoint=_CHECKPOINT_FOR_DOC,
- output_type=LongformerTokenClassifierOutput,
- config_class=_CONFIG_FOR_DOC,
- )
- def forward(
- self,
- input_ids=None,
- attention_mask=None,
- global_attention_mask=None,
- head_mask=None,
- token_type_ids=None,
- position_ids=None,
- inputs_embeds=None,
- labels=None,
- output_attentions=None,
- output_hidden_states=None,
- return_dict=None,
- ):
- r"""
- labels (:obj:`torch.LongTensor` of shape :obj:`(batch_size, sequence_length)`, `optional`):
- Labels for computing the token classification loss. Indices should be in ``[0, ..., config.num_labels -
- 1]``.
- """
- return_dict = return_dict if return_dict is not None else self.config.use_return_dict
-
- outputs = self.longformer(
- input_ids,
- attention_mask=attention_mask,
- global_attention_mask=global_attention_mask,
- head_mask=head_mask,
- token_type_ids=token_type_ids,
- position_ids=position_ids,
- inputs_embeds=inputs_embeds,
- output_attentions=output_attentions,
- output_hidden_states=output_hidden_states,
- return_dict=return_dict,
- )
-
- sequence_output = outputs[0]
-
- sequence_output = self.dropout(sequence_output)
- logits = self.classifier(sequence_output)
-
- loss = None
- if labels is not None:
- loss_fct = CrossEntropyLoss()
- # Only keep active parts of the loss
- if attention_mask is not None:
- active_loss = attention_mask.view(-1) == 1
- active_logits = logits.view(-1, self.num_labels)
- active_labels = torch.where(
- active_loss, labels.view(-1), torch.tensor(
- loss_fct.ignore_index).type_as(labels)
- )
- loss = loss_fct(active_logits, active_labels)
- else:
- loss = loss_fct(
- logits.view(-1, self.num_labels), labels.view(-1))
-
- if not return_dict:
- output = (logits,) + outputs[2:]
- return ((loss,) + output) if loss is not None else output
-
- return LongformerTokenClassifierOutput(
- loss=loss,
- logits=logits,
- hidden_states=outputs.hidden_states,
- attentions=outputs.attentions,
- global_attentions=outputs.global_attentions,
- )
-
-
-@add_start_docstrings(
- """
- Longformer Model with a multiple choice classification head on top (a linear layer on top of the pooled output and
- a softmax) e.g. for RocStories/SWAG tasks.
- """,
- LONGFORMER_START_DOCSTRING,
-)
-class LongformerForMultipleChoice(LongformerPreTrainedModel):
- def __init__(self, config):
- super().__init__(config)
-
- self.longformer = LongformerModel(config)
- self.dropout = nn.Dropout(config.hidden_dropout_prob)
- self.classifier = nn.Linear(config.hidden_size, 1)
-
- self.init_weights()
-
- @add_start_docstrings_to_model_forward(
- LONGFORMER_INPUTS_DOCSTRING.format(
- "batch_size, num_choices, sequence_length")
- )
- @add_code_sample_docstrings(
- processor_class=_TOKENIZER_FOR_DOC,
- checkpoint=_CHECKPOINT_FOR_DOC,
- output_type=LongformerMultipleChoiceModelOutput,
- config_class=_CONFIG_FOR_DOC,
- )
- def forward(
- self,
- input_ids=None,
- token_type_ids=None,
- attention_mask=None,
- global_attention_mask=None,
- head_mask=None,
- labels=None,
- position_ids=None,
- inputs_embeds=None,
- output_attentions=None,
- output_hidden_states=None,
- return_dict=None,
- ):
- r"""
- labels (:obj:`torch.LongTensor` of shape :obj:`(batch_size,)`, `optional`):
- Labels for computing the multiple choice classification loss. Indices should be in ``[0, ...,
- num_choices-1]`` where :obj:`num_choices` is the size of the second dimension of the input tensors. (See
- :obj:`input_ids` above)
- """
- num_choices = input_ids.shape[1] if input_ids is not None else inputs_embeds.shape[1]
- return_dict = return_dict if return_dict is not None else self.config.use_return_dict
-
- # set global attention on question tokens
- if global_attention_mask is None and input_ids is not None:
- logger.info("Initializing global attention on multiple choice...")
- # put global attention on all tokens after `config.sep_token_id`
- global_attention_mask = torch.stack(
- [
- _compute_global_attention_mask(
- input_ids[:, i], self.config.sep_token_id, before_sep_token=False)
- for i in range(num_choices)
- ],
- dim=1,
- )
-
- flat_input_ids = input_ids.view(-1, input_ids.size(-1)
- ) if input_ids is not None else None
- flat_position_ids = position_ids.view(
- -1, position_ids.size(-1)) if position_ids is not None else None
- flat_token_type_ids = token_type_ids.view(
- -1, token_type_ids.size(-1)) if token_type_ids is not None else None
- flat_attention_mask = attention_mask.view(
- -1, attention_mask.size(-1)) if attention_mask is not None else None
- flat_global_attention_mask = (
- global_attention_mask.view(-1, global_attention_mask.size(-1))
- if global_attention_mask is not None
- else None
- )
- flat_inputs_embeds = (
- inputs_embeds.view(-1, inputs_embeds.size(-2),
- inputs_embeds.size(-1))
- if inputs_embeds is not None
- else None
- )
-
- outputs = self.longformer(
- flat_input_ids,
- position_ids=flat_position_ids,
- token_type_ids=flat_token_type_ids,
- attention_mask=flat_attention_mask,
- global_attention_mask=flat_global_attention_mask,
- head_mask=head_mask,
- inputs_embeds=flat_inputs_embeds,
- output_attentions=output_attentions,
- output_hidden_states=output_hidden_states,
- return_dict=return_dict,
- )
- pooled_output = outputs[1]
-
- pooled_output = self.dropout(pooled_output)
- logits = self.classifier(pooled_output)
- reshaped_logits = logits.view(-1, num_choices)
-
- loss = None
- if labels is not None:
- loss_fct = CrossEntropyLoss()
- loss = loss_fct(reshaped_logits, labels)
-
- if not return_dict:
- output = (reshaped_logits,) + outputs[2:]
- return ((loss,) + output) if loss is not None else output
-
- return LongformerMultipleChoiceModelOutput(
- loss=loss,
- logits=reshaped_logits,
- hidden_states=outputs.hidden_states,
- attentions=outputs.attentions,
- global_attentions=outputs.global_attentions,
- )
diff --git a/spaces/HarryLee/eCommerceImageCaptioning/fairseq/tests/gpu/__init__.py b/spaces/HarryLee/eCommerceImageCaptioning/fairseq/tests/gpu/__init__.py
deleted file mode 100644
index e69de29bb2d1d6434b8b29ae775ad8c2e48c5391..0000000000000000000000000000000000000000
diff --git a/spaces/Harveenchadha/Vakyansh-Odia-TTS/ttsv/src/glow_tts/texttospeech.py b/spaces/Harveenchadha/Vakyansh-Odia-TTS/ttsv/src/glow_tts/texttospeech.py
deleted file mode 100644
index 3c88925cac0c56e52d35acfa5d6d7e5ce51329c7..0000000000000000000000000000000000000000
--- a/spaces/Harveenchadha/Vakyansh-Odia-TTS/ttsv/src/glow_tts/texttospeech.py
+++ /dev/null
@@ -1,146 +0,0 @@
-from __future__ import absolute_import, division, print_function, unicode_literals
-from typing import Tuple
-
-from scipy.io.wavfile import write
-from hifi.env import AttrDict
-from hifi.models import Generator
-
-import numpy as np
-import os
-import json
-
-import torch
-from text import text_to_sequence
-import commons
-import models
-import utils
-import sys
-from argparse import ArgumentParser
-
-
-def check_directory(dir):
- if not os.path.exists(dir):
- sys.exit("Error: {} directory does not exist".format(dir))
-
-
-class TextToMel:
- def __init__(self, glow_model_dir, device="cuda"):
- self.glow_model_dir = glow_model_dir
- check_directory(self.glow_model_dir)
- self.device = device
- self.hps, self.glow_tts_model = self.load_glow_tts()
- pass
-
- def load_glow_tts(self):
- hps = utils.get_hparams_from_dir(self.glow_model_dir)
- checkpoint_path = utils.latest_checkpoint_path(self.glow_model_dir)
- symbols = list(hps.data.punc) + list(hps.data.chars)
- glow_tts_model = models.FlowGenerator(
- len(symbols) + getattr(hps.data, "add_blank", False),
- out_channels=hps.data.n_mel_channels,
- **hps.model
- ) # .to(self.device)
-
- if self.device == "cuda":
- glow_tts_model.to("cuda")
-
- utils.load_checkpoint(checkpoint_path, glow_tts_model)
- glow_tts_model.decoder.store_inverse()
- _ = glow_tts_model.eval()
-
- return hps, glow_tts_model
-
- def generate_mel(self, text, noise_scale=0.667, length_scale=1.0):
- symbols = list(self.hps.data.punc) + list(self.hps.data.chars)
- cleaner = self.hps.data.text_cleaners
- if getattr(self.hps.data, "add_blank", False):
- text_norm = text_to_sequence(text, symbols, cleaner)
- text_norm = commons.intersperse(text_norm, len(symbols))
- else: # If not using "add_blank" option during training, adding spaces at the beginning and the end of utterance improves quality
- text = " " + text.strip() + " "
- text_norm = text_to_sequence(text, symbols, cleaner)
-
- sequence = np.array(text_norm)[None, :]
-
- if self.device == "cuda":
- x_tst = torch.autograd.Variable(torch.from_numpy(sequence)).cuda().long()
- x_tst_lengths = torch.tensor([x_tst.shape[1]]).cuda()
- else:
- x_tst = torch.autograd.Variable(torch.from_numpy(sequence)).long()
- x_tst_lengths = torch.tensor([x_tst.shape[1]])
-
- with torch.no_grad():
- (y_gen_tst, *_), *_, (attn_gen, *_) = self.glow_tts_model(
- x_tst,
- x_tst_lengths,
- gen=True,
- noise_scale=noise_scale,
- length_scale=length_scale,
- )
-
- return y_gen_tst
- #return y_gen_tst.cpu().detach().numpy()
-
-
-class MelToWav:
- def __init__(self, hifi_model_dir, device="cuda"):
- self.hifi_model_dir = hifi_model_dir
- check_directory(self.hifi_model_dir)
- self.device = device
- self.h, self.hifi_gan_generator = self.load_hifi_gan()
- pass
-
- def load_hifi_gan(self):
- checkpoint_path = utils.latest_checkpoint_path(self.hifi_model_dir, regex="g_*")
- config_file = os.path.join(self.hifi_model_dir, "config.json")
- data = open(config_file).read()
- json_config = json.loads(data)
- h = AttrDict(json_config)
- torch.manual_seed(h.seed)
-
- generator = Generator(h).to(self.device)
-
- assert os.path.isfile(checkpoint_path)
- print("Loading '{}'".format(checkpoint_path))
- state_dict_g = torch.load(checkpoint_path, map_location=self.device)
- print("Complete.")
-
- generator.load_state_dict(state_dict_g["generator"])
-
- generator.eval()
- generator.remove_weight_norm()
-
- return h, generator
-
- def generate_wav(self, mel):
- #mel = torch.FloatTensor(mel).to(self.device)
-
- y_g_hat = self.hifi_gan_generator(mel.to(self.device)) # passing through vocoder
- audio = y_g_hat.squeeze()
- audio = audio * 32768.0
- audio = audio.cpu().detach().numpy().astype("int16")
-
- return audio, self.h.sampling_rate
-
-
-
-
-
-if __name__ == "__main__":
-
- parser = ArgumentParser()
- parser.add_argument("-m", "--model", required=True, type=str)
- parser.add_argument("-g", "--gan", required=True, type=str)
- parser.add_argument("-d", "--device", type=str, default="cpu")
- parser.add_argument("-t", "--text", type=str, required=True)
- parser.add_argument("-w", "--wav", type=str, required=True)
-
- args = parser.parse_args()
-
- text_to_mel = TextToMel(glow_model_dir=args.model, device=args.device)
- mel_to_wav = MelToWav(hifi_model_dir=args.gan, device=args.device)
-
- mel = text_to_mel.generate_mel(args.text)
- audio, sr = mel_to_wav.generate_wav(mel)
-
- write(filename=args.wav, rate=sr, data=audio)
\ No newline at end of file
diff --git a/spaces/HenryCarle/your_sport_picker/info.md b/spaces/HenryCarle/your_sport_picker/info.md
deleted file mode 100644
index d6143037589c1791a1a313571b9582029bc8c2cb..0000000000000000000000000000000000000000
--- a/spaces/HenryCarle/your_sport_picker/info.md
+++ /dev/null
@@ -1,18 +0,0 @@
-# 😌 [Edit info.md - Sport Recomender]
-
-### 🧐 Problem Statement and Research Summary
-[Our goal is to make it easier for anyone who wants to play a sport to find a sport they can play and enjoy.]
-
-### 🎣 Data Collection Plan
-[Edit info.md - We collected our data by creating a form that has all of the crucial questions to consider what sport you would like to play, then handed it out to our peers]
-
-### 💥 Ethical Considerations (Data Privacy and Bias)
-* Data privacy: [Edit info.md - Your data would only be used for the sake of improving our AI and creating better recomendations for yourself.]
-* Bias: [Edit info.md - Our AI has no known bias that we know of.]
-
-### 👻 Our Team
-[Edit info.md - Erik: I love life, the outdoors, skiing, and chemistry and physics.
-Grady: soccer player, bassoonist, video games.
-Henry: I like to play games, science, & animals.]
-
-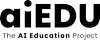
diff --git a/spaces/HighCWu/GFPGAN-1.3/tests/test_ffhq_degradation_dataset.py b/spaces/HighCWu/GFPGAN-1.3/tests/test_ffhq_degradation_dataset.py
deleted file mode 100644
index fa56c03fb8e23df26aa6ed8442a86b3c676eec78..0000000000000000000000000000000000000000
--- a/spaces/HighCWu/GFPGAN-1.3/tests/test_ffhq_degradation_dataset.py
+++ /dev/null
@@ -1,96 +0,0 @@
-import pytest
-import yaml
-
-from gfpgan.data.ffhq_degradation_dataset import FFHQDegradationDataset
-
-
-def test_ffhq_degradation_dataset():
-
- with open('tests/data/test_ffhq_degradation_dataset.yml', mode='r') as f:
- opt = yaml.load(f, Loader=yaml.FullLoader)
-
- dataset = FFHQDegradationDataset(opt)
- assert dataset.io_backend_opt['type'] == 'disk' # io backend
- assert len(dataset) == 1 # whether to read correct meta info
- assert dataset.kernel_list == ['iso', 'aniso'] # correct initialization the degradation configurations
- assert dataset.color_jitter_prob == 1
-
- # test __getitem__
- result = dataset.__getitem__(0)
- # check returned keys
- expected_keys = ['gt', 'lq', 'gt_path']
- assert set(expected_keys).issubset(set(result.keys()))
- # check shape and contents
- assert result['gt'].shape == (3, 512, 512)
- assert result['lq'].shape == (3, 512, 512)
- assert result['gt_path'] == 'tests/data/gt/00000000.png'
-
- # ------------------ test with probability = 0 -------------------- #
- opt['color_jitter_prob'] = 0
- opt['color_jitter_pt_prob'] = 0
- opt['gray_prob'] = 0
- opt['io_backend'] = dict(type='disk')
- dataset = FFHQDegradationDataset(opt)
- assert dataset.io_backend_opt['type'] == 'disk' # io backend
- assert len(dataset) == 1 # whether to read correct meta info
- assert dataset.kernel_list == ['iso', 'aniso'] # correct initialization the degradation configurations
- assert dataset.color_jitter_prob == 0
-
- # test __getitem__
- result = dataset.__getitem__(0)
- # check returned keys
- expected_keys = ['gt', 'lq', 'gt_path']
- assert set(expected_keys).issubset(set(result.keys()))
- # check shape and contents
- assert result['gt'].shape == (3, 512, 512)
- assert result['lq'].shape == (3, 512, 512)
- assert result['gt_path'] == 'tests/data/gt/00000000.png'
-
- # ------------------ test lmdb backend -------------------- #
- opt['dataroot_gt'] = 'tests/data/ffhq_gt.lmdb'
- opt['io_backend'] = dict(type='lmdb')
-
- dataset = FFHQDegradationDataset(opt)
- assert dataset.io_backend_opt['type'] == 'lmdb' # io backend
- assert len(dataset) == 1 # whether to read correct meta info
- assert dataset.kernel_list == ['iso', 'aniso'] # correct initialization the degradation configurations
- assert dataset.color_jitter_prob == 0
-
- # test __getitem__
- result = dataset.__getitem__(0)
- # check returned keys
- expected_keys = ['gt', 'lq', 'gt_path']
- assert set(expected_keys).issubset(set(result.keys()))
- # check shape and contents
- assert result['gt'].shape == (3, 512, 512)
- assert result['lq'].shape == (3, 512, 512)
- assert result['gt_path'] == '00000000'
-
- # ------------------ test with crop_components -------------------- #
- opt['crop_components'] = True
- opt['component_path'] = 'tests/data/test_eye_mouth_landmarks.pth'
- opt['eye_enlarge_ratio'] = 1.4
- opt['gt_gray'] = True
- opt['io_backend'] = dict(type='lmdb')
-
- dataset = FFHQDegradationDataset(opt)
- assert dataset.crop_components is True
-
- # test __getitem__
- result = dataset.__getitem__(0)
- # check returned keys
- expected_keys = ['gt', 'lq', 'gt_path', 'loc_left_eye', 'loc_right_eye', 'loc_mouth']
- assert set(expected_keys).issubset(set(result.keys()))
- # check shape and contents
- assert result['gt'].shape == (3, 512, 512)
- assert result['lq'].shape == (3, 512, 512)
- assert result['gt_path'] == '00000000'
- assert result['loc_left_eye'].shape == (4, )
- assert result['loc_right_eye'].shape == (4, )
- assert result['loc_mouth'].shape == (4, )
-
- # ------------------ lmdb backend should have paths ends with lmdb -------------------- #
- with pytest.raises(ValueError):
- opt['dataroot_gt'] = 'tests/data/gt'
- opt['io_backend'] = dict(type='lmdb')
- dataset = FFHQDegradationDataset(opt)
diff --git a/spaces/Hila/RobustViT/SegmentationTest/utils/iou.py b/spaces/Hila/RobustViT/SegmentationTest/utils/iou.py
deleted file mode 100644
index 4135e15892849edf40a5cdde95e49bb501cf876f..0000000000000000000000000000000000000000
--- a/spaces/Hila/RobustViT/SegmentationTest/utils/iou.py
+++ /dev/null
@@ -1,93 +0,0 @@
-import torch
-import numpy as np
-from . import metric
-from .confusionmatrix import ConfusionMatrix
-
-
-class IoU(metric.Metric):
- """Computes the intersection over union (IoU) per class and corresponding
- mean (mIoU).
-
- Intersection over union (IoU) is a common evaluation metric for semantic
- segmentation. The predictions are first accumulated in a confusion matrix
- and the IoU is computed from it as follows:
-
- IoU = true_positive / (true_positive + false_positive + false_negative).
-
- Keyword arguments:
- - num_classes (int): number of classes in the classification problem
- - normalized (boolean, optional): Determines whether or not the confusion
- matrix is normalized or not. Default: False.
- - ignore_index (int or iterable, optional): Index of the classes to ignore
- when computing the IoU. Can be an int, or any iterable of ints.
- """
-
- def __init__(self, num_classes, normalized=False, ignore_index=None):
- super().__init__()
- self.conf_metric = ConfusionMatrix(num_classes, normalized)
-
- if ignore_index is None:
- self.ignore_index = None
- elif isinstance(ignore_index, int):
- self.ignore_index = (ignore_index,)
- else:
- try:
- self.ignore_index = tuple(ignore_index)
- except TypeError:
- raise ValueError("'ignore_index' must be an int or iterable")
-
- def reset(self):
- self.conf_metric.reset()
-
- def add(self, predicted, target):
- """Adds the predicted and target pair to the IoU metric.
-
- Keyword arguments:
- - predicted (Tensor): Can be a (N, K, H, W) tensor of
- predicted scores obtained from the model for N examples and K classes,
- or (N, H, W) tensor of integer values between 0 and K-1.
- - target (Tensor): Can be a (N, K, H, W) tensor of
- target scores for N examples and K classes, or (N, H, W) tensor of
- integer values between 0 and K-1.
