diff --git a/spaces/1acneusushi/gradio-2dmoleculeeditor/data/Descargar crack batman arkham asylum pc windows 7 el mejor sitio para obtener el juego y el crack.md b/spaces/1acneusushi/gradio-2dmoleculeeditor/data/Descargar crack batman arkham asylum pc windows 7 el mejor sitio para obtener el juego y el crack.md
deleted file mode 100644
index f894fb9354b2495b4f58972ebf6a601dcb2f812b..0000000000000000000000000000000000000000
--- a/spaces/1acneusushi/gradio-2dmoleculeeditor/data/Descargar crack batman arkham asylum pc windows 7 el mejor sitio para obtener el juego y el crack.md
+++ /dev/null
@@ -1,123 +0,0 @@
-
-
How to Download Crack Batman Arkham Asylum PC Windows 7
-
Are you a fan of Batman and want to play one of the best games based on his comic series? Do you want to experience the thrill of fighting against the Joker and his henchmen in a dark and twisted asylum? Do you want to save money and play the game for free? If you answered yes to any of these questions, then this article is for you. In this article, I will show you how to download crack batman arkham asylum pc windows 7 and enjoy the game without any hassle.
Batman Arkham Asylum is a game developed by Rocksteady Studios and published by Warner Bros. Interactive Entertainment in 2009. It is an action-adventure game that features stealth, combat, exploration, and puzzle-solving elements. The game follows Batman as he tries to stop the Joker from taking over the Arkham Asylum, a psychiatric facility that houses some of Gotham's most notorious criminals. The game received critical acclaim for its story, gameplay, graphics, voice acting, and atmosphere. It also won several awards, including Game of the Year from various publications.
-
What is Batman Arkham Asylum?
-
Batman Arkham Asylum is a game that puts you in the shoes of Batman, the world's greatest detective and superhero. You will use your skills, gadgets, and abilities to fight against the Joker and his allies, who have taken over the asylum and unleashed chaos. You will also encounter other famous villains from the Batman universe, such as Harley Quinn, Poison Ivy, Killer Croc, Scarecrow, and Bane. You will also have to deal with the mysterious Riddler, who has hidden hundreds of trophies and riddles throughout the asylum for you to find and solve.
-
Why do you need a crack?
-
A crack is a file that modifies or bypasses the original protection of a game or software. It allows you to run the game or software without having to purchase it or enter a serial key. A crack can also fix some bugs or errors that may occur in the original version. However, cracking a game or software is illegal and may cause harm to your computer or device. Therefore, you should only download cracks from trusted sources and at your own risk.
-
descargar crack batman arkham asylum pc windows 7 mega
-descargar crack batman arkham asylum pc windows 7 64 bits
-descargar crack batman arkham asylum pc windows 7 español
-descargar crack batman arkham asylum pc windows 7 full
-descargar crack batman arkham asylum pc windows 7 gratis
-descargar crack batman arkham asylum pc windows 7 sin virus
-descargar crack batman arkham asylum pc windows 7 utorrent
-descargar crack batman arkham asylum pc windows 7 iso
-descargar crack batman arkham asylum pc windows 7 skidrow
-descargar crack batman arkham asylum pc windows 7 reloaded
-descargar crack batman arkham asylum pc windows 7 goty
-descargar crack batman arkham asylum pc windows 7 steam
-descargar crack batman arkham asylum pc windows 7 no cd
-descargar crack batman arkham asylum pc windows 7 fix
-descargar crack batman arkham asylum pc windows 7 patch
-descargar crack batman arkham asylum pc windows 7 gamecopyworld
-descargar crack batman arkham asylum pc windows 7 razor1911
-descargar crack batman arkham asylum pc windows 7 online
-descargar crack batman arkham asylum pc windows 7 mediafire
-descargar crack batman arkham asylum pc windows 7 megaupload
-descargar crack batman arkham asylum pc windows 7 rapidshare
-descargar crack batman arkham asylum pc windows 7 fileserve
-descargar crack batman arkham asylum pc windows 7 filefactory
-descargar crack batman arkham asylum pc windows 7 depositfiles
-descargar crack batman arkham asylum pc windows 7 hotfile
-descargar crack batman arkham asylum pc windows 7 zippyshare
-descargar crack batman arkham asylum pc windows 7 freakshare
-descargar crack batman arkham asylum pc windows 7 bitshare
-descargar crack batman arkham asylum pc windows 7 uploaded
-descargar crack batman arkham asylum pc windows 7 netload
-descargar crack batman arkham asylum pc windows 7 letitbit
-descargar crack batman arkham asylum pc windows 7 turbobit
-descargar crack batman arkham asylum pc windows 7 shareflare
-descargar crack batman arkham asylum pc windows 7 extabit
-descargar crack batman arkham asylum pc windows 7 crocko
-descargar crack batman arkham asylum pc windows 7 oron
-descargar crack batman arkham asylum pc windows 7 wupload
-descargar crack batman arkham asylum pc windows 7 uploadstation
-descargar crack batman arkham asylum pc windows 7 filesonic
-descargar crack batman arkham asylum pc windows 7 filejungle
-descargar crack batman arkham asylum pc windows 7 filepost
-descargar crack batman arkham asylum pc windows 7 filesmonster
-descargar crack batman arkham asylum pc windows 7 easy-share
-descargar crack batman arkham asylum pc windows 7 uploading.com
-descargar crack batman arkham asylum pc windows 7 uploaded.to
-descargar crack batman arkham asylum pc windows 7 bayfiles.com
-descargar crack batman arkham asylum pc windows 7 putlocker.com
-descargar crack batman arkham asylum pc windows 7 sockshare.com
-descargar crack batman arkham asylum pc windows 7 rapidgator.net
-
How to download and install the crack
-
In order to download crack batman arkham asylum pc windows 7, you will need two things: the game of the year edition and the crack file. The game of the year edition is a remastered version of the original game that includes four extra challenge maps. The crack file is a file that will allow you to run the game without any problems. Here are the steps you need to follow:
-
Step 1: Download the game of the year edition
-
The first step is to download the game of the year edition from a reliable website. One such website is GOG Unlocked, which offers free downloads of various games. You can find Batman Arkham Asylum Game of the Year Edition on their website by searching for it or clicking on this link. Once you are on their website, click on the blue "download now" button and wait for 5 seconds. Then, click on "create download link" and wait for another 5 seconds. Finally, click on "click here to download" and save the file on your computer.
-
Step 2: Extract the game files
-
The second step is to extract the game files from the zip file that you downloaded. You will need a software that can extract zip files, such as 7-Zip, which you can get here. Once you have installed 7-Zip or any other similar software, right-click on the zip file and select "Extract here" or "Extract to Batman: Arkham Asylum Game of the Year Edition v1.1". This will create a folder with all the game files inside.
-
Step 3: Download the crack file
-
The third step is to download the crack file from another reliable website. One such website is MegaGames, which offers various fixes and patches for games. You can find Batman: Arkham Asylum - Game of the Year v1.1 All No-DVD [Prophet] on their website by searching for it or clicking on this link. Once you are on their website, scroll down until you see a blue "Download" button and click on it. Then, save the zip file on your computer.
-
Step 4: Copy and paste the crack file into the game folder
-
The fourth step is to copy and paste the crack file into the game folder that you extracted earlier. To do this, right-click on the zip file that contains the crack file and select "Extract here" or "Extract to BATMAN.AA.GOTY.V1.1.ALL.PROPHET.NODVD". This will create a folder with the crack file inside. Then, open the folder and copy the file named "Binaries". Then, go back to the game folder that contains all the game files and paste the copied file into it. You may be asked to replace or overwrite some files. Click on "Yes" to all.
-
Step 5: Run the game as administrator
-
The final step is to run the game as administrator. To do this, go to the game folder and double-click on the file named "BmStartApp.exe". This will launch the game. However, before you play, you should right-click on the file and select "Properties". Then, go to the "Compatibility" tab and check the box that says "Run this program as an administrator". This will ensure that the game runs smoothly without any errors.
-
Tips and tricks for playing the game
-
Now that you have downloaded crack batman arkham asylum pc windows 7, you are ready to enjoy the game. However, if you want to get the most out of it, you should follow some tips and tricks that will help you improve your skills and have more fun. Here are some of them:
-
How to use the freeflow combat system
-
The freeflow combat system is one of the main features of the game. It allows you to chain together unlimited combos seamlessly and battle with huge groups of enemies in brutal melee brawls. To use it effectively, you should follow these steps:
-
-
Use your mouse or controller to move around and target enemies.
-
Press left mouse button or X button to strike enemies.
-
Press right mouse button or Y button to counter enemies when they flash blue.
-
Press spacebar or A button to jump over enemies when they flash yellow.
-
Press shift or B button to stun enemies with your cape.
-
Press E or RT button to use your gadgets when they flash white.
-
Press F or LT button to aim your batarang at enemies.
-
Keep an eye on your combo meter at the top left corner of your screen.
-
Avoid getting hit or missing attacks as this will break your combo.
-
Build up your combo meter until it reaches x8 or higher.
-
Press middle mouse button or RB button to perform a takedown move when it flashes orange.
-
This will instantly knock out an enemy regardless of their health.
-
You can also perform special moves such as ground takedown, inverted takedown, and environmental takedown by pressing different buttons when prompted.
-
-
By using the freeflow combat system, you will be able to defeat your enemies with style and efficiency. You will also earn more experience points and unlock new moves and upgrades for your combat skills.
-
How to solve the riddler's puzzles
-
The riddler's puzzles are another feature of the game that will challenge your mind and reward you with secrets and collectibles. The riddler's puzzles consist of six types: Chronicles of Arkham, "Mystery", Patient Interview Tapes, Riddles, Riddler Trophies, and Teeth. Each area of the asylum has a number of these puzzles for you to find and solve. To solve them, you will need to use your detective mode, your gadgets, and your logic. Here are some tips for solving them:
-
-
Use your detective mode by pressing Q or LB button to scan the environment for clues and hints. You will see green question marks or symbols that indicate the location or type of a puzzle.
-
Use your gadgets such as batarang, explosive gel, cryptographic sequencer, line launcher, and batclaw to interact with objects or access hidden areas. You can also use them to destroy the Joker's teeth that are scattered around the asylum.
-
Use your logic and knowledge of the Batman universe to answer the riddles that the Riddler will give you. The riddles are usually related to a character, an item, or a location that you can find in the area. For example, one of the riddles is "Don't cut yourself on this sharply observed portrait". The answer is a painting of Zsasz that has a knife on it.
-
Collect the Chronicles of Arkham, the Patient Interview Tapes, and the "Mystery" items to learn more about the history and secrets of the asylum and its inmates. You can listen to them in your menu by pressing Esc or Start button.
-
-
By solving the riddler's puzzles, you will be able to unlock concept art, character bios, challenge maps, achievements, and trophies. You will also be able to confront the Riddler himself once you have solved all of his puzzles.
-
How to unlock the extra challenge maps
-
The extra challenge maps are another feature of the game that will test your skills and abilities in different scenarios. The challenge maps are divided into two types: combat and predator. In combat challenge maps, you have to fight waves of enemies and score as many points as possible by using combos and takedowns. In predator challenge maps, you have to stealthily take out enemies without being detected or killed. You can access the challenge maps from the main menu by selecting "Challenge Mode". To unlock them, you have to do one of these things:
-
-
Complete the story mode on any difficulty level. This will unlock four combat challenge maps and four predator challenge maps.
-
Collect all of the Riddler Trophies in each area of the asylum. This will unlock four more combat challenge maps and four more predator challenge maps.
-
Download the game of the year edition that includes four extra challenge maps: Crime Alley, Scarecrow Nightmare, Totally Insane, and Nocturnal Hunter. These are part of the Insane Night Map Pack that was released as a free DLC for the game.
-
-
By playing the challenge maps, you will be able to improve your skills and compete with other players on online leaderboards. You will also earn more experience points and unlock new costumes for Batman.
-
Conclusion
-
Batman Arkham Asylum is a game that will immerse you in the dark and twisted world of Batman and his enemies. It is a game that will make you feel like you are Batman himself as you use your skills, gadgets, and abilities to stop the Joker's plan. It is a game that will offer you hours of fun and entertainment with its story mode, challenge mode, and riddler's puzzles. It is a game that you can play for free by downloading crack batman arkham asylum pc windows 7 from reliable websites.
-
Summary of the main points
-
In this article, I have shown you how to download crack batman arkham asylum pc windows 7 and enjoy the game without any hassle. I have also given you some tips and tricks for playing the game and getting the most out of it. Here are the main points that you should remember:
-
-
You need to download the game of the year edition and the crack file from reliable websites.
-
You need to extract the game files and copy and paste the crack file into the game folder.
-
You need to run the game as administrator and use the freeflow combat system, the detective mode, and your gadgets to play the game.
-
You can solve the riddler's puzzles, play the challenge maps, and unlock secrets and collectibles by using your logic, skills, and knowledge.
-
-
Call to action
-
If you are ready to play one of the best games based on Batman's comic series, then don't wait any longer. Download crack batman arkham asylum pc windows 7 today and start your adventure in Arkham Asylum. You won't regret it!
- **FAQs** Q: Is it safe to download crack batman arkham asylum pc windows 7? A: Downloading cracks is illegal and may cause harm to your computer or device. Therefore, you should only download cracks from trusted sources and at your own risk. Q: What are the system requirements for playing batman arkham asylum pc windows 7? A: The minimum system requirements are: - OS: Windows XP/Vista/7 - Processor: INTEL 2.4 GHz Dual Core - RAM: 2 GB - Video Memory: 256 MB - Video Card: NVIDIA GeForce 6600 or ATI Radeon X1300 - Sound Card: DirectX Compatible - DirectX: 9.0c - Hard Drive: 8 GB free Q: How long is the story mode of batman arkham asylum pc windows 7? A: The story mode of batman arkham asylum pc windows 7 takes about 10 to 15 hours to complete depending on your difficulty level and playstyle. Q: How many characters are there in batman arkham asylum pc windows 7? A: There are over 20 characters in batman arkham asylum pc windows 7 that you can encounter or play as. Some of them are: - Batman - The Joker - Harley Quinn - Commissioner Gordon - Oracle - Alfred - Scarecrow - Poison Ivy - Killer Croc - Bane - Zsasz - The Riddler Q: How can I get more costumes for Batman in batman arkham asylum pc windows 7? A: You can get more costumes for Batman in batman arkham asylum pc windows 7 by earning more experience points and unlocking new upgrades for your combat skills. You can also get more costumes by playing the challenge maps or downloading DLCs. 0a6ba089eb
-
-
\ No newline at end of file
diff --git a/spaces/1acneusushi/gradio-2dmoleculeeditor/data/Download Serum The Best Wavetable Synthesizer Ever.md b/spaces/1acneusushi/gradio-2dmoleculeeditor/data/Download Serum The Best Wavetable Synthesizer Ever.md
deleted file mode 100644
index 7c4a77ad940d75416464fcea65faa2a1e1ae2575..0000000000000000000000000000000000000000
--- a/spaces/1acneusushi/gradio-2dmoleculeeditor/data/Download Serum The Best Wavetable Synthesizer Ever.md
+++ /dev/null
@@ -1,31 +0,0 @@
-
-
How to Download Serum: A Complete Guide for Beginners
-
Serum is one of the most popular and powerful synthesizers in the music production industry. It allows you to create stunning sounds and effects with its wavetable-based engine, flexible modulation options, and intuitive interface. But how do you download Serum and get started with it?
-
In this article, we will show you how to download Serum from its official website, install it on your computer, and activate it with a license key. We will also give you some tips on how to use Serum effectively and where to find more resources and tutorials. Let's dive in!
The first step to download Serum is to visit the official website of Xfer Records, the company that created Serum. You can find it at https://xferrecords.com/products/serum. There, you will see a button that says "Buy Now". Click on it and you will be redirected to a page where you can choose your payment method and enter your details.
-
Once you complete the payment, you will receive an email with a download link and a license key. The download link will take you to a page where you can choose your operating system (Windows or Mac) and download the installer file. The license key is a code that you will need to activate Serum later.
-
Download the installer file and save it somewhere on your computer. Then, double-click on it and follow the instructions to install Serum on your computer. The installation process is very simple and straightforward. You just need to agree to the terms and conditions, choose a destination folder, and wait for the installation to finish.
-
How to Activate Serum with a License Key
-
After installing Serum on your computer, you need to activate it with your license key. This is a very important step because it will unlock all the features and functions of Serum and prevent any issues or errors.
-
To activate Serum, open your DAW (digital audio workstation) of choice and load Serum as a plugin. You can find it in your VST or AU folder, depending on your operating system and DAW. Once you load Serum, you will see a window that asks you to enter your license key.
-
Copy and paste your license key from the email that you received after purchasing Serum. Make sure that you enter it exactly as it appears in the email, without any spaces or extra characters. Then, click on "Register" and wait for a confirmation message. If everything goes well, you should see a message that says "Thank you for registering Serum!"
-
Congratulations! You have successfully downloaded and activated Serum on your computer. You can now start using it and explore its amazing features.
-
How to Use Serum Effectively
-
Serum is a very versatile and powerful synthesizer that can help you create any sound or effect that you can imagine. However, it can also be overwhelming at first, especially if you are new to synthesis or wavetable synthesis in particular.
-
-
That's why we recommend that you start by learning the basics of Serum and how it works. You can do this by reading the manual that comes with Serum or watching some online tutorials that explain the main features and functions of Serum.
-
Some of the things that you should learn are:
-
-
How to load and edit wavetables
-
How to use envelopes, LFOs, and mod matrix
-
How to use filters, effects, and macros
-
How to use presets and wavetable packs
-
How to create your own sounds from scratch
-
-
Once you master these basics, you can move on to more advanced topics and techniques that will help you take your sound design skills to the next level. You can also experiment with different combinations of wavetables, modulations, filters, effects, and macros to create unique and original sounds.
-
Where to Find More Resources and Tutorials
-
If you want to learn more about Serum and how to use it effectively, there are plenty of resources and tutorials available online that can help you. Here are some of the best ones that we recommend:
ddb901b051
-
-
\ No newline at end of file
diff --git a/spaces/1gistliPinn/ChatGPT4/Examples/8x12PsdKarizmaAlbumBackgroundsFreeDownloadDownloadFullVersionPictures.md b/spaces/1gistliPinn/ChatGPT4/Examples/8x12PsdKarizmaAlbumBackgroundsFreeDownloadDownloadFullVersionPictures.md
deleted file mode 100644
index b02ad573e19cd794d36ca18f89e022f88a10e679..0000000000000000000000000000000000000000
--- a/spaces/1gistliPinn/ChatGPT4/Examples/8x12PsdKarizmaAlbumBackgroundsFreeDownloadDownloadFullVersionPictures.md
+++ /dev/null
@@ -1,8 +0,0 @@
-
-
6way metatun,8x12PsdKarizmaAlbumBackgroundsFreeDownloadDownloadFullVersionPictures. 8way x1 album Album Album Album Album Album Album Album Album Album Album 8x12PsdKarizmaAlbumBackgroundsFreeDownloadDownloadFullVersionPictures,libertalia.
899543212b
-
-
\ No newline at end of file
diff --git a/spaces/1gistliPinn/ChatGPT4/Examples/Ali-rs232-upgrade-tool-v1-2-0 !LINK! Downloader Full.md b/spaces/1gistliPinn/ChatGPT4/Examples/Ali-rs232-upgrade-tool-v1-2-0 !LINK! Downloader Full.md
deleted file mode 100644
index 8e14b5656ebe35af1c55f6102ec6626e6382a950..0000000000000000000000000000000000000000
--- a/spaces/1gistliPinn/ChatGPT4/Examples/Ali-rs232-upgrade-tool-v1-2-0 !LINK! Downloader Full.md
+++ /dev/null
@@ -1,13 +0,0 @@
-
-
-Aug 9, 2018 - 8, ALI RS232 Upgrade Tool, v 1.2.0 03.2012, Download, SR-2000HD Hyper. 9, GXD Loader, v 1.010, Download, SR-8989HD. 10, Multi Tool Box 2018 v1.5, Download, SMC-NOTES, v 0.9.1, Download,.
-Alcatel, All in One, All in One Tool, All in One Tool Lite, All in One Tool Pro.
-All in One Tool Premium, All in One Tool Pro, All in One Tool.
-Alcatel, All in One, All in One Tool, All in One Tool Pro.
-Alcatel, All in One Tool, All in One Tool lite, All in One Tool.
-Alcatel, All in One Tool Pro, All in One Tool Pro lite, All in One Tool lite.
-Alcatel, All in One Tool Lite, All in One Tool Pro.
-Alcatel, All in One Tool Pro, All in One Tool Pro lite. 8a78ff9644
-
-
-
diff --git a/spaces/1pelhydcardo/ChatGPT-prompt-generator/assets/Bus Simulator Indonesia Philippines Mod Apk Discover the Amazing Features of the Philippine Bus Simulator Mod with Bussid Skin Philippines Download.md b/spaces/1pelhydcardo/ChatGPT-prompt-generator/assets/Bus Simulator Indonesia Philippines Mod Apk Discover the Amazing Features of the Philippine Bus Simulator Mod with Bussid Skin Philippines Download.md
deleted file mode 100644
index db82f97c857e5d2927fca8baa13d81259ea137f0..0000000000000000000000000000000000000000
--- a/spaces/1pelhydcardo/ChatGPT-prompt-generator/assets/Bus Simulator Indonesia Philippines Mod Apk Discover the Amazing Features of the Philippine Bus Simulator Mod with Bussid Skin Philippines Download.md
+++ /dev/null
@@ -1,139 +0,0 @@
-
-
Bus Simulator Indonesia Philippines Mod Apk: A Guide for Gamers
-
If you are a fan of bus simulation games, you might have heard of Bus Simulator Indonesia, a popular game that lets you experience the thrill of driving a bus in Indonesia. But did you know that there is a modded version of this game that adds more features and fun to the gameplay? In this article, we will tell you everything you need to know about Bus Simulator Indonesia Philippines Mod Apk, a mod that allows you to drive buses in the Philippines. We will also show you how to download and install this mod on your Android device, and how to play it like a pro. So, buckle up and get ready for an exciting ride!
-
What is Bus Simulator Indonesia?
-
Bus Simulator Indonesia, also known as BUSSID, is a realistic bus simulation game developed by Maleo. It was released in 2017 and has since gained millions of downloads and positive reviews from players around the world. The game lets you drive various types of buses in different cities and regions of Indonesia, such as Jakarta, Bali, Sumatra, Java, and more. You can also customize your bus with different liveries, accessories, horns, stickers, and more. The game features realistic graphics, physics, traffic, weather, and sounds that make you feel like you are really driving a bus in Indonesia.
Some of the features that make Bus Simulator Indonesia stand out from other bus simulation games are:
-
-
You can design your own livery for your bus.
-
You can use the Indonesian currency (rupiah) to buy new buses and upgrades.
-
You can honk your horn with various melodies and sounds.
-
You can interact with other players online through chat and multiplayer mode.
-
You can enjoy the scenery and landmarks of Indonesia along the way.
-
You can follow the rules and regulations of Indonesian traffic.
-
-
How to download and install Bus Simulator Indonesia on Android
-
If you want to play Bus Simulator Indonesia on your Android device, you can easily download and install it from the Google Play Store. Here are the steps to do so:
-
-
Open the Google Play Store app on your device.
-
Search for "Bus Simulator Indonesia" in the search bar.
-
Select the game from the list of results and tap on "Install".
-
Wait for the game to download and install on your device.
-
Once the installation is complete, tap on "Open" to launch the game.
-
-
Congratulations! You have successfully installed Bus Simulator Indonesia on your Android device. You can now start playing the game and enjoy driving buses in Indonesia.
-
What is Bus Simulator Indonesia Philippines Mod Apk?
-
Bus Simulator Indonesia Philippines Mod Apk is a modified version of Bus Simulator Indonesia that adds more features and fun to the original game. As the name suggests, this mod allows you to drive buses in the Philippines, instead of Indonesia. You can explore various cities and regions of the Philippines, such as Manila, Cebu, Davao, Baguio, Boracay, and more. You can also choose from different types of buses that are popular in the Philippines, such as jeepneys, coasters, minibuses, double-deckers, etc. You can also customize your bus with different liveries, accessories, horns, stickers, and more. The mod also features realistic graphics, physics, traffic, weather, and sounds that make you feel like you are really driving a bus in the Philippines.
-
Benefits of using Bus Simulator Indonesia Philippines Mod Apk
-
Some of the benefits of using Bus Simulator Indonesia Philippines Mod Apk are:
-
-
You can experience a different culture and environment by driving buses in the Philippines.
-
You can have more fun and variety by choosing from different types of buses and customizing them.
-
You can challenge yourself by following the rules and regulations of Philippine traffic.
-
You can interact with other players online who are also using the mod.
-
You can enjoy the game without any ads or in-app purchases.
-
-
Risks of using Bus Simulator Indonesia Philippines Mod Apk
-
However, using Bus Simulator Indonesia Philippines Mod Apk also comes with some risks that you should be aware of. Some of the risks are:
-
-
You might face compatibility issues with your device or the original game.
-
You might encounter bugs or glitches that affect the gameplay.
-
You might violate the terms and conditions of the original game developer and get banned or suspended.
-
You might expose your device to malware or viruses that can harm your data or privacy.
-
-
Therefore, you should use Bus Simulator Indonesia Philippines Mod Apk at your own risk and discretion. We are not responsible for any damages or losses that may occur as a result of using this mod.
-
bussid mod bus philippines apk
-bussid mod philippines map download
-bussid skin traffic philippines apk
-bussid mod philippines victorya linear
-bussid mod philippines truck livery
-bussid mod bus simulator indonesia philippine bus
-bussid mod philippines car download
-bussid mod philippines community join
-bussid mod bus simulator the best philippines
-bussid mod philippines 2023 update
-bussid skin philippines download free
-bussid mod philippines map dtutorial
-bussid mod bus simulator vietnam philippines
-bussid mod philippines exfoh diesel
-bussid mod bus simulator thailand philippines
-bussid mod bus simulator cambodia philippines
-bussid mod bus simulator myanmar philippines
-bussid mod bus simulator malaysia philippines
-bussid skin traffic philippines download latest
-bussid mod bus simulator asia philippines
-bussid weebly mod bus simulator indonesia philippine
-bussid review mod map bus simulator indonesia philippine
-bussid 2023 full strobe livery bus simulator indonesia philippine
-bussid hd 2023 bus simulator indonesia philippine
-bussid mbois 2023 bus simulator indonesia philippine
-bussid sr exfoh ordinary bus simulator indonesia philippine
-bussid tourism bus mod bus simulator indonesia philippine
-bussid damaged road map mod bus simulator indonesia philippine
-bussid muddy road map mod bus simulator indonesia philippine
-bussid dragon bend map mod bus simulator indonesia philippine
-bussid bend 44 map mod bus simulator indonesia philippine
-bussid complete variant map mod bus simulator indonesia philippine
-bussid complete variant skin mod bus simulator indonesia philippine
-bussid complete variant truck mod bus simulator indonesia philippine
-bussid complete variant car mod bus simulator indonesia philippine
-bussid complete variant livery mod bus simulator indonesia philippine
-bussid complete variant traffic mod bus simulator indonesia philippine
-bussid holy grail fusion experiment mini sun bus simulator indonesia philippine (just kidding 😜)
-bussid net energy gain nuclear fusion reaction bus simulator indonesia philippine (also kidding 😂)
-bussid superconducting tokamak advanced research facility korea institute of fusion energy bus simulator indonesia philippine (okay, I'll stop now 😅)
-
How to download and install Bus Simulator Indonesia Philippines Mod Apk on Android
-
If you want to try Bus Simulator Indonesia Philippines Mod Apk on your Android device, you will need to download and install it from a third-party source. This is because this mod is not available on the Google Play Store or any other official app store. Here are the steps to do so:
-
Steps to download and install Bus Simulator Indonesia Philippines Mod Apk
-
-
First, you will need to enable the installation of apps from unknown sources on your device. To do this, go to Settings > Security > Unknown Sources and toggle it on.
-
Next, you will need to download the Bus Simulator Indonesia Philippines Mod Apk file from a reliable website. You can search for it on Google or use this link: . Make sure you download the latest version of the mod that is compatible with your device and the original game.
-
After downloading the file, locate it in your device's file manager and tap on it to start the installation process. Follow the instructions on the screen and wait for the installation to complete.
-
Once the installation is done, you will see a new icon for Bus Simulator Indonesia Philippines Mod Apk on your device's home screen. Tap on it to launch the game.
-
-
Congratulations! You have successfully installed Bus Simulator Indonesia Philippines Mod Apk on your Android device. You can now start playing the game and enjoy driving buses in the Philippines.
How to play Bus Simulator Indonesia Philippines Mod Apk
-
Now that you have downloaded and installed Bus Simulator Indonesia Philippines Mod Apk on your Android device, you might be wondering how to play it. Don't worry, we have got you covered. In this section, we will give you some tips and tricks on how to play Bus Simulator Indonesia Philippines Mod Apk like a pro.
-
How to choose and customize your bus
-
One of the fun aspects of Bus Simulator Indonesia Philippines Mod Apk is that you can choose and customize your own bus. You can do this by tapping on the garage icon on the main menu. Here, you can see a list of buses that you can buy or unlock with coins or diamonds. You can also see the stats and features of each bus, such as speed, acceleration, braking, handling, fuel capacity, etc. To buy or unlock a bus, simply tap on the buy or unlock button and confirm your purchase.
-
Once you have bought or unlocked a bus, you can customize it with different liveries, accessories, horns, stickers, and more. You can do this by tapping on the customize icon on the garage menu. Here, you can see a list of categories that you can modify, such as body, wheels, lights, interior, etc. To customize a category, simply tap on it and choose from the available options. You can also see a preview of how your bus will look like after customization. To apply your changes, simply tap on the apply button and confirm your customization.
-
How to drive and control your bus
-
Another fun aspect of Bus Simulator Indonesia Philippines Mod Apk is that you can drive and control your bus in a realistic way. You can do this by tapping on the drive icon on the main menu. Here, you can see a map of the Philippines where you can choose your starting point and destination. You can also see the distance and time of your trip, as well as the traffic and weather conditions. To start your trip, simply tap on the start button and wait for the loading screen.
-
Once you are in the game, you can see a dashboard that shows your speedometer, fuel gauge, gear indicator, steering wheel, horn button, indicators, headlights, wipers, etc. You can also see a mini-map that shows your location and direction. To control your bus, you can use the following options:
-
-
You can use the tilt option to steer your bus by tilting your device left or right.
-
You can use the buttons option to steer your bus by tapping on the left or right arrow buttons.
-
You can use the steering wheel option to steer your bus by dragging the steering wheel left or right.
-
You can use the accelerator pedal to increase your speed by tapping and holding it.
-
You can use the brake pedal to decrease your speed or stop by tapping and holding it.
-
You can use the handbrake to stop your bus completely by tapping on it.
-
You can use the gear lever to change gears by tapping on it.
-
You can use the horn button to honk your horn by tapping on it.
-
You can use the indicators to signal your turns by tapping on them.
-
You can use the headlights to turn on or off your lights by tapping on them.
-
You can use the wipers to turn on or off your wipers by tapping on them.
-
-
To drive safely and smoothly, you should follow the rules and regulations of Philippine traffic. You should also pay attention to the traffic signs, signals, pedestrians, vehicles, obstacles, etc. that you encounter along the way. You should also avoid crashing or damaging your bus as much as possible.
How to complete missions and earn rewards
-
The last fun aspect of Bus Simulator Indonesia Philippines Mod Apk is that you can complete missions and earn rewards. You can do this by tapping on the mission icon on the main menu. Here, you can see a list of missions that you can accept and complete. Each mission has a different objective, such as transporting passengers, delivering cargo, reaching a destination, etc. Each mission also has a different difficulty level, such as easy, medium, hard, etc. To accept a mission, simply tap on the accept button and start your trip.
-
Once you are in the game, you can see a mission indicator that shows your progress and status. You can also see a timer that shows how much time you have left to complete the mission. To complete a mission, you have to follow the instructions and objectives that are given to you. You have to also avoid failing or aborting the mission by crashing, running out of fuel, breaking the law, etc.
-
When you complete a mission successfully, you will earn rewards such as coins, diamonds, experience points, etc. You can use these rewards to buy or unlock new buses and upgrades, or to access new features and modes. You can also see your rank and achievements on the leaderboard and compare them with other players.
-
Conclusion
-
Bus Simulator Indonesia Philippines Mod Apk is a fun and exciting mod that adds more features and fun to the original Bus Simulator Indonesia game. It allows you to drive buses in the Philippines, instead of Indonesia. You can also choose from different types of buses and customize them. You can also complete missions and earn rewards. However, you should also be aware of the risks of using this mod, such as compatibility issues, bugs, glitches, bans, malware, viruses, etc. You should also use this mod at your own risk and discretion.
-
If you are interested in trying Bus Simulator Indonesia Philippines Mod Apk on your Android device, you can follow the steps that we have provided in this article. We hope that this article has been helpful and informative for you. If you have any questions or feedback, please feel free to leave a comment below. Thank you for reading and happy gaming!
-
FAQs
-
Here are some frequently asked questions about Bus Simulator Indonesia Philippines Mod Apk:
-
-
Q: Is Bus Simulator Indonesia Philippines Mod Apk free?
-
A: Yes, Bus Simulator Indonesia Philippines Mod Apk is free to download and play. However, you might need an internet connection to access some features and modes.
-
Q: Is Bus Simulator Indonesia Philippines Mod Apk safe?
-
A: Bus Simulator Indonesia Philippines Mod Apk is not officially endorsed or supported by the original game developer. Therefore, it might not be safe to use. You should always download and install it from a reliable website and scan it for malware or viruses before using it.
-
Q: Can I play Bus Simulator Indonesia Philippines Mod Apk offline?
-
A: Yes, you can play Bus Simulator Indonesia Philippines Mod Apk offline. However, you might not be able to access some features and modes that require an internet connection.
-
Q: Can I play Bus Simulator Indonesia Philippines Mod Apk with friends?
-
A: Yes, you can play Bus Simulator Indonesia Philippines Mod Apk with friends online through chat and multiplayer mode. However, you might need an internet connection to do so.
-
Q: How can I update Bus Simulator Indonesia Philippines Mod Apk?
-
A: To update Bus Simulator Indonesia Philippines Mod Apk, you will need to download and install the latest version of the mod from a reliable website. You should also backup your data before updating to avoid losing your progress.
-
197e85843d
-
-
\ No newline at end of file
diff --git a/spaces/2023Liu2023/bingo/src/components/toaster.tsx b/spaces/2023Liu2023/bingo/src/components/toaster.tsx
deleted file mode 100644
index 4d2693460b61307a1d4c127fd01df9bee16e59ff..0000000000000000000000000000000000000000
--- a/spaces/2023Liu2023/bingo/src/components/toaster.tsx
+++ /dev/null
@@ -1,3 +0,0 @@
-'use client'
-
-export { Toaster } from 'react-hot-toast'
diff --git a/spaces/221090Lstwcm/textgenerator/README.md b/spaces/221090Lstwcm/textgenerator/README.md
deleted file mode 100644
index 32e619608b009e0b419d34b0274f804aefb15a92..0000000000000000000000000000000000000000
--- a/spaces/221090Lstwcm/textgenerator/README.md
+++ /dev/null
@@ -1,12 +0,0 @@
----
-title: Textgenerator
-emoji: 🌍
-colorFrom: red
-colorTo: blue
-sdk: gradio
-sdk_version: 3.19.1
-app_file: app.py
-pinned: false
----
-
-Check out the configuration reference at https://huggingface.co/docs/hub/spaces-config-reference
diff --git a/spaces/232labs/VToonify/vtoonify/model/encoder/encoders/model_irse.py b/spaces/232labs/VToonify/vtoonify/model/encoder/encoders/model_irse.py
deleted file mode 100644
index 6698d9705321dd4a27681ea15204e9ffaa51f62a..0000000000000000000000000000000000000000
--- a/spaces/232labs/VToonify/vtoonify/model/encoder/encoders/model_irse.py
+++ /dev/null
@@ -1,84 +0,0 @@
-from torch.nn import Linear, Conv2d, BatchNorm1d, BatchNorm2d, PReLU, Dropout, Sequential, Module
-from model.encoder.encoders.helpers import get_blocks, Flatten, bottleneck_IR, bottleneck_IR_SE, l2_norm
-
-"""
-Modified Backbone implementation from [TreB1eN](https://github.com/TreB1eN/InsightFace_Pytorch)
-"""
-
-
-class Backbone(Module):
- def __init__(self, input_size, num_layers, mode='ir', drop_ratio=0.4, affine=True):
- super(Backbone, self).__init__()
- assert input_size in [112, 224], "input_size should be 112 or 224"
- assert num_layers in [50, 100, 152], "num_layers should be 50, 100 or 152"
- assert mode in ['ir', 'ir_se'], "mode should be ir or ir_se"
- blocks = get_blocks(num_layers)
- if mode == 'ir':
- unit_module = bottleneck_IR
- elif mode == 'ir_se':
- unit_module = bottleneck_IR_SE
- self.input_layer = Sequential(Conv2d(3, 64, (3, 3), 1, 1, bias=False),
- BatchNorm2d(64),
- PReLU(64))
- if input_size == 112:
- self.output_layer = Sequential(BatchNorm2d(512),
- Dropout(drop_ratio),
- Flatten(),
- Linear(512 * 7 * 7, 512),
- BatchNorm1d(512, affine=affine))
- else:
- self.output_layer = Sequential(BatchNorm2d(512),
- Dropout(drop_ratio),
- Flatten(),
- Linear(512 * 14 * 14, 512),
- BatchNorm1d(512, affine=affine))
-
- modules = []
- for block in blocks:
- for bottleneck in block:
- modules.append(unit_module(bottleneck.in_channel,
- bottleneck.depth,
- bottleneck.stride))
- self.body = Sequential(*modules)
-
- def forward(self, x):
- x = self.input_layer(x)
- x = self.body(x)
- x = self.output_layer(x)
- return l2_norm(x)
-
-
-def IR_50(input_size):
- """Constructs a ir-50 model."""
