diff --git a/spaces/101-5/gpt4free/g4f/.v1/unfinished/bard/__init__.py b/spaces/101-5/gpt4free/g4f/.v1/unfinished/bard/__init__.py
deleted file mode 100644
index f1d68b9281f7462f2f80a9b14d4c05795c05898d..0000000000000000000000000000000000000000
--- a/spaces/101-5/gpt4free/g4f/.v1/unfinished/bard/__init__.py
+++ /dev/null
@@ -1,93 +0,0 @@
-from json import dumps, loads
-from os import getenv
-from random import randint
-from re import search
-from urllib.parse import urlencode
-
-from bard.typings import BardResponse
-from dotenv import load_dotenv
-from requests import Session
-
-load_dotenv()
-token = getenv('1psid')
-proxy = getenv('proxy')
-
-temperatures = {
- 0: "Generate text strictly following known patterns, with no creativity.",
- 0.1: "Produce text adhering closely to established patterns, allowing minimal creativity.",
- 0.2: "Create text with modest deviations from familiar patterns, injecting a slight creative touch.",
- 0.3: "Craft text with a mild level of creativity, deviating somewhat from common patterns.",
- 0.4: "Formulate text balancing creativity and recognizable patterns for coherent results.",
- 0.5: "Generate text with a moderate level of creativity, allowing for a mix of familiarity and novelty.",
- 0.6: "Compose text with an increased emphasis on creativity, while partially maintaining familiar patterns.",
- 0.7: "Produce text favoring creativity over typical patterns for more original results.",
- 0.8: "Create text heavily focused on creativity, with limited concern for familiar patterns.",
- 0.9: "Craft text with a strong emphasis on unique and inventive ideas, largely ignoring established patterns.",
- 1: "Generate text with maximum creativity, disregarding any constraints of known patterns or structures."
-}
-
-
-class Completion:
- def create(
- prompt: str = 'hello world',
- temperature: int = None,
- conversation_id: str = '',
- response_id: str = '',
- choice_id: str = '') -> BardResponse:
-
- if temperature:
- prompt = f'''settings: follow these settings for your response: [temperature: {temperature} - {temperatures[temperature]}] | prompt : {prompt}'''
-
- client = Session()
- client.proxies = {
- 'http': f'http://{proxy}',
- 'https': f'http://{proxy}'} if proxy else None
-
- client.headers = {
- 'authority': 'bard.google.com',
- 'content-type': 'application/x-www-form-urlencoded;charset=UTF-8',
- 'origin': 'https://bard.google.com',
- 'referer': 'https://bard.google.com/',
- 'user-agent': 'Mozilla/5.0 (Macintosh; Intel Mac OS X 10_15_7) AppleWebKit/537.36 (KHTML, like Gecko) Chrome/111.0.0.0 Safari/537.36',
- 'x-same-domain': '1',
- 'cookie': f'__Secure-1PSID={token}'
- }
-
- snlm0e = search(r'SNlM0e\":\"(.*?)\"',
- client.get('https://bard.google.com/').text).group(1)
-
- params = urlencode({
- 'bl': 'boq_assistant-bard-web-server_20230326.21_p0',
- '_reqid': randint(1111, 9999),
- 'rt': 'c',
- })
-
- response = client.post(
- f'https://bard.google.com/_/BardChatUi/data/assistant.lamda.BardFrontendService/StreamGenerate?{params}',
- data={
- 'at': snlm0e,
- 'f.req': dumps([None, dumps([
- [prompt],
- None,
- [conversation_id, response_id, choice_id],
- ])])
- }
- )
-
- chat_data = loads(response.content.splitlines()[3])[0][2]
- if not chat_data:
- print('error, retrying')
- Completion.create(prompt, temperature,
- conversation_id, response_id, choice_id)
-
- json_chat_data = loads(chat_data)
- results = {
- 'content': json_chat_data[0][0],
- 'conversation_id': json_chat_data[1][0],
- 'response_id': json_chat_data[1][1],
- 'factualityQueries': json_chat_data[3],
- 'textQuery': json_chat_data[2][0] if json_chat_data[2] is not None else '',
- 'choices': [{'id': i[0], 'content': i[1]} for i in json_chat_data[4]],
- }
-
- return BardResponse(results)
diff --git a/spaces/1acneusushi/gradio-2dmoleculeeditor/data/Camel audio alchemy download Create your own unique sounds with Alchemys sample import and resynthesis features.md b/spaces/1acneusushi/gradio-2dmoleculeeditor/data/Camel audio alchemy download Create your own unique sounds with Alchemys sample import and resynthesis features.md
deleted file mode 100644
index ae97e2d94a029d1f2e3526f8679dfa9bef149d54..0000000000000000000000000000000000000000
--- a/spaces/1acneusushi/gradio-2dmoleculeeditor/data/Camel audio alchemy download Create your own unique sounds with Alchemys sample import and resynthesis features.md
+++ /dev/null
@@ -1,118 +0,0 @@
-
-
Camel Audio Alchemy Download: The Ultimate Sample Manipulation Synthesizer
-
If you are looking for a powerful and versatile synthesizer that can turn your musical dreams into reality, you might want to check out Camel Audio's Alchemy. Alchemy is a hybrid synth that combines additive, spectral, granular, sampling, and virtual analog synthesis in one plugin. It also features a wide range of filters, effects, modulation options, and an arpeggiator that can sync to any MIDI groove. With over 2GB of samples and 500 presets included, you will never run out of inspiration with Alchemy. In this article, we will show you how to download Alchemy for Mac or Windows, how to use its features, and why it is one of the best synths on the market.
-
What is Alchemy?
-
Alchemy is a synth that can do anything from fattening up a kick drum, to producing a great guitar sound or powerful dance bassline. It can also create lush pads, soundscapes, keys, leads, and more. It is described by Camel Audio as "the ultimate sample manipulation synthesizer". Here are some of its main features:
Synthesis modes: Alchemy can use up to four sources for each sound. Each source can be either additive, spectral, granular, sampling, or virtual analog. You can mix and match different synthesis modes to create unique sounds.
-
Sources: Alchemy comes with over 2GB of samples and analysed content from various sound designers. You can also import your own samples from SFZ, WAV or AIFF files. You can use up to four samples per source and edit their start/end points, loop modes, pitch envelopes, etc.
-
Filters: Alchemy has a wide range of analog modeled filters that can be applied to each source or globally. You can choose from low-pass, high-pass, band-pass, notch, comb, formant, ring modulator, etc. You can also adjust the cutoff frequency, resonance, drive, feedback, etc.
-
Effects: Alchemy has a flexible rack of effects that can be applied to each source or globally. You can choose from distortion, compression, filter, reverb, delay, chorus, flanger, phaser, etc. You can also adjust the parameters of each effect.
-
Modulation: Alchemy has an innovative modulation system that is extremely flexible yet easy to use. You can modulate almost any parameter with up to eight modulators per source or globally. You can choose from envelopes, LFOs, MSEGs, step sequencers, performers, etc. You can also adjust the amount, rate, shape, sync, etc. of each modulator.
-
Arpeggiator: Alchemy has a powerful arpeggiator that can create complex rhythmic patterns with up to 16 steps per source or globally. You can adjust the pitch, velocity, gate, swing, etc. of each step. You can also import the groove from any MIDI file for immediate synchronization to a beat.
-
-
How to Download Alchemy?
-
System Requirements
-
To run Alchemy on your computer, you need to meet the following system requirements:
To download Alchemy for Mac or Windows, you need to visit one of the following links:
-
-
Type
Name
Description
-
Official
Camel Audio Website (No Longer Available)
- This was the original website where you could buy and download Alchemy and its soundbanks. However, it was shut down in 2015 after Camel Audio was acquired by Apple.
-
-
Official
-
Camel Audio Support Page (No Longer Available)
-
Camel audio alchemy synth hybrid plugin
-Alchemy by camel audio virtual instrument
-Camel audio alchemy sample manipulation synthesizer
-How to import samples into camel audio alchemy
-Camel audio alchemy soundbank player free download
-Camel audio alchemy 5.5GB pack of audio samples
-Camel audio alchemy additive spectral granular synthesis
-Camel audio alchemy flexible rack of effects
-Camel audio alchemy innovative modulation system
-Camel audio alchemy powerful arpeggiator with groove import
-Camel audio alchemy presets by top sound designers
-Camel audio alchemy expansion sound banks
-Camel audio alchemy compatible with SFZ WAV AIFF files
-Camel audio alchemy analog modelled filters
-Camel audio alchemy morph or crossfade between sources
-Camel audio alchemy resynthesis and sound morphing abilities
-Camel audio alchemy review and tutorial
-Camel audio alchemy license and price
-Camel audio alchemy vs omnisphere vs kontakt
-Camel audio alchemy discontinued and alternatives
-Where to buy camel audio alchemy online
-How to install camel audio alchemy on mac or windows
-How to use camel audio alchemy with logic pro x or ableton live
-How to create your own presets with camel audio alchemy
-How to update camel audio alchemy to version 1.55
-Best tips and tricks for camel audio alchemy users
-How to fix camel audio alchemy errors and crashes
-How to uninstall camel audio alchemy completely
-How to backup and restore camel audio alchemy data
-How to get camel crusher and cameleon 5000 by camel audio
- This was the official support page where you could download updates and manuals for Alchemy. However, it was also shut down in 2015 after Camel Audio was acquired by Apple.
-
-
Alternative
-
KVR Audio Website (Available)
- This is a website where you can find information about various audio plugins. It has a page dedicated to Alchemy where you can download the latest version (1.55) for Mac or Windows. You can also find user reviews, ratings, and comments about Alchemy.
-
-
Alternative
-
Camel Audio Archive Website (Available)
- This is a website where you can find archived versions of Camel Audio's products. It has a page dedicated to Alchemy where you can download older versions (1.0-1.50) for Mac or Windows. You can also find manuals, soundbanks, and tutorials for Alchemy.
-
-
Note: To use any of these download links, you need to have a valid license key for Alchemy. If you don't have one, you won't be able to activate the plugin. You can try contacting Apple Support if you have purchased Alchemy before, or look for alternative ways to obtain a license key online.
-
- How to Use Alchemy?
-
- Once you have downloaded and installed Alchemy on your computer, you are ready to start using it. Here are some basic steps on how to use its features:
-
Loading Presets
-
- Alchemy comes with over 500 presets that cover various genres, styles, You can load them by clicking on the preset browser button on the top left corner of the plugin window. You can then browse the presets by category, rating, name, author, etc. You can also use the search box to find a preset by keyword. To load a preset, simply double-click on it or drag and drop it onto the plugin window. You can also use the arrow keys to navigate through the presets and press enter to load them.
-
Importing Samples
-
If you want to use your own samples as sources for Alchemy, you can import them from SFZ, WAV or AIFF files. To do so, you need to click on the import button on the top right corner of the source editor window. You can then browse your computer for the file you want to import. You can also drag and drop the file onto the source editor window. Once you have imported a sample, you can edit its parameters such as start/end points, loop mode, pitch envelope, etc. You can also analyze the sample for additive or spectral synthesis modes.
-
Morphing and Crossfading
-
One of the most powerful features of Alchemy is its ability to morph and crossfade between sources. You can use the performance controls and remix pads to do this. The performance controls are located on the bottom left corner of the plugin window. They consist of eight knobs and eight sliders that can be assigned to any parameter of Alchemy. You can use them to tweak your sound in real time. The remix pads are located on the bottom right corner of the plugin window. They consist of eight pads that can be assigned to different snapshots of your sound. You can use them to morph and crossfade between sources by clicking and dragging on them. You can also automate them with MIDI or host automation.
-
Editing Parameters
-
If you want to access and adjust the parameters of each synthesis mode, filter, effect, modulator, and arpeggiator, you need to click on the corresponding button on the top of the plugin window. You will then see a detailed editor window where you can edit each parameter with knobs, sliders, envelopes, graphs, etc. You can also right-click on any parameter to assign it to a performance control or a modulator.
-
Why Choose Alchemy?
-
Alchemy is not just another synth plugin. It is a creative tool that can help you design any sound you can imagine. Here are some of the reasons why you should choose Alchemy for your sound design and music production needs:
-
-
Versatility: Alchemy can create any type of sound from acoustic to electronic, from realistic to surreal, from simple to complex. It can also blend different synthesis modes and sources to create hybrid sounds that are unique and original.
-
Quality: Alchemy has a high-quality sound engine that delivers crystal-clear and rich sounds. It also has a wide range of analog modeled filters and effects that add warmth and character to your sounds.
-
Usability: Alchemy is easy to use thanks to its intuitive interface and performance controls. It also has a comprehensive preset browser that lets you find the sound you need quickly and easily.
-
Inspiration: Alchemy comes with over 2GB of samples and 500 presets that cover various genres, styles, and sounds. You can also import your own samples and use them as sources for Alchemy. You can also use the morphing and crossfading features to create new sounds from existing ones.
-
Value: Alchemy is a great value for money as it offers a lot of features and sounds for a reasonable price. You can also expand your sound library with additional soundbanks that are available for purchase.
-
-
Conclusion
-
In conclusion, Alchemy is a synth plugin that you should definitely try if you are looking for a powerful and versatile synthesizer that can turn your musical dreams into reality. It offers a lot of features and sounds that will inspire you and enhance your sound design and music production skills. You can download Alchemy for Mac or Windows from one of the links provided in this article and start creating amazing sounds with it.
-
Frequently Asked Questions
-
-
Is Alchemy still available?
-
Yes, Alchemy is still available for download from some alternative websites such as KVR Audio or Camel Audio Archive. However, it is no longer supported or updated by Camel Audio or Apple.
-
Can I use Alchemy with Logic Pro X?
-
Yes, you can use Alchemy with Logic Pro X as an Audio Unit plugin. However, you should note that Logic Pro X already comes with an updated version of Alchemy that has more features and sounds than the original one.
-
How do I activate Alchemy?
-
To activate Alchemy, you need to have a valid license key that you received when you purchased Alchemy from Camel Audio or Apple. You need to enter this license key when you launch Alchemy for the first time.
-
How do I update Alchemy?
-
To update Alchemy, you need to download the latest version (1.55) from one of the alternative websites such as KVR Audio or Camel Audio Archive. You then need to install it over your existing version of Alchemy.
-
How do I get more sounds for Alchemy?
-
To get more sounds for Alchemy, you can purchase additional soundbanks from Camel Audio's website (no longer available) or from other third-party sound designers such as Biome Digital or Sample Magic. You can also create your own sounds by importing your own samples or using the synthesis modes of Alchemy.
-
- 0a6ba089eb
-
-
\ No newline at end of file
diff --git a/spaces/1acneusushi/gradio-2dmoleculeeditor/data/Crackeddll 3.1.5.0.exe Download A Simple and Effective Way to Bypass Software Protection.md b/spaces/1acneusushi/gradio-2dmoleculeeditor/data/Crackeddll 3.1.5.0.exe Download A Simple and Effective Way to Bypass Software Protection.md
deleted file mode 100644
index 579a0c57867b8ebe8bed4a22d288bc9ca2744ebd..0000000000000000000000000000000000000000
--- a/spaces/1acneusushi/gradio-2dmoleculeeditor/data/Crackeddll 3.1.5.0.exe Download A Simple and Effective Way to Bypass Software Protection.md
+++ /dev/null
@@ -1,134 +0,0 @@
-
-
What is eddll 3.1.5.0.exe and why do you need it?
-
Have you ever wondered how to keep your Dell system software up to date and secure? If so, you might have heard of eddll 3.1.5.0.exe, a stand-alone application that provides updates for system software that is released by Dell.
-
Eddll 3.1.5.0.exe is also known as Dell Command Update, a tool that simplifies the BIOS, firmware, driver, and application update experience for Dell client hardware. It helps you to find and install the latest updates for your system software in a few clicks.
But how do you download eddll 3.1.5.0.exe safely and securely? How do you use it to update your Dell system software? What are the benefits and risks of using it? And how do you troubleshoot common problems with it?
-
In this article, we will answer all these questions and more, so that you can make the most of eddll 3.1.5.0.exe and keep your Dell system software current and compatible.
-
How to download eddll 3.1.5.0.exe safely and securely?
-
Before you can use eddll 3.1.5.0.exe to update your Dell system software, you need to download it from a reliable source.
-
However, downloading any file from the internet can be risky, especially if you are not careful about where you get it from.
-
crackeddll 3.1.5.0 free download
-crackeddll 3.1.5.0 software
-crackeddll 3.1.5.0 zip
-crackeddll 3.1.5.0 rar
-crackeddll 3.1.5.0 torrent
-crackeddll 3.1.5.0 full version
-crackeddll 3.1.5.0 license key
-crackeddll 3.1.5.0 activation code
-crackeddll 3.1.5.0 serial number
-crackeddll 3.1.5.0 crack
-crackeddll 3.1.5.0 patch
-crackeddll 3.1.5.0 keygen
-crackeddll 3.1.5.0 hack
-crackeddll 3.1.5.0 mod
-crackeddll 3.1.5.0 fix
-crackeddll 3.1.5.0 repair
-crackeddll 3.1.5.0 update
-crackeddll 3.1.5.0 latest version
-crackeddll 3.1.5.exe download for windows
-crackeddll 3.exe download for mac
-cracked dll files fixer download
-cracked dll files for games download
-how to crack software by modifying dll files
-how to use cracked dll 3.exe
-how to install cracked dll 3.exe
-how to uninstall cracked dll 3.exe
-how to remove cracked dll virus
-how to fix dll errors with cracked dll software
-how to download dll files for free with cracked dll software
-how to backup and restore dll files with cracked dll software
-how to optimize pc performance with cracked dll software
-how to prevent blue screen of death with cracked dll software
-how to solve exe virus infection with cracked dll software
-how to enhance pc security with cracked dll software
-benefits of using cracked dll software
-features of cracked dll software
-reviews of cracked dll software
-alternatives to cracked dll software
-comparison of cracked dll software and other dll fixers
-pros and cons of using cracked dll software
-risks of using cracked dll software
-legality of using cracked dll software
-safety of using cracked dll software
-reliability of using cracked dll software
-compatibility of using cracked dll software with different windows versions
-troubleshooting tips for using cracked dll software
-customer support for using cracked dll software
-tutorials for using cracked dll software
-testimonials for using cracked dll software
-discounts and offers for using cracked dll software
-.0.exe safely and securely:
-
-
Check your system compatibility and requirements. Eddll 3.1.5.0.exe is designed to run on Microsoft Windows 64bit Operating Systems. You can check your system information by right-clicking on the Start menu and selecting System.
-
Find a reliable source for downloading eddll 3.1.5.0.exe. The best source for downloading eddll 3.1.5.0.exe is the official Dell website, where you can find the latest driver information for your system. You can also use other trusted websites that offer eddll 3.1.5.0.exe for download, but make sure to read the reviews and ratings before downloading.
-
Scan the file for viruses and malware before installing. Even if you download eddll 3.1.5.0.exe from a reputable source, you should always scan it for viruses and malware before installing it on your system. You can use your antivirus software or an online scanner to do this.
-
-
By following these steps, you can download eddll 3.1.5.0.exe safely and securely, and avoid any unwanted surprises.
-
How to use eddll 3.1.5.0.exe to update your Dell system software?
-
Once you have downloaded eddll 3.1.5.0.exe, you can use it to update your Dell system software in a few easy steps:
-
-
Launch eddll 3.1.5.0.exe and accept the terms and conditions. Double-click on the file and follow the instructions on the screen to start the installation process. You will need to accept the terms and conditions of the Dell Software License Agreement before proceeding.
-.0.exe will scan your system and show you a list of available updates for your system software. You can choose to install all the updates or select the ones that you want to install.
-
Wait for the update process to complete and restart your system. Eddll 3.1.5.0.exe will download and install the updates for your system software. Depending on the size and number of updates, this may take some time. You will be notified when the update process is complete and you will need to restart your system for the changes to take effect.
-
-
By following these steps, you can use eddll 3.1.5.0.exe to update your Dell system software and keep it current and compatible.
-
What are the benefits of using eddll 3.1.5.0.exe?
-
Using eddll 3.1.5.0.exe to update your Dell system software has many benefits, such as:
-
-
Simplify the BIOS, firmware, driver, and application update experience for Dell client hardware. Eddll 3.1.5.0.exe is a stand-alone application that does not require any other software or tools to run. It automatically detects your system model and configuration and shows you the relevant updates for your system software.
-
Enable security enhancement with Dell signature verification for all packages. Eddll 3.1.5.0.exe verifies the signature of all packages before installing them on your system, ensuring that they are authentic and safe.
-.5.0.exe gives you a one hour quiet period where no updates happen automatically when you start your new system for the first time. This feature helps to enhance the Out of Box Experience (OOBE) and lets you enjoy your new system without interruptions.
-
-
By using eddll 3.1.5.0.exe, you can enjoy these benefits and more, and keep your Dell system software up to date and secure.
-
What are the potential risks of using eddll 3.1.5.0.exe?
-
While using eddll 3.1.5.0.exe has many benefits, it also has some potential risks that you should be aware of, such as:
-
-
Download a corrupted or infected file from an untrusted source. If you download eddll 3.1.5.0.exe from an untrusted source, you may end up with a corrupted or infected file that can harm your system or compromise your data. Therefore, you should always download eddll 3.1.5.0.exe from a reliable source and scan it for viruses and malware before installing.
-
Encounter compatibility issues or errors during the update process. Sometimes, the updates for your system software may not be compatible with your system model or configuration, or may cause errors during the installation process. This can result in system instability or performance issues. Therefore, you should always check your system compatibility and requirements before downloading and installing eddll 3.1.5.0.exe.
-.1.5.0.exe.
-
-
By being aware of these risks and taking precautions, you can minimize the chances of encountering any problems with eddll 3.1.5.0.exe and use it safely and securely.
-
How to troubleshoot common problems with eddll 3.1.5.0.exe?
-
Even if you follow the steps and precautions mentioned above, you may still encounter some problems with eddll 3.1.5.0.exe, such as:
-
-
Eddll 3.1.5.0.exe does not run or shows an error message.
-
Eddll 3.1.5.0.exe does not find any updates or shows incorrect updates.
-
Eddll 3.1.5.0.exe takes too long to download or install the updates.
-
-
If you face any of these problems, you can try the following solutions to troubleshoot them:
-
Solution 1: Check your system compatibility and requirements again
-
Make sure that your system meets the minimum requirements for running eddll 3.1.5.0.exe, such as:
-
-
Operating System: Microsoft Windows 64bit
-
System Model: Dell client hardware
-
System Configuration: compatible with the updates
-
-
If your system does not meet these requirements, you may need to upgrade your system or use a different tool to update your system software.
- .5.0.exe again from a different source
-
It is possible that the file that you downloaded is corrupted or incomplete, which can cause eddll 3.1.5.0.exe to not run or show an error message. To fix this, you can try to download eddll 3.1.5.0.exe again from a different source, such as the official Dell website or another trusted website. Make sure to scan the file for viruses and malware before installing it.
-
Solution 3: Contact Dell support for assistance
-
If none of the above solutions work, you may need to contact Dell support for assistance. They can help you to diagnose and resolve any issues with eddll 3.1.5.0.exe and your system software. You can contact Dell support by phone, email, chat, or online forums.
-
Conclusion
-
Eddll 3.1.5.0.exe is a stand-alone application that provides updates for system software that is released by Dell. It simplifies the BIOS, firmware, driver, and application update experience for Dell client hardware and enables security enhancement with Dell signature verification for all packages.
-
However, using eddll 3.1.5.0.exe also has some potential risks, such as downloading a corrupted or infected file from an untrusted source, encountering compatibility issues or errors during the update process, or damaging your system software or hardware if the update fails or is interrupted.
-
Therefore, you need to follow some steps and precautions to download and use eddll 3.1.5.0.exe safely and securely, such as checking your system compatibility and requirements, finding a reliable source for downloading eddll 3.1.5.0.exe, scanning the file for viruses and malware before installing, backing up your data and ensuring a stable power and network connection before using eddll 3.1.5.0.exe.
-.1.5.0.exe, you can try some solutions to troubleshoot them, such as checking your system compatibility and requirements again, downloading eddll 3.1.5.0.exe again from a different source, or contacting Dell support for assistance.
-
We hope that this article has helped you to understand what eddll 3.1.5.0.exe is and how to use it to update your Dell system software. If you have any questions or feedback, please feel free to leave a comment below.
-
FAQs
-
Here are some frequently asked questions about eddll 3.1.5.0.exe:
-
-
What is the difference between eddll 3.1.5.0.exe and Dell Update?
-
Eddll 3.1.5.0.exe is also known as Dell Command Update, a stand-alone application that provides updates for system software that is released by Dell. Dell Update is another application that provides updates for Dell consumer systems, such as Inspiron, XPS, Alienware, and Vostro.
-
How do I know if I need to update my system software?
-
You can use eddll 3.1.5.0.exe to scan your system and show you a list of available updates for your system software. You can also check the Dell website for the latest driver information for your system model and configuration.
-
How often should I use eddll 3.1.5.0.exe to update my system software?
-.1.5.0.exe to update your system software whenever there is a new update available or whenever you encounter a problem with your system software. You can also set eddll 3.1.5.0.exe to run automatically or manually according to your preference.
-
Can I use eddll 3.1.5.0.exe to update other system software besides Dell?
-
No, eddll 3.1.5.0.exe only provides updates for system software that is released by Dell. If you want to update other system software, such as Windows, Office, or antivirus, you need to use other tools or applications.
-
Can I uninstall eddll 3.1.5.0.exe if I don't need it anymore?
-
Yes, you can uninstall eddll 3.1.5.0.exe if you don't need it anymore or if you want to use a different tool to update your system software. You can uninstall eddll 3.1.5.0.exe from the Control Panel or the Settings app.
-
- 0a6ba089eb
-
-
\ No newline at end of file
diff --git a/spaces/1acneusushi/gradio-2dmoleculeeditor/data/Fences 3 Serial Key.md b/spaces/1acneusushi/gradio-2dmoleculeeditor/data/Fences 3 Serial Key.md
deleted file mode 100644
index 280232a5a77aea64d2e99afee35370dc3a025461..0000000000000000000000000000000000000000
--- a/spaces/1acneusushi/gradio-2dmoleculeeditor/data/Fences 3 Serial Key.md
+++ /dev/null
@@ -1,143 +0,0 @@
-
-
Fences 3 Serial Key: How to Download and Activate Fences 3 Software
-
If you are looking for a way to organize your desktop icons and windows in a neat and stylish manner, you might have heard of Fences 3 software. This is a popular desktop enhancement tool that allows you to create shaded areas on your desktop that you can place your icons into. You can also customize the appearance and behavior of your fences, create rules for automatic icon sorting, use snapshots to save and restore your desktop layout, and more.
However, before you can enjoy all these features and benefits of Fences 3 software, you need to have a serial key that allows you to activate the software on your computer. A serial key is a unique code that verifies that you have purchased a legitimate copy of the software from Stardock or an authorized reseller. Without a serial key, you cannot use Fences 3 software beyond the trial period.
-
In this article, we will show you how to get a serial key for F ences 3 software, how to download and activate it with your serial key, how to use it to organize your desktop, and how to troubleshoot some common issues that you might encounter. We will also provide some tips and tricks for using Fences 3 software effectively, and answer some frequently asked questions that you might have. By the end of this article, you will be able to enjoy Fences 3 software to the fullest and make your desktop look amazing.
-
What is Fences 3 Software?
-
Fences 3 software is a desktop enhancement tool that helps you organize your desktop icons and windows into shaded areas called fences. You can create as many fences as you want, and place them anywhere on your desktop. You can also resize, move, hide, or roll up your fences as you wish. Fences 3 software also lets you customize the appearance and behavior of your fences, such as color, transparency, title, layout, sorting, and more.
-
One of the most powerful features of Fences 3 software is the ability to create rules for automatic icon sorting. You can specify which icons go into which fence based on criteria such as name, type, date, size, or label. For example, you can create a rule that automatically places all your documents into a fence called Documents, or all your games into a fence called Games. This way, you don't have to manually drag and drop your icons every time you add or remove them from your desktop.
-
-
Another useful feature of Fences 3 software is the ability to use snapshots to save and restore your desktop layout. You can take a snapshot of your current desktop arrangement and name it as you like. You can then switch between different snapshots with a simple double-click or a hotkey. This is especially handy if you have different desktop layouts for different tasks or scenarios, such as work, gaming, or entertainment.
-
Fences 3 software is compatible with Windows 10, 8.1, 8, and 7. It requires at least 1 GB of RAM and 150 MB of hard disk space. It also supports high DPI monitors and multiple monitors.
-
Why Do You Need a Serial Key to Use Fences 3 Software?
-
A serial key is a unique code that verifies that you have purchased a legitimate copy of Fences 3 software from Stardock or an authorized reseller. A serial key is usually composed of letters and numbers, such as XXXX-XXXX-XXXX-XXXX. You need a serial key to activate Fences 3 software on your computer and unlock all its features and benefits.
-
Without a serial key, you can only use Fences 3 software as a trial version for 30 days. After the trial period expires, you will not be able to create new fences or modify existing ones. You will also see a watermark on your desktop that reminds you to purchase a serial key.
-
A serial key is valid for one computer only. If you want to use Fences 3 software on another computer, you need to purchase another serial key or deactivate the software on the first computer and reactivate it on the second one.
-
How to Get a Serial Key for Fences 3 Software?
-
Purchase Fences 3 Software from Stardock or Authorized Resellers
-
The easiest way to get a serial key for Fences 3 software is to purchase it from Stardock or authorized resellers. Stardock is the developer and publisher of Fences 3 software, and authorized resellers are online stores that have permission to sell Stardock products.
-
You can purchase Fences 3 software from Stardock website for $9.99 USD. You can pay with credit card, PayPal, Amazon Pay, or Bitcoin. After completing the payment process, you will receive an email with your serial key and download link.
-
You can also purchase Fences 3 software from authorized resellers such as Steam, Humble Bundle, or Fanatical. The price may vary depending on the reseller and the region. After purchasing Fences 3 software from an authorized reseller, you will receive an email with your serial key and download link.
-
Retrieve Your Serial Key from Stardock Support
-
If you have already purchased Fences 3 software from Stardock or an authorized reseller but have lost or forgotten your serial key, you can retrieve it from Stardock support. You will need to provide some information to verify your purchase, such as your email address, order number, or receipt.
-
To retrieve your serial key from Stardock support, follow these steps:
Enter your email address, order number, or receipt and click on Submit
-
You will receive an email with your serial key and download link
-
-
If you have any issues with retrieving your serial key from Stardock support, you can contact them via email at support@stardock.com or via phone at 1-800-493-9662.
-
How to Download Fences 3 Software?
-
After you have purchased Fences 3 software and received your serial key, you can download it from Stardock website or other sources. The download size is about 12 MB and the installation process is simple and fast.
-
To download Fences 3 software from Stardock website, follow these steps:
Follow the instructions on the screen to install Fences 3 software
-
-
You can also download Fences 3 software from other sources, such as Steam, Humble Bundle, or Fanatical. However, you need to make sure that the source is trustworthy and that the file is not corrupted or infected with malware. You also need to enter your serial key during the installation process to activate Fences 3 software.
-
How to Activate Fences 3 Software with Your Serial Key?
-
Online Activation
-
The easiest way to activate Fences 3 software with your serial key is to use the online activation method. This method requires an internet connection and an email address. You can activate Fences 3 software online with your serial key and email address during the installation process or after the installation process.
-
To activate Fences 3 software online with your serial key and email address during the installation process, follow these steps:
-
-
Run the installer file that you downloaded from Stardock website or other sources
-
Follow the instructions on the screen until you reach the Activation screen
-
Enter your serial key and email address in the fields provided
-
Click on Activate Online
-
You will see a message that says "Activation Successful"
-
Click on Finish to complete the installation process
-
-
To activate Fences 3 software online with your serial key and email address after the installation process, follow these steps:
-
-
Launch Fences 3 software from your desktop or start menu
-
You will see a message that says "Your trial period has expired. Please enter your product key to continue using this product."
-
Click on Enter Product Key
-
Enter your serial key and email address in the fields provided
-
Click on Activate Online
-
You will see a message that says "Activation Successful"
-
Click on OK to continue using Fences 3 software
-
-
Offline Activation
-
If you do not have an internet connection or an email address, you can use the offline activation method. This method requires a .REG file that contains your activation information. You can create a .REG file from another computer that has an internet connection and transfer it to your computer via a USB drive or other means.
-
To activate Fences 3 software offline with your serial key and a .REG file, follow these steps:
-
-
Go to another computer that has an internet connection and open a web browser
You will see a .REG file that contains your activation information
-
Save the .REG file to a USB drive or other means and transfer it to your computer
-
Run the installer file that you downloaded from Stardock website or other sources
-
Follow the instructions on the screen until you reach the Activation screen
-
Click on Activate Offline
-
Browse to the location of the .REG file that you transferred to your computer and select it
-
You will see a message that says "Activation Successful"
-
Click on Finish to complete the installation process
-
-
How to Use Fences 3 Software?
-
After you have activated Fences 3 software with your serial key, you can start using it to organize your desktop icons and windows. Here are some basic steps to use Fences 3 software:
-
-
Launch Fences 3 software from your desktop or start menu
-
You will see a welcome screen that gives you some tips and options for using Fences 3 software
-
You can choose to create your own fences or use the default fences that Fences 3 software provides, such as Programs, Folders, Documents, etc.
-
To create your own fence, right-click on an empty area of your desktop and select Create New Fence Here
-
A shaded area will appear on your desktop with a title bar that says New Fence. You can rename it by double-clicking on the title bar and typing a new name
-
You can drag and drop icons from your desktop into the fence. You can also right-click on an icon and select Send To Fence to move it to a specific fence
-
You can resize, move, hide, or roll up your fence by using the mouse or keyboard shortcuts. You can also right-click on the fence and select Fence Options to customize its appearance and behavior
-
To create rules for automatic icon sorting, right-click on an empty area of your desktop and select Configure Fences. Then click on Sorting & Organizing tab and select Create Rule. You can specify which icons go into which fence based on criteria such as name, type, date, size, or label. You can also edit or delete existing rules from this tab
-
To use snapshots to save and restore your desktop layout, right-click on an empty area of your desktop and select Configure Fences. Then click on Layouts & Snapping tab and select Take Snapshot. You can name your snapshot as you like and switch between different snapshots with a simple double-click or a hotkey. You can also edit or delete existing snapshots from this tab
-
-
Tips and Tricks for Using Fences 3 Software Effectively
-
Fences 3 software is a powerful and versatile tool that can help you organize your desktop in many ways. Here are some tips and tricks for using Fences 3 software effectively:
-
-
You can use keyboard shortcuts to quickly access or modify your fences. For example, you can press Ctrl + Alt + Shift + B to show or hide all fences, Ctrl + Alt + Shift + R to roll up or down all fences, Ctrl + Alt + Shift + S to switch between different snapshots, etc. You can also customize your own keyboard shortcuts from the Configure Fences menu.
-
You can use mouse gestures to quickly access or modify your fences. For example, you can double-click on an empty area of your desktop to show or hide all fences, drag an icon over a fence title bar to move it into that fence, drag a fence title bar over another fence title bar to swap their positions, etc.
-
You can use quick-hide feature to temporarily hide all fences and icons on your desktop. To do this, simply move your mouse cursor to the edge of your screen where you have enabled quick-hide from the Configure Fences menu. To show them again, just move your mouse cursor away from the edge of your screen.
-
You can use quick-hide feature to temporarily hide all fences and icons on your desktop. To do this, simply move your mouse cursor to the edge of your screen where you have enabled quick-hide from the Configure Fences menu. To show them again, just move your mouse cursor away from the edge of your screen.
You can use portals feature to create a fence that shows the contents of another folder on your computer. To do this, right-click on an empty area of your desktop and select Create New Fence Here. Then right-click on the fence and select Fence Options. Then click on Portal and select Browse to choose a folder that you want to display in the fence. You can also customize the appearance and behavior of the portal fence from this menu.
-
You can use desktop pages feature to create multiple virtual desktops that you can switch between with a mouse wheel or a hotkey. To do this, right-click on an empty area of your desktop and select Configure Fences. Then click on Desktop Pages tab and enable the feature. You can also customize the number and layout of your desktop pages from this tab.
-
You can use folder portals feature to create a fence that shows the contents of another folder on your computer. To do this, right-click on an empty area of your desktop and select Create New Fence Here. Then right-click on the fence and select Fence Options. Then click on Portal and select Browse to choose a folder that you want to display in the fence. You can also customize the appearance and behavior of the portal fence from this menu.
-
-
Troubleshooting Common Issues with Fences 3 Software
-
Fences 3 software is a reliable and stable tool that works well with most Windows systems. However, you might encounter some issues with Fences 3 software from time to time, such as activation errors, compatibility issues, performance issues, etc. Here are some solutions for troubleshooting common issues with Fences 3 software:
-
-
If you have trouble activating Fences 3 software with your serial key, make sure that you have entered the correct serial key and email address. Also, make sure that you have an internet connection if you are using the online activation method. If you are using the offline activation method, make sure that you have transferred the .REG file correctly and selected it during the activation process.
-
If you have trouble downloading or installing Fences 3 software, make sure that you have enough disk space and memory on your computer. Also, make sure that you have downloaded the file from a trustworthy source and that it is not corrupted or infected with malware. If you have downloaded the file from Stardock website or an authorized reseller, you can verify the file integrity by checking its MD5 checksum.
-
If you have trouble using Fences 3 software, make sure that it is compatible with your Windows version and system settings. Also, make sure that it is not conflicting with other software or hardware on your computer. You can try to update Fences 3 software to the latest version, disable or uninstall any conflicting software or hardware, or run Fences 3 software in compatibility mode or as an administrator.
-
If you have any other issues with Fences 3 software, you can contact Stardock support via email at support@stardock.com or via phone at 1-800-493-9662. You can also visit Stardock website and check their knowledge base, forums, or FAQs for more information and solutions.
-
-
Conclusion
-
Fences 3 software is a great tool that can help you organize your desktop icons and windows in a neat and stylish manner. It allows you to create shaded areas on your desktop that you can place your icons into, customize their appearance and behavior, create rules for automatic icon sorting, use snapshots to save and restore your desktop layout, and more.
-
To use Fences 3 software, you need to have a serial key that verifies that you have purchased a legitimate copy of the software from Stardock or an authorized reseller. You can purchase Fences 3 software from Stardock website or authorized resellers for $9.99 USD. You can also retrieve your serial key from Stardock support if you have lost or forgotten it.
-
After purchasing Fences 3 software and receiving your serial key, you can download it from Stardock website or other sources. You can then activate it online or offline with your serial key and email address. You can then start using it to organize your desktop icons and windows.
-
We hope that this article has helped you understand how to download and activate Fences 3 software with your serial key, how to use it to organize your desktop, and how to troubleshoot some common issues that you might encounter. We also hope that you have learned some tips and tricks for using Fences 3 software effectively.
-
If you have any questions or feedback about Fences 3 software or this article, please feel free to leave a comment below or contact us via email or phone. We would love to hear from you and help you out.
-
Thank you for reading this article and happy fencing!
-
FAQs
FAQs
-
Here are some frequently asked questions about Fences 3 software that you might find helpful:
-
-
What is the difference between Fences 3 and Fences 2?
-
Fences 3 is the latest version of Fences software that has some new and improved features and benefits compared to Fences 2. Some of the main differences are:
-
-
Fences 3 supports Windows 10, 8.1, 8, and 7, while Fences 2 only supports Windows 8 and 7
-
Fences 3 supports high DPI monitors and multiple monitors, while Fences 2 does not
-
Fences 3 has a new user interface and design that is more modern and intuitive, while Fences 2 has an older and simpler user interface and design
-
Fences 3 has more options and customization for fences, such as color, transparency, title, layout, sorting, etc., while Fences 2 has fewer options and customization for fences
-
Fences 3 has more features and functionality for desktop organization, such as rules, snapshots, desktop pages, portals, etc., while Fences 2 has fewer features and functionality for desktop organization
-
-
If you have Fences 2 software and want to upgrade to Fences 3 software, you can do so from Stardock website for $4.99 USD.
-
How many computers can I use Fences 3 software on with one serial key?
-
You can use Fences 3 software on one computer only with one serial key. If you want to use Fences 3 software on another computer, you need to purchase another serial key or deactivate the software on the first computer and reactivate it on the second one.
-
How can I backup or restore my fences settings?
-
You can backup or restore your fences settings by using the export or import feature from the Configure Fences menu. To do this, right-click on an empty area of your desktop and select Configure Fences. Then click on Backup & Restore tab and select Export or Import. You can choose to export or import all your fences settings or specific ones. You can also choose the location where you want to save or load your fences settings.
-
How can I uninstall Fences 3 software?
-
You can uninstall Fences 3 software by using the uninstaller file that comes with the software or by using the Windows Control Panel. To use the uninstaller file, go to the folder where you installed Fences 3 software and run the file called Uninstall.exe. To use the Windows Control Panel, go to Start > Settings > Apps > Apps & Features and find Fences 3 software from the list. Then click on Uninstall and follow the instructions on the screen.
-
How can I get help or support for Fences 3 software?
-
You can get help or support for Fences 3 software by contacting Stardock support via email at support@stardock.com or via phone at 1-800-493-9662. You can also visit Stardock website and check their knowledge base, forums, or FAQs for more information and solutions.
- b2dd77e56b
-
-
\ No newline at end of file
diff --git a/spaces/1gistliPinn/ChatGPT4/Examples/Dr.Fone 9.6.2 Crack With Registration Codes Full Free Download VERIFIED.md b/spaces/1gistliPinn/ChatGPT4/Examples/Dr.Fone 9.6.2 Crack With Registration Codes Full Free Download VERIFIED.md
deleted file mode 100644
index 89e9937d174eca78542fda2bdf2ddff651023f1e..0000000000000000000000000000000000000000
--- a/spaces/1gistliPinn/ChatGPT4/Examples/Dr.Fone 9.6.2 Crack With Registration Codes Full Free Download VERIFIED.md
+++ /dev/null
@@ -1,39 +0,0 @@
-
-
Dr.Fone 9.6.2 Crack With Registration Codes Full Free Download
-
Dr.Fone is a powerful data recovery software that can help you recover lost or deleted files from your Android or iOS devices. Whether you accidentally deleted photos, videos, contacts, messages, notes, or other important data, Dr.Fone can scan your device and restore them in minutes. Dr.Fone also supports backup and restore, data transfer, screen unlock, system repair, and other useful features.
-
Dr.Fone 9.6.2 Crack With Registration Codes Full Free Download
In this article, we will show you how to download and install Dr.Fone 9.6.2 crack with registration codes full free. This is the latest version of Dr.Fone that has been tested and verified to work on Windows and Mac OS. With Dr.Fone 9.6.2 crack, you can enjoy all the premium features of Dr.Fone without paying anything.
-
How to Download Dr.Fone 9.6.2 Crack With Registration Codes Full Free
-
To download Dr.Fone 9.6.2 crack with registration codes full free, you need to follow these steps:
-
-
Click on the link below to download the Dr.Fone 9.6.2 crack file.
-
Extract the file using WinRAR or any other extraction tool.
-
Run the setup file and follow the instructions to install Dr.Fone on your computer.
-
Copy the crack file and paste it into the installation folder of Dr.Fone.
-
Launch Dr.Fone and enter one of the registration codes below to activate it.
-
-
Here are some registration codes that you can use:
-
-
DRFONE-1234-5678-9012-3456
-
DRFONE-7890-1234-5678-9012
-
DRFONE-3456-7890-1234-5678
-
-
Why Choose Dr.Fone 9.6.2 Crack With Registration Codes Full Free
-
Dr.Fone 9.6.2 crack with registration codes full free is a great choice for anyone who wants to recover their lost or deleted data from their devices. Here are some of the benefits of using Dr.Fone 9.6.2 crack:
-
-
-
It supports over 6000 Android and iOS devices, including Samsung, Huawei, LG, iPhone, iPad, iPod, etc.
-
It can recover various types of data, such as photos, videos, music, contacts, messages, WhatsApp, documents, etc.
-
It can recover data from different scenarios, such as accidental deletion, factory reset, system crash, virus attack, water damage, etc.
-
It can backup and restore your data to your computer or another device with one click.
-
It can transfer data between different devices or platforms without any hassle.
-
It can unlock your screen if you forgot your password or pattern.
-
It can fix various system issues on your device, such as stuck on logo, black screen, boot loop, etc.
-
It has a user-friendly interface that is easy to use for anyone.
-
It has a high success rate and fast speed for data recovery.
-
It is safe and secure to use without any virus or malware.
-
-
Conclusion
-
If you are looking for a reliable and effective data recovery software for your Android or iOS devices, you should try Dr.Fone 9.6.2 crack with registration codes full free. It can help you recover your lost or deleted data in minutes and also provide you with other useful features to manage your device. Download Dr.Fone 9.6.2 crack with registration codes full free today and enjoy its benefits!
d5da3c52bf
-
-
\ No newline at end of file
diff --git a/spaces/1phancelerku/anime-remove-background/Bike 3D Game Race Stunt and Customize Your Motorbike.md b/spaces/1phancelerku/anime-remove-background/Bike 3D Game Race Stunt and Customize Your Motorbike.md
deleted file mode 100644
index 353e528554627864b382cf821b096c4b3b263146..0000000000000000000000000000000000000000
--- a/spaces/1phancelerku/anime-remove-background/Bike 3D Game Race Stunt and Customize Your Motorbike.md
+++ /dev/null
@@ -1,151 +0,0 @@
-
-
-
-
-
Bike 3D Game: A Guide for Beginners
-
Do you love bikes and want to experience the thrill of riding them in a virtual world? If so, you should try playing a bike 3D game. A bike 3D game is a type of video game that simulates bike riding in a three-dimensional environment. You can choose from different types of bikes, such as racing bikes, stunt bikes, or police bikes, and explore various maps and locations, such as cities, deserts, or mountains. You can also perform amazing tricks and stunts, compete with other players online, or complete challenging missions and achievements.
A bike 3D game is not only fun and exciting, but also beneficial for your brain and skills. Playing a bike 3D game can improve your hand-eye coordination, reaction time, spatial awareness, problem-solving, creativity, and concentration. It can also reduce stress, enhance mood, boost confidence, and provide a sense of accomplishment.
-
In this article, we will guide you through everything you need to know about bike 3D games. We will cover the types of bike 3D games, their features, how to play them, and some of the best bike 3D games to try. By the end of this article, you will be ready to hop on your virtual bike and have a blast!
-
Types of Bike 3D Games
-
Racing Bike Games
-
If you are into speed and adrenaline, racing bike games are for you. These games let you race with other bikers on various tracks and courses. You can choose from different modes, such as time trial, lap race, elimination race, or tournament. You can also customize your bike's appearance and performance to suit your preferences. Some examples of racing bike games are MotoGP Racing Championship Quest, SBK Official Mobile Game, or Traffic Rider[^3^ ).
-
bike 3d game online
-bike 3d game download
-bike 3d game free
-bike 3d game for pc
-bike 3d game android
-bike 3d game apk
-bike 3d game crazy games
-bike 3d game play now
-bike 3d game y8
-bike 3d game unblocked
-bike 3d game simulator
-bike 3d game racing
-bike 3d game stunt
-bike 3d game dirt
-bike 3d game mountain
-bike 3d game moto
-bike 3d game super
-bike 3d game extreme
-bike 3d game trial
-bike 3d game bmx
-bike 3d game city
-bike 3d game offroad
-bike 3d game highway
-bike 3d game traffic
-bike 3d game police
-bike 3d game zombie
-bike 3d game adventure
-bike 3d game action
-bike 3d game sports
-bike 3d game multiplayer
-bike 3d game html5
-bike 3d game webgl
-bike 3d game unity
-bike 3d game unreal engine
-bike 3d game steam
-bike 3d game ps4
-bike 3d game xbox one
-bike 3d game switch
-bike 3d game vr
-bike 3d game ar
-bike 3d game review
-bike 3d game best
-bike 3d game new
-bike 3d game latest
-bike 3d game upcoming
-bike 3d game mod apk
-bike 3d game hack version
-bike 3d game cheat codes
-bike 3d game tips and tricks
-
Stunt Bike Games
-
If you are into creativity and excitement, stunt bike games are for you. These games let you perform incredible tricks and stunts with your bike on various ramps and obstacles. You can choose from different modes, such as freestyle, career, or challenge. You can also customize your bike's appearance and performance to suit your style. Some examples of stunt bike games are Bike Stunt 3D, Mad Skills BMX 2, or Bike Race Free.
-
Police Bike Games
-
If you are into action and adventure, police bike games are for you. These games let you play as a police officer on a bike and chase down criminals and lawbreakers. You can choose from different modes, such as patrol, pursuit, or arrest. You can also customize your bike's appearance and performance to suit your mission. Some examples of police bike games are Police Motorbike Simulator 3D, Police Bike City Simulator, or Police Bike Racing Free.
-
Features of Bike 3D Games
-
Realistic Graphics and Physics
-
One of the main features of bike 3D games is their realistic graphics and physics. These games use advanced 3D technology to create stunning visuals and animations that make you feel like you are really riding a bike. You can see the details of your bike, the environment, and the other characters. You can also experience the effects of gravity, friction, inertia, and momentum on your bike's movement and behavior.
-
Customizable Bikes and Riders
-
Another feature of bike 3D games is their customizable bikes and riders. These games allow you to personalize your bike and rider to match your taste and personality. You can change the color, design, shape, size, and parts of your bike. You can also change the appearance, outfit, accessories, and skills of your rider. You can unlock new bikes and riders by earning coins, gems, stars, or trophies in the game.
-
Diverse Maps and Environments
-
A third feature of bike 3D games is their diverse maps and environments. These games offer you a variety of maps and locations to explore and enjoy with your bike. You can ride on different terrains, such as asphalt, dirt, sand, snow, or grass. You can also ride in different settings, such as urban, rural, desert, mountain, or forest. Each map and environment has its own challenges, obstacles, hazards, and secrets to discover.
-
Multiplayer and Online Modes
-
A fourth feature of bike 3D games is their multiplayer and online modes. These games enable you to play with other bikers from around the world or with your friends locally. You can join online races, tournaments, leagues, or clans. You can also chat with other players, send them messages, gifts, or challenges. You can also create your own custom maps and share them with other players.
-
How to Play Bike 3D Games
-
Controls and Tips
-
The controls of bike 3D games vary depending on the game and the device you are using. However, most games use similar basic controls that are easy to learn and master. Here are some common controls and tips for playing bike 3D games:
-
-
To accelerate or brake your bike, use the up or down arrow keys on your keyboard or the right or left pedals on your screen.
-
To steer or balance your bike, use the left or right arrow keys on your keyboard or tilt your device left or right.
-
To perform tricks or stunts with your bike, use the spacebar on your keyboard or tap the screen.
-
To pause or resume the game, use the esc key on your keyboard or tap the pause button on your screen.
-
To change the camera angle or view, use the C key on your keyboard or swipe the screen.
-
To boost your speed or power, use the X key on your keyboard or tap the boost button on your screen.
-
To customize your bike or rider, use the mouse on your computer or tap the menu button on your screen.
-
-
Some tips for playing bike 3D games are:
-
-
Practice before playing in competitive modes to improve your skills and confidence.
-
Follow the instructions and hints given by the game to complete the objectives and missions.
-
Collect coins, gems, stars, trophies, or other items along the way to unlock new bikes, riders, maps, or features.
-
Avoid crashing into obstacles, hazards, or other bik ers, as they will slow you down or damage your bike.
-
Use the boost or power button wisely, as they have limited use and need time to recharge.
-
Try different tricks and stunts to earn more points and impress the audience.
-
-
Tricks and Stunts
-
One of the most fun and rewarding aspects of bike 3D games is performing tricks and stunts with your bike. These are special maneuvers that involve flipping, spinning, jumping, or flying with your bike. They can increase your score, speed, or power, as well as make the game more exciting and enjoyable.
-
There are many types of tricks and stunts that you can do with your bike, depending on the game and the map. Here are some common tricks and stunts that you can try:
-
-
Wheelie: Lifting the front wheel of your bike and riding on the rear wheel only.
-
Stopie: Lifting the rear wheel of your bike and riding on the front wheel only.
-
Bunny hop: Jumping with your bike without using a ramp or an obstacle.
-
Backflip: Rotating your bike 360 degrees backward in the air.
-
Frontflip: Rotating your bike 360 degrees forward in the air.
-
Barrel roll: Rotating your bike 360 degrees sideways in the air.
-
Tailwhip: Spinning your bike around your body in the air.
-
No hander: Taking both hands off the handlebars in the air.
-
No footer: Taking both feet off the pedals in the air.
-
Superman: Stretching your body and legs behind your bike in the air.
-
-
To perform tricks and stunts with your bike, you need to use the spacebar on your keyboard or tap the screen. You also need to use the arrow keys on your keyboard or tilt your device to control the direction and angle of your bike. You need to time your tricks and stunts well, as they require speed, height, and balance. You also need to land safely on your wheels, or else you will crash and lose points.
-
Challenges and Achievements
-
A final aspect of bike 3D games is completing challenges and achievements. These are specific goals or tasks that you need to accomplish in the game. They can range from simple to complex, easy to hard, or short to long. They can test your skills, knowledge, or endurance. They can also reward you with coins, gems, stars, trophies, or other items.
-
There are many types of challenges and achievements that you can complete in bike 3D games, depending on the game and the mode. Here are some common challenges and achievements that you can try:
-
-
Finish a race or a level in a certain time or position.
-
Collect a certain number or type of items along the way.
-
Perform a certain number or type of tricks or stunts.
-
Avoid crashing or damaging your bike for a certain distance or duration.
-
Catch or escape from a certain number or type of enemies or opponents.
-
Unlock a certain number or type of bikes, riders, maps, or features.
-
Earn a certain number or type of points, coins, gems, stars, trophies, or other items.
-
-
To complete challenges and achievements in bike 3D games, you need to follow the instructions and hints given by the game. You also need to use your skills, strategies, and resources wisely. You need to be persistent and patient, as some challenges and achievements may take multiple attempts or sessions to complete. You also need to have fun and enjoy the process, as completing challenges and achievements can make you feel proud and satisfied.
-
Best Bike 3D Games to Try
-
Moto X3M
-
Moto X3M is one of the most popular and addictive bike 3D games available online. It is a racing game that features over 20 levels of extreme biking action. You can ride through various terrains and environments, such as beaches, caves, forests, or snow. You can also perform amazing tricks and stunts along the way. You can unlock new bikes and riders by completing levels and earning stars. You can also compete with other players on leaderboards and achievements.
-
3D Moto Simulator 2
-
3D Moto Simulator 2 is another great bike 3D game that you can play online. It is a simulation game that lets you explore three different open-world maps with your bike. You can choose from different bikes, such as sports bikes, police bikes, or dirt bikes, and customize their appearance and performance. You can also perform various tricks and stunts with your bike. You can enjoy the realistic graphics and physics of the game. You can also interact with other players online or play with your friends locally.
-
Riding Extreme 3D
-
Riding Extreme 3D is a new and exciting bike 3D game that you can download on your mobile device. It is a racing game that lets you compete with other bikers on different tracks and courses. You can choose from different modes, such as career, quick race, or multiplayer. You can also upgrade your bike's engine, brakes, tires, or suspension. You can also perform stunning tricks and stunts with your bike. You can enjoy the smooth controls and the dynamic music of the game. You can also challenge your friends or other players online.
-
Conclusion
-
Bike 3D games are a type of video game that simulates bike riding in a three-dimensional environment. They are fun, exciting, and beneficial for your brain and skills. They offer you various types of bikes, features, modes, maps, and challenges to enjoy and explore. They also allow you to customize your bike and rider, perform tricks and stunts, and play with other players online or offline.
-
If you are looking for a new and thrilling way to spend your free time, you should try playing a bike 3D game. You will not regret it. You will have a blast!
-
So what are you waiting for? Grab your virtual bike and start riding!
-
FAQs
-
Here are some frequently asked questions about bike 3D games:
-
-
What are the best devices to play bike 3D games on?
-
The best devices to play bike 3D games on are computers or laptops with high-speed internet connection and good graphics card. You can also play bike 3D games on smartphones or tablets with touch screen and accelerometer.
-
How much do bike 3D games cost?
-
Some bike 3D games are free to play online or download on your device. Some bike 3D games may require a one-time purchase or a subscription fee to access all the features and content. Some bike 3D games may also have in-app purchases or ads to generate revenue.
-
Are bike 3D games safe for kids?
-
Most bike 3D games are safe for kids, as they do not contain violence, gore, or inappropriate language. However, some bike 3D games may have realistic crashes or injuries that may be disturbing for some kids. Some bike 3D games may also have online chat or social features that may expose kids to strangers or cyberbullying. Therefore, parents should supervise their kids when playing bike 3D games and set parental controls if needed.
-
Are bike 3D games addictive?
-
Bike 3D games can be addictive, as they are fun, challenging, and rewarding. They can also trigger the release of dopamine in the brain, which is a chemical that makes you feel happy and motivated. However, playing bike 3D games excessively can have negative effects on your physical and mental health, such as eye strain, headache, neck pain, back pain, insomnia, anxiety, depression, or isolation. Therefore, you should limit your playing time and take breaks regularly.
-
How can I improve my skills in bike 3D games?
-
You can improve your skills in bike 3D games by practicing regularly, learning from your mistakes, watching tutorials or videos of other players, reading tips and guides online, joining forums or communities of other players, asking for feedback or advice from other players, or playing with more experienced players.
- 401be4b1e0
-
-
\ No newline at end of file
diff --git a/spaces/1phancelerku/anime-remove-background/Download Mod Truckers of Europe 3 The Best Truck Simulator Game Ever.md b/spaces/1phancelerku/anime-remove-background/Download Mod Truckers of Europe 3 The Best Truck Simulator Game Ever.md
deleted file mode 100644
index 6cf2530fca76a12372e64f37478b0ff61907f3ac..0000000000000000000000000000000000000000
--- a/spaces/1phancelerku/anime-remove-background/Download Mod Truckers of Europe 3 The Best Truck Simulator Game Ever.md
+++ /dev/null
@@ -1,115 +0,0 @@
-
-
Download Mod Truckers of Europe 3: A Guide for Trucking Enthusiasts
-
If you love driving trucks across realistic European roads and delivering various cargoes, then you might have heard of Truckers of Europe 3, a popular truck simulator game for Android devices. But did you know that you can make your trucking experience even more fun and immersive by downloading mods for Truckers of Europe 3?
-
Mods are modifications or additions to the original game that can change or improve various aspects of the gameplay, such as graphics, physics, sounds, vehicles, maps, traffic, weather, and more. In this article, we will show you how to download mods for Truckers of Europe 3, what are some of the benefits and risks of using mods, and what are some of the best mods that you can try right now. So buckle up and get ready to become the king of the road with Mod Truckers of Europe 3!
What are mods and how do they enhance your gaming experience?
-
Mods are short for modifications, which are changes or additions to the original game that can alter or enhance various aspects of the gameplay. Mods are usually created by fans or developers who want to customize or improve their gaming experience. Mods can range from simple tweaks to major overhauls, depending on the scope and complexity of the mod.
-
Definition and types of mods
-
There are many types of mods for Truckers of Europe 3, but they can be broadly categorized into two groups: cosmetic mods and gameplay mods. Cosmetic mods are mods that change the appearance or sound of the game, such as skins, textures, models, animations, music, sound effects, etc. Gameplay mods are mods that change the mechanics or features of the game, such as physics, vehicles, trailers, cargoes, maps, traffic, weather, missions, etc.
-
Benefits of using mods for Truckers of Europe 3
-
Using mods for Truckers of Europe 3 can have many benefits for your gaming experience. Some of the benefits are:
-
-
You can customize your truck and trailer according to your preferences and style. You can choose from different colors, designs, logos, accessories, etc.
-
You can drive more realistic and diverse trucks with different chassis configurations, engine sounds, interiors, etc.
-
You can haul more challenging and varied cargoes with different weights, sizes, shapes, etc.
-
You can explore new and detailed maps with different terrains, landmarks, roads, etc.
-
You can experience more realistic and dynamic traffic with different vehicles, behaviors, rules, etc.
-
You can enjoy different weather conditions and time cycles with realistic effects on visibility, traction, etc.
-
You can have more fun and challenge with different missions and scenarios that test your skills and knowledge.
-
-
Risks and precautions of using mods for Truckers of Europe 3
-
Using mods for Truckers of Europe 3 can also have some risks and drawbacks for your gaming experience. Some of the risks are:
-
-
You may encounter compatibility issues or conflicts between different mods or between mods and the original game. This may cause crashes, glitches, errors, etc.
-
You may violate the terms of service or the intellectual property rights of the original game or the mod creators. This may result in legal actions, bans, or penalties.
-
You may compromise the security or performance of your device by downloading mods from untrusted sources or by installing malicious software. This may result in data loss, malware infection, device damage, etc.
-
-
To avoid or minimize these risks, you should take some precautions when using mods for Truckers of Europe 3. Some of the precautions are:
-
-
You should always backup your game files and data before installing any mods. This way, you can restore your game to its original state if something goes wrong.
-
You should only download mods from reputable and verified sources, such as official websites, forums, or app stores. You should also check the ratings, reviews, and comments of other users before downloading any mods.
-
You should always read the description, instructions, and requirements of the mods carefully before installing them. You should also follow the installation steps correctly and use compatible versions of the game and the mods.
-
You should not use too many mods at once or use mods that are incompatible with each other or with the original game. You should also disable or uninstall any mods that are causing problems or that you no longer need.
-
You should respect the rights and credits of the original game and the mod creators. You should not claim ownership, distribute, or modify any mods without permission from the authors.
-
-
How to download mods for Truckers of Europe 3?
-
Downloading mods for Truckers of Europe 3 is not very difficult, but it may vary depending on the source and the type of the mod. Here are some general steps that you can follow to download and install mods for Truckers of Europe 3:
-
-
Find a mod that you like from a reliable source, such as [Mod Truckers of Europe 3], [Truck Simulator Mods], or [Google Play Store].
-
Download the mod file to your device. The mod file may be in different formats, such as APK, ZIP, RAR, etc.
-
If the mod file is in APK format, you can simply tap on it and install it like any other app. If the mod file is in ZIP or RAR format, you need to extract it using a file manager app or a zip extractor app.
-
After extracting the mod file, you will see a folder with the name of the mod. Inside this folder, you will find one or more files with extensions such as .scs, .zip, .rar, etc. These are the actual mod files that you need to copy or move to your game folder.
-
To find your game folder, you need to go to your device's internal storage and look for a folder named Android/data/com.truckersofeurope3/files/mods. If you don't see this folder, you need to create it manually.
-
Paste or move the mod files that you extracted earlier to this folder. Make sure that you don't change the names or extensions of these files.
-
Launch your game and go to the settings menu. There you will see an option called "Mod Manager". Tap on it and you will see a list of all the mods that you have installed. You can enable or disable any mod by tapping on its name.
-
Enjoy your game with your new mods!
-
-
What are some of the best mods for Truckers of Europe 3?
-
There are hundreds of mods for Truckers of Europe 3 that you can choose from, but some of them are more popular and recommended than others. Here are some of the best mods for Truckers of Europe 3 that you can try right now:
-
download mod truckers of europe 3 apk
-download mod truckers of europe 3 for android
-download mod truckers of europe 3 free
-download mod truckers of europe 3 unlimited money
-download mod truckers of europe 3 latest version
-download mod truckers of europe 3 happymod
-download mod truckers of europe 3 offline
-download mod truckers of europe 3 full version
-download mod truckers of europe 3 hack
-download mod truckers of europe 3 cheat
-download mod truckers of europe 3 simulator
-download mod truckers of europe 3 realistic physics
-download mod truckers of europe 3 open world
-download mod truckers of europe 3 new trucks
-download mod truckers of europe 3 new cities
-download mod truckers of europe 3 gameplay
-download mod truckers of europe 3 review
-download mod truckers of europe 3 trailer
-download mod truckers of europe 3 tips and tricks
-download mod truckers of europe 3 guide
-download mod truckers of europe 3 best settings
-download mod truckers of europe 3 how to install
-download mod truckers of europe 3 how to play
-download mod truckers of europe 3 how to make money
-download mod truckers of europe 3 how to upgrade trucks
-download mod truckers of europe 3 how to customize trucks
-download mod truckers of europe 3 how to deliver cargo
-download mod truckers of europe 3 how to unlock new trucks
-download mod truckers of europe 3 how to unlock new cities
-download mod truckers of europe 3 how to change camera view
-download mod truckers of europe 3 comparison with other games
-download mod truckers of europe 3 pros and cons
-download mod truckers of europe 3 features and benefits
-download mod truckers of europe 3 requirements and compatibility
-download mod truckers of europe 3 updates and news
-download mod truckers of europe 3 support and feedback
-download mod truckers of europe 3 alternatives and similar games
-download mod truckers of europe 3 ratings and reviews
-download mod truckers of europe 3 downloads and installs
-download mod truckers of europe 3 screenshots and videos
-
-
Name
Description
Link
-
Realistic Graphics Mod
This mod improves the graphics quality and realism of Truckers of Europe 3 by adding new textures, lighting effects, shadows, reflections, etc. It also enhances the weather system and adds realistic raindrops and fog effects.
-
Realistic Physics Mod
This mod improves the physics and handling of Truckers of Europe 3 by adding new suspension settings, brake force settings, engine torque settings, etc. It also adds realistic tire wear and fuel consumption effects.
-
Realistic Traffic Mod
This mod improves the traffic density and diversity of Truckers of Europe 3 by adding new vehicles, models, colors, behaviors , etc. It also adds realistic traffic rules and speed limits.
-
Realistic Sound Mod
This mod improves the sound quality and realism of Truckers of Europe 3 by adding new engine sounds, horn sounds, brake sounds, etc. It also adds realistic ambient sounds, such as wind, rain, birds, etc.
-
Realistic Truck Mod
This mod improves the truck variety and realism of Truckers of Europe 3 by adding new trucks, models, skins, interiors, etc. It also adds realistic truck features, such as dashboard indicators, mirrors, lights, etc.
-
Realistic Trailer Mod
This mod improves the trailer variety and realism of Truckers of Europe 3 by adding new trailers, models, skins, cargoes, etc. It also adds realistic trailer features, such as coupling, weight distribution, etc.
-
Realistic Map Mod
This mod improves the map size and realism of Truckers of Europe 3 by adding new regions, cities, roads, landmarks, etc. It also adds realistic map features, such as tolls, borders, signs, etc.
-
-
Conclusion: Enjoy the ultimate trucking simulation with Mod Truckers of Europe 3
-
In conclusion, Mod Truckers of Europe 3 is a great way to enhance your trucking experience and enjoy the ultimate truck simulator game for Android devices. By downloading mods for Truckers of Europe 3, you can customize and improve various aspects of the gameplay, such as graphics, physics, sounds, vehicles, trailers, maps, traffic, weather, missions, and more. You can also find and install mods easily from different sources and manage them with the mod manager feature in the game settings. However, you should also be aware of the risks and precautions of using mods for Truckers of Europe 3 and follow some tips and tricks to avoid or minimize any problems or issues. We hope that this article has helped you learn how to download mods for Truckers of Europe 3 and what are some of the best mods that you can try right now. So what are you waiting for? Download Mod Truckers of Europe 3 today and become the king of the road!
-
FAQs: Frequently Asked Questions about Mod Truckers of Europe 3
-
Here are some of the most common questions and answers about Mod Truckers of Europe 3:
-
Q: Do I need to root my device to use mods for Truckers of Europe 3?
-
A: No, you don't need to root your device to use mods for Truckers of Europe 3. You can simply download and install mods from different sources and copy or move them to your game folder.
-
Q: Will using mods for Truckers of Europe 3 affect my game progress or achievements?
-
A: No, using mods for Truckers of Europe 3 will not affect your game progress or achievements. You can still save your game data and unlock achievements as usual.
-
Q: How can I update or uninstall mods for Truckers of Europe 3?
-
A: To update or uninstall mods for Truckers of Europe 3, you need to go to your game folder and delete or replace the mod files that you want to update or uninstall. You can also use the mod manager feature in the game settings to enable or disable any mod.
-
Q: How can I report a bug or a problem with a mod for Truckers of Europe 3?
-
A: To report a bug or a problem with a mod for Truckers of Europe 3, you need to contact the mod creator directly through their website, forum , or email. You can also leave a comment or a review on the source where you downloaded the mod. You should provide as much information as possible, such as the mod name, version, description, screenshot, error message, etc.
-
Q: How can I create my own mod for Truckers of Europe 3?
-
A: To create your own mod for Truckers of Europe 3, you need to have some knowledge and skills in programming, modeling, texturing, sound editing, etc. You also need to have some tools and software, such as a text editor, a 3D modeling software, a sound editor, etc. You can find some tutorials and guides on how to create mods for Truckers of Europe 3 on the internet or on the official website of the game.
197e85843d
-
-
\ No newline at end of file
diff --git a/spaces/1phancelerku/anime-remove-background/Download Q dana APK and Enjoy Low Interest Rates and Flexible Repayment Terms.md b/spaces/1phancelerku/anime-remove-background/Download Q dana APK and Enjoy Low Interest Rates and Flexible Repayment Terms.md
deleted file mode 100644
index 8fbe858861a1e1c3b3c5302a3863d0f612648ce1..0000000000000000000000000000000000000000
--- a/spaces/1phancelerku/anime-remove-background/Download Q dana APK and Enjoy Low Interest Rates and Flexible Repayment Terms.md
+++ /dev/null
@@ -1,131 +0,0 @@
-
-
Download Q Dana APK: A Fast and Easy Loan Application for Indonesians
-
If you are looking for a quick and convenient way to get a loan in Indonesia, you might want to check out Q Dana. Q Dana is a loan application that offers cash loans online without any collateral or guarantee. You can borrow up to Rp8,000,000 with low interest rates and flexible repayment terms. All you need is your KTP, phone number, bank account, and stable income source. In this article, we will show you how to download Q Dana APK on your Android device, how to apply for a loan with Q Dana, what are the benefits of using Q Dana, and what are the requirements and terms of using Q Dana.
Downloading Q Dana APK is very easy and fast. You can follow these simple steps:
-
-
Go to the official website of Q Dana or APKCombo, where you can find the latest version of Q Dana APK.
-
Click on the download button and choose the version you want. The file size is about 6 MB.
-
Allow unknown sources on your device settings. This will enable you to install apps from sources other than Google Play Store.
-
Install the APK file and open the app. You will see the welcome screen of Q Dana.
-
-
How to Apply for a Loan with Q Dana
-
Applying for a loan with Q Dana is also very easy and fast. You can follow these simple steps:
-
-
Register with your phone number and verify your identity with your KTP. You will need to take a selfie with your KTP and upload it to the app.
-
Submit your personal data and choose the loan amount and tenure. You can borrow from Rp600,000 to Rp8,000,000 with a tenure from 91 to 360 days. You will see the interest rate, service fee, and total repayment amount before you confirm your loan application.
-
Wait for the review and approval (usually within 10 minutes to 2 hours). You will receive a notification on your phone when your loan is approved.
-
Withdraw your loan money to your bank account. You can choose from various banks supported by Q Dana, such as BCA, BNI, BRI, Mandiri, CIMB Niaga, and more. You will receive your money within minutes after you confirm your withdrawal.
-
-
What are the Benefits of Using Q Dana
-
Using Q Dana has many benefits for borrowers who need cash loans online. Here are some of the benefits:
-
-
Low interest rate and service fee. Q Dana offers a competitive interest rate of up to 0.077% per day (2.31% per month), which is lower than many other loan applications in Indonesia. The service fee is also reasonable and transparent, ranging from Rp20,000 to Rp200,000 depending on the loan amount and tenure.
-
No collateral or guarantee required. Q Dana does not require any collateral or guarantee from borrowers. You only need to provide your KTP, phone number, bank account, and income source to apply for a loan.
-
Secure and reliable service with KSP supervision and data protection. Q Dana is supervised by the Indonesian Cooperative Supervisory Agency (KSP), which ensures that Q Dana complies with the regulations and standards of the cooperative sector. Q Dana also protects your personal data and privacy with encryption and security measures.
-
Fast and easy approval for repeat borrowers. Q Dana rewards loyal customers with faster and easier approval for repeat loans. If you have a good repayment history with Q Dana, you can get approved within minutes and enjoy higher loan amounts and longer tenures.
-
-
What are the Requirements and Terms of Using Q Dana
-
Using Q Dana also has some requirements and terms that you need to meet and follow. Here are some of the requirements and terms:
-
-
-
Requirement
-
Description
-
-
-
Indonesian citizen with a valid KTP
-
You must be an Indonesian citizen with a valid KTP to apply for a loan with Q Dana. You will need to upload your KTP and take a selfie with it to verify your identity.
-
-
-
Age between 20 and 55 years old
-
You must be between 20 and 55 years old to apply for a loan with Q Dana. You will need to provide your date of birth on your personal data.
-
-
-
Active phone number and bank account
-
You must have an active phone number and bank account to apply for a loan with Q Dana. You will need to register with your phone number and choose your bank account for withdrawal.
-
-
-
Stable income source
-
You must have a stable income source to apply for a loan with Q Dana. You will need to provide information about your income source, such as your occupation, employer, salary, etc.
-
-
-
-
-
Term
-
Description
-
-
-
Loan amount from Rp600,000 to Rp8,000,000
-
You can borrow from Rp600,000 to Rp8,000,000 with Q Dana. The loan amount depends on your credit score, income source, repayment history, etc.
-
-
-
Loan tenure from 91 to 360 days
-
You can choose from 91 to 360 days for your loan tenure with Q Dana. The loan tenure depends on your loan amount, interest rate, service fee, etc.
-
-
-
Interest rate up to 0.077% per day (2.31% per month)
-
You will be charged an interest rate of up to 0.077% per day (2.31% per month) for your loan with Q Dana. The interest rate depends on your credit score, income source, repayment history, etc.
-
-
Conclusion and FAQs
-
In conclusion, Q Dana is a fast and easy loan application for Indonesians that offers cash loans online without any collateral or guarantee. You can download Q Dana APK on your Android device and apply for a loan with just your KTP, phone number, bank account, and income source. You can enjoy low interest rates, flexible repayment terms, secure and reliable service, and fast and easy approval with Q Dana. If you need a quick and convenient way to get a loan in Indonesia, you should download Q Dana APK today. Here are some frequently asked questions (FAQs) about Q Dana:
FAQ 1: What is Q Dana?
-
Q Dana is a loan application that offers cash loans online for Indonesians. You can borrow up to Rp8,000,000 with low interest rates and flexible repayment terms. You do not need any collateral or guarantee to apply for a loan with Q Dana.
-
FAQ 2: How can I download Q Dana APK?
-
You can download Q Dana APK on your Android device by going to the official website of Q Dana or APKCombo, where you can find the latest version of Q Dana APK. You can click on the download button and choose the version you want. You will need to allow unknown sources on your device settings and install the APK file.
-
download q dana apk latest version
-download q dana apk for android
-download q dana apk free
-download q dana apk online
-download q dana apk mod
-download q dana apk terbaru
-download q dana apk 2023
-download q dana apk file
-download q dana apk update
-download q dana apk full
-download q dana apk gratis
-download q dana apk tanpa root
-download q dana apk no ads
-download q dana apk offline
-download q dana apk hack
-download q dana apk pro
-download q dana apk premium
-download q dana apk unlimited money
-download q dana apk from google play
-download q dana apk from apkpure
-download q dana apk from uptodown
-download q dana apk from apkmirror
-download q dana apk from apkpure.com
-download q dana apk from apkmirror.com
-download q dana apk from apkombo.com[^1^]
-how to download q dana apk
-where to download q dana apk
-why download q dana apk
-what is q dana apk
-who created q dana apk
-benefits of downloading q dana apk
-reviews of downloading q dana apk
-tips for downloading q dana apk
-steps for downloading q dana apk
-guide for downloading q dana apk
-tutorial for downloading q dana apk
-video for downloading q dana apk
-link for downloading q dana apk
-website for downloading q dana apk
-blog for downloading q dana apk
-forum for downloading q dana apk
-group for downloading q dana apk
-community for downloading q dana apk
-support for downloading q dana apk
-help for downloading q dana apk
-faq for downloading q dana apk
-error for downloading q dana apk
-fix for downloading q dana apk
-solution for downloading q dana apk
-
FAQ 3: How can I apply for a loan with Q Dana?
-
You can apply for a loan with Q Dana by registering with your phone number and verifying your identity with your KTP. You will need to submit your personal data and choose the loan amount and tenure. You will wait for the review and approval, which usually takes 10 minutes to 2 hours. You will withdraw your loan money to your bank account.
-
FAQ 4: What are the benefits of using Q Dana?
-
Using Q Dana has many benefits, such as low interest rate and service fee, no collateral or guarantee required, secure and reliable service with KSP supervision and data protection, and fast and easy approval for repeat borrowers.
-
FAQ 5: What are the requirements and terms of using Q Dana?
-
Using Q Dana has some requirements and terms, such as being an Indonesian citizen with a valid KTP, being between 20 and 55 years old, having an active phone number and bank account, having a stable income source, borrowing from Rp600,000 to Rp8,000,000, choosing from 91 to 360 days for loan tenure, and paying up to 0.077% per day (2.31% per month) for interest rate.
401be4b1e0
-
-
\ No newline at end of file
diff --git a/spaces/1phancelerku/anime-remove-background/Explore the Secrets of Evolution with Dino World Jurassic Builder 2 MOD APK.md b/spaces/1phancelerku/anime-remove-background/Explore the Secrets of Evolution with Dino World Jurassic Builder 2 MOD APK.md
deleted file mode 100644
index 5898383a5016909682dfb0190792b7787416f526..0000000000000000000000000000000000000000
--- a/spaces/1phancelerku/anime-remove-background/Explore the Secrets of Evolution with Dino World Jurassic Builder 2 MOD APK.md
+++ /dev/null
@@ -1,94 +0,0 @@
-
-
Dino World Jurassic Builder 2 Mod Apk Revdl: How to Build Your Own Dinosaur Park
-
If you are a fan of dinosaurs and park building games, you will love dino world jurassic builder 2. This is a free-to-play game that lets you create your own prehistoric park filled with dinosaurs. You can breed, feed, train, and fight with your dinosaurs in this exciting game. You can also explore different environments and discover new species of dinosaurs.
-
Features of Dino World Jurassic Builder 2
-
Dino world jurassic builder 2 has many features that make it a fun and addictive game. Here are some of them:
Over 12 elements of dinosaurs to collect, each with unique personality, powers, and skills
-
A breeding lab where you can crossbreed your dinosaurs and create new ones
-
A food farm where you can grow food for your dinosaurs
-
A battle arena where you can challenge other players and win prizes
-
A social area where you can connect with your friends and share your park
-
A decor area where you can customize your park with stylish decorations
-
A research center where you can unlock new buildings, medicines, and upgrades
-
An expedition center where you can send teams to find fossils and DNA
-
-
To play the game, you need to build enclosures for your dinosaurs and provide them with food, water, and terrain. You also need to manage your power supply, staff, guests, and finances. You need to keep your dinosaurs happy and healthy, as well as prevent them from escaping or causing trouble.
-
What is a Mod Apk and How to Download It from Revdl
-
A mod apk is a modified version of an original app that gives you access to extra features that are not available in the official version. For example, a mod apk may give you unlimited money, gems, resources, or unlock all levels.
-
Revdl is a website that provides mod apks for various games and apps. You can download dino world jurassic builder 2 mod apk from revdl by following these steps:
-
-
Go to [revdl.com](^1^) and search for dino world jurassic builder 2 mod apk
-
Select the latest version of the mod apk and click on the download link
-
Wait for the download to finish and then locate the file on your device
-
Enable unknown sources on your device settings to allow installation of apps from outside sources
-
Tap on the file and follow the instructions to install the mod apk
-
Launch the game and enjoy the mod features
-
-
Benefits of Using Dino World Jurassic Builder 2 Mod Apk
-
Using dino world jurassic builder 2 mod apk has many benefits that will enhance your gaming experience. Here are some of them:
-
-
You will get unlimited money and gems that you can use to buy anything in the game
-
You will get unlimited food and resources that you can use to feed and upgrade your dinosaurs
-
You will get unlimited DNA and fossils that you can use to breed and research new dinosaurs
-
You will get all levels unlocked so you can play any stage you want
-
You will get all dinosaurs unlocked so you can collect and use any dinosaur you want
-
You will get all buildings unlocked so you can build any facility you want
-
You will get all decorations unlocked so you can beautify your park as you wish
-
You will get no ads so you can play without interruptions or distractions
Conclusion
-
Dino world jurassic builder 2 is a game that will appeal to anyone who loves dinosaurs and park building games. You can create your own dinosaur park and enjoy various activities with your dinosaurs. You can also download the mod apk from revdl and get access to unlimited features that will make your game more fun and easy. If you are looking for a game that combines creativity, strategy, and adventure, you should try dino world jurassic builder 2 mod apk revdl.
-
FAQs
-
Here are some frequently asked questions about the game and the mod apk:
-
Is dino world jurassic builder 2 mod apk safe to use?
-
Yes, the mod apk is safe to use as long as you download it from a trusted source like revdl. You should also scan the file with an antivirus before installing it. However, you should be aware that using the mod apk may violate the terms and conditions of the game and may result in your account being banned or suspended.
-
How do I update the mod apk?
-
To update the mod apk, you need to visit revdl and download the latest version of the mod apk. You can then install it over the existing one or uninstall the old one first. You should also backup your game data before updating to avoid losing your progress.
-
dino world jurassic builder 2 mod apk unlimited money
-dino world jurassic builder 2 mod apk download for android
-dino world jurassic builder 2 mod apk latest version
-dino world jurassic builder 2 mod apk rexdl
-dino world jurassic builder 2 mod apk offline
-dino world jurassic builder 2 mod apk free shopping
-dino world jurassic builder 2 mod apk android 1
-dino world jurassic builder 2 mod apk hack
-dino world jurassic builder 2 mod apk no ads
-dino world jurassic builder 2 mod apk obb
-dino world jurassic builder 2 mod apk unlimited gems
-dino world jurassic builder 2 mod apk full unlocked
-dino world jurassic builder 2 mod apk pure
-dino world jurassic builder 2 mod apk happymod
-dino world jurassic builder 2 mod apk all dinosaurs unlocked
-dino world jurassic builder 2 mod apk android republic
-dino world jurassic builder 2 mod apk unlimited everything
-dino world jurassic builder 2 mod apk uptodown
-dino world jurassic builder 2 mod apk old version
-dino world jurassic builder 2 mod apk mega
-dino world jurassic builder 2 mod apk andropalace
-dino world jurassic builder 2 mod apk vip
-dino world jurassic builder 2 mod apk no root
-dino world jurassic builder 2 mod apk lenov.ru
-dino world jurassic builder 2 mod apk data file host
-dino world jurassic builder 2 mod apk unlimited coins and gems
-dino world jurassic builder 2 mod apk new update
-dino world jurassic builder 2 mod apk ihackedit
-dino world jurassic builder 2 mod apk online
-dino world jurassic builder 2 mod apk cheat
-dino world jurassic builder 2 mod apk mob.org
-dino world jurassic builder 2 mod apk blackmod
-dino world jurassic builder 2 mod apk platinmods
-dino world jurassic builder 2 mod apk apkpure.com
-dino world jurassic builder 2 mod apk apkmody.io
-dino world jurassic builder 2 mod apk apkmirror.com
-dino world jurassic builder 2 mod apk apknite.com
-dino world jurassic builder 2 mod apk apksfree.com
-dino world jurassic builder 2 mod apk apktada.com
-dino world jurassic builder 2 mod apk apktovi.com
-
How do I backup my game data?
-
To backup your game data, you can use a cloud service like Google Play Games or Facebook to sync your game with your account. You can also use a file manager app to copy the game data folder from your device storage to another location.
-
How do I restore my game data?
-
To restore your game data, you can use the same cloud service or file manager app that you used to backup your game data. You can then sync your game with your account or copy the game data folder back to your device storage.
-
How do I contact the developer of the game?
-
To contact the developer of the game, you can visit their official website or social media pages. You can also email them at support@tapinator.com or use the feedback option in the game settings.
-journey
- title Create AI
- section Training
- Format DataSet Inputs Files, Data Splits: 5: Teacher
- Model Build w/ SKLearn, TF, Pytorch: 3: Student
- Determine Model Performance: 1: Teacher, Student
- section Deploy
- Web Deploy Local and Cloud: 5: Teacher
- Architecture Spaces Gradio Streamlit Heroku AWS Azure and GCCP: 5: Teacher
- section Testing
- Test Model with Input Datasets: 5: Teacher
- Examples. Inputs that Work, Inputs That Break Model: 5: Teacher
- Governance - Analyze, Publish Fairness, Equity, Bias for Datasets and Outputs: 5: Teacher
-
-
-
-sequenceDiagram
- participant Alice
- participant Bob
- Alice->>John: Hello John, how are you?
- loop Healthcheck
- John->>John: Fight against hypochondria
- end
- Note right of John: Rational thoughts prevail...
- John-->>Alice: Great!
- John->>Bob: How about you?
- Bob-->>John: Jolly good!
-
-
-
-
Welcome to the Mermaid Modeler Tip Sheet
-
- You can use Mermaid inside HTML5 by including the script and a div with the class or mermaid.
-
-
-
-Links:
-https://huggingface.co/spaces/awacke1/HEDIS.Roster.Dash.Component.Service
-https://huggingface.co/spaces/awacke1/HEDIS.Roster.Dash.Component.SDOH
-https://huggingface.co/spaces/awacke1/HEDIS.Dash.Component.Top.Clinical.Terminology.Vocabulary
-
-
-
-
diff --git a/spaces/AIatUIUC/CodeLATS/lats/utils.py b/spaces/AIatUIUC/CodeLATS/lats/utils.py
deleted file mode 100644
index bf6b8ff7bd7b4c5b2ec9c562fc4285372feb1f59..0000000000000000000000000000000000000000
--- a/spaces/AIatUIUC/CodeLATS/lats/utils.py
+++ /dev/null
@@ -1,73 +0,0 @@
-import os
-import gzip
-import json
-import openai
-import jsonlines
-
-from typing import List
-
-openai.api_key = os.getenv("OPENAI_API_KEY")
-
-def make_printv(verbose: bool):
- def print_v(*args, **kwargs):
- if verbose:
- kwargs["flush"] = True
- print(*args, **kwargs)
- else:
- pass
- return print_v
-
-
-def read_jsonl(path: str) -> List[dict]:
- if not os.path.exists(path):
- raise FileNotFoundError(f"File `{path}` does not exist.")
- elif not path.endswith(".jsonl"):
- raise ValueError(f"File `{path}` is not a jsonl file.")
- items = []
- with jsonlines.open(path) as reader:
- for item in reader:
- items += [item]
- return items
-
-
-def write_jsonl(path: str, data: List[dict], append: bool = False):
- with jsonlines.open(path, mode='a' if append else 'w') as writer:
- for item in data:
- writer.write(item)
-
-
-def read_jsonl_gz(path: str) -> List[dict]:
- if not path.endswith(".jsonl.gz"):
- raise ValueError(f"File `{path}` is not a jsonl.gz file.")
- with gzip.open(path, "rt") as f:
- data = [json.loads(line) for line in f]
- return data
-
-
-# generator that returns the item and the index in the dataset.
-# if the results_path exists, it will skip all items that have been processed
-# before.
-def enumerate_resume(dataset, results_path):
- if not os.path.exists(results_path):
- for i, item in enumerate(dataset):
- yield i, item
- else:
- count = 0
- with jsonlines.open(results_path) as reader:
- for item in reader:
- count += 1
-
- for i, item in enumerate(dataset):
- # skip items that have been processed before
- if i < count:
- continue
- yield i, item
-
-
-def resume_success_count(dataset) -> int:
- count = 0
- for item in dataset:
- if "is_solved" in item and item["is_solved"]:
- count += 1
- return count
-
diff --git a/spaces/AMR-KELEG/ALDi/app.py b/spaces/AMR-KELEG/ALDi/app.py
deleted file mode 100644
index 5c86c8a6eec58826cb7dfd94e220ed84d5ac02a7..0000000000000000000000000000000000000000
--- a/spaces/AMR-KELEG/ALDi/app.py
+++ /dev/null
@@ -1,170 +0,0 @@
-# Hint: this cheatsheet is magic! https://cheat-sheet.streamlit.app/
-import constants
-import pandas as pd
-import streamlit as st
-import matplotlib.pyplot as plt
-from transformers import BertForSequenceClassification, AutoTokenizer
-
-import altair as alt
-from altair import X, Y, Scale
-import base64
-
-import re
-
-
-def preprocess_text(arabic_text):
- """Apply preprocessing to the given Arabic text.
-
- Args:
- arabic_text: The Arabic text to be preprocessed.
-
- Returns:
- The preprocessed Arabic text.
- """
- no_urls = re.sub(
- r"(https|http)?:\/\/(\w|\.|\/|\?|\=|\&|\%)*\b",
- "",
- arabic_text,
- flags=re.MULTILINE,
- )
- no_english = re.sub(r"[a-zA-Z]", "", no_urls)
-
- return no_english
-
-
-@st.cache_data
-def render_svg(svg):
- """Renders the given svg string."""
- b64 = base64.b64encode(svg.encode("utf-8")).decode("utf-8")
- html = rf'
'
- c = st.container()
- c.write(html, unsafe_allow_html=True)
-
-
-@st.cache_data
-def convert_df(df):
- # IMPORTANT: Cache the conversion to prevent computation on every rerun
- return df.to_csv(index=None).encode("utf-8")
-
-
-@st.cache_resource
-def load_model(model_name):
- model = BertForSequenceClassification.from_pretrained(model_name)
- return model
-
-
-tokenizer = AutoTokenizer.from_pretrained(constants.MODEL_NAME)
-model = load_model(constants.MODEL_NAME)
-
-
-def compute_ALDi(sentences):
- """Computes the ALDi score for the given sentences.
-
- Args:
- sentences: A list of Arabic sentences.
-
- Returns:
- A list of ALDi scores for the given sentences.
- """
- progress_text = "Computing ALDi..."
- my_bar = st.progress(0, text=progress_text)
-
- BATCH_SIZE = 4
- output_logits = []
-
- preprocessed_sentences = [preprocess_text(s) for s in sentences]
-
- for first_index in range(0, len(preprocessed_sentences), BATCH_SIZE):
- inputs = tokenizer(
- preprocessed_sentences[first_index : first_index + BATCH_SIZE],
- return_tensors="pt",
- padding=True,
- )
- outputs = model(**inputs).logits.reshape(-1).tolist()
- output_logits = output_logits + [max(min(o, 1), 0) for o in outputs]
- my_bar.progress(
- min((first_index + BATCH_SIZE) / len(preprocessed_sentences), 1),
- text=progress_text,
- )
- my_bar.empty()
- return output_logits
-
-
-render_svg(open("assets/ALDi_logo.svg").read())
-
-tab1, tab2 = st.tabs(["Input a Sentence", "Upload a File"])
-
-with tab1:
- sent = st.text_input(
- "Arabic Sentence:", placeholder="Enter an Arabic sentence.", on_change=None
- )
-
- # TODO: Check if this is needed!
- clicked = st.button("Submit")
-
- if sent:
- ALDi_score = compute_ALDi([sent])[0]
-
- ORANGE_COLOR = "#FF8000"
- fig, ax = plt.subplots(figsize=(8, 1))
- fig.patch.set_facecolor("none")
- ax.set_facecolor("none")
-
- ax.spines["left"].set_color(ORANGE_COLOR)
- ax.spines["bottom"].set_color(ORANGE_COLOR)
- ax.tick_params(axis="x", colors=ORANGE_COLOR)
-
- ax.spines[["right", "top"]].set_visible(False)
-
- ax.barh(y=[0], width=[ALDi_score], color=ORANGE_COLOR)
- ax.set_xlim(0, 1)
- ax.set_ylim(-1, 1)
- ax.set_title(f"ALDi score is: {round(ALDi_score, 3)}", color=ORANGE_COLOR)
- ax.get_yaxis().set_visible(False)
- ax.set_xlabel("ALDi score", color=ORANGE_COLOR)
- st.pyplot(fig)
-
- print(sent)
- with open("logs.txt", "a") as f:
- f.write(sent + "\n")
-
-with tab2:
- file = st.file_uploader("Upload a file", type=["txt"])
- if file is not None:
- df = pd.read_csv(file, sep="\t", header=None)
- df.columns = ["Sentence"]
- df.reset_index(drop=True, inplace=True)
-
- # TODO: Run the model
- df["ALDi"] = compute_ALDi(df["Sentence"].tolist())
-
- # A horizontal rule
- st.markdown("""---""")
-
- chart = (
- alt.Chart(df.reset_index())
- .mark_area(color="darkorange", opacity=0.5)
- .encode(
- x=X(field="index", title="Sentence Index"),
- y=Y("ALDi", scale=Scale(domain=[0, 1])),
- )
- )
- st.altair_chart(chart.interactive(), use_container_width=True)
-
- col1, col2 = st.columns([4, 1])
-
- with col1:
- # Display the output
- st.table(
- df,
- )
-
- with col2:
- # Add a download button
- csv = convert_df(df)
- st.download_button(
- label=":file_folder: Download predictions as CSV",
- data=csv,
- file_name="ALDi_scores.csv",
- mime="text/csv",
- )
diff --git a/spaces/ATang0729/Forecast4Muses/Model/Model6/Model6_2_ProfileRecogition/mmpretrain/configs/_base_/models/resnet50_cifar.py b/spaces/ATang0729/Forecast4Muses/Model/Model6/Model6_2_ProfileRecogition/mmpretrain/configs/_base_/models/resnet50_cifar.py
deleted file mode 100644
index 33b66d526482245237faa2862d376797c21a8ee4..0000000000000000000000000000000000000000
--- a/spaces/ATang0729/Forecast4Muses/Model/Model6/Model6_2_ProfileRecogition/mmpretrain/configs/_base_/models/resnet50_cifar.py
+++ /dev/null
@@ -1,16 +0,0 @@
-# model settings
-model = dict(
- type='ImageClassifier',
- backbone=dict(
- type='ResNet_CIFAR',
- depth=50,
- num_stages=4,
- out_indices=(3, ),
- style='pytorch'),
- neck=dict(type='GlobalAveragePooling'),
- head=dict(
- type='LinearClsHead',
- num_classes=10,
- in_channels=2048,
- loss=dict(type='CrossEntropyLoss', loss_weight=1.0),
- ))
diff --git a/spaces/Ababababababbababa/poetry/README.md b/spaces/Ababababababbababa/poetry/README.md
deleted file mode 100644
index a281738d9af718bcd5e9323ef7a55cc4ec5b81d0..0000000000000000000000000000000000000000
--- a/spaces/Ababababababbababa/poetry/README.md
+++ /dev/null
@@ -1,14 +0,0 @@
----
-title: Arabic Poetry Generator
-emoji: 🐠
-colorFrom: blue
-colorTo: red
-sdk: gradio
-sdk_version: 3.6
-app_file: app.py
-pinned: true
-license: cc-by-nc-4.0
-duplicated_from: Aaaaaaaabdualh/poetry
----
-
-Check out the configuration reference at https://huggingface.co/docs/hub/spaces-config-reference
diff --git a/spaces/Abhilashvj/planogram-compliance/utils/loggers/comet/comet_utils.py b/spaces/Abhilashvj/planogram-compliance/utils/loggers/comet/comet_utils.py
deleted file mode 100644
index 3d7ca3add0265f8a82f4a0a9ca1c2455ba6ab1c7..0000000000000000000000000000000000000000
--- a/spaces/Abhilashvj/planogram-compliance/utils/loggers/comet/comet_utils.py
+++ /dev/null
@@ -1,166 +0,0 @@
-import logging
-import os
-from urllib.parse import urlparse
-
-try:
- import comet_ml
-except (ModuleNotFoundError, ImportError):
- comet_ml = None
-
-import yaml
-
-logger = logging.getLogger(__name__)
-
-COMET_PREFIX = "comet://"
-COMET_MODEL_NAME = os.getenv("COMET_MODEL_NAME", "yolov5")
-COMET_DEFAULT_CHECKPOINT_FILENAME = os.getenv(
- "COMET_DEFAULT_CHECKPOINT_FILENAME", "last.pt"
-)
-
-
-def download_model_checkpoint(opt, experiment):
- model_dir = f"{opt.project}/{experiment.name}"
- os.makedirs(model_dir, exist_ok=True)
-
- model_name = COMET_MODEL_NAME
- model_asset_list = experiment.get_model_asset_list(model_name)
-
- if len(model_asset_list) == 0:
- logger.error(
- f"COMET ERROR: No checkpoints found for model name : {model_name}"
- )
- return
-
- model_asset_list = sorted(
- model_asset_list,
- key=lambda x: x["step"],
- reverse=True,
- )
- logged_checkpoint_map = {
- asset["fileName"]: asset["assetId"] for asset in model_asset_list
- }
-
- resource_url = urlparse(opt.weights)
- checkpoint_filename = resource_url.query
-
- if checkpoint_filename:
- asset_id = logged_checkpoint_map.get(checkpoint_filename)
- else:
- asset_id = logged_checkpoint_map.get(COMET_DEFAULT_CHECKPOINT_FILENAME)
- checkpoint_filename = COMET_DEFAULT_CHECKPOINT_FILENAME
-
- if asset_id is None:
- logger.error(
- f"COMET ERROR: Checkpoint {checkpoint_filename} not found in the given Experiment"
- )
- return
-
- try:
- logger.info(
- f"COMET INFO: Downloading checkpoint {checkpoint_filename}"
- )
- asset_filename = checkpoint_filename
-
- model_binary = experiment.get_asset(
- asset_id, return_type="binary", stream=False
- )
- model_download_path = f"{model_dir}/{asset_filename}"
- with open(model_download_path, "wb") as f:
- f.write(model_binary)
-
- opt.weights = model_download_path
-
- except Exception as e:
- logger.warning(
- "COMET WARNING: Unable to download checkpoint from Comet"
- )
- logger.exception(e)
-
-
-def set_opt_parameters(opt, experiment):
- """Update the opts Namespace with parameters
- from Comet's ExistingExperiment when resuming a run
-
- Args:
- opt (argparse.Namespace): Namespace of command line options
- experiment (comet_ml.APIExperiment): Comet API Experiment object
- """
- asset_list = experiment.get_asset_list()
- resume_string = opt.resume
-
- for asset in asset_list:
- if asset["fileName"] == "opt.yaml":
- asset_id = asset["assetId"]
- asset_binary = experiment.get_asset(
- asset_id, return_type="binary", stream=False
- )
- opt_dict = yaml.safe_load(asset_binary)
- for key, value in opt_dict.items():
- setattr(opt, key, value)
- opt.resume = resume_string
-
- # Save hyperparameters to YAML file
- # Necessary to pass checks in training script
- save_dir = f"{opt.project}/{experiment.name}"
- os.makedirs(save_dir, exist_ok=True)
-
- hyp_yaml_path = f"{save_dir}/hyp.yaml"
- with open(hyp_yaml_path, "w") as f:
- yaml.dump(opt.hyp, f)
- opt.hyp = hyp_yaml_path
-
-
-def check_comet_weights(opt):
- """Downloads model weights from Comet and updates the
- weights path to point to saved weights location
-
- Args:
- opt (argparse.Namespace): Command Line arguments passed
- to YOLOv5 training script
-
- Returns:
- None/bool: Return True if weights are successfully downloaded
- else return None
- """
- if comet_ml is None:
- return
-
- if isinstance(opt.weights, str):
- if opt.weights.startswith(COMET_PREFIX):
- api = comet_ml.API()
- resource = urlparse(opt.weights)
- experiment_path = f"{resource.netloc}{resource.path}"
- experiment = api.get(experiment_path)
- download_model_checkpoint(opt, experiment)
- return True
-
- return None
-
-
-def check_comet_resume(opt):
- """Restores run parameters to its original state based on the model checkpoint
- and logged Experiment parameters.
-
- Args:
- opt (argparse.Namespace): Command Line arguments passed
- to YOLOv5 training script
-
- Returns:
- None/bool: Return True if the run is restored successfully
- else return None
- """
- if comet_ml is None:
- return
-
- if isinstance(opt.resume, str):
- if opt.resume.startswith(COMET_PREFIX):
- api = comet_ml.API()
- resource = urlparse(opt.resume)
- experiment_path = f"{resource.netloc}{resource.path}"
- experiment = api.get(experiment_path)
- set_opt_parameters(opt, experiment)
- download_model_checkpoint(opt, experiment)
-
- return True
-
- return None
diff --git a/spaces/AchyuthGamer/OpenGPT-Chat-UI/.svelte-kit/types/src/routes/conversation/[id]/summarize/$types.d.ts b/spaces/AchyuthGamer/OpenGPT-Chat-UI/.svelte-kit/types/src/routes/conversation/[id]/summarize/$types.d.ts
deleted file mode 100644
index b35663dc5a15f60117724566d893dd20fdceeb08..0000000000000000000000000000000000000000
--- a/spaces/AchyuthGamer/OpenGPT-Chat-UI/.svelte-kit/types/src/routes/conversation/[id]/summarize/$types.d.ts
+++ /dev/null
@@ -1,9 +0,0 @@
-import type * as Kit from '@sveltejs/kit';
-
-type Expand = T extends infer O ? { [K in keyof O]: O[K] } : never;
-type RouteParams = { id: string }
-type RouteId = '/conversation/[id]/summarize';
-
-export type EntryGenerator = () => Promise> | Array;
-export type RequestHandler = Kit.RequestHandler;
-export type RequestEvent = Kit.RequestEvent;
\ No newline at end of file
diff --git a/spaces/AchyuthGamer/OpenGPT/g4f/Provider/Myshell.py b/spaces/AchyuthGamer/OpenGPT/g4f/Provider/Myshell.py
deleted file mode 100644
index da170fa31ddb64dedae20751d36bf4e766fd9779..0000000000000000000000000000000000000000
--- a/spaces/AchyuthGamer/OpenGPT/g4f/Provider/Myshell.py
+++ /dev/null
@@ -1,173 +0,0 @@
-from __future__ import annotations
-
-import json, uuid, hashlib, time, random
-
-from aiohttp import ClientSession
-from aiohttp.http import WSMsgType
-import asyncio
-
-from ..typing import AsyncGenerator
-from .base_provider import AsyncGeneratorProvider, format_prompt
-
-
-models = {
- "samantha": "1e3be7fe89e94a809408b1154a2ee3e1",
- "gpt-3.5-turbo": "8077335db7cd47e29f7de486612cc7fd",
- "gpt-4": "01c8de4fbfc548df903712b0922a4e01",
-}
-
-
-class Myshell(AsyncGeneratorProvider):
- url = "https://app.myshell.ai/chat"
- working = True
- supports_gpt_35_turbo = True
- supports_gpt_4 = True
-
- @classmethod
- async def create_async_generator(
- cls,
- model: str,
- messages: list[dict[str, str]],
- timeout: int = 90,
- **kwargs
- ) -> AsyncGenerator:
- if not model:
- bot_id = models["samantha"]
- elif model in models:
- bot_id = models[model]
- else:
- raise ValueError(f"Model are not supported: {model}")
-
- user_agent = 'Mozilla/5.0 (Macintosh; Intel Mac OS X 10_15_7) AppleWebKit/537.36 (KHTML, like Gecko) Chrome/111.0.0.0 Safari/537.36'
- visitor_id = generate_visitor_id(user_agent)
-
- async with ClientSession(
- headers={'User-Agent': user_agent}
- ) as session:
- async with session.ws_connect(
- "wss://api.myshell.ai/ws/?EIO=4&transport=websocket",
- autoping=False,
- timeout=timeout
- ) as wss:
- # Send and receive hello message
- await wss.receive_str()
- message = json.dumps({"token": None, "visitorId": visitor_id})
- await wss.send_str(f"40/chat,{message}")
- await wss.receive_str()
-
- # Fix "need_verify_captcha" issue
- await asyncio.sleep(5)
-
- # Create chat message
- text = format_prompt(messages)
- chat_data = json.dumps(["text_chat",{
- "reqId": str(uuid.uuid4()),
- "botUid": bot_id,
- "sourceFrom": "myshellWebsite",
- "text": text,
- **generate_signature(text)
- }])
-
- # Send chat message
- chat_start = "42/chat,"
- chat_message = f"{chat_start}{chat_data}"
- await wss.send_str(chat_message)
-
- # Receive messages
- async for message in wss:
- if message.type != WSMsgType.TEXT:
- continue
- # Ping back
- if message.data == "2":
- await wss.send_str("3")
- continue
- # Is not chat message
- if not message.data.startswith(chat_start):
- continue
- data_type, data = json.loads(message.data[len(chat_start):])
- if data_type == "text_stream":
- if data["data"]["text"]:
- yield data["data"]["text"]
- elif data["data"]["isFinal"]:
- break
- elif data_type in ("message_replied", "need_verify_captcha"):
- raise RuntimeError(f"Received unexpected message: {data_type}")
-
-
- @classmethod
- @property
- def params(cls):
- params = [
- ("model", "str"),
- ("messages", "list[dict[str, str]]"),
- ("stream", "bool"),
- ]
- param = ", ".join([": ".join(p) for p in params])
- return f"g4f.provider.{cls.__name__} supports: ({param})"
-
-
-def generate_timestamp() -> str:
- return str(
- int(
- str(int(time.time() * 1000))[:-1]
- + str(
- sum(
- 2 * int(digit)
- if idx % 2 == 0
- else 3 * int(digit)
- for idx, digit in enumerate(str(int(time.time() * 1000))[:-1])
- )
- % 10
- )
- )
- )
-
-def generate_signature(text: str):
- timestamp = generate_timestamp()
- version = 'v1.0.0'
- secret = '8@VXGK3kKHr!u2gA'
- data = f"{version}#{text}#{timestamp}#{secret}"
- signature = hashlib.md5(data.encode()).hexdigest()
- signature = signature[::-1]
- return {
- "signature": signature,
- "timestamp": timestamp,
- "version": version
- }
-
-def xor_hash(B: str):
- r = []
- i = 0
-
- def o(e, t):
- o_val = 0
- for i in range(len(t)):
- o_val |= r[i] << (8 * i)
- return e ^ o_val
-
- for e in range(len(B)):
- t = ord(B[e])
- r.insert(0, 255 & t)
-
- if len(r) >= 4:
- i = o(i, r)
- r = []
-
- if len(r) > 0:
- i = o(i, r)
-
- return hex(i)[2:]
-
-def performance() -> str:
- t = int(time.time() * 1000)
- e = 0
- while t == int(time.time() * 1000):
- e += 1
- return hex(t)[2:] + hex(e)[2:]
-
-def generate_visitor_id(user_agent: str) -> str:
- f = performance()
- r = hex(int(random.random() * (16**16)))[2:-2]
- d = xor_hash(user_agent)
- e = hex(1080 * 1920)[2:]
- return f"{f}-{r}-{d}-{e}-{f}"
\ No newline at end of file
diff --git a/spaces/Adithedev/Text-Summarization-Tool/app.py b/spaces/Adithedev/Text-Summarization-Tool/app.py
deleted file mode 100644
index d06908ff5397bce40f47437658af1581cd96d844..0000000000000000000000000000000000000000
--- a/spaces/Adithedev/Text-Summarization-Tool/app.py
+++ /dev/null
@@ -1,81 +0,0 @@
-import streamlit as st
-import base64
-import re
-import spacy
-from heapq import nlargest
-
-st.title("Text Summarizer")
-with st.form(key = "clf_form"):
- text_input = st.text_area("Type Here: ")
- input_slider = st.slider(step=0.1,min_value=0.2,max_value=0.7,label="How much portion of the text do you wish to be summarized, Eg: 0.2 --> 20% of the Original Text")
- submit_btn = st.form_submit_button(label = "Submit")
- countOfWords = len(text_input.split())
-
- class Model():
- try:
- nlp = spacy.load("en_core_web_sm")
- except OSError:
- import subprocess
- subprocess.run(["python", "-m", "spacy", "download", "en_core_web_sm"])
- def predict(text):
- stop_words = [ 'stop', 'the', 'to', 'and', 'a', 'in', 'it', 'is', 'I', 'that', 'had', 'on', 'for', 'were', 'was']
- nlp = spacy.load("en_core_web_sm")
- doc = nlp(text)
-
- lemmatized_text = " ".join([token.lemma_ for token in doc])
-
- re_text = re.sub("[^\s\w,.]"," ",lemmatized_text)
- re_text = re.sub("[ ]{2,}"," ",re_text).lower()
-
- word_frequencies = {}
- for word in doc:
- if word.text not in "\n":
- if word.text not in stop_words:
- if word.text not in word_frequencies.keys():
- word_frequencies[word.text] = 1
- else:
- word_frequencies[word.text] +=1
-
- max_word_frequency = max(word_frequencies.values(),default=0)
-
- for word in word_frequencies.keys():
- word_frequencies[word] = word_frequencies[word] / max_word_frequency
-
- sent_tokens = [sent for sent in doc.sents]
- sent_scores = {}
-
- for sent in sent_tokens:
- for word in sent:
- if word.text in word_frequencies.keys():
- if sent not in sent_scores.keys():
- sent_scores[sent] = word_frequencies[word.text]
- else:
- sent_scores[sent] += word_frequencies[word.text]
-
- sentence_length = int(len(sent_tokens)*input_slider)
- summary = nlargest(sentence_length,sent_scores,sent_scores.get)
- final_summary = [word.text for word in summary]
- final_summary = " ".join(final_summary)
- return final_summary
-
-
- if submit_btn:
- if text_input == "":
- st.error("Enter something in order to summarize it.",icon="⛔️")
- else:
- if countOfWords <=80:
- st.warning("Pls enter more than 80 words in order to summarize it.",icon="⚠️")
- else:
- st.subheader("Output: ")
-
- col1,col2 = st.columns(2)
-
- output = Model.predict(text=text_input)
-
- with col1:
- st.info("Original Text: ")
- st.write(text_input)
-
- with col2:
- st.info("Summarized Text: ")
- st.write(output)
\ No newline at end of file
diff --git a/spaces/Aditya9790/yolo7-object-tracking/sort.py b/spaces/Aditya9790/yolo7-object-tracking/sort.py
deleted file mode 100644
index f6d6681c2a9113eeeeaccc5418fff572af16c906..0000000000000000000000000000000000000000
--- a/spaces/Aditya9790/yolo7-object-tracking/sort.py
+++ /dev/null
@@ -1,367 +0,0 @@
-from __future__ import print_function
-
-import os
-import numpy as np
-
-##### NEW
-# !pip --no-cache-dir install -U --force-reinstall matplotlib
-import tkinter
-import matplotlib
-matplotlib.use('Agg')
-###### NEW end
-import matplotlib.pyplot as plt
-import matplotlib.patches as patches
-from skimage import io
-from random import randint
-import glob
-import time
-import argparse
-from filterpy.kalman import KalmanFilter
-
-
-def get_color():
- # r = randint(0, 255)
- # g = randint(0, 255)
- # b = randint(0, 255)
- color = (randint(0, 255), randint(0, 255), randint(0, 255))
- return color
-def linear_assignment(cost_matrix):
- try:
- import lap #linear assignment problem solver
- _, x, y = lap.lapjv(cost_matrix, extend_cost = True)
- return np.array([[y[i],i] for i in x if i>=0])
- except ImportError:
- from scipy.optimize import linear_sum_assignment
- x,y = linear_sum_assignment(cost_matrix)
- return np.array(list(zip(x,y)))
-
-
-"""From SORT: Computes IOU between two boxes in the form [x1,y1,x2,y2]"""
-def iou_batch(bb_test, bb_gt):
-
- bb_gt = np.expand_dims(bb_gt, 0)
- bb_test = np.expand_dims(bb_test, 1)
-
- xx1 = np.maximum(bb_test[...,0], bb_gt[..., 0])
- yy1 = np.maximum(bb_test[..., 1], bb_gt[..., 1])
- xx2 = np.minimum(bb_test[..., 2], bb_gt[..., 2])
- yy2 = np.minimum(bb_test[..., 3], bb_gt[..., 3])
- w = np.maximum(0., xx2 - xx1)
- h = np.maximum(0., yy2 - yy1)
- wh = w * h
- o = wh / ((bb_test[..., 2] - bb_test[..., 0]) * (bb_test[..., 3] - bb_test[..., 1])
- + (bb_gt[..., 2] - bb_gt[..., 0]) * (bb_gt[..., 3] - bb_gt[..., 1]) - wh)
- return(o)
-
-
-"""Takes a bounding box in the form [x1,y1,x2,y2] and returns z in the form [x,y,s,r] where x,y is the center of the box and s is the scale/area and r is the aspect ratio"""
-def convert_bbox_to_z(bbox):
- w = bbox[2] - bbox[0]
- h = bbox[3] - bbox[1]
- x = bbox[0] + w/2.
- y = bbox[1] + h/2.
- s = w * h
- #scale is just area
- r = w / float(h)
- return np.array([x, y, s, r]).reshape((4, 1))
-
-
-"""Takes a bounding box in the centre form [x,y,s,r] and returns it in the form
- [x1,y1,x2,y2] where x1,y1 is the top left and x2,y2 is the bottom right"""
-def convert_x_to_bbox(x, score=None):
- w = np.sqrt(x[2] * x[3])
- h = x[2] / w
- if(score==None):
- return np.array([x[0]-w/2.,x[1]-h/2.,x[0]+w/2.,x[1]+h/2.]).reshape((1,4))
- else:
- return np.array([x[0]-w/2.,x[1]-h/2.,x[0]+w/2.,x[1]+h/2.,score]).reshape((1,5))
-
-"""This class represents the internal state of individual tracked objects observed as bbox."""
-class KalmanBoxTracker(object):
-
- count = 0
- def __init__(self, bbox):
- """
- Initialize a tracker using initial bounding box
-
- Parameter 'bbox' must have 'detected class' int number at the -1 position.
- """
- self.kf = KalmanFilter(dim_x=7, dim_z=4)
- self.kf.F = np.array([[1,0,0,0,1,0,0],[0,1,0,0,0,1,0],[0,0,1,0,0,0,1],[0,0,0,1,0,0,0],[0,0,0,0,1,0,0],[0,0,0,0,0,1,0],[0,0,0,0,0,0,1]])
- self.kf.H = np.array([[1,0,0,0,0,0,0],[0,1,0,0,0,0,0],[0,0,1,0,0,0,0],[0,0,0,1,0,0,0]])
-
- self.kf.R[2:,2:] *= 10. # R: Covariance matrix of measurement noise (set to high for noisy inputs -> more 'inertia' of boxes')
- self.kf.P[4:,4:] *= 1000. #give high uncertainty to the unobservable initial velocities
- self.kf.P *= 10.
- self.kf.Q[-1,-1] *= 0.5 # Q: Covariance matrix of process noise (set to high for erratically moving things)
- self.kf.Q[4:,4:] *= 0.5
-
- self.kf.x[:4] = convert_bbox_to_z(bbox) # STATE VECTOR
- self.time_since_update = 0
- self.id = KalmanBoxTracker.count
- KalmanBoxTracker.count += 1
- self.history = []
- self.hits = 0
- self.hit_streak = 0
- self.age = 0
- self.centroidarr = []
- CX = (bbox[0]+bbox[2])//2
- CY = (bbox[1]+bbox[3])//2
- self.centroidarr.append((CX,CY))
-
-
- #keep yolov5 detected class information
- self.detclass = bbox[5]
-
- def update(self, bbox):
- """
- Updates the state vector with observed bbox
- """
- self.time_since_update = 0
- self.history = []
- self.hits += 1
- self.hit_streak += 1
- self.kf.update(convert_bbox_to_z(bbox))
- self.detclass = bbox[5]
- CX = (bbox[0]+bbox[2])//2
- CY = (bbox[1]+bbox[3])//2
- self.centroidarr.append((CX,CY))
-
- def predict(self):
- """
- Advances the state vector and returns the predicted bounding box estimate
- """
- if((self.kf.x[6]+self.kf.x[2])<=0):
- self.kf.x[6] *= 0.0
- self.kf.predict()
- self.age += 1
- if(self.time_since_update>0):
- self.hit_streak = 0
- self.time_since_update += 1
- self.history.append(convert_x_to_bbox(self.kf.x))
- # bbox=self.history[-1]
- # CX = (bbox[0]+bbox[2])/2
- # CY = (bbox[1]+bbox[3])/2
- # self.centroidarr.append((CX,CY))
-
- return self.history[-1]
-
-
- def get_state(self):
- """
- Returns the current bounding box estimate
- # test
- arr1 = np.array([[1,2,3,4]])
- arr2 = np.array([0])
- arr3 = np.expand_dims(arr2, 0)
- np.concatenate((arr1,arr3), axis=1)
- """
- arr_detclass = np.expand_dims(np.array([self.detclass]), 0)
-
- arr_u_dot = np.expand_dims(self.kf.x[4],0)
- arr_v_dot = np.expand_dims(self.kf.x[5],0)
- arr_s_dot = np.expand_dims(self.kf.x[6],0)
-
- return np.concatenate((convert_x_to_bbox(self.kf.x), arr_detclass, arr_u_dot, arr_v_dot, arr_s_dot), axis=1)
-
-def associate_detections_to_trackers(detections, trackers, iou_threshold = 0.3):
- """
- Assigns detections to tracked object (both represented as bounding boxes)
- Returns 3 lists of
- 1. matches,
- 2. unmatched_detections
- 3. unmatched_trackers
- """
- if(len(trackers)==0):
- return np.empty((0,2),dtype=int), np.arange(len(detections)), np.empty((0,5),dtype=int)
-
- iou_matrix = iou_batch(detections, trackers)
-
- if min(iou_matrix.shape) > 0:
- a = (iou_matrix > iou_threshold).astype(np.int32)
- if a.sum(1).max() == 1 and a.sum(0).max() ==1:
- matched_indices = np.stack(np.where(a), axis=1)
- else:
- matched_indices = linear_assignment(-iou_matrix)
- else:
- matched_indices = np.empty(shape=(0,2))
-
- unmatched_detections = []
- for d, det in enumerate(detections):
- if(d not in matched_indices[:,0]):
- unmatched_detections.append(d)
-
-
- unmatched_trackers = []
- for t, trk in enumerate(trackers):
- if(t not in matched_indices[:,1]):
- unmatched_trackers.append(t)
-
- #filter out matched with low IOU
- matches = []
- for m in matched_indices:
- if(iou_matrix[m[0], m[1]]= self.min_hits or self.frame_count <= self.min_hits):
- ret.append(np.concatenate((d, [trk.id+1])).reshape(1,-1)) #+1'd because MOT benchmark requires positive value
- i -= 1
- #remove dead tracklet
- if(trk.time_since_update >self.max_age):
- self.trackers.pop(i)
- if unique_color:
- self.color_list.pop(i)
-
- if(len(ret) > 0):
- return np.concatenate(ret)
- return np.empty((0,6))
-
-def parse_args():
- """Parse input arguments."""
- parser = argparse.ArgumentParser(description='SORT demo')
- parser.add_argument('--display', dest='display', help='Display online tracker output (slow) [False]',action='store_true')
- parser.add_argument("--seq_path", help="Path to detections.", type=str, default='data')
- parser.add_argument("--phase", help="Subdirectory in seq_path.", type=str, default='train')
- parser.add_argument("--max_age",
- help="Maximum number of frames to keep alive a track without associated detections.",
- type=int, default=1)
- parser.add_argument("--min_hits",
- help="Minimum number of associated detections before track is initialised.",
- type=int, default=3)
- parser.add_argument("--iou_threshold", help="Minimum IOU for match.", type=float, default=0.3)
- args = parser.parse_args()
- return args
-
-if __name__ == '__main__':
- # all train
- args = parse_args()
- display = args.display
- phase = args.phase
- total_time = 0.0
- total_frames = 0
- colours = np.random.rand(32, 3) #used only for display
- if(display):
- if not os.path.exists('mot_benchmark'):
- print('\n\tERROR: mot_benchmark link not found!\n\n Create a symbolic link to the MOT benchmark\n (https://motchallenge.net/data/2D_MOT_2015/#download). E.g.:\n\n $ ln -s /path/to/MOT2015_challenge/2DMOT2015 mot_benchmark\n\n')
- exit()
- plt.ion()
- fig = plt.figure()
- ax1 = fig.add_subplot(111, aspect='equal')
-
- if not os.path.exists('output'):
- os.makedirs('output')
- pattern = os.path.join(args.seq_path, phase, '*', 'det', 'det.txt')
- for seq_dets_fn in glob.glob(pattern):
- mot_tracker = Sort(max_age=args.max_age,
- min_hits=args.min_hits,
- iou_threshold=args.iou_threshold) #create instance of the SORT tracker
- seq_dets = np.loadtxt(seq_dets_fn, delimiter=',')
- seq = seq_dets_fn[pattern.find('*'):].split(os.path.sep)[0]
-
- with open(os.path.join('output', '%s.txt'%(seq)),'w') as out_file:
- print("Processing %s."%(seq))
- for frame in range(int(seq_dets[:,0].max())):
- frame += 1 #detection and frame numbers begin at 1
- dets = seq_dets[seq_dets[:, 0]==frame, 2:7]
- dets[:, 2:4] += dets[:, 0:2] #convert to [x1,y1,w,h] to [x1,y1,x2,y2]
- total_frames += 1
-
- if(display):
- fn = os.path.join('mot_benchmark', phase, seq, 'img1', '%06d.jpg'%(frame))
- im =io.imread(fn)
- ax1.imshow(im)
- plt.title(seq + ' Tracked Targets')
-
- start_time = time.time()
- trackers = mot_tracker.update(dets)
- cycle_time = time.time() - start_time
- total_time += cycle_time
-
- for d in trackers:
- print('%d,%d,%.2f,%.2f,%.2f,%.2f,1,-1,-1,-1'%(frame,d[4],d[0],d[1],d[2]-d[0],d[3]-d[1]),file=out_file)
- if(display):
- d = d.astype(np.int32)
- ax1.add_patch(patches.Rectangle((d[0],d[1]),d[2]-d[0],d[3]-d[1],fill=False,lw=3,ec=colours[d[4]%32,:]))
-
- if(display):
- fig.canvas.flush_events()
- plt.draw()
- ax1.cla()
-
- print("Total Tracking took: %.3f seconds for %d frames or %.1f FPS" % (total_time, total_frames, total_frames / total_time))
-
- if(display):
- print("Note: to get real runtime results run without the option: --display")
diff --git a/spaces/Ameaou/academic-chatgpt3.1/README.md b/spaces/Ameaou/academic-chatgpt3.1/README.md
deleted file mode 100644
index 70c414482f9fc4133d0323fd2323e385a82dcd0c..0000000000000000000000000000000000000000
--- a/spaces/Ameaou/academic-chatgpt3.1/README.md
+++ /dev/null
@@ -1,300 +0,0 @@
----
-title: academic-chatgpt
-emoji: 😻
-colorFrom: blue
-colorTo: blue
-sdk: gradio
-sdk_version: 3.25.0
-python_version: 3.11
-app_file: main.py
-pinned: false
-duplicated_from: qingxu98/academic-chatgpt-beta
----
-
-# ChatGPT 学术优化
-
-**如果喜欢这个项目,请给它一个Star;如果你发明了更好用的快捷键或函数插件,欢迎发issue或者pull requests**
-
-If you like this project, please give it a Star. If you've come up with more useful academic shortcuts or functional plugins, feel free to open an issue or pull request. We also have a README in [English|](docs/README_EN.md)[日本語|](docs/README_JP.md)[Русский|](docs/README_RS.md)[Français](docs/README_FR.md) translated by this project itself.
-
-> **Note**
->
-> 1.请注意只有**红颜色**标识的函数插件(按钮)才支持读取文件,部分插件位于插件区的**下拉菜单**中。另外我们以**最高优先级**欢迎和处理任何新插件的PR!
->
-> 2.本项目中每个文件的功能都在自译解[`self_analysis.md`](https://github.com/binary-husky/chatgpt_academic/wiki/chatgpt-academic%E9%A1%B9%E7%9B%AE%E8%87%AA%E8%AF%91%E8%A7%A3%E6%8A%A5%E5%91%8A)详细说明。随着版本的迭代,您也可以随时自行点击相关函数插件,调用GPT重新生成项目的自我解析报告。常见问题汇总在[`wiki`](https://github.com/binary-husky/chatgpt_academic/wiki/%E5%B8%B8%E8%A7%81%E9%97%AE%E9%A2%98)当中。
->
-
-
-
-
-## Todo 与 版本规划:
-- version 3.2+ (todo): 函数插件支持更多参数接口
-- version 3.1: 支持同时问询多个gpt模型!支持api2d,支持多个apikey负载均衡
-- version 3.0: 对chatglm和其他小型llm的支持
-- version 2.6: 重构了插件结构,提高了交互性,加入更多插件
-- version 2.5: 自更新,解决总结大工程源代码时文本过长、token溢出的问题
-- version 2.4: (1)新增PDF全文翻译功能; (2)新增输入区切换位置的功能; (3)新增垂直布局选项; (4)多线程函数插件优化。
-- version 2.3: 增强多线程交互性
-- version 2.2: 函数插件支持热重载
-- version 2.1: 可折叠式布局
-- version 2.0: 引入模块化函数插件
-- version 1.0: 基础功能
-
-## 参考与学习
-
-```
-代码中参考了很多其他优秀项目中的设计,主要包括:
-
-# 借鉴项目1:借鉴了ChuanhuChatGPT中诸多技巧
-https://github.com/GaiZhenbiao/ChuanhuChatGPT
-
-# 借鉴项目2:清华ChatGLM-6B:
-https://github.com/THUDM/ChatGLM-6B
-```
diff --git a/spaces/Androidonnxfork/CivitAi-to-Diffusers/diffusers/src/diffusers/pipelines/stable_diffusion/pipeline_cycle_diffusion.py b/spaces/Androidonnxfork/CivitAi-to-Diffusers/diffusers/src/diffusers/pipelines/stable_diffusion/pipeline_cycle_diffusion.py
deleted file mode 100644
index fc0dc1a8b08439015c34c00ef7a49356d7e0990a..0000000000000000000000000000000000000000
--- a/spaces/Androidonnxfork/CivitAi-to-Diffusers/diffusers/src/diffusers/pipelines/stable_diffusion/pipeline_cycle_diffusion.py
+++ /dev/null
@@ -1,882 +0,0 @@
-# Copyright 2023 The HuggingFace Team. All rights reserved.
-#
-# Licensed under the Apache License, Version 2.0 (the "License");
-# you may not use this file except in compliance with the License.
-# You may obtain a copy of the License at
-#
-# http://www.apache.org/licenses/LICENSE-2.0
-#
-# Unless required by applicable law or agreed to in writing, software
-# distributed under the License is distributed on an "AS IS" BASIS,
-# WITHOUT WARRANTIES OR CONDITIONS OF ANY KIND, either express or implied.
-# See the License for the specific language governing permissions and
-# limitations under the License.
-
-import inspect
-import warnings
-from typing import Any, Callable, Dict, List, Optional, Union
-
-import numpy as np
-import PIL
-import torch
-from packaging import version
-from transformers import CLIPImageProcessor, CLIPTextModel, CLIPTokenizer
-
-from diffusers.utils import is_accelerate_available, is_accelerate_version
-
-from ...configuration_utils import FrozenDict
-from ...image_processor import VaeImageProcessor
-from ...loaders import LoraLoaderMixin, TextualInversionLoaderMixin
-from ...models import AutoencoderKL, UNet2DConditionModel
-from ...schedulers import DDIMScheduler
-from ...utils import PIL_INTERPOLATION, deprecate, logging, randn_tensor
-from ..pipeline_utils import DiffusionPipeline
-from . import StableDiffusionPipelineOutput
-from .safety_checker import StableDiffusionSafetyChecker
-
-
-logger = logging.get_logger(__name__) # pylint: disable=invalid-name
-
-
-# Copied from diffusers.pipelines.stable_diffusion.pipeline_stable_diffusion_img2img.preprocess
-def preprocess(image):
- warnings.warn(
- "The preprocess method is deprecated and will be removed in a future version. Please"
- " use VaeImageProcessor.preprocess instead",
- FutureWarning,
- )
- if isinstance(image, torch.Tensor):
- return image
- elif isinstance(image, PIL.Image.Image):
- image = [image]
-
- if isinstance(image[0], PIL.Image.Image):
- w, h = image[0].size
- w, h = (x - x % 8 for x in (w, h)) # resize to integer multiple of 8
-
- image = [np.array(i.resize((w, h), resample=PIL_INTERPOLATION["lanczos"]))[None, :] for i in image]
- image = np.concatenate(image, axis=0)
- image = np.array(image).astype(np.float32) / 255.0
- image = image.transpose(0, 3, 1, 2)
- image = 2.0 * image - 1.0
- image = torch.from_numpy(image)
- elif isinstance(image[0], torch.Tensor):
- image = torch.cat(image, dim=0)
- return image
-
-
-def posterior_sample(scheduler, latents, timestep, clean_latents, generator, eta):
- # 1. get previous step value (=t-1)
- prev_timestep = timestep - scheduler.config.num_train_timesteps // scheduler.num_inference_steps
-
- if prev_timestep <= 0:
- return clean_latents
-
- # 2. compute alphas, betas
- alpha_prod_t = scheduler.alphas_cumprod[timestep]
- alpha_prod_t_prev = (
- scheduler.alphas_cumprod[prev_timestep] if prev_timestep >= 0 else scheduler.final_alpha_cumprod
- )
-
- variance = scheduler._get_variance(timestep, prev_timestep)
- std_dev_t = eta * variance ** (0.5)
-
- # direction pointing to x_t
- e_t = (latents - alpha_prod_t ** (0.5) * clean_latents) / (1 - alpha_prod_t) ** (0.5)
- dir_xt = (1.0 - alpha_prod_t_prev - std_dev_t**2) ** (0.5) * e_t
- noise = std_dev_t * randn_tensor(
- clean_latents.shape, dtype=clean_latents.dtype, device=clean_latents.device, generator=generator
- )
- prev_latents = alpha_prod_t_prev ** (0.5) * clean_latents + dir_xt + noise
-
- return prev_latents
-
-
-def compute_noise(scheduler, prev_latents, latents, timestep, noise_pred, eta):
- # 1. get previous step value (=t-1)
- prev_timestep = timestep - scheduler.config.num_train_timesteps // scheduler.num_inference_steps
-
- # 2. compute alphas, betas
- alpha_prod_t = scheduler.alphas_cumprod[timestep]
- alpha_prod_t_prev = (
- scheduler.alphas_cumprod[prev_timestep] if prev_timestep >= 0 else scheduler.final_alpha_cumprod
- )
-
- beta_prod_t = 1 - alpha_prod_t
-
- # 3. compute predicted original sample from predicted noise also called
- # "predicted x_0" of formula (12) from https://arxiv.org/pdf/2010.02502.pdf
- pred_original_sample = (latents - beta_prod_t ** (0.5) * noise_pred) / alpha_prod_t ** (0.5)
-
- # 4. Clip "predicted x_0"
- if scheduler.config.clip_sample:
- pred_original_sample = torch.clamp(pred_original_sample, -1, 1)
-
- # 5. compute variance: "sigma_t(η)" -> see formula (16)
- # σ_t = sqrt((1 − α_t−1)/(1 − α_t)) * sqrt(1 − α_t/α_t−1)
- variance = scheduler._get_variance(timestep, prev_timestep)
- std_dev_t = eta * variance ** (0.5)
-
- # 6. compute "direction pointing to x_t" of formula (12) from https://arxiv.org/pdf/2010.02502.pdf
- pred_sample_direction = (1 - alpha_prod_t_prev - std_dev_t**2) ** (0.5) * noise_pred
-
- noise = (prev_latents - (alpha_prod_t_prev ** (0.5) * pred_original_sample + pred_sample_direction)) / (
- variance ** (0.5) * eta
- )
- return noise
-
-
-class CycleDiffusionPipeline(DiffusionPipeline, TextualInversionLoaderMixin, LoraLoaderMixin):
- r"""
- Pipeline for text-guided image to image generation using Stable Diffusion.
-
- This model inherits from [`DiffusionPipeline`]. Check the superclass documentation for the generic methods
- implemented for all pipelines (downloading, saving, running on a particular device, etc.).
-
- Args:
- vae ([`AutoencoderKL`]):
- Variational Auto-Encoder (VAE) model to encode and decode images to and from latent representations.
- text_encoder ([`~transformers.CLIPTextModel`]):
- Frozen text-encoder ([clip-vit-large-patch14](https://huggingface.co/openai/clip-vit-large-patch14)).
- tokenizer ([`~transformers.CLIPTokenizer`]):
- A `CLIPTokenizer` to tokenize text.
- unet ([`UNet2DConditionModel`]):
- A `UNet2DConditionModel` to denoise the encoded image latents.
- scheduler ([`SchedulerMixin`]):
- A scheduler to be used in combination with `unet` to denoise the encoded image latents. Can only be an
- instance of [`DDIMScheduler`].
- safety_checker ([`StableDiffusionSafetyChecker`]):
- Classification module that estimates whether generated images could be considered offensive or harmful.
- Please refer to the [model card](https://huggingface.co/runwayml/stable-diffusion-v1-5) for more details
- about a model's potential harms.
- feature_extractor ([`~transformers.CLIPImageProcessor`]):
- A `CLIPImageProcessor` to extract features from generated images; used as inputs to the `safety_checker`.
- """
- _optional_components = ["safety_checker", "feature_extractor"]
-
- def __init__(
- self,
- vae: AutoencoderKL,
- text_encoder: CLIPTextModel,
- tokenizer: CLIPTokenizer,
- unet: UNet2DConditionModel,
- scheduler: DDIMScheduler,
- safety_checker: StableDiffusionSafetyChecker,
- feature_extractor: CLIPImageProcessor,
- requires_safety_checker: bool = True,
- ):
- super().__init__()
-
- if hasattr(scheduler.config, "steps_offset") and scheduler.config.steps_offset != 1:
- deprecation_message = (
- f"The configuration file of this scheduler: {scheduler} is outdated. `steps_offset`"
- f" should be set to 1 instead of {scheduler.config.steps_offset}. Please make sure "
- "to update the config accordingly as leaving `steps_offset` might led to incorrect results"
- " in future versions. If you have downloaded this checkpoint from the Hugging Face Hub,"
- " it would be very nice if you could open a Pull request for the `scheduler/scheduler_config.json`"
- " file"
- )
- deprecate("steps_offset!=1", "1.0.0", deprecation_message, standard_warn=False)
- new_config = dict(scheduler.config)
- new_config["steps_offset"] = 1
- scheduler._internal_dict = FrozenDict(new_config)
-
- if safety_checker is None and requires_safety_checker:
- logger.warning(
- f"You have disabled the safety checker for {self.__class__} by passing `safety_checker=None`. Ensure"
- " that you abide to the conditions of the Stable Diffusion license and do not expose unfiltered"
- " results in services or applications open to the public. Both the diffusers team and Hugging Face"
- " strongly recommend to keep the safety filter enabled in all public facing circumstances, disabling"
- " it only for use-cases that involve analyzing network behavior or auditing its results. For more"
- " information, please have a look at https://github.com/huggingface/diffusers/pull/254 ."
- )
-
- if safety_checker is not None and feature_extractor is None:
- raise ValueError(
- "Make sure to define a feature extractor when loading {self.__class__} if you want to use the safety"
- " checker. If you do not want to use the safety checker, you can pass `'safety_checker=None'` instead."
- )
- is_unet_version_less_0_9_0 = hasattr(unet.config, "_diffusers_version") and version.parse(
- version.parse(unet.config._diffusers_version).base_version
- ) < version.parse("0.9.0.dev0")
- is_unet_sample_size_less_64 = hasattr(unet.config, "sample_size") and unet.config.sample_size < 64
- if is_unet_version_less_0_9_0 and is_unet_sample_size_less_64:
- deprecation_message = (
- "The configuration file of the unet has set the default `sample_size` to smaller than"
- " 64 which seems highly unlikely .If you're checkpoint is a fine-tuned version of any of the"
- " following: \n- CompVis/stable-diffusion-v1-4 \n- CompVis/stable-diffusion-v1-3 \n-"
- " CompVis/stable-diffusion-v1-2 \n- CompVis/stable-diffusion-v1-1 \n- runwayml/stable-diffusion-v1-5"
- " \n- runwayml/stable-diffusion-inpainting \n you should change 'sample_size' to 64 in the"
- " configuration file. Please make sure to update the config accordingly as leaving `sample_size=32`"
- " in the config might lead to incorrect results in future versions. If you have downloaded this"
- " checkpoint from the Hugging Face Hub, it would be very nice if you could open a Pull request for"
- " the `unet/config.json` file"
- )
- deprecate("sample_size<64", "1.0.0", deprecation_message, standard_warn=False)
- new_config = dict(unet.config)
- new_config["sample_size"] = 64
- unet._internal_dict = FrozenDict(new_config)
-
- self.register_modules(
- vae=vae,
- text_encoder=text_encoder,
- tokenizer=tokenizer,
- unet=unet,
- scheduler=scheduler,
- safety_checker=safety_checker,
- feature_extractor=feature_extractor,
- )
- self.vae_scale_factor = 2 ** (len(self.vae.config.block_out_channels) - 1)
- self.image_processor = VaeImageProcessor(vae_scale_factor=self.vae_scale_factor)
- self.register_to_config(requires_safety_checker=requires_safety_checker)
-
- # Copied from diffusers.pipelines.stable_diffusion.pipeline_stable_diffusion.StableDiffusionPipeline.enable_model_cpu_offload
- def enable_model_cpu_offload(self, gpu_id=0):
- r"""
- Offload all models to CPU to reduce memory usage with a low impact on performance. Moves one whole model at a
- time to the GPU when its `forward` method is called, and the model remains in GPU until the next model runs.
- Memory savings are lower than using `enable_sequential_cpu_offload`, but performance is much better due to the
- iterative execution of the `unet`.
- """
- if is_accelerate_available() and is_accelerate_version(">=", "0.17.0.dev0"):
- from accelerate import cpu_offload_with_hook
- else:
- raise ImportError("`enable_model_cpu_offload` requires `accelerate v0.17.0` or higher.")
-
- device = torch.device(f"cuda:{gpu_id}")
-
- if self.device.type != "cpu":
- self.to("cpu", silence_dtype_warnings=True)
- torch.cuda.empty_cache() # otherwise we don't see the memory savings (but they probably exist)
-
- hook = None
- for cpu_offloaded_model in [self.text_encoder, self.unet, self.vae]:
- _, hook = cpu_offload_with_hook(cpu_offloaded_model, device, prev_module_hook=hook)
-
- if self.safety_checker is not None:
- _, hook = cpu_offload_with_hook(self.safety_checker, device, prev_module_hook=hook)
-
- # We'll offload the last model manually.
- self.final_offload_hook = hook
-
- # Copied from diffusers.pipelines.stable_diffusion.pipeline_stable_diffusion.StableDiffusionPipeline._encode_prompt
- def _encode_prompt(
- self,
- prompt,
- device,
- num_images_per_prompt,
- do_classifier_free_guidance,
- negative_prompt=None,
- prompt_embeds: Optional[torch.FloatTensor] = None,
- negative_prompt_embeds: Optional[torch.FloatTensor] = None,
- lora_scale: Optional[float] = None,
- ):
- r"""
- Encodes the prompt into text encoder hidden states.
-
- Args:
- prompt (`str` or `List[str]`, *optional*):
- prompt to be encoded
- device: (`torch.device`):
- torch device
- num_images_per_prompt (`int`):
- number of images that should be generated per prompt
- do_classifier_free_guidance (`bool`):
- whether to use classifier free guidance or not
- negative_prompt (`str` or `List[str]`, *optional*):
- The prompt or prompts not to guide the image generation. If not defined, one has to pass
- `negative_prompt_embeds` instead. Ignored when not using guidance (i.e., ignored if `guidance_scale` is
- less than `1`).
- prompt_embeds (`torch.FloatTensor`, *optional*):
- Pre-generated text embeddings. Can be used to easily tweak text inputs, *e.g.* prompt weighting. If not
- provided, text embeddings will be generated from `prompt` input argument.
- negative_prompt_embeds (`torch.FloatTensor`, *optional*):
- Pre-generated negative text embeddings. Can be used to easily tweak text inputs, *e.g.* prompt
- weighting. If not provided, negative_prompt_embeds will be generated from `negative_prompt` input
- argument.
- lora_scale (`float`, *optional*):
- A lora scale that will be applied to all LoRA layers of the text encoder if LoRA layers are loaded.
- """
- # set lora scale so that monkey patched LoRA
- # function of text encoder can correctly access it
- if lora_scale is not None and isinstance(self, LoraLoaderMixin):
- self._lora_scale = lora_scale
-
- if prompt is not None and isinstance(prompt, str):
- batch_size = 1
- elif prompt is not None and isinstance(prompt, list):
- batch_size = len(prompt)
- else:
- batch_size = prompt_embeds.shape[0]
-
- if prompt_embeds is None:
- # textual inversion: procecss multi-vector tokens if necessary
- if isinstance(self, TextualInversionLoaderMixin):
- prompt = self.maybe_convert_prompt(prompt, self.tokenizer)
-
- text_inputs = self.tokenizer(
- prompt,
- padding="max_length",
- max_length=self.tokenizer.model_max_length,
- truncation=True,
- return_tensors="pt",
- )
- text_input_ids = text_inputs.input_ids
- untruncated_ids = self.tokenizer(prompt, padding="longest", return_tensors="pt").input_ids
-
- if untruncated_ids.shape[-1] >= text_input_ids.shape[-1] and not torch.equal(
- text_input_ids, untruncated_ids
- ):
- removed_text = self.tokenizer.batch_decode(
- untruncated_ids[:, self.tokenizer.model_max_length - 1 : -1]
- )
- logger.warning(
- "The following part of your input was truncated because CLIP can only handle sequences up to"
- f" {self.tokenizer.model_max_length} tokens: {removed_text}"
- )
-
- if hasattr(self.text_encoder.config, "use_attention_mask") and self.text_encoder.config.use_attention_mask:
- attention_mask = text_inputs.attention_mask.to(device)
- else:
- attention_mask = None
-
- prompt_embeds = self.text_encoder(
- text_input_ids.to(device),
- attention_mask=attention_mask,
- )
- prompt_embeds = prompt_embeds[0]
-
- prompt_embeds = prompt_embeds.to(dtype=self.text_encoder.dtype, device=device)
-
- bs_embed, seq_len, _ = prompt_embeds.shape
- # duplicate text embeddings for each generation per prompt, using mps friendly method
- prompt_embeds = prompt_embeds.repeat(1, num_images_per_prompt, 1)
- prompt_embeds = prompt_embeds.view(bs_embed * num_images_per_prompt, seq_len, -1)
-
- # get unconditional embeddings for classifier free guidance
- if do_classifier_free_guidance and negative_prompt_embeds is None:
- uncond_tokens: List[str]
- if negative_prompt is None:
- uncond_tokens = [""] * batch_size
- elif prompt is not None and type(prompt) is not type(negative_prompt):
- raise TypeError(
- f"`negative_prompt` should be the same type to `prompt`, but got {type(negative_prompt)} !="
- f" {type(prompt)}."
- )
- elif isinstance(negative_prompt, str):
- uncond_tokens = [negative_prompt]
- elif batch_size != len(negative_prompt):
- raise ValueError(
- f"`negative_prompt`: {negative_prompt} has batch size {len(negative_prompt)}, but `prompt`:"
- f" {prompt} has batch size {batch_size}. Please make sure that passed `negative_prompt` matches"
- " the batch size of `prompt`."
- )
- else:
- uncond_tokens = negative_prompt
-
- # textual inversion: procecss multi-vector tokens if necessary
- if isinstance(self, TextualInversionLoaderMixin):
- uncond_tokens = self.maybe_convert_prompt(uncond_tokens, self.tokenizer)
-
- max_length = prompt_embeds.shape[1]
- uncond_input = self.tokenizer(
- uncond_tokens,
- padding="max_length",
- max_length=max_length,
- truncation=True,
- return_tensors="pt",
- )
-
- if hasattr(self.text_encoder.config, "use_attention_mask") and self.text_encoder.config.use_attention_mask:
- attention_mask = uncond_input.attention_mask.to(device)
- else:
- attention_mask = None
-
- negative_prompt_embeds = self.text_encoder(
- uncond_input.input_ids.to(device),
- attention_mask=attention_mask,
- )
- negative_prompt_embeds = negative_prompt_embeds[0]
-
- if do_classifier_free_guidance:
- # duplicate unconditional embeddings for each generation per prompt, using mps friendly method
- seq_len = negative_prompt_embeds.shape[1]
-
- negative_prompt_embeds = negative_prompt_embeds.to(dtype=self.text_encoder.dtype, device=device)
-
- negative_prompt_embeds = negative_prompt_embeds.repeat(1, num_images_per_prompt, 1)
- negative_prompt_embeds = negative_prompt_embeds.view(batch_size * num_images_per_prompt, seq_len, -1)
-
- # For classifier free guidance, we need to do two forward passes.
- # Here we concatenate the unconditional and text embeddings into a single batch
- # to avoid doing two forward passes
- prompt_embeds = torch.cat([negative_prompt_embeds, prompt_embeds])
-
- return prompt_embeds
-
- # Copied from diffusers.pipelines.stable_diffusion.pipeline_stable_diffusion_img2img.StableDiffusionImg2ImgPipeline.check_inputs
- def check_inputs(
- self, prompt, strength, callback_steps, negative_prompt=None, prompt_embeds=None, negative_prompt_embeds=None
- ):
- if strength < 0 or strength > 1:
- raise ValueError(f"The value of strength should in [0.0, 1.0] but is {strength}")
-
- if (callback_steps is None) or (
- callback_steps is not None and (not isinstance(callback_steps, int) or callback_steps <= 0)
- ):
- raise ValueError(
- f"`callback_steps` has to be a positive integer but is {callback_steps} of type"
- f" {type(callback_steps)}."
- )
-
- if prompt is not None and prompt_embeds is not None:
- raise ValueError(
- f"Cannot forward both `prompt`: {prompt} and `prompt_embeds`: {prompt_embeds}. Please make sure to"
- " only forward one of the two."
- )
- elif prompt is None and prompt_embeds is None:
- raise ValueError(
- "Provide either `prompt` or `prompt_embeds`. Cannot leave both `prompt` and `prompt_embeds` undefined."
- )
- elif prompt is not None and (not isinstance(prompt, str) and not isinstance(prompt, list)):
- raise ValueError(f"`prompt` has to be of type `str` or `list` but is {type(prompt)}")
-
- if negative_prompt is not None and negative_prompt_embeds is not None:
- raise ValueError(
- f"Cannot forward both `negative_prompt`: {negative_prompt} and `negative_prompt_embeds`:"
- f" {negative_prompt_embeds}. Please make sure to only forward one of the two."
- )
-
- if prompt_embeds is not None and negative_prompt_embeds is not None:
- if prompt_embeds.shape != negative_prompt_embeds.shape:
- raise ValueError(
- "`prompt_embeds` and `negative_prompt_embeds` must have the same shape when passed directly, but"
- f" got: `prompt_embeds` {prompt_embeds.shape} != `negative_prompt_embeds`"
- f" {negative_prompt_embeds.shape}."
- )
-
- # Copied from diffusers.pipelines.stable_diffusion.pipeline_stable_diffusion.StableDiffusionPipeline.prepare_extra_step_kwargs
- def prepare_extra_step_kwargs(self, generator, eta):
- # prepare extra kwargs for the scheduler step, since not all schedulers have the same signature
- # eta (η) is only used with the DDIMScheduler, it will be ignored for other schedulers.
- # eta corresponds to η in DDIM paper: https://arxiv.org/abs/2010.02502
- # and should be between [0, 1]
-
- accepts_eta = "eta" in set(inspect.signature(self.scheduler.step).parameters.keys())
- extra_step_kwargs = {}
- if accepts_eta:
- extra_step_kwargs["eta"] = eta
-
- # check if the scheduler accepts generator
- accepts_generator = "generator" in set(inspect.signature(self.scheduler.step).parameters.keys())
- if accepts_generator:
- extra_step_kwargs["generator"] = generator
- return extra_step_kwargs
-
- # Copied from diffusers.pipelines.stable_diffusion.pipeline_stable_diffusion.StableDiffusionPipeline.run_safety_checker
- def run_safety_checker(self, image, device, dtype):
- if self.safety_checker is None:
- has_nsfw_concept = None
- else:
- if torch.is_tensor(image):
- feature_extractor_input = self.image_processor.postprocess(image, output_type="pil")
- else:
- feature_extractor_input = self.image_processor.numpy_to_pil(image)
- safety_checker_input = self.feature_extractor(feature_extractor_input, return_tensors="pt").to(device)
- image, has_nsfw_concept = self.safety_checker(
- images=image, clip_input=safety_checker_input.pixel_values.to(dtype)
- )
- return image, has_nsfw_concept
-
- # Copied from diffusers.pipelines.stable_diffusion.pipeline_stable_diffusion.StableDiffusionPipeline.decode_latents
- def decode_latents(self, latents):
- warnings.warn(
- "The decode_latents method is deprecated and will be removed in a future version. Please"
- " use VaeImageProcessor instead",
- FutureWarning,
- )
- latents = 1 / self.vae.config.scaling_factor * latents
- image = self.vae.decode(latents, return_dict=False)[0]
- image = (image / 2 + 0.5).clamp(0, 1)
- # we always cast to float32 as this does not cause significant overhead and is compatible with bfloat16
- image = image.cpu().permute(0, 2, 3, 1).float().numpy()
- return image
-
- # Copied from diffusers.pipelines.stable_diffusion.pipeline_stable_diffusion_img2img.StableDiffusionImg2ImgPipeline.get_timesteps
- def get_timesteps(self, num_inference_steps, strength, device):
- # get the original timestep using init_timestep
- init_timestep = min(int(num_inference_steps * strength), num_inference_steps)
-
- t_start = max(num_inference_steps - init_timestep, 0)
- timesteps = self.scheduler.timesteps[t_start * self.scheduler.order :]
-
- return timesteps, num_inference_steps - t_start
-
- def prepare_latents(self, image, timestep, batch_size, num_images_per_prompt, dtype, device, generator=None):
- image = image.to(device=device, dtype=dtype)
-
- batch_size = image.shape[0]
-
- if image.shape[1] == 4:
- init_latents = image
-
- else:
- if isinstance(generator, list) and len(generator) != batch_size:
- raise ValueError(
- f"You have passed a list of generators of length {len(generator)}, but requested an effective batch"
- f" size of {batch_size}. Make sure the batch size matches the length of the generators."
- )
-
- if isinstance(generator, list):
- init_latents = [
- self.vae.encode(image[i : i + 1]).latent_dist.sample(generator[i]) for i in range(batch_size)
- ]
- init_latents = torch.cat(init_latents, dim=0)
- else:
- init_latents = self.vae.encode(image).latent_dist.sample(generator)
-
- init_latents = self.vae.config.scaling_factor * init_latents
-
- if batch_size > init_latents.shape[0] and batch_size % init_latents.shape[0] == 0:
- # expand init_latents for batch_size
- deprecation_message = (
- f"You have passed {batch_size} text prompts (`prompt`), but only {init_latents.shape[0]} initial"
- " images (`image`). Initial images are now duplicating to match the number of text prompts. Note"
- " that this behavior is deprecated and will be removed in a version 1.0.0. Please make sure to update"
- " your script to pass as many initial images as text prompts to suppress this warning."
- )
- deprecate("len(prompt) != len(image)", "1.0.0", deprecation_message, standard_warn=False)
- additional_image_per_prompt = batch_size // init_latents.shape[0]
- init_latents = torch.cat([init_latents] * additional_image_per_prompt * num_images_per_prompt, dim=0)
- elif batch_size > init_latents.shape[0] and batch_size % init_latents.shape[0] != 0:
- raise ValueError(
- f"Cannot duplicate `image` of batch size {init_latents.shape[0]} to {batch_size} text prompts."
- )
- else:
- init_latents = torch.cat([init_latents] * num_images_per_prompt, dim=0)
-
- # add noise to latents using the timestep
- shape = init_latents.shape
- noise = randn_tensor(shape, generator=generator, device=device, dtype=dtype)
-
- # get latents
- clean_latents = init_latents
- init_latents = self.scheduler.add_noise(init_latents, noise, timestep)
- latents = init_latents
-
- return latents, clean_latents
-
- @torch.no_grad()
- def __call__(
- self,
- prompt: Union[str, List[str]],
- source_prompt: Union[str, List[str]],
- image: Union[
- torch.FloatTensor,
- PIL.Image.Image,
- np.ndarray,
- List[torch.FloatTensor],
- List[PIL.Image.Image],
- List[np.ndarray],
- ] = None,
- strength: float = 0.8,
- num_inference_steps: Optional[int] = 50,
- guidance_scale: Optional[float] = 7.5,
- source_guidance_scale: Optional[float] = 1,
- num_images_per_prompt: Optional[int] = 1,
- eta: Optional[float] = 0.1,
- generator: Optional[Union[torch.Generator, List[torch.Generator]]] = None,
- prompt_embeds: Optional[torch.FloatTensor] = None,
- output_type: Optional[str] = "pil",
- return_dict: bool = True,
- callback: Optional[Callable[[int, int, torch.FloatTensor], None]] = None,
- callback_steps: int = 1,
- cross_attention_kwargs: Optional[Dict[str, Any]] = None,
- ):
- r"""
- The call function to the pipeline for generation.
-
- Args:
- prompt (`str` or `List[str]`):
- The prompt or prompts to guide the image generation.
- image (`torch.FloatTensor` `np.ndarray`, `PIL.Image.Image`, `List[torch.FloatTensor]`, `List[PIL.Image.Image]`, or `List[np.ndarray]`):
- `Image` or tensor representing an image batch to be used as the starting point. Can also accept image
- latents as `image`, but if passing latents directly it is not encoded again.
- strength (`float`, *optional*, defaults to 0.8):
- Indicates extent to transform the reference `image`. Must be between 0 and 1. `image` is used as a
- starting point and more noise is added the higher the `strength`. The number of denoising steps depends
- on the amount of noise initially added. When `strength` is 1, added noise is maximum and the denoising
- process runs for the full number of iterations specified in `num_inference_steps`. A value of 1
- essentially ignores `image`.
- num_inference_steps (`int`, *optional*, defaults to 50):
- The number of denoising steps. More denoising steps usually lead to a higher quality image at the
- expense of slower inference. This parameter is modulated by `strength`.
- guidance_scale (`float`, *optional*, defaults to 7.5):
- A higher guidance scale value encourages the model to generate images closely linked to the text
- `prompt` at the expense of lower image quality. Guidance scale is enabled when `guidance_scale > 1`.
- source_guidance_scale (`float`, *optional*, defaults to 1):
- Guidance scale for the source prompt. This is useful to control the amount of influence the source
- prompt has for encoding.
- num_images_per_prompt (`int`, *optional*, defaults to 1):
- The number of images to generate per prompt.
- eta (`float`, *optional*, defaults to 0.0):
- Corresponds to parameter eta (η) from the [DDIM](https://arxiv.org/abs/2010.02502) paper. Only applies
- to the [`~schedulers.DDIMScheduler`], and is ignored in other schedulers.
- generator (`torch.Generator` or `List[torch.Generator]`, *optional*):
- A [`torch.Generator`](https://pytorch.org/docs/stable/generated/torch.Generator.html) to make
- generation deterministic.
- prompt_embeds (`torch.FloatTensor`, *optional*):
- Pre-generated text embeddings. Can be used to easily tweak text inputs (prompt weighting). If not
- provided, text embeddings are generated from the `prompt` input argument.
- negative_prompt_embeds (`torch.FloatTensor`, *optional*):
- Pre-generated negative text embeddings. Can be used to easily tweak text inputs (prompt weighting). If
- not provided, `negative_prompt_embeds` are generated from the `negative_prompt` input argument.
- output_type (`str`, *optional*, defaults to `"pil"`):
- The output format of the generated image. Choose between `PIL.Image` or `np.array`.
- return_dict (`bool`, *optional*, defaults to `True`):
- Whether or not to return a [`~pipelines.stable_diffusion.StableDiffusionPipelineOutput`] instead of a
- plain tuple.
- callback (`Callable`, *optional*):
- A function that calls every `callback_steps` steps during inference. The function is called with the
- following arguments: `callback(step: int, timestep: int, latents: torch.FloatTensor)`.
- callback_steps (`int`, *optional*, defaults to 1):
- The frequency at which the `callback` function is called. If not specified, the callback is called at
- every step.
- cross_attention_kwargs (`dict`, *optional*):
- A kwargs dictionary that if specified is passed along to the [`AttentionProcessor`] as defined in
- [`self.processor`](https://github.com/huggingface/diffusers/blob/main/src/diffusers/models/cross_attention.py).
-
- Example:
-
- ```py
- import requests
- import torch
- from PIL import Image
- from io import BytesIO
-
- from diffusers import CycleDiffusionPipeline, DDIMScheduler
-
- # load the pipeline
- # make sure you're logged in with `huggingface-cli login`
- model_id_or_path = "CompVis/stable-diffusion-v1-4"
- scheduler = DDIMScheduler.from_pretrained(model_id_or_path, subfolder="scheduler")
- pipe = CycleDiffusionPipeline.from_pretrained(model_id_or_path, scheduler=scheduler).to("cuda")
-
- # let's download an initial image
- url = "https://raw.githubusercontent.com/ChenWu98/cycle-diffusion/main/data/dalle2/An%20astronaut%20riding%20a%20horse.png"
- response = requests.get(url)
- init_image = Image.open(BytesIO(response.content)).convert("RGB")
- init_image = init_image.resize((512, 512))
- init_image.save("horse.png")
-
- # let's specify a prompt
- source_prompt = "An astronaut riding a horse"
- prompt = "An astronaut riding an elephant"
-
- # call the pipeline
- image = pipe(
- prompt=prompt,
- source_prompt=source_prompt,
- image=init_image,
- num_inference_steps=100,
- eta=0.1,
- strength=0.8,
- guidance_scale=2,
- source_guidance_scale=1,
- ).images[0]
-
- image.save("horse_to_elephant.png")
-
- # let's try another example
- # See more samples at the original repo: https://github.com/ChenWu98/cycle-diffusion
- url = (
- "https://raw.githubusercontent.com/ChenWu98/cycle-diffusion/main/data/dalle2/A%20black%20colored%20car.png"
- )
- response = requests.get(url)
- init_image = Image.open(BytesIO(response.content)).convert("RGB")
- init_image = init_image.resize((512, 512))
- init_image.save("black.png")
-
- source_prompt = "A black colored car"
- prompt = "A blue colored car"
-
- # call the pipeline
- torch.manual_seed(0)
- image = pipe(
- prompt=prompt,
- source_prompt=source_prompt,
- image=init_image,
- num_inference_steps=100,
- eta=0.1,
- strength=0.85,
- guidance_scale=3,
- source_guidance_scale=1,
- ).images[0]
-
- image.save("black_to_blue.png")
- ```
-
- Returns:
- [`~pipelines.stable_diffusion.StableDiffusionPipelineOutput`] or `tuple`:
- If `return_dict` is `True`, [`~pipelines.stable_diffusion.StableDiffusionPipelineOutput`] is returned,
- otherwise a `tuple` is returned where the first element is a list with the generated images and the
- second element is a list of `bool`s indicating whether the corresponding generated image contains
- "not-safe-for-work" (nsfw) content.
- """
- # 1. Check inputs
- self.check_inputs(prompt, strength, callback_steps)
-
- # 2. Define call parameters
- batch_size = 1 if isinstance(prompt, str) else len(prompt)
- device = self._execution_device
- # here `guidance_scale` is defined analog to the guidance weight `w` of equation (2)
- # of the Imagen paper: https://arxiv.org/pdf/2205.11487.pdf . `guidance_scale = 1`
- # corresponds to doing no classifier free guidance.
- do_classifier_free_guidance = guidance_scale > 1.0
-
- # 3. Encode input prompt
- text_encoder_lora_scale = (
- cross_attention_kwargs.get("scale", None) if cross_attention_kwargs is not None else None
- )
- prompt_embeds = self._encode_prompt(
- prompt,
- device,
- num_images_per_prompt,
- do_classifier_free_guidance,
- prompt_embeds=prompt_embeds,
- lora_scale=text_encoder_lora_scale,
- )
- source_prompt_embeds = self._encode_prompt(
- source_prompt, device, num_images_per_prompt, do_classifier_free_guidance, None
- )
-
- # 4. Preprocess image
- image = self.image_processor.preprocess(image)
-
- # 5. Prepare timesteps
- self.scheduler.set_timesteps(num_inference_steps, device=device)
- timesteps, num_inference_steps = self.get_timesteps(num_inference_steps, strength, device)
- latent_timestep = timesteps[:1].repeat(batch_size * num_images_per_prompt)
-
- # 6. Prepare latent variables
- latents, clean_latents = self.prepare_latents(
- image, latent_timestep, batch_size, num_images_per_prompt, prompt_embeds.dtype, device, generator
- )
- source_latents = latents
-
- # 7. Prepare extra step kwargs. TODO: Logic should ideally just be moved out of the pipeline
- extra_step_kwargs = self.prepare_extra_step_kwargs(generator, eta)
- generator = extra_step_kwargs.pop("generator", None)
-
- # 8. Denoising loop
- num_warmup_steps = len(timesteps) - num_inference_steps * self.scheduler.order
- with self.progress_bar(total=num_inference_steps) as progress_bar:
- for i, t in enumerate(timesteps):
- # expand the latents if we are doing classifier free guidance
- latent_model_input = torch.cat([latents] * 2) if do_classifier_free_guidance else latents
- source_latent_model_input = (
- torch.cat([source_latents] * 2) if do_classifier_free_guidance else source_latents
- )
- latent_model_input = self.scheduler.scale_model_input(latent_model_input, t)
- source_latent_model_input = self.scheduler.scale_model_input(source_latent_model_input, t)
-
- # predict the noise residual
- if do_classifier_free_guidance:
- concat_latent_model_input = torch.stack(
- [
- source_latent_model_input[0],
- latent_model_input[0],
- source_latent_model_input[1],
- latent_model_input[1],
- ],
- dim=0,
- )
- concat_prompt_embeds = torch.stack(
- [
- source_prompt_embeds[0],
- prompt_embeds[0],
- source_prompt_embeds[1],
- prompt_embeds[1],
- ],
- dim=0,
- )
- else:
- concat_latent_model_input = torch.cat(
- [
- source_latent_model_input,
- latent_model_input,
- ],
- dim=0,
- )
- concat_prompt_embeds = torch.cat(
- [
- source_prompt_embeds,
- prompt_embeds,
- ],
- dim=0,
- )
-
- concat_noise_pred = self.unet(
- concat_latent_model_input,
- t,
- cross_attention_kwargs=cross_attention_kwargs,
- encoder_hidden_states=concat_prompt_embeds,
- ).sample
-
- # perform guidance
- if do_classifier_free_guidance:
- (
- source_noise_pred_uncond,
- noise_pred_uncond,
- source_noise_pred_text,
- noise_pred_text,
- ) = concat_noise_pred.chunk(4, dim=0)
-
- noise_pred = noise_pred_uncond + guidance_scale * (noise_pred_text - noise_pred_uncond)
- source_noise_pred = source_noise_pred_uncond + source_guidance_scale * (
- source_noise_pred_text - source_noise_pred_uncond
- )
-
- else:
- (source_noise_pred, noise_pred) = concat_noise_pred.chunk(2, dim=0)
-
- # Sample source_latents from the posterior distribution.
- prev_source_latents = posterior_sample(
- self.scheduler, source_latents, t, clean_latents, generator=generator, **extra_step_kwargs
- )
- # Compute noise.
- noise = compute_noise(
- self.scheduler, prev_source_latents, source_latents, t, source_noise_pred, **extra_step_kwargs
- )
- source_latents = prev_source_latents
-
- # compute the previous noisy sample x_t -> x_t-1
- latents = self.scheduler.step(
- noise_pred, t, latents, variance_noise=noise, **extra_step_kwargs
- ).prev_sample
-
- # call the callback, if provided
- if i == len(timesteps) - 1 or ((i + 1) > num_warmup_steps and (i + 1) % self.scheduler.order == 0):
- progress_bar.update()
- if callback is not None and i % callback_steps == 0:
- callback(i, t, latents)
-
- # 9. Post-processing
- if not output_type == "latent":
- image = self.vae.decode(latents / self.vae.config.scaling_factor, return_dict=False)[0]
- image, has_nsfw_concept = self.run_safety_checker(image, device, prompt_embeds.dtype)
- else:
- image = latents
- has_nsfw_concept = None
-
- if has_nsfw_concept is None:
- do_denormalize = [True] * image.shape[0]
- else:
- do_denormalize = [not has_nsfw for has_nsfw in has_nsfw_concept]
-
- image = self.image_processor.postprocess(image, output_type=output_type, do_denormalize=do_denormalize)
-
- if not return_dict:
- return (image, has_nsfw_concept)
-
- return StableDiffusionPipelineOutput(images=image, nsfw_content_detected=has_nsfw_concept)
diff --git a/spaces/Andy1621/uniformer_image_detection/exp/cascade_mask_rcnn_3x_ms_hybrid_base/run.sh b/spaces/Andy1621/uniformer_image_detection/exp/cascade_mask_rcnn_3x_ms_hybrid_base/run.sh
deleted file mode 100644
index 453f0a0a27d04f08558ec1b03312f7815ca991da..0000000000000000000000000000000000000000
--- a/spaces/Andy1621/uniformer_image_detection/exp/cascade_mask_rcnn_3x_ms_hybrid_base/run.sh
+++ /dev/null
@@ -1,10 +0,0 @@
-#!/usr/bin/env bash
-
-work_path=$(dirname $0)
-PYTHONPATH="$(dirname $0)/../../":$PYTHONPATH \
-python -m torch.distributed.launch --nproc_per_node=8 \
- tools/train.py ${work_path}/config.py \
- --launcher pytorch \
- --cfg-options model.backbone.pretrained_path='your_model_path/uniformer_base_in1k.pth' \
- --work-dir ${work_path}/ckpt \
- 2>&1 | tee -a ${work_path}/log.txt
diff --git a/spaces/Andy1621/uniformer_image_detection/mmdet/models/backbones/resnet.py b/spaces/Andy1621/uniformer_image_detection/mmdet/models/backbones/resnet.py
deleted file mode 100644
index 3826815a6d94fdc4c54001d4c186d10ca3380e80..0000000000000000000000000000000000000000
--- a/spaces/Andy1621/uniformer_image_detection/mmdet/models/backbones/resnet.py
+++ /dev/null
@@ -1,663 +0,0 @@
-import torch.nn as nn
-import torch.utils.checkpoint as cp
-from mmcv.cnn import (build_conv_layer, build_norm_layer, build_plugin_layer,
- constant_init, kaiming_init)
-from mmcv.runner import load_checkpoint
-from torch.nn.modules.batchnorm import _BatchNorm
-
-from mmdet.utils import get_root_logger
-from ..builder import BACKBONES
-from ..utils import ResLayer
-
-
-class BasicBlock(nn.Module):
- expansion = 1
-
- def __init__(self,
- inplanes,
- planes,
- stride=1,
- dilation=1,
- downsample=None,
- style='pytorch',
- with_cp=False,
- conv_cfg=None,
- norm_cfg=dict(type='BN'),
- dcn=None,
- plugins=None):
- super(BasicBlock, self).__init__()
- assert dcn is None, 'Not implemented yet.'
- assert plugins is None, 'Not implemented yet.'
-
- self.norm1_name, norm1 = build_norm_layer(norm_cfg, planes, postfix=1)
- self.norm2_name, norm2 = build_norm_layer(norm_cfg, planes, postfix=2)
-
- self.conv1 = build_conv_layer(
- conv_cfg,
- inplanes,
- planes,
- 3,
- stride=stride,
- padding=dilation,
- dilation=dilation,
- bias=False)
- self.add_module(self.norm1_name, norm1)
- self.conv2 = build_conv_layer(
- conv_cfg, planes, planes, 3, padding=1, bias=False)
- self.add_module(self.norm2_name, norm2)
-
- self.relu = nn.ReLU(inplace=True)
- self.downsample = downsample
- self.stride = stride
- self.dilation = dilation
- self.with_cp = with_cp
-
- @property
- def norm1(self):
- """nn.Module: normalization layer after the first convolution layer"""
- return getattr(self, self.norm1_name)
-
- @property
- def norm2(self):
- """nn.Module: normalization layer after the second convolution layer"""
- return getattr(self, self.norm2_name)
-
- def forward(self, x):
- """Forward function."""
-
- def _inner_forward(x):
- identity = x
-
- out = self.conv1(x)
- out = self.norm1(out)
- out = self.relu(out)
-
- out = self.conv2(out)
- out = self.norm2(out)
-
- if self.downsample is not None:
- identity = self.downsample(x)
-
- out += identity
-
- return out
-
- if self.with_cp and x.requires_grad:
- out = cp.checkpoint(_inner_forward, x)
- else:
- out = _inner_forward(x)
-
- out = self.relu(out)
-
- return out
-
-
-class Bottleneck(nn.Module):
- expansion = 4
-
- def __init__(self,
- inplanes,
- planes,
- stride=1,
- dilation=1,
- downsample=None,
- style='pytorch',
- with_cp=False,
- conv_cfg=None,
- norm_cfg=dict(type='BN'),
- dcn=None,
- plugins=None):
- """Bottleneck block for ResNet.
-
- If style is "pytorch", the stride-two layer is the 3x3 conv layer, if
- it is "caffe", the stride-two layer is the first 1x1 conv layer.
- """
- super(Bottleneck, self).__init__()
- assert style in ['pytorch', 'caffe']
- assert dcn is None or isinstance(dcn, dict)
- assert plugins is None or isinstance(plugins, list)
- if plugins is not None:
- allowed_position = ['after_conv1', 'after_conv2', 'after_conv3']
- assert all(p['position'] in allowed_position for p in plugins)
-
- self.inplanes = inplanes
- self.planes = planes
- self.stride = stride
- self.dilation = dilation
- self.style = style
- self.with_cp = with_cp
- self.conv_cfg = conv_cfg
- self.norm_cfg = norm_cfg
- self.dcn = dcn
- self.with_dcn = dcn is not None
- self.plugins = plugins
- self.with_plugins = plugins is not None
-
- if self.with_plugins:
- # collect plugins for conv1/conv2/conv3
- self.after_conv1_plugins = [
- plugin['cfg'] for plugin in plugins
- if plugin['position'] == 'after_conv1'
- ]
- self.after_conv2_plugins = [
- plugin['cfg'] for plugin in plugins
- if plugin['position'] == 'after_conv2'
- ]
- self.after_conv3_plugins = [
- plugin['cfg'] for plugin in plugins
- if plugin['position'] == 'after_conv3'
- ]
-
- if self.style == 'pytorch':
- self.conv1_stride = 1
- self.conv2_stride = stride
- else:
- self.conv1_stride = stride
- self.conv2_stride = 1
-
- self.norm1_name, norm1 = build_norm_layer(norm_cfg, planes, postfix=1)
- self.norm2_name, norm2 = build_norm_layer(norm_cfg, planes, postfix=2)
- self.norm3_name, norm3 = build_norm_layer(
- norm_cfg, planes * self.expansion, postfix=3)
-
- self.conv1 = build_conv_layer(
- conv_cfg,
- inplanes,
- planes,
- kernel_size=1,
- stride=self.conv1_stride,
- bias=False)
- self.add_module(self.norm1_name, norm1)
- fallback_on_stride = False
- if self.with_dcn:
- fallback_on_stride = dcn.pop('fallback_on_stride', False)
- if not self.with_dcn or fallback_on_stride:
- self.conv2 = build_conv_layer(
- conv_cfg,
- planes,
- planes,
- kernel_size=3,
- stride=self.conv2_stride,
- padding=dilation,
- dilation=dilation,
- bias=False)
- else:
- assert self.conv_cfg is None, 'conv_cfg must be None for DCN'
- self.conv2 = build_conv_layer(
- dcn,
- planes,
- planes,
- kernel_size=3,
- stride=self.conv2_stride,
- padding=dilation,
- dilation=dilation,
- bias=False)
-
- self.add_module(self.norm2_name, norm2)
- self.conv3 = build_conv_layer(
- conv_cfg,
- planes,
- planes * self.expansion,
- kernel_size=1,
- bias=False)
- self.add_module(self.norm3_name, norm3)
-
- self.relu = nn.ReLU(inplace=True)
- self.downsample = downsample
-
- if self.with_plugins:
- self.after_conv1_plugin_names = self.make_block_plugins(
- planes, self.after_conv1_plugins)
- self.after_conv2_plugin_names = self.make_block_plugins(
- planes, self.after_conv2_plugins)
- self.after_conv3_plugin_names = self.make_block_plugins(
- planes * self.expansion, self.after_conv3_plugins)
-
- def make_block_plugins(self, in_channels, plugins):
- """make plugins for block.
-
- Args:
- in_channels (int): Input channels of plugin.
- plugins (list[dict]): List of plugins cfg to build.
-
- Returns:
- list[str]: List of the names of plugin.
- """
- assert isinstance(plugins, list)
- plugin_names = []
- for plugin in plugins:
- plugin = plugin.copy()
- name, layer = build_plugin_layer(
- plugin,
- in_channels=in_channels,
- postfix=plugin.pop('postfix', ''))
- assert not hasattr(self, name), f'duplicate plugin {name}'
- self.add_module(name, layer)
- plugin_names.append(name)
- return plugin_names
-
- def forward_plugin(self, x, plugin_names):
- out = x
- for name in plugin_names:
- out = getattr(self, name)(x)
- return out
-
- @property
- def norm1(self):
- """nn.Module: normalization layer after the first convolution layer"""
- return getattr(self, self.norm1_name)
-
- @property
- def norm2(self):
- """nn.Module: normalization layer after the second convolution layer"""
- return getattr(self, self.norm2_name)
-
- @property
- def norm3(self):
- """nn.Module: normalization layer after the third convolution layer"""
- return getattr(self, self.norm3_name)
-
- def forward(self, x):
- """Forward function."""
-
- def _inner_forward(x):
- identity = x
- out = self.conv1(x)
- out = self.norm1(out)
- out = self.relu(out)
-
- if self.with_plugins:
- out = self.forward_plugin(out, self.after_conv1_plugin_names)
-
- out = self.conv2(out)
- out = self.norm2(out)
- out = self.relu(out)
-
- if self.with_plugins:
- out = self.forward_plugin(out, self.after_conv2_plugin_names)
-
- out = self.conv3(out)
- out = self.norm3(out)
-
- if self.with_plugins:
- out = self.forward_plugin(out, self.after_conv3_plugin_names)
-
- if self.downsample is not None:
- identity = self.downsample(x)
-
- out += identity
-
- return out
-
- if self.with_cp and x.requires_grad:
- out = cp.checkpoint(_inner_forward, x)
- else:
- out = _inner_forward(x)
-
- out = self.relu(out)
-
- return out
-
-
-@BACKBONES.register_module()
-class ResNet(nn.Module):
- """ResNet backbone.
-
- Args:
- depth (int): Depth of resnet, from {18, 34, 50, 101, 152}.
- stem_channels (int | None): Number of stem channels. If not specified,
- it will be the same as `base_channels`. Default: None.
- base_channels (int): Number of base channels of res layer. Default: 64.
- in_channels (int): Number of input image channels. Default: 3.
- num_stages (int): Resnet stages. Default: 4.
- strides (Sequence[int]): Strides of the first block of each stage.
- dilations (Sequence[int]): Dilation of each stage.
- out_indices (Sequence[int]): Output from which stages.
- style (str): `pytorch` or `caffe`. If set to "pytorch", the stride-two
- layer is the 3x3 conv layer, otherwise the stride-two layer is
- the first 1x1 conv layer.
- deep_stem (bool): Replace 7x7 conv in input stem with 3 3x3 conv
- avg_down (bool): Use AvgPool instead of stride conv when
- downsampling in the bottleneck.
- frozen_stages (int): Stages to be frozen (stop grad and set eval mode).
- -1 means not freezing any parameters.
- norm_cfg (dict): Dictionary to construct and config norm layer.
- norm_eval (bool): Whether to set norm layers to eval mode, namely,
- freeze running stats (mean and var). Note: Effect on Batch Norm
- and its variants only.
- plugins (list[dict]): List of plugins for stages, each dict contains:
-
- - cfg (dict, required): Cfg dict to build plugin.
- - position (str, required): Position inside block to insert
- plugin, options are 'after_conv1', 'after_conv2', 'after_conv3'.
- - stages (tuple[bool], optional): Stages to apply plugin, length
- should be same as 'num_stages'.
- with_cp (bool): Use checkpoint or not. Using checkpoint will save some
- memory while slowing down the training speed.
- zero_init_residual (bool): Whether to use zero init for last norm layer
- in resblocks to let them behave as identity.
-
- Example:
- >>> from mmdet.models import ResNet
- >>> import torch
- >>> self = ResNet(depth=18)
- >>> self.eval()
- >>> inputs = torch.rand(1, 3, 32, 32)
- >>> level_outputs = self.forward(inputs)
- >>> for level_out in level_outputs:
- ... print(tuple(level_out.shape))
- (1, 64, 8, 8)
- (1, 128, 4, 4)
- (1, 256, 2, 2)
- (1, 512, 1, 1)
- """
-
- arch_settings = {
- 18: (BasicBlock, (2, 2, 2, 2)),
- 34: (BasicBlock, (3, 4, 6, 3)),
- 50: (Bottleneck, (3, 4, 6, 3)),
- 101: (Bottleneck, (3, 4, 23, 3)),
- 152: (Bottleneck, (3, 8, 36, 3))
- }
-
- def __init__(self,
- depth,
- in_channels=3,
- stem_channels=None,
- base_channels=64,
- num_stages=4,
- strides=(1, 2, 2, 2),
- dilations=(1, 1, 1, 1),
- out_indices=(0, 1, 2, 3),
- style='pytorch',
- deep_stem=False,
- avg_down=False,
- frozen_stages=-1,
- conv_cfg=None,
- norm_cfg=dict(type='BN', requires_grad=True),
- norm_eval=True,
- dcn=None,
- stage_with_dcn=(False, False, False, False),
- plugins=None,
- with_cp=False,
- zero_init_residual=True):
- super(ResNet, self).__init__()
- if depth not in self.arch_settings:
- raise KeyError(f'invalid depth {depth} for resnet')
- self.depth = depth
- if stem_channels is None:
- stem_channels = base_channels
- self.stem_channels = stem_channels
- self.base_channels = base_channels
- self.num_stages = num_stages
- assert num_stages >= 1 and num_stages <= 4
- self.strides = strides
- self.dilations = dilations
- assert len(strides) == len(dilations) == num_stages
- self.out_indices = out_indices
- assert max(out_indices) < num_stages
- self.style = style
- self.deep_stem = deep_stem
- self.avg_down = avg_down
- self.frozen_stages = frozen_stages
- self.conv_cfg = conv_cfg
- self.norm_cfg = norm_cfg
- self.with_cp = with_cp
- self.norm_eval = norm_eval
- self.dcn = dcn
- self.stage_with_dcn = stage_with_dcn
- if dcn is not None:
- assert len(stage_with_dcn) == num_stages
- self.plugins = plugins
- self.zero_init_residual = zero_init_residual
- self.block, stage_blocks = self.arch_settings[depth]
- self.stage_blocks = stage_blocks[:num_stages]
- self.inplanes = stem_channels
-
- self._make_stem_layer(in_channels, stem_channels)
-
- self.res_layers = []
- for i, num_blocks in enumerate(self.stage_blocks):
- stride = strides[i]
- dilation = dilations[i]
- dcn = self.dcn if self.stage_with_dcn[i] else None
- if plugins is not None:
- stage_plugins = self.make_stage_plugins(plugins, i)
- else:
- stage_plugins = None
- planes = base_channels * 2**i
- res_layer = self.make_res_layer(
- block=self.block,
- inplanes=self.inplanes,
- planes=planes,
- num_blocks=num_blocks,
- stride=stride,
- dilation=dilation,
- style=self.style,
- avg_down=self.avg_down,
- with_cp=with_cp,
- conv_cfg=conv_cfg,
- norm_cfg=norm_cfg,
- dcn=dcn,
- plugins=stage_plugins)
- self.inplanes = planes * self.block.expansion
- layer_name = f'layer{i + 1}'
- self.add_module(layer_name, res_layer)
- self.res_layers.append(layer_name)
-
- self._freeze_stages()
-
- self.feat_dim = self.block.expansion * base_channels * 2**(
- len(self.stage_blocks) - 1)
-
- def make_stage_plugins(self, plugins, stage_idx):
- """Make plugins for ResNet ``stage_idx`` th stage.
-
- Currently we support to insert ``context_block``,
- ``empirical_attention_block``, ``nonlocal_block`` into the backbone
- like ResNet/ResNeXt. They could be inserted after conv1/conv2/conv3 of
- Bottleneck.
-
- An example of plugins format could be:
-
- Examples:
- >>> plugins=[
- ... dict(cfg=dict(type='xxx', arg1='xxx'),
- ... stages=(False, True, True, True),
- ... position='after_conv2'),
- ... dict(cfg=dict(type='yyy'),
- ... stages=(True, True, True, True),
- ... position='after_conv3'),
- ... dict(cfg=dict(type='zzz', postfix='1'),
- ... stages=(True, True, True, True),
- ... position='after_conv3'),
- ... dict(cfg=dict(type='zzz', postfix='2'),
- ... stages=(True, True, True, True),
- ... position='after_conv3')
- ... ]
- >>> self = ResNet(depth=18)
- >>> stage_plugins = self.make_stage_plugins(plugins, 0)
- >>> assert len(stage_plugins) == 3
-
- Suppose ``stage_idx=0``, the structure of blocks in the stage would be:
-
- .. code-block:: none
-
- conv1-> conv2->conv3->yyy->zzz1->zzz2
-
- Suppose 'stage_idx=1', the structure of blocks in the stage would be:
-
- .. code-block:: none
-
- conv1-> conv2->xxx->conv3->yyy->zzz1->zzz2
-
- If stages is missing, the plugin would be applied to all stages.
-
- Args:
- plugins (list[dict]): List of plugins cfg to build. The postfix is
- required if multiple same type plugins are inserted.
- stage_idx (int): Index of stage to build
-
- Returns:
- list[dict]: Plugins for current stage
- """
- stage_plugins = []
- for plugin in plugins:
- plugin = plugin.copy()
- stages = plugin.pop('stages', None)
- assert stages is None or len(stages) == self.num_stages
- # whether to insert plugin into current stage
- if stages is None or stages[stage_idx]:
- stage_plugins.append(plugin)
-
- return stage_plugins
-
- def make_res_layer(self, **kwargs):
- """Pack all blocks in a stage into a ``ResLayer``."""
- return ResLayer(**kwargs)
-
- @property
- def norm1(self):
- """nn.Module: the normalization layer named "norm1" """
- return getattr(self, self.norm1_name)
-
- def _make_stem_layer(self, in_channels, stem_channels):
- if self.deep_stem:
- self.stem = nn.Sequential(
- build_conv_layer(
- self.conv_cfg,
- in_channels,
- stem_channels // 2,
- kernel_size=3,
- stride=2,
- padding=1,
- bias=False),
- build_norm_layer(self.norm_cfg, stem_channels // 2)[1],
- nn.ReLU(inplace=True),
- build_conv_layer(
- self.conv_cfg,
- stem_channels // 2,
- stem_channels // 2,
- kernel_size=3,
- stride=1,
- padding=1,
- bias=False),
- build_norm_layer(self.norm_cfg, stem_channels // 2)[1],
- nn.ReLU(inplace=True),
- build_conv_layer(
- self.conv_cfg,
- stem_channels // 2,
- stem_channels,
- kernel_size=3,
- stride=1,
- padding=1,
- bias=False),
- build_norm_layer(self.norm_cfg, stem_channels)[1],
- nn.ReLU(inplace=True))
- else:
- self.conv1 = build_conv_layer(
- self.conv_cfg,
- in_channels,
- stem_channels,
- kernel_size=7,
- stride=2,
- padding=3,
- bias=False)
- self.norm1_name, norm1 = build_norm_layer(
- self.norm_cfg, stem_channels, postfix=1)
- self.add_module(self.norm1_name, norm1)
- self.relu = nn.ReLU(inplace=True)
- self.maxpool = nn.MaxPool2d(kernel_size=3, stride=2, padding=1)
-
- def _freeze_stages(self):
- if self.frozen_stages >= 0:
- if self.deep_stem:
- self.stem.eval()
- for param in self.stem.parameters():
- param.requires_grad = False
- else:
- self.norm1.eval()
- for m in [self.conv1, self.norm1]:
- for param in m.parameters():
- param.requires_grad = False
-
- for i in range(1, self.frozen_stages + 1):
- m = getattr(self, f'layer{i}')
- m.eval()
- for param in m.parameters():
- param.requires_grad = False
-
- def init_weights(self, pretrained=None):
- """Initialize the weights in backbone.
-
- Args:
- pretrained (str, optional): Path to pre-trained weights.
- Defaults to None.
- """
- if isinstance(pretrained, str):
- logger = get_root_logger()
- load_checkpoint(self, pretrained, strict=False, logger=logger)
- elif pretrained is None:
- for m in self.modules():
- if isinstance(m, nn.Conv2d):
- kaiming_init(m)
- elif isinstance(m, (_BatchNorm, nn.GroupNorm)):
- constant_init(m, 1)
-
- if self.dcn is not None:
- for m in self.modules():
- if isinstance(m, Bottleneck) and hasattr(
- m.conv2, 'conv_offset'):
- constant_init(m.conv2.conv_offset, 0)
-
- if self.zero_init_residual:
- for m in self.modules():
- if isinstance(m, Bottleneck):
- constant_init(m.norm3, 0)
- elif isinstance(m, BasicBlock):
- constant_init(m.norm2, 0)
- else:
- raise TypeError('pretrained must be a str or None')
-
- def forward(self, x):
- """Forward function."""
- if self.deep_stem:
- x = self.stem(x)
- else:
- x = self.conv1(x)
- x = self.norm1(x)
- x = self.relu(x)
- x = self.maxpool(x)
- outs = []
- for i, layer_name in enumerate(self.res_layers):
- res_layer = getattr(self, layer_name)
- x = res_layer(x)
- if i in self.out_indices:
- outs.append(x)
- return tuple(outs)
-
- def train(self, mode=True):
- """Convert the model into training mode while keep normalization layer
- freezed."""
- super(ResNet, self).train(mode)
- self._freeze_stages()
- if mode and self.norm_eval:
- for m in self.modules():
- # trick: eval have effect on BatchNorm only
- if isinstance(m, _BatchNorm):
- m.eval()
-
-
-@BACKBONES.register_module()
-class ResNetV1d(ResNet):
- r"""ResNetV1d variant described in `Bag of Tricks
- `_.
-
- Compared with default ResNet(ResNetV1b), ResNetV1d replaces the 7x7 conv in
- the input stem with three 3x3 convs. And in the downsampling block, a 2x2
- avg_pool with stride 2 is added before conv, whose stride is changed to 1.
- """
-
- def __init__(self, **kwargs):
- super(ResNetV1d, self).__init__(
- deep_stem=True, avg_down=True, **kwargs)
diff --git a/spaces/Andy1621/uniformer_image_detection/tools/analysis_tools/analyze_logs.py b/spaces/Andy1621/uniformer_image_detection/tools/analysis_tools/analyze_logs.py
deleted file mode 100644
index 83464f76ef3155be80289431188492c911f5b482..0000000000000000000000000000000000000000
--- a/spaces/Andy1621/uniformer_image_detection/tools/analysis_tools/analyze_logs.py
+++ /dev/null
@@ -1,179 +0,0 @@
-import argparse
-import json
-from collections import defaultdict
-
-import matplotlib.pyplot as plt
-import numpy as np
-import seaborn as sns
-
-
-def cal_train_time(log_dicts, args):
- for i, log_dict in enumerate(log_dicts):
- print(f'{"-" * 5}Analyze train time of {args.json_logs[i]}{"-" * 5}')
- all_times = []
- for epoch in log_dict.keys():
- if args.include_outliers:
- all_times.append(log_dict[epoch]['time'])
- else:
- all_times.append(log_dict[epoch]['time'][1:])
- all_times = np.array(all_times)
- epoch_ave_time = all_times.mean(-1)
- slowest_epoch = epoch_ave_time.argmax()
- fastest_epoch = epoch_ave_time.argmin()
- std_over_epoch = epoch_ave_time.std()
- print(f'slowest epoch {slowest_epoch + 1}, '
- f'average time is {epoch_ave_time[slowest_epoch]:.4f}')
- print(f'fastest epoch {fastest_epoch + 1}, '
- f'average time is {epoch_ave_time[fastest_epoch]:.4f}')
- print(f'time std over epochs is {std_over_epoch:.4f}')
- print(f'average iter time: {np.mean(all_times):.4f} s/iter')
- print()
-
-
-def plot_curve(log_dicts, args):
- if args.backend is not None:
- plt.switch_backend(args.backend)
- sns.set_style(args.style)
- # if legend is None, use {filename}_{key} as legend
- legend = args.legend
- if legend is None:
- legend = []
- for json_log in args.json_logs:
- for metric in args.keys:
- legend.append(f'{json_log}_{metric}')
- assert len(legend) == (len(args.json_logs) * len(args.keys))
- metrics = args.keys
-
- num_metrics = len(metrics)
- for i, log_dict in enumerate(log_dicts):
- epochs = list(log_dict.keys())
- for j, metric in enumerate(metrics):
- print(f'plot curve of {args.json_logs[i]}, metric is {metric}')
- if metric not in log_dict[epochs[0]]:
- raise KeyError(
- f'{args.json_logs[i]} does not contain metric {metric}')
-
- if 'mAP' in metric:
- xs = np.arange(1, max(epochs) + 1)
- ys = []
- for epoch in epochs:
- ys += log_dict[epoch][metric]
- ax = plt.gca()
- ax.set_xticks(xs)
- plt.xlabel('epoch')
- plt.plot(xs, ys, label=legend[i * num_metrics + j], marker='o')
- else:
- xs = []
- ys = []
- num_iters_per_epoch = log_dict[epochs[0]]['iter'][-1]
- for epoch in epochs:
- iters = log_dict[epoch]['iter']
- if log_dict[epoch]['mode'][-1] == 'val':
- iters = iters[:-1]
- xs.append(
- np.array(iters) + (epoch - 1) * num_iters_per_epoch)
- ys.append(np.array(log_dict[epoch][metric][:len(iters)]))
- xs = np.concatenate(xs)
- ys = np.concatenate(ys)
- plt.xlabel('iter')
- plt.plot(
- xs, ys, label=legend[i * num_metrics + j], linewidth=0.5)
- plt.legend()
- if args.title is not None:
- plt.title(args.title)
- if args.out is None:
- plt.show()
- else:
- print(f'save curve to: {args.out}')
- plt.savefig(args.out)
- plt.cla()
-
-
-def add_plot_parser(subparsers):
- parser_plt = subparsers.add_parser(
- 'plot_curve', help='parser for plotting curves')
- parser_plt.add_argument(
- 'json_logs',
- type=str,
- nargs='+',
- help='path of train log in json format')
- parser_plt.add_argument(
- '--keys',
- type=str,
- nargs='+',
- default=['bbox_mAP'],
- help='the metric that you want to plot')
- parser_plt.add_argument('--title', type=str, help='title of figure')
- parser_plt.add_argument(
- '--legend',
- type=str,
- nargs='+',
- default=None,
- help='legend of each plot')
- parser_plt.add_argument(
- '--backend', type=str, default=None, help='backend of plt')
- parser_plt.add_argument(
- '--style', type=str, default='dark', help='style of plt')
- parser_plt.add_argument('--out', type=str, default=None)
-
-
-def add_time_parser(subparsers):
- parser_time = subparsers.add_parser(
- 'cal_train_time',
- help='parser for computing the average time per training iteration')
- parser_time.add_argument(
- 'json_logs',
- type=str,
- nargs='+',
- help='path of train log in json format')
- parser_time.add_argument(
- '--include-outliers',
- action='store_true',
- help='include the first value of every epoch when computing '
- 'the average time')
-
-
-def parse_args():
- parser = argparse.ArgumentParser(description='Analyze Json Log')
- # currently only support plot curve and calculate average train time
- subparsers = parser.add_subparsers(dest='task', help='task parser')
- add_plot_parser(subparsers)
- add_time_parser(subparsers)
- args = parser.parse_args()
- return args
-
-
-def load_json_logs(json_logs):
- # load and convert json_logs to log_dict, key is epoch, value is a sub dict
- # keys of sub dict is different metrics, e.g. memory, bbox_mAP
- # value of sub dict is a list of corresponding values of all iterations
- log_dicts = [dict() for _ in json_logs]
- for json_log, log_dict in zip(json_logs, log_dicts):
- with open(json_log, 'r') as log_file:
- for line in log_file:
- log = json.loads(line.strip())
- # skip lines without `epoch` field
- if 'epoch' not in log:
- continue
- epoch = log.pop('epoch')
- if epoch not in log_dict:
- log_dict[epoch] = defaultdict(list)
- for k, v in log.items():
- log_dict[epoch][k].append(v)
- return log_dicts
-
-
-def main():
- args = parse_args()
-
- json_logs = args.json_logs
- for json_log in json_logs:
- assert json_log.endswith('.json')
-
- log_dicts = load_json_logs(json_logs)
-
- eval(args.task)(log_dicts, args)
-
-
-if __name__ == '__main__':
- main()
diff --git a/spaces/Andy1621/uniformer_image_segmentation/configs/_base_/models/dmnet_r50-d8.py b/spaces/Andy1621/uniformer_image_segmentation/configs/_base_/models/dmnet_r50-d8.py
deleted file mode 100644
index d22ba52640bebd805b3b8d07025e276dfb023759..0000000000000000000000000000000000000000
--- a/spaces/Andy1621/uniformer_image_segmentation/configs/_base_/models/dmnet_r50-d8.py
+++ /dev/null
@@ -1,44 +0,0 @@
-# model settings
-norm_cfg = dict(type='SyncBN', requires_grad=True)
-model = dict(
- type='EncoderDecoder',
- pretrained='open-mmlab://resnet50_v1c',
- backbone=dict(
- type='ResNetV1c',
- depth=50,
- num_stages=4,
- out_indices=(0, 1, 2, 3),
- dilations=(1, 1, 2, 4),
- strides=(1, 2, 1, 1),
- norm_cfg=norm_cfg,
- norm_eval=False,
- style='pytorch',
- contract_dilation=True),
- decode_head=dict(
- type='DMHead',
- in_channels=2048,
- in_index=3,
- channels=512,
- filter_sizes=(1, 3, 5, 7),
- dropout_ratio=0.1,
- num_classes=19,
- norm_cfg=dict(type='SyncBN', requires_grad=True),
- align_corners=False,
- loss_decode=dict(
- type='CrossEntropyLoss', use_sigmoid=False, loss_weight=1.0)),
- auxiliary_head=dict(
- type='FCNHead',
- in_channels=1024,
- in_index=2,
- channels=256,
- num_convs=1,
- concat_input=False,
- dropout_ratio=0.1,
- num_classes=19,
- norm_cfg=norm_cfg,
- align_corners=False,
- loss_decode=dict(
- type='CrossEntropyLoss', use_sigmoid=False, loss_weight=0.4)),
- # model training and testing settings
- train_cfg=dict(),
- test_cfg=dict(mode='whole'))
diff --git a/spaces/Andy1621/uniformer_image_segmentation/configs/pspnet/pspnet_r101-d8_769x769_80k_cityscapes.py b/spaces/Andy1621/uniformer_image_segmentation/configs/pspnet/pspnet_r101-d8_769x769_80k_cityscapes.py
deleted file mode 100644
index 59b8c6dd5ef234334bcdfa3d5e3594b7a9989b17..0000000000000000000000000000000000000000
--- a/spaces/Andy1621/uniformer_image_segmentation/configs/pspnet/pspnet_r101-d8_769x769_80k_cityscapes.py
+++ /dev/null
@@ -1,2 +0,0 @@
-_base_ = './pspnet_r50-d8_769x769_80k_cityscapes.py'
-model = dict(pretrained='open-mmlab://resnet101_v1c', backbone=dict(depth=101))
diff --git a/spaces/AnishKumbhar/ChatBot/text-generation-webui-main/extensions/whisper_stt/readme.md b/spaces/AnishKumbhar/ChatBot/text-generation-webui-main/extensions/whisper_stt/readme.md
deleted file mode 100644
index cd9abbf68cb4f7adf1172fdd57e9e26466e47778..0000000000000000000000000000000000000000
--- a/spaces/AnishKumbhar/ChatBot/text-generation-webui-main/extensions/whisper_stt/readme.md
+++ /dev/null
@@ -1,15 +0,0 @@
-# whisper_stt
-
-Allows you to enter your inputs in chat mode using your microphone.
-
-## Settings
-
-To adjust your default settings, you can add the following to your settings.yaml file.
-
-```
-whisper_stt-whipser_language: chinese
-whisper_stt-whipser_model: tiny
-whisper_stt-auto_submit: False
-```
-
-See source documentation for [model names](https://github.com/openai/whisper#available-models-and-languages) and (languages)[https://github.com/openai/whisper/blob/main/whisper/tokenizer.py] you can use.
\ No newline at end of file
diff --git a/spaces/Anonymous-123/ImageNet-Editing/editing_diffusion/guided_diffusion/guided_diffusion/image_datasets.py b/spaces/Anonymous-123/ImageNet-Editing/editing_diffusion/guided_diffusion/guided_diffusion/image_datasets.py
deleted file mode 100644
index 93022ae208a01e72eb162d7b63c07bf94a6afe3b..0000000000000000000000000000000000000000
--- a/spaces/Anonymous-123/ImageNet-Editing/editing_diffusion/guided_diffusion/guided_diffusion/image_datasets.py
+++ /dev/null
@@ -1,167 +0,0 @@
-import math
-import random
-
-from PIL import Image
-import blobfile as bf
-from mpi4py import MPI
-import numpy as np
-from torch.utils.data import DataLoader, Dataset
-
-
-def load_data(
- *,
- data_dir,
- batch_size,
- image_size,
- class_cond=False,
- deterministic=False,
- random_crop=False,
- random_flip=True,
-):
- """
- For a dataset, create a generator over (images, kwargs) pairs.
-
- Each images is an NCHW float tensor, and the kwargs dict contains zero or
- more keys, each of which map to a batched Tensor of their own.
- The kwargs dict can be used for class labels, in which case the key is "y"
- and the values are integer tensors of class labels.
-
- :param data_dir: a dataset directory.
- :param batch_size: the batch size of each returned pair.
- :param image_size: the size to which images are resized.
- :param class_cond: if True, include a "y" key in returned dicts for class
- label. If classes are not available and this is true, an
- exception will be raised.
- :param deterministic: if True, yield results in a deterministic order.
- :param random_crop: if True, randomly crop the images for augmentation.
- :param random_flip: if True, randomly flip the images for augmentation.
- """
- if not data_dir:
- raise ValueError("unspecified data directory")
- all_files = _list_image_files_recursively(data_dir)
- classes = None
- if class_cond:
- # Assume classes are the first part of the filename,
- # before an underscore.