-
- """
- # Dimensions check
- assert predicted.size(0) == target.size(0), \
- 'number of targets and predicted outputs do not match'
- assert predicted.dim() == 3 or predicted.dim() == 4, \
- "predictions must be of dimension (N, H, W) or (N, K, H, W)"
- assert target.dim() == 3 or target.dim() == 4, \
- "targets must be of dimension (N, H, W) or (N, K, H, W)"
-
- # If the tensor is in categorical format convert it to integer format
- if predicted.dim() == 4:
- _, predicted = predicted.max(1)
- if target.dim() == 4:
- _, target = target.max(1)
-
- self.conf_metric.add(predicted.view(-1), target.view(-1))
-
- def value(self):
- """Computes the IoU and mean IoU.
-
- The mean computation ignores NaN elements of the IoU array.
-
- Returns:
- Tuple: (IoU, mIoU). The first output is the per class IoU,
- for K classes it's numpy.ndarray with K elements. The second output,
- is the mean IoU.
- """
- conf_matrix = self.conf_metric.value()
- if self.ignore_index is not None:
- for index in self.ignore_index:
- conf_matrix[:, self.ignore_index] = 0
- conf_matrix[self.ignore_index, :] = 0
- true_positive = np.diag(conf_matrix)
- false_positive = np.sum(conf_matrix, 0) - true_positive
- false_negative = np.sum(conf_matrix, 1) - true_positive
-
- # Just in case we get a division by 0, ignore/hide the error
- with np.errstate(divide='ignore', invalid='ignore'):
- iou = true_positive / (true_positive + false_positive + false_negative)
-
- return iou, np.nanmean(iou)
\ No newline at end of file
diff --git a/spaces/Hua626/QQsign/README.md b/spaces/Hua626/QQsign/README.md
deleted file mode 100644
index bd56881a2a7709591343e2f15af9a6a8133e115b..0000000000000000000000000000000000000000
--- a/spaces/Hua626/QQsign/README.md
+++ /dev/null
@@ -1,11 +0,0 @@
----
-title: QQsign
-emoji: 🦀
-colorFrom: blue
-colorTo: purple
-sdk: docker
-pinned: false
-license: mit
----
-
-Check out the configuration reference at https://huggingface.co/docs/hub/spaces-config-reference
\ No newline at end of file
diff --git a/spaces/HuangLab/CELL-E_2-Image_Prediction/taming/modules/diffusionmodules/model.py b/spaces/HuangLab/CELL-E_2-Image_Prediction/taming/modules/diffusionmodules/model.py
deleted file mode 100644
index d3a5db6aa2ef915e270f1ae135e4a9918fdd884c..0000000000000000000000000000000000000000
--- a/spaces/HuangLab/CELL-E_2-Image_Prediction/taming/modules/diffusionmodules/model.py
+++ /dev/null
@@ -1,776 +0,0 @@
-# pytorch_diffusion + derived encoder decoder
-import math
-import torch
-import torch.nn as nn
-import numpy as np
-
-
-def get_timestep_embedding(timesteps, embedding_dim):
- """
- This matches the implementation in Denoising Diffusion Probabilistic Models:
- From Fairseq.
- Build sinusoidal embeddings.
- This matches the implementation in tensor2tensor, but differs slightly
- from the description in Section 3.5 of "Attention Is All You Need".
- """
- assert len(timesteps.shape) == 1
-
- half_dim = embedding_dim // 2
- emb = math.log(10000) / (half_dim - 1)
- emb = torch.exp(torch.arange(half_dim, dtype=torch.float32) * -emb)
- emb = emb.to(device=timesteps.device)
- emb = timesteps.float()[:, None] * emb[None, :]
- emb = torch.cat([torch.sin(emb), torch.cos(emb)], dim=1)
- if embedding_dim % 2 == 1: # zero pad
- emb = torch.nn.functional.pad(emb, (0,1,0,0))
- return emb
-
-
-def nonlinearity(x):
- # swish
- return x*torch.sigmoid(x)
-
-
-def Normalize(in_channels):
- return torch.nn.GroupNorm(num_groups=32, num_channels=in_channels, eps=1e-6, affine=True)
-
-
-class Upsample(nn.Module):
- def __init__(self, in_channels, with_conv):
- super().__init__()
- self.with_conv = with_conv
- if self.with_conv:
- self.conv = torch.nn.Conv2d(in_channels,
- in_channels,
- kernel_size=3,
- stride=1,
- padding=1)
-
- def forward(self, x):
- x = torch.nn.functional.interpolate(x, scale_factor=2.0, mode="nearest")
- if self.with_conv:
- x = self.conv(x)
- return x
-
-
-class Downsample(nn.Module):
- def __init__(self, in_channels, with_conv):
- super().__init__()
- self.with_conv = with_conv
- if self.with_conv:
- # no asymmetric padding in torch conv, must do it ourselves
- self.conv = torch.nn.Conv2d(in_channels,
- in_channels,
- kernel_size=3,
- stride=2,
- padding=0)
-
- def forward(self, x):
- if self.with_conv:
- pad = (0,1,0,1)
- x = torch.nn.functional.pad(x, pad, mode="constant", value=0)
- x = self.conv(x)
- else:
- x = torch.nn.functional.avg_pool2d(x, kernel_size=2, stride=2)
- return x
-
-
-class ResnetBlock(nn.Module):
- def __init__(self, *, in_channels, out_channels=None, conv_shortcut=False,
- dropout, temb_channels=512):
- super().__init__()
- self.in_channels = in_channels
- out_channels = in_channels if out_channels is None else out_channels
- self.out_channels = out_channels
- self.use_conv_shortcut = conv_shortcut
-
- self.norm1 = Normalize(in_channels)
- self.conv1 = torch.nn.Conv2d(in_channels,
- out_channels,
- kernel_size=3,
- stride=1,
- padding=1)
- if temb_channels > 0:
- self.temb_proj = torch.nn.Linear(temb_channels,
- out_channels)
- self.norm2 = Normalize(out_channels)
- self.dropout = torch.nn.Dropout(dropout)
- self.conv2 = torch.nn.Conv2d(out_channels,
- out_channels,
- kernel_size=3,
- stride=1,
- padding=1)
- if self.in_channels != self.out_channels:
- if self.use_conv_shortcut:
- self.conv_shortcut = torch.nn.Conv2d(in_channels,
- out_channels,
- kernel_size=3,
- stride=1,
- padding=1)
- else:
- self.nin_shortcut = torch.nn.Conv2d(in_channels,
- out_channels,
- kernel_size=1,
- stride=1,
- padding=0)
-
- def forward(self, x, temb):
- h = x
- h = self.norm1(h)
- h = nonlinearity(h)
- h = self.conv1(h)
-
- if temb is not None:
- h = h + self.temb_proj(nonlinearity(temb))[:,:,None,None]
-
- h = self.norm2(h)
- h = nonlinearity(h)
- h = self.dropout(h)
- h = self.conv2(h)
-
- if self.in_channels != self.out_channels:
- if self.use_conv_shortcut:
- x = self.conv_shortcut(x)
- else:
- x = self.nin_shortcut(x)
-
- return x+h
-
-
-class AttnBlock(nn.Module):
- def __init__(self, in_channels):
- super().__init__()
- self.in_channels = in_channels
-
- self.norm = Normalize(in_channels)
- self.q = torch.nn.Conv2d(in_channels,
- in_channels,
- kernel_size=1,
- stride=1,
- padding=0)
- self.k = torch.nn.Conv2d(in_channels,
- in_channels,
- kernel_size=1,
- stride=1,
- padding=0)
- self.v = torch.nn.Conv2d(in_channels,
- in_channels,
- kernel_size=1,
- stride=1,
- padding=0)
- self.proj_out = torch.nn.Conv2d(in_channels,
- in_channels,
- kernel_size=1,
- stride=1,
- padding=0)
-
-
- def forward(self, x):
- h_ = x
- h_ = self.norm(h_)
- q = self.q(h_)
- k = self.k(h_)
- v = self.v(h_)
-
- # compute attention
- b,c,h,w = q.shape
- q = q.reshape(b,c,h*w)
- q = q.permute(0,2,1) # b,hw,c
- k = k.reshape(b,c,h*w) # b,c,hw
- w_ = torch.bmm(q,k) # b,hw,hw w[b,i,j]=sum_c q[b,i,c]k[b,c,j]
- w_ = w_ * (int(c)**(-0.5))
- w_ = torch.nn.functional.softmax(w_, dim=2)
-
- # attend to values
- v = v.reshape(b,c,h*w)
- w_ = w_.permute(0,2,1) # b,hw,hw (first hw of k, second of q)
- h_ = torch.bmm(v,w_) # b, c,hw (hw of q) h_[b,c,j] = sum_i v[b,c,i] w_[b,i,j]
- h_ = h_.reshape(b,c,h,w)
-
- h_ = self.proj_out(h_)
-
- return x+h_
-
-
-class Model(nn.Module):
- def __init__(self, *, ch, out_ch, ch_mult=(1,2,4,8), num_res_blocks,
- attn_resolutions, dropout=0.0, resamp_with_conv=True, in_channels,
- resolution, use_timestep=True):
- super().__init__()
- self.ch = ch
- self.temb_ch = self.ch*4
- self.num_resolutions = len(ch_mult)
- self.num_res_blocks = num_res_blocks
- self.resolution = resolution
- self.in_channels = in_channels
-
- self.use_timestep = use_timestep
- if self.use_timestep:
- # timestep embedding
- self.temb = nn.Module()
- self.temb.dense = nn.ModuleList([
- torch.nn.Linear(self.ch,
- self.temb_ch),
- torch.nn.Linear(self.temb_ch,
- self.temb_ch),
- ])
-
- # downsampling
- self.conv_in = torch.nn.Conv2d(in_channels,
- self.ch,
- kernel_size=3,
- stride=1,
- padding=1)
-
- curr_res = resolution
- in_ch_mult = (1,)+tuple(ch_mult)
- self.down = nn.ModuleList()
- for i_level in range(self.num_resolutions):
- block = nn.ModuleList()
- attn = nn.ModuleList()
- block_in = ch*in_ch_mult[i_level]
- block_out = ch*ch_mult[i_level]
- for i_block in range(self.num_res_blocks):
- block.append(ResnetBlock(in_channels=block_in,
- out_channels=block_out,
- temb_channels=self.temb_ch,
- dropout=dropout))
- block_in = block_out
- if curr_res in attn_resolutions:
- attn.append(AttnBlock(block_in))
- down = nn.Module()
- down.block = block
- down.attn = attn
- if i_level != self.num_resolutions-1:
- down.downsample = Downsample(block_in, resamp_with_conv)
- curr_res = curr_res // 2
- self.down.append(down)
-
- # middle
- self.mid = nn.Module()
- self.mid.block_1 = ResnetBlock(in_channels=block_in,
- out_channels=block_in,
- temb_channels=self.temb_ch,
- dropout=dropout)
- self.mid.attn_1 = AttnBlock(block_in)
- self.mid.block_2 = ResnetBlock(in_channels=block_in,
- out_channels=block_in,
- temb_channels=self.temb_ch,
- dropout=dropout)
-
- # upsampling
- self.up = nn.ModuleList()
- for i_level in reversed(range(self.num_resolutions)):
- block = nn.ModuleList()
- attn = nn.ModuleList()
- block_out = ch*ch_mult[i_level]
- skip_in = ch*ch_mult[i_level]
- for i_block in range(self.num_res_blocks+1):
- if i_block == self.num_res_blocks:
- skip_in = ch*in_ch_mult[i_level]
- block.append(ResnetBlock(in_channels=block_in+skip_in,
- out_channels=block_out,
- temb_channels=self.temb_ch,
- dropout=dropout))
- block_in = block_out
- if curr_res in attn_resolutions:
- attn.append(AttnBlock(block_in))
- up = nn.Module()
- up.block = block
- up.attn = attn
- if i_level != 0:
- up.upsample = Upsample(block_in, resamp_with_conv)
- curr_res = curr_res * 2
- self.up.insert(0, up) # prepend to get consistent order
-
- # end
- self.norm_out = Normalize(block_in)
- self.conv_out = torch.nn.Conv2d(block_in,
- out_ch,
- kernel_size=3,
- stride=1,
- padding=1)
-
-
- def forward(self, x, t=None):
- #assert x.shape[2] == x.shape[3] == self.resolution
-
- if self.use_timestep:
- # timestep embedding
- assert t is not None
- temb = get_timestep_embedding(t, self.ch)
- temb = self.temb.dense[0](temb)
- temb = nonlinearity(temb)
- temb = self.temb.dense[1](temb)
- else:
- temb = None
-
- # downsampling
- hs = [self.conv_in(x)]
- for i_level in range(self.num_resolutions):
- for i_block in range(self.num_res_blocks):
- h = self.down[i_level].block[i_block](hs[-1], temb)
- if len(self.down[i_level].attn) > 0:
- h = self.down[i_level].attn[i_block](h)
- hs.append(h)
- if i_level != self.num_resolutions-1:
- hs.append(self.down[i_level].downsample(hs[-1]))
-
- # middle
- h = hs[-1]
- h = self.mid.block_1(h, temb)
- h = self.mid.attn_1(h)
- h = self.mid.block_2(h, temb)
-
- # upsampling
- for i_level in reversed(range(self.num_resolutions)):
- for i_block in range(self.num_res_blocks+1):
- h = self.up[i_level].block[i_block](
- torch.cat([h, hs.pop()], dim=1), temb)
- if len(self.up[i_level].attn) > 0:
- h = self.up[i_level].attn[i_block](h)
- if i_level != 0:
- h = self.up[i_level].upsample(h)
-
- # end
- h = self.norm_out(h)
- h = nonlinearity(h)
- h = self.conv_out(h)
- return h
-
-
-class Encoder(nn.Module):
- def __init__(self, *, ch, out_ch, ch_mult=(1,2,4,8), num_res_blocks,
- attn_resolutions, dropout=0.0, resamp_with_conv=True, in_channels,
- resolution, z_channels, double_z=True, **ignore_kwargs):
- super().__init__()
- self.ch = ch
- self.temb_ch = 0
- self.num_resolutions = len(ch_mult)
- self.num_res_blocks = num_res_blocks
- self.resolution = resolution
- self.in_channels = in_channels
-
- # downsampling
- self.conv_in = torch.nn.Conv2d(in_channels,
- self.ch,
- kernel_size=3,
- stride=1,
- padding=1)
-
- curr_res = resolution
- in_ch_mult = (1,)+tuple(ch_mult)
- self.down = nn.ModuleList()
- for i_level in range(self.num_resolutions):
- block = nn.ModuleList()
- attn = nn.ModuleList()
- block_in = ch*in_ch_mult[i_level]
- block_out = ch*ch_mult[i_level]
- for i_block in range(self.num_res_blocks):
- block.append(ResnetBlock(in_channels=block_in,
- out_channels=block_out,
- temb_channels=self.temb_ch,
- dropout=dropout))
- block_in = block_out
- if curr_res in attn_resolutions:
- attn.append(AttnBlock(block_in))
- down = nn.Module()
- down.block = block
- down.attn = attn
- if i_level != self.num_resolutions-1:
- down.downsample = Downsample(block_in, resamp_with_conv)
- curr_res = curr_res // 2
- self.down.append(down)
-
- # middle
- self.mid = nn.Module()
- self.mid.block_1 = ResnetBlock(in_channels=block_in,
- out_channels=block_in,
- temb_channels=self.temb_ch,
- dropout=dropout)
- self.mid.attn_1 = AttnBlock(block_in)
- self.mid.block_2 = ResnetBlock(in_channels=block_in,
- out_channels=block_in,
- temb_channels=self.temb_ch,
- dropout=dropout)
-
- # end
- self.norm_out = Normalize(block_in)
- self.conv_out = torch.nn.Conv2d(block_in,
- 2*z_channels if double_z else z_channels,
- kernel_size=3,
- stride=1,
- padding=1)
-
-
- def forward(self, x):
- #assert x.shape[2] == x.shape[3] == self.resolution, "{}, {}, {}".format(x.shape[2], x.shape[3], self.resolution)
-
- # timestep embedding
- temb = None
-
- # downsampling
- hs = [self.conv_in(x)]
- for i_level in range(self.num_resolutions):
- for i_block in range(self.num_res_blocks):
- h = self.down[i_level].block[i_block](hs[-1], temb)
- if len(self.down[i_level].attn) > 0:
- h = self.down[i_level].attn[i_block](h)
- hs.append(h)
- if i_level != self.num_resolutions-1:
- hs.append(self.down[i_level].downsample(hs[-1]))
-
- # middle
- h = hs[-1]
- h = self.mid.block_1(h, temb)
- h = self.mid.attn_1(h)
- h = self.mid.block_2(h, temb)
-
- # end
- h = self.norm_out(h)
- h = nonlinearity(h)
- h = self.conv_out(h)
- return h
-
-
-class Decoder(nn.Module):
- def __init__(self, *, ch, out_ch, ch_mult=(1,2,4,8), num_res_blocks,
- attn_resolutions, dropout=0.0, resamp_with_conv=True, in_channels,
- resolution, z_channels, give_pre_end=False, **ignorekwargs):
- super().__init__()
- self.ch = ch
- self.temb_ch = 0
- self.num_resolutions = len(ch_mult)
- self.num_res_blocks = num_res_blocks
- self.resolution = resolution
- self.in_channels = in_channels
- self.give_pre_end = give_pre_end
-
- # compute in_ch_mult, block_in and curr_res at lowest res
- in_ch_mult = (1,)+tuple(ch_mult)
- block_in = ch*ch_mult[self.num_resolutions-1]
- curr_res = resolution // 2**(self.num_resolutions-1)
- self.z_shape = (1,z_channels,curr_res,curr_res)
- print("Working with z of shape {} = {} dimensions.".format(
- self.z_shape, np.prod(self.z_shape)))
-
- # z to block_in
- self.conv_in = torch.nn.Conv2d(z_channels,
- block_in,
- kernel_size=3,
- stride=1,
- padding=1)
-
- # middle
- self.mid = nn.Module()
- self.mid.block_1 = ResnetBlock(in_channels=block_in,
- out_channels=block_in,
- temb_channels=self.temb_ch,
- dropout=dropout)
- self.mid.attn_1 = AttnBlock(block_in)
- self.mid.block_2 = ResnetBlock(in_channels=block_in,
- out_channels=block_in,
- temb_channels=self.temb_ch,
- dropout=dropout)
-
- # upsampling
- self.up = nn.ModuleList()
- for i_level in reversed(range(self.num_resolutions)):
- block = nn.ModuleList()
- attn = nn.ModuleList()
- block_out = ch*ch_mult[i_level]
- for i_block in range(self.num_res_blocks+1):
- block.append(ResnetBlock(in_channels=block_in,
- out_channels=block_out,
- temb_channels=self.temb_ch,
- dropout=dropout))
- block_in = block_out
- if curr_res in attn_resolutions:
- attn.append(AttnBlock(block_in))
- up = nn.Module()
- up.block = block
- up.attn = attn
- if i_level != 0:
- up.upsample = Upsample(block_in, resamp_with_conv)
- curr_res = curr_res * 2
- self.up.insert(0, up) # prepend to get consistent order
-
- # end
- self.norm_out = Normalize(block_in)
- self.conv_out = torch.nn.Conv2d(block_in,
- out_ch,
- kernel_size=3,
- stride=1,
- padding=1)
-
- def forward(self, z):
- #assert z.shape[1:] == self.z_shape[1:]
- self.last_z_shape = z.shape
-
- # timestep embedding
- temb = None
-
- # z to block_in
- h = self.conv_in(z)
-
- # middle
- h = self.mid.block_1(h, temb)
- h = self.mid.attn_1(h)
- h = self.mid.block_2(h, temb)
-
- # upsampling
- for i_level in reversed(range(self.num_resolutions)):
- for i_block in range(self.num_res_blocks+1):
- h = self.up[i_level].block[i_block](h, temb)
- if len(self.up[i_level].attn) > 0:
- h = self.up[i_level].attn[i_block](h)
- if i_level != 0:
- h = self.up[i_level].upsample(h)
-
- # end
- if self.give_pre_end:
- return h
-
- h = self.norm_out(h)
- h = nonlinearity(h)
- h = self.conv_out(h)
- return h
-
-
-class VUNet(nn.Module):
- def __init__(self, *, ch, out_ch, ch_mult=(1,2,4,8), num_res_blocks,
- attn_resolutions, dropout=0.0, resamp_with_conv=True,
- in_channels, c_channels,
- resolution, z_channels, use_timestep=False, **ignore_kwargs):