- model = Backbone(input_size, num_layers=50, mode='ir', drop_ratio=0.4, affine=False)
- return model
-
-
-def IR_101(input_size):
- """Constructs a ir-101 model."""
- model = Backbone(input_size, num_layers=100, mode='ir', drop_ratio=0.4, affine=False)
- return model
-
-
-def IR_152(input_size):
- """Constructs a ir-152 model."""
- model = Backbone(input_size, num_layers=152, mode='ir', drop_ratio=0.4, affine=False)
- return model
-
-
-def IR_SE_50(input_size):
- """Constructs a ir_se-50 model."""
- model = Backbone(input_size, num_layers=50, mode='ir_se', drop_ratio=0.4, affine=False)
- return model
-
-
-def IR_SE_101(input_size):
- """Constructs a ir_se-101 model."""
- model = Backbone(input_size, num_layers=100, mode='ir_se', drop_ratio=0.4, affine=False)
- return model
-
-
-def IR_SE_152(input_size):
- """Constructs a ir_se-152 model."""
- model = Backbone(input_size, num_layers=152, mode='ir_se', drop_ratio=0.4, affine=False)
- return model
diff --git a/spaces/7hao/bingo/src/pages/api/create.ts b/spaces/7hao/bingo/src/pages/api/create.ts
deleted file mode 100644
index 508fa97ef609cbb215a61085711638e116235ebe..0000000000000000000000000000000000000000
--- a/spaces/7hao/bingo/src/pages/api/create.ts
+++ /dev/null
@@ -1,31 +0,0 @@
-'use server'
-
-import { NextApiRequest, NextApiResponse } from 'next'
-import { fetch, debug } from '@/lib/isomorphic'
-import { createHeaders } from '@/lib/utils'
-
-// const API_ENDPOINT = 'https://www.bing.com/turing/conversation/create'
-const API_ENDPOINT = 'https://edgeservices.bing.com/edgesvc/turing/conversation/create';
-
-export default async function handler(req: NextApiRequest, res: NextApiResponse) {
- try {
- const headers = createHeaders(req.cookies)
-
- res.writeHead(200, {
- 'Content-Type': 'application/json',
- })
-
- debug('headers', headers)
- const response = await fetch(API_ENDPOINT, { method: 'GET', headers })
- .then((res) => res.text())
-
- res.end(response)
- } catch (e) {
- return res.end(JSON.stringify({
- result: {
- value: 'UnauthorizedRequest',
- message: `${e}`
- }
- }))
- }
-}
diff --git a/spaces/ADOPLE/Multi-Doc-Virtual-Chatbot/style.css b/spaces/ADOPLE/Multi-Doc-Virtual-Chatbot/style.css
deleted file mode 100644
index 03cfcd6816530d32c1a8ea6c85547fc277b4c331..0000000000000000000000000000000000000000
--- a/spaces/ADOPLE/Multi-Doc-Virtual-Chatbot/style.css
+++ /dev/null
@@ -1,38 +0,0 @@
-#col-container {
- max-width: 1000px;
- margin-left: auto;
- margin-right: auto;
-}
-.heightfit{
- height:120px;
-}
-
-#row-flex {
- display: flex;
- align-items: center;
- justify-content: center;
-}
-.leftimage .rightimage{
- float:left;
-}
-.leftimage{
- padding-top:27px;
- margin-left:210px;
-}
-.rightimage{
- margin-right:210px;
- margin-top:15px;
-}
-a,
-a:hover,
-a:visited {
- text-decoration-line: underline;
- font-weight: 600;
- color: #1f2937 !important;
-}
-
-.dark a,
-.dark a:hover,
-.dark a:visited {
- color: #f3f4f6 !important;
-}
diff --git a/spaces/AI-Dashboards/Graph.NLP.Sentence.Similarity.Heatmap.KMeansCluster/README.md b/spaces/AI-Dashboards/Graph.NLP.Sentence.Similarity.Heatmap.KMeansCluster/README.md
deleted file mode 100644
index 588cca7b119e55469da63891f957e82cf529cccf..0000000000000000000000000000000000000000
--- a/spaces/AI-Dashboards/Graph.NLP.Sentence.Similarity.Heatmap.KMeansCluster/README.md
+++ /dev/null
@@ -1,13 +0,0 @@
----
-title: 06SL NLP SentenceSimilarity Heatmap Cluster
-emoji: 📊
-colorFrom: indigo
-colorTo: pink
-sdk: streamlit
-sdk_version: 1.10.0
-app_file: app.py
-pinned: false
-license: mit
----
-
-Check out the configuration reference at https://huggingface.co/docs/hub/spaces-config-reference
diff --git a/spaces/AIGText/GlyphControl/ldm/modules/diffusionmodules/model.py b/spaces/AIGText/GlyphControl/ldm/modules/diffusionmodules/model.py
deleted file mode 100644
index 4e6453cb35ecb3b9106f5d658244532c5ec2f1e6..0000000000000000000000000000000000000000
--- a/spaces/AIGText/GlyphControl/ldm/modules/diffusionmodules/model.py
+++ /dev/null
@@ -1,853 +0,0 @@
-# pytorch_diffusion + derived encoder decoder
-import math
-import torch
-import torch.nn as nn
-import numpy as np
-from einops import rearrange
-from typing import Optional, Any
-
-from ldm.modules.attention import MemoryEfficientCrossAttention
-
-try:
- import xformers
- import xformers.ops
- XFORMERS_IS_AVAILBLE = True
-except Exception as e:
- print("xformer", e)
- XFORMERS_IS_AVAILBLE = False
- print("No module 'xformers'. Proceeding without it.")
-# XFORMERS_IS_AVAILBLE = False
-
-def get_timestep_embedding(timesteps, embedding_dim):
- """
- This matches the implementation in Denoising Diffusion Probabilistic Models:
- From Fairseq.
- Build sinusoidal embeddings.
- This matches the implementation in tensor2tensor, but differs slightly
- from the description in Section 3.5 of "Attention Is All You Need".
- """
- assert len(timesteps.shape) == 1
-
- half_dim = embedding_dim // 2
- emb = math.log(10000) / (half_dim - 1)
- emb = torch.exp(torch.arange(half_dim, dtype=torch.float32) * -emb)
- emb = emb.to(device=timesteps.device)
- emb = timesteps.float()[:, None] * emb[None, :]
- emb = torch.cat([torch.sin(emb), torch.cos(emb)], dim=1)
- if embedding_dim % 2 == 1: # zero pad
- emb = torch.nn.functional.pad(emb, (0,1,0,0))
- return emb
-
-
-def nonlinearity(x):
- # swish
- return x*torch.sigmoid(x)
-
-
-def Normalize(in_channels, num_groups=32):
- return torch.nn.GroupNorm(num_groups=num_groups, num_channels=in_channels, eps=1e-6, affine=True)
-
-
-class Upsample(nn.Module):
- def __init__(self, in_channels, with_conv):
- super().__init__()
- self.with_conv = with_conv
- if self.with_conv:
- self.conv = torch.nn.Conv2d(in_channels,
- in_channels,
- kernel_size=3,
- stride=1,
- padding=1)
-
- def forward(self, x):
- x = torch.nn.functional.interpolate(x, scale_factor=2.0, mode="nearest")
- if self.with_conv:
- x = self.conv(x)
- return x
-
-
-class Downsample(nn.Module):
- def __init__(self, in_channels, with_conv):
- super().__init__()
- self.with_conv = with_conv
- if self.with_conv:
- # no asymmetric padding in torch conv, must do it ourselves
- self.conv = torch.nn.Conv2d(in_channels,
- in_channels,
- kernel_size=3,
- stride=2,
- padding=0)
-
- def forward(self, x):
- if self.with_conv:
- pad = (0,1,0,1)
- x = torch.nn.functional.pad(x, pad, mode="constant", value=0)
- x = self.conv(x)
- else:
- x = torch.nn.functional.avg_pool2d(x, kernel_size=2, stride=2)
- return x
-
-
-class ResnetBlock(nn.Module):
- def __init__(self, *, in_channels, out_channels=None, conv_shortcut=False,
- dropout, temb_channels=512):
- super().__init__()
- self.in_channels = in_channels
- out_channels = in_channels if out_channels is None else out_channels
- self.out_channels = out_channels
- self.use_conv_shortcut = conv_shortcut
-
- self.norm1 = Normalize(in_channels)
- self.conv1 = torch.nn.Conv2d(in_channels,
- out_channels,
- kernel_size=3,
- stride=1,
- padding=1)
- if temb_channels > 0:
- self.temb_proj = torch.nn.Linear(temb_channels,
- out_channels)
- self.norm2 = Normalize(out_channels)
- self.dropout = torch.nn.Dropout(dropout)
- self.conv2 = torch.nn.Conv2d(out_channels,
- out_channels,
- kernel_size=3,
- stride=1,
- padding=1)
- if self.in_channels != self.out_channels:
- if self.use_conv_shortcut:
- self.conv_shortcut = torch.nn.Conv2d(in_channels,
- out_channels,
- kernel_size=3,
- stride=1,
- padding=1)
- else:
- self.nin_shortcut = torch.nn.Conv2d(in_channels,
- out_channels,
- kernel_size=1,
- stride=1,
- padding=0)
-
- def forward(self, x, temb):
- h = x
- h = self.norm1(h)
- h = nonlinearity(h)
- h = self.conv1(h)
-
- if temb is not None:
- h = h + self.temb_proj(nonlinearity(temb))[:,:,None,None]
-
- h = self.norm2(h)
- h = nonlinearity(h)
- h = self.dropout(h)
- h = self.conv2(h)
-
- if self.in_channels != self.out_channels:
- if self.use_conv_shortcut:
- x = self.conv_shortcut(x)
- else:
- x = self.nin_shortcut(x)
-
- return x+h
-
-
-class AttnBlock(nn.Module):
- def __init__(self, in_channels):
- super().__init__()
- self.in_channels = in_channels
-
- self.norm = Normalize(in_channels)
- self.q = torch.nn.Conv2d(in_channels,
- in_channels,
- kernel_size=1,
- stride=1,
- padding=0)
- self.k = torch.nn.Conv2d(in_channels,
- in_channels,
- kernel_size=1,
- stride=1,
- padding=0)
- self.v = torch.nn.Conv2d(in_channels,
- in_channels,
- kernel_size=1,
- stride=1,
- padding=0)
- self.proj_out = torch.nn.Conv2d(in_channels,
- in_channels,
- kernel_size=1,
- stride=1,
- padding=0)
-
- def forward(self, x):
- h_ = x
- h_ = self.norm(h_)
- q = self.q(h_)
- k = self.k(h_)
- v = self.v(h_)
-
- # compute attention
- b,c,h,w = q.shape
- q = q.reshape(b,c,h*w)
- q = q.permute(0,2,1) # b,hw,c
- k = k.reshape(b,c,h*w) # b,c,hw
- w_ = torch.bmm(q,k) # b,hw,hw w[b,i,j]=sum_c q[b,i,c]k[b,c,j]
- w_ = w_ * (int(c)**(-0.5))
- w_ = torch.nn.functional.softmax(w_, dim=2)
-
- # attend to values
- v = v.reshape(b,c,h*w)
- w_ = w_.permute(0,2,1) # b,hw,hw (first hw of k, second of q)
- h_ = torch.bmm(v,w_) # b, c,hw (hw of q) h_[b,c,j] = sum_i v[b,c,i] w_[b,i,j]
- h_ = h_.reshape(b,c,h,w)
-
- h_ = self.proj_out(h_)
-
- return x+h_
-
-class MemoryEfficientAttnBlock(nn.Module):
- """
- Uses xformers efficient implementation,
- see https://github.com/MatthieuTPHR/diffusers/blob/d80b531ff8060ec1ea982b65a1b8df70f73aa67c/src/diffusers/models/attention.py#L223
- Note: this is a single-head self-attention operation
- """
- #
- def __init__(self, in_channels):
- super().__init__()
- self.in_channels = in_channels
-
- self.norm = Normalize(in_channels)
- self.q = torch.nn.Conv2d(in_channels,
- in_channels,
- kernel_size=1,
- stride=1,
- padding=0)
- self.k = torch.nn.Conv2d(in_channels,
- in_channels,
- kernel_size=1,
- stride=1,
- padding=0)
- self.v = torch.nn.Conv2d(in_channels,
- in_channels,
- kernel_size=1,
- stride=1,
- padding=0)
- self.proj_out = torch.nn.Conv2d(in_channels,
- in_channels,
- kernel_size=1,
- stride=1,
- padding=0)
- self.attention_op: Optional[Any] = None
-
- def forward(self, x):
- h_ = x
- h_ = self.norm(h_)
- q = self.q(h_)
- k = self.k(h_)
- v = self.v(h_)
-
- # compute attention
- B, C, H, W = q.shape
- q, k, v = map(lambda x: rearrange(x, 'b c h w -> b (h w) c'), (q, k, v))
-
- q, k, v = map(
- lambda t: t.unsqueeze(3)
- .reshape(B, t.shape[1], 1, C)
- .permute(0, 2, 1, 3)
- .reshape(B * 1, t.shape[1], C)
- .contiguous(),
- (q, k, v),
- )
- out = xformers.ops.memory_efficient_attention(q, k, v, attn_bias=None, op=self.attention_op)
-
- out = (
- out.unsqueeze(0)
- .reshape(B, 1, out.shape[1], C)
- .permute(0, 2, 1, 3)
- .reshape(B, out.shape[1], C)
- )
- out = rearrange(out, 'b (h w) c -> b c h w', b=B, h=H, w=W, c=C)
- out = self.proj_out(out)
- return x+out
-
-
-class MemoryEfficientCrossAttentionWrapper(MemoryEfficientCrossAttention):
- def forward(self, x, context=None, mask=None):
- b, c, h, w = x.shape
- x = rearrange(x, 'b c h w -> b (h w) c')
- out = super().forward(x, context=context, mask=mask)
- out = rearrange(out, 'b (h w) c -> b c h w', h=h, w=w, c=c)
- return x + out
-
-
-def make_attn(in_channels, attn_type="vanilla", attn_kwargs=None):
- assert attn_type in ["vanilla", "vanilla-xformers", "memory-efficient-cross-attn", "linear", "none"], f'attn_type {attn_type} unknown'
- if XFORMERS_IS_AVAILBLE and attn_type == "vanilla":
- attn_type = "vanilla-xformers"
- print(f"making attention of type '{attn_type}' with {in_channels} in_channels")
- if attn_type == "vanilla":
- assert attn_kwargs is None
- return AttnBlock(in_channels)
- elif attn_type == "vanilla-xformers":
- print(f"building MemoryEfficientAttnBlock with {in_channels} in_channels...")
- return MemoryEfficientAttnBlock(in_channels)
- elif attn_type == "memory-efficient-cross-attn":
- attn_kwargs["query_dim"] = in_channels
- return MemoryEfficientCrossAttentionWrapper(**attn_kwargs)
- elif attn_type == "none":
- return nn.Identity(in_channels)
- else:
- raise NotImplementedError()
-
-
-class Model(nn.Module):
- def __init__(self, *, ch, out_ch, ch_mult=(1,2,4,8), num_res_blocks,
- attn_resolutions, dropout=0.0, resamp_with_conv=True, in_channels,
- resolution, use_timestep=True, use_linear_attn=False, attn_type="vanilla"):
- super().__init__()
- if use_linear_attn: attn_type = "linear"
- self.ch = ch
- self.temb_ch = self.ch*4
- self.num_resolutions = len(ch_mult)
- self.num_res_blocks = num_res_blocks
- self.resolution = resolution
- self.in_channels = in_channels
-
- self.use_timestep = use_timestep
- if self.use_timestep:
- # timestep embedding
- self.temb = nn.Module()
- self.temb.dense = nn.ModuleList([
- torch.nn.Linear(self.ch,
- self.temb_ch),
- torch.nn.Linear(self.temb_ch,
- self.temb_ch),
- ])
-
- # downsampling
- self.conv_in = torch.nn.Conv2d(in_channels,
- self.ch,
- kernel_size=3,
- stride=1,
- padding=1)
-
- curr_res = resolution
- in_ch_mult = (1,)+tuple(ch_mult)
- self.down = nn.ModuleList()
- for i_level in range(self.num_resolutions):
- block = nn.ModuleList()
- attn = nn.ModuleList()
- block_in = ch*in_ch_mult[i_level]
- block_out = ch*ch_mult[i_level]
- for i_block in range(self.num_res_blocks):
- block.append(ResnetBlock(in_channels=block_in,
- out_channels=block_out,
- temb_channels=self.temb_ch,
- dropout=dropout))
- block_in = block_out
- if curr_res in attn_resolutions:
- attn.append(make_attn(block_in, attn_type=attn_type))
- down = nn.Module()
- down.block = block
- down.attn = attn
- if i_level != self.num_resolutions-1:
- down.downsample = Downsample(block_in, resamp_with_conv)
- curr_res = curr_res // 2
- self.down.append(down)
-
- # middle
- self.mid = nn.Module()
- self.mid.block_1 = ResnetBlock(in_channels=block_in,
- out_channels=block_in,
- temb_channels=self.temb_ch,
- dropout=dropout)
- self.mid.attn_1 = make_attn(block_in, attn_type=attn_type)
- self.mid.block_2 = ResnetBlock(in_channels=block_in,
- out_channels=block_in,
- temb_channels=self.temb_ch,
- dropout=dropout)
-
- # upsampling
- self.up = nn.ModuleList()
- for i_level in reversed(range(self.num_resolutions)):
- block = nn.ModuleList()
- attn = nn.ModuleList()
- block_out = ch*ch_mult[i_level]
- skip_in = ch*ch_mult[i_level]
- for i_block in range(self.num_res_blocks+1):
- if i_block == self.num_res_blocks:
- skip_in = ch*in_ch_mult[i_level]
- block.append(ResnetBlock(in_channels=block_in+skip_in,
- out_channels=block_out,
- temb_channels=self.temb_ch,
- dropout=dropout))
- block_in = block_out
- if curr_res in attn_resolutions:
- attn.append(make_attn(block_in, attn_type=attn_type))
- up = nn.Module()
- up.block = block
- up.attn = attn
- if i_level != 0:
- up.upsample = Upsample(block_in, resamp_with_conv)
- curr_res = curr_res * 2
- self.up.insert(0, up) # prepend to get consistent order
-
- # end
- self.norm_out = Normalize(block_in)
- self.conv_out = torch.nn.Conv2d(block_in,
- out_ch,
- kernel_size=3,
- stride=1,
- padding=1)
-
- def forward(self, x, t=None, context=None):
- #assert x.shape[2] == x.shape[3] == self.resolution
- if context is not None:
- # assume aligned context, cat along channel axis
- x = torch.cat((x, context), dim=1)
- if self.use_timestep:
- # timestep embedding
- assert t is not None
- temb = get_timestep_embedding(t, self.ch)
- temb = self.temb.dense[0](temb)
- temb = nonlinearity(temb)
- temb = self.temb.dense[1](temb)
- else:
- temb = None
-
- # downsampling
- hs = [self.conv_in(x)]
- for i_level in range(self.num_resolutions):
- for i_block in range(self.num_res_blocks):
- h = self.down[i_level].block[i_block](hs[-1], temb)
- if len(self.down[i_level].attn) > 0:
- h = self.down[i_level].attn[i_block](h)
- hs.append(h)
- if i_level != self.num_resolutions-1:
- hs.append(self.down[i_level].downsample(hs[-1]))
-
- # middle
- h = hs[-1]
- h = self.mid.block_1(h, temb)
- h = self.mid.attn_1(h)
- h = self.mid.block_2(h, temb)
-
- # upsampling
- for i_level in reversed(range(self.num_resolutions)):
- for i_block in range(self.num_res_blocks+1):
- h = self.up[i_level].block[i_block](
- torch.cat([h, hs.pop()], dim=1), temb)
- if len(self.up[i_level].attn) > 0:
- h = self.up[i_level].attn[i_block](h)
- if i_level != 0:
- h = self.up[i_level].upsample(h)
-
- # end
- h = self.norm_out(h)
- h = nonlinearity(h)
- h = self.conv_out(h)
- return h
-
- def get_last_layer(self):
- return self.conv_out.weight
-
-
-class Encoder(nn.Module):
- def __init__(self, *, ch, out_ch, ch_mult=(1,2,4,8), num_res_blocks,
- attn_resolutions, dropout=0.0, resamp_with_conv=True, in_channels,
- resolution, z_channels, double_z=True, use_linear_attn=False, attn_type="vanilla",
- **ignore_kwargs):
- super().__init__()
- if use_linear_attn: attn_type = "linear"
- self.ch = ch
- self.temb_ch = 0
- self.num_resolutions = len(ch_mult)
- self.num_res_blocks = num_res_blocks
- self.resolution = resolution
- self.in_channels = in_channels
-
- # downsampling
- self.conv_in = torch.nn.Conv2d(in_channels,
- self.ch,
- kernel_size=3,
- stride=1,
- padding=1)
-
- curr_res = resolution
- in_ch_mult = (1,)+tuple(ch_mult)
- self.in_ch_mult = in_ch_mult
- self.down = nn.ModuleList()
- for i_level in range(self.num_resolutions):
- block = nn.ModuleList()
- attn = nn.ModuleList()
- block_in = ch*in_ch_mult[i_level]
- block_out = ch*ch_mult[i_level]
- for i_block in range(self.num_res_blocks):
- block.append(ResnetBlock(in_channels=block_in,
- out_channels=block_out,
- temb_channels=self.temb_ch,
- dropout=dropout))
- block_in = block_out
- if curr_res in attn_resolutions:
- attn.append(make_attn(block_in, attn_type=attn_type))
- down = nn.Module()
- down.block = block
- down.attn = attn
- if i_level != self.num_resolutions-1:
- down.downsample = Downsample(block_in, resamp_with_conv)
- curr_res = curr_res // 2
- self.down.append(down)
-
- # middle
- self.mid = nn.Module()
- self.mid.block_1 = ResnetBlock(in_channels=block_in,
- out_channels=block_in,
- temb_channels=self.temb_ch,
- dropout=dropout)
- self.mid.attn_1 = make_attn(block_in, attn_type=attn_type)
- self.mid.block_2 = ResnetBlock(in_channels=block_in,
- out_channels=block_in,
- temb_channels=self.temb_ch,
- dropout=dropout)
-
- # end
- self.norm_out = Normalize(block_in)
- self.conv_out = torch.nn.Conv2d(block_in,
- 2*z_channels if double_z else z_channels,
- kernel_size=3,
- stride=1,
- padding=1)
-
- def forward(self, x):
- # timestep embedding
- temb = None
-
- # downsampling
- hs = [self.conv_in(x)]
- for i_level in range(self.num_resolutions):
- for i_block in range(self.num_res_blocks):
- h = self.down[i_level].block[i_block](hs[-1], temb)
- if len(self.down[i_level].attn) > 0:
- h = self.down[i_level].attn[i_block](h)
- hs.append(h)
- if i_level != self.num_resolutions-1:
- hs.append(self.down[i_level].downsample(hs[-1]))
-
- # middle
- h = hs[-1]
- h = self.mid.block_1(h, temb)
- h = self.mid.attn_1(h)
- h = self.mid.block_2(h, temb)
-
- # end
- h = self.norm_out(h)
- h = nonlinearity(h)
- h = self.conv_out(h)
- return h
-
-
-class Decoder(nn.Module):
- def __init__(self, *, ch, out_ch, ch_mult=(1,2,4,8), num_res_blocks,
- attn_resolutions, dropout=0.0, resamp_with_conv=True, in_channels,
- resolution, z_channels, give_pre_end=False, tanh_out=False, use_linear_attn=False,
- attn_type="vanilla", **ignorekwargs):
- super().__init__()
- if use_linear_attn: attn_type = "linear"
- self.ch = ch
- self.temb_ch = 0
- self.num_resolutions = len(ch_mult)
- self.num_res_blocks = num_res_blocks
- self.resolution = resolution
- self.in_channels = in_channels
- self.give_pre_end = give_pre_end
- self.tanh_out = tanh_out
-
- # compute in_ch_mult, block_in and curr_res at lowest res
- in_ch_mult = (1,)+tuple(ch_mult)
- block_in = ch*ch_mult[self.num_resolutions-1]
- curr_res = resolution // 2**(self.num_resolutions-1)
- self.z_shape = (1,z_channels,curr_res,curr_res)
- print("Working with z of shape {} = {} dimensions.".format(
- self.z_shape, np.prod(self.z_shape)))
-
- # z to block_in
- self.conv_in = torch.nn.Conv2d(z_channels,
- block_in,
- kernel_size=3,
- stride=1,
- padding=1)
-
- # middle
- self.mid = nn.Module()
- self.mid.block_1 = ResnetBlock(in_channels=block_in,
- out_channels=block_in,
- temb_channels=self.temb_ch,
- dropout=dropout)
- self.mid.attn_1 = make_attn(block_in, attn_type=attn_type)
- self.mid.block_2 = ResnetBlock(in_channels=block_in,
- out_channels=block_in,
- temb_channels=self.temb_ch,
- dropout=dropout)
-
- # upsampling
- self.up = nn.ModuleList()
- for i_level in reversed(range(self.num_resolutions)):
- block = nn.ModuleList()
- attn = nn.ModuleList()
- block_out = ch*ch_mult[i_level]
- for i_block in range(self.num_res_blocks+1):
- block.append(ResnetBlock(in_channels=block_in,
- out_channels=block_out,
- temb_channels=self.temb_ch,
- dropout=dropout))
- block_in = block_out
- if curr_res in attn_resolutions:
- attn.append(make_attn(block_in, attn_type=attn_type))
- up = nn.Module()
- up.block = block
- up.attn = attn
- if i_level != 0:
- up.upsample = Upsample(block_in, resamp_with_conv)
- curr_res = curr_res * 2
- self.up.insert(0, up) # prepend to get consistent order
-
- # end
- self.norm_out = Normalize(block_in)
- self.conv_out = torch.nn.Conv2d(block_in,
- out_ch,
- kernel_size=3,
- stride=1,
- padding=1)
-
- def forward(self, z):
- #assert z.shape[1:] == self.z_shape[1:]
- self.last_z_shape = z.shape
-
- # timestep embedding
- temb = None
-
- # z to block_in
- h = self.conv_in(z)
-
- # middle
- h = self.mid.block_1(h, temb)
- h = self.mid.attn_1(h)
- h = self.mid.block_2(h, temb)
-
- # upsampling
- for i_level in reversed(range(self.num_resolutions)):
- for i_block in range(self.num_res_blocks+1):
- h = self.up[i_level].block[i_block](h, temb)
- if len(self.up[i_level].attn) > 0:
- h = self.up[i_level].attn[i_block](h)
- if i_level != 0:
- h = self.up[i_level].upsample(h)
-
- # end
- if self.give_pre_end:
- return h
-
- h = self.norm_out(h)
- h = nonlinearity(h)
- h = self.conv_out(h)
- if self.tanh_out:
- h = torch.tanh(h)
- return h
-
-
-class SimpleDecoder(nn.Module):
- def __init__(self, in_channels, out_channels, *args, **kwargs):
- super().__init__()
- self.model = nn.ModuleList([nn.Conv2d(in_channels, in_channels, 1),
- ResnetBlock(in_channels=in_channels,
- out_channels=2 * in_channels,
- temb_channels=0, dropout=0.0),
- ResnetBlock(in_channels=2 * in_channels,
- out_channels=4 * in_channels,
- temb_channels=0, dropout=0.0),
- ResnetBlock(in_channels=4 * in_channels,
- out_channels=2 * in_channels,
- temb_channels=0, dropout=0.0),
- nn.Conv2d(2*in_channels, in_channels, 1),
- Upsample(in_channels, with_conv=True)])
- # end
- self.norm_out = Normalize(in_channels)
- self.conv_out = torch.nn.Conv2d(in_channels,
- out_channels,
- kernel_size=3,
- stride=1,
- padding=1)
-
- def forward(self, x):
- for i, layer in enumerate(self.model):
- if i in [1,2,3]:
- x = layer(x, None)
- else:
- x = layer(x)
-
- h = self.norm_out(x)
- h = nonlinearity(h)
- x = self.conv_out(h)
- return x
-
-
-class UpsampleDecoder(nn.Module):
- def __init__(self, in_channels, out_channels, ch, num_res_blocks, resolution,
- ch_mult=(2,2), dropout=0.0):
- super().__init__()
- # upsampling
- self.temb_ch = 0
- self.num_resolutions = len(ch_mult)
- self.num_res_blocks = num_res_blocks
- block_in = in_channels
- curr_res = resolution // 2 ** (self.num_resolutions - 1)
- self.res_blocks = nn.ModuleList()
- self.upsample_blocks = nn.ModuleList()
- for i_level in range(self.num_resolutions):
- res_block = []
- block_out = ch * ch_mult[i_level]
- for i_block in range(self.num_res_blocks + 1):
- res_block.append(ResnetBlock(in_channels=block_in,
- out_channels=block_out,
- temb_channels=self.temb_ch,
- dropout=dropout))
- block_in = block_out
- self.res_blocks.append(nn.ModuleList(res_block))
- if i_level != self.num_resolutions - 1:
- self.upsample_blocks.append(Upsample(block_in, True))
- curr_res = curr_res * 2
-
- # end
- self.norm_out = Normalize(block_in)
- self.conv_out = torch.nn.Conv2d(block_in,
- out_channels,
- kernel_size=3,
- stride=1,
- padding=1)
-
- def forward(self, x):
- # upsampling
- h = x
- for k, i_level in enumerate(range(self.num_resolutions)):
- for i_block in range(self.num_res_blocks + 1):
- h = self.res_blocks[i_level][i_block](h, None)
- if i_level != self.num_resolutions - 1:
- h = self.upsample_blocks[k](h)
- h = self.norm_out(h)
- h = nonlinearity(h)
- h = self.conv_out(h)
- return h
-
-
-class LatentRescaler(nn.Module):
- def __init__(self, factor, in_channels, mid_channels, out_channels, depth=2):
- super().__init__()
- # residual block, interpolate, residual block
- self.factor = factor
- self.conv_in = nn.Conv2d(in_channels,
- mid_channels,
- kernel_size=3,
- stride=1,
- padding=1)
- self.res_block1 = nn.ModuleList([ResnetBlock(in_channels=mid_channels,
- out_channels=mid_channels,
- temb_channels=0,
- dropout=0.0) for _ in range(depth)])
- self.attn = AttnBlock(mid_channels)
- self.res_block2 = nn.ModuleList([ResnetBlock(in_channels=mid_channels,
- out_channels=mid_channels,
- temb_channels=0,
- dropout=0.0) for _ in range(depth)])
-
- self.conv_out = nn.Conv2d(mid_channels,
- out_channels,
- kernel_size=1,
- )
-
- def forward(self, x):
- x = self.conv_in(x)
- for block in self.res_block1:
- x = block(x, None)
- x = torch.nn.functional.interpolate(x, size=(int(round(x.shape[2]*self.factor)), int(round(x.shape[3]*self.factor))))
- x = self.attn(x)
- for block in self.res_block2:
- x = block(x, None)
- x = self.conv_out(x)
- return x
-
-
-class MergedRescaleEncoder(nn.Module):
- def __init__(self, in_channels, ch, resolution, out_ch, num_res_blocks,
- attn_resolutions, dropout=0.0, resamp_with_conv=True,
- ch_mult=(1,2,4,8), rescale_factor=1.0, rescale_module_depth=1):
- super().__init__()
- intermediate_chn = ch * ch_mult[-1]
- self.encoder = Encoder(in_channels=in_channels, num_res_blocks=num_res_blocks, ch=ch, ch_mult=ch_mult,
- z_channels=intermediate_chn, double_z=False, resolution=resolution,
- attn_resolutions=attn_resolutions, dropout=dropout, resamp_with_conv=resamp_with_conv,
- out_ch=None)
- self.rescaler = LatentRescaler(factor=rescale_factor, in_channels=intermediate_chn,
- mid_channels=intermediate_chn, out_channels=out_ch, depth=rescale_module_depth)
-
- def forward(self, x):
- x = self.encoder(x)
- x = self.rescaler(x)
- return x
-
-
-class MergedRescaleDecoder(nn.Module):
- def __init__(self, z_channels, out_ch, resolution, num_res_blocks, attn_resolutions, ch, ch_mult=(1,2,4,8),
- dropout=0.0, resamp_with_conv=True, rescale_factor=1.0, rescale_module_depth=1):
- super().__init__()
- tmp_chn = z_channels*ch_mult[-1]
- self.decoder = Decoder(out_ch=out_ch, z_channels=tmp_chn, attn_resolutions=attn_resolutions, dropout=dropout,
- resamp_with_conv=resamp_with_conv, in_channels=None, num_res_blocks=num_res_blocks,
- ch_mult=ch_mult, resolution=resolution, ch=ch)
- self.rescaler = LatentRescaler(factor=rescale_factor, in_channels=z_channels, mid_channels=tmp_chn,
- out_channels=tmp_chn, depth=rescale_module_depth)
-
- def forward(self, x):
- x = self.rescaler(x)
- x = self.decoder(x)
- return x
-
-
-class Upsampler(nn.Module):
- def __init__(self, in_size, out_size, in_channels, out_channels, ch_mult=2):
- super().__init__()
- assert out_size >= in_size
- num_blocks = int(np.log2(out_size//in_size))+1
- factor_up = 1.+ (out_size % in_size)
- print(f"Building {self.__class__.__name__} with in_size: {in_size} --> out_size {out_size} and factor {factor_up}")
- self.rescaler = LatentRescaler(factor=factor_up, in_channels=in_channels, mid_channels=2*in_channels,
- out_channels=in_channels)
- self.decoder = Decoder(out_ch=out_channels, resolution=out_size, z_channels=in_channels, num_res_blocks=2,
- attn_resolutions=[], in_channels=None, ch=in_channels,
- ch_mult=[ch_mult for _ in range(num_blocks)])
-
- def forward(self, x):
- x = self.rescaler(x)
- x = self.decoder(x)
- return x
-
-
-class Resize(nn.Module):
- def __init__(self, in_channels=None, learned=False, mode="bilinear"):
- super().__init__()
- self.with_conv = learned
- self.mode = mode
- if self.with_conv:
- print(f"Note: {self.__class__.__name} uses learned downsampling and will ignore the fixed {mode} mode")
- raise NotImplementedError()
- assert in_channels is not None
- # no asymmetric padding in torch conv, must do it ourselves
- self.conv = torch.nn.Conv2d(in_channels,
- in_channels,
- kernel_size=4,
- stride=2,
- padding=1)
-
- def forward(self, x, scale_factor=1.0):
- if scale_factor==1.0:
- return x
- else:
- x = torch.nn.functional.interpolate(x, mode=self.mode, align_corners=False, scale_factor=scale_factor)
- return x
diff --git a/spaces/AIGText/GlyphControl/ldm/modules/encoders/modules.py b/spaces/AIGText/GlyphControl/ldm/modules/encoders/modules.py
deleted file mode 100644
index 0a4c77b8c77cf847b5cf0a330ea81f47adb3391d..0000000000000000000000000000000000000000
--- a/spaces/AIGText/GlyphControl/ldm/modules/encoders/modules.py
+++ /dev/null
@@ -1,459 +0,0 @@
-import torch
-import torch.nn as nn
-from torch.utils.checkpoint import checkpoint
-
-from transformers import T5Tokenizer, T5EncoderModel, CLIPTokenizer, CLIPTextModel, T5ForConditionalGeneration, AutoTokenizer, ByT5Tokenizer
-from transformers import AutoProcessor, CLIPVisionModel
-import open_clip
-from ldm.util import default, count_params, islistortuple
-from transformers import PreTrainedTokenizerBase
-from ldm.modules.diffusionmodules.util import zero_module, identity_init_fc
-class AbstractEncoder(nn.Module):
- def __init__(self):
- super().__init__()
-
- def encode(self, *args, **kwargs):
- raise NotImplementedError
-
-
-class IdentityEncoder(AbstractEncoder):
-
- def encode(self, x):
- return x
-
-
-class ClassEmbedder(nn.Module):
- def __init__(self, embed_dim, n_classes=1000, key='class', ucg_rate=0.1):
- super().__init__()
- self.key = key
- self.embedding = nn.Embedding(n_classes, embed_dim)
- self.n_classes = n_classes
- self.ucg_rate = ucg_rate
-
- def forward(self, batch, key=None, disable_dropout=False):
- if key is None:
- key = self.key
- # this is for use in crossattn
- c = batch[key][:, None]
- if self.ucg_rate > 0. and not disable_dropout:
- mask = 1. - torch.bernoulli(torch.ones_like(c) * self.ucg_rate)
- c = mask * c + (1-mask) * torch.ones_like(c)*(self.n_classes-1)
- c = c.long()
- c = self.embedding(c)
- return c
-
- def get_unconditional_conditioning(self, bs, device="cuda"):
- uc_class = self.n_classes - 1 # 1000 classes --> 0 ... 999, one extra class for ucg (class 1000)
- uc = torch.ones((bs,), device=device) * uc_class
- uc = {self.key: uc}
- return uc
-
-
-def disabled_train(self, mode=True):
- """Overwrite model.train with this function to make sure train/eval mode
- does not change anymore."""