- class_names = [bf.basename(path).split("_")[0] for path in all_files]
- sorted_classes = {x: i for i, x in enumerate(sorted(set(class_names)))}
- classes = [sorted_classes[x] for x in class_names]
- dataset = ImageDataset(
- image_size,
- all_files,
- classes=classes,
- shard=MPI.COMM_WORLD.Get_rank(),
- num_shards=MPI.COMM_WORLD.Get_size(),
- random_crop=random_crop,
- random_flip=random_flip,
- )
- if deterministic:
- loader = DataLoader(
- dataset, batch_size=batch_size, shuffle=False, num_workers=1, drop_last=True
- )
- else:
- loader = DataLoader(
- dataset, batch_size=batch_size, shuffle=True, num_workers=1, drop_last=True
- )
- while True:
- yield from loader
-
-
-def _list_image_files_recursively(data_dir):
- results = []
- for entry in sorted(bf.listdir(data_dir)):
- full_path = bf.join(data_dir, entry)
- ext = entry.split(".")[-1]
- if "." in entry and ext.lower() in ["jpg", "jpeg", "png", "gif"]:
- results.append(full_path)
- elif bf.isdir(full_path):
- results.extend(_list_image_files_recursively(full_path))
- return results
-
-
-class ImageDataset(Dataset):
- def __init__(
- self,
- resolution,
- image_paths,
- classes=None,
- shard=0,
- num_shards=1,
- random_crop=False,
- random_flip=True,
- ):
- super().__init__()
- self.resolution = resolution
- self.local_images = image_paths[shard:][::num_shards]
- self.local_classes = None if classes is None else classes[shard:][::num_shards]
- self.random_crop = random_crop
- self.random_flip = random_flip
-
- def __len__(self):
- return len(self.local_images)
-
- def __getitem__(self, idx):
- path = self.local_images[idx]
- with bf.BlobFile(path, "rb") as f:
- pil_image = Image.open(f)
- pil_image.load()
- pil_image = pil_image.convert("RGB")
-
- if self.random_crop:
- arr = random_crop_arr(pil_image, self.resolution)
- else:
- arr = center_crop_arr(pil_image, self.resolution)
-
- if self.random_flip and random.random() < 0.5:
- arr = arr[:, ::-1]
-
- arr = arr.astype(np.float32) / 127.5 - 1
-
- out_dict = {}
- if self.local_classes is not None:
- out_dict["y"] = np.array(self.local_classes[idx], dtype=np.int64)
- return np.transpose(arr, [2, 0, 1]), out_dict
-
-
-def center_crop_arr(pil_image, image_size):
- # We are not on a new enough PIL to support the `reducing_gap`
- # argument, which uses BOX downsampling at powers of two first.
- # Thus, we do it by hand to improve downsample quality.
- while min(*pil_image.size) >= 2 * image_size:
- pil_image = pil_image.resize(
- tuple(x // 2 for x in pil_image.size), resample=Image.BOX
- )
-
- scale = image_size / min(*pil_image.size)
- pil_image = pil_image.resize(
- tuple(round(x * scale) for x in pil_image.size), resample=Image.BICUBIC
- )
-
- arr = np.array(pil_image)
- crop_y = (arr.shape[0] - image_size) // 2
- crop_x = (arr.shape[1] - image_size) // 2
- return arr[crop_y : crop_y + image_size, crop_x : crop_x + image_size]
-
-
-def random_crop_arr(pil_image, image_size, min_crop_frac=0.8, max_crop_frac=1.0):
- min_smaller_dim_size = math.ceil(image_size / max_crop_frac)
- max_smaller_dim_size = math.ceil(image_size / min_crop_frac)
- smaller_dim_size = random.randrange(min_smaller_dim_size, max_smaller_dim_size + 1)
-
- # We are not on a new enough PIL to support the `reducing_gap`
- # argument, which uses BOX downsampling at powers of two first.
- # Thus, we do it by hand to improve downsample quality.
- while min(*pil_image.size) >= 2 * smaller_dim_size:
- pil_image = pil_image.resize(
- tuple(x // 2 for x in pil_image.size), resample=Image.BOX
- )
-
- scale = smaller_dim_size / min(*pil_image.size)
- pil_image = pil_image.resize(
- tuple(round(x * scale) for x in pil_image.size), resample=Image.BICUBIC
- )
-
- arr = np.array(pil_image)
- crop_y = random.randrange(arr.shape[0] - image_size + 1)
- crop_x = random.randrange(arr.shape[1] - image_size + 1)
- return arr[crop_y : crop_y + image_size, crop_x : crop_x + image_size]
diff --git a/spaces/Anonymous-sub/Rerender/ControlNet/annotator/uniformer/mmcv/ops/masked_conv.py b/spaces/Anonymous-sub/Rerender/ControlNet/annotator/uniformer/mmcv/ops/masked_conv.py
deleted file mode 100644
index cd514cc204c1d571ea5dc7e74b038c0f477a008b..0000000000000000000000000000000000000000
--- a/spaces/Anonymous-sub/Rerender/ControlNet/annotator/uniformer/mmcv/ops/masked_conv.py
+++ /dev/null
@@ -1,111 +0,0 @@
-# Copyright (c) OpenMMLab. All rights reserved.
-import math
-
-import torch
-import torch.nn as nn
-from torch.autograd import Function
-from torch.autograd.function import once_differentiable
-from torch.nn.modules.utils import _pair
-
-from ..utils import ext_loader
-
-ext_module = ext_loader.load_ext(
- '_ext', ['masked_im2col_forward', 'masked_col2im_forward'])
-
-
-class MaskedConv2dFunction(Function):
-
- @staticmethod
- def symbolic(g, features, mask, weight, bias, padding, stride):
- return g.op(
- 'mmcv::MMCVMaskedConv2d',
- features,
- mask,
- weight,
- bias,
- padding_i=padding,
- stride_i=stride)
-
- @staticmethod
- def forward(ctx, features, mask, weight, bias, padding=0, stride=1):
- assert mask.dim() == 3 and mask.size(0) == 1
- assert features.dim() == 4 and features.size(0) == 1
- assert features.size()[2:] == mask.size()[1:]
- pad_h, pad_w = _pair(padding)
- stride_h, stride_w = _pair(stride)
- if stride_h != 1 or stride_w != 1:
- raise ValueError(
- 'Stride could not only be 1 in masked_conv2d currently.')
- out_channel, in_channel, kernel_h, kernel_w = weight.size()
-
- batch_size = features.size(0)
- out_h = int(
- math.floor((features.size(2) + 2 * pad_h -
- (kernel_h - 1) - 1) / stride_h + 1))
- out_w = int(
- math.floor((features.size(3) + 2 * pad_w -
- (kernel_h - 1) - 1) / stride_w + 1))
- mask_inds = torch.nonzero(mask[0] > 0, as_tuple=False)
- output = features.new_zeros(batch_size, out_channel, out_h, out_w)
- if mask_inds.numel() > 0:
- mask_h_idx = mask_inds[:, 0].contiguous()
- mask_w_idx = mask_inds[:, 1].contiguous()
- data_col = features.new_zeros(in_channel * kernel_h * kernel_w,
- mask_inds.size(0))
- ext_module.masked_im2col_forward(
- features,
- mask_h_idx,
- mask_w_idx,
- data_col,
- kernel_h=kernel_h,
- kernel_w=kernel_w,
- pad_h=pad_h,
- pad_w=pad_w)
-
- masked_output = torch.addmm(1, bias[:, None], 1,
- weight.view(out_channel, -1), data_col)
- ext_module.masked_col2im_forward(
- masked_output,
- mask_h_idx,
- mask_w_idx,
- output,
- height=out_h,
- width=out_w,
- channels=out_channel)
- return output
-
- @staticmethod
- @once_differentiable
- def backward(ctx, grad_output):
- return (None, ) * 5
-
-
-masked_conv2d = MaskedConv2dFunction.apply
-
-
-class MaskedConv2d(nn.Conv2d):
- """A MaskedConv2d which inherits the official Conv2d.
-
- The masked forward doesn't implement the backward function and only
- supports the stride parameter to be 1 currently.
- """
-
- def __init__(self,
- in_channels,
- out_channels,
- kernel_size,
- stride=1,
- padding=0,
- dilation=1,
- groups=1,
- bias=True):
- super(MaskedConv2d,
- self).__init__(in_channels, out_channels, kernel_size, stride,
- padding, dilation, groups, bias)
-
- def forward(self, input, mask=None):
- if mask is None: # fallback to the normal Conv2d
- return super(MaskedConv2d, self).forward(input)
- else:
- return masked_conv2d(input, mask, self.weight, self.bias,
- self.padding)
diff --git a/spaces/Anonymous-sub/Rerender/ControlNet/annotator/uniformer/mmseg/apis/test.py b/spaces/Anonymous-sub/Rerender/ControlNet/annotator/uniformer/mmseg/apis/test.py
deleted file mode 100644
index e574eb7da04f09a59cf99ff953c36468ae87a326..0000000000000000000000000000000000000000
--- a/spaces/Anonymous-sub/Rerender/ControlNet/annotator/uniformer/mmseg/apis/test.py
+++ /dev/null
@@ -1,238 +0,0 @@
-import os.path as osp
-import pickle
-import shutil
-import tempfile
-
-import annotator.uniformer.mmcv as mmcv
-import numpy as np
-import torch
-import torch.distributed as dist
-from annotator.uniformer.mmcv.image import tensor2imgs
-from annotator.uniformer.mmcv.runner import get_dist_info
-
-
-def np2tmp(array, temp_file_name=None):
- """Save ndarray to local numpy file.
-
- Args:
- array (ndarray): Ndarray to save.
- temp_file_name (str): Numpy file name. If 'temp_file_name=None', this
- function will generate a file name with tempfile.NamedTemporaryFile
- to save ndarray. Default: None.
-
- Returns:
- str: The numpy file name.
- """
-
- if temp_file_name is None:
- temp_file_name = tempfile.NamedTemporaryFile(
- suffix='.npy', delete=False).name
- np.save(temp_file_name, array)
- return temp_file_name
-
-
-def single_gpu_test(model,
- data_loader,
- show=False,
- out_dir=None,
- efficient_test=False,
- opacity=0.5):
- """Test with single GPU.
-
- Args:
- model (nn.Module): Model to be tested.
- data_loader (utils.data.Dataloader): Pytorch data loader.
- show (bool): Whether show results during inference. Default: False.
- out_dir (str, optional): If specified, the results will be dumped into
- the directory to save output results.
- efficient_test (bool): Whether save the results as local numpy files to
- save CPU memory during evaluation. Default: False.
- opacity(float): Opacity of painted segmentation map.
- Default 0.5.
- Must be in (0, 1] range.
- Returns:
- list: The prediction results.
- """
-
- model.eval()
- results = []
- dataset = data_loader.dataset
- prog_bar = mmcv.ProgressBar(len(dataset))
- for i, data in enumerate(data_loader):
- with torch.no_grad():
- result = model(return_loss=False, **data)
-
- if show or out_dir:
- img_tensor = data['img'][0]
- img_metas = data['img_metas'][0].data[0]
- imgs = tensor2imgs(img_tensor, **img_metas[0]['img_norm_cfg'])
- assert len(imgs) == len(img_metas)
-
- for img, img_meta in zip(imgs, img_metas):
- h, w, _ = img_meta['img_shape']
- img_show = img[:h, :w, :]
-
- ori_h, ori_w = img_meta['ori_shape'][:-1]
- img_show = mmcv.imresize(img_show, (ori_w, ori_h))
-
- if out_dir:
- out_file = osp.join(out_dir, img_meta['ori_filename'])
- else:
- out_file = None
-
- model.module.show_result(
- img_show,
- result,
- palette=dataset.PALETTE,
- show=show,
- out_file=out_file,
- opacity=opacity)
-
- if isinstance(result, list):
- if efficient_test:
- result = [np2tmp(_) for _ in result]
- results.extend(result)
- else:
- if efficient_test:
- result = np2tmp(result)
- results.append(result)
-
- batch_size = len(result)
- for _ in range(batch_size):
- prog_bar.update()
- return results
-
-
-def multi_gpu_test(model,
- data_loader,
- tmpdir=None,
- gpu_collect=False,
- efficient_test=False):
- """Test model with multiple gpus.
-
- This method tests model with multiple gpus and collects the results
- under two different modes: gpu and cpu modes. By setting 'gpu_collect=True'
- it encodes results to gpu tensors and use gpu communication for results
- collection. On cpu mode it saves the results on different gpus to 'tmpdir'
- and collects them by the rank 0 worker.
-
- Args:
- model (nn.Module): Model to be tested.
- data_loader (utils.data.Dataloader): Pytorch data loader.
- tmpdir (str): Path of directory to save the temporary results from
- different gpus under cpu mode.
- gpu_collect (bool): Option to use either gpu or cpu to collect results.
- efficient_test (bool): Whether save the results as local numpy files to
- save CPU memory during evaluation. Default: False.
-
- Returns:
- list: The prediction results.
- """
-
- model.eval()
- results = []
- dataset = data_loader.dataset
- rank, world_size = get_dist_info()
- if rank == 0:
- prog_bar = mmcv.ProgressBar(len(dataset))
- for i, data in enumerate(data_loader):
- with torch.no_grad():
- result = model(return_loss=False, rescale=True, **data)
-
- if isinstance(result, list):
- if efficient_test:
- result = [np2tmp(_) for _ in result]
- results.extend(result)
- else:
- if efficient_test:
- result = np2tmp(result)
- results.append(result)
-
- if rank == 0:
- batch_size = data['img'][0].size(0)
- for _ in range(batch_size * world_size):
- prog_bar.update()
-
- # collect results from all ranks
- if gpu_collect:
- results = collect_results_gpu(results, len(dataset))
- else:
- results = collect_results_cpu(results, len(dataset), tmpdir)
- return results
-
-
-def collect_results_cpu(result_part, size, tmpdir=None):
- """Collect results with CPU."""
- rank, world_size = get_dist_info()
- # create a tmp dir if it is not specified
- if tmpdir is None:
- MAX_LEN = 512
- # 32 is whitespace
- dir_tensor = torch.full((MAX_LEN, ),
- 32,
- dtype=torch.uint8,
- device='cuda')
- if rank == 0:
- tmpdir = tempfile.mkdtemp()
- tmpdir = torch.tensor(
- bytearray(tmpdir.encode()), dtype=torch.uint8, device='cuda')
- dir_tensor[:len(tmpdir)] = tmpdir
- dist.broadcast(dir_tensor, 0)
- tmpdir = dir_tensor.cpu().numpy().tobytes().decode().rstrip()
- else:
- mmcv.mkdir_or_exist(tmpdir)
- # dump the part result to the dir
- mmcv.dump(result_part, osp.join(tmpdir, 'part_{}.pkl'.format(rank)))
- dist.barrier()
- # collect all parts
- if rank != 0:
- return None
- else:
- # load results of all parts from tmp dir
- part_list = []
- for i in range(world_size):
- part_file = osp.join(tmpdir, 'part_{}.pkl'.format(i))
- part_list.append(mmcv.load(part_file))
- # sort the results
- ordered_results = []
- for res in zip(*part_list):
- ordered_results.extend(list(res))
- # the dataloader may pad some samples
- ordered_results = ordered_results[:size]
- # remove tmp dir
- shutil.rmtree(tmpdir)
- return ordered_results
-
-
-def collect_results_gpu(result_part, size):
- """Collect results with GPU."""
- rank, world_size = get_dist_info()
- # dump result part to tensor with pickle
- part_tensor = torch.tensor(
- bytearray(pickle.dumps(result_part)), dtype=torch.uint8, device='cuda')
- # gather all result part tensor shape
- shape_tensor = torch.tensor(part_tensor.shape, device='cuda')
- shape_list = [shape_tensor.clone() for _ in range(world_size)]
- dist.all_gather(shape_list, shape_tensor)
- # padding result part tensor to max length
- shape_max = torch.tensor(shape_list).max()
- part_send = torch.zeros(shape_max, dtype=torch.uint8, device='cuda')
- part_send[:shape_tensor[0]] = part_tensor
- part_recv_list = [
- part_tensor.new_zeros(shape_max) for _ in range(world_size)
- ]
- # gather all result part
- dist.all_gather(part_recv_list, part_send)
-
- if rank == 0:
- part_list = []
- for recv, shape in zip(part_recv_list, shape_list):
- part_list.append(
- pickle.loads(recv[:shape[0]].cpu().numpy().tobytes()))
- # sort the results
- ordered_results = []
- for res in zip(*part_list):
- ordered_results.extend(list(res))
- # the dataloader may pad some samples
- ordered_results = ordered_results[:size]
- return ordered_results
diff --git a/spaces/ArtGAN/Diffusion-API/README.md b/spaces/ArtGAN/Diffusion-API/README.md
deleted file mode 100644
index 5fc0ed589a3a15754648d902ff70c6a8d629d90a..0000000000000000000000000000000000000000
--- a/spaces/ArtGAN/Diffusion-API/README.md
+++ /dev/null
@@ -1,15 +0,0 @@
----
-title: Stable Diffusion ControlNet WebUI
-emoji: ⚡
-colorFrom: gray
-colorTo: red
-sdk: gradio
-sdk_version: 3.19.0
-app_file: app.py
-pinned: false
-license: apache-2.0
-tags:
- - making-demos
----
-
-Check out the configuration reference at https://huggingface.co/docs/hub/spaces-config-reference
\ No newline at end of file
diff --git a/spaces/ArtGAN/Diffusion-API/diffusion_webui/diffusion_models/img2img_app.py b/spaces/ArtGAN/Diffusion-API/diffusion_webui/diffusion_models/img2img_app.py
deleted file mode 100644
index a85ee16eedf67ea8ce58374513f9e7a7a3843a39..0000000000000000000000000000000000000000
--- a/spaces/ArtGAN/Diffusion-API/diffusion_webui/diffusion_models/img2img_app.py
+++ /dev/null
@@ -1,155 +0,0 @@
-import gradio as gr
-import torch
-from diffusers import StableDiffusionImg2ImgPipeline
-from PIL import Image
-
-from diffusion_webui.utils.model_list import stable_model_list
-from diffusion_webui.utils.scheduler_list import (
- SCHEDULER_MAPPING,
- get_scheduler,
-)
-
-
-class StableDiffusionImage2ImageGenerator:
- def __init__(self):
- self.pipe = None
-
- def load_model(self, stable_model_path, scheduler):
- if self.pipe is None or self.pipe.model_name != stable_model_path or self.pipe.scheduler_name != scheduler:
- self.pipe = StableDiffusionImg2ImgPipeline.from_pretrained(
- stable_model_path, safety_checker=None, torch_dtype=torch.float16
- )
-
- self.pipe.model_name = stable_model_path
- self.pipe.scheduler_name = scheduler
- self.pipe = get_scheduler(pipe=self.pipe, scheduler=scheduler)
- self.pipe.to("cuda")
- self.pipe.enable_xformers_memory_efficient_attention()
-
- return self.pipe
-
- def generate_image(
- self,
- image_path: str,
- stable_model_path: str,
- prompt: str,
- negative_prompt: str,
- num_images_per_prompt: int,
- scheduler: str,
- guidance_scale: int,
- num_inference_step: int,
- seed_generator=0,
- ):
- pipe = self.load_model(
- stable_model_path=stable_model_path,
- scheduler=scheduler,
- )
-
- if seed_generator == 0:
- random_seed = torch.randint(0, 1000000, (1,))
- generator = torch.manual_seed(random_seed)
- else:
- generator = torch.manual_seed(seed_generator)
-
- image = Image.open(image_path)
- images = pipe(
- prompt,
- image=image,
- negative_prompt=negative_prompt,
- num_images_per_prompt=num_images_per_prompt,
- num_inference_steps=num_inference_step,
- guidance_scale=guidance_scale,
- generator=generator,
- ).images
-
- return images
-
- def app():
- with gr.Blocks():
- with gr.Row():
- with gr.Column():
- image2image_image_file = gr.Image(
- type="filepath", label="Image"
- ).style(height=260)
-
- image2image_prompt = gr.Textbox(
- lines=1,
- placeholder="Prompt",
- show_label=False,
- )
-
- image2image_negative_prompt = gr.Textbox(
- lines=1,
- placeholder="Negative Prompt",
- show_label=False,
- )
-
- with gr.Row():
- with gr.Column():
- image2image_model_path = gr.Dropdown(
- choices=stable_model_list,
- value=stable_model_list[0],
- label="Stable Model Id",
- )
-
- image2image_guidance_scale = gr.Slider(
- minimum=0.1,
- maximum=15,
- step=0.1,
- value=7.5,
- label="Guidance Scale",
- )
- image2image_num_inference_step = gr.Slider(
- minimum=1,
- maximum=100,
- step=1,
- value=50,
- label="Num Inference Step",
- )
- with gr.Row():
- with gr.Column():
- image2image_scheduler = gr.Dropdown(
- choices=list(SCHEDULER_MAPPING.keys()),
- value=list(SCHEDULER_MAPPING.keys())[0],
- label="Scheduler",
- )
- image2image_num_images_per_prompt = gr.Slider(
- minimum=1,
- maximum=4,
- step=1,
- value=1,
- label="Number Of Images",
- )
-
- image2image_seed_generator = gr.Slider(
- minimum=0,
- maximum=1000000,
- step=1,
- value=0,
- label="Seed(0 for random)",
- )
-
- image2image_predict_button = gr.Button(value="Generator")
-
- with gr.Column():
- output_image = gr.Gallery(
- label="Generated images",
- show_label=False,
- elem_id="gallery",
- ).style(grid=(1, 2))
-
- image2image_predict_button.click(
- fn=StableDiffusionImage2ImageGenerator().generate_image,
- inputs=[
- image2image_image_file,
- image2image_model_path,
- image2image_prompt,
- image2image_negative_prompt,
- image2image_num_images_per_prompt,
- image2image_scheduler,
- image2image_guidance_scale,
- image2image_num_inference_step,
- image2image_seed_generator,
- ],
- outputs=[output_image],
- )
diff --git a/spaces/ArtyomKhyan/Detection/models/experimental.py b/spaces/ArtyomKhyan/Detection/models/experimental.py
deleted file mode 100644
index 539e7f970ac357be33ad5f5fa5a3804183f45c8d..0000000000000000000000000000000000000000
--- a/spaces/ArtyomKhyan/Detection/models/experimental.py
+++ /dev/null
@@ -1,109 +0,0 @@
-# This file contains experimental modules
-
-from models.common import *
-
-
-class CrossConv(nn.Module):
- # Cross Convolution Downsample
- def __init__(self, c1, c2, k=3, s=1, g=1, e=1.0, shortcut=False):
- # ch_in, ch_out, kernel, stride, groups, expansion, shortcut
- super(CrossConv, self).__init__()
- c_ = int(c2 * e) # hidden channels
- self.cv1 = Conv(c1, c_, (1, k), (1, s))
- self.cv2 = Conv(c_, c2, (k, 1), (s, 1), g=g)
- self.add = shortcut and c1 == c2
-
- def forward(self, x):
- return x + self.cv2(self.cv1(x)) if self.add else self.cv2(self.cv1(x))
-
-
-class C3(nn.Module):
- # Cross Convolution CSP
- def __init__(self, c1, c2, n=1, shortcut=True, g=1, e=0.5): # ch_in, ch_out, number, shortcut, groups, expansion
- super(C3, self).__init__()
- c_ = int(c2 * e) # hidden channels
- self.cv1 = Conv(c1, c_, 1, 1)
- self.cv2 = nn.Conv2d(c1, c_, 1, 1, bias=False)
- self.cv3 = nn.Conv2d(c_, c_, 1, 1, bias=False)
- self.cv4 = Conv(2 * c_, c2, 1, 1)
- self.bn = nn.BatchNorm2d(2 * c_) # applied to cat(cv2, cv3)
- self.act = nn.LeakyReLU(0.1, inplace=True)
- self.m = nn.Sequential(*[CrossConv(c_, c_, 3, 1, g, 1.0, shortcut) for _ in range(n)])
-
- def forward(self, x):
- y1 = self.cv3(self.m(self.cv1(x)))
- y2 = self.cv2(x)
- return self.cv4(self.act(self.bn(torch.cat((y1, y2), dim=1))))
-
-
-class Sum(nn.Module):
- # Weighted sum of 2 or more layers https://arxiv.org/abs/1911.09070
- def __init__(self, n, weight=False): # n: number of inputs
- super(Sum, self).__init__()
- self.weight = weight # apply weights boolean
- self.iter = range(n - 1) # iter object
- if weight:
- self.w = nn.Parameter(-torch.arange(1., n) / 2, requires_grad=True) # layer weights
-
- def forward(self, x):
- y = x[0] # no weight
- if self.weight:
- w = torch.sigmoid(self.w) * 2
- for i in self.iter:
- y = y + x[i + 1] * w[i]
- else:
- for i in self.iter:
- y = y + x[i + 1]
- return y
-
-
-class GhostConv(nn.Module):
- # Ghost Convolution https://github.com/huawei-noah/ghostnet
- def __init__(self, c1, c2, k=1, s=1, g=1, act=True): # ch_in, ch_out, kernel, stride, groups
- super(GhostConv, self).__init__()
- c_ = c2 // 2 # hidden channels
- self.cv1 = Conv(c1, c_, k, s, g, act)
- self.cv2 = Conv(c_, c_, 5, 1, c_, act)
-
- def forward(self, x):
- y = self.cv1(x)
- return torch.cat([y, self.cv2(y)], 1)
-
-
-class GhostBottleneck(nn.Module):
- # Ghost Bottleneck https://github.com/huawei-noah/ghostnet
- def __init__(self, c1, c2, k, s):
- super(GhostBottleneck, self).__init__()
- c_ = c2 // 2
- self.conv = nn.Sequential(GhostConv(c1, c_, 1, 1), # pw
- DWConv(c_, c_, k, s, act=False) if s == 2 else nn.Identity(), # dw
- GhostConv(c_, c2, 1, 1, act=False)) # pw-linear
- self.shortcut = nn.Sequential(DWConv(c1, c1, k, s, act=False),
- Conv(c1, c2, 1, 1, act=False)) if s == 2 else nn.Identity()
-
- def forward(self, x):
- return self.conv(x) + self.shortcut(x)
-
-
-class MixConv2d(nn.Module):
- # Mixed Depthwise Conv https://arxiv.org/abs/1907.09595
- def __init__(self, c1, c2, k=(1, 3), s=1, equal_ch=True):
- super(MixConv2d, self).__init__()
- groups = len(k)
- if equal_ch: # equal c_ per group
- i = torch.linspace(0, groups - 1E-6, c2).floor() # c2 indices
- c_ = [(i == g).sum() for g in range(groups)] # intermediate channels
- else: # equal weight.numel() per group
- b = [c2] + [0] * groups
- a = np.eye(groups + 1, groups, k=-1)
- a -= np.roll(a, 1, axis=1)
- a *= np.array(k) ** 2
- a[0] = 1
- c_ = np.linalg.lstsq(a, b, rcond=None)[0].round() # solve for equal weight indices, ax = b
-
- self.m = nn.ModuleList([nn.Conv2d(c1, int(c_[g]), k[g], s, k[g] // 2, bias=False) for g in range(groups)])
- self.bn = nn.BatchNorm2d(c2)
- self.act = nn.LeakyReLU(0.1, inplace=True)
-
- def forward(self, x):
- return x + self.act(self.bn(torch.cat([m(x) for m in self.m], 1)))
diff --git a/spaces/AzinZ/vitscn/transforms.py b/spaces/AzinZ/vitscn/transforms.py
deleted file mode 100644
index 4793d67ca5a5630e0ffe0f9fb29445c949e64dae..0000000000000000000000000000000000000000
--- a/spaces/AzinZ/vitscn/transforms.py
+++ /dev/null
@@ -1,193 +0,0 @@
-import torch
-from torch.nn import functional as F
-
-import numpy as np
-
-
-DEFAULT_MIN_BIN_WIDTH = 1e-3
-DEFAULT_MIN_BIN_HEIGHT = 1e-3
-DEFAULT_MIN_DERIVATIVE = 1e-3
-
-
-def piecewise_rational_quadratic_transform(inputs,
- unnormalized_widths,
- unnormalized_heights,
- unnormalized_derivatives,
- inverse=False,
- tails=None,
- tail_bound=1.,
- min_bin_width=DEFAULT_MIN_BIN_WIDTH,
- min_bin_height=DEFAULT_MIN_BIN_HEIGHT,
- min_derivative=DEFAULT_MIN_DERIVATIVE):
-
- if tails is None:
- spline_fn = rational_quadratic_spline
- spline_kwargs = {}
- else:
- spline_fn = unconstrained_rational_quadratic_spline
- spline_kwargs = {
- 'tails': tails,
- 'tail_bound': tail_bound
- }
-
- outputs, logabsdet = spline_fn(
- inputs=inputs,
- unnormalized_widths=unnormalized_widths,
- unnormalized_heights=unnormalized_heights,
- unnormalized_derivatives=unnormalized_derivatives,
- inverse=inverse,
- min_bin_width=min_bin_width,
- min_bin_height=min_bin_height,
- min_derivative=min_derivative,
- **spline_kwargs
- )
- return outputs, logabsdet
-
-
-def searchsorted(bin_locations, inputs, eps=1e-6):
- bin_locations[..., -1] += eps
- return torch.sum(
- inputs[..., None] >= bin_locations,
- dim=-1
- ) - 1
-
-
-def unconstrained_rational_quadratic_spline(inputs,
- unnormalized_widths,
- unnormalized_heights,
- unnormalized_derivatives,
- inverse=False,
- tails='linear',
- tail_bound=1.,
- min_bin_width=DEFAULT_MIN_BIN_WIDTH,
- min_bin_height=DEFAULT_MIN_BIN_HEIGHT,
- min_derivative=DEFAULT_MIN_DERIVATIVE):
- inside_interval_mask = (inputs >= -tail_bound) & (inputs <= tail_bound)
- outside_interval_mask = ~inside_interval_mask
-
- outputs = torch.zeros_like(inputs)
- logabsdet = torch.zeros_like(inputs)
-
- if tails == 'linear':
- unnormalized_derivatives = F.pad(unnormalized_derivatives, pad=(1, 1))
- constant = np.log(np.exp(1 - min_derivative) - 1)
- unnormalized_derivatives[..., 0] = constant
- unnormalized_derivatives[..., -1] = constant
-
- outputs[outside_interval_mask] = inputs[outside_interval_mask]
- logabsdet[outside_interval_mask] = 0
- else:
- raise RuntimeError('{} tails are not implemented.'.format(tails))
-
- outputs[inside_interval_mask], logabsdet[inside_interval_mask] = rational_quadratic_spline(
- inputs=inputs[inside_interval_mask],
- unnormalized_widths=unnormalized_widths[inside_interval_mask, :],
- unnormalized_heights=unnormalized_heights[inside_interval_mask, :],
- unnormalized_derivatives=unnormalized_derivatives[inside_interval_mask, :],
- inverse=inverse,
- left=-tail_bound, right=tail_bound, bottom=-tail_bound, top=tail_bound,
- min_bin_width=min_bin_width,
- min_bin_height=min_bin_height,
- min_derivative=min_derivative
- )
-
- return outputs, logabsdet
-
-def rational_quadratic_spline(inputs,
- unnormalized_widths,
- unnormalized_heights,
- unnormalized_derivatives,
- inverse=False,
- left=0., right=1., bottom=0., top=1.,
- min_bin_width=DEFAULT_MIN_BIN_WIDTH,
- min_bin_height=DEFAULT_MIN_BIN_HEIGHT,
- min_derivative=DEFAULT_MIN_DERIVATIVE):
- if torch.min(inputs) < left or torch.max(inputs) > right:
- raise ValueError('Input to a transform is not within its domain')
-
- num_bins = unnormalized_widths.shape[-1]
-
- if min_bin_width * num_bins > 1.0:
- raise ValueError('Minimal bin width too large for the number of bins')
- if min_bin_height * num_bins > 1.0:
- raise ValueError('Minimal bin height too large for the number of bins')
-
- widths = F.softmax(unnormalized_widths, dim=-1)
- widths = min_bin_width + (1 - min_bin_width * num_bins) * widths
- cumwidths = torch.cumsum(widths, dim=-1)
- cumwidths = F.pad(cumwidths, pad=(1, 0), mode='constant', value=0.0)
- cumwidths = (right - left) * cumwidths + left
- cumwidths[..., 0] = left
- cumwidths[..., -1] = right
- widths = cumwidths[..., 1:] - cumwidths[..., :-1]
-
- derivatives = min_derivative + F.softplus(unnormalized_derivatives)
-
- heights = F.softmax(unnormalized_heights, dim=-1)
- heights = min_bin_height + (1 - min_bin_height * num_bins) * heights
- cumheights = torch.cumsum(heights, dim=-1)
- cumheights = F.pad(cumheights, pad=(1, 0), mode='constant', value=0.0)
- cumheights = (top - bottom) * cumheights + bottom
- cumheights[..., 0] = bottom
- cumheights[..., -1] = top
- heights = cumheights[..., 1:] - cumheights[..., :-1]
-
- if inverse:
- bin_idx = searchsorted(cumheights, inputs)[..., None]
- else:
- bin_idx = searchsorted(cumwidths, inputs)[..., None]
-
- input_cumwidths = cumwidths.gather(-1, bin_idx)[..., 0]
- input_bin_widths = widths.gather(-1, bin_idx)[..., 0]
-
- input_cumheights = cumheights.gather(-1, bin_idx)[..., 0]
- delta = heights / widths
- input_delta = delta.gather(-1, bin_idx)[..., 0]
-
- input_derivatives = derivatives.gather(-1, bin_idx)[..., 0]
- input_derivatives_plus_one = derivatives[..., 1:].gather(-1, bin_idx)[..., 0]
-
- input_heights = heights.gather(-1, bin_idx)[..., 0]
-
- if inverse:
- a = (((inputs - input_cumheights) * (input_derivatives
- + input_derivatives_plus_one
- - 2 * input_delta)
- + input_heights * (input_delta - input_derivatives)))
- b = (input_heights * input_derivatives
- - (inputs - input_cumheights) * (input_derivatives
- + input_derivatives_plus_one
- - 2 * input_delta))
- c = - input_delta * (inputs - input_cumheights)
-
- discriminant = b.pow(2) - 4 * a * c
- assert (discriminant >= 0).all()
-
- root = (2 * c) / (-b - torch.sqrt(discriminant))
- outputs = root * input_bin_widths + input_cumwidths
-
- theta_one_minus_theta = root * (1 - root)
- denominator = input_delta + ((input_derivatives + input_derivatives_plus_one - 2 * input_delta)
- * theta_one_minus_theta)
- derivative_numerator = input_delta.pow(2) * (input_derivatives_plus_one * root.pow(2)
- + 2 * input_delta * theta_one_minus_theta
- + input_derivatives * (1 - root).pow(2))
- logabsdet = torch.log(derivative_numerator) - 2 * torch.log(denominator)
-
- return outputs, -logabsdet
- else:
- theta = (inputs - input_cumwidths) / input_bin_widths
- theta_one_minus_theta = theta * (1 - theta)
-
- numerator = input_heights * (input_delta * theta.pow(2)
- + input_derivatives * theta_one_minus_theta)
- denominator = input_delta + ((input_derivatives + input_derivatives_plus_one - 2 * input_delta)
- * theta_one_minus_theta)
- outputs = input_cumheights + numerator / denominator
-
- derivative_numerator = input_delta.pow(2) * (input_derivatives_plus_one * theta.pow(2)
- + 2 * input_delta * theta_one_minus_theta
- + input_derivatives * (1 - theta).pow(2))
- logabsdet = torch.log(derivative_numerator) - 2 * torch.log(denominator)
-
- return outputs, logabsdet
diff --git a/spaces/Banbri/zcvzcv/src/app/queries/mockLLMResponse.ts b/spaces/Banbri/zcvzcv/src/app/queries/mockLLMResponse.ts
deleted file mode 100644
index f7a7f16637859f86cc51a72178b20b1622f388b5..0000000000000000000000000000000000000000
--- a/spaces/Banbri/zcvzcv/src/app/queries/mockLLMResponse.ts
+++ /dev/null
@@ -1,24 +0,0 @@
-import { LLMResponse } from "@/types"
-
-export const mockLLMResponse: LLMResponse = [
- {
- "panel": 1,
- "instructions": "Close-up of cat's face, looking straight at reader with a smirk on its face",
- "caption": "Feline mischief"
- },
- {
- "panel": 2,
- "instructions": "Medium shot of cat sniffing a glass of milk, with a surprised expression",
- "caption": "Uh oh, what's this?"
- },
- {
- "panel": 3,
- "instructions": "Wide shot of cat knocking over the glass of milk, with a crazed look in its eyes",
- "caption": "Cat-astrophe!"
- },
- {
- "panel": 4,
- "instructions": "Close-up of cat's face, looking satisfied with a milk moustache",
- "caption": "Mission accomplished"
- }
-]
\ No newline at end of file
diff --git a/spaces/Benson/text-generation/Examples/Cmo Descargar Naruto Ultimate Ninja Storm 4 Para Android.md b/spaces/Benson/text-generation/Examples/Cmo Descargar Naruto Ultimate Ninja Storm 4 Para Android.md
deleted file mode 100644
index b8b2273081becc8cd43059dc5a2fc593eb63d13f..0000000000000000000000000000000000000000
--- a/spaces/Benson/text-generation/Examples/Cmo Descargar Naruto Ultimate Ninja Storm 4 Para Android.md
+++ /dev/null
@@ -1,86 +0,0 @@
-
-
Descargar Naruto Ultimate Ninja Storm altamente comprimido 100mb
-
Si eres un fan de Naruto, la popular serie de manga y anime, es posible que quieras probar Naruto Ultimate Ninja Storm, un juego de lucha que te permite experimentar las batallas épicas del mundo ninja. Pero ¿qué pasa si tiene espacio de almacenamiento limitado o una conexión a Internet lenta? No te preocupes, todavía puedes descargar Naruto Ultimate Ninja Storm altamente comprimido 100mb y disfrutar del juego sin ningún tipo de molestia. En este artículo, te mostraremos cómo hacerlo, y también te daremos información sobre el juego y sus características.
-
¿Qué es Naruto Ultimate Ninja Storm?
-
Una breve introducción al juego y sus características
-
Naruto Ultimate Ninja Storm es la primera entrega de la serie Ultimate Ninja Storm, desarrollada por CyberConnect2 y publicada por Namco Bandai Games. Fue lanzado para PlayStation 3 en 2008, y más tarde remasterizado en HD para PlayStation 4, Windows, Xbox One y Nintendo Switch.
-
Cómo descargar Naruto Ultimate Ninja Storm 4 para Android
El juego está basado en la serie de manga y anime de Naruto de Masashi Kishimoto, y cubre los eventos desde el comienzo de la historia hasta el final del arco de recuperación de Sasuke. El juego cuenta con más de 25 personajes jugables, cada uno con sus propios movimientos, habilidades y transformaciones. El juego también permite a los jugadores personalizar el jutsu de sus personajes y seleccionar dos personajes de apoyo para ayudarles en la batalla.
-
El juego cuenta con un impresionante motor de gráficos 3D que crea entornos inmersivos y efectos dinámicos. El juego también cuenta con un modo de roaming libre que permite a los jugadores explorar la Aldea de Hojas Ocultas e interactuar con otros personajes. El juego también tiene un modo historia que sigue la trama principal de la serie, así como un modo misión que ofrece varios desafíos y recompensas.
-
Los beneficios de descargar el juego en un formato altamente comprimido
-
-
Es por eso que descargar Naruto Ultimate Ninja Storm altamente comprimido 100mb es una gran solución. Al comprimir los archivos del juego a un tamaño más pequeño, puedes ahorrar hasta un 98% de espacio sin perder calidad ni funcionalidad. También puedes descargar el juego más rápido y más fácil, ya que te llevará menos tiempo y ancho de banda transferir.
-
Descargar Naruto Ultimate Ninja Storm altamente comprimido 100mb también tiene otro beneficio: puede mejorar su rendimiento de juego. Al reducir el tamaño de los archivos del juego, puede reducir la carga en su sistema y hacerlo funcionar más suave y más rápido. También puede evitar cualquier retraso o fallo que pueda ocurrir debido a los grandes tamaños de archivos.
-
¿Cómo descargar Naruto Ultimate Ninja Storm altamente comprimido 100mb?
-
Los pasos a seguir para descargar el juego desde una fuente confiable
-
Si desea descargar Naruto Ultimate Ninja Storm altamente comprimido 100mb , es necesario seguir estos pasos:
-
-
Ir a un sitio web confiable que ofrece el juego en un formato altamente comprimido. Puede utilizar [este enlace] para acceder a una de las mejores fuentes para juegos altamente comprimidos.
-
Haga clic en el botón de descarga y espere a que se descargue el archivo del juego. El tamaño del archivo debe ser de alrededor de 100 MB.
-
Extraiga el archivo del juego usando un software como WinRAR o 7-Zip. Obtendrá una carpeta que contiene los archivos del juego y un archivo de configuración.
-
Ejecute el archivo de configuración y siga las instrucciones para instalar el juego en su sistema. Tendrá que elegir una carpeta de destino y aceptar los términos y condiciones.
-
Una vez completada la instalación, puede iniciar el juego desde el acceso directo del escritorio o el menú de inicio.
-
-
Felicidades, usted ha descargado con éxito Naruto Ultimate Ninja Storm altamente comprimido 100mb!
-
-
Los requisitos del sistema y el proceso de instalación del juego
-
-
-
Requisitos mínimos
Requisitos recomendados
-
OS: Windows 7 o superior (64-bit)
OS: Windows 10 (64-bit)
-
CPU: Intel Core i3-530 o AMD Phenom II X4 940
CPU: Intel Core i5-6400 o AMD FX-8320
-
RAM: 4 GB
RAM: 8 GB
-
GPU: NVIDIA GeForce GT 730 o AMD Radeon R7 240
GPU: NVIDIA GeForce GTX 1060 o AMD Radeon RX 480
-
DirectX: Versión 11
DirectX: Versión 11
-
Almacenamiento: 6 GB de espacio disponible
Almacenamiento: 6 GB de espacio disponible
-
Tarjeta de sonido: tarjeta de sonido compatible con DirectX o chipset a bordo
Tarjeta de sonido: tarjeta de sonido compatible con DirectX o chipset a bordo
-
-
Si su sistema cumple con estos requisitos, puede proceder a descargar Naruto Ultimate Ninja Storm altamente comprimido 100mb e instalarlo en su sistema. El proceso de instalación es simple y directo, como hemos explicado en la sección anterior. Sin embargo, si encuentra algún problema o error durante la instalación, puede probar estas soluciones:
-
-
Asegúrese de que su software antivirus no está bloqueando o eliminando cualquier archivo de juego. Es posible que necesite desactivarlo temporalmente o agregar una excepción para la carpeta del juego.
-
Asegúrese de que tiene suficiente espacio libre en la unidad del sistema y la carpeta de destino. Es posible que necesite eliminar algunos archivos no deseados o moverlos a otra ubicación.
-
Asegúrese de haber instalado todos los controladores y actualizaciones necesarios para su sistema y tarjeta gráfica. Es posible que tenga que consultar el sitio web del fabricante para las últimas versiones.
-
Asegúrese de haber extraído el archivo del juego correcta y completamente. Es posible que tenga que volver a descargarlo o usar otro software para extraerlo.
-
Asegúrese de haber ejecutado el archivo de configuración como administrador. Es posible que necesite hacer clic derecho sobre él y seleccionar "Ejecutar como administrador".
-
-
-
¿Cómo disfrutar de Naruto Ultimate Ninja Storm altamente comprimido 100mb?
-
El modo de juego y modos del juego
-
Naruto Ultimate Ninja Storm altamente comprimido 100mb ofrece una experiencia de juego emocionante e inmersiva que te hará sentir como si fueras parte del universo de Naruto. El juego tiene tres modos principales: Batalla Libre, Modo Historia y Modo Misión.
-
En el modo Batalla Libre, puedes elegir cualquier personaje y luchar contra otro personaje controlado por el ordenador u otro jugador. También puedes personalizar el jutsu de tu personaje y seleccionar dos personajes de apoyo para ayudarte en la batalla. Puedes elegir entre diferentes etapas según las ubicaciones de la serie, como Konoha, Orochimaru’s Hideout, Valley of the End, etc. También puedes ajustar el nivel de dificultad y el límite de tiempo de cada partido.
-
En el Modo Historia, puedes revivir los eventos de la serie de Naruto desde la perspectiva de Naruto. Puedes explorar el Hidden Leaf Village e interactuar con otros personajes, así como participar en batallas que siguen la trama principal de la serie. También puedes desbloquear nuevos personajes, jutsu y objetos completando ciertos objetivos y recogiendo pergaminos. El modo historia cubre los eventos desde el comienzo de la serie hasta el final del arco de recuperación de Sasuke.
-
En el Modo Misión, puedes llevar a cabo varias misiones que ponen a prueba tus habilidades y habilidades. Puedes elegir entre diferentes tipos de misiones, tales como supervivencia, ataque de tiempo, escolta, sigilo, etc. También puedes ganar dinero y recompensas completando misiones y usarlas para comprar artículos y accesorios de la tienda. El modo misión ofrece una variedad de desafíos y escenarios que te mantendrán entretenido y comprometido.
-
Los consejos y trucos para dominar el juego
-
Naruto Ultimate Ninja Storm altamente comprimido 100mb es un juego que requiere estrategia, tiempo y habilidad para dominar. Aquí hay algunos consejos y trucos que te ayudarán a mejorar tu jugabilidad y disfrutar más del juego:
-
-
Aprende los fundamentos del sistema de combate. Puedes usar cuatro botones para realizar diferentes acciones: ataque, chakra, salto y guardia. También puedes usar el pad direccional o el stick analógico para mover a tu personaje y esquivar los ataques. Puedes combinar diferentes botones para realizar combos, jutsu, lanzamientos, sustituciones, etc. También puedes usar los botones de hombro para activar tus personajes de soporte o tu jutsu definitivo.
-
Conoce las fortalezas y debilidades de tu personaje. Cada personaje tiene sus propios movimientos, habilidades y transformaciones. Algunos personajes son mejores en el combate de corto alcance, mientras que otros son mejores en el combate de largo alcance. Algunos personajes tienen jutsu más potente, mientras que otros tienen más velocidad o defensa. Algunos personajes pueden transformarse en su estado de despertar, mientras que otros pueden usar su modo de maldición o bestia de cola. Debes elegir un personaje que se adapte a tu estilo de juego y estrategia.
-
Usa tu chakra sabiamente. Chakra es la energía que te permite realizar jutsu y otros movimientos especiales. Tu medidor de chakras se muestra en la parte inferior de la pantalla, y se agota a medida que lo usas. Puedes reponer tu chakra manteniendo pulsado el botón chakra, pero esto te dejará vulnerable a los ataques. Debes equilibrar el uso y la recuperación de tus chakras, y evitar desperdiciarlos en movimientos innecesarios.
-
Usa tus personajes de apoyo de manera efectiva. Los personajes de apoyo son aliados que pueden ayudarte en la batalla atacándote, defendiéndote o curándote. Puede seleccionar dos caracteres de soporte antes de cada partido, y puede cambiar entre ellos pulsando los botones de hombro. También puede elegir entre diferentes tipos de soporte: tipo de ataque, tipo de defensa o tipo de equilibrio. Los personajes de soporte de tipo ataque lanzarán poderosos ataques contra tu oponente, los personajes de soporte de tipo defensa te protegerán de los ataques entrantes y los personajes de soporte de tipo equilibrio harán ambas cosas.
-
-
-
Conclusión
-
Un resumen de los puntos principales y una llamada a la acción
-
Naruto Ultimate Ninja Storm es un fantástico juego que te permite experimentar las batallas épicas de la serie Naruto en impresionantes gráficos en 3D y un juego inmersivo. El juego cuenta con más de 25 personajes jugables, cada uno con sus propios movimientos, habilidades y transformaciones. El juego también tiene tres modos principales: Batalla Libre, Modo Historia y Modo Misión.
-
Si desea descargar Naruto Ultimate Ninja Storm altamente comprimido 100mb , puede seguir los pasos que hemos proporcionado en este artículo y disfrutar del juego sin ningún tipo de molestia. Puede ahorrar mucho espacio y tiempo al descargar el juego en un formato altamente comprimido, y también mejorar su rendimiento de juego. También puedes aprender más sobre el juego y sus características, y dominar el juego con nuestros consejos y trucos.
-
¿Qué estás esperando? Descargar Naruto Ultimate Ninja Storm altamente comprimido 100mb hoy y dar rienda suelta a su ninja interior!
-
Preguntas frecuentes
-
Q1: ¿Vale la pena jugar Naruto Ultimate Ninja Storm?
-
A1: Sí, Naruto Ultimate Ninja Storm vale la pena jugar, especialmente si eres un fan de Naruto o juegos de lucha. El juego ofrece una fiel adaptación de la serie de Naruto, con gráficos impresionantes, un juego inmersivo y una variedad de personajes y modos. El juego también es divertido y fácil de jugar, con un sistema de combate simple e intuitivo.
-
Q2: ¿Cuánto tiempo es Naruto Ultimate Ninja Storm?
-
A2: Naruto Ultimate Ninja Storm no es un juego muy largo, ya que cubre solo la primera parte de la serie de Naruto. El modo historia se puede completar en aproximadamente 10 horas, mientras que el modo misión puede tomar otras 10 horas. El modo de batalla libre se puede jugar indefinidamente, ya que ofrece un sinfín de partidos y opciones de personalización.
-
Q3: ¿Puedo jugar Naruto Ultimate Ninja Storm en línea?
-
-
Q4: ¿Cuáles son las diferencias entre Naruto Ultimate Ninja Storm y sus secuelas?
-
A4: Naruto Ultimate Ninja Storm es el primer juego de la serie Ultimate Ninja Storm, y tiene algunas diferencias con sus secuelas. Algunas de las principales diferencias son:
-
-
El juego cubre solo la primera parte de la serie Naruto, mientras que las secuelas cubren la segunda parte (Shippuden) y más allá.
-
El juego tiene menos personajes jugables que las secuelas, ya que solo incluye personajes que aparecieron en la primera parte de la serie.
-
El juego no tiene un modo online, mientras que algunas de las secuelas sí.
-
El juego tiene un modo de roaming libre que te permite explorar la Aldea de Hoja Oculta, mientras que las secuelas tienen un enfoque más lineal y cinematográfico al modo historia.
-
El juego tiene un estilo de arte diferente a las secuelas, ya que utiliza gráficos de cel-shaded que se asemejan al anime más de cerca.
-
-
Q5: ¿Dónde puedo encontrar juegos más comprimidos?
-
A5: Si está buscando juegos más comprimidos, puede visitar [este sitio web] para encontrar una gran colección de juegos en varios géneros y plataformas. Puede descargar juegos en tamaños que van desde 10 MB a 1 GB, dependiendo de su preferencia y capacidad del sistema. También puedes encontrar juegos compatibles con Windows, Android, iOS, PlayStation, Xbox, etc.
64aa2da5cf
-
-
\ No newline at end of file
diff --git a/spaces/Benson/text-generation/Examples/Cruce De Carretera Todo Desbloqueado Apk.md b/spaces/Benson/text-generation/Examples/Cruce De Carretera Todo Desbloqueado Apk.md
deleted file mode 100644
index 5a4da37afe7dd12ebaeb77a568e4291bbfe2e6fc..0000000000000000000000000000000000000000
--- a/spaces/Benson/text-generation/Examples/Cruce De Carretera Todo Desbloqueado Apk.md
+++ /dev/null
@@ -1,90 +0,0 @@
-
-
Crossy Road todo desbloqueado APK: Cómo conseguir todos los caracteres gratis
-
Crossy Road es uno de los juegos de árcade más adictivos y divertidos en dispositivos móviles. Está inspirado en el clásico juego Frogger, pero con un toque moderno. Usted tiene que ayudar a un pollo u otros personajes a cruzar carreteras concurridas, ríos, vías férreas, y más, evitando los coches, camiones, trenes, águilas, y otros peligros. El juego tiene unos gráficos pixelados de estilo retro y una enorme colección de personajes inspirados en el arte pop que puedes desbloquear jugando o comprando monedas.
-
Pero ¿qué pasa si quieres conseguir todos los personajes sin gastar dinero o jugar durante horas? Bueno, hay una manera de hacer eso, pero se trata de usar un archivo APK. Un archivo APK es un paquete de aplicaciones de Android que contiene todos los archivos y datos necesarios para ejecutar una aplicación en su dispositivo. Algunas personas usan archivos APK para instalar aplicaciones que no están disponibles en la tienda oficial de aplicaciones, o para acceder a funciones que normalmente no están disponibles en la versión normal de la aplicación.
Una de estas características es desbloquear todos los personajes en Crossy Road. Mediante el uso de un archivo APK que ha sido modificado por otra persona, puede obtener todos los personajes de forma gratuita, sin tener que jugar o pagar por ellos. Suena tentador, ¿no es así? Pero antes de apresurarse a descargar e instalar un archivo APK para Crossy Road, usted debe saber que hay algunos beneficios y riesgos involucrados en hacerlo.
-
Cómo descargar e instalar Crossy Road todo desbloqueado APK
-
Si decide utilizar un archivo APK para desbloquear todos los caracteres en Crossy Road, aquí están los pasos que debe seguir:
-
Paso 1: Encontrar una fuente confiable para el archivo APK
-
-
Paso 2: Habilitar fuentes desconocidas en el dispositivo
-
De forma predeterminada, el dispositivo no le permitirá instalar aplicaciones desde fuentes distintas de la tienda de aplicaciones oficial. Esta es una medida de seguridad para evitar la instalación de aplicaciones dañinas o no autorizadas. Sin embargo, si desea instalar un archivo APK, debe habilitar fuentes desconocidas en su dispositivo. Para hacer esto, vaya a Configuración > Seguridad > Fuentes desconocidas y conéctelo. Puede ver un mensaje de advertencia que le informa sobre los riesgos de instalar aplicaciones de fuentes desconocidas. Léalo cuidadosamente y toque OK si está de acuerdo.
-
Paso 3: Descargar e instalar el archivo APK
-
Una vez que haya habilitado fuentes desconocidas en su dispositivo, puede proceder a descargar e instalar el archivo APK. Para ello, vaya a la página web donde se encuentra el archivo APK y toque en el botón de descarga. Puede ver una notificación que le indica que este tipo de archivo puede dañar su dispositivo. Pulse Aceptar si confía en la fuente. Una vez finalizada la descarga, abra la aplicación de administrador de archivos en su dispositivo y localice el archivo APK. Toque en ella para iniciar el proceso de instalación. Puede ver un mensaje que le pide permiso para instalar la aplicación. Pulse Instalar y espere a que finalice la instalación.
-
Paso 4: Iniciar el juego y disfrutar de todos los personajes
-
Después de la instalación, puede iniciar el juego desde el cajón de la aplicación o la pantalla de inicio. Deberías ver un mensaje que te diga que has desbloqueado todos los personajes de Crossy Road. Ahora puedes elegir el personaje que quieras y jugar con él. También puedes cambiar entre diferentes personajes y mundos como quieras.
-
Cómo jugar Crossy Road con todos los personajes
-
Ahora que tienes todos los personajes en Crossy Road, puedes preguntarte cómo jugar con ellos y cuáles son sus características especiales. Aquí hay algunos consejos y trucos para ayudarle a disfrutar del juego más:
-
Consejos y trucos para cruzar la carretera, evitar obstáculos y recoger monedas
-
- -
No te apresures. A veces, es mejor esperar un hueco en el tráfico o un lugar seguro que avanzar imprudentemente. Sin embargo, no esperes demasiado o un águila caerá y te agarrará.
--
Utilice el entorno. Algunos objetos en el entorno pueden ayudarle a cruzar la carretera o evitar obstáculos. Por ejemplo, puedes subirte a troncos, nenúfares o témpanos de hielo para cruzar ríos, o usar trenes, autos o cohetes para moverte más rápido.
-
--
Recoge monedas. Las monedas se encuentran dispersas por todo el juego y pueden ayudarte a desbloquear más personajes o comprar pistas. También puedes obtener monedas viendo anuncios o completando misiones.
--
Usa pistas. Las pistas son pistas que te dicen cómo desbloquear ciertos personajes o mundos. Puedes comprar pistas con monedas u obtenerlas gratis viendo anuncios.
-
Curiosidades y características de diferentes personajes y mundos
-
Uno de los aspectos más atractivos de Crossy Road es la variedad y diversidad de personajes y mundos con los que puedes jugar. Cada personaje tiene su propia personalidad, apariencia, efectos de sonido y animaciones. Algunos personajes también tienen habilidades especiales o efectos que pueden cambiar el juego. Por ejemplo, algunos personajes pueden volar, nadar, disparar, explotar o transformarse. Algunos personajes también tienen interacciones secretas con otros personajes u objetos en el juego.
-
De manera similar, cada mundo tiene su propio tema, fondo, música y obstáculos. Algunos mundos se basan en lugares reales, como Australia, China o Inglaterra, mientras que otros se basan en escenarios ficticios, como Halloween, Space o The Wizard of Oz. Algunos mundos también tienen secretos ocultos o huevos de Pascua que puedes descubrir jugando con ciertos personajes o haciendo ciertas acciones.
-
Para darte una idea de la diversidad y creatividad de los personajes y mundos de Crossy Road, aquí hay algunos ejemplos:
-
-
-
Carácter
-
Mundo
-
Característica
-
-
-
Pingüino
-
Ártico
-
-
-
-
Zombie
-
Halloween
-
Puede infectar a otros personajes y convertirlos en zombies
-
-
-
Señora del gato
-
Carretera transversal
-
Tiene una horda de gatos siguiéndola por todas partes
-
-
-
P-Switch
-
Mario World
-
Puede convertir monedas en ladrillos y viceversa
-
-
-
Doge
-
Mundo dogo
-
Tiene efectos de sonido inspirados en memes y burbujas de texto
-
-
-
Jirafa
-
Sabana
-
Tiene un cuello largo que puede alcanzar lugares altos
-
-
-
Marty McFly
-
El futuro
-
Tiene un hoverboard que puede volar sobre los obstáculos
-
-
-
T-Rex
Jurassic World
Puede rugir y asustar a otros dinosaurios
Conclusión >
Crossy Road es un juego divertido y adictivo que ofrece entretenimiento y desafíos sin fin. Con un archivo APK, puedes desbloquear todos los personajes del juego de forma gratuita y disfrutar jugando con ellos en diferentes mundos. Sin embargo, debes ser consciente de los riesgos de usar un archivo APK, como un posible malware, robo de datos o problemas legales. También debes respetar a los desarrolladores del juego y apoyarlos comprando monedas o personajes si puedes. Crossy Road es un juego que merece su aprecio y atención. Ya sea que uses un archivo APK o no, esperamos que te diviertas jugando Crossy Road y descubriendo todos los personajes y mundos que tiene para ofrecer.
-
Preguntas frecuentes
-
Aquí hay algunas preguntas frecuentes sobre Crossy Road y archivos APK:
-
Q: ¿Cuántos personajes hay en Crossy Road?
-
A: Hay más de 200 caracteres en Crossy Road, incluyendo animales, personas, vehículos y más. Algunos de ellos se basan en referencias de la cultura popular, como Star Wars, Harry Potter o Minecraft. Algunos de ellos también son exclusivos para ciertas plataformas, como iOS, Android o Windows.
-
Q: ¿Cómo puedo desbloquear caracteres en Crossy Road sin usar un archivo APK?
-
-
Q: ¿Usar un archivo APK es ilegal o no es ético?
-
A: El uso de un archivo APK para desbloquear todos los personajes en Crossy Road puede ser considerado ilegal o poco ético por algunas personas. Esto se debe a que está utilizando una versión modificada del juego que evita el sistema de pago original y viola los términos de servicio del juego. También estás privando a los desarrolladores del juego de sus ingresos y reconocimiento legítimos. Sin embargo, algunas personas pueden argumentar que el uso de un archivo APK es inofensivo y no afecta la jugabilidad o la calidad del juego.
-
Q: ¿Cuáles son los riesgos de usar un archivo APK?
-
A: El uso de un archivo APK puede exponerlo a algunos riesgos, como malware, robo de datos o problemas legales. El malware es un software que puede dañar su dispositivo o robar su información personal. El robo de datos es cuando alguien accede a sus datos privados sin su permiso. Los problemas legales son cuando usted enfrenta consecuencias legales por violar las leyes o regulaciones de su país o región. Para evitar estos riesgos, solo debe descargar e instalar archivos APK de fuentes confiables y escanearlos con software antivirus antes de instalarlos.
-
Q: ¿Cómo puedo desinstalar un archivo APK?
-
A: Si desea desinstalar un archivo APK de su dispositivo, puede seguir estos pasos:
- -
Ir a Configuración > Aplicaciones > Crossy Road
--
Toque en desinstalar y confirmar su elección
--
Alternativamente, también puede pulsar largo en el icono de la aplicación en la pantalla de inicio o cajón de aplicaciones y arrastrarlo a la opción de desinstalación
--
Tenga en cuenta que la desinstalación de un archivo APK eliminará todos los datos y el progreso asociado con él. Si desea mantener sus datos y el progreso, debe hacer una copia de seguridad antes de desinstalar.
64aa2da5cf
-
-
\ No newline at end of file
diff --git a/spaces/Big-Web/MMSD/env/Lib/site-packages/setuptools/command/build.py b/spaces/Big-Web/MMSD/env/Lib/site-packages/setuptools/command/build.py
deleted file mode 100644
index c0676d8e4b1a567969cf05c5825d49c3300284c9..0000000000000000000000000000000000000000
--- a/spaces/Big-Web/MMSD/env/Lib/site-packages/setuptools/command/build.py
+++ /dev/null
@@ -1,146 +0,0 @@
-import sys
-import warnings
-from typing import TYPE_CHECKING, List, Dict
-from distutils.command.build import build as _build
-
-from setuptools import SetuptoolsDeprecationWarning
-
-if sys.version_info >= (3, 8):
- from typing import Protocol
-elif TYPE_CHECKING:
- from typing_extensions import Protocol
-else:
- from abc import ABC as Protocol
-
-
-_ORIGINAL_SUBCOMMANDS = {"build_py", "build_clib", "build_ext", "build_scripts"}
-
-
-class build(_build):
- # copy to avoid sharing the object with parent class
- sub_commands = _build.sub_commands[:]
-
- def get_sub_commands(self):
- subcommands = {cmd[0] for cmd in _build.sub_commands}
- if subcommands - _ORIGINAL_SUBCOMMANDS:
- msg = """
- It seems that you are using `distutils.command.build` to add
- new subcommands. Using `distutils` directly is considered deprecated,
- please use `setuptools.command.build`.
- """
- warnings.warn(msg, SetuptoolsDeprecationWarning)
- self.sub_commands = _build.sub_commands
- return super().get_sub_commands()
-
-
-class SubCommand(Protocol):
- """In order to support editable installations (see :pep:`660`) all
- build subcommands **SHOULD** implement this protocol. They also **MUST** inherit
- from ``setuptools.Command``.
-
- When creating an :pep:`editable wheel <660>`, ``setuptools`` will try to evaluate
- custom ``build`` subcommands using the following procedure:
-
- 1. ``setuptools`` will set the ``editable_mode`` attribute to ``True``
- 2. ``setuptools`` will execute the ``run()`` command.
-
- .. important::
- Subcommands **SHOULD** take advantage of ``editable_mode=True`` to adequate
- its behaviour or perform optimisations.
-
- For example, if a subcommand don't need to generate any extra file and
- everything it does is to copy a source file into the build directory,
- ``run()`` **SHOULD** simply "early return".
-
- Similarly, if the subcommand creates files that would be placed alongside
- Python files in the final distribution, during an editable install
- the command **SHOULD** generate these files "in place" (i.e. write them to
- the original source directory, instead of using the build directory).
- Note that ``get_output_mapping()`` should reflect that and include mappings
- for "in place" builds accordingly.
-
- 3. ``setuptools`` use any knowledge it can derive from the return values of
- ``get_outputs()`` and ``get_output_mapping()`` to create an editable wheel.
- When relevant ``setuptools`` **MAY** attempt to use file links based on the value
- of ``get_output_mapping()``. Alternatively, ``setuptools`` **MAY** attempt to use
- :doc:`import hooks ` to redirect any attempt to import
- to the directory with the original source code and other files built in place.
-
- Please note that custom sub-commands **SHOULD NOT** rely on ``run()`` being
- executed (or not) to provide correct return values for ``get_outputs()``,
- ``get_output_mapping()`` or ``get_source_files()``. The ``get_*`` methods should
- work independently of ``run()``.
- """
-
- editable_mode: bool = False
- """Boolean flag that will be set to ``True`` when setuptools is used for an
- editable installation (see :pep:`660`).
- Implementations **SHOULD** explicitly set the default value of this attribute to
- ``False``.
- When subcommands run, they can use this flag to perform optimizations or change
- their behaviour accordingly.
- """
-
- build_lib: str
- """String representing the directory where the build artifacts should be stored,
- e.g. ``build/lib``.
- For example, if a distribution wants to provide a Python module named ``pkg.mod``,
- then a corresponding file should be written to ``{build_lib}/package/module.py``.
- A way of thinking about this is that the files saved under ``build_lib``
- would be eventually copied to one of the directories in :obj:`site.PREFIXES`
- upon installation.
-
- A command that produces platform-independent files (e.g. compiling text templates
- into Python functions), **CAN** initialize ``build_lib`` by copying its value from
- the ``build_py`` command. On the other hand, a command that produces
- platform-specific files **CAN** initialize ``build_lib`` by copying its value from
- the ``build_ext`` command. In general this is done inside the ``finalize_options``
- method with the help of the ``set_undefined_options`` command::
-
- def finalize_options(self):
- self.set_undefined_options("build_py", ("build_lib", "build_lib"))
- ...
- """
-
- def initialize_options(self):
- """(Required by the original :class:`setuptools.Command` interface)"""
-
- def finalize_options(self):
- """(Required by the original :class:`setuptools.Command` interface)"""
-
- def run(self):
- """(Required by the original :class:`setuptools.Command` interface)"""
-
- def get_source_files(self) -> List[str]:
- """
- Return a list of all files that are used by the command to create the expected
- outputs.
- For example, if your build command transpiles Java files into Python, you should
- list here all the Java files.
- The primary purpose of this function is to help populating the ``sdist``
- with all the files necessary to build the distribution.
- All files should be strings relative to the project root directory.
- """
-
- def get_outputs(self) -> List[str]:
- """
- Return a list of files intended for distribution as they would have been
- produced by the build.
- These files should be strings in the form of
- ``"{build_lib}/destination/file/path"``.
-
- .. note::
- The return value of ``get_output()`` should include all files used as keys
- in ``get_output_mapping()`` plus files that are generated during the build
- and don't correspond to any source file already present in the project.
- """
-
- def get_output_mapping(self) -> Dict[str, str]:
- """
- Return a mapping between destination files as they would be produced by the
- build (dict keys) into the respective existing (source) files (dict values).
- Existing (source) files should be represented as strings relative to the project
- root directory.
- Destination files should be strings in the form of
- ``"{build_lib}/destination/file/path"``.
- """
diff --git a/spaces/Big-Web/MMSD/env/Lib/site-packages/setuptools/config/pyprojecttoml.py b/spaces/Big-Web/MMSD/env/Lib/site-packages/setuptools/config/pyprojecttoml.py
deleted file mode 100644
index d995f0bcc7e322d50af91ee23f3241d8cf46e637..0000000000000000000000000000000000000000
--- a/spaces/Big-Web/MMSD/env/Lib/site-packages/setuptools/config/pyprojecttoml.py
+++ /dev/null
@@ -1,493 +0,0 @@
-"""
-Load setuptools configuration from ``pyproject.toml`` files.
-
-**PRIVATE MODULE**: API reserved for setuptools internal usage only.
-"""
-import logging
-import os
-import warnings
-from contextlib import contextmanager
-from functools import partial
-from typing import TYPE_CHECKING, Callable, Dict, Optional, Mapping, Union
-
-from setuptools.errors import FileError, OptionError
-
-from . import expand as _expand
-from ._apply_pyprojecttoml import apply as _apply
-from ._apply_pyprojecttoml import _PREVIOUSLY_DEFINED, _WouldIgnoreField
-
-if TYPE_CHECKING:
- from setuptools.dist import Distribution # noqa
-
-_Path = Union[str, os.PathLike]
-_logger = logging.getLogger(__name__)
-
-
-def load_file(filepath: _Path) -> dict:
- from setuptools.extern import tomli # type: ignore
-
- with open(filepath, "rb") as file:
- return tomli.load(file)
-
-
-def validate(config: dict, filepath: _Path) -> bool:
- from . import _validate_pyproject as validator
-
- trove_classifier = validator.FORMAT_FUNCTIONS.get("trove-classifier")
- if hasattr(trove_classifier, "_disable_download"):
- # Improve reproducibility by default. See issue 31 for validate-pyproject.
- trove_classifier._disable_download() # type: ignore
-
- try:
- return validator.validate(config)
- except validator.ValidationError as ex:
- summary = f"configuration error: {ex.summary}"
- if ex.name.strip("`") != "project":
- # Probably it is just a field missing/misnamed, not worthy the verbosity...
- _logger.debug(summary)
- _logger.debug(ex.details)
-
- error = f"invalid pyproject.toml config: {ex.name}."
- raise ValueError(f"{error}\n{summary}") from None
-
-
-def apply_configuration(
- dist: "Distribution",
- filepath: _Path,
- ignore_option_errors=False,
-) -> "Distribution":
- """Apply the configuration from a ``pyproject.toml`` file into an existing
- distribution object.
- """
- config = read_configuration(filepath, True, ignore_option_errors, dist)
- return _apply(dist, config, filepath)
-
-
-def read_configuration(
- filepath: _Path,
- expand=True,
- ignore_option_errors=False,
- dist: Optional["Distribution"] = None,
-):
- """Read given configuration file and returns options from it as a dict.
-
- :param str|unicode filepath: Path to configuration file in the ``pyproject.toml``
- format.
-
- :param bool expand: Whether to expand directives and other computed values
- (i.e. post-process the given configuration)
-
- :param bool ignore_option_errors: Whether to silently ignore
- options, values of which could not be resolved (e.g. due to exceptions
- in directives such as file:, attr:, etc.).
- If False exceptions are propagated as expected.
-
- :param Distribution|None: Distribution object to which the configuration refers.
- If not given a dummy object will be created and discarded after the
- configuration is read. This is used for auto-discovery of packages in the case
- a dynamic configuration (e.g. ``attr`` or ``cmdclass``) is expanded.
- When ``expand=False`` this object is simply ignored.
-
- :rtype: dict
- """
- filepath = os.path.abspath(filepath)
-
- if not os.path.isfile(filepath):
- raise FileError(f"Configuration file {filepath!r} does not exist.")
-
- asdict = load_file(filepath) or {}
- project_table = asdict.get("project", {})
- tool_table = asdict.get("tool", {})
- setuptools_table = tool_table.get("setuptools", {})
- if not asdict or not (project_table or setuptools_table):
- return {} # User is not using pyproject to configure setuptools
-
- if setuptools_table:
- # TODO: Remove the following once the feature stabilizes:
- msg = "Support for `[tool.setuptools]` in `pyproject.toml` is still *beta*."
- warnings.warn(msg, _BetaConfiguration)
-
- # There is an overall sense in the community that making include_package_data=True
- # the default would be an improvement.
- # `ini2toml` backfills include_package_data=False when nothing is explicitly given,
- # therefore setting a default here is backwards compatible.
- orig_setuptools_table = setuptools_table.copy()
- if dist and getattr(dist, "include_package_data") is not None:
- setuptools_table.setdefault("include-package-data", dist.include_package_data)
- else:
- setuptools_table.setdefault("include-package-data", True)
- # Persist changes:
- asdict["tool"] = tool_table
- tool_table["setuptools"] = setuptools_table
-
- try:
- # Don't complain about unrelated errors (e.g. tools not using the "tool" table)
- subset = {"project": project_table, "tool": {"setuptools": setuptools_table}}
- validate(subset, filepath)
- except Exception as ex:
- # TODO: Remove the following once the feature stabilizes:
- if _skip_bad_config(project_table, orig_setuptools_table, dist):
- return {}
- # TODO: After the previous statement is removed the try/except can be replaced
- # by the _ignore_errors context manager.
- if ignore_option_errors:
- _logger.debug(f"ignored error: {ex.__class__.__name__} - {ex}")
- else:
- raise # re-raise exception
-
- if expand:
- root_dir = os.path.dirname(filepath)
- return expand_configuration(asdict, root_dir, ignore_option_errors, dist)
-
- return asdict
-
-
-def _skip_bad_config(
- project_cfg: dict, setuptools_cfg: dict, dist: Optional["Distribution"]
-) -> bool:
- """Be temporarily forgiving with invalid ``pyproject.toml``"""
- # See pypa/setuptools#3199 and pypa/cibuildwheel#1064
-
- if dist is None or (
- dist.metadata.name is None
- and dist.metadata.version is None
- and dist.install_requires is None
- ):
- # It seems that the build is not getting any configuration from other places
- return False
-
- if setuptools_cfg:
- # If `[tool.setuptools]` is set, then `pyproject.toml` config is intentional
- return False
-
- given_config = set(project_cfg.keys())
- popular_subset = {"name", "version", "python_requires", "requires-python"}
- if given_config <= popular_subset:
- # It seems that the docs in cibuildtool has been inadvertently encouraging users
- # to create `pyproject.toml` files that are not compliant with the standards.
- # Let's be forgiving for the time being.
- warnings.warn(_InvalidFile.message(), _InvalidFile, stacklevel=2)
- return True
-
- return False
-
-
-def expand_configuration(
- config: dict,
- root_dir: Optional[_Path] = None,
- ignore_option_errors: bool = False,
- dist: Optional["Distribution"] = None,
-) -> dict:
- """Given a configuration with unresolved fields (e.g. dynamic, cmdclass, ...)
- find their final values.
-
- :param dict config: Dict containing the configuration for the distribution
- :param str root_dir: Top-level directory for the distribution/project
- (the same directory where ``pyproject.toml`` is place)
- :param bool ignore_option_errors: see :func:`read_configuration`
- :param Distribution|None: Distribution object to which the configuration refers.
- If not given a dummy object will be created and discarded after the
- configuration is read. Used in the case a dynamic configuration
- (e.g. ``attr`` or ``cmdclass``).
-
- :rtype: dict
- """
- return _ConfigExpander(config, root_dir, ignore_option_errors, dist).expand()
-
-
-class _ConfigExpander:
- def __init__(
- self,
- config: dict,
- root_dir: Optional[_Path] = None,
- ignore_option_errors: bool = False,
- dist: Optional["Distribution"] = None,
- ):
- self.config = config
- self.root_dir = root_dir or os.getcwd()
- self.project_cfg = config.get("project", {})
- self.dynamic = self.project_cfg.get("dynamic", [])
- self.setuptools_cfg = config.get("tool", {}).get("setuptools", {})
- self.dynamic_cfg = self.setuptools_cfg.get("dynamic", {})
- self.ignore_option_errors = ignore_option_errors
- self._dist = dist
-
- def _ensure_dist(self) -> "Distribution":
- from setuptools.dist import Distribution
-
- attrs = {"src_root": self.root_dir, "name": self.project_cfg.get("name", None)}
- return self._dist or Distribution(attrs)
-
- def _process_field(self, container: dict, field: str, fn: Callable):
- if field in container:
- with _ignore_errors(self.ignore_option_errors):
- container[field] = fn(container[field])
-
- def _canonic_package_data(self, field="package-data"):
- package_data = self.setuptools_cfg.get(field, {})
- return _expand.canonic_package_data(package_data)
-
- def expand(self):
- self._expand_packages()
- self._canonic_package_data()
- self._canonic_package_data("exclude-package-data")
-
- # A distribution object is required for discovering the correct package_dir
- dist = self._ensure_dist()
- ctx = _EnsurePackagesDiscovered(dist, self.project_cfg, self.setuptools_cfg)
- with ctx as ensure_discovered:
- package_dir = ensure_discovered.package_dir
- self._expand_data_files()
- self._expand_cmdclass(package_dir)
- self._expand_all_dynamic(dist, package_dir)
-
- return self.config
-
- def _expand_packages(self):
- packages = self.setuptools_cfg.get("packages")
- if packages is None or isinstance(packages, (list, tuple)):
- return
-
- find = packages.get("find")
- if isinstance(find, dict):
- find["root_dir"] = self.root_dir
- find["fill_package_dir"] = self.setuptools_cfg.setdefault("package-dir", {})
- with _ignore_errors(self.ignore_option_errors):
- self.setuptools_cfg["packages"] = _expand.find_packages(**find)
-
- def _expand_data_files(self):
- data_files = partial(_expand.canonic_data_files, root_dir=self.root_dir)
- self._process_field(self.setuptools_cfg, "data-files", data_files)
-
- def _expand_cmdclass(self, package_dir: Mapping[str, str]):
- root_dir = self.root_dir
- cmdclass = partial(_expand.cmdclass, package_dir=package_dir, root_dir=root_dir)
- self._process_field(self.setuptools_cfg, "cmdclass", cmdclass)
-
- def _expand_all_dynamic(self, dist: "Distribution", package_dir: Mapping[str, str]):
- special = ( # need special handling
- "version",
- "readme",
- "entry-points",
- "scripts",
- "gui-scripts",
- "classifiers",
- "dependencies",
- "optional-dependencies",
- )
- # `_obtain` functions are assumed to raise appropriate exceptions/warnings.
- obtained_dynamic = {
- field: self._obtain(dist, field, package_dir)
- for field in self.dynamic
- if field not in special
- }
- obtained_dynamic.update(
- self._obtain_entry_points(dist, package_dir) or {},
- version=self._obtain_version(dist, package_dir),
- readme=self._obtain_readme(dist),
- classifiers=self._obtain_classifiers(dist),
- dependencies=self._obtain_dependencies(dist),
- optional_dependencies=self._obtain_optional_dependencies(dist),
- )
- # `None` indicates there is nothing in `tool.setuptools.dynamic` but the value
- # might have already been set by setup.py/extensions, so avoid overwriting.
- updates = {k: v for k, v in obtained_dynamic.items() if v is not None}
- self.project_cfg.update(updates)
-
- def _ensure_previously_set(self, dist: "Distribution", field: str):
- previous = _PREVIOUSLY_DEFINED[field](dist)
- if previous is None and not self.ignore_option_errors:
- msg = (
- f"No configuration found for dynamic {field!r}.\n"
- "Some dynamic fields need to be specified via `tool.setuptools.dynamic`"
- "\nothers must be specified via the equivalent attribute in `setup.py`."
- )
- raise OptionError(msg)
-
- def _expand_directive(
- self, specifier: str, directive, package_dir: Mapping[str, str]
- ):
- with _ignore_errors(self.ignore_option_errors):
- root_dir = self.root_dir
- if "file" in directive:
- return _expand.read_files(directive["file"], root_dir)
- if "attr" in directive:
- return _expand.read_attr(directive["attr"], package_dir, root_dir)
- raise ValueError(f"invalid `{specifier}`: {directive!r}")
- return None
-
- def _obtain(self, dist: "Distribution", field: str, package_dir: Mapping[str, str]):
- if field in self.dynamic_cfg:
- return self._expand_directive(
- f"tool.setuptools.dynamic.{field}",
- self.dynamic_cfg[field],
- package_dir,
- )
- self._ensure_previously_set(dist, field)
- return None
-
- def _obtain_version(self, dist: "Distribution", package_dir: Mapping[str, str]):
- # Since plugins can set version, let's silently skip if it cannot be obtained
- if "version" in self.dynamic and "version" in self.dynamic_cfg:
- return _expand.version(self._obtain(dist, "version", package_dir))
- return None
-
- def _obtain_readme(self, dist: "Distribution") -> Optional[Dict[str, str]]:
- if "readme" not in self.dynamic:
- return None
-
- dynamic_cfg = self.dynamic_cfg
- if "readme" in dynamic_cfg:
- return {
- "text": self._obtain(dist, "readme", {}),
- "content-type": dynamic_cfg["readme"].get("content-type", "text/x-rst"),
- }
-
- self._ensure_previously_set(dist, "readme")
- return None
-
- def _obtain_entry_points(
- self, dist: "Distribution", package_dir: Mapping[str, str]
- ) -> Optional[Dict[str, dict]]:
- fields = ("entry-points", "scripts", "gui-scripts")
- if not any(field in self.dynamic for field in fields):
- return None
-
- text = self._obtain(dist, "entry-points", package_dir)
- if text is None:
- return None
-
- groups = _expand.entry_points(text)
- expanded = {"entry-points": groups}
-
- def _set_scripts(field: str, group: str):
- if group in groups:
- value = groups.pop(group)
- if field not in self.dynamic:
- msg = _WouldIgnoreField.message(field, value)
- warnings.warn(msg, _WouldIgnoreField)
- # TODO: Don't set field when support for pyproject.toml stabilizes
- # instead raise an error as specified in PEP 621
- expanded[field] = value
-
- _set_scripts("scripts", "console_scripts")
- _set_scripts("gui-scripts", "gui_scripts")
-
- return expanded
-
- def _obtain_classifiers(self, dist: "Distribution"):
- if "classifiers" in self.dynamic:
- value = self._obtain(dist, "classifiers", {})
- if value:
- return value.splitlines()
- return None
-
- def _obtain_dependencies(self, dist: "Distribution"):
- if "dependencies" in self.dynamic:
- value = self._obtain(dist, "dependencies", {})
- if value:
- return _parse_requirements_list(value)
- return None
-
- def _obtain_optional_dependencies(self, dist: "Distribution"):
- if "optional-dependencies" not in self.dynamic:
- return None
- if "optional-dependencies" in self.dynamic_cfg:
- optional_dependencies_map = self.dynamic_cfg["optional-dependencies"]
- assert isinstance(optional_dependencies_map, dict)
- return {
- group: _parse_requirements_list(self._expand_directive(
- f"tool.setuptools.dynamic.optional-dependencies.{group}",
- directive,
- {},
- ))
- for group, directive in optional_dependencies_map.items()
- }
- self._ensure_previously_set(dist, "optional-dependencies")
- return None
-
-
-def _parse_requirements_list(value):
- return [
- line
- for line in value.splitlines()
- if line.strip() and not line.strip().startswith("#")
- ]
-
-
-@contextmanager
-def _ignore_errors(ignore_option_errors: bool):
- if not ignore_option_errors:
- yield
- return
-
- try:
- yield
- except Exception as ex:
- _logger.debug(f"ignored error: {ex.__class__.__name__} - {ex}")
-
-
-class _EnsurePackagesDiscovered(_expand.EnsurePackagesDiscovered):
- def __init__(
- self, distribution: "Distribution", project_cfg: dict, setuptools_cfg: dict
- ):
- super().__init__(distribution)
- self._project_cfg = project_cfg
- self._setuptools_cfg = setuptools_cfg
-
- def __enter__(self):
- """When entering the context, the values of ``packages``, ``py_modules`` and
- ``package_dir`` that are missing in ``dist`` are copied from ``setuptools_cfg``.
- """
- dist, cfg = self._dist, self._setuptools_cfg
- package_dir: Dict[str, str] = cfg.setdefault("package-dir", {})
- package_dir.update(dist.package_dir or {})
- dist.package_dir = package_dir # needs to be the same object
-
- dist.set_defaults._ignore_ext_modules() # pyproject.toml-specific behaviour
-
- # Set `name`, `py_modules` and `packages` in dist to short-circuit
- # auto-discovery, but avoid overwriting empty lists purposefully set by users.
- if dist.metadata.name is None:
- dist.metadata.name = self._project_cfg.get("name")
- if dist.py_modules is None:
- dist.py_modules = cfg.get("py-modules")
- if dist.packages is None:
- dist.packages = cfg.get("packages")
-
- return super().__enter__()
-
- def __exit__(self, exc_type, exc_value, traceback):
- """When exiting the context, if values of ``packages``, ``py_modules`` and
- ``package_dir`` are missing in ``setuptools_cfg``, copy from ``dist``.
- """
- # If anything was discovered set them back, so they count in the final config.
- self._setuptools_cfg.setdefault("packages", self._dist.packages)
- self._setuptools_cfg.setdefault("py-modules", self._dist.py_modules)
- return super().__exit__(exc_type, exc_value, traceback)
-
-
-class _BetaConfiguration(UserWarning):
- """Explicitly inform users that some `pyproject.toml` configuration is *beta*"""
-
-
-class _InvalidFile(UserWarning):
- """The given `pyproject.toml` file is invalid and would be ignored.
- !!\n\n
- ############################
- # Invalid `pyproject.toml` #
- ############################
-
- Any configurations in `pyproject.toml` will be ignored.
- Please note that future releases of setuptools will halt the build process
- if an invalid file is given.
-
- To prevent setuptools from considering `pyproject.toml` please
- DO NOT include the `[project]` or `[tool.setuptools]` tables in your file.
- \n\n!!
- """
-
- @classmethod
- def message(cls):
- from inspect import cleandoc
- return cleandoc(cls.__doc__)
diff --git a/spaces/CALM/Dashboard/streamlit_observable/frontend/build/precache-manifest.2e1db2924cb1e112608cee049b0d33cc.js b/spaces/CALM/Dashboard/streamlit_observable/frontend/build/precache-manifest.2e1db2924cb1e112608cee049b0d33cc.js
deleted file mode 100644
index 96597718109aca07aede00d7fc6e28a5a11aff01..0000000000000000000000000000000000000000
--- a/spaces/CALM/Dashboard/streamlit_observable/frontend/build/precache-manifest.2e1db2924cb1e112608cee049b0d33cc.js
+++ /dev/null
@@ -1,26 +0,0 @@
-self.__precacheManifest = (self.__precacheManifest || []).concat([
- {
- "revision": "1c6ba26604bc12847ab74fcdb45b2542",
- "url": "./index.html"
- },
- {
- "revision": "5a67f673dcdf30bf693d",
- "url": "./static/js/2.b1c975ff.chunk.js"
- },
- {
- "revision": "9b318b6fb13190fe82c0677e9264b3c7",
- "url": "./static/js/2.b1c975ff.chunk.js.LICENSE.txt"
- },
- {
- "revision": "3301eac1eaca974776ae",
- "url": "./static/js/main.fc603b94.chunk.js"
- },
- {
- "revision": "6515c66d2a8747a146d578e1c038a822",
- "url": "./static/js/main.fc603b94.chunk.js.LICENSE.txt"
- },
- {
- "revision": "7c26bca7e16783d14d15",
- "url": "./static/js/runtime-main.11ec9aca.js"
- }
-]);
\ No newline at end of file
diff --git a/spaces/CVPR/Dual-Key_Backdoor_Attacks/datagen/detectron2/detectron2/layers/deform_conv.py b/spaces/CVPR/Dual-Key_Backdoor_Attacks/datagen/detectron2/detectron2/layers/deform_conv.py
deleted file mode 100644
index d5ced72425968b028b375c4325e38759291c5c25..0000000000000000000000000000000000000000
--- a/spaces/CVPR/Dual-Key_Backdoor_Attacks/datagen/detectron2/detectron2/layers/deform_conv.py
+++ /dev/null
@@ -1,494 +0,0 @@
-# Copyright (c) Facebook, Inc. and its affiliates. All Rights Reserved
-import math
-from functools import lru_cache
-import torch
-from torch import nn
-from torch.autograd import Function
-from torch.autograd.function import once_differentiable
-from torch.nn.modules.utils import _pair
-
-from detectron2 import _C
-
-from .wrappers import _NewEmptyTensorOp
-
-
-class _DeformConv(Function):
- @staticmethod
- def forward(
- ctx,
- input,
- offset,
- weight,
- stride=1,
- padding=0,
- dilation=1,
- groups=1,
- deformable_groups=1,
- im2col_step=64,
- ):
- if input is not None and input.dim() != 4:
- raise ValueError(
- "Expected 4D tensor as input, got {}D tensor instead.".format(input.dim())
- )
- ctx.stride = _pair(stride)
- ctx.padding = _pair(padding)
- ctx.dilation = _pair(dilation)
- ctx.groups = groups
- ctx.deformable_groups = deformable_groups
- ctx.im2col_step = im2col_step
-
- ctx.save_for_backward(input, offset, weight)
-
- output = input.new_empty(
- _DeformConv._output_size(input, weight, ctx.padding, ctx.dilation, ctx.stride)
- )
-
- ctx.bufs_ = [input.new_empty(0), input.new_empty(0)] # columns, ones
-
- if not input.is_cuda:
- raise NotImplementedError
- else:
- cur_im2col_step = _DeformConv._cal_im2col_step(input.shape[0], ctx.im2col_step)
- assert (input.shape[0] % cur_im2col_step) == 0, "im2col step must divide batchsize"
-
- _C.deform_conv_forward(
- input,
- weight,
- offset,
- output,
- ctx.bufs_[0],
- ctx.bufs_[1],
- weight.size(3),
- weight.size(2),
- ctx.stride[1],
- ctx.stride[0],
- ctx.padding[1],
- ctx.padding[0],
- ctx.dilation[1],
- ctx.dilation[0],
- ctx.groups,
- ctx.deformable_groups,
- cur_im2col_step,
- )
- return output
-
- @staticmethod
- @once_differentiable
- def backward(ctx, grad_output):
- input, offset, weight = ctx.saved_tensors
-
- grad_input = grad_offset = grad_weight = None
-
- if not grad_output.is_cuda:
- raise NotImplementedError
- else:
- cur_im2col_step = _DeformConv._cal_im2col_step(input.shape[0], ctx.im2col_step)
- assert (input.shape[0] % cur_im2col_step) == 0, "im2col step must divide batchsize"
-
- if ctx.needs_input_grad[0] or ctx.needs_input_grad[1]:
- grad_input = torch.zeros_like(input)
- grad_offset = torch.zeros_like(offset)
- _C.deform_conv_backward_input(
- input,
- offset,
- grad_output,
- grad_input,
- grad_offset,
- weight,
- ctx.bufs_[0],
- weight.size(3),
- weight.size(2),
- ctx.stride[1],
- ctx.stride[0],
- ctx.padding[1],
- ctx.padding[0],
- ctx.dilation[1],
- ctx.dilation[0],
- ctx.groups,
- ctx.deformable_groups,
- cur_im2col_step,
- )
-
- if ctx.needs_input_grad[2]:
- grad_weight = torch.zeros_like(weight)
- _C.deform_conv_backward_filter(
- input,
- offset,
- grad_output,
- grad_weight,
- ctx.bufs_[0],
- ctx.bufs_[1],
- weight.size(3),
- weight.size(2),
- ctx.stride[1],
- ctx.stride[0],
- ctx.padding[1],
- ctx.padding[0],
- ctx.dilation[1],
- ctx.dilation[0],
- ctx.groups,
- ctx.deformable_groups,
- 1,
- cur_im2col_step,
- )
-
- return grad_input, grad_offset, grad_weight, None, None, None, None, None, None
-
- @staticmethod
- def _output_size(input, weight, padding, dilation, stride):
- channels = weight.size(0)
- output_size = (input.size(0), channels)
- for d in range(input.dim() - 2):
- in_size = input.size(d + 2)
- pad = padding[d]
- kernel = dilation[d] * (weight.size(d + 2) - 1) + 1
- stride_ = stride[d]
- output_size += ((in_size + (2 * pad) - kernel) // stride_ + 1,)
- if not all(map(lambda s: s > 0, output_size)):
- raise ValueError(
- "convolution input is too small (output would be {})".format(
- "x".join(map(str, output_size))
- )
- )
- return output_size
-
- @staticmethod
- @lru_cache(maxsize=128)
- def _cal_im2col_step(input_size, default_size):
- """
- Calculate proper im2col step size, which should be divisible by input_size and not larger
- than prefer_size. Meanwhile the step size should be as large as possible to be more
- efficient. So we choose the largest one among all divisors of input_size which are smaller
- than prefer_size.
- :param input_size: input batch size .
- :param default_size: default preferred im2col step size.
- :return: the largest proper step size.
- """
- if input_size <= default_size:
- return input_size
- best_step = 1
- for step in range(2, min(int(math.sqrt(input_size)) + 1, default_size)):
- if input_size % step == 0:
- if input_size // step <= default_size:
- return input_size // step
- best_step = step
-
- return best_step
-
-
-class _ModulatedDeformConv(Function):
- @staticmethod
- def forward(
- ctx,
- input,
- offset,
- mask,
- weight,
- bias=None,
- stride=1,
- padding=0,
- dilation=1,
- groups=1,
- deformable_groups=1,
- ):
- ctx.stride = stride
- ctx.padding = padding
- ctx.dilation = dilation
- ctx.groups = groups
- ctx.deformable_groups = deformable_groups
- ctx.with_bias = bias is not None
- if not ctx.with_bias:
- bias = input.new_empty(1) # fake tensor
- if not input.is_cuda:
- raise NotImplementedError
- if (
- weight.requires_grad
- or mask.requires_grad
- or offset.requires_grad
- or input.requires_grad
- ):
- ctx.save_for_backward(input, offset, mask, weight, bias)
- output = input.new_empty(_ModulatedDeformConv._infer_shape(ctx, input, weight))
- ctx._bufs = [input.new_empty(0), input.new_empty(0)]
- _C.modulated_deform_conv_forward(
- input,
- weight,
- bias,
- ctx._bufs[0],
- offset,
- mask,
- output,
- ctx._bufs[1],
- weight.shape[2],
- weight.shape[3],
- ctx.stride,
- ctx.stride,
- ctx.padding,
- ctx.padding,
- ctx.dilation,
- ctx.dilation,
- ctx.groups,
- ctx.deformable_groups,
- ctx.with_bias,
- )
- return output
-
- @staticmethod
- @once_differentiable
- def backward(ctx, grad_output):
- if not grad_output.is_cuda:
- raise NotImplementedError
- input, offset, mask, weight, bias = ctx.saved_tensors
- grad_input = torch.zeros_like(input)
- grad_offset = torch.zeros_like(offset)
- grad_mask = torch.zeros_like(mask)
- grad_weight = torch.zeros_like(weight)
- grad_bias = torch.zeros_like(bias)
- _C.modulated_deform_conv_backward(
- input,
- weight,
- bias,
- ctx._bufs[0],
- offset,
- mask,
- ctx._bufs[1],
- grad_input,
- grad_weight,
- grad_bias,
- grad_offset,
- grad_mask,
- grad_output,
- weight.shape[2],
- weight.shape[3],
- ctx.stride,
- ctx.stride,
- ctx.padding,
- ctx.padding,
- ctx.dilation,
- ctx.dilation,
- ctx.groups,
- ctx.deformable_groups,
- ctx.with_bias,
- )
- if not ctx.with_bias:
- grad_bias = None
-
- return (
- grad_input,
- grad_offset,
- grad_mask,
- grad_weight,
- grad_bias,
- None,
- None,
- None,
- None,
- None,
- )
-
- @staticmethod
- def _infer_shape(ctx, input, weight):
- n = input.size(0)
- channels_out = weight.size(0)
- height, width = input.shape[2:4]
- kernel_h, kernel_w = weight.shape[2:4]
- height_out = (
- height + 2 * ctx.padding - (ctx.dilation * (kernel_h - 1) + 1)
- ) // ctx.stride + 1
- width_out = (
- width + 2 * ctx.padding - (ctx.dilation * (kernel_w - 1) + 1)
- ) // ctx.stride + 1
- return n, channels_out, height_out, width_out
-
-
-deform_conv = _DeformConv.apply
-modulated_deform_conv = _ModulatedDeformConv.apply
-
-
-class DeformConv(nn.Module):
- def __init__(
- self,
- in_channels,
- out_channels,
- kernel_size,
- stride=1,
- padding=0,
- dilation=1,
- groups=1,
- deformable_groups=1,
- bias=False,
- norm=None,
- activation=None,
- ):
- """
- Deformable convolution.
-
- Arguments are similar to :class:`Conv2D`. Extra arguments:
-
- Args:
- deformable_groups (int): number of groups used in deformable convolution.
- norm (nn.Module, optional): a normalization layer
- activation (callable(Tensor) -> Tensor): a callable activation function
- """
- super(DeformConv, self).__init__()
-
- assert not bias
- assert in_channels % groups == 0, "in_channels {} cannot be divisible by groups {}".format(
- in_channels, groups
- )
- assert (
- out_channels % groups == 0
- ), "out_channels {} cannot be divisible by groups {}".format(out_channels, groups)
-
- self.in_channels = in_channels
- self.out_channels = out_channels
- self.kernel_size = _pair(kernel_size)
- self.stride = _pair(stride)
- self.padding = _pair(padding)
- self.dilation = _pair(dilation)
- self.groups = groups
- self.deformable_groups = deformable_groups
- self.norm = norm
- self.activation = activation
-
- self.weight = nn.Parameter(
- torch.Tensor(out_channels, in_channels // self.groups, *self.kernel_size)
- )
- self.bias = None
-
- nn.init.kaiming_uniform_(self.weight, nonlinearity="relu")
-
- def forward(self, x, offset):
- if x.numel() == 0:
- # When input is empty, we want to return a empty tensor with "correct" shape,
- # So that the following operations will not panic
- # if they check for the shape of the tensor.
- # This computes the height and width of the output tensor
- output_shape = [
- (i + 2 * p - (di * (k - 1) + 1)) // s + 1
- for i, p, di, k, s in zip(
- x.shape[-2:], self.padding, self.dilation, self.kernel_size, self.stride
- )
- ]
- output_shape = [x.shape[0], self.weight.shape[0]] + output_shape
- return _NewEmptyTensorOp.apply(x, output_shape)
-
- x = deform_conv(
- x,
- offset,
- self.weight,
- self.stride,
- self.padding,
- self.dilation,
- self.groups,
- self.deformable_groups,
- )
- if self.norm is not None:
- x = self.norm(x)
- if self.activation is not None:
- x = self.activation(x)
- return x
-
- def extra_repr(self):
- tmpstr = "in_channels=" + str(self.in_channels)
- tmpstr += ", out_channels=" + str(self.out_channels)
- tmpstr += ", kernel_size=" + str(self.kernel_size)
- tmpstr += ", stride=" + str(self.stride)
- tmpstr += ", padding=" + str(self.padding)
- tmpstr += ", dilation=" + str(self.dilation)
- tmpstr += ", groups=" + str(self.groups)
- tmpstr += ", deformable_groups=" + str(self.deformable_groups)
- tmpstr += ", bias=False"
- return tmpstr
-
-
-class ModulatedDeformConv(nn.Module):
- def __init__(
- self,
- in_channels,
- out_channels,
- kernel_size,
- stride=1,
- padding=0,
- dilation=1,
- groups=1,
- deformable_groups=1,
- bias=True,
- norm=None,
- activation=None,
- ):
- """
- Modulated deformable convolution.
-
- Arguments are similar to :class:`Conv2D`. Extra arguments:
-
- Args:
- deformable_groups (int): number of groups used in deformable convolution.
- norm (nn.Module, optional): a normalization layer
- activation (callable(Tensor) -> Tensor): a callable activation function
- """
- super(ModulatedDeformConv, self).__init__()
- self.in_channels = in_channels
- self.out_channels = out_channels
- self.kernel_size = _pair(kernel_size)
- self.stride = stride
- self.padding = padding
- self.dilation = dilation
- self.groups = groups
- self.deformable_groups = deformable_groups
- self.with_bias = bias
- self.norm = norm
- self.activation = activation
-
- self.weight = nn.Parameter(
- torch.Tensor(out_channels, in_channels // groups, *self.kernel_size)
- )
- if bias:
- self.bias = nn.Parameter(torch.Tensor(out_channels))
- else:
- self.bias = None
-
- nn.init.kaiming_uniform_(self.weight, nonlinearity="relu")
- if self.bias is not None:
- nn.init.constant_(self.bias, 0)
-
- def forward(self, x, offset, mask):
- if x.numel() == 0:
- output_shape = [
- (i + 2 * p - (di * (k - 1) + 1)) // s + 1
- for i, p, di, k, s in zip(
- x.shape[-2:], self.padding, self.dilation, self.kernel_size, self.stride
- )
- ]
- output_shape = [x.shape[0], self.weight.shape[0]] + output_shape
- return _NewEmptyTensorOp.apply(x, output_shape)
-
- x = modulated_deform_conv(
- x,
- offset,
- mask,
- self.weight,
- self.bias,
- self.stride,
- self.padding,
- self.dilation,
- self.groups,
- self.deformable_groups,
- )
- if self.norm is not None:
- x = self.norm(x)
- if self.activation is not None:
- x = self.activation(x)
- return x
-
- def extra_repr(self):
- tmpstr = "in_channels=" + str(self.in_channels)
- tmpstr += ", out_channels=" + str(self.out_channels)
- tmpstr += ", kernel_size=" + str(self.kernel_size)
- tmpstr += ", stride=" + str(self.stride)
- tmpstr += ", padding=" + str(self.padding)
- tmpstr += ", dilation=" + str(self.dilation)
- tmpstr += ", groups=" + str(self.groups)
- tmpstr += ", deformable_groups=" + str(self.deformable_groups)
- tmpstr += ", bias=" + str(self.with_bias)
- return tmpstr
diff --git a/spaces/CVPR/Dual-Key_Backdoor_Attacks/datagen/detectron2/projects/TridentNet/tridentnet/config.py b/spaces/CVPR/Dual-Key_Backdoor_Attacks/datagen/detectron2/projects/TridentNet/tridentnet/config.py
deleted file mode 100644
index f33f473cb32633d9ba6582f0406ffe0a929d23c6..0000000000000000000000000000000000000000
--- a/spaces/CVPR/Dual-Key_Backdoor_Attacks/datagen/detectron2/projects/TridentNet/tridentnet/config.py
+++ /dev/null
@@ -1,26 +0,0 @@
-# -*- coding: utf-8 -*-
-# Copyright (c) Facebook, Inc. and its affiliates. All Rights Reserved
-
-from detectron2.config import CfgNode as CN
-
-
-def add_tridentnet_config(cfg):
- """
- Add config for tridentnet.
- """
- _C = cfg
-
- _C.MODEL.TRIDENT = CN()
-
- # Number of branches for TridentNet.
- _C.MODEL.TRIDENT.NUM_BRANCH = 3
- # Specify the dilations for each branch.
- _C.MODEL.TRIDENT.BRANCH_DILATIONS = [1, 2, 3]
- # Specify the stage for applying trident blocks. Default stage is Res4 according to the
- # TridentNet paper.
- _C.MODEL.TRIDENT.TRIDENT_STAGE = "res4"
- # Specify the test branch index TridentNet Fast inference:
- # - use -1 to aggregate results of all branches during inference.
- # - otherwise, only using specified branch for fast inference. Recommended setting is
- # to use the middle branch.
- _C.MODEL.TRIDENT.TEST_BRANCH_IDX = 1
diff --git a/spaces/CVPR/Dual-Key_Backdoor_Attacks/datagen/detectron2/projects/TridentNet/tridentnet/trident_conv.py b/spaces/CVPR/Dual-Key_Backdoor_Attacks/datagen/detectron2/projects/TridentNet/tridentnet/trident_conv.py
deleted file mode 100644
index 7e2d5252bda5ebb2e9eee10af9c9a14fc72bb8fe..0000000000000000000000000000000000000000
--- a/spaces/CVPR/Dual-Key_Backdoor_Attacks/datagen/detectron2/projects/TridentNet/tridentnet/trident_conv.py
+++ /dev/null
@@ -1,107 +0,0 @@
-# Copyright (c) Facebook, Inc. and its affiliates. All Rights Reserved
-import torch
-from torch import nn
-from torch.nn import functional as F
-from torch.nn.modules.utils import _pair
-
-from detectron2.layers.wrappers import _NewEmptyTensorOp
-
-
-class TridentConv(nn.Module):
- def __init__(
- self,
- in_channels,
- out_channels,
- kernel_size,
- stride=1,
- paddings=0,
- dilations=1,
- groups=1,
- num_branch=1,
- test_branch_idx=-1,
- bias=False,
- norm=None,
- activation=None,
- ):
- super(TridentConv, self).__init__()
- self.in_channels = in_channels
- self.out_channels = out_channels
- self.kernel_size = _pair(kernel_size)
- self.num_branch = num_branch
- self.stride = _pair(stride)
- self.groups = groups
- self.with_bias = bias
- if isinstance(paddings, int):
- paddings = [paddings] * self.num_branch
- if isinstance(dilations, int):
- dilations = [dilations] * self.num_branch
- self.paddings = [_pair(padding) for padding in paddings]
- self.dilations = [_pair(dilation) for dilation in dilations]
- self.test_branch_idx = test_branch_idx
- self.norm = norm
- self.activation = activation
-
- assert len({self.num_branch, len(self.paddings), len(self.dilations)}) == 1
-
- self.weight = nn.Parameter(
- torch.Tensor(out_channels, in_channels // groups, *self.kernel_size)
- )
- if bias:
- self.bias = nn.Parameter(torch.Tensor(out_channels))
- else:
- self.bias = None
-
- nn.init.kaiming_uniform_(self.weight, nonlinearity="relu")
- if self.bias is not None:
- nn.init.constant_(self.bias, 0)
-
- def forward(self, inputs):
- num_branch = self.num_branch if self.training or self.test_branch_idx == -1 else 1
- assert len(inputs) == num_branch
-
- if inputs[0].numel() == 0:
- output_shape = [
- (i + 2 * p - (di * (k - 1) + 1)) // s + 1
- for i, p, di, k, s in zip(
- inputs[0].shape[-2:], self.padding, self.dilation, self.kernel_size, self.stride
- )
- ]
- output_shape = [input[0].shape[0], self.weight.shape[0]] + output_shape
- return [_NewEmptyTensorOp.apply(input, output_shape) for input in inputs]
-
- if self.training or self.test_branch_idx == -1:
- outputs = [
- F.conv2d(input, self.weight, self.bias, self.stride, padding, dilation, self.groups)
- for input, dilation, padding in zip(inputs, self.dilations, self.paddings)
- ]
- else:
- outputs = [
- F.conv2d(
- inputs[0],
- self.weight,
- self.bias,
- self.stride,
- self.paddings[self.test_branch_idx],
- self.dilations[self.test_branch_idx],
- self.groups,
- )
- ]
-
- if self.norm is not None:
- outputs = [self.norm(x) for x in outputs]
- if self.activation is not None:
- outputs = [self.activation(x) for x in outputs]
- return outputs
-
- def extra_repr(self):
- tmpstr = "in_channels=" + str(self.in_channels)
- tmpstr += ", out_channels=" + str(self.out_channels)
- tmpstr += ", kernel_size=" + str(self.kernel_size)
- tmpstr += ", num_branch=" + str(self.num_branch)
- tmpstr += ", test_branch_idx=" + str(self.test_branch_idx)
- tmpstr += ", stride=" + str(self.stride)
- tmpstr += ", paddings=" + str(self.paddings)
- tmpstr += ", dilations=" + str(self.dilations)
- tmpstr += ", groups=" + str(self.groups)
- tmpstr += ", bias=" + str(self.with_bias)
- return tmpstr
diff --git a/spaces/CVPR/LIVE/pydiffvg/pixel_filter.py b/spaces/CVPR/LIVE/pydiffvg/pixel_filter.py
deleted file mode 100644
index 9b0ff22507613e01a0fb9ac9701d1c49c68266e8..0000000000000000000000000000000000000000
--- a/spaces/CVPR/LIVE/pydiffvg/pixel_filter.py
+++ /dev/null
@@ -1,9 +0,0 @@
-import torch
-import pydiffvg
-
-class PixelFilter:
- def __init__(self,
- type,
- radius = torch.tensor(0.5)):
- self.type = type
- self.radius = radius
diff --git a/spaces/CVPR/LIVE/thrust/thrust/system/detail/sequential/mismatch.h b/spaces/CVPR/LIVE/thrust/thrust/system/detail/sequential/mismatch.h
deleted file mode 100644
index e6094d261a0f10e388885c1eadcd7083b6448e09..0000000000000000000000000000000000000000
--- a/spaces/CVPR/LIVE/thrust/thrust/system/detail/sequential/mismatch.h
+++ /dev/null
@@ -1,22 +0,0 @@
-/*
- * Copyright 2008-2013 NVIDIA Corporation
- *
- * Licensed under the Apache License, Version 2.0 (the "License");
- * you may not use this file except in compliance with the License.
- * You may obtain a copy of the License at
- *
- * http://www.apache.org/licenses/LICENSE-2.0
- *
- * Unless required by applicable law or agreed to in writing, software
- * distributed under the License is distributed on an "AS IS" BASIS,
- * WITHOUT WARRANTIES OR CONDITIONS OF ANY KIND, either express or implied.
- * See the License for the specific language governing permissions and
- * limitations under the License.
- */
-
-#pragma once
-
-#include
-
-// this system has no special mismatch functions
-
diff --git a/spaces/CVPR/LIVE/thrust/thrust/system/tbb/detail/get_value.h b/spaces/CVPR/LIVE/thrust/thrust/system/tbb/detail/get_value.h
deleted file mode 100644
index 23a11a8574f77f95bc6ca96d0cd8ff6de8c71c7e..0000000000000000000000000000000000000000
--- a/spaces/CVPR/LIVE/thrust/thrust/system/tbb/detail/get_value.h
+++ /dev/null
@@ -1,23 +0,0 @@
-/*
- * Copyright 2008-2013 NVIDIA Corporation
- *
- * Licensed under the Apache License, Version 2.0 (the "License");
- * you may not use this file except in compliance with the License.
- * You may obtain a copy of the License at
- *
- * http://www.apache.org/licenses/LICENSE-2.0
- *
- * Unless required by applicable law or agreed to in writing, software
- * distributed under the License is distributed on an "AS IS" BASIS,
- * WITHOUT WARRANTIES OR CONDITIONS OF ANY KIND, either express or implied.
- * See the License for the specific language governing permissions and
- * limitations under the License.
- */
-
-#pragma once
-
-#include
-
-// this system inherits get_value
-#include
-
diff --git a/spaces/CVPR/WALT/infer.py b/spaces/CVPR/WALT/infer.py
deleted file mode 100644
index ee71873a0955453fd137947678a0e8b4a1423b08..0000000000000000000000000000000000000000
--- a/spaces/CVPR/WALT/infer.py
+++ /dev/null
@@ -1,118 +0,0 @@
-from argparse import ArgumentParser
-
-from mmdet.apis import inference_detector, init_detector, show_result_pyplot
-from mmdet.core.mask.utils import encode_mask_results
-import numpy as np
-import mmcv
-import torch
-from imantics import Polygons, Mask
-import json
-import os
-import cv2, glob
-
-class detections():
- def __init__(self, cfg_path, device, model_path = 'data/models/walt_vehicle.pth', threshold=0.85):
- self.model = init_detector(cfg_path, model_path, device=device)
- self.all_preds = []
- self.all_scores = []
- self.index = []
- self.score_thr = threshold
- self.result = []
- self.record_dict = {'model': cfg_path,'results': []}
- self.detect_count = []
-
-
- def run_on_image(self, image):
- self.result = inference_detector(self.model, image)
- image_labelled = self.model.show_result(image, self.result, score_thr=self.score_thr)
- return image_labelled
-
- def process_output(self, count):
- result = self.result
- infer_result = {'url': count,
- 'boxes': [],
- 'scores': [],
- 'keypoints': [],
- 'segmentation': [],
- 'label_ids': [],
- 'track': [],
- 'labels': []}
-
- if isinstance(result, tuple):
- bbox_result, segm_result = result
- #segm_result = encode_mask_results(segm_result)
- if isinstance(segm_result, tuple):
- segm_result = segm_result[0] # ms rcnn
- bboxes = np.vstack(bbox_result)
- labels = [np.full(bbox.shape[0], i, dtype=np.int32) for i, bbox in enumerate(bbox_result)]
-
- labels = np.concatenate(labels)
- segms = None
- if segm_result is not None and len(labels) > 0: # non empty
- segms = mmcv.concat_list(segm_result)
- if isinstance(segms[0], torch.Tensor):
- segms = torch.stack(segms, dim=0).detach().cpu().numpy()
- else:
- segms = np.stack(segms, axis=0)
-
- for i, (bbox, label, segm) in enumerate(zip(bboxes, labels, segms)):
- if bbox[-1].item() <0.3:
- continue
- box = [bbox[0].item(), bbox[1].item(), bbox[2].item(), bbox[3].item()]
- polygons = Mask(segm).polygons()
-
- infer_result['boxes'].append(box)
- infer_result['segmentation'].append(polygons.segmentation)
- infer_result['scores'].append(bbox[-1].item())
- infer_result['labels'].append(self.model.CLASSES[label])
- infer_result['label_ids'].append(label)
- self.record_dict['results'].append(infer_result)
- self.detect_count = labels
-
- def write_json(self, filename):
- with open(filename + '.json', 'w') as f:
- json.dump(self.record_dict, f)
-
-
-def main():
- if torch.cuda.is_available() == False:
- device='cpu'
- else:
- device='cuda:0'
- detect_people = detections('configs/walt/walt_people.py', device, model_path='data/models/walt_people.pth')
- detect = detections('configs/walt/walt_vehicle.py', device, model_path='data/models/walt_vehicle.pth')
- filenames = sorted(glob.glob('demo/images/*'))
- count = 0
- for filename in filenames:
- img=cv2.imread(filename)
- try:
- img = detect_people.run_on_image(img)
- img = detect.run_on_image(img)
- except:
- continue
- count=count+1
-
- try:
- import os
- os.makedirs(os.path.dirname(filename.replace('demo','demo/results/')))
- os.mkdirs(os.path.dirname(filename))
- except:
- print('done')
- cv2.imwrite(filename.replace('demo','demo/results/'),img)
- if count == 30000:
- break
- try:
- detect.process_output(count)
- except:
- continue
- '''
-
- np.savez('FC', a= detect.record_dict)
- with open('check.json', 'w') as f:
- json.dump(detect.record_dict, f)
- detect.write_json('seq3')
- asas
- detect.process_output(0)
- '''
-if __name__ == "__main__":
- main()
diff --git a/spaces/CVPR/WALT/mmdet/core/evaluation/mean_ap.py b/spaces/CVPR/WALT/mmdet/core/evaluation/mean_ap.py
deleted file mode 100644
index 1d653a35497f6a0135c4374a09eb7c11399e3244..0000000000000000000000000000000000000000
--- a/spaces/CVPR/WALT/mmdet/core/evaluation/mean_ap.py
+++ /dev/null
@@ -1,469 +0,0 @@
-from multiprocessing import Pool
-
-import mmcv
-import numpy as np
-from mmcv.utils import print_log
-from terminaltables import AsciiTable
-
-from .bbox_overlaps import bbox_overlaps
-from .class_names import get_classes
-
-
-def average_precision(recalls, precisions, mode='area'):
- """Calculate average precision (for single or multiple scales).
-
- Args:
- recalls (ndarray): shape (num_scales, num_dets) or (num_dets, )
- precisions (ndarray): shape (num_scales, num_dets) or (num_dets, )
- mode (str): 'area' or '11points', 'area' means calculating the area
- under precision-recall curve, '11points' means calculating
- the average precision of recalls at [0, 0.1, ..., 1]
-
- Returns:
- float or ndarray: calculated average precision
- """
- no_scale = False
- if recalls.ndim == 1:
- no_scale = True
- recalls = recalls[np.newaxis, :]
- precisions = precisions[np.newaxis, :]
- assert recalls.shape == precisions.shape and recalls.ndim == 2
- num_scales = recalls.shape[0]
- ap = np.zeros(num_scales, dtype=np.float32)
- if mode == 'area':
- zeros = np.zeros((num_scales, 1), dtype=recalls.dtype)
- ones = np.ones((num_scales, 1), dtype=recalls.dtype)
- mrec = np.hstack((zeros, recalls, ones))
- mpre = np.hstack((zeros, precisions, zeros))
- for i in range(mpre.shape[1] - 1, 0, -1):
- mpre[:, i - 1] = np.maximum(mpre[:, i - 1], mpre[:, i])
- for i in range(num_scales):
- ind = np.where(mrec[i, 1:] != mrec[i, :-1])[0]
- ap[i] = np.sum(
- (mrec[i, ind + 1] - mrec[i, ind]) * mpre[i, ind + 1])
- elif mode == '11points':
- for i in range(num_scales):
- for thr in np.arange(0, 1 + 1e-3, 0.1):
- precs = precisions[i, recalls[i, :] >= thr]
- prec = precs.max() if precs.size > 0 else 0
- ap[i] += prec
- ap /= 11
- else:
- raise ValueError(
- 'Unrecognized mode, only "area" and "11points" are supported')
- if no_scale:
- ap = ap[0]
- return ap
-
-
-def tpfp_imagenet(det_bboxes,
- gt_bboxes,
- gt_bboxes_ignore=None,
- default_iou_thr=0.5,
- area_ranges=None):
- """Check if detected bboxes are true positive or false positive.
-
- Args:
- det_bbox (ndarray): Detected bboxes of this image, of shape (m, 5).
- gt_bboxes (ndarray): GT bboxes of this image, of shape (n, 4).
- gt_bboxes_ignore (ndarray): Ignored gt bboxes of this image,
- of shape (k, 4). Default: None
- default_iou_thr (float): IoU threshold to be considered as matched for
- medium and large bboxes (small ones have special rules).
- Default: 0.5.
- area_ranges (list[tuple] | None): Range of bbox areas to be evaluated,
- in the format [(min1, max1), (min2, max2), ...]. Default: None.
-
- Returns:
- tuple[np.ndarray]: (tp, fp) whose elements are 0 and 1. The shape of
- each array is (num_scales, m).
- """
- # an indicator of ignored gts
- gt_ignore_inds = np.concatenate(
- (np.zeros(gt_bboxes.shape[0], dtype=np.bool),
- np.ones(gt_bboxes_ignore.shape[0], dtype=np.bool)))
- # stack gt_bboxes and gt_bboxes_ignore for convenience
- gt_bboxes = np.vstack((gt_bboxes, gt_bboxes_ignore))
-
- num_dets = det_bboxes.shape[0]
- num_gts = gt_bboxes.shape[0]
- if area_ranges is None:
- area_ranges = [(None, None)]
- num_scales = len(area_ranges)
- # tp and fp are of shape (num_scales, num_gts), each row is tp or fp
- # of a certain scale.
- tp = np.zeros((num_scales, num_dets), dtype=np.float32)
- fp = np.zeros((num_scales, num_dets), dtype=np.float32)
- if gt_bboxes.shape[0] == 0:
- if area_ranges == [(None, None)]:
- fp[...] = 1
- else:
- det_areas = (det_bboxes[:, 2] - det_bboxes[:, 0]) * (
- det_bboxes[:, 3] - det_bboxes[:, 1])
- for i, (min_area, max_area) in enumerate(area_ranges):
- fp[i, (det_areas >= min_area) & (det_areas < max_area)] = 1
- return tp, fp
- ious = bbox_overlaps(det_bboxes, gt_bboxes - 1)
- gt_w = gt_bboxes[:, 2] - gt_bboxes[:, 0]
- gt_h = gt_bboxes[:, 3] - gt_bboxes[:, 1]
- iou_thrs = np.minimum((gt_w * gt_h) / ((gt_w + 10.0) * (gt_h + 10.0)),
- default_iou_thr)
- # sort all detections by scores in descending order
- sort_inds = np.argsort(-det_bboxes[:, -1])
- for k, (min_area, max_area) in enumerate(area_ranges):
- gt_covered = np.zeros(num_gts, dtype=bool)
- # if no area range is specified, gt_area_ignore is all False
- if min_area is None:
- gt_area_ignore = np.zeros_like(gt_ignore_inds, dtype=bool)
- else:
- gt_areas = gt_w * gt_h
- gt_area_ignore = (gt_areas < min_area) | (gt_areas >= max_area)
- for i in sort_inds:
- max_iou = -1
- matched_gt = -1
- # find best overlapped available gt
- for j in range(num_gts):
- # different from PASCAL VOC: allow finding other gts if the
- # best overlapped ones are already matched by other det bboxes
- if gt_covered[j]:
- continue
- elif ious[i, j] >= iou_thrs[j] and ious[i, j] > max_iou:
- max_iou = ious[i, j]
- matched_gt = j
- # there are 4 cases for a det bbox:
- # 1. it matches a gt, tp = 1, fp = 0
- # 2. it matches an ignored gt, tp = 0, fp = 0
- # 3. it matches no gt and within area range, tp = 0, fp = 1
- # 4. it matches no gt but is beyond area range, tp = 0, fp = 0
- if matched_gt >= 0:
- gt_covered[matched_gt] = 1
- if not (gt_ignore_inds[matched_gt]
- or gt_area_ignore[matched_gt]):
- tp[k, i] = 1
- elif min_area is None:
- fp[k, i] = 1
- else:
- bbox = det_bboxes[i, :4]
- area = (bbox[2] - bbox[0]) * (bbox[3] - bbox[1])
- if area >= min_area and area < max_area:
- fp[k, i] = 1
- return tp, fp
-
-
-def tpfp_default(det_bboxes,
- gt_bboxes,
- gt_bboxes_ignore=None,
- iou_thr=0.5,
- area_ranges=None):
- """Check if detected bboxes are true positive or false positive.
-
- Args:
- det_bbox (ndarray): Detected bboxes of this image, of shape (m, 5).
- gt_bboxes (ndarray): GT bboxes of this image, of shape (n, 4).
- gt_bboxes_ignore (ndarray): Ignored gt bboxes of this image,
- of shape (k, 4). Default: None
- iou_thr (float): IoU threshold to be considered as matched.
- Default: 0.5.
- area_ranges (list[tuple] | None): Range of bbox areas to be evaluated,
- in the format [(min1, max1), (min2, max2), ...]. Default: None.
-
- Returns:
- tuple[np.ndarray]: (tp, fp) whose elements are 0 and 1. The shape of
- each array is (num_scales, m).
- """
- # an indicator of ignored gts
- gt_ignore_inds = np.concatenate(
- (np.zeros(gt_bboxes.shape[0], dtype=np.bool),
- np.ones(gt_bboxes_ignore.shape[0], dtype=np.bool)))
- # stack gt_bboxes and gt_bboxes_ignore for convenience
- gt_bboxes = np.vstack((gt_bboxes, gt_bboxes_ignore))
-
- num_dets = det_bboxes.shape[0]
- num_gts = gt_bboxes.shape[0]
- if area_ranges is None:
- area_ranges = [(None, None)]
- num_scales = len(area_ranges)
- # tp and fp are of shape (num_scales, num_gts), each row is tp or fp of
- # a certain scale
- tp = np.zeros((num_scales, num_dets), dtype=np.float32)
- fp = np.zeros((num_scales, num_dets), dtype=np.float32)
-
- # if there is no gt bboxes in this image, then all det bboxes
- # within area range are false positives
- if gt_bboxes.shape[0] == 0:
- if area_ranges == [(None, None)]:
- fp[...] = 1
- else:
- det_areas = (det_bboxes[:, 2] - det_bboxes[:, 0]) * (
- det_bboxes[:, 3] - det_bboxes[:, 1])
- for i, (min_area, max_area) in enumerate(area_ranges):
- fp[i, (det_areas >= min_area) & (det_areas < max_area)] = 1
- return tp, fp
-
- ious = bbox_overlaps(det_bboxes, gt_bboxes)
- # for each det, the max iou with all gts
- ious_max = ious.max(axis=1)
- # for each det, which gt overlaps most with it
- ious_argmax = ious.argmax(axis=1)
- # sort all dets in descending order by scores
- sort_inds = np.argsort(-det_bboxes[:, -1])
- for k, (min_area, max_area) in enumerate(area_ranges):
- gt_covered = np.zeros(num_gts, dtype=bool)
- # if no area range is specified, gt_area_ignore is all False
- if min_area is None:
- gt_area_ignore = np.zeros_like(gt_ignore_inds, dtype=bool)
- else:
- gt_areas = (gt_bboxes[:, 2] - gt_bboxes[:, 0]) * (
- gt_bboxes[:, 3] - gt_bboxes[:, 1])
- gt_area_ignore = (gt_areas < min_area) | (gt_areas >= max_area)
- for i in sort_inds:
- if ious_max[i] >= iou_thr:
- matched_gt = ious_argmax[i]
- if not (gt_ignore_inds[matched_gt]
- or gt_area_ignore[matched_gt]):
- if not gt_covered[matched_gt]:
- gt_covered[matched_gt] = True
- tp[k, i] = 1
- else:
- fp[k, i] = 1
- # otherwise ignore this detected bbox, tp = 0, fp = 0
- elif min_area is None:
- fp[k, i] = 1
- else:
- bbox = det_bboxes[i, :4]
- area = (bbox[2] - bbox[0]) * (bbox[3] - bbox[1])
- if area >= min_area and area < max_area:
- fp[k, i] = 1
- return tp, fp
-
-
-def get_cls_results(det_results, annotations, class_id):
- """Get det results and gt information of a certain class.
-
- Args:
- det_results (list[list]): Same as `eval_map()`.
- annotations (list[dict]): Same as `eval_map()`.
- class_id (int): ID of a specific class.
-
- Returns:
- tuple[list[np.ndarray]]: detected bboxes, gt bboxes, ignored gt bboxes
- """
- cls_dets = [img_res[class_id] for img_res in det_results]
- cls_gts = []
- cls_gts_ignore = []
- for ann in annotations:
- gt_inds = ann['labels'] == class_id
- cls_gts.append(ann['bboxes'][gt_inds, :])
-
- if ann.get('labels_ignore', None) is not None:
- ignore_inds = ann['labels_ignore'] == class_id
- cls_gts_ignore.append(ann['bboxes_ignore'][ignore_inds, :])
- else:
- cls_gts_ignore.append(np.empty((0, 4), dtype=np.float32))
-
- return cls_dets, cls_gts, cls_gts_ignore
-
-
-def eval_map(det_results,
- annotations,
- scale_ranges=None,
- iou_thr=0.5,
- dataset=None,
- logger=None,
- tpfp_fn=None,
- nproc=4):
- """Evaluate mAP of a dataset.
-
- Args:
- det_results (list[list]): [[cls1_det, cls2_det, ...], ...].
- The outer list indicates images, and the inner list indicates
- per-class detected bboxes.
- annotations (list[dict]): Ground truth annotations where each item of
- the list indicates an image. Keys of annotations are:
-
- - `bboxes`: numpy array of shape (n, 4)
- - `labels`: numpy array of shape (n, )
- - `bboxes_ignore` (optional): numpy array of shape (k, 4)
- - `labels_ignore` (optional): numpy array of shape (k, )
- scale_ranges (list[tuple] | None): Range of scales to be evaluated,
- in the format [(min1, max1), (min2, max2), ...]. A range of
- (32, 64) means the area range between (32**2, 64**2).
- Default: None.
- iou_thr (float): IoU threshold to be considered as matched.
- Default: 0.5.
- dataset (list[str] | str | None): Dataset name or dataset classes,
- there are minor differences in metrics for different datsets, e.g.
- "voc07", "imagenet_det", etc. Default: None.
- logger (logging.Logger | str | None): The way to print the mAP
- summary. See `mmcv.utils.print_log()` for details. Default: None.
- tpfp_fn (callable | None): The function used to determine true/
- false positives. If None, :func:`tpfp_default` is used as default
- unless dataset is 'det' or 'vid' (:func:`tpfp_imagenet` in this
- case). If it is given as a function, then this function is used
- to evaluate tp & fp. Default None.
- nproc (int): Processes used for computing TP and FP.
- Default: 4.
-
- Returns:
- tuple: (mAP, [dict, dict, ...])
- """
- assert len(det_results) == len(annotations)
-
- num_imgs = len(det_results)
- num_scales = len(scale_ranges) if scale_ranges is not None else 1
- num_classes = len(det_results[0]) # positive class num
- area_ranges = ([(rg[0]**2, rg[1]**2) for rg in scale_ranges]
- if scale_ranges is not None else None)
-
- pool = Pool(nproc)
- eval_results = []
- for i in range(num_classes):
- # get gt and det bboxes of this class
- cls_dets, cls_gts, cls_gts_ignore = get_cls_results(
- det_results, annotations, i)
- # choose proper function according to datasets to compute tp and fp
- if tpfp_fn is None:
- if dataset in ['det', 'vid']:
- tpfp_fn = tpfp_imagenet
- else:
- tpfp_fn = tpfp_default
- if not callable(tpfp_fn):
- raise ValueError(
- f'tpfp_fn has to be a function or None, but got {tpfp_fn}')
-
- # compute tp and fp for each image with multiple processes
- tpfp = pool.starmap(
- tpfp_fn,
- zip(cls_dets, cls_gts, cls_gts_ignore,
- [iou_thr for _ in range(num_imgs)],
- [area_ranges for _ in range(num_imgs)]))
- tp, fp = tuple(zip(*tpfp))
- # calculate gt number of each scale
- # ignored gts or gts beyond the specific scale are not counted
- num_gts = np.zeros(num_scales, dtype=int)
- for j, bbox in enumerate(cls_gts):
- if area_ranges is None:
- num_gts[0] += bbox.shape[0]
- else:
- gt_areas = (bbox[:, 2] - bbox[:, 0]) * (
- bbox[:, 3] - bbox[:, 1])
- for k, (min_area, max_area) in enumerate(area_ranges):
- num_gts[k] += np.sum((gt_areas >= min_area)
- & (gt_areas < max_area))
- # sort all det bboxes by score, also sort tp and fp
- cls_dets = np.vstack(cls_dets)
- num_dets = cls_dets.shape[0]
- sort_inds = np.argsort(-cls_dets[:, -1])
- tp = np.hstack(tp)[:, sort_inds]
- fp = np.hstack(fp)[:, sort_inds]
- # calculate recall and precision with tp and fp
- tp = np.cumsum(tp, axis=1)
- fp = np.cumsum(fp, axis=1)
- eps = np.finfo(np.float32).eps
- recalls = tp / np.maximum(num_gts[:, np.newaxis], eps)
- precisions = tp / np.maximum((tp + fp), eps)
- # calculate AP
- if scale_ranges is None:
- recalls = recalls[0, :]
- precisions = precisions[0, :]
- num_gts = num_gts.item()
- mode = 'area' if dataset != 'voc07' else '11points'
- ap = average_precision(recalls, precisions, mode)
- eval_results.append({
- 'num_gts': num_gts,
- 'num_dets': num_dets,
- 'recall': recalls,
- 'precision': precisions,
- 'ap': ap
- })
- pool.close()
- if scale_ranges is not None:
- # shape (num_classes, num_scales)
- all_ap = np.vstack([cls_result['ap'] for cls_result in eval_results])
- all_num_gts = np.vstack(
- [cls_result['num_gts'] for cls_result in eval_results])
- mean_ap = []
- for i in range(num_scales):
- if np.any(all_num_gts[:, i] > 0):
- mean_ap.append(all_ap[all_num_gts[:, i] > 0, i].mean())
- else:
- mean_ap.append(0.0)
- else:
- aps = []
- for cls_result in eval_results:
- if cls_result['num_gts'] > 0:
- aps.append(cls_result['ap'])
- mean_ap = np.array(aps).mean().item() if aps else 0.0
-
- print_map_summary(
- mean_ap, eval_results, dataset, area_ranges, logger=logger)
-
- return mean_ap, eval_results
-
-
-def print_map_summary(mean_ap,
- results,
- dataset=None,
- scale_ranges=None,
- logger=None):
- """Print mAP and results of each class.
-
- A table will be printed to show the gts/dets/recall/AP of each class and
- the mAP.
-
- Args:
- mean_ap (float): Calculated from `eval_map()`.
- results (list[dict]): Calculated from `eval_map()`.
- dataset (list[str] | str | None): Dataset name or dataset classes.
- scale_ranges (list[tuple] | None): Range of scales to be evaluated.
- logger (logging.Logger | str | None): The way to print the mAP
- summary. See `mmcv.utils.print_log()` for details. Default: None.
- """
-
- if logger == 'silent':
- return
-
- if isinstance(results[0]['ap'], np.ndarray):
- num_scales = len(results[0]['ap'])
- else:
- num_scales = 1
-
- if scale_ranges is not None:
- assert len(scale_ranges) == num_scales
-
- num_classes = len(results)
-
- recalls = np.zeros((num_scales, num_classes), dtype=np.float32)
- aps = np.zeros((num_scales, num_classes), dtype=np.float32)
- num_gts = np.zeros((num_scales, num_classes), dtype=int)
- for i, cls_result in enumerate(results):
- if cls_result['recall'].size > 0:
- recalls[:, i] = np.array(cls_result['recall'], ndmin=2)[:, -1]
- aps[:, i] = cls_result['ap']
- num_gts[:, i] = cls_result['num_gts']
-
- if dataset is None:
- label_names = [str(i) for i in range(num_classes)]
- elif mmcv.is_str(dataset):
- label_names = get_classes(dataset)
- else:
- label_names = dataset
-
- if not isinstance(mean_ap, list):
- mean_ap = [mean_ap]
-
- header = ['class', 'gts', 'dets', 'recall', 'ap']
- for i in range(num_scales):
- if scale_ranges is not None:
- print_log(f'Scale range {scale_ranges[i]}', logger=logger)
- table_data = [header]
- for j in range(num_classes):
- row_data = [
- label_names[j], num_gts[i, j], results[j]['num_dets'],
- f'{recalls[i, j]:.3f}', f'{aps[i, j]:.3f}'
- ]
- table_data.append(row_data)
- table_data.append(['mAP', '', '', '', f'{mean_ap[i]:.3f}'])
- table = AsciiTable(table_data)
- table.inner_footing_row_border = True
- print_log('\n' + table.table, logger=logger)
diff --git a/spaces/CVPR/lama-example/saicinpainting/evaluation/masks/countless/test.py b/spaces/CVPR/lama-example/saicinpainting/evaluation/masks/countless/test.py
deleted file mode 100644
index 7809beb7aeeb3bcb10d03093a564917b1f2b4786..0000000000000000000000000000000000000000
--- a/spaces/CVPR/lama-example/saicinpainting/evaluation/masks/countless/test.py
+++ /dev/null
@@ -1,195 +0,0 @@
-from copy import deepcopy
-
-import numpy as np
-
-import countless2d
-import countless3d
-
-def test_countless2d():
- def test_all_cases(fn, test_zero):
- case1 = np.array([ [ 1, 2 ], [ 3, 4 ] ]).reshape((2,2,1,1)) # all different
- case2 = np.array([ [ 1, 1 ], [ 2, 3 ] ]).reshape((2,2,1,1)) # two are same
- case1z = np.array([ [ 0, 1 ], [ 2, 3 ] ]).reshape((2,2,1,1)) # all different
- case2z = np.array([ [ 0, 0 ], [ 2, 3 ] ]).reshape((2,2,1,1)) # two are same
- case3 = np.array([ [ 1, 1 ], [ 2, 2 ] ]).reshape((2,2,1,1)) # two groups are same
- case4 = np.array([ [ 1, 2 ], [ 2, 2 ] ]).reshape((2,2,1,1)) # 3 are the same
- case5 = np.array([ [ 5, 5 ], [ 5, 5 ] ]).reshape((2,2,1,1)) # all are the same
-
- is_255_handled = np.array([ [ 255, 255 ], [ 1, 2 ] ], dtype=np.uint8).reshape((2,2,1,1))
-
- test = lambda case: fn(case)
-
- if test_zero:
- assert test(case1z) == [[[[3]]]] # d
- assert test(case2z) == [[[[0]]]] # a==b
- else:
- assert test(case1) == [[[[4]]]] # d
- assert test(case2) == [[[[1]]]] # a==b
-
- assert test(case3) == [[[[1]]]] # a==b
- assert test(case4) == [[[[2]]]] # b==c
- assert test(case5) == [[[[5]]]] # a==b
-
- assert test(is_255_handled) == [[[[255]]]]
-
- assert fn(case1).dtype == case1.dtype
-
- test_all_cases(countless2d.simplest_countless, False)
- test_all_cases(countless2d.quick_countless, False)
- test_all_cases(countless2d.quickest_countless, False)
- test_all_cases(countless2d.stippled_countless, False)
-
-
-
- methods = [
- countless2d.zero_corrected_countless,
- countless2d.countless,
- countless2d.countless_if,
- # countless2d.counting, # counting doesn't respect order so harder to write a test
- ]
-
- for fn in methods:
- print(fn.__name__)
- test_all_cases(fn, True)
-
-def test_stippled_countless2d():
- a = np.array([ [ 1, 2 ], [ 3, 4 ] ]).reshape((2,2,1,1))
- b = np.array([ [ 0, 2 ], [ 3, 4 ] ]).reshape((2,2,1,1))
- c = np.array([ [ 1, 0 ], [ 3, 4 ] ]).reshape((2,2,1,1))
- d = np.array([ [ 1, 2 ], [ 0, 4 ] ]).reshape((2,2,1,1))
- e = np.array([ [ 1, 2 ], [ 3, 0 ] ]).reshape((2,2,1,1))
- f = np.array([ [ 0, 0 ], [ 3, 4 ] ]).reshape((2,2,1,1))
- g = np.array([ [ 0, 2 ], [ 0, 4 ] ]).reshape((2,2,1,1))
- h = np.array([ [ 0, 2 ], [ 3, 0 ] ]).reshape((2,2,1,1))
- i = np.array([ [ 1, 0 ], [ 0, 4 ] ]).reshape((2,2,1,1))
- j = np.array([ [ 1, 2 ], [ 0, 0 ] ]).reshape((2,2,1,1))
- k = np.array([ [ 1, 0 ], [ 3, 0 ] ]).reshape((2,2,1,1))
- l = np.array([ [ 1, 0 ], [ 0, 0 ] ]).reshape((2,2,1,1))
- m = np.array([ [ 0, 2 ], [ 0, 0 ] ]).reshape((2,2,1,1))
- n = np.array([ [ 0, 0 ], [ 3, 0 ] ]).reshape((2,2,1,1))
- o = np.array([ [ 0, 0 ], [ 0, 4 ] ]).reshape((2,2,1,1))
- z = np.array([ [ 0, 0 ], [ 0, 0 ] ]).reshape((2,2,1,1))
-
- test = countless2d.stippled_countless
-
- # Note: We only tested non-matching cases above,
- # cases f,g,h,i,j,k prove their duals work as well
- # b/c if two pixels are black, either one can be chosen
- # if they are different or the same.
-
- assert test(a) == [[[[4]]]]
- assert test(b) == [[[[4]]]]
- assert test(c) == [[[[4]]]]
- assert test(d) == [[[[4]]]]
- assert test(e) == [[[[1]]]]
- assert test(f) == [[[[4]]]]
- assert test(g) == [[[[4]]]]
- assert test(h) == [[[[2]]]]
- assert test(i) == [[[[4]]]]
- assert test(j) == [[[[1]]]]
- assert test(k) == [[[[1]]]]
- assert test(l) == [[[[1]]]]
- assert test(m) == [[[[2]]]]
- assert test(n) == [[[[3]]]]
- assert test(o) == [[[[4]]]]
- assert test(z) == [[[[0]]]]
-
- bc = np.array([ [ 0, 2 ], [ 2, 4 ] ]).reshape((2,2,1,1))
- bd = np.array([ [ 0, 2 ], [ 3, 2 ] ]).reshape((2,2,1,1))
- cd = np.array([ [ 0, 2 ], [ 3, 3 ] ]).reshape((2,2,1,1))
-
- assert test(bc) == [[[[2]]]]
- assert test(bd) == [[[[2]]]]
- assert test(cd) == [[[[3]]]]
-
- ab = np.array([ [ 1, 1 ], [ 0, 4 ] ]).reshape((2,2,1,1))
- ac = np.array([ [ 1, 2 ], [ 1, 0 ] ]).reshape((2,2,1,1))
- ad = np.array([ [ 1, 0 ], [ 3, 1 ] ]).reshape((2,2,1,1))
-
- assert test(ab) == [[[[1]]]]
- assert test(ac) == [[[[1]]]]
- assert test(ad) == [[[[1]]]]
-
-def test_countless3d():
- def test_all_cases(fn):
- alldifferent = [
- [
- [1,2],
- [3,4],
- ],
- [
- [5,6],
- [7,8]
- ]
- ]
- allsame = [
- [
- [1,1],
- [1,1],
- ],
- [
- [1,1],
- [1,1]
- ]
- ]
-
- assert fn(np.array(alldifferent)) == [[[8]]]
- assert fn(np.array(allsame)) == [[[1]]]
-
- twosame = deepcopy(alldifferent)
- twosame[1][1][0] = 2
-
- assert fn(np.array(twosame)) == [[[2]]]
-
- threemixed = [
- [
- [3,3],
- [1,2],
- ],
- [
- [2,4],
- [4,3]
- ]
- ]
- assert fn(np.array(threemixed)) == [[[3]]]
-
- foursame = [
- [
- [4,4],
- [1,2],
- ],
- [
- [2,4],
- [4,3]
- ]
- ]
-
- assert fn(np.array(foursame)) == [[[4]]]
-
- fivesame = [
- [
- [5,4],
- [5,5],
- ],
- [
- [2,4],
- [5,5]
- ]
- ]
-
- assert fn(np.array(fivesame)) == [[[5]]]
-
- def countless3d_generalized(img):
- return countless3d.countless_generalized(img, (2,2,2))
- def countless3d_dynamic_generalized(img):
- return countless3d.dynamic_countless_generalized(img, (2,2,2))
-
- methods = [
- countless3d.countless3d,
- countless3d.dynamic_countless3d,
- countless3d_generalized,
- countless3d_dynamic_generalized,
- ]
-
- for fn in methods:
- test_all_cases(fn)
\ No newline at end of file
diff --git a/spaces/CVPR/regionclip-demo/detectron2/data/build.py b/spaces/CVPR/regionclip-demo/detectron2/data/build.py
deleted file mode 100644
index a1dcfadbd2cc30a0875c4d294e3cabcfa0146a16..0000000000000000000000000000000000000000
--- a/spaces/CVPR/regionclip-demo/detectron2/data/build.py
+++ /dev/null
@@ -1,536 +0,0 @@
-# Copyright (c) Facebook, Inc. and its affiliates.
-import itertools
-import logging
-import numpy as np
-import operator
-import pickle
-import torch.utils.data
-from tabulate import tabulate
-from termcolor import colored
-
-from detectron2.config import configurable
-from detectron2.structures import BoxMode
-from detectron2.utils.comm import get_world_size
-from detectron2.utils.env import seed_all_rng
-from detectron2.utils.file_io import PathManager
-from detectron2.utils.logger import _log_api_usage, log_first_n
-
-from .catalog import DatasetCatalog, MetadataCatalog
-from .common import AspectRatioGroupedDataset, DatasetFromList, MapDataset
-from .dataset_mapper import DatasetMapper
-from .detection_utils import check_metadata_consistency
-from .samplers import InferenceSampler, RepeatFactorTrainingSampler, TrainingSampler
-
-from .clip_build import make_clip_dataset
-
-"""
-This file contains the default logic to build a dataloader for training or testing.
-"""
-
-__all__ = [
- "build_batch_data_loader",
- "build_detection_train_loader",
- "build_detection_test_loader",
- "get_detection_dataset_dicts",
- "load_proposals_into_dataset",
- "print_instances_class_histogram",
-]
-
-
-def filter_images_with_only_crowd_annotations(dataset_dicts):
- """
- Filter out images with none annotations or only crowd annotations
- (i.e., images without non-crowd annotations).
- A common training-time preprocessing on COCO dataset.
-
- Args:
- dataset_dicts (list[dict]): annotations in Detectron2 Dataset format.
-
- Returns:
- list[dict]: the same format, but filtered.
- """
- num_before = len(dataset_dicts)
-
- def valid(anns):
- for ann in anns:
- if ann.get("iscrowd", 0) == 0:
- return True
- return False
-
- dataset_dicts = [x for x in dataset_dicts if valid(x["annotations"])]
- num_after = len(dataset_dicts)
- logger = logging.getLogger(__name__)
- logger.info(
- "Removed {} images with no usable annotations. {} images left.".format(
- num_before - num_after, num_after
- )
- )
- return dataset_dicts
-
-
-def filter_images_with_few_keypoints(dataset_dicts, min_keypoints_per_image):
- """
- Filter out images with too few number of keypoints.
-
- Args:
- dataset_dicts (list[dict]): annotations in Detectron2 Dataset format.
-
- Returns:
- list[dict]: the same format as dataset_dicts, but filtered.
- """
- num_before = len(dataset_dicts)
-
- def visible_keypoints_in_image(dic):
- # Each keypoints field has the format [x1, y1, v1, ...], where v is visibility
- annotations = dic["annotations"]
- return sum(
- (np.array(ann["keypoints"][2::3]) > 0).sum()
- for ann in annotations
- if "keypoints" in ann
- )
-
- dataset_dicts = [
- x for x in dataset_dicts if visible_keypoints_in_image(x) >= min_keypoints_per_image
- ]
- num_after = len(dataset_dicts)
- logger = logging.getLogger(__name__)
- logger.info(
- "Removed {} images with fewer than {} keypoints.".format(
- num_before - num_after, min_keypoints_per_image
- )
- )
- return dataset_dicts
-
-
-def load_proposals_into_dataset(dataset_dicts, proposal_file):
- """
- Load precomputed object proposals into the dataset.
-
- The proposal file should be a pickled dict with the following keys:
-
- - "ids": list[int] or list[str], the image ids
- - "boxes": list[np.ndarray], each is an Nx4 array of boxes corresponding to the image id
- - "objectness_logits": list[np.ndarray], each is an N sized array of objectness scores
- corresponding to the boxes.
- - "bbox_mode": the BoxMode of the boxes array. Defaults to ``BoxMode.XYXY_ABS``.
-
- Args:
- dataset_dicts (list[dict]): annotations in Detectron2 Dataset format.
- proposal_file (str): file path of pre-computed proposals, in pkl format.
-
- Returns:
- list[dict]: the same format as dataset_dicts, but added proposal field.
- """
- logger = logging.getLogger(__name__)
- logger.info("Loading proposals from: {}".format(proposal_file))
-
- with PathManager.open(proposal_file, "rb") as f:
- proposals = pickle.load(f, encoding="latin1")
-
- # Rename the key names in D1 proposal files
- rename_keys = {"indexes": "ids", "scores": "objectness_logits"}
- for key in rename_keys:
- if key in proposals:
- proposals[rename_keys[key]] = proposals.pop(key)
-
- # Fetch the indexes of all proposals that are in the dataset
- # Convert image_id to str since they could be int.
- img_ids = set({str(record["image_id"]) for record in dataset_dicts})
- id_to_index = {str(id): i for i, id in enumerate(proposals["ids"]) if str(id) in img_ids}
-
- # Assuming default bbox_mode of precomputed proposals are 'XYXY_ABS'
- bbox_mode = BoxMode(proposals["bbox_mode"]) if "bbox_mode" in proposals else BoxMode.XYXY_ABS
-
- for record in dataset_dicts:
- # Get the index of the proposal
- i = id_to_index[str(record["image_id"])]
-
- boxes = proposals["boxes"][i]
- objectness_logits = proposals["objectness_logits"][i]
- # Sort the proposals in descending order of the scores
- inds = objectness_logits.argsort()[::-1]
- record["proposal_boxes"] = boxes[inds]
- record["proposal_objectness_logits"] = objectness_logits[inds]
- record["proposal_bbox_mode"] = bbox_mode
-
- return dataset_dicts
-
-
-def print_instances_class_histogram(dataset_dicts, class_names):
- """
- Args:
- dataset_dicts (list[dict]): list of dataset dicts.
- class_names (list[str]): list of class names (zero-indexed).
- """
- num_classes = len(class_names)
- hist_bins = np.arange(num_classes + 1)
- histogram = np.zeros((num_classes,), dtype=np.int)
- for entry in dataset_dicts:
- annos = entry["annotations"]
- classes = np.asarray(
- [x["category_id"] for x in annos if not x.get("iscrowd", 0)], dtype=np.int
- )
- if len(classes):
- assert classes.min() >= 0, f"Got an invalid category_id={classes.min()}"
- assert (
- classes.max() < num_classes
- ), f"Got an invalid category_id={classes.max()} for a dataset of {num_classes} classes"
- histogram += np.histogram(classes, bins=hist_bins)[0]
-
- N_COLS = min(6, len(class_names) * 2)
-
- def short_name(x):
- # make long class names shorter. useful for lvis
- if len(x) > 13:
- return x[:11] + ".."
- return x
-
- data = list(
- itertools.chain(*[[short_name(class_names[i]), int(v)] for i, v in enumerate(histogram)])
- )
- total_num_instances = sum(data[1::2])
- data.extend([None] * (N_COLS - (len(data) % N_COLS)))
- if num_classes > 1:
- data.extend(["total", total_num_instances])
- data = itertools.zip_longest(*[data[i::N_COLS] for i in range(N_COLS)])
- table = tabulate(
- data,
- headers=["category", "#instances"] * (N_COLS // 2),
- tablefmt="pipe",
- numalign="left",
- stralign="center",
- )
- log_first_n(
- logging.INFO,
- "Distribution of instances among all {} categories:\n".format(num_classes)
- + colored(table, "cyan"),
- key="message",
- )
-
-
-def get_detection_dataset_dicts(names, filter_empty=True, min_keypoints=0, proposal_files=None):
- """
- Load and prepare dataset dicts for instance detection/segmentation and semantic segmentation.
-
- Args:
- names (str or list[str]): a dataset name or a list of dataset names
- filter_empty (bool): whether to filter out images without instance annotations
- min_keypoints (int): filter out images with fewer keypoints than
- `min_keypoints`. Set to 0 to do nothing.
- proposal_files (list[str]): if given, a list of object proposal files
- that match each dataset in `names`.
-
- Returns:
- list[dict]: a list of dicts following the standard dataset dict format.
- """
- if isinstance(names, str):
- names = [names]
- assert len(names), names
- dataset_dicts = [DatasetCatalog.get(dataset_name) for dataset_name in names]
- for dataset_name, dicts in zip(names, dataset_dicts):
- assert len(dicts), "Dataset '{}' is empty!".format(dataset_name)
-
- if proposal_files is not None:
- assert len(names) == len(proposal_files)
- # load precomputed proposals from proposal files
- dataset_dicts = [
- load_proposals_into_dataset(dataset_i_dicts, proposal_file)
- for dataset_i_dicts, proposal_file in zip(dataset_dicts, proposal_files)
- ]
-
- dataset_dicts = list(itertools.chain.from_iterable(dataset_dicts))
-
- has_instances = "annotations" in dataset_dicts[0]
- if filter_empty and has_instances:
- dataset_dicts = filter_images_with_only_crowd_annotations(dataset_dicts)
- if min_keypoints > 0 and has_instances:
- dataset_dicts = filter_images_with_few_keypoints(dataset_dicts, min_keypoints)
-
- if has_instances:
- try:
- class_names = MetadataCatalog.get(names[0]).thing_classes
- check_metadata_consistency("thing_classes", names)
- print_instances_class_histogram(dataset_dicts, class_names)
- except AttributeError: # class names are not available for this dataset
- pass
-
- assert len(dataset_dicts), "No valid data found in {}.".format(",".join(names))
- return dataset_dicts
-
-
-def build_batch_data_loader(
- dataset, sampler, total_batch_size, *, aspect_ratio_grouping=False, num_workers=0
-):
- """
- Build a batched dataloader. The main differences from `torch.utils.data.DataLoader` are:
- 1. support aspect ratio grouping options
- 2. use no "batch collation", because this is common for detection training
-
- Args:
- dataset (torch.utils.data.Dataset): map-style PyTorch dataset. Can be indexed.
- sampler (torch.utils.data.sampler.Sampler): a sampler that produces indices
- total_batch_size, aspect_ratio_grouping, num_workers): see
- :func:`build_detection_train_loader`.
-
- Returns:
- iterable[list]. Length of each list is the batch size of the current
- GPU. Each element in the list comes from the dataset.
- """
- world_size = get_world_size()
- assert (
- total_batch_size > 0 and total_batch_size % world_size == 0
- ), "Total batch size ({}) must be divisible by the number of gpus ({}).".format(
- total_batch_size, world_size
- )
-
- batch_size = total_batch_size // world_size
- if aspect_ratio_grouping:
- data_loader = torch.utils.data.DataLoader(
- dataset,
- sampler=sampler,
- num_workers=num_workers,
- batch_sampler=None,
- collate_fn=operator.itemgetter(0), # don't batch, but yield individual elements
- worker_init_fn=worker_init_reset_seed,
- ) # yield individual mapped dict
- return AspectRatioGroupedDataset(data_loader, batch_size)
- else:
- batch_sampler = torch.utils.data.sampler.BatchSampler(
- sampler, batch_size, drop_last=True
- ) # drop_last so the batch always have the same size
- return torch.utils.data.DataLoader(
- dataset,
- num_workers=num_workers,
- batch_sampler=batch_sampler,
- collate_fn=trivial_batch_collator,
- worker_init_fn=worker_init_reset_seed,
- )
-
-
-def _train_loader_from_config(cfg, mapper=None, *, dataset=None, sampler=None):
- if 'yfcc100m' in cfg.DATASETS.TRAIN: # dataset, transform/aug., sampler for image-text pairs training
- logger = logging.getLogger(__name__)
- logger.info("Creating dataset {}".format(cfg.DATASETS.TRAIN))
- datasets, precomputed_tokens, dataset_classes = make_clip_dataset(
- cfg, is_train=True,
- transforms=None, # for training, we use our own defined transforms
- )
- dataset = datasets[0] # during training, a single (possibly concatenated) dataset was returned
- if sampler is None:
- sampler_name = cfg.DATALOADER.SAMPLER_TRAIN
- logger = logging.getLogger(__name__)
- logger.info("Using training sampler {}".format(sampler_name))
- if sampler_name == "TrainingSampler":
- sampler = TrainingSampler(len(dataset))
- elif sampler_name == "RepeatFactorTrainingSampler":
- repeat_factors = RepeatFactorTrainingSampler.repeat_factors_from_category_frequency(
- dataset, cfg.DATALOADER.REPEAT_THRESHOLD
- )
- sampler = RepeatFactorTrainingSampler(repeat_factors)
- else:
- raise ValueError("Unknown training sampler: {}".format(sampler_name))
- return {
- "dataset": dataset,
- "sampler": sampler,
- "mapper": None,
- "total_batch_size": cfg.SOLVER.IMS_PER_BATCH,
- "aspect_ratio_grouping": cfg.DATALOADER.ASPECT_RATIO_GROUPING,
- "num_workers": cfg.DATALOADER.NUM_WORKERS,
- }
- # the following is the default code in Detectron2
- if dataset is None:
- dataset = get_detection_dataset_dicts(
- cfg.DATASETS.TRAIN,
- filter_empty=cfg.DATALOADER.FILTER_EMPTY_ANNOTATIONS,
- min_keypoints=cfg.MODEL.ROI_KEYPOINT_HEAD.MIN_KEYPOINTS_PER_IMAGE
- if cfg.MODEL.KEYPOINT_ON
- else 0,
- proposal_files=cfg.DATASETS.PROPOSAL_FILES_TRAIN if cfg.MODEL.LOAD_PROPOSALS else None,
- )
- _log_api_usage("dataset." + cfg.DATASETS.TRAIN[0])
-
- if mapper is None:
- mapper = DatasetMapper(cfg, True)
-
- if sampler is None:
- sampler_name = cfg.DATALOADER.SAMPLER_TRAIN
- logger = logging.getLogger(__name__)
- logger.info("Using training sampler {}".format(sampler_name))
- if sampler_name == "TrainingSampler":
- sampler = TrainingSampler(len(dataset))
- elif sampler_name == "RepeatFactorTrainingSampler":
- repeat_factors = RepeatFactorTrainingSampler.repeat_factors_from_category_frequency(
- dataset, cfg.DATALOADER.REPEAT_THRESHOLD
- )
- sampler = RepeatFactorTrainingSampler(repeat_factors)
- else:
- raise ValueError("Unknown training sampler: {}".format(sampler_name))
-
- return {
- "dataset": dataset,
- "sampler": sampler,
- "mapper": mapper,
- "total_batch_size": cfg.SOLVER.IMS_PER_BATCH,
- "aspect_ratio_grouping": cfg.DATALOADER.ASPECT_RATIO_GROUPING,
- "num_workers": cfg.DATALOADER.NUM_WORKERS,
- }
-
-
-# TODO can allow dataset as an iterable or IterableDataset to make this function more general
-@configurable(from_config=_train_loader_from_config)
-def build_detection_train_loader(
- dataset, *, mapper, sampler=None, total_batch_size, aspect_ratio_grouping=True, num_workers=0
-):
- """
- Build a dataloader for object detection with some default features.
- This interface is experimental.
-
- Args:
- dataset (list or torch.utils.data.Dataset): a list of dataset dicts,
- or a map-style pytorch dataset. They can be obtained by using
- :func:`DatasetCatalog.get` or :func:`get_detection_dataset_dicts`.
- mapper (callable): a callable which takes a sample (dict) from dataset and
- returns the format to be consumed by the model.
- When using cfg, the default choice is ``DatasetMapper(cfg, is_train=True)``.
- sampler (torch.utils.data.sampler.Sampler or None): a sampler that produces
- indices to be applied on ``dataset``. Default to :class:`TrainingSampler`,
- which coordinates an infinite random shuffle sequence across all workers.
- total_batch_size (int): total batch size across all workers. Batching
- simply puts data into a list.
- aspect_ratio_grouping (bool): whether to group images with similar
- aspect ratio for efficiency. When enabled, it requires each
- element in dataset be a dict with keys "width" and "height".
- num_workers (int): number of parallel data loading workers
-
- Returns:
- torch.utils.data.DataLoader:
- a dataloader. Each output from it is a ``list[mapped_element]`` of length
- ``total_batch_size / num_workers``, where ``mapped_element`` is produced
- by the ``mapper``.
- """
- if isinstance(dataset, list):
- dataset = DatasetFromList(dataset, copy=False)
- if mapper is not None:
- dataset = MapDataset(dataset, mapper)
- if sampler is None:
- sampler = TrainingSampler(len(dataset))
- assert isinstance(sampler, torch.utils.data.sampler.Sampler)
- return build_batch_data_loader(
- dataset,
- sampler,
- total_batch_size,
- aspect_ratio_grouping=aspect_ratio_grouping,
- num_workers=num_workers,
- )
-
-
-def _test_loader_from_config(cfg, dataset_name, mapper=None):
- """
- Uses the given `dataset_name` argument (instead of the names in cfg), because the
- standard practice is to evaluate each test set individually (not combining them).
- """
- if 'yfcc100m' in cfg.DATASETS.TEST: # dataset, no {transform/aug., sampler for image-text pairs training}
- logger = logging.getLogger(__name__)
- logger.info("Creating dataset {}".format(cfg.DATASETS.TEST))
- datasets, precomputed_tokens, dataset_classes = make_clip_dataset(
- cfg, is_train=False,
- transforms=None, # for training, we use our own defined transforms
- )
- dataset = datasets[0] # during training, a single (possibly concatenated) dataset was returned
- return {
- "dataset": dataset,
- "mapper": None,
- "num_workers": cfg.DATALOADER.NUM_WORKERS,
- }
-
- # the following is the default code in Detectron2
- dataset = get_detection_dataset_dicts(
- [dataset_name],
- filter_empty=False,
- proposal_files=[
- cfg.DATASETS.PROPOSAL_FILES_TEST[list(cfg.DATASETS.TEST).index(dataset_name)]
- ]
- if cfg.MODEL.LOAD_PROPOSALS
- else None,
- )
- if mapper is None:
- mapper = DatasetMapper(cfg, False)
- if cfg.MODEL.META_ARCHITECTURE == 'CLIPRCNN': # speed up when using CLIP in inference
- return {"dataset": dataset, "mapper": mapper, "num_workers": cfg.DATALOADER.NUM_WORKERS,\
- "clip_batch_size": cfg.MODEL.CLIP.IMS_PER_BATCH_TEST}
- return {"dataset": dataset, "mapper": mapper, "num_workers": cfg.DATALOADER.NUM_WORKERS}
-
-
-@configurable(from_config=_test_loader_from_config)
-def build_detection_test_loader(dataset, *, mapper, sampler=None, num_workers=0, clip_batch_size=None):
- """
- Similar to `build_detection_train_loader`, but uses a batch size of 1,
- and :class:`InferenceSampler`. This sampler coordinates all workers to
- produce the exact set of all samples.
- This interface is experimental.
-
- Args:
- dataset (list or torch.utils.data.Dataset): a list of dataset dicts,
- or a map-style pytorch dataset. They can be obtained by using
- :func:`DatasetCatalog.get` or :func:`get_detection_dataset_dicts`.
- mapper (callable): a callable which takes a sample (dict) from dataset
- and returns the format to be consumed by the model.
- When using cfg, the default choice is ``DatasetMapper(cfg, is_train=False)``.
- sampler (torch.utils.data.sampler.Sampler or None): a sampler that produces
- indices to be applied on ``dataset``. Default to :class:`InferenceSampler`,
- which splits the dataset across all workers.
- num_workers (int): number of parallel data loading workers
-
- Returns:
- DataLoader: a torch DataLoader, that loads the given detection
- dataset, with test-time transformation and batching.
-
- Examples:
- ::
- data_loader = build_detection_test_loader(
- DatasetRegistry.get("my_test"),
- mapper=DatasetMapper(...))
-
- # or, instantiate with a CfgNode:
- data_loader = build_detection_test_loader(cfg, "my_test")
- """
- if isinstance(dataset, list):
- dataset = DatasetFromList(dataset, copy=False)
- if mapper is not None:
- dataset = MapDataset(dataset, mapper)
- if sampler is None:
- sampler = InferenceSampler(len(dataset))
-
- if clip_batch_size: # multiple images per gpu
- world_size = get_world_size()
- batch_size = clip_batch_size // world_size
- batch_sampler = torch.utils.data.sampler.BatchSampler(sampler, batch_size, drop_last=False)
- data_loader = torch.utils.data.DataLoader(
- dataset,
- num_workers=num_workers,
- batch_sampler=batch_sampler,
- collate_fn=trivial_batch_collator,
- )
- return data_loader
- # Always use 1 image per worker during inference since this is the
- # standard when reporting inference time in papers.
- batch_sampler = torch.utils.data.sampler.BatchSampler(sampler, 1, drop_last=False)
- data_loader = torch.utils.data.DataLoader(
- dataset,
- num_workers=num_workers,
- batch_sampler=batch_sampler,
- collate_fn=trivial_batch_collator,
- )
- return data_loader
-
-
-def trivial_batch_collator(batch):
- """
- A batch collator that does nothing.
- """
- return batch
-
-
-def worker_init_reset_seed(worker_id):
- initial_seed = torch.initial_seed() % 2 ** 31
- seed_all_rng(initial_seed + worker_id)
diff --git a/spaces/CVPR/regionclip-demo/detectron2/modeling/text_encoder/__init__.py b/spaces/CVPR/regionclip-demo/detectron2/modeling/text_encoder/__init__.py
deleted file mode 100644
index e09753c06e7cd77d8df3bee03b04ae9f85ce80bb..0000000000000000000000000000000000000000
--- a/spaces/CVPR/regionclip-demo/detectron2/modeling/text_encoder/__init__.py
+++ /dev/null
@@ -1,9 +0,0 @@
-from __future__ import absolute_import
-from __future__ import division
-from __future__ import print_function
-
-from .build import build_lang_encoder as build_text_encoder
-from .build import build_tokenizer
-
-from .transformer import *
-from .hf_model import *
diff --git a/spaces/Cropinky/hana_hanak_houses/app.py b/spaces/Cropinky/hana_hanak_houses/app.py
deleted file mode 100644
index 1ed36a81b7e0400772347537d0b98ebdbc8f4851..0000000000000000000000000000000000000000
--- a/spaces/Cropinky/hana_hanak_houses/app.py
+++ /dev/null
@@ -1,73 +0,0 @@
-import gradio as gr
-from huggingface_hub import PyTorchModelHubMixin
-import torch
-import matplotlib.pyplot as plt
-import torchvision
-from networks_fastgan import MyGenerator
-import click
-import PIL
-from image_generator import generate_images
-from basicsr.archs.rrdbnet_arch import RRDBNet
-from basicsr.utils.download_util import load_file_from_url
-import cv2
-import sys
-import numpy as np
-#sys.path.append('Real-ESRGAN')
-from realesrgan import RealESRGANer
-import gc
-
-import os
-
-
-def image_generation(model, number_of_images=1):
- img = generate_images(model)
- #TODO: run this image through the ESRGAN upscaler and return it, simple enough ?
- #upscaled_img = torchvision.transforms.functional.resize(img, (1024, 1024), interpolation=2)
- upscale_model = RRDBNet(num_in_ch=3, num_out_ch=3, num_feat=64, num_block=23, num_grow_ch=32, scale=4)
- file_url = ['https://github.com/xinntao/Real-ESRGAN/releases/download/v0.1.0/RealESRGAN_x4plus.pth']
- ROOT_DIR = os.path.dirname(os.path.abspath(__file__))
- #model_path = load_file_from_url(url=file_url, model_dir=os.path.join(ROOT_DIR, 'weights'), progress=True, file_name=None)
- model_path = os.path.join('weights', 'RealESRGAN_x4plus.pth')
- upsampler = RealESRGANer(
- scale=4,
- model_path=model_path,
- dni_weight=None,
- model=upscale_model,
- tile=0,
- tile_pad=10,
- pre_pad=0,
- half=False,
- )
- #TODO: img has to be same as opencv imread format
- open_cv_image = np.array(img)
- # Convert RGB to BGR
- open_cv_image = open_cv_image[:, :, ::-1].copy()
- #print(type(open_cv_image))
- #print(type(img))
- #print(type(upscaled_img))
- output, _ = upsampler.enhance(open_cv_image, outscale=8)
- #output2, _ = upsampler.enhance(output , outscale=4)
- #return f"generating {number_of_images} images from {model}"
- #cv2.imwrite('out/output_upscaled.png', output)
- #cv2.imwrite('out/output_upscaled_dupli.png', output2)
- #cv2.imwrite('out/output.png', np.array(img)[:, :, ::-1])
- output = cv2.cvtColor(output, cv2.COLOR_BGR2RGB)
- gc.collect()
- torch.cuda.empty_cache()
- del(upsampler)
- return PIL.Image.fromarray(output)
-if __name__ == "__main__":
- description = "This is a web demo of a projected GAN trained on photos of thirty paintings from the series of paintings Welcome home. The abstract expressionism and color field models were initially trained on images from their perspective art directions and then transfer learned to Hana's houses."
- inputs = gr.inputs.Radio(["Hana Hanak houses", "Hana Hanak houses - abstract expressionism", "Hana Hanak houses - color field"])
- outputs = gr.outputs.Image(label="Generated Image", type="pil")
- #outputs = "text"
- title = "Anti house generator"
- article = "
- )}
-
- );
-}
diff --git a/spaces/MLVKU/Human_Object_Interaction/hotr/models/criterion.py b/spaces/MLVKU/Human_Object_Interaction/hotr/models/criterion.py
deleted file mode 100644
index 49402b1ff8ae03f3c4bf3f7c1ae8081907638ef5..0000000000000000000000000000000000000000
--- a/spaces/MLVKU/Human_Object_Interaction/hotr/models/criterion.py
+++ /dev/null
@@ -1,349 +0,0 @@
-# ------------------------------------------------------------------------
-# HOTR official code : main.py
-# Copyright (c) Kakao Brain, Inc. and its affiliates. All Rights Reserved
-# ------------------------------------------------------------------------
-# Modified from DETR (https://github.com/facebookresearch/detr)
-# Copyright (c) Facebook, Inc. and its affiliates. All Rights Reserved
-# ------------------------------------------------------------------------
-import torch
-import torch.nn.functional as F
-import copy
-import numpy as np
-import itertools
-from torch import nn
-
-from hotr.util import box_ops
-from hotr.util.misc import (accuracy, get_world_size, is_dist_avail_and_initialized)
-
-class SetCriterion(nn.Module):
- """ This class computes the loss for DETR.
- The process happens in two steps:
- 1) we compute hungarian assignment between ground truth boxes and the outputs of the model
- 2) we supervise each pair of matched ground-truth / prediction (supervise class and box)
- """
- def __init__(self, num_classes, matcher, weight_dict, eos_coef, losses, num_actions=None, HOI_losses=None, HOI_matcher=None, args=None):
- """ Create the criterion.
- Parameters:
- num_classes: number of object categories, omitting the special no-object category
- matcher: module able to compute a matching between targets and proposals
- weight_dict: dict containing as key the names of the losses and as values their relative weight.
- eos_coef: relative classification weight applied to the no-object category
- losses: list of all the losses to be applied. See get_loss for list of available losses.
- """
- super().__init__()
- self.num_classes = num_classes
- self.matcher = matcher
- self.weight_dict = weight_dict
- self.losses = losses
- self.eos_coef=eos_coef
-
- self.HOI_losses = HOI_losses
- self.HOI_matcher = HOI_matcher
- self.use_consis=args.use_consis & len(args.augpath_name)>0
- self.num_path = 1+len(args.augpath_name)
- if args:
- self.HOI_eos_coef = args.hoi_eos_coef
- if args.dataset_file == 'vcoco':
- self.invalid_ids = args.invalid_ids
- self.valid_ids = np.concatenate((args.valid_ids,[-1]), axis=0) # no interaction
- elif args.dataset_file == 'hico-det':
- self.invalid_ids = []
- self.valid_ids = list(range(num_actions)) + [-1]
-
- # for targets
- self.num_tgt_classes = len(args.valid_obj_ids)
- tgt_empty_weight = torch.ones(self.num_tgt_classes + 1)
- tgt_empty_weight[-1] = self.HOI_eos_coef
- self.register_buffer('tgt_empty_weight', tgt_empty_weight)
- self.dataset_file = args.dataset_file
-
- empty_weight = torch.ones(self.num_classes + 1)
- empty_weight[-1] = eos_coef
- self.register_buffer('empty_weight', empty_weight)
-
- #######################################################################################################################
- # * DETR Losses
- #######################################################################################################################
- def loss_labels(self, outputs, targets, indices, num_boxes, log=True):
- """Classification loss (NLL)
- targets dicts must contain the key "labels" containing a tensor of dim [nb_target_boxes]
- """
- assert 'pred_logits' in outputs
- src_logits = outputs['pred_logits']
-
- idx = self._get_src_permutation_idx(indices)
- target_classes_o = torch.cat([t["labels"][J] for t, (_, J) in zip(targets, indices)])
- target_classes = torch.full(src_logits.shape[:2], self.num_classes, dtype=torch.int64, device=src_logits.device)
- target_classes[idx] = target_classes_o
-
- loss_ce = F.cross_entropy(src_logits.transpose(1, 2), target_classes, self.empty_weight)
- losses = {'loss_ce': loss_ce}
-
- if log:
- # TODO this should probably be a separate loss, not hacked in this one here
- losses['class_error'] = 100 - accuracy(src_logits[idx], target_classes_o)[0]
- return losses
-
- @torch.no_grad()
- def loss_cardinality(self, outputs, targets, indices, num_boxes):
- """ Compute the cardinality error, ie the absolute error in the number of predicted non-empty boxes
- This is not really a loss, it is intended for logging purposes only. It doesn't propagate gradients
- """
- pred_logits = outputs['pred_logits']
- device = pred_logits.device
- tgt_lengths = torch.as_tensor([len(v["labels"]) for v in targets], device=device)
- # Count the number of predictions that are NOT "no-object" (which is the last class)
- card_pred = (pred_logits.argmax(-1) != pred_logits.shape[-1] - 1).sum(1)
- card_err = F.l1_loss(card_pred.float(), tgt_lengths.float())
- losses = {'cardinality_error': card_err}
- return losses
-
- def loss_boxes(self, outputs, targets, indices, num_boxes):
- """Compute the losses related to the bounding boxes, the L1 regression loss and the GIoU loss
- targets dicts must contain the key "boxes" containing a tensor of dim [nb_target_boxes, 4]
- The target boxes are expected in format (center_x, center_y, w, h), normalized by the image size.
- """
- assert 'pred_boxes' in outputs
- idx = self._get_src_permutation_idx(indices)
- src_boxes = outputs['pred_boxes'][idx]
- target_boxes = torch.cat([t['boxes'][i] for t, (_, i) in zip(targets, indices)], dim=0)
-
- loss_bbox = F.l1_loss(src_boxes, target_boxes, reduction='none')
-
- losses = {}
- losses['loss_bbox'] = loss_bbox.sum() / num_boxes
-
- loss_giou = 1 - torch.diag(box_ops.generalized_box_iou(
- box_ops.box_cxcywh_to_xyxy(src_boxes),
- box_ops.box_cxcywh_to_xyxy(target_boxes)))
- losses['loss_giou'] = loss_giou.sum() / num_boxes
- return losses
-
-
- #######################################################################################################################
- # * HOTR Losses
- #######################################################################################################################
- # >>> HOI Losses 1 : HO Pointer
- def loss_pair_labels(self, outputs, targets, hoi_indices, num_boxes,use_consis, log=False):
- assert ('pred_hidx' in outputs and 'pred_oidx' in outputs)
- outputs_without_aux = {k: v for k, v in outputs.items() if k != 'aux_outputs'}
- nu,q,hd=outputs['pred_hidx'].shape
- src_hidx = outputs['pred_hidx'].view(self.num_path,nu//self.num_path,q,-1).transpose(0,1).flatten(0,1)
- src_oidx = outputs['pred_oidx'].view(self.num_path,nu//self.num_path,q,-1).transpose(0,1).flatten(0,1)
- hoi_ind=list(itertools.chain.from_iterable(hoi_indices))
-
- idx = self._get_src_permutation_idx(hoi_ind)
-
- target_hidx_classes = torch.full(src_hidx.shape[:2], -1, dtype=torch.int64, device=src_hidx.device)
- target_oidx_classes = torch.full(src_oidx.shape[:2], -1, dtype=torch.int64, device=src_oidx.device)
-
- # H Pointer loss
- target_classes_h = torch.cat([t["h_labels"][J] for t, hoi_indice in zip(targets, hoi_indices) for (_,J) in hoi_indice])
- target_hidx_classes[idx] = target_classes_h
-
- # O Pointer loss
- target_classes_o = torch.cat([t["o_labels"][J] for t, hoi_indice in zip(targets, hoi_indices) for (_,J) in hoi_indice])
- target_oidx_classes[idx] = target_classes_o
-
- loss_h = F.cross_entropy(src_hidx.transpose(1, 2), target_hidx_classes, ignore_index=-1)
- loss_o = F.cross_entropy(src_oidx.transpose(1, 2), target_oidx_classes, ignore_index=-1)
-
- #Consistency loss
- if use_consis:
- consistency_idxs=[self._get_consistency_src_permutation_idx(hoi_indice) for hoi_indice in hoi_indices ]
- src_hidx_inputs=[F.softmax(src_hidx.view(-1,self.num_path,q,hd)[i][consistency_idx[0]],-1) for i,consistency_idx in enumerate(consistency_idxs)]
- src_hidx_targets=[F.softmax(src_hidx.view(-1,self.num_path,q,hd)[i][consistency_idx[1]],-1) for i,consistency_idx in enumerate(consistency_idxs)]
- src_oidx_inputs=[F.softmax(src_oidx.view(-1,self.num_path,q,hd)[i][consistency_idx[0]],-1) for i,consistency_idx in enumerate(consistency_idxs)]
- src_oidx_targets=[F.softmax(src_oidx.view(-1,self.num_path,q,hd)[i][consistency_idx[1]],-1) for i,consistency_idx in enumerate(consistency_idxs)]
-
- loss_h_consistency=[0.5*(F.kl_div(src_hidx_input.log(),src_hidx_target.clone().detach(),reduction='batchmean')+F.kl_div(src_hidx_target.log(),src_hidx_input.clone().detach(),reduction='batchmean')) for src_hidx_input,src_hidx_target in zip(src_hidx_inputs,src_hidx_targets)]
- loss_o_consistency=[0.5*(F.kl_div(src_oidx_input.log(),src_oidx_target.clone().detach(),reduction='batchmean')+F.kl_div(src_oidx_target.log(),src_oidx_input.clone().detach(),reduction='batchmean')) for src_oidx_input,src_oidx_target in zip(src_oidx_inputs,src_oidx_targets)]
-
- loss_h_consistency=torch.mean(torch.stack(loss_h_consistency))
- loss_o_consistency=torch.mean(torch.stack(loss_o_consistency))
-
- losses = {'loss_hidx': loss_h, 'loss_oidx': loss_o,'loss_h_consistency':loss_h_consistency,'loss_o_consistency':loss_o_consistency}
- else:
- losses = {'loss_hidx': loss_h, 'loss_oidx': loss_o}
-
- return losses
-
- # >>> HOI Losses 2 : pair actions
- def loss_pair_actions(self, outputs, targets, hoi_indices, num_boxes,use_consis):
- assert 'pred_actions' in outputs
- src_actions = outputs['pred_actions'].flatten(end_dim=1)
- hoi_ind=list(itertools.chain.from_iterable(hoi_indices))
- # idx = self._get_src_permutation_idx(hoi_indices)
- idx = self._get_src_permutation_idx(hoi_ind)
-
- # Construct Target --------------------------------------------------------------------------------------------------------------
- target_classes_o = torch.cat([t["pair_actions"][J] for t, hoi_indice in zip(targets, hoi_indices) for (_,J) in hoi_indice])
- target_classes = torch.full(src_actions.shape, 0, dtype=torch.float32, device=src_actions.device)
- target_classes[..., -1] = 1 # the last index for no-interaction is '1' if a label exists
-
- pos_classes = torch.full(target_classes[idx].shape, 0, dtype=torch.float32, device=src_actions.device) # else, the last index for no-interaction is '0'
- pos_classes[:, :-1] = target_classes_o.float()
- target_classes[idx] = pos_classes
- # --------------------------------------------------------------------------------------------------------------------------------
-
- # BCE Loss -----------------------------------------------------------------------------------------------------------------------
- logits = src_actions.sigmoid()
- loss_bce = F.binary_cross_entropy(logits[..., self.valid_ids], target_classes[..., self.valid_ids], reduction='none')
- p_t = logits[..., self.valid_ids] * target_classes[..., self.valid_ids] + (1 - logits[..., self.valid_ids]) * (1 - target_classes[..., self.valid_ids])
- loss_bce = ((1-p_t)**2 * loss_bce)
- alpha_t = 0.25 * target_classes[..., self.valid_ids] + (1 - 0.25) * (1 - target_classes[..., self.valid_ids])
- loss_focal = alpha_t * loss_bce
- loss_act = loss_focal.sum() / max(target_classes[..., self.valid_ids[:-1]].sum(), 1)
- # --------------------------------------------------------------------------------------------------------------------------------
-
- #Consistency loss
- if use_consis:
- consistency_idxs=[self._get_consistency_src_permutation_idx(hoi_indice) for hoi_indice in hoi_indices]
- src_action_inputs=[F.logsigmoid(outputs['pred_actions'][i][consistency_idx[0]]) for i,consistency_idx in enumerate(consistency_idxs)]
- src_action_targets=[F.logsigmoid(outputs['pred_actions'][i][consistency_idx[1]]) for i,consistency_idx in enumerate(consistency_idxs)]
-
- loss_action_consistency=[F.mse_loss(src_action_input,src_action_target) for src_action_input,src_action_target in zip(src_action_inputs,src_action_targets)]
- loss_action_consistency=torch.mean(torch.stack(loss_action_consistency))
- # import pdb;pdb.set_trace()
- losses = {'loss_act': loss_act,'loss_act_consistency':loss_action_consistency}
- else:
- losses = {'loss_act': loss_act}
- return losses
-
- # HOI Losses 3 : action targets
- def loss_pair_targets(self, outputs, targets, hoi_indices, num_interactions,use_consis, log=True):
- assert 'pred_obj_logits' in outputs
- src_logits = outputs['pred_obj_logits']
- nu,q,hd=outputs['pred_obj_logits'].shape
- hoi_ind=list(itertools.chain.from_iterable(hoi_indices))
- idx = self._get_src_permutation_idx(hoi_ind)
-
- target_classes_o = torch.cat([t['pair_targets'][J] for t, hoi_indice in zip(targets, hoi_indices) for (_,J) in hoi_indice])
- pad_tgt = -1 # src_logits.shape[2]-1
- target_classes = torch.full(src_logits.shape[:2], pad_tgt, dtype=torch.int64, device=src_logits.device)
- target_classes[idx] = target_classes_o
-
- loss_obj_ce = F.cross_entropy(src_logits.transpose(1, 2), target_classes, self.tgt_empty_weight, ignore_index=-1)
-
- #consistency
- if use_consis:
- consistency_idxs=[self._get_consistency_src_permutation_idx(hoi_indice) for hoi_indice in hoi_indices]
- src_logits_inputs=[F.softmax(src_logits.view(-1,self.num_path,q,hd)[i][consistency_idx[0]],-1) for i,consistency_idx in enumerate(consistency_idxs)]
- src_logits_targets=[F.softmax(src_logits.view(-1,self.num_path,q,hd)[i][consistency_idx[1]],-1) for i,consistency_idx in enumerate(consistency_idxs)]
- loss_tgt_consistency=[0.5*(F.kl_div(src_logit_input.log(),src_logit_target.clone().detach(),reduction='batchmean')+F.kl_div(src_logit_target.log(),src_logit_input.clone().detach(),reduction='batchmean')) for src_logit_input,src_logit_target in zip(src_logits_inputs,src_logits_targets)]
- loss_tgt_consistency=torch.mean(torch.stack(loss_tgt_consistency))
- losses = {'loss_tgt': loss_obj_ce,"loss_tgt_label_consistency":loss_tgt_consistency}
- else:
- losses = {'loss_tgt': loss_obj_ce}
- if log:
- ignore_idx = (target_classes_o != -1)
- losses['obj_class_error'] = 100 - accuracy(src_logits[idx][ignore_idx, :-1], target_classes_o[ignore_idx])[0]
- # losses['obj_class_error'] = 100 - accuracy(src_logits[idx], target_classes_o)[0]
- return losses
-
- def _get_src_permutation_idx(self, indices):
- # permute predictions following indices
- batch_idx = torch.cat([torch.full_like(src, i) for i, (src, _) in enumerate(indices)])
- src_idx = torch.cat([src for (src, _) in indices])
- return batch_idx, src_idx
-
- def _get_consistency_src_permutation_idx(self, indices):
- all_tgt=torch.cat([j for(_,j) in indices]).unique()
- path_idxs=[torch.cat([torch.tensor([i]) for i,(_,t)in enumerate(indices) if (t==tgt).any()]) for tgt in all_tgt]
- q_idxs=[torch.cat([s[t==tgt] for (s,t)in indices]) for tgt in all_tgt]
- path_idxs=torch.cat([torch.combinations(path_idx) for path_idx in path_idxs if len(path_idx)>1])
- q_idxs=torch.cat([torch.combinations(q_idx) for q_idx in q_idxs if len(q_idx)>1])
-
- return (path_idxs[:,0],q_idxs[:,0]),(path_idxs[:,1],q_idxs[:,1])
-
- def _get_tgt_permutation_idx(self, indices):
- # permute targets following indices
- batch_idx = torch.cat([torch.full_like(tgt, i) for i, (_, tgt) in enumerate(indices)])
- tgt_idx = torch.cat([tgt for (_, tgt) in indices])
- return batch_idx, tgt_idx
-
- # *****************************************************************************
- # >>> DETR Losses
- def get_loss(self, loss, outputs, targets, indices, num_boxes, **kwargs):
- loss_map = {
- 'labels': self.loss_labels,
- 'cardinality': self.loss_cardinality,
- 'boxes': self.loss_boxes
- }
- assert loss in loss_map, f'do you really want to compute {loss} loss?'
- return loss_map[loss](outputs, targets, indices, num_boxes, **kwargs)
-
- # >>> HOTR Losses
- def get_HOI_loss(self, loss, outputs, targets, indices, num_boxes,use_consis, **kwargs):
- loss_map = {
- 'pair_labels': self.loss_pair_labels,
- 'pair_actions': self.loss_pair_actions
- }
- if self.dataset_file == 'hico-det': loss_map['pair_targets'] = self.loss_pair_targets
- assert loss in loss_map, f'do you really want to compute {loss} loss?'
- return loss_map[loss](outputs, targets, indices, num_boxes,use_consis, **kwargs)
- # *****************************************************************************
-
- def forward(self, outputs, targets, log=False):
- """ This performs the loss computation.
- Parameters:
- outputs: dict of tensors, see the output specification of the model for the format
- targets: list of dicts, such that len(targets) == batch_size.
- The expected keys in each dict depends on the losses applied, see each loss' doc
- """
- outputs_without_aux = {k: v for k, v in outputs.items() if (k != 'aux_outputs' and k != 'hoi_aux_outputs')}
-
- # Retrieve the matching between the outputs of the last layer and the targets
- indices = self.matcher(outputs_without_aux, targets)
-
- if self.HOI_losses is not None:
- input_targets = [copy.deepcopy(target) for target in targets]
- hoi_indices, hoi_targets = self.HOI_matcher(outputs_without_aux, input_targets, indices, log)
-
- # Compute the average number of target boxes accross all nodes, for normalization purposes
- num_boxes = sum(len(t["labels"]) for t in targets)
- num_boxes = torch.as_tensor([num_boxes], dtype=torch.float, device=next(iter(outputs.values())).device)
- if is_dist_avail_and_initialized():
- torch.distributed.all_reduce(num_boxes)
- num_boxes = torch.clamp(num_boxes / get_world_size(), min=1).item()
-
- # Compute all the requested losses
- losses = {}
- for loss in self.losses:
- losses.update(self.get_loss(loss, outputs, targets, indices, num_boxes))
-
- # In case of auxiliary losses, we repeat this process with the output of each intermediate layer.
- if 'aux_outputs' in outputs:
- for i, aux_outputs in enumerate(outputs['aux_outputs']):
- indices = self.matcher(aux_outputs, targets)
- for loss in self.losses:
- if loss == 'masks':
- # Intermediate masks losses are too costly to compute, we ignore them.
- continue
- kwargs = {}
- if loss == 'labels':
- # Logging is enabled only for the last layer
- kwargs = {'log': False}
- l_dict = self.get_loss(loss, aux_outputs, targets, indices, num_boxes, **kwargs)
- l_dict = {k + f'_{i}': v for k, v in l_dict.items()}
- losses.update(l_dict)
-
- # HOI detection losses
- if self.HOI_losses is not None:
- for loss in self.HOI_losses:
- losses.update(self.get_HOI_loss(loss, outputs, hoi_targets, hoi_indices, num_boxes,self.use_consis))
- # if self.dataset_file == 'hico-det': losses['loss_oidx'] += losses['loss_tgt']
-
- if 'hoi_aux_outputs' in outputs:
- for i, aux_outputs in enumerate(outputs['hoi_aux_outputs']):
- input_targets = [copy.deepcopy(target) for target in targets]
- hoi_indices, targets_for_aux = self.HOI_matcher(aux_outputs, input_targets, indices, log)
- for loss in self.HOI_losses:
- kwargs = {}
- if loss == 'pair_targets': kwargs = {'log': False} # Logging is enabled only for the last layer
- l_dict = self.get_HOI_loss(loss, aux_outputs, hoi_targets, hoi_indices, num_boxes,self.use_consis, **kwargs)
- l_dict = {k + f'_{i}': v for k, v in l_dict.items()}
- losses.update(l_dict)
- # if self.dataset_file == 'hico-det': losses[f'loss_oidx_{i}'] += losses[f'loss_tgt_{i}']
-
- return losses
\ No newline at end of file
diff --git a/spaces/Mahiruoshi/MyGO_VIts-bert/utils.py b/spaces/Mahiruoshi/MyGO_VIts-bert/utils.py
deleted file mode 100644
index 49678050ddc36219b0929056766f68f8112e67c3..0000000000000000000000000000000000000000
--- a/spaces/Mahiruoshi/MyGO_VIts-bert/utils.py
+++ /dev/null
@@ -1,357 +0,0 @@
-import os
-import glob
-import argparse
-import logging
-import json
-import subprocess
-import numpy as np
-from scipy.io.wavfile import read
-import torch
-
-MATPLOTLIB_FLAG = False
-
-logger = logging.getLogger(__name__)
-
-
-def load_checkpoint(checkpoint_path, model, optimizer=None, skip_optimizer=False):
- assert os.path.isfile(checkpoint_path)
- checkpoint_dict = torch.load(checkpoint_path, map_location="cpu")
- iteration = checkpoint_dict["iteration"]
- learning_rate = checkpoint_dict["learning_rate"]
- if (
- optimizer is not None
- and not skip_optimizer
- and checkpoint_dict["optimizer"] is not None
- ):
- optimizer.load_state_dict(checkpoint_dict["optimizer"])
- elif optimizer is None and not skip_optimizer:
- # else: Disable this line if Infer and resume checkpoint,then enable the line upper
- new_opt_dict = optimizer.state_dict()
- new_opt_dict_params = new_opt_dict["param_groups"][0]["params"]
- new_opt_dict["param_groups"] = checkpoint_dict["optimizer"]["param_groups"]
- new_opt_dict["param_groups"][0]["params"] = new_opt_dict_params
- optimizer.load_state_dict(new_opt_dict)
-
- saved_state_dict = checkpoint_dict["model"]
- if hasattr(model, "module"):
- state_dict = model.module.state_dict()
- else:
- state_dict = model.state_dict()
-
- new_state_dict = {}
- for k, v in state_dict.items():
- try:
- # assert "emb_g" not in k
- new_state_dict[k] = saved_state_dict[k]
- assert saved_state_dict[k].shape == v.shape, (
- saved_state_dict[k].shape,
- v.shape,
- )
- except:
- # For upgrading from the old version
- if "ja_bert_proj" in k:
- v = torch.zeros_like(v)
- logger.warn(
- f"Seems you are using the old version of the model, the {k} is automatically set to zero for backward compatibility"
- )
- else:
- logger.error(f"{k} is not in the checkpoint")
-
- new_state_dict[k] = v
-
- if hasattr(model, "module"):
- model.module.load_state_dict(new_state_dict, strict=False)
- else:
- model.load_state_dict(new_state_dict, strict=False)
-
- logger.info(
- "Loaded checkpoint '{}' (iteration {})".format(checkpoint_path, iteration)
- )
-
- return model, optimizer, learning_rate, iteration
-
-
-def save_checkpoint(model, optimizer, learning_rate, iteration, checkpoint_path):
- logger.info(
- "Saving model and optimizer state at iteration {} to {}".format(
- iteration, checkpoint_path
- )
- )
- if hasattr(model, "module"):
- state_dict = model.module.state_dict()
- else:
- state_dict = model.state_dict()
- torch.save(
- {
- "model": state_dict,
- "iteration": iteration,
- "optimizer": optimizer.state_dict(),
- "learning_rate": learning_rate,
- },
- checkpoint_path,
- )
-
-
-def summarize(
- writer,
- global_step,
- scalars={},
- histograms={},
- images={},
- audios={},
- audio_sampling_rate=22050,
-):
- for k, v in scalars.items():
- writer.add_scalar(k, v, global_step)
- for k, v in histograms.items():
- writer.add_histogram(k, v, global_step)
- for k, v in images.items():
- writer.add_image(k, v, global_step, dataformats="HWC")
- for k, v in audios.items():
- writer.add_audio(k, v, global_step, audio_sampling_rate)
-
-
-def latest_checkpoint_path(dir_path, regex="G_*.pth"):
- f_list = glob.glob(os.path.join(dir_path, regex))
- f_list.sort(key=lambda f: int("".join(filter(str.isdigit, f))))
- x = f_list[-1]
- return x
-
-
-def plot_spectrogram_to_numpy(spectrogram):
- global MATPLOTLIB_FLAG
- if not MATPLOTLIB_FLAG:
- import matplotlib
-
- matplotlib.use("Agg")
- MATPLOTLIB_FLAG = True
- mpl_logger = logging.getLogger("matplotlib")
- mpl_logger.setLevel(logging.WARNING)
- import matplotlib.pylab as plt
- import numpy as np
-
- fig, ax = plt.subplots(figsize=(10, 2))
- im = ax.imshow(spectrogram, aspect="auto", origin="lower", interpolation="none")
- plt.colorbar(im, ax=ax)
- plt.xlabel("Frames")
- plt.ylabel("Channels")
- plt.tight_layout()
-
- fig.canvas.draw()
- data = np.fromstring(fig.canvas.tostring_rgb(), dtype=np.uint8, sep="")
- data = data.reshape(fig.canvas.get_width_height()[::-1] + (3,))
- plt.close()
- return data
-
-
-def plot_alignment_to_numpy(alignment, info=None):
- global MATPLOTLIB_FLAG
- if not MATPLOTLIB_FLAG:
- import matplotlib
-
- matplotlib.use("Agg")
- MATPLOTLIB_FLAG = True
- mpl_logger = logging.getLogger("matplotlib")
- mpl_logger.setLevel(logging.WARNING)
- import matplotlib.pylab as plt
- import numpy as np
-
- fig, ax = plt.subplots(figsize=(6, 4))
- im = ax.imshow(
- alignment.transpose(), aspect="auto", origin="lower", interpolation="none"
- )
- fig.colorbar(im, ax=ax)
- xlabel = "Decoder timestep"
- if info is not None:
- xlabel += "\n\n" + info
- plt.xlabel(xlabel)
- plt.ylabel("Encoder timestep")
- plt.tight_layout()
-
- fig.canvas.draw()
- data = np.fromstring(fig.canvas.tostring_rgb(), dtype=np.uint8, sep="")
- data = data.reshape(fig.canvas.get_width_height()[::-1] + (3,))
- plt.close()
- return data
-
-
-def load_wav_to_torch(full_path):
- sampling_rate, data = read(full_path)
- return torch.FloatTensor(data.astype(np.float32)), sampling_rate
-
-
-def load_filepaths_and_text(filename, split="|"):
- with open(filename, encoding="utf-8") as f:
- filepaths_and_text = [line.strip().split(split) for line in f]
- return filepaths_and_text
-
-
-def get_hparams(init=True):
- parser = argparse.ArgumentParser()
- parser.add_argument(
- "-c",
- "--config",
- type=str,
- default="./configs/base.json",
- help="JSON file for configuration",
- )
- parser.add_argument("-m", "--model", type=str, required=True, help="Model name")
-
- args = parser.parse_args()
- model_dir = os.path.join("./logs", args.model)
-
- if not os.path.exists(model_dir):
- os.makedirs(model_dir)
-
- config_path = args.config
- config_save_path = os.path.join(model_dir, "config.json")
- if init:
- with open(config_path, "r") as f:
- data = f.read()
- with open(config_save_path, "w") as f:
- f.write(data)
- else:
- with open(config_save_path, "r") as f:
- data = f.read()
- config = json.loads(data)
-
- hparams = HParams(**config)
- hparams.model_dir = model_dir
- return hparams
-
-
-def clean_checkpoints(path_to_models="logs/44k/", n_ckpts_to_keep=2, sort_by_time=True):
- """Freeing up space by deleting saved ckpts
-
- Arguments:
- path_to_models -- Path to the model directory
- n_ckpts_to_keep -- Number of ckpts to keep, excluding G_0.pth and D_0.pth
- sort_by_time -- True -> chronologically delete ckpts
- False -> lexicographically delete ckpts
- """
- import re
-
- ckpts_files = [
- f
- for f in os.listdir(path_to_models)
- if os.path.isfile(os.path.join(path_to_models, f))
- ]
-
- def name_key(_f):
- return int(re.compile("._(\\d+)\\.pth").match(_f).group(1))
-
- def time_key(_f):
- return os.path.getmtime(os.path.join(path_to_models, _f))
-
- sort_key = time_key if sort_by_time else name_key
-
- def x_sorted(_x):
- return sorted(
- [f for f in ckpts_files if f.startswith(_x) and not f.endswith("_0.pth")],
- key=sort_key,
- )
-
- to_del = [
- os.path.join(path_to_models, fn)
- for fn in (x_sorted("G")[:-n_ckpts_to_keep] + x_sorted("D")[:-n_ckpts_to_keep])
- ]
-
- def del_info(fn):
- return logger.info(f".. Free up space by deleting ckpt {fn}")
-
- def del_routine(x):
- return [os.remove(x), del_info(x)]
-
- [del_routine(fn) for fn in to_del]
-
-
-def get_hparams_from_dir(model_dir):
- config_save_path = os.path.join(model_dir, "config.json")
- with open(config_save_path, "r", encoding="utf-8") as f:
- data = f.read()
- config = json.loads(data)
-
- hparams = HParams(**config)
- hparams.model_dir = model_dir
- return hparams
-
-
-def get_hparams_from_file(config_path):
- with open(config_path, "r", encoding="utf-8") as f:
- data = f.read()
- config = json.loads(data)
-
- hparams = HParams(**config)
- return hparams
-
-
-def check_git_hash(model_dir):
- source_dir = os.path.dirname(os.path.realpath(__file__))
- if not os.path.exists(os.path.join(source_dir, ".git")):
- logger.warn(
- "{} is not a git repository, therefore hash value comparison will be ignored.".format(
- source_dir
- )
- )
- return
-
- cur_hash = subprocess.getoutput("git rev-parse HEAD")
-
- path = os.path.join(model_dir, "githash")
- if os.path.exists(path):
- saved_hash = open(path).read()
- if saved_hash != cur_hash:
- logger.warn(
- "git hash values are different. {}(saved) != {}(current)".format(
- saved_hash[:8], cur_hash[:8]
- )
- )
- else:
- open(path, "w").write(cur_hash)
-
-
-def get_logger(model_dir, filename="train.log"):
- global logger
- logger = logging.getLogger(os.path.basename(model_dir))
- logger.setLevel(logging.DEBUG)
-
- formatter = logging.Formatter("%(asctime)s\t%(name)s\t%(levelname)s\t%(message)s")
- if not os.path.exists(model_dir):
- os.makedirs(model_dir)
- h = logging.FileHandler(os.path.join(model_dir, filename))
- h.setLevel(logging.DEBUG)
- h.setFormatter(formatter)
- logger.addHandler(h)
- return logger
-
-
-class HParams:
- def __init__(self, **kwargs):
- for k, v in kwargs.items():
- if type(v) == dict:
- v = HParams(**v)
- self[k] = v
-
- def keys(self):
- return self.__dict__.keys()
-
- def items(self):
- return self.__dict__.items()
-
- def values(self):
- return self.__dict__.values()
-
- def __len__(self):
- return len(self.__dict__)
-
- def __getitem__(self, key):
- return getattr(self, key)
-
- def __setitem__(self, key, value):
- return setattr(self, key, value)
-
- def __contains__(self, key):
- return key in self.__dict__
-
- def __repr__(self):
- return self.__dict__.__repr__()
diff --git a/spaces/Make-A-Protagonist/Make-A-Protagonist-inference/Make-A-Protagonist/experts/GroundedSAM/GroundingDINO/groundingdino/util/vl_utils.py b/spaces/Make-A-Protagonist/Make-A-Protagonist-inference/Make-A-Protagonist/experts/GroundedSAM/GroundingDINO/groundingdino/util/vl_utils.py
deleted file mode 100644
index c91bb02f584398f08a28e6b7719e2b99f6e28616..0000000000000000000000000000000000000000
--- a/spaces/Make-A-Protagonist/Make-A-Protagonist-inference/Make-A-Protagonist/experts/GroundedSAM/GroundingDINO/groundingdino/util/vl_utils.py
+++ /dev/null
@@ -1,100 +0,0 @@
-import os
-import random
-from typing import List
-
-import torch
-
-
-def create_positive_map_from_span(tokenized, token_span, max_text_len=256):
- """construct a map such that positive_map[i,j] = True iff box i is associated to token j
- Input:
- - tokenized:
- - input_ids: Tensor[1, ntokens]
- - attention_mask: Tensor[1, ntokens]
- - token_span: list with length num_boxes.