- super().__init__()
- self.ch = ch
- self.temb_ch = self.ch*4
- self.num_resolutions = len(ch_mult)
- self.num_res_blocks = num_res_blocks
- self.resolution = resolution
-
- self.use_timestep = use_timestep
- if self.use_timestep:
- # timestep embedding
- self.temb = nn.Module()
- self.temb.dense = nn.ModuleList([
- torch.nn.Linear(self.ch,
- self.temb_ch),
- torch.nn.Linear(self.temb_ch,
- self.temb_ch),
- ])
-
- # downsampling
- self.conv_in = torch.nn.Conv2d(c_channels,
- self.ch,
- kernel_size=3,
- stride=1,
- padding=1)
-
- curr_res = resolution
- in_ch_mult = (1,)+tuple(ch_mult)
- self.down = nn.ModuleList()
- for i_level in range(self.num_resolutions):
- block = nn.ModuleList()
- attn = nn.ModuleList()
- block_in = ch*in_ch_mult[i_level]
- block_out = ch*ch_mult[i_level]
- for i_block in range(self.num_res_blocks):
- block.append(ResnetBlock(in_channels=block_in,
- out_channels=block_out,
- temb_channels=self.temb_ch,
- dropout=dropout))
- block_in = block_out
- if curr_res in attn_resolutions:
- attn.append(AttnBlock(block_in))
- down = nn.Module()
- down.block = block
- down.attn = attn
- if i_level != self.num_resolutions-1:
- down.downsample = Downsample(block_in, resamp_with_conv)
- curr_res = curr_res // 2
- self.down.append(down)
-
- self.z_in = torch.nn.Conv2d(z_channels,
- block_in,
- kernel_size=1,
- stride=1,
- padding=0)
- # middle
- self.mid = nn.Module()
- self.mid.block_1 = ResnetBlock(in_channels=2*block_in,
- out_channels=block_in,
- temb_channels=self.temb_ch,
- dropout=dropout)
- self.mid.attn_1 = AttnBlock(block_in)
- self.mid.block_2 = ResnetBlock(in_channels=block_in,
- out_channels=block_in,
- temb_channels=self.temb_ch,
- dropout=dropout)
-
- # upsampling
- self.up = nn.ModuleList()
- for i_level in reversed(range(self.num_resolutions)):
- block = nn.ModuleList()
- attn = nn.ModuleList()
- block_out = ch*ch_mult[i_level]
- skip_in = ch*ch_mult[i_level]
- for i_block in range(self.num_res_blocks+1):
- if i_block == self.num_res_blocks:
- skip_in = ch*in_ch_mult[i_level]
- block.append(ResnetBlock(in_channels=block_in+skip_in,
- out_channels=block_out,
- temb_channels=self.temb_ch,
- dropout=dropout))
- block_in = block_out
- if curr_res in attn_resolutions:
- attn.append(AttnBlock(block_in))
- up = nn.Module()
- up.block = block
- up.attn = attn
- if i_level != 0:
- up.upsample = Upsample(block_in, resamp_with_conv)
- curr_res = curr_res * 2
- self.up.insert(0, up) # prepend to get consistent order
-
- # end
- self.norm_out = Normalize(block_in)
- self.conv_out = torch.nn.Conv2d(block_in,
- out_ch,
- kernel_size=3,
- stride=1,
- padding=1)
-
-
- def forward(self, x, z):
- #assert x.shape[2] == x.shape[3] == self.resolution
-
- if self.use_timestep:
- # timestep embedding
- assert t is not None
- temb = get_timestep_embedding(t, self.ch)
- temb = self.temb.dense[0](temb)
- temb = nonlinearity(temb)
- temb = self.temb.dense[1](temb)
- else:
- temb = None
-
- # downsampling
- hs = [self.conv_in(x)]
- for i_level in range(self.num_resolutions):
- for i_block in range(self.num_res_blocks):
- h = self.down[i_level].block[i_block](hs[-1], temb)
- if len(self.down[i_level].attn) > 0:
- h = self.down[i_level].attn[i_block](h)
- hs.append(h)
- if i_level != self.num_resolutions-1:
- hs.append(self.down[i_level].downsample(hs[-1]))
-
- # middle
- h = hs[-1]
- z = self.z_in(z)
- h = torch.cat((h,z),dim=1)
- h = self.mid.block_1(h, temb)
- h = self.mid.attn_1(h)
- h = self.mid.block_2(h, temb)
-
- # upsampling
- for i_level in reversed(range(self.num_resolutions)):
- for i_block in range(self.num_res_blocks+1):
- h = self.up[i_level].block[i_block](
- torch.cat([h, hs.pop()], dim=1), temb)
- if len(self.up[i_level].attn) > 0:
- h = self.up[i_level].attn[i_block](h)
- if i_level != 0:
- h = self.up[i_level].upsample(h)
-
- # end
- h = self.norm_out(h)
- h = nonlinearity(h)
- h = self.conv_out(h)
- return h
-
-
-class SimpleDecoder(nn.Module):
- def __init__(self, in_channels, out_channels, *args, **kwargs):
- super().__init__()
- self.model = nn.ModuleList([nn.Conv2d(in_channels, in_channels, 1),
- ResnetBlock(in_channels=in_channels,
- out_channels=2 * in_channels,
- temb_channels=0, dropout=0.0),
- ResnetBlock(in_channels=2 * in_channels,
- out_channels=4 * in_channels,
- temb_channels=0, dropout=0.0),
- ResnetBlock(in_channels=4 * in_channels,
- out_channels=2 * in_channels,
- temb_channels=0, dropout=0.0),
- nn.Conv2d(2*in_channels, in_channels, 1),
- Upsample(in_channels, with_conv=True)])
- # end
- self.norm_out = Normalize(in_channels)
- self.conv_out = torch.nn.Conv2d(in_channels,
- out_channels,
- kernel_size=3,
- stride=1,
- padding=1)
-
- def forward(self, x):
- for i, layer in enumerate(self.model):
- if i in [1,2,3]:
- x = layer(x, None)
- else:
- x = layer(x)
-
- h = self.norm_out(x)
- h = nonlinearity(h)
- x = self.conv_out(h)
- return x
-
-
-class UpsampleDecoder(nn.Module):
- def __init__(self, in_channels, out_channels, ch, num_res_blocks, resolution,
- ch_mult=(2,2), dropout=0.0):
- super().__init__()
- # upsampling
- self.temb_ch = 0
- self.num_resolutions = len(ch_mult)
- self.num_res_blocks = num_res_blocks
- block_in = in_channels
- curr_res = resolution // 2 ** (self.num_resolutions - 1)
- self.res_blocks = nn.ModuleList()
- self.upsample_blocks = nn.ModuleList()
- for i_level in range(self.num_resolutions):
- res_block = []
- block_out = ch * ch_mult[i_level]
- for i_block in range(self.num_res_blocks + 1):
- res_block.append(ResnetBlock(in_channels=block_in,
- out_channels=block_out,
- temb_channels=self.temb_ch,
- dropout=dropout))
- block_in = block_out
- self.res_blocks.append(nn.ModuleList(res_block))
- if i_level != self.num_resolutions - 1:
- self.upsample_blocks.append(Upsample(block_in, True))
- curr_res = curr_res * 2
-
- # end
- self.norm_out = Normalize(block_in)
- self.conv_out = torch.nn.Conv2d(block_in,
- out_channels,
- kernel_size=3,
- stride=1,
- padding=1)
-
- def forward(self, x):
- # upsampling
- h = x
- for k, i_level in enumerate(range(self.num_resolutions)):
- for i_block in range(self.num_res_blocks + 1):
- h = self.res_blocks[i_level][i_block](h, None)
- if i_level != self.num_resolutions - 1:
- h = self.upsample_blocks[k](h)
- h = self.norm_out(h)
- h = nonlinearity(h)
- h = self.conv_out(h)
- return h
-
diff --git a/spaces/HuangLab/CELL-E_2-Sequence_Prediction/taming/lr_scheduler.py b/spaces/HuangLab/CELL-E_2-Sequence_Prediction/taming/lr_scheduler.py
deleted file mode 100644
index e598ed120159c53da6820a55ad86b89f5c70c82d..0000000000000000000000000000000000000000
--- a/spaces/HuangLab/CELL-E_2-Sequence_Prediction/taming/lr_scheduler.py
+++ /dev/null
@@ -1,34 +0,0 @@
-import numpy as np
-
-
-class LambdaWarmUpCosineScheduler:
- """
- note: use with a base_lr of 1.0
- """
- def __init__(self, warm_up_steps, lr_min, lr_max, lr_start, max_decay_steps, verbosity_interval=0):
- self.lr_warm_up_steps = warm_up_steps
- self.lr_start = lr_start
- self.lr_min = lr_min
- self.lr_max = lr_max
- self.lr_max_decay_steps = max_decay_steps
- self.last_lr = 0.
- self.verbosity_interval = verbosity_interval
-
- def schedule(self, n):
- if self.verbosity_interval > 0:
- if n % self.verbosity_interval == 0: print(f"current step: {n}, recent lr-multiplier: {self.last_lr}")
- if n < self.lr_warm_up_steps:
- lr = (self.lr_max - self.lr_start) / self.lr_warm_up_steps * n + self.lr_start
- self.last_lr = lr
- return lr
- else:
- t = (n - self.lr_warm_up_steps) / (self.lr_max_decay_steps - self.lr_warm_up_steps)
- t = min(t, 1.0)
- lr = self.lr_min + 0.5 * (self.lr_max - self.lr_min) * (
- 1 + np.cos(t * np.pi))
- self.last_lr = lr
- return lr
-
- def __call__(self, n):
- return self.schedule(n)
-
diff --git a/spaces/ICML2022/OFA/fairseq/fairseq/modules/same_pad.py b/spaces/ICML2022/OFA/fairseq/fairseq/modules/same_pad.py
deleted file mode 100644
index 4c04990ea6fdb291f162ee8ac3d17a92483daf8e..0000000000000000000000000000000000000000
--- a/spaces/ICML2022/OFA/fairseq/fairseq/modules/same_pad.py
+++ /dev/null
@@ -1,21 +0,0 @@
-# Copyright (c) Facebook, Inc. and its affiliates.
-#
-# This source code is licensed under the MIT license found in the
-# LICENSE file in the root directory of this source tree.
-
-
-from torch import nn
-
-
-class SamePad(nn.Module):
- def __init__(self, kernel_size, causal=False):
- super().__init__()
- if causal:
- self.remove = kernel_size - 1
- else:
- self.remove = 1 if kernel_size % 2 == 0 else 0
-
- def forward(self, x):
- if self.remove > 0:
- x = x[:, :, : -self.remove]
- return x
diff --git a/spaces/Izal887/Konci887/infer_pack/commons.py b/spaces/Izal887/Konci887/infer_pack/commons.py
deleted file mode 100644
index 54470986f37825b35d90d7efa7437d1c26b87215..0000000000000000000000000000000000000000
--- a/spaces/Izal887/Konci887/infer_pack/commons.py
+++ /dev/null
@@ -1,166 +0,0 @@
-import math
-import numpy as np
-import torch
-from torch import nn
-from torch.nn import functional as F
-
-
-def init_weights(m, mean=0.0, std=0.01):
- classname = m.__class__.__name__
- if classname.find("Conv") != -1:
- m.weight.data.normal_(mean, std)
-
-
-def get_padding(kernel_size, dilation=1):
- return int((kernel_size * dilation - dilation) / 2)
-
-
-def convert_pad_shape(pad_shape):
- l = pad_shape[::-1]
- pad_shape = [item for sublist in l for item in sublist]
- return pad_shape
-
-
-def kl_divergence(m_p, logs_p, m_q, logs_q):
- """KL(P||Q)"""
- kl = (logs_q - logs_p) - 0.5
- kl += (
- 0.5 * (torch.exp(2.0 * logs_p) + ((m_p - m_q) ** 2)) * torch.exp(-2.0 * logs_q)
- )
- return kl
-
-
-def rand_gumbel(shape):
- """Sample from the Gumbel distribution, protect from overflows."""
- uniform_samples = torch.rand(shape) * 0.99998 + 0.00001
- return -torch.log(-torch.log(uniform_samples))
-
-
-def rand_gumbel_like(x):
- g = rand_gumbel(x.size()).to(dtype=x.dtype, device=x.device)
- return g
-
-
-def slice_segments(x, ids_str, segment_size=4):
- ret = torch.zeros_like(x[:, :, :segment_size])
- for i in range(x.size(0)):
- idx_str = ids_str[i]
- idx_end = idx_str + segment_size
- ret[i] = x[i, :, idx_str:idx_end]
- return ret
-
-
-def slice_segments2(x, ids_str, segment_size=4):
- ret = torch.zeros_like(x[:, :segment_size])
- for i in range(x.size(0)):
- idx_str = ids_str[i]
- idx_end = idx_str + segment_size
- ret[i] = x[i, idx_str:idx_end]
- return ret
-
-
-def rand_slice_segments(x, x_lengths=None, segment_size=4):
- b, d, t = x.size()
- if x_lengths is None:
- x_lengths = t
- ids_str_max = x_lengths - segment_size + 1
- ids_str = (torch.rand([b]).to(device=x.device) * ids_str_max).to(dtype=torch.long)
- ret = slice_segments(x, ids_str, segment_size)
- return ret, ids_str
-
-
-def get_timing_signal_1d(length, channels, min_timescale=1.0, max_timescale=1.0e4):
- position = torch.arange(length, dtype=torch.float)
- num_timescales = channels // 2
- log_timescale_increment = math.log(float(max_timescale) / float(min_timescale)) / (
- num_timescales - 1
- )
- inv_timescales = min_timescale * torch.exp(
- torch.arange(num_timescales, dtype=torch.float) * -log_timescale_increment
- )
- scaled_time = position.unsqueeze(0) * inv_timescales.unsqueeze(1)
- signal = torch.cat([torch.sin(scaled_time), torch.cos(scaled_time)], 0)
- signal = F.pad(signal, [0, 0, 0, channels % 2])
- signal = signal.view(1, channels, length)
- return signal
-
-
-def add_timing_signal_1d(x, min_timescale=1.0, max_timescale=1.0e4):
- b, channels, length = x.size()
- signal = get_timing_signal_1d(length, channels, min_timescale, max_timescale)
- return x + signal.to(dtype=x.dtype, device=x.device)
-
-
-def cat_timing_signal_1d(x, min_timescale=1.0, max_timescale=1.0e4, axis=1):
- b, channels, length = x.size()
- signal = get_timing_signal_1d(length, channels, min_timescale, max_timescale)
- return torch.cat([x, signal.to(dtype=x.dtype, device=x.device)], axis)
-
-
-def subsequent_mask(length):
- mask = torch.tril(torch.ones(length, length)).unsqueeze(0).unsqueeze(0)
- return mask
-
-
-@torch.jit.script
-def fused_add_tanh_sigmoid_multiply(input_a, input_b, n_channels):
- n_channels_int = n_channels[0]
- in_act = input_a + input_b
- t_act = torch.tanh(in_act[:, :n_channels_int, :])
- s_act = torch.sigmoid(in_act[:, n_channels_int:, :])
- acts = t_act * s_act
- return acts
-
-
-def convert_pad_shape(pad_shape):
- l = pad_shape[::-1]
- pad_shape = [item for sublist in l for item in sublist]
- return pad_shape
-
-
-def shift_1d(x):
- x = F.pad(x, convert_pad_shape([[0, 0], [0, 0], [1, 0]]))[:, :, :-1]
- return x
-
-
-def sequence_mask(length, max_length=None):
- if max_length is None:
- max_length = length.max()
- x = torch.arange(max_length, dtype=length.dtype, device=length.device)
- return x.unsqueeze(0) < length.unsqueeze(1)
-
-
-def generate_path(duration, mask):
- """
- duration: [b, 1, t_x]
- mask: [b, 1, t_y, t_x]
- """
- device = duration.device
-
- b, _, t_y, t_x = mask.shape
- cum_duration = torch.cumsum(duration, -1)
-
- cum_duration_flat = cum_duration.view(b * t_x)
- path = sequence_mask(cum_duration_flat, t_y).to(mask.dtype)
- path = path.view(b, t_x, t_y)
- path = path - F.pad(path, convert_pad_shape([[0, 0], [1, 0], [0, 0]]))[:, :-1]
- path = path.unsqueeze(1).transpose(2, 3) * mask
- return path
-
-
-def clip_grad_value_(parameters, clip_value, norm_type=2):
- if isinstance(parameters, torch.Tensor):
- parameters = [parameters]
- parameters = list(filter(lambda p: p.grad is not None, parameters))
- norm_type = float(norm_type)
- if clip_value is not None:
- clip_value = float(clip_value)
-
- total_norm = 0
- for p in parameters:
- param_norm = p.grad.data.norm(norm_type)
- total_norm += param_norm.item() ** norm_type
- if clip_value is not None:
- p.grad.data.clamp_(min=-clip_value, max=clip_value)
- total_norm = total_norm ** (1.0 / norm_type)
- return total_norm
diff --git a/spaces/JLD/image-search/README.md b/spaces/JLD/image-search/README.md
deleted file mode 100644
index 5350cf5bd95beac262842fa0cae6b7701d95dba8..0000000000000000000000000000000000000000
--- a/spaces/JLD/image-search/README.md
+++ /dev/null
@@ -1,37 +0,0 @@
----
-title: Image Search
-emoji: 🌖
-colorFrom: blue
-colorTo: pink
-sdk: streamlit
-app_file: app.py
-pinned: false
----
-
-# Configuration
-
-`title`: _string_
-Display title for the Space
-
-`emoji`: _string_
-Space emoji (emoji-only character allowed)
-
-`colorFrom`: _string_
-Color for Thumbnail gradient (red, yellow, green, blue, indigo, purple, pink, gray)
-
-`colorTo`: _string_
-Color for Thumbnail gradient (red, yellow, green, blue, indigo, purple, pink, gray)
-
-`sdk`: _string_
-Can be either `gradio` or `streamlit`
-
-`sdk_version` : _string_
-Only applicable for `streamlit` SDK.
-See [doc](https://hf.co/docs/hub/spaces) for more info on supported versions.
-
-`app_file`: _string_
-Path to your main application file (which contains either `gradio` or `streamlit` Python code).
-Path is relative to the root of the repository.
-
-`pinned`: _boolean_
-Whether the Space stays on top of your list.
diff --git a/spaces/Jamel887/Rv-percobaan887/lib/infer_pack/modules/F0Predictor/F0Predictor.py b/spaces/Jamel887/Rv-percobaan887/lib/infer_pack/modules/F0Predictor/F0Predictor.py
deleted file mode 100644
index f56e49e7f0e6eab3babf0711cae2933371b9f9cc..0000000000000000000000000000000000000000
--- a/spaces/Jamel887/Rv-percobaan887/lib/infer_pack/modules/F0Predictor/F0Predictor.py
+++ /dev/null
@@ -1,16 +0,0 @@
-class F0Predictor(object):
- def compute_f0(self, wav, p_len):
- """
- input: wav:[signal_length]
- p_len:int
- output: f0:[signal_length//hop_length]
- """
- pass
-
- def compute_f0_uv(self, wav, p_len):
- """
- input: wav:[signal_length]
- p_len:int
- output: f0:[signal_length//hop_length],uv:[signal_length//hop_length]
- """
- pass
diff --git a/spaces/JohnSmith9982/VITS-Umamusume-voice-synthesizer/monotonic_align/core.c b/spaces/JohnSmith9982/VITS-Umamusume-voice-synthesizer/monotonic_align/core.c
deleted file mode 100644
index 5631d20a9a00db29e143a6e8e4e5c378d6bb850a..0000000000000000000000000000000000000000
--- a/spaces/JohnSmith9982/VITS-Umamusume-voice-synthesizer/monotonic_align/core.c
+++ /dev/null
@@ -1,21299 +0,0 @@
-/* Generated by Cython 0.29.21 */
-
-/* BEGIN: Cython Metadata
-{
- "distutils": {
- "name": "monotonic_align.core",
- "sources": [
- "core.pyx"
- ]
- },
- "module_name": "monotonic_align.core"
-}
-END: Cython Metadata */
-
-#define PY_SSIZE_T_CLEAN
-#include "Python.h"
-#ifndef Py_PYTHON_H
- #error Python headers needed to compile C extensions, please install development version of Python.
-#elif PY_VERSION_HEX < 0x02060000 || (0x03000000 <= PY_VERSION_HEX && PY_VERSION_HEX < 0x03030000)
- #error Cython requires Python 2.6+ or Python 3.3+.