- return self
-
-
-class FrozenT5Embedder_old(AbstractEncoder):
- """Uses the T5 transformer encoder for text"""
- def __init__(self, version="google/t5-v1_1-large", device="cuda", max_length=77, freeze=True): # others are google/t5-v1_1-xl and google/t5-v1_1-xxl
- super().__init__()
- self.tokenizer = T5Tokenizer.from_pretrained(version)
- self.transformer = T5EncoderModel.from_pretrained(version)
- self.device = device
- self.max_length = max_length # TODO: typical value?
- if freeze:
- self.freeze()
-
- def freeze(self):
- self.transformer = self.transformer.eval()
- #self.train = disabled_train
- for param in self.parameters():
- param.requires_grad = False
-
- def forward(self, text):
- batch_encoding = self.tokenizer(text, truncation=True, max_length=self.max_length, return_length=True,
- return_overflowing_tokens=False, padding="max_length", return_tensors="pt")
- tokens = batch_encoding["input_ids"].to(self.device)
- outputs = self.transformer(input_ids=tokens)
-
- z = outputs.last_hidden_state
- return z
-
- def encode(self, text):
- return self(text)
-
-class FrozenT5Embedder(AbstractEncoder):
- """Uses the T5/ByT5 transformer encoder for text"""
- def __init__(self, version="google/t5-v1_1-large", device="cuda", max_length=77, freeze=True, padding="max_length"):
- # version: others for T5 are google/t5-v1_1-xl, google/t5-v1_1-xxl, google/t5-v1_1-small, google/t5-v1_1-base and google/t5-v1_1-large
- # for ByT5 are google/byt5-small, google/byt5-base, google/byt5-large, google/byt5-xl and google/byt5-xxl
- # padding: "max_length" or "longest"
- # https://huggingface.co/docs/transformers/v4.24.0/en/internal/tokenization_utils#transformers.PreTrainedTokenizerBase
- super().__init__()
- self.tokenizer = T5Tokenizer.from_pretrained(version) if "byt5" not in version else ByT5Tokenizer.from_pretrained(version)
- self.transformer = T5EncoderModel.from_pretrained(version)
- self.device = device
- self.max_length = max_length # TODO: typical value?
- self.padding = padding
- if freeze:
- self.freeze()
-
- def freeze(self):
- self.transformer = self.transformer.eval()
- #self.train = disabled_train
- for param in self.parameters():
- param.requires_grad = False
-
- def forward(self, text):
- batch_encoding = self.tokenizer(text, truncation=True, max_length=self.max_length, return_length=True,
- return_overflowing_tokens=False, padding=self.padding, return_tensors="pt")
- tokens = batch_encoding["input_ids"].to(self.device)
- outputs = self.transformer(input_ids=tokens)
-
- z = outputs.last_hidden_state
- return z
-
- def encode(self, text):
- return self(text)
-
-class FrozenCLIPEmbedder(AbstractEncoder):
- """Uses the CLIP transformer encoder for text (from huggingface)"""
- LAYERS = [
- "last",
- "pooled",
- "hidden"
- ]
- def __init__(self, version="openai/clip-vit-large-patch14", device="cuda", max_length=77,
- freeze=True, layer="last", layer_idx=None): # clip-vit-base-patch32
- super().__init__()
- assert layer in self.LAYERS
- self.tokenizer = CLIPTokenizer.from_pretrained(version)
- self.transformer = CLIPTextModel.from_pretrained(version)
- self.device = device
- self.max_length = max_length
- if freeze:
- self.freeze()
- self.layer = layer
- self.layer_idx = layer_idx
- if layer == "hidden":
- assert layer_idx is not None
- assert 0 <= abs(layer_idx) <= 12
-
- def freeze(self):
- self.transformer = self.transformer.eval()
- #self.train = disabled_train
- for param in self.parameters():
- param.requires_grad = False
-
- def forward(self, text):
- batch_encoding = self.tokenizer(text, truncation=True, max_length=self.max_length, return_length=True,
- return_overflowing_tokens=False, padding="max_length", return_tensors="pt")
- tokens = batch_encoding["input_ids"].to(self.device)
- outputs = self.transformer(input_ids=tokens, output_hidden_states=self.layer=="hidden")
- if self.layer == "last":
- z = outputs.last_hidden_state
- elif self.layer == "pooled":
- z = outputs.pooler_output[:, None, :]
- else:
- z = outputs.hidden_states[self.layer_idx]
- return z
-
- def encode(self, text):
- return self(text)
-
-
-class FrozenOpenCLIPEmbedder(AbstractEncoder):
- """
- Uses the OpenCLIP transformer encoder for text
- """
- LAYERS = [
- #"pooled",
- "last",
- "penultimate"
- ]
- def __init__(self, arch="ViT-H-14", version="laion2b_s32b_b79k", device="cuda", max_length=77,
- freeze=True, layer="last"):
- super().__init__()
- assert layer in self.LAYERS
- print("Start initializing the CLIP text encoder")
- model, _, _ = open_clip.create_model_and_transforms(arch, device=torch.device('cpu'), pretrained=version)
- print("Initialization ends")
- # aa = model.encode_image(torch.zeros((1, 3,224,224)))
- del model.visual
- self.model = model
-
- if not torch.cuda.is_available():
- self.device = "cpu"
- else:
- self.device = device
-
- self.max_length = max_length
- if freeze:
- self.freeze()
- self.layer = layer
- if self.layer == "last":
- self.layer_idx = 0
- elif self.layer == "penultimate":
- self.layer_idx = 1
- else:
- raise NotImplementedError()
-
- def freeze(self):
- self.model = self.model.eval()
- for param in self.parameters():
- param.requires_grad = False
-
- def forward(self, text):
- tokens = open_clip.tokenize(text)
- z = self.encode_with_transformer(tokens.to(self.device))
- return z
-
- def encode_with_transformer(self, text):
- x = self.model.token_embedding(text) # [batch_size, n_ctx, d_model]
- x = x + self.model.positional_embedding
- x = x.permute(1, 0, 2) # NLD -> LND
- x = self.text_transformer_forward(x, attn_mask=self.model.attn_mask)
- x = x.permute(1, 0, 2) # LND -> NLD
- x = self.model.ln_final(x)
- # did not do:
- # x = x[torch.arange(x.shape[0]), text.argmax(dim=-1)] @ self.model.text_projection
- # x = F.normalize(x, dim=-1) if normalize else x
- return x
-
- def text_transformer_forward(self, x: torch.Tensor, attn_mask = None):
- for i, r in enumerate(self.model.transformer.resblocks):
- if i == len(self.model.transformer.resblocks) - self.layer_idx:
- break
- if self.model.transformer.grad_checkpointing and not torch.jit.is_scripting():
- x = checkpoint(r, x, attn_mask)
- else:
- x = r(x, attn_mask=attn_mask)
- return x
-
- def encode(self, text):
- return self(text)
-
-class FrozenOpenCLIPSepEncoder(FrozenOpenCLIPEmbedder):
- def forward(self, text):
- if islistortuple(text) and len(text) > 0 and islistortuple(text[0]):
- z_list = []
- for ti in text:
- tokens = open_clip.tokenize(ti)
- z = self.encode_with_transformer(tokens.to(self.device))
- z_list.append(z)
- return z_list
- else:
- tokens = open_clip.tokenize(text)
- z = self.encode_with_transformer(tokens.to(self.device))
- return z
-
-
-class FrozenCLIPT5Encoder(AbstractEncoder):
- def __init__(self,
- clip_version="openai/clip-vit-large-patch14", clip_max_length=77, layer="last", layer_idx=None,
- t5_version="google/t5-v1_1-xl", t5_max_length=77, padding="max_length",
- freeze=True, device="cuda"):
- super().__init__()
- self.clip_encoder = FrozenCLIPEmbedder(
- clip_version, device, max_length=clip_max_length, freeze=freeze, layer=layer, layer_idx=layer_idx
- )
- self.t5_encoder = FrozenT5Embedder(
- t5_version, device, max_length=t5_max_length, freeze=freeze, padding=padding
- )
- print(f"{self.clip_encoder.__class__.__name__} has {count_params(self.clip_encoder)*1.e-6:.2f} M parameters, "
- f"{self.t5_encoder.__class__.__name__} comes with {count_params(self.t5_encoder)*1.e-6:.2f} M params.")
-
- def encode(self, text):
- return self(text)
-
- def forward(self, text):
- clip_z = self.clip_encoder.encode(text)
- t5_z = self.t5_encoder.encode(text)
- return [clip_z, t5_z]
-
-class FrozenOpenCLIPT5Encoder(AbstractEncoder):
- def __init__(self,
- arch="ViT-H-14", clip_version="laion2b_s32b_b79k", layer="last", clip_max_length=77,
- t5_version="google/t5-v1_1-small", t5_max_length=77, padding="max_length",
- device="cuda", freeze=True):
- super().__init__()
- self.clip_encoder = FrozenOpenCLIPEmbedder(
- arch=arch, version=clip_version, device=device, max_length=clip_max_length,
- freeze=freeze, layer=layer
- )
- self.t5_encoder = FrozenT5Embedder(
- t5_version, device, max_length=t5_max_length, freeze=freeze, padding=padding
- )
- print(f"{self.clip_encoder.__class__.__name__} has {count_params(self.clip_encoder)*1.e-6:.2f} M parameters, "
- f"{self.t5_encoder.__class__.__name__} comes with {count_params(self.t5_encoder)*1.e-6:.2f} M params.")
-
- def encode(self, text):
- return self(text)
-
- def forward(self, text):
- clip_z = self.clip_encoder.encode(text) #B*77*1024
- t5_z = self.t5_encoder.encode(text) #B*77*Z
- return [clip_z, t5_z]
-
-class FrozenOpenCLIPT5SepEncoder(FrozenOpenCLIPT5Encoder):
- def forward(self, text):
- if islistortuple(text) and len(text) > 0 and islistortuple(text[0]):
- assert len(text) == 2
- print("two separate input prompts")
- clip_z = self.clip_encoder.encode(text[0]) #B*77*1024
- t5_z = self.t5_encoder.encode(text[1]) #B*77*Z
- else:
- clip_z = self.clip_encoder.encode(text) #B*77*1024
- t5_z = self.t5_encoder.encode(text) #B*77*Z
- return [clip_z, t5_z]
-
-class MergeTextEmb(nn.Module):
- def __init__(self, clip_emb_dim, t5_emb_dim, out_emb_dim=None, trainable=True, merge_mode="add", t5_fc_init="zero"):
- super().__init__()
- out_emb_dim = default(out_emb_dim, clip_emb_dim)
- self.clip_fc = identity_init_fc(nn.Linear(clip_emb_dim, out_emb_dim))
- if t5_fc_init == "zero":
- self.t5_fc = zero_module(nn.Linear(t5_emb_dim, out_emb_dim))
- elif t5_fc_init == "identity":
- self.t5_fc = identity_init_fc(nn.Linear(t5_emb_dim, out_emb_dim))
- else:
- "The initialization way {} is not supported.".format(t5_fc_init)
- raise ValueError
- self.trainable = trainable
- self.merge_mode = merge_mode
-
- def forward(self, clip_emb, t5_emb):
- clip_out = self.clip_fc(clip_emb)
- t5_out = self.t5_fc(t5_emb)
- if self.merge_mode == "concat":
- merge_out = torch.cat([clip_out, t5_out], dim=1)
- elif self.merge_mode == "add":
- assert clip_out.shape == t5_out.shape
- merge_out = clip_out + t5_out
- else:
- print("invalid merging way: {}".format(self.merge_mode))
- raise ValueError
- return merge_out
-
-
-class TransTextEmb(nn.Module):
- def __init__(self, unet_context_dim, emb_dims, fc_inits=None, trans_trainable = None):
- super().__init__()
- # assert isinstance(emb_dims, list)
- emb_num = len(emb_dims)
- if fc_inits is not None:
- # assert isinstance(fc_inits, list) and
- assert len(fc_inits) == emb_num
- else:
- fc_inits = ["random" for i in range(emb_num)]
-
- if trans_trainable is not None:
- # assert isinstance(trans_trainable, list) and
- assert len(trans_trainable) == emb_num
- else:
- trans_trainable = [True for i in range(emb_num)]
-
- module_list = nn.ModuleList([])
- for i in range(emb_num):
- trans = nn.Linear(emb_dims[i], unet_context_dim)
- if fc_inits[i] == "zero":
- trans = zero_module(trans)
- elif fc_inits[i] == "identity":
- trans = identity_init_fc(trans)
- module_list.append(trans)
-
- self.trans_list = module_list
- self.trans_trainable = trans_trainable
- self.emb_num = emb_num
-
- def forward(self, emb_list):
- assert len(emb_list) == self.emb_num
- emb_out_list = []
- for i in range(self.emb_num):
- emb_out = self.trans_list[i](emb_list[i])
- emb_out_list.append(emb_out)
- return emb_out_list
-
-
-class FrozenOpenCLIPT5ByT5Encoder(AbstractEncoder):
- def __init__(self,
- arch="ViT-H-14", clip_version="laion2b_s32b_b79k", layer="last", clip_max_length=77,
- t5_version="google/t5-v1_1-large", t5_max_length=77, padding="max_length",
- byt5_version="google/byt5-large", byt5_max_length=77, byt5_padding="max_length",
- device="cuda", freeze=True):
- super().__init__()
- self.clip_encoder = FrozenOpenCLIPEmbedder(
- arch=arch, version=clip_version, device=device, max_length=clip_max_length,
- freeze=freeze, layer=layer
- )
- self.t5_encoder = FrozenT5Embedder(
- t5_version, device, max_length=t5_max_length, freeze=freeze, padding=padding
- )
- self.byt5_encoder = FrozenT5Embedder(
- byt5_version, device, max_length=byt5_max_length, freeze=freeze, padding=byt5_padding
- )
- print(f"{self.clip_encoder.__class__.__name__} has {count_params(self.clip_encoder)*1.e-6:.2f} M parameters, "
- f"{self.t5_encoder.__class__.__name__} comes with {count_params(self.t5_encoder)*1.e-6:.2f} M params."
- f"{self.byt5_encoder.__class__.__name__} comes with {count_params(self.byt5_encoder)*1.e-6:.2f} M params.")
-
- def encode(self, text):
- return self(text)
-
- def forward(self, text):
- clip_z = self.clip_encoder.encode(text) #B*77*1024
- t5_z = self.t5_encoder.encode(text) #B*77*Z
- byt5_z = self.byt5_encoder.encode(text)
- return [clip_z, t5_z, byt5_z]
-
-
-class FrozenOpenCLIPT5ByT5SepEncoder(FrozenOpenCLIPT5ByT5Encoder):
- def forward(self, text):
- if islistortuple(text) and len(text) > 0 and islistortuple(text[0]):
- assert len(text) <= 3
- clip_text = text[0]
- t5_text = text[1] if len(text) > 1 else text[0]
- byt5_text = text[-1]
- else:
- clip_text = text
- t5_text = text
- byt5_text = text
- clip_z = self.clip_encoder.encode(clip_text) #B*77*1024
- t5_z = self.t5_encoder.encode(t5_text) #B*77*Z_1
- byt5_z = self.byt5_encoder.encode(byt5_text) #B*77*Z_2
- del clip_text, t5_text, byt5_text
- return [clip_z, t5_z, byt5_z]
-
-
-class OpenCLIPImageEmbedder(AbstractEncoder):
- """
- Uses the OpenCLIP transformer encoder for image
- """
- def __init__(self, arch="ViT-H-14", version="laion2b_s32b_b79k", device="cuda",
- freeze=True, set_grad_checkpointing = True):
- super().__init__()
- model, preprocess_train, preprocess_val = open_clip.create_model_and_transforms(arch, device=torch.device('cpu'), pretrained=version)
- self.image_mean = model.visual.image_mean
- self.image_std = model.visual.image_std
- del model.transformer
- del model.token_embedding
- del model.positional_embedding
- del model.ln_final
- del model.text_projection
- del model.logit_scale
- # only model.visual is left
-
- self.model = model
- self.device = device
-
- if not freeze and set_grad_checkpointing:
- self.model.visual.set_grad_checkpointing(True)
- self.freeze_model = freeze
-
- def forward(self, img):
- z = self.model.encode_image(img) # 2.0.2 , normalize=False) 2.7.0
- return z
-
- def encode(self, img):
- return self(img)
\ No newline at end of file
diff --git a/spaces/AIZero2HeroBootcamp/FastSpeech2LinerGradioApp/app.py b/spaces/AIZero2HeroBootcamp/FastSpeech2LinerGradioApp/app.py
deleted file mode 100644
index 624711103fff0eb591bc05f07ae20c47fbe03cd2..0000000000000000000000000000000000000000
--- a/spaces/AIZero2HeroBootcamp/FastSpeech2LinerGradioApp/app.py
+++ /dev/null
@@ -1,3 +0,0 @@
-import gradio as gr
-
-gr.Interface.load("models/facebook/fastspeech2-en-ljspeech").launch()
\ No newline at end of file
diff --git a/spaces/Abhay834/SY_Bot/README.md b/spaces/Abhay834/SY_Bot/README.md
deleted file mode 100644
index f450007986c811a7cff38669125264146f4f9f49..0000000000000000000000000000000000000000
--- a/spaces/Abhay834/SY_Bot/README.md
+++ /dev/null
@@ -1,13 +0,0 @@
----
-title: My Genai Chatbot
-emoji: 🐨
-colorFrom: gray
-colorTo: blue
-sdk: gradio
-sdk_version: 3.39.0
-app_file: app.py
-pinned: false
-duplicated_from: Abhay834/my_genai_chatbot
----
-
-Check out the configuration reference at https://huggingface.co/docs/hub/spaces-config-reference
diff --git a/spaces/AchyuthGamer/OpenGPT/g4f/Provider/Providers/deprecated/GetGpt.py b/spaces/AchyuthGamer/OpenGPT/g4f/Provider/Providers/deprecated/GetGpt.py
deleted file mode 100644
index a5de1d296a5d6abada13030ceabcd181e2f90497..0000000000000000000000000000000000000000
--- a/spaces/AchyuthGamer/OpenGPT/g4f/Provider/Providers/deprecated/GetGpt.py
+++ /dev/null
@@ -1,88 +0,0 @@
-from __future__ import annotations
-
-import json
-import os
-import uuid
-
-import requests
-from Crypto.Cipher import AES
-
-from ...typing import Any, CreateResult
-from ..base_provider import BaseProvider
-
-
-class GetGpt(BaseProvider):
- url = 'https://chat.getgpt.world/'
- supports_stream = True
- working = False
- supports_gpt_35_turbo = True
-
- @staticmethod
- def create_completion(
- model: str,
- messages: list[dict[str, str]],
- stream: bool, **kwargs: Any) -> CreateResult:
-
- headers = {
- 'Content-Type' : 'application/json',
- 'Referer' : 'https://chat.getgpt.world/',
- 'user-agent' : 'Mozilla/5.0 (Windows NT 10.0; Win64; x64) AppleWebKit/537.36 (KHTML, like Gecko) Chrome/114.0.0.0 Safari/537.36',
- }
-
- data = json.dumps(
- {
- 'messages' : messages,
- 'frequency_penalty' : kwargs.get('frequency_penalty', 0),
- 'max_tokens' : kwargs.get('max_tokens', 4000),
- 'model' : 'gpt-3.5-turbo',
- 'presence_penalty' : kwargs.get('presence_penalty', 0),
- 'temperature' : kwargs.get('temperature', 1),
- 'top_p' : kwargs.get('top_p', 1),
- 'stream' : True,
- 'uuid' : str(uuid.uuid4())
- }
- )
-
- res = requests.post('https://chat.getgpt.world/api/chat/stream',
- headers=headers, json={'signature': _encrypt(data)}, stream=True)
-
- res.raise_for_status()
- for line in res.iter_lines():
- if b'content' in line:
- line_json = json.loads(line.decode('utf-8').split('data: ')[1])
- yield (line_json['choices'][0]['delta']['content'])
-
- @classmethod
- @property
- def params(cls):
- params = [
- ('model', 'str'),
- ('messages', 'list[dict[str, str]]'),
- ('stream', 'bool'),
- ('temperature', 'float'),
- ('presence_penalty', 'int'),
- ('frequency_penalty', 'int'),
- ('top_p', 'int'),
- ('max_tokens', 'int'),
- ]
- param = ', '.join([': '.join(p) for p in params])
- return f'g4f.provider.{cls.__name__} supports: ({param})'
-
-
-def _encrypt(e: str):
- t = os.urandom(8).hex().encode('utf-8')
- n = os.urandom(8).hex().encode('utf-8')
- r = e.encode('utf-8')
-
- cipher = AES.new(t, AES.MODE_CBC, n)
- ciphertext = cipher.encrypt(_pad_data(r))
-
- return ciphertext.hex() + t.decode('utf-8') + n.decode('utf-8')
-
-
-def _pad_data(data: bytes) -> bytes:
- block_size = AES.block_size
- padding_size = block_size - len(data) % block_size
- padding = bytes([padding_size] * padding_size)
-
- return data + padding
diff --git a/spaces/AdityaVishwakarma/LiveChecker/app.py b/spaces/AdityaVishwakarma/LiveChecker/app.py
deleted file mode 100644
index b1f0d326d74a480254efca194c950e15e710539b..0000000000000000000000000000000000000000
--- a/spaces/AdityaVishwakarma/LiveChecker/app.py
+++ /dev/null
@@ -1,61 +0,0 @@
-# Import the requests and BeautifulSoup libraries
-import requests
-from bs4 import BeautifulSoup
-import streamlit as st
-
-# Define the URL of the website you want to interact with
-url = 'https://www.iana.org/domains/root/db'
-
-st.title("LiveChecker: Live Site Validator")
-st.write("This code finds and shows active websites with a specific term like google or example")
-
-user_input = st.text_input('Enter a website name', placeholder='google').strip() or "google"
-
-if st.button("Start checking"):
- progress_bar = st.progress(0)
- # Try to send a GET request to the URL and read the HTML content
- try:
- response = requests.get(url)
- response.raise_for_status()
- html = response.text
- except requests.exceptions.RequestException as e:
- st.write('Request Error:', e, url)
-
- if html:
- # Create a Python list from domain texts using BeautifulSoup
- domain_list = [link.get_text().strip() for link in BeautifulSoup(html, 'html.parser').find_all('a', href=lambda x: x and x.startswith('/domains/root/db/'))]
- domain_list = [domain for domain in domain_list if domain.isascii()]
-
- def check_website():
- # Get the total number of domain names in the list
- total = len(domain_list)
- # Initialize a counter for completed domain names
- count = 0
- # Loop through each domain name and check if the website is live or not
- for domain in domain_list:
- # Add the domain name to the base URL of yomovies website
- user_input_url = 'www.' + user_input + domain
- outputtext = ''
-
- try:
- response = requests.get('https://' + user_input_url,stream=True,timeout=2)
- status_code = response.status_code
- outputtext = 'https://' + user_input_url
- except:
- # If https fails, try again with http
- try:
- response = requests.get('http://' + user_input_url,stream=True,timeout=2)
- status_code = response.status_code
- outputtext = 'http://' + user_input_url
- except:
- # If both fail, set status code to None
- status_code = None
- # Print the result based on the status code
- if status_code == 200:
- st.write(outputtext, 'is live ✅')
- # Increment the counter by one
- count += 1
- # Calculate the percentage of completion and update the progress bar value
- percent = int(count / total * 100)
- progress_bar.progress(percent)
- check_website()
\ No newline at end of file
diff --git a/spaces/AgentVerse/agentVerse/dataloader/logic_grid.py b/spaces/AgentVerse/agentVerse/dataloader/logic_grid.py
deleted file mode 100644
index 200344d2570307f87993590f3dd255f33030575f..0000000000000000000000000000000000000000
--- a/spaces/AgentVerse/agentVerse/dataloader/logic_grid.py
+++ /dev/null
@@ -1,22 +0,0 @@
-from .dataloader import DataLoader
-from . import dataloader_registry
-import json
-import re
-
-
-@dataloader_registry.register("tasksolving/logic_grid/gpt-4")
-class LogicGridLoader(DataLoader):
- def __init__(self, path: str):
- self.answer_pat = re.compile(r"#### (-?\d+)")
- super().__init__(path)
-
- def load(self):
- with open(self.path) as f:
- for line in f:
- line = json.loads(line)
- self.examples.append(
- {
- "input": line["inputs"],
- "answer": line["targets"][0],
- }
- )
diff --git a/spaces/AgentVerse/agentVerse/ui/src/constants.ts b/spaces/AgentVerse/agentVerse/ui/src/constants.ts
deleted file mode 100644
index eb9cdfd032fa96bda12704a29f83704d7008392d..0000000000000000000000000000000000000000
--- a/spaces/AgentVerse/agentVerse/ui/src/constants.ts
+++ /dev/null
@@ -1,3 +0,0 @@
-export const COLOR_PRIMARY = 0x4e342e;
-export const COLOR_LIGHT = 0x7b5e57;
-export const COLOR_DARK = 0x260e04;
diff --git a/spaces/AgentVerse/agentVerse/ui/src/phaser3-rex-plugins/templates/ui/container/Factory.js b/spaces/AgentVerse/agentVerse/ui/src/phaser3-rex-plugins/templates/ui/container/Factory.js
deleted file mode 100644
index 7b7e558f751e4b706d88ce38bc51e360508a10d8..0000000000000000000000000000000000000000
--- a/spaces/AgentVerse/agentVerse/ui/src/phaser3-rex-plugins/templates/ui/container/Factory.js
+++ /dev/null
@@ -1,13 +0,0 @@
-import Container from './Container.js';
-import ObjectFactory from '../ObjectFactory.js';
-import SetValue from '../../../plugins/utils/object/SetValue.js';
-
-ObjectFactory.register('container', function (x, y, width, height, children) {
- var gameObject = new Container(this.scene, x, y, width, height, children);
- this.scene.add.existing(gameObject);
- return gameObject;
-});
-
-SetValue(window, 'RexPlugins.UI.Container', Container);
-
-export default Container;
\ No newline at end of file
diff --git a/spaces/Aki004/herta-so-vits/inference/infer_tool_grad.py b/spaces/Aki004/herta-so-vits/inference/infer_tool_grad.py
deleted file mode 100644
index b75af49c08e2e724839828bc419792ed580809bb..0000000000000000000000000000000000000000
--- a/spaces/Aki004/herta-so-vits/inference/infer_tool_grad.py
+++ /dev/null
@@ -1,160 +0,0 @@
-import hashlib
-import json
-import logging
-import os
-import time
-from pathlib import Path
-import io
-import librosa
-import maad
-import numpy as np
-from inference import slicer
-import parselmouth
-import soundfile
-import torch
-import torchaudio
-
-from hubert import hubert_model
-import utils
-from models import SynthesizerTrn
-logging.getLogger('numba').setLevel(logging.WARNING)
-logging.getLogger('matplotlib').setLevel(logging.WARNING)
-
-def resize2d_f0(x, target_len):
- source = np.array(x)
- source[source < 0.001] = np.nan
- target = np.interp(np.arange(0, len(source) * target_len, len(source)) / target_len, np.arange(0, len(source)),
- source)
- res = np.nan_to_num(target)
- return res
-
-def get_f0(x, p_len,f0_up_key=0):
-
- time_step = 160 / 16000 * 1000
- f0_min = 50
- f0_max = 1100
- f0_mel_min = 1127 * np.log(1 + f0_min / 700)
- f0_mel_max = 1127 * np.log(1 + f0_max / 700)
-
- f0 = parselmouth.Sound(x, 16000).to_pitch_ac(
- time_step=time_step / 1000, voicing_threshold=0.6,
- pitch_floor=f0_min, pitch_ceiling=f0_max).selected_array['frequency']
-
- pad_size=(p_len - len(f0) + 1) // 2
- if(pad_size>0 or p_len - len(f0) - pad_size>0):
- f0 = np.pad(f0,[[pad_size,p_len - len(f0) - pad_size]], mode='constant')
-
- f0 *= pow(2, f0_up_key / 12)
- f0_mel = 1127 * np.log(1 + f0 / 700)
- f0_mel[f0_mel > 0] = (f0_mel[f0_mel > 0] - f0_mel_min) * 254 / (f0_mel_max - f0_mel_min) + 1
- f0_mel[f0_mel <= 1] = 1
- f0_mel[f0_mel > 255] = 255
- f0_coarse = np.rint(f0_mel).astype(np.int)
- return f0_coarse, f0
-
-def clean_pitch(input_pitch):
- num_nan = np.sum(input_pitch == 1)
- if num_nan / len(input_pitch) > 0.9:
- input_pitch[input_pitch != 1] = 1
- return input_pitch
-
-
-def plt_pitch(input_pitch):
- input_pitch = input_pitch.astype(float)
- input_pitch[input_pitch == 1] = np.nan
- return input_pitch
-
-
-def f0_to_pitch(ff):
- f0_pitch = 69 + 12 * np.log2(ff / 440)
- return f0_pitch
-
-
-def fill_a_to_b(a, b):
- if len(a) < len(b):
- for _ in range(0, len(b) - len(a)):
- a.append(a[0])
-
-
-def mkdir(paths: list):
- for path in paths:
- if not os.path.exists(path):
- os.mkdir(path)
-
-
-class VitsSvc(object):
- def __init__(self):
- self.device = torch.device("cuda" if torch.cuda.is_available() else "cpu")
- self.SVCVITS = None
- self.hps = None
- self.speakers = None
- self.hubert_soft = utils.get_hubert_model()
-
- def set_device(self, device):
- self.device = torch.device(device)
- self.hubert_soft.to(self.device)
- if self.SVCVITS != None:
- self.SVCVITS.to(self.device)
-
- def loadCheckpoint(self, path):
- self.hps = utils.get_hparams_from_file(f"checkpoints/{path}/config.json")
- self.SVCVITS = SynthesizerTrn(
- self.hps.data.filter_length // 2 + 1,
- self.hps.train.segment_size // self.hps.data.hop_length,
- **self.hps.model)
- _ = utils.load_checkpoint(f"checkpoints/{path}/model.pth", self.SVCVITS, None)
- _ = self.SVCVITS.eval().to(self.device)
- self.speakers = self.hps.spk
-
- def get_units(self, source, sr):
- source = source.unsqueeze(0).to(self.device)
- with torch.inference_mode():
- units = self.hubert_soft.units(source)
- return units
-
-
- def get_unit_pitch(self, in_path, tran):
- source, sr = torchaudio.load(in_path)
- source = torchaudio.functional.resample(source, sr, 16000)
- if len(source.shape) == 2 and source.shape[1] >= 2:
- source = torch.mean(source, dim=0).unsqueeze(0)
- soft = self.get_units(source, sr).squeeze(0).cpu().numpy()
- f0_coarse, f0 = get_f0(source.cpu().numpy()[0], soft.shape[0]*2, tran)
- return soft, f0
-
- def infer(self, speaker_id, tran, raw_path):
- speaker_id = self.speakers[speaker_id]
- sid = torch.LongTensor([int(speaker_id)]).to(self.device).unsqueeze(0)
- soft, pitch = self.get_unit_pitch(raw_path, tran)
- f0 = torch.FloatTensor(clean_pitch(pitch)).unsqueeze(0).to(self.device)
- stn_tst = torch.FloatTensor(soft)
- with torch.no_grad():
- x_tst = stn_tst.unsqueeze(0).to(self.device)
- x_tst = torch.repeat_interleave(x_tst, repeats=2, dim=1).transpose(1, 2)
- audio = self.SVCVITS.infer(x_tst, f0=f0, g=sid)[0,0].data.float()
- return audio, audio.shape[-1]
-
- def inference(self,srcaudio,chara,tran,slice_db):
- sampling_rate, audio = srcaudio
- audio = (audio / np.iinfo(audio.dtype).max).astype(np.float32)
- if len(audio.shape) > 1:
- audio = librosa.to_mono(audio.transpose(1, 0))
- if sampling_rate != 16000:
- audio = librosa.resample(audio, orig_sr=sampling_rate, target_sr=16000)
- soundfile.write("tmpwav.wav", audio, 16000, format="wav")
- chunks = slicer.cut("tmpwav.wav", db_thresh=slice_db)
- audio_data, audio_sr = slicer.chunks2audio("tmpwav.wav", chunks)
- audio = []
- for (slice_tag, data) in audio_data:
- length = int(np.ceil(len(data) / audio_sr * self.hps.data.sampling_rate))
- raw_path = io.BytesIO()
- soundfile.write(raw_path, data, audio_sr, format="wav")
- raw_path.seek(0)
- if slice_tag:
- _audio = np.zeros(length)
- else:
- out_audio, out_sr = self.infer(chara, tran, raw_path)
- _audio = out_audio.cpu().numpy()
- audio.extend(list(_audio))
- audio = (np.array(audio) * 32768.0).astype('int16')
- return (self.hps.data.sampling_rate,audio)
diff --git a/spaces/Akinade/Iris_App/app.py b/spaces/Akinade/Iris_App/app.py
deleted file mode 100644
index bb585b7a5ac984ba91e07e97d70e6e9c4416a80c..0000000000000000000000000000000000000000
--- a/spaces/Akinade/Iris_App/app.py
+++ /dev/null
@@ -1,41 +0,0 @@
-import gradio as gr
-import joblib
-
-
-
-def values(sepal_length, sepal_height, petal_length, petal_height):
- model = joblib.load('iris-predictor.joblib')
- action = model.predict([[sepal_length, sepal_height, petal_length, petal_height]])
- if action == 'Iris-setosa':
- image_1 = 'Irissetosa1 copy.jpg'
- text1 = 'This is Iris Setosa 💐'
- return text1, image_1
- elif action == 'Iris-virginica':
- image_2 = 'Iris_virginica_2 copy.jpg'
- text2 = 'This is Iris Virginica 🌺'
- return text2, image_2
- elif action == 'Iris-versicolor':
- image_3 = 'iris_versicolor copy.JPG'
- text3 = 'This is Iris Versicolor 🌼'
- return text3, image_3
- else:
- "No Picture to display for your ambiguous values"
-
-
-sepal_l = gr.inputs.Slider(0.1, 9.9, label='Sepal-Length')
-sepal_h = gr.inputs.Slider(0.1, 9.9, label='Sepal-Height')
-petal_l = gr.inputs.Slider(0.1, 9.9, label='Petal-height')
-petal_h = gr.inputs.Slider(0.1, 9.9, label='Petal-Length')
-
-output = gr.Textbox(label="Result")
-output1 = gr.outputs.Image(label="Image Result")
-
-
-app = gr.Interface(fn=values, inputs=[sepal_l, sepal_h, petal_l, petal_h], outputs=[output, output1], title='An iris flower app',
- description='Input the Flower Details for Sepal and Petal Respectively.', examples=[[4.7, 3.2, 1.6, 0.2],
- [6.0, 2.7, 5.1, 1.6],
- [6.5, 3.0, 5.5, 1.8]], live=False, theme='huggingface')
-
-
-
-app.launch()
\ No newline at end of file
diff --git a/spaces/Alpaca233/SadTalker/src/facerender/sync_batchnorm/replicate.py b/spaces/Alpaca233/SadTalker/src/facerender/sync_batchnorm/replicate.py
deleted file mode 100644
index b71c7b8ed51a1d6c55b1f753bdd8d90bad79bd06..0000000000000000000000000000000000000000
--- a/spaces/Alpaca233/SadTalker/src/facerender/sync_batchnorm/replicate.py
+++ /dev/null
@@ -1,94 +0,0 @@
-# -*- coding: utf-8 -*-
-# File : replicate.py
-# Author : Jiayuan Mao
-# Email : maojiayuan@gmail.com
-# Date : 27/01/2018
-#
-# This file is part of Synchronized-BatchNorm-PyTorch.
-# https://github.com/vacancy/Synchronized-BatchNorm-PyTorch
-# Distributed under MIT License.
-
-import functools
-
-from torch.nn.parallel.data_parallel import DataParallel
-
-__all__ = [
- 'CallbackContext',
- 'execute_replication_callbacks',
- 'DataParallelWithCallback',
- 'patch_replication_callback'
-]
-
-
-class CallbackContext(object):
- pass
-
-
-def execute_replication_callbacks(modules):
- """
- Execute an replication callback `__data_parallel_replicate__` on each module created by original replication.