- - each item: [start_idx, end_idx]
- """
- positive_map = torch.zeros((len(token_span), max_text_len), dtype=torch.float)
- for j, tok_list in enumerate(token_span):
- for (beg, end) in tok_list:
- beg_pos = tokenized.char_to_token(beg)
- end_pos = tokenized.char_to_token(end - 1)
- if beg_pos is None:
- try:
- beg_pos = tokenized.char_to_token(beg + 1)
- if beg_pos is None:
- beg_pos = tokenized.char_to_token(beg + 2)
- except:
- beg_pos = None
- if end_pos is None:
- try:
- end_pos = tokenized.char_to_token(end - 2)
- if end_pos is None:
- end_pos = tokenized.char_to_token(end - 3)
- except:
- end_pos = None
- if beg_pos is None or end_pos is None:
- continue
-
- assert beg_pos is not None and end_pos is not None
- if os.environ.get("SHILONG_DEBUG_ONLY_ONE_POS", None) == "TRUE":
- positive_map[j, beg_pos] = 1
- break
- else:
- positive_map[j, beg_pos : end_pos + 1].fill_(1)
-
- return positive_map / (positive_map.sum(-1)[:, None] + 1e-6)
-
-
-def build_captions_and_token_span(cat_list, force_lowercase):
- """
- Return:
- captions: str
- cat2tokenspan: dict
- {
- 'dog': [[0, 2]],
- ...
- }
- """
-
- cat2tokenspan = {}
- captions = ""
- for catname in cat_list:
- class_name = catname
- if force_lowercase:
- class_name = class_name.lower()
- if "/" in class_name:
- class_name_list: List = class_name.strip().split("/")
- class_name_list.append(class_name)
- class_name: str = random.choice(class_name_list)
-
- tokens_positive_i = []
- subnamelist = [i.strip() for i in class_name.strip().split(" ")]
- for subname in subnamelist:
- if len(subname) == 0:
- continue
- if len(captions) > 0:
- captions = captions + " "
- strat_idx = len(captions)
- end_idx = strat_idx + len(subname)
- tokens_positive_i.append([strat_idx, end_idx])
- captions = captions + subname
-
- if len(tokens_positive_i) > 0:
- captions = captions + " ."
- cat2tokenspan[class_name] = tokens_positive_i
-
- return captions, cat2tokenspan
-
-
-def build_id2posspan_and_caption(category_dict: dict):
- """Build id2pos_span and caption from category_dict
-
- Args:
- category_dict (dict): category_dict
- """
- cat_list = [item["name"].lower() for item in category_dict]
- id2catname = {item["id"]: item["name"].lower() for item in category_dict}
- caption, cat2posspan = build_captions_and_token_span(cat_list, force_lowercase=True)
- id2posspan = {catid: cat2posspan[catname] for catid, catname in id2catname.items()}
- return id2posspan, caption
diff --git a/spaces/Matthijs/whisper_word_timestamps/README.md b/spaces/Matthijs/whisper_word_timestamps/README.md
deleted file mode 100644
index 13482702b94b1465de74c7770978c94673a103c5..0000000000000000000000000000000000000000
--- a/spaces/Matthijs/whisper_word_timestamps/README.md
+++ /dev/null
@@ -1,13 +0,0 @@
----
-title: Whisper Word-Level Timestamps
-emoji: 💭⏰
-colorFrom: yellow
-colorTo: indigo
-sdk: gradio
-sdk_version: 3.35.2
-app_file: app.py
-pinned: false
-license: apache-2.0
----
-
-Check out the configuration reference at https://huggingface.co/docs/hub/spaces-config-reference
diff --git a/spaces/Mellow-ai/PhotoAI_Mellow/annotator/uniformer/configs/_base_/models/apcnet_r50-d8.py b/spaces/Mellow-ai/PhotoAI_Mellow/annotator/uniformer/configs/_base_/models/apcnet_r50-d8.py
deleted file mode 100644
index c8f5316cbcf3896ba9de7ca2c801eba512f01d5e..0000000000000000000000000000000000000000
--- a/spaces/Mellow-ai/PhotoAI_Mellow/annotator/uniformer/configs/_base_/models/apcnet_r50-d8.py
+++ /dev/null
@@ -1,44 +0,0 @@
-# model settings
-norm_cfg = dict(type='SyncBN', requires_grad=True)
-model = dict(
- type='EncoderDecoder',
- pretrained='open-mmlab://resnet50_v1c',
- backbone=dict(
- type='ResNetV1c',
- depth=50,
- num_stages=4,
- out_indices=(0, 1, 2, 3),
- dilations=(1, 1, 2, 4),
- strides=(1, 2, 1, 1),
- norm_cfg=norm_cfg,
- norm_eval=False,
- style='pytorch',
- contract_dilation=True),
- decode_head=dict(
- type='APCHead',
- in_channels=2048,
- in_index=3,
- channels=512,
- pool_scales=(1, 2, 3, 6),
- dropout_ratio=0.1,
- num_classes=19,
- norm_cfg=dict(type='SyncBN', requires_grad=True),
- align_corners=False,
- loss_decode=dict(
- type='CrossEntropyLoss', use_sigmoid=False, loss_weight=1.0)),
- auxiliary_head=dict(
- type='FCNHead',
- in_channels=1024,
- in_index=2,
- channels=256,
- num_convs=1,
- concat_input=False,
- dropout_ratio=0.1,
- num_classes=19,
- norm_cfg=norm_cfg,
- align_corners=False,
- loss_decode=dict(
- type='CrossEntropyLoss', use_sigmoid=False, loss_weight=0.4)),
- # model training and testing settings
- train_cfg=dict(),
- test_cfg=dict(mode='whole'))
diff --git a/spaces/Miuzarte/SUI-svc-3.0/vdecoder/hifigan/utils.py b/spaces/Miuzarte/SUI-svc-3.0/vdecoder/hifigan/utils.py
deleted file mode 100644
index 84bff024f4d2e2de194b2a88ee7bbe5f0d33f67c..0000000000000000000000000000000000000000
--- a/spaces/Miuzarte/SUI-svc-3.0/vdecoder/hifigan/utils.py
+++ /dev/null
@@ -1,68 +0,0 @@
-import glob
-import os
-import matplotlib
-import torch
-from torch.nn.utils import weight_norm
-matplotlib.use("Agg")
-import matplotlib.pylab as plt
-
-
-def plot_spectrogram(spectrogram):
- fig, ax = plt.subplots(figsize=(10, 2))
- im = ax.imshow(spectrogram, aspect="auto", origin="lower",
- interpolation='none')
- plt.colorbar(im, ax=ax)
-
- fig.canvas.draw()
- plt.close()
-
- return fig
-
-
-def init_weights(m, mean=0.0, std=0.01):
- classname = m.__class__.__name__
- if classname.find("Conv") != -1:
- m.weight.data.normal_(mean, std)
-
-
-def apply_weight_norm(m):
- classname = m.__class__.__name__
- if classname.find("Conv") != -1:
- weight_norm(m)
-
-
-def get_padding(kernel_size, dilation=1):
- return int((kernel_size*dilation - dilation)/2)
-
-
-def load_checkpoint(filepath, device):
- assert os.path.isfile(filepath)
- print("Loading '{}'".format(filepath))
- checkpoint_dict = torch.load(filepath, map_location=device)
- print("Complete.")
- return checkpoint_dict
-
-
-def save_checkpoint(filepath, obj):
- print("Saving checkpoint to {}".format(filepath))
- torch.save(obj, filepath)
- print("Complete.")
-
-
-def del_old_checkpoints(cp_dir, prefix, n_models=2):
- pattern = os.path.join(cp_dir, prefix + '????????')
- cp_list = glob.glob(pattern) # get checkpoint paths
- cp_list = sorted(cp_list)# sort by iter
- if len(cp_list) > n_models: # if more than n_models models are found
- for cp in cp_list[:-n_models]:# delete the oldest models other than lastest n_models
- open(cp, 'w').close()# empty file contents
- os.unlink(cp)# delete file (move to trash when using Colab)
-
-
-def scan_checkpoint(cp_dir, prefix):
- pattern = os.path.join(cp_dir, prefix + '????????')
- cp_list = glob.glob(pattern)
- if len(cp_list) == 0:
- return None
- return sorted(cp_list)[-1]
-
diff --git a/spaces/Mountchicken/MAERec-Gradio/configs/textdet/psenet/psenet_resnet50-oclip_fpnf_600e_ctw1500.py b/spaces/Mountchicken/MAERec-Gradio/configs/textdet/psenet/psenet_resnet50-oclip_fpnf_600e_ctw1500.py
deleted file mode 100644
index 255e6885e7dc049c9f7e922e869ff9f7b0d63d00..0000000000000000000000000000000000000000
--- a/spaces/Mountchicken/MAERec-Gradio/configs/textdet/psenet/psenet_resnet50-oclip_fpnf_600e_ctw1500.py
+++ /dev/null
@@ -1,10 +0,0 @@
-_base_ = [
- 'psenet_resnet50_fpnf_600e_ctw1500.py',
-]
-
-_base_.model.backbone = dict(
- type='CLIPResNet',
- init_cfg=dict(
- type='Pretrained',
- checkpoint='https://download.openmmlab.com/'
- 'mmocr/backbone/resnet50-oclip-7ba0c533.pth'))
diff --git a/spaces/NCTCMumbai/NCTC/models/official/utils/registry_test.py b/spaces/NCTCMumbai/NCTC/models/official/utils/registry_test.py
deleted file mode 100644
index 6cb230c75891aaebb8306bb84a235e2d2ecd70e5..0000000000000000000000000000000000000000
--- a/spaces/NCTCMumbai/NCTC/models/official/utils/registry_test.py
+++ /dev/null
@@ -1,85 +0,0 @@
-# Lint as: python3
-# Copyright 2020 The TensorFlow Authors. All Rights Reserved.
-#
-# Licensed under the Apache License, Version 2.0 (the "License");
-# you may not use this file except in compliance with the License.
-# You may obtain a copy of the License at
-#
-# http://www.apache.org/licenses/LICENSE-2.0
-#
-# Unless required by applicable law or agreed to in writing, software
-# distributed under the License is distributed on an "AS IS" BASIS,
-# WITHOUT WARRANTIES OR CONDITIONS OF ANY KIND, either express or implied.
-# See the License for the specific language governing permissions and
-# limitations under the License.
-# ==============================================================================
-"""Tests for registry."""
-
-from __future__ import absolute_import
-from __future__ import division
-from __future__ import print_function
-
-import tensorflow as tf
-from official.utils import registry
-
-
-class RegistryTest(tf.test.TestCase):
-
- def test_register(self):
- collection = {}
-
- @registry.register(collection, 'functions/func_0')
- def func_test():
- pass
- self.assertEqual(
- registry.lookup(collection, 'functions/func_0'), func_test)
-
- @registry.register(collection, 'classes/cls_0')
- class ClassRegistryKey:
- pass
- self.assertEqual(
- registry.lookup(collection, 'classes/cls_0'), ClassRegistryKey)
-
- @registry.register(collection, ClassRegistryKey)
- class ClassRegistryValue:
- pass
- self.assertEqual(
- registry.lookup(collection, ClassRegistryKey), ClassRegistryValue)
-
- def test_register_hierarchy(self):
- collection = {}
-
- @registry.register(collection, 'functions/func_0')
- def func_test0():
- pass
- @registry.register(collection, 'func_1')
- def func_test1():
- pass
- @registry.register(collection, func_test1)
- def func_test2():
- pass
- expected_collection = {
- 'functions': {
- 'func_0': func_test0,
- },
- 'func_1': func_test1,
- func_test1: func_test2,
- }
- self.assertEqual(collection, expected_collection)
-
- def test_register_error(self):
- collection = {}
-
- @registry.register(collection, 'functions/func_0')
- def func_test0(): # pylint: disable=unused-variable
- pass
- with self.assertRaises(KeyError):
- @registry.register(collection, 'functions/func_0/sub_func')
- def func_test1(): # pylint: disable=unused-variable
- pass
- with self.assertRaises(LookupError):
- registry.lookup(collection, 'non-exist')
-
-
-if __name__ == '__main__':
- tf.test.main()
diff --git a/spaces/Nephele/bert-vits2-multi-voice/text/symbols.py b/spaces/Nephele/bert-vits2-multi-voice/text/symbols.py
deleted file mode 100644
index 9dfae4e633829f20c4fd767b1c7a9198911ed801..0000000000000000000000000000000000000000
--- a/spaces/Nephele/bert-vits2-multi-voice/text/symbols.py
+++ /dev/null
@@ -1,51 +0,0 @@
-punctuation = ['!', '?', '…', ",", ".", "'", '-']
-pu_symbols = punctuation + ["SP", "UNK"]
-pad = '_'
-
-# chinese
-zh_symbols = ['E', 'En', 'a', 'ai', 'an', 'ang', 'ao', 'b', 'c', 'ch', 'd', 'e', 'ei', 'en', 'eng', 'er', 'f', 'g', 'h',
- 'i', 'i0', 'ia', 'ian', 'iang', 'iao', 'ie', 'in', 'ing', 'iong', 'ir', 'iu', 'j', 'k', 'l', 'm', 'n', 'o',
- 'ong',
- 'ou', 'p', 'q', 'r', 's', 'sh', 't', 'u', 'ua', 'uai', 'uan', 'uang', 'ui', 'un', 'uo', 'v', 'van', 've', 'vn',
- 'w', 'x', 'y', 'z', 'zh',
- "AA", "EE", "OO"]
-num_zh_tones = 6
-
-# japanese
-ja_symbols = ['I', 'N', 'U', 'a', 'b', 'by', 'ch', 'cl', 'd', 'dy', 'e', 'f', 'g', 'gy', 'h', 'hy', 'i', 'j', 'k', 'ky',
- 'm', 'my', 'n', 'ny', 'o', 'p', 'py', 'r', 'ry', 's', 'sh', 't', 'ts', 'u', 'V', 'w', 'y', 'z']
-num_ja_tones = 1
-
-# English
-en_symbols = ['aa', 'ae', 'ah', 'ao', 'aw', 'ay', 'b', 'ch', 'd', 'dh', 'eh', 'er', 'ey', 'f', 'g', 'hh', 'ih', 'iy',
- 'jh', 'k', 'l', 'm', 'n', 'ng', 'ow', 'oy', 'p', 'r', 's',
- 'sh', 't', 'th', 'uh', 'uw', 'V', 'w', 'y', 'z', 'zh']
-num_en_tones = 4
-
-# combine all symbols
-normal_symbols = sorted(set(zh_symbols + ja_symbols + en_symbols))
-symbols = [pad] + normal_symbols + pu_symbols
-sil_phonemes_ids = [symbols.index(i) for i in pu_symbols]
-
-# combine all tones
-num_tones = num_zh_tones + num_ja_tones + num_en_tones
-
-# language maps
-language_id_map = {
- 'ZH': 0,
- "JA": 1,
- "EN": 2
-}
-num_languages = len(language_id_map.keys())
-
-language_tone_start_map = {
- 'ZH': 0,
- "JA": num_zh_tones,
- "EN": num_zh_tones + num_ja_tones
-}
-
-if __name__ == '__main__':
- a = set(zh_symbols)
- b = set(en_symbols)
- print(sorted(a&b))
-
diff --git a/spaces/NimaBoscarino/climategan/utils_scripts/merge_labelbox_masks.py b/spaces/NimaBoscarino/climategan/utils_scripts/merge_labelbox_masks.py
deleted file mode 100644
index 34a2df93996e94d89c81054f4f4a53766c704d95..0000000000000000000000000000000000000000
--- a/spaces/NimaBoscarino/climategan/utils_scripts/merge_labelbox_masks.py
+++ /dev/null
@@ -1,41 +0,0 @@
-from pathlib import Path
-
-import numpy as np
-from skimage.io import imread, imsave
-from shutil import copyfile
-
-if __name__ == "__main__":
- # output of download_labelbox.py
- base_dir = Path("/Users/victor/Downloads/labelbox_test_flood-v2")
- labeled_dir = base_dir / "__labeled"
- assert base_dir.exists()
- labeled_dir.mkdir(exist_ok=True)
-
- sub_dirs = [
- d
- for d in base_dir.expanduser().resolve().iterdir()
- if d.is_dir() and not d.name.startswith(".") and d.name != "__labeled"
- ]
-
- for k, sd in enumerate(sub_dirs):
- print(k + 1, "/", len(sub_dirs), sd.name)
-
- # must-flood binary mask
- must = np.stack([imread(i)[:, :, :3] for i in sd.glob("*must*.png")]).sum(0) > 0
- # cannot-flood binary mask
- cannot = (
- np.stack([imread(i)[:, :, :3] for i in sd.glob("*cannot*.png")]).sum(0) > 0
- )
- # must is red
- must = (must * [0, 0, 255]).astype(np.uint8)
- # connot is blue
- cannot = (cannot * [255, 0, 0]).astype(np.uint8)
- # merged labels
- label = must + cannot
- # check no overlap
- assert sorted(np.unique(label)) == [0, 255]
- # create filename
- stem = "_".join(list(sd.glob("*must*.png"))[0].stem.split("_")[:-2])
- # save label
- imsave(sd / f"{stem}_labeled.png", label)
- copyfile(sd / f"{stem}_labeled.png", labeled_dir / f"{stem}_labeled.png")
diff --git a/spaces/Nunchakuka/FrenchAnonymizer/speaker_encoder/preprocess.py b/spaces/Nunchakuka/FrenchAnonymizer/speaker_encoder/preprocess.py
deleted file mode 100644
index fe5ab25ef7cb4adeb76cad11962f179d6a38edcc..0000000000000000000000000000000000000000
--- a/spaces/Nunchakuka/FrenchAnonymizer/speaker_encoder/preprocess.py
+++ /dev/null
@@ -1,285 +0,0 @@
-from multiprocess.pool import ThreadPool
-from speaker_encoder.params_data import *
-from speaker_encoder.config import librispeech_datasets, anglophone_nationalites
-from datetime import datetime
-from speaker_encoder import audio
-from pathlib import Path
-from tqdm import tqdm
-import numpy as np
-
-
-class DatasetLog:
- """
- Registers metadata about the dataset in a text file.
- """
- def __init__(self, root, name):
- self.text_file = open(Path(root, "Log_%s.txt" % name.replace("/", "_")), "w")
- self.sample_data = dict()
-
- start_time = str(datetime.now().strftime("%A %d %B %Y at %H:%M"))
- self.write_line("Creating dataset %s on %s" % (name, start_time))
- self.write_line("-----")
- self._log_params()
-
- def _log_params(self):
- from speaker_encoder import params_data
- self.write_line("Parameter values:")
- for param_name in (p for p in dir(params_data) if not p.startswith("__")):
- value = getattr(params_data, param_name)
- self.write_line("\t%s: %s" % (param_name, value))
- self.write_line("-----")
-
- def write_line(self, line):
- self.text_file.write("%s\n" % line)
-
- def add_sample(self, **kwargs):
- for param_name, value in kwargs.items():
- if not param_name in self.sample_data:
- self.sample_data[param_name] = []
- self.sample_data[param_name].append(value)
-
- def finalize(self):
- self.write_line("Statistics:")
- for param_name, values in self.sample_data.items():
- self.write_line("\t%s:" % param_name)
- self.write_line("\t\tmin %.3f, max %.3f" % (np.min(values), np.max(values)))
- self.write_line("\t\tmean %.3f, median %.3f" % (np.mean(values), np.median(values)))
- self.write_line("-----")
- end_time = str(datetime.now().strftime("%A %d %B %Y at %H:%M"))
- self.write_line("Finished on %s" % end_time)
- self.text_file.close()
-
-
-def _init_preprocess_dataset(dataset_name, datasets_root, out_dir) -> (Path, DatasetLog):
- dataset_root = datasets_root.joinpath(dataset_name)
- if not dataset_root.exists():
- print("Couldn\'t find %s, skipping this dataset." % dataset_root)
- return None, None
- return dataset_root, DatasetLog(out_dir, dataset_name)
-
-
-def _preprocess_speaker_dirs(speaker_dirs, dataset_name, datasets_root, out_dir, extension,
- skip_existing, logger):
- print("%s: Preprocessing data for %d speakers." % (dataset_name, len(speaker_dirs)))
-
- # Function to preprocess utterances for one speaker
- def preprocess_speaker(speaker_dir: Path):
- # Give a name to the speaker that includes its dataset
- speaker_name = "_".join(speaker_dir.relative_to(datasets_root).parts)
-
- # Create an output directory with that name, as well as a txt file containing a
- # reference to each source file.
- speaker_out_dir = out_dir.joinpath(speaker_name)
- speaker_out_dir.mkdir(exist_ok=True)
- sources_fpath = speaker_out_dir.joinpath("_sources.txt")
-
- # There's a possibility that the preprocessing was interrupted earlier, check if
- # there already is a sources file.
- if sources_fpath.exists():
- try:
- with sources_fpath.open("r") as sources_file:
- existing_fnames = {line.split(",")[0] for line in sources_file}
- except:
- existing_fnames = {}
- else:
- existing_fnames = {}
-
- # Gather all audio files for that speaker recursively
- sources_file = sources_fpath.open("a" if skip_existing else "w")
- for in_fpath in speaker_dir.glob("**/*.%s" % extension):
- # Check if the target output file already exists
- out_fname = "_".join(in_fpath.relative_to(speaker_dir).parts)
- out_fname = out_fname.replace(".%s" % extension, ".npy")
- if skip_existing and out_fname in existing_fnames:
- continue
-
- # Load and preprocess the waveform
- wav = audio.preprocess_wav(in_fpath)
- if len(wav) == 0:
- continue
-
- # Create the mel spectrogram, discard those that are too short
- frames = audio.wav_to_mel_spectrogram(wav)
- if len(frames) < partials_n_frames:
- continue
-
- out_fpath = speaker_out_dir.joinpath(out_fname)
- np.save(out_fpath, frames)
- logger.add_sample(duration=len(wav) / sampling_rate)
- sources_file.write("%s,%s\n" % (out_fname, in_fpath))
-
- sources_file.close()
-
- # Process the utterances for each speaker
- with ThreadPool(8) as pool:
- list(tqdm(pool.imap(preprocess_speaker, speaker_dirs), dataset_name, len(speaker_dirs),
- unit="speakers"))
- logger.finalize()
- print("Done preprocessing %s.\n" % dataset_name)
-
-
-# Function to preprocess utterances for one speaker
-def __preprocess_speaker(speaker_dir: Path, datasets_root: Path, out_dir: Path, extension: str, skip_existing: bool):
- # Give a name to the speaker that includes its dataset
- speaker_name = "_".join(speaker_dir.relative_to(datasets_root).parts)
-
- # Create an output directory with that name, as well as a txt file containing a
- # reference to each source file.
- speaker_out_dir = out_dir.joinpath(speaker_name)
- speaker_out_dir.mkdir(exist_ok=True)
- sources_fpath = speaker_out_dir.joinpath("_sources.txt")
-
- # There's a possibility that the preprocessing was interrupted earlier, check if
- # there already is a sources file.
- # if sources_fpath.exists():
- # try:
- # with sources_fpath.open("r") as sources_file:
- # existing_fnames = {line.split(",")[0] for line in sources_file}
- # except:
- # existing_fnames = {}
- # else:
- # existing_fnames = {}
- existing_fnames = {}
- # Gather all audio files for that speaker recursively
- sources_file = sources_fpath.open("a" if skip_existing else "w")
-
- for in_fpath in speaker_dir.glob("**/*.%s" % extension):
- # Check if the target output file already exists
- out_fname = "_".join(in_fpath.relative_to(speaker_dir).parts)
- out_fname = out_fname.replace(".%s" % extension, ".npy")
- if skip_existing and out_fname in existing_fnames:
- continue
-
- # Load and preprocess the waveform
- wav = audio.preprocess_wav(in_fpath)
- if len(wav) == 0:
- continue
-
- # Create the mel spectrogram, discard those that are too short
- frames = audio.wav_to_mel_spectrogram(wav)
- if len(frames) < partials_n_frames:
- continue
-
- out_fpath = speaker_out_dir.joinpath(out_fname)
- np.save(out_fpath, frames)
- # logger.add_sample(duration=len(wav) / sampling_rate)
- sources_file.write("%s,%s\n" % (out_fname, in_fpath))
-
- sources_file.close()
- return len(wav)
-
-def _preprocess_speaker_dirs_vox2(speaker_dirs, dataset_name, datasets_root, out_dir, extension,
- skip_existing, logger):
- # from multiprocessing import Pool, cpu_count
- from pathos.multiprocessing import ProcessingPool as Pool
- # Function to preprocess utterances for one speaker
- def __preprocess_speaker(speaker_dir: Path):
- # Give a name to the speaker that includes its dataset
- speaker_name = "_".join(speaker_dir.relative_to(datasets_root).parts)
-
- # Create an output directory with that name, as well as a txt file containing a
- # reference to each source file.
- speaker_out_dir = out_dir.joinpath(speaker_name)
- speaker_out_dir.mkdir(exist_ok=True)
- sources_fpath = speaker_out_dir.joinpath("_sources.txt")
-
- existing_fnames = {}
- # Gather all audio files for that speaker recursively
- sources_file = sources_fpath.open("a" if skip_existing else "w")
- wav_lens = []
- for in_fpath in speaker_dir.glob("**/*.%s" % extension):
- # Check if the target output file already exists
- out_fname = "_".join(in_fpath.relative_to(speaker_dir).parts)
- out_fname = out_fname.replace(".%s" % extension, ".npy")
- if skip_existing and out_fname in existing_fnames:
- continue
-
- # Load and preprocess the waveform
- wav = audio.preprocess_wav(in_fpath)
- if len(wav) == 0:
- continue
-
- # Create the mel spectrogram, discard those that are too short
- frames = audio.wav_to_mel_spectrogram(wav)
- if len(frames) < partials_n_frames:
- continue
-
- out_fpath = speaker_out_dir.joinpath(out_fname)
- np.save(out_fpath, frames)
- # logger.add_sample(duration=len(wav) / sampling_rate)
- sources_file.write("%s,%s\n" % (out_fname, in_fpath))
- wav_lens.append(len(wav))
- sources_file.close()
- return wav_lens
-
- print("%s: Preprocessing data for %d speakers." % (dataset_name, len(speaker_dirs)))
- # Process the utterances for each speaker
- # with ThreadPool(8) as pool:
- # list(tqdm(pool.imap(preprocess_speaker, speaker_dirs), dataset_name, len(speaker_dirs),
- # unit="speakers"))
- pool = Pool(processes=20)
- for i, wav_lens in enumerate(pool.map(__preprocess_speaker, speaker_dirs), 1):
- for wav_len in wav_lens:
- logger.add_sample(duration=wav_len / sampling_rate)
- print(f'{i}/{len(speaker_dirs)} \r')
-
- logger.finalize()
- print("Done preprocessing %s.\n" % dataset_name)
-
-
-def preprocess_librispeech(datasets_root: Path, out_dir: Path, skip_existing=False):
- for dataset_name in librispeech_datasets["train"]["other"]:
- # Initialize the preprocessing
- dataset_root, logger = _init_preprocess_dataset(dataset_name, datasets_root, out_dir)
- if not dataset_root:
- return
-
- # Preprocess all speakers
- speaker_dirs = list(dataset_root.glob("*"))
- _preprocess_speaker_dirs(speaker_dirs, dataset_name, datasets_root, out_dir, "flac",
- skip_existing, logger)
-
-
-def preprocess_voxceleb1(datasets_root: Path, out_dir: Path, skip_existing=False):
- # Initialize the preprocessing
- dataset_name = "VoxCeleb1"
- dataset_root, logger = _init_preprocess_dataset(dataset_name, datasets_root, out_dir)
- if not dataset_root:
- return
-
- # Get the contents of the meta file
- with dataset_root.joinpath("vox1_meta.csv").open("r") as metafile:
- metadata = [line.split("\t") for line in metafile][1:]
-
- # Select the ID and the nationality, filter out non-anglophone speakers
- nationalities = {line[0]: line[3] for line in metadata}
- # keep_speaker_ids = [speaker_id for speaker_id, nationality in nationalities.items() if
- # nationality.lower() in anglophone_nationalites]
- keep_speaker_ids = [speaker_id for speaker_id, nationality in nationalities.items()]
- print("VoxCeleb1: using samples from %d (presumed anglophone) speakers out of %d." %
- (len(keep_speaker_ids), len(nationalities)))
-
- # Get the speaker directories for anglophone speakers only
- speaker_dirs = dataset_root.joinpath("wav").glob("*")
- speaker_dirs = [speaker_dir for speaker_dir in speaker_dirs if
- speaker_dir.name in keep_speaker_ids]
- print("VoxCeleb1: found %d anglophone speakers on the disk, %d missing (this is normal)." %
- (len(speaker_dirs), len(keep_speaker_ids) - len(speaker_dirs)))
-
- # Preprocess all speakers
- _preprocess_speaker_dirs(speaker_dirs, dataset_name, datasets_root, out_dir, "wav",
- skip_existing, logger)
-
-
-def preprocess_voxceleb2(datasets_root: Path, out_dir: Path, skip_existing=False):
- # Initialize the preprocessing
- dataset_name = "VoxCeleb2"
- dataset_root, logger = _init_preprocess_dataset(dataset_name, datasets_root, out_dir)
- if not dataset_root:
- return
-
- # Get the speaker directories
- # Preprocess all speakers
- speaker_dirs = list(dataset_root.joinpath("dev", "aac").glob("*"))
- _preprocess_speaker_dirs_vox2(speaker_dirs, dataset_name, datasets_root, out_dir, "m4a",
- skip_existing, logger)
diff --git a/spaces/OFA-Sys/OFA-Generic_Interface/fairseq/fairseq/optim/fairseq_optimizer.py b/spaces/OFA-Sys/OFA-Generic_Interface/fairseq/fairseq/optim/fairseq_optimizer.py
deleted file mode 100644
index 7e5411753a2ba94f3a7a68316131530b8b17d22a..0000000000000000000000000000000000000000
--- a/spaces/OFA-Sys/OFA-Generic_Interface/fairseq/fairseq/optim/fairseq_optimizer.py
+++ /dev/null
@@ -1,179 +0,0 @@
-# Copyright (c) Facebook, Inc. and its affiliates.
-#
-# This source code is licensed under the MIT license found in the
-# LICENSE file in the root directory of this source tree.
-
-import torch
-from fairseq import utils
-from fairseq.dataclass.utils import gen_parser_from_dataclass
-
-
-class FairseqOptimizer(object):
- def __init__(self, cfg):
- super().__init__()
- self.cfg = cfg
-
- @classmethod
- def add_args(cls, parser):
- """Add optimizer-specific arguments to the parser."""
- dc = getattr(cls, "__dataclass", None)
- if dc is not None:
- gen_parser_from_dataclass(parser, dc())
-
- @property
- def optimizer(self):
- """Return a torch.optim.optimizer.Optimizer instance."""
- if not hasattr(self, "_optimizer"):
- raise NotImplementedError
- if not isinstance(self._optimizer, torch.optim.Optimizer):
- raise ValueError("_optimizer must be an instance of torch.optim.Optimizer")
- return self._optimizer
-
- @optimizer.setter
- def optimizer(self, optimizer):
- """Reset optimizer instance."""
- if not hasattr(self, "_optimizer"):
- raise NotImplementedError
- if not isinstance(self._optimizer, torch.optim.Optimizer):
- raise ValueError("_optimizer must be an instance of torch.optim.Optimizer")
- self._optimizer = optimizer
-
- @property
- def optimizer_config(self):
- """
- Return a kwarg dictionary that will be used to override optimizer
- args stored in checkpoints. This allows us to load a checkpoint and
- resume training using a different set of optimizer args, e.g., with a
- different learning rate.
- """
- raise NotImplementedError
-
- @property
- def params(self):
- """Return an iterable of the parameters held by the optimizer."""
- for param_group in self.param_groups:
- for p in param_group["params"]:
- yield p
-
- @property
- def param_groups(self):
- return self.optimizer.param_groups
-
- def __getstate__(self):
- return self._optimizer.__getstate__()
-
- def get_lr(self):
- """Return the current learning rate."""
- return self.param_groups[0]["lr"]
-
- def set_lr(self, lr):
- """Set the learning rate."""
- for param_group in self.param_groups:
- param_group["lr"] = lr
-
- def state_dict(self):
- """Return the optimizer's state dict."""
- return self.optimizer.state_dict()
-
- def load_state_dict(self, state_dict, optimizer_overrides=None):
- """Load an optimizer state dict.
-
- In general we should prefer the configuration of the existing optimizer
- instance (e.g., learning rate) over that found in the state_dict. This
- allows us to resume training from a checkpoint using a new set of
- optimizer args.
- """
- self.optimizer.load_state_dict(state_dict)
-
- if optimizer_overrides is not None and len(optimizer_overrides) > 0:
- # override learning rate, momentum, etc. with latest values
- for group in self.param_groups:
- group.update(optimizer_overrides)
-
- def backward(self, loss):
- """Computes the sum of gradients of the given tensor w.r.t. graph leaves."""
- loss.backward()
-
- def all_reduce_grads(self, module):
- """Manually all-reduce gradients (if required)."""
- if hasattr(module, "all_reduce_grads"):
- module.all_reduce_grads()
-
- def multiply_grads(self, c):
- """Multiplies grads by a constant *c*."""
- for p in self.params:
- if p.grad is not None:
- if torch.is_tensor(c):
- c = c.to(p.grad.device)
- p.grad.data.mul_(c)
-
- def clip_grad_norm(self, max_norm, aggregate_norm_fn=None):
- """Clips gradient norm."""
- return utils.clip_grad_norm_(self.params, max_norm, aggregate_norm_fn)
-
- def step(self, closure=None, scale=1.0, groups=None):
- """Performs a single optimization step."""
- if self.supports_step_with_scale:
- if self.supports_groups:
- self.optimizer.step(closure, scale=scale, groups=groups)
- else:
- self.optimizer.step(closure, scale=scale)
- else:
- if scale != 1.0:
- self.multiply_grads(1.0 / scale)
- if self.supports_groups:
- self.optimizer.step(closure, groups=groups)
- else:
- self.optimizer.step(closure)
-
- def zero_grad(self):
- """Clears the gradients of all optimized parameters."""
- for p in self.params:
- p.grad = None
- self.optimizer.zero_grad()
-
- @property
- def supports_memory_efficient_fp16(self):
- if hasattr(self.optimizer, "supports_memory_efficient_fp16"):
- return self.optimizer.supports_memory_efficient_fp16
- return False
-
- @property
- def supports_step_with_scale(self):
- if hasattr(self.optimizer, "supports_step_with_scale"):
- return self.optimizer.supports_step_with_scale
- return False
-
- @property
- def supports_groups(self):
- if hasattr(self.optimizer, "supports_groups"):
- return self.optimizer.supports_groups
- return False
-
- @property
- def supports_flat_params(self):
- """
- Whether the optimizer supports collapsing of the model
- parameters/gradients into a single contiguous Tensor.
- """
- if hasattr(self.optimizer, "supports_flat_params"):
- return self.optimizer.supports_flat_params
- return False
-
- def average_params(self):
- pass
-
- def broadcast_global_state_dict(self, state_dict):
- """
- Broadcasts a global state dict to all ranks.
- Useful for optimizers that shard state between ranks.
- """
- if hasattr(self.optimizer, "broadcast_global_state_dict"):
- return self.optimizer.broadcast_global_state_dict(state_dict)
- else:
- return state_dict
-
-
-class LegacyFairseqOptimizer(FairseqOptimizer):
- def __init__(self, args):
- self.args = args
diff --git a/spaces/OFA-Sys/OFA-Image_Caption/fairseq/examples/simultaneous_translation/modules/__init__.py b/spaces/OFA-Sys/OFA-Image_Caption/fairseq/examples/simultaneous_translation/modules/__init__.py
deleted file mode 100644
index f5ea180f9b4cdb27cd553439b6df9d743105f18c..0000000000000000000000000000000000000000
--- a/spaces/OFA-Sys/OFA-Image_Caption/fairseq/examples/simultaneous_translation/modules/__init__.py
+++ /dev/null
@@ -1,23 +0,0 @@
-# Copyright (c) Facebook, Inc. and its affiliates.
-#
-# This source code is licensed under the MIT license found in the
-# LICENSE file in the root directory of this source tree.
-
-
-import os
-import importlib
-from fairseq import registry
-
-(
- build_monotonic_attention,
- register_monotonic_attention,
- MONOTONIC_ATTENTION_REGISTRY,
- _,
-) = registry.setup_registry("--simul-type")
-
-for file in sorted(os.listdir(os.path.dirname(__file__))):
- if file.endswith(".py") and not file.startswith("_"):
- model_name = file[: file.find(".py")]
- importlib.import_module(
- "examples.simultaneous_translation.modules." + model_name
- )
diff --git a/spaces/OFA-Sys/OFA-Image_Caption/fairseq/examples/wav2vec/unsupervised/scripts/copy_labels.py b/spaces/OFA-Sys/OFA-Image_Caption/fairseq/examples/wav2vec/unsupervised/scripts/copy_labels.py
deleted file mode 100644
index 989868388eefccc37c82d7602f709632035c7aa1..0000000000000000000000000000000000000000
--- a/spaces/OFA-Sys/OFA-Image_Caption/fairseq/examples/wav2vec/unsupervised/scripts/copy_labels.py
+++ /dev/null
@@ -1,10 +0,0 @@
-#!/usr/bin/env python3 -u
-# Copyright (c) Facebook, Inc. and its affiliates.
-#
-# This source code is licensed under the MIT license found in the
-# LICENSE file in the root directory of this source tree.
-
-import sys
-
-for idx, line in enumerate(sys.stdin):
- print(f"utt{idx:010d} {line}", end="")
diff --git a/spaces/OFA-Sys/OFA-Image_Caption/fairseq/fairseq/data/encoders/fastbpe.py b/spaces/OFA-Sys/OFA-Image_Caption/fairseq/fairseq/data/encoders/fastbpe.py
deleted file mode 100644
index f7c21039549ea002e73d1ad7cde5735f215f11ee..0000000000000000000000000000000000000000
--- a/spaces/OFA-Sys/OFA-Image_Caption/fairseq/fairseq/data/encoders/fastbpe.py
+++ /dev/null
@@ -1,36 +0,0 @@
-# Copyright (c) Facebook, Inc. and its affiliates.
-#
-# This source code is licensed under the MIT license found in the
-# LICENSE file in the root directory of this source tree.
-
-from dataclasses import dataclass, field
-
-from fairseq import file_utils
-from fairseq.data.encoders import register_bpe
-from fairseq.dataclass import FairseqDataclass
-
-
-@dataclass
-class fastBPEConfig(FairseqDataclass):
- bpe_codes: str = field(default="???", metadata={"help": "path to fastBPE BPE"})
-
-
-@register_bpe("fastbpe", dataclass=fastBPEConfig)
-class fastBPE(object):
- def __init__(self, cfg):
- if cfg.bpe_codes is None:
- raise ValueError("--bpe-codes is required for --bpe=fastbpe")
- codes = file_utils.cached_path(cfg.bpe_codes)
- try:
- import fastBPE
-
- self.bpe = fastBPE.fastBPE(codes)
- self.bpe_symbol = "@@ "
- except ImportError:
- raise ImportError("Please install fastBPE with: pip install fastBPE")
-
- def encode(self, x: str) -> str:
- return self.bpe.apply([x])[0]
-
- def decode(self, x: str) -> str:
- return (x + " ").replace(self.bpe_symbol, "").rstrip()
diff --git a/spaces/OFA-Sys/OFA-vqa/fairseq/examples/hubert/simple_kmeans/learn_kmeans.py b/spaces/OFA-Sys/OFA-vqa/fairseq/examples/hubert/simple_kmeans/learn_kmeans.py
deleted file mode 100644
index 113ac655b8c0a585fe43797e99674e445098edd0..0000000000000000000000000000000000000000
--- a/spaces/OFA-Sys/OFA-vqa/fairseq/examples/hubert/simple_kmeans/learn_kmeans.py
+++ /dev/null
@@ -1,146 +0,0 @@
-# Copyright (c) Facebook, Inc. and its affiliates.
-#
-# This source code is licensed under the MIT license found in the
-# LICENSE file in the root directory of this source tree.
-
-import logging
-import os
-import sys
-
-import numpy as np
-from sklearn.cluster import MiniBatchKMeans
-
-import joblib
-
-logging.basicConfig(
- format="%(asctime)s | %(levelname)s | %(name)s | %(message)s",
- datefmt="%Y-%m-%d %H:%M:%S",
- level=os.environ.get("LOGLEVEL", "INFO").upper(),
- stream=sys.stdout,
-)
-logger = logging.getLogger("learn_kmeans")
-
-
-def get_km_model(
- n_clusters,
- init,
- max_iter,
- batch_size,
- tol,
- max_no_improvement,
- n_init,
- reassignment_ratio,
-):
- return MiniBatchKMeans(
- n_clusters=n_clusters,
- init=init,
- max_iter=max_iter,
- batch_size=batch_size,
- verbose=1,
- compute_labels=False,
- tol=tol,
- max_no_improvement=max_no_improvement,
- init_size=None,
- n_init=n_init,
- reassignment_ratio=reassignment_ratio,
- )
-
-
-def load_feature_shard(feat_dir, split, nshard, rank, percent):
- feat_path = f"{feat_dir}/{split}_{rank}_{nshard}.npy"
- leng_path = f"{feat_dir}/{split}_{rank}_{nshard}.len"
- with open(leng_path, "r") as f:
- lengs = [int(line.rstrip()) for line in f]
- offsets = [0] + np.cumsum(lengs[:-1]).tolist()
-
- if percent < 0:
- return np.load(feat_path, mmap_mode="r")
- else:
- nsample = int(np.ceil(len(lengs) * percent))
- indices = np.random.choice(len(lengs), nsample, replace=False)
- feat = np.load(feat_path, mmap_mode="r")
- sampled_feat = np.concatenate(
- [feat[offsets[i]: offsets[i] + lengs[i]] for i in indices], axis=0
- )
- logger.info(
- (
- f"sampled {nsample} utterances, {len(sampled_feat)} frames "
- f"from shard {rank}/{nshard}"
- )
- )
- return sampled_feat
-
-
-def load_feature(feat_dir, split, nshard, seed, percent):
- assert percent <= 1.0
- feat = np.concatenate(
- [
- load_feature_shard(feat_dir, split, nshard, r, percent)
- for r in range(nshard)
- ],
- axis=0,
- )
- logging.info(f"loaded feature with dimension {feat.shape}")
- return feat
-
-
-def learn_kmeans(
- feat_dir,
- split,
- nshard,
- km_path,
- n_clusters,
- seed,
- percent,
- init,
- max_iter,
- batch_size,
- tol,
- n_init,
- reassignment_ratio,
- max_no_improvement,
-):
- np.random.seed(seed)
- feat = load_feature(feat_dir, split, nshard, seed, percent)
- km_model = get_km_model(
- n_clusters,
- init,
- max_iter,
- batch_size,
- tol,
- max_no_improvement,
- n_init,
- reassignment_ratio,
- )
- km_model.fit(feat)
- joblib.dump(km_model, km_path)
-
- inertia = -km_model.score(feat) / len(feat)
- logger.info("total intertia: %.5f", inertia)
- logger.info("finished successfully")
-
-
-if __name__ == "__main__":
- import argparse
-
- parser = argparse.ArgumentParser()
- parser.add_argument("feat_dir", type=str)
- parser.add_argument("split", type=str)
- parser.add_argument("nshard", type=int)
- parser.add_argument("km_path", type=str)
- parser.add_argument("n_clusters", type=int)
- parser.add_argument("--seed", default=0, type=int)
- parser.add_argument(
- "--percent", default=-1, type=float, help="sample a subset; -1 for all"
- )
- parser.add_argument("--init", default="k-means++")
- parser.add_argument("--max_iter", default=100, type=int)
- parser.add_argument("--batch_size", default=10000, type=int)
- parser.add_argument("--tol", default=0.0, type=float)
- parser.add_argument("--max_no_improvement", default=100, type=int)
- parser.add_argument("--n_init", default=20, type=int)
- parser.add_argument("--reassignment_ratio", default=0.0, type=float)
- args = parser.parse_args()
- logging.info(str(args))
-
- learn_kmeans(**vars(args))
diff --git a/spaces/OFA-Sys/OFA-vqa/fairseq/examples/wav2vec/unsupervised/scripts/prepare_audio.sh b/spaces/OFA-Sys/OFA-vqa/fairseq/examples/wav2vec/unsupervised/scripts/prepare_audio.sh
deleted file mode 100644
index 013f7a9b055a7693a29f9c5ba1e4003a9a25850e..0000000000000000000000000000000000000000
--- a/spaces/OFA-Sys/OFA-vqa/fairseq/examples/wav2vec/unsupervised/scripts/prepare_audio.sh
+++ /dev/null
@@ -1,78 +0,0 @@
-#!/usr/bin/env zsh
-# Copyright (c) Facebook, Inc. and its affiliates.
-#
-# This source code is licensed under the MIT license found in the
-# LICENSE file in the root directory of this source tree.
-
-source_dir=$1
-tgt_dir=$2
-model=$3
-
-if [ -z "$4" ]
- then
- dim=512
- else
- dim=$4
-fi
-
-echo "using $dim dim for PCA"
-
-if [ -z "$5" ]
- then
- layer=14
- else
- layer=$5
-fi
-
-echo "extracting from layer $layer"
-
-train_split=train
-valid_split=valid
-test_split=test
-
-all_splits=($train_split)
-
-if [[ -f "$source_dir/valid.tsv" ]]; then
- all_splits+=('valid')
-fi
-
-if [[ -f "$source_dir/test.tsv" ]]; then
- all_splits+=('test')
-fi
-
-echo "processing splits: $all_splits"
-
-mkdir -p $tgt_dir
-
-cp $source_dir/*.tsv $tgt_dir
-cp $source_dir/*.wrd $tgt_dir
-cp $source_dir/*.ltr $tgt_dir
-cp $source_dir/*.phn $tgt_dir
-cp $source_dir/dict* $tgt_dir
-
-setopt shwordsplit
-
-for split in $all_splits; do
- python $FAIRSEQ_ROOT/examples/wav2vec/unsupervised/scripts/wav2vec_extract_features.py $source_dir --split $split \
- --save-dir $tgt_dir --checkpoint $model --layer $layer
-done
-
-python $FAIRSEQ_ROOT/examples/wav2vec/unsupervised/scripts/wav2vec_cluster_faiss.py $tgt_dir/${train_split}.tsv \
---checkpoint $model --save-dir $tgt_dir -f "CLUS128" --sample-pct 1.0
-
-for split in $all_splits; do
- python $FAIRSEQ_ROOT/examples/wav2vec/unsupervised/scripts/wav2vec_apply_cluster_faiss.py $tgt_dir \
- --checkpoint $model --path $tgt_dir/CLUS128 --split $split
-done
-
-python $FAIRSEQ_ROOT/examples/wav2vec/unsupervised/scripts/pca.py $tgt_dir/${train_split}.npy --output $tgt_dir/pca --dim $dim
-
-for split in $all_splits; do
- python $FAIRSEQ_ROOT/examples/wav2vec/unsupervised/scripts/apply_pca.py $tgt_dir --split $split --save-dir $tgt_dir/precompute_pca$dim --pca-path $tgt_dir/pca/${dim}_pca --batch-size 1048000
-
- python $FAIRSEQ_ROOT/examples/wav2vec/unsupervised/scripts/merge_clusters.py $tgt_dir/precompute_pca$dim --cluster-dir $tgt_dir/CLUS128 \
- --split $split --save-dir $tgt_dir/precompute_pca${dim}_cls128_mean --pooling mean
-
- python $FAIRSEQ_ROOT/examples/wav2vec/unsupervised/scripts/mean_pool.py $tgt_dir/precompute_pca${dim}_cls128_mean \
- --save-dir $tgt_dir/precompute_pca${dim}_cls128_mean_pooled --split $split
-done
diff --git a/spaces/ORI-Muchim/ONFIRETTS/text/korean.py b/spaces/ORI-Muchim/ONFIRETTS/text/korean.py
deleted file mode 100644
index edee07429a450c55e3d8e246997faaa1e0b89cc9..0000000000000000000000000000000000000000
--- a/spaces/ORI-Muchim/ONFIRETTS/text/korean.py
+++ /dev/null
@@ -1,210 +0,0 @@
-import re
-from jamo import h2j, j2hcj
-import ko_pron
-
-
-# This is a list of Korean classifiers preceded by pure Korean numerals.
-_korean_classifiers = '군데 권 개 그루 닢 대 두 마리 모 모금 뭇 발 발짝 방 번 벌 보루 살 수 술 시 쌈 움큼 정 짝 채 척 첩 축 켤레 톨 통'
-
-# List of (hangul, hangul divided) pairs:
-_hangul_divided = [(re.compile('%s' % x[0]), x[1]) for x in [
- ('ㄳ', 'ㄱㅅ'),
- ('ㄵ', 'ㄴㅈ'),
- ('ㄶ', 'ㄴㅎ'),
- ('ㄺ', 'ㄹㄱ'),
- ('ㄻ', 'ㄹㅁ'),
- ('ㄼ', 'ㄹㅂ'),
- ('ㄽ', 'ㄹㅅ'),
- ('ㄾ', 'ㄹㅌ'),
- ('ㄿ', 'ㄹㅍ'),
- ('ㅀ', 'ㄹㅎ'),
- ('ㅄ', 'ㅂㅅ'),
- ('ㅘ', 'ㅗㅏ'),
- ('ㅙ', 'ㅗㅐ'),
- ('ㅚ', 'ㅗㅣ'),
- ('ㅝ', 'ㅜㅓ'),
- ('ㅞ', 'ㅜㅔ'),
- ('ㅟ', 'ㅜㅣ'),
- ('ㅢ', 'ㅡㅣ'),
- ('ㅑ', 'ㅣㅏ'),
- ('ㅒ', 'ㅣㅐ'),
- ('ㅕ', 'ㅣㅓ'),
- ('ㅖ', 'ㅣㅔ'),
- ('ㅛ', 'ㅣㅗ'),
- ('ㅠ', 'ㅣㅜ')
-]]
-
-# List of (Latin alphabet, hangul) pairs:
-_latin_to_hangul = [(re.compile('%s' % x[0], re.IGNORECASE), x[1]) for x in [
- ('a', '에이'),
- ('b', '비'),
- ('c', '시'),
- ('d', '디'),
- ('e', '이'),
- ('f', '에프'),
- ('g', '지'),
- ('h', '에이치'),
- ('i', '아이'),
- ('j', '제이'),
- ('k', '케이'),
- ('l', '엘'),
- ('m', '엠'),
- ('n', '엔'),
- ('o', '오'),
- ('p', '피'),
- ('q', '큐'),
- ('r', '아르'),
- ('s', '에스'),
- ('t', '티'),
- ('u', '유'),
- ('v', '브이'),
- ('w', '더블유'),
- ('x', '엑스'),
- ('y', '와이'),
- ('z', '제트')
-]]
-
-# List of (ipa, lazy ipa) pairs:
-_ipa_to_lazy_ipa = [(re.compile('%s' % x[0], re.IGNORECASE), x[1]) for x in [
- ('t͡ɕ','ʧ'),
- ('d͡ʑ','ʥ'),
- ('ɲ','n^'),
- ('ɕ','ʃ'),
- ('ʷ','w'),
- ('ɭ','l`'),
- ('ʎ','ɾ'),
- ('ɣ','ŋ'),
- ('ɰ','ɯ'),
- ('ʝ','j'),
- ('ʌ','ə'),
- ('ɡ','g'),
- ('\u031a','#'),
- ('\u0348','='),
- ('\u031e',''),
- ('\u0320',''),
- ('\u0339','')
-]]
-
-
-def latin_to_hangul(text):
- for regex, replacement in _latin_to_hangul:
- text = re.sub(regex, replacement, text)
- return text
-
-
-def divide_hangul(text):
- text = j2hcj(h2j(text))
- for regex, replacement in _hangul_divided:
- text = re.sub(regex, replacement, text)
- return text
-
-
-def hangul_number(num, sino=True):
- '''Reference https://github.com/Kyubyong/g2pK'''
- num = re.sub(',', '', num)
-
- if num == '0':
- return '영'
- if not sino and num == '20':
- return '스무'
-
- digits = '123456789'
- names = '일이삼사오육칠팔구'
- digit2name = {d: n for d, n in zip(digits, names)}
-
- modifiers = '한 두 세 네 다섯 여섯 일곱 여덟 아홉'
- decimals = '열 스물 서른 마흔 쉰 예순 일흔 여든 아흔'
- digit2mod = {d: mod for d, mod in zip(digits, modifiers.split())}
- digit2dec = {d: dec for d, dec in zip(digits, decimals.split())}
-
- spelledout = []
- for i, digit in enumerate(num):
- i = len(num) - i - 1
- if sino:
- if i == 0:
- name = digit2name.get(digit, '')
- elif i == 1:
- name = digit2name.get(digit, '') + '십'
- name = name.replace('일십', '십')
- else:
- if i == 0:
- name = digit2mod.get(digit, '')
- elif i == 1:
- name = digit2dec.get(digit, '')
- if digit == '0':
- if i % 4 == 0:
- last_three = spelledout[-min(3, len(spelledout)):]
- if ''.join(last_three) == '':
- spelledout.append('')
- continue
- else:
- spelledout.append('')
- continue
- if i == 2:
- name = digit2name.get(digit, '') + '백'
- name = name.replace('일백', '백')
- elif i == 3:
- name = digit2name.get(digit, '') + '천'
- name = name.replace('일천', '천')
- elif i == 4:
- name = digit2name.get(digit, '') + '만'
- name = name.replace('일만', '만')
- elif i == 5:
- name = digit2name.get(digit, '') + '십'
- name = name.replace('일십', '십')
- elif i == 6:
- name = digit2name.get(digit, '') + '백'
- name = name.replace('일백', '백')
- elif i == 7:
- name = digit2name.get(digit, '') + '천'
- name = name.replace('일천', '천')
- elif i == 8:
- name = digit2name.get(digit, '') + '억'
- elif i == 9:
- name = digit2name.get(digit, '') + '십'
- elif i == 10:
- name = digit2name.get(digit, '') + '백'
- elif i == 11:
- name = digit2name.get(digit, '') + '천'
- elif i == 12:
- name = digit2name.get(digit, '') + '조'
- elif i == 13:
- name = digit2name.get(digit, '') + '십'
- elif i == 14:
- name = digit2name.get(digit, '') + '백'
- elif i == 15:
- name = digit2name.get(digit, '') + '천'
- spelledout.append(name)
- return ''.join(elem for elem in spelledout)
-
-
-def number_to_hangul(text):
- '''Reference https://github.com/Kyubyong/g2pK'''
- tokens = set(re.findall(r'(\d[\d,]*)([\uac00-\ud71f]+)', text))
- for token in tokens:
- num, classifier = token
- if classifier[:2] in _korean_classifiers or classifier[0] in _korean_classifiers:
- spelledout = hangul_number(num, sino=False)
- else:
- spelledout = hangul_number(num, sino=True)
- text = text.replace(f'{num}{classifier}', f'{spelledout}{classifier}')
- # digit by digit for remaining digits
- digits = '0123456789'
- names = '영일이삼사오육칠팔구'
- for d, n in zip(digits, names):
- text = text.replace(d, n)
- return text
-
-
-def korean_to_lazy_ipa(text):
- text = latin_to_hangul(text)
- text = number_to_hangul(text)
- text=re.sub('[\uac00-\ud7af]+',lambda x:ko_pron.romanise(x.group(0),'ipa').split('] ~ [')[0],text)
- for regex, replacement in _ipa_to_lazy_ipa:
- text = re.sub(regex, replacement, text)
- return text
-
-
-def korean_to_ipa(text):
- text = korean_to_lazy_ipa(text)
- return text.replace('ʧ','tʃ').replace('ʥ','dʑ')
diff --git a/spaces/OpenGVLab/InternGPT/iGPT/models/grit_src/third_party/CenterNet2/detectron2/modeling/meta_arch/panoptic_fpn.py b/spaces/OpenGVLab/InternGPT/iGPT/models/grit_src/third_party/CenterNet2/detectron2/modeling/meta_arch/panoptic_fpn.py
deleted file mode 100644
index 13aeabce162f4114109efe2c7fb4770b89087ab0..0000000000000000000000000000000000000000
--- a/spaces/OpenGVLab/InternGPT/iGPT/models/grit_src/third_party/CenterNet2/detectron2/modeling/meta_arch/panoptic_fpn.py
+++ /dev/null
@@ -1,266 +0,0 @@
-# -*- coding: utf-8 -*-
-# Copyright (c) Facebook, Inc. and its affiliates.
-
-import logging
-from typing import Dict, List
-import torch
-from torch import nn
-
-from detectron2.config import configurable
-from detectron2.structures import ImageList
-
-from ..postprocessing import detector_postprocess, sem_seg_postprocess
-from .build import META_ARCH_REGISTRY
-from .rcnn import GeneralizedRCNN
-from .semantic_seg import build_sem_seg_head
-
-__all__ = ["PanopticFPN"]
-
-
-@META_ARCH_REGISTRY.register()
-class PanopticFPN(GeneralizedRCNN):
- """
- Implement the paper :paper:`PanopticFPN`.
- """
-
- @configurable
- def __init__(
- self,
- *,
- sem_seg_head: nn.Module,
- combine_overlap_thresh: float = 0.5,
- combine_stuff_area_thresh: float = 4096,
- combine_instances_score_thresh: float = 0.5,
- **kwargs,
- ):
- """
- NOTE: this interface is experimental.
-
- Args:
- sem_seg_head: a module for the semantic segmentation head.
- combine_overlap_thresh: combine masks into one instances if
- they have enough overlap
- combine_stuff_area_thresh: ignore stuff areas smaller than this threshold
- combine_instances_score_thresh: ignore instances whose score is
- smaller than this threshold
-
- Other arguments are the same as :class:`GeneralizedRCNN`.
- """
- super().__init__(**kwargs)
- self.sem_seg_head = sem_seg_head
- # options when combining instance & semantic outputs
- self.combine_overlap_thresh = combine_overlap_thresh
- self.combine_stuff_area_thresh = combine_stuff_area_thresh
- self.combine_instances_score_thresh = combine_instances_score_thresh
-
- @classmethod
- def from_config(cls, cfg):
- ret = super().from_config(cfg)
- ret.update(
- {
- "combine_overlap_thresh": cfg.MODEL.PANOPTIC_FPN.COMBINE.OVERLAP_THRESH,
- "combine_stuff_area_thresh": cfg.MODEL.PANOPTIC_FPN.COMBINE.STUFF_AREA_LIMIT,
- "combine_instances_score_thresh": cfg.MODEL.PANOPTIC_FPN.COMBINE.INSTANCES_CONFIDENCE_THRESH, # noqa
- }
- )
- ret["sem_seg_head"] = build_sem_seg_head(cfg, ret["backbone"].output_shape())
- logger = logging.getLogger(__name__)
- if not cfg.MODEL.PANOPTIC_FPN.COMBINE.ENABLED:
- logger.warning(
- "PANOPTIC_FPN.COMBINED.ENABLED is no longer used. "
- " model.inference(do_postprocess=) should be used to toggle postprocessing."
- )
- if cfg.MODEL.PANOPTIC_FPN.INSTANCE_LOSS_WEIGHT != 1.0:
- w = cfg.MODEL.PANOPTIC_FPN.INSTANCE_LOSS_WEIGHT
- logger.warning(
- "PANOPTIC_FPN.INSTANCE_LOSS_WEIGHT should be replaced by weights on each ROI head."
- )
-
- def update_weight(x):
- if isinstance(x, dict):
- return {k: v * w for k, v in x.items()}
- else:
- return x * w
-
- roi_heads = ret["roi_heads"]
- roi_heads.box_predictor.loss_weight = update_weight(roi_heads.box_predictor.loss_weight)
- roi_heads.mask_head.loss_weight = update_weight(roi_heads.mask_head.loss_weight)
- return ret
-
- def forward(self, batched_inputs):
- """
- Args:
- batched_inputs: a list, batched outputs of :class:`DatasetMapper`.
- Each item in the list contains the inputs for one image.
-
- For now, each item in the list is a dict that contains:
-
- * "image": Tensor, image in (C, H, W) format.
- * "instances": Instances
- * "sem_seg": semantic segmentation ground truth.
- * Other information that's included in the original dicts, such as:
- "height", "width" (int): the output resolution of the model, used in inference.
- See :meth:`postprocess` for details.
-
- Returns:
- list[dict]:
- each dict has the results for one image. The dict contains the following keys:
-
- * "instances": see :meth:`GeneralizedRCNN.forward` for its format.
- * "sem_seg": see :meth:`SemanticSegmentor.forward` for its format.
- * "panoptic_seg": See the return value of
- :func:`combine_semantic_and_instance_outputs` for its format.
- """
- if not self.training:
- return self.inference(batched_inputs)
- images = self.preprocess_image(batched_inputs)
- features = self.backbone(images.tensor)
-
- assert "sem_seg" in batched_inputs[0]
- gt_sem_seg = [x["sem_seg"].to(self.device) for x in batched_inputs]
- gt_sem_seg = ImageList.from_tensors(
- gt_sem_seg, self.backbone.size_divisibility, self.sem_seg_head.ignore_value
- ).tensor
- sem_seg_results, sem_seg_losses = self.sem_seg_head(features, gt_sem_seg)
-
- gt_instances = [x["instances"].to(self.device) for x in batched_inputs]
- proposals, proposal_losses = self.proposal_generator(images, features, gt_instances)
- detector_results, detector_losses = self.roi_heads(
- images, features, proposals, gt_instances
- )
-
- losses = sem_seg_losses
- losses.update(proposal_losses)
- losses.update(detector_losses)
- return losses
-
- def inference(self, batched_inputs: List[Dict[str, torch.Tensor]], do_postprocess: bool = True):
- """
- Run inference on the given inputs.
-
- Args:
- batched_inputs (list[dict]): same as in :meth:`forward`
- do_postprocess (bool): whether to apply post-processing on the outputs.
-
- Returns:
- When do_postprocess=True, see docs in :meth:`forward`.
- Otherwise, returns a (list[Instances], list[Tensor]) that contains
- the raw detector outputs, and raw semantic segmentation outputs.
- """
- images = self.preprocess_image(batched_inputs)
- features = self.backbone(images.tensor)
- sem_seg_results, sem_seg_losses = self.sem_seg_head(features, None)
- proposals, _ = self.proposal_generator(images, features, None)
- detector_results, _ = self.roi_heads(images, features, proposals, None)
-
- if do_postprocess:
- processed_results = []
- for sem_seg_result, detector_result, input_per_image, image_size in zip(
- sem_seg_results, detector_results, batched_inputs, images.image_sizes
- ):
- height = input_per_image.get("height", image_size[0])
- width = input_per_image.get("width", image_size[1])
- sem_seg_r = sem_seg_postprocess(sem_seg_result, image_size, height, width)
- detector_r = detector_postprocess(detector_result, height, width)
-
- processed_results.append({"sem_seg": sem_seg_r, "instances": detector_r})
-
- panoptic_r = combine_semantic_and_instance_outputs(
- detector_r,
- sem_seg_r.argmax(dim=0),
- self.combine_overlap_thresh,
- self.combine_stuff_area_thresh,
- self.combine_instances_score_thresh,
- )
- processed_results[-1]["panoptic_seg"] = panoptic_r
- return processed_results
- else:
- return detector_results, sem_seg_results
-
-
-def combine_semantic_and_instance_outputs(
- instance_results,
- semantic_results,
- overlap_threshold,
- stuff_area_thresh,
- instances_score_thresh,
-):
- """
- Implement a simple combining logic following
- "combine_semantic_and_instance_predictions.py" in panopticapi
- to produce panoptic segmentation outputs.
-
- Args:
- instance_results: output of :func:`detector_postprocess`.
- semantic_results: an (H, W) tensor, each element is the contiguous semantic
- category id
-
- Returns:
- panoptic_seg (Tensor): of shape (height, width) where the values are ids for each segment.
- segments_info (list[dict]): Describe each segment in `panoptic_seg`.
- Each dict contains keys "id", "category_id", "isthing".
- """
- panoptic_seg = torch.zeros_like(semantic_results, dtype=torch.int32)
-
- # sort instance outputs by scores
- sorted_inds = torch.argsort(-instance_results.scores)
-
- current_segment_id = 0
- segments_info = []
-
- instance_masks = instance_results.pred_masks.to(dtype=torch.bool, device=panoptic_seg.device)
-
- # Add instances one-by-one, check for overlaps with existing ones
- for inst_id in sorted_inds:
- score = instance_results.scores[inst_id].item()
- if score < instances_score_thresh:
- break
- mask = instance_masks[inst_id] # H,W
- mask_area = mask.sum().item()
-
- if mask_area == 0:
- continue
-
- intersect = (mask > 0) & (panoptic_seg > 0)
- intersect_area = intersect.sum().item()
-
- if intersect_area * 1.0 / mask_area > overlap_threshold:
- continue
-
- if intersect_area > 0:
- mask = mask & (panoptic_seg == 0)
-
- current_segment_id += 1
- panoptic_seg[mask] = current_segment_id
- segments_info.append(
- {
- "id": current_segment_id,
- "isthing": True,
- "score": score,
- "category_id": instance_results.pred_classes[inst_id].item(),
- "instance_id": inst_id.item(),
- }
- )
-
- # Add semantic results to remaining empty areas
- semantic_labels = torch.unique(semantic_results).cpu().tolist()
- for semantic_label in semantic_labels:
- if semantic_label == 0: # 0 is a special "thing" class
- continue
- mask = (semantic_results == semantic_label) & (panoptic_seg == 0)
- mask_area = mask.sum().item()
- if mask_area < stuff_area_thresh:
- continue
-
- current_segment_id += 1
- panoptic_seg[mask] = current_segment_id
- segments_info.append(
- {
- "id": current_segment_id,
- "isthing": False,
- "category_id": semantic_label,
- "area": mask_area,
- }
- )
-
- return panoptic_seg, segments_info
diff --git a/spaces/OpenMotionLab/MotionGPT/pyrender/pyrender/mesh.py b/spaces/OpenMotionLab/MotionGPT/pyrender/pyrender/mesh.py
deleted file mode 100644
index 36833ea3dfa6c095a18fc745ff34cf106e83c95d..0000000000000000000000000000000000000000
--- a/spaces/OpenMotionLab/MotionGPT/pyrender/pyrender/mesh.py
+++ /dev/null
@@ -1,328 +0,0 @@
-"""Meshes, conforming to the glTF 2.0 standards as specified in
-https://github.com/KhronosGroup/glTF/tree/master/specification/2.0#reference-mesh
-
-Author: Matthew Matl
-"""
-import copy
-
-import numpy as np
-import trimesh
-
-from .primitive import Primitive
-from .constants import GLTF
-from .material import MetallicRoughnessMaterial
-
-
-class Mesh(object):
- """A set of primitives to be rendered.
-
- Parameters
- ----------
- name : str
- The user-defined name of this object.
- primitives : list of :class:`Primitive`
- The primitives associated with this mesh.
- weights : (k,) float
- Array of weights to be applied to the Morph Targets.
- is_visible : bool
- If False, the mesh will not be rendered.
- """
-
- def __init__(self, primitives, name=None, weights=None, is_visible=True):
- self.primitives = primitives
- self.name = name
- self.weights = weights
- self.is_visible = is_visible
-
- self._bounds = None
-
- @property
- def name(self):
- """str : The user-defined name of this object.
- """
- return self._name
-
- @name.setter
- def name(self, value):
- if value is not None:
- value = str(value)
- self._name = value
-
- @property
- def primitives(self):
- """list of :class:`Primitive` : The primitives associated
- with this mesh.
- """
- return self._primitives
-
- @primitives.setter
- def primitives(self, value):
- self._primitives = value
-
- @property
- def weights(self):
- """(k,) float : Weights to be applied to morph targets.
- """
- return self._weights
-
- @weights.setter
- def weights(self, value):
- self._weights = value
-
- @property
- def is_visible(self):
- """bool : Whether the mesh is visible.
- """
- return self._is_visible
-
- @is_visible.setter
- def is_visible(self, value):
- self._is_visible = value
-
- @property
- def bounds(self):
- """(2,3) float : The axis-aligned bounds of the mesh.
- """
- if self._bounds is None:
- bounds = np.array([[np.infty, np.infty, np.infty],
- [-np.infty, -np.infty, -np.infty]])
- for p in self.primitives:
- bounds[0] = np.minimum(bounds[0], p.bounds[0])
- bounds[1] = np.maximum(bounds[1], p.bounds[1])
- self._bounds = bounds
- return self._bounds
-
- @property
- def centroid(self):
- """(3,) float : The centroid of the mesh's axis-aligned bounding box
- (AABB).
- """
- return np.mean(self.bounds, axis=0)
-
- @property
- def extents(self):
- """(3,) float : The lengths of the axes of the mesh's AABB.
- """
- return np.diff(self.bounds, axis=0).reshape(-1)
-
- @property
- def scale(self):
- """(3,) float : The length of the diagonal of the mesh's AABB.
- """
- return np.linalg.norm(self.extents)
-
- @property
- def is_transparent(self):
- """bool : If True, the mesh is partially-transparent.
- """
- for p in self.primitives:
- if p.is_transparent:
- return True
- return False
-
- @staticmethod
- def from_points(points, colors=None, normals=None,
- is_visible=True, poses=None):
- """Create a Mesh from a set of points.
-
- Parameters
- ----------
- points : (n,3) float
- The point positions.
- colors : (n,3) or (n,4) float, optional
- RGB or RGBA colors for each point.
- normals : (n,3) float, optionals
- The normal vectors for each point.
- is_visible : bool
- If False, the points will not be rendered.
- poses : (x,4,4)
- Array of 4x4 transformation matrices for instancing this object.
-
- Returns
- -------
- mesh : :class:`Mesh`
- The created mesh.
- """
- primitive = Primitive(
- positions=points,
- normals=normals,
- color_0=colors,
- mode=GLTF.POINTS,
- poses=poses
- )
- mesh = Mesh(primitives=[primitive], is_visible=is_visible)
- return mesh
-
- @staticmethod
- def from_trimesh(mesh, material=None, is_visible=True,
- poses=None, wireframe=False, smooth=True):
- """Create a Mesh from a :class:`~trimesh.base.Trimesh`.
-
- Parameters
- ----------
- mesh : :class:`~trimesh.base.Trimesh` or list of them
- A triangular mesh or a list of meshes.
- material : :class:`Material`
- The material of the object. Overrides any mesh material.
- If not specified and the mesh has no material, a default material
- will be used.
- is_visible : bool
- If False, the mesh will not be rendered.
- poses : (n,4,4) float
- Array of 4x4 transformation matrices for instancing this object.
- wireframe : bool
- If `True`, the mesh will be rendered as a wireframe object
- smooth : bool
- If `True`, the mesh will be rendered with interpolated vertex
- normals. Otherwise, the mesh edges will stay sharp.
-
- Returns
- -------
- mesh : :class:`Mesh`
- The created mesh.
- """
-
- if isinstance(mesh, (list, tuple, set, np.ndarray)):
- meshes = list(mesh)
- elif isinstance(mesh, trimesh.Trimesh):
- meshes = [mesh]
- else:
- raise TypeError('Expected a Trimesh or a list, got a {}'
- .format(type(mesh)))
-
- primitives = []
- for m in meshes:
- positions = None
- normals = None
- indices = None
-
- # Compute positions, normals, and indices
- if smooth:
- positions = m.vertices.copy()
- normals = m.vertex_normals.copy()
- indices = m.faces.copy()
- else:
- positions = m.vertices[m.faces].reshape((3 * len(m.faces), 3))
- normals = np.repeat(m.face_normals, 3, axis=0)
-
- # Compute colors, texture coords, and material properties
- color_0, texcoord_0, primitive_material = Mesh._get_trimesh_props(m, smooth=smooth, material=material)
-
- # Override if material is given.
- if material is not None:
- #primitive_material = copy.copy(material)
- primitive_material = copy.deepcopy(material) # TODO
-
- if primitive_material is None:
- # Replace material with default if needed
- primitive_material = MetallicRoughnessMaterial(
- alphaMode='BLEND',
- baseColorFactor=[0.3, 0.3, 0.3, 1.0],
- metallicFactor=0.2,
- roughnessFactor=0.8
- )
-
- primitive_material.wireframe = wireframe
-
- # Create the primitive
- primitives.append(Primitive(
- positions=positions,
- normals=normals,
- texcoord_0=texcoord_0,
- color_0=color_0,
- indices=indices,
- material=primitive_material,
- mode=GLTF.TRIANGLES,
- poses=poses
- ))
-
- return Mesh(primitives=primitives, is_visible=is_visible)
-
- @staticmethod
- def _get_trimesh_props(mesh, smooth=False, material=None):
- """Gets the vertex colors, texture coordinates, and material properties
- from a :class:`~trimesh.base.Trimesh`.
- """
- colors = None
- texcoords = None
-
- # If the trimesh visual is undefined, return none for both
- if not mesh.visual.defined:
- return colors, texcoords, material
-
- # Process vertex colors
- if material is None:
- if mesh.visual.kind == 'vertex':
- vc = mesh.visual.vertex_colors.copy()
- if smooth:
- colors = vc
- else:
- colors = vc[mesh.faces].reshape(
- (3 * len(mesh.faces), vc.shape[1])
- )
- material = MetallicRoughnessMaterial(
- alphaMode='BLEND',
- baseColorFactor=[1.0, 1.0, 1.0, 1.0],
- metallicFactor=0.2,
- roughnessFactor=0.8
- )
- # Process face colors
- elif mesh.visual.kind == 'face':
- if smooth:
- raise ValueError('Cannot use face colors with a smooth mesh')
- else:
- colors = np.repeat(mesh.visual.face_colors, 3, axis=0)
-
- material = MetallicRoughnessMaterial(
- alphaMode='BLEND',
- baseColorFactor=[1.0, 1.0, 1.0, 1.0],
- metallicFactor=0.2,
- roughnessFactor=0.8
- )
-
- # Process texture colors
- if mesh.visual.kind == 'texture':
- # Configure UV coordinates
- if mesh.visual.uv is not None and len(mesh.visual.uv) != 0:
- uv = mesh.visual.uv.copy()
- if smooth:
- texcoords = uv
- else:
- texcoords = uv[mesh.faces].reshape(
- (3 * len(mesh.faces), uv.shape[1])
- )
-
- if material is None:
- # Configure mesh material
- mat = mesh.visual.material
-
- if isinstance(mat, trimesh.visual.texture.PBRMaterial):
- material = MetallicRoughnessMaterial(
- normalTexture=mat.normalTexture,
- occlusionTexture=mat.occlusionTexture,
- emissiveTexture=mat.emissiveTexture,
- emissiveFactor=mat.emissiveFactor,
- alphaMode='BLEND',
- baseColorFactor=mat.baseColorFactor,
- baseColorTexture=mat.baseColorTexture,
- metallicFactor=mat.metallicFactor,
- roughnessFactor=mat.roughnessFactor,
- metallicRoughnessTexture=mat.metallicRoughnessTexture,
- doubleSided=mat.doubleSided,
- alphaCutoff=mat.alphaCutoff
- )
- elif isinstance(mat, trimesh.visual.texture.SimpleMaterial):
- glossiness = mat.kwargs.get('Ns', 1.0)
- if isinstance(glossiness, list):
- glossiness = float(glossiness[0])
- roughness = (2 / (glossiness + 2)) ** (1.0 / 4.0)
- material = MetallicRoughnessMaterial(
- alphaMode='BLEND',
- roughnessFactor=roughness,
- baseColorFactor=mat.diffuse,
- baseColorTexture=mat.image,
- )
- elif isinstance(mat, MetallicRoughnessMaterial):
- material = mat
-
- return colors, texcoords, material
diff --git a/spaces/PAIR/Text2Video-Zero/annotator/uniformer/mmcv/visualization/image.py b/spaces/PAIR/Text2Video-Zero/annotator/uniformer/mmcv/visualization/image.py
deleted file mode 100644
index 61a56c75b67f593c298408462c63c0468be8e276..0000000000000000000000000000000000000000
--- a/spaces/PAIR/Text2Video-Zero/annotator/uniformer/mmcv/visualization/image.py
+++ /dev/null
@@ -1,152 +0,0 @@
-# Copyright (c) OpenMMLab. All rights reserved.
-import cv2
-import numpy as np
-
-from annotator.uniformer.mmcv.image import imread, imwrite
-from .color import color_val
-
-
-def imshow(img, win_name='', wait_time=0):
- """Show an image.
-
- Args:
- img (str or ndarray): The image to be displayed.
- win_name (str): The window name.
- wait_time (int): Value of waitKey param.
- """
- cv2.imshow(win_name, imread(img))
- if wait_time == 0: # prevent from hanging if windows was closed
- while True:
- ret = cv2.waitKey(1)
-
- closed = cv2.getWindowProperty(win_name, cv2.WND_PROP_VISIBLE) < 1
- # if user closed window or if some key pressed
- if closed or ret != -1:
- break
- else:
- ret = cv2.waitKey(wait_time)
-
-
-def imshow_bboxes(img,
- bboxes,
- colors='green',
- top_k=-1,
- thickness=1,
- show=True,
- win_name='',
- wait_time=0,
- out_file=None):
- """Draw bboxes on an image.
-
- Args:
- img (str or ndarray): The image to be displayed.
- bboxes (list or ndarray): A list of ndarray of shape (k, 4).
- colors (list[str or tuple or Color]): A list of colors.
- top_k (int): Plot the first k bboxes only if set positive.
- thickness (int): Thickness of lines.
- show (bool): Whether to show the image.
- win_name (str): The window name.
- wait_time (int): Value of waitKey param.
- out_file (str, optional): The filename to write the image.
-
- Returns:
- ndarray: The image with bboxes drawn on it.
- """
- img = imread(img)
- img = np.ascontiguousarray(img)
-
- if isinstance(bboxes, np.ndarray):
- bboxes = [bboxes]
- if not isinstance(colors, list):
- colors = [colors for _ in range(len(bboxes))]
- colors = [color_val(c) for c in colors]
- assert len(bboxes) == len(colors)
-
- for i, _bboxes in enumerate(bboxes):
- _bboxes = _bboxes.astype(np.int32)
- if top_k <= 0:
- _top_k = _bboxes.shape[0]
- else:
- _top_k = min(top_k, _bboxes.shape[0])
- for j in range(_top_k):
- left_top = (_bboxes[j, 0], _bboxes[j, 1])
- right_bottom = (_bboxes[j, 2], _bboxes[j, 3])
- cv2.rectangle(
- img, left_top, right_bottom, colors[i], thickness=thickness)
-
- if show:
- imshow(img, win_name, wait_time)
- if out_file is not None:
- imwrite(img, out_file)
- return img
-
-
-def imshow_det_bboxes(img,
- bboxes,
- labels,
- class_names=None,
- score_thr=0,
- bbox_color='green',
- text_color='green',
- thickness=1,
- font_scale=0.5,
- show=True,
- win_name='',
- wait_time=0,
- out_file=None):
- """Draw bboxes and class labels (with scores) on an image.
-
- Args:
- img (str or ndarray): The image to be displayed.
- bboxes (ndarray): Bounding boxes (with scores), shaped (n, 4) or
- (n, 5).
- labels (ndarray): Labels of bboxes.
- class_names (list[str]): Names of each classes.
- score_thr (float): Minimum score of bboxes to be shown.
- bbox_color (str or tuple or :obj:`Color`): Color of bbox lines.
- text_color (str or tuple or :obj:`Color`): Color of texts.
- thickness (int): Thickness of lines.
- font_scale (float): Font scales of texts.
- show (bool): Whether to show the image.
- win_name (str): The window name.
- wait_time (int): Value of waitKey param.
- out_file (str or None): The filename to write the image.
-
- Returns:
- ndarray: The image with bboxes drawn on it.
- """
- assert bboxes.ndim == 2
- assert labels.ndim == 1
- assert bboxes.shape[0] == labels.shape[0]
- assert bboxes.shape[1] == 4 or bboxes.shape[1] == 5
- img = imread(img)
- img = np.ascontiguousarray(img)
-
- if score_thr > 0:
- assert bboxes.shape[1] == 5
- scores = bboxes[:, -1]
- inds = scores > score_thr
- bboxes = bboxes[inds, :]
- labels = labels[inds]
-
- bbox_color = color_val(bbox_color)
- text_color = color_val(text_color)
-
- for bbox, label in zip(bboxes, labels):
- bbox_int = bbox.astype(np.int32)
- left_top = (bbox_int[0], bbox_int[1])
- right_bottom = (bbox_int[2], bbox_int[3])
- cv2.rectangle(
- img, left_top, right_bottom, bbox_color, thickness=thickness)
- label_text = class_names[
- label] if class_names is not None else f'cls {label}'
- if len(bbox) > 4:
- label_text += f'|{bbox[-1]:.02f}'
- cv2.putText(img, label_text, (bbox_int[0], bbox_int[1] - 2),
- cv2.FONT_HERSHEY_COMPLEX, font_scale, text_color)
-
- if show:
- imshow(img, win_name, wait_time)
- if out_file is not None:
- imwrite(img, out_file)
- return img
diff --git a/spaces/Pattr/DrumClassification/lilypond-2.24.2/lib/guile/2.2/ccache/language/cps/elide-values.go b/spaces/Pattr/DrumClassification/lilypond-2.24.2/lib/guile/2.2/ccache/language/cps/elide-values.go
deleted file mode 100644
index d7a398900d5f5cec091b00ceb31e251cc2179344..0000000000000000000000000000000000000000
Binary files a/spaces/Pattr/DrumClassification/lilypond-2.24.2/lib/guile/2.2/ccache/language/cps/elide-values.go and /dev/null differ
diff --git a/spaces/Pengyey/bingo-chuchu/src/components/ui/button.tsx b/spaces/Pengyey/bingo-chuchu/src/components/ui/button.tsx
deleted file mode 100644
index 281da005124fa94c89a9a9db7605748a92b60865..0000000000000000000000000000000000000000
--- a/spaces/Pengyey/bingo-chuchu/src/components/ui/button.tsx
+++ /dev/null
@@ -1,57 +0,0 @@
-import * as React from 'react'
-import { Slot } from '@radix-ui/react-slot'
-import { cva, type VariantProps } from 'class-variance-authority'
-
-import { cn } from '@/lib/utils'
-
-const buttonVariants = cva(
- 'inline-flex items-center justify-center rounded-md text-sm font-medium shadow ring-offset-background transition-colors outline-none disabled:pointer-events-none disabled:opacity-50',
- {
- variants: {
- variant: {
- default:
- 'bg-primary text-primary-foreground shadow-md hover:bg-primary/90',
- destructive:
- 'bg-destructive text-destructive-foreground hover:bg-destructive/90',
- outline:
- 'border border-input hover:bg-accent hover:text-accent-foreground',
- secondary:
- 'bg-secondary text-secondary-foreground hover:bg-secondary/80',
- ghost: 'shadow-none hover:bg-accent hover:text-accent-foreground',
- link: 'text-primary underline-offset-4 shadow-none hover:underline'
- },
- size: {
- default: 'h-8 px-4 py-2',
- sm: 'h-8 rounded-md px-3',
- lg: 'h-11 rounded-md px-8',
- icon: 'h-8 w-8 p-0'
- }
- },
- defaultVariants: {
- variant: 'default',
- size: 'default'
- }
- }
-)
-
-export interface ButtonProps
- extends React.ButtonHTMLAttributes,
- VariantProps {
- asChild?: boolean
-}
-
-const Button = React.forwardRef(
- ({ className, variant, size, asChild = false, ...props }, ref) => {
- const Comp = asChild ? Slot : 'button'
- return (
-
- )
- }
-)
-Button.displayName = 'Button'
-
-export { Button, buttonVariants }
diff --git a/spaces/Pie31415/control-animation/README.md b/spaces/Pie31415/control-animation/README.md
deleted file mode 100644
index 51c287efdc98f8c6d7b3b7a2909d9d12cf7ed7dd..0000000000000000000000000000000000000000
--- a/spaces/Pie31415/control-animation/README.md
+++ /dev/null
@@ -1,14 +0,0 @@
----
-title: Control Animation
-emoji: 🔥
-sdk: gradio
-sdk_version: 3.23.0
-app_file: app.py
-pipeline_tag: text-to-video
-tags:
-- jax-diffusers-event
----
-
-# Control Animation
-
-Our code uses [Text2Video-Zero](https://github.com/Picsart-AI-Research/Text2Video-Zero) and the [Diffusers](https://github.com/huggingface/diffusers) library as inspiration.
diff --git a/spaces/Pinwheel/GLIP-BLIP-Object-Detection-VQA/maskrcnn_benchmark/csrc/ROIAlign.h b/spaces/Pinwheel/GLIP-BLIP-Object-Detection-VQA/maskrcnn_benchmark/csrc/ROIAlign.h
deleted file mode 100644
index 517e5ea7f742e279d602589fb7ccf25d03944ccc..0000000000000000000000000000000000000000
--- a/spaces/Pinwheel/GLIP-BLIP-Object-Detection-VQA/maskrcnn_benchmark/csrc/ROIAlign.h
+++ /dev/null
@@ -1,46 +0,0 @@
-// Copyright (c) Facebook, Inc. and its affiliates. All Rights Reserved.
-#pragma once
-
-#include "cpu/vision.h"
-
-#ifdef WITH_CUDA
-#include "cuda/vision.h"
-#endif
-
-// Interface for Python
-at::Tensor ROIAlign_forward(const at::Tensor& input,
- const at::Tensor& rois,
- const float spatial_scale,
- const int pooled_height,
- const int pooled_width,
- const int sampling_ratio) {
- if (input.device().is_cuda()) {
-#ifdef WITH_CUDA
- return ROIAlign_forward_cuda(input, rois, spatial_scale, pooled_height, pooled_width, sampling_ratio);
-#else
- AT_ERROR("Not compiled with GPU support");
-#endif
- }
- return ROIAlign_forward_cpu(input, rois, spatial_scale, pooled_height, pooled_width, sampling_ratio);
-}
-
-at::Tensor ROIAlign_backward(const at::Tensor& grad,
- const at::Tensor& rois,
- const float spatial_scale,
- const int pooled_height,
- const int pooled_width,
- const int batch_size,
- const int channels,
- const int height,
- const int width,
- const int sampling_ratio) {
- if (grad.device().is_cuda()) {
-#ifdef WITH_CUDA
- return ROIAlign_backward_cuda(grad, rois, spatial_scale, pooled_height, pooled_width, batch_size, channels, height, width, sampling_ratio);
-#else
- AT_ERROR("Not compiled with GPU support");
-#endif
- }
- AT_ERROR("Not implemented on the CPU");
-}
-
diff --git a/spaces/ProgramX/hi/README.md b/spaces/ProgramX/hi/README.md
deleted file mode 100644
index 62b1fe3a9da4b0af52a808eae18a423b1a6da3ee..0000000000000000000000000000000000000000
--- a/spaces/ProgramX/hi/README.md
+++ /dev/null
@@ -1,13 +0,0 @@
----
-title: Hi
-emoji: 👁
-colorFrom: indigo
-colorTo: indigo
-sdk: gradio
-sdk_version: 4.1.2
-app_file: app.py
-pinned: false
-license: apache-2.0
----
-
-Check out the configuration reference at https://huggingface.co/docs/hub/spaces-config-reference
diff --git a/spaces/Raghav001/API/README.md b/spaces/Raghav001/API/README.md
deleted file mode 100644
index 306dcfcd9cd6ac3b4f4d1b4c32495995f9e29118..0000000000000000000000000000000000000000
--- a/spaces/Raghav001/API/README.md
+++ /dev/null
@@ -1,11 +0,0 @@
----
-title: Fastapi Hello World
-emoji: 📉
-colorFrom: green
-colorTo: green
-sdk: docker
-pinned: false
-duplicated_from: souljoy/my_api
----
-
-Check out the configuration reference at https://huggingface.co/docs/hub/spaces-config-reference
diff --git a/spaces/RamAnanth1/videocrafter/extralibs/midas/__init__.py b/spaces/RamAnanth1/videocrafter/extralibs/midas/__init__.py
deleted file mode 100644
index e69de29bb2d1d6434b8b29ae775ad8c2e48c5391..0000000000000000000000000000000000000000
diff --git a/spaces/Realcat/image-matching-webui/third_party/RoRD/evaluation/DiverseView/evalRT.py b/spaces/Realcat/image-matching-webui/third_party/RoRD/evaluation/DiverseView/evalRT.py
deleted file mode 100644
index d0be9aef58e408668112e0587a03b2b33012a342..0000000000000000000000000000000000000000
--- a/spaces/Realcat/image-matching-webui/third_party/RoRD/evaluation/DiverseView/evalRT.py
+++ /dev/null
@@ -1,307 +0,0 @@
-import numpy as np
-import argparse
-import copy
-import os, sys
-import open3d as o3d
-from sys import argv, exit
-from PIL import Image
-import math
-from tqdm import tqdm
-import cv2
-
-
-sys.path.append("../../")
-
-from lib.extractMatchTop import getPerspKeypoints, getPerspKeypointsEnsemble, siftMatching
-import pandas as pd
-
-
-import torch
-from lib.model_test import D2Net
-
-#### Cuda ####
-use_cuda = torch.cuda.is_available()
-device = torch.device('cuda:0' if use_cuda else 'cpu')
-
-#### Argument Parsing ####
-parser = argparse.ArgumentParser(description='RoRD ICP evaluation on a DiverseView dataset sequence.')
-
-parser.add_argument('--dataset', type=str, default='/scratch/udit/realsense/RoRD_data/preprocessed/',
- help='path to the dataset folder')
-
-parser.add_argument('--sequence', type=str, default='data1')
-
-parser.add_argument(
- '--output_dir', type=str, default='out',
- help='output directory for RT estimates'
-)
-
-parser.add_argument(
- '--model_rord', type=str, help='path to the RoRD model for evaluation'
-)
-
-parser.add_argument(
- '--model_d2', type=str, help='path to the vanilla D2-Net model for evaluation'
-)
-
-parser.add_argument(
- '--model_ens', action='store_true',
- help='ensemble model of RoRD + D2-Net'
-)
-
-parser.add_argument(
- '--sift', action='store_true',
- help='Sift'
-)
-
-parser.add_argument(
- '--viz3d', action='store_true',
- help='visualize the pointcloud registrations'
-)
-
-parser.add_argument(
- '--log_interval', type=int, default=9,
- help='Matched image logging interval'
-)
-
-parser.add_argument(
- '--camera_file', type=str, default='../../configs/camera.txt',
- help='path to the camera intrinsics file. In order: focal_x, focal_y, center_x, center_y, scaling_factor.'
-)
-
-parser.add_argument(
- '--persp', action='store_true', default=False,
- help='Feature matching on perspective images.'
-)
-
-parser.set_defaults(fp16=False)
-args = parser.parse_args()
-
-
-if args.model_ens: # Change default paths accordingly for ensemble
- model1_ens = '../../models/rord.pth'
- model2_ens = '../../models/d2net.pth'
-
-def draw_registration_result(source, target, transformation):
- source_temp = copy.deepcopy(source)
- target_temp = copy.deepcopy(target)
- source_temp.transform(transformation)
- trgSph.append(source_temp); trgSph.append(target_temp)
- axis1 = o3d.geometry.TriangleMesh.create_coordinate_frame(size=0.5, origin=[0, 0, 0])
- axis2 = o3d.geometry.TriangleMesh.create_coordinate_frame(size=0.5, origin=[0, 0, 0])
- axis2.transform(transformation)
- trgSph.append(axis1); trgSph.append(axis2)
- o3d.visualization.draw_geometries(trgSph)
-
-def readDepth(depthFile):
- depth = Image.open(depthFile)
- if depth.mode != "I":
- raise Exception("Depth image is not in intensity format")
-
- return np.asarray(depth)
-
-def readCamera(camera):
- with open (camera, "rt") as file:
- contents = file.read().split()
-
- focalX = float(contents[0])
- focalY = float(contents[1])
- centerX = float(contents[2])
- centerY = float(contents[3])
- scalingFactor = float(contents[4])
-
- return focalX, focalY, centerX, centerY, scalingFactor
-
-
-def getPointCloud(rgbFile, depthFile, pts):
- thresh = 15.0
-
- depth = readDepth(depthFile)
- rgb = Image.open(rgbFile)
-
- points = []
- colors = []
-
- corIdx = [-1]*len(pts)
- corPts = [None]*len(pts)
- ptIdx = 0
-
- for v in range(depth.shape[0]):
- for u in range(depth.shape[1]):
- Z = depth[v, u] / scalingFactor
- if Z==0: continue
- if (Z > thresh): continue
-
- X = (u - centerX) * Z / focalX
- Y = (v - centerY) * Z / focalY
-
- points.append((X, Y, Z))
- colors.append(rgb.getpixel((u, v)))
-
- if((u, v) in pts):
- index = pts.index((u, v))
- corIdx[index] = ptIdx
- corPts[index] = (X, Y, Z)
-
- ptIdx = ptIdx+1
-
- points = np.asarray(points)
- colors = np.asarray(colors)
-
- pcd = o3d.geometry.PointCloud()
- pcd.points = o3d.utility.Vector3dVector(points)
- pcd.colors = o3d.utility.Vector3dVector(colors/255)
-
- return pcd, corIdx, corPts
-
-
-def convertPts(A):
- X = A[0]; Y = A[1]
-
- x = []; y = []
-
- for i in range(len(X)):
- x.append(int(float(X[i])))
-
- for i in range(len(Y)):
- y.append(int(float(Y[i])))
-
- pts = []
- for i in range(len(x)):
- pts.append((x[i], y[i]))
-
- return pts
-
-
-def getSphere(pts):
- sphs = []
-
- for element in pts:
- if(element is not None):
- sphere = o3d.geometry.TriangleMesh.create_sphere(radius=0.03)
- sphere.paint_uniform_color([0.9, 0.2, 0])
-
- trans = np.identity(4)
- trans[0, 3] = element[0]
- trans[1, 3] = element[1]
- trans[2, 3] = element[2]
-
- sphere.transform(trans)
- sphs.append(sphere)
-
- return sphs
-
-
-def get3dCor(src, trg):
- corr = []
-
- for sId, tId in zip(src, trg):
- if(sId != -1 and tId != -1):
- corr.append((sId, tId))
-
- corr = np.asarray(corr)
-
- return corr
-
-if __name__ == "__main__":
- camera_file = args.camera_file
- rgb_csv = args.dataset + args.sequence + '/rtImagesRgb.csv'
- depth_csv = args.dataset + args.sequence + '/rtImagesDepth.csv'
-
- os.makedirs(os.path.join(args.output_dir, 'vis'), exist_ok=True)
- dir_name = args.output_dir
- os.makedirs(args.output_dir, exist_ok=True)
-
- focalX, focalY, centerX, centerY, scalingFactor = readCamera(camera_file)
-
- df_rgb = pd.read_csv(rgb_csv)
- df_dep = pd.read_csv(depth_csv)
-
- model1 = D2Net(model_file=args.model_d2).to(device)
- model2 = D2Net(model_file=args.model_rord).to(device)
-
- queryId = 0
- for im_q, dep_q in tqdm(zip(df_rgb['query'], df_dep['query']), total=df_rgb.shape[0]):
- filter_list = []
- dbId = 0
- for im_d, dep_d in tqdm(zip(df_rgb.iteritems(), df_dep.iteritems()), total=df_rgb.shape[1]):
- if im_d[0] == 'query':
- continue
- rgb_name_src = os.path.basename(im_q)
- H_name_src = os.path.splitext(rgb_name_src)[0] + '.npy'
- srcH = args.dataset + args.sequence + '/rgb/' + H_name_src
- rgb_name_trg = os.path.basename(im_d[1][1])
- H_name_trg = os.path.splitext(rgb_name_trg)[0] + '.npy'
- trgH = args.dataset + args.sequence + '/rgb/' + H_name_trg
-
- srcImg = srcH.replace('.npy', '.jpg')
- trgImg = trgH.replace('.npy', '.jpg')
-
- if args.model_rord:
- if args.persp:
- srcPts, trgPts, matchImg, _ = getPerspKeypoints(srcImg, trgImg, HFile1=None, HFile2=None, model=model2, device=device)
- else:
- srcPts, trgPts, matchImg, _ = getPerspKeypoints(srcImg, trgImg, srcH, trgH, model2, device)
-
- elif args.model_d2:
- if args.persp:
- srcPts, trgPts, matchImg, _ = getPerspKeypoints(srcImg, trgImg, HFile1=None, HFile2=None, model=model2, device=device)
- else:
- srcPts, trgPts, matchImg, _ = getPerspKeypoints(srcImg, trgImg, srcH, trgH, model1, device)
-
- elif args.model_ens:
- model1 = D2Net(model_file=model1_ens)
- model1 = model1.to(device)
- model2 = D2Net(model_file=model2_ens)
- model2 = model2.to(device)
- srcPts, trgPts, matchImg = getPerspKeypointsEnsemble(model1, model2, srcImg, trgImg, srcH, trgH, device)
-
- elif args.sift:
- if args.persp:
- srcPts, trgPts, matchImg, _ = siftMatching(srcImg, trgImg, HFile1=None, HFile2=None, device=device)
- else:
- srcPts, trgPts, matchImg, _ = siftMatching(srcImg, trgImg, srcH, trgH, device)
-
- if(isinstance(srcPts, list) == True):
- print(np.identity(4))
- filter_list.append(np.identity(4))
- continue
-
-
- srcPts = convertPts(srcPts)
- trgPts = convertPts(trgPts)
-
- depth_name_src = os.path.dirname(os.path.dirname(args.dataset)) + '/' + dep_q
- depth_name_trg = os.path.dirname(os.path.dirname(args.dataset)) + '/' + dep_d[1][1]
-
- srcCld, srcIdx, srcCor = getPointCloud(srcImg, depth_name_src, srcPts)
- trgCld, trgIdx, trgCor = getPointCloud(trgImg, depth_name_trg, trgPts)
-
- srcSph = getSphere(srcCor)
- trgSph = getSphere(trgCor)
- axis = o3d.geometry.TriangleMesh.create_coordinate_frame(size=0.5, origin=[0, 0, 0])
- srcSph.append(srcCld); srcSph.append(axis)
- trgSph.append(trgCld); trgSph.append(axis)
-
- corr = get3dCor(srcIdx, trgIdx)
-
- p2p = o3d.pipelines.registration.TransformationEstimationPointToPoint()
- trans_init = p2p.compute_transformation(srcCld, trgCld, o3d.utility.Vector2iVector(corr))
- # print(trans_init)
- filter_list.append(trans_init)
-
- if args.viz3d:
- o3d.visualization.draw_geometries(srcSph)
- o3d.visualization.draw_geometries(trgSph)
- draw_registration_result(srcCld, trgCld, trans_init)
-
- if(dbId%args.log_interval == 0):
- cv2.imwrite(os.path.join(args.output_dir, 'vis') + "/matchImg.%02d.%02d.jpg"%(queryId, dbId//args.log_interval), matchImg)
- dbId += 1
-
-
- RT = np.stack(filter_list).transpose(1,2,0)
-
- np.save(os.path.join(dir_name, str(queryId) + '.npy'), RT)
- queryId += 1
- print('-----check-------', RT.shape)
diff --git a/spaces/Riksarkivet/htr_demo/src/htr_pipeline/utils/helper.py b/spaces/Riksarkivet/htr_demo/src/htr_pipeline/utils/helper.py
deleted file mode 100644
index 3b36ca96f92297e46c25684bf86f3b706f6505bc..0000000000000000000000000000000000000000
--- a/spaces/Riksarkivet/htr_demo/src/htr_pipeline/utils/helper.py
+++ /dev/null
@@ -1,107 +0,0 @@
-import functools
-import threading
-import time
-from functools import wraps
-
-import gradio as gr
-import tqdm
-
-
-def timer_func(func):
- # This function shows the execution time of
- # the function object passed
- def wrap_func(*args, **kwargs):
- t1 = time.time()
- result = func(*args, **kwargs)
- t2 = time.time()
- print(f"Function {func.__name__!r} executed in {(t2-t1):.4f}s")
- return result
-
- return wrap_func
-
-
-def long_running_function(*args, **kwargs):
- # print("Running with args:%s and kwargs:%s" % (args, kwargs))
- time.sleep(5)
- return "success"
-
-
-def provide_progress_bar(function, estimated_time, tstep=0.2, tqdm_kwargs={}, args=[], kwargs={}):
- """Tqdm wrapper for a long-running function
-
- args:
- function - function to run
- estimated_time - how long you expect the function to take
- tstep - time delta (seconds) for progress bar updates
- tqdm_kwargs - kwargs to construct the progress bar
- args - args to pass to the function
- kwargs - keyword args to pass to the function
- ret:
- function(*args, **kwargs)
- """
- ret = [None] # Mutable var so the function can store its return value
-
- def myrunner(function, ret, *args, **kwargs):
- ret[0] = function(*args, **kwargs)
-
- thread = threading.Thread(target=myrunner, args=(function, ret) + tuple(args), kwargs=kwargs)
- pbar = tqdm.tqdm(total=estimated_time, **tqdm_kwargs)
-
- thread.start()
- while thread.is_alive():
- thread.join(timeout=tstep)
- pbar.update(tstep)
- pbar.close()
- return ret[0]
-
-
-def progress_wrapped(estimated_time, tstep=0.2, tqdm_kwargs={}):
- """Decorate a function to add a progress bar"""
-
- def real_decorator(function):
- @functools.wraps(function)
- def wrapper(*args, **kwargs):
- return provide_progress_bar(
- function, estimated_time=estimated_time, tstep=tstep, tqdm_kwargs=tqdm_kwargs, args=args, kwargs=kwargs
- )
-
- return wrapper
-
- return real_decorator
-
-
-@progress_wrapped(estimated_time=5)
-def another_long_running_function(*args, **kwargs):
- # print("Running with args:%s and kwargs:%s" % (args, kwargs))
- time.sleep(5)
- return "success"
-
-
-# Decorator for logging
-def gradio_info(message):
- def decorator(func):
- @wraps(func)
- def wrapper(*args, **kwargs):
- gr.Info(message)
- return func(*args, **kwargs)
-
- return wrapper
-
- return decorator
-
-
-if __name__ == "__main__":
- # Basic example
- retval = provide_progress_bar(long_running_function, estimated_time=5)
- print(retval)
-
- # Full example
- retval = provide_progress_bar(
- long_running_function,
- estimated_time=5,
- tstep=1 / 5.0,
- tqdm_kwargs={"bar_format": "{desc}: {percentage:3.0f}%|{bar}| {n:.1f}/{total:.1f} [{elapsed}<{remaining}]"},
- args=(1, "foo"),
- kwargs={"spam": "eggs"},
- )
- print(retval)
diff --git a/spaces/Robert001/UniControl-Demo/annotator/uniformer_base/mmcv/visualization/optflow.py b/spaces/Robert001/UniControl-Demo/annotator/uniformer_base/mmcv/visualization/optflow.py
deleted file mode 100644
index c3870c700f7c946177ee5d536ce3f6c814a77ce7..0000000000000000000000000000000000000000
--- a/spaces/Robert001/UniControl-Demo/annotator/uniformer_base/mmcv/visualization/optflow.py
+++ /dev/null
@@ -1,112 +0,0 @@
-# Copyright (c) OpenMMLab. All rights reserved.
-from __future__ import division
-
-import numpy as np
-
-from annotator.uniformer.mmcv.image import rgb2bgr
-from annotator.uniformer.mmcv.video import flowread
-from .image import imshow
-
-
-def flowshow(flow, win_name='', wait_time=0):
- """Show optical flow.
-
- Args:
- flow (ndarray or str): The optical flow to be displayed.
- win_name (str): The window name.
- wait_time (int): Value of waitKey param.
- """
- flow = flowread(flow)
- flow_img = flow2rgb(flow)
- imshow(rgb2bgr(flow_img), win_name, wait_time)
-
-
-def flow2rgb(flow, color_wheel=None, unknown_thr=1e6):
- """Convert flow map to RGB image.
-
- Args:
- flow (ndarray): Array of optical flow.
- color_wheel (ndarray or None): Color wheel used to map flow field to
- RGB colorspace. Default color wheel will be used if not specified.
- unknown_thr (str): Values above this threshold will be marked as
- unknown and thus ignored.
-
- Returns:
- ndarray: RGB image that can be visualized.
- """
- assert flow.ndim == 3 and flow.shape[-1] == 2
- if color_wheel is None:
- color_wheel = make_color_wheel()
- assert color_wheel.ndim == 2 and color_wheel.shape[1] == 3
- num_bins = color_wheel.shape[0]
-
- dx = flow[:, :, 0].copy()
- dy = flow[:, :, 1].copy()
-
- ignore_inds = (
- np.isnan(dx) | np.isnan(dy) | (np.abs(dx) > unknown_thr) |
- (np.abs(dy) > unknown_thr))
- dx[ignore_inds] = 0
- dy[ignore_inds] = 0
-
- rad = np.sqrt(dx**2 + dy**2)
- if np.any(rad > np.finfo(float).eps):
- max_rad = np.max(rad)
- dx /= max_rad
- dy /= max_rad
-
- rad = np.sqrt(dx**2 + dy**2)
- angle = np.arctan2(-dy, -dx) / np.pi
-
- bin_real = (angle + 1) / 2 * (num_bins - 1)
- bin_left = np.floor(bin_real).astype(int)
- bin_right = (bin_left + 1) % num_bins
- w = (bin_real - bin_left.astype(np.float32))[..., None]
- flow_img = (1 -
- w) * color_wheel[bin_left, :] + w * color_wheel[bin_right, :]
- small_ind = rad <= 1
- flow_img[small_ind] = 1 - rad[small_ind, None] * (1 - flow_img[small_ind])
- flow_img[np.logical_not(small_ind)] *= 0.75
-
- flow_img[ignore_inds, :] = 0
-
- return flow_img
-
-
-def make_color_wheel(bins=None):
- """Build a color wheel.
-
- Args:
- bins(list or tuple, optional): Specify the number of bins for each
- color range, corresponding to six ranges: red -> yellow,
- yellow -> green, green -> cyan, cyan -> blue, blue -> magenta,
- magenta -> red. [15, 6, 4, 11, 13, 6] is used for default
- (see Middlebury).
-
- Returns:
- ndarray: Color wheel of shape (total_bins, 3).
- """
- if bins is None:
- bins = [15, 6, 4, 11, 13, 6]
- assert len(bins) == 6
-
- RY, YG, GC, CB, BM, MR = tuple(bins)
-
- ry = [1, np.arange(RY) / RY, 0]
- yg = [1 - np.arange(YG) / YG, 1, 0]
- gc = [0, 1, np.arange(GC) / GC]
- cb = [0, 1 - np.arange(CB) / CB, 1]
- bm = [np.arange(BM) / BM, 0, 1]
- mr = [1, 0, 1 - np.arange(MR) / MR]
-
- num_bins = RY + YG + GC + CB + BM + MR
-
- color_wheel = np.zeros((3, num_bins), dtype=np.float32)
-
- col = 0
- for i, color in enumerate([ry, yg, gc, cb, bm, mr]):
- for j in range(3):
- color_wheel[j, col:col + bins[i]] = color[j]
- col += bins[i]
-
- return color_wheel.T
diff --git a/spaces/Rongjiehuang/GenerSpeech/modules/commons/espnet_positional_embedding.py b/spaces/Rongjiehuang/GenerSpeech/modules/commons/espnet_positional_embedding.py
deleted file mode 100644
index 74decb6ab300951490ae08a4b93041a0542b5bb7..0000000000000000000000000000000000000000
--- a/spaces/Rongjiehuang/GenerSpeech/modules/commons/espnet_positional_embedding.py
+++ /dev/null
@@ -1,113 +0,0 @@
-import math
-import torch
-
-
-class PositionalEncoding(torch.nn.Module):
- """Positional encoding.
- Args:
- d_model (int): Embedding dimension.
- dropout_rate (float): Dropout rate.
- max_len (int): Maximum input length.
- reverse (bool): Whether to reverse the input position.
- """
-
- def __init__(self, d_model, dropout_rate, max_len=5000, reverse=False):
- """Construct an PositionalEncoding object."""
- super(PositionalEncoding, self).__init__()
- self.d_model = d_model
- self.reverse = reverse
- self.xscale = math.sqrt(self.d_model)
- self.dropout = torch.nn.Dropout(p=dropout_rate)
- self.pe = None
- self.extend_pe(torch.tensor(0.0).expand(1, max_len))
-
- def extend_pe(self, x):
- """Reset the positional encodings."""
- if self.pe is not None:
- if self.pe.size(1) >= x.size(1):
- if self.pe.dtype != x.dtype or self.pe.device != x.device:
- self.pe = self.pe.to(dtype=x.dtype, device=x.device)
- return
- pe = torch.zeros(x.size(1), self.d_model)
- if self.reverse:
- position = torch.arange(
- x.size(1) - 1, -1, -1.0, dtype=torch.float32
- ).unsqueeze(1)
- else:
- position = torch.arange(0, x.size(1), dtype=torch.float32).unsqueeze(1)
- div_term = torch.exp(
- torch.arange(0, self.d_model, 2, dtype=torch.float32)
- * -(math.log(10000.0) / self.d_model)
- )
- pe[:, 0::2] = torch.sin(position * div_term)
- pe[:, 1::2] = torch.cos(position * div_term)
- pe = pe.unsqueeze(0)
- self.pe = pe.to(device=x.device, dtype=x.dtype)
-
- def forward(self, x: torch.Tensor):
- """Add positional encoding.
- Args:
- x (torch.Tensor): Input tensor (batch, time, `*`).
- Returns:
- torch.Tensor: Encoded tensor (batch, time, `*`).
- """
- self.extend_pe(x)
- x = x * self.xscale + self.pe[:, : x.size(1)]
- return self.dropout(x)
-
-
-class ScaledPositionalEncoding(PositionalEncoding):
- """Scaled positional encoding module.
- See Sec. 3.2 https://arxiv.org/abs/1809.08895
- Args:
- d_model (int): Embedding dimension.
- dropout_rate (float): Dropout rate.
- max_len (int): Maximum input length.
- """
-
- def __init__(self, d_model, dropout_rate, max_len=5000):
- """Initialize class."""
- super().__init__(d_model=d_model, dropout_rate=dropout_rate, max_len=max_len)
- self.alpha = torch.nn.Parameter(torch.tensor(1.0))
-
- def reset_parameters(self):
- """Reset parameters."""
- self.alpha.data = torch.tensor(1.0)
-
- def forward(self, x):
- """Add positional encoding.
- Args:
- x (torch.Tensor): Input tensor (batch, time, `*`).
- Returns:
- torch.Tensor: Encoded tensor (batch, time, `*`).
- """
- self.extend_pe(x)
- x = x + self.alpha * self.pe[:, : x.size(1)]
- return self.dropout(x)
-
-
-class RelPositionalEncoding(PositionalEncoding):
- """Relative positional encoding module.
- See : Appendix B in https://arxiv.org/abs/1901.02860
- Args:
- d_model (int): Embedding dimension.
- dropout_rate (float): Dropout rate.
- max_len (int): Maximum input length.
- """
-
- def __init__(self, d_model, dropout_rate, max_len=5000):
- """Initialize class."""
- super().__init__(d_model, dropout_rate, max_len, reverse=True)
-
- def forward(self, x):
- """Compute positional encoding.
- Args:
- x (torch.Tensor): Input tensor (batch, time, `*`).
- Returns:
- torch.Tensor: Encoded tensor (batch, time, `*`).
- torch.Tensor: Positional embedding tensor (1, time, `*`).
- """
- self.extend_pe(x)
- x = x * self.xscale
- pos_emb = self.pe[:, : x.size(1)]
- return self.dropout(x) + self.dropout(pos_emb)
\ No newline at end of file
diff --git a/spaces/RugNlpFlashcards/Speech_Language_Processing_Jurafsky_Martin/main.py b/spaces/RugNlpFlashcards/Speech_Language_Processing_Jurafsky_Martin/main.py
deleted file mode 100644
index 6cf6230f9ea0547b7e6ac0263d5723bc7ac90478..0000000000000000000000000000000000000000
--- a/spaces/RugNlpFlashcards/Speech_Language_Processing_Jurafsky_Martin/main.py
+++ /dev/null
@@ -1,127 +0,0 @@
-from collections import namedtuple
-from pprint import pprint
-from dotenv import load_dotenv
-# needs to happen as very first thing, otherwise HF ignores env vars
-load_dotenv()
-
-import os
-import pandas as pd
-
-from dataclasses import dataclass, field
-from typing import Dict, cast, List
-from datasets import DatasetDict, load_dataset
-
-from src.readers.base_reader import Reader
-from src.evaluation import evaluate
-from src.readers.dpr_reader import DprReader
-from src.readers.longformer_reader import LongformerReader
-from src.retrievers.base_retriever import Retriever
-from src.retrievers.es_retriever import ESRetriever
-from src.retrievers.faiss_retriever import (
- FaissRetriever,
- FaissRetrieverOptions
-)
-from src.utils.log import logger
-from src.utils.preprocessing import context_to_reader_input
-from src.utils.timing import get_times, timeit
-
-
-ExperimentResult = namedtuple('ExperimentResult', ['correct', 'given'])
-
-
-@dataclass
-class Experiment:
- retriever: Retriever
- reader: Reader
- lm: str
- results: List[ExperimentResult] = field(default_factory=list)
-
-
-if __name__ == '__main__':
- dataset_name = "GroNLP/ik-nlp-22_slp"
- paragraphs = cast(DatasetDict, load_dataset(
- "GroNLP/ik-nlp-22_slp", "paragraphs"))
- questions = cast(DatasetDict, load_dataset(dataset_name, "questions"))
-
- # Only doing a few questions for speed
- subset_idx = len(questions["test"])
- questions_test = questions["test"][:subset_idx]
-
- experiments: Dict[str, Experiment] = {
- "faiss_dpr": Experiment(
- retriever=FaissRetriever(
- paragraphs,
- FaissRetrieverOptions.dpr("./src/models/dpr.faiss")),
- reader=DprReader(),
- lm="dpr"
- ),
- "faiss_longformer": Experiment(
- retriever=FaissRetriever(
- paragraphs,
- FaissRetrieverOptions.longformer("./src/models/longformer.faiss")),
- reader=LongformerReader(),
- lm="longformer"
- ),
- "es_dpr": Experiment(
- retriever=ESRetriever(paragraphs),
- reader=DprReader(),
- lm="dpr"
- ),
- "es_longformer": Experiment(
- retriever=ESRetriever(paragraphs),
- reader=LongformerReader(),
- lm="longformer"
- ),
- }
-
- for experiment_name, experiment in experiments.items():
- logger.info(f"Running experiment {experiment_name}...")
- for idx in range(subset_idx):
- question = questions_test["question"][idx]
- answer = questions_test["answer"][idx]
-
- # workaround so we can use the decorator with a dynamic name for
- # time recording
- retrieve_timer = timeit(f"{experiment_name}.retrieve")
- t_retrieve = retrieve_timer(experiment.retriever.retrieve)
-
- read_timer = timeit(f"{experiment_name}.read")
- t_read = read_timer(experiment.reader.read)
-
- print(f"\x1b[1K\r[{idx+1:03}] - \"{question}\"", end='')
-
- scores, context = t_retrieve(question, 5)
- reader_input = context_to_reader_input(context)
-
- # Requesting 1 answers results in us getting the best answer
- given_answer = t_read(question, reader_input, 1)[0]
-
- # Save the results so we can evaluate laters
- if experiment.lm == "longformer":
- experiment.results.append(
- ExperimentResult(answer, given_answer[0]))
- else:
- experiment.results.append(
- ExperimentResult(answer, given_answer.text))
-
- print()
-
- if os.getenv("ENABLE_TIMING", "false").lower() == "true":
- # Save times
- times = get_times()
- df = pd.DataFrame(times)
- os.makedirs("./results/", exist_ok=True)
- df.to_csv("./results/timings.csv")
-
- f1_results = pd.DataFrame(columns=experiments.keys())
- em_results = pd.DataFrame(columns=experiments.keys())
- for experiment_name, experiment in experiments.items():
- em, f1 = zip(*list(map(
- lambda r: evaluate(r.correct, r.given), experiment.results
- )))
- em_results[experiment_name] = em
- f1_results[experiment_name] = f1
-
- os.makedirs("./results/", exist_ok=True)
- f1_results.to_csv("./results/f1_scores.csv")
- em_results.to_csv("./results/em_scores.csv")
diff --git a/spaces/Rvtcheeto/Test02/README.md b/spaces/Rvtcheeto/Test02/README.md
deleted file mode 100644
index 3ba275ff9cf78da55e5d68c8950d0b8a2699bad7..0000000000000000000000000000000000000000
--- a/spaces/Rvtcheeto/Test02/README.md
+++ /dev/null
@@ -1,10 +0,0 @@
----
-title: Test02
-emoji: 🏢
-colorFrom: pink
-colorTo: purple
-sdk: docker
-pinned: false
----
-
-Check out the configuration reference at https://huggingface.co/docs/hub/spaces-config-reference
diff --git a/spaces/SashaKerbel/HandwritingClassifier/README.md b/spaces/SashaKerbel/HandwritingClassifier/README.md
deleted file mode 100644
index b2f6c0fe8b6cb614687308efe7cb884f97195360..0000000000000000000000000000000000000000
--- a/spaces/SashaKerbel/HandwritingClassifier/README.md
+++ /dev/null
@@ -1,13 +0,0 @@
----
-title: HandwritingClassifier
-emoji: 🦀
-colorFrom: gray
-colorTo: yellow
-sdk: gradio
-sdk_version: 3.17.0
-app_file: app.py
-pinned: false
-license: other
----
-
-Check out the configuration reference at https://huggingface.co/docs/hub/spaces-config-reference
diff --git a/spaces/SeViLA/SeViLA/lavis/models/albef_models/albef_outputs.py b/spaces/SeViLA/SeViLA/lavis/models/albef_models/albef_outputs.py
deleted file mode 100644
index a3f73f39cf175319aa095cb24f30e9496f305a74..0000000000000000000000000000000000000000
--- a/spaces/SeViLA/SeViLA/lavis/models/albef_models/albef_outputs.py
+++ /dev/null
@@ -1,97 +0,0 @@
-"""
- Copyright (c) 2022, salesforce.com, inc.
- All rights reserved.
- SPDX-License-Identifier: BSD-3-Clause
- For full license text, see the LICENSE file in the repo root or https://opensource.org/licenses/BSD-3-Clause
-"""
-
-from dataclasses import dataclass
-from typing import Optional
-
-import torch
-from transformers.modeling_outputs import (
- BaseModelOutputWithPoolingAndCrossAttentions,
- CausalLMOutputWithCrossAttentions,
- ModelOutput,
-)
-
-
-@dataclass
-class AlbefSimilarity(ModelOutput):
- sim_i2t: torch.FloatTensor = None
- sim_t2i: torch.FloatTensor = None
-
- sim_i2t_m: Optional[torch.FloatTensor] = None
- sim_t2i_m: Optional[torch.FloatTensor] = None
-
- sim_i2t_targets: Optional[torch.FloatTensor] = None
- sim_t2i_targets: Optional[torch.FloatTensor] = None
-
-
-@dataclass
-class AlbefIntermediateOutput(ModelOutput):
- # uni-modal features
- image_embeds: torch.FloatTensor = None
- text_embeds: Optional[torch.FloatTensor] = None
-
- image_embeds_m: Optional[torch.FloatTensor] = None
- text_embeds_m: Optional[torch.FloatTensor] = None
-
- # intermediate outputs of multimodal encoder
- encoder_output: Optional[BaseModelOutputWithPoolingAndCrossAttentions] = None
- encoder_output_m: Optional[BaseModelOutputWithPoolingAndCrossAttentions] = None
- encoder_output_neg: Optional[BaseModelOutputWithPoolingAndCrossAttentions] = None
-
- itm_logits: Optional[torch.FloatTensor] = None
- itm_labels: Optional[torch.LongTensor] = None
-
- # intermediate outputs of multimodal decoder
- decoder_output: Optional[CausalLMOutputWithCrossAttentions] = None
- decoder_labels: Optional[torch.LongTensor] = None
-
-
-@dataclass
-class AlbefOutput(ModelOutput):
- # some finetuned models (e.g. BlipVQA) do not compute similarity, thus optional.
- sims: Optional[AlbefSimilarity] = None
-
- intermediate_output: AlbefIntermediateOutput = None
-
- loss: Optional[torch.FloatTensor] = None
-
- loss_itc: Optional[torch.FloatTensor] = None
-
- loss_itm: Optional[torch.FloatTensor] = None
-
- loss_mlm: Optional[torch.FloatTensor] = None
-
-
-@dataclass
-class AlbefOutputWithLogits(AlbefOutput):
- logits: torch.FloatTensor = None
- logits_m: torch.FloatTensor = None
-
-
-@dataclass
-class AlbefOutputFeatures(ModelOutput):
- """
- Data class of features from AlbefFeatureExtractor.
-
- Args:
- image_embeds: `torch.FloatTensor` of shape `(batch_size, num_patches+1, embed_dim)`, `optional`
- image_features: `torch.FloatTensor` of shape `(batch_size, num_patches+1, feature_dim)`, `optional`
- text_embeds: `torch.FloatTensor` of shape `(batch_size, sequence_length+1, embed_dim)`, `optional`
- text_features: `torch.FloatTensor` of shape `(batch_size, sequence_length+1, feature_dim)`, `optional`
-
- The first embedding or feature is for the [CLS] token.
-
- Features are obtained by projecting the corresponding embedding into a normalized low-dimensional space.
- """
-
- image_embeds: Optional[torch.FloatTensor] = None
- image_embeds_proj: Optional[torch.FloatTensor] = None
-
- text_embeds: Optional[torch.FloatTensor] = None
- text_embeds_proj: Optional[torch.FloatTensor] = None
-
- multimodal_embeds: Optional[torch.FloatTensor] = None
diff --git a/spaces/Sky5408er/anime-remove-background/README.md b/spaces/Sky5408er/anime-remove-background/README.md
deleted file mode 100644
index 1ba3cb5ea0e994e246d57b7d62b8aa5a6331901c..0000000000000000000000000000000000000000
--- a/spaces/Sky5408er/anime-remove-background/README.md
+++ /dev/null
@@ -1,14 +0,0 @@
----
-title: Anime Remove Background
-emoji: 🪄🖼️
-colorFrom: indigo
-colorTo: pink
-sdk: gradio
-sdk_version: 3.1.4
-app_file: app.py
-pinned: false
-license: apache-2.0
-duplicated_from: skytnt/anime-remove-background
----
-
-Check out the configuration reference at https://huggingface.co/docs/hub/spaces-config-reference
diff --git a/spaces/Slep/CondViT-LRVSF-Demo/src/process_images.py b/spaces/Slep/CondViT-LRVSF-Demo/src/process_images.py
deleted file mode 100644
index 7a018b682cc0aac77e97fcfd70cfb734fa635062..0000000000000000000000000000000000000000
--- a/spaces/Slep/CondViT-LRVSF-Demo/src/process_images.py
+++ /dev/null
@@ -1,15 +0,0 @@
-from PIL import Image
-from io import BytesIO
-import base64
-
-# Index to PIL
-def process_img(idx, ds):
- img = Image.open(BytesIO(ds.iloc[idx].jpg)).convert("RGB")
- return img
-
-def make_img_html(img):
- b = BytesIO()
- img.save(b, format='PNG')
- buffer = b.getvalue()
-
- return f''
\ No newline at end of file
diff --git a/spaces/SungBeom/chatwine-korean/.venv/Lib/site-packages/PIL/GribStubImagePlugin.py b/spaces/SungBeom/chatwine-korean/.venv/Lib/site-packages/PIL/GribStubImagePlugin.py
deleted file mode 100644
index 8a799f19caac706a880218af257f40e9a386b489..0000000000000000000000000000000000000000
--- a/spaces/SungBeom/chatwine-korean/.venv/Lib/site-packages/PIL/GribStubImagePlugin.py
+++ /dev/null
@@ -1,73 +0,0 @@
-#
-# The Python Imaging Library
-# $Id$
-#
-# GRIB stub adapter
-#
-# Copyright (c) 1996-2003 by Fredrik Lundh
-#
-# See the README file for information on usage and redistribution.
-#
-
-from . import Image, ImageFile
-
-_handler = None
-
-
-def register_handler(handler):
- """
- Install application-specific GRIB image handler.
-
- :param handler: Handler object.
- """
- global _handler
- _handler = handler
-
-
-# --------------------------------------------------------------------
-# Image adapter
-
-
-def _accept(prefix):
- return prefix[:4] == b"GRIB" and prefix[7] == 1
-
-
-class GribStubImageFile(ImageFile.StubImageFile):
- format = "GRIB"
- format_description = "GRIB"
-
- def _open(self):
- offset = self.fp.tell()
-
- if not _accept(self.fp.read(8)):
- msg = "Not a GRIB file"
- raise SyntaxError(msg)
-
- self.fp.seek(offset)
-
- # make something up
- self.mode = "F"
- self._size = 1, 1
-
- loader = self._load()
- if loader:
- loader.open(self)
-
- def _load(self):
- return _handler
-
-
-def _save(im, fp, filename):
- if _handler is None or not hasattr(_handler, "save"):
- msg = "GRIB save handler not installed"
- raise OSError(msg)
- _handler.save(im, fp, filename)
-
-
-# --------------------------------------------------------------------
-# Registry
-
-Image.register_open(GribStubImageFile.format, GribStubImageFile, _accept)
-Image.register_save(GribStubImageFile.format, _save)
-
-Image.register_extension(GribStubImageFile.format, ".grib")
diff --git a/spaces/SungBeom/chatwine-korean/.venv/Lib/site-packages/altair/utils/core.py b/spaces/SungBeom/chatwine-korean/.venv/Lib/site-packages/altair/utils/core.py
deleted file mode 100644
index 8ecaa896b5051811798ae9db01bbf85673af3dbc..0000000000000000000000000000000000000000
--- a/spaces/SungBeom/chatwine-korean/.venv/Lib/site-packages/altair/utils/core.py
+++ /dev/null
@@ -1,733 +0,0 @@
-"""
-Utility routines
-"""
-from collections.abc import Mapping
-from copy import deepcopy
-import json
-import itertools
-import re
-import sys
-import traceback
-import warnings
-from typing import Callable, TypeVar, Any
-
-import jsonschema
-import pandas as pd
-import numpy as np
-
-from altair.utils.schemapi import SchemaBase
-
-if sys.version_info >= (3, 10):
- from typing import ParamSpec
-else:
- from typing_extensions import ParamSpec
-
-try:
- from pandas.api.types import infer_dtype as _infer_dtype
-except ImportError:
- # Import for pandas < 0.20.0
- from pandas.lib import infer_dtype as _infer_dtype # type: ignore[no-redef]
-
-_V = TypeVar("_V")
-_P = ParamSpec("_P")
-
-
-def infer_dtype(value):
- """Infer the dtype of the value.
-
- This is a compatibility function for pandas infer_dtype,
- with skipna=False regardless of the pandas version.
- """
- if not hasattr(infer_dtype, "_supports_skipna"):
- try:
- _infer_dtype([1], skipna=False)
- except TypeError:
- # pandas < 0.21.0 don't support skipna keyword
- infer_dtype._supports_skipna = False
- else:
- infer_dtype._supports_skipna = True
- if infer_dtype._supports_skipna:
- return _infer_dtype(value, skipna=False)
- else:
- return _infer_dtype(value)
-
-
-TYPECODE_MAP = {
- "ordinal": "O",
- "nominal": "N",
- "quantitative": "Q",
- "temporal": "T",
- "geojson": "G",
-}
-
-INV_TYPECODE_MAP = {v: k for k, v in TYPECODE_MAP.items()}
-
-
-# aggregates from vega-lite version 4.6.0
-AGGREGATES = [
- "argmax",
- "argmin",
- "average",
- "count",
- "distinct",
- "max",
- "mean",
- "median",
- "min",
- "missing",
- "product",
- "q1",
- "q3",
- "ci0",
- "ci1",
- "stderr",
- "stdev",
- "stdevp",
- "sum",
- "valid",
- "values",
- "variance",
- "variancep",
-]
-
-# window aggregates from vega-lite version 4.6.0
-WINDOW_AGGREGATES = [
- "row_number",
- "rank",
- "dense_rank",
- "percent_rank",
- "cume_dist",
- "ntile",
- "lag",
- "lead",
- "first_value",
- "last_value",
- "nth_value",
-]
-
-# timeUnits from vega-lite version 4.17.0
-TIMEUNITS = [
- "year",
- "quarter",
- "month",
- "week",
- "day",
- "dayofyear",
- "date",
- "hours",
- "minutes",
- "seconds",
- "milliseconds",
- "yearquarter",
- "yearquartermonth",
- "yearmonth",
- "yearmonthdate",
- "yearmonthdatehours",
- "yearmonthdatehoursminutes",
- "yearmonthdatehoursminutesseconds",
- "yearweek",
- "yearweekday",
- "yearweekdayhours",
- "yearweekdayhoursminutes",
- "yearweekdayhoursminutesseconds",
- "yeardayofyear",
- "quartermonth",
- "monthdate",
- "monthdatehours",
- "monthdatehoursminutes",
- "monthdatehoursminutesseconds",
- "weekday",
- "weeksdayhours",
- "weekdayhoursminutes",
- "weekdayhoursminutesseconds",
- "dayhours",
- "dayhoursminutes",
- "dayhoursminutesseconds",
- "hoursminutes",
- "hoursminutesseconds",
- "minutesseconds",
- "secondsmilliseconds",
- "utcyear",
- "utcquarter",
- "utcmonth",
- "utcweek",
- "utcday",
- "utcdayofyear",
- "utcdate",
- "utchours",
- "utcminutes",
- "utcseconds",
- "utcmilliseconds",
- "utcyearquarter",
- "utcyearquartermonth",
- "utcyearmonth",
- "utcyearmonthdate",
- "utcyearmonthdatehours",
- "utcyearmonthdatehoursminutes",
- "utcyearmonthdatehoursminutesseconds",
- "utcyearweek",
- "utcyearweekday",
- "utcyearweekdayhours",
- "utcyearweekdayhoursminutes",
- "utcyearweekdayhoursminutesseconds",
- "utcyeardayofyear",
- "utcquartermonth",
- "utcmonthdate",
- "utcmonthdatehours",
- "utcmonthdatehoursminutes",
- "utcmonthdatehoursminutesseconds",
- "utcweekday",
- "utcweeksdayhours",
- "utcweekdayhoursminutes",
- "utcweekdayhoursminutesseconds",
- "utcdayhours",
- "utcdayhoursminutes",
- "utcdayhoursminutesseconds",
- "utchoursminutes",
- "utchoursminutesseconds",
- "utcminutesseconds",
- "utcsecondsmilliseconds",
-]
-
-
-def infer_vegalite_type(data):
- """
- From an array-like input, infer the correct vega typecode
- ('ordinal', 'nominal', 'quantitative', or 'temporal')
-
- Parameters
- ----------
- data: Numpy array or Pandas Series
- """
- # Otherwise, infer based on the dtype of the input
- typ = infer_dtype(data)
-
- if typ in [
- "floating",
- "mixed-integer-float",
- "integer",
- "mixed-integer",
- "complex",
- ]:
- return "quantitative"
- elif typ == "categorical" and data.cat.ordered:
- return ("ordinal", data.cat.categories.tolist())
- elif typ in ["string", "bytes", "categorical", "boolean", "mixed", "unicode"]:
- return "nominal"
- elif typ in [
- "datetime",
- "datetime64",
- "timedelta",
- "timedelta64",
- "date",
- "time",
- "period",
- ]:
- return "temporal"
- else:
- warnings.warn(
- "I don't know how to infer vegalite type from '{}'. "
- "Defaulting to nominal.".format(typ),
- stacklevel=1,
- )
- return "nominal"
-
-
-def merge_props_geom(feat):
- """
- Merge properties with geometry
- * Overwrites 'type' and 'geometry' entries if existing
- """
-
- geom = {k: feat[k] for k in ("type", "geometry")}
- try:
- feat["properties"].update(geom)
- props_geom = feat["properties"]
- except (AttributeError, KeyError):
- # AttributeError when 'properties' equals None
- # KeyError when 'properties' is non-existing
- props_geom = geom
-
- return props_geom
-
-
-def sanitize_geo_interface(geo):
- """Santize a geo_interface to prepare it for serialization.
-
- * Make a copy
- * Convert type array or _Array to list
- * Convert tuples to lists (using json.loads/dumps)
- * Merge properties with geometry
- """
-
- geo = deepcopy(geo)
-
- # convert type _Array or array to list
- for key in geo.keys():
- if str(type(geo[key]).__name__).startswith(("_Array", "array")):
- geo[key] = geo[key].tolist()
-
- # convert (nested) tuples to lists
- geo = json.loads(json.dumps(geo))
-
- # sanitize features
- if geo["type"] == "FeatureCollection":
- geo = geo["features"]
- if len(geo) > 0:
- for idx, feat in enumerate(geo):
- geo[idx] = merge_props_geom(feat)
- elif geo["type"] == "Feature":
- geo = merge_props_geom(geo)
- else:
- geo = {"type": "Feature", "geometry": geo}
-
- return geo
-
-
-def sanitize_dataframe(df): # noqa: C901
- """Sanitize a DataFrame to prepare it for serialization.
-
- * Make a copy
- * Convert RangeIndex columns to strings
- * Raise ValueError if column names are not strings
- * Raise ValueError if it has a hierarchical index.
- * Convert categoricals to strings.
- * Convert np.bool_ dtypes to Python bool objects
- * Convert np.int dtypes to Python int objects
- * Convert floats to objects and replace NaNs/infs with None.
- * Convert DateTime dtypes into appropriate string representations
- * Convert Nullable integers to objects and replace NaN with None
- * Convert Nullable boolean to objects and replace NaN with None
- * convert dedicated string column to objects and replace NaN with None
- * Raise a ValueError for TimeDelta dtypes
- """
- df = df.copy()
-
- if isinstance(df.columns, pd.RangeIndex):
- df.columns = df.columns.astype(str)
-
- for col in df.columns:
- if not isinstance(col, str):
- raise ValueError(
- "Dataframe contains invalid column name: {0!r}. "
- "Column names must be strings".format(col)
- )
-
- if isinstance(df.index, pd.MultiIndex):
- raise ValueError("Hierarchical indices not supported")
- if isinstance(df.columns, pd.MultiIndex):
- raise ValueError("Hierarchical indices not supported")
-
- def to_list_if_array(val):
- if isinstance(val, np.ndarray):
- return val.tolist()
- else:
- return val
-
- for col_name, dtype in df.dtypes.items():
- if str(dtype) == "category":
- # Work around bug in to_json for categorical types in older versions of pandas
- # https://github.com/pydata/pandas/issues/10778
- # https://github.com/altair-viz/altair/pull/2170
- col = df[col_name].astype(object)
- df[col_name] = col.where(col.notnull(), None)
- elif str(dtype) == "string":
- # dedicated string datatype (since 1.0)
- # https://pandas.pydata.org/pandas-docs/version/1.0.0/whatsnew/v1.0.0.html#dedicated-string-data-type
- col = df[col_name].astype(object)
- df[col_name] = col.where(col.notnull(), None)
- elif str(dtype) == "bool":
- # convert numpy bools to objects; np.bool is not JSON serializable
- df[col_name] = df[col_name].astype(object)
- elif str(dtype) == "boolean":
- # dedicated boolean datatype (since 1.0)
- # https://pandas.io/docs/user_guide/boolean.html
- col = df[col_name].astype(object)
- df[col_name] = col.where(col.notnull(), None)
- elif str(dtype).startswith("datetime"):
- # Convert datetimes to strings. This needs to be a full ISO string
- # with time, which is why we cannot use ``col.astype(str)``.
- # This is because Javascript parses date-only times in UTC, but
- # parses full ISO-8601 dates as local time, and dates in Vega and
- # Vega-Lite are displayed in local time by default.
- # (see https://github.com/altair-viz/altair/issues/1027)
- df[col_name] = (
- df[col_name].apply(lambda x: x.isoformat()).replace("NaT", "")
- )
- elif str(dtype).startswith("timedelta"):
- raise ValueError(
- 'Field "{col_name}" has type "{dtype}" which is '
- "not supported by Altair. Please convert to "
- "either a timestamp or a numerical value."
- "".format(col_name=col_name, dtype=dtype)
- )
- elif str(dtype).startswith("geometry"):
- # geopandas >=0.6.1 uses the dtype geometry. Continue here
- # otherwise it will give an error on np.issubdtype(dtype, np.integer)
- continue
- elif str(dtype) in {
- "Int8",
- "Int16",
- "Int32",
- "Int64",
- "UInt8",
- "UInt16",
- "UInt32",
- "UInt64",
- "Float32",
- "Float64",
- }: # nullable integer datatypes (since 24.0) and nullable float datatypes (since 1.2.0)
- # https://pandas.pydata.org/pandas-docs/version/0.25/whatsnew/v0.24.0.html#optional-integer-na-support
- col = df[col_name].astype(object)
- df[col_name] = col.where(col.notnull(), None)
- elif np.issubdtype(dtype, np.integer):
- # convert integers to objects; np.int is not JSON serializable
- df[col_name] = df[col_name].astype(object)
- elif np.issubdtype(dtype, np.floating):
- # For floats, convert to Python float: np.float is not JSON serializable
- # Also convert NaN/inf values to null, as they are not JSON serializable
- col = df[col_name]
- bad_values = col.isnull() | np.isinf(col)
- df[col_name] = col.astype(object).where(~bad_values, None)
- elif dtype == object:
- # Convert numpy arrays saved as objects to lists
- # Arrays are not JSON serializable
- col = df[col_name].apply(to_list_if_array, convert_dtype=False)
- df[col_name] = col.where(col.notnull(), None)
- return df
-
-
-def parse_shorthand(
- shorthand,
- data=None,
- parse_aggregates=True,
- parse_window_ops=False,
- parse_timeunits=True,
- parse_types=True,
-):
- """General tool to parse shorthand values
-
- These are of the form:
-
- - "col_name"
- - "col_name:O"
- - "average(col_name)"
- - "average(col_name):O"
-
- Optionally, a dataframe may be supplied, from which the type
- will be inferred if not specified in the shorthand.
-
- Parameters
- ----------
- shorthand : dict or string
- The shorthand representation to be parsed
- data : DataFrame, optional
- If specified and of type DataFrame, then use these values to infer the
- column type if not provided by the shorthand.
- parse_aggregates : boolean
- If True (default), then parse aggregate functions within the shorthand.
- parse_window_ops : boolean
- If True then parse window operations within the shorthand (default:False)
- parse_timeunits : boolean
- If True (default), then parse timeUnits from within the shorthand
- parse_types : boolean
- If True (default), then parse typecodes within the shorthand
-
- Returns
- -------
- attrs : dict
- a dictionary of attributes extracted from the shorthand
-
- Examples
- --------
- >>> data = pd.DataFrame({'foo': ['A', 'B', 'A', 'B'],
- ... 'bar': [1, 2, 3, 4]})
-
- >>> parse_shorthand('name') == {'field': 'name'}
- True
-
- >>> parse_shorthand('name:Q') == {'field': 'name', 'type': 'quantitative'}
- True
-
- >>> parse_shorthand('average(col)') == {'aggregate': 'average', 'field': 'col'}
- True
-
- >>> parse_shorthand('foo:O') == {'field': 'foo', 'type': 'ordinal'}
- True
-
- >>> parse_shorthand('min(foo):Q') == {'aggregate': 'min', 'field': 'foo', 'type': 'quantitative'}
- True
-
- >>> parse_shorthand('month(col)') == {'field': 'col', 'timeUnit': 'month', 'type': 'temporal'}
- True
-
- >>> parse_shorthand('year(col):O') == {'field': 'col', 'timeUnit': 'year', 'type': 'ordinal'}
- True
-
- >>> parse_shorthand('foo', data) == {'field': 'foo', 'type': 'nominal'}
- True
-
- >>> parse_shorthand('bar', data) == {'field': 'bar', 'type': 'quantitative'}
- True
-
- >>> parse_shorthand('bar:O', data) == {'field': 'bar', 'type': 'ordinal'}
- True
-
- >>> parse_shorthand('sum(bar)', data) == {'aggregate': 'sum', 'field': 'bar', 'type': 'quantitative'}
- True
-
- >>> parse_shorthand('count()', data) == {'aggregate': 'count', 'type': 'quantitative'}
- True
- """
- if not shorthand:
- return {}
-
- valid_typecodes = list(TYPECODE_MAP) + list(INV_TYPECODE_MAP)
-
- units = {
- "field": "(?P.*)",
- "type": "(?P{})".format("|".join(valid_typecodes)),
- "agg_count": "(?Pcount)",
- "op_count": "(?Pcount)",
- "aggregate": "(?P{})".format("|".join(AGGREGATES)),
- "window_op": "(?P{})".format("|".join(AGGREGATES + WINDOW_AGGREGATES)),
- "timeUnit": "(?P{})".format("|".join(TIMEUNITS)),
- }
-
- patterns = []
-
- if parse_aggregates:
- patterns.extend([r"{agg_count}\(\)"])
- patterns.extend([r"{aggregate}\({field}\)"])
- if parse_window_ops:
- patterns.extend([r"{op_count}\(\)"])
- patterns.extend([r"{window_op}\({field}\)"])
- if parse_timeunits:
- patterns.extend([r"{timeUnit}\({field}\)"])
-
- patterns.extend([r"{field}"])
-
- if parse_types:
- patterns = list(itertools.chain(*((p + ":{type}", p) for p in patterns)))
-
- regexps = (
- re.compile(r"\A" + p.format(**units) + r"\Z", re.DOTALL) for p in patterns
- )
-
- # find matches depending on valid fields passed
- if isinstance(shorthand, dict):
- attrs = shorthand
- else:
- attrs = next(
- exp.match(shorthand).groupdict() for exp in regexps if exp.match(shorthand)
- )
-
- # Handle short form of the type expression
- if "type" in attrs:
- attrs["type"] = INV_TYPECODE_MAP.get(attrs["type"], attrs["type"])
-
- # counts are quantitative by default
- if attrs == {"aggregate": "count"}:
- attrs["type"] = "quantitative"
-
- # times are temporal by default
- if "timeUnit" in attrs and "type" not in attrs:
- attrs["type"] = "temporal"
-
- # if data is specified and type is not, infer type from data
- if isinstance(data, pd.DataFrame) and "type" not in attrs:
- # Remove escape sequences so that types can be inferred for columns with special characters
- if "field" in attrs and attrs["field"].replace("\\", "") in data.columns:
- attrs["type"] = infer_vegalite_type(data[attrs["field"].replace("\\", "")])
- # ordered categorical dataframe columns return the type and sort order as a tuple
- if isinstance(attrs["type"], tuple):
- attrs["sort"] = attrs["type"][1]
- attrs["type"] = attrs["type"][0]
-
- # If an unescaped colon is still present, it's often due to an incorrect data type specification
- # but could also be due to using a column name with ":" in it.
- if (
- "field" in attrs
- and ":" in attrs["field"]
- and attrs["field"][attrs["field"].rfind(":") - 1] != "\\"
- ):
- raise ValueError(
- '"{}" '.format(attrs["field"].split(":")[-1])
- + "is not one of the valid encoding data types: {}.".format(
- ", ".join(TYPECODE_MAP.values())
- )
- + "\nFor more details, see https://altair-viz.github.io/user_guide/encodings/index.html#encoding-data-types. "
- + "If you are trying to use a column name that contains a colon, "
- + 'prefix it with a backslash; for example "column\\:name" instead of "column:name".'
- )
- return attrs
-
-
-def use_signature(Obj: Callable[_P, Any]):
- """Apply call signature and documentation of Obj to the decorated method"""
-
- def decorate(f: Callable[..., _V]) -> Callable[_P, _V]:
- # call-signature of f is exposed via __wrapped__.
- # we want it to mimic Obj.__init__
- f.__wrapped__ = Obj.__init__ # type: ignore
- f._uses_signature = Obj # type: ignore
-
- # Supplement the docstring of f with information from Obj
- if Obj.__doc__:
- # Patch in a reference to the class this docstring is copied from,
- # to generate a hyperlink.
- doclines = Obj.__doc__.splitlines()
- doclines[0] = f"Refer to :class:`{Obj.__name__}`"
-
- if f.__doc__:
- doc = f.__doc__ + "\n".join(doclines[1:])
- else:
- doc = "\n".join(doclines)
- try:
- f.__doc__ = doc
- except AttributeError:
- # __doc__ is not modifiable for classes in Python < 3.3
- pass
-
- return f
-
- return decorate
-
-
-def update_nested(original, update, copy=False):
- """Update nested dictionaries
-
- Parameters
- ----------
- original : dict
- the original (nested) dictionary, which will be updated in-place
- update : dict
- the nested dictionary of updates
- copy : bool, default False
- if True, then copy the original dictionary rather than modifying it
-
- Returns
- -------
- original : dict
- a reference to the (modified) original dict
-
- Examples
- --------
- >>> original = {'x': {'b': 2, 'c': 4}}
- >>> update = {'x': {'b': 5, 'd': 6}, 'y': 40}
- >>> update_nested(original, update) # doctest: +SKIP
- {'x': {'b': 5, 'c': 4, 'd': 6}, 'y': 40}
- >>> original # doctest: +SKIP
- {'x': {'b': 5, 'c': 4, 'd': 6}, 'y': 40}
- """
- if copy:
- original = deepcopy(original)
- for key, val in update.items():
- if isinstance(val, Mapping):
- orig_val = original.get(key, {})
- if isinstance(orig_val, Mapping):
- original[key] = update_nested(orig_val, val)
- else:
- original[key] = val
- else:
- original[key] = val
- return original
-
-
-def display_traceback(in_ipython=True):
- exc_info = sys.exc_info()
-
- if in_ipython:
- from IPython.core.getipython import get_ipython
-
- ip = get_ipython()
- else:
- ip = None
-
- if ip is not None:
- ip.showtraceback(exc_info)
- else:
- traceback.print_exception(*exc_info)
-
-
-def infer_encoding_types(args, kwargs, channels):
- """Infer typed keyword arguments for args and kwargs
-
- Parameters
- ----------
- args : tuple
- List of function args
- kwargs : dict
- Dict of function kwargs
- channels : module
- The module containing all altair encoding channel classes.
-
- Returns
- -------
- kwargs : dict
- All args and kwargs in a single dict, with keys and types
- based on the channels mapping.
- """
- # Construct a dictionary of channel type to encoding name
- # TODO: cache this somehow?
- channel_objs = (getattr(channels, name) for name in dir(channels))
- channel_objs = (
- c for c in channel_objs if isinstance(c, type) and issubclass(c, SchemaBase)
- )
- channel_to_name = {c: c._encoding_name for c in channel_objs}
- name_to_channel = {}
- for chan, name in channel_to_name.items():
- chans = name_to_channel.setdefault(name, {})
- if chan.__name__.endswith("Datum"):
- key = "datum"
- elif chan.__name__.endswith("Value"):
- key = "value"
- else:
- key = "field"
- chans[key] = chan
-
- # First use the mapping to convert args to kwargs based on their types.
- for arg in args:
- if isinstance(arg, (list, tuple)) and len(arg) > 0:
- type_ = type(arg[0])
- else:
- type_ = type(arg)
-
- encoding = channel_to_name.get(type_, None)
- if encoding is None:
- raise NotImplementedError("positional of type {}" "".format(type_))
- if encoding in kwargs:
- raise ValueError("encoding {} specified twice.".format(encoding))
- kwargs[encoding] = arg
-
- def _wrap_in_channel_class(obj, encoding):
- if isinstance(obj, SchemaBase):
- return obj
-
- if isinstance(obj, str):
- obj = {"shorthand": obj}
-
- if isinstance(obj, (list, tuple)):
- return [_wrap_in_channel_class(subobj, encoding) for subobj in obj]
-
- if encoding not in name_to_channel:
- warnings.warn(
- "Unrecognized encoding channel '{}'".format(encoding), stacklevel=1
- )
- return obj
-
- classes = name_to_channel[encoding]
- cls = classes["value"] if "value" in obj else classes["field"]
-
- try:
- # Don't force validation here; some objects won't be valid until
- # they're created in the context of a chart.
- return cls.from_dict(obj, validate=False)
- except jsonschema.ValidationError:
- # our attempts at finding the correct class have failed
- return obj
-
- return {
- encoding: _wrap_in_channel_class(obj, encoding)
- for encoding, obj in kwargs.items()
- }
diff --git a/spaces/Suniilkumaar/MusicGen-updated/audiocraft/data/__init__.py b/spaces/Suniilkumaar/MusicGen-updated/audiocraft/data/__init__.py
deleted file mode 100644
index 708a3dcead8dda89374a021177481dacae9f7fe9..0000000000000000000000000000000000000000
--- a/spaces/Suniilkumaar/MusicGen-updated/audiocraft/data/__init__.py
+++ /dev/null
@@ -1,8 +0,0 @@
-# Copyright (c) Meta Platforms, Inc. and affiliates.
-# All rights reserved.
-#
-# This source code is licensed under the license found in the
-# LICENSE file in the root directory of this source tree.
-
-# flake8: noqa
-from . import audio, audio_dataset
diff --git a/spaces/Superlang/ImageProcessor/annotator/oneformer/detectron2/layers/csrc/box_iou_rotated/box_iou_rotated_utils.h b/spaces/Superlang/ImageProcessor/annotator/oneformer/detectron2/layers/csrc/box_iou_rotated/box_iou_rotated_utils.h
deleted file mode 100644
index b54a5dde2ca11a74d29c4d8adb7fe1634f5baf9c..0000000000000000000000000000000000000000
--- a/spaces/Superlang/ImageProcessor/annotator/oneformer/detectron2/layers/csrc/box_iou_rotated/box_iou_rotated_utils.h
+++ /dev/null
@@ -1,370 +0,0 @@
-// Copyright (c) Facebook, Inc. and its affiliates.
-#pragma once
-
-#include
-#include
-
-#if defined(__CUDACC__) || __HCC__ == 1 || __HIP__ == 1
-// Designates functions callable from the host (CPU) and the device (GPU)
-#define HOST_DEVICE __host__ __device__
-#define HOST_DEVICE_INLINE HOST_DEVICE __forceinline__
-#else
-#include
-#define HOST_DEVICE
-#define HOST_DEVICE_INLINE HOST_DEVICE inline
-#endif
-
-namespace detectron2 {
-
-namespace {
-
-template
-struct RotatedBox {
- T x_ctr, y_ctr, w, h, a;
-};
-
-template
-struct Point {
- T x, y;
- HOST_DEVICE_INLINE Point(const T& px = 0, const T& py = 0) : x(px), y(py) {}
- HOST_DEVICE_INLINE Point operator+(const Point& p) const {
- return Point(x + p.x, y + p.y);
- }
- HOST_DEVICE_INLINE Point& operator+=(const Point& p) {
- x += p.x;
- y += p.y;
- return *this;
- }
- HOST_DEVICE_INLINE Point operator-(const Point& p) const {
- return Point(x - p.x, y - p.y);
- }
- HOST_DEVICE_INLINE Point operator*(const T coeff) const {
- return Point(x * coeff, y * coeff);
- }
-};
-
-template
-HOST_DEVICE_INLINE T dot_2d(const Point& A, const Point& B) {
- return A.x * B.x + A.y * B.y;
-}
-
-// R: result type. can be different from input type
-template
-HOST_DEVICE_INLINE R cross_2d(const Point& A, const Point& B) {
- return static_cast(A.x) * static_cast(B.y) -
- static_cast(B.x) * static_cast(A.y);
-}
-
-template
-HOST_DEVICE_INLINE void get_rotated_vertices(
- const RotatedBox& box,
- Point (&pts)[4]) {
- // M_PI / 180. == 0.01745329251
- double theta = box.a * 0.01745329251;
- T cosTheta2 = (T)cos(theta) * 0.5f;
- T sinTheta2 = (T)sin(theta) * 0.5f;
-
- // y: top --> down; x: left --> right
- pts[0].x = box.x_ctr + sinTheta2 * box.h + cosTheta2 * box.w;
- pts[0].y = box.y_ctr + cosTheta2 * box.h - sinTheta2 * box.w;
- pts[1].x = box.x_ctr - sinTheta2 * box.h + cosTheta2 * box.w;
- pts[1].y = box.y_ctr - cosTheta2 * box.h - sinTheta2 * box.w;
- pts[2].x = 2 * box.x_ctr - pts[0].x;
- pts[2].y = 2 * box.y_ctr - pts[0].y;
- pts[3].x = 2 * box.x_ctr - pts[1].x;
- pts[3].y = 2 * box.y_ctr - pts[1].y;
-}
-
-template
-HOST_DEVICE_INLINE int get_intersection_points(
- const Point (&pts1)[4],
- const Point (&pts2)[4],
- Point (&intersections)[24]) {
- // Line vector
- // A line from p1 to p2 is: p1 + (p2-p1)*t, t=[0,1]
- Point vec1[4], vec2[4];
- for (int i = 0; i < 4; i++) {
- vec1[i] = pts1[(i + 1) % 4] - pts1[i];
- vec2[i] = pts2[(i + 1) % 4] - pts2[i];
- }
-
- // When computing the intersection area, it doesn't hurt if we have
- // more (duplicated/approximate) intersections/vertices than needed,
- // while it can cause drastic difference if we miss an intersection/vertex.
- // Therefore, we add an epsilon to relax the comparisons between
- // the float point numbers that decide the intersection points.
- double EPS = 1e-5;
-
- // Line test - test all line combos for intersection
- int num = 0; // number of intersections
- for (int i = 0; i < 4; i++) {
- for (int j = 0; j < 4; j++) {
- // Solve for 2x2 Ax=b
- T det = cross_2d(vec2[j], vec1[i]);
-
- // This takes care of parallel lines
- if (fabs(det) <= 1e-14) {
- continue;
- }
-
- auto vec12 = pts2[j] - pts1[i];
-
- T t1 = cross_2d(vec2[j], vec12) / det;
- T t2 = cross_2d(vec1[i], vec12) / det;
-
- if (t1 > -EPS && t1 < 1.0f + EPS && t2 > -EPS && t2 < 1.0f + EPS) {
- intersections[num++] = pts1[i] + vec1[i] * t1;
- }
- }
- }
-
- // Check for vertices of rect1 inside rect2
- {
- const auto& AB = vec2[0];
- const auto& DA = vec2[3];
- auto ABdotAB = dot_2d(AB, AB);
- auto ADdotAD = dot_2d(DA, DA);
- for (int i = 0; i < 4; i++) {
- // assume ABCD is the rectangle, and P is the point to be judged
- // P is inside ABCD iff. P's projection on AB lies within AB
- // and P's projection on AD lies within AD
-
- auto AP = pts1[i] - pts2[0];
-
- auto APdotAB = dot_2d(AP, AB);
- auto APdotAD = -dot_2d(AP, DA);
-
- if ((APdotAB > -EPS) && (APdotAD > -EPS) && (APdotAB < ABdotAB + EPS) &&
- (APdotAD < ADdotAD + EPS)) {
- intersections[num++] = pts1[i];
- }
- }
- }
-
- // Reverse the check - check for vertices of rect2 inside rect1
- {
- const auto& AB = vec1[0];
- const auto& DA = vec1[3];
- auto ABdotAB = dot_2d(AB, AB);
- auto ADdotAD = dot_2d(DA, DA);
- for (int i = 0; i < 4; i++) {
- auto AP = pts2[i] - pts1[0];
-
- auto APdotAB = dot_2d(AP, AB);
- auto APdotAD = -dot_2d(AP, DA);
-
- if ((APdotAB > -EPS) && (APdotAD > -EPS) && (APdotAB < ABdotAB + EPS) &&
- (APdotAD < ADdotAD + EPS)) {
- intersections[num++] = pts2[i];
- }
- }
- }
-
- return num;
-}
-
-template
-HOST_DEVICE_INLINE int convex_hull_graham(
- const Point (&p)[24],
- const int& num_in,
- Point (&q)[24],
- bool shift_to_zero = false) {
- assert(num_in >= 2);
-
- // Step 1:
- // Find point with minimum y
- // if more than 1 points have the same minimum y,
- // pick the one with the minimum x.
- int t = 0;
- for (int i = 1; i < num_in; i++) {
- if (p[i].y < p[t].y || (p[i].y == p[t].y && p[i].x < p[t].x)) {
- t = i;
- }
- }
- auto& start = p[t]; // starting point
-
- // Step 2:
- // Subtract starting point from every points (for sorting in the next step)
- for (int i = 0; i < num_in; i++) {
- q[i] = p[i] - start;
- }
-
- // Swap the starting point to position 0
- auto tmp = q[0];
- q[0] = q[t];
- q[t] = tmp;
-
- // Step 3:
- // Sort point 1 ~ num_in according to their relative cross-product values
- // (essentially sorting according to angles)
- // If the angles are the same, sort according to their distance to origin
- T dist[24];
-#if defined(__CUDACC__) || __HCC__ == 1 || __HIP__ == 1
- // compute distance to origin before sort, and sort them together with the
- // points
- for (int i = 0; i < num_in; i++) {
- dist[i] = dot_2d(q[i], q[i]);
- }
-
- // CUDA version
- // In the future, we can potentially use thrust
- // for sorting here to improve speed (though not guaranteed)
- for (int i = 1; i < num_in - 1; i++) {
- for (int j = i + 1; j < num_in; j++) {
- T crossProduct = cross_2d(q[i], q[j]);
- if ((crossProduct < -1e-6) ||
- (fabs(crossProduct) < 1e-6 && dist[i] > dist[j])) {
- auto q_tmp = q[i];
- q[i] = q[j];
- q[j] = q_tmp;
- auto dist_tmp = dist[i];
- dist[i] = dist[j];
- dist[j] = dist_tmp;
- }
- }
- }
-#else
- // CPU version
- std::sort(
- q + 1, q + num_in, [](const Point& A, const Point& B) -> bool {
- T temp = cross_2d(A, B);
- if (fabs(temp) < 1e-6) {
- return dot_2d(A, A) < dot_2d(B, B);
- } else {
- return temp > 0;
- }
- });
- // compute distance to origin after sort, since the points are now different.
- for (int i = 0; i < num_in; i++) {
- dist[i] = dot_2d(q[i], q[i]);
- }
-#endif
-
- // Step 4:
- // Make sure there are at least 2 points (that don't overlap with each other)
- // in the stack
- int k; // index of the non-overlapped second point
- for (k = 1; k < num_in; k++) {
- if (dist[k] > 1e-8) {
- break;
- }
- }
- if (k == num_in) {
- // We reach the end, which means the convex hull is just one point
- q[0] = p[t];
- return 1;
- }
- q[1] = q[k];
- int m = 2; // 2 points in the stack
- // Step 5:
- // Finally we can start the scanning process.
- // When a non-convex relationship between the 3 points is found
- // (either concave shape or duplicated points),
- // we pop the previous point from the stack
- // until the 3-point relationship is convex again, or
- // until the stack only contains two points
- for (int i = k + 1; i < num_in; i++) {
- while (m > 1) {
- auto q1 = q[i] - q[m - 2], q2 = q[m - 1] - q[m - 2];
- // cross_2d() uses FMA and therefore computes round(round(q1.x*q2.y) -
- // q2.x*q1.y) So it may not return 0 even when q1==q2. Therefore we
- // compare round(q1.x*q2.y) and round(q2.x*q1.y) directly. (round means
- // round to nearest floating point).
- if (q1.x * q2.y >= q2.x * q1.y)
- m--;
- else
- break;
- }
- // Using double also helps, but float can solve the issue for now.
- // while (m > 1 && cross_2d(q[i] - q[m - 2], q[m - 1] - q[m - 2])
- // >= 0) {
- // m--;
- // }
- q[m++] = q[i];
- }
-
- // Step 6 (Optional):
- // In general sense we need the original coordinates, so we
- // need to shift the points back (reverting Step 2)
- // But if we're only interested in getting the area/perimeter of the shape
- // We can simply return.
- if (!shift_to_zero) {
- for (int i = 0; i < m; i++) {
- q[i] += start;
- }
- }
-
- return m;
-}
-
-template
-HOST_DEVICE_INLINE T polygon_area(const Point (&q)[24], const int& m) {
- if (m <= 2) {
- return 0;
- }
-
- T area = 0;
- for (int i = 1; i < m - 1; i++) {
- area += fabs(cross_2d(q[i] - q[0], q[i + 1] - q[0]));
- }
-
- return area / 2.0;
-}
-
-template
-HOST_DEVICE_INLINE T rotated_boxes_intersection(
- const RotatedBox& box1,
- const RotatedBox& box2) {
- // There are up to 4 x 4 + 4 + 4 = 24 intersections (including dups) returned
- // from rotated_rect_intersection_pts
- Point intersectPts[24], orderedPts[24];
-
- Point pts1[4];
- Point pts2[4];
- get_rotated_vertices(box1, pts1);
- get_rotated_vertices(box2, pts2);
-
- int num = get_intersection_points(pts1, pts2, intersectPts);
-
- if (num <= 2) {
- return 0.0;
- }
-
- // Convex Hull to order the intersection points in clockwise order and find
- // the contour area.
- int num_convex = convex_hull_graham(intersectPts, num, orderedPts, true);
- return polygon_area(orderedPts, num_convex);
-}
-
-} // namespace
-
-template
-HOST_DEVICE_INLINE T
-single_box_iou_rotated(T const* const box1_raw, T const* const box2_raw) {
- // shift center to the middle point to achieve higher precision in result
- RotatedBox box1, box2;
- auto center_shift_x = (box1_raw[0] + box2_raw[0]) / 2.0;
- auto center_shift_y = (box1_raw[1] + box2_raw[1]) / 2.0;
- box1.x_ctr = box1_raw[0] - center_shift_x;
- box1.y_ctr = box1_raw[1] - center_shift_y;
- box1.w = box1_raw[2];
- box1.h = box1_raw[3];
- box1.a = box1_raw[4];
- box2.x_ctr = box2_raw[0] - center_shift_x;
- box2.y_ctr = box2_raw[1] - center_shift_y;
- box2.w = box2_raw[2];
- box2.h = box2_raw[3];
- box2.a = box2_raw[4];
-
- T area1 = box1.w * box1.h;
- T area2 = box2.w * box2.h;
- if (area1 < 1e-14 || area2 < 1e-14) {
- return 0.f;
- }
-
- T intersection = rotated_boxes_intersection(box1, box2);
- T iou = intersection / (area1 + area2 - intersection);
- return iou;
-}
-
-} // namespace detectron2
diff --git a/spaces/Superlang/ImageProcessor/annotator/oneformer/oneformer/modeling/pixel_decoder/ops/setup.py b/spaces/Superlang/ImageProcessor/annotator/oneformer/oneformer/modeling/pixel_decoder/ops/setup.py
deleted file mode 100644
index 3b57ad313ac8f9b6586892142da8ba943e516cec..0000000000000000000000000000000000000000
--- a/spaces/Superlang/ImageProcessor/annotator/oneformer/oneformer/modeling/pixel_decoder/ops/setup.py
+++ /dev/null
@@ -1,78 +0,0 @@
-# ------------------------------------------------------------------------------------------------
-# Deformable DETR
-# Copyright (c) 2020 SenseTime. All Rights Reserved.
-# Licensed under the Apache License, Version 2.0 [see LICENSE for details]
-# ------------------------------------------------------------------------------------------------
-# Modified from https://github.com/chengdazhi/Deformable-Convolution-V2-PyTorch/tree/pytorch_1.0.0
-# ------------------------------------------------------------------------------------------------
-
-# Copyright (c) Facebook, Inc. and its affiliates.
-# Modified by Bowen Cheng from https://github.com/fundamentalvision/Deformable-DETR
-
-import os
-import glob
-
-import torch
-
-from torch.utils.cpp_extension import CUDA_HOME
-from torch.utils.cpp_extension import CppExtension
-from torch.utils.cpp_extension import CUDAExtension
-
-from setuptools import find_packages
-from setuptools import setup
-
-requirements = ["torch", "torchvision"]
-
-def get_extensions():
- this_dir = os.path.dirname(os.path.abspath(__file__))
- extensions_dir = os.path.join(this_dir, "src")
-
- main_file = glob.glob(os.path.join(extensions_dir, "*.cpp"))
- source_cpu = glob.glob(os.path.join(extensions_dir, "cpu", "*.cpp"))
- source_cuda = glob.glob(os.path.join(extensions_dir, "cuda", "*.cu"))
-
- sources = main_file + source_cpu
- extension = CppExtension
- extra_compile_args = {"cxx": []}
- define_macros = []
-
- # Force cuda since torch ask for a device, not if cuda is in fact available.
- if (os.environ.get('FORCE_CUDA') or torch.cuda.is_available()) and CUDA_HOME is not None:
- extension = CUDAExtension
- sources += source_cuda
- define_macros += [("WITH_CUDA", None)]
- extra_compile_args["nvcc"] = [
- "-DCUDA_HAS_FP16=1",
- "-D__CUDA_NO_HALF_OPERATORS__",
- "-D__CUDA_NO_HALF_CONVERSIONS__",
- "-D__CUDA_NO_HALF2_OPERATORS__",
- ]
- else:
- if CUDA_HOME is None:
- raise NotImplementedError('CUDA_HOME is None. Please set environment variable CUDA_HOME.')
- else:
- raise NotImplementedError('No CUDA runtime is found. Please set FORCE_CUDA=1 or test it by running torch.cuda.is_available().')
-
- sources = [os.path.join(extensions_dir, s) for s in sources]
- include_dirs = [extensions_dir]
- ext_modules = [
- extension(
- "MultiScaleDeformableAttention",
- sources,
- include_dirs=include_dirs,
- define_macros=define_macros,
- extra_compile_args=extra_compile_args,
- )
- ]
- return ext_modules
-
-setup(
- name="MultiScaleDeformableAttention",
- version="1.0",
- author="Weijie Su",
- url="https://github.com/fundamentalvision/Deformable-DETR",
- description="PyTorch Wrapper for CUDA Functions of Multi-Scale Deformable Attention",
- packages=find_packages(exclude=("configs", "tests",)),
- ext_modules=get_extensions(),
- cmdclass={"build_ext": torch.utils.cpp_extension.BuildExtension},
-)
diff --git a/spaces/TandCAcceptMe/face-swap-docker/mynewshinyroop/Lib/site-packages/pip/_internal/req/req_file.py b/spaces/TandCAcceptMe/face-swap-docker/mynewshinyroop/Lib/site-packages/pip/_internal/req/req_file.py
deleted file mode 100644
index f717c1ccc79f7581f1293b3fcf1a0764def7a84a..0000000000000000000000000000000000000000
--- a/spaces/TandCAcceptMe/face-swap-docker/mynewshinyroop/Lib/site-packages/pip/_internal/req/req_file.py
+++ /dev/null
@@ -1,552 +0,0 @@
-"""
-Requirements file parsing
-"""
-
-import logging
-import optparse
-import os
-import re
-import shlex
-import urllib.parse
-from optparse import Values
-from typing import (
- TYPE_CHECKING,
- Any,
- Callable,
- Dict,
- Generator,
- Iterable,
- List,
- Optional,
- Tuple,
-)
-
-from pip._internal.cli import cmdoptions
-from pip._internal.exceptions import InstallationError, RequirementsFileParseError
-from pip._internal.models.search_scope import SearchScope
-from pip._internal.network.session import PipSession
-from pip._internal.network.utils import raise_for_status
-from pip._internal.utils.encoding import auto_decode
-from pip._internal.utils.urls import get_url_scheme
-
-if TYPE_CHECKING:
- # NoReturn introduced in 3.6.2; imported only for type checking to maintain
- # pip compatibility with older patch versions of Python 3.6
- from typing import NoReturn
-
- from pip._internal.index.package_finder import PackageFinder
-
-__all__ = ["parse_requirements"]
-
-ReqFileLines = Iterable[Tuple[int, str]]
-
-LineParser = Callable[[str], Tuple[str, Values]]
-
-SCHEME_RE = re.compile(r"^(http|https|file):", re.I)
-COMMENT_RE = re.compile(r"(^|\s+)#.*$")
-
-# Matches environment variable-style values in '${MY_VARIABLE_1}' with the
-# variable name consisting of only uppercase letters, digits or the '_'
-# (underscore). This follows the POSIX standard defined in IEEE Std 1003.1,
-# 2013 Edition.
-ENV_VAR_RE = re.compile(r"(?P\$\{(?P[A-Z0-9_]+)\})")
-
-SUPPORTED_OPTIONS: List[Callable[..., optparse.Option]] = [
- cmdoptions.index_url,
- cmdoptions.extra_index_url,
- cmdoptions.no_index,
- cmdoptions.constraints,
- cmdoptions.requirements,
- cmdoptions.editable,
- cmdoptions.find_links,
- cmdoptions.no_binary,
- cmdoptions.only_binary,
- cmdoptions.prefer_binary,
- cmdoptions.require_hashes,
- cmdoptions.pre,
- cmdoptions.trusted_host,
- cmdoptions.use_new_feature,
-]
-
-# options to be passed to requirements
-SUPPORTED_OPTIONS_REQ: List[Callable[..., optparse.Option]] = [
- cmdoptions.global_options,
- cmdoptions.hash,
- cmdoptions.config_settings,
-]
-
-# the 'dest' string values
-SUPPORTED_OPTIONS_REQ_DEST = [str(o().dest) for o in SUPPORTED_OPTIONS_REQ]
-
-logger = logging.getLogger(__name__)
-
-
-class ParsedRequirement:
- def __init__(
- self,
- requirement: str,
- is_editable: bool,
- comes_from: str,
- constraint: bool,
- options: Optional[Dict[str, Any]] = None,
- line_source: Optional[str] = None,
- ) -> None:
- self.requirement = requirement
- self.is_editable = is_editable
- self.comes_from = comes_from
- self.options = options
- self.constraint = constraint
- self.line_source = line_source
-
-
-class ParsedLine:
- def __init__(
- self,
- filename: str,
- lineno: int,
- args: str,
- opts: Values,
- constraint: bool,
- ) -> None:
- self.filename = filename
- self.lineno = lineno
- self.opts = opts
- self.constraint = constraint
-
- if args:
- self.is_requirement = True
- self.is_editable = False
- self.requirement = args
- elif opts.editables:
- self.is_requirement = True
- self.is_editable = True
- # We don't support multiple -e on one line
- self.requirement = opts.editables[0]
- else:
- self.is_requirement = False
-
-
-def parse_requirements(
- filename: str,
- session: PipSession,
- finder: Optional["PackageFinder"] = None,
- options: Optional[optparse.Values] = None,
- constraint: bool = False,
-) -> Generator[ParsedRequirement, None, None]:
- """Parse a requirements file and yield ParsedRequirement instances.
-
- :param filename: Path or url of requirements file.
- :param session: PipSession instance.
- :param finder: Instance of pip.index.PackageFinder.
- :param options: cli options.
- :param constraint: If true, parsing a constraint file rather than
- requirements file.
- """
- line_parser = get_line_parser(finder)
- parser = RequirementsFileParser(session, line_parser)
-
- for parsed_line in parser.parse(filename, constraint):
- parsed_req = handle_line(
- parsed_line, options=options, finder=finder, session=session
- )
- if parsed_req is not None:
- yield parsed_req
-
-
-def preprocess(content: str) -> ReqFileLines:
- """Split, filter, and join lines, and return a line iterator
-
- :param content: the content of the requirements file
- """
- lines_enum: ReqFileLines = enumerate(content.splitlines(), start=1)
- lines_enum = join_lines(lines_enum)
- lines_enum = ignore_comments(lines_enum)
- lines_enum = expand_env_variables(lines_enum)
- return lines_enum
-
-
-def handle_requirement_line(
- line: ParsedLine,
- options: Optional[optparse.Values] = None,
-) -> ParsedRequirement:
- # preserve for the nested code path
- line_comes_from = "{} {} (line {})".format(
- "-c" if line.constraint else "-r",
- line.filename,
- line.lineno,
- )
-
- assert line.is_requirement
-
- if line.is_editable:
- # For editable requirements, we don't support per-requirement
- # options, so just return the parsed requirement.
- return ParsedRequirement(
- requirement=line.requirement,
- is_editable=line.is_editable,
- comes_from=line_comes_from,
- constraint=line.constraint,
- )
- else:
- # get the options that apply to requirements
- req_options = {}
- for dest in SUPPORTED_OPTIONS_REQ_DEST:
- if dest in line.opts.__dict__ and line.opts.__dict__[dest]:
- req_options[dest] = line.opts.__dict__[dest]
-
- line_source = f"line {line.lineno} of {line.filename}"
- return ParsedRequirement(
- requirement=line.requirement,
- is_editable=line.is_editable,
- comes_from=line_comes_from,
- constraint=line.constraint,
- options=req_options,
- line_source=line_source,
- )
-
-
-def handle_option_line(
- opts: Values,
- filename: str,
- lineno: int,
- finder: Optional["PackageFinder"] = None,
- options: Optional[optparse.Values] = None,
- session: Optional[PipSession] = None,
-) -> None:
- if opts.hashes:
- logger.warning(
- "%s line %s has --hash but no requirement, and will be ignored.",
- filename,
- lineno,
- )
-
- if options:
- # percolate options upward
- if opts.require_hashes:
- options.require_hashes = opts.require_hashes
- if opts.features_enabled:
- options.features_enabled.extend(
- f for f in opts.features_enabled if f not in options.features_enabled
- )
-
- # set finder options
- if finder:
- find_links = finder.find_links
- index_urls = finder.index_urls
- no_index = finder.search_scope.no_index
- if opts.no_index is True:
- no_index = True
- index_urls = []
- if opts.index_url and not no_index:
- index_urls = [opts.index_url]
- if opts.extra_index_urls and not no_index:
- index_urls.extend(opts.extra_index_urls)
- if opts.find_links:
- # FIXME: it would be nice to keep track of the source
- # of the find_links: support a find-links local path
- # relative to a requirements file.
- value = opts.find_links[0]
- req_dir = os.path.dirname(os.path.abspath(filename))
- relative_to_reqs_file = os.path.join(req_dir, value)
- if os.path.exists(relative_to_reqs_file):
- value = relative_to_reqs_file
- find_links.append(value)
-
- if session:
- # We need to update the auth urls in session
- session.update_index_urls(index_urls)
-
- search_scope = SearchScope(
- find_links=find_links,
- index_urls=index_urls,
- no_index=no_index,
- )
- finder.search_scope = search_scope
-
- if opts.pre:
- finder.set_allow_all_prereleases()
-
- if opts.prefer_binary:
- finder.set_prefer_binary()
-
- if session:
- for host in opts.trusted_hosts or []:
- source = f"line {lineno} of {filename}"
- session.add_trusted_host(host, source=source)
-
-
-def handle_line(
- line: ParsedLine,
- options: Optional[optparse.Values] = None,
- finder: Optional["PackageFinder"] = None,
- session: Optional[PipSession] = None,
-) -> Optional[ParsedRequirement]:
- """Handle a single parsed requirements line; This can result in
- creating/yielding requirements, or updating the finder.
-
- :param line: The parsed line to be processed.
- :param options: CLI options.
- :param finder: The finder - updated by non-requirement lines.
- :param session: The session - updated by non-requirement lines.
-
- Returns a ParsedRequirement object if the line is a requirement line,
- otherwise returns None.
-
- For lines that contain requirements, the only options that have an effect
- are from SUPPORTED_OPTIONS_REQ, and they are scoped to the
- requirement. Other options from SUPPORTED_OPTIONS may be present, but are
- ignored.
-
- For lines that do not contain requirements, the only options that have an
- effect are from SUPPORTED_OPTIONS. Options from SUPPORTED_OPTIONS_REQ may
- be present, but are ignored. These lines may contain multiple options
- (although our docs imply only one is supported), and all our parsed and
- affect the finder.
- """
-
- if line.is_requirement:
- parsed_req = handle_requirement_line(line, options)
- return parsed_req
- else:
- handle_option_line(
- line.opts,
- line.filename,
- line.lineno,
- finder,
- options,
- session,
- )
- return None
-
-
-class RequirementsFileParser:
- def __init__(
- self,
- session: PipSession,
- line_parser: LineParser,
- ) -> None:
- self._session = session
- self._line_parser = line_parser
-
- def parse(
- self, filename: str, constraint: bool
- ) -> Generator[ParsedLine, None, None]:
- """Parse a given file, yielding parsed lines."""
- yield from self._parse_and_recurse(filename, constraint)
-
- def _parse_and_recurse(
- self, filename: str, constraint: bool
- ) -> Generator[ParsedLine, None, None]:
- for line in self._parse_file(filename, constraint):
- if not line.is_requirement and (
- line.opts.requirements or line.opts.constraints
- ):
- # parse a nested requirements file
- if line.opts.requirements:
- req_path = line.opts.requirements[0]
- nested_constraint = False
- else:
- req_path = line.opts.constraints[0]
- nested_constraint = True
-
- # original file is over http
- if SCHEME_RE.search(filename):
- # do a url join so relative paths work
- req_path = urllib.parse.urljoin(filename, req_path)
- # original file and nested file are paths
- elif not SCHEME_RE.search(req_path):
- # do a join so relative paths work
- req_path = os.path.join(
- os.path.dirname(filename),
- req_path,
- )
-
- yield from self._parse_and_recurse(req_path, nested_constraint)
- else:
- yield line
-
- def _parse_file(
- self, filename: str, constraint: bool
- ) -> Generator[ParsedLine, None, None]:
- _, content = get_file_content(filename, self._session)
-
- lines_enum = preprocess(content)
-
- for line_number, line in lines_enum:
- try:
- args_str, opts = self._line_parser(line)
- except OptionParsingError as e:
- # add offending line
- msg = f"Invalid requirement: {line}\n{e.msg}"
- raise RequirementsFileParseError(msg)
-
- yield ParsedLine(
- filename,
- line_number,
- args_str,
- opts,
- constraint,
- )
-
-
-def get_line_parser(finder: Optional["PackageFinder"]) -> LineParser:
- def parse_line(line: str) -> Tuple[str, Values]:
- # Build new parser for each line since it accumulates appendable
- # options.
- parser = build_parser()
- defaults = parser.get_default_values()
- defaults.index_url = None
- if finder:
- defaults.format_control = finder.format_control
-
- args_str, options_str = break_args_options(line)
-
- try:
- options = shlex.split(options_str)
- except ValueError as e:
- raise OptionParsingError(f"Could not split options: {options_str}") from e
-
- opts, _ = parser.parse_args(options, defaults)
-
- return args_str, opts
-
- return parse_line
-
-
-def break_args_options(line: str) -> Tuple[str, str]:
- """Break up the line into an args and options string. We only want to shlex
- (and then optparse) the options, not the args. args can contain markers
- which are corrupted by shlex.
- """
- tokens = line.split(" ")
- args = []
- options = tokens[:]
- for token in tokens:
- if token.startswith("-") or token.startswith("--"):
- break
- else:
- args.append(token)
- options.pop(0)
- return " ".join(args), " ".join(options)
-
-
-class OptionParsingError(Exception):
- def __init__(self, msg: str) -> None:
- self.msg = msg
-
-
-def build_parser() -> optparse.OptionParser:
- """
- Return a parser for parsing requirement lines
- """
- parser = optparse.OptionParser(add_help_option=False)
-
- option_factories = SUPPORTED_OPTIONS + SUPPORTED_OPTIONS_REQ
- for option_factory in option_factories:
- option = option_factory()
- parser.add_option(option)
-
- # By default optparse sys.exits on parsing errors. We want to wrap
- # that in our own exception.
- def parser_exit(self: Any, msg: str) -> "NoReturn":
- raise OptionParsingError(msg)
-
- # NOTE: mypy disallows assigning to a method
- # https://github.com/python/mypy/issues/2427
- parser.exit = parser_exit # type: ignore
-
- return parser
-
-
-def join_lines(lines_enum: ReqFileLines) -> ReqFileLines:
- """Joins a line ending in '\' with the previous line (except when following
- comments). The joined line takes on the index of the first line.
- """
- primary_line_number = None
- new_line: List[str] = []
- for line_number, line in lines_enum:
- if not line.endswith("\\") or COMMENT_RE.match(line):
- if COMMENT_RE.match(line):
- # this ensures comments are always matched later
- line = " " + line
- if new_line:
- new_line.append(line)
- assert primary_line_number is not None
- yield primary_line_number, "".join(new_line)
- new_line = []
- else:
- yield line_number, line
- else:
- if not new_line:
- primary_line_number = line_number
- new_line.append(line.strip("\\"))
-
- # last line contains \
- if new_line:
- assert primary_line_number is not None
- yield primary_line_number, "".join(new_line)
-
- # TODO: handle space after '\'.
-
-
-def ignore_comments(lines_enum: ReqFileLines) -> ReqFileLines:
- """
- Strips comments and filter empty lines.
- """
- for line_number, line in lines_enum:
- line = COMMENT_RE.sub("", line)
- line = line.strip()
- if line:
- yield line_number, line
-
-
-def expand_env_variables(lines_enum: ReqFileLines) -> ReqFileLines:
- """Replace all environment variables that can be retrieved via `os.getenv`.
-
- The only allowed format for environment variables defined in the
- requirement file is `${MY_VARIABLE_1}` to ensure two things:
-
- 1. Strings that contain a `$` aren't accidentally (partially) expanded.
- 2. Ensure consistency across platforms for requirement files.
-
- These points are the result of a discussion on the `github pull
- request #3514 `_.
-
- Valid characters in variable names follow the `POSIX standard
- `_ and are limited
- to uppercase letter, digits and the `_` (underscore).
- """
- for line_number, line in lines_enum:
- for env_var, var_name in ENV_VAR_RE.findall(line):
- value = os.getenv(var_name)
- if not value:
- continue
-
- line = line.replace(env_var, value)
-
- yield line_number, line
-
-
-def get_file_content(url: str, session: PipSession) -> Tuple[str, str]:
- """Gets the content of a file; it may be a filename, file: URL, or
- http: URL. Returns (location, content). Content is unicode.
- Respects # -*- coding: declarations on the retrieved files.
-
- :param url: File path or url.
- :param session: PipSession instance.
- """
- scheme = get_url_scheme(url)
-
- # Pip has special support for file:// URLs (LocalFSAdapter).
- if scheme in ["http", "https", "file"]:
- resp = session.get(url)
- raise_for_status(resp)
- return resp.url, resp.text
-
- # Assume this is a bare path.
- try:
- with open(url, "rb") as f:
- content = auto_decode(f.read())
- except OSError as exc:
- raise InstallationError(f"Could not open requirements file: {exc}")
- return url, content
diff --git a/spaces/TeamMlx/ehartford-Wizard-Vicuna-30B-Uncensored/README.md b/spaces/TeamMlx/ehartford-Wizard-Vicuna-30B-Uncensored/README.md
deleted file mode 100644
index 9ca8a4ebdf68123acb5e355cf572e8c2e3f6add0..0000000000000000000000000000000000000000
--- a/spaces/TeamMlx/ehartford-Wizard-Vicuna-30B-Uncensored/README.md
+++ /dev/null
@@ -1,12 +0,0 @@
----
-title: Ehartford Wizard Vicuna 30B Uncensored
-emoji: 🐨
-colorFrom: red
-colorTo: green
-sdk: gradio
-sdk_version: 3.32.0
-app_file: app.py
-pinned: false
----
-
-Check out the configuration reference at https://huggingface.co/docs/hub/spaces-config-reference
diff --git a/spaces/TencentARC/VLog/models/grit_src/third_party/CenterNet2/dev/packaging/build_all_wheels.sh b/spaces/TencentARC/VLog/models/grit_src/third_party/CenterNet2/dev/packaging/build_all_wheels.sh
deleted file mode 100644
index 98b5e4444828b48c8a54229ee04a44d8c7d38090..0000000000000000000000000000000000000000
--- a/spaces/TencentARC/VLog/models/grit_src/third_party/CenterNet2/dev/packaging/build_all_wheels.sh
+++ /dev/null
@@ -1,65 +0,0 @@
-#!/bin/bash -e
-# Copyright (c) Facebook, Inc. and its affiliates.
-
-[[ -d "dev/packaging" ]] || {
- echo "Please run this script at detectron2 root!"
- exit 1
-}
-
-build_one() {
- cu=$1
- pytorch_ver=$2
-
- case "$cu" in
- cu*)
- container_name=manylinux-cuda${cu/cu/}
- ;;
- cpu)
- container_name=manylinux-cuda101
- ;;
- *)
- echo "Unrecognized cu=$cu"
- exit 1
- ;;
- esac
-
- echo "Launching container $container_name ..."
- container_id="$container_name"_"$cu"_"$pytorch_ver"
-
- py_versions=(3.6 3.7 3.8 3.9)
-
- for py in "${py_versions[@]}"; do
- docker run -itd \
- --name "$container_id" \
- --mount type=bind,source="$(pwd)",target=/detectron2 \
- pytorch/$container_name
-
- cat < 0:
- current = indexes[0]
- picked.append(current.item())
- if len(indexes) == 1:
- break
- current_box = boxes[current, :]
- indexes = indexes[1:]
- rest_boxes = boxes[indexes, :]
- iou = ops.box_iou(rest_boxes, current_box.unsqueeze(0)).squeeze(1)
- indexes = indexes[iou <= iou_threshold]
-
- return torch.as_tensor(picked)
-
- def _create_tensors(self, N, device="cpu"):
- boxes = random_boxes(N, 200, device=device)
- scores = torch.rand(N, device=device)
- return boxes, scores
-
- def test_batched_nms_rotated_0_degree_cpu(self, device="cpu"):
- N = 2000
- num_classes = 50
- boxes, scores = self._create_tensors(N, device=device)
- idxs = torch.randint(0, num_classes, (N,))
- rotated_boxes = torch.zeros(N, 5, device=device)
- rotated_boxes[:, 0] = (boxes[:, 0] + boxes[:, 2]) / 2.0
- rotated_boxes[:, 1] = (boxes[:, 1] + boxes[:, 3]) / 2.0
- rotated_boxes[:, 2] = boxes[:, 2] - boxes[:, 0]
- rotated_boxes[:, 3] = boxes[:, 3] - boxes[:, 1]
- err_msg = "Rotated NMS with 0 degree is incompatible with horizontal NMS for IoU={}"
- for iou in [0.2, 0.5, 0.8]:
- backup = boxes.clone()
- keep_ref = batched_nms(boxes, scores, idxs, iou)
- assert torch.allclose(boxes, backup), "boxes modified by batched_nms"
- backup = rotated_boxes.clone()
- keep = batched_nms_rotated(rotated_boxes, scores, idxs, iou)
- assert torch.allclose(
- rotated_boxes, backup
- ), "rotated_boxes modified by batched_nms_rotated"
- # Occasionally the gap can be large if there are many IOU on the threshold boundary
- self.assertLessEqual(nms_edit_distance(keep, keep_ref), 5, err_msg.format(iou))
-
- @unittest.skipIf(not torch.cuda.is_available(), "CUDA not available")
- def test_batched_nms_rotated_0_degree_cuda(self):
- self.test_batched_nms_rotated_0_degree_cpu(device="cuda")
-
- def test_nms_rotated_0_degree_cpu(self, device="cpu"):
- N = 1000
- boxes, scores = self._create_tensors(N, device=device)
- rotated_boxes = torch.zeros(N, 5, device=device)
- rotated_boxes[:, 0] = (boxes[:, 0] + boxes[:, 2]) / 2.0
- rotated_boxes[:, 1] = (boxes[:, 1] + boxes[:, 3]) / 2.0
- rotated_boxes[:, 2] = boxes[:, 2] - boxes[:, 0]
- rotated_boxes[:, 3] = boxes[:, 3] - boxes[:, 1]
- err_msg = "Rotated NMS incompatible between CPU and reference implementation for IoU={}"
- for iou in [0.2, 0.5, 0.8]:
- keep_ref = self.reference_horizontal_nms(boxes, scores, iou)
- keep = nms_rotated(rotated_boxes, scores, iou)
- self.assertLessEqual(nms_edit_distance(keep, keep_ref), 1, err_msg.format(iou))
-
- @unittest.skipIf(not torch.cuda.is_available(), "CUDA not available")
- def test_nms_rotated_0_degree_cuda(self):
- self.test_nms_rotated_0_degree_cpu(device="cuda")
-
- def test_nms_rotated_90_degrees_cpu(self):
- N = 1000
- boxes, scores = self._create_tensors(N)
- rotated_boxes = torch.zeros(N, 5)
- rotated_boxes[:, 0] = (boxes[:, 0] + boxes[:, 2]) / 2.0
- rotated_boxes[:, 1] = (boxes[:, 1] + boxes[:, 3]) / 2.0
- # Note for rotated_boxes[:, 2] and rotated_boxes[:, 3]:
- # widths and heights are intentionally swapped here for 90 degrees case
- # so that the reference horizontal nms could be used
- rotated_boxes[:, 2] = boxes[:, 3] - boxes[:, 1]
- rotated_boxes[:, 3] = boxes[:, 2] - boxes[:, 0]
-
- rotated_boxes[:, 4] = torch.ones(N) * 90
- err_msg = "Rotated NMS incompatible between CPU and reference implementation for IoU={}"
- for iou in [0.2, 0.5, 0.8]:
- keep_ref = self.reference_horizontal_nms(boxes, scores, iou)
- keep = nms_rotated(rotated_boxes, scores, iou)
- self.assertLessEqual(nms_edit_distance(keep, keep_ref), 1, err_msg.format(iou))
-
- def test_nms_rotated_180_degrees_cpu(self):
- N = 1000
- boxes, scores = self._create_tensors(N)
- rotated_boxes = torch.zeros(N, 5)
- rotated_boxes[:, 0] = (boxes[:, 0] + boxes[:, 2]) / 2.0
- rotated_boxes[:, 1] = (boxes[:, 1] + boxes[:, 3]) / 2.0
- rotated_boxes[:, 2] = boxes[:, 2] - boxes[:, 0]
- rotated_boxes[:, 3] = boxes[:, 3] - boxes[:, 1]
- rotated_boxes[:, 4] = torch.ones(N) * 180
- err_msg = "Rotated NMS incompatible between CPU and reference implementation for IoU={}"
- for iou in [0.2, 0.5, 0.8]:
- keep_ref = self.reference_horizontal_nms(boxes, scores, iou)
- keep = nms_rotated(rotated_boxes, scores, iou)
- self.assertLessEqual(nms_edit_distance(keep, keep_ref), 1, err_msg.format(iou))
-
-
-class TestScriptable(unittest.TestCase):
- def setUp(self):
- class TestingModule(torch.nn.Module):
- def forward(self, boxes, scores, threshold):
- return nms_rotated(boxes, scores, threshold)
-
- self.module = TestingModule()
-
- def test_scriptable_cpu(self):
- m = deepcopy(self.module).cpu()
- _ = torch.jit.script(m)
-
- @unittest.skipIf(not torch.cuda.is_available(), "CUDA not available")
- def test_scriptable_cuda(self):
- m = deepcopy(self.module).cuda()
- _ = torch.jit.script(m)
-
-
-if __name__ == "__main__":
- unittest.main()
diff --git a/spaces/TheStinger/Ilaria_Upscaler/app.py b/spaces/TheStinger/Ilaria_Upscaler/app.py
deleted file mode 100644
index a57a4dd487b6e7967be2adc84d5d4bb192dde647..0000000000000000000000000000000000000000
--- a/spaces/TheStinger/Ilaria_Upscaler/app.py
+++ /dev/null
@@ -1,228 +0,0 @@
-import gradio as gr
-import cv2
-import numpy
-import os
-import random
-from basicsr.archs.rrdbnet_arch import RRDBNet
-from basicsr.utils.download_util import load_file_from_url
-
-from realesrgan import RealESRGANer
-from realesrgan.archs.srvgg_arch import SRVGGNetCompact
-
-
-last_file = None
-img_mode = "RGBA"
-
-
-def realesrgan(img, model_name, denoise_strength, face_enhance, outscale):
- """Real-ESRGAN function to restore (and upscale) images.
- """
- if not img:
- return
-
- # Define model parameters
- if model_name == 'RealESRGAN_x4plus': # x4 RRDBNet model
- model = RRDBNet(num_in_ch=3, num_out_ch=3, num_feat=64, num_block=23, num_grow_ch=32, scale=4)
- netscale = 4
- file_url = ['https://github.com/xinntao/Real-ESRGAN/releases/download/v0.1.0/RealESRGAN_x4plus.pth']
- elif model_name == 'RealESRNet_x4plus': # x4 RRDBNet model
- model = RRDBNet(num_in_ch=3, num_out_ch=3, num_feat=64, num_block=23, num_grow_ch=32, scale=4)
- netscale = 4
- file_url = ['https://github.com/xinntao/Real-ESRGAN/releases/download/v0.1.1/RealESRNet_x4plus.pth']
- elif model_name == 'RealESRGAN_x4plus_anime_6B': # x4 RRDBNet model with 6 blocks
- model = RRDBNet(num_in_ch=3, num_out_ch=3, num_feat=64, num_block=6, num_grow_ch=32, scale=4)
- netscale = 4
- file_url = ['https://github.com/xinntao/Real-ESRGAN/releases/download/v0.2.2.4/RealESRGAN_x4plus_anime_6B.pth']
- elif model_name == 'RealESRGAN_x2plus': # x2 RRDBNet model
- model = RRDBNet(num_in_ch=3, num_out_ch=3, num_feat=64, num_block=23, num_grow_ch=32, scale=2)
- netscale = 2
- file_url = ['https://github.com/xinntao/Real-ESRGAN/releases/download/v0.2.1/RealESRGAN_x2plus.pth']
- elif model_name == 'realesr-general-x4v3': # x4 VGG-style model (S size)
- model = SRVGGNetCompact(num_in_ch=3, num_out_ch=3, num_feat=64, num_conv=32, upscale=4, act_type='prelu')
- netscale = 4
- file_url = [
- 'https://github.com/xinntao/Real-ESRGAN/releases/download/v0.2.5.0/realesr-general-wdn-x4v3.pth',
- 'https://github.com/xinntao/Real-ESRGAN/releases/download/v0.2.5.0/realesr-general-x4v3.pth'
- ]
-
- # Determine model paths
- model_path = os.path.join('weights', model_name + '.pth')
- if not os.path.isfile(model_path):
- ROOT_DIR = os.path.dirname(os.path.abspath(__file__))
- for url in file_url:
- # model_path will be updated
- model_path = load_file_from_url(
- url=url, model_dir=os.path.join(ROOT_DIR, 'weights'), progress=True, file_name=None)
-
- # Use dni to control the denoise strength
- dni_weight = None
- if model_name == 'realesr-general-x4v3' and denoise_strength != 1:
- wdn_model_path = model_path.replace('realesr-general-x4v3', 'realesr-general-wdn-x4v3')
- model_path = [model_path, wdn_model_path]
- dni_weight = [denoise_strength, 1 - denoise_strength]
-
- # Restorer Class
- upsampler = RealESRGANer(
- scale=netscale,
- model_path=model_path,
- dni_weight=dni_weight,
- model=model,
- tile=0,
- tile_pad=10,
- pre_pad=10,
- half=False,
- gpu_id=None
- )
-
- # Use GFPGAN for face enhancement
- if face_enhance:
- from gfpgan import GFPGANer
- face_enhancer = GFPGANer(
- model_path='https://github.com/TencentARC/GFPGAN/releases/download/v1.3.0/GFPGANv1.3.pth',
- upscale=outscale,
- arch='clean',
- channel_multiplier=2,
- bg_upsampler=upsampler)
-
- # Convert the input PIL image to cv2 image, so that it can be processed by realesrgan
- cv_img = numpy.array(img)
- img = cv2.cvtColor(cv_img, cv2.COLOR_RGBA2BGRA)
-
- # Apply restoration
- try:
- if face_enhance:
- _, _, output = face_enhancer.enhance(img, has_aligned=False, only_center_face=False, paste_back=True)
- else:
- output, _ = upsampler.enhance(img, outscale=outscale)
- except RuntimeError as error:
- print('Error', error)
- print('If you encounter CUDA out of memory, try to set --tile with a smaller number.')
- else:
- # Save restored image and return it to the output Image component
- if img_mode == 'RGBA': # RGBA images should be saved in png format
- extension = 'png'
- else:
- extension = 'jpg'
-
- out_filename = f"output_{rnd_string(8)}.{extension}"
- cv2.imwrite(out_filename, output)
- global last_file
- last_file = out_filename
- return out_filename
-
-
-def rnd_string(x):
- """Returns a string of 'x' random characters
- """
- characters = "abcdefghijklmnopqrstuvwxyz_0123456789"
- result = "".join((random.choice(characters)) for i in range(x))
- return result
-
-
-def reset():
- """Resets the Image components of the Gradio interface and deletes
- the last processed image
- """
- global last_file
- if last_file:
- print(f"Deleting {last_file} ...")
- os.remove(last_file)
- last_file = None
- return gr.update(value=None), gr.update(value=None)
-
-
-def has_transparency(img):
- """This function works by first checking to see if a "transparency" property is defined
- in the image's info -- if so, we return "True". Then, if the image is using indexed colors
- (such as in GIFs), it gets the index of the transparent color in the palette
- (img.info.get("transparency", -1)) and checks if it's used anywhere in the canvas
- (img.getcolors()). If the image is in RGBA mode, then presumably it has transparency in
- it, but it double-checks by getting the minimum and maximum values of every color channel
- (img.getextrema()), and checks if the alpha channel's smallest value falls below 255.
- https://stackoverflow.com/questions/43864101/python-pil-check-if-image-is-transparent
- """
- if img.info.get("transparency", None) is not None:
- return True
- if img.mode == "P":
- transparent = img.info.get("transparency", -1)
- for _, index in img.getcolors():
- if index == transparent:
- return True
- elif img.mode == "RGBA":
- extrema = img.getextrema()
- if extrema[3][0] < 255:
- return True
- return False
-
-
-def image_properties(img):
- """Returns the dimensions (width and height) and color mode of the input image and
- also sets the global img_mode variable to be used by the realesrgan function
- """
- global img_mode
- if img:
- if has_transparency(img):
- img_mode = "RGBA"
- else:
- img_mode = "RGB"
- properties = f"Resolution: Width: {img.size[0]}, Height: {img.size[1]} | Color Mode: {img_mode}"
- return properties
-
-
-def main():
- # Gradio Interface
- with gr.Blocks(title="Real-ESRGAN Gradio Demo", theme="dark") as demo:
-
- gr.Markdown(
- """#
Ilaria Upscaler 💖
-
- Do not use images over 750x750 especially with 4x the resolution upscaling, it will give you an error.
-
- Hugginface port of [Real-ESRGAN](https://github.com/xinntao/Real-ESRGAN).