-#else
-#define CYTHON_ABI "0_29_21"
-#define CYTHON_HEX_VERSION 0x001D15F0
-#define CYTHON_FUTURE_DIVISION 0
-#include
-#ifndef offsetof
- #define offsetof(type, member) ( (size_t) & ((type*)0) -> member )
-#endif
-#if !defined(WIN32) && !defined(MS_WINDOWS)
- #ifndef __stdcall
- #define __stdcall
- #endif
- #ifndef __cdecl
- #define __cdecl
- #endif
- #ifndef __fastcall
- #define __fastcall
- #endif
-#endif
-#ifndef DL_IMPORT
- #define DL_IMPORT(t) t
-#endif
-#ifndef DL_EXPORT
- #define DL_EXPORT(t) t
-#endif
-#define __PYX_COMMA ,
-#ifndef HAVE_LONG_LONG
- #if PY_VERSION_HEX >= 0x02070000
- #define HAVE_LONG_LONG
- #endif
-#endif
-#ifndef PY_LONG_LONG
- #define PY_LONG_LONG LONG_LONG
-#endif
-#ifndef Py_HUGE_VAL
- #define Py_HUGE_VAL HUGE_VAL
-#endif
-#ifdef PYPY_VERSION
- #define CYTHON_COMPILING_IN_PYPY 1
- #define CYTHON_COMPILING_IN_PYSTON 0
- #define CYTHON_COMPILING_IN_CPYTHON 0
- #undef CYTHON_USE_TYPE_SLOTS
- #define CYTHON_USE_TYPE_SLOTS 0
- #undef CYTHON_USE_PYTYPE_LOOKUP
- #define CYTHON_USE_PYTYPE_LOOKUP 0
- #if PY_VERSION_HEX < 0x03050000
- #undef CYTHON_USE_ASYNC_SLOTS
- #define CYTHON_USE_ASYNC_SLOTS 0
- #elif !defined(CYTHON_USE_ASYNC_SLOTS)
- #define CYTHON_USE_ASYNC_SLOTS 1
- #endif
- #undef CYTHON_USE_PYLIST_INTERNALS
- #define CYTHON_USE_PYLIST_INTERNALS 0
- #undef CYTHON_USE_UNICODE_INTERNALS
- #define CYTHON_USE_UNICODE_INTERNALS 0
- #undef CYTHON_USE_UNICODE_WRITER
- #define CYTHON_USE_UNICODE_WRITER 0
- #undef CYTHON_USE_PYLONG_INTERNALS
- #define CYTHON_USE_PYLONG_INTERNALS 0
- #undef CYTHON_AVOID_BORROWED_REFS
- #define CYTHON_AVOID_BORROWED_REFS 1
- #undef CYTHON_ASSUME_SAFE_MACROS
- #define CYTHON_ASSUME_SAFE_MACROS 0
- #undef CYTHON_UNPACK_METHODS
- #define CYTHON_UNPACK_METHODS 0
- #undef CYTHON_FAST_THREAD_STATE
- #define CYTHON_FAST_THREAD_STATE 0
- #undef CYTHON_FAST_PYCALL
- #define CYTHON_FAST_PYCALL 0
- #undef CYTHON_PEP489_MULTI_PHASE_INIT
- #define CYTHON_PEP489_MULTI_PHASE_INIT 0
- #undef CYTHON_USE_TP_FINALIZE
- #define CYTHON_USE_TP_FINALIZE 0
- #undef CYTHON_USE_DICT_VERSIONS
- #define CYTHON_USE_DICT_VERSIONS 0
- #undef CYTHON_USE_EXC_INFO_STACK
- #define CYTHON_USE_EXC_INFO_STACK 0
-#elif defined(PYSTON_VERSION)
- #define CYTHON_COMPILING_IN_PYPY 0
- #define CYTHON_COMPILING_IN_PYSTON 1
- #define CYTHON_COMPILING_IN_CPYTHON 0
- #ifndef CYTHON_USE_TYPE_SLOTS
- #define CYTHON_USE_TYPE_SLOTS 1
- #endif
- #undef CYTHON_USE_PYTYPE_LOOKUP
- #define CYTHON_USE_PYTYPE_LOOKUP 0
- #undef CYTHON_USE_ASYNC_SLOTS
- #define CYTHON_USE_ASYNC_SLOTS 0
- #undef CYTHON_USE_PYLIST_INTERNALS
- #define CYTHON_USE_PYLIST_INTERNALS 0
- #ifndef CYTHON_USE_UNICODE_INTERNALS
- #define CYTHON_USE_UNICODE_INTERNALS 1
- #endif
- #undef CYTHON_USE_UNICODE_WRITER
- #define CYTHON_USE_UNICODE_WRITER 0
- #undef CYTHON_USE_PYLONG_INTERNALS
- #define CYTHON_USE_PYLONG_INTERNALS 0
- #ifndef CYTHON_AVOID_BORROWED_REFS
- #define CYTHON_AVOID_BORROWED_REFS 0
- #endif
- #ifndef CYTHON_ASSUME_SAFE_MACROS
- #define CYTHON_ASSUME_SAFE_MACROS 1
- #endif
- #ifndef CYTHON_UNPACK_METHODS
- #define CYTHON_UNPACK_METHODS 1
- #endif
- #undef CYTHON_FAST_THREAD_STATE
- #define CYTHON_FAST_THREAD_STATE 0
- #undef CYTHON_FAST_PYCALL
- #define CYTHON_FAST_PYCALL 0
- #undef CYTHON_PEP489_MULTI_PHASE_INIT
- #define CYTHON_PEP489_MULTI_PHASE_INIT 0
- #undef CYTHON_USE_TP_FINALIZE
- #define CYTHON_USE_TP_FINALIZE 0
- #undef CYTHON_USE_DICT_VERSIONS
- #define CYTHON_USE_DICT_VERSIONS 0
- #undef CYTHON_USE_EXC_INFO_STACK
- #define CYTHON_USE_EXC_INFO_STACK 0
-#else
- #define CYTHON_COMPILING_IN_PYPY 0
- #define CYTHON_COMPILING_IN_PYSTON 0
- #define CYTHON_COMPILING_IN_CPYTHON 1
- #ifndef CYTHON_USE_TYPE_SLOTS
- #define CYTHON_USE_TYPE_SLOTS 1
- #endif
- #if PY_VERSION_HEX < 0x02070000
- #undef CYTHON_USE_PYTYPE_LOOKUP
- #define CYTHON_USE_PYTYPE_LOOKUP 0
- #elif !defined(CYTHON_USE_PYTYPE_LOOKUP)
- #define CYTHON_USE_PYTYPE_LOOKUP 1
- #endif
- #if PY_MAJOR_VERSION < 3
- #undef CYTHON_USE_ASYNC_SLOTS
- #define CYTHON_USE_ASYNC_SLOTS 0
- #elif !defined(CYTHON_USE_ASYNC_SLOTS)
- #define CYTHON_USE_ASYNC_SLOTS 1
- #endif
- #if PY_VERSION_HEX < 0x02070000
- #undef CYTHON_USE_PYLONG_INTERNALS
- #define CYTHON_USE_PYLONG_INTERNALS 0
- #elif !defined(CYTHON_USE_PYLONG_INTERNALS)
- #define CYTHON_USE_PYLONG_INTERNALS 1
- #endif
- #ifndef CYTHON_USE_PYLIST_INTERNALS
- #define CYTHON_USE_PYLIST_INTERNALS 1
- #endif
- #ifndef CYTHON_USE_UNICODE_INTERNALS
- #define CYTHON_USE_UNICODE_INTERNALS 1
- #endif
- #if PY_VERSION_HEX < 0x030300F0
- #undef CYTHON_USE_UNICODE_WRITER
- #define CYTHON_USE_UNICODE_WRITER 0
- #elif !defined(CYTHON_USE_UNICODE_WRITER)
- #define CYTHON_USE_UNICODE_WRITER 1
- #endif
- #ifndef CYTHON_AVOID_BORROWED_REFS
- #define CYTHON_AVOID_BORROWED_REFS 0
- #endif
- #ifndef CYTHON_ASSUME_SAFE_MACROS
- #define CYTHON_ASSUME_SAFE_MACROS 1
- #endif
- #ifndef CYTHON_UNPACK_METHODS
- #define CYTHON_UNPACK_METHODS 1
- #endif
- #ifndef CYTHON_FAST_THREAD_STATE
- #define CYTHON_FAST_THREAD_STATE 1
- #endif
- #ifndef CYTHON_FAST_PYCALL
- #define CYTHON_FAST_PYCALL 1
- #endif
- #ifndef CYTHON_PEP489_MULTI_PHASE_INIT
- #define CYTHON_PEP489_MULTI_PHASE_INIT (PY_VERSION_HEX >= 0x03050000)
- #endif
- #ifndef CYTHON_USE_TP_FINALIZE
- #define CYTHON_USE_TP_FINALIZE (PY_VERSION_HEX >= 0x030400a1)
- #endif
- #ifndef CYTHON_USE_DICT_VERSIONS
- #define CYTHON_USE_DICT_VERSIONS (PY_VERSION_HEX >= 0x030600B1)
- #endif
- #ifndef CYTHON_USE_EXC_INFO_STACK
- #define CYTHON_USE_EXC_INFO_STACK (PY_VERSION_HEX >= 0x030700A3)
- #endif
-#endif
-#if !defined(CYTHON_FAST_PYCCALL)
-#define CYTHON_FAST_PYCCALL (CYTHON_FAST_PYCALL && PY_VERSION_HEX >= 0x030600B1)
-#endif
-#if CYTHON_USE_PYLONG_INTERNALS
- #include "longintrepr.h"
- #undef SHIFT
- #undef BASE
- #undef MASK
- #ifdef SIZEOF_VOID_P
- enum { __pyx_check_sizeof_voidp = 1 / (int)(SIZEOF_VOID_P == sizeof(void*)) };
- #endif
-#endif
-#ifndef __has_attribute
- #define __has_attribute(x) 0
-#endif
-#ifndef __has_cpp_attribute
- #define __has_cpp_attribute(x) 0
-#endif
-#ifndef CYTHON_RESTRICT
- #if defined(__GNUC__)
- #define CYTHON_RESTRICT __restrict__
- #elif defined(_MSC_VER) && _MSC_VER >= 1400
- #define CYTHON_RESTRICT __restrict
- #elif defined (__STDC_VERSION__) && __STDC_VERSION__ >= 199901L
- #define CYTHON_RESTRICT restrict
- #else
- #define CYTHON_RESTRICT
- #endif
-#endif
-#ifndef CYTHON_UNUSED
-# if defined(__GNUC__)
-# if !(defined(__cplusplus)) || (__GNUC__ > 3 || (__GNUC__ == 3 && __GNUC_MINOR__ >= 4))
-# define CYTHON_UNUSED __attribute__ ((__unused__))
-# else
-# define CYTHON_UNUSED
-# endif
-# elif defined(__ICC) || (defined(__INTEL_COMPILER) && !defined(_MSC_VER))
-# define CYTHON_UNUSED __attribute__ ((__unused__))
-# else
-# define CYTHON_UNUSED
-# endif
-#endif
-#ifndef CYTHON_MAYBE_UNUSED_VAR
-# if defined(__cplusplus)
- template void CYTHON_MAYBE_UNUSED_VAR( const T& ) { }
-# else
-# define CYTHON_MAYBE_UNUSED_VAR(x) (void)(x)
-# endif
-#endif
-#ifndef CYTHON_NCP_UNUSED
-# if CYTHON_COMPILING_IN_CPYTHON
-# define CYTHON_NCP_UNUSED
-# else
-# define CYTHON_NCP_UNUSED CYTHON_UNUSED
-# endif
-#endif
-#define __Pyx_void_to_None(void_result) ((void)(void_result), Py_INCREF(Py_None), Py_None)
-#ifdef _MSC_VER
- #ifndef _MSC_STDINT_H_
- #if _MSC_VER < 1300
- typedef unsigned char uint8_t;
- typedef unsigned int uint32_t;
- #else
- typedef unsigned __int8 uint8_t;
- typedef unsigned __int32 uint32_t;
- #endif
- #endif
-#else
- #include
-#endif
-#ifndef CYTHON_FALLTHROUGH
- #if defined(__cplusplus) && __cplusplus >= 201103L
- #if __has_cpp_attribute(fallthrough)
- #define CYTHON_FALLTHROUGH [[fallthrough]]
- #elif __has_cpp_attribute(clang::fallthrough)
- #define CYTHON_FALLTHROUGH [[clang::fallthrough]]
- #elif __has_cpp_attribute(gnu::fallthrough)
- #define CYTHON_FALLTHROUGH [[gnu::fallthrough]]
- #endif
- #endif
- #ifndef CYTHON_FALLTHROUGH
- #if __has_attribute(fallthrough)
- #define CYTHON_FALLTHROUGH __attribute__((fallthrough))
- #else
- #define CYTHON_FALLTHROUGH
- #endif
- #endif
- #if defined(__clang__ ) && defined(__apple_build_version__)
- #if __apple_build_version__ < 7000000
- #undef CYTHON_FALLTHROUGH
- #define CYTHON_FALLTHROUGH
- #endif
- #endif
-#endif
-
-#ifndef CYTHON_INLINE
- #if defined(__clang__)
- #define CYTHON_INLINE __inline__ __attribute__ ((__unused__))
- #elif defined(__GNUC__)
- #define CYTHON_INLINE __inline__
- #elif defined(_MSC_VER)
- #define CYTHON_INLINE __inline
- #elif defined (__STDC_VERSION__) && __STDC_VERSION__ >= 199901L
- #define CYTHON_INLINE inline
- #else
- #define CYTHON_INLINE
- #endif
-#endif
-
-#if CYTHON_COMPILING_IN_PYPY && PY_VERSION_HEX < 0x02070600 && !defined(Py_OptimizeFlag)
- #define Py_OptimizeFlag 0
-#endif
-#define __PYX_BUILD_PY_SSIZE_T "n"
-#define CYTHON_FORMAT_SSIZE_T "z"
-#if PY_MAJOR_VERSION < 3
- #define __Pyx_BUILTIN_MODULE_NAME "__builtin__"
- #define __Pyx_PyCode_New(a, k, l, s, f, code, c, n, v, fv, cell, fn, name, fline, lnos)\
- PyCode_New(a+k, l, s, f, code, c, n, v, fv, cell, fn, name, fline, lnos)
- #define __Pyx_DefaultClassType PyClass_Type
-#else
- #define __Pyx_BUILTIN_MODULE_NAME "builtins"
-#if PY_VERSION_HEX >= 0x030800A4 && PY_VERSION_HEX < 0x030800B2
- #define __Pyx_PyCode_New(a, k, l, s, f, code, c, n, v, fv, cell, fn, name, fline, lnos)\
- PyCode_New(a, 0, k, l, s, f, code, c, n, v, fv, cell, fn, name, fline, lnos)
-#else
- #define __Pyx_PyCode_New(a, k, l, s, f, code, c, n, v, fv, cell, fn, name, fline, lnos)\
- PyCode_New(a, k, l, s, f, code, c, n, v, fv, cell, fn, name, fline, lnos)
-#endif
- #define __Pyx_DefaultClassType PyType_Type
-#endif
-#ifndef Py_TPFLAGS_CHECKTYPES
- #define Py_TPFLAGS_CHECKTYPES 0
-#endif
-#ifndef Py_TPFLAGS_HAVE_INDEX
- #define Py_TPFLAGS_HAVE_INDEX 0
-#endif
-#ifndef Py_TPFLAGS_HAVE_NEWBUFFER
- #define Py_TPFLAGS_HAVE_NEWBUFFER 0
-#endif
-#ifndef Py_TPFLAGS_HAVE_FINALIZE
- #define Py_TPFLAGS_HAVE_FINALIZE 0
-#endif
-#ifndef METH_STACKLESS
- #define METH_STACKLESS 0
-#endif
-#if PY_VERSION_HEX <= 0x030700A3 || !defined(METH_FASTCALL)
- #ifndef METH_FASTCALL
- #define METH_FASTCALL 0x80
- #endif
- typedef PyObject *(*__Pyx_PyCFunctionFast) (PyObject *self, PyObject *const *args, Py_ssize_t nargs);
- typedef PyObject *(*__Pyx_PyCFunctionFastWithKeywords) (PyObject *self, PyObject *const *args,
- Py_ssize_t nargs, PyObject *kwnames);
-#else
- #define __Pyx_PyCFunctionFast _PyCFunctionFast
- #define __Pyx_PyCFunctionFastWithKeywords _PyCFunctionFastWithKeywords
-#endif
-#if CYTHON_FAST_PYCCALL
-#define __Pyx_PyFastCFunction_Check(func)\
- ((PyCFunction_Check(func) && (METH_FASTCALL == (PyCFunction_GET_FLAGS(func) & ~(METH_CLASS | METH_STATIC | METH_COEXIST | METH_KEYWORDS | METH_STACKLESS)))))
-#else
-#define __Pyx_PyFastCFunction_Check(func) 0
-#endif
-#if CYTHON_COMPILING_IN_PYPY && !defined(PyObject_Malloc)
- #define PyObject_Malloc(s) PyMem_Malloc(s)
- #define PyObject_Free(p) PyMem_Free(p)
- #define PyObject_Realloc(p) PyMem_Realloc(p)
-#endif
-#if CYTHON_COMPILING_IN_CPYTHON && PY_VERSION_HEX < 0x030400A1
- #define PyMem_RawMalloc(n) PyMem_Malloc(n)
- #define PyMem_RawRealloc(p, n) PyMem_Realloc(p, n)
- #define PyMem_RawFree(p) PyMem_Free(p)
-#endif
-#if CYTHON_COMPILING_IN_PYSTON
- #define __Pyx_PyCode_HasFreeVars(co) PyCode_HasFreeVars(co)
- #define __Pyx_PyFrame_SetLineNumber(frame, lineno) PyFrame_SetLineNumber(frame, lineno)
-#else
- #define __Pyx_PyCode_HasFreeVars(co) (PyCode_GetNumFree(co) > 0)
- #define __Pyx_PyFrame_SetLineNumber(frame, lineno) (frame)->f_lineno = (lineno)
-#endif
-#if !CYTHON_FAST_THREAD_STATE || PY_VERSION_HEX < 0x02070000
- #define __Pyx_PyThreadState_Current PyThreadState_GET()
-#elif PY_VERSION_HEX >= 0x03060000
- #define __Pyx_PyThreadState_Current _PyThreadState_UncheckedGet()
-#elif PY_VERSION_HEX >= 0x03000000
- #define __Pyx_PyThreadState_Current PyThreadState_GET()
-#else
- #define __Pyx_PyThreadState_Current _PyThreadState_Current
-#endif
-#if PY_VERSION_HEX < 0x030700A2 && !defined(PyThread_tss_create) && !defined(Py_tss_NEEDS_INIT)
-#include "pythread.h"
-#define Py_tss_NEEDS_INIT 0
-typedef int Py_tss_t;
-static CYTHON_INLINE int PyThread_tss_create(Py_tss_t *key) {
- *key = PyThread_create_key();
- return 0;
-}
-static CYTHON_INLINE Py_tss_t * PyThread_tss_alloc(void) {
- Py_tss_t *key = (Py_tss_t *)PyObject_Malloc(sizeof(Py_tss_t));
- *key = Py_tss_NEEDS_INIT;
- return key;
-}
-static CYTHON_INLINE void PyThread_tss_free(Py_tss_t *key) {
- PyObject_Free(key);
-}
-static CYTHON_INLINE int PyThread_tss_is_created(Py_tss_t *key) {
- return *key != Py_tss_NEEDS_INIT;
-}
-static CYTHON_INLINE void PyThread_tss_delete(Py_tss_t *key) {
- PyThread_delete_key(*key);
- *key = Py_tss_NEEDS_INIT;
-}
-static CYTHON_INLINE int PyThread_tss_set(Py_tss_t *key, void *value) {
- return PyThread_set_key_value(*key, value);
-}
-static CYTHON_INLINE void * PyThread_tss_get(Py_tss_t *key) {
- return PyThread_get_key_value(*key);
-}
-#endif
-#if CYTHON_COMPILING_IN_CPYTHON || defined(_PyDict_NewPresized)
-#define __Pyx_PyDict_NewPresized(n) ((n <= 8) ? PyDict_New() : _PyDict_NewPresized(n))
-#else
-#define __Pyx_PyDict_NewPresized(n) PyDict_New()
-#endif
-#if PY_MAJOR_VERSION >= 3 || CYTHON_FUTURE_DIVISION
- #define __Pyx_PyNumber_Divide(x,y) PyNumber_TrueDivide(x,y)
- #define __Pyx_PyNumber_InPlaceDivide(x,y) PyNumber_InPlaceTrueDivide(x,y)
-#else
- #define __Pyx_PyNumber_Divide(x,y) PyNumber_Divide(x,y)
- #define __Pyx_PyNumber_InPlaceDivide(x,y) PyNumber_InPlaceDivide(x,y)
-#endif
-#if CYTHON_COMPILING_IN_CPYTHON && PY_VERSION_HEX >= 0x030500A1 && CYTHON_USE_UNICODE_INTERNALS
-#define __Pyx_PyDict_GetItemStr(dict, name) _PyDict_GetItem_KnownHash(dict, name, ((PyASCIIObject *) name)->hash)
-#else
-#define __Pyx_PyDict_GetItemStr(dict, name) PyDict_GetItem(dict, name)
-#endif
-#if PY_VERSION_HEX > 0x03030000 && defined(PyUnicode_KIND)
- #define CYTHON_PEP393_ENABLED 1
- #define __Pyx_PyUnicode_READY(op) (likely(PyUnicode_IS_READY(op)) ?\
- 0 : _PyUnicode_Ready((PyObject *)(op)))
- #define __Pyx_PyUnicode_GET_LENGTH(u) PyUnicode_GET_LENGTH(u)
- #define __Pyx_PyUnicode_READ_CHAR(u, i) PyUnicode_READ_CHAR(u, i)
- #define __Pyx_PyUnicode_MAX_CHAR_VALUE(u) PyUnicode_MAX_CHAR_VALUE(u)
- #define __Pyx_PyUnicode_KIND(u) PyUnicode_KIND(u)
- #define __Pyx_PyUnicode_DATA(u) PyUnicode_DATA(u)
- #define __Pyx_PyUnicode_READ(k, d, i) PyUnicode_READ(k, d, i)
- #define __Pyx_PyUnicode_WRITE(k, d, i, ch) PyUnicode_WRITE(k, d, i, ch)
- #if defined(PyUnicode_IS_READY) && defined(PyUnicode_GET_SIZE)
- #define __Pyx_PyUnicode_IS_TRUE(u) (0 != (likely(PyUnicode_IS_READY(u)) ? PyUnicode_GET_LENGTH(u) : PyUnicode_GET_SIZE(u)))
- #else
- #define __Pyx_PyUnicode_IS_TRUE(u) (0 != PyUnicode_GET_LENGTH(u))
- #endif
-#else
- #define CYTHON_PEP393_ENABLED 0