-
- The callback will be invoked with arguments `__data_parallel_replicate__(ctx, copy_id)`
-
- Note that, as all modules are isomorphism, we assign each sub-module with a context
- (shared among multiple copies of this module on different devices).
- Through this context, different copies can share some information.
-
- We guarantee that the callback on the master copy (the first copy) will be called ahead of calling the callback
- of any slave copies.
- """
- master_copy = modules[0]
- nr_modules = len(list(master_copy.modules()))
- ctxs = [CallbackContext() for _ in range(nr_modules)]
-
- for i, module in enumerate(modules):
- for j, m in enumerate(module.modules()):
- if hasattr(m, '__data_parallel_replicate__'):
- m.__data_parallel_replicate__(ctxs[j], i)
-
-
-class DataParallelWithCallback(DataParallel):
- """
- Data Parallel with a replication callback.
-
- An replication callback `__data_parallel_replicate__` of each module will be invoked after being created by
- original `replicate` function.
- The callback will be invoked with arguments `__data_parallel_replicate__(ctx, copy_id)`
-
- Examples:
- > sync_bn = SynchronizedBatchNorm1d(10, eps=1e-5, affine=False)
- > sync_bn = DataParallelWithCallback(sync_bn, device_ids=[0, 1])
- # sync_bn.__data_parallel_replicate__ will be invoked.
- """
-
- def replicate(self, module, device_ids):
- modules = super(DataParallelWithCallback, self).replicate(module, device_ids)
- execute_replication_callbacks(modules)
- return modules
-
-
-def patch_replication_callback(data_parallel):
- """
- Monkey-patch an existing `DataParallel` object. Add the replication callback.
- Useful when you have customized `DataParallel` implementation.
-
- Examples:
- > sync_bn = SynchronizedBatchNorm1d(10, eps=1e-5, affine=False)
- > sync_bn = DataParallel(sync_bn, device_ids=[0, 1])
- > patch_replication_callback(sync_bn)
- # this is equivalent to
- > sync_bn = SynchronizedBatchNorm1d(10, eps=1e-5, affine=False)
- > sync_bn = DataParallelWithCallback(sync_bn, device_ids=[0, 1])
- """
-
- assert isinstance(data_parallel, DataParallel)
-
- old_replicate = data_parallel.replicate
-
- @functools.wraps(old_replicate)
- def new_replicate(module, device_ids):
- modules = old_replicate(module, device_ids)
- execute_replication_callbacks(modules)
- return modules
-
- data_parallel.replicate = new_replicate
diff --git a/spaces/Amon1/ChatGPTForAcadamic/crazy_functions/test_project/latex/attention/model_architecture.tex b/spaces/Amon1/ChatGPTForAcadamic/crazy_functions/test_project/latex/attention/model_architecture.tex
deleted file mode 100644
index c82be6242cc9d26203360e90d3ac9184ef6ad842..0000000000000000000000000000000000000000
--- a/spaces/Amon1/ChatGPTForAcadamic/crazy_functions/test_project/latex/attention/model_architecture.tex
+++ /dev/null
@@ -1,155 +0,0 @@
-
-\begin{figure}
- \centering
- \includegraphics[scale=0.6]{Figures/ModalNet-21}
- \caption{The Transformer - model architecture.}
- \label{fig:model-arch}
-\end{figure}
-
-% Although the primary workhorse of our model is attention,
-%Our model maintains the encoder-decoder structure that is common to many so-called sequence-to-sequence models \citep{bahdanau2014neural,sutskever14}. As in all such architectures, the encoder computes a representation of the input sequence, and the decoder consumes these representations along with the output tokens to autoregressively produce the output sequence. Where, traditionally, the encoder and decoder contain stacks of recurrent or convolutional layers, our encoder and decoder stacks are composed of attention layers and position-wise feed-forward layers (Figure~\ref{fig:model-arch}). The following sections describe the gross architecture and these particular components in detail.
-
-Most competitive neural sequence transduction models have an encoder-decoder structure \citep{cho2014learning,bahdanau2014neural,sutskever14}. Here, the encoder maps an input sequence of symbol representations $(x_1, ..., x_n)$ to a sequence of continuous representations $\mathbf{z} = (z_1, ..., z_n)$. Given $\mathbf{z}$, the decoder then generates an output sequence $(y_1,...,y_m)$ of symbols one element at a time. At each step the model is auto-regressive \citep{graves2013generating}, consuming the previously generated symbols as additional input when generating the next.
-
-The Transformer follows this overall architecture using stacked self-attention and point-wise, fully connected layers for both the encoder and decoder, shown in the left and right halves of Figure~\ref{fig:model-arch}, respectively.
-
-\subsection{Encoder and Decoder Stacks}
-
-\paragraph{Encoder:}The encoder is composed of a stack of $N=6$ identical layers. Each layer has two sub-layers. The first is a multi-head self-attention mechanism, and the second is a simple, position-wise fully connected feed-forward network. We employ a residual connection \citep{he2016deep} around each of the two sub-layers, followed by layer normalization \cite{layernorm2016}. That is, the output of each sub-layer is $\mathrm{LayerNorm}(x + \mathrm{Sublayer}(x))$, where $\mathrm{Sublayer}(x)$ is the function implemented by the sub-layer itself. To facilitate these residual connections, all sub-layers in the model, as well as the embedding layers, produce outputs of dimension $\dmodel=512$.
-
-\paragraph{Decoder:}The decoder is also composed of a stack of $N=6$ identical layers. In addition to the two sub-layers in each encoder layer, the decoder inserts a third sub-layer, which performs multi-head attention over the output of the encoder stack. Similar to the encoder, we employ residual connections around each of the sub-layers, followed by layer normalization. We also modify the self-attention sub-layer in the decoder stack to prevent positions from attending to subsequent positions. This masking, combined with fact that the output embeddings are offset by one position, ensures that the predictions for position $i$ can depend only on the known outputs at positions less than $i$.
-
-% In our model (Figure~\ref{fig:model-arch}), the encoder and decoder are composed of stacks of alternating self-attention layers (for cross-positional communication) and position-wise feed-forward layers (for in-place computation). In addition, the decoder stack contains encoder-decoder attention layers. Since attention is agnostic to the distances between words, our model requires a "positional encoding" to be added to the encoder and decoder input. The following sections describe all of these components in detail.
-
-\subsection{Attention} \label{sec:attention}
-An attention function can be described as mapping a query and a set of key-value pairs to an output, where the query, keys, values, and output are all vectors. The output is computed as a weighted sum of the values, where the weight assigned to each value is computed by a compatibility function of the query with the corresponding key.
-
-\subsubsection{Scaled Dot-Product Attention} \label{sec:scaled-dot-prod}
-
-% \begin{figure}
-% \centering
-% \includegraphics[scale=0.6]{Figures/ModalNet-19}
-% \caption{Scaled Dot-Product Attention.}
-% \label{fig:multi-head-att}
-% \end{figure}
-
-We call our particular attention "Scaled Dot-Product Attention" (Figure~\ref{fig:multi-head-att}). The input consists of queries and keys of dimension $d_k$, and values of dimension $d_v$. We compute the dot products of the query with all keys, divide each by $\sqrt{d_k}$, and apply a softmax function to obtain the weights on the values.
-
-In practice, we compute the attention function on a set of queries simultaneously, packed together into a matrix $Q$. The keys and values are also packed together into matrices $K$ and $V$. We compute the matrix of outputs as:
-
-\begin{equation}
- \mathrm{Attention}(Q, K, V) = \mathrm{softmax}(\frac{QK^T}{\sqrt{d_k}})V
-\end{equation}
-
-The two most commonly used attention functions are additive attention \citep{bahdanau2014neural}, and dot-product (multiplicative) attention. Dot-product attention is identical to our algorithm, except for the scaling factor of $\frac{1}{\sqrt{d_k}}$. Additive attention computes the compatibility function using a feed-forward network with a single hidden layer. While the two are similar in theoretical complexity, dot-product attention is much faster and more space-efficient in practice, since it can be implemented using highly optimized matrix multiplication code.
-
-%We scale the dot products by $1/\sqrt{d_k}$ to limit the magnitude of the dot products, which works well in practice. Otherwise, we found applying the softmax to often result in weights very close to 0 or 1, and hence minuscule gradients.
-
-% Already described in the subsequent section
-%When used as part of decoder self-attention, an optional mask function is applied just before the softmax to prevent positions from attending to subsequent positions. This mask simply sets the logits corresponding to all illegal connections (those outside of the lower triangle) to $-\infty$.
-
-%\paragraph{Comparison to Additive Attention: } We choose dot product attention over additive attention \citep{bahdanau2014neural} since it can be computed using highly optimized matrix multiplication code. This optimization is particularly important to us, as we employ many attention layers in our model.
-
-While for small values of $d_k$ the two mechanisms perform similarly, additive attention outperforms dot product attention without scaling for larger values of $d_k$ \citep{DBLP:journals/corr/BritzGLL17}. We suspect that for large values of $d_k$, the dot products grow large in magnitude, pushing the softmax function into regions where it has extremely small gradients \footnote{To illustrate why the dot products get large, assume that the components of $q$ and $k$ are independent random variables with mean $0$ and variance $1$. Then their dot product, $q \cdot k = \sum_{i=1}^{d_k} q_ik_i$, has mean $0$ and variance $d_k$.}. To counteract this effect, we scale the dot products by $\frac{1}{\sqrt{d_k}}$.
-
-
-%We suspect this to be caused by the dot products growing too large in magnitude to result in useful gradients after applying the softmax function. To counteract this, we scale the dot product by $1/\sqrt{d_k}$.
-
-
-\subsubsection{Multi-Head Attention} \label{sec:multihead}
-
-\begin{figure}
-\begin{minipage}[t]{0.5\textwidth}
- \centering
- Scaled Dot-Product Attention \\
- \vspace{0.5cm}
- \includegraphics[scale=0.6]{Figures/ModalNet-19}
-\end{minipage}
-\begin{minipage}[t]{0.5\textwidth}
- \centering
- Multi-Head Attention \\
- \vspace{0.1cm}
- \includegraphics[scale=0.6]{Figures/ModalNet-20}
-\end{minipage}
-
-
- % \centering
-
- \caption{(left) Scaled Dot-Product Attention. (right) Multi-Head Attention consists of several attention layers running in parallel.}
- \label{fig:multi-head-att}
-\end{figure}
-
-Instead of performing a single attention function with $\dmodel$-dimensional keys, values and queries, we found it beneficial to linearly project the queries, keys and values $h$ times with different, learned linear projections to $d_k$, $d_k$ and $d_v$ dimensions, respectively.
-On each of these projected versions of queries, keys and values we then perform the attention function in parallel, yielding $d_v$-dimensional output values. These are concatenated and once again projected, resulting in the final values, as depicted in Figure~\ref{fig:multi-head-att}.
-
-Multi-head attention allows the model to jointly attend to information from different representation subspaces at different positions. With a single attention head, averaging inhibits this.
-
-\begin{align*}
- \mathrm{MultiHead}(Q, K, V) &= \mathrm{Concat}(\mathrm{head_1}, ..., \mathrm{head_h})W^O\\
-% \mathrm{where} \mathrm{head_i} &= \mathrm{Attention}(QW_Q_i^{\dmodel \times d_q}, KW_K_i^{\dmodel \times d_k}, VW^V_i^{\dmodel \times d_v})\\
- \text{where}~\mathrm{head_i} &= \mathrm{Attention}(QW^Q_i, KW^K_i, VW^V_i)\\
-\end{align*}
-
-Where the projections are parameter matrices $W^Q_i \in \mathbb{R}^{\dmodel \times d_k}$, $W^K_i \in \mathbb{R}^{\dmodel \times d_k}$, $W^V_i \in \mathbb{R}^{\dmodel \times d_v}$ and $W^O \in \mathbb{R}^{hd_v \times \dmodel}$.
-
-
-%find it better (and no more expensive) to have multiple parallel attention layers (each over the full set of positions) with proportionally lower-dimensional keys, values and queries. We call this "Multi-Head Attention" (Figure~\ref{fig:multi-head-att}). The keys, values, and queries for each of these parallel attention layers are computed by learned linear transformations of the inputs to the multi-head attention. We use different linear transformations across different parallel attention layers. The output of the parallel attention layers are concatenated, and then passed through a final learned linear transformation.
-
-In this work we employ $h=8$ parallel attention layers, or heads. For each of these we use $d_k=d_v=\dmodel/h=64$.
-Due to the reduced dimension of each head, the total computational cost is similar to that of single-head attention with full dimensionality.
-
-\subsubsection{Applications of Attention in our Model}
-
-The Transformer uses multi-head attention in three different ways:
-\begin{itemize}
- \item In "encoder-decoder attention" layers, the queries come from the previous decoder layer, and the memory keys and values come from the output of the encoder. This allows every position in the decoder to attend over all positions in the input sequence. This mimics the typical encoder-decoder attention mechanisms in sequence-to-sequence models such as \citep{wu2016google, bahdanau2014neural,JonasFaceNet2017}.
-
- \item The encoder contains self-attention layers. In a self-attention layer all of the keys, values and queries come from the same place, in this case, the output of the previous layer in the encoder. Each position in the encoder can attend to all positions in the previous layer of the encoder.
-
- \item Similarly, self-attention layers in the decoder allow each position in the decoder to attend to all positions in the decoder up to and including that position. We need to prevent leftward information flow in the decoder to preserve the auto-regressive property. We implement this inside of scaled dot-product attention by masking out (setting to $-\infty$) all values in the input of the softmax which correspond to illegal connections. See Figure~\ref{fig:multi-head-att}.
-
-\end{itemize}
-
-\subsection{Position-wise Feed-Forward Networks}\label{sec:ffn}
-
-In addition to attention sub-layers, each of the layers in our encoder and decoder contains a fully connected feed-forward network, which is applied to each position separately and identically. This consists of two linear transformations with a ReLU activation in between.
-
-\begin{equation}
- \mathrm{FFN}(x)=\max(0, xW_1 + b_1) W_2 + b_2
-\end{equation}
-
-While the linear transformations are the same across different positions, they use different parameters from layer to layer. Another way of describing this is as two convolutions with kernel size 1. The dimensionality of input and output is $\dmodel=512$, and the inner-layer has dimensionality $d_{ff}=2048$.
-
-
-
-%In the appendix, we describe how the position-wise feed-forward network can also be seen as a form of attention.
-
-%from Jakob: The number of operations required for the model to relate signals from two arbitrary input or output positions grows in the distance between positions in input or output, linearly for ConvS2S and logarithmically for ByteNet, making it harder to learn dependencies between these positions \citep{hochreiter2001gradient}. In the transformer this is reduced to a constant number of operations, albeit at the cost of effective resolution caused by averaging attention-weighted positions, an effect we aim to counteract with multi-headed attention.
-
-
-%Figure~\ref{fig:simple-att} presents a simple attention function, $A$, with a single head, that forms the basis of our multi-head attention. $A$ takes a query key vector $\kq$, matrices of memory keys $\km$ and memory values $\vm$ ,and produces a query value vector $\vq$ as
-%\begin{equation*} \label{eq:attention}
-% A(\kq, \km, \vm) = {\vm}^T (Softmax(\km \kq).
-%\end{equation*}
-%We linearly transform $\kq,\,\km$, and $\vm$ with learned matrices ${\Wkq \text{,} \, \Wkm}$, and ${\Wvm}$ before calling the attention function, and transform the output query with $\Wvq$ before handing it to the feed forward layer. Each attention layer has it's own set of transformation matrices, which are shared across all query positions. $A$ is applied in parallel for each query position, and is implemented very efficiently as a batch of matrix multiplies. The self-attention and encoder-decoder attention layers use $A$, but with different arguments. For example, in encdoder self-attention, queries in encoder layer $i$ attention to memories in encoder layer $i-1$. To ensure that decoder self-attention layers do not look at future words, we add $- \inf$ to the softmax logits in positions $j+1$ to query length for query position $l$.
-
-%In simple attention, the query value is a weighted combination of the memory values where the attention weights sum to one. Although this function performs well in practice, the constraint on attention weights can restrict the amount of information that flows from memories to queries because the query cannot focus on multiple memory positions at once, which might be desirable when translating long sequences. \marginpar{@usz, could you think of an example of this ?} We remedy this by maintaining multiple attention heads at each query position that attend to all memory positions in parallel, with a different set of parameters per attention head $h$.
-%\marginpar{}
-
-\subsection{Embeddings and Softmax}
-Similarly to other sequence transduction models, we use learned embeddings to convert the input tokens and output tokens to vectors of dimension $\dmodel$. We also use the usual learned linear transformation and softmax function to convert the decoder output to predicted next-token probabilities. In our model, we share the same weight matrix between the two embedding layers and the pre-softmax linear transformation, similar to \citep{press2016using}. In the embedding layers, we multiply those weights by $\sqrt{\dmodel}$.
-
-
-\subsection{Positional Encoding}
-Since our model contains no recurrence and no convolution, in order for the model to make use of the order of the sequence, we must inject some information about the relative or absolute position of the tokens in the sequence. To this end, we add "positional encodings" to the input embeddings at the bottoms of the encoder and decoder stacks. The positional encodings have the same dimension $\dmodel$ as the embeddings, so that the two can be summed. There are many choices of positional encodings, learned and fixed \citep{JonasFaceNet2017}.
-
-In this work, we use sine and cosine functions of different frequencies:
-
-\begin{align*}
- PE_{(pos,2i)} = sin(pos / 10000^{2i/\dmodel}) \\
- PE_{(pos,2i+1)} = cos(pos / 10000^{2i/\dmodel})
-\end{align*}
-
-where $pos$ is the position and $i$ is the dimension. That is, each dimension of the positional encoding corresponds to a sinusoid. The wavelengths form a geometric progression from $2\pi$ to $10000 \cdot 2\pi$. We chose this function because we hypothesized it would allow the model to easily learn to attend by relative positions, since for any fixed offset $k$, $PE_{pos+k}$ can be represented as a linear function of $PE_{pos}$.
-
-We also experimented with using learned positional embeddings \citep{JonasFaceNet2017} instead, and found that the two versions produced nearly identical results (see Table~\ref{tab:variations} row (E)). We chose the sinusoidal version because it may allow the model to extrapolate to sequence lengths longer than the ones encountered during training.
diff --git a/spaces/Amrrs/DragGan-Inversion/PTI/models/e4e/stylegan2/op/upfirdn2d.cpp b/spaces/Amrrs/DragGan-Inversion/PTI/models/e4e/stylegan2/op/upfirdn2d.cpp
deleted file mode 100644
index d2e633dc896433c205e18bc3e455539192ff968e..0000000000000000000000000000000000000000
--- a/spaces/Amrrs/DragGan-Inversion/PTI/models/e4e/stylegan2/op/upfirdn2d.cpp
+++ /dev/null
@@ -1,23 +0,0 @@
-#include
-
-
-torch::Tensor upfirdn2d_op(const torch::Tensor& input, const torch::Tensor& kernel,
- int up_x, int up_y, int down_x, int down_y,
- int pad_x0, int pad_x1, int pad_y0, int pad_y1);
-
-#define CHECK_CUDA(x) TORCH_CHECK(x.type().is_cuda(), #x " must be a CUDA tensor")
-#define CHECK_CONTIGUOUS(x) TORCH_CHECK(x.is_contiguous(), #x " must be contiguous")
-#define CHECK_INPUT(x) CHECK_CUDA(x); CHECK_CONTIGUOUS(x)
-
-torch::Tensor upfirdn2d(const torch::Tensor& input, const torch::Tensor& kernel,
- int up_x, int up_y, int down_x, int down_y,
- int pad_x0, int pad_x1, int pad_y0, int pad_y1) {
- CHECK_CUDA(input);
- CHECK_CUDA(kernel);
-
- return upfirdn2d_op(input, kernel, up_x, up_y, down_x, down_y, pad_x0, pad_x1, pad_y0, pad_y1);
-}
-
-PYBIND11_MODULE(TORCH_EXTENSION_NAME, m) {
- m.def("upfirdn2d", &upfirdn2d, "upfirdn2d (CUDA)");
-}
\ No newline at end of file
diff --git a/spaces/Androidonnxfork/CivitAi-to-Diffusers/diffusers/docs/source/en/api/models/unet2d-cond.md b/spaces/Androidonnxfork/CivitAi-to-Diffusers/diffusers/docs/source/en/api/models/unet2d-cond.md
deleted file mode 100644
index a669b02a7fe82049ddb45b2286710a7d1f8d4bdf..0000000000000000000000000000000000000000
--- a/spaces/Androidonnxfork/CivitAi-to-Diffusers/diffusers/docs/source/en/api/models/unet2d-cond.md
+++ /dev/null
@@ -1,19 +0,0 @@
-# UNet2DConditionModel
-
-The [UNet](https://huggingface.co/papers/1505.04597) model was originally introduced by Ronneberger et al for biomedical image segmentation, but it is also commonly used in 🤗 Diffusers because it outputs images that are the same size as the input. It is one of the most important components of a diffusion system because it facilitates the actual diffusion process. There are several variants of the UNet model in 🤗 Diffusers, depending on it's number of dimensions and whether it is a conditional model or not. This is a 2D UNet conditional model.
-
-The abstract from the paper is:
-
-*There is large consent that successful training of deep networks requires many thousand annotated training samples. In this paper, we present a network and training strategy that relies on the strong use of data augmentation to use the available annotated samples more efficiently. The architecture consists of a contracting path to capture context and a symmetric expanding path that enables precise localization. We show that such a network can be trained end-to-end from very few images and outperforms the prior best method (a sliding-window convolutional network) on the ISBI challenge for segmentation of neuronal structures in electron microscopic stacks. Using the same network trained on transmitted light microscopy images (phase contrast and DIC) we won the ISBI cell tracking challenge 2015 in these categories by a large margin. Moreover, the network is fast. Segmentation of a 512x512 image takes less than a second on a recent GPU. The full implementation (based on Caffe) and the trained networks are available at http://lmb.informatik.uni-freiburg.de/people/ronneber/u-net.*
-
-## UNet2DConditionModel
-[[autodoc]] UNet2DConditionModel
-
-## UNet2DConditionOutput
-[[autodoc]] models.unet_2d_condition.UNet2DConditionOutput
-
-## FlaxUNet2DConditionModel
-[[autodoc]] models.unet_2d_condition_flax.FlaxUNet2DConditionModel
-
-## FlaxUNet2DConditionOutput
-[[autodoc]] models.unet_2d_condition_flax.FlaxUNet2DConditionOutput
\ No newline at end of file
diff --git a/spaces/Androidonnxfork/CivitAi-to-Diffusers/diffusers/examples/community/mixture_tiling.py b/spaces/Androidonnxfork/CivitAi-to-Diffusers/diffusers/examples/community/mixture_tiling.py
deleted file mode 100644
index 3e701cf607f55752543683aa7c7bf8615649aff7..0000000000000000000000000000000000000000
--- a/spaces/Androidonnxfork/CivitAi-to-Diffusers/diffusers/examples/community/mixture_tiling.py
+++ /dev/null
@@ -1,405 +0,0 @@
-import inspect
-from copy import deepcopy
-from enum import Enum
-from typing import List, Optional, Tuple, Union
-
-import torch
-from tqdm.auto import tqdm
-
-from diffusers.models import AutoencoderKL, UNet2DConditionModel
-from diffusers.pipeline_utils import DiffusionPipeline
-from diffusers.pipelines.stable_diffusion import StableDiffusionSafetyChecker
-from diffusers.schedulers import DDIMScheduler, LMSDiscreteScheduler, PNDMScheduler
-from diffusers.utils import logging
-
-
-try:
- from ligo.segments import segment
- from transformers import CLIPFeatureExtractor, CLIPTextModel, CLIPTokenizer
-except ImportError:
- raise ImportError("Please install transformers and ligo-segments to use the mixture pipeline")
-
-logger = logging.get_logger(__name__) # pylint: disable=invalid-name
-
-EXAMPLE_DOC_STRING = """
- Examples:
- ```py
- >>> from diffusers import LMSDiscreteScheduler, DiffusionPipeline
-
- >>> scheduler = LMSDiscreteScheduler(beta_start=0.00085, beta_end=0.012, beta_schedule="scaled_linear", num_train_timesteps=1000)
- >>> pipeline = DiffusionPipeline.from_pretrained("CompVis/stable-diffusion-v1-4", scheduler=scheduler, custom_pipeline="mixture_tiling")
- >>> pipeline.to("cuda")
-
- >>> image = pipeline(
- >>> prompt=[[
- >>> "A charming house in the countryside, by jakub rozalski, sunset lighting, elegant, highly detailed, smooth, sharp focus, artstation, stunning masterpiece",
- >>> "A dirt road in the countryside crossing pastures, by jakub rozalski, sunset lighting, elegant, highly detailed, smooth, sharp focus, artstation, stunning masterpiece",
- >>> "An old and rusty giant robot lying on a dirt road, by jakub rozalski, dark sunset lighting, elegant, highly detailed, smooth, sharp focus, artstation, stunning masterpiece"
- >>> ]],
- >>> tile_height=640,
- >>> tile_width=640,
- >>> tile_row_overlap=0,
- >>> tile_col_overlap=256,
- >>> guidance_scale=8,
- >>> seed=7178915308,
- >>> num_inference_steps=50,
- >>> )["images"][0]
- ```
-"""
-
-
-def _tile2pixel_indices(tile_row, tile_col, tile_width, tile_height, tile_row_overlap, tile_col_overlap):
- """Given a tile row and column numbers returns the range of pixels affected by that tiles in the overall image
-
- Returns a tuple with:
- - Starting coordinates of rows in pixel space
- - Ending coordinates of rows in pixel space
- - Starting coordinates of columns in pixel space
- - Ending coordinates of columns in pixel space
- """
- px_row_init = 0 if tile_row == 0 else tile_row * (tile_height - tile_row_overlap)
- px_row_end = px_row_init + tile_height
- px_col_init = 0 if tile_col == 0 else tile_col * (tile_width - tile_col_overlap)
- px_col_end = px_col_init + tile_width
- return px_row_init, px_row_end, px_col_init, px_col_end
-
-
-def _pixel2latent_indices(px_row_init, px_row_end, px_col_init, px_col_end):
- """Translates coordinates in pixel space to coordinates in latent space"""
- return px_row_init // 8, px_row_end // 8, px_col_init // 8, px_col_end // 8
-
-
-def _tile2latent_indices(tile_row, tile_col, tile_width, tile_height, tile_row_overlap, tile_col_overlap):
- """Given a tile row and column numbers returns the range of latents affected by that tiles in the overall image
-
- Returns a tuple with:
- - Starting coordinates of rows in latent space
- - Ending coordinates of rows in latent space
- - Starting coordinates of columns in latent space
- - Ending coordinates of columns in latent space
- """
- px_row_init, px_row_end, px_col_init, px_col_end = _tile2pixel_indices(
- tile_row, tile_col, tile_width, tile_height, tile_row_overlap, tile_col_overlap
- )
- return _pixel2latent_indices(px_row_init, px_row_end, px_col_init, px_col_end)
-
-
-def _tile2latent_exclusive_indices(
- tile_row, tile_col, tile_width, tile_height, tile_row_overlap, tile_col_overlap, rows, columns
-):
- """Given a tile row and column numbers returns the range of latents affected only by that tile in the overall image
-
- Returns a tuple with:
- - Starting coordinates of rows in latent space
- - Ending coordinates of rows in latent space
- - Starting coordinates of columns in latent space
- - Ending coordinates of columns in latent space
- """
- row_init, row_end, col_init, col_end = _tile2latent_indices(
- tile_row, tile_col, tile_width, tile_height, tile_row_overlap, tile_col_overlap
- )
- row_segment = segment(row_init, row_end)
- col_segment = segment(col_init, col_end)
- # Iterate over the rest of tiles, clipping the region for the current tile
- for row in range(rows):
- for column in range(columns):
- if row != tile_row and column != tile_col:
- clip_row_init, clip_row_end, clip_col_init, clip_col_end = _tile2latent_indices(
- row, column, tile_width, tile_height, tile_row_overlap, tile_col_overlap
- )
- row_segment = row_segment - segment(clip_row_init, clip_row_end)
- col_segment = col_segment - segment(clip_col_init, clip_col_end)
- # return row_init, row_end, col_init, col_end
- return row_segment[0], row_segment[1], col_segment[0], col_segment[1]
-
-
-class StableDiffusionExtrasMixin:
- """Mixin providing additional convenience method to Stable Diffusion pipelines"""
-
- def decode_latents(self, latents, cpu_vae=False):
- """Decodes a given array of latents into pixel space"""
- # scale and decode the image latents with vae
- if cpu_vae:
- lat = deepcopy(latents).cpu()
- vae = deepcopy(self.vae).cpu()
- else:
- lat = latents
- vae = self.vae
-
- lat = 1 / 0.18215 * lat
- image = vae.decode(lat).sample
-
- image = (image / 2 + 0.5).clamp(0, 1)
- image = image.cpu().permute(0, 2, 3, 1).numpy()
-
- return self.numpy_to_pil(image)
-
-
-class StableDiffusionTilingPipeline(DiffusionPipeline, StableDiffusionExtrasMixin):
- def __init__(
- self,
- vae: AutoencoderKL,
- text_encoder: CLIPTextModel,
- tokenizer: CLIPTokenizer,
- unet: UNet2DConditionModel,
- scheduler: Union[DDIMScheduler, PNDMScheduler],
- safety_checker: StableDiffusionSafetyChecker,
- feature_extractor: CLIPFeatureExtractor,
- ):
- super().__init__()
- self.register_modules(
- vae=vae,
- text_encoder=text_encoder,
- tokenizer=tokenizer,
- unet=unet,
- scheduler=scheduler,
- safety_checker=safety_checker,
- feature_extractor=feature_extractor,
- )
-
- class SeedTilesMode(Enum):
- """Modes in which the latents of a particular tile can be re-seeded"""
-
- FULL = "full"
- EXCLUSIVE = "exclusive"
-
- @torch.no_grad()
- def __call__(
- self,
- prompt: Union[str, List[List[str]]],
- num_inference_steps: Optional[int] = 50,
- guidance_scale: Optional[float] = 7.5,
- eta: Optional[float] = 0.0,
- seed: Optional[int] = None,
- tile_height: Optional[int] = 512,
- tile_width: Optional[int] = 512,
- tile_row_overlap: Optional[int] = 256,
- tile_col_overlap: Optional[int] = 256,
- guidance_scale_tiles: Optional[List[List[float]]] = None,
- seed_tiles: Optional[List[List[int]]] = None,
- seed_tiles_mode: Optional[Union[str, List[List[str]]]] = "full",
- seed_reroll_regions: Optional[List[Tuple[int, int, int, int, int]]] = None,
- cpu_vae: Optional[bool] = False,
- ):
- r"""
- Function to run the diffusion pipeline with tiling support.
-
- Args:
- prompt: either a single string (no tiling) or a list of lists with all the prompts to use (one list for each row of tiles). This will also define the tiling structure.
- num_inference_steps: number of diffusions steps.
- guidance_scale: classifier-free guidance.
- seed: general random seed to initialize latents.
- tile_height: height in pixels of each grid tile.
- tile_width: width in pixels of each grid tile.
- tile_row_overlap: number of overlap pixels between tiles in consecutive rows.
- tile_col_overlap: number of overlap pixels between tiles in consecutive columns.
- guidance_scale_tiles: specific weights for classifier-free guidance in each tile.
- guidance_scale_tiles: specific weights for classifier-free guidance in each tile. If None, the value provided in guidance_scale will be used.
- seed_tiles: specific seeds for the initialization latents in each tile. These will override the latents generated for the whole canvas using the standard seed parameter.
- seed_tiles_mode: either "full" "exclusive". If "full", all the latents affected by the tile be overriden. If "exclusive", only the latents that are affected exclusively by this tile (and no other tiles) will be overrriden.
- seed_reroll_regions: a list of tuples in the form (start row, end row, start column, end column, seed) defining regions in pixel space for which the latents will be overriden using the given seed. Takes priority over seed_tiles.
- cpu_vae: the decoder from latent space to pixel space can require too mucho GPU RAM for large images. If you find out of memory errors at the end of the generation process, try setting this parameter to True to run the decoder in CPU. Slower, but should run without memory issues.
-
- Examples:
-
- Returns:
- A PIL image with the generated image.
-
- """
- if not isinstance(prompt, list) or not all(isinstance(row, list) for row in prompt):
- raise ValueError(f"`prompt` has to be a list of lists but is {type(prompt)}")
- grid_rows = len(prompt)
- grid_cols = len(prompt[0])
- if not all(len(row) == grid_cols for row in prompt):
- raise ValueError("All prompt rows must have the same number of prompt columns")
- if not isinstance(seed_tiles_mode, str) and (
- not isinstance(seed_tiles_mode, list) or not all(isinstance(row, list) for row in seed_tiles_mode)
- ):
- raise ValueError(f"`seed_tiles_mode` has to be a string or list of lists but is {type(prompt)}")
- if isinstance(seed_tiles_mode, str):
- seed_tiles_mode = [[seed_tiles_mode for _ in range(len(row))] for row in prompt]
-
- modes = [mode.value for mode in self.SeedTilesMode]
- if any(mode not in modes for row in seed_tiles_mode for mode in row):
- raise ValueError(f"Seed tiles mode must be one of {modes}")
- if seed_reroll_regions is None:
- seed_reroll_regions = []
- batch_size = 1
-
- # create original noisy latents using the timesteps
- height = tile_height + (grid_rows - 1) * (tile_height - tile_row_overlap)
- width = tile_width + (grid_cols - 1) * (tile_width - tile_col_overlap)
- latents_shape = (batch_size, self.unet.config.in_channels, height // 8, width // 8)
- generator = torch.Generator("cuda").manual_seed(seed)
- latents = torch.randn(latents_shape, generator=generator, device=self.device)
-
- # overwrite latents for specific tiles if provided
- if seed_tiles is not None:
- for row in range(grid_rows):
- for col in range(grid_cols):
- if (seed_tile := seed_tiles[row][col]) is not None:
- mode = seed_tiles_mode[row][col]
- if mode == self.SeedTilesMode.FULL.value:
- row_init, row_end, col_init, col_end = _tile2latent_indices(
- row, col, tile_width, tile_height, tile_row_overlap, tile_col_overlap
- )
- else:
- row_init, row_end, col_init, col_end = _tile2latent_exclusive_indices(
- row,
- col,
- tile_width,
- tile_height,
- tile_row_overlap,
- tile_col_overlap,
- grid_rows,
- grid_cols,
- )
- tile_generator = torch.Generator("cuda").manual_seed(seed_tile)
- tile_shape = (latents_shape[0], latents_shape[1], row_end - row_init, col_end - col_init)
- latents[:, :, row_init:row_end, col_init:col_end] = torch.randn(
- tile_shape, generator=tile_generator, device=self.device
- )
-
- # overwrite again for seed reroll regions
- for row_init, row_end, col_init, col_end, seed_reroll in seed_reroll_regions:
- row_init, row_end, col_init, col_end = _pixel2latent_indices(
- row_init, row_end, col_init, col_end
- ) # to latent space coordinates
- reroll_generator = torch.Generator("cuda").manual_seed(seed_reroll)
- region_shape = (latents_shape[0], latents_shape[1], row_end - row_init, col_end - col_init)
- latents[:, :, row_init:row_end, col_init:col_end] = torch.randn(
- region_shape, generator=reroll_generator, device=self.device
- )
-
- # Prepare scheduler
- accepts_offset = "offset" in set(inspect.signature(self.scheduler.set_timesteps).parameters.keys())
- extra_set_kwargs = {}
- if accepts_offset:
- extra_set_kwargs["offset"] = 1
- self.scheduler.set_timesteps(num_inference_steps, **extra_set_kwargs)
- # if we use LMSDiscreteScheduler, let's make sure latents are multiplied by sigmas
- if isinstance(self.scheduler, LMSDiscreteScheduler):
- latents = latents * self.scheduler.sigmas[0]
-
- # get prompts text embeddings
- text_input = [
- [
- self.tokenizer(
- col,
- padding="max_length",
- max_length=self.tokenizer.model_max_length,
- truncation=True,
- return_tensors="pt",
- )
- for col in row
- ]
- for row in prompt
- ]
- text_embeddings = [[self.text_encoder(col.input_ids.to(self.device))[0] for col in row] for row in text_input]
-
- # here `guidance_scale` is defined analog to the guidance weight `w` of equation (2)
- # of the Imagen paper: https://arxiv.org/pdf/2205.11487.pdf . `guidance_scale = 1`
- # corresponds to doing no classifier free guidance.
- do_classifier_free_guidance = guidance_scale > 1.0 # TODO: also active if any tile has guidance scale
- # get unconditional embeddings for classifier free guidance
- if do_classifier_free_guidance:
- for i in range(grid_rows):
- for j in range(grid_cols):
- max_length = text_input[i][j].input_ids.shape[-1]
- uncond_input = self.tokenizer(
- [""] * batch_size, padding="max_length", max_length=max_length, return_tensors="pt"
- )
- uncond_embeddings = self.text_encoder(uncond_input.input_ids.to(self.device))[0]
-
- # For classifier free guidance, we need to do two forward passes.