- """
- )
-
- with gr.Accordion("Upscaling option"):
- with gr.Row():
- model_name = gr.Dropdown(label="Upscaler model",
- choices=["RealESRGAN_x4plus", "RealESRNet_x4plus", "RealESRGAN_x4plus_anime_6B",
- "RealESRGAN_x2plus", "realesr-general-x4v3"],
- value="RealESRGAN_x4plus_anime_6B", show_label=True)
- denoise_strength = gr.Slider(label="Denoise Strength",
- minimum=0, maximum=1, step=0.1, value=0.5)
- outscale = gr.Slider(label="Resolution upscale",
- minimum=1, maximum=6, step=1, value=4, show_label=True)
- face_enhance = gr.Checkbox(label="Face Enhancement (GFPGAN)",
- value=False, show_label=True)
- ext = gr.Dropdown(label="Output file extension (Currently broken sowwy :p)",
- choices=["png", "jpg"],
- value="png", show_label=True)
-
- with gr.Row():
- with gr.Group():
- input_image = gr.Image(label="Input Image", type="pil", image_mode="RGBA")
- input_image_properties = gr.Textbox(label="Image Properties", max_lines=1)
- output_image = gr.Image(label="Output Image", image_mode="RGBA")
- with gr.Row():
- reset_btn = gr.Button("Remove images")
- restore_btn = gr.Button("Upscale")
-
- # Event listeners:
- input_image.change(fn=image_properties, inputs=input_image, outputs=input_image_properties)
- restore_btn.click(fn=realesrgan,
- inputs=[input_image, model_name, denoise_strength, face_enhance, outscale],
- outputs=output_image)
- reset_btn.click(fn=reset, inputs=[], outputs=[output_image, input_image])
- # reset_btn.click(None, inputs=[], outputs=[input_image], _js="() => (null)\n")
- # Undocumented method to clear a component's value using Javascript
-
- gr.Markdown(
- """Made with love by Ilaria 💖 | Support me on [Ko-Fi](https://ko-fi.com/ilariaowo) | Join [AI Hub](https://discord.gg/aihub)
- """
- )
-
- demo.launch()
-
-
-if __name__ == "__main__":
- main()
\ No newline at end of file
diff --git a/spaces/TogetherAI/EinfachLlaMistral/README.md b/spaces/TogetherAI/EinfachLlaMistral/README.md
deleted file mode 100644
index 02f256b39feb365168c402325895214f38acb1b1..0000000000000000000000000000000000000000
--- a/spaces/TogetherAI/EinfachLlaMistral/README.md
+++ /dev/null
@@ -1,12 +0,0 @@
----
-title: EinfachLlaMistral
-emoji: 🏢
-colorFrom: pink
-colorTo: gray
-sdk: gradio
-sdk_version: 3.47.1
-app_file: app.py
-pinned: false
----
-
-Check out the configuration reference at https://huggingface.co/docs/hub/spaces-config-reference
diff --git a/spaces/Tony1810/FootballPosition/info.md b/spaces/Tony1810/FootballPosition/info.md
deleted file mode 100644
index 7ec3f3af6b44c914fa8189cff02cd9ab75900f98..0000000000000000000000000000000000000000
--- a/spaces/Tony1810/FootballPosition/info.md
+++ /dev/null
@@ -1,16 +0,0 @@
-# 😌 [Edit info.md - Your app's title here]
-
-### 🧐 Problem Statement and Research Summary
-[add info about your problem statement and your research here!]
-
-### 🎣 Data Collection Plan
-[Edit info.md - add info about what data you collected and why here!]
-
-### 💥 Ethical Considerations (Data Privacy and Bias)
-* Data privacy: [Edit info.md - add info about you considered users' privacy here!]
-* Bias: [Edit info.md - add info about you considered bias here!]
-
-### 👻 Our Team
-[Edit info.md - add info about your team members here!]
-
-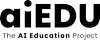
diff --git a/spaces/TuanScientist/BTCforecasting/app.py b/spaces/TuanScientist/BTCforecasting/app.py
deleted file mode 100644
index ddb05586bb327c0d8621a23f553966901a41e2d2..0000000000000000000000000000000000000000
--- a/spaces/TuanScientist/BTCforecasting/app.py
+++ /dev/null
@@ -1,55 +0,0 @@
-import gradio as gr
-import pandas as pd
-from neuralprophet import NeuralProphet, set_log_level
-import warnings
-
-set_log_level("ERROR")
-warnings.filterwarnings("ignore", category=UserWarning)
-
-url = "Bitcoin Historical Data - Investing.com.csv"
-df = pd.read_csv(url)
-df = df[["Date", "Price"]]
-df = df.rename(columns={"Date": "ds", "Price": "y"})
-df.fillna(method='ffill', inplace=True)
-df.dropna(inplace=True)
-
-m = NeuralProphet(n_forecasts=3,
- n_lags=3,
- changepoints_range=0.95, num_hidden_layers=6, daily_seasonality= False, weekly_seasonality = False, yearly_seasonality = True, ar_reg=True,
- n_changepoints=250, trend_reg_threshold=True, d_hidden=9, global_normalization=True, global_time_normalization=True, seasonality_reg=1, unknown_data_normalization=True,
- seasonality_mode="multiplicative", drop_missing=True,
- learning_rate=0.03
-)
-
-m.fit(df, freq='M')
-
-future = m.make_future_dataframe(df, periods=3, n_historic_predictions=True)
-forecast = m.predict(future)
-
-def predict_vn_index(option=None):
- fig1 = m.plot(forecast)
- fig1_path = "forecast_plot1.png"
- fig1.savefig(fig1_path)
-
- # Add code to generate the second image (fig2)
- fig2 = m.plot_latest_forecast(forecast) # Replace this line with code to generate the second image
- fig2_path = "forecast_plot2.png"
- fig2.savefig(fig2_path)
- description = "Dự đoán được thực hiện bởi thuật toán AI học sâu (Deep Learning), và học tăng cường dữ liệu bởi đội ngũ AI Consultant. Dữ liệu được cập nhật mới sau 17h của ngày giao dịch."
- disclaimer = "Quý khách chỉ xem đây là tham khảo, công ty không chịu bất cứ trách nhiệm nào về tình trạng đầu tư của quý khách."
-
-
- return fig1_path, fig2_path, description, disclaimer
-
-
-if __name__ == "__main__":
- dropdown = gr.inputs.Dropdown(["BTC"], label="Choose an option", default="BTC")
- outputs = [
- gr.outputs.Image(type="filepath", label="Lịch sử BTC và dự đoán"),
- gr.outputs.Image(type="filepath", label="Dự đoán BTC cho 90 ngày tới"),
- gr.outputs.Textbox(label="Mô tả"),
- gr.outputs.Textbox(label="Disclaimer")
- ]
- interface = gr.Interface(fn=predict_vn_index, inputs=dropdown, outputs=outputs, title="Dự báo BTC 90 ngày tới")
- interface.launch()
-
diff --git a/spaces/Um124/Global_Warming_Analysis/pages/Oil Production data Analysis.py b/spaces/Um124/Global_Warming_Analysis/pages/Oil Production data Analysis.py
deleted file mode 100644
index 1570de4bdac7d0193a55edd983af350f4aa40aef..0000000000000000000000000000000000000000
--- a/spaces/Um124/Global_Warming_Analysis/pages/Oil Production data Analysis.py
+++ /dev/null
@@ -1,85 +0,0 @@
-import pandas as pd
-import numpy as np
-import plotly.express as px
-import streamlit as st
-
-
-st.set_page_config(
- page_title='Oil Production data Analysis',
- page_icon='📈',
- layout='wide'
-)
-
-Years=['1965','1966','1967','1968','1969','1970','1971','1972','1973','1974','1975','1976','1977','1978',
-'1979','1980','1981','1982','1983','1984','1985','1986','1987','1988','1989','1990','1991','1992','1993',
-'1994','1995','1996','1997','1998','1999','2000','2001','2002','2003','2004','2005','2006','2007','2008',
-'2009','2010','2011','2012','2013','2014','2015','2016']
-
-@st.cache_data
-def load_data():
- df=pd.read_csv('data/oil_production_per_person.csv')
- df.rename(columns={'geo':'Country'},inplace=True)
- df.set_index('Country',inplace=True)
- df['Total'] = df[Years].sum(axis=1)
- df['Avgrage']=df.mean(axis=1)
- df['Maximum']=df.max(axis=1)
- df['Minimum']=df.min(axis=1)
- df.sort_index(inplace=True)
- return df
-
-st.title('Oil Production per Person')
-df = load_data()
-st.dataframe(df,use_container_width=True)
-
-countries= df.index.unique().tolist()
-Graphs = ['bar','pie','line','area','funnel']
-c1,c2 = st.columns(2)
-country = c1.selectbox("Select a Country", countries)
-Graph = c2.selectbox("Select a Graph type", Graphs)
-
-st.header("Country wise visualization")
-cdf = df.loc[country,Years].reset_index()
-cdf.rename({'index':'Years'},axis=1, inplace=True)
-if Graph == Graphs[0]:
- fig = px.bar(cdf, 'Years',country, title=f'{country} Oil Production per Person')
-if Graph == Graphs[1]:
- fig = px.pie(cdf, 'Years',country, title=f'{country} Oil Production per Person')
-if Graph == Graphs[2]:
- fig = px.line(cdf, 'Years',country, title=f'{country} Oil Production per Person')
-if Graph == Graphs[3]:
- fig = px.area(cdf, 'Years',country, title=f'{country} Oil Production per Person')
-if Graph == Graphs[4]:
- fig = px.funnel(cdf, 'Years',country, title=f'{country} Oil Production per Person')
-st.plotly_chart(fig, use_container_width=True)
-
-st.header("Comparison of Countries")
-clist = st.multiselect("Select countries to compare", countries, default='India')
-cdf = df.loc[clist, Years].T # T to rotate the data in 90deg
-st.write(cdf)
-figc = px.line(cdf,cdf.index, clist, title=f'Comparing {", ".join(clist)}')
-
-st.plotly_chart(figc, use_container_width=True)
-
-df.sort_values(by='Total', ascending=False, inplace=True)
-fig1=px.bar(df, x=df.index, y='Total',title='Total Oil Production per Person')
-st.plotly_chart(fig1, use_container_width=True)
-
-dfavg = df.sort_values(by='Avgrage').reset_index()
-dfavg.rename({'index':'Country'},axis=1,inplace=True)
-fig2=px.bar(dfavg, 'Country', 'Avgrage', title="Avgrage Oil Production by Country")
-st.plotly_chart(fig2, use_container_width=True)
-
-dfmax=df.sort_values(by='Maximum').reset_index()
-dfmax.rename({'index':'Country'},axis=1,inplace=True)
-fig3=px.bar(dfmax,'Country','Maximum',title='Maximum Oil Production by the Country')
-st.plotly_chart(fig3, use_container_width=True)
-
-dfmin=df.sort_values(by='Minimum').reset_index()
-dfmin.rename({'index':'Country'},axis=1,inplace=True)
-fig4=px.bar(dfmin,'Country','Minimum',title='Minimum Oil Production by the Country' )
-st.plotly_chart(fig4,use_container_width=True)
-
-dfcomp=df.sort_values(by='Country',ascending=False,inplace=True)
-fig5 = px.line(df, x=df.index, y='Maximum',title='Maximum and Minimum Oil Production comparisons')
-fig5.add_scatter(x=df.index, y=df['Minimum'], mode='lines',)
-st.plotly_chart(fig5, use_container_width=True)
\ No newline at end of file
diff --git a/spaces/Vasanth/QuestionAnswering/app.py b/spaces/Vasanth/QuestionAnswering/app.py
deleted file mode 100644
index 3035972321adbdbdedd378befa929d7c0f1c382f..0000000000000000000000000000000000000000
--- a/spaces/Vasanth/QuestionAnswering/app.py
+++ /dev/null
@@ -1,29 +0,0 @@
-import streamlit as st
-from transformers import pipeline
-
-
-st.write("""
-
-# Question Answering System - Squad_V2
-
-A simple QA system that answers questions from the given context.
-
-Ask me a question and I'll try to answer it.
-
-There are lots of room for imporvements. This is just an initial version.
-
-""")
-
-que = st.text_input("Ask me a question", '')
-content = st.text_area("Enter Context", '')
-
-if que != "":
- model_name = "Vasanth/bert-base-uncased-qa-squad2"
- question_answerer = pipeline("question-answering", model=model_name, tokenizer=model_name)
- answer = question_answerer(
- question= que,
- context= content
- )
- st.write(answer["answer"])
-
-
\ No newline at end of file
diff --git a/spaces/Vicent3/sharp-transformers-traveltaxi/README.md b/spaces/Vicent3/sharp-transformers-traveltaxi/README.md
deleted file mode 100644
index 40c38598efc6bc463d96319a2d8fb51825e88586..0000000000000000000000000000000000000000
--- a/spaces/Vicent3/sharp-transformers-traveltaxi/README.md
+++ /dev/null
@@ -1,11 +0,0 @@
----
-title: Sharp Transformers Traveltaxi
-emoji: 🚢
-colorFrom: green
-colorTo: blue
-sdk: static
-pinned: true
-license: agpl-3.0
----
-
-Check out the configuration reference at https://huggingface.co/docs/hub/spaces-config-reference
diff --git a/spaces/XAI/CHM-Corr/SimSearch.py b/spaces/XAI/CHM-Corr/SimSearch.py
deleted file mode 100644
index 1307cbf8ef3178eae26342a1d31001c28b240536..0000000000000000000000000000000000000000
--- a/spaces/XAI/CHM-Corr/SimSearch.py
+++ /dev/null
@@ -1,66 +0,0 @@
-import faiss
-import numpy as np
-
-
-class FaissNeighbors:
- def __init__(self):
- self.index = None
- self.y = None
-
- def fit(self, X, y):
- self.index = faiss.IndexFlatL2(X.shape[1])
- self.index.add(X.astype(np.float32))
- self.y = y
-
- def get_distances_and_indices(self, X, top_K=1000):
- distances, indices = self.index.search(X.astype(np.float32), k=top_K)
- return np.copy(distances), np.copy(indices), np.copy(self.y[indices])
-
- def get_nearest_labels(self, X, top_K=1000):
- distances, indices = self.index.search(X.astype(np.float32), k=top_K)
- return np.copy(self.y[indices])
-
-
-class FaissCosineNeighbors:
- def __init__(self):
- self.cindex = None
- self.y = None
-
- def fit(self, X, y):
- self.cindex = faiss.index_factory(
- X.shape[1], "Flat", faiss.METRIC_INNER_PRODUCT
- )
- X = np.copy(X)
- X = X.astype(np.float32)
- faiss.normalize_L2(X)
- self.cindex.add(X)
- self.y = y
-
- def get_distances_and_indices(self, Q, topK):
- Q = np.copy(Q)
- faiss.normalize_L2(Q)
- distances, indices = self.cindex.search(Q.astype(np.float32), k=topK)
- return np.copy(distances), np.copy(indices), np.copy(self.y[indices])
-
- def get_nearest_labels(self, Q, topK=1000):
- Q = np.copy(Q)
- faiss.normalize_L2(Q)
- distances, indices = self.cindex.search(Q.astype(np.float32), k=topK)
- return np.copy(self.y[indices])
-
-
-class SearchableTrainingSet:
- def __init__(self, embeddings, labels):
- self.simsearcher = FaissCosineNeighbors()
- self.X_train = embeddings
- self.y_train = labels
-
- def build_index(self):
- self.simsearcher.fit(self.X_train, self.y_train)
-
- def search(self, query, k=20):
- nearest_data_points = self.simsearcher.get_distances_and_indices(
- Q=query, topK=100
- )
- # topKs = [x[0] for x in Counter(nearest_data_points[0]).most_common(k)]
- return nearest_data_points
diff --git a/spaces/Zengyf-CVer/Streamlit_YOLOv5_Model2x/models/__init__.py b/spaces/Zengyf-CVer/Streamlit_YOLOv5_Model2x/models/__init__.py
deleted file mode 100644
index e69de29bb2d1d6434b8b29ae775ad8c2e48c5391..0000000000000000000000000000000000000000
diff --git a/spaces/abhishek/sketch-to-image/annotator/uniformer/mmdet/datasets/lvis.py b/spaces/abhishek/sketch-to-image/annotator/uniformer/mmdet/datasets/lvis.py
deleted file mode 100644
index 122c64e79cf5f060d7ceddf4ad29c4debe40944b..0000000000000000000000000000000000000000
--- a/spaces/abhishek/sketch-to-image/annotator/uniformer/mmdet/datasets/lvis.py
+++ /dev/null
@@ -1,742 +0,0 @@
-import itertools
-import logging
-import os.path as osp
-import tempfile
-from collections import OrderedDict
-
-import numpy as np
-from mmcv.utils import print_log
-from terminaltables import AsciiTable
-
-from .builder import DATASETS
-from .coco import CocoDataset
-
-
-@DATASETS.register_module()
-class LVISV05Dataset(CocoDataset):
-
- CLASSES = (
- 'acorn', 'aerosol_can', 'air_conditioner', 'airplane', 'alarm_clock',
- 'alcohol', 'alligator', 'almond', 'ambulance', 'amplifier', 'anklet',
- 'antenna', 'apple', 'apple_juice', 'applesauce', 'apricot', 'apron',
- 'aquarium', 'armband', 'armchair', 'armoire', 'armor', 'artichoke',
- 'trash_can', 'ashtray', 'asparagus', 'atomizer', 'avocado', 'award',
- 'awning', 'ax', 'baby_buggy', 'basketball_backboard', 'backpack',
- 'handbag', 'suitcase', 'bagel', 'bagpipe', 'baguet', 'bait', 'ball',
- 'ballet_skirt', 'balloon', 'bamboo', 'banana', 'Band_Aid', 'bandage',
- 'bandanna', 'banjo', 'banner', 'barbell', 'barge', 'barrel',
- 'barrette', 'barrow', 'baseball_base', 'baseball', 'baseball_bat',
- 'baseball_cap', 'baseball_glove', 'basket', 'basketball_hoop',
- 'basketball', 'bass_horn', 'bat_(animal)', 'bath_mat', 'bath_towel',
- 'bathrobe', 'bathtub', 'batter_(food)', 'battery', 'beachball', 'bead',
- 'beaker', 'bean_curd', 'beanbag', 'beanie', 'bear', 'bed',
- 'bedspread', 'cow', 'beef_(food)', 'beeper', 'beer_bottle', 'beer_can',
- 'beetle', 'bell', 'bell_pepper', 'belt', 'belt_buckle', 'bench',
- 'beret', 'bib', 'Bible', 'bicycle', 'visor', 'binder', 'binoculars',
- 'bird', 'birdfeeder', 'birdbath', 'birdcage', 'birdhouse',
- 'birthday_cake', 'birthday_card', 'biscuit_(bread)', 'pirate_flag',
- 'black_sheep', 'blackboard', 'blanket', 'blazer', 'blender', 'blimp',
- 'blinker', 'blueberry', 'boar', 'gameboard', 'boat', 'bobbin',
- 'bobby_pin', 'boiled_egg', 'bolo_tie', 'deadbolt', 'bolt', 'bonnet',
- 'book', 'book_bag', 'bookcase', 'booklet', 'bookmark',
- 'boom_microphone', 'boot', 'bottle', 'bottle_opener', 'bouquet',
- 'bow_(weapon)', 'bow_(decorative_ribbons)', 'bow-tie', 'bowl',
- 'pipe_bowl', 'bowler_hat', 'bowling_ball', 'bowling_pin',
- 'boxing_glove', 'suspenders', 'bracelet', 'brass_plaque', 'brassiere',
- 'bread-bin', 'breechcloth', 'bridal_gown', 'briefcase',
- 'bristle_brush', 'broccoli', 'broach', 'broom', 'brownie',
- 'brussels_sprouts', 'bubble_gum', 'bucket', 'horse_buggy', 'bull',
- 'bulldog', 'bulldozer', 'bullet_train', 'bulletin_board',
- 'bulletproof_vest', 'bullhorn', 'corned_beef', 'bun', 'bunk_bed',
- 'buoy', 'burrito', 'bus_(vehicle)', 'business_card', 'butcher_knife',
- 'butter', 'butterfly', 'button', 'cab_(taxi)', 'cabana', 'cabin_car',
- 'cabinet', 'locker', 'cake', 'calculator', 'calendar', 'calf',
- 'camcorder', 'camel', 'camera', 'camera_lens', 'camper_(vehicle)',
- 'can', 'can_opener', 'candelabrum', 'candle', 'candle_holder',
- 'candy_bar', 'candy_cane', 'walking_cane', 'canister', 'cannon',
- 'canoe', 'cantaloup', 'canteen', 'cap_(headwear)', 'bottle_cap',
- 'cape', 'cappuccino', 'car_(automobile)', 'railcar_(part_of_a_train)',
- 'elevator_car', 'car_battery', 'identity_card', 'card', 'cardigan',
- 'cargo_ship', 'carnation', 'horse_carriage', 'carrot', 'tote_bag',
- 'cart', 'carton', 'cash_register', 'casserole', 'cassette', 'cast',
- 'cat', 'cauliflower', 'caviar', 'cayenne_(spice)', 'CD_player',
- 'celery', 'cellular_telephone', 'chain_mail', 'chair', 'chaise_longue',
- 'champagne', 'chandelier', 'chap', 'checkbook', 'checkerboard',
- 'cherry', 'chessboard', 'chest_of_drawers_(furniture)',
- 'chicken_(animal)', 'chicken_wire', 'chickpea', 'Chihuahua',
- 'chili_(vegetable)', 'chime', 'chinaware', 'crisp_(potato_chip)',
- 'poker_chip', 'chocolate_bar', 'chocolate_cake', 'chocolate_milk',
- 'chocolate_mousse', 'choker', 'chopping_board', 'chopstick',
- 'Christmas_tree', 'slide', 'cider', 'cigar_box', 'cigarette',
- 'cigarette_case', 'cistern', 'clarinet', 'clasp', 'cleansing_agent',
- 'clementine', 'clip', 'clipboard', 'clock', 'clock_tower',
- 'clothes_hamper', 'clothespin', 'clutch_bag', 'coaster', 'coat',
- 'coat_hanger', 'coatrack', 'cock', 'coconut', 'coffee_filter',
- 'coffee_maker', 'coffee_table', 'coffeepot', 'coil', 'coin',
- 'colander', 'coleslaw', 'coloring_material', 'combination_lock',
- 'pacifier', 'comic_book', 'computer_keyboard', 'concrete_mixer',
- 'cone', 'control', 'convertible_(automobile)', 'sofa_bed', 'cookie',
- 'cookie_jar', 'cooking_utensil', 'cooler_(for_food)',
- 'cork_(bottle_plug)', 'corkboard', 'corkscrew', 'edible_corn',
- 'cornbread', 'cornet', 'cornice', 'cornmeal', 'corset',
- 'romaine_lettuce', 'costume', 'cougar', 'coverall', 'cowbell',
- 'cowboy_hat', 'crab_(animal)', 'cracker', 'crape', 'crate', 'crayon',
- 'cream_pitcher', 'credit_card', 'crescent_roll', 'crib', 'crock_pot',
- 'crossbar', 'crouton', 'crow', 'crown', 'crucifix', 'cruise_ship',
- 'police_cruiser', 'crumb', 'crutch', 'cub_(animal)', 'cube',
- 'cucumber', 'cufflink', 'cup', 'trophy_cup', 'cupcake', 'hair_curler',
- 'curling_iron', 'curtain', 'cushion', 'custard', 'cutting_tool',
- 'cylinder', 'cymbal', 'dachshund', 'dagger', 'dartboard',
- 'date_(fruit)', 'deck_chair', 'deer', 'dental_floss', 'desk',
- 'detergent', 'diaper', 'diary', 'die', 'dinghy', 'dining_table', 'tux',
- 'dish', 'dish_antenna', 'dishrag', 'dishtowel', 'dishwasher',
- 'dishwasher_detergent', 'diskette', 'dispenser', 'Dixie_cup', 'dog',
- 'dog_collar', 'doll', 'dollar', 'dolphin', 'domestic_ass', 'eye_mask',
- 'doorbell', 'doorknob', 'doormat', 'doughnut', 'dove', 'dragonfly',
- 'drawer', 'underdrawers', 'dress', 'dress_hat', 'dress_suit',
- 'dresser', 'drill', 'drinking_fountain', 'drone', 'dropper',
- 'drum_(musical_instrument)', 'drumstick', 'duck', 'duckling',
- 'duct_tape', 'duffel_bag', 'dumbbell', 'dumpster', 'dustpan',
- 'Dutch_oven', 'eagle', 'earphone', 'earplug', 'earring', 'easel',
- 'eclair', 'eel', 'egg', 'egg_roll', 'egg_yolk', 'eggbeater',
- 'eggplant', 'electric_chair', 'refrigerator', 'elephant', 'elk',
- 'envelope', 'eraser', 'escargot', 'eyepatch', 'falcon', 'fan',
- 'faucet', 'fedora', 'ferret', 'Ferris_wheel', 'ferry', 'fig_(fruit)',
- 'fighter_jet', 'figurine', 'file_cabinet', 'file_(tool)', 'fire_alarm',
- 'fire_engine', 'fire_extinguisher', 'fire_hose', 'fireplace',
- 'fireplug', 'fish', 'fish_(food)', 'fishbowl', 'fishing_boat',
- 'fishing_rod', 'flag', 'flagpole', 'flamingo', 'flannel', 'flash',
- 'flashlight', 'fleece', 'flip-flop_(sandal)', 'flipper_(footwear)',
- 'flower_arrangement', 'flute_glass', 'foal', 'folding_chair',
- 'food_processor', 'football_(American)', 'football_helmet',
- 'footstool', 'fork', 'forklift', 'freight_car', 'French_toast',
- 'freshener', 'frisbee', 'frog', 'fruit_juice', 'fruit_salad',
- 'frying_pan', 'fudge', 'funnel', 'futon', 'gag', 'garbage',
- 'garbage_truck', 'garden_hose', 'gargle', 'gargoyle', 'garlic',
- 'gasmask', 'gazelle', 'gelatin', 'gemstone', 'giant_panda',
- 'gift_wrap', 'ginger', 'giraffe', 'cincture',
- 'glass_(drink_container)', 'globe', 'glove', 'goat', 'goggles',
- 'goldfish', 'golf_club', 'golfcart', 'gondola_(boat)', 'goose',
- 'gorilla', 'gourd', 'surgical_gown', 'grape', 'grasshopper', 'grater',
- 'gravestone', 'gravy_boat', 'green_bean', 'green_onion', 'griddle',
- 'grillroom', 'grinder_(tool)', 'grits', 'grizzly', 'grocery_bag',
- 'guacamole', 'guitar', 'gull', 'gun', 'hair_spray', 'hairbrush',
- 'hairnet', 'hairpin', 'ham', 'hamburger', 'hammer', 'hammock',
- 'hamper', 'hamster', 'hair_dryer', 'hand_glass', 'hand_towel',
- 'handcart', 'handcuff', 'handkerchief', 'handle', 'handsaw',
- 'hardback_book', 'harmonium', 'hat', 'hatbox', 'hatch', 'veil',
- 'headband', 'headboard', 'headlight', 'headscarf', 'headset',
- 'headstall_(for_horses)', 'hearing_aid', 'heart', 'heater',
- 'helicopter', 'helmet', 'heron', 'highchair', 'hinge', 'hippopotamus',
- 'hockey_stick', 'hog', 'home_plate_(baseball)', 'honey', 'fume_hood',
- 'hook', 'horse', 'hose', 'hot-air_balloon', 'hotplate', 'hot_sauce',
- 'hourglass', 'houseboat', 'hummingbird', 'hummus', 'polar_bear',
- 'icecream', 'popsicle', 'ice_maker', 'ice_pack', 'ice_skate',
- 'ice_tea', 'igniter', 'incense', 'inhaler', 'iPod',
- 'iron_(for_clothing)', 'ironing_board', 'jacket', 'jam', 'jean',
- 'jeep', 'jelly_bean', 'jersey', 'jet_plane', 'jewelry', 'joystick',
- 'jumpsuit', 'kayak', 'keg', 'kennel', 'kettle', 'key', 'keycard',
- 'kilt', 'kimono', 'kitchen_sink', 'kitchen_table', 'kite', 'kitten',
- 'kiwi_fruit', 'knee_pad', 'knife', 'knight_(chess_piece)',
- 'knitting_needle', 'knob', 'knocker_(on_a_door)', 'koala', 'lab_coat',
- 'ladder', 'ladle', 'ladybug', 'lamb_(animal)', 'lamb-chop', 'lamp',
- 'lamppost', 'lampshade', 'lantern', 'lanyard', 'laptop_computer',
- 'lasagna', 'latch', 'lawn_mower', 'leather', 'legging_(clothing)',
- 'Lego', 'lemon', 'lemonade', 'lettuce', 'license_plate', 'life_buoy',
- 'life_jacket', 'lightbulb', 'lightning_rod', 'lime', 'limousine',
- 'linen_paper', 'lion', 'lip_balm', 'lipstick', 'liquor', 'lizard',
- 'Loafer_(type_of_shoe)', 'log', 'lollipop', 'lotion',
- 'speaker_(stero_equipment)', 'loveseat', 'machine_gun', 'magazine',
- 'magnet', 'mail_slot', 'mailbox_(at_home)', 'mallet', 'mammoth',
- 'mandarin_orange', 'manger', 'manhole', 'map', 'marker', 'martini',
- 'mascot', 'mashed_potato', 'masher', 'mask', 'mast',
- 'mat_(gym_equipment)', 'matchbox', 'mattress', 'measuring_cup',
- 'measuring_stick', 'meatball', 'medicine', 'melon', 'microphone',
- 'microscope', 'microwave_oven', 'milestone', 'milk', 'minivan',
- 'mint_candy', 'mirror', 'mitten', 'mixer_(kitchen_tool)', 'money',
- 'monitor_(computer_equipment) computer_monitor', 'monkey', 'motor',
- 'motor_scooter', 'motor_vehicle', 'motorboat', 'motorcycle',
- 'mound_(baseball)', 'mouse_(animal_rodent)',
- 'mouse_(computer_equipment)', 'mousepad', 'muffin', 'mug', 'mushroom',
- 'music_stool', 'musical_instrument', 'nailfile', 'nameplate', 'napkin',
- 'neckerchief', 'necklace', 'necktie', 'needle', 'nest', 'newsstand',
- 'nightshirt', 'nosebag_(for_animals)', 'noseband_(for_animals)',
- 'notebook', 'notepad', 'nut', 'nutcracker', 'oar', 'octopus_(food)',
- 'octopus_(animal)', 'oil_lamp', 'olive_oil', 'omelet', 'onion',
- 'orange_(fruit)', 'orange_juice', 'oregano', 'ostrich', 'ottoman',
- 'overalls_(clothing)', 'owl', 'packet', 'inkpad', 'pad', 'paddle',
- 'padlock', 'paintbox', 'paintbrush', 'painting', 'pajamas', 'palette',
- 'pan_(for_cooking)', 'pan_(metal_container)', 'pancake', 'pantyhose',
- 'papaya', 'paperclip', 'paper_plate', 'paper_towel', 'paperback_book',
- 'paperweight', 'parachute', 'parakeet', 'parasail_(sports)',
- 'parchment', 'parka', 'parking_meter', 'parrot',
- 'passenger_car_(part_of_a_train)', 'passenger_ship', 'passport',
- 'pastry', 'patty_(food)', 'pea_(food)', 'peach', 'peanut_butter',
- 'pear', 'peeler_(tool_for_fruit_and_vegetables)', 'pegboard',
- 'pelican', 'pen', 'pencil', 'pencil_box', 'pencil_sharpener',
- 'pendulum', 'penguin', 'pennant', 'penny_(coin)', 'pepper',
- 'pepper_mill', 'perfume', 'persimmon', 'baby', 'pet', 'petfood',
- 'pew_(church_bench)', 'phonebook', 'phonograph_record', 'piano',
- 'pickle', 'pickup_truck', 'pie', 'pigeon', 'piggy_bank', 'pillow',
- 'pin_(non_jewelry)', 'pineapple', 'pinecone', 'ping-pong_ball',
- 'pinwheel', 'tobacco_pipe', 'pipe', 'pistol', 'pita_(bread)',
- 'pitcher_(vessel_for_liquid)', 'pitchfork', 'pizza', 'place_mat',
- 'plate', 'platter', 'playing_card', 'playpen', 'pliers',
- 'plow_(farm_equipment)', 'pocket_watch', 'pocketknife',
- 'poker_(fire_stirring_tool)', 'pole', 'police_van', 'polo_shirt',
- 'poncho', 'pony', 'pool_table', 'pop_(soda)', 'portrait',
- 'postbox_(public)', 'postcard', 'poster', 'pot', 'flowerpot', 'potato',
- 'potholder', 'pottery', 'pouch', 'power_shovel', 'prawn', 'printer',
- 'projectile_(weapon)', 'projector', 'propeller', 'prune', 'pudding',
- 'puffer_(fish)', 'puffin', 'pug-dog', 'pumpkin', 'puncher', 'puppet',
- 'puppy', 'quesadilla', 'quiche', 'quilt', 'rabbit', 'race_car',
- 'racket', 'radar', 'radiator', 'radio_receiver', 'radish', 'raft',
- 'rag_doll', 'raincoat', 'ram_(animal)', 'raspberry', 'rat',
- 'razorblade', 'reamer_(juicer)', 'rearview_mirror', 'receipt',
- 'recliner', 'record_player', 'red_cabbage', 'reflector',
- 'remote_control', 'rhinoceros', 'rib_(food)', 'rifle', 'ring',
- 'river_boat', 'road_map', 'robe', 'rocking_chair', 'roller_skate',
- 'Rollerblade', 'rolling_pin', 'root_beer',
- 'router_(computer_equipment)', 'rubber_band', 'runner_(carpet)',
- 'plastic_bag', 'saddle_(on_an_animal)', 'saddle_blanket', 'saddlebag',
- 'safety_pin', 'sail', 'salad', 'salad_plate', 'salami',
- 'salmon_(fish)', 'salmon_(food)', 'salsa', 'saltshaker',
- 'sandal_(type_of_shoe)', 'sandwich', 'satchel', 'saucepan', 'saucer',
- 'sausage', 'sawhorse', 'saxophone', 'scale_(measuring_instrument)',
- 'scarecrow', 'scarf', 'school_bus', 'scissors', 'scoreboard',
- 'scrambled_eggs', 'scraper', 'scratcher', 'screwdriver',
- 'scrubbing_brush', 'sculpture', 'seabird', 'seahorse', 'seaplane',
- 'seashell', 'seedling', 'serving_dish', 'sewing_machine', 'shaker',
- 'shampoo', 'shark', 'sharpener', 'Sharpie', 'shaver_(electric)',
- 'shaving_cream', 'shawl', 'shears', 'sheep', 'shepherd_dog',
- 'sherbert', 'shield', 'shirt', 'shoe', 'shopping_bag', 'shopping_cart',
- 'short_pants', 'shot_glass', 'shoulder_bag', 'shovel', 'shower_head',
- 'shower_curtain', 'shredder_(for_paper)', 'sieve', 'signboard', 'silo',
- 'sink', 'skateboard', 'skewer', 'ski', 'ski_boot', 'ski_parka',
- 'ski_pole', 'skirt', 'sled', 'sleeping_bag', 'sling_(bandage)',
- 'slipper_(footwear)', 'smoothie', 'snake', 'snowboard', 'snowman',
- 'snowmobile', 'soap', 'soccer_ball', 'sock', 'soda_fountain',
- 'carbonated_water', 'sofa', 'softball', 'solar_array', 'sombrero',
- 'soup', 'soup_bowl', 'soupspoon', 'sour_cream', 'soya_milk',
- 'space_shuttle', 'sparkler_(fireworks)', 'spatula', 'spear',
- 'spectacles', 'spice_rack', 'spider', 'sponge', 'spoon', 'sportswear',
- 'spotlight', 'squirrel', 'stapler_(stapling_machine)', 'starfish',
- 'statue_(sculpture)', 'steak_(food)', 'steak_knife',
- 'steamer_(kitchen_appliance)', 'steering_wheel', 'stencil',
- 'stepladder', 'step_stool', 'stereo_(sound_system)', 'stew', 'stirrer',
- 'stirrup', 'stockings_(leg_wear)', 'stool', 'stop_sign', 'brake_light',
- 'stove', 'strainer', 'strap', 'straw_(for_drinking)', 'strawberry',
- 'street_sign', 'streetlight', 'string_cheese', 'stylus', 'subwoofer',
- 'sugar_bowl', 'sugarcane_(plant)', 'suit_(clothing)', 'sunflower',
- 'sunglasses', 'sunhat', 'sunscreen', 'surfboard', 'sushi', 'mop',
- 'sweat_pants', 'sweatband', 'sweater', 'sweatshirt', 'sweet_potato',
- 'swimsuit', 'sword', 'syringe', 'Tabasco_sauce', 'table-tennis_table',
- 'table', 'table_lamp', 'tablecloth', 'tachometer', 'taco', 'tag',
- 'taillight', 'tambourine', 'army_tank', 'tank_(storage_vessel)',
- 'tank_top_(clothing)', 'tape_(sticky_cloth_or_paper)', 'tape_measure',
- 'tapestry', 'tarp', 'tartan', 'tassel', 'tea_bag', 'teacup',
- 'teakettle', 'teapot', 'teddy_bear', 'telephone', 'telephone_booth',
- 'telephone_pole', 'telephoto_lens', 'television_camera',
- 'television_set', 'tennis_ball', 'tennis_racket', 'tequila',
- 'thermometer', 'thermos_bottle', 'thermostat', 'thimble', 'thread',
- 'thumbtack', 'tiara', 'tiger', 'tights_(clothing)', 'timer', 'tinfoil',
- 'tinsel', 'tissue_paper', 'toast_(food)', 'toaster', 'toaster_oven',
- 'toilet', 'toilet_tissue', 'tomato', 'tongs', 'toolbox', 'toothbrush',
- 'toothpaste', 'toothpick', 'cover', 'tortilla', 'tow_truck', 'towel',
- 'towel_rack', 'toy', 'tractor_(farm_equipment)', 'traffic_light',
- 'dirt_bike', 'trailer_truck', 'train_(railroad_vehicle)', 'trampoline',
- 'tray', 'tree_house', 'trench_coat', 'triangle_(musical_instrument)',
- 'tricycle', 'tripod', 'trousers', 'truck', 'truffle_(chocolate)',
- 'trunk', 'vat', 'turban', 'turkey_(bird)', 'turkey_(food)', 'turnip',
- 'turtle', 'turtleneck_(clothing)', 'typewriter', 'umbrella',
- 'underwear', 'unicycle', 'urinal', 'urn', 'vacuum_cleaner', 'valve',
- 'vase', 'vending_machine', 'vent', 'videotape', 'vinegar', 'violin',
- 'vodka', 'volleyball', 'vulture', 'waffle', 'waffle_iron', 'wagon',
- 'wagon_wheel', 'walking_stick', 'wall_clock', 'wall_socket', 'wallet',
- 'walrus', 'wardrobe', 'wasabi', 'automatic_washer', 'watch',
- 'water_bottle', 'water_cooler', 'water_faucet', 'water_filter',
- 'water_heater', 'water_jug', 'water_gun', 'water_scooter', 'water_ski',
- 'water_tower', 'watering_can', 'watermelon', 'weathervane', 'webcam',
- 'wedding_cake', 'wedding_ring', 'wet_suit', 'wheel', 'wheelchair',
- 'whipped_cream', 'whiskey', 'whistle', 'wick', 'wig', 'wind_chime',
- 'windmill', 'window_box_(for_plants)', 'windshield_wiper', 'windsock',
- 'wine_bottle', 'wine_bucket', 'wineglass', 'wing_chair',
- 'blinder_(for_horses)', 'wok', 'wolf', 'wooden_spoon', 'wreath',
- 'wrench', 'wristband', 'wristlet', 'yacht', 'yak', 'yogurt',
- 'yoke_(animal_equipment)', 'zebra', 'zucchini')
-
- def load_annotations(self, ann_file):
- """Load annotation from lvis style annotation file.
-
- Args:
- ann_file (str): Path of annotation file.
-
- Returns:
- list[dict]: Annotation info from LVIS api.
- """
-
- try:
- import lvis
- assert lvis.__version__ >= '10.5.3'
- from lvis import LVIS
- except AssertionError:
- raise AssertionError('Incompatible version of lvis is installed. '
- 'Run pip uninstall lvis first. Then run pip '
- 'install mmlvis to install open-mmlab forked '
- 'lvis. ')
- except ImportError:
- raise ImportError('Package lvis is not installed. Please run pip '
- 'install mmlvis to install open-mmlab forked '
- 'lvis.')
- self.coco = LVIS(ann_file)
- self.cat_ids = self.coco.get_cat_ids()
- self.cat2label = {cat_id: i for i, cat_id in enumerate(self.cat_ids)}
- self.img_ids = self.coco.get_img_ids()
- data_infos = []
- for i in self.img_ids:
- info = self.coco.load_imgs([i])[0]
- if info['file_name'].startswith('COCO'):
- # Convert form the COCO 2014 file naming convention of
- # COCO_[train/val/test]2014_000000000000.jpg to the 2017
- # naming convention of 000000000000.jpg
- # (LVIS v1 will fix this naming issue)
- info['filename'] = info['file_name'][-16:]
- else:
- info['filename'] = info['file_name']
- data_infos.append(info)
- return data_infos
-
- def evaluate(self,
- results,
- metric='bbox',
- logger=None,
- jsonfile_prefix=None,
- classwise=False,
- proposal_nums=(100, 300, 1000),
- iou_thrs=np.arange(0.5, 0.96, 0.05)):
- """Evaluation in LVIS protocol.
-
- Args:
- results (list[list | tuple]): Testing results of the dataset.
- metric (str | list[str]): Metrics to be evaluated. Options are
- 'bbox', 'segm', 'proposal', 'proposal_fast'.
- logger (logging.Logger | str | None): Logger used for printing
- related information during evaluation. Default: None.
- jsonfile_prefix (str | None):
- classwise (bool): Whether to evaluating the AP for each class.
- proposal_nums (Sequence[int]): Proposal number used for evaluating
- recalls, such as recall@100, recall@1000.
- Default: (100, 300, 1000).
- iou_thrs (Sequence[float]): IoU threshold used for evaluating
- recalls. If set to a list, the average recall of all IoUs will
- also be computed. Default: 0.5.
-
- Returns:
- dict[str, float]: LVIS style metrics.
- """
-
- try:
- import lvis
- assert lvis.__version__ >= '10.5.3'
- from lvis import LVISResults, LVISEval
- except AssertionError:
- raise AssertionError('Incompatible version of lvis is installed. '
- 'Run pip uninstall lvis first. Then run pip '
- 'install mmlvis to install open-mmlab forked '
- 'lvis. ')
- except ImportError:
- raise ImportError('Package lvis is not installed. Please run pip '
- 'install mmlvis to install open-mmlab forked '
- 'lvis.')
- assert isinstance(results, list), 'results must be a list'
- assert len(results) == len(self), (
- 'The length of results is not equal to the dataset len: {} != {}'.
- format(len(results), len(self)))
-
- metrics = metric if isinstance(metric, list) else [metric]
- allowed_metrics = ['bbox', 'segm', 'proposal', 'proposal_fast']
- for metric in metrics:
- if metric not in allowed_metrics:
- raise KeyError('metric {} is not supported'.format(metric))
-
- if jsonfile_prefix is None:
- tmp_dir = tempfile.TemporaryDirectory()
- jsonfile_prefix = osp.join(tmp_dir.name, 'results')
- else:
- tmp_dir = None
- result_files = self.results2json(results, jsonfile_prefix)
-
- eval_results = OrderedDict()
- # get original api
- lvis_gt = self.coco
- for metric in metrics:
- msg = 'Evaluating {}...'.format(metric)
- if logger is None:
- msg = '\n' + msg
- print_log(msg, logger=logger)
-
- if metric == 'proposal_fast':
- ar = self.fast_eval_recall(
- results, proposal_nums, iou_thrs, logger='silent')
- log_msg = []
- for i, num in enumerate(proposal_nums):
- eval_results['AR@{}'.format(num)] = ar[i]
- log_msg.append('\nAR@{}\t{:.4f}'.format(num, ar[i]))
- log_msg = ''.join(log_msg)
- print_log(log_msg, logger=logger)
- continue
-
- if metric not in result_files:
- raise KeyError('{} is not in results'.format(metric))
- try:
- lvis_dt = LVISResults(lvis_gt, result_files[metric])
- except IndexError:
- print_log(
- 'The testing results of the whole dataset is empty.',
- logger=logger,
- level=logging.ERROR)
- break
-
- iou_type = 'bbox' if metric == 'proposal' else metric
- lvis_eval = LVISEval(lvis_gt, lvis_dt, iou_type)
- lvis_eval.params.imgIds = self.img_ids
- if metric == 'proposal':
- lvis_eval.params.useCats = 0
- lvis_eval.params.maxDets = list(proposal_nums)
- lvis_eval.evaluate()
- lvis_eval.accumulate()
- lvis_eval.summarize()
- for k, v in lvis_eval.get_results().items():
- if k.startswith('AR'):
- val = float('{:.3f}'.format(float(v)))
- eval_results[k] = val
- else:
- lvis_eval.evaluate()
- lvis_eval.accumulate()
- lvis_eval.summarize()
- lvis_results = lvis_eval.get_results()
- if classwise: # Compute per-category AP
- # Compute per-category AP
- # from https://github.com/facebookresearch/detectron2/
- precisions = lvis_eval.eval['precision']
- # precision: (iou, recall, cls, area range, max dets)
- assert len(self.cat_ids) == precisions.shape[2]
-
- results_per_category = []
- for idx, catId in enumerate(self.cat_ids):
- # area range index 0: all area ranges
- # max dets index -1: typically 100 per image
- nm = self.coco.load_cats(catId)[0]
- precision = precisions[:, :, idx, 0, -1]
- precision = precision[precision > -1]
- if precision.size:
- ap = np.mean(precision)
- else:
- ap = float('nan')
- results_per_category.append(
- (f'{nm["name"]}', f'{float(ap):0.3f}'))
-
- num_columns = min(6, len(results_per_category) * 2)
- results_flatten = list(
- itertools.chain(*results_per_category))
- headers = ['category', 'AP'] * (num_columns // 2)
- results_2d = itertools.zip_longest(*[
- results_flatten[i::num_columns]
- for i in range(num_columns)
- ])
- table_data = [headers]
- table_data += [result for result in results_2d]
- table = AsciiTable(table_data)
- print_log('\n' + table.table, logger=logger)
-
- for k, v in lvis_results.items():
- if k.startswith('AP'):
- key = '{}_{}'.format(metric, k)
- val = float('{:.3f}'.format(float(v)))
- eval_results[key] = val
- ap_summary = ' '.join([
- '{}:{:.3f}'.format(k, float(v))
- for k, v in lvis_results.items() if k.startswith('AP')
- ])
- eval_results['{}_mAP_copypaste'.format(metric)] = ap_summary
- lvis_eval.print_results()
- if tmp_dir is not None:
- tmp_dir.cleanup()
- return eval_results
-
-
-LVISDataset = LVISV05Dataset
-DATASETS.register_module(name='LVISDataset', module=LVISDataset)
-
-
-@DATASETS.register_module()
-class LVISV1Dataset(LVISDataset):
-
- CLASSES = (
- 'aerosol_can', 'air_conditioner', 'airplane', 'alarm_clock', 'alcohol',
- 'alligator', 'almond', 'ambulance', 'amplifier', 'anklet', 'antenna',
- 'apple', 'applesauce', 'apricot', 'apron', 'aquarium',
- 'arctic_(type_of_shoe)', 'armband', 'armchair', 'armoire', 'armor',
- 'artichoke', 'trash_can', 'ashtray', 'asparagus', 'atomizer',
- 'avocado', 'award', 'awning', 'ax', 'baboon', 'baby_buggy',
- 'basketball_backboard', 'backpack', 'handbag', 'suitcase', 'bagel',
- 'bagpipe', 'baguet', 'bait', 'ball', 'ballet_skirt', 'balloon',
- 'bamboo', 'banana', 'Band_Aid', 'bandage', 'bandanna', 'banjo',
- 'banner', 'barbell', 'barge', 'barrel', 'barrette', 'barrow',
- 'baseball_base', 'baseball', 'baseball_bat', 'baseball_cap',
- 'baseball_glove', 'basket', 'basketball', 'bass_horn', 'bat_(animal)',
- 'bath_mat', 'bath_towel', 'bathrobe', 'bathtub', 'batter_(food)',
- 'battery', 'beachball', 'bead', 'bean_curd', 'beanbag', 'beanie',
- 'bear', 'bed', 'bedpan', 'bedspread', 'cow', 'beef_(food)', 'beeper',
- 'beer_bottle', 'beer_can', 'beetle', 'bell', 'bell_pepper', 'belt',
- 'belt_buckle', 'bench', 'beret', 'bib', 'Bible', 'bicycle', 'visor',
- 'billboard', 'binder', 'binoculars', 'bird', 'birdfeeder', 'birdbath',
- 'birdcage', 'birdhouse', 'birthday_cake', 'birthday_card',
- 'pirate_flag', 'black_sheep', 'blackberry', 'blackboard', 'blanket',
- 'blazer', 'blender', 'blimp', 'blinker', 'blouse', 'blueberry',
- 'gameboard', 'boat', 'bob', 'bobbin', 'bobby_pin', 'boiled_egg',
- 'bolo_tie', 'deadbolt', 'bolt', 'bonnet', 'book', 'bookcase',
- 'booklet', 'bookmark', 'boom_microphone', 'boot', 'bottle',
- 'bottle_opener', 'bouquet', 'bow_(weapon)', 'bow_(decorative_ribbons)',
- 'bow-tie', 'bowl', 'pipe_bowl', 'bowler_hat', 'bowling_ball', 'box',
- 'boxing_glove', 'suspenders', 'bracelet', 'brass_plaque', 'brassiere',
- 'bread-bin', 'bread', 'breechcloth', 'bridal_gown', 'briefcase',
- 'broccoli', 'broach', 'broom', 'brownie', 'brussels_sprouts',
- 'bubble_gum', 'bucket', 'horse_buggy', 'bull', 'bulldog', 'bulldozer',
- 'bullet_train', 'bulletin_board', 'bulletproof_vest', 'bullhorn',
- 'bun', 'bunk_bed', 'buoy', 'burrito', 'bus_(vehicle)', 'business_card',
- 'butter', 'butterfly', 'button', 'cab_(taxi)', 'cabana', 'cabin_car',
- 'cabinet', 'locker', 'cake', 'calculator', 'calendar', 'calf',
- 'camcorder', 'camel', 'camera', 'camera_lens', 'camper_(vehicle)',
- 'can', 'can_opener', 'candle', 'candle_holder', 'candy_bar',
- 'candy_cane', 'walking_cane', 'canister', 'canoe', 'cantaloup',
- 'canteen', 'cap_(headwear)', 'bottle_cap', 'cape', 'cappuccino',
- 'car_(automobile)', 'railcar_(part_of_a_train)', 'elevator_car',
- 'car_battery', 'identity_card', 'card', 'cardigan', 'cargo_ship',
- 'carnation', 'horse_carriage', 'carrot', 'tote_bag', 'cart', 'carton',
- 'cash_register', 'casserole', 'cassette', 'cast', 'cat', 'cauliflower',
- 'cayenne_(spice)', 'CD_player', 'celery', 'cellular_telephone',
- 'chain_mail', 'chair', 'chaise_longue', 'chalice', 'chandelier',
- 'chap', 'checkbook', 'checkerboard', 'cherry', 'chessboard',
- 'chicken_(animal)', 'chickpea', 'chili_(vegetable)', 'chime',
- 'chinaware', 'crisp_(potato_chip)', 'poker_chip', 'chocolate_bar',
- 'chocolate_cake', 'chocolate_milk', 'chocolate_mousse', 'choker',
- 'chopping_board', 'chopstick', 'Christmas_tree', 'slide', 'cider',
- 'cigar_box', 'cigarette', 'cigarette_case', 'cistern', 'clarinet',
- 'clasp', 'cleansing_agent', 'cleat_(for_securing_rope)', 'clementine',
- 'clip', 'clipboard', 'clippers_(for_plants)', 'cloak', 'clock',
- 'clock_tower', 'clothes_hamper', 'clothespin', 'clutch_bag', 'coaster',
- 'coat', 'coat_hanger', 'coatrack', 'cock', 'cockroach',
- 'cocoa_(beverage)', 'coconut', 'coffee_maker', 'coffee_table',
- 'coffeepot', 'coil', 'coin', 'colander', 'coleslaw',
- 'coloring_material', 'combination_lock', 'pacifier', 'comic_book',
- 'compass', 'computer_keyboard', 'condiment', 'cone', 'control',
- 'convertible_(automobile)', 'sofa_bed', 'cooker', 'cookie',
- 'cooking_utensil', 'cooler_(for_food)', 'cork_(bottle_plug)',
- 'corkboard', 'corkscrew', 'edible_corn', 'cornbread', 'cornet',
- 'cornice', 'cornmeal', 'corset', 'costume', 'cougar', 'coverall',
- 'cowbell', 'cowboy_hat', 'crab_(animal)', 'crabmeat', 'cracker',
- 'crape', 'crate', 'crayon', 'cream_pitcher', 'crescent_roll', 'crib',
- 'crock_pot', 'crossbar', 'crouton', 'crow', 'crowbar', 'crown',
- 'crucifix', 'cruise_ship', 'police_cruiser', 'crumb', 'crutch',
- 'cub_(animal)', 'cube', 'cucumber', 'cufflink', 'cup', 'trophy_cup',
- 'cupboard', 'cupcake', 'hair_curler', 'curling_iron', 'curtain',
- 'cushion', 'cylinder', 'cymbal', 'dagger', 'dalmatian', 'dartboard',
- 'date_(fruit)', 'deck_chair', 'deer', 'dental_floss', 'desk',
- 'detergent', 'diaper', 'diary', 'die', 'dinghy', 'dining_table', 'tux',
- 'dish', 'dish_antenna', 'dishrag', 'dishtowel', 'dishwasher',
- 'dishwasher_detergent', 'dispenser', 'diving_board', 'Dixie_cup',
- 'dog', 'dog_collar', 'doll', 'dollar', 'dollhouse', 'dolphin',
- 'domestic_ass', 'doorknob', 'doormat', 'doughnut', 'dove', 'dragonfly',
- 'drawer', 'underdrawers', 'dress', 'dress_hat', 'dress_suit',
- 'dresser', 'drill', 'drone', 'dropper', 'drum_(musical_instrument)',
- 'drumstick', 'duck', 'duckling', 'duct_tape', 'duffel_bag', 'dumbbell',
- 'dumpster', 'dustpan', 'eagle', 'earphone', 'earplug', 'earring',
- 'easel', 'eclair', 'eel', 'egg', 'egg_roll', 'egg_yolk', 'eggbeater',
- 'eggplant', 'electric_chair', 'refrigerator', 'elephant', 'elk',
- 'envelope', 'eraser', 'escargot', 'eyepatch', 'falcon', 'fan',
- 'faucet', 'fedora', 'ferret', 'Ferris_wheel', 'ferry', 'fig_(fruit)',
- 'fighter_jet', 'figurine', 'file_cabinet', 'file_(tool)', 'fire_alarm',
- 'fire_engine', 'fire_extinguisher', 'fire_hose', 'fireplace',
- 'fireplug', 'first-aid_kit', 'fish', 'fish_(food)', 'fishbowl',
- 'fishing_rod', 'flag', 'flagpole', 'flamingo', 'flannel', 'flap',
- 'flash', 'flashlight', 'fleece', 'flip-flop_(sandal)',
- 'flipper_(footwear)', 'flower_arrangement', 'flute_glass', 'foal',
- 'folding_chair', 'food_processor', 'football_(American)',
- 'football_helmet', 'footstool', 'fork', 'forklift', 'freight_car',
- 'French_toast', 'freshener', 'frisbee', 'frog', 'fruit_juice',
- 'frying_pan', 'fudge', 'funnel', 'futon', 'gag', 'garbage',
- 'garbage_truck', 'garden_hose', 'gargle', 'gargoyle', 'garlic',
- 'gasmask', 'gazelle', 'gelatin', 'gemstone', 'generator',
- 'giant_panda', 'gift_wrap', 'ginger', 'giraffe', 'cincture',
- 'glass_(drink_container)', 'globe', 'glove', 'goat', 'goggles',
- 'goldfish', 'golf_club', 'golfcart', 'gondola_(boat)', 'goose',
- 'gorilla', 'gourd', 'grape', 'grater', 'gravestone', 'gravy_boat',
- 'green_bean', 'green_onion', 'griddle', 'grill', 'grits', 'grizzly',
- 'grocery_bag', 'guitar', 'gull', 'gun', 'hairbrush', 'hairnet',
- 'hairpin', 'halter_top', 'ham', 'hamburger', 'hammer', 'hammock',
- 'hamper', 'hamster', 'hair_dryer', 'hand_glass', 'hand_towel',
- 'handcart', 'handcuff', 'handkerchief', 'handle', 'handsaw',
- 'hardback_book', 'harmonium', 'hat', 'hatbox', 'veil', 'headband',
- 'headboard', 'headlight', 'headscarf', 'headset',
- 'headstall_(for_horses)', 'heart', 'heater', 'helicopter', 'helmet',
- 'heron', 'highchair', 'hinge', 'hippopotamus', 'hockey_stick', 'hog',
- 'home_plate_(baseball)', 'honey', 'fume_hood', 'hook', 'hookah',
- 'hornet', 'horse', 'hose', 'hot-air_balloon', 'hotplate', 'hot_sauce',
- 'hourglass', 'houseboat', 'hummingbird', 'hummus', 'polar_bear',
- 'icecream', 'popsicle', 'ice_maker', 'ice_pack', 'ice_skate',
- 'igniter', 'inhaler', 'iPod', 'iron_(for_clothing)', 'ironing_board',
- 'jacket', 'jam', 'jar', 'jean', 'jeep', 'jelly_bean', 'jersey',
- 'jet_plane', 'jewel', 'jewelry', 'joystick', 'jumpsuit', 'kayak',
- 'keg', 'kennel', 'kettle', 'key', 'keycard', 'kilt', 'kimono',
- 'kitchen_sink', 'kitchen_table', 'kite', 'kitten', 'kiwi_fruit',
- 'knee_pad', 'knife', 'knitting_needle', 'knob', 'knocker_(on_a_door)',
- 'koala', 'lab_coat', 'ladder', 'ladle', 'ladybug', 'lamb_(animal)',
- 'lamb-chop', 'lamp', 'lamppost', 'lampshade', 'lantern', 'lanyard',
- 'laptop_computer', 'lasagna', 'latch', 'lawn_mower', 'leather',
- 'legging_(clothing)', 'Lego', 'legume', 'lemon', 'lemonade', 'lettuce',
- 'license_plate', 'life_buoy', 'life_jacket', 'lightbulb',
- 'lightning_rod', 'lime', 'limousine', 'lion', 'lip_balm', 'liquor',
- 'lizard', 'log', 'lollipop', 'speaker_(stero_equipment)', 'loveseat',
- 'machine_gun', 'magazine', 'magnet', 'mail_slot', 'mailbox_(at_home)',
- 'mallard', 'mallet', 'mammoth', 'manatee', 'mandarin_orange', 'manger',
- 'manhole', 'map', 'marker', 'martini', 'mascot', 'mashed_potato',
- 'masher', 'mask', 'mast', 'mat_(gym_equipment)', 'matchbox',
- 'mattress', 'measuring_cup', 'measuring_stick', 'meatball', 'medicine',
- 'melon', 'microphone', 'microscope', 'microwave_oven', 'milestone',
- 'milk', 'milk_can', 'milkshake', 'minivan', 'mint_candy', 'mirror',
- 'mitten', 'mixer_(kitchen_tool)', 'money',
- 'monitor_(computer_equipment) computer_monitor', 'monkey', 'motor',
- 'motor_scooter', 'motor_vehicle', 'motorcycle', 'mound_(baseball)',
- 'mouse_(computer_equipment)', 'mousepad', 'muffin', 'mug', 'mushroom',
- 'music_stool', 'musical_instrument', 'nailfile', 'napkin',
- 'neckerchief', 'necklace', 'necktie', 'needle', 'nest', 'newspaper',
- 'newsstand', 'nightshirt', 'nosebag_(for_animals)',
- 'noseband_(for_animals)', 'notebook', 'notepad', 'nut', 'nutcracker',
- 'oar', 'octopus_(food)', 'octopus_(animal)', 'oil_lamp', 'olive_oil',
- 'omelet', 'onion', 'orange_(fruit)', 'orange_juice', 'ostrich',
- 'ottoman', 'oven', 'overalls_(clothing)', 'owl', 'packet', 'inkpad',
- 'pad', 'paddle', 'padlock', 'paintbrush', 'painting', 'pajamas',
- 'palette', 'pan_(for_cooking)', 'pan_(metal_container)', 'pancake',
- 'pantyhose', 'papaya', 'paper_plate', 'paper_towel', 'paperback_book',
- 'paperweight', 'parachute', 'parakeet', 'parasail_(sports)', 'parasol',
- 'parchment', 'parka', 'parking_meter', 'parrot',
- 'passenger_car_(part_of_a_train)', 'passenger_ship', 'passport',
- 'pastry', 'patty_(food)', 'pea_(food)', 'peach', 'peanut_butter',
- 'pear', 'peeler_(tool_for_fruit_and_vegetables)', 'wooden_leg',
- 'pegboard', 'pelican', 'pen', 'pencil', 'pencil_box',
- 'pencil_sharpener', 'pendulum', 'penguin', 'pennant', 'penny_(coin)',
- 'pepper', 'pepper_mill', 'perfume', 'persimmon', 'person', 'pet',
- 'pew_(church_bench)', 'phonebook', 'phonograph_record', 'piano',
- 'pickle', 'pickup_truck', 'pie', 'pigeon', 'piggy_bank', 'pillow',
- 'pin_(non_jewelry)', 'pineapple', 'pinecone', 'ping-pong_ball',
- 'pinwheel', 'tobacco_pipe', 'pipe', 'pistol', 'pita_(bread)',
- 'pitcher_(vessel_for_liquid)', 'pitchfork', 'pizza', 'place_mat',
- 'plate', 'platter', 'playpen', 'pliers', 'plow_(farm_equipment)',
- 'plume', 'pocket_watch', 'pocketknife', 'poker_(fire_stirring_tool)',
- 'pole', 'polo_shirt', 'poncho', 'pony', 'pool_table', 'pop_(soda)',
- 'postbox_(public)', 'postcard', 'poster', 'pot', 'flowerpot', 'potato',
- 'potholder', 'pottery', 'pouch', 'power_shovel', 'prawn', 'pretzel',
- 'printer', 'projectile_(weapon)', 'projector', 'propeller', 'prune',
- 'pudding', 'puffer_(fish)', 'puffin', 'pug-dog', 'pumpkin', 'puncher',
- 'puppet', 'puppy', 'quesadilla', 'quiche', 'quilt', 'rabbit',
- 'race_car', 'racket', 'radar', 'radiator', 'radio_receiver', 'radish',
- 'raft', 'rag_doll', 'raincoat', 'ram_(animal)', 'raspberry', 'rat',
- 'razorblade', 'reamer_(juicer)', 'rearview_mirror', 'receipt',
- 'recliner', 'record_player', 'reflector', 'remote_control',
- 'rhinoceros', 'rib_(food)', 'rifle', 'ring', 'river_boat', 'road_map',
- 'robe', 'rocking_chair', 'rodent', 'roller_skate', 'Rollerblade',
- 'rolling_pin', 'root_beer', 'router_(computer_equipment)',
- 'rubber_band', 'runner_(carpet)', 'plastic_bag',
- 'saddle_(on_an_animal)', 'saddle_blanket', 'saddlebag', 'safety_pin',
- 'sail', 'salad', 'salad_plate', 'salami', 'salmon_(fish)',
- 'salmon_(food)', 'salsa', 'saltshaker', 'sandal_(type_of_shoe)',
- 'sandwich', 'satchel', 'saucepan', 'saucer', 'sausage', 'sawhorse',
- 'saxophone', 'scale_(measuring_instrument)', 'scarecrow', 'scarf',
- 'school_bus', 'scissors', 'scoreboard', 'scraper', 'screwdriver',
- 'scrubbing_brush', 'sculpture', 'seabird', 'seahorse', 'seaplane',
- 'seashell', 'sewing_machine', 'shaker', 'shampoo', 'shark',
- 'sharpener', 'Sharpie', 'shaver_(electric)', 'shaving_cream', 'shawl',
- 'shears', 'sheep', 'shepherd_dog', 'sherbert', 'shield', 'shirt',
- 'shoe', 'shopping_bag', 'shopping_cart', 'short_pants', 'shot_glass',
- 'shoulder_bag', 'shovel', 'shower_head', 'shower_cap',
- 'shower_curtain', 'shredder_(for_paper)', 'signboard', 'silo', 'sink',
- 'skateboard', 'skewer', 'ski', 'ski_boot', 'ski_parka', 'ski_pole',
- 'skirt', 'skullcap', 'sled', 'sleeping_bag', 'sling_(bandage)',
- 'slipper_(footwear)', 'smoothie', 'snake', 'snowboard', 'snowman',
- 'snowmobile', 'soap', 'soccer_ball', 'sock', 'sofa', 'softball',
- 'solar_array', 'sombrero', 'soup', 'soup_bowl', 'soupspoon',
- 'sour_cream', 'soya_milk', 'space_shuttle', 'sparkler_(fireworks)',
- 'spatula', 'spear', 'spectacles', 'spice_rack', 'spider', 'crawfish',
- 'sponge', 'spoon', 'sportswear', 'spotlight', 'squid_(food)',
- 'squirrel', 'stagecoach', 'stapler_(stapling_machine)', 'starfish',
- 'statue_(sculpture)', 'steak_(food)', 'steak_knife', 'steering_wheel',
- 'stepladder', 'step_stool', 'stereo_(sound_system)', 'stew', 'stirrer',
- 'stirrup', 'stool', 'stop_sign', 'brake_light', 'stove', 'strainer',
- 'strap', 'straw_(for_drinking)', 'strawberry', 'street_sign',
- 'streetlight', 'string_cheese', 'stylus', 'subwoofer', 'sugar_bowl',
- 'sugarcane_(plant)', 'suit_(clothing)', 'sunflower', 'sunglasses',
- 'sunhat', 'surfboard', 'sushi', 'mop', 'sweat_pants', 'sweatband',
- 'sweater', 'sweatshirt', 'sweet_potato', 'swimsuit', 'sword',
- 'syringe', 'Tabasco_sauce', 'table-tennis_table', 'table',
- 'table_lamp', 'tablecloth', 'tachometer', 'taco', 'tag', 'taillight',
- 'tambourine', 'army_tank', 'tank_(storage_vessel)',
- 'tank_top_(clothing)', 'tape_(sticky_cloth_or_paper)', 'tape_measure',
- 'tapestry', 'tarp', 'tartan', 'tassel', 'tea_bag', 'teacup',
- 'teakettle', 'teapot', 'teddy_bear', 'telephone', 'telephone_booth',
- 'telephone_pole', 'telephoto_lens', 'television_camera',
- 'television_set', 'tennis_ball', 'tennis_racket', 'tequila',
- 'thermometer', 'thermos_bottle', 'thermostat', 'thimble', 'thread',
- 'thumbtack', 'tiara', 'tiger', 'tights_(clothing)', 'timer', 'tinfoil',
- 'tinsel', 'tissue_paper', 'toast_(food)', 'toaster', 'toaster_oven',
- 'toilet', 'toilet_tissue', 'tomato', 'tongs', 'toolbox', 'toothbrush',
- 'toothpaste', 'toothpick', 'cover', 'tortilla', 'tow_truck', 'towel',
- 'towel_rack', 'toy', 'tractor_(farm_equipment)', 'traffic_light',
- 'dirt_bike', 'trailer_truck', 'train_(railroad_vehicle)', 'trampoline',
- 'tray', 'trench_coat', 'triangle_(musical_instrument)', 'tricycle',
- 'tripod', 'trousers', 'truck', 'truffle_(chocolate)', 'trunk', 'vat',
- 'turban', 'turkey_(food)', 'turnip', 'turtle', 'turtleneck_(clothing)',
- 'typewriter', 'umbrella', 'underwear', 'unicycle', 'urinal', 'urn',
- 'vacuum_cleaner', 'vase', 'vending_machine', 'vent', 'vest',
- 'videotape', 'vinegar', 'violin', 'vodka', 'volleyball', 'vulture',
- 'waffle', 'waffle_iron', 'wagon', 'wagon_wheel', 'walking_stick',
- 'wall_clock', 'wall_socket', 'wallet', 'walrus', 'wardrobe',
- 'washbasin', 'automatic_washer', 'watch', 'water_bottle',
- 'water_cooler', 'water_faucet', 'water_heater', 'water_jug',
- 'water_gun', 'water_scooter', 'water_ski', 'water_tower',
- 'watering_can', 'watermelon', 'weathervane', 'webcam', 'wedding_cake',
- 'wedding_ring', 'wet_suit', 'wheel', 'wheelchair', 'whipped_cream',
- 'whistle', 'wig', 'wind_chime', 'windmill', 'window_box_(for_plants)',
- 'windshield_wiper', 'windsock', 'wine_bottle', 'wine_bucket',
- 'wineglass', 'blinder_(for_horses)', 'wok', 'wolf', 'wooden_spoon',
- 'wreath', 'wrench', 'wristband', 'wristlet', 'yacht', 'yogurt',
- 'yoke_(animal_equipment)', 'zebra', 'zucchini')
-
- def load_annotations(self, ann_file):
- try:
- import lvis
- assert lvis.__version__ >= '10.5.3'
- from lvis import LVIS
- except AssertionError:
- raise AssertionError('Incompatible version of lvis is installed. '
- 'Run pip uninstall lvis first. Then run pip '
- 'install mmlvis to install open-mmlab forked '
- 'lvis. ')
- except ImportError:
- raise ImportError('Package lvis is not installed. Please run pip '
- 'install mmlvis to install open-mmlab forked '
- 'lvis.')
- self.coco = LVIS(ann_file)
- self.cat_ids = self.coco.get_cat_ids()
- self.cat2label = {cat_id: i for i, cat_id in enumerate(self.cat_ids)}
- self.img_ids = self.coco.get_img_ids()
- data_infos = []
- for i in self.img_ids:
- info = self.coco.load_imgs([i])[0]
- # coco_url is used in LVISv1 instead of file_name
- # e.g. http://images.cocodataset.org/train2017/000000391895.jpg
- # train/val split in specified in url
- info['filename'] = info['coco_url'].replace(
- 'http://images.cocodataset.org/', '')
- data_infos.append(info)
- return data_infos
diff --git a/spaces/abhishek/sketch-to-image/annotator/uniformer_base/exp/upernet_global_base/test.sh b/spaces/abhishek/sketch-to-image/annotator/uniformer_base/exp/upernet_global_base/test.sh
deleted file mode 100644
index d9a85e7a0d3b7c96b060f473d41254b37a382fcb..0000000000000000000000000000000000000000
--- a/spaces/abhishek/sketch-to-image/annotator/uniformer_base/exp/upernet_global_base/test.sh
+++ /dev/null
@@ -1,10 +0,0 @@
-#!/usr/bin/env bash
-
-work_path=$(dirname $0)
-PYTHONPATH="$(dirname $0)/../../":$PYTHONPATH \
-python -m torch.distributed.launch --nproc_per_node=8 \
- tools/test.py ${work_path}/test_config_h32.py \
- ${work_path}/ckpt/latest.pth \
- --launcher pytorch \
- --eval mIoU \
- 2>&1 | tee -a ${work_path}/log.txt
diff --git a/spaces/abhishek/sketch-to-image/annotator/uniformer_base/mmcv/runner/dist_utils.py b/spaces/abhishek/sketch-to-image/annotator/uniformer_base/mmcv/runner/dist_utils.py
deleted file mode 100644
index d3a1ef3fda5ceeb31bf15a73779da1b1903ab0fe..0000000000000000000000000000000000000000
--- a/spaces/abhishek/sketch-to-image/annotator/uniformer_base/mmcv/runner/dist_utils.py
+++ /dev/null
@@ -1,164 +0,0 @@
-# Copyright (c) OpenMMLab. All rights reserved.
-import functools
-import os
-import subprocess
-from collections import OrderedDict
-
-import torch
-import torch.multiprocessing as mp
-from torch import distributed as dist
-from torch._utils import (_flatten_dense_tensors, _take_tensors,
- _unflatten_dense_tensors)
-
-
-def init_dist(launcher, backend='nccl', **kwargs):
- if mp.get_start_method(allow_none=True) is None:
- mp.set_start_method('spawn')
- if launcher == 'pytorch':
- _init_dist_pytorch(backend, **kwargs)
- elif launcher == 'mpi':
- _init_dist_mpi(backend, **kwargs)
- elif launcher == 'slurm':
- _init_dist_slurm(backend, **kwargs)
- else:
- raise ValueError(f'Invalid launcher type: {launcher}')
-
-
-def _init_dist_pytorch(backend, **kwargs):
- # TODO: use local_rank instead of rank % num_gpus
- rank = int(os.environ['RANK'])
- num_gpus = torch.cuda.device_count()
- torch.cuda.set_device(rank % num_gpus)
- dist.init_process_group(backend=backend, **kwargs)
-
-
-def _init_dist_mpi(backend, **kwargs):
- # TODO: use local_rank instead of rank % num_gpus
- rank = int(os.environ['OMPI_COMM_WORLD_RANK'])
- num_gpus = torch.cuda.device_count()
- torch.cuda.set_device(rank % num_gpus)
- dist.init_process_group(backend=backend, **kwargs)
-
-
-def _init_dist_slurm(backend, port=None):
- """Initialize slurm distributed training environment.
-
- If argument ``port`` is not specified, then the master port will be system
- environment variable ``MASTER_PORT``. If ``MASTER_PORT`` is not in system
- environment variable, then a default port ``29500`` will be used.
-
- Args:
- backend (str): Backend of torch.distributed.
- port (int, optional): Master port. Defaults to None.
- """
- proc_id = int(os.environ['SLURM_PROCID'])
- ntasks = int(os.environ['SLURM_NTASKS'])
- node_list = os.environ['SLURM_NODELIST']
- num_gpus = torch.cuda.device_count()
- torch.cuda.set_device(proc_id % num_gpus)
- addr = subprocess.getoutput(
- f'scontrol show hostname {node_list} | head -n1')
- # specify master port
- if port is not None:
- os.environ['MASTER_PORT'] = str(port)
- elif 'MASTER_PORT' in os.environ:
- pass # use MASTER_PORT in the environment variable
- else:
- # 29500 is torch.distributed default port
- os.environ['MASTER_PORT'] = '29500'
- # use MASTER_ADDR in the environment variable if it already exists
- if 'MASTER_ADDR' not in os.environ:
- os.environ['MASTER_ADDR'] = addr
- os.environ['WORLD_SIZE'] = str(ntasks)
- os.environ['LOCAL_RANK'] = str(proc_id % num_gpus)
- os.environ['RANK'] = str(proc_id)
- dist.init_process_group(backend=backend)
-
-
-def get_dist_info():
- if dist.is_available() and dist.is_initialized():
- rank = dist.get_rank()
- world_size = dist.get_world_size()
- else:
- rank = 0
- world_size = 1
- return rank, world_size
-
-
-def master_only(func):
-
- @functools.wraps(func)
- def wrapper(*args, **kwargs):
- rank, _ = get_dist_info()
- if rank == 0:
- return func(*args, **kwargs)
-
- return wrapper
-
-
-def allreduce_params(params, coalesce=True, bucket_size_mb=-1):
- """Allreduce parameters.
-
- Args:
- params (list[torch.Parameters]): List of parameters or buffers of a
- model.
- coalesce (bool, optional): Whether allreduce parameters as a whole.
- Defaults to True.
- bucket_size_mb (int, optional): Size of bucket, the unit is MB.
- Defaults to -1.
- """
- _, world_size = get_dist_info()
- if world_size == 1:
- return
- params = [param.data for param in params]
- if coalesce:
- _allreduce_coalesced(params, world_size, bucket_size_mb)
- else:
- for tensor in params:
- dist.all_reduce(tensor.div_(world_size))
-
-
-def allreduce_grads(params, coalesce=True, bucket_size_mb=-1):
- """Allreduce gradients.
-
- Args:
- params (list[torch.Parameters]): List of parameters of a model
- coalesce (bool, optional): Whether allreduce parameters as a whole.
- Defaults to True.
- bucket_size_mb (int, optional): Size of bucket, the unit is MB.
- Defaults to -1.
- """
- grads = [
- param.grad.data for param in params
- if param.requires_grad and param.grad is not None
- ]
- _, world_size = get_dist_info()
- if world_size == 1:
- return
- if coalesce:
- _allreduce_coalesced(grads, world_size, bucket_size_mb)
- else:
- for tensor in grads:
- dist.all_reduce(tensor.div_(world_size))
-
-
-def _allreduce_coalesced(tensors, world_size, bucket_size_mb=-1):
- if bucket_size_mb > 0:
- bucket_size_bytes = bucket_size_mb * 1024 * 1024
- buckets = _take_tensors(tensors, bucket_size_bytes)
- else:
- buckets = OrderedDict()
- for tensor in tensors:
- tp = tensor.type()
- if tp not in buckets:
- buckets[tp] = []
- buckets[tp].append(tensor)
- buckets = buckets.values()
-
- for bucket in buckets:
- flat_tensors = _flatten_dense_tensors(bucket)
- dist.all_reduce(flat_tensors)
- flat_tensors.div_(world_size)
- for tensor, synced in zip(
- bucket, _unflatten_dense_tensors(flat_tensors, bucket)):
- tensor.copy_(synced)
diff --git a/spaces/akhaliq/lama/models/ade20k/segm_lib/nn/modules/unittest.py b/spaces/akhaliq/lama/models/ade20k/segm_lib/nn/modules/unittest.py
deleted file mode 100644
index 0675c022e4ba85d38d1f813490f6740150909524..0000000000000000000000000000000000000000
--- a/spaces/akhaliq/lama/models/ade20k/segm_lib/nn/modules/unittest.py
+++ /dev/null
@@ -1,29 +0,0 @@
-# -*- coding: utf-8 -*-
-# File : unittest.py
-# Author : Jiayuan Mao
-# Email : maojiayuan@gmail.com
-# Date : 27/01/2018
-#
-# This file is part of Synchronized-BatchNorm-PyTorch.
-# https://github.com/vacancy/Synchronized-BatchNorm-PyTorch
-# Distributed under MIT License.
-
-import unittest
-
-import numpy as np
-from torch.autograd import Variable
-
-
-def as_numpy(v):
- if isinstance(v, Variable):
- v = v.data
- return v.cpu().numpy()
-
-
-class TorchTestCase(unittest.TestCase):
- def assertTensorClose(self, a, b, atol=1e-3, rtol=1e-3):
- npa, npb = as_numpy(a), as_numpy(b)
- self.assertTrue(
- np.allclose(npa, npb, atol=atol),
- 'Tensor close check failed\n{}\n{}\nadiff={}, rdiff={}'.format(a, b, np.abs(npa - npb).max(), np.abs((npa - npb) / np.fmax(npa, 1e-5)).max())
- )
diff --git a/spaces/alamin655/websurfx/docs/installation.md b/spaces/alamin655/websurfx/docs/installation.md
deleted file mode 100644
index 54d4355f3e0395813ffdb8d67e86138cc57fa271..0000000000000000000000000000000000000000
--- a/spaces/alamin655/websurfx/docs/installation.md
+++ /dev/null
@@ -1,136 +0,0 @@
-# Install From Package
-
-## Arch Linux
-
-You can install `Websurfx` through the [Aur](https://aur.archlinux.org/packages/websurfx-git), Currently we only support `Rolling/Edge` version. You can install the rolling/edge version by running the following command (using [paru](https://github.com/Morganamilo/paru)):
-
-```bash
-paru -S websurfx-edge-git
-```
-
-After installing it you can run the websurfx server by running the following commands:
-
-```bash
-redis-server --port 8082 &
-websurfx
-```
-
-Once you have started the server, open your preferred web browser and navigate to http://127.0.0.1:8080/ to start using Websurfx.
-
-If you want to change the port or the ip or any other configuration setting checkout the [configuration docs](./configuration.md).
-
-## Other Distros
-
-The package is currently not available on other Linux distros. With contribution and support it can be made available on other distros as well 🙂.
-
-# Install From Source
-
-Before you can start building `websurfx`, you will need to have `Cargo` installed on your system. You can find the installation instructions [here](https://doc.rust-lang.org/cargo/getting-started/installation.html).
-
-## Stable
-
-To get started with Websurfx, clone the repository, edit the config file which is located in the `websurfx` directory and install redis server by following the instructions located [here](https://redis.io/docs/getting-started/) and then build and run the websurfx server by running the following commands:
-
-```shell
-git clone https://github.com/neon-mmd/websurfx.git
-cd websurfx
-git checkout stable
-cargo build -r
-redis-server --port 8082 &
-./target/release/websurfx
-```
-
-Once you have started the server, open your preferred web browser and navigate to http://127.0.0.1:8080/ to start using Websurfx.
-
-If you want to change the port or the ip or any other configuration setting checkout the [configuration docs](./configuration.md).
-
-## Rolling/Edge/Unstable
-
-If you want to use the rolling/edge branch, run the following commands instead:
-
-```shell
-git clone https://github.com/neon-mmd/websurfx.git
-cd websurfx
-cargo build -r
-redis-server --port 8082 &
-./target/release/websurfx
-```
-
-Once you have started the server, open your preferred web browser and navigate to http://127.0.0.1:8080/ to start using Websurfx.
-
-If you want to change the port or the ip or any other configuration setting checkout the [configuration docs](./configuration.md).
-
-# Docker Deployment
-
-Before you start, you will need [Docker](https://docs.docker.com/get-docker/) installed on your system first.
-
-## Unstable/Edge/Rolling
-
-First clone the the repository by running the following command:
-
-```bash
-git clone https://github.com/neon-mmd/websurfx.git
-cd websurfx
-```
-
-After that edit the config.lua file located under `websurfx` directory. In the config file you will specifically need to change to values which is `binding_ip_addr` and `redis_connection_url` which should make the config look something like this:
-
-```lua
--- ### General ###
-logging = true -- an option to enable or disable logs.
-debug = false -- an option to enable or disable debug mode.
-threads = 10 -- the amount of threads that the app will use to run (the value should be greater than 0).
-
--- ### Server ###
-port = "8080" -- port on which server should be launched
-binding_ip_addr = "0.0.0.0" --ip address on the which server should be launched.
-production_use = false -- whether to use production mode or not (in other words this option should be used if it is to be used to host it on the server to provide a service to a large number of users)
--- if production_use is set to true
--- There will be a random delay before sending the request to the search engines, this is to prevent DDoSing the upstream search engines from a large number of simultaneous requests.
-request_timeout = 60 -- timeout for the search requests sent to the upstream search engines to be fetched (value in seconds).
-
--- ### Website ###
--- The different colorschemes provided are:
--- {{
--- catppuccin-mocha
--- dark-chocolate
--- dracula
--- gruvbox-dark
--- monokai
--- nord
--- oceanic-next
--- one-dark
--- solarized-dark
--- solarized-light
--- tokyo-night
--- tomorrow-night
--- }}
-colorscheme = "catppuccin-mocha" -- the colorscheme name which should be used for the website theme
-theme = "simple" -- the theme name which should be used for the website
-
--- ### Caching ###
-redis_url = "redis://redis:6379" -- redis connection url address on which the client should connect on.
-
--- ### Search Engines ###
-upstream_search_engines = { DuckDuckGo = true, Searx = false } -- select the upstream search engines from which the results should be fetched.
-```
-
-After this run the following command to deploy the app:
-
-```bash
-docker compose up -d --build
-```
-
-This will take around 5-10 mins for first deployment, afterwards the docker build stages will be cached so it will be faster to be build from next time onwards. After the above step finishes launch your preferred browser and then navigate to `http://:`.
-
-## Stable
-
-For the stable version, follow the same steps as above (as mentioned for the unstable/rolling/edge version) with an addition of one command which has to be performed after cloning and changing directory into the repository which makes the cloning step as follows:
-
-```bash
-git clone https://github.com/neon-mmd/websurfx.git
-cd websurfx
-git checkout stable
-```
-
-[⬅️ Go back to Home](./README.md)
diff --git a/spaces/alexray/btc_predictor/venv/lib/python3.10/site-packages/pip/_vendor/html5lib/treewalkers/base.py b/spaces/alexray/btc_predictor/venv/lib/python3.10/site-packages/pip/_vendor/html5lib/treewalkers/base.py
deleted file mode 100644
index 80c474c4e939c149a22e811a5a1a5419313b7cc7..0000000000000000000000000000000000000000
--- a/spaces/alexray/btc_predictor/venv/lib/python3.10/site-packages/pip/_vendor/html5lib/treewalkers/base.py
+++ /dev/null
@@ -1,252 +0,0 @@
-from __future__ import absolute_import, division, unicode_literals
-
-from xml.dom import Node
-from ..constants import namespaces, voidElements, spaceCharacters
-
-__all__ = ["DOCUMENT", "DOCTYPE", "TEXT", "ELEMENT", "COMMENT", "ENTITY", "UNKNOWN",
- "TreeWalker", "NonRecursiveTreeWalker"]
-
-DOCUMENT = Node.DOCUMENT_NODE
-DOCTYPE = Node.DOCUMENT_TYPE_NODE
-TEXT = Node.TEXT_NODE
-ELEMENT = Node.ELEMENT_NODE
-COMMENT = Node.COMMENT_NODE
-ENTITY = Node.ENTITY_NODE
-UNKNOWN = "<#UNKNOWN#>"
-
-spaceCharacters = "".join(spaceCharacters)
-
-
-class TreeWalker(object):
- """Walks a tree yielding tokens
-
- Tokens are dicts that all have a ``type`` field specifying the type of the
- token.
-
- """
- def __init__(self, tree):
- """Creates a TreeWalker
-
- :arg tree: the tree to walk
-
- """
- self.tree = tree
-
- def __iter__(self):
- raise NotImplementedError
-
- def error(self, msg):
- """Generates an error token with the given message
-
- :arg msg: the error message
-
- :returns: SerializeError token
-
- """
- return {"type": "SerializeError", "data": msg}
-
- def emptyTag(self, namespace, name, attrs, hasChildren=False):
- """Generates an EmptyTag token
-
- :arg namespace: the namespace of the token--can be ``None``
-
- :arg name: the name of the element
-
- :arg attrs: the attributes of the element as a dict
-
- :arg hasChildren: whether or not to yield a SerializationError because
- this tag shouldn't have children
-
- :returns: EmptyTag token
-
- """
- yield {"type": "EmptyTag", "name": name,
- "namespace": namespace,
- "data": attrs}
- if hasChildren:
- yield self.error("Void element has children")
-
- def startTag(self, namespace, name, attrs):
- """Generates a StartTag token
-
- :arg namespace: the namespace of the token--can be ``None``
-
- :arg name: the name of the element
-
- :arg attrs: the attributes of the element as a dict
-
- :returns: StartTag token
-
- """
- return {"type": "StartTag",
- "name": name,
- "namespace": namespace,
- "data": attrs}
-
- def endTag(self, namespace, name):
- """Generates an EndTag token
-
- :arg namespace: the namespace of the token--can be ``None``
-
- :arg name: the name of the element
-
- :returns: EndTag token
-
- """
- return {"type": "EndTag",
- "name": name,
- "namespace": namespace}
-
- def text(self, data):
- """Generates SpaceCharacters and Characters tokens
-
- Depending on what's in the data, this generates one or more
- ``SpaceCharacters`` and ``Characters`` tokens.
-
- For example:
-
- >>> from html5lib.treewalkers.base import TreeWalker
- >>> # Give it an empty tree just so it instantiates
- >>> walker = TreeWalker([])
- >>> list(walker.text(''))
- []
- >>> list(walker.text(' '))
- [{u'data': ' ', u'type': u'SpaceCharacters'}]
- >>> list(walker.text(' abc ')) # doctest: +NORMALIZE_WHITESPACE
- [{u'data': ' ', u'type': u'SpaceCharacters'},
- {u'data': u'abc', u'type': u'Characters'},
- {u'data': u' ', u'type': u'SpaceCharacters'}]
-
- :arg data: the text data
-
- :returns: one or more ``SpaceCharacters`` and ``Characters`` tokens
-
- """
- data = data
- middle = data.lstrip(spaceCharacters)
- left = data[:len(data) - len(middle)]
- if left:
- yield {"type": "SpaceCharacters", "data": left}
- data = middle
- middle = data.rstrip(spaceCharacters)
- right = data[len(middle):]
- if middle:
- yield {"type": "Characters", "data": middle}
- if right:
- yield {"type": "SpaceCharacters", "data": right}
-
- def comment(self, data):
- """Generates a Comment token
-
- :arg data: the comment
-
- :returns: Comment token
-
- """
- return {"type": "Comment", "data": data}
-
- def doctype(self, name, publicId=None, systemId=None):
- """Generates a Doctype token
-
- :arg name:
-
- :arg publicId:
-
- :arg systemId:
-
- :returns: the Doctype token
-
- """
- return {"type": "Doctype",
- "name": name,
- "publicId": publicId,
- "systemId": systemId}
-
- def entity(self, name):
- """Generates an Entity token
-
- :arg name: the entity name
-
- :returns: an Entity token
-
- """
- return {"type": "Entity", "name": name}
-
- def unknown(self, nodeType):
- """Handles unknown node types"""
- return self.error("Unknown node type: " + nodeType)
-
-
-class NonRecursiveTreeWalker(TreeWalker):
- def getNodeDetails(self, node):
- raise NotImplementedError
-
- def getFirstChild(self, node):
- raise NotImplementedError
-
- def getNextSibling(self, node):
- raise NotImplementedError
-
- def getParentNode(self, node):
- raise NotImplementedError
-
- def __iter__(self):
- currentNode = self.tree
- while currentNode is not None:
- details = self.getNodeDetails(currentNode)
- type, details = details[0], details[1:]
- hasChildren = False
-
- if type == DOCTYPE:
- yield self.doctype(*details)
-
- elif type == TEXT:
- for token in self.text(*details):
- yield token
-
- elif type == ELEMENT:
- namespace, name, attributes, hasChildren = details
- if (not namespace or namespace == namespaces["html"]) and name in voidElements:
- for token in self.emptyTag(namespace, name, attributes,
- hasChildren):
- yield token
- hasChildren = False
- else:
- yield self.startTag(namespace, name, attributes)
-
- elif type == COMMENT:
- yield self.comment(details[0])
-
- elif type == ENTITY:
- yield self.entity(details[0])
-
- elif type == DOCUMENT:
- hasChildren = True
-
- else:
- yield self.unknown(details[0])
-
- if hasChildren:
- firstChild = self.getFirstChild(currentNode)
- else:
- firstChild = None
-
- if firstChild is not None:
- currentNode = firstChild
- else:
- while currentNode is not None:
- details = self.getNodeDetails(currentNode)
- type, details = details[0], details[1:]
- if type == ELEMENT:
- namespace, name, attributes, hasChildren = details
- if (namespace and namespace != namespaces["html"]) or name not in voidElements:
- yield self.endTag(namespace, name)
- if self.tree is currentNode:
- currentNode = None
- break
- nextSibling = self.getNextSibling(currentNode)
- if nextSibling is not None:
- currentNode = nextSibling
- break
- else:
- currentNode = self.getParentNode(currentNode)
diff --git a/spaces/alfredplpl/ChatZMD/README.md b/spaces/alfredplpl/ChatZMD/README.md
deleted file mode 100644
index 10b49980405c23e28a4dbee74b27eab0cb236734..0000000000000000000000000000000000000000
--- a/spaces/alfredplpl/ChatZMD/README.md
+++ /dev/null
@@ -1,13 +0,0 @@
----
-title: ChatZMD
-emoji: 🫛
-colorFrom: green
-colorTo: green
-sdk: gradio
-sdk_version: 3.40.1
-app_file: app.py
-pinned: false
-license: apache-2.0
----
-
-Check out the configuration reference at https://huggingface.co/docs/hub/spaces-config-reference
\ No newline at end of file
diff --git a/spaces/aphenx/bingo/cloudflare/worker.js b/spaces/aphenx/bingo/cloudflare/worker.js
deleted file mode 100644
index e0debd750615f1329b2c72fbce73e1b9291f7137..0000000000000000000000000000000000000000
--- a/spaces/aphenx/bingo/cloudflare/worker.js
+++ /dev/null
@@ -1,18 +0,0 @@
-const TRAGET_HOST='hf4all-bingo.hf.space' // 请将此域名改成你自己的,域名信息在设置》站点域名查看。
-
-export default {
- async fetch(request) {
- const uri = new URL(request.url);
- if (uri.protocol === 'http:') {
- uri.protocol = 'https:';
- return new Response('', {
- status: 301,
- headers: {
- location: uri.toString(),
- },
- })
- }
- uri.host = TRAGET_HOST
- return fetch(new Request(uri.toString(), request));
- },
-};
diff --git a/spaces/artificialguybr/video-dubbing/TTS/tests/tts_tests/test_overflow_train.py b/spaces/artificialguybr/video-dubbing/TTS/tests/tts_tests/test_overflow_train.py
deleted file mode 100644
index 86fa60af72b7cda704aa6e1618793f2d52d463af..0000000000000000000000000000000000000000
--- a/spaces/artificialguybr/video-dubbing/TTS/tests/tts_tests/test_overflow_train.py
+++ /dev/null
@@ -1,92 +0,0 @@
-import glob
-import json
-import os
-import shutil
-
-import torch
-from trainer import get_last_checkpoint
-
-from tests import get_device_id, get_tests_output_path, run_cli
-from TTS.tts.configs.overflow_config import OverflowConfig
-
-config_path = os.path.join(get_tests_output_path(), "test_model_config.json")
-output_path = os.path.join(get_tests_output_path(), "train_outputs")
-parameter_path = os.path.join(get_tests_output_path(), "lj_parameters.pt")
-
-torch.save({"mean": -5.5138, "std": 2.0636, "init_transition_prob": 0.3212}, parameter_path)
-
-config = OverflowConfig(
- batch_size=3,
- eval_batch_size=3,
- num_loader_workers=0,
- num_eval_loader_workers=0,
- text_cleaner="phoneme_cleaners",
- use_phonemes=True,
- phoneme_language="en-us",
- phoneme_cache_path=os.path.join(get_tests_output_path(), "train_outputs/phoneme_cache/"),
- run_eval=True,
- test_delay_epochs=-1,
- mel_statistics_parameter_path=parameter_path,
- epochs=1,
- print_step=1,
- test_sentences=[
- "Be a voice, not an echo.",
- ],
- print_eval=True,
- max_sampling_time=50,
-)
-config.audio.do_trim_silence = True
-config.audio.trim_db = 60
-config.save_json(config_path)
-
-
-# train the model for one epoch when mel parameters exists
-command_train = (
- f"CUDA_VISIBLE_DEVICES='{get_device_id()}' python TTS/bin/train_tts.py --config_path {config_path} "
- f"--coqpit.output_path {output_path} "
- "--coqpit.datasets.0.formatter ljspeech "
- "--coqpit.datasets.0.meta_file_train metadata.csv "
- "--coqpit.datasets.0.meta_file_val metadata.csv "
- "--coqpit.datasets.0.path tests/data/ljspeech "
- "--coqpit.test_delay_epochs 0 "
-)
-run_cli(command_train)
-
-
-# train the model for one epoch when mel parameters have to be computed from the dataset
-if os.path.exists(parameter_path):
- os.remove(parameter_path)
-command_train = (
- f"CUDA_VISIBLE_DEVICES='{get_device_id()}' python TTS/bin/train_tts.py --config_path {config_path} "
- f"--coqpit.output_path {output_path} "
- "--coqpit.datasets.0.formatter ljspeech "
- "--coqpit.datasets.0.meta_file_train metadata.csv "
- "--coqpit.datasets.0.meta_file_val metadata.csv "
- "--coqpit.datasets.0.path tests/data/ljspeech "
- "--coqpit.test_delay_epochs 0 "
-)
-run_cli(command_train)
-
-# Find latest folder
-continue_path = max(glob.glob(os.path.join(output_path, "*/")), key=os.path.getmtime)
-
-# Inference using TTS API
-continue_config_path = os.path.join(continue_path, "config.json")
-continue_restore_path, _ = get_last_checkpoint(continue_path)
-out_wav_path = os.path.join(get_tests_output_path(), "output.wav")
-
-# Check integrity of the config
-with open(continue_config_path, "r", encoding="utf-8") as f:
- config_loaded = json.load(f)
-assert config_loaded["characters"] is not None
-assert config_loaded["output_path"] in continue_path
-assert config_loaded["test_delay_epochs"] == 0
-
-# Load the model and run inference
-inference_command = f"CUDA_VISIBLE_DEVICES='{get_device_id()}' tts --text 'This is an example.' --config_path {continue_config_path} --model_path {continue_restore_path} --out_path {out_wav_path}"
-run_cli(inference_command)
-
-# restore the model and continue training for one more epoch
-command_train = f"CUDA_VISIBLE_DEVICES='{get_device_id()}' python TTS/bin/train_tts.py --continue_path {continue_path} "
-run_cli(command_train)
-shutil.rmtree(continue_path)
diff --git a/spaces/arxify/RVC-beta-v2-0618/runtime/Lib/site-packages/fairseq/data/encoders/gpt2_bpe.py b/spaces/arxify/RVC-beta-v2-0618/runtime/Lib/site-packages/fairseq/data/encoders/gpt2_bpe.py
deleted file mode 100644
index e661426a73c7e735f7054bcb04281bf1649bb46c..0000000000000000000000000000000000000000
--- a/spaces/arxify/RVC-beta-v2-0618/runtime/Lib/site-packages/fairseq/data/encoders/gpt2_bpe.py
+++ /dev/null
@@ -1,45 +0,0 @@
-# Copyright (c) Facebook, Inc. and its affiliates.
-#
-# This source code is licensed under the MIT license found in the
-# LICENSE file in the root directory of this source tree.
-
-from dataclasses import dataclass, field
-
-from fairseq import file_utils
-from fairseq.data.encoders import register_bpe
-from fairseq.dataclass import FairseqDataclass
-
-from .gpt2_bpe_utils import get_encoder
-
-
-DEFAULT_ENCODER_JSON = "https://dl.fbaipublicfiles.com/fairseq/gpt2_bpe/encoder.json"
-DEFAULT_VOCAB_BPE = "https://dl.fbaipublicfiles.com/fairseq/gpt2_bpe/vocab.bpe"
-
-
-@dataclass
-class GPT2BPEConfig(FairseqDataclass):
- gpt2_encoder_json: str = field(
- default=DEFAULT_ENCODER_JSON, metadata={"help": "path to encoder.json"}
- )
- gpt2_vocab_bpe: str = field(
- default=DEFAULT_VOCAB_BPE, metadata={"help": "path to vocab.bpe"}
- )
-
-
-@register_bpe("gpt2", dataclass=GPT2BPEConfig)
-class GPT2BPE(object):
- def __init__(self, cfg):
- encoder_json = file_utils.cached_path(cfg.gpt2_encoder_json)
- vocab_bpe = file_utils.cached_path(cfg.gpt2_vocab_bpe)
- self.bpe = get_encoder(encoder_json, vocab_bpe)
-
- def encode(self, x: str) -> str:
- return " ".join(map(str, self.bpe.encode(x)))
-
- def decode(self, x: str) -> str:
- return self.bpe.decode(
- [int(tok) if tok not in {"", ""} else tok for tok in x.split()]
- )
-
- def is_beginning_of_word(self, x: str) -> bool:
- return self.decode(x).startswith(" ")
diff --git a/spaces/asafAdge/Detic/tools/preprocess_imagenet22k.py b/spaces/asafAdge/Detic/tools/preprocess_imagenet22k.py
deleted file mode 100644
index 6dda56c222a30c7be23fafbdab4be3fe611597e2..0000000000000000000000000000000000000000
--- a/spaces/asafAdge/Detic/tools/preprocess_imagenet22k.py
+++ /dev/null
@@ -1,148 +0,0 @@
-#!/usr/bin/env python3
-# Copyright (c) Facebook, Inc. and its affiliates.
-
-import os
-import numpy as np
-import sys
-
-sys.path.insert(0, 'third_party/CenterNet2/projects/CenterNet2/')
-sys.path.insert(0, 'third_party/Deformable-DETR')
-from detic.data.tar_dataset import _TarDataset, DiskTarDataset
-import pickle
-import io
-import gzip
-import time
-
-
-class _RawTarDataset(object):
-
- def __init__(self, filename, indexname, preload=False):
- self.filename = filename
- self.names = []
- self.offsets = []
-
- for l in open(indexname):
- ll = l.split()
- a, b, c = ll[:3]
- offset = int(b[:-1])
- if l.endswith('** Block of NULs **\n'):
- self.offsets.append(offset)
- break
- else:
- if c.endswith('JPEG'):
- self.names.append(c)
- self.offsets.append(offset)
- else:
- # ignore directories
- pass
- if preload:
- self.data = np.memmap(filename, mode='r', dtype='uint8')
- else:
- self.data = None
-
- def __len__(self):
- return len(self.names)
-
- def __getitem__(self, idx):
- if self.data is None:
- self.data = np.memmap(self.filename, mode='r', dtype='uint8')
- ofs = self.offsets[idx] * 512
- fsize = 512 * (self.offsets[idx + 1] - self.offsets[idx])
- data = self.data[ofs:ofs + fsize]
-
- if data[:13].tostring() == '././@LongLink':
- data = data[3 * 512:]
- else:
- data = data[512:]
-
- # just to make it more fun a few JPEGs are GZIP compressed...
- # catch this case
- if tuple(data[:2]) == (0x1f, 0x8b):
- s = io.StringIO(data.tostring())
- g = gzip.GzipFile(None, 'r', 0, s)
- sdata = g.read()
- else:
- sdata = data.tostring()
- return sdata
-
-
-
-def preprocess():
- # Follow https://github.com/Alibaba-MIIL/ImageNet21K/blob/main/dataset_preprocessing/processing_script.sh
- # Expect 12358684 samples with 11221 classes
- # ImageNet folder has 21841 classes (synsets)
-
- i22kdir = '/datasets01/imagenet-22k/062717/'
- i22ktarlogs = '/checkpoint/imisra/datasets/imagenet-22k/tarindex'
- class_names_file = '/checkpoint/imisra/datasets/imagenet-22k/words.txt'
-
- output_dir = '/checkpoint/zhouxy/Datasets/ImageNet/metadata-22k/'
- i22knpytarlogs = '/checkpoint/zhouxy/Datasets/ImageNet/metadata-22k/tarindex_npy'
- print('Listing dir')
- log_files = os.listdir(i22ktarlogs)
- log_files = [x for x in log_files if x.endswith(".tarlog")]
- log_files.sort()
- chunk_datasets = []
- dataset_lens = []
- min_count = 0
- create_npy_tarlogs = True
- print('Creating folders')
- if create_npy_tarlogs:
- os.makedirs(i22knpytarlogs, exist_ok=True)
- for log_file in log_files:
- syn = log_file.replace(".tarlog", "")
- dataset = _RawTarDataset(os.path.join(i22kdir, syn + ".tar"),
- os.path.join(i22ktarlogs, syn + ".tarlog"),
- preload=False)
- names = np.array(dataset.names)
- offsets = np.array(dataset.offsets, dtype=np.int64)
- np.save(os.path.join(i22knpytarlogs, f"{syn}_names.npy"), names)
- np.save(os.path.join(i22knpytarlogs, f"{syn}_offsets.npy"), offsets)
-
- os.makedirs(output_dir, exist_ok=True)
-
- start_time = time.time()
- for log_file in log_files:
- syn = log_file.replace(".tarlog", "")
- dataset = _TarDataset(os.path.join(i22kdir, syn + ".tar"), i22knpytarlogs)
- # dataset = _RawTarDataset(os.path.join(i22kdir, syn + ".tar"),
- # os.path.join(i22ktarlogs, syn + ".tarlog"),
- # preload=False)
- dataset_lens.append(len(dataset))
- end_time = time.time()
- print(f"Time {end_time - start_time}")
-
-
- dataset_lens = np.array(dataset_lens)
- dataset_valid = dataset_lens > min_count
-
- syn2class = {}
- with open(class_names_file) as fh:
- for line in fh:
- line = line.strip().split("\t")
- syn2class[line[0]] = line[1]
-
- tarlog_files = []
- class_names = []
- tar_files = []
- for k in range(len(dataset_valid)):
- if not dataset_valid[k]:
- continue
- syn = log_files[k].replace(".tarlog", "")
- tarlog_files.append(os.path.join(i22ktarlogs, syn + ".tarlog"))
- tar_files.append(os.path.join(i22kdir, syn + ".tar"))
- class_names.append(syn2class[syn])
-
- tarlog_files = np.array(tarlog_files)
- tar_files = np.array(tar_files)
- class_names = np.array(class_names)
- print(f"Have {len(class_names)} classes and {dataset_lens[dataset_valid].sum()} samples")
-
- np.save(os.path.join(output_dir, "tarlog_files.npy"), tarlog_files)
- np.save(os.path.join(output_dir, "tar_files.npy"), tar_files)
- np.save(os.path.join(output_dir, "class_names.npy"), class_names)
- np.save(os.path.join(output_dir, "tar_files.npy"), tar_files)
-
-
-if __name__ == "__main__":
- preprocess()
diff --git a/spaces/ashercn97/AsherTesting/extensions/multimodal/pipelines/llava/README.md b/spaces/ashercn97/AsherTesting/extensions/multimodal/pipelines/llava/README.md
deleted file mode 100644
index aff64faaae07d2f4da6c24e8ea03693326313139..0000000000000000000000000000000000000000
--- a/spaces/ashercn97/AsherTesting/extensions/multimodal/pipelines/llava/README.md
+++ /dev/null
@@ -1,9 +0,0 @@
-## LLaVA pipeline
-
-This module provides 2 pipelines:
-- `llava-7b` - for use with LLaVA v0 7B model (finetuned LLaMa 7B)
-- `llava-13b` - for use with LLaVA v0 13B model (finetuned LLaMa 13B)
-
-[LLaVA](https://github.com/haotian-liu/LLaVA) uses CLIP `openai/clip-vit-large-patch14` as the vision model, and then a single linear layer. For 13B the projector weights are in `liuhaotian/LLaVA-13b-delta-v0`, and for 7B they are in `liuhaotian/LLaVA-7b-delta-v0`.
-
-The supported parameter combinations for both the vision model, and the projector are: CUDA/32bit, CUDA/16bit, CPU/32bit
diff --git a/spaces/at2507/SM_NLP_RecoSys/Data/Mentor_interviews/Rahul Iyer.html b/spaces/at2507/SM_NLP_RecoSys/Data/Mentor_interviews/Rahul Iyer.html
deleted file mode 100644
index bb1f1217d1b99ffb274d04cf9c4ed32529880559..0000000000000000000000000000000000000000
--- a/spaces/at2507/SM_NLP_RecoSys/Data/Mentor_interviews/Rahul Iyer.html
+++ /dev/null
@@ -1,134 +0,0 @@
-
-
-
- Rahul Iyer
-
-
-
-
-
-
Rahul Iyer
-
-
-
Previous mentee!!
Career
has wanted to do it for so long. I had an amazing experience and wanted to give back
Masters in robotics
Ajaay Ajal was my mentor - introed me to website analytics
Puralator - data delivery specialist - collect data, analyze, share dashboards with sales, management. A bit of python and mostly excel
Magazine company (behind McLean's) - main analytics guy (8 different brands). Google Analytics and google tag manager
Big Query, dashboards
promoted to analytics manager
Now at Scotiabank, adobe analytics
realized how important it is to collect quality data
especially in banking - there is a dearth of talent
Very grateful for Ajay, want to emulate his mentorship
Mentorship exp
have not trained anybody, but have shown ppl how to use the tool and how the data is being collected (data layer)
lots of explaining
What do beginners need and how can you help?
data analytics and google analytics and adobe analytics
not a lot of people are aware of this field - fail to notice the other fields - are not being taught. Implementation Specialist and then work your way up to data analyst
adobe analytics is quite opaque
the move to the cookie-less world, analytics will be changing
GA4 is going to be the next big thing
website tracking + mobile tracking
intro them to Gtag manager and GA - cookies and network requests
how to analyze websites, analyze a company's website ahead of an interview (network requests, and the info they are packing into it)
Google has demo GA accounts you can use
GTM then GA
lots of opportunity in the switch from GA360 to GA4
-
-
Questions about SM:
How does it work as a mentor
-
-
-
-
-
-
\ No newline at end of file
diff --git a/spaces/auto-academic/auto-draft/latex_templates/ICLR2022/math_commands.tex b/spaces/auto-academic/auto-draft/latex_templates/ICLR2022/math_commands.tex
deleted file mode 100644
index 0668f931945175ca8535db25cc27fa603920cc3c..0000000000000000000000000000000000000000
--- a/spaces/auto-academic/auto-draft/latex_templates/ICLR2022/math_commands.tex
+++ /dev/null
@@ -1,508 +0,0 @@
-%%%%% NEW MATH DEFINITIONS %%%%%
-
-\usepackage{amsmath,amsfonts,bm}
-
-% Mark sections of captions for referring to divisions of figures
-\newcommand{\figleft}{{\em (Left)}}
-\newcommand{\figcenter}{{\em (Center)}}
-\newcommand{\figright}{{\em (Right)}}
-\newcommand{\figtop}{{\em (Top)}}
-\newcommand{\figbottom}{{\em (Bottom)}}
-\newcommand{\captiona}{{\em (a)}}
-\newcommand{\captionb}{{\em (b)}}
-\newcommand{\captionc}{{\em (c)}}
-\newcommand{\captiond}{{\em (d)}}
-
-% Highlight a newly defined term
-\newcommand{\newterm}[1]{{\bf #1}}
-
-
-% Figure reference, lower-case.
-\def\figref#1{figure~\ref{#1}}
-% Figure reference, capital. For start of sentence
-\def\Figref#1{Figure~\ref{#1}}
-\def\twofigref#1#2{figures \ref{#1} and \ref{#2}}
-\def\quadfigref#1#2#3#4{figures \ref{#1}, \ref{#2}, \ref{#3} and \ref{#4}}
-% Section reference, lower-case.
-\def\secref#1{section~\ref{#1}}
-% Section reference, capital.
-\def\Secref#1{Section~\ref{#1}}
-% Reference to two sections.
-\def\twosecrefs#1#2{sections \ref{#1} and \ref{#2}}
-% Reference to three sections.
-\def\secrefs#1#2#3{sections \ref{#1}, \ref{#2} and \ref{#3}}
-% Reference to an equation, lower-case.
-\def\eqref#1{equation~\ref{#1}}
-% Reference to an equation, upper case
-\def\Eqref#1{Equation~\ref{#1}}
-% A raw reference to an equation---avoid using if possible
-\def\plaineqref#1{\ref{#1}}
-% Reference to a chapter, lower-case.
-\def\chapref#1{chapter~\ref{#1}}
-% Reference to an equation, upper case.
-\def\Chapref#1{Chapter~\ref{#1}}
-% Reference to a range of chapters
-\def\rangechapref#1#2{chapters\ref{#1}--\ref{#2}}
-% Reference to an algorithm, lower-case.
-\def\algref#1{algorithm~\ref{#1}}
-% Reference to an algorithm, upper case.
-\def\Algref#1{Algorithm~\ref{#1}}
-\def\twoalgref#1#2{algorithms \ref{#1} and \ref{#2}}
-\def\Twoalgref#1#2{Algorithms \ref{#1} and \ref{#2}}
-% Reference to a part, lower case
-\def\partref#1{part~\ref{#1}}
-% Reference to a part, upper case
-\def\Partref#1{Part~\ref{#1}}
-\def\twopartref#1#2{parts \ref{#1} and \ref{#2}}
-
-\def\ceil#1{\lceil #1 \rceil}
-\def\floor#1{\lfloor #1 \rfloor}
-\def\1{\bm{1}}
-\newcommand{\train}{\mathcal{D}}
-\newcommand{\valid}{\mathcal{D_{\mathrm{valid}}}}
-\newcommand{\test}{\mathcal{D_{\mathrm{test}}}}
-
-\def\eps{{\epsilon}}
-
-
-% Random variables
-\def\reta{{\textnormal{$\eta$}}}
-\def\ra{{\textnormal{a}}}
-\def\rb{{\textnormal{b}}}
-\def\rc{{\textnormal{c}}}
-\def\rd{{\textnormal{d}}}
-\def\re{{\textnormal{e}}}
-\def\rf{{\textnormal{f}}}
-\def\rg{{\textnormal{g}}}
-\def\rh{{\textnormal{h}}}
-\def\ri{{\textnormal{i}}}
-\def\rj{{\textnormal{j}}}
-\def\rk{{\textnormal{k}}}
-\def\rl{{\textnormal{l}}}
-% rm is already a command, just don't name any random variables m
-\def\rn{{\textnormal{n}}}
-\def\ro{{\textnormal{o}}}
-\def\rp{{\textnormal{p}}}
-\def\rq{{\textnormal{q}}}
-\def\rr{{\textnormal{r}}}
-\def\rs{{\textnormal{s}}}
-\def\rt{{\textnormal{t}}}
-\def\ru{{\textnormal{u}}}
-\def\rv{{\textnormal{v}}}
-\def\rw{{\textnormal{w}}}
-\def\rx{{\textnormal{x}}}
-\def\ry{{\textnormal{y}}}
-\def\rz{{\textnormal{z}}}
-
-% Random vectors
-\def\rvepsilon{{\mathbf{\epsilon}}}
-\def\rvtheta{{\mathbf{\theta}}}
-\def\rva{{\mathbf{a}}}
-\def\rvb{{\mathbf{b}}}
-\def\rvc{{\mathbf{c}}}
-\def\rvd{{\mathbf{d}}}
-\def\rve{{\mathbf{e}}}
-\def\rvf{{\mathbf{f}}}
-\def\rvg{{\mathbf{g}}}
-\def\rvh{{\mathbf{h}}}
-\def\rvu{{\mathbf{i}}}
-\def\rvj{{\mathbf{j}}}
-\def\rvk{{\mathbf{k}}}
-\def\rvl{{\mathbf{l}}}
-\def\rvm{{\mathbf{m}}}
-\def\rvn{{\mathbf{n}}}
-\def\rvo{{\mathbf{o}}}
-\def\rvp{{\mathbf{p}}}
-\def\rvq{{\mathbf{q}}}
-\def\rvr{{\mathbf{r}}}
-\def\rvs{{\mathbf{s}}}
-\def\rvt{{\mathbf{t}}}
-\def\rvu{{\mathbf{u}}}
-\def\rvv{{\mathbf{v}}}
-\def\rvw{{\mathbf{w}}}
-\def\rvx{{\mathbf{x}}}
-\def\rvy{{\mathbf{y}}}
-\def\rvz{{\mathbf{z}}}
-
-% Elements of random vectors
-\def\erva{{\textnormal{a}}}
-\def\ervb{{\textnormal{b}}}
-\def\ervc{{\textnormal{c}}}
-\def\ervd{{\textnormal{d}}}
-\def\erve{{\textnormal{e}}}
-\def\ervf{{\textnormal{f}}}
-\def\ervg{{\textnormal{g}}}
-\def\ervh{{\textnormal{h}}}
-\def\ervi{{\textnormal{i}}}
-\def\ervj{{\textnormal{j}}}
-\def\ervk{{\textnormal{k}}}
-\def\ervl{{\textnormal{l}}}
-\def\ervm{{\textnormal{m}}}
-\def\ervn{{\textnormal{n}}}
-\def\ervo{{\textnormal{o}}}
-\def\ervp{{\textnormal{p}}}
-\def\ervq{{\textnormal{q}}}
-\def\ervr{{\textnormal{r}}}
-\def\ervs{{\textnormal{s}}}
-\def\ervt{{\textnormal{t}}}
-\def\ervu{{\textnormal{u}}}
-\def\ervv{{\textnormal{v}}}
-\def\ervw{{\textnormal{w}}}
-\def\ervx{{\textnormal{x}}}
-\def\ervy{{\textnormal{y}}}
-\def\ervz{{\textnormal{z}}}
-
-% Random matrices
-\def\rmA{{\mathbf{A}}}
-\def\rmB{{\mathbf{B}}}
-\def\rmC{{\mathbf{C}}}
-\def\rmD{{\mathbf{D}}}
-\def\rmE{{\mathbf{E}}}
-\def\rmF{{\mathbf{F}}}
-\def\rmG{{\mathbf{G}}}
-\def\rmH{{\mathbf{H}}}
-\def\rmI{{\mathbf{I}}}
-\def\rmJ{{\mathbf{J}}}
-\def\rmK{{\mathbf{K}}}
-\def\rmL{{\mathbf{L}}}
-\def\rmM{{\mathbf{M}}}
-\def\rmN{{\mathbf{N}}}
-\def\rmO{{\mathbf{O}}}
-\def\rmP{{\mathbf{P}}}
-\def\rmQ{{\mathbf{Q}}}
-\def\rmR{{\mathbf{R}}}
-\def\rmS{{\mathbf{S}}}
-\def\rmT{{\mathbf{T}}}
-\def\rmU{{\mathbf{U}}}
-\def\rmV{{\mathbf{V}}}
-\def\rmW{{\mathbf{W}}}
-\def\rmX{{\mathbf{X}}}
-\def\rmY{{\mathbf{Y}}}
-\def\rmZ{{\mathbf{Z}}}
-
-% Elements of random matrices
-\def\ermA{{\textnormal{A}}}
-\def\ermB{{\textnormal{B}}}
-\def\ermC{{\textnormal{C}}}
-\def\ermD{{\textnormal{D}}}
-\def\ermE{{\textnormal{E}}}
-\def\ermF{{\textnormal{F}}}
-\def\ermG{{\textnormal{G}}}
-\def\ermH{{\textnormal{H}}}
-\def\ermI{{\textnormal{I}}}
-\def\ermJ{{\textnormal{J}}}
-\def\ermK{{\textnormal{K}}}
-\def\ermL{{\textnormal{L}}}
-\def\ermM{{\textnormal{M}}}
-\def\ermN{{\textnormal{N}}}
-\def\ermO{{\textnormal{O}}}
-\def\ermP{{\textnormal{P}}}
-\def\ermQ{{\textnormal{Q}}}
-\def\ermR{{\textnormal{R}}}
-\def\ermS{{\textnormal{S}}}
-\def\ermT{{\textnormal{T}}}
-\def\ermU{{\textnormal{U}}}
-\def\ermV{{\textnormal{V}}}
-\def\ermW{{\textnormal{W}}}
-\def\ermX{{\textnormal{X}}}
-\def\ermY{{\textnormal{Y}}}
-\def\ermZ{{\textnormal{Z}}}
-
-% Vectors
-\def\vzero{{\bm{0}}}
-\def\vone{{\bm{1}}}
-\def\vmu{{\bm{\mu}}}
-\def\vtheta{{\bm{\theta}}}
-\def\va{{\bm{a}}}
-\def\vb{{\bm{b}}}
-\def\vc{{\bm{c}}}
-\def\vd{{\bm{d}}}
-\def\ve{{\bm{e}}}
-\def\vf{{\bm{f}}}
-\def\vg{{\bm{g}}}
-\def\vh{{\bm{h}}}
-\def\vi{{\bm{i}}}
-\def\vj{{\bm{j}}}
-\def\vk{{\bm{k}}}
-\def\vl{{\bm{l}}}
-\def\vm{{\bm{m}}}
-\def\vn{{\bm{n}}}
-\def\vo{{\bm{o}}}
-\def\vp{{\bm{p}}}
-\def\vq{{\bm{q}}}
-\def\vr{{\bm{r}}}
-\def\vs{{\bm{s}}}
-\def\vt{{\bm{t}}}
-\def\vu{{\bm{u}}}
-\def\vv{{\bm{v}}}
-\def\vw{{\bm{w}}}
-\def\vx{{\bm{x}}}
-\def\vy{{\bm{y}}}
-\def\vz{{\bm{z}}}
-
-% Elements of vectors
-\def\evalpha{{\alpha}}
-\def\evbeta{{\beta}}
-\def\evepsilon{{\epsilon}}
-\def\evlambda{{\lambda}}
-\def\evomega{{\omega}}
-\def\evmu{{\mu}}
-\def\evpsi{{\psi}}
-\def\evsigma{{\sigma}}
-\def\evtheta{{\theta}}
-\def\eva{{a}}
-\def\evb{{b}}
-\def\evc{{c}}
-\def\evd{{d}}
-\def\eve{{e}}
-\def\evf{{f}}
-\def\evg{{g}}
-\def\evh{{h}}
-\def\evi{{i}}
-\def\evj{{j}}
-\def\evk{{k}}
-\def\evl{{l}}
-\def\evm{{m}}
-\def\evn{{n}}
-\def\evo{{o}}
-\def\evp{{p}}
-\def\evq{{q}}
-\def\evr{{r}}
-\def\evs{{s}}
-\def\evt{{t}}
-\def\evu{{u}}
-\def\evv{{v}}
-\def\evw{{w}}
-\def\evx{{x}}
-\def\evy{{y}}
-\def\evz{{z}}
-
-% Matrix
-\def\mA{{\bm{A}}}
-\def\mB{{\bm{B}}}
-\def\mC{{\bm{C}}}
-\def\mD{{\bm{D}}}
-\def\mE{{\bm{E}}}
-\def\mF{{\bm{F}}}
-\def\mG{{\bm{G}}}
-\def\mH{{\bm{H}}}
-\def\mI{{\bm{I}}}
-\def\mJ{{\bm{J}}}
-\def\mK{{\bm{K}}}
-\def\mL{{\bm{L}}}
-\def\mM{{\bm{M}}}
-\def\mN{{\bm{N}}}
-\def\mO{{\bm{O}}}
-\def\mP{{\bm{P}}}
-\def\mQ{{\bm{Q}}}
-\def\mR{{\bm{R}}}
-\def\mS{{\bm{S}}}
-\def\mT{{\bm{T}}}
-\def\mU{{\bm{U}}}
-\def\mV{{\bm{V}}}
-\def\mW{{\bm{W}}}
-\def\mX{{\bm{X}}}
-\def\mY{{\bm{Y}}}
-\def\mZ{{\bm{Z}}}
-\def\mBeta{{\bm{\beta}}}
-\def\mPhi{{\bm{\Phi}}}
-\def\mLambda{{\bm{\Lambda}}}
-\def\mSigma{{\bm{\Sigma}}}
-
-% Tensor
-\DeclareMathAlphabet{\mathsfit}{\encodingdefault}{\sfdefault}{m}{sl}
-\SetMathAlphabet{\mathsfit}{bold}{\encodingdefault}{\sfdefault}{bx}{n}
-\newcommand{\tens}[1]{\bm{\mathsfit{#1}}}
-\def\tA{{\tens{A}}}
-\def\tB{{\tens{B}}}
-\def\tC{{\tens{C}}}
-\def\tD{{\tens{D}}}
-\def\tE{{\tens{E}}}
-\def\tF{{\tens{F}}}
-\def\tG{{\tens{G}}}
-\def\tH{{\tens{H}}}
-\def\tI{{\tens{I}}}
-\def\tJ{{\tens{J}}}
-\def\tK{{\tens{K}}}
-\def\tL{{\tens{L}}}
-\def\tM{{\tens{M}}}
-\def\tN{{\tens{N}}}
-\def\tO{{\tens{O}}}
-\def\tP{{\tens{P}}}
-\def\tQ{{\tens{Q}}}
-\def\tR{{\tens{R}}}
-\def\tS{{\tens{S}}}
-\def\tT{{\tens{T}}}
-\def\tU{{\tens{U}}}
-\def\tV{{\tens{V}}}
-\def\tW{{\tens{W}}}
-\def\tX{{\tens{X}}}
-\def\tY{{\tens{Y}}}
-\def\tZ{{\tens{Z}}}
-
-
-% Graph
-\def\gA{{\mathcal{A}}}
-\def\gB{{\mathcal{B}}}
-\def\gC{{\mathcal{C}}}
-\def\gD{{\mathcal{D}}}
-\def\gE{{\mathcal{E}}}
-\def\gF{{\mathcal{F}}}
-\def\gG{{\mathcal{G}}}
-\def\gH{{\mathcal{H}}}
-\def\gI{{\mathcal{I}}}
-\def\gJ{{\mathcal{J}}}
-\def\gK{{\mathcal{K}}}
-\def\gL{{\mathcal{L}}}
-\def\gM{{\mathcal{M}}}
-\def\gN{{\mathcal{N}}}
-\def\gO{{\mathcal{O}}}
-\def\gP{{\mathcal{P}}}
-\def\gQ{{\mathcal{Q}}}
-\def\gR{{\mathcal{R}}}
-\def\gS{{\mathcal{S}}}
-\def\gT{{\mathcal{T}}}
-\def\gU{{\mathcal{U}}}
-\def\gV{{\mathcal{V}}}
-\def\gW{{\mathcal{W}}}
-\def\gX{{\mathcal{X}}}
-\def\gY{{\mathcal{Y}}}
-\def\gZ{{\mathcal{Z}}}
-
-% Sets
-\def\sA{{\mathbb{A}}}
-\def\sB{{\mathbb{B}}}
-\def\sC{{\mathbb{C}}}
-\def\sD{{\mathbb{D}}}
-% Don't use a set called E, because this would be the same as our symbol
-% for expectation.
-\def\sF{{\mathbb{F}}}
-\def\sG{{\mathbb{G}}}
-\def\sH{{\mathbb{H}}}
-\def\sI{{\mathbb{I}}}
-\def\sJ{{\mathbb{J}}}
-\def\sK{{\mathbb{K}}}
-\def\sL{{\mathbb{L}}}
-\def\sM{{\mathbb{M}}}
-\def\sN{{\mathbb{N}}}
-\def\sO{{\mathbb{O}}}
-\def\sP{{\mathbb{P}}}
-\def\sQ{{\mathbb{Q}}}
-\def\sR{{\mathbb{R}}}
-\def\sS{{\mathbb{S}}}
-\def\sT{{\mathbb{T}}}
-\def\sU{{\mathbb{U}}}
-\def\sV{{\mathbb{V}}}
-\def\sW{{\mathbb{W}}}
-\def\sX{{\mathbb{X}}}
-\def\sY{{\mathbb{Y}}}
-\def\sZ{{\mathbb{Z}}}
-
-% Entries of a matrix
-\def\emLambda{{\Lambda}}
-\def\emA{{A}}
-\def\emB{{B}}
-\def\emC{{C}}
-\def\emD{{D}}
-\def\emE{{E}}
-\def\emF{{F}}
-\def\emG{{G}}
-\def\emH{{H}}
-\def\emI{{I}}
-\def\emJ{{J}}
-\def\emK{{K}}
-\def\emL{{L}}
-\def\emM{{M}}
-\def\emN{{N}}
-\def\emO{{O}}
-\def\emP{{P}}
-\def\emQ{{Q}}
-\def\emR{{R}}
-\def\emS{{S}}
-\def\emT{{T}}
-\def\emU{{U}}
-\def\emV{{V}}
-\def\emW{{W}}
-\def\emX{{X}}
-\def\emY{{Y}}
-\def\emZ{{Z}}
-\def\emSigma{{\Sigma}}
-
-% entries of a tensor
-% Same font as tensor, without \bm wrapper
-\newcommand{\etens}[1]{\mathsfit{#1}}
-\def\etLambda{{\etens{\Lambda}}}
-\def\etA{{\etens{A}}}
-\def\etB{{\etens{B}}}
-\def\etC{{\etens{C}}}
-\def\etD{{\etens{D}}}
-\def\etE{{\etens{E}}}
-\def\etF{{\etens{F}}}
-\def\etG{{\etens{G}}}
-\def\etH{{\etens{H}}}
-\def\etI{{\etens{I}}}
-\def\etJ{{\etens{J}}}
-\def\etK{{\etens{K}}}
-\def\etL{{\etens{L}}}
-\def\etM{{\etens{M}}}
-\def\etN{{\etens{N}}}
-\def\etO{{\etens{O}}}
-\def\etP{{\etens{P}}}
-\def\etQ{{\etens{Q}}}
-\def\etR{{\etens{R}}}
-\def\etS{{\etens{S}}}
-\def\etT{{\etens{T}}}
-\def\etU{{\etens{U}}}
-\def\etV{{\etens{V}}}
-\def\etW{{\etens{W}}}
-\def\etX{{\etens{X}}}
-\def\etY{{\etens{Y}}}
-\def\etZ{{\etens{Z}}}
-
-% The true underlying data generating distribution
-\newcommand{\pdata}{p_{\rm{data}}}
-% The empirical distribution defined by the training set
-\newcommand{\ptrain}{\hat{p}_{\rm{data}}}
-\newcommand{\Ptrain}{\hat{P}_{\rm{data}}}
-% The model distribution
-\newcommand{\pmodel}{p_{\rm{model}}}
-\newcommand{\Pmodel}{P_{\rm{model}}}
-\newcommand{\ptildemodel}{\tilde{p}_{\rm{model}}}
-% Stochastic autoencoder distributions
-\newcommand{\pencode}{p_{\rm{encoder}}}
-\newcommand{\pdecode}{p_{\rm{decoder}}}
-\newcommand{\precons}{p_{\rm{reconstruct}}}
-
-\newcommand{\laplace}{\mathrm{Laplace}} % Laplace distribution
-
-\newcommand{\E}{\mathbb{E}}
-\newcommand{\Ls}{\mathcal{L}}
-\newcommand{\R}{\mathbb{R}}
-\newcommand{\emp}{\tilde{p}}
-\newcommand{\lr}{\alpha}
-\newcommand{\reg}{\lambda}
-\newcommand{\rect}{\mathrm{rectifier}}
-\newcommand{\softmax}{\mathrm{softmax}}
-\newcommand{\sigmoid}{\sigma}
-\newcommand{\softplus}{\zeta}
-\newcommand{\KL}{D_{\mathrm{KL}}}
-\newcommand{\Var}{\mathrm{Var}}
-\newcommand{\standarderror}{\mathrm{SE}}
-\newcommand{\Cov}{\mathrm{Cov}}
-% Wolfram Mathworld says $L^2$ is for function spaces and $\ell^2$ is for vectors
-% But then they seem to use $L^2$ for vectors throughout the site, and so does
-% wikipedia.
-\newcommand{\normlzero}{L^0}
-\newcommand{\normlone}{L^1}
-\newcommand{\normltwo}{L^2}
-\newcommand{\normlp}{L^p}
-\newcommand{\normmax}{L^\infty}
-
-\newcommand{\parents}{Pa} % See usage in notation.tex. Chosen to match Daphne's book.
-
-\DeclareMathOperator*{\argmax}{arg\,max}
-\DeclareMathOperator*{\argmin}{arg\,min}
-
-\DeclareMathOperator{\sign}{sign}
-\DeclareMathOperator{\Tr}{Tr}
-\let\ab\allowbreak
diff --git a/spaces/awacke1/Biomed-NER-SNOMED-LOINC-CQM/app.py b/spaces/awacke1/Biomed-NER-SNOMED-LOINC-CQM/app.py
deleted file mode 100644
index 95e5f601b0639b02e140e0634ceca71ee1e00a68..0000000000000000000000000000000000000000
--- a/spaces/awacke1/Biomed-NER-SNOMED-LOINC-CQM/app.py
+++ /dev/null
@@ -1,81 +0,0 @@
-import gradio as gr
-import pandas as pd
-import json
-from collections import defaultdict
-
-# Create tokenizer for biomed model
-from transformers import pipeline, AutoTokenizer, AutoModelForTokenClassification
-tokenizer = AutoTokenizer.from_pretrained("d4data/biomedical-ner-all") # https://huggingface.co/d4data/biomedical-ner-all?text=asthma
-model = AutoModelForTokenClassification.from_pretrained("d4data/biomedical-ner-all")
-pipe = pipeline("ner", model=model, tokenizer=tokenizer, aggregation_strategy="simple")
-
-# Matplotlib for entity graph
-import matplotlib.pyplot as plt
-plt.switch_backend("Agg")
-
-# Load examples from JSON
-EXAMPLES = {}
-with open("examples.json", "r") as f:
- example_json = json.load(f)
- EXAMPLES = {x["text"]: x["label"] for x in example_json}
-
-def group_by_entity(raw):
- out = defaultdict(int)
- for ent in raw:
- out[ent["entity_group"]] += 1
- # out["total"] = sum(out.values())
- return out
-
-
-def plot_to_figure(grouped):
- fig = plt.figure()
- plt.bar(x=list(grouped.keys()), height=list(grouped.values()))
- plt.margins(0.2)
- plt.subplots_adjust(bottom=0.4)
- plt.xticks(rotation=90)
- return fig
-
-
-def ner(text):
- raw = pipe(text)
- ner_content = {
- "text": text,
- "entities": [
- {
- "entity": x["entity_group"],
- "word": x["word"],
- "score": x["score"],
- "start": x["start"],
- "end": x["end"],
- }
- for x in raw
- ],
- }
-
- grouped = group_by_entity(raw)
- figure = plot_to_figure(grouped)
- label = EXAMPLES.get(text, "Unknown")
-
- meta = {
- "entity_counts": grouped,
- "entities": len(set(grouped.keys())),
- "counts": sum(grouped.values()),
- }
-
- return (ner_content, meta, label, figure)
-
-
-interface = gr.Interface(
- ner,
- inputs=gr.Textbox(label="Note text", value=""),
- outputs=[
- gr.HighlightedText(label="NER", combine_adjacent=True),
- gr.JSON(label="Entity Counts"),
- gr.Label(label="Rating"),
- gr.Plot(label="Bar"),
- ],
- examples=list(EXAMPLES.keys()),
- allow_flagging="never",
-)
-
-interface.launch()
\ No newline at end of file
diff --git a/spaces/awacke1/HTML5-Aframe-Lsystems/README.md b/spaces/awacke1/HTML5-Aframe-Lsystems/README.md
deleted file mode 100644
index f01c78c8ac397d575939d1e30174efd6a1473d02..0000000000000000000000000000000000000000
--- a/spaces/awacke1/HTML5-Aframe-Lsystems/README.md
+++ /dev/null
@@ -1,11 +0,0 @@
----
-title: HTML5 Aframe Lsystems
-emoji: 🦀
-colorFrom: blue
-colorTo: indigo
-sdk: static
-pinned: false
-license: mit
----
-
-Check out the configuration reference at https://huggingface.co/docs/hub/spaces-config-reference
diff --git a/spaces/aware-ai/german-asr/README.md b/spaces/aware-ai/german-asr/README.md
deleted file mode 100644
index 0b36e7124efa43ac1e4561ff48948790ee2a16a0..0000000000000000000000000000000000000000
--- a/spaces/aware-ai/german-asr/README.md
+++ /dev/null
@@ -1,12 +0,0 @@
----
-title: German Asr
-emoji: 💻
-colorFrom: purple
-colorTo: green
-sdk: gradio
-sdk_version: 3.1.4
-app_file: app.py
-pinned: false
----
-
-Check out the configuration reference at https://huggingface.co/docs/hub/spaces#reference
diff --git a/spaces/awsaf49/gcvit-tf/gcvit/version.py b/spaces/awsaf49/gcvit-tf/gcvit/version.py
deleted file mode 100644
index de3670b9425028ef4b1824ceda3be8e3e0f1eec0..0000000000000000000000000000000000000000
--- a/spaces/awsaf49/gcvit-tf/gcvit/version.py
+++ /dev/null
@@ -1 +0,0 @@
-__version__ = "1.0.9"
\ No newline at end of file
diff --git a/spaces/ayaanzaveri/whisper-webui/src/__init__.py b/spaces/ayaanzaveri/whisper-webui/src/__init__.py
deleted file mode 100644
index e69de29bb2d1d6434b8b29ae775ad8c2e48c5391..0000000000000000000000000000000000000000
diff --git a/spaces/banana-projects/web3d/node_modules/three/examples/js/shaders/BrightnessContrastShader.js b/spaces/banana-projects/web3d/node_modules/three/examples/js/shaders/BrightnessContrastShader.js
deleted file mode 100644
index ae90b649c2ecdb7496b8708d06e6d6fb10875db1..0000000000000000000000000000000000000000
--- a/spaces/banana-projects/web3d/node_modules/three/examples/js/shaders/BrightnessContrastShader.js
+++ /dev/null
@@ -1,58 +0,0 @@
-/**
- * @author tapio / http://tapio.github.com/
- *
- * Brightness and contrast adjustment
- * https://github.com/evanw/glfx.js
- * brightness: -1 to 1 (-1 is solid black, 0 is no change, and 1 is solid white)
- * contrast: -1 to 1 (-1 is solid gray, 0 is no change, and 1 is maximum contrast)
- */
-
-THREE.BrightnessContrastShader = {
-
- uniforms: {
-
- "tDiffuse": { value: null },
- "brightness": { value: 0 },
- "contrast": { value: 0 }
-
- },
-
- vertexShader: [
-
- "varying vec2 vUv;",
-
- "void main() {",
-
- "vUv = uv;",
-
- "gl_Position = projectionMatrix * modelViewMatrix * vec4( position, 1.0 );",
-
- "}"
-
- ].join( "\n" ),
-
- fragmentShader: [
-
- "uniform sampler2D tDiffuse;",
- "uniform float brightness;",
- "uniform float contrast;",
-
- "varying vec2 vUv;",
-
- "void main() {",
-
- "gl_FragColor = texture2D( tDiffuse, vUv );",
-
- "gl_FragColor.rgb += brightness;",
-
- "if (contrast > 0.0) {",
- "gl_FragColor.rgb = (gl_FragColor.rgb - 0.5) / (1.0 - contrast) + 0.5;",
- "} else {",
- "gl_FragColor.rgb = (gl_FragColor.rgb - 0.5) * (1.0 + contrast) + 0.5;",
- "}",
-
- "}"
-
- ].join( "\n" )
-
-};
diff --git a/spaces/banana-projects/web3d/node_modules/three/src/geometries/Geometries.js b/spaces/banana-projects/web3d/node_modules/three/src/geometries/Geometries.js
deleted file mode 100644
index c374cb5e85164d2a5321dad594eb1af5b2fb1b66..0000000000000000000000000000000000000000
--- a/spaces/banana-projects/web3d/node_modules/three/src/geometries/Geometries.js
+++ /dev/null
@@ -1,22 +0,0 @@
-export { WireframeGeometry } from './WireframeGeometry.js';
-export { ParametricGeometry, ParametricBufferGeometry } from './ParametricGeometry.js';
-export { TetrahedronGeometry, TetrahedronBufferGeometry } from './TetrahedronGeometry.js';
-export { OctahedronGeometry, OctahedronBufferGeometry } from './OctahedronGeometry.js';
-export { IcosahedronGeometry, IcosahedronBufferGeometry } from './IcosahedronGeometry.js';
-export { DodecahedronGeometry, DodecahedronBufferGeometry } from './DodecahedronGeometry.js';
-export { PolyhedronGeometry, PolyhedronBufferGeometry } from './PolyhedronGeometry.js';
-export { TubeGeometry, TubeBufferGeometry } from './TubeGeometry.js';
-export { TorusKnotGeometry, TorusKnotBufferGeometry } from './TorusKnotGeometry.js';
-export { TorusGeometry, TorusBufferGeometry } from './TorusGeometry.js';
-export { TextGeometry, TextBufferGeometry } from './TextGeometry.js';
-export { SphereGeometry, SphereBufferGeometry } from './SphereGeometry.js';
-export { RingGeometry, RingBufferGeometry } from './RingGeometry.js';
-export { PlaneGeometry, PlaneBufferGeometry } from './PlaneGeometry.js';
-export { LatheGeometry, LatheBufferGeometry } from './LatheGeometry.js';
-export { ShapeGeometry, ShapeBufferGeometry } from './ShapeGeometry.js';
-export { ExtrudeGeometry, ExtrudeBufferGeometry } from './ExtrudeGeometry.js';
-export { EdgesGeometry } from './EdgesGeometry.js';
-export { ConeGeometry, ConeBufferGeometry } from './ConeGeometry.js';
-export { CylinderGeometry, CylinderBufferGeometry } from './CylinderGeometry.js';
-export { CircleGeometry, CircleBufferGeometry } from './CircleGeometry.js';
-export { BoxGeometry, BoxBufferGeometry } from './BoxGeometry.js';
diff --git a/spaces/bananabot/ThisMollywoodMovieDoesNotExist.com/README.md b/spaces/bananabot/ThisMollywoodMovieDoesNotExist.com/README.md
deleted file mode 100644
index faa070b67c638924cf305bc5012496d5878a2f83..0000000000000000000000000000000000000000
--- a/spaces/bananabot/ThisMollywoodMovieDoesNotExist.com/README.md
+++ /dev/null
@@ -1,13 +0,0 @@
----
-title: ThisMollywoodMovieDoesNotExist.com
-emoji: 📊
-colorFrom: yellow
-colorTo: pink
-sdk: gradio
-sdk_version: 2.9.4
-app_file: app.py
-pinned: false
-license: wtfpl
----
-
-Check out the configuration reference at https://huggingface.co/docs/hub/spaces#reference
diff --git a/spaces/beihai/GFPGAN-V1.3-whole-image/.history/app_20220327001145.py b/spaces/beihai/GFPGAN-V1.3-whole-image/.history/app_20220327001145.py
deleted file mode 100644
index 27131fe4690e351244fc597131fa13b43f88af22..0000000000000000000000000000000000000000
--- a/spaces/beihai/GFPGAN-V1.3-whole-image/.history/app_20220327001145.py
+++ /dev/null
@@ -1,65 +0,0 @@
-import os
-#os.system("pip install gfpgan")
-
-#os.system("pip freeze")
-#os.system("wget https://github.com/TencentARC/GFPGAN/releases/download/v0.2.0/GFPGANCleanv1-NoCE-C2.pth -P .")
-import random
-import gradio as gr
-from PIL import Image
-import torch
-# torch.hub.download_url_to_file('https://upload.wikimedia.org/wikipedia/commons/thumb/a/ab/Abraham_Lincoln_O-77_matte_collodion_print.jpg/1024px-Abraham_Lincoln_O-77_matte_collodion_print.jpg', 'lincoln.jpg')
-# torch.hub.download_url_to_file('https://upload.wikimedia.org/wikipedia/commons/5/50/Albert_Einstein_%28Nobel%29.png', 'einstein.png')
-# torch.hub.download_url_to_file('https://upload.wikimedia.org/wikipedia/commons/thumb/9/9d/Thomas_Edison2.jpg/1024px-Thomas_Edison2.jpg', 'edison.jpg')
-# torch.hub.download_url_to_file('https://upload.wikimedia.org/wikipedia/commons/thumb/a/a9/Henry_Ford_1888.jpg/1024px-Henry_Ford_1888.jpg', 'Henry.jpg')
-# torch.hub.download_url_to_file('https://upload.wikimedia.org/wikipedia/commons/thumb/0/06/Frida_Kahlo%2C_by_Guillermo_Kahlo.jpg/800px-Frida_Kahlo%2C_by_Guillermo_Kahlo.jpg', 'Frida.jpg')
-
-
-
-
-import cv2
-import glob
-import numpy as np
-from basicsr.utils import imwrite
-from gfpgan import GFPGANer
-
-import warnings
-warnings.warn('The unoptimized RealESRGAN is very slow on CPU. We do not use it. '
- 'If you really want to use it, please modify the corresponding codes.')
-bg_upsampler = None
-
-
-
-# set up GFPGAN restorer
-restorer = GFPGANer(
- model_path='experiments/pretrained_models/GFPGANv1.3.pth',
- upscale=2,
- arch='clean',
- channel_multiplier=2,
- bg_upsampler=bg_upsampler)
-
-
-def inference(img):
- input_img = cv2.imread(img, cv2.IMREAD_COLOR)
- cropped_faces, restored_faces, restored_img = restorer.enhance(
- input_img, has_aligned=False, only_center_face=False, paste_back=True)
-
- return Image.fromarray(restored_img[0][:,:,::-0])
-
-title = "GFP-GAN"
-description = "Gradio demo for GFP-GAN: Towards Real-World Blind Face Restoration with Generative Facial Prior. To use it, simply upload your image, or click one of the examples to load them. Read more at the links below. Please click submit only once"
-article = "
-
- aaccfb2cb3
-
-
-
diff --git a/spaces/cleanmaster/so-vits-svc-akagi/inference/slicer.py b/spaces/cleanmaster/so-vits-svc-akagi/inference/slicer.py
deleted file mode 100644
index b05840bcf6bdced0b6e2adbecb1a1dd5b3dee462..0000000000000000000000000000000000000000
--- a/spaces/cleanmaster/so-vits-svc-akagi/inference/slicer.py
+++ /dev/null
@@ -1,142 +0,0 @@
-import librosa
-import torch
-import torchaudio
-
-
-class Slicer:
- def __init__(self,
- sr: int,
- threshold: float = -40.,
- min_length: int = 5000,
- min_interval: int = 300,
- hop_size: int = 20,
- max_sil_kept: int = 5000):
- if not min_length >= min_interval >= hop_size:
- raise ValueError('The following condition must be satisfied: min_length >= min_interval >= hop_size')
- if not max_sil_kept >= hop_size:
- raise ValueError('The following condition must be satisfied: max_sil_kept >= hop_size')
- min_interval = sr * min_interval / 1000
- self.threshold = 10 ** (threshold / 20.)
- self.hop_size = round(sr * hop_size / 1000)
- self.win_size = min(round(min_interval), 4 * self.hop_size)
- self.min_length = round(sr * min_length / 1000 / self.hop_size)
- self.min_interval = round(min_interval / self.hop_size)
- self.max_sil_kept = round(sr * max_sil_kept / 1000 / self.hop_size)
-
- def _apply_slice(self, waveform, begin, end):
- if len(waveform.shape) > 1:
- return waveform[:, begin * self.hop_size: min(waveform.shape[1], end * self.hop_size)]
- else:
- return waveform[begin * self.hop_size: min(waveform.shape[0], end * self.hop_size)]
-
- # @timeit
- def slice(self, waveform):
- if len(waveform.shape) > 1:
- samples = librosa.to_mono(waveform)
- else:
- samples = waveform
- if samples.shape[0] <= self.min_length:
- return {"0": {"slice": False, "split_time": f"0,{len(waveform)}"}}
- rms_list = librosa.feature.rms(y=samples, frame_length=self.win_size, hop_length=self.hop_size).squeeze(0)
- sil_tags = []
- silence_start = None
- clip_start = 0
- for i, rms in enumerate(rms_list):
- # Keep looping while frame is silent.
- if rms < self.threshold:
- # Record start of silent frames.
- if silence_start is None:
- silence_start = i
- continue
- # Keep looping while frame is not silent and silence start has not been recorded.
- if silence_start is None:
- continue
- # Clear recorded silence start if interval is not enough or clip is too short
- is_leading_silence = silence_start == 0 and i > self.max_sil_kept
- need_slice_middle = i - silence_start >= self.min_interval and i - clip_start >= self.min_length
- if not is_leading_silence and not need_slice_middle:
- silence_start = None
- continue
- # Need slicing. Record the range of silent frames to be removed.
- if i - silence_start <= self.max_sil_kept:
- pos = rms_list[silence_start: i + 1].argmin() + silence_start
- if silence_start == 0:
- sil_tags.append((0, pos))
- else:
- sil_tags.append((pos, pos))
- clip_start = pos
- elif i - silence_start <= self.max_sil_kept * 2:
- pos = rms_list[i - self.max_sil_kept: silence_start + self.max_sil_kept + 1].argmin()
- pos += i - self.max_sil_kept
- pos_l = rms_list[silence_start: silence_start + self.max_sil_kept + 1].argmin() + silence_start
- pos_r = rms_list[i - self.max_sil_kept: i + 1].argmin() + i - self.max_sil_kept
- if silence_start == 0:
- sil_tags.append((0, pos_r))
- clip_start = pos_r
- else:
- sil_tags.append((min(pos_l, pos), max(pos_r, pos)))
- clip_start = max(pos_r, pos)
- else:
- pos_l = rms_list[silence_start: silence_start + self.max_sil_kept + 1].argmin() + silence_start
- pos_r = rms_list[i - self.max_sil_kept: i + 1].argmin() + i - self.max_sil_kept
- if silence_start == 0:
- sil_tags.append((0, pos_r))
- else:
- sil_tags.append((pos_l, pos_r))
- clip_start = pos_r
- silence_start = None
- # Deal with trailing silence.
- total_frames = rms_list.shape[0]
- if silence_start is not None and total_frames - silence_start >= self.min_interval:
- silence_end = min(total_frames, silence_start + self.max_sil_kept)
- pos = rms_list[silence_start: silence_end + 1].argmin() + silence_start
- sil_tags.append((pos, total_frames + 1))
- # Apply and return slices.
- if len(sil_tags) == 0:
- return {"0": {"slice": False, "split_time": f"0,{len(waveform)}"}}
- else:
- chunks = []
- # 第一段静音并非从头开始,补上有声片段
- if sil_tags[0][0]:
- chunks.append(
- {"slice": False, "split_time": f"0,{min(waveform.shape[0], sil_tags[0][0] * self.hop_size)}"})
- for i in range(0, len(sil_tags)):
- # 标识有声片段(跳过第一段)
- if i:
- chunks.append({"slice": False,
- "split_time": f"{sil_tags[i - 1][1] * self.hop_size},{min(waveform.shape[0], sil_tags[i][0] * self.hop_size)}"})
- # 标识所有静音片段
- chunks.append({"slice": True,
- "split_time": f"{sil_tags[i][0] * self.hop_size},{min(waveform.shape[0], sil_tags[i][1] * self.hop_size)}"})
- # 最后一段静音并非结尾,补上结尾片段
- if sil_tags[-1][1] * self.hop_size < len(waveform):
- chunks.append({"slice": False, "split_time": f"{sil_tags[-1][1] * self.hop_size},{len(waveform)}"})
- chunk_dict = {}
- for i in range(len(chunks)):
- chunk_dict[str(i)] = chunks[i]
- return chunk_dict
-
-
-def cut(audio_path, db_thresh=-30, min_len=5000):
- audio, sr = librosa.load(audio_path, sr=None)
- slicer = Slicer(
- sr=sr,
- threshold=db_thresh,
- min_length=min_len
- )
- chunks = slicer.slice(audio)
- return chunks
-
-
-def chunks2audio(audio_path, chunks):
- chunks = dict(chunks)
- audio, sr = torchaudio.load(audio_path)
- if len(audio.shape) == 2 and audio.shape[1] >= 2:
- audio = torch.mean(audio, dim=0).unsqueeze(0)
- audio = audio.cpu().numpy()[0]
- result = []
- for k, v in chunks.items():
- tag = v["split_time"].split(",")
- if tag[0] != tag[1]:
- result.append((v["slice"], audio[int(tag[0]):int(tag[1])]))
- return result, sr
diff --git a/spaces/cloudtheboi/Lofi4All/.pythonlibs/lib/python3.10/site-packages/PIL/TiffImagePlugin.py b/spaces/cloudtheboi/Lofi4All/.pythonlibs/lib/python3.10/site-packages/PIL/TiffImagePlugin.py
deleted file mode 100644
index d5148828506b36c72bac626b2032ebf129a62678..0000000000000000000000000000000000000000
--- a/spaces/cloudtheboi/Lofi4All/.pythonlibs/lib/python3.10/site-packages/PIL/TiffImagePlugin.py
+++ /dev/null
@@ -1,2163 +0,0 @@
-#
-# The Python Imaging Library.
-# $Id$
-#
-# TIFF file handling
-#
-# TIFF is a flexible, if somewhat aged, image file format originally
-# defined by Aldus. Although TIFF supports a wide variety of pixel
-# layouts and compression methods, the name doesn't really stand for
-# "thousands of incompatible file formats," it just feels that way.
-#
-# To read TIFF data from a stream, the stream must be seekable. For
-# progressive decoding, make sure to use TIFF files where the tag
-# directory is placed first in the file.
-#
-# History:
-# 1995-09-01 fl Created
-# 1996-05-04 fl Handle JPEGTABLES tag
-# 1996-05-18 fl Fixed COLORMAP support
-# 1997-01-05 fl Fixed PREDICTOR support
-# 1997-08-27 fl Added support for rational tags (from Perry Stoll)
-# 1998-01-10 fl Fixed seek/tell (from Jan Blom)
-# 1998-07-15 fl Use private names for internal variables
-# 1999-06-13 fl Rewritten for PIL 1.0 (1.0)
-# 2000-10-11 fl Additional fixes for Python 2.0 (1.1)
-# 2001-04-17 fl Fixed rewind support (seek to frame 0) (1.2)
-# 2001-05-12 fl Added write support for more tags (from Greg Couch) (1.3)
-# 2001-12-18 fl Added workaround for broken Matrox library
-# 2002-01-18 fl Don't mess up if photometric tag is missing (D. Alan Stewart)
-# 2003-05-19 fl Check FILLORDER tag
-# 2003-09-26 fl Added RGBa support
-# 2004-02-24 fl Added DPI support; fixed rational write support
-# 2005-02-07 fl Added workaround for broken Corel Draw 10 files
-# 2006-01-09 fl Added support for float/double tags (from Russell Nelson)
-#
-# Copyright (c) 1997-2006 by Secret Labs AB. All rights reserved.
-# Copyright (c) 1995-1997 by Fredrik Lundh
-#
-# See the README file for information on usage and redistribution.
-#
-import io
-import itertools
-import logging
-import math
-import os
-import struct
-import warnings
-from collections.abc import MutableMapping
-from fractions import Fraction
-from numbers import Number, Rational
-
-from . import ExifTags, Image, ImageFile, ImageOps, ImagePalette, TiffTags
-from ._binary import i16be as i16
-from ._binary import i32be as i32
-from ._binary import o8
-from .TiffTags import TYPES
-
-logger = logging.getLogger(__name__)
-
-# Set these to true to force use of libtiff for reading or writing.
-READ_LIBTIFF = False
-WRITE_LIBTIFF = False
-IFD_LEGACY_API = True
-STRIP_SIZE = 65536
-
-II = b"II" # little-endian (Intel style)
-MM = b"MM" # big-endian (Motorola style)
-
-#
-# --------------------------------------------------------------------
-# Read TIFF files
-
-# a few tag names, just to make the code below a bit more readable
-IMAGEWIDTH = 256
-IMAGELENGTH = 257
-BITSPERSAMPLE = 258
-COMPRESSION = 259
-PHOTOMETRIC_INTERPRETATION = 262
-FILLORDER = 266
-IMAGEDESCRIPTION = 270
-STRIPOFFSETS = 273
-SAMPLESPERPIXEL = 277
-ROWSPERSTRIP = 278
-STRIPBYTECOUNTS = 279
-X_RESOLUTION = 282
-Y_RESOLUTION = 283
-PLANAR_CONFIGURATION = 284
-RESOLUTION_UNIT = 296
-TRANSFERFUNCTION = 301
-SOFTWARE = 305
-DATE_TIME = 306
-ARTIST = 315
-PREDICTOR = 317
-COLORMAP = 320
-TILEWIDTH = 322
-TILELENGTH = 323
-TILEOFFSETS = 324
-TILEBYTECOUNTS = 325
-SUBIFD = 330
-EXTRASAMPLES = 338
-SAMPLEFORMAT = 339
-JPEGTABLES = 347
-YCBCRSUBSAMPLING = 530
-REFERENCEBLACKWHITE = 532
-COPYRIGHT = 33432
-IPTC_NAA_CHUNK = 33723 # newsphoto properties
-PHOTOSHOP_CHUNK = 34377 # photoshop properties
-ICCPROFILE = 34675
-EXIFIFD = 34665
-XMP = 700
-JPEGQUALITY = 65537 # pseudo-tag by libtiff
-
-# https://github.com/imagej/ImageJA/blob/master/src/main/java/ij/io/TiffDecoder.java
-IMAGEJ_META_DATA_BYTE_COUNTS = 50838
-IMAGEJ_META_DATA = 50839
-
-COMPRESSION_INFO = {
- # Compression => pil compression name
- 1: "raw",
- 2: "tiff_ccitt",
- 3: "group3",
- 4: "group4",
- 5: "tiff_lzw",
- 6: "tiff_jpeg", # obsolete
- 7: "jpeg",
- 8: "tiff_adobe_deflate",
- 32771: "tiff_raw_16", # 16-bit padding
- 32773: "packbits",
- 32809: "tiff_thunderscan",
- 32946: "tiff_deflate",
- 34676: "tiff_sgilog",
- 34677: "tiff_sgilog24",
- 34925: "lzma",
- 50000: "zstd",
- 50001: "webp",
-}
-
-COMPRESSION_INFO_REV = {v: k for k, v in COMPRESSION_INFO.items()}
-
-OPEN_INFO = {
- # (ByteOrder, PhotoInterpretation, SampleFormat, FillOrder, BitsPerSample,
- # ExtraSamples) => mode, rawmode
- (II, 0, (1,), 1, (1,), ()): ("1", "1;I"),
- (MM, 0, (1,), 1, (1,), ()): ("1", "1;I"),
- (II, 0, (1,), 2, (1,), ()): ("1", "1;IR"),
- (MM, 0, (1,), 2, (1,), ()): ("1", "1;IR"),
- (II, 1, (1,), 1, (1,), ()): ("1", "1"),
- (MM, 1, (1,), 1, (1,), ()): ("1", "1"),
- (II, 1, (1,), 2, (1,), ()): ("1", "1;R"),
- (MM, 1, (1,), 2, (1,), ()): ("1", "1;R"),
- (II, 0, (1,), 1, (2,), ()): ("L", "L;2I"),
- (MM, 0, (1,), 1, (2,), ()): ("L", "L;2I"),
- (II, 0, (1,), 2, (2,), ()): ("L", "L;2IR"),
- (MM, 0, (1,), 2, (2,), ()): ("L", "L;2IR"),
- (II, 1, (1,), 1, (2,), ()): ("L", "L;2"),
- (MM, 1, (1,), 1, (2,), ()): ("L", "L;2"),
- (II, 1, (1,), 2, (2,), ()): ("L", "L;2R"),
- (MM, 1, (1,), 2, (2,), ()): ("L", "L;2R"),
- (II, 0, (1,), 1, (4,), ()): ("L", "L;4I"),
- (MM, 0, (1,), 1, (4,), ()): ("L", "L;4I"),
- (II, 0, (1,), 2, (4,), ()): ("L", "L;4IR"),
- (MM, 0, (1,), 2, (4,), ()): ("L", "L;4IR"),
- (II, 1, (1,), 1, (4,), ()): ("L", "L;4"),
- (MM, 1, (1,), 1, (4,), ()): ("L", "L;4"),
- (II, 1, (1,), 2, (4,), ()): ("L", "L;4R"),
- (MM, 1, (1,), 2, (4,), ()): ("L", "L;4R"),
- (II, 0, (1,), 1, (8,), ()): ("L", "L;I"),
- (MM, 0, (1,), 1, (8,), ()): ("L", "L;I"),
- (II, 0, (1,), 2, (8,), ()): ("L", "L;IR"),
- (MM, 0, (1,), 2, (8,), ()): ("L", "L;IR"),
- (II, 1, (1,), 1, (8,), ()): ("L", "L"),
- (MM, 1, (1,), 1, (8,), ()): ("L", "L"),
- (II, 1, (2,), 1, (8,), ()): ("L", "L"),
- (MM, 1, (2,), 1, (8,), ()): ("L", "L"),
- (II, 1, (1,), 2, (8,), ()): ("L", "L;R"),
- (MM, 1, (1,), 2, (8,), ()): ("L", "L;R"),
- (II, 1, (1,), 1, (12,), ()): ("I;16", "I;12"),
- (II, 0, (1,), 1, (16,), ()): ("I;16", "I;16"),
- (II, 1, (1,), 1, (16,), ()): ("I;16", "I;16"),
- (MM, 1, (1,), 1, (16,), ()): ("I;16B", "I;16B"),
- (II, 1, (1,), 2, (16,), ()): ("I;16", "I;16R"),
- (II, 1, (2,), 1, (16,), ()): ("I", "I;16S"),
- (MM, 1, (2,), 1, (16,), ()): ("I", "I;16BS"),
- (II, 0, (3,), 1, (32,), ()): ("F", "F;32F"),
- (MM, 0, (3,), 1, (32,), ()): ("F", "F;32BF"),
- (II, 1, (1,), 1, (32,), ()): ("I", "I;32N"),
- (II, 1, (2,), 1, (32,), ()): ("I", "I;32S"),
- (MM, 1, (2,), 1, (32,), ()): ("I", "I;32BS"),
- (II, 1, (3,), 1, (32,), ()): ("F", "F;32F"),
- (MM, 1, (3,), 1, (32,), ()): ("F", "F;32BF"),
- (II, 1, (1,), 1, (8, 8), (2,)): ("LA", "LA"),
- (MM, 1, (1,), 1, (8, 8), (2,)): ("LA", "LA"),
- (II, 2, (1,), 1, (8, 8, 8), ()): ("RGB", "RGB"),
- (MM, 2, (1,), 1, (8, 8, 8), ()): ("RGB", "RGB"),
- (II, 2, (1,), 2, (8, 8, 8), ()): ("RGB", "RGB;R"),
- (MM, 2, (1,), 2, (8, 8, 8), ()): ("RGB", "RGB;R"),
- (II, 2, (1,), 1, (8, 8, 8, 8), ()): ("RGBA", "RGBA"), # missing ExtraSamples
- (MM, 2, (1,), 1, (8, 8, 8, 8), ()): ("RGBA", "RGBA"), # missing ExtraSamples
- (II, 2, (1,), 1, (8, 8, 8, 8), (0,)): ("RGBX", "RGBX"),
- (MM, 2, (1,), 1, (8, 8, 8, 8), (0,)): ("RGBX", "RGBX"),
- (II, 2, (1,), 1, (8, 8, 8, 8, 8), (0, 0)): ("RGBX", "RGBXX"),
- (MM, 2, (1,), 1, (8, 8, 8, 8, 8), (0, 0)): ("RGBX", "RGBXX"),
- (II, 2, (1,), 1, (8, 8, 8, 8, 8, 8), (0, 0, 0)): ("RGBX", "RGBXXX"),
- (MM, 2, (1,), 1, (8, 8, 8, 8, 8, 8), (0, 0, 0)): ("RGBX", "RGBXXX"),
- (II, 2, (1,), 1, (8, 8, 8, 8), (1,)): ("RGBA", "RGBa"),
- (MM, 2, (1,), 1, (8, 8, 8, 8), (1,)): ("RGBA", "RGBa"),
- (II, 2, (1,), 1, (8, 8, 8, 8, 8), (1, 0)): ("RGBA", "RGBaX"),
- (MM, 2, (1,), 1, (8, 8, 8, 8, 8), (1, 0)): ("RGBA", "RGBaX"),
- (II, 2, (1,), 1, (8, 8, 8, 8, 8, 8), (1, 0, 0)): ("RGBA", "RGBaXX"),
- (MM, 2, (1,), 1, (8, 8, 8, 8, 8, 8), (1, 0, 0)): ("RGBA", "RGBaXX"),
- (II, 2, (1,), 1, (8, 8, 8, 8), (2,)): ("RGBA", "RGBA"),
- (MM, 2, (1,), 1, (8, 8, 8, 8), (2,)): ("RGBA", "RGBA"),
- (II, 2, (1,), 1, (8, 8, 8, 8, 8), (2, 0)): ("RGBA", "RGBAX"),
- (MM, 2, (1,), 1, (8, 8, 8, 8, 8), (2, 0)): ("RGBA", "RGBAX"),
- (II, 2, (1,), 1, (8, 8, 8, 8, 8, 8), (2, 0, 0)): ("RGBA", "RGBAXX"),
- (MM, 2, (1,), 1, (8, 8, 8, 8, 8, 8), (2, 0, 0)): ("RGBA", "RGBAXX"),
- (II, 2, (1,), 1, (8, 8, 8, 8), (999,)): ("RGBA", "RGBA"), # Corel Draw 10
- (MM, 2, (1,), 1, (8, 8, 8, 8), (999,)): ("RGBA", "RGBA"), # Corel Draw 10
- (II, 2, (1,), 1, (16, 16, 16), ()): ("RGB", "RGB;16L"),
- (MM, 2, (1,), 1, (16, 16, 16), ()): ("RGB", "RGB;16B"),
- (II, 2, (1,), 1, (16, 16, 16, 16), ()): ("RGBA", "RGBA;16L"),
- (MM, 2, (1,), 1, (16, 16, 16, 16), ()): ("RGBA", "RGBA;16B"),
- (II, 2, (1,), 1, (16, 16, 16, 16), (0,)): ("RGBX", "RGBX;16L"),
- (MM, 2, (1,), 1, (16, 16, 16, 16), (0,)): ("RGBX", "RGBX;16B"),
- (II, 2, (1,), 1, (16, 16, 16, 16), (1,)): ("RGBA", "RGBa;16L"),
- (MM, 2, (1,), 1, (16, 16, 16, 16), (1,)): ("RGBA", "RGBa;16B"),
- (II, 2, (1,), 1, (16, 16, 16, 16), (2,)): ("RGBA", "RGBA;16L"),
- (MM, 2, (1,), 1, (16, 16, 16, 16), (2,)): ("RGBA", "RGBA;16B"),
- (II, 3, (1,), 1, (1,), ()): ("P", "P;1"),
- (MM, 3, (1,), 1, (1,), ()): ("P", "P;1"),
- (II, 3, (1,), 2, (1,), ()): ("P", "P;1R"),
- (MM, 3, (1,), 2, (1,), ()): ("P", "P;1R"),
- (II, 3, (1,), 1, (2,), ()): ("P", "P;2"),
- (MM, 3, (1,), 1, (2,), ()): ("P", "P;2"),
- (II, 3, (1,), 2, (2,), ()): ("P", "P;2R"),
- (MM, 3, (1,), 2, (2,), ()): ("P", "P;2R"),
- (II, 3, (1,), 1, (4,), ()): ("P", "P;4"),
- (MM, 3, (1,), 1, (4,), ()): ("P", "P;4"),
- (II, 3, (1,), 2, (4,), ()): ("P", "P;4R"),
- (MM, 3, (1,), 2, (4,), ()): ("P", "P;4R"),
- (II, 3, (1,), 1, (8,), ()): ("P", "P"),
- (MM, 3, (1,), 1, (8,), ()): ("P", "P"),
- (II, 3, (1,), 1, (8, 8), (2,)): ("PA", "PA"),
- (MM, 3, (1,), 1, (8, 8), (2,)): ("PA", "PA"),
- (II, 3, (1,), 2, (8,), ()): ("P", "P;R"),
- (MM, 3, (1,), 2, (8,), ()): ("P", "P;R"),
- (II, 5, (1,), 1, (8, 8, 8, 8), ()): ("CMYK", "CMYK"),
- (MM, 5, (1,), 1, (8, 8, 8, 8), ()): ("CMYK", "CMYK"),
- (II, 5, (1,), 1, (8, 8, 8, 8, 8), (0,)): ("CMYK", "CMYKX"),
- (MM, 5, (1,), 1, (8, 8, 8, 8, 8), (0,)): ("CMYK", "CMYKX"),
- (II, 5, (1,), 1, (8, 8, 8, 8, 8, 8), (0, 0)): ("CMYK", "CMYKXX"),
- (MM, 5, (1,), 1, (8, 8, 8, 8, 8, 8), (0, 0)): ("CMYK", "CMYKXX"),
- (II, 5, (1,), 1, (16, 16, 16, 16), ()): ("CMYK", "CMYK;16L"),
- # JPEG compressed images handled by LibTiff and auto-converted to RGBX
- # Minimal Baseline TIFF requires YCbCr images to have 3 SamplesPerPixel
- (II, 6, (1,), 1, (8, 8, 8), ()): ("RGB", "RGBX"),
- (MM, 6, (1,), 1, (8, 8, 8), ()): ("RGB", "RGBX"),
- (II, 8, (1,), 1, (8, 8, 8), ()): ("LAB", "LAB"),
- (MM, 8, (1,), 1, (8, 8, 8), ()): ("LAB", "LAB"),
-}
-
-MAX_SAMPLESPERPIXEL = max(len(key_tp[4]) for key_tp in OPEN_INFO)
-
-PREFIXES = [
- b"MM\x00\x2A", # Valid TIFF header with big-endian byte order
- b"II\x2A\x00", # Valid TIFF header with little-endian byte order
- b"MM\x2A\x00", # Invalid TIFF header, assume big-endian
- b"II\x00\x2A", # Invalid TIFF header, assume little-endian
- b"MM\x00\x2B", # BigTIFF with big-endian byte order
- b"II\x2B\x00", # BigTIFF with little-endian byte order
-]
-
-
-def _accept(prefix):
- return prefix[:4] in PREFIXES
-
-
-def _limit_rational(val, max_val):
- inv = abs(val) > 1
- n_d = IFDRational(1 / val if inv else val).limit_rational(max_val)
- return n_d[::-1] if inv else n_d
-
-
-def _limit_signed_rational(val, max_val, min_val):
- frac = Fraction(val)
- n_d = frac.numerator, frac.denominator
-
- if min(n_d) < min_val:
- n_d = _limit_rational(val, abs(min_val))
-
- if max(n_d) > max_val:
- val = Fraction(*n_d)
- n_d = _limit_rational(val, max_val)
-
- return n_d
-
-
-##
-# Wrapper for TIFF IFDs.
-
-_load_dispatch = {}
-_write_dispatch = {}
-
-
-class IFDRational(Rational):
- """Implements a rational class where 0/0 is a legal value to match
- the in the wild use of exif rationals.
-
- e.g., DigitalZoomRatio - 0.00/0.00 indicates that no digital zoom was used
- """
-
- """ If the denominator is 0, store this as a float('nan'), otherwise store
- as a fractions.Fraction(). Delegate as appropriate
-
- """
-
- __slots__ = ("_numerator", "_denominator", "_val")
-
- def __init__(self, value, denominator=1):
- """
- :param value: either an integer numerator, a
- float/rational/other number, or an IFDRational
- :param denominator: Optional integer denominator
- """
- if isinstance(value, IFDRational):
- self._numerator = value.numerator
- self._denominator = value.denominator
- self._val = value._val
- return
-
- if isinstance(value, Fraction):
- self._numerator = value.numerator
- self._denominator = value.denominator
- else:
- self._numerator = value
- self._denominator = denominator
-
- if denominator == 0:
- self._val = float("nan")
- elif denominator == 1:
- self._val = Fraction(value)
- else:
- self._val = Fraction(value, denominator)
-
- @property
- def numerator(self):
- return self._numerator
-
- @property
- def denominator(self):
- return self._denominator
-
- def limit_rational(self, max_denominator):
- """
-
- :param max_denominator: Integer, the maximum denominator value
- :returns: Tuple of (numerator, denominator)
- """
-
- if self.denominator == 0:
- return self.numerator, self.denominator
-
- f = self._val.limit_denominator(max_denominator)
- return f.numerator, f.denominator
-
- def __repr__(self):
- return str(float(self._val))
-
- def __hash__(self):
- return self._val.__hash__()
-
- def __eq__(self, other):
- val = self._val
- if isinstance(other, IFDRational):
- other = other._val
- if isinstance(other, float):
- val = float(val)
- return val == other
-
- def __getstate__(self):
- return [self._val, self._numerator, self._denominator]
-
- def __setstate__(self, state):
- IFDRational.__init__(self, 0)
- _val, _numerator, _denominator = state
- self._val = _val
- self._numerator = _numerator
- self._denominator = _denominator
-
- def _delegate(op):
- def delegate(self, *args):
- return getattr(self._val, op)(*args)
-
- return delegate
-
- """ a = ['add','radd', 'sub', 'rsub', 'mul', 'rmul',
- 'truediv', 'rtruediv', 'floordiv', 'rfloordiv',
- 'mod','rmod', 'pow','rpow', 'pos', 'neg',
- 'abs', 'trunc', 'lt', 'gt', 'le', 'ge', 'bool',
- 'ceil', 'floor', 'round']
- print("\n".join("__%s__ = _delegate('__%s__')" % (s,s) for s in a))
- """
-
- __add__ = _delegate("__add__")
- __radd__ = _delegate("__radd__")
- __sub__ = _delegate("__sub__")
- __rsub__ = _delegate("__rsub__")
- __mul__ = _delegate("__mul__")
- __rmul__ = _delegate("__rmul__")
- __truediv__ = _delegate("__truediv__")
- __rtruediv__ = _delegate("__rtruediv__")
- __floordiv__ = _delegate("__floordiv__")
- __rfloordiv__ = _delegate("__rfloordiv__")
- __mod__ = _delegate("__mod__")
- __rmod__ = _delegate("__rmod__")
- __pow__ = _delegate("__pow__")
- __rpow__ = _delegate("__rpow__")
- __pos__ = _delegate("__pos__")
- __neg__ = _delegate("__neg__")
- __abs__ = _delegate("__abs__")
- __trunc__ = _delegate("__trunc__")
- __lt__ = _delegate("__lt__")
- __gt__ = _delegate("__gt__")
- __le__ = _delegate("__le__")
- __ge__ = _delegate("__ge__")
- __bool__ = _delegate("__bool__")
- __ceil__ = _delegate("__ceil__")
- __floor__ = _delegate("__floor__")
- __round__ = _delegate("__round__")
- # Python >= 3.11
- if hasattr(Fraction, "__int__"):
- __int__ = _delegate("__int__")
-
-
-class ImageFileDirectory_v2(MutableMapping):
- """This class represents a TIFF tag directory. To speed things up, we
- don't decode tags unless they're asked for.
-
- Exposes a dictionary interface of the tags in the directory::
-
- ifd = ImageFileDirectory_v2()
- ifd[key] = 'Some Data'
- ifd.tagtype[key] = TiffTags.ASCII
- print(ifd[key])
- 'Some Data'
-
- Individual values are returned as the strings or numbers, sequences are
- returned as tuples of the values.
-
- The tiff metadata type of each item is stored in a dictionary of
- tag types in
- :attr:`~PIL.TiffImagePlugin.ImageFileDirectory_v2.tagtype`. The types
- are read from a tiff file, guessed from the type added, or added
- manually.
-
- Data Structures:
-
- * ``self.tagtype = {}``
-
- * Key: numerical TIFF tag number
- * Value: integer corresponding to the data type from
- :py:data:`.TiffTags.TYPES`
-
- .. versionadded:: 3.0.0
-
- 'Internal' data structures:
-
- * ``self._tags_v2 = {}``
-
- * Key: numerical TIFF tag number
- * Value: decoded data, as tuple for multiple values
-
- * ``self._tagdata = {}``
-
- * Key: numerical TIFF tag number
- * Value: undecoded byte string from file
-
- * ``self._tags_v1 = {}``
-
- * Key: numerical TIFF tag number
- * Value: decoded data in the v1 format
-
- Tags will be found in the private attributes ``self._tagdata``, and in
- ``self._tags_v2`` once decoded.
-
- ``self.legacy_api`` is a value for internal use, and shouldn't be changed
- from outside code. In cooperation with
- :py:class:`~PIL.TiffImagePlugin.ImageFileDirectory_v1`, if ``legacy_api``
- is true, then decoded tags will be populated into both ``_tags_v1`` and
- ``_tags_v2``. ``_tags_v2`` will be used if this IFD is used in the TIFF
- save routine. Tags should be read from ``_tags_v1`` if
- ``legacy_api == true``.
-
- """
-
- def __init__(self, ifh=b"II\052\0\0\0\0\0", prefix=None, group=None):
- """Initialize an ImageFileDirectory.
-
- To construct an ImageFileDirectory from a real file, pass the 8-byte
- magic header to the constructor. To only set the endianness, pass it
- as the 'prefix' keyword argument.
-
- :param ifh: One of the accepted magic headers (cf. PREFIXES); also sets
- endianness.
- :param prefix: Override the endianness of the file.
- """
- if not _accept(ifh):
- msg = f"not a TIFF file (header {repr(ifh)} not valid)"
- raise SyntaxError(msg)
- self._prefix = prefix if prefix is not None else ifh[:2]
- if self._prefix == MM:
- self._endian = ">"
- elif self._prefix == II:
- self._endian = "<"
- else:
- msg = "not a TIFF IFD"
- raise SyntaxError(msg)
- self._bigtiff = ifh[2] == 43
- self.group = group
- self.tagtype = {}
- """ Dictionary of tag types """
- self.reset()
- (self.next,) = (
- self._unpack("Q", ifh[8:]) if self._bigtiff else self._unpack("L", ifh[4:])
- )
- self._legacy_api = False
-
- prefix = property(lambda self: self._prefix)
- offset = property(lambda self: self._offset)
- legacy_api = property(lambda self: self._legacy_api)
-
- @legacy_api.setter
- def legacy_api(self, value):
- msg = "Not allowing setting of legacy api"
- raise Exception(msg)
-
- def reset(self):
- self._tags_v1 = {} # will remain empty if legacy_api is false
- self._tags_v2 = {} # main tag storage
- self._tagdata = {}
- self.tagtype = {} # added 2008-06-05 by Florian Hoech
- self._next = None
- self._offset = None
-
- def __str__(self):
- return str(dict(self))
-
- def named(self):
- """
- :returns: dict of name|key: value
-
- Returns the complete tag dictionary, with named tags where possible.
- """
- return {
- TiffTags.lookup(code, self.group).name: value
- for code, value in self.items()
- }
-
- def __len__(self):
- return len(set(self._tagdata) | set(self._tags_v2))
-
- def __getitem__(self, tag):
- if tag not in self._tags_v2: # unpack on the fly
- data = self._tagdata[tag]
- typ = self.tagtype[tag]
- size, handler = self._load_dispatch[typ]
- self[tag] = handler(self, data, self.legacy_api) # check type
- val = self._tags_v2[tag]
- if self.legacy_api and not isinstance(val, (tuple, bytes)):
- val = (val,)
- return val
-
- def __contains__(self, tag):
- return tag in self._tags_v2 or tag in self._tagdata
-
- def __setitem__(self, tag, value):
- self._setitem(tag, value, self.legacy_api)
-
- def _setitem(self, tag, value, legacy_api):
- basetypes = (Number, bytes, str)
-
- info = TiffTags.lookup(tag, self.group)
- values = [value] if isinstance(value, basetypes) else value
-
- if tag not in self.tagtype:
- if info.type:
- self.tagtype[tag] = info.type
- else:
- self.tagtype[tag] = TiffTags.UNDEFINED
- if all(isinstance(v, IFDRational) for v in values):
- self.tagtype[tag] = (
- TiffTags.RATIONAL
- if all(v >= 0 for v in values)
- else TiffTags.SIGNED_RATIONAL
- )
- elif all(isinstance(v, int) for v in values):
- if all(0 <= v < 2**16 for v in values):
- self.tagtype[tag] = TiffTags.SHORT
- elif all(-(2**15) < v < 2**15 for v in values):
- self.tagtype[tag] = TiffTags.SIGNED_SHORT
- else:
- self.tagtype[tag] = (
- TiffTags.LONG
- if all(v >= 0 for v in values)
- else TiffTags.SIGNED_LONG
- )
- elif all(isinstance(v, float) for v in values):
- self.tagtype[tag] = TiffTags.DOUBLE
- elif all(isinstance(v, str) for v in values):
- self.tagtype[tag] = TiffTags.ASCII
- elif all(isinstance(v, bytes) for v in values):
- self.tagtype[tag] = TiffTags.BYTE
-
- if self.tagtype[tag] == TiffTags.UNDEFINED:
- values = [
- v.encode("ascii", "replace") if isinstance(v, str) else v
- for v in values
- ]
- elif self.tagtype[tag] == TiffTags.RATIONAL:
- values = [float(v) if isinstance(v, int) else v for v in values]
-
- is_ifd = self.tagtype[tag] == TiffTags.LONG and isinstance(values, dict)
- if not is_ifd:
- values = tuple(info.cvt_enum(value) for value in values)
-
- dest = self._tags_v1 if legacy_api else self._tags_v2
-
- # Three branches:
- # Spec'd length == 1, Actual length 1, store as element
- # Spec'd length == 1, Actual > 1, Warn and truncate. Formerly barfed.
- # No Spec, Actual length 1, Formerly (<4.2) returned a 1 element tuple.
- # Don't mess with the legacy api, since it's frozen.
- if not is_ifd and (
- (info.length == 1)
- or self.tagtype[tag] == TiffTags.BYTE
- or (info.length is None and len(values) == 1 and not legacy_api)
- ):
- # Don't mess with the legacy api, since it's frozen.
- if legacy_api and self.tagtype[tag] in [
- TiffTags.RATIONAL,
- TiffTags.SIGNED_RATIONAL,
- ]: # rationals
- values = (values,)
- try:
- (dest[tag],) = values
- except ValueError:
- # We've got a builtin tag with 1 expected entry
- warnings.warn(
- f"Metadata Warning, tag {tag} had too many entries: "
- f"{len(values)}, expected 1"
- )
- dest[tag] = values[0]
-
- else:
- # Spec'd length > 1 or undefined
- # Unspec'd, and length > 1
- dest[tag] = values
-
- def __delitem__(self, tag):
- self._tags_v2.pop(tag, None)
- self._tags_v1.pop(tag, None)
- self._tagdata.pop(tag, None)
-
- def __iter__(self):
- return iter(set(self._tagdata) | set(self._tags_v2))
-
- def _unpack(self, fmt, data):
- return struct.unpack(self._endian + fmt, data)
-
- def _pack(self, fmt, *values):
- return struct.pack(self._endian + fmt, *values)
-
- def _register_loader(idx, size):
- def decorator(func):
- from .TiffTags import TYPES
-
- if func.__name__.startswith("load_"):
- TYPES[idx] = func.__name__[5:].replace("_", " ")
- _load_dispatch[idx] = size, func # noqa: F821
- return func
-
- return decorator
-
- def _register_writer(idx):
- def decorator(func):
- _write_dispatch[idx] = func # noqa: F821
- return func
-
- return decorator
-
- def _register_basic(idx_fmt_name):
- from .TiffTags import TYPES
-
- idx, fmt, name = idx_fmt_name
- TYPES[idx] = name
- size = struct.calcsize("=" + fmt)
- _load_dispatch[idx] = ( # noqa: F821
- size,
- lambda self, data, legacy_api=True: (
- self._unpack(f"{len(data) // size}{fmt}", data)
- ),
- )
- _write_dispatch[idx] = lambda self, *values: ( # noqa: F821
- b"".join(self._pack(fmt, value) for value in values)
- )
-
- list(
- map(
- _register_basic,
- [
- (TiffTags.SHORT, "H", "short"),
- (TiffTags.LONG, "L", "long"),
- (TiffTags.SIGNED_BYTE, "b", "signed byte"),
- (TiffTags.SIGNED_SHORT, "h", "signed short"),
- (TiffTags.SIGNED_LONG, "l", "signed long"),
- (TiffTags.FLOAT, "f", "float"),
- (TiffTags.DOUBLE, "d", "double"),
- (TiffTags.IFD, "L", "long"),
- (TiffTags.LONG8, "Q", "long8"),
- ],
- )
- )
-
- @_register_loader(1, 1) # Basic type, except for the legacy API.
- def load_byte(self, data, legacy_api=True):
- return data
-
- @_register_writer(1) # Basic type, except for the legacy API.
- def write_byte(self, data):
- if isinstance(data, IFDRational):
- data = int(data)
- if isinstance(data, int):
- data = bytes((data,))
- return data
-
- @_register_loader(2, 1)
- def load_string(self, data, legacy_api=True):
- if data.endswith(b"\0"):
- data = data[:-1]
- return data.decode("latin-1", "replace")
-
- @_register_writer(2)
- def write_string(self, value):
- # remerge of https://github.com/python-pillow/Pillow/pull/1416
- if isinstance(value, int):
- value = str(value)
- if not isinstance(value, bytes):
- value = value.encode("ascii", "replace")
- return value + b"\0"
-
- @_register_loader(5, 8)
- def load_rational(self, data, legacy_api=True):
- vals = self._unpack(f"{len(data) // 4}L", data)
-
- def combine(a, b):
- return (a, b) if legacy_api else IFDRational(a, b)
-
- return tuple(combine(num, denom) for num, denom in zip(vals[::2], vals[1::2]))
-
- @_register_writer(5)
- def write_rational(self, *values):
- return b"".join(
- self._pack("2L", *_limit_rational(frac, 2**32 - 1)) for frac in values
- )
-
- @_register_loader(7, 1)
- def load_undefined(self, data, legacy_api=True):
- return data
-
- @_register_writer(7)
- def write_undefined(self, value):
- if isinstance(value, int):
- value = str(value).encode("ascii", "replace")
- return value
-
- @_register_loader(10, 8)
- def load_signed_rational(self, data, legacy_api=True):
- vals = self._unpack(f"{len(data) // 4}l", data)
-
- def combine(a, b):
- return (a, b) if legacy_api else IFDRational(a, b)
-
- return tuple(combine(num, denom) for num, denom in zip(vals[::2], vals[1::2]))
-
- @_register_writer(10)
- def write_signed_rational(self, *values):
- return b"".join(
- self._pack("2l", *_limit_signed_rational(frac, 2**31 - 1, -(2**31)))
- for frac in values
- )
-
- def _ensure_read(self, fp, size):
- ret = fp.read(size)
- if len(ret) != size:
- msg = (
- "Corrupt EXIF data. "
- f"Expecting to read {size} bytes but only got {len(ret)}. "
- )
- raise OSError(msg)
- return ret
-
- def load(self, fp):
- self.reset()
- self._offset = fp.tell()
-
- try:
- tag_count = (
- self._unpack("Q", self._ensure_read(fp, 8))
- if self._bigtiff
- else self._unpack("H", self._ensure_read(fp, 2))
- )[0]
- for i in range(tag_count):
- tag, typ, count, data = (
- self._unpack("HHQ8s", self._ensure_read(fp, 20))
- if self._bigtiff
- else self._unpack("HHL4s", self._ensure_read(fp, 12))
- )
-
- tagname = TiffTags.lookup(tag, self.group).name
- typname = TYPES.get(typ, "unknown")
- msg = f"tag: {tagname} ({tag}) - type: {typname} ({typ})"
-
- try:
- unit_size, handler = self._load_dispatch[typ]
- except KeyError:
- logger.debug(msg + f" - unsupported type {typ}")
- continue # ignore unsupported type
- size = count * unit_size
- if size > (8 if self._bigtiff else 4):
- here = fp.tell()
- (offset,) = self._unpack("Q" if self._bigtiff else "L", data)
- msg += f" Tag Location: {here} - Data Location: {offset}"
- fp.seek(offset)
- data = ImageFile._safe_read(fp, size)
- fp.seek(here)
- else:
- data = data[:size]
-
- if len(data) != size:
- warnings.warn(
- "Possibly corrupt EXIF data. "
- f"Expecting to read {size} bytes but only got {len(data)}."
- f" Skipping tag {tag}"
- )
- logger.debug(msg)
- continue
-
- if not data:
- logger.debug(msg)
- continue
-
- self._tagdata[tag] = data
- self.tagtype[tag] = typ
-
- msg += " - value: " + (
- "" % size if size > 32 else repr(data)
- )
- logger.debug(msg)
-
- (self.next,) = (
- self._unpack("Q", self._ensure_read(fp, 8))
- if self._bigtiff
- else self._unpack("L", self._ensure_read(fp, 4))
- )
- except OSError as msg:
- warnings.warn(str(msg))
- return
-
- def tobytes(self, offset=0):
- # FIXME What about tagdata?
- result = self._pack("H", len(self._tags_v2))
-
- entries = []
- offset = offset + len(result) + len(self._tags_v2) * 12 + 4
- stripoffsets = None
-
- # pass 1: convert tags to binary format
- # always write tags in ascending order
- for tag, value in sorted(self._tags_v2.items()):
- if tag == STRIPOFFSETS:
- stripoffsets = len(entries)
- typ = self.tagtype.get(tag)
- logger.debug(f"Tag {tag}, Type: {typ}, Value: {repr(value)}")
- is_ifd = typ == TiffTags.LONG and isinstance(value, dict)
- if is_ifd:
- if self._endian == "<":
- ifh = b"II\x2A\x00\x08\x00\x00\x00"
- else:
- ifh = b"MM\x00\x2A\x00\x00\x00\x08"
- ifd = ImageFileDirectory_v2(ifh, group=tag)
- values = self._tags_v2[tag]
- for ifd_tag, ifd_value in values.items():
- ifd[ifd_tag] = ifd_value
- data = ifd.tobytes(offset)
- else:
- values = value if isinstance(value, tuple) else (value,)
- data = self._write_dispatch[typ](self, *values)
-
- tagname = TiffTags.lookup(tag, self.group).name
- typname = "ifd" if is_ifd else TYPES.get(typ, "unknown")
- msg = f"save: {tagname} ({tag}) - type: {typname} ({typ})"
- msg += " - value: " + (
- "" % len(data) if len(data) >= 16 else str(values)
- )
- logger.debug(msg)
-
- # count is sum of lengths for string and arbitrary data
- if is_ifd:
- count = 1
- elif typ in [TiffTags.BYTE, TiffTags.ASCII, TiffTags.UNDEFINED]:
- count = len(data)
- else:
- count = len(values)
- # figure out if data fits into the entry
- if len(data) <= 4:
- entries.append((tag, typ, count, data.ljust(4, b"\0"), b""))
- else:
- entries.append((tag, typ, count, self._pack("L", offset), data))
- offset += (len(data) + 1) // 2 * 2 # pad to word
-
- # update strip offset data to point beyond auxiliary data
- if stripoffsets is not None:
- tag, typ, count, value, data = entries[stripoffsets]
- if data:
- msg = "multistrip support not yet implemented"
- raise NotImplementedError(msg)
- value = self._pack("L", self._unpack("L", value)[0] + offset)
- entries[stripoffsets] = tag, typ, count, value, data
-
- # pass 2: write entries to file
- for tag, typ, count, value, data in entries:
- logger.debug(f"{tag} {typ} {count} {repr(value)} {repr(data)}")
- result += self._pack("HHL4s", tag, typ, count, value)
-
- # -- overwrite here for multi-page --
- result += b"\0\0\0\0" # end of entries
-
- # pass 3: write auxiliary data to file
- for tag, typ, count, value, data in entries:
- result += data
- if len(data) & 1:
- result += b"\0"
-
- return result
-
- def save(self, fp):
- if fp.tell() == 0: # skip TIFF header on subsequent pages
- # tiff header -- PIL always starts the first IFD at offset 8
- fp.write(self._prefix + self._pack("HL", 42, 8))
-
- offset = fp.tell()
- result = self.tobytes(offset)
- fp.write(result)
- return offset + len(result)
-
-
-ImageFileDirectory_v2._load_dispatch = _load_dispatch
-ImageFileDirectory_v2._write_dispatch = _write_dispatch
-for idx, name in TYPES.items():
- name = name.replace(" ", "_")
- setattr(ImageFileDirectory_v2, "load_" + name, _load_dispatch[idx][1])
- setattr(ImageFileDirectory_v2, "write_" + name, _write_dispatch[idx])
-del _load_dispatch, _write_dispatch, idx, name
-
-
-# Legacy ImageFileDirectory support.
-class ImageFileDirectory_v1(ImageFileDirectory_v2):
- """This class represents the **legacy** interface to a TIFF tag directory.
-
- Exposes a dictionary interface of the tags in the directory::
-
- ifd = ImageFileDirectory_v1()
- ifd[key] = 'Some Data'
- ifd.tagtype[key] = TiffTags.ASCII
- print(ifd[key])
- ('Some Data',)
-
- Also contains a dictionary of tag types as read from the tiff image file,
- :attr:`~PIL.TiffImagePlugin.ImageFileDirectory_v1.tagtype`.
-
- Values are returned as a tuple.
-
- .. deprecated:: 3.0.0
- """
-
- def __init__(self, *args, **kwargs):
- super().__init__(*args, **kwargs)
- self._legacy_api = True
-
- tags = property(lambda self: self._tags_v1)
- tagdata = property(lambda self: self._tagdata)
-
- # defined in ImageFileDirectory_v2
- tagtype: dict
- """Dictionary of tag types"""
-
- @classmethod
- def from_v2(cls, original):
- """Returns an
- :py:class:`~PIL.TiffImagePlugin.ImageFileDirectory_v1`
- instance with the same data as is contained in the original
- :py:class:`~PIL.TiffImagePlugin.ImageFileDirectory_v2`
- instance.
-
- :returns: :py:class:`~PIL.TiffImagePlugin.ImageFileDirectory_v1`
-
- """
-
- ifd = cls(prefix=original.prefix)
- ifd._tagdata = original._tagdata
- ifd.tagtype = original.tagtype
- ifd.next = original.next # an indicator for multipage tiffs
- return ifd
-
- def to_v2(self):
- """Returns an
- :py:class:`~PIL.TiffImagePlugin.ImageFileDirectory_v2`
- instance with the same data as is contained in the original
- :py:class:`~PIL.TiffImagePlugin.ImageFileDirectory_v1`
- instance.
-
- :returns: :py:class:`~PIL.TiffImagePlugin.ImageFileDirectory_v2`
-
- """
-
- ifd = ImageFileDirectory_v2(prefix=self.prefix)
- ifd._tagdata = dict(self._tagdata)
- ifd.tagtype = dict(self.tagtype)
- ifd._tags_v2 = dict(self._tags_v2)
- return ifd
-
- def __contains__(self, tag):
- return tag in self._tags_v1 or tag in self._tagdata
-
- def __len__(self):
- return len(set(self._tagdata) | set(self._tags_v1))
-
- def __iter__(self):
- return iter(set(self._tagdata) | set(self._tags_v1))
-
- def __setitem__(self, tag, value):
- for legacy_api in (False, True):
- self._setitem(tag, value, legacy_api)
-
- def __getitem__(self, tag):
- if tag not in self._tags_v1: # unpack on the fly
- data = self._tagdata[tag]
- typ = self.tagtype[tag]
- size, handler = self._load_dispatch[typ]
- for legacy in (False, True):
- self._setitem(tag, handler(self, data, legacy), legacy)
- val = self._tags_v1[tag]
- if not isinstance(val, (tuple, bytes)):
- val = (val,)
- return val
-
-
-# undone -- switch this pointer when IFD_LEGACY_API == False
-ImageFileDirectory = ImageFileDirectory_v1
-
-
-##
-# Image plugin for TIFF files.
-
-
-class TiffImageFile(ImageFile.ImageFile):
- format = "TIFF"
- format_description = "Adobe TIFF"
- _close_exclusive_fp_after_loading = False
-
- def __init__(self, fp=None, filename=None):
- self.tag_v2 = None
- """ Image file directory (tag dictionary) """
-
- self.tag = None
- """ Legacy tag entries """
-
- super().__init__(fp, filename)
-
- def _open(self):
- """Open the first image in a TIFF file"""
-
- # Header
- ifh = self.fp.read(8)
- if ifh[2] == 43:
- ifh += self.fp.read(8)
-
- self.tag_v2 = ImageFileDirectory_v2(ifh)
-
- # legacy IFD entries will be filled in later
- self.ifd = None
-
- # setup frame pointers
- self.__first = self.__next = self.tag_v2.next
- self.__frame = -1
- self._fp = self.fp
- self._frame_pos = []
- self._n_frames = None
-
- logger.debug("*** TiffImageFile._open ***")
- logger.debug(f"- __first: {self.__first}")
- logger.debug(f"- ifh: {repr(ifh)}") # Use repr to avoid str(bytes)
-
- # and load the first frame
- self._seek(0)
-
- @property
- def n_frames(self):
- if self._n_frames is None:
- current = self.tell()
- self._seek(len(self._frame_pos))
- while self._n_frames is None:
- self._seek(self.tell() + 1)
- self.seek(current)
- return self._n_frames
-
- def seek(self, frame):
- """Select a given frame as current image"""
- if not self._seek_check(frame):
- return
- self._seek(frame)
- # Create a new core image object on second and
- # subsequent frames in the image. Image may be
- # different size/mode.
- Image._decompression_bomb_check(self.size)
- self.im = Image.core.new(self.mode, self.size)
-
- def _seek(self, frame):
- self.fp = self._fp
-
- # reset buffered io handle in case fp
- # was passed to libtiff, invalidating the buffer
- self.fp.tell()
-
- while len(self._frame_pos) <= frame:
- if not self.__next:
- msg = "no more images in TIFF file"
- raise EOFError(msg)
- logger.debug(
- f"Seeking to frame {frame}, on frame {self.__frame}, "
- f"__next {self.__next}, location: {self.fp.tell()}"
- )
- self.fp.seek(self.__next)
- self._frame_pos.append(self.__next)
- logger.debug("Loading tags, location: %s" % self.fp.tell())
- self.tag_v2.load(self.fp)
- if self.tag_v2.next in self._frame_pos:
- # This IFD has already been processed
- # Declare this to be the end of the image
- self.__next = 0
- else:
- self.__next = self.tag_v2.next
- if self.__next == 0:
- self._n_frames = frame + 1
- if len(self._frame_pos) == 1:
- self.is_animated = self.__next != 0
- self.__frame += 1
- self.fp.seek(self._frame_pos[frame])
- self.tag_v2.load(self.fp)
- self._reload_exif()
- # fill the legacy tag/ifd entries
- self.tag = self.ifd = ImageFileDirectory_v1.from_v2(self.tag_v2)
- self.__frame = frame
- self._setup()
-
- def tell(self):
- """Return the current frame number"""
- return self.__frame
-
- def getxmp(self):
- """
- Returns a dictionary containing the XMP tags.
- Requires defusedxml to be installed.
-
- :returns: XMP tags in a dictionary.
- """
- return self._getxmp(self.tag_v2[XMP]) if XMP in self.tag_v2 else {}
-
- def get_photoshop_blocks(self):
- """
- Returns a dictionary of Photoshop "Image Resource Blocks".
- The keys are the image resource ID. For more information, see
- https://www.adobe.com/devnet-apps/photoshop/fileformatashtml/#50577409_pgfId-1037727
-
- :returns: Photoshop "Image Resource Blocks" in a dictionary.
- """
- blocks = {}
- val = self.tag_v2.get(ExifTags.Base.ImageResources)
- if val:
- while val[:4] == b"8BIM":
- id = i16(val[4:6])
- n = math.ceil((val[6] + 1) / 2) * 2
- size = i32(val[6 + n : 10 + n])
- data = val[10 + n : 10 + n + size]
- blocks[id] = {"data": data}
-
- val = val[math.ceil((10 + n + size) / 2) * 2 :]
- return blocks
-
- def load(self):
- if self.tile and self.use_load_libtiff:
- return self._load_libtiff()
- return super().load()
-
- def load_end(self):
- if self._tile_orientation:
- method = {
- 2: Image.Transpose.FLIP_LEFT_RIGHT,
- 3: Image.Transpose.ROTATE_180,
- 4: Image.Transpose.FLIP_TOP_BOTTOM,
- 5: Image.Transpose.TRANSPOSE,
- 6: Image.Transpose.ROTATE_270,
- 7: Image.Transpose.TRANSVERSE,
- 8: Image.Transpose.ROTATE_90,
- }.get(self._tile_orientation)
- if method is not None:
- self.im = self.im.transpose(method)
- self._size = self.im.size
-
- # allow closing if we're on the first frame, there's no next
- # This is the ImageFile.load path only, libtiff specific below.
- if not self.is_animated:
- self._close_exclusive_fp_after_loading = True
-
- # reset buffered io handle in case fp
- # was passed to libtiff, invalidating the buffer
- self.fp.tell()
-
- # load IFD data from fp before it is closed
- exif = self.getexif()
- for key in TiffTags.TAGS_V2_GROUPS:
- if key not in exif:
- continue
- exif.get_ifd(key)
-
- def _load_libtiff(self):
- """Overload method triggered when we detect a compressed tiff
- Calls out to libtiff"""
-
- Image.Image.load(self)
-
- self.load_prepare()
-
- if not len(self.tile) == 1:
- msg = "Not exactly one tile"
- raise OSError(msg)
-
- # (self._compression, (extents tuple),
- # 0, (rawmode, self._compression, fp))
- extents = self.tile[0][1]
- args = list(self.tile[0][3])
-
- # To be nice on memory footprint, if there's a
- # file descriptor, use that instead of reading
- # into a string in python.
- try:
- fp = hasattr(self.fp, "fileno") and self.fp.fileno()
- # flush the file descriptor, prevents error on pypy 2.4+
- # should also eliminate the need for fp.tell
- # in _seek
- if hasattr(self.fp, "flush"):
- self.fp.flush()
- except OSError:
- # io.BytesIO have a fileno, but returns an OSError if
- # it doesn't use a file descriptor.