- #define PyUnicode_1BYTE_KIND 1
- #define PyUnicode_2BYTE_KIND 2
- #define PyUnicode_4BYTE_KIND 4
- #define __Pyx_PyUnicode_READY(op) (0)
- #define __Pyx_PyUnicode_GET_LENGTH(u) PyUnicode_GET_SIZE(u)
- #define __Pyx_PyUnicode_READ_CHAR(u, i) ((Py_UCS4)(PyUnicode_AS_UNICODE(u)[i]))
- #define __Pyx_PyUnicode_MAX_CHAR_VALUE(u) ((sizeof(Py_UNICODE) == 2) ? 65535 : 1114111)
- #define __Pyx_PyUnicode_KIND(u) (sizeof(Py_UNICODE))
- #define __Pyx_PyUnicode_DATA(u) ((void*)PyUnicode_AS_UNICODE(u))
- #define __Pyx_PyUnicode_READ(k, d, i) ((void)(k), (Py_UCS4)(((Py_UNICODE*)d)[i]))
- #define __Pyx_PyUnicode_WRITE(k, d, i, ch) (((void)(k)), ((Py_UNICODE*)d)[i] = ch)
- #define __Pyx_PyUnicode_IS_TRUE(u) (0 != PyUnicode_GET_SIZE(u))
-#endif
-#if CYTHON_COMPILING_IN_PYPY
- #define __Pyx_PyUnicode_Concat(a, b) PyNumber_Add(a, b)
- #define __Pyx_PyUnicode_ConcatSafe(a, b) PyNumber_Add(a, b)
-#else
- #define __Pyx_PyUnicode_Concat(a, b) PyUnicode_Concat(a, b)
- #define __Pyx_PyUnicode_ConcatSafe(a, b) ((unlikely((a) == Py_None) || unlikely((b) == Py_None)) ?\
- PyNumber_Add(a, b) : __Pyx_PyUnicode_Concat(a, b))
-#endif
-#if CYTHON_COMPILING_IN_PYPY && !defined(PyUnicode_Contains)
- #define PyUnicode_Contains(u, s) PySequence_Contains(u, s)
-#endif
-#if CYTHON_COMPILING_IN_PYPY && !defined(PyByteArray_Check)
- #define PyByteArray_Check(obj) PyObject_TypeCheck(obj, &PyByteArray_Type)
-#endif
-#if CYTHON_COMPILING_IN_PYPY && !defined(PyObject_Format)
- #define PyObject_Format(obj, fmt) PyObject_CallMethod(obj, "__format__", "O", fmt)
-#endif
-#define __Pyx_PyString_FormatSafe(a, b) ((unlikely((a) == Py_None || (PyString_Check(b) && !PyString_CheckExact(b)))) ? PyNumber_Remainder(a, b) : __Pyx_PyString_Format(a, b))
-#define __Pyx_PyUnicode_FormatSafe(a, b) ((unlikely((a) == Py_None || (PyUnicode_Check(b) && !PyUnicode_CheckExact(b)))) ? PyNumber_Remainder(a, b) : PyUnicode_Format(a, b))
-#if PY_MAJOR_VERSION >= 3
- #define __Pyx_PyString_Format(a, b) PyUnicode_Format(a, b)
-#else
- #define __Pyx_PyString_Format(a, b) PyString_Format(a, b)
-#endif
-#if PY_MAJOR_VERSION < 3 && !defined(PyObject_ASCII)
- #define PyObject_ASCII(o) PyObject_Repr(o)
-#endif
-#if PY_MAJOR_VERSION >= 3
- #define PyBaseString_Type PyUnicode_Type
- #define PyStringObject PyUnicodeObject
- #define PyString_Type PyUnicode_Type
- #define PyString_Check PyUnicode_Check
- #define PyString_CheckExact PyUnicode_CheckExact
-#ifndef PyObject_Unicode
- #define PyObject_Unicode PyObject_Str
-#endif
-#endif
-#if PY_MAJOR_VERSION >= 3
- #define __Pyx_PyBaseString_Check(obj) PyUnicode_Check(obj)
- #define __Pyx_PyBaseString_CheckExact(obj) PyUnicode_CheckExact(obj)
-#else
- #define __Pyx_PyBaseString_Check(obj) (PyString_Check(obj) || PyUnicode_Check(obj))
- #define __Pyx_PyBaseString_CheckExact(obj) (PyString_CheckExact(obj) || PyUnicode_CheckExact(obj))
-#endif
-#ifndef PySet_CheckExact
- #define PySet_CheckExact(obj) (Py_TYPE(obj) == &PySet_Type)
-#endif
-#if PY_VERSION_HEX >= 0x030900A4
- #define __Pyx_SET_REFCNT(obj, refcnt) Py_SET_REFCNT(obj, refcnt)
- #define __Pyx_SET_SIZE(obj, size) Py_SET_SIZE(obj, size)
-#else
- #define __Pyx_SET_REFCNT(obj, refcnt) Py_REFCNT(obj) = (refcnt)
- #define __Pyx_SET_SIZE(obj, size) Py_SIZE(obj) = (size)
-#endif
-#if CYTHON_ASSUME_SAFE_MACROS
- #define __Pyx_PySequence_SIZE(seq) Py_SIZE(seq)
-#else
- #define __Pyx_PySequence_SIZE(seq) PySequence_Size(seq)
-#endif
-#if PY_MAJOR_VERSION >= 3
- #define PyIntObject PyLongObject
- #define PyInt_Type PyLong_Type
- #define PyInt_Check(op) PyLong_Check(op)
- #define PyInt_CheckExact(op) PyLong_CheckExact(op)
- #define PyInt_FromString PyLong_FromString
- #define PyInt_FromUnicode PyLong_FromUnicode
- #define PyInt_FromLong PyLong_FromLong
- #define PyInt_FromSize_t PyLong_FromSize_t
- #define PyInt_FromSsize_t PyLong_FromSsize_t
- #define PyInt_AsLong PyLong_AsLong
- #define PyInt_AS_LONG PyLong_AS_LONG
- #define PyInt_AsSsize_t PyLong_AsSsize_t
- #define PyInt_AsUnsignedLongMask PyLong_AsUnsignedLongMask
- #define PyInt_AsUnsignedLongLongMask PyLong_AsUnsignedLongLongMask
- #define PyNumber_Int PyNumber_Long
-#endif
-#if PY_MAJOR_VERSION >= 3
- #define PyBoolObject PyLongObject
-#endif
-#if PY_MAJOR_VERSION >= 3 && CYTHON_COMPILING_IN_PYPY
- #ifndef PyUnicode_InternFromString
- #define PyUnicode_InternFromString(s) PyUnicode_FromString(s)
- #endif
-#endif
-#if PY_VERSION_HEX < 0x030200A4
- typedef long Py_hash_t;
- #define __Pyx_PyInt_FromHash_t PyInt_FromLong
- #define __Pyx_PyInt_AsHash_t PyInt_AsLong
-#else
- #define __Pyx_PyInt_FromHash_t PyInt_FromSsize_t
- #define __Pyx_PyInt_AsHash_t PyInt_AsSsize_t
-#endif
-#if PY_MAJOR_VERSION >= 3
- #define __Pyx_PyMethod_New(func, self, klass) ((self) ? ((void)(klass), PyMethod_New(func, self)) : __Pyx_NewRef(func))
-#else
- #define __Pyx_PyMethod_New(func, self, klass) PyMethod_New(func, self, klass)
-#endif
-#if CYTHON_USE_ASYNC_SLOTS
- #if PY_VERSION_HEX >= 0x030500B1
- #define __Pyx_PyAsyncMethodsStruct PyAsyncMethods
- #define __Pyx_PyType_AsAsync(obj) (Py_TYPE(obj)->tp_as_async)
- #else
- #define __Pyx_PyType_AsAsync(obj) ((__Pyx_PyAsyncMethodsStruct*) (Py_TYPE(obj)->tp_reserved))
- #endif
-#else
- #define __Pyx_PyType_AsAsync(obj) NULL
-#endif
-#ifndef __Pyx_PyAsyncMethodsStruct
- typedef struct {
- unaryfunc am_await;
- unaryfunc am_aiter;
- unaryfunc am_anext;
- } __Pyx_PyAsyncMethodsStruct;
-#endif
-
-#if defined(WIN32) || defined(MS_WINDOWS)
- #define _USE_MATH_DEFINES
-#endif
-#include
-#ifdef NAN
-#define __PYX_NAN() ((float) NAN)
-#else
-static CYTHON_INLINE float __PYX_NAN() {
- float value;
- memset(&value, 0xFF, sizeof(value));
- return value;
-}
-#endif
-#if defined(__CYGWIN__) && defined(_LDBL_EQ_DBL)
-#define __Pyx_truncl trunc
-#else
-#define __Pyx_truncl truncl
-#endif
-
-#define __PYX_MARK_ERR_POS(f_index, lineno) \
- { __pyx_filename = __pyx_f[f_index]; (void)__pyx_filename; __pyx_lineno = lineno; (void)__pyx_lineno; __pyx_clineno = __LINE__; (void)__pyx_clineno; }
-#define __PYX_ERR(f_index, lineno, Ln_error) \
- { __PYX_MARK_ERR_POS(f_index, lineno) goto Ln_error; }
-
-#ifndef __PYX_EXTERN_C
- #ifdef __cplusplus
- #define __PYX_EXTERN_C extern "C"
- #else
- #define __PYX_EXTERN_C extern
- #endif
-#endif
-
-#define __PYX_HAVE__monotonic_align__core
-#define __PYX_HAVE_API__monotonic_align__core
-/* Early includes */
-#include "pythread.h"
-#include
-#include
-#include
-#include "pystate.h"
-#ifdef _OPENMP
-#include
-#endif /* _OPENMP */
-
-#if defined(PYREX_WITHOUT_ASSERTIONS) && !defined(CYTHON_WITHOUT_ASSERTIONS)
-#define CYTHON_WITHOUT_ASSERTIONS
-#endif
-
-typedef struct {PyObject **p; const char *s; const Py_ssize_t n; const char* encoding;
- const char is_unicode; const char is_str; const char intern; } __Pyx_StringTabEntry;
-
-#define __PYX_DEFAULT_STRING_ENCODING_IS_ASCII 0
-#define __PYX_DEFAULT_STRING_ENCODING_IS_UTF8 0
-#define __PYX_DEFAULT_STRING_ENCODING_IS_DEFAULT (PY_MAJOR_VERSION >= 3 && __PYX_DEFAULT_STRING_ENCODING_IS_UTF8)
-#define __PYX_DEFAULT_STRING_ENCODING ""
-#define __Pyx_PyObject_FromString __Pyx_PyBytes_FromString
-#define __Pyx_PyObject_FromStringAndSize __Pyx_PyBytes_FromStringAndSize
-#define __Pyx_uchar_cast(c) ((unsigned char)c)
-#define __Pyx_long_cast(x) ((long)x)
-#define __Pyx_fits_Py_ssize_t(v, type, is_signed) (\
- (sizeof(type) < sizeof(Py_ssize_t)) ||\
- (sizeof(type) > sizeof(Py_ssize_t) &&\
- likely(v < (type)PY_SSIZE_T_MAX ||\
- v == (type)PY_SSIZE_T_MAX) &&\
- (!is_signed || likely(v > (type)PY_SSIZE_T_MIN ||\
- v == (type)PY_SSIZE_T_MIN))) ||\
- (sizeof(type) == sizeof(Py_ssize_t) &&\
- (is_signed || likely(v < (type)PY_SSIZE_T_MAX ||\
- v == (type)PY_SSIZE_T_MAX))) )
-static CYTHON_INLINE int __Pyx_is_valid_index(Py_ssize_t i, Py_ssize_t limit) {
- return (size_t) i < (size_t) limit;
-}
-#if defined (__cplusplus) && __cplusplus >= 201103L
- #include
- #define __Pyx_sst_abs(value) std::abs(value)
-#elif SIZEOF_INT >= SIZEOF_SIZE_T
- #define __Pyx_sst_abs(value) abs(value)
-#elif SIZEOF_LONG >= SIZEOF_SIZE_T
- #define __Pyx_sst_abs(value) labs(value)
-#elif defined (_MSC_VER)
- #define __Pyx_sst_abs(value) ((Py_ssize_t)_abs64(value))
-#elif defined (__STDC_VERSION__) && __STDC_VERSION__ >= 199901L
- #define __Pyx_sst_abs(value) llabs(value)
-#elif defined (__GNUC__)
- #define __Pyx_sst_abs(value) __builtin_llabs(value)
-#else
- #define __Pyx_sst_abs(value) ((value<0) ? -value : value)
-#endif
-static CYTHON_INLINE const char* __Pyx_PyObject_AsString(PyObject*);
-static CYTHON_INLINE const char* __Pyx_PyObject_AsStringAndSize(PyObject*, Py_ssize_t* length);
-#define __Pyx_PyByteArray_FromString(s) PyByteArray_FromStringAndSize((const char*)s, strlen((const char*)s))
-#define __Pyx_PyByteArray_FromStringAndSize(s, l) PyByteArray_FromStringAndSize((const char*)s, l)
-#define __Pyx_PyBytes_FromString PyBytes_FromString
-#define __Pyx_PyBytes_FromStringAndSize PyBytes_FromStringAndSize
-static CYTHON_INLINE PyObject* __Pyx_PyUnicode_FromString(const char*);
-#if PY_MAJOR_VERSION < 3
- #define __Pyx_PyStr_FromString __Pyx_PyBytes_FromString
- #define __Pyx_PyStr_FromStringAndSize __Pyx_PyBytes_FromStringAndSize
-#else
- #define __Pyx_PyStr_FromString __Pyx_PyUnicode_FromString
- #define __Pyx_PyStr_FromStringAndSize __Pyx_PyUnicode_FromStringAndSize
-#endif
-#define __Pyx_PyBytes_AsWritableString(s) ((char*) PyBytes_AS_STRING(s))
-#define __Pyx_PyBytes_AsWritableSString(s) ((signed char*) PyBytes_AS_STRING(s))
-#define __Pyx_PyBytes_AsWritableUString(s) ((unsigned char*) PyBytes_AS_STRING(s))
-#define __Pyx_PyBytes_AsString(s) ((const char*) PyBytes_AS_STRING(s))
-#define __Pyx_PyBytes_AsSString(s) ((const signed char*) PyBytes_AS_STRING(s))
-#define __Pyx_PyBytes_AsUString(s) ((const unsigned char*) PyBytes_AS_STRING(s))
-#define __Pyx_PyObject_AsWritableString(s) ((char*) __Pyx_PyObject_AsString(s))
-#define __Pyx_PyObject_AsWritableSString(s) ((signed char*) __Pyx_PyObject_AsString(s))
-#define __Pyx_PyObject_AsWritableUString(s) ((unsigned char*) __Pyx_PyObject_AsString(s))
-#define __Pyx_PyObject_AsSString(s) ((const signed char*) __Pyx_PyObject_AsString(s))
-#define __Pyx_PyObject_AsUString(s) ((const unsigned char*) __Pyx_PyObject_AsString(s))
-#define __Pyx_PyObject_FromCString(s) __Pyx_PyObject_FromString((const char*)s)
-#define __Pyx_PyBytes_FromCString(s) __Pyx_PyBytes_FromString((const char*)s)
-#define __Pyx_PyByteArray_FromCString(s) __Pyx_PyByteArray_FromString((const char*)s)
-#define __Pyx_PyStr_FromCString(s) __Pyx_PyStr_FromString((const char*)s)
-#define __Pyx_PyUnicode_FromCString(s) __Pyx_PyUnicode_FromString((const char*)s)
-static CYTHON_INLINE size_t __Pyx_Py_UNICODE_strlen(const Py_UNICODE *u) {
- const Py_UNICODE *u_end = u;
- while (*u_end++) ;
- return (size_t)(u_end - u - 1);
-}
-#define __Pyx_PyUnicode_FromUnicode(u) PyUnicode_FromUnicode(u, __Pyx_Py_UNICODE_strlen(u))
-#define __Pyx_PyUnicode_FromUnicodeAndLength PyUnicode_FromUnicode
-#define __Pyx_PyUnicode_AsUnicode PyUnicode_AsUnicode
-#define __Pyx_NewRef(obj) (Py_INCREF(obj), obj)
-#define __Pyx_Owned_Py_None(b) __Pyx_NewRef(Py_None)
-static CYTHON_INLINE PyObject * __Pyx_PyBool_FromLong(long b);
-static CYTHON_INLINE int __Pyx_PyObject_IsTrue(PyObject*);
-static CYTHON_INLINE int __Pyx_PyObject_IsTrueAndDecref(PyObject*);
-static CYTHON_INLINE PyObject* __Pyx_PyNumber_IntOrLong(PyObject* x);
-#define __Pyx_PySequence_Tuple(obj)\
- (likely(PyTuple_CheckExact(obj)) ? __Pyx_NewRef(obj) : PySequence_Tuple(obj))
-static CYTHON_INLINE Py_ssize_t __Pyx_PyIndex_AsSsize_t(PyObject*);
-static CYTHON_INLINE PyObject * __Pyx_PyInt_FromSize_t(size_t);
-#if CYTHON_ASSUME_SAFE_MACROS
-#define __pyx_PyFloat_AsDouble(x) (PyFloat_CheckExact(x) ? PyFloat_AS_DOUBLE(x) : PyFloat_AsDouble(x))
-#else
-#define __pyx_PyFloat_AsDouble(x) PyFloat_AsDouble(x)
-#endif
-#define __pyx_PyFloat_AsFloat(x) ((float) __pyx_PyFloat_AsDouble(x))
-#if PY_MAJOR_VERSION >= 3
-#define __Pyx_PyNumber_Int(x) (PyLong_CheckExact(x) ? __Pyx_NewRef(x) : PyNumber_Long(x))
-#else
-#define __Pyx_PyNumber_Int(x) (PyInt_CheckExact(x) ? __Pyx_NewRef(x) : PyNumber_Int(x))
-#endif
-#define __Pyx_PyNumber_Float(x) (PyFloat_CheckExact(x) ? __Pyx_NewRef(x) : PyNumber_Float(x))
-#if PY_MAJOR_VERSION < 3 && __PYX_DEFAULT_STRING_ENCODING_IS_ASCII
-static int __Pyx_sys_getdefaultencoding_not_ascii;
-static int __Pyx_init_sys_getdefaultencoding_params(void) {
- PyObject* sys;
- PyObject* default_encoding = NULL;
- PyObject* ascii_chars_u = NULL;
- PyObject* ascii_chars_b = NULL;
- const char* default_encoding_c;
- sys = PyImport_ImportModule("sys");
- if (!sys) goto bad;
- default_encoding = PyObject_CallMethod(sys, (char*) "getdefaultencoding", NULL);
- Py_DECREF(sys);
- if (!default_encoding) goto bad;
- default_encoding_c = PyBytes_AsString(default_encoding);
- if (!default_encoding_c) goto bad;
- if (strcmp(default_encoding_c, "ascii") == 0) {
- __Pyx_sys_getdefaultencoding_not_ascii = 0;
- } else {
- char ascii_chars[128];
- int c;
- for (c = 0; c < 128; c++) {
- ascii_chars[c] = c;
- }
- __Pyx_sys_getdefaultencoding_not_ascii = 1;
- ascii_chars_u = PyUnicode_DecodeASCII(ascii_chars, 128, NULL);
- if (!ascii_chars_u) goto bad;
- ascii_chars_b = PyUnicode_AsEncodedString(ascii_chars_u, default_encoding_c, NULL);
- if (!ascii_chars_b || !PyBytes_Check(ascii_chars_b) || memcmp(ascii_chars, PyBytes_AS_STRING(ascii_chars_b), 128) != 0) {
- PyErr_Format(
- PyExc_ValueError,
- "This module compiled with c_string_encoding=ascii, but default encoding '%.200s' is not a superset of ascii.",
- default_encoding_c);
- goto bad;
- }
- Py_DECREF(ascii_chars_u);
- Py_DECREF(ascii_chars_b);
- }
- Py_DECREF(default_encoding);
- return 0;
-bad:
- Py_XDECREF(default_encoding);
- Py_XDECREF(ascii_chars_u);
- Py_XDECREF(ascii_chars_b);
- return -1;
-}
-#endif
-#if __PYX_DEFAULT_STRING_ENCODING_IS_DEFAULT && PY_MAJOR_VERSION >= 3
-#define __Pyx_PyUnicode_FromStringAndSize(c_str, size) PyUnicode_DecodeUTF8(c_str, size, NULL)
-#else
-#define __Pyx_PyUnicode_FromStringAndSize(c_str, size) PyUnicode_Decode(c_str, size, __PYX_DEFAULT_STRING_ENCODING, NULL)
-#if __PYX_DEFAULT_STRING_ENCODING_IS_DEFAULT
-static char* __PYX_DEFAULT_STRING_ENCODING;
-static int __Pyx_init_sys_getdefaultencoding_params(void) {
- PyObject* sys;
- PyObject* default_encoding = NULL;
- char* default_encoding_c;
- sys = PyImport_ImportModule("sys");
- if (!sys) goto bad;
- default_encoding = PyObject_CallMethod(sys, (char*) (const char*) "getdefaultencoding", NULL);
- Py_DECREF(sys);
- if (!default_encoding) goto bad;
- default_encoding_c = PyBytes_AsString(default_encoding);
- if (!default_encoding_c) goto bad;
- __PYX_DEFAULT_STRING_ENCODING = (char*) malloc(strlen(default_encoding_c) + 1);