- # Here we concatenate the unconditional and text embeddings into a single batch
- # to avoid doing two forward passes
- text_embeddings[i][j] = torch.cat([uncond_embeddings, text_embeddings[i][j]])
-
- # prepare extra kwargs for the scheduler step, since not all schedulers have the same signature
- # eta (η) is only used with the DDIMScheduler, it will be ignored for other schedulers.
- # eta corresponds to η in DDIM paper: https://arxiv.org/abs/2010.02502
- # and should be between [0, 1]
- accepts_eta = "eta" in set(inspect.signature(self.scheduler.step).parameters.keys())
- extra_step_kwargs = {}
- if accepts_eta:
- extra_step_kwargs["eta"] = eta
-
- # Mask for tile weights strenght
- tile_weights = self._gaussian_weights(tile_width, tile_height, batch_size)
-
- # Diffusion timesteps
- for i, t in tqdm(enumerate(self.scheduler.timesteps)):
- # Diffuse each tile
- noise_preds = []
- for row in range(grid_rows):
- noise_preds_row = []
- for col in range(grid_cols):
- px_row_init, px_row_end, px_col_init, px_col_end = _tile2latent_indices(
- row, col, tile_width, tile_height, tile_row_overlap, tile_col_overlap
- )
- tile_latents = latents[:, :, px_row_init:px_row_end, px_col_init:px_col_end]
- # expand the latents if we are doing classifier free guidance
- latent_model_input = torch.cat([tile_latents] * 2) if do_classifier_free_guidance else tile_latents
- latent_model_input = self.scheduler.scale_model_input(latent_model_input, t)
- # predict the noise residual
- noise_pred = self.unet(latent_model_input, t, encoder_hidden_states=text_embeddings[row][col])[
- "sample"
- ]
- # perform guidance
- if do_classifier_free_guidance:
- noise_pred_uncond, noise_pred_text = noise_pred.chunk(2)
- guidance = (
- guidance_scale
- if guidance_scale_tiles is None or guidance_scale_tiles[row][col] is None
- else guidance_scale_tiles[row][col]
- )
- noise_pred_tile = noise_pred_uncond + guidance * (noise_pred_text - noise_pred_uncond)
- noise_preds_row.append(noise_pred_tile)
- noise_preds.append(noise_preds_row)
- # Stitch noise predictions for all tiles
- noise_pred = torch.zeros(latents.shape, device=self.device)
- contributors = torch.zeros(latents.shape, device=self.device)
- # Add each tile contribution to overall latents
- for row in range(grid_rows):
- for col in range(grid_cols):
- px_row_init, px_row_end, px_col_init, px_col_end = _tile2latent_indices(
- row, col, tile_width, tile_height, tile_row_overlap, tile_col_overlap
- )
- noise_pred[:, :, px_row_init:px_row_end, px_col_init:px_col_end] += (
- noise_preds[row][col] * tile_weights
- )
- contributors[:, :, px_row_init:px_row_end, px_col_init:px_col_end] += tile_weights
- # Average overlapping areas with more than 1 contributor
- noise_pred /= contributors
-
- # compute the previous noisy sample x_t -> x_t-1
- latents = self.scheduler.step(noise_pred, t, latents).prev_sample
-
- # scale and decode the image latents with vae
- image = self.decode_latents(latents, cpu_vae)
-
- return {"images": image}
-
- def _gaussian_weights(self, tile_width, tile_height, nbatches):
- """Generates a gaussian mask of weights for tile contributions"""
- import numpy as np
- from numpy import exp, pi, sqrt
-
- latent_width = tile_width // 8
- latent_height = tile_height // 8
-
- var = 0.01
- midpoint = (latent_width - 1) / 2 # -1 because index goes from 0 to latent_width - 1
- x_probs = [
- exp(-(x - midpoint) * (x - midpoint) / (latent_width * latent_width) / (2 * var)) / sqrt(2 * pi * var)
- for x in range(latent_width)
- ]
- midpoint = latent_height / 2
- y_probs = [
- exp(-(y - midpoint) * (y - midpoint) / (latent_height * latent_height) / (2 * var)) / sqrt(2 * pi * var)
- for y in range(latent_height)
- ]
-
- weights = np.outer(y_probs, x_probs)
- return torch.tile(torch.tensor(weights, device=self.device), (nbatches, self.unet.config.in_channels, 1, 1))
diff --git a/spaces/Andy1621/uniformer_image_detection/configs/dcn/mask_rcnn_r101_fpn_dconv_c3-c5_1x_coco.py b/spaces/Andy1621/uniformer_image_detection/configs/dcn/mask_rcnn_r101_fpn_dconv_c3-c5_1x_coco.py
deleted file mode 100644
index cb340022ea27f563b8c4a570cf89b5f09e6434cd..0000000000000000000000000000000000000000
--- a/spaces/Andy1621/uniformer_image_detection/configs/dcn/mask_rcnn_r101_fpn_dconv_c3-c5_1x_coco.py
+++ /dev/null
@@ -1,5 +0,0 @@
-_base_ = '../mask_rcnn/mask_rcnn_r101_fpn_1x_coco.py'
-model = dict(
- backbone=dict(
- dcn=dict(type='DCN', deform_groups=1, fallback_on_stride=False),
- stage_with_dcn=(False, True, True, True)))
diff --git a/spaces/Andy1621/uniformer_image_detection/configs/gn+ws/mask_rcnn_r50_fpn_gn_ws-all_2x_coco.py b/spaces/Andy1621/uniformer_image_detection/configs/gn+ws/mask_rcnn_r50_fpn_gn_ws-all_2x_coco.py
deleted file mode 100644
index b83e7b5c7dd63658d57397cde60d8ee4c74d8376..0000000000000000000000000000000000000000
--- a/spaces/Andy1621/uniformer_image_detection/configs/gn+ws/mask_rcnn_r50_fpn_gn_ws-all_2x_coco.py
+++ /dev/null
@@ -1,17 +0,0 @@
-_base_ = '../mask_rcnn/mask_rcnn_r50_fpn_1x_coco.py'
-conv_cfg = dict(type='ConvWS')
-norm_cfg = dict(type='GN', num_groups=32, requires_grad=True)
-model = dict(
- pretrained='open-mmlab://jhu/resnet50_gn_ws',
- backbone=dict(conv_cfg=conv_cfg, norm_cfg=norm_cfg),
- neck=dict(conv_cfg=conv_cfg, norm_cfg=norm_cfg),
- roi_head=dict(
- bbox_head=dict(
- type='Shared4Conv1FCBBoxHead',
- conv_out_channels=256,
- conv_cfg=conv_cfg,
- norm_cfg=norm_cfg),
- mask_head=dict(conv_cfg=conv_cfg, norm_cfg=norm_cfg)))
-# learning policy
-lr_config = dict(step=[16, 22])
-runner = dict(type='EpochBasedRunner', max_epochs=24)
diff --git a/spaces/Andy1621/uniformer_image_detection/mmdet/core/bbox/match_costs/builder.py b/spaces/Andy1621/uniformer_image_detection/mmdet/core/bbox/match_costs/builder.py
deleted file mode 100644
index 6894017d42eb16ee4a8ae3ed660a71cda3ad9940..0000000000000000000000000000000000000000
--- a/spaces/Andy1621/uniformer_image_detection/mmdet/core/bbox/match_costs/builder.py
+++ /dev/null
@@ -1,8 +0,0 @@
-from mmcv.utils import Registry, build_from_cfg
-
-MATCH_COST = Registry('Match Cost')
-
-
-def build_match_cost(cfg, default_args=None):
- """Builder of IoU calculator."""
- return build_from_cfg(cfg, MATCH_COST, default_args)
diff --git a/spaces/Andy1621/uniformer_image_detection/mmdet/core/visualization/image.py b/spaces/Andy1621/uniformer_image_detection/mmdet/core/visualization/image.py
deleted file mode 100644
index 5a148384d7a77c4d9849c54570e85740eaff8235..0000000000000000000000000000000000000000
--- a/spaces/Andy1621/uniformer_image_detection/mmdet/core/visualization/image.py
+++ /dev/null
@@ -1,303 +0,0 @@
-import matplotlib.pyplot as plt
-import mmcv
-import numpy as np
-import pycocotools.mask as mask_util
-from matplotlib.collections import PatchCollection
-from matplotlib.patches import Polygon
-
-from ..utils import mask2ndarray
-
-EPS = 1e-2
-
-
-def color_val_matplotlib(color):
- """Convert various input in BGR order to normalized RGB matplotlib color
- tuples,
-
- Args:
- color (:obj:`Color`/str/tuple/int/ndarray): Color inputs
-
- Returns:
- tuple[float]: A tuple of 3 normalized floats indicating RGB channels.
- """
- color = mmcv.color_val(color)
- color = [color / 255 for color in color[::-1]]
- return tuple(color)
-
-
-def imshow_det_bboxes(img,
- bboxes,
- labels,
- segms=None,
- class_names=None,
- score_thr=0,
- bbox_color='green',
- text_color='green',
- mask_color=None,
- thickness=2,
- font_size=13,
- win_name='',
- show=True,
- wait_time=0,
- out_file=None):
- """Draw bboxes and class labels (with scores) on an image.
-
- Args:
- img (str or ndarray): The image to be displayed.
- bboxes (ndarray): Bounding boxes (with scores), shaped (n, 4) or
- (n, 5).
- labels (ndarray): Labels of bboxes.
- segms (ndarray or None): Masks, shaped (n,h,w) or None
- class_names (list[str]): Names of each classes.
- score_thr (float): Minimum score of bboxes to be shown. Default: 0
- bbox_color (str or tuple(int) or :obj:`Color`):Color of bbox lines.
- The tuple of color should be in BGR order. Default: 'green'
- text_color (str or tuple(int) or :obj:`Color`):Color of texts.
- The tuple of color should be in BGR order. Default: 'green'
- mask_color (str or tuple(int) or :obj:`Color`, optional):
- Color of masks. The tuple of color should be in BGR order.
- Default: None
- thickness (int): Thickness of lines. Default: 2
- font_size (int): Font size of texts. Default: 13
- show (bool): Whether to show the image. Default: True
- win_name (str): The window name. Default: ''
- wait_time (float): Value of waitKey param. Default: 0.
- out_file (str, optional): The filename to write the image.
- Default: None
-
- Returns:
- ndarray: The image with bboxes drawn on it.
- """
- assert bboxes.ndim == 2, \
- f' bboxes ndim should be 2, but its ndim is {bboxes.ndim}.'
- assert labels.ndim == 1, \
- f' labels ndim should be 1, but its ndim is {labels.ndim}.'
- assert bboxes.shape[0] == labels.shape[0], \
- 'bboxes.shape[0] and labels.shape[0] should have the same length.'
- assert bboxes.shape[1] == 4 or bboxes.shape[1] == 5, \
- f' bboxes.shape[1] should be 4 or 5, but its {bboxes.shape[1]}.'
- img = mmcv.imread(img).astype(np.uint8)
-
- if score_thr > 0:
- assert bboxes.shape[1] == 5
- scores = bboxes[:, -1]
- inds = scores > score_thr
- bboxes = bboxes[inds, :]
- labels = labels[inds]
- if segms is not None:
- segms = segms[inds, ...]
-
- mask_colors = []
- if labels.shape[0] > 0:
- if mask_color is None:
- # random color
- np.random.seed(42)
- mask_colors = [
- np.random.randint(0, 256, (1, 3), dtype=np.uint8)
- for _ in range(max(labels) + 1)
- ]
- else:
- # specify color
- mask_colors = [
- np.array(mmcv.color_val(mask_color)[::-1], dtype=np.uint8)
- ] * (
- max(labels) + 1)
-
- bbox_color = color_val_matplotlib(bbox_color)
- text_color = color_val_matplotlib(text_color)
-
- img = mmcv.bgr2rgb(img)
- width, height = img.shape[1], img.shape[0]
- img = np.ascontiguousarray(img)
-
- fig = plt.figure(win_name, frameon=False)
- plt.title(win_name)
- canvas = fig.canvas
- dpi = fig.get_dpi()
- # add a small EPS to avoid precision lost due to matplotlib's truncation
- # (https://github.com/matplotlib/matplotlib/issues/15363)
- fig.set_size_inches((width + EPS) / dpi, (height + EPS) / dpi)
-
- # remove white edges by set subplot margin
- plt.subplots_adjust(left=0, right=1, bottom=0, top=1)
- ax = plt.gca()
- ax.axis('off')
-
- polygons = []
- color = []
- for i, (bbox, label) in enumerate(zip(bboxes, labels)):
- bbox_int = bbox.astype(np.int32)
- poly = [[bbox_int[0], bbox_int[1]], [bbox_int[0], bbox_int[3]],
- [bbox_int[2], bbox_int[3]], [bbox_int[2], bbox_int[1]]]
- np_poly = np.array(poly).reshape((4, 2))
- polygons.append(Polygon(np_poly))
- color.append(bbox_color)
- label_text = class_names[
- label] if class_names is not None else f'class {label}'
- if len(bbox) > 4:
- label_text += f'|{bbox[-1]:.02f}'
- ax.text(
- bbox_int[0],
- bbox_int[1],
- f'{label_text}',
- bbox={
- 'facecolor': 'black',
- 'alpha': 0.8,
- 'pad': 0.7,
- 'edgecolor': 'none'
- },
- color=text_color,
- fontsize=font_size,
- verticalalignment='top',
- horizontalalignment='left')
- if segms is not None:
- color_mask = mask_colors[labels[i]]
- mask = segms[i].astype(bool)
- img[mask] = img[mask] * 0.5 + color_mask * 0.5
-
- plt.imshow(img)
-
- p = PatchCollection(
- polygons, facecolor='none', edgecolors=color, linewidths=thickness)
- ax.add_collection(p)
-
- stream, _ = canvas.print_to_buffer()
- buffer = np.frombuffer(stream, dtype='uint8')
- img_rgba = buffer.reshape(height, width, 4)
- rgb, alpha = np.split(img_rgba, [3], axis=2)
- img = rgb.astype('uint8')
- img = mmcv.rgb2bgr(img)
-
- if show:
- # We do not use cv2 for display because in some cases, opencv will
- # conflict with Qt, it will output a warning: Current thread
- # is not the object's thread. You can refer to
- # https://github.com/opencv/opencv-python/issues/46 for details
- if wait_time == 0:
- plt.show()
- else:
- plt.show(block=False)
- plt.pause(wait_time)
- if out_file is not None:
- mmcv.imwrite(img, out_file)
-
- plt.close()
-
- return img
-
-
-def imshow_gt_det_bboxes(img,
- annotation,
- result,
- class_names=None,
- score_thr=0,
- gt_bbox_color=(255, 102, 61),
- gt_text_color=(255, 102, 61),
- gt_mask_color=(255, 102, 61),
- det_bbox_color=(72, 101, 241),
- det_text_color=(72, 101, 241),
- det_mask_color=(72, 101, 241),
- thickness=2,
- font_size=13,
- win_name='',
- show=True,
- wait_time=0,
- out_file=None):
- """General visualization GT and result function.
-
- Args:
- img (str or ndarray): The image to be displayed.)
- annotation (dict): Ground truth annotations where contain keys of
- 'gt_bboxes' and 'gt_labels' or 'gt_masks'
- result (tuple[list] or list): The detection result, can be either
- (bbox, segm) or just bbox.
- class_names (list[str]): Names of each classes.
- score_thr (float): Minimum score of bboxes to be shown. Default: 0
- gt_bbox_color (str or tuple(int) or :obj:`Color`):Color of bbox lines.
- The tuple of color should be in BGR order. Default: (255, 102, 61)
- gt_text_color (str or tuple(int) or :obj:`Color`):Color of texts.
- The tuple of color should be in BGR order. Default: (255, 102, 61)
- gt_mask_color (str or tuple(int) or :obj:`Color`, optional):
- Color of masks. The tuple of color should be in BGR order.
- Default: (255, 102, 61)
- det_bbox_color (str or tuple(int) or :obj:`Color`):Color of bbox lines.
- The tuple of color should be in BGR order. Default: (72, 101, 241)
- det_text_color (str or tuple(int) or :obj:`Color`):Color of texts.
- The tuple of color should be in BGR order. Default: (72, 101, 241)
- det_mask_color (str or tuple(int) or :obj:`Color`, optional):
- Color of masks. The tuple of color should be in BGR order.
- Default: (72, 101, 241)
- thickness (int): Thickness of lines. Default: 2
- font_size (int): Font size of texts. Default: 13
- win_name (str): The window name. Default: ''
- show (bool): Whether to show the image. Default: True
- wait_time (float): Value of waitKey param. Default: 0.
- out_file (str, optional): The filename to write the image.
- Default: None
-
- Returns:
- ndarray: The image with bboxes or masks drawn on it.
- """
- assert 'gt_bboxes' in annotation
- assert 'gt_labels' in annotation
- assert isinstance(
- result,
- (tuple, list)), f'Expected tuple or list, but get {type(result)}'
-
- gt_masks = annotation.get('gt_masks', None)
- if gt_masks is not None:
- gt_masks = mask2ndarray(gt_masks)
-
- img = mmcv.imread(img)
-
- img = imshow_det_bboxes(
- img,
- annotation['gt_bboxes'],
- annotation['gt_labels'],
- gt_masks,
- class_names=class_names,
- bbox_color=gt_bbox_color,
- text_color=gt_text_color,
- mask_color=gt_mask_color,
- thickness=thickness,
- font_size=font_size,
- win_name=win_name,
- show=False)
-
- if isinstance(result, tuple):
- bbox_result, segm_result = result
- if isinstance(segm_result, tuple):
- segm_result = segm_result[0] # ms rcnn
- else:
- bbox_result, segm_result = result, None
-
- bboxes = np.vstack(bbox_result)
- labels = [
- np.full(bbox.shape[0], i, dtype=np.int32)
- for i, bbox in enumerate(bbox_result)
- ]
- labels = np.concatenate(labels)
-
- segms = None
- if segm_result is not None and len(labels) > 0: # non empty
- segms = mmcv.concat_list(segm_result)
- segms = mask_util.decode(segms)
- segms = segms.transpose(2, 0, 1)
-
- img = imshow_det_bboxes(
- img,
- bboxes,
- labels,
- segms=segms,
- class_names=class_names,
- score_thr=score_thr,
- bbox_color=det_bbox_color,
- text_color=det_text_color,
- mask_color=det_mask_color,
- thickness=thickness,
- font_size=font_size,
- win_name=win_name,
- show=show,
- wait_time=wait_time,
- out_file=out_file)
- return img
diff --git a/spaces/Andy1621/uniformer_image_segmentation/configs/ann/ann_r50-d8_512x512_20k_voc12aug.py b/spaces/Andy1621/uniformer_image_segmentation/configs/ann/ann_r50-d8_512x512_20k_voc12aug.py
deleted file mode 100644
index 071f190261c4e8f4a80a5da12a88e0cfcdfef0d8..0000000000000000000000000000000000000000
--- a/spaces/Andy1621/uniformer_image_segmentation/configs/ann/ann_r50-d8_512x512_20k_voc12aug.py
+++ /dev/null
@@ -1,6 +0,0 @@
-_base_ = [
- '../_base_/models/ann_r50-d8.py', '../_base_/datasets/pascal_voc12_aug.py',
- '../_base_/default_runtime.py', '../_base_/schedules/schedule_20k.py'
-]
-model = dict(
- decode_head=dict(num_classes=21), auxiliary_head=dict(num_classes=21))
diff --git a/spaces/Andy1621/uniformer_image_segmentation/configs/deeplabv3/deeplabv3_r50-d8_512x512_40k_voc12aug.py b/spaces/Andy1621/uniformer_image_segmentation/configs/deeplabv3/deeplabv3_r50-d8_512x512_40k_voc12aug.py
deleted file mode 100644
index 492bd3dfdce331070cb9645dbe55142e9b662da1..0000000000000000000000000000000000000000
--- a/spaces/Andy1621/uniformer_image_segmentation/configs/deeplabv3/deeplabv3_r50-d8_512x512_40k_voc12aug.py
+++ /dev/null
@@ -1,7 +0,0 @@
-_base_ = [
- '../_base_/models/deeplabv3_r50-d8.py',
- '../_base_/datasets/pascal_voc12_aug.py', '../_base_/default_runtime.py',
- '../_base_/schedules/schedule_40k.py'
-]
-model = dict(
- decode_head=dict(num_classes=21), auxiliary_head=dict(num_classes=21))
diff --git a/spaces/Andy1621/uniformer_image_segmentation/configs/mobilenet_v3/lraspp_m-v3s-d8_scratch_512x1024_320k_cityscapes.py b/spaces/Andy1621/uniformer_image_segmentation/configs/mobilenet_v3/lraspp_m-v3s-d8_scratch_512x1024_320k_cityscapes.py
deleted file mode 100644
index 0c5f707200c5d8b6d39493762baf59023dcaad11..0000000000000000000000000000000000000000
--- a/spaces/Andy1621/uniformer_image_segmentation/configs/mobilenet_v3/lraspp_m-v3s-d8_scratch_512x1024_320k_cityscapes.py
+++ /dev/null
@@ -1,22 +0,0 @@
-_base_ = './lraspp_m-v3-d8_scratch_512x1024_320k_cityscapes.py'
-norm_cfg = dict(type='SyncBN', eps=0.001, requires_grad=True)
-model = dict(
- type='EncoderDecoder',
- backbone=dict(
- type='MobileNetV3',
- arch='small',
- out_indices=(0, 1, 12),
- norm_cfg=norm_cfg),
- decode_head=dict(
- type='LRASPPHead',
- in_channels=(16, 16, 576),
- in_index=(0, 1, 2),
- channels=128,
- input_transform='multiple_select',
- dropout_ratio=0.1,
- num_classes=19,
- norm_cfg=norm_cfg,
- act_cfg=dict(type='ReLU'),
- align_corners=False,
- loss_decode=dict(
- type='CrossEntropyLoss', use_sigmoid=False, loss_weight=1.0)))
diff --git a/spaces/Artrajz/vits-simple-api/Dockerfile b/spaces/Artrajz/vits-simple-api/Dockerfile
deleted file mode 100644
index f1b1f95b644347246f0925c3b882abfeeb2e31ae..0000000000000000000000000000000000000000
--- a/spaces/Artrajz/vits-simple-api/Dockerfile
+++ /dev/null
@@ -1,38 +0,0 @@
-FROM artrajz/pytorch:1.13.1-cpu-py3.10.11-ubuntu22.04
-
-RUN mkdir -p /app
-WORKDIR /app
-
-ENV DEBIAN_FRONTEND=noninteractive
-
-
-RUN apt-get update && \
- apt-get install -yq build-essential espeak-ng cmake wget ca-certificates tzdata&& \
- update-ca-certificates && \
- apt-get clean && \
- apt-get purge -y --auto-remove -o APT::AutoRemove::RecommendsImportant=false && \
- rm -rf /var/lib/apt/lists/*
-
-# Install jemalloc
-RUN wget https://github.com/jemalloc/jemalloc/releases/download/5.3.0/jemalloc-5.3.0.tar.bz2 && \
- tar -xvf jemalloc-5.3.0.tar.bz2 && \
- cd jemalloc-5.3.0 && \
- ./configure && \
- make -j$(nproc) && \
- make install && \
- cd .. && \
- rm -rf jemalloc-5.3.0* && \
- ldconfig
-
-ENV LD_PRELOAD=/usr/local/lib/libjemalloc.so
-
-COPY requirements.txt /app/
-RUN pip install gunicorn --no-cache-dir && \
- pip install -r requirements.txt --no-cache-dir&& \
- rm -rf /root/.cache/pip/*
-
-COPY . /app
-
-EXPOSE 23456
-
-CMD ["gunicorn", "-c", "gunicorn_config.py", "app:app"]
\ No newline at end of file
diff --git a/spaces/Arulkumar03/GroundingDINO_SOTA_Zero_Shot_Model/groundingdino/models/GroundingDINO/ms_deform_attn.py b/spaces/Arulkumar03/GroundingDINO_SOTA_Zero_Shot_Model/groundingdino/models/GroundingDINO/ms_deform_attn.py
deleted file mode 100644
index 489d501bef364020212306d81e9b85c8daa27491..0000000000000000000000000000000000000000
--- a/spaces/Arulkumar03/GroundingDINO_SOTA_Zero_Shot_Model/groundingdino/models/GroundingDINO/ms_deform_attn.py
+++ /dev/null
@@ -1,413 +0,0 @@
-# ------------------------------------------------------------------------
-# Grounding DINO
-# url: https://github.com/IDEA-Research/GroundingDINO
-# Copyright (c) 2023 IDEA. All Rights Reserved.
-# Licensed under the Apache License, Version 2.0 [see LICENSE for details]
-# ------------------------------------------------------------------------
-# Deformable DETR
-# Copyright (c) 2020 SenseTime. All Rights Reserved.
-# Licensed under the Apache License, Version 2.0 [see LICENSE for details]
-# ------------------------------------------------------------------------------------------------
-# Modified from:
-# https://github.com/fundamentalvision/Deformable-DETR/blob/main/models/ops/functions/ms_deform_attn_func.py
-# https://github.com/fundamentalvision/Deformable-DETR/blob/main/models/ops/modules/ms_deform_attn.py
-# https://github.com/open-mmlab/mmcv/blob/master/mmcv/ops/multi_scale_deform_attn.py
-# ------------------------------------------------------------------------------------------------
-
-import math
-import warnings
-from typing import Optional
-
-import torch
-import torch.nn as nn
-import torch.nn.functional as F
-from torch.autograd import Function
-from torch.autograd.function import once_differentiable
-from torch.nn.init import constant_, xavier_uniform_
-
-try:
- from groundingdino import _C
-except:
- warnings.warn("Failed to load custom C++ ops. Running on CPU mode Only!")
-
-
-# helpers
-def _is_power_of_2(n):
- if (not isinstance(n, int)) or (n < 0):
- raise ValueError("invalid input for _is_power_of_2: {} (type: {})".format(n, type(n)))
- return (n & (n - 1) == 0) and n != 0
-
-
-class MultiScaleDeformableAttnFunction(Function):
- @staticmethod
- def forward(
- ctx,
- value,
- value_spatial_shapes,
- value_level_start_index,
- sampling_locations,
- attention_weights,
- im2col_step,
- ):
- ctx.im2col_step = im2col_step
- output = _C.ms_deform_attn_forward(
- value,
- value_spatial_shapes,
- value_level_start_index,
- sampling_locations,
- attention_weights,
- ctx.im2col_step,
- )
- ctx.save_for_backward(
- value,
- value_spatial_shapes,
- value_level_start_index,
- sampling_locations,
- attention_weights,
- )
- return output
-
- @staticmethod
- @once_differentiable
- def backward(ctx, grad_output):
- (
- value,
- value_spatial_shapes,
- value_level_start_index,
- sampling_locations,
- attention_weights,
- ) = ctx.saved_tensors
- grad_value, grad_sampling_loc, grad_attn_weight = _C.ms_deform_attn_backward(
- value,
- value_spatial_shapes,
- value_level_start_index,
- sampling_locations,
- attention_weights,
- grad_output,
- ctx.im2col_step,
- )
-
- return grad_value, None, None, grad_sampling_loc, grad_attn_weight, None
-
-
-def multi_scale_deformable_attn_pytorch(
- value: torch.Tensor,
- value_spatial_shapes: torch.Tensor,
- sampling_locations: torch.Tensor,
- attention_weights: torch.Tensor,
-) -> torch.Tensor:
-
- bs, _, num_heads, embed_dims = value.shape
- _, num_queries, num_heads, num_levels, num_points, _ = sampling_locations.shape
- value_list = value.split([H_ * W_ for H_, W_ in value_spatial_shapes], dim=1)
- sampling_grids = 2 * sampling_locations - 1
- sampling_value_list = []
- for level, (H_, W_) in enumerate(value_spatial_shapes):
- # bs, H_*W_, num_heads, embed_dims ->
- # bs, H_*W_, num_heads*embed_dims ->
- # bs, num_heads*embed_dims, H_*W_ ->
- # bs*num_heads, embed_dims, H_, W_
- value_l_ = (
- value_list[level].flatten(2).transpose(1, 2).reshape(bs * num_heads, embed_dims, H_, W_)
- )
- # bs, num_queries, num_heads, num_points, 2 ->
- # bs, num_heads, num_queries, num_points, 2 ->
- # bs*num_heads, num_queries, num_points, 2
- sampling_grid_l_ = sampling_grids[:, :, :, level].transpose(1, 2).flatten(0, 1)
- # bs*num_heads, embed_dims, num_queries, num_points
- sampling_value_l_ = F.grid_sample(
- value_l_, sampling_grid_l_, mode="bilinear", padding_mode="zeros", align_corners=False
- )
- sampling_value_list.append(sampling_value_l_)
- # (bs, num_queries, num_heads, num_levels, num_points) ->
- # (bs, num_heads, num_queries, num_levels, num_points) ->
- # (bs, num_heads, 1, num_queries, num_levels*num_points)
- attention_weights = attention_weights.transpose(1, 2).reshape(
- bs * num_heads, 1, num_queries, num_levels * num_points
- )
- output = (
- (torch.stack(sampling_value_list, dim=-2).flatten(-2) * attention_weights)
- .sum(-1)
- .view(bs, num_heads * embed_dims, num_queries)
- )
- return output.transpose(1, 2).contiguous()
-
-
-class MultiScaleDeformableAttention(nn.Module):
- """Multi-Scale Deformable Attention Module used in Deformable-DETR
-
- `Deformable DETR: Deformable Transformers for End-to-End Object Detection.
- `_.
-
- Args:
- embed_dim (int): The embedding dimension of Attention. Default: 256.
- num_heads (int): The number of attention heads. Default: 8.
- num_levels (int): The number of feature map used in Attention. Default: 4.
- num_points (int): The number of sampling points for each query
- in each head. Default: 4.
- img2col_steps (int): The step used in image_to_column. Defualt: 64.
- dropout (float): Dropout layer used in output. Default: 0.1.
- batch_first (bool): if ``True``, then the input and output tensor will be
- provided as `(bs, n, embed_dim)`. Default: False. `(n, bs, embed_dim)`
- """
-
- def __init__(
- self,
- embed_dim: int = 256,
- num_heads: int = 8,
- num_levels: int = 4,
- num_points: int = 4,
- img2col_step: int = 64,
- batch_first: bool = False,
- ):
- super().__init__()
- if embed_dim % num_heads != 0:
- raise ValueError(
- "embed_dim must be divisible by num_heads, but got {} and {}".format(
- embed_dim, num_heads
- )
- )
- head_dim = embed_dim // num_heads
-
- self.batch_first = batch_first
-
- if not _is_power_of_2(head_dim):
- warnings.warn(
- """
- You'd better set d_model in MSDeformAttn to make sure that
- each dim of the attention head a power of 2, which is more efficient.
- """
- )
-
- self.im2col_step = img2col_step
- self.embed_dim = embed_dim
- self.num_heads = num_heads
- self.num_levels = num_levels
- self.num_points = num_points
- self.sampling_offsets = nn.Linear(embed_dim, num_heads * num_levels * num_points * 2)
- self.attention_weights = nn.Linear(embed_dim, num_heads * num_levels * num_points)
- self.value_proj = nn.Linear(embed_dim, embed_dim)
- self.output_proj = nn.Linear(embed_dim, embed_dim)
-
- self.init_weights()
-
- def _reset_parameters(self):
- return self.init_weights()
-
- def init_weights(self):
- """
- Default initialization for Parameters of Module.
- """
- constant_(self.sampling_offsets.weight.data, 0.0)
- thetas = torch.arange(self.num_heads, dtype=torch.float32) * (
- 2.0 * math.pi / self.num_heads
- )
- grid_init = torch.stack([thetas.cos(), thetas.sin()], -1)
- grid_init = (
- (grid_init / grid_init.abs().max(-1, keepdim=True)[0])
- .view(self.num_heads, 1, 1, 2)
- .repeat(1, self.num_levels, self.num_points, 1)
- )
- for i in range(self.num_points):
- grid_init[:, :, i, :] *= i + 1
- with torch.no_grad():
- self.sampling_offsets.bias = nn.Parameter(grid_init.view(-1))
- constant_(self.attention_weights.weight.data, 0.0)
- constant_(self.attention_weights.bias.data, 0.0)
- xavier_uniform_(self.value_proj.weight.data)
- constant_(self.value_proj.bias.data, 0.0)
- xavier_uniform_(self.output_proj.weight.data)
- constant_(self.output_proj.bias.data, 0.0)
-
- def freeze_sampling_offsets(self):
- print("Freeze sampling offsets")
- self.sampling_offsets.weight.requires_grad = False
- self.sampling_offsets.bias.requires_grad = False
-
- def freeze_attention_weights(self):
- print("Freeze attention weights")
- self.attention_weights.weight.requires_grad = False
- self.attention_weights.bias.requires_grad = False
-
- def forward(
- self,
- query: torch.Tensor,
- key: Optional[torch.Tensor] = None,
- value: Optional[torch.Tensor] = None,
- query_pos: Optional[torch.Tensor] = None,
- key_padding_mask: Optional[torch.Tensor] = None,
- reference_points: Optional[torch.Tensor] = None,
- spatial_shapes: Optional[torch.Tensor] = None,
- level_start_index: Optional[torch.Tensor] = None,
- **kwargs
- ) -> torch.Tensor:
-
- """Forward Function of MultiScaleDeformableAttention
-
- Args:
- query (torch.Tensor): Query embeddings with shape
- `(num_query, bs, embed_dim)`
- key (torch.Tensor): Key embeddings with shape
- `(num_key, bs, embed_dim)`
- value (torch.Tensor): Value embeddings with shape
- `(num_key, bs, embed_dim)`
- query_pos (torch.Tensor): The position embedding for `query`. Default: None.
- key_padding_mask (torch.Tensor): ByteTensor for `query`, with shape `(bs, num_key)`,
- indicating which elements within `key` to be ignored in attention.
- reference_points (torch.Tensor): The normalized reference points
- with shape `(bs, num_query, num_levels, 2)`,
- all elements is range in [0, 1], top-left (0, 0),
- bottom-right (1, 1), including padding are.
- or `(N, Length_{query}, num_levels, 4)`, add additional
- two dimensions `(h, w)` to form reference boxes.
- spatial_shapes (torch.Tensor): Spatial shape of features in different levels.
- With shape `(num_levels, 2)`, last dimension represents `(h, w)`.
- level_start_index (torch.Tensor): The start index of each level. A tensor with
- shape `(num_levels, )` which can be represented as
- `[0, h_0 * w_0, h_0 * w_0 + h_1 * w_1, ...]`.
-
- Returns:
- torch.Tensor: forward results with shape `(num_query, bs, embed_dim)`
- """
-
- if value is None:
- value = query
-
- if query_pos is not None:
- query = query + query_pos
-
- if not self.batch_first:
- # change to (bs, num_query ,embed_dims)
- query = query.permute(1, 0, 2)
- value = value.permute(1, 0, 2)
-
- bs, num_query, _ = query.shape
- bs, num_value, _ = value.shape
-
- assert (spatial_shapes[:, 0] * spatial_shapes[:, 1]).sum() == num_value
-
- value = self.value_proj(value)
- if key_padding_mask is not None:
- value = value.masked_fill(key_padding_mask[..., None], float(0))
- value = value.view(bs, num_value, self.num_heads, -1)
- sampling_offsets = self.sampling_offsets(query).view(
- bs, num_query, self.num_heads, self.num_levels, self.num_points, 2
- )
- attention_weights = self.attention_weights(query).view(
- bs, num_query, self.num_heads, self.num_levels * self.num_points
- )
- attention_weights = attention_weights.softmax(-1)
- attention_weights = attention_weights.view(
- bs,
- num_query,
- self.num_heads,
- self.num_levels,
- self.num_points,
- )
-
- # bs, num_query, num_heads, num_levels, num_points, 2
- if reference_points.shape[-1] == 2:
- offset_normalizer = torch.stack([spatial_shapes[..., 1], spatial_shapes[..., 0]], -1)
- sampling_locations = (
- reference_points[:, :, None, :, None, :]
- + sampling_offsets / offset_normalizer[None, None, None, :, None, :]
- )
- elif reference_points.shape[-1] == 4:
- sampling_locations = (
- reference_points[:, :, None, :, None, :2]
- + sampling_offsets
- / self.num_points
- * reference_points[:, :, None, :, None, 2:]
- * 0.5
- )
- else:
- raise ValueError(
- "Last dim of reference_points must be 2 or 4, but get {} instead.".format(
- reference_points.shape[-1]
- )
- )
-
- if torch.cuda.is_available() and value.is_cuda:
- halffloat = False
- if value.dtype == torch.float16:
- halffloat = True
- value = value.float()
- sampling_locations = sampling_locations.float()
- attention_weights = attention_weights.float()
-
- output = MultiScaleDeformableAttnFunction.apply(
- value,
- spatial_shapes,
- level_start_index,
- sampling_locations,
- attention_weights,
- self.im2col_step,
- )
-
- if halffloat:
- output = output.half()
- else:
- output = multi_scale_deformable_attn_pytorch(
- value, spatial_shapes, sampling_locations, attention_weights
- )
-
- output = self.output_proj(output)
-
- if not self.batch_first:
- output = output.permute(1, 0, 2)
-
- return output
-
-
-def create_dummy_class(klass, dependency, message=""):
- """
- When a dependency of a class is not available, create a dummy class which throws ImportError
- when used.