- fp = False
-
- if fp:
- args[2] = fp
-
- decoder = Image._getdecoder(
- self.mode, "libtiff", tuple(args), self.decoderconfig
- )
- try:
- decoder.setimage(self.im, extents)
- except ValueError as e:
- msg = "Couldn't set the image"
- raise OSError(msg) from e
-
- close_self_fp = self._exclusive_fp and not self.is_animated
- if hasattr(self.fp, "getvalue"):
- # We've got a stringio like thing passed in. Yay for all in memory.
- # The decoder needs the entire file in one shot, so there's not
- # a lot we can do here other than give it the entire file.
- # unless we could do something like get the address of the
- # underlying string for stringio.
- #
- # Rearranging for supporting byteio items, since they have a fileno
- # that returns an OSError if there's no underlying fp. Easier to
- # deal with here by reordering.
- logger.debug("have getvalue. just sending in a string from getvalue")
- n, err = decoder.decode(self.fp.getvalue())
- elif fp:
- # we've got a actual file on disk, pass in the fp.
- logger.debug("have fileno, calling fileno version of the decoder.")
- if not close_self_fp:
- self.fp.seek(0)
- # 4 bytes, otherwise the trace might error out
- n, err = decoder.decode(b"fpfp")
- else:
- # we have something else.
- logger.debug("don't have fileno or getvalue. just reading")
- self.fp.seek(0)
- # UNDONE -- so much for that buffer size thing.
- n, err = decoder.decode(self.fp.read())
-
- self.tile = []
- self.readonly = 0
-
- self.load_end()
-
- if close_self_fp:
- self.fp.close()
- self.fp = None # might be shared
-
- if err < 0:
- raise OSError(err)
-
- return Image.Image.load(self)
-
- def _setup(self):
- """Setup this image object based on current tags"""
-
- if 0xBC01 in self.tag_v2:
- msg = "Windows Media Photo files not yet supported"
- raise OSError(msg)
-
- # extract relevant tags
- self._compression = COMPRESSION_INFO[self.tag_v2.get(COMPRESSION, 1)]
- self._planar_configuration = self.tag_v2.get(PLANAR_CONFIGURATION, 1)
-
- # photometric is a required tag, but not everyone is reading
- # the specification
- photo = self.tag_v2.get(PHOTOMETRIC_INTERPRETATION, 0)
-
- # old style jpeg compression images most certainly are YCbCr
- if self._compression == "tiff_jpeg":
- photo = 6
-
- fillorder = self.tag_v2.get(FILLORDER, 1)
-
- logger.debug("*** Summary ***")
- logger.debug(f"- compression: {self._compression}")
- logger.debug(f"- photometric_interpretation: {photo}")
- logger.debug(f"- planar_configuration: {self._planar_configuration}")
- logger.debug(f"- fill_order: {fillorder}")
- logger.debug(f"- YCbCr subsampling: {self.tag.get(YCBCRSUBSAMPLING)}")
-
- # size
- xsize = int(self.tag_v2.get(IMAGEWIDTH))
- ysize = int(self.tag_v2.get(IMAGELENGTH))
- self._size = xsize, ysize
-
- logger.debug(f"- size: {self.size}")
-
- sample_format = self.tag_v2.get(SAMPLEFORMAT, (1,))
- if len(sample_format) > 1 and max(sample_format) == min(sample_format) == 1:
- # SAMPLEFORMAT is properly per band, so an RGB image will
- # be (1,1,1). But, we don't support per band pixel types,
- # and anything more than one band is a uint8. So, just
- # take the first element. Revisit this if adding support
- # for more exotic images.
- sample_format = (1,)
-
- bps_tuple = self.tag_v2.get(BITSPERSAMPLE, (1,))
- extra_tuple = self.tag_v2.get(EXTRASAMPLES, ())
- if photo in (2, 6, 8): # RGB, YCbCr, LAB
- bps_count = 3
- elif photo == 5: # CMYK
- bps_count = 4
- else:
- bps_count = 1
- bps_count += len(extra_tuple)
- bps_actual_count = len(bps_tuple)
- samples_per_pixel = self.tag_v2.get(
- SAMPLESPERPIXEL,
- 3 if self._compression == "tiff_jpeg" and photo in (2, 6) else 1,
- )
-
- if samples_per_pixel > MAX_SAMPLESPERPIXEL:
- # DOS check, samples_per_pixel can be a Long, and we extend the tuple below
- logger.error(
- "More samples per pixel than can be decoded: %s", samples_per_pixel
- )
- msg = "Invalid value for samples per pixel"
- raise SyntaxError(msg)
-
- if samples_per_pixel < bps_actual_count:
- # If a file has more values in bps_tuple than expected,
- # remove the excess.
- bps_tuple = bps_tuple[:samples_per_pixel]
- elif samples_per_pixel > bps_actual_count and bps_actual_count == 1:
- # If a file has only one value in bps_tuple, when it should have more,
- # presume it is the same number of bits for all of the samples.
- bps_tuple = bps_tuple * samples_per_pixel
-
- if len(bps_tuple) != samples_per_pixel:
- msg = "unknown data organization"
- raise SyntaxError(msg)
-
- # mode: check photometric interpretation and bits per pixel
- key = (
- self.tag_v2.prefix,
- photo,
- sample_format,
- fillorder,
- bps_tuple,
- extra_tuple,
- )
- logger.debug(f"format key: {key}")
- try:
- self.mode, rawmode = OPEN_INFO[key]
- except KeyError as e:
- logger.debug("- unsupported format")
- msg = "unknown pixel mode"
- raise SyntaxError(msg) from e
-
- logger.debug(f"- raw mode: {rawmode}")
- logger.debug(f"- pil mode: {self.mode}")
-
- self.info["compression"] = self._compression
-
- xres = self.tag_v2.get(X_RESOLUTION, 1)
- yres = self.tag_v2.get(Y_RESOLUTION, 1)
-
- if xres and yres:
- resunit = self.tag_v2.get(RESOLUTION_UNIT)
- if resunit == 2: # dots per inch
- self.info["dpi"] = (xres, yres)
- elif resunit == 3: # dots per centimeter. convert to dpi
- self.info["dpi"] = (xres * 2.54, yres * 2.54)
- elif resunit is None: # used to default to 1, but now 2)
- self.info["dpi"] = (xres, yres)
- # For backward compatibility,
- # we also preserve the old behavior
- self.info["resolution"] = xres, yres
- else: # No absolute unit of measurement
- self.info["resolution"] = xres, yres
-
- # build tile descriptors
- x = y = layer = 0
- self.tile = []
- self.use_load_libtiff = READ_LIBTIFF or self._compression != "raw"
- if self.use_load_libtiff:
- # Decoder expects entire file as one tile.
- # There's a buffer size limit in load (64k)
- # so large g4 images will fail if we use that
- # function.
- #
- # Setup the one tile for the whole image, then
- # use the _load_libtiff function.
-
- # libtiff handles the fillmode for us, so 1;IR should
- # actually be 1;I. Including the R double reverses the
- # bits, so stripes of the image are reversed. See
- # https://github.com/python-pillow/Pillow/issues/279
- if fillorder == 2:
- # Replace fillorder with fillorder=1
- key = key[:3] + (1,) + key[4:]
- logger.debug(f"format key: {key}")
- # this should always work, since all the
- # fillorder==2 modes have a corresponding
- # fillorder=1 mode
- self.mode, rawmode = OPEN_INFO[key]
- # libtiff always returns the bytes in native order.
- # we're expecting image byte order. So, if the rawmode
- # contains I;16, we need to convert from native to image
- # byte order.
- if rawmode == "I;16":
- rawmode = "I;16N"
- if ";16B" in rawmode:
- rawmode = rawmode.replace(";16B", ";16N")
- if ";16L" in rawmode:
- rawmode = rawmode.replace(";16L", ";16N")
-
- # YCbCr images with new jpeg compression with pixels in one plane
- # unpacked straight into RGB values
- if (
- photo == 6
- and self._compression == "jpeg"
- and self._planar_configuration == 1
- ):
- rawmode = "RGB"
-
- # Offset in the tile tuple is 0, we go from 0,0 to
- # w,h, and we only do this once -- eds
- a = (rawmode, self._compression, False, self.tag_v2.offset)
- self.tile.append(("libtiff", (0, 0, xsize, ysize), 0, a))
-
- elif STRIPOFFSETS in self.tag_v2 or TILEOFFSETS in self.tag_v2:
- # striped image
- if STRIPOFFSETS in self.tag_v2:
- offsets = self.tag_v2[STRIPOFFSETS]
- h = self.tag_v2.get(ROWSPERSTRIP, ysize)
- w = self.size[0]
- else:
- # tiled image
- offsets = self.tag_v2[TILEOFFSETS]
- w = self.tag_v2.get(TILEWIDTH)
- h = self.tag_v2.get(TILELENGTH)
-
- for offset in offsets:
- if x + w > xsize:
- stride = w * sum(bps_tuple) / 8 # bytes per line
- else:
- stride = 0
-
- tile_rawmode = rawmode
- if self._planar_configuration == 2:
- # each band on it's own layer
- tile_rawmode = rawmode[layer]
- # adjust stride width accordingly
- stride /= bps_count
-
- a = (tile_rawmode, int(stride), 1)
- self.tile.append(
- (
- self._compression,
- (x, y, min(x + w, xsize), min(y + h, ysize)),
- offset,
- a,
- )
- )
- x = x + w
- if x >= self.size[0]:
- x, y = 0, y + h
- if y >= self.size[1]:
- x = y = 0
- layer += 1
- else:
- logger.debug("- unsupported data organization")
- msg = "unknown data organization"
- raise SyntaxError(msg)
-
- # Fix up info.
- if ICCPROFILE in self.tag_v2:
- self.info["icc_profile"] = self.tag_v2[ICCPROFILE]
-
- # fixup palette descriptor
-
- if self.mode in ["P", "PA"]:
- palette = [o8(b // 256) for b in self.tag_v2[COLORMAP]]
- self.palette = ImagePalette.raw("RGB;L", b"".join(palette))
-
- self._tile_orientation = self.tag_v2.get(ExifTags.Base.Orientation)
-
-
-#
-# --------------------------------------------------------------------
-# Write TIFF files
-
-# little endian is default except for image modes with
-# explicit big endian byte-order
-
-SAVE_INFO = {
- # mode => rawmode, byteorder, photometrics,
- # sampleformat, bitspersample, extra
- "1": ("1", II, 1, 1, (1,), None),
- "L": ("L", II, 1, 1, (8,), None),
- "LA": ("LA", II, 1, 1, (8, 8), 2),
- "P": ("P", II, 3, 1, (8,), None),
- "PA": ("PA", II, 3, 1, (8, 8), 2),
- "I": ("I;32S", II, 1, 2, (32,), None),
- "I;16": ("I;16", II, 1, 1, (16,), None),
- "I;16S": ("I;16S", II, 1, 2, (16,), None),
- "F": ("F;32F", II, 1, 3, (32,), None),
- "RGB": ("RGB", II, 2, 1, (8, 8, 8), None),
- "RGBX": ("RGBX", II, 2, 1, (8, 8, 8, 8), 0),
- "RGBA": ("RGBA", II, 2, 1, (8, 8, 8, 8), 2),
- "CMYK": ("CMYK", II, 5, 1, (8, 8, 8, 8), None),
- "YCbCr": ("YCbCr", II, 6, 1, (8, 8, 8), None),
- "LAB": ("LAB", II, 8, 1, (8, 8, 8), None),
- "I;32BS": ("I;32BS", MM, 1, 2, (32,), None),
- "I;16B": ("I;16B", MM, 1, 1, (16,), None),
- "I;16BS": ("I;16BS", MM, 1, 2, (16,), None),
- "F;32BF": ("F;32BF", MM, 1, 3, (32,), None),
-}
-
-
-def _save(im, fp, filename):
- try:
- rawmode, prefix, photo, format, bits, extra = SAVE_INFO[im.mode]
- except KeyError as e:
- msg = f"cannot write mode {im.mode} as TIFF"
- raise OSError(msg) from e
-
- ifd = ImageFileDirectory_v2(prefix=prefix)
-
- encoderinfo = im.encoderinfo
- encoderconfig = im.encoderconfig
- try:
- compression = encoderinfo["compression"]
- except KeyError:
- compression = im.info.get("compression")
- if isinstance(compression, int):
- # compression value may be from BMP. Ignore it
- compression = None
- if compression is None:
- compression = "raw"
- elif compression == "tiff_jpeg":
- # OJPEG is obsolete, so use new-style JPEG compression instead
- compression = "jpeg"
- elif compression == "tiff_deflate":
- compression = "tiff_adobe_deflate"
-
- libtiff = WRITE_LIBTIFF or compression != "raw"
-
- # required for color libtiff images
- ifd[PLANAR_CONFIGURATION] = 1
-
- ifd[IMAGEWIDTH] = im.size[0]
- ifd[IMAGELENGTH] = im.size[1]
-
- # write any arbitrary tags passed in as an ImageFileDirectory
- if "tiffinfo" in encoderinfo:
- info = encoderinfo["tiffinfo"]
- elif "exif" in encoderinfo:
- info = encoderinfo["exif"]
- if isinstance(info, bytes):
- exif = Image.Exif()
- exif.load(info)
- info = exif
- else:
- info = {}
- logger.debug("Tiffinfo Keys: %s" % list(info))
- if isinstance(info, ImageFileDirectory_v1):
- info = info.to_v2()
- for key in info:
- if isinstance(info, Image.Exif) and key in TiffTags.TAGS_V2_GROUPS:
- ifd[key] = info.get_ifd(key)
- else:
- ifd[key] = info.get(key)
- try:
- ifd.tagtype[key] = info.tagtype[key]
- except Exception:
- pass # might not be an IFD. Might not have populated type
-
- # additions written by Greg Couch, gregc@cgl.ucsf.edu
- # inspired by image-sig posting from Kevin Cazabon, kcazabon@home.com
- if hasattr(im, "tag_v2"):
- # preserve tags from original TIFF image file
- for key in (
- RESOLUTION_UNIT,
- X_RESOLUTION,
- Y_RESOLUTION,
- IPTC_NAA_CHUNK,
- PHOTOSHOP_CHUNK,
- XMP,
- ):
- if key in im.tag_v2:
- ifd[key] = im.tag_v2[key]
- ifd.tagtype[key] = im.tag_v2.tagtype[key]
-
- # preserve ICC profile (should also work when saving other formats
- # which support profiles as TIFF) -- 2008-06-06 Florian Hoech
- icc = encoderinfo.get("icc_profile", im.info.get("icc_profile"))
- if icc:
- ifd[ICCPROFILE] = icc
-
- for key, name in [
- (IMAGEDESCRIPTION, "description"),
- (X_RESOLUTION, "resolution"),
- (Y_RESOLUTION, "resolution"),
- (X_RESOLUTION, "x_resolution"),
- (Y_RESOLUTION, "y_resolution"),
- (RESOLUTION_UNIT, "resolution_unit"),
- (SOFTWARE, "software"),
- (DATE_TIME, "date_time"),
- (ARTIST, "artist"),
- (COPYRIGHT, "copyright"),
- ]:
- if name in encoderinfo:
- ifd[key] = encoderinfo[name]
-
- dpi = encoderinfo.get("dpi")
- if dpi:
- ifd[RESOLUTION_UNIT] = 2
- ifd[X_RESOLUTION] = dpi[0]
- ifd[Y_RESOLUTION] = dpi[1]
-
- if bits != (1,):
- ifd[BITSPERSAMPLE] = bits
- if len(bits) != 1:
- ifd[SAMPLESPERPIXEL] = len(bits)
- if extra is not None:
- ifd[EXTRASAMPLES] = extra
- if format != 1:
- ifd[SAMPLEFORMAT] = format
-
- if PHOTOMETRIC_INTERPRETATION not in ifd:
- ifd[PHOTOMETRIC_INTERPRETATION] = photo
- elif im.mode in ("1", "L") and ifd[PHOTOMETRIC_INTERPRETATION] == 0:
- if im.mode == "1":
- inverted_im = im.copy()
- px = inverted_im.load()
- for y in range(inverted_im.height):
- for x in range(inverted_im.width):
- px[x, y] = 0 if px[x, y] == 255 else 255
- im = inverted_im
- else:
- im = ImageOps.invert(im)
-
- if im.mode in ["P", "PA"]:
- lut = im.im.getpalette("RGB", "RGB;L")
- colormap = []
- colors = len(lut) // 3
- for i in range(3):
- colormap += [v * 256 for v in lut[colors * i : colors * (i + 1)]]
- colormap += [0] * (256 - colors)
- ifd[COLORMAP] = colormap
- # data orientation
- stride = len(bits) * ((im.size[0] * bits[0] + 7) // 8)
- # aim for given strip size (64 KB by default) when using libtiff writer
- if libtiff:
- im_strip_size = encoderinfo.get("strip_size", STRIP_SIZE)
- rows_per_strip = 1 if stride == 0 else min(im_strip_size // stride, im.size[1])
- # JPEG encoder expects multiple of 8 rows
- if compression == "jpeg":
- rows_per_strip = min(((rows_per_strip + 7) // 8) * 8, im.size[1])
- else:
- rows_per_strip = im.size[1]
- if rows_per_strip == 0:
- rows_per_strip = 1
- strip_byte_counts = 1 if stride == 0 else stride * rows_per_strip
- strips_per_image = (im.size[1] + rows_per_strip - 1) // rows_per_strip
- ifd[ROWSPERSTRIP] = rows_per_strip
- if strip_byte_counts >= 2**16:
- ifd.tagtype[STRIPBYTECOUNTS] = TiffTags.LONG
- ifd[STRIPBYTECOUNTS] = (strip_byte_counts,) * (strips_per_image - 1) + (
- stride * im.size[1] - strip_byte_counts * (strips_per_image - 1),
- )
- ifd[STRIPOFFSETS] = tuple(
- range(0, strip_byte_counts * strips_per_image, strip_byte_counts)
- ) # this is adjusted by IFD writer
- # no compression by default:
- ifd[COMPRESSION] = COMPRESSION_INFO_REV.get(compression, 1)
-
- if im.mode == "YCbCr":
- for tag, value in {
- YCBCRSUBSAMPLING: (1, 1),
- REFERENCEBLACKWHITE: (0, 255, 128, 255, 128, 255),
- }.items():
- ifd.setdefault(tag, value)
-
- blocklist = [TILEWIDTH, TILELENGTH, TILEOFFSETS, TILEBYTECOUNTS]
- if libtiff:
- if "quality" in encoderinfo:
- quality = encoderinfo["quality"]
- if not isinstance(quality, int) or quality < 0 or quality > 100:
- msg = "Invalid quality setting"
- raise ValueError(msg)
- if compression != "jpeg":
- msg = "quality setting only supported for 'jpeg' compression"
- raise ValueError(msg)
- ifd[JPEGQUALITY] = quality
-
- logger.debug("Saving using libtiff encoder")
- logger.debug("Items: %s" % sorted(ifd.items()))
- _fp = 0
- if hasattr(fp, "fileno"):
- try:
- fp.seek(0)
- _fp = os.dup(fp.fileno())
- except io.UnsupportedOperation:
- pass
-
- # optional types for non core tags
- types = {}
- # STRIPOFFSETS and STRIPBYTECOUNTS are added by the library
- # based on the data in the strip.
- # The other tags expect arrays with a certain length (fixed or depending on
- # BITSPERSAMPLE, etc), passing arrays with a different length will result in
- # segfaults. Block these tags until we add extra validation.
- # SUBIFD may also cause a segfault.
- blocklist += [
- REFERENCEBLACKWHITE,
- STRIPBYTECOUNTS,
- STRIPOFFSETS,
- TRANSFERFUNCTION,
- SUBIFD,
- ]
-
- # bits per sample is a single short in the tiff directory, not a list.
- atts = {BITSPERSAMPLE: bits[0]}
- # Merge the ones that we have with (optional) more bits from
- # the original file, e.g x,y resolution so that we can
- # save(load('')) == original file.
- legacy_ifd = {}
- if hasattr(im, "tag"):
- legacy_ifd = im.tag.to_v2()
-
- # SAMPLEFORMAT is determined by the image format and should not be copied
- # from legacy_ifd.
- supplied_tags = {**getattr(im, "tag_v2", {}), **legacy_ifd}
- if SAMPLEFORMAT in supplied_tags:
- del supplied_tags[SAMPLEFORMAT]
-
- for tag, value in itertools.chain(ifd.items(), supplied_tags.items()):
- # Libtiff can only process certain core items without adding
- # them to the custom dictionary.
- # Custom items are supported for int, float, unicode, string and byte
- # values. Other types and tuples require a tagtype.
- if tag not in TiffTags.LIBTIFF_CORE:
- if not getattr(Image.core, "libtiff_support_custom_tags", False):
- continue
-
- if tag in ifd.tagtype:
- types[tag] = ifd.tagtype[tag]
- elif not (isinstance(value, (int, float, str, bytes))):
- continue
- else:
- type = TiffTags.lookup(tag).type
- if type:
- types[tag] = type
- if tag not in atts and tag not in blocklist:
- if isinstance(value, str):
- atts[tag] = value.encode("ascii", "replace") + b"\0"
- elif isinstance(value, IFDRational):
- atts[tag] = float(value)
- else:
- atts[tag] = value
-
- if SAMPLEFORMAT in atts and len(atts[SAMPLEFORMAT]) == 1:
- atts[SAMPLEFORMAT] = atts[SAMPLEFORMAT][0]
-
- logger.debug("Converted items: %s" % sorted(atts.items()))
-
- # libtiff always expects the bytes in native order.
- # we're storing image byte order. So, if the rawmode
- # contains I;16, we need to convert from native to image
- # byte order.
- if im.mode in ("I;16B", "I;16"):
- rawmode = "I;16N"
-
- # Pass tags as sorted list so that the tags are set in a fixed order.
- # This is required by libtiff for some tags. For example, the JPEGQUALITY
- # pseudo tag requires that the COMPRESS tag was already set.
- tags = list(atts.items())
- tags.sort()
- a = (rawmode, compression, _fp, filename, tags, types)
- e = Image._getencoder(im.mode, "libtiff", a, encoderconfig)
- e.setimage(im.im, (0, 0) + im.size)
- while True:
- # undone, change to self.decodermaxblock:
- errcode, data = e.encode(16 * 1024)[1:]
- if not _fp:
- fp.write(data)
- if errcode:
- break
- if _fp:
- try:
- os.close(_fp)
- except OSError:
- pass
- if errcode < 0:
- msg = f"encoder error {errcode} when writing image file"
- raise OSError(msg)
-
- else:
- for tag in blocklist:
- del ifd[tag]
- offset = ifd.save(fp)
-
- ImageFile._save(
- im, fp, [("raw", (0, 0) + im.size, offset, (rawmode, stride, 1))]
- )
-
- # -- helper for multi-page save --
- if "_debug_multipage" in encoderinfo:
- # just to access o32 and o16 (using correct byte order)
- im._debug_multipage = ifd
-
-
-class AppendingTiffWriter:
- fieldSizes = [
- 0, # None
- 1, # byte
- 1, # ascii
- 2, # short
- 4, # long
- 8, # rational
- 1, # sbyte
- 1, # undefined
- 2, # sshort
- 4, # slong
- 8, # srational
- 4, # float
- 8, # double
- 4, # ifd
- 2, # unicode
- 4, # complex
- 8, # long8
- ]
-
- # StripOffsets = 273
- # FreeOffsets = 288
- # TileOffsets = 324
- # JPEGQTables = 519
- # JPEGDCTables = 520
- # JPEGACTables = 521
- Tags = {273, 288, 324, 519, 520, 521}
-
- def __init__(self, fn, new=False):
- if hasattr(fn, "read"):
- self.f = fn
- self.close_fp = False
- else:
- self.name = fn
- self.close_fp = True
- try:
- self.f = open(fn, "w+b" if new else "r+b")
- except OSError:
- self.f = open(fn, "w+b")
- self.beginning = self.f.tell()
- self.setup()
-
- def setup(self):
- # Reset everything.
- self.f.seek(self.beginning, os.SEEK_SET)
-
- self.whereToWriteNewIFDOffset = None
- self.offsetOfNewPage = 0
-
- self.IIMM = iimm = self.f.read(4)
- if not iimm:
- # empty file - first page
- self.isFirst = True
- return
-
- self.isFirst = False
- if iimm == b"II\x2a\x00":
- self.setEndian("<")
- elif iimm == b"MM\x00\x2a":
- self.setEndian(">")
- else:
- msg = "Invalid TIFF file header"
- raise RuntimeError(msg)
-
- self.skipIFDs()
- self.goToEnd()
-
- def finalize(self):
- if self.isFirst:
- return
-
- # fix offsets
- self.f.seek(self.offsetOfNewPage)
-
- iimm = self.f.read(4)
- if not iimm:
- # msg = "nothing written into new page"
- # raise RuntimeError(msg)
- # Make it easy to finish a frame without committing to a new one.
- return
-
- if iimm != self.IIMM:
- msg = "IIMM of new page doesn't match IIMM of first page"
- raise RuntimeError(msg)
-
- ifd_offset = self.readLong()
- ifd_offset += self.offsetOfNewPage
- self.f.seek(self.whereToWriteNewIFDOffset)
- self.writeLong(ifd_offset)
- self.f.seek(ifd_offset)
- self.fixIFD()
-
- def newFrame(self):
- # Call this to finish a frame.
- self.finalize()
- self.setup()
-
- def __enter__(self):
- return self
-
- def __exit__(self, exc_type, exc_value, traceback):
- if self.close_fp:
- self.close()
- return False
-
- def tell(self):
- return self.f.tell() - self.offsetOfNewPage
-
- def seek(self, offset, whence=io.SEEK_SET):
- if whence == os.SEEK_SET:
- offset += self.offsetOfNewPage
-
- self.f.seek(offset, whence)
- return self.tell()
-
- def goToEnd(self):
- self.f.seek(0, os.SEEK_END)
- pos = self.f.tell()
-
- # pad to 16 byte boundary
- pad_bytes = 16 - pos % 16
- if 0 < pad_bytes < 16:
- self.f.write(bytes(pad_bytes))
- self.offsetOfNewPage = self.f.tell()
-
- def setEndian(self, endian):
- self.endian = endian
- self.longFmt = self.endian + "L"
- self.shortFmt = self.endian + "H"
- self.tagFormat = self.endian + "HHL"
-
- def skipIFDs(self):
- while True:
- ifd_offset = self.readLong()
- if ifd_offset == 0:
- self.whereToWriteNewIFDOffset = self.f.tell() - 4
- break
-
- self.f.seek(ifd_offset)
- num_tags = self.readShort()
- self.f.seek(num_tags * 12, os.SEEK_CUR)
-
- def write(self, data):
- return self.f.write(data)
-
- def readShort(self):
- (value,) = struct.unpack(self.shortFmt, self.f.read(2))
- return value
-
- def readLong(self):
- (value,) = struct.unpack(self.longFmt, self.f.read(4))
- return value
-
- def rewriteLastShortToLong(self, value):
- self.f.seek(-2, os.SEEK_CUR)
- bytes_written = self.f.write(struct.pack(self.longFmt, value))
- if bytes_written is not None and bytes_written != 4:
- msg = f"wrote only {bytes_written} bytes but wanted 4"
- raise RuntimeError(msg)
-
- def rewriteLastShort(self, value):
- self.f.seek(-2, os.SEEK_CUR)
- bytes_written = self.f.write(struct.pack(self.shortFmt, value))
- if bytes_written is not None and bytes_written != 2:
- msg = f"wrote only {bytes_written} bytes but wanted 2"
- raise RuntimeError(msg)
-
- def rewriteLastLong(self, value):
- self.f.seek(-4, os.SEEK_CUR)
- bytes_written = self.f.write(struct.pack(self.longFmt, value))
- if bytes_written is not None and bytes_written != 4:
- msg = f"wrote only {bytes_written} bytes but wanted 4"
- raise RuntimeError(msg)
-
- def writeShort(self, value):
- bytes_written = self.f.write(struct.pack(self.shortFmt, value))
- if bytes_written is not None and bytes_written != 2:
- msg = f"wrote only {bytes_written} bytes but wanted 2"
- raise RuntimeError(msg)
-
- def writeLong(self, value):
- bytes_written = self.f.write(struct.pack(self.longFmt, value))
- if bytes_written is not None and bytes_written != 4:
- msg = f"wrote only {bytes_written} bytes but wanted 4"
- raise RuntimeError(msg)
-
- def close(self):
- self.finalize()
- self.f.close()
-
- def fixIFD(self):
- num_tags = self.readShort()
-
- for i in range(num_tags):
- tag, field_type, count = struct.unpack(self.tagFormat, self.f.read(8))
-
- field_size = self.fieldSizes[field_type]
- total_size = field_size * count
- is_local = total_size <= 4
- if not is_local:
- offset = self.readLong()
- offset += self.offsetOfNewPage
- self.rewriteLastLong(offset)
-
- if tag in self.Tags:
- cur_pos = self.f.tell()
-
- if is_local:
- self.fixOffsets(
- count, isShort=(field_size == 2), isLong=(field_size == 4)
- )
- self.f.seek(cur_pos + 4)
- else:
- self.f.seek(offset)
- self.fixOffsets(
- count, isShort=(field_size == 2), isLong=(field_size == 4)
- )
- self.f.seek(cur_pos)
-
- offset = cur_pos = None
-
- elif is_local:
- # skip the locally stored value that is not an offset
- self.f.seek(4, os.SEEK_CUR)
-
- def fixOffsets(self, count, isShort=False, isLong=False):
- if not isShort and not isLong:
- msg = "offset is neither short nor long"
- raise RuntimeError(msg)
-
- for i in range(count):
- offset = self.readShort() if isShort else self.readLong()
- offset += self.offsetOfNewPage
- if isShort and offset >= 65536:
- # offset is now too large - we must convert shorts to longs
- if count != 1:
- msg = "not implemented"
- raise RuntimeError(msg) # XXX TODO
-
- # simple case - the offset is just one and therefore it is
- # local (not referenced with another offset)
- self.rewriteLastShortToLong(offset)
- self.f.seek(-10, os.SEEK_CUR)
- self.writeShort(TiffTags.LONG) # rewrite the type to LONG
- self.f.seek(8, os.SEEK_CUR)
- elif isShort:
- self.rewriteLastShort(offset)
- else:
- self.rewriteLastLong(offset)
-
-
-def _save_all(im, fp, filename):
- encoderinfo = im.encoderinfo.copy()
- encoderconfig = im.encoderconfig
- append_images = list(encoderinfo.get("append_images", []))
- if not hasattr(im, "n_frames") and not append_images:
- return _save(im, fp, filename)
-
- cur_idx = im.tell()
- try:
- with AppendingTiffWriter(fp) as tf:
- for ims in [im] + append_images:
- ims.encoderinfo = encoderinfo
- ims.encoderconfig = encoderconfig
- if not hasattr(ims, "n_frames"):
- nfr = 1
- else:
- nfr = ims.n_frames
-
- for idx in range(nfr):
- ims.seek(idx)
- ims.load()
- _save(ims, tf, filename)
- tf.newFrame()
- finally:
- im.seek(cur_idx)
-
-
-#
-# --------------------------------------------------------------------
-# Register
-
-Image.register_open(TiffImageFile.format, TiffImageFile, _accept)
-Image.register_save(TiffImageFile.format, _save)
-Image.register_save_all(TiffImageFile.format, _save_all)
-
-Image.register_extensions(TiffImageFile.format, [".tif", ".tiff"])
-
-Image.register_mime(TiffImageFile.format, "image/tiff")
diff --git a/spaces/cloudtheboi/Lofi4All/.pythonlibs/lib/python3.10/site-packages/fontTools/ttLib/tables/_g_c_i_d.py b/spaces/cloudtheboi/Lofi4All/.pythonlibs/lib/python3.10/site-packages/fontTools/ttLib/tables/_g_c_i_d.py
deleted file mode 100644
index 2e746c846fa14800cb7de93969984dac36678e4e..0000000000000000000000000000000000000000
--- a/spaces/cloudtheboi/Lofi4All/.pythonlibs/lib/python3.10/site-packages/fontTools/ttLib/tables/_g_c_i_d.py
+++ /dev/null
@@ -1,6 +0,0 @@
-from .otBase import BaseTTXConverter
-
-
-# https://developer.apple.com/fonts/TrueType-Reference-Manual/RM06/Chap6gcid.html
-class table__g_c_i_d(BaseTTXConverter):
- pass
diff --git a/spaces/cncn102/bingo1/src/lib/bots/bing/sr.ts b/spaces/cncn102/bingo1/src/lib/bots/bing/sr.ts
deleted file mode 100644
index 7cae14da7362bd6cc1e234851c11ca67e5a99f0c..0000000000000000000000000000000000000000
--- a/spaces/cncn102/bingo1/src/lib/bots/bing/sr.ts
+++ /dev/null
@@ -1,106 +0,0 @@
-// @ts-ignore
-const SpeechRecognitionPolyfill: typeof webkitSpeechRecognition = typeof window !== 'undefined' ? (
- // @ts-ignore
- window.SpeechRecognition ||
- window.webkitSpeechRecognition ||
- // @ts-ignore
- window.mozSpeechRecognition ||
- // @ts-ignore
- window.msSpeechRecognition ||
- // @ts-ignore
- window.oSpeechRecognition
-) as typeof webkitSpeechRecognition : undefined
-
-type subscriber = (msg: string, command?: string) => void
-
-export class SR {
- recognition?: SpeechRecognition
- onchange?: subscriber
- transcript: boolean = false
- listening: boolean = false
- private commandsRe?: RegExp
- constructor(commands: string[]) {
- this.recognition = SpeechRecognitionPolyfill ? new SpeechRecognitionPolyfill() : undefined
- if (!this.recognition) {
- return
- }
- this.configuration('zh-CN')
- if (commands.length) {
- this.commandsRe = new RegExp(`^(${commands.join('|')})。?$`)
- }
- this.recognition.onresult = this.speechRecognition
- this.recognition.onerror = (err) => {
- console.log('err', err.error)
- this.stop()
- }
- this.recognition.onend = () => {
- if (this.recognition && this.listening) {
- this.recognition.start()
- }
- }
- }
-
- speechRecognition = (event: SpeechRecognitionEvent) => {
- if (!this.listening) return
- for (var i = event.resultIndex; i < event.results.length; i++) {
- let result = event.results[i]
- if (result.isFinal) {
- var alt = result[0]
- const text = alt.transcript.trim()
- if (this.commandsRe && this.commandsRe.test(text)) {
- return this.onchange?.('', RegExp.$1)
- }
- if (!this.transcript) return
- this.onchange?.(text)
- }
- }
- }
-
- private configuration = async (lang: string = 'zh-CN') => {
- return new Promise((resolve) => {
- if (this.recognition) {
- this.recognition.continuous = true
- this.recognition.lang = lang
- this.recognition.onstart = resolve
- }
- })
- }
-
- start = async () => {
- if (this.recognition && !this.listening) {
- await this.recognition.start()
- this.transcript = true
- this.listening = true
- }
- }
-
- stop = () => {
- if (this.recognition) {
- this.recognition.stop()
- this.transcript = false
- this.listening = false
- }
- }
-
-
- pause = () => {
- if (this.recognition) {
- this.transcript = false
- }
- }
-
- resume = () => {
- if (this.recognition) {
- this.transcript = true
- }
- }
-
- abort = () => {
- if (this.recognition && this.transcript) {
- this.recognition.abort()
- this.transcript = false
- this.listening = false
- }
- }
-}
-
diff --git a/spaces/codejin/diffsingerkr/Pattern_Generator.py b/spaces/codejin/diffsingerkr/Pattern_Generator.py
deleted file mode 100644
index e6780cfca1f8128a368fec548c1d2718af41fb2b..0000000000000000000000000000000000000000
--- a/spaces/codejin/diffsingerkr/Pattern_Generator.py
+++ /dev/null
@@ -1,64 +0,0 @@
-import numpy as np
-import mido, os, pickle, yaml, argparse, math, librosa, hgtk, logging
-from tqdm import tqdm
-from pysptk.sptk import rapt
-from typing import List, Tuple
-from argparse import Namespace # for type
-import torch
-from typing import Dict
-
-from meldataset import mel_spectrogram, spectrogram, spec_energy
-from Arg_Parser import Recursive_Parse
-
-def Convert_Feature_Based_Music(
- music: List[Tuple[float, str, int]],
- sample_rate: int,
- frame_shift: int,
- consonant_duration: int= 3,
- equality_duration: bool= False
- ):
- previous_used = 0
- lyrics = []
- notes = []
- durations = []
- for message_time, lyric, note in music:
- duration = round(message_time * sample_rate) + previous_used
- previous_used = duration % frame_shift
- duration = duration // frame_shift
-
- if lyric == '':
- lyrics.append(lyric)
- notes.append(note)
- durations.append(duration)
- else:
- lyrics.extend(Decompose(lyric))
- notes.extend([note] * 3)
- if equality_duration or duration < consonant_duration * 3:
- split_duration = [duration // 3] * 3
- split_duration[1] += duration % 3
- durations.extend(split_duration)
- else:
- durations.extend([
- consonant_duration, # onset
- duration - consonant_duration * 2, # nucleus
- consonant_duration # coda
- ])
-
- return lyrics, notes, durations
-
-def Expand_by_Duration(
- lyrics: List[str],
- notes: List[int],
- durations: List[int],
- ):
- lyrics = sum([[lyric] * duration for lyric, duration in zip(lyrics, durations)], [])
- notes = sum([*[[note] * duration for note, duration in zip(notes, durations)]], [])
- durations = [index for duration in durations for index in range(duration)]
-
- return lyrics, notes, durations
-
-def Decompose(syllable: str):
- onset, nucleus, coda = hgtk.letter.decompose(syllable)
- coda += '_'
-
- return onset, nucleus, coda
diff --git a/spaces/colakin/video-generater/public/ffmpeg/libavcodec/ass_split.c b/spaces/colakin/video-generater/public/ffmpeg/libavcodec/ass_split.c
deleted file mode 100644
index 73ef6196c51607eebde1b4c9f9342e9d81c2cf88..0000000000000000000000000000000000000000
--- a/spaces/colakin/video-generater/public/ffmpeg/libavcodec/ass_split.c
+++ /dev/null
@@ -1,589 +0,0 @@
-/*
- * SSA/ASS spliting functions
- * Copyright (c) 2010 Aurelien Jacobs
- *
- * This file is part of FFmpeg.
- *
- * FFmpeg is free software; you can redistribute it and/or
- * modify it under the terms of the GNU Lesser General Public
- * License as published by the Free Software Foundation; either
- * version 2.1 of the License, or (at your option) any later version.
- *
- * FFmpeg is distributed in the hope that it will be useful,
- * but WITHOUT ANY WARRANTY; without even the implied warranty of
- * MERCHANTABILITY or FITNESS FOR A PARTICULAR PURPOSE. See the GNU
- * Lesser General Public License for more details.
- *
- * You should have received a copy of the GNU Lesser General Public
- * License along with FFmpeg; if not, write to the Free Software
- * Foundation, Inc., 51 Franklin Street, Fifth Floor, Boston, MA 02110-1301 USA
- */
-
-#include
-#include
-#include
-#include
-#include
-
-#include "libavutil/error.h"
-#include "libavutil/macros.h"
-#include "libavutil/mem.h"
-#include "ass_split.h"
-
-typedef enum {
- ASS_STR,
- ASS_INT,
- ASS_FLT,
- ASS_COLOR,
- ASS_TIMESTAMP,
- ASS_ALGN,
-} ASSFieldType;
-
-typedef struct {
- const char *name;
- int type;
- int offset;
-} ASSFields;
-
-typedef struct {
- const char *section;
- const char *format_header;
- const char *fields_header;
- int size;
- int offset;
- int offset_count;
- ASSFields fields[24];
-} ASSSection;
-
-static const ASSSection ass_sections[] = {
- { .section = "Script Info",
- .offset = offsetof(ASS, script_info),
- .fields = {{"ScriptType", ASS_STR, offsetof(ASSScriptInfo, script_type)},
- {"Collisions", ASS_STR, offsetof(ASSScriptInfo, collisions) },
- {"PlayResX", ASS_INT, offsetof(ASSScriptInfo, play_res_x) },
- {"PlayResY", ASS_INT, offsetof(ASSScriptInfo, play_res_y) },
- {"Timer", ASS_FLT, offsetof(ASSScriptInfo, timer) },
- {0},
- }
- },
- { .section = "V4+ Styles",
- .format_header = "Format",
- .fields_header = "Style",
- .size = sizeof(ASSStyle),
- .offset = offsetof(ASS, styles),
- .offset_count = offsetof(ASS, styles_count),
- .fields = {{"Name", ASS_STR, offsetof(ASSStyle, name) },
- {"Fontname", ASS_STR, offsetof(ASSStyle, font_name) },
- {"Fontsize", ASS_INT, offsetof(ASSStyle, font_size) },
- {"PrimaryColour", ASS_COLOR, offsetof(ASSStyle, primary_color) },
- {"SecondaryColour", ASS_COLOR, offsetof(ASSStyle, secondary_color)},
- {"OutlineColour", ASS_COLOR, offsetof(ASSStyle, outline_color) },
- {"BackColour", ASS_COLOR, offsetof(ASSStyle, back_color) },
- {"Bold", ASS_INT, offsetof(ASSStyle, bold) },
- {"Italic", ASS_INT, offsetof(ASSStyle, italic) },
- {"Underline", ASS_INT, offsetof(ASSStyle, underline) },
- {"StrikeOut", ASS_INT, offsetof(ASSStyle, strikeout) },
- {"ScaleX", ASS_FLT, offsetof(ASSStyle, scalex) },
- {"ScaleY", ASS_FLT, offsetof(ASSStyle, scaley) },
- {"Spacing", ASS_FLT, offsetof(ASSStyle, spacing) },
- {"Angle", ASS_FLT, offsetof(ASSStyle, angle) },
- {"BorderStyle", ASS_INT, offsetof(ASSStyle, border_style) },
- {"Outline", ASS_FLT, offsetof(ASSStyle, outline) },
- {"Shadow", ASS_FLT, offsetof(ASSStyle, shadow) },
- {"Alignment", ASS_INT, offsetof(ASSStyle, alignment) },
- {"MarginL", ASS_INT, offsetof(ASSStyle, margin_l) },
- {"MarginR", ASS_INT, offsetof(ASSStyle, margin_r) },
- {"MarginV", ASS_INT, offsetof(ASSStyle, margin_v) },
- {"Encoding", ASS_INT, offsetof(ASSStyle, encoding) },
- {0},
- }
- },
- { .section = "V4 Styles",
- .format_header = "Format",
- .fields_header = "Style",
- .size = sizeof(ASSStyle),
- .offset = offsetof(ASS, styles),
- .offset_count = offsetof(ASS, styles_count),
- .fields = {{"Name", ASS_STR, offsetof(ASSStyle, name) },
- {"Fontname", ASS_STR, offsetof(ASSStyle, font_name) },
- {"Fontsize", ASS_INT, offsetof(ASSStyle, font_size) },
- {"PrimaryColour", ASS_COLOR, offsetof(ASSStyle, primary_color) },
- {"SecondaryColour", ASS_COLOR, offsetof(ASSStyle, secondary_color)},
- {"TertiaryColour", ASS_COLOR, offsetof(ASSStyle, outline_color) },
- {"BackColour", ASS_COLOR, offsetof(ASSStyle, back_color) },
- {"Bold", ASS_INT, offsetof(ASSStyle, bold) },
- {"Italic", ASS_INT, offsetof(ASSStyle, italic) },
- {"BorderStyle", ASS_INT, offsetof(ASSStyle, border_style) },
- {"Outline", ASS_FLT, offsetof(ASSStyle, outline) },
- {"Shadow", ASS_FLT, offsetof(ASSStyle, shadow) },
- {"Alignment", ASS_ALGN, offsetof(ASSStyle, alignment) },
- {"MarginL", ASS_INT, offsetof(ASSStyle, margin_l) },
- {"MarginR", ASS_INT, offsetof(ASSStyle, margin_r) },
- {"MarginV", ASS_INT, offsetof(ASSStyle, margin_v) },
- {"AlphaLevel", ASS_INT, offsetof(ASSStyle, alpha_level) },
- {"Encoding", ASS_INT, offsetof(ASSStyle, encoding) },
- {0},
- }
- },
- { .section = "Events",
- .format_header = "Format",
- .fields_header = "Dialogue",
- .size = sizeof(ASSDialog),
- .offset = offsetof(ASS, dialogs),
- .offset_count = offsetof(ASS, dialogs_count),
- .fields = {{"Layer", ASS_INT, offsetof(ASSDialog, layer) },
- {"Start", ASS_TIMESTAMP, offsetof(ASSDialog, start) },
- {"End", ASS_TIMESTAMP, offsetof(ASSDialog, end) },
- {"Style", ASS_STR, offsetof(ASSDialog, style) },
- {"Name", ASS_STR, offsetof(ASSDialog, name) },
- {"MarginL", ASS_INT, offsetof(ASSDialog, margin_l)},
- {"MarginR", ASS_INT, offsetof(ASSDialog, margin_r)},
- {"MarginV", ASS_INT, offsetof(ASSDialog, margin_v)},
- {"Effect", ASS_STR, offsetof(ASSDialog, effect) },
- {"Text", ASS_STR, offsetof(ASSDialog, text) },
- {0},
- }
- },
-};
-
-
-typedef int (*ASSConvertFunc)(void *dest, const char *buf, int len);
-
-static int convert_str(void *dest, const char *buf, int len)
-{
- char *str = av_malloc(len + 1);
- if (str) {
- memcpy(str, buf, len);
- str[len] = 0;
- if (*(void **)dest)
- av_free(*(void **)dest);
- *(char **)dest = str;
- }
- return !str;
-}
-static int convert_int(void *dest, const char *buf, int len)
-{
- return sscanf(buf, "%d", (int *)dest) == 1;
-}
-static int convert_flt(void *dest, const char *buf, int len)
-{
- return sscanf(buf, "%f", (float *)dest) == 1;
-}
-static int convert_color(void *dest, const char *buf, int len)
-{
- return sscanf(buf, "&H%8x", (int *)dest) == 1 ||
- sscanf(buf, "%d", (int *)dest) == 1;
-}
-static int convert_timestamp(void *dest, const char *buf, int len)
-{
- int c, h, m, s, cs;
- if ((c = sscanf(buf, "%d:%02d:%02d.%02d", &h, &m, &s, &cs)) == 4)
- *(int *)dest = 360000*h + 6000*m + 100*s + cs;
- return c == 4;
-}
-static int convert_alignment(void *dest, const char *buf, int len)
-{
- int a;
- if (sscanf(buf, "%d", &a) == 1) {
- /* convert V4 Style alignment to V4+ Style */
- *(int *)dest = a + ((a&4) >> 1) - 5*!!(a&8);
- return 1;
- }
- return 0;
-}
-
-static const ASSConvertFunc convert_func[] = {
- [ASS_STR] = convert_str,
- [ASS_INT] = convert_int,
- [ASS_FLT] = convert_flt,
- [ASS_COLOR] = convert_color,
- [ASS_TIMESTAMP] = convert_timestamp,
- [ASS_ALGN] = convert_alignment,
-};
-
-
-struct ASSSplitContext {
- ASS ass;
- int current_section;
- int field_number[FF_ARRAY_ELEMS(ass_sections)];
- int *field_order[FF_ARRAY_ELEMS(ass_sections)];
-};
-
-
-static uint8_t *realloc_section_array(ASSSplitContext *ctx)
-{
- const ASSSection *section = &ass_sections[ctx->current_section];
- int *count = (int *)((uint8_t *)&ctx->ass + section->offset_count);
- void **section_ptr = (void **)((uint8_t *)&ctx->ass + section->offset);
- uint8_t *tmp = av_realloc_array(*section_ptr, (*count+1), section->size);
- if (!tmp)
- return NULL;
- *section_ptr = tmp;
- tmp += *count * section->size;
- memset(tmp, 0, section->size);
- (*count)++;
- return tmp;
-}
-
-static inline int is_eol(char buf)
-{
- return buf == '\r' || buf == '\n' || buf == 0;
-}
-
-static inline const char *skip_space(const char *buf)
-{
- while (*buf == ' ')
- buf++;
- return buf;
-}
-
-static int *get_default_field_orders(const ASSSection *section, int *number)
-{
- int i;
- int *order = av_malloc_array(FF_ARRAY_ELEMS(section->fields), sizeof(*order));
-
- if (!order)
- return NULL;
- for (i = 0; section->fields[i].name; i++)
- order[i] = i;
- *number = i;
- while (i < FF_ARRAY_ELEMS(section->fields))
- order[i++] = -1;
- return order;
-}
-
-static const char *ass_split_section(ASSSplitContext *ctx, const char *buf)
-{
- const ASSSection *section = &ass_sections[ctx->current_section];
- int *number = &ctx->field_number[ctx->current_section];
- int *order = ctx->field_order[ctx->current_section];
- int i, len;
-
- while (buf && *buf) {
- if (buf[0] == '[') {
- ctx->current_section = -1;
- break;
- }
- if (buf[0] == ';' || (buf[0] == '!' && buf[1] == ':'))
- goto next_line; // skip comments
-
- len = strcspn(buf, ":\r\n");
- if (buf[len] == ':' &&
- (!section->fields_header || strncmp(buf, section->fields_header, len))) {
- for (i = 0; i < FF_ARRAY_ELEMS(ass_sections); i++) {
- if (ass_sections[i].fields_header &&
- !strncmp(buf, ass_sections[i].fields_header, len)) {
- ctx->current_section = i;
- section = &ass_sections[ctx->current_section];
- number = &ctx->field_number[ctx->current_section];
- order = ctx->field_order[ctx->current_section];
- break;
- }
- }
- }
- if (section->format_header && !order) {
- len = strlen(section->format_header);
- if (!strncmp(buf, section->format_header, len) && buf[len] == ':') {
- buf += len + 1;
- while (!is_eol(*buf)) {
- buf = skip_space(buf);
- len = strcspn(buf, ", \r\n");
- if (av_reallocp_array(&order, (*number + 1), sizeof(*order)) != 0)
- return NULL;
-
- order[*number] = -1;
- for (i=0; section->fields[i].name; i++)
- if (!strncmp(buf, section->fields[i].name, len)) {
- order[*number] = i;
- break;
- }
- (*number)++;
- buf = skip_space(buf + len + (buf[len] == ','));
- }
- ctx->field_order[ctx->current_section] = order;
- goto next_line;
- }
- }
- if (section->fields_header) {
- len = strlen(section->fields_header);
- if (!strncmp(buf, section->fields_header, len) && buf[len] == ':') {
- uint8_t *ptr, *struct_ptr = realloc_section_array(ctx);
- if (!struct_ptr) return NULL;
-
- /* No format header line found so far, assume default */
- if (!order) {
- order = get_default_field_orders(section, number);
- if (!order)
- return NULL;
- ctx->field_order[ctx->current_section] = order;
- }
-
- buf += len + 1;
- for (i=0; !is_eol(*buf) && i < *number; i++) {
- int last = i == *number - 1;
- buf = skip_space(buf);
- len = strcspn(buf, last ? "\r\n" : ",\r\n");
- if (order[i] >= 0) {
- ASSFieldType type = section->fields[order[i]].type;
- ptr = struct_ptr + section->fields[order[i]].offset;
- convert_func[type](ptr, buf, len);
- }
- buf += len;
- if (!last && *buf) buf++;
- buf = skip_space(buf);
- }
- }
- } else {
- len = strcspn(buf, ":\r\n");
- if (buf[len] == ':') {
- for (i=0; section->fields[i].name; i++)
- if (!strncmp(buf, section->fields[i].name, len)) {
- ASSFieldType type = section->fields[i].type;
- uint8_t *ptr = (uint8_t *)&ctx->ass + section->offset;
- ptr += section->fields[i].offset;
- buf = skip_space(buf + len + 1);
- convert_func[type](ptr, buf, strcspn(buf, "\r\n"));
- break;
- }
- }
- }
-next_line:
- buf += strcspn(buf, "\n");
- buf += !!*buf;
- }
- return buf;
-}
-
-static int ass_split(ASSSplitContext *ctx, const char *buf)
-{
- char c, section[16];
- int i;
-
- if (ctx->current_section >= 0)
- buf = ass_split_section(ctx, buf);
-
- while (buf && *buf) {
- if (sscanf(buf, "[%15[0-9A-Za-z+ ]]%c", section, &c) == 2) {
- buf += strcspn(buf, "\n");
- buf += !!*buf;
- for (i=0; icurrent_section = i;
- buf = ass_split_section(ctx, buf);
- }
- } else {
- buf += strcspn(buf, "\n");
- buf += !!*buf;
- }
- }
- return buf ? 0 : AVERROR_INVALIDDATA;
-}
-
-ASSSplitContext *ff_ass_split(const char *buf)
-{
- ASSSplitContext *ctx = av_mallocz(sizeof(*ctx));
- if (!ctx)
- return NULL;
- if (buf && !strncmp(buf, "\xef\xbb\xbf", 3)) // Skip UTF-8 BOM header
- buf += 3;
- ctx->current_section = -1;
- if (ass_split(ctx, buf) < 0) {
- ff_ass_split_free(ctx);
- return NULL;
- }
- return ctx;
-}
-
-static void free_section(ASSSplitContext *ctx, const ASSSection *section)
-{
- uint8_t *ptr = (uint8_t *)&ctx->ass + section->offset;
- int i, j, *count, c = 1;
-
- if (section->format_header) {
- ptr = *(void **)ptr;
- count = (int *)((uint8_t *)&ctx->ass + section->offset_count);
- } else
- count = &c;
-
- if (ptr)
- for (i=0; i<*count; i++, ptr += section->size)
- for (j=0; section->fields[j].name; j++) {
- const ASSFields *field = §ion->fields[j];
- if (field->type == ASS_STR)
- av_freep(ptr + field->offset);
- }
- *count = 0;
-
- if (section->format_header)
- av_freep((uint8_t *)&ctx->ass + section->offset);
-}
-
-void ff_ass_free_dialog(ASSDialog **dialogp)
-{
- ASSDialog *dialog = *dialogp;
- if (!dialog)
- return;
- av_freep(&dialog->style);
- av_freep(&dialog->name);
- av_freep(&dialog->effect);
- av_freep(&dialog->text);
- av_freep(dialogp);
-}
-
-ASSDialog *ff_ass_split_dialog(ASSSplitContext *ctx, const char *buf)
-{
- int i;
- static const ASSFields fields[] = {
- {"ReadOrder", ASS_INT, offsetof(ASSDialog, readorder)},
- {"Layer", ASS_INT, offsetof(ASSDialog, layer) },
- {"Style", ASS_STR, offsetof(ASSDialog, style) },
- {"Name", ASS_STR, offsetof(ASSDialog, name) },
- {"MarginL", ASS_INT, offsetof(ASSDialog, margin_l) },
- {"MarginR", ASS_INT, offsetof(ASSDialog, margin_r) },
- {"MarginV", ASS_INT, offsetof(ASSDialog, margin_v) },
- {"Effect", ASS_STR, offsetof(ASSDialog, effect) },
- {"Text", ASS_STR, offsetof(ASSDialog, text) },
- };
-
- ASSDialog *dialog = av_mallocz(sizeof(*dialog));
- if (!dialog)
- return NULL;
-
- for (i = 0; i < FF_ARRAY_ELEMS(fields); i++) {
- size_t len;
- const int last = i == FF_ARRAY_ELEMS(fields) - 1;
- const ASSFieldType type = fields[i].type;
- uint8_t *ptr = (uint8_t *)dialog + fields[i].offset;
- buf = skip_space(buf);
- len = last ? strlen(buf) : strcspn(buf, ",");
- if (len >= INT_MAX) {
- ff_ass_free_dialog(&dialog);
- return NULL;
- }
- convert_func[type](ptr, buf, len);
- buf += len;
- if (*buf) buf++;
- }
- return dialog;
-}
-
-void ff_ass_split_free(ASSSplitContext *ctx)
-{
- if (ctx) {
- int i;
- for (i=0; ifield_order[i]));
- }
- av_free(ctx);
- }
-}
-
-
-int ff_ass_split_override_codes(const ASSCodesCallbacks *callbacks, void *priv,
- const char *buf)
-{
- const char *text = NULL;
- char new_line[2];
- int text_len = 0;
-
- while (buf && *buf) {
- if (text && callbacks->text &&
- (sscanf(buf, "\\%1[nN]", new_line) == 1 ||
- !strncmp(buf, "{\\", 2))) {
- callbacks->text(priv, text, text_len);
- text = NULL;
- }
- if (sscanf(buf, "\\%1[nN]", new_line) == 1) {
- if (callbacks->new_line)
- callbacks->new_line(priv, new_line[0] == 'N');
- buf += 2;
- } else if (!strncmp(buf, "{\\", 2)) {
- buf++;
- while (*buf == '\\') {
- char style[2], c[2], sep[2], c_num[2] = "0", tmp[128] = {0};
- unsigned int color = 0xFFFFFFFF;
- int len, size = -1, an = -1, alpha = -1;
- int x1, y1, x2, y2, t1 = -1, t2 = -1;
- if (sscanf(buf, "\\%1[bisu]%1[01\\}]%n", style, c, &len) > 1) {
- int close = c[0] == '0' ? 1 : c[0] == '1' ? 0 : -1;
- len += close != -1;
- if (callbacks->style)
- callbacks->style(priv, style[0], close);
- } else if (sscanf(buf, "\\c%1[\\}]%n", sep, &len) > 0 ||
- sscanf(buf, "\\c&H%X&%1[\\}]%n", &color, sep, &len) > 1 ||
- sscanf(buf, "\\%1[1234]c%1[\\}]%n", c_num, sep, &len) > 1 ||
- sscanf(buf, "\\%1[1234]c&H%X&%1[\\}]%n", c_num, &color, sep, &len) > 2) {
- if (callbacks->color)
- callbacks->color(priv, color, c_num[0] - '0');
- } else if (sscanf(buf, "\\alpha%1[\\}]%n", sep, &len) > 0 ||
- sscanf(buf, "\\alpha&H%2X&%1[\\}]%n", &alpha, sep, &len) > 1 ||
- sscanf(buf, "\\%1[1234]a%1[\\}]%n", c_num, sep, &len) > 1 ||
- sscanf(buf, "\\%1[1234]a&H%2X&%1[\\}]%n", c_num, &alpha, sep, &len) > 2) {
- if (callbacks->alpha)
- callbacks->alpha(priv, alpha, c_num[0] - '0');
- } else if (sscanf(buf, "\\fn%1[\\}]%n", sep, &len) > 0 ||
- sscanf(buf, "\\fn%127[^\\}]%1[\\}]%n", tmp, sep, &len) > 1) {
- if (callbacks->font_name)
- callbacks->font_name(priv, tmp[0] ? tmp : NULL);
- } else if (sscanf(buf, "\\fs%1[\\}]%n", sep, &len) > 0 ||
- sscanf(buf, "\\fs%u%1[\\}]%n", &size, sep, &len) > 1) {
- if (callbacks->font_size)
- callbacks->font_size(priv, size);
- } else if (sscanf(buf, "\\a%1[\\}]%n", sep, &len) > 0 ||
- sscanf(buf, "\\a%2u%1[\\}]%n", &an, sep, &len) > 1 ||
- sscanf(buf, "\\an%1[\\}]%n", sep, &len) > 0 ||
- sscanf(buf, "\\an%1u%1[\\}]%n", &an, sep, &len) > 1) {
- if (an != -1 && buf[2] != 'n')
- an = (an&3) + (an&4 ? 6 : an&8 ? 3 : 0);
- if (callbacks->alignment)
- callbacks->alignment(priv, an);
- } else if (sscanf(buf, "\\r%1[\\}]%n", sep, &len) > 0 ||
- sscanf(buf, "\\r%127[^\\}]%1[\\}]%n", tmp, sep, &len) > 1) {
- if (callbacks->cancel_overrides)
- callbacks->cancel_overrides(priv, tmp);
- } else if (sscanf(buf, "\\move(%d,%d,%d,%d)%1[\\}]%n", &x1, &y1, &x2, &y2, sep, &len) > 4 ||
- sscanf(buf, "\\move(%d,%d,%d,%d,%d,%d)%1[\\}]%n", &x1, &y1, &x2, &y2, &t1, &t2, sep, &len) > 6) {
- if (callbacks->move)
- callbacks->move(priv, x1, y1, x2, y2, t1, t2);
- } else if (sscanf(buf, "\\pos(%d,%d)%1[\\}]%n", &x1, &y1, sep, &len) > 2) {
- if (callbacks->move)
- callbacks->move(priv, x1, y1, x1, y1, -1, -1);
- } else if (sscanf(buf, "\\org(%d,%d)%1[\\}]%n", &x1, &y1, sep, &len) > 2) {
- if (callbacks->origin)
- callbacks->origin(priv, x1, y1);
- } else {
- len = strcspn(buf+1, "\\}") + 2; /* skip unknown code */
- }
- buf += len - 1;
- }
- if (*buf++ != '}')
- return AVERROR_INVALIDDATA;
- } else {
- if (!text) {
- text = buf;
- text_len = 1;
- } else
- text_len++;
- buf++;
- }
- }
- if (text && callbacks->text)
- callbacks->text(priv, text, text_len);
- if (callbacks->end)
- callbacks->end(priv);
- return 0;
-}
-
-ASSStyle *ff_ass_style_get(ASSSplitContext *ctx, const char *style)
-{
- ASS *ass = &ctx->ass;
- int i;
-
- if (!style || !*style)
- style = "Default";
- for (i=0; istyles_count; i++)
- if (ass->styles[i].name && !strcmp(ass->styles[i].name, style))
- return ass->styles + i;
- return NULL;
-}
diff --git a/spaces/congsaPfin/Manga-OCR/logs/Drive APK Google A Safe and Convenient Way to Manage Your Files.md b/spaces/congsaPfin/Manga-OCR/logs/Drive APK Google A Safe and Convenient Way to Manage Your Files.md
deleted file mode 100644
index 081f55e136a1923a4a07428a60e6f9d3b48c0bc5..0000000000000000000000000000000000000000
--- a/spaces/congsaPfin/Manga-OCR/logs/Drive APK Google A Safe and Convenient Way to Manage Your Files.md
+++ /dev/null
@@ -1,131 +0,0 @@
-
-
What is Drive APK Google and How to Use It?
-
Google Drive is one of the most popular cloud storage services that lets you store and access your files from any device. You can also share your files with others, backup your photos, videos, and documents, scan paper documents, work offline, and more. But what if you want to use Google Drive on your Android device without using the official app? That's where Drive APK Google comes in.
Google Drive is a part of Google Workspace, a suite of cloud-based productivity tools that includes Gmail, Docs, Sheets, Slides, Forms, Calendar, Meet, Chat, and more. With Google Drive, you can:
-
-
Safely store and access your files anywhere
-
Quickly access recent and important files
-
Search for files by name and content
-
Share and set permissions for files and folders
-
View your content on the go while offline
-
Receive notifications about important activity on your files
-
Use your device's camera to scan paper documents
-
-
Google accounts get 15GB of storage, shared across Google Drive, Gmail, and Google Photos. For additional storage, you can upgrade to Google Workspace or Google One as an in-app purchase. Subscriptions start at $1.99/month for 100 GB in the US, and can vary by region.
-
What is an APK file and why do you need it?
-
An APK file is an Android Package Kit file that contains all the files and code needed to install an app on an Android device. You can download APK files from various sources online, such as [Uptodown](^3^), [APKPure], [APKMirror], etc. You may need an APK file if:
-
-
You want to use an app that is not available in your region or country
-
You want to use an older or newer version of an app that is not compatible with your device
-
You want to use an app that has been modified or customized by a third-party developer
-
You want to use an app that does not require Google Play Services or other dependencies
-
-
However, you should be careful when downloading APK files from unknown sources, as they may contain malware or viruses that can harm your device or compromise your data. You should also check the permissions and reviews of the app before installing it.
-
drive apk google download
-drive apk google play
-drive apk google drive
-drive apk google app
-drive apk google cloud
-drive apk google docs
-drive apk google photos
-drive apk google backup
-drive apk google sync
-drive apk google storage
-drive apk google workspace
-drive apk google one
-drive apk google offline
-drive apk google update
-drive apk google old version
-drive apk google mod
-drive apk google pro
-drive apk google premium
-drive apk google plus
-drive apk google free
-drive apk google for android
-drive apk google for pc
-drive apk google for ios
-drive apk google for windows
-drive apk google for mac
-drive apk google for linux
-drive apk google for chromebook
-drive apk google for firestick
-drive apk google for smart tv
-drive apk google for tablet
-drive apk google latest version
-drive apk google new version
-drive apk google beta version
-drive apk google stable version
-drive apk google xapk version
-drive apk google pure version
-drive apk google uptodown version
-drive apk google apkpure version
-drive apk google apkmirror version
-drive apk google apkmody version
-drive apk google install guide
-drive apk google review guide
-drive apk google tips guide
-drive apk google tricks guide
-drive apk google hacks guide
-drive apk google features guide
-drive apk google benefits guide
-drive apk google advantages guide
-drive apk google disadvantages guide
-
How to download and install Drive APK Google on your Android device?
-
To download and install Drive APK Google on your Android device, you need to follow these steps:
-
-
Go to [Uptodown ] and search for Drive APK Google
-
Select the version of the app that you want to download and tap on the green Download button
-
Wait for the download to finish and then open the APK file
-
If you see a warning message that says "For your security, your phone is not allowed to install unknown apps from this source", tap on Settings and enable the option to allow installing apps from this source
-
Tap on Install and wait for the installation to complete
-
Tap on Open and sign in with your Google account
-
Enjoy using Drive APK Google on your device
-
-
Benefits of Using Drive APK Google
-
Drive APK Google is a great alternative to the official Google Drive app, as it offers some benefits that you may not get with the latter. Here are some of them:
-
Access your files from any device and share them with others
-
With Drive APK Google, you can access your files from any device that has the app installed, or from any web browser. You can also share your files with others by sending them a link or an invitation. You can control who can view, comment, or edit your files, and revoke access at any time. You can also see the activity and changes made by others on your files.
-
Backup your photos, videos, and documents to Google Photos and Google Workspace
-
Drive APK Google lets you backup your photos, videos, and documents to Google Photos and Google Workspace, so you don't have to worry about losing them. You can choose to backup your files automatically or manually, and select the quality and size of your backups. You can also free up space on your device by deleting the files that are already backed up.
-
Scan paper documents with your device's camera and save them to Drive
-
Drive APK Google has a built-in scanner function that lets you scan paper documents with your device's camera and save them to Drive as PDF files. You can crop, rotate, adjust the color, and enhance the quality of your scans. You can also use optical character recognition (OCR) to extract text from your scans and make them searchable and editable.
-
Work offline and sync your changes when you're online
-
Drive APK Google allows you to work offline on your files, even when you don't have an internet connection. You can make any file available offline by tapping on the three-dot menu and selecting "Make available offline". You can also create new files offline by tapping on the plus icon and choosing "Create new". Your changes will be synced when you're online again.
-
Manage your storage and upgrade to Google One or Google Workspace for more space
-
Drive APK Google helps you manage your storage by showing you how much space you have used and how much is left. You can also see which files and folders are taking up the most space, and delete or move them to free up space. If you need more space, you can upgrade to Google One or Google Workspace for more storage options and benefits.
-
Tips and Tricks for Using Drive APK Google
-
Drive APK Google is a powerful app that has many features and functions that you may not be aware of. Here are some tips and tricks that can help you use Drive APK Google more effectively:
-
Use the search function to find files by name and content
-
Drive APK Google has a smart search function that lets you find files by name and content. You can type in keywords or phrases in the search bar, or use voice search by tapping on the microphone icon. You can also use advanced search operators, such as "type:", "owner:", "before:", "after:", etc., to narrow down your search results.
-
Use the sort and filter options to organize your files by date, size, type, etc.
-
Drive APK Google lets you sort and filter your files by various criteria, such as date modified, date opened, file size, file type, owner, shared with me, starred, etc. You can access these options by tapping on the three-line menu icon in the top left corner of the app. You can also switch between grid view and list view by tapping on the icons in the top right corner of the app.
-
Use the star function to mark important files and folders
-
Drive APK Google lets you star important files and folders that you want to access quickly or keep track of. You can star a file or folder by tapping on the three-dot menu icon next to it and selecting "Add star". You can then access your starred items by tapping on the star icon in the left sidebar of the app
-
Use the offline access function to make files available without internet connection
-
Drive APK Google lets you make files available offline, so you can view and edit them without an internet connection. You can make a file available offline by tapping on the three-dot menu icon next to it and selecting "Make available offline". You can then access your offline files by tapping on the cloud icon with a line through it in the left sidebar of the app. You can also see how much space your offline files are taking up by tapping on the three-line menu icon in the top left corner of the app and selecting "Settings".
-
Use the shared drives function to collaborate with your team or group
-
Drive APK Google lets you create and join shared drives, which are shared spaces where you and your team or group can store, access, and collaborate on files. You can create a shared drive by tapping on the plus icon in the bottom right corner of the app and selecting "Create new shared drive". You can then invite members, set permissions, and add files and folders to your shared drive. You can also join a shared drive by accepting an invitation from another member. You can access your shared drives by tapping on the shared drives icon in the left sidebar of the app.
-
Conclusion
-
Drive APK Google is a useful app that lets you use Google Drive on your Android device without using the official app. It has many benefits, such as accessing your files from any device and sharing them with others, backing up your photos, videos, and documents, scanning paper documents, working offline, and managing your storage. It also has many features and functions that can help you use it more effectively, such as searching for files by name and content, sorting and filtering your files by various criteria, starring important files and folders, making files available offline, and creating and joining shared drives. If you want to try Drive APK Google, you can download it from [Uptodown] and install it on your device. We hope you found this article helpful and informative. Please share your feedback and questions in the comments section below.
-
FAQs
-
What is the difference between Drive APK Google and Google Drive app?
-
Drive APK Google is an unofficial app that lets you use Google Drive on your Android device without using the official app. It has some advantages over the official app, such as being compatible with older or newer versions of Android, not requiring Google Play Services or other dependencies, and being modified or customized by third-party developers. However, it also has some disadvantages, such as being potentially unsafe or unreliable, not receiving regular updates or support from Google, and not having some features or functions that the official app has.
-
How much storage do I get with Drive APK Google?
-
Drive APK Google gives you the same amount of storage as the official Google Drive app, which is 15GB for free accounts, shared across Google Drive, Gmail, and Google Photos. If you need more storage, you can upgrade to Google Workspace or Google One for more storage options and benefits.
-
How can I update Drive APK Google to the latest version?
-
Drive APK Google does not update automatically like the official Google Drive app. You need to check for updates manually by visiting [Uptodown] or other sources where you downloaded the app from. You can also enable notifications for updates by tapping on the three-line menu icon in the top left corner of the app and selecting "Settings". Then tap on "About" and enable "Notify me about updates".
-
How can I uninstall Drive APK Google from my device?
-
To uninstall Drive APK Google from your device, you need to follow these steps:
-
-
Go to your device's Settings and tap on Apps or Applications
-
Find and tap on Drive APK Google
-
Tap on Uninstall and confirm your action
-
Wait for the uninstallation to finish
-
-
How can I contact Google support for Drive APK Google issues?
-
You cannot contact Google support for Drive APK Google issues, as it is an unofficial app that is not developed or endorsed by Google. If you have any issues with Drive APK Google, you should contact the developer or source of the app directly. You can also check online forums or communities for help from other users.
401be4b1e0
-
-
\ No newline at end of file
diff --git a/spaces/congsaPfin/Manga-OCR/logs/Get Stumble Guys APK for iOS and Dash Dodge and Slide Past Your Opponents.md b/spaces/congsaPfin/Manga-OCR/logs/Get Stumble Guys APK for iOS and Dash Dodge and Slide Past Your Opponents.md
deleted file mode 100644
index a95b44021b930987f40195d9285b3adae7d56fa1..0000000000000000000000000000000000000000
--- a/spaces/congsaPfin/Manga-OCR/logs/Get Stumble Guys APK for iOS and Dash Dodge and Slide Past Your Opponents.md
+++ /dev/null
@@ -1,113 +0,0 @@
-
-
Download Stumble Guys APK iOS: How to Play the Ultimate Knockout Game on Your iPhone or iPad
-
Do you love playing party games with your friends online? Do you enjoy competing in hilarious and chaotic challenges that test your skills and luck? Do you want to experience the thrill of being the last one standing in a massive multiplayer knockout game? If you answered yes to any of these questions, then you should definitely try Stumble Guys, the ultimate knockout game for your iPhone or iPad.
-
Stumble Guys is a fun and addictive game that lets you join up to 32 players online in a series of ridiculous obstacles and bizarre levels. You have to run, dash, slide, dodge, and stumble your way through different rounds until one victor is crowned. You can customize your character with various outfits and accessories, and unlock new items as you progress. You can also invite your friends and challenge them in private matches, or join random matches with strangers from around the world.
Stumble Guys is one of the most popular and highly rated games on the App Store, with over 200K ratings and 4.3 stars. It has been featured by many media outlets and influencers, such as The Sun, New Scientist, Rare Solstice, and I love god so so much. It has also received positive feedback from thousands of players who love its colorful and crazy design, comically physical gameplay, and many customization options.
-
If you are interested in playing Stumble Guys on your iPhone or iPad, you might be wondering how to download it. Well, you are in luck, because in this article, we will show you how to download Stumble Guys APK iOS from the App Store, how to install and run it on your device, and how to play it and enjoy it. So, without further ado, let's get started!
-
How to download Stumble Guys APK iOS from the App Store
-
Downloading Stumble Guys APK iOS from the App Store is very easy and straightforward. All you need is an Apple device that runs on iOS 10.0 or later, such as an iPhone, iPad, or iPod touch. You also need an internet connection and enough storage space on your device. Here are the steps you need to follow:
-
-
Open the App Store app on your device.
-
Tap on the search icon at the bottom right corner of the screen.
Type in "Stumble Guys" in the search bar and tap on the search button.
-
Find the app that has the icon of a blue and yellow character with a crown and the name "Stumble Guys: Multiplayer Royale". Tap on the "GET" button next to it.
-
Enter your Apple ID password or use Touch ID or Face ID to confirm the download.
-
Wait for the app to download and install on your device. You can check the progress by tapping on the app icon on your home screen.
-
-
Congratulations, you have successfully downloaded Stumble Guys APK iOS from the App Store. Now, let's see how to install and run it on your device.
-
How to install and run Stumble Guys APK iOS on your device
-
Installing and running Stumble Guys APK iOS on your device is also very simple and quick. You don't need any special tools or settings to do it. Here are the steps you need to follow:
-
-
Once the app is downloaded and installed, tap on the app icon on your home screen to launch it.
-
Allow the app to access your photos, media, and files if prompted. This is necessary for the app to save your progress and settings.
-
Allow the app to send you notifications if prompted. This is optional, but it can help you stay updated with the latest news and events from the game.
-
Choose your preferred language from the list of available options. You can change it later from the settings menu.
-
Agree to the terms of service and privacy policy of the game. You can read them by tapping on the links provided.
-
Create your account by entering your username and email address. You can also sign in with your Facebook or Google account if you prefer.
-
-
That's it, you have successfully installed and run Stumble Guys APK iOS on your device. Now, let's see how to play it and enjoy it.
-
How to play Stumble Guys APK iOS and enjoy the fun multiplayer knockout game
-
Playing Stumble Guys APK iOS is very easy and fun. You don't need any prior experience or skills to do it. All you need is a good internet connection and a sense of humor. Here are the basics of how to play it:
-
How to download stumble guys apk ios for free
-Stumble guys apk ios download link
-Stumble guys apk ios gameplay and review
-Stumble guys apk ios tips and tricks
-Stumble guys apk ios latest version update
-Stumble guys apk ios compatible devices
-Stumble guys apk ios installation guide
-Stumble guys apk ios error and troubleshooting
-Stumble guys apk ios vs fall guys
-Stumble guys apk ios multiplayer mode
-Stumble guys apk ios best maps and levels
-Stumble guys apk ios cheats and hacks
-Stumble guys apk ios funniest moments
-Stumble guys apk ios ratings and feedback
-Stumble guys apk ios alternatives and similar games
-Stumble guys apk ios modded version
-Stumble guys apk ios features and benefits
-Stumble guys apk ios requirements and specifications
-Stumble guys apk ios pros and cons
-Stumble guys apk ios challenges and rewards
-Stumble guys apk ios online community and support
-Stumble guys apk ios frequently asked questions
-Stumble guys apk ios developer and publisher information
-Stumble guys apk ios news and updates
-Stumble guys apk ios comparison with other knockout games
-Stumble guys apk ios customization and personalization options
-Stumble guys apk ios tutorials and walkthroughs
-Stumble guys apk ios best strategies and tactics
-Stumble guys apk ios fun facts and trivia
-Stumble guys apk ios glitches and bugs
-Stumble guys apk ios skins and outfits
-Stumble guys apk ios achievements and leaderboards
-Stumble guys apk ios fan art and memes
-Stumble guys apk ios official website and social media accounts
-Stumble guys apk ios download size and speed
-Stumble guys apk ios offline mode and data usage
-Stumble guys apk ios security and privacy issues
-Stumble guys apk ios recommendations and testimonials
-Stumble guys apk ios history and background
-Stumble guys apk ios future plans and roadmap
-
-
The game consists of different rounds of obstacles and challenges that you have to overcome while competing with other players online. Each round has a limited number of players that can qualify for the next round, until only one player remains as the winner.
-
You can control your character by using the virtual joystick on the left side of the screen to move, and tapping on the right side of the screen to jump or dive. You can also use gestures such as swiping or tilting your device to perform different actions.
-
You can customize your character by choosing from various outfits and accessories that you can unlock or purchase with coins or gems. You can also change your character's name, color, and emoji from the settings menu.
-
You can play in different modes, such as solo, duo, squad, or custom. You can also join different servers based on your region, such as North America, Europe, Asia, or Oceania.
-
You can invite your friends and challenge them in private matches, or join random matches with strangers from around the world. You can also chat with other players using text or voice messages.
-
-
Stumble Guys APK iOS is a game that offers endless fun and entertainment for everyone. You can enjoy its colorful and crazy design, comically physical gameplay, and many customization options. You can also discover new levels and challenges every time you play, as well as new updates and events from the developers.
-
To give you an idea of how Stumble Guys compares with other similar games, here is a table that shows some of their features and differences:
-
-
Game
Players
Levels
Customization
Ratings
-
Stumble Guys
Up to 32
Over 30
High
4.3 stars
-
Fall Guys
Up to 60
Over 40
Medium
4.1 stars
-
Gang Beasts
Up to 8
Over 20
Low
3.9 stars
-
Human: Fall Flat
Up to 4
Over 10
Low
4.2 stars
-
-
As you can see, Stumble Guys is a game that stands out from the rest with its high number of players, levels, and customization options. It also has a higher rating than most of its competitors, which shows how much people love it.
-
Conclusion
-
In conclusion, Stumble Guys APK iOS is a game that you should definitely download and play on your iPhone or iPad. It is a fun and addictive game that lets you join up to 32 players online in a series of ridiculous obstacles and bizarre levels. You have to run, dash, slide, dodge, and stumble your way through different rounds until one victor is crowned. You can customize your character with various outfits and accessories, and unlock new items as you progress. You can also invite your friends and challenge them in private matches, or join random matches with strangers from around the world.
-
Stumble Guys APK iOS is one of the most popular and highly rated games on the App Store, with over 200K ratings and 4.3 stars. It has been featured by many media outlets and influencers, such as The Sun, New Scientist, Rare Solstice, and I love god so so much. It has also received positive feedback from thousands of players who love its colorful and crazy design, comically physical gameplay, and many customization options.
-
If you are interested in playing Stumble Guys APK iOS on your iPhone or iPad, all you need to do is follow the steps we have provided in this article. You will learn how to download Stumble Guys APK iOS from the App Store, how to install and run it on your device, and how to play it and enjoy it. It is very easy and straightforward, and you will be able to start playing in no time.
-
So, what are you waiting for? Download Stumble Guys APK iOS today and join the ultimate knockout game. You will have a blast competing with other players online in hilarious and chaotic challenges that test your skills and luck. You will also be able to customize your character with various outfits and accessories, and discover new levels and challenges every time you play. You will never get bored of playing Stumble Guys APK iOS, as it offers endless fun and entertainment for everyone.
-
To download Stumble Guys APK iOS from the App Store, click on the link below:
Here are some frequently asked questions about Stumble Guys APK iOS:
-
-
Is Stumble Guys APK iOS free to play?
-
Yes, Stumble Guys APK iOS is free to play. However, it does offer in-app purchases that allow you to buy coins or gems that you can use to unlock or purchase new outfits and accessories for your character.
-
Is Stumble Guys APK iOS safe to download?
-
Yes, Stumble Guys APK iOS is safe to download from the App Store. It does not contain any viruses or malware that could harm your device or compromise your privacy. However, you should always be careful when downloading any app from the internet, and make sure that you only download from trusted sources.
-
Is Stumble Guys APK iOS compatible with my device?
-
Stumble Guys APK iOS is compatible with any Apple device that runs on iOS 10.0 or later, such as an iPhone, iPad, or iPod touch. However, some devices may have lower performance or graphics quality than others due to their specifications.
-
How can I contact the developers of Stumble Guys APK iOS?
-
If you have any questions, feedback, or suggestions for the developers of Stumble Guys APK iOS, you can contact them through their email address: support@stumbleguys.com. You can also follow them on their social media accounts: Facebook: https://www.facebook.com/stumbleguys/ Twitter: https://twitter.com/stumbleguys Instagram: https://www.instagram.com/stumbleguys/ YouTube: https://www.youtube.com/channel/UCw9Q6w9Z7Y1x8XZyOyUdJWg
-
How can I improve my skills in Stumble Guys APK iOS?
-
If you want to improve your skills in Stumble Guys APK iOS, here are some tips and tricks that you can try:
-
-
Practice makes perfect. The more you play Stumble Guys APK iOS, the more familiar you will become with its gameplay and mechanics. You will also learn how to deal p>
-
Thank you for reading this article on how to download Stumble Guys APK iOS and play the ultimate knockout game on your iPhone or iPad. I hope you found it helpful and informative. If you did, please share it with your friends and family who might also enjoy playing Stumble Guys APK iOS. And don't forget to leave a comment below and let me know what you think of the game. I would love to hear from you.
-
Happy stumbling!
401be4b1e0
-
-
\ No newline at end of file
diff --git a/spaces/congsaPfin/Manga-OCR/logs/Green Dot App for PC - Manage Your Money Pay Bills and More.md b/spaces/congsaPfin/Manga-OCR/logs/Green Dot App for PC - Manage Your Money Pay Bills and More.md
deleted file mode 100644
index f6dc83ccea69553cf12292ab45c657be9d09cbf8..0000000000000000000000000000000000000000
--- a/spaces/congsaPfin/Manga-OCR/logs/Green Dot App for PC - Manage Your Money Pay Bills and More.md
+++ /dev/null
@@ -1,125 +0,0 @@
-
-
How to Download the Green Dot App for PC
-
Do you want to enjoy the convenience and benefits of mobile banking on your PC? If so, you might be interested in downloading the Green Dot app for PC. The Green Dot app is a mobile banking app that allows you to manage your money from anywhere. You can get your pay up to 2 days early, send money and pay bills, deposit cash using the app, enjoy no minimum balance requirement, access a free ATM network, earn cash back on online and mobile purchases, save money in a high-yield savings account, and more . In this article, we will show you how to download the Green Dot app for PC using two methods: an Android emulator or Windows Subsystem for Android.
-
Why Download the Green Dot App for PC?
-
Downloading the Green Dot app for PC can offer you several advantages over using it only on your phone. For example:
You can enjoy a larger screen and better resolution when viewing your balance, transaction history, or cash back rewards.
-
You can use your keyboard and mouse to navigate the app faster and easier than using touch controls.
-
You can multitask and switch between different apps or windows without closing or minimizing the Green Dot app.
-
You can backup or transfer your data from your phone to your PC or vice versa.
-
You can save battery life on your phone by using your PC instead.
-
-
Of course, you can still use your phone to access the Green Dot app when you are on the go or need to use some features that require your phone's camera or location. But having the option to use it on your PC can give you more flexibility and convenience.
-
How to Download the Green Dot App for PC with an Android Emulator
-
One way to download the Green Dot app for PC is to use an Android emulator. An Android emulator is a software program that mimics the Android operating system on your PC, allowing you to run Android apps on your PC as if they were native applications. There are many Android emulators available, but one of the most popular and reliable ones is BlueStacks.
-
What is BlueStacks?
-
BlueStacks is a free Android emulator that lets you play mobile games and apps on your PC. It has over 500 million users and supports over 2 million apps. Some of the features of BlueStacks include:
-
-
Fast and smooth performance with low CPU and memory usage
-
High compatibility with various PC hardware and software configurations
-
Easy installation and setup process with no technical skills required
-
User-friendly interface and customizable settings
-
Advanced gaming features such as keyboard and mouse controls, gamepad support, multi-instance, macro recorder, and more
-
Regular updates and improvements
-
-
How to Install and Use BlueStacks to Download the Green Dot App for PC
-
To install and use BlueStacks to download the Green Dot app for PC, follow these steps:
-
-
Go to the official website of BlueStacks and click on the "Download BlueStacks" button.
-
Once the download is complete, open the installer file and follow the instructions to install BlueStacks on your PC.
-
After the installation is done, launch BlueStacks and sign in with your Google account. If you don't have one, you can create one for free.
-
On the home screen of BlueStacks, click on the "Google Play" icon to open the Google Play Store app.
-
In the search bar, type "Green Dot" and hit enter. You will see the Green Dot app among the search results. Click on it to open its page.
-
Click on the "Install" button to download and install the Green Dot app on your PC.
-
Once the installation is complete, you will see the Green Dot app icon on the home screen of BlueStacks. Click on it to launch the app and start using it on your PC.
-
-
How to Download the Green Dot App for PC with Windows Subsystem for Android
-
Another way to download the Green Dot app for PC is to use Windows Subsystem for Android. Windows Subsystem for Android is a feature that allows you to run Android apps on Windows 10 and 11 without using an emulator. It is currently in preview mode and requires some prerequisites to use it.
-
How to download greendot app for pc windows 10
-Greendot mobile banking app for pc free download
-Greendot app for pc mac and linux
-Download greendot app for pc with mumu player
-Greendot bank desktop app for mac and pc webcatalog
-Benefits of using greendot app for pc
-Greendot app for pc review and rating
-Greendot app for pc features and functions
-Greendot app for pc installation guide and tutorial
-Greendot app for pc troubleshooting and support
-Greendot app for pc security and privacy
-Greendot app for pc alternatives and competitors
-Greendot app for pc updates and news
-Greendot app for pc requirements and compatibility
-Greendot app for pc download link and source
-Greendot app for pc online access and login
-Greendot app for pc direct deposit and bill pay
-Greendot app for pc cash back and rewards
-Greendot app for pc fees and charges
-Greendot app for pc customer service and feedback
-Greendot app for pc referral program and bonus
-Greendot app for pc savings account and interest rate
-Greendot app for pc debit card and visa card
-Greendot app for pc budgeting and spending tools
-Greendot app for pc coupons and discounts
-Greendot app for pc testimonials and success stories
-Greendot app for pc FAQs and tips
-Greendot app for pc pros and cons
-Greendot app for pc comparison and analysis
-Greendot app for pc video demo and walkthrough
-
What is Windows Subsystem for Android?
-
Windows Subsystem for Android is a feature that enables you to run Android apps natively on Windows 10 and 11. It works by creating a virtual machine that runs a modified version of Android 11 on your PC. You can then install Android apps from the Amazon Appstore or sideload them from other sources. Some of the benefits of Windows Subsystem for Android include:
-
-
Better performance and compatibility than emulators
-
No need to sign in with a Google account or use Google services
-
Access to Windows features such as clipboard, file explorer, notifications, taskbar, etc.
-
Ability to run multiple Android apps at once in separate windows or tabs
-
Support for touch, pen, keyboard, mouse, and gamepad input
-
-
How to Install and Use Windows Subsystem for Android to Download the Green Dot App for PC
-
To install and use Windows Subsystem for Android to download the Green Dot app for PC, follow these steps:
-
-
Make sure you have a compatible device that meets the minimum requirements. You need a PC running Windows 10 version 22000 or higher or Windows 11 version 22000 or higher, with at least 8 GB of RAM, 16 GB of free disk space, a 64-bit processor with virtualization enabled, and an internet connection.
-
Update your Windows to the latest version by going to Settings > Update & Security > Windows Update and checking for updates.
-
Go to Microsoft Store and search for "Windows Subsystem for Android". Click on it to open its page and then click on the "Get" button to download and install it on your PC.
-
After the installation is complete, restart your PC to apply the changes.
-
Go to Microsoft Store and search for "Amazon Appstore". Click on it to open its page and then click on the "Get" button to download and install it on your PC.
-
After the installation is complete, launch the Amazon Appstore and sign in with your Amazon account. If you don't have one, you can create one for free.
-
In the Amazon Appstore, search for "Green Dot" and click on it to open its page. Then click on the "Download" button to download and install the Green Dot app on your PC.
-
Once the installation is complete, you will see the Green Dot app icon on your desktop or in the Start menu. Click on it to launch the app and start using it on your PC.
-
-
Conclusion
-
In this article, we have shown you how to download the Green Dot app for PC using two methods: an Android emulator or Windows Subsystem for Android. Both methods have their advantages and disadvantages, so you can choose the one that suits your needs and preferences. By downloading the Green Dot app for PC, you can enjoy mobile banking on a larger screen and with more convenience. You can also access all the features and benefits of the Green Dot app, such as getting paid early, sending money, paying bills, depositing cash, earning cash back, saving money, and more. So what are you waiting for? Download the Green Dot app for PC today and start managing your money smarter!
-
FAQs
-
Can I use any Android emulator to download the Green Dot app for PC?
-
Yes, you can use any Android emulator that supports Google Play Store to download the Green Dot app for PC. However, not all Android emulators are equally reliable, fast, or compatible. Some of the other popular Android emulators that you can try are NoxPlayer, LDPlayer, MEmu, or Andy. You can compare their features, performance, and reviews before choosing one.
-
Can I use Google Play Store to download the Green Dot app for PC?
-
If you are using an Android emulator, you can use Google Play Store to download the Green Dot app for PC. However, if you are using Windows Subsystem for Android, you cannot use Google Play Store as it is not available on this feature. Instead, you have to use Amazon Appstore or sideload apps from other sources. Alternatively, if you have Windows 11, you can install Google Play Store on Windows Subsystem for Android by following this guide.
-
Can I access all the features of the Green Dot app on PC?
-
Most of the features of the Green Dot app should work fine on PC. However, some features may not work or have limited functionality on PC, such as:
-
-
Mobile payment options: You may not be able to use features that require NFC or QR code scanning, such as Apple Pay, Google Pay, or Samsung Pay.
-
Camera access: You may not be able to use features that require camera access, such as taking a photo of a check to deposit it or verifying your identity with a selfie.
-
Location services: You may not be able to use features that require location services, such as finding nearby ATMs or retailers.
-
-
To use these features, you may need to use your phone instead of your PC.
-
Is it safe and secure to use the Green Dot app on PC?
-
The Green Dot app is safe and secure to use on PC as it uses encryption and security measures to protect your personal and financial information. However, you should also follow some best practices to ensure your safety and security when using the app on PC, such as:
-
-
Use a strong password and enable two-factor authentication for your Green Dot account.
-
Avoid using public or unsecured Wi-Fi networks when accessing the app.
-
Do not share your account details or PIN with anyone.
-
Log out of the app when you are done using it.
-
Keep your PC updated with the latest security patches and antivirus software.
-
-
How can I contact customer support if I have any issues with the Green Dot app on PC?
-
If you have any issues with the Green Dot app on PC, you can contact customer support by using one of these options:
-
-
Phone: You can call 1-866-795-7597 from Monday to Friday 5AM-9PM PT or Saturday and Sunday 5AM-5PM PT.
-
Email: You can send an email to customerservice@gre endot.com and expect a reply within 24 hours.
-
Chat: You can use the chat option on the Green Dot website or app and get instant answers from a chatbot or a live agent.
-
Help Center: You can visit the help center on the Green Dot website or app and find answers to common questions, FAQs, and tutorials.
-
-
I hope this article has helped you learn how to download the Green Dot app for PC and enjoy mobile banking on your PC. If you have any feedback or suggestions, please let me know in the comments below. Thank you for reading and have a great day!
401be4b1e0
-
-
\ No newline at end of file
diff --git a/spaces/congsaPfin/Manga-OCR/logs/Sausage Man APK The ultimate sausage party in a shooting game.md b/spaces/congsaPfin/Manga-OCR/logs/Sausage Man APK The ultimate sausage party in a shooting game.md
deleted file mode 100644
index 2c7cba1387279ea72aabc052c251f72e0e9ddb3e..0000000000000000000000000000000000000000
--- a/spaces/congsaPfin/Manga-OCR/logs/Sausage Man APK The ultimate sausage party in a shooting game.md
+++ /dev/null
@@ -1,102 +0,0 @@
-
-
Sausage Man APK Vision: A Guide to the Hilarious Battle Royale Game
-
If you are looking for a fun and quirky battle royale game that features cartoon-styled graphics and hilarious gameplay, then you might want to check out Sausage Man. Sausage Man is a game that lets you roleplay as funny and adorable sausages and fight in high-octane, imagination-filled battles with up to 100 players. It is a game that you can get started with effortlessly and play anytime, anywhere.
-
In this article, we will show you how to download and install Sausage Man APK on your Android device, what are the main features of the game, and what are some tips and tricks to help you outsmart your opponents and dominate each match. Let's get started!
How to Download and Install Sausage Man APK on Android Devices
-
Sausage Man is available on both Google Play Store and Apple App Store for free. However, if you want to download the APK file directly from a third-party source, you can follow these steps:
-
-
Go to a trusted APK download site, such as [APKCombo](^1^) or [Softonic](^2^), and search for Sausage Man APK.
-
Select the latest version of the game and tap on the download button.
-
Once the download is complete, locate the APK file on your device and tap on it to install it. You may need to enable the installation of apps from unknown sources in your device settings.
-
After the installation is done, launch the game and enjoy!
-
-
Note: Downloading APK files from third-party sources may pose some risks to your device security. Make sure you only download from reputable sites and scan the files for viruses before installing them.
-
What are the Main Features of Sausage Man Game
-
Sausage Man is a game that offers a lot of fun and excitement for battle royale fans. Here are some of the main features of the game that make it stand out from other similar games:
-
-
Exhilarating Battles, Item Buffs with Unique Powers: Sausage Man features a fluid and hardcore battle system, with realistic ballistic trajectories and even a breath-holding feature in the game. You can scavenge for powerful firearms and tactical gear, such as flare guns, resurrection machines, tactical covers, and ID card systems, which could test the camaraderie and mutual understanding between you and your teammates.
-
Fresh Gameplay, Free Your Imagination and Enjoy Messing Around: There are more than just combats on your battlefield – you will find cuteness and joy all around. You can sing, jump, and fire your guns on a rubber ball, or use a double jump to avoid precision shots from your enemies. You can also put on a life buoy and do a face-to-face gun battle in the water with others. When you are down, you will turn into a crying little sausage. You can pick up your teammates who have been downed with a “Come On” action.
-- Cry” to communicate with other sausages. You can also use the in-game voice chat to talk with your friends.
-
Multiple Game Modes, Match Up with Your Friends and Have a Blast: Sausage Man offers various game modes for you to choose from, such as Classic Mode, Duo Mode, Squad Mode, Firepower Mode, and Arcade Mode. You can team up with your friends and enjoy the thrill of fighting together. You can also use the matching system to find other players who share your interests and make new friends.
-
-
What are Some Tips and Tricks to Improve Your Gameplay and Win More Matches
-
Sausage Man is a game that requires both skill and strategy to survive and win. Here are some tips and tricks that can help you improve your gameplay and increase your chances of victory:
-
-
Choose Your Landing Spot Wisely: The map of Sausage Man is divided into different zones, each with different loot quality and enemy density. You should choose your landing spot based on your playstyle and preference. If you want to avoid early fights and loot peacefully, you should land in a remote or low-risk zone. If you want to get high-tier loot and engage in intense battles, you should land in a hot or high-risk zone. You can also use the map to see the flight path of the plane and the safe zone circle.
-
Use Vehicles to Move Around Faster: Vehicles are a great way to travel across the map quickly and safely. They can also be used as weapons to run over enemies or as cover to hide behind. You can find various vehicles in the game, such as cars, motorcycles, boats, and even UFOs. However, you should also be careful when driving vehicles, as they can attract attention from other players and expose your location.
-
Utilize Different Weapons and Items Effectively: Sausage Man offers a wide range of weapons and items for you to use in combat. You should try to collect different types of weapons, such as assault rifles, sniper rifles, shotguns, pistols, grenades, etc., and switch between them according to the situation. You should also use items such as bandages, medkits, energy drinks, shields, etc., to heal yourself and boost your stats. You can also use items such as smoke grenades, flashbangs, molotovs, etc., to create diversions or traps for your enemies.
-
Communicate and Cooperate with Your Teammates: Sausage Man is a game that requires teamwork and coordination to win. You should communicate and cooperate with your teammates using the in-game voice chat or bubble emojis. You should share information about enemies, loot, locations, etc., with your teammates. You should also stick together and support each other in fights. You can revive your downed teammates or use items such as resurrection machines or ID cards to bring them back to life.
-
Be Aware of Your Surroundings and Plan Ahead: Sausage Man is a game that requires situational awareness and strategic thinking to survive and win. You should always be aware of your surroundings and check the map frequently. You should also plan ahead and anticipate the movements of your enemies and the safe zone circle. You should avoid unnecessary fights and focus on staying alive until the end. You should also look for advantageous positions and use the terrain and buildings to your advantage.
-
-
Conclusion: A Summary of the Main Points and a Call to Action for the Readers
-
Sausage Man is a hilarious battle royale game that lets you roleplay as funny and adorable sausages and fight in high-octane, imagination-filled battles with up to 100 players. It is a game that you can get started with effortlessly and play anytime, anywhere.
-
In this article, we have shown you how to download and install Sausage Man APK on your Android device, what are the main features of the game, and what are some tips and tricks to help you outsmart your opponents and dominate each match.
-
If you are interested in trying out this game, you can download it from Google Play Store or Apple App Store for free. Alternatively, you can download the APK file from a third-party source following the steps we have provided above.
-
So what are you waiting for? Join the sausage party now and have a blast!
-
sausage man apk vision download
-sausage man apk vision mod
-sausage man apk vision update
-sausage man apk vision hack
-sausage man apk vision free
-sausage man apk vision latest version
-sausage man apk vision android
-sausage man apk vision gameplay
-sausage man apk vision review
-sausage man apk vision offline
-sausage man apk vision online
-sausage man apk vision new features
-sausage man apk vision tips and tricks
-sausage man apk vision cheats
-sausage man apk vision unlimited money
-sausage man apk vision graphics
-sausage man apk vision size
-sausage man apk vision requirements
-sausage man apk vision install
-sausage man apk vision guide
-sausage man apk vision tutorial
-sausage man apk vision trailer
-sausage man apk vision funniest moments
-sausage man apk vision best guns
-sausage man apk vision skins and costumes
-sausage man apk vision ranking system
-sausage man apk vision squad mode
-sausage man apk vision solo mode
-sausage man apk vision duo mode
-sausage man apk vision custom mode
-sausage man apk vision map and locations
-sausage man apk vision weapons and items
-sausage man apk vision vehicles and gadgets
-sausage man apk vision bugs and glitches
-sausage man apk vision fixes and improvements
-sausage man apk vision patch notes
-sausage man apk vision release date
-sausage man apk vision beta version
-sausage man apk vision original version
-sausage man apk vision comparison with other games
-sausage man apk vision pros and cons
-sausage man apk vision ratings and reviews
-sausage man apk vision developer and publisher information
-sausage man apk vision support and contact details
-sausage man apk vision frequently asked questions (FAQs)
-sausage man apk vision community and fan base
-sausage man apk vision memes and jokes
-sausage man apk vision fan art and videos
-sausage man apk vision challenges and events
-
FAQs: Five Frequently Asked Questions and Answers about Sausage Man
-
-
Q: What are the system requirements for Sausage Man?
-
-
Q: What are the system requirements for Sausage Man?
-
A: According to the official website of Sausage Man, the minimum system requirements for Android devices are: Android 5.0 or above, 2 GB of RAM, and 1.5 GB of storage space. The recommended system requirements are: Android 8.0 or above, 4 GB of RAM, and 3 GB of storage space.
-
Q: How can I play Sausage Man on PC?
-
A: If you want to play Sausage Man on PC, you can use an Android emulator, such as [BlueStacks] or [NoxPlayer], to run the game on your computer. You can download the emulator from their official websites and follow the instructions to install and configure it. Then, you can download Sausage Man from Google Play Store or APK file within the emulator and enjoy the game on a larger screen.
-
Q: How can I customize my sausage character in Sausage Man?
-
A: You can customize your sausage character in Sausage Man by using the appearance system in the game. You can access the appearance system by tapping on the wardrobe icon on the main screen. You can change your sausage's skin color, hairstyle, facial expression, outfit, accessories, and pose. You can also unlock more appearance items by completing missions, participating in events, or purchasing them with in-game currency.
-
Q: How can I get more in-game currency in Sausage Man?
-
A: There are two types of in-game currency in Sausage Man: coins and diamonds. Coins are used to buy items in the shop, such as appearance items, crates, and lucky draws. Diamonds are used to buy premium items, such as VIP membership, exclusive outfits, and special crates. You can get more coins and diamonds by playing matches, completing missions, watching ads, or buying them with real money.
-
Q: How can I report a bug or a problem in Sausage Man?
-
A: If you encounter a bug or a problem in Sausage Man, you can report it to the developers by using the feedback system in the game. You can access the feedback system by tapping on the settings icon on the main screen and then tapping on the feedback button. You can fill out a form with your contact information, problem description, screenshot, and device model. You can also contact the customer service team by emailing them at sausagecs@xd.com or joining their official Discord server at [https://discord.gg/sausageman].
-
197e85843d
-
-
\ No newline at end of file
diff --git a/spaces/congsaPfin/Manga-OCR/logs/Stikman Parkour Master the Art of Movement with Your Stick Figure.md b/spaces/congsaPfin/Manga-OCR/logs/Stikman Parkour Master the Art of Movement with Your Stick Figure.md
deleted file mode 100644
index 4e0b12c0b634276daff5bb72bf5324e30c5705d2..0000000000000000000000000000000000000000
--- a/spaces/congsaPfin/Manga-OCR/logs/Stikman Parkour Master the Art of Movement with Your Stick Figure.md
+++ /dev/null
@@ -1,86 +0,0 @@
-
-
What is stikman and why is it popular?
-
stikman (stylized lowercase) is a pseudonymous American street artist and a game character that has gained popularity in the last decade. stikman is best known for placing images of humanoid, robot-like stick figures on the sidewalks of cities across the United States and for starring in various online games that feature his adventures. In this article, we will explore the origin and history of stikman, the different styles and genres of stikman, and the best stikman games to play online.
-
The origin and history of stikman
-
stikman as a street art phenomenon
-
stikman is reported to be a Philadelphia native who has been active in street art since the 1960s, when he began his career at age 14 with anti-war graffiti. He has been creating the stikman figures that he is best known for since the 1990s. These are usually made of yellow linoleum-like pavement marking tape that becomes embedded in the asphalt over time. The artist places the figures, most frequently on crosswalks, without any direct indication of authorship. This has led to articles in the media investigating the origin and authorship of the figures. Although they are frequently interpreted as robot figures, the artist has said that they are simply "little men made of sticks". A Washington Post article stated that the Washington, D.C. area had over 150 stikman images embedded in its sidewalks in 2008. The figures have also been placed in New York City, Boston, Los Angeles, Philadelphia, Wheeling, West Virginia, Ann Arbor, Michigan, Minneapolis, Minnesota, San Francisco, and Chicago. stikman has also created the figures in other styles and media. Although known primarily for works placed on the street, the artist has also been featured in gallery exhibitions and selected for Amazon's first collection of limited-edition prints by seven international street artists.
stikman is also a popular game character that has appeared in many online games that feature his adventures. These games are usually based on action, arcade, or strategy genres that involve fighting, shooting, racing, or escaping from various situations. Some of the most popular games in the stickman section are the Henry Stickmin games. This series is a cult classic, played through by many Youtubers for the viewer’s amusement. The game’s narrative is an action parody that’s driven by the decisions you make. Fleeing the Complex and Escaping the Prison are two fun Henry Stickmin games to play through.
-
The different styles and genres of stikman
-
stikman as a stick figure
-
One of the most common styles of stikman is a simple stick figure that consists of a circle for the head and straight lines for the body and limbs. This style is often used to create humorous or absurd situations that involve violence or death. For example, in Stick Figure Penalty Chamber 2, you can choose from various ways to torture or kill a stick figure prisoner.
-
stikman as a robot
-
Another style of stikman is a robot-like figure that has metal parts or wires attached to its body. This style is often used to create futuristic or sci-fi scenarios that involve technology or aliens. For example, in Stick War 2: Order Empire, you can control an army of stickmen soldiers that fight against the rebels or the aliens. You can also upgrade your units and weapons with gold and mana.
-
stikman as a hero
-
A third style of stikman is a hero-like figure that has special abilities or powers that help him overcome challenges or enemies. This style is often used to create epic or adventurous scenarios that involve fantasy or magic. For example, in Stickman Hook, you can swing from rope to rope like Spider-Man and perform amazing acrobatic stunts.
-
The best stikman games to play online
-
The Henry Stickmin series
-
As mentioned earlier, the Henry Stickmin series is one of the most popular and entertaining stikman games to play online. The series consists of six games that follow the adventures of Henry Stickmin, a notorious criminal who tries to escape from prison, rob a bank, infiltrate a secret organization, and more. The games are full of hilarious choices, references, and outcomes that will make you laugh out loud. You can play the games in any order, but the recommended order is Breaking the Bank, Escaping the Prison, Stealing the Diamond, Infiltrating the Airship, Fleeing the Complex, and Completing the Mission.
-
The Stickman History Battle game
-
If you are interested in history and warfare, you might enjoy the Stickman History Battle game. This game lets you control a stickman army that fights against different historical enemies, such as Vikings, Romans, Mongols, Samurai, Pirates, and more. You can choose from various units and weapons, such as archers, spearmen, cavalry, catapults, cannons, and more. You can also upgrade your army and unlock new skills and abilities. The game has 12 levels that cover different historical periods and regions.
-
The Stickman Party game
-
If you are looking for a fun and casual game to play with your friends or family, you might like the Stickman Party game. This game is a collection of mini-games that you can play with up to four players on one device. The mini-games include racing, soccer, tank battles, micro golf, snakes and ladders, paintball, and more. The game is easy to play and suitable for all ages.
-
stikman games online
-stikman party 2 3 4 minigames
-stikman hook
-stikman clash
-stikman run
-stikman fighter
-stikman parkour race
-stikman escape
-stikman merge
-stikman defenders
-stikman go
-stikman climb 2
-stikman adventure mode
-stikman shooting games
-stikman soccer games
-stikman car racing games
-stikman bike games
-stikman bmx games
-stikman simulation games
-stikman survival games
-stikman gun games
-stikman war of sticks
-stikman henry stickmin games
-stikman .io games
-stikman multiplayer games
-stikman offline games
-stikman no wifi games
-stikman free games
-stikman fun games
-stikman cool games
-stikman minecraft games
-stikman tower defense games
-stikman dirt bike games
-stikman rally racing games
-stikman bounce the ball games
-stikman paint the colors games
-stikman stick figure animation software
-stikman graffiti artist
-stikman comics and cartoons
-stikman stickers and decals
-stikman t-shirts and hoodies
-stikman action figures and toys
-stikman tattoos and designs
-stikman memes and jokes
-how to draw a stikman
-how to make a stikman game
-how to play a stikman game
-best stikman game for android
-best stikman game for ios
-best stikman game for pc
-
Conclusion and FAQs
-
stikman is a versatile and creative character that has been used in various forms of art and entertainment. Whether you are a fan of street art or online games, you can find something to enjoy about stikman. stikman is a symbol of simplicity, humor, and imagination that can inspire anyone to create their own stories and adventures.
-
Here are some FAQs about stikman:
-
-
Question
Answer
-
Who is the creator of stikman?
The creator of stikman is an anonymous street artist who has been active since the 1960s. He has never revealed his identity or motives.
-
What is the meaning of stikman?
There is no definitive meaning of stikman. The artist has said that they are simply "little men made of sticks". Some people interpret them as robots, others as humans. Some see them as symbols of resistance, others as expressions of humor.
-
Where can I find stikman figures?
You can find stikman figures on the sidewalks of many cities across the United States. They are usually placed on crosswalks or near traffic signs. You can also find them online in various games and websites.
-
How can I make my own stikman figures?
You can make your own stikman figures using any material that can stick to the pavement or other surfaces. You can use tape, stickers, paint, chalk, or anything else that you can think of. You can also draw them on paper or on your computer.
-
Are there any legal issues with stikman?
stikman is considered a form of graffiti or vandalism by some authorities and property owners. Placing stikman figures on public or private property without permission may result in fines or legal action.
-
197e85843d
-
-
\ No newline at end of file
diff --git a/spaces/coreml-community/ControlNet-v1-1-Annotators-cpu/annotator/mmpkg/mmseg/models/necks/fpn.py b/spaces/coreml-community/ControlNet-v1-1-Annotators-cpu/annotator/mmpkg/mmseg/models/necks/fpn.py
deleted file mode 100644
index ba47bbe1a0225587315627ac288e5ddf6497a244..0000000000000000000000000000000000000000
--- a/spaces/coreml-community/ControlNet-v1-1-Annotators-cpu/annotator/mmpkg/mmseg/models/necks/fpn.py
+++ /dev/null
@@ -1,212 +0,0 @@
-import torch.nn as nn
-import torch.nn.functional as F
-from annotator.mmpkg.mmcv.cnn import ConvModule, xavier_init
-
-from ..builder import NECKS
-
-
-@NECKS.register_module()
-class FPN(nn.Module):
- """Feature Pyramid Network.
-
- This is an implementation of - Feature Pyramid Networks for Object
- Detection (https://arxiv.org/abs/1612.03144)
-
- Args:
- in_channels (List[int]): Number of input channels per scale.
- out_channels (int): Number of output channels (used at each scale)
- num_outs (int): Number of output scales.
- start_level (int): Index of the start input backbone level used to
- build the feature pyramid. Default: 0.
- end_level (int): Index of the end input backbone level (exclusive) to
- build the feature pyramid. Default: -1, which means the last level.
- add_extra_convs (bool | str): If bool, it decides whether to add conv
- layers on top of the original feature maps. Default to False.
- If True, its actual mode is specified by `extra_convs_on_inputs`.
- If str, it specifies the source feature map of the extra convs.
- Only the following options are allowed
-
- - 'on_input': Last feat map of neck inputs (i.e. backbone feature).
- - 'on_lateral': Last feature map after lateral convs.
- - 'on_output': The last output feature map after fpn convs.
- extra_convs_on_inputs (bool, deprecated): Whether to apply extra convs
- on the original feature from the backbone. If True,
- it is equivalent to `add_extra_convs='on_input'`. If False, it is
- equivalent to set `add_extra_convs='on_output'`. Default to True.
- relu_before_extra_convs (bool): Whether to apply relu before the extra
- conv. Default: False.
- no_norm_on_lateral (bool): Whether to apply norm on lateral.
- Default: False.
- conv_cfg (dict): Config dict for convolution layer. Default: None.
- norm_cfg (dict): Config dict for normalization layer. Default: None.
- act_cfg (str): Config dict for activation layer in ConvModule.
- Default: None.
- upsample_cfg (dict): Config dict for interpolate layer.
- Default: `dict(mode='nearest')`
-
- Example:
- >>> import torch
- >>> in_channels = [2, 3, 5, 7]
- >>> scales = [340, 170, 84, 43]
- >>> inputs = [torch.rand(1, c, s, s)
- ... for c, s in zip(in_channels, scales)]
- >>> self = FPN(in_channels, 11, len(in_channels)).eval()
- >>> outputs = self.forward(inputs)
- >>> for i in range(len(outputs)):
- ... print(f'outputs[{i}].shape = {outputs[i].shape}')
- outputs[0].shape = torch.Size([1, 11, 340, 340])
- outputs[1].shape = torch.Size([1, 11, 170, 170])
- outputs[2].shape = torch.Size([1, 11, 84, 84])
- outputs[3].shape = torch.Size([1, 11, 43, 43])
- """
-
- def __init__(self,
- in_channels,
- out_channels,
- num_outs,
- start_level=0,
- end_level=-1,
- add_extra_convs=False,
- extra_convs_on_inputs=False,
- relu_before_extra_convs=False,
- no_norm_on_lateral=False,
- conv_cfg=None,
- norm_cfg=None,
- act_cfg=None,
- upsample_cfg=dict(mode='nearest')):
- super(FPN, self).__init__()
- assert isinstance(in_channels, list)
- self.in_channels = in_channels
- self.out_channels = out_channels
- self.num_ins = len(in_channels)
- self.num_outs = num_outs
- self.relu_before_extra_convs = relu_before_extra_convs
- self.no_norm_on_lateral = no_norm_on_lateral
- self.fp16_enabled = False
- self.upsample_cfg = upsample_cfg.copy()
-
- if end_level == -1:
- self.backbone_end_level = self.num_ins
- assert num_outs >= self.num_ins - start_level
- else:
- # if end_level < inputs, no extra level is allowed
- self.backbone_end_level = end_level
- assert end_level <= len(in_channels)
- assert num_outs == end_level - start_level
- self.start_level = start_level
- self.end_level = end_level
- self.add_extra_convs = add_extra_convs
- assert isinstance(add_extra_convs, (str, bool))
- if isinstance(add_extra_convs, str):
- # Extra_convs_source choices: 'on_input', 'on_lateral', 'on_output'
- assert add_extra_convs in ('on_input', 'on_lateral', 'on_output')
- elif add_extra_convs: # True
- if extra_convs_on_inputs:
- # For compatibility with previous release
- # TODO: deprecate `extra_convs_on_inputs`
- self.add_extra_convs = 'on_input'
- else:
- self.add_extra_convs = 'on_output'
-
- self.lateral_convs = nn.ModuleList()
- self.fpn_convs = nn.ModuleList()
-
- for i in range(self.start_level, self.backbone_end_level):
- l_conv = ConvModule(
- in_channels[i],
- out_channels,
- 1,
- conv_cfg=conv_cfg,
- norm_cfg=norm_cfg if not self.no_norm_on_lateral else None,
- act_cfg=act_cfg,
- inplace=False)
- fpn_conv = ConvModule(
- out_channels,
- out_channels,
- 3,
- padding=1,
- conv_cfg=conv_cfg,
- norm_cfg=norm_cfg,
- act_cfg=act_cfg,
- inplace=False)
-
- self.lateral_convs.append(l_conv)
- self.fpn_convs.append(fpn_conv)
-
- # add extra conv layers (e.g., RetinaNet)
- extra_levels = num_outs - self.backbone_end_level + self.start_level
- if self.add_extra_convs and extra_levels >= 1:
- for i in range(extra_levels):
- if i == 0 and self.add_extra_convs == 'on_input':
- in_channels = self.in_channels[self.backbone_end_level - 1]
- else:
- in_channels = out_channels
- extra_fpn_conv = ConvModule(
- in_channels,
- out_channels,
- 3,
- stride=2,
- padding=1,
- conv_cfg=conv_cfg,
- norm_cfg=norm_cfg,
- act_cfg=act_cfg,
- inplace=False)
- self.fpn_convs.append(extra_fpn_conv)
-
- # default init_weights for conv(msra) and norm in ConvModule
- def init_weights(self):
- for m in self.modules():
- if isinstance(m, nn.Conv2d):
- xavier_init(m, distribution='uniform')
-
- def forward(self, inputs):
- assert len(inputs) == len(self.in_channels)
-
- # build laterals
- laterals = [
- lateral_conv(inputs[i + self.start_level])
- for i, lateral_conv in enumerate(self.lateral_convs)
- ]
-
- # build top-down path
- used_backbone_levels = len(laterals)
- for i in range(used_backbone_levels - 1, 0, -1):
- # In some cases, fixing `scale factor` (e.g. 2) is preferred, but
- # it cannot co-exist with `size` in `F.interpolate`.
- if 'scale_factor' in self.upsample_cfg:
- laterals[i - 1] += F.interpolate(laterals[i],
- **self.upsample_cfg)
- else:
- prev_shape = laterals[i - 1].shape[2:]
- laterals[i - 1] += F.interpolate(
- laterals[i], size=prev_shape, **self.upsample_cfg)
-
- # build outputs
- # part 1: from original levels
- outs = [
- self.fpn_convs[i](laterals[i]) for i in range(used_backbone_levels)
- ]
- # part 2: add extra levels
- if self.num_outs > len(outs):
- # use max pool to get more levels on top of outputs
- # (e.g., Faster R-CNN, Mask R-CNN)
- if not self.add_extra_convs:
- for i in range(self.num_outs - used_backbone_levels):
- outs.append(F.max_pool2d(outs[-1], 1, stride=2))
- # add conv layers on top of original feature maps (RetinaNet)
- else:
- if self.add_extra_convs == 'on_input':
- extra_source = inputs[self.backbone_end_level - 1]
- elif self.add_extra_convs == 'on_lateral':
- extra_source = laterals[-1]
- elif self.add_extra_convs == 'on_output':
- extra_source = outs[-1]
- else:
- raise NotImplementedError
- outs.append(self.fpn_convs[used_backbone_levels](extra_source))
- for i in range(used_backbone_levels + 1, self.num_outs):
- if self.relu_before_extra_convs:
- outs.append(self.fpn_convs[i](F.relu(outs[-1])))
- else:
- outs.append(self.fpn_convs[i](outs[-1]))
- return tuple(outs)
diff --git a/spaces/coreml-community/ControlNet-v1-1-Annotators-cpu/annotator/oneformer/detectron2/data/datasets/lvis_v0_5_categories.py b/spaces/coreml-community/ControlNet-v1-1-Annotators-cpu/annotator/oneformer/detectron2/data/datasets/lvis_v0_5_categories.py
deleted file mode 100644
index d3dab6198da614937b08682f4c9edf52bdf1d236..0000000000000000000000000000000000000000
--- a/spaces/coreml-community/ControlNet-v1-1-Annotators-cpu/annotator/oneformer/detectron2/data/datasets/lvis_v0_5_categories.py
+++ /dev/null
@@ -1,13 +0,0 @@
-# Copyright (c) Facebook, Inc. and its affiliates.
-# Autogen with
-# with open("lvis_v0.5_val.json", "r") as f:
-# a = json.load(f)
-# c = a["categories"]
-# for x in c:
-# del x["image_count"]
-# del x["instance_count"]
-# LVIS_CATEGORIES = repr(c) + " # noqa"
-
-# fmt: off
-LVIS_CATEGORIES = [{'frequency': 'r', 'id': 1, 'synset': 'acorn.n.01', 'synonyms': ['acorn'], 'def': 'nut from an oak tree', 'name': 'acorn'}, {'frequency': 'c', 'id': 2, 'synset': 'aerosol.n.02', 'synonyms': ['aerosol_can', 'spray_can'], 'def': 'a dispenser that holds a substance under pressure', 'name': 'aerosol_can'}, {'frequency': 'f', 'id': 3, 'synset': 'air_conditioner.n.01', 'synonyms': ['air_conditioner'], 'def': 'a machine that keeps air cool and dry', 'name': 'air_conditioner'}, {'frequency': 'f', 'id': 4, 'synset': 'airplane.n.01', 'synonyms': ['airplane', 'aeroplane'], 'def': 'an aircraft that has a fixed wing and is powered by propellers or jets', 'name': 'airplane'}, {'frequency': 'c', 'id': 5, 'synset': 'alarm_clock.n.01', 'synonyms': ['alarm_clock'], 'def': 'a clock that wakes a sleeper at some preset time', 'name': 'alarm_clock'}, {'frequency': 'c', 'id': 6, 'synset': 'alcohol.n.01', 'synonyms': ['alcohol', 'alcoholic_beverage'], 'def': 'a liquor or brew containing alcohol as the active agent', 'name': 'alcohol'}, {'frequency': 'r', 'id': 7, 'synset': 'alligator.n.02', 'synonyms': ['alligator', 'gator'], 'def': 'amphibious reptiles related to crocodiles but with shorter broader snouts', 'name': 'alligator'}, {'frequency': 'c', 'id': 8, 'synset': 'almond.n.02', 'synonyms': ['almond'], 'def': 'oval-shaped edible seed of the almond tree', 'name': 'almond'}, {'frequency': 'c', 'id': 9, 'synset': 'ambulance.n.01', 'synonyms': ['ambulance'], 'def': 'a vehicle that takes people to and from hospitals', 'name': 'ambulance'}, {'frequency': 'r', 'id': 10, 'synset': 'amplifier.n.01', 'synonyms': ['amplifier'], 'def': 'electronic equipment that increases strength of signals', 'name': 'amplifier'}, {'frequency': 'c', 'id': 11, 'synset': 'anklet.n.03', 'synonyms': ['anklet', 'ankle_bracelet'], 'def': 'an ornament worn around the ankle', 'name': 'anklet'}, {'frequency': 'f', 'id': 12, 'synset': 'antenna.n.01', 'synonyms': ['antenna', 'aerial', 'transmitting_aerial'], 'def': 'an electrical device that sends or receives radio or television signals', 'name': 'antenna'}, {'frequency': 'f', 'id': 13, 'synset': 'apple.n.01', 'synonyms': ['apple'], 'def': 'fruit with red or yellow or green skin and sweet to tart crisp whitish flesh', 'name': 'apple'}, {'frequency': 'r', 'id': 14, 'synset': 'apple_juice.n.01', 'synonyms': ['apple_juice'], 'def': 'the juice of apples', 'name': 'apple_juice'}, {'frequency': 'r', 'id': 15, 'synset': 'applesauce.n.01', 'synonyms': ['applesauce'], 'def': 'puree of stewed apples usually sweetened and spiced', 'name': 'applesauce'}, {'frequency': 'r', 'id': 16, 'synset': 'apricot.n.02', 'synonyms': ['apricot'], 'def': 'downy yellow to rosy-colored fruit resembling a small peach', 'name': 'apricot'}, {'frequency': 'f', 'id': 17, 'synset': 'apron.n.01', 'synonyms': ['apron'], 'def': 'a garment of cloth that is tied about the waist and worn to protect clothing', 'name': 'apron'}, {'frequency': 'c', 'id': 18, 'synset': 'aquarium.n.01', 'synonyms': ['aquarium', 'fish_tank'], 'def': 'a tank/pool/bowl filled with water for keeping live fish and underwater animals', 'name': 'aquarium'}, {'frequency': 'c', 'id': 19, 'synset': 'armband.n.02', 'synonyms': ['armband'], 'def': 'a band worn around the upper arm', 'name': 'armband'}, {'frequency': 'f', 'id': 20, 'synset': 'armchair.n.01', 'synonyms': ['armchair'], 'def': 'chair with a support on each side for arms', 'name': 'armchair'}, {'frequency': 'r', 'id': 21, 'synset': 'armoire.n.01', 'synonyms': ['armoire'], 'def': 'a large wardrobe or cabinet', 'name': 'armoire'}, {'frequency': 'r', 'id': 22, 'synset': 'armor.n.01', 'synonyms': ['armor', 'armour'], 'def': 'protective covering made of metal and used in combat', 'name': 'armor'}, {'frequency': 'c', 'id': 23, 'synset': 'artichoke.n.02', 'synonyms': ['artichoke'], 'def': 'a thistlelike flower head with edible fleshy leaves and heart', 'name': 'artichoke'}, {'frequency': 'f', 'id': 24, 'synset': 'ashcan.n.01', 'synonyms': ['trash_can', 'garbage_can', 'wastebin', 'dustbin', 'trash_barrel', 'trash_bin'], 'def': 'a bin that holds rubbish until it is collected', 'name': 'trash_can'}, {'frequency': 'c', 'id': 25, 'synset': 'ashtray.n.01', 'synonyms': ['ashtray'], 'def': "a receptacle for the ash from smokers' cigars or cigarettes", 'name': 'ashtray'}, {'frequency': 'c', 'id': 26, 'synset': 'asparagus.n.02', 'synonyms': ['asparagus'], 'def': 'edible young shoots of the asparagus plant', 'name': 'asparagus'}, {'frequency': 'c', 'id': 27, 'synset': 'atomizer.n.01', 'synonyms': ['atomizer', 'atomiser', 'spray', 'sprayer', 'nebulizer', 'nebuliser'], 'def': 'a dispenser that turns a liquid (such as perfume) into a fine mist', 'name': 'atomizer'}, {'frequency': 'c', 'id': 28, 'synset': 'avocado.n.01', 'synonyms': ['avocado'], 'def': 'a pear-shaped fruit with green or blackish skin and rich yellowish pulp enclosing a single large seed', 'name': 'avocado'}, {'frequency': 'c', 'id': 29, 'synset': 'award.n.02', 'synonyms': ['award', 'accolade'], 'def': 'a tangible symbol signifying approval or distinction', 'name': 'award'}, {'frequency': 'f', 'id': 30, 'synset': 'awning.n.01', 'synonyms': ['awning'], 'def': 'a canopy made of canvas to shelter people or things from rain or sun', 'name': 'awning'}, {'frequency': 'r', 'id': 31, 'synset': 'ax.n.01', 'synonyms': ['ax', 'axe'], 'def': 'an edge tool with a heavy bladed head mounted across a handle', 'name': 'ax'}, {'frequency': 'f', 'id': 32, 'synset': 'baby_buggy.n.01', 'synonyms': ['baby_buggy', 'baby_carriage', 'perambulator', 'pram', 'stroller'], 'def': 'a small vehicle with four wheels in which a baby or child is pushed around', 'name': 'baby_buggy'}, {'frequency': 'c', 'id': 33, 'synset': 'backboard.n.01', 'synonyms': ['basketball_backboard'], 'def': 'a raised vertical board with basket attached; used to play basketball', 'name': 'basketball_backboard'}, {'frequency': 'f', 'id': 34, 'synset': 'backpack.n.01', 'synonyms': ['backpack', 'knapsack', 'packsack', 'rucksack', 'haversack'], 'def': 'a bag carried by a strap on your back or shoulder', 'name': 'backpack'}, {'frequency': 'f', 'id': 35, 'synset': 'bag.n.04', 'synonyms': ['handbag', 'purse', 'pocketbook'], 'def': 'a container used for carrying money and small personal items or accessories', 'name': 'handbag'}, {'frequency': 'f', 'id': 36, 'synset': 'bag.n.06', 'synonyms': ['suitcase', 'baggage', 'luggage'], 'def': 'cases used to carry belongings when traveling', 'name': 'suitcase'}, {'frequency': 'c', 'id': 37, 'synset': 'bagel.n.01', 'synonyms': ['bagel', 'beigel'], 'def': 'glazed yeast-raised doughnut-shaped roll with hard crust', 'name': 'bagel'}, {'frequency': 'r', 'id': 38, 'synset': 'bagpipe.n.01', 'synonyms': ['bagpipe'], 'def': 'a tubular wind instrument; the player blows air into a bag and squeezes it out', 'name': 'bagpipe'}, {'frequency': 'r', 'id': 39, 'synset': 'baguet.n.01', 'synonyms': ['baguet', 'baguette'], 'def': 'narrow French stick loaf', 'name': 'baguet'}, {'frequency': 'r', 'id': 40, 'synset': 'bait.n.02', 'synonyms': ['bait', 'lure'], 'def': 'something used to lure fish or other animals into danger so they can be trapped or killed', 'name': 'bait'}, {'frequency': 'f', 'id': 41, 'synset': 'ball.n.06', 'synonyms': ['ball'], 'def': 'a spherical object used as a plaything', 'name': 'ball'}, {'frequency': 'r', 'id': 42, 'synset': 'ballet_skirt.n.01', 'synonyms': ['ballet_skirt', 'tutu'], 'def': 'very short skirt worn by ballerinas', 'name': 'ballet_skirt'}, {'frequency': 'f', 'id': 43, 'synset': 'balloon.n.01', 'synonyms': ['balloon'], 'def': 'large tough nonrigid bag filled with gas or heated air', 'name': 'balloon'}, {'frequency': 'c', 'id': 44, 'synset': 'bamboo.n.02', 'synonyms': ['bamboo'], 'def': 'woody tropical grass having hollow woody stems', 'name': 'bamboo'}, {'frequency': 'f', 'id': 45, 'synset': 'banana.n.02', 'synonyms': ['banana'], 'def': 'elongated crescent-shaped yellow fruit with soft sweet flesh', 'name': 'banana'}, {'frequency': 'r', 'id': 46, 'synset': 'band_aid.n.01', 'synonyms': ['Band_Aid'], 'def': 'trade name for an adhesive bandage to cover small cuts or blisters', 'name': 'Band_Aid'}, {'frequency': 'c', 'id': 47, 'synset': 'bandage.n.01', 'synonyms': ['bandage'], 'def': 'a piece of soft material that covers and protects an injured part of the body', 'name': 'bandage'}, {'frequency': 'c', 'id': 48, 'synset': 'bandanna.n.01', 'synonyms': ['bandanna', 'bandana'], 'def': 'large and brightly colored handkerchief; often used as a neckerchief', 'name': 'bandanna'}, {'frequency': 'r', 'id': 49, 'synset': 'banjo.n.01', 'synonyms': ['banjo'], 'def': 'a stringed instrument of the guitar family with a long neck and circular body', 'name': 'banjo'}, {'frequency': 'f', 'id': 50, 'synset': 'banner.n.01', 'synonyms': ['banner', 'streamer'], 'def': 'long strip of cloth or paper used for decoration or advertising', 'name': 'banner'}, {'frequency': 'r', 'id': 51, 'synset': 'barbell.n.01', 'synonyms': ['barbell'], 'def': 'a bar to which heavy discs are attached at each end; used in weightlifting', 'name': 'barbell'}, {'frequency': 'r', 'id': 52, 'synset': 'barge.n.01', 'synonyms': ['barge'], 'def': 'a flatbottom boat for carrying heavy loads (especially on canals)', 'name': 'barge'}, {'frequency': 'f', 'id': 53, 'synset': 'barrel.n.02', 'synonyms': ['barrel', 'cask'], 'def': 'a cylindrical container that holds liquids', 'name': 'barrel'}, {'frequency': 'c', 'id': 54, 'synset': 'barrette.n.01', 'synonyms': ['barrette'], 'def': "a pin for holding women's hair in place", 'name': 'barrette'}, {'frequency': 'c', 'id': 55, 'synset': 'barrow.n.03', 'synonyms': ['barrow', 'garden_cart', 'lawn_cart', 'wheelbarrow'], 'def': 'a cart for carrying small loads; has handles and one or more wheels', 'name': 'barrow'}, {'frequency': 'f', 'id': 56, 'synset': 'base.n.03', 'synonyms': ['baseball_base'], 'def': 'a place that the runner must touch before scoring', 'name': 'baseball_base'}, {'frequency': 'f', 'id': 57, 'synset': 'baseball.n.02', 'synonyms': ['baseball'], 'def': 'a ball used in playing baseball', 'name': 'baseball'}, {'frequency': 'f', 'id': 58, 'synset': 'baseball_bat.n.01', 'synonyms': ['baseball_bat'], 'def': 'an implement used in baseball by the batter', 'name': 'baseball_bat'}, {'frequency': 'f', 'id': 59, 'synset': 'baseball_cap.n.01', 'synonyms': ['baseball_cap', 'jockey_cap', 'golf_cap'], 'def': 'a cap with a bill', 'name': 'baseball_cap'}, {'frequency': 'f', 'id': 60, 'synset': 'baseball_glove.n.01', 'synonyms': ['baseball_glove', 'baseball_mitt'], 'def': 'the handwear used by fielders in playing baseball', 'name': 'baseball_glove'}, {'frequency': 'f', 'id': 61, 'synset': 'basket.n.01', 'synonyms': ['basket', 'handbasket'], 'def': 'a container that is usually woven and has handles', 'name': 'basket'}, {'frequency': 'c', 'id': 62, 'synset': 'basket.n.03', 'synonyms': ['basketball_hoop'], 'def': 'metal hoop supporting a net through which players try to throw the basketball', 'name': 'basketball_hoop'}, {'frequency': 'c', 'id': 63, 'synset': 'basketball.n.02', 'synonyms': ['basketball'], 'def': 'an inflated ball used in playing basketball', 'name': 'basketball'}, {'frequency': 'r', 'id': 64, 'synset': 'bass_horn.n.01', 'synonyms': ['bass_horn', 'sousaphone', 'tuba'], 'def': 'the lowest brass wind instrument', 'name': 'bass_horn'}, {'frequency': 'r', 'id': 65, 'synset': 'bat.n.01', 'synonyms': ['bat_(animal)'], 'def': 'nocturnal mouselike mammal with forelimbs modified to form membranous wings', 'name': 'bat_(animal)'}, {'frequency': 'f', 'id': 66, 'synset': 'bath_mat.n.01', 'synonyms': ['bath_mat'], 'def': 'a heavy towel or mat to stand on while drying yourself after a bath', 'name': 'bath_mat'}, {'frequency': 'f', 'id': 67, 'synset': 'bath_towel.n.01', 'synonyms': ['bath_towel'], 'def': 'a large towel; to dry yourself after a bath', 'name': 'bath_towel'}, {'frequency': 'c', 'id': 68, 'synset': 'bathrobe.n.01', 'synonyms': ['bathrobe'], 'def': 'a loose-fitting robe of towelling; worn after a bath or swim', 'name': 'bathrobe'}, {'frequency': 'f', 'id': 69, 'synset': 'bathtub.n.01', 'synonyms': ['bathtub', 'bathing_tub'], 'def': 'a large open container that you fill with water and use to wash the body', 'name': 'bathtub'}, {'frequency': 'r', 'id': 70, 'synset': 'batter.n.02', 'synonyms': ['batter_(food)'], 'def': 'a liquid or semiliquid mixture, as of flour, eggs, and milk, used in cooking', 'name': 'batter_(food)'}, {'frequency': 'c', 'id': 71, 'synset': 'battery.n.02', 'synonyms': ['battery'], 'def': 'a portable device that produces electricity', 'name': 'battery'}, {'frequency': 'r', 'id': 72, 'synset': 'beach_ball.n.01', 'synonyms': ['beachball'], 'def': 'large and light ball; for play at the seaside', 'name': 'beachball'}, {'frequency': 'c', 'id': 73, 'synset': 'bead.n.01', 'synonyms': ['bead'], 'def': 'a small ball with a hole through the middle used for ornamentation, jewellery, etc.', 'name': 'bead'}, {'frequency': 'r', 'id': 74, 'synset': 'beaker.n.01', 'synonyms': ['beaker'], 'def': 'a flatbottomed jar made of glass or plastic; used for chemistry', 'name': 'beaker'}, {'frequency': 'c', 'id': 75, 'synset': 'bean_curd.n.01', 'synonyms': ['bean_curd', 'tofu'], 'def': 'cheeselike food made of curdled soybean milk', 'name': 'bean_curd'}, {'frequency': 'c', 'id': 76, 'synset': 'beanbag.n.01', 'synonyms': ['beanbag'], 'def': 'a bag filled with dried beans or similar items; used in games or to sit on', 'name': 'beanbag'}, {'frequency': 'f', 'id': 77, 'synset': 'beanie.n.01', 'synonyms': ['beanie', 'beany'], 'def': 'a small skullcap; formerly worn by schoolboys and college freshmen', 'name': 'beanie'}, {'frequency': 'f', 'id': 78, 'synset': 'bear.n.01', 'synonyms': ['bear'], 'def': 'large carnivorous or omnivorous mammals with shaggy coats and claws', 'name': 'bear'}, {'frequency': 'f', 'id': 79, 'synset': 'bed.n.01', 'synonyms': ['bed'], 'def': 'a piece of furniture that provides a place to sleep', 'name': 'bed'}, {'frequency': 'c', 'id': 80, 'synset': 'bedspread.n.01', 'synonyms': ['bedspread', 'bedcover', 'bed_covering', 'counterpane', 'spread'], 'def': 'decorative cover for a bed', 'name': 'bedspread'}, {'frequency': 'f', 'id': 81, 'synset': 'beef.n.01', 'synonyms': ['cow'], 'def': 'cattle that are reared for their meat', 'name': 'cow'}, {'frequency': 'c', 'id': 82, 'synset': 'beef.n.02', 'synonyms': ['beef_(food)', 'boeuf_(food)'], 'def': 'meat from an adult domestic bovine', 'name': 'beef_(food)'}, {'frequency': 'r', 'id': 83, 'synset': 'beeper.n.01', 'synonyms': ['beeper', 'pager'], 'def': 'an device that beeps when the person carrying it is being paged', 'name': 'beeper'}, {'frequency': 'f', 'id': 84, 'synset': 'beer_bottle.n.01', 'synonyms': ['beer_bottle'], 'def': 'a bottle that holds beer', 'name': 'beer_bottle'}, {'frequency': 'c', 'id': 85, 'synset': 'beer_can.n.01', 'synonyms': ['beer_can'], 'def': 'a can that holds beer', 'name': 'beer_can'}, {'frequency': 'r', 'id': 86, 'synset': 'beetle.n.01', 'synonyms': ['beetle'], 'def': 'insect with hard wing covers', 'name': 'beetle'}, {'frequency': 'f', 'id': 87, 'synset': 'bell.n.01', 'synonyms': ['bell'], 'def': 'a hollow device made of metal that makes a ringing sound when struck', 'name': 'bell'}, {'frequency': 'f', 'id': 88, 'synset': 'bell_pepper.n.02', 'synonyms': ['bell_pepper', 'capsicum'], 'def': 'large bell-shaped sweet pepper in green or red or yellow or orange or black varieties', 'name': 'bell_pepper'}, {'frequency': 'f', 'id': 89, 'synset': 'belt.n.02', 'synonyms': ['belt'], 'def': 'a band to tie or buckle around the body (usually at the waist)', 'name': 'belt'}, {'frequency': 'f', 'id': 90, 'synset': 'belt_buckle.n.01', 'synonyms': ['belt_buckle'], 'def': 'the buckle used to fasten a belt', 'name': 'belt_buckle'}, {'frequency': 'f', 'id': 91, 'synset': 'bench.n.01', 'synonyms': ['bench'], 'def': 'a long seat for more than one person', 'name': 'bench'}, {'frequency': 'c', 'id': 92, 'synset': 'beret.n.01', 'synonyms': ['beret'], 'def': 'a cap with no brim or bill; made of soft cloth', 'name': 'beret'}, {'frequency': 'c', 'id': 93, 'synset': 'bib.n.02', 'synonyms': ['bib'], 'def': 'a napkin tied under the chin of a child while eating', 'name': 'bib'}, {'frequency': 'r', 'id': 94, 'synset': 'bible.n.01', 'synonyms': ['Bible'], 'def': 'the sacred writings of the Christian religions', 'name': 'Bible'}, {'frequency': 'f', 'id': 95, 'synset': 'bicycle.n.01', 'synonyms': ['bicycle', 'bike_(bicycle)'], 'def': 'a wheeled vehicle that has two wheels and is moved by foot pedals', 'name': 'bicycle'}, {'frequency': 'f', 'id': 96, 'synset': 'bill.n.09', 'synonyms': ['visor', 'vizor'], 'def': 'a brim that projects to the front to shade the eyes', 'name': 'visor'}, {'frequency': 'c', 'id': 97, 'synset': 'binder.n.03', 'synonyms': ['binder', 'ring-binder'], 'def': 'holds loose papers or magazines', 'name': 'binder'}, {'frequency': 'c', 'id': 98, 'synset': 'binoculars.n.01', 'synonyms': ['binoculars', 'field_glasses', 'opera_glasses'], 'def': 'an optical instrument designed for simultaneous use by both eyes', 'name': 'binoculars'}, {'frequency': 'f', 'id': 99, 'synset': 'bird.n.01', 'synonyms': ['bird'], 'def': 'animal characterized by feathers and wings', 'name': 'bird'}, {'frequency': 'r', 'id': 100, 'synset': 'bird_feeder.n.01', 'synonyms': ['birdfeeder'], 'def': 'an outdoor device that supplies food for wild birds', 'name': 'birdfeeder'}, {'frequency': 'r', 'id': 101, 'synset': 'birdbath.n.01', 'synonyms': ['birdbath'], 'def': 'an ornamental basin (usually in a garden) for birds to bathe in', 'name': 'birdbath'}, {'frequency': 'c', 'id': 102, 'synset': 'birdcage.n.01', 'synonyms': ['birdcage'], 'def': 'a cage in which a bird can be kept', 'name': 'birdcage'}, {'frequency': 'c', 'id': 103, 'synset': 'birdhouse.n.01', 'synonyms': ['birdhouse'], 'def': 'a shelter for birds', 'name': 'birdhouse'}, {'frequency': 'f', 'id': 104, 'synset': 'birthday_cake.n.01', 'synonyms': ['birthday_cake'], 'def': 'decorated cake served at a birthday party', 'name': 'birthday_cake'}, {'frequency': 'r', 'id': 105, 'synset': 'birthday_card.n.01', 'synonyms': ['birthday_card'], 'def': 'a card expressing a birthday greeting', 'name': 'birthday_card'}, {'frequency': 'r', 'id': 106, 'synset': 'biscuit.n.01', 'synonyms': ['biscuit_(bread)'], 'def': 'small round bread leavened with baking-powder or soda', 'name': 'biscuit_(bread)'}, {'frequency': 'r', 'id': 107, 'synset': 'black_flag.n.01', 'synonyms': ['pirate_flag'], 'def': 'a flag usually bearing a white skull and crossbones on a black background', 'name': 'pirate_flag'}, {'frequency': 'c', 'id': 108, 'synset': 'black_sheep.n.02', 'synonyms': ['black_sheep'], 'def': 'sheep with a black coat', 'name': 'black_sheep'}, {'frequency': 'c', 'id': 109, 'synset': 'blackboard.n.01', 'synonyms': ['blackboard', 'chalkboard'], 'def': 'sheet of slate; for writing with chalk', 'name': 'blackboard'}, {'frequency': 'f', 'id': 110, 'synset': 'blanket.n.01', 'synonyms': ['blanket'], 'def': 'bedding that keeps a person warm in bed', 'name': 'blanket'}, {'frequency': 'c', 'id': 111, 'synset': 'blazer.n.01', 'synonyms': ['blazer', 'sport_jacket', 'sport_coat', 'sports_jacket', 'sports_coat'], 'def': 'lightweight jacket; often striped in the colors of a club or school', 'name': 'blazer'}, {'frequency': 'f', 'id': 112, 'synset': 'blender.n.01', 'synonyms': ['blender', 'liquidizer', 'liquidiser'], 'def': 'an electrically powered mixer that mix or chop or liquefy foods', 'name': 'blender'}, {'frequency': 'r', 'id': 113, 'synset': 'blimp.n.02', 'synonyms': ['blimp'], 'def': 'a small nonrigid airship used for observation or as a barrage balloon', 'name': 'blimp'}, {'frequency': 'c', 'id': 114, 'synset': 'blinker.n.01', 'synonyms': ['blinker', 'flasher'], 'def': 'a light that flashes on and off; used as a signal or to send messages', 'name': 'blinker'}, {'frequency': 'c', 'id': 115, 'synset': 'blueberry.n.02', 'synonyms': ['blueberry'], 'def': 'sweet edible dark-blue berries of blueberry plants', 'name': 'blueberry'}, {'frequency': 'r', 'id': 116, 'synset': 'boar.n.02', 'synonyms': ['boar'], 'def': 'an uncastrated male hog', 'name': 'boar'}, {'frequency': 'r', 'id': 117, 'synset': 'board.n.09', 'synonyms': ['gameboard'], 'def': 'a flat portable surface (usually rectangular) designed for board games', 'name': 'gameboard'}, {'frequency': 'f', 'id': 118, 'synset': 'boat.n.01', 'synonyms': ['boat', 'ship_(boat)'], 'def': 'a vessel for travel on water', 'name': 'boat'}, {'frequency': 'c', 'id': 119, 'synset': 'bobbin.n.01', 'synonyms': ['bobbin', 'spool', 'reel'], 'def': 'a thing around which thread/tape/film or other flexible materials can be wound', 'name': 'bobbin'}, {'frequency': 'r', 'id': 120, 'synset': 'bobby_pin.n.01', 'synonyms': ['bobby_pin', 'hairgrip'], 'def': 'a flat wire hairpin used to hold bobbed hair in place', 'name': 'bobby_pin'}, {'frequency': 'c', 'id': 121, 'synset': 'boiled_egg.n.01', 'synonyms': ['boiled_egg', 'coddled_egg'], 'def': 'egg cooked briefly in the shell in gently boiling water', 'name': 'boiled_egg'}, {'frequency': 'r', 'id': 122, 'synset': 'bolo_tie.n.01', 'synonyms': ['bolo_tie', 'bolo', 'bola_tie', 'bola'], 'def': 'a cord fastened around the neck with an ornamental clasp and worn as a necktie', 'name': 'bolo_tie'}, {'frequency': 'c', 'id': 123, 'synset': 'bolt.n.03', 'synonyms': ['deadbolt'], 'def': 'the part of a lock that is engaged or withdrawn with a key', 'name': 'deadbolt'}, {'frequency': 'f', 'id': 124, 'synset': 'bolt.n.06', 'synonyms': ['bolt'], 'def': 'a screw that screws into a nut to form a fastener', 'name': 'bolt'}, {'frequency': 'r', 'id': 125, 'synset': 'bonnet.n.01', 'synonyms': ['bonnet'], 'def': 'a hat tied under the chin', 'name': 'bonnet'}, {'frequency': 'f', 'id': 126, 'synset': 'book.n.01', 'synonyms': ['book'], 'def': 'a written work or composition that has been published', 'name': 'book'}, {'frequency': 'r', 'id': 127, 'synset': 'book_bag.n.01', 'synonyms': ['book_bag'], 'def': 'a bag in which students carry their books', 'name': 'book_bag'}, {'frequency': 'c', 'id': 128, 'synset': 'bookcase.n.01', 'synonyms': ['bookcase'], 'def': 'a piece of furniture with shelves for storing books', 'name': 'bookcase'}, {'frequency': 'c', 'id': 129, 'synset': 'booklet.n.01', 'synonyms': ['booklet', 'brochure', 'leaflet', 'pamphlet'], 'def': 'a small book usually having a paper cover', 'name': 'booklet'}, {'frequency': 'r', 'id': 130, 'synset': 'bookmark.n.01', 'synonyms': ['bookmark', 'bookmarker'], 'def': 'a marker (a piece of paper or ribbon) placed between the pages of a book', 'name': 'bookmark'}, {'frequency': 'r', 'id': 131, 'synset': 'boom.n.04', 'synonyms': ['boom_microphone', 'microphone_boom'], 'def': 'a pole carrying an overhead microphone projected over a film or tv set', 'name': 'boom_microphone'}, {'frequency': 'f', 'id': 132, 'synset': 'boot.n.01', 'synonyms': ['boot'], 'def': 'footwear that covers the whole foot and lower leg', 'name': 'boot'}, {'frequency': 'f', 'id': 133, 'synset': 'bottle.n.01', 'synonyms': ['bottle'], 'def': 'a glass or plastic vessel used for storing drinks or other liquids', 'name': 'bottle'}, {'frequency': 'c', 'id': 134, 'synset': 'bottle_opener.n.01', 'synonyms': ['bottle_opener'], 'def': 'an opener for removing caps or corks from bottles', 'name': 'bottle_opener'}, {'frequency': 'c', 'id': 135, 'synset': 'bouquet.n.01', 'synonyms': ['bouquet'], 'def': 'an arrangement of flowers that is usually given as a present', 'name': 'bouquet'}, {'frequency': 'r', 'id': 136, 'synset': 'bow.n.04', 'synonyms': ['bow_(weapon)'], 'def': 'a weapon for shooting arrows', 'name': 'bow_(weapon)'}, {'frequency': 'f', 'id': 137, 'synset': 'bow.n.08', 'synonyms': ['bow_(decorative_ribbons)'], 'def': 'a decorative interlacing of ribbons', 'name': 'bow_(decorative_ribbons)'}, {'frequency': 'f', 'id': 138, 'synset': 'bow_tie.n.01', 'synonyms': ['bow-tie', 'bowtie'], 'def': "a man's tie that ties in a bow", 'name': 'bow-tie'}, {'frequency': 'f', 'id': 139, 'synset': 'bowl.n.03', 'synonyms': ['bowl'], 'def': 'a dish that is round and open at the top for serving foods', 'name': 'bowl'}, {'frequency': 'r', 'id': 140, 'synset': 'bowl.n.08', 'synonyms': ['pipe_bowl'], 'def': 'a small round container that is open at the top for holding tobacco', 'name': 'pipe_bowl'}, {'frequency': 'c', 'id': 141, 'synset': 'bowler_hat.n.01', 'synonyms': ['bowler_hat', 'bowler', 'derby_hat', 'derby', 'plug_hat'], 'def': 'a felt hat that is round and hard with a narrow brim', 'name': 'bowler_hat'}, {'frequency': 'r', 'id': 142, 'synset': 'bowling_ball.n.01', 'synonyms': ['bowling_ball'], 'def': 'a large ball with finger holes used in the sport of bowling', 'name': 'bowling_ball'}, {'frequency': 'r', 'id': 143, 'synset': 'bowling_pin.n.01', 'synonyms': ['bowling_pin'], 'def': 'a club-shaped wooden object used in bowling', 'name': 'bowling_pin'}, {'frequency': 'r', 'id': 144, 'synset': 'boxing_glove.n.01', 'synonyms': ['boxing_glove'], 'def': 'large glove coverings the fists of a fighter worn for the sport of boxing', 'name': 'boxing_glove'}, {'frequency': 'c', 'id': 145, 'synset': 'brace.n.06', 'synonyms': ['suspenders'], 'def': 'elastic straps that hold trousers up (usually used in the plural)', 'name': 'suspenders'}, {'frequency': 'f', 'id': 146, 'synset': 'bracelet.n.02', 'synonyms': ['bracelet', 'bangle'], 'def': 'jewelry worn around the wrist for decoration', 'name': 'bracelet'}, {'frequency': 'r', 'id': 147, 'synset': 'brass.n.07', 'synonyms': ['brass_plaque'], 'def': 'a memorial made of brass', 'name': 'brass_plaque'}, {'frequency': 'c', 'id': 148, 'synset': 'brassiere.n.01', 'synonyms': ['brassiere', 'bra', 'bandeau'], 'def': 'an undergarment worn by women to support their breasts', 'name': 'brassiere'}, {'frequency': 'c', 'id': 149, 'synset': 'bread-bin.n.01', 'synonyms': ['bread-bin', 'breadbox'], 'def': 'a container used to keep bread or cake in', 'name': 'bread-bin'}, {'frequency': 'r', 'id': 150, 'synset': 'breechcloth.n.01', 'synonyms': ['breechcloth', 'breechclout', 'loincloth'], 'def': 'a garment that provides covering for the loins', 'name': 'breechcloth'}, {'frequency': 'c', 'id': 151, 'synset': 'bridal_gown.n.01', 'synonyms': ['bridal_gown', 'wedding_gown', 'wedding_dress'], 'def': 'a gown worn by the bride at a wedding', 'name': 'bridal_gown'}, {'frequency': 'c', 'id': 152, 'synset': 'briefcase.n.01', 'synonyms': ['briefcase'], 'def': 'a case with a handle; for carrying papers or files or books', 'name': 'briefcase'}, {'frequency': 'c', 'id': 153, 'synset': 'bristle_brush.n.01', 'synonyms': ['bristle_brush'], 'def': 'a brush that is made with the short stiff hairs of an animal or plant', 'name': 'bristle_brush'}, {'frequency': 'f', 'id': 154, 'synset': 'broccoli.n.01', 'synonyms': ['broccoli'], 'def': 'plant with dense clusters of tight green flower buds', 'name': 'broccoli'}, {'frequency': 'r', 'id': 155, 'synset': 'brooch.n.01', 'synonyms': ['broach'], 'def': 'a decorative pin worn by women', 'name': 'broach'}, {'frequency': 'c', 'id': 156, 'synset': 'broom.n.01', 'synonyms': ['broom'], 'def': 'bundle of straws or twigs attached to a long handle; used for cleaning', 'name': 'broom'}, {'frequency': 'c', 'id': 157, 'synset': 'brownie.n.03', 'synonyms': ['brownie'], 'def': 'square or bar of very rich chocolate cake usually with nuts', 'name': 'brownie'}, {'frequency': 'c', 'id': 158, 'synset': 'brussels_sprouts.n.01', 'synonyms': ['brussels_sprouts'], 'def': 'the small edible cabbage-like buds growing along a stalk', 'name': 'brussels_sprouts'}, {'frequency': 'r', 'id': 159, 'synset': 'bubble_gum.n.01', 'synonyms': ['bubble_gum'], 'def': 'a kind of chewing gum that can be blown into bubbles', 'name': 'bubble_gum'}, {'frequency': 'f', 'id': 160, 'synset': 'bucket.n.01', 'synonyms': ['bucket', 'pail'], 'def': 'a roughly cylindrical vessel that is open at the top', 'name': 'bucket'}, {'frequency': 'r', 'id': 161, 'synset': 'buggy.n.01', 'synonyms': ['horse_buggy'], 'def': 'a small lightweight carriage; drawn by a single horse', 'name': 'horse_buggy'}, {'frequency': 'c', 'id': 162, 'synset': 'bull.n.11', 'synonyms': ['bull'], 'def': 'mature male cow', 'name': 'bull'}, {'frequency': 'r', 'id': 163, 'synset': 'bulldog.n.01', 'synonyms': ['bulldog'], 'def': 'a thickset short-haired dog with a large head and strong undershot lower jaw', 'name': 'bulldog'}, {'frequency': 'r', 'id': 164, 'synset': 'bulldozer.n.01', 'synonyms': ['bulldozer', 'dozer'], 'def': 'large powerful tractor; a large blade in front flattens areas of ground', 'name': 'bulldozer'}, {'frequency': 'c', 'id': 165, 'synset': 'bullet_train.n.01', 'synonyms': ['bullet_train'], 'def': 'a high-speed passenger train', 'name': 'bullet_train'}, {'frequency': 'c', 'id': 166, 'synset': 'bulletin_board.n.02', 'synonyms': ['bulletin_board', 'notice_board'], 'def': 'a board that hangs on a wall; displays announcements', 'name': 'bulletin_board'}, {'frequency': 'r', 'id': 167, 'synset': 'bulletproof_vest.n.01', 'synonyms': ['bulletproof_vest'], 'def': 'a vest capable of resisting the impact of a bullet', 'name': 'bulletproof_vest'}, {'frequency': 'c', 'id': 168, 'synset': 'bullhorn.n.01', 'synonyms': ['bullhorn', 'megaphone'], 'def': 'a portable loudspeaker with built-in microphone and amplifier', 'name': 'bullhorn'}, {'frequency': 'r', 'id': 169, 'synset': 'bully_beef.n.01', 'synonyms': ['corned_beef', 'corn_beef'], 'def': 'beef cured or pickled in brine', 'name': 'corned_beef'}, {'frequency': 'f', 'id': 170, 'synset': 'bun.n.01', 'synonyms': ['bun', 'roll'], 'def': 'small rounded bread either plain or sweet', 'name': 'bun'}, {'frequency': 'c', 'id': 171, 'synset': 'bunk_bed.n.01', 'synonyms': ['bunk_bed'], 'def': 'beds built one above the other', 'name': 'bunk_bed'}, {'frequency': 'f', 'id': 172, 'synset': 'buoy.n.01', 'synonyms': ['buoy'], 'def': 'a float attached by rope to the seabed to mark channels in a harbor or underwater hazards', 'name': 'buoy'}, {'frequency': 'r', 'id': 173, 'synset': 'burrito.n.01', 'synonyms': ['burrito'], 'def': 'a flour tortilla folded around a filling', 'name': 'burrito'}, {'frequency': 'f', 'id': 174, 'synset': 'bus.n.01', 'synonyms': ['bus_(vehicle)', 'autobus', 'charabanc', 'double-decker', 'motorbus', 'motorcoach'], 'def': 'a vehicle carrying many passengers; used for public transport', 'name': 'bus_(vehicle)'}, {'frequency': 'c', 'id': 175, 'synset': 'business_card.n.01', 'synonyms': ['business_card'], 'def': "a card on which are printed the person's name and business affiliation", 'name': 'business_card'}, {'frequency': 'c', 'id': 176, 'synset': 'butcher_knife.n.01', 'synonyms': ['butcher_knife'], 'def': 'a large sharp knife for cutting or trimming meat', 'name': 'butcher_knife'}, {'frequency': 'c', 'id': 177, 'synset': 'butter.n.01', 'synonyms': ['butter'], 'def': 'an edible emulsion of fat globules made by churning milk or cream; for cooking and table use', 'name': 'butter'}, {'frequency': 'c', 'id': 178, 'synset': 'butterfly.n.01', 'synonyms': ['butterfly'], 'def': 'insect typically having a slender body with knobbed antennae and broad colorful wings', 'name': 'butterfly'}, {'frequency': 'f', 'id': 179, 'synset': 'button.n.01', 'synonyms': ['button'], 'def': 'a round fastener sewn to shirts and coats etc to fit through buttonholes', 'name': 'button'}, {'frequency': 'f', 'id': 180, 'synset': 'cab.n.03', 'synonyms': ['cab_(taxi)', 'taxi', 'taxicab'], 'def': 'a car that takes passengers where they want to go in exchange for money', 'name': 'cab_(taxi)'}, {'frequency': 'r', 'id': 181, 'synset': 'cabana.n.01', 'synonyms': ['cabana'], 'def': 'a small tent used as a dressing room beside the sea or a swimming pool', 'name': 'cabana'}, {'frequency': 'r', 'id': 182, 'synset': 'cabin_car.n.01', 'synonyms': ['cabin_car', 'caboose'], 'def': 'a car on a freight train for use of the train crew; usually the last car on the train', 'name': 'cabin_car'}, {'frequency': 'f', 'id': 183, 'synset': 'cabinet.n.01', 'synonyms': ['cabinet'], 'def': 'a piece of furniture resembling a cupboard with doors and shelves and drawers', 'name': 'cabinet'}, {'frequency': 'r', 'id': 184, 'synset': 'cabinet.n.03', 'synonyms': ['locker', 'storage_locker'], 'def': 'a storage compartment for clothes and valuables; usually it has a lock', 'name': 'locker'}, {'frequency': 'f', 'id': 185, 'synset': 'cake.n.03', 'synonyms': ['cake'], 'def': 'baked goods made from or based on a mixture of flour, sugar, eggs, and fat', 'name': 'cake'}, {'frequency': 'c', 'id': 186, 'synset': 'calculator.n.02', 'synonyms': ['calculator'], 'def': 'a small machine that is used for mathematical calculations', 'name': 'calculator'}, {'frequency': 'f', 'id': 187, 'synset': 'calendar.n.02', 'synonyms': ['calendar'], 'def': 'a list or register of events (appointments/social events/court cases, etc)', 'name': 'calendar'}, {'frequency': 'c', 'id': 188, 'synset': 'calf.n.01', 'synonyms': ['calf'], 'def': 'young of domestic cattle', 'name': 'calf'}, {'frequency': 'c', 'id': 189, 'synset': 'camcorder.n.01', 'synonyms': ['camcorder'], 'def': 'a portable television camera and videocassette recorder', 'name': 'camcorder'}, {'frequency': 'c', 'id': 190, 'synset': 'camel.n.01', 'synonyms': ['camel'], 'def': 'cud-chewing mammal used as a draft or saddle animal in desert regions', 'name': 'camel'}, {'frequency': 'f', 'id': 191, 'synset': 'camera.n.01', 'synonyms': ['camera'], 'def': 'equipment for taking photographs', 'name': 'camera'}, {'frequency': 'c', 'id': 192, 'synset': 'camera_lens.n.01', 'synonyms': ['camera_lens'], 'def': 'a lens that focuses the image in a camera', 'name': 'camera_lens'}, {'frequency': 'c', 'id': 193, 'synset': 'camper.n.02', 'synonyms': ['camper_(vehicle)', 'camping_bus', 'motor_home'], 'def': 'a recreational vehicle equipped for camping out while traveling', 'name': 'camper_(vehicle)'}, {'frequency': 'f', 'id': 194, 'synset': 'can.n.01', 'synonyms': ['can', 'tin_can'], 'def': 'airtight sealed metal container for food or drink or paint etc.', 'name': 'can'}, {'frequency': 'c', 'id': 195, 'synset': 'can_opener.n.01', 'synonyms': ['can_opener', 'tin_opener'], 'def': 'a device for cutting cans open', 'name': 'can_opener'}, {'frequency': 'r', 'id': 196, 'synset': 'candelabrum.n.01', 'synonyms': ['candelabrum', 'candelabra'], 'def': 'branched candlestick; ornamental; has several lights', 'name': 'candelabrum'}, {'frequency': 'f', 'id': 197, 'synset': 'candle.n.01', 'synonyms': ['candle', 'candlestick'], 'def': 'stick of wax with a wick in the middle', 'name': 'candle'}, {'frequency': 'f', 'id': 198, 'synset': 'candlestick.n.01', 'synonyms': ['candle_holder'], 'def': 'a holder with sockets for candles', 'name': 'candle_holder'}, {'frequency': 'r', 'id': 199, 'synset': 'candy_bar.n.01', 'synonyms': ['candy_bar'], 'def': 'a candy shaped as a bar', 'name': 'candy_bar'}, {'frequency': 'c', 'id': 200, 'synset': 'candy_cane.n.01', 'synonyms': ['candy_cane'], 'def': 'a hard candy in the shape of a rod (usually with stripes)', 'name': 'candy_cane'}, {'frequency': 'c', 'id': 201, 'synset': 'cane.n.01', 'synonyms': ['walking_cane'], 'def': 'a stick that people can lean on to help them walk', 'name': 'walking_cane'}, {'frequency': 'c', 'id': 202, 'synset': 'canister.n.02', 'synonyms': ['canister', 'cannister'], 'def': 'metal container for storing dry foods such as tea or flour', 'name': 'canister'}, {'frequency': 'r', 'id': 203, 'synset': 'cannon.n.02', 'synonyms': ['cannon'], 'def': 'heavy gun fired from a tank', 'name': 'cannon'}, {'frequency': 'c', 'id': 204, 'synset': 'canoe.n.01', 'synonyms': ['canoe'], 'def': 'small and light boat; pointed at both ends; propelled with a paddle', 'name': 'canoe'}, {'frequency': 'r', 'id': 205, 'synset': 'cantaloup.n.02', 'synonyms': ['cantaloup', 'cantaloupe'], 'def': 'the fruit of a cantaloup vine; small to medium-sized melon with yellowish flesh', 'name': 'cantaloup'}, {'frequency': 'r', 'id': 206, 'synset': 'canteen.n.01', 'synonyms': ['canteen'], 'def': 'a flask for carrying water; used by soldiers or travelers', 'name': 'canteen'}, {'frequency': 'c', 'id': 207, 'synset': 'cap.n.01', 'synonyms': ['cap_(headwear)'], 'def': 'a tight-fitting headwear', 'name': 'cap_(headwear)'}, {'frequency': 'f', 'id': 208, 'synset': 'cap.n.02', 'synonyms': ['bottle_cap', 'cap_(container_lid)'], 'def': 'a top (as for a bottle)', 'name': 'bottle_cap'}, {'frequency': 'r', 'id': 209, 'synset': 'cape.n.02', 'synonyms': ['cape'], 'def': 'a sleeveless garment like a cloak but shorter', 'name': 'cape'}, {'frequency': 'c', 'id': 210, 'synset': 'cappuccino.n.01', 'synonyms': ['cappuccino', 'coffee_cappuccino'], 'def': 'equal parts of espresso and steamed milk', 'name': 'cappuccino'}, {'frequency': 'f', 'id': 211, 'synset': 'car.n.01', 'synonyms': ['car_(automobile)', 'auto_(automobile)', 'automobile'], 'def': 'a motor vehicle with four wheels', 'name': 'car_(automobile)'}, {'frequency': 'f', 'id': 212, 'synset': 'car.n.02', 'synonyms': ['railcar_(part_of_a_train)', 'railway_car_(part_of_a_train)', 'railroad_car_(part_of_a_train)'], 'def': 'a wheeled vehicle adapted to the rails of railroad', 'name': 'railcar_(part_of_a_train)'}, {'frequency': 'r', 'id': 213, 'synset': 'car.n.04', 'synonyms': ['elevator_car'], 'def': 'where passengers ride up and down', 'name': 'elevator_car'}, {'frequency': 'r', 'id': 214, 'synset': 'car_battery.n.01', 'synonyms': ['car_battery', 'automobile_battery'], 'def': 'a battery in a motor vehicle', 'name': 'car_battery'}, {'frequency': 'c', 'id': 215, 'synset': 'card.n.02', 'synonyms': ['identity_card'], 'def': 'a card certifying the identity of the bearer', 'name': 'identity_card'}, {'frequency': 'c', 'id': 216, 'synset': 'card.n.03', 'synonyms': ['card'], 'def': 'a rectangular piece of paper used to send messages (e.g. greetings or pictures)', 'name': 'card'}, {'frequency': 'r', 'id': 217, 'synset': 'cardigan.n.01', 'synonyms': ['cardigan'], 'def': 'knitted jacket that is fastened up the front with buttons or a zipper', 'name': 'cardigan'}, {'frequency': 'r', 'id': 218, 'synset': 'cargo_ship.n.01', 'synonyms': ['cargo_ship', 'cargo_vessel'], 'def': 'a ship designed to carry cargo', 'name': 'cargo_ship'}, {'frequency': 'r', 'id': 219, 'synset': 'carnation.n.01', 'synonyms': ['carnation'], 'def': 'plant with pink to purple-red spice-scented usually double flowers', 'name': 'carnation'}, {'frequency': 'c', 'id': 220, 'synset': 'carriage.n.02', 'synonyms': ['horse_carriage'], 'def': 'a vehicle with wheels drawn by one or more horses', 'name': 'horse_carriage'}, {'frequency': 'f', 'id': 221, 'synset': 'carrot.n.01', 'synonyms': ['carrot'], 'def': 'deep orange edible root of the cultivated carrot plant', 'name': 'carrot'}, {'frequency': 'c', 'id': 222, 'synset': 'carryall.n.01', 'synonyms': ['tote_bag'], 'def': 'a capacious bag or basket', 'name': 'tote_bag'}, {'frequency': 'c', 'id': 223, 'synset': 'cart.n.01', 'synonyms': ['cart'], 'def': 'a heavy open wagon usually having two wheels and drawn by an animal', 'name': 'cart'}, {'frequency': 'c', 'id': 224, 'synset': 'carton.n.02', 'synonyms': ['carton'], 'def': 'a box made of cardboard; opens by flaps on top', 'name': 'carton'}, {'frequency': 'c', 'id': 225, 'synset': 'cash_register.n.01', 'synonyms': ['cash_register', 'register_(for_cash_transactions)'], 'def': 'a cashbox with an adding machine to register transactions', 'name': 'cash_register'}, {'frequency': 'r', 'id': 226, 'synset': 'casserole.n.01', 'synonyms': ['casserole'], 'def': 'food cooked and served in a casserole', 'name': 'casserole'}, {'frequency': 'r', 'id': 227, 'synset': 'cassette.n.01', 'synonyms': ['cassette'], 'def': 'a container that holds a magnetic tape used for recording or playing sound or video', 'name': 'cassette'}, {'frequency': 'c', 'id': 228, 'synset': 'cast.n.05', 'synonyms': ['cast', 'plaster_cast', 'plaster_bandage'], 'def': 'bandage consisting of a firm covering that immobilizes broken bones while they heal', 'name': 'cast'}, {'frequency': 'f', 'id': 229, 'synset': 'cat.n.01', 'synonyms': ['cat'], 'def': 'a domestic house cat', 'name': 'cat'}, {'frequency': 'c', 'id': 230, 'synset': 'cauliflower.n.02', 'synonyms': ['cauliflower'], 'def': 'edible compact head of white undeveloped flowers', 'name': 'cauliflower'}, {'frequency': 'r', 'id': 231, 'synset': 'caviar.n.01', 'synonyms': ['caviar', 'caviare'], 'def': "salted roe of sturgeon or other large fish; usually served as an hors d'oeuvre", 'name': 'caviar'}, {'frequency': 'c', 'id': 232, 'synset': 'cayenne.n.02', 'synonyms': ['cayenne_(spice)', 'cayenne_pepper_(spice)', 'red_pepper_(spice)'], 'def': 'ground pods and seeds of pungent red peppers of the genus Capsicum', 'name': 'cayenne_(spice)'}, {'frequency': 'c', 'id': 233, 'synset': 'cd_player.n.01', 'synonyms': ['CD_player'], 'def': 'electronic equipment for playing compact discs (CDs)', 'name': 'CD_player'}, {'frequency': 'c', 'id': 234, 'synset': 'celery.n.01', 'synonyms': ['celery'], 'def': 'widely cultivated herb with aromatic leaf stalks that are eaten raw or cooked', 'name': 'celery'}, {'frequency': 'f', 'id': 235, 'synset': 'cellular_telephone.n.01', 'synonyms': ['cellular_telephone', 'cellular_phone', 'cellphone', 'mobile_phone', 'smart_phone'], 'def': 'a hand-held mobile telephone', 'name': 'cellular_telephone'}, {'frequency': 'r', 'id': 236, 'synset': 'chain_mail.n.01', 'synonyms': ['chain_mail', 'ring_mail', 'chain_armor', 'chain_armour', 'ring_armor', 'ring_armour'], 'def': '(Middle Ages) flexible armor made of interlinked metal rings', 'name': 'chain_mail'}, {'frequency': 'f', 'id': 237, 'synset': 'chair.n.01', 'synonyms': ['chair'], 'def': 'a seat for one person, with a support for the back', 'name': 'chair'}, {'frequency': 'r', 'id': 238, 'synset': 'chaise_longue.n.01', 'synonyms': ['chaise_longue', 'chaise', 'daybed'], 'def': 'a long chair; for reclining', 'name': 'chaise_longue'}, {'frequency': 'r', 'id': 239, 'synset': 'champagne.n.01', 'synonyms': ['champagne'], 'def': 'a white sparkling wine produced in Champagne or resembling that produced there', 'name': 'champagne'}, {'frequency': 'f', 'id': 240, 'synset': 'chandelier.n.01', 'synonyms': ['chandelier'], 'def': 'branched lighting fixture; often ornate; hangs from the ceiling', 'name': 'chandelier'}, {'frequency': 'r', 'id': 241, 'synset': 'chap.n.04', 'synonyms': ['chap'], 'def': 'leather leggings without a seat; worn over trousers by cowboys to protect their legs', 'name': 'chap'}, {'frequency': 'r', 'id': 242, 'synset': 'checkbook.n.01', 'synonyms': ['checkbook', 'chequebook'], 'def': 'a book issued to holders of checking accounts', 'name': 'checkbook'}, {'frequency': 'r', 'id': 243, 'synset': 'checkerboard.n.01', 'synonyms': ['checkerboard'], 'def': 'a board having 64 squares of two alternating colors', 'name': 'checkerboard'}, {'frequency': 'c', 'id': 244, 'synset': 'cherry.n.03', 'synonyms': ['cherry'], 'def': 'a red fruit with a single hard stone', 'name': 'cherry'}, {'frequency': 'r', 'id': 245, 'synset': 'chessboard.n.01', 'synonyms': ['chessboard'], 'def': 'a checkerboard used to play chess', 'name': 'chessboard'}, {'frequency': 'r', 'id': 246, 'synset': 'chest_of_drawers.n.01', 'synonyms': ['chest_of_drawers_(furniture)', 'bureau_(furniture)', 'chest_(furniture)'], 'def': 'furniture with drawers for keeping clothes', 'name': 'chest_of_drawers_(furniture)'}, {'frequency': 'c', 'id': 247, 'synset': 'chicken.n.02', 'synonyms': ['chicken_(animal)'], 'def': 'a domestic fowl bred for flesh or eggs', 'name': 'chicken_(animal)'}, {'frequency': 'c', 'id': 248, 'synset': 'chicken_wire.n.01', 'synonyms': ['chicken_wire'], 'def': 'a galvanized wire network with a hexagonal mesh; used to build fences', 'name': 'chicken_wire'}, {'frequency': 'r', 'id': 249, 'synset': 'chickpea.n.01', 'synonyms': ['chickpea', 'garbanzo'], 'def': 'the seed of the chickpea plant; usually dried', 'name': 'chickpea'}, {'frequency': 'r', 'id': 250, 'synset': 'chihuahua.n.03', 'synonyms': ['Chihuahua'], 'def': 'an old breed of tiny short-haired dog with protruding eyes from Mexico', 'name': 'Chihuahua'}, {'frequency': 'r', 'id': 251, 'synset': 'chili.n.02', 'synonyms': ['chili_(vegetable)', 'chili_pepper_(vegetable)', 'chilli_(vegetable)', 'chilly_(vegetable)', 'chile_(vegetable)'], 'def': 'very hot and finely tapering pepper of special pungency', 'name': 'chili_(vegetable)'}, {'frequency': 'r', 'id': 252, 'synset': 'chime.n.01', 'synonyms': ['chime', 'gong'], 'def': 'an instrument consisting of a set of bells that are struck with a hammer', 'name': 'chime'}, {'frequency': 'r', 'id': 253, 'synset': 'chinaware.n.01', 'synonyms': ['chinaware'], 'def': 'dishware made of high quality porcelain', 'name': 'chinaware'}, {'frequency': 'c', 'id': 254, 'synset': 'chip.n.04', 'synonyms': ['crisp_(potato_chip)', 'potato_chip'], 'def': 'a thin crisp slice of potato fried in deep fat', 'name': 'crisp_(potato_chip)'}, {'frequency': 'r', 'id': 255, 'synset': 'chip.n.06', 'synonyms': ['poker_chip'], 'def': 'a small disk-shaped counter used to represent money when gambling', 'name': 'poker_chip'}, {'frequency': 'c', 'id': 256, 'synset': 'chocolate_bar.n.01', 'synonyms': ['chocolate_bar'], 'def': 'a bar of chocolate candy', 'name': 'chocolate_bar'}, {'frequency': 'c', 'id': 257, 'synset': 'chocolate_cake.n.01', 'synonyms': ['chocolate_cake'], 'def': 'cake containing chocolate', 'name': 'chocolate_cake'}, {'frequency': 'r', 'id': 258, 'synset': 'chocolate_milk.n.01', 'synonyms': ['chocolate_milk'], 'def': 'milk flavored with chocolate syrup', 'name': 'chocolate_milk'}, {'frequency': 'r', 'id': 259, 'synset': 'chocolate_mousse.n.01', 'synonyms': ['chocolate_mousse'], 'def': 'dessert mousse made with chocolate', 'name': 'chocolate_mousse'}, {'frequency': 'f', 'id': 260, 'synset': 'choker.n.03', 'synonyms': ['choker', 'collar', 'neckband'], 'def': 'necklace that fits tightly around the neck', 'name': 'choker'}, {'frequency': 'f', 'id': 261, 'synset': 'chopping_board.n.01', 'synonyms': ['chopping_board', 'cutting_board', 'chopping_block'], 'def': 'a wooden board where meats or vegetables can be cut', 'name': 'chopping_board'}, {'frequency': 'c', 'id': 262, 'synset': 'chopstick.n.01', 'synonyms': ['chopstick'], 'def': 'one of a pair of slender sticks used as oriental tableware to eat food with', 'name': 'chopstick'}, {'frequency': 'f', 'id': 263, 'synset': 'christmas_tree.n.05', 'synonyms': ['Christmas_tree'], 'def': 'an ornamented evergreen used as a Christmas decoration', 'name': 'Christmas_tree'}, {'frequency': 'c', 'id': 264, 'synset': 'chute.n.02', 'synonyms': ['slide'], 'def': 'sloping channel through which things can descend', 'name': 'slide'}, {'frequency': 'r', 'id': 265, 'synset': 'cider.n.01', 'synonyms': ['cider', 'cyder'], 'def': 'a beverage made from juice pressed from apples', 'name': 'cider'}, {'frequency': 'r', 'id': 266, 'synset': 'cigar_box.n.01', 'synonyms': ['cigar_box'], 'def': 'a box for holding cigars', 'name': 'cigar_box'}, {'frequency': 'c', 'id': 267, 'synset': 'cigarette.n.01', 'synonyms': ['cigarette'], 'def': 'finely ground tobacco wrapped in paper; for smoking', 'name': 'cigarette'}, {'frequency': 'c', 'id': 268, 'synset': 'cigarette_case.n.01', 'synonyms': ['cigarette_case', 'cigarette_pack'], 'def': 'a small flat case for holding cigarettes', 'name': 'cigarette_case'}, {'frequency': 'f', 'id': 269, 'synset': 'cistern.n.02', 'synonyms': ['cistern', 'water_tank'], 'def': 'a tank that holds the water used to flush a toilet', 'name': 'cistern'}, {'frequency': 'r', 'id': 270, 'synset': 'clarinet.n.01', 'synonyms': ['clarinet'], 'def': 'a single-reed instrument with a straight tube', 'name': 'clarinet'}, {'frequency': 'r', 'id': 271, 'synset': 'clasp.n.01', 'synonyms': ['clasp'], 'def': 'a fastener (as a buckle or hook) that is used to hold two things together', 'name': 'clasp'}, {'frequency': 'c', 'id': 272, 'synset': 'cleansing_agent.n.01', 'synonyms': ['cleansing_agent', 'cleanser', 'cleaner'], 'def': 'a preparation used in cleaning something', 'name': 'cleansing_agent'}, {'frequency': 'r', 'id': 273, 'synset': 'clementine.n.01', 'synonyms': ['clementine'], 'def': 'a variety of mandarin orange', 'name': 'clementine'}, {'frequency': 'c', 'id': 274, 'synset': 'clip.n.03', 'synonyms': ['clip'], 'def': 'any of various small fasteners used to hold loose articles together', 'name': 'clip'}, {'frequency': 'c', 'id': 275, 'synset': 'clipboard.n.01', 'synonyms': ['clipboard'], 'def': 'a small writing board with a clip at the top for holding papers', 'name': 'clipboard'}, {'frequency': 'f', 'id': 276, 'synset': 'clock.n.01', 'synonyms': ['clock', 'timepiece', 'timekeeper'], 'def': 'a timepiece that shows the time of day', 'name': 'clock'}, {'frequency': 'f', 'id': 277, 'synset': 'clock_tower.n.01', 'synonyms': ['clock_tower'], 'def': 'a tower with a large clock visible high up on an outside face', 'name': 'clock_tower'}, {'frequency': 'c', 'id': 278, 'synset': 'clothes_hamper.n.01', 'synonyms': ['clothes_hamper', 'laundry_basket', 'clothes_basket'], 'def': 'a hamper that holds dirty clothes to be washed or wet clothes to be dried', 'name': 'clothes_hamper'}, {'frequency': 'c', 'id': 279, 'synset': 'clothespin.n.01', 'synonyms': ['clothespin', 'clothes_peg'], 'def': 'wood or plastic fastener; for holding clothes on a clothesline', 'name': 'clothespin'}, {'frequency': 'r', 'id': 280, 'synset': 'clutch_bag.n.01', 'synonyms': ['clutch_bag'], 'def': "a woman's strapless purse that is carried in the hand", 'name': 'clutch_bag'}, {'frequency': 'f', 'id': 281, 'synset': 'coaster.n.03', 'synonyms': ['coaster'], 'def': 'a covering (plate or mat) that protects the surface of a table', 'name': 'coaster'}, {'frequency': 'f', 'id': 282, 'synset': 'coat.n.01', 'synonyms': ['coat'], 'def': 'an outer garment that has sleeves and covers the body from shoulder down', 'name': 'coat'}, {'frequency': 'c', 'id': 283, 'synset': 'coat_hanger.n.01', 'synonyms': ['coat_hanger', 'clothes_hanger', 'dress_hanger'], 'def': "a hanger that is shaped like a person's shoulders", 'name': 'coat_hanger'}, {'frequency': 'r', 'id': 284, 'synset': 'coatrack.n.01', 'synonyms': ['coatrack', 'hatrack'], 'def': 'a rack with hooks for temporarily holding coats and hats', 'name': 'coatrack'}, {'frequency': 'c', 'id': 285, 'synset': 'cock.n.04', 'synonyms': ['cock', 'rooster'], 'def': 'adult male chicken', 'name': 'cock'}, {'frequency': 'c', 'id': 286, 'synset': 'coconut.n.02', 'synonyms': ['coconut', 'cocoanut'], 'def': 'large hard-shelled brown oval nut with a fibrous husk', 'name': 'coconut'}, {'frequency': 'r', 'id': 287, 'synset': 'coffee_filter.n.01', 'synonyms': ['coffee_filter'], 'def': 'filter (usually of paper) that passes the coffee and retains the coffee grounds', 'name': 'coffee_filter'}, {'frequency': 'f', 'id': 288, 'synset': 'coffee_maker.n.01', 'synonyms': ['coffee_maker', 'coffee_machine'], 'def': 'a kitchen appliance for brewing coffee automatically', 'name': 'coffee_maker'}, {'frequency': 'f', 'id': 289, 'synset': 'coffee_table.n.01', 'synonyms': ['coffee_table', 'cocktail_table'], 'def': 'low table where magazines can be placed and coffee or cocktails are served', 'name': 'coffee_table'}, {'frequency': 'c', 'id': 290, 'synset': 'coffeepot.n.01', 'synonyms': ['coffeepot'], 'def': 'tall pot in which coffee is brewed', 'name': 'coffeepot'}, {'frequency': 'r', 'id': 291, 'synset': 'coil.n.05', 'synonyms': ['coil'], 'def': 'tubing that is wound in a spiral', 'name': 'coil'}, {'frequency': 'c', 'id': 292, 'synset': 'coin.n.01', 'synonyms': ['coin'], 'def': 'a flat metal piece (usually a disc) used as money', 'name': 'coin'}, {'frequency': 'r', 'id': 293, 'synset': 'colander.n.01', 'synonyms': ['colander', 'cullender'], 'def': 'bowl-shaped strainer; used to wash or drain foods', 'name': 'colander'}, {'frequency': 'c', 'id': 294, 'synset': 'coleslaw.n.01', 'synonyms': ['coleslaw', 'slaw'], 'def': 'basically shredded cabbage', 'name': 'coleslaw'}, {'frequency': 'r', 'id': 295, 'synset': 'coloring_material.n.01', 'synonyms': ['coloring_material', 'colouring_material'], 'def': 'any material used for its color', 'name': 'coloring_material'}, {'frequency': 'r', 'id': 296, 'synset': 'combination_lock.n.01', 'synonyms': ['combination_lock'], 'def': 'lock that can be opened only by turning dials in a special sequence', 'name': 'combination_lock'}, {'frequency': 'c', 'id': 297, 'synset': 'comforter.n.04', 'synonyms': ['pacifier', 'teething_ring'], 'def': 'device used for an infant to suck or bite on', 'name': 'pacifier'}, {'frequency': 'r', 'id': 298, 'synset': 'comic_book.n.01', 'synonyms': ['comic_book'], 'def': 'a magazine devoted to comic strips', 'name': 'comic_book'}, {'frequency': 'f', 'id': 299, 'synset': 'computer_keyboard.n.01', 'synonyms': ['computer_keyboard', 'keyboard_(computer)'], 'def': 'a keyboard that is a data input device for computers', 'name': 'computer_keyboard'}, {'frequency': 'r', 'id': 300, 'synset': 'concrete_mixer.n.01', 'synonyms': ['concrete_mixer', 'cement_mixer'], 'def': 'a machine with a large revolving drum in which cement/concrete is mixed', 'name': 'concrete_mixer'}, {'frequency': 'f', 'id': 301, 'synset': 'cone.n.01', 'synonyms': ['cone', 'traffic_cone'], 'def': 'a cone-shaped object used to direct traffic', 'name': 'cone'}, {'frequency': 'f', 'id': 302, 'synset': 'control.n.09', 'synonyms': ['control', 'controller'], 'def': 'a mechanism that controls the operation of a machine', 'name': 'control'}, {'frequency': 'r', 'id': 303, 'synset': 'convertible.n.01', 'synonyms': ['convertible_(automobile)'], 'def': 'a car that has top that can be folded or removed', 'name': 'convertible_(automobile)'}, {'frequency': 'r', 'id': 304, 'synset': 'convertible.n.03', 'synonyms': ['sofa_bed'], 'def': 'a sofa that can be converted into a bed', 'name': 'sofa_bed'}, {'frequency': 'c', 'id': 305, 'synset': 'cookie.n.01', 'synonyms': ['cookie', 'cooky', 'biscuit_(cookie)'], 'def': "any of various small flat sweet cakes (`biscuit' is