- if (!__PYX_DEFAULT_STRING_ENCODING) goto bad;
- strcpy(__PYX_DEFAULT_STRING_ENCODING, default_encoding_c);
- Py_DECREF(default_encoding);
- return 0;
-bad:
- Py_XDECREF(default_encoding);
- return -1;
-}
-#endif
-#endif
-
-
-/* Test for GCC > 2.95 */
-#if defined(__GNUC__) && (__GNUC__ > 2 || (__GNUC__ == 2 && (__GNUC_MINOR__ > 95)))
- #define likely(x) __builtin_expect(!!(x), 1)
- #define unlikely(x) __builtin_expect(!!(x), 0)
-#else /* !__GNUC__ or GCC < 2.95 */
- #define likely(x) (x)
- #define unlikely(x) (x)
-#endif /* __GNUC__ */
-static CYTHON_INLINE void __Pyx_pretend_to_initialize(void* ptr) { (void)ptr; }
-
-static PyObject *__pyx_m = NULL;
-static PyObject *__pyx_d;
-static PyObject *__pyx_b;
-static PyObject *__pyx_cython_runtime = NULL;
-static PyObject *__pyx_empty_tuple;
-static PyObject *__pyx_empty_bytes;
-static PyObject *__pyx_empty_unicode;
-static int __pyx_lineno;
-static int __pyx_clineno = 0;
-static const char * __pyx_cfilenm= __FILE__;
-static const char *__pyx_filename;
-
-
-static const char *__pyx_f[] = {
- "core.pyx",
- "stringsource",
-};
-/* NoFastGil.proto */
-#define __Pyx_PyGILState_Ensure PyGILState_Ensure
-#define __Pyx_PyGILState_Release PyGILState_Release
-#define __Pyx_FastGIL_Remember()
-#define __Pyx_FastGIL_Forget()
-#define __Pyx_FastGilFuncInit()
-
-/* MemviewSliceStruct.proto */
-struct __pyx_memoryview_obj;
-typedef struct {
- struct __pyx_memoryview_obj *memview;
- char *data;
- Py_ssize_t shape[8];
- Py_ssize_t strides[8];
- Py_ssize_t suboffsets[8];
-} __Pyx_memviewslice;
-#define __Pyx_MemoryView_Len(m) (m.shape[0])
-
-/* Atomics.proto */
-#include
-#ifndef CYTHON_ATOMICS
- #define CYTHON_ATOMICS 1
-#endif
-#define __pyx_atomic_int_type int
-#if CYTHON_ATOMICS && __GNUC__ >= 4 && (__GNUC_MINOR__ > 1 ||\
- (__GNUC_MINOR__ == 1 && __GNUC_PATCHLEVEL >= 2)) &&\
- !defined(__i386__)
- #define __pyx_atomic_incr_aligned(value, lock) __sync_fetch_and_add(value, 1)
- #define __pyx_atomic_decr_aligned(value, lock) __sync_fetch_and_sub(value, 1)
- #ifdef __PYX_DEBUG_ATOMICS
- #warning "Using GNU atomics"
- #endif
-#elif CYTHON_ATOMICS && defined(_MSC_VER) && 0
- #include
- #undef __pyx_atomic_int_type
- #define __pyx_atomic_int_type LONG
- #define __pyx_atomic_incr_aligned(value, lock) InterlockedIncrement(value)
- #define __pyx_atomic_decr_aligned(value, lock) InterlockedDecrement(value)
- #ifdef __PYX_DEBUG_ATOMICS
- #pragma message ("Using MSVC atomics")
- #endif
-#elif CYTHON_ATOMICS && (defined(__ICC) || defined(__INTEL_COMPILER)) && 0
- #define __pyx_atomic_incr_aligned(value, lock) _InterlockedIncrement(value)
- #define __pyx_atomic_decr_aligned(value, lock) _InterlockedDecrement(value)
- #ifdef __PYX_DEBUG_ATOMICS
- #warning "Using Intel atomics"
- #endif
-#else
- #undef CYTHON_ATOMICS
- #define CYTHON_ATOMICS 0
- #ifdef __PYX_DEBUG_ATOMICS
- #warning "Not using atomics"
- #endif
-#endif
-typedef volatile __pyx_atomic_int_type __pyx_atomic_int;
-#if CYTHON_ATOMICS
- #define __pyx_add_acquisition_count(memview)\
- __pyx_atomic_incr_aligned(__pyx_get_slice_count_pointer(memview), memview->lock)
- #define __pyx_sub_acquisition_count(memview)\
- __pyx_atomic_decr_aligned(__pyx_get_slice_count_pointer(memview), memview->lock)
-#else
- #define __pyx_add_acquisition_count(memview)\
- __pyx_add_acquisition_count_locked(__pyx_get_slice_count_pointer(memview), memview->lock)
- #define __pyx_sub_acquisition_count(memview)\
- __pyx_sub_acquisition_count_locked(__pyx_get_slice_count_pointer(memview), memview->lock)
-#endif
-
-/* ForceInitThreads.proto */
-#ifndef __PYX_FORCE_INIT_THREADS
- #define __PYX_FORCE_INIT_THREADS 0
-#endif
-
-/* BufferFormatStructs.proto */
-#define IS_UNSIGNED(type) (((type) -1) > 0)
-struct __Pyx_StructField_;
-#define __PYX_BUF_FLAGS_PACKED_STRUCT (1 << 0)
-typedef struct {
- const char* name;
- struct __Pyx_StructField_* fields;
- size_t size;
- size_t arraysize[8];
- int ndim;
- char typegroup;
- char is_unsigned;
- int flags;
-} __Pyx_TypeInfo;
-typedef struct __Pyx_StructField_ {
- __Pyx_TypeInfo* type;
- const char* name;
- size_t offset;
-} __Pyx_StructField;
-typedef struct {
- __Pyx_StructField* field;
- size_t parent_offset;
-} __Pyx_BufFmt_StackElem;
-typedef struct {
- __Pyx_StructField root;
- __Pyx_BufFmt_StackElem* head;
- size_t fmt_offset;
- size_t new_count, enc_count;
- size_t struct_alignment;
- int is_complex;
- char enc_type;
- char new_packmode;
- char enc_packmode;
- char is_valid_array;
-} __Pyx_BufFmt_Context;
-
-
-/*--- Type declarations ---*/
-struct __pyx_array_obj;
-struct __pyx_MemviewEnum_obj;
-struct __pyx_memoryview_obj;
-struct __pyx_memoryviewslice_obj;
-struct __pyx_opt_args_15monotonic_align_4core_maximum_path_each;
-
-/* "monotonic_align/core.pyx":7
- * @cython.boundscheck(False)
- * @cython.wraparound(False)
- * cdef void maximum_path_each(int[:,::1] path, float[:,::1] value, int t_y, int t_x, float max_neg_val=-1e9) nogil: # <<<<<<<<<<<<<<
- * cdef int x
- * cdef int y
- */
-struct __pyx_opt_args_15monotonic_align_4core_maximum_path_each {
- int __pyx_n;
- float max_neg_val;
-};
-
-/* "View.MemoryView":105
- *
- * @cname("__pyx_array")
- * cdef class array: # <<<<<<<<<<<<<<
- *
- * cdef:
- */
-struct __pyx_array_obj {
- PyObject_HEAD
- struct __pyx_vtabstruct_array *__pyx_vtab;
- char *data;
- Py_ssize_t len;
- char *format;
- int ndim;
- Py_ssize_t *_shape;
- Py_ssize_t *_strides;
- Py_ssize_t itemsize;
- PyObject *mode;
- PyObject *_format;
- void (*callback_free_data)(void *);
- int free_data;
- int dtype_is_object;
-};
-
-
-/* "View.MemoryView":279
- *
- * @cname('__pyx_MemviewEnum')
- * cdef class Enum(object): # <<<<<<<<<<<<<<
- * cdef object name
- * def __init__(self, name):
- */
-struct __pyx_MemviewEnum_obj {
- PyObject_HEAD
- PyObject *name;
-};
-
-
-/* "View.MemoryView":330
- *
- * @cname('__pyx_memoryview')
- * cdef class memoryview(object): # <<<<<<<<<<<<<<
- *
- * cdef object obj
- */
-struct __pyx_memoryview_obj {
- PyObject_HEAD
- struct __pyx_vtabstruct_memoryview *__pyx_vtab;
- PyObject *obj;
- PyObject *_size;
- PyObject *_array_interface;
- PyThread_type_lock lock;
- __pyx_atomic_int acquisition_count[2];
- __pyx_atomic_int *acquisition_count_aligned_p;
- Py_buffer view;
- int flags;
- int dtype_is_object;
- __Pyx_TypeInfo *typeinfo;
-};
-
-
-/* "View.MemoryView":965
- *
- * @cname('__pyx_memoryviewslice')
- * cdef class _memoryviewslice(memoryview): # <<<<<<<<<<<<<<
- * "Internal class for passing memoryview slices to Python"
- *
- */
-struct __pyx_memoryviewslice_obj {
- struct __pyx_memoryview_obj __pyx_base;
- __Pyx_memviewslice from_slice;
- PyObject *from_object;
- PyObject *(*to_object_func)(char *);
- int (*to_dtype_func)(char *, PyObject *);
-};
-
-
-
-/* "View.MemoryView":105
- *
- * @cname("__pyx_array")
- * cdef class array: # <<<<<<<<<<<<<<
- *
- * cdef:
- */
-
-struct __pyx_vtabstruct_array {
- PyObject *(*get_memview)(struct __pyx_array_obj *);
-};
-static struct __pyx_vtabstruct_array *__pyx_vtabptr_array;
-
-
-/* "View.MemoryView":330
- *
- * @cname('__pyx_memoryview')
- * cdef class memoryview(object): # <<<<<<<<<<<<<<
- *
- * cdef object obj
- */
-
-struct __pyx_vtabstruct_memoryview {
- char *(*get_item_pointer)(struct __pyx_memoryview_obj *, PyObject *);
- PyObject *(*is_slice)(struct __pyx_memoryview_obj *, PyObject *);
- PyObject *(*setitem_slice_assignment)(struct __pyx_memoryview_obj *, PyObject *, PyObject *);
- PyObject *(*setitem_slice_assign_scalar)(struct __pyx_memoryview_obj *, struct __pyx_memoryview_obj *, PyObject *);
- PyObject *(*setitem_indexed)(struct __pyx_memoryview_obj *, PyObject *, PyObject *);
- PyObject *(*convert_item_to_object)(struct __pyx_memoryview_obj *, char *);
- PyObject *(*assign_item_from_object)(struct __pyx_memoryview_obj *, char *, PyObject *);
-};
-static struct __pyx_vtabstruct_memoryview *__pyx_vtabptr_memoryview;
-
-
-/* "View.MemoryView":965
- *
- * @cname('__pyx_memoryviewslice')
- * cdef class _memoryviewslice(memoryview): # <<<<<<<<<<<<<<
- * "Internal class for passing memoryview slices to Python"
- *
- */
-
-struct __pyx_vtabstruct__memoryviewslice {
- struct __pyx_vtabstruct_memoryview __pyx_base;
-};
-static struct __pyx_vtabstruct__memoryviewslice *__pyx_vtabptr__memoryviewslice;
-
-/* --- Runtime support code (head) --- */
-/* Refnanny.proto */
-#ifndef CYTHON_REFNANNY
- #define CYTHON_REFNANNY 0
-#endif
-#if CYTHON_REFNANNY
- typedef struct {
- void (*INCREF)(void*, PyObject*, int);
- void (*DECREF)(void*, PyObject*, int);
- void (*GOTREF)(void*, PyObject*, int);
- void (*GIVEREF)(void*, PyObject*, int);
- void* (*SetupContext)(const char*, int, const char*);
- void (*FinishContext)(void**);
- } __Pyx_RefNannyAPIStruct;
- static __Pyx_RefNannyAPIStruct *__Pyx_RefNanny = NULL;
- static __Pyx_RefNannyAPIStruct *__Pyx_RefNannyImportAPI(const char *modname);
- #define __Pyx_RefNannyDeclarations void *__pyx_refnanny = NULL;
-#ifdef WITH_THREAD
- #define __Pyx_RefNannySetupContext(name, acquire_gil)\
- if (acquire_gil) {\
- PyGILState_STATE __pyx_gilstate_save = PyGILState_Ensure();\
- __pyx_refnanny = __Pyx_RefNanny->SetupContext((name), __LINE__, __FILE__);\
- PyGILState_Release(__pyx_gilstate_save);\
- } else {\
- __pyx_refnanny = __Pyx_RefNanny->SetupContext((name), __LINE__, __FILE__);\
- }
-#else
- #define __Pyx_RefNannySetupContext(name, acquire_gil)\
- __pyx_refnanny = __Pyx_RefNanny->SetupContext((name), __LINE__, __FILE__)
-#endif
- #define __Pyx_RefNannyFinishContext()\
- __Pyx_RefNanny->FinishContext(&__pyx_refnanny)
- #define __Pyx_INCREF(r) __Pyx_RefNanny->INCREF(__pyx_refnanny, (PyObject *)(r), __LINE__)
- #define __Pyx_DECREF(r) __Pyx_RefNanny->DECREF(__pyx_refnanny, (PyObject *)(r), __LINE__)
- #define __Pyx_GOTREF(r) __Pyx_RefNanny->GOTREF(__pyx_refnanny, (PyObject *)(r), __LINE__)
- #define __Pyx_GIVEREF(r) __Pyx_RefNanny->GIVEREF(__pyx_refnanny, (PyObject *)(r), __LINE__)
- #define __Pyx_XINCREF(r) do { if((r) != NULL) {__Pyx_INCREF(r); }} while(0)
- #define __Pyx_XDECREF(r) do { if((r) != NULL) {__Pyx_DECREF(r); }} while(0)
- #define __Pyx_XGOTREF(r) do { if((r) != NULL) {__Pyx_GOTREF(r); }} while(0)
- #define __Pyx_XGIVEREF(r) do { if((r) != NULL) {__Pyx_GIVEREF(r);}} while(0)
-#else
- #define __Pyx_RefNannyDeclarations
- #define __Pyx_RefNannySetupContext(name, acquire_gil)
- #define __Pyx_RefNannyFinishContext()
- #define __Pyx_INCREF(r) Py_INCREF(r)
- #define __Pyx_DECREF(r) Py_DECREF(r)
- #define __Pyx_GOTREF(r)
- #define __Pyx_GIVEREF(r)
- #define __Pyx_XINCREF(r) Py_XINCREF(r)
- #define __Pyx_XDECREF(r) Py_XDECREF(r)
- #define __Pyx_XGOTREF(r)
- #define __Pyx_XGIVEREF(r)
-#endif
-#define __Pyx_XDECREF_SET(r, v) do {\
- PyObject *tmp = (PyObject *) r;\
- r = v; __Pyx_XDECREF(tmp);\
- } while (0)
-#define __Pyx_DECREF_SET(r, v) do {\
- PyObject *tmp = (PyObject *) r;\
- r = v; __Pyx_DECREF(tmp);\
- } while (0)
-#define __Pyx_CLEAR(r) do { PyObject* tmp = ((PyObject*)(r)); r = NULL; __Pyx_DECREF(tmp);} while(0)
-#define __Pyx_XCLEAR(r) do { if((r) != NULL) {PyObject* tmp = ((PyObject*)(r)); r = NULL; __Pyx_DECREF(tmp);}} while(0)
-
-/* PyObjectGetAttrStr.proto */
-#if CYTHON_USE_TYPE_SLOTS
-static CYTHON_INLINE PyObject* __Pyx_PyObject_GetAttrStr(PyObject* obj, PyObject* attr_name);
-#else
-#define __Pyx_PyObject_GetAttrStr(o,n) PyObject_GetAttr(o,n)
-#endif
-
-/* GetBuiltinName.proto */
-static PyObject *__Pyx_GetBuiltinName(PyObject *name);
-
-/* MemviewSliceInit.proto */
-#define __Pyx_BUF_MAX_NDIMS %(BUF_MAX_NDIMS)d
-#define __Pyx_MEMVIEW_DIRECT 1
-#define __Pyx_MEMVIEW_PTR 2
-#define __Pyx_MEMVIEW_FULL 4
-#define __Pyx_MEMVIEW_CONTIG 8
-#define __Pyx_MEMVIEW_STRIDED 16
-#define __Pyx_MEMVIEW_FOLLOW 32
-#define __Pyx_IS_C_CONTIG 1
-#define __Pyx_IS_F_CONTIG 2
-static int __Pyx_init_memviewslice(
- struct __pyx_memoryview_obj *memview,
- int ndim,
- __Pyx_memviewslice *memviewslice,
- int memview_is_new_reference);
-static CYTHON_INLINE int __pyx_add_acquisition_count_locked(
- __pyx_atomic_int *acquisition_count, PyThread_type_lock lock);
-static CYTHON_INLINE int __pyx_sub_acquisition_count_locked(
- __pyx_atomic_int *acquisition_count, PyThread_type_lock lock);
-#define __pyx_get_slice_count_pointer(memview) (memview->acquisition_count_aligned_p)
-#define __pyx_get_slice_count(memview) (*__pyx_get_slice_count_pointer(memview))
-#define __PYX_INC_MEMVIEW(slice, have_gil) __Pyx_INC_MEMVIEW(slice, have_gil, __LINE__)
-#define __PYX_XDEC_MEMVIEW(slice, have_gil) __Pyx_XDEC_MEMVIEW(slice, have_gil, __LINE__)
-static CYTHON_INLINE void __Pyx_INC_MEMVIEW(__Pyx_memviewslice *, int, int);
-static CYTHON_INLINE void __Pyx_XDEC_MEMVIEW(__Pyx_memviewslice *, int, int);
-
-/* RaiseArgTupleInvalid.proto */
-static void __Pyx_RaiseArgtupleInvalid(const char* func_name, int exact,
- Py_ssize_t num_min, Py_ssize_t num_max, Py_ssize_t num_found);
-
-/* RaiseDoubleKeywords.proto */
-static void __Pyx_RaiseDoubleKeywordsError(const char* func_name, PyObject* kw_name);
-
-/* ParseKeywords.proto */
-static int __Pyx_ParseOptionalKeywords(PyObject *kwds, PyObject **argnames[],\
- PyObject *kwds2, PyObject *values[], Py_ssize_t num_pos_args,\
- const char* function_name);
-
-/* None.proto */
-static CYTHON_INLINE void __Pyx_RaiseUnboundLocalError(const char *varname);
-
-/* ArgTypeTest.proto */
-#define __Pyx_ArgTypeTest(obj, type, none_allowed, name, exact)\
- ((likely((Py_TYPE(obj) == type) | (none_allowed && (obj == Py_None)))) ? 1 :\
- __Pyx__ArgTypeTest(obj, type, name, exact))
-static int __Pyx__ArgTypeTest(PyObject *obj, PyTypeObject *type, const char *name, int exact);
-
-/* PyObjectCall.proto */
-#if CYTHON_COMPILING_IN_CPYTHON
-static CYTHON_INLINE PyObject* __Pyx_PyObject_Call(PyObject *func, PyObject *arg, PyObject *kw);
-#else
-#define __Pyx_PyObject_Call(func, arg, kw) PyObject_Call(func, arg, kw)
-#endif
-
-/* PyThreadStateGet.proto */
-#if CYTHON_FAST_THREAD_STATE
-#define __Pyx_PyThreadState_declare PyThreadState *__pyx_tstate;
-#define __Pyx_PyThreadState_assign __pyx_tstate = __Pyx_PyThreadState_Current;
-#define __Pyx_PyErr_Occurred() __pyx_tstate->curexc_type
-#else
-#define __Pyx_PyThreadState_declare
-#define __Pyx_PyThreadState_assign
-#define __Pyx_PyErr_Occurred() PyErr_Occurred()
-#endif
-
-/* PyErrFetchRestore.proto */
-#if CYTHON_FAST_THREAD_STATE
-#define __Pyx_PyErr_Clear() __Pyx_ErrRestore(NULL, NULL, NULL)
-#define __Pyx_ErrRestoreWithState(type, value, tb) __Pyx_ErrRestoreInState(PyThreadState_GET(), type, value, tb)
-#define __Pyx_ErrFetchWithState(type, value, tb) __Pyx_ErrFetchInState(PyThreadState_GET(), type, value, tb)
-#define __Pyx_ErrRestore(type, value, tb) __Pyx_ErrRestoreInState(__pyx_tstate, type, value, tb)
-#define __Pyx_ErrFetch(type, value, tb) __Pyx_ErrFetchInState(__pyx_tstate, type, value, tb)