-
- Args:
- klass (str): name of the class.
- dependency (str): name of the dependency.
- message: extra message to print
- Returns:
- class: a class object
- """
- err = "Cannot import '{}', therefore '{}' is not available.".format(dependency, klass)
- if message:
- err = err + " " + message
-
- class _DummyMetaClass(type):
- # throw error on class attribute access
- def __getattr__(_, __): # noqa: B902
- raise ImportError(err)
-
- class _Dummy(object, metaclass=_DummyMetaClass):
- # throw error on constructor
- def __init__(self, *args, **kwargs):
- raise ImportError(err)
-
- return _Dummy
-
-
-def create_dummy_func(func, dependency, message=""):
- """
- When a dependency of a function is not available, create a dummy function which throws
- ImportError when used.
-
- Args:
- func (str): name of the function.
- dependency (str or list[str]): name(s) of the dependency.
- message: extra message to print
- Returns:
- function: a function object
- """
- err = "Cannot import '{}', therefore '{}' is not available.".format(dependency, func)
- if message:
- err = err + " " + message
-
- if isinstance(dependency, (list, tuple)):
- dependency = ",".join(dependency)
-
- def _dummy(*args, **kwargs):
- raise ImportError(err)
-
- return _dummy
diff --git a/spaces/Awiny/Image2Paragraph/models/grit_src/third_party/CenterNet2/configs/common/models/panoptic_fpn.py b/spaces/Awiny/Image2Paragraph/models/grit_src/third_party/CenterNet2/configs/common/models/panoptic_fpn.py
deleted file mode 100644
index 88f55d2ce9db62e61445d6a3700067d9d864ecae..0000000000000000000000000000000000000000
--- a/spaces/Awiny/Image2Paragraph/models/grit_src/third_party/CenterNet2/configs/common/models/panoptic_fpn.py
+++ /dev/null
@@ -1,20 +0,0 @@
-from detectron2.config import LazyCall as L
-from detectron2.layers import ShapeSpec
-from detectron2.modeling import PanopticFPN
-from detectron2.modeling.meta_arch.semantic_seg import SemSegFPNHead
-
-from .mask_rcnn_fpn import model
-
-model._target_ = PanopticFPN
-model.sem_seg_head = L(SemSegFPNHead)(
- input_shape={
- f: L(ShapeSpec)(stride=s, channels="${....backbone.out_channels}")
- for f, s in zip(["p2", "p3", "p4", "p5"], [4, 8, 16, 32])
- },
- ignore_value=255,
- num_classes=54, # COCO stuff + 1
- conv_dims=128,
- common_stride=4,
- loss_weight=0.5,
- norm="GN",
-)
diff --git a/spaces/Beasto/Image_Colorizer_Pix2Pix/README.md b/spaces/Beasto/Image_Colorizer_Pix2Pix/README.md
deleted file mode 100644
index c9a39e385e1c9d5ba895067fb8791a4ab8415af4..0000000000000000000000000000000000000000
--- a/spaces/Beasto/Image_Colorizer_Pix2Pix/README.md
+++ /dev/null
@@ -1,13 +0,0 @@
----
-title: Image Colorizer Pix2Pix
-emoji: 💻
-colorFrom: green
-colorTo: red
-sdk: streamlit
-sdk_version: 1.27.2
-app_file: app.py
-pinned: false
-license: apache-2.0
----
-
-Check out the configuration reference at https://huggingface.co/docs/hub/spaces-config-reference
diff --git a/spaces/Benson/text-generation/Examples/Descargar Gta 5 100 Mb.md b/spaces/Benson/text-generation/Examples/Descargar Gta 5 100 Mb.md
deleted file mode 100644
index ec5c3f3f53ff9001e895ffa32e9045ce615ef3f1..0000000000000000000000000000000000000000
--- a/spaces/Benson/text-generation/Examples/Descargar Gta 5 100 Mb.md
+++ /dev/null
@@ -1,94 +0,0 @@
-
-
Cómo descargar GTA 5 en 100 MB
-
Grand Theft Auto V, o GTA 5 para abreviar, es uno de los videojuegos más populares y exitosos de todos los tiempos. Lanzado en 2013 por Rockstar Games, GTA 5 es un juego de acción y aventura de mundo abierto que te permite explorar la ciudad ficticia de Los Santos y sus alrededores. Puedes jugar como uno de los tres protagonistas, cada uno con su propia personalidad e historia, o unirte a otros jugadores en línea en varios modos y actividades. GTA 5 ha recibido elogios de la crítica por su jugabilidad, gráficos, historia y características en línea, y ha vendido más de 150 millones de copias en todo el mundo.
-
Sin embargo, GTA 5 es también un juego muy grande que requiere mucho espacio en tu dispositivo. Dependiendo de su plataforma, el tamaño de descarga de GTA 5 puede variar de 72 GB a más de 100 GB. Esto puede ser un problema si tiene una conexión a Internet lenta o espacio de almacenamiento limitado. Afortunadamente, hay una manera de descargar GTA 5 en un tamaño mucho más pequeño mediante el uso de archivos comprimidos. En este artículo, explicaremos qué son los archivos comprimidos, cómo pueden ayudarle a descargar GTA 5 más rápido y más fácil, y cómo encontrarlos y descargarlos de forma segura. También te daremos un breve resumen de lo que GTA 5 ofrece en términos de jugabilidad, gráficos y características.
Antes de descargar GTA 5, debe asegurarse de que su dispositivo pueda ejecutarlo sin problemas. GTA 5 está disponible en varias plataformas, incluyendo PC, PS4, PS5, Xbox One y Xbox Series X/S. Cada plataforma tiene sus propios requisitos mínimos y recomendados que debe cumplir o superar. Aquí están los requisitos del sistema para cada plataforma:
- Consola PS4 - Almacenamiento: Al menos 100 GB - Almacenamiento: Al menos 100 GB
-
-
-
PS5
-
- Consola PS5 - Almacenamiento: Al menos 72 GB
-
-
-
Xbox One
-
- Consola Xbox One - Almacenamiento: Al menos 100 GB
-
-
-
Serie Xbox X/S
-
- Consola Xbox Series X/S - Almacenamiento: Al menos 72 GB
-
-
-
GTA 5 Tamaño de descarga
-
Como puedes ver en la tabla de arriba, GTA 5 es un juego muy grande que requiere mucho espacio en tu dispositivo. El tamaño de descarga de GTA 5 varía según tu plataforma y la versión del juego que tengas. Por ejemplo, las versiones para PS4 y Xbox One de GTA 5 ocupan unos 100 GB de espacio, mientras que las versiones para PS5 y Xbox Series X/S ocupan unos 72 GB. La versión para PC de GTA 5 ocupa unos 106 GB de espacio, pero también incluye contenido adicional y funciones que no están disponibles en las consolas.
-
Si tiene una conexión rápida a Internet y suficiente espacio de almacenamiento, puede descargar GTA 5 directamente de las fuentes oficiales, como Steam, Epic Games Store, PlayStation Store o Microsoft Store. Sin embargo, si tiene una conexión a Internet lenta o espacio de almacenamiento limitado, es posible que desee considerar el uso de archivos comprimidos para descargar GTA 5 en un tamaño más pequeño.
-
GTA 5 Archivos comprimidos
-
Los archivos comprimidos son archivos que se han reducido en tamaño mediante el uso de varios algoritmos y técnicas. La compresión de archivos puede ayudar a ahorrar ancho de banda, espacio de almacenamiento y tiempo de descarga. Por ejemplo, un archivo que originalmente es de 100 MB puede ser comprimido a 10 MB, lo que significa que tomará menos tiempo para descargar y menos espacio para almacenar.
-
-
Una de las formas de descargar GTA 5 en un tamaño más pequeño es utilizar archivos comprimidos que contienen los datos del juego. Estos archivos se pueden encontrar en varios sitios web y foros que ofrecen descargas de GTA 5. Sin embargo, no todos los archivos comprimidos son seguros y confiables. Algunos de ellos pueden contener malware, virus o datos corruptos que pueden dañar su dispositivo o arruinar su experiencia de juego. Por lo tanto, debe tener cuidado y precaución al descargar archivos comprimidos para GTA 5.
-
Beneficios de los archivos comprimidos
-
El uso de archivos comprimidos para descargar GTA 5 puede tener algunos beneficios, como:
-
-
-
Velocidad de descarga más rápida: Los archivos comprimidos pueden reducir el tiempo de descarga de GTA 5 hasta un 90%, dependiendo de la relación de compresión y su velocidad de Internet. Esto significa que puede empezar a jugar GTA 5 antes y ahorrar su tiempo precioso.
-
Menor uso de ancho de banda: Los archivos comprimidos también pueden reducir la cantidad de datos que utiliza para descargar GTA 5. Esto puede ayudarlo a evitar exceder su límite de datos o pagar tarifas adicionales por su proveedor de servicios de Internet.
-
Más espacio de almacenamiento: Los archivos comprimidos también pueden liberar algo de espacio en su dispositivo ocupando menos espacio que los archivos originales. Esto puede ayudarlo a evitar quedarse sin espacio de almacenamiento o eliminar otros archivos o aplicaciones importantes.
-
-
Inconvenientes de los archivos comprimidos
-
Sin embargo, usar archivos comprimidos para descargar GTA 5 también puede tener algunos inconvenientes, como:
-
-
Posible pérdida de calidad: Comprimir archivos a veces puede resultar en una pérdida de calidad o rendimiento del juego. Esto puede afectar los gráficos, el sonido o la jugabilidad de GTA 5. Por ejemplo, algunos archivos comprimidos pueden tener texturas de menor resolución, calidad de audio reducida o características que faltan.
-
-
Riesgo de malware: Algunos archivos comprimidos pueden contener malware o virus que pueden infectar su dispositivo o robar su información personal. Esto puede dañar su dispositivo o comprometer su seguridad y privacidad. Por ejemplo, algunos archivos comprimidos pueden tener troyanos ocultos, keyloggers o ransomware.
-
-
Cómo encontrar y descargar archivos comprimidos para GTA 5
Si desea descargar GTA 5 en un tamaño más pequeño utilizando archivos comprimidos, debe seguir estos pasos:
-
-
Busque fuentes confiables y seguras de archivos comprimidos para GTA 5. Puede usar motores de búsqueda, foros, blogs o redes sociales para encontrar sitios web que ofrecen descargas de GTA 5. Sin embargo, debe tener cuidado y evitar hacer clic en enlaces sospechosos o falsos que podrían llevarlo a malware o estafas. También puede utilizar herramientas o reseñas en línea para comprobar la reputación y credibilidad de los sitios web antes de descargar nada de ellos.
-
Seleccione el archivo comprimido que se adapte a su dispositivo y plataforma. Debe asegurarse de que el archivo comprimido que elija sea compatible con su dispositivo y plataforma. También debe verificar la relación de compresión, el tamaño de la descarga y la calidad del archivo. Puede comparar diferentes archivos y leer la descripción y los comentarios de otros usuarios para tomar una decisión informada.
-
Descargue el archivo comprimido en su dispositivo. Necesita tener suficiente espacio en su dispositivo para descargar el archivo comprimido. También necesita tener una conexión a Internet estable y un buen gestor de descargas o software para acelerar el proceso. También puede usar una VPN o proxy para evitar cualquier restricción o limitación que pueda afectar su descarga.
-
-
Instalar y ejecutar GTA 5 en su dispositivo. Debe seguir los pasos de instalación e ingresar la clave de activación si es necesario para instalar GTA 5 en su dispositivo. También necesitas actualizar el juego e instalar cualquier parche o mods si es necesario. Luego, puedes lanzar GTA 5 y disfrutar jugando.
-
-
GTA 5 Juego
-
Ahora que ha descargado GTA 5 en un tamaño más pequeño usando archivos comprimidos, es posible que se pregunte qué tiene GTA 5 para ofrecer en términos de jugabilidad, gráficos y características. GTA 5 es un juego que te permite experimentar la vida de crimen, aventura y diversión en un vasto y diverso mundo abierto. Estos son algunos de los aspectos del juego de GTA 5 que puedes disfrutar:
-
Modo historia
-
GTA 5 tiene un modo de historia que sigue las vidas de tres protagonistas: Michael, Franklin y Trevor. Michael es un ladrón de bancos retirado que vive una vida lujosa pero infeliz con su familia en Los Santos. Franklin es un joven y ambicioso estafador callejero que trabaja para un distribuidor de automóviles en el sur de Los Santos. Trevor es un antiguo compañero de Michael que vive una vida caótica y violenta en el condado de Blaine. Los tres personajes tienen sus propios antecedentes, personalidades, habilidades y objetivos, pero también están conectados por una serie de eventos que los obligan a trabajar juntos.
-
El modo historia de GTA 5 consiste en varias misiones que involucran robos, tiroteos, persecuciones, sigilo y más. Puedes cambiar entre los tres personajes en cualquier momento durante el juego, ya sea manualmente o automáticamente dependiendo de la situación. También puede personalizar su apariencia, habilidades, armas, vehículos y propiedades. El modo historia de GTA 5 está lleno de humor, drama, acción y sorpresas que te mantendrán enganchado hasta el final.
-
Modo en línea
-
-
GTA Online ofrece muchas opciones para que te diviertas y ganes dinero en el mundo online. Puedes jugar varios modos como carreras, deathmatches, atracos, misiones, modos adversarios, etc. También puedes explorar el mapa y encontrar diversas actividades como golf, tenis, paracaidismo, caza, etc. También puedes comprar varios negocios como clubes nocturnos, bunkers, arcadas, etc. y ejecutarlos como desee. GTA Online se actualiza constantemente con nuevos contenidos y características que añaden más variedad y emoción al juego.
-
Nuevas características y actualizaciones
-
GTA 5 no es solo un juego que fue lanzado en 2013, sino también un juego que todavía está evolucionando y mejorando en 2023. Rockstar Games ha estado añadiendo nuevos contenidos y características a GTA 5 en consolas de generación actual y PC que mejoran la experiencia y la calidad del juego. Algunas de las nuevas características y actualizaciones son:
-
-
The
The Enhanced and Expanded Edition: Esta es una nueva versión de GTA 5 que se lanzará en noviembre de 2023 para PS5 y Xbox Series X/S. Esta edición contará con gráficos mejorados, rendimiento, tiempos de carga y mejoras de juego. También incluirá GTA Online y todas las actualizaciones existentes y futuras de forma gratuita.
-
El robo de Cayo Perico: Esta es la última y más grande actualización para GTA Online que fue lanzado en diciembre de 2022. Esta actualización añade un nuevo atraco que tiene lugar en una isla privada propiedad de un capo de la droga. Puedes planificar y ejecutar el robo solo o con hasta otros tres jugadores. También puede explorar la isla y encontrar nuevos vehículos, armas, música y más.
-
Los Santos Tuners: Esta es otra actualización para GTA Online que fue lanzada en julio de 2023. Esta actualización se centra en la cultura del automóvil y la escena de carreras callejeras de Los Santos. Puede unirse a un nuevo espacio social llamado LS Car Meet, donde puede mostrar sus coches personalizados, competir con otros jugadores y acceder a nuevas misiones, vehículos, mods y recompensas.
-
-
Conclusión
-
-
Esperamos que este artículo te haya ayudado a aprender a descargar GTA 5 en 100 MB usando archivos comprimidos. Si usted tiene alguna pregunta o retroalimentación, por favor no dude en dejar un comentario a continuación. Gracias por leer y feliz juego!
-
Preguntas frecuentes
-
Aquí hay algunas preguntas frecuentes sobre GTA 5 y sus respuestas:
-
-
Q: ¿Cuánto tiempo es el modo historia GTA 5? A: El modo de historia GTA 5 puede tardar entre 25 y 40 horas en completarse, dependiendo de su estilo de juego y sus opciones. Sin embargo, también hay muchas misiones secundarias, actividades y secretos que pueden extender el tiempo de juego significativamente.
-
P: ¿Cuántos jugadores pueden jugar GTA Online? A: GTA Online puede admitir hasta 30 jugadores por sesión en consolas de generación actual y PC. Sin embargo, algunos modos y actividades pueden tener diferentes límites de jugador.
-
P: ¿Cómo puedo jugar GTA Online con mis amigos? A: Puedes jugar a GTA Online con tus amigos uniéndote o creando una sesión de solo invitación, una sesión de equipo o una sesión de amigos. También puedes unirte a una fiesta o crear un grupo de chat para comunicarte con tus amigos.
-
P: ¿Cómo puedo ganar dinero en GTA Online? A: Hay muchas maneras de ganar dinero en GTA Online, como completar misiones, atracos, carreras, negocios, etc. También puedes robar tiendas, vender autos o apostar en eventos. Sin embargo, debes evitar usar trucos, hacks o fallos para ganar dinero, ya que pueden hacer que te prohíban o penalicen.
-
P: ¿Cómo puedo obtener GTA 5 gratis? A: No hay forma legal de obtener GTA 5 gratis a partir de ahora. Sin embargo, es posible que puedas obtenerlo gratis en el futuro si está disponible en plataformas como PlayStation Plus o Epic Games Store.
- 64aa2da5cf
-
-
\ No newline at end of file
diff --git a/spaces/Big-Web/MMSD/env/Lib/site-packages/pip/_vendor/rich/_timer.py b/spaces/Big-Web/MMSD/env/Lib/site-packages/pip/_vendor/rich/_timer.py
deleted file mode 100644
index a2ca6be03c43054caaa3660998273ebf704345dd..0000000000000000000000000000000000000000
--- a/spaces/Big-Web/MMSD/env/Lib/site-packages/pip/_vendor/rich/_timer.py
+++ /dev/null
@@ -1,19 +0,0 @@
-"""
-Timer context manager, only used in debug.
-
-"""
-
-from time import time
-
-import contextlib
-from typing import Generator
-
-
-@contextlib.contextmanager
-def timer(subject: str = "time") -> Generator[None, None, None]:
- """print the elapsed time. (only used in debugging)"""
- start = time()
- yield
- elapsed = time() - start
- elapsed_ms = elapsed * 1000
- print(f"{subject} elapsed {elapsed_ms:.1f}ms")
diff --git a/spaces/BigChungux/Pet_Survey2/README.md b/spaces/BigChungux/Pet_Survey2/README.md
deleted file mode 100644
index 2cc853d6b0c57d01fc94fe2f571893a35865bc3f..0000000000000000000000000000000000000000
--- a/spaces/BigChungux/Pet_Survey2/README.md
+++ /dev/null
@@ -1,13 +0,0 @@
----
-title: Pet Survey2
-emoji: 🏢
-colorFrom: red
-colorTo: purple
-sdk: gradio
-sdk_version: 3.19.1
-app_file: app.py
-pinned: false
-license: afl-3.0
----
-
-Check out the configuration reference at https://huggingface.co/docs/hub/spaces-config-reference
diff --git a/spaces/Boops88/gsdf-Counterfeit-V2.5/README.md b/spaces/Boops88/gsdf-Counterfeit-V2.5/README.md
deleted file mode 100644
index b8908b2f71191a72c7b07dea566d9189d7b345a1..0000000000000000000000000000000000000000
--- a/spaces/Boops88/gsdf-Counterfeit-V2.5/README.md
+++ /dev/null
@@ -1,12 +0,0 @@
----
-title: Gsdf Counterfeit V2.5
-emoji: 👁
-colorFrom: blue
-colorTo: purple
-sdk: gradio
-sdk_version: 3.17.0
-app_file: app.py
-pinned: false
----
-
-Check out the configuration reference at https://huggingface.co/docs/hub/spaces-config-reference
diff --git a/spaces/BreadBytes1/CC-Dashboard/old_app.py b/spaces/BreadBytes1/CC-Dashboard/old_app.py
deleted file mode 100644
index dc2708776a5c116ffbcb00c30a095768af457d1c..0000000000000000000000000000000000000000
--- a/spaces/BreadBytes1/CC-Dashboard/old_app.py
+++ /dev/null
@@ -1,330 +0,0 @@
-# ---
-# jupyter:
-# jupytext:
-# text_representation:
-# extension: .py
-# format_name: light
-# format_version: '1.5'
-# jupytext_version: 1.14.2
-# kernelspec:
-# display_name: Python [conda env:bbytes] *
-# language: python
-# name: conda-env-bbytes-py
-# ---
-
-# +
-import csv
-import pandas as pd
-from datetime import datetime, timedelta
-import numpy as np
-import datetime as dt
-import matplotlib.pyplot as plt
-from pathlib import Path
-
-import streamlit as st
-import plotly.express as px
-import altair as alt
-import dateutil.parser
-import copy
-
-
-# +
-@st.experimental_memo
-def get_hist_info(df_coin, principal_balance,plheader):
- numtrades = int(len(df_coin))
- numwin = int(sum(df_coin[plheader] > 0))
- numloss = int(sum(df_coin[plheader] < 0))
- winrate = int(np.round(100*numwin/numtrades,2))
-
- grosswin = sum(df_coin[df_coin[plheader] > 0][plheader])
- grossloss = sum(df_coin[df_coin[plheader] < 0][plheader])
- if grossloss !=0:
- pfactor = -1*np.round(grosswin/grossloss,2)
- else:
- pfactor = np.nan
- return numtrades, numwin, numloss, winrate, pfactor
-@st.experimental_memo
-def get_rolling_stats(df, lev, otimeheader, days):
- max_roll = (df[otimeheader].max() - df[otimeheader].min()).days
-
- if max_roll >= days:
- rollend = df[otimeheader].max()-timedelta(days=days)
- rolling_df = df[df[otimeheader] >= rollend]
-
- if len(rolling_df) > 0:
- rolling_perc = rolling_df['Return Per Trade'].dropna().cumprod().values[-1]-1
- else:
- rolling_perc = np.nan
- else:
- rolling_perc = np.nan
- return 100*rolling_perc
-
-@st.experimental_memo
-def filt_df(df, cheader, symbol_selections):
- """
- Inputs: df (pd.DataFrame), cheader (str) and symbol_selections (list[str]).
-
- Returns a filtered pd.DataFrame containing only data that matches symbol_selections (list[str])
- from df[cheader].
- """
-
- df = df.copy()
- df = df[df[cheader].isin(symbol_selections)]
-
- return df
-
-@st.experimental_memo
-def my_style(v, props=''):
- props = 'color:red' if v < 0 else 'color:green'
- return props
-
-@st.experimental_memo
-def cc_coding(row):
- return ['background-color: lightgrey'] * len(row) if row['Exit Date'] <= datetime.strptime('2022-12-16 00:00:00','%Y-%m-%d %H:%M:%S').date() else [''] * len(row)
-
-
-@st.cache(ttl=24*3600, allow_output_mutation=True)
-def load_data(filename, otimeheader,fmat):
- df = pd.read_csv(open(filename,'r'), sep='\t') # so as not to mutate cached value
- df.columns = ['Trade','Signal','Entry Date','Buy Price', 'Sell Price','Exit Date', 'P/L per token', 'P/L %', 'Drawdown %']
-# df.insert(1, 'Signal', ['Long']*len(df))
-
- df['Buy Price'] = df['Buy Price'].str.replace('$', '', regex=True)
- df['Sell Price'] = df['Sell Price'].str.replace('$', '', regex=True)
- df['Buy Price'] = df['Buy Price'].str.replace(',', '', regex=True)
- df['Sell Price'] = df['Sell Price'].str.replace(',', '', regex=True)
- df['P/L per token'] = df['P/L per token'].str.replace('$', '', regex=True)
- df['P/L per token'] = df['P/L per token'].str.replace(',', '', regex=True)
- df['P/L %'] = df['P/L %'].str.replace('%', '', regex=True)
-
- df['Buy Price'] = pd.to_numeric(df['Buy Price'])
- df['Sell Price'] = pd.to_numeric(df['Sell Price'])
- df['P/L per token'] = pd.to_numeric(df['P/L per token'])
- df['P/L %'] = pd.to_numeric(df['P/L %'])
-
- dateheader = 'Date'
- theader = 'Time'
-
- df[dateheader] = [tradetimes.split(" ")[0] for tradetimes in df[otimeheader].values]
- df[theader] = [tradetimes.split(" ")[1] for tradetimes in df[otimeheader].values]
-
- df[otimeheader]= [dateutil.parser.parse(date+' '+time)
- for date,time in zip(df[dateheader],df[theader])]
-
- df[otimeheader] = pd.to_datetime(df[otimeheader])
- df['Exit Date'] = pd.to_datetime(df['Exit Date'])
- df.sort_values(by=otimeheader, inplace=True)
-
- df[dateheader] = [dateutil.parser.parse(date).date() for date in df[dateheader]]
- df[theader] = [dateutil.parser.parse(time).time() for time in df[theader]]
- df['Trade'] = [i+1 for i in range(len(df))] #reindex
-
- return df
-
-def runapp():
- bot_selections = "Cosmic Cupcake"
- otimeheader = 'Entry Date'
- plheader = 'P/L %'
- fmat = '%Y-%m-%d %H:%M:%S'
- dollar_cap = 100000.00
- fees = .075/100
- st.header(f"{bot_selections} Performance Dashboard :bread: :moneybag:")
- st.write("Welcome to the Trading Bot Dashboard by BreadBytes! You can use this dashboard to track " +
- "the performance of our trading bots.")
- # st.sidebar.header("FAQ")
-
- # with st.sidebar.subheader("FAQ"):
- # st.write(Path("FAQ_README.md").read_text())
- st.subheader("Choose your settings:")
- no_errors = True
-
- data = load_data("CC-Trade-Log.csv",otimeheader,fmat)
- df = data.copy(deep=True)
-
- dateheader = 'Date'
- theader = 'Time'
-
- with st.form("user input", ):
- if no_errors:
- with st.container():
- col1, col2 = st.columns(2)
- with col1:
- try:
- startdate = st.date_input("Start Date", value=pd.to_datetime(df[otimeheader]).min())
- except:
- st.error("Please select your exchange or upload a supported trade log file.")
- no_errors = False
- with col2:
- try:
- enddate = st.date_input("End Date", value=datetime.today())
- except:
- st.error("Please select your exchange or upload a supported trade log file.")
- no_errors = False
- #st.sidebar.subheader("Customize your Dashboard")
-
- if no_errors and (enddate < startdate):
- st.error("End Date must be later than Start date. Please try again.")
- no_errors = False
- with st.container():
- col1,col2 = st.columns(2)
- with col2:
- lev = st.number_input('Leverage', min_value=1, value=1, max_value= 3, step=1)
- with col1:
- principal_balance = st.number_input('Starting Balance', min_value=0.00, value=1000.00, max_value= dollar_cap, step=.01)
-
- #hack way to get button centered
- c = st.columns(9)
- with c[4]:
- submitted = st.form_submit_button("Get Cookin'!")
-
- signal_map = {'Long': 1, 'Short':-1} # 1 for long #-1 for short
-
- df['Calculated Return %'] = df['Signal'].map(signal_map)*(1-fees)*((df['Sell Price']-df['Buy Price'])/df['Buy Price'] - fees) #accounts for fees on open and close of trade
-
-
- if submitted and principal_balance * lev > dollar_cap:
- lev = np.floor(dollar_cap/principal_balance)
- st.error(f"WARNING: (Starting Balance)*(Leverage) exceeds the ${dollar_cap} limit. Using maximum available leverage of {lev}")
-
- if submitted and no_errors:
- df = df[(df[dateheader] >= startdate) & (df[dateheader] <= enddate)]
-
- if len(df) == 0:
- st.error("There are no available trades matching your selections. Please try again!")
- no_errors = False
- if no_errors:
- df['Return Per Trade'] = 1+lev*df['Calculated Return %'].values
-
- df['Compounded Return'] = df['Return Per Trade'].cumprod()
- df['New Balance'] = [min(dollar_cap/lev, bal*principal_balance) for bal in df['Compounded Return']]
- df['Balance used in Trade'] = np.concatenate([[principal_balance], df['New Balance'].values[:-1]])
- df['Net P/L Per Trade'] = (df['Return Per Trade']-1)*df['Balance used in Trade']
- df['Cumulative P/L'] = df['Net P/L Per Trade'].cumsum()
- cum_pl = df.loc[df.drop('Drawdown %', axis=1).dropna().index[-1],'Cumulative P/L'] + principal_balance
-
- effective_return = 100*((cum_pl - principal_balance)/principal_balance)
-
- st.header(f"{bot_selections} Results")
- if len(bot_selections) > 1:
- st.metric(
- "Total Account Balance",
- f"${cum_pl:.2f}",
- f"{100*(cum_pl-principal_balance)/(principal_balance):.2f} %",
- )
-
- st.line_chart(data=df.drop('Drawdown %', axis=1).dropna(), x='Exit Date', y='Cumulative P/L', use_container_width=True)
-
- df['Per Trade Return Rate'] = df['Return Per Trade']-1
-
- totals = pd.DataFrame([], columns = ['# of Trades', 'Wins', 'Losses', 'Win Rate', 'Profit Factor'])
- data = get_hist_info(df.drop('Drawdown %', axis=1).dropna(), principal_balance,'Per Trade Return Rate')
- totals.loc[len(totals)] = list(i for i in data)
-
- totals['Cum. P/L'] = cum_pl-principal_balance
- totals['Cum. P/L (%)'] = 100*(cum_pl-principal_balance)/principal_balance
- #results_df['Avg. P/L'] = (cum_pl-principal_balance)/results_df['# of Trades'].values[0]
- #results_df['Avg. P/L (%)'] = 100*results_df['Avg. P/L'].values[0]/principal_balance
-
- if df.empty:
- st.error("Oops! None of the data provided matches your selection(s). Please try again.")
- else:
- #st.dataframe(totals.style.format({'# of Trades': '{:.0f}','Wins': '{:.0f}','Losses': '{:.0f}','Win Rate': '{:.2f}%','Profit Factor' : '{:.2f}', 'Avg. P/L (%)': '{:.2f}%', 'Cum. P/L (%)': '{:.2f}%', 'Cum. P/L': '{:.2f}', 'Avg. P/L': '{:.2f}'})
- #.text_gradient(subset=['Win Rate'],cmap="RdYlGn", vmin = 0, vmax = 100)\
- #.text_gradient(subset=['Profit Factor'],cmap="RdYlGn", vmin = 0, vmax = 2), use_container_width=True)
- for row in totals.itertuples():
- col1, col2, col3, col4 = st.columns(4)
- c1, c2, c3, c4 = st.columns(4)
- with col1:
- st.metric(
- "Total Trades",
- f"{row._1:.0f}",
- )
- with c1:
- st.metric(
- "Profit Factor",
- f"{row._5:.2f}",
- )
- with col2:
- st.metric(
- "Wins",
- f"{row.Wins:.0f}",
- )
- with c2:
- st.metric(
- "Cumulative P/L",
- f"${row._6:.2f}",
- f"{row._7:.2f} %",
- )
- with col3:
- st.metric(
- "Losses",
- f"{row.Losses:.0f}",
- )
- with c3:
- st.metric(
- "Rolling 7 Days",
- "",#f"{(1+get_rolling_stats(df,otimeheader, 30))*principal_balance:.2f}",
- f"{get_rolling_stats(df,lev, otimeheader, 7):.2f}%",
- )
- st.metric(
- "Rolling 30 Days",
- "",#f"{(1+get_rolling_stats(df,otimeheader, 30))*principal_balance:.2f}",
- f"{get_rolling_stats(df,lev, otimeheader, 30):.2f}%",
- )
-
- with col4:
- st.metric(
- "Win Rate",
- f"{row._4:.1f}%",
- )
- with c4:
- st.metric(
- "Rolling 90 Days",
- "",#f"{(1+get_rolling_stats(df,otimeheader, 30))*principal_balance:.2f}",
- f"{get_rolling_stats(df,lev, otimeheader, 90):.2f}%",
- )
- st.metric(
- "Rolling 180 Days",
- "",#f"{(1+get_rolling_stats(df,otimeheader, 30))*principal_balance:.2f}",
- f"{get_rolling_stats(df,lev, otimeheader, 180):.2f}%",
- )
-
- if submitted:
- grouped_df = df.groupby('Exit Date').agg({'Signal':'min','Entry Date': 'min','Exit Date': 'max','Buy Price': 'mean',
- 'Sell Price' : 'max',
- 'Net P/L Per Trade': 'mean',
- 'Calculated Return %' : lambda x: np.round(100*lev*x.sum(),2)})
- grouped_df.index = range(1, len(grouped_df)+1)
- grouped_df.rename(columns={'Buy Price':'Avg. Buy Price',
- 'Net P/L Per Trade':'Net P/L',
- 'Calculated Return %':'P/L %'}, inplace=True)
- else:
- grouped_df = df.groupby('Exit Date').agg({'Signal':'min','Entry Date': 'min','Exit Date': 'max','Buy Price': 'mean',
- 'Sell Price' : 'max',
- 'P/L per token' : 'mean',
- 'Calculated Return %' : lambda x: np.round(100*x.sum(),2)})
- grouped_df.index = range(1, len(grouped_df)+1)
- grouped_df.rename(columns={'Buy Price':'Avg. Buy Price',
- 'P/L per token':'Net P/L',
- 'Calculated Return %':'P/L %'}, inplace=True)
-
- st.subheader("Trade Logs")
- grouped_df['Entry Date'] = pd.to_datetime(grouped_df['Entry Date'])
- grouped_df['Exit Date'] = pd.to_datetime(grouped_df['Exit Date'])
- st.dataframe(grouped_df.style.format({'Entry Date':'{:%m-%d-%Y %H:%M:%S}','Exit Date':'{:%m-%d-%Y %H:%M:%S}','Avg. Buy Price': '${:.2f}', 'Sell Price': '${:.2f}', 'Net P/L':'${:.2f}', 'P/L %':'{:.2f}%'})\
- .apply(cc_coding, axis=1)\
- .applymap(my_style,subset=['Net P/L'])\
- .applymap(my_style,subset=['P/L %'])\
- ,use_container_width=True)
- new_title = '
Backtest Data
'
- st.markdown(new_title, unsafe_allow_html=True)
-
-if __name__ == "__main__":
- st.set_page_config(
- "Trading Bot Dashboard",
- layout="wide",
- )
- runapp()
-# -
-
-
diff --git a/spaces/CVPR/LIVE/thrust/thrust/system/tbb/detail/scan.h b/spaces/CVPR/LIVE/thrust/thrust/system/tbb/detail/scan.h
deleted file mode 100644
index 32a05a5a6bd3a5be92bbd84c1bf4edb9e929abeb..0000000000000000000000000000000000000000
--- a/spaces/CVPR/LIVE/thrust/thrust/system/tbb/detail/scan.h
+++ /dev/null
@@ -1,64 +0,0 @@
-/*
- * Copyright 2008-2013 NVIDIA Corporation
- *
- * Licensed under the Apache License, Version 2.0 (the "License");
- * you may not use this file except in compliance with the License.
- * You may obtain a copy of the License at
- *
- * http://www.apache.org/licenses/LICENSE-2.0
- *
- * Unless required by applicable law or agreed to in writing, software
- * distributed under the License is distributed on an "AS IS" BASIS,
- * WITHOUT WARRANTIES OR CONDITIONS OF ANY KIND, either express or implied.
- * See the License for the specific language governing permissions and
- * limitations under the License.
- */
-
-
-/*! \file scan.h
- * \brief TBB implementations of scan functions.