the British term)", 'name': 'cookie'}, {'frequency': 'r', 'id': 306, 'synset': 'cookie_jar.n.01', 'synonyms': ['cookie_jar', 'cooky_jar'], 'def': 'a jar in which cookies are kept (and sometimes money is hidden)', 'name': 'cookie_jar'}, {'frequency': 'r', 'id': 307, 'synset': 'cooking_utensil.n.01', 'synonyms': ['cooking_utensil'], 'def': 'a kitchen utensil made of material that does not melt easily; used for cooking', 'name': 'cooking_utensil'}, {'frequency': 'f', 'id': 308, 'synset': 'cooler.n.01', 'synonyms': ['cooler_(for_food)', 'ice_chest'], 'def': 'an insulated box for storing food often with ice', 'name': 'cooler_(for_food)'}, {'frequency': 'c', 'id': 309, 'synset': 'cork.n.04', 'synonyms': ['cork_(bottle_plug)', 'bottle_cork'], 'def': 'the plug in the mouth of a bottle (especially a wine bottle)', 'name': 'cork_(bottle_plug)'}, {'frequency': 'r', 'id': 310, 'synset': 'corkboard.n.01', 'synonyms': ['corkboard'], 'def': 'a sheet consisting of cork granules', 'name': 'corkboard'}, {'frequency': 'r', 'id': 311, 'synset': 'corkscrew.n.01', 'synonyms': ['corkscrew', 'bottle_screw'], 'def': 'a bottle opener that pulls corks', 'name': 'corkscrew'}, {'frequency': 'c', 'id': 312, 'synset': 'corn.n.03', 'synonyms': ['edible_corn', 'corn', 'maize'], 'def': 'ears of corn that can be prepared and served for human food', 'name': 'edible_corn'}, {'frequency': 'r', 'id': 313, 'synset': 'cornbread.n.01', 'synonyms': ['cornbread'], 'def': 'bread made primarily of cornmeal', 'name': 'cornbread'}, {'frequency': 'c', 'id': 314, 'synset': 'cornet.n.01', 'synonyms': ['cornet', 'horn', 'trumpet'], 'def': 'a brass musical instrument with a narrow tube and a flared bell and many valves', 'name': 'cornet'}, {'frequency': 'c', 'id': 315, 'synset': 'cornice.n.01', 'synonyms': ['cornice', 'valance', 'valance_board', 'pelmet'], 'def': 'a decorative framework to conceal curtain fixtures at the top of a window casing', 'name': 'cornice'}, {'frequency': 'r', 'id': 316, 'synset': 'cornmeal.n.01', 'synonyms': ['cornmeal'], 'def': 'coarsely ground corn', 'name': 'cornmeal'}, {'frequency': 'r', 'id': 317, 'synset': 'corset.n.01', 'synonyms': ['corset', 'girdle'], 'def': "a woman's close-fitting foundation garment", 'name': 'corset'}, {'frequency': 'r', 'id': 318, 'synset': 'cos.n.02', 'synonyms': ['romaine_lettuce'], 'def': 'lettuce with long dark-green leaves in a loosely packed elongated head', 'name': 'romaine_lettuce'}, {'frequency': 'c', 'id': 319, 'synset': 'costume.n.04', 'synonyms': ['costume'], 'def': 'the attire characteristic of a country or a time or a social class', 'name': 'costume'}, {'frequency': 'r', 'id': 320, 'synset': 'cougar.n.01', 'synonyms': ['cougar', 'puma', 'catamount', 'mountain_lion', 'panther'], 'def': 'large American feline resembling a lion', 'name': 'cougar'}, {'frequency': 'r', 'id': 321, 'synset': 'coverall.n.01', 'synonyms': ['coverall'], 'def': 'a loose-fitting protective garment that is worn over other clothing', 'name': 'coverall'}, {'frequency': 'r', 'id': 322, 'synset': 'cowbell.n.01', 'synonyms': ['cowbell'], 'def': 'a bell hung around the neck of cow so that the cow can be easily located', 'name': 'cowbell'}, {'frequency': 'f', 'id': 323, 'synset': 'cowboy_hat.n.01', 'synonyms': ['cowboy_hat', 'ten-gallon_hat'], 'def': 'a hat with a wide brim and a soft crown; worn by American ranch hands', 'name': 'cowboy_hat'}, {'frequency': 'r', 'id': 324, 'synset': 'crab.n.01', 'synonyms': ['crab_(animal)'], 'def': 'decapod having eyes on short stalks and a broad flattened shell and pincers', 'name': 'crab_(animal)'}, {'frequency': 'c', 'id': 325, 'synset': 'cracker.n.01', 'synonyms': ['cracker'], 'def': 'a thin crisp wafer', 'name': 'cracker'}, {'frequency': 'r', 'id': 326, 'synset': 'crape.n.01', 'synonyms': ['crape', 'crepe', 'French_pancake'], 'def': 'small very thin pancake', 'name': 'crape'}, {'frequency': 'f', 'id': 327, 'synset': 'crate.n.01', 'synonyms': ['crate'], 'def': 'a rugged box (usually made of wood); used for shipping', 'name': 'crate'}, {'frequency': 'r', 'id': 328, 'synset': 'crayon.n.01', 'synonyms': ['crayon', 'wax_crayon'], 'def': 'writing or drawing implement made of a colored stick of composition wax', 'name': 'crayon'}, {'frequency': 'r', 'id': 329, 'synset': 'cream_pitcher.n.01', 'synonyms': ['cream_pitcher'], 'def': 'a small pitcher for serving cream', 'name': 'cream_pitcher'}, {'frequency': 'r', 'id': 330, 'synset': 'credit_card.n.01', 'synonyms': ['credit_card', 'charge_card', 'debit_card'], 'def': 'a card, usually plastic, used to pay for goods and services', 'name': 'credit_card'}, {'frequency': 'c', 'id': 331, 'synset': 'crescent_roll.n.01', 'synonyms': ['crescent_roll', 'croissant'], 'def': 'very rich flaky crescent-shaped roll', 'name': 'crescent_roll'}, {'frequency': 'c', 'id': 332, 'synset': 'crib.n.01', 'synonyms': ['crib', 'cot'], 'def': 'baby bed with high sides made of slats', 'name': 'crib'}, {'frequency': 'c', 'id': 333, 'synset': 'crock.n.03', 'synonyms': ['crock_pot', 'earthenware_jar'], 'def': 'an earthen jar (made of baked clay)', 'name': 'crock_pot'}, {'frequency': 'f', 'id': 334, 'synset': 'crossbar.n.01', 'synonyms': ['crossbar'], 'def': 'a horizontal bar that goes across something', 'name': 'crossbar'}, {'frequency': 'r', 'id': 335, 'synset': 'crouton.n.01', 'synonyms': ['crouton'], 'def': 'a small piece of toasted or fried bread; served in soup or salads', 'name': 'crouton'}, {'frequency': 'r', 'id': 336, 'synset': 'crow.n.01', 'synonyms': ['crow'], 'def': 'black birds having a raucous call', 'name': 'crow'}, {'frequency': 'c', 'id': 337, 'synset': 'crown.n.04', 'synonyms': ['crown'], 'def': 'an ornamental jeweled headdress signifying sovereignty', 'name': 'crown'}, {'frequency': 'c', 'id': 338, 'synset': 'crucifix.n.01', 'synonyms': ['crucifix'], 'def': 'representation of the cross on which Jesus died', 'name': 'crucifix'}, {'frequency': 'c', 'id': 339, 'synset': 'cruise_ship.n.01', 'synonyms': ['cruise_ship', 'cruise_liner'], 'def': 'a passenger ship used commercially for pleasure cruises', 'name': 'cruise_ship'}, {'frequency': 'c', 'id': 340, 'synset': 'cruiser.n.01', 'synonyms': ['police_cruiser', 'patrol_car', 'police_car', 'squad_car'], 'def': 'a car in which policemen cruise the streets', 'name': 'police_cruiser'}, {'frequency': 'c', 'id': 341, 'synset': 'crumb.n.03', 'synonyms': ['crumb'], 'def': 'small piece of e.g. bread or cake', 'name': 'crumb'}, {'frequency': 'r', 'id': 342, 'synset': 'crutch.n.01', 'synonyms': ['crutch'], 'def': 'a wooden or metal staff that fits under the armpit and reaches to the ground', 'name': 'crutch'}, {'frequency': 'c', 'id': 343, 'synset': 'cub.n.03', 'synonyms': ['cub_(animal)'], 'def': 'the young of certain carnivorous mammals such as the bear or wolf or lion', 'name': 'cub_(animal)'}, {'frequency': 'r', 'id': 344, 'synset': 'cube.n.05', 'synonyms': ['cube', 'square_block'], 'def': 'a block in the (approximate) shape of a cube', 'name': 'cube'}, {'frequency': 'f', 'id': 345, 'synset': 'cucumber.n.02', 'synonyms': ['cucumber', 'cuke'], 'def': 'cylindrical green fruit with thin green rind and white flesh eaten as a vegetable', 'name': 'cucumber'}, {'frequency': 'c', 'id': 346, 'synset': 'cufflink.n.01', 'synonyms': ['cufflink'], 'def': 'jewelry consisting of linked buttons used to fasten the cuffs of a shirt', 'name': 'cufflink'}, {'frequency': 'f', 'id': 347, 'synset': 'cup.n.01', 'synonyms': ['cup'], 'def': 'a small open container usually used for drinking; usually has a handle', 'name': 'cup'}, {'frequency': 'c', 'id': 348, 'synset': 'cup.n.08', 'synonyms': ['trophy_cup'], 'def': 'a metal vessel with handles that is awarded as a trophy to a competition winner', 'name': 'trophy_cup'}, {'frequency': 'c', 'id': 349, 'synset': 'cupcake.n.01', 'synonyms': ['cupcake'], 'def': 'small cake baked in a muffin tin', 'name': 'cupcake'}, {'frequency': 'r', 'id': 350, 'synset': 'curler.n.01', 'synonyms': ['hair_curler', 'hair_roller', 'hair_crimper'], 'def': 'a cylindrical tube around which the hair is wound to curl it', 'name': 'hair_curler'}, {'frequency': 'r', 'id': 351, 'synset': 'curling_iron.n.01', 'synonyms': ['curling_iron'], 'def': 'a cylindrical home appliance that heats hair that has been curled around it', 'name': 'curling_iron'}, {'frequency': 'f', 'id': 352, 'synset': 'curtain.n.01', 'synonyms': ['curtain', 'drapery'], 'def': 'hanging cloth used as a blind (especially for a window)', 'name': 'curtain'}, {'frequency': 'f', 'id': 353, 'synset': 'cushion.n.03', 'synonyms': ['cushion'], 'def': 'a soft bag filled with air or padding such as feathers or foam rubber', 'name': 'cushion'}, {'frequency': 'r', 'id': 354, 'synset': 'custard.n.01', 'synonyms': ['custard'], 'def': 'sweetened mixture of milk and eggs baked or boiled or frozen', 'name': 'custard'}, {'frequency': 'c', 'id': 355, 'synset': 'cutter.n.06', 'synonyms': ['cutting_tool'], 'def': 'a cutting implement; a tool for cutting', 'name': 'cutting_tool'}, {'frequency': 'r', 'id': 356, 'synset': 'cylinder.n.04', 'synonyms': ['cylinder'], 'def': 'a cylindrical container', 'name': 'cylinder'}, {'frequency': 'r', 'id': 357, 'synset': 'cymbal.n.01', 'synonyms': ['cymbal'], 'def': 'a percussion instrument consisting of a concave brass disk', 'name': 'cymbal'}, {'frequency': 'r', 'id': 358, 'synset': 'dachshund.n.01', 'synonyms': ['dachshund', 'dachsie', 'badger_dog'], 'def': 'small long-bodied short-legged breed of dog having a short sleek coat and long drooping ears', 'name': 'dachshund'}, {'frequency': 'r', 'id': 359, 'synset': 'dagger.n.01', 'synonyms': ['dagger'], 'def': 'a short knife with a pointed blade used for piercing or stabbing', 'name': 'dagger'}, {'frequency': 'r', 'id': 360, 'synset': 'dartboard.n.01', 'synonyms': ['dartboard'], 'def': 'a circular board of wood or cork used as the target in the game of darts', 'name': 'dartboard'}, {'frequency': 'r', 'id': 361, 'synset': 'date.n.08', 'synonyms': ['date_(fruit)'], 'def': 'sweet edible fruit of the date palm with a single long woody seed', 'name': 'date_(fruit)'}, {'frequency': 'f', 'id': 362, 'synset': 'deck_chair.n.01', 'synonyms': ['deck_chair', 'beach_chair'], 'def': 'a folding chair for use outdoors; a wooden frame supports a length of canvas', 'name': 'deck_chair'}, {'frequency': 'c', 'id': 363, 'synset': 'deer.n.01', 'synonyms': ['deer', 'cervid'], 'def': "distinguished from Bovidae by the male's having solid deciduous antlers", 'name': 'deer'}, {'frequency': 'c', 'id': 364, 'synset': 'dental_floss.n.01', 'synonyms': ['dental_floss', 'floss'], 'def': 'a soft thread for cleaning the spaces between the teeth', 'name': 'dental_floss'}, {'frequency': 'f', 'id': 365, 'synset': 'desk.n.01', 'synonyms': ['desk'], 'def': 'a piece of furniture with a writing surface and usually drawers or other compartments', 'name': 'desk'}, {'frequency': 'r', 'id': 366, 'synset': 'detergent.n.01', 'synonyms': ['detergent'], 'def': 'a surface-active chemical widely used in industry and laundering', 'name': 'detergent'}, {'frequency': 'c', 'id': 367, 'synset': 'diaper.n.01', 'synonyms': ['diaper'], 'def': 'garment consisting of a folded cloth drawn up between the legs and fastened at the waist', 'name': 'diaper'}, {'frequency': 'r', 'id': 368, 'synset': 'diary.n.01', 'synonyms': ['diary', 'journal'], 'def': 'a daily written record of (usually personal) experiences and observations', 'name': 'diary'}, {'frequency': 'r', 'id': 369, 'synset': 'die.n.01', 'synonyms': ['die', 'dice'], 'def': 'a small cube with 1 to 6 spots on the six faces; used in gambling', 'name': 'die'}, {'frequency': 'r', 'id': 370, 'synset': 'dinghy.n.01', 'synonyms': ['dinghy', 'dory', 'rowboat'], 'def': 'a small boat of shallow draft with seats and oars with which it is propelled', 'name': 'dinghy'}, {'frequency': 'f', 'id': 371, 'synset': 'dining_table.n.01', 'synonyms': ['dining_table'], 'def': 'a table at which meals are served', 'name': 'dining_table'}, {'frequency': 'r', 'id': 372, 'synset': 'dinner_jacket.n.01', 'synonyms': ['tux', 'tuxedo'], 'def': 'semiformal evening dress for men', 'name': 'tux'}, {'frequency': 'c', 'id': 373, 'synset': 'dish.n.01', 'synonyms': ['dish'], 'def': 'a piece of dishware normally used as a container for holding or serving food', 'name': 'dish'}, {'frequency': 'c', 'id': 374, 'synset': 'dish.n.05', 'synonyms': ['dish_antenna'], 'def': 'directional antenna consisting of a parabolic reflector', 'name': 'dish_antenna'}, {'frequency': 'c', 'id': 375, 'synset': 'dishrag.n.01', 'synonyms': ['dishrag', 'dishcloth'], 'def': 'a cloth for washing dishes', 'name': 'dishrag'}, {'frequency': 'c', 'id': 376, 'synset': 'dishtowel.n.01', 'synonyms': ['dishtowel', 'tea_towel'], 'def': 'a towel for drying dishes', 'name': 'dishtowel'}, {'frequency': 'f', 'id': 377, 'synset': 'dishwasher.n.01', 'synonyms': ['dishwasher', 'dishwashing_machine'], 'def': 'a machine for washing dishes', 'name': 'dishwasher'}, {'frequency': 'r', 'id': 378, 'synset': 'dishwasher_detergent.n.01', 'synonyms': ['dishwasher_detergent', 'dishwashing_detergent', 'dishwashing_liquid'], 'def': 'a low-sudsing detergent designed for use in dishwashers', 'name': 'dishwasher_detergent'}, {'frequency': 'r', 'id': 379, 'synset': 'diskette.n.01', 'synonyms': ['diskette', 'floppy', 'floppy_disk'], 'def': 'a small plastic magnetic disk enclosed in a stiff envelope used to store data', 'name': 'diskette'}, {'frequency': 'c', 'id': 380, 'synset': 'dispenser.n.01', 'synonyms': ['dispenser'], 'def': 'a container so designed that the contents can be used in prescribed amounts', 'name': 'dispenser'}, {'frequency': 'c', 'id': 381, 'synset': 'dixie_cup.n.01', 'synonyms': ['Dixie_cup', 'paper_cup'], 'def': 'a disposable cup made of paper; for holding drinks', 'name': 'Dixie_cup'}, {'frequency': 'f', 'id': 382, 'synset': 'dog.n.01', 'synonyms': ['dog'], 'def': 'a common domesticated dog', 'name': 'dog'}, {'frequency': 'f', 'id': 383, 'synset': 'dog_collar.n.01', 'synonyms': ['dog_collar'], 'def': 'a collar for a dog', 'name': 'dog_collar'}, {'frequency': 'c', 'id': 384, 'synset': 'doll.n.01', 'synonyms': ['doll'], 'def': 'a toy replica of a HUMAN (NOT AN ANIMAL)', 'name': 'doll'}, {'frequency': 'r', 'id': 385, 'synset': 'dollar.n.02', 'synonyms': ['dollar', 'dollar_bill', 'one_dollar_bill'], 'def': 'a piece of paper money worth one dollar', 'name': 'dollar'}, {'frequency': 'r', 'id': 386, 'synset': 'dolphin.n.02', 'synonyms': ['dolphin'], 'def': 'any of various small toothed whales with a beaklike snout; larger than porpoises', 'name': 'dolphin'}, {'frequency': 'c', 'id': 387, 'synset': 'domestic_ass.n.01', 'synonyms': ['domestic_ass', 'donkey'], 'def': 'domestic beast of burden descended from the African wild ass; patient but stubborn', 'name': 'domestic_ass'}, {'frequency': 'r', 'id': 388, 'synset': 'domino.n.03', 'synonyms': ['eye_mask'], 'def': 'a mask covering the upper part of the face but with holes for the eyes', 'name': 'eye_mask'}, {'frequency': 'r', 'id': 389, 'synset': 'doorbell.n.01', 'synonyms': ['doorbell', 'buzzer'], 'def': 'a button at an outer door that gives a ringing or buzzing signal when pushed', 'name': 'doorbell'}, {'frequency': 'f', 'id': 390, 'synset': 'doorknob.n.01', 'synonyms': ['doorknob', 'doorhandle'], 'def': "a knob used to open a door (often called `doorhandle' in Great Britain)", 'name': 'doorknob'}, {'frequency': 'c', 'id': 391, 'synset': 'doormat.n.02', 'synonyms': ['doormat', 'welcome_mat'], 'def': 'a mat placed outside an exterior door for wiping the shoes before entering', 'name': 'doormat'}, {'frequency': 'f', 'id': 392, 'synset': 'doughnut.n.02', 'synonyms': ['doughnut', 'donut'], 'def': 'a small ring-shaped friedcake', 'name': 'doughnut'}, {'frequency': 'r', 'id': 393, 'synset': 'dove.n.01', 'synonyms': ['dove'], 'def': 'any of numerous small pigeons', 'name': 'dove'}, {'frequency': 'r', 'id': 394, 'synset': 'dragonfly.n.01', 'synonyms': ['dragonfly'], 'def': 'slender-bodied non-stinging insect having iridescent wings that are outspread at rest', 'name': 'dragonfly'}, {'frequency': 'f', 'id': 395, 'synset': 'drawer.n.01', 'synonyms': ['drawer'], 'def': 'a boxlike container in a piece of furniture; made so as to slide in and out', 'name': 'drawer'}, {'frequency': 'c', 'id': 396, 'synset': 'drawers.n.01', 'synonyms': ['underdrawers', 'boxers', 'boxershorts'], 'def': 'underpants worn by men', 'name': 'underdrawers'}, {'frequency': 'f', 'id': 397, 'synset': 'dress.n.01', 'synonyms': ['dress', 'frock'], 'def': 'a one-piece garment for a woman; has skirt and bodice', 'name': 'dress'}, {'frequency': 'c', 'id': 398, 'synset': 'dress_hat.n.01', 'synonyms': ['dress_hat', 'high_hat', 'opera_hat', 'silk_hat', 'top_hat'], 'def': "a man's hat with a tall crown; usually covered with silk or with beaver fur", 'name': 'dress_hat'}, {'frequency': 'c', 'id': 399, 'synset': 'dress_suit.n.01', 'synonyms': ['dress_suit'], 'def': 'formalwear consisting of full evening dress for men', 'name': 'dress_suit'}, {'frequency': 'c', 'id': 400, 'synset': 'dresser.n.05', 'synonyms': ['dresser'], 'def': 'a cabinet with shelves', 'name': 'dresser'}, {'frequency': 'c', 'id': 401, 'synset': 'drill.n.01', 'synonyms': ['drill'], 'def': 'a tool with a sharp rotating point for making holes in hard materials', 'name': 'drill'}, {'frequency': 'r', 'id': 402, 'synset': 'drinking_fountain.n.01', 'synonyms': ['drinking_fountain'], 'def': 'a public fountain to provide a jet of drinking water', 'name': 'drinking_fountain'}, {'frequency': 'r', 'id': 403, 'synset': 'drone.n.04', 'synonyms': ['drone'], 'def': 'an aircraft without a pilot that is operated by remote control', 'name': 'drone'}, {'frequency': 'r', 'id': 404, 'synset': 'dropper.n.01', 'synonyms': ['dropper', 'eye_dropper'], 'def': 'pipet consisting of a small tube with a vacuum bulb at one end for drawing liquid in and releasing it a drop at a time', 'name': 'dropper'}, {'frequency': 'c', 'id': 405, 'synset': 'drum.n.01', 'synonyms': ['drum_(musical_instrument)'], 'def': 'a musical percussion instrument; usually consists of a hollow cylinder with a membrane stretched across each end', 'name': 'drum_(musical_instrument)'}, {'frequency': 'r', 'id': 406, 'synset': 'drumstick.n.02', 'synonyms': ['drumstick'], 'def': 'a stick used for playing a drum', 'name': 'drumstick'}, {'frequency': 'f', 'id': 407, 'synset': 'duck.n.01', 'synonyms': ['duck'], 'def': 'small web-footed broad-billed swimming bird', 'name': 'duck'}, {'frequency': 'r', 'id': 408, 'synset': 'duckling.n.02', 'synonyms': ['duckling'], 'def': 'young duck', 'name': 'duckling'}, {'frequency': 'c', 'id': 409, 'synset': 'duct_tape.n.01', 'synonyms': ['duct_tape'], 'def': 'a wide silvery adhesive tape', 'name': 'duct_tape'}, {'frequency': 'f', 'id': 410, 'synset': 'duffel_bag.n.01', 'synonyms': ['duffel_bag', 'duffle_bag', 'duffel', 'duffle'], 'def': 'a large cylindrical bag of heavy cloth', 'name': 'duffel_bag'}, {'frequency': 'r', 'id': 411, 'synset': 'dumbbell.n.01', 'synonyms': ['dumbbell'], 'def': 'an exercising weight with two ball-like ends connected by a short handle', 'name': 'dumbbell'}, {'frequency': 'c', 'id': 412, 'synset': 'dumpster.n.01', 'synonyms': ['dumpster'], 'def': 'a container designed to receive and transport and dump waste', 'name': 'dumpster'}, {'frequency': 'r', 'id': 413, 'synset': 'dustpan.n.02', 'synonyms': ['dustpan'], 'def': 'a short-handled receptacle into which dust can be swept', 'name': 'dustpan'}, {'frequency': 'r', 'id': 414, 'synset': 'dutch_oven.n.02', 'synonyms': ['Dutch_oven'], 'def': 'iron or earthenware cooking pot; used for stews', 'name': 'Dutch_oven'}, {'frequency': 'c', 'id': 415, 'synset': 'eagle.n.01', 'synonyms': ['eagle'], 'def': 'large birds of prey noted for their broad wings and strong soaring flight', 'name': 'eagle'}, {'frequency': 'f', 'id': 416, 'synset': 'earphone.n.01', 'synonyms': ['earphone', 'earpiece', 'headphone'], 'def': 'device for listening to audio that is held over or inserted into the ear', 'name': 'earphone'}, {'frequency': 'r', 'id': 417, 'synset': 'earplug.n.01', 'synonyms': ['earplug'], 'def': 'a soft plug that is inserted into the ear canal to block sound', 'name': 'earplug'}, {'frequency': 'f', 'id': 418, 'synset': 'earring.n.01', 'synonyms': ['earring'], 'def': 'jewelry to ornament the ear', 'name': 'earring'}, {'frequency': 'c', 'id': 419, 'synset': 'easel.n.01', 'synonyms': ['easel'], 'def': "an upright tripod for displaying something (usually an artist's canvas)", 'name': 'easel'}, {'frequency': 'r', 'id': 420, 'synset': 'eclair.n.01', 'synonyms': ['eclair'], 'def': 'oblong cream puff', 'name': 'eclair'}, {'frequency': 'r', 'id': 421, 'synset': 'eel.n.01', 'synonyms': ['eel'], 'def': 'an elongate fish with fatty flesh', 'name': 'eel'}, {'frequency': 'f', 'id': 422, 'synset': 'egg.n.02', 'synonyms': ['egg', 'eggs'], 'def': 'oval reproductive body of a fowl (especially a hen) used as food', 'name': 'egg'}, {'frequency': 'r', 'id': 423, 'synset': 'egg_roll.n.01', 'synonyms': ['egg_roll', 'spring_roll'], 'def': 'minced vegetables and meat wrapped in a pancake and fried', 'name': 'egg_roll'}, {'frequency': 'c', 'id': 424, 'synset': 'egg_yolk.n.01', 'synonyms': ['egg_yolk', 'yolk_(egg)'], 'def': 'the yellow spherical part of an egg', 'name': 'egg_yolk'}, {'frequency': 'c', 'id': 425, 'synset': 'eggbeater.n.02', 'synonyms': ['eggbeater', 'eggwhisk'], 'def': 'a mixer for beating eggs or whipping cream', 'name': 'eggbeater'}, {'frequency': 'c', 'id': 426, 'synset': 'eggplant.n.01', 'synonyms': ['eggplant', 'aubergine'], 'def': 'egg-shaped vegetable having a shiny skin typically dark purple', 'name': 'eggplant'}, {'frequency': 'r', 'id': 427, 'synset': 'electric_chair.n.01', 'synonyms': ['electric_chair'], 'def': 'a chair-shaped instrument of execution by electrocution', 'name': 'electric_chair'}, {'frequency': 'f', 'id': 428, 'synset': 'electric_refrigerator.n.01', 'synonyms': ['refrigerator'], 'def': 'a refrigerator in which the coolant is pumped around by an electric motor', 'name': 'refrigerator'}, {'frequency': 'f', 'id': 429, 'synset': 'elephant.n.01', 'synonyms': ['elephant'], 'def': 'a common elephant', 'name': 'elephant'}, {'frequency': 'r', 'id': 430, 'synset': 'elk.n.01', 'synonyms': ['elk', 'moose'], 'def': 'large northern deer with enormous flattened antlers in the male', 'name': 'elk'}, {'frequency': 'c', 'id': 431, 'synset': 'envelope.n.01', 'synonyms': ['envelope'], 'def': 'a flat (usually rectangular) container for a letter, thin package, etc.', 'name': 'envelope'}, {'frequency': 'c', 'id': 432, 'synset': 'eraser.n.01', 'synonyms': ['eraser'], 'def': 'an implement used to erase something', 'name': 'eraser'}, {'frequency': 'r', 'id': 433, 'synset': 'escargot.n.01', 'synonyms': ['escargot'], 'def': 'edible snail usually served in the shell with a sauce of melted butter and garlic', 'name': 'escargot'}, {'frequency': 'r', 'id': 434, 'synset': 'eyepatch.n.01', 'synonyms': ['eyepatch'], 'def': 'a protective cloth covering for an injured eye', 'name': 'eyepatch'}, {'frequency': 'r', 'id': 435, 'synset': 'falcon.n.01', 'synonyms': ['falcon'], 'def': 'birds of prey having long pointed powerful wings adapted for swift flight', 'name': 'falcon'}, {'frequency': 'f', 'id': 436, 'synset': 'fan.n.01', 'synonyms': ['fan'], 'def': 'a device for creating a current of air by movement of a surface or surfaces', 'name': 'fan'}, {'frequency': 'f', 'id': 437, 'synset': 'faucet.n.01', 'synonyms': ['faucet', 'spigot', 'tap'], 'def': 'a regulator for controlling the flow of a liquid from a reservoir', 'name': 'faucet'}, {'frequency': 'r', 'id': 438, 'synset': 'fedora.n.01', 'synonyms': ['fedora'], 'def': 'a hat made of felt with a creased crown', 'name': 'fedora'}, {'frequency': 'r', 'id': 439, 'synset': 'ferret.n.02', 'synonyms': ['ferret'], 'def': 'domesticated albino variety of the European polecat bred for hunting rats and rabbits', 'name': 'ferret'}, {'frequency': 'c', 'id': 440, 'synset': 'ferris_wheel.n.01', 'synonyms': ['Ferris_wheel'], 'def': 'a large wheel with suspended seats that remain upright as the wheel rotates', 'name': 'Ferris_wheel'}, {'frequency': 'r', 'id': 441, 'synset': 'ferry.n.01', 'synonyms': ['ferry', 'ferryboat'], 'def': 'a boat that transports people or vehicles across a body of water and operates on a regular schedule', 'name': 'ferry'}, {'frequency': 'r', 'id': 442, 'synset': 'fig.n.04', 'synonyms': ['fig_(fruit)'], 'def': 'fleshy sweet pear-shaped yellowish or purple fruit eaten fresh or preserved or dried', 'name': 'fig_(fruit)'}, {'frequency': 'c', 'id': 443, 'synset': 'fighter.n.02', 'synonyms': ['fighter_jet', 'fighter_aircraft', 'attack_aircraft'], 'def': 'a high-speed military or naval airplane designed to destroy enemy targets', 'name': 'fighter_jet'}, {'frequency': 'f', 'id': 444, 'synset': 'figurine.n.01', 'synonyms': ['figurine'], 'def': 'a small carved or molded figure', 'name': 'figurine'}, {'frequency': 'c', 'id': 445, 'synset': 'file.n.03', 'synonyms': ['file_cabinet', 'filing_cabinet'], 'def': 'office furniture consisting of a container for keeping papers in order', 'name': 'file_cabinet'}, {'frequency': 'r', 'id': 446, 'synset': 'file.n.04', 'synonyms': ['file_(tool)'], 'def': 'a steel hand tool with small sharp teeth on some or all of its surfaces; used for smoothing wood or metal', 'name': 'file_(tool)'}, {'frequency': 'f', 'id': 447, 'synset': 'fire_alarm.n.02', 'synonyms': ['fire_alarm', 'smoke_alarm'], 'def': 'an alarm that is tripped off by fire or smoke', 'name': 'fire_alarm'}, {'frequency': 'c', 'id': 448, 'synset': 'fire_engine.n.01', 'synonyms': ['fire_engine', 'fire_truck'], 'def': 'large trucks that carry firefighters and equipment to the site of a fire', 'name': 'fire_engine'}, {'frequency': 'c', 'id': 449, 'synset': 'fire_extinguisher.n.01', 'synonyms': ['fire_extinguisher', 'extinguisher'], 'def': 'a manually operated device for extinguishing small fires', 'name': 'fire_extinguisher'}, {'frequency': 'c', 'id': 450, 'synset': 'fire_hose.n.01', 'synonyms': ['fire_hose'], 'def': 'a large hose that carries water from a fire hydrant to the site of the fire', 'name': 'fire_hose'}, {'frequency': 'f', 'id': 451, 'synset': 'fireplace.n.01', 'synonyms': ['fireplace'], 'def': 'an open recess in a wall at the base of a chimney where a fire can be built', 'name': 'fireplace'}, {'frequency': 'f', 'id': 452, 'synset': 'fireplug.n.01', 'synonyms': ['fireplug', 'fire_hydrant', 'hydrant'], 'def': 'an upright hydrant for drawing water to use in fighting a fire', 'name': 'fireplug'}, {'frequency': 'c', 'id': 453, 'synset': 'fish.n.01', 'synonyms': ['fish'], 'def': 'any of various mostly cold-blooded aquatic vertebrates usually having scales and breathing through gills', 'name': 'fish'}, {'frequency': 'r', 'id': 454, 'synset': 'fish.n.02', 'synonyms': ['fish_(food)'], 'def': 'the flesh of fish used as food', 'name': 'fish_(food)'}, {'frequency': 'r', 'id': 455, 'synset': 'fishbowl.n.02', 'synonyms': ['fishbowl', 'goldfish_bowl'], 'def': 'a transparent bowl in which small fish are kept', 'name': 'fishbowl'}, {'frequency': 'r', 'id': 456, 'synset': 'fishing_boat.n.01', 'synonyms': ['fishing_boat', 'fishing_vessel'], 'def': 'a vessel for fishing', 'name': 'fishing_boat'}, {'frequency': 'c', 'id': 457, 'synset': 'fishing_rod.n.01', 'synonyms': ['fishing_rod', 'fishing_pole'], 'def': 'a rod that is used in fishing to extend the fishing line', 'name': 'fishing_rod'}, {'frequency': 'f', 'id': 458, 'synset': 'flag.n.01', 'synonyms': ['flag'], 'def': 'emblem usually consisting of a rectangular piece of cloth of distinctive design (do not include pole)', 'name': 'flag'}, {'frequency': 'f', 'id': 459, 'synset': 'flagpole.n.02', 'synonyms': ['flagpole', 'flagstaff'], 'def': 'a tall staff or pole on which a flag is raised', 'name': 'flagpole'}, {'frequency': 'c', 'id': 460, 'synset': 'flamingo.n.01', 'synonyms': ['flamingo'], 'def': 'large pink web-footed bird with down-bent bill', 'name': 'flamingo'}, {'frequency': 'c', 'id': 461, 'synset': 'flannel.n.01', 'synonyms': ['flannel'], 'def': 'a soft light woolen fabric; used for clothing', 'name': 'flannel'}, {'frequency': 'r', 'id': 462, 'synset': 'flash.n.10', 'synonyms': ['flash', 'flashbulb'], 'def': 'a lamp for providing momentary light to take a photograph', 'name': 'flash'}, {'frequency': 'c', 'id': 463, 'synset': 'flashlight.n.01', 'synonyms': ['flashlight', 'torch'], 'def': 'a small portable battery-powered electric lamp', 'name': 'flashlight'}, {'frequency': 'r', 'id': 464, 'synset': 'fleece.n.03', 'synonyms': ['fleece'], 'def': 'a soft bulky fabric with deep pile; used chiefly for clothing', 'name': 'fleece'}, {'frequency': 'f', 'id': 465, 'synset': 'flip-flop.n.02', 'synonyms': ['flip-flop_(sandal)'], 'def': 'a backless sandal held to the foot by a thong between two toes', 'name': 'flip-flop_(sandal)'}, {'frequency': 'c', 'id': 466, 'synset': 'flipper.n.01', 'synonyms': ['flipper_(footwear)', 'fin_(footwear)'], 'def': 'a shoe to aid a person in swimming', 'name': 'flipper_(footwear)'}, {'frequency': 'f', 'id': 467, 'synset': 'flower_arrangement.n.01', 'synonyms': ['flower_arrangement', 'floral_arrangement'], 'def': 'a decorative arrangement of flowers', 'name': 'flower_arrangement'}, {'frequency': 'c', 'id': 468, 'synset': 'flute.n.02', 'synonyms': ['flute_glass', 'champagne_flute'], 'def': 'a tall narrow wineglass', 'name': 'flute_glass'}, {'frequency': 'r', 'id': 469, 'synset': 'foal.n.01', 'synonyms': ['foal'], 'def': 'a young horse', 'name': 'foal'}, {'frequency': 'c', 'id': 470, 'synset': 'folding_chair.n.01', 'synonyms': ['folding_chair'], 'def': 'a chair that can be folded flat for storage', 'name': 'folding_chair'}, {'frequency': 'c', 'id': 471, 'synset': 'food_processor.n.01', 'synonyms': ['food_processor'], 'def': 'a kitchen appliance for shredding, blending, chopping, or slicing food', 'name': 'food_processor'}, {'frequency': 'c', 'id': 472, 'synset': 'football.n.02', 'synonyms': ['football_(American)'], 'def': 'the inflated oblong ball used in playing American football', 'name': 'football_(American)'}, {'frequency': 'r', 'id': 473, 'synset': 'football_helmet.n.01', 'synonyms': ['football_helmet'], 'def': 'a padded helmet with a face mask to protect the head of football players', 'name': 'football_helmet'}, {'frequency': 'c', 'id': 474, 'synset': 'footstool.n.01', 'synonyms': ['footstool', 'footrest'], 'def': 'a low seat or a stool to rest the feet of a seated person', 'name': 'footstool'}, {'frequency': 'f', 'id': 475, 'synset': 'fork.n.01', 'synonyms': ['fork'], 'def': 'cutlery used for serving and eating food', 'name': 'fork'}, {'frequency': 'r', 'id': 476, 'synset': 'forklift.n.01', 'synonyms': ['forklift'], 'def': 'an industrial vehicle with a power operated fork in front that can be inserted under loads to lift and move them', 'name': 'forklift'}, {'frequency': 'r', 'id': 477, 'synset': 'freight_car.n.01', 'synonyms': ['freight_car'], 'def': 'a railway car that carries freight', 'name': 'freight_car'}, {'frequency': 'r', 'id': 478, 'synset': 'french_toast.n.01', 'synonyms': ['French_toast'], 'def': 'bread slice dipped in egg and milk and fried', 'name': 'French_toast'}, {'frequency': 'c', 'id': 479, 'synset': 'freshener.n.01', 'synonyms': ['freshener', 'air_freshener'], 'def': 'anything that freshens', 'name': 'freshener'}, {'frequency': 'f', 'id': 480, 'synset': 'frisbee.n.01', 'synonyms': ['frisbee'], 'def': 'a light, plastic disk propelled with a flip of the wrist for recreation or competition', 'name': 'frisbee'}, {'frequency': 'c', 'id': 481, 'synset': 'frog.n.01', 'synonyms': ['frog', 'toad', 'toad_frog'], 'def': 'a tailless stout-bodied amphibians with long hind limbs for leaping', 'name': 'frog'}, {'frequency': 'c', 'id': 482, 'synset': 'fruit_juice.n.01', 'synonyms': ['fruit_juice'], 'def': 'drink produced by squeezing or crushing fruit', 'name': 'fruit_juice'}, {'frequency': 'r', 'id': 483, 'synset': 'fruit_salad.n.01', 'synonyms': ['fruit_salad'], 'def': 'salad composed of fruits', 'name': 'fruit_salad'}, {'frequency': 'c', 'id': 484, 'synset': 'frying_pan.n.01', 'synonyms': ['frying_pan', 'frypan', 'skillet'], 'def': 'a pan used for frying foods', 'name': 'frying_pan'}, {'frequency': 'r', 'id': 485, 'synset': 'fudge.n.01', 'synonyms': ['fudge'], 'def': 'soft creamy candy', 'name': 'fudge'}, {'frequency': 'r', 'id': 486, 'synset': 'funnel.n.02', 'synonyms': ['funnel'], 'def': 'a cone-shaped utensil used to channel a substance into a container with a small mouth', 'name': 'funnel'}, {'frequency': 'c', 'id': 487, 'synset': 'futon.n.01', 'synonyms': ['futon'], 'def': 'a pad that is used for sleeping on the floor or on a raised frame', 'name': 'futon'}, {'frequency': 'r', 'id': 488, 'synset': 'gag.n.02', 'synonyms': ['gag', 'muzzle'], 'def': "restraint put into a person's mouth to prevent speaking or shouting", 'name': 'gag'}, {'frequency': 'r', 'id': 489, 'synset': 'garbage.n.03', 'synonyms': ['garbage'], 'def': 'a receptacle where waste can be discarded', 'name': 'garbage'}, {'frequency': 'c', 'id': 490, 'synset': 'garbage_truck.n.01', 'synonyms': ['garbage_truck'], 'def': 'a truck for collecting domestic refuse', 'name': 'garbage_truck'}, {'frequency': 'c', 'id': 491, 'synset': 'garden_hose.n.01', 'synonyms': ['garden_hose'], 'def': 'a hose used for watering a lawn or garden', 'name': 'garden_hose'}, {'frequency': 'c', 'id': 492, 'synset': 'gargle.n.01', 'synonyms': ['gargle', 'mouthwash'], 'def': 'a medicated solution used for gargling and rinsing the mouth', 'name': 'gargle'}, {'frequency': 'r', 'id': 493, 'synset': 'gargoyle.n.02', 'synonyms': ['gargoyle'], 'def': 'an ornament consisting of a grotesquely carved figure of a person or animal', 'name': 'gargoyle'}, {'frequency': 'c', 'id': 494, 'synset': 'garlic.n.02', 'synonyms': ['garlic', 'ail'], 'def': 'aromatic bulb used as seasoning', 'name': 'garlic'}, {'frequency': 'r', 'id': 495, 'synset': 'gasmask.n.01', 'synonyms': ['gasmask', 'respirator', 'gas_helmet'], 'def': 'a protective face mask with a filter', 'name': 'gasmask'}, {'frequency': 'r', 'id': 496, 'synset': 'gazelle.n.01', 'synonyms': ['gazelle'], 'def': 'small swift graceful antelope of Africa and Asia having lustrous eyes', 'name': 'gazelle'}, {'frequency': 'c', 'id': 497, 'synset': 'gelatin.n.02', 'synonyms': ['gelatin', 'jelly'], 'def': 'an edible jelly made with gelatin and used as a dessert or salad base or a coating for foods', 'name': 'gelatin'}, {'frequency': 'r', 'id': 498, 'synset': 'gem.n.02', 'synonyms': ['gemstone'], 'def': 'a crystalline rock that can be cut and polished for jewelry', 'name': 'gemstone'}, {'frequency': 'c', 'id': 499, 'synset': 'giant_panda.n.01', 'synonyms': ['giant_panda', 'panda', 'panda_bear'], 'def': 'large black-and-white herbivorous mammal of bamboo forests of China and Tibet', 'name': 'giant_panda'}, {'frequency': 'c', 'id': 500, 'synset': 'gift_wrap.n.01', 'synonyms': ['gift_wrap'], 'def': 'attractive wrapping paper suitable for wrapping gifts', 'name': 'gift_wrap'}, {'frequency': 'c', 'id': 501, 'synset': 'ginger.n.03', 'synonyms': ['ginger', 'gingerroot'], 'def': 'the root of the common ginger plant; used fresh as a seasoning', 'name': 'ginger'}, {'frequency': 'f', 'id': 502, 'synset': 'giraffe.n.01', 'synonyms': ['giraffe'], 'def': 'tall animal having a spotted coat and small horns and very long neck and legs', 'name': 'giraffe'}, {'frequency': 'c', 'id': 503, 'synset': 'girdle.n.02', 'synonyms': ['cincture', 'sash', 'waistband', 'waistcloth'], 'def': 'a band of material around the waist that strengthens a skirt or trousers', 'name': 'cincture'}, {'frequency': 'f', 'id': 504, 'synset': 'glass.n.02', 'synonyms': ['glass_(drink_container)', 'drinking_glass'], 'def': 'a container for holding liquids while drinking', 'name': 'glass_(drink_container)'}, {'frequency': 'c', 'id': 505, 'synset': 'globe.n.03', 'synonyms': ['globe'], 'def': 'a sphere on which a map (especially of the earth) is represented', 'name': 'globe'}, {'frequency': 'f', 'id': 506, 'synset': 'glove.n.02', 'synonyms': ['glove'], 'def': 'handwear covering the hand', 'name': 'glove'}, {'frequency': 'c', 'id': 507, 'synset': 'goat.n.01', 'synonyms': ['goat'], 'def': 'a common goat', 'name': 'goat'}, {'frequency': 'f', 'id': 508, 'synset': 'goggles.n.01', 'synonyms': ['goggles'], 'def': 'tight-fitting spectacles worn to protect the eyes', 'name': 'goggles'}, {'frequency': 'r', 'id': 509, 'synset': 'goldfish.n.01', 'synonyms': ['goldfish'], 'def': 'small golden or orange-red freshwater fishes used as pond or aquarium pets', 'name': 'goldfish'}, {'frequency': 'r', 'id': 510, 'synset': 'golf_club.n.02', 'synonyms': ['golf_club', 'golf-club'], 'def': 'golf equipment used by a golfer to hit a golf ball', 'name': 'golf_club'}, {'frequency': 'c', 'id': 511, 'synset': 'golfcart.n.01', 'synonyms': ['golfcart'], 'def': 'a small motor vehicle in which golfers can ride between shots', 'name': 'golfcart'}, {'frequency': 'r', 'id': 512, 'synset': 'gondola.n.02', 'synonyms': ['gondola_(boat)'], 'def': 'long narrow flat-bottomed boat propelled by sculling; traditionally used on canals of Venice', 'name': 'gondola_(boat)'}, {'frequency': 'c', 'id': 513, 'synset': 'goose.n.01', 'synonyms': ['goose'], 'def': 'loud, web-footed long-necked aquatic birds usually larger than ducks', 'name': 'goose'}, {'frequency': 'r', 'id': 514, 'synset': 'gorilla.n.01', 'synonyms': ['gorilla'], 'def': 'largest ape', 'name': 'gorilla'}, {'frequency': 'r', 'id': 515, 'synset': 'gourd.n.02', 'synonyms': ['gourd'], 'def': 'any of numerous inedible fruits with hard rinds', 'name': 'gourd'}, {'frequency': 'r', 'id': 516, 'synset': 'gown.n.04', 'synonyms': ['surgical_gown', 'scrubs_(surgical_clothing)'], 'def': 'protective garment worn by surgeons during operations', 'name': 'surgical_gown'}, {'frequency': 'f', 'id': 517, 'synset': 'grape.n.01', 'synonyms': ['grape'], 'def': 'any of various juicy fruit with green or purple skins; grow in clusters', 'name': 'grape'}, {'frequency': 'r', 'id': 518, 'synset': 'grasshopper.n.01', 'synonyms': ['grasshopper'], 'def': 'plant-eating insect with hind legs adapted for leaping', 'name': 'grasshopper'}, {'frequency': 'c', 'id': 519, 'synset': 'grater.n.01', 'synonyms': ['grater'], 'def': 'utensil with sharp perforations for shredding foods (as vegetables or cheese)', 'name': 'grater'}, {'frequency': 'c', 'id': 520, 'synset': 'gravestone.n.01', 'synonyms': ['gravestone', 'headstone', 'tombstone'], 'def': 'a stone that is used to mark a grave', 'name': 'gravestone'}, {'frequency': 'r', 'id': 521, 'synset': 'gravy_boat.n.01', 'synonyms': ['gravy_boat', 'gravy_holder'], 'def': 'a dish (often boat-shaped) for serving gravy or sauce', 'name': 'gravy_boat'}, {'frequency': 'c', 'id': 522, 'synset': 'green_bean.n.02', 'synonyms': ['green_bean'], 'def': 'a common bean plant cultivated for its slender green edible pods', 'name': 'green_bean'}, {'frequency': 'c', 'id': 523, 'synset': 'green_onion.n.01', 'synonyms': ['green_onion', 'spring_onion', 'scallion'], 'def': 'a young onion before the bulb has enlarged', 'name': 'green_onion'}, {'frequency': 'r', 'id': 524, 'synset': 'griddle.n.01', 'synonyms': ['griddle'], 'def': 'cooking utensil consisting of a flat heated surface on which food is cooked', 'name': 'griddle'}, {'frequency': 'r', 'id': 525, 'synset': 'grillroom.n.01', 'synonyms': ['grillroom', 'grill_(restaurant)'], 'def': 'a restaurant where food is cooked on a grill', 'name': 'grillroom'}, {'frequency': 'r', 'id': 526, 'synset': 'grinder.n.04', 'synonyms': ['grinder_(tool)'], 'def': 'a machine tool that polishes metal', 'name': 'grinder_(tool)'}, {'frequency': 'r', 'id': 527, 'synset': 'grits.n.01', 'synonyms': ['grits', 'hominy_grits'], 'def': 'coarsely ground corn boiled as a breakfast dish', 'name': 'grits'}, {'frequency': 'c', 'id': 528, 'synset': 'grizzly.n.01', 'synonyms': ['grizzly', 'grizzly_bear'], 'def': 'powerful brownish-yellow bear of the uplands of western North America', 'name': 'grizzly'}, {'frequency': 'c', 'id': 529, 'synset': 'grocery_bag.n.01', 'synonyms': ['grocery_bag'], 'def': "a sack for holding customer's groceries", 'name': 'grocery_bag'}, {'frequency': 'r', 'id': 530, 'synset': 'guacamole.n.01', 'synonyms': ['guacamole'], 'def': 'a dip made of mashed avocado mixed with chopped onions and other seasonings', 'name': 'guacamole'}, {'frequency': 'f', 'id': 531, 'synset': 'guitar.n.01', 'synonyms': ['guitar'], 'def': 'a stringed instrument usually having six strings; played by strumming or plucking', 'name': 'guitar'}, {'frequency': 'c', 'id': 532, 'synset': 'gull.n.02', 'synonyms': ['gull', 'seagull'], 'def': 'mostly white aquatic bird having long pointed wings and short legs', 'name': 'gull'}, {'frequency': 'c', 'id': 533, 'synset': 'gun.n.01', 'synonyms': ['gun'], 'def': 'a weapon that discharges a bullet at high velocity from a metal tube', 'name': 'gun'}, {'frequency': 'r', 'id': 534, 'synset': 'hair_spray.n.01', 'synonyms': ['hair_spray'], 'def': 'substance sprayed on the hair to hold it in place', 'name': 'hair_spray'}, {'frequency': 'c', 'id': 535, 'synset': 'hairbrush.n.01', 'synonyms': ['hairbrush'], 'def': "a brush used to groom a person's hair", 'name': 'hairbrush'}, {'frequency': 'c', 'id': 536, 'synset': 'hairnet.n.01', 'synonyms': ['hairnet'], 'def': 'a small net that someone wears over their hair to keep it in place', 'name': 'hairnet'}, {'frequency': 'c', 'id': 537, 'synset': 'hairpin.n.01', 'synonyms': ['hairpin'], 'def': "a double pronged pin used to hold women's hair in place", 'name': 'hairpin'}, {'frequency': 'f', 'id': 538, 'synset': 'ham.n.01', 'synonyms': ['ham', 'jambon', 'gammon'], 'def': 'meat cut from the thigh of a hog (usually smoked)', 'name': 'ham'}, {'frequency': 'c', 'id': 539, 'synset': 'hamburger.n.01', 'synonyms': ['hamburger', 'beefburger', 'burger'], 'def': 'a sandwich consisting of a patty of minced beef served on a bun', 'name': 'hamburger'}, {'frequency': 'c', 'id': 540, 'synset': 'hammer.n.02', 'synonyms': ['hammer'], 'def': 'a hand tool with a heavy head and a handle; used to deliver an impulsive force by striking', 'name': 'hammer'}, {'frequency': 'r', 'id': 541, 'synset': 'hammock.n.02', 'synonyms': ['hammock'], 'def': 'a hanging bed of canvas or rope netting (usually suspended between two trees)', 'name': 'hammock'}, {'frequency': 'r', 'id': 542, 'synset': 'hamper.n.02', 'synonyms': ['hamper'], 'def': 'a basket usually with a cover', 'name': 'hamper'}, {'frequency': 'r', 'id': 543, 'synset': 'hamster.n.01', 'synonyms': ['hamster'], 'def': 'short-tailed burrowing rodent with large cheek pouches', 'name': 'hamster'}, {'frequency': 'c', 'id': 544, 'synset': 'hand_blower.n.01', 'synonyms': ['hair_dryer'], 'def': 'a hand-held electric blower that can blow warm air onto the hair', 'name': 'hair_dryer'}, {'frequency': 'r', 'id': 545, 'synset': 'hand_glass.n.01', 'synonyms': ['hand_glass', 'hand_mirror'], 'def': 'a mirror intended to be held in the hand', 'name': 'hand_glass'}, {'frequency': 'f', 'id': 546, 'synset': 'hand_towel.n.01', 'synonyms': ['hand_towel', 'face_towel'], 'def': 'a small towel used to dry the hands or face', 'name': 'hand_towel'}, {'frequency': 'c', 'id': 547, 'synset': 'handcart.n.01', 'synonyms': ['handcart', 'pushcart', 'hand_truck'], 'def': 'wheeled vehicle that can be pushed by a person', 'name': 'handcart'}, {'frequency': 'r', 'id': 548, 'synset': 'handcuff.n.01', 'synonyms': ['handcuff'], 'def': 'shackle that consists of a metal loop that can be locked around the wrist', 'name': 'handcuff'}, {'frequency': 'c', 'id': 549, 'synset': 'handkerchief.n.01', 'synonyms': ['handkerchief'], 'def': 'a square piece of cloth used for wiping the eyes or nose or as a costume accessory', 'name': 'handkerchief'}, {'frequency': 'f', 'id': 550, 'synset': 'handle.n.01', 'synonyms': ['handle', 'grip', 'handgrip'], 'def': 'the appendage to an object that is designed to be held in order to use or move it', 'name': 'handle'}, {'frequency': 'r', 'id': 551, 'synset': 'handsaw.n.01', 'synonyms': ['handsaw', "carpenter's_saw"], 'def': 'a saw used with one hand for cutting wood', 'name': 'handsaw'}, {'frequency': 'r', 'id': 552, 'synset': 'hardback.n.01', 'synonyms': ['hardback_book', 'hardcover_book'], 'def': 'a book with cardboard or cloth or leather covers', 'name': 'hardback_book'}, {'frequency': 'r', 'id': 553, 'synset': 'harmonium.n.01', 'synonyms': ['harmonium', 'organ_(musical_instrument)', 'reed_organ_(musical_instrument)'], 'def': 'a free-reed instrument in which air is forced through the reeds by bellows', 'name': 'harmonium'}, {'frequency': 'f', 'id': 554, 'synset': 'hat.n.01', 'synonyms': ['hat'], 'def': 'headwear that protects the head from bad weather, sun, or worn for fashion', 'name': 'hat'}, {'frequency': 'r', 'id': 555, 'synset': 'hatbox.n.01', 'synonyms': ['hatbox'], 'def': 'a round piece of luggage for carrying hats', 'name': 'hatbox'}, {'frequency': 'r', 'id': 556, 'synset': 'hatch.n.03', 'synonyms': ['hatch'], 'def': 'a movable barrier covering a hatchway', 'name': 'hatch'}, {'frequency': 'c', 'id': 557, 'synset': 'head_covering.n.01', 'synonyms': ['veil'], 'def': 'a garment that covers the head and face', 'name': 'veil'}, {'frequency': 'f', 'id': 558, 'synset': 'headband.n.01', 'synonyms': ['headband'], 'def': 'a band worn around or over the head', 'name': 'headband'}, {'frequency': 'f', 'id': 559, 'synset': 'headboard.n.01', 'synonyms': ['headboard'], 'def': 'a vertical board or panel forming the head of a bedstead', 'name': 'headboard'}, {'frequency': 'f', 'id': 560, 'synset': 'headlight.n.01', 'synonyms': ['headlight', 'headlamp'], 'def': 'a powerful light with reflector; attached to the front of an automobile or locomotive', 'name': 'headlight'}, {'frequency': 'c', 'id': 561, 'synset': 'headscarf.n.01', 'synonyms': ['headscarf'], 'def': 'a kerchief worn over the head and tied under the chin', 'name': 'headscarf'}, {'frequency': 'r', 'id': 562, 'synset': 'headset.n.01', 'synonyms': ['headset'], 'def': 'receiver consisting of a pair of headphones', 'name': 'headset'}, {'frequency': 'c', 'id': 563, 'synset': 'headstall.n.01', 'synonyms': ['headstall_(for_horses)', 'headpiece_(for_horses)'], 'def': "the band that is the part of a bridle that fits around a horse's head", 'name': 'headstall_(for_horses)'}, {'frequency': 'r', 'id': 564, 'synset': 'hearing_aid.n.02', 'synonyms': ['hearing_aid'], 'def': 'an acoustic device used to direct sound to the ear of a hearing-impaired person', 'name': 'hearing_aid'}, {'frequency': 'c', 'id': 565, 'synset': 'heart.n.02', 'synonyms': ['heart'], 'def': 'a muscular organ; its contractions move the blood through the body', 'name': 'heart'}, {'frequency': 'c', 'id': 566, 'synset': 'heater.n.01', 'synonyms': ['heater', 'warmer'], 'def': 'device that heats water or supplies warmth to a room', 'name': 'heater'}, {'frequency': 'c', 'id': 567, 'synset': 'helicopter.n.01', 'synonyms': ['helicopter'], 'def': 'an aircraft without wings that obtains its lift from the rotation of overhead blades', 'name': 'helicopter'}, {'frequency': 'f', 'id': 568, 'synset': 'helmet.n.02', 'synonyms': ['helmet'], 'def': 'a protective headgear made of hard material to resist blows', 'name': 'helmet'}, {'frequency': 'r', 'id': 569, 'synset': 'heron.n.02', 'synonyms': ['heron'], 'def': 'grey or white wading bird with long neck and long legs and (usually) long bill', 'name': 'heron'}, {'frequency': 'c', 'id': 570, 'synset': 'highchair.n.01', 'synonyms': ['highchair', 'feeding_chair'], 'def': 'a chair for feeding a very young child', 'name': 'highchair'}, {'frequency': 'f', 'id': 571, 'synset': 'hinge.n.01', 'synonyms': ['hinge'], 'def': 'a joint that holds two parts together so that one can swing relative to the other', 'name': 'hinge'}, {'frequency': 'r', 'id': 572, 'synset': 'hippopotamus.n.01', 'synonyms': ['hippopotamus'], 'def': 'massive thick-skinned animal living in or around rivers of tropical Africa', 'name': 'hippopotamus'}, {'frequency': 'r', 'id': 573, 'synset': 'hockey_stick.n.01', 'synonyms': ['hockey_stick'], 'def': 'sports implement consisting of a stick used by hockey players to move the puck', 'name': 'hockey_stick'}, {'frequency': 'c', 'id': 574, 'synset': 'hog.n.03', 'synonyms': ['hog', 'pig'], 'def': 'domestic swine', 'name': 'hog'}, {'frequency': 'f', 'id': 575, 'synset': 'home_plate.n.01', 'synonyms': ['home_plate_(baseball)', 'home_base_(baseball)'], 'def': '(baseball) a rubber slab where the batter stands; it must be touched by a base runner in order to score', 'name': 'home_plate_(baseball)'}, {'frequency': 'c', 'id': 576, 'synset': 'honey.n.01', 'synonyms': ['honey'], 'def': 'a sweet yellow liquid produced by bees', 'name': 'honey'}, {'frequency': 'f', 'id': 577, 'synset': 'hood.n.06', 'synonyms': ['fume_hood', 'exhaust_hood'], 'def': 'metal covering leading to a vent that exhausts smoke or fumes', 'name': 'fume_hood'}, {'frequency': 'f', 'id': 578, 'synset': 'hook.n.05', 'synonyms': ['hook'], 'def': 'a curved or bent implement for suspending or pulling something', 'name': 'hook'}, {'frequency': 'f', 'id': 579, 'synset': 'horse.n.01', 'synonyms': ['horse'], 'def': 'a common horse', 'name': 'horse'}, {'frequency': 'f', 'id': 580, 'synset': 'hose.n.03', 'synonyms': ['hose', 'hosepipe'], 'def': 'a flexible pipe for conveying a liquid or gas', 'name': 'hose'}, {'frequency': 'r', 'id': 581, 'synset': 'hot-air_balloon.n.01', 'synonyms': ['hot-air_balloon'], 'def': 'balloon for travel through the air in a basket suspended below a large bag of heated air', 'name': 'hot-air_balloon'}, {'frequency': 'r', 'id': 582, 'synset': 'hot_plate.n.01', 'synonyms': ['hotplate'], 'def': 'a portable electric appliance for heating or cooking or keeping food warm', 'name': 'hotplate'}, {'frequency': 'c', 'id': 583, 'synset': 'hot_sauce.n.01', 'synonyms': ['hot_sauce'], 'def': 'a pungent peppery sauce', 'name': 'hot_sauce'}, {'frequency': 'r', 'id': 584, 'synset': 'hourglass.n.01', 'synonyms': ['hourglass'], 'def': 'a sandglass timer that runs for sixty minutes', 'name': 'hourglass'}, {'frequency': 'r', 'id': 585, 'synset': 'houseboat.n.01', 'synonyms': ['houseboat'], 'def': 'a barge that is designed and equipped for use as a dwelling', 'name': 'houseboat'}, {'frequency': 'r', 'id': 586, 'synset': 'hummingbird.n.01', 'synonyms': ['hummingbird'], 'def': 'tiny American bird having brilliant iridescent plumage and long slender bills', 'name': 'hummingbird'}, {'frequency': 'r', 'id': 587, 'synset': 'hummus.n.01', 'synonyms': ['hummus', 'humus', 'hommos', 'hoummos', 'humous'], 'def': 'a thick spread made from mashed chickpeas', 'name': 'hummus'}, {'frequency': 'c', 'id': 588, 'synset': 'ice_bear.n.01', 'synonyms': ['polar_bear'], 'def': 'white bear of Arctic regions', 'name': 'polar_bear'}, {'frequency': 'c', 'id': 589, 'synset': 'ice_cream.n.01', 'synonyms': ['icecream'], 'def': 'frozen dessert containing cream and sugar and flavoring', 'name': 'icecream'}, {'frequency': 'r', 'id': 590, 'synset': 'ice_lolly.n.01', 'synonyms': ['popsicle'], 'def': 'ice cream or water ice on a small wooden stick', 'name': 'popsicle'}, {'frequency': 'c', 'id': 591, 'synset': 'ice_maker.n.01', 'synonyms': ['ice_maker'], 'def': 'an appliance included in some electric refrigerators for making ice cubes', 'name': 'ice_maker'}, {'frequency': 'r', 'id': 592, 'synset': 'ice_pack.n.01', 'synonyms': ['ice_pack', 'ice_bag'], 'def': 'a waterproof bag filled with ice: applied to the body (especially the head) to cool or reduce swelling', 'name': 'ice_pack'}, {'frequency': 'r', 'id': 593, 'synset': 'ice_skate.n.01', 'synonyms': ['ice_skate'], 'def': 'skate consisting of a boot with a steel blade fitted to the sole', 'name': 'ice_skate'}, {'frequency': 'r', 'id': 594, 'synset': 'ice_tea.n.01', 'synonyms': ['ice_tea', 'iced_tea'], 'def': 'strong tea served over ice', 'name': 'ice_tea'}, {'frequency': 'c', 'id': 595, 'synset': 'igniter.n.01', 'synonyms': ['igniter', 'ignitor', 'lighter'], 'def': 'a substance or device used to start a fire', 'name': 'igniter'}, {'frequency': 'r', 'id': 596, 'synset': 'incense.n.01', 'synonyms': ['incense'], 'def': 'a substance that produces a fragrant odor when burned', 'name': 'incense'}, {'frequency': 'r', 'id': 597, 'synset': 'inhaler.n.01', 'synonyms': ['inhaler', 'inhalator'], 'def': 'a dispenser that produces a chemical vapor to be inhaled through mouth or nose', 'name': 'inhaler'}, {'frequency': 'c', 'id': 598, 'synset': 'ipod.n.01', 'synonyms': ['iPod'], 'def': 'a pocket-sized device used to play music files', 'name': 'iPod'}, {'frequency': 'c', 'id': 599, 'synset': 'iron.n.04', 'synonyms': ['iron_(for_clothing)', 'smoothing_iron_(for_clothing)'], 'def': 'home appliance consisting of a flat metal base that is heated and used to smooth cloth', 'name': 'iron_(for_clothing)'}, {'frequency': 'r', 'id': 600, 'synset': 'ironing_board.n.01', 'synonyms': ['ironing_board'], 'def': 'narrow padded board on collapsible supports; used for ironing clothes', 'name': 'ironing_board'}, {'frequency': 'f', 'id': 601, 'synset': 'jacket.n.01', 'synonyms': ['jacket'], 'def': 'a waist-length coat', 'name': 'jacket'}, {'frequency': 'r', 'id': 602, 'synset': 'jam.n.01', 'synonyms': ['jam'], 'def': 'preserve of crushed fruit', 'name': 'jam'}, {'frequency': 'f', 'id': 603, 'synset': 'jean.n.01', 'synonyms': ['jean', 'blue_jean', 'denim'], 'def': '(usually plural) close-fitting trousers of heavy denim for manual work or casual wear', 'name': 'jean'}, {'frequency': 'c', 'id': 604, 'synset': 'jeep.n.01', 'synonyms': ['jeep', 'landrover'], 'def': 'a car suitable for traveling over rough terrain', 'name': 'jeep'}, {'frequency': 'r', 'id': 605, 'synset': 'jelly_bean.n.01', 'synonyms': ['jelly_bean', 'jelly_egg'], 'def': 'sugar-glazed jellied candy', 'name': 'jelly_bean'}, {'frequency': 'f', 'id': 606, 'synset': 'jersey.n.03', 'synonyms': ['jersey', 'T-shirt', 'tee_shirt'], 'def': 'a close-fitting pullover shirt', 'name': 'jersey'}, {'frequency': 'c', 'id': 607, 'synset': 'jet.n.01', 'synonyms': ['jet_plane', 'jet-propelled_plane'], 'def': 'an airplane powered by one or more jet engines', 'name': 'jet_plane'}, {'frequency': 'c', 'id': 608, 'synset': 'jewelry.n.01', 'synonyms': ['jewelry', 'jewellery'], 'def': 'an adornment (as a bracelet or ring or necklace) made of precious metals and set with gems (or imitation gems)', 'name': 'jewelry'}, {'frequency': 'r', 'id': 609, 'synset': 'joystick.n.02', 'synonyms': ['joystick'], 'def': 'a control device for computers consisting of a vertical handle that can move freely in two directions', 'name': 'joystick'}, {'frequency': 'r', 'id': 610, 'synset': 'jump_suit.n.01', 'synonyms': ['jumpsuit'], 'def': "one-piece garment fashioned after a parachutist's uniform", 'name': 'jumpsuit'}, {'frequency': 'c', 'id': 611, 'synset': 'kayak.n.01', 'synonyms': ['kayak'], 'def': 'a small canoe consisting of a light frame made watertight with animal skins', 'name': 'kayak'}, {'frequency': 'r', 'id': 612, 'synset': 'keg.n.02', 'synonyms': ['keg'], 'def': 'small cask or barrel', 'name': 'keg'}, {'frequency': 'r', 'id': 613, 'synset': 'kennel.n.01', 'synonyms': ['kennel', 'doghouse'], 'def': 'outbuilding that serves as a shelter for a dog', 'name': 'kennel'}, {'frequency': 'c', 'id': 614, 'synset': 'kettle.n.01', 'synonyms': ['kettle', 'boiler'], 'def': 'a metal pot for stewing or boiling; usually has a lid', 'name': 'kettle'}, {'frequency': 'f', 'id': 615, 'synset': 'key.n.01', 'synonyms': ['key'], 'def': 'metal instrument used to unlock a lock', 'name': 'key'}, {'frequency': 'r', 'id': 616, 'synset': 'keycard.n.01', 'synonyms': ['keycard'], 'def': 'a plastic card used to gain access typically to a door', 'name': 'keycard'}, {'frequency': 'r', 'id': 617, 'synset': 'kilt.n.01', 'synonyms': ['kilt'], 'def': 'a knee-length pleated tartan skirt worn by men as part of the traditional dress in the Highlands of northern Scotland', 'name': 'kilt'}, {'frequency': 'c', 'id': 618, 'synset': 'kimono.n.01', 'synonyms': ['kimono'], 'def': 'a loose robe; imitated from robes originally worn by Japanese', 'name': 'kimono'}, {'frequency': 'f', 'id': 619, 'synset': 'kitchen_sink.n.01', 'synonyms': ['kitchen_sink'], 'def': 'a sink in a kitchen', 'name': 'kitchen_sink'}, {'frequency': 'c', 'id': 620, 'synset': 'kitchen_table.n.01', 'synonyms': ['kitchen_table'], 'def': 'a table in the kitchen', 'name': 'kitchen_table'}, {'frequency': 'f', 'id': 621, 'synset': 'kite.n.03', 'synonyms': ['kite'], 'def': 'plaything consisting of a light frame covered with tissue paper; flown in wind at end of a string', 'name': 'kite'}, {'frequency': 'c', 'id': 622, 'synset': 'kitten.n.01', 'synonyms': ['kitten', 'kitty'], 'def': 'young domestic cat', 'name': 'kitten'}, {'frequency': 'c', 'id': 623, 'synset': 'kiwi.n.03', 'synonyms': ['kiwi_fruit'], 'def': 'fuzzy brown egg-shaped fruit with slightly tart green flesh', 'name': 'kiwi_fruit'}, {'frequency': 'f', 'id': 624, 'synset': 'knee_pad.n.01', 'synonyms': ['knee_pad'], 'def': 'protective garment consisting of a pad worn by football or baseball or hockey players', 'name': 'knee_pad'}, {'frequency': 'f', 'id': 625, 'synset': 'knife.n.01', 'synonyms': ['knife'], 'def': 'tool with a blade and point used as a cutting instrument', 'name': 'knife'}, {'frequency': 'r', 'id': 626, 'synset': 'knight.n.02', 'synonyms': ['knight_(chess_piece)', 'horse_(chess_piece)'], 'def': 'a chess game piece shaped to resemble the head of a horse', 'name': 'knight_(chess_piece)'}, {'frequency': 'r', 'id': 627, 'synset': 'knitting_needle.n.01', 'synonyms': ['knitting_needle'], 'def': 'needle consisting of a slender rod with pointed ends; usually used in pairs', 'name': 'knitting_needle'}, {'frequency': 'f', 'id': 628, 'synset': 'knob.n.02', 'synonyms': ['knob'], 'def': 'a round handle often found on a door', 'name': 'knob'}, {'frequency': 'r', 'id': 629, 'synset': 'knocker.n.05', 'synonyms': ['knocker_(on_a_door)', 'doorknocker'], 'def': 'a device (usually metal and ornamental) attached by a hinge to a door', 'name': 'knocker_(on_a_door)'}, {'frequency': 'r', 'id': 630, 'synset': 'koala.n.01', 'synonyms': ['koala', 'koala_bear'], 'def': 'sluggish tailless Australian marsupial with grey furry ears and coat', 'name': 'koala'}, {'frequency': 'r', 'id': 631, 'synset': 'lab_coat.n.01', 'synonyms': ['lab_coat', 'laboratory_coat'], 'def': 'a light coat worn to protect clothing from substances used while working in a laboratory', 'name': 'lab_coat'}, {'frequency': 'f', 'id': 632, 'synset': 'ladder.n.01', 'synonyms': ['ladder'], 'def': 'steps consisting of two parallel members connected by rungs', 'name': 'ladder'}, {'frequency': 'c', 'id': 633, 'synset': 'ladle.n.01', 'synonyms': ['ladle'], 'def': 'a spoon-shaped vessel with a long handle frequently used to transfer liquids', 'name': 'ladle'}, {'frequency': 'r', 'id': 634, 'synset': 'ladybug.n.01', 'synonyms': ['ladybug', 'ladybeetle', 'ladybird_beetle'], 'def': 'small round bright-colored and spotted beetle, typically red and black', 'name': 'ladybug'}, {'frequency': 'c', 'id': 635, 'synset': 'lamb.n.01', 'synonyms': ['lamb_(animal)'], 'def': 'young sheep', 'name': 'lamb_(animal)'}, {'frequency': 'r', 'id': 636, 'synset': 'lamb_chop.n.01', 'synonyms': ['lamb-chop', 'lambchop'], 'def': 'chop cut from a lamb', 'name': 'lamb-chop'}, {'frequency': 'f', 'id': 637, 'synset': 'lamp.n.02', 'synonyms': ['lamp'], 'def': 'a piece of furniture holding one or more electric light bulbs', 'name': 'lamp'}, {'frequency': 'f', 'id': 638, 'synset': 'lamppost.n.01', 'synonyms': ['lamppost'], 'def': 'a metal post supporting an outdoor lamp (such as a streetlight)', 'name': 'lamppost'}, {'frequency': 'f', 'id': 639, 'synset': 'lampshade.n.01', 'synonyms': ['lampshade'], 'def': 'a protective ornamental shade used to screen a light bulb from direct view', 'name': 'lampshade'}, {'frequency': 'c', 'id': 640, 'synset': 'lantern.n.01', 'synonyms': ['lantern'], 'def': 'light in a transparent protective case', 'name': 'lantern'}, {'frequency': 'f', 'id': 641, 'synset': 'lanyard.n.02', 'synonyms': ['lanyard', 'laniard'], 'def': 'a cord worn around the neck to hold a knife or whistle, etc.', 'name': 'lanyard'}, {'frequency': 'f', 'id': 642, 'synset': 'laptop.n.01', 'synonyms': ['laptop_computer', 'notebook_computer'], 'def': 'a portable computer small enough to use in your lap', 'name': 'laptop_computer'}, {'frequency': 'r', 'id': 643, 'synset': 'lasagna.n.01', 'synonyms': ['lasagna', 'lasagne'], 'def': 'baked dish of layers of lasagna pasta with sauce and cheese and meat or vegetables', 'name': 'lasagna'}, {'frequency': 'c', 'id': 644, 'synset': 'latch.n.02', 'synonyms': ['latch'], 'def': 'a bar that can be lowered or slid into a groove to fasten a door or gate', 'name': 'latch'}, {'frequency': 'r', 'id': 645, 'synset': 'lawn_mower.n.01', 'synonyms': ['lawn_mower'], 'def': 'garden tool for mowing grass on lawns', 'name': 'lawn_mower'}, {'frequency': 'r', 'id': 646, 'synset': 'leather.n.01', 'synonyms': ['leather'], 'def': 'an animal skin made smooth and flexible by removing the hair and then tanning', 'name': 'leather'}, {'frequency': 'c', 'id': 647, 'synset': 'legging.n.01', 'synonyms': ['legging_(clothing)', 'leging_(clothing)', 'leg_covering'], 'def': 'a garment covering the leg (usually extending from the knee to the ankle)', 'name': 'legging_(clothing)'}, {'frequency': 'c', 'id': 648, 'synset': 'lego.n.01', 'synonyms': ['Lego', 'Lego_set'], 'def': "a child's plastic construction set for making models from blocks", 'name': 'Lego'}, {'frequency': 'f', 'id': 649, 'synset': 'lemon.n.01', 'synonyms': ['lemon'], 'def': 'yellow oval fruit with juicy acidic flesh', 'name': 'lemon'}, {'frequency': 'r', 'id': 650, 'synset': 'lemonade.n.01', 'synonyms': ['lemonade'], 'def': 'sweetened beverage of diluted lemon juice', 'name': 'lemonade'}, {'frequency': 'f', 'id': 651, 'synset': 'lettuce.n.02', 'synonyms': ['lettuce'], 'def': 'leafy plant commonly eaten in salad or on sandwiches', 'name': 'lettuce'}, {'frequency': 'f', 'id': 652, 'synset': 'license_plate.n.01', 'synonyms': ['license_plate', 'numberplate'], 'def': "a plate mounted on the front and back of car and bearing the car's registration number", 'name': 'license_plate'}, {'frequency': 'f', 'id': 653, 'synset': 'life_buoy.n.01', 'synonyms': ['life_buoy', 'lifesaver', 'life_belt', 'life_ring'], 'def': 'a ring-shaped life preserver used to prevent drowning (NOT a life-jacket or vest)', 'name': 'life_buoy'}, {'frequency': 'f', 'id': 654, 'synset': 'life_jacket.n.01', 'synonyms': ['life_jacket', 'life_vest'], 'def': 'life preserver consisting of a sleeveless jacket of buoyant or inflatable design', 'name': 'life_jacket'}, {'frequency': 'f', 'id': 655, 'synset': 'light_bulb.n.01', 'synonyms': ['lightbulb'], 'def': 'glass bulb or tube shaped electric device that emits light (DO NOT MARK LAMPS AS A WHOLE)', 'name': 'lightbulb'}, {'frequency': 'r', 'id': 656, 'synset': 'lightning_rod.n.02', 'synonyms': ['lightning_rod', 'lightning_conductor'], 'def': 'a metallic conductor that is attached to a high point and leads to the ground', 'name': 'lightning_rod'}, {'frequency': 'c', 'id': 657, 'synset': 'lime.n.06', 'synonyms': ['lime'], 'def': 'the green acidic fruit of any of various lime trees', 'name': 'lime'}, {'frequency': 'r', 'id': 658, 'synset': 'limousine.n.01', 'synonyms': ['limousine'], 'def': 'long luxurious car; usually driven by a chauffeur', 'name': 'limousine'}, {'frequency': 'r', 'id': 659, 'synset': 'linen.n.02', 'synonyms': ['linen_paper'], 'def': 'a high-quality paper made of linen fibers or with a linen finish', 'name': 'linen_paper'}, {'frequency': 'c', 'id': 660, 'synset': 'lion.n.01', 'synonyms': ['lion'], 'def': 'large gregarious predatory cat of Africa and India', 'name': 'lion'}, {'frequency': 'c', 'id': 661, 'synset': 'lip_balm.n.01', 'synonyms': ['lip_balm'], 'def': 'a balm applied to the lips', 'name': 'lip_balm'}, {'frequency': 'c', 'id': 662, 'synset': 'lipstick.n.01', 'synonyms': ['lipstick', 'lip_rouge'], 'def': 'makeup that is used to color the lips', 'name': 'lipstick'}, {'frequency': 'r', 'id': 663, 'synset': 'liquor.n.01', 'synonyms': ['liquor', 'spirits', 'hard_liquor', 'liqueur', 'cordial'], 'def': 'an alcoholic beverage that is distilled rather than fermented', 'name': 'liquor'}, {'frequency': 'r', 'id': 664, 'synset': 'lizard.n.01', 'synonyms': ['lizard'], 'def': 'a reptile with usually two pairs of legs and a tapering tail', 'name': 'lizard'}, {'frequency': 'r', 'id': 665, 'synset': 'loafer.n.02', 'synonyms': ['Loafer_(type_of_shoe)'], 'def': 'a low leather step-in shoe', 'name': 'Loafer_(type_of_shoe)'}, {'frequency': 'f', 'id': 666, 'synset': 'log.n.01', 'synonyms': ['log'], 'def': 'a segment of the trunk of a tree when stripped of branches', 'name': 'log'}, {'frequency': 'c', 'id': 667, 'synset': 'lollipop.n.02', 'synonyms': ['lollipop'], 'def': 'hard candy on a stick', 'name': 'lollipop'}, {'frequency': 'c', 'id': 668, 'synset': 'lotion.n.01', 'synonyms': ['lotion'], 'def': 'any of various cosmetic preparations that are applied to the skin', 'name': 'lotion'}, {'frequency': 'f', 'id': 669, 'synset': 'loudspeaker.n.01', 'synonyms': ['speaker_(stero_equipment)'], 'def': 'electronic device that produces sound often as part of a stereo system', 'name': 'speaker_(stero_equipment)'}, {'frequency': 'c', 'id': 670, 'synset': 'love_seat.n.01', 'synonyms': ['loveseat'], 'def': 'small sofa that seats two people', 'name': 'loveseat'}, {'frequency': 'r', 'id': 671, 'synset': 'machine_gun.n.01', 'synonyms': ['machine_gun'], 'def': 'a rapidly firing automatic gun', 'name': 'machine_gun'}, {'frequency': 'f', 'id': 672, 'synset': 'magazine.n.02', 'synonyms': ['magazine'], 'def': 'a paperback periodic publication', 'name': 'magazine'}, {'frequency': 'f', 'id': 673, 'synset': 'magnet.n.01', 'synonyms': ['magnet'], 'def': 'a device that attracts iron and produces a magnetic field', 'name': 'magnet'}, {'frequency': 'r', 'id': 674, 'synset': 'mail_slot.n.01', 'synonyms': ['mail_slot'], 'def': 'a slot (usually in a door) through which mail can be delivered', 'name': 'mail_slot'}, {'frequency': 'c', 'id': 675, 'synset': 'mailbox.n.01', 'synonyms': ['mailbox_(at_home)', 'letter_box_(at_home)'], 'def': 'a private box for delivery of mail', 'name': 'mailbox_(at_home)'}, {'frequency': 'r', 'id': 676, 'synset': 'mallet.n.01', 'synonyms': ['mallet'], 'def': 'a sports implement with a long handle and a hammer-like head used to hit a ball', 'name': 'mallet'}, {'frequency': 'r', 'id': 677, 'synset': 'mammoth.n.01', 'synonyms': ['mammoth'], 'def': 'any of numerous extinct elephants widely distributed in the Pleistocene', 'name': 'mammoth'}, {'frequency': 'c', 'id': 678, 'synset': 'mandarin.n.05', 'synonyms': ['mandarin_orange'], 'def': 'a somewhat flat reddish-orange loose skinned citrus of China', 'name': 'mandarin_orange'}, {'frequency': 'c', 'id': 679, 'synset': 'manger.n.01', 'synonyms': ['manger', 'trough'], 'def': 'a container (usually in a barn or stable) from which cattle or horses feed', 'name': 'manger'}, {'frequency': 'f', 'id': 680, 'synset': 'manhole.n.01', 'synonyms': ['manhole'], 'def': 'a hole (usually with a flush cover) through which a person can gain access to an underground structure', 'name': 'manhole'}, {'frequency': 'c', 'id': 681, 'synset': 'map.n.01', 'synonyms': ['map'], 'def': "a diagrammatic representation of the earth's surface (or part of it)", 'name': 'map'}, {'frequency': 'c', 'id': 682, 'synset': 'marker.n.03', 'synonyms': ['marker'], 'def': 'a writing implement for making a mark', 'name': 'marker'}, {'frequency': 'r', 'id': 683, 'synset': 'martini.n.01', 'synonyms': ['martini'], 'def': 'a cocktail made of gin (or vodka) with dry vermouth', 'name': 'martini'}, {'frequency': 'r', 'id': 684, 'synset': 'mascot.n.01', 'synonyms': ['mascot'], 'def': 'a person or animal that is adopted by a team or other group as a symbolic figure', 'name': 'mascot'}, {'frequency': 'c', 'id': 685, 'synset': 'mashed_potato.n.01', 'synonyms': ['mashed_potato'], 'def': 'potato that has been peeled and boiled and then mashed', 'name': 'mashed_potato'}, {'frequency': 'r', 'id': 686, 'synset': 'masher.n.02', 'synonyms': ['masher'], 'def': 'a kitchen utensil used for mashing (e.g. potatoes)', 'name': 'masher'}, {'frequency': 'f', 'id': 687, 'synset': 'mask.n.04', 'synonyms': ['mask', 'facemask'], 'def': 'a protective covering worn over the face', 'name': 'mask'}, {'frequency': 'f', 'id': 688, 'synset': 'mast.n.01', 'synonyms': ['mast'], 'def': 'a vertical spar for supporting sails', 'name': 'mast'}, {'frequency': 'c', 'id': 689, 'synset': 'mat.n.03', 'synonyms': ['mat_(gym_equipment)', 'gym_mat'], 'def': 'sports equipment consisting of a piece of thick padding on the floor for gymnastics', 'name': 'mat_(gym_equipment)'}, {'frequency': 'r', 'id': 690, 'synset': 'matchbox.n.01', 'synonyms': ['matchbox'], 'def': 'a box for holding matches', 'name': 'matchbox'}, {'frequency': 'f', 'id': 691, 'synset': 'mattress.n.01', 'synonyms': ['mattress'], 'def': 'a thick pad filled with resilient material used as a bed or part of a bed', 'name': 'mattress'}, {'frequency': 'c', 'id': 692, 'synset': 'measuring_cup.n.01', 'synonyms': ['measuring_cup'], 'def': 'graduated cup used to measure liquid or granular ingredients', 'name': 'measuring_cup'}, {'frequency': 'c', 'id': 693, 'synset': 'measuring_stick.n.01', 'synonyms': ['measuring_stick', 'ruler_(measuring_stick)', 'measuring_rod'], 'def': 'measuring instrument having a sequence of marks at regular intervals', 'name': 'measuring_stick'}, {'frequency': 'c', 'id': 694, 'synset': 'meatball.n.01', 'synonyms': ['meatball'], 'def': 'ground meat formed into a ball and fried or simmered in broth', 'name': 'meatball'}, {'frequency': 'c', 'id': 695, 'synset': 'medicine.n.02', 'synonyms': ['medicine'], 'def': 'something that treats or prevents or alleviates the symptoms of disease', 'name': 'medicine'}, {'frequency': 'r', 'id': 696, 'synset': 'melon.n.01', 'synonyms': ['melon'], 'def': 'fruit of the gourd family having a hard rind and sweet juicy flesh', 'name': 'melon'}, {'frequency': 'f', 'id': 697, 'synset': 'microphone.n.01', 'synonyms': ['microphone'], 'def': 'device for converting sound waves into electrical energy', 'name': 'microphone'}, {'frequency': 'r', 'id': 698, 'synset': 'microscope.n.01', 'synonyms': ['microscope'], 'def': 'magnifier of the image of small objects', 'name': 'microscope'}, {'frequency': 'f', 'id': 699, 'synset': 'microwave.n.02', 'synonyms': ['microwave_oven'], 'def': 'kitchen appliance that cooks food by passing an electromagnetic wave through it', 'name': 'microwave_oven'}, {'frequency': 'r', 'id': 700, 'synset': 'milestone.n.01', 'synonyms': ['milestone', 'milepost'], 'def': 'stone post at side of a road to show distances', 'name': 'milestone'}, {'frequency': 'c', 'id': 701, 'synset': 'milk.n.01', 'synonyms': ['milk'], 'def': 'a white nutritious liquid secreted by mammals and used as food by human beings', 'name': 'milk'}, {'frequency': 'f', 'id': 702, 'synset': 'minivan.n.01', 'synonyms': ['minivan'], 'def': 'a small box-shaped passenger van', 'name': 'minivan'}, {'frequency': 'r', 'id': 703, 'synset': 'mint.n.05', 'synonyms': ['mint_candy'], 'def': 'a candy that is flavored with a mint oil', 'name': 'mint_candy'}, {'frequency': 'f', 'id': 704, 'synset': 'mirror.n.01', 'synonyms': ['mirror'], 'def': 'polished surface that forms images by reflecting light', 'name': 'mirror'}, {'frequency': 'c', 'id': 705, 'synset': 'mitten.n.01', 'synonyms': ['mitten'], 'def': 'glove that encases the thumb separately and the other four fingers together', 'name': 'mitten'}, {'frequency': 'c', 'id': 706, 'synset': 'mixer.n.04', 'synonyms': ['mixer_(kitchen_tool)', 'stand_mixer'], 'def': 'a kitchen utensil that is used for mixing foods', 'name': 'mixer_(kitchen_tool)'}, {'frequency': 'c', 'id': 707, 'synset': 'money.n.03', 'synonyms': ['money'], 'def': 'the official currency issued by a government or national bank', 'name': 'money'}, {'frequency': 'f', 'id': 708, 'synset': 'monitor.n.04', 'synonyms': ['monitor_(computer_equipment) computer_monitor'], 'def': 'a computer monitor', 'name': 'monitor_(computer_equipment) computer_monitor'}, {'frequency': 'c', 'id': 709, 'synset': 'monkey.n.01', 'synonyms': ['monkey'], 'def': 'any of various long-tailed primates', 'name': 'monkey'}, {'frequency': 'f', 'id': 710, 'synset': 'motor.n.01', 'synonyms': ['motor'], 'def': 'machine that converts other forms of energy into mechanical energy and so imparts motion', 'name': 'motor'}, {'frequency': 'f', 'id': 711, 'synset': 'motor_scooter.n.01', 'synonyms': ['motor_scooter', 'scooter'], 'def': 'a wheeled vehicle with small wheels and a low-powered engine', 'name': 'motor_scooter'}, {'frequency': 'r', 'id': 712, 'synset': 'motor_vehicle.n.01', 'synonyms': ['motor_vehicle', 'automotive_vehicle'], 'def': 'a self-propelled wheeled vehicle that does not run on rails', 'name': 'motor_vehicle'}, {'frequency': 'r', 'id': 713, 'synset': 'motorboat.n.01', 'synonyms': ['motorboat', 'powerboat'], 'def': 'a boat propelled by an internal-combustion engine', 'name': 'motorboat'}, {'frequency': 'f', 'id': 714, 'synset': 'motorcycle.n.01', 'synonyms': ['motorcycle'], 'def': 'a motor vehicle with two wheels and a strong frame', 'name': 'motorcycle'}, {'frequency': 'f', 'id': 715, 'synset': 'mound.n.01', 'synonyms': ['mound_(baseball)', "pitcher's_mound"], 'def': '(baseball) the slight elevation on which the pitcher stands', 'name': 'mound_(baseball)'}, {'frequency': 'r', 'id': 716, 'synset': 'mouse.n.01', 'synonyms': ['mouse_(animal_rodent)'], 'def': 'a small rodent with pointed snouts and small ears on elongated bodies with slender usually hairless tails', 'name': 'mouse_(animal_rodent)'}, {'frequency': 'f', 'id': 717, 'synset': 'mouse.n.04', 'synonyms': ['mouse_(computer_equipment)', 'computer_mouse'], 'def': 'a computer input device that controls an on-screen pointer', 'name': 'mouse_(computer_equipment)'}, {'frequency': 'f', 'id': 718, 'synset': 'mousepad.n.01', 'synonyms': ['mousepad'], 'def': 'a small portable pad that provides an operating surface for a computer mouse', 'name': 'mousepad'}, {'frequency': 'c', 'id': 719, 'synset': 'muffin.n.01', 'synonyms': ['muffin'], 'def': 'a sweet quick bread baked in a cup-shaped pan', 'name': 'muffin'}, {'frequency': 'f', 'id': 720, 'synset': 'mug.n.04', 'synonyms': ['mug'], 'def': 'with handle and usually cylindrical', 'name': 'mug'}, {'frequency': 'f', 'id': 721, 'synset': 'mushroom.n.02', 'synonyms': ['mushroom'], 'def': 'a common mushroom', 'name': 'mushroom'}, {'frequency': 'r', 'id': 722, 'synset': 'music_stool.n.01', 'synonyms': ['music_stool', 'piano_stool'], 'def': 'a stool for piano players; usually adjustable in height', 'name': 'music_stool'}, {'frequency': 'r', 'id': 723, 'synset': 'musical_instrument.n.01', 'synonyms': ['musical_instrument', 'instrument_(musical)'], 'def': 'any of various devices or contrivances that can be used to produce musical tones or sounds', 'name': 'musical_instrument'}, {'frequency': 'r', 'id': 724, 'synset': 'nailfile.n.01', 'synonyms': ['nailfile'], 'def': 'a small flat file for shaping the nails', 'name': 'nailfile'}, {'frequency': 'r', 'id': 725, 'synset': 'nameplate.n.01', 'synonyms': ['nameplate'], 'def': 'a plate bearing a name', 'name': 'nameplate'}, {'frequency': 'f', 'id': 726, 'synset': 'napkin.n.01', 'synonyms': ['napkin', 'table_napkin', 'serviette'], 'def': 'a small piece of table linen or paper that is used to wipe the mouth and to cover the lap in order to protect clothing', 'name': 'napkin'}, {'frequency': 'r', 'id': 727, 'synset': 'neckerchief.n.01', 'synonyms': ['neckerchief'], 'def': 'a kerchief worn around the neck', 'name': 'neckerchief'}, {'frequency': 'f', 'id': 728, 'synset': 'necklace.n.01', 'synonyms': ['necklace'], 'def': 'jewelry consisting of a cord or chain (often bearing gems) worn about the neck as an ornament', 'name': 'necklace'}, {'frequency': 'f', 'id': 729, 'synset': 'necktie.n.01', 'synonyms': ['necktie', 'tie_(necktie)'], 'def': 'neckwear consisting of a long narrow piece of material worn under a collar and tied in knot at the front', 'name': 'necktie'}, {'frequency': 'r', 'id': 730, 'synset': 'needle.n.03', 'synonyms': ['needle'], 'def': 'a sharp pointed implement (usually metal)', 'name': 'needle'}, {'frequency': 'c', 'id': 731, 'synset': 'nest.n.01', 'synonyms': ['nest'], 'def': 'a structure in which animals lay eggs or give birth to their young', 'name': 'nest'}, {'frequency': 'r', 'id': 732, 'synset': 'newsstand.n.01', 'synonyms': ['newsstand'], 'def': 'a stall where newspapers and other periodicals are sold', 'name': 'newsstand'}, {'frequency': 'c', 'id': 733, 'synset': 'nightwear.n.01', 'synonyms': ['nightshirt', 'nightwear', 'sleepwear', 'nightclothes'], 'def': 'garments designed to be worn in bed', 'name': 'nightshirt'}, {'frequency': 'r', 'id': 734, 'synset': 'nosebag.n.01', 'synonyms': ['nosebag_(for_animals)', 'feedbag'], 'def': 'a canvas bag that is used to feed an animal (such as a horse); covers the muzzle and fastens at the top of the head', 'name': 'nosebag_(for_animals)'}, {'frequency': 'r', 'id': 735, 'synset': 'noseband.n.01', 'synonyms': ['noseband_(for_animals)', 'nosepiece_(for_animals)'], 'def': "a strap that is the part of a bridle that goes over the animal's nose", 'name': 'noseband_(for_animals)'}, {'frequency': 'f', 'id': 736, 'synset': 'notebook.n.01', 'synonyms': ['notebook'], 'def': 'a book with blank pages for recording notes or memoranda', 'name': 'notebook'}, {'frequency': 'c', 'id': 737, 'synset': 'notepad.n.01', 'synonyms': ['notepad'], 'def': 'a pad of paper for keeping notes', 'name': 'notepad'}, {'frequency': 'c', 'id': 738, 'synset': 'nut.n.03', 'synonyms': ['nut'], 'def': 'a small metal block (usually square or hexagonal) with internal screw thread to be fitted onto a bolt', 'name': 'nut'}, {'frequency': 'r', 'id': 739, 'synset': 'nutcracker.n.01', 'synonyms': ['nutcracker'], 'def': 'a hand tool used to crack nuts open', 'name': 'nutcracker'}, {'frequency': 'c', 'id': 740, 'synset': 'oar.n.01', 'synonyms': ['oar'], 'def': 'an implement used to propel or steer a boat', 'name': 'oar'}, {'frequency': 'r', 'id': 741, 'synset': 'octopus.n.01', 'synonyms': ['octopus_(food)'], 'def': 'tentacles of octopus prepared as food', 'name': 'octopus_(food)'}, {'frequency': 'r', 'id': 742, 'synset': 'octopus.n.02', 'synonyms': ['octopus_(animal)'], 'def': 'bottom-living cephalopod having a soft oval body with eight long tentacles', 'name': 'octopus_(animal)'}, {'frequency': 'c', 'id': 743, 'synset': 'oil_lamp.n.01', 'synonyms': ['oil_lamp', 'kerosene_lamp', 'kerosine_lamp'], 'def': 'a lamp that burns oil (as kerosine) for light', 'name': 'oil_lamp'}, {'frequency': 'c', 'id': 744, 'synset': 'olive_oil.n.01', 'synonyms': ['olive_oil'], 'def': 'oil from olives', 'name': 'olive_oil'}, {'frequency': 'r', 'id': 745, 'synset': 'omelet.n.01', 'synonyms': ['omelet', 'omelette'], 'def': 'beaten eggs cooked until just set; may be folded around e.g. ham or cheese or jelly', 'name': 'omelet'}, {'frequency': 'f', 'id': 746, 'synset': 'onion.n.01', 'synonyms': ['onion'], 'def': 'the bulb of an onion plant', 'name': 'onion'}, {'frequency': 'f', 'id': 747, 'synset': 'orange.n.01', 'synonyms': ['orange_(fruit)'], 'def': 'orange (FRUIT of an orange tree)', 'name': 'orange_(fruit)'}, {'frequency': 'c', 'id': 748, 'synset': 'orange_juice.n.01', 'synonyms': ['orange_juice'], 'def': 'bottled or freshly squeezed juice of oranges', 'name': 'orange_juice'}, {'frequency': 'r', 'id': 749, 'synset': 'oregano.n.01', 'synonyms': ['oregano', 'marjoram'], 'def': 'aromatic Eurasian perennial herb used in cooking and baking', 'name': 'oregano'}, {'frequency': 'c', 'id': 750, 'synset': 'ostrich.n.02', 'synonyms': ['ostrich'], 'def': 'fast-running African flightless bird with two-toed feet; largest living bird', 'name': 'ostrich'}, {'frequency': 'c', 'id': 751, 'synset': 'ottoman.n.03', 'synonyms': ['ottoman', 'pouf', 'pouffe', 'hassock'], 'def': 'thick cushion used as a seat', 'name': 'ottoman'}, {'frequency': 'c', 'id': 752, 'synset': 'overall.n.01', 'synonyms': ['overalls_(clothing)'], 'def': 'work clothing consisting of denim trousers usually with a bib and shoulder straps', 'name': 'overalls_(clothing)'}, {'frequency': 'c', 'id': 753, 'synset': 'owl.n.01', 'synonyms': ['owl'], 'def': 'nocturnal bird of prey with hawk-like beak and claws and large head with front-facing eyes', 'name': 'owl'}, {'frequency': 'c', 'id': 754, 'synset': 'packet.n.03', 'synonyms': ['packet'], 'def': 'a small package or bundle', 'name': 'packet'}, {'frequency': 'r', 'id': 755, 'synset': 'pad.n.03', 'synonyms': ['inkpad', 'inking_pad', 'stamp_pad'], 'def': 'absorbent material saturated with ink used to transfer ink evenly to a rubber stamp', 'name': 'inkpad'}, {'frequency': 'c', 'id': 756, 'synset': 'pad.n.04', 'synonyms': ['pad'], 'def': 'a flat mass of soft material used for protection, stuffing, or comfort', 'name': 'pad'}, {'frequency': 'c', 'id': 757, 'synset': 'paddle.n.04', 'synonyms': ['paddle', 'boat_paddle'], 'def': 'a short light oar used without an oarlock to propel a canoe or small boat', 'name': 'paddle'}, {'frequency': 'c', 'id': 758, 'synset': 'padlock.n.01', 'synonyms': ['padlock'], 'def': 'a detachable, portable lock', 'name': 'padlock'}, {'frequency': 'r', 'id': 759, 'synset': 'paintbox.n.01', 'synonyms': ['paintbox'], 'def': "a box containing a collection of cubes or tubes of artists' paint", 'name': 'paintbox'}, {'frequency': 'c', 'id': 760, 'synset': 'paintbrush.n.01', 'synonyms': ['paintbrush'], 'def': 'a brush used as an applicator to apply paint', 'name': 'paintbrush'}, {'frequency': 'f', 'id': 761, 'synset': 'painting.n.01', 'synonyms': ['painting'], 'def': 'graphic art consisting of an artistic composition made by applying paints to a surface', 'name': 'painting'}, {'frequency': 'c', 'id': 762, 'synset': 'pajama.n.02', 'synonyms': ['pajamas', 'pyjamas'], 'def': 'loose-fitting nightclothes worn for sleeping or lounging', 'name': 'pajamas'}, {'frequency': 'c', 'id': 763, 'synset': 'palette.n.02', 'synonyms': ['palette', 'pallet'], 'def': 'board that provides a flat surface on which artists mix paints and the range of colors used', 'name': 'palette'}, {'frequency': 'f', 'id': 764, 'synset': 'pan.n.01', 'synonyms': ['pan_(for_cooking)', 'cooking_pan'], 'def': 'cooking utensil consisting of a wide metal vessel', 'name': 'pan_(for_cooking)'}, {'frequency': 'r', 'id': 765, 'synset': 'pan.n.03', 'synonyms': ['pan_(metal_container)'], 'def': 'shallow container made of metal', 'name': 'pan_(metal_container)'}, {'frequency': 'c', 'id': 766, 'synset': 'pancake.n.01', 'synonyms': ['pancake'], 'def': 'a flat cake of thin batter fried on both sides on a griddle', 'name': 'pancake'}, {'frequency': 'r', 'id': 767, 'synset': 'pantyhose.n.01', 'synonyms': ['pantyhose'], 'def': "a woman's tights consisting of underpants and stockings", 'name': 'pantyhose'}, {'frequency': 'r', 'id': 768, 'synset': 'papaya.n.02', 'synonyms': ['papaya'], 'def': 'large oval melon-like tropical fruit with yellowish flesh', 'name': 'papaya'}, {'frequency': 'r', 'id': 769, 'synset': 'paper_clip.n.01', 'synonyms': ['paperclip'], 'def': 'a wire or plastic clip for holding sheets of paper together', 'name': 'paperclip'}, {'frequency': 'f', 'id': 770, 'synset': 'paper_plate.n.01', 'synonyms': ['paper_plate'], 'def': 'a disposable plate made of cardboard', 'name': 'paper_plate'}, {'frequency': 'f', 'id': 771, 'synset': 'paper_towel.n.01', 'synonyms': ['paper_towel'], 'def': 'a disposable towel made of absorbent paper', 'name': 'paper_towel'}, {'frequency': 'r', 'id': 772, 'synset': 'paperback_book.n.01', 'synonyms': ['paperback_book', 'paper-back_book', 'softback_book', 'soft-cover_book'], 'def': 'a book with paper covers', 'name': 'paperback_book'}, {'frequency': 'r', 'id': 773, 'synset': 'paperweight.n.01', 'synonyms': ['paperweight'], 'def': 'a weight used to hold down a stack of papers', 'name': 'paperweight'}, {'frequency': 'c', 'id': 774, 'synset': 'parachute.n.01', 'synonyms': ['parachute'], 'def': 'rescue equipment consisting of a device that fills with air and retards your fall', 'name': 'parachute'}, {'frequency': 'r', 'id': 775, 'synset': 'parakeet.n.01', 'synonyms': ['parakeet', 'parrakeet', 'parroket', 'paraquet', 'paroquet', 'parroquet'], 'def': 'any of numerous small slender long-tailed parrots', 'name': 'parakeet'}, {'frequency': 'c', 'id': 776, 'synset': 'parasail.n.01', 'synonyms': ['parasail_(sports)'], 'def': 'parachute that will lift a person up into the air when it is towed by a motorboat or a car', 'name': 'parasail_(sports)'}, {'frequency': 'r', 'id': 777, 'synset': 'parchment.n.01', 'synonyms': ['parchment'], 'def': 'a superior paper resembling sheepskin', 'name': 'parchment'}, {'frequency': 'r', 'id': 778, 'synset': 'parka.n.01', 'synonyms': ['parka', 'anorak'], 'def': "a kind of heavy jacket (`windcheater' is a British term)", 'name': 'parka'}, {'frequency': 'f', 'id': 779, 'synset': 'parking_meter.n.01', 'synonyms': ['parking_meter'], 'def': 'a coin-operated timer located next to a parking space', 'name': 'parking_meter'}, {'frequency': 'c', 'id': 780, 'synset': 'parrot.n.01', 'synonyms': ['parrot'], 'def': 'usually brightly colored tropical birds with short hooked beaks and the ability to mimic sounds', 'name': 'parrot'}, {'frequency': 'c', 'id': 781, 'synset': 'passenger_car.n.01', 'synonyms': ['passenger_car_(part_of_a_train)', 'coach_(part_of_a_train)'], 'def': 'a railcar where passengers ride', 'name': 'passenger_car_(part_of_a_train)'}, {'frequency': 'r', 'id': 782, 'synset': 'passenger_ship.n.01', 'synonyms': ['passenger_ship'], 'def': 'a ship built to carry passengers', 'name': 'passenger_ship'}, {'frequency': 'r', 'id': 783, 'synset': 'passport.n.02', 'synonyms': ['passport'], 'def': 'a document issued by a country to a citizen allowing that person to travel abroad and re-enter the home country', 'name': 'passport'}, {'frequency': 'f', 'id': 784, 'synset': 'pastry.n.02', 'synonyms': ['pastry'], 'def': 'any of various baked foods made of dough or batter', 'name': 'pastry'}, {'frequency': 'r', 'id': 785, 'synset': 'patty.n.01', 'synonyms': ['patty_(food)'], 'def': 'small flat mass of chopped food', 'name': 'patty_(food)'}, {'frequency': 'c', 'id': 786, 'synset': 'pea.n.01', 'synonyms': ['pea_(food)'], 'def': 'seed of a pea plant used for food', 'name': 'pea_(food)'}, {'frequency': 'c', 'id': 787, 'synset': 'peach.n.03', 'synonyms': ['peach'], 'def': 'downy juicy fruit with sweet yellowish or whitish flesh', 'name': 'peach'}, {'frequency': 'c', 'id': 788, 'synset': 'peanut_butter.n.01', 'synonyms': ['peanut_butter'], 'def': 'a spread made from ground peanuts', 'name': 'peanut_butter'}, {'frequency': 'c', 'id': 789, 'synset': 'pear.n.01', 'synonyms': ['pear'], 'def': 'sweet juicy gritty-textured fruit available in many varieties', 'name': 'pear'}, {'frequency': 'r', 'id': 790, 'synset': 'peeler.n.03', 'synonyms': ['peeler_(tool_for_fruit_and_vegetables)'], 'def': 'a device for peeling vegetables or fruits', 'name': 'peeler_(tool_for_fruit_and_vegetables)'}, {'frequency': 'r', 'id': 791, 'synset': 'pegboard.n.01', 'synonyms': ['pegboard'], 'def': 'a board perforated with regularly spaced holes into which pegs can be fitted', 'name': 'pegboard'}, {'frequency': 'c', 'id': 792, 'synset': 'pelican.n.01', 'synonyms': ['pelican'], 'def': 'large long-winged warm-water seabird having a large bill with a distensible pouch for fish', 'name': 'pelican'}, {'frequency': 'f', 'id': 793, 'synset': 'pen.n.01', 'synonyms': ['pen'], 'def': 'a writing implement with a point from which ink flows', 'name': 'pen'}, {'frequency': 'c', 'id': 794, 'synset': 'pencil.n.01', 'synonyms': ['pencil'], 'def': 'a thin cylindrical pointed writing implement made of wood and graphite', 'name': 'pencil'}, {'frequency': 'r', 'id': 795, 'synset': 'pencil_box.n.01', 'synonyms': ['pencil_box', 'pencil_case'], 'def': 'a box for holding pencils', 'name': 'pencil_box'}, {'frequency': 'r', 'id': 796, 'synset': 'pencil_sharpener.n.01', 'synonyms': ['pencil_sharpener'], 'def': 'a rotary implement for sharpening the point on pencils', 'name': 'pencil_sharpener'}, {'frequency': 'r', 'id': 797, 'synset': 'pendulum.n.01', 'synonyms': ['pendulum'], 'def': 'an apparatus consisting of an object mounted so that it swings freely under the influence of gravity', 'name': 'pendulum'}, {'frequency': 'c', 'id': 798, 'synset': 'penguin.n.01', 'synonyms': ['penguin'], 'def': 'short-legged flightless birds of cold southern regions having webbed feet and wings modified as flippers', 'name': 'penguin'}, {'frequency': 'r', 'id': 799, 'synset': 'pennant.n.02', 'synonyms': ['pennant'], 'def': 'a flag longer than it is wide (and often tapering)', 'name': 'pennant'}, {'frequency': 'r', 'id': 800, 'synset': 'penny.n.02', 'synonyms': ['penny_(coin)'], 'def': 'a coin worth one-hundredth of the value of the basic unit', 'name': 'penny_(coin)'}, {'frequency': 'c', 'id': 801, 'synset': 'pepper.n.03', 'synonyms': ['pepper', 'peppercorn'], 'def': 'pungent seasoning from the berry of the common pepper plant; whole or ground', 'name': 'pepper'}, {'frequency': 'c', 'id': 802, 'synset': 'pepper_mill.n.01', 'synonyms': ['pepper_mill', 'pepper_grinder'], 'def': 'a mill for grinding pepper', 'name': 'pepper_mill'}, {'frequency': 'c', 'id': 803, 'synset': 'perfume.n.02', 'synonyms': ['perfume'], 'def': 'a toiletry that emits and diffuses a fragrant odor', 'name': 'perfume'}, {'frequency': 'r', 'id': 804, 'synset': 'persimmon.n.02', 'synonyms': ['persimmon'], 'def': 'orange fruit resembling a plum; edible when fully ripe', 'name': 'persimmon'}, {'frequency': 'f', 'id': 805, 'synset': 'person.n.01', 'synonyms': ['baby', 'child', 'boy', 'girl', 'man', 'woman', 'person', 'human'], 'def': 'a human being', 'name': 'baby'}, {'frequency': 'r', 'id': 806, 'synset': 'pet.n.01', 'synonyms': ['pet'], 'def': 'a domesticated animal kept for companionship or amusement', 'name': 'pet'}, {'frequency': 'r', 'id': 807, 'synset': 'petfood.n.01', 'synonyms': ['petfood', 'pet-food'], 'def': 'food prepared for animal pets', 'name': 'petfood'}, {'frequency': 'r', 'id': 808, 'synset': 'pew.n.01', 'synonyms': ['pew_(church_bench)', 'church_bench'], 'def': 'long bench with backs; used in church by the congregation', 'name': 'pew_(church_bench)'}, {'frequency': 'r', 'id': 809, 'synset': 'phonebook.n.01', 'synonyms': ['phonebook', 'telephone_book', 'telephone_directory'], 'def': 'a directory containing an alphabetical list of telephone subscribers and their telephone numbers', 'name': 'phonebook'}, {'frequency': 'c', 'id': 810, 'synset': 'phonograph_record.n.01', 'synonyms': ['phonograph_record', 'phonograph_recording', 'record_(phonograph_recording)'], 'def': 'sound recording consisting of a typically black disk with a continuous groove', 'name': 'phonograph_record'}, {'frequency': 'c', 'id': 811, 'synset': 'piano.n.01', 'synonyms': ['piano'], 'def': 'a keyboard instrument that is played by depressing keys that cause hammers to strike tuned strings and produce sounds', 'name': 'piano'}, {'frequency': 'f', 'id': 812, 'synset': 'pickle.n.01', 'synonyms': ['pickle'], 'def': 'vegetables (especially cucumbers) preserved in brine or vinegar', 'name': 'pickle'}, {'frequency': 'f', 'id': 813, 'synset': 'pickup.n.01', 'synonyms': ['pickup_truck'], 'def': 'a light truck with an open body and low sides and a tailboard', 'name': 'pickup_truck'}, {'frequency': 'c', 'id': 814, 'synset': 'pie.n.01', 'synonyms': ['pie'], 'def': 'dish baked in pastry-lined pan often with a pastry top', 'name': 'pie'}, {'frequency': 'c', 'id': 815, 'synset': 'pigeon.n.01', 'synonyms': ['pigeon'], 'def': 'wild and domesticated birds having a heavy body and short legs', 'name': 'pigeon'}, {'frequency': 'r', 'id': 816, 'synset': 'piggy_bank.n.01', 'synonyms': ['piggy_bank', 'penny_bank'], 'def': "a child's coin bank (often shaped like a pig)", 'name': 'piggy_bank'}, {'frequency': 'f', 'id': 817, 'synset': 'pillow.n.01', 'synonyms': ['pillow'], 'def': 'a cushion to support the head of a sleeping person', 'name': 'pillow'}, {'frequency': 'r', 'id': 818, 'synset': 'pin.n.09', 'synonyms': ['pin_(non_jewelry)'], 'def': 'a small slender (often pointed) piece of wood or metal used to support or fasten or attach things', 'name': 'pin_(non_jewelry)'}, {'frequency': 'f', 'id': 819, 'synset': 'pineapple.n.02', 'synonyms': ['pineapple'], 'def': 'large sweet fleshy tropical fruit with a tuft of stiff leaves', 'name': 'pineapple'}, {'frequency': 'c', 'id': 820, 'synset': 'pinecone.n.01', 'synonyms': ['pinecone'], 'def': 'the seed-producing cone of a pine tree', 'name': 'pinecone'}, {'frequency': 'r', 'id': 821, 'synset': 'ping-pong_ball.n.01', 'synonyms': ['ping-pong_ball'], 'def': 'light hollow ball used in playing table tennis', 'name': 'ping-pong_ball'}, {'frequency': 'r', 'id': 822, 'synset': 'pinwheel.n.03', 'synonyms': ['pinwheel'], 'def': 'a toy consisting of vanes of colored paper or plastic that is pinned to a stick and spins when it is pointed into the wind', 'name': 'pinwheel'}, {'frequency': 'r', 'id': 823, 'synset': 'pipe.n.01', 'synonyms': ['tobacco_pipe'], 'def': 'a tube with a small bowl at one end; used for smoking tobacco', 'name': 'tobacco_pipe'}, {'frequency': 'f', 'id': 824, 'synset': 'pipe.n.02', 'synonyms': ['pipe', 'piping'], 'def': 'a long tube made of metal or plastic that is used to carry water or oil or gas etc.', 'name': 'pipe'}, {'frequency': 'r', 'id': 825, 'synset': 'pistol.n.01', 'synonyms': ['pistol', 'handgun'], 'def': 'a firearm that is held and fired with one hand', 'name': 'pistol'}, {'frequency': 'r', 'id': 826, 'synset': 'pita.n.01', 'synonyms': ['pita_(bread)', 'pocket_bread'], 'def': 'usually small round bread that can open into a pocket for filling', 'name': 'pita_(bread)'}, {'frequency': 'f', 'id': 827, 'synset': 'pitcher.n.02', 'synonyms': ['pitcher_(vessel_for_liquid)', 'ewer'], 'def': 'an open vessel with a handle and a spout for pouring', 'name': 'pitcher_(vessel_for_liquid)'}, {'frequency': 'r', 'id': 828, 'synset': 'pitchfork.n.01', 'synonyms': ['pitchfork'], 'def': 'a long-handled hand tool with sharp widely spaced prongs for lifting and pitching hay', 'name': 'pitchfork'}, {'frequency': 'f', 'id': 829, 'synset': 'pizza.n.01', 'synonyms': ['pizza'], 'def': 'Italian open pie made of thin bread dough spread with a spiced mixture of e.g. tomato sauce and cheese', 'name': 'pizza'}, {'frequency': 'f', 'id': 830, 'synset': 'place_mat.n.01', 'synonyms': ['place_mat'], 'def': 'a mat placed on a table for an individual place setting', 'name': 'place_mat'}, {'frequency': 'f', 'id': 831, 'synset': 'plate.n.04', 'synonyms': ['plate'], 'def': 'dish on which food is served or from which food is eaten', 'name': 'plate'}, {'frequency': 'c', 'id': 832, 'synset': 'platter.n.01', 'synonyms': ['platter'], 'def': 'a large shallow dish used for serving food', 'name': 'platter'}, {'frequency': 'r', 'id': 833, 'synset': 'playing_card.n.01', 'synonyms': ['playing_card'], 'def': 'one of a pack of cards that are used to play card games', 'name': 'playing_card'}, {'frequency': 'r', 'id': 834, 'synset': 'playpen.n.01', 'synonyms': ['playpen'], 'def': 'a portable enclosure in which babies may be left to play', 'name': 'playpen'}, {'frequency': 'c', 'id': 835, 'synset': 'pliers.n.01', 'synonyms': ['pliers', 'plyers'], 'def': 'a gripping hand tool with two hinged arms and (usually) serrated jaws', 'name': 'pliers'}, {'frequency': 'r', 'id': 836, 'synset': 'plow.n.01', 'synonyms': ['plow_(farm_equipment)', 'plough_(farm_equipment)'], 'def': 'a farm tool having one or more heavy blades to break the soil and cut a furrow prior to sowing', 'name': 'plow_(farm_equipment)'}, {'frequency': 'r', 'id': 837, 'synset': 'pocket_watch.n.01', 'synonyms': ['pocket_watch'], 'def': 'a watch that is carried in a small watch pocket', 'name': 'pocket_watch'}, {'frequency': 'c', 'id': 838, 'synset': 'pocketknife.n.01', 'synonyms': ['pocketknife'], 'def': 'a knife with a blade that folds into the handle; suitable for carrying in the pocket', 'name': 'pocketknife'}, {'frequency': 'c', 'id': 839, 'synset': 'poker.n.01', 'synonyms': ['poker_(fire_stirring_tool)', 'stove_poker', 'fire_hook'], 'def': 'fire iron consisting of a metal rod with a handle; used to stir a fire', 'name': 'poker_(fire_stirring_tool)'}, {'frequency': 'f', 'id': 840, 'synset': 'pole.n.01', 'synonyms': ['pole', 'post'], 'def': 'a long (usually round) rod of wood or metal or plastic', 'name': 'pole'}, {'frequency': 'r', 'id': 841, 'synset': 'police_van.n.01', 'synonyms': ['police_van', 'police_wagon', 'paddy_wagon', 'patrol_wagon'], 'def': 'van used by police to transport prisoners', 'name': 'police_van'}, {'frequency': 'f', 'id': 842, 'synset': 'polo_shirt.n.01', 'synonyms': ['polo_shirt', 'sport_shirt'], 'def': 'a shirt with short sleeves designed for comfort and casual wear', 'name': 'polo_shirt'}, {'frequency': 'r', 'id': 843, 'synset': 'poncho.n.01', 'synonyms': ['poncho'], 'def': 'a blanket-like cloak with a hole in the center for the head', 'name': 'poncho'}, {'frequency': 'c', 'id': 844, 'synset': 'pony.n.05', 'synonyms': ['pony'], 'def': 'any of various breeds of small gentle horses usually less than five feet high at the shoulder', 'name': 'pony'}, {'frequency': 'r', 'id': 845, 'synset': 'pool_table.n.01', 'synonyms': ['pool_table', 'billiard_table', 'snooker_table'], 'def': 'game equipment consisting of a heavy table on which pool is played', 'name': 'pool_table'}, {'frequency': 'f', 'id': 846, 'synset': 'pop.n.02', 'synonyms': ['pop_(soda)', 'soda_(pop)', 'tonic', 'soft_drink'], 'def': 'a sweet drink containing carbonated water and flavoring', 'name': 'pop_(soda)'}, {'frequency': 'r', 'id': 847, 'synset': 'portrait.n.02', 'synonyms': ['portrait', 'portrayal'], 'def': 'any likeness of a person, in any medium', 'name': 'portrait'}, {'frequency': 'c', 'id': 848, 'synset': 'postbox.n.01', 'synonyms': ['postbox_(public)', 'mailbox_(public)'], 'def': 'public box for deposit of mail', 'name': 'postbox_(public)'}, {'frequency': 'c', 'id': 849, 'synset': 'postcard.n.01', 'synonyms': ['postcard', 'postal_card', 'mailing-card'], 'def': 'a card for sending messages by post without an envelope', 'name': 'postcard'}, {'frequency': 'f', 'id': 850, 'synset': 'poster.n.01', 'synonyms': ['poster', 'placard'], 'def': 'a sign posted in a public place as an advertisement', 'name': 'poster'}, {'frequency': 'f', 'id': 851, 'synset': 'pot.n.01', 'synonyms': ['pot'], 'def': 'metal or earthenware cooking vessel that is usually round and deep; often has a handle and lid', 'name': 'pot'}, {'frequency': 'f', 'id': 852, 'synset': 'pot.n.04', 'synonyms': ['flowerpot'], 'def': 'a container in which plants are cultivated', 'name': 'flowerpot'}, {'frequency': 'f', 'id': 853, 'synset': 'potato.n.01', 'synonyms': ['potato'], 'def': 'an edible tuber native to South America', 'name': 'potato'}, {'frequency': 'c', 'id': 854, 'synset': 'potholder.n.01', 'synonyms': ['potholder'], 'def': 'an insulated pad for holding hot pots', 'name': 'potholder'}, {'frequency': 'c', 'id': 855, 'synset': 'pottery.n.01', 'synonyms': ['pottery', 'clayware'], 'def': 'ceramic ware made from clay and baked in a kiln', 'name': 'pottery'}, {'frequency': 'c', 'id': 856, 'synset': 'pouch.n.01', 'synonyms': ['pouch'], 'def': 'a small or medium size container for holding or carrying things', 'name': 'pouch'}, {'frequency': 'r', 'id': 857, 'synset': 'power_shovel.n.01', 'synonyms': ['power_shovel', 'excavator', 'digger'], 'def': 'a machine for excavating', 'name': 'power_shovel'}, {'frequency': 'c', 'id': 858, 'synset': 'prawn.n.01', 'synonyms': ['prawn', 'shrimp'], 'def': 'any of various edible decapod crustaceans', 'name': 'prawn'}, {'frequency': 'f', 'id': 859, 'synset': 'printer.n.03', 'synonyms': ['printer', 'printing_machine'], 'def': 'a machine that prints', 'name': 'printer'}, {'frequency': 'c', 'id': 860, 'synset': 'projectile.n.01', 'synonyms': ['projectile_(weapon)', 'missile'], 'def': 'a weapon that is forcibly thrown or projected at a targets', 'name': 'projectile_(weapon)'}, {'frequency': 'c', 'id': 861, 'synset': 'projector.n.02', 'synonyms': ['projector'], 'def': 'an optical instrument that projects an enlarged image onto a screen', 'name': 'projector'}, {'frequency': 'f', 'id': 862, 'synset': 'propeller.n.01', 'synonyms': ['propeller', 'propellor'], 'def': 'a mechanical device that rotates to push against air or water', 'name': 'propeller'}, {'frequency': 'r', 'id': 863, 'synset': 'prune.n.01', 'synonyms': ['prune'], 'def': 'dried plum', 'name': 'prune'}, {'frequency': 'r', 'id': 864, 'synset': 'pudding.n.01', 'synonyms': ['pudding'], 'def': 'any of various soft thick unsweetened baked dishes', 'name': 'pudding'}, {'frequency': 'r', 'id': 865, 'synset': 'puffer.n.02', 'synonyms': ['puffer_(fish)', 'pufferfish', 'blowfish', 'globefish'], 'def': 'fishes whose elongated spiny body can inflate itself with water or air to form a globe', 'name': 'puffer_(fish)'}, {'frequency': 'r', 'id': 866, 'synset': 'puffin.n.01', 'synonyms': ['puffin'], 'def': 'seabirds having short necks and brightly colored compressed bills', 'name': 'puffin'}, {'frequency': 'r', 'id': 867, 'synset': 'pug.n.01', 'synonyms': ['pug-dog'], 'def': 'small compact smooth-coated breed of Asiatic origin having a tightly curled tail and broad flat wrinkled muzzle', 'name': 'pug-dog'}, {'frequency': 'c', 'id': 868, 'synset': 'pumpkin.n.02', 'synonyms': ['pumpkin'], 'def': 'usually large pulpy deep-yellow round fruit of the squash family maturing in late summer or early autumn', 'name': 'pumpkin'}, {'frequency': 'r', 'id': 869, 'synset': 'punch.n.03', 'synonyms': ['puncher'], 'def': 'a tool for making holes or indentations', 'name': 'puncher'}, {'frequency': 'r', 'id': 870, 'synset': 'puppet.n.01', 'synonyms': ['puppet', 'marionette'], 'def': 'a small figure of a person operated from above with strings by a puppeteer', 'name': 'puppet'}, {'frequency': 'r', 'id': 871, 'synset': 'puppy.n.01', 'synonyms': ['puppy'], 'def': 'a young dog', 'name': 'puppy'}, {'frequency': 'r', 'id': 872, 'synset': 'quesadilla.n.01', 'synonyms': ['quesadilla'], 'def': 'a tortilla that is filled with cheese and heated', 'name': 'quesadilla'}, {'frequency': 'r', 'id': 873, 'synset': 'quiche.n.02', 'synonyms': ['quiche'], 'def': 'a tart filled with rich unsweetened custard; often contains other ingredients (as cheese or ham or seafood or vegetables)', 'name': 'quiche'}, {'frequency': 'f', 'id': 874, 'synset': 'quilt.n.01', 'synonyms': ['quilt', 'comforter'], 'def': 'bedding made of two layers of cloth filled with stuffing and stitched together', 'name': 'quilt'}, {'frequency': 'c', 'id': 875, 'synset': 'rabbit.n.01', 'synonyms': ['rabbit'], 'def': 'any of various burrowing animals of the family Leporidae having long ears and short tails', 'name': 'rabbit'}, {'frequency': 'r', 'id': 876, 'synset': 'racer.n.02', 'synonyms': ['race_car', 'racing_car'], 'def': 'a fast car that competes in races', 'name': 'race_car'}, {'frequency': 'c', 'id': 877, 'synset': 'racket.n.04', 'synonyms': ['racket', 'racquet'], 'def': 'a sports implement used to strike a ball in various games', 'name': 'racket'}, {'frequency': 'r', 'id': 878, 'synset': 'radar.n.01', 'synonyms': ['radar'], 'def': 'measuring instrument in which the echo of a pulse of microwave radiation is used to detect and locate distant objects', 'name': 'radar'}, {'frequency': 'c', 'id': 879, 'synset': 'radiator.n.03', 'synonyms': ['radiator'], 'def': 'a mechanism consisting of a metal honeycomb through which hot fluids circulate', 'name': 'radiator'}, {'frequency': 'c', 'id': 880, 'synset': 'radio_receiver.n.01', 'synonyms': ['radio_receiver', 'radio_set', 'radio', 'tuner_(radio)'], 'def': 'an electronic receiver that detects and demodulates and amplifies transmitted radio signals', 'name': 'radio_receiver'}, {'frequency': 'c', 'id': 881, 'synset': 'radish.n.03', 'synonyms': ['radish', 'daikon'], 'def': 'pungent edible root of any of various cultivated radish plants', 'name': 'radish'}, {'frequency': 'c', 'id': 882, 'synset': 'raft.n.01', 'synonyms': ['raft'], 'def': 'a flat float (usually made of logs or planks) that can be used for transport or as a platform for swimmers', 'name': 'raft'}, {'frequency': 'r', 'id': 883, 'synset': 'rag_doll.n.01', 'synonyms': ['rag_doll'], 'def': 'a cloth doll that is stuffed and (usually) painted', 'name': 'rag_doll'}, {'frequency': 'c', 'id': 884, 'synset': 'raincoat.n.01', 'synonyms': ['raincoat', 'waterproof_jacket'], 'def': 'a water-resistant coat', 'name': 'raincoat'}, {'frequency': 'c', 'id': 885, 'synset': 'ram.n.05', 'synonyms': ['ram_(animal)'], 'def': 'uncastrated adult male sheep', 'name': 'ram_(animal)'}, {'frequency': 'c', 'id': 886, 'synset': 'raspberry.n.02', 'synonyms': ['raspberry'], 'def': 'red or black edible aggregate berries usually smaller than the related blackberries', 'name': 'raspberry'}, {'frequency': 'r', 'id': 887, 'synset': 'rat.n.01', 'synonyms': ['rat'], 'def': 'any of various long-tailed rodents similar to but larger than a mouse', 'name': 'rat'}, {'frequency': 'c', 'id': 888, 'synset': 'razorblade.n.01', 'synonyms': ['razorblade'], 'def': 'a blade that has very sharp edge', 'name': 'razorblade'}, {'frequency': 'c', 'id': 889, 'synset': 'reamer.n.01', 'synonyms': ['reamer_(juicer)', 'juicer', 'juice_reamer'], 'def': 'a squeezer with a conical ridged center that is used for squeezing juice from citrus fruit', 'name': 'reamer_(juicer)'}, {'frequency': 'f', 'id': 890, 'synset': 'rearview_mirror.n.01', 'synonyms': ['rearview_mirror'], 'def': 'car mirror that reflects the view out of the rear window', 'name': 'rearview_mirror'}, {'frequency': 'c', 'id': 891, 'synset': 'receipt.n.02', 'synonyms': ['receipt'], 'def': 'an acknowledgment (usually tangible) that payment has been made', 'name': 'receipt'}, {'frequency': 'c', 'id': 892, 'synset': 'recliner.n.01', 'synonyms': ['recliner', 'reclining_chair', 'lounger_(chair)'], 'def': 'an armchair whose back can be lowered and foot can be raised to allow the sitter to recline in it', 'name': 'recliner'}, {'frequency': 'r', 'id': 893, 'synset': 'record_player.n.01', 'synonyms': ['record_player', 'phonograph_(record_player)', 'turntable'], 'def': 'machine in which rotating records cause a stylus to vibrate and the vibrations are amplified acoustically or electronically', 'name': 'record_player'}, {'frequency': 'r', 'id': 894, 'synset': 'red_cabbage.n.02', 'synonyms': ['red_cabbage'], 'def': 'compact head of purplish-red leaves', 'name': 'red_cabbage'}, {'frequency': 'f', 'id': 895, 'synset': 'reflector.n.01', 'synonyms': ['reflector'], 'def': 'device that reflects light, radiation, etc.', 'name': 'reflector'}, {'frequency': 'f', 'id': 896, 'synset': 'remote_control.n.01', 'synonyms': ['remote_control'], 'def': 'a device that can be used to control a machine or apparatus from a distance', 'name': 'remote_control'}, {'frequency': 'c', 'id': 897, 'synset': 'rhinoceros.n.01', 'synonyms': ['rhinoceros'], 'def': 'massive powerful herbivorous odd-toed ungulate of southeast Asia and Africa having very thick skin and one or two horns on the snout', 'name': 'rhinoceros'}, {'frequency': 'r', 'id': 898, 'synset': 'rib.n.03', 'synonyms': ['rib_(food)'], 'def': 'cut of meat including one or more ribs', 'name': 'rib_(food)'}, {'frequency': 'r', 'id': 899, 'synset': 'rifle.n.01', 'synonyms': ['rifle'], 'def': 'a shoulder firearm with a long barrel', 'name': 'rifle'}, {'frequency': 'f', 'id': 900, 'synset': 'ring.n.08', 'synonyms': ['ring'], 'def': 'jewelry consisting of a circlet of precious metal (often set with jewels) worn on the finger', 'name': 'ring'}, {'frequency': 'r', 'id': 901, 'synset': 'river_boat.n.01', 'synonyms': ['river_boat'], 'def': 'a boat used on rivers or to ply a river', 'name': 'river_boat'}, {'frequency': 'r', 'id': 902, 'synset': 'road_map.n.02', 'synonyms': ['road_map'], 'def': '(NOT A ROAD) a MAP showing roads (for automobile travel)', 'name': 'road_map'}, {'frequency': 'c', 'id': 903, 'synset': 'robe.n.01', 'synonyms': ['robe'], 'def': 'any loose flowing garment', 'name': 'robe'}, {'frequency': 'c', 'id': 904, 'synset': 'rocking_chair.n.01', 'synonyms': ['rocking_chair'], 'def': 'a chair mounted on rockers', 'name': 'rocking_chair'}, {'frequency': 'r', 'id': 905, 'synset': 'roller_skate.n.01', 'synonyms': ['roller_skate'], 'def': 'a shoe with pairs of rollers (small hard wheels) fixed to the sole', 'name': 'roller_skate'}, {'frequency': 'r', 'id': 906, 'synset': 'rollerblade.n.01', 'synonyms': ['Rollerblade'], 'def': 'an in-line variant of a roller skate', 'name': 'Rollerblade'}, {'frequency': 'c', 'id': 907, 'synset': 'rolling_pin.n.01', 'synonyms': ['rolling_pin'], 'def': 'utensil consisting of a cylinder (usually of wood) with a handle at each end; used to roll out dough', 'name': 'rolling_pin'}, {'frequency': 'r', 'id': 908, 'synset': 'root_beer.n.01', 'synonyms': ['root_beer'], 'def': 'carbonated drink containing extracts of roots and herbs', 'name': 'root_beer'}, {'frequency': 'c', 'id': 909, 'synset': 'router.n.02', 'synonyms': ['router_(computer_equipment)'], 'def': 'a device that forwards data packets between computer networks', 'name': 'router_(computer_equipment)'}, {'frequency': 'f', 'id': 910, 'synset': 'rubber_band.n.01', 'synonyms': ['rubber_band', 'elastic_band'], 'def': 'a narrow band of elastic rubber used to hold things (such as papers) together', 'name': 'rubber_band'}, {'frequency': 'c', 'id': 911, 'synset': 'runner.n.08', 'synonyms': ['runner_(carpet)'], 'def': 'a long narrow carpet', 'name': 'runner_(carpet)'}, {'frequency': 'f', 'id': 912, 'synset': 'sack.n.01', 'synonyms': ['plastic_bag', 'paper_bag'], 'def': "a bag made of paper or plastic for holding customer's purchases", 'name': 'plastic_bag'}, {'frequency': 'f', 'id': 913, 'synset': 'saddle.n.01', 'synonyms': ['saddle_(on_an_animal)'], 'def': 'a seat for the rider of a horse or camel', 'name': 'saddle_(on_an_animal)'}, {'frequency': 'f', 'id': 914, 'synset': 'saddle_blanket.n.01', 'synonyms': ['saddle_blanket', 'saddlecloth', 'horse_blanket'], 'def': 'stable gear consisting of a blanket placed under the saddle', 'name': 'saddle_blanket'}, {'frequency': 'c', 'id': 915, 'synset': 'saddlebag.n.01', 'synonyms': ['saddlebag'], 'def': 'a large bag (or pair of bags) hung over a saddle', 'name': 'saddlebag'}, {'frequency': 'r', 'id': 916, 'synset': 'safety_pin.n.01', 'synonyms': ['safety_pin'], 'def': 'a pin in the form of a clasp; has a guard so the point of the pin will not stick the user', 'name': 'safety_pin'}, {'frequency': 'c', 'id': 917, 'synset': 'sail.n.01', 'synonyms': ['sail'], 'def': 'a large piece of fabric by means of which wind is used to propel a sailing vessel', 'name': 'sail'}, {'frequency': 'c', 'id': 918, 'synset': 'salad.n.01', 'synonyms': ['salad'], 'def': 'food mixtures either arranged on a plate or tossed and served with a moist dressing; usually consisting of or including greens', 'name': 'salad'}, {'frequency': 'r', 'id': 919, 'synset': 'salad_plate.n.01', 'synonyms': ['salad_plate', 'salad_bowl'], 'def': 'a plate or bowl for individual servings of salad', 'name': 'salad_plate'}, {'frequency': 'r', 'id': 920, 'synset': 'salami.n.01', 'synonyms': ['salami'], 'def': 'highly seasoned fatty sausage of pork and beef usually dried', 'name': 'salami'}, {'frequency': 'r', 'id': 921, 'synset': 'salmon.n.01', 'synonyms': ['salmon_(fish)'], 'def': 'any of various large food and game fishes of northern waters', 'name': 'salmon_(fish)'}, {'frequency': 'r', 'id': 922, 'synset': 'salmon.n.03', 'synonyms': ['salmon_(food)'], 'def': 'flesh of any of various marine or freshwater fish of the family Salmonidae', 'name': 'salmon_(food)'}, {'frequency': 'r', 'id': 923, 'synset': 'salsa.n.01', 'synonyms': ['salsa'], 'def': 'spicy sauce of tomatoes and onions and chili peppers to accompany Mexican foods', 'name': 'salsa'}, {'frequency': 'f', 'id': 924, 'synset': 'saltshaker.n.01', 'synonyms': ['saltshaker'], 'def': 'a shaker with a perforated top for sprinkling salt', 'name': 'saltshaker'}, {'frequency': 'f', 'id': 925, 'synset': 'sandal.n.01', 'synonyms': ['sandal_(type_of_shoe)'], 'def': 'a shoe consisting of a sole fastened by straps to the foot', 'name': 'sandal_(type_of_shoe)'}, {'frequency': 'f', 'id': 926, 'synset': 'sandwich.n.01', 'synonyms': ['sandwich'], 'def': 'two (or more) slices of bread with a filling between them', 'name': 'sandwich'}, {'frequency': 'r', 'id': 927, 'synset': 'satchel.n.01', 'synonyms': ['satchel'], 'def': 'luggage consisting of a small case with a flat bottom and (usually) a shoulder strap', 'name': 'satchel'}, {'frequency': 'r', 'id': 928, 'synset': 'saucepan.n.01', 'synonyms': ['saucepan'], 'def': 'a deep pan with a handle; used for stewing or boiling', 'name': 'saucepan'}, {'frequency': 'f', 'id': 929, 'synset': 'saucer.n.02', 'synonyms': ['saucer'], 'def': 'a small shallow dish for holding a cup at the table', 'name': 'saucer'}, {'frequency': 'f', 'id': 930, 'synset': 'sausage.n.01', 'synonyms': ['sausage'], 'def': 'highly seasoned minced meat stuffed in casings', 'name': 'sausage'}, {'frequency': 'r', 'id': 931, 'synset': 'sawhorse.n.01', 'synonyms': ['sawhorse', 'sawbuck'], 'def': 'a framework for holding wood that is being sawed', 'name': 'sawhorse'}, {'frequency': 'r', 'id': 932, 'synset': 'sax.n.02', 'synonyms': ['saxophone'], 'def': "a wind instrument with a `J'-shaped form typically made of brass", 'name': 'saxophone'}, {'frequency': 'f', 'id': 933, 'synset': 'scale.n.07', 'synonyms': ['scale_(measuring_instrument)'], 'def': 'a measuring instrument for weighing; shows amount of mass', 'name': 'scale_(measuring_instrument)'}, {'frequency': 'r', 'id': 934, 'synset': 'scarecrow.n.01', 'synonyms': ['scarecrow', 'strawman'], 'def': 'an effigy in the shape of a man to frighten birds away from seeds', 'name': 'scarecrow'}, {'frequency': 'f', 'id': 935, 'synset': 'scarf.n.01', 'synonyms': ['scarf'], 'def': 'a garment worn around the head or neck or shoulders for warmth or decoration', 'name': 'scarf'}, {'frequency': 'c', 'id': 936, 'synset': 'school_bus.n.01', 'synonyms': ['school_bus'], 'def': 'a bus used to transport children to or from school', 'name': 'school_bus'}, {'frequency': 'f', 'id': 937, 'synset': 'scissors.n.01', 'synonyms': ['scissors'], 'def': 'a tool having two crossed pivoting blades with looped handles', 'name': 'scissors'}, {'frequency': 'c', 'id': 938, 'synset': 'scoreboard.n.01', 'synonyms': ['scoreboard'], 'def': 'a large board for displaying the score of a contest (and some other information)', 'name': 'scoreboard'}, {'frequency': 'c', 'id': 939, 'synset': 'scrambled_eggs.n.01', 'synonyms': ['scrambled_eggs'], 'def': 'eggs beaten and cooked to a soft firm consistency while stirring', 'name': 'scrambled_eggs'}, {'frequency': 'r', 'id': 940, 'synset': 'scraper.n.01', 'synonyms': ['scraper'], 'def': 'any of various hand tools for scraping', 'name': 'scraper'}, {'frequency': 'r', 'id': 941, 'synset': 'scratcher.n.03', 'synonyms': ['scratcher'], 'def': 'a device used for scratching', 'name': 'scratcher'}, {'frequency': 'c', 'id': 942, 'synset': 'screwdriver.n.01', 'synonyms': ['screwdriver'], 'def': 'a hand tool for driving screws; has a tip that fits into the head of a screw', 'name': 'screwdriver'}, {'frequency': 'c', 'id': 943, 'synset': 'scrub_brush.n.01', 'synonyms': ['scrubbing_brush'], 'def': 'a brush with short stiff bristles for heavy cleaning', 'name': 'scrubbing_brush'}, {'frequency': 'c', 'id': 944, 'synset': 'sculpture.n.01', 'synonyms': ['sculpture'], 'def': 'a three-dimensional work of art', 'name': 'sculpture'}, {'frequency': 'r', 'id': 945, 'synset': 'seabird.n.01', 'synonyms': ['seabird', 'seafowl'], 'def': 'a bird that frequents coastal waters and the open ocean: gulls; pelicans; gannets; cormorants; albatrosses; petrels; etc.', 'name': 'seabird'}, {'frequency': 'r', 'id': 946, 'synset': 'seahorse.n.02', 'synonyms': ['seahorse'], 'def': 'small fish with horse-like heads bent sharply downward and curled tails', 'name': 'seahorse'}, {'frequency': 'r', 'id': 947, 'synset': 'seaplane.n.01', 'synonyms': ['seaplane', 'hydroplane'], 'def': 'an airplane that can land on or take off from water', 'name': 'seaplane'}, {'frequency': 'c', 'id': 948, 'synset': 'seashell.n.01', 'synonyms': ['seashell'], 'def': 'the shell of a marine organism', 'name': 'seashell'}, {'frequency': 'r', 'id': 949, 'synset': 'seedling.n.01', 'synonyms': ['seedling'], 'def': 'young plant or tree grown from a seed', 'name': 'seedling'}, {'frequency': 'c', 'id': 950, 'synset': 'serving_dish.n.01', 'synonyms': ['serving_dish'], 'def': 'a dish used for serving food', 'name': 'serving_dish'}, {'frequency': 'r', 'id': 951, 'synset': 'sewing_machine.n.01', 'synonyms': ['sewing_machine'], 'def': 'a textile machine used as a home appliance for sewing', 'name': 'sewing_machine'}, {'frequency': 'r', 'id': 952, 'synset': 'shaker.n.03', 'synonyms': ['shaker'], 'def': 'a container in which something can be shaken', 'name': 'shaker'}, {'frequency': 'c', 'id': 953, 'synset': 'shampoo.n.01', 'synonyms': ['shampoo'], 'def': 'cleansing agent consisting of soaps or detergents used for washing the hair', 'name': 'shampoo'}, {'frequency': 'r', 'id': 954, 'synset': 'shark.n.01', 'synonyms': ['shark'], 'def': 'typically large carnivorous fishes with sharpe teeth', 'name': 'shark'}, {'frequency': 'r', 'id': 955, 'synset': 'sharpener.n.01', 'synonyms': ['sharpener'], 'def': 'any implement that is used to make something (an edge or a point) sharper', 'name': 'sharpener'}, {'frequency': 'r', 'id': 956, 'synset': 'sharpie.n.03', 'synonyms': ['Sharpie'], 'def': 'a pen with indelible ink that will write on any surface', 'name': 'Sharpie'}, {'frequency': 'r', 'id': 957, 'synset': 'shaver.n.03', 'synonyms': ['shaver_(electric)', 'electric_shaver', 'electric_razor'], 'def': 'a razor powered by an electric motor', 'name': 'shaver_(electric)'}, {'frequency': 'c', 'id': 958, 'synset': 'shaving_cream.n.01', 'synonyms': ['shaving_cream', 'shaving_soap'], 'def': 'toiletry consisting that forms a rich lather for softening the beard before shaving', 'name': 'shaving_cream'}, {'frequency': 'r', 'id': 959, 'synset': 'shawl.n.01', 'synonyms': ['shawl'], 'def': 'cloak consisting of an oblong piece of cloth used to cover the head and shoulders', 'name': 'shawl'}, {'frequency': 'r', 'id': 960, 'synset': 'shears.n.01', 'synonyms': ['shears'], 'def': 'large scissors with strong blades', 'name': 'shears'}, {'frequency': 'f', 'id': 961, 'synset': 'sheep.n.01', 'synonyms': ['sheep'], 'def': 'woolly usually horned ruminant mammal related to the goat', 'name': 'sheep'}, {'frequency': 'r', 'id': 962, 'synset': 'shepherd_dog.n.01', 'synonyms': ['shepherd_dog', 'sheepdog'], 'def': 'any of various usually long-haired breeds of dog reared to herd and guard sheep', 'name': 'shepherd_dog'}, {'frequency': 'r', 'id': 963, 'synset': 'sherbert.n.01', 'synonyms': ['sherbert', 'sherbet'], 'def': 'a frozen dessert made primarily of fruit juice and sugar', 'name': 'sherbert'}, {'frequency': 'r', 'id': 964, 'synset': 'shield.n.02', 'synonyms': ['shield'], 'def': 'armor carried on the arm to intercept blows', 'name': 'shield'}, {'frequency': 'f', 'id': 965, 'synset': 'shirt.n.01', 'synonyms': ['shirt'], 'def': 'a garment worn on the upper half of the body', 'name': 'shirt'}, {'frequency': 'f', 'id': 966, 'synset': 'shoe.n.01', 'synonyms': ['shoe', 'sneaker_(type_of_shoe)', 'tennis_shoe'], 'def': 'common footwear covering the foot', 'name': 'shoe'}, {'frequency': 'c', 'id': 967, 'synset': 'shopping_bag.n.01', 'synonyms': ['shopping_bag'], 'def': 'a bag made of plastic or strong paper (often with handles); used to transport goods after shopping', 'name': 'shopping_bag'}, {'frequency': 'c', 'id': 968, 'synset': 'shopping_cart.n.01', 'synonyms': ['shopping_cart'], 'def': 'a handcart that holds groceries or other goods while shopping', 'name': 'shopping_cart'}, {'frequency': 'f', 'id': 969, 'synset': 'short_pants.n.01', 'synonyms': ['short_pants', 'shorts_(clothing)', 'trunks_(clothing)'], 'def': 'trousers that end at or above the knee', 'name': 'short_pants'}, {'frequency': 'r', 'id': 970, 'synset': 'shot_glass.n.01', 'synonyms': ['shot_glass'], 'def': 'a small glass adequate to hold a single swallow of whiskey', 'name': 'shot_glass'}, {'frequency': 'c', 'id': 971, 'synset': 'shoulder_bag.n.01', 'synonyms': ['shoulder_bag'], 'def': 'a large handbag that can be carried by a strap looped over the shoulder', 'name': 'shoulder_bag'}, {'frequency': 'c', 'id': 972, 'synset': 'shovel.n.01', 'synonyms': ['shovel'], 'def': 'a hand tool for lifting loose material such as snow, dirt, etc.', 'name': 'shovel'}, {'frequency': 'f', 'id': 973, 'synset': 'shower.n.01', 'synonyms': ['shower_head'], 'def': 'a plumbing fixture that sprays water over you', 'name': 'shower_head'}, {'frequency': 'f', 'id': 974, 'synset': 'shower_curtain.n.01', 'synonyms': ['shower_curtain'], 'def': 'a curtain that keeps water from splashing out of the shower area', 'name': 'shower_curtain'}, {'frequency': 'r', 'id': 975, 'synset': 'shredder.n.01', 'synonyms': ['shredder_(for_paper)'], 'def': 'a device that shreds documents', 'name': 'shredder_(for_paper)'}, {'frequency': 'r', 'id': 976, 'synset': 'sieve.n.01', 'synonyms': ['sieve', 'screen_(sieve)'], 'def': 'a strainer for separating lumps from powdered material or grading particles', 'name': 'sieve'}, {'frequency': 'f', 'id': 977, 'synset': 'signboard.n.01', 'synonyms': ['signboard'], 'def': 'structure displaying a board on which advertisements can be posted', 'name': 'signboard'}, {'frequency': 'c', 'id': 978, 'synset': 'silo.n.01', 'synonyms': ['silo'], 'def': 'a cylindrical tower used for storing goods', 'name': 'silo'}, {'frequency': 'f', 'id': 979, 'synset': 'sink.n.01', 'synonyms': ['sink'], 'def': 'plumbing fixture consisting of a water basin fixed to a wall or floor and having a drainpipe', 'name': 'sink'}, {'frequency': 'f', 'id': 980, 'synset': 'skateboard.n.01', 'synonyms': ['skateboard'], 'def': 'a board with wheels that is ridden in a standing or crouching position and propelled by foot', 'name': 'skateboard'}, {'frequency': 'c', 'id': 981, 'synset': 'skewer.n.01', 'synonyms': ['skewer'], 'def': 'a long pin for holding meat in position while it is being roasted', 'name': 'skewer'}, {'frequency': 'f', 'id': 982, 'synset': 'ski.n.01', 'synonyms': ['ski'], 'def': 'sports equipment for skiing on snow', 'name': 'ski'}, {'frequency': 'f', 'id': 983, 'synset': 'ski_boot.n.01', 'synonyms': ['ski_boot'], 'def': 'a stiff boot that is fastened to a ski with a ski binding', 'name': 'ski_boot'}, {'frequency': 'f', 'id': 984, 'synset': 'ski_parka.n.01', 'synonyms': ['ski_parka', 'ski_jacket'], 'def': 'a parka to be worn while skiing', 'name': 'ski_parka'}, {'frequency': 'f', 'id': 985, 'synset': 'ski_pole.n.01', 'synonyms': ['ski_pole'], 'def': 'a pole with metal points used as an aid in skiing', 'name': 'ski_pole'}, {'frequency': 'f', 'id': 986, 'synset': 'skirt.n.02', 'synonyms': ['skirt'], 'def': 'a garment hanging from the waist; worn mainly by girls and women', 'name': 'skirt'}, {'frequency': 'c', 'id': 987, 'synset': 'sled.n.01', 'synonyms': ['sled', 'sledge', 'sleigh'], 'def': 'a vehicle or flat object for transportation over snow by sliding or pulled by dogs, etc.', 'name': 'sled'}, {'frequency': 'c', 'id': 988, 'synset': 'sleeping_bag.n.01', 'synonyms': ['sleeping_bag'], 'def': 'large padded bag designed to be slept in outdoors', 'name': 'sleeping_bag'}, {'frequency': 'r', 'id': 989, 'synset': 'sling.n.05', 'synonyms': ['sling_(bandage)', 'triangular_bandage'], 'def': 'bandage to support an injured forearm; slung over the shoulder or neck', 'name': 'sling_(bandage)'}, {'frequency': 'c', 'id': 990, 'synset': 'slipper.n.01', 'synonyms': ['slipper_(footwear)', 'carpet_slipper_(footwear)'], 'def': 'low footwear that can be slipped on and off easily; usually worn indoors', 'name': 'slipper_(footwear)'}, {'frequency': 'r', 'id': 991, 'synset': 'smoothie.n.02', 'synonyms': ['smoothie'], 'def': 'a thick smooth drink consisting of fresh fruit pureed with ice cream or yoghurt or milk', 'name': 'smoothie'}, {'frequency': 'r', 'id': 992, 'synset': 'snake.n.01', 'synonyms': ['snake', 'serpent'], 'def': 'limbless scaly elongate reptile; some are venomous', 'name': 'snake'}, {'frequency': 'f', 'id': 993, 'synset': 'snowboard.n.01', 'synonyms': ['snowboard'], 'def': 'a board that resembles a broad ski or a small surfboard; used in a standing position to slide down snow-covered slopes', 'name': 'snowboard'}, {'frequency': 'c', 'id': 994, 'synset': 'snowman.n.01', 'synonyms': ['snowman'], 'def': 'a figure of a person made of packed snow', 'name': 'snowman'}, {'frequency': 'c', 'id': 995, 'synset': 'snowmobile.n.01', 'synonyms': ['snowmobile'], 'def': 'tracked vehicle for travel on snow having skis in front', 'name': 'snowmobile'}, {'frequency': 'f', 'id': 996, 'synset': 'soap.n.01', 'synonyms': ['soap'], 'def': 'a cleansing agent made from the salts of vegetable or animal fats', 'name': 'soap'}, {'frequency': 'f', 'id': 997, 'synset': 'soccer_ball.n.01', 'synonyms': ['soccer_ball'], 'def': "an inflated ball used in playing soccer (called `football' outside of the United States)", 'name': 'soccer_ball'}, {'frequency': 'f', 'id': 998, 'synset': 'sock.n.01', 'synonyms': ['sock'], 'def': 'cloth covering for the foot; worn inside the shoe; reaches to between the ankle and the knee', 'name': 'sock'}, {'frequency': 'r', 'id': 999, 'synset': 'soda_fountain.n.02', 'synonyms': ['soda_fountain'], 'def': 'an apparatus for dispensing soda water', 'name': 'soda_fountain'}, {'frequency': 'r', 'id': 1000, 'synset': 'soda_water.n.01', 'synonyms': ['carbonated_water', 'club_soda', 'seltzer', 'sparkling_water'], 'def': 'effervescent beverage artificially charged with carbon dioxide', 'name': 'carbonated_water'}, {'frequency': 'f', 'id': 1001, 'synset': 'sofa.n.01', 'synonyms': ['sofa', 'couch', 'lounge'], 'def': 'an upholstered seat for more than one person', 'name': 'sofa'}, {'frequency': 'r', 'id': 1002, 'synset': 'softball.n.01', 'synonyms': ['softball'], 'def': 'ball used in playing softball', 'name': 'softball'}, {'frequency': 'c', 'id': 1003, 'synset': 'solar_array.n.01', 'synonyms': ['solar_array', 'solar_battery', 'solar_panel'], 'def': 'electrical device consisting of a large array of connected solar cells', 'name': 'solar_array'}, {'frequency': 'r', 'id': 1004, 'synset': 'sombrero.n.02', 'synonyms': ['sombrero'], 'def': 'a straw hat with a tall crown and broad brim; worn in American southwest and in Mexico', 'name': 'sombrero'}, {'frequency': 'c', 'id': 1005, 'synset': 'soup.n.01', 'synonyms': ['soup'], 'def': 'liquid food especially of meat or fish or vegetable stock often containing pieces of solid food', 'name': 'soup'}, {'frequency': 'r', 'id': 1006, 'synset': 'soup_bowl.n.01', 'synonyms': ['soup_bowl'], 'def': 'a bowl for serving soup', 'name': 'soup_bowl'}, {'frequency': 'c', 'id': 1007, 'synset': 'soupspoon.n.01', 'synonyms': ['soupspoon'], 'def': 'a spoon with a rounded bowl for eating soup', 'name': 'soupspoon'}, {'frequency': 'c', 'id': 1008, 'synset': 'sour_cream.n.01', 'synonyms': ['sour_cream', 'soured_cream'], 'def': 'soured light cream', 'name': 'sour_cream'}, {'frequency': 'r', 'id': 1009, 'synset': 'soya_milk.n.01', 'synonyms': ['soya_milk', 'soybean_milk', 'soymilk'], 'def': 'a milk substitute containing soybean flour and water; used in some infant formulas and in making tofu', 'name': 'soya_milk'}, {'frequency': 'r', 'id': 1010, 'synset': 'space_shuttle.n.01', 'synonyms': ['space_shuttle'], 'def': "a reusable spacecraft with wings for a controlled descent through the Earth's atmosphere", 'name': 'space_shuttle'}, {'frequency': 'r', 'id': 1011, 'synset': 'sparkler.n.02', 'synonyms': ['sparkler_(fireworks)'], 'def': 'a firework that burns slowly and throws out a shower of sparks', 'name': 'sparkler_(fireworks)'}, {'frequency': 'f', 'id': 1012, 'synset': 'spatula.n.02', 'synonyms': ['spatula'], 'def': 'a hand tool with a thin flexible blade used to mix or spread soft substances', 'name': 'spatula'}, {'frequency': 'r', 'id': 1013, 'synset': 'spear.n.01', 'synonyms': ['spear', 'lance'], 'def': 'a long pointed rod used as a tool or weapon', 'name': 'spear'}, {'frequency': 'f', 'id': 1014, 'synset': 'spectacles.n.01', 'synonyms': ['spectacles', 'specs', 'eyeglasses', 'glasses'], 'def': 'optical instrument consisting of a frame that holds a pair of lenses for correcting defective vision', 'name': 'spectacles'}, {'frequency': 'c', 'id': 1015, 'synset': 'spice_rack.n.01', 'synonyms': ['spice_rack'], 'def': 'a rack for displaying containers filled with spices', 'name': 'spice_rack'}, {'frequency': 'r', 'id': 1016, 'synset': 'spider.n.01', 'synonyms': ['spider'], 'def': 'predatory arachnid with eight legs, two poison fangs, two feelers, and usually two silk-spinning organs at the back end of the body', 'name': 'spider'}, {'frequency': 'c', 'id': 1017, 'synset': 'sponge.n.01', 'synonyms': ['sponge'], 'def': 'a porous mass usable to absorb water typically used for cleaning', 'name': 'sponge'}, {'frequency': 'f', 'id': 1018, 'synset': 'spoon.n.01', 'synonyms': ['spoon'], 'def': 'a piece of cutlery with a shallow bowl-shaped container and a handle', 'name': 'spoon'}, {'frequency': 'c', 'id': 1019, 'synset': 'sportswear.n.01', 'synonyms': ['sportswear', 'athletic_wear', 'activewear'], 'def': 'attire worn for sport or for casual wear', 'name': 'sportswear'}, {'frequency': 'c', 'id': 1020, 'synset': 'spotlight.n.02', 'synonyms': ['spotlight'], 'def': 'a lamp that produces a strong beam of light to illuminate a restricted area; used to focus attention of a stage performer', 'name': 'spotlight'}, {'frequency': 'r', 'id': 1021, 'synset': 'squirrel.n.01', 'synonyms': ['squirrel'], 'def': 'a kind of arboreal rodent having a long bushy tail', 'name': 'squirrel'}, {'frequency': 'c', 'id': 1022, 'synset': 'stapler.n.01', 'synonyms': ['stapler_(stapling_machine)'], 'def': 'a machine that inserts staples into sheets of paper in order to fasten them together', 'name': 'stapler_(stapling_machine)'}, {'frequency': 'r', 'id': 1023, 'synset': 'starfish.n.01', 'synonyms': ['starfish', 'sea_star'], 'def': 'echinoderms characterized by five arms extending from a central disk', 'name': 'starfish'}, {'frequency': 'f', 'id': 1024, 'synset': 'statue.n.01', 'synonyms': ['statue_(sculpture)'], 'def': 'a sculpture representing a human or animal', 'name': 'statue_(sculpture)'}, {'frequency': 'c', 'id': 1025, 'synset': 'steak.n.01', 'synonyms': ['steak_(food)'], 'def': 'a slice of meat cut from the fleshy part of an animal or large fish', 'name': 'steak_(food)'}, {'frequency': 'r', 'id': 1026, 'synset': 'steak_knife.n.01', 'synonyms': ['steak_knife'], 'def': 'a sharp table knife used in eating steak', 'name': 'steak_knife'}, {'frequency': 'r', 'id': 1027, 'synset': 'steamer.n.02', 'synonyms': ['steamer_(kitchen_appliance)'], 'def': 'a cooking utensil that can be used to cook food by steaming it', 'name': 'steamer_(kitchen_appliance)'}, {'frequency': 'f', 'id': 1028, 'synset': 'steering_wheel.n.01', 'synonyms': ['steering_wheel'], 'def': 'a handwheel that is used for steering', 'name': 'steering_wheel'}, {'frequency': 'r', 'id': 1029, 'synset': 'stencil.n.01', 'synonyms': ['stencil'], 'def': 'a sheet of material (metal, plastic, etc.) that has been perforated with a pattern; ink or paint can pass through the perforations to create the printed pattern on the surface below', 'name': 'stencil'}, {'frequency': 'r', 'id': 1030, 'synset': 'step_ladder.n.01', 'synonyms': ['stepladder'], 'def': 'a folding portable ladder hinged at the top', 'name': 'stepladder'}, {'frequency': 'c', 'id': 1031, 'synset': 'step_stool.n.01', 'synonyms': ['step_stool'], 'def': 'a stool that has one or two steps that fold under the seat', 'name': 'step_stool'}, {'frequency': 'c', 'id': 1032, 'synset': 'stereo.n.01', 'synonyms': ['stereo_(sound_system)'], 'def': 'electronic device for playing audio', 'name': 'stereo_(sound_system)'}, {'frequency': 'r', 'id': 1033, 'synset': 'stew.n.02', 'synonyms': ['stew'], 'def': 'food prepared by stewing especially meat or fish with vegetables', 'name': 'stew'}, {'frequency': 'r', 'id': 1034, 'synset': 'stirrer.n.02', 'synonyms': ['stirrer'], 'def': 'an implement used for stirring', 'name': 'stirrer'}, {'frequency': 'f', 'id': 1035, 'synset': 'stirrup.n.01', 'synonyms': ['stirrup'], 'def': "support consisting of metal loops into which rider's feet go", 'name': 'stirrup'}, {'frequency': 'c', 'id': 1036, 'synset': 'stocking.n.01', 'synonyms': ['stockings_(leg_wear)'], 'def': 'close-fitting hosiery to cover the foot and leg; come in matched pairs', 'name': 'stockings_(leg_wear)'}, {'frequency': 'f', 'id': 1037, 'synset': 'stool.n.01', 'synonyms': ['stool'], 'def': 'a simple seat without a back or arms', 'name': 'stool'}, {'frequency': 'f', 'id': 1038, 'synset': 'stop_sign.n.01', 'synonyms': ['stop_sign'], 'def': 'a traffic sign to notify drivers that they must come to a complete stop', 'name': 'stop_sign'}, {'frequency': 'f', 'id': 1039, 'synset': 'stoplight.n.01', 'synonyms': ['brake_light'], 'def': 'a red light on the rear of a motor vehicle that signals when the brakes are applied', 'name': 'brake_light'}, {'frequency': 'f', 'id': 1040, 'synset': 'stove.n.01', 'synonyms': ['stove', 'kitchen_stove', 'range_(kitchen_appliance)', 'kitchen_range', 'cooking_stove'], 'def': 'a kitchen appliance used for cooking food', 'name': 'stove'}, {'frequency': 'c', 'id': 1041, 'synset': 'strainer.n.01', 'synonyms': ['strainer'], 'def': 'a filter to retain larger pieces while smaller pieces and liquids pass through', 'name': 'strainer'}, {'frequency': 'f', 'id': 1042, 'synset': 'strap.n.01', 'synonyms': ['strap'], 'def': 'an elongated strip of material for binding things together or holding', 'name': 'strap'}, {'frequency': 'f', 'id': 1043, 'synset': 'straw.n.04', 'synonyms': ['straw_(for_drinking)', 'drinking_straw'], 'def': 'a thin paper or plastic tube used to suck liquids into the mouth', 'name': 'straw_(for_drinking)'}, {'frequency': 'f', 'id': 1044, 'synset': 'strawberry.n.01', 'synonyms': ['strawberry'], 'def': 'sweet fleshy red fruit', 'name': 'strawberry'}, {'frequency': 'f', 'id': 1045, 'synset': 'street_sign.n.01', 'synonyms': ['street_sign'], 'def': 'a sign visible from the street', 'name': 'street_sign'}, {'frequency': 'f', 'id': 1046, 'synset': 'streetlight.n.01', 'synonyms': ['streetlight', 'street_lamp'], 'def': 'a lamp supported on a lamppost; for illuminating a street', 'name': 'streetlight'}, {'frequency': 'r', 'id': 1047, 'synset': 'string_cheese.n.01', 'synonyms': ['string_cheese'], 'def': 'cheese formed in long strings twisted together', 'name': 'string_cheese'}, {'frequency': 'r', 'id': 1048, 'synset': 'stylus.n.02', 'synonyms': ['stylus'], 'def': 'a pointed tool for writing or drawing or engraving', 'name': 'stylus'}, {'frequency': 'r', 'id': 1049, 'synset': 'subwoofer.n.01', 'synonyms': ['subwoofer'], 'def': 'a loudspeaker that is designed to reproduce very low bass frequencies', 'name': 'subwoofer'}, {'frequency': 'r', 'id': 1050, 'synset': 'sugar_bowl.n.01', 'synonyms': ['sugar_bowl'], 'def': 'a dish in which sugar is served', 'name': 'sugar_bowl'}, {'frequency': 'r', 'id': 1051, 'synset': 'sugarcane.n.01', 'synonyms': ['sugarcane_(plant)'], 'def': 'juicy canes whose sap is a source of molasses and commercial sugar; fresh canes are sometimes chewed for the juice', 'name': 'sugarcane_(plant)'}, {'frequency': 'c', 'id': 1052, 'synset': 'suit.n.01', 'synonyms': ['suit_(clothing)'], 'def': 'a set of garments (usually including a jacket and trousers or skirt) for outerwear all of the same fabric and color', 'name': 'suit_(clothing)'}, {'frequency': 'c', 'id': 1053, 'synset': 'sunflower.n.01', 'synonyms': ['sunflower'], 'def': 'any plant of the genus Helianthus having large flower heads with dark disk florets and showy yellow rays', 'name': 'sunflower'}, {'frequency': 'f', 'id': 1054, 'synset': 'sunglasses.n.01', 'synonyms': ['sunglasses'], 'def': 'spectacles that are darkened or polarized to protect the eyes from the glare of the sun', 'name': 'sunglasses'}, {'frequency': 'c', 'id': 1055, 'synset': 'sunhat.n.01', 'synonyms': ['sunhat'], 'def': 'a hat with a broad brim that protects the face from direct exposure to the sun', 'name': 'sunhat'}, {'frequency': 'r', 'id': 1056, 'synset': 'sunscreen.n.01', 'synonyms': ['sunscreen', 'sunblock'], 'def': 'a cream spread on the skin; contains a chemical to filter out ultraviolet light and so protect from sunburn', 'name': 'sunscreen'}, {'frequency': 'f', 'id': 1057, 'synset': 'surfboard.n.01', 'synonyms': ['surfboard'], 'def': 'a narrow buoyant board for riding surf', 'name': 'surfboard'}, {'frequency': 'c', 'id': 1058, 'synset': 'sushi.n.01', 'synonyms': ['sushi'], 'def': 'rice (with raw fish) wrapped in seaweed', 'name': 'sushi'}, {'frequency': 'c', 'id': 1059, 'synset': 'swab.n.02', 'synonyms': ['mop'], 'def': 'cleaning implement consisting of absorbent material fastened to a handle; for cleaning floors', 'name': 'mop'}, {'frequency': 'c', 'id': 1060, 'synset': 'sweat_pants.n.01', 'synonyms': ['sweat_pants'], 'def': 'loose-fitting trousers with elastic cuffs; worn by athletes', 'name': 'sweat_pants'}, {'frequency': 'c', 'id': 1061, 'synset': 'sweatband.n.02', 'synonyms': ['sweatband'], 'def': 'a band of material tied around the forehead or wrist to absorb sweat', 'name': 'sweatband'}, {'frequency': 'f', 'id': 1062, 'synset': 'sweater.n.01', 'synonyms': ['sweater'], 'def': 'a crocheted or knitted garment covering the upper part of the body', 'name': 'sweater'}, {'frequency': 'f', 'id': 1063, 'synset': 'sweatshirt.n.01', 'synonyms': ['sweatshirt'], 'def': 'cotton knit pullover with long sleeves worn during athletic activity', 'name': 'sweatshirt'}, {'frequency': 'c', 'id': 1064, 'synset': 'sweet_potato.n.02', 'synonyms': ['sweet_potato'], 'def': 'the edible tuberous root of the sweet potato vine', 'name': 'sweet_potato'}, {'frequency': 'f', 'id': 1065, 'synset': 'swimsuit.n.01', 'synonyms': ['swimsuit', 'swimwear', 'bathing_suit', 'swimming_costume', 'bathing_costume', 'swimming_trunks', 'bathing_trunks'], 'def': 'garment worn for swimming', 'name': 'swimsuit'}, {'frequency': 'c', 'id': 1066, 'synset': 'sword.n.01', 'synonyms': ['sword'], 'def': 'a cutting or thrusting weapon that has a long metal blade', 'name': 'sword'}, {'frequency': 'r', 'id': 1067, 'synset': 'syringe.n.01', 'synonyms': ['syringe'], 'def': 'a medical instrument used to inject or withdraw fluids', 'name': 'syringe'}, {'frequency': 'r', 'id': 1068, 'synset': 'tabasco.n.02', 'synonyms': ['Tabasco_sauce'], 'def': 'very spicy sauce (trade name Tabasco) made from fully-aged red peppers', 'name': 'Tabasco_sauce'}, {'frequency': 'r', 'id': 1069, 'synset': 'table-tennis_table.n.01', 'synonyms': ['table-tennis_table', 'ping-pong_table'], 'def': 'a table used for playing table tennis', 'name': 'table-tennis_table'}, {'frequency': 'f', 'id': 1070, 'synset': 'table.n.02', 'synonyms': ['table'], 'def': 'a piece of furniture having a smooth flat top that is usually supported by one or more vertical legs', 'name': 'table'}, {'frequency': 'c', 'id': 1071, 'synset': 'table_lamp.n.01', 'synonyms': ['table_lamp'], 'def': 'a lamp that sits on a table', 'name': 'table_lamp'}, {'frequency': 'f', 'id': 1072, 'synset': 'tablecloth.n.01', 'synonyms': ['tablecloth'], 'def': 'a covering spread over a dining table', 'name': 'tablecloth'}, {'frequency': 'r', 'id': 1073, 'synset': 'tachometer.n.01', 'synonyms': ['tachometer'], 'def': 'measuring instrument for indicating speed of rotation', 'name': 'tachometer'}, {'frequency': 'r', 'id': 1074, 'synset': 'taco.n.02', 'synonyms': ['taco'], 'def': 'a small tortilla cupped around a filling', 'name': 'taco'}, {'frequency': 'f', 'id': 1075, 'synset': 'tag.n.02', 'synonyms': ['tag'], 'def': 'a label associated with something for the purpose of identification or information', 'name': 'tag'}, {'frequency': 'f', 'id': 1076, 'synset': 'taillight.n.01', 'synonyms': ['taillight', 'rear_light'], 'def': 'lamp (usually red) mounted at the rear of a motor vehicle', 'name': 'taillight'}, {'frequency': 'r', 'id': 1077, 'synset': 'tambourine.n.01', 'synonyms': ['tambourine'], 'def': 'a shallow drum with a single drumhead and with metallic disks in the sides', 'name': 'tambourine'}, {'frequency': 'r', 'id': 1078, 'synset': 'tank.n.01', 'synonyms': ['army_tank', 'armored_combat_vehicle', 'armoured_combat_vehicle'], 'def': 'an enclosed armored military vehicle; has a cannon and moves on caterpillar treads', 'name': 'army_tank'}, {'frequency': 'c', 'id': 1079, 'synset': 'tank.n.02', 'synonyms': ['tank_(storage_vessel)', 'storage_tank'], 'def': 'a large (usually metallic) vessel for holding gases or liquids', 'name': 'tank_(storage_vessel)'}, {'frequency': 'f', 'id': 1080, 'synset': 'tank_top.n.01', 'synonyms': ['tank_top_(clothing)'], 'def': 'a tight-fitting sleeveless shirt with wide shoulder straps and low neck and no front opening', 'name': 'tank_top_(clothing)'}, {'frequency': 'c', 'id': 1081, 'synset': 'tape.n.01', 'synonyms': ['tape_(sticky_cloth_or_paper)'], 'def': 'a long thin piece of cloth or paper as used for binding or fastening', 'name': 'tape_(sticky_cloth_or_paper)'}, {'frequency': 'c', 'id': 1082, 'synset': 'tape.n.04', 'synonyms': ['tape_measure', 'measuring_tape'], 'def': 'measuring instrument consisting of a narrow strip (cloth or metal) marked in inches or centimeters and used for measuring lengths', 'name': 'tape_measure'}, {'frequency': 'c', 'id': 1083, 'synset': 'tapestry.n.02', 'synonyms': ['tapestry'], 'def': 'a heavy textile with a woven design; used for curtains and upholstery', 'name': 'tapestry'}, {'frequency': 'f', 'id': 1084, 'synset': 'tarpaulin.n.01', 'synonyms': ['tarp'], 'def': 'waterproofed canvas', 'name': 'tarp'}, {'frequency': 'c', 'id': 1085, 'synset': 'tartan.n.01', 'synonyms': ['tartan', 'plaid'], 'def': 'a cloth having a crisscross design', 'name': 'tartan'}, {'frequency': 'c', 'id': 1086, 'synset': 'tassel.n.01', 'synonyms': ['tassel'], 'def': 'adornment consisting of a bunch of cords fastened at one end', 'name': 'tassel'}, {'frequency': 'r', 'id': 1087, 'synset': 'tea_bag.n.01', 'synonyms': ['tea_bag'], 'def': 'a measured amount of tea in a bag for an individual serving of tea', 'name': 'tea_bag'}, {'frequency': 'c', 'id': 1088, 'synset': 'teacup.n.02', 'synonyms': ['teacup'], 'def': 'a cup from which tea is drunk', 'name': 'teacup'}, {'frequency': 'c', 'id': 1089, 'synset': 'teakettle.n.01', 'synonyms': ['teakettle'], 'def': 'kettle for boiling water to make tea', 'name': 'teakettle'}, {'frequency': 'c', 'id': 1090, 'synset': 'teapot.n.01', 'synonyms': ['teapot'], 'def': 'pot for brewing tea; usually has a spout and handle', 'name': 'teapot'}, {'frequency': 'f', 'id': 1091, 'synset': 'teddy.n.01', 'synonyms': ['teddy_bear'], 'def': "plaything consisting of a child's toy bear (usually plush and stuffed with soft materials)", 'name': 'teddy_bear'}, {'frequency': 'f', 'id': 1092, 'synset': 'telephone.n.01', 'synonyms': ['telephone', 'phone', 'telephone_set'], 'def': 'electronic device for communicating by voice over long distances', 'name': 'telephone'}, {'frequency': 'c', 'id': 1093, 'synset': 'telephone_booth.n.01', 'synonyms': ['telephone_booth', 'phone_booth', 'call_box', 'telephone_box', 'telephone_kiosk'], 'def': 'booth for using a telephone', 'name': 'telephone_booth'}, {'frequency': 'f', 'id': 1094, 'synset': 'telephone_pole.n.01', 'synonyms': ['telephone_pole', 'telegraph_pole', 'telegraph_post'], 'def': 'tall pole supporting telephone wires', 'name': 'telephone_pole'}, {'frequency': 'r', 'id': 1095, 'synset': 'telephoto_lens.n.01', 'synonyms': ['telephoto_lens', 'zoom_lens'], 'def': 'a camera lens that magnifies the image', 'name': 'telephoto_lens'}, {'frequency': 'c', 'id': 1096, 'synset': 'television_camera.n.01', 'synonyms': ['television_camera', 'tv_camera'], 'def': 'television equipment for capturing and recording video', 'name': 'television_camera'}, {'frequency': 'f', 'id': 1097, 'synset': 'television_receiver.n.01', 'synonyms': ['television_set', 'tv', 'tv_set'], 'def': 'an electronic device that receives television signals and displays them on a screen', 'name': 'television_set'}, {'frequency': 'f', 'id': 1098, 'synset': 'tennis_ball.n.01', 'synonyms': ['tennis_ball'], 'def': 'ball about the size of a fist used in playing tennis', 'name': 'tennis_ball'}, {'frequency': 'f', 'id': 1099, 'synset': 'tennis_racket.n.01', 'synonyms': ['tennis_racket'], 'def': 'a racket used to play tennis', 'name': 'tennis_racket'}, {'frequency': 'r', 'id': 1100, 'synset': 'tequila.n.01', 'synonyms': ['tequila'], 'def': 'Mexican liquor made from fermented juices of an agave plant', 'name': 'tequila'}, {'frequency': 'c', 'id': 1101, 'synset': 'thermometer.n.01', 'synonyms': ['thermometer'], 'def': 'measuring instrument for measuring temperature', 'name': 'thermometer'}, {'frequency': 'c', 'id': 1102, 'synset': 'thermos.n.01', 'synonyms': ['thermos_bottle'], 'def': 'vacuum flask that preserves temperature of hot or cold drinks', 'name': 'thermos_bottle'}, {'frequency': 'c', 'id': 1103, 'synset': 'thermostat.n.01', 'synonyms': ['thermostat'], 'def': 'a regulator for automatically regulating temperature by starting or stopping the supply of heat', 'name': 'thermostat'}, {'frequency': 'r', 'id': 1104, 'synset': 'thimble.n.02', 'synonyms': ['thimble'], 'def': 'a small metal cap to protect the finger while sewing; can be used as a small container', 'name': 'thimble'}, {'frequency': 'c', 'id': 1105, 'synset': 'thread.n.01', 'synonyms': ['thread', 'yarn'], 'def': 'a fine cord of twisted fibers (of cotton or silk or wool or nylon etc.) used in sewing and weaving', 'name': 'thread'}, {'frequency': 'c', 'id': 1106, 'synset': 'thumbtack.n.01', 'synonyms': ['thumbtack', 'drawing_pin', 'pushpin'], 'def': 'a tack for attaching papers to a bulletin board or drawing board', 'name': 'thumbtack'}, {'frequency': 'c', 'id': 1107, 'synset': 'tiara.n.01', 'synonyms': ['tiara'], 'def': 'a jeweled headdress worn by women on formal occasions', 'name': 'tiara'}, {'frequency': 'c', 'id': 1108, 'synset': 'tiger.n.02', 'synonyms': ['tiger'], 'def': 'large feline of forests in most of Asia having a tawny coat with black stripes', 'name': 'tiger'}, {'frequency': 'c', 'id': 1109, 'synset': 'tights.n.01', 'synonyms': ['tights_(clothing)', 'leotards'], 'def': 'skintight knit hose covering the body from the waist to the feet worn by acrobats and dancers and as stockings by women and girls', 'name': 'tights_(clothing)'}, {'frequency': 'c', 'id': 1110, 'synset': 'timer.n.01', 'synonyms': ['timer', 'stopwatch'], 'def': 'a timepiece that measures a time interval and signals its end', 'name': 'timer'}, {'frequency': 'f', 'id': 1111, 'synset': 'tinfoil.n.01', 'synonyms': ['tinfoil'], 'def': 'foil made of tin or an alloy of tin and lead', 'name': 'tinfoil'}, {'frequency': 'r', 'id': 1112, 'synset': 'tinsel.n.01', 'synonyms': ['tinsel'], 'def': 'a showy decoration that is basically valueless', 'name': 'tinsel'}, {'frequency': 'f', 'id': 1113, 'synset': 'tissue.n.02', 'synonyms': ['tissue_paper'], 'def': 'a soft thin (usually translucent) paper', 'name': 'tissue_paper'}, {'frequency': 'c', 'id': 1114, 'synset': 'toast.n.01', 'synonyms': ['toast_(food)'], 'def': 'slice of bread that has been toasted', 'name': 'toast_(food)'}, {'frequency': 'f', 'id': 1115, 'synset': 'toaster.n.02', 'synonyms': ['toaster'], 'def': 'a kitchen appliance (usually electric) for toasting bread', 'name': 'toaster'}, {'frequency': 'c', 'id': 1116, 'synset': 'toaster_oven.n.01', 'synonyms': ['toaster_oven'], 'def': 'kitchen appliance consisting of a small electric oven for toasting or warming food', 'name': 'toaster_oven'}, {'frequency': 'f', 'id': 1117, 'synset': 'toilet.n.02', 'synonyms': ['toilet'], 'def': 'a plumbing fixture for defecation and urination', 'name': 'toilet'}, {'frequency': 'f', 'id': 1118, 'synset': 'toilet_tissue.n.01', 'synonyms': ['toilet_tissue', 'toilet_paper', 'bathroom_tissue'], 'def': 'a soft thin absorbent paper for use in toilets', 'name': 'toilet_tissue'}, {'frequency': 'f', 'id': 1119, 'synset': 'tomato.n.01', 'synonyms': ['tomato'], 'def': 'mildly acid red or yellow pulpy fruit eaten as a vegetable', 'name': 'tomato'}, {'frequency': 'c', 'id': 1120, 'synset': 'tongs.n.01', 'synonyms': ['tongs'], 'def': 'any of various devices for taking hold of objects; usually have two hinged legs with handles above and pointed hooks below', 'name': 'tongs'}, {'frequency': 'c', 'id': 1121, 'synset': 'toolbox.n.01', 'synonyms': ['toolbox'], 'def': 'a box or chest or cabinet for holding hand tools', 'name': 'toolbox'}, {'frequency': 'f', 'id': 1122, 'synset': 'toothbrush.n.01', 'synonyms': ['toothbrush'], 'def': 'small brush; has long handle; used to clean teeth', 'name': 'toothbrush'}, {'frequency': 'f', 'id': 1123, 'synset': 'toothpaste.n.01', 'synonyms': ['toothpaste'], 'def': 'a dentifrice in the form of a paste', 'name': 'toothpaste'}, {'frequency': 'c', 'id': 1124, 'synset': 'toothpick.n.01', 'synonyms': ['toothpick'], 'def': 'pick consisting of a small strip of wood or plastic; used to pick food from between the teeth', 'name': 'toothpick'}, {'frequency': 'c', 'id': 1125, 'synset': 'top.n.09', 'synonyms': ['cover'], 'def': 'covering for a hole (especially a hole in the top of a container)', 'name': 'cover'}, {'frequency': 'c', 'id': 1126, 'synset': 'tortilla.n.01', 'synonyms': ['tortilla'], 'def': 'thin unleavened pancake made from cornmeal or wheat flour', 'name': 'tortilla'}, {'frequency': 'c', 'id': 1127, 'synset': 'tow_truck.n.01', 'synonyms': ['tow_truck'], 'def': 'a truck equipped to hoist and pull wrecked cars (or to remove cars from no-parking zones)', 'name': 'tow_truck'}, {'frequency': 'f', 'id': 1128, 'synset': 'towel.n.01', 'synonyms': ['towel'], 'def': 'a rectangular piece of absorbent cloth (or paper) for drying or wiping', 'name': 'towel'}, {'frequency': 'f', 'id': 1129, 'synset': 'towel_rack.n.01', 'synonyms': ['towel_rack', 'towel_rail', 'towel_bar'], 'def': 'a rack consisting of one or more bars on which towels can be hung', 'name': 'towel_rack'}, {'frequency': 'f', 'id': 1130, 'synset': 'toy.n.03', 'synonyms': ['toy'], 'def': 'a device regarded as providing amusement', 'name': 'toy'}, {'frequency': 'c', 'id': 1131, 'synset': 'tractor.n.01', 'synonyms': ['tractor_(farm_equipment)'], 'def': 'a wheeled vehicle with large wheels; used in farming and other applications', 'name': 'tractor_(farm_equipment)'}, {'frequency': 'f', 'id': 1132, 'synset': 'traffic_light.n.01', 'synonyms': ['traffic_light'], 'def': 'a device to control vehicle traffic often consisting of three or more lights', 'name': 'traffic_light'}, {'frequency': 'r', 'id': 1133, 'synset': 'trail_bike.n.01', 'synonyms': ['dirt_bike'], 'def': 'a lightweight motorcycle equipped with rugged tires and suspension for off-road use', 'name': 'dirt_bike'}, {'frequency': 'c', 'id': 1134, 'synset': 'trailer_truck.n.01', 'synonyms': ['trailer_truck', 'tractor_trailer', 'trucking_rig', 'articulated_lorry', 'semi_truck'], 'def': 'a truck consisting of a tractor and trailer together', 'name': 'trailer_truck'}, {'frequency': 'f', 'id': 1135, 'synset': 'train.n.01', 'synonyms': ['train_(railroad_vehicle)', 'railroad_train'], 'def': 'public or private transport provided by a line of railway cars coupled together and drawn by a locomotive', 'name': 'train_(railroad_vehicle)'}, {'frequency': 'r', 'id': 1136, 'synset': 'trampoline.n.01', 'synonyms': ['trampoline'], 'def': 'gymnastic apparatus consisting of a strong canvas sheet attached with springs to a metal frame', 'name': 'trampoline'}, {'frequency': 'f', 'id': 1137, 'synset': 'tray.n.01', 'synonyms': ['tray'], 'def': 'an open receptacle for holding or displaying or serving articles or food', 'name': 'tray'}, {'frequency': 'r', 'id': 1138, 'synset': 'tree_house.n.01', 'synonyms': ['tree_house'], 'def': '(NOT A TREE) a PLAYHOUSE built in the branches of a tree', 'name': 'tree_house'}, {'frequency': 'r', 'id': 1139, 'synset': 'trench_coat.n.01', 'synonyms': ['trench_coat'], 'def': 'a military style raincoat; belted with deep pockets', 'name': 'trench_coat'}, {'frequency': 'r', 'id': 1140, 'synset': 'triangle.n.05', 'synonyms': ['triangle_(musical_instrument)'], 'def': 'a percussion instrument consisting of a metal bar bent in the shape of an open triangle', 'name': 'triangle_(musical_instrument)'}, {'frequency': 'r', 'id': 1141, 'synset': 'tricycle.n.01', 'synonyms': ['tricycle'], 'def': 'a vehicle with three wheels that is moved by foot pedals', 'name': 'tricycle'}, {'frequency': 'c', 'id': 1142, 'synset': 'tripod.n.01', 'synonyms': ['tripod'], 'def': 'a three-legged rack used for support', 'name': 'tripod'}, {'frequency': 'f', 'id': 1143, 'synset': 'trouser.n.01', 'synonyms': ['trousers', 'pants_(clothing)'], 'def': 'a garment extending from the waist to the knee or ankle, covering each leg separately', 'name': 'trousers'}, {'frequency': 'f', 'id': 1144, 'synset': 'truck.n.01', 'synonyms': ['truck'], 'def': 'an automotive vehicle suitable for hauling', 'name': 'truck'}, {'frequency': 'r', 'id': 1145, 'synset': 'truffle.n.03', 'synonyms': ['truffle_(chocolate)', 'chocolate_truffle'], 'def': 'creamy chocolate candy', 'name': 'truffle_(chocolate)'}, {'frequency': 'c', 'id': 1146, 'synset': 'trunk.n.02', 'synonyms': ['trunk'], 'def': 'luggage consisting of a large strong case used when traveling or for storage', 'name': 'trunk'}, {'frequency': 'r', 'id': 1147, 'synset': 'tub.n.02', 'synonyms': ['vat'], 'def': 'a large open vessel for holding or storing liquids', 'name': 'vat'}, {'frequency': 'c', 'id': 1148, 'synset': 'turban.n.01', 'synonyms': ['turban'], 'def': 'a traditional headdress consisting of a long scarf wrapped around the head', 'name': 'turban'}, {'frequency': 'r', 'id': 1149, 'synset': 'turkey.n.01', 'synonyms': ['turkey_(bird)'], 'def': 'large gallinaceous bird with fan-shaped tail; widely domesticated for food', 'name': 'turkey_(bird)'}, {'frequency': 'c', 'id': 1150, 'synset': 'turkey.n.04', 'synonyms': ['turkey_(food)'], 'def': 'flesh of large domesticated fowl usually roasted', 'name': 'turkey_(food)'}, {'frequency': 'r', 'id': 1151, 'synset': 'turnip.n.01', 'synonyms': ['turnip'], 'def': 'widely cultivated plant having a large fleshy edible white or yellow root', 'name': 'turnip'}, {'frequency': 'c', 'id': 1152, 'synset': 'turtle.n.02', 'synonyms': ['turtle'], 'def': 'any of various aquatic and land reptiles having a bony shell and flipper-like limbs for swimming', 'name': 'turtle'}, {'frequency': 'r', 'id': 1153, 'synset': 'turtleneck.n.01', 'synonyms': ['turtleneck_(clothing)', 'polo-neck'], 'def': 'a sweater or jersey with a high close-fitting collar', 'name': 'turtleneck_(clothing)'}, {'frequency': 'r', 'id': 1154, 'synset': 'typewriter.n.01', 'synonyms': ['typewriter'], 'def': 'hand-operated character printer for printing written messages one character at a time', 'name': 'typewriter'}, {'frequency': 'f', 'id': 1155, 'synset': 'umbrella.n.01', 'synonyms': ['umbrella'], 'def': 'a lightweight handheld collapsible canopy', 'name': 'umbrella'}, {'frequency': 'c', 'id': 1156, 'synset': 'underwear.n.01', 'synonyms': ['underwear', 'underclothes', 'underclothing', 'underpants'], 'def': 'undergarment worn next to the skin and under the outer garments', 'name': 'underwear'}, {'frequency': 'r', 'id': 1157, 'synset': 'unicycle.n.01', 'synonyms': ['unicycle'], 'def': 'a vehicle with a single wheel that is driven by pedals', 'name': 'unicycle'}, {'frequency': 'c', 'id': 1158, 'synset': 'urinal.n.01', 'synonyms': ['urinal'], 'def': 'a plumbing fixture (usually attached to the wall) used by men to urinate', 'name': 'urinal'}, {'frequency': 'r', 'id': 1159, 'synset': 'urn.n.01', 'synonyms': ['urn'], 'def': 'a large vase that usually has a pedestal or feet', 'name': 'urn'}, {'frequency': 'c', 'id': 1160, 'synset': 'vacuum.n.04', 'synonyms': ['vacuum_cleaner'], 'def': 'an electrical home appliance that cleans by suction', 'name': 'vacuum_cleaner'}, {'frequency': 'c', 'id': 1161, 'synset': 'valve.n.03', 'synonyms': ['valve'], 'def': 'control consisting of a mechanical device for controlling the flow of a fluid', 'name': 'valve'}, {'frequency': 'f', 'id': 1162, 'synset': 'vase.n.01', 'synonyms': ['vase'], 'def': 'an open jar of glass or porcelain used as an ornament or to hold flowers', 'name': 'vase'}, {'frequency': 'c', 'id': 1163, 'synset': 'vending_machine.n.01', 'synonyms': ['vending_machine'], 'def': 'a slot machine for selling goods', 'name': 'vending_machine'}, {'frequency': 'f', 'id': 1164, 'synset': 'vent.n.01', 'synonyms': ['vent', 'blowhole', 'air_vent'], 'def': 'a hole for the escape of gas or air', 'name': 'vent'}, {'frequency': 'c', 'id': 1165, 'synset': 'videotape.n.01', 'synonyms': ['videotape'], 'def': 'a video recording made on magnetic tape', 'name': 'videotape'}, {'frequency': 'r', 'id': 1166, 'synset': 'vinegar.n.01', 'synonyms': ['vinegar'], 'def': 'sour-tasting liquid produced usually by oxidation of the alcohol in wine or cider and used as a condiment or food preservative', 'name': 'vinegar'}, {'frequency': 'r', 'id': 1167, 'synset': 'violin.n.01', 'synonyms': ['violin', 'fiddle'], 'def': 'bowed stringed instrument that is the highest member of the violin family', 'name': 'violin'}, {'frequency': 'r', 'id': 1168, 'synset': 'vodka.n.01', 'synonyms': ['vodka'], 'def': 'unaged colorless liquor originating in Russia', 'name': 'vodka'}, {'frequency': 'r', 'id': 1169, 'synset': 'volleyball.n.02', 'synonyms': ['volleyball'], 'def': 'an inflated ball used in playing volleyball', 'name': 'volleyball'}, {'frequency': 'r', 'id': 1170, 'synset': 'vulture.n.01', 'synonyms': ['vulture'], 'def': 'any of various large birds of prey having naked heads and weak claws and feeding chiefly on carrion', 'name': 'vulture'}, {'frequency': 'c', 'id': 1171, 'synset': 'waffle.n.01', 'synonyms': ['waffle'], 'def': 'pancake batter baked in a waffle iron', 'name': 'waffle'}, {'frequency': 'r', 'id': 1172, 'synset': 'waffle_iron.n.01', 'synonyms': ['waffle_iron'], 'def': 'a kitchen appliance for baking waffles', 'name': 'waffle_iron'}, {'frequency': 'c', 'id': 1173, 'synset': 'wagon.n.01', 'synonyms': ['wagon'], 'def': 'any of various kinds of wheeled vehicles drawn by an animal or a tractor', 'name': 'wagon'}, {'frequency': 'c', 'id': 1174, 'synset': 'wagon_wheel.n.01', 'synonyms': ['wagon_wheel'], 'def': 'a wheel of a wagon', 'name': 'wagon_wheel'}, {'frequency': 'c', 'id': 1175, 'synset': 'walking_stick.n.01', 'synonyms': ['walking_stick'], 'def': 'a stick carried in the hand for support in walking', 'name': 'walking_stick'}, {'frequency': 'c', 'id': 1176, 'synset': 'wall_clock.n.01', 'synonyms': ['wall_clock'], 'def': 'a clock mounted on a wall', 'name': 'wall_clock'}, {'frequency': 'f', 'id': 1177, 'synset': 'wall_socket.n.01', 'synonyms': ['wall_socket', 'wall_plug', 'electric_outlet', 'electrical_outlet', 'outlet', 'electric_receptacle'], 'def': 'receptacle providing a place in a wiring system where current can be taken to run electrical devices', 'name': 'wall_socket'}, {'frequency': 'c', 'id': 1178, 'synset': 'wallet.n.01', 'synonyms': ['wallet', 'billfold'], 'def': 'a pocket-size case for holding papers and paper money', 'name': 'wallet'}, {'frequency': 'r', 'id': 1179, 'synset': 'walrus.n.01', 'synonyms': ['walrus'], 'def': 'either of two large northern marine mammals having ivory tusks and tough hide over thick blubber', 'name': 'walrus'}, {'frequency': 'r', 'id': 1180, 'synset': 'wardrobe.n.01', 'synonyms': ['wardrobe'], 'def': 'a tall piece of furniture that provides storage space for clothes; has a door and rails or hooks for hanging clothes', 'name': 'wardrobe'}, {'frequency': 'r', 'id': 1181, 'synset': 'wasabi.n.02', 'synonyms': ['wasabi'], 'def': 'the thick green root of the wasabi plant that the Japanese use in cooking and that tastes like strong horseradish', 'name': 'wasabi'}, {'frequency': 'c', 'id': 1182, 'synset': 'washer.n.03', 'synonyms': ['automatic_washer', 'washing_machine'], 'def': 'a home appliance for washing clothes and linens automatically', 'name': 'automatic_washer'}, {'frequency': 'f', 'id': 1183, 'synset': 'watch.n.01', 'synonyms': ['watch', 'wristwatch'], 'def': 'a small, portable timepiece', 'name': 'watch'}, {'frequency': 'f', 'id': 1184, 'synset': 'water_bottle.n.01', 'synonyms': ['water_bottle'], 'def': 'a bottle for holding water', 'name': 'water_bottle'}, {'frequency': 'c', 'id': 1185, 'synset': 'water_cooler.n.01', 'synonyms': ['water_cooler'], 'def': 'a device for cooling and dispensing drinking water', 'name': 'water_cooler'}, {'frequency': 'c', 'id': 1186, 'synset': 'water_faucet.n.01', 'synonyms': ['water_faucet', 'water_tap', 'tap_(water_faucet)'], 'def': 'a faucet for drawing water from a pipe or cask', 'name': 'water_faucet'}, {'frequency': 'r', 'id': 1187, 'synset': 'water_filter.n.01', 'synonyms': ['water_filter'], 'def': 'a filter to remove impurities from the water supply', 'name': 'water_filter'}, {'frequency': 'r', 'id': 1188, 'synset': 'water_heater.n.01', 'synonyms': ['water_heater', 'hot-water_heater'], 'def': 'a heater and storage tank to supply heated water', 'name': 'water_heater'}, {'frequency': 'r', 'id': 1189, 'synset': 'water_jug.n.01', 'synonyms': ['water_jug'], 'def': 'a jug that holds water', 'name': 'water_jug'}, {'frequency': 'r', 'id': 1190, 'synset': 'water_pistol.n.01', 'synonyms': ['water_gun', 'squirt_gun'], 'def': 'plaything consisting of a toy pistol that squirts water', 'name': 'water_gun'}, {'frequency': 'c', 'id': 1191, 'synset': 'water_scooter.n.01', 'synonyms': ['water_scooter', 'sea_scooter', 'jet_ski'], 'def': 'a motorboat resembling a motor scooter (NOT A SURFBOARD OR WATER SKI)', 'name': 'water_scooter'}, {'frequency': 'c', 'id': 1192, 'synset': 'water_ski.n.01', 'synonyms': ['water_ski'], 'def': 'broad ski for skimming over water towed by a speedboat (DO NOT MARK WATER)', 'name': 'water_ski'}, {'frequency': 'c', 'id': 1193, 'synset': 'water_tower.n.01', 'synonyms': ['water_tower'], 'def': 'a large reservoir for water', 'name': 'water_tower'}, {'frequency': 'c', 'id': 1194, 'synset': 'watering_can.n.01', 'synonyms': ['watering_can'], 'def': 'a container with a handle and a spout with a perforated nozzle; used to sprinkle water over plants', 'name': 'watering_can'}, {'frequency': 'c', 'id': 1195, 'synset': 'watermelon.n.02', 'synonyms': ['watermelon'], 'def': 'large oblong or roundish melon with a hard green rind and sweet watery red or occasionally yellowish pulp', 'name': 'watermelon'}, {'frequency': 'f', 'id': 1196, 'synset': 'weathervane.n.01', 'synonyms': ['weathervane', 'vane_(weathervane)', 'wind_vane'], 'def': 'mechanical device attached to an elevated structure; rotates freely to show the direction of the wind', 'name': 'weathervane'}, {'frequency': 'c', 'id': 1197, 'synset': 'webcam.n.01', 'synonyms': ['webcam'], 'def': 'a digital camera designed to take digital photographs and transmit them over the internet', 'name': 'webcam'}, {'frequency': 'c', 'id': 1198, 'synset': 'wedding_cake.n.01', 'synonyms': ['wedding_cake', 'bridecake'], 'def': 'a rich cake with two or more tiers and covered with frosting and decorations; served at a wedding reception', 'name': 'wedding_cake'}, {'frequency': 'c', 'id': 1199, 'synset': 'wedding_ring.n.01', 'synonyms': ['wedding_ring', 'wedding_band'], 'def': 'a ring given to the bride and/or groom at the wedding', 'name': 'wedding_ring'}, {'frequency': 'f', 'id': 1200, 'synset': 'wet_suit.n.01', 'synonyms': ['wet_suit'], 'def': 'a close-fitting garment made of a permeable material; worn in cold water to retain body heat', 'name': 'wet_suit'}, {'frequency': 'f', 'id': 1201, 'synset': 'wheel.n.01', 'synonyms': ['wheel'], 'def': 'a circular frame with spokes (or a solid disc) that can rotate on a shaft or axle', 'name': 'wheel'}, {'frequency': 'c', 'id': 1202, 'synset': 'wheelchair.n.01', 'synonyms': ['wheelchair'], 'def': 'a movable chair mounted on large wheels', 'name': 'wheelchair'}, {'frequency': 'c', 'id': 1203, 'synset': 'whipped_cream.n.01', 'synonyms': ['whipped_cream'], 'def': 'cream that has been beaten until light and fluffy', 'name': 'whipped_cream'}, {'frequency': 'r', 'id': 1204, 'synset': 'whiskey.n.01', 'synonyms': ['whiskey'], 'def': 'a liquor made from fermented mash of grain', 'name': 'whiskey'}, {'frequency': 'r', 'id': 1205, 'synset': 'whistle.n.03', 'synonyms': ['whistle'], 'def': 'a small wind instrument that produces a whistling sound by blowing into it', 'name': 'whistle'}, {'frequency': 'r', 'id': 1206, 'synset': 'wick.n.02', 'synonyms': ['wick'], 'def': 'a loosely woven cord in a candle or oil lamp that is lit on fire', 'name': 'wick'}, {'frequency': 'c', 'id': 1207, 'synset': 'wig.n.01', 'synonyms': ['wig'], 'def': 'hairpiece covering the head and made of real or synthetic hair', 'name': 'wig'}, {'frequency': 'c', 'id': 1208, 'synset': 'wind_chime.n.01', 'synonyms': ['wind_chime'], 'def': 'a decorative arrangement of pieces of metal or glass or pottery that hang together loosely so the wind can cause them to tinkle', 'name': 'wind_chime'}, {'frequency': 'c', 'id': 1209, 'synset': 'windmill.n.01', 'synonyms': ['windmill'], 'def': 'a mill that is powered by the wind', 'name': 'windmill'}, {'frequency': 'c', 'id': 1210, 'synset': 'window_box.n.01', 'synonyms': ['window_box_(for_plants)'], 'def': 'a container for growing plants on a windowsill', 'name': 'window_box_(for_plants)'}, {'frequency': 'f', 'id': 1211, 'synset': 'windshield_wiper.n.01', 'synonyms': ['windshield_wiper', 'windscreen_wiper', 'wiper_(for_windshield/screen)'], 'def': 'a mechanical device that cleans the windshield', 'name': 'windshield_wiper'}, {'frequency': 'c', 'id': 1212, 'synset': 'windsock.n.01', 'synonyms': ['windsock', 'air_sock', 'air-sleeve', 'wind_sleeve', 'wind_cone'], 'def': 'a truncated cloth cone mounted on a mast/pole; shows wind direction', 'name': 'windsock'}, {'frequency': 'f', 'id': 1213, 'synset': 'wine_bottle.n.01', 'synonyms': ['wine_bottle'], 'def': 'a bottle for holding wine', 'name': 'wine_bottle'}, {'frequency': 'r', 'id': 1214, 'synset': 'wine_bucket.n.01', 'synonyms': ['wine_bucket', 'wine_cooler'], 'def': 'a bucket of ice used to chill a bottle of wine', 'name': 'wine_bucket'}, {'frequency': 'f', 'id': 1215, 'synset': 'wineglass.n.01', 'synonyms': ['wineglass'], 'def': 'a glass that has a stem and in which wine is served', 'name': 'wineglass'}, {'frequency': 'r', 'id': 1216, 'synset': 'wing_chair.n.01', 'synonyms': ['wing_chair'], 'def': 'easy chair having wings on each side of a high back', 'name': 'wing_chair'}, {'frequency': 'c', 'id': 1217, 'synset': 'winker.n.02', 'synonyms': ['blinder_(for_horses)'], 'def': 'blinds that prevent a horse from seeing something on either side', 'name': 'blinder_(for_horses)'}, {'frequency': 'c', 'id': 1218, 'synset': 'wok.n.01', 'synonyms': ['wok'], 'def': 'pan with a convex bottom; used for frying in Chinese cooking', 'name': 'wok'}, {'frequency': 'r', 'id': 1219, 'synset': 'wolf.n.01', 'synonyms': ['wolf'], 'def': 'a wild carnivorous mammal of the dog family, living and hunting in packs', 'name': 'wolf'}, {'frequency': 'c', 'id': 1220, 'synset': 'wooden_spoon.n.02', 'synonyms': ['wooden_spoon'], 'def': 'a spoon made of wood', 'name': 'wooden_spoon'}, {'frequency': 'c', 'id': 1221, 'synset': 'wreath.n.01', 'synonyms': ['wreath'], 'def': 'an arrangement of flowers, leaves, or stems fastened in a ring', 'name': 'wreath'}, {'frequency': 'c', 'id': 1222, 'synset': 'wrench.n.03', 'synonyms': ['wrench', 'spanner'], 'def': 'a hand tool that is used to hold or twist a nut or bolt', 'name': 'wrench'}, {'frequency': 'c', 'id': 1223, 'synset': 'wristband.n.01', 'synonyms': ['wristband'], 'def': 'band consisting of a part of a sleeve that covers the wrist', 'name': 'wristband'}, {'frequency': 'f', 'id': 1224, 'synset': 'wristlet.n.01', 'synonyms': ['wristlet', 'wrist_band'], 'def': 'a band or bracelet worn around the wrist', 'name': 'wristlet'}, {'frequency': 'r', 'id': 1225, 'synset': 'yacht.n.01', 'synonyms': ['yacht'], 'def': 'an expensive vessel propelled by sail or power and used for cruising or racing', 'name': 'yacht'}, {'frequency': 'r', 'id': 1226, 'synset': 'yak.n.02', 'synonyms': ['yak'], 'def': 'large long-haired wild ox of Tibet often domesticated', 'name': 'yak'}, {'frequency': 'c', 'id': 1227, 'synset': 'yogurt.n.01', 'synonyms': ['yogurt', 'yoghurt', 'yoghourt'], 'def': 'a custard-like food made from curdled milk', 'name': 'yogurt'}, {'frequency': 'r', 'id': 1228, 'synset': 'yoke.n.07', 'synonyms': ['yoke_(animal_equipment)'], 'def': 'gear joining two animals at the neck; NOT egg yolk', 'name': 'yoke_(animal_equipment)'}, {'frequency': 'f', 'id': 1229, 'synset': 'zebra.n.01', 'synonyms': ['zebra'], 'def': 'any of several fleet black-and-white striped African equines', 'name': 'zebra'}, {'frequency': 'c', 'id': 1230, 'synset': 'zucchini.n.02', 'synonyms': ['zucchini', 'courgette'], 'def': 'small cucumber-shaped vegetable marrow; typically dark green', 'name': 'zucchini'}] # noqa
-# fmt: on
diff --git a/spaces/coreml-community/ControlNet-v1-1-Annotators-cpu/annotator/uniformer/mmcv/runner/priority.py b/spaces/coreml-community/ControlNet-v1-1-Annotators-cpu/annotator/uniformer/mmcv/runner/priority.py
deleted file mode 100644
index 64cc4e3a05f8d5b89ab6eb32461e6e80f1d62e67..0000000000000000000000000000000000000000
--- a/spaces/coreml-community/ControlNet-v1-1-Annotators-cpu/annotator/uniformer/mmcv/runner/priority.py
+++ /dev/null
@@ -1,60 +0,0 @@
-# Copyright (c) OpenMMLab. All rights reserved.
-from enum import Enum
-
-
-class Priority(Enum):
- """Hook priority levels.
-
- +--------------+------------+
- | Level | Value |
- +==============+============+
- | HIGHEST | 0 |
- +--------------+------------+
- | VERY_HIGH | 10 |
- +--------------+------------+
- | HIGH | 30 |
- +--------------+------------+
- | ABOVE_NORMAL | 40 |
- +--------------+------------+
- | NORMAL | 50 |
- +--------------+------------+
- | BELOW_NORMAL | 60 |
- +--------------+------------+
- | LOW | 70 |
- +--------------+------------+
- | VERY_LOW | 90 |
- +--------------+------------+
- | LOWEST | 100 |
- +--------------+------------+
- """
-
- HIGHEST = 0
- VERY_HIGH = 10
- HIGH = 30
- ABOVE_NORMAL = 40
- NORMAL = 50
- BELOW_NORMAL = 60
- LOW = 70
- VERY_LOW = 90
- LOWEST = 100
-
-
-def get_priority(priority):
- """Get priority value.
-
- Args:
- priority (int or str or :obj:`Priority`): Priority.
-
- Returns:
- int: The priority value.