-static CYTHON_INLINE void __Pyx_ErrRestoreInState(PyThreadState *tstate, PyObject *type, PyObject *value, PyObject *tb);
-static CYTHON_INLINE void __Pyx_ErrFetchInState(PyThreadState *tstate, PyObject **type, PyObject **value, PyObject **tb);
-#if CYTHON_COMPILING_IN_CPYTHON
-#define __Pyx_PyErr_SetNone(exc) (Py_INCREF(exc), __Pyx_ErrRestore((exc), NULL, NULL))
-#else
-#define __Pyx_PyErr_SetNone(exc) PyErr_SetNone(exc)
-#endif
-#else
-#define __Pyx_PyErr_Clear() PyErr_Clear()
-#define __Pyx_PyErr_SetNone(exc) PyErr_SetNone(exc)
-#define __Pyx_ErrRestoreWithState(type, value, tb) PyErr_Restore(type, value, tb)
-#define __Pyx_ErrFetchWithState(type, value, tb) PyErr_Fetch(type, value, tb)
-#define __Pyx_ErrRestoreInState(tstate, type, value, tb) PyErr_Restore(type, value, tb)
-#define __Pyx_ErrFetchInState(tstate, type, value, tb) PyErr_Fetch(type, value, tb)
-#define __Pyx_ErrRestore(type, value, tb) PyErr_Restore(type, value, tb)
-#define __Pyx_ErrFetch(type, value, tb) PyErr_Fetch(type, value, tb)
-#endif
-
-/* RaiseException.proto */
-static void __Pyx_Raise(PyObject *type, PyObject *value, PyObject *tb, PyObject *cause);
-
-/* PyCFunctionFastCall.proto */
-#if CYTHON_FAST_PYCCALL
-static CYTHON_INLINE PyObject *__Pyx_PyCFunction_FastCall(PyObject *func, PyObject **args, Py_ssize_t nargs);
-#else
-#define __Pyx_PyCFunction_FastCall(func, args, nargs) (assert(0), NULL)
-#endif
-
-/* PyFunctionFastCall.proto */
-#if CYTHON_FAST_PYCALL
-#define __Pyx_PyFunction_FastCall(func, args, nargs)\
- __Pyx_PyFunction_FastCallDict((func), (args), (nargs), NULL)
-#if 1 || PY_VERSION_HEX < 0x030600B1
-static PyObject *__Pyx_PyFunction_FastCallDict(PyObject *func, PyObject **args, Py_ssize_t nargs, PyObject *kwargs);
-#else
-#define __Pyx_PyFunction_FastCallDict(func, args, nargs, kwargs) _PyFunction_FastCallDict(func, args, nargs, kwargs)
-#endif
-#define __Pyx_BUILD_ASSERT_EXPR(cond)\
- (sizeof(char [1 - 2*!(cond)]) - 1)
-#ifndef Py_MEMBER_SIZE
-#define Py_MEMBER_SIZE(type, member) sizeof(((type *)0)->member)
-#endif
- static size_t __pyx_pyframe_localsplus_offset = 0;
- #include "frameobject.h"
- #define __Pxy_PyFrame_Initialize_Offsets()\
- ((void)__Pyx_BUILD_ASSERT_EXPR(sizeof(PyFrameObject) == offsetof(PyFrameObject, f_localsplus) + Py_MEMBER_SIZE(PyFrameObject, f_localsplus)),\
- (void)(__pyx_pyframe_localsplus_offset = ((size_t)PyFrame_Type.tp_basicsize) - Py_MEMBER_SIZE(PyFrameObject, f_localsplus)))
- #define __Pyx_PyFrame_GetLocalsplus(frame)\
- (assert(__pyx_pyframe_localsplus_offset), (PyObject **)(((char *)(frame)) + __pyx_pyframe_localsplus_offset))
-#endif
-
-/* PyObjectCall2Args.proto */
-static CYTHON_UNUSED PyObject* __Pyx_PyObject_Call2Args(PyObject* function, PyObject* arg1, PyObject* arg2);
-
-/* PyObjectCallMethO.proto */
-#if CYTHON_COMPILING_IN_CPYTHON
-static CYTHON_INLINE PyObject* __Pyx_PyObject_CallMethO(PyObject *func, PyObject *arg);
-#endif
-
-/* PyObjectCallOneArg.proto */
-static CYTHON_INLINE PyObject* __Pyx_PyObject_CallOneArg(PyObject *func, PyObject *arg);
-
-/* IncludeStringH.proto */
-#include
-
-/* BytesEquals.proto */
-static CYTHON_INLINE int __Pyx_PyBytes_Equals(PyObject* s1, PyObject* s2, int equals);
-
-/* UnicodeEquals.proto */
-static CYTHON_INLINE int __Pyx_PyUnicode_Equals(PyObject* s1, PyObject* s2, int equals);
-
-/* StrEquals.proto */
-#if PY_MAJOR_VERSION >= 3
-#define __Pyx_PyString_Equals __Pyx_PyUnicode_Equals
-#else
-#define __Pyx_PyString_Equals __Pyx_PyBytes_Equals
-#endif
-
-/* None.proto */
-static CYTHON_INLINE Py_ssize_t __Pyx_div_Py_ssize_t(Py_ssize_t, Py_ssize_t);
-
-/* UnaryNegOverflows.proto */
-#define UNARY_NEG_WOULD_OVERFLOW(x)\
- (((x) < 0) & ((unsigned long)(x) == 0-(unsigned long)(x)))
-
-static CYTHON_UNUSED int __pyx_array_getbuffer(PyObject *__pyx_v_self, Py_buffer *__pyx_v_info, int __pyx_v_flags); /*proto*/
-static PyObject *__pyx_array_get_memview(struct __pyx_array_obj *); /*proto*/
-/* GetAttr.proto */
-static CYTHON_INLINE PyObject *__Pyx_GetAttr(PyObject *, PyObject *);
-
-/* GetItemInt.proto */
-#define __Pyx_GetItemInt(o, i, type, is_signed, to_py_func, is_list, wraparound, boundscheck)\
- (__Pyx_fits_Py_ssize_t(i, type, is_signed) ?\
- __Pyx_GetItemInt_Fast(o, (Py_ssize_t)i, is_list, wraparound, boundscheck) :\
- (is_list ? (PyErr_SetString(PyExc_IndexError, "list index out of range"), (PyObject*)NULL) :\
- __Pyx_GetItemInt_Generic(o, to_py_func(i))))
-#define __Pyx_GetItemInt_List(o, i, type, is_signed, to_py_func, is_list, wraparound, boundscheck)\
- (__Pyx_fits_Py_ssize_t(i, type, is_signed) ?\
- __Pyx_GetItemInt_List_Fast(o, (Py_ssize_t)i, wraparound, boundscheck) :\
- (PyErr_SetString(PyExc_IndexError, "list index out of range"), (PyObject*)NULL))
-static CYTHON_INLINE PyObject *__Pyx_GetItemInt_List_Fast(PyObject *o, Py_ssize_t i,
- int wraparound, int boundscheck);
-#define __Pyx_GetItemInt_Tuple(o, i, type, is_signed, to_py_func, is_list, wraparound, boundscheck)\
- (__Pyx_fits_Py_ssize_t(i, type, is_signed) ?\
- __Pyx_GetItemInt_Tuple_Fast(o, (Py_ssize_t)i, wraparound, boundscheck) :\
- (PyErr_SetString(PyExc_IndexError, "tuple index out of range"), (PyObject*)NULL))
-static CYTHON_INLINE PyObject *__Pyx_GetItemInt_Tuple_Fast(PyObject *o, Py_ssize_t i,
- int wraparound, int boundscheck);
-static PyObject *__Pyx_GetItemInt_Generic(PyObject *o, PyObject* j);
-static CYTHON_INLINE PyObject *__Pyx_GetItemInt_Fast(PyObject *o, Py_ssize_t i,
- int is_list, int wraparound, int boundscheck);
-
-/* ObjectGetItem.proto */
-#if CYTHON_USE_TYPE_SLOTS
-static CYTHON_INLINE PyObject *__Pyx_PyObject_GetItem(PyObject *obj, PyObject* key);
-#else
-#define __Pyx_PyObject_GetItem(obj, key) PyObject_GetItem(obj, key)
-#endif
-
-/* decode_c_string_utf16.proto */
-static CYTHON_INLINE PyObject *__Pyx_PyUnicode_DecodeUTF16(const char *s, Py_ssize_t size, const char *errors) {
- int byteorder = 0;
- return PyUnicode_DecodeUTF16(s, size, errors, &byteorder);
-}
-static CYTHON_INLINE PyObject *__Pyx_PyUnicode_DecodeUTF16LE(const char *s, Py_ssize_t size, const char *errors) {
- int byteorder = -1;
- return PyUnicode_DecodeUTF16(s, size, errors, &byteorder);
-}
-static CYTHON_INLINE PyObject *__Pyx_PyUnicode_DecodeUTF16BE(const char *s, Py_ssize_t size, const char *errors) {
- int byteorder = 1;
- return PyUnicode_DecodeUTF16(s, size, errors, &byteorder);
-}
-
-/* decode_c_string.proto */
-static CYTHON_INLINE PyObject* __Pyx_decode_c_string(
- const char* cstring, Py_ssize_t start, Py_ssize_t stop,
- const char* encoding, const char* errors,
- PyObject* (*decode_func)(const char *s, Py_ssize_t size, const char *errors));
-
-/* PyErrExceptionMatches.proto */
-#if CYTHON_FAST_THREAD_STATE
-#define __Pyx_PyErr_ExceptionMatches(err) __Pyx_PyErr_ExceptionMatchesInState(__pyx_tstate, err)
-static CYTHON_INLINE int __Pyx_PyErr_ExceptionMatchesInState(PyThreadState* tstate, PyObject* err);
-#else
-#define __Pyx_PyErr_ExceptionMatches(err) PyErr_ExceptionMatches(err)
-#endif
-
-/* GetAttr3.proto */
-static CYTHON_INLINE PyObject *__Pyx_GetAttr3(PyObject *, PyObject *, PyObject *);
-
-/* PyDictVersioning.proto */
-#if CYTHON_USE_DICT_VERSIONS && CYTHON_USE_TYPE_SLOTS
-#define __PYX_DICT_VERSION_INIT ((PY_UINT64_T) -1)
-#define __PYX_GET_DICT_VERSION(dict) (((PyDictObject*)(dict))->ma_version_tag)
-#define __PYX_UPDATE_DICT_CACHE(dict, value, cache_var, version_var)\
- (version_var) = __PYX_GET_DICT_VERSION(dict);\
- (cache_var) = (value);
-#define __PYX_PY_DICT_LOOKUP_IF_MODIFIED(VAR, DICT, LOOKUP) {\
- static PY_UINT64_T __pyx_dict_version = 0;\
- static PyObject *__pyx_dict_cached_value = NULL;\
- if (likely(__PYX_GET_DICT_VERSION(DICT) == __pyx_dict_version)) {\
- (VAR) = __pyx_dict_cached_value;\
- } else {\
- (VAR) = __pyx_dict_cached_value = (LOOKUP);\
- __pyx_dict_version = __PYX_GET_DICT_VERSION(DICT);\
- }\
-}
-static CYTHON_INLINE PY_UINT64_T __Pyx_get_tp_dict_version(PyObject *obj);
-static CYTHON_INLINE PY_UINT64_T __Pyx_get_object_dict_version(PyObject *obj);
-static CYTHON_INLINE int __Pyx_object_dict_version_matches(PyObject* obj, PY_UINT64_T tp_dict_version, PY_UINT64_T obj_dict_version);
-#else
-#define __PYX_GET_DICT_VERSION(dict) (0)
-#define __PYX_UPDATE_DICT_CACHE(dict, value, cache_var, version_var)
-#define __PYX_PY_DICT_LOOKUP_IF_MODIFIED(VAR, DICT, LOOKUP) (VAR) = (LOOKUP);
-#endif
-
-/* GetModuleGlobalName.proto */
-#if CYTHON_USE_DICT_VERSIONS
-#define __Pyx_GetModuleGlobalName(var, name) {\
- static PY_UINT64_T __pyx_dict_version = 0;\
- static PyObject *__pyx_dict_cached_value = NULL;\
- (var) = (likely(__pyx_dict_version == __PYX_GET_DICT_VERSION(__pyx_d))) ?\
- (likely(__pyx_dict_cached_value) ? __Pyx_NewRef(__pyx_dict_cached_value) : __Pyx_GetBuiltinName(name)) :\
- __Pyx__GetModuleGlobalName(name, &__pyx_dict_version, &__pyx_dict_cached_value);\
-}
-#define __Pyx_GetModuleGlobalNameUncached(var, name) {\
- PY_UINT64_T __pyx_dict_version;\
- PyObject *__pyx_dict_cached_value;\
- (var) = __Pyx__GetModuleGlobalName(name, &__pyx_dict_version, &__pyx_dict_cached_value);\
-}
-static PyObject *__Pyx__GetModuleGlobalName(PyObject *name, PY_UINT64_T *dict_version, PyObject **dict_cached_value);
-#else
-#define __Pyx_GetModuleGlobalName(var, name) (var) = __Pyx__GetModuleGlobalName(name)
-#define __Pyx_GetModuleGlobalNameUncached(var, name) (var) = __Pyx__GetModuleGlobalName(name)
-static CYTHON_INLINE PyObject *__Pyx__GetModuleGlobalName(PyObject *name);
-#endif
-
-/* RaiseTooManyValuesToUnpack.proto */
-static CYTHON_INLINE void __Pyx_RaiseTooManyValuesError(Py_ssize_t expected);
-
-/* RaiseNeedMoreValuesToUnpack.proto */
-static CYTHON_INLINE void __Pyx_RaiseNeedMoreValuesError(Py_ssize_t index);
-
-/* RaiseNoneIterError.proto */
-static CYTHON_INLINE void __Pyx_RaiseNoneNotIterableError(void);
-
-/* ExtTypeTest.proto */
-static CYTHON_INLINE int __Pyx_TypeTest(PyObject *obj, PyTypeObject *type);
-
-/* GetTopmostException.proto */
-#if CYTHON_USE_EXC_INFO_STACK
-static _PyErr_StackItem * __Pyx_PyErr_GetTopmostException(PyThreadState *tstate);
-#endif
-
-/* SaveResetException.proto */
-#if CYTHON_FAST_THREAD_STATE
-#define __Pyx_ExceptionSave(type, value, tb) __Pyx__ExceptionSave(__pyx_tstate, type, value, tb)
-static CYTHON_INLINE void __Pyx__ExceptionSave(PyThreadState *tstate, PyObject **type, PyObject **value, PyObject **tb);
-#define __Pyx_ExceptionReset(type, value, tb) __Pyx__ExceptionReset(__pyx_tstate, type, value, tb)
-static CYTHON_INLINE void __Pyx__ExceptionReset(PyThreadState *tstate, PyObject *type, PyObject *value, PyObject *tb);
-#else
-#define __Pyx_ExceptionSave(type, value, tb) PyErr_GetExcInfo(type, value, tb)
-#define __Pyx_ExceptionReset(type, value, tb) PyErr_SetExcInfo(type, value, tb)
-#endif
-
-/* GetException.proto */
-#if CYTHON_FAST_THREAD_STATE
-#define __Pyx_GetException(type, value, tb) __Pyx__GetException(__pyx_tstate, type, value, tb)
-static int __Pyx__GetException(PyThreadState *tstate, PyObject **type, PyObject **value, PyObject **tb);
-#else
-static int __Pyx_GetException(PyObject **type, PyObject **value, PyObject **tb);
-#endif
-
-/* SwapException.proto */
-#if CYTHON_FAST_THREAD_STATE
-#define __Pyx_ExceptionSwap(type, value, tb) __Pyx__ExceptionSwap(__pyx_tstate, type, value, tb)
-static CYTHON_INLINE void __Pyx__ExceptionSwap(PyThreadState *tstate, PyObject **type, PyObject **value, PyObject **tb);
-#else
-static CYTHON_INLINE void __Pyx_ExceptionSwap(PyObject **type, PyObject **value, PyObject **tb);
-#endif
-
-/* Import.proto */
-static PyObject *__Pyx_Import(PyObject *name, PyObject *from_list, int level);
-
-/* FastTypeChecks.proto */
-#if CYTHON_COMPILING_IN_CPYTHON
-#define __Pyx_TypeCheck(obj, type) __Pyx_IsSubtype(Py_TYPE(obj), (PyTypeObject *)type)
-static CYTHON_INLINE int __Pyx_IsSubtype(PyTypeObject *a, PyTypeObject *b);
-static CYTHON_INLINE int __Pyx_PyErr_GivenExceptionMatches(PyObject *err, PyObject *type);
-static CYTHON_INLINE int __Pyx_PyErr_GivenExceptionMatches2(PyObject *err, PyObject *type1, PyObject *type2);
-#else
-#define __Pyx_TypeCheck(obj, type) PyObject_TypeCheck(obj, (PyTypeObject *)type)
-#define __Pyx_PyErr_GivenExceptionMatches(err, type) PyErr_GivenExceptionMatches(err, type)
-#define __Pyx_PyErr_GivenExceptionMatches2(err, type1, type2) (PyErr_GivenExceptionMatches(err, type1) || PyErr_GivenExceptionMatches(err, type2))
-#endif
-#define __Pyx_PyException_Check(obj) __Pyx_TypeCheck(obj, PyExc_Exception)
-
-static CYTHON_UNUSED int __pyx_memoryview_getbuffer(PyObject *__pyx_v_self, Py_buffer *__pyx_v_info, int __pyx_v_flags); /*proto*/
-/* ListCompAppend.proto */
-#if CYTHON_USE_PYLIST_INTERNALS && CYTHON_ASSUME_SAFE_MACROS
-static CYTHON_INLINE int __Pyx_ListComp_Append(PyObject* list, PyObject* x) {
- PyListObject* L = (PyListObject*) list;
- Py_ssize_t len = Py_SIZE(list);
- if (likely(L->allocated > len)) {
- Py_INCREF(x);
- PyList_SET_ITEM(list, len, x);
- __Pyx_SET_SIZE(list, len + 1);
- return 0;
- }
- return PyList_Append(list, x);
-}
-#else
-#define __Pyx_ListComp_Append(L,x) PyList_Append(L,x)
-#endif
-
-/* PyIntBinop.proto */
-#if !CYTHON_COMPILING_IN_PYPY
-static PyObject* __Pyx_PyInt_AddObjC(PyObject *op1, PyObject *op2, long intval, int inplace, int zerodivision_check);
-#else
-#define __Pyx_PyInt_AddObjC(op1, op2, intval, inplace, zerodivision_check)\
- (inplace ? PyNumber_InPlaceAdd(op1, op2) : PyNumber_Add(op1, op2))
-#endif
-
-/* ListExtend.proto */
-static CYTHON_INLINE int __Pyx_PyList_Extend(PyObject* L, PyObject* v) {
-#if CYTHON_COMPILING_IN_CPYTHON
- PyObject* none = _PyList_Extend((PyListObject*)L, v);
- if (unlikely(!none))
- return -1;
- Py_DECREF(none);
- return 0;
-#else
- return PyList_SetSlice(L, PY_SSIZE_T_MAX, PY_SSIZE_T_MAX, v);
-#endif
-}
-
-/* ListAppend.proto */
-#if CYTHON_USE_PYLIST_INTERNALS && CYTHON_ASSUME_SAFE_MACROS
-static CYTHON_INLINE int __Pyx_PyList_Append(PyObject* list, PyObject* x) {
- PyListObject* L = (PyListObject*) list;
- Py_ssize_t len = Py_SIZE(list);
- if (likely(L->allocated > len) & likely(len > (L->allocated >> 1))) {
- Py_INCREF(x);
- PyList_SET_ITEM(list, len, x);
- __Pyx_SET_SIZE(list, len + 1);
- return 0;
- }
- return PyList_Append(list, x);
-}
-#else
-#define __Pyx_PyList_Append(L,x) PyList_Append(L,x)
-#endif
-
-/* None.proto */
-static CYTHON_INLINE long __Pyx_div_long(long, long);
-
-/* ImportFrom.proto */
-static PyObject* __Pyx_ImportFrom(PyObject* module, PyObject* name);
-
-/* HasAttr.proto */
-static CYTHON_INLINE int __Pyx_HasAttr(PyObject *, PyObject *);
-
-/* PyObject_GenericGetAttrNoDict.proto */
-#if CYTHON_USE_TYPE_SLOTS && CYTHON_USE_PYTYPE_LOOKUP && PY_VERSION_HEX < 0x03070000
-static CYTHON_INLINE PyObject* __Pyx_PyObject_GenericGetAttrNoDict(PyObject* obj, PyObject* attr_name);