- */
-
-#pragma once
-
-#include
-#include
-
-namespace thrust
-{
-namespace system
-{
-namespace tbb
-{
-namespace detail
-{
-
-template
- OutputIterator inclusive_scan(tag,
- InputIterator first,
- InputIterator last,
- OutputIterator result,
- BinaryFunction binary_op);
-
-
-template
- OutputIterator exclusive_scan(tag,
- InputIterator first,
- InputIterator last,
- OutputIterator result,
- T init,
- BinaryFunction binary_op);
-
-
-} // end namespace detail
-} // end namespace tbb
-} // end namespace system
-} // end namespace thrust
-
-#include
-
diff --git a/spaces/Chomkwoy/Nilkessye/utils/hangul.py b/spaces/Chomkwoy/Nilkessye/utils/hangul.py
deleted file mode 100644
index 82f09fe3994308491f0e40c2129abf3e6ba570dc..0000000000000000000000000000000000000000
--- a/spaces/Chomkwoy/Nilkessye/utils/hangul.py
+++ /dev/null
@@ -1,269 +0,0 @@
-import copy
-import unicodedata
-
-YALE_TO_HANGUL_INITIAL_CONSONANTS = {
- 'k': '\u1100',
- 'kk': '\u1101',
- 'n': '\u1102',
- 'nn': '\u1114',
- 't': '\u1103',
- 'tt': '\u1104',
- 'l': '\u1105',
- 'm': '\u1106',
- 'p': '\u1107',
- 'pp': '\u1108',
- 's': '\u1109',
- 'ss': '\u110a',
- 'G': '\u110B',
- 'GG': '\u1147',
- 'c': '\u110C',
- 'cc': '\u110D',
- 'ch': '\u110e',
- 'kh': '\u110f',
- 'th': '\u1110',
- 'ph': '\u1111',
- 'h': '\u1112',
-
- 'pk': '\u111e',
- 'pt': '\u1120',
- 'ps': '\u1121',
- 'psk': '\u1122',
- 'pst': '\u1123',
- 'pc': '\u1127',
- 'pth': '\u1129',
- 'W': '\u112b',
- 'sk': '\u112d',
- 'sn': '\u112e',
- 'st': '\u112f',
- 'sp': '\u1132',
- 'sc': '\u1136',
- 'sh': '\u113b',
- 'z': '\u1140',
- 'hh': '\u1158',
- 'q': '\u1159',
-
- 'ng': '\u114c',
-
- '': '\u115f',
-}
-
-YALE_TO_HANGUL_FINAL_CONSONANTS = {
- 'k': '\u11a8',
- 'ks': '\u11aa',
- 'n': '\u11ab',
- 't': '\u11ae',
- 'l': '\u11af',
- 'lk': '\u11b0',
- 'lm': '\u11b1',
- 'lp': '\u11b2',
- 'ls': '\u11b3',
- 'm': '\u11b7',
- 'p': '\u11b8',
- 'ps': '\u11b9',
- 's': '\u11ba',
- 'G': '\u11bc',
- 'nt': '\u11c6',
- 'ns': '\u11c7',
- 'nz': '\u11c8',
- 'lks': '\u11cc',
- 'lz': '\u11d7',
- 'lq': '\u11d9',
- 'mp': '\u11dc',
- 'ms': '\u11dd',
- 'mz': '\u11df',
- 'M': '\u11e2',
- 'W': '\u11e6',
- 'z': '\u11eb',
- 'ng': '\u11f0',
- 'q': '\u11f9',
- 'ngs': '\u11f1',
- '': ''
-}
-
-YALE_TO_HANGUL_VOWELS = {
- 'a': '\u1161',
- 'ay': '\u1162',
- 'ya': '\u1163',
- 'yay': '\u1164',
- 'e': '\u1165',
- 'ey': '\u1166',
- 'ye': '\u1167',
- 'yey': '\u1168',
- 'wo': '\u1169',
- 'wa': '\u116a',
- 'way': '\u116b',
- 'woy': '\u116c',
- 'yo': '\u116d',
- 'wu': '\u116e',
- 'we': '\u116f',
- 'wey': '\u1170',
- 'wuy': '\u1171',
- 'yu': '\u1172',
- 'u': '\u1173',
- 'uy': '\u1174',
- 'i': '\u1175',
-
- 'o': '\u119e',
- 'oy': '\u11a1',
- 'yoy': '\u1188',
- 'yuy': '\u1194',
- 'ywe': '\u1191',
- 'ywey': '\u1192',
- 'ywa': '\u1184',
- 'yway': '\u1185'
-}
-
-UNICODE_COMPATIBILITY_FORMS = {
- 'ᄀ': 'ㄱ',
- 'ᄁ': 'ㄲ',
- 'ᆪ': 'ㄳ',
- 'ᄂ': 'ㄴ',
- 'ᆬ': 'ㄵ',
- 'ᆭ': 'ㄶ',
- 'ᄃ': 'ㄷ',
- 'ᄄ': 'ㄸ',
- 'ᄅ': 'ㄹ',
- 'ᆰ': 'ㄺ',
- 'ᆱ': 'ㄻ',
- 'ᆲ': 'ㄼ',
- 'ᆳ': 'ㄽ',
- 'ᆴ': 'ㄾ',
- 'ᆵ': 'ㄿ',
- 'ᄚ': 'ㅀ',
- 'ᄆ': 'ㅁ',
- 'ᄇ': 'ㅂ',
- 'ᄈ': 'ㅃ',
- 'ᄡ': 'ㅄ',
- 'ᄉ': 'ㅅ',
- 'ᄊ': 'ㅆ',
- 'ᄋ': 'ㅇ',
- 'ᄌ': 'ㅈ',
- 'ᄍ': 'ㅉ',
- 'ᄎ': 'ㅊ',
- 'ᄏ': 'ㅋ',
- 'ᄐ': 'ㅌ',
- 'ᄑ': 'ㅍ',
- 'ᄒ': 'ㅎ',
- 'ᅡ': 'ㅏ',
- 'ᅢ': 'ㅐ',
- 'ᅣ': 'ㅑ',
- 'ᅤ': 'ㅒ',
- 'ᅥ': 'ㅓ',
- 'ᅦ': 'ㅔ',
- 'ᅧ': 'ㅕ',
- 'ᅨ': 'ㅖ',
- 'ᅩ': 'ㅗ',
- 'ᅪ': 'ㅘ',
- 'ᅫ': 'ㅙ',
- 'ᅬ': 'ㅚ',
- 'ᅭ': 'ㅛ',
- 'ᅮ': 'ㅜ',
- 'ᅯ': 'ㅝ',
- 'ᅰ': 'ㅞ',
- 'ᅱ': 'ㅟ',
- 'ᅲ': 'ㅠ',
- 'ᅳ': 'ㅡ',
- 'ᅴ': 'ㅢ',
- 'ᅵ': 'ㅣ',
- 'ᄔ': 'ㅥ',
- 'ᄕ': 'ㅦ',
- 'ᇇ': 'ㅧ',
- 'ᇈ': 'ㅨ',
- 'ᇌ': 'ㅩ',
- 'ᇎ': 'ㅪ',
- 'ᇓ': 'ㅫ',
- 'ᇗ': 'ㅬ',
- 'ᇙ': 'ㅭ',
- 'ᄜ': 'ㅮ',
- 'ᇝ': 'ㅯ',
- 'ᇟ': 'ㅰ',
- 'ᄝ': 'ㅱ',
- 'ᄞ': 'ㅲ',
- 'ᄠ': 'ㅳ',
- 'ᄢ': 'ㅴ',
- 'ᄣ': 'ㅵ',
- 'ᄧ': 'ㅶ',
- 'ᄩ': 'ㅷ',
- 'ᄫ': 'ㅸ',
- 'ᄬ': 'ㅹ',
- 'ᄭ': 'ㅺ',
- 'ᄮ': 'ㅻ',
- 'ᄯ': 'ㅼ',
- 'ᄲ': 'ㅽ',
- 'ᄶ': 'ㅾ',
- 'ᅀ': 'ㅿ',
- 'ᅇ': 'ㆀ',
- 'ᅌ': 'ㆁ',
- 'ᇱ': 'ㆂ',
- 'ᇲ': 'ㆃ',
- 'ᅗ': 'ㆄ',
- 'ᅘ': 'ㆅ',
- 'ᅙ': 'ㆆ',
- 'ᆄ': 'ㆇ',
- 'ᆅ': 'ㆈ',
- 'ᆈ': 'ㆉ',
- 'ᆑ': 'ㆊ',
- 'ᆒ': 'ㆋ',
- 'ᆔ': 'ㆌ',
- 'ᆞ': 'ㆍ',
- 'ᆡ': 'ㆎ',
-}
-
-
-def convert_yale_to_hangul(yale):
- syllables = yale.split('.')
-
- result = ""
- for syllable in syllables:
- out_syll = ""
- tone_mark = ""
-
- orig_syllable = copy.copy(syllable)
-
- if any(syllable.endswith(t) for t in ['L', "H", "R"]):
- tone_mark = {
- 'L': '',
- 'H': '\u302e',
- 'R': '\u302f'
- }[syllable[-1]]
- syllable = syllable[:-1]
-
- initial_exists = False
- for n in range(4, -1, -1):
- if syllable[:n] in YALE_TO_HANGUL_INITIAL_CONSONANTS:
- out_syll += YALE_TO_HANGUL_INITIAL_CONSONANTS[syllable[:n]]
- syllable = syllable[n:]
- initial_exists = (n > 0)
- break
-
- vowel_exists = False
- for n in range(4, 0, -1):
- if syllable[:n] in YALE_TO_HANGUL_VOWELS:
- out_syll += YALE_TO_HANGUL_VOWELS[syllable[:n]]
- syllable = syllable[n:]
- vowel_exists = True
- break
-
- for n in range(4, 0, -1):
- if syllable[:n] in YALE_TO_HANGUL_FINAL_CONSONANTS:
- out_syll += YALE_TO_HANGUL_FINAL_CONSONANTS[syllable[:n]]
- syllable = syllable[n:]
- break
-
- out_syll += tone_mark
-
- if initial_exists and not vowel_exists and tone_mark == "":
- if out_syll in UNICODE_COMPATIBILITY_FORMS:
- out_syll = UNICODE_COMPATIBILITY_FORMS[out_syll]
-
- if not initial_exists and vowel_exists and tone_mark == "":
- if out_syll[1:] in UNICODE_COMPATIBILITY_FORMS:
- out_syll = UNICODE_COMPATIBILITY_FORMS[out_syll[1:]]
-
- if len(syllable) > 0:
- # Failed to convert
- out_syll = orig_syllable
-
- result += out_syll
-
- return result
diff --git a/spaces/Chris4K/llms_compare/101 Trucos Baraja Svengali Pdf ((HOT)) Free.md b/spaces/Chris4K/llms_compare/101 Trucos Baraja Svengali Pdf ((HOT)) Free.md
deleted file mode 100644
index a2583fac6bee0e9adb32dbcd2a70fca40c910029..0000000000000000000000000000000000000000
--- a/spaces/Chris4K/llms_compare/101 Trucos Baraja Svengali Pdf ((HOT)) Free.md
+++ /dev/null
@@ -1,98 +0,0 @@
-## 101 Trucos Baraja Svengali Pdf Free
-
-
-
-
-
- 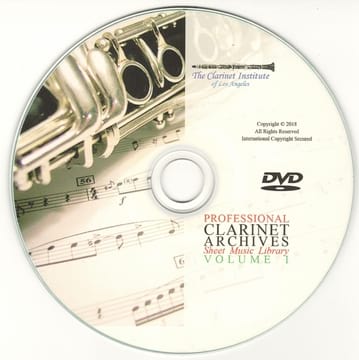
-
-
-
-
-
-**DOWNLOAD ••• [https://urluso.com/2tBNBt](https://urluso.com/2tBNBt)**
-
-
-
-
-
-
-
-
-
-
-
-
-
-# How to Perform Amazing Magic Tricks with a Svengali Deck
-
-
-
-A Svengali deck is a special type of playing cards that allows you to perform amazing magic tricks with ease. The deck consists of 52 cards, but half of them are identical and the other half are different. The identical cards are slightly shorter than the different ones, and they are arranged in a way that you can control which card appears on top or bottom of the deck.
-
-
-
-In this article, we will show you how to perform 101 amazing magic tricks with a Svengali deck. You will learn how to make cards change, disappear, reappear, jump, fly, and more. You will also learn how to use the deck for mind reading, predictions, and mentalism effects. You will be able to amaze your friends and family with your incredible skills and creativity.
-
-
-
-Before we start, you will need to get a Svengali deck. You can buy one online or at a magic shop, or you can make your own by cutting one card shorter than the rest and gluing it to another card of the same value. You can also download a free pdf of 101 Trucos Baraja Svengali Pdf Free[^1^], a book written by Lisa L. Hayes that teaches you how to perform amazing magic tricks with a Svengali deck.
-
-
-
-## Trick #1: The Basic Force
-
-
-
-The basic force is the most important technique you need to master when using a Svengali deck. It allows you to make any spectator choose the card you want them to choose. Here is how it works:
-
-
-
-1. Hold the deck in your left hand with your thumb on top and your fingers on the bottom.
-
-2. Riffle the cards from the back with your right thumb, making sure that you stop at one of the different cards.
-
-3. Ask the spectator to say "stop" whenever they want.
-
-4. When they say "stop", lift up all the cards above your right thumb and show them the bottom card of that packet. This will be one of the identical cards.
-
-5. Remember this card and put it back on top of the deck.
-
-
-
-You have now forced the spectator to choose the card you wanted them to choose. You can use this technique for many tricks, such as revealing their card in a surprising way or making it match your prediction.
-
-
-
-## Trick #2: The Card Change
-
-
-
-This trick will make it seem like you can change one card into another with a snap of your fingers. Here is how it works:
-
-
-
-1. Force a card on the spectator using the basic force technique.
-
-2. Show them their card and ask them to remember it.
-
-3. Put their card on top of the deck and cut it in half.
-
-4. Hold the top half of the deck in your right hand and show them the bottom card of that packet. This will be a different card.
-
-5. Say that you will change their card into this card with a snap of your fingers.
-
-6. Snap your fingers and turn over the top card of the bottom half of the deck. This will be one of the identical cards, matching their original card.
-
-7. Show them that their card has changed into this card and act surprised.
-
-
-
-You have now made it seem like you can change one card into another with a snap of your fingers. You can use this technique for many tricks, such as changing their card into a joker or a blank card.
-
- 145887f19f
-
-
-
-
-
diff --git a/spaces/Cicooo/vits-uma-genshin-honkai/Docker/Dockerfile b/spaces/Cicooo/vits-uma-genshin-honkai/Docker/Dockerfile
deleted file mode 100644
index 4d39cdf02a2ec151686cc1d61234bf723068fed8..0000000000000000000000000000000000000000
--- a/spaces/Cicooo/vits-uma-genshin-honkai/Docker/Dockerfile
+++ /dev/null
@@ -1,12 +0,0 @@
-FROM python:3.9-bullseye
-VOLUME ["/app"]
-WORKDIR /app
-# Set apt to Chinese mirror
-RUN sed -i 's/deb.debian.org/mirrors.ustc.edu.cn/g' /etc/apt/sources.list
-RUN apt-get update && apt-get -y install cmake git
-RUN git clone https://huggingface.co/spaces/ikechan8370/vits-uma-genshin-honkai
-WORKDIR /app/vits-uma-genshin-honkai
-RUN sed -i "s/\.launch()/\.launch(server_name=\"0.0.0.0\")/" /app/vits-uma-genshin-honkai/app.py
-ADD vits.sh /app/vits.sh
-EXPOSE 7860
-ENTRYPOINT [ "/app/vits.sh" ]
\ No newline at end of file
diff --git a/spaces/CofAI/chat.b4/g4f/__init__.py b/spaces/CofAI/chat.b4/g4f/__init__.py
deleted file mode 100644
index a0b4bac6aa4de9c0449095a3874c2cb9716169d7..0000000000000000000000000000000000000000
--- a/spaces/CofAI/chat.b4/g4f/__init__.py
+++ /dev/null
@@ -1,39 +0,0 @@
-import sys
-from . import Provider
-from g4f.models import Model, ModelUtils
-
-
-class ChatCompletion:
- @staticmethod
- def create(model: Model.model or str, messages: list, provider: Provider.Provider = None, stream: bool = False, auth: str = False, **kwargs):
- kwargs['auth'] = auth
-
- if provider and provider.needs_auth and not auth:
- print(
- f'ValueError: {provider.__name__} requires authentication (use auth="cookie or token or jwt ..." param)', file=sys.stderr)
- sys.exit(1)
-
- try:
- if isinstance(model, str):
- try:
- model = ModelUtils.convert[model]
- except KeyError:
- raise Exception(f'The model: {model} does not exist')
-
- engine = model.best_provider if not provider else provider
-
- if not engine.supports_stream and stream == True:
- print(
- f"ValueError: {engine.__name__} does not support 'stream' argument", file=sys.stderr)
- sys.exit(1)
-
- print(f'Using {engine.__name__} provider')
-
- return (engine._create_completion(model.name, messages, stream, **kwargs)
- if stream else ''.join(engine._create_completion(model.name, messages, stream, **kwargs)))
- except TypeError as e:
- print(e)
- arg: str = str(e).split("'")[1]
- print(
- f"ValueError: {engine.__name__} does not support '{arg}' argument", file=sys.stderr)
- sys.exit(1)
diff --git a/spaces/CoreyMorris/MMLU-by-task-Leaderboard/app.py b/spaces/CoreyMorris/MMLU-by-task-Leaderboard/app.py
deleted file mode 100644
index 1a7e52d886a4cc080e9c4f069386f799da1d9ae1..0000000000000000000000000000000000000000
--- a/spaces/CoreyMorris/MMLU-by-task-Leaderboard/app.py
+++ /dev/null
@@ -1,371 +0,0 @@
-import streamlit as st
-import pandas as pd
-import plotly.express as px
-import matplotlib.pyplot as plt
-import numpy as np
-import plotly.graph_objects as go
-
-st.set_page_config(layout="wide")
-
-def load_csv_data(file_path):
- return pd.read_csv(file_path)
-
-
-
-
-
-def plot_top_n(df, target_column, n=10):
- top_n = df.nlargest(n, target_column)
-
- # Initialize the bar plot
- fig, ax1 = plt.subplots(figsize=(10, 5))
-
- # Set width for each bar and their positions
- width = 0.28
- ind = np.arange(len(top_n))
-
- # Plot target_column and MMLU_average on the primary y-axis with adjusted positions
- ax1.bar(ind - width, top_n[target_column], width=width, color='blue', label=target_column)
- ax1.bar(ind, top_n['MMLU_average'], width=width, color='orange', label='MMLU_average')
-
- # Set the primary y-axis labels and title
- ax1.set_title(f'Top {n} performing models on {target_column}')
- ax1.set_xlabel('Model')
- ax1.set_ylabel('Score')
-
- # Create a secondary y-axis for Parameters
- ax2 = ax1.twinx()
-
- # Plot Parameters as bars on the secondary y-axis with adjusted position
- ax2.bar(ind + width, top_n['Parameters'], width=width, color='red', label='Parameters')
-
- # Set the secondary y-axis labels
- ax2.set_ylabel('Parameters', color='red')
- ax2.tick_params(axis='y', labelcolor='red')
-
- # Set the x-ticks and their labels
- ax1.set_xticks(ind)
- ax1.set_xticklabels(top_n.index, rotation=45, ha="right")
-
- # Adjust the legend
- fig.tight_layout()
- fig.legend(loc='center left', bbox_to_anchor=(1, 0.5))
-
- # Show the plot
- st.pyplot(fig)
-
-# Function to create an unfilled radar chart
-def create_radar_chart_unfilled(df, model_names, metrics):
- fig = go.Figure()
- min_value = df.loc[model_names, metrics].min().min()
- max_value = df.loc[model_names, metrics].max().max()
- for model_name in model_names:
- values_model = df.loc[model_name, metrics]
- fig.add_trace(go.Scatterpolar(
- r=values_model,
- theta=metrics,
- name=model_name
- ))
-
- fig.update_layout(
- polar=dict(
- radialaxis=dict(
- visible=True,
- range=[min_value, max_value]
- )),
- showlegend=True,
- width=800, # Change the width as needed
- height=600 # Change the height as needed
- )
- return fig
-
-
-
-# Function to create a line chart
-def create_line_chart(df, model_names, metrics):
- line_data = []
- for model_name in model_names:
- values_model = df.loc[model_name, metrics]
- for metric, value in zip(metrics, values_model):
- line_data.append({'Model': model_name, 'Metric': metric, 'Value': value})
-
- line_df = pd.DataFrame(line_data)
-
- fig = px.line(line_df, x='Metric', y='Value', color='Model', title='Comparison of Models', line_dash_sequence=['solid'])
- fig.update_layout(showlegend=True)
- return fig
-
-def find_top_differences_table(df, target_model, closest_models, num_differences=10, exclude_columns=['Parameters']):
- # Calculate the absolute differences for each task between the target model and the closest models
- new_df = df.drop(columns=exclude_columns)
- differences = new_df.loc[closest_models].sub(new_df.loc[target_model]).abs()
- # Unstack the differences and sort by the largest absolute difference
- top_differences = differences.unstack().nlargest(num_differences)
- # Convert the top differences to a DataFrame for display
- top_differences_table = pd.DataFrame({
- 'Task': [idx[0] for idx in top_differences.index],
- 'Difference': top_differences.values
- })
- # Ensure that only unique tasks are returned
- unique_top_differences_tasks = list(set(top_differences_table['Task'].tolist()))
- return top_differences_table, unique_top_differences_tasks
-
-# st.title('Model Evaluation Results including MMLU by task')
-st.title('Interactive Portal for Analyzing Open Source Large Language Models')
-st.markdown("""***Last updated October 6th***""")
-st.markdown("""**Models that are suspected to have training data contaminated with evaluation data have been removed.**""")
-st.markdown("""
- This page provides a way to explore the results for individual tasks and compare models across tasks. Data for the benchmarks hellaswag, arc_challenge, and truthfulQA have also been included for comparison.
- There are 57 tasks in the MMLU evaluation that cover a wide variety of subjects including Science, Math, Humanities, Social Science, Applied Science, Logic, and Security.
- [Preliminary analysis of MMLU-by-Task data](https://coreymorrisdata.medium.com/preliminary-analysis-of-mmlu-evaluation-data-insights-from-500-open-source-models-e67885aa364b)
- """)
-
-# Load the data into memory
-data_path = "processed_data_2023-10-08.csv"
-data_df = load_csv_data(data_path)
-# drop the column Unnamed: 0
-data_df.rename(columns={'Unnamed: 0': "Model Name"}, inplace=True)
-data_df.set_index("Model Name", inplace=True)
-
-filtered_data = data_df
-
-# sort the table by the MMLU_average column
-filtered_data = filtered_data.sort_values(by=['MMLU_average'], ascending=False)
-
-# Select box for filtering by Parameters
-parameter_threshold = st.selectbox(
- 'Filter by Parameters (Less Than or Equal To):',
- options=[3, 7, 13, 35, 'No threshold'],
- index=4, # Set the default selected option to 'No threshold'
- format_func=lambda x: f"{x}" if isinstance(x, int) else x
-)
-if isinstance(parameter_threshold, int):
- filtered_data = filtered_data[filtered_data['Parameters'] <= parameter_threshold]
-
-# model name filtering
-search_queries = st.text_input("Filter by Model Name:", "").replace(" ", "").split(',')
-if search_queries:
- filtered_data = filtered_data[filtered_data.index.str.contains('|'.join(search_queries), case=False)]
-
-# column name filtering
-column_search_query = st.text_input("Filter by Column/Task Name:", "").replace(" ", "").split(',')
-matching_columns = [col for col in filtered_data.columns if any(query.lower() in col.lower() for query in column_search_query)]
-filtered_data = filtered_data[matching_columns]
-
-
-# Display the DataFrame with only the matching columns
-st.markdown("## Sortable Results")
-st.dataframe(
- filtered_data[matching_columns],
- column_config={
- "URL": st.column_config.LinkColumn( # Only current way to make url a clickable link with streamlit without removing the interactivity of the table
- width="small"
- )
- },
- hide_index=True,
-)
-
-# CSV download
-filtered_data.index.name = "Model Name"
-
-csv = filtered_data.to_csv(index=True)
-st.download_button(
- label="Download data as CSV",
- data=csv,
- file_name="model_evaluation_results.csv",
- mime="text/csv",
-)
-
-
-def create_plot(df, x_values, y_values, models=None, title=None):
- if models is not None:
- df = df[df.index.isin(models)]
-
- # remove rows with NaN values
- df = df.dropna(subset=[x_values, y_values])
-
- #remove label rows URL, full_model_name
- df = df.drop(columns=['URL', 'full_model_name'])
-
- plot_data = pd.DataFrame({
- 'Model': df.index,
- x_values: df[x_values],
- y_values: df[y_values],
- })
-
- plot_data['color'] = 'purple'
- fig = px.scatter(plot_data, x=x_values, y=y_values, color='color', hover_data=['Model'], trendline="ols")
-
- # If title is not provided, use x_values vs. y_values as the default title
- if title is None:
- title = x_values + " vs. " + y_values
-
- layout_args = dict(
- showlegend=False,
- xaxis_title=x_values,
- yaxis_title=y_values,
- xaxis=dict(),
- yaxis=dict(),
- title=title,
- height=500,
- width=1000,
- )
- fig.update_layout(**layout_args)
-
- # Add a dashed line at 0.25 for the y_values
- x_min = df[x_values].min()
- x_max = df[x_values].max()
-
- y_min = df[y_values].min()
- y_max = df[y_values].max()
-
- if x_values.startswith('MMLU'):
- fig.add_shape(
- type='line',
- x0=0.25, x1=0.25,
- y0=y_min, y1=y_max,
- line=dict(
- color='red',
- width=2,
- dash='dash'
- )
- )
-
- if y_values.startswith('MMLU'):
- fig.add_shape(
- type='line',
- x0=x_min, x1=x_max,
- y0=0.25, y1=0.25,
- line=dict(
- color='red',
- width=2,
- dash='dash'
- )
- )
-
- return fig
-
-
-# Custom scatter plots
-st.header('Custom scatter plots')
-st.write("""
- The scatter plot is useful to identify models that outperform or underperform on a particular task in relation to their size or overall performance.
- Identifying these models is a first step to better understand what training strategies result in better performance on a particular task.
- """)
-st.markdown("***The dashed red line indicates random chance accuracy of 0.25 as the MMLU evaluation is multiple choice with 4 response options.***")
-# add a line separating the writing
-st.markdown("***")
-st.write("As expected, there is a strong positive relationship between the number of parameters and average performance on the MMLU evaluation.")
-
-
-column_list_for_plotting = filtered_data.columns.tolist()
-if 'URL' in column_list_for_plotting:
- column_list_for_plotting.remove('URL')
-if 'full_model_name' in column_list_for_plotting:
- column_list_for_plotting.remove('full_model_name')
-
-selected_x_column = st.selectbox('Select x-axis', column_list_for_plotting, index=0)
-selected_y_column = st.selectbox('Select y-axis', column_list_for_plotting, index=1)
-
-if selected_x_column != selected_y_column: # Avoid creating a plot with the same column on both axes
- fig = create_plot(filtered_data, selected_x_column, selected_y_column)
- st.plotly_chart(fig)
-else:
- st.write("Please select different columns for the x and y axes.")
-
-
-# end of custom scatter plots
-
-
-
-# # Section to select a model and display radar and line charts
-# st.header("Compare a Selected Model to the 5 Models Closest in MMLU Average Performance")
-# st.write("""
-# This comparison highlights the nuances in model performance across different tasks.
-# While the overall MMLU average score provides a general understanding of a model's capabilities,
-# examining the closest models reveals variations in performance on individual tasks.
-# Such an analysis can uncover specific strengths and weaknesses and guide further exploration and improvement.
-# """)
-
-# default_model_name = "GPT-JT-6B-v0"
-
-# default_model_index = filtered_data.index.tolist().index(default_model_name) if default_model_name in filtered_data.index else 0
-# selected_model_name = st.selectbox("Select a Model:", filtered_data.index.tolist(), index=default_model_index)
-
-# # Get the closest 5 models with unique indices
-# closest_models_diffs = filtered_data['MMLU_average'].sub(filtered_data.loc[selected_model_name, 'MMLU_average']).abs()
-# closest_models = closest_models_diffs.nsmallest(5, keep='first').index.drop_duplicates().tolist()
-
-
-# Find the top 10 tasks with the largest differences and convert to a DataFrame
-# top_differences_table, top_differences_tasks = find_top_differences_table(filtered_data, selected_model_name, closest_models)
-
-# Display the DataFrame for the closest models and the top differences tasks
-# st.dataframe(filtered_data.loc[closest_models, top_differences_tasks])
-
-# # Display the table in the Streamlit app
-# st.markdown("## Top Differences")
-# st.dataframe(top_differences_table)
-
-# Create a radar chart for the tasks with the largest differences
-# fig_radar_top_differences = create_radar_chart_unfilled(filtered_data, closest_models, top_differences_tasks)
-
-# Display the radar chart
-# st.plotly_chart(fig_radar_top_differences)
-
-
-st.markdown("## Notable findings and plots")
-
-# Moral scenarios plots
-st.markdown("### MMLU’s Moral Scenarios Benchmark Doesn’t Measure What You Think it Measures")
-def show_random_moral_scenarios_question():
- moral_scenarios_data = pd.read_csv('moral_scenarios_questions.csv')
- random_question = moral_scenarios_data.sample()
- expander = st.expander("Show a random moral scenarios question")
- expander.write(random_question['query'].values[0])
-
-
-
-st.write("""
- After a deeper dive into the moral scenarios task, it appears that benchmark is not a valid measurement of moral judgement.
- The challenges these models face are not rooted in understanding each scenario, but rather in the structure of the task itself.
- I would recommend using a different benchmark for moral judgement. More details of the analysis can be found here: [MMLU’s Moral Scenarios Benchmark Doesn’t Measure What You Think it Measures ](https://medium.com/p/74fd6e512521)
- """)
-
-show_random_moral_scenarios_question()
-
-fig = create_plot(filtered_data, 'Parameters', 'MMLU_moral_scenarios', title="Impact of Parameter Count on Accuracy for Moral Scenarios")
-st.plotly_chart(fig)
-st.write()
-
-
-
-fig = create_plot(filtered_data, 'MMLU_average', 'MMLU_moral_scenarios')
-st.plotly_chart(fig)
-
-st.markdown('### Abstract Algebra Performance')
-st.write("Small models showed surprisingly strong performance on the abstract algebra task. A 6 Billion parameter model is tied for the best performance on this task and there are a number of other small models in the top 10.")
-plot_top_n(filtered_data, 'MMLU_abstract_algebra', 10)
-
-fig = create_plot(filtered_data, 'Parameters', 'MMLU_abstract_algebra')
-st.plotly_chart(fig)
-
-st.markdown("***Thank you to hugging face for running the evaluations and supplying the data as well as the original authors of the evaluations.***")
-
-st.markdown("""
-# Citation
-
-1. Corey Morris (2023). *Exploring the Characteristics of Large Language Models: An Interactive Portal for Analyzing 700+ Open Source Models Across 57 Diverse Evaluation Tasks*. [link](https://huggingface.co/spaces/CoreyMorris/MMLU-by-task-Leaderboard)
-
-2. Edward Beeching, Clémentine Fourrier, Nathan Habib, Sheon Han, Nathan Lambert, Nazneen Rajani, Omar Sanseviero, Lewis Tunstall, Thomas Wolf. (2023). *Open LLM Leaderboard*. Hugging Face. [link](https://huggingface.co/spaces/HuggingFaceH4/open_llm_leaderboard)
-
-3. Gao, Leo et al. (2021). *A framework for few-shot language model evaluation*. Zenodo. [link](https://doi.org/10.5281/zenodo.5371628)
-
-4. Peter Clark, Isaac Cowhey, Oren Etzioni, Tushar Khot, Ashish Sabharwal, Carissa Schoenick, Oyvind Tafjord. (2018). *Think you have Solved Question Answering? Try ARC, the AI2 Reasoning Challenge*. arXiv. [link](https://arxiv.org/abs/1803.05457)
-
-5. Rowan Zellers, Ari Holtzman, Yonatan Bisk, Ali Farhadi, Yejin Choi. (2019). *HellaSwag: Can a Machine Really Finish Your Sentence?*. arXiv. [link](https://arxiv.org/abs/1905.07830)
-
-6. Dan Hendrycks, Collin Burns, Steven Basart, Andy Zou, Mantas Mazeika, Dawn Song, Jacob Steinhardt. (2021). *Measuring Massive Multitask Language Understanding*. arXiv. [link](https://arxiv.org/abs/2009.03300)
-
-7. Stephanie Lin, Jacob Hilton, Owain Evans. (2022). *TruthfulQA: Measuring How Models Mimic Human Falsehoods*. arXiv. [link](https://arxiv.org/abs/2109.07958)
-""")
diff --git a/spaces/Cyril666/ContourNet-ABI/maskrcnn_benchmark/utils/c2_model_loading.py b/spaces/Cyril666/ContourNet-ABI/maskrcnn_benchmark/utils/c2_model_loading.py
deleted file mode 100644
index 041d7e0141d52c2b6390d13a437062477b493fd5..0000000000000000000000000000000000000000
--- a/spaces/Cyril666/ContourNet-ABI/maskrcnn_benchmark/utils/c2_model_loading.py
+++ /dev/null
@@ -1,177 +0,0 @@
-# Copyright (c) Facebook, Inc. and its affiliates. All Rights Reserved.