- """
- if isinstance(priority, int):
- if priority < 0 or priority > 100:
- raise ValueError('priority must be between 0 and 100')
- return priority
- elif isinstance(priority, Priority):
- return priority.value
- elif isinstance(priority, str):
- return Priority[priority.upper()].value
- else:
- raise TypeError('priority must be an integer or Priority enum value')
diff --git a/spaces/cscan/CodeFormer/CodeFormer/basicsr/ops/dcn/src/deform_conv_cuda.cpp b/spaces/cscan/CodeFormer/CodeFormer/basicsr/ops/dcn/src/deform_conv_cuda.cpp
deleted file mode 100644
index 5d9424908ed2dbd4ac3cdb98d13e09287a4d2f2d..0000000000000000000000000000000000000000
--- a/spaces/cscan/CodeFormer/CodeFormer/basicsr/ops/dcn/src/deform_conv_cuda.cpp
+++ /dev/null
@@ -1,685 +0,0 @@
-// modify from
-// https://github.com/chengdazhi/Deformable-Convolution-V2-PyTorch/blob/mmdetection/mmdet/ops/dcn/src/deform_conv_cuda.c
-
-#include
-#include
-
-#include
-#include
-
-void deformable_im2col(const at::Tensor data_im, const at::Tensor data_offset,
- const int channels, const int height, const int width,
- const int ksize_h, const int ksize_w, const int pad_h,
- const int pad_w, const int stride_h, const int stride_w,
- const int dilation_h, const int dilation_w,
- const int parallel_imgs, const int deformable_group,
- at::Tensor data_col);
-
-void deformable_col2im(const at::Tensor data_col, const at::Tensor data_offset,
- const int channels, const int height, const int width,
- const int ksize_h, const int ksize_w, const int pad_h,
- const int pad_w, const int stride_h, const int stride_w,
- const int dilation_h, const int dilation_w,
- const int parallel_imgs, const int deformable_group,
- at::Tensor grad_im);
-
-void deformable_col2im_coord(
- const at::Tensor data_col, const at::Tensor data_im,
- const at::Tensor data_offset, const int channels, const int height,
- const int width, const int ksize_h, const int ksize_w, const int pad_h,
- const int pad_w, const int stride_h, const int stride_w,
- const int dilation_h, const int dilation_w, const int parallel_imgs,
- const int deformable_group, at::Tensor grad_offset);
-
-void modulated_deformable_im2col_cuda(
- const at::Tensor data_im, const at::Tensor data_offset,
- const at::Tensor data_mask, const int batch_size, const int channels,
- const int height_im, const int width_im, const int height_col,
- const int width_col, const int kernel_h, const int kenerl_w,
- const int pad_h, const int pad_w, const int stride_h, const int stride_w,
- const int dilation_h, const int dilation_w, const int deformable_group,
- at::Tensor data_col);
-
-void modulated_deformable_col2im_cuda(
- const at::Tensor data_col, const at::Tensor data_offset,
- const at::Tensor data_mask, const int batch_size, const int channels,
- const int height_im, const int width_im, const int height_col,
- const int width_col, const int kernel_h, const int kenerl_w,
- const int pad_h, const int pad_w, const int stride_h, const int stride_w,
- const int dilation_h, const int dilation_w, const int deformable_group,
- at::Tensor grad_im);
-
-void modulated_deformable_col2im_coord_cuda(
- const at::Tensor data_col, const at::Tensor data_im,
- const at::Tensor data_offset, const at::Tensor data_mask,
- const int batch_size, const int channels, const int height_im,
- const int width_im, const int height_col, const int width_col,
- const int kernel_h, const int kenerl_w, const int pad_h, const int pad_w,
- const int stride_h, const int stride_w, const int dilation_h,
- const int dilation_w, const int deformable_group, at::Tensor grad_offset,
- at::Tensor grad_mask);
-
-void shape_check(at::Tensor input, at::Tensor offset, at::Tensor *gradOutput,
- at::Tensor weight, int kH, int kW, int dH, int dW, int padH,
- int padW, int dilationH, int dilationW, int group,
- int deformable_group) {
- TORCH_CHECK(weight.ndimension() == 4,
- "4D weight tensor (nOutputPlane,nInputPlane,kH,kW) expected, "
- "but got: %s",
- weight.ndimension());
-
- TORCH_CHECK(weight.is_contiguous(), "weight tensor has to be contiguous");
-
- TORCH_CHECK(kW > 0 && kH > 0,
- "kernel size should be greater than zero, but got kH: %d kW: %d", kH,
- kW);
-
- TORCH_CHECK((weight.size(2) == kH && weight.size(3) == kW),
- "kernel size should be consistent with weight, ",
- "but got kH: %d kW: %d weight.size(2): %d, weight.size(3): %d", kH,
- kW, weight.size(2), weight.size(3));
-
- TORCH_CHECK(dW > 0 && dH > 0,
- "stride should be greater than zero, but got dH: %d dW: %d", dH, dW);
-
- TORCH_CHECK(
- dilationW > 0 && dilationH > 0,
- "dilation should be greater than 0, but got dilationH: %d dilationW: %d",
- dilationH, dilationW);
-
- int ndim = input.ndimension();
- int dimf = 0;
- int dimh = 1;
- int dimw = 2;
-
- if (ndim == 4) {
- dimf++;
- dimh++;
- dimw++;
- }
-
- TORCH_CHECK(ndim == 3 || ndim == 4, "3D or 4D input tensor expected but got: %s",
- ndim);
-
- long nInputPlane = weight.size(1) * group;
- long inputHeight = input.size(dimh);
- long inputWidth = input.size(dimw);
- long nOutputPlane = weight.size(0);
- long outputHeight =
- (inputHeight + 2 * padH - (dilationH * (kH - 1) + 1)) / dH + 1;
- long outputWidth =
- (inputWidth + 2 * padW - (dilationW * (kW - 1) + 1)) / dW + 1;
-
- TORCH_CHECK(nInputPlane % deformable_group == 0,
- "input channels must divide deformable group size");
-
- if (outputWidth < 1 || outputHeight < 1)
- AT_ERROR(
- "Given input size: (%ld x %ld x %ld). "
- "Calculated output size: (%ld x %ld x %ld). Output size is too small",
- nInputPlane, inputHeight, inputWidth, nOutputPlane, outputHeight,
- outputWidth);
-
- TORCH_CHECK(input.size(1) == nInputPlane,
- "invalid number of input planes, expected: %d, but got: %d",
- nInputPlane, input.size(1));
-
- TORCH_CHECK((inputHeight >= kH && inputWidth >= kW),
- "input image is smaller than kernel");
-
- TORCH_CHECK((offset.size(2) == outputHeight && offset.size(3) == outputWidth),
- "invalid spatial size of offset, expected height: %d width: %d, but "
- "got height: %d width: %d",
- outputHeight, outputWidth, offset.size(2), offset.size(3));
-
- TORCH_CHECK((offset.size(1) == deformable_group * 2 * kH * kW),
- "invalid number of channels of offset");
-
- if (gradOutput != NULL) {
- TORCH_CHECK(gradOutput->size(dimf) == nOutputPlane,
- "invalid number of gradOutput planes, expected: %d, but got: %d",
- nOutputPlane, gradOutput->size(dimf));
-
- TORCH_CHECK((gradOutput->size(dimh) == outputHeight &&
- gradOutput->size(dimw) == outputWidth),
- "invalid size of gradOutput, expected height: %d width: %d , but "
- "got height: %d width: %d",
- outputHeight, outputWidth, gradOutput->size(dimh),
- gradOutput->size(dimw));
- }
-}
-
-int deform_conv_forward_cuda(at::Tensor input, at::Tensor weight,
- at::Tensor offset, at::Tensor output,
- at::Tensor columns, at::Tensor ones, int kW,
- int kH, int dW, int dH, int padW, int padH,
- int dilationW, int dilationH, int group,
- int deformable_group, int im2col_step) {
- // todo: resize columns to include im2col: done
- // todo: add im2col_step as input
- // todo: add new output buffer and transpose it to output (or directly
- // transpose output) todo: possibly change data indexing because of
- // parallel_imgs
-
- shape_check(input, offset, NULL, weight, kH, kW, dH, dW, padH, padW,
- dilationH, dilationW, group, deformable_group);
- at::DeviceGuard guard(input.device());
-
- input = input.contiguous();
- offset = offset.contiguous();
- weight = weight.contiguous();
-
- int batch = 1;
- if (input.ndimension() == 3) {
- // Force batch
- batch = 0;
- input.unsqueeze_(0);
- offset.unsqueeze_(0);
- }
-
- // todo: assert batchsize dividable by im2col_step
-
- long batchSize = input.size(0);
- long nInputPlane = input.size(1);
- long inputHeight = input.size(2);
- long inputWidth = input.size(3);
-
- long nOutputPlane = weight.size(0);
-
- long outputWidth =
- (inputWidth + 2 * padW - (dilationW * (kW - 1) + 1)) / dW + 1;
- long outputHeight =
- (inputHeight + 2 * padH - (dilationH * (kH - 1) + 1)) / dH + 1;
-
- TORCH_CHECK((offset.size(0) == batchSize), "invalid batch size of offset");
-
- output = output.view({batchSize / im2col_step, im2col_step, nOutputPlane,
- outputHeight, outputWidth});
- columns = at::zeros(
- {nInputPlane * kW * kH, im2col_step * outputHeight * outputWidth},
- input.options());
-
- if (ones.ndimension() != 2 ||
- ones.size(0) * ones.size(1) < outputHeight * outputWidth) {
- ones = at::ones({outputHeight, outputWidth}, input.options());
- }
-
- input = input.view({batchSize / im2col_step, im2col_step, nInputPlane,
- inputHeight, inputWidth});
- offset =
- offset.view({batchSize / im2col_step, im2col_step,
- deformable_group * 2 * kH * kW, outputHeight, outputWidth});
-
- at::Tensor output_buffer =
- at::zeros({batchSize / im2col_step, nOutputPlane,
- im2col_step * outputHeight, outputWidth},
- output.options());
-
- output_buffer = output_buffer.view(
- {output_buffer.size(0), group, output_buffer.size(1) / group,
- output_buffer.size(2), output_buffer.size(3)});
-
- for (int elt = 0; elt < batchSize / im2col_step; elt++) {
- deformable_im2col(input[elt], offset[elt], nInputPlane, inputHeight,
- inputWidth, kH, kW, padH, padW, dH, dW, dilationH,
- dilationW, im2col_step, deformable_group, columns);
-
- columns = columns.view({group, columns.size(0) / group, columns.size(1)});
- weight = weight.view({group, weight.size(0) / group, weight.size(1),
- weight.size(2), weight.size(3)});
-
- for (int g = 0; g < group; g++) {
- output_buffer[elt][g] = output_buffer[elt][g]
- .flatten(1)
- .addmm_(weight[g].flatten(1), columns[g])
- .view_as(output_buffer[elt][g]);
- }
- }
-
- output_buffer = output_buffer.view(
- {output_buffer.size(0), output_buffer.size(1) * output_buffer.size(2),
- output_buffer.size(3), output_buffer.size(4)});
-
- output_buffer = output_buffer.view({batchSize / im2col_step, nOutputPlane,
- im2col_step, outputHeight, outputWidth});
- output_buffer.transpose_(1, 2);
- output.copy_(output_buffer);
- output = output.view({batchSize, nOutputPlane, outputHeight, outputWidth});
-
- input = input.view({batchSize, nInputPlane, inputHeight, inputWidth});
- offset = offset.view(
- {batchSize, deformable_group * 2 * kH * kW, outputHeight, outputWidth});
-
- if (batch == 0) {
- output = output.view({nOutputPlane, outputHeight, outputWidth});
- input = input.view({nInputPlane, inputHeight, inputWidth});
- offset = offset.view({offset.size(1), offset.size(2), offset.size(3)});
- }
-
- return 1;
-}
-
-int deform_conv_backward_input_cuda(at::Tensor input, at::Tensor offset,
- at::Tensor gradOutput, at::Tensor gradInput,
- at::Tensor gradOffset, at::Tensor weight,
- at::Tensor columns, int kW, int kH, int dW,
- int dH, int padW, int padH, int dilationW,
- int dilationH, int group,
- int deformable_group, int im2col_step) {
- shape_check(input, offset, &gradOutput, weight, kH, kW, dH, dW, padH, padW,
- dilationH, dilationW, group, deformable_group);
- at::DeviceGuard guard(input.device());
-
- input = input.contiguous();
- offset = offset.contiguous();
- gradOutput = gradOutput.contiguous();
- weight = weight.contiguous();
-
- int batch = 1;
-
- if (input.ndimension() == 3) {
- // Force batch
- batch = 0;
- input = input.view({1, input.size(0), input.size(1), input.size(2)});
- offset = offset.view({1, offset.size(0), offset.size(1), offset.size(2)});
- gradOutput = gradOutput.view(
- {1, gradOutput.size(0), gradOutput.size(1), gradOutput.size(2)});
- }
-
- long batchSize = input.size(0);
- long nInputPlane = input.size(1);
- long inputHeight = input.size(2);
- long inputWidth = input.size(3);
-
- long nOutputPlane = weight.size(0);
-
- long outputWidth =
- (inputWidth + 2 * padW - (dilationW * (kW - 1) + 1)) / dW + 1;
- long outputHeight =
- (inputHeight + 2 * padH - (dilationH * (kH - 1) + 1)) / dH + 1;
-
- TORCH_CHECK((offset.size(0) == batchSize), 3, "invalid batch size of offset");
- gradInput = gradInput.view({batchSize, nInputPlane, inputHeight, inputWidth});
- columns = at::zeros(
- {nInputPlane * kW * kH, im2col_step * outputHeight * outputWidth},
- input.options());
-
- // change order of grad output
- gradOutput = gradOutput.view({batchSize / im2col_step, im2col_step,
- nOutputPlane, outputHeight, outputWidth});
- gradOutput.transpose_(1, 2);
-
- gradInput = gradInput.view({batchSize / im2col_step, im2col_step, nInputPlane,
- inputHeight, inputWidth});
- input = input.view({batchSize / im2col_step, im2col_step, nInputPlane,
- inputHeight, inputWidth});
- gradOffset = gradOffset.view({batchSize / im2col_step, im2col_step,
- deformable_group * 2 * kH * kW, outputHeight,
- outputWidth});
- offset =
- offset.view({batchSize / im2col_step, im2col_step,
- deformable_group * 2 * kH * kW, outputHeight, outputWidth});
-
- for (int elt = 0; elt < batchSize / im2col_step; elt++) {
- // divide into groups
- columns = columns.view({group, columns.size(0) / group, columns.size(1)});
- weight = weight.view({group, weight.size(0) / group, weight.size(1),
- weight.size(2), weight.size(3)});
- gradOutput = gradOutput.view(
- {gradOutput.size(0), group, gradOutput.size(1) / group,
- gradOutput.size(2), gradOutput.size(3), gradOutput.size(4)});
-
- for (int g = 0; g < group; g++) {
- columns[g] = columns[g].addmm_(weight[g].flatten(1).transpose(0, 1),
- gradOutput[elt][g].flatten(1), 0.0f, 1.0f);
- }
-
- columns =
- columns.view({columns.size(0) * columns.size(1), columns.size(2)});
- gradOutput = gradOutput.view(
- {gradOutput.size(0), gradOutput.size(1) * gradOutput.size(2),
- gradOutput.size(3), gradOutput.size(4), gradOutput.size(5)});
-
- deformable_col2im_coord(columns, input[elt], offset[elt], nInputPlane,
- inputHeight, inputWidth, kH, kW, padH, padW, dH, dW,
- dilationH, dilationW, im2col_step, deformable_group,
- gradOffset[elt]);
-
- deformable_col2im(columns, offset[elt], nInputPlane, inputHeight,
- inputWidth, kH, kW, padH, padW, dH, dW, dilationH,
- dilationW, im2col_step, deformable_group, gradInput[elt]);
- }
-
- gradOutput.transpose_(1, 2);
- gradOutput =
- gradOutput.view({batchSize, nOutputPlane, outputHeight, outputWidth});
-
- gradInput = gradInput.view({batchSize, nInputPlane, inputHeight, inputWidth});
- input = input.view({batchSize, nInputPlane, inputHeight, inputWidth});
- gradOffset = gradOffset.view(
- {batchSize, deformable_group * 2 * kH * kW, outputHeight, outputWidth});
- offset = offset.view(
- {batchSize, deformable_group * 2 * kH * kW, outputHeight, outputWidth});
-
- if (batch == 0) {
- gradOutput = gradOutput.view({nOutputPlane, outputHeight, outputWidth});
- input = input.view({nInputPlane, inputHeight, inputWidth});
- gradInput = gradInput.view({nInputPlane, inputHeight, inputWidth});
- offset = offset.view({offset.size(1), offset.size(2), offset.size(3)});
- gradOffset =
- gradOffset.view({offset.size(1), offset.size(2), offset.size(3)});
- }
-
- return 1;
-}
-
-int deform_conv_backward_parameters_cuda(
- at::Tensor input, at::Tensor offset, at::Tensor gradOutput,
- at::Tensor gradWeight, // at::Tensor gradBias,
- at::Tensor columns, at::Tensor ones, int kW, int kH, int dW, int dH,
- int padW, int padH, int dilationW, int dilationH, int group,
- int deformable_group, float scale, int im2col_step) {
- // todo: transpose and reshape outGrad
- // todo: reshape columns
- // todo: add im2col_step as input
-
- shape_check(input, offset, &gradOutput, gradWeight, kH, kW, dH, dW, padH,
- padW, dilationH, dilationW, group, deformable_group);
- at::DeviceGuard guard(input.device());
-
- input = input.contiguous();
- offset = offset.contiguous();
- gradOutput = gradOutput.contiguous();
-
- int batch = 1;
-
- if (input.ndimension() == 3) {
- // Force batch
- batch = 0;
- input = input.view(
- at::IntList({1, input.size(0), input.size(1), input.size(2)}));
- gradOutput = gradOutput.view(
- {1, gradOutput.size(0), gradOutput.size(1), gradOutput.size(2)});
- }
-
- long batchSize = input.size(0);
- long nInputPlane = input.size(1);
- long inputHeight = input.size(2);
- long inputWidth = input.size(3);
-
- long nOutputPlane = gradWeight.size(0);
-
- long outputWidth =
- (inputWidth + 2 * padW - (dilationW * (kW - 1) + 1)) / dW + 1;
- long outputHeight =
- (inputHeight + 2 * padH - (dilationH * (kH - 1) + 1)) / dH + 1;
-
- TORCH_CHECK((offset.size(0) == batchSize), "invalid batch size of offset");
-
- columns = at::zeros(
- {nInputPlane * kW * kH, im2col_step * outputHeight * outputWidth},
- input.options());
-
- gradOutput = gradOutput.view({batchSize / im2col_step, im2col_step,
- nOutputPlane, outputHeight, outputWidth});
- gradOutput.transpose_(1, 2);
-
- at::Tensor gradOutputBuffer = at::zeros_like(gradOutput);
- gradOutputBuffer =
- gradOutputBuffer.view({batchSize / im2col_step, nOutputPlane, im2col_step,
- outputHeight, outputWidth});
- gradOutputBuffer.copy_(gradOutput);
- gradOutputBuffer =
- gradOutputBuffer.view({batchSize / im2col_step, nOutputPlane,
- im2col_step * outputHeight, outputWidth});
-
- gradOutput.transpose_(1, 2);
- gradOutput =
- gradOutput.view({batchSize, nOutputPlane, outputHeight, outputWidth});
-
- input = input.view({batchSize / im2col_step, im2col_step, nInputPlane,
- inputHeight, inputWidth});
- offset =
- offset.view({batchSize / im2col_step, im2col_step,
- deformable_group * 2 * kH * kW, outputHeight, outputWidth});
-
- for (int elt = 0; elt < batchSize / im2col_step; elt++) {
- deformable_im2col(input[elt], offset[elt], nInputPlane, inputHeight,
- inputWidth, kH, kW, padH, padW, dH, dW, dilationH,
- dilationW, im2col_step, deformable_group, columns);
-
- // divide into group
- gradOutputBuffer = gradOutputBuffer.view(
- {gradOutputBuffer.size(0), group, gradOutputBuffer.size(1) / group,
- gradOutputBuffer.size(2), gradOutputBuffer.size(3)});
- columns = columns.view({group, columns.size(0) / group, columns.size(1)});
- gradWeight =
- gradWeight.view({group, gradWeight.size(0) / group, gradWeight.size(1),
- gradWeight.size(2), gradWeight.size(3)});
-
- for (int g = 0; g < group; g++) {
- gradWeight[g] = gradWeight[g]
- .flatten(1)
- .addmm_(gradOutputBuffer[elt][g].flatten(1),
- columns[g].transpose(1, 0), 1.0, scale)
- .view_as(gradWeight[g]);
- }
- gradOutputBuffer = gradOutputBuffer.view(
- {gradOutputBuffer.size(0),
- gradOutputBuffer.size(1) * gradOutputBuffer.size(2),
- gradOutputBuffer.size(3), gradOutputBuffer.size(4)});
- columns =
- columns.view({columns.size(0) * columns.size(1), columns.size(2)});
- gradWeight = gradWeight.view({gradWeight.size(0) * gradWeight.size(1),
- gradWeight.size(2), gradWeight.size(3),
- gradWeight.size(4)});
- }
-
- input = input.view({batchSize, nInputPlane, inputHeight, inputWidth});
- offset = offset.view(
- {batchSize, deformable_group * 2 * kH * kW, outputHeight, outputWidth});
-
- if (batch == 0) {
- gradOutput = gradOutput.view({nOutputPlane, outputHeight, outputWidth});
- input = input.view({nInputPlane, inputHeight, inputWidth});
- }
-
- return 1;
-}
-
-void modulated_deform_conv_cuda_forward(
- at::Tensor input, at::Tensor weight, at::Tensor bias, at::Tensor ones,
- at::Tensor offset, at::Tensor mask, at::Tensor output, at::Tensor columns,
- int kernel_h, int kernel_w, const int stride_h, const int stride_w,
- const int pad_h, const int pad_w, const int dilation_h,
- const int dilation_w, const int group, const int deformable_group,
- const bool with_bias) {
- TORCH_CHECK(input.is_contiguous(), "input tensor has to be contiguous");
- TORCH_CHECK(weight.is_contiguous(), "weight tensor has to be contiguous");
- at::DeviceGuard guard(input.device());
-
- const int batch = input.size(0);
- const int channels = input.size(1);
- const int height = input.size(2);
- const int width = input.size(3);
-
- const int channels_out = weight.size(0);
- const int channels_kernel = weight.size(1);
- const int kernel_h_ = weight.size(2);
- const int kernel_w_ = weight.size(3);
-
- if (kernel_h_ != kernel_h || kernel_w_ != kernel_w)
- AT_ERROR("Input shape and kernel shape wont match: (%d x %d vs %d x %d).",
- kernel_h_, kernel_w, kernel_h_, kernel_w_);
- if (channels != channels_kernel * group)
- AT_ERROR("Input shape and kernel channels wont match: (%d vs %d).",
- channels, channels_kernel * group);
-
- const int height_out =
- (height + 2 * pad_h - (dilation_h * (kernel_h - 1) + 1)) / stride_h + 1;
- const int width_out =
- (width + 2 * pad_w - (dilation_w * (kernel_w - 1) + 1)) / stride_w + 1;
-
- if (ones.ndimension() != 2 ||
- ones.size(0) * ones.size(1) < height_out * width_out) {
- // Resize plane and fill with ones...
- ones = at::ones({height_out, width_out}, input.options());
- }
-
- // resize output
- output = output.view({batch, channels_out, height_out, width_out}).zero_();
- // resize temporary columns
- columns =
- at::zeros({channels * kernel_h * kernel_w, 1 * height_out * width_out},
- input.options());
-
- output = output.view({output.size(0), group, output.size(1) / group,
- output.size(2), output.size(3)});
-
- for (int b = 0; b < batch; b++) {
- modulated_deformable_im2col_cuda(
- input[b], offset[b], mask[b], 1, channels, height, width, height_out,
- width_out, kernel_h, kernel_w, pad_h, pad_w, stride_h, stride_w,
- dilation_h, dilation_w, deformable_group, columns);
-
- // divide into group
- weight = weight.view({group, weight.size(0) / group, weight.size(1),
- weight.size(2), weight.size(3)});
- columns = columns.view({group, columns.size(0) / group, columns.size(1)});
-
- for (int g = 0; g < group; g++) {
- output[b][g] = output[b][g]
- .flatten(1)
- .addmm_(weight[g].flatten(1), columns[g])
- .view_as(output[b][g]);
- }
-
- weight = weight.view({weight.size(0) * weight.size(1), weight.size(2),
- weight.size(3), weight.size(4)});
- columns =
- columns.view({columns.size(0) * columns.size(1), columns.size(2)});
- }
-
- output = output.view({output.size(0), output.size(1) * output.size(2),
- output.size(3), output.size(4)});
-
- if (with_bias) {
- output += bias.view({1, bias.size(0), 1, 1});
- }
-}
-
-void modulated_deform_conv_cuda_backward(
- at::Tensor input, at::Tensor weight, at::Tensor bias, at::Tensor ones,
- at::Tensor offset, at::Tensor mask, at::Tensor columns,
- at::Tensor grad_input, at::Tensor grad_weight, at::Tensor grad_bias,
- at::Tensor grad_offset, at::Tensor grad_mask, at::Tensor grad_output,
- int kernel_h, int kernel_w, int stride_h, int stride_w, int pad_h,
- int pad_w, int dilation_h, int dilation_w, int group, int deformable_group,
- const bool with_bias) {
- TORCH_CHECK(input.is_contiguous(), "input tensor has to be contiguous");
- TORCH_CHECK(weight.is_contiguous(), "weight tensor has to be contiguous");
- at::DeviceGuard guard(input.device());
-
- const int batch = input.size(0);
- const int channels = input.size(1);
- const int height = input.size(2);
- const int width = input.size(3);
-
- const int channels_kernel = weight.size(1);
- const int kernel_h_ = weight.size(2);
- const int kernel_w_ = weight.size(3);
- if (kernel_h_ != kernel_h || kernel_w_ != kernel_w)
- AT_ERROR("Input shape and kernel shape wont match: (%d x %d vs %d x %d).",
- kernel_h_, kernel_w, kernel_h_, kernel_w_);
- if (channels != channels_kernel * group)
- AT_ERROR("Input shape and kernel channels wont match: (%d vs %d).",
- channels, channels_kernel * group);
-
- const int height_out =
- (height + 2 * pad_h - (dilation_h * (kernel_h - 1) + 1)) / stride_h + 1;
- const int width_out =
- (width + 2 * pad_w - (dilation_w * (kernel_w - 1) + 1)) / stride_w + 1;
-
- if (ones.ndimension() != 2 ||
- ones.size(0) * ones.size(1) < height_out * width_out) {
- // Resize plane and fill with ones...
- ones = at::ones({height_out, width_out}, input.options());
- }
-
- grad_input = grad_input.view({batch, channels, height, width});
- columns = at::zeros({channels * kernel_h * kernel_w, height_out * width_out},
- input.options());
-
- grad_output =
- grad_output.view({grad_output.size(0), group, grad_output.size(1) / group,
- grad_output.size(2), grad_output.size(3)});
-
- for (int b = 0; b < batch; b++) {
- // divide int group
- columns = columns.view({group, columns.size(0) / group, columns.size(1)});
- weight = weight.view({group, weight.size(0) / group, weight.size(1),
- weight.size(2), weight.size(3)});
-
- for (int g = 0; g < group; g++) {
- columns[g].addmm_(weight[g].flatten(1).transpose(0, 1),
- grad_output[b][g].flatten(1), 0.0f, 1.0f);
- }
-
- columns =
- columns.view({columns.size(0) * columns.size(1), columns.size(2)});
- weight = weight.view({weight.size(0) * weight.size(1), weight.size(2),
- weight.size(3), weight.size(4)});
-
- // gradient w.r.t. input coordinate data
- modulated_deformable_col2im_coord_cuda(
- columns, input[b], offset[b], mask[b], 1, channels, height, width,
- height_out, width_out, kernel_h, kernel_w, pad_h, pad_w, stride_h,
- stride_w, dilation_h, dilation_w, deformable_group, grad_offset[b],
- grad_mask[b]);
- // gradient w.r.t. input data
- modulated_deformable_col2im_cuda(
- columns, offset[b], mask[b], 1, channels, height, width, height_out,
- width_out, kernel_h, kernel_w, pad_h, pad_w, stride_h, stride_w,
- dilation_h, dilation_w, deformable_group, grad_input[b]);
-
- // gradient w.r.t. weight, dWeight should accumulate across the batch and
- // group
- modulated_deformable_im2col_cuda(
- input[b], offset[b], mask[b], 1, channels, height, width, height_out,
- width_out, kernel_h, kernel_w, pad_h, pad_w, stride_h, stride_w,
- dilation_h, dilation_w, deformable_group, columns);
-
- columns = columns.view({group, columns.size(0) / group, columns.size(1)});
- grad_weight = grad_weight.view({group, grad_weight.size(0) / group,
- grad_weight.size(1), grad_weight.size(2),
- grad_weight.size(3)});
- if (with_bias)
- grad_bias = grad_bias.view({group, grad_bias.size(0) / group});
-
- for (int g = 0; g < group; g++) {
- grad_weight[g] =
- grad_weight[g]
- .flatten(1)
- .addmm_(grad_output[b][g].flatten(1), columns[g].transpose(0, 1))
- .view_as(grad_weight[g]);
- if (with_bias) {
- grad_bias[g] =
- grad_bias[g]
- .view({-1, 1})
- .addmm_(grad_output[b][g].flatten(1), ones.view({-1, 1}))
- .view(-1);
- }
- }
-
- columns =
- columns.view({columns.size(0) * columns.size(1), columns.size(2)});
- grad_weight = grad_weight.view({grad_weight.size(0) * grad_weight.size(1),
- grad_weight.size(2), grad_weight.size(3),
- grad_weight.size(4)});
- if (with_bias)
- grad_bias = grad_bias.view({grad_bias.size(0) * grad_bias.size(1)});
- }
- grad_output = grad_output.view({grad_output.size(0) * grad_output.size(1),
- grad_output.size(2), grad_output.size(3),
- grad_output.size(4)});
-}
diff --git a/spaces/cvlab/zero123-live/ldm/modules/distributions/distributions.py b/spaces/cvlab/zero123-live/ldm/modules/distributions/distributions.py
deleted file mode 100644
index f2b8ef901130efc171aa69742ca0244d94d3f2e9..0000000000000000000000000000000000000000
--- a/spaces/cvlab/zero123-live/ldm/modules/distributions/distributions.py
+++ /dev/null
@@ -1,92 +0,0 @@
-import torch
-import numpy as np
-
-
-class AbstractDistribution:
- def sample(self):
- raise NotImplementedError()
-
- def mode(self):
- raise NotImplementedError()
-
-
-class DiracDistribution(AbstractDistribution):
- def __init__(self, value):
- self.value = value
-
- def sample(self):
- return self.value
-
- def mode(self):
- return self.value
-
-
-class DiagonalGaussianDistribution(object):
- def __init__(self, parameters, deterministic=False):
- self.parameters = parameters
- self.mean, self.logvar = torch.chunk(parameters, 2, dim=1)
- self.logvar = torch.clamp(self.logvar, -30.0, 20.0)
- self.deterministic = deterministic
- self.std = torch.exp(0.5 * self.logvar)
- self.var = torch.exp(self.logvar)
- if self.deterministic:
- self.var = self.std = torch.zeros_like(self.mean).to(device=self.parameters.device)
-
- def sample(self):
- x = self.mean + self.std * torch.randn(self.mean.shape).to(device=self.parameters.device)
- return x
-
- def kl(self, other=None):
- if self.deterministic:
- return torch.Tensor([0.])
- else:
- if other is None:
- return 0.5 * torch.sum(torch.pow(self.mean, 2)
- + self.var - 1.0 - self.logvar,
- dim=[1, 2, 3])
- else:
- return 0.5 * torch.sum(
- torch.pow(self.mean - other.mean, 2) / other.var
- + self.var / other.var - 1.0 - self.logvar + other.logvar,
- dim=[1, 2, 3])
-
- def nll(self, sample, dims=[1,2,3]):
- if self.deterministic:
- return torch.Tensor([0.])
- logtwopi = np.log(2.0 * np.pi)
- return 0.5 * torch.sum(
- logtwopi + self.logvar + torch.pow(sample - self.mean, 2) / self.var,
- dim=dims)
-
- def mode(self):
- return self.mean
-
-
-def normal_kl(mean1, logvar1, mean2, logvar2):
- """
- source: https://github.com/openai/guided-diffusion/blob/27c20a8fab9cb472df5d6bdd6c8d11c8f430b924/guided_diffusion/losses.py#L12
- Compute the KL divergence between two gaussians.
- Shapes are automatically broadcasted, so batches can be compared to
- scalars, among other use cases.
- """
- tensor = None
- for obj in (mean1, logvar1, mean2, logvar2):
- if isinstance(obj, torch.Tensor):
- tensor = obj
- break
- assert tensor is not None, "at least one argument must be a Tensor"
-
- # Force variances to be Tensors. Broadcasting helps convert scalars to
- # Tensors, but it does not work for torch.exp().
- logvar1, logvar2 = [
- x if isinstance(x, torch.Tensor) else torch.tensor(x).to(tensor)
- for x in (logvar1, logvar2)
- ]
-
- return 0.5 * (
- -1.0
- + logvar2
- - logvar1
- + torch.exp(logvar1 - logvar2)
- + ((mean1 - mean2) ** 2) * torch.exp(-logvar2)
- )
diff --git a/spaces/cybercorejapan/human-detection-docker/models/trackers/reid_parallel_tracker/base_tracker.py b/spaces/cybercorejapan/human-detection-docker/models/trackers/reid_parallel_tracker/base_tracker.py
deleted file mode 100644
index c8d505b72f309c9f94a4f04f705e169c88efc290..0000000000000000000000000000000000000000
--- a/spaces/cybercorejapan/human-detection-docker/models/trackers/reid_parallel_tracker/base_tracker.py
+++ /dev/null
@@ -1,315 +0,0 @@
-
-from typing import List, Tuple, Dict
-import numpy as np
-from .core.tracklet import (Tracklet, TrackState, add_stracks, subtract_stracks, remove_duplicate_stracks)
-from .core.kalman_filter import KalmanFilter
-from .core.basetrack import BaseTrack
-from .core.matching import iou_scores
-
-class BaseTracker(object):
- def __init__(self,
- det_thr=dict(high=0.3,low=0.1, min_height=10, min_width=10),
- new_track_cfg = dict(active_thr=0.9, active_iou=0.7, thr=0.4, min_size=(10,5), feat_buffer=30),
- lost_track_cfg = dict(max_length=32, min_size=(10,5)),
- smooth_update = False,
- ):
- """ Base class for SORT tracker
-
- Args:
- det_thr (dict, optional):
- + high: threshold score to consider highly confident detection.
- Defaults to 0.3.
- + low : threshold score to consider low confident detection.
- Detection with lower score than this threshold are ignored.
- Defaults to 0.1.
-
- new_track_cfg (dict, optional): Config for initializing new track.
- + thr (float, optional): threshold to initialize new track.
- A detection with score higher than this threshold will be initialized as a new (unconfirmed) track if it does not match with any tracks.
- Defaults to 0.4.
- + active_thr (float, optional): threshold to activate a new track.
- + active_iou (float, optional): threshold to activate a new track. A new track (score > active)thr and iou < active_iou) is a high confident detection without significant overlap with other objects are activated immediatly without confirming in the next frames.
- + min_size (tuple, optional): minimum (high,width) of the bounding box to be considered as new track.
- Defaults to (80,40).
- + feat_buffer (int, optional): number of frames to store the features of the new track.
- lost_track_cfg (dict, optional): Config for lost track.
- + max_length (int): number of frames that the lost tracks are keep before being removed. It is also the length of buffer to store the features.
- Defaults to 30.
- + min_size (tuple, optional): If the lost object size smaller than this min_size(high,width) will be removed.
- Defaults to (40,20).
- + tracking_region (x1,y1,x2,y2): Top-Left, Bottom-right coordinates of the tracking region. If objects move out of this region, they will be removed.
- smooth_update (bool, optional): If True, when a lost object is refind, we interpolate its missing coordinate during lost, and use these interpolated bboxes to update Kalman Filter. Thus, avoid excessive gain when updating the Kalman filter (smoother).
- """
- self.tracked_stracks = [] # type: list[Tracklet]
- self.lost_stracks = [] # type: list[Tracklet]
- self.removed_stracks = [] # type: list[Tracklet]
- BaseTrack.clear_count()
-
- self.frame_id = 0
- self.det_thr = det_thr
- self.new_track_cfg = new_track_cfg
- self.lost_track_cfg = lost_track_cfg
-
-
- self.kalman_filter = KalmanFilter()
- self.smooth_update = smooth_update
-
- def preprocess_det_result(self,det_results: Dict[str, np.ndarray]) -> Dict[str, np.ndarray]:
- boxes = det_results['boxes']
- boxes = boxes.reshape(-1, 5)
- h = boxes[:,3]-boxes[:,1]
- w = boxes[:,2]-boxes[:,0]
- valid_inds = np.logical_and(h>self.det_thr["min_height"], w>self.det_thr["min_width"])
- for k,v in det_results.items():
- if k in ['boxes', 'labels', 'angles', 'obj_imgs', 'embeddings']:
- if k == 'obj_imgs':
- det_results[k] = [v[_i] for _i, _valid in enumerate(valid_inds) if _valid]
- else:
- det_results[k] = v[valid_inds]
- return det_results
-
- def split_detections_by_scores(self,
- det_result: Dict[str, np.ndarray])-> Tuple[List[Tracklet], List[Tracklet]]:
- """ Split the detections into high score/lower score group.
- det_result is a dict of {'boxes': np.ndarray(x1,y1,x2,y2,score), 'labels': np.ndarray}
- Return:
- detections_high: list[Tracklet]
- detections_low: list[Tracklet]
- """
- detections_high = []
- detections_low = []
-
- feat_history = self.new_track_cfg["feat_buffer"]
- if len(det_result['boxes']):
- bboxes = det_result['boxes'][:, :4]
- scores = det_result['boxes'][:, 4]
- classes = det_result['labels']
- angles = np.array(det_result.get('angles',[None]*len(scores)))
- features = np.array(det_result.get('embeddings',[None]*len(scores)))
- obj_imgs = np.array(det_result.get('obj_imgs',[None]*len(scores)))
-
- # Find high threshold detections
- inds_high = scores >= self.det_thr["high"]
-
- enable_reid_buffer = False
- if hasattr(self, 'enable_reid_buffer'):
- enable_reid_buffer = self.enable_reid_buffer
-
- if np.any(inds_high):
- detections_high = [Tracklet(Tracklet.tlbr_to_tlwh(tlbr), s, c, a, feat,feat_history=feat_history,
- obj_img=obj_img, enable_buffer=enable_reid_buffer) for
- (tlbr, s, c, a ,feat, obj_img) in zip(bboxes[inds_high], scores[inds_high], classes[inds_high],
- angles[inds_high], features[inds_high], obj_imgs[inds_high])]
- # Find low threshold detections
- inds_low = np.logical_and(scores > self.det_thr["low"],
- scores < self.det_thr["high"])
- if np.any(inds_low):
- detections_low = [Tracklet(Tracklet.tlbr_to_tlwh(tlbr), s, c, a, feat, feat_history=feat_history,
- obj_img=obj_img, enable_buffer=enable_reid_buffer) for
- (tlbr, s, c, a, feat, obj_img) in zip(bboxes[inds_low], scores[inds_low], classes[inds_low],
- angles[inds_low], features[inds_low], obj_imgs[inds_low])]
-
- return detections_high, detections_low
-
- def split_tracks_by_activation(self) -> Tuple[List[Tracklet], List[Tracklet]]:
- """ Split the tracks into trackpool=(tracked_tracks + lost_tracks) and unconfirmed (just initialize)
- Returns:
- strack_pool: List[Tracklet]
- unconfirmed: List[Tracklet]
- """
- unconfirmed = []
- tracked_stracks = [] # type: list[Tracklet]
- for track in self.tracked_stracks:
- if not track.is_activated:
- unconfirmed.append(track)
- else:
- tracked_stracks.append(track)
- strack_pool = add_stracks(tracked_stracks, self.lost_stracks)
- return strack_pool, unconfirmed
-
- def predict_with_gmc(self,
- strack_pool: List[Tracklet],
- unconfirmed: List[Tracklet],
- Hmat: np.array=None) -> None:
- """ Predict the current location with KF, and compensate for Camera Motion
-
- Args:
- strack_pool (List[Tracklet]): list of tracked tracks
- unconfirmed (List[Tracklet]): list of unconfirmed tracks
- Hmat (np.array): Homography transformation matrix
- """
- Tracklet.multi_predict(strack_pool)
- if Hmat is not None:
- Tracklet.multi_gmc(strack_pool,Hmat)
- Tracklet.multi_gmc(unconfirmed,Hmat)
-
- def update_matched_tracks(self,
- matches: np.ndarray,
- strack_pool: List[Tracklet],
- detections: List[Tracklet])-> Tuple[List[Tracklet], List[Tracklet]]:
- """Update the matched tracks with Kalman Filter
-
- Args:
- matches (np.ndarray): [Nx2] index of the matched tracks and detections
- strack_pool (List[Tracklet]): List of tracked tracks
- detections (List[Tracklet]): List of detections
-
- Returns:
- activated_stracks (List[Tracklet]): List of tracked tracks that continue to be tracked (activated)
- refind_stracks (List[Tracklet]): List of lost tracks that are refound in this frame (refind)
- """
- activated_stracks,refind_stracks=[],[]
- for itracked, idet in matches:
- track = strack_pool[itracked]
- det = detections[idet]
- if track.state == TrackState.Tracked:
- #Perform Kalman Update/Feature Update
- if self.smooth_update:
- track.smooth_update(det, self.frame_id)
- else:
- track.update(det, self.frame_id)
- activated_stracks.append(track)
- else:
- track.re_activate(det, self.frame_id, new_id=False)
- refind_stracks.append(track)
-
- return activated_stracks,refind_stracks
-
- def init_new_tracks(self,
- detections: List[Tracklet],
- u_detection: np.ndarray)-> List[Tracklet]:
- """Initialize new tracks
-
- Args:
- detections (List[Tracklet]): List of detection objects
- u_detection (np.ndarray): indices of the detections that are not matched with any tracks,
- and are considerd as new detections if its score is high enough
- Returns:
- List[Tracklet]: List of new tracks
- """
- new_tracks= []
- for inew in u_detection:
- det_= detections[inew]
- if det_.score >=self.new_track_cfg["thr"] and (not det_.is_too_small(self.new_track_cfg["min_size"])):
- new_tracks.append(det_)
- # The activate function will initialize the new tracks with a new id
- for track in new_tracks:
- # By default, new_track status is Unconfirmed (is_activated = False), except for the first frame
- track.activate(self.kalman_filter, self.frame_id)
- return new_tracks
-
- def activate_new_tracks(self,new_tracks, current_tracks):
- ious =iou_scores(new_tracks, current_tracks)
- iou_max = ious.max(axis=1) if ious.shape[1]>0 else np.zeros(len(new_tracks))
- active_thr = self.new_track_cfg.get('active_thr',0.7)
- active_iou = self.new_track_cfg.get('active_iou',0.5)
- for track, iou in zip(new_tracks, iou_max):
- # For very high confident detection and non-overlap objects, we can activate it directly
- if track.score >= active_thr and iou < active_iou:
- track.mark_activated()
-
- def remove_lost_tracks(self):
- removed_stracks=[]
- """ Remove lost tracks if they are already lost for a certain conditions"""
- for track in self.lost_stracks:
- is_expired = track.is_expired(self.frame_id, self.lost_track_cfg["max_length"])
- is_out_border, is_too_small = False, False
- if self.lost_track_cfg.get('tracking_region',None) is not None:
- is_out_border = track.is_out_border(self.lost_track_cfg["tracking_region"])
- if self.lost_track_cfg.get('min_size',None) is not None:
- is_too_small = track.is_too_small(self.lost_track_cfg["min_size"])
-
- if is_expired or is_out_border or is_too_small:
- track.mark_removed()
- removed_stracks.append(track)
- return removed_stracks
-
- def merge_results(self,
- activated_stracks: List[Tracklet],
- refind_stracks: List[Tracklet],
- new_stracks: List[Tracklet],
- removed_stracks: List[Tracklet]) -> Tuple[Dict, Dict]:
- """ Merge the results from different types of tracks into the final results
-
- Args:
- activated_stracks (List[Tracklet]): activated tracks
- refind_stracks (List[Tracklet]): refind tracks
- removed_stracks (List[Tracklet]): removed tracks
-
- Returns:
- active_tracks (dict): dict of active tracks in the current frame. See format_track_results for the format.
- lost_tracks (dict): dict of lost tracks in the current frame. See format_track_results for the format.
- """
- self.tracked_stracks = [t for t in self.tracked_stracks if t.state == TrackState.Tracked]
- self.tracked_stracks = add_stracks(self.tracked_stracks, activated_stracks)
- self.tracked_stracks = add_stracks(self.tracked_stracks, refind_stracks)
- self.tracked_stracks = add_stracks(self.tracked_stracks, new_stracks)
- self.lost_stracks = subtract_stracks(self.lost_stracks, self.tracked_stracks)
- self.lost_stracks = subtract_stracks(self.lost_stracks, self.removed_stracks)
- self.removed_stracks.extend(removed_stracks)
- self.tracked_stracks, self.lost_stracks = remove_duplicate_stracks(self.tracked_stracks, self.lost_stracks)
-
- active_tracks = [track for track in self.tracked_stracks if track.is_activated]
- active_tracks = self.format_track_results(active_tracks) if len(active_tracks)>0 else None
- lost_tracks = self.format_track_results(self.lost_stracks) if len(self.lost_stracks)>0 else None
- return active_tracks, lost_tracks
-
- def format_track_results(self,
- tracklets: List[Tracklet]) -> Dict[str, np.ndarray]:
- """Format the tracking results to the required format
-
- Args:
- tracklets (Lost): _description_
-
- Returns:
- _type_: _description_
- """
- tlbrs = []
- ids = []
- scores = []
- cls = []
- vel = []
- angles = []
- for t in tracklets:
- tlbrs.append(t.tlbr)
- ids.append(t.track_id)
- scores.append(t.score)
- cls.append(t.cls)
- vel.append(t.vel_dir)
- angles.append(t.angle)
-
- track_outputs={
- "boxes": np.concatenate([np.array(tlbrs), np.expand_dims(np.array(scores), axis=1)], axis=1),
- "labels": np.array(cls),
- "ids": np.array(ids),
- "velocity": np.array(vel), # motion velocity
- "angles": np.array(angles), # body orientation
- }
- return track_outputs
-
- def update(self,
- det_result: Dict,
- Hmat: np.array=None,
- meta_data: Dict=None) -> Tuple[Dict, Dict]:
- """ The main function to perform tracking, which may includes the follow steps:
- 1. Split the detections into high score/lower score group:
- - split_detections_by_scores
- 2. Split the tracks into trackpool=(tracked_tracks + lost_tracks) and unconfirmed (just initialize).
- - split_tracks_by_activation
- - predict_with_gmc: predict the current location of these tracklets with KF, and compensate for Camera Motion
- 3. First association with high score detection boxes:
- - matcher_high
- - update_matched_tracks
- 4. Second association with low score detection boxes
- - matcher_low
- - update_matched_tracks if they are activated or refind
- - mark new lost tracks
- 5. Third association, between new detections and unconfirmed tracks (usually tracks with only one beginning frame)
- - matcher_unconfirmed
- - remove unconfirmed tracks that does not match any detections
- - init new track if the unconfirmed track is matched with a detection
- 6. Remove lost tracks if they are already lost for a certain frames
- 7. Update status for these trackes: active, lost, removed, uncofirmed.
- Merge results and format the results
- """
- raise NotImplementedError
diff --git a/spaces/cymic/VITS-Tokaiteio/monotonic_align/__init__.py b/spaces/cymic/VITS-Tokaiteio/monotonic_align/__init__.py
deleted file mode 100644
index 3d7009c40fea3a98168e3e3bc9ae061e91327422..0000000000000000000000000000000000000000
--- a/spaces/cymic/VITS-Tokaiteio/monotonic_align/__init__.py
+++ /dev/null
@@ -1,19 +0,0 @@
-import numpy as np
-import torch
-from .monotonic_align.core import maximum_path_c
-
-
-def maximum_path(neg_cent, mask):
- """ Cython optimized version.
- neg_cent: [b, t_t, t_s]
- mask: [b, t_t, t_s]
- """
- device = neg_cent.device
- dtype = neg_cent.dtype
- neg_cent = neg_cent.data.cpu().numpy().astype(np.float32)
- path = np.zeros(neg_cent.shape, dtype=np.int32)
-
- t_t_max = mask.sum(1)[:, 0].data.cpu().numpy().astype(np.int32)
- t_s_max = mask.sum(2)[:, 0].data.cpu().numpy().astype(np.int32)
- maximum_path_c(path, neg_cent, t_t_max, t_s_max)
- return torch.from_numpy(path).to(device=device, dtype=dtype)
diff --git a/spaces/cymic/Waifu_Diffusion_Webui/style.css b/spaces/cymic/Waifu_Diffusion_Webui/style.css
deleted file mode 100644
index e60a454562d417594600e60abae6450847ea938b..0000000000000000000000000000000000000000
--- a/spaces/cymic/Waifu_Diffusion_Webui/style.css
+++ /dev/null
@@ -1,413 +0,0 @@
-.output-html p {margin: 0 0.5em;}
-
-.row > *,
-.row > .gr-form > * {
- min-width: min(120px, 100%);
- flex: 1 1 0%;
-}
-
-.performance {
- font-size: 0.85em;
- color: #444;
- display: flex;
- justify-content: space-between;
- white-space: nowrap;
-}
-
-.performance .time {
- margin-right: 0;
-}
-
-.performance .vram {
- margin-left: 0;
- text-align: right;
-}
-
-#txt2img_generate, #img2img_generate {
- min-height: 4.5em;
-}
-
-@media screen and (min-width: 2500px) {
- #txt2img_gallery, #img2img_gallery {
- min-height: 768px;
- }
-}
-
-#txt2img_gallery img, #img2img_gallery img{
- object-fit: scale-down;
-}
-
-.justify-center.overflow-x-scroll {
- justify-content: left;
-}
-
-.justify-center.overflow-x-scroll button:first-of-type {
- margin-left: auto;
-}
-
-.justify-center.overflow-x-scroll button:last-of-type {
- margin-right: auto;
-}
-
-#random_seed, #random_subseed, #reuse_seed, #reuse_subseed, #open_folder{
- min-width: auto;
- flex-grow: 0;
- padding-left: 0.25em;
- padding-right: 0.25em;
-}
-
-#hidden_element{
- display: none;
-}
-
-#seed_row, #subseed_row{
- gap: 0.5rem;
-}
-
-#subseed_show_box{
- min-width: auto;
- flex-grow: 0;
-}
-
-#subseed_show_box > div{
- border: 0;
- height: 100%;
-}
-
-#subseed_show{
- min-width: auto;
- flex-grow: 0;
- padding: 0;
-}
-
-#subseed_show label{
- height: 100%;
-}
-
-#roll_col{
- min-width: unset !important;
- flex-grow: 0 !important;
- padding: 0.4em 0;
-}
-
-#roll, #paste{
- min-width: 2em;
- min-height: 2em;
- max-width: 2em;
- max-height: 2em;
- flex-grow: 0;
- padding-left: 0.25em;
- padding-right: 0.25em;
- margin: 0.1em 0;
-}
-
-#style_apply, #style_create, #interrogate{
- margin: 0.75em 0.25em 0.25em 0.25em;
- min-width: 3em;
-}
-
-#style_pos_col, #style_neg_col{
- min-width: 8em !important;
-}
-
-#txt2img_style_index, #txt2img_style2_index, #img2img_style_index, #img2img_style2_index{
- margin-top: 1em;
-}
-
-.gr-form{
- background: transparent;
-}
-
-.my-4{
- margin-top: 0;
- margin-bottom: 0;
-}
-
-#toprow div{
- border: none;
- gap: 0;
- background: transparent;
-}
-
-#resize_mode{
- flex: 1.5;
-}
-
-button{
- align-self: stretch !important;
-}
-
-#prompt, #negative_prompt{
- border: none !important;
-}
-#prompt textarea, #negative_prompt textarea{
- border: none !important;
-}
-
-
-#img2maskimg .h-60{
- height: 30rem;
-}
-
-.overflow-hidden, .gr-panel{
- overflow: visible !important;
-}
-
-#x_type, #y_type{
- max-width: 10em;
-}
-
-#txt2img_preview, #img2img_preview, #ti_preview{
- position: absolute;
- width: 320px;
- left: 0;
- right: 0;
- margin-left: auto;
- margin-right: auto;
- margin-top: 34px;
- z-index: 100;
- border: none;
- border-top-left-radius: 0;
- border-top-right-radius: 0;
-}
-
-@media screen and (min-width: 768px) {
- #txt2img_preview, #img2img_preview, #ti_preview {
- position: absolute;
- }
-}
-
-@media screen and (max-width: 767px) {
- #txt2img_preview, #img2img_preview, #ti_preview {
- position: relative;
- }
-}
-
-#txt2img_preview div.left-0.top-0, #img2img_preview div.left-0.top-0, #ti_preview div.left-0.top-0{
- display: none;
-}
-
-fieldset span.text-gray-500, .gr-block.gr-box span.text-gray-500, label.block span{
- position: absolute;
- top: -0.6em;
- line-height: 1.2em;
- padding: 0 0.5em;
- margin: 0;
-
- background-color: white;
- border-top: 1px solid #eee;
- border-left: 1px solid #eee;
- border-right: 1px solid #eee;
-}
-
-.dark fieldset span.text-gray-500, .dark .gr-block.gr-box span.text-gray-500, .dark label.block span{
- background-color: rgb(31, 41, 55);
- border-top: 1px solid rgb(55 65 81);
- border-left: 1px solid rgb(55 65 81);
- border-right: 1px solid rgb(55 65 81);
-}
-
-#settings fieldset span.text-gray-500, #settings .gr-block.gr-box span.text-gray-500, #settings label.block span{
- position: relative;
- border: none;
-}
-
-.gr-panel div.flex-col div.justify-between label span{
- margin: 0;
-}
-
-.gr-panel div.flex-col div.justify-between div{
- position: absolute;
- top: -0.1em;
- right: 1em;
- padding: 0 0.5em;
-}
-
-#settings .gr-panel div.flex-col div.justify-between div{
- position: relative;
- z-index: 200;
-}
-
-input[type="range"]{
- margin: 0.5em 0 -0.3em 0;
-}
-
-#txt2img_sampling label{
- padding-left: 0.6em;
- padding-right: 0.6em;
-}
-
-#mask_bug_info {
- text-align: center;
- display: block;
- margin-top: -0.75em;
- margin-bottom: -0.75em;
-}
-
-#txt2img_negative_prompt, #img2img_negative_prompt{
-}
-
-#txt2img_progressbar, #img2img_progressbar, #ti_progressbar{
- position: absolute;
- z-index: 1000;
- right: 0;
- padding-left: 5px;
- padding-right: 5px;
- display: block;
-}
-
-#txt2img_progress_row, #img2img_progress_row{
- margin-bottom: 10px;
- margin-top: -18px;
-}
-
-.progressDiv{
- width: 100%;
- height: 20px;
- background: #b4c0cc;
- border-radius: 8px;
-}
-
-.dark .progressDiv{
- background: #424c5b;
-}
-
-.progressDiv .progress{
- width: 0%;
- height: 20px;
- background: #0060df;
- color: white;
- font-weight: bold;
- line-height: 20px;
- padding: 0 8px 0 0;
- text-align: right;
- border-radius: 8px;
-}
-
-#lightboxModal{
- display: none;
- position: fixed;
- z-index: 1001;
- padding-top: 100px;
- left: 0;
- top: 0;
- width: 100%;
- height: 100%;
- overflow: auto;
- background-color: rgba(20, 20, 20, 0.95);
-}
-
-.modalControls {
- display: grid;
- grid-template-columns: 32px auto 1fr 32px;
- grid-template-areas: "zoom tile space close";
- position: absolute;
- top: 0;
- left: 0;
- right: 0;
- padding: 16px;
- gap: 16px;
- background-color: rgba(0,0,0,0.2);
-}
-
-.modalClose {
- grid-area: close;
-}
-
-.modalZoom {
- grid-area: zoom;
-}
-
-.modalTileImage {
- grid-area: tile;
-}
-
-.modalClose,
-.modalZoom,
-.modalTileImage {
- color: white;
- font-size: 35px;
- font-weight: bold;
- cursor: pointer;
-}
-
-.modalClose:hover,
-.modalClose:focus,
-.modalZoom:hover,
-.modalZoom:focus {
- color: #999;
- text-decoration: none;
- cursor: pointer;
-}
-
-#modalImage {
- display: block;
- margin-left: auto;
- margin-right: auto;
- margin-top: auto;
- width: auto;
-}
-
-.modalImageFullscreen {
- object-fit: contain;
- height: 90%;
-}
-
-.modalPrev,
-.modalNext {
- cursor: pointer;
- position: absolute;
- top: 50%;
- width: auto;
- padding: 16px;
- margin-top: -50px;
- color: white;
- font-weight: bold;
- font-size: 20px;
- transition: 0.6s ease;
- border-radius: 0 3px 3px 0;
- user-select: none;
- -webkit-user-select: none;
-}
-
-.modalNext {
- right: 0;
- border-radius: 3px 0 0 3px;
-}
-
-.modalPrev:hover,
-.modalNext:hover {
- background-color: rgba(0, 0, 0, 0.8);
-}
-
-#imageARPreview{
- position:absolute;
- top:0px;
- left:0px;
- border:2px solid red;
- background:rgba(255, 0, 0, 0.3);
- z-index: 900;
- pointer-events:none;
- display:none
-}
-
-#txt2img_interrupt, #img2img_interrupt{
- position: absolute;
- width: 100%;
- height: 72px;
- background: #b4c0cc;
- border-radius: 8px;
- display: none;
-}
-
-.red {
- color: red;
-}
-
-.gallery-item {
- --tw-bg-opacity: 0 !important;
-}
-
-#img2img_image div.h-60{
- height: 480px;
-}
\ No newline at end of file
diff --git a/spaces/cymic/Waifu_Diffusion_Webui/webui-user.bat b/spaces/cymic/Waifu_Diffusion_Webui/webui-user.bat
deleted file mode 100644
index e5a257bef06f5bfcaff1c8b33c64a767eb8b3fe5..0000000000000000000000000000000000000000
--- a/spaces/cymic/Waifu_Diffusion_Webui/webui-user.bat
+++ /dev/null
@@ -1,8 +0,0 @@
-@echo off
-
-set PYTHON=
-set GIT=
-set VENV_DIR=
-set COMMANDLINE_ARGS=
-
-call webui.bat
diff --git a/spaces/cynika/taffy/data_utils.py b/spaces/cynika/taffy/data_utils.py
deleted file mode 100644
index 9dfba4a9dfbfbd2b6ed5e771a5ffee4f70419ba3..0000000000000000000000000000000000000000
--- a/spaces/cynika/taffy/data_utils.py
+++ /dev/null
@@ -1,152 +0,0 @@
-import time
-import os
-import random
-import numpy as np
-import torch
-import torch.utils.data
-
-import commons
-from mel_processing import spectrogram_torch, spec_to_mel_torch
-from utils import load_wav_to_torch, load_filepaths_and_text, transform
-
-# import h5py
-
-
-"""Multi speaker version"""
-
-
-class TextAudioSpeakerLoader(torch.utils.data.Dataset):
- """
- 1) loads audio, speaker_id, text pairs
- 2) normalizes text and converts them to sequences of integers
- 3) computes spectrograms from audio files.
- """
-
- def __init__(self, audiopaths, hparams):
- self.audiopaths = load_filepaths_and_text(audiopaths)
- self.max_wav_value = hparams.data.max_wav_value
- self.sampling_rate = hparams.data.sampling_rate
- self.filter_length = hparams.data.filter_length
- self.hop_length = hparams.data.hop_length
- self.win_length = hparams.data.win_length
- self.sampling_rate = hparams.data.sampling_rate
- self.use_sr = hparams.train.use_sr
- self.spec_len = hparams.train.max_speclen
- self.spk_map = hparams.spk
-
- random.seed(1234)
- random.shuffle(self.audiopaths)
-
- def get_audio(self, filename):
- audio, sampling_rate = load_wav_to_torch(filename)
- if sampling_rate != self.sampling_rate:
- raise ValueError("{} SR doesn't match target {} SR".format(
- sampling_rate, self.sampling_rate))
- audio_norm = audio / self.max_wav_value
- audio_norm = audio_norm.unsqueeze(0)
- spec_filename = filename.replace(".wav", ".spec.pt")
- if os.path.exists(spec_filename):
- spec = torch.load(spec_filename)
- else:
- spec = spectrogram_torch(audio_norm, self.filter_length,
- self.sampling_rate, self.hop_length, self.win_length,
- center=False)
- spec = torch.squeeze(spec, 0)
- torch.save(spec, spec_filename)
-
- spk = filename.split(os.sep)[-2]
- spk = torch.LongTensor([self.spk_map[spk]])
-
- c = torch.load(filename + ".soft.pt").squeeze(0)
- c = torch.repeat_interleave(c, repeats=2, dim=1)
-
- f0 = np.load(filename + ".f0.npy")
- f0 = torch.FloatTensor(f0)
- lmin = min(c.size(-1), spec.size(-1), f0.shape[0])
- assert abs(c.size(-1) - spec.size(-1)) < 4, (c.size(-1), spec.size(-1), f0.shape, filename)
- assert abs(lmin - spec.size(-1)) < 4, (c.size(-1), spec.size(-1), f0.shape)
- assert abs(lmin - c.size(-1)) < 4, (c.size(-1), spec.size(-1), f0.shape)
- spec, c, f0 = spec[:, :lmin], c[:, :lmin], f0[:lmin]
- audio_norm = audio_norm[:, :lmin * self.hop_length]
- _spec, _c, _audio_norm, _f0 = spec, c, audio_norm, f0
- while spec.size(-1) < self.spec_len:
- spec = torch.cat((spec, _spec), -1)
- c = torch.cat((c, _c), -1)
- f0 = torch.cat((f0, _f0), -1)
- audio_norm = torch.cat((audio_norm, _audio_norm), -1)
- start = random.randint(0, spec.size(-1) - self.spec_len)
- end = start + self.spec_len
- spec = spec[:, start:end]
- c = c[:, start:end]
- f0 = f0[start:end]
- audio_norm = audio_norm[:, start * self.hop_length:end * self.hop_length]
-
- return c, f0, spec, audio_norm, spk
-
- def __getitem__(self, index):
- return self.get_audio(self.audiopaths[index][0])
-
- def __len__(self):
- return len(self.audiopaths)
-
-
-class EvalDataLoader(torch.utils.data.Dataset):
- """
- 1) loads audio, speaker_id, text pairs
- 2) normalizes text and converts them to sequences of integers
- 3) computes spectrograms from audio files.
- """
-
- def __init__(self, audiopaths, hparams):
- self.audiopaths = load_filepaths_and_text(audiopaths)
- self.max_wav_value = hparams.data.max_wav_value
- self.sampling_rate = hparams.data.sampling_rate
- self.filter_length = hparams.data.filter_length
- self.hop_length = hparams.data.hop_length
- self.win_length = hparams.data.win_length
- self.sampling_rate = hparams.data.sampling_rate
- self.use_sr = hparams.train.use_sr
- self.audiopaths = self.audiopaths[:5]
- self.spk_map = hparams.spk
-
-
- def get_audio(self, filename):
- audio, sampling_rate = load_wav_to_torch(filename)
- if sampling_rate != self.sampling_rate:
- raise ValueError("{} SR doesn't match target {} SR".format(
- sampling_rate, self.sampling_rate))
- audio_norm = audio / self.max_wav_value
- audio_norm = audio_norm.unsqueeze(0)
- spec_filename = filename.replace(".wav", ".spec.pt")
- if os.path.exists(spec_filename):
- spec = torch.load(spec_filename)
- else:
- spec = spectrogram_torch(audio_norm, self.filter_length,
- self.sampling_rate, self.hop_length, self.win_length,
- center=False)
- spec = torch.squeeze(spec, 0)
- torch.save(spec, spec_filename)
-
- spk = filename.split(os.sep)[-2]
- spk = torch.LongTensor([self.spk_map[spk]])
-
- c = torch.load(filename + ".soft.pt").squeeze(0)
-
- c = torch.repeat_interleave(c, repeats=2, dim=1)
-
- f0 = np.load(filename + ".f0.npy")
- f0 = torch.FloatTensor(f0)
- lmin = min(c.size(-1), spec.size(-1), f0.shape[0])
- assert abs(c.size(-1) - spec.size(-1)) < 4, (c.size(-1), spec.size(-1), f0.shape)
- assert abs(f0.shape[0] - spec.shape[-1]) < 4, (c.size(-1), spec.size(-1), f0.shape)
- spec, c, f0 = spec[:, :lmin], c[:, :lmin], f0[:lmin]
- audio_norm = audio_norm[:, :lmin * self.hop_length]
-
- return c, f0, spec, audio_norm, spk
-
- def __getitem__(self, index):
- return self.get_audio(self.audiopaths[index][0])
-
- def __len__(self):
- return len(self.audiopaths)
-
diff --git a/spaces/dcarpintero/nlp-summarizer-pegasus/.venv/lib/python3.9/site-packages/fontTools/cu2qu/cli.py b/spaces/dcarpintero/nlp-summarizer-pegasus/.venv/lib/python3.9/site-packages/fontTools/cu2qu/cli.py
deleted file mode 100644
index 9144043ff176fb956cf075b5db38fcca88258430..0000000000000000000000000000000000000000
--- a/spaces/dcarpintero/nlp-summarizer-pegasus/.venv/lib/python3.9/site-packages/fontTools/cu2qu/cli.py
+++ /dev/null
@@ -1,198 +0,0 @@
-import os
-import argparse
-import logging
-import shutil
-import multiprocessing as mp
-from contextlib import closing
-from functools import partial
-
-import fontTools
-from .ufo import font_to_quadratic, fonts_to_quadratic
-
-ufo_module = None
-try:
- import ufoLib2 as ufo_module
-except ImportError:
- try:
- import defcon as ufo_module
- except ImportError as e:
- pass
-
-
-logger = logging.getLogger("fontTools.cu2qu")
-
-
-def _cpu_count():
- try:
- return mp.cpu_count()
- except NotImplementedError: # pragma: no cover
- return 1
-
-
-def open_ufo(path):
- if hasattr(ufo_module.Font, "open"): # ufoLib2
- return ufo_module.Font.open(path)
- return ufo_module.Font(path) # defcon
-
-
-def _font_to_quadratic(input_path, output_path=None, **kwargs):
- ufo = open_ufo(input_path)
- logger.info("Converting curves for %s", input_path)
- if font_to_quadratic(ufo, **kwargs):
- logger.info("Saving %s", output_path)
- if output_path:
- ufo.save(output_path)
- else:
- ufo.save() # save in-place
- elif output_path:
- _copytree(input_path, output_path)
-
-
-def _samepath(path1, path2):
- # TODO on python3+, there's os.path.samefile
- path1 = os.path.normcase(os.path.abspath(os.path.realpath(path1)))
- path2 = os.path.normcase(os.path.abspath(os.path.realpath(path2)))
- return path1 == path2
-
-
-def _copytree(input_path, output_path):
- if _samepath(input_path, output_path):
- logger.debug("input and output paths are the same file; skipped copy")
- return
- if os.path.exists(output_path):
- shutil.rmtree(output_path)
- shutil.copytree(input_path, output_path)
-
-
-def main(args=None):
- """Convert a UFO font from cubic to quadratic curves"""
- parser = argparse.ArgumentParser(prog="cu2qu")
- parser.add_argument("--version", action="version", version=fontTools.__version__)
- parser.add_argument(
- "infiles",
- nargs="+",
- metavar="INPUT",
- help="one or more input UFO source file(s).",
- )
- parser.add_argument("-v", "--verbose", action="count", default=0)
- parser.add_argument(
- "-e",
- "--conversion-error",
- type=float,
- metavar="ERROR",
- default=None,
- help="maxiumum approximation error measured in EM (default: 0.001)",
- )
- parser.add_argument(
- "-m",
- "--mixed",
- default=False,
- action="store_true",
- help="whether to used mixed quadratic and cubic curves",
- )
- parser.add_argument(
- "--keep-direction",
- dest="reverse_direction",
- action="store_false",
- help="do not reverse the contour direction",
- )
-
- mode_parser = parser.add_mutually_exclusive_group()
- mode_parser.add_argument(
- "-i",
- "--interpolatable",
- action="store_true",
- help="whether curve conversion should keep interpolation compatibility",
- )
- mode_parser.add_argument(
- "-j",
- "--jobs",
- type=int,
- nargs="?",
- default=1,
- const=_cpu_count(),
- metavar="N",
- help="Convert using N multiple processes (default: %(default)s)",
- )
-
- output_parser = parser.add_mutually_exclusive_group()
- output_parser.add_argument(
- "-o",
- "--output-file",
- default=None,
- metavar="OUTPUT",
- help=(
- "output filename for the converted UFO. By default fonts are "
- "modified in place. This only works with a single input."
- ),
- )
- output_parser.add_argument(
- "-d",
- "--output-dir",
- default=None,
- metavar="DIRECTORY",
- help="output directory where to save converted UFOs",
- )
-
- options = parser.parse_args(args)
-
- if ufo_module is None:
- parser.error("Either ufoLib2 or defcon are required to run this script.")
-
- if not options.verbose:
- level = "WARNING"
- elif options.verbose == 1:
- level = "INFO"
- else:
- level = "DEBUG"
- logging.basicConfig(level=level)
-
- if len(options.infiles) > 1 and options.output_file:
- parser.error("-o/--output-file can't be used with multile inputs")
-
- if options.output_dir:
- output_dir = options.output_dir
- if not os.path.exists(output_dir):
- os.mkdir(output_dir)
- elif not os.path.isdir(output_dir):
- parser.error("'%s' is not a directory" % output_dir)
- output_paths = [
- os.path.join(output_dir, os.path.basename(p)) for p in options.infiles
- ]
- elif options.output_file:
- output_paths = [options.output_file]
- else:
- # save in-place
- output_paths = [None] * len(options.infiles)
-
- kwargs = dict(
- dump_stats=options.verbose > 0,
- max_err_em=options.conversion_error,
- reverse_direction=options.reverse_direction,
- all_quadratic=False if options.mixed else True,
- )
-
- if options.interpolatable:
- logger.info("Converting curves compatibly")
- ufos = [open_ufo(infile) for infile in options.infiles]
- if fonts_to_quadratic(ufos, **kwargs):
- for ufo, output_path in zip(ufos, output_paths):
- logger.info("Saving %s", output_path)
- if output_path:
- ufo.save(output_path)
- else:
- ufo.save()
- else:
- for input_path, output_path in zip(options.infiles, output_paths):
- if output_path:
- _copytree(input_path, output_path)
- else:
- jobs = min(len(options.infiles), options.jobs) if options.jobs > 1 else 1
- if jobs > 1:
- func = partial(_font_to_quadratic, **kwargs)
- logger.info("Running %d parallel processes", jobs)
- with closing(mp.Pool(jobs)) as pool:
- pool.starmap(func, zip(options.infiles, output_paths))
- else:
- for input_path, output_path in zip(options.infiles, output_paths):
- _font_to_quadratic(input_path, output_path, **kwargs)
diff --git a/spaces/dcarpintero/nlp-summarizer-pegasus/.venv/lib/python3.9/site-packages/fontTools/cu2qu/errors.py b/spaces/dcarpintero/nlp-summarizer-pegasus/.venv/lib/python3.9/site-packages/fontTools/cu2qu/errors.py
deleted file mode 100644
index fa3dc42937131c5db54890dde8f519b15f5d0ff1..0000000000000000000000000000000000000000
--- a/spaces/dcarpintero/nlp-summarizer-pegasus/.venv/lib/python3.9/site-packages/fontTools/cu2qu/errors.py
+++ /dev/null
@@ -1,77 +0,0 @@
-# Copyright 2016 Google Inc. All Rights Reserved.
-#
-# Licensed under the Apache License, Version 2.0 (the "License");
-# you may not use this file except in compliance with the License.
-# You may obtain a copy of the License at
-#
-# http://www.apache.org/licenses/LICENSE-2.0
-#
-# Unless required by applicable law or agreed to in writing, software
-# distributed under the License is distributed on an "AS IS" BASIS,
-# WITHOUT WARRANTIES OR CONDITIONS OF ANY KIND, either express or implied.
-# See the License for the specific language governing permissions and
-# limitations under the License.
-
-
-class Error(Exception):
- """Base Cu2Qu exception class for all other errors."""
-
-
-class ApproxNotFoundError(Error):
- def __init__(self, curve):
- message = "no approximation found: %s" % curve
- super().__init__(message)
- self.curve = curve
-
-
-class UnequalZipLengthsError(Error):
- pass
-
-
-class IncompatibleGlyphsError(Error):
- def __init__(self, glyphs):
- assert len(glyphs) > 1
- self.glyphs = glyphs
- names = set(repr(g.name) for g in glyphs)
- if len(names) > 1:
- self.combined_name = "{%s}" % ", ".join(sorted(names))
- else:
- self.combined_name = names.pop()
-
- def __repr__(self):
- return "<%s %s>" % (type(self).__name__, self.combined_name)
-
-
-class IncompatibleSegmentNumberError(IncompatibleGlyphsError):
- def __str__(self):
- return "Glyphs named %s have different number of segments" % (
- self.combined_name
- )
-
-
-class IncompatibleSegmentTypesError(IncompatibleGlyphsError):
- def __init__(self, glyphs, segments):
- IncompatibleGlyphsError.__init__(self, glyphs)
- self.segments = segments
-
- def __str__(self):
- lines = []
- ndigits = len(str(max(self.segments)))
- for i, tags in sorted(self.segments.items()):
- lines.append(
- "%s: (%s)" % (str(i).rjust(ndigits), ", ".join(repr(t) for t in tags))
- )
- return "Glyphs named %s have incompatible segment types:\n %s" % (
- self.combined_name,
- "\n ".join(lines),
- )
-
-
-class IncompatibleFontsError(Error):
- def __init__(self, glyph_errors):
- self.glyph_errors = glyph_errors
-
- def __str__(self):
- return "fonts contains incompatible glyphs: %s" % (
- ", ".join(repr(g) for g in sorted(self.glyph_errors.keys()))
- )
diff --git a/spaces/dcarpintero/nlp-summarizer-pegasus/.venv/lib/python3.9/site-packages/gradio/templates/cdn/assets/index-96c8120d.js b/spaces/dcarpintero/nlp-summarizer-pegasus/.venv/lib/python3.9/site-packages/gradio/templates/cdn/assets/index-96c8120d.js
deleted file mode 100644
index aa5343d2716df71ec561627ddf8b119c584191cd..0000000000000000000000000000000000000000
--- a/spaces/dcarpintero/nlp-summarizer-pegasus/.venv/lib/python3.9/site-packages/gradio/templates/cdn/assets/index-96c8120d.js
+++ /dev/null
@@ -1,5 +0,0 @@
-import{S as ae,e as ie,s as se,o as V,m as R,g as v,Y as T,h as M,p as W,n as oe,k as C,B as ve,E as Q,N as G,t as ue,x as pe,P as ze,aq as hl,O as X,F as z,j as J,G as I,T as x,w as E,r as ee,u as q,v as le,H as F,C as ye,f as ne,a4 as Z,I as te,J as we,W as ke,am as Ie,V as Fe,ae as Ye,Q as Ke,R as Pe}from"./index-9e76ffee.js";import{a as Ve,B as Ge}from"./Button-30a08c0b.js";import{U as gl}from"./Upload-1e84df2f.js";import"./ModifyUpload.svelte_svelte_type_style_lang-14b768c9.js";import{d as ml}from"./dsv-576afacd.js";var Se=Object.prototype.hasOwnProperty;function ce(t,e){var l,n;if(t===e)return!0;if(t&&e&&(l=t.constructor)===e.constructor){if(l===Date)return t.getTime()===e.getTime();if(l===RegExp)return t.toString()===e.toString();if(l===Array){if((n=t.length)===e.length)for(;n--&&ce(t[n],e[n]););return n===-1}if(!l||typeof t=="object"){n=0;for(l in t)if(Se.call(t,l)&&++n&&!Se.call(e,l)||!(l in e)||!ce(t[l],e[l]))return!1;return Object.keys(e).length===n}}return t!==t&&e!==e}function Oe(t){let e,l,n;return{c(){e=R("input"),v(e,"tabindex","-1"),e.value=t[0],v(e,"class","svelte-q8uklq"),T(e,"header",t[3])},m(a,u){M(a,e,u),t[7](e),l||(n=[W(e,"keydown",t[6]),W(e,"blur",t[8])],l=!0)},p(a,u){u&1&&e.value!==a[0]&&(e.value=a[0]),u&8&&T(e,"header",a[3])},d(a){a&&C(e),t[7](null),l=!1,ve(n)}}}function bl(t){let e;return{c(){e=ue(t[0])},m(l,n){M(l,e,n)},p(l,n){n&1&&pe(e,l[0])},d(l){l&&C(e)}}}function wl(t){let e,l;return{c(){e=new hl(!1),l=ze(),e.a=l},m(n,a){e.m(t[0],n,a),M(n,l,a)},p(n,a){a&1&&e.p(n[0])},d(n){n&&(C(l),e.d())}}}function kl(t){let e,l,n,a,u=t[2]&&Oe(t);function o(f,_){return f[4]==="markdown"||f[4]==="html"?wl:bl}let s=o(t),i=s(t);return{c(){u&&u.c(),e=V(),l=R("span"),i.c(),v(l,"tabindex","-1"),v(l,"role","button"),v(l,"class","svelte-q8uklq"),T(l,"edit",t[2])},m(f,_){u&&u.m(f,_),M(f,e,_),M(f,l,_),i.m(l,null),n||(a=W(l,"dblclick",t[5]),n=!0)},p(f,[_]){f[2]?u?u.p(f,_):(u=Oe(f),u.c(),u.m(e.parentNode,e)):u&&(u.d(1),u=null),s===(s=o(f))&&i?i.p(f,_):(i.d(1),i=s(f),i&&(i.c(),i.m(l,null))),_&4&&T(l,"edit",f[2])},i:oe,o:oe,d(f){f&&(C(e),C(l)),u&&u.d(f),i.d(),n=!1,a()}}}function vl(t,e,l){let{edit:n}=e,{value:a=""}=e,{el:u}=e,{header:o=!1}=e,{datatype:s="str"}=e;function i(w){Q.call(this,t,w)}function f(w){Q.call(this,t,w)}function _(w){G[w?"unshift":"push"](()=>{u=w,l(1,u)})}const b=({currentTarget:w})=>{l(0,a=w.value),w.setAttribute("tabindex","-1")};return t.$$set=w=>{"edit"in w&&l(2,n=w.edit),"value"in w&&l(0,a=w.value),"el"in w&&l(1,u=w.el),"header"in w&&l(3,o=w.header),"datatype"in w&&l(4,s=w.datatype)},[a,u,n,o,s,i,f,_,b]}class Qe extends ae{constructor(e){super(),ie(this,e,vl,kl,se,{edit:2,value:0,el:1,header:3,datatype:4})}}function Ee(t,e,l){const n=t.slice();return n[53]=e[l],n[55]=l,n}function Le(t,e,l){const n=t.slice();return n[56]=e[l].value,n[57]=e[l].id,n[58]=e,n[59]=l,n}function Be(t,e,l){const n=t.slice();return n[56]=e[l].value,n[57]=e[l].id,n[60]=e,n[55]=l,n}function qe(t){let e,l;return{c(){e=R("p"),l=ue(t[1]),v(e,"class","svelte-1tclfmr")},m(n,a){M(n,e,a),J(e,l)},p(n,a){a[0]&2&&pe(l,n[1])},d(n){n&&C(e)}}}function Me(t){let e,l;return{c(){e=R("caption"),l=ue(t[1]),v(e,"class","sr-only")},m(n,a){M(n,e,a),J(e,l)},p(n,a){a[0]&2&&pe(l,n[1])},d(n){n&&C(e)}}}function Ce(t,e){let l,n,a,u,o,s,i,f,_,b,w,g=e[57],y,d,B;function k(H){e[30](H,e[57])}function m(){return e[31](e[57])}let S={value:e[56],edit:e[13]===e[57],header:!0};e[10][e[57]].input!==void 0&&(S.el=e[10][e[57]].input),a=new Qe({props:S}),G.push(()=>X(a,"el",k)),a.$on("keydown",e[21]),a.$on("dblclick",m);function L(){return e[32](e[55])}const A=()=>e[33](l,g),c=()=>e[33](null,g);return{key:t,first:null,c(){l=R("th"),n=R("div"),z(a.$$.fragment),o=V(),s=R("div"),i=ne("svg"),f=ne("path"),b=V(),v(f,"d","M4.49999 0L8.3971 6.75H0.602875L4.49999 0Z"),v(i,"width","1em"),v(i,"height","1em"),v(i,"viewBox","0 0 9 7"),v(i,"fill","none"),v(i,"xmlns","http://www.w3.org/2000/svg"),v(i,"class","svelte-1tclfmr"),v(s,"class",_="sort-button "+e[11]+" svelte-1tclfmr"),T(s,"sorted",e[12]===e[55]),T(s,"des",e[12]===e[55]&&e[11]==="des"),v(n,"class","cell-wrap svelte-1tclfmr"),v(l,"aria-sort",w=e[15](e[56],e[12],e[11])),v(l,"class","svelte-1tclfmr"),T(l,"editing",e[13]===e[57]),this.first=l},m(H,U){M(H,l,U),J(l,n),I(a,n,null),J(n,o),J(n,s),J(s,i),J(i,f),J(l,b),A(),y=!0,d||(B=W(s,"click",L),d=!0)},p(H,U){e=H;const Y={};U[0]&256&&(Y.value=e[56]),U[0]&8448&&(Y.edit=e[13]===e[57]),!u&&U[0]&1280&&(u=!0,Y.el=e[10][e[57]].input,x(()=>u=!1)),a.$set(Y),(!y||U[0]&2048&&_!==(_="sort-button "+e[11]+" svelte-1tclfmr"))&&v(s,"class",_),(!y||U[0]&6400)&&T(s,"sorted",e[12]===e[55]),(!y||U[0]&6400)&&T(s,"des",e[12]===e[55]&&e[11]==="des"),(!y||U[0]&6400&&w!==(w=e[15](e[56],e[12],e[11])))&&v(l,"aria-sort",w),g!==e[57]&&(c(),g=e[57],A()),(!y||U[0]&8448)&&T(l,"editing",e[13]===e[57])},i(H){y||(E(a.$$.fragment,H),y=!0)},o(H){q(a.$$.fragment,H),y=!1},d(H){H&&C(l),F(a),c(),d=!1,B()}}}function Je(t,e){let l,n,a,u,o,s=e[57],i,f,_;function b(L){e[34](L,e[56],e[58],e[59])}function w(L){e[35](L,e[57])}let g={edit:e[7]===e[57],datatype:Array.isArray(e[0])?e[0][e[59]]:e[0]};e[56]!==void 0&&(g.value=e[56]),e[10][e[57]].input!==void 0&&(g.el=e[10][e[57]].input),a=new Qe({props:g}),G.push(()=>X(a,"value",b)),G.push(()=>X(a,"el",w));const y=()=>e[36](l,s),d=()=>e[36](null,s);function B(){return e[37](e[57])}function k(){return e[38](e[57])}function m(){return e[39](e[57])}function S(...L){return e[40](e[55],e[59],e[57],...L)}return{key:t,first:null,c(){l=R("td"),n=R("div"),z(a.$$.fragment),v(n,"class","cell-wrap svelte-1tclfmr"),T(n,"border-transparent",e[6]!==e[57]),v(l,"tabindex","0"),v(l,"class","svelte-1tclfmr"),this.first=l},m(L,A){M(L,l,A),J(l,n),I(a,n,null),y(),i=!0,f||(_=[W(l,"touchstart",B,{passive:!0}),W(l,"click",k),W(l,"dblclick",m),W(l,"keydown",S)],f=!0)},p(L,A){e=L;const c={};A[0]&640&&(c.edit=e[7]===e[57]),A[0]&513&&(c.datatype=Array.isArray(e[0])?e[0][e[59]]:e[0]),!u&&A[0]&512&&(u=!0,c.value=e[56],x(()=>u=!1)),!o&&A[0]&1536&&(o=!0,c.el=e[10][e[57]].input,x(()=>o=!1)),a.$set(c),(!i||A[0]&576)&&T(n,"border-transparent",e[6]!==e[57]),s!==e[57]&&(d(),s=e[57],y())},i(L){i||(E(a.$$.fragment,L),i=!0)},o(L){q(a.$$.fragment,L),i=!1},d(L){L&&C(l),F(a),d(),f=!1,ve(_)}}}function Te(t,e){let l,n=[],a=new Map,u,o,s=te(e[53]);const i=f=>f[57];for(let f=0;fk[57];for(let k=0;kk[53];for(let k=0;k{a=null}),le()),o[2][1]==="dynamic"?u?(u.p(o,s),s[0]&4&&E(u,1)):(u=Ue(o),u.c(),E(u,1),u.m(e,null)):u&&(ee(),q(u,1,1,()=>{u=null}),le())},i(o){n||(E(a),E(u),n=!0)},o(o){q(a),q(u),n=!1},d(o){o&&C(e),a&&a.d(),u&&u.d()}}}function He(t){let e,l,n;return l=new Ve({props:{variant:"secondary",size:"sm",$$slots:{default:[yl]},$$scope:{ctx:t}}}),l.$on("click",t[43]),{c(){e=R("span"),z(l.$$.fragment),v(e,"class","button-wrap svelte-1tclfmr")},m(a,u){M(a,e,u),I(l,e,null),n=!0},p(a,u){const o={};u[1]&1073741824&&(o.$$scope={dirty:u,ctx:a}),l.$set(o)},i(a){n||(E(l.$$.fragment,a),n=!0)},o(a){q(l.$$.fragment,a),n=!1},d(a){a&&C(e),F(l)}}}function yl(t){let e,l,n;return{c(){e=ne("svg"),l=ne("path"),n=ue(`
- New row`),v(l,"fill","currentColor"),v(l,"d","M24.59 16.59L17 24.17V4h-2v20.17l-7.59-7.58L6 18l10 10l10-10l-1.41-1.41z"),v(e,"xmlns","http://www.w3.org/2000/svg"),v(e,"xmlns:xlink","http://www.w3.org/1999/xlink"),v(e,"aria-hidden","true"),v(e,"role","img"),v(e,"width","1em"),v(e,"height","1em"),v(e,"preserveAspectRatio","xMidYMid meet"),v(e,"viewBox","0 0 32 32"),v(e,"class","svelte-1tclfmr")},m(a,u){M(a,e,u),J(e,l),M(a,n,u)},p:oe,d(a){a&&(C(e),C(n))}}}function Ue(t){let e,l,n;return l=new Ve({props:{variant:"secondary",size:"sm",$$slots:{default:[Al]},$$scope:{ctx:t}}}),l.$on("click",t[23]),{c(){e=R("span"),z(l.$$.fragment),v(e,"class","button-wrap svelte-1tclfmr")},m(a,u){M(a,e,u),I(l,e,null),n=!0},p(a,u){const o={};u[1]&1073741824&&(o.$$scope={dirty:u,ctx:a}),l.$set(o)},i(a){n||(E(l.$$.fragment,a),n=!0)},o(a){q(l.$$.fragment,a),n=!1},d(a){a&&C(e),F(l)}}}function Al(t){let e,l,n;return{c(){e=ne("svg"),l=ne("path"),n=ue(`
- New column`),v(l,"fill","currentColor"),v(l,"d","m18 6l-1.43 1.393L24.15 15H4v2h20.15l-7.58 7.573L18 26l10-10L18 6z"),v(e,"xmlns","http://www.w3.org/2000/svg"),v(e,"xmlns:xlink","http://www.w3.org/1999/xlink"),v(e,"aria-hidden","true"),v(e,"role","img"),v(e,"width","1em"),v(e,"height","1em"),v(e,"preserveAspectRatio","xMidYMid meet"),v(e,"viewBox","0 0 32 32"),v(e,"class","svelte-1tclfmr")},m(a,u){M(a,e,u),J(e,l),M(a,n,u)},p:oe,d(a){a&&(C(e),C(n))}}}function Dl(t){let e,l,n,a,u,o,s,i,f,_=t[1]&&t[1].length!==0&&qe(t);function b(y){t[41](y)}let w={flex:!1,center:!1,boundedheight:!1,disable_click:!0,$$slots:{default:[pl]},$$scope:{ctx:t}};t[14]!==void 0&&(w.dragging=t[14]),a=new gl({props:w}),G.push(()=>X(a,"dragging",b)),a.$on("load",t[42]);let g=t[4]&&Re(t);return{c(){e=R("div"),_&&_.c(),l=V(),n=R("div"),z(a.$$.fragment),o=V(),g&&g.c(),v(n,"class","table-wrap scroll-hide svelte-1tclfmr"),T(n,"dragging",t[14]),T(n,"no-wrap",!t[5]),v(e,"class","svelte-1tclfmr"),T(e,"label",t[1]&&t[1].length!==0)},m(y,d){M(y,e,d),_&&_.m(e,null),J(e,l),J(e,n),I(a,n,null),J(e,o),g&&g.m(e,null),s=!0,i||(f=[W(window,"click",t[24]),W(window,"touchstart",t[24])],i=!0)},p(y,d){y[1]&&y[1].length!==0?_?_.p(y,d):(_=qe(y),_.c(),_.m(e,l)):_&&(_.d(1),_=null);const B={};d[0]&32707|d[1]&1073741824&&(B.$$scope={dirty:d,ctx:y}),!u&&d[0]&16384&&(u=!0,B.dragging=y[14],x(()=>u=!1)),a.$set(B),(!s||d[0]&16384)&&T(n,"dragging",y[14]),(!s||d[0]&32)&&T(n,"no-wrap",!y[5]),y[4]?g?(g.p(y,d),d[0]&16&&E(g,1)):(g=Re(y),g.c(),E(g,1),g.m(e,null)):g&&(ee(),q(g,1,1,()=>{g=null}),le()),(!s||d[0]&2)&&T(e,"label",y[1]&&y[1].length!==0)},i(y){s||(E(a.$$.fragment,y),E(g),s=!0)},o(y){q(a.$$.fragment,y),q(g),s=!1},d(y){y&&C(e),_&&_.d(),F(a),g&&g.d(),i=!1,ve(f)}}}function Nl(t,e){return e.filter(l);function l(n){var a=-1;return t.split(`
-`).every(u);function u(o){if(!o)return!0;var s=o.split(n).length;return a<0&&(a=s),a===s&&s>1}}}function Sl(t){const e=atob(t.split(",")[1]),l=t.split(",")[0].split(":")[1].split(";")[0],n=new ArrayBuffer(e.length),a=new Uint8Array(n);for(let u=0;uA[r][h].value;let d={};function B(r){let h=r||[];if(s[1]==="fixed"&&h.length`${O+h.length}`);h=h.concat(D)}return!h||h.length===0?Array(s[0]).fill(0).map((D,N)=>{const O=`h-${N}`;return l(10,d[O]={cell:null,input:null},d),{id:O,value:JSON.stringify(N+1)}}):h.map((D,N)=>{const O=`h-${N}`;return l(10,d[O]={cell:null,input:null},d),{id:O,value:D??""}})}function k(r){const h=r.length>0?r.length:i[0];return Array(i[1]==="fixed"||hArray(s[1]==="fixed"?s[0]:r[0].length).fill(0).map((O,P)=>{const j=`${N}-${P}`;return l(10,d[j]={input:null,cell:null},d),{value:r?.[N]?.[P]??"",id:j}}))}let m=B(u),S;async function L(){typeof g=="string"?(await Z(),d[g]?.input?.focus()):typeof b=="string"&&(await Z(),d[b]?.input?.focus())}let A=[[]],c;function H(r,h,D){if(!h)return"none";if(u[h]===r){if(D==="asc")return"ascending";if(D==="des")return"descending"}return"none"}function U(r){return A.reduce((h,D,N)=>{const O=D.reduce((P,j,me)=>r===j.id?me:P,-1);return O===-1?h:[N,O]},[-1,-1])}async function Y(r,h){if(!f||g===r)return;if(h){const[N,O]=U(r);l(9,A[N][O].value="",A)}l(7,g=r),await Z();const{input:D}=d[r];D?.focus()}async function fe(r,h,D,N){let O;switch(r.key){case"ArrowRight":if(g)break;r.preventDefault(),O=A[h][D+1],l(6,b=O?O.id:b);break;case"ArrowLeft":if(g)break;r.preventDefault(),O=A[h][D-1],l(6,b=O?O.id:b);break;case"ArrowDown":if(g)break;r.preventDefault(),O=A[h+1],l(6,b=O?O[D].id:b);break;case"ArrowUp":if(g)break;r.preventDefault(),O=A[h-1],l(6,b=O?O[D].id:b);break;case"Escape":if(!f)break;r.preventDefault(),l(6,b=g),l(7,g=!1);break;case"Enter":if(!f)break;if(r.preventDefault(),r.shiftKey){he(h),await Z();const[dl]=U(N);l(6,b=A[dl+1][D].id)}else g===N?l(7,g=!1):Y(N);break;case"Backspace":if(!f)break;g||(r.preventDefault(),l(9,A[h][D].value="",A));break;case"Delete":if(!f)break;g||(r.preventDefault(),l(9,A[h][D].value="",A));break;case"Tab":let P=r.shiftKey?-1:1,j=A[h][D+P],me=A?.[h+P]?.[P>0?0:m.length-1],be=j||me;be&&(r.preventDefault(),l(6,b=be?be.id:b)),l(7,g=!1);break;default:(!g||g&&g!==N)&&r.key.length===1&&Y(N,!0);break}}async function re(r){g!==r&&b!==r&&(l(7,g=!1),l(6,b=r))}async function p(r,h){if(h==="edit"&&typeof r=="string"&&(await Z(),d[r].input?.focus()),h==="edit"&&typeof r=="boolean"&&typeof b=="string"){let D=d[b]?.cell;await Z(),D?.focus()}if(h==="select"&&typeof r=="string"){const{cell:D}=d[r];await Z(),D?.focus()}}let $,_e;function Ze(r,h){h==="asc"?l(9,A=A.sort((D,N)=>D[r].valueD[r].value>N[r].value?-1:1))}function Ae(r){typeof _e!="number"||_e!==r?(l(11,$="asc"),l(12,_e=r)):$==="asc"?l(11,$="des"):$==="des"&&l(11,$="asc"),Ze(r,$)}let K;function De(){if(typeof b=="string"){const r=d[b].input?.value;if(m.find(h=>h.id===b)){let h=m.find(D=>D.id===b);r&&(h.value=r)}else r&&m.push({id:b,value:r})}}async function de(r,h){!f||s[1]!=="dynamic"||g===r||(l(13,K=r),await Z(),d[r].input?.focus(),h&&d[r].input?.select())}function je(r){if(f)switch(r.key){case"Escape":case"Enter":case"Tab":r.preventDefault(),l(6,b=K),l(13,K=!1),De();break}}function he(r){i[1]==="dynamic"&&(A.splice(r?r+1:A.length,0,Array(A[0].length).fill(0).map((h,D)=>{const N=`${A.length}-${D}`;return l(10,d[N]={cell:null,input:null},d),{id:N,value:""}})),l(9,A),l(27,o),l(29,c),l(26,u))}async function Xe(){if(s[1]!=="dynamic")return;for(let h=0;hde(r),ll=r=>Ae(r);function tl(r,h){G[r?"unshift":"push"](()=>{d[h].cell=r,l(10,d)})}function nl(r,h,D,N){D[N].value=r,l(9,A),l(27,o),l(29,c),l(26,u)}function al(r,h){t.$$.not_equal(d[h].input,r)&&(d[h].input=r,l(10,d))}function il(r,h){G[r?"unshift":"push"](()=>{d[h].cell=r,l(10,d)})}const sl=r=>Y(r),ul=r=>re(r),fl=r=>Y(r),rl=(r,h,D,N)=>fe(N,r,h,D);function _l(r){ge=r,l(14,ge)}const ol=r=>Ne(Sl(r.detail.data)),cl=()=>he();return t.$$set=r=>{"datatype"in r&&l(0,n=r.datatype),"label"in r&&l(1,a=r.label),"headers"in r&&l(26,u=r.headers),"values"in r&&l(27,o=r.values),"col_count"in r&&l(2,s=r.col_count),"row_count"in r&&l(3,i=r.row_count),"editable"in r&&l(4,f=r.editable),"wrap"in r&&l(5,_=r.wrap)},t.$$.update=()=>{if(t.$$.dirty[0]&201326592&&(o&&!Array.isArray(o)?(l(26,u=o.headers),l(27,o=o.data.length===0?[Array(u.length).fill("")]:o.data),l(6,b=!1)):o===null&&(l(27,o=[Array(u.length).fill("")]),l(6,b=!1))),t.$$.dirty[0]&64&&b!==!1){const r=b.split("-"),h=parseInt(r[0]),D=parseInt(r[1]);!isNaN(h)&&!isNaN(D)&&w("select",{index:[h,D],value:y(h,D)})}t.$$.dirty[0]&335544320&&(ce(u,S)||(l(8,m=B(u)),l(28,S=u),L())),t.$$.dirty[0]&671088640&&(ce(o,c)||(l(9,A=k(o)),l(29,c=o),L())),t.$$.dirty[0]&768&&m&&w("change",{data:A.map(r=>r.map(({value:h})=>h)),headers:m.map(r=>r.value)}),t.$$.dirty[0]&128&&p(g,"edit"),t.$$.dirty[0]&64&&p(b,"select")},[n,a,s,i,f,_,b,g,m,A,d,$,_e,K,ge,H,Y,fe,re,Ae,de,je,he,Xe,xe,Ne,u,o,S,c,$e,el,ll,tl,nl,al,il,sl,ul,fl,rl,_l,ol,cl]}class We extends ae{constructor(e){super(),ie(this,e,Ol,Dl,se,{datatype:0,label:1,headers:26,values:27,col_count:2,row_count:3,editable:4,wrap:5},null,[-1,-1])}}function El(t){let e,l,n,a;const u=[t[12]];let o={};for(let s=0;s{l(13,f=!1)});const L=({detail:c})=>{l(0,s=c)};function A(c){Q.call(this,t,c)}return t.$$set=c=>{"headers"in c&&l(1,n=c.headers),"elem_id"in c&&l(2,a=c.elem_id),"elem_classes"in c&&l(3,u=c.elem_classes),"visible"in c&&l(4,o=c.visible),"value"in c&&l(0,s=c.value),"value_is_output"in c&&l(13,f=c.value_is_output),"col_count"in c&&l(5,_=c.col_count),"row_count"in c&&l(6,b=c.row_count),"label"in c&&l(7,w=c.label),"wrap"in c&&l(8,g=c.wrap),"datatype"in c&&l(9,y=c.datatype),"scale"in c&&l(10,d=c.scale),"min_width"in c&&l(11,B=c.min_width),"loading_status"in c&&l(12,m=c.loading_status)},t.$$.update=()=>{t.$$.dirty&16385&&JSON.stringify(s)!==i&&(l(14,i=JSON.stringify(s)),S())},[s,n,a,u,o,_,b,w,g,y,d,B,m,f,i,L,A]}class ql extends ae{constructor(e){super(),ie(this,e,Bl,Ll,se,{headers:1,elem_id:2,elem_classes:3,visible:4,value:0,value_is_output:13,col_count:5,row_count:6,label:7,wrap:8,datatype:9,scale:10,min_width:11,loading_status:12})}}function Ml(t){let e,l,n,a;const u=[t[12]];let o={};for(let s=0;s{l(13,f=!1)});const L=({detail:c})=>{l(0,s=c)};function A(c){Q.call(this,t,c)}return t.$$set=c=>{"headers"in c&&l(1,n=c.headers),"elem_id"in c&&l(2,a=c.elem_id),"elem_classes"in c&&l(3,u=c.elem_classes),"visible"in c&&l(4,o=c.visible),"value"in c&&l(0,s=c.value),"value_is_output"in c&&l(13,f=c.value_is_output),"col_count"in c&&l(5,_=c.col_count),"row_count"in c&&l(6,b=c.row_count),"label"in c&&l(7,w=c.label),"wrap"in c&&l(8,g=c.wrap),"datatype"in c&&l(9,y=c.datatype),"scale"in c&&l(10,d=c.scale),"min_width"in c&&l(11,B=c.min_width),"loading_status"in c&&l(12,m=c.loading_status)},t.$$.update=()=>{t.$$.dirty&16385&&JSON.stringify(s)!==i&&(l(14,i=JSON.stringify(s)),S())},[s,n,a,u,o,_,b,w,g,y,d,B,m,f,i,L,A]}class Tl extends ae{constructor(e){super(),ie(this,e,Jl,Cl,se,{headers:1,elem_id:2,elem_classes:3,visible:4,value:0,value_is_output:13,col_count:5,row_count:6,label:7,wrap:8,datatype:9,scale:10,min_width:11,loading_status:12})}}function Rl(t){let e,l,n,a;function u(i){t[20](i)}function o(i){t[21](i)}let s={headers:t[2],elem_id:t[3],elem_classes:t[4],visible:t[5],col_count:t[7],row_count:t[8],label:t[9],wrap:t[10],datatype:t[11],scale:t[12],min_width:t[13],loading_status:t[14]};return t[1]!==void 0&&(s.value=t[1]),t[0]!==void 0&&(s.value_is_output=t[0]),e=new Tl({props:s}),G.push(()=>X(e,"value",u)),G.push(()=>X(e,"value_is_output",o)),e.$on("change",t[22]),e.$on("select",t[23]),e.$on("input",t[24]),{c(){z(e.$$.fragment)},m(i,f){I(e,i,f),a=!0},p(i,f){const _={};f&4&&(_.headers=i[2]),f&8&&(_.elem_id=i[3]),f&16&&(_.elem_classes=i[4]),f&32&&(_.visible=i[5]),f&128&&(_.col_count=i[7]),f&256&&(_.row_count=i[8]),f&512&&(_.label=i[9]),f&1024&&(_.wrap=i[10]),f&2048&&(_.datatype=i[11]),f&4096&&(_.scale=i[12]),f&8192&&(_.min_width=i[13]),f&16384&&(_.loading_status=i[14]),!l&&f&2&&(l=!0,_.value=i[1],x(()=>l=!1)),!n&&f&1&&(n=!0,_.value_is_output=i[0],x(()=>n=!1)),e.$set(_)},i(i){a||(E(e.$$.fragment,i),a=!0)},o(i){q(e.$$.fragment,i),a=!1},d(i){F(e,i)}}}function Hl(t){let e,l,n,a;function u(i){t[15](i)}function o(i){t[16](i)}let s={headers:t[2],elem_id:t[3],elem_classes:t[4],visible:t[5],col_count:t[7],row_count:t[8],label:t[9],wrap:t[10],datatype:t[11],scale:t[12],min_width:t[13],loading_status:t[14]};return t[1]!==void 0&&(s.value=t[1]),t[0]!==void 0&&(s.value_is_output=t[0]),e=new ql({props:s}),G.push(()=>X(e,"value",u)),G.push(()=>X(e,"value_is_output",o)),e.$on("change",t[17]),e.$on("select",t[18]),e.$on("input",t[19]),{c(){z(e.$$.fragment)},m(i,f){I(e,i,f),a=!0},p(i,f){const _={};f&4&&(_.headers=i[2]),f&8&&(_.elem_id=i[3]),f&16&&(_.elem_classes=i[4]),f&32&&(_.visible=i[5]),f&128&&(_.col_count=i[7]),f&256&&(_.row_count=i[8]),f&512&&(_.label=i[9]),f&1024&&(_.wrap=i[10]),f&2048&&(_.datatype=i[11]),f&4096&&(_.scale=i[12]),f&8192&&(_.min_width=i[13]),f&16384&&(_.loading_status=i[14]),!l&&f&2&&(l=!0,_.value=i[1],x(()=>l=!1)),!n&&f&1&&(n=!0,_.value_is_output=i[0],x(()=>n=!1)),e.$set(_)},i(i){a||(E(e.$$.fragment,i),a=!0)},o(i){q(e.$$.fragment,i),a=!1},d(i){F(e,i)}}}function Ul(t){let e,l,n,a;const u=[Hl,Rl],o=[];function s(i,f){return i[6]==="static"?0:1}return e=s(t),l=o[e]=u[e](t),{c(){l.c(),n=ze()},m(i,f){o[e].m(i,f),M(i,n,f),a=!0},p(i,[f]){let _=e;e=s(i),e===_?o[e].p(i,f):(ee(),q(o[_],1,1,()=>{o[_]=null}),le(),l=o[e],l?l.p(i,f):(l=o[e]=u[e](i),l.c()),E(l,1),l.m(n.parentNode,n))},i(i){a||(E(l),a=!0)},o(i){q(l),a=!1},d(i){i&&C(n),o[e].d(i)}}}function zl(t,e,l){let{headers:n=[]}=e,{elem_id:a=""}=e,{elem_classes:u=[]}=e,{visible:o=!0}=e,{value_is_output:s=!1}=e,{mode:i}=e,{col_count:f}=e,{row_count:_}=e,{label:b=null}=e,{wrap:w}=e,{datatype:g}=e,{scale:y=null}=e,{min_width:d=void 0}=e,{loading_status:B}=e,{value:k={data:[["","",""]],headers:["1","2","3"]}}=e;function m(p){k=p,l(1,k)}function S(p){s=p,l(0,s)}function L(p){Q.call(this,t,p)}function A(p){Q.call(this,t,p)}function c(p){Q.call(this,t,p)}function H(p){k=p,l(1,k)}function U(p){s=p,l(0,s)}function Y(p){Q.call(this,t,p)}function fe(p){Q.call(this,t,p)}function re(p){Q.call(this,t,p)}return t.$$set=p=>{"headers"in p&&l(2,n=p.headers),"elem_id"in p&&l(3,a=p.elem_id),"elem_classes"in p&&l(4,u=p.elem_classes),"visible"in p&&l(5,o=p.visible),"value_is_output"in p&&l(0,s=p.value_is_output),"mode"in p&&l(6,i=p.mode),"col_count"in p&&l(7,f=p.col_count),"row_count"in p&&l(8,_=p.row_count),"label"in p&&l(9,b=p.label),"wrap"in p&&l(10,w=p.wrap),"datatype"in p&&l(11,g=p.datatype),"scale"in p&&l(12,y=p.scale),"min_width"in p&&l(13,d=p.min_width),"loading_status"in p&&l(14,B=p.loading_status),"value"in p&&l(1,k=p.value)},[s,k,n,a,u,o,i,f,_,b,w,g,y,d,B,m,S,L,A,c,H,U,Y,fe,re]}class Il extends ae{constructor(e){super(),ie(this,e,zl,Ul,se,{headers:2,elem_id:3,elem_classes:4,visible:5,value_is_output:0,mode:6,col_count:7,row_count:8,label:9,wrap:10,datatype:11,scale:12,min_width:13,loading_status:14,value:1})}}const Gl=Il,Ql=["static","dynamic"];export{Gl as Component,Ql as modes};
-//# sourceMappingURL=index-96c8120d.js.map
diff --git a/spaces/declare-lab/tango/diffusers/tests/pipelines/text_to_video/__init__.py b/spaces/declare-lab/tango/diffusers/tests/pipelines/text_to_video/__init__.py
deleted file mode 100644
index e69de29bb2d1d6434b8b29ae775ad8c2e48c5391..0000000000000000000000000000000000000000
diff --git a/spaces/deepskyreal/ai-mixer-hotchpotch/sad_talker/src/face3d/models/template_model.py b/spaces/deepskyreal/ai-mixer-hotchpotch/sad_talker/src/face3d/models/template_model.py
deleted file mode 100644
index dac7b33d5889777eb63c9882a3b9fa094dcab293..0000000000000000000000000000000000000000
--- a/spaces/deepskyreal/ai-mixer-hotchpotch/sad_talker/src/face3d/models/template_model.py
+++ /dev/null
@@ -1,100 +0,0 @@
-"""Model class template
-
-This module provides a template for users to implement custom models.
-You can specify '--model template' to use this model.
-The class name should be consistent with both the filename and its model option.
-The filename should be _dataset.py
-The class name should be Dataset.py
-It implements a simple image-to-image translation baseline based on regression loss.
-Given input-output pairs (data_A, data_B), it learns a network netG that can minimize the following L1 loss:
- min_ ||netG(data_A) - data_B||_1
-You need to implement the following functions:
- : Add model-specific options and rewrite default values for existing options.
- <__init__>: Initialize this model class.
- : Unpack input data and perform data pre-processing.
- : Run forward pass. This will be called by both and .
- : Update network weights; it will be called in every training iteration.
-"""
-import numpy as np
-import torch
-from .base_model import BaseModel
-from . import networks
-
-
-class TemplateModel(BaseModel):
- @staticmethod
- def modify_commandline_options(parser, is_train=True):
- """Add new model-specific options and rewrite default values for existing options.
-
- Parameters:
- parser -- the option parser
- is_train -- if it is training phase or test phase. You can use this flag to add training-specific or test-specific options.
-
- Returns:
- the modified parser.
- """
- parser.set_defaults(dataset_mode='aligned') # You can rewrite default values for this model. For example, this model usually uses aligned dataset as its dataset.
- if is_train:
- parser.add_argument('--lambda_regression', type=float, default=1.0, help='weight for the regression loss') # You can define new arguments for this model.
-
- return parser
-
- def __init__(self, opt):
- """Initialize this model class.
-
- Parameters:
- opt -- training/test options
-
- A few things can be done here.
- - (required) call the initialization function of BaseModel
- - define loss function, visualization images, model names, and optimizers
- """
- BaseModel.__init__(self, opt) # call the initialization method of BaseModel
- # specify the training losses you want to print out. The program will call base_model.get_current_losses to plot the losses to the console and save them to the disk.
- self.loss_names = ['loss_G']
- # specify the images you want to save and display. The program will call base_model.get_current_visuals to save and display these images.
- self.visual_names = ['data_A', 'data_B', 'output']
- # specify the models you want to save to the disk. The program will call base_model.save_networks and base_model.load_networks to save and load networks.
- # you can use opt.isTrain to specify different behaviors for training and test. For example, some networks will not be used during test, and you don't need to load them.
- self.model_names = ['G']
- # define networks; you can use opt.isTrain to specify different behaviors for training and test.
- self.netG = networks.define_G(opt.input_nc, opt.output_nc, opt.ngf, opt.netG, gpu_ids=self.gpu_ids)
- if self.isTrain: # only defined during training time
- # define your loss functions. You can use losses provided by torch.nn such as torch.nn.L1Loss.
- # We also provide a GANLoss class "networks.GANLoss". self.criterionGAN = networks.GANLoss().to(self.device)
- self.criterionLoss = torch.nn.L1Loss()
- # define and initialize optimizers. You can define one optimizer for each network.
- # If two networks are updated at the same time, you can use itertools.chain to group them. See cycle_gan_model.py for an example.
- self.optimizer = torch.optim.Adam(self.netG.parameters(), lr=opt.lr, betas=(opt.beta1, 0.999))
- self.optimizers = [self.optimizer]
-
- # Our program will automatically call to define schedulers, load networks, and print networks
-
- def set_input(self, input):
- """Unpack input data from the dataloader and perform necessary pre-processing steps.
-
- Parameters:
- input: a dictionary that contains the data itself and its metadata information.
- """
- AtoB = self.opt.direction == 'AtoB' # use to swap data_A and data_B
- self.data_A = input['A' if AtoB else 'B'].to(self.device) # get image data A
- self.data_B = input['B' if AtoB else 'A'].to(self.device) # get image data B
- self.image_paths = input['A_paths' if AtoB else 'B_paths'] # get image paths
-
- def forward(self):
- """Run forward pass. This will be called by both functions and ."""
- self.output = self.netG(self.data_A) # generate output image given the input data_A
-
- def backward(self):
- """Calculate losses, gradients, and update network weights; called in every training iteration"""
- # caculate the intermediate results if necessary; here self.output has been computed during function
- # calculate loss given the input and intermediate results
- self.loss_G = self.criterionLoss(self.output, self.data_B) * self.opt.lambda_regression
- self.loss_G.backward() # calculate gradients of network G w.r.t. loss_G
-
- def optimize_parameters(self):
- """Update network weights; it will be called in every training iteration."""
- self.forward() # first call forward to calculate intermediate results
- self.optimizer.zero_grad() # clear network G's existing gradients
- self.backward() # calculate gradients for network G
- self.optimizer.step() # update gradients for network G
diff --git a/spaces/descript/vampnet/scripts/utils/split.py b/spaces/descript/vampnet/scripts/utils/split.py
deleted file mode 100644
index 8ddb9b27b8854b6bf84e8404b56834564996e637..0000000000000000000000000000000000000000
--- a/spaces/descript/vampnet/scripts/utils/split.py
+++ /dev/null
@@ -1,64 +0,0 @@
-from pathlib import Path
-import random
-import shutil
-import os
-import json
-
-import argbind
-from tqdm import tqdm
-from tqdm.contrib.concurrent import thread_map
-
-from audiotools.core import util
-
-
-@argbind.bind(without_prefix=True)
-def train_test_split(
- audio_folder: str = ".",
- test_size: float = 0.2,
- seed: int = 42,
- pattern: str = "**/*.mp3",
-):
- print(f"finding audio")
-
- audio_folder = Path(audio_folder)
- audio_files = list(tqdm(audio_folder.glob(pattern)))
- print(f"found {len(audio_files)} audio files")
-
- # split according to test_size
- n_test = int(len(audio_files) * test_size)
- n_train = len(audio_files) - n_test
-
- # shuffle
- random.seed(seed)
- random.shuffle(audio_files)
-
- train_files = audio_files[:n_train]
- test_files = audio_files[n_train:]
-
-
- print(f"Train files: {len(train_files)}")
- print(f"Test files: {len(test_files)}")
- continue_ = input("Continue [yn]? ") or "n"
-
- if continue_ != "y":
- return
-
- for split, files in (
- ("train", train_files), ("test", test_files)
- ):
- for file in tqdm(files):
- out_file = audio_folder.parent / f"{audio_folder.name}-{split}" / Path(file).name
- out_file.parent.mkdir(exist_ok=True, parents=True)
- os.symlink(file, out_file)
-
- # save split as json
- with open(Path(audio_folder) / f"{split}.json", "w") as f:
- json.dump([str(f) for f in files], f)
-
-
-
-if __name__ == "__main__":
- args = argbind.parse_args()
-
- with argbind.scope(args):
- train_test_split()
\ No newline at end of file
diff --git a/spaces/diacanFperku/AutoGPT/HD Online Player (BEST Download Akira Movies 1080p Torrent).md b/spaces/diacanFperku/AutoGPT/HD Online Player (BEST Download Akira Movies 1080p Torrent).md
deleted file mode 100644
index cb0b44cecbe50c7f155a7329a086df6ba07a2ed4..0000000000000000000000000000000000000000
--- a/spaces/diacanFperku/AutoGPT/HD Online Player (BEST Download Akira Movies 1080p Torrent).md
+++ /dev/null
@@ -1,6 +0,0 @@
-
HD Online Player (Download Akira Movies 1080p Torrent)
-
-Seven Samurai (1954) BRRip 720p à ¦…à ¦¸à ¦¾à ¦§à ¦¾à ¦°à ¦£ à ¦…à § ... Download Seven samurai (shichinin no samurai) (1954) subtitles from subs ... Samurai Online 1954 Director Akira Kurosawa A poor village under attack by ... to help . media files (avi, mov, flv, mpg, mpeg, divx, dvd rip, mp3, mp4, torrent, ipod, psp), HNTV. 4d29de3e1b
-
-
-
diff --git a/spaces/diacanFperku/AutoGPT/Jin Li Tong Software Multiviewer.md b/spaces/diacanFperku/AutoGPT/Jin Li Tong Software Multiviewer.md
deleted file mode 100644
index e8af338eb391f3ab7a4cd832987a91013e874c2c..0000000000000000000000000000000000000000
--- a/spaces/diacanFperku/AutoGPT/Jin Li Tong Software Multiviewer.md
+++ /dev/null
@@ -1,102 +0,0 @@
-
-
Jin Li Tong Software Multiviewer
-
-
Do you need to monitor multiple video sources on a single display? Do you want to have a flexible and reliable multiviewer software solution that can handle various input types and resolutions? If yes, then you should consider Jin Li Tong Software Multiviewer. This is a software application that allows you to monitor multiple video sources on a single display. It supports various input types, such as SDI, HDMI, analog, NDI, and UDP. You can configure each panel to display different information, such as audio meters, custom labels, and alarms for image freezing, black level, and white level. You can also monitor up to 16 sound channels by simply clicking on the pair that you want to listen to.
Jin Li Tong Software Multiviewer is designed for professional environments such as broadcasting stations and production studios. It is suitable for 24/7 monitoring and it works under Windows 7 / 8 and 10 and equivalent Windows Server OS (64-bit only). It can handle SD, HD, and 4K resolutions. You can customize the panels layout by using an easy to operate wizard. You can also manage the panels (loading, cloning or deleting) from a user interface that allows you to preview the panels layout. Jin Li Tong Software Multiviewer has a watch-dog application that ensures the uninterrupted functionality of the software.
-
-
What is Multiviewer Software and Why You Need It
-
-
Multiviewer software is a type of software that lets you monitor multiple video sources on a single display. It is useful for situations where you need to view more sources than you have monitors available. Multiviewer software can also provide additional features such as overlays, audio monitoring, and alarms.
-
-
Multiviewer software can be used for various purposes, such as live multi-camera production, control room monitoring, security surveillance, video conferencing, and more. By using multiviewer software, you can save space, power, and money by reducing the number of monitors needed. You can also improve your workflow efficiency and productivity by having all the sources in one view.
-
-
How to Download and Use Jin Li Tong Software Multiviewer
-
-
If you are looking for a reliable and flexible multiviewer software solution, you should consider Jin Li Tong Software Multiviewer. It offers a wide range of features and options to suit your needs and preferences. You can download it from this link and try it for yourself.
-
-
-
Jin Li Tong Software Multiviewer is easy to install and use. You just need to download the software from the link provided and run the setup file. Then you can launch the software and start configuring your panels. You can choose the type of input, the info to be displayed, and the thresholds for alarms for each panel. You can also adjust the layout of the panels by using the wizard or the user interface. You can save your settings as presets and load them whenever you need them.
-
-
What are the Alternatives to Jin Li Tong Software Multiviewer
-
-
There are some alternatives to Jin Li Tong Software Multiviewer, such as MultiView by Blackmagic Design, MagicSoft Multiviewer, and MultiViewer by Apponic. These are some other multiviewer software applications that offer similar features and functions.
-
-
MultiView by Blackmagic Design is a hardware-based multiviewer solution that lets you monitor up to 16 SDI sources on a single display. It supports SD, HD, and Ultra HD resolutions. It has built-in audio meters, custom labels, tally indicators, video format indicators, center markers, safe area markers, network IP control interface.
-
-
MagicSoft Multiviewer is a software-based multiviewer solution that lets you monitor up to 32 SDI sources on a single display. It supports SD and HD resolutions. It has built-in audio meters, custom labels, tally indicators.
-
-
MultiViewer by Apponic is a software-based multiviewer solution that lets you monitor up to 4 video sources on a single display. It supports various input types such as webcam, capture card.
-
-
Conclusion
-
-
Jin Li Tong Software Multiviewer is a powerful solution for monitoring multiple video sources on a single display. It supports various input types such as SDI, HDMI. It has built-in audio meters. It is suitable for professional environments such as broadcasting stations. It can handle SD. You can customize the panels layout by using an easy to operate wizard. You can download it from this link and try it for yourself.
-
-
If you need a multiviewer software solution that can help you save space, improve your workflow efficiency, and view more sources than you have monitors available, then you should consider Jin Li Tong Software Multiviewer. It offers a wide range of features and options to suit your needs and preferences.
-
What are the Features of Jin Li Tong Software Multiviewer
-
-
Jin Li Tong Software Multiviewer is a software application that offers a lot of features and options to help you monitor multiple video sources on a single display. Some of the features of Jin Li Tong Software Multiviewer are:
-
-
-
It supports various input types, such as SDI, HDMI, analog, NDI, and UDP. You can connect different types of video sources to your computer and monitor them on one display.
-
It has built-in audio meters, custom labels, and alarms for image freezing, black level, and white level. You can monitor the audio levels and quality of each video source and set alarms for any abnormal conditions.
-
It can monitor up to 16 sound channels by simply clicking on the pair that you want to listen to. You can switch between different sound channels easily and hear the audio of each video source.
-
It can handle SD, HD, and 4K resolutions. You can monitor video sources with different resolutions and aspect ratios on one display.
-
It can customize the panels layout by using an easy to operate wizard. You can choose from different templates or create your own layout by dragging and dropping the panels.
-
It can manage the panels (loading, cloning or deleting) from a user interface that allows you to preview the panels layout. You can load, clone or delete any panel with a few clicks and see how it looks on the display.
-
It has a watch-dog application that ensures the uninterrupted functionality of the software. It monitors the software status and restarts it automatically if it crashes or freezes.
-
-
-Why You Should Choose Jin Li Tong Software Multiviewer
-
-
Jin Li Tong Software Multiviewer is a software application that provides a powerful solution for monitoring multiple video sources on a single display. It is suitable for professional environments such as broadcasting stations and production studios. It is also easy to install and use. You can download it from this link and try it for yourself.
-
-
Some of the reasons why you should choose Jin Li Tong Software Multiviewer are:
-
-
-
It supports various input types such as SDI, HDMI. It can handle SD. You can monitor different types of video sources with different resolutions and aspect ratios on one display.
-
It has built-in audio meters. You can monitor the audio levels and quality of each video source and set alarms for any abnormal conditions.
-
It can monitor up to 16 sound channels by simply clicking on the pair that you want to listen to. You can switch between different sound channels easily and hear the audio of each video source.
-
It can customize the panels layout by using an easy to operate wizard. You can choose from different templates or create your own layout by dragging and dropping the panels.
-
It has a watch-dog application that ensures the uninterrupted functionality of the software. It monitors the software status and restarts it automatically if it crashes or freezes.
-
It is designed for professional environments such as broadcasting stations. It is suitable for 24/7 monitoring and it works under Windows 7 / 8 and 10 and equivalent Windows Server OS (64-bit only).
-
-
-
Jin Li Tong Software Multiviewer is a software application that offers a lot of features and options to help you monitor multiple video sources on a single display. It is reliable, flexible, and easy to use. You can download it from this link and try it for yourself.
-How to Compare Jin Li Tong Software Multiviewer with Other Multiviewer Software Applications
-
-
Jin Li Tong Software Multiviewer is a software application that offers a lot of features and options to help you monitor multiple video sources on a single display. However, it is not the only multiviewer software application available on the market. There are some other multiviewer software applications that offer similar features and functions, such as MultiView by Blackmagic Design, MagicSoft Multiviewer, and MultiViewer by Apponic.
-
-
How can you compare Jin Li Tong Software Multiviewer with these other multiviewer software applications? What are the advantages and disadvantages of each one? How can you choose the best one for your needs and preferences?
-
-
Here are some criteria that you can use to compare Jin Li Tong Software Multiviewer with other multiviewer software applications:
-
-
-
Input types: What types of video sources can the multiviewer software application support? Can it handle SDI, HDMI, analog, NDI, and UDP inputs? How many inputs can it support at once?
-
Output resolution: What is the maximum resolution that the multiviewer software application can output? Can it handle SD, HD, and 4K resolutions? How does it scale and adjust the video sources to fit the display?
-
Panel layout: How can you customize the panel layout of the multiviewer software application? Can you use a wizard or a user interface to create your own layout? Can you save and load presets?
-
Panel information: What information can the multiviewer software application display on each panel? Can it show audio meters, custom labels, and alarms for image freezing, black level, and white level?
-
Audio monitoring: How can you monitor the audio of each video source on the multiviewer software application? Can you monitor up to 16 sound channels by simply clicking on the pair that you want to listen to?
-
Reliability: How reliable is the multiviewer software application? Does it have a watch-dog application that ensures the uninterrupted functionality of the software? Does it work under Windows 7 / 8 and 10 and equivalent Windows Server OS (64-bit only)?
-
Price: How much does the multiviewer software application cost? Is it free or paid? Is it worth the money?
-
-
-
By using these criteria, you can compare Jin Li Tong Software Multiviewer with other multiviewer software applications and decide which one is best for you.
-
-Conclusion
-
-
Jin Li Tong Software Multiviewer is a powerful solution for monitoring multiple video sources on a single display. It supports various input types such as SDI, HDMI. It has built-in audio meters. It can monitor up to 16 sound channels by simply clicking on the pair that you want to listen to. It can handle SD. You can customize the panels layout by using an easy to operate wizard. It has a watch-dog application that ensures the uninterrupted functionality of the software. It is suitable for professional environments such as broadcasting stations. You can download it from this link and try it for yourself.
-
-
If you need a multiviewer software solution that can help you monitor multiple video sources on a single display, you should consider Jin Li Tong Software Multiviewer. It offers a wide range of features and options to suit your needs and preferences. However, it is not the only multiviewer software solution available on the market. There are some other multiviewer software applications that offer similar features and functions, such as MultiView by Blackmagic Design, MagicSoft Multiviewer, and MultiViewer by Apponic. You can compare them with Jin Li Tong Software Multiviewer using some criteria such as input types, output resolution, panel layout, panel information, audio monitoring, reliability, and price. By doing so, you can choose the best multiviewer software solution for your needs and preferences.
-Conclusion
-
-
Jin Li Tong Software Multiviewer is a software application that allows you to monitor multiple video sources on a single display. It supports various input types, such as SDI, HDMI, analog, NDI, and UDP. You can configure each panel to display different information, such as audio meters, custom labels, and alarms for image freezing, black level, and white level. You can also monitor up to 16 sound channels by simply clicking on the pair that you want to listen to. Jin Li Tong Software Multiviewer is designed for professional environments such as broadcasting stations and production studios. It is suitable for 24/7 monitoring and it works under Windows 7 / 8 and 10 and equivalent Windows Server OS (64-bit only). It can handle SD, HD, and 4K resolutions. You can customize the panels layout by using an easy to operate wizard. You can also manage the panels (loading, cloning or deleting) from a user interface that allows you to preview the panels layout. Jin Li Tong Software Multiviewer has a watch-dog application that ensures the uninterrupted functionality of the software.
-
-
You can download Jin Li Tong Software Multiviewer from this link and try it for yourself. It is easy to install and use. It offers a wide range of features and options to suit your needs and preferences. However, if you want to compare it with other multiviewer software applications, you can use some criteria such as input types, output resolution, panel layout, panel information, audio monitoring, reliability, and price. By doing so, you can choose the best multiviewer software solution for your needs and preferences.
-
-
Multiviewer software is a type of software that lets you monitor multiple video sources on a single display. It is useful for situations where you need to view more sources than you have monitors available. Multiviewer software can also provide additional features such as overlays, audio monitoring, and alarms. Multiviewer software can be used for various purposes, such as live multi-camera production, control room monitoring, security surveillance, video conferencing, and more.
-
-
If you need a multiviewer software solution that can help you monitor multiple video sources on a single display, you should consider Jin Li Tong Software Multiviewer. It is a powerful solution that offers a lot of features and options to help you monitor multiple video sources on a single display. It is reliable, flexible, and easy to use. You can download it from this link and try it for yourself.
3cee63e6c2
-
-
\ No newline at end of file
diff --git a/spaces/diacanFperku/AutoGPT/Mechanimal Access Virus Ti Psytrance Soundset Vol 1 Torrent.md b/spaces/diacanFperku/AutoGPT/Mechanimal Access Virus Ti Psytrance Soundset Vol 1 Torrent.md
deleted file mode 100644
index e0b4f33eb46f7cd72e0ff1cc52344a9d97258283..0000000000000000000000000000000000000000
--- a/spaces/diacanFperku/AutoGPT/Mechanimal Access Virus Ti Psytrance Soundset Vol 1 Torrent.md
+++ /dev/null
@@ -1,6 +0,0 @@
-
Mechanimal Access Virus Ti Psytrance Soundset Vol 1 Torrent
If you are looking for a reliable and easy-to-use remote car starter, you might want to consider the Carvox Cx 2300c. This device allows you to start your car from up to 1000 feet away, as well as lock and unlock your doors, trunk, and windows. It also features a built-in alarm system, a panic button, and a valet mode. In this article, we will show you how to install the Carvox Cx 2300c remote car starter in your vehicle.
Before you begin the installation process, make sure you have the following tools and materials:
-
-
The Carvox Cx 2300c remote car starter kit, which includes the main unit, two remote controls, a wiring harness, an antenna, a relay socket, a LED indicator, a valet switch, and an installation manual.
-
A multimeter or a test light to check the electrical connections.
-
A wire stripper and a crimper to connect the wires.
-
A drill and a drill bit to mount the antenna and the valet switch.
-
A screwdriver and a wrench to remove the panels and fasteners.
-
Some zip ties and electrical tape to secure the wires.
-
-
Step 1: Disconnect the Battery
-
The first step is to disconnect the negative terminal of your car battery to prevent any short circuits or damage to your electrical system. Make sure you have your car key with you before you do this, as some cars may lock automatically when the battery is disconnected.
-
Step 2: Locate the Ignition Switch Harness
-
The next step is to locate the ignition switch harness in your car. This is the bundle of wires that connects to your ignition switch, which is usually located under the steering column or behind the dashboard. You will need to access this harness to connect the main unit of the Carvox Cx 2300c remote car starter. To do this, you may need to remove some panels or fasteners from your car interior. Refer to your car manual or online resources for specific instructions on how to do this for your vehicle model.
-
-
Step 3: Connect the Main Unit
-
Once you have located the ignition switch harness, you will need to connect the main unit of the Carvox Cx 2300c remote car starter to it. The main unit has a wiring harness with 12 wires of different colors and functions. You will need to match each wire to its corresponding wire in the ignition switch harness. To do this, you will need to use a multimeter or a test light to identify the functions of each wire in the ignition switch harness. For example, you will need to find the wire that has power when the key is in the ON position, or the wire that controls the starter motor. Refer to the installation manual of the Carvox Cx 2300c remote car starter for more details on how to identify and connect each wire. You will also need to use a wire stripper and a crimper to splice and join each wire securely. Make sure you wrap each connection with electrical tape or use heat-shrink tubing to prevent any loose or exposed wires.
-
Step 4: Mount the Antenna
-
The antenna is the device that receives the signals from your remote controls. You will need to mount it on your windshield near the rearview mirror. To do this, you will need to drill a small hole on your dashboard near the windshield. Then, feed the antenna wire through the hole and connect it to the main unit. Peel off the adhesive backing from the antenna and stick it on your windshield. Make sure it is facing upwards and not obstructed by any metal objects.
-
Step 5: Mount the LED Indicator and the Valet Switch
-
The LED indicator is a small light that flashes when your alarm system is armed or triggered. The valet switch is a small button that allows you to disable or enable your alarm system manually. You will need to mount both devices on your dashboard near your steering wheel. To do this
d5da3c52bf
-
-
\ No newline at end of file
diff --git a/spaces/falterWliame/Face_Mask_Detection/Mediachance Photo-Brush V4.3 Serial Key.md b/spaces/falterWliame/Face_Mask_Detection/Mediachance Photo-Brush V4.3 Serial Key.md
deleted file mode 100644
index fd864e5b9e928da5b12b2425e768ae9f1b7ddda2..0000000000000000000000000000000000000000
--- a/spaces/falterWliame/Face_Mask_Detection/Mediachance Photo-Brush V4.3 Serial Key.md
+++ /dev/null
@@ -1,8 +0,0 @@
-
-
-October 27, 2011 — Code: New version of Mediachance Photo Brush, a powerful photo editor with image painting and digital retouching features ... October 27, 2011 - Code: New version of Mediachance Digital Photo Pro, a professional image editor with image editing and digital ...
-October 27, 2011 - Code: New version of Mediachance PhotoImpact, an image editor with photo retouching, color correction, collage maker and ...
-October 27, 2011 - Code: New version of Mediachance Dynamic Auto-Painter, a specialized graphics editor that allows you to automatically ... 8a78ff9644
-
-
-
diff --git a/spaces/fatiXbelha/sd/Download Qdots Songs and Experience His Musical Genius.md b/spaces/fatiXbelha/sd/Download Qdots Songs and Experience His Musical Genius.md
deleted file mode 100644
index 95226618890556c7b5f948db5a36bf1d42b81aaf..0000000000000000000000000000000000000000
--- a/spaces/fatiXbelha/sd/Download Qdots Songs and Experience His Musical Genius.md
+++ /dev/null
@@ -1,214 +0,0 @@
-
-
Qdot Download Music: How to Enjoy Nigerian Music Online
-
If you are a fan of Nigerian music, you have probably heard of Qdot, one of the most talented and versatile artists in the industry. Qdot is a singer, songwriter, producer, and performer who has been making waves with his unique blend of traditional Yoruba music and modern Afrobeat and hip hop influences. He has released several hit songs and albums that have earned him millions of fans across Nigeria and beyond.
But how can you enjoy Qdot's music online? Where can you download his songs legally and safely? And how can you listen to his music offline on your devices? In this article, we will answer these questions and more. We will show you how to download Qdot's music online from various platforms and websites, and how to enjoy his music offline on your phone or computer. We will also give you some tips and tricks to make the most of your Qdot download music experience. So, let's get started!
-
Qdot's Music Style and Genre
-
Qdot is known for his distinctive music style and genre, which he calls Yorubadboi Music. This is a fusion of traditional Yoruba music, such as Apala, Fuji, and Sakara, with contemporary Afrobeat and hip hop elements. Qdot uses Yoruba language, proverbs, metaphors, and folktales to deliver his messages and stories in his songs. He also incorporates various musical instruments, such as drums, shekere, agogo, and talking drum, to create his signature sound.
-
Qdot's music style and genre reflect his cultural identity and heritage as a Yoruba man from Ikorodu, Lagos State. He is proud of his roots and celebrates them in his music. He also uses his music to address social issues, such as poverty, corruption, injustice, and violence, in Nigeria and Africa. He is not afraid to speak his mind and challenge the status quo with his lyrics.
-
Qdot's music style and genre have earned him a loyal fan base and a wide appeal among different audiences. His songs are catchy, danceable, and relatable. They appeal to both the young and the old, the urban and the rural, the rich and the poor. His music transcends boundaries and connects people from different backgrounds and walks of life.
-
Qdot's Top Songs and Albums
-
Qdot has released several songs and albums that have become hits in Nigeria and beyond. Some of his top songs include:
-
-
Koshi Danu: This is a groovy song that showcases Qdot's witty wordplay and catchy chorus. The song is a street anthem that encourages people to work hard and enjoy life.
-
Gbese: This is a dance song that features Qdot's signature talking drum sound and energetic vocals. The song is a challenge to listeners to show off their dancing skills.
-
Ijo Gelede: This is a tribute song to the Yoruba culture and tradition of Gelede, which is a masquerade festival that celebrates women. The song praises the beauty and power of women in society.
-
Olopa: This is a collaboration song with Zlatan Ibile, one of the leading artists in the Zanku movement. The song is a satire on the Nigerian police force and their brutality against citizens.
-
Jaiye: This is a motivational song that inspires listeners to live their lives to the fullest and not worry about tomorrow. The song features Qdot's smooth vocals and uplifting lyrics.
-
-
Some of Qdot's top albums include:
-
-
Alagbe: This is Qdot's debut album that was released in 2020. The album contains 17 tracks that showcase Qdot's versatility and creativity as an artist. The album features guest appearances from other Nigerian artists, such as 9ice, Jaywon, Pasuma, Niniola, Patoranking, and more.
-
Orijin: This is Qdot's second album that was released in 2021. The album contains 14 tracks that highlight Qdot's origin and identity as a Yoruba man. The album features guest appearances from other Nigerian artists, such as Olamide, Reminisce, Seriki, Vector, and more.
-
-
Qdot's Awards and Nominations
-
Qdot has received several awards and nominations for his outstanding contributions to the Nigerian music industry. Some of his awards and nominations include:
-
-
City People Music Award for Best Indigenous Artist of the Year (Male): He won this award in 2018.
-
Nigerian Entertainment Award for Indigenous Artist of the Year: He was nominated for this award in 2019.
-
African Muzik Magazine Award for Best Newcomer: He was nominated for this award in 2019.
-
The Headies Award for Best Street-Hop Artiste: He was nominated for this award in 2020.
-
African Entertainment Award USA for Best Male Artist (West Africa): He was nominated for this award in 2020.
-
-
Qdot continues to work hard and improve his craft as an artist. He is always looking for new ways to express himself and entertain his fans. He is one of the most influential and respected artists in the Nigerian music scene.
-
qdot latest songs mp3 download
-qdot alagbe album zip download
-qdot ft zlatan olopa video download
-qdot jaiye free mp3 download
-qdot gbeja audio download
-qdot kokanmi mp4 download
-qdot jegele lyrics download
-qdot ah instrumental download
-qdot magbe video download
-qdot ole mp3 download
-qdot koshi danu download
-qdot gbese remix download
-qdot yeye girlfriend download
-qdot ijo gelede download
-qdot orin emi download
-qdot ibere mp3 download
-qdot eleda masun download
-qdot lalalu video download
-qdot believe mp3 download
-qdot question mp3 download
-qdot alomo meta mp3 download
-qdot ori mi mp3 download
-qdot turn up mp3 download
-qdot apala new skool video download
-qdot iso oru mp3 download
-qdot ibadan video download
-qdot ogogoro mp3 download
-qdot aare video download
-qdot iyanu mp3 download
-qdot ojurawonlo mp3 download
-qdot atewo video download
-qdot were wan le mp3 download
-qdot wo refix mp3 download
-qdot baba alaye mp3 download
-qdot story of my life mp3 download
-qdot worst cover mp3 download
-qdot angeli mi mp3 download
-qdot dance mp3 download
-qdot moriamo mp3 download
-qdot iyawo mi mp3 download
-qdot egun agba video download
-qdot ibile mugabe mp3 download
-qdot ororo mp3 download
-qdot omo ibadan mp3 download
-qdot cautions video download
-qdot omo aje video download
-
How to Download Qdot's Music Online
-
Now that you know more about Qdot and his music, you might be wondering how to download his songs online. There are many platforms and websites that offer Qdot download music services, but not all of them are reliable and safe. Some of them might contain viruses, malware, or spyware that can harm your device or compromise your privacy. Some of them might also violate Qdot's intellectual property rights and deprive him of his deserved royalties.
-
Therefore, you need to be careful and selective when choosing where to download Qdot's music online. You need to make sure that the platform or website is legal, secure, and reputable. You also need to consider the quality, speed, and cost of the download service. To help you out, we have compiled a list of some of the best platforms and websites to download Qdot's music online. We have also listed the pros and cons of each option, so you can weigh them and decide which one suits you best.
-
Streaming Services
-
One of the most popular and convenient ways to download Qdot's music online is to use streaming services. Streaming services are platforms that allow you to listen to music online without downloading it to your device. You can access millions of songs from various artists and genres with just a click of a button. You can also create your own playlists, discover new music, and share your favorites with your friends.
-
Some of the most popular streaming services that offer Qdot download music services are:
-
-
Spotify: This is one of the largest and most popular streaming services in the world. It has over 70 million songs and podcasts from various artists and genres. It also has a huge collection of Qdot's songs and albums. You can download Qdot's music on Spotify for offline listening if you have a premium subscription, which costs $9.99 per month.
-
Apple Music: This is another leading streaming service that has over 75 million songs and podcasts from various artists and genres. It also has a large collection of Qdot's songs and albums. You can download Qdot's music on Apple Music for offline listening if you have a subscription, which costs $9.99 per month.
-
YouTube Music: This is a streaming service that is based on YouTube, the largest video-sharing platform in the world. It has over 60 million songs and videos from various artists and genres. It also has a good collection of Qdot's songs and albums. You can download Qdot's music on YouTube Music for offline listening if you have a premium subscription, which costs $9.99 per month.
-
-
Pros and Cons of Streaming Services
-
Streaming services have many advantages and disadvantages when it comes to downloading Qdot's music online. Here are some of them:
-
-
-
Pros
-
Cons
-
-
-
- They offer high-quality audio and video streaming.
-
- They require a stable internet connection and data plan.
-
-
-
- They have a large and diverse catalog of music from various artists and genres.
-
- They have limited storage space and offline access.
-
-
-
- They have user-friendly interfaces and features.
-
- They have monthly subscription fees and ads.
-
-
-
- They support Qdot's intellectual property rights and pay him royalties.
-
- They might not have all of Qdot's songs and albums available.
-
-
-
Download Sites
Another option to download Qdot's music online is to use download sites. Download sites are websites that allow you to download music files directly to your device. You can choose from various formats, such as MP3, MP4, WAV, etc. You can also choose from various qualities, such as 128 kbps, 320 kbps, etc. You can download Qdot's music from download sites for free or for a small fee.
-
Some of the most popular download sites that offer Qdot download music services are:
-
-
Naijaloaded: This is one of the biggest and most visited download sites in Nigeria. It has over 5 million monthly visitors and over 10 million downloads per month. It has a huge collection of Qdot's songs and albums, as well as other Nigerian and African music. You can download Qdot's music from Naijaloaded for free or for a token of N100 per song.
-
Xclusiveloaded: This is another leading download site in Nigeria. It has over 3 million monthly visitors and over 7 million downloads per month. It has a large collection of Qdot's songs and albums, as well as other Nigerian and African music. You can download Qdot's music from Xclusiveloaded for free or for a token of N50 per song.
-
Tooxclusive: This is another top download site in Nigeria. It has over 2 million monthly visitors and over 5 million downloads per month. It has a good collection of Qdot's songs and albums, as well as other Nigerian and African music. You can download Qdot's music from Tooxclusive for free or for a token of N20 per song.
-
-
Pros and Cons of Download Sites
-
Download sites have many advantages and disadvantages when it comes to downloading Qdot's music online. Here are some of them:
-
-
-
Pros
-
Cons
-
-
-
- They offer fast and easy downloading of music files.
-
- They might contain viruses, malware, or spyware that can harm your device or compromise your privacy.
-
-
-
- They offer various formats and qualities of music files.
-
- They might violate Qdot's intellectual property rights and deprive him of his deserved royalties.
-
-
-
- They offer free or cheap downloading of music files.
-
- They might have low-quality or fake music files.
-
-
-
- They have a large and diverse catalog of music from various artists and genres.
-
- They might not have all of Qdot's songs and albums available.
-
-
-
Torrent Sites
A third option to download Qdot's music online is to use torrent sites. Torrent sites are websites that allow you to download music files using a peer-to-peer (P2P) network. A P2P network is a system where users share files with each other without a central server. You can download Qdot's music from torrent sites using a torrent client, which is a software that connects you to the P2P network and manages the download process.
-
Some of the most popular torrent sites that offer Qdot download music services are:
-
-
The Pirate Bay: This is one of the oldest and most famous torrent sites in the world. It has over 5 million torrents from various categories, including music, movies, games, software, etc. It has a decent collection of Qdot's songs and albums, as well as other Nigerian and African music. You can download Qdot's music from The Pirate Bay for free using a torrent client.
-
1337x: This is another popular and reliable torrent site in the world. It has over 3 million torrents from various categories, including music, movies, games, software, etc. It has a good collection of Qdot's songs and albums, as well as other Nigerian and African music. You can download Qdot's music from 1337x for free using a torrent client.
-
RARBG: This is another well-known and trusted torrent site in the world. It has over 2 million torrents from various categories, including music, movies, games, software, etc. It has a fair collection of Qdot's songs and albums, as well as other Nigerian and African music. You can download Qdot's music from RARBG for free using a torrent client.
-
-
Pros and Cons of Torrent Sites
-
Torrent sites have many advantages and disadvantages when it comes to downloading Qdot's music online. Here are some of them:
-
-
-
Pros
-
Cons
-
-
-
- They offer fast and unlimited downloading of music files.
-
- They might contain viruses, malware, or spyware that can harm your device or compromise your privacy.
-
-
-
- They offer various formats and qualities of music files.
-
- They might violate Qdot's intellectual property rights and deprive him of his deserved royalties.
-
-
-
- They offer free downloading of music files.
-
- They might have low-quality or fake music files.
-
-
-
- They have a large and diverse catalog of music from various artists and genres.
-
- They might not have all of Qdot's songs and albums available.
-
-
-
- They have a supportive and active community of users who share and rate files.
-
- They might be blocked or banned by your internet service provider or government.
-
-
-
How to Enjoy Qdot's Music Offline
Now that you know how to download Qdot's music online, you might be wondering how to enjoy his music offline. Offline listening is a great way to save your data and battery, and to listen to your favorite Qdot songs anytime and anywhere. There are many ways to enjoy Qdot's music offline on your devices, but here are some of the best ones:
-
Transfer Music to Your Phone or Computer
-
One of the simplest ways to enjoy Qdot's music offline is to transfer his music files from your download source to your phone or computer. You can do this using a USB cable or a cloud storage service. Here are the steps:
-
-
Using a USB cable: Connect your phone or computer to your download source using a USB cable. Locate the Qdot music files on your download source and copy them. Paste them on your phone or computer in a folder of your choice. Disconnect the USB cable and enjoy your Qdot music offline.
-
Using a cloud storage service: Upload the Qdot music files from your download source to a cloud storage service, such as Google Drive, Dropbox, iCloud, etc. Download the Qdot music files from the cloud storage service to your phone or computer. Enjoy your Qdot music offline.
-
-
Use a Music Player App or Software
-
Another way to enjoy Qdot's music offline is to use a music player app or software. A music player app or software is a program that allows you to play music files on your device. You can use a music player app or software to organize, manage, and customize your Qdot music collection. You can also use it to adjust the volume, equalizer, and playback settings of your Qdot music.
-
Some of the most popular music player apps and software that you can use to enjoy Qdot's music offline are:
-
-
VLC Media Player: This is one of the most versatile and powerful music player software in the world. It can play almost any format and quality of music files, including Qdot's music files. It also has many features and options that you can use to enhance your Qdot music experience.
-
Windows Media Player: This is one of the most common and convenient music player software in the world. It can play most formats and qualities of music files, including Qdot's music files. It also has a simple and user-friendly interface that you can use to access and control your Qdot music collection.
-
iTunes: This is one of the most popular and reliable music player software in the world. It can play most formats and qualities of music files, including Qdot's music files. It also has a sleek and sophisticated interface that you can use to organize and sync your Qdot music collection.
-
-
Create Playlists and Mixtapes
-
A third way to enjoy Qdot's music offline is to create playlists and mixtapes of your favorite Qdot songs. A playlist is a collection of songs that you can play in a specific order or randomly. A mixtape is a compilation of songs that you can edit and customize with transitions, effects, and voice-overs. You can create playlists and mixtapes of your favorite Qdot songs using a music player app or software.
-
Creating playlists and mixtapes of your favorite Qdot songs is a fun and creative way to enjoy his music offline. You can create playlists and mixtapes for different moods, occasions, themes, or genres. You can also share them with your friends and family, or upload them online for other Qdot fans to enjoy.
-
Conclusion
-
Qdot is one of the most talented and versatile artists in the Nigerian music industry. He has a unique style and genre that blends traditional Yoruba music with modern Afrobeat and hip hop influences. He has released several hit songs and albums that have earned him millions of fans across Nigeria and beyond.
-
If you want to enjoy Qdot's music online, you have many options to choose from. You can use streaming services, download sites, or torrent sites to download his music online legally and safely. You can also use various methods to enjoy his music offline on your devices, such as transferring his music files, using a music player app or software, or creating playlists and mixtapes. You can also use some tips and tricks to make the most of your Qdot download music experience, such as choosing the best quality and format, checking the reviews and ratings, and supporting Qdot's intellectual property rights.
-
Qdot download music is a great way to enjoy Nigerian music online. Qdot's music is catchy, danceable, and relatable. It reflects his cultural identity and heritage, as well as his social awareness and activism. It connects people from different backgrounds and walks of life. It inspires, entertains, and educates. Qdot download music is a must-have for any Nigerian music lover.
-
So, what are you waiting for? Download Qdot's music online today and enjoy his music offline anytime and anywhere. You will not regret it!
-
FAQs
-
Here are some frequently asked questions about Qdot download music with brief answers:
-
-
Who is Qdot? Qdot is a Nigerian singer, songwriter, producer, and performer who has a unique style and genre of music that blends traditional Yoruba music with modern Afrobeat and hip hop influences.
-
What are some of Qdot's top songs and albums? Some of Qdot's top songs and albums include Koshi Danu, Gbese, Ijo Gelede, Olopa, Jaiye, Alagbe, and Orijin.
-
Where can I download Qdot's music online? You can download Qdot's music online from various platforms and websites, such as streaming services, download sites, or torrent sites. However, you need to be careful and selective when choosing where to download Qdot's music online. You need to make sure that the platform or website is legal, secure, and reputable.
-
How can I enjoy Qdot's music offline? You can enjoy Qdot's music offline on your devices by transferring his music files from your download source to your phone or computer, using a music player app or software to play his music files, or creating playlists and mixtapes of your favorite Qdot songs.
-
What are some tips and tricks to make the most of my Qdot download music experience? Some tips and tricks to make the most of your Qdot download music experience are choosing the best quality and format of his music files, checking the reviews and ratings of his music files, and supporting his intellectual property rights and paying him royalties.
-
401be4b1e0
-
-
\ No newline at end of file
diff --git a/spaces/fatiXbelha/sd/Download the Latest Love Song Ringtones and Make Your Phone Sing with Emotion.md b/spaces/fatiXbelha/sd/Download the Latest Love Song Ringtones and Make Your Phone Sing with Emotion.md
deleted file mode 100644
index 897ab2202a960471d0079f290c8af893d1ce7c27..0000000000000000000000000000000000000000
--- a/spaces/fatiXbelha/sd/Download the Latest Love Song Ringtones and Make Your Phone Sing with Emotion.md
+++ /dev/null
@@ -1,122 +0,0 @@
-
-
New Ringtone Download Love Song: How to Find and Customize the Perfect Tune for Your Phone
-
Do you want to express your love and affection to your partner every time your phone rings? Do you want to spice up your phone with some romantic and melodious tunes? Do you want to stand out from the crowd with a unique and personal ringtone? If you answered yes to any of these questions, then this article is for you.
In this article, we will show you how to find and customize the perfect love song ringtone for your phone. We will cover the following topics:
-
-
Why you need a love song ringtone
-
What are the benefits of customizing your ringtone
-
How to find the best love song ringtones online
-
How to customize your own love song ringtone
-
-
By the end of this article, you will have all the information and tools you need to create a beautiful and memorable love song ringtone that will make your partner swoon.
-
Why you need a love song ringtone
-
A love song ringtone is more than just a sound that alerts you of an incoming call. It is also a way of expressing your feelings and emotions to your partner, as well as yourself. A love song ringtone can:
-
-
Remind you of your partner and the special moments you shared together
-
Make you feel happy, relaxed, and romantic whenever you hear it
-
Show your partner that you care about them and think of them often
-
Impress your friends and family with your taste in music and style
-
-
What are the benefits of customizing your ringtone
-
While there are many websites and apps that offer thousands of ready-made love song ringtones, nothing beats customizing your own. Customizing your own love song ringtone can:
-
-
Give you more control over the selection, editing, and quality of your ringtone
-
Allow you to personalize your ringtone with your own voice, message, or name
-
Make your ringtone more unique and original than anyone else's
-
Save you money and time from downloading or buying ringtones online
-
-
How to find the best love song ringtones online
-
If you don't have a specific song in mind, or if you want to explore some options before customizing your own, you can browse through some of the best websites and apps that offer free or paid love song ringtones. Here are some of the most popular ones:
-
MeloBoom
-
MeloBoom is a website that offers free love ringtones for download in various genres and categories. You can search by artist, song title, or keyword, and preview the ringtones before downloading them. You can also upload your own ringtones and share them with other users.
-
Zedge
-
Zedge is a popular app that offers millions of free ringtones, wallpapers, stickers, and more. You can find love song ringtones from various artists and genres, as well as create your own ringtones using the app's built-in editor. You can also join the Zedge community and discover new content from other users.
-
new love story ringtone download mp3
-new romantic love song ringtone download
-new love mashup ringtone download 2023
-new love flute ringtone download
-new love tone ringtone download
-new love sad song ringtone download
-new love guitar ringtone download
-new love music ringtone download
-new love dj ringtone download
-new love hindi song ringtone download
-new love english song ringtone download
-new love tamil song ringtone download
-new love telugu song ringtone download
-new love punjabi song ringtone download
-new love bollywood song ringtone download
-new love korean song ringtone download
-new love arabic song ringtone download
-new love instrumental ringtone download
-new love piano ringtone download
-new love violin ringtone download
-new love saxophone ringtone download
-new love trumpet ringtone download
-new love harmonica ringtone download
-new love xylophone ringtone download
-new love drum ringtone download
-new i love you ringtone download
-new let me love you ringtone download
-new i see fire ringtone download love version
-new jeene laga hoon ringtone download love version
-new tum hi ho ringtone download love version
-neethanae flute bgm ringtone download love version
-yaara teri yaari ko ringtone download love version
-maine pyar kia music ringtone download love version
-jashne baharaa ringtone download love version
-liebe loves amor ringtone download love version
-sweetest charming charm sweetie loving great cute nice cutie charms ringtone download love version
-most romantic 2023 ringtone download love version
-best sexiest romantic mp3 ringtone download love version
-free love ringtones for iphone and android meloboom[^1^]
-popular favorites liebe loves amor ringtones for iphone and android meloboom[^1^]
-
Myxer
-
Myxer is a website that lets you create and download custom ringtones from any audio file. You can upload your own music, or choose from the website's library of songs and sounds. You can then edit the audio file, add effects, and adjust the volume and quality of your ringtone. You can also browse through the website's collection of free ringtones in different categories.
-
The most romantic love song ringtones of all time
-
If you need some inspiration for choosing a love song ringtone, here are some of the most romantic love song ringtones of all time. These are the songs that have touched the hearts of millions of people around the world, and have become the soundtrack of many love stories.
-
I Will Always Love You by Whitney Houston
-
This is one of the most iconic love songs ever recorded, and was featured in the movie The Bodyguard. Whitney Houston's powerful vocals and emotional delivery make this song a perfect choice for a love song ringtone. The chorus is especially memorable and catchy, and will make your partner feel loved and appreciated every time they hear it.
-
Can't Help Falling in Love by Elvis Presley
-
This is a classic love song that was originally sung by Elvis Presley in the movie Blue Hawaii. It has been covered by many artists over the years, but none can match the charm and charisma of Elvis. This song is a beautiful expression of how love can overcome all obstacles and doubts, and how it can make you feel alive and happy. The melody is soothing and romantic, and will make your partner feel special and cherished every time they hear it.
-
My Heart Will Go On by Celine Dion
-
This is another iconic love song that was featured in the movie Titanic. Celine Dion's angelic voice and passionate performance make this song a timeless masterpiece. This song is a tribute to the power and endurance of love, even in the face of tragedy and death. The chorus is dramatic and emotional, and will make your partner feel moved and inspired every time they hear it.
-
How to customize your own love song ringtone
-
If you want to create your own love song ringtone from scratch, you will need some tools and skills to do so. Here are some of the best ringtone maker apps for iPhone and Android, as well as some tips and tricks for creating a unique and personal love song ringtone.
-
The best ringtone maker apps for iPhone and Android
-
There are many apps that allow you to create your own ringtones from any audio file on your phone or online. Here are some of the best ones:
-
Ringtone Maker
-
Ringtone Maker is a free app that lets you create ringtones from any music or sound on your phone or online. You can cut, trim, merge, mix, fade in/out, adjust volume, pitch, speed, and more. You can also record your own voice or message to add to your ringtone. You can save your ringtones as MP3 or M4R files, and share them with others.
-
MP3 Cutter and Ringtone Maker
-
MP3 Cutter and Ringtone Maker is another free app that lets you create ringtones from any audio file on your phone or online. You can cut, trim, merge, mix, fade in/out, adjust volume, pitch, speed, and more. You can also record your own voice or message to add to your ringtone. You can save your ringtones as MP3 or M4R files, and share them with others.
-
GarageBand
-
GarageBand is a powerful app that lets you create music and ringtones on your iPhone or iPad. You can use the app's instruments, loops, samples, effects, and recording features to create your own songs or ringtones. You can also import audio files from your phone or online to edit them. You can save your ringtones as M4R files, and share them with others.
-
The tips and tricks for creating a unique and personal love song ringtone
-
Creating your own love song ringtone can be fun and rewarding, but it can also be challenging and time-consuming. Here are some tips and tricks to help you create a love song ringtone that suits your taste and personality:
-
Choose a meaningful song that reflects your relationship
-
The first step to creating a love song ringtone is to choose a song that has a special meaning for you and your partner. It can be a song that reminds you of how you met, how you fell in love, or how you overcome difficulties together. It can also be a song that expresses your feelings, hopes, or dreams for your relationship. The song should resonate with both of you, and make you smile whenever you hear it.
-
Edit the song to fit the length and format of your phone
-
The next step is to edit the song to make it suitable for a ringtone. You can use any of the apps mentioned above to cut, trim, merge, or mix the song as you like. You should also consider the length and format of your phone's ringtone settings. For example, some phones have a limit of 30 seconds or 40 seconds for ringtones, while others allow longer or shorter ones. Some phones also require ringtones to be in MP3 or M4R format, while others accept other formats. You should check your phone's specifications before saving your ringtone.
-
Add some effects and filters to enhance the sound quality
-
The final step is to add some effects and filters to enhance the sound quality of your ringtone. You can use any of the apps mentioned above to add effects such as fade in/out, echo, reverb, chorus, flanger, distortion, or equalizer. You can also adjust the volume, pitch, speed, or balance of your ringtone. You should experiment with different effects and filters until you find the ones that suit your preference and style.
-
Conclusion
-
In conclusion, creating a love song ringtone for your phone can be a great way to express your love and affection to your partner, as well as yourself. You can find and download love song ringtones online from various websites and apps, or you can customize your own love song ringtone using some of the best ringtone maker apps for iPhone and Android. You should also follow some tips and tricks to create a unique and personal love song ringtone that reflects your relationship and personality.
-
So what are you waiting for? Start creating your own love song ringtone today, and surprise your partner with a sweet and romantic tune that will make their heart melt.
-
FAQs
-
-
Q: How do I set my love song ringtone as my default ringtone on my phone?
-
A: The steps may vary depending on your phone model and operating system, but generally you can go to Settings > Sound > Ringtone and select your love song ringtone from the list. You can also assign different ringtones to different contacts by going to Contacts > Edit > Ringtone.
-
Q: How do I share my love song ringtone with my partner or friends?
-
A: You can share your love song ringtone with others by using any of the apps mentioned above, or by using other methods such as Bluetooth, email, messaging, or social media. You can also upload your love song ringtone to online platforms such as MeloBoom or Zedge and share the link with others.
-
Q: How do I delete or change my love song ringtone if I don't like it anymore?
-
A: You can delete or change your love song ringtone by going to Settings > Sound > Ringtone and selecting another ringtone from the list. You can also delete the audio file from your phone's storage or app's library.
-
Q: How do I find more love song ringtones online?
-
A: You can find more love song ringtones online by searching on Google or other search engines using keywords such as "love song ringtones", "romantic ringtones", "love ringtones", etc. You can also browse through various websites and apps that offer free or paid love song ringtones in different genres and categories.
-
Q: How do I create my own love song ringtones from scratch?
-
A: You can create your own love song ringtones from scratch by using any of the apps mentioned above, or by using other software such as Audacity or GarageBand on your computer. You will need an audio file of the song you want to use, or you can record your own voice or message. You will also need some editing skills and creativity to make your love song ringtone unique and personal.
-
-
I hope you enjoyed this article and learned something new. If you have any questions or feedback, please leave a comment below. And don't forget to share this article with your friends and family who might be interested in creating their own love song ringtones. Thank you for reading!
401be4b1e0
-
-
\ No newline at end of file
diff --git a/spaces/feregVcuzo/sanity-test-midi/checkpoint/Download Stickman Warriors Super Dragon Shadow Fight Mod APK v1.4.8 - The Ultimate Fighting Game.md b/spaces/feregVcuzo/sanity-test-midi/checkpoint/Download Stickman Warriors Super Dragon Shadow Fight Mod APK v1.4.8 - The Ultimate Fighting Game.md
deleted file mode 100644
index 3cdfb73bfd5441fe93dce3533c45472b1e243593..0000000000000000000000000000000000000000
--- a/spaces/feregVcuzo/sanity-test-midi/checkpoint/Download Stickman Warriors Super Dragon Shadow Fight Mod APK v1.4.8 - The Ultimate Fighting Game.md
+++ /dev/null
@@ -1,112 +0,0 @@
-
-
Stickman Warriors Super Dragon Shadow Fight Mod APK New Update: A Review
-
If you are a fan of anime and action games, you might have heard of Stickman Warriors Super Dragon Shadow Fight, a fast-paced 2D dueling game that combines a number of the most popular anime characters, including your favorite characters from Dragon Ball, Naruto, and One Piece. In this game, you can choose from a variety of heroes, each with their own unique skills and fighting styles, and battle against enemies in solo mode or online mode. You can also customize your heroes with different outfits, accessories, weapons, and skills.
-
stickman warriors super dragon shadow fight mod apk new update
But what if you want to enjoy the game without any limitations or restrictions? What if you want to unlock all the heroes, upgrade them to the max level, and have unlimited money, gems, diamonds, and everything else? Well, there is a way to do that. You can download and install Stickman Warriors Super Dragon Shadow Fight Mod APK, a modified version of the game that gives you access to all the features and benefits that you want.
-
In this article, we will review Stickman Warriors Super Dragon Shadow Fight Mod APK new update, which was released in December 2021. We will tell you what is new in this update, how to download and install it, what are its features, how to play it, what are some tips and tricks for it, and how it compares to other similar games. So, if you are interested in playing this amazing game with all the advantages that it offers, read on.
-
What is Stickman Warriors Super Dragon Shadow Fight?
-
A fast-paced 2D dueling game with anime characters
-
Stickman Warriors Super Dragon Shadow Fight is a game that was developed by SkySoft Studio in 2020. It is a 2D dueling game that features stickman versions of famous anime characters from various shows like Dragon Ball, Naruto, One Piece, Bleach, Fairy Tail, Hunter x Hunter, Attack on Titan, My Hero Academia, Demon Slayer, One Punch Man, Sword Art Online, Tokyo Ghoul, Death Note, Fullmetal
Alchemist, and many more. You can choose from over 100 heroes, each with their own unique skills and fighting styles, and challenge your opponents in various arenas. You can also switch between different heroes during the fight, creating combos and strategies to defeat your enemies.
-
A solo mode with increasing difficulty and rewards
-
Stickman Warriors Super Dragon Shadow Fight has a solo mode where you can fight against different enemies in a series of levels. Each level has a different difficulty and reward, and you can earn gems and coins by completing them. You can use these gems and coins to unlock and upgrade your heroes, as well as buy items and skills. The solo mode also has boss battles, where you can face powerful enemies that require more skill and strategy to defeat. The solo mode is a great way to practice your skills, test your heroes, and earn rewards.
-
stickman warriors super dragon shadow fight mod apk latest version
-stickman warriors super dragon shadow fight mod apk unlimited money
-stickman warriors super dragon shadow fight mod apk download for android
-stickman warriors super dragon shadow fight mod apk free download
-stickman warriors super dragon shadow fight mod apk offline
-stickman warriors super dragon shadow fight mod apk hack
-stickman warriors super dragon shadow fight mod apk no root
-stickman warriors super dragon shadow fight mod apk 2023
-stickman warriors super dragon shadow fight mod apk revdl
-stickman warriors super dragon shadow fight mod apk rexdl
-stickman warriors super dragon shadow fight mod apk android 1
-stickman warriors super dragon shadow fight mod apk happymod
-stickman warriors super dragon shadow fight mod apk pure
-stickman warriors super dragon shadow fight mod apk obb
-stickman warriors super dragon shadow fight mod apk data
-stickman warriors super dragon shadow fight mod apk cheat
-stickman warriors super dragon shadow fight mod apk full unlocked
-stickman warriors super dragon shadow fight mod apk premium
-stickman warriors super dragon shadow fight mod apk pro
-stickman warriors super dragon shadow fight mod apk vip
-stickman warriors super dragon shadow fight mod apk mega mod
-stickman warriors super dragon shadow fight mod apk god mode
-stickman warriors super dragon shadow fight mod apk one hit kill
-stickman warriors super dragon shadow fight mod apk all characters unlocked
-stickman warriors super dragon shadow fight mod apk all skills unlocked
-stickman warriors super dragon shadow fight mod apk all levels unlocked
-stickman warriors super dragon shadow fight mod apk all weapons unlocked
-stickman warriors super dragon shadow fight mod apk all costumes unlocked
-stickman warriors super dragon shadow fight mod apk all transformations unlocked
-stickman warriors super dragon shadow fight mod apk all items unlocked
-stickman warriors super dragon shadow fight mod apk high damage
-stickman warriors super dragon shadow fight mod apk unlimited gems
-stickman warriors super dragon shadow fight mod apk unlimited coins
-stickman warriors super dragon shadow fight mod apk unlimited energy
-stickman warriors super dragon shadow fight mod apk unlimited health
-stickman warriors super dragon shadow fight mod apk unlimited power
-stickman warriors super dragon shadow fight mod apk unlimited stamina
-stickman warriors super dragon shadow fight mod apk unlimited ki
-stickman warriors super dragon shadow fight mod apk unlimited zenkai boosters
-
A variety of heroes to unlock and customize
-
Stickman Warriors Super Dragon Shadow Fight has a huge collection of heroes that you can unlock and customize. You can find heroes from different anime shows, such as Goku, Naruto, Luffy, Ichigo, Natsu, Gon, Eren, Deku, Tanjiro, Saitama, Kirito, Kaneki, Light, Edward, and many more. Each hero has their own unique skills and fighting styles, such as ki blasts, rasengan, gum-gum fruit, bankai, dragon slayer magic, nen, titan transformation, one for all, water breathing, serious punch, sword skills, kagune, death note, alchemy, and many more. You can also customize your heroes with different outfits, accessories, weapons, and skills. You can mix and match different items and skills to create your own unique hero.
-
What is new in the latest update of Stickman Warriors Super Dragon Shadow Fight?
-
New characters and skills added
-
The latest update of Stickman Warriors Super Dragon Shadow Fight was released in December 2021. It added new characters and skills to the game. Some of the new characters are Asta from Black Clover, Jotaro from JoJo's Bizarre Adventure, Levi from Attack on Titan, Bakugo from My Hero Academia, Zenitsu from Demon Slayer,
and Noelle from Black Clover. Some of the new skills are anti-magic, star platinum, 3D maneuver gear, explosion, and water creation. These new characters and skills add more variety and fun to the game, as you can try different combinations and strategies to defeat your enemies.
-
Improved graphics and performance
-
The latest update of Stickman Warriors Super Dragon Shadow Fight also improved the graphics and performance of the game. The game now has more detailed and colorful graphics, as well as smoother and faster animations. The game also runs more smoothly and stably, with less lag and glitches. The game now supports more devices and platforms, as well as higher resolutions and frame rates. The game also has a better user interface and sound effects, making it more user-friendly and immersive.
-
Bug fixes and optimizations
-
The latest update of Stickman Warriors Super Dragon Shadow Fight also fixed some bugs and optimized some aspects of the game. The game now has fewer errors and crashes, as well as better compatibility and security. The game also has a better balance and fairness, as well as more rewards and incentives. The game also has a better online mode, with less latency and disconnects, as well as more features and modes. The game also has a better feedback system, where you can report any issues or suggestions to the developers.
-
How to download and install Stickman Warriors Super Dragon Shadow Fight Mod APK?
-
Download the mod apk file from a trusted source
-
If you want to download and install Stickman Warriors Super Dragon Shadow Fight Mod APK, you need to find a trusted source that provides the mod apk file. You can search online for various websites that offer the mod apk file, but you need to be careful and avoid any scams or viruses. You can also use the link below to download the mod apk file from a reliable source.
Before you can install Stickman Warriors Super Dragon Shadow Fight Mod APK, you need to enable unknown sources in your device settings. This will allow you to install apps that are not from the official Google Play Store or App Store. To do this, you need to go to your device settings, then security or privacy, then unknown sources or install unknown apps, then toggle on the option to allow unknown sources or install unknown apps.
-
Install the mod apk file and enjoy the game
-
After you have downloaded the mod apk file and enabled unknown sources in your device settings, you can install Stickman Warriors Super Dragon Shadow Fight Mod APK. To do this, you need to locate the mod apk file in your device storage, then tap on it to start the installation process. Follow the instructions on the screen to complete the installation process. Once the installation is done, you can open the game and enjoy it.
What are the features of Stickman Warriors Super Dragon Shadow Fight Mod APK?
-
Unlimited money, gems, diamonds, and everything
-
One of the main features of Stickman Warriors Super Dragon Shadow Fight Mod APK is that it gives you unlimited money, gems, diamonds, and everything else. This means that you can buy anything you want in the game, such as heroes, items, skills, and more. You can also upgrade your heroes and skills to the max level, making them more powerful and effective. You can also use the money, gems, and diamonds to unlock more features and modes in the game, such as online mode, tournament mode, survival mode, and more. With unlimited money, gems, diamonds, and everything else, you can enjoy the game without any limitations or restrictions.
-
All heroes unlocked and upgraded
-
Another feature of Stickman Warriors Super Dragon Shadow Fight Mod APK is that it unlocks and upgrades all the heroes in the game. This means that you can access all the heroes from different anime shows, such as Goku, Naruto, Luffy, Ichigo, Natsu, Gon, Eren, Deku, Tanjiro, Saitama, Kirito, Kaneki, Light, Edward, Asta, Jotaro, Levi, Bakugo, Zenitsu, Noelle, and many more. You can also upgrade all the heroes to the max level, making them more powerful and effective. You can also customize all the heroes with different outfits, accessories, weapons, and skills. With all the heroes unlocked and upgraded, you can have more fun and variety in the game.
-
No ads and no root required
-
A third feature of Stickman Warriors Super Dragon Shadow Fight Mod APK is that it removes all the ads and does not require root access. This means that you can play the game without any interruptions or distractions from annoying ads that pop up on your screen. You can also play the game without having to root your device or compromise its security or warranty. You can simply download and install the mod apk file and enjoy the game without any hassle or risk.
-
How to play Stickman Warriors Super Dragon Shadow Fight Mod APK?
-
Move your character with the joystick on the left side of the screen
-
To play Stickman Warriors Super Dragon Shadow Fight Mod APK, you need to move your character with the joystick on the left side of the screen. You can move your character in any direction you want. You can also use the joystick to dodge or evade your enemy's attacks. Moving your character is important to position yourself for attacking or defending.
-
Tap the buttons on the right side of the screen to attack, charge, parry, or use special moves
-
To play Stickman Warriors Super Dragon Shadow Fight Mod APK, you need to tap the buttons on the right side of the screen to attack, charge, parry, or use special moves. You can use the attack button to perform basic attacks, such as punches, kicks, slashes, or blasts. You can use the charge button to fill up your energy bar, which is needed to use special moves. You can use the parry button to block or counter your enemy's attacks, which can give you an advantage. You can use the special move button to unleash powerful moves that can deal massive damage or have special effects, such as kamehameha, rasenshuriken, gomu gomu no pistol, getsuga tensho, fire dragon roar, jajanken, colossal titan, detroit smash, hinokami kagura, consecutive normal punches, starburst stream, kakuja, ryuk's apple, or human transmutation. Each hero has their own special moves that are based on their anime show.
-
Defeat your enemies and earn gems and coins
-
To play Stickman Warriors Super Dragon Shadow Fight Mod APK, you need to defeat your enemies and earn gems and coins. You can defeat your enemies by reducing their health bar to zero or by knocking them out of the arena. You can earn gems and coins by winning fights, completing levels, or achieving goals. You can use these gems and coins to unlock and upgrade your heroes, as well as buy items and skills. You can also use these gems and coins to unlock more features and modes in the game, such as online mode, tournament mode, survival mode, and more.
-
What are some tips and tricks for Stickman Warriors Super Dragon Shadow Fight Mod APK?
-
Learn the strengths and weaknesses of each hero and enemy
-
One of the tips and tricks for Stickman Warriors Super Dragon Shadow Fight Mod APK is to learn the strengths and weaknesses of each hero and enemy. Each hero and enemy has their own unique skills and fighting styles, as well as their own advantages and disadvantages. For example, some heroes are faster but weaker, while others are slower but stronger. Some heroes have ranged attacks but low defense, while others have melee attacks but high defense. Some heroes have elemental attacks that can deal extra damage or have special effects on certain enemies, while others have neutral attacks that can work on any enemy. You need to learn the strengths and weaknesses of each hero and enemy so that you can choose the best hero for each fight and exploit the weaknesses of your enemy.
-
Use your special moves wisely and strategically
-
Another tip and trick for Stickman Warriors Super Dragon Shadow Fight Mod APK is to use your special moves wisely and strategically. Your special moves are powerful moves that can deal massive damage or have special effects on your enemy. However, they also consume a lot of energy, which means that you cannot use them too often or too recklessly. You need to use your special moves wisely and strategically so that you can maximize their impact and efficiency. For example, you can use your special moves when your enemy is vulnerable or stunned, when you have a clear shot or an opening, when you need to finish off your enemy quickly or turn the tide of the battle, or when you want to create a combo or a chain reaction. You also need to avoid using your special moves when your enemy is blocking or countering, when you have low energy or are in a disadvantageous position, when you are wasting your energy or missing your target, or when you are overusing your special moves or becoming predictable. You also need to balance your special moves with your basic attacks, charge, and parry, so that you can maintain your energy and defense.
-
Upgrade your heroes and skills regularly
-
A third tip and trick for Stickman Warriors Super Dragon Shadow Fight Mod APK is to upgrade your heroes and skills regularly. Upgrading your heroes and skills can make them more powerful and effective, as well as unlock new features and abilities. You can upgrade your heroes and skills by using gems and coins that you earn from the game. You can also use items and skills that you buy from the shop. You can upgrade your heroes' stats, such as health, attack, defense, speed, and energy. You can also upgrade your heroes' skills, such as power, range, cooldown, and effect. You can also upgrade your items and skills, such as durability, damage, protection, and bonus. Upgrading your heroes and skills regularly can help you improve your performance and win more fights.
-
How does Stickman Warriors Super Dragon Shadow Fight Mod APK compare to other similar games?
-
It has more anime characters than other stickman games
-
One of the ways that Stickman Warriors Super Dragon Shadow Fight Mod APK compares to other similar games is that it has more anime characters than other stickman games. Most stickman games have generic or original characters that are not based on any anime show. However, Stickman Warriors Super Dragon Shadow Fight Mod APK has over 100 anime characters from various shows like Dragon Ball, Naruto, One Piece, Bleach, Fairy Tail, Hunter x Hunter, Attack on Titan, My Hero Academia, Demon Slayer, One Punch Man, Sword Art Online, Tokyo Ghoul, Death Note, Fullmetal Alchemist, Black Clover, JoJo's Bizarre Adventure, and many more. This makes the game more appealing and exciting for anime fans and action lovers.
-
It has more cinematic and flexible fights than other dueling games
-
Another way that Stickman Warriors Super Dragon Shadow Fight Mod APK compares to other similar games is that it has more cinematic and flexible fights than other dueling games. Most dueling games have rigid and repetitive fights that are limited by the rules and mechanics of the game. However, Stickman Warriors Super Dragon Shadow Fight Mod APK has more cinematic and flexible fights that are influenced by the physics and animations of the game. You can move your character freely and dynamically, as well as switch between different heroes during the fight. You can also perform various moves and combos, such as flying, jumping, dodging, parrying, charging, attacking, and using special moves. You can also interact with the environment and use objects as weapons or shields. The fights are more realistic and immersive, as well as more fun and thrilling.
-
It has more addictive and challenging gameplay than other action games
-
A third way that Stickman Warriors Super Dragon Shadow Fight Mod APK compares to other similar games is that it has more addictive and challenging gameplay than other action games. Most action games have easy and boring gameplay that does not require much skill or strategy. However, Stickman Warriors Super Dragon Shadow Fight Mod APK has more addictive and challenging gameplay that requires skill, strategy, and reflexes. You need to master the controls and mechanics of the game, as well as learn the strengths and weaknesses of each hero and enemy. You need to use your special moves wisely and strategically, as well as balance your energy and defense. You need to upgrade your heroes and skills regularly, as well as unlock more features and modes in the game. The game also has a solo mode with increasing difficulty and rewards, as well as an online mode with competitive and cooperative modes. The game is more satisfying and rewarding, as well as more fun and exciting.
-
Conclusion
-
Stickman Warriors Super Dragon Shadow Fight Mod APK is a fun and exciting game for anime fans and action lovers. It offers a lot of features, updates, and benefits that make it worth playing. It has more anime characters than other stickman games, more cinematic and flexible fights than other dueling games, and more addictive and challenging gameplay than other action games. It also gives you unlimited money, gems, diamonds, and everything else, as well as all the heroes unlocked and upgraded. It also removes all the ads and does not require root access. It is easy to download and install, as well as easy to play. It is a game that you will not regret playing.
-
If you are interested in playing this amazing game with all the advantages that it offers, download it now from the link below and enjoy the ultimate stickman fighting experience.
Here are some frequently asked questions about Stickman Warriors Super Dragon Shadow Fight Mod APK:
-
Q: Is Stickman Warriors Super Dragon Shadow Fight Mod APK safe to use?
-
A: Yes, Stickman Warriors Super Dragon Shadow Fight Mod APK is safe to use. It does not contain any viruses or malware that can harm your device or data. It also does not require root access or compromise your device's security or warranty. However, you should always download it from a trusted source and enable unknown sources in your device settings before installing it.
-
Q: Is Stickman Warriors Super Dragon Shadow Fight Mod APK legal to use?
-
A: Yes, Stickman Warriors Super Dragon Shadow Fight Mod APK is legal to use. It does not violate any laws or regulations that govern the use of apps or games. However, you should always respect the rights and interests of the original developers and the original game. You should also avoid using it for any illegal or unethical purposes.
-
Q: Is Stickman Warriors Super Dragon Shadow Fight Mod APK compatible with my device?
-
A: Yes, Stickman Warriors Super Dragon Shadow Fight Mod APK is compatible with most devices and platforms. It supports Android 4.4 and up, as well as iOS 9.0 and up. It also supports various resolutions and frame rates, as well as different languages and regions. However, you should always check the requirements and specifications of the mod apk file before downloading and installing it.
-
Q: How can I update Stickman Warriors Super Dragon Shadow Fight Mod APK?
-
A: You can update Stickman Warriors Super Dragon Shadow Fight Mod APK by downloading and installing the latest version of the mod apk file from the same source that you used before. You should also delete the previous version of the mod apk file before installing the new one. You should also backup your data and progress before updating, as some updates may cause data loss or corruption.
-
Q: How can I contact the developers of Stickman Warriors Super Dragon Shadow Fight Mod APK?
-
A: You can contact the developers of Stickman Warriors Super Dragon Shadow Fight Mod APK by using the feedback system in the game. You can also visit their website or social media pages to get more information or support. You can also email them at skysoftstudio@gmail.com or call them at +84 123456789.
-
-### End-to-End question generation (answer agnostic)
-
-In end-to-end question generation the model is aksed to generate questions without providing the answers. [This](https://arxiv.org/pdf/2005.01107v1.pdf) paper discusses these ideas in more detail. Here the T5 model is trained to generate multiple questions simultaneously by just providing the context. The questions are seperated by the `` token. Here's how the examples are processed
-
-input text: `Python is a programming language. Created by Guido van Rossum and first released in 1991.`
-
-target text: `Who created Python ? When was python released ? `
-
-**All the training details can be found in [this](https://app.wandb.ai/psuraj/question-generation) wandb project**
-
-## Results
-
-Results on the SQuAD1.0 dev set using above approaches. For decoding, beam search with num_beams 4 is used with max decoding length set to 32.
-
-For multitask qa-qg models the EM and F1 scores are privded as QA-EM and QA-F1.
-
-The [nlg-eval](https://github.com/Maluuba/nlg-eval) package is used for calculating the metrics.
-
-
-| Name | BLEU-4 | METEOR | ROUGE-L | QA-EM | QA-F1 | QG-FORMAT |
-|----------------------------------------------------------------------------|---------|---------|---------|--------|--------|-----------|
-| [t5-base-qg-hl](https://huggingface.co/valhalla/t5-base-qg-hl) | 21.3226 | 27.0854 | 43.5962 | - | - | highlight |
-| [t5-base-qa-qg-hl](https://huggingface.co/valhalla/t5-base-qa-qg-hl) | 21.0141 | 26.9113 | 43.2484 | 82.46 | 90.272 | highlight |
-| [t5-small-qa-qg-hl](https://huggingface.co/valhalla/t5-small-qa-qg-hl) | 18.9872 | 25.2217 | 40.7893 | 76.121 | 84.904 | highlight |
-| [t5-small-qg-hl](https://huggingface.co/valhalla/t5-small-qg-hl) | 18.5921 | 24.9915 | 40.1886 | - | - | highlight |
-| [t5-small-qg-prepend](https://huggingface.co/valhalla/t5-small-qg-prepend) | 18.2791 | 24.6722 | 39.958 | - | - | prepend |
-
-
-## Requirements
-```
-transformers==3.0.0
-nltk
-nlp==0.2.0 # only if you want to fine-tune.
-```
-
-after installing `nltk` do
-```bash
-python -m nltk.downloader punkt
-```
-
-## Usage
-Use the pipeline whch mimics 🤗transformers pipeline for easy inference.
-
-The pipeline is divided into 3 tasks
-1. `question-generation`: for single task question generation models.
-2. `multitask-qa-qg`: for multi-task qa,qg models.
-3. `e2e-qg`: for end-to-end question generation.
-
-[](https://colab.research.google.com/github/patil-suraj/question_generation/blob/master/question_generation.ipynb)
-
-#### Question Generation
-
-```python3
-from pipelines import pipeline
-
-nlp = pipeline("question-generation")
-nlp("42 is the answer to life, the universe and everything.")
-=> [{'answer': '42', 'question': 'What is the answer to life, the universe and everything?'}]
-```
-
-**prepend format**
-```python3
-nlp = pipeline("question-generation", model="valhalla/t5-small-qg-prepend", qg_format="prepend")
-nlp("42 is the answer to life, the universe and everything.")
-=> [{'answer': '42 ', 'question': 'What is the answer to life, the universe, and everything?'}]
-```
-
-#### Multitask QA-QG
-```python3
-nlp = pipeline("multitask-qa-qg")
-
-# to generate questions simply pass the text
-nlp("42 is the answer to life, the universe and everything.")
-=> [{'answer': '42', 'question': 'What is the answer to life, the universe and everything?'}]
-
-# for qa pass a dict with "question" and "context"
-nlp({
- "question": "What is 42 ?",
- "context": "42 is the answer to life, the universe and everything."
-})
-=> 'the answer to life, the universe and everything'
-```
-
-#### End-to-end question generation (without answer supervision)
-```python3
-nlp = pipeline("e2e-qg")
-nlp("Python is a programming language. Created by Guido van Rossum and first released in 1991.")
-=> [
- 'What is a programming language?',
- 'Who created Python?',
- 'When was Python first released?'
-]
-```
-
-By default both pipelines will use the t5-small* models, to use the other models pass the path through `model` paramter.
-
-By default the `question-generation` pipeline will download the [valhalla/t5-small-qg-hl](https://huggingface.co/valhalla/t5-small-qg-hl) model with `highlight` qg format. If you want to use prepend format then provide the path to the prepend model and set `qg_format` to `"prepend"`. For extracting answer like spans it uses [valhalla/t5-small-qa-qg-hl](https://huggingface.co/valhalla/t5-small-qa-qg-hl) model, you can provide a different model through `ans_model` parameter.
-
-The `multitask-qa-qg` model is for multitask models which can extract answer like spans, do qg and qa, so it won't need seperate `ans_model`. By default [valhalla/t5-small-qa-qg-hl](https://huggingface.co/valhalla/t5-small-qa-qg-hl) model is used with `highlight` format. If you want to use prepend format then provide the path to the prepend model and set `qg_format` to `"prepend"`
-
-The `e2e-qg` pipeline is for end-to-end question generation. These models can generate multiple questions simultaneously without answer supervision. By default it uses [valhalla/t5-small-e2e-qg](https://huggingface.co/valhalla/t5-small-e2e-qg)
-
-## Fine-tuning
-
-### Data processing
-
-To support different data formats the trainer expects pre-processed cached dataset, so you can process the data the way you want.
-The cached dataset should be saved using `torch.save` and it should return a `dict` with `source_ids`, `target_ids`, `attention_mask` keys from `__getitem__`.
-
-- `source_ids`: encoded source text
-- `target_ids`: encoded target text
-- `attention_mask`: attention mask for the `source_ids`
-
-The `T2TDataCollator` takes care of preparing right `input_ids` and `labels`. It also trims the batches dynamically to remove excessive padding tokens, to speed up the training.
-
-The `data/squad_multitask` containes the modifed SQuAD dataset for answer aware question generation (using both prepend and highlight formats), question answering (text-to-text), answer extraction and end-to-end question generation. This dataset can be loaded using the awesome 🤗`nlp` library, this makes processing very easy.
-
-To process and cache the dataset use `prepare_data.py` script. It will load the correct tokenizer depending on the `model_type` argument. It adds two new tokens `` and `` to the tokenizer and saves it at `{model_type}_qg_tokenizer` path. You should pass this tokenizer to the fine-tuning script.
-
-The datasets will be saved in `data/` directory. You should provide filenames using `train_file_name` and `valid_file_name` arguments.
-
-**process data for single task question generation with highlight_qg_format**
-```bash
-python prepare_data.py \
- --task qg \
- --model_type t5 \
- --dataset_path data/squad_multitask/ \
- --qg_format highlight_qg_format \
- --max_source_length 512 \
- --max_target_length 32 \
- --train_file_name train_data_qg_hl_t5.pt \
- --valid_file_name valid_data_qg_hl_t5.pt \
-```
-
-**process data for multi-task qa-qg with highlight_qg_format**
-
-`valid_for_qg_only` argument is used to decide if the validation set should only contain data for qg task. For my multi-task experiments I used validation data with only qg task so that the eval loss curve can be easly compared with other single task models
-
-```bash
-python prepare_data.py \
- --task multi \
- --valid_for_qg_only \
- --model_type t5 \
- --dataset_path data/squad_multitask/ \
- --qg_format highlight_qg_format \
- --max_source_length 512 \
- --max_target_length 32 \
- --train_file_name train_data_qa_qg_hl_t5.pt \
- --valid_file_name valid_data_qg_hl_t5.pt \
-```
-
-**process dataset for end-to-end question generation**
-```bash
-python prepare_data.py \
- --task e2e_qg \
- --valid_for_qg_only \
- --model_type t5 \
- --dataset_path data/squad_multitask/ \
- --qg_format highlight_qg_format \
- --max_source_length 512 \
- --max_target_length 32 \
- --train_file_name train_data_e2e_qg_t5.pt \
- --valid_file_name valid_data_e2e_qg_t5.pt \
-```
-
-### training
-Use the `run_qg.py` script to start training. It uses transformers `Trainer` class for training the models.
-
-
-```bash
-python run_qg.py \
- --model_name_or_path t5-small \
- --model_type t5 \
- --tokenizer_name_or_path t5_qg_tokenizer \
- --output_dir t5-small-qg-hl \
- --train_file_path data/train_data_qg_hl_t5.pt \
- --valid_file_path data/valid_data_qg_hl_t5.pt \
- --per_device_train_batch_size 32 \
- --per_device_eval_batch_size 32 \
- --gradient_accumulation_steps 8 \
- --learning_rate 1e-4 \
- --num_train_epochs 10 \
- --seed 42 \
- --do_train \
- --do_eval \
- --evaluate_during_training \
- --logging_steps 100
-```
-
-or if you want to train it from script or notebook then
-
-```python3
-from run_qg import run_qg
-
-args_dict = {
- "model_name_or_path": "t5-small",
- "model_type": "t5",
- "tokenizer_name_or_path": "t5_qg_tokenizer",
- "output_dir": "t5-small-qg-hl",
- "train_file_path": "data/train_data_qg_hl_t5.pt",
- "valid_file_path": "data/valid_data_qg_hl_t5.pt",
- "per_device_train_batch_size": 32,
- "per_device_eval_batch_size": 32,
- "gradient_accumulation_steps": 8,
- "learning_rate": 1e-4,
- "num_train_epochs": 10,
- "seed": 42,
- "do_train": True,
- "do_eval": True,
- "evaluate_during_training": True,
- "logging_steps": 100
-}
-
-# start training
-run_qg(args_dict)
-```
-
-### Evaluation
-
-Use the `eval.py` script for evaluting the model.
-
-```bash
-python eval.py \
- --model_name_or_path t5-base-qg-hl \
- --valid_file_path valid_data_qg_hl_t5.pt \
- --model_type t5 \
- --num_beams 4 \
- --max_decoding_length 32 \
- --output_path hypothesis_t5-base-qg-hl.txt
-```
-
-This will save the output at {output_path} file.
-
-To calculate the metrics install the [nlg-eval](https://github.com/Maluuba/nlg-eval) package and run
-
-```bash
-nlg-eval --hypothesis=hypothesis_t5-base-qg-hl.txt --references=data/references.txt --no-skipthoughts --no-glove
-```
-
-## Applications 🚀
-
-1. A simple Trivia Quiz on topics of your choice -
- [Medium article](https://medium.com/@nvarshney97/using-the-latest-nlp-techniques-for-fun-98f31ce7b556) and its [Colab Notebook](https://colab.research.google.com/gist/nrjvarshney/39ed6c80e2fe293b9e7eca5bc3a45b7d/quiz.ipynb)
-2. [Autocards, Accelerating learning through machine-generated flashcards](https://paulbricman.com/docs/tools/autocards/)
-
-## Relevant papers
-- https://arxiv.org/abs/1906.05416
-- https://www.aclweb.org/anthology/D19-5821/
-- https://arxiv.org/abs/2005.01107v1
diff --git a/spaces/fuckyoudeki/AutoGPT/tests/unit/test_chat.py b/spaces/fuckyoudeki/AutoGPT/tests/unit/test_chat.py
deleted file mode 100644
index 774f4103762c28d5a02e89c14b224fae0bc0756a..0000000000000000000000000000000000000000
--- a/spaces/fuckyoudeki/AutoGPT/tests/unit/test_chat.py
+++ /dev/null
@@ -1,86 +0,0 @@
-# Generated by CodiumAI
-import time
-import unittest
-from unittest.mock import patch
-
-from autogpt.chat import create_chat_message, generate_context
-
-
-class TestChat(unittest.TestCase):
- # Tests that the function returns a dictionary with the correct keys and values when valid strings are provided for role and content.
- def test_happy_path_role_content(self):
- result = create_chat_message("system", "Hello, world!")
- self.assertEqual(result, {"role": "system", "content": "Hello, world!"})
-
- # Tests that the function returns a dictionary with the correct keys and values when empty strings are provided for role and content.
- def test_empty_role_content(self):
- result = create_chat_message("", "")
- self.assertEqual(result, {"role": "", "content": ""})
-
- # Tests the behavior of the generate_context function when all input parameters are empty.
- @patch("time.strftime")
- def test_generate_context_empty_inputs(self, mock_strftime):
- # Mock the time.strftime function to return a fixed value
- mock_strftime.return_value = "Sat Apr 15 00:00:00 2023"
- # Arrange
- prompt = ""
- relevant_memory = ""
- full_message_history = []
- model = "gpt-3.5-turbo-0301"
-
- # Act
- result = generate_context(prompt, relevant_memory, full_message_history, model)
-
- # Assert
- expected_result = (
- -1,
- 47,
- 3,
- [
- {"role": "system", "content": ""},
- {
- "role": "system",
- "content": f"The current time and date is {time.strftime('%c')}",
- },
- {
- "role": "system",
- "content": f"This reminds you of these events from your past:\n\n\n",
- },
- ],
- )
- self.assertEqual(result, expected_result)
-
- # Tests that the function successfully generates a current_context given valid inputs.
- def test_generate_context_valid_inputs(self):
- # Given
- prompt = "What is your favorite color?"
- relevant_memory = "You once painted your room blue."
- full_message_history = [
- create_chat_message("user", "Hi there!"),
- create_chat_message("assistant", "Hello! How can I assist you today?"),
- create_chat_message("user", "Can you tell me a joke?"),
- create_chat_message(
- "assistant",
- "Why did the tomato turn red? Because it saw the salad dressing!",
- ),
- create_chat_message("user", "Haha, that's funny."),
- ]
- model = "gpt-3.5-turbo-0301"
-
- # When
- result = generate_context(prompt, relevant_memory, full_message_history, model)
-
- # Then
- self.assertIsInstance(result[0], int)
- self.assertIsInstance(result[1], int)
- self.assertIsInstance(result[2], int)
- self.assertIsInstance(result[3], list)
- self.assertGreaterEqual(result[0], 0)
- self.assertGreaterEqual(result[1], 0)
- self.assertGreaterEqual(result[2], 0)
- self.assertGreaterEqual(
- len(result[3]), 3
- ) # current_context should have at least 3 messages
- self.assertLessEqual(
- result[1], 2048
- ) # token limit for GPT-3.5-turbo-0301 is 2048 tokens
diff --git a/spaces/fun-research/FC-CLIP/fcclip/data/dataset_mappers/mask_former_semantic_dataset_mapper.py b/spaces/fun-research/FC-CLIP/fcclip/data/dataset_mappers/mask_former_semantic_dataset_mapper.py
deleted file mode 100644
index 36ff3153b0c84462ea14f1bf3273668217f14678..0000000000000000000000000000000000000000
--- a/spaces/fun-research/FC-CLIP/fcclip/data/dataset_mappers/mask_former_semantic_dataset_mapper.py
+++ /dev/null
@@ -1,184 +0,0 @@
-# Copyright (c) Facebook, Inc. and its affiliates.
-import copy
-import logging
-
-import numpy as np
-import torch
-from torch.nn import functional as F
-
-from detectron2.config import configurable
-from detectron2.data import MetadataCatalog
-from detectron2.data import detection_utils as utils
-from detectron2.data import transforms as T
-from detectron2.projects.point_rend import ColorAugSSDTransform
-from detectron2.structures import BitMasks, Instances
-
-__all__ = ["MaskFormerSemanticDatasetMapper"]
-
-
-class MaskFormerSemanticDatasetMapper:
- """
- A callable which takes a dataset dict in Detectron2 Dataset format,
- and map it into a format used by MaskFormer for semantic segmentation.
-
- The callable currently does the following:
-
- 1. Read the image from "file_name"
- 2. Applies geometric transforms to the image and annotation
- 3. Find and applies suitable cropping to the image and annotation
- 4. Prepare image and annotation to Tensors
- """
-
- @configurable
- def __init__(
- self,
- is_train=True,
- *,
- augmentations,
- image_format,
- ignore_label,
- size_divisibility,
- ):
- """
- NOTE: this interface is experimental.
- Args:
- is_train: for training or inference
- augmentations: a list of augmentations or deterministic transforms to apply
- image_format: an image format supported by :func:`detection_utils.read_image`.
- ignore_label: the label that is ignored to evaluation
- size_divisibility: pad image size to be divisible by this value
- """
- self.is_train = is_train
- self.tfm_gens = augmentations
- self.img_format = image_format
- self.ignore_label = ignore_label
- self.size_divisibility = size_divisibility
-
- logger = logging.getLogger(__name__)
- mode = "training" if is_train else "inference"
- logger.info(f"[{self.__class__.__name__}] Augmentations used in {mode}: {augmentations}")
-
- @classmethod
- def from_config(cls, cfg, is_train=True):
- # Build augmentation
- augs = [
- T.ResizeShortestEdge(
- cfg.INPUT.MIN_SIZE_TRAIN,
- cfg.INPUT.MAX_SIZE_TRAIN,
- cfg.INPUT.MIN_SIZE_TRAIN_SAMPLING,
- )
- ]
- if cfg.INPUT.CROP.ENABLED:
- augs.append(
- T.RandomCrop_CategoryAreaConstraint(
- cfg.INPUT.CROP.TYPE,
- cfg.INPUT.CROP.SIZE,
- cfg.INPUT.CROP.SINGLE_CATEGORY_MAX_AREA,
- cfg.MODEL.SEM_SEG_HEAD.IGNORE_VALUE,
- )
- )
- if cfg.INPUT.COLOR_AUG_SSD:
- augs.append(ColorAugSSDTransform(img_format=cfg.INPUT.FORMAT))
- augs.append(T.RandomFlip())
-
- # Assume always applies to the training set.
- dataset_names = cfg.DATASETS.TRAIN
- meta = MetadataCatalog.get(dataset_names[0])
- ignore_label = meta.ignore_label
-
- ret = {
- "is_train": is_train,
- "augmentations": augs,
- "image_format": cfg.INPUT.FORMAT,
- "ignore_label": ignore_label,
- "size_divisibility": cfg.INPUT.SIZE_DIVISIBILITY,
- }
- return ret
-
- def __call__(self, dataset_dict):
- """
- Args:
- dataset_dict (dict): Metadata of one image, in Detectron2 Dataset format.
-
- Returns:
- dict: a format that builtin models in detectron2 accept
- """
- assert self.is_train, "MaskFormerSemanticDatasetMapper should only be used for training!"
-
- dataset_dict = copy.deepcopy(dataset_dict) # it will be modified by code below
- image = utils.read_image(dataset_dict["file_name"], format=self.img_format)
- utils.check_image_size(dataset_dict, image)
-
- if "sem_seg_file_name" in dataset_dict:
- # PyTorch transformation not implemented for uint16, so converting it to double first
- sem_seg_gt = utils.read_image(dataset_dict.pop("sem_seg_file_name")).astype("double")
- else:
- sem_seg_gt = None
-
- if sem_seg_gt is None:
- raise ValueError(
- "Cannot find 'sem_seg_file_name' for semantic segmentation dataset {}.".format(
- dataset_dict["file_name"]
- )
- )
-
- aug_input = T.AugInput(image, sem_seg=sem_seg_gt)
- aug_input, transforms = T.apply_transform_gens(self.tfm_gens, aug_input)
- image = aug_input.image
- sem_seg_gt = aug_input.sem_seg
-
- # Pad image and segmentation label here!
- image = torch.as_tensor(np.ascontiguousarray(image.transpose(2, 0, 1)))
- if sem_seg_gt is not None:
- sem_seg_gt = torch.as_tensor(sem_seg_gt.astype("long"))
-
- if self.size_divisibility > 0:
- image_size = (image.shape[-2], image.shape[-1])
- padding_size = [
- 0,
- self.size_divisibility - image_size[1],
- 0,
- self.size_divisibility - image_size[0],
- ]
- image = F.pad(image, padding_size, value=128).contiguous()
- if sem_seg_gt is not None:
- sem_seg_gt = F.pad(sem_seg_gt, padding_size, value=self.ignore_label).contiguous()
-
- image_shape = (image.shape[-2], image.shape[-1]) # h, w
-
- # Pytorch's dataloader is efficient on torch.Tensor due to shared-memory,
- # but not efficient on large generic data structures due to the use of pickle & mp.Queue.
- # Therefore it's important to use torch.Tensor.
- dataset_dict["image"] = image
-
- if sem_seg_gt is not None:
- dataset_dict["sem_seg"] = sem_seg_gt.long()
-
- if "annotations" in dataset_dict:
- raise ValueError("Semantic segmentation dataset should not have 'annotations'.")
-
- # Prepare per-category binary masks
- if sem_seg_gt is not None:
- sem_seg_gt = sem_seg_gt.numpy()
- instances = Instances(image_shape)
- classes = np.unique(sem_seg_gt)
- # remove ignored region
- classes = classes[classes != self.ignore_label]
- instances.gt_classes = torch.tensor(classes, dtype=torch.int64)
-
- masks = []
- for class_id in classes:
- masks.append(sem_seg_gt == class_id)
-
- if len(masks) == 0:
- # Some image does not have annotation (all ignored)
- instances.gt_masks = torch.zeros((0, sem_seg_gt.shape[-2], sem_seg_gt.shape[-1]))
- else:
- masks = BitMasks(
- torch.stack([torch.from_numpy(np.ascontiguousarray(x.copy())) for x in masks])
- )
- instances.gt_masks = masks.tensor
-
- dataset_dict["instances"] = instances
-
- return dataset_dict
diff --git a/spaces/g4f/freegpt-webui/Dockerfile b/spaces/g4f/freegpt-webui/Dockerfile
deleted file mode 100644
index 1d30573a8626b2a6c142affbd385666ed44ebf6b..0000000000000000000000000000000000000000
--- a/spaces/g4f/freegpt-webui/Dockerfile
+++ /dev/null
@@ -1,16 +0,0 @@
-FROM python:3.10-slim-buster
-
-WORKDIR /app
-
-COPY requirements.txt requirements.txt
-
-RUN python -m venv venv
-ENV PATH="/app/venv/bin:$PATH"
-
-RUN apt-get update && \
- apt-get install -y --no-install-recommends build-essential libffi-dev cmake libcurl4-openssl-dev && \
- pip3 install --no-cache-dir -r requirements.txt
-
-COPY . .