-#else
-#define __Pyx_PyObject_GenericGetAttrNoDict PyObject_GenericGetAttr
-#endif
-
-/* PyObject_GenericGetAttr.proto */
-#if CYTHON_USE_TYPE_SLOTS && CYTHON_USE_PYTYPE_LOOKUP && PY_VERSION_HEX < 0x03070000
-static PyObject* __Pyx_PyObject_GenericGetAttr(PyObject* obj, PyObject* attr_name);
-#else
-#define __Pyx_PyObject_GenericGetAttr PyObject_GenericGetAttr
-#endif
-
-/* SetVTable.proto */
-static int __Pyx_SetVtable(PyObject *dict, void *vtable);
-
-/* PyObjectGetAttrStrNoError.proto */
-static CYTHON_INLINE PyObject* __Pyx_PyObject_GetAttrStrNoError(PyObject* obj, PyObject* attr_name);
-
-/* SetupReduce.proto */
-static int __Pyx_setup_reduce(PyObject* type_obj);
-
-/* CLineInTraceback.proto */
-#ifdef CYTHON_CLINE_IN_TRACEBACK
-#define __Pyx_CLineForTraceback(tstate, c_line) (((CYTHON_CLINE_IN_TRACEBACK)) ? c_line : 0)
-#else
-static int __Pyx_CLineForTraceback(PyThreadState *tstate, int c_line);
-#endif
-
-/* CodeObjectCache.proto */
-typedef struct {
- PyCodeObject* code_object;
- int code_line;
-} __Pyx_CodeObjectCacheEntry;
-struct __Pyx_CodeObjectCache {
- int count;
- int max_count;
- __Pyx_CodeObjectCacheEntry* entries;
-};
-static struct __Pyx_CodeObjectCache __pyx_code_cache = {0,0,NULL};
-static int __pyx_bisect_code_objects(__Pyx_CodeObjectCacheEntry* entries, int count, int code_line);
-static PyCodeObject *__pyx_find_code_object(int code_line);
-static void __pyx_insert_code_object(int code_line, PyCodeObject* code_object);
-
-/* AddTraceback.proto */
-static void __Pyx_AddTraceback(const char *funcname, int c_line,
- int py_line, const char *filename);
-
-#if PY_MAJOR_VERSION < 3
- static int __Pyx_GetBuffer(PyObject *obj, Py_buffer *view, int flags);
- static void __Pyx_ReleaseBuffer(Py_buffer *view);
-#else
- #define __Pyx_GetBuffer PyObject_GetBuffer
- #define __Pyx_ReleaseBuffer PyBuffer_Release
-#endif
-
-
-/* BufferStructDeclare.proto */
-typedef struct {
- Py_ssize_t shape, strides, suboffsets;
-} __Pyx_Buf_DimInfo;
-typedef struct {
- size_t refcount;
- Py_buffer pybuffer;
-} __Pyx_Buffer;
-typedef struct {
- __Pyx_Buffer *rcbuffer;
- char *data;
- __Pyx_Buf_DimInfo diminfo[8];
-} __Pyx_LocalBuf_ND;
-
-/* MemviewSliceIsContig.proto */
-static int __pyx_memviewslice_is_contig(const __Pyx_memviewslice mvs, char order, int ndim);
-
-/* OverlappingSlices.proto */
-static int __pyx_slices_overlap(__Pyx_memviewslice *slice1,
- __Pyx_memviewslice *slice2,
- int ndim, size_t itemsize);
-
-/* Capsule.proto */
-static CYTHON_INLINE PyObject *__pyx_capsule_create(void *p, const char *sig);
-
-/* IsLittleEndian.proto */
-static CYTHON_INLINE int __Pyx_Is_Little_Endian(void);
-
-/* BufferFormatCheck.proto */
-static const char* __Pyx_BufFmt_CheckString(__Pyx_BufFmt_Context* ctx, const char* ts);
-static void __Pyx_BufFmt_Init(__Pyx_BufFmt_Context* ctx,
- __Pyx_BufFmt_StackElem* stack,
- __Pyx_TypeInfo* type);
-
-/* TypeInfoCompare.proto */
-static int __pyx_typeinfo_cmp(__Pyx_TypeInfo *a, __Pyx_TypeInfo *b);
-
-/* MemviewSliceValidateAndInit.proto */
-static int __Pyx_ValidateAndInit_memviewslice(
- int *axes_specs,
- int c_or_f_flag,
- int buf_flags,
- int ndim,
- __Pyx_TypeInfo *dtype,
- __Pyx_BufFmt_StackElem stack[],
- __Pyx_memviewslice *memviewslice,
- PyObject *original_obj);
-
-/* ObjectToMemviewSlice.proto */
-static CYTHON_INLINE __Pyx_memviewslice __Pyx_PyObject_to_MemoryviewSlice_d_d_dc_int(PyObject *, int writable_flag);
-
-/* ObjectToMemviewSlice.proto */
-static CYTHON_INLINE __Pyx_memviewslice __Pyx_PyObject_to_MemoryviewSlice_d_d_dc_float(PyObject *, int writable_flag);
-
-/* ObjectToMemviewSlice.proto */
-static CYTHON_INLINE __Pyx_memviewslice __Pyx_PyObject_to_MemoryviewSlice_dc_int(PyObject *, int writable_flag);
-
-/* CIntToPy.proto */
-static CYTHON_INLINE PyObject* __Pyx_PyInt_From_int(int value);
-
-/* CIntToPy.proto */
-static CYTHON_INLINE PyObject* __Pyx_PyInt_From_long(long value);
-
-/* MemviewSliceCopyTemplate.proto */
-static __Pyx_memviewslice
-__pyx_memoryview_copy_new_contig(const __Pyx_memviewslice *from_mvs,
- const char *mode, int ndim,
- size_t sizeof_dtype, int contig_flag,
- int dtype_is_object);
-
-/* CIntFromPy.proto */
-static CYTHON_INLINE int __Pyx_PyInt_As_int(PyObject *);
-
-/* CIntFromPy.proto */
-static CYTHON_INLINE long __Pyx_PyInt_As_long(PyObject *);
-
-/* CIntFromPy.proto */
-static CYTHON_INLINE char __Pyx_PyInt_As_char(PyObject *);
-
-/* CheckBinaryVersion.proto */
-static int __Pyx_check_binary_version(void);
-
-/* InitStrings.proto */
-static int __Pyx_InitStrings(__Pyx_StringTabEntry *t);
-
-static PyObject *__pyx_array_get_memview(struct __pyx_array_obj *__pyx_v_self); /* proto*/
-static char *__pyx_memoryview_get_item_pointer(struct __pyx_memoryview_obj *__pyx_v_self, PyObject *__pyx_v_index); /* proto*/
-static PyObject *__pyx_memoryview_is_slice(struct __pyx_memoryview_obj *__pyx_v_self, PyObject *__pyx_v_obj); /* proto*/
-static PyObject *__pyx_memoryview_setitem_slice_assignment(struct __pyx_memoryview_obj *__pyx_v_self, PyObject *__pyx_v_dst, PyObject *__pyx_v_src); /* proto*/
-static PyObject *__pyx_memoryview_setitem_slice_assign_scalar(struct __pyx_memoryview_obj *__pyx_v_self, struct __pyx_memoryview_obj *__pyx_v_dst, PyObject *__pyx_v_value); /* proto*/
-static PyObject *__pyx_memoryview_setitem_indexed(struct __pyx_memoryview_obj *__pyx_v_self, PyObject *__pyx_v_index, PyObject *__pyx_v_value); /* proto*/
-static PyObject *__pyx_memoryview_convert_item_to_object(struct __pyx_memoryview_obj *__pyx_v_self, char *__pyx_v_itemp); /* proto*/
-static PyObject *__pyx_memoryview_assign_item_from_object(struct __pyx_memoryview_obj *__pyx_v_self, char *__pyx_v_itemp, PyObject *__pyx_v_value); /* proto*/
-static PyObject *__pyx_memoryviewslice_convert_item_to_object(struct __pyx_memoryviewslice_obj *__pyx_v_self, char *__pyx_v_itemp); /* proto*/
-static PyObject *__pyx_memoryviewslice_assign_item_from_object(struct __pyx_memoryviewslice_obj *__pyx_v_self, char *__pyx_v_itemp, PyObject *__pyx_v_value); /* proto*/
-
-/* Module declarations from 'cython.view' */
-
-/* Module declarations from 'cython' */
-
-/* Module declarations from 'monotonic_align.core' */
-static PyTypeObject *__pyx_array_type = 0;
-static PyTypeObject *__pyx_MemviewEnum_type = 0;
-static PyTypeObject *__pyx_memoryview_type = 0;
-static PyTypeObject *__pyx_memoryviewslice_type = 0;
-static PyObject *generic = 0;
-static PyObject *strided = 0;
-static PyObject *indirect = 0;
-static PyObject *contiguous = 0;
-static PyObject *indirect_contiguous = 0;
-static int __pyx_memoryview_thread_locks_used;
-static PyThread_type_lock __pyx_memoryview_thread_locks[8];
-static void __pyx_f_15monotonic_align_4core_maximum_path_each(__Pyx_memviewslice, __Pyx_memviewslice, int, int, struct __pyx_opt_args_15monotonic_align_4core_maximum_path_each *__pyx_optional_args); /*proto*/
-static void __pyx_f_15monotonic_align_4core_maximum_path_c(__Pyx_memviewslice, __Pyx_memviewslice, __Pyx_memviewslice, __Pyx_memviewslice, int __pyx_skip_dispatch); /*proto*/
-static struct __pyx_array_obj *__pyx_array_new(PyObject *, Py_ssize_t, char *, char *, char *); /*proto*/
-static void *__pyx_align_pointer(void *, size_t); /*proto*/
-static PyObject *__pyx_memoryview_new(PyObject *, int, int, __Pyx_TypeInfo *); /*proto*/
-static CYTHON_INLINE int __pyx_memoryview_check(PyObject *); /*proto*/
-static PyObject *_unellipsify(PyObject *, int); /*proto*/
-static PyObject *assert_direct_dimensions(Py_ssize_t *, int); /*proto*/
-static struct __pyx_memoryview_obj *__pyx_memview_slice(struct __pyx_memoryview_obj *, PyObject *); /*proto*/
-static int __pyx_memoryview_slice_memviewslice(__Pyx_memviewslice *, Py_ssize_t, Py_ssize_t, Py_ssize_t, int, int, int *, Py_ssize_t, Py_ssize_t, Py_ssize_t, int, int, int, int); /*proto*/
-static char *__pyx_pybuffer_index(Py_buffer *, char *, Py_ssize_t, Py_ssize_t); /*proto*/
-static int __pyx_memslice_transpose(__Pyx_memviewslice *); /*proto*/
-static PyObject *__pyx_memoryview_fromslice(__Pyx_memviewslice, int, PyObject *(*)(char *), int (*)(char *, PyObject *), int); /*proto*/
-static __Pyx_memviewslice *__pyx_memoryview_get_slice_from_memoryview(struct __pyx_memoryview_obj *, __Pyx_memviewslice *); /*proto*/
-static void __pyx_memoryview_slice_copy(struct __pyx_memoryview_obj *, __Pyx_memviewslice *); /*proto*/
-static PyObject *__pyx_memoryview_copy_object(struct __pyx_memoryview_obj *); /*proto*/
-static PyObject *__pyx_memoryview_copy_object_from_slice(struct __pyx_memoryview_obj *, __Pyx_memviewslice *); /*proto*/
-static Py_ssize_t abs_py_ssize_t(Py_ssize_t); /*proto*/
-static char __pyx_get_best_slice_order(__Pyx_memviewslice *, int); /*proto*/
-static void _copy_strided_to_strided(char *, Py_ssize_t *, char *, Py_ssize_t *, Py_ssize_t *, Py_ssize_t *, int, size_t); /*proto*/
-static void copy_strided_to_strided(__Pyx_memviewslice *, __Pyx_memviewslice *, int, size_t); /*proto*/
-static Py_ssize_t __pyx_memoryview_slice_get_size(__Pyx_memviewslice *, int); /*proto*/
-static Py_ssize_t __pyx_fill_contig_strides_array(Py_ssize_t *, Py_ssize_t *, Py_ssize_t, int, char); /*proto*/
-static void *__pyx_memoryview_copy_data_to_temp(__Pyx_memviewslice *, __Pyx_memviewslice *, char, int); /*proto*/
-static int __pyx_memoryview_err_extents(int, Py_ssize_t, Py_ssize_t); /*proto*/
-static int __pyx_memoryview_err_dim(PyObject *, char *, int); /*proto*/
-static int __pyx_memoryview_err(PyObject *, char *); /*proto*/
-static int __pyx_memoryview_copy_contents(__Pyx_memviewslice, __Pyx_memviewslice, int, int, int); /*proto*/
-static void __pyx_memoryview_broadcast_leading(__Pyx_memviewslice *, int, int); /*proto*/
-static void __pyx_memoryview_refcount_copying(__Pyx_memviewslice *, int, int, int); /*proto*/
-static void __pyx_memoryview_refcount_objects_in_slice_with_gil(char *, Py_ssize_t *, Py_ssize_t *, int, int); /*proto*/
-static void __pyx_memoryview_refcount_objects_in_slice(char *, Py_ssize_t *, Py_ssize_t *, int, int); /*proto*/
-static void __pyx_memoryview_slice_assign_scalar(__Pyx_memviewslice *, int, size_t, void *, int); /*proto*/
-static void __pyx_memoryview__slice_assign_scalar(char *, Py_ssize_t *, Py_ssize_t *, int, size_t, void *); /*proto*/
-static PyObject *__pyx_unpickle_Enum__set_state(struct __pyx_MemviewEnum_obj *, PyObject *); /*proto*/
-static __Pyx_TypeInfo __Pyx_TypeInfo_int = { "int", NULL, sizeof(int), { 0 }, 0, IS_UNSIGNED(int) ? 'U' : 'I', IS_UNSIGNED(int), 0 };
-static __Pyx_TypeInfo __Pyx_TypeInfo_float = { "float", NULL, sizeof(float), { 0 }, 0, 'R', 0, 0 };
-#define __Pyx_MODULE_NAME "monotonic_align.core"
-extern int __pyx_module_is_main_monotonic_align__core;
-int __pyx_module_is_main_monotonic_align__core = 0;
-
-/* Implementation of 'monotonic_align.core' */
-static PyObject *__pyx_builtin_range;
-static PyObject *__pyx_builtin_ValueError;
-static PyObject *__pyx_builtin_MemoryError;
-static PyObject *__pyx_builtin_enumerate;
-static PyObject *__pyx_builtin_TypeError;
-static PyObject *__pyx_builtin_Ellipsis;
-static PyObject *__pyx_builtin_id;
-static PyObject *__pyx_builtin_IndexError;
-static const char __pyx_k_O[] = "O";
-static const char __pyx_k_c[] = "c";
-static const char __pyx_k_id[] = "id";
-static const char __pyx_k_new[] = "__new__";
-static const char __pyx_k_obj[] = "obj";
-static const char __pyx_k_base[] = "base";
-static const char __pyx_k_dict[] = "__dict__";
-static const char __pyx_k_main[] = "__main__";
-static const char __pyx_k_mode[] = "mode";
-static const char __pyx_k_name[] = "name";
-static const char __pyx_k_ndim[] = "ndim";
-static const char __pyx_k_pack[] = "pack";
-static const char __pyx_k_size[] = "size";
-static const char __pyx_k_step[] = "step";
-static const char __pyx_k_stop[] = "stop";
-static const char __pyx_k_t_xs[] = "t_xs";
-static const char __pyx_k_t_ys[] = "t_ys";
-static const char __pyx_k_test[] = "__test__";
-static const char __pyx_k_ASCII[] = "ASCII";
-static const char __pyx_k_class[] = "__class__";
-static const char __pyx_k_error[] = "error";
-static const char __pyx_k_flags[] = "flags";
-static const char __pyx_k_paths[] = "paths";
-static const char __pyx_k_range[] = "range";
-static const char __pyx_k_shape[] = "shape";
-static const char __pyx_k_start[] = "start";
-static const char __pyx_k_encode[] = "encode";
-static const char __pyx_k_format[] = "format";
-static const char __pyx_k_import[] = "__import__";
-static const char __pyx_k_name_2[] = "__name__";
-static const char __pyx_k_pickle[] = "pickle";
-static const char __pyx_k_reduce[] = "__reduce__";
-static const char __pyx_k_struct[] = "struct";
-static const char __pyx_k_unpack[] = "unpack";
-static const char __pyx_k_update[] = "update";
-static const char __pyx_k_values[] = "values";
-static const char __pyx_k_fortran[] = "fortran";
-static const char __pyx_k_memview[] = "memview";
-static const char __pyx_k_Ellipsis[] = "Ellipsis";
-static const char __pyx_k_getstate[] = "__getstate__";
-static const char __pyx_k_itemsize[] = "itemsize";
-static const char __pyx_k_pyx_type[] = "__pyx_type";
-static const char __pyx_k_setstate[] = "__setstate__";
-static const char __pyx_k_TypeError[] = "TypeError";
-static const char __pyx_k_enumerate[] = "enumerate";
-static const char __pyx_k_pyx_state[] = "__pyx_state";
-static const char __pyx_k_reduce_ex[] = "__reduce_ex__";
-static const char __pyx_k_IndexError[] = "IndexError";
-static const char __pyx_k_ValueError[] = "ValueError";
-static const char __pyx_k_pyx_result[] = "__pyx_result";
-static const char __pyx_k_pyx_vtable[] = "__pyx_vtable__";
-static const char __pyx_k_MemoryError[] = "MemoryError";
-static const char __pyx_k_PickleError[] = "PickleError";
-static const char __pyx_k_pyx_checksum[] = "__pyx_checksum";
-static const char __pyx_k_stringsource[] = "stringsource";
-static const char __pyx_k_pyx_getbuffer[] = "__pyx_getbuffer";
-static const char __pyx_k_reduce_cython[] = "__reduce_cython__";
-static const char __pyx_k_View_MemoryView[] = "View.MemoryView";
-static const char __pyx_k_allocate_buffer[] = "allocate_buffer";
-static const char __pyx_k_dtype_is_object[] = "dtype_is_object";
-static const char __pyx_k_pyx_PickleError[] = "__pyx_PickleError";
-static const char __pyx_k_setstate_cython[] = "__setstate_cython__";
-static const char __pyx_k_pyx_unpickle_Enum[] = "__pyx_unpickle_Enum";
-static const char __pyx_k_cline_in_traceback[] = "cline_in_traceback";
-static const char __pyx_k_strided_and_direct[] = "";
-static const char __pyx_k_strided_and_indirect[] = "";
-static const char __pyx_k_contiguous_and_direct[] = "";
-static const char __pyx_k_MemoryView_of_r_object[] = "";
-static const char __pyx_k_MemoryView_of_r_at_0x_x[] = "