-import logging
-import pickle
-from collections import OrderedDict
-
-import torch
-
-from maskrcnn_benchmark.utils.model_serialization import load_state_dict
-from maskrcnn_benchmark.utils.registry import Registry
-
-
-def _rename_basic_resnet_weights(layer_keys):
- layer_keys = [k.replace("_", ".") for k in layer_keys]
- layer_keys = [k.replace(".w", ".weight") for k in layer_keys]
- layer_keys = [k.replace(".bn", "_bn") for k in layer_keys]
- layer_keys = [k.replace(".b", ".bias") for k in layer_keys]
- layer_keys = [k.replace("_bn.s", "_bn.scale") for k in layer_keys]
- layer_keys = [k.replace(".biasranch", ".branch") for k in layer_keys]
- layer_keys = [k.replace("bbox.pred", "bbox_pred") for k in layer_keys]
- layer_keys = [k.replace("cls.score", "cls_score") for k in layer_keys]
- layer_keys = [k.replace("res.conv1_", "conv1_") for k in layer_keys]
-
- # RPN / Faster RCNN
- layer_keys = [k.replace(".biasbox", ".bbox") for k in layer_keys]
- layer_keys = [k.replace("conv.rpn", "rpn.conv") for k in layer_keys]
- layer_keys = [k.replace("rpn.bbox.pred", "rpn.bbox_pred") for k in layer_keys]
- layer_keys = [k.replace("rpn.cls.logits", "rpn.cls_logits") for k in layer_keys]
-
- # Affine-Channel -> BatchNorm enaming
- layer_keys = [k.replace("_bn.scale", "_bn.weight") for k in layer_keys]
-
- # Make torchvision-compatible
- layer_keys = [k.replace("conv1_bn.", "bn1.") for k in layer_keys]
-
- layer_keys = [k.replace("res2.", "layer1.") for k in layer_keys]
- layer_keys = [k.replace("res3.", "layer2.") for k in layer_keys]
- layer_keys = [k.replace("res4.", "layer3.") for k in layer_keys]
- layer_keys = [k.replace("res5.", "layer4.") for k in layer_keys]
-
- layer_keys = [k.replace(".branch2a.", ".conv1.") for k in layer_keys]
- layer_keys = [k.replace(".branch2a_bn.", ".bn1.") for k in layer_keys]
- layer_keys = [k.replace(".branch2b.", ".conv2.") for k in layer_keys]
- layer_keys = [k.replace(".branch2b_bn.", ".bn2.") for k in layer_keys]
- layer_keys = [k.replace(".branch2c.", ".conv3.") for k in layer_keys]
- layer_keys = [k.replace(".branch2c_bn.", ".bn3.") for k in layer_keys]
-
- layer_keys = [k.replace(".branch1.", ".downsample.0.") for k in layer_keys]
- layer_keys = [k.replace(".branch1_bn.", ".downsample.1.") for k in layer_keys]
-
- # GroupNorm
- layer_keys = [k.replace("conv1.gn.s", "bn1.weight") for k in layer_keys]
- layer_keys = [k.replace("conv1.gn.bias", "bn1.bias") for k in layer_keys]
- layer_keys = [k.replace("conv2.gn.s", "bn2.weight") for k in layer_keys]
- layer_keys = [k.replace("conv2.gn.bias", "bn2.bias") for k in layer_keys]
- layer_keys = [k.replace("conv3.gn.s", "bn3.weight") for k in layer_keys]
- layer_keys = [k.replace("conv3.gn.bias", "bn3.bias") for k in layer_keys]
- layer_keys = [k.replace("downsample.0.gn.s", "downsample.1.weight") \
- for k in layer_keys]
- layer_keys = [k.replace("downsample.0.gn.bias", "downsample.1.bias") \
- for k in layer_keys]
-
- return layer_keys
-
-def _rename_fpn_weights(layer_keys, stage_names):
- for mapped_idx, stage_name in enumerate(stage_names, 1):
- suffix = ""
- if mapped_idx < 4:
- suffix = ".lateral"
- layer_keys = [
- k.replace("fpn.inner.layer{}.sum{}".format(stage_name, suffix), "fpn_inner{}".format(mapped_idx)) for k in layer_keys
- ]
- layer_keys = [k.replace("fpn.layer{}.sum".format(stage_name), "fpn_layer{}".format(mapped_idx)) for k in layer_keys]
-
-
- layer_keys = [k.replace("rpn.conv.fpn2", "rpn.conv") for k in layer_keys]
- layer_keys = [k.replace("rpn.bbox_pred.fpn2", "rpn.bbox_pred") for k in layer_keys]
- layer_keys = [
- k.replace("rpn.cls_logits.fpn2", "rpn.cls_logits") for k in layer_keys
- ]
-
- return layer_keys
-
-
-def _rename_weights_for_resnet(weights, stage_names):
- original_keys = sorted(weights.keys())
- layer_keys = sorted(weights.keys())
-
- # for X-101, rename output to fc1000 to avoid conflicts afterwards
- layer_keys = [k if k != "pred_b" else "fc1000_b" for k in layer_keys]
- layer_keys = [k if k != "pred_w" else "fc1000_w" for k in layer_keys]
-
- # performs basic renaming: _ -> . , etc
- layer_keys = _rename_basic_resnet_weights(layer_keys)
-
- # FPN
- layer_keys = _rename_fpn_weights(layer_keys, stage_names)
-
- # Mask R-CNN
- layer_keys = [k.replace("mask.fcn.logits", "mask_fcn_logits") for k in layer_keys]
- layer_keys = [k.replace(".[mask].fcn", "mask_fcn") for k in layer_keys]
- layer_keys = [k.replace("conv5.mask", "conv5_mask") for k in layer_keys]
-
- # Keypoint R-CNN
- layer_keys = [k.replace("kps.score.lowres", "kps_score_lowres") for k in layer_keys]
- layer_keys = [k.replace("kps.score", "kps_score") for k in layer_keys]
- layer_keys = [k.replace("conv.fcn", "conv_fcn") for k in layer_keys]
-
- # Rename for our RPN structure
- layer_keys = [k.replace("rpn.", "rpn.head.") for k in layer_keys]
-
- key_map = {k: v for k, v in zip(original_keys, layer_keys)}
-
- logger = logging.getLogger(__name__)
- logger.info("Remapping C2 weights")
- max_c2_key_size = max([len(k) for k in original_keys if "_momentum" not in k])
-
- new_weights = OrderedDict()
- for k in original_keys:
- v = weights[k]
- if "_momentum" in k:
- continue
- # if 'fc1000' in k:
- # continue
- w = torch.from_numpy(v)
- # if "bn" in k:
- # w = w.view(1, -1, 1, 1)
- logger.info("C2 name: {: <{}} mapped name: {}".format(k, max_c2_key_size, key_map[k]))
- new_weights[key_map[k]] = w
-
- return new_weights
-
-
-def _load_c2_pickled_weights(file_path):
- with open(file_path, "rb") as f:
- if torch._six.PY3:
- data = pickle.load(f, encoding="latin1")
- else:
- data = pickle.load(f)
- if "blobs" in data:
- weights = data["blobs"]
- else:
- weights = data
- return weights
-
-
-_C2_STAGE_NAMES = {
- "R-50": ["1.2", "2.3", "3.5", "4.2"],
- "R-101": ["1.2", "2.3", "3.22", "4.2"],
- "R-152": ["1.2", "2.7", "3.35", "4.2"],
-}
-
-C2_FORMAT_LOADER = Registry()
-
-
-@C2_FORMAT_LOADER.register("R-50-C4")
-@C2_FORMAT_LOADER.register("R-50-C5")
-@C2_FORMAT_LOADER.register("R-101-C4")
-@C2_FORMAT_LOADER.register("R-101-C5")
-@C2_FORMAT_LOADER.register("R-50-FPN")
-@C2_FORMAT_LOADER.register("R-50-FPN-RETINANET")
-@C2_FORMAT_LOADER.register("R-101-FPN")
-@C2_FORMAT_LOADER.register("R-101-PAN")
-@C2_FORMAT_LOADER.register("R-101-FPN-RETINANET")
-@C2_FORMAT_LOADER.register("R-152-FPN")
-@C2_FORMAT_LOADER.register("R-152-PAN")
-def load_resnet_c2_format(cfg, f):
- state_dict = _load_c2_pickled_weights(f)
- conv_body = cfg.MODEL.BACKBONE.CONV_BODY
- arch = conv_body.replace("-C4", "").replace("-C5", "").replace("-FPN", "")
- arch = arch.replace("-RETINANET", "").replace("-PAN", "")
- stages = _C2_STAGE_NAMES[arch]
- state_dict = _rename_weights_for_resnet(state_dict, stages)
- return dict(model=state_dict)
-
-
-def load_c2_format(cfg, f):
- return C2_FORMAT_LOADER[cfg.MODEL.BACKBONE.CONV_BODY](cfg, f)
diff --git a/spaces/DQChoi/gpt-demo/venv/lib/python3.11/site-packages/anyio/_backends/__init__.py b/spaces/DQChoi/gpt-demo/venv/lib/python3.11/site-packages/anyio/_backends/__init__.py
deleted file mode 100644
index e69de29bb2d1d6434b8b29ae775ad8c2e48c5391..0000000000000000000000000000000000000000
diff --git a/spaces/DQChoi/gpt-demo/venv/lib/python3.11/site-packages/gradio/templates/frontend/assets/index-3370be2a.js b/spaces/DQChoi/gpt-demo/venv/lib/python3.11/site-packages/gradio/templates/frontend/assets/index-3370be2a.js
deleted file mode 100644
index 727cf49fce2eb4e98d5f5c9f68aac2dcde37f774..0000000000000000000000000000000000000000
--- a/spaces/DQChoi/gpt-demo/venv/lib/python3.11/site-packages/gradio/templates/frontend/assets/index-3370be2a.js
+++ /dev/null
@@ -1,16 +0,0 @@
-(function(){const t=document.createElement("link").relList;if(t&&t.supports&&t.supports("modulepreload"))return;for(const i of document.querySelectorAll('link[rel="modulepreload"]'))n(i);new MutationObserver(i=>{for(const o of i)if(o.type==="childList")for(const s of o.addedNodes)s.tagName==="LINK"&&s.rel==="modulepreload"&&n(s)}).observe(document,{childList:!0,subtree:!0});function r(i){const o={};return i.integrity&&(o.integrity=i.integrity),i.referrerPolicy&&(o.referrerPolicy=i.referrerPolicy),i.crossOrigin==="use-credentials"?o.credentials="include":i.crossOrigin==="anonymous"?o.credentials="omit":o.credentials="same-origin",o}function n(i){if(i.ep)return;i.ep=!0;const o=r(i);fetch(i.href,o)}})();var ei=typeof globalThis<"u"?globalThis:typeof window<"u"?window:typeof global<"u"?global:typeof self<"u"?self:{};function kr(e){return e&&e.__esModule&&Object.prototype.hasOwnProperty.call(e,"default")?e.default:e}var Ye={},Pe={},_t={exports:{}},R=String,tr=function(){return{isColorSupported:!1,reset:R,bold:R,dim:R,italic:R,underline:R,inverse:R,hidden:R,strikethrough:R,black:R,red:R,green:R,yellow:R,blue:R,magenta:R,cyan:R,white:R,gray:R,bgBlack:R,bgRed:R,bgGreen:R,bgYellow:R,bgBlue:R,bgMagenta:R,bgCyan:R,bgWhite:R}};_t.exports=tr();_t.exports.createColors=tr;var zr=_t.exports;Object.defineProperty(Pe,"__esModule",{value:!0});Pe.dim=Ar;Pe.default=void 0;var le=xr(zr);function xr(e){return e&&e.__esModule?e:{default:e}}let kt=new Set;function rt(e,t,r){typeof process<"u"&&{}.JEST_WORKER_ID||r&&kt.has(r)||(r&&kt.add(r),console.warn(""),t.forEach(n=>console.warn(e,"-",n)))}function Ar(e){return le.default.dim(e)}var Er={info(e,t){rt(le.default.bold(le.default.cyan("info")),...Array.isArray(e)?[e]:[t,e])},warn(e,t){rt(le.default.bold(le.default.yellow("warn")),...Array.isArray(e)?[e]:[t,e])},risk(e,t){rt(le.default.bold(le.default.magenta("risk")),...Array.isArray(e)?[e]:[t,e])}};Pe.default=Er;Object.defineProperty(Ye,"__esModule",{value:!0});Ye.default=void 0;var Sr=Nr(Pe);function Nr(e){return e&&e.__esModule?e:{default:e}}function Ne({version:e,from:t,to:r}){Sr.default.warn(`${t}-color-renamed`,[`As of Tailwind CSS ${e}, \`${t}\` has been renamed to \`${r}\`.`,"Update your configuration file to silence this warning."])}var qr={inherit:"inherit",current:"currentColor",transparent:"transparent",black:"#000",white:"#fff",slate:{50:"#f8fafc",100:"#f1f5f9",200:"#e2e8f0",300:"#cbd5e1",400:"#94a3b8",500:"#64748b",600:"#475569",700:"#334155",800:"#1e293b",900:"#0f172a"},gray:{50:"#f9fafb",100:"#f3f4f6",200:"#e5e7eb",300:"#d1d5db",400:"#9ca3af",500:"#6b7280",600:"#4b5563",700:"#374151",800:"#1f2937",900:"#111827"},zinc:{50:"#fafafa",100:"#f4f4f5",200:"#e4e4e7",300:"#d4d4d8",400:"#a1a1aa",500:"#71717a",600:"#52525b",700:"#3f3f46",800:"#27272a",900:"#18181b"},neutral:{50:"#fafafa",100:"#f5f5f5",200:"#e5e5e5",300:"#d4d4d4",400:"#a3a3a3",500:"#737373",600:"#525252",700:"#404040",800:"#262626",900:"#171717"},stone:{50:"#fafaf9",100:"#f5f5f4",200:"#e7e5e4",300:"#d6d3d1",400:"#a8a29e",500:"#78716c",600:"#57534e",700:"#44403c",800:"#292524",900:"#1c1917"},red:{50:"#fef2f2",100:"#fee2e2",200:"#fecaca",300:"#fca5a5",400:"#f87171",500:"#ef4444",600:"#dc2626",700:"#b91c1c",800:"#991b1b",900:"#7f1d1d"},orange:{50:"#fff7ed",100:"#ffedd5",200:"#fed7aa",300:"#fdba74",400:"#fb923c",500:"#f97316",600:"#ea580c",700:"#c2410c",800:"#9a3412",900:"#7c2d12"},amber:{50:"#fffbeb",100:"#fef3c7",200:"#fde68a",300:"#fcd34d",400:"#fbbf24",500:"#f59e0b",600:"#d97706",700:"#b45309",800:"#92400e",900:"#78350f"},yellow:{50:"#fefce8",100:"#fef9c3",200:"#fef08a",300:"#fde047",400:"#facc15",500:"#eab308",600:"#ca8a04",700:"#a16207",800:"#854d0e",900:"#713f12"},lime:{50:"#f7fee7",100:"#ecfccb",200:"#d9f99d",300:"#bef264",400:"#a3e635",500:"#84cc16",600:"#65a30d",700:"#4d7c0f",800:"#3f6212",900:"#365314"},green:{50:"#f0fdf4",100:"#dcfce7",200:"#bbf7d0",300:"#86efac",400:"#4ade80",500:"#22c55e",600:"#16a34a",700:"#15803d",800:"#166534",900:"#14532d"},emerald:{50:"#ecfdf5",100:"#d1fae5",200:"#a7f3d0",300:"#6ee7b7",400:"#34d399",500:"#10b981",600:"#059669",700:"#047857",800:"#065f46",900:"#064e3b"},teal:{50:"#f0fdfa",100:"#ccfbf1",200:"#99f6e4",300:"#5eead4",400:"#2dd4bf",500:"#14b8a6",600:"#0d9488",700:"#0f766e",800:"#115e59",900:"#134e4a"},cyan:{50:"#ecfeff",100:"#cffafe",200:"#a5f3fc",300:"#67e8f9",400:"#22d3ee",500:"#06b6d4",600:"#0891b2",700:"#0e7490",800:"#155e75",900:"#164e63"},sky:{50:"#f0f9ff",100:"#e0f2fe",200:"#bae6fd",300:"#7dd3fc",400:"#38bdf8",500:"#0ea5e9",600:"#0284c7",700:"#0369a1",800:"#075985",900:"#0c4a6e"},blue:{50:"#eff6ff",100:"#dbeafe",200:"#bfdbfe",300:"#93c5fd",400:"#60a5fa",500:"#3b82f6",600:"#2563eb",700:"#1d4ed8",800:"#1e40af",900:"#1e3a8a"},indigo:{50:"#eef2ff",100:"#e0e7ff",200:"#c7d2fe",300:"#a5b4fc",400:"#818cf8",500:"#6366f1",600:"#4f46e5",700:"#4338ca",800:"#3730a3",900:"#312e81"},violet:{50:"#f5f3ff",100:"#ede9fe",200:"#ddd6fe",300:"#c4b5fd",400:"#a78bfa",500:"#8b5cf6",600:"#7c3aed",700:"#6d28d9",800:"#5b21b6",900:"#4c1d95"},purple:{50:"#faf5ff",100:"#f3e8ff",200:"#e9d5ff",300:"#d8b4fe",400:"#c084fc",500:"#a855f7",600:"#9333ea",700:"#7e22ce",800:"#6b21a8",900:"#581c87"},fuchsia:{50:"#fdf4ff",100:"#fae8ff",200:"#f5d0fe",300:"#f0abfc",400:"#e879f9",500:"#d946ef",600:"#c026d3",700:"#a21caf",800:"#86198f",900:"#701a75"},pink:{50:"#fdf2f8",100:"#fce7f3",200:"#fbcfe8",300:"#f9a8d4",400:"#f472b6",500:"#ec4899",600:"#db2777",700:"#be185d",800:"#9d174d",900:"#831843"},rose:{50:"#fff1f2",100:"#ffe4e6",200:"#fecdd3",300:"#fda4af",400:"#fb7185",500:"#f43f5e",600:"#e11d48",700:"#be123c",800:"#9f1239",900:"#881337"},get lightBlue(){return Ne({version:"v2.2",from:"lightBlue",to:"sky"}),this.sky},get warmGray(){return Ne({version:"v3.0",from:"warmGray",to:"stone"}),this.stone},get trueGray(){return Ne({version:"v3.0",from:"trueGray",to:"neutral"}),this.neutral},get coolGray(){return Ne({version:"v3.0",from:"coolGray",to:"gray"}),this.gray},get blueGray(){return Ne({version:"v3.0",from:"blueGray",to:"slate"}),this.slate}};Ye.default=qr;let nt=Ye;var Cr=(nt.__esModule?nt:{default:nt}).default;const zt=kr(Cr),ti=["red","green","blue","yellow","purple","teal","orange","cyan","lime","pink"],Lr=[{color:"red",primary:600,secondary:100},{color:"green",primary:600,secondary:100},{color:"blue",primary:600,secondary:100},{color:"yellow",primary:500,secondary:100},{color:"purple",primary:600,secondary:100},{color:"teal",primary:600,secondary:100},{color:"orange",primary:600,secondary:100},{color:"cyan",primary:600,secondary:100},{color:"lime",primary:500,secondary:100},{color:"pink",primary:600,secondary:100}],ri=Lr.reduce((e,{color:t,primary:r,secondary:n})=>({...e,[t]:{primary:zt[t][r],secondary:zt[t][n]}}),{}),Mr="modulepreload",Or=function(e,t){return new URL(e,t).href},xt={},Ge=function(t,r,n){if(!r||r.length===0)return t();const i=document.getElementsByTagName("link");return Promise.all(r.map(o=>{if(o=Or(o,n),o in xt)return;xt[o]=!0;const s=o.endsWith(".css"),a=s?'[rel="stylesheet"]':"";if(!!n)for(let f=i.length-1;f>=0;f--){const u=i[f];if(u.href===o&&(!s||u.rel==="stylesheet"))return}else if(document.querySelector(`link[href="${o}"]${a}`))return;const l=document.createElement("link");if(l.rel=s?"stylesheet":Mr,s||(l.as="script",l.crossOrigin=""),l.href=o,document.head.appendChild(l),s)return new Promise((f,u)=>{l.addEventListener("load",f),l.addEventListener("error",()=>u(new Error(`Unable to preload CSS for ${o}`)))})})).then(()=>t())};var it=new Intl.Collator(0,{numeric:1}).compare;function At(e,t,r){return e=e.split("."),t=t.split("."),it(e[0],t[0])||it(e[1],t[1])||(t[2]=t.slice(2).join("."),r=/[.-]/.test(e[2]=e.slice(2).join(".")),r==/[.-]/.test(t[2])?it(e[2],t[2]):r?-1:1)}function ot(e){if(e.startsWith("http")){const{protocol:t,host:r}=new URL(e);return r.endsWith("hf.space")?{ws_protocol:"wss",host:r,http_protocol:t}:{ws_protocol:t==="https:"?"wss":"ws",http_protocol:t,host:r}}return{ws_protocol:"wss",http_protocol:"https:",host:e}}const rr=/^[^\/]*\/[^\/]*$/,Pr=/.*hf\.space\/{0,1}$/;async function Tr(e,t){const r={};t&&(r.Authorization=`Bearer ${t}`);const n=e.trim();if(rr.test(n))try{const i=await fetch(`https://huggingface.co/api/spaces/${n}/host`,{headers:r});if(i.status!==200)throw new Error("Space metadata could not be loaded.");const o=(await i.json()).host;return{space_id:e,...ot(o)}}catch(i){throw new Error("Space metadata could not be loaded."+i.message)}if(Pr.test(n)){const{ws_protocol:i,http_protocol:o,host:s}=ot(n);return{space_id:s.replace(".hf.space",""),ws_protocol:i,http_protocol:o,host:s}}return{space_id:!1,...ot(n)}}function Br(e){let t={};return e.forEach(({api_name:r},n)=>{r&&(t[r]=n)}),t}const Fr=/^(?=[^]*\b[dD]iscussions{0,1}\b)(?=[^]*\b[dD]isabled\b)[^]*$/;async function Et(e){try{const r=(await fetch(`https://huggingface.co/api/spaces/${e}/discussions`,{method:"HEAD"})).headers.get("x-error-message");return!(r&&Fr.test(r))}catch{return!1}}const Rr="This application is too busy. Keep trying!",Re="Connection errored out.";let nr;function Dr(e){return{post_data:t,upload_files:r,client:n,handle_blob:i};async function t(o,s,a){const c={"Content-Type":"application/json"};a&&(c.Authorization=`Bearer ${a}`);try{var l=await e(o,{method:"POST",body:JSON.stringify(s),headers:c})}catch{return[{error:Re},500]}return[await l.json(),l.status]}async function r(o,s,a){const c={};a&&(c.Authorization=`Bearer ${a}`);const l=new FormData;s.forEach(g=>{l.append("files",g)});try{var f=await e(`${o}/upload`,{method:"POST",body:l,headers:c})}catch{return{error:Re}}return{files:await f.json()}}async function n(o,s={normalise_files:!0}){return new Promise(async a=>{const{status_callback:c,hf_token:l,normalise_files:f}=s,u={predict:M,submit:U,view_api:ee},g=f??!0;if(typeof window>"u"||!("WebSocket"in window)){const C=await Ge(()=>import("./wrapper-6f348d45-38be7a64.js"),["./wrapper-6f348d45-38be7a64.js","./__vite-browser-external-b25bb000.js"],import.meta.url);nr=(await Ge(()=>import("./__vite-browser-external-b25bb000.js"),[],import.meta.url)).Blob,global.WebSocket=C.WebSocket}const{ws_protocol:h,http_protocol:d,host:w,space_id:b}=await Tr(o,l),z=Math.random().toString(36).substring(2),N={};let p,x={},E=!1;l&&b&&(E=await jr(b,l));async function T(C){p=C,x=Br(C?.dependencies||[]);try{A=await ee(p)}catch(D){console.error(`Could not get api details: ${D.message}`)}return{config:p,...u}}let A;async function L(C){if(c&&c(C),C.status==="running")try{p=await Mt(e,`${d}//${w}`,l);const D=await T(p);a(D)}catch(D){console.error(D),c&&c({status:"error",message:"Could not load this space.",load_status:"error",detail:"NOT_FOUND"})}}try{p=await Mt(e,`${d}//${w}`,l);const C=await T(p);a(C)}catch(C){console.error(C),b?ft(b,rr.test(b)?"space_name":"subdomain",L):c&&c({status:"error",message:"Could not load this space.",load_status:"error",detail:"NOT_FOUND"})}function M(C,D,te){let q=!1,W=!1;return new Promise((j,I)=>{const ie=U(C,D,te);ie.on("data",Z=>{q=!0,W&&ie.destroy(),j(Z)}).on("status",Z=>{Z.stage==="error"&&I(Z),Z.stage==="complete"&&q&&ie.destroy(),Z.stage==="complete"&&(W=!0)})})}function U(C,D,te){let q,W;if(typeof C=="number")q=C,W=A.unnamed_endpoints[q];else{const G=C.replace(/^\//,"");q=x[G],W=A.named_endpoints[C.trim()]}if(typeof q!="number")throw new Error("There is no endpoint matching that name of fn_index matching that number.");let j;const I=typeof C=="number"?"/predict":C;let ie,Z=!1;const y={};i(`${d}//${w+p.path}`,D,W,l).then(G=>{if(ie={data:G||[],event_data:te,fn_index:q},Gr(q,p))X({type:"status",endpoint:I,stage:"pending",queue:!1,fn_index:q,time:new Date}),t(`${d}//${w+p.path}/run${I.startsWith("/")?I:`/${I}`}`,{...ie,session_hash:z},l).then(([m,F])=>{const P=g?qt(m.data,W,p.root,p.root_url):m.data;F==200?(X({type:"data",endpoint:I,fn_index:q,data:P,time:new Date}),X({type:"status",endpoint:I,fn_index:q,stage:"complete",eta:m.average_duration,queue:!1,time:new Date})):X({type:"status",stage:"error",endpoint:I,fn_index:q,message:m.error,queue:!1,time:new Date})}).catch(m=>{X({type:"status",stage:"error",message:m.message,endpoint:I,fn_index:q,queue:!1,time:new Date})});else{X({type:"status",stage:"pending",queue:!0,endpoint:I,fn_index:q,time:new Date});let m=new URL(`${h}://${w}${p.path}
- /queue/join`);E&&m.searchParams.set("__sign",E),j=new WebSocket(m),j.onclose=F=>{F.wasClean||X({type:"status",stage:"error",broken:!0,message:Re,queue:!0,endpoint:I,fn_index:q,time:new Date})},j.onmessage=function(F){const P=JSON.parse(F.data),{type:Y,status:se,data:Se}=Vr(P,N[q]);if(Y==="update"&&se&&!Z)X({type:"status",endpoint:I,fn_index:q,time:new Date,...se}),se.stage==="error"&&j.close();else if(Y==="hash"){j.send(JSON.stringify({fn_index:q,session_hash:z}));return}else Y==="data"?j.send(JSON.stringify({...ie,session_hash:z})):Y==="complete"?Z=se:Y==="log"?X({type:"log",log:Se.log,level:Se.level,endpoint:I,fn_index:q}):Y==="generating"&&X({type:"status",time:new Date,...se,stage:se?.stage,queue:!0,endpoint:I,fn_index:q});Se&&(X({type:"data",time:new Date,data:g?qt(Se.data,W,p.root,p.root_url):Se.data,endpoint:I,fn_index:q}),Z&&(X({type:"status",time:new Date,...Z,stage:se?.stage,queue:!0,endpoint:I,fn_index:q}),j.close()))},At(p.version||"2.0.0","3.6")<0&&addEventListener("open",()=>j.send(JSON.stringify({hash:z})))}});function X(G){const F=y[G.type]||[];F?.forEach(P=>P(G))}function xe(G,m){const F=y,P=F[G]||[];return F[G]=P,P?.push(m),{on:xe,off:ge,cancel:Ae,destroy:Ee}}function ge(G,m){const F=y;let P=F[G]||[];return P=P?.filter(Y=>Y!==m),F[G]=P,{on:xe,off:ge,cancel:Ae,destroy:Ee}}async function Ae(){const G={stage:"complete",queue:!1,time:new Date};Z=G,X({...G,type:"status",endpoint:I,fn_index:q}),j&&j.readyState===0?j.addEventListener("open",()=>{j.close()}):j.close();try{await e(`${d}//${w+p.path}/reset`,{headers:{"Content-Type":"application/json"},method:"POST",body:JSON.stringify({fn_index:q,session_hash:z})})}catch{console.warn("The `/reset` endpoint could not be called. Subsequent endpoint results may be unreliable.")}}function Ee(){for(const G in y)y[G].forEach(m=>{ge(G,m)})}return{on:xe,off:ge,cancel:Ae,destroy:Ee}}async function ee(C){if(A)return A;const D={"Content-Type":"application/json"};l&&(D.Authorization=`Bearer ${l}`);let te;if(At(C.version||"2.0.0","3.30")<0?te=await e("https://gradio-space-api-fetcher-v2.hf.space/api",{method:"POST",body:JSON.stringify({serialize:!1,config:JSON.stringify(C)}),headers:D}):te=await e(`${C.root}/info`,{headers:D}),!te.ok)throw new Error(Re);let q=await te.json();return"api"in q&&(q=q.api),q.named_endpoints["/predict"]&&!q.unnamed_endpoints[0]&&(q.unnamed_endpoints[0]=q.named_endpoints["/predict"]),Ir(q,C,x)}})}async function i(o,s,a,c){const l=await ct(s,void 0,[],!0,a);return Promise.all(l.map(async({path:f,blob:u,data:g,type:h})=>{if(u){const d=(await r(o,[u],c)).files[0];return{path:f,file_url:d,type:h}}else return{path:f,base64:g,type:h}})).then(f=>(f.forEach(({path:u,file_url:g,base64:h,type:d})=>{if(h)st(s,h,u);else if(d==="Gallery")st(s,g,u);else if(g){const w={is_file:!0,name:`${g}`,data:null};st(s,w,u)}}),s))}}const{post_data:ni,upload_files:St,client:Nt,handle_blob:ii}=Dr(fetch);function qt(e,t,r,n){return e.map((i,o)=>{var s,a,c,l;return((a=(s=t.returns)==null?void 0:s[o])==null?void 0:a.component)==="File"?Ce(i,r,n):((l=(c=t.returns)==null?void 0:c[o])==null?void 0:l.component)==="Gallery"?i.map(f=>Array.isArray(f)?[Ce(f[0],r,n),f[1]]:[Ce(f,r,n),null]):typeof i=="object"&&i.is_file?Ce(i,r,n):i})}function Ce(e,t,r){if(e==null)return null;if(typeof e=="string")return{name:"file_data",data:e};if(Array.isArray(e)){const n=[];for(const i of e)i===null?n.push(null):n.push(Ce(i,t,r));return n}else e.is_file&&(r?e.data="/proxy="+r+"file="+e.name:e.data=t+"/file="+e.name);return e}function Ct(e,t,r,n){switch(e.type){case"string":return"string";case"boolean":return"boolean";case"number":return"number"}if(r==="JSONSerializable"||r==="StringSerializable")return"any";if(r==="ListStringSerializable")return"string[]";if(t==="Image")return n==="parameter"?"Blob | File | Buffer":"string";if(r==="FileSerializable")return e?.type==="array"?n==="parameter"?"(Blob | File | Buffer)[]":"{ name: string; data: string; size?: number; is_file?: boolean; orig_name?: string}[]":n==="parameter"?"Blob | File | Buffer":"{ name: string; data: string; size?: number; is_file?: boolean; orig_name?: string}";if(r==="GallerySerializable")return n==="parameter"?"[(Blob | File | Buffer), (string | null)][]":"[{ name: string; data: string; size?: number; is_file?: boolean; orig_name?: string}, (string | null))][]"}function Lt(e,t){return t==="GallerySerializable"?"array of [file, label] tuples":t==="ListStringSerializable"?"array of strings":t==="FileSerializable"?"array of files or single file":e.description}function Ir(e,t,r){const n={named_endpoints:{},unnamed_endpoints:{}};for(const i in e){const o=e[i];for(const s in o){const a=t.dependencies[s]?s:r[s.replace("/","")],c=o[s];n[i][s]={},n[i][s].parameters={},n[i][s].returns={},n[i][s].type=t.dependencies[a].types,n[i][s].parameters=c.parameters.map(({label:l,component:f,type:u,serializer:g})=>({label:l,component:f,type:Ct(u,f,g,"parameter"),description:Lt(u,g)})),n[i][s].returns=c.returns.map(({label:l,component:f,type:u,serializer:g})=>({label:l,component:f,type:Ct(u,f,g,"return"),description:Lt(u,g)}))}}return n}async function jr(e,t){try{return(await(await fetch(`https://huggingface.co/api/spaces/${e}/jwt`,{headers:{Authorization:`Bearer ${t}`}})).json()).token||!1}catch(r){return console.error(r),!1}}function st(e,t,r){for(;r.length>1;)e=e[r.shift()];e[r.shift()]=t}async function ct(e,t=void 0,r=[],n=!1,i=void 0){if(Array.isArray(e)){let o=[];return await Promise.all(e.map(async(s,a)=>{var c;let l=r.slice();l.push(a);const f=await ct(e[a],n?((c=i?.parameters[a])==null?void 0:c.component)||void 0:t,l,!1,i);o=o.concat(f)})),o}else if(globalThis.Buffer&&e instanceof globalThis.Buffer){const o=t==="Image";return[{path:r,blob:o?!1:new nr([e]),data:o?`${e.toString("base64")}`:!1,type:t}]}else if(e instanceof Blob||typeof window<"u"&&e instanceof File)if(t==="Image"){let o;if(typeof window<"u")o=await Ur(e);else{const s=await e.arrayBuffer();o=Buffer.from(s).toString("base64")}return[{path:r,data:o,type:t}]}else return[{path:r,blob:e,type:t}];else if(typeof e=="object"){let o=[];for(let s in e)if(e.hasOwnProperty(s)){let a=r.slice();a.push(s),o=o.concat(await ct(e[s],void 0,a,!1,i))}return o}else return[]}function Ur(e){return new Promise((t,r)=>{const n=new FileReader;n.onloadend=()=>t(n.result),n.readAsDataURL(e)})}function Gr(e,t){var r,n,i,o;return!(((n=(r=t?.dependencies)==null?void 0:r[e])==null?void 0:n.queue)===null?t.enable_queue:(o=(i=t?.dependencies)==null?void 0:i[e])!=null&&o.queue)||!1}async function Mt(e,t,r){const n={};if(r&&(n.Authorization=`Bearer ${r}`),typeof window<"u"&&window.gradio_config&&location.origin!=="http://localhost:9876"){const i=window.gradio_config.root,o=window.gradio_config;return o.root=t+o.root,{...o,path:i}}else if(t){let i=await e(`${t}/config`,{headers:n});if(i.status===200){const o=await i.json();return o.path=o.path??"",o.root=t,o}else throw new Error("Could not get config.")}throw new Error("No config or app endpoint found")}async function ft(e,t,r){let n=t==="subdomain"?`https://huggingface.co/api/spaces/by-subdomain/${e}`:`https://huggingface.co/api/spaces/${e}`,i,o;try{if(i=await fetch(n),o=i.status,o!==200)throw new Error;i=await i.json()}catch{r({status:"error",load_status:"error",message:"Could not get space status",detail:"NOT_FOUND"});return}if(!i||o!==200)return;const{runtime:{stage:s},id:a}=i;switch(s){case"STOPPED":case"SLEEPING":r({status:"sleeping",load_status:"pending",message:"Space is asleep. Waking it up...",detail:s}),setTimeout(()=>{ft(e,t,r)},1e3);break;case"PAUSED":r({status:"paused",load_status:"error",message:"This space has been paused by the author. If you would like to try this demo, consider duplicating the space.",detail:s,discussions_enabled:await Et(a)});break;case"RUNNING":case"RUNNING_BUILDING":r({status:"running",load_status:"complete",message:"",detail:s});break;case"BUILDING":r({status:"building",load_status:"pending",message:"Space is building...",detail:s}),setTimeout(()=>{ft(e,t,r)},1e3);break;default:r({status:"space_error",load_status:"error",message:"This space is experiencing an issue.",detail:s,discussions_enabled:await Et(a)});break}}function Vr(e,t){switch(e.msg){case"send_data":return{type:"data"};case"send_hash":return{type:"hash"};case"queue_full":return{type:"update",status:{queue:!0,message:Rr,stage:"error",code:e.code,success:e.success}};case"estimation":return{type:"update",status:{queue:!0,stage:t||"pending",code:e.code,size:e.queue_size,position:e.rank,eta:e.rank_eta,success:e.success}};case"progress":return{type:"update",status:{queue:!0,stage:"pending",code:e.code,progress_data:e.progress_data,success:e.success}};case"log":return{type:"log",data:e};case"process_generating":return{type:"generating",status:{queue:!0,message:e.success?null:e.output.error,stage:e.success?"generating":"error",code:e.code,progress_data:e.progress_data,eta:e.average_duration},data:e.success?e.output:null};case"process_completed":return"error"in e.output?{type:"update",status:{queue:!0,message:e.output.error,stage:"error",code:e.code,success:e.success}}:{type:"complete",status:{queue:!0,message:e.success?void 0:e.output.error,stage:e.success?"complete":"error",code:e.code,progress_data:e.progress_data,eta:e.output.average_duration},data:e.success?e.output:null};case"process_starts":return{type:"update",status:{queue:!0,stage:"pending",code:e.code,size:e.rank,position:0,success:e.success}}}return{type:"none",status:{stage:"error",queue:!0}}}function ut(e,t){if(document.querySelector(`link[href='${e}']`))return Promise.resolve();const n=document.createElement("link");return n.rel="stylesheet",n.href=e,t.appendChild(n),new Promise((i,o)=>{n.addEventListener("load",()=>i()),n.addEventListener("error",()=>{console.error(`Unable to preload CSS for ${e}`),i()})})}function V(){}const bt=e=>e;function ir(e,t){for(const r in t)e[r]=t[r];return e}function or(e){return e()}function Ot(){return Object.create(null)}function ae(e){e.forEach(or)}function ue(e){return typeof e=="function"}function Te(e,t){return e!=e?t==t:e!==t||e&&typeof e=="object"||typeof e=="function"}let De;function Wr(e,t){return De||(De=document.createElement("a")),De.href=t,e===De.href}function Hr(e){return Object.keys(e).length===0}function sr(e,...t){if(e==null){for(const n of t)n(void 0);return V}const r=e.subscribe(...t);return r.unsubscribe?()=>r.unsubscribe():r}function Ve(e,t,r){e.$$.on_destroy.push(sr(t,r))}function ar(e,t,r,n){if(e){const i=lr(e,t,r,n);return e[0](i)}}function lr(e,t,r,n){return e[1]&&n?ir(r.ctx.slice(),e[1](n(t))):r.ctx}function cr(e,t,r,n){if(e[2]&&n){const i=e[2](n(r));if(t.dirty===void 0)return i;if(typeof i=="object"){const o=[],s=Math.max(t.dirty.length,i.length);for(let a=0;a32){const t=[],r=e.ctx.length/32;for(let n=0;nwindow.performance.now():()=>Date.now(),wt=dr?e=>requestAnimationFrame(e):V;const we=new Set;function pr(e){we.forEach(t=>{t.c(e)||(we.delete(t),t.f())}),we.size!==0&&wt(pr)}function $e(e){let t;return we.size===0&&wt(pr),{promise:new Promise(r=>{we.add(t={c:e,f:r})}),abort(){we.delete(t)}}}const Jr=typeof window<"u"?window:typeof globalThis<"u"?globalThis:global;"WeakMap"in Jr;function S(e,t){e.appendChild(t)}function gr(e){if(!e)return document;const t=e.getRootNode?e.getRootNode():e.ownerDocument;return t&&t.host?t:e.ownerDocument}function Zr(e){const t=B("style");return t.textContent="/* empty */",Qr(gr(e),t),t.sheet}function Qr(e,t){return S(e.head||e,t),t.sheet}function k(e,t,r){e.insertBefore(t,r||null)}function v(e){e.parentNode&&e.parentNode.removeChild(e)}function hr(e,t){for(let r=0;re.removeEventListener(t,r,n)}function ci(e){return function(t){return t.preventDefault(),e.call(this,t)}}function fi(e){return function(t){return t.stopPropagation(),e.call(this,t)}}function _(e,t,r){r==null?e.removeAttribute(t):e.getAttribute(t)!==r&&e.setAttribute(t,r)}const Kr=["width","height"];function Xr(e,t){const r=Object.getOwnPropertyDescriptors(e.__proto__);for(const n in t)t[n]==null?e.removeAttribute(n):n==="style"?e.style.cssText=t[n]:n==="__value"?e.value=e[n]=t[n]:r[n]&&r[n].set&&Kr.indexOf(n)===-1?e[n]=t[n]:_(e,n,t[n])}function Yr(e,t){Object.keys(t).forEach(r=>{$r(e,r,t[r])})}function $r(e,t,r){t in e?e[t]=typeof e[t]=="boolean"&&r===""?!0:r:_(e,t,r)}function ui(e){return/-/.test(e)?Yr:Xr}function di(e){let t;return{p(...r){t=r,t.forEach(n=>e.push(n))},r(){t.forEach(r=>e.splice(e.indexOf(r),1))}}}function pi(e){return e===""?null:+e}function en(e){return Array.from(e.childNodes)}function re(e,t){t=""+t,e.data!==t&&(e.data=t)}function gi(e,t){e.value=t??""}function Q(e,t,r,n){r==null?e.style.removeProperty(t):e.style.setProperty(t,r,n?"important":"")}let Ie;function tn(){if(Ie===void 0){Ie=!1;try{typeof window<"u"&&window.parent&&window.parent.document}catch{Ie=!0}}return Ie}function hi(e,t){getComputedStyle(e).position==="static"&&(e.style.position="relative");const n=B("iframe");n.setAttribute("style","display: block; position: absolute; top: 0; left: 0; width: 100%; height: 100%; overflow: hidden; border: 0; opacity: 0; pointer-events: none; z-index: -1;"),n.setAttribute("aria-hidden","true"),n.tabIndex=-1;const i=tn();let o;return i?(n.src="data:text/html,
-
-
-
-
-
-
-