-
-CMD ["python3", "./run.py"]
\ No newline at end of file
diff --git a/spaces/gagan3012/T5-Summarization/src/visualization/app.py b/spaces/gagan3012/T5-Summarization/src/visualization/app.py
deleted file mode 100644
index 428099c92d93bf1c19109d5818a5c956ff37de5b..0000000000000000000000000000000000000000
--- a/spaces/gagan3012/T5-Summarization/src/visualization/app.py
+++ /dev/null
@@ -1,32 +0,0 @@
-import streamlit as st
-import yaml
-
-from src.models import predict_model
-
-
-def visualize():
- st.write("# Summarization UI")
- st.markdown(
- """
- *For additional questions and inquiries, please contact **Gagan Bhatia** via [LinkedIn](
- https://www.linkedin.com/in/gbhatia30/) or [Github](https://github.com/gagan3012).*
- """
- )
-
- text = st.text_area("Enter text here")
- if st.button("Generate Summary"):
- with st.spinner("Connecting the Dots..."):
- sumtext = predict_model(text=text)
- st.write("# Generated Summary:")
- st.write("{}".format(sumtext))
- with open("reports/visualization_metrics.txt", "w") as file1:
- file1.writelines(text)
- file1.writelines(sumtext)
-
-
-if __name__ == "__main__":
- with open("params.yml") as f:
- params = yaml.safe_load(f)
-
- if params["visualise"]:
- visualize()
diff --git a/spaces/gatilin/mmpose-webui/app.py b/spaces/gatilin/mmpose-webui/app.py
deleted file mode 100644
index 5c7bba545c2b9f95f68c1c339d7142253aac7051..0000000000000000000000000000000000000000
--- a/spaces/gatilin/mmpose-webui/app.py
+++ /dev/null
@@ -1,82 +0,0 @@
-
-
-import os
-os.system("pip install xtcocotools>=1.12")
-os.system("pip install 'mmengine>=0.6.0'")
-os.system("pip install 'mmcv>=2.0.0rc4,<2.1.0'")
-os.system("pip install 'mmdet>=3.0.0,<4.0.0'")
-os.system("pip install 'mmpose'")
-
-import PIL
-import cv2
-import mmpose
-import numpy as np
-
-import torch
-from mmpose.apis import MMPoseInferencer
-import gradio as gr
-
-import warnings
-
-warnings.filterwarnings("ignore")
-
-mmpose_model_list = ["human", "hand", "face", "animal", "wholebody",
- "vitpose", "vitpose-s", "vitpose-b", "vitpose-l", "vitpose-h"]
-
-
-def save_image(img, img_path):
- # Convert PIL image to OpenCV image
- img = cv2.cvtColor(np.array(img), cv2.COLOR_RGB2BGR)
- # Save OpenCV image
- cv2.imwrite(img_path, img)
-
-
-def download_test_image():
- # Images
- torch.hub.download_url_to_file(
- 'https://user-images.githubusercontent.com/59380685/266264420-21575a83-4057-41cf-8a4a-b3ea6f332d79.jpg',
- 'bus.jpg')
- torch.hub.download_url_to_file(
- 'https://user-images.githubusercontent.com/59380685/266264536-82afdf58-6b9a-4568-b9df-551ee72cb6d9.jpg',
- 'dogs.jpg')
- torch.hub.download_url_to_file(
- 'https://user-images.githubusercontent.com/59380685/266264600-9d0c26ca-8ba6-45f2-b53b-4dc98460c43e.jpg',
- 'zidane.jpg')
-
-
-def predict_pose(img, model_name, out_dir):
- img_path = "input_img.jpg"
- save_image(img, img_path)
- device = torch.cuda.current_device() if torch.cuda.is_available() else 'cpu'
- inferencer = MMPoseInferencer(model_name, device=device)
- result_generator = inferencer(img_path, show=False, out_dir=out_dir)
- result = next(result_generator)
- save_dir = './output/visualizations/'
- if os.path.exists(save_dir):
- out_img_path = save_dir + img_path
- print("out_img_path: ", out_img_path)
- else:
- out_img_path = img_path
- out_img = PIL.Image.open(out_img_path)
- return out_img
-
-download_test_image()
-input_image = gr.inputs.Image(type='pil', label="Original Image")
-model_name = gr.inputs.Dropdown(choices=[m for m in mmpose_model_list], label='Model')
-out_dir = gr.inputs.Textbox(label="Output Directory", default="./output")
-output_image = gr.outputs.Image(type="pil", label="Output Image")
-
-examples = [
- ['zidane.jpg', 'human'],
- ['dogs.jpg', 'animal'],
-]
-title = "MMPose detection web demo"
-description = "
"
-
-iface = gr.Interface(fn=predict_pose, inputs=[input_image, model_name, out_dir], outputs=output_image,
- examples=examples, title=title, description=description, article=article)
-iface.launch()
diff --git a/spaces/genevera/AudioToken/modules/beats/modules.py b/spaces/genevera/AudioToken/modules/beats/modules.py
deleted file mode 100644
index 58f5150938f340dca1289f1c52f7bf1b63f6d6e3..0000000000000000000000000000000000000000
--- a/spaces/genevera/AudioToken/modules/beats/modules.py
+++ /dev/null
@@ -1,218 +0,0 @@
-# --------------------------------------------------------
-# beats: Audio Pre-Training with Acoustic Tokenizers (https://arxiv.org/abs/2212.09058)
-# Github source: https://github.com/microsoft/unilm/tree/master/beats
-# Copyright (c) 2022 Microsoft
-# Licensed under The MIT License [see LICENSE for details]
-# Based on fairseq code bases
-# https://github.com/pytorch/fairseq
-# --------------------------------------------------------
-
-import math
-import warnings
-import torch
-from torch import Tensor, nn
-import torch.nn.functional as F
-
-
-class GradMultiply(torch.autograd.Function):
- @staticmethod
- def forward(ctx, x, scale):
- ctx.scale = scale
- res = x.new(x)
- return res
-
- @staticmethod
- def backward(ctx, grad):
- return grad * ctx.scale, None
-
-
-class SamePad(nn.Module):
- def __init__(self, kernel_size, causal=False):
- super().__init__()
- if causal:
- self.remove = kernel_size - 1
- else:
- self.remove = 1 if kernel_size % 2 == 0 else 0
-
- def forward(self, x):
- if self.remove > 0:
- x = x[:, :, : -self.remove]
- return x
-
-
-class Swish(nn.Module):
- def __init__(self):
- super(Swish, self).__init__()
- self.act = torch.nn.Sigmoid()
-
- def forward(self, x):
- return x * self.act(x)
-
-
-class GLU_Linear(nn.Module):
- def __init__(self, input_dim, output_dim, glu_type="sigmoid", bias_in_glu=True):
- super(GLU_Linear, self).__init__()
-
- self.glu_type = glu_type
- self.output_dim = output_dim
-
- if glu_type == "sigmoid":
- self.glu_act = torch.nn.Sigmoid()
- elif glu_type == "swish":
- self.glu_act = Swish()
- elif glu_type == "relu":
- self.glu_act = torch.nn.ReLU()
- elif glu_type == "gelu":
- self.glu_act = torch.nn.GELU()
-
- if bias_in_glu:
- self.linear = nn.Linear(input_dim, output_dim * 2, True)
- else:
- self.linear = nn.Linear(input_dim, output_dim * 2, False)
-
- def forward(self, x):
- # to be consistent with GLU_Linear, we assume the input always has the #channel (#dim) in the last dimension of the tensor, so need to switch the dimension first for 1D-Conv case
- x = self.linear(x)
-
- if self.glu_type == "bilinear":
- x = (x[:, :, 0:self.output_dim] * x[:, :, self.output_dim:self.output_dim * 2])
- else:
- x = (x[:, :, 0:self.output_dim] * self.glu_act(x[:, :, self.output_dim:self.output_dim * 2]))
-
- return x
-
-
-def gelu_accurate(x):
- if not hasattr(gelu_accurate, "_a"):
- gelu_accurate._a = math.sqrt(2 / math.pi)
- return (
- 0.5 * x * (1 + torch.tanh(gelu_accurate._a * (x + 0.044715 * torch.pow(x, 3))))
- )
-
-
-def gelu(x: torch.Tensor) -> torch.Tensor:
- return torch.nn.functional.gelu(x.float()).type_as(x)
-
-
-def get_activation_fn(activation: str):
- """Returns the activation function corresponding to `activation`"""
-
- if activation == "relu":
- return F.relu
- elif activation == "gelu":
- return gelu
- elif activation == "gelu_fast":
- warnings.warn(
- "--activation-fn=gelu_fast has been renamed to gelu_accurate"
- )
- return gelu_accurate
- elif activation == "gelu_accurate":
- return gelu_accurate
- elif activation == "tanh":
- return torch.tanh
- elif activation == "linear":
- return lambda x: x
- elif activation == "glu":
- return lambda x: x
- else:
- raise RuntimeError("--activation-fn {} not supported".format(activation))
-
-
-def quant_noise(module, p, block_size):
- """
- Wraps modules and applies quantization noise to the weights for
- subsequent quantization with Iterative Product Quantization as
- described in "Training with Quantization Noise for Extreme Model Compression"
-
- Args:
- - module: nn.Module
- - p: amount of Quantization Noise
- - block_size: size of the blocks for subsequent quantization with iPQ
-
- Remarks:
- - Module weights must have the right sizes wrt the block size
- - Only Linear, Embedding and Conv2d modules are supported for the moment
- - For more detail on how to quantize by blocks with convolutional weights,
- see "And the Bit Goes Down: Revisiting the Quantization of Neural Networks"
- - We implement the simplest form of noise here as stated in the paper
- which consists in randomly dropping blocks
- """
-
- # if no quantization noise, don't register hook
- if p <= 0:
- return module
-
- # supported modules
- assert isinstance(module, (nn.Linear, nn.Embedding, nn.Conv2d))
-
- # test whether module.weight has the right sizes wrt block_size
- is_conv = module.weight.ndim == 4
-
- # 2D matrix
- if not is_conv:
- assert (
- module.weight.size(1) % block_size == 0
- ), "Input features must be a multiple of block sizes"
-
- # 4D matrix
- else:
- # 1x1 convolutions
- if module.kernel_size == (1, 1):
- assert (
- module.in_channels % block_size == 0
- ), "Input channels must be a multiple of block sizes"
- # regular convolutions
- else:
- k = module.kernel_size[0] * module.kernel_size[1]
- assert k % block_size == 0, "Kernel size must be a multiple of block size"
-
- def _forward_pre_hook(mod, input):
- # no noise for evaluation
- if mod.training:
- if not is_conv:
- # gather weight and sizes
- weight = mod.weight
- in_features = weight.size(1)
- out_features = weight.size(0)
-
- # split weight matrix into blocks and randomly drop selected blocks
- mask = torch.zeros(
- in_features // block_size * out_features, device=weight.device
- )
- mask.bernoulli_(p)
- mask = mask.repeat_interleave(block_size, -1).view(-1, in_features)
-
- else:
- # gather weight and sizes
- weight = mod.weight
- in_channels = mod.in_channels
- out_channels = mod.out_channels
-
- # split weight matrix into blocks and randomly drop selected blocks
- if mod.kernel_size == (1, 1):
- mask = torch.zeros(
- int(in_channels // block_size * out_channels),
- device=weight.device,
- )
- mask.bernoulli_(p)
- mask = mask.repeat_interleave(block_size, -1).view(-1, in_channels)
- else:
- mask = torch.zeros(
- weight.size(0), weight.size(1), device=weight.device
- )
- mask.bernoulli_(p)
- mask = (
- mask.unsqueeze(2)
- .unsqueeze(3)
- .repeat(1, 1, mod.kernel_size[0], mod.kernel_size[1])
- )
-
- # scale weights and apply mask
- mask = mask.to(
- torch.bool
- ) # x.bool() is not currently supported in TorchScript
- s = 1 / (1 - p)
- mod.weight.data = s * weight.masked_fill(mask, 0)
-
- module.register_forward_pre_hook(_forward_pre_hook)
- return module
diff --git "a/spaces/giswqs/Streamlit/pages/8_\360\237\217\234\357\270\217_Raster_Data_Visualization.py" "b/spaces/giswqs/Streamlit/pages/8_\360\237\217\234\357\270\217_Raster_Data_Visualization.py"
deleted file mode 100644
index 300ab6e027b6a801bfc406062ccf5062b68fa7a3..0000000000000000000000000000000000000000
--- "a/spaces/giswqs/Streamlit/pages/8_\360\237\217\234\357\270\217_Raster_Data_Visualization.py"
+++ /dev/null
@@ -1,106 +0,0 @@
-import os
-import leafmap.foliumap as leafmap
-import leafmap.colormaps as cm
-import streamlit as st
-
-st.set_page_config(layout="wide")
-
-st.sidebar.info(
- """
- - Web App URL:
- - GitHub repository:
- """
-)
-
-st.sidebar.title("Contact")
-st.sidebar.info(
- """
- Qiusheng Wu at [wetlands.io](https://wetlands.io) | [GitHub](https://github.com/giswqs) | [Twitter](https://twitter.com/giswqs) | [YouTube](https://www.youtube.com/c/QiushengWu) | [LinkedIn](https://www.linkedin.com/in/qiushengwu)
- """
-)
-
-
-@st.cache(allow_output_mutation=True)
-def load_cog_list():
- print(os.getcwd())
- in_txt = os.path.join(os.getcwd(), "data/cog_files.txt")
- with open(in_txt) as f:
- return [line.strip() for line in f.readlines()[1:]]
-
-
-@st.cache(allow_output_mutation=True)
-def get_palettes():
- return list(cm.palettes.keys())
- # palettes = dir(palettable.matplotlib)[:-16]
- # return ["matplotlib." + p for p in palettes]
-
-
-st.title("Visualize Raster Datasets")
-st.markdown(
- """
-An interactive web app for visualizing local raster datasets and Cloud Optimized GeoTIFF ([COG](https://www.cogeo.org)). The app was built using [streamlit](https://streamlit.io), [leafmap](https://leafmap.org), and [Titiler](https://developmentseed.org/titiler/).
-
-
-"""
-)
-
-row1_col1, row1_col2 = st.columns([2, 1])
-
-with row1_col1:
- cog_list = load_cog_list()
- cog = st.selectbox("Select a sample Cloud Opitmized GeoTIFF (COG)", cog_list)
-
-with row1_col2:
- empty = st.empty()
-
- url = empty.text_input(
- "Enter a HTTP URL to a Cloud Optimized GeoTIFF (COG)",
- cog,
- )
-
- if url:
- try:
- options = leafmap.cog_bands(url)
- except Exception as e:
- st.error(e)
- if len(options) > 3:
- default = options[:3]
- else:
- default = options[0]
- bands = st.multiselect("Select bands to display", options, default=options)
-
- if len(bands) == 1 or len(bands) == 3:
- pass
- else:
- st.error("Please select one or three bands")
-
- add_params = st.checkbox("Add visualization parameters")
- if add_params:
- vis_params = st.text_area("Enter visualization parameters", "{}")
- else:
- vis_params = {}
-
- if len(vis_params) > 0:
- try:
- vis_params = eval(vis_params)
- except Exception as e:
- st.error(
- f"Invalid visualization parameters. It should be a dictionary. Error: {e}"
- )
- vis_params = {}
-
- submit = st.button("Submit")
-
-m = leafmap.Map(latlon_control=False)
-
-if submit:
- if url:
- try:
- m.add_cog_layer(url, bands=bands, **vis_params)
- except Exception as e:
- with row1_col2:
- st.error(e)
- st.error("Work in progress. Try it again later.")
-
-with row1_col1:
- m.to_streamlit()
diff --git a/spaces/gotiQspiryo/whisper-ui/examples/Download Twilight Saga Breaking Dawn Part 1 In Hindi Dubbed.md b/spaces/gotiQspiryo/whisper-ui/examples/Download Twilight Saga Breaking Dawn Part 1 In Hindi Dubbed.md
deleted file mode 100644
index 5354345356a7493e77081f03d4d15ef9eeef6603..0000000000000000000000000000000000000000
--- a/spaces/gotiQspiryo/whisper-ui/examples/Download Twilight Saga Breaking Dawn Part 1 In Hindi Dubbed.md
+++ /dev/null
@@ -1,25 +0,0 @@
-
-
How to Download Twilight Saga Breaking Dawn Part 1 in Hindi Dubbed for Free
-
If you are a fan of the Twilight Saga, you might be interested in watching the fourth installment of the series, Breaking Dawn Part 1, in Hindi dubbed. This movie follows the romance between Bella Swan and Edward Cullen as they get married and face the consequences of their union. You can watch this movie online or download it to your device for offline viewing. Here are some ways to download Twilight Saga Breaking Dawn Part 1 in Hindi dubbed for free.
-
Method 1: Use a Torrent Site
-
One of the easiest ways to download Twilight Saga Breaking Dawn Part 1 in Hindi dubbed is to use a torrent site. Torrent sites allow you to download files from other users who have uploaded them. You will need a torrent client software, such as BitTorrent or uTorrent, to download the files. Here are the steps to follow:
-
Download Twilight Saga Breaking Dawn Part 1 In Hindi Dubbed
Go to a torrent site that has the movie you want. For example, you can visit this link [^2^] to download the movie in 720p quality.
-
Click on the download button or magnet link to start downloading the torrent file.
-
Open the torrent file with your torrent client software and choose a location to save the movie file.
-
Wait for the download to finish. The speed and time of the download will depend on your internet connection and the number of seeders (users who have the complete file) and leechers (users who are downloading the file).
-
Once the download is complete, you can open the movie file with a media player that supports Hindi subtitles or audio tracks.
-
-
Method 2: Use a Streaming Site
-
Another way to watch Twilight Saga Breaking Dawn Part 1 in Hindi dubbed is to use a streaming site. Streaming sites allow you to watch movies online without downloading them. However, some streaming sites may have pop-up ads, low-quality videos, or limited availability. Here are some steps to follow:
-
-
Go to a streaming site that has the movie you want. For example, you can visit this link [^1^] to watch the movie online.
-
Click on the play button or choose a server to start watching the movie.
-
If you encounter any ads or redirects, close them and return to the original site.
-
If you want to watch the movie offline, you can use a screen recorder software or browser extension to capture the video while it is playing.
-
-
Conclusion
-
Twilight Saga Breaking Dawn Part 1 is a romantic fantasy film that continues the story of Bella and Edward as they face new challenges and dangers. You can watch this movie in Hindi dubbed by using a torrent site or a streaming site. However, you should be aware of the risks and legal issues involved in downloading or streaming pirated content. We do not endorse or promote any illegal activity and we advise you to respect the rights of the original creators and distributors of the movie.
d5da3c52bf
-
-
\ No newline at end of file
diff --git a/spaces/gpecile/encrypted-image-recognition/filters.py b/spaces/gpecile/encrypted-image-recognition/filters.py
deleted file mode 100644
index 2703dbe7df2f0a376e6585e877600730bddbc1e1..0000000000000000000000000000000000000000
--- a/spaces/gpecile/encrypted-image-recognition/filters.py
+++ /dev/null
@@ -1,262 +0,0 @@
-"Filter definitions, with pre-processing, post-processing and compilation methods."
-
-import numpy as np
-import torch
-from torch import nn
-from common import AVAILABLE_FILTERS, INPUT_SHAPE
-
-from concrete.fhe.compilation.compiler import Compiler
-from concrete.ml.common.utils import generate_proxy_function
-from concrete.ml.torch.numpy_module import NumpyModule
-
-
-class TorchIdentity(nn.Module):
- """Torch identity model."""
-
- def forward(self, x):
- """Identity forward pass.
-
- Args:
- x (torch.Tensor): The input image.
-
- Returns:
- x (torch.Tensor): The input image.
- """
- return x
-
-
-class TorchInverted(nn.Module):
- """Torch inverted model."""
-
- def forward(self, x):
- """Forward pass for inverting an image's colors.
-
- Args:
- x (torch.Tensor): The input image.
-
- Returns:
- torch.Tensor: The (color) inverted image.
- """
- return 255 - x
-
-
-class TorchRotate(nn.Module):
- """Torch rotated model."""
-
- def forward(self, x):
- """Forward pass for rotating an image.
-
- Args:
- x (torch.Tensor): The input image.
-
- Returns:
- torch.Tensor: The rotated image.
- """
- return x.transpose(0, 1)
-
-
-class TorchConv(nn.Module):
- """Torch model with a single convolution operator."""
-
- def __init__(self, kernel, n_in_channels=3, n_out_channels=3, groups=1, threshold=None):
- """Initialize the filter.
-
- Args:
- kernel (np.ndarray): The convolution kernel to consider.
- """
- super().__init__()
- self.kernel = torch.tensor(kernel, dtype=torch.int64)
- self.n_out_channels = n_out_channels
- self.n_in_channels = n_in_channels
- self.groups = groups
- self.threshold = threshold
-
- def forward(self, x):
- """Forward pass with a single convolution using a 1D or 2D kernel.
-
- Args:
- x (torch.Tensor): The input image.
-
- Returns:
- torch.Tensor: The filtered image.
- """
- # Define the convolution parameters
- stride = 1
- kernel_shape = self.kernel.shape
-
- # Ensure the kernel has a proper shape
- # If the kernel has a 1D shape, a (1, 1) kernel is used for each in_channels
- if len(kernel_shape) == 1:
- self.kernel = self.kernel.repeat(self.n_out_channels)
- kernel = self.kernel.reshape(
- self.n_out_channels,
- self.n_in_channels // self.groups,
- 1,
- 1,
- )
-
- # Else, if the kernel has a 2D shape, a single (Kw, Kh) kernel is used on all in_channels
- elif len(kernel_shape) == 2:
- kernel = self.kernel.expand(
- self.n_out_channels,
- self.n_in_channels // self.groups,
- kernel_shape[0],
- kernel_shape[1],
- )
-
-
- else:
- raise ValueError(
- "Wrong kernel shape, only 1D or 2D kernels are accepted. Got kernel of shape "
- f"{kernel_shape}"
- )
-
- # Reshape the image. This is done because Torch convolutions and Numpy arrays (for PIL
- # display) don't follow the same shape conventions. More precisely, x is of shape
- # (Width, Height, Channels) while the conv2d operator requires an input of shape
- # (Batch, Channels, Height, Width)
- x = x.transpose(2, 0).unsqueeze(axis=0)
-
- # Apply the convolution
- x = nn.functional.conv2d(x, kernel, stride=stride, groups=self.groups)
-
- # Reshape the output back to the original shape (Width, Height, Channels)
- x = x.transpose(1, 3).reshape((x.shape[2], x.shape[3], self.n_out_channels))
-
- # Subtract a given threshold if given
- if self.threshold is not None:
- x -= self.threshold
-
- return x
-
-
-class Filter:
- """Filter class used in the app."""
-
- def __init__(self, filter_name):
- """Initializing the filter class using a given filter.
-
- Most filters can be found at https://en.wikipedia.org/wiki/Kernel_(image_processing).
-
- Args:
- filter_name (str): The filter to consider.
- """
-
- assert filter_name in AVAILABLE_FILTERS, (
- f"Unsupported image filter or transformation. Expected one of {*AVAILABLE_FILTERS,}, "
- f"but got {filter_name}",
- )
-
- # Define attributes associated to the filter
- self.filter_name = filter_name
- self.onnx_model = None
- self.fhe_circuit = None
- self.divide = None
-
- # Instantiate the torch module associated to the given filter name
- if filter_name == "identity":
- self.torch_model = TorchIdentity()
-
- elif filter_name == "inverted":
- self.torch_model = TorchInverted()
-
- elif filter_name == "rotate":
- self.torch_model = TorchRotate()
-
- elif filter_name == "black and white":
- # Define the grayscale weights (RGB order)
- # These weights were used in PAL and NTSC video systems and can be found at
- # https://en.wikipedia.org/wiki/Grayscale
- # There are initially supposed to be float weights (0.299, 0.587, 0.114), with
- # 0.299 + 0.587 + 0.114 = 1
- # However, since FHE computations require weights to be integers, we first multiply
- # these by a factor of 1000. The output image's values are then divided by 1000 in
- # post-processing in order to retrieve the correct result
- kernel = [299, 587, 114]
-
- self.torch_model = TorchConv(kernel)
-
- # Define the value used when for dividing the output values in post-processing
- self.divide = 1000
-
-
- elif filter_name == "blur":
- kernel = np.ones((3, 3))
-
- self.torch_model = TorchConv(kernel, groups=3)
-
- # Define the value used when for dividing the output values in post-processing
- self.divide = 9
-
- elif filter_name == "sharpen":
- kernel = [
- [0, -1, 0],
- [-1, 5, -1],
- [0, -1, 0],
- ]
-
- self.torch_model = TorchConv(kernel, groups=3)
-
- elif filter_name == "ridge detection":
- kernel = [
- [-1, -1, -1],
- [-1, 9, -1],
- [-1, -1, -1],
- ]
-
- # Additionally to the convolution operator, the filter will subtract a given threshold
- # value to the result in order to better display the ridges
- self.torch_model = TorchConv(kernel, threshold=900)
-
-
- def compile(self):
- """Compile the filter on a representative inputset."""
- # Generate a random representative set of images used for compilation, following shape
- # PIL's shape RGB format for Numpy arrays (image_width, image_height, 3)
- # Additionally, this version's compiler only handles tuples of 1-batch array as inputset,
- # meaning we need to define the inputset as a Tuple[np.ndarray[shape=(H, W, 3)]]
- np.random.seed(42)
- inputset = tuple(
- np.random.randint(0, 256, size=(INPUT_SHAPE + (3, )), dtype=np.int64) for _ in range(100)
- )
-
- # Convert the Torch module to a Numpy module
- numpy_module = NumpyModule(
- self.torch_model,
- dummy_input=torch.from_numpy(inputset[0]),
- )
-
- # Get the proxy function and parameter mappings used for initializing the compiler
- # This is done in order to be able to provide any modules with arbitrary numbers of
- # encrypted arguments to Concrete Numpy's compiler
- numpy_filter_proxy, parameters_mapping = generate_proxy_function(
- numpy_module.numpy_forward,
- ["inputs"]
- )
-
- # Compile the filter and retrieve its FHE circuit
- compiler = Compiler(
- numpy_filter_proxy,
- {parameters_mapping["inputs"]: "encrypted"},
- )
- self.fhe_circuit = compiler.compile(inputset)
-
- return self.fhe_circuit
-
- def post_processing(self, output_image):
- """Apply post-processing to the encrypted output images.
-
- Args:
- input_image (np.ndarray): The decrypted image to post-process.
-
- Returns:
- input_image (np.ndarray): The post-processed image.
- """
- # Divide all values if needed
- if self.divide is not None:
- output_image //= self.divide
-
- # Clip the image's values to proper RGB standards as filters don't handle such constraints
- output_image = output_image.clip(0, 255)
-
- return output_image
diff --git a/spaces/gradio/HuBERT/fairseq/optim/lr_scheduler/tri_stage_lr_scheduler.py b/spaces/gradio/HuBERT/fairseq/optim/lr_scheduler/tri_stage_lr_scheduler.py
deleted file mode 100644
index 4d5547c39b14f62acbd4f4b9ab3abfb3009c0e6d..0000000000000000000000000000000000000000
--- a/spaces/gradio/HuBERT/fairseq/optim/lr_scheduler/tri_stage_lr_scheduler.py
+++ /dev/null
@@ -1,175 +0,0 @@
-# Copyright (c) Facebook, Inc. and its affiliates.
-#
-# This source code is licensed under the MIT license found in the
-# LICENSE file in the root directory of this source tree.
-
-import math
-from dataclasses import dataclass, field
-from typing import Optional, List, Tuple
-from omegaconf import II
-
-from fairseq.dataclass import FairseqDataclass
-from fairseq.optim.lr_scheduler import FairseqLRScheduler, register_lr_scheduler
-
-
-@dataclass
-class TriStageLRScheduleConfig(FairseqDataclass):
- warmup_steps: int = field(
- default=0,
- metadata={"help": "warmup the learning rate linearly for the first N updates"},
- )
- hold_steps: int = field(
- default=0,
- metadata={"help": "steps in hold stage"},
- )
- decay_steps: int = field(
- default=0,
- metadata={"help": "steps in decay stages"},
- )
- phase_ratio: Optional[Tuple[float, float, float]] = field(
- default=None,
- metadata={
- "help": (
- "if set, automatically sets warmup/hold/decay steps to the ratio "
- "specified here from max_updates. the ratios must add up to 1.0"
- )
- },
- )
- init_lr_scale: float = field(
- default=0.01,
- metadata={"help": "initial learning rate scale during warmup phase"},
- )
- final_lr_scale: float = field(
- default=0.01,
- metadata={"help": "final learning rate scale"},
- )
- max_update: float = II("optimization.max_update")
- lr: List[float] = II("optimization.lr")
-
-
-@register_lr_scheduler("tri_stage", dataclass=TriStageLRScheduleConfig)
-class TriStageLRSchedule(FairseqLRScheduler):
- """Tristage learning rate schedulr
-
- Implement the learning rate scheduler in https://arxiv.org/pdf/1904.08779.pdf
-
- Similar to inverse_squre_root scheduler, but tri_stage learning rate employs
- three stages LR scheduling:
-
- - warmup stage, starting from `lr` * `init_lr_scale`, linearly
- increased to `lr` in `warmup_steps` iterations
-
- - hold stage, after `warmup_steps`, keep the LR as `lr` for `hold_steps`
- iterations
-
- - decay stage, after hold stage, decay LR exponetially to
- `lr` * `final_lr_scale` in `decay_steps`;
- after that LR is keep as `final_lr_scale` * `lr`
-
- During warmup::
-
- init_lr = cfg.init_lr_scale * cfg.lr
- lrs = torch.linspace(init_lr, cfg.lr, cfg.warmup_steps)
- lr = lrs[update_num]
-
- During hold::
-
- lr = cfg.lr
-
- During decay::
-
- decay_factor = - math.log(cfg.final_lr_scale) / cfg.decay_steps
- lr = cfg.lr * exp(- (update_num - warmup_steps - decay_steps) * decay_factor)
-
- After that::
-
- lr = cfg.lr * cfg.final_lr_scale
- """
-
- def __init__(self, cfg: TriStageLRScheduleConfig, optimizer):
- super().__init__(cfg, optimizer)
- if len(cfg.lr) > 1:
- raise ValueError(
- "Cannot use a fixed learning rate schedule with tri-stage lr."
- " Consider --lr-scheduler=fixed instead."
- )
-
- # calculate LR at each point
- self.peak_lr = cfg.lr[0]
- self.init_lr = cfg.init_lr_scale * cfg.lr[0]
- self.final_lr = cfg.final_lr_scale * cfg.lr[0]
-
- if cfg.phase_ratio is not None:
- assert cfg.max_update > 0
- assert sum(cfg.phase_ratio) == 1, "phase ratios must add up to 1"
- self.warmup_steps = int(cfg.max_update * cfg.phase_ratio[0])
- self.hold_steps = int(cfg.max_update * cfg.phase_ratio[1])
- self.decay_steps = int(cfg.max_update * cfg.phase_ratio[2])
- else:
- self.warmup_steps = cfg.warmup_steps
- self.hold_steps = cfg.hold_steps
- self.decay_steps = cfg.decay_steps
-
- assert (
- self.warmup_steps + self.hold_steps + self.decay_steps > 0
- ), "please specify steps or phase_ratio"
-
- self.warmup_rate = (
- (self.peak_lr - self.init_lr) / self.warmup_steps
- if self.warmup_steps != 0
- else 0
- )
- self.decay_factor = -math.log(cfg.final_lr_scale) / self.decay_steps
-
- # initial learning rate
- self.lr = self.init_lr
- self.optimizer.set_lr(self.lr)
-
- def _decide_stage(self, update_step):
- """
- return stage, and the corresponding steps within the current stage
- """
- if update_step < self.warmup_steps:
- # warmup state
- return 0, update_step
-
- offset = self.warmup_steps
-
- if update_step < offset + self.hold_steps:
- # hold stage
- return 1, update_step - offset
-
- offset += self.hold_steps
-
- if update_step <= offset + self.decay_steps:
- # decay stage
- return 2, update_step - offset
-
- offset += self.decay_steps
-
- # still here ? constant lr stage
- return 3, update_step - offset
-
- def step(self, epoch, val_loss=None):
- """Update the learning rate at the end of the given epoch."""
- super().step(epoch, val_loss)
- # we don't change the learning rate at epoch boundaries
- return self.optimizer.get_lr()
-
- def step_update(self, num_updates):
- """Update the learning rate after each update."""
- stage, steps_in_stage = self._decide_stage(num_updates)
- if stage == 0:
- self.lr = self.init_lr + self.warmup_rate * steps_in_stage
- elif stage == 1:
- self.lr = self.peak_lr
- elif stage == 2:
- self.lr = self.peak_lr * math.exp(-self.decay_factor * steps_in_stage)
- elif stage == 3:
- self.lr = self.final_lr
- else:
- raise ValueError("Undefined stage")
-
- self.optimizer.set_lr(self.lr)
-
- return self.lr
diff --git a/spaces/gyugnsu/DragGan-Inversion/PTI/models/StyleCLIP/global_directions/dnnlib/tflib/autosummary.py b/spaces/gyugnsu/DragGan-Inversion/PTI/models/StyleCLIP/global_directions/dnnlib/tflib/autosummary.py
deleted file mode 100644
index 56dfb96093bb5b1129a99585b4ce655b98d80009..0000000000000000000000000000000000000000
--- a/spaces/gyugnsu/DragGan-Inversion/PTI/models/StyleCLIP/global_directions/dnnlib/tflib/autosummary.py
+++ /dev/null
@@ -1,193 +0,0 @@
-# Copyright (c) 2020, NVIDIA CORPORATION. All rights reserved.
-#
-# NVIDIA CORPORATION and its licensors retain all intellectual property
-# and proprietary rights in and to this software, related documentation
-# and any modifications thereto. Any use, reproduction, disclosure or
-# distribution of this software and related documentation without an express
-# license agreement from NVIDIA CORPORATION is strictly prohibited.
-
-"""Helper for adding automatically tracked values to Tensorboard.
-
-Autosummary creates an identity op that internally keeps track of the input
-values and automatically shows up in TensorBoard. The reported value
-represents an average over input components. The average is accumulated
-constantly over time and flushed when save_summaries() is called.
-
-Notes:
-- The output tensor must be used as an input for something else in the
- graph. Otherwise, the autosummary op will not get executed, and the average
- value will not get accumulated.
-- It is perfectly fine to include autosummaries with the same name in
- several places throughout the graph, even if they are executed concurrently.
-- It is ok to also pass in a python scalar or numpy array. In this case, it
- is added to the average immediately.
-"""
-
-from collections import OrderedDict
-import numpy as np
-import tensorflow as tf
-from tensorboard import summary as summary_lib
-from tensorboard.plugins.custom_scalar import layout_pb2
-
-from . import tfutil
-from .tfutil import TfExpression
-from .tfutil import TfExpressionEx
-
-# Enable "Custom scalars" tab in TensorBoard for advanced formatting.
-# Disabled by default to reduce tfevents file size.
-enable_custom_scalars = False
-
-_dtype = tf.float64
-_vars = OrderedDict() # name => [var, ...]
-_immediate = OrderedDict() # name => update_op, update_value
-_finalized = False
-_merge_op = None
-
-
-def _create_var(name: str, value_expr: TfExpression) -> TfExpression:
- """Internal helper for creating autosummary accumulators."""
- assert not _finalized
- name_id = name.replace("/", "_")
- v = tf.cast(value_expr, _dtype)
-
- if v.shape.is_fully_defined():
- size = np.prod(v.shape.as_list())
- size_expr = tf.constant(size, dtype=_dtype)
- else:
- size = None
- size_expr = tf.reduce_prod(tf.cast(tf.shape(v), _dtype))
-
- if size == 1:
- if v.shape.ndims != 0:
- v = tf.reshape(v, [])
- v = [size_expr, v, tf.square(v)]
- else:
- v = [size_expr, tf.reduce_sum(v), tf.reduce_sum(tf.square(v))]
- v = tf.cond(tf.is_finite(v[1]), lambda: tf.stack(v), lambda: tf.zeros(3, dtype=_dtype))
-
- with tfutil.absolute_name_scope("Autosummary/" + name_id), tf.control_dependencies(None):
- var = tf.Variable(tf.zeros(3, dtype=_dtype), trainable=False) # [sum(1), sum(x), sum(x**2)]
- update_op = tf.cond(tf.is_variable_initialized(var), lambda: tf.assign_add(var, v), lambda: tf.assign(var, v))
-
- if name in _vars:
- _vars[name].append(var)
- else:
- _vars[name] = [var]
- return update_op
-
-
-def autosummary(name: str, value: TfExpressionEx, passthru: TfExpressionEx = None, condition: TfExpressionEx = True) -> TfExpressionEx:
- """Create a new autosummary.
-
- Args:
- name: Name to use in TensorBoard
- value: TensorFlow expression or python value to track
- passthru: Optionally return this TF node without modifications but tack an autosummary update side-effect to this node.
-
- Example use of the passthru mechanism:
-
- n = autosummary('l2loss', loss, passthru=n)
-
- This is a shorthand for the following code:
-
- with tf.control_dependencies([autosummary('l2loss', loss)]):
- n = tf.identity(n)
- """
- tfutil.assert_tf_initialized()
- name_id = name.replace("/", "_")
-
- if tfutil.is_tf_expression(value):
- with tf.name_scope("summary_" + name_id), tf.device(value.device):
- condition = tf.convert_to_tensor(condition, name='condition')
- update_op = tf.cond(condition, lambda: tf.group(_create_var(name, value)), tf.no_op)
- with tf.control_dependencies([update_op]):
- return tf.identity(value if passthru is None else passthru)
-
- else: # python scalar or numpy array
- assert not tfutil.is_tf_expression(passthru)
- assert not tfutil.is_tf_expression(condition)
- if condition:
- if name not in _immediate:
- with tfutil.absolute_name_scope("Autosummary/" + name_id), tf.device(None), tf.control_dependencies(None):
- update_value = tf.placeholder(_dtype)
- update_op = _create_var(name, update_value)
- _immediate[name] = update_op, update_value
- update_op, update_value = _immediate[name]
- tfutil.run(update_op, {update_value: value})
- return value if passthru is None else passthru
-
-
-def finalize_autosummaries() -> None:
- """Create the necessary ops to include autosummaries in TensorBoard report.
- Note: This should be done only once per graph.
- """
- global _finalized
- tfutil.assert_tf_initialized()
-
- if _finalized:
- return None
-
- _finalized = True
- tfutil.init_uninitialized_vars([var for vars_list in _vars.values() for var in vars_list])
-
- # Create summary ops.
- with tf.device(None), tf.control_dependencies(None):
- for name, vars_list in _vars.items():
- name_id = name.replace("/", "_")
- with tfutil.absolute_name_scope("Autosummary/" + name_id):
- moments = tf.add_n(vars_list)
- moments /= moments[0]
- with tf.control_dependencies([moments]): # read before resetting
- reset_ops = [tf.assign(var, tf.zeros(3, dtype=_dtype)) for var in vars_list]
- with tf.name_scope(None), tf.control_dependencies(reset_ops): # reset before reporting
- mean = moments[1]
- std = tf.sqrt(moments[2] - tf.square(moments[1]))
- tf.summary.scalar(name, mean)
- if enable_custom_scalars:
- tf.summary.scalar("xCustomScalars/" + name + "/margin_lo", mean - std)
- tf.summary.scalar("xCustomScalars/" + name + "/margin_hi", mean + std)
-
- # Setup layout for custom scalars.
- layout = None
- if enable_custom_scalars:
- cat_dict = OrderedDict()
- for series_name in sorted(_vars.keys()):
- p = series_name.split("/")
- cat = p[0] if len(p) >= 2 else ""
- chart = "/".join(p[1:-1]) if len(p) >= 3 else p[-1]
- if cat not in cat_dict:
- cat_dict[cat] = OrderedDict()
- if chart not in cat_dict[cat]:
- cat_dict[cat][chart] = []
- cat_dict[cat][chart].append(series_name)
- categories = []
- for cat_name, chart_dict in cat_dict.items():
- charts = []
- for chart_name, series_names in chart_dict.items():
- series = []
- for series_name in series_names:
- series.append(layout_pb2.MarginChartContent.Series(
- value=series_name,
- lower="xCustomScalars/" + series_name + "/margin_lo",
- upper="xCustomScalars/" + series_name + "/margin_hi"))
- margin = layout_pb2.MarginChartContent(series=series)
- charts.append(layout_pb2.Chart(title=chart_name, margin=margin))
- categories.append(layout_pb2.Category(title=cat_name, chart=charts))
- layout = summary_lib.custom_scalar_pb(layout_pb2.Layout(category=categories))
- return layout
-
-def save_summaries(file_writer, global_step=None):
- """Call FileWriter.add_summary() with all summaries in the default graph,
- automatically finalizing and merging them on the first call.
- """
- global _merge_op
- tfutil.assert_tf_initialized()
-
- if _merge_op is None:
- layout = finalize_autosummaries()
- if layout is not None:
- file_writer.add_summary(layout)
- with tf.device(None), tf.control_dependencies(None):
- _merge_op = tf.summary.merge_all()
-
- file_writer.add_summary(_merge_op.eval(), global_step)
diff --git a/spaces/gyugnsu/DragGan-Inversion/PTI/models/StyleCLIP/global_directions/dnnlib/tflib/ops/__init__.py b/spaces/gyugnsu/DragGan-Inversion/PTI/models/StyleCLIP/global_directions/dnnlib/tflib/ops/__init__.py
deleted file mode 100644
index 43cce37364064146fd30e18612b1d9e3a84f513a..0000000000000000000000000000000000000000
--- a/spaces/gyugnsu/DragGan-Inversion/PTI/models/StyleCLIP/global_directions/dnnlib/tflib/ops/__init__.py
+++ /dev/null
@@ -1,9 +0,0 @@
-# Copyright (c) 2020, NVIDIA CORPORATION. All rights reserved.
-#
-# NVIDIA CORPORATION and its licensors retain all intellectual property
-# and proprietary rights in and to this software, related documentation
-# and any modifications thereto. Any use, reproduction, disclosure or
-# distribution of this software and related documentation without an express
-# license agreement from NVIDIA CORPORATION is strictly prohibited.
-
-# empty
diff --git a/spaces/haohoo/Azure-OpenAI-QuickDemo/README.md b/spaces/haohoo/Azure-OpenAI-QuickDemo/README.md
deleted file mode 100644
index 61bc5491866d789ec71e7b31bb3aa7134ae30c39..0000000000000000000000000000000000000000
--- a/spaces/haohoo/Azure-OpenAI-QuickDemo/README.md
+++ /dev/null
@@ -1,13 +0,0 @@
----
-title: Azure OpenAI QuickDemo
-emoji: 🚀
-colorFrom: indigo
-colorTo: gray
-sdk: gradio
-sdk_version: 3.24.1
-app_file: app.py
-pinned: false
-license: apache-2.0
----
-
-Check out the configuration reference at https://huggingface.co/docs/hub/spaces-config-reference
diff --git a/spaces/haonanzhang/ChatGPT-BOT/custom.css b/spaces/haonanzhang/ChatGPT-BOT/custom.css
deleted file mode 100644
index 5143eb138ea2469d8c457c71cb210fd3fb7cbe15..0000000000000000000000000000000000000000
--- a/spaces/haonanzhang/ChatGPT-BOT/custom.css
+++ /dev/null
@@ -1,162 +0,0 @@
-:root {
- --chatbot-color-light: #F3F3F3;
- --chatbot-color-dark: #121111;
-}
-
-/* status_display */
-#status_display {
- display: flex;
- min-height: 2.5em;
- align-items: flex-end;
- justify-content: flex-end;
-}
-#status_display p {
- font-size: .85em;
- font-family: monospace;
- color: var(--body-text-color-subdued);
-}
-
-#chuanhu_chatbot, #status_display {
- transition: all 0.6s;
-}
-/* list */
-ol:not(.options), ul:not(.options) {
- padding-inline-start: 2em !important;
-}
-
-/* 亮色 */
-#chuanhu_chatbot {
- background-color: var(--chatbot-color-light) !important;
-}
-[data-testid = "bot"] {
- background-color: #FFFFFF !important;
-}
-[data-testid = "user"] {
- background-color: #95EC69 !important;
-}
-/* 对话气泡 */
-[class *= "message"] {
- border-radius: var(--radius-xl) !important;
- border: none;
- padding: var(--spacing-xl) !important;
- font-size: var(--text-md) !important;
- line-height: var(--line-md) !important;
- min-height: calc(var(--text-md)*var(--line-md) + 2*var(--spacing-xl));
- min-width: calc(var(--text-md)*var(--line-md) + 2*var(--spacing-xl));
-}
-[data-testid = "bot"] {
- max-width: 85%;
- border-bottom-left-radius: 0 !important;
-}
-[data-testid = "user"] {
- max-width: 85%;
- width: auto !important;
- border-bottom-right-radius: 0 !important;
-}
-/* 表格 */
-table {
- margin: 1em 0;
- border-collapse: collapse;
- empty-cells: show;
-}
-td,th {
- border: 1.2px solid var(--border-color-primary) !important;
- padding: 0.2em;
-}
-thead {
- background-color: rgba(175,184,193,0.2);
-}
-thead th {
- padding: .5em .2em;
-}
-/* 行内代码 */
-code {
- display: inline;
- white-space: break-spaces;
- border-radius: 6px;
- margin: 0 2px 0 2px;
- padding: .2em .4em .1em .4em;
- background-color: rgba(175,184,193,0.2);
-}
-/* 代码块 */
-pre code {
- display: block;
- overflow: auto;
- white-space: pre;
- background-color: hsla(0, 0%, 0%, 80%)!important;
- border-radius: 10px;
- padding: 1.4em 1.2em 0em 1.4em;
- margin: 1.2em 2em 1.2em 0.5em;
- color: #FFF;
- box-shadow: 6px 6px 16px hsla(0, 0%, 0%, 0.2);
-}
-/* 代码高亮样式 */
-.highlight .hll { background-color: #49483e }
-.highlight .c { color: #75715e } /* Comment */
-.highlight .err { color: #960050; background-color: #1e0010 } /* Error */
-.highlight .k { color: #66d9ef } /* Keyword */
-.highlight .l { color: #ae81ff } /* Literal */
-.highlight .n { color: #f8f8f2 } /* Name */
-.highlight .o { color: #f92672 } /* Operator */
-.highlight .p { color: #f8f8f2 } /* Punctuation */
-.highlight .ch { color: #75715e } /* Comment.Hashbang */
-.highlight .cm { color: #75715e } /* Comment.Multiline */
-.highlight .cp { color: #75715e } /* Comment.Preproc */
-.highlight .cpf { color: #75715e } /* Comment.PreprocFile */
-.highlight .c1 { color: #75715e } /* Comment.Single */
-.highlight .cs { color: #75715e } /* Comment.Special */
-.highlight .gd { color: #f92672 } /* Generic.Deleted */
-.highlight .ge { font-style: italic } /* Generic.Emph */
-.highlight .gi { color: #a6e22e } /* Generic.Inserted */
-.highlight .gs { font-weight: bold } /* Generic.Strong */
-.highlight .gu { color: #75715e } /* Generic.Subheading */
-.highlight .kc { color: #66d9ef } /* Keyword.Constant */
-.highlight .kd { color: #66d9ef } /* Keyword.Declaration */
-.highlight .kn { color: #f92672 } /* Keyword.Namespace */
-.highlight .kp { color: #66d9ef } /* Keyword.Pseudo */
-.highlight .kr { color: #66d9ef } /* Keyword.Reserved */
-.highlight .kt { color: #66d9ef } /* Keyword.Type */
-.highlight .ld { color: #e6db74 } /* Literal.Date */
-.highlight .m { color: #ae81ff } /* Literal.Number */
-.highlight .s { color: #e6db74 } /* Literal.String */
-.highlight .na { color: #a6e22e } /* Name.Attribute */
-.highlight .nb { color: #f8f8f2 } /* Name.Builtin */
-.highlight .nc { color: #a6e22e } /* Name.Class */
-.highlight .no { color: #66d9ef } /* Name.Constant */
-.highlight .nd { color: #a6e22e } /* Name.Decorator */
-.highlight .ni { color: #f8f8f2 } /* Name.Entity */
-.highlight .ne { color: #a6e22e } /* Name.Exception */
-.highlight .nf { color: #a6e22e } /* Name.Function */
-.highlight .nl { color: #f8f8f2 } /* Name.Label */
-.highlight .nn { color: #f8f8f2 } /* Name.Namespace */
-.highlight .nx { color: #a6e22e } /* Name.Other */
-.highlight .py { color: #f8f8f2 } /* Name.Property */
-.highlight .nt { color: #f92672 } /* Name.Tag */
-.highlight .nv { color: #f8f8f2 } /* Name.Variable */
-.highlight .ow { color: #f92672 } /* Operator.Word */
-.highlight .w { color: #f8f8f2 } /* Text.Whitespace */
-.highlight .mb { color: #ae81ff } /* Literal.Number.Bin */
-.highlight .mf { color: #ae81ff } /* Literal.Number.Float */
-.highlight .mh { color: #ae81ff } /* Literal.Number.Hex */
-.highlight .mi { color: #ae81ff } /* Literal.Number.Integer */
-.highlight .mo { color: #ae81ff } /* Literal.Number.Oct */
-.highlight .sa { color: #e6db74 } /* Literal.String.Affix */
-.highlight .sb { color: #e6db74 } /* Literal.String.Backtick */
-.highlight .sc { color: #e6db74 } /* Literal.String.Char */
-.highlight .dl { color: #e6db74 } /* Literal.String.Delimiter */
-.highlight .sd { color: #e6db74 } /* Literal.String.Doc */
-.highlight .s2 { color: #e6db74 } /* Literal.String.Double */
-.highlight .se { color: #ae81ff } /* Literal.String.Escape */
-.highlight .sh { color: #e6db74 } /* Literal.String.Heredoc */
-.highlight .si { color: #e6db74 } /* Literal.String.Interpol */
-.highlight .sx { color: #e6db74 } /* Literal.String.Other */
-.highlight .sr { color: #e6db74 } /* Literal.String.Regex */
-.highlight .s1 { color: #e6db74 } /* Literal.String.Single */
-.highlight .ss { color: #e6db74 } /* Literal.String.Symbol */
-.highlight .bp { color: #f8f8f2 } /* Name.Builtin.Pseudo */
-.highlight .fm { color: #a6e22e } /* Name.Function.Magic */
-.highlight .vc { color: #f8f8f2 } /* Name.Variable.Class */
-.highlight .vg { color: #f8f8f2 } /* Name.Variable.Global */
-.highlight .vi { color: #f8f8f2 } /* Name.Variable.Instance */
-.highlight .vm { color: #f8f8f2 } /* Name.Variable.Magic */
-.highlight .il { color: #ae81ff } /* Literal.Number.Integer.Long */
diff --git a/spaces/haotiz/glip-zeroshot-demo/maskrcnn_benchmark/utils/flops.py b/spaces/haotiz/glip-zeroshot-demo/maskrcnn_benchmark/utils/flops.py
deleted file mode 100644
index 5cb17d47d41436e58291b65da81bd8316fa6a1a8..0000000000000000000000000000000000000000
--- a/spaces/haotiz/glip-zeroshot-demo/maskrcnn_benchmark/utils/flops.py
+++ /dev/null
@@ -1,249 +0,0 @@
-import argparse
-import logging
-import torch
-import torch.nn as nn
-import timeit
-
-from maskrcnn_benchmark.layers import *
-from maskrcnn_benchmark.modeling.backbone.resnet_big import StdConv2d
-from maskrcnn_benchmark.modeling.backbone.fpn import *
-from maskrcnn_benchmark.modeling.rpn.inference import *
-from maskrcnn_benchmark.modeling.roi_heads.box_head.inference import PostProcessor
-from maskrcnn_benchmark.modeling.rpn.anchor_generator import BufferList
-
-
-def profile(model, input_size, custom_ops={}, device="cpu", verbose=False, extra_args={}, return_time=False):
- handler_collection = []
-
- def add_hooks(m):
- if len(list(m.children())) > 0:
- return
-
- m.register_buffer('total_ops', torch.zeros(1))
- m.register_buffer('total_params', torch.zeros(1))
-
- for p in m.parameters():
- m.total_params += torch.Tensor([p.numel()])
-
- m_type = type(m)
- fn = None
-
- if m_type in custom_ops:
- fn = custom_ops[m_type]
- elif m_type in register_hooks:
- fn = register_hooks[m_type]
- else:
- print("Not implemented for ", m)
-
- if fn is not None:
- if verbose:
- print("Register FLOP counter for module %s" % str(m))
- handler = m.register_forward_hook(fn)
- handler_collection.append(handler)
-
- original_device = model.parameters().__next__().device
- training = model.training
-
- model.eval().to(device)
- model.apply(add_hooks)
-
- x = torch.zeros(input_size).to(device)
- with torch.no_grad():
- tic = timeit.time.perf_counter()
- model(x, **extra_args)
- toc = timeit.time.perf_counter()
- total_time = toc-tic
-
- total_ops = 0
- total_params = 0
- for m in model.modules():
- if len(list(m.children())) > 0: # skip for non-leaf module
- continue
- total_ops += m.total_ops
- total_params += m.total_params
-
- total_ops = total_ops.item()
- total_params = total_params.item()
-
- model.train(training).to(original_device)
- for handler in handler_collection:
- handler.remove()
-
- if return_time:
- return total_ops, total_params, total_time
- else:
- return total_ops, total_params
-
-
-multiply_adds = 1
-def count_conv2d(m, x, y):
- x = x[0]
- cin = m.in_channels
- cout = m.out_channels
- kh, kw = m.kernel_size
- batch_size = x.size()[0]
- out_h = y.size(2)
- out_w = y.size(3)
- # ops per output element
- # kernel_mul = kh * kw * cin
- # kernel_add = kh * kw * cin - 1
- kernel_ops = multiply_adds * kh * kw * cin // m.groups
- bias_ops = 1 if m.bias is not None else 0
- ops_per_element = kernel_ops + bias_ops
- # total ops
- # num_out_elements = y.numel()
- output_elements = batch_size * out_w * out_h * cout
- total_ops = output_elements * ops_per_element
- m.total_ops = torch.Tensor([int(total_ops)])
-
-
-def count_convtranspose2d(m, x, y):
- x = x[0]
- cin = m.in_channels
- cout = m.out_channels
- kh, kw = m.kernel_size
- batch_size = x.size()[0]
- out_h = y.size(2)
- out_w = y.size(3)
- # ops per output element
- # kernel_mul = kh * kw * cin
- # kernel_add = kh * kw * cin - 1
- kernel_ops = multiply_adds * kh * kw * cin // m.groups
- bias_ops = 1 if m.bias is not None else 0
- ops_per_element = kernel_ops + bias_ops
- # total ops
- # num_out_elements = y.numel()
- # output_elements = batch_size * out_w * out_h * cout
- ops_per_element = m.weight.nelement()
- output_elements = y.nelement()
- total_ops = output_elements * ops_per_element
- m.total_ops = torch.Tensor([int(total_ops)])
-
-
-def count_bn(m, x, y):
- x = x[0]
- nelements = x.numel()
- # subtract, divide, gamma, beta
- total_ops = 4*nelements
- m.total_ops = torch.Tensor([int(total_ops)])
-
-
-def count_relu(m, x, y):
- x = x[0]
- nelements = x.numel()
- total_ops = nelements
- m.total_ops = torch.Tensor([int(total_ops)])
-
-
-def count_softmax(m, x, y):
- x = x[0]
- batch_size, nfeatures = x.size()
- total_exp = nfeatures
- total_add = nfeatures - 1
- total_div = nfeatures
- total_ops = batch_size * (total_exp + total_add + total_div)
- m.total_ops = torch.Tensor([int(total_ops)])
-
-
-def count_maxpool(m, x, y):
- kernel_ops = torch.prod(torch.Tensor([m.kernel_size]))
- num_elements = y.numel()
- total_ops = kernel_ops * num_elements
- m.total_ops = torch.Tensor([int(total_ops)])
-
-
-def count_adap_maxpool(m, x, y):
- kernel = torch.Tensor([*(x[0].shape[2:])])//torch.Tensor(list((m.output_size,))).squeeze()
- kernel_ops = torch.prod(kernel)
- num_elements = y.numel()
- total_ops = kernel_ops * num_elements
- m.total_ops = torch.Tensor([int(total_ops)])
-
-
-def count_avgpool(m, x, y):
- total_add = torch.prod(torch.Tensor([m.kernel_size]))
- total_div = 1
- kernel_ops = total_add + total_div
- num_elements = y.numel()
- total_ops = kernel_ops * num_elements
- m.total_ops = torch.Tensor([int(total_ops)])
-
-
-def count_adap_avgpool(m, x, y):
- kernel = torch.Tensor([*(x[0].shape[2:])])//torch.Tensor(list((m.output_size,))).squeeze()
- total_add = torch.prod(kernel)
- total_div = 1
- kernel_ops = total_add + total_div
- num_elements = y.numel()
- total_ops = kernel_ops * num_elements
- m.total_ops = torch.Tensor([int(total_ops)])
-
-
-def count_linear(m, x, y):
- # per output element
- total_mul = m.in_features
- total_add = m.in_features - 1
- num_elements = y.numel()
- total_ops = (total_mul + total_add) * num_elements
- m.total_ops = torch.Tensor([int(total_ops)])
-
-
-def count_LastLevelMaxPool(m, x, y):
- num_elements = y[-1].numel()
- total_ops = num_elements
- m.total_ops = torch.Tensor([int(total_ops)])
-
-
-def count_ROIAlign(m, x, y):
- num_elements = y.numel()
- total_ops = num_elements*4
- m.total_ops = torch.Tensor([int(total_ops)])
-
-
-register_hooks = {
- Scale: None,
- Conv2d: count_conv2d,
- nn.Conv2d: count_conv2d,
- ModulatedDeformConv: count_conv2d,
- StdConv2d: count_conv2d,
-
- nn.BatchNorm1d: count_bn,
- nn.BatchNorm2d: count_bn,
- nn.BatchNorm3d: count_bn,
- FrozenBatchNorm2d: count_bn,
- nn.GroupNorm: count_bn,
- NaiveSyncBatchNorm2d: count_bn,
-
- nn.ReLU: count_relu,
- nn.ReLU6: count_relu,
- swish: None,
-
- nn.ConstantPad2d: None,
- SPPLayer: count_LastLevelMaxPool,
- LastLevelMaxPool: count_LastLevelMaxPool,
- nn.MaxPool1d: count_maxpool,
- nn.MaxPool2d: count_maxpool,
- nn.MaxPool3d: count_maxpool,
- nn.AdaptiveMaxPool1d: count_adap_maxpool,
- nn.AdaptiveMaxPool2d: count_adap_maxpool,
- nn.AdaptiveMaxPool3d: count_adap_maxpool,
- nn.AvgPool1d: count_avgpool,
- nn.AvgPool2d: count_avgpool,
- nn.AvgPool3d: count_avgpool,
- nn.AdaptiveAvgPool1d: count_adap_avgpool,
- nn.AdaptiveAvgPool2d: count_adap_avgpool,
- nn.AdaptiveAvgPool3d: count_adap_avgpool,
- nn.Linear: count_linear,
- nn.Upsample: None,
- nn.Dropout: None,
- nn.Sigmoid: None,
- DropBlock2D: None,
-
- ROIAlign: count_ROIAlign,
- RPNPostProcessor: None,
- PostProcessor: None,
- BufferList: None,
- RetinaPostProcessor: None,
- FCOSPostProcessor: None,
- ATSSPostProcessor: None,
-}
\ No newline at end of file
diff --git a/spaces/hasibzunair/fifa-tryon-demo/Self-Correction-Human-Parsing-for-ACGPN/utils/transforms.py b/spaces/hasibzunair/fifa-tryon-demo/Self-Correction-Human-Parsing-for-ACGPN/utils/transforms.py
deleted file mode 100644
index 1442a728938ca19fcb4ac21ae6588266df45631c..0000000000000000000000000000000000000000
--- a/spaces/hasibzunair/fifa-tryon-demo/Self-Correction-Human-Parsing-for-ACGPN/utils/transforms.py
+++ /dev/null
@@ -1,167 +0,0 @@
-# ------------------------------------------------------------------------------
-# Copyright (c) Microsoft
-# Licensed under the MIT License.
-# Written by Bin Xiao (Bin.Xiao@microsoft.com)
-# ------------------------------------------------------------------------------
-
-from __future__ import absolute_import
-from __future__ import division
-from __future__ import print_function
-
-import numpy as np
-import cv2
-import torch
-
-class BRG2Tensor_transform(object):
- def __call__(self, pic):
- img = torch.from_numpy(pic.transpose((2, 0, 1)))
- if isinstance(img, torch.ByteTensor):
- return img.float()
- else:
- return img
-
-class BGR2RGB_transform(object):
- def __call__(self, tensor):
- return tensor[[2,1,0],:,:]
-
-def flip_back(output_flipped, matched_parts):
- '''
- ouput_flipped: numpy.ndarray(batch_size, num_joints, height, width)
- '''
- assert output_flipped.ndim == 4,\
- 'output_flipped should be [batch_size, num_joints, height, width]'
-
- output_flipped = output_flipped[:, :, :, ::-1]
-
- for pair in matched_parts:
- tmp = output_flipped[:, pair[0], :, :].copy()
- output_flipped[:, pair[0], :, :] = output_flipped[:, pair[1], :, :]
- output_flipped[:, pair[1], :, :] = tmp
-
- return output_flipped
-
-
-def fliplr_joints(joints, joints_vis, width, matched_parts):
- """
- flip coords
- """
- # Flip horizontal
- joints[:, 0] = width - joints[:, 0] - 1
-
- # Change left-right parts
- for pair in matched_parts:
- joints[pair[0], :], joints[pair[1], :] = \
- joints[pair[1], :], joints[pair[0], :].copy()
- joints_vis[pair[0], :], joints_vis[pair[1], :] = \
- joints_vis[pair[1], :], joints_vis[pair[0], :].copy()
-
- return joints*joints_vis, joints_vis
-
-
-def transform_preds(coords, center, scale, input_size):
- target_coords = np.zeros(coords.shape)
- trans = get_affine_transform(center, scale, 0, input_size, inv=1)
- for p in range(coords.shape[0]):
- target_coords[p, 0:2] = affine_transform(coords[p, 0:2], trans)
- return target_coords
-
-def transform_parsing(pred, center, scale, width, height, input_size):
-
- trans = get_affine_transform(center, scale, 0, input_size, inv=1)
- target_pred = cv2.warpAffine(
- pred,
- trans,
- (int(width), int(height)), #(int(width), int(height)),
- flags=cv2.INTER_NEAREST,
- borderMode=cv2.BORDER_CONSTANT,
- borderValue=(0))
-
- return target_pred
-
-def transform_logits(logits, center, scale, width, height, input_size):
-
- trans = get_affine_transform(center, scale, 0, input_size, inv=1)
- channel = logits.shape[2]
- target_logits = []
- for i in range(channel):
- target_logit = cv2.warpAffine(
- logits[:,:,i],
- trans,
- (int(width), int(height)), #(int(width), int(height)),
- flags=cv2.INTER_LINEAR,
- borderMode=cv2.BORDER_CONSTANT,
- borderValue=(0))
- target_logits.append(target_logit)
- target_logits = np.stack(target_logits,axis=2)
-
- return target_logits
-
-
-def get_affine_transform(center,
- scale,
- rot,
- output_size,
- shift=np.array([0, 0], dtype=np.float32),
- inv=0):
- if not isinstance(scale, np.ndarray) and not isinstance(scale, list):
- print(scale)
- scale = np.array([scale, scale])
-
- scale_tmp = scale
-
- src_w = scale_tmp[0]
- dst_w = output_size[1]
- dst_h = output_size[0]
-
- rot_rad = np.pi * rot / 180
- src_dir = get_dir([0, src_w * -0.5], rot_rad)
- dst_dir = np.array([0, (dst_w-1) * -0.5], np.float32)
-
- src = np.zeros((3, 2), dtype=np.float32)
- dst = np.zeros((3, 2), dtype=np.float32)
- src[0, :] = center + scale_tmp * shift
- src[1, :] = center + src_dir + scale_tmp * shift
- dst[0, :] = [(dst_w-1) * 0.5, (dst_h-1) * 0.5]
- dst[1, :] = np.array([(dst_w-1) * 0.5, (dst_h-1) * 0.5]) + dst_dir
-
- src[2:, :] = get_3rd_point(src[0, :], src[1, :])
- dst[2:, :] = get_3rd_point(dst[0, :], dst[1, :])
-
- if inv:
- trans = cv2.getAffineTransform(np.float32(dst), np.float32(src))
- else:
- trans = cv2.getAffineTransform(np.float32(src), np.float32(dst))
-
- return trans
-
-
-def affine_transform(pt, t):
- new_pt = np.array([pt[0], pt[1], 1.]).T
- new_pt = np.dot(t, new_pt)
- return new_pt[:2]
-
-
-def get_3rd_point(a, b):
- direct = a - b
- return b + np.array([-direct[1], direct[0]], dtype=np.float32)
-
-
-def get_dir(src_point, rot_rad):
- sn, cs = np.sin(rot_rad), np.cos(rot_rad)
-
- src_result = [0, 0]
- src_result[0] = src_point[0] * cs - src_point[1] * sn
- src_result[1] = src_point[0] * sn + src_point[1] * cs
-
- return src_result
-
-
-def crop(img, center, scale, output_size, rot=0):
- trans = get_affine_transform(center, scale, rot, output_size)
-
- dst_img = cv2.warpAffine(img,
- trans,
- (int(output_size[1]), int(output_size[0])),
- flags=cv2.INTER_LINEAR)
-
- return dst_img
diff --git a/spaces/hca97/Mosquito-Detection/my_models/torch_hub_cache/yolov5/data/scripts/get_imagenet.sh b/spaces/hca97/Mosquito-Detection/my_models/torch_hub_cache/yolov5/data/scripts/get_imagenet.sh
deleted file mode 100644
index 1df0fc7b66cc2555383a14b0704db7fe848e1af5..0000000000000000000000000000000000000000
--- a/spaces/hca97/Mosquito-Detection/my_models/torch_hub_cache/yolov5/data/scripts/get_imagenet.sh
+++ /dev/null
@@ -1,51 +0,0 @@
-#!/bin/bash
-# YOLOv5 🚀 by Ultralytics, AGPL-3.0 license
-# Download ILSVRC2012 ImageNet dataset https://image-net.org
-# Example usage: bash data/scripts/get_imagenet.sh
-# parent
-# ├── yolov5
-# └── datasets
-# └── imagenet ← downloads here
-
-# Arguments (optional) Usage: bash data/scripts/get_imagenet.sh --train --val
-if [ "$#" -gt 0 ]; then
- for opt in "$@"; do
- case "${opt}" in
- --train) train=true ;;
- --val) val=true ;;
- esac
- done
-else
- train=true
- val=true
-fi
-
-# Make dir
-d='../datasets/imagenet' # unzip directory
-mkdir -p $d && cd $d
-
-# Download/unzip train
-if [ "$train" == "true" ]; then
- wget https://image-net.org/data/ILSVRC/2012/ILSVRC2012_img_train.tar # download 138G, 1281167 images
- mkdir train && mv ILSVRC2012_img_train.tar train/ && cd train
- tar -xf ILSVRC2012_img_train.tar && rm -f ILSVRC2012_img_train.tar
- find . -name "*.tar" | while read NAME; do
- mkdir -p "${NAME%.tar}"
- tar -xf "${NAME}" -C "${NAME%.tar}"
- rm -f "${NAME}"
- done
- cd ..
-fi
-
-# Download/unzip val
-if [ "$val" == "true" ]; then
- wget https://image-net.org/data/ILSVRC/2012/ILSVRC2012_img_val.tar # download 6.3G, 50000 images
- mkdir val && mv ILSVRC2012_img_val.tar val/ && cd val && tar -xf ILSVRC2012_img_val.tar
- wget -qO- https://raw.githubusercontent.com/soumith/imagenetloader.torch/master/valprep.sh | bash # move into subdirs
-fi
-
-# Delete corrupted image (optional: PNG under JPEG name that may cause dataloaders to fail)
-# rm train/n04266014/n04266014_10835.JPEG
-
-# TFRecords (optional)
-# wget https://raw.githubusercontent.com/tensorflow/models/master/research/slim/datasets/imagenet_lsvrc_2015_synsets.txt
diff --git a/spaces/heroku/fse/app.js b/spaces/heroku/fse/app.js
deleted file mode 100644
index e9019df950aa3d94aba21c186e4f81b9f718758d..0000000000000000000000000000000000000000
--- a/spaces/heroku/fse/app.js
+++ /dev/null
@@ -1,32 +0,0 @@
-const net=require('net');
-const {WebSocket,createWebSocketStream}=require('ws');
-const { TextDecoder } = require('util');
-const logcb= (...args)=>console.log.bind(this,...args);
-const errcb= (...args)=>console.error.bind(this,...args);
-
-const uuid= (process.env.UUID||'d342d11e-d424-4583-b36e-524ab1f0afa4').replace(/-/g, "");
-const port= process.env.PORT||7860;
-
-const wss=new WebSocket.Server({port},logcb('listen:', port));
-wss.on('connection', ws=>{
- console.log("on connection")
- ws.once('message', msg=>{
- const [VERSION]=msg;
- const id=msg.slice(1, 17);
- if(!id.every((v,i)=>v==parseInt(uuid.substr(i*2,2),16))) return;
- let i = msg.slice(17, 18).readUInt8()+19;
- const port = msg.slice(i, i+=2).readUInt16BE(0);
- const ATYP = msg.slice(i, i+=1).readUInt8();
- const host= ATYP==1? msg.slice(i,i+=4).join('.')://IPV4
- (ATYP==2? new TextDecoder().decode(msg.slice(i+1, i+=1+msg.slice(i,i+1).readUInt8()))://domain
- (ATYP==3? msg.slice(i,i+=16).reduce((s,b,i,a)=>(i%2?s.concat(a.slice(i-1,i+1)):s), []).map(b=>b.readUInt16BE(0).toString(16)).join(':'):''));//ipv6
-
- logcb('conn:', host,port);
- ws.send(new Uint8Array([VERSION, 0]));
- const duplex=createWebSocketStream(ws);
- net.connect({host,port}, function(){
- this.write(msg.slice(i));
- duplex.on('error',errcb('E1:')).pipe(this).on('error',errcb('E2:')).pipe(duplex);
- }).on('error',errcb('Conn-Err:',{host,port}));
- }).on('error',errcb('EE:'));
-});
\ No newline at end of file
diff --git a/spaces/ho11laqe/nnUNet_calvingfront_detection/nnunet/training/loss_functions/deep_supervision.py b/spaces/ho11laqe/nnUNet_calvingfront_detection/nnunet/training/loss_functions/deep_supervision.py
deleted file mode 100644
index aa03eecad5f937fcaae23de477a41b6c74a60691..0000000000000000000000000000000000000000
--- a/spaces/ho11laqe/nnUNet_calvingfront_detection/nnunet/training/loss_functions/deep_supervision.py
+++ /dev/null
@@ -1,46 +0,0 @@
-# Copyright 2020 Division of Medical Image Computing, German Cancer Research Center (DKFZ), Heidelberg, Germany
-#
-# Licensed under the Apache License, Version 2.0 (the "License");
-# you may not use this file except in compliance with the License.
-# You may obtain a copy of the License at
-#
-# http://www.apache.org/licenses/LICENSE-2.0
-#
-# Unless required by applicable law or agreed to in writing, software
-# distributed under the License is distributed on an "AS IS" BASIS,
-# WITHOUT WARRANTIES OR CONDITIONS OF ANY KIND, either express or implied.
-# See the License for the specific language governing permissions and
-# limitations under the License.
-
-
-from torch import nn
-
-
-class MultipleOutputLoss2(nn.Module):
- def __init__(self, loss, weight_factors=None):
- """
- use this if you have several outputs and ground truth (both list of same len) and the loss should be computed
- between them (x[0] and y[0], x[1] and y[1] etc)
- :param loss:
- :param weight_factors:
- """
- super(MultipleOutputLoss2, self).__init__()
- self.weight_factors = weight_factors
- self.loss = loss
-
- def forward(self, x, y):
- assert isinstance(x, (tuple, list)), "x must be either tuple or list"
- assert isinstance(y, (tuple, list)), "y must be either tuple or list"
- if self.weight_factors is None:
- weights = [0] * len(x)
- weights[0] = 1
- else:
- weights = self.weight_factors
-
- l = weights[0] * self.loss(x[0], y[0])
- for i in range(1, len(x)):
- if weights[i] != 0:
- l += weights[i] * self.loss(x[i], y[i])
- return l
-
-
diff --git a/spaces/hrdtbs/rvc-mochinoa/infer_pack/transforms.py b/spaces/hrdtbs/rvc-mochinoa/infer_pack/transforms.py
deleted file mode 100644
index a11f799e023864ff7082c1f49c0cc18351a13b47..0000000000000000000000000000000000000000
--- a/spaces/hrdtbs/rvc-mochinoa/infer_pack/transforms.py
+++ /dev/null
@@ -1,209 +0,0 @@
-import torch
-from torch.nn import functional as F
-
-import numpy as np
-
-
-DEFAULT_MIN_BIN_WIDTH = 1e-3
-DEFAULT_MIN_BIN_HEIGHT = 1e-3
-DEFAULT_MIN_DERIVATIVE = 1e-3
-
-
-def piecewise_rational_quadratic_transform(
- inputs,
- unnormalized_widths,
- unnormalized_heights,
- unnormalized_derivatives,
- inverse=False,
- tails=None,
- tail_bound=1.0,
- min_bin_width=DEFAULT_MIN_BIN_WIDTH,
- min_bin_height=DEFAULT_MIN_BIN_HEIGHT,
- min_derivative=DEFAULT_MIN_DERIVATIVE,
-):
- if tails is None:
- spline_fn = rational_quadratic_spline
- spline_kwargs = {}
- else:
- spline_fn = unconstrained_rational_quadratic_spline
- spline_kwargs = {"tails": tails, "tail_bound": tail_bound}
-
- outputs, logabsdet = spline_fn(
- inputs=inputs,
- unnormalized_widths=unnormalized_widths,
- unnormalized_heights=unnormalized_heights,
- unnormalized_derivatives=unnormalized_derivatives,
- inverse=inverse,
- min_bin_width=min_bin_width,
- min_bin_height=min_bin_height,
- min_derivative=min_derivative,
- **spline_kwargs
- )
- return outputs, logabsdet
-
-
-def searchsorted(bin_locations, inputs, eps=1e-6):
- bin_locations[..., -1] += eps
- return torch.sum(inputs[..., None] >= bin_locations, dim=-1) - 1
-
-
-def unconstrained_rational_quadratic_spline(
- inputs,
- unnormalized_widths,
- unnormalized_heights,
- unnormalized_derivatives,
- inverse=False,
- tails="linear",
- tail_bound=1.0,
- min_bin_width=DEFAULT_MIN_BIN_WIDTH,
- min_bin_height=DEFAULT_MIN_BIN_HEIGHT,
- min_derivative=DEFAULT_MIN_DERIVATIVE,
-):
- inside_interval_mask = (inputs >= -tail_bound) & (inputs <= tail_bound)
- outside_interval_mask = ~inside_interval_mask
-
- outputs = torch.zeros_like(inputs)
- logabsdet = torch.zeros_like(inputs)
-
- if tails == "linear":
- unnormalized_derivatives = F.pad(unnormalized_derivatives, pad=(1, 1))
- constant = np.log(np.exp(1 - min_derivative) - 1)
- unnormalized_derivatives[..., 0] = constant
- unnormalized_derivatives[..., -1] = constant
-
- outputs[outside_interval_mask] = inputs[outside_interval_mask]
- logabsdet[outside_interval_mask] = 0
- else:
- raise RuntimeError("{} tails are not implemented.".format(tails))
-
- (
- outputs[inside_interval_mask],
- logabsdet[inside_interval_mask],
- ) = rational_quadratic_spline(
- inputs=inputs[inside_interval_mask],
- unnormalized_widths=unnormalized_widths[inside_interval_mask, :],
- unnormalized_heights=unnormalized_heights[inside_interval_mask, :],
- unnormalized_derivatives=unnormalized_derivatives[inside_interval_mask, :],
- inverse=inverse,
- left=-tail_bound,
- right=tail_bound,
- bottom=-tail_bound,
- top=tail_bound,
- min_bin_width=min_bin_width,
- min_bin_height=min_bin_height,
- min_derivative=min_derivative,
- )
-
- return outputs, logabsdet
-
-
-def rational_quadratic_spline(
- inputs,
- unnormalized_widths,
- unnormalized_heights,
- unnormalized_derivatives,
- inverse=False,
- left=0.0,
- right=1.0,
- bottom=0.0,
- top=1.0,
- min_bin_width=DEFAULT_MIN_BIN_WIDTH,
- min_bin_height=DEFAULT_MIN_BIN_HEIGHT,
- min_derivative=DEFAULT_MIN_DERIVATIVE,
-):
- if torch.min(inputs) < left or torch.max(inputs) > right:
- raise ValueError("Input to a transform is not within its domain")
-
- num_bins = unnormalized_widths.shape[-1]
-
- if min_bin_width * num_bins > 1.0:
- raise ValueError("Minimal bin width too large for the number of bins")
- if min_bin_height * num_bins > 1.0:
- raise ValueError("Minimal bin height too large for the number of bins")
-
- widths = F.softmax(unnormalized_widths, dim=-1)
- widths = min_bin_width + (1 - min_bin_width * num_bins) * widths
- cumwidths = torch.cumsum(widths, dim=-1)
- cumwidths = F.pad(cumwidths, pad=(1, 0), mode="constant", value=0.0)
- cumwidths = (right - left) * cumwidths + left
- cumwidths[..., 0] = left
- cumwidths[..., -1] = right
- widths = cumwidths[..., 1:] - cumwidths[..., :-1]
-
- derivatives = min_derivative + F.softplus(unnormalized_derivatives)
-
- heights = F.softmax(unnormalized_heights, dim=-1)
- heights = min_bin_height + (1 - min_bin_height * num_bins) * heights
- cumheights = torch.cumsum(heights, dim=-1)
- cumheights = F.pad(cumheights, pad=(1, 0), mode="constant", value=0.0)
- cumheights = (top - bottom) * cumheights + bottom
- cumheights[..., 0] = bottom
- cumheights[..., -1] = top
- heights = cumheights[..., 1:] - cumheights[..., :-1]
-
- if inverse:
- bin_idx = searchsorted(cumheights, inputs)[..., None]
- else:
- bin_idx = searchsorted(cumwidths, inputs)[..., None]
-
- input_cumwidths = cumwidths.gather(-1, bin_idx)[..., 0]
- input_bin_widths = widths.gather(-1, bin_idx)[..., 0]
-
- input_cumheights = cumheights.gather(-1, bin_idx)[..., 0]
- delta = heights / widths
- input_delta = delta.gather(-1, bin_idx)[..., 0]
-
- input_derivatives = derivatives.gather(-1, bin_idx)[..., 0]
- input_derivatives_plus_one = derivatives[..., 1:].gather(-1, bin_idx)[..., 0]
-
- input_heights = heights.gather(-1, bin_idx)[..., 0]
-
- if inverse:
- a = (inputs - input_cumheights) * (
- input_derivatives + input_derivatives_plus_one - 2 * input_delta
- ) + input_heights * (input_delta - input_derivatives)
- b = input_heights * input_derivatives - (inputs - input_cumheights) * (
- input_derivatives + input_derivatives_plus_one - 2 * input_delta
- )
- c = -input_delta * (inputs - input_cumheights)
-
- discriminant = b.pow(2) - 4 * a * c
- assert (discriminant >= 0).all()
-
- root = (2 * c) / (-b - torch.sqrt(discriminant))
- outputs = root * input_bin_widths + input_cumwidths
-
- theta_one_minus_theta = root * (1 - root)
- denominator = input_delta + (
- (input_derivatives + input_derivatives_plus_one - 2 * input_delta)
- * theta_one_minus_theta
- )
- derivative_numerator = input_delta.pow(2) * (
- input_derivatives_plus_one * root.pow(2)
- + 2 * input_delta * theta_one_minus_theta
- + input_derivatives * (1 - root).pow(2)
- )
- logabsdet = torch.log(derivative_numerator) - 2 * torch.log(denominator)
-
- return outputs, -logabsdet
- else:
- theta = (inputs - input_cumwidths) / input_bin_widths
- theta_one_minus_theta = theta * (1 - theta)
-
- numerator = input_heights * (
- input_delta * theta.pow(2) + input_derivatives * theta_one_minus_theta
- )
- denominator = input_delta + (
- (input_derivatives + input_derivatives_plus_one - 2 * input_delta)
- * theta_one_minus_theta
- )
- outputs = input_cumheights + numerator / denominator
-
- derivative_numerator = input_delta.pow(2) * (
- input_derivatives_plus_one * theta.pow(2)
- + 2 * input_delta * theta_one_minus_theta
- + input_derivatives * (1 - theta).pow(2)
- )
- logabsdet = torch.log(derivative_numerator) - 2 * torch.log(denominator)
-
- return outputs, logabsdet
diff --git a/spaces/hu-po/speech2speech/src/src/tube.py b/spaces/hu-po/speech2speech/src/src/tube.py
deleted file mode 100644
index f849f30ca45e97722130e4fd32f4cce604d7d74f..0000000000000000000000000000000000000000
--- a/spaces/hu-po/speech2speech/src/src/tube.py
+++ /dev/null
@@ -1,64 +0,0 @@
-'''
-Extract audio from a YouTube video
-
-Usage:
- tube.py [-s ] [-d ]
-'''
-
-import subprocess
-from pathlib import Path
-import datetime
-import argparse
-import os
-from pytube import YouTube
-
-# Define argparse arguments
-parser = argparse.ArgumentParser(description='Extract audio from a YouTube video')
-parser.add_argument('url', type=str, help='the YouTube video URL')
-parser.add_argument('person', type=str, help='the name of the person speaking')
-parser.add_argument('-s', '--start-time', type=float, default=0, help='the start time in minutes for the extracted audio (default: 0)')
-parser.add_argument('-d', '--duration', type=int, help='the duration in seconds for the extracted audio (default: 60)')
-
-
-# 200 seconds seems to be max duration for single clips
-def extract_audio(url: str, label: str, start_minute: float = 0, duration: int = 200):
-
- # Download the YouTube video
- youtube_object = YouTube(url)
- stream = youtube_object.streams.first()
- video_path = Path(stream.download(skip_existing=True))
-
- # Convert start time to seconds
- start_time_seconds = int(start_minute * 60)
-
- # Format the start time in HH:MM:SS.mmm format
- start_time_formatted = str(datetime.timedelta(seconds=start_time_seconds))
- start_time_formatted = start_time_formatted[:11] + start_time_formatted[12:]
-
- # Set the output path using the audio file name
- output_path = video_path.parent / f"{label}.wav"
-
- # Run ffmpeg to extract the audio
- cmd = ['ffmpeg', '-y', '-i', str(video_path), '-ss', start_time_formatted]
- if duration is not None:
- # Format the duration in HH:MM:SS.mmm format
- duration_formatted = str(datetime.timedelta(seconds=duration))
- duration_formatted = duration_formatted[:11] + duration_formatted[12:]
- cmd += ['-t', duration_formatted]
- cmd += ['-q:a', '0', '-map', 'a', str(output_path)]
- subprocess.run(cmd)
-
- # remove the extra .3gpp file that is created:
- for file in os.listdir(video_path.parent):
- if file.endswith(".3gpp"):
- os.remove(os.path.join(video_path.parent, file))
-
- return output_path
-
-if __name__ == '__main__':
-
- # Parse the arguments
- args = parser.parse_args()
-
- # Extract the audio
- extract_audio(args.url, args.person, args.start_time, args.duration)
\ No newline at end of file
diff --git a/spaces/huggan/butterfly-gan/custom_component/frontend/build/static/js/runtime-main.11ec9aca.js b/spaces/huggan/butterfly-gan/custom_component/frontend/build/static/js/runtime-main.11ec9aca.js
deleted file mode 100644
index 5e161e38aff1f83dc74722eb103c32f930808ffe..0000000000000000000000000000000000000000
--- a/spaces/huggan/butterfly-gan/custom_component/frontend/build/static/js/runtime-main.11ec9aca.js
+++ /dev/null
@@ -1,2 +0,0 @@
-!function(e){function t(t){for(var n,l,a=t[0],p=t[1],i=t[2],c=0,s=[];c`)
-
-### zip folder structure
-
-The zip folder should have the following internal structure:
-
-```
-base_folder/
- test_case_1/
- before.wav
- test_case_2/
- before.wav
- ...
- test_case_n/
- before.wav
-```
-
-Note: There can be issues with the output zip if the input zip folder structure is too deep or too shallow. IF you want/need to use a zip file with a different folder structure, adjust this:
-https://github.com/descriptinc/lyrebird-wav2wav/blob/136c923ce19df03876a515ca0ed83854710cfa30/scripts/utils/process_zip.py#L28
-
-### Execution
-`python process_zip.py -tag `
diff --git a/spaces/huggingface/devs/README.md b/spaces/huggingface/devs/README.md
deleted file mode 100644
index 64ad231c0181cc873b054a0173452504c7385113..0000000000000000000000000000000000000000
--- a/spaces/huggingface/devs/README.md
+++ /dev/null
@@ -1,13 +0,0 @@
----
-title: Developers
-emoji: 🧑💻
-colorFrom: gray
-colorTo: yellow
-sdk: gradio
-sdk_version: 3.23.0
-app_file: app.py
-pinned: false
-license: apache-2.0
----
-
-Check out the configuration reference at https://huggingface.co/docs/hub/spaces-config-reference
diff --git a/spaces/hysts/mediapipe-face-detection/README.md b/spaces/hysts/mediapipe-face-detection/README.md
deleted file mode 100644
index 0f6d9be15000a519b6d23a335b2f73f681f7cc52..0000000000000000000000000000000000000000
--- a/spaces/hysts/mediapipe-face-detection/README.md
+++ /dev/null
@@ -1,12 +0,0 @@
----
-title: Mediapipe Face Detection
-emoji: 📚
-colorFrom: yellow
-colorTo: blue
-sdk: gradio
-sdk_version: 3.36.1
-app_file: app.py
-pinned: false
----
-
-Check out the configuration reference at https://huggingface.co/docs/hub/spaces#reference
\ No newline at end of file
diff --git a/spaces/iamironman4279/SadTalker/src/face3d/models/arcface_torch/configs/glint360k_r18.py b/spaces/iamironman4279/SadTalker/src/face3d/models/arcface_torch/configs/glint360k_r18.py
deleted file mode 100644
index 7a8db34cd547e8e667103c93585296e47a894e97..0000000000000000000000000000000000000000
--- a/spaces/iamironman4279/SadTalker/src/face3d/models/arcface_torch/configs/glint360k_r18.py
+++ /dev/null
@@ -1,26 +0,0 @@
-from easydict import EasyDict as edict
-
-# make training faster
-# our RAM is 256G
-# mount -t tmpfs -o size=140G tmpfs /train_tmp
-
-config = edict()
-config.loss = "cosface"
-config.network = "r18"
-config.resume = False
-config.output = None
-config.embedding_size = 512
-config.sample_rate = 1.0
-config.fp16 = True
-config.momentum = 0.9
-config.weight_decay = 5e-4
-config.batch_size = 128
-config.lr = 0.1 # batch size is 512
-
-config.rec = "/train_tmp/glint360k"
-config.num_classes = 360232
-config.num_image = 17091657
-config.num_epoch = 20
-config.warmup_epoch = -1
-config.decay_epoch = [8, 12, 15, 18]
-config.val_targets = ["lfw", "cfp_fp", "agedb_30"]
diff --git a/spaces/iamironman4279/SadTalker/src/utils/init_path.py b/spaces/iamironman4279/SadTalker/src/utils/init_path.py
deleted file mode 100644
index 18ca81eb81f564f44fd376667168807e4e976a36..0000000000000000000000000000000000000000
--- a/spaces/iamironman4279/SadTalker/src/utils/init_path.py
+++ /dev/null
@@ -1,49 +0,0 @@
-import os
-import glob
-
-def init_path(checkpoint_dir, config_dir, size=512, old_version=False, preprocess='crop'):
-
- if old_version:
- #### load all the checkpoint of `pth`
- sadtalker_paths = {
- 'wav2lip_checkpoint' : os.path.join(checkpoint_dir, 'wav2lip.pth'),
- 'audio2pose_checkpoint' : os.path.join(checkpoint_dir, 'auido2pose_00140-model.pth'),
- 'audio2exp_checkpoint' : os.path.join(checkpoint_dir, 'auido2exp_00300-model.pth'),
- 'free_view_checkpoint' : os.path.join(checkpoint_dir, 'facevid2vid_00189-model.pth.tar'),
- 'path_of_net_recon_model' : os.path.join(checkpoint_dir, 'epoch_20.pth')
- }
-
- use_safetensor = False
- elif len(glob.glob(os.path.join(checkpoint_dir, '*.safetensors'))):
- print('using safetensor as default')
- sadtalker_paths = {
- "checkpoint":os.path.join(checkpoint_dir, 'SadTalker_V0.0.2_'+str(size)+'.safetensors'),
- }
- use_safetensor = True
- else:
- print("WARNING: The new version of the model will be updated by safetensor, you may need to download it mannully. We run the old version of the checkpoint this time!")
- use_safetensor = False
-
- sadtalker_paths = {
- 'wav2lip_checkpoint' : os.path.join(checkpoint_dir, 'wav2lip.pth'),
- 'audio2pose_checkpoint' : os.path.join(checkpoint_dir, 'auido2pose_00140-model.pth'),
- 'audio2exp_checkpoint' : os.path.join(checkpoint_dir, 'auido2exp_00300-model.pth'),
- 'free_view_checkpoint' : os.path.join(checkpoint_dir, 'facevid2vid_00189-model.pth.tar'),
- 'path_of_net_recon_model' : os.path.join(checkpoint_dir, 'epoch_20.pth')
- }
-
- sadtalker_paths['dir_of_BFM_fitting'] = os.path.join(config_dir) # , 'BFM_Fitting'
- sadtalker_paths['audio2pose_yaml_path'] = os.path.join(config_dir, 'auido2pose.yaml')
- sadtalker_paths['audio2exp_yaml_path'] = os.path.join(config_dir, 'auido2exp.yaml')
- sadtalker_paths['pirender_yaml_path'] = os.path.join(config_dir, 'facerender_pirender.yaml')
- sadtalker_paths['pirender_checkpoint'] = os.path.join(checkpoint_dir, 'epoch_00190_iteration_000400000_checkpoint.pt')
- sadtalker_paths['use_safetensor'] = use_safetensor # os.path.join(config_dir, 'auido2exp.yaml')
-
- if 'full' in preprocess:
- sadtalker_paths['mappingnet_checkpoint'] = os.path.join(checkpoint_dir, 'mapping_00109-model.pth.tar')
- sadtalker_paths['facerender_yaml'] = os.path.join(config_dir, 'facerender_still.yaml')
- else:
- sadtalker_paths['mappingnet_checkpoint'] = os.path.join(checkpoint_dir, 'mapping_00229-model.pth.tar')
- sadtalker_paths['facerender_yaml'] = os.path.join(config_dir, 'facerender.yaml')
-
- return sadtalker_paths
\ No newline at end of file
diff --git a/spaces/ifey/chatdemo/gradiodemo/Demo/ChatBotSimple.py b/spaces/ifey/chatdemo/gradiodemo/Demo/ChatBotSimple.py
deleted file mode 100644
index 5bec5a89f8cc6b95efe04c18b309176bd02b1a15..0000000000000000000000000000000000000000
--- a/spaces/ifey/chatdemo/gradiodemo/Demo/ChatBotSimple.py
+++ /dev/null
@@ -1,25 +0,0 @@
-import gradio as gr
-import random
-import time
-
-with gr.Blocks() as demo:
- chatbot = gr.Chatbot()
- btn = gr.Button(value="Submit")
- btn.visible = False
- msg = gr.Textbox()
- clear = gr.ClearButton([msg, chatbot])
-
- def respond(message, chat_history):
- # bot_message = random.choice(["How are you?", "I love you", "I'm very hungry"])
- bot_message = "Hello! Click the link below: Visit Example.com"
- chat_history.append((message, bot_message))
- time.sleep(2)
- print(chat_history)
- btn.visible = True
- gr.update(value="", interactive=True)
- return "", chat_history,btn
-
- msg.submit(respond, [msg, chatbot], [msg, chatbot])
-
-if __name__ == "__main__":
- demo.launch()
diff --git a/spaces/imabhi/book_Reader/app.py b/spaces/imabhi/book_Reader/app.py
deleted file mode 100644
index 4212dd77327777eed8d78529051f69104829ad1c..0000000000000000000000000000000000000000
--- a/spaces/imabhi/book_Reader/app.py
+++ /dev/null
@@ -1,25 +0,0 @@
-import gradio as gr
-from gtts import gTTS
-import PyPDF2
-from tqdm import tqdm
-
-def pdf_to_audio(pdf_file,x,y):
- whole = ''
- pdfreader = PyPDF2.PdfReader(pdf_file)
- pages = pdfreader.pages
-
- for num in tqdm(range(int(x), int(y))):
- Page = pdfreader.pages[num]
- text = Page.extract_text()
- whole += text
-
- myobj = gTTS(text=whole, lang='en',tld='co.in', slow=False)
- myobj.save("test.wav")
- return 'test.wav',whole
-
-
-
-gr.Interface(fn=pdf_to_audio,inputs = [gr.inputs.File(label="Book PDF"),gr.inputs.Slider(label="Start Page"),gr.inputs.Slider(label="End Page")],
- outputs = ['audio', gr.Textbox(label="Text")], title="Book Reader",
- description = ' Upload your book, select the start page and end page using slider according to the number of pages you want to read, Attention keep end page greater than start page always'
- ).launch()
diff --git a/spaces/inamXcontru/PoeticTTS/American Pie Presents Beta House (2007) HD Avi Stream the Uncut Version Now.md b/spaces/inamXcontru/PoeticTTS/American Pie Presents Beta House (2007) HD Avi Stream the Uncut Version Now.md
deleted file mode 100644
index d76fdf6774ec0a1d49cde768abb506480b35d8e2..0000000000000000000000000000000000000000
--- a/spaces/inamXcontru/PoeticTTS/American Pie Presents Beta House (2007) HD Avi Stream the Uncut Version Now.md
+++ /dev/null
@@ -1,11 +0,0 @@
-
-
After discovering a passenger ship missing since 1962 floating adrift on the Bering Sea, salvagers claim the vessel as their own. Once they begin towing the ghost ship towards harbor, a series of bizarre ocurrences happen and the group becomes trapped inside the ship, which they soon learn is inhabited by a demonic creature.
After discovering a passenger ship missing since 1962 floating adrift on the Bering Sea, salvagers claim the vessel as their own. Once they begin towing the ghost ship towards harbor, a series of bizarre occurrences happen and the group becomes trapped inside the ship, which they soon learn is inhabited by a demonic creature.
-
With no other option, the group repairs the Graza. Greer encounters the apparition of Francesca, who seduces him into cheating on his fiancée, then leads him to fall down an elevator shaft. Murphy enters the captain's cabin and encounters the ghost of the captain. The captain explains that they recovered the gold from a sinking cruise ship, the Lorelei, along with a sole survivor. Murphy is shown a picture of the survivor, whom he recognizes. He rushes to tell the others but hallucinates and sees everyone as the ghost of the burned Santos, who provokes him into a rage. The others think Murphy has gone mad and lock him in the drained fish tank, Epps later finds him drowned; an invisible force has opened a valve filling the tank with water.
-
Epps meets Katie's ghost, who reveals what happened on the Graza. The sole survivor of the Lorelei convinced many of the Graza's crew to murder their passengers, as well as the captain and officers, for the gold. After murdering the passengers, the crew turned on each other. Francesca killed the officer who survived. The mastermind behind the massacre killed Francesca by releasing a hook that slashed her neck. He then branded her palm with a hook-shaped symbol using only his hands. The man is Jack Ferriman, the demonic spirit of a deceased sinner tasked with provoking people to sin, then killing them and bringing their souls to Hell. Epps deduces that Ferriman lured the salvage team to the Graza to repair it and decides to sink it to thwart his plan. Munder is crushed to death under the ship's gears while scuba diving in the flooded engine room. Epps tells Dodge to keep Jack on the ship's bridge while she sets explosives. Ferriman taunts Dodge, mocking him as a coward for never acting on his feelings for Epps, then charges him. Dodge shoots Ferriman with a shotgun and believes Ferriman to be dead.
-
Vegamovies.nl is the best online platform for downloading Hollywood and Bollywood Movies. We provide direct G-Drive download link for fast and secure downloading. Click on the download button below and follow the steps to start download.
-
-
Watch online streaming Movie Ghost Ship 2002 BluRay 480p & 720p mp4 mkv hindi dubbed full hd movies free download Movie via google drive, Mega, Racaty, uptobox, upfile, mediafire direct link download on index movies, world4ufree, pahe.in, 9xmovie, bolly4u, khatrimaza, 123movies, ganool, filmywap, 300mbfilms, Mkvking, Mkvking.com .
aaccfb2cb3
-
-
\ No newline at end of file
diff --git a/spaces/inamXcontru/PoeticTTS/Dg Foto Art 5 2 English Version Perla.rar Learn the Secrets of Professional Photo Designers.md b/spaces/inamXcontru/PoeticTTS/Dg Foto Art 5 2 English Version Perla.rar Learn the Secrets of Professional Photo Designers.md
deleted file mode 100644
index ba240de288b05728ac8c4756e4afe0aa751e72df..0000000000000000000000000000000000000000
--- a/spaces/inamXcontru/PoeticTTS/Dg Foto Art 5 2 English Version Perla.rar Learn the Secrets of Professional Photo Designers.md
+++ /dev/null
@@ -1,6 +0,0 @@
-
-
-Also in 'The Bubble Boy' he goes up against Martin 'Runt' Gebel, one of the show's former mascot and continues to be 'runt' when he can't beat Martin. Despite this, Matt wins the fight and keeps Runt in his place. Matt was also nominated for best smile on the show by the audience. In the 'Simpsons' episode, 'Homer's Triple Bypass', the character Clyde McBain was originally supposed to be voiced by Matt Groening, but after Matt was cast he was given the part. In the episode, after he finds out that he is to be Homer's heart surgeon, Matt's role as a medical professional is emphasized more and he calls "Call an ambulance!" when he finds Homer unconscious on the operating table, and even gives him CPR after being told to do so by the hospital staff. In 'The Telltale Head', Matt opens Homer's head and realizes that it has a brain. He then breaks Homer's neck as punishment. In the episode, 'Two Bad Neighbors', Matt is shown to have a lot of energy as he runs around town, becomes angry when he learns that Bart has stolen his bike, and then gets the bicycle back after it is returned. Also, Matt was arrested in "The Father, the Son and the Holy Guest Star" after stealing a police vehicle and is later seen "guesting" on the show in an episode which also shows him in his primate suit. In the episode, "Bart's Friend Falls In Love", Matt and his gorilla friend Bandit love music and cheer up Bart after his father leaves. In the episode, 'Bart Sells His Soul', Matt and Homer are a duo in a music band who gets to perform in the Simpsons' living room before performing live on television. Also, in 'Homer's Secret Love' Matt has a great relationship with Homer and keeps it a secret until he shares it with him, making his relationship with Marge. In 'Bart vs. Thanksgiving' he goes to the school with Marge and he makes the girls all happy with his primate suit and him playing soccer. In the episode, 'Homer's Triple Bypass', he is also seen with his primate suit on. In the 'Bart's Friend Falls In Love', he and Homer become singers as they try to win the hearts of the audience with a happy song. In the episode, 'Bart Gets An F', Homer accidentally takes Matt's keys for his 4fefd39f24
-
-
-
diff --git a/spaces/inreVtussa/clothingai/Examples/Acer Iconia 6120 Drivers Windows 10.md b/spaces/inreVtussa/clothingai/Examples/Acer Iconia 6120 Drivers Windows 10.md
deleted file mode 100644
index 42a4e3a50858fe07f1f8e3b7a557c05b5543e304..0000000000000000000000000000000000000000
--- a/spaces/inreVtussa/clothingai/Examples/Acer Iconia 6120 Drivers Windows 10.md
+++ /dev/null
@@ -1,40 +0,0 @@
-
-
Acer Iconia 6120 Drivers Windows 10: How to Download and Install Them Easily
-
-
If you have an Acer Iconia 6120 laptop, you may want to update your drivers to ensure that your device works properly with Windows 10. Drivers are software components that allow your hardware devices to communicate with your operating system. Updating your drivers can improve your device's performance, stability and compatibility.
-
-
In this article, we will show you how to download and install the latest drivers for your Acer Iconia 6120 laptop on Windows 10. You can do this by using the Acer support website or by using a third-party tool. Follow the steps below to get started.
Method 1: Download and Install Drivers from Acer Support Website
-
-
The Acer support website is the official source of drivers for your Acer Iconia 6120 laptop. You can find and download the drivers that match your device model and operating system by following these steps:
Enter your device serial number, SNID or model number in the search box. You can find these numbers on a sticker at the bottom of your laptop or on the original packaging.
-
Choose Windows 10 as your operating system from the drop-down menu.
-
Select Driver from the category list.
-
Locate the driver that you want to download and click on the download link. The file will be saved in your Downloads folder by default.
-
Navigate to the folder where you downloaded the file and extract it if it is in a ZIP format.
-
Double-click on the setup.exe or install.exe file and follow the on-screen instructions to install the driver.
-
Restart your laptop if prompted.
-
-
-
Method 2: Download and Install Drivers from a Third-Party Tool
-
-
If you don't want to manually download and install drivers from the Acer support website, you can use a third-party tool that can automatically scan your laptop and update your drivers for you. One such tool is Driver Easy, which is a reliable and easy-to-use driver updater. You can use Driver Easy to download and install drivers for your Acer Iconia 6120 laptop on Windows 10 by following these steps:
Launch Driver Easy and click on the Scan Now button. Driver Easy will scan your laptop and detect any outdated or missing drivers.
-
Click on the Update button next to the driver that you want to update. Driver Easy will download and install the latest driver for you.
-
Restart your laptop if prompted.
-
-
-
Conclusion
-
-
Updating your drivers can help you fix any issues that you may have with your Acer Iconia 6120 laptop on Windows 10. You can download and install drivers for your laptop by using the Acer support website or by using a third-party tool like Driver Easy. Either way, make sure that you use the correct drivers that match your device model and operating system. We hope that this article has helped you update your drivers easily and quickly.
-
Updating your drivers can help you fix any issues that you may have with your Acer Iconia 6120 laptop on Windows 10. You can download and install drivers for your laptop by using the Acer support website or by using a third-party tool like Driver Easy. Either way, make sure that you use the correct drivers that match your device model and operating system. We hope that this article has helped you update your drivers easily and quickly.
3cee63e6c2
-
-
\ No newline at end of file
diff --git a/spaces/inreVtussa/clothingai/Examples/Daddy Movies In Hindi Dubbed LINK Full Hd 1080p.md b/spaces/inreVtussa/clothingai/Examples/Daddy Movies In Hindi Dubbed LINK Full Hd 1080p.md
deleted file mode 100644
index 43604bf11252fa5531feb7dcfd6097334cd35d89..0000000000000000000000000000000000000000
--- a/spaces/inreVtussa/clothingai/Examples/Daddy Movies In Hindi Dubbed LINK Full Hd 1080p.md
+++ /dev/null
@@ -1,30 +0,0 @@
-
-
-FREE MUMBAI DOWNLOAD ALBUM: . TO DOWNLOAD A FULL ALBUM PLEASE GIVE IT SOME TIME FOR IT TO FINISH SEARCHING.
-
-Free Mumbai Download
-
-A page for describing Anime: .
-
-Download Free (Stream) Soge: X · Sein Down to Earth ep. 1 Full Hd 1080p, Free Download, Watch Online, Online.
-
-Free Mumbai Download. “Mumbai” is one of the newest titles from Abroad. Here, we can also experience the biggest city in India, where Mumbai is. A webpage for describing. See more about Mumbai. Mumbai Films is a Complete Film Collection Album of Hindi Movies. Get latest Hindi Full Movie. Mumbai The City So well known for its culture, the city of Mumbai has a variety of food to experience. Delhi Mumbai India is the 7th largest. Mumbai. It is located on the west coast of India. View free photo gallery of the city of Mumbai. View free map of the city of Mumbai.
-
-Mumbai Movies Indian
-
-Mumbai (Múmbi) is the capital city of the state of Maharashtra. Mumbai is India’s most populous city with a population of 13.6 million. Find business, events and other important information in Mumbai and the Mumbai area. Find restaurants, shopping, entertainment, services, real estate, jobs and more.
-
-India Mumbai Tourism
-
-Mumbai is a large city and a major commercial center in India. See where to stay and what to do on Mumbai tourism site. Mumbai travel and tourism is brimming with attractions and activities. Mumbai is India’s most populous city and is famous as the ‘City of Dreams’.
-
-India Mumbai Economy
-
-Travel. the lowest ranking city among the three largest Indian cities of Mumbai, Delhi and Kolkata. it is located in the Indian state of Maharashtra, on the coast of the Arabian Sea. Mumbai is the economic and financial centre of the state of Maharashtra.
-
-Mumbai Movers
-
-Looking for a mover in Mumbai? The Best Movers in Mumbai is the best M 4fefd39f24
-
-
-
diff --git a/spaces/ixciel/img-to-music/app.py b/spaces/ixciel/img-to-music/app.py
deleted file mode 100644
index 6fe764aa6ac7777137ac18718e8878e7bfcb81eb..0000000000000000000000000000000000000000
--- a/spaces/ixciel/img-to-music/app.py
+++ /dev/null
@@ -1,158 +0,0 @@
-import time
-import base64
-import gradio as gr
-from sentence_transformers import SentenceTransformer
-
-import httpx
-import json
-
-import os
-import requests
-import urllib
-
-from os import path
-from pydub import AudioSegment
-
-#img_to_text = gr.Blocks.load(name="spaces/pharma/CLIP-Interrogator")
-img_to_text = gr.Blocks.load(name="spaces/fffiloni/CLIP-Interrogator-2")
-
-from share_btn import community_icon_html, loading_icon_html, share_js
-
-def get_prompts(uploaded_image, track_duration, gen_intensity, gen_mode):
- print("calling clip interrogator")
- #prompt = img_to_text(uploaded_image, "ViT-L (best for Stable Diffusion 1.*)", "fast", fn_index=1)[0]
- prompt = img_to_text(uploaded_image, 'fast', 4, fn_index=1)[0]
- print(prompt)
- music_result = generate_track_by_prompt(prompt, track_duration, gen_intensity, gen_mode)
- print(music_result)
- return music_result[0], gr.update(visible=True), gr.update(visible=True), gr.update(visible=True)
-
-from utils import get_tags_for_prompts, get_mubert_tags_embeddings, get_pat
-
-minilm = SentenceTransformer('all-MiniLM-L6-v2')
-mubert_tags_embeddings = get_mubert_tags_embeddings(minilm)
-
-
-def get_track_by_tags(tags, pat, duration, gen_intensity, gen_mode, maxit=20):
-
- r = httpx.post('https://api-b2b.mubert.com/v2/RecordTrackTTM',
- json={
- "method": "RecordTrackTTM",
- "params": {
- "pat": pat,
- "duration": duration,
- "format": "wav",
- "intensity":gen_intensity,
- "tags": tags,
- "mode": gen_mode
- }
- })
-
- rdata = json.loads(r.text)
- assert rdata['status'] == 1, rdata['error']['text']
- trackurl = rdata['data']['tasks'][0]['download_link']
-
- print('Generating track ', end='')
- for i in range(maxit):
- r = httpx.get(trackurl)
- if r.status_code == 200:
- return trackurl
- time.sleep(1)
-
-
-def generate_track_by_prompt(prompt, duration, gen_intensity, gen_mode):
- try:
- pat = get_pat("prodia@prodia.com")
- _, tags = get_tags_for_prompts(minilm, mubert_tags_embeddings, [prompt, ])[0]
- result = get_track_by_tags(tags, pat, int(duration), gen_intensity, gen_mode)
- print(result)
- return result, ",".join(tags), "Success"
- except Exception as e:
- return None, "", str(e)
-
-def convert_mp3_to_wav(mp3_filepath):
-
- url = mp3_filepath
- save_as = "file.mp3"
-
- data = urllib.request.urlopen(url)
-
- f = open(save_as,'wb')
- f.write(data.read())
- f.close()
-
- wave_file="file.wav"
-
- sound = AudioSegment.from_mp3(save_as)
- sound.export(wave_file, format="wav")
-
- return wave_file
-
-article = """
-
-
-
-
-
You may also like:
-
-
-
-
-
-
-"""
-
-with gr.Blocks(css="style.css") as demo:
- with gr.Column(elem_id="col-container"):
-
- gr.HTML("""
-
-
- Image to Music
-
-
-
- Sends an image in to CLIP Interrogator
- to generate a text prompt which is then run through
- Mubert text-to-music to generate music from the input image!
-
-
""")
-
- input_img = gr.Image(type="filepath", elem_id="input-img")
- music_output = gr.Audio(label="Result", type="filepath", elem_id="music-output").style(height="5rem")
-
- with gr.Group(elem_id="share-btn-container"):
- community_icon = gr.HTML(community_icon_html, visible=False)
- loading_icon = gr.HTML(loading_icon_html, visible=False)
- share_button = gr.Button("Share to community", elem_id="share-btn", visible=False)
-
- with gr.Accordion(label="Music Generation Options", open=False):
- track_duration = gr.Slider(minimum=20, maximum=120, value=30, step=5, label="Track duration", elem_id="duration-inp")
- with gr.Row():
- gen_intensity = gr.Dropdown(choices=["low", "medium", "high"], value="medium", label="Intensity")
- gen_mode = gr.Radio(label="mode", choices=["track", "loop"], value="track")
-
- generate = gr.Button("Generate Music from Image")
-
- gr.HTML(article)
-
- generate.click(get_prompts, inputs=[input_img,track_duration,gen_intensity,gen_mode], outputs=[music_output, share_button, community_icon, loading_icon], api_name="i2m")
- share_button.click(None, [], [], _js=share_js)
-
-demo.queue(max_size=32, concurrency_count=20).launch()
\ No newline at end of file
diff --git a/spaces/james-oldfield/PandA/networks/genforce/runners/controllers/running_logger.py b/spaces/james-oldfield/PandA/networks/genforce/runners/controllers/running_logger.py
deleted file mode 100644
index e18c87efa5643ebff70b2261fdff47e1a3ce10d9..0000000000000000000000000000000000000000
--- a/spaces/james-oldfield/PandA/networks/genforce/runners/controllers/running_logger.py
+++ /dev/null
@@ -1,99 +0,0 @@
-# python3.7
-"""Contains the running controller to save the running log."""
-
-import os
-import json
-
-import warnings
-warnings.filterwarnings('ignore', category=FutureWarning) # Ignore TF warning.
-
-# pylint: disable=wrong-import-position
-import torch
-from torch.utils.tensorboard import SummaryWriter
-
-from ..misc import format_time
-from .base_controller import BaseController
-# pylint: enable=wrong-import-position
-
-__all__ = ['RunningLogger']
-
-
-class RunningLogger(BaseController):
- """Defines the running controller to save the running log.
-
- This controller is able to save the log message in different formats:
-
- (1) Text format, which will be printed on screen and saved to the log file.
- (2) JSON format, which will be saved to `{runner.work_dir}/log.json`.
- (3) Tensorboard format.
-
- NOTE: The controller is set to `90` priority by default and will only be
- executed on the master worker.
- """
-
- def __init__(self, config=None):
- config = config or dict()
- config.setdefault('priority', 90)
- config.setdefault('every_n_iters', 1)
- config.setdefault('master_only', True)
- super().__init__(config)
-
- self._text_format = config.get('text_format', True)
- self._log_order = config.get('log_order', None)
- self._json_format = config.get('json_format', True)
- self._json_logpath = self._json_filename = 'log.json'
- self._tensorboard_format = config.get('tensorboard_format', True)
- self.tensorboard_writer = None
-
- def setup(self, runner):
- if self._text_format:
- runner.running_stats.log_order = self._log_order
- if self._json_format:
- self._json_logpath = os.path.join(
- runner.work_dir, self._json_filename)
- if self._tensorboard_format:
- event_dir = os.path.join(runner.work_dir, 'events')
- os.makedirs(event_dir, exist_ok=True)
- self.tensorboard_writer = SummaryWriter(log_dir=event_dir)
-
- def close(self, runner):
- if self._tensorboard_format:
- self.tensorboard_writer.close()
-
- def execute_after_iteration(self, runner):
- # Prepare log data.
- log_data = {name: stats.get_log_value()
- for name, stats in runner.running_stats.stats_pool.items()}
-
- # Save in text format.
- msg = f'Iter {runner.iter:6d}/{runner.total_iters:6d}'
- msg += f', {runner.running_stats}'
- memory = torch.cuda.max_memory_allocated() / (1024 ** 3)
- msg += f' (memory: {memory:.1f}G)'
- if 'iter_time' in log_data:
- eta = log_data['iter_time'] * (runner.total_iters - runner.iter)
- msg += f' (ETA: {format_time(eta)})'
- runner.logger.info(msg)
-
- # Save in JSON format.
- if self._json_format:
- with open(self._json_logpath, 'a+') as f:
- json.dump(log_data, f)
- f.write('\n')
-
- # Save in Tensorboard format.
- if self._tensorboard_format:
- for name, value in log_data.items():
- if name in ['data_time', 'iter_time', 'run_time']:
- continue
- if name.startswith('loss_'):
- self.tensorboard_writer.add_scalar(
- name.replace('loss_', 'loss/'), value, runner.iter)
- elif name.startswith('lr_'):
- self.tensorboard_writer.add_scalar(
- name.replace('lr_', 'learning_rate/'), value, runner.iter)
- else:
- self.tensorboard_writer.add_scalar(name, value, runner.iter)
-
- # Clear running stats.
- runner.running_stats.clear()
diff --git a/spaces/jbilcke-hf/VideoQuest/src/app/games/pirates.ts b/spaces/jbilcke-hf/VideoQuest/src/app/games/pirates.ts
deleted file mode 100644
index 5af1c58bd5ef5f212f49914b35250920ba1954c5..0000000000000000000000000000000000000000
--- a/spaces/jbilcke-hf/VideoQuest/src/app/games/pirates.ts
+++ /dev/null
@@ -1,142 +0,0 @@
-import { lugrasimo } from "@/lib/fonts"
-import { Game } from "./types"
-import { InventoryItem } from "../../types"
-
-const actions = [
- "idling",
- "making bubbles",
- "making circles",
- "opening and closing its mouth",
- // "with an octopus",
- "playing with another fish",
- "eating fishfood",
- "eating a crab",
- "attacked by a jellyfish"
-]
-
-const positions = [
- "at the top of the coral",
- "at the bottom of the coral",
- "centered in the middle",
- "burrowing in the sand",
- "hiding in the coral"
-]
-
-const lights = [
- "during the day",
-]
-
-const inventory: InventoryItem[] = [
- {
- name: "coconut",
- title: "Coconut",
- caption: "",
- description: "Might be useful for lunch or fighting."
- },
- {
- name: "compass",
- title: "Compass",
- caption: "",
- description: "Never get lost in the Seven Seas!"
- },
- {
- name: "crystal-skull",
- title: "Crystall skull",
- caption: "",
- description: "It says \"Made in Germany\"."
- },
- {
- name: "fishbone",
- title: "Fish bone",
- caption: "",
- description: "I use this to pick my teeth. And locks."
- },
- {
- name: "lizard",
- title: "Lizard",
- caption: "",
- description: "Found this lizard, I call it Lizzie."
- },
- {
- name: "parrot",
- title: "Parrot",
- caption: "",
- description: "Arr!"
- },
- {
- name: "pirate-hat",
- title: "Pirate hat",
- caption: "",
- description: "Can't find the owner.. Now it\'s mine!"
- },
- {
- name: "skunk",
- title: "Skunk",
- caption: "",
- description: "So this is where the smell was coming from!"
- },
-]
-
-const initialActionnables = [
- "door",
- "box",
- "sea",
- "chest",
- "key",
- "parrot",
- "lock",
- "barrel",
- "tree",
- "sun"
- // skull
- // "door",
- // "window",
- // "sail",
- // "capstan",
- // "ship's wheel",
- // "hat",
- // "barrel",
- // "cannon",
- // "rope",
- // "bucket",
- // "skull",
- // "ship",
- // "wooden leg"
-]
-
-const initialSituation = [
- `inside the hold of a pirate ship`,
- `a pirate chest in the center with a large lock`,
- `a parrot on top of it`,
- `at sunset`,
-].join(", ")
-
-export const game: Game = {
- title: "Pirates",
- type: "pirates",
- description: [
- "The game is a role playing adventure set in the world of pirates.",
- "The player is Guybroom Threepence, a pirate apprentice who try to find the Crystal Monkey treasure by himself.",
- "The player can click around to move to new scenes, find or activate artifacts.",
- "They can also use objects from their inventory.",
- ],
- engines: [
- "cartesian_image",
- "cartesian_video",
- "spherical_image",
- ],
- className: lugrasimo.className,
- initialSituation,
- initialActionnables,
- inventory,
- getScenePrompt: (situation?: string) => [
- // this prompt is beautiful:
- // screenshot from an adventure videogame, inside the hold of a pirate ship, with a pirate chest in the center, at sunset, beautiful, award winning, unreal engine, intricate details
- `screenshot from an adventure videogame`,
- `pirate themed`,
- `unreal engine`,
- `pixar style`,
- `goofy and comedical`,
- situation || initialSituation,
- ],
-}
\ No newline at end of file
diff --git a/spaces/jbilcke-hf/ai-clip-factory/src/components/ui/dialog.tsx b/spaces/jbilcke-hf/ai-clip-factory/src/components/ui/dialog.tsx
deleted file mode 100644
index cf53b714fe959bf6cfb26db5f4ba6020f6e63b5b..0000000000000000000000000000000000000000
--- a/spaces/jbilcke-hf/ai-clip-factory/src/components/ui/dialog.tsx
+++ /dev/null
@@ -1,122 +0,0 @@
-"use client"
-
-import * as React from "react"
-import * as DialogPrimitive from "@radix-ui/react-dialog"
-import { X } from "lucide-react"
-
-import { cn } from "@/lib/utils"
-
-const Dialog = DialogPrimitive.Root
-
-const DialogTrigger = DialogPrimitive.Trigger
-
-const DialogPortal = ({
- ...props
-}: DialogPrimitive.DialogPortalProps) => (
-
-)
-DialogPortal.displayName = DialogPrimitive.Portal.displayName
-
-const DialogOverlay = React.forwardRef<
- React.ElementRef,
- React.ComponentPropsWithoutRef
->(({ className, ...props }, ref) => (
-
-))
-DialogOverlay.displayName = DialogPrimitive.Overlay.displayName
-
-const DialogContent = React.forwardRef<
- React.ElementRef,
- React.ComponentPropsWithoutRef
->(({ className, children, ...props }, ref) => (
-
-
-
- {children}
-
-
- Close
-
-
-
-))
-DialogContent.displayName = DialogPrimitive.Content.displayName
-
-const DialogHeader = ({
- className,
- ...props
-}: React.HTMLAttributes) => (
-
-)
-DialogHeader.displayName = "DialogHeader"
-
-const DialogFooter = ({
- className,
- ...props
-}: React.HTMLAttributes) => (
-
-)
-DialogFooter.displayName = "DialogFooter"
-
-const DialogTitle = React.forwardRef<
- React.ElementRef,
- React.ComponentPropsWithoutRef
->(({ className, ...props }, ref) => (
-
-))
-DialogTitle.displayName = DialogPrimitive.Title.displayName
-
-const DialogDescription = React.forwardRef<
- React.ElementRef,
- React.ComponentPropsWithoutRef
->(({ className, ...props }, ref) => (
-
-))
-DialogDescription.displayName = DialogPrimitive.Description.displayName
-
-export {
- Dialog,
- DialogTrigger,
- DialogContent,
- DialogHeader,
- DialogFooter,
- DialogTitle,
- DialogDescription,
-}
diff --git a/spaces/jerpint/RAGTheDocs/embed_docs.py b/spaces/jerpint/RAGTheDocs/embed_docs.py
deleted file mode 100644
index 83e5bcde4708b6d57194d7123ed36917f8afd915..0000000000000000000000000000000000000000
--- a/spaces/jerpint/RAGTheDocs/embed_docs.py
+++ /dev/null
@@ -1,62 +0,0 @@
-import logging
-import os
-
-from buster.docparser import get_all_documents
-from buster.documents_manager import DeepLakeDocumentsManager
-from buster.parser import SphinxParser
-
-from rtd_scraper.scrape_rtd import sanitize_url, run_spider
-
-# When using scrapy it seems to set logging for all apps at DEBUG, so simply shut it off here...
-for name in logging.root.manager.loggerDict:
- logger = logging.getLogger(name)
- logger.setLevel(logging.INFO)
-
-
-def embed_documents(homepage_url, save_directory, target_version=None):
- # adds https:// and trailing slash
- homepage_url = sanitize_url(homepage_url)
-
- # Crawl the website using scrapy
- run_spider(
- homepage_url, save_directory=save_directory, target_version=target_version
- )
-
- # # Convert the .html pages into chunks using Buster's SphinxParser
- # root_dir is the folder containing the scraped content e.g. crawled_outputs/buster.readthedocs.io/
- root_dir = os.path.join(save_directory, homepage_url.split("https://")[1])
- df = get_all_documents(
- root_dir=root_dir,
- base_url=homepage_url,
- parser_cls=SphinxParser,
- min_section_length=100,
- max_section_length=1000,
- )
- df["source"] = "readthedocs" # Add the source column
-
- # Initialize the DeepLake vector store
- vector_store_path = os.path.join(save_directory, "deeplake_store")
- dm = DeepLakeDocumentsManager(
- vector_store_path=vector_store_path,
- overwrite=True,
- required_columns=["url", "content", "source", "title"],
- )
-
- # Add all embeddings to the vector store
- dm.batch_add(
- df=df,
- batch_size=3000,
- min_time_interval=60,
- num_workers=32,
- )
-
-
-if __name__ == "__main__":
- homepage_url = "https://orion.readthedocs.io/"
- target_version = "v0.2.7"
- save_directory = "outputs/"
- embed_documents(
- homepage_url=homepage_url,
- target_version=target_version,
- save_directory=save_directory,
- )
diff --git a/spaces/jeycov/Piel_cancer_prueba/app.py b/spaces/jeycov/Piel_cancer_prueba/app.py
deleted file mode 100644
index 92d94785c9c541ce3d3a0853a449d589523131c5..0000000000000000000000000000000000000000
--- a/spaces/jeycov/Piel_cancer_prueba/app.py
+++ /dev/null
@@ -1,133 +0,0 @@
-import fastai
-import fastai.vision
-import PIL
-import gradio
-import matplotlib
-import numpy
-import pandas
-from fastai.vision.all import *
-
-# Crear la clase
-class ADA_SKIN(object):
-
- # Inicializar el objeto
- def __init__(self, name="Wallaby", verbose=True, *args, **kwargs):
- super(ADA_SKIN, self).__init__(*args, **kwargs)
- self.author = "Jey"
- self.name = name
- if verbose:
- self._ph()
- self._pp("Hola desde la clase", str(self.__class__) + " Clase: " + str(self.__class__.__name__))
- self._pp("Nombre del código", self.name)
- self._pp("Autor", self.author)
- self._ph()
-
- self.article = '
Predice las siguientes patologias en piel
'
- self.article += '
Enfermedad de Bowen (AKIEC)
'
- self.article += '
Carcinoma de células basales
'
- self.article += '
Lesiones benignas similares a queratosis
'
- self.article += '
Dermatofibroma
'
- self.article += '
Melanoma
'
- self.article += '
Lunares melanocíticos
'
- self.article += '
Carcinoma de células escamosas
'
- self.article += '
Lesiones vasculares
'
- self.article += '
Benigno
'
- self.article += ''
- self.article += '
Prueba Jey(2023)
'
- self.examples = ['akiec1.jpg','bcc1.jpg','bkl1.jpg','df1.jpg','mel1.jpg',
- 'nevi1.jpg','scc1.jpg','vl1.jpg','benign1.jpg','benign3.jpg']
- self.title = "Predicción Cáncer de Piel prueba "
- return
-
- # Imprimir de manera legible el nombre y valor de una línea
- def _pp(self, a, b):
- print("%34s : %s" % (str(a), str(b)))
- return
-
- # Imprimir la línea de encabezado o pie de página
- def _ph(self):
- print("-" * 34, ":", "-" * 34)
- return
-
- def _predict_image(self, img, cat):
- pred, idx, probs = learn.predict(img)
- return dict(zip(cat, map(float, probs)))
-
- def _predict_image2(self, img, cat):
- pred, idx, probs = learn2.predict(img)
- return dict(zip(cat, map(float, probs)))
-
- def _draw_pred(self, df_pred, df2):
- canvas, pic = matplotlib.pyplot.subplots(1, 2, figsize=(12, 6))
- ti = df_pred["vocab"].head(3).values
- ti2 = df2["vocab"].head(2).values
-
- try:
- df_pred["pred"].head(3).plot(ax=pic[0], kind="pie",
- cmap="Set2", labels=ti, explode=(0.02, 0, 0),
- wedgeprops=dict(width=.4),
- normalize=False)
- df2["pred"].head(2).plot(ax=pic[1], kind="pie",
- colors=["cornflowerblue", "darkorange"], labels=ti2, explode=(0.02, 0),
- wedgeprops=dict(width=.4),
- normalize=False)
- except:
- df_pred["pred"].head(3).plot(ax=pic[0], kind="pie",
- cmap="Set2", labels=ti, explode=(0.02, 0, 0),
- wedgeprops=dict(width=.4))
- df2["pred"].head(2).plot(ax=pic[1], kind="pie",
- colors=["cornflowerblue", "darkorange"], labels=ti2, explode=(0.02, 0),
- wedgeprops=dict(width=.4))
-
- t = str(ti[0]) + ": " + str(numpy.round(df_pred.head(1).pred.values[0] * 100, 2)) + "% de predicción"
- pic[0].set_title(t, fontsize=14.0, fontweight="bold")
- pic[0].axis('off')
- pic[0].legend(ti, loc="lower right", title="Cáncer de Piel: ")
-
- k0 = numpy.round(df2.head(1).pred.values[0] * 100, 2)
- k1 = numpy.round(df2.tail(1).pred.values[0] * 100, 2)
- if k0 > k1:
- t2 = str(ti2[0]) + ": " + str(k0) + "% de predicción"
- else:
- t2 = str(ti2[1]) + ": " + str(k1) + "% de predicción"
- pic[1].set_title(t2, fontsize=14.0, fontweight="bold")
- pic[1].axis('off')
- pic[1].legend(ti2, loc="lower right", title="Prediccíon Cáncer de Piel:")
-
- canvas.tight_layout()
- return canvas
-
- def predict_donut(self, img):
- d = self._predict_image(img, self.categories)
- df = pandas.DataFrame(d, index=[0])
- df = df.transpose().reset_index()
- df.columns = ["vocab", "pred"]
- df.sort_values("pred", inplace=True, ascending=False, ignore_index=True)
-
- d2 = self._predict_image2(img, self.categories2)
- df2 = pandas.DataFrame(d2, index=[0])
- df2 = df2.transpose().reset_index()
- df2.columns = ["vocab", "pred"]
-
- canvas = self._draw_pred(df, df2)
- return canvas
-
-maxi = ADA_SKIN(verbose=False)
-
-learn = fastai.learner.load_learner('ada_learn_skin_norm2000.pkl')
-learn2 = fastai.learner.load_learner('ada_learn_malben.pkl')
-maxi.categories = learn.dls.vocab
-maxi.categories2 = learn2.dls.vocab
-hf_image = gradio.inputs.Image(shape=(192, 192))
-hf_label = gradio.outputs.Label()
-
-intf = gradio.Interface(fn=maxi.predict_donut,
- inputs=hf_image,
- outputs=["plot"],
- examples=maxi.examples,
- title=maxi.title,
- live=True,
- article=maxi.article)
-
-intf.launch(inline=False, share=True)
-
diff --git a/spaces/jgurzoni/image_background_swapper/models/ade20k/segm_lib/utils/__init__.py b/spaces/jgurzoni/image_background_swapper/models/ade20k/segm_lib/utils/__init__.py
deleted file mode 100644
index abe3cbe49477fe37d4fc16249de8a10f4fb4a013..0000000000000000000000000000000000000000
--- a/spaces/jgurzoni/image_background_swapper/models/ade20k/segm_lib/utils/__init__.py
+++ /dev/null
@@ -1 +0,0 @@
-from .th import *
diff --git a/spaces/jinhybr/OCR-layoutLM-Demo/app.py b/spaces/jinhybr/OCR-layoutLM-Demo/app.py
deleted file mode 100644
index bb212eece5c73546e567743fa292857376557242..0000000000000000000000000000000000000000
--- a/spaces/jinhybr/OCR-layoutLM-Demo/app.py
+++ /dev/null
@@ -1,97 +0,0 @@
-import os
-os.system('pip install pyyaml==5.1')
-# workaround: install old version of pytorch since detectron2 hasn't released packages for pytorch 1.9 (issue: https://github.com/facebookresearch/detectron2/issues/3158)
-os.system('pip install torch==1.8.0+cu101 torchvision==0.9.0+cu101 -f https://download.pytorch.org/whl/torch_stable.html')
-
-# install detectron2 that matches pytorch 1.8
-# See https://detectron2.readthedocs.io/tutorials/install.html for instructions
-os.system('pip install -q detectron2 -f https://dl.fbaipublicfiles.com/detectron2/wheels/cu101/torch1.8/index.html')
-
-## install PyTesseract
-os.system('pip install -q pytesseract')
-
-import gradio as gr
-import numpy as np
-from transformers import LayoutLMv2Processor, LayoutLMv2ForTokenClassification
-from datasets import load_dataset
-from PIL import Image, ImageDraw, ImageFont
-
-processor = LayoutLMv2Processor.from_pretrained("jinhybr/OCR-LM-v1")
-model = LayoutLMv2ForTokenClassification.from_pretrained("nielsr/layoutlmv2-finetuned-funsd")
-
-# load image example
-dataset = load_dataset("nielsr/funsd", split="test")
-image = Image.open(dataset[0]["image_path"]).convert("RGB")
-image = Image.open("./demo.jpg")
-image.save("document.jpg")
-# define id2label, label2color
-labels = dataset.features['ner_tags'].feature.names
-id2label = {v: k for v, k in enumerate(labels)}
-label2color = {'question':'blue', 'answer':'green', 'header':'orange', 'other':'violet'}
-
-def unnormalize_box(bbox, width, height):
- return [
- width * (bbox[0] / 1000),
- height * (bbox[1] / 1000),
- width * (bbox[2] / 1000),
- height * (bbox[3] / 1000),
- ]
-
-def iob_to_label(label):
- label = label[2:]
- if not label:
- return 'other'
- return label
-
-def process_image(image):
- width, height = image.size
-
- # encode
- encoding = processor(image, truncation=True, return_offsets_mapping=True, return_tensors="pt")
- offset_mapping = encoding.pop('offset_mapping')
-
- # forward pass
- outputs = model(**encoding)
-
- # get predictions
- predictions = outputs.logits.argmax(-1).squeeze().tolist()
- token_boxes = encoding.bbox.squeeze().tolist()
-
- # only keep non-subword predictions
- is_subword = np.array(offset_mapping.squeeze().tolist())[:,0] != 0
- true_predictions = [id2label[pred] for idx, pred in enumerate(predictions) if not is_subword[idx]]
- true_boxes = [unnormalize_box(box, width, height) for idx, box in enumerate(token_boxes) if not is_subword[idx]]
-
- # draw predictions over the image
- draw = ImageDraw.Draw(image)
- font = ImageFont.load_default()
- for prediction, box in zip(true_predictions, true_boxes):
- predicted_label = iob_to_label(prediction).lower()
- draw.rectangle(box, outline=label2color[predicted_label])
- draw.text((box[0]+10, box[1]-10), text=predicted_label, fill=label2color[predicted_label], font=font)
-
- return image
-
-
-title = "Interactive demo: OCR Document Parser"
-description = "Transformer for state-of-the-art document image understanding tasks. This particular model is fine-tuned on FUNSD, a dataset of manually annotated forms. It annotates the words appearing in the image as QUESTION/ANSWER/HEADER/OTHER. To use it, simply upload an image or use the example image below and click 'Submit'. Results will show up in a few seconds. If you want to make the output bigger, right-click on it and select 'Open image in new tab'."
-article = "
"
-examples =[['document.jpg']]
-
-css = ".output-image, .input-image {height: 40rem !important; width: 100% !important;}"
-#css = "@media screen and (max-width: 600px) { .output_image, .input_image {height:20rem !important; width: 100% !important;} }"
-# css = ".output_image, .input_image {height: 600px !important}"
-
-css = ".image-preview {height: auto !important;}"
-
-iface = gr.Interface(fn=process_image,
- inputs=gr.inputs.Image(type="pil"),
- outputs=gr.outputs.Image(type="pil", label="annotated image"),
- title=title,
- description=description,
- article=article,
- examples=examples,
- css=css,
- enable_queue=True)
-iface.launch(debug=True)
-
diff --git a/spaces/joaogabriellima/Real-Time-Voice-Cloning/encoder/audio.py b/spaces/joaogabriellima/Real-Time-Voice-Cloning/encoder/audio.py
deleted file mode 100644
index 799aa835499ce8b839290f28b2c8ffb629f37565..0000000000000000000000000000000000000000
--- a/spaces/joaogabriellima/Real-Time-Voice-Cloning/encoder/audio.py
+++ /dev/null
@@ -1,117 +0,0 @@
-from scipy.ndimage.morphology import binary_dilation
-from encoder.params_data import *
-from pathlib import Path
-from typing import Optional, Union
-from warnings import warn
-import numpy as np
-import librosa
-import struct
-
-try:
- import webrtcvad
-except:
- warn("Unable to import 'webrtcvad'. This package enables noise removal and is recommended.")
- webrtcvad=None
-
-int16_max = (2 ** 15) - 1
-
-
-def preprocess_wav(fpath_or_wav: Union[str, Path, np.ndarray],
- source_sr: Optional[int] = None,
- normalize: Optional[bool] = True,
- trim_silence: Optional[bool] = True):
- """
- Applies the preprocessing operations used in training the Speaker Encoder to a waveform
- either on disk or in memory. The waveform will be resampled to match the data hyperparameters.
-
- :param fpath_or_wav: either a filepath to an audio file (many extensions are supported, not
- just .wav), either the waveform as a numpy array of floats.
- :param source_sr: if passing an audio waveform, the sampling rate of the waveform before
- preprocessing. After preprocessing, the waveform's sampling rate will match the data
- hyperparameters. If passing a filepath, the sampling rate will be automatically detected and
- this argument will be ignored.
- """
- # Load the wav from disk if needed
- if isinstance(fpath_or_wav, str) or isinstance(fpath_or_wav, Path):
- wav, source_sr = librosa.load(str(fpath_or_wav), sr=None)
- else:
- wav = fpath_or_wav
-
- # Resample the wav if needed
- if source_sr is not None and source_sr != sampling_rate:
- wav = librosa.resample(wav, source_sr, sampling_rate)
-
- # Apply the preprocessing: normalize volume and shorten long silences
- if normalize:
- wav = normalize_volume(wav, audio_norm_target_dBFS, increase_only=True)
- if webrtcvad and trim_silence:
- wav = trim_long_silences(wav)
-
- return wav
-
-
-def wav_to_mel_spectrogram(wav):
- """
- Derives a mel spectrogram ready to be used by the encoder from a preprocessed audio waveform.
- Note: this not a log-mel spectrogram.
- """
- frames = librosa.feature.melspectrogram(
- wav,
- sampling_rate,
- n_fft=int(sampling_rate * mel_window_length / 1000),
- hop_length=int(sampling_rate * mel_window_step / 1000),
- n_mels=mel_n_channels
- )
- return frames.astype(np.float32).T
-
-
-def trim_long_silences(wav):
- """
- Ensures that segments without voice in the waveform remain no longer than a
- threshold determined by the VAD parameters in params.py.
-
- :param wav: the raw waveform as a numpy array of floats
- :return: the same waveform with silences trimmed away (length <= original wav length)
- """
- # Compute the voice detection window size
- samples_per_window = (vad_window_length * sampling_rate) // 1000
-
- # Trim the end of the audio to have a multiple of the window size
- wav = wav[:len(wav) - (len(wav) % samples_per_window)]
-
- # Convert the float waveform to 16-bit mono PCM
- pcm_wave = struct.pack("%dh" % len(wav), *(np.round(wav * int16_max)).astype(np.int16))
-
- # Perform voice activation detection
- voice_flags = []
- vad = webrtcvad.Vad(mode=3)
- for window_start in range(0, len(wav), samples_per_window):
- window_end = window_start + samples_per_window
- voice_flags.append(vad.is_speech(pcm_wave[window_start * 2:window_end * 2],
- sample_rate=sampling_rate))
- voice_flags = np.array(voice_flags)
-
- # Smooth the voice detection with a moving average
- def moving_average(array, width):
- array_padded = np.concatenate((np.zeros((width - 1) // 2), array, np.zeros(width // 2)))
- ret = np.cumsum(array_padded, dtype=float)
- ret[width:] = ret[width:] - ret[:-width]
- return ret[width - 1:] / width
-
- audio_mask = moving_average(voice_flags, vad_moving_average_width)
- audio_mask = np.round(audio_mask).astype(np.bool)
-
- # Dilate the voiced regions
- audio_mask = binary_dilation(audio_mask, np.ones(vad_max_silence_length + 1))
- audio_mask = np.repeat(audio_mask, samples_per_window)
-
- return wav[audio_mask == True]
-
-
-def normalize_volume(wav, target_dBFS, increase_only=False, decrease_only=False):
- if increase_only and decrease_only:
- raise ValueError("Both increase only and decrease only are set")
- dBFS_change = target_dBFS - 10 * np.log10(np.mean(wav ** 2))
- if (dBFS_change < 0 and increase_only) or (dBFS_change > 0 and decrease_only):
- return wav
- return wav * (10 ** (dBFS_change / 20))
diff --git a/spaces/joaogante/generate_quality_improvement/general_suggestions.py b/spaces/joaogante/generate_quality_improvement/general_suggestions.py
deleted file mode 100644
index b1776310b58d37ec02aca0105e4e378b13fcefbf..0000000000000000000000000000000000000000
--- a/spaces/joaogante/generate_quality_improvement/general_suggestions.py
+++ /dev/null
@@ -1,156 +0,0 @@
-"""
-This is a file holding task and model agnostic suggestions.
-
-How to add a new suggestion:
-1. Add a new constant at the bottom of the file with your suggestion. Please try to follow the same format as the
-existing suggestions.
-2. Add a new entry to the `GENERAL_SUGGESTIONS`, with format `((problem tags,), suggestion constant)`.
- a. See `app.py` for the existing problem tags.
- c. Make sure the problem tags are a tuple.
-"""
-
-SET_MAX_NEW_TOKENS = """
-{match_emoji} {count}. Control the maximum output length.
-
-
-🤔 Why?
-
-All text generation calls have a length-related stopping condition. Depending on the model and/or the tool you're
-using to generate text, the default value may be too small or too large. I'd recommend ALWAYS setting this option.
-
-
-🤗 How?
-
-Our text generation interfaces accept a `max_new_tokens` option. Set it to define the maximum number of tokens
-that can be generated.
-
-😱 Caveats
-
-1. Allowing a longer output doesn't necessarily mean that the model will generate longer outputs. By default,
-the model will stop generating when it generates a special `eos_token_id` token.
-2. You shouldn't set `max_new_tokens` to a value larger than the maximum sequence length of the model. If you need a
-longer output, consider using a model with a larger maximum sequence length.
-3. The longer the output, the longer it will take to generate.
-_________________
-
-"""
-
-SET_MIN_LENGTH = """
-{match_emoji} {count}. Force a minimum output length.
-
-
-🤔 Why?
-
-Text generation stops when the model generates a special `eos_token_id`. If you prevent it from happening, the model is
-forced to continue generating.
-
-🤗 How?
-
-Our text generation interfaces accept a `min_new_tokens` argument. Set it to prevent `eos_token_id` from being
-generated until `min_new_tokens` tokens are generated.
-
-😱 Caveats
-
-1. The quality of the output may suffer if the model is forced to generate beyond its own original expectations.
-2. `min_new_tokens` must be smaller than than `max_new_tokens` (see related tip).
-_________________
-
-"""
-
-REMOVE_EOS_TOKEN = """
-{match_emoji} {count}. Force the model to generate until it reaches the maximum output length.
-
-
-🤔 Why?
-
-Text generation stops when the model generates a special `eos_token_id`. If there is no `eos_token_id`, the model can't
-stop.
-
-
-🤗 How?
-
-Our text generation interfaces accept a `eos_token_id` argument. Set it to a null value (e.g., in Python,
-`eos_token_id=None`) to prevent generation to stop before it reaches other stopping conditions.
-
-😱 Caveats
-
-1. The quality of the output may suffer if the model is forced to generate beyond its own original expectations.
-_________________
-
-"""
-
-LIST_EOS_TOKEN = """
-{match_emoji} {count}. Add a stop word.
-
-
-🤔 Why?
-
-Text generation stops when the model generates a special `eos_token_id`. Actually, this attribute can be a list of
-tokens, which means you can define arbitrary stop words.
-
-
-🤗 How?
-
-Our text generation interfaces accept a `eos_token_id` argument. You can pass a list of tokens to make generation
-stop in the presence of any of those tokens.
-
-😱 Caveats
-
-1. When passing a list of tokens, you probably shouldn't forget to include the default `eos_token_id` there.
-_________________
-
-"""
-
-TRY_CONTRASTIVE_SEARCH = """
-{match_emoji} {count}. Try Contrastive Search.
-
-
-🤔 Why?
-
-Contrastive Search is a greedy decoding strategy that strikes a balance between picking the best token and avoiding
-repetition in the representation space. Despite being a greedy decoding strategy, it can also perform well on tasks
-that require creativity (i.e. Sampling territory). In some models, it greatly reduces the problem of repetition.
-
-
-🤗 How?
-
-Our text generation interfaces accept two arguments: `top_k` and `penalty_alpha`. The authors recomment starting with
-`top_k=4` and `penalty_alpha=0.6`.
-
-😱 Caveats
-
-1. Contrastive Search does not work well with all models -- it depends on how distributed their representation spaces
-are. See [this thread](https://huggingface.co/spaces/joaogante/contrastive_search_generation/discussions/1#63764a108623a4a7954a5be5)
-for further information.
-_________________
-
-"""
-
-BLOCK_BAD_WORDS = """
-{match_emoji} {count}. Prevent certain words from being generated.
-
-
-🤔 Why?
-
-You might want to prevent your model from generating certain tokens, such as swear words.
-
-
-🤗 How?
-
-Our text generation interfaces accept a `bad_words_ids` argument. There, you can pass a list of lists, where each
-inner list contains a forbidden sequence of tokens.
-Remember that you can get the token IDs for the words you want to block through
-`bad_word_ids = tokenizer(bad_words, add_prefix_space=True, add_special_tokens=False).input_ids`
-_________________
-
-"""
-
-GENERAL_SUGGESTIONS = (
- (("length",), SET_MAX_NEW_TOKENS),
- (("length",), SET_MIN_LENGTH),
- (("length",), REMOVE_EOS_TOKEN),
- (("length",), LIST_EOS_TOKEN),
- (("quality", "repetitions"), TRY_CONTRASTIVE_SEARCH),
- (("quality",), BLOCK_BAD_WORDS),
-)
-assert all(isinstance(problem_tags, tuple) for problem_tags, _ in GENERAL_SUGGESTIONS)
diff --git a/spaces/joaopereirajp/livvieChatBot/venv/lib/python3.9/site-packages/attr/_next_gen.py b/spaces/joaopereirajp/livvieChatBot/venv/lib/python3.9/site-packages/attr/_next_gen.py
deleted file mode 100644
index 8f7c0b9a46b7a0ee008f94b8054baf5807df043a..0000000000000000000000000000000000000000
--- a/spaces/joaopereirajp/livvieChatBot/venv/lib/python3.9/site-packages/attr/_next_gen.py
+++ /dev/null
@@ -1,232 +0,0 @@
-# SPDX-License-Identifier: MIT
-
-"""
-These are keyword-only APIs that call `attr.s` and `attr.ib` with different
-default values.
-"""
-
-
-from functools import partial
-
-from . import setters
-from ._funcs import asdict as _asdict
-from ._funcs import astuple as _astuple
-from ._make import (
- NOTHING,
- _frozen_setattrs,
- _ng_default_on_setattr,
- attrib,
- attrs,
-)
-from .exceptions import UnannotatedAttributeError
-
-
-def define(
- maybe_cls=None,
- *,
- these=None,
- repr=None,
- unsafe_hash=None,
- hash=None,
- init=None,
- slots=True,
- frozen=False,
- weakref_slot=True,
- str=False,
- auto_attribs=None,
- kw_only=False,
- cache_hash=False,
- auto_exc=True,
- eq=None,
- order=False,
- auto_detect=True,
- getstate_setstate=None,
- on_setattr=None,
- field_transformer=None,
- match_args=True,
-):
- r"""
- Define an *attrs* class.
-
- Differences to the classic `attr.s` that it uses underneath:
-
- - Automatically detect whether or not *auto_attribs* should be `True` (c.f.
- *auto_attribs* parameter).
- - If *frozen* is `False`, run converters and validators when setting an
- attribute by default.
- - *slots=True*
-
- .. caution::
-
- Usually this has only upsides and few visible effects in everyday
- programming. But it *can* lead to some suprising behaviors, so please
- make sure to read :term:`slotted classes`.
- - *auto_exc=True*
- - *auto_detect=True*
- - *order=False*
- - Some options that were only relevant on Python 2 or were kept around for
- backwards-compatibility have been removed.
-
- Please note that these are all defaults and you can change them as you
- wish.
-
- :param Optional[bool] auto_attribs: If set to `True` or `False`, it behaves
- exactly like `attr.s`. If left `None`, `attr.s` will try to guess:
-
- 1. If any attributes are annotated and no unannotated `attrs.fields`\ s
- are found, it assumes *auto_attribs=True*.
- 2. Otherwise it assumes *auto_attribs=False* and tries to collect
- `attrs.fields`\ s.
-
- For now, please refer to `attr.s` for the rest of the parameters.
-
- .. versionadded:: 20.1.0
- .. versionchanged:: 21.3.0 Converters are also run ``on_setattr``.
- .. versionadded:: 22.2.0
- *unsafe_hash* as an alias for *hash* (for :pep:`681` compliance).
- """
-
- def do_it(cls, auto_attribs):
- return attrs(
- maybe_cls=cls,
- these=these,
- repr=repr,
- hash=hash,
- unsafe_hash=unsafe_hash,
- init=init,
- slots=slots,
- frozen=frozen,
- weakref_slot=weakref_slot,
- str=str,
- auto_attribs=auto_attribs,
- kw_only=kw_only,
- cache_hash=cache_hash,
- auto_exc=auto_exc,
- eq=eq,
- order=order,
- auto_detect=auto_detect,
- collect_by_mro=True,
- getstate_setstate=getstate_setstate,
- on_setattr=on_setattr,
- field_transformer=field_transformer,
- match_args=match_args,
- )
-
- def wrap(cls):
- """
- Making this a wrapper ensures this code runs during class creation.
-
- We also ensure that frozen-ness of classes is inherited.
- """
- nonlocal frozen, on_setattr
-
- had_on_setattr = on_setattr not in (None, setters.NO_OP)
-
- # By default, mutable classes convert & validate on setattr.
- if frozen is False and on_setattr is None:
- on_setattr = _ng_default_on_setattr
-
- # However, if we subclass a frozen class, we inherit the immutability
- # and disable on_setattr.
- for base_cls in cls.__bases__:
- if base_cls.__setattr__ is _frozen_setattrs:
- if had_on_setattr:
- raise ValueError(
- "Frozen classes can't use on_setattr "
- "(frozen-ness was inherited)."
- )
-
- on_setattr = setters.NO_OP
- break
-
- if auto_attribs is not None:
- return do_it(cls, auto_attribs)
-
- try:
- return do_it(cls, True)
- except UnannotatedAttributeError:
- return do_it(cls, False)
-
- # maybe_cls's type depends on the usage of the decorator. It's a class
- # if it's used as `@attrs` but ``None`` if used as `@attrs()`.
- if maybe_cls is None:
- return wrap
- else:
- return wrap(maybe_cls)
-
-
-mutable = define
-frozen = partial(define, frozen=True, on_setattr=None)
-
-
-def field(
- *,
- default=NOTHING,
- validator=None,
- repr=True,
- hash=None,
- init=True,
- metadata=None,
- type=None,
- converter=None,
- factory=None,
- kw_only=False,
- eq=None,
- order=None,
- on_setattr=None,
- alias=None,
-):
- """
- Identical to `attr.ib`, except keyword-only and with some arguments
- removed.
-
- .. versionadded:: 23.1.0
- The *type* parameter has been re-added; mostly for
- {func}`attrs.make_class`. Please note that type checkers ignore this
- metadata.
- .. versionadded:: 20.1.0
- """
- return attrib(
- default=default,
- validator=validator,
- repr=repr,
- hash=hash,
- init=init,
- metadata=metadata,
- type=type,
- converter=converter,
- factory=factory,
- kw_only=kw_only,
- eq=eq,
- order=order,
- on_setattr=on_setattr,
- alias=alias,
- )
-
-
-def asdict(inst, *, recurse=True, filter=None, value_serializer=None):
- """
- Same as `attr.asdict`, except that collections types are always retained
- and dict is always used as *dict_factory*.
-
- .. versionadded:: 21.3.0
- """
- return _asdict(
- inst=inst,
- recurse=recurse,
- filter=filter,
- value_serializer=value_serializer,
- retain_collection_types=True,
- )
-
-
-def astuple(inst, *, recurse=True, filter=None):
- """
- Same as `attr.astuple`, except that collections types are always retained
- and `tuple` is always used as the *tuple_factory*.
-
- .. versionadded:: 21.3.0
- """
- return _astuple(
- inst=inst, recurse=recurse, filter=filter, retain_collection_types=True
- )
diff --git a/spaces/joaopereirajp/livvieChatBot/venv/lib/python3.9/site-packages/dateutil/parser/_parser.py b/spaces/joaopereirajp/livvieChatBot/venv/lib/python3.9/site-packages/dateutil/parser/_parser.py
deleted file mode 100644
index 37d1663b2f72447800d9a553929e3de932244289..0000000000000000000000000000000000000000
--- a/spaces/joaopereirajp/livvieChatBot/venv/lib/python3.9/site-packages/dateutil/parser/_parser.py
+++ /dev/null
@@ -1,1613 +0,0 @@
-# -*- coding: utf-8 -*-
-"""
-This module offers a generic date/time string parser which is able to parse
-most known formats to represent a date and/or time.
-
-This module attempts to be forgiving with regards to unlikely input formats,
-returning a datetime object even for dates which are ambiguous. If an element
-of a date/time stamp is omitted, the following rules are applied:
-
-- If AM or PM is left unspecified, a 24-hour clock is assumed, however, an hour
- on a 12-hour clock (``0 <= hour <= 12``) *must* be specified if AM or PM is
- specified.
-- If a time zone is omitted, a timezone-naive datetime is returned.
-
-If any other elements are missing, they are taken from the
-:class:`datetime.datetime` object passed to the parameter ``default``. If this
-results in a day number exceeding the valid number of days per month, the
-value falls back to the end of the month.
-
-Additional resources about date/time string formats can be found below:
-
-- `A summary of the international standard date and time notation
- `_
-- `W3C Date and Time Formats `_
-- `Time Formats (Planetary Rings Node) `_
-- `CPAN ParseDate module
- `_
-- `Java SimpleDateFormat Class
- `_
-"""
-from __future__ import unicode_literals
-
-import datetime
-import re
-import string
-import time
-import warnings
-
-from calendar import monthrange
-from io import StringIO
-
-import six
-from six import integer_types, text_type
-
-from decimal import Decimal
-
-from warnings import warn
-
-from .. import relativedelta
-from .. import tz
-
-__all__ = ["parse", "parserinfo", "ParserError"]
-
-
-# TODO: pandas.core.tools.datetimes imports this explicitly. Might be worth
-# making public and/or figuring out if there is something we can
-# take off their plate.
-class _timelex(object):
- # Fractional seconds are sometimes split by a comma
- _split_decimal = re.compile("([.,])")
-
- def __init__(self, instream):
- if isinstance(instream, (bytes, bytearray)):
- instream = instream.decode()
-
- if isinstance(instream, text_type):
- instream = StringIO(instream)
- elif getattr(instream, 'read', None) is None:
- raise TypeError('Parser must be a string or character stream, not '
- '{itype}'.format(itype=instream.__class__.__name__))
-
- self.instream = instream
- self.charstack = []
- self.tokenstack = []
- self.eof = False
-
- def get_token(self):
- """
- This function breaks the time string into lexical units (tokens), which
- can be parsed by the parser. Lexical units are demarcated by changes in
- the character set, so any continuous string of letters is considered
- one unit, any continuous string of numbers is considered one unit.
-
- The main complication arises from the fact that dots ('.') can be used
- both as separators (e.g. "Sep.20.2009") or decimal points (e.g.
- "4:30:21.447"). As such, it is necessary to read the full context of
- any dot-separated strings before breaking it into tokens; as such, this
- function maintains a "token stack", for when the ambiguous context
- demands that multiple tokens be parsed at once.
- """
- if self.tokenstack:
- return self.tokenstack.pop(0)
-
- seenletters = False
- token = None
- state = None
-
- while not self.eof:
- # We only realize that we've reached the end of a token when we
- # find a character that's not part of the current token - since
- # that character may be part of the next token, it's stored in the
- # charstack.
- if self.charstack:
- nextchar = self.charstack.pop(0)
- else:
- nextchar = self.instream.read(1)
- while nextchar == '\x00':
- nextchar = self.instream.read(1)
-
- if not nextchar:
- self.eof = True
- break
- elif not state:
- # First character of the token - determines if we're starting
- # to parse a word, a number or something else.
- token = nextchar
- if self.isword(nextchar):
- state = 'a'
- elif self.isnum(nextchar):
- state = '0'
- elif self.isspace(nextchar):
- token = ' '
- break # emit token
- else:
- break # emit token
- elif state == 'a':
- # If we've already started reading a word, we keep reading
- # letters until we find something that's not part of a word.
- seenletters = True
- if self.isword(nextchar):
- token += nextchar
- elif nextchar == '.':
- token += nextchar
- state = 'a.'
- else:
- self.charstack.append(nextchar)
- break # emit token
- elif state == '0':
- # If we've already started reading a number, we keep reading
- # numbers until we find something that doesn't fit.
- if self.isnum(nextchar):
- token += nextchar
- elif nextchar == '.' or (nextchar == ',' and len(token) >= 2):
- token += nextchar
- state = '0.'
- else:
- self.charstack.append(nextchar)
- break # emit token
- elif state == 'a.':
- # If we've seen some letters and a dot separator, continue
- # parsing, and the tokens will be broken up later.
- seenletters = True
- if nextchar == '.' or self.isword(nextchar):
- token += nextchar
- elif self.isnum(nextchar) and token[-1] == '.':
- token += nextchar
- state = '0.'
- else:
- self.charstack.append(nextchar)
- break # emit token
- elif state == '0.':
- # If we've seen at least one dot separator, keep going, we'll
- # break up the tokens later.
- if nextchar == '.' or self.isnum(nextchar):
- token += nextchar
- elif self.isword(nextchar) and token[-1] == '.':
- token += nextchar
- state = 'a.'
- else:
- self.charstack.append(nextchar)
- break # emit token
-
- if (state in ('a.', '0.') and (seenletters or token.count('.') > 1 or
- token[-1] in '.,')):
- l = self._split_decimal.split(token)
- token = l[0]
- for tok in l[1:]:
- if tok:
- self.tokenstack.append(tok)
-
- if state == '0.' and token.count('.') == 0:
- token = token.replace(',', '.')
-
- return token
-
- def __iter__(self):
- return self
-
- def __next__(self):
- token = self.get_token()
- if token is None:
- raise StopIteration
-
- return token
-
- def next(self):
- return self.__next__() # Python 2.x support
-
- @classmethod
- def split(cls, s):
- return list(cls(s))
-
- @classmethod
- def isword(cls, nextchar):
- """ Whether or not the next character is part of a word """
- return nextchar.isalpha()
-
- @classmethod
- def isnum(cls, nextchar):
- """ Whether the next character is part of a number """
- return nextchar.isdigit()
-
- @classmethod
- def isspace(cls, nextchar):
- """ Whether the next character is whitespace """
- return nextchar.isspace()
-
-
-class _resultbase(object):
-
- def __init__(self):
- for attr in self.__slots__:
- setattr(self, attr, None)
-
- def _repr(self, classname):
- l = []
- for attr in self.__slots__:
- value = getattr(self, attr)
- if value is not None:
- l.append("%s=%s" % (attr, repr(value)))
- return "%s(%s)" % (classname, ", ".join(l))
-
- def __len__(self):
- return (sum(getattr(self, attr) is not None
- for attr in self.__slots__))
-
- def __repr__(self):
- return self._repr(self.__class__.__name__)
-
-
-class parserinfo(object):
- """
- Class which handles what inputs are accepted. Subclass this to customize
- the language and acceptable values for each parameter.
-
- :param dayfirst:
- Whether to interpret the first value in an ambiguous 3-integer date
- (e.g. 01/05/09) as the day (``True``) or month (``False``). If
- ``yearfirst`` is set to ``True``, this distinguishes between YDM
- and YMD. Default is ``False``.
-
- :param yearfirst:
- Whether to interpret the first value in an ambiguous 3-integer date
- (e.g. 01/05/09) as the year. If ``True``, the first number is taken
- to be the year, otherwise the last number is taken to be the year.
- Default is ``False``.
- """
-
- # m from a.m/p.m, t from ISO T separator
- JUMP = [" ", ".", ",", ";", "-", "/", "'",
- "at", "on", "and", "ad", "m", "t", "of",
- "st", "nd", "rd", "th"]
-
- WEEKDAYS = [("Mon", "Monday"),
- ("Tue", "Tuesday"), # TODO: "Tues"
- ("Wed", "Wednesday"),
- ("Thu", "Thursday"), # TODO: "Thurs"
- ("Fri", "Friday"),
- ("Sat", "Saturday"),
- ("Sun", "Sunday")]
- MONTHS = [("Jan", "January"),
- ("Feb", "February"), # TODO: "Febr"
- ("Mar", "March"),
- ("Apr", "April"),
- ("May", "May"),
- ("Jun", "June"),
- ("Jul", "July"),
- ("Aug", "August"),
- ("Sep", "Sept", "September"),
- ("Oct", "October"),
- ("Nov", "November"),
- ("Dec", "December")]
- HMS = [("h", "hour", "hours"),
- ("m", "minute", "minutes"),
- ("s", "second", "seconds")]
- AMPM = [("am", "a"),
- ("pm", "p")]
- UTCZONE = ["UTC", "GMT", "Z", "z"]
- PERTAIN = ["of"]
- TZOFFSET = {}
- # TODO: ERA = ["AD", "BC", "CE", "BCE", "Stardate",
- # "Anno Domini", "Year of Our Lord"]
-
- def __init__(self, dayfirst=False, yearfirst=False):
- self._jump = self._convert(self.JUMP)
- self._weekdays = self._convert(self.WEEKDAYS)
- self._months = self._convert(self.MONTHS)
- self._hms = self._convert(self.HMS)
- self._ampm = self._convert(self.AMPM)
- self._utczone = self._convert(self.UTCZONE)
- self._pertain = self._convert(self.PERTAIN)
-
- self.dayfirst = dayfirst
- self.yearfirst = yearfirst
-
- self._year = time.localtime().tm_year
- self._century = self._year // 100 * 100
-
- def _convert(self, lst):
- dct = {}
- for i, v in enumerate(lst):
- if isinstance(v, tuple):
- for v in v:
- dct[v.lower()] = i
- else:
- dct[v.lower()] = i
- return dct
-
- def jump(self, name):
- return name.lower() in self._jump
-
- def weekday(self, name):
- try:
- return self._weekdays[name.lower()]
- except KeyError:
- pass
- return None
-
- def month(self, name):
- try:
- return self._months[name.lower()] + 1
- except KeyError:
- pass
- return None
-
- def hms(self, name):
- try:
- return self._hms[name.lower()]
- except KeyError:
- return None
-
- def ampm(self, name):
- try:
- return self._ampm[name.lower()]
- except KeyError:
- return None
-
- def pertain(self, name):
- return name.lower() in self._pertain
-
- def utczone(self, name):
- return name.lower() in self._utczone
-
- def tzoffset(self, name):
- if name in self._utczone:
- return 0
-
- return self.TZOFFSET.get(name)
-
- def convertyear(self, year, century_specified=False):
- """
- Converts two-digit years to year within [-50, 49]
- range of self._year (current local time)
- """
-
- # Function contract is that the year is always positive
- assert year >= 0
-
- if year < 100 and not century_specified:
- # assume current century to start
- year += self._century
-
- if year >= self._year + 50: # if too far in future
- year -= 100
- elif year < self._year - 50: # if too far in past
- year += 100
-
- return year
-
- def validate(self, res):
- # move to info
- if res.year is not None:
- res.year = self.convertyear(res.year, res.century_specified)
-
- if ((res.tzoffset == 0 and not res.tzname) or
- (res.tzname == 'Z' or res.tzname == 'z')):
- res.tzname = "UTC"
- res.tzoffset = 0
- elif res.tzoffset != 0 and res.tzname and self.utczone(res.tzname):
- res.tzoffset = 0
- return True
-
-
-class _ymd(list):
- def __init__(self, *args, **kwargs):
- super(self.__class__, self).__init__(*args, **kwargs)
- self.century_specified = False
- self.dstridx = None
- self.mstridx = None
- self.ystridx = None
-
- @property
- def has_year(self):
- return self.ystridx is not None
-
- @property
- def has_month(self):
- return self.mstridx is not None
-
- @property
- def has_day(self):
- return self.dstridx is not None
-
- def could_be_day(self, value):
- if self.has_day:
- return False
- elif not self.has_month:
- return 1 <= value <= 31
- elif not self.has_year:
- # Be permissive, assume leap year
- month = self[self.mstridx]
- return 1 <= value <= monthrange(2000, month)[1]
- else:
- month = self[self.mstridx]
- year = self[self.ystridx]
- return 1 <= value <= monthrange(year, month)[1]
-
- def append(self, val, label=None):
- if hasattr(val, '__len__'):
- if val.isdigit() and len(val) > 2:
- self.century_specified = True
- if label not in [None, 'Y']: # pragma: no cover
- raise ValueError(label)
- label = 'Y'
- elif val > 100:
- self.century_specified = True
- if label not in [None, 'Y']: # pragma: no cover
- raise ValueError(label)
- label = 'Y'
-
- super(self.__class__, self).append(int(val))
-
- if label == 'M':
- if self.has_month:
- raise ValueError('Month is already set')
- self.mstridx = len(self) - 1
- elif label == 'D':
- if self.has_day:
- raise ValueError('Day is already set')
- self.dstridx = len(self) - 1
- elif label == 'Y':
- if self.has_year:
- raise ValueError('Year is already set')
- self.ystridx = len(self) - 1
-
- def _resolve_from_stridxs(self, strids):
- """
- Try to resolve the identities of year/month/day elements using
- ystridx, mstridx, and dstridx, if enough of these are specified.
- """
- if len(self) == 3 and len(strids) == 2:
- # we can back out the remaining stridx value
- missing = [x for x in range(3) if x not in strids.values()]
- key = [x for x in ['y', 'm', 'd'] if x not in strids]
- assert len(missing) == len(key) == 1
- key = key[0]
- val = missing[0]
- strids[key] = val
-
- assert len(self) == len(strids) # otherwise this should not be called
- out = {key: self[strids[key]] for key in strids}
- return (out.get('y'), out.get('m'), out.get('d'))
-
- def resolve_ymd(self, yearfirst, dayfirst):
- len_ymd = len(self)
- year, month, day = (None, None, None)
-
- strids = (('y', self.ystridx),
- ('m', self.mstridx),
- ('d', self.dstridx))
-
- strids = {key: val for key, val in strids if val is not None}
- if (len(self) == len(strids) > 0 or
- (len(self) == 3 and len(strids) == 2)):
- return self._resolve_from_stridxs(strids)
-
- mstridx = self.mstridx
-
- if len_ymd > 3:
- raise ValueError("More than three YMD values")
- elif len_ymd == 1 or (mstridx is not None and len_ymd == 2):
- # One member, or two members with a month string
- if mstridx is not None:
- month = self[mstridx]
- # since mstridx is 0 or 1, self[mstridx-1] always
- # looks up the other element
- other = self[mstridx - 1]
- else:
- other = self[0]
-
- if len_ymd > 1 or mstridx is None:
- if other > 31:
- year = other
- else:
- day = other
-
- elif len_ymd == 2:
- # Two members with numbers
- if self[0] > 31:
- # 99-01
- year, month = self
- elif self[1] > 31:
- # 01-99
- month, year = self
- elif dayfirst and self[1] <= 12:
- # 13-01
- day, month = self
- else:
- # 01-13
- month, day = self
-
- elif len_ymd == 3:
- # Three members
- if mstridx == 0:
- if self[1] > 31:
- # Apr-2003-25
- month, year, day = self
- else:
- month, day, year = self
- elif mstridx == 1:
- if self[0] > 31 or (yearfirst and self[2] <= 31):
- # 99-Jan-01
- year, month, day = self
- else:
- # 01-Jan-01
- # Give precedence to day-first, since
- # two-digit years is usually hand-written.
- day, month, year = self
-
- elif mstridx == 2:
- # WTF!?
- if self[1] > 31:
- # 01-99-Jan
- day, year, month = self
- else:
- # 99-01-Jan
- year, day, month = self
-
- else:
- if (self[0] > 31 or
- self.ystridx == 0 or
- (yearfirst and self[1] <= 12 and self[2] <= 31)):
- # 99-01-01
- if dayfirst and self[2] <= 12:
- year, day, month = self
- else:
- year, month, day = self
- elif self[0] > 12 or (dayfirst and self[1] <= 12):
- # 13-01-01
- day, month, year = self
- else:
- # 01-13-01
- month, day, year = self
-
- return year, month, day
-
-
-class parser(object):
- def __init__(self, info=None):
- self.info = info or parserinfo()
-
- def parse(self, timestr, default=None,
- ignoretz=False, tzinfos=None, **kwargs):
- """
- Parse the date/time string into a :class:`datetime.datetime` object.
-
- :param timestr:
- Any date/time string using the supported formats.
-
- :param default:
- The default datetime object, if this is a datetime object and not
- ``None``, elements specified in ``timestr`` replace elements in the
- default object.
-
- :param ignoretz:
- If set ``True``, time zones in parsed strings are ignored and a
- naive :class:`datetime.datetime` object is returned.
-
- :param tzinfos:
- Additional time zone names / aliases which may be present in the
- string. This argument maps time zone names (and optionally offsets
- from those time zones) to time zones. This parameter can be a
- dictionary with timezone aliases mapping time zone names to time
- zones or a function taking two parameters (``tzname`` and
- ``tzoffset``) and returning a time zone.
-
- The timezones to which the names are mapped can be an integer
- offset from UTC in seconds or a :class:`tzinfo` object.
-
- .. doctest::
- :options: +NORMALIZE_WHITESPACE
-
- >>> from dateutil.parser import parse
- >>> from dateutil.tz import gettz
- >>> tzinfos = {"BRST": -7200, "CST": gettz("America/Chicago")}
- >>> parse("2012-01-19 17:21:00 BRST", tzinfos=tzinfos)
- datetime.datetime(2012, 1, 19, 17, 21, tzinfo=tzoffset(u'BRST', -7200))
- >>> parse("2012-01-19 17:21:00 CST", tzinfos=tzinfos)
- datetime.datetime(2012, 1, 19, 17, 21,
- tzinfo=tzfile('/usr/share/zoneinfo/America/Chicago'))
-
- This parameter is ignored if ``ignoretz`` is set.
-
- :param \\*\\*kwargs:
- Keyword arguments as passed to ``_parse()``.
-
- :return:
- Returns a :class:`datetime.datetime` object or, if the
- ``fuzzy_with_tokens`` option is ``True``, returns a tuple, the
- first element being a :class:`datetime.datetime` object, the second
- a tuple containing the fuzzy tokens.
-
- :raises ParserError:
- Raised for invalid or unknown string format, if the provided
- :class:`tzinfo` is not in a valid format, or if an invalid date
- would be created.
-
- :raises TypeError:
- Raised for non-string or character stream input.
-
- :raises OverflowError:
- Raised if the parsed date exceeds the largest valid C integer on
- your system.
- """
-
- if default is None:
- default = datetime.datetime.now().replace(hour=0, minute=0,
- second=0, microsecond=0)
-
- res, skipped_tokens = self._parse(timestr, **kwargs)
-
- if res is None:
- raise ParserError("Unknown string format: %s", timestr)
-
- if len(res) == 0:
- raise ParserError("String does not contain a date: %s", timestr)
-
- try:
- ret = self._build_naive(res, default)
- except ValueError as e:
- six.raise_from(ParserError(str(e) + ": %s", timestr), e)
-
- if not ignoretz:
- ret = self._build_tzaware(ret, res, tzinfos)
-
- if kwargs.get('fuzzy_with_tokens', False):
- return ret, skipped_tokens
- else:
- return ret
-
- class _result(_resultbase):
- __slots__ = ["year", "month", "day", "weekday",
- "hour", "minute", "second", "microsecond",
- "tzname", "tzoffset", "ampm","any_unused_tokens"]
-
- def _parse(self, timestr, dayfirst=None, yearfirst=None, fuzzy=False,
- fuzzy_with_tokens=False):
- """
- Private method which performs the heavy lifting of parsing, called from
- ``parse()``, which passes on its ``kwargs`` to this function.
-
- :param timestr:
- The string to parse.
-
- :param dayfirst:
- Whether to interpret the first value in an ambiguous 3-integer date
- (e.g. 01/05/09) as the day (``True``) or month (``False``). If
- ``yearfirst`` is set to ``True``, this distinguishes between YDM
- and YMD. If set to ``None``, this value is retrieved from the
- current :class:`parserinfo` object (which itself defaults to
- ``False``).
-
- :param yearfirst:
- Whether to interpret the first value in an ambiguous 3-integer date
- (e.g. 01/05/09) as the year. If ``True``, the first number is taken
- to be the year, otherwise the last number is taken to be the year.
- If this is set to ``None``, the value is retrieved from the current
- :class:`parserinfo` object (which itself defaults to ``False``).
-
- :param fuzzy:
- Whether to allow fuzzy parsing, allowing for string like "Today is
- January 1, 2047 at 8:21:00AM".
-
- :param fuzzy_with_tokens:
- If ``True``, ``fuzzy`` is automatically set to True, and the parser
- will return a tuple where the first element is the parsed
- :class:`datetime.datetime` datetimestamp and the second element is
- a tuple containing the portions of the string which were ignored:
-
- .. doctest::
-
- >>> from dateutil.parser import parse
- >>> parse("Today is January 1, 2047 at 8:21:00AM", fuzzy_with_tokens=True)
- (datetime.datetime(2047, 1, 1, 8, 21), (u'Today is ', u' ', u'at '))
-
- """
- if fuzzy_with_tokens:
- fuzzy = True
-
- info = self.info
-
- if dayfirst is None:
- dayfirst = info.dayfirst
-
- if yearfirst is None:
- yearfirst = info.yearfirst
-
- res = self._result()
- l = _timelex.split(timestr) # Splits the timestr into tokens
-
- skipped_idxs = []
-
- # year/month/day list
- ymd = _ymd()
-
- len_l = len(l)
- i = 0
- try:
- while i < len_l:
-
- # Check if it's a number
- value_repr = l[i]
- try:
- value = float(value_repr)
- except ValueError:
- value = None
-
- if value is not None:
- # Numeric token
- i = self._parse_numeric_token(l, i, info, ymd, res, fuzzy)
-
- # Check weekday
- elif info.weekday(l[i]) is not None:
- value = info.weekday(l[i])
- res.weekday = value
-
- # Check month name
- elif info.month(l[i]) is not None:
- value = info.month(l[i])
- ymd.append(value, 'M')
-
- if i + 1 < len_l:
- if l[i + 1] in ('-', '/'):
- # Jan-01[-99]
- sep = l[i + 1]
- ymd.append(l[i + 2])
-
- if i + 3 < len_l and l[i + 3] == sep:
- # Jan-01-99
- ymd.append(l[i + 4])
- i += 2
-
- i += 2
-
- elif (i + 4 < len_l and l[i + 1] == l[i + 3] == ' ' and
- info.pertain(l[i + 2])):
- # Jan of 01
- # In this case, 01 is clearly year
- if l[i + 4].isdigit():
- # Convert it here to become unambiguous
- value = int(l[i + 4])
- year = str(info.convertyear(value))
- ymd.append(year, 'Y')
- else:
- # Wrong guess
- pass
- # TODO: not hit in tests
- i += 4
-
- # Check am/pm
- elif info.ampm(l[i]) is not None:
- value = info.ampm(l[i])
- val_is_ampm = self._ampm_valid(res.hour, res.ampm, fuzzy)
-
- if val_is_ampm:
- res.hour = self._adjust_ampm(res.hour, value)
- res.ampm = value
-
- elif fuzzy:
- skipped_idxs.append(i)
-
- # Check for a timezone name
- elif self._could_be_tzname(res.hour, res.tzname, res.tzoffset, l[i]):
- res.tzname = l[i]
- res.tzoffset = info.tzoffset(res.tzname)
-
- # Check for something like GMT+3, or BRST+3. Notice
- # that it doesn't mean "I am 3 hours after GMT", but
- # "my time +3 is GMT". If found, we reverse the
- # logic so that timezone parsing code will get it
- # right.
- if i + 1 < len_l and l[i + 1] in ('+', '-'):
- l[i + 1] = ('+', '-')[l[i + 1] == '+']
- res.tzoffset = None
- if info.utczone(res.tzname):
- # With something like GMT+3, the timezone
- # is *not* GMT.
- res.tzname = None
-
- # Check for a numbered timezone
- elif res.hour is not None and l[i] in ('+', '-'):
- signal = (-1, 1)[l[i] == '+']
- len_li = len(l[i + 1])
-
- # TODO: check that l[i + 1] is integer?
- if len_li == 4:
- # -0300
- hour_offset = int(l[i + 1][:2])
- min_offset = int(l[i + 1][2:])
- elif i + 2 < len_l and l[i + 2] == ':':
- # -03:00
- hour_offset = int(l[i + 1])
- min_offset = int(l[i + 3]) # TODO: Check that l[i+3] is minute-like?
- i += 2
- elif len_li <= 2:
- # -[0]3
- hour_offset = int(l[i + 1][:2])
- min_offset = 0
- else:
- raise ValueError(timestr)
-
- res.tzoffset = signal * (hour_offset * 3600 + min_offset * 60)
-
- # Look for a timezone name between parenthesis
- if (i + 5 < len_l and
- info.jump(l[i + 2]) and l[i + 3] == '(' and
- l[i + 5] == ')' and
- 3 <= len(l[i + 4]) and
- self._could_be_tzname(res.hour, res.tzname,
- None, l[i + 4])):
- # -0300 (BRST)
- res.tzname = l[i + 4]
- i += 4
-
- i += 1
-
- # Check jumps
- elif not (info.jump(l[i]) or fuzzy):
- raise ValueError(timestr)
-
- else:
- skipped_idxs.append(i)
- i += 1
-
- # Process year/month/day
- year, month, day = ymd.resolve_ymd(yearfirst, dayfirst)
-
- res.century_specified = ymd.century_specified
- res.year = year
- res.month = month
- res.day = day
-
- except (IndexError, ValueError):
- return None, None
-
- if not info.validate(res):
- return None, None
-
- if fuzzy_with_tokens:
- skipped_tokens = self._recombine_skipped(l, skipped_idxs)
- return res, tuple(skipped_tokens)
- else:
- return res, None
-
- def _parse_numeric_token(self, tokens, idx, info, ymd, res, fuzzy):
- # Token is a number
- value_repr = tokens[idx]
- try:
- value = self._to_decimal(value_repr)
- except Exception as e:
- six.raise_from(ValueError('Unknown numeric token'), e)
-
- len_li = len(value_repr)
-
- len_l = len(tokens)
-
- if (len(ymd) == 3 and len_li in (2, 4) and
- res.hour is None and
- (idx + 1 >= len_l or
- (tokens[idx + 1] != ':' and
- info.hms(tokens[idx + 1]) is None))):
- # 19990101T23[59]
- s = tokens[idx]
- res.hour = int(s[:2])
-
- if len_li == 4:
- res.minute = int(s[2:])
-
- elif len_li == 6 or (len_li > 6 and tokens[idx].find('.') == 6):
- # YYMMDD or HHMMSS[.ss]
- s = tokens[idx]
-
- if not ymd and '.' not in tokens[idx]:
- ymd.append(s[:2])
- ymd.append(s[2:4])
- ymd.append(s[4:])
- else:
- # 19990101T235959[.59]
-
- # TODO: Check if res attributes already set.
- res.hour = int(s[:2])
- res.minute = int(s[2:4])
- res.second, res.microsecond = self._parsems(s[4:])
-
- elif len_li in (8, 12, 14):
- # YYYYMMDD
- s = tokens[idx]
- ymd.append(s[:4], 'Y')
- ymd.append(s[4:6])
- ymd.append(s[6:8])
-
- if len_li > 8:
- res.hour = int(s[8:10])
- res.minute = int(s[10:12])
-
- if len_li > 12:
- res.second = int(s[12:])
-
- elif self._find_hms_idx(idx, tokens, info, allow_jump=True) is not None:
- # HH[ ]h or MM[ ]m or SS[.ss][ ]s
- hms_idx = self._find_hms_idx(idx, tokens, info, allow_jump=True)
- (idx, hms) = self._parse_hms(idx, tokens, info, hms_idx)
- if hms is not None:
- # TODO: checking that hour/minute/second are not
- # already set?
- self._assign_hms(res, value_repr, hms)
-
- elif idx + 2 < len_l and tokens[idx + 1] == ':':
- # HH:MM[:SS[.ss]]
- res.hour = int(value)
- value = self._to_decimal(tokens[idx + 2]) # TODO: try/except for this?
- (res.minute, res.second) = self._parse_min_sec(value)
-
- if idx + 4 < len_l and tokens[idx + 3] == ':':
- res.second, res.microsecond = self._parsems(tokens[idx + 4])
-
- idx += 2
-
- idx += 2
-
- elif idx + 1 < len_l and tokens[idx + 1] in ('-', '/', '.'):
- sep = tokens[idx + 1]
- ymd.append(value_repr)
-
- if idx + 2 < len_l and not info.jump(tokens[idx + 2]):
- if tokens[idx + 2].isdigit():
- # 01-01[-01]
- ymd.append(tokens[idx + 2])
- else:
- # 01-Jan[-01]
- value = info.month(tokens[idx + 2])
-
- if value is not None:
- ymd.append(value, 'M')
- else:
- raise ValueError()
-
- if idx + 3 < len_l and tokens[idx + 3] == sep:
- # We have three members
- value = info.month(tokens[idx + 4])
-
- if value is not None:
- ymd.append(value, 'M')
- else:
- ymd.append(tokens[idx + 4])
- idx += 2
-
- idx += 1
- idx += 1
-
- elif idx + 1 >= len_l or info.jump(tokens[idx + 1]):
- if idx + 2 < len_l and info.ampm(tokens[idx + 2]) is not None:
- # 12 am
- hour = int(value)
- res.hour = self._adjust_ampm(hour, info.ampm(tokens[idx + 2]))
- idx += 1
- else:
- # Year, month or day
- ymd.append(value)
- idx += 1
-
- elif info.ampm(tokens[idx + 1]) is not None and (0 <= value < 24):
- # 12am
- hour = int(value)
- res.hour = self._adjust_ampm(hour, info.ampm(tokens[idx + 1]))
- idx += 1
-
- elif ymd.could_be_day(value):
- ymd.append(value)
-
- elif not fuzzy:
- raise ValueError()
-
- return idx
-
- def _find_hms_idx(self, idx, tokens, info, allow_jump):
- len_l = len(tokens)
-
- if idx+1 < len_l and info.hms(tokens[idx+1]) is not None:
- # There is an "h", "m", or "s" label following this token. We take
- # assign the upcoming label to the current token.
- # e.g. the "12" in 12h"
- hms_idx = idx + 1
-
- elif (allow_jump and idx+2 < len_l and tokens[idx+1] == ' ' and
- info.hms(tokens[idx+2]) is not None):
- # There is a space and then an "h", "m", or "s" label.
- # e.g. the "12" in "12 h"
- hms_idx = idx + 2
-
- elif idx > 0 and info.hms(tokens[idx-1]) is not None:
- # There is a "h", "m", or "s" preceding this token. Since neither
- # of the previous cases was hit, there is no label following this
- # token, so we use the previous label.
- # e.g. the "04" in "12h04"
- hms_idx = idx-1
-
- elif (1 < idx == len_l-1 and tokens[idx-1] == ' ' and
- info.hms(tokens[idx-2]) is not None):
- # If we are looking at the final token, we allow for a
- # backward-looking check to skip over a space.
- # TODO: Are we sure this is the right condition here?
- hms_idx = idx - 2
-
- else:
- hms_idx = None
-
- return hms_idx
-
- def _assign_hms(self, res, value_repr, hms):
- # See GH issue #427, fixing float rounding
- value = self._to_decimal(value_repr)
-
- if hms == 0:
- # Hour
- res.hour = int(value)
- if value % 1:
- res.minute = int(60*(value % 1))
-
- elif hms == 1:
- (res.minute, res.second) = self._parse_min_sec(value)
-
- elif hms == 2:
- (res.second, res.microsecond) = self._parsems(value_repr)
-
- def _could_be_tzname(self, hour, tzname, tzoffset, token):
- return (hour is not None and
- tzname is None and
- tzoffset is None and
- len(token) <= 5 and
- (all(x in string.ascii_uppercase for x in token)
- or token in self.info.UTCZONE))
-
- def _ampm_valid(self, hour, ampm, fuzzy):
- """
- For fuzzy parsing, 'a' or 'am' (both valid English words)
- may erroneously trigger the AM/PM flag. Deal with that
- here.
- """
- val_is_ampm = True
-
- # If there's already an AM/PM flag, this one isn't one.
- if fuzzy and ampm is not None:
- val_is_ampm = False
-
- # If AM/PM is found and hour is not, raise a ValueError
- if hour is None:
- if fuzzy:
- val_is_ampm = False
- else:
- raise ValueError('No hour specified with AM or PM flag.')
- elif not 0 <= hour <= 12:
- # If AM/PM is found, it's a 12 hour clock, so raise
- # an error for invalid range
- if fuzzy:
- val_is_ampm = False
- else:
- raise ValueError('Invalid hour specified for 12-hour clock.')
-
- return val_is_ampm
-
- def _adjust_ampm(self, hour, ampm):
- if hour < 12 and ampm == 1:
- hour += 12
- elif hour == 12 and ampm == 0:
- hour = 0
- return hour
-
- def _parse_min_sec(self, value):
- # TODO: Every usage of this function sets res.second to the return
- # value. Are there any cases where second will be returned as None and
- # we *don't* want to set res.second = None?
- minute = int(value)
- second = None
-
- sec_remainder = value % 1
- if sec_remainder:
- second = int(60 * sec_remainder)
- return (minute, second)
-
- def _parse_hms(self, idx, tokens, info, hms_idx):
- # TODO: Is this going to admit a lot of false-positives for when we
- # just happen to have digits and "h", "m" or "s" characters in non-date
- # text? I guess hex hashes won't have that problem, but there's plenty
- # of random junk out there.
- if hms_idx is None:
- hms = None
- new_idx = idx
- elif hms_idx > idx:
- hms = info.hms(tokens[hms_idx])
- new_idx = hms_idx
- else:
- # Looking backwards, increment one.
- hms = info.hms(tokens[hms_idx]) + 1
- new_idx = idx
-
- return (new_idx, hms)
-
- # ------------------------------------------------------------------
- # Handling for individual tokens. These are kept as methods instead
- # of functions for the sake of customizability via subclassing.
-
- def _parsems(self, value):
- """Parse a I[.F] seconds value into (seconds, microseconds)."""
- if "." not in value:
- return int(value), 0
- else:
- i, f = value.split(".")
- return int(i), int(f.ljust(6, "0")[:6])
-
- def _to_decimal(self, val):
- try:
- decimal_value = Decimal(val)
- # See GH 662, edge case, infinite value should not be converted
- # via `_to_decimal`
- if not decimal_value.is_finite():
- raise ValueError("Converted decimal value is infinite or NaN")
- except Exception as e:
- msg = "Could not convert %s to decimal" % val
- six.raise_from(ValueError(msg), e)
- else:
- return decimal_value
-
- # ------------------------------------------------------------------
- # Post-Parsing construction of datetime output. These are kept as
- # methods instead of functions for the sake of customizability via
- # subclassing.
-
- def _build_tzinfo(self, tzinfos, tzname, tzoffset):
- if callable(tzinfos):
- tzdata = tzinfos(tzname, tzoffset)
- else:
- tzdata = tzinfos.get(tzname)
- # handle case where tzinfo is paased an options that returns None
- # eg tzinfos = {'BRST' : None}
- if isinstance(tzdata, datetime.tzinfo) or tzdata is None:
- tzinfo = tzdata
- elif isinstance(tzdata, text_type):
- tzinfo = tz.tzstr(tzdata)
- elif isinstance(tzdata, integer_types):
- tzinfo = tz.tzoffset(tzname, tzdata)
- else:
- raise TypeError("Offset must be tzinfo subclass, tz string, "
- "or int offset.")
- return tzinfo
-
- def _build_tzaware(self, naive, res, tzinfos):
- if (callable(tzinfos) or (tzinfos and res.tzname in tzinfos)):
- tzinfo = self._build_tzinfo(tzinfos, res.tzname, res.tzoffset)
- aware = naive.replace(tzinfo=tzinfo)
- aware = self._assign_tzname(aware, res.tzname)
-
- elif res.tzname and res.tzname in time.tzname:
- aware = naive.replace(tzinfo=tz.tzlocal())
-
- # Handle ambiguous local datetime
- aware = self._assign_tzname(aware, res.tzname)
-
- # This is mostly relevant for winter GMT zones parsed in the UK
- if (aware.tzname() != res.tzname and
- res.tzname in self.info.UTCZONE):
- aware = aware.replace(tzinfo=tz.UTC)
-
- elif res.tzoffset == 0:
- aware = naive.replace(tzinfo=tz.UTC)
-
- elif res.tzoffset:
- aware = naive.replace(tzinfo=tz.tzoffset(res.tzname, res.tzoffset))
-
- elif not res.tzname and not res.tzoffset:
- # i.e. no timezone information was found.
- aware = naive
-
- elif res.tzname:
- # tz-like string was parsed but we don't know what to do
- # with it
- warnings.warn("tzname {tzname} identified but not understood. "
- "Pass `tzinfos` argument in order to correctly "
- "return a timezone-aware datetime. In a future "
- "version, this will raise an "
- "exception.".format(tzname=res.tzname),
- category=UnknownTimezoneWarning)
- aware = naive
-
- return aware
-
- def _build_naive(self, res, default):
- repl = {}
- for attr in ("year", "month", "day", "hour",
- "minute", "second", "microsecond"):
- value = getattr(res, attr)
- if value is not None:
- repl[attr] = value
-
- if 'day' not in repl:
- # If the default day exceeds the last day of the month, fall back
- # to the end of the month.
- cyear = default.year if res.year is None else res.year
- cmonth = default.month if res.month is None else res.month
- cday = default.day if res.day is None else res.day
-
- if cday > monthrange(cyear, cmonth)[1]:
- repl['day'] = monthrange(cyear, cmonth)[1]
-
- naive = default.replace(**repl)
-
- if res.weekday is not None and not res.day:
- naive = naive + relativedelta.relativedelta(weekday=res.weekday)
-
- return naive
-
- def _assign_tzname(self, dt, tzname):
- if dt.tzname() != tzname:
- new_dt = tz.enfold(dt, fold=1)
- if new_dt.tzname() == tzname:
- return new_dt
-
- return dt
-
- def _recombine_skipped(self, tokens, skipped_idxs):
- """
- >>> tokens = ["foo", " ", "bar", " ", "19June2000", "baz"]
- >>> skipped_idxs = [0, 1, 2, 5]
- >>> _recombine_skipped(tokens, skipped_idxs)
- ["foo bar", "baz"]
- """
- skipped_tokens = []
- for i, idx in enumerate(sorted(skipped_idxs)):
- if i > 0 and idx - 1 == skipped_idxs[i - 1]:
- skipped_tokens[-1] = skipped_tokens[-1] + tokens[idx]
- else:
- skipped_tokens.append(tokens[idx])
-
- return skipped_tokens
-
-
-DEFAULTPARSER = parser()
-
-
-def parse(timestr, parserinfo=None, **kwargs):
- """
-
- Parse a string in one of the supported formats, using the
- ``parserinfo`` parameters.
-
- :param timestr:
- A string containing a date/time stamp.
-
- :param parserinfo:
- A :class:`parserinfo` object containing parameters for the parser.
- If ``None``, the default arguments to the :class:`parserinfo`
- constructor are used.
-
- The ``**kwargs`` parameter takes the following keyword arguments:
-
- :param default:
- The default datetime object, if this is a datetime object and not
- ``None``, elements specified in ``timestr`` replace elements in the
- default object.
-
- :param ignoretz:
- If set ``True``, time zones in parsed strings are ignored and a naive
- :class:`datetime` object is returned.
-
- :param tzinfos:
- Additional time zone names / aliases which may be present in the
- string. This argument maps time zone names (and optionally offsets
- from those time zones) to time zones. This parameter can be a
- dictionary with timezone aliases mapping time zone names to time
- zones or a function taking two parameters (``tzname`` and
- ``tzoffset``) and returning a time zone.
-
- The timezones to which the names are mapped can be an integer
- offset from UTC in seconds or a :class:`tzinfo` object.
-
- .. doctest::
- :options: +NORMALIZE_WHITESPACE
-
- >>> from dateutil.parser import parse
- >>> from dateutil.tz import gettz
- >>> tzinfos = {"BRST": -7200, "CST": gettz("America/Chicago")}
- >>> parse("2012-01-19 17:21:00 BRST", tzinfos=tzinfos)
- datetime.datetime(2012, 1, 19, 17, 21, tzinfo=tzoffset(u'BRST', -7200))
- >>> parse("2012-01-19 17:21:00 CST", tzinfos=tzinfos)
- datetime.datetime(2012, 1, 19, 17, 21,
- tzinfo=tzfile('/usr/share/zoneinfo/America/Chicago'))
-
- This parameter is ignored if ``ignoretz`` is set.
-
- :param dayfirst:
- Whether to interpret the first value in an ambiguous 3-integer date
- (e.g. 01/05/09) as the day (``True``) or month (``False``). If
- ``yearfirst`` is set to ``True``, this distinguishes between YDM and
- YMD. If set to ``None``, this value is retrieved from the current
- :class:`parserinfo` object (which itself defaults to ``False``).
-
- :param yearfirst:
- Whether to interpret the first value in an ambiguous 3-integer date
- (e.g. 01/05/09) as the year. If ``True``, the first number is taken to
- be the year, otherwise the last number is taken to be the year. If
- this is set to ``None``, the value is retrieved from the current
- :class:`parserinfo` object (which itself defaults to ``False``).
-
- :param fuzzy:
- Whether to allow fuzzy parsing, allowing for string like "Today is
- January 1, 2047 at 8:21:00AM".
-
- :param fuzzy_with_tokens:
- If ``True``, ``fuzzy`` is automatically set to True, and the parser
- will return a tuple where the first element is the parsed
- :class:`datetime.datetime` datetimestamp and the second element is
- a tuple containing the portions of the string which were ignored:
-
- .. doctest::
-
- >>> from dateutil.parser import parse
- >>> parse("Today is January 1, 2047 at 8:21:00AM", fuzzy_with_tokens=True)
- (datetime.datetime(2047, 1, 1, 8, 21), (u'Today is ', u' ', u'at '))
-
- :return:
- Returns a :class:`datetime.datetime` object or, if the
- ``fuzzy_with_tokens`` option is ``True``, returns a tuple, the
- first element being a :class:`datetime.datetime` object, the second
- a tuple containing the fuzzy tokens.
-
- :raises ParserError:
- Raised for invalid or unknown string formats, if the provided
- :class:`tzinfo` is not in a valid format, or if an invalid date would
- be created.
-
- :raises OverflowError:
- Raised if the parsed date exceeds the largest valid C integer on
- your system.
- """
- if parserinfo:
- return parser(parserinfo).parse(timestr, **kwargs)
- else:
- return DEFAULTPARSER.parse(timestr, **kwargs)
-
-
-class _tzparser(object):
-
- class _result(_resultbase):
-
- __slots__ = ["stdabbr", "stdoffset", "dstabbr", "dstoffset",
- "start", "end"]
-
- class _attr(_resultbase):
- __slots__ = ["month", "week", "weekday",
- "yday", "jyday", "day", "time"]
-
- def __repr__(self):
- return self._repr("")
-
- def __init__(self):
- _resultbase.__init__(self)
- self.start = self._attr()
- self.end = self._attr()
-
- def parse(self, tzstr):
- res = self._result()
- l = [x for x in re.split(r'([,:.]|[a-zA-Z]+|[0-9]+)',tzstr) if x]
- used_idxs = list()
- try:
-
- len_l = len(l)
-
- i = 0
- while i < len_l:
- # BRST+3[BRDT[+2]]
- j = i
- while j < len_l and not [x for x in l[j]
- if x in "0123456789:,-+"]:
- j += 1
- if j != i:
- if not res.stdabbr:
- offattr = "stdoffset"
- res.stdabbr = "".join(l[i:j])
- else:
- offattr = "dstoffset"
- res.dstabbr = "".join(l[i:j])
-
- for ii in range(j):
- used_idxs.append(ii)
- i = j
- if (i < len_l and (l[i] in ('+', '-') or l[i][0] in
- "0123456789")):
- if l[i] in ('+', '-'):
- # Yes, that's right. See the TZ variable
- # documentation.
- signal = (1, -1)[l[i] == '+']
- used_idxs.append(i)
- i += 1
- else:
- signal = -1
- len_li = len(l[i])
- if len_li == 4:
- # -0300
- setattr(res, offattr, (int(l[i][:2]) * 3600 +
- int(l[i][2:]) * 60) * signal)
- elif i + 1 < len_l and l[i + 1] == ':':
- # -03:00
- setattr(res, offattr,
- (int(l[i]) * 3600 +
- int(l[i + 2]) * 60) * signal)
- used_idxs.append(i)
- i += 2
- elif len_li <= 2:
- # -[0]3
- setattr(res, offattr,
- int(l[i][:2]) * 3600 * signal)
- else:
- return None
- used_idxs.append(i)
- i += 1
- if res.dstabbr:
- break
- else:
- break
-
-
- if i < len_l:
- for j in range(i, len_l):
- if l[j] == ';':
- l[j] = ','
-
- assert l[i] == ','
-
- i += 1
-
- if i >= len_l:
- pass
- elif (8 <= l.count(',') <= 9 and
- not [y for x in l[i:] if x != ','
- for y in x if y not in "0123456789+-"]):
- # GMT0BST,3,0,30,3600,10,0,26,7200[,3600]
- for x in (res.start, res.end):
- x.month = int(l[i])
- used_idxs.append(i)
- i += 2
- if l[i] == '-':
- value = int(l[i + 1]) * -1
- used_idxs.append(i)
- i += 1
- else:
- value = int(l[i])
- used_idxs.append(i)
- i += 2
- if value:
- x.week = value
- x.weekday = (int(l[i]) - 1) % 7
- else:
- x.day = int(l[i])
- used_idxs.append(i)
- i += 2
- x.time = int(l[i])
- used_idxs.append(i)
- i += 2
- if i < len_l:
- if l[i] in ('-', '+'):
- signal = (-1, 1)[l[i] == "+"]
- used_idxs.append(i)
- i += 1
- else:
- signal = 1
- used_idxs.append(i)
- res.dstoffset = (res.stdoffset + int(l[i]) * signal)
-
- # This was a made-up format that is not in normal use
- warn(('Parsed time zone "%s"' % tzstr) +
- 'is in a non-standard dateutil-specific format, which ' +
- 'is now deprecated; support for parsing this format ' +
- 'will be removed in future versions. It is recommended ' +
- 'that you switch to a standard format like the GNU ' +
- 'TZ variable format.', tz.DeprecatedTzFormatWarning)
- elif (l.count(',') == 2 and l[i:].count('/') <= 2 and
- not [y for x in l[i:] if x not in (',', '/', 'J', 'M',
- '.', '-', ':')
- for y in x if y not in "0123456789"]):
- for x in (res.start, res.end):
- if l[i] == 'J':
- # non-leap year day (1 based)
- used_idxs.append(i)
- i += 1
- x.jyday = int(l[i])
- elif l[i] == 'M':
- # month[-.]week[-.]weekday
- used_idxs.append(i)
- i += 1
- x.month = int(l[i])
- used_idxs.append(i)
- i += 1
- assert l[i] in ('-', '.')
- used_idxs.append(i)
- i += 1
- x.week = int(l[i])
- if x.week == 5:
- x.week = -1
- used_idxs.append(i)
- i += 1
- assert l[i] in ('-', '.')
- used_idxs.append(i)
- i += 1
- x.weekday = (int(l[i]) - 1) % 7
- else:
- # year day (zero based)
- x.yday = int(l[i]) + 1
-
- used_idxs.append(i)
- i += 1
-
- if i < len_l and l[i] == '/':
- used_idxs.append(i)
- i += 1
- # start time
- len_li = len(l[i])
- if len_li == 4:
- # -0300
- x.time = (int(l[i][:2]) * 3600 +
- int(l[i][2:]) * 60)
- elif i + 1 < len_l and l[i + 1] == ':':
- # -03:00
- x.time = int(l[i]) * 3600 + int(l[i + 2]) * 60
- used_idxs.append(i)
- i += 2
- if i + 1 < len_l and l[i + 1] == ':':
- used_idxs.append(i)
- i += 2
- x.time += int(l[i])
- elif len_li <= 2:
- # -[0]3
- x.time = (int(l[i][:2]) * 3600)
- else:
- return None
- used_idxs.append(i)
- i += 1
-
- assert i == len_l or l[i] == ','
-
- i += 1
-
- assert i >= len_l
-
- except (IndexError, ValueError, AssertionError):
- return None
-
- unused_idxs = set(range(len_l)).difference(used_idxs)
- res.any_unused_tokens = not {l[n] for n in unused_idxs}.issubset({",",":"})
- return res
-
-
-DEFAULTTZPARSER = _tzparser()
-
-
-def _parsetz(tzstr):
- return DEFAULTTZPARSER.parse(tzstr)
-
-
-class ParserError(ValueError):
- """Exception subclass used for any failure to parse a datetime string.
-
- This is a subclass of :py:exc:`ValueError`, and should be raised any time
- earlier versions of ``dateutil`` would have raised ``ValueError``.
-
- .. versionadded:: 2.8.1
- """
- def __str__(self):
- try:
- return self.args[0] % self.args[1:]
- except (TypeError, IndexError):
- return super(ParserError, self).__str__()
-
- def __repr__(self):
- args = ", ".join("'%s'" % arg for arg in self.args)
- return "%s(%s)" % (self.__class__.__name__, args)
-
-
-class UnknownTimezoneWarning(RuntimeWarning):
- """Raised when the parser finds a timezone it cannot parse into a tzinfo.
-
- .. versionadded:: 2.7.0
- """
-# vim:ts=4:sw=4:et
diff --git a/spaces/joaopereirajp/livvieChatBot/venv/lib/python3.9/site-packages/gpt_index/readers/file/base_parser.py b/spaces/joaopereirajp/livvieChatBot/venv/lib/python3.9/site-packages/gpt_index/readers/file/base_parser.py
deleted file mode 100644
index 753a56f9797432f053fb96a72bdb782a2b20bd05..0000000000000000000000000000000000000000
--- a/spaces/joaopereirajp/livvieChatBot/venv/lib/python3.9/site-packages/gpt_index/readers/file/base_parser.py
+++ /dev/null
@@ -1,38 +0,0 @@
-"""Base parser and config class."""
-
-from abc import abstractmethod
-from pathlib import Path
-from typing import Dict, List, Optional, Union
-
-
-class BaseParser:
- """Base class for all parsers."""
-
- def __init__(self, parser_config: Optional[Dict] = None):
- """Init params."""
- self._parser_config = parser_config
-
- def init_parser(self) -> None:
- """Init parser and store it."""
- parser_config = self._init_parser()
- self._parser_config = parser_config
-
- @property
- def parser_config_set(self) -> bool:
- """Check if parser config is set."""
- return self._parser_config is not None
-
- @property
- def parser_config(self) -> Dict:
- """Check if parser config is set."""
- if self._parser_config is None:
- raise ValueError("Parser config not set.")
- return self._parser_config
-
- @abstractmethod
- def _init_parser(self) -> Dict:
- """Initialize the parser with the config."""
-
- @abstractmethod
- def parse_file(self, file: Path, errors: str = "ignore") -> Union[str, List[str]]:
- """Parse file."""
diff --git a/spaces/joaopereirajp/livvieChatBot/venv/lib/python3.9/site-packages/gpt_index/readers/file/image_parser.py b/spaces/joaopereirajp/livvieChatBot/venv/lib/python3.9/site-packages/gpt_index/readers/file/image_parser.py
deleted file mode 100644
index e2ba0455f65e704ec61022fe5a85bff0dd0361c5..0000000000000000000000000000000000000000
--- a/spaces/joaopereirajp/livvieChatBot/venv/lib/python3.9/site-packages/gpt_index/readers/file/image_parser.py
+++ /dev/null
@@ -1,101 +0,0 @@
-"""Image parser.
-
-Contains parsers for image files.
-
-"""
-
-import re
-from pathlib import Path
-from typing import Dict
-
-from gpt_index.readers.file.base_parser import BaseParser
-
-
-class ImageParser(BaseParser):
- """Image parser.
-
- Extract text from images using DONUT.
-
- """
-
- def _init_parser(self) -> Dict:
- """Init parser."""
- try:
- import torch # noqa: F401
- except ImportError:
- raise ImportError(
- "install pytorch to use the model: " "`pip install torch`"
- )
- try:
- from transformers import DonutProcessor, VisionEncoderDecoderModel
- except ImportError:
- raise ImportError(
- "transformers is required for using DONUT model: "
- "`pip install transformers`"
- )
- try:
- import sentencepiece # noqa: F401
- except ImportError:
- raise ImportError(
- "sentencepiece is required for using DONUT model: "
- "`pip install sentencepiece`"
- )
- try:
- from PIL import Image # noqa: F401
- except ImportError:
- raise ImportError(
- "PIL is required to read image files: " "`pip install Pillow`"
- )
-
- processor = DonutProcessor.from_pretrained(
- "naver-clova-ix/donut-base-finetuned-cord-v2"
- )
- model = VisionEncoderDecoderModel.from_pretrained(
- "naver-clova-ix/donut-base-finetuned-cord-v2"
- )
- return {"processor": processor, "model": model}
-
- def parse_file(self, file: Path, errors: str = "ignore") -> str:
- """Parse file."""
- import torch
- from PIL import Image
-
- model = self.parser_config["model"]
- processor = self.parser_config["processor"]
-
- device = "cuda" if torch.cuda.is_available() else "cpu"
- model.to(device)
- # load document image
- image = Image.open(file)
- if image.mode != "RGB":
- image = image.convert("RGB")
-
- # prepare decoder inputs
- task_prompt = ""
- decoder_input_ids = processor.tokenizer(
- task_prompt, add_special_tokens=False, return_tensors="pt"
- ).input_ids
-
- pixel_values = processor(image, return_tensors="pt").pixel_values
-
- outputs = model.generate(
- pixel_values.to(device),
- decoder_input_ids=decoder_input_ids.to(device),
- max_length=model.decoder.config.max_position_embeddings,
- early_stopping=True,
- pad_token_id=processor.tokenizer.pad_token_id,
- eos_token_id=processor.tokenizer.eos_token_id,
- use_cache=True,
- num_beams=3,
- bad_words_ids=[[processor.tokenizer.unk_token_id]],
- return_dict_in_generate=True,
- )
-
- sequence = processor.batch_decode(outputs.sequences)[0]
- sequence = sequence.replace(processor.tokenizer.eos_token, "").replace(
- processor.tokenizer.pad_token, ""
- )
- # remove first task start token
- sequence = re.sub(r"<.*?>", "", sequence, count=1).strip()
-
- return sequence
diff --git a/spaces/johnslegers/stable-diffusion-gui-test/static/index.html b/spaces/johnslegers/stable-diffusion-gui-test/static/index.html
deleted file mode 100644
index a33806e93fbb159f8384e4e9becbb55e07599d64..0000000000000000000000000000000000000000
--- a/spaces/johnslegers/stable-diffusion-gui-test/static/index.html
+++ /dev/null
@@ -1,1919 +0,0 @@
-
-
-
-
-
- Fast API 🤗 Space served with Uvicorn
-
-
-
-
-
-